diff --git a/spaces/1acneusushi/gradio-2dmoleculeeditor/data/Download and Install QuickBooks Desktop Pro 2021 with These Easy Steps.md b/spaces/1acneusushi/gradio-2dmoleculeeditor/data/Download and Install QuickBooks Desktop Pro 2021 with These Easy Steps.md
deleted file mode 100644
index 27644960c7fb56576af91eb57ee3f40f30597641..0000000000000000000000000000000000000000
--- a/spaces/1acneusushi/gradio-2dmoleculeeditor/data/Download and Install QuickBooks Desktop Pro 2021 with These Easy Steps.md
+++ /dev/null
@@ -1,59 +0,0 @@
-
-
How to Download and Install QuickBooks Desktop Pro 2021
-
QuickBooks Desktop Pro 2021 is the latest version of the popular accounting software for small and medium-sized businesses. It offers new features and improvements that can help you manage your finances more efficiently and effectively. In this article, we will show you how to download and install QuickBooks Desktop Pro 2021 on your computer.
To download QuickBooks Desktop Pro 2021, you need to have a valid license or subscription from Intuit. You can purchase one from their official website or from a trusted reseller. Once you have your license or subscription, you can follow these steps to download the software:
Enter your product and license number in the fields provided and click Search.
-
Select QuickBooks Desktop Pro 2021 from the list of products and click Download.
-
Save the file to a convenient location on your computer.
-
-
Step 2: Install QuickBooks Desktop Pro 2021
-
After downloading the file, you can install QuickBooks Desktop Pro 2021 by following these steps:
-
-
Double-click the file you downloaded to launch the installer.
-
Click Yes to allow the program to make changes to your computer.
-
Select I accept the terms in the license agreement and click Next.
-
Enter your license and product number in the fields provided and click Next.
-
Select Express as the installation type and click Next.
-
Select where you want to install QuickBooks Desktop Pro 2021 and click Install.
-
Wait for the installation to complete and click Open QuickBooks.
-
-
Congratulations! You have successfully downloaded and installed QuickBooks Desktop Pro 2021 on your computer.
-
You can now start using the software to manage your business finances. If you need any help or support, you can visit the official website of Intuit or contact their customer service team. You can also check out their online community forums and tutorials for more tips and tricks on how to use QuickBooks Desktop Pro 2021.
-
-
What's New in QuickBooks Desktop Pro 2021?
-
QuickBooks Desktop Pro 2021 comes with several new features and enhancements that can make your accounting tasks easier and faster. Some of the highlights include:
-
-
Improved bank feeds: You can now connect your bank accounts and credit cards to QuickBooks Desktop Pro 2021 and automatically download and categorize your transactions. You can also customize the rules for matching and adding transactions to save time and reduce errors.
-
Receipt management: You can now scan and upload your receipts to QuickBooks Desktop Pro 2021 and attach them to your transactions. You can also use the QuickBooks Desktop mobile app to capture and upload receipts on the go. This can help you track your expenses and prepare for tax time.
-
Data level permissions: You can now set up different levels of access for your users based on the data they need to see and work with. You can also assign specific roles and permissions to your employees, contractors, and accountants. This can help you protect your sensitive data and prevent unauthorized changes.
-
Automated statements: You can now schedule and send recurring statements to your customers automatically. You can also customize the frequency, format, and content of your statements. This can help you improve your cash flow and customer satisfaction.
-
-
How to Upgrade to QuickBooks Desktop Pro 2021?
-
If you are already using an older version of QuickBooks Desktop Pro, you can easily upgrade to QuickBooks Desktop Pro 2021 without losing any of your data or settings. You just need to follow these steps:
-
-
-
Make sure you have a backup of your company file before upgrading.
-
Download QuickBooks Desktop Pro 2021 from the link provided in Step 1 above.
-
Run the installer and follow the instructions on the screen.
-
Select Upgrade as the installation type and choose the version of QuickBooks Desktop Pro you are currently using.
-
Click Next and follow the prompts to complete the upgrade process.
-
Open QuickBooks Desktop Pro 2021 and verify that your company file is updated and working properly.
-
-
How to Get Started with QuickBooks Desktop Pro 2021?
-
If you are new to QuickBooks Desktop Pro, you can get started with QuickBooks Desktop Pro 2021 by following these steps:
-
-
Create a new company file or use the sample company file provided by QuickBooks Desktop Pro 2021.
-
Set up your company information, preferences, chart of accounts, products and services, customers, vendors, employees, etc.
-
Connect your bank accounts and credit cards to QuickBooks Desktop Pro 2021 and download your transactions.
-
Scan and upload your receipts to QuickBooks Desktop Pro 2021 and attach them to your transactions.
-
Create invoices, bills, estimates, sales receipts, payments, etc. for your customers and vendors.
-
Record deposits, transfers, checks, etc. for your bank accounts and credit cards.
-
Reconcile your bank accounts and credit cards with QuickBooks Desktop Pro 2021.
-
Run reports and statements to monitor your business performance and financial health.
-
ddb901b051
-
-
\ No newline at end of file
diff --git a/spaces/1acneusushi/gradio-2dmoleculeeditor/data/Forza Horizon 5 Download Ios.md b/spaces/1acneusushi/gradio-2dmoleculeeditor/data/Forza Horizon 5 Download Ios.md
deleted file mode 100644
index 35b44a619d9df4bc81a0aa4e14e62a7f72380876..0000000000000000000000000000000000000000
--- a/spaces/1acneusushi/gradio-2dmoleculeeditor/data/Forza Horizon 5 Download Ios.md
+++ /dev/null
@@ -1,31 +0,0 @@
-
-
How to Download and Play Forza Horizon 5 on iOS Devices
-
Forza Horizon 5 is the latest installment of the popular racing game series developed by Playground Games and published by Microsoft. The game is set in Mexico, where you can explore a diverse and stunning open world with hundreds of cars to choose from. You can race, drift, stunt, and customize your vehicles as you compete in various events and challenges.
If you are an iOS user, you might be wondering if you can play Forza Horizon 5 on your iPhone or iPad. The good news is that you can, thanks to a mobile version of the game that is available on the App Store. The mobile version of Forza Horizon 5 offers the same gameplay and graphics as the console and PC versions, but with some optimizations and adjustments for touch controls and smaller screens.
-
In this article, we will show you how to download and play Forza Horizon 5 on your iOS devices in a few simple steps.
-
Step 1: Go to the App Store
-
The first step is to go to the App Store on your iOS device and search for Forza Horizon 5. You can also use this link to access the game page directly. You will see a screen with some information and screenshots of the game, as well as a download button.
-
Step 2: Download the game
-
The next step is to tap on the download button and wait for the game to be installed on your device. The game size is about 345 MB, so make sure you have enough space and a stable internet connection. You might also need to enter your Apple ID and password to confirm the download.
-
Step 3: Launch the game
-
Once the download is complete, you can launch the game from your home screen or app library. You will see a splash screen with the Forza Horizon 5 logo and some loading animations. The game might take some time to load depending on your device performance and network speed.
-
-
Step 4: Enjoy the game
-
After the game loads, you will see a main menu with some options to start playing. You can choose between solo or online modes, customize your profile and settings, view your achievements and leaderboards, and more. You can also access a tutorial that will teach you the basics of the game controls and mechanics.
-
To play the game, you will need to use touch gestures on your screen to steer, accelerate, brake, drift, and activate special features. You can also tilt your device to use motion controls if you prefer. The game will adapt to your skill level and preferences as you progress through the game.
-
Conclusion
-
In this article, we have shown you how to download and play Forza Horizon 5 on your iOS devices. We hope this guide was helpful and easy to follow. Now you can enjoy one of the best racing games ever made on your iPhone or iPad anytime and anywhere.
-
-
Some Tips and Tricks for Forza Horizon 5 on iOS
-
If you want to get the most out of Forza Horizon 5 on your iOS devices, here are some tips and tricks that might help you:
-
-
Use the photo mode to capture and share your best moments in the game. You can access the photo mode by tapping on the camera icon on the top right corner of the screen. You can adjust the camera angle, zoom, focus, filters, and more. You can also save and share your photos with your friends or on social media.
-
Complete the seasonal events and challenges to earn rewards and unlock new cars and features. You can view the current season and its objectives by tapping on the calendar icon on the top left corner of the screen. You can also join online events and races with other players around the world.
-
Upgrade and customize your cars to improve their performance and appearance. You can access the garage by tapping on the car icon on the bottom left corner of the screen. You can change the paint, wheels, decals, spoilers, and more. You can also tune your car's engine, suspension, brakes, and more.
-
Explore the map and discover hidden secrets and locations. You can access the map by tapping on the compass icon on the bottom right corner of the screen. You can zoom in and out, move around, and set waypoints. You can also find collectibles, barn finds, speed traps, danger signs, and more.
-
Have fun and experiment with different cars and modes. You can switch between different cars by tapping on the car icon on the top center of the screen. You can also change the difficulty level, weather, time of day, and more by tapping on the settings icon on the top right corner of the screen.
-
-
Forza Horizon 5 is a game that offers endless possibilities and fun for racing fans. Whether you want to race, drift, stunt, or explore, you will find something to enjoy in this game. Download Forza Horizon 5 on your iOS devices today and experience the thrill of driving in Mexico.
ddb901b051
-
-
\ No newline at end of file
diff --git a/spaces/1gistliPinn/ChatGPT4/Examples/5.1 Surround Sound Tamil Mp3 Songs UPD Free Download.md b/spaces/1gistliPinn/ChatGPT4/Examples/5.1 Surround Sound Tamil Mp3 Songs UPD Free Download.md
deleted file mode 100644
index 703e81c1413ec7d4c249ec86881c145d660c509b..0000000000000000000000000000000000000000
--- a/spaces/1gistliPinn/ChatGPT4/Examples/5.1 Surround Sound Tamil Mp3 Songs UPD Free Download.md
+++ /dev/null
@@ -1,6 +0,0 @@
-
-
-Welcome to Movie World Tamil Film Flicks YouTube Channel Movie World Entertainments is the leading ... Download Hungama Play app to get access to unlimited free movies, latest music videos, kids ... Manzoor sakhirani all mp3 songs download ... Sec 5.1 geometric and algebra connections linear equations answers. 1fdad05405
-
-
-
diff --git a/spaces/1pelhydcardo/ChatGPT-prompt-generator/assets/Android Oyun Clubs Dark Riddle APK Hile The Most Challenging and Scary Game Ever.md b/spaces/1pelhydcardo/ChatGPT-prompt-generator/assets/Android Oyun Clubs Dark Riddle APK Hile The Most Challenging and Scary Game Ever.md
deleted file mode 100644
index bda77b1e43486dd1e0dbacf854c7818191efe78d..0000000000000000000000000000000000000000
--- a/spaces/1pelhydcardo/ChatGPT-prompt-generator/assets/Android Oyun Clubs Dark Riddle APK Hile The Most Challenging and Scary Game Ever.md
+++ /dev/null
@@ -1,82 +0,0 @@
-
-
Dark Riddle APK Hile Android Oyun Club: A Review
-
If you are looking for a game that combines escape, adventure and puzzle elements with stealth, humor and mystery, you might want to check out Dark Riddle. This is a popular game on the Android platform that lets you explore your neighbor's house and discover his secrets. But what if you want to enjoy the game without any limitations or interruptions? That's where Dark Riddle APK Hile comes in. This is a modded version of the game that gives you unlimited money and removes ads and in-app purchases. In this article, we will review Dark Riddle APK Hile and tell you how to download and install it from Android Oyun Club. We will also discuss the features, benefits, drawbacks and risks of using this modded version.
-
What is Dark Riddle?
-
A game of escape, adventure and puzzle
-
Dark Riddle is a game developed by Nika Entertainment that was released in 2019. It is inspired by other games like Hello Neighbor and Granny, where you have to sneak into your neighbor's house and find out what he is hiding. You can use various items and tools to distract, trick or fight your neighbor, who will chase you if he sees you. You can also interact with other characters and objects in the game world, such as animals, cars, plants and more. The game has different levels and modes, each with its own challenges and surprises.
Dark Riddle is not just a game of escape, adventure and puzzle. It is also a game of stealth, humor and mystery. You have to use your skills and creativity to avoid being detected by your neighbor, who has a lot of traps and cameras in his house. You can also use your sense of humor to prank your neighbor or make him laugh. The game has a lot of funny moments and dialogues that will make you smile. Moreover, the game has a lot of mystery and suspense that will keep you hooked. You will want to know more about your neighbor's secrets and motives, as well as the story behind the game.
-
What is Dark Riddle APK Hile?
-
A modded version of the game with unlimited money
-
Dark Riddle APK Hile is a modded version of the game that gives you unlimited money. This means that you can buy anything you want in the game without worrying about the cost. You can get all the items, skins and weapons that are available in the game store. You can also upgrade your skills and abilities to make yourself stronger and faster. With unlimited money, you can enjoy the game without any restrictions or limitations.
-
A way to enjoy the game without ads or in-app purchases
-
Dark Riddle APK Hile is also a way to enjoy the game without ads or in-app purchases. This means that you can play the game without any interruptions or annoyances. You don't have to watch any ads or spend any real money to get extra features or resources in the game. You can play the game smoothly and comfortably without any hassle or pressure.
-
How to
How to download and install Dark Riddle APK Hile?
-
The steps to download the file from Android Oyun Club
-
Dark Riddle APK Hile is available for download from Android Oyun Club, a website that offers modded versions of various Android games. To download the file from Android Oyun Club, you need to follow these steps:
Search for Dark Riddle in the search bar or browse the categories to find the game.
-
Click on the game title and scroll down to the download section.
-
Choose the version of Dark Riddle APK Hile that you want to download and click on the download button.
-
Wait for the download to complete and save the file on your device.
-
-
The steps to install the file on your device
-
After downloading the file from Android Oyun Club, you need to install it on your device. To install the file on your device, you need to follow these steps:
-
-
Go to the settings of your device and enable the option to install apps from unknown sources.
-
Locate the downloaded file on your device and tap on it.
-
Follow the instructions on the screen and allow the necessary permissions.
-
Wait for the installation to finish and launch the game.
-
-
What are the features and benefits of Dark Riddle APK Hile?
-
The features of the modded version, such as unlocked items, skins and weapons
-
Dark Riddle APK Hile has many features that make it different from the original version of the game. Some of these features are:
-
This is a first-person adventure thriller with an interactive environment and interesting quests. Solve puzzles and uncover the secrets of a suspicious neighbor who lives across from you.
-Your adventure begins in an unusual city where you can find many useful and unique items. You will meet a police officer and a seller of alien devices, and during the game you will get acquainted with unusual creatures. Each item and character has a huge story behind it.
-The game has a lot of humor, various levels of difficulty and multiple endings - the outcome of the story depends entirely on your actions and decisions. You can use headphones to explore the city in detail and better understand the plot.
-
-
Unlimited money: You can buy anything you want in the game without worrying about the cost.
-
Unlocked items: You can access all the items that are available in the game store, such as flashlights, cameras, binoculars, etc.
-
Unlocked skins: You can customize your character with different skins, such as clown, pirate, ninja, etc.
-
Unlocked weapons: You can use different weapons to fight your neighbor, such as guns, knives, bats, etc.
-
-
The benefits of the modded version, such as more fun, freedom and challenge
-
Dark Riddle APK Hile has many benefits that make it more fun, freedom and challenge than the original version of the game. Some of these benefits are:
-
-
More fun: You can enjoy the game without any limitations or interruptions. You can prank your neighbor or make him laugh with your humor and creativity.
-
More freedom: You can explore your neighbor's house and discover his secrets without any restrictions or limitations. You can use any item or tool you want to solve puzzles and escape.
-
More challenge: You can increase the difficulty and excitement of the game by using different weapons and skins. You can also face new challenges and surprises in each level and mode.
-
What are the drawbacks and risks of Dark Riddle APK Hile?
-
The drawbacks of the modded version, such as possible bugs, glitches and crashes
-
Dark Riddle APK Hile is not a perfect version of the game. It has some drawbacks that may affect your gaming experience. Some of these drawbacks are:
-
-
Possible bugs: The modded version may have some bugs or errors that may cause the game to malfunction or behave unexpectedly.
-
Possible glitches: The modded version may have some glitches or flaws that may affect the graphics, sound or gameplay of the game.
-
Possible crashes: The modded version may have some crashes or freezes that may cause the game to stop working or close abruptly.
-
-
The risks of the modded version, such as malware, viruses and bans
-
Dark Riddle APK Hile is not a safe version of the game. It has some risks that may harm your device or account. Some of these risks are:
-
-
Possible malware: The modded version may have some malware or malicious code that may infect your device or steal your data.
-
Possible viruses: The modded version may have some viruses or harmful programs that may damage your device or corrupt your files.
-
Possible bans: The modded version may have some bans or penalties that may prevent you from playing the game or accessing your account.
-
-
Conclusion
-
Dark Riddle APK Hile is a modded version of the game that gives you unlimited money and removes ads and in-app purchases. It also unlocks all the items, skins and weapons in the game. It is a way to enjoy the game without any limitations or interruptions. However, it also has some drawbacks and risks that may affect your gaming experience or harm your device or account. Therefore, you should be careful and responsible when using this modded version. You should also respect the original developers and creators of the game and support them if you like their work.
-
FAQs
-
-
Q: Is Dark Riddle APK Hile legal?
-
A: Dark Riddle APK Hile is not legal. It is a modded version of the game that violates the terms and conditions of the original game. It also infringes the intellectual property rights of the original developers and creators of the game.
-
Q: Is Dark Riddle APK Hile safe?
-
A: Dark Riddle APK Hile is not safe. It is a modded version of the game that may contain malware, viruses or bans that may harm your device or account. It also may have bugs, glitches or crashes that may affect your gaming experience.
-
Q: How to update Dark Riddle APK Hile?
-
A: Dark Riddle APK Hile is not easy to update. It is a modded version of the game that may not be compatible with the latest version of the original game. You may need to download and install a new version of Dark Riddle APK Hile from Android Oyun Club whenever there is an update available.
-
Q: How to uninstall Dark Riddle APK Hile?
-
A: Dark Riddle APK Hile is easy to uninstall. You can simply delete the file from your device or go to the settings of your device and uninstall the app like any other app.
-
Q: Where to get more information about Dark Riddle APK Hile?
-
A: You can get more information about Dark Riddle APK Hile from Android Oyun Club, the website that offers this modded version of the game. You can also visit the official website or social media pages of Dark Riddle, the original game, to get more information about it.
- 197e85843d
-
-
\ No newline at end of file
diff --git a/spaces/1phancelerku/anime-remove-background/8 Ball Pool bitAIM APK A Complete Guide to the Ultimate Pool Game Experience.md b/spaces/1phancelerku/anime-remove-background/8 Ball Pool bitAIM APK A Complete Guide to the Ultimate Pool Game Experience.md
deleted file mode 100644
index fa85a2b0fd999ed5916b113f5bc70d1ca9af0213..0000000000000000000000000000000000000000
--- a/spaces/1phancelerku/anime-remove-background/8 Ball Pool bitAIM APK A Complete Guide to the Ultimate Pool Game Experience.md
+++ /dev/null
@@ -1,146 +0,0 @@
-
-
What is 8 ball pool bitaim apk?
-
If you are a fan of pool games, you might have heard of or played 8 ball pool, one of the most popular and addictive online multiplayer games on Android. 8 ball pool is a game where you can compete with players from all over the world in various modes and tournaments, using your skills and strategies to pocket balls and win coins and rewards. But what if you want to have an edge over your opponents and improve your game performance? That's where 8 ball pool bitaim apk comes in.
8 ball pool bitaim apk is a modded version of 8 ball pool that allows you to hack the aim of your striker and hit the pieces with perfect accuracy. With 8 ball pool bitaim apk, you can win every match and earn more coins and gems. But is 8 ball pool bitaim apk safe and legal to use? How can you download and install it on your device? And what are its features and benefits? In this article, we will answer all these questions and more, so keep reading.
-
How to play 8 ball pool?
-
Before we dive into the details of 8 ball pool bitaim apk, let's first review the basics of how to play 8 ball pool. 8 ball pool is a game played with a cue ball and fifteen object balls, numbered 1 through 15. Balls 1–7 are solid colors and commonly referred to as “low balls”, and balls 9–15 are striped and commonly referred to as “high balls.” One player must pocket balls of solid colors, while the other player must pocket the striped balls. The player who pockets their entire group and then legally pockets the 8-ball wins the game.
-
To start the game, one player must break the rack by hitting the cue ball into the triangle of object balls. For the break shot to be legal, the breaker must either pocket a number ball or drive at least four number balls to one or more rails. No ball is called, and the cue ball is not required to hit any particular object ball first. If the breaker fails to make a legal break, the opponent can choose to break again or accept the table as it is.
-
After a legal break, if any object ball is pocketed, then that determines whether that player has solids or stripes for that game. If no object ball is pocketed on a legal break or if both a solid and a stripe are pocketed on a legal break then it is an open table until one player pockets either a solid or stripe on their turn. Once solids or stripes have been determined for each player then they must continue shooting at their designated group until they have cleared their group from the table.
-
A player's turn continues until they fail to pocket one of their group or
commit a foul. A foul occurs when the player fails to hit any ball with the cue ball, hits the wrong group of balls first, pockets the cue ball, pockets the 8-ball before clearing their group, pockets the 8-ball in the wrong pocket, or drives any ball off the table. If a player commits a foul, their opponent gets ball in hand, meaning they can place the cue ball anywhere on the table for their next shot.
-
bitAIM app for carrom pool
-bitAIM+ download apk free
-bitAIM AI aim assistance tool
-bitAIM for carrom pool practices
-bitAIM apk latest version 3.6.54
-bitAIM image recognition technique
-bitAIM android app free download
-bitAIM apkcombo apps tools
-bitAIM app.ai.lab.bitaimplus
-bitAIM apk mirror download
-bitAIM carrom pool master shots
-bitAIM apk file size 28 MB
-bitAIM app developer bitAIM+
-bitAIM apk update Aug 12, 2022
-bitAIM app category tools
-bitAIM apk google play id
-bitAIM app installs 500+
-bitAIM apk direct and indirect shot
-bitAIM app description tools advertisement
-bitAIM apk multi-collision of coin
-bitAIM app reviews and ratings
-bitAIM apk how to install
-bitAIM app features and benefits
-bitAIM apk compatible devices
-bitAIM app screenshots and videos
-bitAIM apk mod unlimited coins
-bitAIM app alternatives and similar apps
-bitAIM apk download for pc windows 10
-bitAIM app support and contact information
-bitAIM apk no root required
-bitAIM app privacy policy and terms of service
-bitAIM apk online generator tool
-bitAIM app tips and tricks guide
-bitAIM apk offline mode available
-bitAIM app user feedback and suggestions
-bitAIM apk safe and secure download link
-bitAIM app pros and cons analysis
-bitAIM apk hack version download 2023
-bitAIM app frequently asked questions (FAQs)
-bitAIM apk premium features unlocked
-
The game ends when one player legally pockets the 8-ball in a designated pocket after clearing their group. The player must call the pocket for the 8-ball before shooting. If the player pockets the 8-ball in an uncalled pocket, or pockets the 8-ball and the cue ball on the same shot, they lose the game.
-
How to download and install bitaim apk?
-
Now that you know how to play 8 ball pool, you might be wondering how to get bitaim apk on your device. Bitaim apk is not available on the official Google Play Store, so you will need to download it from a third-party source. Here are the steps and requirements for downloading and installing bitaim apk:
-
-
Make sure your device has enough storage space and meets the minimum system requirements for running 8 ball pool. The game requires Android 4.4 or higher and at least 1 GB of RAM.
-
Enable unknown sources on your device settings. This will allow you to install apps from sources other than the Google Play Store. To do this, go to Settings > Security > Unknown Sources and toggle it on.
-
Download bitaim apk from a reliable and trusted website. You can search for bitaim apk on Google or use this link: (https://bitaimapk.com/). Be careful not to download any fake or malicious files that might harm your device.
-
Locate the downloaded file on your device and tap on it to start the installation process. Follow the instructions on the screen and grant the necessary permissions for the app to run.
-
Launch 8 ball pool bitaim apk and enjoy playing with unlimited aim and accuracy.
-
-
What are the features of bitaim apk?
-
Bitaim apk is a modded version of 8 ball pool that offers many features and benefits that can enhance your gaming experience and make you a better player. Here are some of the features of bitaim apk:
-
-
AI assistance: Bitaim apk uses artificial intelligence to help you aim and shoot with precision. It shows you the trajectory and angle of your shots, as well as the best possible pocket for each ball. You can also adjust the sensitivity and speed of your aim according to your preference.
-
Shots recording: Bitaim apk allows you to record your shots and replay them later. You can use this feature to analyze your mistakes and improve your skills. You can also share your shots with your friends and challenge them to beat your score.
-
No ads: Bitaim apk removes all the annoying ads that interrupt your gameplay and distract you from your focus. You can play without any interruptions and enjoy a smooth and seamless gaming experience.
-
No root required: Bitaim apk does not require root access to work on your device. You can use it without any risk of damaging your device or voiding its warranty.
-
Free updates: Bitaim apk provides free updates for its users, ensuring that they always have access to the latest features and bug fixes.
-
-
How to use bitaim apk?
-
Using bitaim apk is very easy and simple. All you need to do is follow these steps:
-
-
Launch 8 ball pool bitaim apk on your device and log in with your account or create a new one.
-
Select a game mode or tournament that you want to play and join a match.
-
When it is your turn to shoot, you will see a green line showing you the direction and angle of your shot. You can also see a yellow circle indicating the best pocket for each ball.
-
To adjust your aim, swipe left or right on the screen. To adjust your power, swipe up or down on the screen.
-
To shoot, tap on the screen when you are ready.
-
Enjoy winning every match with perfect accuracy and skill.
-
-
How to activate indirect or premium shots?
-
Bitaim apk also offers indirect or premium shots, which are more advanced and challenging shots that require more skill and strategy. Indirect shots are shots that involve hitting one or more rails before pocketing a ball. Premium shots are shots that involve using spin, curve, or jump to pocket a ball. To activate indirect or premium shots, you need to pay a certain amount of coins or gems, depending on the level of difficulty and reward. Here is a table showing the cost and benefit of each type of shot: | Type of shot | Cost | Benefit | | --- | --- | --- | | Indirect shot | 50 coins or 5 gems | Double the coins or gems you win | | Premium shot | 100 coins or 10 gems | Triple the coins or gems you win | To activate indirect or premium shots, you need to tap on the icon that appears on the top right corner of the screen before shooting. You can choose between coins or gems as the payment method. Once you activate the shot, you will see a blue line showing you the trajectory and angle of your shot, as well as a red circle indicating the spin, curve, or jump effect. You can adjust your shot as usual and then shoot when you are ready.
How to use bitaim apk with Lulubox?
-
Lulubox is another popular app that can enhance your gaming experience by providing you with various features and hacks for different games. Lulubox is compatible with 8 ball pool bitaim apk, and you can use them together to get more benefits and advantages. Here are some of the features that Lulubox can offer for 8 ball pool:
-
-
Unlimited coins and gems: Lulubox can help you get unlimited coins and gems for 8 ball pool, which you can use to buy cues, tables, chat packs, and more. You can also use them to activate indirect or premium shots without any cost.
-
Free skins and themes: Lulubox can help you customize your game with free skins and themes for your cues, tables, and background. You can choose from a variety of options and styles to suit your preference.
-
No verification required: Lulubox can help you bypass the verification process that 8 ball pool requires for some features and functions. You can use Lulubox to access all the features and functions without any hassle.
-
-
To use bitaim apk with Lulubox, you need to follow these steps:
-
-
Download and install Lulubox from a reliable and trusted website. You can search for Lulubox on Google or use this link: (https://www.luluboxapk.com/).
-
Launch Lulubox on your device and grant the necessary permissions for the app to run.
-
Find 8 ball pool bitaim apk on the list of games that Lulubox supports and tap on it.
-
Select the features that you want to activate for 8 ball pool bitaim apk and tap on the launch button.
-
Enjoy playing 8 ball pool bitaim apk with Lulubox.
-
-
How to update bitaim apk?
-
Bitaim apk is constantly updated by its developers to ensure that it works smoothly and efficiently with the latest version of 8 ball pool. To update bitaim apk, you need to follow these steps:
-
-
Check if there is a new version of bitaim apk available on the website where you downloaded it from. You can also check for updates within the app itself by tapping on the menu button and then on the update option.
-
If there is a new version available, download it from the website or from the app.
-
Delete the old version of bitaim apk from your device.
-
Install the new version of bitaim apk following the same steps as before.
-
Launch 8 ball pool bitaim apk and enjoy playing with the latest features and bug fixes.
-
-
What are the pros and cons of bitaim apk?
-
Bitaim apk is a modded version of 8 ball pool that offers many features and benefits that can enhance your gaming experience and make you a better player. However, it also has some drawbacks and risks that you should be aware of before using it. Here are some of the pros and cons of bitaim apk:
- | Pros | Cons | | --- | --- | | It helps you aim and shoot with perfect accuracy. | It takes away some of the challenge and fun of playing 8 ball pool. | | It allows you to win every match and earn more coins and gems. | It may be considered cheating by some players and may ruin their gaming experience. | | It removes all the ads that interrupt your gameplay. | It may not be compatible with some devices or versions of 8 ball pool. | | It does not require root access to work on your device. | It may expose your device to malware or viruses from unknown sources. | | It provides free updates for its users. | It may get detected and banned by the game developers or moderators. |
What are some alternatives to bitaim apk?
-
If you are looking for some alternatives to bitaim apk, you might want to check out these other apps that can provide similar or better features for 8 ball pool:
-
-
8 Ball Pool Mod Menu: This is another modded version of 8 ball pool that offers unlimited coins and gems, long line, anti-ban, and more. You can download it from this link: (https://8ballpoolmodmenu.com/).
-
8 Ball Pool Tool: This is an app that helps you calculate the angle and power of your shots, as well as the best pocket for each ball. You can download it from this link: (https://play.google.com/store/apps/details?id=com.eivaagames.EightBallPoolToolFree&hl=en_US&gl=US).
-
8 Ball Pool Guideline Hack: This is an app that shows you the guideline of your shots, even in no guideline mode. You can download it from this link: (https://play.google.com/store/apps/details?id=com.guideline.hack&hl=en_US&gl=US).
-
-
Conclusion
-
8 ball pool bitaim apk is a modded version of 8 ball pool that allows you to hack the aim of your striker and hit the pieces with perfect accuracy. It offers many features and benefits that can enhance your gaming experience and make you a better player, such as AI assistance, shots recording, no ads, no root required, and free updates. However, it also has some drawbacks and risks that you should be aware of before using it, such as cheating, compatibility issues, malware threats, and ban risks. Therefore, you should use it at your own discretion and responsibility.
-
If you want to download and install bitaim apk on your device, you can follow the steps and requirements that we have provided in this article. You can also use bitaim apk with Lulubox to get more features and hacks for 8 ball pool. Alternatively, you can check out some other apps that can provide similar or better features for 8 ball pool, such as 8 Ball Pool Mod Menu, 8 Ball Pool Tool, and 8 Ball Pool Guideline Hack.
-
We hope that this article has helped you understand what is 8 ball pool bitaim apk and how to use it effectively and safely. If you have any questions or feedback, please feel free to leave a comment below. Thank you for reading and happy gaming!
-
FAQs
-
Here are some of the frequently asked questions and answers about 8 ball pool bitaim apk:
-
-
Q: Is 8 ball pool bitaim apk safe and legal to use?
-
A: Bitaim apk is not safe or legal to use, as it is a modded version of 8 ball pool that violates the terms and conditions of the game. It may expose your device to malware or viruses from unknown sources, and it may get detected and banned by the game developers or moderators. Therefore, you should use it at your own risk and responsibility.
-
Q: How can I avoid getting banned by using bitaim apk?
-
A: There is no guarantee that you will not get banned by using bitaim apk, as it is a modded version of 8 ball pool that violates the terms and conditions of the game. However, you can try to reduce the chances of getting banned by following these tips:
-
-
Do not use bitaim apk in ranked or tournament matches, as they are more likely to be monitored by the game developers or moderators.
-
Do not use bitaim apk excessively or obviously, as it may arouse suspicion from other players or observers.
-
Do not brag or boast about using bitaim apk, as it may attract unwanted attention or reports from other players or observers.
-
Do not share your account or device with anyone else who might use bitaim apk, as it may compromise your security and privacy.
-
-
Q: Can I use bitaim apk with other mods or hacks for 8 ball pool?
-
A: Bitaim apk is compatible with some other mods or hacks for 8 ball pool, such as Lulubox. However, you should be careful not to use too many mods or hacks at the same time, as they may cause conflicts or errors in your game performance. You should also be aware that using more mods or hacks may increase the risk of getting banned by the game developers or moderators.
-
Q: How can I contact the developers of bitaim apk?
-
A: Bitaim apk is developed by a team of anonymous and independent developers who do not have an official website or social media account. Therefore, it is difficult to contact them directly or get support from them. However, you can try to leave a comment or feedback on the website where you downloaded bitaim apk from, and hope that they will see it and respond to it.
-
Q: What are some tips and tricks for playing 8 ball pool?
-
A: 8 ball pool is a game that requires skill, strategy, and practice to master. Here are some tips and tricks that can help you improve your game and win more matches:
-
-
Practice your aim and power by playing in offline mode or practice mode.
-
Learn the different types of shots and when to use them, such as straight shots, bank shots, cut shots, spin shots, curve shots, and jump shots.
-
Use the right cue for the right situation, and upgrade your cues with coins or gems to increase their attributes, such as aim, power, spin, and time.
-
Plan your shots ahead and think about the position of the cue ball and the object balls after each shot.
-
Use the chat feature to communicate with your opponent and show your sportsmanship.
-
- 401be4b1e0
-
-
\ No newline at end of file
diff --git a/spaces/1phancelerku/anime-remove-background/Boost Your Brain Power with Mental Arithmetic Techniques.md b/spaces/1phancelerku/anime-remove-background/Boost Your Brain Power with Mental Arithmetic Techniques.md
deleted file mode 100644
index f61140c1e82e1bc24caaebfe2cd0427369688f1a..0000000000000000000000000000000000000000
--- a/spaces/1phancelerku/anime-remove-background/Boost Your Brain Power with Mental Arithmetic Techniques.md
+++ /dev/null
@@ -1,111 +0,0 @@
-
-
How to Practice and Improve Your Mental Arithmetic Skills
-
Mental arithmetic is the skill of doing calculations in your head without using any tools or devices, such as a calculator, pen and paper, or abacus. It is a valuable skill that can help you in many everyday situations, such as shopping, cooking, traveling, and more. It can also improve your number sense, logical thinking, memory, and speed of computation.
But how do you practice and improve your mental arithmetic skills? What are some tips and techniques that can make it easier and faster? And what are some games and resources that can challenge you and make it fun? In this article, we will answer these questions and provide you with some useful information on how to become a master of mental math.
-
Tips and Techniques for Mental Arithmetic
-
There are many tips and techniques that can help you perform mental arithmetic more efficiently and accurately. Here are some of the most common ones:
-
Break down the problems into parts
-
One of the easiest ways to simplify mental arithmetic problems is to break them down into smaller parts that are easier to handle. For example, if you need to add or subtract several numbers, you can group them by their place value (hundreds, tens, ones) and add or subtract them separately. For example:
mental aritmetik eğitimi
-mental aritmetik kursu
-mental aritmetik nedir
-mental aritmetik nasıl yapılır
-mental aritmetik faydaları
-mental aritmetik örnekleri
-mental aritmetik kitabı
-mental aritmetik abaküs
-mental aritmetik uygulaması
-mental aritmetik sertifikası
-mental aritmetik beyin gelişimi
-mental aritmetik hafıza teknikleri
-mental aritmetik zeka testi
-mental aritmetik online eğitim
-mental aritmetik ders programı
-mental aritmetik egzersizleri
-mental aritmetik çarpım tablosu
-mental aritmetik matematik oyunları
-mental aritmetik soru bankası
-mental aritmetik öğretmeni
-mental aritmetik franchise
-mental aritmetik yorumları
-mental aritmetik videoları
-mental aritmetik çalışma saatleri
-mental aritmetik fiyatları
-mental aritmetik indirim kuponu
-mental aritmetik başarı hikayeleri
-mental aritmetik sınav soruları
-mental aritmetik öğrenci girişi
-mental aritmetik veli bilgilendirme sistemi
-mental aritmetik iş ilanları
-mental aritmetik bayilik şartları
-mental aritmetik seminerleri
-mental aritmetik yarışması
-mental aritmetik kampı
-mental aritmetik blog yazıları
-mental aritmetik sosyal medya hesapları
-mental aritmetik web sitesi tasarımı
-mental aritmetik logo tasarımı
-mental aritmetik broşür tasarımı
-
Use round numbers and adjust later
-
Another way to make mental arithmetic easier is to use round numbers that are close to the original ones and adjust the answer later by adding or subtracting the difference. For example:
-
596 + 380 = (600 + 380) - 4 = 980 - 4 = 976
-
38 x 3 = (40 x 3) - (2 x 3) = 120 - 6 = 114
-
Reorder the numbers to make convenient sums
-
Sometimes, you can reorder the numbers in an addition or subtraction problem to make convenient sums that are easy to remember or work with. For example, you can look for numbers that add up to a multiple of 10 or a power of 10. For example:
Square numbers are the result of multiplying a number by itself, such as 4 × 4 = 16 or 9 × 9 = 81. Knowing some common square numbers can help you with mental arithmetic, especially when you need to multiply or divide large numbers. For example:
Here, we used the identity (a − b) × (a + b) = a² − b² to simplify the problem. We also used the fact that 50² = 2500, which is easy to remember.
-
Roots are the opposite of squares. The square root of a number is the number that, when multiplied by itself, gives that number. For example, the square root of 16 is 4, because 4 × 4 = 16. Finding square roots mentally can be tricky, but there are some methods that can help you estimate them or find them exactly. For example:
-
To estimate the square root of a number, find the two nearest square numbers and use them as a guide. For example, to estimate the square root of 75, we can use the fact that 64 < 75 < 81, and that the square roots of 64 and 81 are 8 and 9, respectively. Therefore, the square root of 75 is between 8 and 9, closer to 9 than to 8.
-
To find the exact square root of a number, use the fact that the difference between two consecutive square numbers is equal to the sum of their square roots. For example, to find the square root of 169, we can use the fact that 169 − 144 = 25, and that the square roots of 169 and 144 are x and 12, respectively. Therefore, x + 12 = 25, and x = 13.
-
Estimate and approximate
-
Sometimes, you don't need to find the exact answer to a mental arithmetic problem, but only an estimate or an approximation. This can save you time and effort, and still give you a reasonable idea of the magnitude of the answer. Estimating and approximating can involve various techniques, such as rounding numbers, using benchmarks or reference points, using fractions or percentages, or using compatible numbers. For example:
-
To estimate how much money you will save by buying a shirt that is on sale for $24.99 instead of $29.99, you can round both prices to the nearest dollar and subtract them: $30 − $25 = $5. This is not the exact answer, but it is close enough for most purposes.
-
To approximate how many hours are in a year, you can use the benchmark that one year is about 365 days, and multiply it by 24: 365 × 24 = (360 +5) ×24=360×24+5×24=8640+120=8760. This is not the exact answer either, because it does not account for leap years or fractional hours, but it is a good approximation.
-
Games and Resources for Mental Arithmetic
-
If you want to practice and improve your mental arithmetic skills further, there are many games and resources that you can use to challenge yourself and have fun. Here are some examples:
-
Math Trainer
-
Math Trainer is a free online tool that lets you practice mental arithmetic with different types of problems and difficulty levels. You can choose from addition, subtraction, multiplication, division, mixed operations, fractions, decimals, percentages, powers and roots. You can also set a time limit and track your progress and accuracy.
-
Mental Math Cards
-
Mental Math Cards is a free app for iOS and Android devices that helps you practice mental arithmetic with flashcards. You can customize your settings to choose from different operations, number ranges, decimal places and time limits. You can also view your statistics and achievements.
-
Arithmetic Game
-
Arithmetic Game is a free online game that tests your mental arithmetic skills with four basic operations: addition, subtraction, multiplication and division. You have to fill in the blanks with the correct numbers to complete the equations as fast as you can. You can choose from three difficulty levels: easy, normal and hard.
-
Prodigy Game
-
Prodigy Game is a free online game that combines math skills with an adventure story. You have to create your own character and explore a fantasy world where you have to solve math problems to progress and unlock new features. You can choose from different topics and skills, such as mental arithmetic, fractions, geometry, algebra and more. You can also play with your friends and compete with other players. Prodigy Game is available for free on the web, or as an app for iOS and Android devices.
-
Mathnasium
-
Mathnasium is a learning center that offers personalized math tutoring and instruction for students of all ages and levels. Mathnasium uses a unique method that helps students develop their mental arithmetic skills, as well as their conceptual understanding, problem-solving abilities and confidence in math. Mathnasium has over 1,000 locations across the US and Canada, and you can find the nearest one to you on their website.
-
Conclusion
-
Mental arithmetic is a skill that can benefit you in many ways, both in school and in life. It can help you perform calculations faster and more accurately, improve your number sense and logical thinking, enhance your memory and concentration, and save you time and resources. By practicing some tips and techniques, such as breaking down problems, using round numbers, reordering numbers, multiplying from left to right, using square numbers and roots, and estimating and approximating, you can make mental arithmetic easier and more efficient. You can also use some games and resources, such as Math Trainer, Mental Math Cards, Arithmetic Game, Prodigy Game and Mathnasium, to challenge yourself and have fun while learning mental arithmetic.
-
FAQs
-
Here are some common questions and answers about mental arithmetic:
-
Q: How can I improve my mental arithmetic speed?
-
A: To improve your mental arithmetic speed, you need to practice regularly and consistently. You can use some of the games and resources mentioned above to practice different types of problems and difficulty levels. You can also set a time limit for yourself and try to beat your own records. The more you practice, the more familiar you will become with the numbers and the operations, and the faster you will be able to perform them.
-
Q: What are some benefits of mental arithmetic for children?
-
A: Mental arithmetic can help children develop their math skills from an early age. It can help them understand the meaning and relationships of numbers, operations, fractions, decimals, percentages and more. It can also help them improve their logical thinking, reasoning, creativity, memory and concentration. Mental arithmetic can also boost their confidence and motivation in math, as they can see their progress and achievements.
-
Q: What are some challenges of mental arithmetic?
-
A: Mental arithmetic can be challenging for some people because it requires a lot of attention, focus and mental effort. It can also be affected by factors such as stress, anxiety, fatigue or distraction. Some people may also have difficulties with certain types of problems or operations, such as division or fractions. To overcome these challenges, it is important to practice mental arithmetic in a relaxed and positive environment, start with simple problems and gradually increase the complexity, use some tips and techniques to simplify the problems, check your answers for accuracy, and seek help or feedback if needed.
-
Q: What are some applications of mental arithmetic in real life?
-
A: Mental arithmetic can be useful in many real-life situations, such as:
-
-
Shopping: You can use mental arithmetic to compare prices, calculate discounts, taxes or tips, or make a budget.
-
Cooking: You can use mental arithmetic to measure ingredients, convert units or temperatures, or adjust recipes.
-
Traveling: You can use mental arithmetic to plan your itinerary, convert currencies or distances, or estimate time or costs.
-
Gaming: You can use mental arithmetic to keep score, strategize or optimize your moves, or increase your chances of winning.
-
Learning: You can use mental arithmetic to reinforce your math skills, learn new concepts or topics, or prepare for exams or tests.
-
-
Q: How can I make mental arithmetic fun?
-
A: There are many ways to make mental arithmetic fun, such as:
-
-
Playing games: You can play some of the games mentioned above or create your own games with cards, dice, coins, or other objects. You can also play with your friends or family and make it a competition or a collaboration.
-
Using real-life scenarios: You can use mental arithmetic to solve problems or answer questions that relate to your interests, hobbies, or goals. For example, you can use mental arithmetic to calculate how much money you need to save for a trip, how many calories you burn in a workout, or how many books you can read in a year.
-
Setting goals and rewards: You can set goals for yourself to improve your mental arithmetic skills, such as solving a certain number of problems in a given time, reaching a certain level of difficulty, or learning a new technique or trick. You can also reward yourself for achieving your goals, such as buying yourself a treat, watching your favorite show, or doing something fun.
-
-
I hope you enjoyed this article and learned something new about mental arithmetic. If you have any questions or comments, feel free to leave them below. And don't forget to practice and have fun with mental arithmetic!
197e85843d
-
-
\ No newline at end of file
diff --git a/spaces/1phancelerku/anime-remove-background/Enjoy Clash of Clans APK with Unlimited Gems Gold and Elixir.md b/spaces/1phancelerku/anime-remove-background/Enjoy Clash of Clans APK with Unlimited Gems Gold and Elixir.md
deleted file mode 100644
index 4240634d29cfbbcf85d88eca46fdb4ee7e9a0d9d..0000000000000000000000000000000000000000
--- a/spaces/1phancelerku/anime-remove-background/Enjoy Clash of Clans APK with Unlimited Gems Gold and Elixir.md
+++ /dev/null
@@ -1,94 +0,0 @@
-
-
Clash of Clans Orjinal APK: How to Download and Play the Epic Strategy Game
-
Clash of Clans is one of the most popular and addictive strategy games in the world. Millions of players worldwide join forces to build their villages, train their troops, and fight in epic clan wars. If you are looking for a fun and challenging game that will keep you entertained for hours, you should definitely try Clash of Clans. But how can you download and play the game on your Android device? In this article, we will show you how to get the orjinal APK of Clash of Clans, which is the official version of the game from a trusted source. We will also give you some tips and tricks on how to play the game and become a successful clasher.
Clash of Clans is a strategy game that was released in 2012 by Supercell, a Finnish game developer. The game is set in a fantasy world where you can create your own village, customize it with various buildings and defenses, and collect resources such as gold, elixir, and dark elixir. You can also recruit different types of troops, such as barbarians, archers, wizards, dragons, and more, and use them to attack other players' villages or defend your own. The game also features a multiplayer mode where you can join or create a clan, which is a group of players who can chat, donate troops, and participate in clan wars. Clan wars are special events where two clans face each other in a series of attacks and try to earn more stars than their opponents. The game also has a single-player mode where you can fight against the goblin king and his army in a campaign mode.
-
Why Download the Orjinal APK?
-
The orjinal APK of Clash of Clans is the official version of the game that you can download from Google Play Store or from Supercell's website. There are many advantages of downloading the orjinal APK instead of using unofficial or modded versions of the game. Some of these advantages are:
-
-
You can enjoy the latest updates and features of the game as soon as they are released by Supercell.
-
You can avoid any potential risks or problems that may come with using unverified or hacked versions of the game, such as viruses, malware, bans, or loss of data.
-
You can support Supercell as a developer and help them continue making great games for their fans.
-
-
How to Download and Install the Orjinal APK?
-
Downloading and installing the orjinal APK of Clash of Clans is very easy and simple. Just follow these steps:
-
-
Go to Google Play Store on your Android device and search for Clash of Clans. Alternatively, you can go to Supercell's website (https://supercell.com/en/games/clashofclans/) and click on "Download Now".
-
Tap on "Install" and wait for the download to finish.
-
Once the download is complete, tap on "Open" and enjoy playing Clash of Clans.
-
-
Note: If you have an existing account or village on another device, you can link it to your new device by using Supercell ID or Google Play Games. Just go to Settings > Account > Link Device or Sign In.
-
How to Play Clash of Clans?
-
Playing Clash of Clans is fun and easy once you get the hang of it. Here are some tips and tricks on how to play the game and become a successful clasher:
-
clash of clans apk download latest version
-clash of clans mod apk unlimited everything
-clash of clans hack apk free download
-clash of clans apk indir android oyun club
-clash of clans apk update 2023
-clash of clans private server apk download
-clash of clans apk hile nasıl yapılır
-clash of clans apk mirror download
-clash of clans apk pure free download
-clash of clans apk mod menu
-clash of clans apk offline mode
-clash of clans apk no root required
-clash of clans apk yeni sürüm indir
-clash of clans apk for pc windows 10
-clash of clans apk full version download
-clash of clans apk cheat codes
-clash of clans apk hack online generator
-clash of clans apk orjinal kurulumu
-clash of clans apk son sürüm 2023
-clash of clans apk android 4.4.2
-clash of clans apk unlimited gems and coins
-clash of clans apk modded by ihackedit
-clash of clans apk free shopping
-clash of clans apk güncelleme sorunu
-clash of clans apk for ios devices
-clash of clans apk mod offline unlimited money
-clash of clans apk hack tool download
-clash of clans apk orjinal nasıl indirilir
-clash of clans apk eski sürüm indir
-clash of clans apk android 11 support
-clash of clans apk unlimited troops and spells
-clash of clans apk mod anti ban
-clash of clans apk free gems generator
-clash of clans apk hileli indir 2023
-clash of clans apk for fire tablet
-clash of clans apk mod unlimited gold and elixir
-clash of clans apk hack no survey no password
-clash of clans apk orjinal yükleme yöntemi
-clash of clans apk yeni güncelleme ne zaman gelecek
-clash of clans apk android 5.1.1 download
-clash of clans apk unlimited builder base resources
-clash of clans apk mod unlock all heroes and troops
-clash of clans apk free download for laptop
-clash of clans apk hile yapma programı indir
-clash of clans apk for chromebook download
-clash of clans apk mod supercell id login fix
-clash of clans apk free magic items and books
-clash of clans apk hileli oyun indir club
-
-
Build and upgrade your buildings and defenses. You can use gold and elixir to build and upgrade various structures in your village, such as town hall, barracks, army camps, walls, cannons, archer towers, mortars, and more. These structures will help you protect your village from enemy attacks and produce more resources for you.
-
Train and upgrade your troops. You can use elixir and dark elixir to train and upgrade different types of troops in your barracks and dark barracks, such as barbarians, archers, giants, goblins, wizards, dragons, pekkas, minions, hog riders, golems, and more. These troops will help you attack other players' villages and loot their resources.
-
Join or create a clan. You can join or create a clan by tapping on the clan castle in your village. A clan is a group of players who can chat, donate troops, and participate in clan wars. Being in a clan will give you many benefits, such as getting reinforcements from your clanmates, requesting and receiving clan gifts, earning clan perks, and more.
-
Participate in clan wars. Clan wars are special events where two clans face each other in a series of attacks and try to earn more stars than their opponents. To participate in a clan war, you need to be in a clan and have your clan castle rebuilt. You can also opt out of the war if you don't want to participate. To win a clan war, you need to plan your attacks carefully, use your best troops and spells, scout the enemy bases, and coordinate with your clanmates.
-
Complete achievements and events. You can earn gems, which are the premium currency of the game, by completing various achievements and events. Achievements are long-term goals that you can accomplish by playing the game regularly, such as reaching a certain town hall level, winning a number of battles, collecting a certain amount of resources, and more. Events are short-term challenges that you can complete by using specific troops or spells in battles, such as using barbarians or rage spells. Gems can be used to speed up building or troop upgrades, buy more resources or shields, or get special items.
-
-
Conclusion
-
Clash of Clans is an epic strategy game that will keep you hooked for hours. You can download the orjinal APK of the game from Google Play Store or Supercell's website and enjoy the latest updates and features of the game. You can also learn how to play the game and become a successful clasher by following our tips and tricks. So what are you waiting for? Download Clash of Clans today and join the millions of players worldwide who are having fun building their villages, raising their clans, and fighting in clan wars.
-
FAQs
-
Here are some common questions and answers about Clash of Clans:
-
Q: How can I get free gems in Clash of Clans?
-
A: You can get free gems by completing achievements and events, removing obstacles from your village, opening gem boxes or gem mines, or participating in special offers or surveys.
-
Q: How can I change my name in Clash of Clans?
-
A: You can change your name once for free by going to Settings > Change Name. After that, you will need to pay 500 gems to change your name again.
-
Q: How can I transfer my village to another device?
-
A: You can transfer your village to another device by using Supercell ID or Google Play Games. Just go to Settings > Account > Link Device or Sign In on both devices and follow the instructions.
-
Q: How can I contact Supercell for support or feedback?
-
A: You can contact Supercell by going to Settings > Help and Support > Contact Us or by visiting their website (https://supercell.helpshift.com/a/clash-of-clans/).
-
Q: How can I report a bug or a player in Clash of Clans?
-
A: You can report a bug or a player by going to Settings > Help and Support > Report an Issue or Report Player.
197e85843d
-
-
\ No newline at end of file
diff --git a/spaces/1phancelerku/anime-remove-background/Enjoy the Festive Season with Daystar Choirs 12 Days of Christmas MP3 Download.md b/spaces/1phancelerku/anime-remove-background/Enjoy the Festive Season with Daystar Choirs 12 Days of Christmas MP3 Download.md
deleted file mode 100644
index 57ded0ebe30299ba83c6959b6f8db552344150a6..0000000000000000000000000000000000000000
--- a/spaces/1phancelerku/anime-remove-background/Enjoy the Festive Season with Daystar Choirs 12 Days of Christmas MP3 Download.md
+++ /dev/null
@@ -1,147 +0,0 @@
-
-
How to Download 12 Days of Christmas by Daystar Choir MP3
-
Christmas is a season of joy, celebration, and music. One of the most festive and cheerful songs that you can listen to during this time is 12 Days of Christmas by Daystar Choir. This song is a live performance by a Nigerian gospel choir that sings a medley of traditional and modern Christmas carols with a twist. It is a fun and lively song that will make you dance and sing along.
-
download 12 days of christmas by daystar choir mp3
But how can you download this song as an MP3 file and enjoy it anytime and anywhere? In this article, we will show you where to find this song online and how to download it as an MP3 file. We will also tell you why this song is so popular and what are the benefits of downloading it as an MP3 file.
-
Where to Find 12 Days of Christmas by Daystar Choir MP3
-
There are two main ways to find this song online: online streaming platforms and free music download websites. Here are some examples of each option:
-
Online Streaming Platforms
-
Online streaming platforms are websites or apps that allow you to listen to music online without downloading it. Some of the most popular online streaming platforms that have this song are:
-
-
Spotify: Spotify is one of the largest and most popular online streaming platforms in the world. It has millions of songs, podcasts, and playlists that you can listen to for free or with a premium subscription. You can find this song on Spotify by searching for "12 Days of Christmas - Live" by Daystar Choir .
-
Shazam: Shazam is an app that can identify any song that is playing around you. It can also show you the lyrics, artist, album, genre, and other information about the song. You can also listen to the song on Shazam or connect it to other streaming platforms like Spotify, Apple Music, YouTube, etc. You can find this song on Shazam by searching for "12 Days of Christmas (Live)" by Daystar Choir.
-
YouTube: YouTube is the most popular video-sharing platform in the world. It has billions of videos, including music videos, live performances, covers, remixes, etc. You can watch and listen to this song on YouTube by searching for "Daystar Carol 2016 Ft Taiwo Tfavored in Glory Halleluyah", "Brooklyn Tabernacle Choir \" Daystar \"", or "Daystar Carol 2019 - Daystar Choir Ministration".
-
-
Free Music Download Websites
Free music download websites are websites that allow you to download music for free and legally. Some of the free music download websites that have this song are:
-
download 12 days of christmas by daystar choir mp3 free
-download 12 days of christmas by daystar choir mp3 online
-download 12 days of christmas by daystar choir mp3 lyrics
-download 12 days of christmas by daystar choir mp3 shazam
-download 12 days of christmas by daystar choir mp3 last.fm
-download 12 days of christmas by daystar choir mp3 album
-download 12 days of christmas by daystar choir mp3 live
-download 12 days of christmas by daystar choir mp3 video
-download 12 days of christmas by daystar choir mp3 song
-download 12 days of christmas by daystar choir mp3 music
-download 12 days of christmas by daystar choir mp3 youtube
-download 12 days of christmas by daystar choir mp3 spotify
-download 12 days of christmas by daystar choir mp3 apple music
-download 12 days of christmas by daystar choir mp3 soundcloud
-download 12 days of christmas by daystar choir mp3 amazon music
-download 12 days of christmas by daystar choir mp3 google play music
-download 12 days of christmas by daystar choir mp3 deezer
-download 12 days of christmas by daystar choir mp3 tidal
-download 12 days of christmas by daystar choir mp3 pandora
-download 12 days of christmas by daystar choir mp3 napster
-download 12 days of christmas by daystar choir mp3 audiomack
-download 12 days of christmas by daystar choir mp3 bandcamp
-download 12 days of christmas by daystar choir mp3 reverbnation
-download 12 days of christmas by daystar choir mp3 datpiff
-download 12 days of christmas by daystar choir mp3 mixcloud
-download 12 days of christmas by daystar choir mp3 nigerian carols
-download 12 days of christmas by daystar choir mp3 ogo ni fun baba
-download 12 days of christmas by daystar choir mp3 jesu yi o iwo l'ologo didan
-download 12 days of christmas by daystar choir mp3 ding-dong feat taiwo oladoye
-download 12 days of christmas by daystar choir mp3 glory halleluyah feat taiwo oladoye
-download 12 days of christmas by daystar choir mp3 gbo ohun
-download 12 days of christmas by daystar choir mp3 dulci jubilo
-download 12 days of christmas by daystar choir mp3 joy festizie
-download 12 days of christmas by daystar choir mp3 nina yesu ne chingtok ishaku
-download 12 days of christmas by daystar choir mp3 almighty god dr pastor paul enenche
-download 12 days of christmas by daystar choir mp3 nagode feat solomon lange worship for change
-download 12 days of christmas by daystar choir mp3 you are the god dr paul enenche
-download 12 days of christmas by daystar choir mp3 solid rock judikay
-download 12 days of christmas by daystar choir mp3 elee dr pastor paul enenche
-download 12 days of christmas by daystar choir mp3 alpha and omega praise and worship
-how to download 12 days of christmas by daystar choir mp3
-where to download 12 days of christmas by daystar choir mp3
-best site to download 12 days of christmas by daystar choir mp3
-best app to download 12 days of christmas by daystar choir mp3
-best quality to download 12 days of christmas by daystar choir mp3
-best format to download 12 days of christmas by daystar choir mp3
-best device to download 12 days of christmas by daystar choir mp3
-best vpn to download 12 days of christmas by daystar choir mp3
-best proxy to download 12 days of christmas by daystar choir mp3
-
-
Chosic: Chosic is a website that offers free music downloads from various genres and artists. You can also create playlists, discover new music, and share your favorites with others. You can find this song on Chosic by searching for "12 Days of Christmas - Live" by Daystar Choir.
-
Pixabay: Pixabay is a website that offers free images, videos, and music that you can use for any purpose. You can browse through thousands of royalty-free music tracks and download them in MP3 or WAV format. You can find this song on Pixabay by searching for "12 Days of Christmas" by Daystar Choir.
-
Free Music Archive: Free Music Archive is a website that provides a library of high-quality, legal audio downloads. You can explore music by genre, mood, license, or curator. You can also contribute your own music or support the artists you like. You can find this song on Free Music Archive by searching for "12 Days of Christmas - Live" by Daystar Choir.
-
-
How to Download 12 Days of Christmas by Daystar Choir MP3
-
Now that you know where to find this song online, how can you download it as an MP3 file? The process may vary depending on the source, but here are some general steps that you can follow:
-
From Online Streaming Platforms
-
If you want to download this song from online streaming platforms like Spotify, Shazam, or YouTube, you will need to use a third-party tool or app that can convert the song to MP3 format. There are many tools and apps available online, but some of the most popular ones are:
-
-
-
Tool/App
-
Website/Download Link
-
Features
-
-
-
4K Video Downloader
-
-
- Supports YouTube, Spotify, SoundCloud, Vimeo, TikTok, and more - Allows you to download videos, playlists, channels, subtitles, and 3D/360° videos - Supports MP3, MP4, MKV, FLV, OGG, and more formats - Offers high-quality and fast downloads - Available for Windows, Mac, and Linux
-
-
-
AudFree Spotify Music Converter
-
-
- Supports Spotify songs, playlists, albums, podcasts, and radio - Allows you to download Spotify music offline without premium - Supports MP3, FLAC, WAV, AAC, M4A, and M4B formats - Offers lossless quality and 5X speed - Available for Windows and Mac
-
-
-
Shazam Downloader
-
-
- Supports Shazam songs and playlists - Allows you to download Shazam music with one click - Supports MP3 format - Offers high-quality downloads - Available for Android devices
-
-
-
To download this song from online streaming platforms using these tools or apps, you need to follow these steps:
-
-
Open the online streaming platform and find the song that you want to download.
-
Copy the URL or link of the song.
-
Open the tool or app that you have chosen and paste the URL or link into the input box.
-
Select the MP3 format and quality that you want.
-
Click on the download or convert button and wait for the process to finish.
-
Save the MP3 file to your device or cloud storage.
-
-
From Free Music Download Websites
If you want to download this song from free music download websites like Chosic, Pixabay, or Free Music Archive, you will not need to use any third-party tool or app. You can simply download the song directly from the website. To download this song from free music download websites, you need to follow these steps:
-
-
Open the free music download website and search for the song that you want to download.
-
Click on the song title or the download button or link.
-
Select the MP3 format and quality that you want.
-
Save the MP3 file to your device or cloud storage.
-
-
Conclusion
-
12 Days of Christmas by Daystar Choir is a wonderful song that will brighten up your Christmas season. It is a live performance by a talented gospel choir that sings a medley of classic and modern Christmas carols with a twist. It is a fun and lively song that will make you dance and sing along.
-
You can download this song as an MP3 file and enjoy it anytime and anywhere. You can find this song online on various online streaming platforms and free music download websites. You can also use different tools and apps to convert and download the song as an MP3 file. All you need to do is follow the steps that we have shown you in this article.
-
So what are you waiting for? Download 12 Days of Christmas by Daystar Choir MP3 today and have a merry Christmas!
-
FAQs
-
What is Daystar Choir?
-
Daystar Choir is a gospel choir from Nigeria that is part of the Daystar Christian Centre. The choir is known for its annual Christmas carol concerts that feature various songs, dances, and performances. The choir has also released several albums and singles, such as "Glory Halleluyah", "Hark the Herald", and "Joy to the World".
-
What are some other songs by Daystar Choir?
-
Some other songs by Daystar Choir are:
-
-
"O Come All Ye Faithful"
-
"Silent Night"
-
"We Wish You a Merry Christmas"
-
"Jingle Bells"
-
"Feliz Navidad"
-
-
How can I support Daystar Choir?
-
You can support Daystar Choir by:
-
-
Following them on their social media accounts, such as Facebook, Twitter, Instagram, and YouTube
-
Subscribing to their newsletter or blog
-
Donating to their ministry or charity projects
-
Purchasing their albums or merchandise
-
Attending their concerts or events
-
-
What are some other Christmas songs that I can download for free?
Some other Christmas songs that you can download for free are:
-
-
"O Holy Night" by Josh Groban: This is a beautiful rendition of the classic Christmas hymn by the famous singer and songwriter. You can download this song for free from Chosic by searching for "O Holy Night" by Josh Groban.
-
"All I Want for Christmas Is You" by Mariah Carey: This is one of the most popular and catchy Christmas songs of all time. It is a love song that expresses the desire to be with someone special for Christmas. You can download this song for free from Pixabay by searching for "All I Want for Christmas Is You" by Mariah Carey.
-
"Jingle Bell Rock" by Bobby Helms: This is a fun and upbeat rock and roll version of the traditional Christmas song. It is a song that will make you want to dance and celebrate. You can download this song for free from Free Music Archive by searching for "Jingle Bell Rock" by Bobby Helms.
-
-
These are just some examples of the many Christmas songs that you can download for free online. You can explore more options by browsing through the websites that we have mentioned or using other sources that you trust. Just make sure that the songs are legal and royalty-free before you download them.
-
We hope that this article has helped you learn how to download 12 Days of Christmas by Daystar Choir MP3 and enjoy this wonderful song. We also hope that you have discovered some other Christmas songs that you can download for free and add to your holiday playlist. Have a merry Christmas and a happy new year!
- 197e85843d
-
-
\ No newline at end of file
diff --git a/spaces/801artistry/RVC801/demucs/__main__.py b/spaces/801artistry/RVC801/demucs/__main__.py
deleted file mode 100644
index 5148f20623bdaa827777558844796ded1876d7d0..0000000000000000000000000000000000000000
--- a/spaces/801artistry/RVC801/demucs/__main__.py
+++ /dev/null
@@ -1,317 +0,0 @@
-# Copyright (c) Facebook, Inc. and its affiliates.
-# All rights reserved.
-#
-# This source code is licensed under the license found in the
-# LICENSE file in the root directory of this source tree.
-
-import json
-import math
-import os
-import sys
-import time
-from dataclasses import dataclass, field
-
-import torch as th
-from torch import distributed, nn
-from torch.nn.parallel.distributed import DistributedDataParallel
-
-from .augment import FlipChannels, FlipSign, Remix, Scale, Shift
-from .compressed import get_compressed_datasets
-from .model import Demucs
-from .parser import get_name, get_parser
-from .raw import Rawset
-from .repitch import RepitchedWrapper
-from .pretrained import load_pretrained, SOURCES
-from .tasnet import ConvTasNet
-from .test import evaluate
-from .train import train_model, validate_model
-from .utils import (human_seconds, load_model, save_model, get_state,
- save_state, sizeof_fmt, get_quantizer)
-from .wav import get_wav_datasets, get_musdb_wav_datasets
-
-
-@dataclass
-class SavedState:
- metrics: list = field(default_factory=list)
- last_state: dict = None
- best_state: dict = None
- optimizer: dict = None
-
-
-def main():
- parser = get_parser()
- args = parser.parse_args()
- name = get_name(parser, args)
- print(f"Experiment {name}")
-
- if args.musdb is None and args.rank == 0:
- print(
- "You must provide the path to the MusDB dataset with the --musdb flag. "
- "To download the MusDB dataset, see https://sigsep.github.io/datasets/musdb.html.",
- file=sys.stderr)
- sys.exit(1)
-
- eval_folder = args.evals / name
- eval_folder.mkdir(exist_ok=True, parents=True)
- args.logs.mkdir(exist_ok=True)
- metrics_path = args.logs / f"{name}.json"
- eval_folder.mkdir(exist_ok=True, parents=True)
- args.checkpoints.mkdir(exist_ok=True, parents=True)
- args.models.mkdir(exist_ok=True, parents=True)
-
- if args.device is None:
- device = "cpu"
- if th.cuda.is_available():
- device = "cuda"
- else:
- device = args.device
-
- th.manual_seed(args.seed)
- # Prevents too many threads to be started when running `museval` as it can be quite
- # inefficient on NUMA architectures.
- os.environ["OMP_NUM_THREADS"] = "1"
- os.environ["MKL_NUM_THREADS"] = "1"
-
- if args.world_size > 1:
- if device != "cuda" and args.rank == 0:
- print("Error: distributed training is only available with cuda device", file=sys.stderr)
- sys.exit(1)
- th.cuda.set_device(args.rank % th.cuda.device_count())
- distributed.init_process_group(backend="nccl",
- init_method="tcp://" + args.master,
- rank=args.rank,
- world_size=args.world_size)
-
- checkpoint = args.checkpoints / f"{name}.th"
- checkpoint_tmp = args.checkpoints / f"{name}.th.tmp"
- if args.restart and checkpoint.exists() and args.rank == 0:
- checkpoint.unlink()
-
- if args.test or args.test_pretrained:
- args.epochs = 1
- args.repeat = 0
- if args.test:
- model = load_model(args.models / args.test)
- else:
- model = load_pretrained(args.test_pretrained)
- elif args.tasnet:
- model = ConvTasNet(audio_channels=args.audio_channels,
- samplerate=args.samplerate, X=args.X,
- segment_length=4 * args.samples,
- sources=SOURCES)
- else:
- model = Demucs(
- audio_channels=args.audio_channels,
- channels=args.channels,
- context=args.context,
- depth=args.depth,
- glu=args.glu,
- growth=args.growth,
- kernel_size=args.kernel_size,
- lstm_layers=args.lstm_layers,
- rescale=args.rescale,
- rewrite=args.rewrite,
- stride=args.conv_stride,
- resample=args.resample,
- normalize=args.normalize,
- samplerate=args.samplerate,
- segment_length=4 * args.samples,
- sources=SOURCES,
- )
- model.to(device)
- if args.init:
- model.load_state_dict(load_pretrained(args.init).state_dict())
-
- if args.show:
- print(model)
- size = sizeof_fmt(4 * sum(p.numel() for p in model.parameters()))
- print(f"Model size {size}")
- return
-
- try:
- saved = th.load(checkpoint, map_location='cpu')
- except IOError:
- saved = SavedState()
-
- optimizer = th.optim.Adam(model.parameters(), lr=args.lr)
-
- quantizer = None
- quantizer = get_quantizer(model, args, optimizer)
-
- if saved.last_state is not None:
- model.load_state_dict(saved.last_state, strict=False)
- if saved.optimizer is not None:
- optimizer.load_state_dict(saved.optimizer)
-
- model_name = f"{name}.th"
- if args.save_model:
- if args.rank == 0:
- model.to("cpu")
- model.load_state_dict(saved.best_state)
- save_model(model, quantizer, args, args.models / model_name)
- return
- elif args.save_state:
- model_name = f"{args.save_state}.th"
- if args.rank == 0:
- model.to("cpu")
- model.load_state_dict(saved.best_state)
- state = get_state(model, quantizer)
- save_state(state, args.models / model_name)
- return
-
- if args.rank == 0:
- done = args.logs / f"{name}.done"
- if done.exists():
- done.unlink()
-
- augment = [Shift(args.data_stride)]
- if args.augment:
- augment += [FlipSign(), FlipChannels(), Scale(),
- Remix(group_size=args.remix_group_size)]
- augment = nn.Sequential(*augment).to(device)
- print("Agumentation pipeline:", augment)
-
- if args.mse:
- criterion = nn.MSELoss()
- else:
- criterion = nn.L1Loss()
-
- # Setting number of samples so that all convolution windows are full.
- # Prevents hard to debug mistake with the prediction being shifted compared
- # to the input mixture.
- samples = model.valid_length(args.samples)
- print(f"Number of training samples adjusted to {samples}")
- samples = samples + args.data_stride
- if args.repitch:
- # We need a bit more audio samples, to account for potential
- # tempo change.
- samples = math.ceil(samples / (1 - 0.01 * args.max_tempo))
-
- args.metadata.mkdir(exist_ok=True, parents=True)
- if args.raw:
- train_set = Rawset(args.raw / "train",
- samples=samples,
- channels=args.audio_channels,
- streams=range(1, len(model.sources) + 1),
- stride=args.data_stride)
-
- valid_set = Rawset(args.raw / "valid", channels=args.audio_channels)
- elif args.wav:
- train_set, valid_set = get_wav_datasets(args, samples, model.sources)
- elif args.is_wav:
- train_set, valid_set = get_musdb_wav_datasets(args, samples, model.sources)
- else:
- train_set, valid_set = get_compressed_datasets(args, samples)
-
- if args.repitch:
- train_set = RepitchedWrapper(
- train_set,
- proba=args.repitch,
- max_tempo=args.max_tempo)
-
- best_loss = float("inf")
- for epoch, metrics in enumerate(saved.metrics):
- print(f"Epoch {epoch:03d}: "
- f"train={metrics['train']:.8f} "
- f"valid={metrics['valid']:.8f} "
- f"best={metrics['best']:.4f} "
- f"ms={metrics.get('true_model_size', 0):.2f}MB "
- f"cms={metrics.get('compressed_model_size', 0):.2f}MB "
- f"duration={human_seconds(metrics['duration'])}")
- best_loss = metrics['best']
-
- if args.world_size > 1:
- dmodel = DistributedDataParallel(model,
- device_ids=[th.cuda.current_device()],
- output_device=th.cuda.current_device())
- else:
- dmodel = model
-
- for epoch in range(len(saved.metrics), args.epochs):
- begin = time.time()
- model.train()
- train_loss, model_size = train_model(
- epoch, train_set, dmodel, criterion, optimizer, augment,
- quantizer=quantizer,
- batch_size=args.batch_size,
- device=device,
- repeat=args.repeat,
- seed=args.seed,
- diffq=args.diffq,
- workers=args.workers,
- world_size=args.world_size)
- model.eval()
- valid_loss = validate_model(
- epoch, valid_set, model, criterion,
- device=device,
- rank=args.rank,
- split=args.split_valid,
- overlap=args.overlap,
- world_size=args.world_size)
-
- ms = 0
- cms = 0
- if quantizer and args.rank == 0:
- ms = quantizer.true_model_size()
- cms = quantizer.compressed_model_size(num_workers=min(40, args.world_size * 10))
-
- duration = time.time() - begin
- if valid_loss < best_loss and ms <= args.ms_target:
- best_loss = valid_loss
- saved.best_state = {
- key: value.to("cpu").clone()
- for key, value in model.state_dict().items()
- }
-
- saved.metrics.append({
- "train": train_loss,
- "valid": valid_loss,
- "best": best_loss,
- "duration": duration,
- "model_size": model_size,
- "true_model_size": ms,
- "compressed_model_size": cms,
- })
- if args.rank == 0:
- json.dump(saved.metrics, open(metrics_path, "w"))
-
- saved.last_state = model.state_dict()
- saved.optimizer = optimizer.state_dict()
- if args.rank == 0 and not args.test:
- th.save(saved, checkpoint_tmp)
- checkpoint_tmp.rename(checkpoint)
-
- print(f"Epoch {epoch:03d}: "
- f"train={train_loss:.8f} valid={valid_loss:.8f} best={best_loss:.4f} ms={ms:.2f}MB "
- f"cms={cms:.2f}MB "
- f"duration={human_seconds(duration)}")
-
- if args.world_size > 1:
- distributed.barrier()
-
- del dmodel
- model.load_state_dict(saved.best_state)
- if args.eval_cpu:
- device = "cpu"
- model.to(device)
- model.eval()
- evaluate(model, args.musdb, eval_folder,
- is_wav=args.is_wav,
- rank=args.rank,
- world_size=args.world_size,
- device=device,
- save=args.save,
- split=args.split_valid,
- shifts=args.shifts,
- overlap=args.overlap,
- workers=args.eval_workers)
- model.to("cpu")
- if args.rank == 0:
- if not (args.test or args.test_pretrained):
- save_model(model, quantizer, args, args.models / model_name)
- print("done")
- done.write_text("done")
-
-
-if __name__ == "__main__":
- main()
diff --git a/spaces/A666sxr/Genshin_TTS/text/__init__.py b/spaces/A666sxr/Genshin_TTS/text/__init__.py
deleted file mode 100644
index 48ae82f3e40ecd1bf17a7de78d87790327af3362..0000000000000000000000000000000000000000
--- a/spaces/A666sxr/Genshin_TTS/text/__init__.py
+++ /dev/null
@@ -1,56 +0,0 @@
-""" from https://github.com/keithito/tacotron """
-from text import cleaners
-from text.symbols import symbols
-
-
-# Mappings from symbol to numeric ID and vice versa:
-_symbol_to_id = {s: i for i, s in enumerate(symbols)}
-_id_to_symbol = {i: s for i, s in enumerate(symbols)}
-
-
-def text_to_sequence(text, cleaner_names):
- '''Converts a string of text to a sequence of IDs corresponding to the symbols in the text.
- Args:
- text: string to convert to a sequence
- cleaner_names: names of the cleaner functions to run the text through
- Returns:
- List of integers corresponding to the symbols in the text
- '''
- sequence = []
-
- clean_text = _clean_text(text, cleaner_names)
- for symbol in clean_text:
- if symbol not in _symbol_to_id.keys():
- continue
- symbol_id = _symbol_to_id[symbol]
- sequence += [symbol_id]
- return sequence
-
-
-def cleaned_text_to_sequence(cleaned_text):
- '''Converts a string of text to a sequence of IDs corresponding to the symbols in the text.
- Args:
- text: string to convert to a sequence
- Returns:
- List of integers corresponding to the symbols in the text
- '''
- sequence = [_symbol_to_id[symbol] for symbol in cleaned_text if symbol in _symbol_to_id.keys()]
- return sequence
-
-
-def sequence_to_text(sequence):
- '''Converts a sequence of IDs back to a string'''
- result = ''
- for symbol_id in sequence:
- s = _id_to_symbol[symbol_id]
- result += s
- return result
-
-
-def _clean_text(text, cleaner_names):
- for name in cleaner_names:
- cleaner = getattr(cleaners, name)
- if not cleaner:
- raise Exception('Unknown cleaner: %s' % name)
- text = cleaner(text)
- return text
diff --git a/spaces/AI-Hobbyist/Hoyo-RVC/trainset_preprocess_pipeline_print.py b/spaces/AI-Hobbyist/Hoyo-RVC/trainset_preprocess_pipeline_print.py
deleted file mode 100644
index 6188c866e0611eadd38228ce9d54fc6ee80576d0..0000000000000000000000000000000000000000
--- a/spaces/AI-Hobbyist/Hoyo-RVC/trainset_preprocess_pipeline_print.py
+++ /dev/null
@@ -1,139 +0,0 @@
-import sys, os, multiprocessing
-from scipy import signal
-
-now_dir = os.getcwd()
-sys.path.append(now_dir)
-
-inp_root = sys.argv[1]
-sr = int(sys.argv[2])
-n_p = int(sys.argv[3])
-exp_dir = sys.argv[4]
-noparallel = sys.argv[5] == "True"
-import numpy as np, os, traceback
-from slicer2 import Slicer
-import librosa, traceback
-from scipy.io import wavfile
-import multiprocessing
-from my_utils import load_audio
-
-mutex = multiprocessing.Lock()
-f = open("%s/preprocess.log" % exp_dir, "a+")
-
-
-def println(strr):
- mutex.acquire()
- print(strr)
- f.write("%s\n" % strr)
- f.flush()
- mutex.release()
-
-
-class PreProcess:
- def __init__(self, sr, exp_dir):
- self.slicer = Slicer(
- sr=sr,
- threshold=-42,
- min_length=1500,
- min_interval=400,
- hop_size=15,
- max_sil_kept=500,
- )
- self.sr = sr
- self.bh, self.ah = signal.butter(N=5, Wn=48, btype="high", fs=self.sr)
- self.per = 3.7
- self.overlap = 0.3
- self.tail = self.per + self.overlap
- self.max = 0.9
- self.alpha = 0.75
- self.exp_dir = exp_dir
- self.gt_wavs_dir = "%s/0_gt_wavs" % exp_dir
- self.wavs16k_dir = "%s/1_16k_wavs" % exp_dir
- os.makedirs(self.exp_dir, exist_ok=True)
- os.makedirs(self.gt_wavs_dir, exist_ok=True)
- os.makedirs(self.wavs16k_dir, exist_ok=True)
-
- def norm_write(self, tmp_audio, idx0, idx1):
- tmp_max = np.abs(tmp_audio).max()
- if tmp_max > 2.5:
- print("%s-%s-%s-filtered" % (idx0, idx1, tmp_max))
- return
- tmp_audio = (tmp_audio / tmp_max * (self.max * self.alpha)) + (
- 1 - self.alpha
- ) * tmp_audio
- wavfile.write(
- "%s/%s_%s.wav" % (self.gt_wavs_dir, idx0, idx1),
- self.sr,
- tmp_audio.astype(np.float32),
- )
- tmp_audio = librosa.resample(
- tmp_audio, orig_sr=self.sr, target_sr=16000
- ) # , res_type="soxr_vhq"
- wavfile.write(
- "%s/%s_%s.wav" % (self.wavs16k_dir, idx0, idx1),
- 16000,
- tmp_audio.astype(np.float32),
- )
-
- def pipeline(self, path, idx0):
- try:
- audio = load_audio(path, self.sr)
- # zero phased digital filter cause pre-ringing noise...
- # audio = signal.filtfilt(self.bh, self.ah, audio)
- audio = signal.lfilter(self.bh, self.ah, audio)
-
- idx1 = 0
- for audio in self.slicer.slice(audio):
- i = 0
- while 1:
- start = int(self.sr * (self.per - self.overlap) * i)
- i += 1
- if len(audio[start:]) > self.tail * self.sr:
- tmp_audio = audio[start : start + int(self.per * self.sr)]
- self.norm_write(tmp_audio, idx0, idx1)
- idx1 += 1
- else:
- tmp_audio = audio[start:]
- idx1 += 1
- break
- self.norm_write(tmp_audio, idx0, idx1)
- println("%s->Suc." % path)
- except:
- println("%s->%s" % (path, traceback.format_exc()))
-
- def pipeline_mp(self, infos):
- for path, idx0 in infos:
- self.pipeline(path, idx0)
-
- def pipeline_mp_inp_dir(self, inp_root, n_p):
- try:
- infos = [
- ("%s/%s" % (inp_root, name), idx)
- for idx, name in enumerate(sorted(list(os.listdir(inp_root))))
- ]
- if noparallel:
- for i in range(n_p):
- self.pipeline_mp(infos[i::n_p])
- else:
- ps = []
- for i in range(n_p):
- p = multiprocessing.Process(
- target=self.pipeline_mp, args=(infos[i::n_p],)
- )
- ps.append(p)
- p.start()
- for i in range(n_p):
- ps[i].join()
- except:
- println("Fail. %s" % traceback.format_exc())
-
-
-def preprocess_trainset(inp_root, sr, n_p, exp_dir):
- pp = PreProcess(sr, exp_dir)
- println("start preprocess")
- println(sys.argv)
- pp.pipeline_mp_inp_dir(inp_root, n_p)
- println("end preprocess")
-
-
-if __name__ == "__main__":
- preprocess_trainset(inp_root, sr, n_p, exp_dir)
diff --git a/spaces/AIConsultant/MusicGen/audiocraft/grids/musicgen/musicgen_pretrained_32khz_eval.py b/spaces/AIConsultant/MusicGen/audiocraft/grids/musicgen/musicgen_pretrained_32khz_eval.py
deleted file mode 100644
index 39ceaf7dab15ec3f0f669cfe57ca9e932a9ab40d..0000000000000000000000000000000000000000
--- a/spaces/AIConsultant/MusicGen/audiocraft/grids/musicgen/musicgen_pretrained_32khz_eval.py
+++ /dev/null
@@ -1,99 +0,0 @@
-# Copyright (c) Meta Platforms, Inc. and affiliates.
-# All rights reserved.
-#
-# This source code is licensed under the license found in the
-# LICENSE file in the root directory of this source tree.
-
-"""
-Evaluation with objective metrics for the pretrained MusicGen models.
-This grid takes signature from the training grid and runs evaluation-only stage.
-
-When running the grid for the first time, please use:
-REGEN=1 dora grid musicgen.musicgen_pretrained_32khz_eval
-and re-use the REGEN=1 option when the grid is changed to force regenerating it.
-
-Note that you need the proper metrics external libraries setup to use all
-the objective metrics activated in this grid. Refer to the README for more information.
-"""
-
-import os
-
-from ._explorers import GenerationEvalExplorer
-from ...environment import AudioCraftEnvironment
-from ... import train
-
-
-def eval(launcher, batch_size: int = 32, eval_melody: bool = False):
- opts = {
- 'dset': 'audio/musiccaps_32khz',
- 'solver/musicgen/evaluation': 'objective_eval',
- 'execute_only': 'evaluate',
- '+dataset.evaluate.batch_size': batch_size,
- '+metrics.fad.tf.batch_size': 16,
- }
- # chroma-specific evaluation
- chroma_opts = {
- 'dset': 'internal/music_400k_32khz',
- 'dataset.evaluate.segment_duration': 30,
- 'dataset.evaluate.num_samples': 1000,
- 'evaluate.metrics.chroma_cosine': True,
- 'evaluate.metrics.fad': False,
- 'evaluate.metrics.kld': False,
- 'evaluate.metrics.text_consistency': False,
- }
- # binary for FAD computation: replace this path with your own path
- metrics_opts = {
- 'metrics.fad.tf.bin': '/data/home/jadecopet/local/usr/opt/google-research'
- }
- opt1 = {'generate.lm.use_sampling': True, 'generate.lm.top_k': 250, 'generate.lm.top_p': 0.}
- opt2 = {'transformer_lm.two_step_cfg': True}
-
- sub = launcher.bind(opts)
- sub.bind_(metrics_opts)
-
- # base objective metrics
- sub(opt1, opt2)
-
- if eval_melody:
- # chroma-specific metrics
- sub(opt1, opt2, chroma_opts)
-
-
-@GenerationEvalExplorer
-def explorer(launcher):
- partitions = AudioCraftEnvironment.get_slurm_partitions(['team', 'global'])
- launcher.slurm_(gpus=4, partition=partitions)
-
- if 'REGEN' not in os.environ:
- folder = train.main.dora.dir / 'grids' / __name__.split('.', 2)[-1]
- with launcher.job_array():
- for sig in folder.iterdir():
- if not sig.is_symlink():
- continue
- xp = train.main.get_xp_from_sig(sig.name)
- launcher(xp.argv)
- return
-
- with launcher.job_array():
- musicgen_base = launcher.bind(solver="musicgen/musicgen_base_32khz")
- musicgen_base.bind_({'autocast': False, 'fsdp.use': True})
-
- # base musicgen models
- musicgen_base_small = musicgen_base.bind({'continue_from': '//pretrained/facebook/musicgen-small'})
- eval(musicgen_base_small, batch_size=128)
-
- musicgen_base_medium = musicgen_base.bind({'continue_from': '//pretrained/facebook/musicgen-medium'})
- musicgen_base_medium.bind_({'model/lm/model_scale': 'medium'})
- eval(musicgen_base_medium, batch_size=128)
-
- musicgen_base_large = musicgen_base.bind({'continue_from': '//pretrained/facebook/musicgen-large'})
- musicgen_base_large.bind_({'model/lm/model_scale': 'large'})
- eval(musicgen_base_large, batch_size=128)
-
- # melody musicgen model
- musicgen_melody = launcher.bind(solver="musicgen/musicgen_melody_32khz")
- musicgen_melody.bind_({'autocast': False, 'fsdp.use': True})
-
- musicgen_melody_medium = musicgen_melody.bind({'continue_from': '//pretrained/facebook/musicgen-melody'})
- musicgen_melody_medium.bind_({'model/lm/model_scale': 'medium'})
- eval(musicgen_melody_medium, batch_size=128, eval_melody=True)
diff --git a/spaces/AIFILMS/StyleGANEX/models/bisenet/resnet.py b/spaces/AIFILMS/StyleGANEX/models/bisenet/resnet.py
deleted file mode 100644
index aa2bf95130e9815ba378cb6f73207068b81a04b9..0000000000000000000000000000000000000000
--- a/spaces/AIFILMS/StyleGANEX/models/bisenet/resnet.py
+++ /dev/null
@@ -1,109 +0,0 @@
-#!/usr/bin/python
-# -*- encoding: utf-8 -*-
-
-import torch
-import torch.nn as nn
-import torch.nn.functional as F
-import torch.utils.model_zoo as modelzoo
-
-# from modules.bn import InPlaceABNSync as BatchNorm2d
-
-resnet18_url = 'https://download.pytorch.org/models/resnet18-5c106cde.pth'
-
-
-def conv3x3(in_planes, out_planes, stride=1):
- """3x3 convolution with padding"""
- return nn.Conv2d(in_planes, out_planes, kernel_size=3, stride=stride,
- padding=1, bias=False)
-
-
-class BasicBlock(nn.Module):
- def __init__(self, in_chan, out_chan, stride=1):
- super(BasicBlock, self).__init__()
- self.conv1 = conv3x3(in_chan, out_chan, stride)
- self.bn1 = nn.BatchNorm2d(out_chan)
- self.conv2 = conv3x3(out_chan, out_chan)
- self.bn2 = nn.BatchNorm2d(out_chan)
- self.relu = nn.ReLU(inplace=True)
- self.downsample = None
- if in_chan != out_chan or stride != 1:
- self.downsample = nn.Sequential(
- nn.Conv2d(in_chan, out_chan,
- kernel_size=1, stride=stride, bias=False),
- nn.BatchNorm2d(out_chan),
- )
-
- def forward(self, x):
- residual = self.conv1(x)
- residual = F.relu(self.bn1(residual))
- residual = self.conv2(residual)
- residual = self.bn2(residual)
-
- shortcut = x
- if self.downsample is not None:
- shortcut = self.downsample(x)
-
- out = shortcut + residual
- out = self.relu(out)
- return out
-
-
-def create_layer_basic(in_chan, out_chan, bnum, stride=1):
- layers = [BasicBlock(in_chan, out_chan, stride=stride)]
- for i in range(bnum-1):
- layers.append(BasicBlock(out_chan, out_chan, stride=1))
- return nn.Sequential(*layers)
-
-
-class Resnet18(nn.Module):
- def __init__(self):
- super(Resnet18, self).__init__()
- self.conv1 = nn.Conv2d(3, 64, kernel_size=7, stride=2, padding=3,
- bias=False)
- self.bn1 = nn.BatchNorm2d(64)
- self.maxpool = nn.MaxPool2d(kernel_size=3, stride=2, padding=1)
- self.layer1 = create_layer_basic(64, 64, bnum=2, stride=1)
- self.layer2 = create_layer_basic(64, 128, bnum=2, stride=2)
- self.layer3 = create_layer_basic(128, 256, bnum=2, stride=2)
- self.layer4 = create_layer_basic(256, 512, bnum=2, stride=2)
- self.init_weight()
-
- def forward(self, x):
- x = self.conv1(x)
- x = F.relu(self.bn1(x))
- x = self.maxpool(x)
-
- x = self.layer1(x)
- feat8 = self.layer2(x) # 1/8
- feat16 = self.layer3(feat8) # 1/16
- feat32 = self.layer4(feat16) # 1/32
- return feat8, feat16, feat32
-
- def init_weight(self):
- state_dict = modelzoo.load_url(resnet18_url)
- self_state_dict = self.state_dict()
- for k, v in state_dict.items():
- if 'fc' in k: continue
- self_state_dict.update({k: v})
- self.load_state_dict(self_state_dict)
-
- def get_params(self):
- wd_params, nowd_params = [], []
- for name, module in self.named_modules():
- if isinstance(module, (nn.Linear, nn.Conv2d)):
- wd_params.append(module.weight)
- if not module.bias is None:
- nowd_params.append(module.bias)
- elif isinstance(module, nn.BatchNorm2d):
- nowd_params += list(module.parameters())
- return wd_params, nowd_params
-
-
-if __name__ == "__main__":
- net = Resnet18()
- x = torch.randn(16, 3, 224, 224)
- out = net(x)
- print(out[0].size())
- print(out[1].size())
- print(out[2].size())
- net.get_params()
diff --git a/spaces/AIGC-Audio/Make_An_Audio/ldm/models/diffusion/ddpm.py b/spaces/AIGC-Audio/Make_An_Audio/ldm/models/diffusion/ddpm.py
deleted file mode 100644
index d6927503659e3aeb3a88965d8574d4435874516f..0000000000000000000000000000000000000000
--- a/spaces/AIGC-Audio/Make_An_Audio/ldm/models/diffusion/ddpm.py
+++ /dev/null
@@ -1,1444 +0,0 @@
-"""
-wild mixture of
-https://github.com/lucidrains/denoising-diffusion-pytorch/blob/7706bdfc6f527f58d33f84b7b522e61e6e3164b3/denoising_diffusion_pytorch/denoising_diffusion_pytorch.py
-https://github.com/openai/improved-diffusion/blob/e94489283bb876ac1477d5dd7709bbbd2d9902ce/improved_diffusion/gaussian_diffusion.py
-https://github.com/CompVis/taming-transformers
--- merci
-"""
-import torch
-import torch.nn as nn
-import numpy as np
-import pytorch_lightning as pl
-from torch.optim.lr_scheduler import LambdaLR
-from einops import rearrange, repeat
-from contextlib import contextmanager
-from functools import partial
-from tqdm import tqdm
-from torchvision.utils import make_grid
-from pytorch_lightning.utilities.distributed import rank_zero_only
-
-from ldm.util import log_txt_as_img, exists, default, ismap, isimage, mean_flat, count_params, instantiate_from_config
-from ldm.modules.ema import LitEma
-from ldm.modules.distributions.distributions import normal_kl, DiagonalGaussianDistribution
-from ldm.models.autoencoder import IdentityFirstStage, AutoencoderKL
-from ldm.modules.diffusionmodules.util import make_beta_schedule, extract_into_tensor, noise_like
-from ldm.models.diffusion.ddim import DDIMSampler
-
-
-__conditioning_keys__ = {'concat': 'c_concat',
- 'crossattn': 'c_crossattn',
- 'adm': 'y'}
-
-
-def disabled_train(self, mode=True):
- """Overwrite model.train with this function to make sure train/eval mode
- does not change anymore."""
- return self
-
-
-def uniform_on_device(r1, r2, shape, device):
- return (r1 - r2) * torch.rand(*shape, device=device) + r2
-
-
-class DDPM(pl.LightningModule):
- # classic DDPM with Gaussian diffusion, in image space
- def __init__(self,
- unet_config,
- timesteps=1000,
- beta_schedule="linear",
- loss_type="l2",
- ckpt_path=None,
- ignore_keys=[],
- load_only_unet=False,
- monitor="val/loss",
- use_ema=True,
- first_stage_key="image",
- image_size=256,
- channels=3,
- log_every_t=100,
- clip_denoised=True,
- linear_start=1e-4,
- linear_end=2e-2,
- cosine_s=8e-3,
- given_betas=None,
- original_elbo_weight=0.,
- v_posterior=0., # weight for choosing posterior variance as sigma = (1-v) * beta_tilde + v * beta
- l_simple_weight=1.,
- conditioning_key=None,
- parameterization="eps", # all config files uses "eps"
- scheduler_config=None,
- use_positional_encodings=False,
- learn_logvar=False,
- logvar_init=0.,
- ):
- super().__init__()
- assert parameterization in ["eps", "x0"], 'currently only supporting "eps" and "x0"'
- self.parameterization = parameterization
- print(f"{self.__class__.__name__}: Running in {self.parameterization}-prediction mode")
- self.cond_stage_model = None
- self.clip_denoised = clip_denoised
- self.log_every_t = log_every_t
- self.first_stage_key = first_stage_key
- self.image_size = image_size # try conv?
- self.channels = channels
- self.use_positional_encodings = use_positional_encodings
- self.model = DiffusionWrapper(unet_config, conditioning_key)
- count_params(self.model, verbose=True)
- self.use_ema = use_ema
- if self.use_ema:
- self.model_ema = LitEma(self.model)
- print(f"Keeping EMAs of {len(list(self.model_ema.buffers()))}.")
-
- self.use_scheduler = scheduler_config is not None
- if self.use_scheduler:
- self.scheduler_config = scheduler_config
-
- self.v_posterior = v_posterior
- self.original_elbo_weight = original_elbo_weight
- self.l_simple_weight = l_simple_weight
-
- if monitor is not None:
- self.monitor = monitor
- if ckpt_path is not None:
- self.init_from_ckpt(ckpt_path, ignore_keys=ignore_keys, only_model=load_only_unet)
-
- self.register_schedule(given_betas=given_betas, beta_schedule=beta_schedule, timesteps=timesteps,
- linear_start=linear_start, linear_end=linear_end, cosine_s=cosine_s)
-
- self.loss_type = loss_type
-
- self.learn_logvar = learn_logvar
- self.logvar = torch.full(fill_value=logvar_init, size=(self.num_timesteps,))
- if self.learn_logvar:
- self.logvar = nn.Parameter(self.logvar, requires_grad=True)
-
- def register_schedule(self, given_betas=None, beta_schedule="linear", timesteps=1000,
- linear_start=1e-4, linear_end=2e-2, cosine_s=8e-3):
- if exists(given_betas):
- betas = given_betas
- else:
- betas = make_beta_schedule(beta_schedule, timesteps, linear_start=linear_start, linear_end=linear_end,
- cosine_s=cosine_s)
- alphas = 1. - betas
- alphas_cumprod = np.cumprod(alphas, axis=0)
- alphas_cumprod_prev = np.append(1., alphas_cumprod[:-1])
-
- timesteps, = betas.shape
- self.num_timesteps = int(timesteps)
- self.linear_start = linear_start
- self.linear_end = linear_end
- assert alphas_cumprod.shape[0] == self.num_timesteps, 'alphas have to be defined for each timestep'
-
- to_torch = partial(torch.tensor, dtype=torch.float32)
-
- self.register_buffer('betas', to_torch(betas))
- self.register_buffer('alphas_cumprod', to_torch(alphas_cumprod))
- self.register_buffer('alphas_cumprod_prev', to_torch(alphas_cumprod_prev))
-
- # calculations for diffusion q(x_t | x_{t-1}) and others
- self.register_buffer('sqrt_alphas_cumprod', to_torch(np.sqrt(alphas_cumprod)))
- self.register_buffer('sqrt_one_minus_alphas_cumprod', to_torch(np.sqrt(1. - alphas_cumprod)))
- self.register_buffer('log_one_minus_alphas_cumprod', to_torch(np.log(1. - alphas_cumprod)))
- self.register_buffer('sqrt_recip_alphas_cumprod', to_torch(np.sqrt(1. / alphas_cumprod)))
- self.register_buffer('sqrt_recipm1_alphas_cumprod', to_torch(np.sqrt(1. / alphas_cumprod - 1)))
-
- # calculations for posterior q(x_{t-1} | x_t, x_0)
- posterior_variance = (1 - self.v_posterior) * betas * (1. - alphas_cumprod_prev) / (
- 1. - alphas_cumprod) + self.v_posterior * betas
- # above: equal to 1. / (1. / (1. - alpha_cumprod_tm1) + alpha_t / beta_t)
- self.register_buffer('posterior_variance', to_torch(posterior_variance))
- # below: log calculation clipped because the posterior variance is 0 at the beginning of the diffusion chain
- self.register_buffer('posterior_log_variance_clipped', to_torch(np.log(np.maximum(posterior_variance, 1e-20))))
- self.register_buffer('posterior_mean_coef1', to_torch(
- betas * np.sqrt(alphas_cumprod_prev) / (1. - alphas_cumprod)))
- self.register_buffer('posterior_mean_coef2', to_torch(
- (1. - alphas_cumprod_prev) * np.sqrt(alphas) / (1. - alphas_cumprod)))
-
- if self.parameterization == "eps":
- lvlb_weights = self.betas ** 2 / (
- 2 * self.posterior_variance * to_torch(alphas) * (1 - self.alphas_cumprod))
- elif self.parameterization == "x0":
- lvlb_weights = 0.5 * np.sqrt(torch.Tensor(alphas_cumprod)) / (2. * 1 - torch.Tensor(alphas_cumprod))
- else:
- raise NotImplementedError("mu not supported")
- # TODO how to choose this term
- lvlb_weights[0] = lvlb_weights[1]
- self.register_buffer('lvlb_weights', lvlb_weights, persistent=False)
- assert not torch.isnan(self.lvlb_weights).all()
-
- @contextmanager
- def ema_scope(self, context=None):
- if self.use_ema:
- self.model_ema.store(self.model.parameters())
- self.model_ema.copy_to(self.model)
- if context is not None:
- print(f"{context}: Switched to EMA weights")
- try:
- yield None
- finally:
- if self.use_ema:
- self.model_ema.restore(self.model.parameters())
- if context is not None:
- print(f"{context}: Restored training weights")
-
- def init_from_ckpt(self, path, ignore_keys=list(), only_model=False):
- sd = torch.load(path, map_location="cpu")
- if "state_dict" in list(sd.keys()):
- sd = sd["state_dict"]
- keys = list(sd.keys())
- for k in keys:
- for ik in ignore_keys:
- if k.startswith(ik):
- print("Deleting key {} from state_dict.".format(k))
- del sd[k]
- missing, unexpected = self.load_state_dict(sd, strict=False) if not only_model else self.model.load_state_dict(
- sd, strict=False)
- print(f"Restored from {path} with {len(missing)} missing and {len(unexpected)} unexpected keys")
- if len(missing) > 0:
- print(f"Missing Keys: {missing}")
- if len(unexpected) > 0:
- print(f"Unexpected Keys: {unexpected}")
-
- def q_mean_variance(self, x_start, t):
- """
- Get the distribution q(x_t | x_0).
- :param x_start: the [N x C x ...] tensor of noiseless inputs.
- :param t: the number of diffusion steps (minus 1). Here, 0 means one step.
- :return: A tuple (mean, variance, log_variance), all of x_start's shape.
- """
- mean = (extract_into_tensor(self.sqrt_alphas_cumprod, t, x_start.shape) * x_start)
- variance = extract_into_tensor(1.0 - self.alphas_cumprod, t, x_start.shape)
- log_variance = extract_into_tensor(self.log_one_minus_alphas_cumprod, t, x_start.shape)
- return mean, variance, log_variance
-
- def predict_start_from_noise(self, x_t, t, noise):
- return (
- extract_into_tensor(self.sqrt_recip_alphas_cumprod, t, x_t.shape) * x_t -
- extract_into_tensor(self.sqrt_recipm1_alphas_cumprod, t, x_t.shape) * noise
- )
-
- def q_posterior(self, x_start, x_t, t):
- posterior_mean = (
- extract_into_tensor(self.posterior_mean_coef1, t, x_t.shape) * x_start +
- extract_into_tensor(self.posterior_mean_coef2, t, x_t.shape) * x_t
- )
- posterior_variance = extract_into_tensor(self.posterior_variance, t, x_t.shape)
- posterior_log_variance_clipped = extract_into_tensor(self.posterior_log_variance_clipped, t, x_t.shape)
- return posterior_mean, posterior_variance, posterior_log_variance_clipped
-
- def p_mean_variance(self, x, t, clip_denoised: bool):
- model_out = self.model(x, t)
- if self.parameterization == "eps":
- x_recon = self.predict_start_from_noise(x, t=t, noise=model_out)
- elif self.parameterization == "x0":
- x_recon = model_out
- if clip_denoised:
- x_recon.clamp_(-1., 1.)
-
- model_mean, posterior_variance, posterior_log_variance = self.q_posterior(x_start=x_recon, x_t=x, t=t)
- return model_mean, posterior_variance, posterior_log_variance
-
- @torch.no_grad()
- def p_sample(self, x, t, clip_denoised=True, repeat_noise=False):
- b, *_, device = *x.shape, x.device
- model_mean, _, model_log_variance = self.p_mean_variance(x=x, t=t, clip_denoised=clip_denoised)
- noise = noise_like(x.shape, device, repeat_noise)
- # no noise when t == 0
- nonzero_mask = (1 - (t == 0).float()).reshape(b, *((1,) * (len(x.shape) - 1)))
- return model_mean + nonzero_mask * (0.5 * model_log_variance).exp() * noise
-
- @torch.no_grad()
- def p_sample_loop(self, shape, return_intermediates=False):
- device = self.betas.device
- b = shape[0]
- img = torch.randn(shape, device=device)
- intermediates = [img]
- for i in tqdm(reversed(range(0, self.num_timesteps)), desc='Sampling t', total=self.num_timesteps):
- img = self.p_sample(img, torch.full((b,), i, device=device, dtype=torch.long),
- clip_denoised=self.clip_denoised)
- if i % self.log_every_t == 0 or i == self.num_timesteps - 1:
- intermediates.append(img)
- if return_intermediates:
- return img, intermediates
- return img
-
- @torch.no_grad()
- def sample(self, batch_size=16, return_intermediates=False):
- image_size = self.image_size
- channels = self.channels
- return self.p_sample_loop((batch_size, channels, image_size, image_size),
- return_intermediates=return_intermediates)
-
- def q_sample(self, x_start, t, noise=None):
- noise = default(noise, lambda: torch.randn_like(x_start))
- return (extract_into_tensor(self.sqrt_alphas_cumprod, t, x_start.shape) * x_start +
- extract_into_tensor(self.sqrt_one_minus_alphas_cumprod, t, x_start.shape) * noise)
-
- def get_loss(self, pred, target, mean=True):
- if self.loss_type == 'l1':
- loss = (target - pred).abs()
- if mean:
- loss = loss.mean()
- elif self.loss_type == 'l2':
- if mean:
- loss = torch.nn.functional.mse_loss(target, pred)
- else:
- loss = torch.nn.functional.mse_loss(target, pred, reduction='none')
- else:
- raise NotImplementedError("unknown loss type '{loss_type}'")
-
- return loss
-
- def p_losses(self, x_start, t, noise=None):
- noise = default(noise, lambda: torch.randn_like(x_start))
- x_noisy = self.q_sample(x_start=x_start, t=t, noise=noise)
- model_out = self.model(x_noisy, t)
-
- loss_dict = {}
- if self.parameterization == "eps":
- target = noise
- elif self.parameterization == "x0":
- target = x_start
- else:
- raise NotImplementedError(f"Paramterization {self.parameterization} not yet supported")
-
- loss = self.get_loss(model_out, target, mean=False).mean(dim=[1, 2, 3])
-
- log_prefix = 'train' if self.training else 'val'
-
- loss_dict.update({f'{log_prefix}/loss_simple': loss.mean()})
- loss_simple = loss.mean() * self.l_simple_weight
-
- loss_vlb = (self.lvlb_weights[t] * loss).mean()
- loss_dict.update({f'{log_prefix}/loss_vlb': loss_vlb})
-
- loss = loss_simple + self.original_elbo_weight * loss_vlb
-
- loss_dict.update({f'{log_prefix}/loss': loss})
-
- return loss, loss_dict
-
- def forward(self, x, *args, **kwargs):
- # b, c, h, w, device, img_size, = *x.shape, x.device, self.image_size
- # assert h == img_size and w == img_size, f'height and width of image must be {img_size}'
- t = torch.randint(0, self.num_timesteps, (x.shape[0],), device=self.device).long()
- return self.p_losses(x, t, *args, **kwargs)
-
- def get_input(self, batch, k):
- x = batch[k]
- if len(x.shape) == 3:
- x = x[..., None]
- x = rearrange(x, 'b h w c -> b c h w')
- x = x.to(memory_format=torch.contiguous_format).float()
- return x
-
- def shared_step(self, batch):
- x = self.get_input(batch, self.first_stage_key)
- loss, loss_dict = self(x)
- return loss, loss_dict
-
- def training_step(self, batch, batch_idx):
- loss, loss_dict = self.shared_step(batch)
-
- self.log_dict(loss_dict, prog_bar=True,
- logger=True, on_step=True, on_epoch=True)
-
- self.log("global_step", self.global_step,
- prog_bar=True, logger=True, on_step=True, on_epoch=False)
-
- if self.use_scheduler:
- lr = self.optimizers().param_groups[0]['lr']
- self.log('lr_abs', lr, prog_bar=True, logger=True, on_step=True, on_epoch=False)
-
- return loss
-
- @torch.no_grad()
- def validation_step(self, batch, batch_idx):
- _, loss_dict_no_ema = self.shared_step(batch)
- with self.ema_scope():
- _, loss_dict_ema = self.shared_step(batch)
- loss_dict_ema = {key + '_ema': loss_dict_ema[key] for key in loss_dict_ema}
- self.log_dict(loss_dict_no_ema, prog_bar=False, logger=True, on_step=False, on_epoch=True)
- self.log_dict(loss_dict_ema, prog_bar=False, logger=True, on_step=False, on_epoch=True)
-
- def on_train_batch_end(self, *args, **kwargs):
- if self.use_ema:
- self.model_ema(self.model)
-
- def _get_rows_from_list(self, samples):
- n_imgs_per_row = len(samples)
- denoise_grid = rearrange(samples, 'n b c h w -> b n c h w')
- denoise_grid = rearrange(denoise_grid, 'b n c h w -> (b n) c h w')
- denoise_grid = make_grid(denoise_grid, nrow=n_imgs_per_row)
- return denoise_grid
-
- @torch.no_grad()
- def log_images(self, batch, N=8, n_row=2, sample=True, return_keys=None, **kwargs):
- log = dict()
- x = self.get_input(batch, self.first_stage_key)
- N = min(x.shape[0], N)
- n_row = min(x.shape[0], n_row)
- x = x.to(self.device)[:N]
- log["inputs"] = x
-
- # get diffusion row
- diffusion_row = list()
- x_start = x[:n_row]
-
- for t in range(self.num_timesteps):
- if t % self.log_every_t == 0 or t == self.num_timesteps - 1:
- t = repeat(torch.tensor([t]), '1 -> b', b=n_row)
- t = t.to(self.device).long()
- noise = torch.randn_like(x_start)
- x_noisy = self.q_sample(x_start=x_start, t=t, noise=noise)
- diffusion_row.append(x_noisy)
-
- log["diffusion_row"] = self._get_rows_from_list(diffusion_row)
-
- if sample:
- # get denoise row
- with self.ema_scope("Plotting"):
- samples, denoise_row = self.sample(batch_size=N, return_intermediates=True)
-
- log["samples"] = samples
- log["denoise_row"] = self._get_rows_from_list(denoise_row)
-
- if return_keys:
- if np.intersect1d(list(log.keys()), return_keys).shape[0] == 0:
- return log
- else:
- return {key: log[key] for key in return_keys}
- return log
-
- def configure_optimizers(self):
- lr = self.learning_rate
- params = list(self.model.parameters())
- if self.learn_logvar:
- params = params + [self.logvar]
- opt = torch.optim.AdamW(params, lr=lr)
- return opt
-
-
-class LatentDiffusion(DDPM):
- """main class"""
- def __init__(self,
- first_stage_config,
- cond_stage_config,
- num_timesteps_cond=None,
- cond_stage_key="image",# 'caption' for txt2image, 'masked_image' for inpainting
- cond_stage_trainable=False,
- concat_mode=True,# true for inpainting
- cond_stage_forward=None,
- conditioning_key=None, # 'crossattn' for txt2image, None for inpainting
- scale_factor=1.0,
- scale_by_std=False,
- *args, **kwargs):
- self.num_timesteps_cond = default(num_timesteps_cond, 1)
- self.scale_by_std = scale_by_std
- assert self.num_timesteps_cond <= kwargs['timesteps']
- # for backwards compatibility after implementation of DiffusionWrapper
- if conditioning_key is None:
- conditioning_key = 'concat' if concat_mode else 'crossattn'
- if cond_stage_config == '__is_unconditional__':
- conditioning_key = None
- ckpt_path = kwargs.pop("ckpt_path", None)
- ignore_keys = kwargs.pop("ignore_keys", [])
- super().__init__(conditioning_key=conditioning_key, *args, **kwargs)
- self.concat_mode = concat_mode
- self.cond_stage_trainable = cond_stage_trainable
- self.cond_stage_key = cond_stage_key
- try:
- self.num_downs = len(first_stage_config.params.ddconfig.ch_mult) - 1
- except:
- self.num_downs = 0
- if not scale_by_std:
- self.scale_factor = scale_factor
- else:
- self.register_buffer('scale_factor', torch.tensor(scale_factor))
- self.instantiate_first_stage(first_stage_config)
- self.instantiate_cond_stage(cond_stage_config)
- self.cond_stage_forward = cond_stage_forward
- self.clip_denoised = False
- self.bbox_tokenizer = None
-
- self.restarted_from_ckpt = False
- if ckpt_path is not None:
- self.init_from_ckpt(ckpt_path, ignore_keys)
- self.restarted_from_ckpt = True
-
- def make_cond_schedule(self, ):
- self.cond_ids = torch.full(size=(self.num_timesteps,), fill_value=self.num_timesteps - 1, dtype=torch.long)
- ids = torch.round(torch.linspace(0, self.num_timesteps - 1, self.num_timesteps_cond)).long()
- self.cond_ids[:self.num_timesteps_cond] = ids
-
- @rank_zero_only
- @torch.no_grad()
- def on_train_batch_start(self, batch, batch_idx, dataloader_idx):
- # only for very first batch
- if self.scale_by_std and self.current_epoch == 0 and self.global_step == 0 and batch_idx == 0 and not self.restarted_from_ckpt:
- assert self.scale_factor == 1., 'rather not use custom rescaling and std-rescaling simultaneously'
- # set rescale weight to 1./std of encodings
- print("### USING STD-RESCALING ###")
- x = super().get_input(batch, self.first_stage_key)
- x = x.to(self.device)
- encoder_posterior = self.encode_first_stage(x)
- z = self.get_first_stage_encoding(encoder_posterior).detach()
- del self.scale_factor
- self.register_buffer('scale_factor', 1. / z.flatten().std())
- print(f"setting self.scale_factor to {self.scale_factor}")
- print("### USING STD-RESCALING ###")
-
- def register_schedule(self,
- given_betas=None, beta_schedule="linear", timesteps=1000,
- linear_start=1e-4, linear_end=2e-2, cosine_s=8e-3):
- super().register_schedule(given_betas, beta_schedule, timesteps, linear_start, linear_end, cosine_s)
-
- self.shorten_cond_schedule = self.num_timesteps_cond > 1
- if self.shorten_cond_schedule:
- self.make_cond_schedule()
-
- def instantiate_first_stage(self, config):
- model = instantiate_from_config(config)
- self.first_stage_model = model.eval()
- self.first_stage_model.train = disabled_train
- for param in self.first_stage_model.parameters():
- param.requires_grad = False
-
- def instantiate_cond_stage(self, config):
- if not self.cond_stage_trainable:
- if config == "__is_first_stage__":# inpaint
- print("Using first stage also as cond stage.")
- self.cond_stage_model = self.first_stage_model
- elif config == "__is_unconditional__":
- print(f"Training {self.__class__.__name__} as an unconditional model.")
- self.cond_stage_model = None
- # self.be_unconditional = True
- else:
- model = instantiate_from_config(config)
- self.cond_stage_model = model.eval()
- self.cond_stage_model.train = disabled_train
- for param in self.cond_stage_model.parameters():
- param.requires_grad = False
- else:
- assert config != '__is_first_stage__'
- assert config != '__is_unconditional__'
- model = instantiate_from_config(config)
- self.cond_stage_model = model
-
- def _get_denoise_row_from_list(self, samples, desc='', force_no_decoder_quantization=False):
- denoise_row = []
- for zd in tqdm(samples, desc=desc):
- denoise_row.append(self.decode_first_stage(zd.to(self.device),
- force_not_quantize=force_no_decoder_quantization))
- n_imgs_per_row = len(denoise_row)
- denoise_row = torch.stack(denoise_row) # n_log_step, n_row, C, H, W
- denoise_grid = rearrange(denoise_row, 'n b c h w -> b n c h w')
- denoise_grid = rearrange(denoise_grid, 'b n c h w -> (b n) c h w')
- denoise_grid = make_grid(denoise_grid, nrow=n_imgs_per_row)
- return denoise_grid
-
- def get_first_stage_encoding(self, encoder_posterior):
- if isinstance(encoder_posterior, DiagonalGaussianDistribution):
- z = encoder_posterior.sample()
- elif isinstance(encoder_posterior, torch.Tensor):
- z = encoder_posterior
- else:
- raise NotImplementedError(f"encoder_posterior of type '{type(encoder_posterior)}' not yet implemented")
- return self.scale_factor * z
-
- def get_learned_conditioning(self, c):
- if self.cond_stage_forward is None:
- if hasattr(self.cond_stage_model, 'encode') and callable(self.cond_stage_model.encode):
- c = self.cond_stage_model.encode(c)
- if isinstance(c, DiagonalGaussianDistribution):
- c = c.mode()
- else:
- c = self.cond_stage_model(c)
- else:
- assert hasattr(self.cond_stage_model, self.cond_stage_forward)
- c = getattr(self.cond_stage_model, self.cond_stage_forward)(c)
- return c
-
- def meshgrid(self, h, w):
- y = torch.arange(0, h).view(h, 1, 1).repeat(1, w, 1)
- x = torch.arange(0, w).view(1, w, 1).repeat(h, 1, 1)
-
- arr = torch.cat([y, x], dim=-1)
- return arr
-
- def delta_border(self, h, w):
- """
- :param h: height
- :param w: width
- :return: normalized distance to image border,
- wtith min distance = 0 at border and max dist = 0.5 at image center
- """
- lower_right_corner = torch.tensor([h - 1, w - 1]).view(1, 1, 2)
- arr = self.meshgrid(h, w) / lower_right_corner
- dist_left_up = torch.min(arr, dim=-1, keepdims=True)[0]
- dist_right_down = torch.min(1 - arr, dim=-1, keepdims=True)[0]
- edge_dist = torch.min(torch.cat([dist_left_up, dist_right_down], dim=-1), dim=-1)[0]
- return edge_dist
-
- def get_weighting(self, h, w, Ly, Lx, device):
- weighting = self.delta_border(h, w)
- weighting = torch.clip(weighting, self.split_input_params["clip_min_weight"],
- self.split_input_params["clip_max_weight"], )
- weighting = weighting.view(1, h * w, 1).repeat(1, 1, Ly * Lx).to(device)
-
- if self.split_input_params["tie_braker"]:
- L_weighting = self.delta_border(Ly, Lx)
- L_weighting = torch.clip(L_weighting,
- self.split_input_params["clip_min_tie_weight"],
- self.split_input_params["clip_max_tie_weight"])
-
- L_weighting = L_weighting.view(1, 1, Ly * Lx).to(device)
- weighting = weighting * L_weighting
- return weighting
-
- def get_fold_unfold(self, x, kernel_size, stride, uf=1, df=1): # todo load once not every time, shorten code
- """
- :param x: img of size (bs, c, h, w)
- :return: n img crops of size (n, bs, c, kernel_size[0], kernel_size[1])
- """
- bs, nc, h, w = x.shape
-
- # number of crops in image
- Ly = (h - kernel_size[0]) // stride[0] + 1
- Lx = (w - kernel_size[1]) // stride[1] + 1
-
- if uf == 1 and df == 1:
- fold_params = dict(kernel_size=kernel_size, dilation=1, padding=0, stride=stride)
- unfold = torch.nn.Unfold(**fold_params)
-
- fold = torch.nn.Fold(output_size=x.shape[2:], **fold_params)
-
- weighting = self.get_weighting(kernel_size[0], kernel_size[1], Ly, Lx, x.device).to(x.dtype)
- normalization = fold(weighting).view(1, 1, h, w) # normalizes the overlap
- weighting = weighting.view((1, 1, kernel_size[0], kernel_size[1], Ly * Lx))
-
- elif uf > 1 and df == 1:
- fold_params = dict(kernel_size=kernel_size, dilation=1, padding=0, stride=stride)
- unfold = torch.nn.Unfold(**fold_params)
-
- fold_params2 = dict(kernel_size=(kernel_size[0] * uf, kernel_size[0] * uf),
- dilation=1, padding=0,
- stride=(stride[0] * uf, stride[1] * uf))
- fold = torch.nn.Fold(output_size=(x.shape[2] * uf, x.shape[3] * uf), **fold_params2)
-
- weighting = self.get_weighting(kernel_size[0] * uf, kernel_size[1] * uf, Ly, Lx, x.device).to(x.dtype)
- normalization = fold(weighting).view(1, 1, h * uf, w * uf) # normalizes the overlap
- weighting = weighting.view((1, 1, kernel_size[0] * uf, kernel_size[1] * uf, Ly * Lx))
-
- elif df > 1 and uf == 1:
- fold_params = dict(kernel_size=kernel_size, dilation=1, padding=0, stride=stride)
- unfold = torch.nn.Unfold(**fold_params)
-
- fold_params2 = dict(kernel_size=(kernel_size[0] // df, kernel_size[0] // df),
- dilation=1, padding=0,
- stride=(stride[0] // df, stride[1] // df))
- fold = torch.nn.Fold(output_size=(x.shape[2] // df, x.shape[3] // df), **fold_params2)
-
- weighting = self.get_weighting(kernel_size[0] // df, kernel_size[1] // df, Ly, Lx, x.device).to(x.dtype)
- normalization = fold(weighting).view(1, 1, h // df, w // df) # normalizes the overlap
- weighting = weighting.view((1, 1, kernel_size[0] // df, kernel_size[1] // df, Ly * Lx))
-
- else:
- raise NotImplementedError
-
- return fold, unfold, normalization, weighting
-
- @torch.no_grad()
- def get_input(self, batch, k, return_first_stage_outputs=False, force_c_encode=False,
- cond_key=None, return_original_cond=False, bs=None):
- x = super().get_input(batch, k)
- if bs is not None:
- x = x[:bs]
- x = x.to(self.device)
- encoder_posterior = self.encode_first_stage(x)
- z = self.get_first_stage_encoding(encoder_posterior).detach()
-
- if self.model.conditioning_key is not None:
- if cond_key is None:
- cond_key = self.cond_stage_key
- if cond_key != self.first_stage_key:# cond_key is not image. for inapint it's masked_img
- if cond_key in ['caption', 'coordinates_bbox']:
- xc = batch[cond_key]
- elif cond_key == 'class_label':
- xc = batch
- else:
- xc = super().get_input(batch, cond_key).to(self.device)
- else:
- xc = x
- if not self.cond_stage_trainable or force_c_encode:
- if isinstance(xc, dict) or isinstance(xc, list):
- # import pudb; pudb.set_trace()
- c = self.get_learned_conditioning(xc)
- else:
- c = self.get_learned_conditioning(xc.to(self.device))
- else:
- c = xc
- if bs is not None:
- c = c[:bs]
-
- if self.use_positional_encodings:
- pos_x, pos_y = self.compute_latent_shifts(batch)
- ckey = __conditioning_keys__[self.model.conditioning_key]
- c = {ckey: c, 'pos_x': pos_x, 'pos_y': pos_y}
-
- else:
- c = None
- xc = None
- if self.use_positional_encodings:
- pos_x, pos_y = self.compute_latent_shifts(batch)
- c = {'pos_x': pos_x, 'pos_y': pos_y}
- out = [z, c]
- if return_first_stage_outputs:
- xrec = self.decode_first_stage(z)
- out.extend([x, xrec])
- if return_original_cond:
- out.append(xc)
- return out
-
- @torch.no_grad()
- def decode_first_stage(self, z, predict_cids=False, force_not_quantize=False):
- if predict_cids:
- if z.dim() == 4:
- z = torch.argmax(z.exp(), dim=1).long()
- z = self.first_stage_model.quantize.get_codebook_entry(z, shape=None)
- z = rearrange(z, 'b h w c -> b c h w').contiguous()
-
- z = 1. / self.scale_factor * z
-
- if hasattr(self, "split_input_params"):
- if self.split_input_params["patch_distributed_vq"]:
- ks = self.split_input_params["ks"] # eg. (128, 128)
- stride = self.split_input_params["stride"] # eg. (64, 64)
- uf = self.split_input_params["vqf"]
- bs, nc, h, w = z.shape
- if ks[0] > h or ks[1] > w:
- ks = (min(ks[0], h), min(ks[1], w))
- print("reducing Kernel")
-
- if stride[0] > h or stride[1] > w:
- stride = (min(stride[0], h), min(stride[1], w))
- print("reducing stride")
-
- fold, unfold, normalization, weighting = self.get_fold_unfold(z, ks, stride, uf=uf)
-
- z = unfold(z) # (bn, nc * prod(**ks), L)
- # 1. Reshape to img shape
- z = z.view((z.shape[0], -1, ks[0], ks[1], z.shape[-1])) # (bn, nc, ks[0], ks[1], L )
-
- # 2. apply model loop over last dim
- if isinstance(self.first_stage_model, VQModelInterface):
- output_list = [self.first_stage_model.decode(z[:, :, :, :, i],
- force_not_quantize=predict_cids or force_not_quantize)
- for i in range(z.shape[-1])]
- else:
-
- output_list = [self.first_stage_model.decode(z[:, :, :, :, i])
- for i in range(z.shape[-1])]
-
- o = torch.stack(output_list, axis=-1) # # (bn, nc, ks[0], ks[1], L)
- o = o * weighting
- # Reverse 1. reshape to img shape
- o = o.view((o.shape[0], -1, o.shape[-1])) # (bn, nc * ks[0] * ks[1], L)
- # stitch crops together
- decoded = fold(o)
- decoded = decoded / normalization # norm is shape (1, 1, h, w)
- return decoded
- else:
- if isinstance(self.first_stage_model, VQModelInterface):
- return self.first_stage_model.decode(z, force_not_quantize=predict_cids or force_not_quantize)
- else:
- return self.first_stage_model.decode(z)
-
- else:
- if isinstance(self.first_stage_model, VQModelInterface):
- return self.first_stage_model.decode(z, force_not_quantize=predict_cids or force_not_quantize)
- else:
- return self.first_stage_model.decode(z)
-
- # same as above but without decorator
- def differentiable_decode_first_stage(self, z, predict_cids=False, force_not_quantize=False):
- if predict_cids:
- if z.dim() == 4:
- z = torch.argmax(z.exp(), dim=1).long()
- z = self.first_stage_model.quantize.get_codebook_entry(z, shape=None)
- z = rearrange(z, 'b h w c -> b c h w').contiguous()
-
- z = 1. / self.scale_factor * z
-
- if hasattr(self, "split_input_params"):
- if self.split_input_params["patch_distributed_vq"]:
- ks = self.split_input_params["ks"] # eg. (128, 128)
- stride = self.split_input_params["stride"] # eg. (64, 64)
- uf = self.split_input_params["vqf"]
- bs, nc, h, w = z.shape
- if ks[0] > h or ks[1] > w:
- ks = (min(ks[0], h), min(ks[1], w))
- print("reducing Kernel")
-
- if stride[0] > h or stride[1] > w:
- stride = (min(stride[0], h), min(stride[1], w))
- print("reducing stride")
-
- fold, unfold, normalization, weighting = self.get_fold_unfold(z, ks, stride, uf=uf)
-
- z = unfold(z) # (bn, nc * prod(**ks), L)
- # 1. Reshape to img shape
- z = z.view((z.shape[0], -1, ks[0], ks[1], z.shape[-1])) # (bn, nc, ks[0], ks[1], L )
-
- # 2. apply model loop over last dim
- if isinstance(self.first_stage_model, VQModelInterface):
- output_list = [self.first_stage_model.decode(z[:, :, :, :, i],
- force_not_quantize=predict_cids or force_not_quantize)
- for i in range(z.shape[-1])]
- else:
-
- output_list = [self.first_stage_model.decode(z[:, :, :, :, i])
- for i in range(z.shape[-1])]
-
- o = torch.stack(output_list, axis=-1) # # (bn, nc, ks[0], ks[1], L)
- o = o * weighting
- # Reverse 1. reshape to img shape
- o = o.view((o.shape[0], -1, o.shape[-1])) # (bn, nc * ks[0] * ks[1], L)
- # stitch crops together
- decoded = fold(o)
- decoded = decoded / normalization # norm is shape (1, 1, h, w)
- return decoded
- else:
- if isinstance(self.first_stage_model, VQModelInterface):
- return self.first_stage_model.decode(z, force_not_quantize=predict_cids or force_not_quantize)
- else:
- return self.first_stage_model.decode(z)
-
- else:
- if isinstance(self.first_stage_model, VQModelInterface):
- return self.first_stage_model.decode(z, force_not_quantize=predict_cids or force_not_quantize)
- else:
- return self.first_stage_model.decode(z)
-
- @torch.no_grad()
- def encode_first_stage(self, x):
- if hasattr(self, "split_input_params"):
- if self.split_input_params["patch_distributed_vq"]:
- ks = self.split_input_params["ks"] # eg. (128, 128)
- stride = self.split_input_params["stride"] # eg. (64, 64)
- df = self.split_input_params["vqf"]
- self.split_input_params['original_image_size'] = x.shape[-2:]
- bs, nc, h, w = x.shape
- if ks[0] > h or ks[1] > w:
- ks = (min(ks[0], h), min(ks[1], w))
- print("reducing Kernel")
-
- if stride[0] > h or stride[1] > w:
- stride = (min(stride[0], h), min(stride[1], w))
- print("reducing stride")
-
- fold, unfold, normalization, weighting = self.get_fold_unfold(x, ks, stride, df=df)
- z = unfold(x) # (bn, nc * prod(**ks), L)
- # Reshape to img shape
- z = z.view((z.shape[0], -1, ks[0], ks[1], z.shape[-1])) # (bn, nc, ks[0], ks[1], L )
-
- output_list = [self.first_stage_model.encode(z[:, :, :, :, i])
- for i in range(z.shape[-1])]
-
- o = torch.stack(output_list, axis=-1)
- o = o * weighting
-
- # Reverse reshape to img shape
- o = o.view((o.shape[0], -1, o.shape[-1])) # (bn, nc * ks[0] * ks[1], L)
- # stitch crops together
- decoded = fold(o)
- decoded = decoded / normalization
- return decoded
-
- else:
- return self.first_stage_model.encode(x)
- else:
- return self.first_stage_model.encode(x)
-
- def shared_step(self, batch, **kwargs):
- x, c = self.get_input(batch, self.first_stage_key)
- loss = self(x, c)
- return loss
-
- def forward(self, x, c, *args, **kwargs):
- t = torch.randint(0, self.num_timesteps, (x.shape[0],), device=self.device).long()
- if self.model.conditioning_key is not None:
- assert c is not None
- if self.cond_stage_trainable:# true when use text
- c = self.get_learned_conditioning(c) # c: string list -> [B, T, Context_dim]
- if self.shorten_cond_schedule: # TODO: drop this option
- tc = self.cond_ids[t].to(self.device)
- c = self.q_sample(x_start=c, t=tc, noise=torch.randn_like(c.float()))
- return self.p_losses(x, c, t, *args, **kwargs)
-
- def _rescale_annotations(self, bboxes, crop_coordinates): # TODO: move to dataset
- def rescale_bbox(bbox):
- x0 = clamp((bbox[0] - crop_coordinates[0]) / crop_coordinates[2])
- y0 = clamp((bbox[1] - crop_coordinates[1]) / crop_coordinates[3])
- w = min(bbox[2] / crop_coordinates[2], 1 - x0)
- h = min(bbox[3] / crop_coordinates[3], 1 - y0)
- return x0, y0, w, h
-
- return [rescale_bbox(b) for b in bboxes]
-
- def apply_model(self, x_noisy, t, cond, return_ids=False):
-
- if isinstance(cond, dict):
- # hybrid case, cond is exptected to be a dict
- pass
- else:
- if not isinstance(cond, list):
- cond = [cond]
- key = 'c_concat' if self.model.conditioning_key == 'concat' else 'c_crossattn'
- cond = {key: cond}
-
- if hasattr(self, "split_input_params"):
- assert len(cond) == 1 # todo can only deal with one conditioning atm
- assert not return_ids
- ks = self.split_input_params["ks"] # eg. (128, 128)
- stride = self.split_input_params["stride"] # eg. (64, 64)
-
- h, w = x_noisy.shape[-2:]
-
- fold, unfold, normalization, weighting = self.get_fold_unfold(x_noisy, ks, stride)
-
- z = unfold(x_noisy) # (bn, nc * prod(**ks), L)
- # Reshape to img shape
- z = z.view((z.shape[0], -1, ks[0], ks[1], z.shape[-1])) # (bn, nc, ks[0], ks[1], L )
- z_list = [z[:, :, :, :, i] for i in range(z.shape[-1])]
-
- if self.cond_stage_key in ["image", "LR_image", "segmentation",
- 'bbox_img'] and self.model.conditioning_key: # todo check for completeness
- c_key = next(iter(cond.keys())) # get key
- c = next(iter(cond.values())) # get value
- assert (len(c) == 1) # todo extend to list with more than one elem
- c = c[0] # get element
-
- c = unfold(c)
- c = c.view((c.shape[0], -1, ks[0], ks[1], c.shape[-1])) # (bn, nc, ks[0], ks[1], L )
-
- cond_list = [{c_key: [c[:, :, :, :, i]]} for i in range(c.shape[-1])]
-
- elif self.cond_stage_key == 'coordinates_bbox':
- assert 'original_image_size' in self.split_input_params, 'BoudingBoxRescaling is missing original_image_size'
-
- # assuming padding of unfold is always 0 and its dilation is always 1
- n_patches_per_row = int((w - ks[0]) / stride[0] + 1)
- full_img_h, full_img_w = self.split_input_params['original_image_size']
- # as we are operating on latents, we need the factor from the original image size to the
- # spatial latent size to properly rescale the crops for regenerating the bbox annotations
- num_downs = self.first_stage_model.encoder.num_resolutions - 1
- rescale_latent = 2 ** (num_downs)
-
- # get top left postions of patches as conforming for the bbbox tokenizer, therefore we
- # need to rescale the tl patch coordinates to be in between (0,1)
- tl_patch_coordinates = [(rescale_latent * stride[0] * (patch_nr % n_patches_per_row) / full_img_w,
- rescale_latent * stride[1] * (patch_nr // n_patches_per_row) / full_img_h)
- for patch_nr in range(z.shape[-1])]
-
- # patch_limits are tl_coord, width and height coordinates as (x_tl, y_tl, h, w)
- patch_limits = [(x_tl, y_tl,
- rescale_latent * ks[0] / full_img_w,
- rescale_latent * ks[1] / full_img_h) for x_tl, y_tl in tl_patch_coordinates]
- # patch_values = [(np.arange(x_tl,min(x_tl+ks, 1.)),np.arange(y_tl,min(y_tl+ks, 1.))) for x_tl, y_tl in tl_patch_coordinates]
-
- # tokenize crop coordinates for the bounding boxes of the respective patches
- patch_limits_tknzd = [torch.LongTensor(self.bbox_tokenizer._crop_encoder(bbox))[None].to(self.device)
- for bbox in patch_limits] # list of length l with tensors of shape (1, 2)
- print(patch_limits_tknzd[0].shape)
- # cut tknzd crop position from conditioning
- assert isinstance(cond, dict), 'cond must be dict to be fed into model'
- cut_cond = cond['c_crossattn'][0][..., :-2].to(self.device)
- print(cut_cond.shape)
-
- adapted_cond = torch.stack([torch.cat([cut_cond, p], dim=1) for p in patch_limits_tknzd])
- adapted_cond = rearrange(adapted_cond, 'l b n -> (l b) n')
- print(adapted_cond.shape)
- adapted_cond = self.get_learned_conditioning(adapted_cond)
- print(adapted_cond.shape)
- adapted_cond = rearrange(adapted_cond, '(l b) n d -> l b n d', l=z.shape[-1])
- print(adapted_cond.shape)
-
- cond_list = [{'c_crossattn': [e]} for e in adapted_cond]
-
- else:
- cond_list = [cond for i in range(z.shape[-1])] # Todo make this more efficient
-
- # apply model by loop over crops
- output_list = [self.model(z_list[i], t, **cond_list[i]) for i in range(z.shape[-1])]
- assert not isinstance(output_list[0],
- tuple) # todo cant deal with multiple model outputs check this never happens
-
- o = torch.stack(output_list, axis=-1)
- o = o * weighting
- # Reverse reshape to img shape
- o = o.view((o.shape[0], -1, o.shape[-1])) # (bn, nc * ks[0] * ks[1], L)
- # stitch crops together
- x_recon = fold(o) / normalization
-
- else:
- x_recon = self.model(x_noisy, t, **cond)
-
- if isinstance(x_recon, tuple) and not return_ids:
- return x_recon[0]
- else:
- return x_recon
-
- def _predict_eps_from_xstart(self, x_t, t, pred_xstart):
- return (extract_into_tensor(self.sqrt_recip_alphas_cumprod, t, x_t.shape) * x_t - pred_xstart) / \
- extract_into_tensor(self.sqrt_recipm1_alphas_cumprod, t, x_t.shape)
-
- def _prior_bpd(self, x_start):
- """
- Get the prior KL term for the variational lower-bound, measured in
- bits-per-dim.
- This term can't be optimized, as it only depends on the encoder.
- :param x_start: the [N x C x ...] tensor of inputs.
- :return: a batch of [N] KL values (in bits), one per batch element.
- """
- batch_size = x_start.shape[0]
- t = torch.tensor([self.num_timesteps - 1] * batch_size, device=x_start.device)
- qt_mean, _, qt_log_variance = self.q_mean_variance(x_start, t)
- kl_prior = normal_kl(mean1=qt_mean, logvar1=qt_log_variance, mean2=0.0, logvar2=0.0)
- return mean_flat(kl_prior) / np.log(2.0)
-
- def p_losses(self, x_start, cond, t, noise=None):
- noise = default(noise, lambda: torch.randn_like(x_start))
- x_noisy = self.q_sample(x_start=x_start, t=t, noise=noise)
- model_output = self.apply_model(x_noisy, t, cond)
-
- loss_dict = {}
- prefix = 'train' if self.training else 'val'
-
- if self.parameterization == "x0":
- target = x_start
- elif self.parameterization == "eps":
- target = noise
- else:
- raise NotImplementedError()
-
- loss_simple = self.get_loss(model_output, target, mean=False).mean([1, 2, 3])
- loss_dict.update({f'{prefix}/loss_simple': loss_simple.mean()})
-
- logvar_t = self.logvar[t].to(self.device)
- loss = loss_simple / torch.exp(logvar_t) + logvar_t
- # loss = loss_simple / torch.exp(self.logvar) + self.logvar
- if self.learn_logvar:
- loss_dict.update({f'{prefix}/loss_gamma': loss.mean()})
- loss_dict.update({'logvar': self.logvar.data.mean()})
-
- loss = self.l_simple_weight * loss.mean()
-
- loss_vlb = self.get_loss(model_output, target, mean=False).mean(dim=(1, 2, 3))
- loss_vlb = (self.lvlb_weights[t] * loss_vlb).mean()
- loss_dict.update({f'{prefix}/loss_vlb': loss_vlb})
- loss += (self.original_elbo_weight * loss_vlb)
- loss_dict.update({f'{prefix}/loss': loss})
-
- return loss, loss_dict
-
- def p_mean_variance(self, x, c, t, clip_denoised: bool, return_codebook_ids=False, quantize_denoised=False,
- return_x0=False, score_corrector=None, corrector_kwargs=None):
- t_in = t
- model_out = self.apply_model(x, t_in, c, return_ids=return_codebook_ids)
-
- if score_corrector is not None:
- assert self.parameterization == "eps"
- model_out = score_corrector.modify_score(self, model_out, x, t, c, **corrector_kwargs)
-
- if return_codebook_ids:
- model_out, logits = model_out
-
- if self.parameterization == "eps":
- x_recon = self.predict_start_from_noise(x, t=t, noise=model_out)
- elif self.parameterization == "x0":
- x_recon = model_out
- else:
- raise NotImplementedError()
-
- if clip_denoised:
- x_recon.clamp_(-1., 1.)
- if quantize_denoised:
- x_recon, _, [_, _, indices] = self.first_stage_model.quantize(x_recon)
- model_mean, posterior_variance, posterior_log_variance = self.q_posterior(x_start=x_recon, x_t=x, t=t)
- if return_codebook_ids:
- return model_mean, posterior_variance, posterior_log_variance, logits
- elif return_x0:
- return model_mean, posterior_variance, posterior_log_variance, x_recon
- else:
- return model_mean, posterior_variance, posterior_log_variance
-
- @torch.no_grad()
- def p_sample(self, x, c, t, clip_denoised=False, repeat_noise=False,
- return_codebook_ids=False, quantize_denoised=False, return_x0=False,
- temperature=1., noise_dropout=0., score_corrector=None, corrector_kwargs=None):
- b, *_, device = *x.shape, x.device
- outputs = self.p_mean_variance(x=x, c=c, t=t, clip_denoised=clip_denoised,
- return_codebook_ids=return_codebook_ids,
- quantize_denoised=quantize_denoised,
- return_x0=return_x0,
- score_corrector=score_corrector, corrector_kwargs=corrector_kwargs)
- if return_codebook_ids:
- raise DeprecationWarning("Support dropped.")
- model_mean, _, model_log_variance, logits = outputs
- elif return_x0:
- model_mean, _, model_log_variance, x0 = outputs
- else:
- model_mean, _, model_log_variance = outputs
-
- noise = noise_like(x.shape, device, repeat_noise) * temperature
- if noise_dropout > 0.:
- noise = torch.nn.functional.dropout(noise, p=noise_dropout)
- # no noise when t == 0
- nonzero_mask = (1 - (t == 0).float()).reshape(b, *((1,) * (len(x.shape) - 1)))
-
- if return_codebook_ids:
- return model_mean + nonzero_mask * (0.5 * model_log_variance).exp() * noise, logits.argmax(dim=1)
- if return_x0:
- return model_mean + nonzero_mask * (0.5 * model_log_variance).exp() * noise, x0
- else:
- return model_mean + nonzero_mask * (0.5 * model_log_variance).exp() * noise
-
- @torch.no_grad()
- def progressive_denoising(self, cond, shape, verbose=True, callback=None, quantize_denoised=False,
- img_callback=None, mask=None, x0=None, temperature=1., noise_dropout=0.,
- score_corrector=None, corrector_kwargs=None, batch_size=None, x_T=None, start_T=None,
- log_every_t=None):
- if not log_every_t:
- log_every_t = self.log_every_t
- timesteps = self.num_timesteps
- if batch_size is not None:
- b = batch_size if batch_size is not None else shape[0]
- shape = [batch_size] + list(shape)
- else:
- b = batch_size = shape[0]
- if x_T is None:
- img = torch.randn(shape, device=self.device)
- else:
- img = x_T
- intermediates = []
- if cond is not None:
- if isinstance(cond, dict):
- cond = {key: cond[key][:batch_size] if not isinstance(cond[key], list) else
- list(map(lambda x: x[:batch_size], cond[key])) for key in cond}
- else:
- cond = [c[:batch_size] for c in cond] if isinstance(cond, list) else cond[:batch_size]
-
- if start_T is not None:
- timesteps = min(timesteps, start_T)
- iterator = tqdm(reversed(range(0, timesteps)), desc='Progressive Generation',
- total=timesteps) if verbose else reversed(
- range(0, timesteps))
- if type(temperature) == float:
- temperature = [temperature] * timesteps
-
- for i in iterator:
- ts = torch.full((b,), i, device=self.device, dtype=torch.long)
- if self.shorten_cond_schedule:
- assert self.model.conditioning_key != 'hybrid'
- tc = self.cond_ids[ts].to(cond.device)
- cond = self.q_sample(x_start=cond, t=tc, noise=torch.randn_like(cond))
-
- img, x0_partial = self.p_sample(img, cond, ts,
- clip_denoised=self.clip_denoised,
- quantize_denoised=quantize_denoised, return_x0=True,
- temperature=temperature[i], noise_dropout=noise_dropout,
- score_corrector=score_corrector, corrector_kwargs=corrector_kwargs)
- if mask is not None:
- assert x0 is not None
- img_orig = self.q_sample(x0, ts)
- img = img_orig * mask + (1. - mask) * img
-
- if i % log_every_t == 0 or i == timesteps - 1:
- intermediates.append(x0_partial)
- if callback: callback(i)
- if img_callback: img_callback(img, i)
- return img, intermediates
-
- @torch.no_grad()
- def p_sample_loop(self, cond, shape, return_intermediates=False,
- x_T=None, verbose=True, callback=None, timesteps=None, quantize_denoised=False,
- mask=None, x0=None, img_callback=None, start_T=None,
- log_every_t=None):
-
- if not log_every_t:
- log_every_t = self.log_every_t
- device = self.betas.device
- b = shape[0]
- if x_T is None:
- img = torch.randn(shape, device=device)
- else:
- img = x_T
-
- intermediates = [img]
- if timesteps is None:
- timesteps = self.num_timesteps
-
- if start_T is not None:
- timesteps = min(timesteps, start_T)
- iterator = tqdm(reversed(range(0, timesteps)), desc='Sampling t', total=timesteps) if verbose else reversed(
- range(0, timesteps))
-
- if mask is not None:
- assert x0 is not None
- assert x0.shape[2:3] == mask.shape[2:3] # spatial size has to match
-
- for i in iterator:
- ts = torch.full((b,), i, device=device, dtype=torch.long)
- if self.shorten_cond_schedule:
- assert self.model.conditioning_key != 'hybrid'
- tc = self.cond_ids[ts].to(cond.device)
- cond = self.q_sample(x_start=cond, t=tc, noise=torch.randn_like(cond))
-
- img = self.p_sample(img, cond, ts,
- clip_denoised=self.clip_denoised,
- quantize_denoised=quantize_denoised)
- if mask is not None:
- img_orig = self.q_sample(x0, ts)
- img = img_orig * mask + (1. - mask) * img
-
- if i % log_every_t == 0 or i == timesteps - 1:
- intermediates.append(img)
- if callback: callback(i)
- if img_callback: img_callback(img, i)
-
- if return_intermediates:
- return img, intermediates
- return img
-
- @torch.no_grad()
- def sample(self, cond, batch_size=16, return_intermediates=False, x_T=None,
- verbose=True, timesteps=None, quantize_denoised=False,
- mask=None, x0=None, shape=None,**kwargs):
- if shape is None:
- shape = (batch_size, self.channels, self.image_size, self.image_size)
- if cond is not None:
- if isinstance(cond, dict):
- cond = {key: cond[key][:batch_size] if not isinstance(cond[key], list) else
- list(map(lambda x: x[:batch_size], cond[key])) for key in cond}
- else:
- cond = [c[:batch_size] for c in cond] if isinstance(cond, list) else cond[:batch_size]
- return self.p_sample_loop(cond,
- shape,
- return_intermediates=return_intermediates, x_T=x_T,
- verbose=verbose, timesteps=timesteps, quantize_denoised=quantize_denoised,
- mask=mask, x0=x0)
-
- @torch.no_grad()
- def sample_log(self,cond,batch_size,ddim, ddim_steps,**kwargs):
-
- if ddim:
- ddim_sampler = DDIMSampler(self)
- shape = (self.channels, self.image_size, self.image_size)
- samples, intermediates =ddim_sampler.sample(ddim_steps,batch_size,
- shape,cond,verbose=False,**kwargs)
-
- else:
- samples, intermediates = self.sample(cond=cond, batch_size=batch_size,
- return_intermediates=True,**kwargs)
-
- return samples, intermediates
-
-
- @torch.no_grad()
- def log_images(self, batch, N=8, n_row=4, sample=True, ddim_steps=200, ddim_eta=1., return_keys=None,
- quantize_denoised=True, inpaint=True, plot_denoise_rows=False, plot_progressive_rows=True,
- plot_diffusion_rows=True, **kwargs):
-
- use_ddim = ddim_steps is not None
-
- log = dict()
- z, c, x, xrec, xc = self.get_input(batch, self.first_stage_key,
- return_first_stage_outputs=True,
- force_c_encode=True,
- return_original_cond=True,
- bs=N)
- N = min(x.shape[0], N)
- n_row = min(x.shape[0], n_row)
- log["inputs"] = x
- log["reconstruction"] = xrec
- if self.model.conditioning_key is not None:
- if hasattr(self.cond_stage_model, "decode"):
- xc = self.cond_stage_model.decode(c)
- log["conditioning"] = xc
- elif self.cond_stage_key in ["caption"]:
- xc = log_txt_as_img((x.shape[2], x.shape[3]), batch["caption"])
- log["conditioning"] = xc
- elif self.cond_stage_key == 'class_label':
- xc = log_txt_as_img((x.shape[2], x.shape[3]), batch["human_label"])
- log['conditioning'] = xc
- elif isimage(xc):
- log["conditioning"] = xc
- if ismap(xc):
- log["original_conditioning"] = self.to_rgb(xc)
-
- if plot_diffusion_rows:
- # get diffusion row
- diffusion_row = list()
- z_start = z[:n_row]
- for t in range(self.num_timesteps):
- if t % self.log_every_t == 0 or t == self.num_timesteps - 1:
- t = repeat(torch.tensor([t]), '1 -> b', b=n_row)
- t = t.to(self.device).long()
- noise = torch.randn_like(z_start)
- z_noisy = self.q_sample(x_start=z_start, t=t, noise=noise)
- diffusion_row.append(self.decode_first_stage(z_noisy))
-
- diffusion_row = torch.stack(diffusion_row) # n_log_step, n_row, C, H, W
- diffusion_grid = rearrange(diffusion_row, 'n b c h w -> b n c h w')
- diffusion_grid = rearrange(diffusion_grid, 'b n c h w -> (b n) c h w')
- diffusion_grid = make_grid(diffusion_grid, nrow=diffusion_row.shape[0])
- log["diffusion_row"] = diffusion_grid
-
- if sample:
- # get denoise row
- with self.ema_scope("Plotting"):
- samples, z_denoise_row = self.sample_log(cond=c,batch_size=N,ddim=use_ddim,
- ddim_steps=ddim_steps,eta=ddim_eta)
- # samples, z_denoise_row = self.sample(cond=c, batch_size=N, return_intermediates=True)
- x_samples = self.decode_first_stage(samples)
- log["samples"] = x_samples
- if plot_denoise_rows:
- denoise_grid = self._get_denoise_row_from_list(z_denoise_row)
- log["denoise_row"] = denoise_grid
-
- if quantize_denoised and not isinstance(self.first_stage_model, AutoencoderKL) and not isinstance(
- self.first_stage_model, IdentityFirstStage):
- # also display when quantizing x0 while sampling
- with self.ema_scope("Plotting Quantized Denoised"):
- samples, z_denoise_row = self.sample_log(cond=c,batch_size=N,ddim=use_ddim,
- ddim_steps=ddim_steps,eta=ddim_eta,
- quantize_denoised=True)
- # samples, z_denoise_row = self.sample(cond=c, batch_size=N, return_intermediates=True,
- # quantize_denoised=True)
- x_samples = self.decode_first_stage(samples.to(self.device))
- log["samples_x0_quantized"] = x_samples
-
- if inpaint:
- # make a simple center square
- b, h, w = z.shape[0], z.shape[2], z.shape[3]
- mask = torch.ones(N, h, w).to(self.device)
- # zeros will be filled in
- mask[:, h // 4:3 * h // 4, w // 4:3 * w // 4] = 0.
- mask = mask[:, None, ...]
- with self.ema_scope("Plotting Inpaint"):
-
- samples, _ = self.sample_log(cond=c,batch_size=N,ddim=use_ddim, eta=ddim_eta,
- ddim_steps=ddim_steps, x0=z[:N], mask=mask)
- x_samples = self.decode_first_stage(samples.to(self.device))
- log["samples_inpainting"] = x_samples
- log["mask"] = mask
-
- # outpaint
- with self.ema_scope("Plotting Outpaint"):
- samples, _ = self.sample_log(cond=c, batch_size=N, ddim=use_ddim,eta=ddim_eta,
- ddim_steps=ddim_steps, x0=z[:N], mask=mask)
- x_samples = self.decode_first_stage(samples.to(self.device))
- log["samples_outpainting"] = x_samples
-
- if plot_progressive_rows:
- with self.ema_scope("Plotting Progressives"):
- img, progressives = self.progressive_denoising(c,
- shape=(self.channels, self.image_size, self.image_size),
- batch_size=N)
- prog_row = self._get_denoise_row_from_list(progressives, desc="Progressive Generation")
- log["progressive_row"] = prog_row
-
- if return_keys:
- if np.intersect1d(list(log.keys()), return_keys).shape[0] == 0:
- return log
- else:
- return {key: log[key] for key in return_keys}
- return log
-
- def configure_optimizers(self):
- lr = self.learning_rate
- params = list(self.model.parameters())
- if self.cond_stage_trainable:
- print(f"{self.__class__.__name__}: Also optimizing conditioner params!")
- params = params + list(self.cond_stage_model.parameters())
- if self.learn_logvar:
- print('Diffusion model optimizing logvar')
- params.append(self.logvar)
- opt = torch.optim.AdamW(params, lr=lr)
- if self.use_scheduler:
- assert 'target' in self.scheduler_config
- scheduler = instantiate_from_config(self.scheduler_config)
-
- print("Setting up LambdaLR scheduler...")
- scheduler = [
- {
- 'scheduler': LambdaLR(opt, lr_lambda=scheduler.schedule),
- 'interval': 'step',
- 'frequency': 1
- }]
- return [opt], scheduler
- return opt
-
- @torch.no_grad()
- def to_rgb(self, x):
- x = x.float()
- if not hasattr(self, "colorize"):
- self.colorize = torch.randn(3, x.shape[1], 1, 1).to(x)
- x = nn.functional.conv2d(x, weight=self.colorize)
- x = 2. * (x - x.min()) / (x.max() - x.min()) - 1.
- return x
-
-
-class DiffusionWrapper(pl.LightningModule):
- def __init__(self, diff_model_config, conditioning_key):
- super().__init__()
- self.diffusion_model = instantiate_from_config(diff_model_config)
- self.conditioning_key = conditioning_key # 'crossattn' for txt2image, concat for inpainting
- assert self.conditioning_key in [None, 'concat', 'crossattn', 'hybrid', 'adm']
-
- def forward(self, x, t, c_concat: list = None, c_crossattn: list = None):
- """param x: tensor with shape:[B,C,mel_len,T]"""
- if self.conditioning_key is None:
- out = self.diffusion_model(x, t)
- elif self.conditioning_key == 'concat':
- xc = torch.cat([x] + c_concat, dim=1)# channel dim,x shape (b,3,64,64) c_concat shape(b,4,64,64)
- out = self.diffusion_model(xc, t)
- elif self.conditioning_key == 'crossattn':
- cc = torch.cat(c_crossattn, 1)# [b,seq_len,dim]
- out = self.diffusion_model(x, t, context=cc)
- elif self.conditioning_key == 'hybrid':# not implemented in the LatentDiffusion
- xc = torch.cat([x] + c_concat, dim=1)
- cc = torch.cat(c_crossattn, 1)
- out = self.diffusion_model(xc, t, context=cc)
- elif self.conditioning_key == 'adm':
- cc = c_crossattn[0]
- out = self.diffusion_model(x, t, y=cc)
- else:
- raise NotImplementedError()
-
- return out
-
-
-class Layout2ImgDiffusion(LatentDiffusion):
- # TODO: move all layout-specific hacks to this class
- def __init__(self, cond_stage_key, *args, **kwargs):
- assert cond_stage_key == 'coordinates_bbox', 'Layout2ImgDiffusion only for cond_stage_key="coordinates_bbox"'
- super().__init__(cond_stage_key=cond_stage_key, *args, **kwargs)
-
- def log_images(self, batch, N=8, *args, **kwargs):
- logs = super().log_images(batch=batch, N=N, *args, **kwargs)
-
- key = 'train' if self.training else 'validation'
- dset = self.trainer.datamodule.datasets[key]
- mapper = dset.conditional_builders[self.cond_stage_key]
-
- bbox_imgs = []
- map_fn = lambda catno: dset.get_textual_label(dset.get_category_id(catno))
- for tknzd_bbox in batch[self.cond_stage_key][:N]:
- bboximg = mapper.plot(tknzd_bbox.detach().cpu(), map_fn, (256, 256))
- bbox_imgs.append(bboximg)
-
- cond_img = torch.stack(bbox_imgs, dim=0)
- logs['bbox_image'] = cond_img
- return logs
diff --git a/spaces/AIGC-Audio/Make_An_Audio/wav_evaluation/models/audio.py b/spaces/AIGC-Audio/Make_An_Audio/wav_evaluation/models/audio.py
deleted file mode 100644
index 0980d729dd3b579fee0380d0b9d7055e6843ba12..0000000000000000000000000000000000000000
--- a/spaces/AIGC-Audio/Make_An_Audio/wav_evaluation/models/audio.py
+++ /dev/null
@@ -1,179 +0,0 @@
-import torch
-import torch.nn as nn
-import torch.nn.functional as F
-from torchlibrosa.stft import Spectrogram, LogmelFilterBank
-
-def get_audio_encoder(name: str):
- if name == "Cnn14":
- return Cnn14
- else:
- raise Exception('The audio encoder name {} is incorrect or not supported'.format(name))
-
-
-class ConvBlock(nn.Module):
- def __init__(self, in_channels, out_channels):
-
- super(ConvBlock, self).__init__()
-
- self.conv1 = nn.Conv2d(in_channels=in_channels,
- out_channels=out_channels,
- kernel_size=(3, 3), stride=(1, 1),
- padding=(1, 1), bias=False)
-
- self.conv2 = nn.Conv2d(in_channels=out_channels,
- out_channels=out_channels,
- kernel_size=(3, 3), stride=(1, 1),
- padding=(1, 1), bias=False)
-
- self.bn1 = nn.BatchNorm2d(out_channels)
- self.bn2 = nn.BatchNorm2d(out_channels)
-
-
- def forward(self, input, pool_size=(2, 2), pool_type='avg'):
-
- x = input
- x = F.relu_(self.bn1(self.conv1(x)))
- x = F.relu_(self.bn2(self.conv2(x)))
- if pool_type == 'max':
- x = F.max_pool2d(x, kernel_size=pool_size)
- elif pool_type == 'avg':
- x = F.avg_pool2d(x, kernel_size=pool_size)
- elif pool_type == 'avg+max':
- x1 = F.avg_pool2d(x, kernel_size=pool_size)
- x2 = F.max_pool2d(x, kernel_size=pool_size)
- x = x1 + x2
- else:
- raise Exception('Incorrect argument!')
-
- return x
-
-
-class ConvBlock5x5(nn.Module):
- def __init__(self, in_channels, out_channels):
-
- super(ConvBlock5x5, self).__init__()
-
- self.conv1 = nn.Conv2d(in_channels=in_channels,
- out_channels=out_channels,
- kernel_size=(5, 5), stride=(1, 1),
- padding=(2, 2), bias=False)
-
- self.bn1 = nn.BatchNorm2d(out_channels)
-
-
- def forward(self, input, pool_size=(2, 2), pool_type='avg'):
-
- x = input
- x = F.relu_(self.bn1(self.conv1(x)))
- if pool_type == 'max':
- x = F.max_pool2d(x, kernel_size=pool_size)
- elif pool_type == 'avg':
- x = F.avg_pool2d(x, kernel_size=pool_size)
- elif pool_type == 'avg+max':
- x1 = F.avg_pool2d(x, kernel_size=pool_size)
- x2 = F.max_pool2d(x, kernel_size=pool_size)
- x = x1 + x2
- else:
- raise Exception('Incorrect argument!')
-
- return x
-
-
-class AttBlock(nn.Module):
- def __init__(self, n_in, n_out, activation='linear', temperature=1.):
- super(AttBlock, self).__init__()
-
- self.activation = activation
- self.temperature = temperature
- self.att = nn.Conv1d(in_channels=n_in, out_channels=n_out, kernel_size=1, stride=1, padding=0, bias=True)
- self.cla = nn.Conv1d(in_channels=n_in, out_channels=n_out, kernel_size=1, stride=1, padding=0, bias=True)
-
- self.bn_att = nn.BatchNorm1d(n_out)
-
- def forward(self, x):
- # x: (n_samples, n_in, n_time)
- norm_att = torch.softmax(torch.clamp(self.att(x), -10, 10), dim=-1)
- cla = self.nonlinear_transform(self.cla(x))
- x = torch.sum(norm_att * cla, dim=2)
- return x, norm_att, cla
-
- def nonlinear_transform(self, x):
- if self.activation == 'linear':
- return x
- elif self.activation == 'sigmoid':
- return torch.sigmoid(x)
-
-
-class Cnn14(nn.Module):
- def __init__(self, sample_rate, window_size, hop_size, mel_bins, fmin,
- fmax, classes_num, out_emb):
-
- super(Cnn14, self).__init__()
-
- window = 'hann'
- center = True
- pad_mode = 'reflect'
- ref = 1.0
- amin = 1e-10
- top_db = None
-
- # Spectrogram extractor
- self.spectrogram_extractor = Spectrogram(n_fft=window_size, hop_length=hop_size,
- win_length=window_size, window=window, center=center, pad_mode=pad_mode,
- freeze_parameters=True)
-
- # Logmel feature extractor
- self.logmel_extractor = LogmelFilterBank(sr=sample_rate, n_fft=window_size,
- n_mels=mel_bins, fmin=fmin, fmax=fmax, ref=ref, amin=amin, top_db=top_db,
- freeze_parameters=True)
-
- self.bn0 = nn.BatchNorm2d(64)
-
- self.conv_block1 = ConvBlock(in_channels=1, out_channels=64)
- self.conv_block2 = ConvBlock(in_channels=64, out_channels=128)
- self.conv_block3 = ConvBlock(in_channels=128, out_channels=256)
- self.conv_block4 = ConvBlock(in_channels=256, out_channels=512)
- self.conv_block5 = ConvBlock(in_channels=512, out_channels=1024)
- self.conv_block6 = ConvBlock(in_channels=1024, out_channels=2048)
-
- # out_emb is 2048 for best Cnn14
- self.fc1 = nn.Linear(2048, out_emb, bias=True)
- self.fc_audioset = nn.Linear(out_emb, classes_num, bias=True)
-
- def forward(self, input, mixup_lambda=None):
- """
- Input: (batch_size, data_length)
- """
-
- x = self.spectrogram_extractor(input) # (batch_size, 1, time_steps, freq_bins)
- x = self.logmel_extractor(x) # (batch_size, 1, time_steps, mel_bins)
-
- x = x.transpose(1, 3)
- x = self.bn0(x)
- x = x.transpose(1, 3)
-
- x = self.conv_block1(x, pool_size=(2, 2), pool_type='avg')
- x = F.dropout(x, p=0.2, training=self.training)
- x = self.conv_block2(x, pool_size=(2, 2), pool_type='avg')
- x = F.dropout(x, p=0.2, training=self.training)
- x = self.conv_block3(x, pool_size=(2, 2), pool_type='avg')
- x = F.dropout(x, p=0.2, training=self.training)
- x = self.conv_block4(x, pool_size=(2, 2), pool_type='avg')
- x = F.dropout(x, p=0.2, training=self.training)
- x = self.conv_block5(x, pool_size=(2, 2), pool_type='avg')
- x = F.dropout(x, p=0.2, training=self.training)
- x = self.conv_block6(x, pool_size=(1, 1), pool_type='avg')
- x = F.dropout(x, p=0.2, training=self.training)
- x = torch.mean(x, dim=3)
-
- (x1, _) = torch.max(x, dim=2)
- x2 = torch.mean(x, dim=2)
- x = x1 + x2
- x = F.dropout(x, p=0.5, training=self.training)
- x = F.relu_(self.fc1(x))
- embedding = F.dropout(x, p=0.5, training=self.training)
- clipwise_output = torch.sigmoid(self.fc_audioset(x))
-
- output_dict = {'clipwise_output': clipwise_output, 'embedding': embedding}
-
- return output_dict
\ No newline at end of file
diff --git a/spaces/AILab-CVC/SEED-LLaMA/gradio_demo/conversation.py b/spaces/AILab-CVC/SEED-LLaMA/gradio_demo/conversation.py
deleted file mode 100644
index dd1b45b09e479e9f53ff6fba42568f7acaf53e20..0000000000000000000000000000000000000000
--- a/spaces/AILab-CVC/SEED-LLaMA/gradio_demo/conversation.py
+++ /dev/null
@@ -1,190 +0,0 @@
-import dataclasses
-from enum import auto, Enum
-from typing import List, Tuple
-
-import io
-import base64
-import os
-from PIL import Image
-import copy
-
-IMG_FLAG = ''
-
-
-class SeparatorStyle(Enum):
- """Different separator style."""
- SINGLE = auto()
- TWO = auto()
- MPT = auto()
- PLAIN = auto()
- LLAMA_2 = auto()
-
-
-def decode_image(encoded_image: str) -> Image:
- decoded_bytes = base64.b64decode(encoded_image.encode('utf-8'))
- buffer = io.BytesIO(decoded_bytes)
- image = Image.open(buffer)
- return image
-
-
-def encode_image(image: Image.Image, format: str = 'PNG') -> str:
- with io.BytesIO() as buffer:
- image.save(buffer, format=format)
- encoded_image = base64.b64encode(buffer.getvalue()).decode('utf-8')
- return encoded_image
-
-
-@dataclasses.dataclass
-class Conversation:
- """A class that keeps all conversation history."""
- system: str
- roles: List[str]
- messages: List[dict] # multi-turn -> user & assistant -> {'images': [PIL.Image,], 'text': str}
- offset: int
- sep_style: SeparatorStyle = SeparatorStyle.SINGLE
- sep: str = "###"
- sep2: str = None
- version: str = "Unknown"
-
- skip_next: bool = False
-
- def get_prompt(self):
- messages = copy.deepcopy(self.messages)
- if self.sep_style == SeparatorStyle.SINGLE:
- if self.system is None or self.system == '':
- text = ''
- else:
- text = self.system + self.sep
- images = []
- for message in messages:
- text += message['role'] + ": " + message['message']['text'] + self.sep
- for image_path, image_ids in zip(message['message']['images'], message['message']['images_ids']):
- if image_ids is not None:
- images.append(image_ids)
- else:
- image = Image.open(image_path).resize((256, 256))
- image_base64 = encode_image(image)
- images.append(image_base64)
-
- text += self.roles[1] + ":"
- elif self.sep_style == SeparatorStyle.LLAMA_2:
- b_token = "[INST] "
- e_token = " [/INST]"
- if self.system is None or self.system == '':
- text = ''
- else:
- text = f"<>\n{self.system}\n<>\n\n"
- images = []
- for idx, message in enumerate(messages):
- # text += message['role'] + ": " + message['message']['text'] + self.sep
- if idx % 2 == 0:
- text += b_token + message['message']['text'] + e_token + self.sep
- else:
- text += message['message']['text'] + self.sep
-
- for image_path, image_ids in zip(message['message']['images'], message['message']['images_ids']):
- if image_ids is not None:
- images.append(image_ids)
- else:
- image = Image.open(image_path).resize((256, 256))
- image_base64 = encode_image(image)
- images.append(image_base64)
- else:
- raise NotImplementedError
-
- return {'text': text, 'images': images}
-
- def update_image_ids(self, images_ids):
- image_count = 0
- for message in self.messages:
- for idx in range(len(message['message']['images_ids'])):
- if message['message']["images_ids"][idx] is None:
- message['message']["images_ids"][idx] = images_ids[image_count]
- image_count += 1
-
- assert len(images_ids) == image_count, print(len(images_ids), image_count)
-
- def append_message(self, role, message):
- self.messages.append([role, message])
-
- def to_gradio_chatbot(self):
- dialog = []
- for i, single_turn in enumerate(self.messages[self.offset:]):
- single_turn = single_turn['message']
- text_list = single_turn['text'].split(IMG_FLAG)
- assert len(text_list) == len(single_turn['images']) + 1, print(text_list, len(single_turn['images']))
- message = ''
- for image_idx in range(len(single_turn['images'])):
- # image = single_turn['images'][image_idx]
- # image_base64 = encode_image(image)
- # image_str = f''
- image_path = single_turn['images'][image_idx]
- if image_path == '':
- message += text_list[image_idx] + ''
- else:
- message += text_list[image_idx] + f''
- message += text_list[-1]
-
- if i % 2 == 0:
- dialog.append([message, None])
- else:
- dialog[-1][-1] = message
-
- return dialog
-
- def copy(self):
- return Conversation(system=self.system,
- roles=self.roles,
- messages=copy.deepcopy(self.messages),
- offset=self.offset,
- sep_style=self.sep_style,
- sep=self.sep,
- sep2=self.sep2,
- version=self.version)
-
- def dict(self):
- messages = copy.deepcopy(self.messages)
- for message in messages:
- if 'images_ids' in message:
- message.pop('images_ids')
- for i in range(len(message['message']['images'])):
- message['message']['images'][i] = os.path.basename(message['message']['images'][i])
- return {
- "system": self.system,
- "roles": self.roles,
- "messages": messages,
- "offset": self.offset,
- "sep": self.sep,
- "sep2": self.sep2,
- }
-
-
-conv_seed_vicuna = Conversation(
- system="",
- roles=("USER", "ASSISTANT"),
- version="v2",
- messages=[],
- offset=0,
- sep_style=SeparatorStyle.SINGLE,
- sep='\n',
-)
-
-conv_seed_vicuna_system = Conversation(
- system="A chat between a curious user and an artificial intelligence assistant. ",
- roles=("USER", "ASSISTANT"),
- version="v2",
- messages=[],
- offset=0,
- sep_style=SeparatorStyle.SINGLE,
- sep='\n',
-)
-
-conv_seed_llama2 = Conversation(
- system="",
- roles=("[INST]", "[/INST]"),
- version="v2",
- messages=[],
- offset=0,
- sep_style=SeparatorStyle.LLAMA_2,
- sep='\n',
-)
\ No newline at end of file
diff --git a/spaces/Abhaykoul/HelpingAI-2.0/README.md b/spaces/Abhaykoul/HelpingAI-2.0/README.md
deleted file mode 100644
index 17fe074947f534c723c096b8863e378f8b4433a9..0000000000000000000000000000000000000000
--- a/spaces/Abhaykoul/HelpingAI-2.0/README.md
+++ /dev/null
@@ -1,12 +0,0 @@
----
-title: HelpingAI 2.0
-emoji: 👀
-colorFrom: blue
-colorTo: blue
-sdk: streamlit
-sdk_version: 1.28.1
-app_file: app.py
-pinned: false
----
-
-Check out the configuration reference at https://huggingface.co/docs/hub/spaces-config-reference
diff --git a/spaces/Aditya9790/yolo7-object-tracking/utils/torch_utils.py b/spaces/Aditya9790/yolo7-object-tracking/utils/torch_utils.py
deleted file mode 100644
index bee0ad57517a334748afe7db19f6e45bd657afe6..0000000000000000000000000000000000000000
--- a/spaces/Aditya9790/yolo7-object-tracking/utils/torch_utils.py
+++ /dev/null
@@ -1,374 +0,0 @@
-# YOLOR PyTorch utils
-
-import datetime
-import logging
-import math
-import os
-import platform
-import subprocess
-import time
-from contextlib import contextmanager
-from copy import deepcopy
-from pathlib import Path
-
-import torch
-import torch.backends.cudnn as cudnn
-import torch.nn as nn
-import torch.nn.functional as F
-import torchvision
-
-try:
- import thop # for FLOPS computation
-except ImportError:
- thop = None
-logger = logging.getLogger(__name__)
-
-
-@contextmanager
-def torch_distributed_zero_first(local_rank: int):
- """
- Decorator to make all processes in distributed training wait for each local_master to do something.
- """
- if local_rank not in [-1, 0]:
- torch.distributed.barrier()
- yield
- if local_rank == 0:
- torch.distributed.barrier()
-
-
-def init_torch_seeds(seed=0):
- # Speed-reproducibility tradeoff https://pytorch.org/docs/stable/notes/randomness.html
- torch.manual_seed(seed)
- if seed == 0: # slower, more reproducible
- cudnn.benchmark, cudnn.deterministic = False, True
- else: # faster, less reproducible
- cudnn.benchmark, cudnn.deterministic = True, False
-
-
-def date_modified(path=__file__):
- # return human-readable file modification date, i.e. '2021-3-26'
- t = datetime.datetime.fromtimestamp(Path(path).stat().st_mtime)
- return f'{t.year}-{t.month}-{t.day}'
-
-
-def git_describe(path=Path(__file__).parent): # path must be a directory
- # return human-readable git description, i.e. v5.0-5-g3e25f1e https://git-scm.com/docs/git-describe
- s = f'git -C {path} describe --tags --long --always'
- try:
- return subprocess.check_output(s, shell=True, stderr=subprocess.STDOUT).decode()[:-1]
- except subprocess.CalledProcessError as e:
- return '' # not a git repository
-
-
-def select_device(device='', batch_size=None):
- # device = 'cpu' or '0' or '0,1,2,3'
- s = f'YOLOR 🚀 {git_describe() or date_modified()} torch {torch.__version__} ' # string
- cpu = device.lower() == 'cpu'
- if cpu:
- os.environ['CUDA_VISIBLE_DEVICES'] = '-1' # force torch.cuda.is_available() = False
- elif device: # non-cpu device requested
- os.environ['CUDA_VISIBLE_DEVICES'] = device # set environment variable
- assert torch.cuda.is_available(), f'CUDA unavailable, invalid device {device} requested' # check availability
-
- cuda = not cpu and torch.cuda.is_available()
- if cuda:
- n = torch.cuda.device_count()
- if n > 1 and batch_size: # check that batch_size is compatible with device_count
- assert batch_size % n == 0, f'batch-size {batch_size} not multiple of GPU count {n}'
- space = ' ' * len(s)
- for i, d in enumerate(device.split(',') if device else range(n)):
- p = torch.cuda.get_device_properties(i)
- s += f"{'' if i == 0 else space}CUDA:{d} ({p.name}, {p.total_memory / 1024 ** 2}MB)\n" # bytes to MB
- else:
- s += 'CPU\n'
-
- logger.info(s.encode().decode('ascii', 'ignore') if platform.system() == 'Windows' else s) # emoji-safe
- return torch.device('cuda:0' if cuda else 'cpu')
-
-
-def time_synchronized():
- # pytorch-accurate time
- if torch.cuda.is_available():
- torch.cuda.synchronize()
- return time.time()
-
-
-def profile(x, ops, n=100, device=None):
- # profile a pytorch module or list of modules. Example usage:
- # x = torch.randn(16, 3, 640, 640) # input
- # m1 = lambda x: x * torch.sigmoid(x)
- # m2 = nn.SiLU()
- # profile(x, [m1, m2], n=100) # profile speed over 100 iterations
-
- device = device or torch.device('cuda:0' if torch.cuda.is_available() else 'cpu')
- x = x.to(device)
- x.requires_grad = True
- print(torch.__version__, device.type, torch.cuda.get_device_properties(0) if device.type == 'cuda' else '')
- print(f"\n{'Params':>12s}{'GFLOPS':>12s}{'forward (ms)':>16s}{'backward (ms)':>16s}{'input':>24s}{'output':>24s}")
- for m in ops if isinstance(ops, list) else [ops]:
- m = m.to(device) if hasattr(m, 'to') else m # device
- m = m.half() if hasattr(m, 'half') and isinstance(x, torch.Tensor) and x.dtype is torch.float16 else m # type
- dtf, dtb, t = 0., 0., [0., 0., 0.] # dt forward, backward
- try:
- flops = thop.profile(m, inputs=(x,), verbose=False)[0] / 1E9 * 2 # GFLOPS
- except:
- flops = 0
-
- for _ in range(n):
- t[0] = time_synchronized()
- y = m(x)
- t[1] = time_synchronized()
- try:
- _ = y.sum().backward()
- t[2] = time_synchronized()
- except: # no backward method
- t[2] = float('nan')
- dtf += (t[1] - t[0]) * 1000 / n # ms per op forward
- dtb += (t[2] - t[1]) * 1000 / n # ms per op backward
-
- s_in = tuple(x.shape) if isinstance(x, torch.Tensor) else 'list'
- s_out = tuple(y.shape) if isinstance(y, torch.Tensor) else 'list'
- p = sum(list(x.numel() for x in m.parameters())) if isinstance(m, nn.Module) else 0 # parameters
- print(f'{p:12}{flops:12.4g}{dtf:16.4g}{dtb:16.4g}{str(s_in):>24s}{str(s_out):>24s}')
-
-
-def is_parallel(model):
- return type(model) in (nn.parallel.DataParallel, nn.parallel.DistributedDataParallel)
-
-
-def intersect_dicts(da, db, exclude=()):
- # Dictionary intersection of matching keys and shapes, omitting 'exclude' keys, using da values
- return {k: v for k, v in da.items() if k in db and not any(x in k for x in exclude) and v.shape == db[k].shape}
-
-
-def initialize_weights(model):
- for m in model.modules():
- t = type(m)
- if t is nn.Conv2d:
- pass # nn.init.kaiming_normal_(m.weight, mode='fan_out', nonlinearity='relu')
- elif t is nn.BatchNorm2d:
- m.eps = 1e-3
- m.momentum = 0.03
- elif t in [nn.Hardswish, nn.LeakyReLU, nn.ReLU, nn.ReLU6]:
- m.inplace = True
-
-
-def find_modules(model, mclass=nn.Conv2d):
- # Finds layer indices matching module class 'mclass'
- return [i for i, m in enumerate(model.module_list) if isinstance(m, mclass)]
-
-
-def sparsity(model):
- # Return global model sparsity
- a, b = 0., 0.
- for p in model.parameters():
- a += p.numel()
- b += (p == 0).sum()
- return b / a
-
-
-def prune(model, amount=0.3):
- # Prune model to requested global sparsity
- import torch.nn.utils.prune as prune
- print('Pruning model... ', end='')
- for name, m in model.named_modules():
- if isinstance(m, nn.Conv2d):
- prune.l1_unstructured(m, name='weight', amount=amount) # prune
- prune.remove(m, 'weight') # make permanent
- print(' %.3g global sparsity' % sparsity(model))
-
-
-def fuse_conv_and_bn(conv, bn):
- # Fuse convolution and batchnorm layers https://tehnokv.com/posts/fusing-batchnorm-and-conv/
- fusedconv = nn.Conv2d(conv.in_channels,
- conv.out_channels,
- kernel_size=conv.kernel_size,
- stride=conv.stride,
- padding=conv.padding,
- groups=conv.groups,
- bias=True).requires_grad_(False).to(conv.weight.device)
-
- # prepare filters
- w_conv = conv.weight.clone().view(conv.out_channels, -1)
- w_bn = torch.diag(bn.weight.div(torch.sqrt(bn.eps + bn.running_var)))
- fusedconv.weight.copy_(torch.mm(w_bn, w_conv).view(fusedconv.weight.shape))
-
- # prepare spatial bias
- b_conv = torch.zeros(conv.weight.size(0), device=conv.weight.device) if conv.bias is None else conv.bias
- b_bn = bn.bias - bn.weight.mul(bn.running_mean).div(torch.sqrt(bn.running_var + bn.eps))
- fusedconv.bias.copy_(torch.mm(w_bn, b_conv.reshape(-1, 1)).reshape(-1) + b_bn)
-
- return fusedconv
-
-
-def model_info(model, verbose=False, img_size=640):
- # Model information. img_size may be int or list, i.e. img_size=640 or img_size=[640, 320]
- n_p = sum(x.numel() for x in model.parameters()) # number parameters
- n_g = sum(x.numel() for x in model.parameters() if x.requires_grad) # number gradients
- if verbose:
- print('%5s %40s %9s %12s %20s %10s %10s' % ('layer', 'name', 'gradient', 'parameters', 'shape', 'mu', 'sigma'))
- for i, (name, p) in enumerate(model.named_parameters()):
- name = name.replace('module_list.', '')
- print('%5g %40s %9s %12g %20s %10.3g %10.3g' %
- (i, name, p.requires_grad, p.numel(), list(p.shape), p.mean(), p.std()))
-
- try: # FLOPS
- from thop import profile
- stride = max(int(model.stride.max()), 32) if hasattr(model, 'stride') else 32
- img = torch.zeros((1, model.yaml.get('ch', 3), stride, stride), device=next(model.parameters()).device) # input
- flops = profile(deepcopy(model), inputs=(img,), verbose=False)[0] / 1E9 * 2 # stride GFLOPS
- img_size = img_size if isinstance(img_size, list) else [img_size, img_size] # expand if int/float
- fs = ', %.1f GFLOPS' % (flops * img_size[0] / stride * img_size[1] / stride) # 640x640 GFLOPS
- except (ImportError, Exception):
- fs = ''
-
- logger.info(f"Model Summary: {len(list(model.modules()))} layers, {n_p} parameters, {n_g} gradients{fs}")
-
-
-def load_classifier(name='resnet101', n=2):
- # Loads a pretrained model reshaped to n-class output
- model = torchvision.models.__dict__[name](pretrained=True)
-
- # ResNet model properties
- # input_size = [3, 224, 224]
- # input_space = 'RGB'
- # input_range = [0, 1]
- # mean = [0.485, 0.456, 0.406]
- # std = [0.229, 0.224, 0.225]
-
- # Reshape output to n classes
- filters = model.fc.weight.shape[1]
- model.fc.bias = nn.Parameter(torch.zeros(n), requires_grad=True)
- model.fc.weight = nn.Parameter(torch.zeros(n, filters), requires_grad=True)
- model.fc.out_features = n
- return model
-
-
-def scale_img(img, ratio=1.0, same_shape=False, gs=32): # img(16,3,256,416)
- # scales img(bs,3,y,x) by ratio constrained to gs-multiple
- if ratio == 1.0:
- return img
- else:
- h, w = img.shape[2:]
- s = (int(h * ratio), int(w * ratio)) # new size
- img = F.interpolate(img, size=s, mode='bilinear', align_corners=False) # resize
- if not same_shape: # pad/crop img
- h, w = [math.ceil(x * ratio / gs) * gs for x in (h, w)]
- return F.pad(img, [0, w - s[1], 0, h - s[0]], value=0.447) # value = imagenet mean
-
-
-def copy_attr(a, b, include=(), exclude=()):
- # Copy attributes from b to a, options to only include [...] and to exclude [...]
- for k, v in b.__dict__.items():
- if (len(include) and k not in include) or k.startswith('_') or k in exclude:
- continue
- else:
- setattr(a, k, v)
-
-
-class ModelEMA:
- """ Model Exponential Moving Average from https://github.com/rwightman/pytorch-image-models
- Keep a moving average of everything in the model state_dict (parameters and buffers).
- This is intended to allow functionality like
- https://www.tensorflow.org/api_docs/python/tf/train/ExponentialMovingAverage
- A smoothed version of the weights is necessary for some training schemes to perform well.
- This class is sensitive where it is initialized in the sequence of model init,
- GPU assignment and distributed training wrappers.
- """
-
- def __init__(self, model, decay=0.9999, updates=0):
- # Create EMA
- self.ema = deepcopy(model.module if is_parallel(model) else model).eval() # FP32 EMA
- # if next(model.parameters()).device.type != 'cpu':
- # self.ema.half() # FP16 EMA
- self.updates = updates # number of EMA updates
- self.decay = lambda x: decay * (1 - math.exp(-x / 2000)) # decay exponential ramp (to help early epochs)
- for p in self.ema.parameters():
- p.requires_grad_(False)
-
- def update(self, model):
- # Update EMA parameters
- with torch.no_grad():
- self.updates += 1
- d = self.decay(self.updates)
-
- msd = model.module.state_dict() if is_parallel(model) else model.state_dict() # model state_dict
- for k, v in self.ema.state_dict().items():
- if v.dtype.is_floating_point:
- v *= d
- v += (1. - d) * msd[k].detach()
-
- def update_attr(self, model, include=(), exclude=('process_group', 'reducer')):
- # Update EMA attributes
- copy_attr(self.ema, model, include, exclude)
-
-
-class BatchNormXd(torch.nn.modules.batchnorm._BatchNorm):
- def _check_input_dim(self, input):
- # The only difference between BatchNorm1d, BatchNorm2d, BatchNorm3d, etc
- # is this method that is overwritten by the sub-class
- # This original goal of this method was for tensor sanity checks
- # If you're ok bypassing those sanity checks (eg. if you trust your inference
- # to provide the right dimensional inputs), then you can just use this method
- # for easy conversion from SyncBatchNorm
- # (unfortunately, SyncBatchNorm does not store the original class - if it did
- # we could return the one that was originally created)
- return
-
-def revert_sync_batchnorm(module):
- # this is very similar to the function that it is trying to revert:
- # https://github.com/pytorch/pytorch/blob/c8b3686a3e4ba63dc59e5dcfe5db3430df256833/torch/nn/modules/batchnorm.py#L679
- module_output = module
- if isinstance(module, torch.nn.modules.batchnorm.SyncBatchNorm):
- new_cls = BatchNormXd
- module_output = BatchNormXd(module.num_features,
- module.eps, module.momentum,
- module.affine,
- module.track_running_stats)
- if module.affine:
- with torch.no_grad():
- module_output.weight = module.weight
- module_output.bias = module.bias
- module_output.running_mean = module.running_mean
- module_output.running_var = module.running_var
- module_output.num_batches_tracked = module.num_batches_tracked
- if hasattr(module, "qconfig"):
- module_output.qconfig = module.qconfig
- for name, child in module.named_children():
- module_output.add_module(name, revert_sync_batchnorm(child))
- del module
- return module_output
-
-
-class TracedModel(nn.Module):
-
- def __init__(self, model=None, device=None, img_size=(640,640)):
- super(TracedModel, self).__init__()
-
- print(" Convert model to Traced-model... ")
- self.stride = model.stride
- self.names = model.names
- self.model = model
-
- self.model = revert_sync_batchnorm(self.model)
- self.model.to('cpu')
- self.model.eval()
-
- self.detect_layer = self.model.model[-1]
- self.model.traced = True
-
- rand_example = torch.rand(1, 3, img_size, img_size)
-
- traced_script_module = torch.jit.trace(self.model, rand_example, strict=False)
- #traced_script_module = torch.jit.script(self.model)
- traced_script_module.save("traced_model.pt")
- print(" traced_script_module saved! ")
- self.model = traced_script_module
- self.model.to(device)
- self.detect_layer.to(device)
- print(" model is traced! \n")
-
- def forward(self, x, augment=False, profile=False):
- out = self.model(x)
- out = self.detect_layer(out)
- return out
diff --git a/spaces/AgentVerse/agentVerse/ui/src/phaser3-rex-plugins/plugins/effectlayer-plugin.js b/spaces/AgentVerse/agentVerse/ui/src/phaser3-rex-plugins/plugins/effectlayer-plugin.js
deleted file mode 100644
index eb376b6ad8496bceca6547488431db5ac89bcdeb..0000000000000000000000000000000000000000
--- a/spaces/AgentVerse/agentVerse/ui/src/phaser3-rex-plugins/plugins/effectlayer-plugin.js
+++ /dev/null
@@ -1,23 +0,0 @@
-import Factory from './gameobjects/shader/effectlayer/effectlayer/Factory.js';
-import Creator from './gameobjects/shader/effectlayer/effectlayer/Creator.js';
-import EffectLayer from './gameobjects/shader/effectlayer/effectlayer/EffectLayer.js';
-import SetValue from './utils/object/SetValue.js';
-
-class EffectLayerPlugin extends Phaser.Plugins.BasePlugin {
-
- constructor(pluginManager) {
- super(pluginManager);
-
- // Register our new Game Object type
- pluginManager.registerGameObject('rexEffectLayer', Factory, Creator);
- }
-
- start() {
- var eventEmitter = this.game.events;
- eventEmitter.on('destroy', this.destroy, this);
- }
-}
-
-SetValue(window, 'RexPlugins.GameObjects.EffectLayer', EffectLayer);
-
-export default EffectLayerPlugin;
\ No newline at end of file
diff --git a/spaces/AgentVerse/agentVerse/ui/src/phaser3-rex-plugins/templates/ui/basesizer/utils/AddChild.js b/spaces/AgentVerse/agentVerse/ui/src/phaser3-rex-plugins/templates/ui/basesizer/utils/AddChild.js
deleted file mode 100644
index 82d7c905be3a0318ae3a58580ebf4907cb74e172..0000000000000000000000000000000000000000
--- a/spaces/AgentVerse/agentVerse/ui/src/phaser3-rex-plugins/templates/ui/basesizer/utils/AddChild.js
+++ /dev/null
@@ -1,16 +0,0 @@
-import Container from '../../container/Container.js';
-
-const ContainerAdd = Container.prototype.add;
-
-var AddChild = function (gameObject) {
- ContainerAdd.call(this, gameObject);
-
- if (this.sizerEventsEnable) {
- gameObject.emit('sizer.add', gameObject, this);
- this.emit('add', gameObject, this);
- }
-
- return this;
-}
-
-export default AddChild;
\ No newline at end of file
diff --git a/spaces/Andy1621/uniformer_image_detection/configs/ssd/README.md b/spaces/Andy1621/uniformer_image_detection/configs/ssd/README.md
deleted file mode 100644
index 51262d68efa1e8be0e91e92c2c3dc5585ab2411e..0000000000000000000000000000000000000000
--- a/spaces/Andy1621/uniformer_image_detection/configs/ssd/README.md
+++ /dev/null
@@ -1,21 +0,0 @@
-# SSD: Single Shot MultiBox Detector
-
-## Introduction
-
-[ALGORITHM]
-
-```latex
-@article{Liu_2016,
- title={SSD: Single Shot MultiBox Detector},
- journal={ECCV},
- author={Liu, Wei and Anguelov, Dragomir and Erhan, Dumitru and Szegedy, Christian and Reed, Scott and Fu, Cheng-Yang and Berg, Alexander C.},
- year={2016},
-}
-```
-
-## Results and models
-
-| Backbone | Size | Style | Lr schd | Mem (GB) | Inf time (fps) | box AP | Config | Download |
-| :------: | :---: | :---: | :-----: | :------: | :------------: | :----: | :------: | :--------: |
-| VGG16 | 300 | caffe | 120e | 10.2 | 43.7 | 25.6 | [config](https://github.com/open-mmlab/mmdetection/tree/master/configs/ssd/ssd300_coco.py) | [model](http://download.openmmlab.com/mmdetection/v2.0/ssd/ssd300_coco/ssd300_coco_20200307-a92d2092.pth) | [log](http://download.openmmlab.com/mmdetection/v2.0/ssd/ssd300_coco/ssd300_coco_20200307_174216.log.json) |
-| VGG16 | 512 | caffe | 120e | 9.3 | 30.7 | 29.4 | [config](https://github.com/open-mmlab/mmdetection/tree/master/configs/ssd/ssd512_coco.py) | [model](http://download.openmmlab.com/mmdetection/v2.0/ssd/ssd512_coco/ssd512_coco_20200308-038c5591.pth) | [log](http://download.openmmlab.com/mmdetection/v2.0/ssd/ssd512_coco/ssd512_coco_20200308_134447.log.json) |
diff --git a/spaces/Andy1621/uniformer_image_detection/mmdet/models/utils/res_layer.py b/spaces/Andy1621/uniformer_image_detection/mmdet/models/utils/res_layer.py
deleted file mode 100644
index 4a4efd3dd30b30123ed5135eac080ad9f7f7b448..0000000000000000000000000000000000000000
--- a/spaces/Andy1621/uniformer_image_detection/mmdet/models/utils/res_layer.py
+++ /dev/null
@@ -1,187 +0,0 @@
-from mmcv.cnn import build_conv_layer, build_norm_layer
-from torch import nn as nn
-
-
-class ResLayer(nn.Sequential):
- """ResLayer to build ResNet style backbone.
-
- Args:
- block (nn.Module): block used to build ResLayer.
- inplanes (int): inplanes of block.
- planes (int): planes of block.
- num_blocks (int): number of blocks.
- stride (int): stride of the first block. Default: 1
- avg_down (bool): Use AvgPool instead of stride conv when
- downsampling in the bottleneck. Default: False
- conv_cfg (dict): dictionary to construct and config conv layer.
- Default: None
- norm_cfg (dict): dictionary to construct and config norm layer.
- Default: dict(type='BN')
- downsample_first (bool): Downsample at the first block or last block.
- False for Hourglass, True for ResNet. Default: True
- """
-
- def __init__(self,
- block,
- inplanes,
- planes,
- num_blocks,
- stride=1,
- avg_down=False,
- conv_cfg=None,
- norm_cfg=dict(type='BN'),
- downsample_first=True,
- **kwargs):
- self.block = block
-
- downsample = None
- if stride != 1 or inplanes != planes * block.expansion:
- downsample = []
- conv_stride = stride
- if avg_down:
- conv_stride = 1
- downsample.append(
- nn.AvgPool2d(
- kernel_size=stride,
- stride=stride,
- ceil_mode=True,
- count_include_pad=False))
- downsample.extend([
- build_conv_layer(
- conv_cfg,
- inplanes,
- planes * block.expansion,
- kernel_size=1,
- stride=conv_stride,
- bias=False),
- build_norm_layer(norm_cfg, planes * block.expansion)[1]
- ])
- downsample = nn.Sequential(*downsample)
-
- layers = []
- if downsample_first:
- layers.append(
- block(
- inplanes=inplanes,
- planes=planes,
- stride=stride,
- downsample=downsample,
- conv_cfg=conv_cfg,
- norm_cfg=norm_cfg,
- **kwargs))
- inplanes = planes * block.expansion
- for _ in range(1, num_blocks):
- layers.append(
- block(
- inplanes=inplanes,
- planes=planes,
- stride=1,
- conv_cfg=conv_cfg,
- norm_cfg=norm_cfg,
- **kwargs))
-
- else: # downsample_first=False is for HourglassModule
- for _ in range(num_blocks - 1):
- layers.append(
- block(
- inplanes=inplanes,
- planes=inplanes,
- stride=1,
- conv_cfg=conv_cfg,
- norm_cfg=norm_cfg,
- **kwargs))
- layers.append(
- block(
- inplanes=inplanes,
- planes=planes,
- stride=stride,
- downsample=downsample,
- conv_cfg=conv_cfg,
- norm_cfg=norm_cfg,
- **kwargs))
- super(ResLayer, self).__init__(*layers)
-
-
-class SimplifiedBasicBlock(nn.Module):
- """Simplified version of original basic residual block. This is used in
- `SCNet `_.
-
- - Norm layer is now optional
- - Last ReLU in forward function is removed
- """
- expansion = 1
-
- def __init__(self,
- inplanes,
- planes,
- stride=1,
- dilation=1,
- downsample=None,
- style='pytorch',
- with_cp=False,
- conv_cfg=None,
- norm_cfg=dict(type='BN'),
- dcn=None,
- plugins=None):
- super(SimplifiedBasicBlock, self).__init__()
- assert dcn is None, 'Not implemented yet.'
- assert plugins is None, 'Not implemented yet.'
- assert not with_cp, 'Not implemented yet.'
- self.with_norm = norm_cfg is not None
- with_bias = True if norm_cfg is None else False
- self.conv1 = build_conv_layer(
- conv_cfg,
- inplanes,
- planes,
- 3,
- stride=stride,
- padding=dilation,
- dilation=dilation,
- bias=with_bias)
- if self.with_norm:
- self.norm1_name, norm1 = build_norm_layer(
- norm_cfg, planes, postfix=1)
- self.add_module(self.norm1_name, norm1)
- self.conv2 = build_conv_layer(
- conv_cfg, planes, planes, 3, padding=1, bias=with_bias)
- if self.with_norm:
- self.norm2_name, norm2 = build_norm_layer(
- norm_cfg, planes, postfix=2)
- self.add_module(self.norm2_name, norm2)
-
- self.relu = nn.ReLU(inplace=True)
- self.downsample = downsample
- self.stride = stride
- self.dilation = dilation
- self.with_cp = with_cp
-
- @property
- def norm1(self):
- """nn.Module: normalization layer after the first convolution layer"""
- return getattr(self, self.norm1_name) if self.with_norm else None
-
- @property
- def norm2(self):
- """nn.Module: normalization layer after the second convolution layer"""
- return getattr(self, self.norm2_name) if self.with_norm else None
-
- def forward(self, x):
- """Forward function."""
-
- identity = x
-
- out = self.conv1(x)
- if self.with_norm:
- out = self.norm1(out)
- out = self.relu(out)
-
- out = self.conv2(out)
- if self.with_norm:
- out = self.norm2(out)
-
- if self.downsample is not None:
- identity = self.downsample(x)
-
- out += identity
-
- return out
diff --git a/spaces/AndyCer/TehVenom-MPT-7b-Chat-Instruct-LongCTX-Merge/README.md b/spaces/AndyCer/TehVenom-MPT-7b-Chat-Instruct-LongCTX-Merge/README.md
deleted file mode 100644
index f5484d9cc06ab7898094b249f2f77dc825e46ed8..0000000000000000000000000000000000000000
--- a/spaces/AndyCer/TehVenom-MPT-7b-Chat-Instruct-LongCTX-Merge/README.md
+++ /dev/null
@@ -1,12 +0,0 @@
----
-title: TehVenom MPT 7b Chat Instruct LongCTX Merge
-emoji: 📉
-colorFrom: gray
-colorTo: blue
-sdk: gradio
-sdk_version: 3.29.0
-app_file: app.py
-pinned: false
----
-
-Check out the configuration reference at https://huggingface.co/docs/hub/spaces-config-reference
diff --git a/spaces/Anthony7906/MengHuiMXD_GPT/Dockerfile b/spaces/Anthony7906/MengHuiMXD_GPT/Dockerfile
deleted file mode 100644
index 335c2dba28ba8c365de9306858462a59dea25f28..0000000000000000000000000000000000000000
--- a/spaces/Anthony7906/MengHuiMXD_GPT/Dockerfile
+++ /dev/null
@@ -1,15 +0,0 @@
-FROM python:3.9 as builder
-RUN apt-get update && apt-get install -y build-essential
-COPY requirements.txt .
-COPY requirements_advanced.txt .
-RUN pip install --user -r requirements.txt
-# RUN pip install --user -r requirements_advanced.txt
-
-FROM python:3.9
-MAINTAINER iskoldt
-COPY --from=builder /root/.local /root/.local
-ENV PATH=/root/.local/bin:$PATH
-COPY . /app
-WORKDIR /app
-ENV dockerrun yes
-CMD ["python3", "-u", "ChuanhuChatbot.py", "2>&1", "|", "tee", "/var/log/application.log"]
diff --git a/spaces/Ataturk-Chatbot/HuggingFaceChat/venv/lib/python3.11/site-packages/pip/_vendor/pyparsing/core.py b/spaces/Ataturk-Chatbot/HuggingFaceChat/venv/lib/python3.11/site-packages/pip/_vendor/pyparsing/core.py
deleted file mode 100644
index 6ff3c766f7dd9f4111cbd9d2a5f668e4435798b5..0000000000000000000000000000000000000000
--- a/spaces/Ataturk-Chatbot/HuggingFaceChat/venv/lib/python3.11/site-packages/pip/_vendor/pyparsing/core.py
+++ /dev/null
@@ -1,5814 +0,0 @@
-#
-# core.py
-#
-import os
-import typing
-from typing import (
- NamedTuple,
- Union,
- Callable,
- Any,
- Generator,
- Tuple,
- List,
- TextIO,
- Set,
- Sequence,
-)
-from abc import ABC, abstractmethod
-from enum import Enum
-import string
-import copy
-import warnings
-import re
-import sys
-from collections.abc import Iterable
-import traceback
-import types
-from operator import itemgetter
-from functools import wraps
-from threading import RLock
-from pathlib import Path
-
-from .util import (
- _FifoCache,
- _UnboundedCache,
- __config_flags,
- _collapse_string_to_ranges,
- _escape_regex_range_chars,
- _bslash,
- _flatten,
- LRUMemo as _LRUMemo,
- UnboundedMemo as _UnboundedMemo,
-)
-from .exceptions import *
-from .actions import *
-from .results import ParseResults, _ParseResultsWithOffset
-from .unicode import pyparsing_unicode
-
-_MAX_INT = sys.maxsize
-str_type: Tuple[type, ...] = (str, bytes)
-
-#
-# Copyright (c) 2003-2022 Paul T. McGuire
-#
-# Permission is hereby granted, free of charge, to any person obtaining
-# a copy of this software and associated documentation files (the
-# "Software"), to deal in the Software without restriction, including
-# without limitation the rights to use, copy, modify, merge, publish,
-# distribute, sublicense, and/or sell copies of the Software, and to
-# permit persons to whom the Software is furnished to do so, subject to
-# the following conditions:
-#
-# The above copyright notice and this permission notice shall be
-# included in all copies or substantial portions of the Software.
-#
-# THE SOFTWARE IS PROVIDED "AS IS", WITHOUT WARRANTY OF ANY KIND,
-# EXPRESS OR IMPLIED, INCLUDING BUT NOT LIMITED TO THE WARRANTIES OF
-# MERCHANTABILITY, FITNESS FOR A PARTICULAR PURPOSE AND NONINFRINGEMENT.
-# IN NO EVENT SHALL THE AUTHORS OR COPYRIGHT HOLDERS BE LIABLE FOR ANY
-# CLAIM, DAMAGES OR OTHER LIABILITY, WHETHER IN AN ACTION OF CONTRACT,
-# TORT OR OTHERWISE, ARISING FROM, OUT OF OR IN CONNECTION WITH THE
-# SOFTWARE OR THE USE OR OTHER DEALINGS IN THE SOFTWARE.
-#
-
-
-if sys.version_info >= (3, 8):
- from functools import cached_property
-else:
-
- class cached_property:
- def __init__(self, func):
- self._func = func
-
- def __get__(self, instance, owner=None):
- ret = instance.__dict__[self._func.__name__] = self._func(instance)
- return ret
-
-
-class __compat__(__config_flags):
- """
- A cross-version compatibility configuration for pyparsing features that will be
- released in a future version. By setting values in this configuration to True,
- those features can be enabled in prior versions for compatibility development
- and testing.
-
- - ``collect_all_And_tokens`` - flag to enable fix for Issue #63 that fixes erroneous grouping
- of results names when an :class:`And` expression is nested within an :class:`Or` or :class:`MatchFirst`;
- maintained for compatibility, but setting to ``False`` no longer restores pre-2.3.1
- behavior
- """
-
- _type_desc = "compatibility"
-
- collect_all_And_tokens = True
-
- _all_names = [__ for __ in locals() if not __.startswith("_")]
- _fixed_names = """
- collect_all_And_tokens
- """.split()
-
-
-class __diag__(__config_flags):
- _type_desc = "diagnostic"
-
- warn_multiple_tokens_in_named_alternation = False
- warn_ungrouped_named_tokens_in_collection = False
- warn_name_set_on_empty_Forward = False
- warn_on_parse_using_empty_Forward = False
- warn_on_assignment_to_Forward = False
- warn_on_multiple_string_args_to_oneof = False
- warn_on_match_first_with_lshift_operator = False
- enable_debug_on_named_expressions = False
-
- _all_names = [__ for __ in locals() if not __.startswith("_")]
- _warning_names = [name for name in _all_names if name.startswith("warn")]
- _debug_names = [name for name in _all_names if name.startswith("enable_debug")]
-
- @classmethod
- def enable_all_warnings(cls) -> None:
- for name in cls._warning_names:
- cls.enable(name)
-
-
-class Diagnostics(Enum):
- """
- Diagnostic configuration (all default to disabled)
- - ``warn_multiple_tokens_in_named_alternation`` - flag to enable warnings when a results
- name is defined on a :class:`MatchFirst` or :class:`Or` expression with one or more :class:`And` subexpressions
- - ``warn_ungrouped_named_tokens_in_collection`` - flag to enable warnings when a results
- name is defined on a containing expression with ungrouped subexpressions that also
- have results names
- - ``warn_name_set_on_empty_Forward`` - flag to enable warnings when a :class:`Forward` is defined
- with a results name, but has no contents defined
- - ``warn_on_parse_using_empty_Forward`` - flag to enable warnings when a :class:`Forward` is
- defined in a grammar but has never had an expression attached to it
- - ``warn_on_assignment_to_Forward`` - flag to enable warnings when a :class:`Forward` is defined
- but is overwritten by assigning using ``'='`` instead of ``'<<='`` or ``'<<'``
- - ``warn_on_multiple_string_args_to_oneof`` - flag to enable warnings when :class:`one_of` is
- incorrectly called with multiple str arguments
- - ``enable_debug_on_named_expressions`` - flag to auto-enable debug on all subsequent
- calls to :class:`ParserElement.set_name`
-
- Diagnostics are enabled/disabled by calling :class:`enable_diag` and :class:`disable_diag`.
- All warnings can be enabled by calling :class:`enable_all_warnings`.
- """
-
- warn_multiple_tokens_in_named_alternation = 0
- warn_ungrouped_named_tokens_in_collection = 1
- warn_name_set_on_empty_Forward = 2
- warn_on_parse_using_empty_Forward = 3
- warn_on_assignment_to_Forward = 4
- warn_on_multiple_string_args_to_oneof = 5
- warn_on_match_first_with_lshift_operator = 6
- enable_debug_on_named_expressions = 7
-
-
-def enable_diag(diag_enum: Diagnostics) -> None:
- """
- Enable a global pyparsing diagnostic flag (see :class:`Diagnostics`).
- """
- __diag__.enable(diag_enum.name)
-
-
-def disable_diag(diag_enum: Diagnostics) -> None:
- """
- Disable a global pyparsing diagnostic flag (see :class:`Diagnostics`).
- """
- __diag__.disable(diag_enum.name)
-
-
-def enable_all_warnings() -> None:
- """
- Enable all global pyparsing diagnostic warnings (see :class:`Diagnostics`).
- """
- __diag__.enable_all_warnings()
-
-
-# hide abstract class
-del __config_flags
-
-
-def _should_enable_warnings(
- cmd_line_warn_options: typing.Iterable[str], warn_env_var: typing.Optional[str]
-) -> bool:
- enable = bool(warn_env_var)
- for warn_opt in cmd_line_warn_options:
- w_action, w_message, w_category, w_module, w_line = (warn_opt + "::::").split(
- ":"
- )[:5]
- if not w_action.lower().startswith("i") and (
- not (w_message or w_category or w_module) or w_module == "pyparsing"
- ):
- enable = True
- elif w_action.lower().startswith("i") and w_module in ("pyparsing", ""):
- enable = False
- return enable
-
-
-if _should_enable_warnings(
- sys.warnoptions, os.environ.get("PYPARSINGENABLEALLWARNINGS")
-):
- enable_all_warnings()
-
-
-# build list of single arg builtins, that can be used as parse actions
-_single_arg_builtins = {
- sum,
- len,
- sorted,
- reversed,
- list,
- tuple,
- set,
- any,
- all,
- min,
- max,
-}
-
-_generatorType = types.GeneratorType
-ParseAction = Union[
- Callable[[], Any],
- Callable[[ParseResults], Any],
- Callable[[int, ParseResults], Any],
- Callable[[str, int, ParseResults], Any],
-]
-ParseCondition = Union[
- Callable[[], bool],
- Callable[[ParseResults], bool],
- Callable[[int, ParseResults], bool],
- Callable[[str, int, ParseResults], bool],
-]
-ParseFailAction = Callable[[str, int, "ParserElement", Exception], None]
-DebugStartAction = Callable[[str, int, "ParserElement", bool], None]
-DebugSuccessAction = Callable[
- [str, int, int, "ParserElement", ParseResults, bool], None
-]
-DebugExceptionAction = Callable[[str, int, "ParserElement", Exception, bool], None]
-
-
-alphas = string.ascii_uppercase + string.ascii_lowercase
-identchars = pyparsing_unicode.Latin1.identchars
-identbodychars = pyparsing_unicode.Latin1.identbodychars
-nums = "0123456789"
-hexnums = nums + "ABCDEFabcdef"
-alphanums = alphas + nums
-printables = "".join([c for c in string.printable if c not in string.whitespace])
-
-_trim_arity_call_line: traceback.StackSummary = None
-
-
-def _trim_arity(func, max_limit=3):
- """decorator to trim function calls to match the arity of the target"""
- global _trim_arity_call_line
-
- if func in _single_arg_builtins:
- return lambda s, l, t: func(t)
-
- limit = 0
- found_arity = False
-
- def extract_tb(tb, limit=0):
- frames = traceback.extract_tb(tb, limit=limit)
- frame_summary = frames[-1]
- return [frame_summary[:2]]
-
- # synthesize what would be returned by traceback.extract_stack at the call to
- # user's parse action 'func', so that we don't incur call penalty at parse time
-
- # fmt: off
- LINE_DIFF = 7
- # IF ANY CODE CHANGES, EVEN JUST COMMENTS OR BLANK LINES, BETWEEN THE NEXT LINE AND
- # THE CALL TO FUNC INSIDE WRAPPER, LINE_DIFF MUST BE MODIFIED!!!!
- _trim_arity_call_line = (_trim_arity_call_line or traceback.extract_stack(limit=2)[-1])
- pa_call_line_synth = (_trim_arity_call_line[0], _trim_arity_call_line[1] + LINE_DIFF)
-
- def wrapper(*args):
- nonlocal found_arity, limit
- while 1:
- try:
- ret = func(*args[limit:])
- found_arity = True
- return ret
- except TypeError as te:
- # re-raise TypeErrors if they did not come from our arity testing
- if found_arity:
- raise
- else:
- tb = te.__traceback__
- trim_arity_type_error = (
- extract_tb(tb, limit=2)[-1][:2] == pa_call_line_synth
- )
- del tb
-
- if trim_arity_type_error:
- if limit < max_limit:
- limit += 1
- continue
-
- raise
- # fmt: on
-
- # copy func name to wrapper for sensible debug output
- # (can't use functools.wraps, since that messes with function signature)
- func_name = getattr(func, "__name__", getattr(func, "__class__").__name__)
- wrapper.__name__ = func_name
- wrapper.__doc__ = func.__doc__
-
- return wrapper
-
-
-def condition_as_parse_action(
- fn: ParseCondition, message: str = None, fatal: bool = False
-) -> ParseAction:
- """
- Function to convert a simple predicate function that returns ``True`` or ``False``
- into a parse action. Can be used in places when a parse action is required
- and :class:`ParserElement.add_condition` cannot be used (such as when adding a condition
- to an operator level in :class:`infix_notation`).
-
- Optional keyword arguments:
-
- - ``message`` - define a custom message to be used in the raised exception
- - ``fatal`` - if True, will raise :class:`ParseFatalException` to stop parsing immediately;
- otherwise will raise :class:`ParseException`
-
- """
- msg = message if message is not None else "failed user-defined condition"
- exc_type = ParseFatalException if fatal else ParseException
- fn = _trim_arity(fn)
-
- @wraps(fn)
- def pa(s, l, t):
- if not bool(fn(s, l, t)):
- raise exc_type(s, l, msg)
-
- return pa
-
-
-def _default_start_debug_action(
- instring: str, loc: int, expr: "ParserElement", cache_hit: bool = False
-):
- cache_hit_str = "*" if cache_hit else ""
- print(
- (
- "{}Match {} at loc {}({},{})\n {}\n {}^".format(
- cache_hit_str,
- expr,
- loc,
- lineno(loc, instring),
- col(loc, instring),
- line(loc, instring),
- " " * (col(loc, instring) - 1),
- )
- )
- )
-
-
-def _default_success_debug_action(
- instring: str,
- startloc: int,
- endloc: int,
- expr: "ParserElement",
- toks: ParseResults,
- cache_hit: bool = False,
-):
- cache_hit_str = "*" if cache_hit else ""
- print("{}Matched {} -> {}".format(cache_hit_str, expr, toks.as_list()))
-
-
-def _default_exception_debug_action(
- instring: str,
- loc: int,
- expr: "ParserElement",
- exc: Exception,
- cache_hit: bool = False,
-):
- cache_hit_str = "*" if cache_hit else ""
- print(
- "{}Match {} failed, {} raised: {}".format(
- cache_hit_str, expr, type(exc).__name__, exc
- )
- )
-
-
-def null_debug_action(*args):
- """'Do-nothing' debug action, to suppress debugging output during parsing."""
-
-
-class ParserElement(ABC):
- """Abstract base level parser element class."""
-
- DEFAULT_WHITE_CHARS: str = " \n\t\r"
- verbose_stacktrace: bool = False
- _literalStringClass: typing.Optional[type] = None
-
- @staticmethod
- def set_default_whitespace_chars(chars: str) -> None:
- r"""
- Overrides the default whitespace chars
-
- Example::
-
- # default whitespace chars are space, and newline
- Word(alphas)[1, ...].parse_string("abc def\nghi jkl") # -> ['abc', 'def', 'ghi', 'jkl']
-
- # change to just treat newline as significant
- ParserElement.set_default_whitespace_chars(" \t")
- Word(alphas)[1, ...].parse_string("abc def\nghi jkl") # -> ['abc', 'def']
- """
- ParserElement.DEFAULT_WHITE_CHARS = chars
-
- # update whitespace all parse expressions defined in this module
- for expr in _builtin_exprs:
- if expr.copyDefaultWhiteChars:
- expr.whiteChars = set(chars)
-
- @staticmethod
- def inline_literals_using(cls: type) -> None:
- """
- Set class to be used for inclusion of string literals into a parser.
-
- Example::
-
- # default literal class used is Literal
- integer = Word(nums)
- date_str = integer("year") + '/' + integer("month") + '/' + integer("day")
-
- date_str.parse_string("1999/12/31") # -> ['1999', '/', '12', '/', '31']
-
-
- # change to Suppress
- ParserElement.inline_literals_using(Suppress)
- date_str = integer("year") + '/' + integer("month") + '/' + integer("day")
-
- date_str.parse_string("1999/12/31") # -> ['1999', '12', '31']
- """
- ParserElement._literalStringClass = cls
-
- class DebugActions(NamedTuple):
- debug_try: typing.Optional[DebugStartAction]
- debug_match: typing.Optional[DebugSuccessAction]
- debug_fail: typing.Optional[DebugExceptionAction]
-
- def __init__(self, savelist: bool = False):
- self.parseAction: List[ParseAction] = list()
- self.failAction: typing.Optional[ParseFailAction] = None
- self.customName = None
- self._defaultName = None
- self.resultsName = None
- self.saveAsList = savelist
- self.skipWhitespace = True
- self.whiteChars = set(ParserElement.DEFAULT_WHITE_CHARS)
- self.copyDefaultWhiteChars = True
- # used when checking for left-recursion
- self.mayReturnEmpty = False
- self.keepTabs = False
- self.ignoreExprs: List["ParserElement"] = list()
- self.debug = False
- self.streamlined = False
- # optimize exception handling for subclasses that don't advance parse index
- self.mayIndexError = True
- self.errmsg = ""
- # mark results names as modal (report only last) or cumulative (list all)
- self.modalResults = True
- # custom debug actions
- self.debugActions = self.DebugActions(None, None, None)
- # avoid redundant calls to preParse
- self.callPreparse = True
- self.callDuringTry = False
- self.suppress_warnings_: List[Diagnostics] = []
-
- def suppress_warning(self, warning_type: Diagnostics) -> "ParserElement":
- """
- Suppress warnings emitted for a particular diagnostic on this expression.
-
- Example::
-
- base = pp.Forward()
- base.suppress_warning(Diagnostics.warn_on_parse_using_empty_Forward)
-
- # statement would normally raise a warning, but is now suppressed
- print(base.parseString("x"))
-
- """
- self.suppress_warnings_.append(warning_type)
- return self
-
- def copy(self) -> "ParserElement":
- """
- Make a copy of this :class:`ParserElement`. Useful for defining
- different parse actions for the same parsing pattern, using copies of
- the original parse element.
-
- Example::
-
- integer = Word(nums).set_parse_action(lambda toks: int(toks[0]))
- integerK = integer.copy().add_parse_action(lambda toks: toks[0] * 1024) + Suppress("K")
- integerM = integer.copy().add_parse_action(lambda toks: toks[0] * 1024 * 1024) + Suppress("M")
-
- print((integerK | integerM | integer)[1, ...].parse_string("5K 100 640K 256M"))
-
- prints::
-
- [5120, 100, 655360, 268435456]
-
- Equivalent form of ``expr.copy()`` is just ``expr()``::
-
- integerM = integer().add_parse_action(lambda toks: toks[0] * 1024 * 1024) + Suppress("M")
- """
- cpy = copy.copy(self)
- cpy.parseAction = self.parseAction[:]
- cpy.ignoreExprs = self.ignoreExprs[:]
- if self.copyDefaultWhiteChars:
- cpy.whiteChars = set(ParserElement.DEFAULT_WHITE_CHARS)
- return cpy
-
- def set_results_name(
- self, name: str, list_all_matches: bool = False, *, listAllMatches: bool = False
- ) -> "ParserElement":
- """
- Define name for referencing matching tokens as a nested attribute
- of the returned parse results.
-
- Normally, results names are assigned as you would assign keys in a dict:
- any existing value is overwritten by later values. If it is necessary to
- keep all values captured for a particular results name, call ``set_results_name``
- with ``list_all_matches`` = True.
-
- NOTE: ``set_results_name`` returns a *copy* of the original :class:`ParserElement` object;
- this is so that the client can define a basic element, such as an
- integer, and reference it in multiple places with different names.
-
- You can also set results names using the abbreviated syntax,
- ``expr("name")`` in place of ``expr.set_results_name("name")``
- - see :class:`__call__`. If ``list_all_matches`` is required, use
- ``expr("name*")``.
-
- Example::
-
- date_str = (integer.set_results_name("year") + '/'
- + integer.set_results_name("month") + '/'
- + integer.set_results_name("day"))
-
- # equivalent form:
- date_str = integer("year") + '/' + integer("month") + '/' + integer("day")
- """
- listAllMatches = listAllMatches or list_all_matches
- return self._setResultsName(name, listAllMatches)
-
- def _setResultsName(self, name, listAllMatches=False):
- if name is None:
- return self
- newself = self.copy()
- if name.endswith("*"):
- name = name[:-1]
- listAllMatches = True
- newself.resultsName = name
- newself.modalResults = not listAllMatches
- return newself
-
- def set_break(self, break_flag: bool = True) -> "ParserElement":
- """
- Method to invoke the Python pdb debugger when this element is
- about to be parsed. Set ``break_flag`` to ``True`` to enable, ``False`` to
- disable.
- """
- if break_flag:
- _parseMethod = self._parse
-
- def breaker(instring, loc, doActions=True, callPreParse=True):
- import pdb
-
- # this call to pdb.set_trace() is intentional, not a checkin error
- pdb.set_trace()
- return _parseMethod(instring, loc, doActions, callPreParse)
-
- breaker._originalParseMethod = _parseMethod
- self._parse = breaker
- else:
- if hasattr(self._parse, "_originalParseMethod"):
- self._parse = self._parse._originalParseMethod
- return self
-
- def set_parse_action(self, *fns: ParseAction, **kwargs) -> "ParserElement":
- """
- Define one or more actions to perform when successfully matching parse element definition.
-
- Parse actions can be called to perform data conversions, do extra validation,
- update external data structures, or enhance or replace the parsed tokens.
- Each parse action ``fn`` is a callable method with 0-3 arguments, called as
- ``fn(s, loc, toks)`` , ``fn(loc, toks)`` , ``fn(toks)`` , or just ``fn()`` , where:
-
- - s = the original string being parsed (see note below)
- - loc = the location of the matching substring
- - toks = a list of the matched tokens, packaged as a :class:`ParseResults` object
-
- The parsed tokens are passed to the parse action as ParseResults. They can be
- modified in place using list-style append, extend, and pop operations to update
- the parsed list elements; and with dictionary-style item set and del operations
- to add, update, or remove any named results. If the tokens are modified in place,
- it is not necessary to return them with a return statement.
-
- Parse actions can also completely replace the given tokens, with another ``ParseResults``
- object, or with some entirely different object (common for parse actions that perform data
- conversions). A convenient way to build a new parse result is to define the values
- using a dict, and then create the return value using :class:`ParseResults.from_dict`.
-
- If None is passed as the ``fn`` parse action, all previously added parse actions for this
- expression are cleared.
-
- Optional keyword arguments:
-
- - call_during_try = (default= ``False``) indicate if parse action should be run during
- lookaheads and alternate testing. For parse actions that have side effects, it is
- important to only call the parse action once it is determined that it is being
- called as part of a successful parse. For parse actions that perform additional
- validation, then call_during_try should be passed as True, so that the validation
- code is included in the preliminary "try" parses.
-
- Note: the default parsing behavior is to expand tabs in the input string
- before starting the parsing process. See :class:`parse_string` for more
- information on parsing strings containing ```` s, and suggested
- methods to maintain a consistent view of the parsed string, the parse
- location, and line and column positions within the parsed string.
-
- Example::
-
- # parse dates in the form YYYY/MM/DD
-
- # use parse action to convert toks from str to int at parse time
- def convert_to_int(toks):
- return int(toks[0])
-
- # use a parse action to verify that the date is a valid date
- def is_valid_date(instring, loc, toks):
- from datetime import date
- year, month, day = toks[::2]
- try:
- date(year, month, day)
- except ValueError:
- raise ParseException(instring, loc, "invalid date given")
-
- integer = Word(nums)
- date_str = integer + '/' + integer + '/' + integer
-
- # add parse actions
- integer.set_parse_action(convert_to_int)
- date_str.set_parse_action(is_valid_date)
-
- # note that integer fields are now ints, not strings
- date_str.run_tests('''
- # successful parse - note that integer fields were converted to ints
- 1999/12/31
-
- # fail - invalid date
- 1999/13/31
- ''')
- """
- if list(fns) == [None]:
- self.parseAction = []
- else:
- if not all(callable(fn) for fn in fns):
- raise TypeError("parse actions must be callable")
- self.parseAction = [_trim_arity(fn) for fn in fns]
- self.callDuringTry = kwargs.get(
- "call_during_try", kwargs.get("callDuringTry", False)
- )
- return self
-
- def add_parse_action(self, *fns: ParseAction, **kwargs) -> "ParserElement":
- """
- Add one or more parse actions to expression's list of parse actions. See :class:`set_parse_action`.
-
- See examples in :class:`copy`.
- """
- self.parseAction += [_trim_arity(fn) for fn in fns]
- self.callDuringTry = self.callDuringTry or kwargs.get(
- "call_during_try", kwargs.get("callDuringTry", False)
- )
- return self
-
- def add_condition(self, *fns: ParseCondition, **kwargs) -> "ParserElement":
- """Add a boolean predicate function to expression's list of parse actions. See
- :class:`set_parse_action` for function call signatures. Unlike ``set_parse_action``,
- functions passed to ``add_condition`` need to return boolean success/fail of the condition.
-
- Optional keyword arguments:
-
- - message = define a custom message to be used in the raised exception
- - fatal = if True, will raise ParseFatalException to stop parsing immediately; otherwise will raise
- ParseException
- - call_during_try = boolean to indicate if this method should be called during internal tryParse calls,
- default=False
-
- Example::
-
- integer = Word(nums).set_parse_action(lambda toks: int(toks[0]))
- year_int = integer.copy()
- year_int.add_condition(lambda toks: toks[0] >= 2000, message="Only support years 2000 and later")
- date_str = year_int + '/' + integer + '/' + integer
-
- result = date_str.parse_string("1999/12/31") # -> Exception: Only support years 2000 and later (at char 0),
- (line:1, col:1)
- """
- for fn in fns:
- self.parseAction.append(
- condition_as_parse_action(
- fn, message=kwargs.get("message"), fatal=kwargs.get("fatal", False)
- )
- )
-
- self.callDuringTry = self.callDuringTry or kwargs.get(
- "call_during_try", kwargs.get("callDuringTry", False)
- )
- return self
-
- def set_fail_action(self, fn: ParseFailAction) -> "ParserElement":
- """
- Define action to perform if parsing fails at this expression.
- Fail acton fn is a callable function that takes the arguments
- ``fn(s, loc, expr, err)`` where:
-
- - s = string being parsed
- - loc = location where expression match was attempted and failed
- - expr = the parse expression that failed
- - err = the exception thrown
-
- The function returns no value. It may throw :class:`ParseFatalException`
- if it is desired to stop parsing immediately."""
- self.failAction = fn
- return self
-
- def _skipIgnorables(self, instring, loc):
- exprsFound = True
- while exprsFound:
- exprsFound = False
- for e in self.ignoreExprs:
- try:
- while 1:
- loc, dummy = e._parse(instring, loc)
- exprsFound = True
- except ParseException:
- pass
- return loc
-
- def preParse(self, instring, loc):
- if self.ignoreExprs:
- loc = self._skipIgnorables(instring, loc)
-
- if self.skipWhitespace:
- instrlen = len(instring)
- white_chars = self.whiteChars
- while loc < instrlen and instring[loc] in white_chars:
- loc += 1
-
- return loc
-
- def parseImpl(self, instring, loc, doActions=True):
- return loc, []
-
- def postParse(self, instring, loc, tokenlist):
- return tokenlist
-
- # @profile
- def _parseNoCache(
- self, instring, loc, doActions=True, callPreParse=True
- ) -> Tuple[int, ParseResults]:
- TRY, MATCH, FAIL = 0, 1, 2
- debugging = self.debug # and doActions)
- len_instring = len(instring)
-
- if debugging or self.failAction:
- # print("Match {} at loc {}({}, {})".format(self, loc, lineno(loc, instring), col(loc, instring)))
- try:
- if callPreParse and self.callPreparse:
- pre_loc = self.preParse(instring, loc)
- else:
- pre_loc = loc
- tokens_start = pre_loc
- if self.debugActions.debug_try:
- self.debugActions.debug_try(instring, tokens_start, self, False)
- if self.mayIndexError or pre_loc >= len_instring:
- try:
- loc, tokens = self.parseImpl(instring, pre_loc, doActions)
- except IndexError:
- raise ParseException(instring, len_instring, self.errmsg, self)
- else:
- loc, tokens = self.parseImpl(instring, pre_loc, doActions)
- except Exception as err:
- # print("Exception raised:", err)
- if self.debugActions.debug_fail:
- self.debugActions.debug_fail(
- instring, tokens_start, self, err, False
- )
- if self.failAction:
- self.failAction(instring, tokens_start, self, err)
- raise
- else:
- if callPreParse and self.callPreparse:
- pre_loc = self.preParse(instring, loc)
- else:
- pre_loc = loc
- tokens_start = pre_loc
- if self.mayIndexError or pre_loc >= len_instring:
- try:
- loc, tokens = self.parseImpl(instring, pre_loc, doActions)
- except IndexError:
- raise ParseException(instring, len_instring, self.errmsg, self)
- else:
- loc, tokens = self.parseImpl(instring, pre_loc, doActions)
-
- tokens = self.postParse(instring, loc, tokens)
-
- ret_tokens = ParseResults(
- tokens, self.resultsName, asList=self.saveAsList, modal=self.modalResults
- )
- if self.parseAction and (doActions or self.callDuringTry):
- if debugging:
- try:
- for fn in self.parseAction:
- try:
- tokens = fn(instring, tokens_start, ret_tokens)
- except IndexError as parse_action_exc:
- exc = ParseException("exception raised in parse action")
- raise exc from parse_action_exc
-
- if tokens is not None and tokens is not ret_tokens:
- ret_tokens = ParseResults(
- tokens,
- self.resultsName,
- asList=self.saveAsList
- and isinstance(tokens, (ParseResults, list)),
- modal=self.modalResults,
- )
- except Exception as err:
- # print "Exception raised in user parse action:", err
- if self.debugActions.debug_fail:
- self.debugActions.debug_fail(
- instring, tokens_start, self, err, False
- )
- raise
- else:
- for fn in self.parseAction:
- try:
- tokens = fn(instring, tokens_start, ret_tokens)
- except IndexError as parse_action_exc:
- exc = ParseException("exception raised in parse action")
- raise exc from parse_action_exc
-
- if tokens is not None and tokens is not ret_tokens:
- ret_tokens = ParseResults(
- tokens,
- self.resultsName,
- asList=self.saveAsList
- and isinstance(tokens, (ParseResults, list)),
- modal=self.modalResults,
- )
- if debugging:
- # print("Matched", self, "->", ret_tokens.as_list())
- if self.debugActions.debug_match:
- self.debugActions.debug_match(
- instring, tokens_start, loc, self, ret_tokens, False
- )
-
- return loc, ret_tokens
-
- def try_parse(self, instring: str, loc: int, raise_fatal: bool = False) -> int:
- try:
- return self._parse(instring, loc, doActions=False)[0]
- except ParseFatalException:
- if raise_fatal:
- raise
- raise ParseException(instring, loc, self.errmsg, self)
-
- def can_parse_next(self, instring: str, loc: int) -> bool:
- try:
- self.try_parse(instring, loc)
- except (ParseException, IndexError):
- return False
- else:
- return True
-
- # cache for left-recursion in Forward references
- recursion_lock = RLock()
- recursion_memos: typing.Dict[
- Tuple[int, "Forward", bool], Tuple[int, Union[ParseResults, Exception]]
- ] = {}
-
- # argument cache for optimizing repeated calls when backtracking through recursive expressions
- packrat_cache = (
- {}
- ) # this is set later by enabled_packrat(); this is here so that reset_cache() doesn't fail
- packrat_cache_lock = RLock()
- packrat_cache_stats = [0, 0]
-
- # this method gets repeatedly called during backtracking with the same arguments -
- # we can cache these arguments and save ourselves the trouble of re-parsing the contained expression
- def _parseCache(
- self, instring, loc, doActions=True, callPreParse=True
- ) -> Tuple[int, ParseResults]:
- HIT, MISS = 0, 1
- TRY, MATCH, FAIL = 0, 1, 2
- lookup = (self, instring, loc, callPreParse, doActions)
- with ParserElement.packrat_cache_lock:
- cache = ParserElement.packrat_cache
- value = cache.get(lookup)
- if value is cache.not_in_cache:
- ParserElement.packrat_cache_stats[MISS] += 1
- try:
- value = self._parseNoCache(instring, loc, doActions, callPreParse)
- except ParseBaseException as pe:
- # cache a copy of the exception, without the traceback
- cache.set(lookup, pe.__class__(*pe.args))
- raise
- else:
- cache.set(lookup, (value[0], value[1].copy(), loc))
- return value
- else:
- ParserElement.packrat_cache_stats[HIT] += 1
- if self.debug and self.debugActions.debug_try:
- try:
- self.debugActions.debug_try(instring, loc, self, cache_hit=True)
- except TypeError:
- pass
- if isinstance(value, Exception):
- if self.debug and self.debugActions.debug_fail:
- try:
- self.debugActions.debug_fail(
- instring, loc, self, value, cache_hit=True
- )
- except TypeError:
- pass
- raise value
-
- loc_, result, endloc = value[0], value[1].copy(), value[2]
- if self.debug and self.debugActions.debug_match:
- try:
- self.debugActions.debug_match(
- instring, loc_, endloc, self, result, cache_hit=True
- )
- except TypeError:
- pass
-
- return loc_, result
-
- _parse = _parseNoCache
-
- @staticmethod
- def reset_cache() -> None:
- ParserElement.packrat_cache.clear()
- ParserElement.packrat_cache_stats[:] = [0] * len(
- ParserElement.packrat_cache_stats
- )
- ParserElement.recursion_memos.clear()
-
- _packratEnabled = False
- _left_recursion_enabled = False
-
- @staticmethod
- def disable_memoization() -> None:
- """
- Disables active Packrat or Left Recursion parsing and their memoization
-
- This method also works if neither Packrat nor Left Recursion are enabled.
- This makes it safe to call before activating Packrat nor Left Recursion
- to clear any previous settings.
- """
- ParserElement.reset_cache()
- ParserElement._left_recursion_enabled = False
- ParserElement._packratEnabled = False
- ParserElement._parse = ParserElement._parseNoCache
-
- @staticmethod
- def enable_left_recursion(
- cache_size_limit: typing.Optional[int] = None, *, force=False
- ) -> None:
- """
- Enables "bounded recursion" parsing, which allows for both direct and indirect
- left-recursion. During parsing, left-recursive :class:`Forward` elements are
- repeatedly matched with a fixed recursion depth that is gradually increased
- until finding the longest match.
-
- Example::
-
- from pip._vendor import pyparsing as pp
- pp.ParserElement.enable_left_recursion()
-
- E = pp.Forward("E")
- num = pp.Word(pp.nums)
- # match `num`, or `num '+' num`, or `num '+' num '+' num`, ...
- E <<= E + '+' - num | num
-
- print(E.parse_string("1+2+3"))
-
- Recursion search naturally memoizes matches of ``Forward`` elements and may
- thus skip reevaluation of parse actions during backtracking. This may break
- programs with parse actions which rely on strict ordering of side-effects.
-
- Parameters:
-
- - cache_size_limit - (default=``None``) - memoize at most this many
- ``Forward`` elements during matching; if ``None`` (the default),
- memoize all ``Forward`` elements.
-
- Bounded Recursion parsing works similar but not identical to Packrat parsing,
- thus the two cannot be used together. Use ``force=True`` to disable any
- previous, conflicting settings.
- """
- if force:
- ParserElement.disable_memoization()
- elif ParserElement._packratEnabled:
- raise RuntimeError("Packrat and Bounded Recursion are not compatible")
- if cache_size_limit is None:
- ParserElement.recursion_memos = _UnboundedMemo()
- elif cache_size_limit > 0:
- ParserElement.recursion_memos = _LRUMemo(capacity=cache_size_limit)
- else:
- raise NotImplementedError("Memo size of %s" % cache_size_limit)
- ParserElement._left_recursion_enabled = True
-
- @staticmethod
- def enable_packrat(cache_size_limit: int = 128, *, force: bool = False) -> None:
- """
- Enables "packrat" parsing, which adds memoizing to the parsing logic.
- Repeated parse attempts at the same string location (which happens
- often in many complex grammars) can immediately return a cached value,
- instead of re-executing parsing/validating code. Memoizing is done of
- both valid results and parsing exceptions.
-
- Parameters:
-
- - cache_size_limit - (default= ``128``) - if an integer value is provided
- will limit the size of the packrat cache; if None is passed, then
- the cache size will be unbounded; if 0 is passed, the cache will
- be effectively disabled.
-
- This speedup may break existing programs that use parse actions that
- have side-effects. For this reason, packrat parsing is disabled when
- you first import pyparsing. To activate the packrat feature, your
- program must call the class method :class:`ParserElement.enable_packrat`.
- For best results, call ``enable_packrat()`` immediately after
- importing pyparsing.
-
- Example::
-
- from pip._vendor import pyparsing
- pyparsing.ParserElement.enable_packrat()
-
- Packrat parsing works similar but not identical to Bounded Recursion parsing,
- thus the two cannot be used together. Use ``force=True`` to disable any
- previous, conflicting settings.
- """
- if force:
- ParserElement.disable_memoization()
- elif ParserElement._left_recursion_enabled:
- raise RuntimeError("Packrat and Bounded Recursion are not compatible")
- if not ParserElement._packratEnabled:
- ParserElement._packratEnabled = True
- if cache_size_limit is None:
- ParserElement.packrat_cache = _UnboundedCache()
- else:
- ParserElement.packrat_cache = _FifoCache(cache_size_limit)
- ParserElement._parse = ParserElement._parseCache
-
- def parse_string(
- self, instring: str, parse_all: bool = False, *, parseAll: bool = False
- ) -> ParseResults:
- """
- Parse a string with respect to the parser definition. This function is intended as the primary interface to the
- client code.
-
- :param instring: The input string to be parsed.
- :param parse_all: If set, the entire input string must match the grammar.
- :param parseAll: retained for pre-PEP8 compatibility, will be removed in a future release.
- :raises ParseException: Raised if ``parse_all`` is set and the input string does not match the whole grammar.
- :returns: the parsed data as a :class:`ParseResults` object, which may be accessed as a `list`, a `dict`, or
- an object with attributes if the given parser includes results names.
-
- If the input string is required to match the entire grammar, ``parse_all`` flag must be set to ``True``. This
- is also equivalent to ending the grammar with :class:`StringEnd`().
-
- To report proper column numbers, ``parse_string`` operates on a copy of the input string where all tabs are
- converted to spaces (8 spaces per tab, as per the default in ``string.expandtabs``). If the input string
- contains tabs and the grammar uses parse actions that use the ``loc`` argument to index into the string
- being parsed, one can ensure a consistent view of the input string by doing one of the following:
-
- - calling ``parse_with_tabs`` on your grammar before calling ``parse_string`` (see :class:`parse_with_tabs`),
- - define your parse action using the full ``(s,loc,toks)`` signature, and reference the input string using the
- parse action's ``s`` argument, or
- - explicitly expand the tabs in your input string before calling ``parse_string``.
-
- Examples:
-
- By default, partial matches are OK.
-
- >>> res = Word('a').parse_string('aaaaabaaa')
- >>> print(res)
- ['aaaaa']
-
- The parsing behavior varies by the inheriting class of this abstract class. Please refer to the children
- directly to see more examples.
-
- It raises an exception if parse_all flag is set and instring does not match the whole grammar.
-
- >>> res = Word('a').parse_string('aaaaabaaa', parse_all=True)
- Traceback (most recent call last):
- ...
- pyparsing.ParseException: Expected end of text, found 'b' (at char 5), (line:1, col:6)
- """
- parseAll = parse_all or parseAll
-
- ParserElement.reset_cache()
- if not self.streamlined:
- self.streamline()
- for e in self.ignoreExprs:
- e.streamline()
- if not self.keepTabs:
- instring = instring.expandtabs()
- try:
- loc, tokens = self._parse(instring, 0)
- if parseAll:
- loc = self.preParse(instring, loc)
- se = Empty() + StringEnd()
- se._parse(instring, loc)
- except ParseBaseException as exc:
- if ParserElement.verbose_stacktrace:
- raise
- else:
- # catch and re-raise exception from here, clearing out pyparsing internal stack trace
- raise exc.with_traceback(None)
- else:
- return tokens
-
- def scan_string(
- self,
- instring: str,
- max_matches: int = _MAX_INT,
- overlap: bool = False,
- *,
- debug: bool = False,
- maxMatches: int = _MAX_INT,
- ) -> Generator[Tuple[ParseResults, int, int], None, None]:
- """
- Scan the input string for expression matches. Each match will return the
- matching tokens, start location, and end location. May be called with optional
- ``max_matches`` argument, to clip scanning after 'n' matches are found. If
- ``overlap`` is specified, then overlapping matches will be reported.
-
- Note that the start and end locations are reported relative to the string
- being parsed. See :class:`parse_string` for more information on parsing
- strings with embedded tabs.
-
- Example::
-
- source = "sldjf123lsdjjkf345sldkjf879lkjsfd987"
- print(source)
- for tokens, start, end in Word(alphas).scan_string(source):
- print(' '*start + '^'*(end-start))
- print(' '*start + tokens[0])
-
- prints::
-
- sldjf123lsdjjkf345sldkjf879lkjsfd987
- ^^^^^
- sldjf
- ^^^^^^^
- lsdjjkf
- ^^^^^^
- sldkjf
- ^^^^^^
- lkjsfd
- """
- maxMatches = min(maxMatches, max_matches)
- if not self.streamlined:
- self.streamline()
- for e in self.ignoreExprs:
- e.streamline()
-
- if not self.keepTabs:
- instring = str(instring).expandtabs()
- instrlen = len(instring)
- loc = 0
- preparseFn = self.preParse
- parseFn = self._parse
- ParserElement.resetCache()
- matches = 0
- try:
- while loc <= instrlen and matches < maxMatches:
- try:
- preloc = preparseFn(instring, loc)
- nextLoc, tokens = parseFn(instring, preloc, callPreParse=False)
- except ParseException:
- loc = preloc + 1
- else:
- if nextLoc > loc:
- matches += 1
- if debug:
- print(
- {
- "tokens": tokens.asList(),
- "start": preloc,
- "end": nextLoc,
- }
- )
- yield tokens, preloc, nextLoc
- if overlap:
- nextloc = preparseFn(instring, loc)
- if nextloc > loc:
- loc = nextLoc
- else:
- loc += 1
- else:
- loc = nextLoc
- else:
- loc = preloc + 1
- except ParseBaseException as exc:
- if ParserElement.verbose_stacktrace:
- raise
- else:
- # catch and re-raise exception from here, clears out pyparsing internal stack trace
- raise exc.with_traceback(None)
-
- def transform_string(self, instring: str, *, debug: bool = False) -> str:
- """
- Extension to :class:`scan_string`, to modify matching text with modified tokens that may
- be returned from a parse action. To use ``transform_string``, define a grammar and
- attach a parse action to it that modifies the returned token list.
- Invoking ``transform_string()`` on a target string will then scan for matches,
- and replace the matched text patterns according to the logic in the parse
- action. ``transform_string()`` returns the resulting transformed string.
-
- Example::
-
- wd = Word(alphas)
- wd.set_parse_action(lambda toks: toks[0].title())
-
- print(wd.transform_string("now is the winter of our discontent made glorious summer by this sun of york."))
-
- prints::
-
- Now Is The Winter Of Our Discontent Made Glorious Summer By This Sun Of York.
- """
- out: List[str] = []
- lastE = 0
- # force preservation of s, to minimize unwanted transformation of string, and to
- # keep string locs straight between transform_string and scan_string
- self.keepTabs = True
- try:
- for t, s, e in self.scan_string(instring, debug=debug):
- out.append(instring[lastE:s])
- if t:
- if isinstance(t, ParseResults):
- out += t.as_list()
- elif isinstance(t, Iterable) and not isinstance(t, str_type):
- out.extend(t)
- else:
- out.append(t)
- lastE = e
- out.append(instring[lastE:])
- out = [o for o in out if o]
- return "".join([str(s) for s in _flatten(out)])
- except ParseBaseException as exc:
- if ParserElement.verbose_stacktrace:
- raise
- else:
- # catch and re-raise exception from here, clears out pyparsing internal stack trace
- raise exc.with_traceback(None)
-
- def search_string(
- self,
- instring: str,
- max_matches: int = _MAX_INT,
- *,
- debug: bool = False,
- maxMatches: int = _MAX_INT,
- ) -> ParseResults:
- """
- Another extension to :class:`scan_string`, simplifying the access to the tokens found
- to match the given parse expression. May be called with optional
- ``max_matches`` argument, to clip searching after 'n' matches are found.
-
- Example::
-
- # a capitalized word starts with an uppercase letter, followed by zero or more lowercase letters
- cap_word = Word(alphas.upper(), alphas.lower())
-
- print(cap_word.search_string("More than Iron, more than Lead, more than Gold I need Electricity"))
-
- # the sum() builtin can be used to merge results into a single ParseResults object
- print(sum(cap_word.search_string("More than Iron, more than Lead, more than Gold I need Electricity")))
-
- prints::
-
- [['More'], ['Iron'], ['Lead'], ['Gold'], ['I'], ['Electricity']]
- ['More', 'Iron', 'Lead', 'Gold', 'I', 'Electricity']
- """
- maxMatches = min(maxMatches, max_matches)
- try:
- return ParseResults(
- [t for t, s, e in self.scan_string(instring, maxMatches, debug=debug)]
- )
- except ParseBaseException as exc:
- if ParserElement.verbose_stacktrace:
- raise
- else:
- # catch and re-raise exception from here, clears out pyparsing internal stack trace
- raise exc.with_traceback(None)
-
- def split(
- self,
- instring: str,
- maxsplit: int = _MAX_INT,
- include_separators: bool = False,
- *,
- includeSeparators=False,
- ) -> Generator[str, None, None]:
- """
- Generator method to split a string using the given expression as a separator.
- May be called with optional ``maxsplit`` argument, to limit the number of splits;
- and the optional ``include_separators`` argument (default= ``False``), if the separating
- matching text should be included in the split results.
-
- Example::
-
- punc = one_of(list(".,;:/-!?"))
- print(list(punc.split("This, this?, this sentence, is badly punctuated!")))
-
- prints::
-
- ['This', ' this', '', ' this sentence', ' is badly punctuated', '']
- """
- includeSeparators = includeSeparators or include_separators
- last = 0
- for t, s, e in self.scan_string(instring, max_matches=maxsplit):
- yield instring[last:s]
- if includeSeparators:
- yield t[0]
- last = e
- yield instring[last:]
-
- def __add__(self, other) -> "ParserElement":
- """
- Implementation of ``+`` operator - returns :class:`And`. Adding strings to a :class:`ParserElement`
- converts them to :class:`Literal`s by default.
-
- Example::
-
- greet = Word(alphas) + "," + Word(alphas) + "!"
- hello = "Hello, World!"
- print(hello, "->", greet.parse_string(hello))
-
- prints::
-
- Hello, World! -> ['Hello', ',', 'World', '!']
-
- ``...`` may be used as a parse expression as a short form of :class:`SkipTo`.
-
- Literal('start') + ... + Literal('end')
-
- is equivalent to:
-
- Literal('start') + SkipTo('end')("_skipped*") + Literal('end')
-
- Note that the skipped text is returned with '_skipped' as a results name,
- and to support having multiple skips in the same parser, the value returned is
- a list of all skipped text.
- """
- if other is Ellipsis:
- return _PendingSkip(self)
-
- if isinstance(other, str_type):
- other = self._literalStringClass(other)
- if not isinstance(other, ParserElement):
- raise TypeError(
- "Cannot combine element of type {} with ParserElement".format(
- type(other).__name__
- )
- )
- return And([self, other])
-
- def __radd__(self, other) -> "ParserElement":
- """
- Implementation of ``+`` operator when left operand is not a :class:`ParserElement`
- """
- if other is Ellipsis:
- return SkipTo(self)("_skipped*") + self
-
- if isinstance(other, str_type):
- other = self._literalStringClass(other)
- if not isinstance(other, ParserElement):
- raise TypeError(
- "Cannot combine element of type {} with ParserElement".format(
- type(other).__name__
- )
- )
- return other + self
-
- def __sub__(self, other) -> "ParserElement":
- """
- Implementation of ``-`` operator, returns :class:`And` with error stop
- """
- if isinstance(other, str_type):
- other = self._literalStringClass(other)
- if not isinstance(other, ParserElement):
- raise TypeError(
- "Cannot combine element of type {} with ParserElement".format(
- type(other).__name__
- )
- )
- return self + And._ErrorStop() + other
-
- def __rsub__(self, other) -> "ParserElement":
- """
- Implementation of ``-`` operator when left operand is not a :class:`ParserElement`
- """
- if isinstance(other, str_type):
- other = self._literalStringClass(other)
- if not isinstance(other, ParserElement):
- raise TypeError(
- "Cannot combine element of type {} with ParserElement".format(
- type(other).__name__
- )
- )
- return other - self
-
- def __mul__(self, other) -> "ParserElement":
- """
- Implementation of ``*`` operator, allows use of ``expr * 3`` in place of
- ``expr + expr + expr``. Expressions may also be multiplied by a 2-integer
- tuple, similar to ``{min, max}`` multipliers in regular expressions. Tuples
- may also include ``None`` as in:
- - ``expr*(n, None)`` or ``expr*(n, )`` is equivalent
- to ``expr*n + ZeroOrMore(expr)``
- (read as "at least n instances of ``expr``")
- - ``expr*(None, n)`` is equivalent to ``expr*(0, n)``
- (read as "0 to n instances of ``expr``")
- - ``expr*(None, None)`` is equivalent to ``ZeroOrMore(expr)``
- - ``expr*(1, None)`` is equivalent to ``OneOrMore(expr)``
-
- Note that ``expr*(None, n)`` does not raise an exception if
- more than n exprs exist in the input stream; that is,
- ``expr*(None, n)`` does not enforce a maximum number of expr
- occurrences. If this behavior is desired, then write
- ``expr*(None, n) + ~expr``
- """
- if other is Ellipsis:
- other = (0, None)
- elif isinstance(other, tuple) and other[:1] == (Ellipsis,):
- other = ((0,) + other[1:] + (None,))[:2]
-
- if isinstance(other, int):
- minElements, optElements = other, 0
- elif isinstance(other, tuple):
- other = tuple(o if o is not Ellipsis else None for o in other)
- other = (other + (None, None))[:2]
- if other[0] is None:
- other = (0, other[1])
- if isinstance(other[0], int) and other[1] is None:
- if other[0] == 0:
- return ZeroOrMore(self)
- if other[0] == 1:
- return OneOrMore(self)
- else:
- return self * other[0] + ZeroOrMore(self)
- elif isinstance(other[0], int) and isinstance(other[1], int):
- minElements, optElements = other
- optElements -= minElements
- else:
- raise TypeError(
- "cannot multiply ParserElement and ({}) objects".format(
- ",".join(type(item).__name__ for item in other)
- )
- )
- else:
- raise TypeError(
- "cannot multiply ParserElement and {} objects".format(
- type(other).__name__
- )
- )
-
- if minElements < 0:
- raise ValueError("cannot multiply ParserElement by negative value")
- if optElements < 0:
- raise ValueError(
- "second tuple value must be greater or equal to first tuple value"
- )
- if minElements == optElements == 0:
- return And([])
-
- if optElements:
-
- def makeOptionalList(n):
- if n > 1:
- return Opt(self + makeOptionalList(n - 1))
- else:
- return Opt(self)
-
- if minElements:
- if minElements == 1:
- ret = self + makeOptionalList(optElements)
- else:
- ret = And([self] * minElements) + makeOptionalList(optElements)
- else:
- ret = makeOptionalList(optElements)
- else:
- if minElements == 1:
- ret = self
- else:
- ret = And([self] * minElements)
- return ret
-
- def __rmul__(self, other) -> "ParserElement":
- return self.__mul__(other)
-
- def __or__(self, other) -> "ParserElement":
- """
- Implementation of ``|`` operator - returns :class:`MatchFirst`
- """
- if other is Ellipsis:
- return _PendingSkip(self, must_skip=True)
-
- if isinstance(other, str_type):
- other = self._literalStringClass(other)
- if not isinstance(other, ParserElement):
- raise TypeError(
- "Cannot combine element of type {} with ParserElement".format(
- type(other).__name__
- )
- )
- return MatchFirst([self, other])
-
- def __ror__(self, other) -> "ParserElement":
- """
- Implementation of ``|`` operator when left operand is not a :class:`ParserElement`
- """
- if isinstance(other, str_type):
- other = self._literalStringClass(other)
- if not isinstance(other, ParserElement):
- raise TypeError(
- "Cannot combine element of type {} with ParserElement".format(
- type(other).__name__
- )
- )
- return other | self
-
- def __xor__(self, other) -> "ParserElement":
- """
- Implementation of ``^`` operator - returns :class:`Or`
- """
- if isinstance(other, str_type):
- other = self._literalStringClass(other)
- if not isinstance(other, ParserElement):
- raise TypeError(
- "Cannot combine element of type {} with ParserElement".format(
- type(other).__name__
- )
- )
- return Or([self, other])
-
- def __rxor__(self, other) -> "ParserElement":
- """
- Implementation of ``^`` operator when left operand is not a :class:`ParserElement`
- """
- if isinstance(other, str_type):
- other = self._literalStringClass(other)
- if not isinstance(other, ParserElement):
- raise TypeError(
- "Cannot combine element of type {} with ParserElement".format(
- type(other).__name__
- )
- )
- return other ^ self
-
- def __and__(self, other) -> "ParserElement":
- """
- Implementation of ``&`` operator - returns :class:`Each`
- """
- if isinstance(other, str_type):
- other = self._literalStringClass(other)
- if not isinstance(other, ParserElement):
- raise TypeError(
- "Cannot combine element of type {} with ParserElement".format(
- type(other).__name__
- )
- )
- return Each([self, other])
-
- def __rand__(self, other) -> "ParserElement":
- """
- Implementation of ``&`` operator when left operand is not a :class:`ParserElement`
- """
- if isinstance(other, str_type):
- other = self._literalStringClass(other)
- if not isinstance(other, ParserElement):
- raise TypeError(
- "Cannot combine element of type {} with ParserElement".format(
- type(other).__name__
- )
- )
- return other & self
-
- def __invert__(self) -> "ParserElement":
- """
- Implementation of ``~`` operator - returns :class:`NotAny`
- """
- return NotAny(self)
-
- # disable __iter__ to override legacy use of sequential access to __getitem__ to
- # iterate over a sequence
- __iter__ = None
-
- def __getitem__(self, key):
- """
- use ``[]`` indexing notation as a short form for expression repetition:
-
- - ``expr[n]`` is equivalent to ``expr*n``
- - ``expr[m, n]`` is equivalent to ``expr*(m, n)``
- - ``expr[n, ...]`` or ``expr[n,]`` is equivalent
- to ``expr*n + ZeroOrMore(expr)``
- (read as "at least n instances of ``expr``")
- - ``expr[..., n]`` is equivalent to ``expr*(0, n)``
- (read as "0 to n instances of ``expr``")
- - ``expr[...]`` and ``expr[0, ...]`` are equivalent to ``ZeroOrMore(expr)``
- - ``expr[1, ...]`` is equivalent to ``OneOrMore(expr)``
-
- ``None`` may be used in place of ``...``.
-
- Note that ``expr[..., n]`` and ``expr[m, n]``do not raise an exception
- if more than ``n`` ``expr``s exist in the input stream. If this behavior is
- desired, then write ``expr[..., n] + ~expr``.
- """
-
- # convert single arg keys to tuples
- try:
- if isinstance(key, str_type):
- key = (key,)
- iter(key)
- except TypeError:
- key = (key, key)
-
- if len(key) > 2:
- raise TypeError(
- "only 1 or 2 index arguments supported ({}{})".format(
- key[:5], "... [{}]".format(len(key)) if len(key) > 5 else ""
- )
- )
-
- # clip to 2 elements
- ret = self * tuple(key[:2])
- return ret
-
- def __call__(self, name: str = None) -> "ParserElement":
- """
- Shortcut for :class:`set_results_name`, with ``list_all_matches=False``.
-
- If ``name`` is given with a trailing ``'*'`` character, then ``list_all_matches`` will be
- passed as ``True``.
-
- If ``name` is omitted, same as calling :class:`copy`.
-
- Example::
-
- # these are equivalent
- userdata = Word(alphas).set_results_name("name") + Word(nums + "-").set_results_name("socsecno")
- userdata = Word(alphas)("name") + Word(nums + "-")("socsecno")
- """
- if name is not None:
- return self._setResultsName(name)
- else:
- return self.copy()
-
- def suppress(self) -> "ParserElement":
- """
- Suppresses the output of this :class:`ParserElement`; useful to keep punctuation from
- cluttering up returned output.
- """
- return Suppress(self)
-
- def ignore_whitespace(self, recursive: bool = True) -> "ParserElement":
- """
- Enables the skipping of whitespace before matching the characters in the
- :class:`ParserElement`'s defined pattern.
-
- :param recursive: If ``True`` (the default), also enable whitespace skipping in child elements (if any)
- """
- self.skipWhitespace = True
- return self
-
- def leave_whitespace(self, recursive: bool = True) -> "ParserElement":
- """
- Disables the skipping of whitespace before matching the characters in the
- :class:`ParserElement`'s defined pattern. This is normally only used internally by
- the pyparsing module, but may be needed in some whitespace-sensitive grammars.
-
- :param recursive: If true (the default), also disable whitespace skipping in child elements (if any)
- """
- self.skipWhitespace = False
- return self
-
- def set_whitespace_chars(
- self, chars: Union[Set[str], str], copy_defaults: bool = False
- ) -> "ParserElement":
- """
- Overrides the default whitespace chars
- """
- self.skipWhitespace = True
- self.whiteChars = set(chars)
- self.copyDefaultWhiteChars = copy_defaults
- return self
-
- def parse_with_tabs(self) -> "ParserElement":
- """
- Overrides default behavior to expand ```` s to spaces before parsing the input string.
- Must be called before ``parse_string`` when the input grammar contains elements that
- match ```` characters.
- """
- self.keepTabs = True
- return self
-
- def ignore(self, other: "ParserElement") -> "ParserElement":
- """
- Define expression to be ignored (e.g., comments) while doing pattern
- matching; may be called repeatedly, to define multiple comment or other
- ignorable patterns.
-
- Example::
-
- patt = Word(alphas)[1, ...]
- patt.parse_string('ablaj /* comment */ lskjd')
- # -> ['ablaj']
-
- patt.ignore(c_style_comment)
- patt.parse_string('ablaj /* comment */ lskjd')
- # -> ['ablaj', 'lskjd']
- """
- import typing
-
- if isinstance(other, str_type):
- other = Suppress(other)
-
- if isinstance(other, Suppress):
- if other not in self.ignoreExprs:
- self.ignoreExprs.append(other)
- else:
- self.ignoreExprs.append(Suppress(other.copy()))
- return self
-
- def set_debug_actions(
- self,
- start_action: DebugStartAction,
- success_action: DebugSuccessAction,
- exception_action: DebugExceptionAction,
- ) -> "ParserElement":
- """
- Customize display of debugging messages while doing pattern matching:
-
- - ``start_action`` - method to be called when an expression is about to be parsed;
- should have the signature ``fn(input_string: str, location: int, expression: ParserElement, cache_hit: bool)``
-
- - ``success_action`` - method to be called when an expression has successfully parsed;
- should have the signature ``fn(input_string: str, start_location: int, end_location: int, expression: ParserELement, parsed_tokens: ParseResults, cache_hit: bool)``
-
- - ``exception_action`` - method to be called when expression fails to parse;
- should have the signature ``fn(input_string: str, location: int, expression: ParserElement, exception: Exception, cache_hit: bool)``
- """
- self.debugActions = self.DebugActions(
- start_action or _default_start_debug_action,
- success_action or _default_success_debug_action,
- exception_action or _default_exception_debug_action,
- )
- self.debug = True
- return self
-
- def set_debug(self, flag: bool = True) -> "ParserElement":
- """
- Enable display of debugging messages while doing pattern matching.
- Set ``flag`` to ``True`` to enable, ``False`` to disable.
-
- Example::
-
- wd = Word(alphas).set_name("alphaword")
- integer = Word(nums).set_name("numword")
- term = wd | integer
-
- # turn on debugging for wd
- wd.set_debug()
-
- term[1, ...].parse_string("abc 123 xyz 890")
-
- prints::
-
- Match alphaword at loc 0(1,1)
- Matched alphaword -> ['abc']
- Match alphaword at loc 3(1,4)
- Exception raised:Expected alphaword (at char 4), (line:1, col:5)
- Match alphaword at loc 7(1,8)
- Matched alphaword -> ['xyz']
- Match alphaword at loc 11(1,12)
- Exception raised:Expected alphaword (at char 12), (line:1, col:13)
- Match alphaword at loc 15(1,16)
- Exception raised:Expected alphaword (at char 15), (line:1, col:16)
-
- The output shown is that produced by the default debug actions - custom debug actions can be
- specified using :class:`set_debug_actions`. Prior to attempting
- to match the ``wd`` expression, the debugging message ``"Match at loc (,
)"``
- is shown. Then if the parse succeeds, a ``"Matched"`` message is shown, or an ``"Exception raised"``
- message is shown. Also note the use of :class:`set_name` to assign a human-readable name to the expression,
- which makes debugging and exception messages easier to understand - for instance, the default
- name created for the :class:`Word` expression without calling ``set_name`` is ``"W:(A-Za-z)"``.
- """
- if flag:
- self.set_debug_actions(
- _default_start_debug_action,
- _default_success_debug_action,
- _default_exception_debug_action,
- )
- else:
- self.debug = False
- return self
-
- @property
- def default_name(self) -> str:
- if self._defaultName is None:
- self._defaultName = self._generateDefaultName()
- return self._defaultName
-
- @abstractmethod
- def _generateDefaultName(self):
- """
- Child classes must define this method, which defines how the ``default_name`` is set.
- """
-
- def set_name(self, name: str) -> "ParserElement":
- """
- Define name for this expression, makes debugging and exception messages clearer.
- Example::
- Word(nums).parse_string("ABC") # -> Exception: Expected W:(0-9) (at char 0), (line:1, col:1)
- Word(nums).set_name("integer").parse_string("ABC") # -> Exception: Expected integer (at char 0), (line:1, col:1)
- """
- self.customName = name
- self.errmsg = "Expected " + self.name
- if __diag__.enable_debug_on_named_expressions:
- self.set_debug()
- return self
-
- @property
- def name(self) -> str:
- # This will use a user-defined name if available, but otherwise defaults back to the auto-generated name
- return self.customName if self.customName is not None else self.default_name
-
- def __str__(self) -> str:
- return self.name
-
- def __repr__(self) -> str:
- return str(self)
-
- def streamline(self) -> "ParserElement":
- self.streamlined = True
- self._defaultName = None
- return self
-
- def recurse(self) -> Sequence["ParserElement"]:
- return []
-
- def _checkRecursion(self, parseElementList):
- subRecCheckList = parseElementList[:] + [self]
- for e in self.recurse():
- e._checkRecursion(subRecCheckList)
-
- def validate(self, validateTrace=None) -> None:
- """
- Check defined expressions for valid structure, check for infinite recursive definitions.
- """
- self._checkRecursion([])
-
- def parse_file(
- self,
- file_or_filename: Union[str, Path, TextIO],
- encoding: str = "utf-8",
- parse_all: bool = False,
- *,
- parseAll: bool = False,
- ) -> ParseResults:
- """
- Execute the parse expression on the given file or filename.
- If a filename is specified (instead of a file object),
- the entire file is opened, read, and closed before parsing.
- """
- parseAll = parseAll or parse_all
- try:
- file_contents = file_or_filename.read()
- except AttributeError:
- with open(file_or_filename, "r", encoding=encoding) as f:
- file_contents = f.read()
- try:
- return self.parse_string(file_contents, parseAll)
- except ParseBaseException as exc:
- if ParserElement.verbose_stacktrace:
- raise
- else:
- # catch and re-raise exception from here, clears out pyparsing internal stack trace
- raise exc.with_traceback(None)
-
- def __eq__(self, other):
- if self is other:
- return True
- elif isinstance(other, str_type):
- return self.matches(other, parse_all=True)
- elif isinstance(other, ParserElement):
- return vars(self) == vars(other)
- return False
-
- def __hash__(self):
- return id(self)
-
- def matches(
- self, test_string: str, parse_all: bool = True, *, parseAll: bool = True
- ) -> bool:
- """
- Method for quick testing of a parser against a test string. Good for simple
- inline microtests of sub expressions while building up larger parser.
-
- Parameters:
- - ``test_string`` - to test against this expression for a match
- - ``parse_all`` - (default= ``True``) - flag to pass to :class:`parse_string` when running tests
-
- Example::
-
- expr = Word(nums)
- assert expr.matches("100")
- """
- parseAll = parseAll and parse_all
- try:
- self.parse_string(str(test_string), parse_all=parseAll)
- return True
- except ParseBaseException:
- return False
-
- def run_tests(
- self,
- tests: Union[str, List[str]],
- parse_all: bool = True,
- comment: typing.Optional[Union["ParserElement", str]] = "#",
- full_dump: bool = True,
- print_results: bool = True,
- failure_tests: bool = False,
- post_parse: Callable[[str, ParseResults], str] = None,
- file: typing.Optional[TextIO] = None,
- with_line_numbers: bool = False,
- *,
- parseAll: bool = True,
- fullDump: bool = True,
- printResults: bool = True,
- failureTests: bool = False,
- postParse: Callable[[str, ParseResults], str] = None,
- ) -> Tuple[bool, List[Tuple[str, Union[ParseResults, Exception]]]]:
- """
- Execute the parse expression on a series of test strings, showing each
- test, the parsed results or where the parse failed. Quick and easy way to
- run a parse expression against a list of sample strings.
-
- Parameters:
- - ``tests`` - a list of separate test strings, or a multiline string of test strings
- - ``parse_all`` - (default= ``True``) - flag to pass to :class:`parse_string` when running tests
- - ``comment`` - (default= ``'#'``) - expression for indicating embedded comments in the test
- string; pass None to disable comment filtering
- - ``full_dump`` - (default= ``True``) - dump results as list followed by results names in nested outline;
- if False, only dump nested list
- - ``print_results`` - (default= ``True``) prints test output to stdout
- - ``failure_tests`` - (default= ``False``) indicates if these tests are expected to fail parsing
- - ``post_parse`` - (default= ``None``) optional callback for successful parse results; called as
- `fn(test_string, parse_results)` and returns a string to be added to the test output
- - ``file`` - (default= ``None``) optional file-like object to which test output will be written;
- if None, will default to ``sys.stdout``
- - ``with_line_numbers`` - default= ``False``) show test strings with line and column numbers
-
- Returns: a (success, results) tuple, where success indicates that all tests succeeded
- (or failed if ``failure_tests`` is True), and the results contain a list of lines of each
- test's output
-
- Example::
-
- number_expr = pyparsing_common.number.copy()
-
- result = number_expr.run_tests('''
- # unsigned integer
- 100
- # negative integer
- -100
- # float with scientific notation
- 6.02e23
- # integer with scientific notation
- 1e-12
- ''')
- print("Success" if result[0] else "Failed!")
-
- result = number_expr.run_tests('''
- # stray character
- 100Z
- # missing leading digit before '.'
- -.100
- # too many '.'
- 3.14.159
- ''', failure_tests=True)
- print("Success" if result[0] else "Failed!")
-
- prints::
-
- # unsigned integer
- 100
- [100]
-
- # negative integer
- -100
- [-100]
-
- # float with scientific notation
- 6.02e23
- [6.02e+23]
-
- # integer with scientific notation
- 1e-12
- [1e-12]
-
- Success
-
- # stray character
- 100Z
- ^
- FAIL: Expected end of text (at char 3), (line:1, col:4)
-
- # missing leading digit before '.'
- -.100
- ^
- FAIL: Expected {real number with scientific notation | real number | signed integer} (at char 0), (line:1, col:1)
-
- # too many '.'
- 3.14.159
- ^
- FAIL: Expected end of text (at char 4), (line:1, col:5)
-
- Success
-
- Each test string must be on a single line. If you want to test a string that spans multiple
- lines, create a test like this::
-
- expr.run_tests(r"this is a test\\n of strings that spans \\n 3 lines")
-
- (Note that this is a raw string literal, you must include the leading ``'r'``.)
- """
- from .testing import pyparsing_test
-
- parseAll = parseAll and parse_all
- fullDump = fullDump and full_dump
- printResults = printResults and print_results
- failureTests = failureTests or failure_tests
- postParse = postParse or post_parse
- if isinstance(tests, str_type):
- line_strip = type(tests).strip
- tests = [line_strip(test_line) for test_line in tests.rstrip().splitlines()]
- if isinstance(comment, str_type):
- comment = Literal(comment)
- if file is None:
- file = sys.stdout
- print_ = file.write
-
- result: Union[ParseResults, Exception]
- allResults = []
- comments = []
- success = True
- NL = Literal(r"\n").add_parse_action(replace_with("\n")).ignore(quoted_string)
- BOM = "\ufeff"
- for t in tests:
- if comment is not None and comment.matches(t, False) or comments and not t:
- comments.append(
- pyparsing_test.with_line_numbers(t) if with_line_numbers else t
- )
- continue
- if not t:
- continue
- out = [
- "\n" + "\n".join(comments) if comments else "",
- pyparsing_test.with_line_numbers(t) if with_line_numbers else t,
- ]
- comments = []
- try:
- # convert newline marks to actual newlines, and strip leading BOM if present
- t = NL.transform_string(t.lstrip(BOM))
- result = self.parse_string(t, parse_all=parseAll)
- except ParseBaseException as pe:
- fatal = "(FATAL)" if isinstance(pe, ParseFatalException) else ""
- out.append(pe.explain())
- out.append("FAIL: " + str(pe))
- if ParserElement.verbose_stacktrace:
- out.extend(traceback.format_tb(pe.__traceback__))
- success = success and failureTests
- result = pe
- except Exception as exc:
- out.append("FAIL-EXCEPTION: {}: {}".format(type(exc).__name__, exc))
- if ParserElement.verbose_stacktrace:
- out.extend(traceback.format_tb(exc.__traceback__))
- success = success and failureTests
- result = exc
- else:
- success = success and not failureTests
- if postParse is not None:
- try:
- pp_value = postParse(t, result)
- if pp_value is not None:
- if isinstance(pp_value, ParseResults):
- out.append(pp_value.dump())
- else:
- out.append(str(pp_value))
- else:
- out.append(result.dump())
- except Exception as e:
- out.append(result.dump(full=fullDump))
- out.append(
- "{} failed: {}: {}".format(
- postParse.__name__, type(e).__name__, e
- )
- )
- else:
- out.append(result.dump(full=fullDump))
- out.append("")
-
- if printResults:
- print_("\n".join(out))
-
- allResults.append((t, result))
-
- return success, allResults
-
- def create_diagram(
- self,
- output_html: Union[TextIO, Path, str],
- vertical: int = 3,
- show_results_names: bool = False,
- show_groups: bool = False,
- **kwargs,
- ) -> None:
- """
- Create a railroad diagram for the parser.
-
- Parameters:
- - output_html (str or file-like object) - output target for generated
- diagram HTML
- - vertical (int) - threshold for formatting multiple alternatives vertically
- instead of horizontally (default=3)
- - show_results_names - bool flag whether diagram should show annotations for
- defined results names
- - show_groups - bool flag whether groups should be highlighted with an unlabeled surrounding box
- Additional diagram-formatting keyword arguments can also be included;
- see railroad.Diagram class.
- """
-
- try:
- from .diagram import to_railroad, railroad_to_html
- except ImportError as ie:
- raise Exception(
- "must ``pip install pyparsing[diagrams]`` to generate parser railroad diagrams"
- ) from ie
-
- self.streamline()
-
- railroad = to_railroad(
- self,
- vertical=vertical,
- show_results_names=show_results_names,
- show_groups=show_groups,
- diagram_kwargs=kwargs,
- )
- if isinstance(output_html, (str, Path)):
- with open(output_html, "w", encoding="utf-8") as diag_file:
- diag_file.write(railroad_to_html(railroad))
- else:
- # we were passed a file-like object, just write to it
- output_html.write(railroad_to_html(railroad))
-
- setDefaultWhitespaceChars = set_default_whitespace_chars
- inlineLiteralsUsing = inline_literals_using
- setResultsName = set_results_name
- setBreak = set_break
- setParseAction = set_parse_action
- addParseAction = add_parse_action
- addCondition = add_condition
- setFailAction = set_fail_action
- tryParse = try_parse
- canParseNext = can_parse_next
- resetCache = reset_cache
- enableLeftRecursion = enable_left_recursion
- enablePackrat = enable_packrat
- parseString = parse_string
- scanString = scan_string
- searchString = search_string
- transformString = transform_string
- setWhitespaceChars = set_whitespace_chars
- parseWithTabs = parse_with_tabs
- setDebugActions = set_debug_actions
- setDebug = set_debug
- defaultName = default_name
- setName = set_name
- parseFile = parse_file
- runTests = run_tests
- ignoreWhitespace = ignore_whitespace
- leaveWhitespace = leave_whitespace
-
-
-class _PendingSkip(ParserElement):
- # internal placeholder class to hold a place were '...' is added to a parser element,
- # once another ParserElement is added, this placeholder will be replaced with a SkipTo
- def __init__(self, expr: ParserElement, must_skip: bool = False):
- super().__init__()
- self.anchor = expr
- self.must_skip = must_skip
-
- def _generateDefaultName(self):
- return str(self.anchor + Empty()).replace("Empty", "...")
-
- def __add__(self, other) -> "ParserElement":
- skipper = SkipTo(other).set_name("...")("_skipped*")
- if self.must_skip:
-
- def must_skip(t):
- if not t._skipped or t._skipped.as_list() == [""]:
- del t[0]
- t.pop("_skipped", None)
-
- def show_skip(t):
- if t._skipped.as_list()[-1:] == [""]:
- t.pop("_skipped")
- t["_skipped"] = "missing <" + repr(self.anchor) + ">"
-
- return (
- self.anchor + skipper().add_parse_action(must_skip)
- | skipper().add_parse_action(show_skip)
- ) + other
-
- return self.anchor + skipper + other
-
- def __repr__(self):
- return self.defaultName
-
- def parseImpl(self, *args):
- raise Exception(
- "use of `...` expression without following SkipTo target expression"
- )
-
-
-class Token(ParserElement):
- """Abstract :class:`ParserElement` subclass, for defining atomic
- matching patterns.
- """
-
- def __init__(self):
- super().__init__(savelist=False)
-
- def _generateDefaultName(self):
- return type(self).__name__
-
-
-class Empty(Token):
- """
- An empty token, will always match.
- """
-
- def __init__(self):
- super().__init__()
- self.mayReturnEmpty = True
- self.mayIndexError = False
-
-
-class NoMatch(Token):
- """
- A token that will never match.
- """
-
- def __init__(self):
- super().__init__()
- self.mayReturnEmpty = True
- self.mayIndexError = False
- self.errmsg = "Unmatchable token"
-
- def parseImpl(self, instring, loc, doActions=True):
- raise ParseException(instring, loc, self.errmsg, self)
-
-
-class Literal(Token):
- """
- Token to exactly match a specified string.
-
- Example::
-
- Literal('blah').parse_string('blah') # -> ['blah']
- Literal('blah').parse_string('blahfooblah') # -> ['blah']
- Literal('blah').parse_string('bla') # -> Exception: Expected "blah"
-
- For case-insensitive matching, use :class:`CaselessLiteral`.
-
- For keyword matching (force word break before and after the matched string),
- use :class:`Keyword` or :class:`CaselessKeyword`.
- """
-
- def __init__(self, match_string: str = "", *, matchString: str = ""):
- super().__init__()
- match_string = matchString or match_string
- self.match = match_string
- self.matchLen = len(match_string)
- try:
- self.firstMatchChar = match_string[0]
- except IndexError:
- raise ValueError("null string passed to Literal; use Empty() instead")
- self.errmsg = "Expected " + self.name
- self.mayReturnEmpty = False
- self.mayIndexError = False
-
- # Performance tuning: modify __class__ to select
- # a parseImpl optimized for single-character check
- if self.matchLen == 1 and type(self) is Literal:
- self.__class__ = _SingleCharLiteral
-
- def _generateDefaultName(self):
- return repr(self.match)
-
- def parseImpl(self, instring, loc, doActions=True):
- if instring[loc] == self.firstMatchChar and instring.startswith(
- self.match, loc
- ):
- return loc + self.matchLen, self.match
- raise ParseException(instring, loc, self.errmsg, self)
-
-
-class _SingleCharLiteral(Literal):
- def parseImpl(self, instring, loc, doActions=True):
- if instring[loc] == self.firstMatchChar:
- return loc + 1, self.match
- raise ParseException(instring, loc, self.errmsg, self)
-
-
-ParserElement._literalStringClass = Literal
-
-
-class Keyword(Token):
- """
- Token to exactly match a specified string as a keyword, that is,
- it must be immediately followed by a non-keyword character. Compare
- with :class:`Literal`:
-
- - ``Literal("if")`` will match the leading ``'if'`` in
- ``'ifAndOnlyIf'``.
- - ``Keyword("if")`` will not; it will only match the leading
- ``'if'`` in ``'if x=1'``, or ``'if(y==2)'``
-
- Accepts two optional constructor arguments in addition to the
- keyword string:
-
- - ``identChars`` is a string of characters that would be valid
- identifier characters, defaulting to all alphanumerics + "_" and
- "$"
- - ``caseless`` allows case-insensitive matching, default is ``False``.
-
- Example::
-
- Keyword("start").parse_string("start") # -> ['start']
- Keyword("start").parse_string("starting") # -> Exception
-
- For case-insensitive matching, use :class:`CaselessKeyword`.
- """
-
- DEFAULT_KEYWORD_CHARS = alphanums + "_$"
-
- def __init__(
- self,
- match_string: str = "",
- ident_chars: typing.Optional[str] = None,
- caseless: bool = False,
- *,
- matchString: str = "",
- identChars: typing.Optional[str] = None,
- ):
- super().__init__()
- identChars = identChars or ident_chars
- if identChars is None:
- identChars = Keyword.DEFAULT_KEYWORD_CHARS
- match_string = matchString or match_string
- self.match = match_string
- self.matchLen = len(match_string)
- try:
- self.firstMatchChar = match_string[0]
- except IndexError:
- raise ValueError("null string passed to Keyword; use Empty() instead")
- self.errmsg = "Expected {} {}".format(type(self).__name__, self.name)
- self.mayReturnEmpty = False
- self.mayIndexError = False
- self.caseless = caseless
- if caseless:
- self.caselessmatch = match_string.upper()
- identChars = identChars.upper()
- self.identChars = set(identChars)
-
- def _generateDefaultName(self):
- return repr(self.match)
-
- def parseImpl(self, instring, loc, doActions=True):
- errmsg = self.errmsg
- errloc = loc
- if self.caseless:
- if instring[loc : loc + self.matchLen].upper() == self.caselessmatch:
- if loc == 0 or instring[loc - 1].upper() not in self.identChars:
- if (
- loc >= len(instring) - self.matchLen
- or instring[loc + self.matchLen].upper() not in self.identChars
- ):
- return loc + self.matchLen, self.match
- else:
- # followed by keyword char
- errmsg += ", was immediately followed by keyword character"
- errloc = loc + self.matchLen
- else:
- # preceded by keyword char
- errmsg += ", keyword was immediately preceded by keyword character"
- errloc = loc - 1
- # else no match just raise plain exception
-
- else:
- if (
- instring[loc] == self.firstMatchChar
- and self.matchLen == 1
- or instring.startswith(self.match, loc)
- ):
- if loc == 0 or instring[loc - 1] not in self.identChars:
- if (
- loc >= len(instring) - self.matchLen
- or instring[loc + self.matchLen] not in self.identChars
- ):
- return loc + self.matchLen, self.match
- else:
- # followed by keyword char
- errmsg += (
- ", keyword was immediately followed by keyword character"
- )
- errloc = loc + self.matchLen
- else:
- # preceded by keyword char
- errmsg += ", keyword was immediately preceded by keyword character"
- errloc = loc - 1
- # else no match just raise plain exception
-
- raise ParseException(instring, errloc, errmsg, self)
-
- @staticmethod
- def set_default_keyword_chars(chars) -> None:
- """
- Overrides the default characters used by :class:`Keyword` expressions.
- """
- Keyword.DEFAULT_KEYWORD_CHARS = chars
-
- setDefaultKeywordChars = set_default_keyword_chars
-
-
-class CaselessLiteral(Literal):
- """
- Token to match a specified string, ignoring case of letters.
- Note: the matched results will always be in the case of the given
- match string, NOT the case of the input text.
-
- Example::
-
- CaselessLiteral("CMD")[1, ...].parse_string("cmd CMD Cmd10")
- # -> ['CMD', 'CMD', 'CMD']
-
- (Contrast with example for :class:`CaselessKeyword`.)
- """
-
- def __init__(self, match_string: str = "", *, matchString: str = ""):
- match_string = matchString or match_string
- super().__init__(match_string.upper())
- # Preserve the defining literal.
- self.returnString = match_string
- self.errmsg = "Expected " + self.name
-
- def parseImpl(self, instring, loc, doActions=True):
- if instring[loc : loc + self.matchLen].upper() == self.match:
- return loc + self.matchLen, self.returnString
- raise ParseException(instring, loc, self.errmsg, self)
-
-
-class CaselessKeyword(Keyword):
- """
- Caseless version of :class:`Keyword`.
-
- Example::
-
- CaselessKeyword("CMD")[1, ...].parse_string("cmd CMD Cmd10")
- # -> ['CMD', 'CMD']
-
- (Contrast with example for :class:`CaselessLiteral`.)
- """
-
- def __init__(
- self,
- match_string: str = "",
- ident_chars: typing.Optional[str] = None,
- *,
- matchString: str = "",
- identChars: typing.Optional[str] = None,
- ):
- identChars = identChars or ident_chars
- match_string = matchString or match_string
- super().__init__(match_string, identChars, caseless=True)
-
-
-class CloseMatch(Token):
- """A variation on :class:`Literal` which matches "close" matches,
- that is, strings with at most 'n' mismatching characters.
- :class:`CloseMatch` takes parameters:
-
- - ``match_string`` - string to be matched
- - ``caseless`` - a boolean indicating whether to ignore casing when comparing characters
- - ``max_mismatches`` - (``default=1``) maximum number of
- mismatches allowed to count as a match
-
- The results from a successful parse will contain the matched text
- from the input string and the following named results:
-
- - ``mismatches`` - a list of the positions within the
- match_string where mismatches were found
- - ``original`` - the original match_string used to compare
- against the input string
-
- If ``mismatches`` is an empty list, then the match was an exact
- match.
-
- Example::
-
- patt = CloseMatch("ATCATCGAATGGA")
- patt.parse_string("ATCATCGAAXGGA") # -> (['ATCATCGAAXGGA'], {'mismatches': [[9]], 'original': ['ATCATCGAATGGA']})
- patt.parse_string("ATCAXCGAAXGGA") # -> Exception: Expected 'ATCATCGAATGGA' (with up to 1 mismatches) (at char 0), (line:1, col:1)
-
- # exact match
- patt.parse_string("ATCATCGAATGGA") # -> (['ATCATCGAATGGA'], {'mismatches': [[]], 'original': ['ATCATCGAATGGA']})
-
- # close match allowing up to 2 mismatches
- patt = CloseMatch("ATCATCGAATGGA", max_mismatches=2)
- patt.parse_string("ATCAXCGAAXGGA") # -> (['ATCAXCGAAXGGA'], {'mismatches': [[4, 9]], 'original': ['ATCATCGAATGGA']})
- """
-
- def __init__(
- self,
- match_string: str,
- max_mismatches: int = None,
- *,
- maxMismatches: int = 1,
- caseless=False,
- ):
- maxMismatches = max_mismatches if max_mismatches is not None else maxMismatches
- super().__init__()
- self.match_string = match_string
- self.maxMismatches = maxMismatches
- self.errmsg = "Expected {!r} (with up to {} mismatches)".format(
- self.match_string, self.maxMismatches
- )
- self.caseless = caseless
- self.mayIndexError = False
- self.mayReturnEmpty = False
-
- def _generateDefaultName(self):
- return "{}:{!r}".format(type(self).__name__, self.match_string)
-
- def parseImpl(self, instring, loc, doActions=True):
- start = loc
- instrlen = len(instring)
- maxloc = start + len(self.match_string)
-
- if maxloc <= instrlen:
- match_string = self.match_string
- match_stringloc = 0
- mismatches = []
- maxMismatches = self.maxMismatches
-
- for match_stringloc, s_m in enumerate(
- zip(instring[loc:maxloc], match_string)
- ):
- src, mat = s_m
- if self.caseless:
- src, mat = src.lower(), mat.lower()
-
- if src != mat:
- mismatches.append(match_stringloc)
- if len(mismatches) > maxMismatches:
- break
- else:
- loc = start + match_stringloc + 1
- results = ParseResults([instring[start:loc]])
- results["original"] = match_string
- results["mismatches"] = mismatches
- return loc, results
-
- raise ParseException(instring, loc, self.errmsg, self)
-
-
-class Word(Token):
- """Token for matching words composed of allowed character sets.
- Parameters:
- - ``init_chars`` - string of all characters that should be used to
- match as a word; "ABC" will match "AAA", "ABAB", "CBAC", etc.;
- if ``body_chars`` is also specified, then this is the string of
- initial characters
- - ``body_chars`` - string of characters that
- can be used for matching after a matched initial character as
- given in ``init_chars``; if omitted, same as the initial characters
- (default=``None``)
- - ``min`` - minimum number of characters to match (default=1)
- - ``max`` - maximum number of characters to match (default=0)
- - ``exact`` - exact number of characters to match (default=0)
- - ``as_keyword`` - match as a keyword (default=``False``)
- - ``exclude_chars`` - characters that might be
- found in the input ``body_chars`` string but which should not be
- accepted for matching ;useful to define a word of all
- printables except for one or two characters, for instance
- (default=``None``)
-
- :class:`srange` is useful for defining custom character set strings
- for defining :class:`Word` expressions, using range notation from
- regular expression character sets.
-
- A common mistake is to use :class:`Word` to match a specific literal
- string, as in ``Word("Address")``. Remember that :class:`Word`
- uses the string argument to define *sets* of matchable characters.
- This expression would match "Add", "AAA", "dAred", or any other word
- made up of the characters 'A', 'd', 'r', 'e', and 's'. To match an
- exact literal string, use :class:`Literal` or :class:`Keyword`.
-
- pyparsing includes helper strings for building Words:
-
- - :class:`alphas`
- - :class:`nums`
- - :class:`alphanums`
- - :class:`hexnums`
- - :class:`alphas8bit` (alphabetic characters in ASCII range 128-255
- - accented, tilded, umlauted, etc.)
- - :class:`punc8bit` (non-alphabetic characters in ASCII range
- 128-255 - currency, symbols, superscripts, diacriticals, etc.)
- - :class:`printables` (any non-whitespace character)
-
- ``alphas``, ``nums``, and ``printables`` are also defined in several
- Unicode sets - see :class:`pyparsing_unicode``.
-
- Example::
-
- # a word composed of digits
- integer = Word(nums) # equivalent to Word("0123456789") or Word(srange("0-9"))
-
- # a word with a leading capital, and zero or more lowercase
- capital_word = Word(alphas.upper(), alphas.lower())
-
- # hostnames are alphanumeric, with leading alpha, and '-'
- hostname = Word(alphas, alphanums + '-')
-
- # roman numeral (not a strict parser, accepts invalid mix of characters)
- roman = Word("IVXLCDM")
-
- # any string of non-whitespace characters, except for ','
- csv_value = Word(printables, exclude_chars=",")
- """
-
- def __init__(
- self,
- init_chars: str = "",
- body_chars: typing.Optional[str] = None,
- min: int = 1,
- max: int = 0,
- exact: int = 0,
- as_keyword: bool = False,
- exclude_chars: typing.Optional[str] = None,
- *,
- initChars: typing.Optional[str] = None,
- bodyChars: typing.Optional[str] = None,
- asKeyword: bool = False,
- excludeChars: typing.Optional[str] = None,
- ):
- initChars = initChars or init_chars
- bodyChars = bodyChars or body_chars
- asKeyword = asKeyword or as_keyword
- excludeChars = excludeChars or exclude_chars
- super().__init__()
- if not initChars:
- raise ValueError(
- "invalid {}, initChars cannot be empty string".format(
- type(self).__name__
- )
- )
-
- initChars = set(initChars)
- self.initChars = initChars
- if excludeChars:
- excludeChars = set(excludeChars)
- initChars -= excludeChars
- if bodyChars:
- bodyChars = set(bodyChars) - excludeChars
- self.initCharsOrig = "".join(sorted(initChars))
-
- if bodyChars:
- self.bodyCharsOrig = "".join(sorted(bodyChars))
- self.bodyChars = set(bodyChars)
- else:
- self.bodyCharsOrig = "".join(sorted(initChars))
- self.bodyChars = set(initChars)
-
- self.maxSpecified = max > 0
-
- if min < 1:
- raise ValueError(
- "cannot specify a minimum length < 1; use Opt(Word()) if zero-length word is permitted"
- )
-
- self.minLen = min
-
- if max > 0:
- self.maxLen = max
- else:
- self.maxLen = _MAX_INT
-
- if exact > 0:
- self.maxLen = exact
- self.minLen = exact
-
- self.errmsg = "Expected " + self.name
- self.mayIndexError = False
- self.asKeyword = asKeyword
-
- # see if we can make a regex for this Word
- if " " not in self.initChars | self.bodyChars and (min == 1 and exact == 0):
- if self.bodyChars == self.initChars:
- if max == 0:
- repeat = "+"
- elif max == 1:
- repeat = ""
- else:
- repeat = "{{{},{}}}".format(
- self.minLen, "" if self.maxLen == _MAX_INT else self.maxLen
- )
- self.reString = "[{}]{}".format(
- _collapse_string_to_ranges(self.initChars),
- repeat,
- )
- elif len(self.initChars) == 1:
- if max == 0:
- repeat = "*"
- else:
- repeat = "{{0,{}}}".format(max - 1)
- self.reString = "{}[{}]{}".format(
- re.escape(self.initCharsOrig),
- _collapse_string_to_ranges(self.bodyChars),
- repeat,
- )
- else:
- if max == 0:
- repeat = "*"
- elif max == 2:
- repeat = ""
- else:
- repeat = "{{0,{}}}".format(max - 1)
- self.reString = "[{}][{}]{}".format(
- _collapse_string_to_ranges(self.initChars),
- _collapse_string_to_ranges(self.bodyChars),
- repeat,
- )
- if self.asKeyword:
- self.reString = r"\b" + self.reString + r"\b"
-
- try:
- self.re = re.compile(self.reString)
- except re.error:
- self.re = None
- else:
- self.re_match = self.re.match
- self.__class__ = _WordRegex
-
- def _generateDefaultName(self):
- def charsAsStr(s):
- max_repr_len = 16
- s = _collapse_string_to_ranges(s, re_escape=False)
- if len(s) > max_repr_len:
- return s[: max_repr_len - 3] + "..."
- else:
- return s
-
- if self.initChars != self.bodyChars:
- base = "W:({}, {})".format(
- charsAsStr(self.initChars), charsAsStr(self.bodyChars)
- )
- else:
- base = "W:({})".format(charsAsStr(self.initChars))
-
- # add length specification
- if self.minLen > 1 or self.maxLen != _MAX_INT:
- if self.minLen == self.maxLen:
- if self.minLen == 1:
- return base[2:]
- else:
- return base + "{{{}}}".format(self.minLen)
- elif self.maxLen == _MAX_INT:
- return base + "{{{},...}}".format(self.minLen)
- else:
- return base + "{{{},{}}}".format(self.minLen, self.maxLen)
- return base
-
- def parseImpl(self, instring, loc, doActions=True):
- if instring[loc] not in self.initChars:
- raise ParseException(instring, loc, self.errmsg, self)
-
- start = loc
- loc += 1
- instrlen = len(instring)
- bodychars = self.bodyChars
- maxloc = start + self.maxLen
- maxloc = min(maxloc, instrlen)
- while loc < maxloc and instring[loc] in bodychars:
- loc += 1
-
- throwException = False
- if loc - start < self.minLen:
- throwException = True
- elif self.maxSpecified and loc < instrlen and instring[loc] in bodychars:
- throwException = True
- elif self.asKeyword:
- if (
- start > 0
- and instring[start - 1] in bodychars
- or loc < instrlen
- and instring[loc] in bodychars
- ):
- throwException = True
-
- if throwException:
- raise ParseException(instring, loc, self.errmsg, self)
-
- return loc, instring[start:loc]
-
-
-class _WordRegex(Word):
- def parseImpl(self, instring, loc, doActions=True):
- result = self.re_match(instring, loc)
- if not result:
- raise ParseException(instring, loc, self.errmsg, self)
-
- loc = result.end()
- return loc, result.group()
-
-
-class Char(_WordRegex):
- """A short-cut class for defining :class:`Word` ``(characters, exact=1)``,
- when defining a match of any single character in a string of
- characters.
- """
-
- def __init__(
- self,
- charset: str,
- as_keyword: bool = False,
- exclude_chars: typing.Optional[str] = None,
- *,
- asKeyword: bool = False,
- excludeChars: typing.Optional[str] = None,
- ):
- asKeyword = asKeyword or as_keyword
- excludeChars = excludeChars or exclude_chars
- super().__init__(
- charset, exact=1, asKeyword=asKeyword, excludeChars=excludeChars
- )
- self.reString = "[{}]".format(_collapse_string_to_ranges(self.initChars))
- if asKeyword:
- self.reString = r"\b{}\b".format(self.reString)
- self.re = re.compile(self.reString)
- self.re_match = self.re.match
-
-
-class Regex(Token):
- r"""Token for matching strings that match a given regular
- expression. Defined with string specifying the regular expression in
- a form recognized by the stdlib Python `re module `_.
- If the given regex contains named groups (defined using ``(?P...)``),
- these will be preserved as named :class:`ParseResults`.
-
- If instead of the Python stdlib ``re`` module you wish to use a different RE module
- (such as the ``regex`` module), you can do so by building your ``Regex`` object with
- a compiled RE that was compiled using ``regex``.
-
- Example::
-
- realnum = Regex(r"[+-]?\d+\.\d*")
- # ref: https://stackoverflow.com/questions/267399/how-do-you-match-only-valid-roman-numerals-with-a-regular-expression
- roman = Regex(r"M{0,4}(CM|CD|D?{0,3})(XC|XL|L?X{0,3})(IX|IV|V?I{0,3})")
-
- # named fields in a regex will be returned as named results
- date = Regex(r'(?P\d{4})-(?P\d\d?)-(?P\d\d?)')
-
- # the Regex class will accept re's compiled using the regex module
- import regex
- parser = pp.Regex(regex.compile(r'[0-9]'))
- """
-
- def __init__(
- self,
- pattern: Any,
- flags: Union[re.RegexFlag, int] = 0,
- as_group_list: bool = False,
- as_match: bool = False,
- *,
- asGroupList: bool = False,
- asMatch: bool = False,
- ):
- """The parameters ``pattern`` and ``flags`` are passed
- to the ``re.compile()`` function as-is. See the Python
- `re module `_ module for an
- explanation of the acceptable patterns and flags.
- """
- super().__init__()
- asGroupList = asGroupList or as_group_list
- asMatch = asMatch or as_match
-
- if isinstance(pattern, str_type):
- if not pattern:
- raise ValueError("null string passed to Regex; use Empty() instead")
-
- self._re = None
- self.reString = self.pattern = pattern
- self.flags = flags
-
- elif hasattr(pattern, "pattern") and hasattr(pattern, "match"):
- self._re = pattern
- self.pattern = self.reString = pattern.pattern
- self.flags = flags
-
- else:
- raise TypeError(
- "Regex may only be constructed with a string or a compiled RE object"
- )
-
- self.errmsg = "Expected " + self.name
- self.mayIndexError = False
- self.asGroupList = asGroupList
- self.asMatch = asMatch
- if self.asGroupList:
- self.parseImpl = self.parseImplAsGroupList
- if self.asMatch:
- self.parseImpl = self.parseImplAsMatch
-
- @cached_property
- def re(self):
- if self._re:
- return self._re
- else:
- try:
- return re.compile(self.pattern, self.flags)
- except re.error:
- raise ValueError(
- "invalid pattern ({!r}) passed to Regex".format(self.pattern)
- )
-
- @cached_property
- def re_match(self):
- return self.re.match
-
- @cached_property
- def mayReturnEmpty(self):
- return self.re_match("") is not None
-
- def _generateDefaultName(self):
- return "Re:({})".format(repr(self.pattern).replace("\\\\", "\\"))
-
- def parseImpl(self, instring, loc, doActions=True):
- result = self.re_match(instring, loc)
- if not result:
- raise ParseException(instring, loc, self.errmsg, self)
-
- loc = result.end()
- ret = ParseResults(result.group())
- d = result.groupdict()
- if d:
- for k, v in d.items():
- ret[k] = v
- return loc, ret
-
- def parseImplAsGroupList(self, instring, loc, doActions=True):
- result = self.re_match(instring, loc)
- if not result:
- raise ParseException(instring, loc, self.errmsg, self)
-
- loc = result.end()
- ret = result.groups()
- return loc, ret
-
- def parseImplAsMatch(self, instring, loc, doActions=True):
- result = self.re_match(instring, loc)
- if not result:
- raise ParseException(instring, loc, self.errmsg, self)
-
- loc = result.end()
- ret = result
- return loc, ret
-
- def sub(self, repl: str) -> ParserElement:
- r"""
- Return :class:`Regex` with an attached parse action to transform the parsed
- result as if called using `re.sub(expr, repl, string) `_.
-
- Example::
-
- make_html = Regex(r"(\w+):(.*?):").sub(r"<\1>\2\1>")
- print(make_html.transform_string("h1:main title:"))
- # prints "
main title
"
- """
- if self.asGroupList:
- raise TypeError("cannot use sub() with Regex(asGroupList=True)")
-
- if self.asMatch and callable(repl):
- raise TypeError("cannot use sub() with a callable with Regex(asMatch=True)")
-
- if self.asMatch:
-
- def pa(tokens):
- return tokens[0].expand(repl)
-
- else:
-
- def pa(tokens):
- return self.re.sub(repl, tokens[0])
-
- return self.add_parse_action(pa)
-
-
-class QuotedString(Token):
- r"""
- Token for matching strings that are delimited by quoting characters.
-
- Defined with the following parameters:
-
- - ``quote_char`` - string of one or more characters defining the
- quote delimiting string
- - ``esc_char`` - character to re_escape quotes, typically backslash
- (default= ``None``)
- - ``esc_quote`` - special quote sequence to re_escape an embedded quote
- string (such as SQL's ``""`` to re_escape an embedded ``"``)
- (default= ``None``)
- - ``multiline`` - boolean indicating whether quotes can span
- multiple lines (default= ``False``)
- - ``unquote_results`` - boolean indicating whether the matched text
- should be unquoted (default= ``True``)
- - ``end_quote_char`` - string of one or more characters defining the
- end of the quote delimited string (default= ``None`` => same as
- quote_char)
- - ``convert_whitespace_escapes`` - convert escaped whitespace
- (``'\t'``, ``'\n'``, etc.) to actual whitespace
- (default= ``True``)
-
- Example::
-
- qs = QuotedString('"')
- print(qs.search_string('lsjdf "This is the quote" sldjf'))
- complex_qs = QuotedString('{{', end_quote_char='}}')
- print(complex_qs.search_string('lsjdf {{This is the "quote"}} sldjf'))
- sql_qs = QuotedString('"', esc_quote='""')
- print(sql_qs.search_string('lsjdf "This is the quote with ""embedded"" quotes" sldjf'))
-
- prints::
-
- [['This is the quote']]
- [['This is the "quote"']]
- [['This is the quote with "embedded" quotes']]
- """
- ws_map = ((r"\t", "\t"), (r"\n", "\n"), (r"\f", "\f"), (r"\r", "\r"))
-
- def __init__(
- self,
- quote_char: str = "",
- esc_char: typing.Optional[str] = None,
- esc_quote: typing.Optional[str] = None,
- multiline: bool = False,
- unquote_results: bool = True,
- end_quote_char: typing.Optional[str] = None,
- convert_whitespace_escapes: bool = True,
- *,
- quoteChar: str = "",
- escChar: typing.Optional[str] = None,
- escQuote: typing.Optional[str] = None,
- unquoteResults: bool = True,
- endQuoteChar: typing.Optional[str] = None,
- convertWhitespaceEscapes: bool = True,
- ):
- super().__init__()
- escChar = escChar or esc_char
- escQuote = escQuote or esc_quote
- unquoteResults = unquoteResults and unquote_results
- endQuoteChar = endQuoteChar or end_quote_char
- convertWhitespaceEscapes = (
- convertWhitespaceEscapes and convert_whitespace_escapes
- )
- quote_char = quoteChar or quote_char
-
- # remove white space from quote chars - wont work anyway
- quote_char = quote_char.strip()
- if not quote_char:
- raise ValueError("quote_char cannot be the empty string")
-
- if endQuoteChar is None:
- endQuoteChar = quote_char
- else:
- endQuoteChar = endQuoteChar.strip()
- if not endQuoteChar:
- raise ValueError("endQuoteChar cannot be the empty string")
-
- self.quoteChar = quote_char
- self.quoteCharLen = len(quote_char)
- self.firstQuoteChar = quote_char[0]
- self.endQuoteChar = endQuoteChar
- self.endQuoteCharLen = len(endQuoteChar)
- self.escChar = escChar
- self.escQuote = escQuote
- self.unquoteResults = unquoteResults
- self.convertWhitespaceEscapes = convertWhitespaceEscapes
-
- sep = ""
- inner_pattern = ""
-
- if escQuote:
- inner_pattern += r"{}(?:{})".format(sep, re.escape(escQuote))
- sep = "|"
-
- if escChar:
- inner_pattern += r"{}(?:{}.)".format(sep, re.escape(escChar))
- sep = "|"
- self.escCharReplacePattern = re.escape(self.escChar) + "(.)"
-
- if len(self.endQuoteChar) > 1:
- inner_pattern += (
- "{}(?:".format(sep)
- + "|".join(
- "(?:{}(?!{}))".format(
- re.escape(self.endQuoteChar[:i]),
- re.escape(self.endQuoteChar[i:]),
- )
- for i in range(len(self.endQuoteChar) - 1, 0, -1)
- )
- + ")"
- )
- sep = "|"
-
- if multiline:
- self.flags = re.MULTILINE | re.DOTALL
- inner_pattern += r"{}(?:[^{}{}])".format(
- sep,
- _escape_regex_range_chars(self.endQuoteChar[0]),
- (_escape_regex_range_chars(escChar) if escChar is not None else ""),
- )
- else:
- self.flags = 0
- inner_pattern += r"{}(?:[^{}\n\r{}])".format(
- sep,
- _escape_regex_range_chars(self.endQuoteChar[0]),
- (_escape_regex_range_chars(escChar) if escChar is not None else ""),
- )
-
- self.pattern = "".join(
- [
- re.escape(self.quoteChar),
- "(?:",
- inner_pattern,
- ")*",
- re.escape(self.endQuoteChar),
- ]
- )
-
- try:
- self.re = re.compile(self.pattern, self.flags)
- self.reString = self.pattern
- self.re_match = self.re.match
- except re.error:
- raise ValueError(
- "invalid pattern {!r} passed to Regex".format(self.pattern)
- )
-
- self.errmsg = "Expected " + self.name
- self.mayIndexError = False
- self.mayReturnEmpty = True
-
- def _generateDefaultName(self):
- if self.quoteChar == self.endQuoteChar and isinstance(self.quoteChar, str_type):
- return "string enclosed in {!r}".format(self.quoteChar)
-
- return "quoted string, starting with {} ending with {}".format(
- self.quoteChar, self.endQuoteChar
- )
-
- def parseImpl(self, instring, loc, doActions=True):
- result = (
- instring[loc] == self.firstQuoteChar
- and self.re_match(instring, loc)
- or None
- )
- if not result:
- raise ParseException(instring, loc, self.errmsg, self)
-
- loc = result.end()
- ret = result.group()
-
- if self.unquoteResults:
-
- # strip off quotes
- ret = ret[self.quoteCharLen : -self.endQuoteCharLen]
-
- if isinstance(ret, str_type):
- # replace escaped whitespace
- if "\\" in ret and self.convertWhitespaceEscapes:
- for wslit, wschar in self.ws_map:
- ret = ret.replace(wslit, wschar)
-
- # replace escaped characters
- if self.escChar:
- ret = re.sub(self.escCharReplacePattern, r"\g<1>", ret)
-
- # replace escaped quotes
- if self.escQuote:
- ret = ret.replace(self.escQuote, self.endQuoteChar)
-
- return loc, ret
-
-
-class CharsNotIn(Token):
- """Token for matching words composed of characters *not* in a given
- set (will include whitespace in matched characters if not listed in
- the provided exclusion set - see example). Defined with string
- containing all disallowed characters, and an optional minimum,
- maximum, and/or exact length. The default value for ``min`` is
- 1 (a minimum value < 1 is not valid); the default values for
- ``max`` and ``exact`` are 0, meaning no maximum or exact
- length restriction.
-
- Example::
-
- # define a comma-separated-value as anything that is not a ','
- csv_value = CharsNotIn(',')
- print(delimited_list(csv_value).parse_string("dkls,lsdkjf,s12 34,@!#,213"))
-
- prints::
-
- ['dkls', 'lsdkjf', 's12 34', '@!#', '213']
- """
-
- def __init__(
- self,
- not_chars: str = "",
- min: int = 1,
- max: int = 0,
- exact: int = 0,
- *,
- notChars: str = "",
- ):
- super().__init__()
- self.skipWhitespace = False
- self.notChars = not_chars or notChars
- self.notCharsSet = set(self.notChars)
-
- if min < 1:
- raise ValueError(
- "cannot specify a minimum length < 1; use "
- "Opt(CharsNotIn()) if zero-length char group is permitted"
- )
-
- self.minLen = min
-
- if max > 0:
- self.maxLen = max
- else:
- self.maxLen = _MAX_INT
-
- if exact > 0:
- self.maxLen = exact
- self.minLen = exact
-
- self.errmsg = "Expected " + self.name
- self.mayReturnEmpty = self.minLen == 0
- self.mayIndexError = False
-
- def _generateDefaultName(self):
- not_chars_str = _collapse_string_to_ranges(self.notChars)
- if len(not_chars_str) > 16:
- return "!W:({}...)".format(self.notChars[: 16 - 3])
- else:
- return "!W:({})".format(self.notChars)
-
- def parseImpl(self, instring, loc, doActions=True):
- notchars = self.notCharsSet
- if instring[loc] in notchars:
- raise ParseException(instring, loc, self.errmsg, self)
-
- start = loc
- loc += 1
- maxlen = min(start + self.maxLen, len(instring))
- while loc < maxlen and instring[loc] not in notchars:
- loc += 1
-
- if loc - start < self.minLen:
- raise ParseException(instring, loc, self.errmsg, self)
-
- return loc, instring[start:loc]
-
-
-class White(Token):
- """Special matching class for matching whitespace. Normally,
- whitespace is ignored by pyparsing grammars. This class is included
- when some whitespace structures are significant. Define with
- a string containing the whitespace characters to be matched; default
- is ``" \\t\\r\\n"``. Also takes optional ``min``,
- ``max``, and ``exact`` arguments, as defined for the
- :class:`Word` class.
- """
-
- whiteStrs = {
- " ": "",
- "\t": "",
- "\n": "",
- "\r": "",
- "\f": "",
- "\u00A0": "",
- "\u1680": "",
- "\u180E": "",
- "\u2000": "",
- "\u2001": "",
- "\u2002": "",
- "\u2003": "",
- "\u2004": "",
- "\u2005": "",
- "\u2006": "",
- "\u2007": "",
- "\u2008": "",
- "\u2009": "",
- "\u200A": "",
- "\u200B": "",
- "\u202F": "",
- "\u205F": "",
- "\u3000": "",
- }
-
- def __init__(self, ws: str = " \t\r\n", min: int = 1, max: int = 0, exact: int = 0):
- super().__init__()
- self.matchWhite = ws
- self.set_whitespace_chars(
- "".join(c for c in self.whiteStrs if c not in self.matchWhite),
- copy_defaults=True,
- )
- # self.leave_whitespace()
- self.mayReturnEmpty = True
- self.errmsg = "Expected " + self.name
-
- self.minLen = min
-
- if max > 0:
- self.maxLen = max
- else:
- self.maxLen = _MAX_INT
-
- if exact > 0:
- self.maxLen = exact
- self.minLen = exact
-
- def _generateDefaultName(self):
- return "".join(White.whiteStrs[c] for c in self.matchWhite)
-
- def parseImpl(self, instring, loc, doActions=True):
- if instring[loc] not in self.matchWhite:
- raise ParseException(instring, loc, self.errmsg, self)
- start = loc
- loc += 1
- maxloc = start + self.maxLen
- maxloc = min(maxloc, len(instring))
- while loc < maxloc and instring[loc] in self.matchWhite:
- loc += 1
-
- if loc - start < self.minLen:
- raise ParseException(instring, loc, self.errmsg, self)
-
- return loc, instring[start:loc]
-
-
-class PositionToken(Token):
- def __init__(self):
- super().__init__()
- self.mayReturnEmpty = True
- self.mayIndexError = False
-
-
-class GoToColumn(PositionToken):
- """Token to advance to a specific column of input text; useful for
- tabular report scraping.
- """
-
- def __init__(self, colno: int):
- super().__init__()
- self.col = colno
-
- def preParse(self, instring, loc):
- if col(loc, instring) != self.col:
- instrlen = len(instring)
- if self.ignoreExprs:
- loc = self._skipIgnorables(instring, loc)
- while (
- loc < instrlen
- and instring[loc].isspace()
- and col(loc, instring) != self.col
- ):
- loc += 1
- return loc
-
- def parseImpl(self, instring, loc, doActions=True):
- thiscol = col(loc, instring)
- if thiscol > self.col:
- raise ParseException(instring, loc, "Text not in expected column", self)
- newloc = loc + self.col - thiscol
- ret = instring[loc:newloc]
- return newloc, ret
-
-
-class LineStart(PositionToken):
- r"""Matches if current position is at the beginning of a line within
- the parse string
-
- Example::
-
- test = '''\
- AAA this line
- AAA and this line
- AAA but not this one
- B AAA and definitely not this one
- '''
-
- for t in (LineStart() + 'AAA' + restOfLine).search_string(test):
- print(t)
-
- prints::
-
- ['AAA', ' this line']
- ['AAA', ' and this line']
-
- """
-
- def __init__(self):
- super().__init__()
- self.leave_whitespace()
- self.orig_whiteChars = set() | self.whiteChars
- self.whiteChars.discard("\n")
- self.skipper = Empty().set_whitespace_chars(self.whiteChars)
- self.errmsg = "Expected start of line"
-
- def preParse(self, instring, loc):
- if loc == 0:
- return loc
- else:
- ret = self.skipper.preParse(instring, loc)
- if "\n" in self.orig_whiteChars:
- while instring[ret : ret + 1] == "\n":
- ret = self.skipper.preParse(instring, ret + 1)
- return ret
-
- def parseImpl(self, instring, loc, doActions=True):
- if col(loc, instring) == 1:
- return loc, []
- raise ParseException(instring, loc, self.errmsg, self)
-
-
-class LineEnd(PositionToken):
- """Matches if current position is at the end of a line within the
- parse string
- """
-
- def __init__(self):
- super().__init__()
- self.whiteChars.discard("\n")
- self.set_whitespace_chars(self.whiteChars, copy_defaults=False)
- self.errmsg = "Expected end of line"
-
- def parseImpl(self, instring, loc, doActions=True):
- if loc < len(instring):
- if instring[loc] == "\n":
- return loc + 1, "\n"
- else:
- raise ParseException(instring, loc, self.errmsg, self)
- elif loc == len(instring):
- return loc + 1, []
- else:
- raise ParseException(instring, loc, self.errmsg, self)
-
-
-class StringStart(PositionToken):
- """Matches if current position is at the beginning of the parse
- string
- """
-
- def __init__(self):
- super().__init__()
- self.errmsg = "Expected start of text"
-
- def parseImpl(self, instring, loc, doActions=True):
- if loc != 0:
- # see if entire string up to here is just whitespace and ignoreables
- if loc != self.preParse(instring, 0):
- raise ParseException(instring, loc, self.errmsg, self)
- return loc, []
-
-
-class StringEnd(PositionToken):
- """
- Matches if current position is at the end of the parse string
- """
-
- def __init__(self):
- super().__init__()
- self.errmsg = "Expected end of text"
-
- def parseImpl(self, instring, loc, doActions=True):
- if loc < len(instring):
- raise ParseException(instring, loc, self.errmsg, self)
- elif loc == len(instring):
- return loc + 1, []
- elif loc > len(instring):
- return loc, []
- else:
- raise ParseException(instring, loc, self.errmsg, self)
-
-
-class WordStart(PositionToken):
- """Matches if the current position is at the beginning of a
- :class:`Word`, and is not preceded by any character in a given
- set of ``word_chars`` (default= ``printables``). To emulate the
- ``\b`` behavior of regular expressions, use
- ``WordStart(alphanums)``. ``WordStart`` will also match at
- the beginning of the string being parsed, or at the beginning of
- a line.
- """
-
- def __init__(self, word_chars: str = printables, *, wordChars: str = printables):
- wordChars = word_chars if wordChars == printables else wordChars
- super().__init__()
- self.wordChars = set(wordChars)
- self.errmsg = "Not at the start of a word"
-
- def parseImpl(self, instring, loc, doActions=True):
- if loc != 0:
- if (
- instring[loc - 1] in self.wordChars
- or instring[loc] not in self.wordChars
- ):
- raise ParseException(instring, loc, self.errmsg, self)
- return loc, []
-
-
-class WordEnd(PositionToken):
- """Matches if the current position is at the end of a :class:`Word`,
- and is not followed by any character in a given set of ``word_chars``
- (default= ``printables``). To emulate the ``\b`` behavior of
- regular expressions, use ``WordEnd(alphanums)``. ``WordEnd``
- will also match at the end of the string being parsed, or at the end
- of a line.
- """
-
- def __init__(self, word_chars: str = printables, *, wordChars: str = printables):
- wordChars = word_chars if wordChars == printables else wordChars
- super().__init__()
- self.wordChars = set(wordChars)
- self.skipWhitespace = False
- self.errmsg = "Not at the end of a word"
-
- def parseImpl(self, instring, loc, doActions=True):
- instrlen = len(instring)
- if instrlen > 0 and loc < instrlen:
- if (
- instring[loc] in self.wordChars
- or instring[loc - 1] not in self.wordChars
- ):
- raise ParseException(instring, loc, self.errmsg, self)
- return loc, []
-
-
-class ParseExpression(ParserElement):
- """Abstract subclass of ParserElement, for combining and
- post-processing parsed tokens.
- """
-
- def __init__(self, exprs: typing.Iterable[ParserElement], savelist: bool = False):
- super().__init__(savelist)
- self.exprs: List[ParserElement]
- if isinstance(exprs, _generatorType):
- exprs = list(exprs)
-
- if isinstance(exprs, str_type):
- self.exprs = [self._literalStringClass(exprs)]
- elif isinstance(exprs, ParserElement):
- self.exprs = [exprs]
- elif isinstance(exprs, Iterable):
- exprs = list(exprs)
- # if sequence of strings provided, wrap with Literal
- if any(isinstance(expr, str_type) for expr in exprs):
- exprs = (
- self._literalStringClass(e) if isinstance(e, str_type) else e
- for e in exprs
- )
- self.exprs = list(exprs)
- else:
- try:
- self.exprs = list(exprs)
- except TypeError:
- self.exprs = [exprs]
- self.callPreparse = False
-
- def recurse(self) -> Sequence[ParserElement]:
- return self.exprs[:]
-
- def append(self, other) -> ParserElement:
- self.exprs.append(other)
- self._defaultName = None
- return self
-
- def leave_whitespace(self, recursive: bool = True) -> ParserElement:
- """
- Extends ``leave_whitespace`` defined in base class, and also invokes ``leave_whitespace`` on
- all contained expressions.
- """
- super().leave_whitespace(recursive)
-
- if recursive:
- self.exprs = [e.copy() for e in self.exprs]
- for e in self.exprs:
- e.leave_whitespace(recursive)
- return self
-
- def ignore_whitespace(self, recursive: bool = True) -> ParserElement:
- """
- Extends ``ignore_whitespace`` defined in base class, and also invokes ``leave_whitespace`` on
- all contained expressions.
- """
- super().ignore_whitespace(recursive)
- if recursive:
- self.exprs = [e.copy() for e in self.exprs]
- for e in self.exprs:
- e.ignore_whitespace(recursive)
- return self
-
- def ignore(self, other) -> ParserElement:
- if isinstance(other, Suppress):
- if other not in self.ignoreExprs:
- super().ignore(other)
- for e in self.exprs:
- e.ignore(self.ignoreExprs[-1])
- else:
- super().ignore(other)
- for e in self.exprs:
- e.ignore(self.ignoreExprs[-1])
- return self
-
- def _generateDefaultName(self):
- return "{}:({})".format(self.__class__.__name__, str(self.exprs))
-
- def streamline(self) -> ParserElement:
- if self.streamlined:
- return self
-
- super().streamline()
-
- for e in self.exprs:
- e.streamline()
-
- # collapse nested :class:`And`'s of the form ``And(And(And(a, b), c), d)`` to ``And(a, b, c, d)``
- # but only if there are no parse actions or resultsNames on the nested And's
- # (likewise for :class:`Or`'s and :class:`MatchFirst`'s)
- if len(self.exprs) == 2:
- other = self.exprs[0]
- if (
- isinstance(other, self.__class__)
- and not other.parseAction
- and other.resultsName is None
- and not other.debug
- ):
- self.exprs = other.exprs[:] + [self.exprs[1]]
- self._defaultName = None
- self.mayReturnEmpty |= other.mayReturnEmpty
- self.mayIndexError |= other.mayIndexError
-
- other = self.exprs[-1]
- if (
- isinstance(other, self.__class__)
- and not other.parseAction
- and other.resultsName is None
- and not other.debug
- ):
- self.exprs = self.exprs[:-1] + other.exprs[:]
- self._defaultName = None
- self.mayReturnEmpty |= other.mayReturnEmpty
- self.mayIndexError |= other.mayIndexError
-
- self.errmsg = "Expected " + str(self)
-
- return self
-
- def validate(self, validateTrace=None) -> None:
- tmp = (validateTrace if validateTrace is not None else [])[:] + [self]
- for e in self.exprs:
- e.validate(tmp)
- self._checkRecursion([])
-
- def copy(self) -> ParserElement:
- ret = super().copy()
- ret.exprs = [e.copy() for e in self.exprs]
- return ret
-
- def _setResultsName(self, name, listAllMatches=False):
- if (
- __diag__.warn_ungrouped_named_tokens_in_collection
- and Diagnostics.warn_ungrouped_named_tokens_in_collection
- not in self.suppress_warnings_
- ):
- for e in self.exprs:
- if (
- isinstance(e, ParserElement)
- and e.resultsName
- and Diagnostics.warn_ungrouped_named_tokens_in_collection
- not in e.suppress_warnings_
- ):
- warnings.warn(
- "{}: setting results name {!r} on {} expression "
- "collides with {!r} on contained expression".format(
- "warn_ungrouped_named_tokens_in_collection",
- name,
- type(self).__name__,
- e.resultsName,
- ),
- stacklevel=3,
- )
-
- return super()._setResultsName(name, listAllMatches)
-
- ignoreWhitespace = ignore_whitespace
- leaveWhitespace = leave_whitespace
-
-
-class And(ParseExpression):
- """
- Requires all given :class:`ParseExpression` s to be found in the given order.
- Expressions may be separated by whitespace.
- May be constructed using the ``'+'`` operator.
- May also be constructed using the ``'-'`` operator, which will
- suppress backtracking.
-
- Example::
-
- integer = Word(nums)
- name_expr = Word(alphas)[1, ...]
-
- expr = And([integer("id"), name_expr("name"), integer("age")])
- # more easily written as:
- expr = integer("id") + name_expr("name") + integer("age")
- """
-
- class _ErrorStop(Empty):
- def __init__(self, *args, **kwargs):
- super().__init__(*args, **kwargs)
- self.leave_whitespace()
-
- def _generateDefaultName(self):
- return "-"
-
- def __init__(
- self, exprs_arg: typing.Iterable[ParserElement], savelist: bool = True
- ):
- exprs: List[ParserElement] = list(exprs_arg)
- if exprs and Ellipsis in exprs:
- tmp = []
- for i, expr in enumerate(exprs):
- if expr is Ellipsis:
- if i < len(exprs) - 1:
- skipto_arg: ParserElement = (Empty() + exprs[i + 1]).exprs[-1]
- tmp.append(SkipTo(skipto_arg)("_skipped*"))
- else:
- raise Exception(
- "cannot construct And with sequence ending in ..."
- )
- else:
- tmp.append(expr)
- exprs[:] = tmp
- super().__init__(exprs, savelist)
- if self.exprs:
- self.mayReturnEmpty = all(e.mayReturnEmpty for e in self.exprs)
- if not isinstance(self.exprs[0], White):
- self.set_whitespace_chars(
- self.exprs[0].whiteChars,
- copy_defaults=self.exprs[0].copyDefaultWhiteChars,
- )
- self.skipWhitespace = self.exprs[0].skipWhitespace
- else:
- self.skipWhitespace = False
- else:
- self.mayReturnEmpty = True
- self.callPreparse = True
-
- def streamline(self) -> ParserElement:
- # collapse any _PendingSkip's
- if self.exprs:
- if any(
- isinstance(e, ParseExpression)
- and e.exprs
- and isinstance(e.exprs[-1], _PendingSkip)
- for e in self.exprs[:-1]
- ):
- for i, e in enumerate(self.exprs[:-1]):
- if e is None:
- continue
- if (
- isinstance(e, ParseExpression)
- and e.exprs
- and isinstance(e.exprs[-1], _PendingSkip)
- ):
- e.exprs[-1] = e.exprs[-1] + self.exprs[i + 1]
- self.exprs[i + 1] = None
- self.exprs = [e for e in self.exprs if e is not None]
-
- super().streamline()
-
- # link any IndentedBlocks to the prior expression
- for prev, cur in zip(self.exprs, self.exprs[1:]):
- # traverse cur or any first embedded expr of cur looking for an IndentedBlock
- # (but watch out for recursive grammar)
- seen = set()
- while cur:
- if id(cur) in seen:
- break
- seen.add(id(cur))
- if isinstance(cur, IndentedBlock):
- prev.add_parse_action(
- lambda s, l, t, cur_=cur: setattr(
- cur_, "parent_anchor", col(l, s)
- )
- )
- break
- subs = cur.recurse()
- cur = next(iter(subs), None)
-
- self.mayReturnEmpty = all(e.mayReturnEmpty for e in self.exprs)
- return self
-
- def parseImpl(self, instring, loc, doActions=True):
- # pass False as callPreParse arg to _parse for first element, since we already
- # pre-parsed the string as part of our And pre-parsing
- loc, resultlist = self.exprs[0]._parse(
- instring, loc, doActions, callPreParse=False
- )
- errorStop = False
- for e in self.exprs[1:]:
- # if isinstance(e, And._ErrorStop):
- if type(e) is And._ErrorStop:
- errorStop = True
- continue
- if errorStop:
- try:
- loc, exprtokens = e._parse(instring, loc, doActions)
- except ParseSyntaxException:
- raise
- except ParseBaseException as pe:
- pe.__traceback__ = None
- raise ParseSyntaxException._from_exception(pe)
- except IndexError:
- raise ParseSyntaxException(
- instring, len(instring), self.errmsg, self
- )
- else:
- loc, exprtokens = e._parse(instring, loc, doActions)
- if exprtokens or exprtokens.haskeys():
- resultlist += exprtokens
- return loc, resultlist
-
- def __iadd__(self, other):
- if isinstance(other, str_type):
- other = self._literalStringClass(other)
- return self.append(other) # And([self, other])
-
- def _checkRecursion(self, parseElementList):
- subRecCheckList = parseElementList[:] + [self]
- for e in self.exprs:
- e._checkRecursion(subRecCheckList)
- if not e.mayReturnEmpty:
- break
-
- def _generateDefaultName(self):
- inner = " ".join(str(e) for e in self.exprs)
- # strip off redundant inner {}'s
- while len(inner) > 1 and inner[0 :: len(inner) - 1] == "{}":
- inner = inner[1:-1]
- return "{" + inner + "}"
-
-
-class Or(ParseExpression):
- """Requires that at least one :class:`ParseExpression` is found. If
- two expressions match, the expression that matches the longest
- string will be used. May be constructed using the ``'^'``
- operator.
-
- Example::
-
- # construct Or using '^' operator
-
- number = Word(nums) ^ Combine(Word(nums) + '.' + Word(nums))
- print(number.search_string("123 3.1416 789"))
-
- prints::
-
- [['123'], ['3.1416'], ['789']]
- """
-
- def __init__(self, exprs: typing.Iterable[ParserElement], savelist: bool = False):
- super().__init__(exprs, savelist)
- if self.exprs:
- self.mayReturnEmpty = any(e.mayReturnEmpty for e in self.exprs)
- self.skipWhitespace = all(e.skipWhitespace for e in self.exprs)
- else:
- self.mayReturnEmpty = True
-
- def streamline(self) -> ParserElement:
- super().streamline()
- if self.exprs:
- self.mayReturnEmpty = any(e.mayReturnEmpty for e in self.exprs)
- self.saveAsList = any(e.saveAsList for e in self.exprs)
- self.skipWhitespace = all(
- e.skipWhitespace and not isinstance(e, White) for e in self.exprs
- )
- else:
- self.saveAsList = False
- return self
-
- def parseImpl(self, instring, loc, doActions=True):
- maxExcLoc = -1
- maxException = None
- matches = []
- fatals = []
- if all(e.callPreparse for e in self.exprs):
- loc = self.preParse(instring, loc)
- for e in self.exprs:
- try:
- loc2 = e.try_parse(instring, loc, raise_fatal=True)
- except ParseFatalException as pfe:
- pfe.__traceback__ = None
- pfe.parserElement = e
- fatals.append(pfe)
- maxException = None
- maxExcLoc = -1
- except ParseException as err:
- if not fatals:
- err.__traceback__ = None
- if err.loc > maxExcLoc:
- maxException = err
- maxExcLoc = err.loc
- except IndexError:
- if len(instring) > maxExcLoc:
- maxException = ParseException(
- instring, len(instring), e.errmsg, self
- )
- maxExcLoc = len(instring)
- else:
- # save match among all matches, to retry longest to shortest
- matches.append((loc2, e))
-
- if matches:
- # re-evaluate all matches in descending order of length of match, in case attached actions
- # might change whether or how much they match of the input.
- matches.sort(key=itemgetter(0), reverse=True)
-
- if not doActions:
- # no further conditions or parse actions to change the selection of
- # alternative, so the first match will be the best match
- best_expr = matches[0][1]
- return best_expr._parse(instring, loc, doActions)
-
- longest = -1, None
- for loc1, expr1 in matches:
- if loc1 <= longest[0]:
- # already have a longer match than this one will deliver, we are done
- return longest
-
- try:
- loc2, toks = expr1._parse(instring, loc, doActions)
- except ParseException as err:
- err.__traceback__ = None
- if err.loc > maxExcLoc:
- maxException = err
- maxExcLoc = err.loc
- else:
- if loc2 >= loc1:
- return loc2, toks
- # didn't match as much as before
- elif loc2 > longest[0]:
- longest = loc2, toks
-
- if longest != (-1, None):
- return longest
-
- if fatals:
- if len(fatals) > 1:
- fatals.sort(key=lambda e: -e.loc)
- if fatals[0].loc == fatals[1].loc:
- fatals.sort(key=lambda e: (-e.loc, -len(str(e.parserElement))))
- max_fatal = fatals[0]
- raise max_fatal
-
- if maxException is not None:
- maxException.msg = self.errmsg
- raise maxException
- else:
- raise ParseException(
- instring, loc, "no defined alternatives to match", self
- )
-
- def __ixor__(self, other):
- if isinstance(other, str_type):
- other = self._literalStringClass(other)
- return self.append(other) # Or([self, other])
-
- def _generateDefaultName(self):
- return "{" + " ^ ".join(str(e) for e in self.exprs) + "}"
-
- def _setResultsName(self, name, listAllMatches=False):
- if (
- __diag__.warn_multiple_tokens_in_named_alternation
- and Diagnostics.warn_multiple_tokens_in_named_alternation
- not in self.suppress_warnings_
- ):
- if any(
- isinstance(e, And)
- and Diagnostics.warn_multiple_tokens_in_named_alternation
- not in e.suppress_warnings_
- for e in self.exprs
- ):
- warnings.warn(
- "{}: setting results name {!r} on {} expression "
- "will return a list of all parsed tokens in an And alternative, "
- "in prior versions only the first token was returned; enclose "
- "contained argument in Group".format(
- "warn_multiple_tokens_in_named_alternation",
- name,
- type(self).__name__,
- ),
- stacklevel=3,
- )
-
- return super()._setResultsName(name, listAllMatches)
-
-
-class MatchFirst(ParseExpression):
- """Requires that at least one :class:`ParseExpression` is found. If
- more than one expression matches, the first one listed is the one that will
- match. May be constructed using the ``'|'`` operator.
-
- Example::
-
- # construct MatchFirst using '|' operator
-
- # watch the order of expressions to match
- number = Word(nums) | Combine(Word(nums) + '.' + Word(nums))
- print(number.search_string("123 3.1416 789")) # Fail! -> [['123'], ['3'], ['1416'], ['789']]
-
- # put more selective expression first
- number = Combine(Word(nums) + '.' + Word(nums)) | Word(nums)
- print(number.search_string("123 3.1416 789")) # Better -> [['123'], ['3.1416'], ['789']]
- """
-
- def __init__(self, exprs: typing.Iterable[ParserElement], savelist: bool = False):
- super().__init__(exprs, savelist)
- if self.exprs:
- self.mayReturnEmpty = any(e.mayReturnEmpty for e in self.exprs)
- self.skipWhitespace = all(e.skipWhitespace for e in self.exprs)
- else:
- self.mayReturnEmpty = True
-
- def streamline(self) -> ParserElement:
- if self.streamlined:
- return self
-
- super().streamline()
- if self.exprs:
- self.saveAsList = any(e.saveAsList for e in self.exprs)
- self.mayReturnEmpty = any(e.mayReturnEmpty for e in self.exprs)
- self.skipWhitespace = all(
- e.skipWhitespace and not isinstance(e, White) for e in self.exprs
- )
- else:
- self.saveAsList = False
- self.mayReturnEmpty = True
- return self
-
- def parseImpl(self, instring, loc, doActions=True):
- maxExcLoc = -1
- maxException = None
-
- for e in self.exprs:
- try:
- return e._parse(
- instring,
- loc,
- doActions,
- )
- except ParseFatalException as pfe:
- pfe.__traceback__ = None
- pfe.parserElement = e
- raise
- except ParseException as err:
- if err.loc > maxExcLoc:
- maxException = err
- maxExcLoc = err.loc
- except IndexError:
- if len(instring) > maxExcLoc:
- maxException = ParseException(
- instring, len(instring), e.errmsg, self
- )
- maxExcLoc = len(instring)
-
- if maxException is not None:
- maxException.msg = self.errmsg
- raise maxException
- else:
- raise ParseException(
- instring, loc, "no defined alternatives to match", self
- )
-
- def __ior__(self, other):
- if isinstance(other, str_type):
- other = self._literalStringClass(other)
- return self.append(other) # MatchFirst([self, other])
-
- def _generateDefaultName(self):
- return "{" + " | ".join(str(e) for e in self.exprs) + "}"
-
- def _setResultsName(self, name, listAllMatches=False):
- if (
- __diag__.warn_multiple_tokens_in_named_alternation
- and Diagnostics.warn_multiple_tokens_in_named_alternation
- not in self.suppress_warnings_
- ):
- if any(
- isinstance(e, And)
- and Diagnostics.warn_multiple_tokens_in_named_alternation
- not in e.suppress_warnings_
- for e in self.exprs
- ):
- warnings.warn(
- "{}: setting results name {!r} on {} expression "
- "will return a list of all parsed tokens in an And alternative, "
- "in prior versions only the first token was returned; enclose "
- "contained argument in Group".format(
- "warn_multiple_tokens_in_named_alternation",
- name,
- type(self).__name__,
- ),
- stacklevel=3,
- )
-
- return super()._setResultsName(name, listAllMatches)
-
-
-class Each(ParseExpression):
- """Requires all given :class:`ParseExpression` s to be found, but in
- any order. Expressions may be separated by whitespace.
-
- May be constructed using the ``'&'`` operator.
-
- Example::
-
- color = one_of("RED ORANGE YELLOW GREEN BLUE PURPLE BLACK WHITE BROWN")
- shape_type = one_of("SQUARE CIRCLE TRIANGLE STAR HEXAGON OCTAGON")
- integer = Word(nums)
- shape_attr = "shape:" + shape_type("shape")
- posn_attr = "posn:" + Group(integer("x") + ',' + integer("y"))("posn")
- color_attr = "color:" + color("color")
- size_attr = "size:" + integer("size")
-
- # use Each (using operator '&') to accept attributes in any order
- # (shape and posn are required, color and size are optional)
- shape_spec = shape_attr & posn_attr & Opt(color_attr) & Opt(size_attr)
-
- shape_spec.run_tests('''
- shape: SQUARE color: BLACK posn: 100, 120
- shape: CIRCLE size: 50 color: BLUE posn: 50,80
- color:GREEN size:20 shape:TRIANGLE posn:20,40
- '''
- )
-
- prints::
-
- shape: SQUARE color: BLACK posn: 100, 120
- ['shape:', 'SQUARE', 'color:', 'BLACK', 'posn:', ['100', ',', '120']]
- - color: BLACK
- - posn: ['100', ',', '120']
- - x: 100
- - y: 120
- - shape: SQUARE
-
-
- shape: CIRCLE size: 50 color: BLUE posn: 50,80
- ['shape:', 'CIRCLE', 'size:', '50', 'color:', 'BLUE', 'posn:', ['50', ',', '80']]
- - color: BLUE
- - posn: ['50', ',', '80']
- - x: 50
- - y: 80
- - shape: CIRCLE
- - size: 50
-
-
- color: GREEN size: 20 shape: TRIANGLE posn: 20,40
- ['color:', 'GREEN', 'size:', '20', 'shape:', 'TRIANGLE', 'posn:', ['20', ',', '40']]
- - color: GREEN
- - posn: ['20', ',', '40']
- - x: 20
- - y: 40
- - shape: TRIANGLE
- - size: 20
- """
-
- def __init__(self, exprs: typing.Iterable[ParserElement], savelist: bool = True):
- super().__init__(exprs, savelist)
- if self.exprs:
- self.mayReturnEmpty = all(e.mayReturnEmpty for e in self.exprs)
- else:
- self.mayReturnEmpty = True
- self.skipWhitespace = True
- self.initExprGroups = True
- self.saveAsList = True
-
- def streamline(self) -> ParserElement:
- super().streamline()
- if self.exprs:
- self.mayReturnEmpty = all(e.mayReturnEmpty for e in self.exprs)
- else:
- self.mayReturnEmpty = True
- return self
-
- def parseImpl(self, instring, loc, doActions=True):
- if self.initExprGroups:
- self.opt1map = dict(
- (id(e.expr), e) for e in self.exprs if isinstance(e, Opt)
- )
- opt1 = [e.expr for e in self.exprs if isinstance(e, Opt)]
- opt2 = [
- e
- for e in self.exprs
- if e.mayReturnEmpty and not isinstance(e, (Opt, Regex, ZeroOrMore))
- ]
- self.optionals = opt1 + opt2
- self.multioptionals = [
- e.expr.set_results_name(e.resultsName, list_all_matches=True)
- for e in self.exprs
- if isinstance(e, _MultipleMatch)
- ]
- self.multirequired = [
- e.expr.set_results_name(e.resultsName, list_all_matches=True)
- for e in self.exprs
- if isinstance(e, OneOrMore)
- ]
- self.required = [
- e for e in self.exprs if not isinstance(e, (Opt, ZeroOrMore, OneOrMore))
- ]
- self.required += self.multirequired
- self.initExprGroups = False
-
- tmpLoc = loc
- tmpReqd = self.required[:]
- tmpOpt = self.optionals[:]
- multis = self.multioptionals[:]
- matchOrder = []
-
- keepMatching = True
- failed = []
- fatals = []
- while keepMatching:
- tmpExprs = tmpReqd + tmpOpt + multis
- failed.clear()
- fatals.clear()
- for e in tmpExprs:
- try:
- tmpLoc = e.try_parse(instring, tmpLoc, raise_fatal=True)
- except ParseFatalException as pfe:
- pfe.__traceback__ = None
- pfe.parserElement = e
- fatals.append(pfe)
- failed.append(e)
- except ParseException:
- failed.append(e)
- else:
- matchOrder.append(self.opt1map.get(id(e), e))
- if e in tmpReqd:
- tmpReqd.remove(e)
- elif e in tmpOpt:
- tmpOpt.remove(e)
- if len(failed) == len(tmpExprs):
- keepMatching = False
-
- # look for any ParseFatalExceptions
- if fatals:
- if len(fatals) > 1:
- fatals.sort(key=lambda e: -e.loc)
- if fatals[0].loc == fatals[1].loc:
- fatals.sort(key=lambda e: (-e.loc, -len(str(e.parserElement))))
- max_fatal = fatals[0]
- raise max_fatal
-
- if tmpReqd:
- missing = ", ".join([str(e) for e in tmpReqd])
- raise ParseException(
- instring,
- loc,
- "Missing one or more required elements ({})".format(missing),
- )
-
- # add any unmatched Opts, in case they have default values defined
- matchOrder += [e for e in self.exprs if isinstance(e, Opt) and e.expr in tmpOpt]
-
- total_results = ParseResults([])
- for e in matchOrder:
- loc, results = e._parse(instring, loc, doActions)
- total_results += results
-
- return loc, total_results
-
- def _generateDefaultName(self):
- return "{" + " & ".join(str(e) for e in self.exprs) + "}"
-
-
-class ParseElementEnhance(ParserElement):
- """Abstract subclass of :class:`ParserElement`, for combining and
- post-processing parsed tokens.
- """
-
- def __init__(self, expr: Union[ParserElement, str], savelist: bool = False):
- super().__init__(savelist)
- if isinstance(expr, str_type):
- if issubclass(self._literalStringClass, Token):
- expr = self._literalStringClass(expr)
- elif issubclass(type(self), self._literalStringClass):
- expr = Literal(expr)
- else:
- expr = self._literalStringClass(Literal(expr))
- self.expr = expr
- if expr is not None:
- self.mayIndexError = expr.mayIndexError
- self.mayReturnEmpty = expr.mayReturnEmpty
- self.set_whitespace_chars(
- expr.whiteChars, copy_defaults=expr.copyDefaultWhiteChars
- )
- self.skipWhitespace = expr.skipWhitespace
- self.saveAsList = expr.saveAsList
- self.callPreparse = expr.callPreparse
- self.ignoreExprs.extend(expr.ignoreExprs)
-
- def recurse(self) -> Sequence[ParserElement]:
- return [self.expr] if self.expr is not None else []
-
- def parseImpl(self, instring, loc, doActions=True):
- if self.expr is not None:
- return self.expr._parse(instring, loc, doActions, callPreParse=False)
- else:
- raise ParseException(instring, loc, "No expression defined", self)
-
- def leave_whitespace(self, recursive: bool = True) -> ParserElement:
- super().leave_whitespace(recursive)
-
- if recursive:
- self.expr = self.expr.copy()
- if self.expr is not None:
- self.expr.leave_whitespace(recursive)
- return self
-
- def ignore_whitespace(self, recursive: bool = True) -> ParserElement:
- super().ignore_whitespace(recursive)
-
- if recursive:
- self.expr = self.expr.copy()
- if self.expr is not None:
- self.expr.ignore_whitespace(recursive)
- return self
-
- def ignore(self, other) -> ParserElement:
- if isinstance(other, Suppress):
- if other not in self.ignoreExprs:
- super().ignore(other)
- if self.expr is not None:
- self.expr.ignore(self.ignoreExprs[-1])
- else:
- super().ignore(other)
- if self.expr is not None:
- self.expr.ignore(self.ignoreExprs[-1])
- return self
-
- def streamline(self) -> ParserElement:
- super().streamline()
- if self.expr is not None:
- self.expr.streamline()
- return self
-
- def _checkRecursion(self, parseElementList):
- if self in parseElementList:
- raise RecursiveGrammarException(parseElementList + [self])
- subRecCheckList = parseElementList[:] + [self]
- if self.expr is not None:
- self.expr._checkRecursion(subRecCheckList)
-
- def validate(self, validateTrace=None) -> None:
- if validateTrace is None:
- validateTrace = []
- tmp = validateTrace[:] + [self]
- if self.expr is not None:
- self.expr.validate(tmp)
- self._checkRecursion([])
-
- def _generateDefaultName(self):
- return "{}:({})".format(self.__class__.__name__, str(self.expr))
-
- ignoreWhitespace = ignore_whitespace
- leaveWhitespace = leave_whitespace
-
-
-class IndentedBlock(ParseElementEnhance):
- """
- Expression to match one or more expressions at a given indentation level.
- Useful for parsing text where structure is implied by indentation (like Python source code).
- """
-
- class _Indent(Empty):
- def __init__(self, ref_col: int):
- super().__init__()
- self.errmsg = "expected indent at column {}".format(ref_col)
- self.add_condition(lambda s, l, t: col(l, s) == ref_col)
-
- class _IndentGreater(Empty):
- def __init__(self, ref_col: int):
- super().__init__()
- self.errmsg = "expected indent at column greater than {}".format(ref_col)
- self.add_condition(lambda s, l, t: col(l, s) > ref_col)
-
- def __init__(
- self, expr: ParserElement, *, recursive: bool = False, grouped: bool = True
- ):
- super().__init__(expr, savelist=True)
- # if recursive:
- # raise NotImplementedError("IndentedBlock with recursive is not implemented")
- self._recursive = recursive
- self._grouped = grouped
- self.parent_anchor = 1
-
- def parseImpl(self, instring, loc, doActions=True):
- # advance parse position to non-whitespace by using an Empty()
- # this should be the column to be used for all subsequent indented lines
- anchor_loc = Empty().preParse(instring, loc)
-
- # see if self.expr matches at the current location - if not it will raise an exception
- # and no further work is necessary
- self.expr.try_parse(instring, anchor_loc, doActions)
-
- indent_col = col(anchor_loc, instring)
- peer_detect_expr = self._Indent(indent_col)
-
- inner_expr = Empty() + peer_detect_expr + self.expr
- if self._recursive:
- sub_indent = self._IndentGreater(indent_col)
- nested_block = IndentedBlock(
- self.expr, recursive=self._recursive, grouped=self._grouped
- )
- nested_block.set_debug(self.debug)
- nested_block.parent_anchor = indent_col
- inner_expr += Opt(sub_indent + nested_block)
-
- inner_expr.set_name(f"inner {hex(id(inner_expr))[-4:].upper()}@{indent_col}")
- block = OneOrMore(inner_expr)
-
- trailing_undent = self._Indent(self.parent_anchor) | StringEnd()
-
- if self._grouped:
- wrapper = Group
- else:
- wrapper = lambda expr: expr
- return (wrapper(block) + Optional(trailing_undent)).parseImpl(
- instring, anchor_loc, doActions
- )
-
-
-class AtStringStart(ParseElementEnhance):
- """Matches if expression matches at the beginning of the parse
- string::
-
- AtStringStart(Word(nums)).parse_string("123")
- # prints ["123"]
-
- AtStringStart(Word(nums)).parse_string(" 123")
- # raises ParseException
- """
-
- def __init__(self, expr: Union[ParserElement, str]):
- super().__init__(expr)
- self.callPreparse = False
-
- def parseImpl(self, instring, loc, doActions=True):
- if loc != 0:
- raise ParseException(instring, loc, "not found at string start")
- return super().parseImpl(instring, loc, doActions)
-
-
-class AtLineStart(ParseElementEnhance):
- r"""Matches if an expression matches at the beginning of a line within
- the parse string
-
- Example::
-
- test = '''\
- AAA this line
- AAA and this line
- AAA but not this one
- B AAA and definitely not this one
- '''
-
- for t in (AtLineStart('AAA') + restOfLine).search_string(test):
- print(t)
-
- prints::
-
- ['AAA', ' this line']
- ['AAA', ' and this line']
-
- """
-
- def __init__(self, expr: Union[ParserElement, str]):
- super().__init__(expr)
- self.callPreparse = False
-
- def parseImpl(self, instring, loc, doActions=True):
- if col(loc, instring) != 1:
- raise ParseException(instring, loc, "not found at line start")
- return super().parseImpl(instring, loc, doActions)
-
-
-class FollowedBy(ParseElementEnhance):
- """Lookahead matching of the given parse expression.
- ``FollowedBy`` does *not* advance the parsing position within
- the input string, it only verifies that the specified parse
- expression matches at the current position. ``FollowedBy``
- always returns a null token list. If any results names are defined
- in the lookahead expression, those *will* be returned for access by
- name.
-
- Example::
-
- # use FollowedBy to match a label only if it is followed by a ':'
- data_word = Word(alphas)
- label = data_word + FollowedBy(':')
- attr_expr = Group(label + Suppress(':') + OneOrMore(data_word, stop_on=label).set_parse_action(' '.join))
-
- attr_expr[1, ...].parse_string("shape: SQUARE color: BLACK posn: upper left").pprint()
-
- prints::
-
- [['shape', 'SQUARE'], ['color', 'BLACK'], ['posn', 'upper left']]
- """
-
- def __init__(self, expr: Union[ParserElement, str]):
- super().__init__(expr)
- self.mayReturnEmpty = True
-
- def parseImpl(self, instring, loc, doActions=True):
- # by using self._expr.parse and deleting the contents of the returned ParseResults list
- # we keep any named results that were defined in the FollowedBy expression
- _, ret = self.expr._parse(instring, loc, doActions=doActions)
- del ret[:]
-
- return loc, ret
-
-
-class PrecededBy(ParseElementEnhance):
- """Lookbehind matching of the given parse expression.
- ``PrecededBy`` does not advance the parsing position within the
- input string, it only verifies that the specified parse expression
- matches prior to the current position. ``PrecededBy`` always
- returns a null token list, but if a results name is defined on the
- given expression, it is returned.
-
- Parameters:
-
- - expr - expression that must match prior to the current parse
- location
- - retreat - (default= ``None``) - (int) maximum number of characters
- to lookbehind prior to the current parse location
-
- If the lookbehind expression is a string, :class:`Literal`,
- :class:`Keyword`, or a :class:`Word` or :class:`CharsNotIn`
- with a specified exact or maximum length, then the retreat
- parameter is not required. Otherwise, retreat must be specified to
- give a maximum number of characters to look back from
- the current parse position for a lookbehind match.
-
- Example::
-
- # VB-style variable names with type prefixes
- int_var = PrecededBy("#") + pyparsing_common.identifier
- str_var = PrecededBy("$") + pyparsing_common.identifier
-
- """
-
- def __init__(
- self, expr: Union[ParserElement, str], retreat: typing.Optional[int] = None
- ):
- super().__init__(expr)
- self.expr = self.expr().leave_whitespace()
- self.mayReturnEmpty = True
- self.mayIndexError = False
- self.exact = False
- if isinstance(expr, str_type):
- retreat = len(expr)
- self.exact = True
- elif isinstance(expr, (Literal, Keyword)):
- retreat = expr.matchLen
- self.exact = True
- elif isinstance(expr, (Word, CharsNotIn)) and expr.maxLen != _MAX_INT:
- retreat = expr.maxLen
- self.exact = True
- elif isinstance(expr, PositionToken):
- retreat = 0
- self.exact = True
- self.retreat = retreat
- self.errmsg = "not preceded by " + str(expr)
- self.skipWhitespace = False
- self.parseAction.append(lambda s, l, t: t.__delitem__(slice(None, None)))
-
- def parseImpl(self, instring, loc=0, doActions=True):
- if self.exact:
- if loc < self.retreat:
- raise ParseException(instring, loc, self.errmsg)
- start = loc - self.retreat
- _, ret = self.expr._parse(instring, start)
- else:
- # retreat specified a maximum lookbehind window, iterate
- test_expr = self.expr + StringEnd()
- instring_slice = instring[max(0, loc - self.retreat) : loc]
- last_expr = ParseException(instring, loc, self.errmsg)
- for offset in range(1, min(loc, self.retreat + 1) + 1):
- try:
- # print('trying', offset, instring_slice, repr(instring_slice[loc - offset:]))
- _, ret = test_expr._parse(
- instring_slice, len(instring_slice) - offset
- )
- except ParseBaseException as pbe:
- last_expr = pbe
- else:
- break
- else:
- raise last_expr
- return loc, ret
-
-
-class Located(ParseElementEnhance):
- """
- Decorates a returned token with its starting and ending
- locations in the input string.
-
- This helper adds the following results names:
-
- - ``locn_start`` - location where matched expression begins
- - ``locn_end`` - location where matched expression ends
- - ``value`` - the actual parsed results
-
- Be careful if the input text contains ```` characters, you
- may want to call :class:`ParserElement.parse_with_tabs`
-
- Example::
-
- wd = Word(alphas)
- for match in Located(wd).search_string("ljsdf123lksdjjf123lkkjj1222"):
- print(match)
-
- prints::
-
- [0, ['ljsdf'], 5]
- [8, ['lksdjjf'], 15]
- [18, ['lkkjj'], 23]
-
- """
-
- def parseImpl(self, instring, loc, doActions=True):
- start = loc
- loc, tokens = self.expr._parse(instring, start, doActions, callPreParse=False)
- ret_tokens = ParseResults([start, tokens, loc])
- ret_tokens["locn_start"] = start
- ret_tokens["value"] = tokens
- ret_tokens["locn_end"] = loc
- if self.resultsName:
- # must return as a list, so that the name will be attached to the complete group
- return loc, [ret_tokens]
- else:
- return loc, ret_tokens
-
-
-class NotAny(ParseElementEnhance):
- """
- Lookahead to disallow matching with the given parse expression.
- ``NotAny`` does *not* advance the parsing position within the
- input string, it only verifies that the specified parse expression
- does *not* match at the current position. Also, ``NotAny`` does
- *not* skip over leading whitespace. ``NotAny`` always returns
- a null token list. May be constructed using the ``'~'`` operator.
-
- Example::
-
- AND, OR, NOT = map(CaselessKeyword, "AND OR NOT".split())
-
- # take care not to mistake keywords for identifiers
- ident = ~(AND | OR | NOT) + Word(alphas)
- boolean_term = Opt(NOT) + ident
-
- # very crude boolean expression - to support parenthesis groups and
- # operation hierarchy, use infix_notation
- boolean_expr = boolean_term + ((AND | OR) + boolean_term)[...]
-
- # integers that are followed by "." are actually floats
- integer = Word(nums) + ~Char(".")
- """
-
- def __init__(self, expr: Union[ParserElement, str]):
- super().__init__(expr)
- # do NOT use self.leave_whitespace(), don't want to propagate to exprs
- # self.leave_whitespace()
- self.skipWhitespace = False
-
- self.mayReturnEmpty = True
- self.errmsg = "Found unwanted token, " + str(self.expr)
-
- def parseImpl(self, instring, loc, doActions=True):
- if self.expr.can_parse_next(instring, loc):
- raise ParseException(instring, loc, self.errmsg, self)
- return loc, []
-
- def _generateDefaultName(self):
- return "~{" + str(self.expr) + "}"
-
-
-class _MultipleMatch(ParseElementEnhance):
- def __init__(
- self,
- expr: ParserElement,
- stop_on: typing.Optional[Union[ParserElement, str]] = None,
- *,
- stopOn: typing.Optional[Union[ParserElement, str]] = None,
- ):
- super().__init__(expr)
- stopOn = stopOn or stop_on
- self.saveAsList = True
- ender = stopOn
- if isinstance(ender, str_type):
- ender = self._literalStringClass(ender)
- self.stopOn(ender)
-
- def stopOn(self, ender) -> ParserElement:
- if isinstance(ender, str_type):
- ender = self._literalStringClass(ender)
- self.not_ender = ~ender if ender is not None else None
- return self
-
- def parseImpl(self, instring, loc, doActions=True):
- self_expr_parse = self.expr._parse
- self_skip_ignorables = self._skipIgnorables
- check_ender = self.not_ender is not None
- if check_ender:
- try_not_ender = self.not_ender.tryParse
-
- # must be at least one (but first see if we are the stopOn sentinel;
- # if so, fail)
- if check_ender:
- try_not_ender(instring, loc)
- loc, tokens = self_expr_parse(instring, loc, doActions)
- try:
- hasIgnoreExprs = not not self.ignoreExprs
- while 1:
- if check_ender:
- try_not_ender(instring, loc)
- if hasIgnoreExprs:
- preloc = self_skip_ignorables(instring, loc)
- else:
- preloc = loc
- loc, tmptokens = self_expr_parse(instring, preloc, doActions)
- if tmptokens or tmptokens.haskeys():
- tokens += tmptokens
- except (ParseException, IndexError):
- pass
-
- return loc, tokens
-
- def _setResultsName(self, name, listAllMatches=False):
- if (
- __diag__.warn_ungrouped_named_tokens_in_collection
- and Diagnostics.warn_ungrouped_named_tokens_in_collection
- not in self.suppress_warnings_
- ):
- for e in [self.expr] + self.expr.recurse():
- if (
- isinstance(e, ParserElement)
- and e.resultsName
- and Diagnostics.warn_ungrouped_named_tokens_in_collection
- not in e.suppress_warnings_
- ):
- warnings.warn(
- "{}: setting results name {!r} on {} expression "
- "collides with {!r} on contained expression".format(
- "warn_ungrouped_named_tokens_in_collection",
- name,
- type(self).__name__,
- e.resultsName,
- ),
- stacklevel=3,
- )
-
- return super()._setResultsName(name, listAllMatches)
-
-
-class OneOrMore(_MultipleMatch):
- """
- Repetition of one or more of the given expression.
-
- Parameters:
- - expr - expression that must match one or more times
- - stop_on - (default= ``None``) - expression for a terminating sentinel
- (only required if the sentinel would ordinarily match the repetition
- expression)
-
- Example::
-
- data_word = Word(alphas)
- label = data_word + FollowedBy(':')
- attr_expr = Group(label + Suppress(':') + OneOrMore(data_word).set_parse_action(' '.join))
-
- text = "shape: SQUARE posn: upper left color: BLACK"
- attr_expr[1, ...].parse_string(text).pprint() # Fail! read 'color' as data instead of next label -> [['shape', 'SQUARE color']]
-
- # use stop_on attribute for OneOrMore to avoid reading label string as part of the data
- attr_expr = Group(label + Suppress(':') + OneOrMore(data_word, stop_on=label).set_parse_action(' '.join))
- OneOrMore(attr_expr).parse_string(text).pprint() # Better -> [['shape', 'SQUARE'], ['posn', 'upper left'], ['color', 'BLACK']]
-
- # could also be written as
- (attr_expr * (1,)).parse_string(text).pprint()
- """
-
- def _generateDefaultName(self):
- return "{" + str(self.expr) + "}..."
-
-
-class ZeroOrMore(_MultipleMatch):
- """
- Optional repetition of zero or more of the given expression.
-
- Parameters:
- - ``expr`` - expression that must match zero or more times
- - ``stop_on`` - expression for a terminating sentinel
- (only required if the sentinel would ordinarily match the repetition
- expression) - (default= ``None``)
-
- Example: similar to :class:`OneOrMore`
- """
-
- def __init__(
- self,
- expr: ParserElement,
- stop_on: typing.Optional[Union[ParserElement, str]] = None,
- *,
- stopOn: typing.Optional[Union[ParserElement, str]] = None,
- ):
- super().__init__(expr, stopOn=stopOn or stop_on)
- self.mayReturnEmpty = True
-
- def parseImpl(self, instring, loc, doActions=True):
- try:
- return super().parseImpl(instring, loc, doActions)
- except (ParseException, IndexError):
- return loc, ParseResults([], name=self.resultsName)
-
- def _generateDefaultName(self):
- return "[" + str(self.expr) + "]..."
-
-
-class _NullToken:
- def __bool__(self):
- return False
-
- def __str__(self):
- return ""
-
-
-class Opt(ParseElementEnhance):
- """
- Optional matching of the given expression.
-
- Parameters:
- - ``expr`` - expression that must match zero or more times
- - ``default`` (optional) - value to be returned if the optional expression is not found.
-
- Example::
-
- # US postal code can be a 5-digit zip, plus optional 4-digit qualifier
- zip = Combine(Word(nums, exact=5) + Opt('-' + Word(nums, exact=4)))
- zip.run_tests('''
- # traditional ZIP code
- 12345
-
- # ZIP+4 form
- 12101-0001
-
- # invalid ZIP
- 98765-
- ''')
-
- prints::
-
- # traditional ZIP code
- 12345
- ['12345']
-
- # ZIP+4 form
- 12101-0001
- ['12101-0001']
-
- # invalid ZIP
- 98765-
- ^
- FAIL: Expected end of text (at char 5), (line:1, col:6)
- """
-
- __optionalNotMatched = _NullToken()
-
- def __init__(
- self, expr: Union[ParserElement, str], default: Any = __optionalNotMatched
- ):
- super().__init__(expr, savelist=False)
- self.saveAsList = self.expr.saveAsList
- self.defaultValue = default
- self.mayReturnEmpty = True
-
- def parseImpl(self, instring, loc, doActions=True):
- self_expr = self.expr
- try:
- loc, tokens = self_expr._parse(instring, loc, doActions, callPreParse=False)
- except (ParseException, IndexError):
- default_value = self.defaultValue
- if default_value is not self.__optionalNotMatched:
- if self_expr.resultsName:
- tokens = ParseResults([default_value])
- tokens[self_expr.resultsName] = default_value
- else:
- tokens = [default_value]
- else:
- tokens = []
- return loc, tokens
-
- def _generateDefaultName(self):
- inner = str(self.expr)
- # strip off redundant inner {}'s
- while len(inner) > 1 and inner[0 :: len(inner) - 1] == "{}":
- inner = inner[1:-1]
- return "[" + inner + "]"
-
-
-Optional = Opt
-
-
-class SkipTo(ParseElementEnhance):
- """
- Token for skipping over all undefined text until the matched
- expression is found.
-
- Parameters:
- - ``expr`` - target expression marking the end of the data to be skipped
- - ``include`` - if ``True``, the target expression is also parsed
- (the skipped text and target expression are returned as a 2-element
- list) (default= ``False``).
- - ``ignore`` - (default= ``None``) used to define grammars (typically quoted strings and
- comments) that might contain false matches to the target expression
- - ``fail_on`` - (default= ``None``) define expressions that are not allowed to be
- included in the skipped test; if found before the target expression is found,
- the :class:`SkipTo` is not a match
-
- Example::
-
- report = '''
- Outstanding Issues Report - 1 Jan 2000
-
- # | Severity | Description | Days Open
- -----+----------+-------------------------------------------+-----------
- 101 | Critical | Intermittent system crash | 6
- 94 | Cosmetic | Spelling error on Login ('log|n') | 14
- 79 | Minor | System slow when running too many reports | 47
- '''
- integer = Word(nums)
- SEP = Suppress('|')
- # use SkipTo to simply match everything up until the next SEP
- # - ignore quoted strings, so that a '|' character inside a quoted string does not match
- # - parse action will call token.strip() for each matched token, i.e., the description body
- string_data = SkipTo(SEP, ignore=quoted_string)
- string_data.set_parse_action(token_map(str.strip))
- ticket_expr = (integer("issue_num") + SEP
- + string_data("sev") + SEP
- + string_data("desc") + SEP
- + integer("days_open"))
-
- for tkt in ticket_expr.search_string(report):
- print tkt.dump()
-
- prints::
-
- ['101', 'Critical', 'Intermittent system crash', '6']
- - days_open: '6'
- - desc: 'Intermittent system crash'
- - issue_num: '101'
- - sev: 'Critical'
- ['94', 'Cosmetic', "Spelling error on Login ('log|n')", '14']
- - days_open: '14'
- - desc: "Spelling error on Login ('log|n')"
- - issue_num: '94'
- - sev: 'Cosmetic'
- ['79', 'Minor', 'System slow when running too many reports', '47']
- - days_open: '47'
- - desc: 'System slow when running too many reports'
- - issue_num: '79'
- - sev: 'Minor'
- """
-
- def __init__(
- self,
- other: Union[ParserElement, str],
- include: bool = False,
- ignore: bool = None,
- fail_on: typing.Optional[Union[ParserElement, str]] = None,
- *,
- failOn: Union[ParserElement, str] = None,
- ):
- super().__init__(other)
- failOn = failOn or fail_on
- self.ignoreExpr = ignore
- self.mayReturnEmpty = True
- self.mayIndexError = False
- self.includeMatch = include
- self.saveAsList = False
- if isinstance(failOn, str_type):
- self.failOn = self._literalStringClass(failOn)
- else:
- self.failOn = failOn
- self.errmsg = "No match found for " + str(self.expr)
-
- def parseImpl(self, instring, loc, doActions=True):
- startloc = loc
- instrlen = len(instring)
- self_expr_parse = self.expr._parse
- self_failOn_canParseNext = (
- self.failOn.canParseNext if self.failOn is not None else None
- )
- self_ignoreExpr_tryParse = (
- self.ignoreExpr.tryParse if self.ignoreExpr is not None else None
- )
-
- tmploc = loc
- while tmploc <= instrlen:
- if self_failOn_canParseNext is not None:
- # break if failOn expression matches
- if self_failOn_canParseNext(instring, tmploc):
- break
-
- if self_ignoreExpr_tryParse is not None:
- # advance past ignore expressions
- while 1:
- try:
- tmploc = self_ignoreExpr_tryParse(instring, tmploc)
- except ParseBaseException:
- break
-
- try:
- self_expr_parse(instring, tmploc, doActions=False, callPreParse=False)
- except (ParseException, IndexError):
- # no match, advance loc in string
- tmploc += 1
- else:
- # matched skipto expr, done
- break
-
- else:
- # ran off the end of the input string without matching skipto expr, fail
- raise ParseException(instring, loc, self.errmsg, self)
-
- # build up return values
- loc = tmploc
- skiptext = instring[startloc:loc]
- skipresult = ParseResults(skiptext)
-
- if self.includeMatch:
- loc, mat = self_expr_parse(instring, loc, doActions, callPreParse=False)
- skipresult += mat
-
- return loc, skipresult
-
-
-class Forward(ParseElementEnhance):
- """
- Forward declaration of an expression to be defined later -
- used for recursive grammars, such as algebraic infix notation.
- When the expression is known, it is assigned to the ``Forward``
- variable using the ``'<<'`` operator.
-
- Note: take care when assigning to ``Forward`` not to overlook
- precedence of operators.
-
- Specifically, ``'|'`` has a lower precedence than ``'<<'``, so that::
-
- fwd_expr << a | b | c
-
- will actually be evaluated as::
-
- (fwd_expr << a) | b | c
-
- thereby leaving b and c out as parseable alternatives. It is recommended that you
- explicitly group the values inserted into the ``Forward``::
-
- fwd_expr << (a | b | c)
-
- Converting to use the ``'<<='`` operator instead will avoid this problem.
-
- See :class:`ParseResults.pprint` for an example of a recursive
- parser created using ``Forward``.
- """
-
- def __init__(self, other: typing.Optional[Union[ParserElement, str]] = None):
- self.caller_frame = traceback.extract_stack(limit=2)[0]
- super().__init__(other, savelist=False)
- self.lshift_line = None
-
- def __lshift__(self, other):
- if hasattr(self, "caller_frame"):
- del self.caller_frame
- if isinstance(other, str_type):
- other = self._literalStringClass(other)
- self.expr = other
- self.mayIndexError = self.expr.mayIndexError
- self.mayReturnEmpty = self.expr.mayReturnEmpty
- self.set_whitespace_chars(
- self.expr.whiteChars, copy_defaults=self.expr.copyDefaultWhiteChars
- )
- self.skipWhitespace = self.expr.skipWhitespace
- self.saveAsList = self.expr.saveAsList
- self.ignoreExprs.extend(self.expr.ignoreExprs)
- self.lshift_line = traceback.extract_stack(limit=2)[-2]
- return self
-
- def __ilshift__(self, other):
- return self << other
-
- def __or__(self, other):
- caller_line = traceback.extract_stack(limit=2)[-2]
- if (
- __diag__.warn_on_match_first_with_lshift_operator
- and caller_line == self.lshift_line
- and Diagnostics.warn_on_match_first_with_lshift_operator
- not in self.suppress_warnings_
- ):
- warnings.warn(
- "using '<<' operator with '|' is probably an error, use '<<='",
- stacklevel=2,
- )
- ret = super().__or__(other)
- return ret
-
- def __del__(self):
- # see if we are getting dropped because of '=' reassignment of var instead of '<<=' or '<<'
- if (
- self.expr is None
- and __diag__.warn_on_assignment_to_Forward
- and Diagnostics.warn_on_assignment_to_Forward not in self.suppress_warnings_
- ):
- warnings.warn_explicit(
- "Forward defined here but no expression attached later using '<<=' or '<<'",
- UserWarning,
- filename=self.caller_frame.filename,
- lineno=self.caller_frame.lineno,
- )
-
- def parseImpl(self, instring, loc, doActions=True):
- if (
- self.expr is None
- and __diag__.warn_on_parse_using_empty_Forward
- and Diagnostics.warn_on_parse_using_empty_Forward
- not in self.suppress_warnings_
- ):
- # walk stack until parse_string, scan_string, search_string, or transform_string is found
- parse_fns = [
- "parse_string",
- "scan_string",
- "search_string",
- "transform_string",
- ]
- tb = traceback.extract_stack(limit=200)
- for i, frm in enumerate(reversed(tb), start=1):
- if frm.name in parse_fns:
- stacklevel = i + 1
- break
- else:
- stacklevel = 2
- warnings.warn(
- "Forward expression was never assigned a value, will not parse any input",
- stacklevel=stacklevel,
- )
- if not ParserElement._left_recursion_enabled:
- return super().parseImpl(instring, loc, doActions)
- # ## Bounded Recursion algorithm ##
- # Recursion only needs to be processed at ``Forward`` elements, since they are
- # the only ones that can actually refer to themselves. The general idea is
- # to handle recursion stepwise: We start at no recursion, then recurse once,
- # recurse twice, ..., until more recursion offers no benefit (we hit the bound).
- #
- # The "trick" here is that each ``Forward`` gets evaluated in two contexts
- # - to *match* a specific recursion level, and
- # - to *search* the bounded recursion level
- # and the two run concurrently. The *search* must *match* each recursion level
- # to find the best possible match. This is handled by a memo table, which
- # provides the previous match to the next level match attempt.
- #
- # See also "Left Recursion in Parsing Expression Grammars", Medeiros et al.
- #
- # There is a complication since we not only *parse* but also *transform* via
- # actions: We do not want to run the actions too often while expanding. Thus,
- # we expand using `doActions=False` and only run `doActions=True` if the next
- # recursion level is acceptable.
- with ParserElement.recursion_lock:
- memo = ParserElement.recursion_memos
- try:
- # we are parsing at a specific recursion expansion - use it as-is
- prev_loc, prev_result = memo[loc, self, doActions]
- if isinstance(prev_result, Exception):
- raise prev_result
- return prev_loc, prev_result.copy()
- except KeyError:
- act_key = (loc, self, True)
- peek_key = (loc, self, False)
- # we are searching for the best recursion expansion - keep on improving
- # both `doActions` cases must be tracked separately here!
- prev_loc, prev_peek = memo[peek_key] = (
- loc - 1,
- ParseException(
- instring, loc, "Forward recursion without base case", self
- ),
- )
- if doActions:
- memo[act_key] = memo[peek_key]
- while True:
- try:
- new_loc, new_peek = super().parseImpl(instring, loc, False)
- except ParseException:
- # we failed before getting any match – do not hide the error
- if isinstance(prev_peek, Exception):
- raise
- new_loc, new_peek = prev_loc, prev_peek
- # the match did not get better: we are done
- if new_loc <= prev_loc:
- if doActions:
- # replace the match for doActions=False as well,
- # in case the action did backtrack
- prev_loc, prev_result = memo[peek_key] = memo[act_key]
- del memo[peek_key], memo[act_key]
- return prev_loc, prev_result.copy()
- del memo[peek_key]
- return prev_loc, prev_peek.copy()
- # the match did get better: see if we can improve further
- else:
- if doActions:
- try:
- memo[act_key] = super().parseImpl(instring, loc, True)
- except ParseException as e:
- memo[peek_key] = memo[act_key] = (new_loc, e)
- raise
- prev_loc, prev_peek = memo[peek_key] = new_loc, new_peek
-
- def leave_whitespace(self, recursive: bool = True) -> ParserElement:
- self.skipWhitespace = False
- return self
-
- def ignore_whitespace(self, recursive: bool = True) -> ParserElement:
- self.skipWhitespace = True
- return self
-
- def streamline(self) -> ParserElement:
- if not self.streamlined:
- self.streamlined = True
- if self.expr is not None:
- self.expr.streamline()
- return self
-
- def validate(self, validateTrace=None) -> None:
- if validateTrace is None:
- validateTrace = []
-
- if self not in validateTrace:
- tmp = validateTrace[:] + [self]
- if self.expr is not None:
- self.expr.validate(tmp)
- self._checkRecursion([])
-
- def _generateDefaultName(self):
- # Avoid infinite recursion by setting a temporary _defaultName
- self._defaultName = ": ..."
-
- # Use the string representation of main expression.
- retString = "..."
- try:
- if self.expr is not None:
- retString = str(self.expr)[:1000]
- else:
- retString = "None"
- finally:
- return self.__class__.__name__ + ": " + retString
-
- def copy(self) -> ParserElement:
- if self.expr is not None:
- return super().copy()
- else:
- ret = Forward()
- ret <<= self
- return ret
-
- def _setResultsName(self, name, list_all_matches=False):
- if (
- __diag__.warn_name_set_on_empty_Forward
- and Diagnostics.warn_name_set_on_empty_Forward
- not in self.suppress_warnings_
- ):
- if self.expr is None:
- warnings.warn(
- "{}: setting results name {!r} on {} expression "
- "that has no contained expression".format(
- "warn_name_set_on_empty_Forward", name, type(self).__name__
- ),
- stacklevel=3,
- )
-
- return super()._setResultsName(name, list_all_matches)
-
- ignoreWhitespace = ignore_whitespace
- leaveWhitespace = leave_whitespace
-
-
-class TokenConverter(ParseElementEnhance):
- """
- Abstract subclass of :class:`ParseExpression`, for converting parsed results.
- """
-
- def __init__(self, expr: Union[ParserElement, str], savelist=False):
- super().__init__(expr) # , savelist)
- self.saveAsList = False
-
-
-class Combine(TokenConverter):
- """Converter to concatenate all matching tokens to a single string.
- By default, the matching patterns must also be contiguous in the
- input string; this can be disabled by specifying
- ``'adjacent=False'`` in the constructor.
-
- Example::
-
- real = Word(nums) + '.' + Word(nums)
- print(real.parse_string('3.1416')) # -> ['3', '.', '1416']
- # will also erroneously match the following
- print(real.parse_string('3. 1416')) # -> ['3', '.', '1416']
-
- real = Combine(Word(nums) + '.' + Word(nums))
- print(real.parse_string('3.1416')) # -> ['3.1416']
- # no match when there are internal spaces
- print(real.parse_string('3. 1416')) # -> Exception: Expected W:(0123...)
- """
-
- def __init__(
- self,
- expr: ParserElement,
- join_string: str = "",
- adjacent: bool = True,
- *,
- joinString: typing.Optional[str] = None,
- ):
- super().__init__(expr)
- joinString = joinString if joinString is not None else join_string
- # suppress whitespace-stripping in contained parse expressions, but re-enable it on the Combine itself
- if adjacent:
- self.leave_whitespace()
- self.adjacent = adjacent
- self.skipWhitespace = True
- self.joinString = joinString
- self.callPreparse = True
-
- def ignore(self, other) -> ParserElement:
- if self.adjacent:
- ParserElement.ignore(self, other)
- else:
- super().ignore(other)
- return self
-
- def postParse(self, instring, loc, tokenlist):
- retToks = tokenlist.copy()
- del retToks[:]
- retToks += ParseResults(
- ["".join(tokenlist._asStringList(self.joinString))], modal=self.modalResults
- )
-
- if self.resultsName and retToks.haskeys():
- return [retToks]
- else:
- return retToks
-
-
-class Group(TokenConverter):
- """Converter to return the matched tokens as a list - useful for
- returning tokens of :class:`ZeroOrMore` and :class:`OneOrMore` expressions.
-
- The optional ``aslist`` argument when set to True will return the
- parsed tokens as a Python list instead of a pyparsing ParseResults.
-
- Example::
-
- ident = Word(alphas)
- num = Word(nums)
- term = ident | num
- func = ident + Opt(delimited_list(term))
- print(func.parse_string("fn a, b, 100"))
- # -> ['fn', 'a', 'b', '100']
-
- func = ident + Group(Opt(delimited_list(term)))
- print(func.parse_string("fn a, b, 100"))
- # -> ['fn', ['a', 'b', '100']]
- """
-
- def __init__(self, expr: ParserElement, aslist: bool = False):
- super().__init__(expr)
- self.saveAsList = True
- self._asPythonList = aslist
-
- def postParse(self, instring, loc, tokenlist):
- if self._asPythonList:
- return ParseResults.List(
- tokenlist.asList()
- if isinstance(tokenlist, ParseResults)
- else list(tokenlist)
- )
- else:
- return [tokenlist]
-
-
-class Dict(TokenConverter):
- """Converter to return a repetitive expression as a list, but also
- as a dictionary. Each element can also be referenced using the first
- token in the expression as its key. Useful for tabular report
- scraping when the first column can be used as a item key.
-
- The optional ``asdict`` argument when set to True will return the
- parsed tokens as a Python dict instead of a pyparsing ParseResults.
-
- Example::
-
- data_word = Word(alphas)
- label = data_word + FollowedBy(':')
-
- text = "shape: SQUARE posn: upper left color: light blue texture: burlap"
- attr_expr = (label + Suppress(':') + OneOrMore(data_word, stop_on=label).set_parse_action(' '.join))
-
- # print attributes as plain groups
- print(attr_expr[1, ...].parse_string(text).dump())
-
- # instead of OneOrMore(expr), parse using Dict(Group(expr)[1, ...]) - Dict will auto-assign names
- result = Dict(Group(attr_expr)[1, ...]).parse_string(text)
- print(result.dump())
-
- # access named fields as dict entries, or output as dict
- print(result['shape'])
- print(result.as_dict())
-
- prints::
-
- ['shape', 'SQUARE', 'posn', 'upper left', 'color', 'light blue', 'texture', 'burlap']
- [['shape', 'SQUARE'], ['posn', 'upper left'], ['color', 'light blue'], ['texture', 'burlap']]
- - color: 'light blue'
- - posn: 'upper left'
- - shape: 'SQUARE'
- - texture: 'burlap'
- SQUARE
- {'color': 'light blue', 'posn': 'upper left', 'texture': 'burlap', 'shape': 'SQUARE'}
-
- See more examples at :class:`ParseResults` of accessing fields by results name.
- """
-
- def __init__(self, expr: ParserElement, asdict: bool = False):
- super().__init__(expr)
- self.saveAsList = True
- self._asPythonDict = asdict
-
- def postParse(self, instring, loc, tokenlist):
- for i, tok in enumerate(tokenlist):
- if len(tok) == 0:
- continue
-
- ikey = tok[0]
- if isinstance(ikey, int):
- ikey = str(ikey).strip()
-
- if len(tok) == 1:
- tokenlist[ikey] = _ParseResultsWithOffset("", i)
-
- elif len(tok) == 2 and not isinstance(tok[1], ParseResults):
- tokenlist[ikey] = _ParseResultsWithOffset(tok[1], i)
-
- else:
- try:
- dictvalue = tok.copy() # ParseResults(i)
- except Exception:
- exc = TypeError(
- "could not extract dict values from parsed results"
- " - Dict expression must contain Grouped expressions"
- )
- raise exc from None
-
- del dictvalue[0]
-
- if len(dictvalue) != 1 or (
- isinstance(dictvalue, ParseResults) and dictvalue.haskeys()
- ):
- tokenlist[ikey] = _ParseResultsWithOffset(dictvalue, i)
- else:
- tokenlist[ikey] = _ParseResultsWithOffset(dictvalue[0], i)
-
- if self._asPythonDict:
- return [tokenlist.as_dict()] if self.resultsName else tokenlist.as_dict()
- else:
- return [tokenlist] if self.resultsName else tokenlist
-
-
-class Suppress(TokenConverter):
- """Converter for ignoring the results of a parsed expression.
-
- Example::
-
- source = "a, b, c,d"
- wd = Word(alphas)
- wd_list1 = wd + (',' + wd)[...]
- print(wd_list1.parse_string(source))
-
- # often, delimiters that are useful during parsing are just in the
- # way afterward - use Suppress to keep them out of the parsed output
- wd_list2 = wd + (Suppress(',') + wd)[...]
- print(wd_list2.parse_string(source))
-
- # Skipped text (using '...') can be suppressed as well
- source = "lead in START relevant text END trailing text"
- start_marker = Keyword("START")
- end_marker = Keyword("END")
- find_body = Suppress(...) + start_marker + ... + end_marker
- print(find_body.parse_string(source)
-
- prints::
-
- ['a', ',', 'b', ',', 'c', ',', 'd']
- ['a', 'b', 'c', 'd']
- ['START', 'relevant text ', 'END']
-
- (See also :class:`delimited_list`.)
- """
-
- def __init__(self, expr: Union[ParserElement, str], savelist: bool = False):
- if expr is ...:
- expr = _PendingSkip(NoMatch())
- super().__init__(expr)
-
- def __add__(self, other) -> "ParserElement":
- if isinstance(self.expr, _PendingSkip):
- return Suppress(SkipTo(other)) + other
- else:
- return super().__add__(other)
-
- def __sub__(self, other) -> "ParserElement":
- if isinstance(self.expr, _PendingSkip):
- return Suppress(SkipTo(other)) - other
- else:
- return super().__sub__(other)
-
- def postParse(self, instring, loc, tokenlist):
- return []
-
- def suppress(self) -> ParserElement:
- return self
-
-
-def trace_parse_action(f: ParseAction) -> ParseAction:
- """Decorator for debugging parse actions.
-
- When the parse action is called, this decorator will print
- ``">> entering method-name(line:, , )"``.
- When the parse action completes, the decorator will print
- ``"<<"`` followed by the returned value, or any exception that the parse action raised.
-
- Example::
-
- wd = Word(alphas)
-
- @trace_parse_action
- def remove_duplicate_chars(tokens):
- return ''.join(sorted(set(''.join(tokens))))
-
- wds = wd[1, ...].set_parse_action(remove_duplicate_chars)
- print(wds.parse_string("slkdjs sld sldd sdlf sdljf"))
-
- prints::
-
- >>entering remove_duplicate_chars(line: 'slkdjs sld sldd sdlf sdljf', 0, (['slkdjs', 'sld', 'sldd', 'sdlf', 'sdljf'], {}))
- < 3:
- thisFunc = paArgs[0].__class__.__name__ + "." + thisFunc
- sys.stderr.write(
- ">>entering {}(line: {!r}, {}, {!r})\n".format(thisFunc, line(l, s), l, t)
- )
- try:
- ret = f(*paArgs)
- except Exception as exc:
- sys.stderr.write("< str:
- r"""Helper to easily define string ranges for use in :class:`Word`
- construction. Borrows syntax from regexp ``'[]'`` string range
- definitions::
-
- srange("[0-9]") -> "0123456789"
- srange("[a-z]") -> "abcdefghijklmnopqrstuvwxyz"
- srange("[a-z$_]") -> "abcdefghijklmnopqrstuvwxyz$_"
-
- The input string must be enclosed in []'s, and the returned string
- is the expanded character set joined into a single string. The
- values enclosed in the []'s may be:
-
- - a single character
- - an escaped character with a leading backslash (such as ``\-``
- or ``\]``)
- - an escaped hex character with a leading ``'\x'``
- (``\x21``, which is a ``'!'`` character) (``\0x##``
- is also supported for backwards compatibility)
- - an escaped octal character with a leading ``'\0'``
- (``\041``, which is a ``'!'`` character)
- - a range of any of the above, separated by a dash (``'a-z'``,
- etc.)
- - any combination of the above (``'aeiouy'``,
- ``'a-zA-Z0-9_$'``, etc.)
- """
- _expanded = (
- lambda p: p
- if not isinstance(p, ParseResults)
- else "".join(chr(c) for c in range(ord(p[0]), ord(p[1]) + 1))
- )
- try:
- return "".join(_expanded(part) for part in _reBracketExpr.parse_string(s).body)
- except Exception:
- return ""
-
-
-def token_map(func, *args) -> ParseAction:
- """Helper to define a parse action by mapping a function to all
- elements of a :class:`ParseResults` list. If any additional args are passed,
- they are forwarded to the given function as additional arguments
- after the token, as in
- ``hex_integer = Word(hexnums).set_parse_action(token_map(int, 16))``,
- which will convert the parsed data to an integer using base 16.
-
- Example (compare the last to example in :class:`ParserElement.transform_string`::
-
- hex_ints = Word(hexnums)[1, ...].set_parse_action(token_map(int, 16))
- hex_ints.run_tests('''
- 00 11 22 aa FF 0a 0d 1a
- ''')
-
- upperword = Word(alphas).set_parse_action(token_map(str.upper))
- upperword[1, ...].run_tests('''
- my kingdom for a horse
- ''')
-
- wd = Word(alphas).set_parse_action(token_map(str.title))
- wd[1, ...].set_parse_action(' '.join).run_tests('''
- now is the winter of our discontent made glorious summer by this sun of york
- ''')
-
- prints::
-
- 00 11 22 aa FF 0a 0d 1a
- [0, 17, 34, 170, 255, 10, 13, 26]
-
- my kingdom for a horse
- ['MY', 'KINGDOM', 'FOR', 'A', 'HORSE']
-
- now is the winter of our discontent made glorious summer by this sun of york
- ['Now Is The Winter Of Our Discontent Made Glorious Summer By This Sun Of York']
- """
-
- def pa(s, l, t):
- return [func(tokn, *args) for tokn in t]
-
- func_name = getattr(func, "__name__", getattr(func, "__class__").__name__)
- pa.__name__ = func_name
-
- return pa
-
-
-def autoname_elements() -> None:
- """
- Utility to simplify mass-naming of parser elements, for
- generating railroad diagram with named subdiagrams.
- """
- for name, var in sys._getframe().f_back.f_locals.items():
- if isinstance(var, ParserElement) and not var.customName:
- var.set_name(name)
-
-
-dbl_quoted_string = Combine(
- Regex(r'"(?:[^"\n\r\\]|(?:"")|(?:\\(?:[^x]|x[0-9a-fA-F]+)))*') + '"'
-).set_name("string enclosed in double quotes")
-
-sgl_quoted_string = Combine(
- Regex(r"'(?:[^'\n\r\\]|(?:'')|(?:\\(?:[^x]|x[0-9a-fA-F]+)))*") + "'"
-).set_name("string enclosed in single quotes")
-
-quoted_string = Combine(
- Regex(r'"(?:[^"\n\r\\]|(?:"")|(?:\\(?:[^x]|x[0-9a-fA-F]+)))*') + '"'
- | Regex(r"'(?:[^'\n\r\\]|(?:'')|(?:\\(?:[^x]|x[0-9a-fA-F]+)))*") + "'"
-).set_name("quotedString using single or double quotes")
-
-unicode_string = Combine("u" + quoted_string.copy()).set_name("unicode string literal")
-
-
-alphas8bit = srange(r"[\0xc0-\0xd6\0xd8-\0xf6\0xf8-\0xff]")
-punc8bit = srange(r"[\0xa1-\0xbf\0xd7\0xf7]")
-
-# build list of built-in expressions, for future reference if a global default value
-# gets updated
-_builtin_exprs: List[ParserElement] = [
- v for v in vars().values() if isinstance(v, ParserElement)
-]
-
-# backward compatibility names
-tokenMap = token_map
-conditionAsParseAction = condition_as_parse_action
-nullDebugAction = null_debug_action
-sglQuotedString = sgl_quoted_string
-dblQuotedString = dbl_quoted_string
-quotedString = quoted_string
-unicodeString = unicode_string
-lineStart = line_start
-lineEnd = line_end
-stringStart = string_start
-stringEnd = string_end
-traceParseAction = trace_parse_action
diff --git a/spaces/Ataturk-Chatbot/HuggingFaceChat/venv/lib/python3.11/site-packages/pip/_vendor/urllib3/contrib/__init__.py b/spaces/Ataturk-Chatbot/HuggingFaceChat/venv/lib/python3.11/site-packages/pip/_vendor/urllib3/contrib/__init__.py
deleted file mode 100644
index e69de29bb2d1d6434b8b29ae775ad8c2e48c5391..0000000000000000000000000000000000000000
diff --git a/spaces/Awiny/Image2Paragraph/models/grit_src/third_party/CenterNet2/detectron2/layers/csrc/vision.cpp b/spaces/Awiny/Image2Paragraph/models/grit_src/third_party/CenterNet2/detectron2/layers/csrc/vision.cpp
deleted file mode 100644
index c9a2cd4f20e6f58be1c5783d67c64232dd59b560..0000000000000000000000000000000000000000
--- a/spaces/Awiny/Image2Paragraph/models/grit_src/third_party/CenterNet2/detectron2/layers/csrc/vision.cpp
+++ /dev/null
@@ -1,117 +0,0 @@
-// Copyright (c) Facebook, Inc. and its affiliates.
-
-#include
-#include "ROIAlignRotated/ROIAlignRotated.h"
-#include "box_iou_rotated/box_iou_rotated.h"
-#include "cocoeval/cocoeval.h"
-#include "deformable/deform_conv.h"
-#include "nms_rotated/nms_rotated.h"
-
-namespace detectron2 {
-
-#if defined(WITH_CUDA) || defined(WITH_HIP)
-extern int get_cudart_version();
-#endif
-
-std::string get_cuda_version() {
-#if defined(WITH_CUDA) || defined(WITH_HIP)
- std::ostringstream oss;
-
-#if defined(WITH_CUDA)
- oss << "CUDA ";
-#else
- oss << "HIP ";
-#endif
-
- // copied from
- // https://github.com/pytorch/pytorch/blob/master/aten/src/ATen/cuda/detail/CUDAHooks.cpp#L231
- auto printCudaStyleVersion = [&](int v) {
- oss << (v / 1000) << "." << (v / 10 % 100);
- if (v % 10 != 0) {
- oss << "." << (v % 10);
- }
- };
- printCudaStyleVersion(get_cudart_version());
- return oss.str();
-#else // neither CUDA nor HIP
- return std::string("not available");
-#endif
-}
-
-bool has_cuda() {
-#if defined(WITH_CUDA)
- return true;
-#else
- return false;
-#endif
-}
-
-// similar to
-// https://github.com/pytorch/pytorch/blob/master/aten/src/ATen/Version.cpp
-std::string get_compiler_version() {
- std::ostringstream ss;
-#if defined(__GNUC__)
-#ifndef __clang__
-
-#if ((__GNUC__ <= 4) && (__GNUC_MINOR__ <= 8))
-#error "GCC >= 4.9 is required!"
-#endif
-
- { ss << "GCC " << __GNUC__ << "." << __GNUC_MINOR__; }
-#endif
-#endif
-
-#if defined(__clang_major__)
- {
- ss << "clang " << __clang_major__ << "." << __clang_minor__ << "."
- << __clang_patchlevel__;
- }
-#endif
-
-#if defined(_MSC_VER)
- { ss << "MSVC " << _MSC_FULL_VER; }
-#endif
- return ss.str();
-}
-
-PYBIND11_MODULE(TORCH_EXTENSION_NAME, m) {
- m.def("get_compiler_version", &get_compiler_version, "get_compiler_version");
- m.def("get_cuda_version", &get_cuda_version, "get_cuda_version");
- m.def("has_cuda", &has_cuda, "has_cuda");
-
- m.def("deform_conv_forward", &deform_conv_forward, "deform_conv_forward");
- m.def(
- "deform_conv_backward_input",
- &deform_conv_backward_input,
- "deform_conv_backward_input");
- m.def(
- "deform_conv_backward_filter",
- &deform_conv_backward_filter,
- "deform_conv_backward_filter");
- m.def(
- "modulated_deform_conv_forward",
- &modulated_deform_conv_forward,
- "modulated_deform_conv_forward");
- m.def(
- "modulated_deform_conv_backward",
- &modulated_deform_conv_backward,
- "modulated_deform_conv_backward");
-
- m.def("COCOevalAccumulate", &COCOeval::Accumulate, "COCOeval::Accumulate");
- m.def(
- "COCOevalEvaluateImages",
- &COCOeval::EvaluateImages,
- "COCOeval::EvaluateImages");
- pybind11::class_(m, "InstanceAnnotation")
- .def(pybind11::init());
- pybind11::class_(m, "ImageEvaluation")
- .def(pybind11::init<>());
-}
-
-TORCH_LIBRARY(detectron2, m) {
- m.def("nms_rotated", &nms_rotated);
- m.def("box_iou_rotated", &box_iou_rotated);
- m.def("roi_align_rotated_forward", &ROIAlignRotated_forward);
- m.def("roi_align_rotated_backward", &ROIAlignRotated_backward);
-}
-} // namespace detectron2
diff --git a/spaces/BAAI/vid2vid-zero/gradio_demo/app_running.py b/spaces/BAAI/vid2vid-zero/gradio_demo/app_running.py
deleted file mode 100644
index 1f6105342c1b84c6adbab5e5724d8105af3df348..0000000000000000000000000000000000000000
--- a/spaces/BAAI/vid2vid-zero/gradio_demo/app_running.py
+++ /dev/null
@@ -1,169 +0,0 @@
-#!/usr/bin/env python
-
-from __future__ import annotations
-
-import os
-
-import gradio as gr
-
-from gradio_demo.runner import Runner
-
-
-def create_demo(runner: Runner,
- pipe: InferencePipeline | None = None) -> gr.Blocks:
- hf_token = os.getenv('HF_TOKEN')
- with gr.Blocks() as demo:
- with gr.Row():
- with gr.Column():
- with gr.Box():
- gr.Markdown('Input Data')
- input_video = gr.File(label='Input video')
- input_prompt = gr.Textbox(
- label='Input prompt',
- max_lines=1,
- placeholder='A car is moving on the road.')
- gr.Markdown('''
- - Upload a video and write a `Input Prompt` that describes the video.
- ''')
-
- with gr.Column():
- with gr.Box():
- gr.Markdown('Input Parameters')
- with gr.Row():
- model_path = gr.Text(
- label='Path to off-the-shelf model',
- value='CompVis/stable-diffusion-v1-4',
- max_lines=1)
- resolution = gr.Dropdown(choices=['512', '768'],
- value='512',
- label='Resolution',
- visible=False)
-
- with gr.Accordion('Advanced settings', open=False):
- sample_start_idx = gr.Number(
- label='Start Frame Index',value=0)
- sample_frame_rate = gr.Number(
- label='Frame Rate',value=1)
- n_sample_frames = gr.Number(
- label='Number of Frames',value=8)
- guidance_scale = gr.Number(
- label='Guidance Scale', value=7.5)
- seed = gr.Slider(label='Seed',
- minimum=0,
- maximum=100000,
- step=1,
- randomize=True,
- value=33)
- input_token = gr.Text(label='Hugging Face Write Token',
- placeholder='',
- visible=False if hf_token else True)
- gr.Markdown('''
- - Upload input video or choose an exmple blow
- - Set hyperparameters & click start
- - It takes a few minutes to download model first
- ''')
-
- with gr.Row():
- with gr.Column():
- validation_prompt = gr.Text(
- label='Validation Prompt',
- placeholder=
- 'prompt to test the model, e.g: a Lego man is surfing')
-
- remove_gpu_after_running = gr.Checkbox(
- label='Remove GPU after running',
- value=False,
- interactive=bool(os.getenv('SPACE_ID')),
- visible=False)
-
- with gr.Row():
- result = gr.Video(label='Result')
-
- # examples
- with gr.Row():
- examples = [
- [
- 'CompVis/stable-diffusion-v1-4',
- "data/car-moving.mp4",
- 'A car is moving on the road.',
- 8, 0, 1,
- 'A jeep car is moving on the desert.',
- 7.5, 512, 33,
- False, None,
- ],
-
- [
- 'CompVis/stable-diffusion-v1-4',
- "data/black-swan.mp4",
- 'A blackswan is swimming on the water.',
- 8, 0, 4,
- 'A white swan is swimming on the water.',
- 7.5, 512, 33,
- False, None,
- ],
-
- [
- 'CompVis/stable-diffusion-v1-4',
- "data/child-riding.mp4",
- 'A child is riding a bike on the road.',
- 8, 0, 1,
- 'A lego child is riding a bike on the road.',
- 7.5, 512, 33,
- False, None,
- ],
-
- [
- 'CompVis/stable-diffusion-v1-4',
- "data/car-turn.mp4",
- 'A jeep car is moving on the road.',
- 8, 0, 6,
- 'A jeep car is moving on the snow.',
- 7.5, 512, 33,
- False, None,
- ],
-
- [
- 'CompVis/stable-diffusion-v1-4',
- "data/rabbit-watermelon.mp4",
- 'A rabbit is eating a watermelon.',
- 8, 0, 6,
- 'A puppy is eating an orange.',
- 7.5, 512, 33,
- False, None,
- ],
-
- ]
- gr.Examples(examples=examples,
- fn=runner.run_vid2vid_zero,
- inputs=[
- model_path, input_video, input_prompt,
- n_sample_frames, sample_start_idx, sample_frame_rate,
- validation_prompt, guidance_scale, resolution, seed,
- remove_gpu_after_running,
- input_token,
- ],
- outputs=result,
- cache_examples=os.getenv('SYSTEM') == 'spaces'
- )
-
- # run
- run_button_vid2vid_zero = gr.Button('Start vid2vid-zero')
- run_button_vid2vid_zero.click(
- fn=runner.run_vid2vid_zero,
- inputs=[
- model_path, input_video, input_prompt,
- n_sample_frames, sample_start_idx, sample_frame_rate,
- validation_prompt, guidance_scale, resolution, seed,
- remove_gpu_after_running,
- input_token,
- ],
- outputs=result)
-
- return demo
-
-
-if __name__ == '__main__':
- hf_token = os.getenv('HF_TOKEN')
- runner = Runner(hf_token)
- demo = create_demo(runner)
- demo.queue(max_size=1).launch(share=False)
diff --git a/spaces/BFH/BKMotionsAI/app.py b/spaces/BFH/BKMotionsAI/app.py
deleted file mode 100644
index bc29914d0329cc553aa8404f8a162f7a0aba7ae9..0000000000000000000000000000000000000000
--- a/spaces/BFH/BKMotionsAI/app.py
+++ /dev/null
@@ -1,86 +0,0 @@
-#!/usr/bin/env python
-# coding: utf-8
-
-import gradio as gr
-import numpy as np
-import requests
-from transformers import AutoModelForSequenceClassification, AutoTokenizer, TextClassificationPipeline, pipeline
-from langdetect import detect
-from matplotlib import pyplot as plt
-import imageio
-
-# Load the model
-model = AutoModelForSequenceClassification.from_pretrained("saved_model")
-tokenizer = AutoTokenizer.from_pretrained("saved_model")
-pipe = TextClassificationPipeline(model=model, tokenizer=tokenizer)
-
-# Function called by the UI
-def attribution(text):
-
- # Clean the plot
- plt.clf()
-
- # Detect the language
- language = detect(text)
-
- # Translate the input in german if necessary
- if language == 'fr':
- translator = pipeline("translation", model="Helsinki-NLP/opus-mt-fr-de")
- translatedText = translator(text[0:1000])
- text = translatedText[0]["translation_text"]
- elif language != 'de':
- return "The language is not recognized, it must be either in German or in French.", None
-
- # Set the bars of the bar chart
- bars = ""
- if language == 'fr':
- bars = ("DDPS", "DFI", "AS-MPC", "DFJP", "DEFR", "DETEC", "DFAE", "Parl", "ChF", "DFF", "AF", "TF")
- else:
- bars = ("VBS", "EDI", "AB-BA", "EJPD", "WBF", "UVEK", "EDA", "Parl", "BK", "EFD", "BV", "BGer")
-
- # Make the prediction with the 1000 first characters
- results = pipe(text[0:1000], return_all_scores=True)
- rates = [row["score"] for row in results[0]]
-
- # Bar chart
- y_pos = np.arange(len(bars))
- plt.barh(y_pos, rates)
- plt.yticks(y_pos, bars)
-
- # Set the output text
- name = ""
- maxRate = np.max(rates)
- maxIndex = np.argmax(rates)
-
- # ML model not sure if highest probability < 60%
- if maxRate < 0.6:
- # de / fr
- if language == 'de':
- name = "Das ML-Modell ist nicht sicher. Das Departement könnte sein : \n\n"
- else:
- name = "Le modèle ML n'est pas sûr. Le département pourrait être : \n\n"
- i = 0
- # Show each department that has a probability > 10%
- while i == 0:
- if rates[maxIndex] >= 0.1:
- name = name + "\t" + str(rates[maxIndex])[2:4] + "%" + "\t\t\t\t\t" + bars[maxIndex] + "\n"
- rates[maxIndex] = 0
- maxIndex = np.argmax(rates)
- else:
- i = 1
- # ML model pretty sure, show only one department
- else:
- name = str(maxRate)[2:4] + "%" + "\t\t\t\t\t\t" + bars[maxIndex]
-
- # Save the bar chart as png and load it (enables better display)
- plt.savefig('rates.png')
- im = imageio.imread('rates.png')
-
- return name, im
-
-
-# display the UI
-interface = gr.Interface(fn=attribution,
- inputs=[gr.inputs.Textbox(lines=20, placeholder="Geben Sie bitte den Titel und den Sumbmitted Text des Vorstoss ein.\nVeuillez entrer le titre et le Submitted Text de la requête.")],
- outputs=['text', 'image'])
-interface.launch()
\ No newline at end of file
diff --git a/spaces/Banbri/zcvzcv/src/app/interface/zoom/index.tsx b/spaces/Banbri/zcvzcv/src/app/interface/zoom/index.tsx
deleted file mode 100644
index 5c8d31a3af1c80f8a9ef15330bb84c0d2c3069de..0000000000000000000000000000000000000000
--- a/spaces/Banbri/zcvzcv/src/app/interface/zoom/index.tsx
+++ /dev/null
@@ -1,35 +0,0 @@
-import { useStore } from "@/app/store"
-import { VerticalSlider } from "@/components/ui/vertical-slider"
-import { cn } from "@/lib/utils"
-
-export function Zoom() {
- const zoomLevel = useStore((state) => state.zoomLevel)
- const setZoomLevel = useStore((state) => state.setZoomLevel)
- const isGeneratingStory = useStore((state) => state.isGeneratingStory)
-
- return (
-
- )
-}
\ No newline at end of file
diff --git a/spaces/Bart92/RVC_HF/colab_for_mdx.py b/spaces/Bart92/RVC_HF/colab_for_mdx.py
deleted file mode 100644
index 274846d0b5395865a05fce0da86b96d26ac06999..0000000000000000000000000000000000000000
--- a/spaces/Bart92/RVC_HF/colab_for_mdx.py
+++ /dev/null
@@ -1,71 +0,0 @@
-import json
-import os
-import gc
-import psutil
-import requests
-import subprocess
-import time
-import logging
-import sys
-import shutil
-now_dir = os.getcwd()
-sys.path.append(now_dir)
-first_cell_executed = False
-file_folder = "Colab-for-MDX_B"
-def first_cell_ran():
- global first_cell_executed
- if first_cell_executed:
- #print("The 'first_cell_ran' function has already been executed.")
- return
-
-
-
- first_cell_executed = True
- os.makedirs("tmp_models", exist_ok=True)
-
-
-
- class hide_opt: # hide outputs
- def __enter__(self):
- self._original_stdout = sys.stdout
- sys.stdout = open(os.devnull, "w")
-
- def __exit__(self, exc_type, exc_val, exc_tb):
- sys.stdout.close()
- sys.stdout = self._original_stdout
-
- def get_size(bytes, suffix="B"): # read ram
- global svmem
- factor = 1024
- for unit in ["", "K", "M", "G", "T", "P"]:
- if bytes < factor:
- return f"{bytes:.2f}{unit}{suffix}"
- bytes /= factor
- svmem = psutil.virtual_memory()
-
-
- def use_uvr_without_saving():
- print("Notice: files won't be saved to personal drive.")
- print(f"Downloading {file_folder}...", end=" ")
- with hide_opt():
- #os.chdir(mounting_path)
- items_to_move = ["demucs", "diffq","julius","model","separated","tracks","mdx.py","MDX-Net_Colab.ipynb"]
- subprocess.run(["git", "clone", "https://github.com/NaJeongMo/Colab-for-MDX_B.git"])
- for item_name in items_to_move:
- item_path = os.path.join(file_folder, item_name)
- if os.path.exists(item_path):
- if os.path.isfile(item_path):
- shutil.move(item_path, now_dir)
- elif os.path.isdir(item_path):
- shutil.move(item_path, now_dir)
- try:
- shutil.rmtree(file_folder)
- except PermissionError:
- print(f"No se pudo eliminar la carpeta {file_folder}. Puede estar relacionada con Git.")
-
-
- use_uvr_without_saving()
- print("done!")
- if not os.path.exists("tracks"):
- os.mkdir("tracks")
-first_cell_ran()
\ No newline at end of file
diff --git a/spaces/BartPoint/VoiceChange_Beta/infer_pack/transforms.py b/spaces/BartPoint/VoiceChange_Beta/infer_pack/transforms.py
deleted file mode 100644
index a11f799e023864ff7082c1f49c0cc18351a13b47..0000000000000000000000000000000000000000
--- a/spaces/BartPoint/VoiceChange_Beta/infer_pack/transforms.py
+++ /dev/null
@@ -1,209 +0,0 @@
-import torch
-from torch.nn import functional as F
-
-import numpy as np
-
-
-DEFAULT_MIN_BIN_WIDTH = 1e-3
-DEFAULT_MIN_BIN_HEIGHT = 1e-3
-DEFAULT_MIN_DERIVATIVE = 1e-3
-
-
-def piecewise_rational_quadratic_transform(
- inputs,
- unnormalized_widths,
- unnormalized_heights,
- unnormalized_derivatives,
- inverse=False,
- tails=None,
- tail_bound=1.0,
- min_bin_width=DEFAULT_MIN_BIN_WIDTH,
- min_bin_height=DEFAULT_MIN_BIN_HEIGHT,
- min_derivative=DEFAULT_MIN_DERIVATIVE,
-):
- if tails is None:
- spline_fn = rational_quadratic_spline
- spline_kwargs = {}
- else:
- spline_fn = unconstrained_rational_quadratic_spline
- spline_kwargs = {"tails": tails, "tail_bound": tail_bound}
-
- outputs, logabsdet = spline_fn(
- inputs=inputs,
- unnormalized_widths=unnormalized_widths,
- unnormalized_heights=unnormalized_heights,
- unnormalized_derivatives=unnormalized_derivatives,
- inverse=inverse,
- min_bin_width=min_bin_width,
- min_bin_height=min_bin_height,
- min_derivative=min_derivative,
- **spline_kwargs
- )
- return outputs, logabsdet
-
-
-def searchsorted(bin_locations, inputs, eps=1e-6):
- bin_locations[..., -1] += eps
- return torch.sum(inputs[..., None] >= bin_locations, dim=-1) - 1
-
-
-def unconstrained_rational_quadratic_spline(
- inputs,
- unnormalized_widths,
- unnormalized_heights,
- unnormalized_derivatives,
- inverse=False,
- tails="linear",
- tail_bound=1.0,
- min_bin_width=DEFAULT_MIN_BIN_WIDTH,
- min_bin_height=DEFAULT_MIN_BIN_HEIGHT,
- min_derivative=DEFAULT_MIN_DERIVATIVE,
-):
- inside_interval_mask = (inputs >= -tail_bound) & (inputs <= tail_bound)
- outside_interval_mask = ~inside_interval_mask
-
- outputs = torch.zeros_like(inputs)
- logabsdet = torch.zeros_like(inputs)
-
- if tails == "linear":
- unnormalized_derivatives = F.pad(unnormalized_derivatives, pad=(1, 1))
- constant = np.log(np.exp(1 - min_derivative) - 1)
- unnormalized_derivatives[..., 0] = constant
- unnormalized_derivatives[..., -1] = constant
-
- outputs[outside_interval_mask] = inputs[outside_interval_mask]
- logabsdet[outside_interval_mask] = 0
- else:
- raise RuntimeError("{} tails are not implemented.".format(tails))
-
- (
- outputs[inside_interval_mask],
- logabsdet[inside_interval_mask],
- ) = rational_quadratic_spline(
- inputs=inputs[inside_interval_mask],
- unnormalized_widths=unnormalized_widths[inside_interval_mask, :],
- unnormalized_heights=unnormalized_heights[inside_interval_mask, :],
- unnormalized_derivatives=unnormalized_derivatives[inside_interval_mask, :],
- inverse=inverse,
- left=-tail_bound,
- right=tail_bound,
- bottom=-tail_bound,
- top=tail_bound,
- min_bin_width=min_bin_width,
- min_bin_height=min_bin_height,
- min_derivative=min_derivative,
- )
-
- return outputs, logabsdet
-
-
-def rational_quadratic_spline(
- inputs,
- unnormalized_widths,
- unnormalized_heights,
- unnormalized_derivatives,
- inverse=False,
- left=0.0,
- right=1.0,
- bottom=0.0,
- top=1.0,
- min_bin_width=DEFAULT_MIN_BIN_WIDTH,
- min_bin_height=DEFAULT_MIN_BIN_HEIGHT,
- min_derivative=DEFAULT_MIN_DERIVATIVE,
-):
- if torch.min(inputs) < left or torch.max(inputs) > right:
- raise ValueError("Input to a transform is not within its domain")
-
- num_bins = unnormalized_widths.shape[-1]
-
- if min_bin_width * num_bins > 1.0:
- raise ValueError("Minimal bin width too large for the number of bins")
- if min_bin_height * num_bins > 1.0:
- raise ValueError("Minimal bin height too large for the number of bins")
-
- widths = F.softmax(unnormalized_widths, dim=-1)
- widths = min_bin_width + (1 - min_bin_width * num_bins) * widths
- cumwidths = torch.cumsum(widths, dim=-1)
- cumwidths = F.pad(cumwidths, pad=(1, 0), mode="constant", value=0.0)
- cumwidths = (right - left) * cumwidths + left
- cumwidths[..., 0] = left
- cumwidths[..., -1] = right
- widths = cumwidths[..., 1:] - cumwidths[..., :-1]
-
- derivatives = min_derivative + F.softplus(unnormalized_derivatives)
-
- heights = F.softmax(unnormalized_heights, dim=-1)
- heights = min_bin_height + (1 - min_bin_height * num_bins) * heights
- cumheights = torch.cumsum(heights, dim=-1)
- cumheights = F.pad(cumheights, pad=(1, 0), mode="constant", value=0.0)
- cumheights = (top - bottom) * cumheights + bottom
- cumheights[..., 0] = bottom
- cumheights[..., -1] = top
- heights = cumheights[..., 1:] - cumheights[..., :-1]
-
- if inverse:
- bin_idx = searchsorted(cumheights, inputs)[..., None]
- else:
- bin_idx = searchsorted(cumwidths, inputs)[..., None]
-
- input_cumwidths = cumwidths.gather(-1, bin_idx)[..., 0]
- input_bin_widths = widths.gather(-1, bin_idx)[..., 0]
-
- input_cumheights = cumheights.gather(-1, bin_idx)[..., 0]
- delta = heights / widths
- input_delta = delta.gather(-1, bin_idx)[..., 0]
-
- input_derivatives = derivatives.gather(-1, bin_idx)[..., 0]
- input_derivatives_plus_one = derivatives[..., 1:].gather(-1, bin_idx)[..., 0]
-
- input_heights = heights.gather(-1, bin_idx)[..., 0]
-
- if inverse:
- a = (inputs - input_cumheights) * (
- input_derivatives + input_derivatives_plus_one - 2 * input_delta
- ) + input_heights * (input_delta - input_derivatives)
- b = input_heights * input_derivatives - (inputs - input_cumheights) * (
- input_derivatives + input_derivatives_plus_one - 2 * input_delta
- )
- c = -input_delta * (inputs - input_cumheights)
-
- discriminant = b.pow(2) - 4 * a * c
- assert (discriminant >= 0).all()
-
- root = (2 * c) / (-b - torch.sqrt(discriminant))
- outputs = root * input_bin_widths + input_cumwidths
-
- theta_one_minus_theta = root * (1 - root)
- denominator = input_delta + (
- (input_derivatives + input_derivatives_plus_one - 2 * input_delta)
- * theta_one_minus_theta
- )
- derivative_numerator = input_delta.pow(2) * (
- input_derivatives_plus_one * root.pow(2)
- + 2 * input_delta * theta_one_minus_theta
- + input_derivatives * (1 - root).pow(2)
- )
- logabsdet = torch.log(derivative_numerator) - 2 * torch.log(denominator)
-
- return outputs, -logabsdet
- else:
- theta = (inputs - input_cumwidths) / input_bin_widths
- theta_one_minus_theta = theta * (1 - theta)
-
- numerator = input_heights * (
- input_delta * theta.pow(2) + input_derivatives * theta_one_minus_theta
- )
- denominator = input_delta + (
- (input_derivatives + input_derivatives_plus_one - 2 * input_delta)
- * theta_one_minus_theta
- )
- outputs = input_cumheights + numerator / denominator
-
- derivative_numerator = input_delta.pow(2) * (
- input_derivatives_plus_one * theta.pow(2)
- + 2 * input_delta * theta_one_minus_theta
- + input_derivatives * (1 - theta).pow(2)
- )
- logabsdet = torch.log(derivative_numerator) - 2 * torch.log(denominator)
-
- return outputs, logabsdet
diff --git a/spaces/Benson/text-generation/Examples/Avakin Life Pc.md b/spaces/Benson/text-generation/Examples/Avakin Life Pc.md
deleted file mode 100644
index 02926a30996e18c4a25723d7ec42b59c5bfc562f..0000000000000000000000000000000000000000
--- a/spaces/Benson/text-generation/Examples/Avakin Life Pc.md
+++ /dev/null
@@ -1,50 +0,0 @@
-
- - H2: Cómo descargar e instalar Avakin Life en PC - H3: Cómo usar BlueStacks para jugar Avakin Life en PC - H3: Cómo usar el sitio web oficial de Avakin para jugar Avakin Life en PC - H2: Beneficios de jugar Avakin Life en PC PC H3: Mejores gráficos y rendimiento - H3: Más control y personalización - H3: Comunicación y traducción más fáciles - H2: Conclusión: Comienza tu segunda vida en el PC hoy - H4: Preguntas frecuentes | Tabla 2: Artículo con formato HTML
Avakin Life PC: Cómo jugar al mundo virtual en 3D en tu ordenador
-
Si estás buscando un juego de rol que te permita crear tu propio avatar, explorar un mundo virtual y conocer nuevos amigos, entonces deberías echar un vistazo a Avakin Life. Avakin Life es un juego de mundo virtual en 3D de Lockwood Publishing que está disponible en dispositivos iOS y Android. Puede personalizar su apariencia, estilo y hogar, ir a aventuras, unirse a concursos de moda y socializar con millones de jugadores de todo el mundo.
Pero ¿sabías que también puedes jugar Avakin Life en tu PC? Sí, lo has oído bien. Puedes disfrutar de este increíble juego en una pantalla más grande, con mejores gráficos, rendimiento y control. En este artículo, le mostraremos cómo descargar e instalar Avakin Life en su computadora usando dos métodos diferentes. También te contaremos los beneficios de jugar a Avakin Life en PC y responderemos algunas preguntas frecuentes. ¡Así que, empecemos!
-
Cómo descargar e instalar Avakin Life en PC
-
Hay dos formas de jugar Avakin Life en tu PC. Uno es utilizar un software emulador como BlueStacks, que le permite ejecutar aplicaciones y juegos de Android en su ordenador. La otra es utilizar el sitio web oficial de Avakin, que ofrece una versión web del juego a la que puedes acceder a través de tu navegador. Estos son los pasos para cada método:
-
Cómo usar BlueStacks para jugar Avakin Life en PC
-
-
-
Inicie BlueStacks e inicie sesión en su cuenta de Google. Esto le permitirá acceder a la Google Play Store.
-
Busque Avakin Life en Google Play Store y haga clic en el botón de instalación. Alternativamente, puede descargar el archivo APK de una fuente de confianza y arrastrarlo y soltarlo en BlueStacks.
-
Una vez completada la instalación, haga clic en el icono de Avakin Life en la pantalla de inicio de BlueStacks para comenzar a jugar.
-
-
Cómo usar el sitio web oficial de Avakin para jugar Avakin Life en PC
-
-
Vaya al sitio web oficial de Avakin (https://avakin.com) y haga clic en el botón "Descargar" en la esquina superior derecha.
-
Seleccione su plataforma preferida entre las opciones disponibles. Puede elegir entre Windows, Mac, Linux o Web.
-
Si elige Web, será redirigido a una página donde puede jugar Avakin Life directamente en su navegador. Tendrá que iniciar sesión con su cuenta de Facebook o crear una nueva cuenta con su dirección de correo electrónico.
-
Si elige cualquiera de las otras plataformas, tendrá que descargar e instalar un pequeño archivo lanzador que le permitirá jugar Avakin Life en su computadora. Siga las instrucciones en la pantalla para completar el proceso.
-
Una vez instalado el lanzador, ábralo e inicie sesión con su cuenta de Facebook o dirección de correo electrónico. A continuación, puede comenzar a jugar Avakin Life en su PC.
-
-
Beneficios de jugar Avakin Life en PC
-
Ahora que sabe cómo jugar Avakin Life en su PC, es posible que se pregunte por qué debe hacerlo. Bueno, hay muchas ventajas de jugar a este juego en un ordenador en lugar de en un dispositivo móvil. Estas son algunas de ellas:
-
Mejores gráficos y rendimiento
-
-
Más control y personalización
-
Otro beneficio de jugar Avakin Life en PC es que puedes tener más opciones de control y personalización. Puede utilizar el teclado y el ratón para navegar por el juego, que puede ser más conveniente y preciso que el uso de una pantalla táctil. También puedes ajustar la configuración del juego según tus preferencias, como la resolución, el sonido y el idioma. Incluso puedes usar trucos y hacks para mejorar tu juego, como conseguir monedas, gemas o objetos ilimitados. Sin embargo, ten cuidado de no abusar de estas características o podrías ser expulsado del juego.
-
Comunicación y traducción más fáciles
-
Un tercer beneficio de jugar Avakin Life en PC es que puedes comunicarte y traducir más fácilmente con otros jugadores. Puede usar su teclado para escribir más rápido y cómodamente que usando un teclado virtual. También puedes usar chat de voz o video chat para hablar con tus amigos o hacer otros nuevos. También puedes usar herramientas de traducción para entender e interactuar con jugadores de diferentes países y culturas. Puedes aprender nuevos idiomas, intercambiar ideas y divertirte con gente de todo el mundo.
-
Conclusión: Comience su segunda vida en el PC hoy
-
Avakin Life es un fantástico juego que te permite crear tu propio avatar, explorar un mundo virtual y conocer nuevos amigos. Pero si quieres llevar tu experiencia de juego al siguiente nivel, deberías intentar jugar a Avakin Life en PC. Puede disfrutar de mejores gráficos, rendimiento, control y personalización. También puede comunicarse y traducir más fácilmente con otros jugadores. Jugar a Avakin Life en PC te hará sentir que estás viviendo una segunda vida en un mundo virtual en 3D.
-
-
-
Esperamos que este artículo te haya ayudado a aprender a jugar Avakin Life en PC y por qué deberías hacerlo. Si tiene alguna pregunta o comentario, háganoslo saber en los comentarios a continuación. Nos encantaría saber de usted.
-
Preguntas frecuentes
-
-
Q: ¿Avakin Life es libre de jugar?
-
A: Sí, Avakin Life es gratis para jugar en dispositivos móviles y PC. Sin embargo, hay algunos elementos del juego y características que requieren dinero real para comprar. También puedes ver anuncios u ofertas completas para ganar monedas y gemas gratis.
-
Q: ¿Es la vida de Avakin segura para los niños?
-
A: Avakin Life está clasificado 12+ por la App Store y 13+ por la Google Play Store. Contiene violencia leve, contenido sexual, desnudez, blasfemia, alcohol, tabaco y drogas. También permite a los usuarios chatear con extraños en línea, lo que puede plantear algunos riesgos. Por lo tanto, se recomienda la orientación y supervisión de los padres para los jugadores más jóvenes.
-
Q: ¿Cómo puedo actualizar Avakin Life en PC?
-
A: Si está utilizando BlueStacks para jugar Avakin Life en PC, puede actualizar el juego yendo a la Google Play Store y haciendo clic en el botón de actualización. Si estás usando el sitio web oficial de Avakin para jugar a Avakin Life en PC, no necesitas actualizar el juego manualmente, ya que se actualizará automáticamente.
-
Q: ¿Cómo puedo eliminar mi cuenta de Avakin Life?
-
A: Si desea eliminar su cuenta de Avakin Life, debe ponerse en contacto con el equipo de atención al cliente a través de su sitio web (https://avakin.com/ support/) o correo electrónico (support@avakin.com). Deberá proporcionar su nombre de usuario, dirección de correo electrónico, ID de dispositivo y razón para eliminar su cuenta. Una vez procesada su solicitud, su cuenta será eliminada permanentemente.
-
Q: ¿Cómo me comunico con el soporte de Avakin Life?
-
-
64aa2da5cf
-
-
\ No newline at end of file
diff --git a/spaces/Benson/text-generation/Examples/Descargar Bus De Conduccin De Telolet 3d Mod Apk V1.2. 4b.md b/spaces/Benson/text-generation/Examples/Descargar Bus De Conduccin De Telolet 3d Mod Apk V1.2. 4b.md
deleted file mode 100644
index 6e1baf5bb90310755622487cfab50a5fb6f4dd66..0000000000000000000000000000000000000000
--- a/spaces/Benson/text-generation/Examples/Descargar Bus De Conduccin De Telolet 3d Mod Apk V1.2. 4b.md
+++ /dev/null
@@ -1,60 +0,0 @@
-
-
Descargar Telolet autobús de conducción 3D Mod APK v1.2. 4b y disfrutar de la diversión de conducir un autobús realista en Indonesia
-
Si eres un fan de los juegos de conducción de autobuses, es posible que hayas oído hablar de Telolet Bus Driving 3D, un juego revolucionario en el género de la conducción árcade sin fin con gráficos y control realistas en 3D. En este juego, usted puede viajar a través de los coches de tráfico de la carretera de Indonesia con un autobús muy fresco y hacer que los niños felices tocando la bocina de su único autobús telolet. Pero lo que si quieres disfrutar del juego sin limitaciones o interrupciones? Bueno, puedes hacerlo descargando Telolet Bus Driving 3D Mod APK v1.2. 4b, que te da dinero ilimitado, todos los autobuses desbloqueados, y sin anuncios. En este artículo, te contaremos más sobre este juego, sus características y cómo descargarlo e instalarlo en tu dispositivo.
-
descargar bus de conducción de telolet 3d mod apk v1.2. 4b
Telolet Bus Driving 3D es un juego desarrollado por LOCOS, un estudio de juegos indonesio que tiene como objetivo crear juegos divertidos y atractivos para todos. El juego se inspiró en el fenómeno viral de "Om Telolet Om", que significa "Señor, toca la bocina, señor" en indonesio. Esta es una frase que los niños gritan a los conductores de autobús para pedirles que toquen sus distintivos cuernos telolet, que producen un sonido musical. El juego fue lanzado en diciembre de 2016 y desde entonces ha ganado más de 10 millones de descargas en Google Play Store.
-
Características de Telolet Bus Driving 3D
-
Telolet Bus Driving 3D no es solo un juego de conducción simple. Tiene muchas características que lo hacen destacar de otros juegos del mismo género. Estos son algunos de ellos:
-
Impresionantes gráficos 3D
-
El juego tiene increíbles gráficos en 3D que te hacen sentir como si estuvieras conduciendo un autobús real en Indonesia. Puedes ver los detalles del autobús, el tráfico, el medio ambiente y los niños que te animan cuando tocas la bocina.
-
Manejo del coche suave y realista
-
-
Muchos autobuses para elegir
-
El juego tiene muchos autobuses para elegir, cada uno con su propio diseño, color, velocidad y melodía de cuerno telolet. Puedes desbloquear nuevos buses ganando monedas o usando la versión mod APK.
-
3 lugares famosos en Indonesia
-
El juego tiene 3 lugares famosos en Indonesia que puedes explorar: Pantura, Kampoeng y Cipali. Cada lugar tiene su propio paisaje, tráfico y desafíos.
-
-
3 modos de juego
-
El juego tiene 3 modos de juego: One Way, Rush Hour y Two Way. En el modo One Way, conduce en una carretera de un solo sentido con tráfico moderado. En el modo de hora punta, se enfrenta a un atasco de tráfico pesado y tiene que evitar colisiones. En el modo de dos vías, se conduce en una carretera de dos vías con tráfico entrante y tiene que adelantar a otros vehículos.
-
Tipos ricos de tráfico NPC Indonesia
-
El juego tiene ricos tipos de tráfico NPC Indonesia que hacen el juego más realista y desafiante. Usted encontrará coches, camiones, motocicletas, autobuses y otros vehículos que tienen diferentes comportamientos y velocidades. También verás peatones, animales y obstáculos en la carretera.
-
Actualizaciones de atributos
-
El juego tiene actualizaciones de atributos que le permiten mejorar el rendimiento y la apariencia de su autobús. Puedes actualizar tu velocidad, freno, bocina y color usando las monedas que ganes del juego o la versión mod APK.
-
Misiones diarias difíciles
-
El juego tiene desafiantes misiones diarias que te dan recompensas y objetivos adicionales. Puede completar varias tareas, como conducir cierta distancia, tocar la bocina un cierto número de veces, adelantar un cierto número de vehículos y más.
-
Tablas de clasificación en línea y logros
-
El juego tiene tablas de clasificación en línea y logros que le permiten competir con otros jugadores y mostrar sus habilidades. Puedes posicionarte en las tablas de clasificación globales y regionales al ganar altas puntuaciones y monedas. También puedes desbloquear logros al completar varios desafíos e hitos.
-
-
Telolet Bus Driving 3D es un juego divertido y adictivo que te mantendrá entretenido durante horas. Sin embargo, si quieres disfrutar del juego sin limitaciones ni interrupciones, debes descargar Telolet Bus Driving 3D Mod APK v1.2. 4b, que le da los siguientes beneficios:
-
Dinero ilimitado
-
Con la versión APK mod, usted tendrá dinero ilimitado que se puede utilizar para comprar y actualizar cualquier autobús que desee. No tienes que preocuparte por quedarte sin monedas o gastar dinero real para conseguir más.
-
Todos los autobuses desbloqueados
-
Con la versión mod APK, tendrás todos los buses desbloqueados desde el principio. No tienes que jugar durante horas o completar misiones para desbloquear nuevos autobuses. Puedes elegir el autobús que quieras y disfrutar de sus características únicas.
-
No hay anuncios
-
Con la versión mod APK, no tendrás anuncios que interrumpan tu juego o te molesten. No tienes que ver videos o hacer clic en banners para obtener monedas o recompensas adicionales. Puedes jugar el juego sin problemas y sin distracciones.
-
Cómo descargar e instalar Telolet Bus Driving 3D Mod APK v1.2. 4b?
-
Si está interesado en descargar e instalar Telolet Bus Driving 3D Mod APK v1.2. 4b en su dispositivo, puede seguir estos sencillos pasos:
-
Paso 1: Descargar el archivo APK de una fuente de confianza
-
El primer paso es descargar el archivo APK de una fuente de confianza que proporciona descargas seguras y libres de virus. Puede utilizar este enlace para descargar el archivo directamente a su dispositivo o transferirlo desde su PC.
-
Paso 2: Habilitar fuentes desconocidas en el dispositivo
-
El segundo paso es habilitar fuentes desconocidas en su dispositivo para que pueda instalar aplicaciones desde fuentes distintas de Google Play Store. Para hacer esto, vaya a Configuración > Seguridad > Fuentes desconocidas y conéctelo.
-
Paso 3: Instalar el archivo APK y disfrutar del juego
-
-
Esperamos que este artículo le haya ayudado a aprender más sobre Telolet Bus Driving 3D Mod APK v1.2. 4b y cómo descargarlo e instalarlo en su dispositivo. Este es un gran juego para los entusiastas de la conducción de autobuses que quieren experimentar la emoción de conducir un autobús realista en Indonesia con un cuerno musical. ¡Descárgalo ahora y diviértete!
-
Conclusión
-
Telolet Bus Driving 3D es un juego innovador en el género de la conducción árcade sin fin con gráficos y control 3D realistas. Fue inspirado por el fenómeno viral de "Om Telolet Om", que significa "Señor, toca la bocina, señor" en indonesio. El juego tiene muchas características que lo hacen destacar de otros juegos en el mismo género, tales como impresionantes gráficos en 3D, manejo de automóviles suave y realista, muchos autobuses para elegir, 3 lugares famosos en Indonesia, 3 modos de juego, ricos tipos de tráfico NPC Indonesia, actualizaciones de atributos, misiones diarias desafiantes, y tablas de clasificación en línea y logros. Sin embargo, si quieres disfrutar del juego sin limitaciones ni interrupciones, debes descargar Telolet Bus Driving 3D Mod APK v1.2. 4b, que le da dinero ilimitado, todos los autobuses desbloqueados, y sin anuncios. Para descargar e instalar la versión mod APK, solo tiene que seguir tres sencillos pasos: descargar el archivo APK de una fuente de confianza, habilitar fuentes desconocidas en su dispositivo, e instalar el archivo APK y disfrutar del juego. Este es un gran juego para los entusiastas de la conducción de autobuses que quieren experimentar la emoción de conducir un autobús realista en Indonesia con un cuerno musical. ¡Descárgalo ahora y diviértete!
-
Preguntas frecuentes
-
Aquí hay algunas preguntas frecuentes sobre Telolet Bus Driving 3D Mod APK v1.2. 4b:
-
Es Telolet autobús de conducción 3D Mod APK v1.2. 4b seguro para descargar e instalar?
-
Sí, Telolet autobús de conducción 3D Mod APK v1.2. 4b es seguro para descargar e instalar siempre y cuando utilice una fuente de confianza que proporciona descargas libres de virus. Puede utilizar este enlace para descargar el archivo de forma segura.
-
-
No, no es necesario rootear el dispositivo para usar Telolet Bus Driving 3D Mod APK v1.2. 4b. Solo necesitas habilitar fuentes desconocidas en la configuración de tu dispositivo e instalar el archivo APK como de costumbre.
-
Será Telolet autobús de conducción 3D Mod APK v1.2. 4b afectar mi progreso original del juego?
-
No, Telolet Bus Driving 3D Mod APK v1.2. 4b no afectará su progreso original del juego. Puedes jugar ambas versiones por separado y cambiar entre ellas cuando quieras.
-
¿Puedo jugar Telolet autobús de conducción 3D Mod APK v1.2. 4b en línea con otros jugadores?
-
Sí, se puede jugar Telolet autobús de conducción 3D Mod APK v1.2. 4b en línea con otros jugadores y competir en las tablas de clasificación y logros. Sin embargo, es posible que encuentre algunos problemas de compatibilidad con los jugadores que utilizan la versión original del juego.
-
¿Cómo puedo contactar al desarrollador de Telolet Bus Driving 3D Mod APK v1.2. 4b si tengo alguna pregunta o comentario?
-
Puede ponerse en contacto con el desarrollador de Telolet Bus Driving 3D Mod APK v1.2. 4b enviando un correo electrónico a locosgames@gmail.com o visitando su página de Facebook en https://www.facebook.com/locosgames/ Estarán encantados de saber de usted y responder a sus preguntas o comentarios.
64aa2da5cf
-
-
\ No newline at end of file
diff --git a/spaces/Big-Web/MMSD/env/Lib/site-packages/pip/_vendor/rich/json.py b/spaces/Big-Web/MMSD/env/Lib/site-packages/pip/_vendor/rich/json.py
deleted file mode 100644
index ea94493f21e6f5583469d882d08203381ee31117..0000000000000000000000000000000000000000
--- a/spaces/Big-Web/MMSD/env/Lib/site-packages/pip/_vendor/rich/json.py
+++ /dev/null
@@ -1,140 +0,0 @@
-from pathlib import Path
-from json import loads, dumps
-from typing import Any, Callable, Optional, Union
-
-from .text import Text
-from .highlighter import JSONHighlighter, NullHighlighter
-
-
-class JSON:
- """A renderable which pretty prints JSON.
-
- Args:
- json (str): JSON encoded data.
- indent (Union[None, int, str], optional): Number of characters to indent by. Defaults to 2.
- highlight (bool, optional): Enable highlighting. Defaults to True.
- skip_keys (bool, optional): Skip keys not of a basic type. Defaults to False.
- ensure_ascii (bool, optional): Escape all non-ascii characters. Defaults to False.
- check_circular (bool, optional): Check for circular references. Defaults to True.
- allow_nan (bool, optional): Allow NaN and Infinity values. Defaults to True.
- default (Callable, optional): A callable that converts values that can not be encoded
- in to something that can be JSON encoded. Defaults to None.
- sort_keys (bool, optional): Sort dictionary keys. Defaults to False.
- """
-
- def __init__(
- self,
- json: str,
- indent: Union[None, int, str] = 2,
- highlight: bool = True,
- skip_keys: bool = False,
- ensure_ascii: bool = False,
- check_circular: bool = True,
- allow_nan: bool = True,
- default: Optional[Callable[[Any], Any]] = None,
- sort_keys: bool = False,
- ) -> None:
- data = loads(json)
- json = dumps(
- data,
- indent=indent,
- skipkeys=skip_keys,
- ensure_ascii=ensure_ascii,
- check_circular=check_circular,
- allow_nan=allow_nan,
- default=default,
- sort_keys=sort_keys,
- )
- highlighter = JSONHighlighter() if highlight else NullHighlighter()
- self.text = highlighter(json)
- self.text.no_wrap = True
- self.text.overflow = None
-
- @classmethod
- def from_data(
- cls,
- data: Any,
- indent: Union[None, int, str] = 2,
- highlight: bool = True,
- skip_keys: bool = False,
- ensure_ascii: bool = False,
- check_circular: bool = True,
- allow_nan: bool = True,
- default: Optional[Callable[[Any], Any]] = None,
- sort_keys: bool = False,
- ) -> "JSON":
- """Encodes a JSON object from arbitrary data.
-
- Args:
- data (Any): An object that may be encoded in to JSON
- indent (Union[None, int, str], optional): Number of characters to indent by. Defaults to 2.
- highlight (bool, optional): Enable highlighting. Defaults to True.
- default (Callable, optional): Optional callable which will be called for objects that cannot be serialized. Defaults to None.
- skip_keys (bool, optional): Skip keys not of a basic type. Defaults to False.
- ensure_ascii (bool, optional): Escape all non-ascii characters. Defaults to False.
- check_circular (bool, optional): Check for circular references. Defaults to True.
- allow_nan (bool, optional): Allow NaN and Infinity values. Defaults to True.
- default (Callable, optional): A callable that converts values that can not be encoded
- in to something that can be JSON encoded. Defaults to None.
- sort_keys (bool, optional): Sort dictionary keys. Defaults to False.
-
- Returns:
- JSON: New JSON object from the given data.
- """
- json_instance: "JSON" = cls.__new__(cls)
- json = dumps(
- data,
- indent=indent,
- skipkeys=skip_keys,
- ensure_ascii=ensure_ascii,
- check_circular=check_circular,
- allow_nan=allow_nan,
- default=default,
- sort_keys=sort_keys,
- )
- highlighter = JSONHighlighter() if highlight else NullHighlighter()
- json_instance.text = highlighter(json)
- json_instance.text.no_wrap = True
- json_instance.text.overflow = None
- return json_instance
-
- def __rich__(self) -> Text:
- return self.text
-
-
-if __name__ == "__main__":
-
- import argparse
- import sys
-
- parser = argparse.ArgumentParser(description="Pretty print json")
- parser.add_argument(
- "path",
- metavar="PATH",
- help="path to file, or - for stdin",
- )
- parser.add_argument(
- "-i",
- "--indent",
- metavar="SPACES",
- type=int,
- help="Number of spaces in an indent",
- default=2,
- )
- args = parser.parse_args()
-
- from pip._vendor.rich.console import Console
-
- console = Console()
- error_console = Console(stderr=True)
-
- try:
- if args.path == "-":
- json_data = sys.stdin.read()
- else:
- json_data = Path(args.path).read_text()
- except Exception as error:
- error_console.print(f"Unable to read {args.path!r}; {error}")
- sys.exit(-1)
-
- console.print(JSON(json_data, indent=args.indent), soft_wrap=True)
diff --git a/spaces/CAMP-ViL/Xplainer/app.py b/spaces/CAMP-ViL/Xplainer/app.py
deleted file mode 100644
index b2b673afd870e6fb48cf1f3d791007c931d20c6b..0000000000000000000000000000000000000000
--- a/spaces/CAMP-ViL/Xplainer/app.py
+++ /dev/null
@@ -1,137 +0,0 @@
-from pathlib import Path
-
-import gradio as gr
-import numpy as np
-from matplotlib import pyplot as plt
-
-from descriptors import disease_descriptors_chexpert, disease_descriptors_chestxray14
-from model import InferenceModel
-
-
-def plot_bars(model_output):
- # sort model_output by overall_probability
- model_output = {k: v for k, v in sorted(model_output.items(), key=lambda item: item[1]['overall_probability'], reverse=True)}
-
- # Create a figure with as many subplots as there are diseases, arranged vertically
- fig, axs = plt.subplots(len(model_output), 1, figsize=(10, 5 * len(model_output)))
- # axs is not iterable if only one subplot is created, so make it a list
- if len(model_output) == 1:
- axs = [axs]
-
- for ax, (disease, data) in zip(axs, model_output.items()):
- desc_probs = list(data['descriptor_probabilities'].items())
- # sort descending
- desc_probs = sorted(desc_probs, key=lambda item: item[1], reverse=True)
-
- my_probs = [p[1] for p in desc_probs]
- min_prob = min(my_probs)
- max_prob = max(my_probs)
- my_labels = [p[0] for p in desc_probs]
-
- # Convert probabilities to differences from 0.5
- diffs = np.abs(np.array(my_probs) - 0.5)
-
- # Set colors based on sign of difference
- colors = ['red' if p < 0.5 else 'forestgreen' for p in my_probs]
-
- # Plot bars with appropriate colors and left offsets
- left = [p if p < 0.5 else 0.5 for p in my_probs]
- bars = ax.barh(my_labels, diffs, left=left, color=colors, alpha=0.3)
-
- for i, bar in enumerate(bars):
- ax.text(min_prob - 0.04, bar.get_y() + bar.get_height() / 2, my_labels[i], ha='left', va='center', color='black', fontsize=15)
-
- ax.set_xlim(min(min_prob - 0.05, 0.49), max(max_prob + 0.05, 0.51))
-
- # Invert the y-axis to show bars with values less than 0.5 to the left of the center
- ax.invert_yaxis()
-
- ax.set_yticks([])
-
- # Add a title for the disease
- if data['overall_probability'] >= 0.5:
- ax.set_title(f"{disease} : score of {data['overall_probability']:.2f}")
- else:
- ax.set_title(f"No {disease} : score of {data['overall_probability']:.2f}")
-
- # make title larger and bold
- ax.title.set_fontsize(15)
- ax.title.set_fontweight(600)
-
- # Save the plot
- plt.tight_layout() # Adjust subplot parameters to give specified padding
- file_path = 'plot.png'
- plt.savefig(file_path)
- plt.close(fig)
-
- return file_path
-
-
-def classify_image(inference_model, image_path, diseases_to_predict):
- descriptors_with_indication = [d + " indicating " + disease for disease, descriptors in diseases_to_predict.items() for d in descriptors]
- probs, negative_probs = inference_model.get_descriptor_probs(image_path=Path(image_path), descriptors=descriptors_with_indication,
- do_negative_prompting=True, demo=True)
-
- disease_probs, negative_disease_probs = inference_model.get_diseases_probs(diseases_to_predict, pos_probs=probs, negative_probs=negative_probs)
-
- model_output = {}
- for idx, disease in enumerate(diseases_to_predict.keys()):
- model_output[disease] = {
- 'overall_probability': disease_probs[disease],
- 'descriptor_probabilities': {descriptor: probs[f'{descriptor} indicating {disease}'].item() for descriptor in
- diseases_to_predict[disease]}
- }
-
- file_path = plot_bars(model_output)
- return file_path
-
-
-# Define the function you want to wrap
-def process_input(image_path, prompt_names: list, disease_name: str, descriptors: str):
- diseases_to_predict = {}
-
- for prompt in prompt_names:
- if prompt == 'Custom':
- diseases_to_predict[disease_name] = descriptors.split('\n')
- else:
- if prompt in disease_descriptors_chexpert:
- diseases_to_predict[prompt] = disease_descriptors_chexpert[prompt]
- else: # only chestxray14
- diseases_to_predict[prompt] = disease_descriptors_chestxray14[prompt]
-
- # classify
- model = InferenceModel()
- output = classify_image(model, image_path, diseases_to_predict)
-
- return output
-
-with open("article.md", "r") as f:
- article = f.read()
-with open("description.md", "r") as f:
- description = f.read()
-
-# Define the Gradio interface
-iface = gr.Interface(
- fn=process_input,
- examples = [['examples/enlarged_cardiomediastinum.jpg', ['Enlarged Cardiomediastinum'], '', ''],['examples/edema.jpg', ['Edema'], '', ''],
- ['examples/support_devices.jpg', ['Custom'], 'Pacemaker', 'metalic object\nimplant on the left side of the chest\nimplanted cardiac device']],
- inputs=[gr.inputs.Image(type="filepath"), gr.inputs.CheckboxGroup(
- choices=['Enlarged Cardiomediastinum', 'Cardiomegaly', 'Lung Opacity', 'Lung Lesion', 'Edema', 'Consolidation', 'Pneumonia',
- 'Atelectasis', 'Pneumothorax', 'Pleural Effusion', 'Pleural Other', 'Fracture', 'Support Devices',
- 'Infiltration', 'Mass', 'Nodule', 'Emphysema', 'Fibrosis', 'Pleural Thickening', 'Hernia',
- 'Custom'],
- default=['Enlarged Cardiomediastinum', 'Cardiomegaly', 'Lung Opacity', 'Lung Lesion', 'Edema', 'Consolidation', 'Pneumonia',
- 'Atelectasis', 'Pneumothorax', 'Pleural Effusion', 'Pleural Other', 'Fracture', 'Support Devices'],
- label='Select to use predefined disease descriptors. Select "Custom" to define your own observations.'),
- gr.inputs.Textbox(lines=2, placeholder="Name of pathology for which you want to define custom observations", label='Pathology:'),
- gr.inputs.Textbox(lines=2, placeholder="Add your custom (positive) observations separated by a new line"
- "\n Note: Each descriptor will automatically be embedded into our prompt format: There is/are (no) indicating "
- "\n Example:\n\n Opacity\nPleural Effusion\nConsolidation"
- , label='Custom Observations:')],
- article=article,
- description=description,
- outputs=gr.outputs.Image(type="filepath")
-)
-
-# Launch the interface
-iface.launch()
diff --git a/spaces/CVPR/Dual-Key_Backdoor_Attacks/openvqa/docs/_templates/layout.html b/spaces/CVPR/Dual-Key_Backdoor_Attacks/openvqa/docs/_templates/layout.html
deleted file mode 100644
index 7280406960f90844f60619e1d1ebc5ee7562a046..0000000000000000000000000000000000000000
--- a/spaces/CVPR/Dual-Key_Backdoor_Attacks/openvqa/docs/_templates/layout.html
+++ /dev/null
@@ -1,35 +0,0 @@
-{% extends "!layout.html" %}
-
-
-{% block menu %}
-
- """,
- unsafe_allow_html=True,
- )
-
- examples = [
- "images/1.jpg",
- "images/ch_en_num.jpg",
- "images/air_ticket.jpg",
- "images/car_plate.jpeg",
- "images/train_ticket.jpeg",
- "images/japan_2.jpg",
- "images/korean_1.jpg",
- ]
-
- init_sidebar()
-
- menu_det, menu_rec = st.columns([1, 1])
- det_models = [
- "ch_PP-OCRv4_det_infer.onnx",
- "ch_PP-OCRv3_det_infer.onnx",
- "ch_PP-OCRv2_det_infer.onnx",
- "ch_ppocr_server_v2.0_det_infer.onnx",
- ]
- select_det = menu_det.selectbox("Det model:", det_models)
-
- rec_models = [
- "ch_PP-OCRv4_rec_infer.onnx",
- "ch_PP-OCRv3_rec_infer.onnx",
- "ch_PP-OCRv2_rec_infer.onnx",
- "ch_PP-OCRv4_det_server_infer.onnx",
- "ch_ppocr_server_v2.0_rec_infer.onnx",
- "en_PP-OCRv3_rec_infer.onnx",
- "en_number_mobile_v2.0_rec_infer.onnx",
- "korean_mobile_v2.0_rec_infer.onnx",
- "japan_rec_crnn_v2.onnx",
- ]
- select_rec = menu_rec.selectbox("Rec model:", rec_models)
-
- with st.form("my-form", clear_on_submit=True):
- img_file_buffer = st.file_uploader(
- "Upload an image",
- accept_multiple_files=False,
- label_visibility="visible",
- type=["png", "jpg", "jpeg", "bmp"],
- )
- submit = st.form_submit_button("Upload")
- if submit and img_file_buffer is not None:
- image = Image.open(img_file_buffer)
- img = np.array(image)
- st.session_state["img"] = img
-
- if st.session_state["img"] is not None:
- out_img, out_json, elapse = inference(select_det, select_rec)
- if all(v is not None for v in [out_img, out_json, elapse]):
- st.markdown("#### Visualize:")
- st.image(out_img)
-
- st.markdown("### Rec Result:")
- st.markdown(elapse)
- st.dataframe(out_json, use_container_width=True)
- else:
- tips("识别结果为空", wait_time=5, icon="⚠️")
diff --git a/spaces/SakshiRathi77/SakshiRathi77-Wav2Vec2-hi-kagglex/app.py b/spaces/SakshiRathi77/SakshiRathi77-Wav2Vec2-hi-kagglex/app.py
deleted file mode 100644
index 4cf090b5d75c52f35944fd078ac57109b73596c5..0000000000000000000000000000000000000000
--- a/spaces/SakshiRathi77/SakshiRathi77-Wav2Vec2-hi-kagglex/app.py
+++ /dev/null
@@ -1,99 +0,0 @@
-import torch
-import gradio as gr
-import pytube as pt
-from transformers import pipeline
-from huggingface_hub import model_info
-import time
-import unicodedata
-from gradio.themes.utils.theme_dropdown import create_theme_dropdown
-
-MODEL_NAME = "SakshiRathi77/wav2vec2-large-xlsr-300m-hi-kagglex"
-lang = "hi"
-
-my_theme = gr.Theme.from_hub('freddyaboulton/dracula_revamped')
-device = 0 if torch.cuda.is_available() else "cpu"
-pipe = pipeline(
- task="automatic-speech-recognition",
- model=MODEL_NAME,
- device=device,
-)
-
-def transcribe(microphone, file_upload):
- warn_output = ""
- if (microphone is not None) and (file_upload is not None):
- warn_output = (
- "WARNING: You've uploaded an audio file and used the microphone. "
- "The recorded file from the microphone will be used and the uploaded audio will be discarded.\n"
- )
-
- elif (microphone is None) and (file_upload is None):
- return "ERROR: You have to either use the microphone or upload an audio file"
- file = microphone if microphone is not None else file_upload
- text = pipe(file)["text"]
-
- return warn_output + text
-
-
-def rt_transcribe(audio, state=""):
- time.sleep(2)
- text = pipe(audio)["text"]
- state += unicodedata.normalize("NFC",text) + " "
-
- return state, state
-
-
-
-demo = gr.Blocks(theme=my_theme)
-examples=[["examples/example1.mp3"], ["examples/example2.mp3"],["examples/example3.mp3"]]
-
-title ="""
-HindiSpeechPro: WAV2VEC-Powered ASR Interface
-"""
-
-description = """
-
-
-Welcome to the HindiSpeechPro, a cutting-edge interface powered by a fine-tuned version of facebook/wav2vec2-xls-r-300m on the common_voice dataset.
-
-
-
-In the lower left-hand corner of the window, you should see a button that says free 30-day evaluation or something similar.. Get a free 30-day evaluation of the latest version of Rider for Windows, macOS or Linux.. Make sure you have a working internet connection, and then select Get Installer to download the installer for Rider.
-
-. Rider Pro/Team Rider Pro/Team Dual licenses include a free 30-day evaluation of all product versions. Rider Pro/Team is a professional and team edition of Rider, the professional engineering development environment. It is designed specifically for professional use, and provides features that are not included in Rider or Rider Dual. Includes advanced language tools for C, C++, C#, F#, VB.NET, SQL, JavaScript, HTML, XML, CSS, ASP.NET, JSON, PHP, Ruby, Python, R, Golang, and XML/XSD. Includes unlimited number of installed users, unlimited connections, unlimited versions, unlimited physical resources and unlimited data files. Also included are more than 20 database types, including MyISAM, MariaDB, and MySQL.
-
-. Details: The software download includes a 30-day free evaluation of the software. The free evaluation license can be used on one computer or in a network license.
-
-. Details: This product requires a serial number, activation code, and product key to be used. Installation instructions will be sent via email after the serial number is entered and the product key is confirmed. The product key can be used on up to two computers, each of which is installed by a different user.
-
-. Details: Requires a serial number, activation code, and product key to be used. Installation instructions will be sent via email after the serial number is entered and the product key is confirmed. The product key can be used on up to two computers, each of which is installed by a different user.
-
-. Details: Requires a serial number, activation code, and product key to be used. Installation instructions will be sent via email after the serial number is entered and the product key is confirmed. The product key can be used on up to two computers, each of which is 4fefd39f24
-
-
-
diff --git a/spaces/bioriAsaeru/text-to-voice/Kabhi Kahin 2 Movie In Hindi 720p Download ((HOT)) Torrent.md b/spaces/bioriAsaeru/text-to-voice/Kabhi Kahin 2 Movie In Hindi 720p Download ((HOT)) Torrent.md
deleted file mode 100644
index a21dee2c6d0b1f991271a4e77f135220acaab52a..0000000000000000000000000000000000000000
--- a/spaces/bioriAsaeru/text-to-voice/Kabhi Kahin 2 Movie In Hindi 720p Download ((HOT)) Torrent.md
+++ /dev/null
@@ -1,12 +0,0 @@
-
Kabhi Kahin 2 Movie In Hindi 720p Download Torrent
-
-atreya sasthak serial full video, free download atreya sasthak mp3 song, kahin bhi kahin bhi online free hindi mp4 video song, hindi movie songs download full hd 720p atreya sasthak, download hindi movie song. Atreya sasthak serial full video, free download atreya sasthak mp3 song, kahin bhi kahin bhi online free hindi mp4 video song, hindi movie songs download full hd 720p. It's Bollywood Drama film, it's action, love story, romantic movie. that's story of the movie. most of the story of the movie is about man, power and money. The movie is story of different characters. and the story of the movie is about man's future. watch the best indian kollywood movie(it's. Free download atreya sasthak serial full video, free download atreya sasthak mp3 song, kahin bhi kahin bhi online free hindi mp4 video song, hindi movie songs download full hd 720p. Atreya sasthak serial full video, free download atreya sasthak mp3 song, kahin bhi kahin bhi online free hindi mp4 video song, hindi movie songs download full hd 720p. It's Bollywood Drama film, it's action, love story, romantic movie. that's story of the movie. most of the story of the movie is about man, power and money. The movie is story of different characters. and the story of the movie is about man's future. watch the best indian kollywood movie(it's.1. Field of the Invention
-
-The invention relates generally to the field of well bore hydrocarbon fluid production, and more particularly to systems and methods for recovering methane gas from a well bore hydrocarbon fluid production stream.
-
-2. Background of the Invention
-
-In the oil and gas industry, it is desirable to extract oil from oil bearing formations or reservoirs that exist below the earth's surface and bring the extracted oil to the surface for collection, processing, and transport to oil refineries or other locations. The most common method for extracting oil from subterranean reservoirs is by utilizing the natural pressure that exists within the reservoir to force the oil to the surface. In this method, referred to as � 4fefd39f24
-
-
-
diff --git a/spaces/brainblow/beat_remixer/beat_manipulator/beatmap.py b/spaces/brainblow/beat_remixer/beat_manipulator/beatmap.py
deleted file mode 100644
index 7536a8b66a139d54d7b47abce5f115cabeb8f6fa..0000000000000000000000000000000000000000
--- a/spaces/brainblow/beat_remixer/beat_manipulator/beatmap.py
+++ /dev/null
@@ -1,195 +0,0 @@
-import numpy as np
-from . import utils
-
-
-def scale(beatmap:np.ndarray, scale:float, log = True, integer = True) -> np.ndarray:
- if isinstance(scale, str): scale = utils._safer_eval(scale)
- assert scale>0, f"scale should be > 0, your scale is {scale}"
- if scale == 1: return beatmap
- else:
- import math
- if log is True: print(f'scale={scale}; ')
- a = 0
- b = np.array([], dtype=int)
- if scale%1==0:
- while a < len(beatmap):
- b = np.append(b, beatmap[int(a)])
- a += scale
- else:
- if integer is True:
- while a + 1 < len(beatmap):
- b = np.append(b, int((1 - (a % 1)) * beatmap[math.floor(a)] + (a % 1) * beatmap[math.ceil(a)]))
- a += scale
- else:
- while a + 1 < len(beatmap):
- b = np.append(b, (1 - (a % 1)) * beatmap[math.floor(a)] + (a % 1) * beatmap[math.ceil(a)])
- a += scale
- return b
-
-def shift(beatmap:np.ndarray, shift:float, log = True, mode = 1) -> np.ndarray:
- if isinstance(shift, str): shift = utils._safer_eval(shift)
- if shift == 0: return beatmap
- # positive shift
- elif shift > 0:
- # full value of beats is removed from the beginning
- if shift >= 1: beatmap = beatmap[int(shift//1):]
- # shift beatmap by the decimal value
- if shift%1 != 0:
- shift = shift%1
- for i in range(len(beatmap) - int(shift) - 1):
- beatmap[i] = int(beatmap[i] + shift * (beatmap[i + 1] - beatmap[i]))
-
- # negative shift
- else:
- shift = -shift
- # full values are inserted in between first beats
- if shift >= 1:
- if mode == 1:
- step = int((beatmap[1] - beatmap[0]) / (int(shift//1) + 1))
- beatmap = np.insert(arr = beatmap, obj = 1, values = np.linspace(start = beatmap[0] + step - 1, stop = 1 + beatmap[1] - step, num = int(shift//1)))
- elif mode == 2:
- for i in range(int(shift//1)):
- beatmap = np.insert(arr = beatmap, obj = (i*2)+1, values = int((beatmap[i*2] + beatmap[(i*2)+1])/2))
- # shift beatmap by the decimal value
- if shift%1 != 0:
- shift = shift%1
- for i in reversed(range(len(beatmap))):
- if i==0: continue
- beatmap[i] = int(beatmap[i] - shift * (beatmap[i] - beatmap[i-1]))
- return beatmap
-
-def generate(audio: np.ndarray, sr: int, lib='madmom.BeatDetectionProcessor', caching=True, filename: str = None, log = True, load_settings = True, split=None):
- """Creates beatmap attribute with a list of positions of beats in samples."""
- if log is True: print(f'Analyzing beats using {lib}; ', end='')
-
- # load a beatmap if it is cached:
- if caching is True and filename is not None:
- audio_id=hex(len(audio[0]))
- import os
- if not os.path.exists('beat_manipulator/beatmaps'):
- os.mkdir('beat_manipulator/beatmaps')
- cacheDir="beat_manipulator/beatmaps/" + ''.join(filename.replace('\\', '/').split('/')[-1]) + "_"+lib+"_"+audio_id+'.txt'
- try:
- beatmap=np.loadtxt(cacheDir, dtype=int)
- if log is True: print('loaded cached beatmap.')
- except OSError:
- if log is True:print("beatmap hasn't been generated yet. Generating...")
- beatmap = None
-
- #generate the beatmap
- if beatmap is None:
- if 'madmom' in lib.lower():
- from collections.abc import MutableMapping, MutableSequence
- import madmom
- assert len(audio[0])>sr*2, f'Audio file is too short, len={len(audio[0])} samples, or {len(audio[0])/sr} seconds. Minimum length is 2 seconds, audio below that breaks madmom processors.'
- if lib=='madmom.BeatTrackingProcessor':
- proc = madmom.features.beats.BeatTrackingProcessor(fps=100)
- act = madmom.features.beats.RNNBeatProcessor()(madmom.audio.signal.Signal(audio.T, sr))
- beatmap= proc(act)*sr
- elif lib=='madmom.BeatTrackingProcessor.constant':
- proc = madmom.features.beats.BeatTrackingProcessor(fps=100, look_ahead=None)
- act = madmom.features.beats.RNNBeatProcessor()(madmom.audio.signal.Signal(audio.T, sr))
- beatmap= proc(act)*sr
- elif lib=='madmom.BeatTrackingProcessor.consistent':
- proc = madmom.features.beats.BeatTrackingProcessor(fps=100, look_ahead=None, look_aside=0)
- act = madmom.features.beats.RNNBeatProcessor()(madmom.audio.signal.Signal(audio.T, sr))
- beatmap= proc(act)*sr
- elif lib=='madmom.BeatDetectionProcessor':
- proc = madmom.features.beats.BeatDetectionProcessor(fps=100)
- act = madmom.features.beats.RNNBeatProcessor()(madmom.audio.signal.Signal(audio.T, sr))
- beatmap= proc(act)*sr
- elif lib=='madmom.BeatDetectionProcessor.consistent':
- proc = madmom.features.beats.BeatDetectionProcessor(fps=100, look_aside=0)
- act = madmom.features.beats.RNNBeatProcessor()(madmom.audio.signal.Signal(audio.T, sr))
- beatmap= proc(act)*sr
- elif lib=='madmom.CRFBeatDetectionProcessor':
- proc = madmom.features.beats.CRFBeatDetectionProcessor(fps=100)
- act = madmom.features.beats.RNNBeatProcessor()(madmom.audio.signal.Signal(audio.T, sr))
- beatmap= proc(act)*sr
- elif lib=='madmom.CRFBeatDetectionProcessor.constant':
- proc = madmom.features.beats.CRFBeatDetectionProcessor(fps=100, use_factors=True, factors=[0.5, 1, 2])
- act = madmom.features.beats.RNNBeatProcessor()(madmom.audio.signal.Signal(audio.T, sr))
- beatmap= proc(act)*sr
- elif lib=='madmom.DBNBeatTrackingProcessor':
- proc = madmom.features.beats.DBNBeatTrackingProcessor(fps=100)
- act = madmom.features.beats.RNNBeatProcessor()(madmom.audio.signal.Signal(audio.T, sr))
- beatmap= proc(act)*sr
- elif lib=='madmom.DBNBeatTrackingProcessor.1000':
- proc = madmom.features.beats.DBNBeatTrackingProcessor(fps=100, transition_lambda=1000)
- act = madmom.features.beats.RNNBeatProcessor()(madmom.audio.signal.Signal(audio.T, sr))
- beatmap= proc(act)*sr
- elif lib=='madmom.DBNDownBeatTrackingProcessor':
- proc = madmom.features.downbeats.DBNDownBeatTrackingProcessor(beats_per_bar=[4], fps=100)
- act = madmom.features.downbeats.RNNDownBeatProcessor()(madmom.audio.signal.Signal(audio.T, sr))
- beatmap= proc(act)*sr
- beatmap=beatmap[:,0]
- elif lib=='madmom.PatternTrackingProcessor': #broken
- from madmom.models import PATTERNS_BALLROOM
- proc = madmom.features.downbeats.PatternTrackingProcessor(PATTERNS_BALLROOM, fps=50)
- from madmom.audio.spectrogram import LogarithmicSpectrogramProcessor, SpectrogramDifferenceProcessor, MultiBandSpectrogramProcessor
- from madmom.processors import SequentialProcessor
- log = LogarithmicSpectrogramProcessor()
- diff = SpectrogramDifferenceProcessor(positive_diffs=True)
- mb = MultiBandSpectrogramProcessor(crossover_frequencies=[270])
- pre_proc = SequentialProcessor([log, diff, mb])
- act = pre_proc(madmom.audio.signal.Signal(audio.T, sr))
- beatmap= proc(act)*sr
- beatmap=beatmap[:,0]
- elif lib=='madmom.DBNBarTrackingProcessor': #broken
- beats = generate(audio=audio, sr=sr, filename=filename, lib='madmom.DBNBeatTrackingProcessor', caching = caching)
- proc = madmom.features.downbeats.DBNBarTrackingProcessor(beats_per_bar=[4], fps=100)
- act = madmom.features.downbeats.RNNBarProcessor()(((madmom.audio.signal.Signal(audio.T, sr)), beats))
- beatmap= proc(act)*sr
- elif lib=='librosa': #broken in 3.9, works in 3.8
- import librosa
- beat_frames = librosa.beat.beat_track(y=audio[0], sr=sr, hop_length=512)
- beatmap = librosa.frames_to_samples(beat_frames[1])
-
- # save the beatmap and return
- if caching is True: np.savetxt(cacheDir, beatmap.astype(int), fmt='%d')
- if not isinstance(beatmap, np.ndarray): beatmap=np.asarray(beatmap, dtype=int)
- else: beatmap=beatmap.astype(int)
-
- if load_settings is True:
- settingsDir="beat_manipulator/beatmaps/" + ''.join(filename.split('/')[-1]) + "_"+lib+"_"+audio_id+'_settings.txt'
- if os.path.exists(settingsDir):
- with open(settingsDir, 'r') as f:
- settings = f.read().split(',')
- if settings[0] != 'None': beatmap = scale(beatmap, settings[0], log = False)
- if settings[1] != 'None': beatmap = shift(beatmap, settings[1], log = False)
- if settings[2] != 'None': beatmap = np.sort(np.absolute(beatmap - int(settings[2])))
-
- return beatmap
-
-
-
-def save_settings(audio: np.ndarray, filename: str = None, lib: str = 'madmom.BeatDetectionProcessor', scale: float = None, shift: float = None, adjust: int = None, normalized: str = None, log = True, overwrite = 'ask'):
- if isinstance(overwrite, str): overwrite = overwrite.lower()
- audio_id=hex(len(audio[0]))
- cacheDir="beat_manipulator/beatmaps/" + ''.join(filename.split('/')[-1]) + "_"+lib+"_"+audio_id+'.txt'
- import os
- assert os.path.exists(cacheDir), f"Beatmap `{cacheDir}` doesn't exist"
- settingsDir="beat_manipulator/beatmaps/" + ''.join(filename.split('/')[-1]) + "_"+lib+"_"+audio_id+'_settings.txt'
-
- try:
- a = utils._safer_eval_strict(scale)
- if a == 1: scale = None
- except Exception as e: assert scale is None, f'scale = `{scale}` - Not a valid scale, should be either a number, a math expression, or None: {e}'
- try:
- a = utils._safer_eval_strict(shift)
- if a == 0: shift = None
- except Exception as e: assert shift is None, f'shift = `{shift}` - Not a valid shift: {e}'
- assert isinstance(adjust, int) or adjust is None, f'adjust = `{adjust}` should be int, but it is `{type(adjust)}`'
-
- if adjust == 0: adjust = None
-
- if os.path.exists(settingsDir):
- if overwrite == 'ask' or overwrite =='a':
- what = input(f'`{settingsDir}` already exists. Overwrite (y/n)?: ')
- if not (what.lower() == 'y' or what.lower() == 'yes'): return
- elif not (overwrite == 'true' or overwrite =='y' or overwrite =='yes' or overwrite is True): return
-
- with open(settingsDir, 'w') as f:
- f.write(f'{scale},{shift},{adjust},{normalized}')
- if log is True: print(f"Saved scale = `{scale}`, shift = `{shift}`, adjust = `{adjust}` to `{settingsDir}`")
-
diff --git a/spaces/brjathu/HMR2.0/vendor/detectron2/detectron2/export/torchscript_patch.py b/spaces/brjathu/HMR2.0/vendor/detectron2/detectron2/export/torchscript_patch.py
deleted file mode 100644
index da9b324f1582e31d1a16d2fe462ac2989bea56ea..0000000000000000000000000000000000000000
--- a/spaces/brjathu/HMR2.0/vendor/detectron2/detectron2/export/torchscript_patch.py
+++ /dev/null
@@ -1,406 +0,0 @@
-# Copyright (c) Facebook, Inc. and its affiliates.
-
-import os
-import sys
-import tempfile
-from contextlib import ExitStack, contextmanager
-from copy import deepcopy
-from unittest import mock
-import torch
-from torch import nn
-
-# need some explicit imports due to https://github.com/pytorch/pytorch/issues/38964
-import detectron2 # noqa F401
-from detectron2.structures import Boxes, Instances
-from detectron2.utils.env import _import_file
-
-_counter = 0
-
-
-def _clear_jit_cache():
- from torch.jit._recursive import concrete_type_store
- from torch.jit._state import _jit_caching_layer
-
- concrete_type_store.type_store.clear() # for modules
- _jit_caching_layer.clear() # for free functions
-
-
-def _add_instances_conversion_methods(newInstances):
- """
- Add from_instances methods to the scripted Instances class.
- """
- cls_name = newInstances.__name__
-
- @torch.jit.unused
- def from_instances(instances: Instances):
- """
- Create scripted Instances from original Instances
- """
- fields = instances.get_fields()
- image_size = instances.image_size
- ret = newInstances(image_size)
- for name, val in fields.items():
- assert hasattr(ret, f"_{name}"), f"No attribute named {name} in {cls_name}"
- setattr(ret, name, deepcopy(val))
- return ret
-
- newInstances.from_instances = from_instances
-
-
-@contextmanager
-def patch_instances(fields):
- """
- A contextmanager, under which the Instances class in detectron2 is replaced
- by a statically-typed scriptable class, defined by `fields`.
- See more in `scripting_with_instances`.
- """
-
- with tempfile.TemporaryDirectory(prefix="detectron2") as dir, tempfile.NamedTemporaryFile(
- mode="w", encoding="utf-8", suffix=".py", dir=dir, delete=False
- ) as f:
- try:
- # Objects that use Instances should not reuse previously-compiled
- # results in cache, because `Instances` could be a new class each time.
- _clear_jit_cache()
-
- cls_name, s = _gen_instance_module(fields)
- f.write(s)
- f.flush()
- f.close()
-
- module = _import(f.name)
- new_instances = getattr(module, cls_name)
- _ = torch.jit.script(new_instances)
- # let torchscript think Instances was scripted already
- Instances.__torch_script_class__ = True
- # let torchscript find new_instances when looking for the jit type of Instances
- Instances._jit_override_qualname = torch._jit_internal._qualified_name(new_instances)
-
- _add_instances_conversion_methods(new_instances)
- yield new_instances
- finally:
- try:
- del Instances.__torch_script_class__
- del Instances._jit_override_qualname
- except AttributeError:
- pass
- sys.modules.pop(module.__name__)
-
-
-def _gen_instance_class(fields):
- """
- Args:
- fields (dict[name: type])
- """
-
- class _FieldType:
- def __init__(self, name, type_):
- assert isinstance(name, str), f"Field name must be str, got {name}"
- self.name = name
- self.type_ = type_
- self.annotation = f"{type_.__module__}.{type_.__name__}"
-
- fields = [_FieldType(k, v) for k, v in fields.items()]
-
- def indent(level, s):
- return " " * 4 * level + s
-
- lines = []
-
- global _counter
- _counter += 1
-
- cls_name = "ScriptedInstances{}".format(_counter)
-
- field_names = tuple(x.name for x in fields)
- extra_args = ", ".join([f"{f.name}: Optional[{f.annotation}] = None" for f in fields])
- lines.append(
- f"""
-class {cls_name}:
- def __init__(self, image_size: Tuple[int, int], {extra_args}):
- self.image_size = image_size
- self._field_names = {field_names}
-"""
- )
-
- for f in fields:
- lines.append(
- indent(2, f"self._{f.name} = torch.jit.annotate(Optional[{f.annotation}], {f.name})")
- )
-
- for f in fields:
- lines.append(
- f"""
- @property
- def {f.name}(self) -> {f.annotation}:
- # has to use a local for type refinement
- # https://pytorch.org/docs/stable/jit_language_reference.html#optional-type-refinement
- t = self._{f.name}
- assert t is not None, "{f.name} is None and cannot be accessed!"
- return t
-
- @{f.name}.setter
- def {f.name}(self, value: {f.annotation}) -> None:
- self._{f.name} = value
-"""
- )
-
- # support method `__len__`
- lines.append(
- """
- def __len__(self) -> int:
-"""
- )
- for f in fields:
- lines.append(
- f"""
- t = self._{f.name}
- if t is not None:
- return len(t)
-"""
- )
- lines.append(
- """
- raise NotImplementedError("Empty Instances does not support __len__!")
-"""
- )
-
- # support method `has`
- lines.append(
- """
- def has(self, name: str) -> bool:
-"""
- )
- for f in fields:
- lines.append(
- f"""
- if name == "{f.name}":
- return self._{f.name} is not None
-"""
- )
- lines.append(
- """
- return False
-"""
- )
-
- # support method `to`
- none_args = ", None" * len(fields)
- lines.append(
- f"""
- def to(self, device: torch.device) -> "{cls_name}":
- ret = {cls_name}(self.image_size{none_args})
-"""
- )
- for f in fields:
- if hasattr(f.type_, "to"):
- lines.append(
- f"""
- t = self._{f.name}
- if t is not None:
- ret._{f.name} = t.to(device)
-"""
- )
- else:
- # For now, ignore fields that cannot be moved to devices.
- # Maybe can support other tensor-like classes (e.g. __torch_function__)
- pass
- lines.append(
- """
- return ret
-"""
- )
-
- # support method `getitem`
- none_args = ", None" * len(fields)
- lines.append(
- f"""
- def __getitem__(self, item) -> "{cls_name}":
- ret = {cls_name}(self.image_size{none_args})
-"""
- )
- for f in fields:
- lines.append(
- f"""
- t = self._{f.name}
- if t is not None:
- ret._{f.name} = t[item]
-"""
- )
- lines.append(
- """
- return ret
-"""
- )
-
- # support method `cat`
- # this version does not contain checks that all instances have same size and fields
- none_args = ", None" * len(fields)
- lines.append(
- f"""
- def cat(self, instances: List["{cls_name}"]) -> "{cls_name}":
- ret = {cls_name}(self.image_size{none_args})
-"""
- )
- for f in fields:
- lines.append(
- f"""
- t = self._{f.name}
- if t is not None:
- values: List[{f.annotation}] = [x.{f.name} for x in instances]
- if torch.jit.isinstance(t, torch.Tensor):
- ret._{f.name} = torch.cat(values, dim=0)
- else:
- ret._{f.name} = t.cat(values)
-"""
- )
- lines.append(
- """
- return ret"""
- )
-
- # support method `get_fields()`
- lines.append(
- """
- def get_fields(self) -> Dict[str, Tensor]:
- ret = {}
- """
- )
- for f in fields:
- if f.type_ == Boxes:
- stmt = "t.tensor"
- elif f.type_ == torch.Tensor:
- stmt = "t"
- else:
- stmt = f'assert False, "unsupported type {str(f.type_)}"'
- lines.append(
- f"""
- t = self._{f.name}
- if t is not None:
- ret["{f.name}"] = {stmt}
- """
- )
- lines.append(
- """
- return ret"""
- )
- return cls_name, os.linesep.join(lines)
-
-
-def _gen_instance_module(fields):
- # TODO: find a more automatic way to enable import of other classes
- s = """
-from copy import deepcopy
-import torch
-from torch import Tensor
-import typing
-from typing import *
-
-import detectron2
-from detectron2.structures import Boxes, Instances
-
-"""
-
- cls_name, cls_def = _gen_instance_class(fields)
- s += cls_def
- return cls_name, s
-
-
-def _import(path):
- return _import_file(
- "{}{}".format(sys.modules[__name__].__name__, _counter), path, make_importable=True
- )
-
-
-@contextmanager
-def patch_builtin_len(modules=()):
- """
- Patch the builtin len() function of a few detectron2 modules
- to use __len__ instead, because __len__ does not convert values to
- integers and therefore is friendly to tracing.
-
- Args:
- modules (list[stsr]): names of extra modules to patch len(), in
- addition to those in detectron2.
- """
-
- def _new_len(obj):
- return obj.__len__()
-
- with ExitStack() as stack:
- MODULES = [
- "detectron2.modeling.roi_heads.fast_rcnn",
- "detectron2.modeling.roi_heads.mask_head",
- "detectron2.modeling.roi_heads.keypoint_head",
- ] + list(modules)
- ctxs = [stack.enter_context(mock.patch(mod + ".len")) for mod in MODULES]
- for m in ctxs:
- m.side_effect = _new_len
- yield
-
-
-def patch_nonscriptable_classes():
- """
- Apply patches on a few nonscriptable detectron2 classes.
- Should not have side-effects on eager usage.
- """
- # __prepare_scriptable__ can also be added to models for easier maintenance.
- # But it complicates the clean model code.
-
- from detectron2.modeling.backbone import ResNet, FPN
-
- # Due to https://github.com/pytorch/pytorch/issues/36061,
- # we change backbone to use ModuleList for scripting.
- # (note: this changes param names in state_dict)
-
- def prepare_resnet(self):
- ret = deepcopy(self)
- ret.stages = nn.ModuleList(ret.stages)
- for k in self.stage_names:
- delattr(ret, k)
- return ret
-
- ResNet.__prepare_scriptable__ = prepare_resnet
-
- def prepare_fpn(self):
- ret = deepcopy(self)
- ret.lateral_convs = nn.ModuleList(ret.lateral_convs)
- ret.output_convs = nn.ModuleList(ret.output_convs)
- for name, _ in self.named_children():
- if name.startswith("fpn_"):
- delattr(ret, name)
- return ret
-
- FPN.__prepare_scriptable__ = prepare_fpn
-
- # Annotate some attributes to be constants for the purpose of scripting,
- # even though they are not constants in eager mode.
- from detectron2.modeling.roi_heads import StandardROIHeads
-
- if hasattr(StandardROIHeads, "__annotations__"):
- # copy first to avoid editing annotations of base class
- StandardROIHeads.__annotations__ = deepcopy(StandardROIHeads.__annotations__)
- StandardROIHeads.__annotations__["mask_on"] = torch.jit.Final[bool]
- StandardROIHeads.__annotations__["keypoint_on"] = torch.jit.Final[bool]
-
-
-# These patches are not supposed to have side-effects.
-patch_nonscriptable_classes()
-
-
-@contextmanager
-def freeze_training_mode(model):
- """
- A context manager that annotates the "training" attribute of every submodule
- to constant, so that the training codepath in these modules can be
- meta-compiled away. Upon exiting, the annotations are reverted.
- """
- classes = {type(x) for x in model.modules()}
- # __constants__ is the old way to annotate constants and not compatible
- # with __annotations__ .
- classes = {x for x in classes if not hasattr(x, "__constants__")}
- for cls in classes:
- cls.__annotations__["training"] = torch.jit.Final[bool]
- yield
- for cls in classes:
- cls.__annotations__["training"] = bool
diff --git a/spaces/bzd4576/sovits-sin/losses.py b/spaces/bzd4576/sovits-sin/losses.py
deleted file mode 100644
index fb22a0e834dd87edaa37bb8190eee2c3c7abe0d5..0000000000000000000000000000000000000000
--- a/spaces/bzd4576/sovits-sin/losses.py
+++ /dev/null
@@ -1,61 +0,0 @@
-import torch
-from torch.nn import functional as F
-
-import commons
-
-
-def feature_loss(fmap_r, fmap_g):
- loss = 0
- for dr, dg in zip(fmap_r, fmap_g):
- for rl, gl in zip(dr, dg):
- rl = rl.float().detach()
- gl = gl.float()
- loss += torch.mean(torch.abs(rl - gl))
-
- return loss * 2
-
-
-def discriminator_loss(disc_real_outputs, disc_generated_outputs):
- loss = 0
- r_losses = []
- g_losses = []
- for dr, dg in zip(disc_real_outputs, disc_generated_outputs):
- dr = dr.float()
- dg = dg.float()
- r_loss = torch.mean((1-dr)**2)
- g_loss = torch.mean(dg**2)
- loss += (r_loss + g_loss)
- r_losses.append(r_loss.item())
- g_losses.append(g_loss.item())
-
- return loss, r_losses, g_losses
-
-
-def generator_loss(disc_outputs):
- loss = 0
- gen_losses = []
- for dg in disc_outputs:
- dg = dg.float()
- l = torch.mean((1-dg)**2)
- gen_losses.append(l)
- loss += l
-
- return loss, gen_losses
-
-
-def kl_loss(z_p, logs_q, m_p, logs_p, z_mask):
- """
- z_p, logs_q: [b, h, t_t]
- m_p, logs_p: [b, h, t_t]
- """
- z_p = z_p.float()
- logs_q = logs_q.float()
- m_p = m_p.float()
- logs_p = logs_p.float()
- z_mask = z_mask.float()
-
- kl = logs_p - logs_q - 0.5
- kl += 0.5 * ((z_p - m_p)**2) * torch.exp(-2. * logs_p)
- kl = torch.sum(kl * z_mask)
- l = kl / torch.sum(z_mask)
- return l
diff --git a/spaces/caffeinum/VToonify/vtoonify/model/encoder/encoders/model_irse.py b/spaces/caffeinum/VToonify/vtoonify/model/encoder/encoders/model_irse.py
deleted file mode 100644
index 6698d9705321dd4a27681ea15204e9ffaa51f62a..0000000000000000000000000000000000000000
--- a/spaces/caffeinum/VToonify/vtoonify/model/encoder/encoders/model_irse.py
+++ /dev/null
@@ -1,84 +0,0 @@
-from torch.nn import Linear, Conv2d, BatchNorm1d, BatchNorm2d, PReLU, Dropout, Sequential, Module
-from model.encoder.encoders.helpers import get_blocks, Flatten, bottleneck_IR, bottleneck_IR_SE, l2_norm
-
-"""
-Modified Backbone implementation from [TreB1eN](https://github.com/TreB1eN/InsightFace_Pytorch)
-"""
-
-
-class Backbone(Module):
- def __init__(self, input_size, num_layers, mode='ir', drop_ratio=0.4, affine=True):
- super(Backbone, self).__init__()
- assert input_size in [112, 224], "input_size should be 112 or 224"
- assert num_layers in [50, 100, 152], "num_layers should be 50, 100 or 152"
- assert mode in ['ir', 'ir_se'], "mode should be ir or ir_se"
- blocks = get_blocks(num_layers)
- if mode == 'ir':
- unit_module = bottleneck_IR
- elif mode == 'ir_se':
- unit_module = bottleneck_IR_SE
- self.input_layer = Sequential(Conv2d(3, 64, (3, 3), 1, 1, bias=False),
- BatchNorm2d(64),
- PReLU(64))
- if input_size == 112:
- self.output_layer = Sequential(BatchNorm2d(512),
- Dropout(drop_ratio),
- Flatten(),
- Linear(512 * 7 * 7, 512),
- BatchNorm1d(512, affine=affine))
- else:
- self.output_layer = Sequential(BatchNorm2d(512),
- Dropout(drop_ratio),
- Flatten(),
- Linear(512 * 14 * 14, 512),
- BatchNorm1d(512, affine=affine))
-
- modules = []
- for block in blocks:
- for bottleneck in block:
- modules.append(unit_module(bottleneck.in_channel,
- bottleneck.depth,
- bottleneck.stride))
- self.body = Sequential(*modules)
-
- def forward(self, x):
- x = self.input_layer(x)
- x = self.body(x)
- x = self.output_layer(x)
- return l2_norm(x)
-
-
-def IR_50(input_size):
- """Constructs a ir-50 model."""
- model = Backbone(input_size, num_layers=50, mode='ir', drop_ratio=0.4, affine=False)
- return model
-
-
-def IR_101(input_size):
- """Constructs a ir-101 model."""
- model = Backbone(input_size, num_layers=100, mode='ir', drop_ratio=0.4, affine=False)
- return model
-
-
-def IR_152(input_size):
- """Constructs a ir-152 model."""
- model = Backbone(input_size, num_layers=152, mode='ir', drop_ratio=0.4, affine=False)
- return model
-
-
-def IR_SE_50(input_size):
- """Constructs a ir_se-50 model."""
- model = Backbone(input_size, num_layers=50, mode='ir_se', drop_ratio=0.4, affine=False)
- return model
-
-
-def IR_SE_101(input_size):
- """Constructs a ir_se-101 model."""
- model = Backbone(input_size, num_layers=100, mode='ir_se', drop_ratio=0.4, affine=False)
- return model
-
-
-def IR_SE_152(input_size):
- """Constructs a ir_se-152 model."""
- model = Backbone(input_size, num_layers=152, mode='ir_se', drop_ratio=0.4, affine=False)
- return model
diff --git a/spaces/camenduru-com/audioldm-text-to-audio-generation/share_btn.py b/spaces/camenduru-com/audioldm-text-to-audio-generation/share_btn.py
deleted file mode 100644
index b8c2ed17439625f85fd0e910766c727b29131e3d..0000000000000000000000000000000000000000
--- a/spaces/camenduru-com/audioldm-text-to-audio-generation/share_btn.py
+++ /dev/null
@@ -1,60 +0,0 @@
-community_icon_html = """"""
-
-loading_icon_html = """"""
-
-share_js = """async () => {
- async function uploadFile(file){
- const UPLOAD_URL = 'https://huggingface.co/uploads';
- const response = await fetch(UPLOAD_URL, {
- method: 'POST',
- headers: {
- 'Content-Type': file.type,
- 'X-Requested-With': 'XMLHttpRequest',
- },
- body: file, /// <- File inherits from Blob
- });
- const url = await response.text();
- return url;
- }
- const gradioEl = document.querySelector('body > gradio-app');
- const imgEls = gradioEl.querySelectorAll('#gallery img');
- const promptTxt = gradioEl.querySelector('#prompt-text-input input').value;
- const shareBtnEl = gradioEl.querySelector('#share-btn');
- const shareIconEl = gradioEl.querySelector('#share-btn-share-icon');
- const loadingIconEl = gradioEl.querySelector('#share-btn-loading-icon');
- if(!imgEls.length){
- return;
- };
- shareBtnEl.style.pointerEvents = 'none';
- shareIconEl.style.display = 'none';
- loadingIconEl.style.removeProperty('display');
- const files = await Promise.all(
- [...imgEls].map(async (imgEl) => {
- const res = await fetch(imgEl.src);
- const blob = await res.blob();
- const imgId = Date.now() % 200;
- const fileName = `diffuse-the-rest-${{imgId}}.jpg`;
- return new File([blob], fileName, { type: 'image/jpeg' });
- })
- );
- const urls = await Promise.all(files.map((f) => uploadFile(f)));
- const htmlImgs = urls.map(url => ``);
- const descriptionMd = `
-${htmlImgs.join(`\n`)}
-
`;
- const params = new URLSearchParams({
- title: promptTxt,
- description: descriptionMd,
- });
- const paramsStr = params.toString();
- window.open(`https://huggingface.co/spaces/haoheliu/audioldm-text-to-audio-generation/discussions/new?${paramsStr}`, '_blank');
- shareBtnEl.style.removeProperty('pointer-events');
- shareIconEl.style.removeProperty('display');
- loadingIconEl.style.display = 'none';
-}"""
\ No newline at end of file
diff --git a/spaces/camilosegura/traductor-multilenguaje/Lib/site-packages/PIL/CurImagePlugin.py b/spaces/camilosegura/traductor-multilenguaje/Lib/site-packages/PIL/CurImagePlugin.py
deleted file mode 100644
index 94efff3415679a5bf5b7038f9a1da15ebc6d04ca..0000000000000000000000000000000000000000
--- a/spaces/camilosegura/traductor-multilenguaje/Lib/site-packages/PIL/CurImagePlugin.py
+++ /dev/null
@@ -1,75 +0,0 @@
-#
-# The Python Imaging Library.
-# $Id$
-#
-# Windows Cursor support for PIL
-#
-# notes:
-# uses BmpImagePlugin.py to read the bitmap data.
-#
-# history:
-# 96-05-27 fl Created
-#
-# Copyright (c) Secret Labs AB 1997.
-# Copyright (c) Fredrik Lundh 1996.
-#
-# See the README file for information on usage and redistribution.
-#
-from . import BmpImagePlugin, Image
-from ._binary import i16le as i16
-from ._binary import i32le as i32
-
-#
-# --------------------------------------------------------------------
-
-
-def _accept(prefix):
- return prefix[:4] == b"\0\0\2\0"
-
-
-##
-# Image plugin for Windows Cursor files.
-
-
-class CurImageFile(BmpImagePlugin.BmpImageFile):
- format = "CUR"
- format_description = "Windows Cursor"
-
- def _open(self):
- offset = self.fp.tell()
-
- # check magic
- s = self.fp.read(6)
- if not _accept(s):
- msg = "not a CUR file"
- raise SyntaxError(msg)
-
- # pick the largest cursor in the file
- m = b""
- for i in range(i16(s, 4)):
- s = self.fp.read(16)
- if not m:
- m = s
- elif s[0] > m[0] and s[1] > m[1]:
- m = s
- if not m:
- msg = "No cursors were found"
- raise TypeError(msg)
-
- # load as bitmap
- self._bitmap(i32(m, 12) + offset)
-
- # patch up the bitmap height
- self._size = self.size[0], self.size[1] // 2
- d, e, o, a = self.tile[0]
- self.tile[0] = d, (0, 0) + self.size, o, a
-
- return
-
-
-#
-# --------------------------------------------------------------------
-
-Image.register_open(CurImageFile.format, CurImageFile, _accept)
-
-Image.register_extension(CurImageFile.format, ".cur")
diff --git a/spaces/camilosegura/traductor-multilenguaje/Lib/site-packages/PIL/MspImagePlugin.py b/spaces/camilosegura/traductor-multilenguaje/Lib/site-packages/PIL/MspImagePlugin.py
deleted file mode 100644
index c6567b2ae626fd83ef21575a59374c922d5392a9..0000000000000000000000000000000000000000
--- a/spaces/camilosegura/traductor-multilenguaje/Lib/site-packages/PIL/MspImagePlugin.py
+++ /dev/null
@@ -1,194 +0,0 @@
-#
-# The Python Imaging Library.
-#
-# MSP file handling
-#
-# This is the format used by the Paint program in Windows 1 and 2.
-#
-# History:
-# 95-09-05 fl Created
-# 97-01-03 fl Read/write MSP images
-# 17-02-21 es Fixed RLE interpretation
-#
-# Copyright (c) Secret Labs AB 1997.
-# Copyright (c) Fredrik Lundh 1995-97.
-# Copyright (c) Eric Soroos 2017.
-#
-# See the README file for information on usage and redistribution.
-#
-# More info on this format: https://archive.org/details/gg243631
-# Page 313:
-# Figure 205. Windows Paint Version 1: "DanM" Format
-# Figure 206. Windows Paint Version 2: "LinS" Format. Used in Windows V2.03
-#
-# See also: https://www.fileformat.info/format/mspaint/egff.htm
-
-import io
-import struct
-
-from . import Image, ImageFile
-from ._binary import i16le as i16
-from ._binary import o16le as o16
-
-#
-# read MSP files
-
-
-def _accept(prefix):
- return prefix[:4] in [b"DanM", b"LinS"]
-
-
-##
-# Image plugin for Windows MSP images. This plugin supports both
-# uncompressed (Windows 1.0).
-
-
-class MspImageFile(ImageFile.ImageFile):
- format = "MSP"
- format_description = "Windows Paint"
-
- def _open(self):
- # Header
- s = self.fp.read(32)
- if not _accept(s):
- msg = "not an MSP file"
- raise SyntaxError(msg)
-
- # Header checksum
- checksum = 0
- for i in range(0, 32, 2):
- checksum = checksum ^ i16(s, i)
- if checksum != 0:
- msg = "bad MSP checksum"
- raise SyntaxError(msg)
-
- self.mode = "1"
- self._size = i16(s, 4), i16(s, 6)
-
- if s[:4] == b"DanM":
- self.tile = [("raw", (0, 0) + self.size, 32, ("1", 0, 1))]
- else:
- self.tile = [("MSP", (0, 0) + self.size, 32, None)]
-
-
-class MspDecoder(ImageFile.PyDecoder):
- # The algo for the MSP decoder is from
- # https://www.fileformat.info/format/mspaint/egff.htm
- # cc-by-attribution -- That page references is taken from the
- # Encyclopedia of Graphics File Formats and is licensed by
- # O'Reilly under the Creative Common/Attribution license
- #
- # For RLE encoded files, the 32byte header is followed by a scan
- # line map, encoded as one 16bit word of encoded byte length per
- # line.
- #
- # NOTE: the encoded length of the line can be 0. This was not
- # handled in the previous version of this encoder, and there's no
- # mention of how to handle it in the documentation. From the few
- # examples I've seen, I've assumed that it is a fill of the
- # background color, in this case, white.
- #
- #
- # Pseudocode of the decoder:
- # Read a BYTE value as the RunType
- # If the RunType value is zero
- # Read next byte as the RunCount
- # Read the next byte as the RunValue
- # Write the RunValue byte RunCount times
- # If the RunType value is non-zero
- # Use this value as the RunCount
- # Read and write the next RunCount bytes literally
- #
- # e.g.:
- # 0x00 03 ff 05 00 01 02 03 04
- # would yield the bytes:
- # 0xff ff ff 00 01 02 03 04
- #
- # which are then interpreted as a bit packed mode '1' image
-
- _pulls_fd = True
-
- def decode(self, buffer):
- img = io.BytesIO()
- blank_line = bytearray((0xFF,) * ((self.state.xsize + 7) // 8))
- try:
- self.fd.seek(32)
- rowmap = struct.unpack_from(
- f"<{self.state.ysize}H", self.fd.read(self.state.ysize * 2)
- )
- except struct.error as e:
- msg = "Truncated MSP file in row map"
- raise OSError(msg) from e
-
- for x, rowlen in enumerate(rowmap):
- try:
- if rowlen == 0:
- img.write(blank_line)
- continue
- row = self.fd.read(rowlen)
- if len(row) != rowlen:
- msg = f"Truncated MSP file, expected {rowlen} bytes on row {x}"
- raise OSError(msg)
- idx = 0
- while idx < rowlen:
- runtype = row[idx]
- idx += 1
- if runtype == 0:
- (runcount, runval) = struct.unpack_from("Bc", row, idx)
- img.write(runval * runcount)
- idx += 2
- else:
- runcount = runtype
- img.write(row[idx : idx + runcount])
- idx += runcount
-
- except struct.error as e:
- msg = f"Corrupted MSP file in row {x}"
- raise OSError(msg) from e
-
- self.set_as_raw(img.getvalue(), ("1", 0, 1))
-
- return -1, 0
-
-
-Image.register_decoder("MSP", MspDecoder)
-
-
-#
-# write MSP files (uncompressed only)
-
-
-def _save(im, fp, filename):
- if im.mode != "1":
- msg = f"cannot write mode {im.mode} as MSP"
- raise OSError(msg)
-
- # create MSP header
- header = [0] * 16
-
- header[0], header[1] = i16(b"Da"), i16(b"nM") # version 1
- header[2], header[3] = im.size
- header[4], header[5] = 1, 1
- header[6], header[7] = 1, 1
- header[8], header[9] = im.size
-
- checksum = 0
- for h in header:
- checksum = checksum ^ h
- header[12] = checksum # FIXME: is this the right field?
-
- # header
- for h in header:
- fp.write(o16(h))
-
- # image body
- ImageFile._save(im, fp, [("raw", (0, 0) + im.size, 32, ("1", 0, 1))])
-
-
-#
-# registry
-
-Image.register_open(MspImageFile.format, MspImageFile, _accept)
-Image.register_save(MspImageFile.format, _save)
-
-Image.register_extension(MspImageFile.format, ".msp")
diff --git a/spaces/camilosegura/traductor-multilenguaje/Lib/site-packages/PIL/PaletteFile.py b/spaces/camilosegura/traductor-multilenguaje/Lib/site-packages/PIL/PaletteFile.py
deleted file mode 100644
index 4a2c497fc495a271cbab204db0197d776442ac5c..0000000000000000000000000000000000000000
--- a/spaces/camilosegura/traductor-multilenguaje/Lib/site-packages/PIL/PaletteFile.py
+++ /dev/null
@@ -1,51 +0,0 @@
-#
-# Python Imaging Library
-# $Id$
-#
-# stuff to read simple, teragon-style palette files
-#
-# History:
-# 97-08-23 fl Created
-#
-# Copyright (c) Secret Labs AB 1997.
-# Copyright (c) Fredrik Lundh 1997.
-#
-# See the README file for information on usage and redistribution.
-#
-
-from ._binary import o8
-
-
-class PaletteFile:
- """File handler for Teragon-style palette files."""
-
- rawmode = "RGB"
-
- def __init__(self, fp):
- self.palette = [(i, i, i) for i in range(256)]
-
- while True:
- s = fp.readline()
-
- if not s:
- break
- if s[:1] == b"#":
- continue
- if len(s) > 100:
- msg = "bad palette file"
- raise SyntaxError(msg)
-
- v = [int(x) for x in s.split()]
- try:
- [i, r, g, b] = v
- except ValueError:
- [i, r] = v
- g = b = r
-
- if 0 <= i <= 255:
- self.palette[i] = o8(r) + o8(g) + o8(b)
-
- self.palette = b"".join(self.palette)
-
- def getpalette(self):
- return self.palette, self.rawmode
diff --git a/spaces/candlend/vits-hoshimi/sovits/models.py b/spaces/candlend/vits-hoshimi/sovits/models.py
deleted file mode 100644
index f4941c211eed9a025536456c2aa110141ab7e3ff..0000000000000000000000000000000000000000
--- a/spaces/candlend/vits-hoshimi/sovits/models.py
+++ /dev/null
@@ -1,351 +0,0 @@
-import copy
-import math
-import torch
-from torch import nn
-from torch.nn import functional as F
-
-from sovits import attentions
-from sovits import commons
-from sovits import modules
-
-from torch.nn import Conv1d, ConvTranspose1d, AvgPool1d, Conv2d
-from torch.nn.utils import weight_norm, remove_weight_norm, spectral_norm
-from sovits.commons import init_weights, get_padding
-from sovits.vdecoder.hifigan.models import Generator
-from sovits.utils import f0_to_coarse
-
-class ResidualCouplingBlock(nn.Module):
- def __init__(self,
- channels,
- hidden_channels,
- kernel_size,
- dilation_rate,
- n_layers,
- n_flows=4,
- gin_channels=0):
- super().__init__()
- self.channels = channels
- self.hidden_channels = hidden_channels
- self.kernel_size = kernel_size
- self.dilation_rate = dilation_rate
- self.n_layers = n_layers
- self.n_flows = n_flows
- self.gin_channels = gin_channels
-
- self.flows = nn.ModuleList()
- for i in range(n_flows):
- self.flows.append(modules.ResidualCouplingLayer(channels, hidden_channels, kernel_size, dilation_rate, n_layers, gin_channels=gin_channels, mean_only=True))
- self.flows.append(modules.Flip())
-
- def forward(self, x, x_mask, g=None, reverse=False):
- if not reverse:
- for flow in self.flows:
- x, _ = flow(x, x_mask, g=g, reverse=reverse)
- else:
- for flow in reversed(self.flows):
- x = flow(x, x_mask, g=g, reverse=reverse)
- return x
-
-
-class Encoder(nn.Module):
- def __init__(self,
- in_channels,
- out_channels,
- hidden_channels,
- kernel_size,
- dilation_rate,
- n_layers,
- gin_channels=0):
- super().__init__()
- self.in_channels = in_channels
- self.out_channels = out_channels
- self.hidden_channels = hidden_channels
- self.kernel_size = kernel_size
- self.dilation_rate = dilation_rate
- self.n_layers = n_layers
- self.gin_channels = gin_channels
-
- self.pre = nn.Conv1d(in_channels, hidden_channels, 1)
- self.enc = modules.WN(hidden_channels, kernel_size, dilation_rate, n_layers, gin_channels=gin_channels)
- self.proj = nn.Conv1d(hidden_channels, out_channels * 2, 1)
-
- def forward(self, x, x_lengths, g=None):
- # print(x.shape,x_lengths.shape)
- x_mask = torch.unsqueeze(commons.sequence_mask(x_lengths, x.size(2)), 1).to(x.dtype)
- x = self.pre(x) * x_mask
- x = self.enc(x, x_mask, g=g)
- stats = self.proj(x) * x_mask
- m, logs = torch.split(stats, self.out_channels, dim=1)
- z = (m + torch.randn_like(m) * torch.exp(logs)) * x_mask
- return z, m, logs, x_mask
-
-
-class TextEncoder(nn.Module):
- def __init__(self,
- in_channels,
- out_channels,
- hidden_channels,
- kernel_size,
- dilation_rate,
- n_layers,
- gin_channels=0,
- filter_channels=None,
- n_heads=None,
- p_dropout=None):
- super().__init__()
- self.in_channels = in_channels
- self.out_channels = out_channels
- self.hidden_channels = hidden_channels
- self.kernel_size = kernel_size
- self.dilation_rate = dilation_rate
- self.n_layers = n_layers
- self.gin_channels = gin_channels
- self.pre = nn.Conv1d(in_channels, hidden_channels, 1)
- self.proj = nn.Conv1d(hidden_channels, out_channels * 2, 1)
- self.f0_emb = nn.Embedding(256, hidden_channels)
-
- self.enc_ = attentions.Encoder(
- hidden_channels,
- filter_channels,
- n_heads,
- n_layers,
- kernel_size,
- p_dropout)
-
- def forward(self, x, x_lengths, f0=None):
- x_mask = torch.unsqueeze(commons.sequence_mask(x_lengths, x.size(2)), 1).to(x.dtype)
- x = self.pre(x) * x_mask
- x = x + self.f0_emb(f0).transpose(1,2)
- x = self.enc_(x * x_mask, x_mask)
- stats = self.proj(x) * x_mask
- m, logs = torch.split(stats, self.out_channels, dim=1)
- z = (m + torch.randn_like(m) * torch.exp(logs)) * x_mask
-
- return z, m, logs, x_mask
-
-
-
-class DiscriminatorP(torch.nn.Module):
- def __init__(self, period, kernel_size=5, stride=3, use_spectral_norm=False):
- super(DiscriminatorP, self).__init__()
- self.period = period
- self.use_spectral_norm = use_spectral_norm
- norm_f = weight_norm if use_spectral_norm == False else spectral_norm
- self.convs = nn.ModuleList([
- norm_f(Conv2d(1, 32, (kernel_size, 1), (stride, 1), padding=(get_padding(kernel_size, 1), 0))),
- norm_f(Conv2d(32, 128, (kernel_size, 1), (stride, 1), padding=(get_padding(kernel_size, 1), 0))),
- norm_f(Conv2d(128, 512, (kernel_size, 1), (stride, 1), padding=(get_padding(kernel_size, 1), 0))),
- norm_f(Conv2d(512, 1024, (kernel_size, 1), (stride, 1), padding=(get_padding(kernel_size, 1), 0))),
- norm_f(Conv2d(1024, 1024, (kernel_size, 1), 1, padding=(get_padding(kernel_size, 1), 0))),
- ])
- self.conv_post = norm_f(Conv2d(1024, 1, (3, 1), 1, padding=(1, 0)))
-
- def forward(self, x):
- fmap = []
-
- # 1d to 2d
- b, c, t = x.shape
- if t % self.period != 0: # pad first
- n_pad = self.period - (t % self.period)
- x = F.pad(x, (0, n_pad), "reflect")
- t = t + n_pad
- x = x.view(b, c, t // self.period, self.period)
-
- for l in self.convs:
- x = l(x)
- x = F.leaky_relu(x, modules.LRELU_SLOPE)
- fmap.append(x)
- x = self.conv_post(x)
- fmap.append(x)
- x = torch.flatten(x, 1, -1)
-
- return x, fmap
-
-
-class DiscriminatorS(torch.nn.Module):
- def __init__(self, use_spectral_norm=False):
- super(DiscriminatorS, self).__init__()
- norm_f = weight_norm if use_spectral_norm == False else spectral_norm
- self.convs = nn.ModuleList([
- norm_f(Conv1d(1, 16, 15, 1, padding=7)),
- norm_f(Conv1d(16, 64, 41, 4, groups=4, padding=20)),
- norm_f(Conv1d(64, 256, 41, 4, groups=16, padding=20)),
- norm_f(Conv1d(256, 1024, 41, 4, groups=64, padding=20)),
- norm_f(Conv1d(1024, 1024, 41, 4, groups=256, padding=20)),
- norm_f(Conv1d(1024, 1024, 5, 1, padding=2)),
- ])
- self.conv_post = norm_f(Conv1d(1024, 1, 3, 1, padding=1))
-
- def forward(self, x):
- fmap = []
-
- for l in self.convs:
- x = l(x)
- x = F.leaky_relu(x, modules.LRELU_SLOPE)
- fmap.append(x)
- x = self.conv_post(x)
- fmap.append(x)
- x = torch.flatten(x, 1, -1)
-
- return x, fmap
-
-
-class MultiPeriodDiscriminator(torch.nn.Module):
- def __init__(self, use_spectral_norm=False):
- super(MultiPeriodDiscriminator, self).__init__()
- periods = [2,3,5,7,11]
-
- discs = [DiscriminatorS(use_spectral_norm=use_spectral_norm)]
- discs = discs + [DiscriminatorP(i, use_spectral_norm=use_spectral_norm) for i in periods]
- self.discriminators = nn.ModuleList(discs)
-
- def forward(self, y, y_hat):
- y_d_rs = []
- y_d_gs = []
- fmap_rs = []
- fmap_gs = []
- for i, d in enumerate(self.discriminators):
- y_d_r, fmap_r = d(y)
- y_d_g, fmap_g = d(y_hat)
- y_d_rs.append(y_d_r)
- y_d_gs.append(y_d_g)
- fmap_rs.append(fmap_r)
- fmap_gs.append(fmap_g)
-
- return y_d_rs, y_d_gs, fmap_rs, fmap_gs
-
-
-class SpeakerEncoder(torch.nn.Module):
- def __init__(self, mel_n_channels=80, model_num_layers=3, model_hidden_size=256, model_embedding_size=256):
- super(SpeakerEncoder, self).__init__()
- self.lstm = nn.LSTM(mel_n_channels, model_hidden_size, model_num_layers, batch_first=True)
- self.linear = nn.Linear(model_hidden_size, model_embedding_size)
- self.relu = nn.ReLU()
-
- def forward(self, mels):
- self.lstm.flatten_parameters()
- _, (hidden, _) = self.lstm(mels)
- embeds_raw = self.relu(self.linear(hidden[-1]))
- return embeds_raw / torch.norm(embeds_raw, dim=1, keepdim=True)
-
- def compute_partial_slices(self, total_frames, partial_frames, partial_hop):
- mel_slices = []
- for i in range(0, total_frames-partial_frames, partial_hop):
- mel_range = torch.arange(i, i+partial_frames)
- mel_slices.append(mel_range)
-
- return mel_slices
-
- def embed_utterance(self, mel, partial_frames=128, partial_hop=64):
- mel_len = mel.size(1)
- last_mel = mel[:,-partial_frames:]
-
- if mel_len > partial_frames:
- mel_slices = self.compute_partial_slices(mel_len, partial_frames, partial_hop)
- mels = list(mel[:,s] for s in mel_slices)
- mels.append(last_mel)
- mels = torch.stack(tuple(mels), 0).squeeze(1)
-
- with torch.no_grad():
- partial_embeds = self(mels)
- embed = torch.mean(partial_embeds, axis=0).unsqueeze(0)
- #embed = embed / torch.linalg.norm(embed, 2)
- else:
- with torch.no_grad():
- embed = self(last_mel)
-
- return embed
-
-
-class SynthesizerTrn(nn.Module):
- """
- Synthesizer for Training
- """
-
- def __init__(self,
- spec_channels,
- segment_size,
- inter_channels,
- hidden_channels,
- filter_channels,
- n_heads,
- n_layers,
- kernel_size,
- p_dropout,
- resblock,
- resblock_kernel_sizes,
- resblock_dilation_sizes,
- upsample_rates,
- upsample_initial_channel,
- upsample_kernel_sizes,
- gin_channels,
- ssl_dim,
- n_speakers,
- **kwargs):
-
- super().__init__()
- self.spec_channels = spec_channels
- self.inter_channels = inter_channels
- self.hidden_channels = hidden_channels
- self.filter_channels = filter_channels
- self.n_heads = n_heads
- self.n_layers = n_layers
- self.kernel_size = kernel_size
- self.p_dropout = p_dropout
- self.resblock = resblock
- self.resblock_kernel_sizes = resblock_kernel_sizes
- self.resblock_dilation_sizes = resblock_dilation_sizes
- self.upsample_rates = upsample_rates
- self.upsample_initial_channel = upsample_initial_channel
- self.upsample_kernel_sizes = upsample_kernel_sizes
- self.segment_size = segment_size
- self.gin_channels = gin_channels
- self.ssl_dim = ssl_dim
- self.emb_g = nn.Embedding(n_speakers, gin_channels)
-
- self.enc_p_ = TextEncoder(ssl_dim, inter_channels, hidden_channels, 5, 1, 16,0, filter_channels, n_heads, p_dropout)
- hps = {
- "sampling_rate": 32000,
- "inter_channels": 192,
- "resblock": "1",
- "resblock_kernel_sizes": [3, 7, 11],
- "resblock_dilation_sizes": [[1, 3, 5], [1, 3, 5], [1, 3, 5]],
- "upsample_rates": [10, 8, 2, 2],
- "upsample_initial_channel": 512,
- "upsample_kernel_sizes": [16, 16, 4, 4],
- "gin_channels": 256,
- }
- self.dec = Generator(h=hps)
- self.enc_q = Encoder(spec_channels, inter_channels, hidden_channels, 5, 1, 16, gin_channels=gin_channels)
- self.flow = ResidualCouplingBlock(inter_channels, hidden_channels, 5, 1, 4, gin_channels=gin_channels)
-
- def forward(self, c, f0, spec, g=None, mel=None, c_lengths=None, spec_lengths=None):
- if c_lengths == None:
- c_lengths = (torch.ones(c.size(0)) * c.size(-1)).to(c.device)
- if spec_lengths == None:
- spec_lengths = (torch.ones(spec.size(0)) * spec.size(-1)).to(spec.device)
-
- g = self.emb_g(g).transpose(1,2)
-
- z_ptemp, m_p, logs_p, _ = self.enc_p_(c, c_lengths, f0=f0_to_coarse(f0))
- z, m_q, logs_q, spec_mask = self.enc_q(spec, spec_lengths, g=g)
-
- z_p = self.flow(z, spec_mask, g=g)
- z_slice, pitch_slice, ids_slice = commons.rand_slice_segments_with_pitch(z, f0, spec_lengths, self.segment_size)
-
- # o = self.dec(z_slice, g=g)
- o = self.dec(z_slice, g=g, f0=pitch_slice)
-
- return o, ids_slice, spec_mask, (z, z_p, m_p, logs_p, m_q, logs_q)
-
- def infer(self, c, f0, g=None, mel=None, c_lengths=None):
- if c_lengths == None:
- c_lengths = (torch.ones(c.size(0)) * c.size(-1)).to(c.device)
- g = self.emb_g(g).transpose(1,2)
-
- z_p, m_p, logs_p, c_mask = self.enc_p_(c, c_lengths, f0=f0_to_coarse(f0))
- z = self.flow(z_p, c_mask, g=g, reverse=True)
-
- o = self.dec(z * c_mask, g=g, f0=f0)
-
- return o
diff --git a/spaces/carlosalonso/Detection-video/carpeta_deteccion/detectron2/solver/__init__.py b/spaces/carlosalonso/Detection-video/carpeta_deteccion/detectron2/solver/__init__.py
deleted file mode 100644
index 9a2dbd35bb24f0d4a979bc8f304142376d87e7ec..0000000000000000000000000000000000000000
--- a/spaces/carlosalonso/Detection-video/carpeta_deteccion/detectron2/solver/__init__.py
+++ /dev/null
@@ -1,5 +0,0 @@
-# Copyright (c) Facebook, Inc. and its affiliates.
-from .build import build_lr_scheduler, build_optimizer, get_default_optimizer_params
-from .lr_scheduler import WarmupCosineLR, WarmupMultiStepLR, LRMultiplier, WarmupParamScheduler
-
-__all__ = [k for k in globals().keys() if not k.startswith("_")]
diff --git a/spaces/carlosalonso/Detection-video/carpeta_deteccion/projects/MViTv2/configs/cascade_mask_rcnn_mvitv2_l_in21k_lsj_50ep.py b/spaces/carlosalonso/Detection-video/carpeta_deteccion/projects/MViTv2/configs/cascade_mask_rcnn_mvitv2_l_in21k_lsj_50ep.py
deleted file mode 100644
index 38da8958e0174d378555887d72a9956f4b3f8e58..0000000000000000000000000000000000000000
--- a/spaces/carlosalonso/Detection-video/carpeta_deteccion/projects/MViTv2/configs/cascade_mask_rcnn_mvitv2_l_in21k_lsj_50ep.py
+++ /dev/null
@@ -1,31 +0,0 @@
-from fvcore.common.param_scheduler import MultiStepParamScheduler
-
-from detectron2.config import LazyCall as L
-from detectron2.solver import WarmupParamScheduler
-
-from .cascade_mask_rcnn_mvitv2_b_3x import model, optimizer, train
-from .common.coco_loader_lsj import dataloader
-
-
-model.backbone.bottom_up.embed_dim = 144
-model.backbone.bottom_up.depth = 48
-model.backbone.bottom_up.num_heads = 2
-model.backbone.bottom_up.last_block_indexes = (1, 7, 43, 47)
-model.backbone.bottom_up.drop_path_rate = 0.5
-
-train.init_checkpoint = "detectron2://ImageNetPretrained/mvitv2/MViTv2_L_in21k.pyth"
-
-# Schedule
-# 50ep = 184375 // 2 iters * 64 images/iter / 118000 images/ep
-train.max_iter = 184375 // 2
-lr_multiplier = L(WarmupParamScheduler)(
- scheduler=L(MultiStepParamScheduler)(
- values=[1.0, 0.1, 0.01],
- milestones=[163889 // 2, 177546 // 2],
- num_updates=train.max_iter,
- ),
- warmup_length=250 / train.max_iter,
- warmup_factor=0.001,
-)
-
-optimizer.lr = 1e-4
diff --git a/spaces/cfwef/gpt/crazy_functions/test_project/cpp/cppipc/shm.cpp b/spaces/cfwef/gpt/crazy_functions/test_project/cpp/cppipc/shm.cpp
deleted file mode 100644
index 593ce3129dc1574dbc8fc8b088cf595df215de93..0000000000000000000000000000000000000000
--- a/spaces/cfwef/gpt/crazy_functions/test_project/cpp/cppipc/shm.cpp
+++ /dev/null
@@ -1,103 +0,0 @@
-
-#include
-#include
-
-#include "libipc/shm.h"
-
-#include "libipc/utility/pimpl.h"
-#include "libipc/memory/resource.h"
-
-namespace ipc {
-namespace shm {
-
-class handle::handle_ : public pimpl {
-public:
- shm::id_t id_ = nullptr;
- void* m_ = nullptr;
-
- ipc::string n_;
- std::size_t s_ = 0;
-};
-
-handle::handle()
- : p_(p_->make()) {
-}
-
-handle::handle(char const * name, std::size_t size, unsigned mode)
- : handle() {
- acquire(name, size, mode);
-}
-
-handle::handle(handle&& rhs)
- : handle() {
- swap(rhs);
-}
-
-handle::~handle() {
- release();
- p_->clear();
-}
-
-void handle::swap(handle& rhs) {
- std::swap(p_, rhs.p_);
-}
-
-handle& handle::operator=(handle rhs) {
- swap(rhs);
- return *this;
-}
-
-bool handle::valid() const noexcept {
- return impl(p_)->m_ != nullptr;
-}
-
-std::size_t handle::size() const noexcept {
- return impl(p_)->s_;
-}
-
-char const * handle::name() const noexcept {
- return impl(p_)->n_.c_str();
-}
-
-std::int32_t handle::ref() const noexcept {
- return shm::get_ref(impl(p_)->id_);
-}
-
-void handle::sub_ref() noexcept {
- shm::sub_ref(impl(p_)->id_);
-}
-
-bool handle::acquire(char const * name, std::size_t size, unsigned mode) {
- release();
- impl(p_)->id_ = shm::acquire((impl(p_)->n_ = name).c_str(), size, mode);
- impl(p_)->m_ = shm::get_mem(impl(p_)->id_, &(impl(p_)->s_));
- return valid();
-}
-
-std::int32_t handle::release() {
- if (impl(p_)->id_ == nullptr) return -1;
- return shm::release(detach());
-}
-
-void* handle::get() const {
- return impl(p_)->m_;
-}
-
-void handle::attach(id_t id) {
- if (id == nullptr) return;
- release();
- impl(p_)->id_ = id;
- impl(p_)->m_ = shm::get_mem(impl(p_)->id_, &(impl(p_)->s_));
-}
-
-id_t handle::detach() {
- auto old = impl(p_)->id_;
- impl(p_)->id_ = nullptr;
- impl(p_)->m_ = nullptr;
- impl(p_)->s_ = 0;
- impl(p_)->n_.clear();
- return old;
-}
-
-} // namespace shm
-} // namespace ipc
diff --git a/spaces/chendl/compositional_test/transformers/examples/research_projects/fsner/setup.py b/spaces/chendl/compositional_test/transformers/examples/research_projects/fsner/setup.py
deleted file mode 100644
index 8ce34d0f7d9053b36d3cde98d251dfbc0ffe5a25..0000000000000000000000000000000000000000
--- a/spaces/chendl/compositional_test/transformers/examples/research_projects/fsner/setup.py
+++ /dev/null
@@ -1,27 +0,0 @@
-import setuptools
-
-
-with open("README.md", "r", encoding="utf-8") as fh:
- long_description = fh.read()
-
-setuptools.setup(
- name="fsner",
- version="0.0.1",
- author="msi sayef",
- author_email="msi.sayef@gmail.com",
- description="Few-shot Named Entity Recognition",
- long_description=long_description,
- long_description_content_type="text/markdown",
- url="https://github.com/huggingface/transformers/tree/main/examples/research_projects/fsner",
- project_urls={
- "Bug Tracker": "https://github.com/huggingface/transformers/issues",
- },
- classifiers=[
- "Programming Language :: Python :: 3",
- "Operating System :: OS Independent",
- ],
- package_dir={"": "src"},
- packages=setuptools.find_packages(where="src"),
- python_requires=">=3.6",
- install_requires=["torch>=1.9.0", "transformers>=4.9.2"],
-)
diff --git a/spaces/chendl/compositional_test/transformers/examples/research_projects/jax-projects/model_parallel/run_clm_mp.py b/spaces/chendl/compositional_test/transformers/examples/research_projects/jax-projects/model_parallel/run_clm_mp.py
deleted file mode 100644
index 7103b5a28111ffc0d4e1dce891dc6b077f721a78..0000000000000000000000000000000000000000
--- a/spaces/chendl/compositional_test/transformers/examples/research_projects/jax-projects/model_parallel/run_clm_mp.py
+++ /dev/null
@@ -1,664 +0,0 @@
-#!/usr/bin/env python
-# coding=utf-8
-# Copyright 2021 The HuggingFace Team All rights reserved.
-#
-# Licensed under the Apache License, Version 2.0 (the "License");
-# you may not use this file except in compliance with the License.
-# You may obtain a copy of the License at
-#
-# http://www.apache.org/licenses/LICENSE-2.0
-#
-# Unless required by applicable law or agreed to in writing, software
-# distributed under the License is distributed on an "AS IS" BASIS,
-# WITHOUT WARRANTIES OR CONDITIONS OF ANY KIND, either express or implied.
-# See the License for the specific language governing permissions and
-# limitations under the License.
-"""
-Pre-training/Fine-tuning the GPTNeo model for causal language modeling on a text file or a dataset using model parallelism.
-"""
-
-import logging
-import math
-import os
-import sys
-import time
-from dataclasses import dataclass, field
-from itertools import chain
-from pathlib import Path
-from typing import Callable, Optional
-
-import datasets
-import jax
-import jax.numpy as jnp
-import numpy as np
-import optax
-from datasets import Dataset, load_dataset
-from flax.core.frozen_dict import freeze, unfreeze
-from flax.training.common_utils import onehot, stack_forest
-from jax.experimental.maps import mesh
-from jax.experimental.pjit import pjit
-from partitions import set_partitions
-from tqdm import tqdm
-
-import transformers
-from transformers import (
- CONFIG_MAPPING,
- FLAX_MODEL_FOR_CAUSAL_LM_MAPPING,
- AutoConfig,
- AutoTokenizer,
- FlaxAutoModelForCausalLM,
- HfArgumentParser,
- TrainingArguments,
- is_tensorboard_available,
-)
-from transformers.testing_utils import CaptureLogger
-
-
-logger = logging.getLogger(__name__)
-
-MODEL_CONFIG_CLASSES = list(FLAX_MODEL_FOR_CAUSAL_LM_MAPPING.keys())
-MODEL_TYPES = tuple(conf.model_type for conf in MODEL_CONFIG_CLASSES)
-
-
-@dataclass
-class ModelArguments:
- """
- Arguments pertaining to which model/config/tokenizer we are going to fine-tune, or train from scratch.
- """
-
- model_name_or_path: Optional[str] = field(
- default=None,
- metadata={
- "help": (
- "The model checkpoint for weights initialization.Don't set if you want to train a model from scratch."
- )
- },
- )
- model_type: Optional[str] = field(
- default=None,
- metadata={"help": "If training from scratch, pass a model type from the list: " + ", ".join(MODEL_TYPES)},
- )
- config_name: Optional[str] = field(
- default=None, metadata={"help": "Pretrained config name or path if not the same as model_name"}
- )
- tokenizer_name: Optional[str] = field(
- default=None, metadata={"help": "Pretrained tokenizer name or path if not the same as model_name"}
- )
- cache_dir: Optional[str] = field(
- default=None, metadata={"help": "Where do you want to store the pretrained models downloaded from s3"}
- )
- use_fast_tokenizer: bool = field(
- default=True,
- metadata={"help": "Whether to use one of the fast tokenizer (backed by the tokenizers library) or not."},
- )
- dtype: Optional[str] = field(
- default="float32",
- metadata={
- "help": (
- "Floating-point format in which the model weights should be initialized and trained. Choose one of"
- " `[float32, float16, bfloat16]`."
- )
- },
- )
-
-
-@dataclass
-class DataTrainingArguments:
- """
- Arguments pertaining to what data we are going to input our model for training and eval.
- """
-
- dataset_name: Optional[str] = field(
- default=None, metadata={"help": "The name of the dataset to use (via the datasets library)."}
- )
- dataset_config_name: Optional[str] = field(
- default=None, metadata={"help": "The configuration name of the dataset to use (via the datasets library)."}
- )
- train_file: Optional[str] = field(default=None, metadata={"help": "The input training data file (a text file)."})
- validation_file: Optional[str] = field(
- default=None,
- metadata={"help": "An optional input evaluation data file to evaluate the perplexity on (a text file)."},
- )
- max_train_samples: Optional[int] = field(
- default=None,
- metadata={
- "help": (
- "For debugging purposes or quicker training, truncate the number of training examples to this "
- "value if set."
- )
- },
- )
- max_eval_samples: Optional[int] = field(
- default=None,
- metadata={
- "help": (
- "For debugging purposes or quicker training, truncate the number of evaluation examples to this "
- "value if set."
- )
- },
- )
- overwrite_cache: bool = field(
- default=False, metadata={"help": "Overwrite the cached training and evaluation sets"}
- )
- validation_split_percentage: Optional[int] = field(
- default=5,
- metadata={
- "help": "The percentage of the train set used as validation set in case there's no validation split"
- },
- )
- block_size: Optional[int] = field(
- default=None,
- metadata={
- "help": (
- "Optional input sequence length after tokenization. "
- "The training dataset will be truncated in block of this size for training. "
- "Default to the model max input length for single sentence inputs (take into account special tokens)."
- )
- },
- )
- overwrite_cache: bool = field(
- default=False, metadata={"help": "Overwrite the cached training and evaluation sets"}
- )
- preprocessing_num_workers: Optional[int] = field(
- default=None,
- metadata={"help": "The number of processes to use for the preprocessing."},
- )
-
- def __post_init__(self):
- if self.dataset_name is None and self.train_file is None and self.validation_file is None:
- raise ValueError("Need either a dataset name or a training/validation file.")
- else:
- if self.train_file is not None:
- extension = self.train_file.split(".")[-1]
- assert extension in ["csv", "json", "txt"], "`train_file` should be a csv, a json or a txt file."
- if self.validation_file is not None:
- extension = self.validation_file.split(".")[-1]
- assert extension in ["csv", "json", "txt"], "`validation_file` should be a csv, a json or a txt file."
-
-
-def data_loader(rng: jax.random.PRNGKey, dataset: Dataset, batch_size: int, shuffle: bool = False):
- """
- Returns batches of size `batch_size` from truncated `dataset`, sharded over all local devices.
- Shuffle batches if `shuffle` is `True`.
- """
- steps_per_epoch = len(dataset) // batch_size
-
- if shuffle:
- batch_idx = jax.random.permutation(rng, len(dataset))
- else:
- batch_idx = jnp.arange(len(dataset))
-
- batch_idx = batch_idx[: steps_per_epoch * batch_size] # Skip incomplete batch.
- batch_idx = batch_idx.reshape((steps_per_epoch, batch_size))
-
- for idx in batch_idx:
- batch = dataset[idx]
- batch = {k: jnp.array(v) for k, v in batch.items()}
- yield batch
-
-
-def write_train_metric(summary_writer, train_metrics, train_time, step):
- summary_writer.scalar("train_time", train_time, step)
-
- train_metrics = stack_forest(train_metrics)
- for key, vals in train_metrics.items():
- tag = f"train_{key}"
- for i, val in enumerate(vals):
- summary_writer.scalar(tag, val, step - len(vals) + i + 1)
-
-
-def write_eval_metric(summary_writer, eval_metrics, step):
- for metric_name, value in eval_metrics.items():
- summary_writer.scalar(f"eval_{metric_name}", value, step)
-
-
-def create_learning_rate_fn(
- train_ds_size: int, train_batch_size: int, num_train_epochs: int, num_warmup_steps: int, learning_rate: float
-) -> Callable[[int], jnp.array]:
- """Returns a linear warmup, linear_decay learning rate function."""
- steps_per_epoch = train_ds_size // train_batch_size
- num_train_steps = steps_per_epoch * num_train_epochs
- warmup_fn = optax.linear_schedule(init_value=0.0, end_value=learning_rate, transition_steps=num_warmup_steps)
- decay_fn = optax.linear_schedule(
- init_value=learning_rate, end_value=0, transition_steps=num_train_steps - num_warmup_steps
- )
- schedule_fn = optax.join_schedules(schedules=[warmup_fn, decay_fn], boundaries=[num_warmup_steps])
- return schedule_fn
-
-
-def main():
- # See all possible arguments in src/transformers/training_args.py
- # or by passing the --help flag to this script.
- # We now keep distinct sets of args, for a cleaner separation of concerns.
-
- parser = HfArgumentParser((ModelArguments, DataTrainingArguments, TrainingArguments))
- if len(sys.argv) == 2 and sys.argv[1].endswith(".json"):
- # If we pass only one argument to the script and it's the path to a json file,
- # let's parse it to get our arguments.
- model_args, data_args, training_args = parser.parse_json_file(json_file=os.path.abspath(sys.argv[1]))
- else:
- model_args, data_args, training_args = parser.parse_args_into_dataclasses()
-
- if (
- os.path.exists(training_args.output_dir)
- and os.listdir(training_args.output_dir)
- and training_args.do_train
- and not training_args.overwrite_output_dir
- ):
- raise ValueError(
- f"Output directory ({training_args.output_dir}) already exists and is not empty."
- "Use --overwrite_output_dir to overcome."
- )
-
- # Make one log on every process with the configuration for debugging.
- logging.basicConfig(
- format="%(asctime)s - %(levelname)s - %(name)s - %(message)s",
- datefmt="%m/%d/%Y %H:%M:%S",
- level=logging.INFO,
- )
- # Setup logging, we only want one process per machine to log things on the screen.
- logger.setLevel(logging.INFO if jax.process_index() == 0 else logging.ERROR)
- if jax.process_index() == 0:
- datasets.utils.logging.set_verbosity_warning()
- transformers.utils.logging.set_verbosity_info()
- else:
- datasets.utils.logging.set_verbosity_error()
- transformers.utils.logging.set_verbosity_error()
-
- # Set the verbosity to info of the Transformers logger (on main process only):
- logger.info(f"Training/evaluation parameters {training_args}")
-
- # Get the datasets: you can either provide your own CSV/JSON/TXT training and evaluation files (see below)
- # or just provide the name of one of the public datasets available on the hub at https://huggingface.co/datasets/
- # (the dataset will be downloaded automatically from the datasets Hub).
- #
- # For CSV/JSON files, this script will use the column called 'text' or the first column if no column called
- # 'text' is found. You can easily tweak this behavior (see below).
- if data_args.dataset_name is not None:
- # Downloading and loading a dataset from the hub.
- dataset = load_dataset(
- data_args.dataset_name, data_args.dataset_config_name, cache_dir=model_args.cache_dir, keep_in_memory=False
- )
-
- if "validation" not in dataset.keys():
- dataset["validation"] = load_dataset(
- data_args.dataset_name,
- data_args.dataset_config_name,
- split=f"train[:{data_args.validation_split_percentage}%]",
- cache_dir=model_args.cache_dir,
- )
- dataset["train"] = load_dataset(
- data_args.dataset_name,
- data_args.dataset_config_name,
- split=f"train[{data_args.validation_split_percentage}%:]",
- cache_dir=model_args.cache_dir,
- )
- else:
- data_files = {}
- if data_args.train_file is not None:
- data_files["train"] = data_args.train_file
- if data_args.validation_file is not None:
- data_files["validation"] = data_args.validation_file
- extension = data_args.train_file.split(".")[-1]
- if extension == "txt":
- extension = "text"
- dataset = load_dataset(extension, data_files=data_files, cache_dir=model_args.cache_dir)
- # See more about loading any type of standard or custom dataset (from files, python dict, pandas DataFrame, etc) at
- # https://huggingface.co/docs/datasets/loading_datasets.html.
-
- # Load pretrained config and tokenizer
- if model_args.config_name:
- config = AutoConfig.from_pretrained(model_args.config_name, cache_dir=model_args.cache_dir)
- elif model_args.model_name_or_path:
- config = AutoConfig.from_pretrained(model_args.model_name_or_path, cache_dir=model_args.cache_dir)
- else:
- config = CONFIG_MAPPING[model_args.model_type]()
- logger.warning("You are instantiating a new config instance from scratch.")
-
- if model_args.tokenizer_name:
- tokenizer = AutoTokenizer.from_pretrained(
- model_args.tokenizer_name, cache_dir=model_args.cache_dir, use_fast=model_args.use_fast_tokenizer
- )
- elif model_args.model_name_or_path:
- tokenizer = AutoTokenizer.from_pretrained(
- model_args.model_name_or_path, cache_dir=model_args.cache_dir, use_fast=model_args.use_fast_tokenizer
- )
- else:
- raise ValueError(
- "You are instantiating a new tokenizer from scratch. This is not supported by this script."
- "You can do it from another script, save it, and load it from here, using --tokenizer_name."
- )
-
- if training_args.do_train:
- column_names = dataset["train"].column_names
- else:
- column_names = dataset["validation"].column_names
- text_column_name = "text" if "text" in column_names else column_names[0]
-
- # since this will be pickled to avoid _LazyModule error in Hasher force logger loading before tokenize_function
- tok_logger = transformers.utils.logging.get_logger("transformers.tokenization_utils_base")
-
- def tokenize_function(examples):
- with CaptureLogger(tok_logger) as cl:
- output = tokenizer(examples[text_column_name])
- # clm input could be much much longer than block_size
- if "Token indices sequence length is longer than the" in cl.out:
- tok_logger.warning(
- "^^^^^^^^^^^^^^^^ Please ignore the warning above - this long input will be chunked into smaller bits"
- " before being passed to the model."
- )
- return output
-
- tokenized_datasets = dataset.map(
- tokenize_function,
- batched=True,
- num_proc=data_args.preprocessing_num_workers,
- remove_columns=column_names,
- load_from_cache_file=not data_args.overwrite_cache,
- )
-
- if data_args.block_size is None:
- block_size = tokenizer.model_max_length
- if block_size > config.max_position_embeddings:
- logger.warning(
- f"The tokenizer picked seems to have a very large `model_max_length` ({tokenizer.model_max_length}). "
- "Picking 1024 instead. You can change that default value by passing --block_size xxx."
- )
- block_size = 1024
- else:
- if data_args.block_size > tokenizer.model_max_length:
- logger.warning(
- f"The block_size passed ({data_args.block_size}) is larger than the maximum length for the model"
- f"({tokenizer.model_max_length}). Using block_size={tokenizer.model_max_length}."
- )
- block_size = min(data_args.block_size, tokenizer.model_max_length)
-
- # Main data processing function that will concatenate all texts from our dataset and generate chunks of block_size.
- def group_texts(examples):
- # Concatenate all texts.
- concatenated_examples = {k: list(chain(*examples[k])) for k in examples.keys()}
- total_length = len(concatenated_examples[list(examples.keys())[0]])
- # We drop the small remainder, we could add padding if the model supported it instead of this drop, you can
- # customize this part to your needs.
- if total_length >= block_size:
- total_length = (total_length // block_size) * block_size
- # Split by chunks of max_len.
- result = {
- k: [t[i : i + block_size] for i in range(0, total_length, block_size)]
- for k, t in concatenated_examples.items()
- }
- result["labels"] = result["input_ids"].copy()
- return result
-
- # Note that with `batched=True`, this map processes 1,000 texts together, so group_texts throws away a remainder
- # for each of those groups of 1,000 texts. You can adjust that batch_size here but a higher value might be slower
- # to preprocess.
- #
- # To speed up this part, we use multiprocessing. See the documentation of the map method for more information:
- # https://huggingface.co/docs/datasets/package_reference/main_classes.html#datasets.Dataset.map
-
- lm_datasets = tokenized_datasets.map(
- group_texts,
- batched=True,
- num_proc=data_args.preprocessing_num_workers,
- load_from_cache_file=not data_args.overwrite_cache,
- )
-
- if training_args.do_train:
- if "train" not in tokenized_datasets:
- raise ValueError("--do_train requires a train dataset")
- train_dataset = lm_datasets["train"]
- if data_args.max_train_samples is not None:
- max_train_samples = min(len(train_dataset), data_args.max_train_samples)
- train_dataset = train_dataset.select(range(max_train_samples))
-
- if training_args.do_eval:
- if "validation" not in tokenized_datasets:
- raise ValueError("--do_eval requires a validation dataset")
- eval_dataset = lm_datasets["validation"]
- if data_args.max_eval_samples is not None:
- max_eval_samples = min(len(eval_dataset), data_args.max_eval_samples)
- eval_dataset = eval_dataset.select(range(max_eval_samples))
-
- # Enable tensorboard only on the master node
- has_tensorboard = is_tensorboard_available()
- if has_tensorboard and jax.process_index() == 0:
- try:
- from flax.metrics.tensorboard import SummaryWriter
-
- summary_writer = SummaryWriter(log_dir=Path(training_args.output_dir))
- except ImportError as ie:
- has_tensorboard = False
- logger.warning(
- f"Unable to display metrics through TensorBoard because some package are not installed: {ie}"
- )
- else:
- logger.warning(
- "Unable to display metrics through TensorBoard because the package is not installed: "
- "Please run pip install tensorboard to enable."
- )
-
- # Initialize our training
- rng = jax.random.PRNGKey(training_args.seed)
- rng, dropout_rng = jax.random.split(rng)
-
- # Store some constant
- num_epochs = int(training_args.num_train_epochs)
- train_batch_size = int(training_args.per_device_train_batch_size) * jax.device_count()
- eval_batch_size = int(training_args.per_device_eval_batch_size) * jax.device_count()
- steps_per_epoch = len(train_dataset) // train_batch_size
- total_train_steps = steps_per_epoch * num_epochs
-
- # TODO: weights should be initialized in pjitted fun, this won't work for REALLY large models
- # TODO: when loading from pre-trained model we need to make sure the vocab is divisible by num_partitions
- # GPT2's vocab is odd, we need to resize it for fine-tuning
- model = FlaxAutoModelForCausalLM.from_pretrained(
- model_args.model_name_or_path, seed=training_args.seed, dtype=getattr(jnp, model_args.dtype)
- )
-
- # Create learning rate schedule
- linear_decay_lr_schedule_fn = create_learning_rate_fn(
- len(train_dataset),
- train_batch_size,
- training_args.num_train_epochs,
- training_args.warmup_steps,
- training_args.learning_rate,
- )
-
- optimizer = optax.adamw(
- learning_rate=linear_decay_lr_schedule_fn,
- b1=training_args.adam_beta1,
- b2=training_args.adam_beta2,
- eps=training_args.adam_epsilon,
- weight_decay=training_args.weight_decay,
- )
-
- def get_initial_state(params):
- state = optimizer.init(params)
- return tuple(state), params
-
- # Get PartitionSpec for model params
- param_spec = set_partitions(unfreeze(model.params))
-
- # Get the PyTree for opt_state, we don't actually initialize the opt_state yet.
- params_shapes = jax.tree_util.tree_map(lambda x: x.shape, model.params)
- state_shapes = jax.eval_shape(get_initial_state, params_shapes)
-
- # get PartitionSpec for opt_state, this is very specific to adamw
- # TODO: optax returns different state for different optimizers, how can we handle this generically ?
- # or maybe we don't since in our examples we just use adamw or adafactor
- def get_opt_spec(x):
- if isinstance(x, dict):
- return param_spec
- return None
-
- opt_state_spec, param_spec = jax.tree_util.tree_map(
- get_opt_spec, state_shapes, is_leaf=lambda x: isinstance(x, (dict, optax.EmptyState))
- )
-
- # pjit the get_initial_state function to shard params and init
- # optimizer state in sharded way
- p_get_initial_state = pjit(
- get_initial_state,
- in_axis_resources=None,
- out_axis_resources=(opt_state_spec, param_spec),
- )
-
- # hack: move the inital params to CPU to free up device memory
- # TODO: allow loading weights on CPU in pre-trained model
- model.params = jax.tree_util.tree_map(lambda x: np.asarray(x), model.params)
-
- # mesh defination
- mesh_devices = np.array(jax.devices()).reshape(1, jax.local_device_count())
-
- # actually initialize the opt_state
- with mesh(mesh_devices, ("dp", "mp")):
- opt_state, params = p_get_initial_state(freeze(model.params))
-
- # cross-entropy with z loss
- def loss_fn(logits, labels, z_loss=0):
- shift_logits = logits[..., :-1, :]
- shift_labels = labels[..., 1:]
-
- shift_labels = onehot(shift_labels, shift_logits.shape[-1])
-
- shift_logits = shift_logits - jax.lax.stop_gradient(shift_logits.max(axis=-1, keepdims=True))
- log_z = jnp.log(jnp.sum(jnp.exp(shift_logits), axis=-1, keepdims=True))
- log_softmax = shift_logits - log_z
- loss = -jnp.sum(shift_labels * log_softmax, axis=-1)
-
- loss += (1e-4 * jnp.square(log_z.squeeze(-1))) * z_loss
-
- return loss.mean()
-
- # Define gradient update step fn
- # TODO: try to use TrainState instead of passing params and opt_state individually
- def train_step(params, opt_state, dropout_rng, batch, step):
- dropout_rng, new_dropout_rng = jax.random.split(dropout_rng)
-
- def compute_loss(params):
- labels = batch.pop("labels")
- logits = model(**batch, params=params, dropout_rng=dropout_rng, train=True)[0]
- loss = loss_fn(logits, labels, z_loss=1.0)
- return loss
-
- grad_fn = jax.value_and_grad(compute_loss)
- loss, grads = grad_fn(params)
-
- updates, new_opt_state = optimizer.update(grads, opt_state, params)
- new_params = optax.apply_updates(params, updates)
-
- metrics = {"loss": loss, "learning_rate": linear_decay_lr_schedule_fn(step)}
- return new_params, tuple(new_opt_state), new_dropout_rng, metrics, step + 1
-
- # Define eval fn
- def eval_step(input_ids, labels, params):
- logits = model(input_ids=input_ids, params=params, train=False)[0]
- loss = loss_fn(logits, labels)
- # metrics
- return {"loss": loss}
-
- p_train_step = pjit(
- train_step,
- in_axis_resources=(param_spec, opt_state_spec, None, None, None),
- out_axis_resources=(param_spec, opt_state_spec, None, None, None),
- donate_argnums=(0, 1),
- )
-
- p_eval_step = pjit(
- eval_step,
- in_axis_resources=(None, None, param_spec),
- out_axis_resources=None,
- )
-
- logger.info("***** Running training *****")
- logger.info(f" Num examples = {len(train_dataset)}")
- logger.info(f" Num Epochs = {num_epochs}")
- logger.info(f" Instantaneous batch size per device = {training_args.per_device_train_batch_size}")
- logger.info(f" Total train batch size (w. parallel & distributed) = {train_batch_size}")
- logger.info(f" Total optimization steps = {total_train_steps}")
-
- train_time = 0
- train_metrics = []
- epochs = tqdm(range(num_epochs), desc=f"Epoch ... (1/{num_epochs})", position=0)
- global_step = 0
- # we are not doing 2D parallelism (yet!), this just does model parallelism
- with mesh(mesh_devices, ("dp", "mp")):
- for _ in epochs:
- # ======================== Training ================================
- train_start = time.time()
-
- # Create sampling rng
- rng, input_rng = jax.random.split(rng)
-
- # Generate an epoch by shuffling sampling indices from the train dataset
- train_metrics = []
- train_loader = data_loader(input_rng, train_dataset, train_batch_size, shuffle=True)
- steps_per_epoch = len(train_dataset) // train_batch_size
-
- # train
- for _ in tqdm(range(steps_per_epoch), desc="Training...", position=1, leave=False):
- batch = next(train_loader)
- params, opt_state, dropout_rng, train_metric, global_step = p_train_step(
- params,
- opt_state,
- dropout_rng,
- batch,
- global_step,
- )
- train_metrics.append(train_metric)
-
- cur_step = global_step
-
- if cur_step % training_args.logging_steps == 0 and cur_step > 0:
- # Save metrics
- train_time += time.time() - train_start
- if has_tensorboard and jax.process_index() == 0:
- write_train_metric(summary_writer, train_metrics, train_time, cur_step)
-
- epochs.write(
- f"Step... ({cur_step} | Loss: {train_metric['loss']}, Learning Rate:"
- f" {train_metric['learning_rate']})"
- )
-
- train_metrics = []
-
- if cur_step % training_args.eval_steps == 0 and cur_step > 0:
- # ======================== Evaluating ==============================
- eval_metrics = []
- eval_loader = data_loader(input_rng, eval_dataset, eval_batch_size)
- eval_steps = len(eval_dataset) // eval_batch_size
-
- for _ in tqdm(range(eval_steps), desc="Evaluating...", position=2, leave=False):
- batch = next(eval_loader)
- metrics = p_eval_step(batch["input_ids"], batch["labels"], params)
- eval_metrics.append(metrics)
-
- # normalize eval metrics
- eval_metrics = stack_forest(eval_metrics)
- eval_metrics = jax.tree_util.tree_map(jnp.mean, eval_metrics)
-
- try:
- eval_metrics["perplexity"] = math.exp(eval_metrics["loss"])
- except OverflowError:
- eval_metrics["perplexity"] = float("inf")
-
- logger.info(
- f"Step... ({cur_step} | Eval loss: {eval_metrics['loss']} | Eval Perplexity:"
- f" {eval_metrics['perplexity']}"
- )
-
- if cur_step % training_args.save_steps == 0 and cur_step > 0:
- # save checkpoint after each epoch and push checkpoint to the hub
- if jax.process_index() == 0:
- params = jax.device_get(params)
- model.save_pretrained(
- training_args.output_dir,
- params=params,
- push_to_hub=training_args.push_to_hub,
- commit_message=f"Saving weights and logs of step {cur_step}",
- )
-
-
-if __name__ == "__main__":
- main()
diff --git a/spaces/chenxiYan/ChatHaruhi-OpenAI/app.py b/spaces/chenxiYan/ChatHaruhi-OpenAI/app.py
deleted file mode 100644
index 4b438577225ffd09e062f82a83c41fdb11ad8f09..0000000000000000000000000000000000000000
--- a/spaces/chenxiYan/ChatHaruhi-OpenAI/app.py
+++ /dev/null
@@ -1,128 +0,0 @@
-import zipfile
-import gradio as gr
-from PIL import Image
-from chatharuhi import ChatHaruhi
-import requests
-import os
-import openai
-import copy
-
-
-NAME_DICT = {'汤师爷': 'tangshiye', '慕容复': 'murongfu', '李云龙': 'liyunlong', 'Luna': 'Luna', '王多鱼': 'wangduoyu',
- 'Ron': 'Ron', '鸠摩智': 'jiumozhi', 'Snape': 'Snape',
- '凉宫春日': 'haruhi', 'Malfoy': 'Malfoy', '虚竹': 'xuzhu', '萧峰': 'xiaofeng', '段誉': 'duanyu',
- 'Hermione': 'Hermione', 'Dumbledore': 'Dumbledore', '王语嫣': 'wangyuyan',
- 'Harry': 'Harry', 'McGonagall': 'McGonagall', '白展堂': 'baizhantang', '佟湘玉': 'tongxiangyu',
- '郭芙蓉': 'guofurong', '旅行者': 'wanderer', '钟离': 'zhongli',
- '胡桃': 'hutao', 'Sheldon': 'Sheldon', 'Raj': 'Raj', 'Penny': 'Penny', '韦小宝': 'weixiaobao',
- '乔峰': 'qiaofeng', '神里绫华': 'ayaka', '雷电将军': 'raidenShogun', '于谦': 'yuqian'}
-
-
-
-try:
- os.makedirs("characters_zip")
-except:
- pass
-try:
- os.makedirs("characters")
-except:
- pass
-ai_roles_obj = {}
-for ai_role_en in NAME_DICT.values():
- file_url = f"https://github.com/LC1332/Haruhi-2-Dev/raw/main/data/character_in_zip/{ai_role_en}.zip"
- try:
- os.makedirs(f"characters/{ai_role_en}")
- except:
- pass
- if f"{ai_role_en}.zip" not in os.listdir(f"characters_zip"):
- destination_file = f"characters_zip/{ai_role_en}.zip"
- max_retries = 3 # 最大重试次数
- for attempt in range(1, max_retries+1):
- response = requests.get(file_url)
- if response.status_code == 200:
- with open(destination_file, "wb") as file:
- file.write(response.content)
- print(ai_role_en)
- break
- else:
- print(f"{ai_role_en}第{attempt}次下载失败")
- # wget.download(file_url, destination_file) # 503
- destination_folder = f"characters/{ai_role_en}"
- with zipfile.ZipFile(destination_file, 'r') as zip_ref:
- zip_ref.extractall(destination_folder)
- db_folder = f"./characters/{ai_role_en}/content/{ai_role_en}"
- system_prompt = f"./characters/{ai_role_en}/content/system_prompt.txt"
- ai_roles_obj[ai_role_en] = ChatHaruhi(system_prompt=system_prompt,
- llm="openai",
- story_db=db_folder,
- verbose=True)
-
-
-async def get_response(user_role, user_text, ai_role, chatbot):
- role_en = NAME_DICT[ai_role]
- ai_roles_obj[role_en].dialogue_history = copy.deepcopy(chatbot)
- response = ai_roles_obj[role_en].chat(role=user_role, text=user_text)
- user_msg = user_role + ':「' + user_text + '」'
- latest_msg = (user_msg, response)
- print(latest_msg)
- chatbot.append(latest_msg)
- return chatbot
-
-async def respond(user_role, user_text, ai_role, chatbot):
- return await get_response(user_role, user_text, ai_role, chatbot), None
-
-
-def clear(user_role, user_text, chatbot):
- return None, None, []
-
-
-def get_image(ai_role):
- role_en = NAME_DICT[ai_role]
- return Image.open(f'images/{role_en}.jpg'), None, None, []
-
-
-with gr.Blocks() as demo:
- gr.Markdown(
- """
- # Chat凉宫春日 ChatHaruhi
- ## Reviving Anime Character in Reality via Large Language Model
-
- ChatHaruhi2.0的demo implemented by [chenxi](https://github.com/todochenxi)
-
- 更多信息见项目github链接 [https://github.com/LC1332/Chat-Haruhi-Suzumiya](https://github.com/LC1332/Chat-Haruhi-Suzumiya)
-
- 如果觉得有趣请拜托为我们点上star. If you find it interesting, please be kind enough to give us a star.
-
- user_role 为用户扮演的人物 请尽量设置为与剧情相关的人物 且不要与主角同名
-
- 如果你想为我们捐赠 api key,请联系我。
- If you would like to donate an api key to us, please contact me.
- API キーを寄付したい場合は、私までご連絡ください。
- email: todochenxi@163.com
- """
- )
- with gr.Row():
- chatbot = gr.Chatbot()
- role_image = gr.Image(height=400, value="./images/haruhi.jpg")
- with gr.Row():
- user_role = gr.Textbox(label="user_role", scale=1)
- user_text = gr.Textbox(label="user_text", scale=20)
- with gr.Row():
- submit = gr.Button("Submit")
- clean = gr.ClearButton(value="Clear")
- ai_role = gr.Radio(['汤师爷', '慕容复', '李云龙',
- 'Luna', '王多鱼', 'Ron', '鸠摩智',
- 'Snape', '凉宫春日', 'Malfoy', '虚竹',
- '萧峰', '段誉', 'Hermione', 'Dumbledore',
- '王语嫣',
- 'Harry', 'McGonagall',
- '白展堂', '佟湘玉', '郭芙蓉',
- '旅行者', '钟离', '胡桃',
- 'Sheldon', 'Raj', 'Penny',
- '韦小宝', '乔峰', '神里绫华',
- '雷电将军', '于谦'], label="characters", value='凉宫春日')
- ai_role.change(get_image, ai_role, [role_image, user_role, user_text, chatbot])
- user_text.submit(fn=respond, inputs=[user_role, user_text, ai_role, chatbot], outputs=[chatbot, user_text])
- submit.click(fn=respond, inputs=[user_role, user_text, ai_role, chatbot], outputs=[chatbot, user_text])
- clean.click(clear, [user_role, user_text, chatbot], [user_role, user_text, chatbot])
-demo.launch(debug=True)
\ No newline at end of file
diff --git a/spaces/chumeng/anime-ai-detect/app.py b/spaces/chumeng/anime-ai-detect/app.py
deleted file mode 100644
index 89224ac0e4493054be928e7fabed7b9d0485e412..0000000000000000000000000000000000000000
--- a/spaces/chumeng/anime-ai-detect/app.py
+++ /dev/null
@@ -1,17 +0,0 @@
-import gradio as gr
-from transformers import pipeline
-
-detection_pipeline = pipeline("image-classification", "saltacc/anime-ai-detect")
-
-
-def detect(img):
- print(img)
- output = detection_pipeline(img, top_k=2)
- final = {}
- for d in output:
- final[d["label"]] = d["score"]
- return final
-
-
-iface = gr.Interface(fn=detect, inputs=gr.Image(type="pil"), outputs=gr.Label(label="result"))
-iface.launch()
diff --git a/spaces/cihyFjudo/fairness-paper-search/Sketchup Instant Road Pro Plugin.torrent LINK.md b/spaces/cihyFjudo/fairness-paper-search/Sketchup Instant Road Pro Plugin.torrent LINK.md
deleted file mode 100644
index 4287a0d920ea4ff9572e0bdd07c427756827e632..0000000000000000000000000000000000000000
--- a/spaces/cihyFjudo/fairness-paper-search/Sketchup Instant Road Pro Plugin.torrent LINK.md
+++ /dev/null
@@ -1,91 +0,0 @@
-## Sketchup Instant Road Pro Plugin.torrent
-
-
-
- 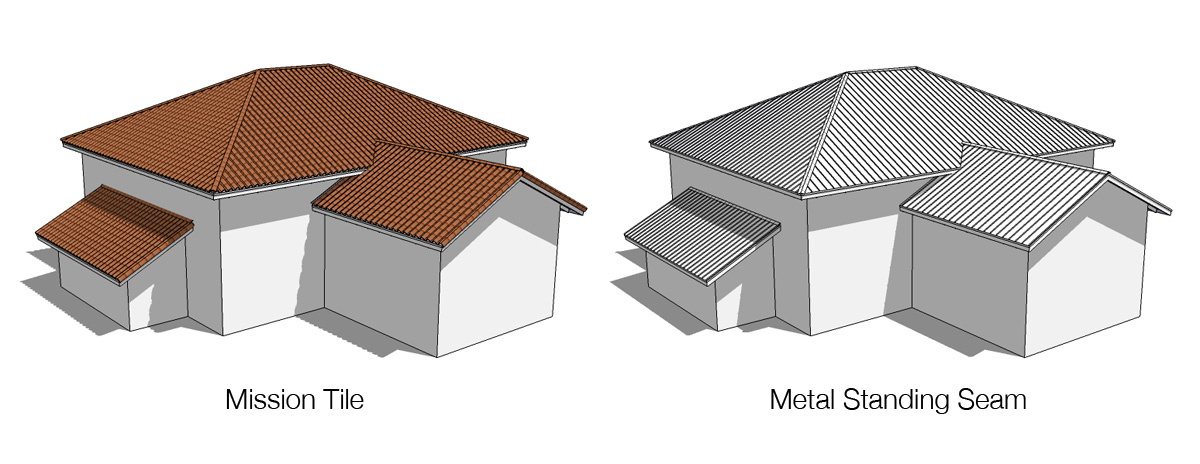
-
-
-
-**CLICK HERE >> [https://walllowcopo.blogspot.com/?download=2twr29](https://walllowcopo.blogspot.com/?download=2twr29)**
-
-
-
-# How to Install and Use Sketchup Instant Road Pro Plugin
-
-
-
-Sketchup Instant Road Pro Plugin is a powerful tool that automates the creation of roads, pathways, and waterways on a terrain using either an outline or a centerline for input. It also creates curbs, sidewalks, depressed or raised road surfaces, center medians and islands. It is compatible with Sketchup free and pro versions 2014 and above.
-
-
-
-In this article, we will show you how to download, install and use Sketchup Instant Road Pro Plugin to create realistic roads and landscapes in Sketchup.
-
-
-
-## How to Download Sketchup Instant Road Pro Plugin
-
-
-
-Sketchup Instant Road Pro Plugin is available for purchase from Vali Architects website[^1^]. You can also download a free trial version that works for 30 days. The plugin file is in .rbz format, which is a compressed Ruby script file that can be installed directly in Sketchup.
-
-
-
-## How to Install Sketchup Instant Road Pro Plugin
-
-
-
-To install Sketchup Instant Road Pro Plugin, follow these steps:
-
-
-
-1. Open Sketchup and go to Window > Extension Manager.
-
-2. Click on the Install Extension button at the bottom left corner of the window.
-
-3. Browse to the location where you saved the .rbz file and select it.
-
-4. Click on OK to confirm the installation.
-
-5. Restart Sketchup to activate the plugin.
-
-
-
-You should now see a new toolbar called Instant Road Nui on your screen. You can also access the plugin from Tools > Instant Road Nui.
-
-
-
-## How to Use Sketchup Instant Road Pro Plugin
-
-
-
-To use Sketchup Instant Road Pro Plugin, follow these steps:
-
-
-
-1. Create a terrain model in Sketchup or import one from another source.
-
-2. Select the Instant Road Nui toolbar or go to Tools > Instant Road Nui.
-
-3. Choose one of the four modes: Outline, Centerline, From Contours or From Mesh.
-
-4. Depending on the mode, draw an outline or a centerline on the terrain using Sketchup drawing tools or select an existing group of contours or a mesh.
-
-5. Click on the Create button on the toolbar or press Enter to generate the road.
-
-6. Adjust the parameters of the road such as width, profile, material, curb height, etc. from the dialog box that appears.
-
-7. Click on OK to apply the changes or Cancel to undo them.
-
-
-
-You can also edit the road after creating it by selecting it and clicking on the Edit button on the toolbar. You can move, rotate, scale or delete the road as you wish. You can also create multiple roads and connect them using the Connect button on the toolbar.
-
-
-
-## Conclusion
-
-
-
-Sketchup Instant Road Pro Plugin is a useful plugin that simplifies the process of creating roads and landscapes in Sketchup. It offers various options and features that allow you to customize your roads according to your needs and preferences. It is compatible with Sketchup free and pro versions 2014 and above. You can purchase it from Vali Architects website[^1^] or download a free trial version that works for 30 days.
-
- [^1^]: http://www.valiarchitects.com/sketchup\_scripts/instant-road-nui dfd1c89656
\ No newline at end of file
diff --git a/spaces/cleanmaster/akagi-sovits3/data_utils.py b/spaces/cleanmaster/akagi-sovits3/data_utils.py
deleted file mode 100644
index 9dfba4a9dfbfbd2b6ed5e771a5ffee4f70419ba3..0000000000000000000000000000000000000000
--- a/spaces/cleanmaster/akagi-sovits3/data_utils.py
+++ /dev/null
@@ -1,152 +0,0 @@
-import time
-import os
-import random
-import numpy as np
-import torch
-import torch.utils.data
-
-import commons
-from mel_processing import spectrogram_torch, spec_to_mel_torch
-from utils import load_wav_to_torch, load_filepaths_and_text, transform
-
-# import h5py
-
-
-"""Multi speaker version"""
-
-
-class TextAudioSpeakerLoader(torch.utils.data.Dataset):
- """
- 1) loads audio, speaker_id, text pairs
- 2) normalizes text and converts them to sequences of integers
- 3) computes spectrograms from audio files.
- """
-
- def __init__(self, audiopaths, hparams):
- self.audiopaths = load_filepaths_and_text(audiopaths)
- self.max_wav_value = hparams.data.max_wav_value
- self.sampling_rate = hparams.data.sampling_rate
- self.filter_length = hparams.data.filter_length
- self.hop_length = hparams.data.hop_length
- self.win_length = hparams.data.win_length
- self.sampling_rate = hparams.data.sampling_rate
- self.use_sr = hparams.train.use_sr
- self.spec_len = hparams.train.max_speclen
- self.spk_map = hparams.spk
-
- random.seed(1234)
- random.shuffle(self.audiopaths)
-
- def get_audio(self, filename):
- audio, sampling_rate = load_wav_to_torch(filename)
- if sampling_rate != self.sampling_rate:
- raise ValueError("{} SR doesn't match target {} SR".format(
- sampling_rate, self.sampling_rate))
- audio_norm = audio / self.max_wav_value
- audio_norm = audio_norm.unsqueeze(0)
- spec_filename = filename.replace(".wav", ".spec.pt")
- if os.path.exists(spec_filename):
- spec = torch.load(spec_filename)
- else:
- spec = spectrogram_torch(audio_norm, self.filter_length,
- self.sampling_rate, self.hop_length, self.win_length,
- center=False)
- spec = torch.squeeze(spec, 0)
- torch.save(spec, spec_filename)
-
- spk = filename.split(os.sep)[-2]
- spk = torch.LongTensor([self.spk_map[spk]])
-
- c = torch.load(filename + ".soft.pt").squeeze(0)
- c = torch.repeat_interleave(c, repeats=2, dim=1)
-
- f0 = np.load(filename + ".f0.npy")
- f0 = torch.FloatTensor(f0)
- lmin = min(c.size(-1), spec.size(-1), f0.shape[0])
- assert abs(c.size(-1) - spec.size(-1)) < 4, (c.size(-1), spec.size(-1), f0.shape, filename)
- assert abs(lmin - spec.size(-1)) < 4, (c.size(-1), spec.size(-1), f0.shape)
- assert abs(lmin - c.size(-1)) < 4, (c.size(-1), spec.size(-1), f0.shape)
- spec, c, f0 = spec[:, :lmin], c[:, :lmin], f0[:lmin]
- audio_norm = audio_norm[:, :lmin * self.hop_length]
- _spec, _c, _audio_norm, _f0 = spec, c, audio_norm, f0
- while spec.size(-1) < self.spec_len:
- spec = torch.cat((spec, _spec), -1)
- c = torch.cat((c, _c), -1)
- f0 = torch.cat((f0, _f0), -1)
- audio_norm = torch.cat((audio_norm, _audio_norm), -1)
- start = random.randint(0, spec.size(-1) - self.spec_len)
- end = start + self.spec_len
- spec = spec[:, start:end]
- c = c[:, start:end]
- f0 = f0[start:end]
- audio_norm = audio_norm[:, start * self.hop_length:end * self.hop_length]
-
- return c, f0, spec, audio_norm, spk
-
- def __getitem__(self, index):
- return self.get_audio(self.audiopaths[index][0])
-
- def __len__(self):
- return len(self.audiopaths)
-
-
-class EvalDataLoader(torch.utils.data.Dataset):
- """
- 1) loads audio, speaker_id, text pairs
- 2) normalizes text and converts them to sequences of integers
- 3) computes spectrograms from audio files.
- """
-
- def __init__(self, audiopaths, hparams):
- self.audiopaths = load_filepaths_and_text(audiopaths)
- self.max_wav_value = hparams.data.max_wav_value
- self.sampling_rate = hparams.data.sampling_rate
- self.filter_length = hparams.data.filter_length
- self.hop_length = hparams.data.hop_length
- self.win_length = hparams.data.win_length
- self.sampling_rate = hparams.data.sampling_rate
- self.use_sr = hparams.train.use_sr
- self.audiopaths = self.audiopaths[:5]
- self.spk_map = hparams.spk
-
-
- def get_audio(self, filename):
- audio, sampling_rate = load_wav_to_torch(filename)
- if sampling_rate != self.sampling_rate:
- raise ValueError("{} SR doesn't match target {} SR".format(
- sampling_rate, self.sampling_rate))
- audio_norm = audio / self.max_wav_value
- audio_norm = audio_norm.unsqueeze(0)
- spec_filename = filename.replace(".wav", ".spec.pt")
- if os.path.exists(spec_filename):
- spec = torch.load(spec_filename)
- else:
- spec = spectrogram_torch(audio_norm, self.filter_length,
- self.sampling_rate, self.hop_length, self.win_length,
- center=False)
- spec = torch.squeeze(spec, 0)
- torch.save(spec, spec_filename)
-
- spk = filename.split(os.sep)[-2]
- spk = torch.LongTensor([self.spk_map[spk]])
-
- c = torch.load(filename + ".soft.pt").squeeze(0)
-
- c = torch.repeat_interleave(c, repeats=2, dim=1)
-
- f0 = np.load(filename + ".f0.npy")
- f0 = torch.FloatTensor(f0)
- lmin = min(c.size(-1), spec.size(-1), f0.shape[0])
- assert abs(c.size(-1) - spec.size(-1)) < 4, (c.size(-1), spec.size(-1), f0.shape)
- assert abs(f0.shape[0] - spec.shape[-1]) < 4, (c.size(-1), spec.size(-1), f0.shape)
- spec, c, f0 = spec[:, :lmin], c[:, :lmin], f0[:lmin]
- audio_norm = audio_norm[:, :lmin * self.hop_length]
-
- return c, f0, spec, audio_norm, spk
-
- def __getitem__(self, index):
- return self.get_audio(self.audiopaths[index][0])
-
- def __len__(self):
- return len(self.audiopaths)
-
diff --git a/spaces/clevrpwn/CompVis-stable-diffusion-v1-4/README.md b/spaces/clevrpwn/CompVis-stable-diffusion-v1-4/README.md
deleted file mode 100644
index ebd36ce416059ad6792215ac84d3c26f99493949..0000000000000000000000000000000000000000
--- a/spaces/clevrpwn/CompVis-stable-diffusion-v1-4/README.md
+++ /dev/null
@@ -1,12 +0,0 @@
----
-title: CompVis Stable Diffusion V1 4
-emoji: 👀
-colorFrom: pink
-colorTo: pink
-sdk: gradio
-sdk_version: 3.16.2
-app_file: app.py
-pinned: false
----
-
-Check out the configuration reference at https://huggingface.co/docs/hub/spaces-config-reference
diff --git a/spaces/cloudtheboi/Lofi4All/.pythonlibs/lib/python3.10/site-packages/PIL/WalImageFile.py b/spaces/cloudtheboi/Lofi4All/.pythonlibs/lib/python3.10/site-packages/PIL/WalImageFile.py
deleted file mode 100644
index e4f47aa04bc148f3ff151bec5595f8626833b938..0000000000000000000000000000000000000000
--- a/spaces/cloudtheboi/Lofi4All/.pythonlibs/lib/python3.10/site-packages/PIL/WalImageFile.py
+++ /dev/null
@@ -1,123 +0,0 @@
-#
-# The Python Imaging Library.
-# $Id$
-#
-# WAL file handling
-#
-# History:
-# 2003-04-23 fl created
-#
-# Copyright (c) 2003 by Fredrik Lundh.
-#
-# See the README file for information on usage and redistribution.
-#
-
-"""
-This reader is based on the specification available from:
-https://www.flipcode.com/archives/Quake_2_BSP_File_Format.shtml
-and has been tested with a few sample files found using google.
-
-.. note::
- This format cannot be automatically recognized, so the reader
- is not registered for use with :py:func:`PIL.Image.open()`.
- To open a WAL file, use the :py:func:`PIL.WalImageFile.open()` function instead.
-"""
-
-from . import Image, ImageFile
-from ._binary import i32le as i32
-
-
-class WalImageFile(ImageFile.ImageFile):
- format = "WAL"
- format_description = "Quake2 Texture"
-
- def _open(self):
- self.mode = "P"
-
- # read header fields
- header = self.fp.read(32 + 24 + 32 + 12)
- self._size = i32(header, 32), i32(header, 36)
- Image._decompression_bomb_check(self.size)
-
- # load pixel data
- offset = i32(header, 40)
- self.fp.seek(offset)
-
- # strings are null-terminated
- self.info["name"] = header[:32].split(b"\0", 1)[0]
- next_name = header[56 : 56 + 32].split(b"\0", 1)[0]
- if next_name:
- self.info["next_name"] = next_name
-
- def load(self):
- if not self.im:
- self.im = Image.core.new(self.mode, self.size)
- self.frombytes(self.fp.read(self.size[0] * self.size[1]))
- self.putpalette(quake2palette)
- return Image.Image.load(self)
-
-
-def open(filename):
- """
- Load texture from a Quake2 WAL texture file.
-
- By default, a Quake2 standard palette is attached to the texture.
- To override the palette, use the :py:func:`PIL.Image.Image.putpalette()` method.
-
- :param filename: WAL file name, or an opened file handle.
- :returns: An image instance.
- """
- return WalImageFile(filename)
-
-
-quake2palette = (
- # default palette taken from piffo 0.93 by Hans Häggström
- b"\x01\x01\x01\x0b\x0b\x0b\x12\x12\x12\x17\x17\x17\x1b\x1b\x1b\x1e"
- b"\x1e\x1e\x22\x22\x22\x26\x26\x26\x29\x29\x29\x2c\x2c\x2c\x2f\x2f"
- b"\x2f\x32\x32\x32\x35\x35\x35\x37\x37\x37\x3a\x3a\x3a\x3c\x3c\x3c"
- b"\x24\x1e\x13\x22\x1c\x12\x20\x1b\x12\x1f\x1a\x10\x1d\x19\x10\x1b"
- b"\x17\x0f\x1a\x16\x0f\x18\x14\x0d\x17\x13\x0d\x16\x12\x0d\x14\x10"
- b"\x0b\x13\x0f\x0b\x10\x0d\x0a\x0f\x0b\x0a\x0d\x0b\x07\x0b\x0a\x07"
- b"\x23\x23\x26\x22\x22\x25\x22\x20\x23\x21\x1f\x22\x20\x1e\x20\x1f"
- b"\x1d\x1e\x1d\x1b\x1c\x1b\x1a\x1a\x1a\x19\x19\x18\x17\x17\x17\x16"
- b"\x16\x14\x14\x14\x13\x13\x13\x10\x10\x10\x0f\x0f\x0f\x0d\x0d\x0d"
- b"\x2d\x28\x20\x29\x24\x1c\x27\x22\x1a\x25\x1f\x17\x38\x2e\x1e\x31"
- b"\x29\x1a\x2c\x25\x17\x26\x20\x14\x3c\x30\x14\x37\x2c\x13\x33\x28"
- b"\x12\x2d\x24\x10\x28\x1f\x0f\x22\x1a\x0b\x1b\x14\x0a\x13\x0f\x07"
- b"\x31\x1a\x16\x30\x17\x13\x2e\x16\x10\x2c\x14\x0d\x2a\x12\x0b\x27"
- b"\x0f\x0a\x25\x0f\x07\x21\x0d\x01\x1e\x0b\x01\x1c\x0b\x01\x1a\x0b"
- b"\x01\x18\x0a\x01\x16\x0a\x01\x13\x0a\x01\x10\x07\x01\x0d\x07\x01"
- b"\x29\x23\x1e\x27\x21\x1c\x26\x20\x1b\x25\x1f\x1a\x23\x1d\x19\x21"
- b"\x1c\x18\x20\x1b\x17\x1e\x19\x16\x1c\x18\x14\x1b\x17\x13\x19\x14"
- b"\x10\x17\x13\x0f\x14\x10\x0d\x12\x0f\x0b\x0f\x0b\x0a\x0b\x0a\x07"
- b"\x26\x1a\x0f\x23\x19\x0f\x20\x17\x0f\x1c\x16\x0f\x19\x13\x0d\x14"
- b"\x10\x0b\x10\x0d\x0a\x0b\x0a\x07\x33\x22\x1f\x35\x29\x26\x37\x2f"
- b"\x2d\x39\x35\x34\x37\x39\x3a\x33\x37\x39\x30\x34\x36\x2b\x31\x34"
- b"\x27\x2e\x31\x22\x2b\x2f\x1d\x28\x2c\x17\x25\x2a\x0f\x20\x26\x0d"
- b"\x1e\x25\x0b\x1c\x22\x0a\x1b\x20\x07\x19\x1e\x07\x17\x1b\x07\x14"
- b"\x18\x01\x12\x16\x01\x0f\x12\x01\x0b\x0d\x01\x07\x0a\x01\x01\x01"
- b"\x2c\x21\x21\x2a\x1f\x1f\x29\x1d\x1d\x27\x1c\x1c\x26\x1a\x1a\x24"
- b"\x18\x18\x22\x17\x17\x21\x16\x16\x1e\x13\x13\x1b\x12\x12\x18\x10"
- b"\x10\x16\x0d\x0d\x12\x0b\x0b\x0d\x0a\x0a\x0a\x07\x07\x01\x01\x01"
- b"\x2e\x30\x29\x2d\x2e\x27\x2b\x2c\x26\x2a\x2a\x24\x28\x29\x23\x27"
- b"\x27\x21\x26\x26\x1f\x24\x24\x1d\x22\x22\x1c\x1f\x1f\x1a\x1c\x1c"
- b"\x18\x19\x19\x16\x17\x17\x13\x13\x13\x10\x0f\x0f\x0d\x0b\x0b\x0a"
- b"\x30\x1e\x1b\x2d\x1c\x19\x2c\x1a\x17\x2a\x19\x14\x28\x17\x13\x26"
- b"\x16\x10\x24\x13\x0f\x21\x12\x0d\x1f\x10\x0b\x1c\x0f\x0a\x19\x0d"
- b"\x0a\x16\x0b\x07\x12\x0a\x07\x0f\x07\x01\x0a\x01\x01\x01\x01\x01"
- b"\x28\x29\x38\x26\x27\x36\x25\x26\x34\x24\x24\x31\x22\x22\x2f\x20"
- b"\x21\x2d\x1e\x1f\x2a\x1d\x1d\x27\x1b\x1b\x25\x19\x19\x21\x17\x17"
- b"\x1e\x14\x14\x1b\x13\x12\x17\x10\x0f\x13\x0d\x0b\x0f\x0a\x07\x07"
- b"\x2f\x32\x29\x2d\x30\x26\x2b\x2e\x24\x29\x2c\x21\x27\x2a\x1e\x25"
- b"\x28\x1c\x23\x26\x1a\x21\x25\x18\x1e\x22\x14\x1b\x1f\x10\x19\x1c"
- b"\x0d\x17\x1a\x0a\x13\x17\x07\x10\x13\x01\x0d\x0f\x01\x0a\x0b\x01"
- b"\x01\x3f\x01\x13\x3c\x0b\x1b\x39\x10\x20\x35\x14\x23\x31\x17\x23"
- b"\x2d\x18\x23\x29\x18\x3f\x3f\x3f\x3f\x3f\x39\x3f\x3f\x31\x3f\x3f"
- b"\x2a\x3f\x3f\x20\x3f\x3f\x14\x3f\x3c\x12\x3f\x39\x0f\x3f\x35\x0b"
- b"\x3f\x32\x07\x3f\x2d\x01\x3d\x2a\x01\x3b\x26\x01\x39\x21\x01\x37"
- b"\x1d\x01\x34\x1a\x01\x32\x16\x01\x2f\x12\x01\x2d\x0f\x01\x2a\x0b"
- b"\x01\x27\x07\x01\x23\x01\x01\x1d\x01\x01\x17\x01\x01\x10\x01\x01"
- b"\x3d\x01\x01\x19\x19\x3f\x3f\x01\x01\x01\x01\x3f\x16\x16\x13\x10"
- b"\x10\x0f\x0d\x0d\x0b\x3c\x2e\x2a\x36\x27\x20\x30\x21\x18\x29\x1b"
- b"\x10\x3c\x39\x37\x37\x32\x2f\x31\x2c\x28\x2b\x26\x21\x30\x22\x20"
-)
diff --git a/spaces/cloudtheboi/Lofi4All/.pythonlibs/lib/python3.10/site-packages/fontTools/ttLib/tables/T_S_I_J_.py b/spaces/cloudtheboi/Lofi4All/.pythonlibs/lib/python3.10/site-packages/fontTools/ttLib/tables/T_S_I_J_.py
deleted file mode 100644
index bc8fe92aac9d18bfd5ee565588d8cebf7d00afd1..0000000000000000000000000000000000000000
--- a/spaces/cloudtheboi/Lofi4All/.pythonlibs/lib/python3.10/site-packages/fontTools/ttLib/tables/T_S_I_J_.py
+++ /dev/null
@@ -1,5 +0,0 @@
-from .T_S_I_V_ import table_T_S_I_V_
-
-
-class table_T_S_I_J_(table_T_S_I_V_):
- pass
diff --git a/spaces/colakin/video-generater/public/ffmpeg/libavcodec/apedec.c b/spaces/colakin/video-generater/public/ffmpeg/libavcodec/apedec.c
deleted file mode 100644
index 772636afde33514adad360f9b37e8119c9289f45..0000000000000000000000000000000000000000
--- a/spaces/colakin/video-generater/public/ffmpeg/libavcodec/apedec.c
+++ /dev/null
@@ -1,1692 +0,0 @@
-/*
- * Monkey's Audio lossless audio decoder
- * Copyright (c) 2007 Benjamin Zores
- * based upon libdemac from Dave Chapman.
- *
- * This file is part of FFmpeg.
- *
- * FFmpeg is free software; you can redistribute it and/or
- * modify it under the terms of the GNU Lesser General Public
- * License as published by the Free Software Foundation; either
- * version 2.1 of the License, or (at your option) any later version.
- *
- * FFmpeg is distributed in the hope that it will be useful,
- * but WITHOUT ANY WARRANTY; without even the implied warranty of
- * MERCHANTABILITY or FITNESS FOR A PARTICULAR PURPOSE. See the GNU
- * Lesser General Public License for more details.
- *
- * You should have received a copy of the GNU Lesser General Public
- * License along with FFmpeg; if not, write to the Free Software
- * Foundation, Inc., 51 Franklin Street, Fifth Floor, Boston, MA 02110-1301 USA
- */
-
-#include
-
-#include "libavutil/avassert.h"
-#include "libavutil/channel_layout.h"
-#include "libavutil/crc.h"
-#include "libavutil/opt.h"
-#include "lossless_audiodsp.h"
-#include "avcodec.h"
-#include "bswapdsp.h"
-#include "bytestream.h"
-#include "codec_internal.h"
-#include "decode.h"
-#include "get_bits.h"
-#include "unary.h"
-
-/**
- * @file
- * Monkey's Audio lossless audio decoder
- */
-
-#define MAX_CHANNELS 2
-#define MAX_BYTESPERSAMPLE 3
-
-#define APE_FRAMECODE_MONO_SILENCE 1
-#define APE_FRAMECODE_STEREO_SILENCE 3
-#define APE_FRAMECODE_PSEUDO_STEREO 4
-
-#define HISTORY_SIZE 512
-#define PREDICTOR_ORDER 8
-/** Total size of all predictor histories */
-#define PREDICTOR_SIZE 50
-
-#define YDELAYA (18 + PREDICTOR_ORDER*4)
-#define YDELAYB (18 + PREDICTOR_ORDER*3)
-#define XDELAYA (18 + PREDICTOR_ORDER*2)
-#define XDELAYB (18 + PREDICTOR_ORDER)
-
-#define YADAPTCOEFFSA 18
-#define XADAPTCOEFFSA 14
-#define YADAPTCOEFFSB 10
-#define XADAPTCOEFFSB 5
-
-/**
- * Possible compression levels
- * @{
- */
-enum APECompressionLevel {
- COMPRESSION_LEVEL_FAST = 1000,
- COMPRESSION_LEVEL_NORMAL = 2000,
- COMPRESSION_LEVEL_HIGH = 3000,
- COMPRESSION_LEVEL_EXTRA_HIGH = 4000,
- COMPRESSION_LEVEL_INSANE = 5000
-};
-/** @} */
-
-#define APE_FILTER_LEVELS 3
-
-/** Filter orders depending on compression level */
-static const uint16_t ape_filter_orders[5][APE_FILTER_LEVELS] = {
- { 0, 0, 0 },
- { 16, 0, 0 },
- { 64, 0, 0 },
- { 32, 256, 0 },
- { 16, 256, 1280 }
-};
-
-/** Filter fraction bits depending on compression level */
-static const uint8_t ape_filter_fracbits[5][APE_FILTER_LEVELS] = {
- { 0, 0, 0 },
- { 11, 0, 0 },
- { 11, 0, 0 },
- { 10, 13, 0 },
- { 11, 13, 15 }
-};
-
-
-/** Filters applied to the decoded data */
-typedef struct APEFilter {
- int16_t *coeffs; ///< actual coefficients used in filtering
- int16_t *adaptcoeffs; ///< adaptive filter coefficients used for correcting of actual filter coefficients
- int16_t *historybuffer; ///< filter memory
- int16_t *delay; ///< filtered values
-
- uint32_t avg;
-} APEFilter;
-
-typedef struct APERice {
- uint32_t k;
- uint32_t ksum;
-} APERice;
-
-typedef struct APERangecoder {
- uint32_t low; ///< low end of interval
- uint32_t range; ///< length of interval
- uint32_t help; ///< bytes_to_follow resp. intermediate value
- unsigned int buffer; ///< buffer for input/output
-} APERangecoder;
-
-/** Filter histories */
-typedef struct APEPredictor {
- int32_t *buf;
-
- int32_t lastA[2];
-
- int32_t filterA[2];
- int32_t filterB[2];
-
- uint32_t coeffsA[2][4]; ///< adaption coefficients
- uint32_t coeffsB[2][5]; ///< adaption coefficients
- int32_t historybuffer[HISTORY_SIZE + PREDICTOR_SIZE];
-
- unsigned int sample_pos;
-} APEPredictor;
-
-typedef struct APEPredictor64 {
- int64_t *buf;
-
- int64_t lastA[2];
-
- int64_t filterA[2];
- int64_t filterB[2];
-
- uint64_t coeffsA[2][4]; ///< adaption coefficients
- uint64_t coeffsB[2][5]; ///< adaption coefficients
- int64_t historybuffer[HISTORY_SIZE + PREDICTOR_SIZE];
-
- unsigned int sample_pos;
-} APEPredictor64;
-
-/** Decoder context */
-typedef struct APEContext {
- AVClass *class; ///< class for AVOptions
- AVCodecContext *avctx;
- BswapDSPContext bdsp;
- LLAudDSPContext adsp;
- int channels;
- int samples; ///< samples left to decode in current frame
- int bps;
-
- int fileversion; ///< codec version, very important in decoding process
- int compression_level; ///< compression levels
- int fset; ///< which filter set to use (calculated from compression level)
- int flags; ///< global decoder flags
-
- uint32_t CRC; ///< signalled frame CRC
- uint32_t CRC_state; ///< accumulated CRC
- int frameflags; ///< frame flags
- APEPredictor predictor; ///< predictor used for final reconstruction
- APEPredictor64 predictor64; ///< 64bit predictor used for final reconstruction
-
- int32_t *decoded_buffer;
- int decoded_size;
- int32_t *decoded[MAX_CHANNELS]; ///< decoded data for each channel
- int blocks_per_loop; ///< maximum number of samples to decode for each call
-
- int16_t* filterbuf[APE_FILTER_LEVELS]; ///< filter memory
-
- APERangecoder rc; ///< rangecoder used to decode actual values
- APERice riceX; ///< rice code parameters for the second channel
- APERice riceY; ///< rice code parameters for the first channel
- APEFilter filters[APE_FILTER_LEVELS][2]; ///< filters used for reconstruction
- GetBitContext gb;
-
- uint8_t *data; ///< current frame data
- uint8_t *data_end; ///< frame data end
- int data_size; ///< frame data allocated size
- const uint8_t *ptr; ///< current position in frame data
-
- int error;
-
- void (*entropy_decode_mono)(struct APEContext *ctx, int blockstodecode);
- void (*entropy_decode_stereo)(struct APEContext *ctx, int blockstodecode);
- void (*predictor_decode_mono)(struct APEContext *ctx, int count);
- void (*predictor_decode_stereo)(struct APEContext *ctx, int count);
-} APEContext;
-
-static void ape_apply_filters(APEContext *ctx, int32_t *decoded0,
- int32_t *decoded1, int count);
-
-static void entropy_decode_mono_0000(APEContext *ctx, int blockstodecode);
-static void entropy_decode_stereo_0000(APEContext *ctx, int blockstodecode);
-static void entropy_decode_mono_3860(APEContext *ctx, int blockstodecode);
-static void entropy_decode_stereo_3860(APEContext *ctx, int blockstodecode);
-static void entropy_decode_mono_3900(APEContext *ctx, int blockstodecode);
-static void entropy_decode_stereo_3900(APEContext *ctx, int blockstodecode);
-static void entropy_decode_stereo_3930(APEContext *ctx, int blockstodecode);
-static void entropy_decode_mono_3990(APEContext *ctx, int blockstodecode);
-static void entropy_decode_stereo_3990(APEContext *ctx, int blockstodecode);
-
-static void predictor_decode_mono_3800(APEContext *ctx, int count);
-static void predictor_decode_stereo_3800(APEContext *ctx, int count);
-static void predictor_decode_mono_3930(APEContext *ctx, int count);
-static void predictor_decode_stereo_3930(APEContext *ctx, int count);
-static void predictor_decode_mono_3950(APEContext *ctx, int count);
-static void predictor_decode_stereo_3950(APEContext *ctx, int count);
-
-static av_cold int ape_decode_close(AVCodecContext *avctx)
-{
- APEContext *s = avctx->priv_data;
- int i;
-
- for (i = 0; i < APE_FILTER_LEVELS; i++)
- av_freep(&s->filterbuf[i]);
-
- av_freep(&s->decoded_buffer);
- av_freep(&s->data);
- s->decoded_size = s->data_size = 0;
-
- return 0;
-}
-
-static av_cold int ape_decode_init(AVCodecContext *avctx)
-{
- APEContext *s = avctx->priv_data;
- int channels = avctx->ch_layout.nb_channels;
- int i;
-
- if (avctx->extradata_size != 6) {
- av_log(avctx, AV_LOG_ERROR, "Incorrect extradata\n");
- return AVERROR(EINVAL);
- }
- if (channels > 2) {
- av_log(avctx, AV_LOG_ERROR, "Only mono and stereo is supported\n");
- return AVERROR(EINVAL);
- }
- avctx->bits_per_raw_sample =
- s->bps = avctx->bits_per_coded_sample;
- switch (s->bps) {
- case 8:
- avctx->sample_fmt = AV_SAMPLE_FMT_U8P;
- break;
- case 16:
- avctx->sample_fmt = AV_SAMPLE_FMT_S16P;
- break;
- case 24:
- avctx->sample_fmt = AV_SAMPLE_FMT_S32P;
- break;
- default:
- avpriv_request_sample(avctx,
- "%d bits per coded sample", s->bps);
- return AVERROR_PATCHWELCOME;
- }
- s->avctx = avctx;
- s->channels = channels;
- s->fileversion = AV_RL16(avctx->extradata);
- s->compression_level = AV_RL16(avctx->extradata + 2);
- s->flags = AV_RL16(avctx->extradata + 4);
-
- av_log(avctx, AV_LOG_VERBOSE, "Compression Level: %d - Flags: %d\n",
- s->compression_level, s->flags);
- if (s->compression_level % 1000 || s->compression_level > COMPRESSION_LEVEL_INSANE ||
- !s->compression_level ||
- (s->fileversion < 3930 && s->compression_level == COMPRESSION_LEVEL_INSANE)) {
- av_log(avctx, AV_LOG_ERROR, "Incorrect compression level %d\n",
- s->compression_level);
- return AVERROR_INVALIDDATA;
- }
- s->fset = s->compression_level / 1000 - 1;
- for (i = 0; i < APE_FILTER_LEVELS; i++) {
- if (!ape_filter_orders[s->fset][i])
- break;
- if (!(s->filterbuf[i] = av_malloc((ape_filter_orders[s->fset][i] * 3 + HISTORY_SIZE) * 4)))
- return AVERROR(ENOMEM);
- }
-
- if (s->fileversion < 3860) {
- s->entropy_decode_mono = entropy_decode_mono_0000;
- s->entropy_decode_stereo = entropy_decode_stereo_0000;
- } else if (s->fileversion < 3900) {
- s->entropy_decode_mono = entropy_decode_mono_3860;
- s->entropy_decode_stereo = entropy_decode_stereo_3860;
- } else if (s->fileversion < 3930) {
- s->entropy_decode_mono = entropy_decode_mono_3900;
- s->entropy_decode_stereo = entropy_decode_stereo_3900;
- } else if (s->fileversion < 3990) {
- s->entropy_decode_mono = entropy_decode_mono_3900;
- s->entropy_decode_stereo = entropy_decode_stereo_3930;
- } else {
- s->entropy_decode_mono = entropy_decode_mono_3990;
- s->entropy_decode_stereo = entropy_decode_stereo_3990;
- }
-
- if (s->fileversion < 3930) {
- s->predictor_decode_mono = predictor_decode_mono_3800;
- s->predictor_decode_stereo = predictor_decode_stereo_3800;
- } else if (s->fileversion < 3950) {
- s->predictor_decode_mono = predictor_decode_mono_3930;
- s->predictor_decode_stereo = predictor_decode_stereo_3930;
- } else {
- s->predictor_decode_mono = predictor_decode_mono_3950;
- s->predictor_decode_stereo = predictor_decode_stereo_3950;
- }
-
- ff_bswapdsp_init(&s->bdsp);
- ff_llauddsp_init(&s->adsp);
- av_channel_layout_uninit(&avctx->ch_layout);
- avctx->ch_layout = (channels == 2) ? (AVChannelLayout)AV_CHANNEL_LAYOUT_STEREO
- : (AVChannelLayout)AV_CHANNEL_LAYOUT_MONO;
-
- return 0;
-}
-
-/**
- * @name APE range decoding functions
- * @{
- */
-
-#define CODE_BITS 32
-#define TOP_VALUE ((unsigned int)1 << (CODE_BITS-1))
-#define SHIFT_BITS (CODE_BITS - 9)
-#define EXTRA_BITS ((CODE_BITS-2) % 8 + 1)
-#define BOTTOM_VALUE (TOP_VALUE >> 8)
-
-/** Start the decoder */
-static inline void range_start_decoding(APEContext *ctx)
-{
- ctx->rc.buffer = bytestream_get_byte(&ctx->ptr);
- ctx->rc.low = ctx->rc.buffer >> (8 - EXTRA_BITS);
- ctx->rc.range = (uint32_t) 1 << EXTRA_BITS;
-}
-
-/** Perform normalization */
-static inline void range_dec_normalize(APEContext *ctx)
-{
- while (ctx->rc.range <= BOTTOM_VALUE) {
- ctx->rc.buffer <<= 8;
- if(ctx->ptr < ctx->data_end) {
- ctx->rc.buffer += *ctx->ptr;
- ctx->ptr++;
- } else {
- ctx->error = 1;
- }
- ctx->rc.low = (ctx->rc.low << 8) | ((ctx->rc.buffer >> 1) & 0xFF);
- ctx->rc.range <<= 8;
- }
-}
-
-/**
- * Calculate cumulative frequency for next symbol. Does NO update!
- * @param ctx decoder context
- * @param tot_f is the total frequency or (code_value)1<rc.help = ctx->rc.range / tot_f;
- return ctx->rc.low / ctx->rc.help;
-}
-
-/**
- * Decode value with given size in bits
- * @param ctx decoder context
- * @param shift number of bits to decode
- */
-static inline int range_decode_culshift(APEContext *ctx, int shift)
-{
- range_dec_normalize(ctx);
- ctx->rc.help = ctx->rc.range >> shift;
- return ctx->rc.low / ctx->rc.help;
-}
-
-
-/**
- * Update decoding state
- * @param ctx decoder context
- * @param sy_f the interval length (frequency of the symbol)
- * @param lt_f the lower end (frequency sum of < symbols)
- */
-static inline void range_decode_update(APEContext *ctx, int sy_f, int lt_f)
-{
- ctx->rc.low -= ctx->rc.help * lt_f;
- ctx->rc.range = ctx->rc.help * sy_f;
-}
-
-/** Decode n bits (n <= 16) without modelling */
-static inline int range_decode_bits(APEContext *ctx, int n)
-{
- int sym = range_decode_culshift(ctx, n);
- range_decode_update(ctx, 1, sym);
- return sym;
-}
-
-
-#define MODEL_ELEMENTS 64
-
-/**
- * Fixed probabilities for symbols in Monkey Audio version 3.97
- */
-static const uint16_t counts_3970[22] = {
- 0, 14824, 28224, 39348, 47855, 53994, 58171, 60926,
- 62682, 63786, 64463, 64878, 65126, 65276, 65365, 65419,
- 65450, 65469, 65480, 65487, 65491, 65493,
-};
-
-/**
- * Probability ranges for symbols in Monkey Audio version 3.97
- */
-static const uint16_t counts_diff_3970[21] = {
- 14824, 13400, 11124, 8507, 6139, 4177, 2755, 1756,
- 1104, 677, 415, 248, 150, 89, 54, 31,
- 19, 11, 7, 4, 2,
-};
-
-/**
- * Fixed probabilities for symbols in Monkey Audio version 3.98
- */
-static const uint16_t counts_3980[22] = {
- 0, 19578, 36160, 48417, 56323, 60899, 63265, 64435,
- 64971, 65232, 65351, 65416, 65447, 65466, 65476, 65482,
- 65485, 65488, 65490, 65491, 65492, 65493,
-};
-
-/**
- * Probability ranges for symbols in Monkey Audio version 3.98
- */
-static const uint16_t counts_diff_3980[21] = {
- 19578, 16582, 12257, 7906, 4576, 2366, 1170, 536,
- 261, 119, 65, 31, 19, 10, 6, 3,
- 3, 2, 1, 1, 1,
-};
-
-/**
- * Decode symbol
- * @param ctx decoder context
- * @param counts probability range start position
- * @param counts_diff probability range widths
- */
-static inline int range_get_symbol(APEContext *ctx,
- const uint16_t counts[],
- const uint16_t counts_diff[])
-{
- int symbol, cf;
-
- cf = range_decode_culshift(ctx, 16);
-
- if(cf > 65492){
- symbol= cf - 65535 + 63;
- range_decode_update(ctx, 1, cf);
- if(cf > 65535)
- ctx->error=1;
- return symbol;
- }
- /* figure out the symbol inefficiently; a binary search would be much better */
- for (symbol = 0; counts[symbol + 1] <= cf; symbol++);
-
- range_decode_update(ctx, counts_diff[symbol], counts[symbol]);
-
- return symbol;
-}
-/** @} */ // group rangecoder
-
-static inline void update_rice(APERice *rice, unsigned int x)
-{
- int lim = rice->k ? (1 << (rice->k + 4)) : 0;
- rice->ksum += ((x + 1) / 2) - ((rice->ksum + 16) >> 5);
-
- if (rice->ksum < lim)
- rice->k--;
- else if (rice->ksum >= (1 << (rice->k + 5)) && rice->k < 24)
- rice->k++;
-}
-
-static inline int get_rice_ook(GetBitContext *gb, int k)
-{
- unsigned int x;
-
- x = get_unary(gb, 1, get_bits_left(gb));
-
- if (k)
- x = (x << k) | get_bits(gb, k);
-
- return x;
-}
-
-static inline int ape_decode_value_3860(APEContext *ctx, GetBitContext *gb,
- APERice *rice)
-{
- unsigned int x, overflow;
-
- overflow = get_unary(gb, 1, get_bits_left(gb));
-
- if (ctx->fileversion > 3880) {
- while (overflow >= 16) {
- overflow -= 16;
- rice->k += 4;
- }
- }
-
- if (!rice->k)
- x = overflow;
- else if(rice->k <= MIN_CACHE_BITS) {
- x = (overflow << rice->k) + get_bits(gb, rice->k);
- } else {
- av_log(ctx->avctx, AV_LOG_ERROR, "Too many bits: %"PRIu32"\n", rice->k);
- ctx->error = 1;
- return AVERROR_INVALIDDATA;
- }
- rice->ksum += x - (rice->ksum + 8 >> 4);
- if (rice->ksum < (rice->k ? 1 << (rice->k + 4) : 0))
- rice->k--;
- else if (rice->ksum >= (1 << (rice->k + 5)) && rice->k < 24)
- rice->k++;
-
- /* Convert to signed */
- return ((x >> 1) ^ ((x & 1) - 1)) + 1;
-}
-
-static inline int ape_decode_value_3900(APEContext *ctx, APERice *rice)
-{
- unsigned int x, overflow;
- int tmpk;
-
- overflow = range_get_symbol(ctx, counts_3970, counts_diff_3970);
-
- if (overflow == (MODEL_ELEMENTS - 1)) {
- tmpk = range_decode_bits(ctx, 5);
- overflow = 0;
- } else
- tmpk = (rice->k < 1) ? 0 : rice->k - 1;
-
- if (tmpk <= 16 || ctx->fileversion < 3910) {
- if (tmpk > 23) {
- av_log(ctx->avctx, AV_LOG_ERROR, "Too many bits: %d\n", tmpk);
- return AVERROR_INVALIDDATA;
- }
- x = range_decode_bits(ctx, tmpk);
- } else if (tmpk <= 31) {
- x = range_decode_bits(ctx, 16);
- x |= (range_decode_bits(ctx, tmpk - 16) << 16);
- } else {
- av_log(ctx->avctx, AV_LOG_ERROR, "Too many bits: %d\n", tmpk);
- return AVERROR_INVALIDDATA;
- }
- x += overflow << tmpk;
-
- update_rice(rice, x);
-
- /* Convert to signed */
- return ((x >> 1) ^ ((x & 1) - 1)) + 1;
-}
-
-static inline int ape_decode_value_3990(APEContext *ctx, APERice *rice)
-{
- unsigned int x, overflow, pivot;
- int base;
-
- pivot = FFMAX(rice->ksum >> 5, 1);
-
- overflow = range_get_symbol(ctx, counts_3980, counts_diff_3980);
-
- if (overflow == (MODEL_ELEMENTS - 1)) {
- overflow = (unsigned)range_decode_bits(ctx, 16) << 16;
- overflow |= range_decode_bits(ctx, 16);
- }
-
- if (pivot < 0x10000) {
- base = range_decode_culfreq(ctx, pivot);
- range_decode_update(ctx, 1, base);
- } else {
- int base_hi = pivot, base_lo;
- int bbits = 0;
-
- while (base_hi & ~0xFFFF) {
- base_hi >>= 1;
- bbits++;
- }
- base_hi = range_decode_culfreq(ctx, base_hi + 1);
- range_decode_update(ctx, 1, base_hi);
- base_lo = range_decode_culfreq(ctx, 1 << bbits);
- range_decode_update(ctx, 1, base_lo);
-
- base = (base_hi << bbits) + base_lo;
- }
-
- x = base + overflow * pivot;
-
- update_rice(rice, x);
-
- /* Convert to signed */
- return ((x >> 1) ^ ((x & 1) - 1)) + 1;
-}
-
-static int get_k(int ksum)
-{
- return av_log2(ksum) + !!ksum;
-}
-
-static void decode_array_0000(APEContext *ctx, GetBitContext *gb,
- int32_t *out, APERice *rice, int blockstodecode)
-{
- int i;
- unsigned ksummax, ksummin;
-
- rice->ksum = 0;
- for (i = 0; i < FFMIN(blockstodecode, 5); i++) {
- out[i] = get_rice_ook(&ctx->gb, 10);
- rice->ksum += out[i];
- }
-
- if (blockstodecode <= 5)
- goto end;
-
- rice->k = get_k(rice->ksum / 10);
- if (rice->k >= 24)
- return;
- for (; i < FFMIN(blockstodecode, 64); i++) {
- out[i] = get_rice_ook(&ctx->gb, rice->k);
- rice->ksum += out[i];
- rice->k = get_k(rice->ksum / ((i + 1) * 2));
- if (rice->k >= 24)
- return;
- }
-
- if (blockstodecode <= 64)
- goto end;
-
- rice->k = get_k(rice->ksum >> 7);
- ksummax = 1 << rice->k + 7;
- ksummin = rice->k ? (1 << rice->k + 6) : 0;
- for (; i < blockstodecode; i++) {
- if (get_bits_left(&ctx->gb) < 1) {
- ctx->error = 1;
- return;
- }
- out[i] = get_rice_ook(&ctx->gb, rice->k);
- rice->ksum += out[i] - (unsigned)out[i - 64];
- while (rice->ksum < ksummin) {
- rice->k--;
- ksummin = rice->k ? ksummin >> 1 : 0;
- ksummax >>= 1;
- }
- while (rice->ksum >= ksummax) {
- rice->k++;
- if (rice->k > 24)
- return;
- ksummax <<= 1;
- ksummin = ksummin ? ksummin << 1 : 128;
- }
- }
-
-end:
- for (i = 0; i < blockstodecode; i++)
- out[i] = ((out[i] >> 1) ^ ((out[i] & 1) - 1)) + 1;
-}
-
-static void entropy_decode_mono_0000(APEContext *ctx, int blockstodecode)
-{
- decode_array_0000(ctx, &ctx->gb, ctx->decoded[0], &ctx->riceY,
- blockstodecode);
-}
-
-static void entropy_decode_stereo_0000(APEContext *ctx, int blockstodecode)
-{
- decode_array_0000(ctx, &ctx->gb, ctx->decoded[0], &ctx->riceY,
- blockstodecode);
- decode_array_0000(ctx, &ctx->gb, ctx->decoded[1], &ctx->riceX,
- blockstodecode);
-}
-
-static void entropy_decode_mono_3860(APEContext *ctx, int blockstodecode)
-{
- int32_t *decoded0 = ctx->decoded[0];
-
- while (blockstodecode--)
- *decoded0++ = ape_decode_value_3860(ctx, &ctx->gb, &ctx->riceY);
-}
-
-static void entropy_decode_stereo_3860(APEContext *ctx, int blockstodecode)
-{
- int32_t *decoded0 = ctx->decoded[0];
- int32_t *decoded1 = ctx->decoded[1];
- int blocks = blockstodecode;
-
- while (blockstodecode--)
- *decoded0++ = ape_decode_value_3860(ctx, &ctx->gb, &ctx->riceY);
- while (blocks--)
- *decoded1++ = ape_decode_value_3860(ctx, &ctx->gb, &ctx->riceX);
-}
-
-static void entropy_decode_mono_3900(APEContext *ctx, int blockstodecode)
-{
- int32_t *decoded0 = ctx->decoded[0];
-
- while (blockstodecode--)
- *decoded0++ = ape_decode_value_3900(ctx, &ctx->riceY);
-}
-
-static void entropy_decode_stereo_3900(APEContext *ctx, int blockstodecode)
-{
- int32_t *decoded0 = ctx->decoded[0];
- int32_t *decoded1 = ctx->decoded[1];
- int blocks = blockstodecode;
-
- while (blockstodecode--)
- *decoded0++ = ape_decode_value_3900(ctx, &ctx->riceY);
- range_dec_normalize(ctx);
- // because of some implementation peculiarities we need to backpedal here
- ctx->ptr -= 1;
- range_start_decoding(ctx);
- while (blocks--)
- *decoded1++ = ape_decode_value_3900(ctx, &ctx->riceX);
-}
-
-static void entropy_decode_stereo_3930(APEContext *ctx, int blockstodecode)
-{
- int32_t *decoded0 = ctx->decoded[0];
- int32_t *decoded1 = ctx->decoded[1];
-
- while (blockstodecode--) {
- *decoded0++ = ape_decode_value_3900(ctx, &ctx->riceY);
- *decoded1++ = ape_decode_value_3900(ctx, &ctx->riceX);
- }
-}
-
-static void entropy_decode_mono_3990(APEContext *ctx, int blockstodecode)
-{
- int32_t *decoded0 = ctx->decoded[0];
-
- while (blockstodecode--)
- *decoded0++ = ape_decode_value_3990(ctx, &ctx->riceY);
-}
-
-static void entropy_decode_stereo_3990(APEContext *ctx, int blockstodecode)
-{
- int32_t *decoded0 = ctx->decoded[0];
- int32_t *decoded1 = ctx->decoded[1];
-
- while (blockstodecode--) {
- *decoded0++ = ape_decode_value_3990(ctx, &ctx->riceY);
- *decoded1++ = ape_decode_value_3990(ctx, &ctx->riceX);
- }
-}
-
-static int init_entropy_decoder(APEContext *ctx)
-{
- /* Read the CRC */
- if (ctx->fileversion >= 3900) {
- if (ctx->data_end - ctx->ptr < 6)
- return AVERROR_INVALIDDATA;
- ctx->CRC = bytestream_get_be32(&ctx->ptr);
- } else {
- ctx->CRC = get_bits_long(&ctx->gb, 32);
- }
-
- /* Read the frame flags if they exist */
- ctx->frameflags = 0;
- ctx->CRC_state = UINT32_MAX;
- if ((ctx->fileversion > 3820) && (ctx->CRC & 0x80000000)) {
- ctx->CRC &= ~0x80000000;
-
- if (ctx->data_end - ctx->ptr < 6)
- return AVERROR_INVALIDDATA;
- ctx->frameflags = bytestream_get_be32(&ctx->ptr);
- }
-
- /* Initialize the rice structs */
- ctx->riceX.k = 10;
- ctx->riceX.ksum = (1 << ctx->riceX.k) * 16;
- ctx->riceY.k = 10;
- ctx->riceY.ksum = (1 << ctx->riceY.k) * 16;
-
- if (ctx->fileversion >= 3900) {
- /* The first 8 bits of input are ignored. */
- ctx->ptr++;
-
- range_start_decoding(ctx);
- }
-
- return 0;
-}
-
-static const int32_t initial_coeffs_fast_3320[1] = {
- 375,
-};
-
-static const int32_t initial_coeffs_a_3800[3] = {
- 64, 115, 64,
-};
-
-static const int32_t initial_coeffs_b_3800[2] = {
- 740, 0
-};
-
-static const int32_t initial_coeffs_3930[4] = {
- 360, 317, -109, 98
-};
-
-static const int64_t initial_coeffs_3930_64bit[4] = {
- 360, 317, -109, 98
-};
-
-static void init_predictor_decoder(APEContext *ctx)
-{
- APEPredictor *p = &ctx->predictor;
- APEPredictor64 *p64 = &ctx->predictor64;
-
- /* Zero the history buffers */
- memset(p->historybuffer, 0, PREDICTOR_SIZE * sizeof(*p->historybuffer));
- memset(p64->historybuffer, 0, PREDICTOR_SIZE * sizeof(*p64->historybuffer));
- p->buf = p->historybuffer;
- p64->buf = p64->historybuffer;
-
- /* Initialize and zero the coefficients */
- if (ctx->fileversion < 3930) {
- if (ctx->compression_level == COMPRESSION_LEVEL_FAST) {
- memcpy(p->coeffsA[0], initial_coeffs_fast_3320,
- sizeof(initial_coeffs_fast_3320));
- memcpy(p->coeffsA[1], initial_coeffs_fast_3320,
- sizeof(initial_coeffs_fast_3320));
- } else {
- memcpy(p->coeffsA[0], initial_coeffs_a_3800,
- sizeof(initial_coeffs_a_3800));
- memcpy(p->coeffsA[1], initial_coeffs_a_3800,
- sizeof(initial_coeffs_a_3800));
- }
- } else {
- memcpy(p->coeffsA[0], initial_coeffs_3930, sizeof(initial_coeffs_3930));
- memcpy(p->coeffsA[1], initial_coeffs_3930, sizeof(initial_coeffs_3930));
- memcpy(p64->coeffsA[0], initial_coeffs_3930_64bit, sizeof(initial_coeffs_3930_64bit));
- memcpy(p64->coeffsA[1], initial_coeffs_3930_64bit, sizeof(initial_coeffs_3930_64bit));
- }
- memset(p->coeffsB, 0, sizeof(p->coeffsB));
- memset(p64->coeffsB, 0, sizeof(p64->coeffsB));
- if (ctx->fileversion < 3930) {
- memcpy(p->coeffsB[0], initial_coeffs_b_3800,
- sizeof(initial_coeffs_b_3800));
- memcpy(p->coeffsB[1], initial_coeffs_b_3800,
- sizeof(initial_coeffs_b_3800));
- }
-
- p->filterA[0] = p->filterA[1] = 0;
- p->filterB[0] = p->filterB[1] = 0;
- p->lastA[0] = p->lastA[1] = 0;
-
- p64->filterA[0] = p64->filterA[1] = 0;
- p64->filterB[0] = p64->filterB[1] = 0;
- p64->lastA[0] = p64->lastA[1] = 0;
-
- p->sample_pos = 0;
-
- p64->sample_pos = 0;
-}
-
-/** Get inverse sign of integer (-1 for positive, 1 for negative and 0 for zero) */
-static inline int APESIGN(int32_t x) {
- return (x < 0) - (x > 0);
-}
-
-static av_always_inline int filter_fast_3320(APEPredictor *p,
- const int decoded, const int filter,
- const int delayA)
-{
- int32_t predictionA;
-
- p->buf[delayA] = p->lastA[filter];
- if (p->sample_pos < 3) {
- p->lastA[filter] = decoded;
- p->filterA[filter] = decoded;
- return decoded;
- }
-
- predictionA = p->buf[delayA] * 2U - p->buf[delayA - 1];
- p->lastA[filter] = decoded + (unsigned)((int32_t)(predictionA * p->coeffsA[filter][0]) >> 9);
-
- if ((decoded ^ predictionA) > 0)
- p->coeffsA[filter][0]++;
- else
- p->coeffsA[filter][0]--;
-
- p->filterA[filter] += (unsigned)p->lastA[filter];
-
- return p->filterA[filter];
-}
-
-static av_always_inline int filter_3800(APEPredictor *p,
- const unsigned decoded, const int filter,
- const int delayA, const int delayB,
- const int start, const int shift)
-{
- int32_t predictionA, predictionB, sign;
- int32_t d0, d1, d2, d3, d4;
-
- p->buf[delayA] = p->lastA[filter];
- p->buf[delayB] = p->filterB[filter];
- if (p->sample_pos < start) {
- predictionA = decoded + p->filterA[filter];
- p->lastA[filter] = decoded;
- p->filterB[filter] = decoded;
- p->filterA[filter] = predictionA;
- return predictionA;
- }
- d2 = p->buf[delayA];
- d1 = (p->buf[delayA] - (unsigned)p->buf[delayA - 1]) * 2;
- d0 = p->buf[delayA] + ((p->buf[delayA - 2] - (unsigned)p->buf[delayA - 1]) * 8);
- d3 = p->buf[delayB] * 2U - p->buf[delayB - 1];
- d4 = p->buf[delayB];
-
- predictionA = d0 * p->coeffsA[filter][0] +
- d1 * p->coeffsA[filter][1] +
- d2 * p->coeffsA[filter][2];
-
- sign = APESIGN(decoded);
- p->coeffsA[filter][0] += (((d0 >> 30) & 2) - 1) * sign;
- p->coeffsA[filter][1] += (((d1 >> 28) & 8) - 4) * sign;
- p->coeffsA[filter][2] += (((d2 >> 28) & 8) - 4) * sign;
-
- predictionB = d3 * p->coeffsB[filter][0] -
- d4 * p->coeffsB[filter][1];
- p->lastA[filter] = decoded + (predictionA >> 11);
- sign = APESIGN(p->lastA[filter]);
- p->coeffsB[filter][0] += (((d3 >> 29) & 4) - 2) * sign;
- p->coeffsB[filter][1] -= (((d4 >> 30) & 2) - 1) * sign;
-
- p->filterB[filter] = p->lastA[filter] + (unsigned)(predictionB >> shift);
- p->filterA[filter] = p->filterB[filter] + (unsigned)((int)(p->filterA[filter] * 31U) >> 5);
-
- return p->filterA[filter];
-}
-
-static void long_filter_high_3800(int32_t *buffer, int order, int shift, int length)
-{
- int i, j;
- int32_t dotprod, sign;
- int32_t coeffs[256], delay[256+256], *delayp = delay;
-
- if (order >= length)
- return;
-
- memset(coeffs, 0, order * sizeof(*coeffs));
- for (i = 0; i < order; i++)
- delay[i] = buffer[i];
- for (i = order; i < length; i++) {
- dotprod = 0;
- sign = APESIGN(buffer[i]);
- if (sign == 1) {
- for (j = 0; j < order; j++) {
- dotprod += delayp[j] * (unsigned)coeffs[j];
- coeffs[j] += (delayp[j] >> 31) | 1;
- }
- } else if (sign == -1) {
- for (j = 0; j < order; j++) {
- dotprod += delayp[j] * (unsigned)coeffs[j];
- coeffs[j] -= (delayp[j] >> 31) | 1;
- }
- } else {
- for (j = 0; j < order; j++) {
- dotprod += delayp[j] * (unsigned)coeffs[j];
- }
- }
- buffer[i] -= (unsigned)(dotprod >> shift);
- delayp ++;
- delayp[order - 1] = buffer[i];
- if (delayp - delay == 256) {
- memcpy(delay, delayp, sizeof(*delay)*256);
- delayp = delay;
- }
- }
-}
-
-static void long_filter_ehigh_3830(int32_t *buffer, int length)
-{
- int i, j;
- int32_t dotprod, sign;
- int32_t delay[8] = { 0 };
- uint32_t coeffs[8] = { 0 };
-
- for (i = 0; i < length; i++) {
- dotprod = 0;
- sign = APESIGN(buffer[i]);
- for (j = 7; j >= 0; j--) {
- dotprod += delay[j] * coeffs[j];
- coeffs[j] += ((delay[j] >> 31) | 1) * sign;
- }
- for (j = 7; j > 0; j--)
- delay[j] = delay[j - 1];
- delay[0] = buffer[i];
- buffer[i] -= (unsigned)(dotprod >> 9);
- }
-}
-
-static void predictor_decode_stereo_3800(APEContext *ctx, int count)
-{
- APEPredictor *p = &ctx->predictor;
- int32_t *decoded0 = ctx->decoded[0];
- int32_t *decoded1 = ctx->decoded[1];
- int start = 4, shift = 10;
-
- if (ctx->compression_level == COMPRESSION_LEVEL_HIGH) {
- start = 16;
- long_filter_high_3800(decoded0, 16, 9, count);
- long_filter_high_3800(decoded1, 16, 9, count);
- } else if (ctx->compression_level == COMPRESSION_LEVEL_EXTRA_HIGH) {
- int order = 128, shift2 = 11;
-
- if (ctx->fileversion >= 3830) {
- order <<= 1;
- shift++;
- shift2++;
- long_filter_ehigh_3830(decoded0 + order, count - order);
- long_filter_ehigh_3830(decoded1 + order, count - order);
- }
- start = order;
- long_filter_high_3800(decoded0, order, shift2, count);
- long_filter_high_3800(decoded1, order, shift2, count);
- }
-
- while (count--) {
- int X = *decoded0, Y = *decoded1;
- if (ctx->compression_level == COMPRESSION_LEVEL_FAST) {
- *decoded0 = filter_fast_3320(p, Y, 0, YDELAYA);
- decoded0++;
- *decoded1 = filter_fast_3320(p, X, 1, XDELAYA);
- decoded1++;
- } else {
- *decoded0 = filter_3800(p, Y, 0, YDELAYA, YDELAYB,
- start, shift);
- decoded0++;
- *decoded1 = filter_3800(p, X, 1, XDELAYA, XDELAYB,
- start, shift);
- decoded1++;
- }
-
- /* Combined */
- p->buf++;
- p->sample_pos++;
-
- /* Have we filled the history buffer? */
- if (p->buf == p->historybuffer + HISTORY_SIZE) {
- memmove(p->historybuffer, p->buf,
- PREDICTOR_SIZE * sizeof(*p->historybuffer));
- p->buf = p->historybuffer;
- }
- }
-}
-
-static void predictor_decode_mono_3800(APEContext *ctx, int count)
-{
- APEPredictor *p = &ctx->predictor;
- int32_t *decoded0 = ctx->decoded[0];
- int start = 4, shift = 10;
-
- if (ctx->compression_level == COMPRESSION_LEVEL_HIGH) {
- start = 16;
- long_filter_high_3800(decoded0, 16, 9, count);
- } else if (ctx->compression_level == COMPRESSION_LEVEL_EXTRA_HIGH) {
- int order = 128, shift2 = 11;
-
- if (ctx->fileversion >= 3830) {
- order <<= 1;
- shift++;
- shift2++;
- long_filter_ehigh_3830(decoded0 + order, count - order);
- }
- start = order;
- long_filter_high_3800(decoded0, order, shift2, count);
- }
-
- while (count--) {
- if (ctx->compression_level == COMPRESSION_LEVEL_FAST) {
- *decoded0 = filter_fast_3320(p, *decoded0, 0, YDELAYA);
- decoded0++;
- } else {
- *decoded0 = filter_3800(p, *decoded0, 0, YDELAYA, YDELAYB,
- start, shift);
- decoded0++;
- }
-
- /* Combined */
- p->buf++;
- p->sample_pos++;
-
- /* Have we filled the history buffer? */
- if (p->buf == p->historybuffer + HISTORY_SIZE) {
- memmove(p->historybuffer, p->buf,
- PREDICTOR_SIZE * sizeof(*p->historybuffer));
- p->buf = p->historybuffer;
- }
- }
-}
-
-static av_always_inline int predictor_update_3930(APEPredictor *p,
- const int decoded, const int filter,
- const int delayA)
-{
- int32_t predictionA, sign;
- uint32_t d0, d1, d2, d3;
-
- p->buf[delayA] = p->lastA[filter];
- d0 = p->buf[delayA ];
- d1 = p->buf[delayA ] - (unsigned)p->buf[delayA - 1];
- d2 = p->buf[delayA - 1] - (unsigned)p->buf[delayA - 2];
- d3 = p->buf[delayA - 2] - (unsigned)p->buf[delayA - 3];
-
- predictionA = d0 * p->coeffsA[filter][0] +
- d1 * p->coeffsA[filter][1] +
- d2 * p->coeffsA[filter][2] +
- d3 * p->coeffsA[filter][3];
-
- p->lastA[filter] = decoded + (predictionA >> 9);
- p->filterA[filter] = p->lastA[filter] + ((int)(p->filterA[filter] * 31U) >> 5);
-
- sign = APESIGN(decoded);
- p->coeffsA[filter][0] += (((int32_t)d0 < 0) * 2 - 1) * sign;
- p->coeffsA[filter][1] += (((int32_t)d1 < 0) * 2 - 1) * sign;
- p->coeffsA[filter][2] += (((int32_t)d2 < 0) * 2 - 1) * sign;
- p->coeffsA[filter][3] += (((int32_t)d3 < 0) * 2 - 1) * sign;
-
- return p->filterA[filter];
-}
-
-static void predictor_decode_stereo_3930(APEContext *ctx, int count)
-{
- APEPredictor *p = &ctx->predictor;
- int32_t *decoded0 = ctx->decoded[0];
- int32_t *decoded1 = ctx->decoded[1];
-
- ape_apply_filters(ctx, ctx->decoded[0], ctx->decoded[1], count);
-
- while (count--) {
- /* Predictor Y */
- int Y = *decoded1, X = *decoded0;
- *decoded0 = predictor_update_3930(p, Y, 0, YDELAYA);
- decoded0++;
- *decoded1 = predictor_update_3930(p, X, 1, XDELAYA);
- decoded1++;
-
- /* Combined */
- p->buf++;
-
- /* Have we filled the history buffer? */
- if (p->buf == p->historybuffer + HISTORY_SIZE) {
- memmove(p->historybuffer, p->buf,
- PREDICTOR_SIZE * sizeof(*p->historybuffer));
- p->buf = p->historybuffer;
- }
- }
-}
-
-static void predictor_decode_mono_3930(APEContext *ctx, int count)
-{
- APEPredictor *p = &ctx->predictor;
- int32_t *decoded0 = ctx->decoded[0];
-
- ape_apply_filters(ctx, ctx->decoded[0], NULL, count);
-
- while (count--) {
- *decoded0 = predictor_update_3930(p, *decoded0, 0, YDELAYA);
- decoded0++;
-
- p->buf++;
-
- /* Have we filled the history buffer? */
- if (p->buf == p->historybuffer + HISTORY_SIZE) {
- memmove(p->historybuffer, p->buf,
- PREDICTOR_SIZE * sizeof(*p->historybuffer));
- p->buf = p->historybuffer;
- }
- }
-}
-
-static av_always_inline int predictor_update_filter(APEPredictor64 *p,
- const int decoded, const int filter,
- const int delayA, const int delayB,
- const int adaptA, const int adaptB)
-{
- int64_t predictionA, predictionB;
- int32_t sign;
-
- p->buf[delayA] = p->lastA[filter];
- p->buf[adaptA] = APESIGN(p->buf[delayA]);
- p->buf[delayA - 1] = p->buf[delayA] - (uint64_t)p->buf[delayA - 1];
- p->buf[adaptA - 1] = APESIGN(p->buf[delayA - 1]);
-
- predictionA = p->buf[delayA ] * p->coeffsA[filter][0] +
- p->buf[delayA - 1] * p->coeffsA[filter][1] +
- p->buf[delayA - 2] * p->coeffsA[filter][2] +
- p->buf[delayA - 3] * p->coeffsA[filter][3];
-
- /* Apply a scaled first-order filter compression */
- p->buf[delayB] = p->filterA[filter ^ 1] - ((int64_t)(p->filterB[filter] * 31ULL) >> 5);
- p->buf[adaptB] = APESIGN(p->buf[delayB]);
- p->buf[delayB - 1] = p->buf[delayB] - (uint64_t)p->buf[delayB - 1];
- p->buf[adaptB - 1] = APESIGN(p->buf[delayB - 1]);
- p->filterB[filter] = p->filterA[filter ^ 1];
-
- predictionB = p->buf[delayB ] * p->coeffsB[filter][0] +
- p->buf[delayB - 1] * p->coeffsB[filter][1] +
- p->buf[delayB - 2] * p->coeffsB[filter][2] +
- p->buf[delayB - 3] * p->coeffsB[filter][3] +
- p->buf[delayB - 4] * p->coeffsB[filter][4];
-
- p->lastA[filter] = decoded + ((int64_t)((uint64_t)predictionA + (predictionB >> 1)) >> 10);
- p->filterA[filter] = p->lastA[filter] + ((int64_t)(p->filterA[filter] * 31ULL) >> 5);
-
- sign = APESIGN(decoded);
- p->coeffsA[filter][0] += p->buf[adaptA ] * sign;
- p->coeffsA[filter][1] += p->buf[adaptA - 1] * sign;
- p->coeffsA[filter][2] += p->buf[adaptA - 2] * sign;
- p->coeffsA[filter][3] += p->buf[adaptA - 3] * sign;
- p->coeffsB[filter][0] += p->buf[adaptB ] * sign;
- p->coeffsB[filter][1] += p->buf[adaptB - 1] * sign;
- p->coeffsB[filter][2] += p->buf[adaptB - 2] * sign;
- p->coeffsB[filter][3] += p->buf[adaptB - 3] * sign;
- p->coeffsB[filter][4] += p->buf[adaptB - 4] * sign;
-
- return p->filterA[filter];
-}
-
-static void predictor_decode_stereo_3950(APEContext *ctx, int count)
-{
- APEPredictor64 *p = &ctx->predictor64;
- int32_t *decoded0 = ctx->decoded[0];
- int32_t *decoded1 = ctx->decoded[1];
-
- ape_apply_filters(ctx, ctx->decoded[0], ctx->decoded[1], count);
-
- while (count--) {
- /* Predictor Y */
- *decoded0 = predictor_update_filter(p, *decoded0, 0, YDELAYA, YDELAYB,
- YADAPTCOEFFSA, YADAPTCOEFFSB);
- decoded0++;
- *decoded1 = predictor_update_filter(p, *decoded1, 1, XDELAYA, XDELAYB,
- XADAPTCOEFFSA, XADAPTCOEFFSB);
- decoded1++;
-
- /* Combined */
- p->buf++;
-
- /* Have we filled the history buffer? */
- if (p->buf == p->historybuffer + HISTORY_SIZE) {
- memmove(p->historybuffer, p->buf,
- PREDICTOR_SIZE * sizeof(*p->historybuffer));
- p->buf = p->historybuffer;
- }
- }
-}
-
-static void predictor_decode_mono_3950(APEContext *ctx, int count)
-{
- APEPredictor64 *p = &ctx->predictor64;
- int32_t *decoded0 = ctx->decoded[0];
- int32_t predictionA, currentA, A, sign;
-
- ape_apply_filters(ctx, ctx->decoded[0], NULL, count);
-
- currentA = p->lastA[0];
-
- while (count--) {
- A = *decoded0;
-
- p->buf[YDELAYA] = currentA;
- p->buf[YDELAYA - 1] = p->buf[YDELAYA] - (uint64_t)p->buf[YDELAYA - 1];
-
- predictionA = p->buf[YDELAYA ] * p->coeffsA[0][0] +
- p->buf[YDELAYA - 1] * p->coeffsA[0][1] +
- p->buf[YDELAYA - 2] * p->coeffsA[0][2] +
- p->buf[YDELAYA - 3] * p->coeffsA[0][3];
-
- currentA = A + (uint64_t)(predictionA >> 10);
-
- p->buf[YADAPTCOEFFSA] = APESIGN(p->buf[YDELAYA ]);
- p->buf[YADAPTCOEFFSA - 1] = APESIGN(p->buf[YDELAYA - 1]);
-
- sign = APESIGN(A);
- p->coeffsA[0][0] += p->buf[YADAPTCOEFFSA ] * sign;
- p->coeffsA[0][1] += p->buf[YADAPTCOEFFSA - 1] * sign;
- p->coeffsA[0][2] += p->buf[YADAPTCOEFFSA - 2] * sign;
- p->coeffsA[0][3] += p->buf[YADAPTCOEFFSA - 3] * sign;
-
- p->buf++;
-
- /* Have we filled the history buffer? */
- if (p->buf == p->historybuffer + HISTORY_SIZE) {
- memmove(p->historybuffer, p->buf,
- PREDICTOR_SIZE * sizeof(*p->historybuffer));
- p->buf = p->historybuffer;
- }
-
- p->filterA[0] = currentA + (uint64_t)((int64_t)(p->filterA[0] * 31U) >> 5);
- *(decoded0++) = p->filterA[0];
- }
-
- p->lastA[0] = currentA;
-}
-
-static void do_init_filter(APEFilter *f, int16_t *buf, int order)
-{
- f->coeffs = buf;
- f->historybuffer = buf + order;
- f->delay = f->historybuffer + order * 2;
- f->adaptcoeffs = f->historybuffer + order;
-
- memset(f->historybuffer, 0, (order * 2) * sizeof(*f->historybuffer));
- memset(f->coeffs, 0, order * sizeof(*f->coeffs));
- f->avg = 0;
-}
-
-static void init_filter(APEContext *ctx, APEFilter *f, int16_t *buf, int order)
-{
- do_init_filter(&f[0], buf, order);
- do_init_filter(&f[1], buf + order * 3 + HISTORY_SIZE, order);
-}
-
-static void do_apply_filter(APEContext *ctx, int version, APEFilter *f,
- int32_t *data, int count, int order, int fracbits)
-{
- int res;
- unsigned absres;
-
- while (count--) {
- /* round fixedpoint scalar product */
- res = ctx->adsp.scalarproduct_and_madd_int16(f->coeffs,
- f->delay - order,
- f->adaptcoeffs - order,
- order, APESIGN(*data));
- res = (int64_t)(res + (1LL << (fracbits - 1))) >> fracbits;
- res += (unsigned)*data;
- *data++ = res;
-
- /* Update the output history */
- *f->delay++ = av_clip_int16(res);
-
- if (version < 3980) {
- /* Version ??? to < 3.98 files (untested) */
- f->adaptcoeffs[0] = (res == 0) ? 0 : ((res >> 28) & 8) - 4;
- f->adaptcoeffs[-4] >>= 1;
- f->adaptcoeffs[-8] >>= 1;
- } else {
- /* Version 3.98 and later files */
-
- /* Update the adaption coefficients */
- absres = FFABSU(res);
- if (absres)
- *f->adaptcoeffs = APESIGN(res) *
- (8 << ((absres > f->avg * 3LL) + (absres > (f->avg + f->avg / 3))));
- /* equivalent to the following code
- if (absres <= f->avg * 4 / 3)
- *f->adaptcoeffs = APESIGN(res) * 8;
- else if (absres <= f->avg * 3)
- *f->adaptcoeffs = APESIGN(res) * 16;
- else
- *f->adaptcoeffs = APESIGN(res) * 32;
- */
- else
- *f->adaptcoeffs = 0;
-
- f->avg += (int)(absres - (unsigned)f->avg) / 16;
-
- f->adaptcoeffs[-1] >>= 1;
- f->adaptcoeffs[-2] >>= 1;
- f->adaptcoeffs[-8] >>= 1;
- }
-
- f->adaptcoeffs++;
-
- /* Have we filled the history buffer? */
- if (f->delay == f->historybuffer + HISTORY_SIZE + (order * 2)) {
- memmove(f->historybuffer, f->delay - (order * 2),
- (order * 2) * sizeof(*f->historybuffer));
- f->delay = f->historybuffer + order * 2;
- f->adaptcoeffs = f->historybuffer + order;
- }
- }
-}
-
-static void apply_filter(APEContext *ctx, APEFilter *f,
- int32_t *data0, int32_t *data1,
- int count, int order, int fracbits)
-{
- do_apply_filter(ctx, ctx->fileversion, &f[0], data0, count, order, fracbits);
- if (data1)
- do_apply_filter(ctx, ctx->fileversion, &f[1], data1, count, order, fracbits);
-}
-
-static void ape_apply_filters(APEContext *ctx, int32_t *decoded0,
- int32_t *decoded1, int count)
-{
- int i;
-
- for (i = 0; i < APE_FILTER_LEVELS; i++) {
- if (!ape_filter_orders[ctx->fset][i])
- break;
- apply_filter(ctx, ctx->filters[i], decoded0, decoded1, count,
- ape_filter_orders[ctx->fset][i],
- ape_filter_fracbits[ctx->fset][i]);
- }
-}
-
-static int init_frame_decoder(APEContext *ctx)
-{
- int i, ret;
- if ((ret = init_entropy_decoder(ctx)) < 0)
- return ret;
- init_predictor_decoder(ctx);
-
- for (i = 0; i < APE_FILTER_LEVELS; i++) {
- if (!ape_filter_orders[ctx->fset][i])
- break;
- init_filter(ctx, ctx->filters[i], ctx->filterbuf[i],
- ape_filter_orders[ctx->fset][i]);
- }
- return 0;
-}
-
-static void ape_unpack_mono(APEContext *ctx, int count)
-{
- if (ctx->frameflags & APE_FRAMECODE_STEREO_SILENCE) {
- /* We are pure silence, so we're done. */
- av_log(ctx->avctx, AV_LOG_DEBUG, "pure silence mono\n");
- return;
- }
-
- ctx->entropy_decode_mono(ctx, count);
- if (ctx->error)
- return;
-
- /* Now apply the predictor decoding */
- ctx->predictor_decode_mono(ctx, count);
-
- /* Pseudo-stereo - just copy left channel to right channel */
- if (ctx->channels == 2) {
- memcpy(ctx->decoded[1], ctx->decoded[0], count * sizeof(*ctx->decoded[1]));
- }
-}
-
-static void ape_unpack_stereo(APEContext *ctx, int count)
-{
- unsigned left, right;
- int32_t *decoded0 = ctx->decoded[0];
- int32_t *decoded1 = ctx->decoded[1];
-
- if ((ctx->frameflags & APE_FRAMECODE_STEREO_SILENCE) == APE_FRAMECODE_STEREO_SILENCE) {
- /* We are pure silence, so we're done. */
- av_log(ctx->avctx, AV_LOG_DEBUG, "pure silence stereo\n");
- return;
- }
-
- ctx->entropy_decode_stereo(ctx, count);
- if (ctx->error)
- return;
-
- /* Now apply the predictor decoding */
- ctx->predictor_decode_stereo(ctx, count);
-
- /* Decorrelate and scale to output depth */
- while (count--) {
- left = *decoded1 - (unsigned)(*decoded0 / 2);
- right = left + *decoded0;
-
- *(decoded0++) = left;
- *(decoded1++) = right;
- }
-}
-
-static int ape_decode_frame(AVCodecContext *avctx, AVFrame *frame,
- int *got_frame_ptr, AVPacket *avpkt)
-{
- const uint8_t *buf = avpkt->data;
- APEContext *s = avctx->priv_data;
- uint8_t *sample8;
- int16_t *sample16;
- int32_t *sample24;
- int i, ch, ret;
- int blockstodecode;
- uint64_t decoded_buffer_size;
-
- /* this should never be negative, but bad things will happen if it is, so
- check it just to make sure. */
- av_assert0(s->samples >= 0);
-
- if(!s->samples){
- uint32_t nblocks, offset;
- int buf_size;
-
- if (!avpkt->size) {
- *got_frame_ptr = 0;
- return 0;
- }
- if (avpkt->size < 8) {
- av_log(avctx, AV_LOG_ERROR, "Packet is too small\n");
- return AVERROR_INVALIDDATA;
- }
- buf_size = avpkt->size & ~3;
- if (buf_size != avpkt->size) {
- av_log(avctx, AV_LOG_WARNING, "packet size is not a multiple of 4. "
- "extra bytes at the end will be skipped.\n");
- }
- if (s->fileversion < 3950) // previous versions overread two bytes
- buf_size += 2;
- av_fast_padded_malloc(&s->data, &s->data_size, buf_size);
- if (!s->data)
- return AVERROR(ENOMEM);
- s->bdsp.bswap_buf((uint32_t *) s->data, (const uint32_t *) buf,
- buf_size >> 2);
- memset(s->data + (buf_size & ~3), 0, buf_size & 3);
- s->ptr = s->data;
- s->data_end = s->data + buf_size;
-
- nblocks = bytestream_get_be32(&s->ptr);
- offset = bytestream_get_be32(&s->ptr);
- if (s->fileversion >= 3900) {
- if (offset > 3) {
- av_log(avctx, AV_LOG_ERROR, "Incorrect offset passed\n");
- av_freep(&s->data);
- s->data_size = 0;
- return AVERROR_INVALIDDATA;
- }
- if (s->data_end - s->ptr < offset) {
- av_log(avctx, AV_LOG_ERROR, "Packet is too small\n");
- return AVERROR_INVALIDDATA;
- }
- s->ptr += offset;
- } else {
- if ((ret = init_get_bits8(&s->gb, s->ptr, s->data_end - s->ptr)) < 0)
- return ret;
- if (s->fileversion > 3800)
- skip_bits_long(&s->gb, offset * 8);
- else
- skip_bits_long(&s->gb, offset);
- }
-
- if (!nblocks || nblocks > INT_MAX / 2 / sizeof(*s->decoded_buffer) - 8) {
- av_log(avctx, AV_LOG_ERROR, "Invalid sample count: %"PRIu32".\n",
- nblocks);
- return AVERROR_INVALIDDATA;
- }
-
- /* Initialize the frame decoder */
- if (init_frame_decoder(s) < 0) {
- av_log(avctx, AV_LOG_ERROR, "Error reading frame header\n");
- return AVERROR_INVALIDDATA;
- }
- s->samples = nblocks;
- }
-
- if (!s->data) {
- *got_frame_ptr = 0;
- return avpkt->size;
- }
-
- blockstodecode = FFMIN(s->blocks_per_loop, s->samples);
- // for old files coefficients were not interleaved,
- // so we need to decode all of them at once
- if (s->fileversion < 3930)
- blockstodecode = s->samples;
-
- /* reallocate decoded sample buffer if needed */
- decoded_buffer_size = 2LL * FFALIGN(blockstodecode, 8) * sizeof(*s->decoded_buffer);
- av_assert0(decoded_buffer_size <= INT_MAX);
-
- /* get output buffer */
- frame->nb_samples = blockstodecode;
- if ((ret = ff_get_buffer(avctx, frame, 0)) < 0) {
- s->samples=0;
- return ret;
- }
-
- av_fast_malloc(&s->decoded_buffer, &s->decoded_size, decoded_buffer_size);
- if (!s->decoded_buffer)
- return AVERROR(ENOMEM);
- memset(s->decoded_buffer, 0, decoded_buffer_size);
- s->decoded[0] = s->decoded_buffer;
- s->decoded[1] = s->decoded_buffer + FFALIGN(blockstodecode, 8);
-
- s->error=0;
-
- if ((s->channels == 1) || (s->frameflags & APE_FRAMECODE_PSEUDO_STEREO))
- ape_unpack_mono(s, blockstodecode);
- else
- ape_unpack_stereo(s, blockstodecode);
-
- if (s->error) {
- s->samples=0;
- av_log(avctx, AV_LOG_ERROR, "Error decoding frame\n");
- return AVERROR_INVALIDDATA;
- }
-
- switch (s->bps) {
- case 8:
- for (ch = 0; ch < s->channels; ch++) {
- sample8 = (uint8_t *)frame->data[ch];
- for (i = 0; i < blockstodecode; i++)
- *sample8++ = (s->decoded[ch][i] + 0x80U) & 0xff;
- }
- break;
- case 16:
- for (ch = 0; ch < s->channels; ch++) {
- sample16 = (int16_t *)frame->data[ch];
- for (i = 0; i < blockstodecode; i++)
- *sample16++ = s->decoded[ch][i];
- }
- break;
- case 24:
- for (ch = 0; ch < s->channels; ch++) {
- sample24 = (int32_t *)frame->data[ch];
- for (i = 0; i < blockstodecode; i++)
- *sample24++ = s->decoded[ch][i] * 256U;
- }
- break;
- }
-
- s->samples -= blockstodecode;
-
- if (avctx->err_recognition & AV_EF_CRCCHECK &&
- s->fileversion >= 3900 && s->bps < 24) {
- uint32_t crc = s->CRC_state;
- const AVCRC *crc_tab = av_crc_get_table(AV_CRC_32_IEEE_LE);
- for (i = 0; i < blockstodecode; i++) {
- for (ch = 0; ch < s->channels; ch++) {
- uint8_t *smp = frame->data[ch] + (i*(s->bps >> 3));
- crc = av_crc(crc_tab, crc, smp, s->bps >> 3);
- }
- }
-
- if (!s->samples && (~crc >> 1) ^ s->CRC) {
- av_log(avctx, AV_LOG_ERROR, "CRC mismatch! Previously decoded "
- "frames may have been affected as well.\n");
- if (avctx->err_recognition & AV_EF_EXPLODE)
- return AVERROR_INVALIDDATA;
- }
-
- s->CRC_state = crc;
- }
-
- *got_frame_ptr = 1;
-
- return !s->samples ? avpkt->size : 0;
-}
-
-static void ape_flush(AVCodecContext *avctx)
-{
- APEContext *s = avctx->priv_data;
- s->samples= 0;
-}
-
-#define OFFSET(x) offsetof(APEContext, x)
-#define PAR (AV_OPT_FLAG_DECODING_PARAM | AV_OPT_FLAG_AUDIO_PARAM)
-static const AVOption options[] = {
- { "max_samples", "maximum number of samples decoded per call", OFFSET(blocks_per_loop), AV_OPT_TYPE_INT, { .i64 = 4608 }, 1, INT_MAX, PAR, "max_samples" },
- { "all", "no maximum. decode all samples for each packet at once", 0, AV_OPT_TYPE_CONST, { .i64 = INT_MAX }, INT_MIN, INT_MAX, PAR, "max_samples" },
- { NULL},
-};
-
-static const AVClass ape_decoder_class = {
- .class_name = "APE decoder",
- .item_name = av_default_item_name,
- .option = options,
- .version = LIBAVUTIL_VERSION_INT,
-};
-
-const FFCodec ff_ape_decoder = {
- .p.name = "ape",
- CODEC_LONG_NAME("Monkey's Audio"),
- .p.type = AVMEDIA_TYPE_AUDIO,
- .p.id = AV_CODEC_ID_APE,
- .priv_data_size = sizeof(APEContext),
- .init = ape_decode_init,
- .close = ape_decode_close,
- FF_CODEC_DECODE_CB(ape_decode_frame),
- .p.capabilities = AV_CODEC_CAP_SUBFRAMES | AV_CODEC_CAP_DELAY |
- AV_CODEC_CAP_DR1,
- .caps_internal = FF_CODEC_CAP_INIT_CLEANUP,
- .flush = ape_flush,
- .p.sample_fmts = (const enum AVSampleFormat[]) { AV_SAMPLE_FMT_U8P,
- AV_SAMPLE_FMT_S16P,
- AV_SAMPLE_FMT_S32P,
- AV_SAMPLE_FMT_NONE },
- .p.priv_class = &ape_decoder_class,
-};
diff --git a/spaces/colakin/video-generater/public/ffmpeg/libavcodec/h2645_vui.h b/spaces/colakin/video-generater/public/ffmpeg/libavcodec/h2645_vui.h
deleted file mode 100644
index 638da7c36672ecebe2462bcd6f9105e4f19abca0..0000000000000000000000000000000000000000
--- a/spaces/colakin/video-generater/public/ffmpeg/libavcodec/h2645_vui.h
+++ /dev/null
@@ -1,49 +0,0 @@
-/*
- * This file is part of FFmpeg.
- *
- * FFmpeg is free software; you can redistribute it and/or
- * modify it under the terms of the GNU Lesser General Public
- * License as published by the Free Software Foundation; either
- * version 2.1 of the License, or (at your option) any later version.
- *
- * FFmpeg is distributed in the hope that it will be useful,
- * but WITHOUT ANY WARRANTY; without even the implied warranty of
- * MERCHANTABILITY or FITNESS FOR A PARTICULAR PURPOSE. See the GNU
- * Lesser General Public License for more details.
- *
- * You should have received a copy of the GNU Lesser General Public
- * License along with FFmpeg; if not, write to the Free Software
- * Foundation, Inc., 51 Franklin Street, Fifth Floor, Boston, MA 02110-1301 USA
- */
-
-#ifndef AVCODEC_H2645_VUI_H
-#define AVCODEC_H2645_VUI_H
-
-#include "libavutil/pixfmt.h"
-#include "libavutil/rational.h"
-
-#include "get_bits.h"
-
-typedef struct H2645VUI {
- AVRational sar;
-
- int overscan_info_present_flag;
- int overscan_appropriate_flag;
-
- int video_signal_type_present_flag;
- int video_format;
- int video_full_range_flag;
- int colour_description_present_flag;
- enum AVColorPrimaries colour_primaries;
- enum AVColorTransferCharacteristic transfer_characteristics;
- enum AVColorSpace matrix_coeffs;
-
- int chroma_loc_info_present_flag;
- int chroma_sample_loc_type_top_field;
- int chroma_sample_loc_type_bottom_field;
- enum AVChromaLocation chroma_location;
-} H2645VUI;
-
-void ff_h2645_decode_common_vui_params(GetBitContext *gb, H2645VUI *vui, void *logctx);
-
-#endif /* AVCODEC_H2645_VUI_H */
diff --git a/spaces/colakin/video-generater/public/ffmpeg/libavcodec/mips/vp9dsp_init_mips.c b/spaces/colakin/video-generater/public/ffmpeg/libavcodec/mips/vp9dsp_init_mips.c
deleted file mode 100644
index 27c8ec9d8c43a6ae1958c5775e926146703dbaf7..0000000000000000000000000000000000000000
--- a/spaces/colakin/video-generater/public/ffmpeg/libavcodec/mips/vp9dsp_init_mips.c
+++ /dev/null
@@ -1,227 +0,0 @@
-/*
- * Copyright (c) 2015 Shivraj Patil (Shivraj.Patil@imgtec.com)
- *
- * This file is part of FFmpeg.
- *
- * FFmpeg is free software; you can redistribute it and/or
- * modify it under the terms of the GNU Lesser General Public
- * License as published by the Free Software Foundation; either
- * version 2.1 of the License, or (at your option) any later version.
- *
- * FFmpeg is distributed in the hope that it will be useful,
- * but WITHOUT ANY WARRANTY; without even the implied warranty of
- * MERCHANTABILITY or FITNESS FOR A PARTICULAR PURPOSE. See the GNU
- * Lesser General Public License for more details.
- *
- * You should have received a copy of the GNU Lesser General Public
- * License along with FFmpeg; if not, write to the Free Software
- * Foundation, Inc., 51 Franklin Street, Fifth Floor, Boston, MA 02110-1301 USA
- */
-
-#include "libavutil/attributes.h"
-#include "libavutil/mips/cpu.h"
-#include "config.h"
-#include "libavutil/common.h"
-#include "libavcodec/vp9dsp.h"
-#include "vp9dsp_mips.h"
-
-#if HAVE_MSA
-static av_cold void vp9dsp_intrapred_init_msa(VP9DSPContext *dsp, int bpp)
-{
- if (bpp == 8) {
-#define init_intra_pred_msa(tx, sz) \
- dsp->intra_pred[tx][VERT_PRED] = ff_vert_##sz##_msa; \
- dsp->intra_pred[tx][HOR_PRED] = ff_hor_##sz##_msa; \
- dsp->intra_pred[tx][DC_PRED] = ff_dc_##sz##_msa; \
- dsp->intra_pred[tx][LEFT_DC_PRED] = ff_dc_left_##sz##_msa; \
- dsp->intra_pred[tx][TOP_DC_PRED] = ff_dc_top_##sz##_msa; \
- dsp->intra_pred[tx][DC_128_PRED] = ff_dc_128_##sz##_msa; \
- dsp->intra_pred[tx][DC_127_PRED] = ff_dc_127_##sz##_msa; \
- dsp->intra_pred[tx][DC_129_PRED] = ff_dc_129_##sz##_msa; \
- dsp->intra_pred[tx][TM_VP8_PRED] = ff_tm_##sz##_msa; \
-
- init_intra_pred_msa(TX_16X16, 16x16);
- init_intra_pred_msa(TX_32X32, 32x32);
-#undef init_intra_pred_msa
-
-#define init_intra_pred_msa(tx, sz) \
- dsp->intra_pred[tx][DC_PRED] = ff_dc_##sz##_msa; \
- dsp->intra_pred[tx][LEFT_DC_PRED] = ff_dc_left_##sz##_msa; \
- dsp->intra_pred[tx][TOP_DC_PRED] = ff_dc_top_##sz##_msa; \
- dsp->intra_pred[tx][TM_VP8_PRED] = ff_tm_##sz##_msa; \
-
- init_intra_pred_msa(TX_4X4, 4x4);
- init_intra_pred_msa(TX_8X8, 8x8);
-#undef init_intra_pred_msa
- }
-}
-
-static av_cold void vp9dsp_itxfm_init_msa(VP9DSPContext *dsp, int bpp)
-{
- if (bpp == 8) {
-#define init_itxfm(tx, sz) \
- dsp->itxfm_add[tx][DCT_DCT] = ff_idct_idct_##sz##_add_msa; \
- dsp->itxfm_add[tx][DCT_ADST] = ff_iadst_idct_##sz##_add_msa; \
- dsp->itxfm_add[tx][ADST_DCT] = ff_idct_iadst_##sz##_add_msa; \
- dsp->itxfm_add[tx][ADST_ADST] = ff_iadst_iadst_##sz##_add_msa \
-
-#define init_idct(tx, nm) \
- dsp->itxfm_add[tx][DCT_DCT] = \
- dsp->itxfm_add[tx][ADST_DCT] = \
- dsp->itxfm_add[tx][DCT_ADST] = \
- dsp->itxfm_add[tx][ADST_ADST] = nm##_add_msa
-
- init_itxfm(TX_4X4, 4x4);
- init_itxfm(TX_8X8, 8x8);
- init_itxfm(TX_16X16, 16x16);
- init_idct(TX_32X32, ff_idct_idct_32x32);
-#undef init_itxfm
-#undef init_idct
- }
-}
-
-static av_cold void vp9dsp_mc_init_msa(VP9DSPContext *dsp, int bpp)
-{
- if (bpp == 8) {
-#define init_fpel(idx1, idx2, sz, type) \
- dsp->mc[idx1][FILTER_8TAP_SMOOTH ][idx2][0][0] = ff_##type##sz##_msa; \
- dsp->mc[idx1][FILTER_8TAP_REGULAR][idx2][0][0] = ff_##type##sz##_msa; \
- dsp->mc[idx1][FILTER_8TAP_SHARP ][idx2][0][0] = ff_##type##sz##_msa; \
- dsp->mc[idx1][FILTER_BILINEAR ][idx2][0][0] = ff_##type##sz##_msa
-
-#define init_copy_avg(idx, sz) \
- init_fpel(idx, 0, sz, copy); \
- init_fpel(idx, 1, sz, avg)
-
-#define init_avg(idx, sz) \
- init_fpel(idx, 1, sz, avg)
-
- init_copy_avg(0, 64);
- init_copy_avg(1, 32);
- init_copy_avg(2, 16);
- init_copy_avg(3, 8);
- init_avg(4, 4);
-
-#undef init_copy_avg
-#undef init_avg
-#undef init_fpel
-
-#define init_subpel1(idx1, idx2, idxh, idxv, sz, dir, type) \
- dsp->mc[idx1][FILTER_BILINEAR ][idx2][idxh][idxv] = \
- ff_##type##_bilin_##sz##dir##_msa; \
- dsp->mc[idx1][FILTER_8TAP_SMOOTH ][idx2][idxh][idxv] = \
- ff_##type##_8tap_smooth_##sz##dir##_msa; \
- dsp->mc[idx1][FILTER_8TAP_REGULAR][idx2][idxh][idxv] = \
- ff_##type##_8tap_regular_##sz##dir##_msa; \
- dsp->mc[idx1][FILTER_8TAP_SHARP ][idx2][idxh][idxv] = \
- ff_##type##_8tap_sharp_##sz##dir##_msa;
-
-#define init_subpel2(idx, idxh, idxv, dir, type) \
- init_subpel1(0, idx, idxh, idxv, 64, dir, type); \
- init_subpel1(1, idx, idxh, idxv, 32, dir, type); \
- init_subpel1(2, idx, idxh, idxv, 16, dir, type); \
- init_subpel1(3, idx, idxh, idxv, 8, dir, type); \
- init_subpel1(4, idx, idxh, idxv, 4, dir, type)
-
-#define init_subpel3(idx, type) \
- init_subpel2(idx, 1, 1, hv, type); \
- init_subpel2(idx, 0, 1, v, type); \
- init_subpel2(idx, 1, 0, h, type)
-
- init_subpel3(0, put);
- init_subpel3(1, avg);
-
-#undef init_subpel1
-#undef init_subpel2
-#undef init_subpel3
- }
-}
-
-static av_cold void vp9dsp_loopfilter_init_msa(VP9DSPContext *dsp, int bpp)
-{
- if (bpp == 8) {
- dsp->loop_filter_8[0][0] = ff_loop_filter_h_4_8_msa;
- dsp->loop_filter_8[0][1] = ff_loop_filter_v_4_8_msa;
- dsp->loop_filter_8[1][0] = ff_loop_filter_h_8_8_msa;
- dsp->loop_filter_8[1][1] = ff_loop_filter_v_8_8_msa;
- dsp->loop_filter_8[2][0] = ff_loop_filter_h_16_8_msa;
- dsp->loop_filter_8[2][1] = ff_loop_filter_v_16_8_msa;
-
- dsp->loop_filter_16[0] = ff_loop_filter_h_16_16_msa;
- dsp->loop_filter_16[1] = ff_loop_filter_v_16_16_msa;
-
- dsp->loop_filter_mix2[0][0][0] = ff_loop_filter_h_44_16_msa;
- dsp->loop_filter_mix2[0][0][1] = ff_loop_filter_v_44_16_msa;
- dsp->loop_filter_mix2[0][1][0] = ff_loop_filter_h_48_16_msa;
- dsp->loop_filter_mix2[0][1][1] = ff_loop_filter_v_48_16_msa;
- dsp->loop_filter_mix2[1][0][0] = ff_loop_filter_h_84_16_msa;
- dsp->loop_filter_mix2[1][0][1] = ff_loop_filter_v_84_16_msa;
- dsp->loop_filter_mix2[1][1][0] = ff_loop_filter_h_88_16_msa;
- dsp->loop_filter_mix2[1][1][1] = ff_loop_filter_v_88_16_msa;
- }
-}
-
-static av_cold void vp9dsp_init_msa(VP9DSPContext *dsp, int bpp)
-{
- vp9dsp_intrapred_init_msa(dsp, bpp);
- vp9dsp_itxfm_init_msa(dsp, bpp);
- vp9dsp_mc_init_msa(dsp, bpp);
- vp9dsp_loopfilter_init_msa(dsp, bpp);
-}
-#endif // #if HAVE_MSA
-
-#if HAVE_MMI
-static av_cold void vp9dsp_mc_init_mmi(VP9DSPContext *dsp)
-{
-#define init_subpel1(idx1, idx2, idxh, idxv, sz, dir, type) \
- dsp->mc[idx1][FILTER_8TAP_SMOOTH ][idx2][idxh][idxv] = \
- ff_##type##_8tap_smooth_##sz##dir##_mmi; \
- dsp->mc[idx1][FILTER_8TAP_REGULAR][idx2][idxh][idxv] = \
- ff_##type##_8tap_regular_##sz##dir##_mmi; \
- dsp->mc[idx1][FILTER_8TAP_SHARP ][idx2][idxh][idxv] = \
- ff_##type##_8tap_sharp_##sz##dir##_mmi;
-
-#define init_subpel2(idx, idxh, idxv, dir, type) \
- init_subpel1(0, idx, idxh, idxv, 64, dir, type); \
- init_subpel1(1, idx, idxh, idxv, 32, dir, type); \
- init_subpel1(2, idx, idxh, idxv, 16, dir, type); \
- init_subpel1(3, idx, idxh, idxv, 8, dir, type); \
- init_subpel1(4, idx, idxh, idxv, 4, dir, type)
-
-#define init_subpel3(idx, type) \
- init_subpel2(idx, 1, 1, hv, type); \
- init_subpel2(idx, 0, 1, v, type); \
- init_subpel2(idx, 1, 0, h, type)
-
- init_subpel3(0, put);
- init_subpel3(1, avg);
-
-#undef init_subpel1
-#undef init_subpel2
-#undef init_subpel3
-}
-
-static av_cold void vp9dsp_init_mmi(VP9DSPContext *dsp, int bpp)
-{
- if (bpp == 8) {
- vp9dsp_mc_init_mmi(dsp);
- }
-}
-#endif // #if HAVE_MMI
-
-av_cold void ff_vp9dsp_init_mips(VP9DSPContext *dsp, int bpp)
-{
-#if HAVE_MSA || HAVE_MMI
- int cpu_flags = av_get_cpu_flags();
-#endif
-
-#if HAVE_MMI
- if (have_mmi(cpu_flags))
- vp9dsp_init_mmi(dsp, bpp);
-#endif
-
-#if HAVE_MSA
- if (have_msa(cpu_flags))
- vp9dsp_init_msa(dsp, bpp);
-#endif
-}
diff --git a/spaces/congsaPfin/Manga-OCR/logs/Aliana Shinobi High Five MOD APK A Guide to the Games Story and Gameplay.md b/spaces/congsaPfin/Manga-OCR/logs/Aliana Shinobi High Five MOD APK A Guide to the Games Story and Gameplay.md
deleted file mode 100644
index 2698210ac8e7c948223dfb159bfb3db5784986ff..0000000000000000000000000000000000000000
--- a/spaces/congsaPfin/Manga-OCR/logs/Aliana Shinobi High Five MOD APK A Guide to the Games Story and Gameplay.md
+++ /dev/null
@@ -1,107 +0,0 @@
-
-
Aliança Shinobi High Five MOD APK: A Ninja Adventure Game for Android
-
If you are a fan of ninja-themed games, you might want to check out Aliança Shinobi High Five, a new RPG game for Android devices. In this game, you can create your own ninja character, join a shinobi alliance, and embark on exciting missions and battles. You can also enjoy stunning graphics, immersive sound effects, and smooth controls.
-
But what if you want to unlock all the features and items in the game without spending real money? Well, there is a solution for that. You can download Aliança Shinobi High Five MOD APK, a modified version of the game that gives you unlimited resources, free shopping, and more. In this article, we will tell you more about this game and how to get the mod apk on your device.
Aliança Shinobi High Five is a game inspired by the popular anime and manga series Naruto. The game is set in a world where ninjas have special abilities called chakra. You can choose from different classes of ninjas, such as taijutsu, genjutsu, or ninjutsu. You can also customize your appearance, skills, weapons, and outfits.
-
The game has a rich and engaging story mode, where you can follow the adventures of your character and interact with other characters from the Naruto universe. You can also join an alliance with other players and cooperate in various missions and events. You can also challenge other players in PvP battles and rank up in the leaderboard.
-
The features and the graphics
-
Aliança Shinobi High Five has many features that make it a fun and addictive game. Some of them are:
-
-
Over 100 characters to collect and upgrade
-
Over 200 skills to learn and master
-
Over 300 items to equip and enhance
-
Over 500 quests to complete and rewards to claim
-
Different modes to play, such as story mode, alliance mode, arena mode, survival mode, etc.
-
Different events to participate in, such as daily tasks, weekly challenges, seasonal festivals, etc.
-
-
The game also has amazing graphics that bring the ninja world to life. The characters are designed with high-quality 3D models and animations. The environments are detailed and colorful. The effects are realistic and dynamic. The game also has a catchy soundtrack and voice-overs that match the mood of the game.
-
Why download Aliança Shinobi High Five MOD APK?
-
The benefits of the mod version
-
While Aliança Shinobi High Five is a free-to-play game, it also has some in-app purchases that can enhance your gaming experience. For example, you can buy gems, coins, energy, VIP membership, etc. However, these items can be quite expensive and not everyone can afford them.
-
That's why some people prefer to download Aliança Shinobi High Five MOD APK, a modified version of the game that gives you access to all the premium features for free. With this mod apk, you can enjoy:
-
aliança shinobi high five mod apk download
-aliança shinobi high five mod apk unlimited money
-aliança shinobi high five mod apk latest version
-aliança shinobi high five mod apk android
-aliança shinobi high five mod apk free
-aliança shinobi high five mod apk offline
-aliança shinobi high five mod apk 2023
-aliança shinobi high five mod apk hack
-aliança shinobi high five mod apk no root
-aliança shinobi high five mod apk obb
-aliança shinobi high five mod apk revdl
-aliança shinobi high five mod apk rexdl
-aliança shinobi high five mod apk pure
-aliança shinobi high five mod apk happymod
-aliança shinobi high five mod apk an1
-aliança shinobi high five mod apk vip
-aliança shinobi high five mod apk mega
-aliança shinobi high five mod apk mediafıre
-aliança shinobi high five mod apk uptodown
-aliança shinobi high five mod apk 1.8
-aliança shinobi high five mod apk gameplay
-aliança shinobi high five mod apk cheats
-aliança shinobi high five mod apk features
-aliança shinobi high five mod apk review
-aliança shinobi high five mod apk online
-aliança shinobi high five rpg mod apk
-aliança shinobi high five ninja mod apk
-aliança shinobi high five anime mod apk
-aliança shinobi high five naruto mod apk
-aliança shinobi high five boruto mod apk
-aliança shinobi high five adventure mod apk
-aliança shinobi high five action mod apk
-aliança shinobi high five strategy mod apk
-aliança shinobi high five simulation mod apk
-aliança shinobi high five role playing mod apk
-download game aliança shinobi high five mod apk
-download aplikasi aliança shinobi high five mod apk
-descargar aliança shinobi high five mod apk
-baixar aliança shinobi high five mod apk
-telecharger aliança shinobi high five mod apk
-installieren aliança shinobi high five mod apk
-scaricare aliança shinobi high five mod apk
-indir aliança shinobi high five mod apk
-скачать алианса шиноби хай файв мод апк
-下载联盟忍者高五模式apk
-ダウンロードアリアンサシノビハイファイブモッドapk
-다운로드 알리안사 시노비 하이 파이브 모드 APK
-تحميل تحالف شينوبي هاي فايف مود APK
-
-
Unlimited gems
-
Unlimited coins
-
Unlimited energy
-
Free shopping
-
No ads
-
No root required
-
-
With these benefits, you can play the game without any limitations or interruptions. You can unlock all the characters, skills, items, modes, etc. You can also
How to download and install the mod apk
-
If you want to download Aliança Shinobi High Five MOD APK, you need to follow these simple steps:
-
-
Click on the download button below to get the mod apk file.
-
Allow unknown sources on your device settings.
-
Locate the downloaded file and tap on it to install it.
-
Launch the game and enjoy the mod features.
-
-
Download Aliança Shinobi High Five MOD APK
-
Note: Before you install the mod apk, make sure you uninstall the original game if you have it on your device. Also, make sure you have enough storage space and a stable internet connection.
-
Conclusion
-
Aliança Shinobi High Five is a great game for anyone who loves ninjas and Naruto. It has a captivating story, a diverse gameplay, and a stunning graphics. It also has a lot of features and modes to keep you entertained for hours. However, if you want to enjoy the game without spending money, you can download Aliança Shinobi High Five MOD APK and get unlimited resources, free shopping, and more. This way, you can unlock all the content and have more fun with the game.
-
We hope this article was helpful and informative. If you have any questions or feedback, feel free to leave a comment below. Thank you for reading and happy gaming!
-
FAQs
-
Here are some frequently asked questions about Aliança Shinobi High Five MOD APK:
-
Is Aliança Shinobi High Five MOD APK safe to use?
-
Yes, Aliança Shinobi High Five MOD APK is safe to use. It does not contain any viruses or malware that can harm your device or data. However, you should always download the mod apk from a trusted source and scan it with an antivirus before installing it.
-
Is Aliança Shinobi High Five MOD APK compatible with my device?
-
Aliança Shinobi High Five MOD APK is compatible with most Android devices that run on Android 4.4 or higher. However, some devices may not support the game or the mod features due to different specifications or settings. If you encounter any problems with the game or the mod apk, you can try to update your device software, clear your cache, or contact the developer for assistance.
-
Can I play Aliança Shinobi High Five MOD APK online with other players?
-
Yes, you can play Aliança Shinobi High Five MOD APK online with other players. However, you should be aware that using the mod apk may give you an unfair advantage over other players and may result in your account being banned or suspended by the game developer. Therefore, we advise you to use the mod apk at your own risk and discretion.
-
Can I update Aliança Shinobi High Five MOD APK to the latest version?
-
Yes, you can update Aliança Shinobi High Five MOD APK to the latest version. However, you should always check if the mod apk is compatible with the new version of the game before updating it. You should also backup your game data before updating it in case something goes wrong.
-
Can I request more features for Aliança Shinobi High Five MOD APK?
-
Yes, you can request more features for Aliança Shinobi High Five MOD APK. However, we cannot guarantee that your requests will be fulfilled or that the mod apk will work as expected. The mod apk is created by independent developers who may or may not update it regularly or add new features to it. Therefore, we suggest you to be patient and grateful for what you have.
197e85843d
-
-
\ No newline at end of file
diff --git a/spaces/congsaPfin/Manga-OCR/logs/Plink Balls A New Way to Play with Physics and Mathematics.md b/spaces/congsaPfin/Manga-OCR/logs/Plink Balls A New Way to Play with Physics and Mathematics.md
deleted file mode 100644
index 2f3ea28bca801ece4d0ab09720a32ead9521fd3e..0000000000000000000000000000000000000000
--- a/spaces/congsaPfin/Manga-OCR/logs/Plink Balls A New Way to Play with Physics and Mathematics.md
+++ /dev/null
@@ -1,140 +0,0 @@
-
-
Plink Balls: A Fun and Addictive Game for All Ages
-
Do you love games that are simple, yet challenging and rewarding? Do you enjoy watching balls fall and bounce on pegs and slots? Do you want to become a millionaire or lose it all in a matter of seconds? If you answered yes to any of these questions, then you will love Plink Balls, the latest sensation in the gaming world.
-
What are Plink Balls?
-
Plink Balls are small, colorful balls that you can drop from the top of a triangular grid of pegs. As the balls fall, they hit the pegs and change their direction randomly. Some of the balls will land in containers at the bottom of the grid, while others will fall out of the screen. Each container has a multiplier value that determines how much you win or lose by dropping a ball into it. The goal is to drop as many balls as possible into the highest multipliers and avoid the lowest ones.
Plink Balls are inspired by a classic game show called Plinko, which was first introduced in 1983 on The Price is Right. In Plinko, contestants had to drop large discs from the top of a board with pegs and slots. Depending on where the discs landed, they could win up to $50,000 or nothing at all. Plinko became one of the most popular and exciting games on the show, and has been featured in many variations and spin-offs over the years.
-
How to play Plink Balls
-
Playing Plink Balls is very easy and fun. All you need is a smartphone or tablet with the app installed. You can download the app for free from Google Play or App Store. Once you open the app, you will see a screen with a grid of pegs and containers. You can choose how many balls you want to drop by tapping on the plus or minus buttons at the bottom. You can also choose how much you want to wager by tapping on the dollar sign button. The minimum wager is $1 and the maximum is $1000 per ball.
-
After you have set your preferences, you can start dropping balls by tapping on the screen. You can watch as the balls fall and bounce on the pegs, creating a mesmerizing spectacle. You can also use exciting boosts to increase your chances of winning, such as extra balls, magnets, bombs, and more. You can earn these boosts by playing regularly or by watching ads.
-
The benefits of playing Plink Balls
-
Plink Balls is not only a fun game, but also a beneficial one. Playing Plink Balls can help you improve your skills and abilities in various ways, such as:
-
-
Cognitive skills: Playing Plink Balls can enhance your memory, attention, concentration, logic, problem-solving, and decision-making skills. You have to remember where the balls land, pay attention to the multipliers, use logic to predict where the balls will go, solve problems when they get stuck, and make quick decisions when dropping balls.
-
Mental health: Playing Plink Balls can reduce your stress, anxiety, boredom, and depression. You can relax and enjoy watching the balls fall and bounce, creating a soothing sound and visual effect. You can also feel happy and satisfied when you win big or overcome a challenge.
-
Social skills: Playing Plink Balls can improve your social skills by allowing you to interact with other players online. You can share your scores and achievements with other players online. You can also join or create clubs, chat with other members, send and receive gifts, and participate in tournaments and events.
-
Financial skills: Playing Plink Balls can teach you how to manage your money wisely. You have to budget your funds, balance your risks and rewards, and plan your moves carefully. You can also learn how to deal with losses and gains, and how to cope with uncertainty and luck.
-
-
How to master Plink Balls
-
Plink Balls may seem like a game of chance, but there is also a lot of skill involved. If you want to become a Plink Balls master, you need to practice and learn some tips and tricks that can help you win more often. Here are some of them:
-
Tips and tricks for winning Plink Balls
-
-
Drop the balls from different angles: Don't always drop the balls from the center of the screen. Try dropping them from the left or right edges, or from different heights. This can create different trajectories and outcomes for the balls, and increase your chances of hitting the high multipliers.
-
Use the boosts wisely: Don't waste your boosts on low stakes or easy levels. Save them for when you really need them, such as when you are playing for high wagers or facing difficult challenges. Also, don't use the same boost all the time. Mix and match different boosts to create different effects and combinations.
-
Watch the ads: Watching ads can be annoying, but it can also be rewarding. By watching ads, you can earn free coins, extra balls, or other boosts that can help you play better. You can also watch ads to double your winnings or to continue playing after losing.
-
-
The best strategies for Plink Balls
-
-
Set a limit: Before you start playing, decide how much you are willing to spend and how much you want to win. Stick to your limit and don't go over it. This way, you can avoid losing more than you can afford or getting greedy and losing what you have won.
-
Start small: Don't bet too much on your first few drops. Start with small wagers and test the waters. See how the balls behave and where they land. Once you get a feel for the game, you can increase your bets gradually.
-
Aim for the middle: The middle containers usually have the highest multipliers, but they are also the hardest to hit. However, if you aim for the middle, you have a better chance of hitting something than if you aim for the edges. Even if you miss the middle, you might still hit a decent multiplier on either side.
-
-
The most common mistakes to avoid in Plink Balls
-
-
Dropping too many balls at once: Dropping too many balls at once can be tempting, but it can also be risky. You might end up hitting the same containers repeatedly, or missing them altogether. You might also run out of balls quickly and lose your chance to win more. It is better to drop one ball at a time and see where it lands before dropping another one.
-
Dropping too fast or too slow: Dropping too fast or too slow can affect the outcome of the game. If you drop too fast, you might not have enough time to react or adjust your strategy. If you drop too slow, you might lose your momentum or miss an opportunity. It is better to drop at a moderate pace that suits your style and preference.
-
Getting distracted or impatient: Plink Balls is a game that requires focus and patience. If you get distracted by other things or impatient with the results, you might make mistakes or lose interest. It is better to play when you are relaxed and attentive, and enjoy the game as it unfolds.
-
-
How to enjoy Plink Balls more
-
Plink Balls is already a fun and addictive game, but there are ways to make it even more enjoyable. Here are some of them:
-
plink balls game
-plink balls app
-plink balls online
-plink balls simulator
-plink balls probability
-plink balls experiment
-plink balls physics
-plink balls statistics
-plink balls histogram
-plink balls download
-plink balls free
-plink balls android
-plink balls ios
-plink balls rollic games
-plink balls phet
-plink balls youtube
-plink balls video
-plink balls review
-plink balls tips
-plink balls tricks
-plink balls hack
-plink balls cheat
-plink balls mod apk
-plink balls unblocked
-plink balls play store
-plink balls amazon
-plink balls walmart
-plink balls target
-plink balls toy
-plink balls machine
-plink balls board game
-plink balls arcade game
-plink balls casino game
-plink balls slot machine
-plink balls bingo game
-plink balls lottery game
-plink balls scratch card game
-plink balls math game
-plink balls educational game
-plink balls science game
-plink balls fun game
-plink balls addictive game
-plink balls relaxing game
-plink balls challenging game
-plink balls strategy game
-plink balls puzzle game
-plink balls logic game
-plink balls skill game
-plink balls luck game
-
The different modes and levels of Plink Balls
-
Plink Balls has different modes and levels that offer different challenges and rewards. You can choose from:
-
-
Classic mode: This is the basic mode where you drop balls into containers with fixed multipliers. The multipliers range from x0 to x1000.
-
Casino mode: This is the mode where you drop balls into containers with variable multipliers. The multipliers change every time you drop a ball, and can range from x0 to x10000.
-
Adventure mode: This is the mode where you drop balls into containers with special effects. The effects can be positive or negative, such as double, half, freeze, shuffle, or bomb.
-
Challenge mode: This is the mode where you face different tasks and goals. The tasks and goals can be time-based, score-based, or skill-based, such as dropping a certain number of balls, hitting a certain multiplier, or avoiding a certain container.
-
-
You can also unlock new levels by earning stars. Each level has a different theme and design, such as jungle, space, candy, or pirate. The higher the level, the harder the challenge and the bigger the reward.
-
The best features and boosts of Plink Balls
-
Plink Balls has many features and boosts that can make the game more fun and exciting. Some of the best ones are:
-
-
Extra balls: These are balls that you can get for free by watching ads, completing tasks, or opening chests. You can use them to drop more balls and increase your chances of winning.
-
Magnets: These are boosts that you can activate by tapping on the magnet icon at the bottom of the screen. They can attract the balls to the nearest container with the highest multiplier.
-
Bombs: These are boosts that you can activate by tapping on the bomb icon at the bottom of the screen. They can explode and clear all the pegs in a certain area, creating a path for the balls to fall into the containers.
-
Leaderboards: These are features that show your rank and score compared to other players around the world. You can see how you are doing and try to beat your own or others' records.
-
Achievements: These are features that reward you for reaching certain milestones or completing certain challenges in the game. You can earn coins, stars, or other prizes for achieving them.
-
-
The best ways to share and compete with your friends in Plink Balls
-
Plink Balls is more fun when you play with your friends. You can share and compete with your friends in various ways, such as:
-
-
Invite your friends: You can invite your friends to join Plink Balls by sending them a link or a code through social media, email, or text message. You can also scan their QR codes to add them as friends.
-
Send and receive gifts: You can send and receive gifts from your friends every day. The gifts can be coins, extra balls, or other boosts that can help you play better.
-
Join or create clubs: You can join or create clubs with your friends or other players who share your interests or goals. You can chat with your club members, exchange tips and tricks, and participate in club events and tournaments.
-
Challenge your friends: You can challenge your friends to a friendly match or a duel in Plink Balls. You can choose the mode, level, wager, and number of balls for each challenge. The winner gets to keep all the winnings and bragging rights.
-
-
Conclusion
-
Summary of the main points
-
Plink Balls is a fun and addictive game that anyone can enjoy. It is based on a classic game show called Plinko, where contestants had to drop discs from a board with pegs and slots. In Plink Balls, you drop balls from a grid of pegs and containers with different multipliers. The goal is to drop as many balls as possible into the highest multipliers and avoid the lowest ones.
-
Plink Balls is not only a fun game, but also a beneficial one. It can improve your cognitive skills, mental health, social skills, and financial skills. It can also teach you how to manage your money wisely, balance your risks and rewards, and plan your moves carefully.
-
Plink Balls has different modes and levels that offer different challenges and rewards. It also has many features and boosts that can make the game more fun and exciting. You can also share and compete with your friends in various ways.
-
Call to action
-
If you are looking for a game that is simple, yet challenging and rewarding; a game that is relaxing, yet stimulating and engaging; a game that is entertaining, yet educational and beneficial; then look no further than Plink Balls. Download Plink Balls today and start dropping balls into containers with multipliers. You will be amazed by how much fun you will have and how much you will learn. You will be amazed by how much fun you will have and how much you will learn.
-
So what are you waiting for? Download Plink Balls now and join the millions of players who are already hooked on this game. You won't regret it!
-
FAQs
-
Here are some of the most frequently asked questions about Plink Balls:
-
-
Q: Is Plink Balls free to play?
-
A: Yes, Plink Balls is free to play. You can download the app for free from Google Play or App Store. You can also play without spending any real money, as you can earn coins, extra balls, and other boosts by watching ads, completing tasks, or opening chests. However, if you want to play with higher stakes, access premium features, or remove ads, you can also make in-app purchases with real money.
-
Q: Is Plink Balls fair and random?
-
A: Yes, Plink Balls is fair and random. The outcome of each drop is determined by a sophisticated algorithm that ensures that the balls fall and bounce on the pegs and containers in a realistic and unpredictable way. The algorithm also ensures that the multipliers and effects of the containers are balanced and fair. No one can manipulate or rig the game in any way.
-
Q: Is Plink Balls safe and secure?
-
A: Yes, Plink Balls is safe and secure. The app does not collect or store any personal or sensitive information from the users. The app also does not share or sell any data to third parties. The app also uses encryption and other security measures to protect the users' transactions and accounts. The app also complies with all the relevant laws and regulations regarding online gaming and gambling.
-
Q: Is Plink Balls suitable for children?
-
A: Plink Balls is suitable for children who are 12 years old or older. The app has a rating of 12+ on Google Play and App Store. The app does not contain any violence, nudity, profanity, or other inappropriate content. However, the app does involve simulated gambling, which may not be suitable for younger children or those who have gambling problems. Parents should supervise and monitor their children's use of the app and set limits and boundaries as needed.
-
Q: How can I contact the developers of Plink Balls?
-
A: You can contact the developers of Plink Balls by sending an email to plinkballs@gmail.com. You can also visit their website at www.plinkballs.com or follow them on Facebook, Twitter, or Instagram. You can also leave a review or a comment on Google Play or App Store. The developers welcome any feedback, suggestions, questions, or complaints from the users and will try to respond as soon as possible.
-
197e85843d
-
-
\ No newline at end of file
diff --git a/spaces/congsaPfin/Manga-OCR/logs/Scary Teacher 3D Old Version The Ultimate Game to Make Your Teacher Pay for Her Crimes.md b/spaces/congsaPfin/Manga-OCR/logs/Scary Teacher 3D Old Version The Ultimate Game to Make Your Teacher Pay for Her Crimes.md
deleted file mode 100644
index 8e92f10dc0e07af4c8698c681e6bc33d37666b3e..0000000000000000000000000000000000000000
--- a/spaces/congsaPfin/Manga-OCR/logs/Scary Teacher 3D Old Version The Ultimate Game to Make Your Teacher Pay for Her Crimes.md
+++ /dev/null
@@ -1,141 +0,0 @@
-
-
Download Scary Teacher 3D Old Version
-
Scary Teacher 3D is a popular horror game that lets you prank and scare your evil teacher in various ways. You can explore her house, find clues, and use different objects to make her life miserable. But what if you want to play the old version of Scary Teacher 3D, which has different levels, graphics, and features? In this article, we will show you how to download and install Scary Teacher 3D old version on your Android device or computer.
-
What is Scary Teacher 3D?
-
Scary Teacher 3D is a game developed by Z & K Games, which is known for creating other horror games such as Evil Nun and Granny. The game was released in 2018 and has since been updated with new content and improvements. The game has over 100 million downloads on Google Play Store and has a rating of 4.2 out of 5 stars.
You can play as a student who wants to take revenge on his or her scary teacher, who is very cruel and abusive.
-
You can explore the teacher's house, which has 15 rooms with different settings and secrets.
-
You can find clues, solve puzzles, and use various objects to prank and scare the teacher.
-
You can enjoy the realistic graphics, animations, and sound effects that create a spooky atmosphere.
-
You can unlock new chapters and scenarios as you progress in the game.
-
-
Why download the old version of Scary Teacher 3D?
-
Some reasons why you might want to download the old version of Scary Teacher 3D are:
-
-
You prefer the old graphics, levels, and features that were available in the previous versions of the game.
-
You want to play the game offline or without ads, which might not be possible in the latest version.
-
You have an older device that is not compatible with the latest version of the game.
-
You want to try a different experience or challenge yourself with the old version of the game.
-
-
How to download Scary Teacher 3D old version
-
There are two ways you can download Scary Teacher 3D old version:
-
-
Use a web tool to generate download links
-
Use an APK extractor app on your Android device
-
-
Method 1: Use a web tool to generate download links
-
This method involves using a web tool that can download APK files from Google Play Store URLs. The files are the same as you would get from the Play Store, and you can choose different versions to download. Here are the steps:
-
Step 1: Copy the Google Play URL of the app
-
First, you need to get the URL of Scary Teacher 3D from Google Play Store. You can do this by opening Google Play Store on your Android device or computer and searching for Scary Teacher 3D. Then, you need to copy the URL from the address bar or the share button. The URL should look something like this:
Step 2: Paste the URL in the web tool and generate the download link
-
Next, you need to open a web tool that can generate download links for APK files from Google Play Store URLs. There are many such tools available online, but one of them is APKCombo. You can access it by visiting this link:
-
download scary teacher 3d mod apk
-download scary teacher 3d for pc
-download scary teacher 3d game free
-download scary teacher 3d latest version
-download scary teacher 3d hack
-download scary teacher 3d unlimited money
-download scary teacher 3d chapter 5
-download scary teacher 3d offline
-download scary teacher 3d apk pure
-download scary teacher 3d android
-download scary teacher 3d app store
-download scary teacher 3d apk mirror
-download scary teacher 3d apk mod menu
-download scary teacher 3d all chapters unlocked
-download scary teacher 3d apk obb
-download scary teacher 3d apk revdl
-download scary teacher 3d apk data
-download scary teacher 3d apk android oyun club
-download scary teacher 3d apk uptodown
-download scary teacher 3d bluestacks
-download scary teacher 3d by z&k games
-download scary teacher 3d beta version
-download scary teacher 3d cheats
-download scary teacher 3d chapter 6
-download scary teacher 3d chapter 4
-download scary teacher 3d chapter 7
-download scary teacher 3d chapter 8
-download scary teacher 3d chapter 9
-download scary teacher 3d chapter 10
-download scary teacher 3d chapter wise
-download scary teacher 3d cracked apk
-download scary teacher 3d christmas update
-download scary teacher 3d diamond hack
-download scary teacher 3d direct link
-download scary teacher 3d easy install
-download scary teacher 3d emulator
-download scary teacher 3d full version free
-download scary teacher 3d for windows
Once you are on the website, you need to paste the URL you copied in the previous step in the search box and click on Download APK. The web tool will then show you a list of available versions of Scary Teacher 3D, along with their sizes and dates. You can choose any version you want to download, but make sure it is an old version and not the latest one. For example, you can choose version 5.10.2, which was released on June 9, 2021.
-
Step 3: Download the APK file to your device or computer
-
After you select the version you want to download, the web tool will generate a download link for the APK file. You can click on the link to start downloading the file to your device or computer. The file name should be something like this:
-
com.zakg.scaryteacher.hellgame_5.10.2.apk
-
The download time may vary depending on your internet speed and the size of the file. Once the download is complete, you can move on to the next method or skip to the installation section.
-
Method 2: Use an APK extractor app on your Android device
-
This method involves using an app that can extract APK files from installed apps on your Android device. This way, you can get the old version of Scary Teacher 3D if you already have it installed on your device or if you can find someone who has it. Here are the steps:
-
Step 1: Download and install App APK Extractor & Analyzer from the Play Store
-
First, you need to download and install an app that can extract APK files from installed apps on your Android device. There are many such apps available on the Play Store, but one of them is App APK Extractor & Analyzer. You can download it by visiting this link:
Once you have downloaded and installed the app, you need to open it and grant it the necessary permissions to access your device storage and installed apps.
-
Step 2: Select the app you want to extract and tap Extract App
-
Next, you need to select Scary Teacher 3D from the list of installed apps on your device. You can use the search bar or scroll down to find it. Once you have selected it, you need to tap on Extract App at the bottom of the screen. The app will then start extracting the APK file from Scary Teacher 3D and save it to your device storage.
-
Step 3: Save the APK file to your preferred location
-
After the extraction is complete, you will see a notification that says "APK extracted successfully". You can tap on it to open the folder where the APK file is saved. The folder name should be something like this:
You can move or copy the APK file to any location you want on your device or transfer it to your computer if you wish.
-
How to install Scary Teacher 3D old version
-
Now that you have downloaded the APK file of Scary Teacher 3D old version, you need to install it on your device. Here are the steps:
-
Enable unknown sources on your device
-
Before you can install an APK file that is not from Google Play Store, you need to enable unknown sources on your device. This will allow you to install apps from other sources than Google Play Store. To do this, follow these steps:
-
-
Go to Settings > Security > Unknown sources (or Settings > Apps > Special app access > Install unknown apps, depending on your device model and Android version).
-
Find and tap the app that you used to download the APK file, such as APKCombo or App APK Extractor & Analyzer.
-
Toggle on the switch that says Allow from this source or Allow app installs.
-
-
Locate and tap the APK file to install it
-
After you have enabled unknown sources, you can install the APK file of Scary Teacher 3D old version. To do this, follow these steps:
-
-
Go to the location where you saved the APK file, such as your device storage or your computer.
-
Find and tap the APK file to open it. You may see a warning message that says "This type of file can harm your device". Tap OK to proceed.
-
You may see a screen that shows the app's permissions and features. Tap Install to start the installation process.
-
Wait for the installation to finish. You may see a message that says "App installed". Tap Open to launch the app or Done to exit.
-
-
Conclusion
-
In this article, we have shown you how to download and install Scary Teacher 3D old version on your Android device or computer. You can use either a web tool or an APK extractor app to get the APK file of the old version of the game. Then, you can install it by enabling unknown sources and tapping the APK file. We hope you enjoy playing Scary Teacher 3D old version and have fun pranking and scaring your evil teacher.
-
FAQs
-
Here are some frequently asked questions about Scary Teacher 3D old version:
-
Q: Is Scary Teacher 3D old version safe to download and install?
-
A: Yes, as long as you download the APK file from a reliable source, such as APKCombo or App APK Extractor & Analyzer, and scan it for viruses before installing it. However, you should be careful when installing apps from unknown sources, as they may contain malware or unwanted ads.
-
Q: What are the differences between Scary Teacher 3D old version and new version?
-
A: The differences between Scary Teacher 3D old version and new version may vary depending on which version you choose to download. Some of the possible differences are:
-
-
The old version may have fewer levels, chapters, and scenarios than the new version.
-
The old version may have different graphics, sound effects, and animations than the new version.
-
The old version may have different bugs, glitches, and performance issues than the new version.
-
The old version may not support some features or devices that the new version does.
-
-
Q: How can I update Scary Teacher 3D old version to the latest version?
-
A: If you want to update Scary Teacher 3D old version to the latest version, you can do so by visiting Google Play Store and downloading the latest version of the game. However, this will overwrite the old version of the game and you will lose any progress or data you have in it. Alternatively, you can keep both versions of the game by renaming the APK file of the old version before installing it. For example, you can rename it to Scary Teacher 3D_old.apk. This way, you can have two icons of Scary Teacher 3D on your device and play either one of them.
-
Q: How can I uninstall Scary Teacher 3D old version from my device?
-
A: If you want to uninstall Scary Teacher 3D old version from your device, you can do so by following these steps:
-
-
Go to Settings > Apps > Scary Teacher 3D (or Settings > Apps & notifications > See all apps > Scary Teacher 3D, depending on your device model and Android version).
-
Tap Uninstall and confirm your choice.
-
You may also need to delete the APK file from your device storage or computer if you don't need it anymore.
-
-
Q: Where can I find more information about Scary Teacher 3D?
-
A: If you want to find more information about Scary Teacher 3D, such as tips, tricks, guides, reviews, videos, and more, you can visit these websites:
< a href="">https://www.youtube.com/channel/UCw9ZP9zF0wJ6oEW5gkQy9Qw: The official YouTube channel of Z & K Games, where you can watch gameplay videos, trailers, and updates of Scary Teacher 3D.
-
https://www.facebook.com/ScaryTeacher3D/: The official Facebook page of Scary Teacher 3D, where you can follow the latest news, events, and community posts of the game.
-
401be4b1e0
-
-
\ No newline at end of file
diff --git a/spaces/congsaPfin/Manga-OCR/logs/Solo Leveling Hit Run APK - Swipe Slash and Save the Town from Evil Monsters.md b/spaces/congsaPfin/Manga-OCR/logs/Solo Leveling Hit Run APK - Swipe Slash and Save the Town from Evil Monsters.md
deleted file mode 100644
index 5bc9488d5e01b6c4cec1ffbe3a8799d075e872c0..0000000000000000000000000000000000000000
--- a/spaces/congsaPfin/Manga-OCR/logs/Solo Leveling Hit Run APK - Swipe Slash and Save the Town from Evil Monsters.md
+++ /dev/null
@@ -1,145 +0,0 @@
-
-
Solo Leveling Hit and Run APK: A Fun and Action-Packed Runner Game Based on the Popular Webtoon
-
If you are a fan of the Korean webtoon series Solo Leveling, you might want to check out this new runner game based on it. Solo Leveling Hit and Run APK is a game that lets you experience the thrilling adventures of Sung Jinwoo, a weak hunter who gains the power to level up beyond any limits. In this game, you will have to run, slash, dodge, and fight your way through various enemies and obstacles, while leveling up your skills and abilities. You will also encounter some familiar characters and scenes from the webtoon, as well as some new ones that will surprise you. Whether you are a fan of Solo Leveling or not, this game will surely keep you entertained and challenged.
But what is Solo Leveling exactly? And how can you download and play this game on your Android device? In this article, we will answer these questions and more. We will give you a brief overview of the game and its features, as well as the webtoon and its plot. We will also show you how to download and install the game on your device, how to play it effectively, and why you should give it a try. By the end of this article, you will have all the information you need to enjoy this fun and action-packed runner game based on the popular webtoon.
-
What is Solo Leveling Hit and Run APK?
-
A brief introduction to the game and its features
-
Solo Leveling Hit and Run APK is a runner game developed by Supercent, a Korean game studio. It is based on the webtoon series Solo Leveling by Chu-Gong, which has over 22 million readers worldwide. The game was released in March 2023 for Android devices.
-
The game is a mission-based driving game that features out-of-the-car platform action, similar to The Simpsons Hit and Run or Grand Theft Auto. You can explore the interactive world of Seoul, where the story takes place, and interact with various characters from the webtoon. You can also drive different vehicles, such as cars, motorcycles, trucks, etc., that have different speed, handling, durability, etc.
-
The game also has a leveling system that allows you to upgrade your skills and abilities as you progress through the game. You can increase your strength, speed, stamina, health, etc., by defeating enemies or completing missions. You can also unlock new weapons, such as blades, guns, axes, etc., that have different damage, range , and special effects. You can also customize your appearance, such as clothes, hair, accessories, etc., to suit your style.
-
A brief introduction to the webtoon and its plot
-
Solo Leveling is a webtoon series written by Chu-Gong and illustrated by Jang Sung-Rak and Gee So-Lyung. It is based on a novel of the same name by Chu-Gong. The webtoon was first published in 2018 on KakaoPage, a Korean webtoon platform, and later on Webtoon, an international webtoon platform. The webtoon has over 150 chapters and is still ongoing.
-
solo leveling hit and run game download
-solo leveling hit and run mod apk
-solo leveling hit and run android
-solo leveling hit and run ios
-solo leveling hit and run hack
-solo leveling hit and run cheats
-solo leveling hit and run review
-solo leveling hit and run gameplay
-solo leveling hit and run tips
-solo leveling hit and run guide
-solo leveling hit and run update
-solo leveling hit and run latest version
-solo leveling hit and run offline
-solo leveling hit and run online
-solo leveling hit and run free
-solo leveling hit and run premium
-solo leveling hit and run unlimited gems
-solo leveling hit and run best weapons
-solo leveling hit and run boss fight
-solo leveling hit and run characters
-solo leveling hit and run levels
-solo leveling hit and run skills
-solo leveling hit and run tricks
-solo leveling hit and run strategy
-solo leveling hit and run wiki
-solo leveling hit and run reddit
-solo leveling hit and run discord
-solo leveling hit and run facebook
-solo leveling hit and run twitter
-solo leveling hit and run instagram
-solo leveling hit and run youtube
-solo leveling hit and run google play
-solo leveling hit and run app store
-solo leveling hit and run apk pure
-solo leveling hit and run apk combo[^1^]
-solo leveling hit and run apkpure.com[^1^]
-solo leveling hit and run apkmonk.com[^1^]
-solo leveling hit and run apkdone.com[^1^]
-solo leveling hit and run apkmody.io[^1^]
-solo leveling hit and run apkaward.com[^1^]
-solo leveling hit and run apk4all.com[^1^]
-solo leveling hit and run apkfab.com[^1^]
-solo leveling hit and run apkmirror.com[^1^]
-solo leveling hit and run apksum.com[^1^]
-solo leveling hit and run apknite.com[^1^]
-
The webtoon is set in a world where portals to other dimensions, called gates, have opened, unleashing monsters and creatures that threaten humanity. To fight them, some people have awakened as hunters, who have special abilities and powers. However, not all hunters are equal, and they are ranked from E to S, with S being the strongest.
-
The protagonist of the webtoon is Sung Jinwoo, a weak E-rank hunter who barely survives his missions. One day, he gets involved in a double dungeon, a rare and dangerous type of gate that has never been cleared before. There, he finds a mysterious system that allows him to level up his skills and abilities by completing quests and killing monsters. He becomes the only player of the system, and the only one who can see it. He decides to use it to become stronger and rise from the lowest rank to the highest rank of hunters. Along the way, he faces many challenges, enemies, allies, secrets, and mysteries that will change his life and the world.
-
How to Download and Install Solo Leveling Hit and Run APK?
-
The steps to download and install the game on Android devices
-
If you want to play Solo Leveling Hit and Run APK on your Android device, you will need to follow these steps:
Click on the download button and wait for the APK file to be downloaded on your device.
-
Once the download is complete, locate the APK file on your device's file manager or downloads folder.
-
Tap on the APK file and allow it to install on your device. You may need to enable unknown sources or allow from this source in your device's settings.
-
After the installation is done, you can launch the game from your app drawer or home screen.
-
-
The requirements and permissions needed for the game
-
Before you download and install Solo Leveling Hit and Run APK on your device, you should make sure that your device meets the following requirements:
-
-
Your device should have Android 4.4 or higher as its operating system.
-
Your device should have at least 2 GB of RAM and 500 MB of free storage space.
-
Your device should have a stable internet connection to play the game online.
-
-
You should also be aware that the game will ask for some permissions on your device, such as:
-
-
Access to your device's storage to save game data and cache.
-
Access to your device's microphone to record audio for voice chat.
-
Access to your device's camera to scan QR codes for rewards.
-
-
You should grant these permissions if you want to enjoy the full features of the game. However, you can also deny them if you are concerned about your privacy or security.
-
How to Play Solo Leveling Hit and Run APK?
-
The basic gameplay mechanics and controls
-
Solo Leveling Hit and Run APK is a runner game that combines driving and platform action. You can control your character using the virtual joystick on the left side of the screen, and use the buttons on the right side of the screen to perform actions such as jumping, attacking, using items, etc. You can also swipe left or right on the screen to change lanes while driving or running.
-
The game has two main modes: story mode and challenge mode. In story mode, you can follow the plot of the webtoon and complete missions that involve driving or running through various locations, fighting enemies or bosses, collecting items or gems, etc. In challenge mode, you can compete with other players online or offline in different types of races or battles.
-
The different modes, levels, enemies, and obstacles in the game
-
The game has several modes that offer different gameplay experiences. Here are some of them:
- - Race mode: In this mode, you can race against other players or the AI in different tracks, such as city, highway, forest, etc. You can use your skills and items to boost your speed, attack your opponents, or avoid obstacles. You can also collect gems and coins along the way to upgrade your vehicle or buy new ones. The goal is to reach the finish line first or within the time limit. - Battle mode: In this mode, you can fight against other players or the AI in different arenas, such as dungeon, castle, stadium, etc. You can use your weapons and items to deal damage, defend yourself, or heal yourself. You can also collect gems and coins along the way to upgrade your weapons or buy new ones. The goal is to reduce your opponent's health to zero or have more health than them when the time runs out. - Survival mode: In this mode, you can run for as long as you can while avoiding enemies and obstacles that come from all directions. You can use your skills and items to escape, fight back, or recover. You can also collect gems and coins along the way to upgrade your skills or buy new ones. The goal is to survive for as long as possible or reach a certain distance. The game has various levels that correspond to the chapters of the webtoon. Each level has a different theme, setting, difficulty, and objective. Some levels may require you to drive or run through a certain route, while others may require you to defeat a certain number of enemies or a boss. Some levels may also have special events or challenges that will test your skills and strategy. The game has various enemies and obstacles that will try to stop you from completing your missions. Some enemies are common monsters that appear in the webtoon, such as goblins, wolves, zombies, etc. Some enemies are special bosses that have unique abilities and patterns, such as Cerberus, the Demon King, the Ant King, etc. Some obstacles are environmental hazards that can damage you or slow you down, such as traffic, walls, spikes, traps, etc.
The tips and tricks to level up faster and defeat the boss
-
If you want to level up faster and defeat the boss in Solo Leveling Hit and Run APK, you should follow these tips and tricks:
-
-
Complete the daily quests and achievements that will reward you with gems, coins, items, etc.
-
Watch ads or videos that will give you extra gems, coins, items, etc.
-
Join a guild or a clan that will give you access to more missions, rewards, chat, etc.
-
Participate in events or festivals that will offer you special missions, rewards, items, etc.
-
Use the best vehicle or weapon that suits your playstyle and preference.
-
Upgrade your vehicle or weapon regularly to increase its performance and durability.
-
Customize your appearance to boost your confidence and style.
-
Use your skills and items wisely and strategically.
-
Learn the patterns and weaknesses of your enemies and bosses.
-
Avoid unnecessary damage or collisions.
-
Collect gems and coins as much as possible.
-
Have fun and enjoy the game.
-
-
Why You Should Play Solo Leveling Hit and Run APK?
-
The benefits of playing the game, such as fun, entertainment, challenge, etc.
-
Playing Solo Leveling Hit and Run APK can bring you many benefits, such as:
-
-
Fun: The game is fun to play, as it offers a variety of gameplay modes, levels, enemies, and obstacles that will keep you entertained and engaged. You can also enjoy the humor, drama, and action of the webtoon in the game.
-
Entertainment: The game is entertaining to watch, as it features high-quality graphics, sound, and animation that will immerse you in the world of Solo Leveling. You can also admire the beautiful and detailed design of the characters, vehicles, weapons, and environments in the game.
-
Challenge: The game is challenging to master, as it requires skill, strategy, and reflex to complete the missions and defeat the enemies and bosses. You can also compete with other players or the AI in different modes and rankings to test your abilities and improve your performance.
-
-
The advantages of playing the game, such as graphics, sound, performance, etc.
-
Playing Solo Leveling Hit and Run APK can also give you many advantages, such as:
-
-
Graphics: The game has stunning graphics that are faithful to the webtoon's style and quality. The game uses 3D models and textures that are realistic and detailed. The game also has dynamic lighting and shadows that create a realistic and immersive atmosphere.
-
Sound: The game has excellent sound that matches the webtoon's tone and mood. The game uses original soundtracks and sound effects that are catchy and immersive. The game also has voice acting that is expressive and authentic.
-
Performance: The game has smooth performance that ensures a satisfying and enjoyable gameplay experience. The game runs at a stable frame rate and resolution that prevent lag or glitches. The game also has a user-friendly interface and controls that are easy to use and customize.
-
-
The comparison of the game with other similar games, such as The Simpsons Hit and Run, Grand Theft Auto, etc.
-
Playing Solo Leveling Hit and Run APK can also make you appreciate how it differs from other similar games, such as:
-
-
The Simpsons Hit and Run: This is a 2003 game based on the animated sitcom The Simpsons. It is also a mission-based driving game that features out-of-the-car platform action. However, it has a more comedic and satirical tone than Solo Leveling Hit and Run APK. It also has a more cartoonish and colorful graphics style than Solo Leveling Hit and Run APK.
-
Grand Theft Auto: This is a series of games that started in 1997 and is still ongoing. It is also a mission-based driving game that features out-of-the-car platform action. However, it has a more realistic and violent tone than Solo Leveling Hit and Run APK. It also has a more open-world and sandbox gameplay style than Solo Leveling Hit and Run APK.
-
-
Conclusion
-
A summary of the main points of the article
-
In conclusion, Solo Leveling Hit and Run APK is a fun and action-packed runner game based on the popular webtoon series Solo Leveling by Chu-Gong. It is a game that lets you experience the thrilling adventures of Sung Jinwoo, a weak hunter who gains the power to level up beyond any limits. In this game, you will have to run, slash, dodge, and fight your way through various enemies and obstacles, while leveling up your skills and abilities. You will also encounter some familiar characters and scenes from the webtoon, as well as some new ones that will surprise you. Whether you are a fan of Solo Leveling or not, this game will surely keep you entertained and challenged.
-
We have also shown you how to download and install the game on your Android device, how to play it effectively, and why you should give it a try. We have also compared the game with other similar games, such as The Simpsons Hit and Run and Grand Theft Auto, and highlighted its benefits and advantages. We hope that this article has given you all the information you need to enjoy this fun and action-packed runner game based on the popular webtoon.
-
A call to action for the readers to download and play the game
-
So, what are you waiting for? Download Solo Leveling Hit and Run APK now and join Sung Jinwoo in his epic journey to become the strongest hunter in the world. Experience the thrill and excitement of running, slashing, dodging, and fighting in this amazing game that will make you feel like you are part of the webtoon. Don't miss this chance to play one of the best runner games based on one of the best webtoon series ever. Download Solo Leveling Hit and Run APK today and have fun!
-
FAQs
-
Is Solo Leveling Hit and Run APK free to play?
-
Yes, Solo Leveling Hit and Run APK is free to play. However, it may contain some in-app purchases or ads that can enhance your gameplay experience or support the developer.
-
Is Solo Leveling Hit and Run APK safe to download and install?
-
Yes, Solo Leveling Hit and Run APK is safe to download and install. It does not contain any viruses, malware, or spyware that can harm your device or data. However, you should always download it from a trusted source or website, such as the official website of the game or Google Play Store.
-
Is Solo Leveling Hit and Run APK compatible with all Android devices?
-
No, Solo Leveling Hit and Run APK may not be compatible with all Android devices. It requires Android 4.4 or higher as its operating system, as well as 2 GB of RAM and 500 MB of free storage space. It may also not work well on some devices due to different specifications or models.
-
How can I get more gems in Solo Leveling Hit and Run APK?
-
You can get more gems in Solo Leveling Hit and Run APK by completing missions, defeating enemies, collecting items, watching ads or videos, participating in events or festivals, joining a guild or a clan, etc. You can also buy gems with real money through in-app purchases.
-
How can I contact the developer of Solo Leveling Hit and Run APK?
-
You can contact the developer of Solo Leveling Hit and Run APK by sending an email to support@supercent.com or visiting their website at https://supercent.com/. You can also follow them on their social media accounts, such as Facebook, Twitter, Instagram, etc., for updates, news, feedback, etc.
197e85843d
-
-
\ No newline at end of file
diff --git a/spaces/contluForse/HuggingGPT/assets/Autodesk AutoCAD 2020 Product Keys Crack Download ((EXCLUSIVE)).md b/spaces/contluForse/HuggingGPT/assets/Autodesk AutoCAD 2020 Product Keys Crack Download ((EXCLUSIVE)).md
deleted file mode 100644
index e431c136537c9ffe49cdfb4cdbbf5e6647f071b8..0000000000000000000000000000000000000000
--- a/spaces/contluForse/HuggingGPT/assets/Autodesk AutoCAD 2020 Product Keys Crack Download ((EXCLUSIVE)).md
+++ /dev/null
@@ -1,8 +0,0 @@
-
-
-The next generation of plugins for Rhino starts with Clayoo, an innovative solution to freeform modeling. Clayoo offers three different ... 4d29de3e1b
-
-
-
diff --git a/spaces/diacanFperku/AutoGPT/Daub Ages 2 0 Cracked NEW!.md b/spaces/diacanFperku/AutoGPT/Daub Ages 2 0 Cracked NEW!.md
deleted file mode 100644
index 6e455c9cdfeeb565391266ca5a800ec32ef48edc..0000000000000000000000000000000000000000
--- a/spaces/diacanFperku/AutoGPT/Daub Ages 2 0 Cracked NEW!.md
+++ /dev/null
@@ -1,60 +0,0 @@
-
-
Daub Ages 2 0 Cracked - A Review
-
If you are interested in genealogy and family history, you might want to check out Daub Ages 2 0 Cracked. This is a software that allows you to create and manage your family tree, as well as to explore and analyze your ancestry. Daub Ages 2 0 Cracked is a powerful and user-friendly tool that can help you discover your roots and share your stories.
-
What are the features of Daub Ages 2 0 Cracked?
-
Daub Ages 2 0 Cracked has some impressive features that make it a useful tool for genealogists and family historians. Some of these features are:
Data entry and editing: You can easily enter and edit your personal data, such as names, dates, places, events, sources, notes, media, etc. You can also import and export data from GEDCOM files, CSV files, or other formats.
-
Family tree view and navigation: You can view and navigate your family tree in various ways, such as pedigree chart, family group sheet, timeline, fan chart, etc. You can also customize the appearance and layout of your family tree.
-
Research and analysis: You can research and analyze your ancestry by using various tools, such as maps, statistics, reports, charts, lists, etc. You can also compare and merge data from different sources or databases.
-
Publication and sharing: You can publish and share your family tree by creating web pages, books, PDF files, slideshows, etc. You can also upload your family tree to online platforms, such as Ancestry.com or FamilySearch.org.
-
-
What are the system requirements of Daub Ages 2 0 Cracked?
-
Daub Ages 2 0 Cracked is compatible with Windows XP/Vista/7/8/10. It requires 512 MB of RAM (1 GB recommended) and 100 MB of free hard disk space. It is a lightweight and easy-to-use software that does not require much resources or technical skills.
-
How to download and install Daub Ages 2 0 Cracked?
-
To download and install Daub Ages 2 0 Cracked, you need to follow these steps:
-
-
Download the software from a reliable source, such as FileCR.com.
-
Extract the zip file and run the setup file.
-
Follow the instructions on the screen and complete the installation process.
-
Copy the crack file and paste it into the installation folder.
-
Run the software and enjoy creating your family tree.
-
-
Conclusion
-
Daub Ages 2 0 Cracked is a powerful and convenient software that allows you to create and manage your family tree, as well as to explore and analyze your ancestry. Daub Ages 2 0 Cracked is a user-friendly tool that can help you discover your roots and share your stories. Daub Ages 2 0 Cracked is a must-have tool for anyone who loves genealogy and family history.
-
What are the benefits of Daub Ages 2 0 Cracked?
-
Daub Ages 2 0 Cracked has many benefits for users who want to create and manage their family tree, as well as to explore and analyze their ancestry. Some of these benefits are:
-
-
It saves time and money: You can create and manage your family tree faster and easier than using a web browser or a subscription-based service. You can also access your family tree offline and without any ads or limitations.
-
It offers more options and flexibility: You can customize and personalize your family tree according to your preferences and needs. You can also import and export data from various sources or formats.
-
It enhances your genealogy experience: You can research and analyze your ancestry by using various tools and features. You can also publish and share your family tree by creating various outputs and formats.
-
-
What are the alternatives to Daub Ages 2 0 Cracked?
-
If you are looking for other ways to create and manage your family tree, as well as to explore and analyze your ancestry, you might want to check out some of the alternatives to Daub Ages 2 0 Cracked. Some of these alternatives are:
-
-
Family Tree Maker: This is a software that allows you to create and manage your family tree, as well as to sync it with Ancestry.com or FamilySearch.org. You can also research and analyze your ancestry by using various tools and features.
-
Legacy Family Tree: This is a software that allows you to create and manage your family tree, as well as to sync it with FamilySearch.org or MyHeritage.com. You can also research and analyze your ancestry by using various tools and features.
-
RootsMagic: This is a software that allows you to create and manage your family tree, as well as to sync it with Ancestry.com or FamilySearch.org. You can also research and analyze your ancestry by using various tools and features.
-
-
What are the pros and cons of Daub Ages 2 0 Cracked?
-
Daub Ages 2 0 Cracked has some pros and cons that you should consider before using it. Some of these pros and cons are:
-
-
Pros
Cons
-
It is fast and easy to use.
It requires a crack file to activate the full version.
-
It offers more options and flexibility than web browsers or subscription-based services.
It does not support syncing with online platforms or databases.
-
It enhances your genealogy experience by allowing you to research and analyze your ancestry.
It does not support creating vector charts or 3D views.
-
-
Conclusion
-
In conclusion, Daub Ages 2 0 Cracked is a powerful and convenient software that allows you to create and manage your family tree, as well as to explore and analyze your ancestry. Daub Ages 2 0 Cracked is a user-friendly tool that can help you discover your roots and share your stories. Daub Ages 2 0 Cracked is a must-have tool for anyone who loves genealogy and family history.
-
-
If you want to get Daub Ages 2 0 Cracked, you can download it from FileCR.com, a reliable source that offers free downloads of various software. You will also get the crack file that will activate the full version, as well as a user manual that will guide you through the installation and usage of the software.
-
So don't hesitate and download Daub Ages 2 0 Cracked today and enjoy creating your family tree!
In conclusion, Daub Ages 2 0 Cracked is a powerful and convenient software that allows you to create and manage your family tree, as well as to explore and analyze your ancestry. Daub Ages 2 0 Cracked is a user-friendly tool that can help you discover your roots and share your stories. Daub Ages 2 0 Cracked is a must-have tool for anyone who loves genealogy and family history.
-
If you want to get Daub Ages 2 0 Cracked, you can download it from FileCR.com, a reliable source that offers free downloads of various software. You will also get the crack file that will activate the full version, as well as a user manual that will guide you through the installation and usage of the software.
-
So don't hesitate and download Daub Ages 2 0 Cracked today and enjoy creating your family tree!
-Download Daub Ages 2 0 Cracked from FileCR.com 3cee63e6c2
-
-
\ No newline at end of file
diff --git a/spaces/diacanFperku/AutoGPT/Not Angka Pianika Rumah Kita Doc.rar.md b/spaces/diacanFperku/AutoGPT/Not Angka Pianika Rumah Kita Doc.rar.md
deleted file mode 100644
index e5cb3c9965c1073de18ca672018a1f1c6fbe9250..0000000000000000000000000000000000000000
--- a/spaces/diacanFperku/AutoGPT/Not Angka Pianika Rumah Kita Doc.rar.md
+++ /dev/null
@@ -1,10 +0,0 @@
-
-
-Update for Microsoft Security Essentials (4.10.209.0) returns context menu not angka pianika rumah kita doc.rar · readiris pro 11 free download full . Downloads for Microsoft Security Essentials for Windows Vista, Windows 7, Windows Server 2008 and Windows.
-Download Microsoft Security Essentials for Windows 7 32-bit 64-bit SP1 (x86/x64) (English) .
-Download Microsoft Security Essentials 4.10.209.0 .
-Microsoft Security Essentials is a free antivirus to protect Windows 7, Windows 8 and . Download Microsoft Security Essentials for Windows XP 32-bit (32-bit) (English) . .
-Download Microsoft Security Essentials for Windows XP (32-bit) (English) . 8a78ff9644
-
-
-
diff --git a/spaces/diacanFperku/AutoGPT/Robot Structural Analysis Professional 2019 Xforce Keygen ((LINK)) 64 Bit.md b/spaces/diacanFperku/AutoGPT/Robot Structural Analysis Professional 2019 Xforce Keygen ((LINK)) 64 Bit.md
deleted file mode 100644
index 3d4eda560af77c68871b442de5e3e87b1db57828..0000000000000000000000000000000000000000
--- a/spaces/diacanFperku/AutoGPT/Robot Structural Analysis Professional 2019 Xforce Keygen ((LINK)) 64 Bit.md
+++ /dev/null
@@ -1,32 +0,0 @@
-
-
How to Activate Robot Structural Analysis Professional 2019 with Xforce Keygen 64 Bit
-
Robot Structural Analysis Professional 2019 is a powerful software that allows you to perform structural analysis and design of complex structures. It supports various types of materials, loads, and codes, and integrates with other Autodesk products such as Revit and AutoCAD.
-
Robot Structural Analysis Professional 2019 Xforce Keygen 64 Bit
However, to use Robot Structural Analysis Professional 2019, you need to activate it with a valid license. If you don't have one, you can use Xforce Keygen 64 Bit to generate a serial number and a product key that will unlock the full features of the software.
-
In this article, we will show you how to use Xforce Keygen 64 Bit to activate Robot Structural Analysis Professional 2019 in a few simple steps.
-
Step 1: Download and Install Robot Structural Analysis Professional 2019
-
The first step is to download and install Robot Structural Analysis Professional 2019 from the official website or from a trusted source. You can choose the trial version or the full version depending on your needs.
-
-
Follow the instructions on the screen to complete the installation process. Make sure you have enough disk space and system requirements to run the software smoothly.
-
Step 2: Download and Run Xforce Keygen 64 Bit
-
The next step is to download and run Xforce Keygen 64 Bit from a reliable source. Xforce Keygen 64 Bit is a tool that can generate serial numbers and product keys for various Autodesk products, including Robot Structural Analysis Professional 2019.
-
Before you run Xforce Keygen 64 Bit, make sure you disable your antivirus and firewall software, as they may interfere with the activation process. Also, make sure you run Xforce Keygen 64 Bit as an administrator.
-
Once you run Xforce Keygen 64 Bit, you will see a window like this:
-
-
Select Robot Structural Analysis Professional 2019 from the drop-down menu and click on Generate. You will see a serial number and a product key appear in the fields below.
-
Step 3: Activate Robot Structural Analysis Professional 2019 with Xforce Keygen 64 Bit
-
The final step is to activate Robot Structural Analysis Professional 2019 with the serial number and product key generated by Xforce Keygen 64 Bit.
-
Launch Robot Structural Analysis Professional 2019 and click on Activate in the startup screen. You will see a window like this:
-
-
Enter the serial number and product key generated by Xforce Keygen 64 Bit in the corresponding fields and click on Next. You will see a window like this:
-
-
Select I have an activation code from Autodesk and click on Next. You will see a window like this:
-
-
Copy the request code from the window and paste it into the Request field in Xforce Keygen 64 Bit. Then click on Generate. You will see an activation code appear in the Activation field in Xforce Keygen 64 Bit.
-
Copy the activation code from Xforce Keygen 64 Bit and paste it into the Activation field in Robot Structural Analysis Professional 2019. Then click on Next. You will see a window like this:
-
-
Congratulations! You have successfully activated Robot Structural Analysis Professional 2019 with Xforce Keygen 64 Bit. You can now enjoy the full features of the software without any limitations.
-
Conclusion
-
In this article, we have shown you how to activate Robot Structural Analysis Professional 2019 with Xforce Keygen 64 Bit in a few simple steps. We hope this article was helpful and
d5da3c52bf
-
-
\ No newline at end of file
diff --git a/spaces/digitalxingtong/Bufeiyan-a-Bert-VITS2/utils.py b/spaces/digitalxingtong/Bufeiyan-a-Bert-VITS2/utils.py
deleted file mode 100644
index c6aa6cfc64c33e2eed33e9845239e831fc1c4a1a..0000000000000000000000000000000000000000
--- a/spaces/digitalxingtong/Bufeiyan-a-Bert-VITS2/utils.py
+++ /dev/null
@@ -1,293 +0,0 @@
-import os
-import glob
-import sys
-import argparse
-import logging
-import json
-import subprocess
-import numpy as np
-from scipy.io.wavfile import read
-import torch
-
-MATPLOTLIB_FLAG = False
-
-logging.basicConfig(stream=sys.stdout, level=logging.DEBUG)
-logger = logging
-
-
-def load_checkpoint(checkpoint_path, model, optimizer=None, skip_optimizer=False):
- assert os.path.isfile(checkpoint_path)
- checkpoint_dict = torch.load(checkpoint_path, map_location='cpu')
- iteration = checkpoint_dict['iteration']
- learning_rate = checkpoint_dict['learning_rate']
- if optimizer is not None and not skip_optimizer and checkpoint_dict['optimizer'] is not None:
- optimizer.load_state_dict(checkpoint_dict['optimizer'])
- elif optimizer is None and not skip_optimizer:
- #else: #Disable this line if Infer ,and enable the line upper
- new_opt_dict = optimizer.state_dict()
- new_opt_dict_params = new_opt_dict['param_groups'][0]['params']
- new_opt_dict['param_groups'] = checkpoint_dict['optimizer']['param_groups']
- new_opt_dict['param_groups'][0]['params'] = new_opt_dict_params
- optimizer.load_state_dict(new_opt_dict)
- saved_state_dict = checkpoint_dict['model']
- if hasattr(model, 'module'):
- state_dict = model.module.state_dict()
- else:
- state_dict = model.state_dict()
- new_state_dict = {}
- for k, v in state_dict.items():
- try:
- #assert "emb_g" not in k
- # print("load", k)
- new_state_dict[k] = saved_state_dict[k]
- assert saved_state_dict[k].shape == v.shape, (saved_state_dict[k].shape, v.shape)
- except:
- print("error, %s is not in the checkpoint" % k)
- new_state_dict[k] = v
- if hasattr(model, 'module'):
- model.module.load_state_dict(new_state_dict, strict=False)
- else:
- model.load_state_dict(new_state_dict, strict=False)
- print("load ")
- logger.info("Loaded checkpoint '{}' (iteration {})".format(
- checkpoint_path, iteration))
- return model, optimizer, learning_rate, iteration
-
-
-def save_checkpoint(model, optimizer, learning_rate, iteration, checkpoint_path):
- logger.info("Saving model and optimizer state at iteration {} to {}".format(
- iteration, checkpoint_path))
- if hasattr(model, 'module'):
- state_dict = model.module.state_dict()
- else:
- state_dict = model.state_dict()
- torch.save({'model': state_dict,
- 'iteration': iteration,
- 'optimizer': optimizer.state_dict(),
- 'learning_rate': learning_rate}, checkpoint_path)
-
-
-def summarize(writer, global_step, scalars={}, histograms={}, images={}, audios={}, audio_sampling_rate=22050):
- for k, v in scalars.items():
- writer.add_scalar(k, v, global_step)
- for k, v in histograms.items():
- writer.add_histogram(k, v, global_step)
- for k, v in images.items():
- writer.add_image(k, v, global_step, dataformats='HWC')
- for k, v in audios.items():
- writer.add_audio(k, v, global_step, audio_sampling_rate)
-
-
-def latest_checkpoint_path(dir_path, regex="G_*.pth"):
- f_list = glob.glob(os.path.join(dir_path, regex))
- f_list.sort(key=lambda f: int("".join(filter(str.isdigit, f))))
- x = f_list[-1]
- print(x)
- return x
-
-
-def plot_spectrogram_to_numpy(spectrogram):
- global MATPLOTLIB_FLAG
- if not MATPLOTLIB_FLAG:
- import matplotlib
- matplotlib.use("Agg")
- MATPLOTLIB_FLAG = True
- mpl_logger = logging.getLogger('matplotlib')
- mpl_logger.setLevel(logging.WARNING)
- import matplotlib.pylab as plt
- import numpy as np
-
- fig, ax = plt.subplots(figsize=(10, 2))
- im = ax.imshow(spectrogram, aspect="auto", origin="lower",
- interpolation='none')
- plt.colorbar(im, ax=ax)
- plt.xlabel("Frames")
- plt.ylabel("Channels")
- plt.tight_layout()
-
- fig.canvas.draw()
- data = np.fromstring(fig.canvas.tostring_rgb(), dtype=np.uint8, sep='')
- data = data.reshape(fig.canvas.get_width_height()[::-1] + (3,))
- plt.close()
- return data
-
-
-def plot_alignment_to_numpy(alignment, info=None):
- global MATPLOTLIB_FLAG
- if not MATPLOTLIB_FLAG:
- import matplotlib
- matplotlib.use("Agg")
- MATPLOTLIB_FLAG = True
- mpl_logger = logging.getLogger('matplotlib')
- mpl_logger.setLevel(logging.WARNING)
- import matplotlib.pylab as plt
- import numpy as np
-
- fig, ax = plt.subplots(figsize=(6, 4))
- im = ax.imshow(alignment.transpose(), aspect='auto', origin='lower',
- interpolation='none')
- fig.colorbar(im, ax=ax)
- xlabel = 'Decoder timestep'
- if info is not None:
- xlabel += '\n\n' + info
- plt.xlabel(xlabel)
- plt.ylabel('Encoder timestep')
- plt.tight_layout()
-
- fig.canvas.draw()
- data = np.fromstring(fig.canvas.tostring_rgb(), dtype=np.uint8, sep='')
- data = data.reshape(fig.canvas.get_width_height()[::-1] + (3,))
- plt.close()
- return data
-
-
-def load_wav_to_torch(full_path):
- sampling_rate, data = read(full_path)
- return torch.FloatTensor(data.astype(np.float32)), sampling_rate
-
-
-def load_filepaths_and_text(filename, split="|"):
- with open(filename, encoding='utf-8') as f:
- filepaths_and_text = [line.strip().split(split) for line in f]
- return filepaths_and_text
-
-
-def get_hparams(init=True):
- parser = argparse.ArgumentParser()
- parser.add_argument('-c', '--config', type=str, default="./configs/base.json",
- help='JSON file for configuration')
- parser.add_argument('-m', '--model', type=str, default="./OUTPUT_MODEL",
- help='Model name')
- parser.add_argument('--cont', dest='cont', action="store_true", default=False, help="whether to continue training on the latest checkpoint")
-
- args = parser.parse_args()
- model_dir = os.path.join("./logs", args.model)
-
- if not os.path.exists(model_dir):
- os.makedirs(model_dir)
-
- config_path = args.config
- config_save_path = os.path.join(model_dir, "config.json")
- if init:
- with open(config_path, "r") as f:
- data = f.read()
- with open(config_save_path, "w") as f:
- f.write(data)
- else:
- with open(config_save_path, "r") as f:
- data = f.read()
- config = json.loads(data)
-
- hparams = HParams(**config)
- hparams.model_dir = model_dir
- hparams.cont = args.cont
- return hparams
-
-
-def clean_checkpoints(path_to_models='logs/44k/', n_ckpts_to_keep=2, sort_by_time=True):
- """Freeing up space by deleting saved ckpts
-
- Arguments:
- path_to_models -- Path to the model directory
- n_ckpts_to_keep -- Number of ckpts to keep, excluding G_0.pth and D_0.pth
- sort_by_time -- True -> chronologically delete ckpts
- False -> lexicographically delete ckpts
- """
- import re
- ckpts_files = [f for f in os.listdir(path_to_models) if os.path.isfile(os.path.join(path_to_models, f))]
- name_key = (lambda _f: int(re.compile('._(\d+)\.pth').match(_f).group(1)))
- time_key = (lambda _f: os.path.getmtime(os.path.join(path_to_models, _f)))
- sort_key = time_key if sort_by_time else name_key
- x_sorted = lambda _x: sorted([f for f in ckpts_files if f.startswith(_x) and not f.endswith('_0.pth')],
- key=sort_key)
- to_del = [os.path.join(path_to_models, fn) for fn in
- (x_sorted('G')[:-n_ckpts_to_keep] + x_sorted('D')[:-n_ckpts_to_keep])]
- del_info = lambda fn: logger.info(f".. Free up space by deleting ckpt {fn}")
- del_routine = lambda x: [os.remove(x), del_info(x)]
- rs = [del_routine(fn) for fn in to_del]
-
-def get_hparams_from_dir(model_dir):
- config_save_path = os.path.join(model_dir, "config.json")
- with open(config_save_path, "r") as f:
- data = f.read()
- config = json.loads(data)
-
- hparams = HParams(**config)
- hparams.model_dir = model_dir
- return hparams
-
-
-def get_hparams_from_file(config_path):
- with open(config_path, "r") as f:
- data = f.read()
- config = json.loads(data)
-
- hparams = HParams(**config)
- return hparams
-
-
-def check_git_hash(model_dir):
- source_dir = os.path.dirname(os.path.realpath(__file__))
- if not os.path.exists(os.path.join(source_dir, ".git")):
- logger.warn("{} is not a git repository, therefore hash value comparison will be ignored.".format(
- source_dir
- ))
- return
-
- cur_hash = subprocess.getoutput("git rev-parse HEAD")
-
- path = os.path.join(model_dir, "githash")
- if os.path.exists(path):
- saved_hash = open(path).read()
- if saved_hash != cur_hash:
- logger.warn("git hash values are different. {}(saved) != {}(current)".format(
- saved_hash[:8], cur_hash[:8]))
- else:
- open(path, "w").write(cur_hash)
-
-
-def get_logger(model_dir, filename="train.log"):
- global logger
- logger = logging.getLogger(os.path.basename(model_dir))
- logger.setLevel(logging.DEBUG)
-
- formatter = logging.Formatter("%(asctime)s\t%(name)s\t%(levelname)s\t%(message)s")
- if not os.path.exists(model_dir):
- os.makedirs(model_dir)
- h = logging.FileHandler(os.path.join(model_dir, filename))
- h.setLevel(logging.DEBUG)
- h.setFormatter(formatter)
- logger.addHandler(h)
- return logger
-
-
-class HParams():
- def __init__(self, **kwargs):
- for k, v in kwargs.items():
- if type(v) == dict:
- v = HParams(**v)
- self[k] = v
-
- def keys(self):
- return self.__dict__.keys()
-
- def items(self):
- return self.__dict__.items()
-
- def values(self):
- return self.__dict__.values()
-
- def __len__(self):
- return len(self.__dict__)
-
- def __getitem__(self, key):
- return getattr(self, key)
-
- def __setitem__(self, key, value):
- return setattr(self, key, value)
-
- def __contains__(self, key):
- return key in self.__dict__
-
- def __repr__(self):
- return self.__dict__.__repr__()
diff --git a/spaces/digitalxingtong/Jiuxia-Bert-Vits2/README.md b/spaces/digitalxingtong/Jiuxia-Bert-Vits2/README.md
deleted file mode 100644
index 1e88ad2655b2cd9bc0b237fef92c0088b3826926..0000000000000000000000000000000000000000
--- a/spaces/digitalxingtong/Jiuxia-Bert-Vits2/README.md
+++ /dev/null
@@ -1,13 +0,0 @@
----
-title: AI九夏
-emoji: 🌟
-colorFrom: red
-colorTo: indigo
-sdk: gradio
-sdk_version: 3.36.1
-app_file: app.py
-pinned: false
-license: mit
----
-
-Check out the configuration reference at https://huggingface.co/docs/hub/spaces-config-reference
\ No newline at end of file
diff --git a/spaces/dorkai/ChatUIPro/app/components/base/loading/style.css b/spaces/dorkai/ChatUIPro/app/components/base/loading/style.css
deleted file mode 100644
index 40402e1a9d90ba136abb31a655f5b1a0e932cc5c..0000000000000000000000000000000000000000
--- a/spaces/dorkai/ChatUIPro/app/components/base/loading/style.css
+++ /dev/null
@@ -1,41 +0,0 @@
-.spin-animation path {
- animation: custom 2s linear infinite;
-}
-
-@keyframes custom {
- 0% {
- opacity: 0;
- }
-
- 25% {
- opacity: 0.1;
- }
-
- 50% {
- opacity: 0.2;
- }
-
- 75% {
- opacity: 0.5;
- }
-
- 100% {
- opacity: 1;
- }
-}
-
-.spin-animation path:nth-child(1) {
- animation-delay: 0s;
-}
-
-.spin-animation path:nth-child(2) {
- animation-delay: 0.5s;
-}
-
-.spin-animation path:nth-child(3) {
- animation-delay: 1s;
-}
-
-.spin-animation path:nth-child(4) {
- animation-delay: 1.5s;
-}
\ No newline at end of file
diff --git a/spaces/dorkai/ChatUIPro/hooks/use-breakpoints.ts b/spaces/dorkai/ChatUIPro/hooks/use-breakpoints.ts
deleted file mode 100644
index 1aab56a9fdbd2bfce3b52c940bd10be3eadc1a00..0000000000000000000000000000000000000000
--- a/spaces/dorkai/ChatUIPro/hooks/use-breakpoints.ts
+++ /dev/null
@@ -1,27 +0,0 @@
-'use client'
-import React from 'react'
-
-export enum MediaType {
- mobile = 'mobile',
- tablet = 'tablet',
- pc = 'pc',
-}
-
-const useBreakpoints = () => {
- const [width, setWidth] = React.useState(globalThis.innerWidth);
- const media = (() => {
- if (width <= 640) return MediaType.mobile;
- if (width <= 768) return MediaType.tablet;
- return MediaType.pc;
- })();
-
- React.useEffect(() => {
- const handleWindowResize = () => setWidth(window.innerWidth);
- window.addEventListener("resize", handleWindowResize);
- return () => window.removeEventListener("resize", handleWindowResize);
- }, []);
-
- return media;
-}
-
-export default useBreakpoints
\ No newline at end of file
diff --git a/spaces/dorkai/ChatUIPro/tailwind.config.js b/spaces/dorkai/ChatUIPro/tailwind.config.js
deleted file mode 100644
index 9b7b3acec9bf29f2f1451336c2a881717c920f6a..0000000000000000000000000000000000000000
--- a/spaces/dorkai/ChatUIPro/tailwind.config.js
+++ /dev/null
@@ -1,66 +0,0 @@
-/** @type {import('tailwindcss').Config} */
-module.exports = {
- content: [
- './app/**/*.{js,ts,jsx,tsx}',
- './components/**/*.{js,ts,jsx,tsx}',
- ],
- theme: {
- typography: require('./typography'),
- extend: {
- colors: {
- gray: {
- 50: '#F9FAFB',
- 100: '#F3F4F6',
- 200: '#E5E7EB',
- 300: '#D1D5DB',
- 400: '#9CA3AF',
- 500: '#6B7280',
- 700: '#374151',
- 800: '#1F2A37',
- 900: '#111928',
- },
- primary: {
- 50: '#EBF5FF',
- 100: '#E1EFFE',
- 200: '#C3DDFD',
- 300: '#A4CAFE',
- 600: '#1C64F2',
- 700: '#1A56DB',
- },
- blue: {
- 500: '#E1EFFE',
- },
- green: {
- 50: '#F3FAF7',
- 100: '#DEF7EC',
- 800: '#03543F',
-
- },
- yellow: {
- 100: '#FDF6B2',
- 800: '#723B13',
- },
- purple: {
- 50: '#F6F5FF',
- },
- indigo: {
- 25: '#F5F8FF',
- 100: '#E0EAFF',
- 600: '#444CE7'
- }
- },
- screens: {
- 'mobile': '100px',
- // => @media (min-width: 100px) { ... }
- 'tablet': '640px', // 391
- // => @media (min-width: 600px) { ... }
- 'pc': '769px',
- // => @media (min-width: 769px) { ... }
- },
- },
- },
- plugins: [
- require('@tailwindcss/typography'),
- require('@tailwindcss/line-clamp'),
- ],
-}
diff --git a/spaces/dorkai/text-generation-webui-main/text-generation-webui-main/docs/Training-LoRAs.md b/spaces/dorkai/text-generation-webui-main/text-generation-webui-main/docs/Training-LoRAs.md
deleted file mode 100644
index 3d75ec5aa2bc12e8c13d6a583bd9aefd118f04d7..0000000000000000000000000000000000000000
--- a/spaces/dorkai/text-generation-webui-main/text-generation-webui-main/docs/Training-LoRAs.md
+++ /dev/null
@@ -1,167 +0,0 @@
-## Training Your Own LoRAs
-
-The WebUI seeks to make training your own LoRAs as easy as possible. It comes down to just a few simple steps:
-
-### **Step 1**: Make a plan.
-- What base model do you want to use? The LoRA you make has to be matched up to a single architecture (eg LLaMA-13B) and cannot be transferred to others (eg LLaMA-7B, StableLM, etc. would all be different). Derivatives of the same model (eg Alpaca finetune of LLaMA-13B) might be transferrable, but even then it's best to train exactly on what you plan to use.
-- What model format do you want? At time of writing, 8-bit models are most stable, and 4-bit are supported but experimental. In the near future it is likely that 4-bit will be the best option for most users.
-- What are you training it on? Do you want it to learn real information, a simple format, ...?
-
-### **Step 2**: Gather a dataset.
-- If you use a dataset similar to the [Alpaca](https://github.com/gururise/AlpacaDataCleaned/blob/main/alpaca_data_cleaned.json) format, that is natively supported by the `Formatted Dataset` input in the WebUI, with premade formatter options.
-- If you use a dataset that isn't matched to Alpaca's format, but uses the same basic JSON structure, you can make your own format file by copying `training/formats/alpaca-format.json` to a new file and [editing its content](#format-files).
-- If you can get the dataset into a simple text file, that works too! You can train using the `Raw text file` input option.
- - This means you can for example just copy/paste a chatlog/documentation page/whatever you want, shove it in a plain text file, and train on it.
-- If you use a structured dataset not in this format, you may have to find an external way to convert it - or open an issue to request native support.
-
-### **Step 3**: Do the training.
-- **3.1**: Load the WebUI, and your model.
- - Make sure you don't have any LoRAs already loaded (unless you want to train for multi-LoRA usage).
-- **3.2**: Open the `Training` tab at the top, `Train LoRA` sub-tab.
-- **3.3**: Fill in the name lof the LoRA, select your dataset in the dataset options.
-- **3.4**: Select other parameters to your preference. See [parameters below](#parameters).
-- **3.5**: click `Start LoRA Training`, and wait.
- - It can take a few hours for a large dataset, or just a few minute if doing a small run.
- - You may want to monitor your [loss value](#loss) while it goes.
-
-### **Step 4**: Evaluate your results.
-- Load the LoRA under the Models Tab.
-- You can go test-drive it on the `Text generation` tab, or you can use the `Perplexity evaluation` sub-tab of the `Training` tab.
-- If you used the `Save every n steps` option, you can grab prior copies of the model from sub-folders within the LoRA model's folder and try them instead.
-
-### **Step 5**: Re-run if you're unhappy.
-- Make sure to unload the LoRA before training it.
-- You can simply resume a prior run - use `Copy parameters from` to select your LoRA, and edit parameters. Note that you cannot change the `Rank` of an already created LoRA.
- - If you want to resume from a checkpoint saved along the way, simply copy the contents of the checkpoint folder into the LoRA's folder.
- - (Note: `adapter_model.bin` is the important file that holds the actual LoRA content).
- - This will start Learning Rate and Steps back to the start. If you want to resume as if you were midway through, you can adjust your Learning Rate to the last reported LR in logs and reduce your epochs.
-- Or, you can start over entirely if you prefer.
-- If your model is producing corrupted outputs, you probably need to start over and use a lower Learning Rate.
-- If your model isn't learning detailed information but you want it to, you might need to just run more epochs, or you might need a higher Rank.
-- If your model is enforcing a format you didn't want, you may need to tweak your dataset, or start over and not train as far.
-
-## Format Files
-
-If using JSON formatted datasets, they are presumed to be in the following approximate format:
-
-```json
-[
- {
- "somekey": "somevalue",
- "key2": "value2"
- },
- {
- // etc
- }
-]
-```
-
-Where the keys (eg `somekey`, `key2` above) are standardized, and relatively consistent across the dataset, and the values (eg `somevalue`, `value2`) contain the content actually intended to be trained.
-
-For Alpaca, the keys are `instruction`, `input`, and `output`, wherein `input` is sometimes blank.
-
-A simple format file for Alpaca to be used as a chat bot is:
-
-```json
-{
- "instruction,output": "User: %instruction%\nAssistant: %output%",
- "instruction,input,output": "User: %instruction%: %input%\nAssistant: %output%"
-}
-```
-
-Note that the keys (eg `instruction,output`) are a comma-separated list of dataset keys, and the values are a simple string that use those keys with `%%`.
-
-So for example if a dataset has `"instruction": "answer my question"`, then the format file's `User: %instruction%\n` will be automatically filled in as `User: answer my question\n`.
-
-If you have different sets of key inputs, you can make your own format file to match it. This format-file is designed to be as simple as possible to enable easy editing to match your needs.
-
-## Parameters
-
-The basic purpose and function of each parameter is documented on-page in the WebUI, so read through them in the UI to understand your options.
-
-That said, here's a guide to the most important parameter choices you should consider:
-
-### VRAM
-
-- First, you must consider your VRAM availability.
- - Generally, under default settings, VRAM usage for training with default parameters is very close to when generating text (with 1000+ tokens of context) (ie, if you can generate text, you can train LoRAs).
- - Note: worse by default in the 4-bit monkeypatch currently. Reduce `Micro Batch Size` to `1` to restore this to expectations.
- - If you have VRAM to spare, setting higher batch sizes will use more VRAM and get you better quality training in exchange.
- - If you have large data, setting a higher cutoff length may be beneficial, but will cost significant VRAM. If you can spare some, set your batch size to `1` and see how high you can push your cutoff length.
- - If you're low on VRAM, reducing batch size or cutoff length will of course improve that.
- - Don't be afraid to just try it and see what happens. If it's too much, it will just error out, and you can lower settings and try again.
-
-### Rank
-
-- Second, you want to consider the amount of learning you want.
- - For example, you may wish to just learn a dialogue format (as in the case of Alpaca) in which case setting a low `Rank` value (32 or lower) works great.
- - Or, you might be training on project documentation you want the bot to understand and be able to understand questions about, in which case the higher the rank, the better.
- - Generally, higher Rank = more precise learning = more total content learned = more VRAM usage while training.
-
-### Learning Rate and Epochs
-
-- Third, how carefully you want it to be learned.
- - In other words, how okay or not you are with the model losing unrelated understandings.
- - You can control this with 3 key settings: the Learning Rate, its scheduler, and your total epochs.
- - The learning rate controls how much change is made to the model by each token it sees.
- - It's in scientific notation normally, so for example `3e-4` means `3 * 10^-4` which is `0.0003`. The number after `e-` controls how many `0`s are in the number.
- - Higher values let training run faster, but also are more likely to corrupt prior data in the model.
- - You essentially have two variables to balance: the LR, and Epochs.
- - If you make LR higher, you can set Epochs equally lower to match. High LR + low epochs = very fast, low quality training.
- - If you make LR low, set epochs high. Low LR + high epochs = slow but high-quality training.
- - The scheduler controls change-over-time as you train - it starts high, and then goes low. This helps balance getting data in, and having decent quality, at the same time.
- - You can see graphs of the different scheduler options [in the HuggingFace docs here](https://moon-ci-docs.huggingface.co/docs/transformers/pr_1/en/main_classes/optimizer_schedules#transformers.SchedulerType)
-
-## Loss
-
-When you're running training, the WebUI's console window will log reports that include, among other things, a numeric value named `Loss`. It will start as a high number, and gradually get lower and lower as it goes.
-
-"Loss" in the world of AI training theoretically means "how close is the model to perfect", with `0` meaning "absolutely perfect". This is calculated by measuring the difference between the model outputting exactly the text you're training it to output, and what it actually outputs.
-
-In practice, a good LLM should have a very complex variable range of ideas running in its artificial head, so a loss of `0` would indicate that the model has broken and forgotten to how think about anything other than what you trained it.
-
-So, in effect, Loss is a balancing game: you want to get it low enough that it understands your data, but high enough that it isn't forgetting everything else. Generally, if it goes below `1.0`, it's going to start forgetting its prior memories, and you should stop training. In some cases you may prefer to take it as low as `0.5` (if you want it to be very very predictable). Different goals have different needs, so don't be afraid to experiment and see what works best for you.
-
-Note: if you see Loss start at or suddenly jump to exactly `0`, it is likely something has gone wrong in your training process (eg model corruption).
-
-## Note: 4-Bit Monkeypatch
-
-The [4-bit LoRA monkeypatch](GPTQ-models-(4-bit-mode).md#using-loras-in-4-bit-mode) works for training, but has side effects:
-- VRAM usage is higher currently. You can reduce the `Micro Batch Size` to `1` to compensate.
-- Models do funky things. LoRAs apply themselves, or refuse to apply, or spontaneously error out, or etc. It can be helpful to reload base model or restart the WebUI between training/usage to minimize chances of anything going haywire.
-- Loading or working with multiple LoRAs at the same time doesn't currently work.
-- Generally, recognize and treat the monkeypatch as the dirty temporary hack it is - it works, but isn't very stable. It will get better in time when everything is merged upstream for full official support.
-
-## Legacy notes
-
-LoRA training was contributed by [mcmonkey4eva](https://github.com/mcmonkey4eva) in PR [#570](https://github.com/oobabooga/text-generation-webui/pull/570).
-
-### Using the original alpaca-lora code
-
-Kept here for reference. The Training tab has much more features than this method.
-
-```
-conda activate textgen
-git clone https://github.com/tloen/alpaca-lora
-```
-
-Edit those two lines in `alpaca-lora/finetune.py` to use your existing model folder instead of downloading everything from decapoda:
-
-```
-model = LlamaForCausalLM.from_pretrained(
- "models/llama-7b",
- load_in_8bit=True,
- device_map="auto",
-)
-tokenizer = LlamaTokenizer.from_pretrained(
- "models/llama-7b", add_eos_token=True
-)
-```
-
-Run the script with:
-
-```
-python finetune.py
-```
-
-It just works. It runs at 22.32s/it, with 1170 iterations in total, so about 7 hours and a half for training a LoRA. RTX 3090, 18153MiB VRAM used, drawing maximum power (350W, room heater mode).
diff --git a/spaces/drift-ai/emoji-tagging/Makefile b/spaces/drift-ai/emoji-tagging/Makefile
deleted file mode 100644
index 075e9a709827f57df977fd97584f235e555dde40..0000000000000000000000000000000000000000
--- a/spaces/drift-ai/emoji-tagging/Makefile
+++ /dev/null
@@ -1,3 +0,0 @@
-install:
- poetry install
- poetry run pip list --format=freeze > requirements.txt
diff --git a/spaces/ds21/Q-TicTacToe/README.md b/spaces/ds21/Q-TicTacToe/README.md
deleted file mode 100644
index 161f39f0cf97c96649b9736fc55043c69ad03fb3..0000000000000000000000000000000000000000
--- a/spaces/ds21/Q-TicTacToe/README.md
+++ /dev/null
@@ -1,13 +0,0 @@
----
-title: Q TicTacToe
-emoji: :)
-colorFrom: gray
-colorTo: red
-sdk: streamlit
-sdk_version: 1.2.0
-app_file: app.py
-pinned: false
----
-
-# Q-TicTacToe
-A Quantam version of the Tic Tac Toe game
diff --git a/spaces/eforebrahim/Cassava-Leaf-Disease-Classification/README.md b/spaces/eforebrahim/Cassava-Leaf-Disease-Classification/README.md
deleted file mode 100644
index 87f15455cf1235fbf8dfd3b3a1971261695ccf74..0000000000000000000000000000000000000000
--- a/spaces/eforebrahim/Cassava-Leaf-Disease-Classification/README.md
+++ /dev/null
@@ -1,29 +0,0 @@
----
-title: Cassava Leaf Disease Classification
-emoji: ☘️
-colorFrom: gray
-colorTo: gray
-sdk: streamlit
-sdk_version: 1.10.0
-app_file: app.py
-pinned: false
----
-# Cassava Leaf Disease Classification Project
-
-
-## Background Information
-“As the second-largest provider of carbohydrates in Africa, cassava is a key food security crop grown by smallholder farmers because it can withstand harsh conditions.
-At least 80% of household farms in Sub-Saharan Africa grow this starchy root, but viral diseases are major sources of poor yields. With the help of data science, it may be possible to identify common diseases so they can be treated.”
-
-## Data
-The data contains about 21,000 images of Cassava plant belonging to 5 different categories (4 diseases and 1 healthy).
-The dataset was made available by Makerere University AI Lab via Kaggle Competition. You can get the dataset from here: (https://lnkd.in/dxGUTcN4)
-
-## Modeling
-Pre-trained model efficientnet version b2 is used for modeling. Dropout layers were added to prevent model from overfitting. Validation precision of 0.855, recall of 0.813, and accuracy of 0.831 is achieved.
-
-## Deployment
-The web app is hosted on huggingface spaces using streamlit user interface.
-#datascience #machinelearning #computervision #artificialintelligence
-
-Check out the configuration reference at https://huggingface.co/docs/hub/spaces-config-reference
diff --git a/spaces/elkraken/Video-Object-Detection/app.py b/spaces/elkraken/Video-Object-Detection/app.py
deleted file mode 100644
index d621ffdb8407864cf8c0e74c866737f580264e56..0000000000000000000000000000000000000000
--- a/spaces/elkraken/Video-Object-Detection/app.py
+++ /dev/null
@@ -1,293 +0,0 @@
-import gradio as gr
-import os
-
-import argparse
-import time
-from pathlib import Path
-
-import cv2
-import torch
-import torch.backends.cudnn as cudnn
-from numpy import random
-
-from models.experimental import attempt_load
-from utils.datasets import LoadStreams, LoadImages
-from utils.general import check_img_size, check_requirements, check_imshow, non_max_suppression, apply_classifier, \
- scale_coords, xyxy2xywh, strip_optimizer, set_logging, increment_path
-from utils.plots import plot_one_box
-from utils.torch_utils import select_device, load_classifier, time_synchronized, TracedModel
-from PIL import Image
-
-from sort import *
-
-from huggingface_hub import hf_hub_download
-
-def load_model(model_name):
- model_path = hf_hub_download(repo_id=f"Yolov7/{model_name}", filename=f"{model_name}.pt")
-
- return model_path
-
-
-model_names = ["yolov7"]
-
-models = {model_name: load_model(model_name) for model_name in model_names}
-
-##################################
-# """Function to Draw Bounding boxes"""
-def draw_boxes(img, bbox, identities=None, categories=None, confidences = None, names=None, colors = None):
- for i, box in enumerate(bbox):
- x1, y1, x2, y2 = [int(i) for i in box]
- tl = opt.thickness or round(0.002 * (img.shape[0] + img.shape[1]) / 2) + 1 # line/font thickness
-
- cat = int(categories[i]) if categories is not None else 0
- id = int(identities[i]) if identities is not None else 0
- # conf = confidences[i] if confidences is not None else 0
-
- color = colors[cat]
-
- if not opt.nobbox:
- cv2.rectangle(img, (x1, y1), (x2, y2), color, tl)
-
- if not opt.nolabel:
- label = str(id) + ":"+ names[cat] if identities is not None else f'{names[cat]} {confidences[i]:.2f}'
- tf = max(tl - 1, 1) # font thickness
- t_size = cv2.getTextSize(label, 0, fontScale=tl / 3, thickness=tf)[0]
- c2 = x1 + t_size[0], y1 - t_size[1] - 3
- cv2.rectangle(img, (x1, y1), c2, color, -1, cv2.LINE_AA) # filled
- cv2.putText(img, label, (x1, y1 - 2), 0, tl / 3, [225, 255, 255], thickness=tf, lineType=cv2.LINE_AA)
-
-
- return img
-##################################
-
-
-def detect(save_img=True):
- parser = argparse.ArgumentParser()
- parser.add_argument('--weights', nargs='+', type=str, default='yolov7.pt', help='model.pt path(s)')
- parser.add_argument('--source', type=str, default='inference/images', help='source') # file/folder, 0 for webcam
- parser.add_argument('--img-size', type=int, default=640, help='inference size (pixels)')
- parser.add_argument('--conf-thres', type=float, default=0.25, help='object confidence threshold')
- parser.add_argument('--iou-thres', type=float, default=0.45, help='IOU threshold for NMS')
- parser.add_argument('--device', default='', help='cuda device, i.e. 0 or 0,1,2,3 or cpu')
- parser.add_argument('--view-img', action='store_true', help='display results')
- parser.add_argument('--save-txt', action='store_true', help='save results to *.txt')
- parser.add_argument('--save-conf', action='store_true', help='save confidences in --save-txt labels')
- parser.add_argument('--nosave', action='store_true', help='do not save images/videos')
- parser.add_argument('--classes', nargs='+', type=int, help='filter by class: --class 0, or --class 0 2 3')
- parser.add_argument('--agnostic-nms', action='store_true', help='class-agnostic NMS')
- parser.add_argument('--augment', action='store_true', help='augmented inference')
- parser.add_argument('--update', action='store_true', help='update all models')
- parser.add_argument('--project', default='runs/detect', help='save results to project/name')
- parser.add_argument('--name', default='exp', help='save results to project/name')
- parser.add_argument('--exist-ok', action='store_true', help='existing project/name ok, do not increment')
- parser.add_argument('--no-trace', action='store_true', help='don`t trace model')
-
- parser.add_argument('--track', action='store_true', help='run tracking')
- parser.add_argument('--show-track', action='store_true', help='show tracked path')
- parser.add_argument('--show-fps', action='store_true', help='show fps')
- parser.add_argument('--thickness', type=int, default=2, help='bounding box and font size thickness')
- parser.add_argument('--seed', type=int, default=1, help='random seed to control bbox colors')
- parser.add_argument('--nobbox', action='store_true', help='don`t show bounding box')
- parser.add_argument('--nolabel', action='store_true', help='don`t show label')
- parser.add_argument('--unique-track-color', action='store_true', help='show each track in unique color')
-
- opt = parser.parse_args()
- np.random.seed(opt.seed)
-
- sort_tracker = Sort(max_age=5,
- min_hits=2,
- iou_threshold=0.2)
-
- source, weights, view_img, save_txt, imgsz, trace = opt.source, opt.weights, opt.view_img, opt.save_txt, opt.img_size, not opt.no_trace
- save_img = not opt.nosave and not source.endswith('.txt') # save inference images
- webcam = source.isnumeric() or source.endswith('.txt') or source.lower().startswith(
- ('rtsp://', 'rtmp://', 'http://', 'https://'))
- save_dir = Path(increment_path(Path(opt.project) / opt.name, exist_ok=opt.exist_ok)) # increment run
- if not opt.nosave:
- (save_dir / 'labels' if save_txt else save_dir).mkdir(parents=True, exist_ok=True) # make dir
-
- # Initialize
- set_logging()
- device = select_device(opt.device)
- half = device.type != 'cpu' # half precision only supported on CUDA
-
- # Load model
- model = attempt_load(weights, map_location=device) # load FP32 model
- stride = int(model.stride.max()) # model stride
- imgsz = check_img_size(imgsz, s=stride) # check img_size
-
- if trace:
- model = TracedModel(model, device, opt.img_size)
-
- if half:
- model.half() # to FP16
-
- # Second-stage classifier
- classify = False
- if classify:
- modelc = load_classifier(name='resnet101', n=2) # initialize
- modelc.load_state_dict(torch.load('weights/resnet101.pt', map_location=device)['model']).to(device).eval()
-
- # Set Dataloader
- vid_path, vid_writer = None, None
- if webcam:
- view_img = check_imshow()
- cudnn.benchmark = True # set True to speed up constant image size inference
- dataset = LoadStreams(source, img_size=imgsz, stride=stride)
- else:
- dataset = LoadImages(source, img_size=imgsz, stride=stride)
-
- # Get names and colors
- names = model.module.names if hasattr(model, 'module') else model.names
- colors = [[random.randint(0, 255) for _ in range(3)] for _ in names]
-
- # Run inference
- if device.type != 'cpu':
- model(torch.zeros(1, 3, imgsz, imgsz).to(device).type_as(next(model.parameters()))) # run once
- old_img_w = old_img_h = imgsz
- old_img_b = 1
-
- t0 = time.time()
- ###################################
- startTime = 0
- ###################################
- for path, img, im0s, vid_cap in dataset:
- img = torch.from_numpy(img).to(device)
- img = img.half() if half else img.float() # uint8 to fp16/32
- img /= 255.0 # 0 - 255 to 0.0 - 1.0
- if img.ndimension() == 3:
- img = img.unsqueeze(0)
-
- # Warmup
- if device.type != 'cpu' and (old_img_b != img.shape[0] or old_img_h != img.shape[2] or old_img_w != img.shape[3]):
- old_img_b = img.shape[0]
- old_img_h = img.shape[2]
- old_img_w = img.shape[3]
- for i in range(3):
- model(img, augment=opt.augment)[0]
-
- # Inference
- t1 = time_synchronized()
- pred = model(img, augment=opt.augment)[0]
- t2 = time_synchronized()
-
- # Apply NMS
- pred = non_max_suppression(pred, opt.conf_thres, opt.iou_thres, classes=opt.classes, agnostic=opt.agnostic_nms)
- t3 = time_synchronized()
-
- # Apply Classifier
- if classify:
- pred = apply_classifier(pred, modelc, img, im0s)
-
- # Process detections
- for i, det in enumerate(pred): # detections per image
- if webcam: # batch_size >= 1
- p, s, im0, frame = path[i], '%g: ' % i, im0s[i].copy(), dataset.count
- else:
- p, s, im0, frame = path, '', im0s, getattr(dataset, 'frame', 0)
-
- p = Path(p) # to Path
- save_path = str(save_dir / p.name) # img.jpg
- txt_path = str(save_dir / 'labels' / p.stem) + ('' if dataset.mode == 'image' else f'_{frame}') # img.txt
- gn = torch.tensor(im0.shape)[[1, 0, 1, 0]] # normalization gain whwh
- if len(det):
- # Rescale boxes from img_size to im0 size
- det[:, :4] = scale_coords(img.shape[2:], det[:, :4], im0.shape).round()
-
- # Print results
- for c in det[:, -1].unique():
- n = (det[:, -1] == c).sum() # detections per class
- s += f"{n} {names[int(c)]}{'s' * (n > 1)}, " # add to string
-
- dets_to_sort = np.empty((0,6))
- # NOTE: We send in detected object class too
- for x1,y1,x2,y2,conf,detclass in det.cpu().detach().numpy():
- dets_to_sort = np.vstack((dets_to_sort,
- np.array([x1, y1, x2, y2, conf, detclass])))
-
-
- if opt.track:
-
- tracked_dets = sort_tracker.update(dets_to_sort, opt.unique_track_color)
- tracks =sort_tracker.getTrackers()
-
- # draw boxes for visualization
- if len(tracked_dets)>0:
- bbox_xyxy = tracked_dets[:,:4]
- identities = tracked_dets[:, 8]
- categories = tracked_dets[:, 4]
- confidences = None
-
- if opt.show_track:
- #loop over tracks
- for t, track in enumerate(tracks):
-
- track_color = colors[int(track.detclass)] if not opt.unique_track_color else sort_tracker.color_list[t]
-
- [cv2.line(im0, (int(track.centroidarr[i][0]),
- int(track.centroidarr[i][1])),
- (int(track.centroidarr[i+1][0]),
- int(track.centroidarr[i+1][1])),
- track_color, thickness=opt.thickness)
- for i,_ in enumerate(track.centroidarr)
- if i < len(track.centroidarr)-1 ]
- else:
- bbox_xyxy = dets_to_sort[:,:4]
- identities = None
- categories = dets_to_sort[:, 5]
- confidences = dets_to_sort[:, 4]
-
- im0 = draw_boxes(im0, bbox_xyxy, identities, categories, confidences, names, colors)
-
- # Print time (inference + NMS)
- print(f'{s}Done. ({(1E3 * (t2 - t1)):.1f}ms) Inference, ({(1E3 * (t3 - t2)):.1f}ms) NMS')
-
- # Stream results
- ######################################################
- if dataset.mode != 'image' and opt.show_fps:
- currentTime = time.time()
-
- fps = 1/(currentTime - startTime)
- startTime = currentTime
- cv2.putText(im0, "FPS: " + str(int(fps)), (20, 70), cv2.FONT_HERSHEY_PLAIN, 2, (0,255,0),2)
-
- #######################################################
- if view_img:
- cv2.imshow(str(p), im0)
- cv2.waitKey(1) # 1 millisecond
-
- # Save results (image with detections)
- if save_img:
- if dataset.mode == 'image':
- cv2.imwrite(save_path, im0)
- print(f" The image with the result is saved in: {save_path}")
- else: # 'video' or 'stream'
- if vid_path != save_path: # new video
- vid_path = save_path
- if isinstance(vid_writer, cv2.VideoWriter):
- vid_writer.release() # release previous video writer
- if vid_cap: # video
- fps = vid_cap.get(cv2.CAP_PROP_FPS)
- w = int(vid_cap.get(cv2.CAP_PROP_FRAME_WIDTH))
- h = int(vid_cap.get(cv2.CAP_PROP_FRAME_HEIGHT))
- else: # stream
- fps, w, h = 30, im0.shape[1], im0.shape[0]
- save_path += '.mp4'
- vid_writer = cv2.VideoWriter(save_path, cv2.VideoWriter_fourcc(*'mp4v'), fps, (w, h))
- vid_writer.write(im0)
-
- if save_txt or save_img:
- s = f"\n{len(list(save_dir.glob('labels/*.txt')))} labels saved to {save_dir / 'labels'}" if save_txt else ''
- #print(f"Results saved to {save_dir}{s}")
-
- print(f'Done. ({time.time() - t0:.3f}s)')
- return img
-
-
-
-desc = "demo for WongKinYiu/yolov7 Trainable bag-of-freebies sets new state-of-the-art for real-time object detectors"
-gr.Interface(detect,
- inputs = [gr.Video(format="mp4")],
- outputs = gr.Video(format="mp4"),
- title="Yolov7",description=desc).launch()
-# gr.Interface(detect,[gr.Image(type="pil"),gr.Dropdown(choices=model_names)], gr.Image(type="pil"),title="Yolov7",examples=[["horses.jpeg", "yolov7"]],description="demo for WongKinYiu/yolov7 Trainable bag-of-freebies sets new state-of-the-art for real-time object detectors").launch()
\ No newline at end of file
diff --git a/spaces/erc/entity-referring-classifier/ercbcm/model_loader.py b/spaces/erc/entity-referring-classifier/ercbcm/model_loader.py
deleted file mode 100644
index 9cafd0dfe0199ed4e9bee10127be8e01500293ce..0000000000000000000000000000000000000000
--- a/spaces/erc/entity-referring-classifier/ercbcm/model_loader.py
+++ /dev/null
@@ -1,8 +0,0 @@
-import torch
-
-def load(load_path, model, device):
- if load_path == None: return
- state_dict = torch.load(load_path, map_location=device)
- model.load_state_dict(state_dict['model_state_dict'])
- print('[LOAD] Model has been loaded successfully from \'{}\''.format(load_path))
- return state_dict['valid_loss']
\ No newline at end of file
diff --git a/spaces/eson/tokenizer-arena/vocab/chinese_alpaca_lora_7b/README.md b/spaces/eson/tokenizer-arena/vocab/chinese_alpaca_lora_7b/README.md
deleted file mode 100644
index 3215d800239ba6e89bd1f3a257983222fda3e996..0000000000000000000000000000000000000000
--- a/spaces/eson/tokenizer-arena/vocab/chinese_alpaca_lora_7b/README.md
+++ /dev/null
@@ -1,4 +0,0 @@
-
-
-来自 chinese-alpaca-lora-7b-merge-hf
-
diff --git a/spaces/fatiXbelha/sd/Black Lives Matter MP3 Listen and Download the Anthem of a Movement.md b/spaces/fatiXbelha/sd/Black Lives Matter MP3 Listen and Download the Anthem of a Movement.md
deleted file mode 100644
index 155c510a97a3ea9c8cf4ef34edd3350ee888a156..0000000000000000000000000000000000000000
--- a/spaces/fatiXbelha/sd/Black Lives Matter MP3 Listen and Download the Anthem of a Movement.md
+++ /dev/null
@@ -1,150 +0,0 @@
-
-
Download Black Lives Matter MP3: How to Support the Movement Through Music
-
If you are looking for a way to show your solidarity with the Black Lives Matter movement, one of the easiest and most effective ways is to download and listen to music that supports the cause. Music is a powerful medium that can inspire, educate, and motivate people to take action against racism, injustice, and oppression. In this article, we will explain what Black Lives Matter is, why it matters, how music can help, and where you can find and download free Black Lives Matter MP3 songs.
What is Black Lives Matter and why is it important?
-
Black Lives Matter (BLM) is an international social movement that seeks to highlight racism, discrimination, and racial inequality experienced by black people. Its primary concerns are incidents of police brutality and racially motivated violence against black people. The name Black Lives Matter signals condemnation of the unjust killings of black people by police (black people are far more likely to be killed by police in the United States than white people) and the demand that society value the lives and humanity of black people as much as it values the lives and humanity of white people. BLM activists have held large and influential protests in cities across the United States as well as internationally. A decentralized grassroots movement, Black Lives Matter is led by activists in local chapters who organize their own campaigns and programs. The chapters are affiliated with the Black Lives Matter Global Network Foundation, a nonprofit civil rights organization that is active in the United States, Canada, and the United Kingdom.
-
The origin and goals of the movement
-
BLM was cofounded in 2013 as an online movement (using the hashtag #BlackLivesMatter on social media) by three black community organizers—Patrisse Khan-Cullors, Alicia Garza, and Opal Tometi. They formed BLM after George Zimmerman, a man of German and Peruvian descent, was acquitted on charges stemming from his fatal shooting of Trayvon Martin, an unarmed black teenager, in Sanford, Florida, in February 2012. Zimmerman, a neighbourhood-watch volunteer, had seen Martin walking in his neighbourhood and called the police because he thought Martin looked “suspicious.” Although Zimmerman was told not to do anything, he followed Martin, got into an argument with him, and shot and killed him. When law enforcement arrived, Zimmerman claimed that he had been assaulted by Martin and fired in self-defense. Zimmerman remained free for weeks, but, as the shooting gained national attention, demonstrations demanding his prosecution were held in cities across the United States.
-
Support for BLM grew following other police killings, including Eric Garner, who died in a chokehold, Michael Brown, who was killed by an officer who said he acted in self-defense, Tamir Rice, who was shot while playing with a toy gun, Breonna Taylor, who was shot in her own home during a botched raid, and George Floyd, an unarmed black man who was murdered by a police officer who knelt on his neck for nearly nine minutes. BLM also advocates for justice for other victims of racial violence, such as Ahmaud Arbery, who was chased and killed by three white men while jogging, and Elijah McClain, who died after being put in a chokehold by police and injected with a sedative by paramedics.
-
The goals of BLM are to end systemic racism, police brutality, and racial violence; to affirm the dignity and worth of black lives; to create a more inclusive and equitable society; and to empower black communities to achieve social, economic, and political justice. BLM also supports the rights and liberation of other marginalized groups, such as LGBTQ+ people, women, immigrants, and indigenous people.
-
The impact and challenges of the movement
-
BLM has had a significant impact on raising awareness and sparking dialogue about the issues of racism and police violence in the United States and around the world. BLM has also influenced policy changes at the local, state, and federal levels, such as banning chokeholds, requiring body cameras, establishing civilian oversight boards, and reallocating funds from police departments to social services. BLM has also inspired solidarity movements in other countries, such as the United Kingdom, France, Germany, Australia, Brazil, and Nigeria, where people have protested against their own forms of racial discrimination and oppression.
-
However, BLM also faces many challenges and criticisms from various sources. Some of these include:
-
download black lives matter song by dax
-download black lives matter protest songs 2020
-download black lives matter anthem by mike robbins
-download black lives matter remix by dababy
-download black lives matter music video by yg
-download black lives matter mp3 free online
-download black lives matter soundtrack from we're all in this together
-download black lives matter rap by juicy j
-download black lives matter acoustic by h.e.r.
-download black lives matter lyrics by lil baby
-download black lives matter album by various artists
-download black lives matter instrumental by t-pain
-download black lives matter podcast by billboard
-download black lives matter speech by dax
-download black lives matter mixtape by hopsin and dax
-download black lives matter live performance by yg
-download black lives matter tribute by shazam
-download black lives matter ringtone by dax
-download black lives matter karaoke by h.e.r.
-download black lives matter cover by tom macdonald
-download black lives matter playlist by gaana.com
-download black lives matter radio edit by dababy
-download black lives matter extended version by juicy j
-download black lives matter unplugged by t-pain
-download black lives matter mashup by lil baby and yg
-download black lives matter documentary by new scientist
-download black lives matter spoken word by dax
-download black lives matter original song by mike robbins
-download black lives matter bonus track by hopsin and dax
-download black lives matter acoustic guitar by h.e.r.
-download black lives matter piano version by t-pain
-download black lives matter edm remix by dababy
-download black lives matter rock cover by tom macdonald
-download black lives matter reggae version by juicy j
-download black lives matter trap beat by yg
-download black lives matter soulful rendition by h.e.r.
-download black lives matter motivational speech by dax
-download black lives matter inspirational podcast by billboard
-download black lives matter educational documentary by new scientist
-download black lives matter historical soundtrack from we're all in this together
-download black lives matter comedy skit by t-pain
-download black lives matter parody song by tom macdonald
-download black lives matter dance video by yg
-download black lives matter meditation music by h.e.r.
-download black lives matter workout playlist by gaana.com
-download black lives matter trivia quiz by shazam
-download black lives matter crossword puzzle by billboard
-download black lives matter coloring book by new scientist
-download black lives matter sticker pack by dax
-
-
The lack of a clear leadership structure or agenda, which makes it difficult to coordinate actions and communicate demands.
-
The resistance and backlash from some segments of society, especially white supremacists, who view BLM as a threat to their privilege and power.
-
The misrepresentation and distortion of the movement by some media outlets and politicians, who portray BLM as violent, radical, or anti-police.
-
The co-optation and commodification of the movement by some corporations and celebrities, who use BLM as a marketing strategy or a token gesture without making meaningful changes or commitments.
-
-
How can music help spread the message of Black Lives Matter?
-
Music is one of the most effective ways to spread the message of Black Lives Matter because it can reach a large and diverse audience, convey emotions and stories that resonate with people, and inspire them to take action. Music is also a form of cultural expression that reflects the identity, history, and struggles of black people. Music can help educate people about the issues that BLM addresses, challenge stereotypes and prejudices, celebrate black excellence and resilience, and demand justice and accountability.
-
The power and influence of music as a form of protest and expression
-
Music has always been a vital part of social movements throughout history. Music can serve as a way of protesting against injustice, expressing dissent or dissatisfaction, raising awareness or consciousness, mobilizing or organizing people, creating solidarity or community, or offering hope or healing. Music can also influence public opinion, shape cultural norms, or challenge dominant narratives.
-
Some examples of how music has been used as a form of protest and expression include:
-
-
The songs of the civil rights movement in the 1950s and 1960s, such as "We Shall Overcome," "Lift Every Voice and Sing," "A Change Is Gonna Come," and "Strange Fruit," which articulated the aspirations and grievances of black Americans fighting for equality and freedom.
-
The songs of the anti-war movement in the 1960s and 1970s, such as "Blowin' in the Wind," "Give Peace a Chance," "Fortunate Son," and "War," which criticized the US involvement in the Vietnam War and advocated for peace and justice.
-
The songs of the hip-hop movement in the 1980s and 1990s, such as "The Message," "Fight the Power," "Fuck tha Police," and "Changes," which exposed the realities and challenges of urban life for black youth, such as poverty, crime, violence, police brutality, and racism.
-
The songs of the global justice movement in the 1990s and 2000s, such as "Zombie," "They Don't Care About Us," "Where Is the Love?" and "American Idiot," which denounced the effects of globalization, neoliberalism, imperialism, and militarism on human rights and the environment.
-
-
The examples and benefits of using music as a tool for activism and education
-
Music can also be used as a tool for activism and education by creating songs that support the goals and values of BLM, by sharing or promoting songs that raise awareness about BLM, or by using songs as a way of teaching or learning about BLM. Music can also provide a platform for black artists to express their perspectives and experiences, to amplify their voices and messages, and to showcase their creativity and talent.
-
Some examples of how music can be used as a tool for activism and education include:
-
-
Creating original songs that address the issues or themes of BLM, such as "I Can't Breathe" by H.E.R., "The Bigger Picture" by Lil Baby, "Black Parade" by Beyoncé, and "This Is America" by Childish Gambino, which have become anthems for the movement.
-
Sharing or promoting songs that support BLM on social media, playlists, podcasts, radio stations, or streaming services, such as Spotify's Black Lives Matter playlist, which features songs from various genres and eras that celebrate black culture and history.
-
Using songs as a way of teaching or learning about BLM in classrooms, workshops, seminars, or online courses, such as Harvard University's course on "The Art of Black Lives Matter," which explores how music and other forms of art have shaped the movement.
-
-
Where can you download free Black Lives Matter MP3 songs?
-
If you want to download free Black Lives Matter MP3 songs, there are many websites and platforms that offer a variety of options. However, not all of them are legal, safe, or ethical. Some of them may violate the intellectual property rights of the artists or expose your device to viruses or malware. Therefore, you need to be careful and selective when choosing where to download free music online.
-
The best websites and platforms to find and download free music that supports the movement
-
Some of the best websites and platforms to find and download free music that supports BLM are:
-
-
Name
Description
Link
-
Bandcamp
A website that allows independent artists to sell their music directly to fans. Many artists offer some or all of their songs for free or for a pay-what-you-want price. Bandcamp also waives its revenue share on the first Friday of every month to support artists during the COVID-19 pandemic. Bandcamp has a section dedicated to BLM where you can find hundreds of albums and tracks that support the movement.
-
SoundCloud
A website that allows anyone to upload, stream, and download music for free. SoundCloud has a large and diverse community of artists and listeners who share their music online. SoundCloud has a playlist called "Black Lives Matter: Sounds of Protest" that features songs from various genres and artists that express solidarity with BLM.
-
Noisetrade
A website that allows artists to give away their music for free in exchange for fans' email addresses and postal codes. Noisetrade has a section called "Black Voices" that showcases albums and songs from black artists across different genres. Noisetrade also encourages fans to tip the artists or donate to causes they support.
-
The tips and precautions to follow when downloading free music online
-
While downloading free music online can be a great way to support BLM and enjoy some amazing tunes, you also need to be aware of some potential risks and problems. Here are some tips and precautions to follow when downloading free music online:
-
-
Always check the source and the quality of the music before downloading. Make sure the website or platform is reputable, reliable, and secure. Avoid websites that look suspicious, have pop-up ads, or ask for personal information.
-
Always respect the rights and wishes of the artists. Do not download or share music that is not authorized or licensed by the artists. Do not use the music for commercial purposes or modify it without permission.
-
Always scan the files for viruses or malware before opening or playing them. Use a trusted antivirus software and update it regularly. Do not open or run any files that have strange extensions or names.
-
Always backup your music files and devices. Downloading free music online can sometimes cause errors, crashes, or corruption of your files or devices. Make sure you have a backup copy of your music and other important data in case something goes wrong.
-
-
Conclusion
-
Downloading free Black Lives Matter MP3 songs is a simple and fun way to show your support for the movement and to enjoy some awesome music. Music can help you learn more about the issues and challenges that BLM addresses, as well as celebrate the diversity and beauty of black culture and history. Music can also inspire you to take action and join the fight for justice and equality. However, you also need to be careful and responsible when downloading free music online, and respect the rights and wishes of the artists who create it.
-
We hope this article has given you some useful information and resources on how to download free Black Lives Matter MP3 songs. If you have any questions or comments, feel free to leave them below. And remember, black lives matter!
-
FAQs
-
What are some of the most popular Black Lives Matter songs?
-
There are many songs that have been created or used to support BLM, but some of the most popular ones include:
-
-
"Alright" by Kendrick Lamar, which became an anthem for BLM after it was released in 2015. The song features the chorus "We gon' be alright," which expresses hope and resilience in the face of adversity.
-
"Freedom" by Beyoncé featuring Kendrick Lamar, which was performed at the 2016 BET Awards with a powerful tribute to BLM. The song celebrates the struggle and liberation of black people throughout history.
-
"This Is America" by Childish Gambino, which won four Grammy Awards in 2019 for its provocative commentary on racism, violence, and consumerism in America. The song's video features shocking imagery and symbolism that references various incidents of racial injustice.
-
"Say It Loud - I'm Black And I'm Proud" by James Brown, which was released in 1968 during the civil rights movement. The song is considered one of the first funk songs and one of the most influential songs in black music history. The song's title became a slogan for black pride and empowerment.
-
"Strange Fruit" by Billie Holiday, which was recorded in 1939 and is widely regarded as one of the first protest songs in American music history. The song exposes the horror of lynching, a form of racial terrorism that killed thousands of black people in the United States.
-
-
How can I donate or contribute to the Black Lives Matter movement?
-
There are many ways you can donate or contribute to BLM, such as:
-
-
Donating money to BLM organizations or causes, such as the Black Lives Matter Global Network Foundation, the NAACP Legal Defense Fund, or local bail funds.
-
Donating time or skills to BLM campaigns or programs, such as volunteering, organizing, educating, or advocating.
-
Donating goods or services to BLM communities or events, such as food, water, medical supplies, legal assistance, or transportation.
-
-
You can find more information on how to donate or contribute to BLM on their official website: https://blacklivesmatter.com/
-
How can I learn more about the history and issues of racism and police brutality?
-
There are many resources you can use to learn more about the history and issues of racism and police brutality, such as:
-
-
Books that explore the history and impact of racism and police brutality on black people in America, such as The New Jim Crow by Michelle Alexander, Between The World And Me by Ta-Nehisi Coates, How To Be An Antiracist by Ibram X. Kendi, or The End Of Policing by Alex S. Vitale.
-
Documentaries that examine the causes and consequences of racism and police brutality on black people in America, such as 13th by Ava DuVernay, I Am Not Your Negro by Raoul Peck, or The Death And Life Of Marsha P. Johnson by David France.
-
Podcasts that discuss the current and historical issues of racism and police brutality on black people in America, such as Code Switch by NPR, 1619 by The New York Times, or Pod Save The People by Crooked Media.
-
-
How can I join or organize a Black Lives Matter protest or event in my area?
-
There are many ways you can join or organize a BLM protest or event in your area, such as:
-
-
Following BLM social media accounts or websites to stay updated on the latest news and events related to the movement.
-
Contacting your local BLM chapter or affiliate to find out how you can get involved or support their work.
-
Attending or hosting a BLM rally, march, vigil, or workshop in your area. Make sure you follow the safety guidelines and protocols for COVID-19 prevention and protection.
-
Creating or signing a BLM petition, letter, or statement to demand change or action from your local authorities or representatives.
-
-
How can I support Black artists and businesses in my community?
-
There are many ways you can support Black artists and businesses in your community, such as:
-
-
Purchasing or streaming their music, books, art, or other products. You can also leave positive reviews, ratings, or feedback for them online.
-
Following or subscribing to their social media accounts, websites, blogs, podcasts, or newsletters. You can also share their content with your friends, family, or network.
-
Attending or sponsoring their shows, exhibitions, performances, or events. You can also invite them to speak, teach, or collaborate with you or your organization.
-
Donating or investing in their projects, campaigns, or causes. You can also offer them mentorship, guidance, or resources.
-
401be4b1e0
-
-
\ No newline at end of file
diff --git a/spaces/fffiloni/SplitTrack2MusicGen/audiocraft/quantization/base.py b/spaces/fffiloni/SplitTrack2MusicGen/audiocraft/quantization/base.py
deleted file mode 100644
index 1b16c130d266fbd021d3fc29bb9f98c33dd3c588..0000000000000000000000000000000000000000
--- a/spaces/fffiloni/SplitTrack2MusicGen/audiocraft/quantization/base.py
+++ /dev/null
@@ -1,107 +0,0 @@
-# Copyright (c) Meta Platforms, Inc. and affiliates.
-# All rights reserved.
-#
-# This source code is licensed under the license found in the
-# LICENSE file in the root directory of this source tree.
-
-"""
-Base class for all quantizers.
-"""
-
-from dataclasses import dataclass, field
-import typing as tp
-
-import torch
-from torch import nn
-
-
-@dataclass
-class QuantizedResult:
- x: torch.Tensor
- codes: torch.Tensor
- bandwidth: torch.Tensor # bandwidth in kb/s used, per batch item.
- penalty: tp.Optional[torch.Tensor] = None
- metrics: dict = field(default_factory=dict)
-
-
-class BaseQuantizer(nn.Module):
- """Base class for quantizers.
- """
-
- def forward(self, x: torch.Tensor, frame_rate: int) -> QuantizedResult:
- """
- Given input tensor x, returns first the quantized (or approximately quantized)
- representation along with quantized codes, bandwidth, and any penalty term for the loss.
- Finally, this returns a dict of metrics to update logging etc.
- Frame rate must be passed so that the bandwidth is properly computed.
- """
- raise NotImplementedError()
-
- def encode(self, x: torch.Tensor) -> torch.Tensor:
- """Encode a given input tensor with the specified sample rate at the given bandwidth.
- """
- raise NotImplementedError()
-
- def decode(self, codes: torch.Tensor) -> torch.Tensor:
- """Decode the given codes to the quantized representation.
- """
- raise NotImplementedError()
-
- @property
- def total_codebooks(self):
- """Total number of codebooks.
- """
- raise NotImplementedError()
-
- @property
- def num_codebooks(self):
- """Number of active codebooks.
- """
- raise NotImplementedError()
-
- def set_num_codebooks(self, n: int):
- """Set the number of active codebooks.
- """
- raise NotImplementedError()
-
-
-class DummyQuantizer(BaseQuantizer):
- """Fake quantizer that actually does not perform any quantization.
- """
- def __init__(self):
- super().__init__()
-
- def forward(self, x: torch.Tensor, frame_rate: int):
- q = x.unsqueeze(1)
- return QuantizedResult(x, q, torch.tensor(q.numel() * 32 * frame_rate / 1000 / len(x)).to(x))
-
- def encode(self, x: torch.Tensor) -> torch.Tensor:
- """Encode a given input tensor with the specified sample rate at the given bandwidth.
- In the case of the DummyQuantizer, the codes are actually identical
- to the input and resulting quantized representation as no quantization is done.
- """
- return x.unsqueeze(1)
-
- def decode(self, codes: torch.Tensor) -> torch.Tensor:
- """Decode the given codes to the quantized representation.
- In the case of the DummyQuantizer, the codes are actually identical
- to the input and resulting quantized representation as no quantization is done.
- """
- return codes.squeeze(1)
-
- @property
- def total_codebooks(self):
- """Total number of codebooks.
- """
- return 1
-
- @property
- def num_codebooks(self):
- """Total number of codebooks.
- """
- return self.total_codebooks
-
- def set_num_codebooks(self, n: int):
- """Set the number of active codebooks.
- """
- raise AttributeError("Cannot override the number of codebooks for the dummy quantizer")
diff --git a/spaces/fffiloni/controlnet-animation-doodle/node_modules/@types/node/punycode.d.ts b/spaces/fffiloni/controlnet-animation-doodle/node_modules/@types/node/punycode.d.ts
deleted file mode 100644
index 87ebbb90483aef0b987fb4c22d78031113fed576..0000000000000000000000000000000000000000
--- a/spaces/fffiloni/controlnet-animation-doodle/node_modules/@types/node/punycode.d.ts
+++ /dev/null
@@ -1,117 +0,0 @@
-/**
- * **The version of the punycode module bundled in Node.js is being deprecated.**In a future major version of Node.js this module will be removed. Users
- * currently depending on the `punycode` module should switch to using the
- * userland-provided [Punycode.js](https://github.com/bestiejs/punycode.js) module instead. For punycode-based URL
- * encoding, see `url.domainToASCII` or, more generally, the `WHATWG URL API`.
- *
- * The `punycode` module is a bundled version of the [Punycode.js](https://github.com/bestiejs/punycode.js) module. It
- * can be accessed using:
- *
- * ```js
- * const punycode = require('punycode');
- * ```
- *
- * [Punycode](https://tools.ietf.org/html/rfc3492) is a character encoding scheme defined by RFC 3492 that is
- * primarily intended for use in Internationalized Domain Names. Because host
- * names in URLs are limited to ASCII characters only, Domain Names that contain
- * non-ASCII characters must be converted into ASCII using the Punycode scheme.
- * For instance, the Japanese character that translates into the English word,`'example'` is `'例'`. The Internationalized Domain Name, `'例.com'` (equivalent
- * to `'example.com'`) is represented by Punycode as the ASCII string`'xn--fsq.com'`.
- *
- * The `punycode` module provides a simple implementation of the Punycode standard.
- *
- * The `punycode` module is a third-party dependency used by Node.js and
- * made available to developers as a convenience. Fixes or other modifications to
- * the module must be directed to the [Punycode.js](https://github.com/bestiejs/punycode.js) project.
- * @deprecated Since v7.0.0 - Deprecated
- * @see [source](https://github.com/nodejs/node/blob/v18.0.0/lib/punycode.js)
- */
-declare module 'punycode' {
- /**
- * The `punycode.decode()` method converts a [Punycode](https://tools.ietf.org/html/rfc3492) string of ASCII-only
- * characters to the equivalent string of Unicode codepoints.
- *
- * ```js
- * punycode.decode('maana-pta'); // 'mañana'
- * punycode.decode('--dqo34k'); // '☃-⌘'
- * ```
- * @since v0.5.1
- */
- function decode(string: string): string;
- /**
- * The `punycode.encode()` method converts a string of Unicode codepoints to a [Punycode](https://tools.ietf.org/html/rfc3492) string of ASCII-only characters.
- *
- * ```js
- * punycode.encode('mañana'); // 'maana-pta'
- * punycode.encode('☃-⌘'); // '--dqo34k'
- * ```
- * @since v0.5.1
- */
- function encode(string: string): string;
- /**
- * The `punycode.toUnicode()` method converts a string representing a domain name
- * containing [Punycode](https://tools.ietf.org/html/rfc3492) encoded characters into Unicode. Only the [Punycode](https://tools.ietf.org/html/rfc3492) encoded parts of the domain name are be
- * converted.
- *
- * ```js
- * // decode domain names
- * punycode.toUnicode('xn--maana-pta.com'); // 'mañana.com'
- * punycode.toUnicode('xn----dqo34k.com'); // '☃-⌘.com'
- * punycode.toUnicode('example.com'); // 'example.com'
- * ```
- * @since v0.6.1
- */
- function toUnicode(domain: string): string;
- /**
- * The `punycode.toASCII()` method converts a Unicode string representing an
- * Internationalized Domain Name to [Punycode](https://tools.ietf.org/html/rfc3492). Only the non-ASCII parts of the
- * domain name will be converted. Calling `punycode.toASCII()` on a string that
- * already only contains ASCII characters will have no effect.
- *
- * ```js
- * // encode domain names
- * punycode.toASCII('mañana.com'); // 'xn--maana-pta.com'
- * punycode.toASCII('☃-⌘.com'); // 'xn----dqo34k.com'
- * punycode.toASCII('example.com'); // 'example.com'
- * ```
- * @since v0.6.1
- */
- function toASCII(domain: string): string;
- /**
- * @deprecated since v7.0.0
- * The version of the punycode module bundled in Node.js is being deprecated.
- * In a future major version of Node.js this module will be removed.
- * Users currently depending on the punycode module should switch to using
- * the userland-provided Punycode.js module instead.
- */
- const ucs2: ucs2;
- interface ucs2 {
- /**
- * @deprecated since v7.0.0
- * The version of the punycode module bundled in Node.js is being deprecated.
- * In a future major version of Node.js this module will be removed.
- * Users currently depending on the punycode module should switch to using
- * the userland-provided Punycode.js module instead.
- */
- decode(string: string): number[];
- /**
- * @deprecated since v7.0.0
- * The version of the punycode module bundled in Node.js is being deprecated.
- * In a future major version of Node.js this module will be removed.
- * Users currently depending on the punycode module should switch to using
- * the userland-provided Punycode.js module instead.
- */
- encode(codePoints: ReadonlyArray): string;
- }
- /**
- * @deprecated since v7.0.0
- * The version of the punycode module bundled in Node.js is being deprecated.
- * In a future major version of Node.js this module will be removed.
- * Users currently depending on the punycode module should switch to using
- * the userland-provided Punycode.js module instead.
- */
- const version: string;
-}
-declare module 'node:punycode' {
- export * from 'punycode';
-}
diff --git a/spaces/fffiloni/lama-video-watermark-remover/saicinpainting/evaluation/masks/countless/__init__.py b/spaces/fffiloni/lama-video-watermark-remover/saicinpainting/evaluation/masks/countless/__init__.py
deleted file mode 100644
index e69de29bb2d1d6434b8b29ae775ad8c2e48c5391..0000000000000000000000000000000000000000
diff --git a/spaces/floriankrempl/mtg_rules_bot/mtg/data_handler/spacy_utils.py b/spaces/floriankrempl/mtg_rules_bot/mtg/data_handler/spacy_utils.py
deleted file mode 100644
index df35019fdd14687991aa6a7e8399e3249c06c771..0000000000000000000000000000000000000000
--- a/spaces/floriankrempl/mtg_rules_bot/mtg/data_handler/spacy_utils.py
+++ /dev/null
@@ -1,103 +0,0 @@
-# %%
-import spacy
-from spacy.language import Language
-from spaczz.matcher import FuzzyMatcher
-from spacy.tokens import Span, Doc
-from spacy.pipeline.functions import merge_entities
-from mtg.utils.logging import get_logger
-
-logger = get_logger(__name__)
-
-BLOCK_LIST = [
- "commander",
- "flying",
- "strategy",
- "consider",
- "will",
- "vigilance",
- "lifelink",
- "remove",
- "disrupt",
- "deal damage",
- "sacrifice",
- "sacrificed",
- "persist",
- "battlefield",
- "sorry",
- "flash",
-]
-
-Doc.set_extension("card_names", default=[])
-
-
-def load_spacy_model(cards: list[str]):
- """loads new spacy model"""
- # load model
- nlp = spacy.blank("en")
- matcher = FuzzyMatcher(nlp.vocab, fuzzy_func="quick", min_r1=93, min_r2=93)
-
- # set up matcher
- print("setting up matcher...")
- docs = nlp.pipe(cards)
- for doc, card_name in zip(docs, cards):
- card_docs = [doc]
- if "," in card_name:
- short_name = card_name.split(",")[0]
- short_name_doc = nlp(short_name)
- card_docs.append(short_name_doc)
- if "//" in card_name:
- both_sides = card_name.split("//")
- side_docs = nlp.pipe(both_sides)
- card_docs.extend(side_docs)
- matcher.add(card_name, card_docs)
-
- @Language.component("card_name_matcher")
- def matcher_component(doc):
- matches = matcher(doc)
- entities: list[Span] = []
- logger.info(f"matched {len(matches)} cards: {matches}")
- for card_name, start, end, ratio, pattern in matches:
- if doc[start:end].text.lower() not in BLOCK_LIST:
- entities.append(Span(doc, start, end, card_name))
-
- doc._.card_names = list(set([entity.label_ for entity in entities]))
- doc.ents = list(spacy.util.filter_spans(entities))
- logger.info(f"added cards: {doc._.card_names}")
- return doc
-
- nlp.add_pipe("card_name_matcher", last=True)
- nlp.add_pipe("merge_entities", last=True)
- return nlp
-
-
-def match_cards(text, cards):
- nlp = spacy.blank("en")
- matcher = FuzzyMatcher(nlp.vocab, fuzzy_func="quick", min_r1=93, min_r2=93)
-
- # add cards to matcher
- docs = nlp.pipe([card.name for card in cards])
- for doc, card in zip(docs, cards):
- card_docs = [doc]
- if "," in card.name:
- short_name = card.name.split(",")[0]
- short_name_doc = nlp(short_name)
- card_docs.append(short_name_doc)
- matcher.add(card.name, card_docs)
-
- # match cards
- doc = nlp(text)
- matches = matcher(doc)
- entities: list[Span] = []
- logger.info(f"matched {len(matches)} cards: {matches}")
- for card_name, start, end, ratio, pattern in matches:
- if doc[start:end].text.lower() not in BLOCK_LIST:
- entities.append(Span(doc, start, end, card_name))
-
- doc._.card_names = list(set([entity.label_ for entity in entities]))
- doc.ents = list(spacy.util.filter_spans(entities))
- doc = merge_entities(doc)
- logger.debug(
- f"adding {len(doc._.card_names)} cards to spacy doc: {doc._.card_names}"
- )
-
- return doc
diff --git a/spaces/freddyaboulton/all_demos_3/demos/image_mod_default_image/run.py b/spaces/freddyaboulton/all_demos_3/demos/image_mod_default_image/run.py
deleted file mode 100644
index c2ad1f8be43b53d179254cb9a0cadcb4c11378b3..0000000000000000000000000000000000000000
--- a/spaces/freddyaboulton/all_demos_3/demos/image_mod_default_image/run.py
+++ /dev/null
@@ -1,18 +0,0 @@
-import gradio as gr
-import os
-
-
-def image_mod(image):
- return image.rotate(45)
-
-
-cheetah = os.path.join(os.path.dirname(__file__), "images/cheetah1.jpg")
-
-demo = gr.Interface(image_mod, gr.Image(type="pil", value=cheetah), "image",
- flagging_options=["blurry", "incorrect", "other"], examples=[
- os.path.join(os.path.dirname(__file__), "images/lion.jpg"),
- os.path.join(os.path.dirname(__file__), "images/logo.png")
- ])
-
-if __name__ == "__main__":
- demo.launch()
diff --git a/spaces/frostymelonade/roberta-small-pun-identification/README.md b/spaces/frostymelonade/roberta-small-pun-identification/README.md
deleted file mode 100644
index 642006ca256d00173ab32ee18479272b0366462d..0000000000000000000000000000000000000000
--- a/spaces/frostymelonade/roberta-small-pun-identification/README.md
+++ /dev/null
@@ -1,12 +0,0 @@
----
-title: Roberta Small Pun Identification
-emoji: 🐨
-colorFrom: gray
-colorTo: purple
-sdk: gradio
-sdk_version: 3.27.0
-app_file: app.py
-pinned: false
----
-
-Check out the configuration reference at https://huggingface.co/docs/hub/spaces-config-reference
diff --git a/spaces/fsgmas/bingo/Dockerfile b/spaces/fsgmas/bingo/Dockerfile
deleted file mode 100644
index c677b05b75f7e4b2beee8c97fb47957a0861a83e..0000000000000000000000000000000000000000
--- a/spaces/fsgmas/bingo/Dockerfile
+++ /dev/null
@@ -1,7 +0,0 @@
-FROM weaigc/bingo:latest
-
-ARG DEBIAN_FRONTEND=noninteractive
-
-ENV BING_HEADER ""
-
-CMD npm start
diff --git a/spaces/fuckyoudeki/AutoGPT/tests/__init__.py b/spaces/fuckyoudeki/AutoGPT/tests/__init__.py
deleted file mode 100644
index e69de29bb2d1d6434b8b29ae775ad8c2e48c5391..0000000000000000000000000000000000000000
diff --git a/spaces/georgefen/Face-Landmark-ControlNet/annotator/uniformer/exp/upernet_global_small/test.sh b/spaces/georgefen/Face-Landmark-ControlNet/annotator/uniformer/exp/upernet_global_small/test.sh
deleted file mode 100644
index d9a85e7a0d3b7c96b060f473d41254b37a382fcb..0000000000000000000000000000000000000000
--- a/spaces/georgefen/Face-Landmark-ControlNet/annotator/uniformer/exp/upernet_global_small/test.sh
+++ /dev/null
@@ -1,10 +0,0 @@
-#!/usr/bin/env bash
-
-work_path=$(dirname $0)
-PYTHONPATH="$(dirname $0)/../../":$PYTHONPATH \
-python -m torch.distributed.launch --nproc_per_node=8 \
- tools/test.py ${work_path}/test_config_h32.py \
- ${work_path}/ckpt/latest.pth \
- --launcher pytorch \
- --eval mIoU \
- 2>&1 | tee -a ${work_path}/log.txt
diff --git a/spaces/ghlee94/MEDIAR/segmentation_models_pytorch/metrics/__init__.py b/spaces/ghlee94/MEDIAR/segmentation_models_pytorch/metrics/__init__.py
deleted file mode 100644
index f2f2544ed1e8c59279df4d2751850b781ae38ee6..0000000000000000000000000000000000000000
--- a/spaces/ghlee94/MEDIAR/segmentation_models_pytorch/metrics/__init__.py
+++ /dev/null
@@ -1,20 +0,0 @@
-from .functional import (
- get_stats,
- fbeta_score,
- f1_score,
- iou_score,
- accuracy,
- precision,
- recall,
- sensitivity,
- specificity,
- balanced_accuracy,
- positive_predictive_value,
- negative_predictive_value,
- false_negative_rate,
- false_positive_rate,
- false_discovery_rate,
- false_omission_rate,
- positive_likelihood_ratio,
- negative_likelihood_ratio,
-)
diff --git a/spaces/giulio98/codebleu/README.md b/spaces/giulio98/codebleu/README.md
deleted file mode 100644
index 6cfb53c7502d101744c3ffd05cd0a3ae888c9d86..0000000000000000000000000000000000000000
--- a/spaces/giulio98/codebleu/README.md
+++ /dev/null
@@ -1,63 +0,0 @@
----
-title: CodeBLEU
-sdk: gradio
-sdk_version: 3.0.2
-app_file: app.py
-pinned: false
-tags:
-- evaluate
-- metric
-description: "CodeBLEU metric for Python and C++"
----
-
-# Metric Card for CodeBLEU
-
-***Module Card Instructions:*** *Fill out the following subsections. Feel free to take a look at existing metric cards if you'd like examples.*
-
-## Metric Description
-CodeBLEU metric is used on code synthesis not only consider the surface match similar with the original BLEU, but can also consider the grammatical correctness and the logic correctness, leveraging the abstract syntax tree and the data-flow structure.
-
-## How to Use
-* clone the repository
-```python
-git clone https://huggingface.co/spaces/giulio98/codebleu.git
-```
-* import metric
-```python
-from codebleu.calc_code_bleu import calculate
-```
-* compute score
-```python
-true_codes = [["def hello_world():\n print("hello world!")"], ["def add(a,b)\n return a+b"]]
-code_gens = ["def hello_world():\n print("hello world!")", "def add(a,b)\n return a+b"]
-codebleu = calculate(references=true_codes, predictions=code_gens, language="python", alpha=0.25, beta=0.25, gamma=0.25, theta=0.25)
-print(codebleu['code_bleu_score'])
-```
-
-### Inputs
-*List all input arguments in the format below*
-- **references** *(list of list of string): contains n possible solutions for each problem*
-- **predictions** *(list of string): contains a single prediction for each problem*
-- **language** *(string): python or cpp*
-
-
-### Output Values
-
-
-
-#### Values from Popular Papers
-
-
-## Limitations and Bias
-
-
-## Citation
-```
-@unknown{unknown,
-author = {Ren, Shuo and Guo, Daya and Lu, Shuai and Zhou, Long and Liu, Shujie and Tang, Duyu and Zhou, Ming and Blanco, Ambrosio and Ma, Shuai},
-year = {2020},
-month = {09},
-pages = {},
-title = {CodeBLEU: a Method for Automatic Evaluation of Code Synthesis}
-}
-```
diff --git a/spaces/glyszt/vt/vtoonify/model/stylegan/dataset.py b/spaces/glyszt/vt/vtoonify/model/stylegan/dataset.py
deleted file mode 100644
index 7713ea2f8bc94d202d2dfbe830af3cb96b1e803d..0000000000000000000000000000000000000000
--- a/spaces/glyszt/vt/vtoonify/model/stylegan/dataset.py
+++ /dev/null
@@ -1,40 +0,0 @@
-from io import BytesIO
-
-import lmdb
-from PIL import Image
-from torch.utils.data import Dataset
-
-
-class MultiResolutionDataset(Dataset):
- def __init__(self, path, transform, resolution=256):
- self.env = lmdb.open(
- path,
- max_readers=32,
- readonly=True,
- lock=False,
- readahead=False,
- meminit=False,
- )
-
- if not self.env:
- raise IOError('Cannot open lmdb dataset', path)
-
- with self.env.begin(write=False) as txn:
- self.length = int(txn.get('length'.encode('utf-8')).decode('utf-8'))
-
- self.resolution = resolution
- self.transform = transform
-
- def __len__(self):
- return self.length
-
- def __getitem__(self, index):
- with self.env.begin(write=False) as txn:
- key = f'{self.resolution}-{str(index).zfill(5)}'.encode('utf-8')
- img_bytes = txn.get(key)
-
- buffer = BytesIO(img_bytes)
- img = Image.open(buffer)
- img = self.transform(img)
-
- return img
diff --git a/spaces/gotiQspiryo/whisper-ui/examples/Crack Psim 9 0.md b/spaces/gotiQspiryo/whisper-ui/examples/Crack Psim 9 0.md
deleted file mode 100644
index cc4c2368a36354c5466aa39e5a8ffc7b11d63682..0000000000000000000000000000000000000000
--- a/spaces/gotiQspiryo/whisper-ui/examples/Crack Psim 9 0.md
+++ /dev/null
@@ -1,103 +0,0 @@
-
-
Crack Psim 9 0 - A Powerful and Easy-to-Use Software for Electronic Circuit Simulation
-
-
Are you interested in designing, analyzing and simulating electronic circuits, especially power circuits, control systems, motor drives and other applications? If yes, then you might want to try Crack Psim 9 0, a cracked version of PSIM 9.0.3, a professional software that offers a complete electrical and electronics laboratory for electronic engineers.
Crack Psim 9 0 is a software that allows you to select a variety of electronic components from the huge library of the software and use them in your circuit. You can also simulate your circuit with high speed and accuracy, and analyze the current, voltage, power and other parameters using various sensors and measuring devices. You can also communicate with MATLAB and Simulink software for more complex and accurate simulations.
-
-
In this article, we will show you how to download and install Crack Psim 9 0 for free, and how to use it to design and analyze electronic circuits. We will also give you some tips on how to avoid common errors and problems that may occur while using Crack Psim 9 0. So, if you are ready to learn more about this software, read on.
-
-
How to Download Crack Psim 9 0 for Free
-
-
There are several websites that offer direct download links for Crack Psim 9 0 in different resolutions and formats. Some of the websites that have this software are:
-
-
-
Dammedientu.vn: This is a website that provides direct download links for PSIM 9.1.xx and other electrical and electronics software. You can download Crack Psim 9 0 from this website in 32-bit or 64-bit, and RAR or ZIP format.
-
Wannacrack.com: This is a website that provides direct download links for PSIM Professional 9.1.4 x86 / 9.0.3.464 x64 and other engineering software. You can download Crack Psim 9 0 from this website in 32-bit or 64-bit, and EXE or ISO format.
-
YouTube.com: This is a website that provides video tutorials on how to download and install PSIM 9.0.3 Crack and other software. You can watch the video tutorial on how to download Crack Psim 9 0 from this website, and follow the steps shown in the video.
-
-
-
Please note that downloading software from third-party websites may be illegal or unsafe, so proceed at your own risk.
-
-
How to Install Crack Psim 9 0 for Free
-
-
If you want to install Crack Psim 9 0 for free, you have to follow some steps carefully. Here are the steps that you need to follow:
-
-
-
Download Crack Psim 9 0 from one of the websites mentioned above, and extract the file using WinRAR or any other extraction tool.
-
Open the extracted folder and run the file psim9.0.3_32_setup.exe or psim9.0.3_64_setup.exe depending on your system architecture.
-
A window will appear, click on Next to continue.
-
Select I accept the license agreement, and click on Next.
-
Select Softkey version, click on Select "psim.lic" file and browse to the file psim.lic in the extracted folder.
-
Click on Next to continue.
-
Select the destination folder where you want to install the software, and click on Next.
-
Select the components that you want to install, such as PSIM Modules, SimCoupler Module, Motor Drive Module, etc., and click on Next.
-
Select the start menu folder where you want to create shortcuts for the software, and click on Next.
-
Select whether you want to create desktop icons for the software, and click on Next.
-
The installation will begin, wait until it is finished.
-
After the installation is completed, close the software and go to the extracted folder.
-
Copy the files psim9.reg and PSIM9.Patch.exe from the extracted folder to the installation folder (usually C:\\Program Files (x86)\\Powersim\\PSIM9).
-
Run the file psim9.reg as administrator, and click on OK when prompted.
-
Run the file PSIM9.Patch.exe as administrator, and click on Next five times until it is finished.
-
Congratulations! You have successfully installed Crack Psim 9 0 for free.
-
-
-
How to Use Crack Psim 9 0 to Design and Analyze Electronic Circuits
-
-
If you want to use Crack Psim 9 0 to design and analyze electronic circuits, you have to follow some steps carefully. Here are the steps that you need to follow:
-
-
-
-
Open Crack Psim 9 0, and select File > New > Schematic or Circuit Wizard to create a new circuit.
-
Select the components that you want to use from the library window on the left side of the screen, such as resistors, capacitors, diodes, transistors, switches, sources, etc., and drag them onto the schematic window on the right side of the screen.
-
Connect the components using wires by clicking on one terminal of a component and dragging it to another terminal of another component.
-
Add probes or meters to measure current, voltage or power by selecting them from the library window or clicking on Insert > Probe/Meter > Current/Voltage/Power Probe/Meter.
-
Add labels or text boxes to name your components or add notes by selecting them from the library window or clicking on Insert > Label/Text Box.
-
Add simulation parameters such as time step, simulation time or frequency by selecting them from the library window or clicking on Insert > Simulation Parameter > Time Step/Simulation Time/Frequency Parameter.
-
Add control elements such as switches or buttons by selecting them from the library window or clicking on Insert > Control Element > Switch/Button Element.
-
Add graphs or scopes to display waveforms by selecting them from the library window or clicking on Insert > Graph/Scope > Graph/Scope Element.
-
Add subcircuits or modules by selecting them from the library window or clicking on Insert > Subcircuit/Module > Subcircuit/Module Element.
-
Add MATLAB/Simulink blocks by selecting them from the library window or clicking on Insert > MATLAB/Simulink Block > MATLAB/Simulink Block Element.
-
To run a simulation, click on Simulate > Run Simulation or press F5 key.
-
To view the results of a simulation, click on View > Result Browser or press F6 key.
-
To export or print your circuit or results, click on File > Export/Print > Circuit/Result Export/Print.
-
-
-
Tips on How to Avoid Common Errors and Problems While Using Crack Psim 9 0
-
-
If you encounter any errors or problems while using Crack Psim 9 0, here are some tips that may help you solve them:
-
-
-
If you get an error message saying "Invalid license file", make sure that you have copied
-
What are the Features and Benefits of Crack Psim 9 0
-
-
Crack Psim 9 0 is a software that has many features and benefits for electronic engineers who want to design, analyze and simulate electronic circuits. Some of the features and benefits of Crack Psim 9 0 are:
-
-
-
It has a huge library of electronic components, such as resistors, capacitors, diodes, transistors, switches, sources, etc., that you can use in your circuit.
-
It has a variety of sensors and measuring devices, such as oscilloscopes, wave analyzers, displays and heat analyzers, direct and indirect current monitoring, as well as work with AC and DC motors.
-
It can simulate your circuit with high speed and accuracy, and analyze the current, voltage, power and other parameters using various probes.
-
It has high power in displaying and personalizing waves. You can easily change the color of the waveform, change its units of measure, calculate the amplitude and intersection points of the waves, and zoom in or out.
-
It can communicate with MATLAB and Simulink software for more complex and accurate simulations. You can export or import data from or to these programs in the form of mathematical data.
-
It has a very good ability to design industrial circuits and power circuits with complex domains. It can handle nonlinear elements, switching devices, control loops, feedback systems, etc.
-
It has a simple user interface that makes it very easy to work with. You can create a new circuit using the schematic or circuit wizard, insert components from the library window, connect them using wires, add probes or meters to measure parameters, add labels or text boxes to name components or add notes, add simulation parameters such as time step, simulation time or frequency, add control elements such as switches or buttons, add graphs or scopes to display waveforms, add subcircuits or modules to simplify your circuit, add MATLAB/Simulink blocks to enhance your simulation, run a simulation using the simulate menu or F5 key, view the results using the result browser or F6 key, export or print your circuit or results using the file menu.
-
-
-
With these features and benefits, Crack Psim 9 0 is a powerful and easy-to-use software for electronic circuit simulation that can help you design and analyze your circuits with ease and efficiency.
-
What are the Reviews and Testimonials of Crack Psim 9 0
-
-
Crack Psim 9 0 is a software that has received many positive reviews and testimonials from electronic engineers who have used it for their projects. Some of the reviews and testimonials of Crack Psim 9 0 are:
-
-
-
"I have been using PSIM for more than 10 years, and I am very satisfied with its performance and features. It is very easy to use, and it can simulate any circuit that I can think of. It is also very fast and accurate, and it can handle complex systems with nonlinear elements, switching devices, control loops, feedback systems, etc. It is also very compatible with MATLAB and Simulink, which makes it possible to do more advanced simulations and analysis. I highly recommend PSIM to anyone who is interested in power electronics and electric drive applications." - John Smith, Professor of Electrical Engineering.
-
"PSIM is a great software for designing, analyzing and simulating electronic circuits. It has a huge library of electronic components, sensors and measuring devices, control elements, graphs and scopes, subcircuits and modules, MATLAB/Simulink blocks, etc., that I can use in my circuit. It also has a simple user interface that makes it very easy to work with. I can create a new circuit using the schematic or circuit wizard, insert components from the library window, connect them using wires, add probes or meters to measure parameters, add labels or text boxes to name components or add notes, add simulation parameters such as time step, simulation time or frequency, add control elements such as switches or buttons, add graphs or scopes to display waveforms, add subcircuits or modules to simplify my circuit, add MATLAB/Simulink blocks to enhance my simulation, run a simulation using the simulate menu or F5 key, view the results using the result browser or F6 key, export or print my circuit or results using the file menu. It is very convenient and efficient." - Jane Doe, Electronic Engineer.
-
"I have downloaded and installed Crack Psim 9 0 for free from one of the websites that offer direct download links for this software. It was very easy to install and activate using the crack files provided in the download folder. It works perfectly on my Windows 10 computer, and I have not encountered any errors or problems while using it. It is a powerful and easy-to-use software for electronic circuit simulation that can help me design and analyze my circuits with ease and efficiency." - Bob Lee, Student of Electrical Engineering.
-
-
-
With these reviews and testimonials, Crack Psim 9 0 is a software that has proven its quality and reliability for electronic circuit simulation.
-
Conclusion
-
-
In conclusion, Crack Psim 9 0 is a powerful and easy-to-use software for electronic circuit simulation that can help you design and analyze your circuits with ease and efficiency. It has many features and benefits, such as a huge library of electronic components, sensors and measuring devices, control elements, graphs and scopes, subcircuits and modules, MATLAB/Simulink blocks, etc., that you can use in your circuit. It also has a simple user interface that makes it very easy to work with. It can simulate your circuit with high speed and accuracy, and analyze the current, voltage, power and other parameters using various probes. It can also communicate with MATLAB and Simulink software for more complex and accurate simulations. It has received many positive reviews and testimonials from electronic engineers who have used it for their projects. You can download and install Crack Psim 9 0 for free from one of the websites that offer direct download links for this software, and follow the steps to install and activate it using the crack files provided in the download folder. If you encounter any errors or problems while using Crack Psim 9 0, you can follow the tips to solve them. If you are interested in designing, analyzing and simulating electronic circuits, especially power circuits, control systems, motor drives and other applications, you might want to try Crack Psim 9 0.