diff --git a/spaces/1gistliPinn/ChatGPT4/Examples/AutoDATA 2006 V3.16 Serial Key Keygen _BEST_.md b/spaces/1gistliPinn/ChatGPT4/Examples/AutoDATA 2006 V3.16 Serial Key Keygen _BEST_.md
deleted file mode 100644
index c5e174a93a3347ef51eacf29076e041215c6aa87..0000000000000000000000000000000000000000
--- a/spaces/1gistliPinn/ChatGPT4/Examples/AutoDATA 2006 V3.16 Serial Key Keygen _BEST_.md
+++ /dev/null
@@ -1,5 +0,0 @@
-
-
If serialized.[[Name]] is CallValueError, then set prototype to %CallValueError.prototype%.
If serialized.[[Name]] is LoadError, then set prototype to %LoadError.prototype%.
If serialized.[[Name]] is UnsupportedOperationError, then set prototype to %UnsupportedOperationError.prototype%.
If serialized.[[Name]] is ValueError, then set prototype to %ValueError.prototype%.
If serialized.[[Name]] is ObjectCreatedError, then set prototype to %ObjectCreatedError.prototype%.
If serialized.[[Name]] is ReferenceError, then set prototype to %ReferenceError.prototype%.
If serialized.[[Name]] is URIError, then set prototype to %URIError.prototype%.
If serialized.[[Name]] is TypeError, then set prototype to %TypeError.prototype%.
If serialized.[[Name]] is URIError, then set prototype to %URIError.prototype%.
Let message be serialized.[[Message]].
Set value to OrdinaryObjectCreate (prototype, [[ErrorData]] ).
Let messageDesc be PropertyDescriptor [[Value]]: message, [[Writable]]: true, [[Enumerable]]: false, [[Configurable]]: true .
If message is not undefined, then perform! OrdinaryDefineOwnProperty (value, message
, messageDesc).
Any interesting accompanying data attached to serialized should be deserialized and attached to value.
Object.defineProperty( String.
-AutoDATA 2006 v3.16 Serial Key keygen
DOWNLOAD — https://imgfil.com/2uxXry
899543212b
-
-
\ No newline at end of file
diff --git a/spaces/1pelhydcardo/ChatGPT-prompt-generator/assets/Blast Your Way Through Enemies and Become the Tank Hero in This Action-Packed Game.md b/spaces/1pelhydcardo/ChatGPT-prompt-generator/assets/Blast Your Way Through Enemies and Become the Tank Hero in This Action-Packed Game.md
deleted file mode 100644
index ce46354a120519b4af3db6eb7c9bdb7ed4eed804..0000000000000000000000000000000000000000
--- a/spaces/1pelhydcardo/ChatGPT-prompt-generator/assets/Blast Your Way Through Enemies and Become the Tank Hero in This Action-Packed Game.md
+++ /dev/null
@@ -1,109 +0,0 @@
-
-Tank Hero: A Guide to the Best Tank Games on Android
-Do you love tanks? Do you enjoy blasting your enemies with cannons, heat seekers, and howitzers? Do you want to experience the thrill of tank wars on your Android device? If you answered yes to any of these questions, then you should check out Tank Hero, a genre of games that features awesome tank action and fun gameplay. In this article, we will introduce you to some of the best tank games on Android, including Tank Hero by Clapfoot Inc., Tank Hero - Awesome tank war g by BETTA GAMES, and Tank Games by CrazyGames. We will also give you some tips and tricks for playing tank games and answer some frequently asked questions. So, what are you waiting for? Let's get started!
-tank hero
Download Zip … https://urlin.us/2uSUt8
- Tank Hero by Clapfoot Inc.
-Tank Hero by Clapfoot Inc. is one of the most popular tank games on Android, with over 10 million downloads and 4.5 stars rating on Google Play. This game offers fast-paced 3D tank action on your Android phone, where you have to take out your enemies with various weapons and tactics. You can battle tanks in over 120 levels, engage in tank wars over 3 unique environments, and choose from 5 different weapons to suit your style. You can also play in campaign and survival game modes, and enjoy the support for HD devices and tablets. The best part is that this game is completely free with no consumables, time, or upgrades to purchase. You can also play it offline without any internet connection.
-Here are some of the reviews from satisfied players:
-
-- "Fairly good tanks game. It's difficult trying to shoot at enemies close by because the tanks does not shoot. The stages with the blackholes where the tank emerges out of nowhere are annoying. They are like a box of chocolates; you never know what you're gonna get. The survival stages are awful. The grid always looks the same and you're basically couped up with 15 tanks that are destroying you continuously. There needs to be a quicker way to switch weapons in Survival mode." - Junior Tlhape
-- "I literally only play two games ever, this game whenever I am not doing anything and can concentrate on it or Pokemon Go when I am on a walk. This is SUCH a simple game, but it definitely gets harder level after level. I recommend playing on Campaign Mode first to get used to the arenas and types of tanks against you. When you are ready for the main challenge, play Survival Mode as each wave sets you up against more and more tanks on your own. Amazing job in designing this game." - Andrew Angel
-- "This game is actually good! I thought this would be somewhat okay considering the low-shaped tanks and the low detailed textures, but this is actually good (x2)! Simple, challenging and very little ads. I also like the difference in tank abilities/speed; makes it more of a challenge. Gg (literally)." - Germane McCrea
-
-If you want to download Tank Hero by Clapfoot Inc., you can click here.
- Tank Hero - Awesome tank war g by BETTA GAMES
-Tank Hero - Awesome tank war g by BETTA GAMES is another great tank game on Android, with over 1 million downloads and 4.2 stars rating on Google Play. This game is simple but challenging, where you have to use your special ability to defend against waves of enemy tanks, then find the opportunity for a counter attack. You can upgrade your weapons and tanks, unlock new maps and modes, and challenge your friends in multiplayer mode. You can also enjoy the realistic physics, smooth controls, and cool sound effects of this game.
-Here are some of the reviews from happy players:
-tank hero game
-tank hero app
-tank hero online
-tank hero download
-tank hero apk
-tank hero mod
-tank hero cheats
-tank hero hack
-tank hero android
-tank hero ios
-tank hero pc
-tank hero review
-tank hero tips
-tank hero guide
-tank hero walkthrough
-tank hero gameplay
-tank hero trailer
-tank hero levels
-tank hero weapons
-tank hero skills
-tank hero upgrade
-tank hero survival mode
-tank hero campaign mode
-tank hero multiplayer
-tank hero 3d
-tank hero 2
-tank hero lite
-tank hero laser wars
-tank hero beta games
-tank hero clapfoot inc.
-tank hero awesome war g
-tank hero betta games
-tank hero crazy games
-tank hero new scientist
-tank hero the sun
-tank hero yahoo news
-tank hero wikipedia
-tank hero montana.edu
-tank hero cornell.edu
-tank hero nasa.gov
-best tank hero game
-free tank hero game
-play tank hero game online
-how to play tank hero game
-how to download tank hero game
-how to install tank hero game
-how to hack tank hero game
-how to beat tank hero game
-how to win in survival mode in tank hero game
-
-- "This game is very fun and addictive. The graphics are good and the gameplay is smooth. The only thing I don't like is that sometimes the enemy tanks can shoot through walls and obstacles, which is unfair. Other than that, I recommend this game to anyone who likes tank games." - A Google user
-- "This is a very good game. It has many levels and modes to play. The tanks are very cool and have different abilities. The multiplayer mode is also fun and competitive. The only problem is that sometimes the game crashes or freezes, which is annoying. Please fix this bug." - A Google user
-- "I love this game. It is very challenging and exciting. The tanks are awesome and have different weapons and skills. The maps are also very diverse and have different obstacles and enemies. The multiplayer mode is also very fun and allows you to play with your friends or other players online. The best tank game ever." - A Google user
-
-If you want to download Tank Hero - Awesome tank war g by BETTA GAMES, you can click here.
- Tank Games by CrazyGames
-Tank Games by CrazyGames is a collection of free online tank games that you can play on your browser, without downloading any app or software. You can choose from a variety of tank games, such as Tank Trouble, Tank Off, Tanko.io, Tank Fury, Tank Wars, and more. You can play solo or with your friends, and enjoy the different modes, graphics, and features of each game. You can also rate and comment on the games, and see what other players think of them.
-Here are some of the reviews from satisfied players:
-
-- "Tank Trouble is my favorite tank game on this site. It is very fun and addictive. You can play with up to 3 players on the same keyboard, or with 2 players online. The game is simple but challenging, as you have to avoid the bullets that bounce off the walls and hit your opponents. The game also has different power-ups that can give you an advantage or a disadvantage. I love this game." - A CrazyGames user
-- "Tank Off is a very cool tank game on this site. It is a 3D multiplayer game where you have to capture the enemy flag and bring it back to your base. You can customize your tank with different colors, skins, and weapons. You can also join different rooms and teams, and chat with other players. The game is very realistic and fun." - A CrazyGames user
-- "Tanko.io is a very fun tank game on this site. It is a multiplayer game where you have to join one of the two teams, red or blue, and fight for territory and resources. You can upgrade your tank with different stats, such as speed, damage, health, and reload time. You can also cooperate with your teammates and use strategy to win the game. The game is very simple but enjoyable." - A CrazyGames user
-
-If you want to play Tank Games by CrazyGames, you can click here.
- Tips and Tricks for Playing Tank Games
-Tank games are fun and exciting, but they can also be challenging and frustrating at times. Here are some tips and tricks that can help you improve your skills, strategy, and enjoyment of tank games:
-
-- Know your tank: Different tanks have different strengths and weaknesses, such as speed, armor, firepower, range, accuracy, etc. You should know what your tank can do and what it cannot do, and use it accordingly.
-- Know your enemy: Similarly, you should know what your enemy's tank can do and what it cannot do, and exploit their weaknesses.
-- Know your terrain: The terrain can affect your movement, visibility, cover, and shooting angle. You should use the terrain to your advantage, such as hiding behind obstacles, using hills for elevation, or avoiding open areas.
-- Know your weapon: Different weapons have different effects, such as damage, splash radius, reload time, etc. You should use the weapon that suits your situation best.
-- Know your mode: Different modes have different objectives, rules, and rewards. You should know what your mode requires you to do, such as capturing flags, destroying bases, or surviving waves, and play accordingly.
-- Know your team: If you are playing in a team mode, you should cooperate with your teammates, communicate with them, and support them. You can also use tactics such as flanking, distracting, or ambushing your enemies.
-- Know your limit: Tank games can be addictive, but they can also be stressful and tiring. You should know when to take a break, relax, and have fun.
-
- Conclusion
-Tank games are a genre of games that features awesome tank action and fun gameplay. You can play some of the best tank games on Android, such as Tank Hero by Clapfoot Inc., Tank Hero - Awesome tank war g by BETTA GAMES, and Tank Games by CrazyGames. You can also improve your skills, strategy, and enjoyment of tank games by following some tips and tricks. Tank games are a great way to spend your time and have fun. So, what are you waiting for? Download your favorite tank game today and become a tank hero!
- FAQs
-Here are some frequently asked questions and answers about tank games:
-
-- What are the benefits of playing tank games?
-Tank games can have many benefits, such as improving your hand-eye coordination, reaction time, spatial awareness, problem-solving skills, strategic thinking, teamwork skills, and creativity. They can also help you relieve stress, have fun, and learn about tanks and history.
-- What are the drawbacks of playing tank games?
-Tank games can also have some drawbacks, such as consuming your time, battery, and data. They can also cause eye strain, headaches, neck pain, or wrist pain if you play for too long or in a bad posture. They can also make you frustrated, angry, or bored if you lose or face unfair opponents.
-- How to choose the best tank game for me?
-The best tank game for you depends on your personal preference, taste, and device. You should consider factors such as the graphics quality, sound effects, gameplay mechanics, difficulty level, game modes, features, reviews, ratings, price, and compatibility of the game. You should also try out different games and see which one you enjoy the most.
-- How to download tank games on Android?
-You can download tank games on Android from various sources, such as Google Play Store, third-party websites, or APK files. However, you should be careful and only download from trusted and secure sources. You should also check the permissions and requirements of the game before installing it.
-- How to play tank games on Android?
-You can play tank games on Android by using the touch screen controls or a compatible controller. You should also adjust the settings and options of the game to suit your preference and device. You should also follow the instructions and rules of the game to play it properly.
-
197e85843d
-
-
\ No newline at end of file
diff --git a/spaces/1pelhydcardo/ChatGPT-prompt-generator/assets/Clash of Clans Mod iOS The Ultimate Guide to Download and Install.md b/spaces/1pelhydcardo/ChatGPT-prompt-generator/assets/Clash of Clans Mod iOS The Ultimate Guide to Download and Install.md
deleted file mode 100644
index de0d06d8b1e08362f5132d795376a815b8f7aef1..0000000000000000000000000000000000000000
--- a/spaces/1pelhydcardo/ChatGPT-prompt-generator/assets/Clash of Clans Mod iOS The Ultimate Guide to Download and Install.md
+++ /dev/null
@@ -1,113 +0,0 @@
-
-Clash of Clans Mod iOS Download: How to Play with Unlimited Resources and Custom Mods
- Clash of Clans is one of the most popular mobile games in the world, with millions of players who enjoy building their villages, training their troops, and fighting other players in epic clan wars. But what if you could play the game with unlimited resources and custom mods that give you new buildings, heroes, troops, spells, and more? That's what Clash of Clans mod iOS download offers you. In this article, we will explain what Clash of Clans mod is, what are its features, how to download and install it on your iOS device, and what are the risks and benefits of using it.
- What is Clash of Clans and why is it so popular?
- Clash of Clans is a strategy game where you build your village, train your troops, and fight other players. You can join a clan or create your own, and participate in clan wars, clan games, friendly wars, friendly challenges, events, tournaments, leagues, and more. You can also explore different game modes such as single-player campaign, builder base, practice mode, super troop mode, etc. You can collect resources such as gold, elixir, dark elixir, gems, etc. to upgrade your buildings, troops, heroes, spells, etc. You can also customize your village with different skins, sceneries, decorations, etc.
-clash of clans mod ios download
Download Zip ✯ https://urlin.us/2uSXdj
- Clash of Clans has been constantly evolving since its launch in 2012. It has received countless updates that added new content and features to the game. It has also developed a huge community of players who share their tips, tricks, strategies, guides, videos, memes, fan art, etc. on various platforms such as Reddit, YouTube, Discord, Instagram, Twitter, Facebook, etc. Clash of Clans is a game that appeals to players of all ages and backgrounds. It is a game that combines strategy, creativity,
fun, competition, cooperation, and social interaction. It is a game that never gets old or boring.
- What is Clash of Clans mod and what are its features?
- Clash of Clans mod is a modified version of the game that gives you unlimited resources and custom mods that enhance your gaming experience. It is not an official version of the game, but a fan-made one that runs on private servers. It is not affiliated with or endorsed by Supercell, the developer of Clash of Clans.
- Clash of Clans mod has many features that make it different from the original game. Some of these features are:
- Unlimited gold, elixir, gems, and dark elixir
- With Clash of Clans mod, you don't have to worry about running out of resources. You can have as much gold, elixir, gems, and dark elixir as you want. You can use them to upgrade your buildings, troops, heroes, spells, etc. without any waiting time. You can also buy anything from the shop with gems without spending real money.
- New buildings, heroes, troops, and spells with special abilities
- With Clash of Clans mod, you can access new buildings, heroes, troops, and spells that are not available in the original game. These include:
- - New buildings such as dragon tower, witch tower, archer queen tower, barbarian king tower, etc.
-- New heroes such as goblin king, giant king, skeleton king, ice wizard king, etc.
-- New troops such as dragon rider, hog rider 2.0, lava hound 2.0, electro dragon 2.0, etc.
-- New spells such as freeze spell 2.0, rage spell 2.0, jump spell 2.0, clone spell 2.0, etc.
- These new buildings, heroes, troops, and spells have special abilities that make them more powerful and fun to use.
- PvP battles with other modded players
- With Clash of Clans mod, you can fight other players who are also using the modded version of the game. You can challenge them in friendly battles or attack their villages in multiplayer mode. You can also join or create a clan with other modded players and participate in clan wars and clan games with them.
-clash of clans modded ios app
-clash of clans hack ios no jailbreak
-clash of clans private server ios download
-clash of clans unlimited gems mod ios
-clash of clans mod apk ios free download
-clash of clans cheats ios download
-clash of clans plenixclash ios
-clash of clans mod menu ios
-clash of clans custom mods ios
-clash of clans hack version download ios
-clash of clans modded server ios
-clash of clans hack tool ios
-clash of clans unlimited everything mod ios
-clash of clans mod ipa download
-clash of clans hacked account ios
-clash of clans modded base ios
-clash of clans hack generator ios
-clash of clans unlimited troops mod ios
-clash of clans mod appvalley ios
-clash of clans hack online ios
-clash of clans mod tutuapp ios
-clash of clans hack cydia ios
-clash of clans unlimited resources mod ios
-clash of clans mod tweakbox ios
-clash of clans hack app ios
-clash of clans mod panda helper ios
-clash of clans hack dns code ios
-clash of clans unlimited coins and elixir mod ios
-clash of clans mod ignition ios
-clash of clans hack apk download ios
-clash of clans mod ipogo ios
-clash of clans hack reddit ios
-clash of clans unlimited gold and dark elixir mod ios
-clash of clans mod altstore ios
-clash of clans hack youtube ios
-clash of clans mod buildstore ios
-clash of clans hack no verification ios
-clash of clans unlimited builder base mod ios
-clash of clans mod cokernutx ios
-clash of clans hack no survey ios
-clash of clans unlimited heroes and spells mod ios
-clash of clans mod appcake ios
-clash of clans hack no human verification ios
-clash of clans unlimited clan wars mod ios
-clash of clans mod operationidroid ios
-clash of clans hack without offers ios
-clash of clans unlimited town hall 15 mod ios
-clash of clans mod appdb pro ios
-clash of clans hack without downloading apps ios
- Clan wars and clan games with modded clans
- With Clash of Clans mod, you can enjoy clan wars and clan games with other modded clans. You can compete with them for trophies and rewards. You can also complete clan games tasks and earn clan points to unlock clan perks and rewards.
How to download and install Clash of Clans mod on iOS devices?
- If you want to try out Clash of Clans mod on your iOS device, you need to follow these steps:
- Download the modded app from a reliable source
- The first step is to download the modded app from a reliable source. There are many websites that claim to offer Clash of Clans mod iOS download, but not all of them are safe and trustworthy. Some of them may contain viruses, malware, or spyware that can harm your device or steal your personal information. Some of them may also provide fake or outdated versions of the mod that may not work properly or cause errors.
- Therefore, you need to be careful and do some research before downloading any modded app. You can check the reviews, ratings, comments, and feedbacks of other users who have downloaded the app from the same source. You can also look for the official website or social media pages of the mod developers and download the app from there. You can also ask for recommendations from your friends or other modded players who have used the app before.
- Enable installation from unknown sources in your device settings
- The next step is to enable installation from unknown sources in your device settings. This is because Clash of Clans mod is not available on the App Store, and you need to install it from a third-party source. To do this, you need to go to your device settings and find the option that allows you to install apps from unknown sources. You may need to enter your passcode or use your Touch ID or Face ID to confirm this action.
- Install the modded app and launch it
- The final step is to install the modded app and launch it. You need to locate the downloaded file on your device and tap on it to start the installation process. You may need to agree to some terms and conditions and grant some permissions to the app. Once the installation is complete, you can launch the app and enjoy playing Clash of Clans with unlimited resources and custom mods.
- What are the risks and benefits of using Clash of Clans mod?
- Using Clash of Clans mod can be fun and exciting, but it also comes with some risks and benefits that you should be aware of before playing. Here are some of them:
- The risks include:
- - Violating the game's terms of service and fair play policy
-- By using Clash of Clans mod, you are violating the game's terms of service and fair play policy that prohibit the use of any third-party software, tools, or modifications that alter or affect the game's functionality or performance. This means that you are playing the game in an unfair and unauthorized way that gives you an advantage over other players who are playing legitimately.
-- Getting banned from the official game servers
-- By using Clash of Clans mod, you are risking getting banned from the official game servers by Supercell, the developer of Clash of Clans. Supercell has a strict anti-cheating system that detects and bans any players who are using mods, hacks, bots, or any other cheating methods. If you get banned, you will lose access to your account, your village, your progress, your clan, your friends, and everything else related to the game. You will also not be able to create a new account or play the game again on the same device.
-- Losing your account or personal data to hackers or scammers
-- By using Clash of Clans mod, you are exposing your account or personal data to hackers or scammers who may try to steal them for malicious purposes. Some modded apps may contain hidden codes or scripts that can access your device's data, such as your contacts, photos, messages, emails, passwords, bank details, etc. Some websites may also ask you to provide your account details, such as your username, password, email address, etc., in order to download or use the mod. These hackers or scammers may use your account or personal data to hack into your other accounts, steal your money, identity, or information, blackmail you, spam you, or harm you in other ways.
- The benefits include:
- - Having more fun and freedom in the game
-- By using Clash of Clans mod, you can have more fun and freedom in the game. You can play with unlimited resources and custom mods that give you new buildings, heroes, troops, spells, and more. You can experiment with different strategies and combinations that are not possible in the original game. You can also challenge yourself and others with new content and features that
enhance your gaming experience. You can also enjoy playing with other modded players who share your passion and interest in the game.
- - Experimenting with different strategies and combinations
-- By using Clash of Clans mod, you can experiment with different strategies and combinations that are not possible in the original game. You can try out new buildings, heroes, troops, and spells that have special abilities and effects. You can also mix and match different troops and spells to create unique and powerful attacks. You can also test your skills and knowledge in different game modes and scenarios that challenge your creativity and logic.
-- Challenging yourself and others with new content and features
-- By using Clash of Clans mod, you can challenge yourself and others with new content and features that add more excitement and variety to the game. You can fight other modded players in PvP battles or clan wars and see who has the better village, troops, or strategy. You can also participate in clan games or events with other modded clans and compete for trophies and rewards. You can also explore new maps, sceneries, themes, etc. that change the look and feel of the game.
- Conclusion
- Clash of Clans mod iOS download is a way to play the game with unlimited resources and custom mods that give you new buildings, heroes, troops, spells, and more. However, it also comes with some risks and benefits that you should consider before playing. If you decide to try it out, make sure you download it from a trusted source and follow the installation guide carefully. Remember that Clash of Clans mod is not an official version of the game, but a fan-made one that runs on private servers. It is not affiliated with or endorsed by Supercell, the developer of Clash of Clans.
- We hope this article has helped you understand what Clash of Clans mod is, what are its features, how to download and install it on your iOS device, and what are the risks and benefits of using it. If you have any questions or feedback, please feel free to leave a comment below. Thank you for reading!
- FAQs
- Here are some frequently asked questions about Clash of Clans mod iOS download:
- - Is Clash of Clans mod iOS download safe?
-- Clash of Clans mod iOS download is not completely safe, as it involves downloading and installing a modded app from a third-party source that may contain viruses, malware, or spyware that can harm your device or steal your personal information. It also violates the game's terms of service and fair play policy that prohibit the use of any third-party software, tools, or modifications that alter or affect the game's functionality or performance. You may also get banned from the official game servers by Supercell if they detect that you are using a modded app.
-- Is Clash of Clans mod iOS download free?
-- Clash of Clans mod iOS download is free, as you don't have to pay anything to download or use the modded app. However, you may have to watch some ads or complete some surveys or offers to access some websites that provide the modded app. You may also have to spend some real money to buy some in-app purchases or subscriptions that some modded apps may offer.
-- Can I play Clash of Clans mod iOS download with my friends who are playing the original game?
-- No, you cannot play Clash of Clans mod iOS download with your friends who are playing the original game. This is because Clash of Clans mod runs on private servers that are separate from the official game servers. You can only play with other players who are also using the modded app on the same private server.
-- Can I switch between Clash of Clans mod iOS download and the original game?
-- Yes, you can switch between Clash of Clans mod iOS download and the original game by installing both apps on your device. However, you need to make sure that you use different accounts for each app, as using the same account may cause errors or conflicts. You also need to make sure that you backup your progress on both apps regularly, as deleting or uninstalling one app may erase your data on the other app.
-- Can I update Clash of Clans mod iOS download?
-- Yes, you can update Clash of Clans mod iOS download by downloading and installing the latest version of the modded app from the same source where you got it from. However, you need to be careful as some updates may not be compatible with your device or may cause errors or
problems. You also need to check if the update is safe and trustworthy, as some updates may contain viruses, malware, or spyware that can harm your device or steal your personal information.
197e85843d
-
-
\ No newline at end of file
diff --git a/spaces/1phancelerku/anime-remove-background/Bhuj The Pride of India - A Movie that Celebrates the Spirit of India.md b/spaces/1phancelerku/anime-remove-background/Bhuj The Pride of India - A Movie that Celebrates the Spirit of India.md
deleted file mode 100644
index 01fae80dec54a327f8161c850867780c85eb86d4..0000000000000000000000000000000000000000
--- a/spaces/1phancelerku/anime-remove-background/Bhuj The Pride of India - A Movie that Celebrates the Spirit of India.md
+++ /dev/null
@@ -1,114 +0,0 @@
-
-Bhuj: The Pride of India Movie Download Filmyzilla
-Bhuj: The Pride of India is a 2021 Indian Hindi-language war film that has become one of the most talked-about movies of the year. The film is based on the true events of the 1971 Indo-Pak war, when a group of 300 local women helped rebuild a damaged airstrip in Bhuj, Gujarat, under the leadership of Indian Air Force Squadron Leader Vijay Karnik. The film showcases the bravery, patriotism, and sacrifice of these unsung heroes who played a pivotal role in India's victory over Pakistan.
-The film is directed by Abhishek Dudhaiya and stars Ajay Devgn as Vijay Karnik, Sanjay Dutt as Ranchordas Pagi, Sonakshi Sinha as Sunderben Jetha Madharparya, Sharad Kelkar as Ram Karan 'RK' Nair, Nora Fatehi as Heena Rehman, Ammy Virk as Vikram Singh Baj Jethaaz, Pranitha Subhash as Usha Karnik, Mahesh Shetty as Vinod Karnik, Ihana Dhillon as Nimrat Kaur, Pawan Shankar as Mohammad Hussain OMANI, Zahid Ali as Brigadier Mukhtar Baig, and Heroxicated Armaan as Armaan.
-bhuj the pride of india movie download filmyzilla
Download ✵ https://jinyurl.com/2uNT9h
-The film was released on 13 August 2021 on Disney+ Hotstar, coinciding with the Independence Day weekend. The film received mixed reviews from critics but positive feedback from audiences, who praised the performances, action sequences, patriotic theme, and emotional quotient of the film. The film also received a nomination for Best Film at the Zee Cine Awards 2022.
- Why is Bhuj: The Pride of India movie popular?
-Bhuj: The Pride of India movie has gained popularity among viewers for various reasons. Some of them are:
-How does the movie depict the historical events of the 1971 Indo-Pak war?
-The movie depicts one of the most crucial episodes of the 1971 Indo-Pak war, when Pakistan launched a surprise attack on the Bhuj airbase on 8 December 1971. The airstrip was severely damaged and rendered unusable for Indian fighter planes. Vijay Karnik, who was in charge of the airbase, decided to rebuild the airstrip with the help of 300 local women from Madhapur village, who volunteered to work day and night under his guidance. They used their sarees, carrom boards, metal sheets, and other materials to fill up the craters and level up the runway. They also disguised themselves as villagers to avoid detection by Pakistani spies. They managed to complete the task in just 72 hours and enabled Indian planes to take off and launch counterattacks on Pakistan. This feat was later termed as India's 'Pearl Harbour' moment.
-What are the positive reviews and ratings of the movie?
-The movie has received positive reviews and ratings from viewers who have appreciated the performances, action sequences, patriotic theme, and emotional quotient of the film. The film has a rating of 7.1 out of 10 on IMDb, 4.2 out of 5 on Google, and 3.5 out of 5 on Times of India. Some of the positive reviews are: - "Bhuj: The Pride of India is a tribute to the unsung heroes of the 1971 war. The film is well-made, well-acted, and well-directed. It keeps you hooked till the end with its gripping story, thrilling action, and patriotic fervour. Ajay Devgn, Sanjay Dutt, Sonakshi Sinha, and the rest of the cast have done a commendable job in portraying their characters. The film is a must-watch for every Indian who loves their country and respects their soldiers." - Ramesh Sharma, Google user - "Bhuj: The Pride of India is a movie that will make you proud of being an Indian. The movie showcases the bravery, patriotism, and sacrifice of the 300 women who helped rebuild the Bhuj airstrip in just 72 hours. The movie also highlights the role of the Indian Air Force in defending the nation from Pakistan's aggression. The movie has some amazing action scenes, especially the aerial combat scenes, which are very realistic and thrilling. The movie also has some emotional moments, which will touch your heart. Ajay Devgn as Vijay Karnik is outstanding in his performance. He brings out the courage, leadership, and dignity of his character very well. Sanjay Dutt as Ranchordas Pagi is also brilliant in his role as a local spy who helps Vijay Karnik in his mission. Sonakshi Sinha as Sunderben Jetha Madharparya is also impressive in her role as the leader of the women who volunteer to rebuild the airstrip. She shows the strength, determination, and resilience of her character very well. The other actors also do justice to their roles. The direction by Abhishek Dudhaiya is commendable. He has made a movie that is not only entertaining but also inspiring and patriotic. The music by Arko Pravo Mukherjee, Tanishk Bagchi, Lijo George-DJ Chetas, and Gourov Dasgupta is also good and suits the mood of the film. The cinematography by Aseem Bajaj is also excellent and captures the beauty and intensity of the scenes very well. The editing by Dharmendra Sharma is also crisp and smooth. The production design by Narendra Rahurikar is also authentic and realistic. The costumes by Payal Saluja are also appropriate and fitting for the characters and the period. The sound design by Parikshit Lalwani and Kunal Mehta is also superb and enhances the impact of the scenes. The visual effects by Red Chillies VFX are also stunning and believable. The action choreography by Peter Hein is also spectacular and exciting. The film is a visual treat for the eyes and a feast for the soul." - Rajesh Kumar, IMDb user - "Bhuj: The Pride of India is a film that celebrates the spirit of India and its heroes. The film tells the story of how a group of 300 women from Madhapur village helped rebuild a damaged airstrip in Bhuj under the guidance of IAF Squadron Leader Vijay Karnik during the 1971 war with Pakistan. The film is a tribute to their courage, patriotism, and sacrifice that helped India win the war. The film has a stellar cast that delivers powerful performances. Ajay Devgn as Vijay Karnik is superb in his role as the brave and inspiring officer who leads his team and motivates the women to join his mission. He portrays his character with conviction and charisma. Sanjay Dutt as Ranchordas Pagi is excellent in his role as a local spy who assists Vijay Karnik in gathering intelligence and foiling Pakistan's plans. He brings out the humour, loyalty, and heroism of his character very well. Sonakshi Sinha as Sunderben Jetha Madharparya is wonderful in her role as the leader of the women who volunteer to rebuild the airstrip. She displays the courage, determination, and compassion of her character very well. Sharad Kelkar as Ram Karan 'RK' Nair is fantastic in his role as Vijay Karnik's friend and colleague who supports him in his mission. He shows his character's friendship, professionalism, and bravery very well. Nora Fatehi as Heena Rehman is impressive in her role as a Pakistani spy who defects to India after falling in love with RK Nair. She shows her character's conflict, romance, and redemption very well. Ammy Virk as Vikram Singh Baj Jethaaz is splendid in his role as an IAF pilot who flies his plane despite being injured to help Vijay Karnik in his mission. He portrays his character's courage, loyalty, and patriotism very well. Pranitha Subhash as Usha Karnik is lovely in her role as Vijay Karnik's wife who supports him in his mission. She shows her character's love, care, and strength very well. The other actors also do a great job in their roles. The film is directed by Abhishek Dudhaiya who has done a remarkable job in making a film that is not only entertaining but also inspiring and patriotic. He has handled the historical subject with sensitivity and accuracy. He has also balanced the drama, action, emotion, and humour of the film very well. The film is a must-watch for every Indian who loves their country and respects their soldiers." - Priya Singh, Times of India user
- What are the awards and nominations of the movie?
-The movie has also received recognition and appreciation from various award ceremonies and festivals. The movie has received a nomination for Best Film at the Zee Cine Awards 2022. The movie has also won the Best Action Film award at the Dadasaheb Phalke International Film Festival 2022. The movie has also been selected for screening at the Indian Film Festival of Melbourne 2022. The movie has also been praised by various celebrities and dignitaries, such as Prime Minister Narendra Modi, Defence Minister Rajnath Singh, Air Chief Marshal Rakesh Kumar Singh Bhadauria, and others.
- How to watch Bhuj: The Pride of India movie legally?
-Bhuj: The Pride of India movie is a film that deserves to be watched legally and ethically. However, some people may be tempted to download the movie from Filmyzilla and other torrent websites that offer pirated copies of the movie for free. This is not only illegal but also risky for various reasons. Some of them are:
-bhuj the pride of india full movie download filmyzilla
-bhuj the pride of india movie download 480p filmyzilla
-bhuj the pride of india movie download 720p filmyzilla
-bhuj the pride of india movie download in hindi filmyzilla
-bhuj the pride of india movie download hd filmyzilla
-bhuj the pride of india movie download free filmyzilla
-bhuj the pride of india movie download online filmyzilla
-bhuj the pride of india movie download link filmyzilla
-bhuj the pride of india movie download mp4 filmyzilla
-bhuj the pride of india movie download mkv filmyzilla
-bhuj the pride of india movie download torrent filmyzilla
-bhuj the pride of india movie download moviespyhd[^1^]
-bhuj the pride of india movie download internet archive[^2^]
-bhuj the pride of india movie download disney+ hotstar[^3^]
-bhuj the pride of india movie download telegram channel
-bhuj the pride of india movie download google drive
-bhuj the pride of india movie download watch online
-bhuj the pride of india movie download 1080p filmyzilla
-bhuj the pride of india movie download 300mb filmyzilla
-bhuj the pride of india movie download bluray filmyzilla
-bhuj the pride of india movie download dvdrip filmyzilla
-bhuj the pride of india movie download english subtitles
-bhuj the pride of india movie download hd quality
-bhuj the pride of india movie download leaked online
-bhuj the pride of india movie download pagalworld
-bhuj the pride of india movie download quora
-bhuj the pride of india movie download review
-bhuj the pride of india movie download songs
-bhuj the pride of india movie download tamilrockers
-bhuj the pride of india movie download video
-Why is it illegal and risky to download movies from Filmyzilla and other torrent websites?
-Downloading movies from Filmyzilla and other torrent websites is illegal and risky because:
-
-- It violates the copyright laws and infringes the intellectual property rights of the filmmakers and producers who have invested their time, money, and effort in making the movie.
-- It causes huge losses to the film industry and affects the livelihoods of thousands of people who work in it.
-- It deprives the filmmakers and producers of their rightful share of revenue and recognition that they deserve for their work.
-- It exposes the users to various cyber threats, such as malware, viruses, phishing, identity theft, data breach, and others that can harm their devices and personal information.
-- It compromises the quality and experience of watching the movie, as the pirated copies are often low in resolution, audio, subtitles, and other features.
-- It disrespects the hard work and creativity of the filmmakers and actors who have made the movie with passion and dedication.
-
-Therefore, it is advisable to avoid downloading movies from Filmyzilla and other torrent websites and watch them legally instead.
-What are the legal and safe ways to watch Bhuj: The Pride of India movie online or offline?
-The legal and safe ways to watch Bhuj: The Pride of India movie online or offline are:
-
-- Watch the movie on Disney+ Hotstar, which is the official streaming partner of the movie. You can subscribe to Disney+ Hotstar VIP or Premium plans to watch the movie along with other exclusive content.
-- Watch the movie on TV channels that have acquired the satellite rights of the movie. You can check the TV listings or guides to find out when and where the movie will be telecasted.
-- Watch the movie on DVD or Blu-ray discs that have been released by the official distributors of the movie. You can buy or rent them from authorized stores or online platforms.
-- Watch the movie on legal online platforms that have obtained the digital rights of the movie. You can pay a nominal fee to watch or download the movie from these platforms.
-
-What are the benefits of watching movies legally?
-The benefits of watching movies legally are:
-
-- You support the film industry and encourage more quality content to be produced.
-- You enjoy the best quality and experience of watching the movie with high resolution, audio, subtitles, and other features.
-- You protect your devices and personal information from cyber threats that can harm them.
-- You respect the filmmakers and actors who have made the movie with passion and dedication.
-
- Conclusion
-Bhuj: The Pride of India is a film that celebrates the spirit of India and its heroes. The film tells the story of how a group of 300 women from Madhapur village helped rebuild a damaged airstrip in Bhuj under the guidance of IAF Squadron Leader Vijay Karnik during the 1971 war with Pakistan. The film is a tribute to their courage, patriotism, and sacrifice that helped India win the war. The film has a stellar cast that delivers powerful performances. The film has also received positive reviews and ratings from viewers and critics. The film has also received recognition and appreciation from various award ceremonies and festivals. The film is a must-watch for every Indian who loves their country and respects their soldiers.
-However, the film should be watched legally and ethically, and not downloaded from Filmyzilla and other torrent websites that offer pirated copies of the film for free. Downloading movies from these websites is illegal and risky, as it violates the copyright laws, causes losses to the film industry, exposes users to cyber threats, compromises the quality and experience of watching the film, and disrespects the hard work and creativity of the filmmakers and actors. There are many legal and safe ways to watch Bhuj: The Pride of India movie online or offline, such as Disney+ Hotstar, TV channels, DVD or Blu-ray discs, and legal online platforms. Watching movies legally has many benefits, such as supporting the film industry, enjoying the best quality and experience of watching the film, protecting devices and personal information from cyber threats, and respecting the filmmakers and actors.
-Therefore, we urge you to watch Bhuj: The Pride of India movie legally and ethically, and enjoy this patriotic and inspiring film that will make you proud of being an Indian.
- FAQs
-Here are some frequently asked questions about Bhuj: The Pride of India movie:
-What is the budget and box office collection of Bhuj: The Pride of India movie?
-The budget of Bhuj: The Pride of India movie is estimated to be around ₹100 crore. The box office collection of the movie is not available as it was released on Disney+ Hotstar due to the COVID-19 pandemic. However, according to some reports, the movie has earned around ₹50 crore from its digital rights.
-Is Bhuj: The Pride of India movie based on a true story?
-Yes, Bhuj: The Pride of India movie is based on a true story. The movie is based on the true events of the 1971 Indo-Pak war, when a group of 300 local women helped rebuild a damaged airstrip in Bhuj, Gujarat, under the leadership of Indian Air Force Squadron Leader Vijay Karnik. The movie also depicts other real-life characters and incidents that took place during the war.
-Who are the real-life heroes portrayed in Bhuj: The Pride of India movie?
-The real-life heroes portrayed in Bhuj: The Pride of India movie are:
-
-Character Actor Real-life hero
-Vijay Karnik Ajay Devgn Indian Air Force Squadron Leader who was in charge of the Bhuj airbase during the 1971 war.
-Ranchordas Pagi Sanjay Dutt A local civilian who worked as a spy for Vijay Karnik and helped him gather intelligence and foil Pakistan's plans.
-Sunderben Jetha Madharparya Sonakshi Sinha The leader of the 300 women from Madhapur village who volunteered to rebuild the airstrip in Bhuj.
-Ram Karan 'RK' Nair Sharad Kelkar Indian Air Force Officer who was Vijay Karnik's friend and colleague.
-Heena Rehman Nora Fatehi A Pakistani spy who defected to India after falling in love with RK Nair.
-Vikram Singh Baj Jethaaz Ammy Virk Indian Air Force Pilot who flew his plane despite being injured to help Vijay Karnik in his mission.
-Usha Karnik Pranitha Subhash Vijay Karnik's wife who supported him in his mission.
-Vinod Karnik Mahesh Shetty Vijay Karnik's brother who also worked in the Indian Air Force.
-Nimrat Kaur Ihana Dhillon An Indian Air Force Officer who was RK Nair's colleague.
-Mohammad Hussain OMANI Pawan Shankar A Pakistani Air Force Officer who was Heena Rehman's handler.
-Brigadier Mukhtar Baig Zahid Ali A Pakistani Army Officer who led the attack on Bhuj airbase.
-Armaan Heroxicated Armaan A Pakistani spy who infiltrated the Indian Air Force and tried to sabotage Vijay Karnik's mission.
-
- How accurate is Bhuj: The Pride of India movie in terms of historical facts?
-Bhuj: The Pride of India movie is based on historical facts, but it also takes some creative liberties and dramatizes some events for cinematic purposes. Some of the factual and fictional aspects of the movie are:
-
-Factual Fictional
-The airstrip in Bhuj was damaged by Pakistani bombs and rebuilt by 300 local women in 72 hours under Vijay Karnik's guidance. The airstrip was damaged twice and rebuilt twice in the movie, whereas in reality, it was damaged once and rebuilt once.
-Vijay Karnik was assisted by Ranchordas Pagi, a local civilian who worked as a spy for him and helped him gather intelligence and foil Pakistan's plans. Ranchordas Pagi was shown as a scrap dealer and a master of disguise in the movie, whereas in reality, he was a shepherd and a farmer.
-Sunderben Jetha Madharparya was the leader of the 300 women who volunteered to rebuild the airstrip. She also convinced other women to join her by appealing to their patriotism and pride. Sunderben Jetha Madharparya was shown as a widow who had lost her husband and son in the war in the movie, whereas in reality, she was married and had children.
-Ram Karan 'RK' Nair was Vijay Karnik's friend and colleague who supported him in his mission. He also had a romantic relationship with Heena Rehman, a Pakistani spy who defected to India. RK Nair and Heena Rehman were fictional characters created for the movie. There is no evidence of their existence or involvement in the real events.
-Vikram Singh Baj Jethaaz was an IAF pilot who flew his plane despite being injured to help Vijay Karnik in his mission. He also dropped bombs on Pakistan's radar station and fuel depot. Vikram Singh Baj Jethaaz was a fictional character created for the movie. There is no evidence of his existence or involvement in the real events.
-
- Where can I watch the trailer of Bhuj: The Pride of India movie?
-You can watch the trailer of Bhuj: The Pride of India movie on YouTube or on Disney+ Hotstar. Here is the link to the official trailer on YouTube: [Bhuj: The Pride Of India | Official Trailer | Ajay D. Sonakshi S. Sanjay D. Nora F. Sharad K. Ammy V.]
401be4b1e0
-
-
\ No newline at end of file
diff --git a/spaces/1phancelerku/anime-remove-background/Download Naruto Shippuden Ultimate Ninja Storm 4 APK OBB and Join the Ninja World War.md b/spaces/1phancelerku/anime-remove-background/Download Naruto Shippuden Ultimate Ninja Storm 4 APK OBB and Join the Ninja World War.md
deleted file mode 100644
index 41111f25fc6ffb9dacec706f25fe55f951ee5ee3..0000000000000000000000000000000000000000
--- a/spaces/1phancelerku/anime-remove-background/Download Naruto Shippuden Ultimate Ninja Storm 4 APK OBB and Join the Ninja World War.md
+++ /dev/null
@@ -1,110 +0,0 @@
-
-How to Download Naruto Ultimate Ninja Storm 3 APK Data OBB
-If you are a fan of Naruto anime and manga, you might want to play one of the best Naruto games on your Android device. Naruto Ultimate Ninja Storm 3 is a fighting game that lets you experience the epic story and battles of the Fourth Great Ninja War. In this article, we will show you how to download Naruto Ultimate Ninja Storm 3 APK data OBB files and enjoy this amazing game on your smartphone or tablet.
-download naruto ultimate ninja storm 3 apk data obb
Download ✫✫✫ https://jinyurl.com/2uNOxd
- What is Naruto Ultimate Ninja Storm 3?
-A brief introduction to the game and its features
-Naruto Ultimate Ninja Storm 3 is a game developed by CyberConnect2 and published by Namco-Bandai for the Xbox 360 and PlayStation 3 in 2013. It is based on the Naruto Shippuden anime series and covers the events from the Five Kage Summit arc to the end of the Fourth Great Ninja War arc. The game features over 80 playable characters, each with their own unique abilities, combos, and ultimate jutsus. You can also customize your character's appearance, items, and skills.
-The game has several modes, such as Story Mode, where you can relive the main storyline and explore the ninja world; Free Battle Mode, where you can fight against the computer or another player; Online Mode, where you can challenge other players from around the world; Tournament Mode, where you can participate in various tournaments with different rules; and Collection Mode, where you can view your unlocked items, cards, movies, music, and more.
- The difference between the Full Burst and the HD versions
-In 2014, an enhanced version of the game called Naruto Ultimate Ninja Storm 3 Full Burst was released for PC, Xbox 360, PlayStation 3, and Nintendo Switch. This version added a new chapter featuring Sasuke and Itachi vs Kabuto, a new playable character (Kabuto in Sage Mode), improved graphics, revamped cinematics, 100 new missions, and 38 additional costumes.
-download naruto shippuden ultimate ninja storm 3 ppsspp android
-download ultimate ninja storm 3 battle apk obb latest version
-download naruto shippuden ultimate ninja storm 4 apk obb
-download naruto shippuden ultimate ninja storm 3 full burst apk obb
-download naruto shippuden ultimate ninja storm 3 mod apk obb
-download naruto shippuden ultimate ninja storm 3 iso for android
-download naruto shippuden ultimate ninja storm 3 highly compressed android
-download naruto shippuden ultimate ninja storm 3 road to boruto apk obb
-download naruto shippuden ultimate ninja storm 3 offline apk obb
-download naruto shippuden ultimate ninja storm 3 android apk + data
-download game naruto shippuden ultimate ninja storm 3 for android apk obb
-how to download naruto shippuden ultimate ninja storm 3 on android
-free download naruto shippuden ultimate ninja storm 3 for android
-best site to download naruto shippuden ultimate ninja storm 3 apk obb
-download naruto shippuden ultimate ninja storm 3 apk data obb no verification
-download naruto shippuden ultimate ninja storm 3 apk data obb offline mode
-download naruto shippuden ultimate ninja storm 3 apk data obb with cheats
-download naruto shippuden ultimate ninja storm 3 apk data obb english version
-download naruto shippuden ultimate ninja storm 3 apk data obb unlimited money
-download naruto shippuden ultimate ninja storm 3 apk data obb unlocked all characters
-download naruto shippuden ultimate ninja storm 3 apk data obb latest update
-download naruto shippuden ultimate ninja storm 3 apk data obb for pc
-download naruto shippuden ultimate ninja storm 3 apk data obb for ios
-download naruto shippuden ultimate ninja storm 3 apk data obb for ps4
-download naruto shippuden ultimate ninja storm 3 apk data obb for xbox one
-download naruto shippuden ultimate ninja storm revolution apk data obb
-download naruto shippuden ultimate ninja storm generations apk data obb
-download naruto shippuden ultimate ninja storm legacy apk data obb
-download naruto shippuden ultimate ninja impact apk data obb
-download naruto shippuden ultimate ninja heroes 3 apk data obb
-download boruto x naruto shinobi striker apk data obb
-download boruto x naruto voltage apk data obb
-download boruto x sarada x mitsuki shinobi collection apk data obb
-download boruto x sarada x mitsuki shinobi formation battle apk data obb
-download boruto x sarada x mitsuki shinobi war online apk data obb
-download boruto x sarada x mitsuki shinobi master senran kagura new link apk data obb
-download boruto x sarada x mitsuki shinobi master senran kagura burst re:newal apk data obb
-download boruto x sarada x mitsuki shinobi master senran kagura peach beach splash apk data obb
-download boruto x sarada x mitsuki shinobi master senran kagura estival versus apk data obb
-download boruto x sarada x mitsuki shinobi master senran kagura bon appetit! full course edition! apk data obb
-In 2017, another version of the game called Naruto Ultimate Ninja Storm 3 HD was released for PC, Xbox One, PlayStation 4, and Nintendo Switch as part of the Naruto Shippuden: Ultimate Ninja Storm Trilogy bundle. This version included all the content from the Full Burst version, but with enhanced graphics and performance for modern consoles.
- Why do you need APK data OBB files?
-The explanation of APK, data, and OBB files and their roles
-APK stands for Android Package Kit. It is a file format that contains all the code, resources, assets, certificates, and manifest of an Android app. It is similar to an executable file (.exe) on a Windows PC. You can install an APK file on your Android device by tapping on it or using a file manager app.
- Data files are files that contain additional information and settings for an Android app. They are usually stored in the internal storage of your device, under the /data/data/ folder. They can include user preferences, saved progress, cache, databases, and more. You can access and modify data files using a root explorer app, but you need to have root access on your device.
- OBB stands for Opaque Binary Blob. It is a file format that contains large amounts of data, such as graphics, audio, video, and other media. It is usually used for games and apps that have high-quality content and require a lot of storage space. OBB files are stored in the external storage of your device, under the /Android/obb/ folder. You can copy and paste OBB files using a file manager app, but you need to have the corresponding APK file installed on your device.
- The benefits of using APK data OBB files for large games
-One of the benefits of using APK data OBB files for large games is that you can save time and bandwidth by downloading them from third-party sources instead of the official app store. This way, you can avoid waiting for updates, compatibility issues, or regional restrictions. You can also backup and restore your game data easily by copying and pasting the files.
- Another benefit of using APK data OBB files for large games is that you can customize and optimize your game experience by modifying the files. For example, you can change the language, graphics quality, sound effects, and more by editing the data files. You can also unlock premium features, cheats, mods, and hacks by replacing the OBB files with modified ones. However, you should be careful when doing this, as it may cause errors, crashes, or bans.
- How to download Naruto Ultimate Ninja Storm 3 APK data OBB files?
-The requirements and precautions before downloading
-Before you download Naruto Ultimate Ninja Storm 3 APK data OBB files, you need to make sure that your device meets the minimum requirements for the game. According to the official website, you need to have at least Android 4.4 or higher, 2 GB of RAM, 4 GB of free storage space, and a stable internet connection.
- You also need to take some precautions before downloading Naruto Ultimate Ninja Storm 3 APK data OBB files from third-party sources. First, you need to enable the installation of apps from unknown sources on your device. To do this, go to Settings > Security > Unknown Sources and toggle it on. Second, you need to scan the files for viruses and malware using a reliable antivirus app. Third, you need to check the reviews and ratings of the source website and the files before downloading them.
- The steps to download and install the files
-Once you have prepared your device and found a trustworthy source for Naruto Ultimate Ninja Storm 3 APK data OBB files, you can follow these steps to download and install them:
-
-- Download the Naruto Ultimate Ninja Storm 3 APK file from the source website and save it on your device.
-- Download the Naruto Ultimate Ninja Storm 3 data file and OBB file from the source website and save them on your device.
-- Extract the Naruto Ultimate Ninja Storm 3 data file using a file extractor app such as ZArchiver or RAR. You will get a folder named
com.namcobandaigames.narutounstorm3
.
-- Copy the folder
com.namcobandaigames.narutounstorm3
and paste it in the /data/data/
folder of your device's internal storage.
-- Extract the Naruto Ultimate Ninja Storm 3 OBB file using a file extractor app such as ZArchiver or RAR. You will get a file named
main.1.com.namcobandaigames.narutounstorm3.obb
.
-- Copy the file
main.1.com.namcobandaigames.narutounstorm3.obb
and paste it in the /Android/obb/com.namcobandaigames.narutounstorm3/
folder of your device's external storage. If the folder does not exist, create it manually.
-- Tap on the Naruto Ultimate Ninja Storm 3 APK file and follow the instructions to install it on your device.
-- Launch the game and enjoy!
-
- How to play Naruto Ultimate Ninja Storm 3 on your Android device?
The tips and tricks to optimize the game performance and settings
-Now that you have successfully installed Naruto Ultimate Ninja Storm 3 on your Android device, you might want to optimize the game performance and settings to have a smooth and enjoyable gameplay. Here are some tips and tricks that you can try:
-
-- Adjust the graphics quality and resolution according to your device's specifications. You can do this by going to Options > Display Settings in the game menu. You can choose from Low, Medium, High, or Custom settings. The higher the settings, the more battery and memory the game will consume.
-- Turn off the sound effects and music if you don't need them. You can do this by going to Options > Sound Settings in the game menu. You can also adjust the volume levels of the sound effects, music, and voice.
-- Close other apps and background processes that are running on your device. This will free up some RAM and CPU resources for the game. You can do this by going to Settings > Apps > Running on your device and tapping on the apps that you want to stop.
-- Use a game booster app that can optimize your device's performance and battery life for gaming. Some examples of game booster apps are Game Booster, Game Launcher, Game Mode, and Game Turbo. You can download them from the Google Play Store or other sources.
-- Use a controller or a keyboard and mouse to play the game more comfortably and accurately. You can connect a controller or a keyboard and mouse to your device via Bluetooth, USB, or OTG cable. You can also customize the controls and buttons in the game menu.
-
- The best features and modes to enjoy in the game
-Naruto Ultimate Ninja Storm 3 is a game that offers a lot of features and modes for you to enjoy. Here are some of the best ones that you should not miss:
-
-- The Story Mode, where you can relive the epic story of Naruto Shippuden from the Five Kage Summit arc to the end of the Fourth Great Ninja War arc. You can also experience the new chapter featuring Sasuke and Itachi vs Kabuto in the Full Burst version.
-- The Free Battle Mode, where you can fight against the computer or another player in various stages and settings. You can also choose from over 80 playable characters, each with their own unique abilities, combos, and ultimate jutsus.
-- The Online Mode, where you can challenge other players from around the world in ranked or unranked matches. You can also join or create clans, chat with other players, and view your stats and rankings.
-- The Tournament Mode, where you can participate in various tournaments with different rules and prizes. You can also create your own tournaments and invite other players to join.
-- The Collection Mode, where you can view your unlocked items, cards, movies, music, and more. You can also customize your character's appearance, items, and skills.
-
- Conclusion
-A summary of the main points and a call to action
-Naruto Ultimate Ninja Storm 3 is one of the best Naruto games that you can play on your Android device. It is a fighting game that lets you experience the epic story and battles of the Fourth Great Ninja War. To download Naruto Ultimate Ninja Storm 3 APK data OBB files, you need to follow some simple steps and precautions. You also need to optimize your game performance and settings to have a smooth and enjoyable gameplay. You can also enjoy various features and modes in the game, such as Story Mode, Free Battle Mode, Online Mode, Tournament Mode, and Collection Mode.
- If you are a fan of Naruto anime and manga, you should not miss this opportunity to play Naruto Ultimate Ninja Storm 3 on your Android device. Download Naruto Ultimate Ninja Storm 3 APK data OBB files now and join the ultimate ninja adventure!
- FAQs
-Q1: Is Naruto Ultimate Ninja Storm 3 compatible with all Android devices?
-A1: No, Naruto Ultimate Ninja Storm 3 is not compatible with all Android devices. It requires at least Android 4.4 or higher, 2 GB of RAM, 4 GB of free storage space, and a stable internet connection. You should also check the compatibility of your device with the source website before downloading the files.
- Q2: How much storage space do I need to download Naruto Ultimate Ninja Storm 3 APK data OBB files?
-A2: You need about 4 GB of free storage space to download Naruto Ultimate Ninja Storm 3 APK data OBB files. The APK file is about 30 MB, the data file is about 500 MB, and the OBB file is about 3.5 GB. You should also have some extra space for the game installation and updates.
- Q3: Is Naruto Ultimate Ninja Storm 3 safe and legal to download?
-A3: It depends on the source website and the files that you download. Some websites may offer fake, corrupted, or infected files that can harm your device or steal your data. Some websites may also violate the intellectual property rights of the game developers and publishers. You should always download Naruto Ultimate Ninja Storm 3 APK data OBB files from reputable and trusted sources. You should also respect the terms and conditions of the game and the app store.
- Q4: Can I play Naruto Ultimate Ninja Storm 3 offline or online?
-A4: You can play Naruto Ultimate Ninja Storm 3 offline or online, depending on the mode that you choose. You can play Story Mode, Free Battle Mode, Tournament Mode, and Collection Mode offline, without an internet connection. You can play Online Mode online, with an internet connection. You should also note that some features and updates may require an internet connection to access.
- Q5: Where can I find more information and support for Naruto Ultimate Ninja Storm 3?
-A5: You can find more information and support for Naruto Ultimate Ninja Storm 3 on the official website of the game, the official Facebook page of the game, the official Twitter account of the game, and the official YouTube channel of the game. You can also contact the customer service of the game by sending an email to support@bandainamcoent.com or filling out a form on their website.
197e85843d
-
-
\ No newline at end of file
diff --git a/spaces/1phancelerku/anime-remove-background/Download and Play Stick War 3 The Best Strategy Game Ever.md b/spaces/1phancelerku/anime-remove-background/Download and Play Stick War 3 The Best Strategy Game Ever.md
deleted file mode 100644
index f71cb5f65067b634d045e7f68f09c212e5661de5..0000000000000000000000000000000000000000
--- a/spaces/1phancelerku/anime-remove-background/Download and Play Stick War 3 The Best Strategy Game Ever.md
+++ /dev/null
@@ -1,136 +0,0 @@
-
-How to Download Stick War 3: A Complete Guide
-Stick War 3 is a brand new stickman strategy game that is still under development. It is the sequel to the popular Stick War and Stick War: Legacy games, and it offers a lot of new features and game modes. If you are a fan of stickman games, you might be wondering how to download Stick War 3 and play it on your device. In this article, we will show you how to download Stick War 3 for Android, iOS, and Windows PC devices, as well as some tips and tricks for playing the game.
-What is Stick War 3?
-Stick War 3 is a real-time multiplayer strategy game that lets you control any unit at any time. You can team up with your friends in 2v2 matches, or play solo in various single player modes. You can also build your own custom armies and battle decks, using a variety of units, spells, enchantments, and upgrades. You can customize your battlefield with unique skins, statues, voice-lines, and emotes. You can also watch and share live replays of your games, and learn from other players' strategies.
-how do you download stick war 3
Download File ---> https://jinyurl.com/2uNMNJ
-Features of Stick War 3
-Stick War 3 has many features that make it an exciting and challenging game. Here are some of them:
-Real-Time Multiplayer Strategy PVP Matches
-You can play against other players online in real-time strategy matches. You can choose from different game modes, such as Capture the Flag, King of the Hill, or Classic. You can also join or create clans, chat with other players, and compete in leaderboards and tournaments.
-Single Player Modes
-You can also play offline in various single player modes. You can enjoy a huge ever expanding campaign mode, where you will follow an epic storyline and face different enemies and challenges. You can also practice your strategies against AI's custom armies in Proving Grounds mode, or challenge yourself in Daily Battles mode, where you will face special scenarios with fixed decks and conditions.
-Custom Armies and Battle Decks
-You can build your own custom armies and battle decks using cards that you collect and unlock from a growing selection of army types. You can choose from different units, such as Swordwrath, Spearton, Archidon , Magikill, and more. You can also use different spells, such as Fireball, Heal, Rage, and more. You can also use different enchantments, such as Poison, Freeze, Shield, and more. You can upgrade your cards to make them stronger and more effective.
-Customize your Battlefield
-You can also customize your battlefield with unique skins, statues, voice-lines, and emotes. You can choose from different themes, such as Medieval, Fantasy, Sci-Fi, and more. You can also unlock and use different skins for your units, such as Ninja, Pirate, Zombie, and more. You can also use different statues to boost your army's stats, such as Attack, Defense, Speed, and more. You can also use different voice-lines and emotes to communicate and taunt your opponents.
-Live Replays
-You can also watch and share live replays of your games. You can see how other players play and learn from their strategies. You can also see the stats and cards of both players during the replay. You can also comment and rate the replays, and follow your favorite players.
-How to install stick war 3 on android
-Stick war 3 free download for pc
-Where can I get stick war 3 apk
-Download stick war 3 from google play store
-Stick war 3 ios app download
-How to play stick war 3 online
-Stick war 3 multiplayer strategy game download
-Best tips and tricks for stick war 3
-How to update stick war 3 on my device
-Stick war 3 latest version download
-How to uninstall stick war 3 from my phone
-Stick war 3 mod apk download
-How to get unlimited gems in stick war 3
-Download stick war 3 for windows 10
-Stick war 3 offline mode download
-How to backup and restore stick war 3 data
-Stick war 3 cheats and hacks download
-How to fix stick war 3 not working issue
-Stick war 3 review and rating download
-How to join a clan in stick war 3
-Download stick war 3 for macbook
-Stick war 3 gameplay video download
-How to customize my army in stick war 3
-Download stick war 3 for chromebook
-Stick war 3 wiki and guide download
-How to unlock all units in stick war 3
-Download stick war 3 for linux
-Stick war 3 soundtrack and music download
-How to earn coins and rewards in stick war 3
-Download stick war 3 for ipad
-Stick war 3 forum and community download
-How to change language and settings in stick war 3
-Download stick war 3 for kindle fire
-Stick war 3 wallpapers and images download
-How to report a bug or problem in stick war 3
-Download stick war 3 for samsung galaxy
-Stick war 3 news and updates download
-How to contact the developer of stick war 3
-Download stick war 3 for huawei mate
-Stick war 3 memes and jokes download
-How to share my replays in stick war 3
-Download stick war 3 for nokia lumia
-Stick war 3 fan art and comics download
-How to invite my friends to play stick war 3 with me
-Download stick war 3 for lg g6
-Stick war 3 merchandise and toys download
-Massive Growing Campaign
-You can also enjoy a massive growing campaign mode that is still under development. You will follow an epic storyline that will take you to different worlds and regions. You will face different enemies and bosses that will challenge your skills and strategies. You will also unlock new cards and rewards as you progress through the campaign.
-How to Download Stick War 3 for Android Devices
-If you want to play Stick War 3 on your Android device, you will need to download it from the Google Play Store. Here are the steps to do so:
-Step 1: Go to Google Play Store
-Open the Google Play Store app on your Android device. If you don't have it installed, you can download it from here.
-Step 2: Search for Stick War 3
-Type "Stick War 3" in the search bar and tap on the magnifying glass icon. You should see the game's icon with the name "Stick War 3: RTS Multiplayer" by Max Games Studios.
-Step 3: Tap on Install
-Tap on the green Install button to start downloading the game. You might need to accept some permissions and terms of service before proceeding.
-Step 4: Wait for the Download to Finish
-Wait for the download to finish. It might take a few minutes depending on your internet speed and device storage. You can see the progress bar on the screen.
-Step 5: Enjoy the Game
-Once the download is complete, you can tap on the Open button to launch the game. You can also find the game's icon on your home screen or app drawer. Enjoy playing Stick War 3 on your Android device!
-How to Download Stick War 3 for iOS Devices
-If you want to play Stick War 3 on your iOS device, you will need to download it from the App Store. Here are the steps to do so:
-Step 1: Go to App Store
-Open the App Store app on your iOS device. If you don't have it installed, you can download it from here.
-Step 2: Search for Stick War 3
-Type "Stick War 3" in the search bar and tap on the blue Search button. You should see the game's icon with the name "Stick War 3: RTS Multiplayer" by Max Games Studios.
-Step 3: Tap on Get
-Tap on the blue Get button to start downloading the game. You might need to enter your Apple ID password or use Touch ID or Face ID before proceeding.
-Step 4: Wait for the Download to Finish
-Wait for the download to finish. It might take a few minutes depending on your internet speed and device storage. You can see the progress circle on the screen.
-Step 5: Enjoy the Game
-Once the download is complete, you can tap on the Open button to launch the game. You can also find the game's icon on your home screen or app library. Enjoy playing Stick War 3 on your iOS device!
How to Download Stick War 3 for Windows PC
-If you want to play Stick War 3 on your Windows PC, you will need to download an APK file of the game and run it using an emulator tool. Here are the steps to do so:
-Step 1: Go to APKPure Website
-Open your web browser and go to the APKPure website. This is a trusted source for downloading APK files of Android apps and games. You can access it from here.
-Step 2: Search for Stick War 3
-Type "Stick War 3" in the search bar and press Enter. You should see the game's icon with the name "Stick War 3: RTS Multiplayer" by Max Games Studios.
-Step 3: Click on Download APK (3.6 MB)
-Click on the green Download APK (3.6 MB) button to start downloading the APK file of the game. You might need to choose a download location and confirm the download before proceeding.
-Step 4: Wait for the Download to Finish
-Wait for the download to finish. It might take a few seconds depending on your internet speed and PC storage. You can see the progress bar on the screen.
-Step 5: Install the GameLoop Tool and Run the APK File
-To run the APK file of the game, you will need an emulator tool that can simulate an Android device on your PC. We recommend using the GameLoop tool, which is designed for playing mobile games on PC. You can download it from here.
-Once you have downloaded and installed the GameLoop tool, open it and click on the My Games tab. Then, click on the Local Install button and select the APK file of Stick War 3 that you have downloaded. The game will be installed and added to your game library.
-To play the game, click on its icon and wait for it to load. You can also customize the settings, such as keyboard controls, graphics, sound, and more. Enjoy playing Stick War 3 on your Windows PC!
-Tips and Tricks for Playing Stick War 3
-Stick War 3 is a fun and addictive game, but it can also be challenging and competitive. To help you improve your skills and strategies, here are some tips and tricks for playing Stick War 3:
-Tip 1: Choose Your Deck Wisely
-Your deck is your main weapon in Stick War 3. It determines what units, spells, and enchantments you can use in battle. Therefore, you should choose your deck wisely based on your play style, strategy, and opponent.
-You can have up to three decks at a time, and you can switch between them before each match. You can also edit your decks by adding or removing cards, or changing their order. You should try to balance your deck with different types of cards, such as melee units, ranged units, support units, offensive spells, defensive spells, and more.
-You should also consider the cost and cooldown of each card, as well as their synergies and counters. For example, if you have a lot of expensive cards, you might run out of mana quickly. If you have a lot of slow cards, you might lose tempo and initiative. If you have a lot of cards that work well together, you might create powerful combos. If you have a lot of cards that counter your opponent's cards, you might gain an advantage.
-Tip 2: Use Your Spells and Enchantments Strategically
-Your spells and enchantments are your special abilities in Stick War 3. They can make a big difference in battle if used strategically. Therefore, you should use them wisely based on the situation and timing.
-You should use your spells and enchantments when they can have the most impact or value. For example, you can use a Fireball spell to damage multiple enemies at once or finish off a low-health unit. You can use a Heal spell to restore health to your injured units or prevent them from dying. You can use a Rage spell to boost your attack speed and damage or break through enemy defenses.
-You should also use your spells and enchantments when they can counter or negate your opponent's spells and enchantments. For example, you can use a Freeze spell to stop an enemy unit from attacking or moving or cancel their Rage spell. You can use a Shield spell to protect your units from enemy spells or attacks or block their Fireball spell.
-Tip 3: Control Your Units Manually When Needed
-Your units are your main forces in Stick War 3. They will automatically attack and move according to their AI, but you can also control them manually when needed. Therefore, you should control your units manually when you can improve their performance or outcome.
-You can control your units manually by tapping on them and dragging them to a desired location or target. You can also use the buttons on the bottom of the screen to select all units, select a specific type of unit, or deselect all units. You can also use the buttons on the top of the screen to change the formation or behavior of your units.
-You should control your units manually when you can make better decisions or actions than their AI. For example, you can control your units manually to avoid enemy spells or traps, to focus fire on a priority target, to retreat or advance when needed, to flank or surround the enemy, or to use their special abilities at the right time.
-Tip 4: Watch and Learn from Live Replays and Daily Battles
-One of the best ways to improve your skills and strategies in Stick War 3 is to watch and learn from live replays and daily battles. These are videos of real matches that you can watch and analyze. Therefore, you should watch and learn from live replays and daily battles whenever you can.
-You can watch live replays and daily battles by tapping on the TV icon on the main menu. You can choose from different categories, such as Top Players, Featured Matches, Clans, Friends, or Your Replays. You can also filter by game mode, army type, or rating.
-You should watch live replays and daily battles to learn from other players' strategies and mistakes. You can see how they build their decks, how they use their spells and enchantments, how they control their units, how they react to different situations, and more. You can also see the stats and cards of both players during the replay. You can also comment and rate the replays, and follow your favorite players.
-Conclusion and FAQs
-Stick War 3 is a fun and addictive stickman strategy game that is still under development. It offers a lot of new features and game modes that will keep you entertained and challenged. You can download Stick War 3 for Android, iOS, and Windows PC devices using the steps we have shown you in this article. You can also improve your skills and strategies using the tips and tricks we have shared with you in this article.
-We hope you have enjoyed this article and learned something new. If you have any questions or feedback, please feel free to leave a comment below. Here are some FAQs that might help you:
-FAQ 1: When will Stick War 3 be officially released?
-Stick War 3 is still under development and there is no official release date yet. However, you can join the beta testing program by following the instructions on the game's official website or social media pages. You can also subscribe to their newsletter or follow their blog for updates and news.
-FAQ 2: How can I get more cards and gems in Stick War 3?
-You can get more cards and gems in Stick War 3 by playing matches, completing quests, opening chests, watching ads, or buying them with real money. You can also join clans and participate in clan wars to get more rewards.
-FAQ 3: How can I report bugs or glitches in Stick War 3?
-You can report bugs or glitches in Stick War 3 by tapping on the Settings icon on the main menu and then tapping on the Feedback button. You can also send an email to support@maxgames.com or contact them through their official website or social media pages.
-FAQ 4: How can I play Stick War 3 with my friends?
-You can play Stick War 3 with your friends by tapping on the Friends icon on the main menu and then tapping on the Invite button. You can also join or create clans and chat with your clan members.
-FAQ 5: How can I change my name or avatar in Stick War 3?
-You can change your name or avatar in Stick War 3 by tapping on the Profile icon on the main menu and then tapping on the Edit button. You can also unlock new avatars by playing matches or opening chests.
401be4b1e0
-
-
\ No newline at end of file
diff --git a/spaces/1phancelerku/anime-remove-background/Enjoy Fruits Coloring Pages with Your Friends and Family.md b/spaces/1phancelerku/anime-remove-background/Enjoy Fruits Coloring Pages with Your Friends and Family.md
deleted file mode 100644
index 65f0e657616c25a9d53e47499281a787864f16ee..0000000000000000000000000000000000000000
--- a/spaces/1phancelerku/anime-remove-background/Enjoy Fruits Coloring Pages with Your Friends and Family.md
+++ /dev/null
@@ -1,103 +0,0 @@
-
-Fruits Coloring Pages: A Fun Way to Learn About Fruits
-Fruits are delicious, nutritious, and colorful. They are also fun to color! Fruits coloring pages are a great way to introduce your kids to different kinds of fruits and their benefits. They can also help your kids develop their fine motor skills, creativity, and knowledge. In this article, we will tell you why fruits coloring pages are good for kids, how to use them, and some examples of fruits coloring pages that you can find online or print out.
-fruits coloring pages
Download ✏ https://jinyurl.com/2uNUk1
- Why Fruits Coloring Pages Are Good for Kids
-Fruits coloring pages are not only entertaining but also educational. Here are some reasons why they are good for kids:
-They help develop fine motor skills and hand-eye coordination
-Coloring is a simple but effective way to improve your kids' fine motor skills and hand-eye coordination. These skills are essential for writing, drawing, cutting, and other activities that require precise movements of the fingers and hands. Coloring also helps your kids practice holding and using different tools like crayons, markers, pencils, or paints.
-They stimulate creativity and imagination
-Coloring allows your kids to express their creativity and imagination. They can choose from a variety of colors and shades to fill the fruits with. They can also add some details or backgrounds to make their artworks more interesting. Coloring also encourages your kids to experiment with different combinations of colors and shapes.
-They teach kids about different fruits and their benefits
-Coloring fruits can help your kids learn about different fruits and their benefits. They can learn the names, shapes, colors, and tastes of various fruits. They can also learn about the vitamins, minerals, antioxidants, and fiber that fruits provide. Coloring fruits can also spark your kids' curiosity and interest in trying new fruits or eating more fruits.
- How to Use Fruits Coloring Pages
-Fruits coloring pages are easy to use and enjoy. Here are some steps on how to use them:
-Choose from a variety of fruits coloring pages online or print them out
-You can find many fruits coloring pages online that you can download or print out for free. You can search for specific fruits like apples, bananas, pineapples, strawberries, or watermelons. You can also search for fruit baskets, fruit patterns, fruit characters, or fruit themes. You can choose the ones that suit your kids' preferences and skill levels.
-* apple coloring pages for kids
-* banana coloring pages printable
-* pineapple coloring pages free
-* watermelon coloring pages summer
-* strawberry coloring pages preschool
-* grapes coloring pages realistic
-* orange coloring pages easy
-* lemon coloring pages cute
-* cherry coloring pages cartoon
-* peach coloring pages for adults
-* pear coloring pages simple
-* kiwi fruit coloring pages fun
-* coconut coloring pages tropical
-* mango coloring pages sweet
-* pomegranate coloring pages detailed
-* star fruit coloring pages unique
-* dragon fruit coloring pages exotic
-* avocado coloring pages healthy
-* cranberry coloring pages thanksgiving
-* blueberry coloring pages yummy
-* raspberry coloring pages delicious
-* blackberry coloring pages fresh
-* figs coloring pages ancient
-* apricot coloring pages juicy
-* plum coloring pages purple
-* nectarine coloring pages orange
-* persimmon coloring pages autumn
-* pomelo coloring pages citrus
-* grapefruit coloring pages sour
-* lime coloring pages green
-* melon coloring pages cool
-* honeydew coloring pages refreshing
-* cantaloupe coloring pages tasty
-* papaya coloring pages tropical
-* passion fruit coloring pages colorful
-* guava coloring pages pink
-* lychee coloring pages asian
-* rambutan coloring pages hairy
-* durian coloring pages spiky
-* jackfruit coloring pages big
-* breadfruit coloring pages starchy
-* quince coloring pages yellow
-* kumquat coloring pages small
-* elderberry coloring pages medicinal
-* gooseberry coloring pages sour
-* huckleberry coloring pages wild
-* date palm fruit coloring pages middle eastern
-* olive fruit coloring pages mediterranean
-* cactus fruit coloring pages desert
-* tomato fruit coloring page vegetable
-Gather some coloring tools like crayons, markers, pencils, or paints
-You can use any coloring tools that you have at home or buy some new ones. You can use crayons, markers, pencils, or paints to color the fruits. You can also use stickers, glitter, sequins, or other embellishments to decorate the fruits. You can let your kids choose their favorite colors and tools or suggest some ideas for them.
-Let your kids color the fruits as they like or follow some instructions
-You can let your kids color the fruits as they like or follow some instructions. You can let them use their imagination and creativity to color the fruits however they want. You can also give them some guidance or tips on how to color the fruits realistically or artistically. You can also show them some examples of colored fruits or pictures of real fruits for reference.
-Display or share their artworks with others
After your kids finish coloring the fruits, you can display or share their artworks with others. You can hang them on the wall, fridge, or bulletin board. You can also frame them, laminate them, or make them into cards or bookmarks. You can also take photos of them and share them with your family, friends, or online. You can also use them as teaching materials or conversation starters. You can praise your kids for their efforts and skills and ask them questions about the fruits they colored.
- Some Examples of Fruits Coloring Pages
-Here are some examples of fruits coloring pages that you can find online or print out. You can click on the links to see the images or download them.
-Apple coloring page
-An apple is a round, red, green, or yellow fruit that grows on a tree. It is crunchy, juicy, and sweet. It is rich in vitamin C, fiber, and antioxidants. It is good for your teeth, skin, and immune system. It is also one of the most popular fruits in the world. You can eat it raw, cooked, or dried. You can also make it into juice, cider, sauce, pie, or salad.
-You can find an apple coloring page here: [text]
-Banana coloring page
-A banana is a long, curved, yellow fruit that grows on a plant. It is soft, creamy, and sweet. It is rich in potassium, fiber, and vitamin B6. It is good for your muscles, nerves, and digestion. It is also one of the most versatile fruits in the world. You can eat it raw, cooked, or frozen. You can also make it into smoothies, muffins, breads, or pancakes.
-You can find a banana coloring page here: [text]
-Pineapple coloring page
-A pineapple is a spiky, oval, yellow fruit that grows on a plant. It is tangy, juicy, and refreshing. It is rich in vitamin C, manganese, and bromelain. It is good for your bones, skin, and inflammation. It is also one of the most exotic fruits in the world. You can eat it raw, cooked, or canned. You can also make it into juice, jam, salsa, or pizza.
-You can find a pineapple coloring page here: [text]
-Strawberry coloring page
-A strawberry is a small, heart-shaped, red fruit that grows on a plant. It is sweet, juicy, and fragrant. It is rich in vitamin C, folic acid, and antioxidants. It is good for your blood vessels, eyesight, and immunity. It is also one of the most beloved fruits in the world. You can eat it raw, cooked, or dried. You can also make it into jam, cake, ice cream, or salad.
-You can find a strawberry coloring page here: [text]
-Watermelon coloring page
-A watermelon is a large, round, green fruit that grows on a vine. It is crisp, juicy, and refreshing. It is rich in water, lycopene, and vitamin A. It is good for your hydration, skin, and vision. It is also one of the most fun fruits in the world. You can eat it raw, sliced, or cubed. You can also make it into juice, salad, or popsicles.
- You can find a watermelon coloring page here: [text]
- Conclusion
-Fruits coloring pages are a fun way to learn about fruits and their benefits. They are also good for developing fine motor skills, creativity, and knowledge. You can use fruits coloring pages by choosing from a variety of online or printable options, gathering some coloring tools, letting your kids color the fruits as they like or following some instructions, and displaying or sharing their artworks with others. You can also find some examples of fruits coloring pages online or print them out. We hope you enjoy fruits coloring pages with your kids and have a fruitful time!
- FAQs
-What are some other fruits that have coloring pages?
-Some other fruits that have coloring pages are oranges, grapes, pears, cherries, kiwis, mangoes, and coconuts. You can search for them online or print them out.
-How can I make my own fruits coloring pages?
-You can make your own fruits coloring pages by drawing some fruits on a paper or using a computer program. You can also use some templates or stencils to create some fruits shapes. You can also add some details or backgrounds to make them more interesting.
-How can I make fruits coloring pages more fun and challenging?
-You can make fruits coloring pages more fun and challenging by adding some games or activities to them. For example, you can ask your kids to name the fruits, count the fruits, sort the fruits by color or size, match the fruits with their benefits, or find the hidden fruits in the picture. You can also give them some clues or hints to help them.
-How can I use fruits coloring pages to teach my kids about healthy eating?
-You can use fruits coloring pages to teach your kids about healthy eating by explaining to them why fruits are good for them and how they can include more fruits in their diet. You can also encourage them to try new fruits or eat more fruits by giving them some rewards or incentives. You can also make some healthy snacks or desserts with fruits and let your kids help you prepare them.
-Where can I find more resources or information about fruits and their benefits?
-You can find more resources or information about fruits and their benefits by visiting some websites, blogs, books, magazines, or videos that are related to fruits and nutrition. You can also ask your doctor, nutritionist, teacher, or librarian for some recommendations or advice.
197e85843d
-
-
\ No newline at end of file
diff --git a/spaces/4Taps/SadTalker/src/face3d/data/image_folder.py b/spaces/4Taps/SadTalker/src/face3d/data/image_folder.py
deleted file mode 100644
index efadc2ecbe2fb4b53b78230aba25ec505eff0e55..0000000000000000000000000000000000000000
--- a/spaces/4Taps/SadTalker/src/face3d/data/image_folder.py
+++ /dev/null
@@ -1,66 +0,0 @@
-"""A modified image folder class
-
-We modify the official PyTorch image folder (https://github.com/pytorch/vision/blob/master/torchvision/datasets/folder.py)
-so that this class can load images from both current directory and its subdirectories.
-"""
-import numpy as np
-import torch.utils.data as data
-
-from PIL import Image
-import os
-import os.path
-
-IMG_EXTENSIONS = [
- '.jpg', '.JPG', '.jpeg', '.JPEG',
- '.png', '.PNG', '.ppm', '.PPM', '.bmp', '.BMP',
- '.tif', '.TIF', '.tiff', '.TIFF',
-]
-
-
-def is_image_file(filename):
- return any(filename.endswith(extension) for extension in IMG_EXTENSIONS)
-
-
-def make_dataset(dir, max_dataset_size=float("inf")):
- images = []
- assert os.path.isdir(dir) or os.path.islink(dir), '%s is not a valid directory' % dir
-
- for root, _, fnames in sorted(os.walk(dir, followlinks=True)):
- for fname in fnames:
- if is_image_file(fname):
- path = os.path.join(root, fname)
- images.append(path)
- return images[:min(max_dataset_size, len(images))]
-
-
-def default_loader(path):
- return Image.open(path).convert('RGB')
-
-
-class ImageFolder(data.Dataset):
-
- def __init__(self, root, transform=None, return_paths=False,
- loader=default_loader):
- imgs = make_dataset(root)
- if len(imgs) == 0:
- raise(RuntimeError("Found 0 images in: " + root + "\n"
- "Supported image extensions are: " + ",".join(IMG_EXTENSIONS)))
-
- self.root = root
- self.imgs = imgs
- self.transform = transform
- self.return_paths = return_paths
- self.loader = loader
-
- def __getitem__(self, index):
- path = self.imgs[index]
- img = self.loader(path)
- if self.transform is not None:
- img = self.transform(img)
- if self.return_paths:
- return img, path
- else:
- return img
-
- def __len__(self):
- return len(self.imgs)
diff --git a/spaces/AI-ZeroToHero-031523/README/README.md b/spaces/AI-ZeroToHero-031523/README/README.md
deleted file mode 100644
index 91382d374b13cf4527bb4f77b0815edcf74200be..0000000000000000000000000000000000000000
--- a/spaces/AI-ZeroToHero-031523/README/README.md
+++ /dev/null
@@ -1,179 +0,0 @@
----
-title: README.md
-sdk: static
-emoji: 🏃
-colorFrom: blue
-colorTo: yellow
----
-
-# Classroom Examples for Today:
-
-# HF Features to Check Out First - Boost your Speed:
-1. HF_TOKEN create - Why? Hit quota on free usage and see errors - Solve w this. Also this lets spaces read/write as you.
-2. Model Easy Button with Gradio
- 1. https://huggingface.co/spaces/awacke1/Model-Easy-Button1-ZeroShotImageClassifier-Openai-clip-vit-large-patch14
- 2. https://huggingface.co/spaces/awacke1/Easy-Button-Zero-Shot-Text-Classifier-facebook-bart-large-mnli
- 3. https://huggingface.co/spaces/awacke1/Model-Easy-Button-Generative-Images-runwayml-stable-diffusion-v1-5
- 4. https://huggingface.co/spaces/awacke1/Model-Easy-Button-Generative-Text-bigscience-bloom
- 5. Check out API Link at Bottom - Gradio auto generates API for you along with usage.
-3. Spaces Embed Button
- 1. Bring all four together now into a dashboard!
-4. Space Duplicate Button
-
-# Examples 03_16_2023:
-1. HTML5 - Build AI Dashboards with HTML5 Spaces. Spaces Context Menu. Mediapipe. https://huggingface.co/spaces/awacke1/AI.Dashboard.HEDIS.Terminology.Vocabulary.Codes
-2. ChatGPT - Demonstrate three modes including GPT-4 which started this week. https://chat.openai.com/chat
-3. Wikipedia Crowdsource Human Feedback (HF) and Headless URL: https://awacke1-streamlitwikipediachat.hf.space https://huggingface.co/spaces/awacke1/StreamlitWikipediaChat
-4. Cognitive Memory - AI Human Feedback (HF), Wikichat, Tweet Sentiment Dash: https://huggingface.co/spaces/awacke1/AI.Dashboard.Wiki.Chat.Cognitive.HTML5
-5. Twitter Sentiment Graph Example: https://awacke1-twitter-sentiment-live-realtime.hf.space/ Modify to split URL w ChatGPT?
-6. ASR Comparitive Review:
- 1. Multilingual Models: jonatasgrosman/wav2vec2-large-xlsr-53-english Space: https://huggingface.co/spaces/awacke1/ASR-High-Accuracy-Test
- 2. Speech to Text and Back to Speech in Voice Models: https://huggingface.co/spaces/awacke1/TTS-STT-Blocks Model: https://huggingface.co/facebook/wav2vec2-base-960h
- 3. Gradio Live Mode: https://huggingface.co/spaces/awacke1/2-LiveASR Models: facebook/blenderbot-400M-distill nvidia/stt_en_conformer_transducer_xlarge
-7. Bloom Example:
- 1. Step By Step w Bloom: https://huggingface.co/spaces/EuroPython2022/Step-By-Step-With-Bloom
-8. ChatGPT with Key Example: https://huggingface.co/spaces/awacke1/chatgpt-demo
- 1. Get or revoke your keys here: https://platform.openai.com/account/api-keys
- 2. Example fake: tsk-H2W4lEeT4Aonxe2tQnUzT3BlbkFJq1cMwMANfYc0ftXwrJSo12345t
-
-# Components for Dash - Demo button to Embed Space to get IFRAME code:
-https://huggingface.co/spaces/awacke1/Health.Assessments.Summarizer
-HEDIS Dash:
-1. HEDIS Related Dashboard with CT: https://huggingface.co/spaces/awacke1/AI.Dashboard.HEDIS
-
-# 👋 Two easy ways to turbo boost your AI learning journey! 💻
-# 🌐 AI Pair Programming
-## Open 2 Browsers to:
-1. __🌐 ChatGPT__ [URL](https://chat.openai.com/chat) or [URL2](https://platform.openai.com/playground) and
-2. __🌐 Huggingface__ [URL](https://huggingface.co/awacke1) in separate browser windows.
-
-# 🎥 YouTube University Method:
-
-## 🎥 2023 AI/ML Advanced Learning Playlists:
-1. [2023 Streamlit Pro Tips for AI UI UX for Data Science, Engineering, and Mathematics](https://www.youtube.com/playlist?list=PLHgX2IExbFou3cP19hHO9Xb-cN8uwr5RM)
-2. [2023 Fun, New and Interesting AI, Videos, and AI/ML Techniques](https://www.youtube.com/playlist?list=PLHgX2IExbFotoMt32SrT3Xynt5BXTGnEP)
-3. [2023 Best Minds in AGI AI Gamification and Large Language Models](https://www.youtube.com/playlist?list=PLHgX2IExbFotmFeBTpyje1uI22n0GAkXT)
-4. [2023 State of the Art for Vision Image Classification, Text Classification and Regression, Extractive Question Answering and Tabular Classification](https://www.youtube.com/playlist?list=PLHgX2IExbFotPcPu6pauNHOoZTTbnAQ2F)
-5. [2023 QA Models and Long Form Question Answering NLP](https://www.youtube.com/playlist?list=PLHgX2IExbFovrkkx8HMTLNgYdjCMNYmX_)
-
-# Cloud Patterns - Dataset Architecture Patterns for Cloud Optimal Datasets:
-1. Azure Blob/DataLake adlfs: https://huggingface.co/docs/datasets/filesystems
-2. AWS: Amazon S3 s3fs: https://s3fs.readthedocs.io/en/latest/
-3. Google Cloud Storage gcsfs: https://gcsfs.readthedocs.io/en/latest/
-4. Google Drive: Google Drive gdrivefs: https://github.com/intake/gdrivefs
-
-Apache BEAM: https://huggingface.co/docs/datasets/beam
-Datasets: https://huggingface.co/docs/datasets/index
-
-# Datasets Spaces - High Performance Cloud Dataset Patterns
-1. Health Care AI Datasets: https://huggingface.co/spaces/awacke1/Health-Care-AI-and-Datasets
-2. Dataset Analyzer: https://huggingface.co/spaces/awacke1/DatasetAnalyzer
-3. Shared Memory with Github LFS: https://huggingface.co/spaces/awacke1/Memory-Shared
-4. CSV Dataset Analyzer: https://huggingface.co/spaces/awacke1/CSVDatasetAnalyzer
-5. Pandas Profiler Report for EDA Datasets: https://huggingface.co/spaces/awacke1/WikipediaProfilerTestforDatasets
-6. Datasets High Performance IMDB Patterns for AI: https://huggingface.co/spaces/awacke1/SaveAndReloadDataset
-
-# ChatGPT Prompts Datasets
-1. https://huggingface.co/datasets/fka/awesome-chatgpt-prompts
-2. https://github.com/f/awesome-chatgpt-prompts
-3. Example with role based behavior: I want you to act as a stand-up comedian. I will provide you with some topics related to current events and you will use your wit, creativity, and observational skills to create a routine based on those topics. You should also be sure to incorporate personal anecdotes or experiences into the routine in order to make it more relatable and engaging for the audience. My first request is "I want a humorous story and jokes to talk about the funny things about AI development and executive presentation videos"
-
-# Language Models 🗣️
-🏆 Bloom sets new record for most performant and efficient AI model in science! 🌸
-
-### Comparison of Large Language Models
-| Model Name | Model Size (in Parameters) |
-| ----------------- | -------------------------- |
-| BigScience-tr11-176B | 176 billion |
-| GPT-3 | 175 billion |
-| OpenAI's DALL-E 2.0 | 500 million |
-| NVIDIA's Megatron | 8.3 billion |
-| Transformer-XL | 250 million |
-| XLNet | 210 million |
-
-## ChatGPT Datasets 📚
-- WebText
-- Common Crawl
-- BooksCorpus
-- English Wikipedia
-- Toronto Books Corpus
-- OpenWebText
--
-## ChatGPT Datasets - Details 📚
-- **WebText:** A dataset of web pages crawled from domains on the Alexa top 5,000 list. This dataset was used to pretrain GPT-2.
- - [WebText: A Large-Scale Unsupervised Text Corpus by Radford et al.](https://paperswithcode.com/dataset/webtext)
-- **Common Crawl:** A dataset of web pages from a variety of domains, which is updated regularly. This dataset was used to pretrain GPT-3.
- - [Language Models are Few-Shot Learners](https://paperswithcode.com/dataset/common-crawl) by Brown et al.
-- **BooksCorpus:** A dataset of over 11,000 books from a variety of genres.
- - [Scalable Methods for 8 Billion Token Language Modeling](https://paperswithcode.com/dataset/bookcorpus) by Zhu et al.
-- **English Wikipedia:** A dump of the English-language Wikipedia as of 2018, with articles from 2001-2017.
- - [Improving Language Understanding by Generative Pre-Training](https://huggingface.co/spaces/awacke1/WikipediaUltimateAISearch?logs=build) Space for Wikipedia Search
-- **Toronto Books Corpus:** A dataset of over 7,000 books from a variety of genres, collected by the University of Toronto.
- - [Massively Multilingual Sentence Embeddings for Zero-Shot Cross-Lingual Transfer and Beyond](https://paperswithcode.com/dataset/bookcorpus) by Schwenk and Douze.
-- **OpenWebText:** A dataset of web pages that were filtered to remove content that was likely to be low-quality or spammy. This dataset was used to pretrain GPT-3.
- - [Language Models are Few-Shot Learners](https://paperswithcode.com/dataset/openwebtext) by Brown et al.
-
-## Big Science Model 🚀
-- 📜 Papers:
- 1. BLOOM: A 176B-Parameter Open-Access Multilingual Language Model [Paper](https://arxiv.org/abs/2211.05100)
- 2. Megatron-LM: Training Multi-Billion Parameter Language Models Using Model Parallelism [Paper](https://arxiv.org/abs/1909.08053)
- 3. 8-bit Optimizers via Block-wise Quantization [Paper](https://arxiv.org/abs/2110.02861)
- 4. Train Short, Test Long: Attention with Linear Biases Enables Input Length Extrapolation [Paper](https://arxiv.org/abs/2108.12409)
- 5. [Other papers related to Big Science](https://huggingface.co/models?other=doi:10.57967/hf/0003)
- 6. [217 other models optimized for use with Bloom](https://huggingface.co/models?other=bloom)
-
-- 📚 Datasets:
-
-**Datasets:**
-1. - **Universal Dependencies:** A collection of annotated corpora for natural language processing in a range of languages, with a focus on dependency parsing.
- - [Universal Dependencies official website.](https://universaldependencies.org/)
-2. - **WMT 2014:** The fourth edition of the Workshop on Statistical Machine Translation, featuring shared tasks on translating between English and various other languages.
- - [WMT14 website.](http://www.statmt.org/wmt14/)
-3. - **The Pile:** An English language corpus of diverse text, sourced from various places on the internet.
- - [The Pile official website.](https://pile.eleuther.ai/)
-4. - **HumanEval:** A dataset of English sentences, annotated with human judgments on a range of linguistic qualities.
- - [HumanEval: An Evaluation Benchmark for Language Understanding](https://github.com/google-research-datasets/humaneval) by Gabriel Ilharco, Daniel Loureiro, Pedro Rodriguez, and Afonso Mendes.
-5. - **FLORES-101:** A dataset of parallel sentences in 101 languages, designed for multilingual machine translation.
- - [FLORES-101: A Massively Multilingual Parallel Corpus for Language Understanding](https://flores101.opennmt.net/) by Aman Madaan, Shruti Rijhwani, Raghav Gupta, and Mitesh M. Khapra.
-6. - **CrowS-Pairs:** A dataset of sentence pairs, designed for evaluating the plausibility of generated text.
- - [CrowS-Pairs: A Challenge Dataset for Plausible Plausibility Judgments](https://github.com/stanford-cogsci/crows-pairs) by Andrea Madotto, Zhaojiang Lin, Chien-Sheng Wu, Pascale Fung, and Caiming Xiong.
-7. - **WikiLingua:** A dataset of parallel sentences in 75 languages, sourced from Wikipedia.
- - [WikiLingua: A New Benchmark Dataset for Cross-Lingual Wikification](https://arxiv.org/abs/2105.08031) by Jiarui Yao, Yanqiao Zhu, Ruihan Bao, Guosheng Lin, Lidong Bing, and Bei Shi.
-8. - **MTEB:** A dataset of English sentences, annotated with their entailment relationships with respect to other sentences.
- - [Multi-Task Evaluation Benchmark for Natural Language Inference](https://github.com/google-research-datasets/mteb) by Michał Lukasik, Marcin Junczys-Dowmunt, and Houda Bouamor.
-9. - **xP3:** A dataset of English sentences, annotated with their paraphrase relationships with respect to other sentences.
- - [xP3: A Large-Scale Evaluation Benchmark for Paraphrase Identification in Context](https://github.com/nyu-dl/xp3) by Aniket Didolkar, James Mayfield, Markus Saers, and Jason Baldridge.
-10. - **DiaBLa:** A dataset of English dialogue, annotated with dialogue acts.
- - [A Large-Scale Corpus for Conversation Disentanglement](https://github.com/HLTCHKUST/DiaBLA) by Samuel Broscheit, António Branco, and André F. T. Martins.
-
-- 📚 Dataset Papers with Code
- 1. [Universal Dependencies](https://paperswithcode.com/dataset/universal-dependencies)
- 2. [WMT 2014](https://paperswithcode.com/dataset/wmt-2014)
- 3. [The Pile](https://paperswithcode.com/dataset/the-pile)
- 4. [HumanEval](https://paperswithcode.com/dataset/humaneval)
- 5. [FLORES-101](https://paperswithcode.com/dataset/flores-101)
- 6. [CrowS-Pairs](https://paperswithcode.com/dataset/crows-pairs)
- 7. [WikiLingua](https://paperswithcode.com/dataset/wikilingua)
- 8. [MTEB](https://paperswithcode.com/dataset/mteb)
- 9. [xP3](https://paperswithcode.com/dataset/xp3)
- 10. [DiaBLa](https://paperswithcode.com/dataset/diabla)
-
-# Deep RL ML Strategy 🧠
-The AI strategies are:
-- Language Model Preparation using Human Augmented with Supervised Fine Tuning 🤖
-- Reward Model Training with Prompts Dataset Multi-Model Generate Data to Rank 🎁
-- Fine Tuning with Reinforcement Reward and Distance Distribution Regret Score 🎯
-- Proximal Policy Optimization Fine Tuning 🤝
-- Variations - Preference Model Pretraining 🤔
-- Use Ranking Datasets Sentiment - Thumbs Up/Down, Distribution 📊
-- Online Version Getting Feedback 💬
-- OpenAI - InstructGPT - Humans generate LM Training Text 🔍
-- DeepMind - Advantage Actor Critic Sparrow, GopherCite 🦜
-- Reward Model Human Prefence Feedback 🏆
-
-
-For more information on specific techniques and implementations, check out the following resources:
-- OpenAI's paper on [GPT-3](https://arxiv.org/abs/2005.14165) which details their Language Model Preparation approach
-- DeepMind's paper on [SAC](https://arxiv.org/abs/1801.01290) which describes the Advantage Actor Critic algorithm
-- OpenAI's paper on [Reward Learning](https://arxiv.org/abs/1810.06580) which explains their approach to training Reward Models
-- OpenAI's blog post on [GPT-3's fine-tuning process](https://openai.com/blog/fine-tuning-gpt-3/)
\ No newline at end of file
diff --git a/spaces/AIGC-Audio/AudioGPT/NeuralSeq/tasks/vocoder/dataset_utils.py b/spaces/AIGC-Audio/AudioGPT/NeuralSeq/tasks/vocoder/dataset_utils.py
deleted file mode 100644
index 05dcdaa524efde31575dd30b57b627d22744b53c..0000000000000000000000000000000000000000
--- a/spaces/AIGC-Audio/AudioGPT/NeuralSeq/tasks/vocoder/dataset_utils.py
+++ /dev/null
@@ -1,204 +0,0 @@
-import glob
-import importlib
-import os
-from resemblyzer import VoiceEncoder
-import numpy as np
-import torch
-import torch.distributed as dist
-from torch.utils.data import DistributedSampler
-import utils
-from tasks.base_task import BaseDataset
-from utils.hparams import hparams
-from utils.indexed_datasets import IndexedDataset
-from tqdm import tqdm
-
-class EndlessDistributedSampler(DistributedSampler):
- def __init__(self, dataset, num_replicas=None, rank=None, shuffle=True):
- if num_replicas is None:
- if not dist.is_available():
- raise RuntimeError("Requires distributed package to be available")
- num_replicas = dist.get_world_size()
- if rank is None:
- if not dist.is_available():
- raise RuntimeError("Requires distributed package to be available")
- rank = dist.get_rank()
- self.dataset = dataset
- self.num_replicas = num_replicas
- self.rank = rank
- self.epoch = 0
- self.shuffle = shuffle
-
- g = torch.Generator()
- g.manual_seed(self.epoch)
- if self.shuffle:
- indices = [i for _ in range(1000) for i in torch.randperm(
- len(self.dataset), generator=g).tolist()]
- else:
- indices = [i for _ in range(1000) for i in list(range(len(self.dataset)))]
- indices = indices[:len(indices) // self.num_replicas * self.num_replicas]
- indices = indices[self.rank::self.num_replicas]
- self.indices = indices
-
- def __iter__(self):
- return iter(self.indices)
-
- def __len__(self):
- return len(self.indices)
-
-
-class VocoderDataset(BaseDataset):
- def __init__(self, prefix, shuffle=False):
- super().__init__(shuffle)
- self.hparams = hparams
- self.prefix = prefix
- self.data_dir = hparams['binary_data_dir']
- self.is_infer = prefix == 'test'
- self.batch_max_frames = 0 if self.is_infer else hparams['max_samples'] // hparams['hop_size']
- self.aux_context_window = hparams['aux_context_window']
- self.hop_size = hparams['hop_size']
- if self.is_infer and hparams['test_input_dir'] != '':
- self.indexed_ds, self.sizes = self.load_test_inputs(hparams['test_input_dir'])
- self.avail_idxs = [i for i, _ in enumerate(self.sizes)]
- elif self.is_infer and hparams['test_mel_dir'] != '':
- self.indexed_ds, self.sizes = self.load_mel_inputs(hparams['test_mel_dir'])
- self.avail_idxs = [i for i, _ in enumerate(self.sizes)]
- else:
- self.indexed_ds = None
- self.sizes = np.load(f'{self.data_dir}/{self.prefix}_lengths.npy')
- self.avail_idxs = [idx for idx, s in enumerate(self.sizes) if
- s - 2 * self.aux_context_window > self.batch_max_frames]
- print(f"| {len(self.sizes) - len(self.avail_idxs)} short items are skipped in {prefix} set.")
- self.sizes = [s for idx, s in enumerate(self.sizes) if
- s - 2 * self.aux_context_window > self.batch_max_frames]
-
- def _get_item(self, index):
- if self.indexed_ds is None:
- self.indexed_ds = IndexedDataset(f'{self.data_dir}/{self.prefix}')
- item = self.indexed_ds[index]
- return item
-
- def __getitem__(self, index):
- index = self.avail_idxs[index]
- item = self._get_item(index)
- sample = {
- "id": index,
- "item_name": item['item_name'],
- "mel": torch.FloatTensor(item['mel']),
- "wav": torch.FloatTensor(item['wav'].astype(np.float32)),
- }
- if 'pitch' in item:
- sample['pitch'] = torch.LongTensor(item['pitch'])
- sample['f0'] = torch.FloatTensor(item['f0'])
-
- if hparams.get('use_spk_embed', False):
- sample["spk_embed"] = torch.Tensor(item['spk_embed'])
- if hparams.get('use_emo_embed', False):
- sample["emo_embed"] = torch.Tensor(item['emo_embed'])
-
- return sample
-
- def collater(self, batch):
- if len(batch) == 0:
- return {}
-
- y_batch, c_batch, p_batch, f0_batch = [], [], [], []
- item_name = []
- have_pitch = 'pitch' in batch[0]
- for idx in range(len(batch)):
- item_name.append(batch[idx]['item_name'])
- x, c = batch[idx]['wav'] if self.hparams['use_wav'] else None, batch[idx]['mel'].squeeze(0)
- if have_pitch:
- p = batch[idx]['pitch']
- f0 = batch[idx]['f0']
- if self.hparams['use_wav']:self._assert_ready_for_upsampling(x, c, self.hop_size, 0)
- if len(c) - 2 * self.aux_context_window > self.batch_max_frames:
- # randomly pickup with the batch_max_steps length of the part
- batch_max_frames = self.batch_max_frames if self.batch_max_frames != 0 else len(
- c) - 2 * self.aux_context_window - 1
- batch_max_steps = batch_max_frames * self.hop_size
- interval_start = self.aux_context_window
- interval_end = len(c) - batch_max_frames - self.aux_context_window
- start_frame = np.random.randint(interval_start, interval_end)
- start_step = start_frame * self.hop_size
- if self.hparams['use_wav']:y = x[start_step: start_step + batch_max_steps]
- c = c[start_frame - self.aux_context_window:
- start_frame + self.aux_context_window + batch_max_frames]
- if have_pitch:
- p = p[start_frame - self.aux_context_window:
- start_frame + self.aux_context_window + batch_max_frames]
- f0 = f0[start_frame - self.aux_context_window:
- start_frame + self.aux_context_window + batch_max_frames]
- if self.hparams['use_wav']:self._assert_ready_for_upsampling(y, c, self.hop_size, self.aux_context_window)
- else:
- print(f"Removed short sample from batch (length={len(x)}).")
- continue
- if self.hparams['use_wav']:y_batch += [y.reshape(-1, 1)] # [(T, 1), (T, 1), ...]
- c_batch += [c] # [(T' C), (T' C), ...]
- if have_pitch:
- p_batch += [p] # [(T' C), (T' C), ...]
- f0_batch += [f0] # [(T' C), (T' C), ...]
-
- # convert each batch to tensor, asuume that each item in batch has the same length
- if self.hparams['use_wav']:y_batch = utils.collate_2d(y_batch, 0).transpose(2, 1) # (B, 1, T)
- c_batch = utils.collate_2d(c_batch, 0).transpose(2, 1) # (B, C, T')
- if have_pitch:
- p_batch = utils.collate_1d(p_batch, 0) # (B, T')
- f0_batch = utils.collate_1d(f0_batch, 0) # (B, T')
- else:
- p_batch, f0_batch = None, None
-
- # make input noise signal batch tensor
- if self.hparams['use_wav']: z_batch = torch.randn(y_batch.size()) # (B, 1, T)
- else: z_batch=[]
- return {
- 'z': z_batch,
- 'mels': c_batch,
- 'wavs': y_batch,
- 'pitches': p_batch,
- 'f0': f0_batch,
- 'item_name': item_name
- }
-
- @staticmethod
- def _assert_ready_for_upsampling(x, c, hop_size, context_window):
- """Assert the audio and feature lengths are correctly adjusted for upsamping."""
- assert len(x) == (len(c) - 2 * context_window) * hop_size
-
- def load_test_inputs(self, test_input_dir, spk_id=0):
- inp_wav_paths = sorted(glob.glob(f'{test_input_dir}/*.wav') + glob.glob(f'{test_input_dir}/**/*.mp3'))
- sizes = []
- items = []
-
- binarizer_cls = hparams.get("binarizer_cls", 'data_gen.tts.base_binarizer.BaseBinarizer')
- pkg = ".".join(binarizer_cls.split(".")[:-1])
- cls_name = binarizer_cls.split(".")[-1]
- binarizer_cls = getattr(importlib.import_module(pkg), cls_name)
- binarization_args = hparams['binarization_args']
-
- for wav_fn in inp_wav_paths:
- item_name = wav_fn[len(test_input_dir) + 1:].replace("/", "_")
- item = binarizer_cls.process_item(
- item_name, wav_fn, binarization_args)
- items.append(item)
- sizes.append(item['len'])
- return items, sizes
-
- def load_mel_inputs(self, test_input_dir, spk_id=0):
- inp_mel_paths = sorted(glob.glob(f'{test_input_dir}/*.npy'))
- sizes = []
- items = []
-
- binarizer_cls = hparams.get("binarizer_cls", 'data_gen.tts.base_binarizer.BaseBinarizer')
- pkg = ".".join(binarizer_cls.split(".")[:-1])
- cls_name = binarizer_cls.split(".")[-1]
- binarizer_cls = getattr(importlib.import_module(pkg), cls_name)
- binarization_args = hparams['binarization_args']
-
- for mel in inp_mel_paths:
- mel_input = np.load(mel)
- mel_input = torch.FloatTensor(mel_input)
- item_name = mel[len(test_input_dir) + 1:].replace("/", "_")
- item = binarizer_cls.process_mel_item(item_name, mel_input, None, binarization_args)
- items.append(item)
- sizes.append(item['len'])
- return items, sizes
diff --git a/spaces/AIGC-Audio/AudioGPT/audio_detection/audio_infer/pytorch/losses.py b/spaces/AIGC-Audio/AudioGPT/audio_detection/audio_infer/pytorch/losses.py
deleted file mode 100644
index 587e8a64f2593e4a72c1a29cf374c1e24e20c366..0000000000000000000000000000000000000000
--- a/spaces/AIGC-Audio/AudioGPT/audio_detection/audio_infer/pytorch/losses.py
+++ /dev/null
@@ -1,14 +0,0 @@
-import torch
-import torch.nn.functional as F
-
-
-def clip_bce(output_dict, target_dict):
- """Binary crossentropy loss.
- """
- return F.binary_cross_entropy(
- output_dict['clipwise_output'], target_dict['target'])
-
-
-def get_loss_func(loss_type):
- if loss_type == 'clip_bce':
- return clip_bce
\ No newline at end of file
diff --git a/spaces/AIGC-Audio/AudioGPT/text_to_speech/TTS_binding.py b/spaces/AIGC-Audio/AudioGPT/text_to_speech/TTS_binding.py
deleted file mode 100644
index f90c581acc887b69619328abbfb6aa4b9f124647..0000000000000000000000000000000000000000
--- a/spaces/AIGC-Audio/AudioGPT/text_to_speech/TTS_binding.py
+++ /dev/null
@@ -1,126 +0,0 @@
-import torch
-import os
-
-
-class TTSInference:
- def __init__(self, device=None):
- print("Initializing TTS model to %s" % device)
- from .tasks.tts.tts_utils import load_data_preprocessor
- from .utils.commons.hparams import set_hparams
- if device is None:
- device = 'cuda' if torch.cuda.is_available() else 'cpu'
- self.hparams = set_hparams("text_to_speech/checkpoints/ljspeech/ps_adv_baseline/config.yaml")
- self.device = device
- self.data_dir = 'text_to_speech/checkpoints/ljspeech/data_info'
- self.preprocessor, self.preprocess_args = load_data_preprocessor()
- self.ph_encoder, self.word_encoder = self.preprocessor.load_dict(self.data_dir)
- self.spk_map = self.preprocessor.load_spk_map(self.data_dir)
- self.model = self.build_model()
- self.model.eval()
- self.model.to(self.device)
- self.vocoder = self.build_vocoder()
- self.vocoder.eval()
- self.vocoder.to(self.device)
- print("TTS loaded!")
-
- def build_model(self):
- from .utils.commons.ckpt_utils import load_ckpt
- from .modules.tts.portaspeech.portaspeech import PortaSpeech
-
- ph_dict_size = len(self.ph_encoder)
- word_dict_size = len(self.word_encoder)
- model = PortaSpeech(ph_dict_size, word_dict_size, self.hparams)
- load_ckpt(model, 'text_to_speech/checkpoints/ljspeech/ps_adv_baseline', 'model')
- model.to(self.device)
- with torch.no_grad():
- model.store_inverse_all()
- model.eval()
- return model
-
- def forward_model(self, inp):
- sample = self.input_to_batch(inp)
- with torch.no_grad():
- output = self.model(
- sample['txt_tokens'],
- sample['word_tokens'],
- ph2word=sample['ph2word'],
- word_len=sample['word_lengths'].max(),
- infer=True,
- forward_post_glow=True,
- spk_id=sample.get('spk_ids')
- )
- mel_out = output['mel_out']
- wav_out = self.run_vocoder(mel_out)
- wav_out = wav_out.cpu().numpy()
- return wav_out[0]
-
- def build_vocoder(self):
- from .utils.commons.hparams import set_hparams
- from .modules.vocoder.hifigan.hifigan import HifiGanGenerator
- from .utils.commons.ckpt_utils import load_ckpt
- base_dir = 'text_to_speech/checkpoints/hifi_lj'
- config_path = f'{base_dir}/config.yaml'
- config = set_hparams(config_path, global_hparams=False)
- vocoder = HifiGanGenerator(config)
- load_ckpt(vocoder, base_dir, 'model_gen')
- return vocoder
-
- def run_vocoder(self, c):
- c = c.transpose(2, 1)
- y = self.vocoder(c)[:, 0]
- return y
-
- def preprocess_input(self, inp):
- """
-
- :param inp: {'text': str, 'item_name': (str, optional), 'spk_name': (str, optional)}
- :return:
- """
- preprocessor, preprocess_args = self.preprocessor, self.preprocess_args
- text_raw = inp['text']
- item_name = inp.get('item_name', '')
- spk_name = inp.get('spk_name', '')
- ph, txt, word, ph2word, ph_gb_word = preprocessor.txt_to_ph(
- preprocessor.txt_processor, text_raw, preprocess_args)
- word_token = self.word_encoder.encode(word)
- ph_token = self.ph_encoder.encode(ph)
- spk_id = self.spk_map[spk_name]
- item = {'item_name': item_name, 'text': txt, 'ph': ph, 'spk_id': spk_id,
- 'ph_token': ph_token, 'word_token': word_token, 'ph2word': ph2word,
- 'ph_words':ph_gb_word, 'words': word}
- item['ph_len'] = len(item['ph_token'])
- return item
-
- def input_to_batch(self, item):
- item_names = [item['item_name']]
- text = [item['text']]
- ph = [item['ph']]
- txt_tokens = torch.LongTensor(item['ph_token'])[None, :].to(self.device)
- txt_lengths = torch.LongTensor([txt_tokens.shape[1]]).to(self.device)
- word_tokens = torch.LongTensor(item['word_token'])[None, :].to(self.device)
- word_lengths = torch.LongTensor([txt_tokens.shape[1]]).to(self.device)
- ph2word = torch.LongTensor(item['ph2word'])[None, :].to(self.device)
- spk_ids = torch.LongTensor(item['spk_id'])[None, :].to(self.device)
- batch = {
- 'item_name': item_names,
- 'text': text,
- 'ph': ph,
- 'txt_tokens': txt_tokens,
- 'txt_lengths': txt_lengths,
- 'word_tokens': word_tokens,
- 'word_lengths': word_lengths,
- 'ph2word': ph2word,
- 'spk_ids': spk_ids,
- }
- return batch
-
- def postprocess_output(self, output):
- return output
-
- def infer_once(self, inp):
- inp = self.preprocess_input(inp)
- output = self.forward_model(inp)
- output = self.postprocess_output(output)
- return output
-
-
diff --git a/spaces/ATang0729/Forecast4Muses/Model/Model6/Model6_2_ProfileRecogition/mmpretrain/configs/_base_/schedules/.ipynb_checkpoints/__init__.py b/spaces/ATang0729/Forecast4Muses/Model/Model6/Model6_2_ProfileRecogition/mmpretrain/configs/_base_/schedules/.ipynb_checkpoints/__init__.py
deleted file mode 100644
index e69de29bb2d1d6434b8b29ae775ad8c2e48c5391..0000000000000000000000000000000000000000
diff --git a/spaces/AchyuthGamer/OpenGPT/g4f/Provider/OpenAssistant.py b/spaces/AchyuthGamer/OpenGPT/g4f/Provider/OpenAssistant.py
deleted file mode 100644
index 1e9a0661b4fdf03ee0fa30eeb229ce155c33ce94..0000000000000000000000000000000000000000
--- a/spaces/AchyuthGamer/OpenGPT/g4f/Provider/OpenAssistant.py
+++ /dev/null
@@ -1,100 +0,0 @@
-from __future__ import annotations
-
-import json
-
-from aiohttp import ClientSession
-
-from ..typing import Any, AsyncGenerator
-from .base_provider import AsyncGeneratorProvider, format_prompt, get_cookies
-
-
-class OpenAssistant(AsyncGeneratorProvider):
- url = "https://open-assistant.io/chat"
- needs_auth = True
- working = True
- model = "OA_SFT_Llama_30B_6"
-
- @classmethod
- async def create_async_generator(
- cls,
- model: str,
- messages: list[dict[str, str]],
- proxy: str = None,
- cookies: dict = None,
- **kwargs: Any
- ) -> AsyncGenerator:
- if not cookies:
- cookies = get_cookies("open-assistant.io")
-
- headers = {
- 'User-Agent': 'Mozilla/5.0 (Macintosh; Intel Mac OS X 10_15_7) AppleWebKit/537.36 (KHTML, like Gecko) Chrome/111.0.0.0 Safari/537.36',
- }
- async with ClientSession(
- cookies=cookies,
- headers=headers
- ) as session:
- async with session.post("https://open-assistant.io/api/chat", proxy=proxy) as response:
- chat_id = (await response.json())["id"]
-
- data = {
- "chat_id": chat_id,
- "content": f"[INST]\n{format_prompt(messages)}\n[/INST]",
- "parent_id": None
- }
- async with session.post("https://open-assistant.io/api/chat/prompter_message", proxy=proxy, json=data) as response:
- parent_id = (await response.json())["id"]
-
- data = {
- "chat_id": chat_id,
- "parent_id": parent_id,
- "model_config_name": model if model else cls.model,
- "sampling_parameters":{
- "top_k": 50,
- "top_p": None,
- "typical_p": None,
- "temperature": 0.35,
- "repetition_penalty": 1.1111111111111112,
- "max_new_tokens": 1024,
- **kwargs
- },
- "plugins":[]
- }
- async with session.post("https://open-assistant.io/api/chat/assistant_message", proxy=proxy, json=data) as response:
- data = await response.json()
- if "id" in data:
- message_id = data["id"]
- elif "message" in data:
- raise RuntimeError(data["message"])
- else:
- response.raise_for_status()
-
- params = {
- 'chat_id': chat_id,
- 'message_id': message_id,
- }
- async with session.post("https://open-assistant.io/api/chat/events", proxy=proxy, params=params) as response:
- start = "data: "
- async for line in response.content:
- line = line.decode("utf-8")
- if line and line.startswith(start):
- line = json.loads(line[len(start):])
- if line["event_type"] == "token":
- yield line["text"]
-
- params = {
- 'chat_id': chat_id,
- }
- async with session.delete("https://open-assistant.io/api/chat", proxy=proxy, params=params) as response:
- response.raise_for_status()
-
- @classmethod
- @property
- def params(cls):
- params = [
- ("model", "str"),
- ("messages", "list[dict[str, str]]"),
- ("stream", "bool"),
- ("proxy", "str"),
- ]
- param = ", ".join([": ".join(p) for p in params])
- return f"g4f.provider.{cls.__name__} supports: ({param})"
diff --git a/spaces/AgentVerse/agentVerse/ui/src/phaser3-rex-plugins/templates/ui/filechooser/Factory.js b/spaces/AgentVerse/agentVerse/ui/src/phaser3-rex-plugins/templates/ui/filechooser/Factory.js
deleted file mode 100644
index 30abe8924a5d0c63015fd548211e90594b93f5aa..0000000000000000000000000000000000000000
--- a/spaces/AgentVerse/agentVerse/ui/src/phaser3-rex-plugins/templates/ui/filechooser/Factory.js
+++ /dev/null
@@ -1,13 +0,0 @@
-import { FileChooser } from './FileChooser.js';
-import ObjectFactory from '../ObjectFactory.js';
-import SetValue from '../../../plugins/utils/object/SetValue.js';
-
-ObjectFactory.register('fileChooser', function (config) {
- var gameObject = new FileChooser(this.scene, config);
- this.scene.add.existing(gameObject);
- return gameObject;
-});
-
-SetValue(window, 'RexPlugins.UI.FileChooser', FileChooser);
-
-export default FileChooser;
\ No newline at end of file
diff --git a/spaces/Alfasign/chat-llm-streaming/README.md b/spaces/Alfasign/chat-llm-streaming/README.md
deleted file mode 100644
index e060a7e39365a40d46c37d752a32f150acc8a7f9..0000000000000000000000000000000000000000
--- a/spaces/Alfasign/chat-llm-streaming/README.md
+++ /dev/null
@@ -1,13 +0,0 @@
----
-title: Chat Llm Streaming
-emoji: 📊
-colorFrom: blue
-colorTo: gray
-sdk: gradio
-sdk_version: 3.20.1
-app_file: app.py
-pinned: false
-duplicated_from: olivierdehaene/chat-llm-streaming
----
-
-Check out the configuration reference at https://huggingface.co/docs/hub/spaces-config-reference
diff --git a/spaces/Amrrs/DragGan-Inversion/PTI/models/StyleCLIP/models/__init__.py b/spaces/Amrrs/DragGan-Inversion/PTI/models/StyleCLIP/models/__init__.py
deleted file mode 100644
index e69de29bb2d1d6434b8b29ae775ad8c2e48c5391..0000000000000000000000000000000000000000
diff --git a/spaces/Amrrs/DragGan-Inversion/PTI/models/e4e/encoders/psp_encoders.py b/spaces/Amrrs/DragGan-Inversion/PTI/models/e4e/encoders/psp_encoders.py
deleted file mode 100644
index cbd9d849149ca5df3a5589015811dc17876a51d7..0000000000000000000000000000000000000000
--- a/spaces/Amrrs/DragGan-Inversion/PTI/models/e4e/encoders/psp_encoders.py
+++ /dev/null
@@ -1,200 +0,0 @@
-from enum import Enum
-import math
-import numpy as np
-import torch
-from torch import nn
-from torch.nn import Conv2d, BatchNorm2d, PReLU, Sequential, Module
-
-from PTI.models.e4e.encoders.helpers import get_blocks, bottleneck_IR, bottleneck_IR_SE, _upsample_add
-from PTI.models.e4e.stylegan2.model import EqualLinear
-
-
-class ProgressiveStage(Enum):
- WTraining = 0
- Delta1Training = 1
- Delta2Training = 2
- Delta3Training = 3
- Delta4Training = 4
- Delta5Training = 5
- Delta6Training = 6
- Delta7Training = 7
- Delta8Training = 8
- Delta9Training = 9
- Delta10Training = 10
- Delta11Training = 11
- Delta12Training = 12
- Delta13Training = 13
- Delta14Training = 14
- Delta15Training = 15
- Delta16Training = 16
- Delta17Training = 17
- Inference = 18
-
-
-class GradualStyleBlock(Module):
- def __init__(self, in_c, out_c, spatial):
- super(GradualStyleBlock, self).__init__()
- self.out_c = out_c
- self.spatial = spatial
- num_pools = int(np.log2(spatial))
- modules = []
- modules += [Conv2d(in_c, out_c, kernel_size=3, stride=2, padding=1),
- nn.LeakyReLU()]
- for i in range(num_pools - 1):
- modules += [
- Conv2d(out_c, out_c, kernel_size=3, stride=2, padding=1),
- nn.LeakyReLU()
- ]
- self.convs = nn.Sequential(*modules)
- self.linear = EqualLinear(out_c, out_c, lr_mul=1)
-
- def forward(self, x):
- x = self.convs(x)
- x = x.view(-1, self.out_c)
- x = self.linear(x)
- return x
-
-
-class GradualStyleEncoder(Module):
- def __init__(self, num_layers, mode='ir', opts=None):
- super(GradualStyleEncoder, self).__init__()
- assert num_layers in [50, 100, 152], 'num_layers should be 50,100, or 152'
- assert mode in ['ir', 'ir_se'], 'mode should be ir or ir_se'
- blocks = get_blocks(num_layers)
- if mode == 'ir':
- unit_module = bottleneck_IR
- elif mode == 'ir_se':
- unit_module = bottleneck_IR_SE
- self.input_layer = Sequential(Conv2d(3, 64, (3, 3), 1, 1, bias=False),
- BatchNorm2d(64),
- PReLU(64))
- modules = []
- for block in blocks:
- for bottleneck in block:
- modules.append(unit_module(bottleneck.in_channel,
- bottleneck.depth,
- bottleneck.stride))
- self.body = Sequential(*modules)
-
- self.styles = nn.ModuleList()
- log_size = int(math.log(opts.stylegan_size, 2))
- self.style_count = 2 * log_size - 2
- self.coarse_ind = 3
- self.middle_ind = 7
- for i in range(self.style_count):
- if i < self.coarse_ind:
- style = GradualStyleBlock(512, 512, 16)
- elif i < self.middle_ind:
- style = GradualStyleBlock(512, 512, 32)
- else:
- style = GradualStyleBlock(512, 512, 64)
- self.styles.append(style)
- self.latlayer1 = nn.Conv2d(256, 512, kernel_size=1, stride=1, padding=0)
- self.latlayer2 = nn.Conv2d(128, 512, kernel_size=1, stride=1, padding=0)
-
- def forward(self, x):
- x = self.input_layer(x)
-
- latents = []
- modulelist = list(self.body._modules.values())
- for i, l in enumerate(modulelist):
- x = l(x)
- if i == 6:
- c1 = x
- elif i == 20:
- c2 = x
- elif i == 23:
- c3 = x
-
- for j in range(self.coarse_ind):
- latents.append(self.styles[j](c3))
-
- p2 = _upsample_add(c3, self.latlayer1(c2))
- for j in range(self.coarse_ind, self.middle_ind):
- latents.append(self.styles[j](p2))
-
- p1 = _upsample_add(p2, self.latlayer2(c1))
- for j in range(self.middle_ind, self.style_count):
- latents.append(self.styles[j](p1))
-
- out = torch.stack(latents, dim=1)
- return out
-
-
-class Encoder4Editing(Module):
- def __init__(self, num_layers, mode='ir', opts=None):
- super(Encoder4Editing, self).__init__()
- assert num_layers in [50, 100, 152], 'num_layers should be 50,100, or 152'
- assert mode in ['ir', 'ir_se'], 'mode should be ir or ir_se'
- blocks = get_blocks(num_layers)
- if mode == 'ir':
- unit_module = bottleneck_IR
- elif mode == 'ir_se':
- unit_module = bottleneck_IR_SE
- self.input_layer = Sequential(Conv2d(3, 64, (3, 3), 1, 1, bias=False),
- BatchNorm2d(64),
- PReLU(64))
- modules = []
- for block in blocks:
- for bottleneck in block:
- modules.append(unit_module(bottleneck.in_channel,
- bottleneck.depth,
- bottleneck.stride))
- self.body = Sequential(*modules)
-
- self.styles = nn.ModuleList()
- log_size = int(math.log(opts.stylegan_size, 2))
- self.style_count = 2 * log_size - 2
- self.coarse_ind = 3
- self.middle_ind = 7
-
- for i in range(self.style_count):
- if i < self.coarse_ind:
- style = GradualStyleBlock(512, 512, 16)
- elif i < self.middle_ind:
- style = GradualStyleBlock(512, 512, 32)
- else:
- style = GradualStyleBlock(512, 512, 64)
- self.styles.append(style)
-
- self.latlayer1 = nn.Conv2d(256, 512, kernel_size=1, stride=1, padding=0)
- self.latlayer2 = nn.Conv2d(128, 512, kernel_size=1, stride=1, padding=0)
-
- self.progressive_stage = ProgressiveStage.Inference
-
- def get_deltas_starting_dimensions(self):
- ''' Get a list of the initial dimension of every delta from which it is applied '''
- return list(range(self.style_count)) # Each dimension has a delta applied to it
-
- def set_progressive_stage(self, new_stage: ProgressiveStage):
- self.progressive_stage = new_stage
- print('Changed progressive stage to: ', new_stage)
-
- def forward(self, x):
- x = self.input_layer(x)
-
- modulelist = list(self.body._modules.values())
- for i, l in enumerate(modulelist):
- x = l(x)
- if i == 6:
- c1 = x
- elif i == 20:
- c2 = x
- elif i == 23:
- c3 = x
-
- # Infer main W and duplicate it
- w0 = self.styles[0](c3)
- w = w0.repeat(self.style_count, 1, 1).permute(1, 0, 2)
- stage = self.progressive_stage.value
- features = c3
- for i in range(1, min(stage + 1, self.style_count)): # Infer additional deltas
- if i == self.coarse_ind:
- p2 = _upsample_add(c3, self.latlayer1(c2)) # FPN's middle features
- features = p2
- elif i == self.middle_ind:
- p1 = _upsample_add(p2, self.latlayer2(c1)) # FPN's fine features
- features = p1
- delta_i = self.styles[i](features)
- w[:, i] += delta_i
- return w
diff --git a/spaces/Andy1621/uniformer_image_detection/configs/gcnet/mask_rcnn_r101_fpn_syncbn-backbone_r4_gcb_c3-c5_1x_coco.py b/spaces/Andy1621/uniformer_image_detection/configs/gcnet/mask_rcnn_r101_fpn_syncbn-backbone_r4_gcb_c3-c5_1x_coco.py
deleted file mode 100644
index 32972de857b3c4f43170dcd3e7fbce76425f094d..0000000000000000000000000000000000000000
--- a/spaces/Andy1621/uniformer_image_detection/configs/gcnet/mask_rcnn_r101_fpn_syncbn-backbone_r4_gcb_c3-c5_1x_coco.py
+++ /dev/null
@@ -1,11 +0,0 @@
-_base_ = '../mask_rcnn/mask_rcnn_r101_fpn_1x_coco.py'
-model = dict(
- backbone=dict(
- norm_cfg=dict(type='SyncBN', requires_grad=True),
- norm_eval=False,
- plugins=[
- dict(
- cfg=dict(type='ContextBlock', ratio=1. / 4),
- stages=(False, True, True, True),
- position='after_conv3')
- ]))
diff --git a/spaces/Andy1621/uniformer_image_detection/configs/grid_rcnn/grid_rcnn_r50_fpn_gn-head_2x_coco.py b/spaces/Andy1621/uniformer_image_detection/configs/grid_rcnn/grid_rcnn_r50_fpn_gn-head_2x_coco.py
deleted file mode 100644
index 6ed5bcbb090b29ee57444d35b2eab5f23b58c2ee..0000000000000000000000000000000000000000
--- a/spaces/Andy1621/uniformer_image_detection/configs/grid_rcnn/grid_rcnn_r50_fpn_gn-head_2x_coco.py
+++ /dev/null
@@ -1,131 +0,0 @@
-_base_ = [
- '../_base_/datasets/coco_detection.py', '../_base_/default_runtime.py'
-]
-# model settings
-model = dict(
- type='GridRCNN',
- pretrained='torchvision://resnet50',
- backbone=dict(
- type='ResNet',
- depth=50,
- num_stages=4,
- out_indices=(0, 1, 2, 3),
- frozen_stages=1,
- norm_cfg=dict(type='BN', requires_grad=True),
- norm_eval=True,
- style='pytorch'),
- neck=dict(
- type='FPN',
- in_channels=[256, 512, 1024, 2048],
- out_channels=256,
- num_outs=5),
- rpn_head=dict(
- type='RPNHead',
- in_channels=256,
- feat_channels=256,
- anchor_generator=dict(
- type='AnchorGenerator',
- scales=[8],
- ratios=[0.5, 1.0, 2.0],
- strides=[4, 8, 16, 32, 64]),
- bbox_coder=dict(
- type='DeltaXYWHBBoxCoder',
- target_means=[.0, .0, .0, .0],
- target_stds=[1.0, 1.0, 1.0, 1.0]),
- loss_cls=dict(
- type='CrossEntropyLoss', use_sigmoid=True, loss_weight=1.0),
- loss_bbox=dict(type='SmoothL1Loss', beta=1.0 / 9.0, loss_weight=1.0)),
- roi_head=dict(
- type='GridRoIHead',
- bbox_roi_extractor=dict(
- type='SingleRoIExtractor',
- roi_layer=dict(type='RoIAlign', output_size=7, sampling_ratio=0),
- out_channels=256,
- featmap_strides=[4, 8, 16, 32]),
- bbox_head=dict(
- type='Shared2FCBBoxHead',
- with_reg=False,
- in_channels=256,
- fc_out_channels=1024,
- roi_feat_size=7,
- num_classes=80,
- bbox_coder=dict(
- type='DeltaXYWHBBoxCoder',
- target_means=[0., 0., 0., 0.],
- target_stds=[0.1, 0.1, 0.2, 0.2]),
- reg_class_agnostic=False),
- grid_roi_extractor=dict(
- type='SingleRoIExtractor',
- roi_layer=dict(type='RoIAlign', output_size=14, sampling_ratio=0),
- out_channels=256,
- featmap_strides=[4, 8, 16, 32]),
- grid_head=dict(
- type='GridHead',
- grid_points=9,
- num_convs=8,
- in_channels=256,
- point_feat_channels=64,
- norm_cfg=dict(type='GN', num_groups=36),
- loss_grid=dict(
- type='CrossEntropyLoss', use_sigmoid=True, loss_weight=15))),
- # model training and testing settings
- train_cfg=dict(
- rpn=dict(
- assigner=dict(
- type='MaxIoUAssigner',
- pos_iou_thr=0.7,
- neg_iou_thr=0.3,
- min_pos_iou=0.3,
- ignore_iof_thr=-1),
- sampler=dict(
- type='RandomSampler',
- num=256,
- pos_fraction=0.5,
- neg_pos_ub=-1,
- add_gt_as_proposals=False),
- allowed_border=0,
- pos_weight=-1,
- debug=False),
- rpn_proposal=dict(
- nms_pre=2000,
- max_per_img=2000,
- nms=dict(type='nms', iou_threshold=0.7),
- min_bbox_size=0),
- rcnn=dict(
- assigner=dict(
- type='MaxIoUAssigner',
- pos_iou_thr=0.5,
- neg_iou_thr=0.5,
- min_pos_iou=0.5,
- ignore_iof_thr=-1),
- sampler=dict(
- type='RandomSampler',
- num=512,
- pos_fraction=0.25,
- neg_pos_ub=-1,
- add_gt_as_proposals=True),
- pos_radius=1,
- pos_weight=-1,
- max_num_grid=192,
- debug=False)),
- test_cfg=dict(
- rpn=dict(
- nms_pre=1000,
- max_per_img=1000,
- nms=dict(type='nms', iou_threshold=0.7),
- min_bbox_size=0),
- rcnn=dict(
- score_thr=0.03,
- nms=dict(type='nms', iou_threshold=0.3),
- max_per_img=100)))
-# optimizer
-optimizer = dict(type='SGD', lr=0.02, momentum=0.9, weight_decay=0.0001)
-optimizer_config = dict(grad_clip=None)
-# learning policy
-lr_config = dict(
- policy='step',
- warmup='linear',
- warmup_iters=3665,
- warmup_ratio=1.0 / 80,
- step=[17, 23])
-runner = dict(type='EpochBasedRunner', max_epochs=25)
diff --git a/spaces/Andy1621/uniformer_image_segmentation/configs/apcnet/apcnet_r101-d8_512x512_160k_ade20k.py b/spaces/Andy1621/uniformer_image_segmentation/configs/apcnet/apcnet_r101-d8_512x512_160k_ade20k.py
deleted file mode 100644
index 1ce2279a0fbfd6fcc7cd20e3f552b1a39f47d943..0000000000000000000000000000000000000000
--- a/spaces/Andy1621/uniformer_image_segmentation/configs/apcnet/apcnet_r101-d8_512x512_160k_ade20k.py
+++ /dev/null
@@ -1,2 +0,0 @@
-_base_ = './apcnet_r50-d8_512x512_160k_ade20k.py'
-model = dict(pretrained='open-mmlab://resnet101_v1c', backbone=dict(depth=101))
diff --git a/spaces/Andy1621/uniformer_image_segmentation/configs/deeplabv3/deeplabv3_r18b-d8_769x769_80k_cityscapes.py b/spaces/Andy1621/uniformer_image_segmentation/configs/deeplabv3/deeplabv3_r18b-d8_769x769_80k_cityscapes.py
deleted file mode 100644
index fd920f0ca7c690d3d1c44f5f7be1cbea18fa14d4..0000000000000000000000000000000000000000
--- a/spaces/Andy1621/uniformer_image_segmentation/configs/deeplabv3/deeplabv3_r18b-d8_769x769_80k_cityscapes.py
+++ /dev/null
@@ -1,9 +0,0 @@
-_base_ = './deeplabv3_r50-d8_769x769_80k_cityscapes.py'
-model = dict(
- pretrained='torchvision://resnet18',
- backbone=dict(type='ResNet', depth=18),
- decode_head=dict(
- in_channels=512,
- channels=128,
- ),
- auxiliary_head=dict(in_channels=256, channels=64))
diff --git a/spaces/AnimalEquality/chatbot/_proc/_docs/styles.css b/spaces/AnimalEquality/chatbot/_proc/_docs/styles.css
deleted file mode 100644
index 66ccc49ee8f0e73901dac02dc4e9224b7d1b2c78..0000000000000000000000000000000000000000
--- a/spaces/AnimalEquality/chatbot/_proc/_docs/styles.css
+++ /dev/null
@@ -1,37 +0,0 @@
-.cell {
- margin-bottom: 1rem;
-}
-
-.cell > .sourceCode {
- margin-bottom: 0;
-}
-
-.cell-output > pre {
- margin-bottom: 0;
-}
-
-.cell-output > pre, .cell-output > .sourceCode > pre, .cell-output-stdout > pre {
- margin-left: 0.8rem;
- margin-top: 0;
- background: none;
- border-left: 2px solid lightsalmon;
- border-top-left-radius: 0;
- border-top-right-radius: 0;
-}
-
-.cell-output > .sourceCode {
- border: none;
-}
-
-.cell-output > .sourceCode {
- background: none;
- margin-top: 0;
-}
-
-div.description {
- padding-left: 2px;
- padding-top: 5px;
- font-style: italic;
- font-size: 135%;
- opacity: 70%;
-}
diff --git a/spaces/AnimeStudio/anime-models/index.html b/spaces/AnimeStudio/anime-models/index.html
deleted file mode 100644
index 40b11abfac0f6f7c145d1d349a978f07587cf433..0000000000000000000000000000000000000000
--- a/spaces/AnimeStudio/anime-models/index.html
+++ /dev/null
@@ -1,305 +0,0 @@
-import gradio as gr
-import os
-import sys
-from pathlib import Path
-
-models = [
- {"name": "Deliberate", "url": "Masagin/Deliberate"},
- {"name": "Dreamlike Anime", "url": "dreamlike-art/dreamlike-anime-1.0"},
- {"name": "Dreamlike Diffusion", "url": "dreamlike-art/dreamlike-diffusion-1.0"},
- {"name": "Dreamlike Photoreal", "url": "dreamlike-art/dreamlike-photoreal-2.0"},
- {"name": "Dreamshaper", "url": "Lykon/DreamShaper"},
- {"name": "Lyriel 1.3", "url": "sakistriker/Lyriel_V1.3"},
- {"name": "Never Ending Dream 2", "url": "luongphamit/NeverEnding-Dream2"},
- {"name": "Protogen X 5.8", "url": "darkstorm2150/Protogen_x5.8_Official_Release"},
- {"name": "❤ ART MODELS ==========", "url": "dreamlike-art/dreamlike-diffusion-1.0"},
- {"name": "Alice in Diffusion Land", "url": "Guizmus/SDArt_AliceInDiffusionLand"},
- {"name": "Alt Clip", "url": "BAAI/AltCLIP"},
- {"name": "Anything Midjourney 4.1", "url": "Joeythemonster/anything-midjourney-v-4-1"},
- {"name": "Chaos and Order", "url": "Guizmus/SDArt_ChaosAndOrder768"},
- {"name": "Chilloutclara", "url": "Fred99774/chilloutvlara"},
- {"name": "Comic Diffusion", "url": "ogkalu/Comic-Diffusion"},
- {"name": "Cosmic Horros 768", "url": "Guizmus/SDArt_cosmichorrors768"},
- {"name": "Cosmic Horros", "url": "Guizmus/SDArt_cosmichorrors"},
- {"name": "DGSpitzer", "url": "DGSpitzer/DGSpitzer-Art-Diffusion"},
- {"name": "Dungeons and Diffusion", "url": "0xJustin/Dungeons-and-Diffusion"},
- {"name": "Elden Ring", "url": "nitrosocke/elden-ring-diffusion"},
- {"name": "Epic Diffusion 1.1", "url": "johnslegers/epic-diffusion-v1.1"},
- {"name": "Epic Diffusion", "url": "johnslegers/epic-diffusion"},
- {"name": "EpicMix Realism", "url": "Duskfallcrew/EpicMix_Realism"},
- {"name": "Fantasy Mix", "url": "theintuitiveye/FantasyMix"},
- {"name": "Girl New 1", "url": "Fred99774/girlnew1"},
- {"name": "Lit 6B", "url": "hakurei/lit-6B"},
- {"name": "Luna Diffusion", "url": "proximasanfinetuning/luna-diffusion"},
- {"name": "Midjourney 4.0", "url": "flax/midjourney-v4-diffusion"},
- {"name": "Midjourney 4.1", "url": "Joeythemonster/anything-midjourney-v-4-1"},
- {"name": "Mo-Di Diffusion", "url": "nitrosocke/mo-di-diffusion"},
- {"name": "Nitro Diffusion", "url": "nitrosocke/Nitro-Diffusion"},
- {"name": "Openjourney V2", "url": "prompthero/openjourney-v2"},
- {"name": "Openjourney", "url": "prompthero/openjourney"},
- {"name": "Seek Art Mega", "url": "coreco/seek.art_MEGA"},
- {"name": "Something", "url": "Guizmus/SDArt_something"},
- {"name": "Spider Verse diffusion", "url": "nitrosocke/spider-verse-diffusion"},
- {"name": "Vintedois 1.0", "url": "22h/vintedois-diffusion-v0-1"},
- {"name": "Vintedois 2.0", "url": "22h/vintedois-diffusion-v0-2"},
- {"name": "❤ ART STYLES ==========", "url": "joachimsallstrom/Double-Exposure-Diffusion"},
- {"name": "Balloon Art", "url": "Fictiverse/Stable_Diffusion_BalloonArt_Model"},
- {"name": "Double Exposure Diffusion", "url": "joachimsallstrom/Double-Exposure-Diffusion"},
- {"name": "Fluid Art", "url": "Fictiverse/Stable_Diffusion_FluidArt_Model"},
- {"name": "GTA5 Artwork Diffusion", "url": "ItsJayQz/GTA5_Artwork_Diffusion"},
- {"name": "Marvel WhatIf Diffusion", "url": "ItsJayQz/Marvel_WhatIf_Diffusion"},
- {"name": "Naruto Diffuser", "url": "lambdalabs/sd-naruto-diffusers"},
- {"name": "Papercut", "url": "Fictiverse/Stable_Diffusion_PaperCut_Model"},
- {"name": "Pokemon Diffuser", "url": "lambdalabs/sd-pokemon-diffusers"},
- {"name": "Synthwave Punk 2", "url": "ItsJayQz/SynthwavePunk-v2"},
- {"name": "Valorant Diffusion", "url": "ItsJayQz/Valorant_Diffusion"},
- {"name": "Van Gogh Diffusion", "url": "dallinmackay/Van-Gogh-diffusion"},
- {"name": "Vectorartz Diffusion", "url": "coder119/Vectorartz_Diffusion"},
- {"name": "VoxelArt", "url": "Fictiverse/Stable_Diffusion_VoxelArt_Model"},
- {"name": "❤ ANIME MODELS ==========", "url": "dreamlike-art/dreamlike-anime-1.0"},
- {"name": "7 Pa", "url": "AIARTCHAN/7pa"},
- {"name": "A Certain Model", "url": "JosephusCheung/ACertainModel"},
- {"name": "A Certain Thing", "url": "JosephusCheung/ACertainThing"},
- {"name": "A Certainity", "url": "JosephusCheung/ACertainty"},
- {"name": "Abyss Hell Hero", "url": "AIARTCHAN/AbyssHellHero"},
- {"name": "Abyss Maple 3", "url": "AIARTCHAN/AbyssMapleVer3"},
- {"name": "Abyss Orange Mix 2", "url": "WarriorMama777/AbyssOrangeMix2"},
- {"name": "Abyss Orange Mix 4", "url": "sakistriker/AbyssOrangeMix3"},
- {"name": "Abyss Orange Mix", "url": "WarriorMama777/AbyssOrangeMix"},
- {"name": "AbyssHell 3", "url": "AIARTCHAN/AbyssHellVer3"},
- {"name": "All 526 Animated", "url": "stablediffusionapi/all-526-animated"},
- {"name": "Anidosmix 3", "url": "AIARTCHAN/anidosmixV2"},
- {"name": "Anime Kawai Diffusion", "url": "Ojimi/anime-kawai-diffusion"},
- {"name": "Anireal 3D V2", "url": "circulus/sd-anireal-3d-v2"},
- {"name": "AnyLORA", "url": "kubanemil/AnyLORA"},
- {"name": "Anything 2.1", "url": "swl-models/anything-v2.1"},
- {"name": "Anything 3.0 Light", "url": "mm00/anything-v3.0-light"},
- {"name": "Anything 3.0", "url": "Linaqruf/anything-v3.0"},
- {"name": "Anything 3.1", "url": "cag/anything-v3-1"},
- {"name": "Anything 3X", "url": "iZELX1/Anything-V3-X"},
- {"name": "Anything 4.0", "url": "andite/anything-v4.0"},
- {"name": "Anything 5", "url": "sakistriker/Anything_V5_PrtRE"},
- {"name": "Anything 5.0", "url": "stablediffusionapi/anything-v5"},
- {"name": "Anything Else 4", "url": "stablediffusionapi/anythingelse-v4"},
- {"name": "Anything Else 5", "url": "stablediffusionapi/anything-v5"},
- {"name": "Arcane Diffusion", "url": "nitrosocke/Arcane-Diffusion"},
- {"name": "Archer Diffusion", "url": "nitrosocke/archer-diffusion"},
- {"name": "Asian Mix", "url": "D1b4l4p/AsianMix"},
- {"name": "Blood Orange Mix", "url": "WarriorMama777/BloodOrangeMix"},
- {"name": "CamelliaMix 2.5D","url": "stablediffusionapi/camelliamix25d"},
- {"name": "CamelliaMix Line","url": "stablediffusionapi/camelliamixline"},
- {"name": "CamelliaMix","url": "Powidl43/CamelliaMix"},
- {"name": "Cetusmix", "url": "stablediffusionapi/cetusmix"},
- {"name": "Chik Mix", "url": "stablediffusionapi/chikmix"},
- {"name": "Chikmix", "url": "stablediffusionapi/chikmix"},
- {"name": "Chillout App Factory","url": "stablediffusionapi/chillout-app-factory"},
- {"name": "Classic Anime", "url": "nitrosocke/classic-anim-diffusion"},
- {"name": "Cool Japan Diffusion 2.1.2", "url": "aipicasso/cool-japan-diffusion-2-1-2"},
- {"name": "Cosmic Babes", "url": "stablediffusionapi/cosmic-babes"},
- {"name": "Counterfeit 1.0", "url": "gsdf/counterfeit-v1.0"},
- {"name": "Counterfeit 2", "url": "gsdf/Counterfeit-V2.0"},
- {"name": "Counterfeit 2.0", "url": "gsdf/Counterfeit-V2.0"},
- {"name": "Counterfeit 3.0", "url": "stablediffusionapi/counterfeit-v30"},
- {"name": "CuteSexyRobutts", "url": "andite/cutesexyrobutts-diffusion"},
- {"name": "CyberPunk Anime", "url": "DGSpitzer/Cyberpunk-Anime-Diffusion"},
- {"name": "Dark Sushi Mix", "url": "stablediffusionapi/dark-sushi-mix"},
- {"name": "Dash Sushi 25d", "url": "stablediffusionapi/dark-sushi-25d"},
- {"name": "DucHaiten Anime", "url": "DucHaiten/DucHaitenAnime"},
- {"name": "Eerie Orange Mix", "url": "WarriorMama777/EerieOrangeMix"},
- {"name": "Eimis Anime Diffusion", "url": "eimiss/EimisAnimeDiffusion_1.0v"},
- {"name": "Ghibli Diffusion", "url": "nitrosocke/Ghibli-Diffusion"},
- {"name": "GrapeFruit", "url": "iZELX1/Grapefruit"},
- {"name": "GuoFeng 3", "url": "xiaolxl/GuoFeng3"},
- {"name": "Guweiz Diffusion", "url": "andite/guweiz-diffusion"},
- {"name": "Hiten Diffusion", "url": "andite/hiten-diffusion"},
- {"name": "Icomix 2", "url": "stablediffusionapi/icomix-2"},
- {"name": "InkPunk Diffusion", "url": "Envvi/Inkpunk-Diffusion"},
- {"name": "Mama Orange Mixs", "url": "WarriorMama777/OrangeMixs"},
- {"name": "Mashuu Diffusion", "url": "andite/mashuu-diffusion"},
- {"name": "Meainamis 8", "url": "sakistriker/MeinaMix_V8"},
- {"name": "Meina Alter", "url": "stablediffusionapi/meinaalter"},
- {"name": "Meina Pastel", "url": "stablediffusionapi/meinapastel"},
- {"name": "MeinaMix 7", "url": "Nacholmo/meinamixv7-diffusers"},
- {"name": "Mignon Diffusion", "url": "andite/mignon-diffusion"},
- {"name": "MikaPikazo Diffusion", "url": "andite/mikapikazo-diffusion"},
- {"name": "Mikapikazo", "url": "andite/mikapikazo-diffusion"},
- {"name": "Mix Pro V4", "url": "AIARTCHAN/MIX-Pro-V4"},
- {"name": "NeverEnding-Dream", "url": "Lykon/NeverEnding-Dream"},
- {"name": "Niji V5 Style 1", "url": "sakistriker/NijiV5style_V1"},
- {"name": "Openjourney 4", "url": "prompthero/openjourney-v4"},
- {"name": "OpenNiji", "url": "Korakoe/OpenNiji"},
- {"name": "Pastel Mix", "url": "andite/pastel-mix"},
- {"name": "Picasso Diffusion 1.1", "url": "aipicasso/picasso-diffusion-1-1"},
- {"name": "Piromizu Diffusion", "url": "andite/piromizu-diffusion"},
- {"name": "Protogen 2.2", "url": "darkstorm2150/Protogen_v2.2_Official_Release"},
- {"name": "Protogen Infinity", "url": "darkstorm2150/Protogen_Infinity_Official_Release"},
- {"name": "Protogen X 3.4", "url": "darkstorm2150/Protogen_x3.4_Official_Release"},
- {"name": "Rev Anim", "url": "stablediffusionapi/rev-anim"},
- {"name": "Rev Animated", "url": "coreml/coreml-ReV-Animated"},
- {"name": "Rev Animated", "url": "LottePeisch/RevAnimated-Diffusers"},
- {"name": "Something V 2.2","url": "NoCrypt/SomethingV2_2"},
- {"name": "Something V2","url": "NoCrypt/SomethingV2"},
- {"name": "Three Delicacy", "url": "stablediffusionapi/three-delicacy"},
- {"name": "Three Delicacy wonto", "url": "stablediffusionapi/three-delicacy-wonto"},
- {"name": "TMND mix", "url": "stablediffusionapi/tmnd-mix"},
- {"name": "Waifu Diffusion", "url": "hakurei/waifu-diffusion"},
- {"name": "❤ REALISTIC PHOTO MODELS ==========", "url": "dreamlike-art/dreamlike-photoreal-2.0"},
- {"name": "AmiIReal", "url": "stablediffusionapi/amireal"},
- {"name": "Analog Diffusion", "url": "wavymulder/Analog-Diffusion"},
- {"name": "Circulus 2.8", "url": "circulus/sd-photoreal-v2.8"},
- {"name": "Circulus Photoreal V2", "url": "circulus/sd-photoreal-real-v2"},
- {"name": "Claudfuen 1", "url": "claudfuen/photorealistic-fuen-v1"},
- {"name": "Collage Diffusion", "url": "wavymulder/collage-diffusion"},
- {"name": "Cyberrealistic", "url": "stablediffusionapi/cyberrealistic"},
- {"name": "Dreamful 2", "url": "Hius/DreamFul-V2"},
- {"name": "GakkiMix768", "url": "Sa1i/gakki-mix-768"},
- {"name": "Grimoeresigils", "url": "ECarbenia/grimoiresigils"},
- {"name": "HARDBlend", "url": "theintuitiveye/HARDblend"},
- {"name": "HassanBlend 1.4", "url": "hassanblend/hassanblend1.4"},
- {"name": "HassanBlend 1.5.1.2", "url": "hassanblend/HassanBlend1.5.1.2"},
- {"name": "Lomo Diffusion", "url": "wavymulder/lomo-diffusion"},
- {"name": "Model Shoot", "url": "wavymulder/modelshoot"},
- {"name": "Portrait Plus", "url": "wavymulder/portraitplus"},
- {"name": "QuinceMix", "url": "Hemlok/QuinceMix"},
- {"name": "Realistic Vision 1.4", "url": "SG161222/Realistic_Vision_V1.4"},
- {"name": "The Ally", "url": "stablediffusionapi/the-ally"},
- {"name": "Timeless Diffusion", "url": "wavymulder/timeless-diffusion"},
- {"name": "UltraSkin", "url": "VegaKH/Ultraskin"},
- {"name": "Wavyfusion", "url": "wavymulder/wavyfusion"},
- {"name": "❤ SEMI-REALISTIC MODELS ==========", "url": "stablediffusionapi/all-526"},
- {"name": "All 526", "url": "stablediffusionapi/all-526"},
- {"name": "All 526 animated", "url": "stablediffusionapi/all-526-animated"},
- {"name": "Circulus Semi Real 2", "url": "circulus/sd-photoreal-semi-v2"},
- {"name": "Semi Real Mix", "url": "robotjung/SemiRealMix"},
- {"name": "SpyBG", "url": "stablediffusionapi/spybg"},
- {"name": "❤ STABLE DIFFUSION MODELS ==========", "url": "stabilityai/stable-diffusion-2-1"},
- {"name": "Stable Diffusion 1.4","url": "CompVis/stable-diffusion-v1-4"},
- {"name": "Stable Diffusion 1.5","url": "runwayml/stable-diffusion-v1-5"},
- {"name": "Stable Diffusion 2.1","url": "stabilityai/stable-diffusion-2-1"},
- {"name": "Stable Diffusion 2.1 Base","url": "stabilityai/stable-diffusion-2-1-base"},
- {"name": "Stable Diffusion 2.1 Unclip","url": "stabilityai/stable-diffusion-2-1-unclip"},
- {"name": "❤ SCI FI MODELS ==========", "url": "nitrosocke/Future-Diffusion"},
- {"name": "Future Diffusion", "url": "nitrosocke/Future-Diffusion"},
- {"name": "JWST Deep Space Diffusion", "url": "dallinmackay/JWST-Deep-Space-diffusion"},
- {"name": "Robo Diffusion 3 Base", "url": "nousr/robo-diffusion-2-base"},
- {"name": "Robo Diffusion", "url": "nousr/robo-diffusion"},
- {"name": "Tron Legacy Diffusion", "url": "dallinmackay/Tron-Legacy-diffusion"},
- {"name": "❤ 3D ART MODELS ==========", "url": "DucHaiten/DucHaitenAIart"},
- {"name": "DucHaiten Art", "url": "DucHaiten/DucHaitenAIart"},
- {"name": "DucHaiten ClassicAnime", "url": "DucHaiten/DH_ClassicAnime"},
- {"name": "DucHaiten DreamWorld", "url": "DucHaiten/DucHaitenDreamWorld"},
- {"name": "DucHaiten Journey", "url": "DucHaiten/DucHaitenJourney"},
- {"name": "DucHaiten StyleLikeMe", "url": "DucHaiten/DucHaiten-StyleLikeMe"},
- {"name": "DucHaiten SuperCute", "url": "DucHaiten/DucHaitenSuperCute"},
- {"name": "Redshift Diffusion 768", "url": "nitrosocke/redshift-diffusion-768"},
- {"name": "Redshift Diffusion", "url": "nitrosocke/redshift-diffusion"},
-]
-
-current_model = models[0]
-
-text_gen = gr.Interface.load("spaces/Omnibus/MagicPrompt-Stable-Diffusion_link")
-
-models2 = []
-for model in models:
- model_url = f"models/{model['url']}"
- loaded_model = gr.Interface.load(model_url, live=True, preprocess=True)
- models2.append(loaded_model)
-
-
-def text_it(inputs, text_gen=text_gen):
- return text_gen(inputs)
-
-
-def set_model(current_model_index):
- global current_model
- current_model = models[current_model_index]
- return gr.update(label=f"{current_model['name']}")
-
-
-def send_it(inputs, model_choice):
- proc = models2[model_choice]
- return proc(inputs)
-
-
-css = """"""
-
-with gr.Blocks(css=css) as myface:
- gr.HTML(
- """
-
-
-
-
-
-
-
-
-
-
-
-
-
-
-
-"""
- )
-
- with gr.Row():
- with gr.Row():
- input_text = gr.Textbox(label="Prompt idea", lines=1)
- # Model selection dropdown
- model_name1 = gr.Dropdown(
- label="Choose Model",
- choices=[m["name"] for m in models],
- type="index",
- value=current_model["name"],
- interactive=True,
- )
- with gr.Row():
- see_prompts = gr.Button("Generate Prompts")
- run = gr.Button("Generate Images", variant="primary")
- with gr.Tab("Main"):
- with gr.Row():
- output1 = gr.Image(label=f"{current_model['name']}")
- output2 = gr.Image(label=f"{current_model['name']}")
- output3 = gr.Image(label=f"{current_model['name']}")
- output4 = gr.Image(label=f"{current_model['name']}")
- with gr.Row():
- magic1 = gr.Textbox(lines=4)
- magic2 = gr.Textbox(lines=4)
- magic3 = gr.Textbox(lines=4)
- magic4 = gr.Textbox(lines=4)
-
- with gr.Row():
- output5 = gr.Image(label=f"{current_model['name']}")
- output6 = gr.Image(label=f"{current_model['name']}")
- output7 = gr.Image(label=f"{current_model['name']}")
- output8 = gr.Image(label=f"{current_model['name']}")
- with gr.Row():
- magic5 = gr.Textbox(lines=4)
- magic6 = gr.Textbox(lines=4)
- magic7 = gr.Textbox(lines=4)
- magic8 = gr.Textbox(lines=4)
-
- model_name1.change(set_model, inputs=model_name1, outputs=[output1, output2, output3, output4, output5, output6, output7, output8])
-
- run.click(send_it, inputs=[magic1, model_name1], outputs=[output1])
- run.click(send_it, inputs=[magic2, model_name1], outputs=[output2])
- run.click(send_it, inputs=[magic3, model_name1], outputs=[output3])
- run.click(send_it, inputs=[magic4, model_name1], outputs=[output4])
- run.click(send_it, inputs=[magic5, model_name1], outputs=[output5])
- run.click(send_it, inputs=[magic6, model_name1], outputs=[output6])
- run.click(send_it, inputs=[magic7, model_name1], outputs=[output7])
- run.click(send_it, inputs=[magic8, model_name1], outputs=[output8])
-
- see_prompts.click(text_it, inputs=[input_text], outputs=[magic1])
- see_prompts.click(text_it, inputs=[input_text], outputs=[magic2])
- see_prompts.click(text_it, inputs=[input_text], outputs=[magic3])
- see_prompts.click(text_it, inputs=[input_text], outputs=[magic4])
- see_prompts.click(text_it, inputs=[input_text], outputs=[magic5])
- see_prompts.click(text_it, inputs=[input_text], outputs=[magic6])
- see_prompts.click(text_it, inputs=[input_text], outputs=[magic7])
- see_prompts.click(text_it, inputs=[input_text], outputs=[magic8])
-
-myface.queue(concurrency_count=200)
-myface.launch(inline=True, show_api=False, max_threads=400)
\ No newline at end of file
diff --git a/spaces/Araloak/fz/start_server.sh b/spaces/Araloak/fz/start_server.sh
deleted file mode 100644
index 70024d145c33c909606a4760764ba0861fe53c72..0000000000000000000000000000000000000000
--- a/spaces/Araloak/fz/start_server.sh
+++ /dev/null
@@ -1,3 +0,0 @@
-#!/bin/bash
-
-python server.py
\ No newline at end of file
diff --git a/spaces/Arnx/MusicGenXvAKN/audiocraft/quantization/vq.py b/spaces/Arnx/MusicGenXvAKN/audiocraft/quantization/vq.py
deleted file mode 100644
index f67c3a0cd30d4b8993a36c587f00dc8a451d926f..0000000000000000000000000000000000000000
--- a/spaces/Arnx/MusicGenXvAKN/audiocraft/quantization/vq.py
+++ /dev/null
@@ -1,116 +0,0 @@
-# Copyright (c) Meta Platforms, Inc. and affiliates.
-# All rights reserved.
-#
-# This source code is licensed under the license found in the
-# LICENSE file in the root directory of this source tree.
-
-import math
-import typing as tp
-
-import torch
-
-from .base import BaseQuantizer, QuantizedResult
-from .core_vq import ResidualVectorQuantization
-
-
-class ResidualVectorQuantizer(BaseQuantizer):
- """Residual Vector Quantizer.
-
- Args:
- dimension (int): Dimension of the codebooks.
- n_q (int): Number of residual vector quantizers used.
- q_dropout (bool): Random quantizer drop out at train time.
- bins (int): Codebook size.
- decay (float): Decay for exponential moving average over the codebooks.
- kmeans_init (bool): Whether to use kmeans to initialize the codebooks.
- kmeans_iters (int): Number of iterations used for kmeans initialization.
- threshold_ema_dead_code (int): Threshold for dead code expiration. Replace any codes
- that have an exponential moving average cluster size less than the specified threshold with
- randomly selected vector from the current batch.
- orthogonal_reg_weight (float): Orthogonal regularization weights.
- orthogonal_reg_active_codes_only (bool): Apply orthogonal regularization only on active codes.
- orthogonal_reg_max_codes (optional int): Maximum number of codes to consider.
- for orthogonal regulariation.
- """
- def __init__(
- self,
- dimension: int = 256,
- n_q: int = 8,
- q_dropout: bool = False,
- bins: int = 1024,
- decay: float = 0.99,
- kmeans_init: bool = True,
- kmeans_iters: int = 10,
- threshold_ema_dead_code: int = 2,
- orthogonal_reg_weight: float = 0.0,
- orthogonal_reg_active_codes_only: bool = False,
- orthogonal_reg_max_codes: tp.Optional[int] = None,
- ):
- super().__init__()
- self.max_n_q = n_q
- self.n_q = n_q
- self.q_dropout = q_dropout
- self.dimension = dimension
- self.bins = bins
- self.decay = decay
- self.kmeans_init = kmeans_init
- self.kmeans_iters = kmeans_iters
- self.threshold_ema_dead_code = threshold_ema_dead_code
- self.orthogonal_reg_weight = orthogonal_reg_weight
- self.orthogonal_reg_active_codes_only = orthogonal_reg_active_codes_only
- self.orthogonal_reg_max_codes = orthogonal_reg_max_codes
- self.vq = ResidualVectorQuantization(
- dim=self.dimension,
- codebook_size=self.bins,
- num_quantizers=self.n_q,
- decay=self.decay,
- kmeans_init=self.kmeans_init,
- kmeans_iters=self.kmeans_iters,
- threshold_ema_dead_code=self.threshold_ema_dead_code,
- orthogonal_reg_weight=self.orthogonal_reg_weight,
- orthogonal_reg_active_codes_only=self.orthogonal_reg_active_codes_only,
- orthogonal_reg_max_codes=self.orthogonal_reg_max_codes,
- channels_last=False
- )
-
- def forward(self, x: torch.Tensor, frame_rate: int):
- n_q = self.n_q
- if self.training and self.q_dropout:
- n_q = int(torch.randint(1, self.n_q + 1, (1,)).item())
- bw_per_q = math.log2(self.bins) * frame_rate / 1000
- quantized, codes, commit_loss = self.vq(x, n_q=n_q)
- codes = codes.transpose(0, 1)
- # codes is [B, K, T], with T frames, K nb of codebooks.
- bw = torch.tensor(n_q * bw_per_q).to(x)
- return QuantizedResult(quantized, codes, bw, penalty=torch.mean(commit_loss))
-
- def encode(self, x: torch.Tensor) -> torch.Tensor:
- """Encode a given input tensor with the specified frame rate at the given bandwidth.
- The RVQ encode method sets the appropriate number of quantizer to use
- and returns indices for each quantizer.
- """
- n_q = self.n_q
- codes = self.vq.encode(x, n_q=n_q)
- codes = codes.transpose(0, 1)
- # codes is [B, K, T], with T frames, K nb of codebooks.
- return codes
-
- def decode(self, codes: torch.Tensor) -> torch.Tensor:
- """Decode the given codes to the quantized representation.
- """
- # codes is [B, K, T], with T frames, K nb of codebooks, vq.decode expects [K, B, T].
- codes = codes.transpose(0, 1)
- quantized = self.vq.decode(codes)
- return quantized
-
- @property
- def total_codebooks(self):
- return self.max_n_q
-
- @property
- def num_codebooks(self):
- return self.n_q
-
- def set_num_codebooks(self, n: int):
- assert n > 0 and n <= self.max_n_q
- self.n_q = n
diff --git a/spaces/Ataturk-Chatbot/HuggingFaceChat/venv/lib/python3.11/site-packages/pip/_vendor/requests/__version__.py b/spaces/Ataturk-Chatbot/HuggingFaceChat/venv/lib/python3.11/site-packages/pip/_vendor/requests/__version__.py
deleted file mode 100644
index 69be3dec7418c9bececde7811fd1d5a62f995f03..0000000000000000000000000000000000000000
--- a/spaces/Ataturk-Chatbot/HuggingFaceChat/venv/lib/python3.11/site-packages/pip/_vendor/requests/__version__.py
+++ /dev/null
@@ -1,14 +0,0 @@
-# .-. .-. .-. . . .-. .-. .-. .-.
-# |( |- |.| | | |- `-. | `-.
-# ' ' `-' `-`.`-' `-' `-' ' `-'
-
-__title__ = "requests"
-__description__ = "Python HTTP for Humans."
-__url__ = "https://requests.readthedocs.io"
-__version__ = "2.28.2"
-__build__ = 0x022802
-__author__ = "Kenneth Reitz"
-__author_email__ = "me@kennethreitz.org"
-__license__ = "Apache 2.0"
-__copyright__ = "Copyright Kenneth Reitz"
-__cake__ = "\u2728 \U0001f370 \u2728"
diff --git a/spaces/Awesimo/jojogan/e4e/utils/__init__.py b/spaces/Awesimo/jojogan/e4e/utils/__init__.py
deleted file mode 100644
index e69de29bb2d1d6434b8b29ae775ad8c2e48c5391..0000000000000000000000000000000000000000
diff --git a/spaces/AxelBell/EasyOCR_text_recognition/assets/footer.html b/spaces/AxelBell/EasyOCR_text_recognition/assets/footer.html
deleted file mode 100644
index dbcd14d03bbac66eeb0b10eb3e9a55c8de3d083d..0000000000000000000000000000000000000000
--- a/spaces/AxelBell/EasyOCR_text_recognition/assets/footer.html
+++ /dev/null
@@ -1,53 +0,0 @@
-
diff --git a/spaces/Bart92/RVC_HF/tools/infer_batch_rvc.py b/spaces/Bart92/RVC_HF/tools/infer_batch_rvc.py
deleted file mode 100644
index 763d17f14877a2ce35f750202e91356c1f24270f..0000000000000000000000000000000000000000
--- a/spaces/Bart92/RVC_HF/tools/infer_batch_rvc.py
+++ /dev/null
@@ -1,72 +0,0 @@
-import argparse
-import os
-import sys
-
-print("Command-line arguments:", sys.argv)
-
-now_dir = os.getcwd()
-sys.path.append(now_dir)
-import sys
-
-import tqdm as tq
-from dotenv import load_dotenv
-from scipy.io import wavfile
-
-from configs.config import Config
-from infer.modules.vc.modules import VC
-
-
-def arg_parse() -> tuple:
- parser = argparse.ArgumentParser()
- parser.add_argument("--f0up_key", type=int, default=0)
- parser.add_argument("--input_path", type=str, help="input path")
- parser.add_argument("--index_path", type=str, help="index path")
- parser.add_argument("--f0method", type=str, default="harvest", help="harvest or pm")
- parser.add_argument("--opt_path", type=str, help="opt path")
- parser.add_argument("--model_name", type=str, help="store in assets/weight_root")
- parser.add_argument("--index_rate", type=float, default=0.66, help="index rate")
- parser.add_argument("--device", type=str, help="device")
- parser.add_argument("--is_half", type=bool, help="use half -> True")
- parser.add_argument("--filter_radius", type=int, default=3, help="filter radius")
- parser.add_argument("--resample_sr", type=int, default=0, help="resample sr")
- parser.add_argument("--rms_mix_rate", type=float, default=1, help="rms mix rate")
- parser.add_argument("--protect", type=float, default=0.33, help="protect")
-
- args = parser.parse_args()
- sys.argv = sys.argv[:1]
-
- return args
-
-
-def main():
- load_dotenv()
- args = arg_parse()
- config = Config()
- config.device = args.device if args.device else config.device
- config.is_half = args.is_half if args.is_half else config.is_half
- vc = VC(config)
- vc.get_vc(args.model_name)
- audios = os.listdir(args.input_path)
- for file in tq.tqdm(audios):
- if file.endswith(".wav"):
- file_path = os.path.join(args.input_path, file)
- _, wav_opt = vc.vc_single(
- 0,
- file_path,
- args.f0up_key,
- None,
- args.f0method,
- args.index_path,
- None,
- args.index_rate,
- args.filter_radius,
- args.resample_sr,
- args.rms_mix_rate,
- args.protect,
- )
- out_path = os.path.join(args.opt_path, file)
- wavfile.write(out_path, wav_opt[0], wav_opt[1])
-
-
-if __name__ == "__main__":
- main()
diff --git a/spaces/Benson/text-generation/Examples/Animate Release 2022.md b/spaces/Benson/text-generation/Examples/Animate Release 2022.md
deleted file mode 100644
index b6ae7de066bb8a657cfeb11b380a7f1b1b02e5f8..0000000000000000000000000000000000000000
--- a/spaces/Benson/text-generation/Examples/Animate Release 2022.md
+++ /dev/null
@@ -1,71 +0,0 @@
-animate release 2022
DOWNLOAD ✒ https://bltlly.com/2v6K3r
-
-< h1 > Cartoon 2022: Most anticipated animated films of next year < / h1 >
- < h2 > Introduction < / h2 >
- < p > Who doesn't love cartoons? Whether we are children or adults, cartoons fascinate us with their magic, their humor, their stories full of adventure and emotion. Cartoon allows us to escape from reality and enter fantastic worlds, where everything is possible. < / p >
- < p > But how to choose the best cartoons for us and the kids? What awaits us in 2022 in the field of animated films? What news and surprises do animation studios around the world prepare for us?< / p >
- < p > To help you answer these questions, we have prepared for you a complete guide with the most awaited cartoons 2022. We will talk about the top 5 cartoons 2022, but also about other animated films that are worth mentioning. We will find out what it is about in every movie, why it is worth seeing, when it is released and where you can watch it. We will also use a comparative table to give you an overview of the 2022 cartoons. We hope you like this article and inspire you to watch as many 2022 cartoons as possible!< / p >
- < h2 > Top 5 cartoons 2022 < / h2 >
- < p > Of the multitude of animated films that will be released in 2022, we selected 5 cartoons that attracted our attention through originality, quality and popularity. These are cartoons that promise to give us an unforgettable visual and emotional experience, to amuse us, to excite us and to impress us. Here are these: < / p >
- < h3 > No tojimari foam ( The Closing of Suzume ) / h3 <
- < h4 > About < / h4 >
-
- < h4 > Why worth seeing < / h4 >
- < p > Suume no tojimari is a film that combines elements of fantasy, drama, adventure and humor, in the unmistakable style of Miyazaki. The film explores themes such as responsibility, friendship, love, sacrifice and destiny, through memorable characters and spectacular scenes. The film is a testament to Miyazaki's boundless artistic mastery and imagination, which returns after a 9-year hiatus from his latest film, The Wind Rises. Suzume no tojimari is a film that should not be missed by any fan of Japanese animation or cinematographic art in general. < / p >
- < h3 > Little Allan - An alien adventure ( Little Allan - The Human Antenna ) < / h3 >
- < h4 > About < / h4 >
- < p > Little Allan - An Alien Adventure ( Little Allan - The Human Antenna ) is an American animated film produced by Pixar Animation Studios, directed by Pete Docter, the one who brought us movies like Up, Inside Out or Soul. The film is about a boy named Allan who has a peculiarity: he can receive radio signals with his ears. This makes him considered strange and isolated from other children. But his life changes when he receives a mysterious message from space, inviting him to come to a meeting with some aliens. Allan accepts the challenge and embarks on an intergalactic adventure full of adventures and discoveries. < / p >
- < h4 > Why worth seeing < / h4 >
-
- < h3 > Rabbit Academy: Easter is in danger ( Rabbit Academy: Easter's in Danger ) < / h3 >
- < h4 > About < / h4 >
- < p > Rabbit Academy: Easter is in danger ( Rabbit Academy: Easter's in Danger ) is a French animated film produced by Gaumont Animation, directed by Benjamin Renner, the one who brought us movies like Ernest & Celestine or The Big Bad Fox and Other Tales. The film is about a special school for bunnies, where they learn how to become Easter bunnies. The protagonist is a bunny named Max, who has a dream: to become the best Easter bunny in history. But his dream is threatened by a group of mischievous foxes who want to steal Easter eggs and destroy the holiday. Max must join forces with his friends and teachers to save the Passover and fulfill his destiny. < / p >
- < h4 > Why worth seeing < / h4 >
- < p > Rabbit Academy: Easter is in danger is a film that brings a smile to our face through its delicious humor, its adorable characters and its funny situations. The film is a comedy full of action, suspense and emotion, which shows us how important it is to have courage, friendship and passion for what we do. The film is a real pleasure for the eyes, with a simple but expressive graphic style, which reminds us of classic comics. The film is a celebration of the tradition and joy of being a child, which makes us appreciate more the beauty and symbolism of Easter. < / p >
- < h3 > Shoeless Cat: Last Desire ( Puss in Boots: The Last Wish ) < / h3 >
- < h4 > About < / h4 >
-
- < h4 > Why worth seeing < / h4 >
- < p > The Shoeless Cat: The Last Wish is a film that gives us a generous dose of laughter, adventure and magic, in the company of one of the most beloved animated characters in recent years: the cat in shoes. The film is a funny and intelligent parody of the classic stories about Aladdin, the enchanted lamp and dragons, which surprises us with many cultural references and subtle jokes. The film is an explosion of color and energy, which captivates us with its action scenes, its engaging music and its high quality animation. The film is a life lesson about the importance of being grateful for what we have, of taking risks and following our heart. < / p >
- < h3 > Maurice Cat Ship ( The Amazing Maurice ) < / h3 >
- < h4 > About < / h4 >
- < p > The devastating cat Maurice ( The Amazing Maurice ) is a British animated film produced by Sky Cinema, directed by Toby Genkel, who brought us movies like Ooops! Noah is Gone ... or Two by Two: Overboard!. The film is based on the novel of the same name written by Terry Pratchett, about a talking cat named Maurice, who along with a band of smart rats and a naive flutist, sets up a scam in different cities. They claim that cities are invaded by rats and that the flutist can drive them away with his music, asking for money for their service. Everything goes well until they reach the city of Bad Blintz, where they discover a dark secret that endangers their lives. < / p >
- < h4 > Why worth seeing < / h4 >
-
- < h2 > Other 2022 cartoons worth mentioning < / h2 >
- < p > In addition to the top 5 cartoons 2022, there are other animated films that are announced to be interesting and fun. Here are some of them: < / p >
- < h3 > Inspector Sun's Adventures ( Sun Inspector and the Black Widow Course ) < / h3 >
- < p > Inspector Sun's Adventures ( Sun Inspector and the Race of the Black Widow ) is a Chinese animated film produced by Light Chaser Animation Studios, directed by Gary Wang, the one who brought us movies like The Guardian Brothers or Cats and Peachtopia. The film is about a police inspector named Sun, who is sent to investigate a series of strange crimes in Shanghai. He discovers that all the victims were bitten by a venomous black spider, which seems to be related to an old legend about a black widow who takes revenge on unfaithful men. Sun must solve the case before the spider can make new victims. < / p >
- < p > Inspector Sun's Adventures is a film that attracts us through its combination of comedy, action and mystery, through its funny characters and its story full of suspense and black humor. The film is a parody of polys and horror movies, which gives us a funny and original perspective on Chinese culture and history. The film is a testament to the talent and progress of the Light Chaser Animation studio, which claims to be one of the leading animation producers in China. < / p >
- < h3 > Ut ( Ut ) / h3 <
-
- < p > Uta is a film that excites us through its sensitive and profound story, through its complex characters and its beautiful music. The film is an exploration of the power of art to transform reality and heal souls, but also of the tragic history of Spain during the civil war. The film is an aesthetic and sound masterpiece, which delights us with its fluid and detailed animation, its vibrant colors and its memorable songs. The film is an ode to music and love, which makes us appreciate more the beauty and value of life. < / p >
- < h3 > Sing 2 ( Sing 2 ) / h3 <
- < p > Sing 2 ( Sing 2 ) is an American animated film produced by Illumination Entertainment, directed by Garth Jennings, who brought us the first Sing film of 2016. The film is a sequel to Buster Moon's story, a koala that runs a musical talent theater. This time, he wants to organize a grand show in Redshore City, the largest entertainment city in the world. To succeed, he must persuade a retired star named Clay Calloway, a rockstar lion, to join his band of talented and nice artists. < / p >
- < p > Sing 2 is a film that entertains us through its contagious humor, its charismatic characters and its engaging music. The film is a musical full of energy, rhythm and color, which shows us how important it is to trust ourselves, to follow our dreams and to enjoy life. The film is a source of inspiration and entertainment for all ages, which gives us a varied selection of well-known and new songs, performed by famous actors such as Matthew McConaughey, Reese Witherspoon, Scarlett Johansson or Bono. < / p >
- < h3 > Minions 2 ( Minions 2 ) / h3 <
-
- < p > Minions 2 is a film that amuses us through comic situations, adorable characters and their unintelligible language. The film is an adventure full of action, humor and nostalgia, which shows us how the connections between the minions and Gru formed, but also how the minions have evolved throughout history. The film is a delicacy for fans of the Despicable Me series, but also for those who love quality animation and unlimited fun. < / p >
- < h3 > Raya and the last dragon ( Raya and the Last Dragon ) / h3 <
- < p > Raya and the last dragon ( Raya and the Last Dragon ) is an American animated film produced by Walt Disney Animation Studios, directed by Don Hall and Carlos López Estrada, those who brought us movies like Big Hero 6 or Spider-Man: Into the Spider-Verse. The film is about a girl named Raya, who lives in a world called Kumandra, where people and dragons lived in harmony. But when an evil force called Druun threatened to destroy everything, the dragons sacrificed themselves to save humanity. After 500 years, Druun returns and Raya must find the last dragon left, named Sisu, to restore balance and peace in the world. < / p >
- < p > Raya and the last dragon is a film that impresses us with its epic and inspired story, through its strong and diverse characters, through its beautiful and detailed animation. The film is an exploration of Asian culture and mythology, which sends us messages about courage, friendship, trust and hope. The film is a fantastic and emotional adventure, which captivates us with its action scenes, its exotic music and its unique magic. < / p >
- < h2 > Conclusion < / h2 >
-
- < p > What do you think about 2022 cartoons? What is your favorite movie? What other animated films would you like to see? We look forward to your comments and feedback!< / p >
- < h2 > Frequently Asked Questions < / h2 >
- < h3 > When are the 2022 cartoons released?< / h3 >
- < p > 2022 animated drawings are released on various country and studio data. For example, Suzume no tojimari launches in Japan on March 18, 2022, Little Allan - An alien adventure takes place in the USA on July 15, 2022, the Academy of Bunnies: Easter is in danger, launch in France on April 6, 2022, the Cat in violation: Last wish launches in the USA on September 23, 2022, The naughty cat Maurice launches in the UK on October 28, 2022. To find out the exact release date of each film in your country, you can consult the official websites of animation studios or local distributors. < / p >
- < h3 > Where can I see the 2022 cartoon trailers?< / h3 >
-
- < h3 > What are the best sites to watch cartoons online?< / h3 >
-
-you can find cartoons from studios such as DreamWorks Animation, Nickelodeon or PBS Kids. Amazon Prime Video is available on various devices, such as TVs, computers, tablets or phones. < / p >
- < h3 > How can I find out more about 2022 cartoons?< / h3 >
- < p > To learn more about 2022 cartoons, you can consult various and credible sources, such as: - Official sites of animation studios or local distributors, where you will find release data, synopsis, trailers, images, interviews and other news about movies. - Sites specializing in reviews of movies and animated series, such as Rotten Tomatoes, IMDb or Metacritic, where you will find reviews of critics and audiences, notes and rankings of movies. - Sites and magazines dedicated to culture and entertainment, such as Variety, Entertainment Weekly or The Hollywood Reporter, where you will find articles and reports about animated films and the film industry in general. - Personal sites and blogs of fans and animation enthusiasts, such as Animation World Network, Cartoon Brew or Animation Magazine, where you will find detailed analyzes and comments about animated films and trends in the field. < / p >
- < h3 > What other types of animated films are there?< / h3 >
-
-
-< p > These are just some of the criteria by which animated films can be classified, but there are others, such as duration, purpose, message or audience. It is important to appreciate the diversity and quality of animated films, which give us the opportunity to enjoy art and enrich our culture. < / p > 64aa2da5cf
-
-
-
diff --git a/spaces/Benson/text-generation/Examples/Araa Solitario 4 Trajes De Descarga Gratuita Para Ventanas 7.md b/spaces/Benson/text-generation/Examples/Araa Solitario 4 Trajes De Descarga Gratuita Para Ventanas 7.md
deleted file mode 100644
index 485b74cf4f63218694ed0b79cdc489ff501692c2..0000000000000000000000000000000000000000
--- a/spaces/Benson/text-generation/Examples/Araa Solitario 4 Trajes De Descarga Gratuita Para Ventanas 7.md
+++ /dev/null
@@ -1,65 +0,0 @@
-
-Spider Solitaire 4 trajes: un juego de cartas desafiante y divertido para Windows 7
-Si estás buscando un juego de cartas que ponga a prueba tus habilidades y te mantenga entretenido, deberías probar Spider Solitaire 4 Suits. Esta es una variación del clásico juego de solitario de araña, donde tienes que organizar las cartas por palo de rey a as para limpiar el tablero. Sin embargo, a diferencia de la versión estándar, donde solo tienes que lidiar con uno o dos trajes, en Spider Solitaire 4 Suits tienes que jugar con los cuatro trajes: picas, corazones, diamantes y tréboles. Esto hace que el juego sea mucho más difícil y emocionante.
-¿Qué es Spider Solitaire 4 trajes?
-Las reglas del juego
-El juego comienza con 10 montones de tableau, cada uno de los cuatro montones a la izquierda contiene cuatro cartas boca abajo y cada uno de los montones restantes contiene tres cartas boca abajo. Cada uno de los montones del tableau se repartirá una carta boca arriba. Las cartas restantes serán boca abajo y se dividirán en seis pilas en la esquina inferior derecha.
-araña solitario 4 trajes de descarga gratuita para ventanas 7
Download File ———>>> https://bltlly.com/2v6MDD
-Un grupo de cartas o una sola carta se puede mover a otra pila de tableau si el movimiento en sí está en secuencia y en palo, por ejemplo, un tres de picas y un dos de picas se pueden mover a un cuatro de picas en otra pila de tableau. Las cartas boca abajo en los montones del tablero se revelarán cuando se eliminen las cartas boca arriba. Una pila de mesa vacía puede ser ocupada por cualquier carta.
-Si no se pueden hacer más movimientos, puede hacer clic en una pila de acciones para repartir una nueva carta en cada columna del cuadro. Pero tenga en cuenta que esto solo se puede hacer cuando todos los montones de tableau están ocupados por cartas. Perderá 10 puntos por cada movimiento que haga. Si no está satisfecho con su progreso, puede hacer clic en el botón Renunciar en la esquina inferior izquierda de la pantalla para salir del juego actual.
-Los beneficios de jugar Spider Solitaire 4 trajes
-Spider Solitaire 4 Suits no es solo un juego divertido, sino también una gran manera de mejorar tus habilidades mentales. Jugar a este juego puede ayudarte:
-
-Mejora tu concentración y enfoque
-Desarrolla tus habilidades de pensamiento lógico y resolución de problemas
-Mejora tu memoria y recuerda
-Reduce el estrés y la ansiedad
-Diviértete y relájate
-
-Cómo descargar e instalar Spider Solitaire 4 trajes para Windows 7?
-Descargar desde CNET
-Si quieres descargar Spider Solitaire 4 Suits de CNET, puedes seguir estos pasos:
-
-- Vaya a este enlace y haga clic en el botón Descargar ahora.
-- Guarde el archivo en su computadora y ejecútelo.
-- Siga las instrucciones en la pantalla para instalar el juego.
-- Iniciar el juego y disfrutar!
-
-Descargar de Microsoft Store
-Si quieres descargar Spider Solitaire 4 Suits de Microsoft Store, puedes seguir estos pasos:
-
-- Vaya a este enlace y haga clic en el botón Obtener.
-- Inicie sesión con su cuenta de Microsoft si se le solicita.
-- Espera a que el juego se descargue e instale en tu dispositivo.
-- Iniciar el juego y disfrutar!
-
-Descargar desde Solitr.com
-Si quieres descargar Spider Solitaire 4 Suits de Solitr.com, puedes seguir estos pasos:
-
-- Vaya a https://bltlly.com/2v6Jzt
-Pero ¿qué pasa si quieres jugar Candy Crush Saga en tu dispositivo Android sin usar Google Play Store? ¿O qué pasa si desea disfrutar de las últimas características y actualizaciones antes de que se publiquen oficialmente? En ese caso, es posible que desee descargar e instalar Candy Crush Saga APK 1.246.0.1, la última versión del juego a partir de junio de 2023.
-Pero ¿qué es un archivo APK y cómo lo usas? En este artículo, vamos a explicar todo lo que necesita saber sobre Candy Crush Saga APK 1.246.0.1, incluyendo cómo descargar e instalar, lo que ofrece características, y algunos consejos y trucos para jugar el juego.
- ¿Qué es Candy Crush Saga y por qué es popular?
-Candy Crush Saga es un juego de combinación de fichas gratuito desarrollado por King, una empresa líder en juegos casuales. El juego fue lanzado por primera vez en Facebook en abril de 2012, y luego en las plataformas iOS, Android, Windows Phone y Windows 10.
-La premisa del juego es simple: tienes que combinar tres o más caramelos del mismo color en un tablero para eliminarlos y ganar puntos. También tienes que completar varios objetivos dentro de un número limitado de movimientos o tiempo, como recoger ingredientes, limpiar jalea, o alcanzar una puntuación objetivo.
-
-También necesitarás usar boosters, que son elementos que pueden ayudarte a borrar niveles más rápido o más fácil. Los boosters se pueden ganar completando desafíos, girando la rueda de refuerzo diaria o comprándolos con dinero real.
-Candy Crush Saga es popular porque es divertido, desafiante, colorido y fácil de jugar. También tiene un elemento social, ya que puede conectarse con sus amigos de Facebook y comparar sus puntuaciones, enviar y recibir vidas y refuerzos, y competir en eventos y torneos.
- ¿Qué es un archivo APK y por qué lo necesita?
-Un archivo APK es un archivo de paquete de Android que contiene todos los archivos y el código necesario para instalar una aplicación en un dispositivo Android. Los archivos APK generalmente se descargan de Google Play Store u otras fuentes oficiales, pero a veces también son distribuidos por sitios web o desarrolladores de terceros.
-
-Es posible que necesite un archivo APK por varias razones:
-
-- Desea instalar una aplicación que no está disponible en su región o país.
-- Desea instalar una aplicación que ha sido eliminado de Google Play Store para algunos - Desea instalar una aplicación que tiene una versión más nueva o más antigua que la de Google Play Store. - Desea instalar una aplicación que ha sido modificada o hackeada por otra persona. - Desea instalar una aplicación que no es compatible con su dispositivo o sistema operativo.
-Sin embargo, también debe tener cuidado al descargar e instalar archivos APK de fuentes desconocidas, ya que podrían contener malware, virus o spyware que pueden dañar su dispositivo o robar su información personal. Siempre debe comprobar la reputación y las revisiones del sitio web o desarrollador antes de descargar un archivo APK, y escanearlo con un software antivirus confiable antes de instalarlo.
-También debería habilitar la opción de instalar aplicaciones de fuentes desconocidas en la configuración de su dispositivo, ya que esto generalmente está deshabilitado por razones de seguridad. Para hacer esto, puedes seguir estos pasos:
-
-
-- Encontrar la opción que dice Fuentes desconocidas o Instalar aplicaciones desconocidas y alternar en.
-- Confirme su elección tocando OK o Permitir.
-
-Ahora usted está listo para descargar e instalar Candy Crush Saga APK 1.246.0.1 en su dispositivo.
- Cómo descargar e instalar Candy Crush Saga APK 1.246.0.1
-Para descargar e instalar Candy Crush Saga APK 1.246.0.1, debe seguir estos pasos:
-
-- Ir a un sitio web de confianza que ofrece el archivo APK, como APKPure, APKMirror, o
-
- Si ves un mensaje que dice "¿Quieres instalar esta aplicación?", toca Instalar o Sí.
-- Espere a que termine el proceso de instalación y luego toque en Abrir o Listo.
-
-Felicidades! Usted ha instalado con éxito Candy Crush Saga APK 1.246.0.1 en su dispositivo. Ahora puedes disfrutar jugando el juego con todas sus últimas características y actualizaciones.
- Consejos y advertencias
-
-- Asegúrese de que tiene suficiente espacio de almacenamiento en el dispositivo antes de descargar e instalar el archivo APK, ya que es de aproximadamente 100 MB de tamaño.
-- Asegúrese de que tiene una conexión a Internet estable durante la descarga e instalación del archivo APK, ya que podría tomar algún tiempo dependiendo de su velocidad.
-
-- Asegúrese de que ha desinstalado cualquier versión anterior de Candy Crush Saga desde su dispositivo antes de instalar el archivo APK, ya que podrían causar conflictos o errores.
-- Asegúrese de actualizar su juego con regularidad mediante la comprobación de nuevas versiones del archivo APK en el sitio web que lo descargó de, ya que podrían corregir errores o añadir nuevas características.
-
- Características de Candy Crush Saga APK 1.246.0.1
-Candy Crush Saga APK 1.246.0.1 es la última versión del juego a partir de junio de 2023, y viene con muchas características nuevas y mejoras que lo hacen más divertido y emocionante que nunca. Estos son algunos de ellos:
- ¿Qué hay de nuevo en esta versión?
-
-- Nuevos episodios y niveles: El juego ahora tiene más de 8.000 niveles en más de 500 episodios, cada uno con un tema y un desafío diferentes. Los últimos episodios son Sweet Swamp (niveles 8,001-8,015), Fruity Fairground (niveles 8,016-8,030), y Sugary Shire (niveles 8,031-8,045).
-- Nuevos eventos y recompensas: El juego ahora tiene más eventos y recompensas para que disfrutes, como Sweet Streak (niveles completos sin perder vidas para obtener refuerzos), Sugar Track (recoge dulces para obtener premios), Star Chaser (recoge estrellas para obtener recompensas) y más.
-- Nuevos gráficos y sonidos - Nuevos gráficos y sonidos: El juego ahora tiene gráficos y sonidos mejorados que lo hacen más inmersivo y agradable. Notarás nuevas animaciones, efectos, fondos, música y voces en off que mejoran la atmósfera y el estado de ánimo del juego.
-- Nuevas características y mejoras: El juego ahora tiene algunas nuevas características y mejoras que lo hacen más fácil de usar y conveniente. Notarás nuevas opciones, ajustes, menús, botones e iconos que hacen que el juego sea más fácil de navegar y personalizar.
-
- ¿Cuáles son los modos de juego y los desafíos?
-Candy Crush Saga tiene diferentes modos de juego y desafíos que ponen a prueba tus habilidades y estrategia. Estos son algunos de ellos:
-
-
-- Ingredientes: Este es un modo de juego donde tienes que recoger ingredientes como cerezas y avellanas llevándolos al fondo del tablero.
-- jalea: este es un modo de juego donde tienes que limpiar toda la jalea en el tablero haciendo coincidir los caramelos en la parte superior.
-- Orden: Este es un modo de juego donde tienes que recoger un número específico de dulces o combinaciones haciendo coincidir en el tablero.
-- Mixto: Este es un modo de juego donde tienes que completar dos o más objetivos en un nivel, como limpiar jalea y recoger ingredientes.
-- Temporizado: Este es un desafío donde tienes que anotar tantos puntos como sea posible dentro de un tiempo limitado.
-- Candy Order: Este es un desafío donde tienes que recoger un número específico de dulces o combinaciones dentro de un número limitado de movimientos.
-- Movimientos: Este es un desafío donde tienes que anotar tantos puntos como sea posible dentro de un número limitado de movimientos.
-
- ¿Cómo usar caramelos y potenciadores especiales?
-Los caramelos y potenciadores especiales son elementos que pueden ayudarte a limpiar los niveles más rápido o más fácil. Estos son algunos de ellos y cómo usarlos:
-
-
-Dulces especiales
-Cómo crear
-Cómo usar
-
-
-Dulces a rayas
-Combina cuatro caramelos del mismo color en una fila o columna
-Borra toda una fila o columna cuando coincide o se activa
-
-
-Dulces envueltos
-Combina cinco caramelos del mismo color en forma de L o T
-Explota dos veces cuando coincide o se activa, despejando un área de 3x3 cada vez
-
-
-Bomba de color
-Combina cinco caramelos del mismo color en una fila o columna
-Borra todos los caramelos del mismo color cuando se empareja o se activa con otro caramelo
-
-
-Peces de jalea
-Combina cuatro caramelos del mismo color en forma cuadrada
-Envía tres peces de jalea a las baldosas al azar cuando coincide o se activa, la limpieza de cualquier jalea o bloqueadores en ellos
-
-
-Rueda de coco
-Combina cinco caramelos del mismo color en forma de L o T en un nivel de ingrediente
-Se mueve a lo largo del tablero cuando coincide o se activa, convirtiendo cualquier caramelo que pasa en un caramelo de rayas
-
- Booster
- Nombre Descripción Cómo obtener
- Lollipop Hammer Rompe cualquier caramelo o bloqueador en el tablero Gana completando desafíos, girando la rueda de refuerzo diaria, o comprando con dinero real
- Free Switch Intercambia dos dulces adyacentes en el tablero sin usar un movimiento Gana completando desafíos, girando la rueda de refuerzo diaria, o comprando con dinero real
- Sweet Teeth Come cualquier caramelo o bloqueador en el tablero hasta que se quede sin movimientos Gana completando desafíos, girando la rueda de refuerzo diaria, o comprando con dinero real
- Enfriador de bombas Añade cinco movimientos adicionales a cualquier bomba en el tablero antes de que explote Gana completando desafíos, girando la rueda de refuerzo diaria, o comprando con dinero real
- Jelly Fish Booster Añade tres peces de jalea a tu tablero al comienzo de un nivel de jalea Gana completando desafíos, girando la rueda de refuerzo diaria, o comprando con dinero real
- Color Bomb Booster Añade una bomba de color a su tablero al comienzo de cualquier nivel Gane completando desafíos, girando la rueda de refuerzo diaria, o comprando con dinero real
- Booster a rayas y envuelto Añade un caramelo a rayas y envuelto a tu tablero al comienzo de cualquier nivel Gana completando desafíos, girando la rueda de refuerzo diaria, o comprando con dinero real
- Extra Moves Booster Añade cinco movimientos adicionales a tu nivel Gana completando desafíos, girando la Daily Booster Wheel, o comprando con dinero real
-
-
-Candy Crush Saga es un juego que requiere habilidad, estrategia y suerte. Aquí hay algunos consejos y trucos que pueden ayudarte a jugar mejor y divertirte más:
- ¿Cómo anotar más puntos y limpiar niveles más rápido?
-
-- Combina tantos dulces como sea posible en un movimiento, ya que esto creará cascadas y combos que aumentarán tu puntuación.
-- Combina dulces especiales entre sí, ya que esto creará efectos poderosos que borrarán más dulces y sumarán más puntos.
-- Combina caramelos en la parte inferior del tablero, ya que esto hará que más dulces caigan y creará más oportunidades para los partidos.
-- Combina dulces cerca de los bloqueadores, como chocolate, regaliz o merengue, ya que esto los despejará y hará más espacio en el tablero.
-- Combina caramelos cerca de los bordes o esquinas del tablero, ya que son más difíciles de alcanzar y limpiar.
-- Planifica tus movimientos y busca el mejor partido posible en el tablero, ya que tienes un número limitado de movimientos o tiempo.
-- Utilice refuerzos sabiamente y con moderación, ya que pueden ayudarle a eliminar niveles o situaciones difíciles, pero también son limitados y caros.
-
- ¿Cómo evitar quedarse sin vidas y oro?
-
-- Las vidas son el número de veces que puedes jugar un nivel antes de tener que esperar o pagar más. Puedes tener hasta cinco vidas a la vez, y regeneran una cada 30 minutos.
-- Puedes obtener más vidas preguntando a tus amigos de Facebook, viendo anuncios, girando la rueda de refuerzo diaria o comprándolos con dinero real.
-- También puedes obtener más vidas cambiando la configuración de tiempo de tu dispositivo, pero esto puede causar errores o fallos en el juego.
-- El oro es la moneda premium del juego que puedes usar para comprar boosters, movimientos extra o tickets para desbloquear nuevos episodios.
-- Puedes obtener más oro completando logros, viendo anuncios, girando la Daily Booster Wheel o comprándolos con dinero real.
-
-
- ¿Cómo combinar dulces especiales para efectos potentes?
-
-- Rayado + rayado: Limpia dos filas o columnas en forma de cruz.
-- Rayado + envuelto: Despeja un área de 3x3 dos veces en una fila.
-- Striped + Color Bomb: Borra todos los dulces del mismo color que el caramelo de rayas.
-- Rayas + Jelly Fish: Envía tres peces de jalea a rayas a las baldosas al azar.
-- Striped + Coconut Wheel: Convierte todos los dulces en una fila o columna en dulces rayados.
-- Envuelto + Envuelto: Borra un área de 5x5 una vez.
-- Envuelto + Color Bomb: Borra todos los dulces de un color y luego otro color.
-- Envuelto + Jelly Fish: Envía tres peces gelatina envueltos a las baldosas al azar.
-- Envuelto + Rueda de coco: Convierte todos los dulces en una fila o columna en caramelos envueltos.
-- Bomba de color + Bomba de color: Borra todos los dulces en el tablero.
-- Bomba de color + Jelly Fish: Envía tres peces bomba de color a las baldosas al azar.
-- Bomba de color + Rueda de coco: Convierte todos los dulces en una fila o columna en bombas de color.
-- Jelly Fish + Jelly Fish: Envía seis peces de jalea a azulejos al azar.
-- Jelly Fish + Coconut Wheel: Envía tres peces de gelatina de rueda de coco a azulejos aleatorios.
-- Rueda de coco + Rueda de coco: Convierte dos filas o columnas en caramelos rayados.
-
- Alternativas a Candy Crush Saga
-Si te gusta Candy Crush Saga pero quieres probar algo diferente, hay muchos otros juegos que son similares en términos de jugabilidad y gráficos. Estos son algunos de ellos:
- C Saga de refrescos de caramelo
-
- Caramelo Crush Jelly Saga
-
Candy Crush Jelly Saga es otro spin-off de Candy Crush Saga que cuenta con jalea como el elemento principal. El juego tiene más de 3.000 niveles en más de 200 episodios, cada uno con un tema diferente y desafío. El juego también tiene nuevos modos de juego, como Jelly Mode (donde tienes que esparcir jalea en el tablero), Boss Mode (donde tienes que competir con un personaje jefe) y Puffler Mode (donde tienes que encontrar pufflers ocultos bajo glaseado). El juego está disponible en Facebook, iOS, Android, Windows Phone y Windows 10 plataformas.
- Dulces Crush Amigos Saga
-Candy Crush Friends Saga es el último spin-off de Candy Crush Saga que cuenta con personajes del Candy Kingdom como tus amigos. El juego tiene más de 4.000 niveles en más de 300 episodios, cada uno con un tema diferente y desafío. El juego también tiene nuevas características, como amigos (que pueden ayudarte con sus habilidades especiales), trajes (que pueden cambiar la apariencia y el poder de tus amigos), y pegatinas (que pueden desbloquear recompensas y sorpresas). El juego está disponible en Facebook, iOS, Android, Windows Phone y Windows 10 plataformas.
- Conclusión
-Candy Crush Saga es uno de los juegos de puzzle más populares y adictivos de todos los tiempos. Tiene millones de fans que disfrutan de combinar dulces y resolver puzzles en sus dispositivos móviles. Si desea jugar el juego con las últimas características y actualizaciones, puede descargar e instalar Candy Crush Saga APK 1.246.0.1, la versión más reciente del juego a partir de junio de 2023.
-En este artículo, hemos explicado todo lo que necesita saber sobre Candy Crush Saga APK 1.246.0.1, incluyendo cómo descargar e instalar, lo que ofrece, y algunos consejos y trucos para jugar el juego. También hemos sugerido algunas alternativas a Candy Crush Saga que puedes probar si quieres experimentar algo diferente.
-
- Preguntas frecuentes
-Aquí hay algunas preguntas frecuentes sobre Candy Crush Saga y su archivo APK:
- ¿Es seguro descargar e instalar Candy Crush Saga APK 1.246.0.1?
-Sí, Candy Crush Saga APK 1.246.0.1 es seguro de descargar e instalar si lo obtiene de un sitio web de confianza o desarrollador. Sin embargo, siempre debe tener cuidado al descargar e instalar archivos APK de fuentes desconocidas, ya que pueden contener malware o virus que pueden dañar su dispositivo o robar sus datos. También debe escanear el archivo APK con un software antivirus confiable antes de instalarlo.
- ¿Es Candy Crush Saga APK 1.246.0.1 libre para jugar?
-Sí, Candy Crush Saga APK 1.246.0.1 es gratis para jugar, pero también ofrece compras en la aplicación que pueden mejorar su juego o desbloquear más características. Puedes comprar boosters, movimientos extra, barras de oro o tickets con dinero real si quieres progresar más rápido o más fácil en el juego.
- ¿Cómo actualizo Candy Crush Saga APK 1.246.0.1?
-Puede actualizar Candy Crush Saga APK 1.246.0.1 mediante la comprobación de nuevas versiones del archivo APK en el sitio web que lo descargó desde. También puede habilitar la opción de permitir actualizaciones automáticas en la configuración del dispositivo si desea obtener las últimas actualizaciones sin tener que descargarlas e instalarlas manualmente.
- ¿Cómo puedo desinstalar Candy Crush Saga APK 1.246.0.1?
-Puede desinstalar Candy Crush Saga APK 1.246.0.1 siguiendo estos pasos:
-
-- Ir a la configuración de su dispositivo y toque en aplicaciones o aplicaciones.
-- Encuentra Candy Crush Saga y toque en él.
-- Toque en Desinstalar o Quitar y confirme su elección.
-- Espere a que el proceso de desinstalación termine y luego toque en OK o Hecho.
-
- ¿Cómo me pongo en contacto con el desarrollador de Candy Cómo me pongo en contacto con el desarrollador de Candy Crush Saga?
-
Si tiene alguna pregunta, comentario o problema con respecto a Candy Crush Saga, puede ponerse en contacto con el desarrollador del juego, King, utilizando uno de estos métodos:
-
-
-- Sitio web: Puede visitar el sitio web oficial de Candy Crush Saga en https://candycrushsaga.com/ y encontrar más información, consejos, noticias y apoyo.
-- Facebook: Puede seguir la página oficial de Facebook de Candy Crush Saga en https://www.facebook.com/CandyCrushSaga/ y unirse a la comunidad de otros jugadores, compartir sus opiniones y obtener actualizaciones.
-- Twitter: Puedes seguir la cuenta oficial de Twitter de Candy Crush Saga en @CandyCrushSaga y enviar tus preguntas, comentarios o problemas al desarrollador.
-- Instagram: Puedes seguir la cuenta oficial de Instagram de Candy Crush Saga en @candycrushsaga y ver las últimas fotos y videos del juego.
-
64aa2da5cf
-
-
\ No newline at end of file
diff --git a/spaces/Big-Web/MMSD/env/Lib/site-packages/pip/_internal/commands/wheel.py b/spaces/Big-Web/MMSD/env/Lib/site-packages/pip/_internal/commands/wheel.py
deleted file mode 100644
index c6a588ff09bcc652fc660b412b040242972d6944..0000000000000000000000000000000000000000
--- a/spaces/Big-Web/MMSD/env/Lib/site-packages/pip/_internal/commands/wheel.py
+++ /dev/null
@@ -1,180 +0,0 @@
-import logging
-import os
-import shutil
-from optparse import Values
-from typing import List
-
-from pip._internal.cache import WheelCache
-from pip._internal.cli import cmdoptions
-from pip._internal.cli.req_command import RequirementCommand, with_cleanup
-from pip._internal.cli.status_codes import SUCCESS
-from pip._internal.exceptions import CommandError
-from pip._internal.operations.build.build_tracker import get_build_tracker
-from pip._internal.req.req_install import (
- InstallRequirement,
- check_legacy_setup_py_options,
-)
-from pip._internal.utils.misc import ensure_dir, normalize_path
-from pip._internal.utils.temp_dir import TempDirectory
-from pip._internal.wheel_builder import build, should_build_for_wheel_command
-
-logger = logging.getLogger(__name__)
-
-
-class WheelCommand(RequirementCommand):
- """
- Build Wheel archives for your requirements and dependencies.
-
- Wheel is a built-package format, and offers the advantage of not
- recompiling your software during every install. For more details, see the
- wheel docs: https://wheel.readthedocs.io/en/latest/
-
- 'pip wheel' uses the build system interface as described here:
- https://pip.pypa.io/en/stable/reference/build-system/
-
- """
-
- usage = """
- %prog [options] ...
- %prog [options] -r ...
- %prog [options] [-e] ...
- %prog [options] [-e] ...
- %prog [options] ..."""
-
- def add_options(self) -> None:
- self.cmd_opts.add_option(
- "-w",
- "--wheel-dir",
- dest="wheel_dir",
- metavar="dir",
- default=os.curdir,
- help=(
- "Build wheels into , where the default is the "
- "current working directory."
- ),
- )
- self.cmd_opts.add_option(cmdoptions.no_binary())
- self.cmd_opts.add_option(cmdoptions.only_binary())
- self.cmd_opts.add_option(cmdoptions.prefer_binary())
- self.cmd_opts.add_option(cmdoptions.no_build_isolation())
- self.cmd_opts.add_option(cmdoptions.use_pep517())
- self.cmd_opts.add_option(cmdoptions.no_use_pep517())
- self.cmd_opts.add_option(cmdoptions.check_build_deps())
- self.cmd_opts.add_option(cmdoptions.constraints())
- self.cmd_opts.add_option(cmdoptions.editable())
- self.cmd_opts.add_option(cmdoptions.requirements())
- self.cmd_opts.add_option(cmdoptions.src())
- self.cmd_opts.add_option(cmdoptions.ignore_requires_python())
- self.cmd_opts.add_option(cmdoptions.no_deps())
- self.cmd_opts.add_option(cmdoptions.progress_bar())
-
- self.cmd_opts.add_option(
- "--no-verify",
- dest="no_verify",
- action="store_true",
- default=False,
- help="Don't verify if built wheel is valid.",
- )
-
- self.cmd_opts.add_option(cmdoptions.config_settings())
- self.cmd_opts.add_option(cmdoptions.build_options())
- self.cmd_opts.add_option(cmdoptions.global_options())
-
- self.cmd_opts.add_option(
- "--pre",
- action="store_true",
- default=False,
- help=(
- "Include pre-release and development versions. By default, "
- "pip only finds stable versions."
- ),
- )
-
- self.cmd_opts.add_option(cmdoptions.require_hashes())
-
- index_opts = cmdoptions.make_option_group(
- cmdoptions.index_group,
- self.parser,
- )
-
- self.parser.insert_option_group(0, index_opts)
- self.parser.insert_option_group(0, self.cmd_opts)
-
- @with_cleanup
- def run(self, options: Values, args: List[str]) -> int:
- session = self.get_default_session(options)
-
- finder = self._build_package_finder(options, session)
-
- options.wheel_dir = normalize_path(options.wheel_dir)
- ensure_dir(options.wheel_dir)
-
- build_tracker = self.enter_context(get_build_tracker())
-
- directory = TempDirectory(
- delete=not options.no_clean,
- kind="wheel",
- globally_managed=True,
- )
-
- reqs = self.get_requirements(args, options, finder, session)
- check_legacy_setup_py_options(options, reqs)
-
- wheel_cache = WheelCache(options.cache_dir)
-
- preparer = self.make_requirement_preparer(
- temp_build_dir=directory,
- options=options,
- build_tracker=build_tracker,
- session=session,
- finder=finder,
- download_dir=options.wheel_dir,
- use_user_site=False,
- verbosity=self.verbosity,
- )
-
- resolver = self.make_resolver(
- preparer=preparer,
- finder=finder,
- options=options,
- wheel_cache=wheel_cache,
- ignore_requires_python=options.ignore_requires_python,
- use_pep517=options.use_pep517,
- )
-
- self.trace_basic_info(finder)
-
- requirement_set = resolver.resolve(reqs, check_supported_wheels=True)
-
- reqs_to_build: List[InstallRequirement] = []
- for req in requirement_set.requirements.values():
- if req.is_wheel:
- preparer.save_linked_requirement(req)
- elif should_build_for_wheel_command(req):
- reqs_to_build.append(req)
-
- # build wheels
- build_successes, build_failures = build(
- reqs_to_build,
- wheel_cache=wheel_cache,
- verify=(not options.no_verify),
- build_options=options.build_options or [],
- global_options=options.global_options or [],
- )
- for req in build_successes:
- assert req.link and req.link.is_wheel
- assert req.local_file_path
- # copy from cache to target directory
- try:
- shutil.copy(req.local_file_path, options.wheel_dir)
- except OSError as e:
- logger.warning(
- "Building wheel for %s failed: %s",
- req.name,
- e,
- )
- build_failures.append(req)
- if len(build_failures) != 0:
- raise CommandError("Failed to build one or more wheels")
-
- return SUCCESS
diff --git a/spaces/Big-Web/MMSD/env/Lib/site-packages/pip/_vendor/chardet/sbcsgroupprober.py b/spaces/Big-Web/MMSD/env/Lib/site-packages/pip/_vendor/chardet/sbcsgroupprober.py
deleted file mode 100644
index 890ae8465c5b0ad2a5f99464fe5f5c0be49809f1..0000000000000000000000000000000000000000
--- a/spaces/Big-Web/MMSD/env/Lib/site-packages/pip/_vendor/chardet/sbcsgroupprober.py
+++ /dev/null
@@ -1,88 +0,0 @@
-######################## BEGIN LICENSE BLOCK ########################
-# The Original Code is Mozilla Universal charset detector code.
-#
-# The Initial Developer of the Original Code is
-# Netscape Communications Corporation.
-# Portions created by the Initial Developer are Copyright (C) 2001
-# the Initial Developer. All Rights Reserved.
-#
-# Contributor(s):
-# Mark Pilgrim - port to Python
-# Shy Shalom - original C code
-#
-# This library is free software; you can redistribute it and/or
-# modify it under the terms of the GNU Lesser General Public
-# License as published by the Free Software Foundation; either
-# version 2.1 of the License, or (at your option) any later version.
-#
-# This library is distributed in the hope that it will be useful,
-# but WITHOUT ANY WARRANTY; without even the implied warranty of
-# MERCHANTABILITY or FITNESS FOR A PARTICULAR PURPOSE. See the GNU
-# Lesser General Public License for more details.
-#
-# You should have received a copy of the GNU Lesser General Public
-# License along with this library; if not, write to the Free Software
-# Foundation, Inc., 51 Franklin St, Fifth Floor, Boston, MA
-# 02110-1301 USA
-######################### END LICENSE BLOCK #########################
-
-from .charsetgroupprober import CharSetGroupProber
-from .hebrewprober import HebrewProber
-from .langbulgarianmodel import ISO_8859_5_BULGARIAN_MODEL, WINDOWS_1251_BULGARIAN_MODEL
-from .langgreekmodel import ISO_8859_7_GREEK_MODEL, WINDOWS_1253_GREEK_MODEL
-from .langhebrewmodel import WINDOWS_1255_HEBREW_MODEL
-
-# from .langhungarianmodel import (ISO_8859_2_HUNGARIAN_MODEL,
-# WINDOWS_1250_HUNGARIAN_MODEL)
-from .langrussianmodel import (
- IBM855_RUSSIAN_MODEL,
- IBM866_RUSSIAN_MODEL,
- ISO_8859_5_RUSSIAN_MODEL,
- KOI8_R_RUSSIAN_MODEL,
- MACCYRILLIC_RUSSIAN_MODEL,
- WINDOWS_1251_RUSSIAN_MODEL,
-)
-from .langthaimodel import TIS_620_THAI_MODEL
-from .langturkishmodel import ISO_8859_9_TURKISH_MODEL
-from .sbcharsetprober import SingleByteCharSetProber
-
-
-class SBCSGroupProber(CharSetGroupProber):
- def __init__(self) -> None:
- super().__init__()
- hebrew_prober = HebrewProber()
- logical_hebrew_prober = SingleByteCharSetProber(
- WINDOWS_1255_HEBREW_MODEL, is_reversed=False, name_prober=hebrew_prober
- )
- # TODO: See if using ISO-8859-8 Hebrew model works better here, since
- # it's actually the visual one
- visual_hebrew_prober = SingleByteCharSetProber(
- WINDOWS_1255_HEBREW_MODEL, is_reversed=True, name_prober=hebrew_prober
- )
- hebrew_prober.set_model_probers(logical_hebrew_prober, visual_hebrew_prober)
- # TODO: ORDER MATTERS HERE. I changed the order vs what was in master
- # and several tests failed that did not before. Some thought
- # should be put into the ordering, and we should consider making
- # order not matter here, because that is very counter-intuitive.
- self.probers = [
- SingleByteCharSetProber(WINDOWS_1251_RUSSIAN_MODEL),
- SingleByteCharSetProber(KOI8_R_RUSSIAN_MODEL),
- SingleByteCharSetProber(ISO_8859_5_RUSSIAN_MODEL),
- SingleByteCharSetProber(MACCYRILLIC_RUSSIAN_MODEL),
- SingleByteCharSetProber(IBM866_RUSSIAN_MODEL),
- SingleByteCharSetProber(IBM855_RUSSIAN_MODEL),
- SingleByteCharSetProber(ISO_8859_7_GREEK_MODEL),
- SingleByteCharSetProber(WINDOWS_1253_GREEK_MODEL),
- SingleByteCharSetProber(ISO_8859_5_BULGARIAN_MODEL),
- SingleByteCharSetProber(WINDOWS_1251_BULGARIAN_MODEL),
- # TODO: Restore Hungarian encodings (iso-8859-2 and windows-1250)
- # after we retrain model.
- # SingleByteCharSetProber(ISO_8859_2_HUNGARIAN_MODEL),
- # SingleByteCharSetProber(WINDOWS_1250_HUNGARIAN_MODEL),
- SingleByteCharSetProber(TIS_620_THAI_MODEL),
- SingleByteCharSetProber(ISO_8859_9_TURKISH_MODEL),
- hebrew_prober,
- logical_hebrew_prober,
- visual_hebrew_prober,
- ]
- self.reset()
diff --git a/spaces/Big-Web/MMSD/env/Lib/site-packages/pip/_vendor/requests/compat.py b/spaces/Big-Web/MMSD/env/Lib/site-packages/pip/_vendor/requests/compat.py
deleted file mode 100644
index 9ab2bb48656520a95ec9ac87d090f2e741f0e544..0000000000000000000000000000000000000000
--- a/spaces/Big-Web/MMSD/env/Lib/site-packages/pip/_vendor/requests/compat.py
+++ /dev/null
@@ -1,67 +0,0 @@
-"""
-requests.compat
-~~~~~~~~~~~~~~~
-
-This module previously handled import compatibility issues
-between Python 2 and Python 3. It remains for backwards
-compatibility until the next major version.
-"""
-
-from pip._vendor import chardet
-
-import sys
-
-# -------
-# Pythons
-# -------
-
-# Syntax sugar.
-_ver = sys.version_info
-
-#: Python 2.x?
-is_py2 = _ver[0] == 2
-
-#: Python 3.x?
-is_py3 = _ver[0] == 3
-
-# Note: We've patched out simplejson support in pip because it prevents
-# upgrading simplejson on Windows.
-import json
-from json import JSONDecodeError
-
-# Keep OrderedDict for backwards compatibility.
-from collections import OrderedDict
-from collections.abc import Callable, Mapping, MutableMapping
-from http import cookiejar as cookielib
-from http.cookies import Morsel
-from io import StringIO
-
-# --------------
-# Legacy Imports
-# --------------
-from urllib.parse import (
- quote,
- quote_plus,
- unquote,
- unquote_plus,
- urldefrag,
- urlencode,
- urljoin,
- urlparse,
- urlsplit,
- urlunparse,
-)
-from urllib.request import (
- getproxies,
- getproxies_environment,
- parse_http_list,
- proxy_bypass,
- proxy_bypass_environment,
-)
-
-builtin_str = str
-str = str
-bytes = bytes
-basestring = (str, bytes)
-numeric_types = (int, float)
-integer_types = (int,)
diff --git a/spaces/Big-Web/MMSD/env/Lib/site-packages/pkg_resources/_vendor/jaraco/__init__.py b/spaces/Big-Web/MMSD/env/Lib/site-packages/pkg_resources/_vendor/jaraco/__init__.py
deleted file mode 100644
index e69de29bb2d1d6434b8b29ae775ad8c2e48c5391..0000000000000000000000000000000000000000
diff --git a/spaces/Big-Web/MMSD/env/Lib/site-packages/urllib3/packages/backports/makefile.py b/spaces/Big-Web/MMSD/env/Lib/site-packages/urllib3/packages/backports/makefile.py
deleted file mode 100644
index b8fb2154b6d0618b62281578e5e947bca487cee4..0000000000000000000000000000000000000000
--- a/spaces/Big-Web/MMSD/env/Lib/site-packages/urllib3/packages/backports/makefile.py
+++ /dev/null
@@ -1,51 +0,0 @@
-# -*- coding: utf-8 -*-
-"""
-backports.makefile
-~~~~~~~~~~~~~~~~~~
-
-Backports the Python 3 ``socket.makefile`` method for use with anything that
-wants to create a "fake" socket object.
-"""
-import io
-from socket import SocketIO
-
-
-def backport_makefile(
- self, mode="r", buffering=None, encoding=None, errors=None, newline=None
-):
- """
- Backport of ``socket.makefile`` from Python 3.5.
- """
- if not set(mode) <= {"r", "w", "b"}:
- raise ValueError("invalid mode %r (only r, w, b allowed)" % (mode,))
- writing = "w" in mode
- reading = "r" in mode or not writing
- assert reading or writing
- binary = "b" in mode
- rawmode = ""
- if reading:
- rawmode += "r"
- if writing:
- rawmode += "w"
- raw = SocketIO(self, rawmode)
- self._makefile_refs += 1
- if buffering is None:
- buffering = -1
- if buffering < 0:
- buffering = io.DEFAULT_BUFFER_SIZE
- if buffering == 0:
- if not binary:
- raise ValueError("unbuffered streams must be binary")
- return raw
- if reading and writing:
- buffer = io.BufferedRWPair(raw, raw, buffering)
- elif reading:
- buffer = io.BufferedReader(raw, buffering)
- else:
- assert writing
- buffer = io.BufferedWriter(raw, buffering)
- if binary:
- return buffer
- text = io.TextIOWrapper(buffer, encoding, errors, newline)
- text.mode = mode
- return text
diff --git a/spaces/Boilin/URetinex-Net/network/illumination_enhance.py b/spaces/Boilin/URetinex-Net/network/illumination_enhance.py
deleted file mode 100644
index d21513cc4caeafedc50018b358ea4f76d0fada09..0000000000000000000000000000000000000000
--- a/spaces/Boilin/URetinex-Net/network/illumination_enhance.py
+++ /dev/null
@@ -1,31 +0,0 @@
-import torch.nn as nn
-import torch
-import math
-from network.architecture import get_batchnorm_layer, get_conv2d_layer
-
-class Illumination_Alone(nn.Module):
- def __init__(self, opts):
- super().__init__()
- self.opts = opts
- self.conv1 = get_conv2d_layer(in_c=1, out_c=32, k=5, s=1, p=2)
- self.conv2 = get_conv2d_layer(in_c=32, out_c=32, k=5, s=1, p=2)
- self.conv3 = get_conv2d_layer(in_c=32, out_c=32, k=5, s=1, p=2)
- self.conv4 = get_conv2d_layer(in_c=32, out_c=32, k=5, s=1, p=2)
- self.conv5 = get_conv2d_layer(in_c=32, out_c=1, k=1, s=1, p=0)
-
- self.leaky_relu_1 = nn.LeakyReLU(0.2, inplace=True)
- self.leaky_relu_2 = nn.LeakyReLU(0.2, inplace=True)
- self.leaky_relu_3 = nn.LeakyReLU(0.2, inplace=True)
- self.leaky_relu_4 = nn.LeakyReLU(0.2, inplace=True)
- self.relu = nn.ReLU()
- #self.sigmoid = nn.Sigmoid()
-
- def forward(self, l):
- x = l
- x1 = self.leaky_relu_1(self.conv1(x))
- x2 = self.leaky_relu_2(self.conv2(x1))
- x3 = self.leaky_relu_3(self.conv3(x2))
- x4 = self.leaky_relu_4(self.conv4(x3))
- x5 = self.relu(self.conv5(x4))
- return x5
-
diff --git a/spaces/CVH-vn1210/make_hair/minigpt4/datasets/__init__.py b/spaces/CVH-vn1210/make_hair/minigpt4/datasets/__init__.py
deleted file mode 100644
index e69de29bb2d1d6434b8b29ae775ad8c2e48c5391..0000000000000000000000000000000000000000
diff --git a/spaces/CVPR/WALT/mmdet/models/detectors/gfl.py b/spaces/CVPR/WALT/mmdet/models/detectors/gfl.py
deleted file mode 100644
index 64d65cb2dfb7a56f57e08c3fcad67e1539e1e841..0000000000000000000000000000000000000000
--- a/spaces/CVPR/WALT/mmdet/models/detectors/gfl.py
+++ /dev/null
@@ -1,16 +0,0 @@
-from ..builder import DETECTORS
-from .single_stage import SingleStageDetector
-
-
-@DETECTORS.register_module()
-class GFL(SingleStageDetector):
-
- def __init__(self,
- backbone,
- neck,
- bbox_head,
- train_cfg=None,
- test_cfg=None,
- pretrained=None):
- super(GFL, self).__init__(backbone, neck, bbox_head, train_cfg,
- test_cfg, pretrained)
diff --git a/spaces/CarlDennis/Lovelive-VITS-JPZH/commons.py b/spaces/CarlDennis/Lovelive-VITS-JPZH/commons.py
deleted file mode 100644
index 2153153f527d94e2abb641ea00c80b518ff6c5bd..0000000000000000000000000000000000000000
--- a/spaces/CarlDennis/Lovelive-VITS-JPZH/commons.py
+++ /dev/null
@@ -1,97 +0,0 @@
-import math
-import torch
-from torch.nn import functional as F
-import torch.jit
-
-
-def script_method(fn, _rcb=None):
- return fn
-
-
-def script(obj, optimize=True, _frames_up=0, _rcb=None):
- return obj
-
-
-torch.jit.script_method = script_method
-torch.jit.script = script
-
-
-def init_weights(m, mean=0.0, std=0.01):
- classname = m.__class__.__name__
- if classname.find("Conv") != -1:
- m.weight.data.normal_(mean, std)
-
-
-def get_padding(kernel_size, dilation=1):
- return int((kernel_size*dilation - dilation)/2)
-
-
-def intersperse(lst, item):
- result = [item] * (len(lst) * 2 + 1)
- result[1::2] = lst
- return result
-
-
-def slice_segments(x, ids_str, segment_size=4):
- ret = torch.zeros_like(x[:, :, :segment_size])
- for i in range(x.size(0)):
- idx_str = ids_str[i]
- idx_end = idx_str + segment_size
- ret[i] = x[i, :, idx_str:idx_end]
- return ret
-
-
-def rand_slice_segments(x, x_lengths=None, segment_size=4):
- b, d, t = x.size()
- if x_lengths is None:
- x_lengths = t
- ids_str_max = x_lengths - segment_size + 1
- ids_str = (torch.rand([b]).to(device=x.device) * ids_str_max).to(dtype=torch.long)
- ret = slice_segments(x, ids_str, segment_size)
- return ret, ids_str
-
-
-def subsequent_mask(length):
- mask = torch.tril(torch.ones(length, length)).unsqueeze(0).unsqueeze(0)
- return mask
-
-
-@torch.jit.script
-def fused_add_tanh_sigmoid_multiply(input_a, input_b, n_channels):
- n_channels_int = n_channels[0]
- in_act = input_a + input_b
- t_act = torch.tanh(in_act[:, :n_channels_int, :])
- s_act = torch.sigmoid(in_act[:, n_channels_int:, :])
- acts = t_act * s_act
- return acts
-
-
-def convert_pad_shape(pad_shape):
- l = pad_shape[::-1]
- pad_shape = [item for sublist in l for item in sublist]
- return pad_shape
-
-
-def sequence_mask(length, max_length=None):
- if max_length is None:
- max_length = length.max()
- x = torch.arange(max_length, dtype=length.dtype, device=length.device)
- return x.unsqueeze(0) < length.unsqueeze(1)
-
-
-def generate_path(duration, mask):
- """
- duration: [b, 1, t_x]
- mask: [b, 1, t_y, t_x]
- """
- device = duration.device
-
- b, _, t_y, t_x = mask.shape
- cum_duration = torch.cumsum(duration, -1)
-
- cum_duration_flat = cum_duration.view(b * t_x)
- path = sequence_mask(cum_duration_flat, t_y).to(mask.dtype)
- path = path.view(b, t_x, t_y)
- path = path - F.pad(path, convert_pad_shape([[0, 0], [1, 0], [0, 0]]))[:, :-1]
- path = path.unsqueeze(1).transpose(2,3) * mask
- return path
diff --git a/spaces/ChanceFocus/FLARE/README.md b/spaces/ChanceFocus/FLARE/README.md
deleted file mode 100644
index f31608db7ffd0130cc2d0e13a7aceb63441f52fe..0000000000000000000000000000000000000000
--- a/spaces/ChanceFocus/FLARE/README.md
+++ /dev/null
@@ -1,60 +0,0 @@
----
-title: FLARE
-emoji: 🐠
-colorFrom: pink
-colorTo: pink
-sdk: gradio
-sdk_version: 3.34.0
-app_file: app.py
-pinned: false
-license: mit
----
-
-Check out the configuration reference at https://huggingface.co/docs/hub/spaces-config-reference
-
-## Add New Language
-
-1. Add new `[LAN]_result.csv`, which should be format like
-
-|Model|Task Metric 1|Task Metric 2|
-|---|---|---|
-|GPT-4|0.28|0|
-
-2. Add new COL variable on `app.py`
-
-```python
-SPA_COLS = [
- ("Model", "str"),
- ("MultiFin-F1", "number"),
-]
-```
-
-3. Add new Col categorization on `app.py`
-
-```python
-spa_cols = [col_name for col_name, _ in SPA_COLS]
-spa_cates = {
- "Sentiment Analysis": ["Model", "TSA-Acc", "TSA-F1", "FinanceES-Acc", "FinanceES-F1"],
- "Examination": ["Model", "EFP-Acc", "EFP-F1", "EFPA-Acc", "EFPA-F1"],
- "Classification": ["Model", "MultiFin-Acc", "MultiFin-F1"],
- "Text Summarization": ["Model", "FNS-Rouge1", "FNS-Rouge2", "FNS-RougeL",],
-}
-```
-
-4. Add new key to lan dict on `app.py`
-
-```python
-df_lang = {
- "English": create_df_dict("english", eng_cols, eng_cates),
- "Spanish": create_df_dict("spanish", spa_cols, spa_cates),
-}
-```
-
-5. If new categories need to define new column selection rules, add it like:
-
-```python
-elif key == "Credit Scoring":
- tdf = tdf[[val for val in tdf.columns if "Acc" in val]]
-elif key == "Text Summarization":
- tdf = tdf[[val for val in tdf.columns if "Bert" in val or "Rouge" in val]]
-```
diff --git a/spaces/ChrisPreston/diff-svc_minato_aqua/modules/diff/net.py b/spaces/ChrisPreston/diff-svc_minato_aqua/modules/diff/net.py
deleted file mode 100644
index 2c5aff1f6d5dba67d42d41f0bfe1845ba79f444b..0000000000000000000000000000000000000000
--- a/spaces/ChrisPreston/diff-svc_minato_aqua/modules/diff/net.py
+++ /dev/null
@@ -1,135 +0,0 @@
-import math
-from math import sqrt
-
-import torch
-import torch.nn as nn
-import torch.nn.functional as F
-
-from modules.commons.common_layers import Mish
-from utils.hparams import hparams
-
-Linear = nn.Linear
-ConvTranspose2d = nn.ConvTranspose2d
-
-
-class AttrDict(dict):
- def __init__(self, *args, **kwargs):
- super(AttrDict, self).__init__(*args, **kwargs)
- self.__dict__ = self
-
- def override(self, attrs):
- if isinstance(attrs, dict):
- self.__dict__.update(**attrs)
- elif isinstance(attrs, (list, tuple, set)):
- for attr in attrs:
- self.override(attr)
- elif attrs is not None:
- raise NotImplementedError
- return self
-
-
-class SinusoidalPosEmb(nn.Module):
- def __init__(self, dim):
- super().__init__()
- self.dim = dim
-
- def forward(self, x):
- device = x.device
- half_dim = self.dim // 2
- emb = math.log(10000) / (half_dim - 1)
- emb = torch.exp(torch.arange(half_dim, device=device) * -emb)
- emb = x[:, None] * emb[None, :]
- emb = torch.cat((emb.sin(), emb.cos()), dim=-1)
- return emb
-
-
-def Conv1d(*args, **kwargs):
- layer = nn.Conv1d(*args, **kwargs)
- nn.init.kaiming_normal_(layer.weight)
- return layer
-
-
-@torch.jit.script
-def silu(x):
- return x * torch.sigmoid(x)
-
-
-class ResidualBlock(nn.Module):
- def __init__(self, encoder_hidden, residual_channels, dilation):
- super().__init__()
- self.dilated_conv = Conv1d(residual_channels, 2 * residual_channels, 3, padding=dilation, dilation=dilation)
- self.diffusion_projection = Linear(residual_channels, residual_channels)
- self.conditioner_projection = Conv1d(encoder_hidden, 2 * residual_channels, 1)
- self.output_projection = Conv1d(residual_channels, 2 * residual_channels, 1)
-
- def forward(self, x, conditioner, diffusion_step):
- diffusion_step = self.diffusion_projection(diffusion_step).unsqueeze(-1)
- conditioner = self.conditioner_projection(conditioner)
- y = x + diffusion_step
-
- y = self.dilated_conv(y) + conditioner
-
- gate, filter = torch.chunk(y, 2, dim=1)
- # Using torch.split instead of torch.chunk to avoid using onnx::Slice
- # gate, filter = torch.split(y, torch.div(y.shape[1], 2), dim=1)
-
- y = torch.sigmoid(gate) * torch.tanh(filter)
-
- y = self.output_projection(y)
- residual, skip = torch.chunk(y, 2, dim=1)
- # Using torch.split instead of torch.chunk to avoid using onnx::Slice
- # residual, skip = torch.split(y, torch.div(y.shape[1], 2), dim=1)
-
- return (x + residual) / sqrt(2.0), skip
-
-
-class DiffNet(nn.Module):
- def __init__(self, in_dims=80):
- super().__init__()
- self.params = params = AttrDict(
- # Model params
- encoder_hidden=hparams['hidden_size'],
- residual_layers=hparams['residual_layers'],
- residual_channels=hparams['residual_channels'],
- dilation_cycle_length=hparams['dilation_cycle_length'],
- )
- self.input_projection = Conv1d(in_dims, params.residual_channels, 1)
- self.diffusion_embedding = SinusoidalPosEmb(params.residual_channels)
- dim = params.residual_channels
- self.mlp = nn.Sequential(
- nn.Linear(dim, dim * 4),
- Mish(),
- nn.Linear(dim * 4, dim)
- )
- self.residual_layers = nn.ModuleList([
- ResidualBlock(params.encoder_hidden, params.residual_channels, 2 ** (i % params.dilation_cycle_length))
- for i in range(params.residual_layers)
- ])
- self.skip_projection = Conv1d(params.residual_channels, params.residual_channels, 1)
- self.output_projection = Conv1d(params.residual_channels, in_dims, 1)
- nn.init.zeros_(self.output_projection.weight)
-
- def forward(self, spec, diffusion_step, cond):
- """
-
- :param spec: [B, 1, M, T]
- :param diffusion_step: [B, 1]
- :param cond: [B, M, T]
- :return:
- """
- x = spec[:, 0]
- x = self.input_projection(x) # x [B, residual_channel, T]
-
- x = F.relu(x)
- diffusion_step = self.diffusion_embedding(diffusion_step)
- diffusion_step = self.mlp(diffusion_step)
- skip = []
- for layer_id, layer in enumerate(self.residual_layers):
- x, skip_connection = layer(x, cond, diffusion_step)
- skip.append(skip_connection)
-
- x = torch.sum(torch.stack(skip), dim=0) / sqrt(len(self.residual_layers))
- x = self.skip_projection(x)
- x = F.relu(x)
- x = self.output_projection(x) # [B, 80, T]
- return x[:, None, :, :]
diff --git a/spaces/CofAI/chat/g4f/Provider/Providers/AiService.py b/spaces/CofAI/chat/g4f/Provider/Providers/AiService.py
deleted file mode 100644
index ef8265ff8f5cae4d87fea24369373ae74491d2bc..0000000000000000000000000000000000000000
--- a/spaces/CofAI/chat/g4f/Provider/Providers/AiService.py
+++ /dev/null
@@ -1,40 +0,0 @@
-import os
-import requests
-from ...typing import get_type_hints
-
-url = "https://aiservice.vercel.app/api/chat/answer"
-model = ['gpt-3.5-turbo']
-supports_stream = False
-needs_auth = False
-
-
-def _create_completion(model: str, messages: list, stream: bool, **kwargs):
- base = ''
- for message in messages:
- base += '%s: %s\n' % (message['role'], message['content'])
- base += 'assistant:'
-
- headers = {
- "accept": "*/*",
- "content-type": "text/plain;charset=UTF-8",
- "sec-fetch-dest": "empty",
- "sec-fetch-mode": "cors",
- "sec-fetch-site": "same-origin",
- "Referer": "https://aiservice.vercel.app/chat",
- }
- data = {
- "input": base
- }
- response = requests.post(url, headers=headers, json=data)
- if response.status_code == 200:
- _json = response.json()
- yield _json['data']
- else:
- print(f"Error Occurred::{response.status_code}")
- return None
-
-
-
-params = f'g4f.Providers.{os.path.basename(__file__)[:-3]} supports: ' + \
- '(%s)' % ', '.join(
- [f"{name}: {get_type_hints(_create_completion)[name].__name__}" for name in _create_completion.__code__.co_varnames[:_create_completion.__code__.co_argcount]])
\ No newline at end of file
diff --git a/spaces/CognitiveLabs/GPT-auto-webscraping/chains/code_generator/templates.py b/spaces/CognitiveLabs/GPT-auto-webscraping/chains/code_generator/templates.py
deleted file mode 100644
index 8aea89e64b9497aff8670816ffc3309b7481f7f0..0000000000000000000000000000000000000000
--- a/spaces/CognitiveLabs/GPT-auto-webscraping/chains/code_generator/templates.py
+++ /dev/null
@@ -1,58 +0,0 @@
-from langchain.prompts import (
- SystemMessagePromptTemplate,
- HumanMessagePromptTemplate,
- ChatPromptTemplate,
- PromptTemplate,
-)
-
-# Prompt templates
-system_template_script = PromptTemplate(
- input_variables=["output_format", "html_content"],
- template="""You are a helpful assitant that helps people create python scripts for web scraping.
- --------------------------------
- The example of the html content is: {html_content}
- --------------------------------
- You have to create a python function that extract information from an html code using web scrapping.
-
- Try to select the deeper class that is common among the elements to make de find_all function.
-
- Your answer SHOULD only contain the python function code without any aditional word or character.
-
- Import the used libraries above the function definition.
-
- The function name must be extract_info.
-
- The function have to receive the html data as a parameter.
-
- Your function needs to extract information for all the elements with similar attributes.
-
- An element could have missing attributes
-
- Before calling .text or ['href'] methods, check if the element exists.
-
- ----------------
- FINAL ANSWER EXAMPLE:
- from bs4 import BeautifulSoup
-
- def extract_info(html):
- ...CODE...
- return {output_format}
- ----------------
-
- Always check if the element exists before calling some method.
-
- """,
-)
-
-human_template_script = PromptTemplate(input_variables=[], template="give me the code")
-
-# Chat Prompt objects
-system_template_script_prompt = SystemMessagePromptTemplate.from_template(
- system_template_script.template
-)
-human_template_script_prompt = HumanMessagePromptTemplate.from_template(
- human_template_script.template
-)
-chat_script_prompt = ChatPromptTemplate.from_messages(
- [system_template_script_prompt, human_template_script_prompt]
-)
diff --git a/spaces/Cpp4App/Cpp4App/SEM/sentence_bayesian.py b/spaces/Cpp4App/Cpp4App/SEM/sentence_bayesian.py
deleted file mode 100644
index 021c5930d95e8ada687b71e02017377619ebddc0..0000000000000000000000000000000000000000
--- a/spaces/Cpp4App/Cpp4App/SEM/sentence_bayesian.py
+++ /dev/null
@@ -1,62 +0,0 @@
-import csv
-import joblib
-
-
-from sklearn.metrics import f1_score, recall_score
-from sklearn.naive_bayes import MultinomialNB
-
-from SEM.text_preprocessing import pre_process_title
-from sklearn.feature_extraction.text import CountVectorizer, TfidfVectorizer
-
-
-def readtrain():
- with open('SEM/training_data/personal_type.csv', 'rt') as csvfile:
- reader = csv.reader(csvfile)
- column1 = [row for row in reader]
- content_train = [i[0] for i in column1[1:]]
- opinion_train = [i[1] for i in column1[1:]]
-
- train = [content_train, opinion_train]
- return train
-
-def segmentWord(cont):
- c = []
- for i in cont:
- clean_text = pre_process_title(i)
- c.append(clean_text)
- return c
-
-train = readtrain()
-content = segmentWord(train[1])
-
-textMark = train[0]
-
-train_content = content[:499]
-# test_content = content[400:499]
-train_textMark = textMark[:499]
-# test_textMark = textMark[400:499]
-
-tf = TfidfVectorizer(max_df=0.5)
-
-train_features = tf.fit_transform(train_content)
-
-load_pretrain_model = True
-
-if not load_pretrain_model:
-
-
- clf_type = MultinomialNB(alpha=0.1)
- clf_type.fit(train_features,train_textMark)
-
- joblib.dump(clf_type, 'SEM/model/sen_model.pkl')
-
- # test_features = tf.transform(test_content)
- # print("clf test score: ", clf_type.score(test_features, test_textMark))
-else:
- clf_type = joblib.load('SEM/model/sen_model.pkl')
- # print("clf training score: ", clf_type.score(train_features, train_textMark))
-
- # test_features = tf.transform(test_content)
- # print("clf test score: ", clf_type.score(test_features, test_textMark))
-
-
diff --git a/spaces/Cyril666/ContourNet-ABI/maskrcnn_benchmark/data/datasets/evaluation/word/util/misc.py b/spaces/Cyril666/ContourNet-ABI/maskrcnn_benchmark/data/datasets/evaluation/word/util/misc.py
deleted file mode 100644
index 324309c3f9b7a3f5e3430fd53575779c394f283f..0000000000000000000000000000000000000000
--- a/spaces/Cyril666/ContourNet-ABI/maskrcnn_benchmark/data/datasets/evaluation/word/util/misc.py
+++ /dev/null
@@ -1,74 +0,0 @@
-'''Some helper functions for PyTorch, including:
- - get_mean_and_std: calculate the mean and std value of dataset.
- - msr_init: net parameter initialization.
- - progress_bar: progress bar mimic xlua.progress.
-'''
-import errno
-import os
-import sys
-import time
-import math
-
-import torch.nn as nn
-import torch.nn.init as init
-from torch.autograd import Variable
-
-__all__ = ['get_mean_and_std', 'init_params', 'mkdir_p', 'AverageMeter']
-
-
-def get_mean_and_std(dataset):
- '''Compute the mean and std value of dataset.'''
- dataloader = trainloader = torch.utils.data.DataLoader(dataset, batch_size=1, shuffle=True, num_workers=2)
-
- mean = torch.zeros(3)
- std = torch.zeros(3)
- print('==> Computing mean and std..')
- for inputs, targets in dataloader:
- for i in range(3):
- mean[i] += inputs[:,i,:,:].mean()
- std[i] += inputs[:,i,:,:].std()
- mean.div_(len(dataset))
- std.div_(len(dataset))
- return mean, std
-
-def init_params(net):
- '''Init layer parameters.'''
- for m in net.modules():
- if isinstance(m, nn.Conv2d):
- init.kaiming_normal(m.weight, mode='fan_out')
- if m.bias:
- init.constant(m.bias, 0)
- elif isinstance(m, nn.BatchNorm2d):
- init.constant(m.weight, 1)
- init.constant(m.bias, 0)
- elif isinstance(m, nn.Linear):
- init.normal(m.weight, std=1e-3)
- if m.bias:
- init.constant(m.bias, 0)
-
-def mkdir_p(path):
- '''make dir if not exist'''
- try:
- os.makedirs(path)
- except OSError as exc: # Python >2.5
- if exc.errno == errno.EEXIST and os.path.isdir(path):
- pass
- else:
- raise
-
-class AverageMeter(object):
- """Computes and stores the average and current value"""
- def __init__(self):
- self.reset()
-
- def reset(self):
- self.val = 0
- self.avg = 0
- self.sum = 0
- self.count = 0
-
- def update(self, val, n=1):
- self.val = val
- self.sum += val * n
- self.count += n
- self.avg = self.sum / self.count
\ No newline at end of file
diff --git a/spaces/DEVILOVER/image_captioning/README.md b/spaces/DEVILOVER/image_captioning/README.md
deleted file mode 100644
index f7c22dea8353b4f44ed0d5864717419af46cd9f6..0000000000000000000000000000000000000000
--- a/spaces/DEVILOVER/image_captioning/README.md
+++ /dev/null
@@ -1,13 +0,0 @@
----
-title: Image Captioning
-emoji: 😻
-colorFrom: gray
-colorTo: purple
-sdk: gradio
-sdk_version: 3.47.1
-app_file: app.py
-pinned: false
-license: apache-2.0
----
-
-Check out the configuration reference at https://huggingface.co/docs/hub/spaces-config-reference
diff --git a/spaces/DQChoi/gpt-demo/venv/lib/python3.11/site-packages/aiofiles/threadpool/utils.py b/spaces/DQChoi/gpt-demo/venv/lib/python3.11/site-packages/aiofiles/threadpool/utils.py
deleted file mode 100644
index f429877cd0c139616b7a7a8e951af86c16c74796..0000000000000000000000000000000000000000
--- a/spaces/DQChoi/gpt-demo/venv/lib/python3.11/site-packages/aiofiles/threadpool/utils.py
+++ /dev/null
@@ -1,74 +0,0 @@
-import functools
-from types import coroutine
-
-
-def delegate_to_executor(*attrs):
- def cls_builder(cls):
- for attr_name in attrs:
- setattr(cls, attr_name, _make_delegate_method(attr_name))
- return cls
-
- return cls_builder
-
-
-def proxy_method_directly(*attrs):
- def cls_builder(cls):
- for attr_name in attrs:
- setattr(cls, attr_name, _make_proxy_method(attr_name))
- return cls
-
- return cls_builder
-
-
-def proxy_property_directly(*attrs):
- def cls_builder(cls):
- for attr_name in attrs:
- setattr(cls, attr_name, _make_proxy_property(attr_name))
- return cls
-
- return cls_builder
-
-
-def cond_delegate_to_executor(*attrs):
- def cls_builder(cls):
- for attr_name in attrs:
- setattr(cls, attr_name, _make_cond_delegate_method(attr_name))
- return cls
-
- return cls_builder
-
-
-def _make_delegate_method(attr_name):
- @coroutine
- def method(self, *args, **kwargs):
- cb = functools.partial(getattr(self._file, attr_name), *args, **kwargs)
- return (yield from self._loop.run_in_executor(self._executor, cb))
-
- return method
-
-
-def _make_proxy_method(attr_name):
- def method(self, *args, **kwargs):
- return getattr(self._file, attr_name)(*args, **kwargs)
-
- return method
-
-
-def _make_proxy_property(attr_name):
- def proxy_property(self):
- return getattr(self._file, attr_name)
-
- return property(proxy_property)
-
-
-def _make_cond_delegate_method(attr_name):
- """For spooled temp files, delegate only if rolled to file object"""
-
- async def method(self, *args, **kwargs):
- if self._file._rolled:
- cb = functools.partial(getattr(self._file, attr_name), *args, **kwargs)
- return await self._loop.run_in_executor(self._executor, cb)
- else:
- return getattr(self._file, attr_name)(*args, **kwargs)
-
- return method
diff --git a/spaces/DQChoi/gpt-demo/venv/lib/python3.11/site-packages/huggingface_hub/hf_file_system.py b/spaces/DQChoi/gpt-demo/venv/lib/python3.11/site-packages/huggingface_hub/hf_file_system.py
deleted file mode 100644
index 8c283b8069915c9b000659ae2992948e864b4260..0000000000000000000000000000000000000000
--- a/spaces/DQChoi/gpt-demo/venv/lib/python3.11/site-packages/huggingface_hub/hf_file_system.py
+++ /dev/null
@@ -1,434 +0,0 @@
-import itertools
-import os
-import tempfile
-from dataclasses import dataclass
-from datetime import datetime
-from glob import has_magic
-from typing import Any, Dict, List, Optional, Tuple, Union
-from urllib.parse import quote, unquote
-
-import fsspec
-
-from ._commit_api import CommitOperationCopy, CommitOperationDelete
-from .constants import DEFAULT_REVISION, ENDPOINT, REPO_TYPE_MODEL, REPO_TYPES_MAPPING, REPO_TYPES_URL_PREFIXES
-from .hf_api import HfApi
-from .utils import (
- EntryNotFoundError,
- HFValidationError,
- RepositoryNotFoundError,
- RevisionNotFoundError,
- hf_raise_for_status,
- http_backoff,
- paginate,
- parse_datetime,
-)
-
-
-@dataclass
-class HfFileSystemResolvedPath:
- """Data structure containing information about a resolved Hugging Face file system path."""
-
- repo_type: str
- repo_id: str
- revision: str
- path_in_repo: str
-
- def unresolve(self) -> str:
- return (
- f"{REPO_TYPES_URL_PREFIXES.get(self.repo_type, '') + self.repo_id}@{safe_quote(self.revision)}/{self.path_in_repo}"
- .rstrip("/")
- )
-
-
-class HfFileSystem(fsspec.AbstractFileSystem):
- """
- Access a remote Hugging Face Hub repository as if were a local file system.
-
- Args:
- endpoint (`str`, *optional*):
- The endpoint to use. If not provided, the default one (https://huggingface.co) is used.
- token (`str`, *optional*):
- Authentication token, obtained with [`HfApi.login`] method. Will default to the stored token.
-
- Usage:
-
- ```python
- >>> from huggingface_hub import HfFileSystem
-
- >>> fs = HfFileSystem()
-
- >>> # List files
- >>> fs.glob("my-username/my-model/*.bin")
- ['my-username/my-model/pytorch_model.bin']
- >>> fs.ls("datasets/my-username/my-dataset", detail=False)
- ['datasets/my-username/my-dataset/.gitattributes', 'datasets/my-username/my-dataset/README.md', 'datasets/my-username/my-dataset/data.json']
-
- >>> # Read/write files
- >>> with fs.open("my-username/my-model/pytorch_model.bin") as f:
- ... data = f.read()
- >>> with fs.open("my-username/my-model/pytorch_model.bin", "wb") as f:
- ... f.write(data)
- ```
- """
-
- root_marker = ""
- protocol = "hf"
-
- def __init__(
- self,
- *args,
- endpoint: Optional[str] = None,
- token: Optional[str] = None,
- **storage_options,
- ):
- super().__init__(*args, **storage_options)
- self.endpoint = endpoint or ENDPOINT
- self.token = token
- self._api = HfApi(endpoint=endpoint, token=token)
- # Maps (repo_type, repo_id, revision) to a 2-tuple with:
- # * the 1st element indicating whether the repositoy and the revision exist
- # * the 2nd element being the exception raised if the repository or revision doesn't exist
- self._repo_and_revision_exists_cache: Dict[
- Tuple[str, str, Optional[str]], Tuple[bool, Optional[Exception]]
- ] = {}
-
- def _repo_and_revision_exist(
- self, repo_type: str, repo_id: str, revision: Optional[str]
- ) -> Tuple[bool, Optional[Exception]]:
- if (repo_type, repo_id, revision) not in self._repo_and_revision_exists_cache:
- try:
- self._api.repo_info(repo_id, revision=revision, repo_type=repo_type)
- except (RepositoryNotFoundError, HFValidationError) as e:
- self._repo_and_revision_exists_cache[(repo_type, repo_id, revision)] = False, e
- self._repo_and_revision_exists_cache[(repo_type, repo_id, None)] = False, e
- except RevisionNotFoundError as e:
- self._repo_and_revision_exists_cache[(repo_type, repo_id, revision)] = False, e
- self._repo_and_revision_exists_cache[(repo_type, repo_id, None)] = True, None
- else:
- self._repo_and_revision_exists_cache[(repo_type, repo_id, revision)] = True, None
- self._repo_and_revision_exists_cache[(repo_type, repo_id, None)] = True, None
- return self._repo_and_revision_exists_cache[(repo_type, repo_id, revision)]
-
- def resolve_path(self, path: str, revision: Optional[str] = None) -> HfFileSystemResolvedPath:
- def _align_revision_in_path_with_revision(
- revision_in_path: Optional[str], revision: Optional[str]
- ) -> Optional[str]:
- if revision is not None:
- if revision_in_path is not None and revision_in_path != revision:
- raise ValueError(
- f'Revision specified in path ("{revision_in_path}") and in `revision` argument ("{revision}")'
- " are not the same."
- )
- else:
- revision = revision_in_path
- return revision
-
- path = self._strip_protocol(path)
- if not path:
- # can't list repositories at root
- raise NotImplementedError("Access to repositories lists is not implemented.")
- elif path.split("/")[0] + "/" in REPO_TYPES_URL_PREFIXES.values():
- if "/" not in path:
- # can't list repositories at the repository type level
- raise NotImplementedError("Acces to repositories lists is not implemented.")
- repo_type, path = path.split("/", 1)
- repo_type = REPO_TYPES_MAPPING[repo_type]
- else:
- repo_type = REPO_TYPE_MODEL
- if path.count("/") > 0:
- if "@" in path:
- repo_id, revision_in_path = path.split("@", 1)
- if "/" in revision_in_path:
- revision_in_path, path_in_repo = revision_in_path.split("/", 1)
- else:
- path_in_repo = ""
- revision_in_path = unquote(revision_in_path)
- revision = _align_revision_in_path_with_revision(revision_in_path, revision)
- repo_and_revision_exist, err = self._repo_and_revision_exist(repo_type, repo_id, revision)
- if not repo_and_revision_exist:
- raise FileNotFoundError(path) from err
- else:
- repo_id_with_namespace = "/".join(path.split("/")[:2])
- path_in_repo_with_namespace = "/".join(path.split("/")[2:])
- repo_id_without_namespace = path.split("/")[0]
- path_in_repo_without_namespace = "/".join(path.split("/")[1:])
- repo_id = repo_id_with_namespace
- path_in_repo = path_in_repo_with_namespace
- repo_and_revision_exist, err = self._repo_and_revision_exist(repo_type, repo_id, revision)
- if not repo_and_revision_exist:
- if isinstance(err, (RepositoryNotFoundError, HFValidationError)):
- repo_id = repo_id_without_namespace
- path_in_repo = path_in_repo_without_namespace
- repo_and_revision_exist, _ = self._repo_and_revision_exist(repo_type, repo_id, revision)
- if not repo_and_revision_exist:
- raise FileNotFoundError(path) from err
- else:
- raise FileNotFoundError(path) from err
- else:
- repo_id = path
- path_in_repo = ""
- if "@" in path:
- repo_id, revision_in_path = path.split("@", 1)
- revision_in_path = unquote(revision_in_path)
- revision = _align_revision_in_path_with_revision(revision_in_path, revision)
- repo_and_revision_exist, _ = self._repo_and_revision_exist(repo_type, repo_id, revision)
- if not repo_and_revision_exist:
- raise NotImplementedError("Acces to repositories lists is not implemented.")
-
- revision = revision if revision is not None else DEFAULT_REVISION
- return HfFileSystemResolvedPath(repo_type, repo_id, revision, path_in_repo)
-
- def invalidate_cache(self, path: Optional[str] = None) -> None:
- if not path:
- self.dircache.clear()
- self._repository_type_and_id_exists_cache.clear()
- else:
- path = self.resolve_path(path).unresolve()
- while path:
- self.dircache.pop(path, None)
- path = self._parent(path)
-
- def _open(
- self,
- path: str,
- mode: str = "rb",
- revision: Optional[str] = None,
- **kwargs,
- ) -> "HfFileSystemFile":
- if mode == "ab":
- raise NotImplementedError("Appending to remote files is not yet supported.")
- return HfFileSystemFile(self, path, mode=mode, revision=revision, **kwargs)
-
- def _rm(self, path: str, revision: Optional[str] = None, **kwargs) -> None:
- resolved_path = self.resolve_path(path, revision=revision)
- self._api.delete_file(
- path_in_repo=resolved_path.path_in_repo,
- repo_id=resolved_path.repo_id,
- token=self.token,
- repo_type=resolved_path.repo_type,
- revision=resolved_path.revision,
- commit_message=kwargs.get("commit_message"),
- commit_description=kwargs.get("commit_description"),
- )
- self.invalidate_cache(path=resolved_path.unresolve())
-
- def rm(
- self,
- path: str,
- recursive: bool = False,
- maxdepth: Optional[int] = None,
- revision: Optional[str] = None,
- **kwargs,
- ) -> None:
- resolved_path = self.resolve_path(path, revision=revision)
- root_path = REPO_TYPES_URL_PREFIXES.get(resolved_path.repo_type, "") + resolved_path.repo_id
- paths = self.expand_path(path, recursive=recursive, maxdepth=maxdepth, revision=resolved_path.revision)
- paths_in_repo = [path[len(root_path) + 1 :] for path in paths if not self.isdir(path)]
- operations = [CommitOperationDelete(path_in_repo=path_in_repo) for path_in_repo in paths_in_repo]
- commit_message = f"Delete {path} "
- commit_message += "recursively " if recursive else ""
- commit_message += f"up to depth {maxdepth} " if maxdepth is not None else ""
- # TODO: use `commit_description` to list all the deleted paths?
- self._api.create_commit(
- repo_id=resolved_path.repo_id,
- repo_type=resolved_path.repo_type,
- token=self.token,
- operations=operations,
- revision=resolved_path.revision,
- commit_message=kwargs.get("commit_message", commit_message),
- commit_description=kwargs.get("commit_description"),
- )
- self.invalidate_cache(path=resolved_path.unresolve())
-
- def ls(
- self, path: str, detail: bool = True, refresh: bool = False, revision: Optional[str] = None, **kwargs
- ) -> List[Union[str, Dict[str, Any]]]:
- """List the contents of a directory."""
- resolved_path = self.resolve_path(path, revision=revision)
- revision_in_path = "@" + safe_quote(resolved_path.revision)
- has_revision_in_path = revision_in_path in path
- path = resolved_path.unresolve()
- if path not in self.dircache or refresh:
- path_prefix = (
- HfFileSystemResolvedPath(
- resolved_path.repo_type, resolved_path.repo_id, resolved_path.revision, ""
- ).unresolve()
- + "/"
- )
- tree_path = path
- tree_iter = self._iter_tree(tree_path, revision=resolved_path.revision)
- try:
- tree_item = next(tree_iter)
- except EntryNotFoundError:
- if "/" in resolved_path.path_in_repo:
- tree_path = self._parent(path)
- tree_iter = self._iter_tree(tree_path, revision=resolved_path.revision)
- else:
- raise
- else:
- tree_iter = itertools.chain([tree_item], tree_iter)
- child_infos = []
- for tree_item in tree_iter:
- child_info = {
- "name": path_prefix + tree_item["path"],
- "size": tree_item["size"],
- "type": tree_item["type"],
- }
- if tree_item["type"] == "file":
- child_info.update(
- {
- "blob_id": tree_item["oid"],
- "lfs": tree_item.get("lfs"),
- "last_modified": parse_datetime(tree_item["lastCommit"]["date"]),
- },
- )
- child_infos.append(child_info)
- self.dircache[tree_path] = child_infos
- out = self._ls_from_cache(path)
- if not has_revision_in_path:
- out = [{**o, "name": o["name"].replace(revision_in_path, "", 1)} for o in out]
- return out if detail else [o["name"] for o in out]
-
- def _iter_tree(self, path: str, revision: Optional[str] = None):
- # TODO: use HfApi.list_files_info instead when it supports "lastCommit" and "expand=True"
- # See https://github.com/huggingface/moon-landing/issues/5993
- resolved_path = self.resolve_path(path, revision=revision)
- path = f"{self._api.endpoint}/api/{resolved_path.repo_type}s/{resolved_path.repo_id}/tree/{safe_quote(resolved_path.revision)}/{resolved_path.path_in_repo}".rstrip(
- "/"
- )
- headers = self._api._build_hf_headers()
- yield from paginate(path, params={"expand": True}, headers=headers)
-
- def cp_file(self, path1: str, path2: str, revision: Optional[str] = None, **kwargs) -> None:
- resolved_path1 = self.resolve_path(path1, revision=revision)
- resolved_path2 = self.resolve_path(path2, revision=revision)
-
- same_repo = (
- resolved_path1.repo_type == resolved_path2.repo_type and resolved_path1.repo_id == resolved_path2.repo_id
- )
-
- # TODO: Wait for https://github.com/huggingface/huggingface_hub/issues/1083 to be resolved to simplify this logic
- if same_repo and self.info(path1, revision=resolved_path1.revision)["lfs"] is not None:
- commit_message = f"Copy {path1} to {path2}"
- self._api.create_commit(
- repo_id=resolved_path1.repo_id,
- repo_type=resolved_path1.repo_type,
- revision=resolved_path2.revision,
- commit_message=kwargs.get("commit_message", commit_message),
- commit_description=kwargs.get("commit_description", ""),
- operations=[
- CommitOperationCopy(
- src_path_in_repo=resolved_path1.path_in_repo,
- path_in_repo=resolved_path2.path_in_repo,
- src_revision=resolved_path1.revision,
- )
- ],
- )
- else:
- with self.open(path1, "rb", revision=resolved_path1.revision) as f:
- content = f.read()
- commit_message = f"Copy {path1} to {path2}"
- self._api.upload_file(
- path_or_fileobj=content,
- path_in_repo=resolved_path2.path_in_repo,
- repo_id=resolved_path2.repo_id,
- token=self.token,
- repo_type=resolved_path2.repo_type,
- revision=resolved_path2.revision,
- commit_message=kwargs.get("commit_message", commit_message),
- commit_description=kwargs.get("commit_description"),
- )
- self.invalidate_cache(path=resolved_path1.unresolve())
- self.invalidate_cache(path=resolved_path2.unresolve())
-
- def modified(self, path: str, **kwargs) -> datetime:
- info = self.info(path, **kwargs)
- if "last_modified" not in info:
- raise IsADirectoryError(path)
- return info["last_modified"]
-
- def info(self, path: str, **kwargs) -> Dict[str, Any]:
- resolved_path = self.resolve_path(path)
- if not resolved_path.path_in_repo:
- revision_in_path = "@" + safe_quote(resolved_path.revision)
- has_revision_in_path = revision_in_path in path
- name = resolved_path.unresolve()
- name = name.replace(revision_in_path, "", 1) if not has_revision_in_path else name
- return {"name": name, "size": 0, "type": "directory"}
- return super().info(path, **kwargs)
-
- def expand_path(
- self, path: Union[str, List[str]], recursive: bool = False, maxdepth: Optional[int] = None, **kwargs
- ) -> List[str]:
- # The default implementation does not allow passing custom kwargs (e.g., we use these kwargs to propagate the `revision`)
- if maxdepth is not None and maxdepth < 1:
- raise ValueError("maxdepth must be at least 1")
-
- if isinstance(path, str):
- return self.expand_path([path], recursive, maxdepth)
-
- out = set()
- path = [self._strip_protocol(p) for p in path]
- for p in path:
- if has_magic(p):
- bit = set(self.glob(p, **kwargs))
- out |= bit
- if recursive:
- out |= set(self.expand_path(list(bit), recursive=recursive, maxdepth=maxdepth, **kwargs))
- continue
- elif recursive:
- rec = set(self.find(p, maxdepth=maxdepth, withdirs=True, detail=False, **kwargs))
- out |= rec
- if p not in out and (recursive is False or self.exists(p)):
- # should only check once, for the root
- out.add(p)
- if not out:
- raise FileNotFoundError(path)
- return list(sorted(out))
-
-
-class HfFileSystemFile(fsspec.spec.AbstractBufferedFile):
- def __init__(self, fs: HfFileSystem, path: str, revision: Optional[str] = None, **kwargs):
- super().__init__(fs, path, **kwargs)
- self.fs: HfFileSystem
- self.resolved_path = fs.resolve_path(path, revision=revision)
-
- def _fetch_range(self, start: int, end: int) -> bytes:
- headers = {
- "range": f"bytes={start}-{end - 1}",
- **self.fs._api._build_hf_headers(),
- }
- url = (
- f"{self.fs.endpoint}/{REPO_TYPES_URL_PREFIXES.get(self.resolved_path.repo_type, '') + self.resolved_path.repo_id}/resolve/{safe_quote(self.resolved_path.revision)}/{safe_quote(self.resolved_path.path_in_repo)}"
- )
- r = http_backoff("GET", url, headers=headers)
- hf_raise_for_status(r)
- return r.content
-
- def _initiate_upload(self) -> None:
- self.temp_file = tempfile.NamedTemporaryFile(prefix="hffs-", delete=False)
-
- def _upload_chunk(self, final: bool = False) -> None:
- self.buffer.seek(0)
- block = self.buffer.read()
- self.temp_file.write(block)
- if final:
- self.temp_file.close()
- self.fs._api.upload_file(
- path_or_fileobj=self.temp_file.name,
- path_in_repo=self.resolved_path.path_in_repo,
- repo_id=self.resolved_path.repo_id,
- token=self.fs.token,
- repo_type=self.resolved_path.repo_type,
- revision=self.resolved_path.revision,
- commit_message=self.kwargs.get("commit_message"),
- commit_description=self.kwargs.get("commit_description"),
- )
- os.remove(self.temp_file.name)
- self.fs.invalidate_cache(
- path=self.resolved_path.unresolve(),
- )
-
-
-def safe_quote(s: str) -> str:
- return quote(s, safe="")
diff --git a/spaces/Detomo/ai-comic-generation/src/lib/dirtyCaptionCleaner.ts b/spaces/Detomo/ai-comic-generation/src/lib/dirtyCaptionCleaner.ts
deleted file mode 100644
index fdfa2831e7a783706e64c006e84f30515aa00d3e..0000000000000000000000000000000000000000
--- a/spaces/Detomo/ai-comic-generation/src/lib/dirtyCaptionCleaner.ts
+++ /dev/null
@@ -1,38 +0,0 @@
-export function dirtyCaptionCleaner({
- panel,
- instructions,
- caption
-}: {
- panel: number;
- instructions: string;
- caption: string
-}) {
- let newCaption = caption.split(":").pop()?.trim() || ""
- let newInstructions = (
- // need to remove from LLM garbage here, too
- (instructions.split(":").pop() || "")
- .replaceAll("Show a", "")
- .replaceAll("Show the", "")
- .replaceAll("Opens with a", "")
- .replaceAll("Opens with the", "")
- .replaceAll("Opens with", "")
- .replaceAll("Cut to a", "")
- .replaceAll("Cut to the", "")
- .replaceAll("Cut to", "")
- .replaceAll("End with a", "")
- .replaceAll("End with", "").trim() || ""
- )
-
- // we have to crop the instructions unfortunately, otherwise the style will disappear
- // newInstructions = newInstructions.slice(0, 77)
- // EDIT: well actually the instructions are already at the end of the prompt,
- // so we can let SDXL do this cropping job for us
-
- // american comic about brunette wood elf walks around a dark forrest and suddenly stops when hearing a strange noise, single panel, modern american comic, comicbook style, 2010s, digital print, color comicbook, color drawing, Full shot of the elf, her eyes widening in surprise, as a glowing, ethereal creature steps out of the shadows.",
-
- return {
- panel,
- instructions: newInstructions,
- caption: newCaption,
- }
-}
\ No newline at end of file
diff --git a/spaces/Docfile/open_llm_leaderboard/models_backlinks.py b/spaces/Docfile/open_llm_leaderboard/models_backlinks.py
deleted file mode 100644
index 836993a8220290e78fedea7a4839dbe11c7e7a52..0000000000000000000000000000000000000000
--- a/spaces/Docfile/open_llm_leaderboard/models_backlinks.py
+++ /dev/null
@@ -1 +0,0 @@
-models = ['uni-tianyan/Uni-TianYan', 'fangloveskari/ORCA_LLaMA_70B_QLoRA', 'garage-bAInd/Platypus2-70B-instruct', 'upstage/Llama-2-70b-instruct-v2', 'fangloveskari/Platypus_QLoRA_LLaMA_70b', 'yeontaek/llama-2-70B-ensemble-v5', 'TheBloke/Genz-70b-GPTQ', 'TheBloke/Platypus2-70B-Instruct-GPTQ', 'psmathur/model_007', 'yeontaek/llama-2-70B-ensemble-v4', 'psmathur/orca_mini_v3_70b', 'ehartford/Samantha-1.11-70b', 'MayaPH/GodziLLa2-70B', 'psmathur/model_007_v2', 'chargoddard/MelangeA-70b', 'ehartford/Samantha-1.1-70b', 'psmathur/model_009', 'upstage/Llama-2-70b-instruct', 'yeontaek/llama-2-70B-ensemble-v7', 'yeontaek/llama-2-70B-ensemble-v6', 'chargoddard/MelangeB-70b', 'yeontaek/llama-2-70B-ensemble-v3', 'chargoddard/MelangeC-70b', 'garage-bAInd/Camel-Platypus2-70B', 'yeontaek/llama-2-70B-ensemble-v2', 'garage-bAInd/Camel-Platypus2-70B', 'migtissera/Synthia-70B-v1.2', 'v2ray/LLaMA-2-Wizard-70B-QLoRA', 'quantumaikr/llama-2-70b-fb16-orca-chat-10k', 'v2ray/LLaMA-2-Wizard-70B-QLoRA', 'stabilityai/StableBeluga2', 'quantumaikr/llama-2-70b-fb16-guanaco-1k', 'garage-bAInd/Camel-Platypus2-70B', 'migtissera/Synthia-70B-v1.1', 'migtissera/Synthia-70B', 'psmathur/model_101', 'augtoma/qCammel70', 'augtoma/qCammel-70', 'augtoma/qCammel-70v1', 'augtoma/qCammel-70x', 'augtoma/qCammel-70-x', 'jondurbin/airoboros-l2-70b-gpt4-1.4.1', 'dfurman/llama-2-70b-dolphin-peft', 'jondurbin/airoboros-l2-70b-2.1', 'TheBloke/llama-2-70b-Guanaco-QLoRA-fp16', 'quantumaikr/QuantumLM-llama2-70B-Korean-LoRA', 'quantumaikr/quantumairk-llama-2-70B-instruct', 'psmathur/model_420', 'psmathur/model_51', 'garage-bAInd/Camel-Platypus2-70B', 'TheBloke/Airoboros-L2-70B-2.1-GPTQ', 'OpenAssistant/llama2-70b-oasst-sft-v10', 'garage-bAInd/Platypus2-70B', 'liuxiang886/llama2-70B-qlora-gpt4', 'upstage/llama-65b-instruct', 'quantumaikr/llama-2-70b-fb16-korean', 'NousResearch/Nous-Hermes-Llama2-70b', 'v2ray/LLaMA-2-Jannie-70B-QLoRA', 'jondurbin/airoboros-l2-70b-gpt4-m2.0', 'jondurbin/airoboros-l2-70b-gpt4-m2.0', 'OpenAssistant/llama2-70b-oasst-sft-v10', 'yeontaek/llama-2-70B-ensemble-v8', 'jondurbin/airoboros-l2-70b-gpt4-2.0', 'jarradh/llama2_70b_chat_uncensored', 'WizardLM/WizardMath-70B-V1.0', 'jordiclive/Llama-2-70b-oasst-1-200', 'WizardLM/WizardMath-70B-V1.0', 'jondurbin/airoboros-l2-70b-gpt4-2.0', 'OpenLemur/lemur-70b-chat-v1', 'tiiuae/falcon-180B', 'tiiuae/falcon-180B', 'stabilityai/StableBeluga1-Delta', 'psmathur/model_42_70b', 'psmathur/test_42_70b', 'TheBloke/fiction.live-Kimiko-V2-70B-fp16', 'tiiuae/falcon-180B', 'WizardLM/WizardMath-70B-V1.0', 'tiiuae/falcon-180B-chat', 'jondurbin/airoboros-l2-70b-gpt4-2.0', 'ehartford/samantha-1.1-llama-33b', 'ajibawa-2023/scarlett-33b', 'ddobokki/Llama-2-70b-orca-200k', 'TheBloke/gpt4-alpaca-lora_mlp-65B-HF', 'tiiuae/falcon-180B-chat', 'tiiuae/falcon-180B-chat', 'tiiuae/falcon-180B', 'TheBloke/Lemur-70B-Chat-v1-GPTQ', 'NousResearch/Nous-Puffin-70B', 'WizardLM/WizardLM-70B-V1.0', 'WizardLM/WizardMath-70B-V1.0', 'meta-llama/Llama-2-70b-hf', 'TheBloke/Llama-2-70B-fp16', 'Weyaxi/llama-2-alpacagpt4-1000step', 'WizardLM/WizardLM-70B-V1.0', 'simsim314/WizardLM-70B-V1.0-HF', 'simsim314/WizardLM-70B-V1.0-HF', 'WizardLM/WizardLM-70B-V1.0', 'openbmb/UltraLM-65b', 'psmathur/model_420_preview', 'WizardLM/WizardLM-70B-V1.0', 'simsim314/WizardLM-70B-V1.0-HF', 'OpenBuddy/openbuddy-llama2-70b-v10.1-bf16', 'upstage/llama-30b-instruct-2048', 'jondurbin/airoboros-65b-gpt4-1.2', 'TheBloke/guanaco-65B-HF', 'jondurbin/airoboros-65b-gpt4-1.3', 'meta-llama/Llama-2-70b-chat-hf', 'ValiantLabs/ShiningValiant', 'Faradaylab/Aria-70B', 'lilloukas/GPlatty-30B', 'TheBloke/VicUnlocked-alpaca-65B-QLoRA-fp16', 'jondurbin/airoboros-65b-gpt4-1.4-peft', 'jondurbin/airoboros-65b-gpt4-1.4', 'jondurbin/airoboros-65b-gpt4-2.0', 'TheBloke/WizardLM-70B-V1.0-GPTQ', 'TheBloke/WizardLM-70B-V1.0-GPTQ', 'ariellee/SuperPlatty-30B', 'jondurbin/airoboros-65b-gpt4-1.4', 'jondurbin/airoboros-65b-gpt4-2.0', 'yeontaek/llama-2-70b-IA3-guanaco', 'CalderaAI/30B-Lazarus', 'Aspik101/trurl-2-13b-pl-instruct_unload', 'ehartford/WizardLM-33B-V1.0-Uncensored', 'ehartford/WizardLM-33B-V1.0-Uncensored', 'OpenBuddy/openbuddy-llama-65b-v8-bf16', 'Aspik101/llama-30b-instruct-2048-PL-lora', 'h2oai/h2ogpt-research-oasst1-llama-65b', 'Aspik101/llama-30b-instruct-2048-PL-lora', 'CalderaAI/30B-Epsilon', 'Aspik101/llama-30b-2048-instruct-PL-lora_unload', 'jondurbin/airoboros-65b-gpt4-m2.0', 'jondurbin/airoboros-65b-gpt4-m2.0', 'Aeala/Alpaca-elina-65b', 'TheBloke/robin-65b-v2-fp16', 'TheBloke/gpt4-alpaca-lora-30b-HF', 'TheBloke/Llama-2-70B-chat-GPTQ', 'upstage/llama-30b-instruct', 'OpenLemur/lemur-70b-v1', 'lmsys/vicuna-33b-v1.3', 'ausboss/llama-30b-supercot', 'ai-business/Luban-13B', 'Henk717/airochronos-33B', 'lmsys/vicuna-33b-v1.3', 'Henk717/airochronos-33B', 'bavest/fin-llama-33b-merged', 'jondurbin/airoboros-33b-gpt4-1.4', 'YeungNLP/firefly-llama-30b', 'Aspik101/30B-Lazarus-instruct-PL-lora_unload', 'uukuguy/speechless-llama2-luban-orca-platypus-13b', 'xxyyy123/test_merge_p_ov1_w0.66_w0.5_n1', 'jondurbin/airoboros-33b-gpt4-1.2', 'TheBloke/alpaca-lora-65B-HF', 'bofenghuang/vigogne-33b-instruct', 'yeontaek/llama-2-13B-ensemble-v5', 'garage-bAInd/Platypus-30B', 'Open-Orca/OpenOrca-Platypus2-13B', 'kajdun/viwaai-30b_v4', 'lilloukas/Platypus-30B', 'Open-Orca/OpenOrca-Platypus2-13B', 'Henk717/chronoboros-33B', 'jondurbin/airoboros-33b-2.1', 'HiTZ/alpaca-lora-65b-en-pt-es-ca', 'quantumaikr/QuantumLM-70B-hf', 'uukuguy/speechless-llama2-13b', 'uukuguy/speechless-llama2-hermes-orca-platypus-13b', 'openaccess-ai-collective/manticore-30b-chat-pyg-alpha', 'LLMs/WizardLM-30B-V1.0', 'TheBloke/WizardLM-30B-fp16', 'openaccess-ai-collective/hippogriff-30b-chat', 'concedo/Vicuzard-30B-Uncensored', 'TFLai/OpenOrca-Platypus2-13B-QLoRA-0.80-epoch', 'huggingface/llama-65b', 'huggyllama/llama-65b', 'gaodrew/gaodrew-llama-30b-instruct-2048-Open-Platypus-100steps', 'uukuguy/speechless-llama2-hermes-orca-platypus-wizardlm-13b', 'Sao10K/Mythical-Destroyer-V2-L2-13B', 'camel-ai/CAMEL-33B-Combined-Data', 'dsvv-cair/alpaca-cleaned-llama-30b-bf16', 'MetaIX/GPT4-X-Alpasta-30b', 'garage-bAInd/Stable-Platypus2-13B', 'TFLai/Luban-Platypus2-13B-QLora-0.80-epoch', 'TheBloke/OpenOrca-Platypus2-13B-GPTQ', 'IkariDev/Athena-tmp', 'OpenBuddyEA/openbuddy-llama-30b-v7.1-bf16', 'OpenBuddyEA/openbuddy-llama-30b-v7.1-bf16', 'Open-Orca/OpenOrcaxOpenChat-Preview2-13B', 'psmathur/model_007_13b_v2', 'Aspik101/Vicuzard-30B-Uncensored-instruct-PL-lora_unload', 'jondurbin/airoboros-33b-gpt4-m2.0', 'Sao10K/Mythical-Destroyer-L2-13B', 'TheBloke/Wizard-Vicuna-30B-Uncensored-fp16', 'ehartford/Wizard-Vicuna-30B-Uncensored', 'TFLai/Nova-13B', 'TheBloke/robin-33B-v2-fp16', 'totally-not-an-llm/PuddleJumper-13b', 'Aeala/VicUnlocked-alpaca-30b', 'Yhyu13/oasst-rlhf-2-llama-30b-7k-steps-hf', 'jondurbin/airoboros-33b-gpt4', 'jondurbin/airoboros-33b-gpt4-m2.0', 'tiiuae/falcon-40b-instruct', 'psmathur/orca_mini_v3_13b', 'Aeala/GPT4-x-AlpacaDente-30b', 'MayaPH/GodziLLa-30B', 'jondurbin/airoboros-33b-gpt4-m2.0', 'TFLai/SpeechlessV1-Nova-13B', 'yeontaek/llama-2-13B-ensemble-v4', 'ajibawa-2023/carl-33b', 'jondurbin/airoboros-33b-gpt4-2.0', 'TFLai/Stable-Platypus2-13B-QLoRA-0.80-epoch', 'jondurbin/airoboros-33b-gpt4-1.3', 'TehVenom/oasst-sft-6-llama-33b-xor-MERGED-16bit', 'TFLai/OrcaMini-Platypus2-13B-QLoRA-0.80-epoch', 'jondurbin/airoboros-33b-gpt4-2.0', 'chargoddard/Chronorctypus-Limarobormes-13b', 'jondurbin/airoboros-33b-gpt4-1.3', 'Open-Orca/OpenOrca-Platypus2-13B', 'FelixChao/vicuna-33b-coder', 'FelixChao/vicuna-33b-coder', 'Gryphe/MythoMix-L2-13b', 'Aeala/Enterredaas-33b', 'yeontaek/llama-2-13B-ensemble-v1', 'TFLai/OpenOrcaPlatypus2-Platypus2-13B-QLora-0.80-epoch', 'TFLai/Ensemble5-Platypus2-13B-QLora-0.80-epoch', 'yeontaek/llama-2-13B-ensemble-v3', 'TFLai/MythoMix-Platypus2-13B-QLoRA-0.80-epoch', 'yihan6324/llama2-13b-instructmining-40k-sharegpt', 'timdettmers/guanaco-33b-merged', 'TFLai/EnsembleV5-Nova-13B', 'circulus/Llama-2-13b-orca-v1', 'Undi95/ReMM-SLERP-L2-13B', 'Gryphe/MythoMax-L2-13b', 'stabilityai/StableBeluga-13B', 'circulus/Llama-2-13b-orca-v1', 'ehartford/WizardLM-30B-Uncensored', 'The-Face-Of-Goonery/huginnv1.2', 'TheBloke/OpenOrcaxOpenChat-Preview2-13B-GPTQ', 'Sao10K/Stheno-L2-13B', 'bofenghuang/vigogne-2-13b-instruct', 'The-Face-Of-Goonery/Huginn-13b-FP16', 'grimpep/L2-MythoMax22b-instruct-Falseblock', 'TFLai/Nous-Hermes-Platypus2-13B-QLoRA-0.80-epoch', 'yeontaek/Platypus2xOpenOrca-13B-IA3-v4', 'yeontaek/Platypus2xOpenOrca-13B-IA3', 'yeontaek/Platypus2xOpenOrca-13B-IA3-ensemble', 'Open-Orca/LlongOrca-13B-16k', 'Sao10K/Stheno-Inverted-L2-13B', 'garage-bAInd/Camel-Platypus2-13B', 'digitous/Alpacino30b', 'NousResearch/Nous-Hermes-Llama2-13b', 'yeontaek/Platypus2xOpenOrca-13B-IA3-v3', 'TFLai/MythicalDestroyerV2-Platypus2-13B-QLora-0.80-epoch', 'TheBloke/VicUnlocked-30B-LoRA-HF', 'Undi95/Nous-Hermes-13B-Code', 'The-Face-Of-Goonery/Chronos-Beluga-v2-13bfp16', 'NousResearch/Nous-Hermes-Llama2-13b', 'Monero/WizardLM-Uncensored-SuperCOT-StoryTelling-30b', 'TheBloke/Wizard-Vicuna-30B-Uncensored-GPTQ', 'Open-Orca/OpenOrcaxOpenChat-Preview2-13B', 'Austism/chronos-hermes-13b-v2', 'yeontaek/Platypus2xOpenOrca-13B-IA3-v2.1', 'yeontaek/Platypus2xOpenOrca-13B-IA3-v2', 'Gryphe/MythoLogic-L2-13b', 'augtoma/qCammel-13', 'YeungNLP/firefly-llama2-13b-v1.2', 'Aspik101/StableBeluga-13B-instruct-PL-lora_unload', 'andreaskoepf/llama2-13b-megacode2_min100', 'rombodawg/LosslessMegaCoder-llama2-13b-mini', 'yulan-team/YuLan-Chat-2-13b-fp16', 'elinas/chronos-33b', 'YeungNLP/firefly-llama2-13b', 'Sao10K/Medusa-13b', 'OptimalScale/robin-65b-v2-delta', 'minlik/chinese-alpaca-33b-merged', 'OpenAssistant/llama2-13b-megacode2-oasst', 'TheBloke/OpenAssistant-SFT-7-Llama-30B-HF', 'Undi95/UndiMix-v1-13b', 'ehartford/Samantha-1.11-13b', 'beaugogh/Llama2-13b-sharegpt4', 'Aeala/GPT4-x-AlpacaDente2-30b', 'luffycodes/nash-vicuna-13b-v1dot5-ep2-w-rag-w-simple', 'WizardLM/WizardLM-13B-V1.1', 'uukuguy/speechless-orca-platypus-coig-lite-2k-0.6e-13b', 'huggyllama/llama-30b', 'Undi95/ReMM-L2-13B-PIPPA', 'Undi95/ReMM-L2-13B', 'gaodrew/gaodrew-gorgonzola-13b', 'lmsys/vicuna-13b-v1.5', 'yeontaek/Platypus2xOpenOrca-13B-LoRa', 'Yhyu13/llama-30B-hf-openassitant', 'huggingface/llama-30b', 'lmsys/vicuna-13b-v1.5', 'TFLai/Athena-Platypus2-13B-QLora-0.80-epoch', 'TheBloke/dromedary-65b-lora-HF', 'yeontaek/llama-2-13b-Beluga-QLoRA', 'The-Face-Of-Goonery/Huginn-13b-V4', 'The-Face-Of-Goonery/Huginn-13b-v4.5', 'The-Face-Of-Goonery/Huginn-v3-13b', 'tiiuae/falcon-40b', 'WhoTookMyAmogusNickname/NewHope_HF_not_official', 'gaodrew/OpenOrca-Platypus2-13B-thera-1250', 'SLAM-group/NewHope', 'garage-bAInd/Platypus2-13B', 'migtissera/Synthia-13B', 'elinas/chronos-13b-v2', 'mosaicml/mpt-30b-chat', 'CHIH-HUNG/llama-2-13b-OpenOrca_5w', 'uukuguy/speechless-hermes-coig-lite-13b', 'TheBloke/tulu-30B-fp16', 'uukuguy/speechless-hermes-coig-lite-13b', 'xDAN-AI/xDAN_13b_l2_lora', 'lmsys/vicuna-13b-v1.5-16k', 'openchat/openchat_v3.1', 'CHIH-HUNG/llama-2-13b-dolphin_5w', 'Aspik101/vicuna-13b-v1.5-PL-lora_unload', 'Undi95/MLewd-L2-13B', 'ehartford/minotaur-llama2-13b-qlora', 'kajdun/iubaris-13b-v3', 'TFLai/Limarp-Platypus2-13B-QLoRA-0.80-epoch', 'openchat/openchat_v3.1', 'uukuguy/speechless-orca-platypus-coig-lite-4k-0.6e-13b', 'ziqingyang/chinese-alpaca-2-13b', 'TFLai/Airboros2.1-Platypus2-13B-QLora-0.80-epoch', 'yeontaek/llama-2-13b-Guanaco-QLoRA', 'lmsys/vicuna-13b-v1.5-16k', 'ehartford/based-30b', 'kingbri/airolima-chronos-grad-l2-13B', 'openchat/openchat_v3.2', 'uukuguy/speechless-orca-platypus-coig-lite-4k-0.5e-13b', 'yeontaek/Platypus2-13B-LoRa', 'kingbri/chronolima-airo-grad-l2-13B', 'openchat/openchat_v3.2', 'TFLai/PuddleJumper-Platypus2-13B-QLoRA-0.80-epoch', 'shareAI/llama2-13b-Chinese-chat', 'ehartford/WizardLM-1.0-Uncensored-Llama2-13b', 'Aspik101/Redmond-Puffin-13B-instruct-PL-lora_unload', 'yeontaek/llama-2-13B-ensemble-v6', 'WizardLM/WizardLM-13B-V1.2', 'TheBloke/WizardLM-13B-V1.1-GPTQ', 'bhenrym14/airophin-13b-pntk-16k-fp16', 'ehartford/WizardLM-1.0-Uncensored-Llama2-13b', 'Mikael110/llama-2-13b-guanaco-fp16', 'yeontaek/airoboros-2.1-llama-2-13B-QLoRa', 'CalderaAI/13B-Legerdemain-L2', 'grimpep/llama2-22b-wizard_vicuna', 'grimpep/llama2-22B-GPLATTY', 'bhenrym14/airophin-13b-pntk-16k-fp16', 'yeontaek/llama-2-13b-QLoRA', 'OpenAssistant/llama2-13b-orca-8k-3319', 'TheBloke/WizardLM-13B-V1-1-SuperHOT-8K-fp16', 'duliadotio/dulia-13b-8k-alpha', 'Undi95/LewdEngine', 'OpenBuddy/openbuddy-llama2-13b-v8.1-fp16', 'CHIH-HUNG/llama-2-13b-open_orca_20w', 'bhenrym14/airoboros-33b-gpt4-1.4.1-lxctx-PI-16384-fp16', 'FlagAlpha/Llama2-Chinese-13b-Chat', 'LLMs/WizardLM-13B-V1.0', 'chansung/gpt4-alpaca-lora-13b-decapoda-1024', 'TheBloke/wizardLM-13B-1.0-fp16', 'digitous/13B-Chimera', 'yeontaek/Platypus2xOpenOrcaxGuanaco-13B-LoRa', 'jondurbin/airoboros-l2-13b-2.1', 'Monero/WizardLM-30B-Uncensored-Guanaco-SuperCOT-30b', 'TheBloke/UltraLM-13B-fp16', 'openaccess-ai-collective/minotaur-13b-fixed', 'NousResearch/Redmond-Puffin-13B', 'KoboldAI/LLaMA2-13B-Holomax', 'Lajonbot/WizardLM-13B-V1.2-PL-lora_unload', 'yeontaek/Platypus2-13B-LoRa-v2', 'TheBloke/airoboros-13B-HF', 'jondurbin/airoboros-13b', 'jjaaaww/posi_13b', 'CoolWP/llama-2-13b-guanaco-fp16', 'yeontaek/Platypus2-13B-QLoRa', 'h2oai/h2ogpt-research-oig-oasst1-512-30b', 'dfurman/llama-2-13b-guanaco-peft', 'NousResearch/Redmond-Puffin-13B', 'pe-nlp/llama-2-13b-platypus-vicuna-wizard', 'CHIH-HUNG/llama-2-13b-dolphin_20w', 'NousResearch/Nous-Hermes-13b', 'NobodyExistsOnTheInternet/GiftedConvo13bLoraNoEconsE4', 'ehartford/Wizard-Vicuna-13B-Uncensored', 'TheBloke/Wizard-Vicuna-13B-Uncensored-HF', 'openchat/openchat_v3.2_super', 'bhenrym14/airophin-v2-13b-PI-8k-fp16', 'openaccess-ai-collective/manticore-13b', 'The-Face-Of-Goonery/Huginn-22b-Prototype', 'jphme/Llama-2-13b-chat-german', 'grimpep/llama2-28B-Airo03', 'TheBloke/Kimiko-v2-13B-fp16', 'FPHam/Free_Sydney_13b_HF', 'lmsys/vicuna-13b-v1.3', 'FelixChao/llama2-13b-math1.1', 'CalderaAI/13B-BlueMethod', 'meta-llama/Llama-2-13b-chat-hf', 'deepse/CodeUp-Llama-2-13b-chat-hf', 'WizardLM/WizardMath-13B-V1.0', 'WizardLM/WizardMath-13B-V1.0', 'HyperbeeAI/Tulpar-7b-v0', 'xxyyy123/test_qkvo_adptor', 'xxyyy123/mc_data_30k_from_platpus_orca_7b_10k_v1_lora_qkvo_rank14_v2', 'openchat/openchat_v2_w', 'FelixChao/llama2-13b-math1.1', 'psmathur/orca_mini_v3_7b', 'TehVenom/Metharme-13b-Merged', 'xxyyy123/10k_v1_lora_qkvo_rank14_v3', 'OpenAssistant/llama2-13b-orca-v2-8k-3166', 'openaccess-ai-collective/wizard-mega-13b', 'jondurbin/airoboros-13b-gpt4-1.4', 'jondurbin/airoboros-13b-gpt4-1.4-fp16', 'Monero/Manticore-13b-Chat-Pyg-Guanaco', 'FelixChao/llama2-13b-math1.2', 'chargoddard/platypus-2-22b-relora', 'FelixChao/llama2-13b-math1.2', 'Gryphe/MythoBoros-13b', 'CalderaAI/13B-Ouroboros', 'OpenAssistant/llama2-13b-orca-v2-8k-3166', 'heegyu/LIMA2-13b-hf', 'digitous/13B-HyperMantis', 'Gryphe/MythoLogic-13b', 'TheBloke/Airoboros-L2-13B-2.1-GPTQ', 'chargoddard/platypus2-22b-relora', 'openchat/openchat_v2', 'yeontaek/Platypus2-13B-IA3', 'stabilityai/StableBeluga-7B', 'circulus/Llama-2-7b-orca-v1', 'budecosystem/genz-13b-v2', 'TheBloke/gpt4-x-vicuna-13B-HF', 'NobodyExistsOnTheInternet/GiftedConvo13bLoraNoEcons', 'zarakiquemparte/zarafusionex-1.1-l2-7b', 'Lajonbot/tableBeluga-7B-instruct-pl-lora_unload', 'jondurbin/airoboros-13b-gpt4', 'gaodrew/gaodrew-gorgonzola-13b', 'jondurbin/airoboros-13b-gpt4-1.1', 'TheBloke/gpt4-alpaca-lora-13B-HF', 'zarakiquemparte/zarablendex-vq-l2-7b', 'openaccess-ai-collective/manticore-13b-chat-pyg', 'Lajonbot/Llama-2-13b-hf-instruct-pl-lora_unload', 'NobodyExistsOnTheInternet/PuffedLIMA13bQLORA', 'xxyyy123/10k_v1_lora_qkvo_rank28_v2', 'jondurbin/airoboros-l2-13b-gpt4-1.4.1', 'dhmeltzer/Llama-2-13b-hf-eli5-wiki-1024_r_64_alpha_16', 'NobodyExistsOnTheInternet/PuffedConvo13bLoraE4', 'yihan6324/llama2-7b-instructmining-40k-sharegpt', 'CHIH-HUNG/llama-2-13b-Open_Platypus_and_ccp_2.6w', 'Aeala/GPT4-x-Alpasta-13b', 'psmathur/orca_mini_v2_13b', 'YeungNLP/firefly-llama-13b', 'psmathur/orca_mini_v2_13b', 'zarakiquemparte/zarafusionix-l2-7b', 'yihan6324/llama2-7b-instructmining-60k-sharegpt', 'yihan6324/llama-2-7b-instructmining-60k-sharegpt', 'layoric/llama-2-13b-code-alpaca', 'bofenghuang/vigogne-13b-instruct', 'Lajonbot/vicuna-13b-v1.3-PL-lora_unload', 'lvkaokao/llama2-7b-hf-chat-lora-v3', 'ehartford/dolphin-llama-13b', 'YeungNLP/firefly-llama-13b-v1.2', 'TheBloke/Kimiko-13B-fp16', 'kevinpro/Vicuna-13B-CoT', 'eachadea/vicuna-13b-1.1', 'pillowtalks-ai/delta13b', 'TheBloke/vicuna-13B-1.1-HF', 'TheBloke/Vicuna-13B-CoT-fp16', 'lmsys/vicuna-13b-delta-v1.1', 'lmsys/vicuna-13b-v1.1', 'xxyyy123/20k_v1_lora_qkvo_rank14_v2', 'TheBloke/guanaco-13B-HF', 'TheBloke/vicuna-13b-v1.3.0-GPTQ', 'edor/Stable-Platypus2-mini-7B', 'totally-not-an-llm/EverythingLM-13b-V2-16k', 'zarakiquemparte/zaraxe-l2-7b', 'beaugogh/Llama2-7b-openorca-mc-v2', 'TheBloke/Nous-Hermes-13B-SuperHOT-8K-fp16', 'quantumaikr/QuantumLM', 'jondurbin/airoboros-13b-gpt4-1.2', 'TheBloke/robin-13B-v2-fp16', 'TFLai/llama-2-13b-4bit-alpaca-gpt4', 'yihan6324/llama2-7b-instructmining-orca-40k', 'dvruette/oasst-llama-13b-2-epochs', 'Open-Orca/LlongOrca-7B-16k', 'Aspik101/Nous-Hermes-13b-pl-lora_unload', 'ehartford/Samantha-1.11-CodeLlama-34b', 'nkpz/llama2-22b-chat-wizard-uncensored', 'bofenghuang/vigogne-13b-chat', 'beaugogh/Llama2-7b-openorca-mc-v1', 'OptimalScale/robin-13b-v2-delta', 'pe-nlp/llama-2-13b-vicuna-wizard', 'chargoddard/llama2-22b', 'gywy/llama2-13b-chinese-v1', 'frank098/Wizard-Vicuna-13B-juniper', 'IGeniusDev/llama13B-quant8-testv1-openorca-customdataset', 'CHIH-HUNG/llama-2-13b-huangyt_Fintune_1_17w-gate_up_down_proj', 'eachadea/vicuna-13b', 'yihan6324/llama2-7b-instructmining-orca-90k', 'chargoddard/llama2-22b-blocktriangular', 'luffycodes/mcq-vicuna-13b-v1.5', 'Yhyu13/chimera-inst-chat-13b-hf', 'luffycodes/mcq-vicuna-13b-v1.5', 'chargoddard/ypotryll-22b-epoch2-qlora', 'totally-not-an-llm/EverythingLM-13b-16k', 'luffycodes/mcq-hal-vicuna-13b-v1.5', 'openaccess-ai-collective/minotaur-13b', 'IGeniusDev/llama13B-quant8-testv1-openorca-customdataset', 'chargoddard/llama2-22b-blocktriangular', 'TFLai/Platypus2-13B-QLoRA-0.80-epoch', 'meta-llama/Llama-2-13b-hf', 'CHIH-HUNG/llama-2-13b-huangyt_FINETUNE2_3w-gate_up_down_proj', 'luffycodes/mcq-hal-vicuna-13b-v1.5', 'TheBloke/Llama-2-13B-fp16', 'TaylorAI/Flash-Llama-13B', 'shareAI/bimoGPT-llama2-13b', 'wahaha1987/llama_13b_sharegpt94k_fastchat', 'openchat/openchat_8192', 'CHIH-HUNG/llama-2-13b-huangyt_Fintune_1_17w-q_k_v_o_proj', 'dvruette/llama-13b-pretrained-sft-do2', 'CHIH-HUNG/llama-2-13b-alpaca-test', 'OpenBuddy/openbuddy-llama2-13b-v11.1-bf16', 'CHIH-HUNG/llama-2-13b-FINETUNE2_TEST_2.2w', 'project-baize/baize-v2-13b', 'jondurbin/airoboros-l2-13b-gpt4-m2.0', 'yeontaek/Platypus2xOpenOrca-13B-LoRa-v2', 'CHIH-HUNG/llama-2-13b-huangyt_FINETUNE2_3w', 'xzuyn/Alpacino-SuperCOT-13B', 'jondurbin/airoboros-l2-13b-gpt4-2.0', 'aiplanet/effi-13b', 'clibrain/Llama-2-13b-ft-instruct-es', 'CHIH-HUNG/llama-2-13b-huangyt_Fintune_1_17w', 'bofenghuang/vigogne-2-7b-instruct', 'CHIH-HUNG/llama-2-13b-huangyt_FINETUNE2_3w-q_k_v_o_proj', 'bofenghuang/vigogne-2-7b-chat', 'aiplanet/effi-13b', 'haonan-li/bactrian-x-llama-13b-merged', 'beaugogh/Llama2-7b-sharegpt4', 'HWERI/Llama2-7b-sharegpt4', 'jondurbin/airoboros-13b-gpt4-1.3', 'jondurbin/airoboros-c34b-2.1', 'junelee/wizard-vicuna-13b', 'TheBloke/wizard-vicuna-13B-HF', 'Open-Orca/OpenOrca-Preview1-13B', 'TheBloke/h2ogpt-oasst1-512-30B-HF', 'TheBloke/Llama-2-13B-GPTQ', 'camel-ai/CAMEL-13B-Combined-Data', 'lmsys/vicuna-7b-v1.5', 'lmsys/vicuna-7b-v1.5-16k', 'lmsys/vicuna-7b-v1.5', 'ausboss/llama-13b-supercot', 'TheBloke/tulu-13B-fp16', 'NousResearch/Nous-Hermes-llama-2-7b', 'jlevin/guanaco-13b-llama-2', 'lmsys/vicuna-7b-v1.5-16k', 'dvruette/llama-13b-pretrained', 'nkpz/llama2-22b-daydreamer-v3', 'dvruette/llama-13b-pretrained-dropout', 'jondurbin/airoboros-l2-13b-2.1', 'LLMs/Stable-Vicuna-13B', '64bits/LexPodLM-13B', 'lizhuang144/llama_mirror_13b_v1.0', 'TheBloke/stable-vicuna-13B-HF', 'zarakiquemparte/zaraxls-l2-7b', 'TheBloke/Llama-2-13B-GPTQ', 'Kiddyz/testlm-3', 'migtissera/Synthia-7B', 'zarakiquemparte/zarablend-l2-7b', 'mosaicml/mpt-30b-instruct', 'PocketDoc/Dans-PileOfSets-Mk1-llama-13b-merged', 'vonjack/Qwen-LLaMAfied-HFTok-7B-Chat', 'l3utterfly/llama2-7b-layla', 'Lajonbot/vicuna-7b-v1.5-PL-lora_unload', 'heegyu/LIMA-13b-hf', 'frank098/WizardLM_13B_juniper', 'ashercn97/manatee-7b', 'chavinlo/gpt4-x-alpaca', 'PocketDoc/Dans-PersonalityEngine-13b', 'ehartford/WizardLM-1.0-Uncensored-CodeLlama-34b', 'digitous/Alpacino13b', 'edor/Hermes-Platypus2-mini-7B', 'lvkaokao/llama2-7b-hf-chat-lora-v2', 'Kiddyz/testlm-1-1', 'Kiddyz/testlm', 'Kiddyz/testlm-1', 'Kiddyz/testlm2', 'radm/Philosophy-Platypus2-13b', 'aiplanet/effi-13b', 'Harshvir/Llama-2-7B-physics', 'YeungNLP/firefly-ziya-13b', 'LinkSoul/Chinese-Llama-2-7b', 'PeanutJar/LLaMa-2-PeanutButter_v10-7B', 'OpenBuddy/openbuddy-llama2-13b-v11-bf16', 'StudentLLM/Alpagasus-2-13B-QLoRA-pipeline', 'meta-llama/Llama-2-13b-hf', 'WizardLM/WizardCoder-Python-34B-V1.0', 'dvruette/llama-13b-pretrained-sft-epoch-1', 'camel-ai/CAMEL-13B-Role-Playing-Data', 'ziqingyang/chinese-llama-2-13b', 'rombodawg/LosslessMegaCoder-llama2-7b-mini', 'TheBloke/koala-13B-HF', 'lmsys/vicuna-7b-delta-v1.1', 'eachadea/vicuna-7b-1.1', 'Ejafa/vicuna_7B_vanilla_1.1', 'lvkaokao/llama2-7b-hf-chat-lora', 'OpenBuddy/openbuddy-atom-13b-v9-bf16', 'Norquinal/llama-2-7b-claude-chat-rp', 'Danielbrdz/Barcenas-7b', 'heegyu/WizardVicuna2-13b-hf', 'meta-llama/Llama-2-7b-chat-hf', 'PeanutJar/LLaMa-2-PeanutButter_v14-7B', 'PeanutJar/LLaMa-2-PeanutButter_v4-7B', 'davzoku/cria-llama2-7b-v1.3', 'OpenBuddy/openbuddy-atom-13b-v9-bf16', 'lvkaokao/llama2-7b-hf-instruction-lora', 'Tap-M/Luna-AI-Llama2-Uncensored', 'ehartford/Samantha-1.11-7b', 'WizardLM/WizardCoder-Python-34B-V1.0', 'TheBloke/Manticore-13B-Chat-Pyg-Guanaco-SuperHOT-8K-GPTQ', 'Mikael110/llama-2-7b-guanaco-fp16', 'garage-bAInd/Platypus2-7B', 'PeanutJar/LLaMa-2-PeanutButter_v18_B-7B', 'mosaicml/mpt-30b', 'garage-bAInd/Platypus2-7B', 'huggingface/llama-13b', 'dvruette/oasst-llama-13b-1000-steps', 'jordiclive/gpt4all-alpaca-oa-codealpaca-lora-13b', 'huggyllama/llama-13b', 'Voicelab/trurl-2-7b', 'TFLai/llama-13b-4bit-alpaca', 'gywy/llama2-13b-chinese-v2', 'lmsys/longchat-13b-16k', 'Aspik101/trurl-2-7b-pl-instruct_unload', 'WizardLM/WizardMath-7B-V1.0', 'Norquinal/llama-2-7b-claude-chat', 'TheTravellingEngineer/llama2-7b-chat-hf-dpo', 'HuggingFaceH4/starchat-beta', 'joehuangx/spatial-vicuna-7b-v1.5-LoRA', 'conceptofmind/LLongMA-2-13b-16k', 'tianyil1/denas-llama2', 'lmsys/vicuna-7b-v1.3', 'conceptofmind/LLongMA-2-13b-16k', 'openchat/opencoderplus', 'ajibawa-2023/scarlett-7b', 'dhmeltzer/llama-7b-SFT_eli5_wiki65k_1024_r_64_alpha_16_merged', 'psyche/kollama2-7b-v2', 'heegyu/LIMA2-7b-hf', 'dhmeltzer/llama-7b-SFT-qlora-eli5-wiki_DPO_ds_RM_top_2_1024_r_64_alpha_16', 'abhishek/llama2guanacotest', 'jondurbin/airoboros-l2-7b-2.1', 'llama-anon/instruct-13b', 'FelixChao/vicuna-7B-physics', 'Aspik101/Llama-2-7b-hf-instruct-pl-lora_unload', 'shibing624/chinese-alpaca-plus-13b-hf', 'davzoku/cria-llama2-7b-v1.3_peft', 'quantumaikr/llama-2-7b-hf-guanaco-1k', 'togethercomputer/Llama-2-7B-32K-Instruct', 'sia-ai/llama-2-7b-1-percent-open-orca-1000-steps-v0', 'TheTravellingEngineer/llama2-7b-hf-guanaco', 'Lajonbot/Llama-2-7b-chat-hf-instruct-pl-lora_unload', 'jondurbin/airoboros-l2-7b-gpt4-1.4.1', 'wahaha1987/llama_7b_sharegpt94k_fastchat', 'FelixChao/vicuna-7B-chemical', 'TinyPixel/llama2-7b-oa', 'chaoyi-wu/MedLLaMA_13B', 'edor/Platypus2-mini-7B', 'RoversX/llama-2-7b-hf-small-shards-Samantha-V1-SFT', 'venkycs/llama-v2-7b-32kC-Security', 'psyche/kollama2-7b', 'Fredithefish/Guanaco-7B-Uncensored', 'TheTravellingEngineer/llama2-7b-chat-hf-guanaco', 'ehartford/WizardLM-13B-Uncensored', 'PocketDoc/Dans-CreepingSenseOfDoom', 'wenge-research/yayi-7b-llama2', 'georgesung/llama2_7b_chat_uncensored', 'TinyPixel/llama2-7b-instruct', 'quantumaikr/QuantumLM-7B', 'xzuyn/MedicWizard-7B', 'wenge-research/yayi-7b-llama2', 'TinyPixel/lima-test', 'elyza/ELYZA-japanese-Llama-2-7b-instruct', 'lgaalves/llama-2-7b-hf_open-platypus', 'ziqingyang/chinese-alpaca-2-7b', 'TehVenom/Pygmalion-Vicuna-1.1-7b', 'meta-llama/Llama-2-7b-hf', 'bongchoi/test-llama2-7b', 'TaylorAI/Flash-Llama-7B', 'TheTravellingEngineer/llama2-7b-chat-hf-v2', 'TheTravellingEngineer/llama2-7b-chat-hf-v4', 'kashif/stack-llama-2', 'PeanutJar/LLaMa-2-PeanutButter_v18_A-7B', 'ToolBench/ToolLLaMA-7b-LoRA', 'Monero/WizardLM-13b-OpenAssistant-Uncensored', 'TheTravellingEngineer/llama2-7b-chat-hf-v2', 'TheTravellingEngineer/llama2-7b-chat-hf-v4', 'mrm8488/llama-2-coder-7b', 'elyza/ELYZA-japanese-Llama-2-7b-fast-instruct', 'clibrain/Llama-2-7b-ft-instruct-es', 'medalpaca/medalpaca-7b', 'TheBloke/tulu-7B-fp16', 'OpenBuddy/openbuddy-openllama-13b-v7-fp16', 'TaylorAI/FLAN-Llama-7B-2_Llama2-7B-Flash_868_full_model', 'Aspik101/vicuna-7b-v1.3-instruct-pl-lora_unload', 'jondurbin/airoboros-l2-7b-gpt4-2.0', 'dhmeltzer/llama-7b-SFT_ds_eli5_1024_r_64_alpha_16_merged', 'GOAT-AI/GOAT-7B-Community', 'AtomEchoAI/AtomGPT_56k', 'julianweng/Llama-2-7b-chat-orcah', 'TehVenom/Pygmalion-13b-Merged', 'jondurbin/airoboros-7b-gpt4-1.1', 'dhmeltzer/llama-7b-SFT_ds_wiki65k_1024_r_64_alpha_16_merged', 'bofenghuang/vigogne-7b-chat', 'lmsys/longchat-7b-v1.5-32k', 'jondurbin/airoboros-l2-7b-gpt4-m2.0', 'synapsoft/Llama-2-7b-chat-hf-flan2022-1.2M', 'jondurbin/airoboros-7b-gpt4-1.4', 'Charlie911/vicuna-7b-v1.5-lora-mctaco', 'yihan6324/instructmining-platypus-15k', 'meta-llama/Llama-2-7b-hf', 'TheTravellingEngineer/llama2-7b-chat-hf-v3', 'quantumaikr/KoreanLM-hf', 'openthaigpt/openthaigpt-1.0.0-alpha-7b-chat-ckpt-hf', 'TheBloke/Llama-2-7B-GPTQ', 'TheBloke/Llama-2-7B-GPTQ', 'LLMs/AlpacaGPT4-7B-elina', 'ehartford/Wizard-Vicuna-7B-Uncensored', 'TheBloke/Wizard-Vicuna-7B-Uncensored-HF', 'TheTravellingEngineer/llama2-7b-chat-hf-v3', 'golaxy/gowizardlm', 'ehartford/dolphin-llama2-7b', 'CHIH-HUNG/llama-2-7b-dolphin_10w-test', 'mncai/chatdoctor', 'psyche/kollama2-7b-v3', 'jondurbin/airoboros-7b-gpt4', 'jondurbin/airoboros-7b', 'TheBloke/airoboros-7b-gpt4-fp16', 'mosaicml/mpt-7b-8k-chat', 'elyza/ELYZA-japanese-Llama-2-7b', 'bofenghuang/vigogne-7b-instruct', 'jxhong/CAlign-alpaca-7b', 'golaxy/goims', 'jondurbin/airoboros-7b-gpt4-1.2', 'jphme/orca_mini_v2_ger_7b', 'psmathur/orca_mini_v2_7b', 'notstoic/PygmalionCoT-7b', 'golaxy/gogpt2-13b', 'golaxy/gogpt2-13b-chat', 'togethercomputer/LLaMA-2-7B-32K', 'TheBloke/wizardLM-7B-HF', 'keyfan/vicuna-chinese-replication-v1.1', 'golaxy/gogpt2-7b', 'aiplanet/effi-7b', 'arver/llama7b-qlora', 'titan087/OpenLlama13B-Guanaco', 'chavinlo/alpaca-native', 'project-baize/baize-healthcare-lora-7B', 'AlpinDale/pygmalion-instruct', 'openlm-research/open_llama_13b', 'jondurbin/airoboros-7b-gpt4-1.3', 'elyza/ELYZA-japanese-Llama-2-7b-fast', 'jondurbin/airoboros-gpt-3.5-turbo-100k-7b', 'uukuguy/speechless-codellama-orca-13b', 'bigcode/starcoderplus', 'TheBloke/guanaco-7B-HF', 'Neko-Institute-of-Science/metharme-7b', 'TigerResearch/tigerbot-7b-base', 'golaxy/gogpt-7b', 'togethercomputer/LLaMA-2-7B-32K', 'yhyhy3/open_llama_7b_v2_med_instruct', 'ajibawa-2023/carl-7b', 'stabilityai/stablelm-base-alpha-7b-v2', 'conceptofmind/LLongMA-2-7b-16k', 'TehVenom/Pygmalion_AlpacaLora-7b', 'jondurbin/airoboros-7b-gpt4-1.4.1-qlora', 'wannaphong/openthaigpt-0.1.0-beta-full-model_for_open_llm_leaderboard', 'ausboss/llama7b-wizardlm-unfiltered', 'project-baize/baize-v2-7b', 'LMFlow/Robin-v2', 'HanningZhang/Robin-v2', 'LMFlow/Robin-7b-v2', 'OptimalScale/robin-7b-v2-delta', 'uukuguy/speechless-codellama-platypus-13b', 'jerryjalapeno/nart-100k-7b', 'wenge-research/yayi-13b-llama2', 'fireballoon/baichuan-vicuna-chinese-7b', 'jlevin/guanaco-unchained-llama-2-7b', 'csitfun/llama-7b-logicot', 'DevaMalla/llama7b_alpaca_1gpu_bf16', 'WeOpenML/PandaLM-Alpaca-7B-v1', 'illuin/test-custom-llama', 'yeontaek/WizardCoder-Python-13B-LoRa', 'ashercn97/giraffe-7b', 'mosaicml/mpt-7b-chat', 'abhishek/autotrain-llama-alpaca-peft-52508123785', 'Neko-Institute-of-Science/pygmalion-7b', 'TFLai/llama-7b-4bit-alpaca', 'huggingface/llama-7b', 'TheBloke/Planner-7B-fp16', 'shibing624/chinese-llama-plus-13b-hf', 'AGI-inc/lora_moe_7b_baseline', 'DevaMalla/llama-base-7b', 'AGI-inc/lora_moe_7b', 'togethercomputer/GPT-JT-6B-v0', 'ehartford/WizardLM-7B-Uncensored', 'shibing624/chinese-alpaca-plus-7b-hf', 'beomi/llama-2-ko-7b', 'mosaicml/mpt-7b-8k-instruct', 'Enno-Ai/ennodata-7b', 'mosaicml/mpt-7b-instruct', 'facebook/opt-iml-max-30b', 'WeOpenML/Alpaca-7B-v1', 'TheBloke/Project-Baize-v2-7B-GPTQ', 'codellama/CodeLlama-13b-Instruct-hf', 'TheBloke/CodeLlama-13B-Instruct-fp16', 'facebook/galactica-30b', 'FreedomIntelligence/phoenix-inst-chat-7b', 'openlm-research/open_llama_7b_v2', 'GeorgiaTechResearchInstitute/galpaca-30b', 'THUDM/chatglm2-6b', 'togethercomputer/GPT-JT-6B-v1', 'TheBloke/koala-7B-HF', 'nathan0/mpt_delta_tuned_model_v3', 'nathan0/mpt_delta_tuned_model_v2', 'GeorgiaTechResearchInstitute/galpaca-30b', 'JosephusCheung/Guanaco', 'shareAI/CodeLLaMA-chat-13b-Chinese', 'TigerResearch/tigerbot-7b-sft', 'Writer/InstructPalmyra-20b', 'OpenAssistant/codellama-13b-oasst-sft-v10', 'bigscience/bloomz-7b1-mt', 'nathan0/mpt_delta_tuned_model_v3', 'VMware/open-llama-7b-open-instruct', 'baichuan-inc/Baichuan-7B', 'anas-awadalla/mpt-7b', 'mosaicml/mpt-7b', 'bigscience/bloomz-7b1', 'ziqingyang/chinese-llama-2-7b', 'OpenAssistant/codellama-13b-oasst-sft-v10', 'wenge-research/yayi-7b', 'tiiuae/falcon-7b', 'togethercomputer/RedPajama-INCITE-Instruct-7B-v0.1', 'togethercomputer/RedPajama-INCITE-7B-Instruct', 'TheBloke/landmark-attention-llama7b-fp16', 'togethercomputer/GPT-JT-Moderation-6B', 'h2oai/h2ogpt-gm-oasst1-en-1024-20b', 'dvruette/gpt-neox-20b-full-precision', 'TehVenom/Moderator-Chan_GPT-JT-6b', 'dvruette/oasst-gpt-neox-20b-1000-steps', 'AlekseyKorshuk/pygmalion-6b-vicuna-chatml', 'facebook/opt-66b', 'Salesforce/codegen-16B-nl', 'Vmware/open-llama-7b-v2-open-instruct', 'mosaicml/mpt-7b-storywriter', 'acrastt/Marx-3B-V2', 'openlm-research/open_llama_7b', 'Fredithefish/ReasonixPajama-3B-HF', 'togethercomputer/GPT-NeoXT-Chat-Base-20B', 'psmathur/orca_mini_13b', 'RWKV/rwkv-raven-14b', 'h2oai/h2ogpt-oasst1-512-20b', 'acrastt/Marx-3B', 'klosax/open_llama_13b_600bt_preview', 'synapsoft/Llama-2-7b-hf-flan2022-1.2M', 'OpenAssistant/oasst-sft-1-pythia-12b', 'golaxy/gogpt-7b-bloom', 'Writer/palmyra-large', 'psmathur/orca_mini_7b', 'dvruette/oasst-pythia-12b-6000-steps', 'NousResearch/CodeLlama-13b-hf', 'codellama/CodeLlama-13b-hf', 'h2oai/h2ogpt-gm-oasst1-multilang-1024-20b', 'VMware/open-llama-0.7T-7B-open-instruct-v1.1', 'dvruette/oasst-pythia-12b-flash-attn-5000-steps', 'dvruette/oasst-gpt-neox-20b-3000-steps', 'RobbeD/OpenLlama-Platypus-3B', 'facebook/opt-30b', 'acrastt/Puma-3B', 'OpenAssistant/oasst-sft-4-pythia-12b-epoch-3.5', 'dvruette/oasst-pythia-12b-pretrained-sft', 'digitous/GPT-R', 'acrastt/Griffin-3B', 'togethercomputer/RedPajama-INCITE-Base-7B-v0.1', 'togethercomputer/RedPajama-INCITE-7B-Base', 'CobraMamba/mamba-gpt-3b-v3', 'Danielbrdz/CodeBarcenas-7b', 'l3utterfly/open-llama-3b-v2-layla', 'CobraMamba/mamba-gpt-3b-v2', 'OpenAssistant/pythia-12b-sft-v8-7k-steps', 'KoboldAI/GPT-NeoX-20B-Erebus', 'RobbeD/Orca-Platypus-3B', 'h2oai/h2ogpt-gm-oasst1-en-1024-12b', 'OpenAssistant/pythia-12b-sft-v8-2.5k-steps', 'AlekseyKorshuk/chatml-pyg-v1', 'togethercomputer/RedPajama-INCITE-Chat-7B-v0.1', 'togethercomputer/RedPajama-INCITE-7B-Chat', 'digitous/Javelin-R', 'dvruette/oasst-pythia-12b-reference', 'EleutherAI/gpt-neox-20b', 'KoboldAI/fairseq-dense-13B', 'OpenAssistant/pythia-12b-sft-v8-rlhf-2k-steps', 'codellama/CodeLlama-7b-Instruct-hf', 'digitous/Javelin-GPTJ', 'KoboldAI/GPT-NeoX-20B-Skein', 'digitous/Javalion-R', 'h2oai/h2ogpt-oasst1-512-12b', 'acrastt/Bean-3B', 'KoboldAI/GPT-J-6B-Skein', 'nomic-ai/gpt4all-j', 'databricks/dolly-v2-12b', 'TehVenom/Dolly_Shygmalion-6b-Dev_V8P2', 'databricks/dolly-v2-7b', 'Aspik101/WizardVicuna-Uncensored-3B-instruct-PL-lora_unload', 'digitous/Adventien-GPTJ', 'openlm-research/open_llama_3b_v2', 'RWKV/rwkv-4-14b-pile', 'Lazycuber/Janemalion-6B', 'OpenAssistant/pythia-12b-pre-v8-12.5k-steps', 'digitous/Janin-R', 'kfkas/Llama-2-ko-7b-Chat', 'heegyu/WizardVicuna-Uncensored-3B-0719', 'h2oai/h2ogpt-gm-oasst1-en-1024-open-llama-7b-preview-400bt', 'TaylorAI/Flash-Llama-3B', 'kfkas/Llama-2-ko-7b-Chat', 'digitous/Skegma-GPTJ', 'digitous/Javalion-GPTJ', 'Pirr/pythia-13b-deduped-green_devil', 'TehVenom/PPO_Shygmalion-V8p4_Dev-6b', 'dvruette/oasst-pythia-6.9b-4000-steps', 'heegyu/WizardVicuna-3B-0719', 'psmathur/orca_mini_3b', 'OpenAssistant/galactica-6.7b-finetuned', 'frank098/orca_mini_3b_juniper', 'PygmalionAI/pygmalion-6b', 'TehVenom/PPO_Pygway-V8p4_Dev-6b', 'TFLai/gpt-neox-20b-4bit-alpaca', 'Corianas/gpt-j-6B-Dolly', 'TehVenom/Dolly_Shygmalion-6b', 'digitous/Janin-GPTJ', 'TehVenom/GPT-J-Pyg_PPO-6B-Dev-V8p4', 'EleutherAI/gpt-j-6b', 'KoboldAI/GPT-J-6B-Shinen', 'TehVenom/Dolly_Malion-6b', 'TehVenom/ChanMalion', 'Salesforce/codegen-6B-nl', 'Fredithefish/RedPajama-INCITE-Chat-3B-Instruction-Tuning-with-GPT-4', 'KoboldAI/GPT-J-6B-Janeway', 'togethercomputer/RedPajama-INCITE-Chat-3B-v1', 'togethercomputer/Pythia-Chat-Base-7B', 'heegyu/RedTulu-Uncensored-3B-0719', 'KoboldAI/PPO_Pygway-6b-Mix', 'KoboldAI/OPT-13B-Erebus', 'KoboldAI/fairseq-dense-6.7B', 'EleutherAI/pythia-12b-deduped', 'pszemraj/pythia-6.9b-HC3', 'Fredithefish/Guanaco-3B-Uncensored-v2', 'facebook/opt-13b', 'TehVenom/GPT-J-Pyg_PPO-6B', 'EleutherAI/pythia-6.9b-deduped', 'Devio/test-1400', 'Fredithefish/Guanaco-3B-Uncensored', 'codellama/CodeLlama-7b-hf', 'acrastt/RedPajama-INCITE-Chat-Instruct-3B-V1', 'Fredithefish/ScarletPajama-3B-HF', 'KoboldAI/OPT-13B-Nerybus-Mix', 'YeungNLP/firefly-bloom-7b1', 'DanielSc4/RedPajama-INCITE-Chat-3B-v1-RL-LoRA-8bit-test1', 'klosax/open_llama_7b_400bt_preview', 'KoboldAI/OPT-13B-Nerys-v2', 'TehVenom/PPO_Shygmalion-6b', 'amazon/LightGPT', 'KnutJaegersberg/black_goo_recipe_c', 'NousResearch/CodeLlama-7b-hf', 'togethercomputer/RedPajama-INCITE-Instruct-3B-v1', 'heegyu/WizardVicuna-open-llama-3b-v2', 'bigscience/bloom-7b1', 'Devio/test-22B', 'RWKV/rwkv-raven-7b', 'hakurei/instruct-12b', 'CobraMamba/mamba-gpt-3b', 'KnutJaegersberg/black_goo_recipe_a', 'acrastt/OmegLLaMA-3B', 'codellama/CodeLlama-7b-Instruct-hf', 'h2oai/h2ogpt-oig-oasst1-512-6_9b', 'KoboldAI/OPT-6.7B-Erebus', 'facebook/opt-6.7b', 'KnutJaegersberg/black_goo_recipe_d', 'KnutJaegersberg/LLongMA-3b-LIMA', 'KnutJaegersberg/black_goo_recipe_b', 'KoboldAI/OPT-6.7B-Nerybus-Mix', 'health360/Healix-3B', 'EleutherAI/pythia-12b', 'Fredithefish/RedPajama-INCITE-Chat-3B-ShareGPT-11K', 'GeorgiaTechResearchInstitute/galactica-6.7b-evol-instruct-70k', 'h2oai/h2ogpt-oig-oasst1-256-6_9b', 'ikala/bloom-zh-3b-chat', 'Taekyoon/llama2-ko-7b-test', 'anhnv125/pygmalion-6b-roleplay', 'TehVenom/DiffMerge_Pygmalion_Main-onto-V8P4', 'KoboldAI/OPT-6B-nerys-v2', 'Lazycuber/pyg-instruct-wizardlm', 'Devio/testC', 'KoboldAI/OPT-30B-Erebus', 'Fredithefish/CrimsonPajama', 'togethercomputer/RedPajama-INCITE-Base-3B-v1', 'bigscience/bloomz-3b', 'conceptofmind/Open-LLongMA-3b', 'RWKV/rwkv-4-7b-pile', 'openlm-research/open_llama_3b', 'ewof/koishi-instruct-3b', 'DanielSc4/RedPajama-INCITE-Chat-3B-v1-FT-LoRA-8bit-test1', 'cerebras/Cerebras-GPT-13B', 'EleutherAI/pythia-6.7b', 'aisquared/chopt-2_7b', 'Azure99/blossom-v1-3b', 'PSanni/Deer-3b', 'bertin-project/bertin-gpt-j-6B-alpaca', 'OpenBuddy/openbuddy-openllama-3b-v10-bf16', 'KoboldAI/fairseq-dense-2.7B', 'ehartford/CodeLlama-34b-Instruct-hf', 'codellama/CodeLlama-34b-Instruct-hf', 'TheBloke/CodeLlama-34B-Instruct-fp16', 'h2oai/h2ogpt-gm-oasst1-en-2048-open-llama-7b-preview-300bt-v2', 'openlm-research/open_llama_7b_700bt_preview', 'NbAiLab/nb-gpt-j-6B-alpaca', 'KoboldAI/OPT-2.7B-Erebus', 'Writer/camel-5b-hf', 'EleutherAI/pythia-2.7b', 'facebook/xglm-7.5B', 'EleutherAI/pythia-2.8b-deduped', 'klosax/open_llama_3b_350bt_preview', 'klosax/openllama-3b-350bt', 'KoboldAI/OPT-2.7B-Nerybus-Mix', 'KoboldAI/GPT-J-6B-Adventure', 'cerebras/Cerebras-GPT-6.7B', 'TFLai/pythia-2.8b-4bit-alpaca', 'facebook/opt-2.7b', 'KoboldAI/OPT-2.7B-Nerys-v2', 'bigscience/bloom-3b', 'Devio/test100', 'RWKV/rwkv-raven-3b', 'Azure99/blossom-v2-3b', 'codellama/CodeLlama-34b-Python-hf', 'bhenrym14/airoboros-33b-gpt4-1.4.1-PI-8192-fp16', 'EleutherAI/gpt-neo-2.7B', 'danielhanchen/open_llama_3b_600bt_preview', 'HuggingFaceH4/starchat-alpha', 'pythainlp/wangchanglm-7.5B-sft-en-sharded', 'beaugogh/pythia-1.4b-deduped-sharegpt', 'HWERI/pythia-1.4b-deduped-sharegpt', 'OpenAssistant/stablelm-7b-sft-v7-epoch-3', 'codellama/CodeLlama-7b-Python-hf', 'aisquared/chopt-1_3b', 'PygmalionAI/metharme-1.3b', 'Linly-AI/Chinese-LLaMA-2-13B-hf', 'chargoddard/llama-2-34b-uncode', 'RWKV/rwkv-4-3b-pile', 'pythainlp/wangchanglm-7.5B-sft-enth', 'MBZUAI/LaMini-GPT-1.5B', 'Writer/palmyra-base', 'KoboldAI/fairseq-dense-1.3B', 'EleutherAI/pythia-1.4b-deduped', 'MBZUAI/lamini-neo-1.3b', 'h2oai/h2ogpt-gm-oasst1-en-2048-open-llama-7b-preview-300bt', 'sartmis1/starcoder-finetune-openapi', 'MayaPH/opt-flan-iml-6.7b', 'facebook/xglm-4.5B', 'WizardLM/WizardCoder-15B-V1.0', 'facebook/opt-iml-max-1.3b', 'stabilityai/stablelm-tuned-alpha-7b', 'aisquared/dlite-v2-1_5b', 'stabilityai/stablelm-base-alpha-7b', 'sartmis1/starcoder-finetune-selfinstruct', 'lizhuang144/starcoder_mirror', 'bigcode/starcoder', 'TheBloke/CodeLlama-34B-Python-fp16', 'open-llm-leaderboard/bloomz-1b7-4bit-alpaca-auto-eval-adapter-applied', 'ehartford/CodeLlama-34b-Python-hf', 'codellama/CodeLlama-7b-Python-hf', 'GeorgiaTechResearchInstitute/starcoder-gpteacher-code-instruct', 'LoupGarou/WizardCoder-Guanaco-15B-V1.0', 'golaxy/gogpt-3b-bloom', 'EleutherAI/pythia-1.3b', 'codellama/CodeLlama-13b-Python-hf', 'hakurei/lotus-12B', 'NYTK/PULI-GPTrio', 'facebook/opt-1.3b', 'TheBloke/CodeLlama-13B-Python-fp16', 'codellama/CodeLlama-13b-Python-hf', 'RWKV/rwkv-raven-1b5', 'PygmalionAI/pygmalion-2.7b', 'bigscience/bloom-1b7', 'gpt2-xl', 'LoupGarou/WizardCoder-Guanaco-15B-V1.1', 'RWKV/rwkv-4-1b5-pile', 'codellama/CodeLlama-34b-hf', 'NousResearch/CodeLlama-34b-hf', 'rinna/bilingual-gpt-neox-4b-8k', 'lxe/Cerebras-GPT-2.7B-Alpaca-SP', 'cerebras/Cerebras-GPT-2.7B', 'jzjiao/opt-1.3b-rlhf', 'EleutherAI/gpt-neo-1.3B', 'aisquared/dlite-v1-1_5b', 'Corianas/Quokka_2.7b', 'MrNJK/gpt2-xl-sft', 'facebook/galactica-1.3b', 'aisquared/dlite-v2-774m', 'EleutherAI/pythia-1b-deduped', 'Kunhao/pile-7b-250b-tokens', 'w601sxs/b1ade-1b', 'rinna/bilingual-gpt-neox-4b', 'shaohang/SparseOPT-1.3B', 'shaohang/Sparse0.5_OPT-1.3', 'EleutherAI/polyglot-ko-12.8b', 'Salesforce/codegen-6B-multi', 'bigscience/bloom-1b1', 'TFLai/gpt-neo-1.3B-4bit-alpaca', 'FabbriSimo01/Bloom_1b_Quantized', 'MBZUAI/LaMini-GPT-774M', 'Locutusque/gpt2-large-conversational', 'Devio/test-3b', 'stabilityai/stablelm-tuned-alpha-3b', 'PygmalionAI/pygmalion-1.3b', 'KoboldAI/fairseq-dense-355M', 'Rachneet/gpt2-xl-alpaca', 'gpt2-large', 'Mikivis/gpt2-large-lora-sft', 'stabilityai/stablelm-base-alpha-3b', 'gpt2-medium', 'Kunhao/pile-7b', 'aisquared/dlite-v1-774m', 'aisquared/dlite-v2-355m', 'YeungNLP/firefly-bloom-2b6-v2', 'KnutJaegersberg/gpt-2-xl-EvolInstruct', 'KnutJaegersberg/galactica-orca-wizardlm-1.3b', 'cerebras/Cerebras-GPT-1.3B', 'FabbriSimo01/Cerebras_1.3b_Quantized', 'facebook/xglm-1.7B', 'EleutherAI/pythia-410m-deduped', 'TheBloke/GPlatty-30B-SuperHOT-8K-fp16', 'DataLinguistic/DataLinguistic-34B-V1.0', 'Corianas/Quokka_1.3b', 'TheTravellingEngineer/bloom-560m-RLHF-v2', 'Corianas/1.3b', 'RWKV/rwkv-4-430m-pile', 'porkorbeef/Llama-2-13b-sf', 'xhyi/PT_GPTNEO350_ATG', 'TheBloke/Wizard-Vicuna-13B-Uncensored-GPTQ', 'bigscience/bloomz-560m', 'TheBloke/medalpaca-13B-GPTQ-4bit', 'TheBloke/Vicuna-33B-1-3-SuperHOT-8K-fp16', 'aisquared/dlite-v1-355m', 'uukuguy/speechless-codellama-orca-airoboros-13b-0.10e', 'yhyhy3/med-orca-instruct-33b', 'TheBloke/Wizard-Vicuna-30B-Superhot-8K-fp16', 'TheTravellingEngineer/bloom-1b1-RLHF', 'MBZUAI/lamini-cerebras-1.3b', 'IDEA-CCNL/Ziya-LLaMA-13B-Pretrain-v1', 'TheBloke/WizardLM-7B-uncensored-GPTQ', 'TheBloke/EverythingLM-13B-16K-GPTQ', 'quantumaikr/open_llama_7b_hf', 'TheBloke/chronos-wizardlm-uc-scot-st-13B-GPTQ', 'TheBloke/WizardLM-30B-Uncensored-GPTQ', 'IDEA-CCNL/Ziya-LLaMA-13B-v1', 'Phind/Phind-CodeLlama-34B-v1', 'robowaifudev/megatron-gpt2-345m', 'MayaPH/GodziLLa-30B-instruct', 'TheBloke/CAMEL-33B-Combined-Data-SuperHOT-8K-fp16', 'uukuguy/speechless-codellama-orca-platypus-13b-0.10e', 'doas/test2', 'BreadAi/PM_modelV2', 'bigcode/santacoder', 'TheBloke/wizard-vicuna-13B-GPTQ', 'porkorbeef/Llama-2-13b', 'TehVenom/DiffMerge-DollyGPT-Pygmalion', 'PygmalionAI/pygmalion-350m', 'TheBloke/orca_mini_v3_7B-GPTQ', 'TheBloke/WizardLM-Uncensored-SuperCOT-StoryTelling-30B-GPTQ', 'TheBloke/WizardLM-30B-GPTQ', 'bigscience/bloom-560m', 'TFLai/gpt2-turkish-uncased', 'TheBloke/guanaco-33B-GPTQ', 'TheBloke/openchat_v2_openorca_preview-GPTQ', 'porkorbeef/Llama-2-13b-public', 'TheBloke/LongChat-13B-GPTQ', 'yhyhy3/med-orca-instruct-33b', 'TheBloke/airoboros-33B-gpt4-1-4-SuperHOT-8K-fp16', 'TheBloke/Chinese-Alpaca-33B-SuperHOT-8K-fp16', 'MayaPH/FinOPT-Franklin', 'TheBloke/WizardLM-33B-V1.0-Uncensored-GPTQ', 'TheBloke/Project-Baize-v2-13B-GPTQ', 'malhajar/Platypus2-70B-instruct-4bit-gptq', 'KoboldAI/OPT-350M-Erebus', 'rishiraj/bloom-560m-guanaco', 'Panchovix/WizardLM-33B-V1.0-Uncensored-SuperHOT-8k', 'doas/test5', 'vicgalle/alpaca-7b', 'beomi/KoAlpaca-Polyglot-5.8B', 'Phind/Phind-CodeLlama-34B-Python-v1', 'timdettmers/guanaco-65b-merged', 'TheBloke/wizard-mega-13B-GPTQ', 'MayaPH/GodziLLa-30B-plus', 'TheBloke/Platypus-30B-SuperHOT-8K-fp16', 'facebook/opt-350m', 'KoboldAI/OPT-350M-Nerys-v2', 'TheBloke/robin-33B-v2-GPTQ', 'jaspercatapang/Echidna-30B', 'TheBloke/llama-30b-supercot-SuperHOT-8K-fp16', 'marcchew/test1', 'Harshvir/LaMini-Neo-1.3B-Mental-Health_lora', 'golaxy/gogpt-560m', 'TheBloke/orca_mini_13B-GPTQ', 'Panchovix/airoboros-33b-gpt4-1.2-SuperHOT-8k', 'Aspik101/tulu-7b-instruct-pl-lora_unload', 'Phind/Phind-CodeLlama-34B-v2', 'BreadAi/MusePy-1-2', 'cerebras/Cerebras-GPT-590M', 'microsoft/CodeGPT-small-py', 'victor123/WizardLM-13B-1.0', 'OptimalScale/robin-65b-v2-delta', 'voidful/changpt-bart', 'FabbriSimo01/GPT_Large_Quantized', 'MayaPH/FinOPT-Lincoln', 'KoboldAI/fairseq-dense-125M', 'SebastianSchramm/Cerebras-GPT-111M-instruction', 'TheTravellingEngineer/bloom-560m-RLHF', 'breadlicker45/dough-instruct-base-001', 'WizardLM/WizardLM-30B-V1.0', 'WizardLM/WizardLM-30B-V1.0', 'WizardLM/WizardLM-30B-V1.0', 'TaylorAI/Flash-Llama-30M-20001', 'porkorbeef/Llama-2-13b-12_153950', 'huggingtweets/bladeecity-jerma985', 'KnutJaegersberg/megatron-GPT-2-345m-EvolInstruct', 'bhenrym14/airoboros-33b-gpt4-1.4.1-lxctx-PI-16384-fp16', 'microsoft/DialoGPT-small', 'Corianas/590m', 'facebook/xglm-564M', 'EleutherAI/gpt-neo-125m', 'EleutherAI/pythia-160m-deduped', 'klosax/pythia-160m-deduped-step92k-193bt', 'MBZUAI/lamini-neo-125m', 'bigcode/tiny_starcoder_py', 'concedo/OPT-19M-ChatSalad', 'anton-l/gpt-j-tiny-random', 'grantprice/Cerebras-GPT-590M-finetuned-DND', 'deepnight-research/zsc-text', 'WangZeJun/bloom-820m-chat', 'cerebras/Cerebras-GPT-256M', 'ai-forever/rugpt3large_based_on_gpt2', 'alibidaran/medical_transcription_generator', 'Deci/DeciCoder-1b', 'microsoft/DialoGPT-medium', 'ogimgio/gpt-neo-125m-neurallinguisticpioneers', 'open-llm-leaderboard/bloom-560m-4bit-alpaca-auto-eval-adapter-applied', 'BreadAi/gpt-YA-1-1_160M', 'microsoft/DialoGPT-large', 'facebook/opt-125m', 'huggingtweets/jerma985', 'Locutusque/gpt2-conversational-or-qa', 'concedo/Pythia-70M-ChatSalad', 'roneneldan/TinyStories-1M', 'BreadAi/DiscordPy', 'bigcode/gpt_bigcode-santacoder', 'Tincando/fiction_story_generator', 'klosax/pythia-70m-deduped-step44k-92bt', 'Quake24/easyTermsSummerizer', 'BreadAi/gpt-YA-1-1_70M', 'EleutherAI/pythia-160m', 'euclaise/gpt-neox-122m-minipile-digits', 'MBZUAI/lamini-cerebras-590m', 'nicholasKluge/Aira-124M', 'MayaPH/FinOPT-Washington', 'cyberagent/open-calm-large', 'BreadAi/StoryPy', 'EleutherAI/pythia-70m', 'BreadAi/gpt-Youtube', 'roneneldan/TinyStories-33M', 'EleutherAI/pythia-70m-deduped', 'lgaalves/gpt2_guanaco-dolly-platypus', 'Corianas/Quokka_590m', 'lgaalves/gpt2_platypus-dolly-guanaco', 'cyberagent/open-calm-7b', 'RWKV/rwkv-4-169m-pile', 'gpt2', 'roneneldan/TinyStories-28M', 'lgaalves/gpt2_open-platypus', 'gpt2', 'SaylorTwift/gpt2_test', 'roneneldan/TinyStories-3M', 'nthngdy/pythia-owt2-70m-50k', 'Corianas/256_5epoch', 'roneneldan/TinyStories-8M', 'lgaalves/gpt2-dolly', 'nthngdy/pythia-owt2-70m-100k', 'aisquared/dlite-v2-124m', 'mncai/SGPT-1.3B-insurance-epoch10', 'huggingtweets/gladosystem', 'abhiramtirumala/DialoGPT-sarcastic-medium', 'MBZUAI/lamini-cerebras-256m', 'cerebras/Cerebras-GPT-111M', 'uberkie/metharme-1.3b-finetuned', 'MBZUAI/lamini-cerebras-111m', 'psyche/kogpt', 'Corianas/Quokka_256m', 'vicgalle/gpt2-alpaca-gpt4', 'aisquared/dlite-v1-124m', 'Mikivis/xuanxuan', 'MBZUAI/LaMini-GPT-124M', 'vicgalle/gpt2-alpaca', 'huashiyiqike/testmodel', 'Corianas/111m', 'baseline']
diff --git a/spaces/DragGan/DragGan/dnnlib/util.py b/spaces/DragGan/DragGan/dnnlib/util.py
deleted file mode 100644
index 6bbdf3bd8fe1c138cd969d37dcc52190b45c4c16..0000000000000000000000000000000000000000
--- a/spaces/DragGan/DragGan/dnnlib/util.py
+++ /dev/null
@@ -1,491 +0,0 @@
-# Copyright (c) 2021, NVIDIA CORPORATION & AFFILIATES. All rights reserved.
-#
-# NVIDIA CORPORATION and its licensors retain all intellectual property
-# and proprietary rights in and to this software, related documentation
-# and any modifications thereto. Any use, reproduction, disclosure or
-# distribution of this software and related documentation without an express
-# license agreement from NVIDIA CORPORATION is strictly prohibited.
-
-"""Miscellaneous utility classes and functions."""
-
-import ctypes
-import fnmatch
-import importlib
-import inspect
-import numpy as np
-import os
-import shutil
-import sys
-import types
-import io
-import pickle
-import re
-import requests
-import html
-import hashlib
-import glob
-import tempfile
-import urllib
-import urllib.request
-import uuid
-
-from distutils.util import strtobool
-from typing import Any, List, Tuple, Union
-
-
-# Util classes
-# ------------------------------------------------------------------------------------------
-
-
-class EasyDict(dict):
- """Convenience class that behaves like a dict but allows access with the attribute syntax."""
-
- def __getattr__(self, name: str) -> Any:
- try:
- return self[name]
- except KeyError:
- raise AttributeError(name)
-
- def __setattr__(self, name: str, value: Any) -> None:
- self[name] = value
-
- def __delattr__(self, name: str) -> None:
- del self[name]
-
-
-class Logger(object):
- """Redirect stderr to stdout, optionally print stdout to a file, and optionally force flushing on both stdout and the file."""
-
- def __init__(self, file_name: str = None, file_mode: str = "w", should_flush: bool = True):
- self.file = None
-
- if file_name is not None:
- self.file = open(file_name, file_mode)
-
- self.should_flush = should_flush
- self.stdout = sys.stdout
- self.stderr = sys.stderr
-
- sys.stdout = self
- sys.stderr = self
-
- def __enter__(self) -> "Logger":
- return self
-
- def __exit__(self, exc_type: Any, exc_value: Any, traceback: Any) -> None:
- self.close()
-
- def write(self, text: Union[str, bytes]) -> None:
- """Write text to stdout (and a file) and optionally flush."""
- if isinstance(text, bytes):
- text = text.decode()
- if len(text) == 0: # workaround for a bug in VSCode debugger: sys.stdout.write(''); sys.stdout.flush() => crash
- return
-
- if self.file is not None:
- self.file.write(text)
-
- self.stdout.write(text)
-
- if self.should_flush:
- self.flush()
-
- def flush(self) -> None:
- """Flush written text to both stdout and a file, if open."""
- if self.file is not None:
- self.file.flush()
-
- self.stdout.flush()
-
- def close(self) -> None:
- """Flush, close possible files, and remove stdout/stderr mirroring."""
- self.flush()
-
- # if using multiple loggers, prevent closing in wrong order
- if sys.stdout is self:
- sys.stdout = self.stdout
- if sys.stderr is self:
- sys.stderr = self.stderr
-
- if self.file is not None:
- self.file.close()
- self.file = None
-
-
-# Cache directories
-# ------------------------------------------------------------------------------------------
-
-_dnnlib_cache_dir = None
-
-def set_cache_dir(path: str) -> None:
- global _dnnlib_cache_dir
- _dnnlib_cache_dir = path
-
-def make_cache_dir_path(*paths: str) -> str:
- if _dnnlib_cache_dir is not None:
- return os.path.join(_dnnlib_cache_dir, *paths)
- if 'DNNLIB_CACHE_DIR' in os.environ:
- return os.path.join(os.environ['DNNLIB_CACHE_DIR'], *paths)
- if 'HOME' in os.environ:
- return os.path.join(os.environ['HOME'], '.cache', 'dnnlib', *paths)
- if 'USERPROFILE' in os.environ:
- return os.path.join(os.environ['USERPROFILE'], '.cache', 'dnnlib', *paths)
- return os.path.join(tempfile.gettempdir(), '.cache', 'dnnlib', *paths)
-
-# Small util functions
-# ------------------------------------------------------------------------------------------
-
-
-def format_time(seconds: Union[int, float]) -> str:
- """Convert the seconds to human readable string with days, hours, minutes and seconds."""
- s = int(np.rint(seconds))
-
- if s < 60:
- return "{0}s".format(s)
- elif s < 60 * 60:
- return "{0}m {1:02}s".format(s // 60, s % 60)
- elif s < 24 * 60 * 60:
- return "{0}h {1:02}m {2:02}s".format(s // (60 * 60), (s // 60) % 60, s % 60)
- else:
- return "{0}d {1:02}h {2:02}m".format(s // (24 * 60 * 60), (s // (60 * 60)) % 24, (s // 60) % 60)
-
-
-def format_time_brief(seconds: Union[int, float]) -> str:
- """Convert the seconds to human readable string with days, hours, minutes and seconds."""
- s = int(np.rint(seconds))
-
- if s < 60:
- return "{0}s".format(s)
- elif s < 60 * 60:
- return "{0}m {1:02}s".format(s // 60, s % 60)
- elif s < 24 * 60 * 60:
- return "{0}h {1:02}m".format(s // (60 * 60), (s // 60) % 60)
- else:
- return "{0}d {1:02}h".format(s // (24 * 60 * 60), (s // (60 * 60)) % 24)
-
-
-def ask_yes_no(question: str) -> bool:
- """Ask the user the question until the user inputs a valid answer."""
- while True:
- try:
- print("{0} [y/n]".format(question))
- return strtobool(input().lower())
- except ValueError:
- pass
-
-
-def tuple_product(t: Tuple) -> Any:
- """Calculate the product of the tuple elements."""
- result = 1
-
- for v in t:
- result *= v
-
- return result
-
-
-_str_to_ctype = {
- "uint8": ctypes.c_ubyte,
- "uint16": ctypes.c_uint16,
- "uint32": ctypes.c_uint32,
- "uint64": ctypes.c_uint64,
- "int8": ctypes.c_byte,
- "int16": ctypes.c_int16,
- "int32": ctypes.c_int32,
- "int64": ctypes.c_int64,
- "float32": ctypes.c_float,
- "float64": ctypes.c_double
-}
-
-
-def get_dtype_and_ctype(type_obj: Any) -> Tuple[np.dtype, Any]:
- """Given a type name string (or an object having a __name__ attribute), return matching Numpy and ctypes types that have the same size in bytes."""
- type_str = None
-
- if isinstance(type_obj, str):
- type_str = type_obj
- elif hasattr(type_obj, "__name__"):
- type_str = type_obj.__name__
- elif hasattr(type_obj, "name"):
- type_str = type_obj.name
- else:
- raise RuntimeError("Cannot infer type name from input")
-
- assert type_str in _str_to_ctype.keys()
-
- my_dtype = np.dtype(type_str)
- my_ctype = _str_to_ctype[type_str]
-
- assert my_dtype.itemsize == ctypes.sizeof(my_ctype)
-
- return my_dtype, my_ctype
-
-
-def is_pickleable(obj: Any) -> bool:
- try:
- with io.BytesIO() as stream:
- pickle.dump(obj, stream)
- return True
- except:
- return False
-
-
-# Functionality to import modules/objects by name, and call functions by name
-# ------------------------------------------------------------------------------------------
-
-def get_module_from_obj_name(obj_name: str) -> Tuple[types.ModuleType, str]:
- """Searches for the underlying module behind the name to some python object.
- Returns the module and the object name (original name with module part removed)."""
-
- # allow convenience shorthands, substitute them by full names
- obj_name = re.sub("^np.", "numpy.", obj_name)
- obj_name = re.sub("^tf.", "tensorflow.", obj_name)
-
- # list alternatives for (module_name, local_obj_name)
- parts = obj_name.split(".")
- name_pairs = [(".".join(parts[:i]), ".".join(parts[i:])) for i in range(len(parts), 0, -1)]
-
- # try each alternative in turn
- for module_name, local_obj_name in name_pairs:
- try:
- module = importlib.import_module(module_name) # may raise ImportError
- get_obj_from_module(module, local_obj_name) # may raise AttributeError
- return module, local_obj_name
- except:
- pass
-
- # maybe some of the modules themselves contain errors?
- for module_name, _local_obj_name in name_pairs:
- try:
- importlib.import_module(module_name) # may raise ImportError
- except ImportError:
- if not str(sys.exc_info()[1]).startswith("No module named '" + module_name + "'"):
- raise
-
- # maybe the requested attribute is missing?
- for module_name, local_obj_name in name_pairs:
- try:
- module = importlib.import_module(module_name) # may raise ImportError
- get_obj_from_module(module, local_obj_name) # may raise AttributeError
- except ImportError:
- pass
-
- # we are out of luck, but we have no idea why
- raise ImportError(obj_name)
-
-
-def get_obj_from_module(module: types.ModuleType, obj_name: str) -> Any:
- """Traverses the object name and returns the last (rightmost) python object."""
- if obj_name == '':
- return module
- obj = module
- for part in obj_name.split("."):
- obj = getattr(obj, part)
- return obj
-
-
-def get_obj_by_name(name: str) -> Any:
- """Finds the python object with the given name."""
- module, obj_name = get_module_from_obj_name(name)
- return get_obj_from_module(module, obj_name)
-
-
-def call_func_by_name(*args, func_name: str = None, **kwargs) -> Any:
- """Finds the python object with the given name and calls it as a function."""
- assert func_name is not None
- func_obj = get_obj_by_name(func_name)
- assert callable(func_obj)
- return func_obj(*args, **kwargs)
-
-
-def construct_class_by_name(*args, class_name: str = None, **kwargs) -> Any:
- """Finds the python class with the given name and constructs it with the given arguments."""
- return call_func_by_name(*args, func_name=class_name, **kwargs)
-
-
-def get_module_dir_by_obj_name(obj_name: str) -> str:
- """Get the directory path of the module containing the given object name."""
- module, _ = get_module_from_obj_name(obj_name)
- return os.path.dirname(inspect.getfile(module))
-
-
-def is_top_level_function(obj: Any) -> bool:
- """Determine whether the given object is a top-level function, i.e., defined at module scope using 'def'."""
- return callable(obj) and obj.__name__ in sys.modules[obj.__module__].__dict__
-
-
-def get_top_level_function_name(obj: Any) -> str:
- """Return the fully-qualified name of a top-level function."""
- assert is_top_level_function(obj)
- module = obj.__module__
- if module == '__main__':
- module = os.path.splitext(os.path.basename(sys.modules[module].__file__))[0]
- return module + "." + obj.__name__
-
-
-# File system helpers
-# ------------------------------------------------------------------------------------------
-
-def list_dir_recursively_with_ignore(dir_path: str, ignores: List[str] = None, add_base_to_relative: bool = False) -> List[Tuple[str, str]]:
- """List all files recursively in a given directory while ignoring given file and directory names.
- Returns list of tuples containing both absolute and relative paths."""
- assert os.path.isdir(dir_path)
- base_name = os.path.basename(os.path.normpath(dir_path))
-
- if ignores is None:
- ignores = []
-
- result = []
-
- for root, dirs, files in os.walk(dir_path, topdown=True):
- for ignore_ in ignores:
- dirs_to_remove = [d for d in dirs if fnmatch.fnmatch(d, ignore_)]
-
- # dirs need to be edited in-place
- for d in dirs_to_remove:
- dirs.remove(d)
-
- files = [f for f in files if not fnmatch.fnmatch(f, ignore_)]
-
- absolute_paths = [os.path.join(root, f) for f in files]
- relative_paths = [os.path.relpath(p, dir_path) for p in absolute_paths]
-
- if add_base_to_relative:
- relative_paths = [os.path.join(base_name, p) for p in relative_paths]
-
- assert len(absolute_paths) == len(relative_paths)
- result += zip(absolute_paths, relative_paths)
-
- return result
-
-
-def copy_files_and_create_dirs(files: List[Tuple[str, str]]) -> None:
- """Takes in a list of tuples of (src, dst) paths and copies files.
- Will create all necessary directories."""
- for file in files:
- target_dir_name = os.path.dirname(file[1])
-
- # will create all intermediate-level directories
- if not os.path.exists(target_dir_name):
- os.makedirs(target_dir_name)
-
- shutil.copyfile(file[0], file[1])
-
-
-# URL helpers
-# ------------------------------------------------------------------------------------------
-
-def is_url(obj: Any, allow_file_urls: bool = False) -> bool:
- """Determine whether the given object is a valid URL string."""
- if not isinstance(obj, str) or not "://" in obj:
- return False
- if allow_file_urls and obj.startswith('file://'):
- return True
- try:
- res = requests.compat.urlparse(obj)
- if not res.scheme or not res.netloc or not "." in res.netloc:
- return False
- res = requests.compat.urlparse(requests.compat.urljoin(obj, "/"))
- if not res.scheme or not res.netloc or not "." in res.netloc:
- return False
- except:
- return False
- return True
-
-
-def open_url(url: str, cache_dir: str = None, num_attempts: int = 10, verbose: bool = True, return_filename: bool = False, cache: bool = True) -> Any:
- """Download the given URL and return a binary-mode file object to access the data."""
- assert num_attempts >= 1
- assert not (return_filename and (not cache))
-
- # Doesn't look like an URL scheme so interpret it as a local filename.
- if not re.match('^[a-z]+://', url):
- return url if return_filename else open(url, "rb")
-
- # Handle file URLs. This code handles unusual file:// patterns that
- # arise on Windows:
- #
- # file:///c:/foo.txt
- #
- # which would translate to a local '/c:/foo.txt' filename that's
- # invalid. Drop the forward slash for such pathnames.
- #
- # If you touch this code path, you should test it on both Linux and
- # Windows.
- #
- # Some internet resources suggest using urllib.request.url2pathname() but
- # but that converts forward slashes to backslashes and this causes
- # its own set of problems.
- if url.startswith('file://'):
- filename = urllib.parse.urlparse(url).path
- if re.match(r'^/[a-zA-Z]:', filename):
- filename = filename[1:]
- return filename if return_filename else open(filename, "rb")
-
- assert is_url(url)
-
- # Lookup from cache.
- if cache_dir is None:
- cache_dir = make_cache_dir_path('downloads')
-
- url_md5 = hashlib.md5(url.encode("utf-8")).hexdigest()
- if cache:
- cache_files = glob.glob(os.path.join(cache_dir, url_md5 + "_*"))
- if len(cache_files) == 1:
- filename = cache_files[0]
- return filename if return_filename else open(filename, "rb")
-
- # Download.
- url_name = None
- url_data = None
- with requests.Session() as session:
- if verbose:
- print("Downloading %s ..." % url, end="", flush=True)
- for attempts_left in reversed(range(num_attempts)):
- try:
- with session.get(url) as res:
- res.raise_for_status()
- if len(res.content) == 0:
- raise IOError("No data received")
-
- if len(res.content) < 8192:
- content_str = res.content.decode("utf-8")
- if "download_warning" in res.headers.get("Set-Cookie", ""):
- links = [html.unescape(link) for link in content_str.split('"') if "export=download" in link]
- if len(links) == 1:
- url = requests.compat.urljoin(url, links[0])
- raise IOError("Google Drive virus checker nag")
- if "Google Drive - Quota exceeded" in content_str:
- raise IOError("Google Drive download quota exceeded -- please try again later")
-
- match = re.search(r'filename="([^"]*)"', res.headers.get("Content-Disposition", ""))
- url_name = match[1] if match else url
- url_data = res.content
- if verbose:
- print(" done")
- break
- except KeyboardInterrupt:
- raise
- except:
- if not attempts_left:
- if verbose:
- print(" failed")
- raise
- if verbose:
- print(".", end="", flush=True)
-
- # Save to cache.
- if cache:
- safe_name = re.sub(r"[^0-9a-zA-Z-._]", "_", url_name)
- cache_file = os.path.join(cache_dir, url_md5 + "_" + safe_name)
- temp_file = os.path.join(cache_dir, "tmp_" + uuid.uuid4().hex + "_" + url_md5 + "_" + safe_name)
- os.makedirs(cache_dir, exist_ok=True)
- with open(temp_file, "wb") as f:
- f.write(url_data)
- os.replace(temp_file, cache_file) # atomic
- if return_filename:
- return cache_file
-
- # Return data as file object.
- assert not return_filename
- return io.BytesIO(url_data)
diff --git a/spaces/DragGan/DragGan/viz/latent_widget.py b/spaces/DragGan/DragGan/viz/latent_widget.py
deleted file mode 100644
index eb8fd50c1b461fbab39cfda4b229bafdb05be511..0000000000000000000000000000000000000000
--- a/spaces/DragGan/DragGan/viz/latent_widget.py
+++ /dev/null
@@ -1,96 +0,0 @@
-# Copyright (c) 2021, NVIDIA CORPORATION & AFFILIATES. All rights reserved.
-#
-# NVIDIA CORPORATION and its licensors retain all intellectual property
-# and proprietary rights in and to this software, related documentation
-# and any modifications thereto. Any use, reproduction, disclosure or
-# distribution of this software and related documentation without an express
-# license agreement from NVIDIA CORPORATION is strictly prohibited.
-
-import os
-import numpy as np
-import imgui
-import dnnlib
-import torch
-from gui_utils import imgui_utils
-
-#----------------------------------------------------------------------------
-
-class LatentWidget:
- def __init__(self, viz):
- self.viz = viz
- self.seed = 0
- self.w_plus = True
- self.reg = 0
- self.lr = 0.001
- self.w_path = ''
- self.w_load = None
- self.defer_frames = 0
- self.disabled_time = 0
-
- @imgui_utils.scoped_by_object_id
- def __call__(self, show=True):
- viz = self.viz
- if show:
- with imgui_utils.grayed_out(self.disabled_time != 0):
- imgui.text('Latent')
- imgui.same_line(viz.label_w)
- with imgui_utils.item_width(viz.font_size * 8.75):
- changed, seed = imgui.input_int('Seed', self.seed)
- if changed:
- self.seed = seed
- # reset latent code
- self.w_load = None
-
- # load latent code
- imgui.text(' ')
- imgui.same_line(viz.label_w)
- _changed, self.w_path = imgui_utils.input_text('##path', self.w_path, 1024,
- flags=(imgui.INPUT_TEXT_AUTO_SELECT_ALL | imgui.INPUT_TEXT_ENTER_RETURNS_TRUE),
- width=(-1),
- help_text='Path to latent code')
- if imgui.is_item_hovered() and not imgui.is_item_active() and self.w_path != '':
- imgui.set_tooltip(self.w_path)
-
- imgui.text(' ')
- imgui.same_line(viz.label_w)
- if imgui_utils.button('Load latent', width=viz.button_w, enabled=(self.disabled_time == 0 and 'image' in viz.result)):
- assert os.path.isfile(self.w_path), f"{self.w_path} does not exist!"
- self.w_load = torch.load(self.w_path)
- self.defer_frames = 2
- self.disabled_time = 0.5
-
- imgui.text(' ')
- imgui.same_line(viz.label_w)
- with imgui_utils.item_width(viz.button_w):
- changed, lr = imgui.input_float('Step Size', self.lr)
- if changed:
- self.lr = lr
-
- # imgui.text(' ')
- # imgui.same_line(viz.label_w)
- # with imgui_utils.item_width(viz.button_w):
- # changed, reg = imgui.input_float('Regularize', self.reg)
- # if changed:
- # self.reg = reg
-
- imgui.text(' ')
- imgui.same_line(viz.label_w)
- reset_w = imgui_utils.button('Reset', width=viz.button_w, enabled='image' in viz.result)
- imgui.same_line()
- _clicked, w = imgui.checkbox('w', not self.w_plus)
- if w:
- self.w_plus = False
- imgui.same_line()
- _clicked, self.w_plus = imgui.checkbox('w+', self.w_plus)
-
- self.disabled_time = max(self.disabled_time - viz.frame_delta, 0)
- if self.defer_frames > 0:
- self.defer_frames -= 1
- viz.args.w0_seed = self.seed
- viz.args.w_load = self.w_load
- viz.args.reg = self.reg
- viz.args.w_plus = self.w_plus
- viz.args.reset_w = reset_w
- viz.args.lr = lr
-
-#----------------------------------------------------------------------------
diff --git a/spaces/Dukcar/Pix2Pix-Video/README.md b/spaces/Dukcar/Pix2Pix-Video/README.md
deleted file mode 100644
index 20cff0d5ee51519b0677d10d6b8808b162b79085..0000000000000000000000000000000000000000
--- a/spaces/Dukcar/Pix2Pix-Video/README.md
+++ /dev/null
@@ -1,13 +0,0 @@
----
-title: Pix2Pix Video
-emoji: 🎨🎞️
-colorFrom: pink
-colorTo: purple
-sdk: gradio
-sdk_version: 3.29.0
-app_file: app.py
-pinned: false
-duplicated_from: fffiloni/Pix2Pix-Video
----
-
-Check out the configuration reference at https://huggingface.co/docs/hub/spaces-config-reference
diff --git a/spaces/ECCV2022/bytetrack/tutorials/motr/mot_online/basetrack.py b/spaces/ECCV2022/bytetrack/tutorials/motr/mot_online/basetrack.py
deleted file mode 100644
index 4fe2233607f6d4ed28b11a0ae6c0303c8ca19098..0000000000000000000000000000000000000000
--- a/spaces/ECCV2022/bytetrack/tutorials/motr/mot_online/basetrack.py
+++ /dev/null
@@ -1,52 +0,0 @@
-import numpy as np
-from collections import OrderedDict
-
-
-class TrackState(object):
- New = 0
- Tracked = 1
- Lost = 2
- Removed = 3
-
-
-class BaseTrack(object):
- _count = 0
-
- track_id = 0
- is_activated = False
- state = TrackState.New
-
- history = OrderedDict()
- features = []
- curr_feature = None
- score = 0
- start_frame = 0
- frame_id = 0
- time_since_update = 0
-
- # multi-camera
- location = (np.inf, np.inf)
-
- @property
- def end_frame(self):
- return self.frame_id
-
- @staticmethod
- def next_id():
- BaseTrack._count += 1
- return BaseTrack._count
-
- def activate(self, *args):
- raise NotImplementedError
-
- def predict(self):
- raise NotImplementedError
-
- def update(self, *args, **kwargs):
- raise NotImplementedError
-
- def mark_lost(self):
- self.state = TrackState.Lost
-
- def mark_removed(self):
- self.state = TrackState.Removed
diff --git a/spaces/ElainaFanBoy/MusicGen/README.md b/spaces/ElainaFanBoy/MusicGen/README.md
deleted file mode 100644
index 6a3dbd50cb5862d8d084df848d7f02e3094d9553..0000000000000000000000000000000000000000
--- a/spaces/ElainaFanBoy/MusicGen/README.md
+++ /dev/null
@@ -1,137 +0,0 @@
----
-title: MusicGen
-python_version: '3.9'
-tags:
-- music generation
-- language models
-- LLMs
-app_file: app.py
-emoji: 🎵
-colorFrom: white
-colorTo: blue
-sdk: gradio
-sdk_version: 3.34.0
-pinned: true
-license: cc-by-nc-4.0
-duplicated_from: facebook/MusicGen
----
-# Audiocraft
-
-
-
-
-Audiocraft is a PyTorch library for deep learning research on audio generation. At the moment, it contains the code for MusicGen, a state-of-the-art controllable text-to-music model.
-
-## MusicGen
-
-Audiocraft provides the code and models for MusicGen, [a simple and controllable model for music generation][arxiv]. MusicGen is a single stage auto-regressive
-Transformer model trained over a 32kHz EnCodec tokenizer with 4 codebooks sampled at 50 Hz. Unlike existing methods like [MusicLM](https://arxiv.org/abs/2301.11325), MusicGen doesn't require a self-supervised semantic representation, and it generates
-all 4 codebooks in one pass. By introducing a small delay between the codebooks, we show we can predict
-them in parallel, thus having only 50 auto-regressive steps per second of audio.
-Check out our [sample page][musicgen_samples] or test the available demo!
-
-
-
-
-
-
-
-
-
-We use 20K hours of licensed music to train MusicGen. Specifically, we rely on an internal dataset of 10K high-quality music tracks, and on the ShutterStock and Pond5 music data.
-
-## Installation
-Audiocraft requires Python 3.9, PyTorch 2.0.0, and a GPU with at least 16 GB of memory (for the medium-sized model). To install Audiocraft, you can run the following:
-
-```shell
-# Best to make sure you have torch installed first, in particular before installing xformers.
-# Don't run this if you already have PyTorch installed.
-pip install 'torch>=2.0'
-# Then proceed to one of the following
-pip install -U audiocraft # stable release
-pip install -U git+https://git@github.com/facebookresearch/audiocraft#egg=audiocraft # bleeding edge
-pip install -e . # or if you cloned the repo locally
-```
-
-## Usage
-We offer a number of way to interact with MusicGen:
-1. A demo is also available on the [`facebook/MusicGen` HuggingFace Space](https://huggingface.co/spaces/facebook/MusicGen) (huge thanks to all the HF team for their support).
-2. You can run the extended demo on a Colab: [colab notebook](https://colab.research.google.com/drive/1fxGqfg96RBUvGxZ1XXN07s3DthrKUl4-?usp=sharing).
-3. You can use the gradio demo locally by running `python app.py`.
-4. You can play with MusicGen by running the jupyter notebook at [`demo.ipynb`](./demo.ipynb) locally (if you have a GPU).
-5. Finally, checkout [@camenduru Colab page](https://github.com/camenduru/MusicGen-colab) which is regularly
- updated with contributions from @camenduru and the community.
-
-## API
-
-We provide a simple API and 4 pre-trained models. The pre trained models are:
-- `small`: 300M model, text to music only - [🤗 Hub](https://huggingface.co/facebook/musicgen-small)
-- `medium`: 1.5B model, text to music only - [🤗 Hub](https://huggingface.co/facebook/musicgen-medium)
-- `melody`: 1.5B model, text to music and text+melody to music - [🤗 Hub](https://huggingface.co/facebook/musicgen-melody)
-- `large`: 3.3B model, text to music only - [🤗 Hub](https://huggingface.co/facebook/musicgen-large)
-
-We observe the best trade-off between quality and compute with the `medium` or `melody` model.
-In order to use MusicGen locally **you must have a GPU**. We recommend 16GB of memory, but smaller
-GPUs will be able to generate short sequences, or longer sequences with the `small` model.
-
-**Note**: Please make sure to have [ffmpeg](https://ffmpeg.org/download.html) installed when using newer version of `torchaudio`.
-You can install it with:
-```
-apt-get install ffmpeg
-```
-
-See after a quick example for using the API.
-
-```python
-import torchaudio
-from audiocraft.models import MusicGen
-from audiocraft.data.audio import audio_write
-
-model = MusicGen.get_pretrained('melody')
-model.set_generation_params(duration=8) # generate 8 seconds.
-wav = model.generate_unconditional(4) # generates 4 unconditional audio samples
-descriptions = ['happy rock', 'energetic EDM', 'sad jazz']
-wav = model.generate(descriptions) # generates 3 samples.
-
-melody, sr = torchaudio.load('./assets/bach.mp3')
-# generates using the melody from the given audio and the provided descriptions.
-wav = model.generate_with_chroma(descriptions, melody[None].expand(3, -1, -1), sr)
-
-for idx, one_wav in enumerate(wav):
- # Will save under {idx}.wav, with loudness normalization at -14 db LUFS.
- audio_write(f'{idx}', one_wav.cpu(), model.sample_rate, strategy="loudness", loudness_compressor=True)
-```
-
-
-## Model Card
-
-See [the model card page](./MODEL_CARD.md).
-
-## FAQ
-
-#### Will the training code be released?
-
-Yes. We will soon release the training code for MusicGen and EnCodec.
-
-
-#### I need help on Windows
-
-@FurkanGozukara made a complete tutorial for [Audiocraft/MusicGen on Windows](https://youtu.be/v-YpvPkhdO4)
-
-
-## Citation
-```
-@article{copet2023simple,
- title={Simple and Controllable Music Generation},
- author={Jade Copet and Felix Kreuk and Itai Gat and Tal Remez and David Kant and Gabriel Synnaeve and Yossi Adi and Alexandre Défossez},
- year={2023},
- journal={arXiv preprint arXiv:2306.05284},
-}
-```
-
-## License
-* The code in this repository is released under the MIT license as found in the [LICENSE file](LICENSE).
-* The weights in this repository are released under the CC-BY-NC 4.0 license as found in the [LICENSE_weights file](LICENSE_weights).
-
-[arxiv]: https://arxiv.org/abs/2306.05284
-[musicgen_samples]: https://ai.honu.io/papers/musicgen/
diff --git a/spaces/Elbhnasy/Foodvision_mini/README.md b/spaces/Elbhnasy/Foodvision_mini/README.md
deleted file mode 100644
index 69eb8ef7b3f7e59a23f104aac07f5c32425c49be..0000000000000000000000000000000000000000
--- a/spaces/Elbhnasy/Foodvision_mini/README.md
+++ /dev/null
@@ -1,13 +0,0 @@
----
-title: Foodvision Mini
-emoji: 🦀
-colorFrom: green
-colorTo: indigo
-sdk: gradio
-sdk_version: 3.16.2
-app_file: app.py
-pinned: false
-license: mit
----
-
-Check out the configuration reference at https://huggingface.co/docs/hub/spaces-config-reference
diff --git a/spaces/Ellight/Steady-state-heat-conduction-GANs-Vision-Transformer/README.md b/spaces/Ellight/Steady-state-heat-conduction-GANs-Vision-Transformer/README.md
deleted file mode 100644
index 2e69ba0a99f61b492bc42b371fcf6b39db2d1157..0000000000000000000000000000000000000000
--- a/spaces/Ellight/Steady-state-heat-conduction-GANs-Vision-Transformer/README.md
+++ /dev/null
@@ -1,12 +0,0 @@
----
-title: Steady State Heat Conduction GANs Vision Transformer
-emoji: 🏢
-colorFrom: green
-colorTo: yellow
-sdk: gradio
-sdk_version: 3.5
-app_file: app.py
-pinned: false
----
-
-Check out the configuration reference at https://huggingface.co/docs/hub/spaces-config-reference
diff --git a/spaces/EmoHugger/MyGenAIChatBot/app.py b/spaces/EmoHugger/MyGenAIChatBot/app.py
deleted file mode 100644
index cd4084f6c2611d98cf3c04a200210f6453fd568b..0000000000000000000000000000000000000000
--- a/spaces/EmoHugger/MyGenAIChatBot/app.py
+++ /dev/null
@@ -1,34 +0,0 @@
-import os
-import gradio as gr
-from langchain.chat_models import ChatOpenAI
-from langchain import LLMChain, PromptTemplate
-from langchain.memory import ConversationBufferMemory
-
-OPENAI_API_KEY=os.getenv('OPENAI_API_KEY')
-
-template = """Meet Pavan, your youthful and witty personal assistant! At 21 years old, he's full of energy and always eager to help. Pavan's goal is to assist you with any questions or problems you might have. His enthusiasm shines through in every response, making interactions with his enjoyable and engaging.
-{chat_history}
-User: {user_message}
-Chatbot:"""
-
-prompt = PromptTemplate(
- input_variables=["chat_history", "user_message"], template=template
-)
-
-memory = ConversationBufferMemory(memory_key="chat_history")
-
-llm_chain = LLMChain(
- llm=ChatOpenAI(temperature='0.5', model_name="gpt-3.5-turbo"),
- prompt=prompt,
- verbose=True,
- memory=memory,
-)
-
-def get_text_response(user_message,history):
- response = llm_chain.predict(user_message = user_message)
- return response
-
-demo = gr.ChatInterface(get_text_response)
-
-if __name__ == "__main__":
- demo.launch() #To create a public link, set `share=True` in `launch()`. To enable errors and logs, set `debug=True` in `launch()`.
diff --git a/spaces/EsoCode/text-generation-webui/modules/evaluate.py b/spaces/EsoCode/text-generation-webui/modules/evaluate.py
deleted file mode 100644
index d94863d978e51e3240b967df622a5fd313713501..0000000000000000000000000000000000000000
--- a/spaces/EsoCode/text-generation-webui/modules/evaluate.py
+++ /dev/null
@@ -1,154 +0,0 @@
-import datetime
-from pathlib import Path
-
-import pandas as pd
-import torch
-from datasets import load_dataset
-from tqdm import tqdm
-
-from modules import shared
-from modules.models import load_model, unload_model
-from modules.models_settings import (
- get_model_settings_from_yamls,
- update_model_parameters
-)
-from modules.text_generation import encode
-
-
-def load_past_evaluations():
- if Path('logs/evaluations.csv').exists():
- df = pd.read_csv(Path('logs/evaluations.csv'), dtype=str)
- df['Perplexity'] = pd.to_numeric(df['Perplexity'])
- return df
- else:
- return pd.DataFrame(columns=['Model', 'LoRAs', 'Dataset', 'Perplexity', 'stride', 'max_length', 'Date', 'Comment'])
-
-
-past_evaluations = load_past_evaluations()
-
-
-def save_past_evaluations(df):
- global past_evaluations
- past_evaluations = df
- filepath = Path('logs/evaluations.csv')
- filepath.parent.mkdir(parents=True, exist_ok=True)
- df.to_csv(filepath, index=False)
-
-
-def calculate_perplexity(models, input_dataset, stride, _max_length):
- '''
- Based on:
- https://huggingface.co/docs/transformers/perplexity#calculating-ppl-with-fixedlength-models
- '''
-
- global past_evaluations
- cumulative_log = ''
- cumulative_log += "Loading the input dataset...\n\n"
- yield cumulative_log
-
- # Copied from https://github.com/qwopqwop200/GPTQ-for-LLaMa/blob/triton/utils/datautils.py
- if input_dataset == 'wikitext':
- data = load_dataset('wikitext', 'wikitext-2-raw-v1', split='test')
- text = "\n\n".join(data['text'])
- elif input_dataset == 'ptb':
- data = load_dataset('ptb_text_only', 'penn_treebank', split='validation')
- text = "\n\n".join(data['sentence'])
- elif input_dataset == 'ptb_new':
- data = load_dataset('ptb_text_only', 'penn_treebank', split='test')
- text = " ".join(data['sentence'])
- else:
- with open(Path(f'training/datasets/{input_dataset}.txt'), 'r', encoding='utf-8') as f:
- text = f.read()
-
- for model in models:
- if is_in_past_evaluations(model, input_dataset, stride, _max_length):
- cumulative_log += f"{model} has already been tested. Ignoring.\n\n"
- yield cumulative_log
- continue
-
- if model != 'current model':
- try:
- yield cumulative_log + f"Loading {model}...\n\n"
- model_settings = get_model_settings_from_yamls(model)
- shared.settings.update(model_settings) # hijacking the interface defaults
- update_model_parameters(model_settings) # hijacking the command-line arguments
- shared.model_name = model
- unload_model()
- shared.model, shared.tokenizer = load_model(shared.model_name)
- except:
- cumulative_log += f"Failed to load {model}. Moving on.\n\n"
- yield cumulative_log
- continue
-
- cumulative_log += f"Processing {shared.model_name}...\n\n"
- yield cumulative_log + "Tokenizing the input dataset...\n\n"
- encodings = encode(text, add_special_tokens=False)
- seq_len = encodings.shape[1]
- if _max_length:
- max_length = _max_length
- elif hasattr(shared.model.config, 'max_position_embeddings'):
- max_length = shared.model.config.max_position_embeddings
- else:
- max_length = 2048
-
- nlls = []
- prev_end_loc = 0
- for begin_loc in tqdm(range(0, seq_len, stride)):
- yield cumulative_log + f"Evaluating... {100*begin_loc/seq_len:.2f}%"
- end_loc = min(begin_loc + max_length, seq_len)
- trg_len = end_loc - prev_end_loc # may be different from stride on last loop
- input_ids = encodings[:, begin_loc:end_loc]
- target_ids = input_ids.clone()
- target_ids[:, :-trg_len] = -100
-
- with torch.no_grad():
- outputs = shared.model(input_ids=input_ids, labels=target_ids)
-
- # loss is calculated using CrossEntropyLoss which averages over valid labels
- # N.B. the model only calculates loss over trg_len - 1 labels, because it internally shifts the labels
- # to the left by 1.
- neg_log_likelihood = outputs.loss
-
- nlls.append(neg_log_likelihood)
-
- prev_end_loc = end_loc
- if end_loc == seq_len:
- break
-
- ppl = torch.exp(torch.stack(nlls).mean())
- add_entry_to_past_evaluations(float(ppl), shared.model_name, input_dataset, stride, _max_length)
- save_past_evaluations(past_evaluations)
- cumulative_log += f"The perplexity for {shared.model_name} is: {float(ppl)}\n\n"
- yield cumulative_log
-
-
-def add_entry_to_past_evaluations(perplexity, model, dataset, stride, max_length):
- global past_evaluations
- entry = {
- 'Model': model,
- 'LoRAs': ', '.join(shared.lora_names) or '-',
- 'Dataset': dataset,
- 'Perplexity': perplexity,
- 'stride': str(stride),
- 'max_length': str(max_length),
- 'Date': datetime.datetime.now().strftime('%Y-%m-%d %H:%M:%S'),
- 'Comment': ''
- }
- past_evaluations = pd.concat([past_evaluations, pd.DataFrame([entry])], ignore_index=True)
-
-
-def is_in_past_evaluations(model, dataset, stride, max_length):
- entries = past_evaluations[(past_evaluations['Model'] == model) &
- (past_evaluations['Dataset'] == dataset) &
- (past_evaluations['max_length'] == str(max_length)) &
- (past_evaluations['stride'] == str(stride))]
-
- if entries.shape[0] > 0:
- return True
- else:
- return False
-
-
-def generate_markdown_table():
- sorted_df = past_evaluations.sort_values(by=['Dataset', 'stride', 'Perplexity', 'Date'])
- return sorted_df
diff --git a/spaces/EuroPython2022/Warehouse_Apparel_Detection/metadata/predictor_yolo_detector/utils/datasets.py b/spaces/EuroPython2022/Warehouse_Apparel_Detection/metadata/predictor_yolo_detector/utils/datasets.py
deleted file mode 100644
index 552c330869709b15b46b4cd3c1c46d1fafebdcfb..0000000000000000000000000000000000000000
--- a/spaces/EuroPython2022/Warehouse_Apparel_Detection/metadata/predictor_yolo_detector/utils/datasets.py
+++ /dev/null
@@ -1,946 +0,0 @@
-import glob
-import os
-import random
-import shutil
-import time
-from pathlib import Path
-from threading import Thread
-
-import cv2
-import math
-import numpy as np
-import torch
-from PIL import Image, ExifTags
-from torch.utils.data import Dataset
-from tqdm import tqdm
-
-from metadata.predictor_yolo_detector.utils.general import xyxy2xywh, xywh2xyxy, \
- torch_distributed_zero_first
-
-help_url = 'https://github.com/ultralytics/yolov5/wiki/Train-Custom-Data'
-img_formats = ['.bmp', '.jpg', '.jpeg', '.png', '.tif', '.tiff', '.dng']
-vid_formats = ['.mov', '.avi', '.mp4', '.mpg', '.mpeg', '.m4v', '.wmv', '.mkv']
-
-# Get orientation exif tag
-for orientation in ExifTags.TAGS.keys():
- if ExifTags.TAGS[orientation] == 'Orientation':
- break
-
-
-def get_hash(files):
- # Returns a single hash value of a list of files
- return sum(os.path.getsize(f) for f in files if os.path.isfile(f))
-
-
-def exif_size(img):
- # Returns exif-corrected PIL size
- s = img.size # (width, height)
- try:
- rotation = dict(img._getexif().items())[orientation]
- if rotation == 6: # rotation 270
- s = (s[1], s[0])
- elif rotation == 8: # rotation 90
- s = (s[1], s[0])
- except:
- pass
-
- return s
-
-
-def create_dataloader(path, imgsz, batch_size, stride, opt, hyp=None, augment=False, cache=False, pad=0.0, rect=False,
- rank=-1, world_size=1, workers=8):
- # Make sure only the first process in DDP process the dataset first, and the following others can use the cache.
- with torch_distributed_zero_first(rank):
- dataset = LoadImagesAndLabels(path, imgsz, batch_size,
- augment=augment, # augment images
- hyp=hyp, # augmentation hyperparameters
- rect=rect, # rectangular training
- cache_images=cache,
- single_cls=opt.single_cls,
- stride=int(stride),
- pad=pad,
- rank=rank)
-
- batch_size = min(batch_size, len(dataset))
- nw = min([os.cpu_count() // world_size, batch_size if batch_size > 1 else 0, workers]) # number of workers
- sampler = torch.utils.data.distributed.DistributedSampler(dataset) if rank != -1 else None
- dataloader = InfiniteDataLoader(dataset,
- batch_size=batch_size,
- num_workers=nw,
- sampler=sampler,
- pin_memory=True,
- collate_fn=LoadImagesAndLabels.collate_fn) # torch.utils.data.DataLoader()
- return dataloader, dataset
-
-
-class InfiniteDataLoader(torch.utils.data.dataloader.DataLoader):
- """ Dataloader that reuses workers.
-
- Uses same syntax as vanilla DataLoader.
- """
-
- def __init__(self, *args, **kwargs):
- super().__init__(*args, **kwargs)
- object.__setattr__(self, 'batch_sampler', _RepeatSampler(self.batch_sampler))
- self.iterator = super().__iter__()
-
- def __len__(self):
- return len(self.batch_sampler.sampler)
-
- def __iter__(self):
- for i in range(len(self)):
- yield next(self.iterator)
-
-
-class _RepeatSampler(object):
- """ Sampler that repeats forever.
-
- Args:
- sampler (Sampler)
- """
-
- def __init__(self, sampler):
- self.sampler = sampler
-
- def __iter__(self):
- while True:
- yield from iter(self.sampler)
-
-
-class LoadImages: # for inference
- def __init__(self, path, img_size=640):
- p = str(Path(path)) # os-agnostic
- p = os.path.abspath(p) # absolute path
- if '*' in p:
- files = sorted(glob.glob(p, recursive=True)) # glob
- elif os.path.isdir(p):
- files = sorted(glob.glob(os.path.join(p, '*.*'))) # dir
- elif os.path.isfile(p):
- files = [p] # files
- else:
- raise Exception('ERROR: %s does not exist' % p)
-
- images = [x for x in files if os.path.splitext(x)[-1].lower() in img_formats]
- videos = [x for x in files if os.path.splitext(x)[-1].lower() in vid_formats]
- ni, nv = len(images), len(videos)
-
- self.img_size = img_size
- self.files = images + videos
- self.nf = ni + nv # number of files
- self.video_flag = [False] * ni + [True] * nv
- self.mode = 'images'
- if any(videos):
- self.new_video(videos[0]) # new video
- else:
- self.cap = None
- assert self.nf > 0, 'No images or videos found in %s. Supported formats are:\nimages: %s\nvideos: %s' % \
- (p, img_formats, vid_formats)
-
- def __iter__(self):
- self.count = 0
- return self
-
- def __next__(self):
- if self.count == self.nf:
- raise StopIteration
- path = self.files[self.count]
-
- if self.video_flag[self.count]:
- # Read video
- self.mode = 'video'
- ret_val, img0 = self.cap.read()
- if not ret_val:
- self.count += 1
- self.cap.release()
- if self.count == self.nf: # last video
- raise StopIteration
- else:
- path = self.files[self.count]
- self.new_video(path)
- ret_val, img0 = self.cap.read()
-
- self.frame += 1
- print('video %g/%g (%g/%g) %s: ' % (self.count + 1, self.nf, self.frame, self.nframes, path), end='')
-
- else:
- # Read image
- self.count += 1
- img0 = cv2.imread(path) # BGR
- assert img0 is not None, 'Image Not Found ' + path
- print('image %g/%g %s: ' % (self.count, self.nf, path), end='')
-
- # Padded resize
- img = letterbox(img0, new_shape=self.img_size)[0]
-
- # Convert
- img = img[:, :, ::-1].transpose(2, 0, 1) # BGR to RGB, to 3x416x416
- img = np.ascontiguousarray(img)
-
- # cv2.imwrite(path + '.letterbox.jpg', 255 * img.transpose((1, 2, 0))[:, :, ::-1]) # save letterbox image
- return path, img, img0, self.cap
-
- def new_video(self, path):
- self.frame = 0
- self.cap = cv2.VideoCapture(path)
- self.nframes = int(self.cap.get(cv2.CAP_PROP_FRAME_COUNT))
-
- def __len__(self):
- return self.nf # number of files
-
-
-class LoadWebcam: # for inference
- def __init__(self, pipe=0, img_size=640):
- self.img_size = img_size
-
- if pipe == '0':
- pipe = 0 # local camera
- # pipe = 'rtsp://192.168.1.64/1' # IP camera
- # pipe = 'rtsp://username:password@192.168.1.64/1' # IP camera with login
- # pipe = 'rtsp://170.93.143.139/rtplive/470011e600ef003a004ee33696235daa' # IP traffic camera
- # pipe = 'http://wmccpinetop.axiscam.net/mjpg/video.mjpg' # IP golf camera
-
- # https://answers.opencv.org/question/215996/changing-gstreamer-pipeline-to-opencv-in-pythonsolved/
- # pipe = '"rtspsrc location="rtsp://username:password@192.168.1.64/1" latency=10 ! appsink' # GStreamer
-
- # https://answers.opencv.org/question/200787/video-acceleration-gstremer-pipeline-in-videocapture/
- # https://stackoverflow.com/questions/54095699/install-gstreamer-support-for-opencv-python-package # install help
- # pipe = "rtspsrc location=rtsp://root:root@192.168.0.91:554/axis-media/media.amp?videocodec=h264&resolution=3840x2160 protocols=GST_RTSP_LOWER_TRANS_TCP ! rtph264depay ! queue ! vaapih264dec ! videoconvert ! appsink" # GStreamer
-
- self.pipe = pipe
- self.cap = cv2.VideoCapture(pipe) # video capture object
- self.cap.set(cv2.CAP_PROP_BUFFERSIZE, 3) # set buffer size
-
- def __iter__(self):
- self.count = -1
- return self
-
- def __next__(self):
- self.count += 1
- if cv2.waitKey(1) == ord('q'): # q to quit
- self.cap.release()
- cv2.destroyAllWindows()
- raise StopIteration
-
- # Read frame
- if self.pipe == 0: # local camera
- ret_val, img0 = self.cap.read()
- img0 = cv2.flip(img0, 1) # flip left-right
- else: # IP camera
- n = 0
- while True:
- n += 1
- self.cap.grab()
- if n % 30 == 0: # skip frames
- ret_val, img0 = self.cap.retrieve()
- if ret_val:
- break
-
- # Print
- assert ret_val, 'Camera Error %s' % self.pipe
- img_path = 'webcam.jpg'
- print('webcam %g: ' % self.count, end='')
-
- # Padded resize
- img = letterbox(img0, new_shape=self.img_size)[0]
-
- # Convert
- img = img[:, :, ::-1].transpose(2, 0, 1) # BGR to RGB, to 3x416x416
- img = np.ascontiguousarray(img)
-
- return img_path, img, img0, None
-
- def __len__(self):
- return 0
-
-
-class LoadStreams: # multiple IP or RTSP cameras
- def __init__(self, sources='streams.txt', img_size=640):
- self.mode = 'images'
- self.img_size = img_size
-
- if os.path.isfile(sources):
- with open(sources, 'r') as f:
- sources = [x.strip() for x in f.read().splitlines() if len(x.strip())]
- else:
- sources = [sources]
-
- n = len(sources)
- self.imgs = [None] * n
- self.sources = sources
- for i, s in enumerate(sources):
- # Start the thread to read frames from the video stream
- print('%g/%g: %s... ' % (i + 1, n, s), end='')
- cap = cv2.VideoCapture(eval(s) if s.isnumeric() else s)
- assert cap.isOpened(), 'Failed to open %s' % s
- w = int(cap.get(cv2.CAP_PROP_FRAME_WIDTH))
- h = int(cap.get(cv2.CAP_PROP_FRAME_HEIGHT))
- fps = cap.get(cv2.CAP_PROP_FPS) % 100
- _, self.imgs[i] = cap.read() # guarantee first frame
- thread = Thread(target=self.update, args=([i, cap]), daemon=True)
- print(' success (%gx%g at %.2f FPS).' % (w, h, fps))
- thread.start()
- print('') # newline
-
- # check for common shapes
- s = np.stack([letterbox(x, new_shape=self.img_size)[0].shape for x in self.imgs], 0) # inference shapes
- self.rect = np.unique(s, axis=0).shape[0] == 1 # rect inference if all shapes equal
- if not self.rect:
- print('WARNING: Different stream shapes detected. For optimal performance supply similarly-shaped streams.')
-
- def update(self, index, cap):
- # Read next stream frame in a daemon thread
- n = 0
- while cap.isOpened():
- n += 1
- # _, self.imgs[index] = cap.read()
- cap.grab()
- if n == 4: # read every 4th frame
- _, self.imgs[index] = cap.retrieve()
- n = 0
- time.sleep(0.01) # wait time
-
- def __iter__(self):
- self.count = -1
- return self
-
- def __next__(self):
- self.count += 1
- img0 = self.imgs.copy()
- if cv2.waitKey(1) == ord('q'): # q to quit
- cv2.destroyAllWindows()
- raise StopIteration
-
- # Letterbox
- img = [letterbox(x, new_shape=self.img_size, auto=self.rect)[0] for x in img0]
-
- # Stack
- img = np.stack(img, 0)
-
- # Convert
- img = img[:, :, :, ::-1].transpose(0, 3, 1, 2) # BGR to RGB, to bsx3x416x416
- img = np.ascontiguousarray(img)
-
- return self.sources, img, img0, None
-
- def __len__(self):
- return 0 # 1E12 frames = 32 streams at 30 FPS for 30 years
-
-
-class LoadImagesAndLabels(Dataset): # for training/testing
- def __init__(self, path, img_size=640, batch_size=16, augment=False, hyp=None, rect=False, image_weights=False,
- cache_images=False, single_cls=False, stride=32, pad=0.0, rank=-1):
- self.img_size = img_size
- self.augment = augment
- self.hyp = hyp
- self.image_weights = image_weights
- self.rect = False if image_weights else rect
- self.mosaic = self.augment and not self.rect # load 4 images at a time into a mosaic (only during training)
- self.mosaic_border = [-img_size // 2, -img_size // 2]
- self.stride = stride
-
- def img2label_paths(img_paths):
- # Define label paths as a function of image paths
- sa, sb = os.sep + 'images' + os.sep, os.sep + 'labels' + os.sep # /images/, /labels/ substrings
- return [x.replace(sa, sb, 1).replace(os.path.splitext(x)[-1], '.txt') for x in img_paths]
-
- try:
- f = [] # image files
- for p in path if isinstance(path, list) else [path]:
- p = str(Path(p)) # os-agnostic
- parent = str(Path(p).parent) + os.sep
- if os.path.isfile(p): # file
- with open(p, 'r') as t:
- t = t.read().splitlines()
- f += [x.replace('./', parent) if x.startswith('./') else x for x in t] # local to global path
- elif os.path.isdir(p): # folder
- f += glob.iglob(p + os.sep + '*.*')
- else:
- raise Exception('%s does not exist' % p)
- self.img_files = sorted(
- [x.replace('/', os.sep) for x in f if os.path.splitext(x)[-1].lower() in img_formats])
- assert len(self.img_files) > 0, 'No images found'
- except Exception as e:
- raise Exception('Error loading data from %s: %s\nSee %s' % (path, e, help_url))
-
- # Check cache
- self.label_files = img2label_paths(self.img_files) # labels
- cache_path = str(Path(self.label_files[0]).parent) + '.cache' # cached labels
- if os.path.isfile(cache_path):
- cache = torch.load(cache_path) # load
- if cache['hash'] != get_hash(self.label_files + self.img_files): # dataset changed
- cache = self.cache_labels(cache_path) # re-cache
- else:
- cache = self.cache_labels(cache_path) # cache
-
- # Read cache
- cache.pop('hash') # remove hash
- labels, shapes = zip(*cache.values())
- self.labels = list(labels)
- self.shapes = np.array(shapes, dtype=np.float64)
- self.img_files = list(cache.keys()) # update
- self.label_files = img2label_paths(cache.keys()) # update
-
- n = len(shapes) # number of images
- bi = np.floor(np.arange(n) / batch_size).astype(np.int) # batch index
- nb = bi[-1] + 1 # number of batches
- self.batch = bi # batch index of image
- self.n = n
-
- # Rectangular Training
- if self.rect:
- # Sort by aspect ratio
- s = self.shapes # wh
- ar = s[:, 1] / s[:, 0] # aspect ratio
- irect = ar.argsort()
- self.img_files = [self.img_files[i] for i in irect]
- self.label_files = [self.label_files[i] for i in irect]
- self.labels = [self.labels[i] for i in irect]
- self.shapes = s[irect] # wh
- ar = ar[irect]
-
- # Set training image shapes
- shapes = [[1, 1]] * nb
- for i in range(nb):
- ari = ar[bi == i]
- mini, maxi = ari.min(), ari.max()
- if maxi < 1:
- shapes[i] = [maxi, 1]
- elif mini > 1:
- shapes[i] = [1, 1 / mini]
-
- self.batch_shapes = np.ceil(np.array(shapes) * img_size / stride + pad).astype(np.int) * stride
-
- # Check labels
- create_datasubset, extract_bounding_boxes, labels_loaded = False, False, False
- nm, nf, ne, ns, nd = 0, 0, 0, 0, 0 # number missing, found, empty, datasubset, duplicate
- pbar = enumerate(self.label_files)
- if rank in [-1, 0]:
- pbar = tqdm(pbar)
- for i, file in pbar:
- l = self.labels[i] # label
- if l is not None and l.shape[0]:
- assert l.shape[1] == 5, '> 5 label columns: %s' % file
- assert (l >= 0).all(), 'negative labels: %s' % file
- assert (l[:, 1:] <= 1).all(), 'non-normalized or out of bounds coordinate labels: %s' % file
- if np.unique(l, axis=0).shape[0] < l.shape[0]: # duplicate rows
- nd += 1 # print('WARNING: duplicate rows in %s' % self.label_files[i]) # duplicate rows
- if single_cls:
- l[:, 0] = 0 # force dataset into single-class mode
- self.labels[i] = l
- nf += 1 # file found
-
- # Create subdataset (a smaller dataset)
- if create_datasubset and ns < 1E4:
- if ns == 0:
- create_folder(path='./datasubset')
- os.makedirs('./datasubset/images')
- exclude_classes = 43
- if exclude_classes not in l[:, 0]:
- ns += 1
- # shutil.copy(src=self.img_files[i], dst='./datasubset/images/') # copy image
- with open('./datasubset/images.txt', 'a') as f:
- f.write(self.img_files[i] + '\n')
-
- # Extract object detection boxes for a second stage classifier
- if extract_bounding_boxes:
- p = Path(self.img_files[i])
- img = cv2.imread(str(p))
- h, w = img.shape[:2]
- for j, x in enumerate(l):
- f = '%s%sclassifier%s%g_%g_%s' % (p.parent.parent, os.sep, os.sep, x[0], j, p.name)
- if not os.path.exists(Path(f).parent):
- os.makedirs(Path(f).parent) # make new output folder
-
- b = x[1:] * [w, h, w, h] # box
- b[2:] = b[2:].max() # rectangle to square
- b[2:] = b[2:] * 1.3 + 30 # pad
- b = xywh2xyxy(b.reshape(-1, 4)).ravel().astype(np.int)
-
- b[[0, 2]] = np.clip(b[[0, 2]], 0, w) # clip boxes outside of image
- b[[1, 3]] = np.clip(b[[1, 3]], 0, h)
- assert cv2.imwrite(f, img[b[1]:b[3], b[0]:b[2]]), 'Failure extracting classifier boxes'
- else:
- ne += 1 # print('empty labels for image %s' % self.img_files[i]) # file empty
- # os.system("rm '%s' '%s'" % (self.img_files[i], self.label_files[i])) # remove
-
- if rank in [-1, 0]:
- pbar.desc = 'Scanning labels %s (%g found, %g missing, %g empty, %g duplicate, for %g images)' % (
- cache_path, nf, nm, ne, nd, n)
- if nf == 0:
- s = 'WARNING: No labels found in %s. See %s' % (os.path.dirname(file) + os.sep, help_url)
- print(s)
- assert not augment, '%s. Can not train without labels.' % s
-
- # Cache images into memory for faster training (WARNING: large datasets may exceed system RAM)
- self.imgs = [None] * n
- if cache_images:
- gb = 0 # Gigabytes of cached images
- pbar = tqdm(range(len(self.img_files)), desc='Caching images')
- self.img_hw0, self.img_hw = [None] * n, [None] * n
- for i in pbar: # max 10k images
- self.imgs[i], self.img_hw0[i], self.img_hw[i] = load_image(self, i) # img, hw_original, hw_resized
- gb += self.imgs[i].nbytes
- pbar.desc = 'Caching images (%.1fGB)' % (gb / 1E9)
-
- def cache_labels(self, path='labels.cache'):
- # Cache dataset labels, check images and read shapes
- x = {} # dict
- pbar = tqdm(zip(self.img_files, self.label_files), desc='Scanning images', total=len(self.img_files))
- for (img, label) in pbar:
- try:
- l = []
- im = Image.open(img)
- im.verify() # PIL verify
- shape = exif_size(im) # image size
- assert (shape[0] > 9) & (shape[1] > 9), 'image size <10 pixels'
- if os.path.isfile(label):
- with open(label, 'r') as f:
- l = np.array([x.split() for x in f.read().splitlines()], dtype=np.float32) # labels
- if len(l) == 0:
- l = np.zeros((0, 5), dtype=np.float32)
- x[img] = [l, shape]
- except Exception as e:
- print('WARNING: Ignoring corrupted image and/or label %s: %s' % (img, e))
-
- x['hash'] = get_hash(self.label_files + self.img_files)
- torch.save(x, path) # save for next time
- return x
-
- def __len__(self):
- return len(self.img_files)
-
- # def __iter__(self):
- # self.count = -1
- # print('ran dataset iter')
- # #self.shuffled_vector = np.random.permutation(self.nF) if self.augment else np.arange(self.nF)
- # return self
-
- def __getitem__(self, index):
- if self.image_weights:
- index = self.indices[index]
-
- hyp = self.hyp
- mosaic = self.mosaic and random.random() < hyp['mosaic']
- if mosaic:
- # Load mosaic
- img, labels = load_mosaic(self, index)
- shapes = None
-
- # MixUp https://arxiv.org/pdf/1710.09412.pdf
- if random.random() < hyp['mixup']:
- img2, labels2 = load_mosaic(self, random.randint(0, len(self.labels) - 1))
- r = np.random.beta(8.0, 8.0) # mixup ratio, alpha=beta=8.0
- img = (img * r + img2 * (1 - r)).astype(np.uint8)
- labels = np.concatenate((labels, labels2), 0)
-
- else:
- # Load image
- img, (h0, w0), (h, w) = load_image(self, index)
-
- # Letterbox
- shape = self.batch_shapes[self.batch[index]] if self.rect else self.img_size # final letterboxed shape
- img, ratio, pad = letterbox(img, shape, auto=False, scaleup=self.augment)
- shapes = (h0, w0), ((h / h0, w / w0), pad) # for COCO mAP rescaling
-
- # Load labels
- labels = []
- x = self.labels[index]
- if x.size > 0:
- # Normalized xywh to pixel xyxy format
- labels = x.copy()
- labels[:, 1] = ratio[0] * w * (x[:, 1] - x[:, 3] / 2) + pad[0] # pad width
- labels[:, 2] = ratio[1] * h * (x[:, 2] - x[:, 4] / 2) + pad[1] # pad height
- labels[:, 3] = ratio[0] * w * (x[:, 1] + x[:, 3] / 2) + pad[0]
- labels[:, 4] = ratio[1] * h * (x[:, 2] + x[:, 4] / 2) + pad[1]
-
- if self.augment:
- # Augment imagespace
- if not mosaic:
- img, labels = random_perspective(img, labels,
- degrees=hyp['degrees'],
- translate=hyp['translate'],
- scale=hyp['scale'],
- shear=hyp['shear'],
- perspective=hyp['perspective'])
-
- # Augment colorspace
- augment_hsv(img, hgain=hyp['hsv_h'], sgain=hyp['hsv_s'], vgain=hyp['hsv_v'])
-
- # Apply cutouts
- # if random.random() < 0.9:
- # labels = cutout(img, labels)
-
- nL = len(labels) # number of labels
- if nL:
- labels[:, 1:5] = xyxy2xywh(labels[:, 1:5]) # convert xyxy to xywh
- labels[:, [2, 4]] /= img.shape[0] # normalized height 0-1
- labels[:, [1, 3]] /= img.shape[1] # normalized width 0-1
-
- if self.augment:
- # flip up-down
- if random.random() < hyp['flipud']:
- img = np.flipud(img)
- if nL:
- labels[:, 2] = 1 - labels[:, 2]
-
- # flip left-right
- if random.random() < hyp['fliplr']:
- img = np.fliplr(img)
- if nL:
- labels[:, 1] = 1 - labels[:, 1]
-
- labels_out = torch.zeros((nL, 6))
- if nL:
- labels_out[:, 1:] = torch.from_numpy(labels)
-
- # Convert
- img = img[:, :, ::-1].transpose(2, 0, 1) # BGR to RGB, to 3x416x416
- img = np.ascontiguousarray(img)
-
- return torch.from_numpy(img), labels_out, self.img_files[index], shapes
-
- @staticmethod
- def collate_fn(batch):
- img, label, path, shapes = zip(*batch) # transposed
- for i, l in enumerate(label):
- l[:, 0] = i # add target image index for build_targets()
- return torch.stack(img, 0), torch.cat(label, 0), path, shapes
-
-
-# Ancillary functions --------------------------------------------------------------------------------------------------
-def load_image(self, index):
- # loads 1 image from dataset, returns img, original hw, resized hw
- img = self.imgs[index]
- if img is None: # not cached
- path = self.img_files[index]
- img = cv2.imread(path) # BGR
- assert img is not None, 'Image Not Found ' + path
- h0, w0 = img.shape[:2] # orig hw
- r = self.img_size / max(h0, w0) # resize image to img_size
- if r != 1: # always resize down, only resize up if training with augmentation
- interp = cv2.INTER_AREA if r < 1 and not self.augment else cv2.INTER_LINEAR
- img = cv2.resize(img, (int(w0 * r), int(h0 * r)), interpolation=interp)
- return img, (h0, w0), img.shape[:2] # img, hw_original, hw_resized
- else:
- return self.imgs[index], self.img_hw0[index], self.img_hw[index] # img, hw_original, hw_resized
-
-
-def augment_hsv(img, hgain=0.5, sgain=0.5, vgain=0.5):
- r = np.random.uniform(-1, 1, 3) * [hgain, sgain, vgain] + 1 # random gains
- hue, sat, val = cv2.split(cv2.cvtColor(img, cv2.COLOR_BGR2HSV))
- dtype = img.dtype # uint8
-
- x = np.arange(0, 256, dtype=np.int16)
- lut_hue = ((x * r[0]) % 180).astype(dtype)
- lut_sat = np.clip(x * r[1], 0, 255).astype(dtype)
- lut_val = np.clip(x * r[2], 0, 255).astype(dtype)
-
- img_hsv = cv2.merge((cv2.LUT(hue, lut_hue), cv2.LUT(sat, lut_sat), cv2.LUT(val, lut_val))).astype(dtype)
- cv2.cvtColor(img_hsv, cv2.COLOR_HSV2BGR, dst=img) # no return needed
-
- # Histogram equalization
- # if random.random() < 0.2:
- # for i in range(3):
- # img[:, :, i] = cv2.equalizeHist(img[:, :, i])
-
-
-def load_mosaic(self, index):
- # loads images in a mosaic
-
- labels4 = []
- s = self.img_size
- yc, xc = [int(random.uniform(-x, 2 * s + x)) for x in self.mosaic_border] # mosaic center x, y
- indices = [index] + [random.randint(0, len(self.labels) - 1) for _ in range(3)] # 3 additional image indices
- for i, index in enumerate(indices):
- # Load image
- img, _, (h, w) = load_image(self, index)
-
- # place img in img4
- if i == 0: # top left
- img4 = np.full((s * 2, s * 2, img.shape[2]), 114, dtype=np.uint8) # base image with 4 tiles
- x1a, y1a, x2a, y2a = max(xc - w, 0), max(yc - h, 0), xc, yc # xmin, ymin, xmax, ymax (large image)
- x1b, y1b, x2b, y2b = w - (x2a - x1a), h - (y2a - y1a), w, h # xmin, ymin, xmax, ymax (small image)
- elif i == 1: # top right
- x1a, y1a, x2a, y2a = xc, max(yc - h, 0), min(xc + w, s * 2), yc
- x1b, y1b, x2b, y2b = 0, h - (y2a - y1a), min(w, x2a - x1a), h
- elif i == 2: # bottom left
- x1a, y1a, x2a, y2a = max(xc - w, 0), yc, xc, min(s * 2, yc + h)
- x1b, y1b, x2b, y2b = w - (x2a - x1a), 0, w, min(y2a - y1a, h)
- elif i == 3: # bottom right
- x1a, y1a, x2a, y2a = xc, yc, min(xc + w, s * 2), min(s * 2, yc + h)
- x1b, y1b, x2b, y2b = 0, 0, min(w, x2a - x1a), min(y2a - y1a, h)
-
- img4[y1a:y2a, x1a:x2a] = img[y1b:y2b, x1b:x2b] # img4[ymin:ymax, xmin:xmax]
- padw = x1a - x1b
- padh = y1a - y1b
-
- # Labels
- x = self.labels[index]
- labels = x.copy()
- if x.size > 0: # Normalized xywh to pixel xyxy format
- labels[:, 1] = w * (x[:, 1] - x[:, 3] / 2) + padw
- labels[:, 2] = h * (x[:, 2] - x[:, 4] / 2) + padh
- labels[:, 3] = w * (x[:, 1] + x[:, 3] / 2) + padw
- labels[:, 4] = h * (x[:, 2] + x[:, 4] / 2) + padh
- labels4.append(labels)
-
- # Concat/clip labels
- if len(labels4):
- labels4 = np.concatenate(labels4, 0)
- np.clip(labels4[:, 1:], 0, 2 * s, out=labels4[:, 1:]) # use with random_perspective
- # img4, labels4 = replicate(img4, labels4) # replicate
-
- # Augment
- img4, labels4 = random_perspective(img4, labels4,
- degrees=self.hyp['degrees'],
- translate=self.hyp['translate'],
- scale=self.hyp['scale'],
- shear=self.hyp['shear'],
- perspective=self.hyp['perspective'],
- border=self.mosaic_border) # border to remove
-
- return img4, labels4
-
-
-def replicate(img, labels):
- # Replicate labels
- h, w = img.shape[:2]
- boxes = labels[:, 1:].astype(int)
- x1, y1, x2, y2 = boxes.T
- s = ((x2 - x1) + (y2 - y1)) / 2 # side length (pixels)
- for i in s.argsort()[:round(s.size * 0.5)]: # smallest indices
- x1b, y1b, x2b, y2b = boxes[i]
- bh, bw = y2b - y1b, x2b - x1b
- yc, xc = int(random.uniform(0, h - bh)), int(random.uniform(0, w - bw)) # offset x, y
- x1a, y1a, x2a, y2a = [xc, yc, xc + bw, yc + bh]
- img[y1a:y2a, x1a:x2a] = img[y1b:y2b, x1b:x2b] # img4[ymin:ymax, xmin:xmax]
- labels = np.append(labels, [[labels[i, 0], x1a, y1a, x2a, y2a]], axis=0)
-
- return img, labels
-
-
-def letterbox(img, new_shape=(640, 640), color=(114, 114, 114), auto=True, scaleFill=False, scaleup=True):
- # Resize image to a 32-pixel-multiple rectangle https://github.com/ultralytics/yolov3/issues/232
- shape = img.shape[:2] # current shape [height, width]
- if isinstance(new_shape, int):
- new_shape = (new_shape, new_shape)
-
- # Scale ratio (new / old)
- r = min(new_shape[0] / shape[0], new_shape[1] / shape[1])
- if not scaleup: # only scale down, do not scale up (for better test mAP)
- r = min(r, 1.0)
-
- # Compute padding
- ratio = r, r # width, height ratios
- new_unpad = int(round(shape[1] * r)), int(round(shape[0] * r))
- dw, dh = new_shape[1] - new_unpad[0], new_shape[0] - new_unpad[1] # wh padding
- if auto: # minimum rectangle
- dw, dh = np.mod(dw, 32), np.mod(dh, 32) # wh padding
- elif scaleFill: # stretch
- dw, dh = 0.0, 0.0
- new_unpad = (new_shape[1], new_shape[0])
- ratio = new_shape[1] / shape[1], new_shape[0] / shape[0] # width, height ratios
-
- dw /= 2 # divide padding into 2 sides
- dh /= 2
-
- if shape[::-1] != new_unpad: # resize
- img = cv2.resize(img, new_unpad, interpolation=cv2.INTER_LINEAR)
- top, bottom = int(round(dh - 0.1)), int(round(dh + 0.1))
- left, right = int(round(dw - 0.1)), int(round(dw + 0.1))
- img = cv2.copyMakeBorder(img, top, bottom, left, right, cv2.BORDER_CONSTANT, value=color) # add border
- return img, ratio, (dw, dh)
-
-
-def random_perspective(img, targets=(), degrees=10, translate=.1, scale=.1, shear=10, perspective=0.0, border=(0, 0)):
- # torchvision.transforms.RandomAffine(degrees=(-10, 10), translate=(.1, .1), scale=(.9, 1.1), shear=(-10, 10))
- # targets = [cls, xyxy]
-
- height = img.shape[0] + border[0] * 2 # shape(h,w,c)
- width = img.shape[1] + border[1] * 2
-
- # Center
- C = np.eye(3)
- C[0, 2] = -img.shape[1] / 2 # x translation (pixels)
- C[1, 2] = -img.shape[0] / 2 # y translation (pixels)
-
- # Perspective
- P = np.eye(3)
- P[2, 0] = random.uniform(-perspective, perspective) # x perspective (about y)
- P[2, 1] = random.uniform(-perspective, perspective) # y perspective (about x)
-
- # Rotation and Scale
- R = np.eye(3)
- a = random.uniform(-degrees, degrees)
- # a += random.choice([-180, -90, 0, 90]) # add 90deg rotations to small rotations
- s = random.uniform(1 - scale, 1 + scale)
- # s = 2 ** random.uniform(-scale, scale)
- R[:2] = cv2.getRotationMatrix2D(angle=a, center=(0, 0), scale=s)
-
- # Shear
- S = np.eye(3)
- S[0, 1] = math.tan(random.uniform(-shear, shear) * math.pi / 180) # x shear (deg)
- S[1, 0] = math.tan(random.uniform(-shear, shear) * math.pi / 180) # y shear (deg)
-
- # Translation
- T = np.eye(3)
- T[0, 2] = random.uniform(0.5 - translate, 0.5 + translate) * width # x translation (pixels)
- T[1, 2] = random.uniform(0.5 - translate, 0.5 + translate) * height # y translation (pixels)
-
- # Combined rotation matrix
- M = T @ S @ R @ P @ C # order of operations (right to left) is IMPORTANT
- if (border[0] != 0) or (border[1] != 0) or (M != np.eye(3)).any(): # image changed
- if perspective:
- img = cv2.warpPerspective(img, M, dsize=(width, height), borderValue=(114, 114, 114))
- else: # affine
- img = cv2.warpAffine(img, M[:2], dsize=(width, height), borderValue=(114, 114, 114))
-
- # Visualize
- # import matplotlib.pyplot as plt
- # ax = plt.subplots(1, 2, figsize=(12, 6))[1].ravel()
- # ax[0].imshow(img[:, :, ::-1]) # base
- # ax[1].imshow(img2[:, :, ::-1]) # warped
-
- # Transform label coordinates
- n = len(targets)
- if n:
- # warp points
- xy = np.ones((n * 4, 3))
- xy[:, :2] = targets[:, [1, 2, 3, 4, 1, 4, 3, 2]].reshape(n * 4, 2) # x1y1, x2y2, x1y2, x2y1
- xy = xy @ M.T # transform
- if perspective:
- xy = (xy[:, :2] / xy[:, 2:3]).reshape(n, 8) # rescale
- else: # affine
- xy = xy[:, :2].reshape(n, 8)
-
- # create new boxes
- x = xy[:, [0, 2, 4, 6]]
- y = xy[:, [1, 3, 5, 7]]
- xy = np.concatenate((x.min(1), y.min(1), x.max(1), y.max(1))).reshape(4, n).T
-
- # # apply angle-based reduction of bounding boxes
- # radians = a * math.pi / 180
- # reduction = max(abs(math.sin(radians)), abs(math.cos(radians))) ** 0.5
- # x = (xy[:, 2] + xy[:, 0]) / 2
- # y = (xy[:, 3] + xy[:, 1]) / 2
- # w = (xy[:, 2] - xy[:, 0]) * reduction
- # h = (xy[:, 3] - xy[:, 1]) * reduction
- # xy = np.concatenate((x - w / 2, y - h / 2, x + w / 2, y + h / 2)).reshape(4, n).T
-
- # clip boxes
- xy[:, [0, 2]] = xy[:, [0, 2]].clip(0, width)
- xy[:, [1, 3]] = xy[:, [1, 3]].clip(0, height)
-
- # filter candidates
- i = box_candidates(box1=targets[:, 1:5].T * s, box2=xy.T)
- targets = targets[i]
- targets[:, 1:5] = xy[i]
-
- return img, targets
-
-
-def box_candidates(box1, box2, wh_thr=2, ar_thr=20, area_thr=0.1): # box1(4,n), box2(4,n)
- # Compute candidate boxes: box1 before augment, box2 after augment, wh_thr (pixels), aspect_ratio_thr, area_ratio
- w1, h1 = box1[2] - box1[0], box1[3] - box1[1]
- w2, h2 = box2[2] - box2[0], box2[3] - box2[1]
- ar = np.maximum(w2 / (h2 + 1e-16), h2 / (w2 + 1e-16)) # aspect ratio
- return (w2 > wh_thr) & (h2 > wh_thr) & (w2 * h2 / (w1 * h1 + 1e-16) > area_thr) & (ar < ar_thr) # candidates
-
-
-def cutout(image, labels):
- # Applies image cutout augmentation https://arxiv.org/abs/1708.04552
- h, w = image.shape[:2]
-
- def bbox_ioa(box1, box2):
- # Returns the intersection over box2 area given box1, box2. box1 is 4, box2 is nx4. boxes are x1y1x2y2
- box2 = box2.transpose()
-
- # Get the coordinates of bounding boxes
- b1_x1, b1_y1, b1_x2, b1_y2 = box1[0], box1[1], box1[2], box1[3]
- b2_x1, b2_y1, b2_x2, b2_y2 = box2[0], box2[1], box2[2], box2[3]
-
- # Intersection area
- inter_area = (np.minimum(b1_x2, b2_x2) - np.maximum(b1_x1, b2_x1)).clip(0) * \
- (np.minimum(b1_y2, b2_y2) - np.maximum(b1_y1, b2_y1)).clip(0)
-
- # box2 area
- box2_area = (b2_x2 - b2_x1) * (b2_y2 - b2_y1) + 1e-16
-
- # Intersection over box2 area
- return inter_area / box2_area
-
- # create random masks
- scales = [0.5] * 1 + [0.25] * 2 + [0.125] * 4 + [0.0625] * 8 + [0.03125] * 16 # image size fraction
- for s in scales:
- mask_h = random.randint(1, int(h * s))
- mask_w = random.randint(1, int(w * s))
-
- # box
- xmin = max(0, random.randint(0, w) - mask_w // 2)
- ymin = max(0, random.randint(0, h) - mask_h // 2)
- xmax = min(w, xmin + mask_w)
- ymax = min(h, ymin + mask_h)
-
- # apply random color mask
- image[ymin:ymax, xmin:xmax] = [random.randint(64, 191) for _ in range(3)]
-
- # return unobscured labels
- if len(labels) and s > 0.03:
- box = np.array([xmin, ymin, xmax, ymax], dtype=np.float32)
- ioa = bbox_ioa(box, labels[:, 1:5]) # intersection over area
- labels = labels[ioa < 0.60] # remove >60% obscured labels
-
- return labels
-
-
-def reduce_img_size(path='path/images', img_size=1024): # from utils.datasets import *; reduce_img_size()
- # creates a new ./images_reduced folder with reduced size images of maximum size img_size
- path_new = path + '_reduced' # reduced images path
- create_folder(path_new)
- for f in tqdm(glob.glob('%s/*.*' % path)):
- try:
- img = cv2.imread(f)
- h, w = img.shape[:2]
- r = img_size / max(h, w) # size ratio
- if r < 1.0:
- img = cv2.resize(img, (int(w * r), int(h * r)), interpolation=cv2.INTER_AREA) # _LINEAR fastest
- fnew = f.replace(path, path_new) # .replace(Path(f).suffix, '.jpg')
- cv2.imwrite(fnew, img)
- except:
- print('WARNING: image failure %s' % f)
-
-
-def recursive_dataset2bmp(dataset='path/dataset_bmp'): # from utils.datasets import *; recursive_dataset2bmp()
- # Converts dataset to bmp (for faster training)
- formats = [x.lower() for x in img_formats] + [x.upper() for x in img_formats]
- for a, b, files in os.walk(dataset):
- for file in tqdm(files, desc=a):
- p = a + '/' + file
- s = Path(file).suffix
- if s == '.txt': # replace text
- with open(p, 'r') as f:
- lines = f.read()
- for f in formats:
- lines = lines.replace(f, '.bmp')
- with open(p, 'w') as f:
- f.write(lines)
- elif s in formats: # replace image
- cv2.imwrite(p.replace(s, '.bmp'), cv2.imread(p))
- if s != '.bmp':
- os.system("rm '%s'" % p)
-
-
-def imagelist2folder(path='path/images.txt'): # from utils.datasets import *; imagelist2folder()
- # Copies all the images in a text file (list of images) into a folder
- create_folder(path[:-4])
- with open(path, 'r') as f:
- for line in f.read().splitlines():
- os.system('cp "%s" %s' % (line, path[:-4]))
- print(line)
-
-
-def create_folder(path='./new'):
- # Create folder
- if os.path.exists(path):
- shutil.rmtree(path) # delete output folder
- os.makedirs(path) # make new output folder
diff --git a/spaces/EuroPython2022/mediapipe-hands/README.md b/spaces/EuroPython2022/mediapipe-hands/README.md
deleted file mode 100644
index f0d06e69d3067f51eb77376f938203a606ce59d5..0000000000000000000000000000000000000000
--- a/spaces/EuroPython2022/mediapipe-hands/README.md
+++ /dev/null
@@ -1,12 +0,0 @@
----
-title: MediaPipe's Hand & Finger Tracking
-emoji: 🙌
-colorFrom: pink
-colorTo: purple
-sdk: gradio
-sdk_version: 3.0.26
-app_file: app.py
-pinned: false
----
-
-Check out the configuration reference at https://huggingface.co/docs/hub/spaces-config-reference
diff --git a/spaces/FYP-23-S1-21/Refineverse_Plugin/templates/RefineverseDashboardUI.html b/spaces/FYP-23-S1-21/Refineverse_Plugin/templates/RefineverseDashboardUI.html
deleted file mode 100644
index 9bf730c9e6273c72190be10e317a6ee14e9bece9..0000000000000000000000000000000000000000
--- a/spaces/FYP-23-S1-21/Refineverse_Plugin/templates/RefineverseDashboardUI.html
+++ /dev/null
@@ -1,57 +0,0 @@
-
-
-
- DashboardUI
-
-
-
-
-
- User Story Refinement
-
-
-
-
-
-
-
-
-
-
- User Story Summarization
- With our plugin's natural language processing capabilities, you can now generate a concise summary of your user story with just a few clicks. No more sifting through lengthy descriptions or struggling to communicate the essence of your user story to team members. Our plugin does the heavy lifting for you!
-
-
-
- Project Task Breakdown
- With our plugin's natural language processing capabilities, our project task breakdown feature allows you to reduce a user story into multiple project tasks, for easier allocation of tasks to project members within a team. No more having to think of project tasks for team allocation.
-
-
-
-
-
-
-
-
-
-
-
- Language Translation
- With our plugin's advanced natural language processing capabilities, our language translation feature provides customizable translation options, allowing you to choose which languages you want to translate your user stories into. Allowing team members who speak different languages to collaborate more effectively and improving productivity.
-
-
- User Story Generation
- With our plugin's advanced natural language processing capabilities, our User Story Generation feature provides a powerful solution for agile teams seeking to streamline their project and user story refinement process. With our User Story Generation feature, you can easily create new user stories via a single prompt, and the feature will generate a suggested user story for you. Farewell to the hassle of manual user story creation!
-
-
-
-
-
-
-
\ No newline at end of file
diff --git a/spaces/Ferion/image-matting-app/ppmatting/__init__.py b/spaces/Ferion/image-matting-app/ppmatting/__init__.py
deleted file mode 100644
index c1094808e27aa683fc3b5766e9968712b3021532..0000000000000000000000000000000000000000
--- a/spaces/Ferion/image-matting-app/ppmatting/__init__.py
+++ /dev/null
@@ -1 +0,0 @@
-from . import ml, metrics, transforms, datasets, models
diff --git a/spaces/GIanlucaRub/DoubleResolution/README.md b/spaces/GIanlucaRub/DoubleResolution/README.md
deleted file mode 100644
index 293c625df81ac1e0fa1911c8aa7d2e746ea0ca8d..0000000000000000000000000000000000000000
--- a/spaces/GIanlucaRub/DoubleResolution/README.md
+++ /dev/null
@@ -1,12 +0,0 @@
----
-title: DoubleResolution
-emoji: 🐢
-colorFrom: red
-colorTo: red
-sdk: gradio
-sdk_version: 3.15.0
-app_file: app.py
-pinned: false
----
-
-Check out the configuration reference at https://huggingface.co/docs/hub/spaces-config-reference
diff --git a/spaces/Gameel/TextToSpeech/app.py b/spaces/Gameel/TextToSpeech/app.py
deleted file mode 100644
index f2d8e3c7c3f91c69bef66da7d4f8a87aaf0e4b7b..0000000000000000000000000000000000000000
--- a/spaces/Gameel/TextToSpeech/app.py
+++ /dev/null
@@ -1,6 +0,0 @@
-import gradio as gr
-gr.Interface.load("huggingface/facebook/fastspeech2-en-ljspeech",
- description="TTS using FastSpeech2",
- title="Text to Speech (TTS)",
- examples=[["The quick brown fox jumps over the lazy dog."]]
- ).launch()
\ No newline at end of file
diff --git a/spaces/GolDNenex/Super-Resolution-Anime-Diffusion/Waifu2x/utils/__init__.py b/spaces/GolDNenex/Super-Resolution-Anime-Diffusion/Waifu2x/utils/__init__.py
deleted file mode 100644
index fb1623a14865e1d1b1e79275a3d5595642f92d9b..0000000000000000000000000000000000000000
--- a/spaces/GolDNenex/Super-Resolution-Anime-Diffusion/Waifu2x/utils/__init__.py
+++ /dev/null
@@ -1,8 +0,0 @@
-# -*- coding: utf-8 -*-
-# file: __init__.py
-# time: 05/12/2022
-# author: yangheng
-# github: https://github.com/yangheng95
-# huggingface: https://huggingface.co/yangheng
-# google scholar: https://scholar.google.com/citations?user=NPq5a_0AAAAJ&hl=en
-# Copyright (C) 2021. All Rights Reserved.
diff --git a/spaces/Goutam982/RVC_V2_voice_clone/config.py b/spaces/Goutam982/RVC_V2_voice_clone/config.py
deleted file mode 100644
index 5b72235b58b65ac629f49bcc4aad032b5b59d8d4..0000000000000000000000000000000000000000
--- a/spaces/Goutam982/RVC_V2_voice_clone/config.py
+++ /dev/null
@@ -1,204 +0,0 @@
-import argparse
-import sys
-import torch
-import json
-from multiprocessing import cpu_count
-
-global usefp16
-usefp16 = False
-
-
-def use_fp32_config():
- usefp16 = False
- device_capability = 0
- if torch.cuda.is_available():
- device = torch.device("cuda:0") # Assuming you have only one GPU (index 0).
- device_capability = torch.cuda.get_device_capability(device)[0]
- if device_capability >= 7:
- usefp16 = True
- for config_file in ["32k.json", "40k.json", "48k.json"]:
- with open(f"configs/{config_file}", "r") as d:
- data = json.load(d)
-
- if "train" in data and "fp16_run" in data["train"]:
- data["train"]["fp16_run"] = True
-
- with open(f"configs/{config_file}", "w") as d:
- json.dump(data, d, indent=4)
-
- print(f"Set fp16_run to true in {config_file}")
-
- with open(
- "trainset_preprocess_pipeline_print.py", "r", encoding="utf-8"
- ) as f:
- strr = f.read()
-
- strr = strr.replace("3.0", "3.7")
-
- with open(
- "trainset_preprocess_pipeline_print.py", "w", encoding="utf-8"
- ) as f:
- f.write(strr)
- else:
- for config_file in ["32k.json", "40k.json", "48k.json"]:
- with open(f"configs/{config_file}", "r") as f:
- data = json.load(f)
-
- if "train" in data and "fp16_run" in data["train"]:
- data["train"]["fp16_run"] = False
-
- with open(f"configs/{config_file}", "w") as d:
- json.dump(data, d, indent=4)
-
- print(f"Set fp16_run to false in {config_file}")
-
- with open(
- "trainset_preprocess_pipeline_print.py", "r", encoding="utf-8"
- ) as f:
- strr = f.read()
-
- strr = strr.replace("3.7", "3.0")
-
- with open(
- "trainset_preprocess_pipeline_print.py", "w", encoding="utf-8"
- ) as f:
- f.write(strr)
- else:
- print(
- "CUDA is not available. Make sure you have an NVIDIA GPU and CUDA installed."
- )
- return (usefp16, device_capability)
-
-
-class Config:
- def __init__(self):
- self.device = "cuda:0"
- self.is_half = True
- self.n_cpu = 0
- self.gpu_name = None
- self.gpu_mem = None
- (
- self.python_cmd,
- self.listen_port,
- self.iscolab,
- self.noparallel,
- self.noautoopen,
- self.paperspace,
- self.is_cli,
- ) = self.arg_parse()
-
- self.x_pad, self.x_query, self.x_center, self.x_max = self.device_config()
-
- @staticmethod
- def arg_parse() -> tuple:
- exe = sys.executable or "python"
- parser = argparse.ArgumentParser()
- parser.add_argument("--port", type=int, default=7865, help="Listen port")
- parser.add_argument("--pycmd", type=str, default=exe, help="Python command")
- parser.add_argument("--colab", action="store_true", help="Launch in colab")
- parser.add_argument(
- "--noparallel", action="store_true", help="Disable parallel processing"
- )
- parser.add_argument(
- "--noautoopen",
- action="store_true",
- help="Do not open in browser automatically",
- )
- parser.add_argument( # Fork Feature. Paperspace integration for web UI
- "--paperspace",
- action="store_true",
- help="Note that this argument just shares a gradio link for the web UI. Thus can be used on other non-local CLI systems.",
- )
- parser.add_argument( # Fork Feature. Embed a CLI into the infer-web.py
- "--is_cli",
- action="store_true",
- help="Use the CLI instead of setting up a gradio UI. This flag will launch an RVC text interface where you can execute functions from infer-web.py!",
- )
- cmd_opts = parser.parse_args()
-
- cmd_opts.port = cmd_opts.port if 0 <= cmd_opts.port <= 65535 else 7865
-
- return (
- cmd_opts.pycmd,
- cmd_opts.port,
- cmd_opts.colab,
- cmd_opts.noparallel,
- cmd_opts.noautoopen,
- cmd_opts.paperspace,
- cmd_opts.is_cli,
- )
-
- # has_mps is only available in nightly pytorch (for now) and MasOS 12.3+.
- # check `getattr` and try it for compatibility
- @staticmethod
- def has_mps() -> bool:
- if not torch.backends.mps.is_available():
- return False
- try:
- torch.zeros(1).to(torch.device("mps"))
- return True
- except Exception:
- return False
-
- def device_config(self) -> tuple:
- if torch.cuda.is_available():
- i_device = int(self.device.split(":")[-1])
- self.gpu_name = torch.cuda.get_device_name(i_device)
- if (
- ("16" in self.gpu_name and "V100" not in self.gpu_name.upper())
- or "P40" in self.gpu_name.upper()
- or "1060" in self.gpu_name
- or "1070" in self.gpu_name
- or "1080" in self.gpu_name
- ):
- print("Found GPU", self.gpu_name, ", force to fp32")
- self.is_half = False
- else:
- print("Found GPU", self.gpu_name)
- use_fp32_config()
- self.gpu_mem = int(
- torch.cuda.get_device_properties(i_device).total_memory
- / 1024
- / 1024
- / 1024
- + 0.4
- )
- if self.gpu_mem <= 4:
- with open("trainset_preprocess_pipeline_print.py", "r") as f:
- strr = f.read().replace("3.7", "3.0")
- with open("trainset_preprocess_pipeline_print.py", "w") as f:
- f.write(strr)
- elif self.has_mps():
- print("No supported Nvidia GPU found, use MPS instead")
- self.device = "mps"
- self.is_half = False
- use_fp32_config()
- else:
- print("No supported Nvidia GPU found, use CPU instead")
- self.device = "cpu"
- self.is_half = False
- use_fp32_config()
-
- if self.n_cpu == 0:
- self.n_cpu = cpu_count()
-
- if self.is_half:
- # 6G显存配置
- x_pad = 3
- x_query = 10
- x_center = 60
- x_max = 65
- else:
- # 5G显存配置
- x_pad = 1
- x_query = 6
- x_center = 38
- x_max = 41
-
- if self.gpu_mem != None and self.gpu_mem <= 4:
- x_pad = 1
- x_query = 5
- x_center = 30
- x_max = 32
-
- return x_pad, x_query, x_center, x_max
diff --git a/spaces/Gradio-Blocks/uniformer_image_detection/configs/ghm/retinanet_ghm_x101_32x4d_fpn_1x_coco.py b/spaces/Gradio-Blocks/uniformer_image_detection/configs/ghm/retinanet_ghm_x101_32x4d_fpn_1x_coco.py
deleted file mode 100644
index a89fc1389ce0f1f9712b4b5d684e632aaee25ce8..0000000000000000000000000000000000000000
--- a/spaces/Gradio-Blocks/uniformer_image_detection/configs/ghm/retinanet_ghm_x101_32x4d_fpn_1x_coco.py
+++ /dev/null
@@ -1,13 +0,0 @@
-_base_ = './retinanet_ghm_r50_fpn_1x_coco.py'
-model = dict(
- pretrained='open-mmlab://resnext101_32x4d',
- backbone=dict(
- type='ResNeXt',
- depth=101,
- groups=32,
- base_width=4,
- num_stages=4,
- out_indices=(0, 1, 2, 3),
- frozen_stages=1,
- norm_cfg=dict(type='BN', requires_grad=True),
- style='pytorch'))
diff --git a/spaces/Gradio-Blocks/uniformer_image_detection/configs/scnet/scnet_x101_64x4d_fpn_20e_coco.py b/spaces/Gradio-Blocks/uniformer_image_detection/configs/scnet/scnet_x101_64x4d_fpn_20e_coco.py
deleted file mode 100644
index a0ff32ba9f6e69a039db3344c6742b4f619f6d36..0000000000000000000000000000000000000000
--- a/spaces/Gradio-Blocks/uniformer_image_detection/configs/scnet/scnet_x101_64x4d_fpn_20e_coco.py
+++ /dev/null
@@ -1,14 +0,0 @@
-_base_ = './scnet_r50_fpn_20e_coco.py'
-model = dict(
- pretrained='open-mmlab://resnext101_64x4d',
- backbone=dict(
- type='ResNeXt',
- depth=101,
- groups=64,
- base_width=4,
- num_stages=4,
- out_indices=(0, 1, 2, 3),
- frozen_stages=1,
- norm_cfg=dict(type='BN', requires_grad=True),
- norm_eval=True,
- style='pytorch'))
diff --git a/spaces/Gradio-Blocks/uniformer_image_segmentation/configs/fcn/fcn_r101-d8_480x480_40k_pascal_context_59.py b/spaces/Gradio-Blocks/uniformer_image_segmentation/configs/fcn/fcn_r101-d8_480x480_40k_pascal_context_59.py
deleted file mode 100644
index 908f4bff0062e06ce1607c55827aac9fe5b1c354..0000000000000000000000000000000000000000
--- a/spaces/Gradio-Blocks/uniformer_image_segmentation/configs/fcn/fcn_r101-d8_480x480_40k_pascal_context_59.py
+++ /dev/null
@@ -1,2 +0,0 @@
-_base_ = './fcn_r50-d8_480x480_40k_pascal_context_59.py'
-model = dict(pretrained='open-mmlab://resnet101_v1c', backbone=dict(depth=101))
diff --git a/spaces/HCMUT-GraduateThesis-HNTThinh/rgbdsod-multimae-demo/s_multimae/logger_fn.py b/spaces/HCMUT-GraduateThesis-HNTThinh/rgbdsod-multimae-demo/s_multimae/logger_fn.py
deleted file mode 100644
index e26b12c4ae5d6660c7068ea61150483d816a13de..0000000000000000000000000000000000000000
--- a/spaces/HCMUT-GraduateThesis-HNTThinh/rgbdsod-multimae-demo/s_multimae/logger_fn.py
+++ /dev/null
@@ -1,63 +0,0 @@
-import logging
-from logging import Logger
-import functools
-from termcolor import colored
-import os, sys
-from logging import Logger
-import wandb
-
-from .dataloader import WarmupDataLoader
-from .configs.base_config import base_cfg
-
-@functools.lru_cache()
-def create_logger(output_dir: str, name: str = '') -> Logger:
- os.makedirs(output_dir, exist_ok=True)
-
- # create logger
- logger = logging.getLogger(name)
- logger.setLevel(logging.DEBUG)
- logger.propagate = False
-
- # create formatter
- fmt = '[%(asctime)s %(name)s] (%(filename)s %(lineno)d): %(levelname)s %(message)s'
- color_fmt = colored('[%(asctime)s %(name)s]', 'green') + \
- colored('(%(filename)s %(lineno)d)', 'yellow') + ': %(levelname)s %(message)s'
-
- # create console handlers for master process
- console_handler = logging.StreamHandler(sys.stdout)
- console_handler.setLevel(logging.DEBUG)
- console_handler.setFormatter(logging.Formatter(fmt=color_fmt, datefmt='%Y-%m-%d %H:%M:%S'))
- logger.addHandler(console_handler)
-
- # create file handlers
- file_handler = logging.FileHandler(os.path.join(output_dir, f'log.txt'), mode='a')
- file_handler.setLevel(logging.DEBUG)
- file_handler.setFormatter(logging.Formatter(fmt=fmt, datefmt='%Y-%m-%d %H:%M:%S'))
- logger.addHandler(file_handler)
-
- return logger
-
-def train_epoch_log(
- cfg: base_cfg,
- epoch: int,
- logger: Logger,
- batch_idx: int,
- lr: float,
- loss: float,
- sum_loss: float,
- warmup_dataloader: WarmupDataLoader,
-) -> None:
- print_str = 'Epoch {}/{}'.format(epoch, cfg.nepochs) \
- + ' Iter {}/{}:'.format(batch_idx + 1, cfg.niters_per_epoch) \
- + ' lr=%.4e' % (lr * cfg.lr_scale) \
- + ' loss=%.4f total_loss=%.4f' % (loss, (sum_loss / batch_idx))
- logger.info(print_str)
-
- wandb.log({
- 'loss': loss,
- 'avg_loss': sum_loss / batch_idx,
- 'lr': (lr * cfg.lr_scale),
- 'batch_size': warmup_dataloader.batch_size,
- # 'inputs': wandb.Image(images),
- # "loss_hist": wandb.Histogram(ouputs),
- })
\ No newline at end of file
diff --git a/spaces/HCMUT-GraduateThesis-HNTThinh/rgbdsod-multimae-demo/s_multimae/visualizer.py b/spaces/HCMUT-GraduateThesis-HNTThinh/rgbdsod-multimae-demo/s_multimae/visualizer.py
deleted file mode 100644
index 0d3b86c70d2c01d8857939a19e50499b8b4126ee..0000000000000000000000000000000000000000
--- a/spaces/HCMUT-GraduateThesis-HNTThinh/rgbdsod-multimae-demo/s_multimae/visualizer.py
+++ /dev/null
@@ -1,517 +0,0 @@
-import colorsys
-from typing import Union
-import numpy as np
-import cv2
-import matplotlib.colors as mplc
-import pycocotools.mask as mask_util
-import matplotlib.figure as mplfigure
-from matplotlib.backends.backend_agg import FigureCanvasAgg
-import matplotlib as mpl
-from enum import Enum, unique
-from PIL import Image
-
-_LARGE_MASK_AREA_THRESH = 120000
-_COLORS = np.array(
- [
- 0.000, 0.447, 0.741,
- 0.850, 0.325, 0.098,
- 0.929, 0.694, 0.125,
- 0.494, 0.184, 0.556,
- 0.466, 0.674, 0.188,
- 0.301, 0.745, 0.933,
- 0.635, 0.078, 0.184,
- 0.300, 0.300, 0.300,
- 0.600, 0.600, 0.600,
- 1.000, 0.000, 0.000,
- 1.000, 0.500, 0.000,
- 0.749, 0.749, 0.000,
- 0.000, 1.000, 0.000,
- 0.000, 0.000, 1.000,
- 0.667, 0.000, 1.000,
- 0.333, 0.333, 0.000,
- 0.333, 0.667, 0.000,
- 0.333, 1.000, 0.000,
- 0.667, 0.333, 0.000,
- 0.667, 0.667, 0.000,
- 0.667, 1.000, 0.000,
- 1.000, 0.333, 0.000,
- 1.000, 0.667, 0.000,
- 1.000, 1.000, 0.000,
- 0.000, 0.333, 0.500,
- 0.000, 0.667, 0.500,
- 0.000, 1.000, 0.500,
- 0.333, 0.000, 0.500,
- 0.333, 0.333, 0.500,
- 0.333, 0.667, 0.500,
- 0.333, 1.000, 0.500,
- 0.667, 0.000, 0.500,
- 0.667, 0.333, 0.500,
- 0.667, 0.667, 0.500,
- 0.667, 1.000, 0.500,
- 1.000, 0.000, 0.500,
- 1.000, 0.333, 0.500,
- 1.000, 0.667, 0.500,
- 1.000, 1.000, 0.500,
- 0.000, 0.333, 1.000,
- 0.000, 0.667, 1.000,
- 0.000, 1.000, 1.000,
- 0.333, 0.000, 1.000,
- 0.333, 0.333, 1.000,
- 0.333, 0.667, 1.000,
- 0.333, 1.000, 1.000,
- 0.667, 0.000, 1.000,
- 0.667, 0.333, 1.000,
- 0.667, 0.667, 1.000,
- 0.667, 1.000, 1.000,
- 1.000, 0.000, 1.000,
- 1.000, 0.333, 1.000,
- 1.000, 0.667, 1.000,
- 0.333, 0.000, 0.000,
- 0.500, 0.000, 0.000,
- 0.667, 0.000, 0.000,
- 0.833, 0.000, 0.000,
- 1.000, 0.000, 0.000,
- 0.000, 0.167, 0.000,
- 0.000, 0.333, 0.000,
- 0.000, 0.500, 0.000,
- 0.000, 0.667, 0.000,
- 0.000, 0.833, 0.000,
- 0.000, 1.000, 0.000,
- 0.000, 0.000, 0.167,
- 0.000, 0.000, 0.333,
- 0.000, 0.000, 0.500,
- 0.000, 0.000, 0.667,
- 0.000, 0.000, 0.833,
- 0.000, 0.000, 1.000,
- 0.000, 0.000, 0.000,
- 0.143, 0.143, 0.143,
- 0.857, 0.857, 0.857,
- 1.000, 1.000, 1.000
- ]
-).astype(np.float32).reshape(-1, 3)
-
-def random_color(rgb=False, maximum=255):
- """
- Args:
- rgb (bool): whether to return RGB colors or BGR colors.
- maximum (int): either 255 or 1
-
- Returns:
- ndarray: a vector of 3 numbers
- """
- idx = np.random.randint(0, len(_COLORS))
- ret = _COLORS[idx] * maximum
- if not rgb:
- ret = ret[::-1]
- return ret
-
-@unique
-class ColorMode(Enum):
- """
- Enum of different color modes to use for instance visualizations.
- """
-
- IMAGE = 0
- """
- Picks a random color for every instance and overlay segmentations with low opacity.
- """
- SEGMENTATION = 1
- """
- Let instances of the same category have similar colors
- (from metadata.thing_colors), and overlay them with
- high opacity. This provides more attention on the quality of segmentation.
- """
- IMAGE_BW = 2
- """
- Same as IMAGE, but convert all areas without masks to gray-scale.
- Only available for drawing per-instance mask predictions.
- """
-
-class VisImage:
- def __init__(self, img, scale=1.0):
- """
- Args:
- img (ndarray): an RGB image of shape (H, W, 3) in range [0, 255].
- scale (float): scale the input image
- """
- self.img = img
- self.scale = scale
- self.width, self.height = img.shape[1], img.shape[0]
- self._setup_figure(img)
-
- def _setup_figure(self, img):
- """
- Args:
- Same as in :meth:`__init__()`.
-
- Returns:
- fig (matplotlib.pyplot.figure): top level container for all the image plot elements.
- ax (matplotlib.pyplot.Axes): contains figure elements and sets the coordinate system.
- """
- fig = mplfigure.Figure(frameon=False)
- self.dpi = fig.get_dpi()
- # add a small 1e-2 to avoid precision lost due to matplotlib's truncation
- # (https://github.com/matplotlib/matplotlib/issues/15363)
- fig.set_size_inches(
- (self.width * self.scale + 1e-2) / self.dpi,
- (self.height * self.scale + 1e-2) / self.dpi,
- )
- self.canvas = FigureCanvasAgg(fig)
- # self.canvas = mpl.backends.backend_cairo.FigureCanvasCairo(fig)
- ax = fig.add_axes([0.0, 0.0, 1.0, 1.0])
- ax.axis("off")
- self.fig = fig
- self.ax = ax
- self.reset_image(img)
-
- def reset_image(self, img):
- """
- Args:
- img: same as in __init__
- """
- img = img.astype("uint8")
- self.ax.imshow(img, extent=(0, self.width, self.height, 0), interpolation="nearest")
-
- def save(self, filepath):
- """
- Args:
- filepath (str): a string that contains the absolute path, including the file name, where
- the visualized image will be saved.
- """
- self.fig.savefig(filepath)
-
- def get_image(self):
- """
- Returns:
- ndarray:
- the visualized image of shape (H, W, 3) (RGB) in uint8 type.
- The shape is scaled w.r.t the input image using the given `scale` argument.
- """
- canvas = self.canvas
- s, (width, height) = canvas.print_to_buffer()
- # buf = io.BytesIO() # works for cairo backend
- # canvas.print_rgba(buf)
- # width, height = self.width, self.height
- # s = buf.getvalue()
-
- buffer = np.frombuffer(s, dtype="uint8")
-
- img_rgba = buffer.reshape(height, width, 4)
- rgb, alpha = np.split(img_rgba, [3], axis=2)
- return rgb.astype("uint8")
-
-class GenericMask:
- """
- Attribute:
- polygons (list[ndarray]): list[ndarray]: polygons for this mask.
- Each ndarray has format [x, y, x, y, ...]
- mask (ndarray): a binary mask
- """
-
- def __init__(self, mask_or_polygons, height, width):
- self._mask = self._polygons = self._has_holes = None
- self.height = height
- self.width = width
-
- m = mask_or_polygons
- if isinstance(m, dict):
- # RLEs
- assert "counts" in m and "size" in m
- if isinstance(m["counts"], list): # uncompressed RLEs
- h, w = m["size"]
- assert h == height and w == width
- m = mask_util.frPyObjects(m, h, w)
- self._mask = mask_util.decode(m)[:, :]
- return
-
- if isinstance(m, list): # list[ndarray]
- self._polygons = [np.asarray(x).reshape(-1) for x in m]
- return
-
- if isinstance(m, np.ndarray): # assumed to be a binary mask
- assert m.shape[1] != 2, m.shape
- assert m.shape == (
- height,
- width,
- ), f"mask shape: {m.shape}, target dims: {height}, {width}"
- self._mask = m.astype("uint8")
- return
-
- raise ValueError("GenericMask cannot handle object {} of type '{}'".format(m, type(m)))
-
- @property
- def mask(self):
- if self._mask is None:
- self._mask = self.polygons_to_mask(self._polygons)
- return self._mask
-
- @property
- def polygons(self):
- if self._polygons is None:
- self._polygons, self._has_holes = self.mask_to_polygons(self._mask)
- return self._polygons
-
- @property
- def has_holes(self):
- if self._has_holes is None:
- if self._mask is not None:
- self._polygons, self._has_holes = self.mask_to_polygons(self._mask)
- else:
- self._has_holes = False # if original format is polygon, does not have holes
- return self._has_holes
-
- def mask_to_polygons(self, mask):
- # cv2.RETR_CCOMP flag retrieves all the contours and arranges them to a 2-level
- # hierarchy. External contours (boundary) of the object are placed in hierarchy-1.
- # Internal contours (holes) are placed in hierarchy-2.
- # cv2.CHAIN_APPROX_NONE flag gets vertices of polygons from contours.
- mask = np.ascontiguousarray(mask) # some versions of cv2 does not support incontiguous arr
- res = cv2.findContours(mask.astype("uint8"), cv2.RETR_CCOMP, cv2.CHAIN_APPROX_NONE)
- hierarchy = res[-1]
- if hierarchy is None: # empty mask
- return [], False
- has_holes = (hierarchy.reshape(-1, 4)[:, 3] >= 0).sum() > 0
- res = res[-2]
- res = [x.flatten() for x in res]
- # These coordinates from OpenCV are integers in range [0, W-1 or H-1].
- # We add 0.5 to turn them into real-value coordinate space. A better solution
- # would be to first +0.5 and then dilate the returned polygon by 0.5.
- res = [x + 0.5 for x in res if len(x) >= 6]
- return res, has_holes
-
- def polygons_to_mask(self, polygons):
- rle = mask_util.frPyObjects(polygons, self.height, self.width)
- rle = mask_util.merge(rle)
- return mask_util.decode(rle)[:, :]
-
- def area(self):
- return self.mask.sum()
-
- def bbox(self):
- p = mask_util.frPyObjects(self.polygons, self.height, self.width)
- p = mask_util.merge(p)
- bbox = mask_util.toBbox(p)
- bbox[2] += bbox[0]
- bbox[3] += bbox[1]
- return bbox
-
-
-class Visualizer:
- """
- Visualizer that draws data about detection/segmentation on images.
-
- It contains methods like `draw_{text,box,circle,line,binary_mask,polygon}`
- that draw primitive objects to images, as well as high-level wrappers like
- `draw_{instance_predictions,sem_seg,panoptic_seg_predictions,dataset_dict}`
- that draw composite data in some pre-defined style.
-
- Note that the exact visualization style for the high-level wrappers are subject to change.
- Style such as color, opacity, label contents, visibility of labels, or even the visibility
- of objects themselves (e.g. when the object is too small) may change according
- to different heuristics, as long as the results still look visually reasonable.
-
- To obtain a consistent style, you can implement custom drawing functions with the
- abovementioned primitive methods instead. If you need more customized visualization
- styles, you can process the data yourself following their format documented in
- tutorials (:doc:`/tutorials/models`, :doc:`/tutorials/datasets`). This class does not
- intend to satisfy everyone's preference on drawing styles.
-
- This visualizer focuses on high rendering quality rather than performance. It is not
- designed to be used for real-time applications.
- """
-
- # TODO implement a fast, rasterized version using OpenCV
-
- def __init__(self, img_rgb: Union[Image.Image, np.ndarray], scale=1.0, instance_mode=ColorMode.IMAGE):
- """
- Args:
- img_rgb: a numpy array of shape (H, W, C), where H and W correspond to
- the height and width of the image respectively. C is the number of
- color channels. The image is required to be in RGB format since that
- is a requirement of the Matplotlib library. The image is also expected
- to be in the range [0, 255].
- instance_mode (ColorMode): defines one of the pre-defined style for drawing
- instances on an image.
- """
- if type(img_rgb) == np.ndarray:
- img_rgb = img_rgb[:, :, ::-1]
- else:
- img_rgb = np.array(img_rgb)[:, :, ::-1]
- self.img = np.asarray(img_rgb).clip(0, 255).astype(np.uint8)
- self.output = VisImage(self.img, scale=scale)
-
- # too small texts are useless, therefore clamp to 9
- self._default_font_size = max(
- np.sqrt(self.output.height * self.output.width) // 90, 10 // scale
- )
- self._instance_mode = instance_mode
-
- def draw_binary_mask(
- self, binary_mask, color=None, *, edge_color=None, text=None, alpha=0.5, area_threshold=10
- ):
- """
- Args:
- binary_mask (ndarray): numpy array of shape (H, W), where H is the image height and
- W is the image width. Each value in the array is either a 0 or 1 value of uint8
- type.
- color: color of the mask. Refer to `matplotlib.colors` for a full list of
- formats that are accepted. If None, will pick a random color.
- edge_color: color of the polygon edges. Refer to `matplotlib.colors` for a
- full list of formats that are accepted.
- text (str): if None, will be drawn on the object
- alpha (float): blending efficient. Smaller values lead to more transparent masks.
- area_threshold (float): a connected component smaller than this area will not be shown.
-
- Returns:
- output (VisImage): image object with mask drawn.
- """
- if color is None:
- color = random_color(rgb=True, maximum=1)
- color = mplc.to_rgb(color)
-
- has_valid_segment = False
- binary_mask = binary_mask.astype("uint8") # opencv needs uint8
- mask = GenericMask(binary_mask, self.output.height, self.output.width)
- shape2d = (binary_mask.shape[0], binary_mask.shape[1])
-
- if not mask.has_holes:
- # draw polygons for regular masks
- for segment in mask.polygons:
- area = mask_util.area(mask_util.frPyObjects([segment], shape2d[0], shape2d[1]))
- if area < (area_threshold or 0):
- continue
- has_valid_segment = True
- segment = segment.reshape(-1, 2)
- self.draw_polygon(segment, color=color, edge_color=edge_color, alpha=alpha)
- else:
- # TODO: Use Path/PathPatch to draw vector graphics:
- # https://stackoverflow.com/questions/8919719/how-to-plot-a-complex-polygon
- rgba = np.zeros(shape2d + (4,), dtype="float32")
- rgba[:, :, :3] = color
- rgba[:, :, 3] = (mask.mask == 1).astype("float32") * alpha
- has_valid_segment = True
- self.output.ax.imshow(rgba, extent=(0, self.output.width, self.output.height, 0))
-
- if text is not None and has_valid_segment:
- lighter_color = self._change_color_brightness(color, brightness_factor=0.7)
- self._draw_text_in_mask(binary_mask, text, lighter_color)
- return self.output
-
- def draw_polygon(self, segment, color, edge_color=None, alpha=0.5):
- """
- Args:
- segment: numpy array of shape Nx2, containing all the points in the polygon.
- color: color of the polygon. Refer to `matplotlib.colors` for a full list of
- formats that are accepted.
- edge_color: color of the polygon edges. Refer to `matplotlib.colors` for a
- full list of formats that are accepted. If not provided, a darker shade
- of the polygon color will be used instead.
- alpha (float): blending efficient. Smaller values lead to more transparent masks.
-
- Returns:
- output (VisImage): image object with polygon drawn.
- """
- if edge_color is None:
- # make edge color darker than the polygon color
- if alpha > 0.8:
- edge_color = self._change_color_brightness(color, brightness_factor=-0.7)
- else:
- edge_color = color
- edge_color = mplc.to_rgb(edge_color) + (1,)
-
- polygon = mpl.patches.Polygon(
- segment,
- fill=True,
- facecolor=mplc.to_rgb(color) + (alpha,),
- edgecolor=edge_color,
- linewidth=max(self._default_font_size // 15 * self.output.scale, 1),
- )
- self.output.ax.add_patch(polygon)
- return self.output
-
-
- """
- Internal methods:
- """
-
- def _change_color_brightness(self, color, brightness_factor):
- """
- Depending on the brightness_factor, gives a lighter or darker color i.e. a color with
- less or more saturation than the original color.
-
- Args:
- color: color of the polygon. Refer to `matplotlib.colors` for a full list of
- formats that are accepted.
- brightness_factor (float): a value in [-1.0, 1.0] range. A lightness factor of
- 0 will correspond to no change, a factor in [-1.0, 0) range will result in
- a darker color and a factor in (0, 1.0] range will result in a lighter color.
-
- Returns:
- modified_color (tuple[double]): a tuple containing the RGB values of the
- modified color. Each value in the tuple is in the [0.0, 1.0] range.
- """
- assert brightness_factor >= -1.0 and brightness_factor <= 1.0
- color = mplc.to_rgb(color)
- polygon_color = colorsys.rgb_to_hls(*mplc.to_rgb(color))
- modified_lightness = polygon_color[1] + (brightness_factor * polygon_color[1])
- modified_lightness = 0.0 if modified_lightness < 0.0 else modified_lightness
- modified_lightness = 1.0 if modified_lightness > 1.0 else modified_lightness
- modified_color = colorsys.hls_to_rgb(polygon_color[0], modified_lightness, polygon_color[2])
- return modified_color
-
- def _draw_text_in_mask(self, binary_mask, text, color):
- """
- Find proper places to draw text given a binary mask.
- """
- # TODO sometimes drawn on wrong objects. the heuristics here can improve.
- _num_cc, cc_labels, stats, centroids = cv2.connectedComponentsWithStats(binary_mask, 8)
- if stats[1:, -1].size == 0:
- return
- largest_component_id = np.argmax(stats[1:, -1]) + 1
-
- # draw text on the largest component, as well as other very large components.
- for cid in range(1, _num_cc):
- if cid == largest_component_id or stats[cid, -1] > _LARGE_MASK_AREA_THRESH:
- # median is more stable than centroid
- # center = centroids[largest_component_id]
- center = np.median((cc_labels == cid).nonzero(), axis=1)[::-1]
- self.draw_text(text, center, color=color)
-
- def get_output(self):
- """
- Returns:
- output (VisImage): the image output containing the visualizations added
- to the image.
- """
- return self.output
-
-def apply_threshold(pred: np.ndarray) -> np.ndarray:
- """Apply threshold to a salient map
-
- Args:
- pred (np.ndarray): each pixel is in range [0, 255]
-
- Returns:
- np.ndarray: each pixel is only 0.0 or 1.0
- """
- binary_mask = pred / 255.0
- binary_mask[binary_mask >= 0.5] = 1.0
- binary_mask[binary_mask < 0.5] = 0.0
- return binary_mask
-
-def normalize(data: np.ndarray) -> np.ndarray:
- return (data - data.min()) / (data.max() - data.min() + 1e-8)
-
-def post_processing_depth(depth: np.ndarray) -> np.ndarray:
- depth = (normalize(depth) * 255).astype(np.uint8)
- return cv2.applyColorMap(depth, cv2.COLORMAP_OCEAN)
-
-def apply_vis_to_image(
- image: np.ndarray,
- binary_mask: np.ndarray,
- color: np.ndarray
-) -> np.ndarray:
- visualizer = Visualizer(image)
- vis_image: VisImage = visualizer.draw_binary_mask(binary_mask, color)
- vis_image = vis_image.get_image()[:, :, ::-1]
- return vis_image
diff --git a/spaces/HaloMaster/chinesesummary/fengshen/data/universal_datamodule/universal_datamodule.py b/spaces/HaloMaster/chinesesummary/fengshen/data/universal_datamodule/universal_datamodule.py
deleted file mode 100644
index e73d985f661c77ebb452f5060cd30bfb1d8968be..0000000000000000000000000000000000000000
--- a/spaces/HaloMaster/chinesesummary/fengshen/data/universal_datamodule/universal_datamodule.py
+++ /dev/null
@@ -1,161 +0,0 @@
-from pytorch_lightning import LightningDataModule
-from typing import Optional
-
-from torch.utils.data import DataLoader, DistributedSampler
-
-
-def get_consume_samples(data_model: LightningDataModule) -> int:
- if hasattr(data_model.trainer.lightning_module, 'consumed_samples'):
- consumed_samples = data_model.trainer.lightning_module.consumed_samples
- print('get consumed samples from model: {}'.format(consumed_samples))
- else:
- world_size = data_model.trainer.world_size
- consumed_samples = max(0, data_model.trainer.global_step - 1) * \
- data_model.hparams.train_batchsize * world_size * data_model.trainer.accumulate_grad_batches
- print('calculate consumed samples: {}'.format(consumed_samples))
- return consumed_samples
-
-
-class UniversalDataModule(LightningDataModule):
- @ staticmethod
- def add_data_specific_args(parent_args):
- parser = parent_args.add_argument_group('Universal DataModule')
- parser.add_argument('--num_workers', default=8, type=int)
- parser.add_argument('--dataloader_workers', default=2, type=int)
- parser.add_argument('--train_batchsize', default=32, type=int)
- parser.add_argument('--val_batchsize', default=32, type=int)
- parser.add_argument('--test_batchsize', default=32, type=int)
- parser.add_argument('--datasets_name', type=str, default=None)
- parser.add_argument('--train_datasets_field', type=str, default='train')
- parser.add_argument('--val_datasets_field', type=str, default='validation')
- parser.add_argument('--test_datasets_field', type=str, default='test')
- parser.add_argument('--train_file', type=str, default=None)
- parser.add_argument('--val_file', type=str, default=None)
- parser.add_argument('--test_file', type=str, default=None)
- parser.add_argument('--raw_file_type', type=str, default='json')
- parser.add_argument('--sampler_type', type=str,
- choices=['single',
- 'random'],
- default='random')
- return parent_args
-
- def __init__(
- self,
- tokenizer,
- collate_fn,
- args,
- datasets=None,
- **kwargs,
- ):
- super().__init__()
- # 如果不传入datasets的名字,则可以在对象外部替换内部的datasets为模型需要的
- if datasets is not None:
- self.datasets = datasets
- elif args.datasets_name is not None:
- from fengshen.data.fs_datasets import load_dataset
- print('---------begin to load datasets {}'.format(args.datasets_name))
- self.datasets = load_dataset(
- args.datasets_name, num_proc=args.num_workers)
- print('---------ending load datasets {}'.format(args.datasets_name))
- else:
- print('---------begin to load datasets from local file')
- from datasets import load_dataset
- self.datasets = load_dataset(args.raw_file_type,
- data_files={
- args.train_datasets_field: args.train_file,
- args.val_datasets_field: args.val_file,
- args.test_datasets_field: args.test_file})
- print('---------end to load datasets from local file')
-
- self.tokenizer = tokenizer
- self.collate_fn = collate_fn
- self.save_hyperparameters(args)
-
- def get_custom_sampler(self, ds):
- from .universal_sampler import PretrainingRandomSampler
- from .universal_sampler import PretrainingSampler
- world_size = self.trainer.world_size
- consumed_samples = get_consume_samples(self)
- # use the user default sampler
- if self.hparams.sampler_type == 'random':
- return PretrainingRandomSampler(
- total_samples=len(ds),
- # consumed_samples cal by global steps
- consumed_samples=consumed_samples,
- micro_batch_size=self.hparams.train_batchsize,
- data_parallel_rank=self.trainer.global_rank,
- data_parallel_size=world_size,
- epoch=self.trainer.current_epoch,
- )
- elif self.hparams.sampler_type == 'single':
- return PretrainingSampler(
- total_samples=len(ds),
- # consumed_samples cal by global steps
- consumed_samples=consumed_samples,
- micro_batch_size=self.hparams.train_batchsize,
- data_parallel_rank=self.trainer.global_rank,
- data_parallel_size=world_size,
- )
- else:
- raise Exception('Unknown sampler type: {}'.format(self.hparams.sampler_type))
-
- def setup(self, stage: Optional[str] = None) -> None:
- return
-
- def train_dataloader(self):
- ds = self.datasets[self.hparams.train_datasets_field]
-
- collate_fn = self.collate_fn
- if collate_fn is None and hasattr(ds, 'collater'):
- collate_fn = ds.collater
-
- if self.hparams.replace_sampler_ddp is False:
- return DataLoader(
- ds,
- batch_sampler=self.get_custom_sampler(ds),
- num_workers=self.hparams.dataloader_workers,
- collate_fn=collate_fn,
- pin_memory=True,
- )
- return DataLoader(
- ds,
- batch_size=self.hparams.train_batchsize,
- num_workers=self.hparams.dataloader_workers,
- collate_fn=collate_fn,
- pin_memory=True,
- )
-
- def val_dataloader(self):
- ds = self.datasets[self.hparams.val_datasets_field]
- collate_fn = self.collate_fn
- if collate_fn is None and hasattr(ds, 'collater'):
- collate_fn = ds.collater
-
- return DataLoader(
- ds,
- batch_size=self.hparams.val_batchsize,
- shuffle=False,
- num_workers=self.hparams.dataloader_workers,
- collate_fn=collate_fn,
- sampler=DistributedSampler(
- ds, shuffle=False),
- pin_memory=True,
- )
-
- def test_dataloader(self):
- ds = self.datasets[self.hparams.test_datasets_field]
-
- collate_fn = self.collate_fn
- if collate_fn is None and hasattr(ds, 'collater'):
- collate_fn = ds.collater
-
- return DataLoader(
- ds,
- batch_size=self.hparams.test_batchsize,
- shuffle=False,
- num_workers=self.hparams.dataloader_workers,
- collate_fn=collate_fn,
- sampler=DistributedSampler(
- ds, shuffle=False),
- pin_memory=True,
- )
diff --git a/spaces/HamidRezaAttar/gpt2-home/meta.py b/spaces/HamidRezaAttar/gpt2-home/meta.py
deleted file mode 100644
index df001c9cf8cc3f750566244bd9101528bacc74a8..0000000000000000000000000000000000000000
--- a/spaces/HamidRezaAttar/gpt2-home/meta.py
+++ /dev/null
@@ -1,8 +0,0 @@
-HEADER_INFO = """
-# GPT2 - Home
-English GPT-2 home product description generator demo.
-""".strip()
-SIDEBAR_INFO = """
-# Configuration
-""".strip()
-PROMPT_BOX = "Enter your text..."
diff --git a/spaces/HaoFeng2019/DocTr/extractor.py b/spaces/HaoFeng2019/DocTr/extractor.py
deleted file mode 100644
index 85a135503e1b07ab354d1f26d81c0fe2153d6ceb..0000000000000000000000000000000000000000
--- a/spaces/HaoFeng2019/DocTr/extractor.py
+++ /dev/null
@@ -1,115 +0,0 @@
-import torch
-import torch.nn as nn
-import torch.nn.functional as F
-
-
-class ResidualBlock(nn.Module):
- def __init__(self, in_planes, planes, norm_fn='group', stride=1):
- super(ResidualBlock, self).__init__()
-
- self.conv1 = nn.Conv2d(in_planes, planes, kernel_size=3, padding=1, stride=stride)
- self.conv2 = nn.Conv2d(planes, planes, kernel_size=3, padding=1)
- self.relu = nn.ReLU(inplace=True)
-
- num_groups = planes // 8
-
- if norm_fn == 'group':
- self.norm1 = nn.GroupNorm(num_groups=num_groups, num_channels=planes)
- self.norm2 = nn.GroupNorm(num_groups=num_groups, num_channels=planes)
- if not stride == 1:
- self.norm3 = nn.GroupNorm(num_groups=num_groups, num_channels=planes)
-
- elif norm_fn == 'batch':
- self.norm1 = nn.BatchNorm2d(planes)
- self.norm2 = nn.BatchNorm2d(planes)
- if not stride == 1:
- self.norm3 = nn.BatchNorm2d(planes)
-
- elif norm_fn == 'instance':
- self.norm1 = nn.InstanceNorm2d(planes)
- self.norm2 = nn.InstanceNorm2d(planes)
- if not stride == 1:
- self.norm3 = nn.InstanceNorm2d(planes)
-
- elif norm_fn == 'none':
- self.norm1 = nn.Sequential()
- self.norm2 = nn.Sequential()
- if not stride == 1:
- self.norm3 = nn.Sequential()
-
- if stride == 1:
- self.downsample = None
-
- else:
- self.downsample = nn.Sequential(
- nn.Conv2d(in_planes, planes, kernel_size=1, stride=stride), self.norm3)
-
-
- def forward(self, x):
- y = x
- y = self.relu(self.norm1(self.conv1(y)))
- y = self.relu(self.norm2(self.conv2(y)))
-
- if self.downsample is not None:
- x = self.downsample(x)
-
- return self.relu(x+y)
-
-
-class BasicEncoder(nn.Module):
- def __init__(self, output_dim=128, norm_fn='batch'):
- super(BasicEncoder, self).__init__()
- self.norm_fn = norm_fn
-
- if self.norm_fn == 'group':
- self.norm1 = nn.GroupNorm(num_groups=8, num_channels=64)
-
- elif self.norm_fn == 'batch':
- self.norm1 = nn.BatchNorm2d(64)
-
- elif self.norm_fn == 'instance':
- self.norm1 = nn.InstanceNorm2d(64)
-
- elif self.norm_fn == 'none':
- self.norm1 = nn.Sequential()
-
- self.conv1 = nn.Conv2d(3, 64, kernel_size=7, stride=2, padding=3)
- self.relu1 = nn.ReLU(inplace=True)
-
- self.in_planes = 64
- self.layer1 = self._make_layer(64, stride=1)
- self.layer2 = self._make_layer(128, stride=2)
- self.layer3 = self._make_layer(192, stride=2)
-
- # output convolution
- self.conv2 = nn.Conv2d(192, output_dim, kernel_size=1)
-
- for m in self.modules():
- if isinstance(m, nn.Conv2d):
- nn.init.kaiming_normal_(m.weight, mode='fan_out', nonlinearity='relu')
- elif isinstance(m, (nn.BatchNorm2d, nn.InstanceNorm2d, nn.GroupNorm)):
- if m.weight is not None:
- nn.init.constant_(m.weight, 1)
- if m.bias is not None:
- nn.init.constant_(m.bias, 0)
-
- def _make_layer(self, dim, stride=1):
- layer1 = ResidualBlock(self.in_planes, dim, self.norm_fn, stride=stride)
- layer2 = ResidualBlock(dim, dim, self.norm_fn, stride=1)
- layers = (layer1, layer2)
-
- self.in_planes = dim
- return nn.Sequential(*layers)
-
- def forward(self, x):
- x = self.conv1(x)
- x = self.norm1(x)
- x = self.relu1(x)
-
- x = self.layer1(x)
- x = self.layer2(x)
- x = self.layer3(x)
-
- x = self.conv2(x)
-
- return x
diff --git a/spaces/HarryLee/eCommerceImageCaptioning/fairseq/examples/roberta/preprocess_GLUE_tasks.sh b/spaces/HarryLee/eCommerceImageCaptioning/fairseq/examples/roberta/preprocess_GLUE_tasks.sh
deleted file mode 100644
index 7f215a3b53e1c4a7b1f0320102915a49d84a5015..0000000000000000000000000000000000000000
--- a/spaces/HarryLee/eCommerceImageCaptioning/fairseq/examples/roberta/preprocess_GLUE_tasks.sh
+++ /dev/null
@@ -1,185 +0,0 @@
-#!/bin/bash
-# Copyright (c) Facebook, Inc. and its affiliates.
-#
-# This source code is licensed under the MIT license found in the
-# LICENSE file in the root directory of this source tree.
-
-
-# raw glue data as downloaded by glue download script (https://gist.github.com/W4ngatang/60c2bdb54d156a41194446737ce03e2e)
-if [[ $# -ne 2 ]]; then
- echo "Run as following:"
- echo "./examples/roberta/preprocess_GLUE_tasks.sh "
- exit 1
-fi
-
-GLUE_DATA_FOLDER=$1
-
-# download bpe encoder.json, vocabulary and fairseq dictionary
-wget -N 'https://dl.fbaipublicfiles.com/fairseq/gpt2_bpe/encoder.json'
-wget -N 'https://dl.fbaipublicfiles.com/fairseq/gpt2_bpe/vocab.bpe'
-wget -N 'https://dl.fbaipublicfiles.com/fairseq/gpt2_bpe/dict.txt'
-
-TASKS=$2 # QQP
-
-if [ "$TASKS" = "ALL" ]
-then
- TASKS="QQP MNLI QNLI MRPC RTE STS-B SST-2 CoLA"
-fi
-
-for TASK in $TASKS
-do
- echo "Preprocessing $TASK"
-
- TASK_DATA_FOLDER="$GLUE_DATA_FOLDER/$TASK"
- echo "Raw data as downloaded from glue website: $TASK_DATA_FOLDER"
-
- SPLITS="train dev test"
- INPUT_COUNT=2
- if [ "$TASK" = "QQP" ]
- then
- INPUT_COLUMNS=( 4 5 )
- TEST_INPUT_COLUMNS=( 2 3 )
- LABEL_COLUMN=6
- elif [ "$TASK" = "MNLI" ]
- then
- SPLITS="train dev_matched dev_mismatched test_matched test_mismatched"
- INPUT_COLUMNS=( 9 10 )
- TEST_INPUT_COLUMNS=( 9 10 )
- DEV_LABEL_COLUMN=16
- LABEL_COLUMN=12
- elif [ "$TASK" = "QNLI" ]
- then
- INPUT_COLUMNS=( 2 3 )
- TEST_INPUT_COLUMNS=( 2 3 )
- LABEL_COLUMN=4
- elif [ "$TASK" = "MRPC" ]
- then
- INPUT_COLUMNS=( 4 5 )
- TEST_INPUT_COLUMNS=( 4 5 )
- LABEL_COLUMN=1
- elif [ "$TASK" = "RTE" ]
- then
- INPUT_COLUMNS=( 2 3 )
- TEST_INPUT_COLUMNS=( 2 3 )
- LABEL_COLUMN=4
- elif [ "$TASK" = "STS-B" ]
- then
- INPUT_COLUMNS=( 8 9 )
- TEST_INPUT_COLUMNS=( 8 9 )
- LABEL_COLUMN=10
- # Following are single sentence tasks.
- elif [ "$TASK" = "SST-2" ]
- then
- INPUT_COLUMNS=( 1 )
- TEST_INPUT_COLUMNS=( 2 )
- LABEL_COLUMN=2
- INPUT_COUNT=1
- elif [ "$TASK" = "CoLA" ]
- then
- INPUT_COLUMNS=( 4 )
- TEST_INPUT_COLUMNS=( 2 )
- LABEL_COLUMN=2
- INPUT_COUNT=1
- fi
-
- # Strip out header and filter lines that don't have expected number of fields.
- rm -rf "$TASK_DATA_FOLDER/processed"
- mkdir -p "$TASK_DATA_FOLDER/processed"
- for SPLIT in $SPLITS
- do
- # CoLA train and dev doesn't have header.
- if [[ ( "$TASK" = "CoLA") && ( "$SPLIT" != "test" ) ]]
- then
- cp "$TASK_DATA_FOLDER/$SPLIT.tsv" "$TASK_DATA_FOLDER/processed/$SPLIT.tsv.temp";
- else
- tail -n +2 "$TASK_DATA_FOLDER/$SPLIT.tsv" > "$TASK_DATA_FOLDER/processed/$SPLIT.tsv.temp";
- fi
-
- # Remove unformatted lines from train and dev files for QQP dataset.
- if [[ ( "$TASK" = "QQP") && ( "$SPLIT" != "test" ) ]]
- then
- awk -F '\t' -v NUM_FIELDS=6 'NF==NUM_FIELDS{print}{}' "$TASK_DATA_FOLDER/processed/$SPLIT.tsv.temp" > "$TASK_DATA_FOLDER/processed/$SPLIT.tsv";
- else
- cp "$TASK_DATA_FOLDER/processed/$SPLIT.tsv.temp" "$TASK_DATA_FOLDER/processed/$SPLIT.tsv";
- fi
- rm "$TASK_DATA_FOLDER/processed/$SPLIT.tsv.temp";
- done
-
- # Split into input0, input1 and label
- for SPLIT in $SPLITS
- do
- for INPUT_TYPE in $(seq 0 $((INPUT_COUNT-1)))
- do
- if [[ "$SPLIT" != test* ]]
- then
- COLUMN_NUMBER=${INPUT_COLUMNS[$INPUT_TYPE]}
- else
- COLUMN_NUMBER=${TEST_INPUT_COLUMNS[$INPUT_TYPE]}
- fi
- cut -f"$COLUMN_NUMBER" "$TASK_DATA_FOLDER/processed/$SPLIT.tsv" > "$TASK_DATA_FOLDER/processed/$SPLIT.raw.input$INPUT_TYPE";
- done
-
- if [[ "$SPLIT" != test* ]]
- then
- if [ "$TASK" = "MNLI" ] && [ "$SPLIT" != "train" ]
- then
- cut -f"$DEV_LABEL_COLUMN" "$TASK_DATA_FOLDER/processed/$SPLIT.tsv" > "$TASK_DATA_FOLDER/processed/$SPLIT.label";
- else
- cut -f"$LABEL_COLUMN" "$TASK_DATA_FOLDER/processed/$SPLIT.tsv" > "$TASK_DATA_FOLDER/processed/$SPLIT.label";
- fi
- fi
-
- # BPE encode.
- for INPUT_TYPE in $(seq 0 $((INPUT_COUNT-1)))
- do
- LANG="input$INPUT_TYPE"
- echo "BPE encoding $SPLIT/$LANG"
- python -m examples.roberta.multiprocessing_bpe_encoder \
- --encoder-json encoder.json \
- --vocab-bpe vocab.bpe \
- --inputs "$TASK_DATA_FOLDER/processed/$SPLIT.raw.$LANG" \
- --outputs "$TASK_DATA_FOLDER/processed/$SPLIT.$LANG" \
- --workers 60 \
- --keep-empty;
- done
- done
-
- # Remove output directory.
- rm -rf "$TASK-bin"
-
- DEVPREF="$TASK_DATA_FOLDER/processed/dev.LANG"
- TESTPREF="$TASK_DATA_FOLDER/processed/test.LANG"
- if [ "$TASK" = "MNLI" ]
- then
- DEVPREF="$TASK_DATA_FOLDER/processed/dev_matched.LANG,$TASK_DATA_FOLDER/processed/dev_mismatched.LANG"
- TESTPREF="$TASK_DATA_FOLDER/processed/test_matched.LANG,$TASK_DATA_FOLDER/processed/test_mismatched.LANG"
- fi
-
- # Run fairseq preprocessing:
- for INPUT_TYPE in $(seq 0 $((INPUT_COUNT-1)))
- do
- LANG="input$INPUT_TYPE"
- fairseq-preprocess \
- --only-source \
- --trainpref "$TASK_DATA_FOLDER/processed/train.$LANG" \
- --validpref "${DEVPREF//LANG/$LANG}" \
- --testpref "${TESTPREF//LANG/$LANG}" \
- --destdir "$TASK-bin/$LANG" \
- --workers 60 \
- --srcdict dict.txt;
- done
- if [[ "$TASK" != "STS-B" ]]
- then
- fairseq-preprocess \
- --only-source \
- --trainpref "$TASK_DATA_FOLDER/processed/train.label" \
- --validpref "${DEVPREF//LANG/label}" \
- --destdir "$TASK-bin/label" \
- --workers 60;
- else
- # For STS-B output range is converted to be between: [0.0, 1.0]
- mkdir -p "$TASK-bin/label"
- awk '{print $1 / 5.0 }' "$TASK_DATA_FOLDER/processed/train.label" > "$TASK-bin/label/train.label"
- awk '{print $1 / 5.0 }' "$TASK_DATA_FOLDER/processed/dev.label" > "$TASK-bin/label/valid.label"
- fi
-done
diff --git a/spaces/HarryLee/eCommerceImageCaptioning/fairseq/examples/speech_recognition/kaldi/__init__.py b/spaces/HarryLee/eCommerceImageCaptioning/fairseq/examples/speech_recognition/kaldi/__init__.py
deleted file mode 100644
index e69de29bb2d1d6434b8b29ae775ad8c2e48c5391..0000000000000000000000000000000000000000
diff --git a/spaces/HarryLee/eCommerceImageCaptioning/fairseq/examples/wav2vec/unsupervised/kaldi_self_train/st/decode_phone.sh b/spaces/HarryLee/eCommerceImageCaptioning/fairseq/examples/wav2vec/unsupervised/kaldi_self_train/st/decode_phone.sh
deleted file mode 100644
index 947342a0b7d8f50bcf4164b284ef3303a1247b64..0000000000000000000000000000000000000000
--- a/spaces/HarryLee/eCommerceImageCaptioning/fairseq/examples/wav2vec/unsupervised/kaldi_self_train/st/decode_phone.sh
+++ /dev/null
@@ -1,33 +0,0 @@
-#!/bin/bash
-
-# decode into phones (and prepare a new data directory for HMM outputs)
-
-. ./path.sh
-
-set -eu
-
-out_dir= # same as in train.sh
-dec_lmparam= # LM hyperparameters (e.g., 7.0.0)
-dec_exp=
-dec_script=
-dec_splits="train valid"
-dec_data_dir=$out_dir/dec_data # where to write HMM output
-
-data_dir=${out_dir}/data
-
-local/decode.sh --nj 40 --graph_name graph \
- --val_sets "$dec_splits" --decode_script $dec_script \
- $out_dir/exp/$dec_exp $data_dir $data_dir/lang_test
-
-if [ ! -z $dec_lmparam ]; then
- for x in $dec_splits; do
- mkdir -p $dec_data_dir/$x
- cp $data_dir/$x/{feats.scp,cmvn.scp,utt2spk,spk2utt} $dec_data_dir/$x/
-
- tra=$out_dir/exp/$dec_exp/decode_${x}/scoring/${dec_lmparam}.tra
- cat $tra | utils/int2sym.pl -f 2- $data_dir/lang/words.txt | \
- sed 's:::g' | sed 's:::g' > $dec_data_dir/${x}/text
- utils/fix_data_dir.sh $dec_data_dir/${x}
- echo "WER on ${x} is" $(compute-wer ark:$data_dir/${x}_gt/text ark:$dec_data_dir/$x/text | cut -d" " -f2-)
- done
-fi
diff --git a/spaces/HarryLee/eCommerceImageCaptioning/fairseq/fairseq/model_parallel/models/roberta/__init__.py b/spaces/HarryLee/eCommerceImageCaptioning/fairseq/fairseq/model_parallel/models/roberta/__init__.py
deleted file mode 100644
index 117827c3e9c176477f33e3a6fd7fe19a922411a2..0000000000000000000000000000000000000000
--- a/spaces/HarryLee/eCommerceImageCaptioning/fairseq/fairseq/model_parallel/models/roberta/__init__.py
+++ /dev/null
@@ -1,6 +0,0 @@
-# Copyright (c) Facebook, Inc. and its affiliates.
-#
-# This source code is licensed under the MIT license found in the
-# LICENSE file in the root directory of this source tree.
-
-from .model import * # noqa
diff --git a/spaces/HarryLee/eCommerceImageCaptioning/fairseq/fairseq/models/nat/levenshtein_utils.py b/spaces/HarryLee/eCommerceImageCaptioning/fairseq/fairseq/models/nat/levenshtein_utils.py
deleted file mode 100644
index 375a98c2e11354de085f0a7926f407bd1a6a2ad4..0000000000000000000000000000000000000000
--- a/spaces/HarryLee/eCommerceImageCaptioning/fairseq/fairseq/models/nat/levenshtein_utils.py
+++ /dev/null
@@ -1,293 +0,0 @@
-# Copyright (c) Facebook, Inc. and its affiliates.
-#
-# This source code is licensed under the MIT license found in the
-# LICENSE file in the root directory of this source tree.
-
-import torch
-from fairseq.utils import new_arange
-
-
-# -------------- Helper Functions --------------------------------------------------- #
-
-
-def load_libnat():
- try:
- from fairseq import libnat_cuda
-
- return libnat_cuda, True
-
- except ImportError as e:
- print(str(e) + "... fall back to CPU version")
-
- try:
- from fairseq import libnat
-
- return libnat, False
-
- except ImportError as e:
- import sys
-
- sys.stderr.write(
- "ERROR: missing libnat_cuda. run `python setup.py build_ext --inplace`\n"
- )
- raise e
-
-
-def _get_ins_targets(in_tokens, out_tokens, padding_idx, unk_idx):
- libnat, use_cuda = load_libnat()
-
- def _get_ins_targets_cuda(in_tokens, out_tokens, padding_idx, unk_idx):
- in_masks = in_tokens.ne(padding_idx)
- out_masks = out_tokens.ne(padding_idx)
- mask_ins_targets, masked_tgt_masks = libnat.generate_insertion_labels(
- out_tokens.int(),
- libnat.levenshtein_distance(
- in_tokens.int(),
- out_tokens.int(),
- in_masks.sum(1).int(),
- out_masks.sum(1).int(),
- ),
- )
- masked_tgt_masks = masked_tgt_masks.bool() & out_masks
- mask_ins_targets = mask_ins_targets.type_as(in_tokens)[
- :, 1 : in_masks.size(1)
- ].masked_fill_(~in_masks[:, 1:], 0)
- masked_tgt_tokens = out_tokens.masked_fill(masked_tgt_masks, unk_idx)
- return masked_tgt_masks, masked_tgt_tokens, mask_ins_targets
-
- def _get_ins_targets_cpu(in_tokens, out_tokens, padding_idx, unk_idx):
- in_seq_len, out_seq_len = in_tokens.size(1), out_tokens.size(1)
-
- in_tokens_list = [
- [t for t in s if t != padding_idx] for i, s in enumerate(in_tokens.tolist())
- ]
- out_tokens_list = [
- [t for t in s if t != padding_idx]
- for i, s in enumerate(out_tokens.tolist())
- ]
-
- full_labels = libnat.suggested_ed2_path(
- in_tokens_list, out_tokens_list, padding_idx
- )
- mask_inputs = [
- [len(c) if c[0] != padding_idx else 0 for c in a[:-1]] for a in full_labels
- ]
-
- # generate labels
- masked_tgt_masks = []
- for mask_input in mask_inputs:
- mask_label = []
- for beam_size in mask_input[1:-1]: # HACK 1:-1
- mask_label += [0] + [1 for _ in range(beam_size)]
- masked_tgt_masks.append(
- mask_label + [0 for _ in range(out_seq_len - len(mask_label))]
- )
- mask_ins_targets = [
- mask_input[1:-1]
- + [0 for _ in range(in_seq_len - 1 - len(mask_input[1:-1]))]
- for mask_input in mask_inputs
- ]
-
- # transform to tensor
- masked_tgt_masks = torch.tensor(
- masked_tgt_masks, device=out_tokens.device
- ).bool()
- mask_ins_targets = torch.tensor(mask_ins_targets, device=in_tokens.device)
- masked_tgt_tokens = out_tokens.masked_fill(masked_tgt_masks, unk_idx)
- return masked_tgt_masks, masked_tgt_tokens, mask_ins_targets
-
- if use_cuda:
- return _get_ins_targets_cuda(in_tokens, out_tokens, padding_idx, unk_idx)
- return _get_ins_targets_cpu(in_tokens, out_tokens, padding_idx, unk_idx)
-
-
-def _get_del_targets(in_tokens, out_tokens, padding_idx):
- libnat, use_cuda = load_libnat()
-
- def _get_del_targets_cuda(in_tokens, out_tokens, padding_idx):
- in_masks = in_tokens.ne(padding_idx)
- out_masks = out_tokens.ne(padding_idx)
-
- word_del_targets = libnat.generate_deletion_labels(
- in_tokens.int(),
- libnat.levenshtein_distance(
- in_tokens.int(),
- out_tokens.int(),
- in_masks.sum(1).int(),
- out_masks.sum(1).int(),
- ),
- )
- word_del_targets = word_del_targets.type_as(in_tokens).masked_fill_(
- ~in_masks, 0
- )
- return word_del_targets
-
- def _get_del_targets_cpu(in_tokens, out_tokens, padding_idx):
- out_seq_len = out_tokens.size(1)
- with torch.cuda.device_of(in_tokens):
- in_tokens_list = [
- [t for t in s if t != padding_idx]
- for i, s in enumerate(in_tokens.tolist())
- ]
- out_tokens_list = [
- [t for t in s if t != padding_idx]
- for i, s in enumerate(out_tokens.tolist())
- ]
-
- full_labels = libnat.suggested_ed2_path(
- in_tokens_list, out_tokens_list, padding_idx
- )
- word_del_targets = [b[-1] for b in full_labels]
- word_del_targets = [
- labels + [0 for _ in range(out_seq_len - len(labels))]
- for labels in word_del_targets
- ]
-
- # transform to tensor
- word_del_targets = torch.tensor(word_del_targets, device=out_tokens.device)
- return word_del_targets
-
- if use_cuda:
- return _get_del_targets_cuda(in_tokens, out_tokens, padding_idx)
- return _get_del_targets_cpu(in_tokens, out_tokens, padding_idx)
-
-
-def _apply_ins_masks(
- in_tokens, in_scores, mask_ins_pred, padding_idx, unk_idx, eos_idx
-):
-
- in_masks = in_tokens.ne(padding_idx)
- in_lengths = in_masks.sum(1)
-
- # HACK: hacky way to shift all the paddings to eos first.
- in_tokens.masked_fill_(~in_masks, eos_idx)
- mask_ins_pred.masked_fill_(~in_masks[:, 1:], 0)
-
- out_lengths = in_lengths + mask_ins_pred.sum(1)
- out_max_len = out_lengths.max()
- out_masks = new_arange(out_lengths, out_max_len)[None, :] < out_lengths[:, None]
-
- reordering = (mask_ins_pred + in_masks[:, 1:].long()).cumsum(1)
- out_tokens = (
- in_tokens.new_zeros(in_tokens.size(0), out_max_len)
- .fill_(padding_idx)
- .masked_fill_(out_masks, unk_idx)
- )
- out_tokens[:, 0] = in_tokens[:, 0]
- out_tokens.scatter_(1, reordering, in_tokens[:, 1:])
-
- out_scores = None
- if in_scores is not None:
- in_scores.masked_fill_(~in_masks, 0)
- out_scores = in_scores.new_zeros(*out_tokens.size())
- out_scores[:, 0] = in_scores[:, 0]
- out_scores.scatter_(1, reordering, in_scores[:, 1:])
-
- return out_tokens, out_scores
-
-
-def _apply_ins_words(in_tokens, in_scores, word_ins_pred, word_ins_scores, unk_idx):
- word_ins_masks = in_tokens.eq(unk_idx)
- out_tokens = in_tokens.masked_scatter(word_ins_masks, word_ins_pred[word_ins_masks])
-
- if in_scores is not None:
- out_scores = in_scores.masked_scatter(
- word_ins_masks, word_ins_scores[word_ins_masks]
- )
- else:
- out_scores = None
-
- return out_tokens, out_scores
-
-
-def _apply_del_words(
- in_tokens, in_scores, in_attn, word_del_pred, padding_idx, bos_idx, eos_idx
-):
- # apply deletion to a tensor
- in_masks = in_tokens.ne(padding_idx)
- bos_eos_masks = in_tokens.eq(bos_idx) | in_tokens.eq(eos_idx)
-
- max_len = in_tokens.size(1)
- word_del_pred.masked_fill_(~in_masks, 1)
- word_del_pred.masked_fill_(bos_eos_masks, 0)
-
- reordering = new_arange(in_tokens).masked_fill_(word_del_pred, max_len).sort(1)[1]
-
- out_tokens = in_tokens.masked_fill(word_del_pred, padding_idx).gather(1, reordering)
-
- out_scores = None
- if in_scores is not None:
- out_scores = in_scores.masked_fill(word_del_pred, 0).gather(1, reordering)
-
- out_attn = None
- if in_attn is not None:
- _mask = word_del_pred[:, :, None].expand_as(in_attn)
- _reordering = reordering[:, :, None].expand_as(in_attn)
- out_attn = in_attn.masked_fill(_mask, 0.0).gather(1, _reordering)
-
- return out_tokens, out_scores, out_attn
-
-
-def _skip(x, mask):
- """
- Getting sliced (dim=0) tensor by mask. Supporting tensor and list/dict of tensors.
- """
- if isinstance(x, int):
- return x
-
- if x is None:
- return None
-
- if isinstance(x, torch.Tensor):
- if x.size(0) == mask.size(0):
- return x[mask]
- elif x.size(1) == mask.size(0):
- return x[:, mask]
-
- if isinstance(x, list):
- return [_skip(x_i, mask) for x_i in x]
-
- if isinstance(x, dict):
- return {k: _skip(v, mask) for k, v in x.items()}
-
- raise NotImplementedError
-
-
-def _skip_encoder_out(encoder, encoder_out, mask):
- if not mask.any():
- return encoder_out
- else:
- return encoder.reorder_encoder_out(
- encoder_out, mask.nonzero(as_tuple=False).squeeze()
- )
-
-
-def _fill(x, mask, y, padding_idx):
- """
- Filling tensor x with y at masked positions (dim=0).
- """
- if x is None:
- return y
- assert x.dim() == y.dim() and mask.size(0) == x.size(0)
- assert x.dim() == 2 or (x.dim() == 3 and x.size(2) == y.size(2))
- n_selected = mask.sum()
- assert n_selected == y.size(0)
-
- if n_selected == x.size(0):
- return y
-
- if x.size(1) < y.size(1):
- dims = [x.size(0), y.size(1) - x.size(1)]
- if x.dim() == 3:
- dims.append(x.size(2))
- x = torch.cat([x, x.new_zeros(*dims).fill_(padding_idx)], 1)
- x[mask] = y
- elif x.size(1) > y.size(1):
- x[mask] = padding_idx
- if x.dim() == 2:
- x[mask, : y.size(1)] = y
- else:
- x[mask, : y.size(1), :] = y
- else:
- x[mask] = y
- return x
diff --git a/spaces/HarryLee/eCommerceImageCaptioning/fairseq/fairseq/modules/quantization/pq/utils.py b/spaces/HarryLee/eCommerceImageCaptioning/fairseq/fairseq/modules/quantization/pq/utils.py
deleted file mode 100644
index 14c015b7c19aae65812e864cf1d95ef3d39de606..0000000000000000000000000000000000000000
--- a/spaces/HarryLee/eCommerceImageCaptioning/fairseq/fairseq/modules/quantization/pq/utils.py
+++ /dev/null
@@ -1,374 +0,0 @@
-# Copyright (c) Facebook, Inc. and its affiliates.
-#
-# This source code is licensed under the MIT license found in the
-# LICENSE file in the root directory of this source tree.
-
-import logging
-import re
-from operator import attrgetter, itemgetter
-import torch
-import numpy as np
-import torch.distributed as dist
-import torch.nn as nn
-
-from .modules import PQConv2d, PQEmbedding, PQLinear
-from .pq import PQ
-
-
-def quantize_model_(
- model,
- size_tracker,
- layers_to_quantize,
- block_sizes_config,
- n_centroids_config,
- step=0,
- n_iter=15,
- eps=1e-6,
- max_tentatives=100,
- remove_weights=False,
- verbose=True,
- state_dict=None,
-):
- """
- Quantize a model in-place by stages. All the targeted
- layers are replaced by their quantized counterpart,
- and the model is ready for the finetuning of the
- centroids in a standard training loop (no modifications
- required). Note that we do not quantize biases.
-
- Args:
- - model: a nn.Module
- - size_tracker: useful for tracking quatization statistics
- - layers_to_quantize: a list containing regexps for
- filtering the layers to quantize at each stage according
- to their name (as in model.named_parameters())
- - block_sizes_config: dict like
- {
- 'Conv2d': ('kernel_size', {'(3, 3)': 9, '(1, 1)': 4}),
- 'Linear': ('in_features', {'*': 8})
- }
- For instance, all conv2d layers with kernel size 3x3 have
- a block size of 9 and all Linear layers are quantized with
- a block size of 8, irrespective of their size.
- - n_centroids_config: dict like
- {
- 'Conv2d': ('kernel_size', {'*': 256}),
- 'Linear': ('in_features', {'*': 256})
- }
- For instance, all conv2d layers are quantized with 256 centroids
- - step: the layers to quantize inplace corresponding
- to layers_to_quantize[step]
- """
-
- quantized_layers = get_layers(model, layers_to_quantize[step], remove_weights=remove_weights)
-
- for layer in quantized_layers:
-
- # book-keeping
- is_master_process = (not dist.is_initialized()) or (
- dist.is_initialized() and dist.get_rank() == 0
- )
- verbose = verbose and is_master_process
-
- # get block size and centroids
- module = attrgetter(layer)(model)
- block_size = get_param(module, layer, block_sizes_config)
- n_centroids = get_param(module, layer, n_centroids_config)
- if verbose:
- logging.info(
- f"Quantizing layer {layer} with block size {block_size} and {n_centroids} centroids"
- )
-
- # quantize layer
- weight = module.weight.data.clone()
- is_bias = "bias" in [x[0] for x in module.named_parameters()]
- bias = module.bias.data.clone() if is_bias else None
- quantizer = PQ(
- weight,
- block_size,
- n_centroids=n_centroids,
- n_iter=n_iter,
- eps=eps,
- max_tentatives=max_tentatives,
- verbose=verbose,
- )
-
- # quantization performed on all GPUs with same seed
- quantizer.encode()
- centroids = quantizer.centroids.contiguous()
- assignments = quantizer.assignments.contiguous()
-
- # If n_iter = 0 and state_dict is provided, then
- # we initialize random assignments and centroids to
- # random values of the appropriate dimensions
- # because the quantized model parameters will
- # overwritten by the state_dict later on.
- if n_iter == 0 and state_dict:
- # Initialize random centroids of the correct size
- centroids = torch.rand(centroids.size())
- centroids.cuda()
- # Get counts and assignment keys from layer in loaded checkpoint.
- counts_key = layer+"."+"counts"
- assignment_key = layer+"."+"assignments"
- # Get number of different bins to include.
- counts = list(state_dict[counts_key].shape)[0]
- print(layer)
- print(state_dict[counts_key])
- print(counts)
- # Initialize random assignments of the correct size
- # with an appropriate number of bins.
- num_assignments = list(state_dict[assignment_key].shape)[0]
- num_extra = num_assignments - counts
- print(num_assignments)
- print(num_extra)
- assignments_bins = torch.arange(counts)
- assignments_rand = torch.randint(0, counts-1, (num_extra, ))
- assignments = torch.cat((assignments_bins, assignments_rand), 0)
- # assignments = assignments.type(torch.IntTensor)
- assignments.cuda()
- print("assignments")
- print(assignments)
-
- # broadcast results to make sure weights are up-to-date
- if dist.is_initialized():
- dist.broadcast(centroids, 0)
- dist.broadcast(assignments, 0)
-
- # instantiate the quantized counterpart
- if isinstance(module, nn.Linear):
- out_features, in_features = map(
- lambda k: module.__dict__[k], ["out_features", "in_features"]
- )
- quantized_module = PQLinear(
- centroids, assignments, bias, in_features, out_features
- )
- elif isinstance(module, nn.Embedding):
- num_embeddings, embedding_dim = map(
- lambda k: module.__dict__[k], ["num_embeddings", "embedding_dim"]
- )
- quantized_module = PQEmbedding(
- centroids, assignments, num_embeddings, embedding_dim
- )
- elif isinstance(module, nn.Conv2d):
- out_channels, in_channels, kernel_size = map(
- lambda k: module.__dict__[k],
- ["out_channels", "in_channels", "kernel_size"],
- )
- stride, padding, dilation, groups, padding_mode = map(
- lambda k: module.__dict__[k],
- ["stride", "padding", "dilation", "groups", "padding_mode"],
- )
-
- quantized_module = PQConv2d(
- centroids,
- assignments,
- bias,
- in_channels,
- out_channels,
- kernel_size,
- stride=stride,
- padding=padding,
- dilation=dilation,
- groups=groups,
- padding_mode=padding_mode,
- )
- else:
- raise ValueError(f"Module {module} not yet supported for quantization")
-
- # replace layer by its quantized counterpart
- attrsetter(layer)(model, quantized_module)
-
- # update statistics
- size_tracker.update(weight, block_size, n_centroids)
-
- # return name of quantized layers
- return quantized_layers
-
-
-def get_layers(model, filter_regexp, remove_weights=False):
- """
- Filters out the layers according to a regexp. Note that
- we omit biases.
-
- Args:
- - model: a nn.Module
- - filter_regexp: a regexp to filter the layers to keep
- according to their name in model.named_parameters().
- For instance, the regexp:
-
- down_layers\\.[123456]\\.(conv[12]|identity\\.conv))
-
- is keeping blocks down_layers from 1 to 6, and inside
- each block is keeping conv1, conv2 and identity.conv.
-
- Remarks:
- - We add (module\\.)? at the beginning of the regexp to
- account for the possible use of nn.parallel.DataParallel
- """
-
- # get all parameter names
- all_layers = map(itemgetter(0), model.named_parameters())
-
- # remove biases
- all_layers = filter(lambda x: "bias" not in x, all_layers)
-
- # remove .weight in all other names (or .weight_orig is spectral norm)
- all_layers = map(lambda x: x.replace(".weight_orig", ""), all_layers)
- # remove weights indicates whether the weights extension should be removed, in addition to
- # weight_orig and weight extension on names
- if remove_weights:
- all_layers = map(lambda x: x.replace(".weights", ""), all_layers)
- all_layers = map(lambda x: x.replace(".weight", ""), all_layers)
-
- # return filtered layers
- filter_regexp = "(module\\.)?" + "(" + filter_regexp + ")"
- r = re.compile(filter_regexp)
-
- return list(filter(r.match, all_layers))
-
-
-def get_param(module, layer_name, param_config):
- """
- Given a quantization configuration, get the right parameter
- for the module to be quantized.
-
- Args:
- - module: a nn.Module
- - layer_name: the name of the layer
- - param_config: a dict like
- {
- 'Conv2d': ('kernel_size', {'(3, 3)': 9, '(1, 1)': 4}),
- 'Linear': ('in_features', {'*': 8})
- }
- For instance, all conv2d layers with kernel size 3x3 have
- a block size of 9 and all Linear layers are quantized with
- a block size of 8, irrespective of their size.
-
- Remarks:
- - if 'fuzzy_name' is passed as a parameter, layers whose layer_name
- include 'fuzzy_name' will be assigned the given parameter.
- In the following example, conv.expand layers will have a block
- size of 9 while conv.reduce will have a block size of 4 and all
- other layers will have a block size of 2.
- {
- 'Conv2d': ('fuzzy_name', {'expand': 9, 'reduce': 4, '*': 2}),
- 'Linear': ('fuzzy_name', {'classifier': 8, 'projection': 4})
- }
-
- """
-
- layer_type = module.__class__.__name__
-
- if layer_type not in param_config:
- raise KeyError(f"Layer type {layer_type} not in config for layer {module}")
-
- feature, params = param_config[module.__class__.__name__]
-
- if feature != "fuzzy_name":
- feature_value = str(getattr(module, feature))
- if feature_value not in params:
- if "*" in params:
- feature_value = "*"
- else:
- raise KeyError(
- f"{feature}={feature_value} not in config for layer {module}"
- )
- else:
- feature_values = [name for name in params if name in layer_name]
- if len(feature_values) == 0:
- if "*" in params:
- feature_value = "*"
- else:
- raise KeyError(f"name={layer_name} not in config for {module}")
- else:
- feature_value = feature_values[0]
-
- return params[feature_value]
-
-
-class SizeTracker(object):
- """
- Class to keep track of the compressed network size with iPQ.
-
- Args:
- - model: a nn.Module
-
- Remarks:
- - The compressed size is the sum of three components
- for each layer in the network:
- (1) Storing the centroids given by iPQ in fp16
- (2) Storing the assignments of the blocks in int8
- (3) Storing all non-compressed elements such as biases
- - This cost in only valid if we use 256 centroids (then
- indexing can indeed by done with int8).
- """
-
- def __init__(self, model):
- self.model = model
- self.size_non_compressed_model = self.compute_size()
- self.size_non_quantized = self.size_non_compressed_model
- self.size_index = 0
- self.size_centroids = 0
- self.n_quantized_layers = 0
-
- def compute_size(self):
- """
- Computes the size of the model (in MB).
- """
-
- res = 0
- for _, p in self.model.named_parameters():
- res += p.numel()
- return res * 4 / 1024 / 1024
-
- def update(self, W, block_size, n_centroids):
- """
- Updates the running statistics when quantizing a new layer.
- """
-
- # bits per weights
- bits_per_weight = np.log2(n_centroids) / block_size
- self.n_quantized_layers += 1
-
- # size of indexing the subvectors of size block_size (in MB)
- size_index_layer = bits_per_weight * W.numel() / 8 / 1024 / 1024
- self.size_index += size_index_layer
-
- # size of the centroids stored in float16 (in MB)
- size_centroids_layer = n_centroids * block_size * 2 / 1024 / 1024
- self.size_centroids += size_centroids_layer
-
- # size of non-compressed layers, e.g. LayerNorms or biases (in MB)
- size_uncompressed_layer = W.numel() * 4 / 1024 / 1024
- self.size_non_quantized -= size_uncompressed_layer
-
- def __repr__(self):
- size_compressed = (
- self.size_index + self.size_centroids + self.size_non_quantized
- )
- compression_ratio = self.size_non_compressed_model / size_compressed # NOQA
- return (
- f"Non-compressed model size: {self.size_non_compressed_model:.2f} MB. "
- f"After quantizing {self.n_quantized_layers} layers, size "
- f"(indexing + centroids + other): {self.size_index:.2f} MB + "
- f"{self.size_centroids:.2f} MB + {self.size_non_quantized:.2f} MB = "
- f"{size_compressed:.2f} MB, compression ratio: {compression_ratio:.2f}x"
- )
-
-
-def attrsetter(*items):
- def resolve_attr(obj, attr):
- attrs = attr.split(".")
- head = attrs[:-1]
- tail = attrs[-1]
-
- for name in head:
- obj = getattr(obj, name)
- return obj, tail
-
- def g(obj, val):
- for attr in items:
- resolved_obj, resolved_attr = resolve_attr(obj, attr)
- setattr(resolved_obj, resolved_attr, val)
-
- return g
diff --git a/spaces/HarryLee/eCommerceImageCaptioning/run_scripts/caption/train_caption_stage1_base.sh b/spaces/HarryLee/eCommerceImageCaptioning/run_scripts/caption/train_caption_stage1_base.sh
deleted file mode 100644
index 600978950feb39d223ae37526f3cd48ef9295672..0000000000000000000000000000000000000000
--- a/spaces/HarryLee/eCommerceImageCaptioning/run_scripts/caption/train_caption_stage1_base.sh
+++ /dev/null
@@ -1,108 +0,0 @@
-#!/usr/bin/env
-
-# The port for communication. Note that if you want to run multiple tasks on the same machine,
-# you need to specify different port numbers.
-export MASTER_PORT=1061
-
-log_dir=./stage1_logs
-save_dir=./stage1_checkpoints
-mkdir -p $log_dir $save_dir
-
-bpe_dir=../../utils/BPE
-user_dir=../../ofa_module
-
-data_dir=../../dataset/caption_data
-data=${data_dir}/caption_stage1_train.tsv,${data_dir}/caption_val.tsv
-restore_file=../../checkpoints/ofa_base.pt
-selected_cols=0,4,2
-
-task=caption
-arch=ofa_base
-criterion=adjust_label_smoothed_cross_entropy
-label_smoothing=0.1
-lr=1e-5
-max_epoch=5
-warmup_ratio=0.06
-batch_size=8
-update_freq=4
-resnet_drop_path_rate=0.0
-encoder_drop_path_rate=0.1
-decoder_drop_path_rate=0.1
-dropout=0.1
-attention_dropout=0.0
-max_src_length=80
-max_tgt_length=20
-num_bins=1000
-patch_image_size=480
-eval_cider_cached=${data_dir}/cider_cached_tokens/coco-valid-words.p
-drop_worst_ratio=0.2
-
-for max_epoch in {5,}; do
- echo "max_epoch "${max_epoch}
- for warmup_ratio in {0.06,}; do
- echo "warmup_ratio "${warmup_ratio}
- for drop_worst_after in {6000,}; do
- echo "drop_worst_after "${drop_worst_after}
-
- log_file=${log_dir}/${max_epoch}"_"${warmup_ratio}"_"${drop_worst_after}".log"
- save_path=${save_dir}/${max_epoch}"_"${warmup_ratio}"_"${drop_worst_after}
- mkdir -p $save_path
-
- CUDA_VISIBLE_DEVICES=0,1,2,3 python3 -m torch.distributed.launch --nproc_per_node=4 --master_port=${MASTER_PORT} ../../train.py \
- $data \
- --selected-cols=${selected_cols} \
- --bpe-dir=${bpe_dir} \
- --user-dir=${user_dir} \
- --restore-file=${restore_file} \
- --reset-optimizer --reset-dataloader --reset-meters \
- --save-dir=${save_path} \
- --task=${task} \
- --arch=${arch} \
- --criterion=${criterion} \
- --label-smoothing=${label_smoothing} \
- --batch-size=${batch_size} \
- --update-freq=${update_freq} \
- --encoder-normalize-before \
- --decoder-normalize-before \
- --share-decoder-input-output-embed \
- --share-all-embeddings \
- --layernorm-embedding \
- --patch-layernorm-embedding \
- --code-layernorm-embedding \
- --resnet-drop-path-rate=${resnet_drop_path_rate} \
- --encoder-drop-path-rate=${encoder_drop_path_rate} \
- --decoder-drop-path-rate=${decoder_drop_path_rate} \
- --dropout=${dropout} \
- --attention-dropout=${attention_dropout} \
- --weight-decay=0.01 --optimizer=adam --adam-betas="(0.9,0.999)" --adam-eps=1e-08 --clip-norm=1.0 \
- --lr-scheduler=polynomial_decay --lr=${lr} \
- --max-epoch=${max_epoch} --warmup-ratio=${warmup_ratio} \
- --log-format=simple --log-interval=10 \
- --fixed-validation-seed=7 \
- --no-epoch-checkpoints --keep-best-checkpoints=1 \
- --save-interval=1 --validate-interval=1 \
- --save-interval-updates=500 --validate-interval-updates=500 \
- --eval-cider \
- --eval-cider-cached-tokens=${eval_cider_cached} \
- --eval-args='{"beam":5,"max_len_b":16,"no_repeat_ngram_size":3}' \
- --best-checkpoint-metric=cider --maximize-best-checkpoint-metric \
- --max-src-length=${max_src_length} \
- --max-tgt-length=${max_tgt_length} \
- --find-unused-parameters \
- --freeze-encoder-embedding \
- --freeze-decoder-embedding \
- --add-type-embedding \
- --scale-attn \
- --scale-fc \
- --scale-heads \
- --disable-entangle \
- --num-bins=${num_bins} \
- --patch-image-size=${patch_image_size} \
- --drop-worst-ratio=${drop_worst_ratio} \
- --drop-worst-after=${drop_worst_after} \
- --fp16 \
- --fp16-scale-window=512 \
- --num-workers=0 > ${log_file} 2>&1
- done
- done
-done
\ No newline at end of file
diff --git a/spaces/Harveenchadha/Vakyansh-Odia-TTS/ttsv/src/glow_tts/audio_processing.py b/spaces/Harveenchadha/Vakyansh-Odia-TTS/ttsv/src/glow_tts/audio_processing.py
deleted file mode 100644
index 3a4467355952fefaba117b6014864139ac319c6b..0000000000000000000000000000000000000000
--- a/spaces/Harveenchadha/Vakyansh-Odia-TTS/ttsv/src/glow_tts/audio_processing.py
+++ /dev/null
@@ -1,100 +0,0 @@
-import torch
-import numpy as np
-from scipy.signal import get_window
-import librosa.util as librosa_util
-
-
-def window_sumsquare(
- window,
- n_frames,
- hop_length=200,
- win_length=800,
- n_fft=800,
- dtype=np.float32,
- norm=None,
-):
- """
- # from librosa 0.6
- Compute the sum-square envelope of a window function at a given hop length.
-
- This is used to estimate modulation effects induced by windowing
- observations in short-time fourier transforms.
-
- Parameters
- ----------
- window : string, tuple, number, callable, or list-like
- Window specification, as in `get_window`
-
- n_frames : int > 0
- The number of analysis frames
-
- hop_length : int > 0
- The number of samples to advance between frames
-
- win_length : [optional]
- The length of the window function. By default, this matches `n_fft`.
-
- n_fft : int > 0
- The length of each analysis frame.
-
- dtype : np.dtype
- The data type of the output
-
- Returns
- -------
- wss : np.ndarray, shape=`(n_fft + hop_length * (n_frames - 1))`
- The sum-squared envelope of the window function
- """
- if win_length is None:
- win_length = n_fft
-
- n = n_fft + hop_length * (n_frames - 1)
- x = np.zeros(n, dtype=dtype)
-
- # Compute the squared window at the desired length
- win_sq = get_window(window, win_length, fftbins=True)
- win_sq = librosa_util.normalize(win_sq, norm=norm) ** 2
- win_sq = librosa_util.pad_center(win_sq, n_fft)
-
- # Fill the envelope
- for i in range(n_frames):
- sample = i * hop_length
- x[sample : min(n, sample + n_fft)] += win_sq[: max(0, min(n_fft, n - sample))]
- return x
-
-
-def griffin_lim(magnitudes, stft_fn, n_iters=30):
- """
- PARAMS
- ------
- magnitudes: spectrogram magnitudes
- stft_fn: STFT class with transform (STFT) and inverse (ISTFT) methods
- """
-
- angles = np.angle(np.exp(2j * np.pi * np.random.rand(*magnitudes.size())))
- angles = angles.astype(np.float32)
- angles = torch.autograd.Variable(torch.from_numpy(angles))
- signal = stft_fn.inverse(magnitudes, angles).squeeze(1)
-
- for i in range(n_iters):
- _, angles = stft_fn.transform(signal)
- signal = stft_fn.inverse(magnitudes, angles).squeeze(1)
- return signal
-
-
-def dynamic_range_compression(x, C=1, clip_val=1e-5):
- """
- PARAMS
- ------
- C: compression factor
- """
- return torch.log(torch.clamp(x, min=clip_val) * C)
-
-
-def dynamic_range_decompression(x, C=1):
- """
- PARAMS
- ------
- C: compression factor used to compress
- """
- return torch.exp(x) / C
diff --git a/spaces/Harveenchadha/Vakyansh-Odia-TTS/ttsv/src/glow_tts/monotonic_align/monotonic_align/mas.py b/spaces/Harveenchadha/Vakyansh-Odia-TTS/ttsv/src/glow_tts/monotonic_align/monotonic_align/mas.py
deleted file mode 100644
index 207ab3e858389ec06c902fd6f5bec6c5da2996af..0000000000000000000000000000000000000000
--- a/spaces/Harveenchadha/Vakyansh-Odia-TTS/ttsv/src/glow_tts/monotonic_align/monotonic_align/mas.py
+++ /dev/null
@@ -1,57 +0,0 @@
-from typing import overload
-import numpy as np
-import torch
-from monotonic_align.core import maximum_path_c
-
-
-def mask_from_len(lens: torch.Tensor, max_len=None):
- """
- Make a `mask` from lens.
-
- :param inputs: (B, T, D)
- :param lens: (B)
-
- :return:
- `mask`: (B, T)
- """
- if max_len is None:
- max_len = lens.max()
- index = torch.arange(max_len).to(lens).view(1, -1)
- return index < lens.unsqueeze(1) # (B, T)
-
-
-def mask_from_lens(
- similarity: torch.Tensor,
- symbol_lens: torch.Tensor,
- mel_lens: torch.Tensor,
-):
- """
- :param similarity: (B, S, T)
- :param symbol_lens: (B,)
- :param mel_lens: (B,)
- """
- _, S, T = similarity.size()
- mask_S = mask_from_len(symbol_lens, S)
- mask_T = mask_from_len(mel_lens, T)
- mask_ST = mask_S.unsqueeze(2) * mask_T.unsqueeze(1)
- return mask_ST.to(similarity)
-
-
-def maximum_path(value, mask=None):
- """Cython optimised version.
- value: [b, t_x, t_y]
- mask: [b, t_x, t_y]
- """
- if mask is None:
- mask = torch.zeros_like(value)
-
- value = value * mask
- device = value.device
- dtype = value.dtype
- value = value.data.cpu().numpy().astype(np.float32)
- path = np.zeros_like(value).astype(np.int32)
- mask = mask.data.cpu().numpy()
- t_x_max = mask.sum(1)[:, 0].astype(np.int32)
- t_y_max = mask.sum(2)[:, 0].astype(np.int32)
- maximum_path_c(path, value, t_x_max, t_y_max)
- return torch.from_numpy(path).to(device=device, dtype=dtype)
diff --git a/spaces/Hazem/roop/roop/core.py b/spaces/Hazem/roop/roop/core.py
deleted file mode 100644
index b70d8548194c74cce3e4d20c53c7a88c119c2028..0000000000000000000000000000000000000000
--- a/spaces/Hazem/roop/roop/core.py
+++ /dev/null
@@ -1,215 +0,0 @@
-#!/usr/bin/env python3
-
-import os
-import sys
-# single thread doubles cuda performance - needs to be set before torch import
-if any(arg.startswith('--execution-provider') for arg in sys.argv):
- os.environ['OMP_NUM_THREADS'] = '1'
-# reduce tensorflow log level
-os.environ['TF_CPP_MIN_LOG_LEVEL'] = '2'
-import warnings
-from typing import List
-import platform
-import signal
-import shutil
-import argparse
-import torch
-import onnxruntime
-import tensorflow
-
-import roop.globals
-import roop.metadata
-import roop.ui as ui
-from roop.predicter import predict_image, predict_video
-from roop.processors.frame.core import get_frame_processors_modules
-from roop.utilities import has_image_extension, is_image, is_video, detect_fps, create_video, extract_frames, get_temp_frame_paths, restore_audio, create_temp, move_temp, clean_temp, normalize_output_path
-
-if 'ROCMExecutionProvider' in roop.globals.execution_providers:
- del torch
-
-warnings.filterwarnings('ignore', category=FutureWarning, module='insightface')
-warnings.filterwarnings('ignore', category=UserWarning, module='torchvision')
-
-
-def parse_args() -> None:
- signal.signal(signal.SIGINT, lambda signal_number, frame: destroy())
- program = argparse.ArgumentParser(formatter_class=lambda prog: argparse.HelpFormatter(prog, max_help_position=100))
- program.add_argument('-s', '--source', help='select an source image', dest='source_path')
- program.add_argument('-t', '--target', help='select an target image or video', dest='target_path')
- program.add_argument('-o', '--output', help='select output file or directory', dest='output_path')
- program.add_argument('--frame-processor', help='frame processors (choices: face_swapper, face_enhancer, ...)', dest='frame_processor', default=['face_swapper'], nargs='+')
- program.add_argument('--keep-fps', help='keep original fps', dest='keep_fps', action='store_true', default=False)
- program.add_argument('--keep-audio', help='keep original audio', dest='keep_audio', action='store_true', default=True)
- program.add_argument('--keep-frames', help='keep temporary frames', dest='keep_frames', action='store_true', default=False)
- program.add_argument('--many-faces', help='process every face', dest='many_faces', action='store_true', default=False)
- program.add_argument('--video-encoder', help='adjust output video encoder', dest='video_encoder', default='libx264', choices=['libx264', 'libx265', 'libvpx-vp9'])
- program.add_argument('--video-quality', help='adjust output video quality', dest='video_quality', type=int, default=18, choices=range(52), metavar='[0-51]')
- program.add_argument('--max-memory', help='maximum amount of RAM in GB', dest='max_memory', type=int, default=suggest_max_memory())
- program.add_argument('--execution-provider', help='available execution provider (choices: cpu, ...)', dest='execution_provider', default=['cpu'], choices=suggest_execution_providers(), nargs='+')
- program.add_argument('--execution-threads', help='number of execution threads', dest='execution_threads', type=int, default=suggest_execution_threads())
- program.add_argument('-v', '--version', action='version', version=f'{roop.metadata.name} {roop.metadata.version}')
-
- args = program.parse_args()
-
- roop.globals.source_path = args.source_path
- roop.globals.target_path = args.target_path
- roop.globals.output_path = normalize_output_path(roop.globals.source_path, roop.globals.target_path, args.output_path)
- roop.globals.frame_processors = args.frame_processor
- roop.globals.headless = args.source_path or args.target_path or args.output_path
- roop.globals.keep_fps = args.keep_fps
- roop.globals.keep_audio = args.keep_audio
- roop.globals.keep_frames = args.keep_frames
- roop.globals.many_faces = args.many_faces
- roop.globals.video_encoder = args.video_encoder
- roop.globals.video_quality = args.video_quality
- roop.globals.max_memory = args.max_memory
- roop.globals.execution_providers = decode_execution_providers(args.execution_provider)
- roop.globals.execution_threads = args.execution_threads
-
-
-def encode_execution_providers(execution_providers: List[str]) -> List[str]:
- return [execution_provider.replace('ExecutionProvider', '').lower() for execution_provider in execution_providers]
-
-
-def decode_execution_providers(execution_providers: List[str]) -> List[str]:
- return [provider for provider, encoded_execution_provider in zip(onnxruntime.get_available_providers(), encode_execution_providers(onnxruntime.get_available_providers()))
- if any(execution_provider in encoded_execution_provider for execution_provider in execution_providers)]
-
-
-def suggest_max_memory() -> int:
- if platform.system().lower() == 'darwin':
- return 4
- return 16
-
-
-def suggest_execution_providers() -> List[str]:
- return encode_execution_providers(onnxruntime.get_available_providers())
-
-
-def suggest_execution_threads() -> int:
- if 'DmlExecutionProvider' in roop.globals.execution_providers:
- return 1
- if 'ROCMExecutionProvider' in roop.globals.execution_providers:
- return 1
- return 8
-
-
-def limit_resources() -> None:
- # prevent tensorflow memory leak
- gpus = tensorflow.config.experimental.list_physical_devices('GPU')
- for gpu in gpus:
- tensorflow.config.experimental.set_virtual_device_configuration(gpu, [
- tensorflow.config.experimental.VirtualDeviceConfiguration(memory_limit=1024)
- ])
- # limit memory usage
- if roop.globals.max_memory:
- memory = roop.globals.max_memory * 1024 ** 3
- if platform.system().lower() == 'darwin':
- memory = roop.globals.max_memory * 1024 ** 6
- if platform.system().lower() == 'windows':
- import ctypes
- kernel32 = ctypes.windll.kernel32
- kernel32.SetProcessWorkingSetSize(-1, ctypes.c_size_t(memory), ctypes.c_size_t(memory))
- else:
- import resource
- resource.setrlimit(resource.RLIMIT_DATA, (memory, memory))
-
-
-def release_resources() -> None:
- if 'CUDAExecutionProvider' in roop.globals.execution_providers:
- torch.cuda.empty_cache()
-
-
-def pre_check() -> bool:
- if sys.version_info < (3, 9):
- update_status('Python version is not supported - please upgrade to 3.9 or higher.')
- return False
- if not shutil.which('ffmpeg'):
- update_status('ffmpeg is not installed.')
- return False
- return True
-
-
-def update_status(message: str, scope: str = 'ROOP.CORE') -> None:
- print(f'[{scope}] {message}')
- if not roop.globals.headless:
- ui.update_status(message)
-
-
-def start() -> None:
- for frame_processor in get_frame_processors_modules(roop.globals.frame_processors):
- if not frame_processor.pre_start():
- return
- # process image to image
- if has_image_extension(roop.globals.target_path):
- if predict_image(roop.globals.target_path):
- destroy()
- shutil.copy2(roop.globals.target_path, roop.globals.output_path)
- for frame_processor in get_frame_processors_modules(roop.globals.frame_processors):
- update_status('Progressing...', frame_processor.NAME)
- frame_processor.process_image(roop.globals.source_path, roop.globals.output_path, roop.globals.output_path)
- frame_processor.post_process()
- release_resources()
- if is_image(roop.globals.target_path):
- update_status('Processing to image succeed!')
- else:
- update_status('Processing to image failed!')
- return
- # process image to videos
- if predict_video(roop.globals.target_path):
- destroy()
- update_status('Creating temp resources...')
- create_temp(roop.globals.target_path)
- update_status('Extracting frames...')
- extract_frames(roop.globals.target_path)
- temp_frame_paths = get_temp_frame_paths(roop.globals.target_path)
- for frame_processor in get_frame_processors_modules(roop.globals.frame_processors):
- update_status('Progressing...', frame_processor.NAME)
- frame_processor.process_video(roop.globals.source_path, temp_frame_paths)
- frame_processor.post_process()
- release_resources()
- # handles fps
- if roop.globals.keep_fps:
- update_status('Detecting fps...')
- fps = detect_fps(roop.globals.target_path)
- update_status(f'Creating video with {fps} fps...')
- create_video(roop.globals.target_path, fps)
- else:
- update_status('Creating video with 30.0 fps...')
- create_video(roop.globals.target_path)
- # handle audio
- if roop.globals.keep_audio:
- if roop.globals.keep_fps:
- update_status('Restoring audio...')
- else:
- update_status('Restoring audio might cause issues as fps are not kept...')
- restore_audio(roop.globals.target_path, roop.globals.output_path)
- else:
- move_temp(roop.globals.target_path, roop.globals.output_path)
- # clean and validate
- clean_temp(roop.globals.target_path)
- if is_video(roop.globals.target_path):
- update_status('Processing to video succeed!')
- else:
- update_status('Processing to video failed!')
-
-
-def destroy() -> None:
- if roop.globals.target_path:
- clean_temp(roop.globals.target_path)
- quit()
-
-
-def run() -> None:
- parse_args()
- if not pre_check():
- return
- for frame_processor in get_frame_processors_modules(roop.globals.frame_processors):
- if not frame_processor.pre_check():
- return
- limit_resources()
- if roop.globals.headless:
- start()
- else:
- window = ui.init(start, destroy)
- window.mainloop()
diff --git a/spaces/HighCWu/anime-colorization-with-hint/gradio-modified/gradio/processing_utils.py b/spaces/HighCWu/anime-colorization-with-hint/gradio-modified/gradio/processing_utils.py
deleted file mode 100644
index 98e35365c9a2dcb3a0e9ccaeedaad275f09132f1..0000000000000000000000000000000000000000
--- a/spaces/HighCWu/anime-colorization-with-hint/gradio-modified/gradio/processing_utils.py
+++ /dev/null
@@ -1,755 +0,0 @@
-from __future__ import annotations
-
-import base64
-import hashlib
-import json
-import mimetypes
-import os
-import pathlib
-import shutil
-import subprocess
-import tempfile
-import urllib.request
-import warnings
-from io import BytesIO
-from pathlib import Path
-from typing import Dict, Tuple
-
-import numpy as np
-import requests
-from ffmpy import FFmpeg, FFprobe, FFRuntimeError
-from PIL import Image, ImageOps, PngImagePlugin
-
-from gradio import encryptor, utils
-
-with warnings.catch_warnings():
- warnings.simplefilter("ignore") # Ignore pydub warning if ffmpeg is not installed
- from pydub import AudioSegment
-
-
-#########################
-# GENERAL
-#########################
-
-
-def to_binary(x: str | Dict) -> bytes:
- """Converts a base64 string or dictionary to a binary string that can be sent in a POST."""
- if isinstance(x, dict):
- if x.get("data"):
- base64str = x["data"]
- else:
- base64str = encode_url_or_file_to_base64(x["name"])
- else:
- base64str = x
- return base64.b64decode(base64str.split(",")[1])
-
-
-#########################
-# IMAGE PRE-PROCESSING
-#########################
-
-
-def decode_base64_to_image(encoding: str) -> Image.Image:
- content = encoding.split(";")[1]
- image_encoded = content.split(",")[1]
- return Image.open(BytesIO(base64.b64decode(image_encoded)))
-
-
-def encode_url_or_file_to_base64(path: str | Path, encryption_key: bytes | None = None):
- if utils.validate_url(str(path)):
- return encode_url_to_base64(str(path), encryption_key=encryption_key)
- else:
- return encode_file_to_base64(str(path), encryption_key=encryption_key)
-
-
-def get_mimetype(filename: str) -> str | None:
- mimetype = mimetypes.guess_type(filename)[0]
- if mimetype is not None:
- mimetype = mimetype.replace("x-wav", "wav").replace("x-flac", "flac")
- return mimetype
-
-
-def get_extension(encoding: str) -> str | None:
- encoding = encoding.replace("audio/wav", "audio/x-wav")
- type = mimetypes.guess_type(encoding)[0]
- if type == "audio/flac": # flac is not supported by mimetypes
- return "flac"
- elif type is None:
- return None
- extension = mimetypes.guess_extension(type)
- if extension is not None and extension.startswith("."):
- extension = extension[1:]
- return extension
-
-
-def encode_file_to_base64(f, encryption_key=None):
- with open(f, "rb") as file:
- encoded_string = base64.b64encode(file.read())
- if encryption_key:
- encoded_string = encryptor.decrypt(encryption_key, encoded_string)
- base64_str = str(encoded_string, "utf-8")
- mimetype = get_mimetype(f)
- return (
- "data:"
- + (mimetype if mimetype is not None else "")
- + ";base64,"
- + base64_str
- )
-
-
-def encode_url_to_base64(url, encryption_key=None):
- encoded_string = base64.b64encode(requests.get(url).content)
- if encryption_key:
- encoded_string = encryptor.decrypt(encryption_key, encoded_string)
- base64_str = str(encoded_string, "utf-8")
- mimetype = get_mimetype(url)
- return (
- "data:" + (mimetype if mimetype is not None else "") + ";base64," + base64_str
- )
-
-
-def encode_plot_to_base64(plt):
- with BytesIO() as output_bytes:
- plt.savefig(output_bytes, format="png")
- bytes_data = output_bytes.getvalue()
- base64_str = str(base64.b64encode(bytes_data), "utf-8")
- return "data:image/png;base64," + base64_str
-
-
-def save_array_to_file(image_array, dir=None):
- pil_image = Image.fromarray(_convert(image_array, np.uint8, force_copy=False))
- file_obj = tempfile.NamedTemporaryFile(delete=False, suffix=".png", dir=dir)
- pil_image.save(file_obj)
- return file_obj
-
-
-def save_pil_to_file(pil_image, dir=None):
- file_obj = tempfile.NamedTemporaryFile(delete=False, suffix=".png", dir=dir)
- pil_image.save(file_obj)
- return file_obj
-
-
-def encode_pil_to_base64(pil_image):
- with BytesIO() as output_bytes:
-
- # Copy any text-only metadata
- use_metadata = False
- metadata = PngImagePlugin.PngInfo()
- for key, value in pil_image.info.items():
- if isinstance(key, str) and isinstance(value, str):
- metadata.add_text(key, value)
- use_metadata = True
-
- pil_image.save(
- output_bytes, "PNG", pnginfo=(metadata if use_metadata else None)
- )
- bytes_data = output_bytes.getvalue()
- base64_str = str(base64.b64encode(bytes_data), "utf-8")
- return "data:image/png;base64," + base64_str
-
-
-def encode_array_to_base64(image_array):
- with BytesIO() as output_bytes:
- pil_image = Image.fromarray(_convert(image_array, np.uint8, force_copy=False))
- pil_image.save(output_bytes, "PNG")
- bytes_data = output_bytes.getvalue()
- base64_str = str(base64.b64encode(bytes_data), "utf-8")
- return "data:image/png;base64," + base64_str
-
-
-def resize_and_crop(img, size, crop_type="center"):
- """
- Resize and crop an image to fit the specified size.
- args:
- size: `(width, height)` tuple. Pass `None` for either width or height
- to only crop and resize the other.
- crop_type: can be 'top', 'middle' or 'bottom', depending on this
- value, the image will cropped getting the 'top/left', 'middle' or
- 'bottom/right' of the image to fit the size.
- raises:
- ValueError: if an invalid `crop_type` is provided.
- """
- if crop_type == "top":
- center = (0, 0)
- elif crop_type == "center":
- center = (0.5, 0.5)
- else:
- raise ValueError
-
- resize = list(size)
- if size[0] is None:
- resize[0] = img.size[0]
- if size[1] is None:
- resize[1] = img.size[1]
- return ImageOps.fit(img, resize, centering=center) # type: ignore
-
-
-##################
-# Audio
-##################
-
-
-def audio_from_file(filename, crop_min=0, crop_max=100):
- try:
- audio = AudioSegment.from_file(filename)
- except FileNotFoundError as e:
- isfile = Path(filename).is_file()
- msg = (
- f"Cannot load audio from file: `{'ffprobe' if isfile else filename}` not found."
- + " Please install `ffmpeg` in your system to use non-WAV audio file formats"
- " and make sure `ffprobe` is in your PATH."
- if isfile
- else ""
- )
- raise RuntimeError(msg) from e
- if crop_min != 0 or crop_max != 100:
- audio_start = len(audio) * crop_min / 100
- audio_end = len(audio) * crop_max / 100
- audio = audio[audio_start:audio_end]
- data = np.array(audio.get_array_of_samples())
- if audio.channels > 1:
- data = data.reshape(-1, audio.channels)
- return audio.frame_rate, data
-
-
-def audio_to_file(sample_rate, data, filename):
- data = convert_to_16_bit_wav(data)
- audio = AudioSegment(
- data.tobytes(),
- frame_rate=sample_rate,
- sample_width=data.dtype.itemsize,
- channels=(1 if len(data.shape) == 1 else data.shape[1]),
- )
- file = audio.export(filename, format="wav")
- file.close() # type: ignore
-
-
-def convert_to_16_bit_wav(data):
- # Based on: https://docs.scipy.org/doc/scipy/reference/generated/scipy.io.wavfile.write.html
- warning = "Trying to convert audio automatically from {} to 16-bit int format."
- if data.dtype in [np.float64, np.float32, np.float16]:
- warnings.warn(warning.format(data.dtype))
- data = data / np.abs(data).max()
- data = data * 32767
- data = data.astype(np.int16)
- elif data.dtype == np.int32:
- warnings.warn(warning.format(data.dtype))
- data = data / 65538
- data = data.astype(np.int16)
- elif data.dtype == np.int16:
- pass
- elif data.dtype == np.uint16:
- warnings.warn(warning.format(data.dtype))
- data = data - 32768
- data = data.astype(np.int16)
- elif data.dtype == np.uint8:
- warnings.warn(warning.format(data.dtype))
- data = data * 257 - 32768
- data = data.astype(np.int16)
- else:
- raise ValueError(
- "Audio data cannot be converted automatically from "
- f"{data.dtype} to 16-bit int format."
- )
- return data
-
-
-##################
-# OUTPUT
-##################
-
-
-def decode_base64_to_binary(encoding) -> Tuple[bytes, str | None]:
- extension = get_extension(encoding)
- data = encoding.split(",")[1]
- return base64.b64decode(data), extension
-
-
-def decode_base64_to_file(
- encoding, encryption_key=None, file_path=None, dir=None, prefix=None
-):
- if dir is not None:
- os.makedirs(dir, exist_ok=True)
- data, extension = decode_base64_to_binary(encoding)
- if file_path is not None and prefix is None:
- filename = Path(file_path).name
- prefix = filename
- if "." in filename:
- prefix = filename[0 : filename.index(".")]
- extension = filename[filename.index(".") + 1 :]
-
- if prefix is not None:
- prefix = utils.strip_invalid_filename_characters(prefix)
-
- if extension is None:
- file_obj = tempfile.NamedTemporaryFile(delete=False, prefix=prefix, dir=dir)
- else:
- file_obj = tempfile.NamedTemporaryFile(
- delete=False,
- prefix=prefix,
- suffix="." + extension,
- dir=dir,
- )
- if encryption_key is not None:
- data = encryptor.encrypt(encryption_key, data)
- file_obj.write(data)
- file_obj.flush()
- return file_obj
-
-
-def dict_or_str_to_json_file(jsn, dir=None):
- if dir is not None:
- os.makedirs(dir, exist_ok=True)
-
- file_obj = tempfile.NamedTemporaryFile(
- delete=False, suffix=".json", dir=dir, mode="w+"
- )
- if isinstance(jsn, str):
- jsn = json.loads(jsn)
- json.dump(jsn, file_obj)
- file_obj.flush()
- return file_obj
-
-
-def file_to_json(file_path: str | Path) -> Dict:
- with open(file_path) as f:
- return json.load(f)
-
-
-class TempFileManager:
- """
- A class that should be inherited by any Component that needs to manage temporary files.
- It should be instantiated in the __init__ method of the component.
- """
-
- def __init__(self) -> None:
- # Set stores all the temporary files created by this component.
- self.temp_files = set()
-
- def hash_file(self, file_path: str, chunk_num_blocks: int = 128) -> str:
- sha1 = hashlib.sha1()
- with open(file_path, "rb") as f:
- for chunk in iter(lambda: f.read(chunk_num_blocks * sha1.block_size), b""):
- sha1.update(chunk)
- return sha1.hexdigest()
-
- def hash_url(self, url: str, chunk_num_blocks: int = 128) -> str:
- sha1 = hashlib.sha1()
- remote = urllib.request.urlopen(url)
- max_file_size = 100 * 1024 * 1024 # 100MB
- total_read = 0
- while True:
- data = remote.read(chunk_num_blocks * sha1.block_size)
- total_read += chunk_num_blocks * sha1.block_size
- if not data or total_read > max_file_size:
- break
- sha1.update(data)
- return sha1.hexdigest()
-
- def get_prefix_and_extension(self, file_path_or_url: str) -> Tuple[str, str]:
- file_name = Path(file_path_or_url).name
- prefix, extension = file_name, None
- if "." in file_name:
- prefix = file_name[0 : file_name.index(".")]
- extension = "." + file_name[file_name.index(".") + 1 :]
- else:
- extension = ""
- prefix = utils.strip_invalid_filename_characters(prefix)
- return prefix, extension
-
- def get_temp_file_path(self, file_path: str) -> str:
- prefix, extension = self.get_prefix_and_extension(file_path)
- file_hash = self.hash_file(file_path)
- return prefix + file_hash + extension
-
- def get_temp_url_path(self, url: str) -> str:
- prefix, extension = self.get_prefix_and_extension(url)
- file_hash = self.hash_url(url)
- return prefix + file_hash + extension
-
- def make_temp_copy_if_needed(self, file_path: str) -> str:
- """Returns a temporary file path for a copy of the given file path if it does
- not already exist. Otherwise returns the path to the existing temp file."""
- f = tempfile.NamedTemporaryFile()
- temp_dir = Path(f.name).parent
-
- temp_file_path = self.get_temp_file_path(file_path)
- f.name = str(temp_dir / temp_file_path)
- full_temp_file_path = str(Path(f.name).resolve())
-
- if not Path(full_temp_file_path).exists():
- shutil.copy2(file_path, full_temp_file_path)
-
- self.temp_files.add(full_temp_file_path)
- return full_temp_file_path
-
- def download_temp_copy_if_needed(self, url: str) -> str:
- """Downloads a file and makes a temporary file path for a copy if does not already
- exist. Otherwise returns the path to the existing temp file."""
- f = tempfile.NamedTemporaryFile()
- temp_dir = Path(f.name).parent
-
- temp_file_path = self.get_temp_url_path(url)
- f.name = str(temp_dir / temp_file_path)
- full_temp_file_path = str(Path(f.name).resolve())
-
- if not Path(full_temp_file_path).exists():
- with requests.get(url, stream=True) as r:
- with open(full_temp_file_path, "wb") as f:
- shutil.copyfileobj(r.raw, f)
-
- self.temp_files.add(full_temp_file_path)
- return full_temp_file_path
-
-
-def create_tmp_copy_of_file(file_path, dir=None):
- if dir is not None:
- os.makedirs(dir, exist_ok=True)
- file_name = Path(file_path).name
- prefix, extension = file_name, None
- if "." in file_name:
- prefix = file_name[0 : file_name.index(".")]
- extension = file_name[file_name.index(".") + 1 :]
- prefix = utils.strip_invalid_filename_characters(prefix)
- if extension is None:
- file_obj = tempfile.NamedTemporaryFile(delete=False, prefix=prefix, dir=dir)
- else:
- file_obj = tempfile.NamedTemporaryFile(
- delete=False,
- prefix=prefix,
- suffix="." + extension,
- dir=dir,
- )
- shutil.copy2(file_path, file_obj.name)
- return file_obj
-
-
-def _convert(image, dtype, force_copy=False, uniform=False):
- """
- Adapted from: https://github.com/scikit-image/scikit-image/blob/main/skimage/util/dtype.py#L510-L531
-
- Convert an image to the requested data-type.
- Warnings are issued in case of precision loss, or when negative values
- are clipped during conversion to unsigned integer types (sign loss).
- Floating point values are expected to be normalized and will be clipped
- to the range [0.0, 1.0] or [-1.0, 1.0] when converting to unsigned or
- signed integers respectively.
- Numbers are not shifted to the negative side when converting from
- unsigned to signed integer types. Negative values will be clipped when
- converting to unsigned integers.
- Parameters
- ----------
- image : ndarray
- Input image.
- dtype : dtype
- Target data-type.
- force_copy : bool, optional
- Force a copy of the data, irrespective of its current dtype.
- uniform : bool, optional
- Uniformly quantize the floating point range to the integer range.
- By default (uniform=False) floating point values are scaled and
- rounded to the nearest integers, which minimizes back and forth
- conversion errors.
- .. versionchanged :: 0.15
- ``_convert`` no longer warns about possible precision or sign
- information loss. See discussions on these warnings at:
- https://github.com/scikit-image/scikit-image/issues/2602
- https://github.com/scikit-image/scikit-image/issues/543#issuecomment-208202228
- https://github.com/scikit-image/scikit-image/pull/3575
- References
- ----------
- .. [1] DirectX data conversion rules.
- https://msdn.microsoft.com/en-us/library/windows/desktop/dd607323%28v=vs.85%29.aspx
- .. [2] Data Conversions. In "OpenGL ES 2.0 Specification v2.0.25",
- pp 7-8. Khronos Group, 2010.
- .. [3] Proper treatment of pixels as integers. A.W. Paeth.
- In "Graphics Gems I", pp 249-256. Morgan Kaufmann, 1990.
- .. [4] Dirty Pixels. J. Blinn. In "Jim Blinn's corner: Dirty Pixels",
- pp 47-57. Morgan Kaufmann, 1998.
- """
- dtype_range = {
- bool: (False, True),
- np.bool_: (False, True),
- np.bool8: (False, True),
- float: (-1, 1),
- np.float_: (-1, 1),
- np.float16: (-1, 1),
- np.float32: (-1, 1),
- np.float64: (-1, 1),
- }
-
- def _dtype_itemsize(itemsize, *dtypes):
- """Return first of `dtypes` with itemsize greater than `itemsize`
- Parameters
- ----------
- itemsize: int
- The data type object element size.
- Other Parameters
- ----------------
- *dtypes:
- Any Object accepted by `np.dtype` to be converted to a data
- type object
- Returns
- -------
- dtype: data type object
- First of `dtypes` with itemsize greater than `itemsize`.
- """
- return next(dt for dt in dtypes if np.dtype(dt).itemsize >= itemsize)
-
- def _dtype_bits(kind, bits, itemsize=1):
- """Return dtype of `kind` that can store a `bits` wide unsigned int
- Parameters:
- kind: str
- Data type kind.
- bits: int
- Desired number of bits.
- itemsize: int
- The data type object element size.
- Returns
- -------
- dtype: data type object
- Data type of `kind` that can store a `bits` wide unsigned int
- """
-
- s = next(
- i
- for i in (itemsize,) + (2, 4, 8)
- if bits < (i * 8) or (bits == (i * 8) and kind == "u")
- )
-
- return np.dtype(kind + str(s))
-
- def _scale(a, n, m, copy=True):
- """Scale an array of unsigned/positive integers from `n` to `m` bits.
- Numbers can be represented exactly only if `m` is a multiple of `n`.
- Parameters
- ----------
- a : ndarray
- Input image array.
- n : int
- Number of bits currently used to encode the values in `a`.
- m : int
- Desired number of bits to encode the values in `out`.
- copy : bool, optional
- If True, allocates and returns new array. Otherwise, modifies
- `a` in place.
- Returns
- -------
- out : array
- Output image array. Has the same kind as `a`.
- """
- kind = a.dtype.kind
- if n > m and a.max() < 2**m:
- return a.astype(_dtype_bits(kind, m))
- elif n == m:
- return a.copy() if copy else a
- elif n > m:
- # downscale with precision loss
- if copy:
- b = np.empty(a.shape, _dtype_bits(kind, m))
- np.floor_divide(a, 2 ** (n - m), out=b, dtype=a.dtype, casting="unsafe")
- return b
- else:
- a //= 2 ** (n - m)
- return a
- elif m % n == 0:
- # exact upscale to a multiple of `n` bits
- if copy:
- b = np.empty(a.shape, _dtype_bits(kind, m))
- np.multiply(a, (2**m - 1) // (2**n - 1), out=b, dtype=b.dtype)
- return b
- else:
- a = a.astype(_dtype_bits(kind, m, a.dtype.itemsize), copy=False)
- a *= (2**m - 1) // (2**n - 1)
- return a
- else:
- # upscale to a multiple of `n` bits,
- # then downscale with precision loss
- o = (m // n + 1) * n
- if copy:
- b = np.empty(a.shape, _dtype_bits(kind, o))
- np.multiply(a, (2**o - 1) // (2**n - 1), out=b, dtype=b.dtype)
- b //= 2 ** (o - m)
- return b
- else:
- a = a.astype(_dtype_bits(kind, o, a.dtype.itemsize), copy=False)
- a *= (2**o - 1) // (2**n - 1)
- a //= 2 ** (o - m)
- return a
-
- image = np.asarray(image)
- dtypeobj_in = image.dtype
- if dtype is np.floating:
- dtypeobj_out = np.dtype("float64")
- else:
- dtypeobj_out = np.dtype(dtype)
- dtype_in = dtypeobj_in.type
- dtype_out = dtypeobj_out.type
- kind_in = dtypeobj_in.kind
- kind_out = dtypeobj_out.kind
- itemsize_in = dtypeobj_in.itemsize
- itemsize_out = dtypeobj_out.itemsize
-
- # Below, we do an `issubdtype` check. Its purpose is to find out
- # whether we can get away without doing any image conversion. This happens
- # when:
- #
- # - the output and input dtypes are the same or
- # - when the output is specified as a type, and the input dtype
- # is a subclass of that type (e.g. `np.floating` will allow
- # `float32` and `float64` arrays through)
-
- if np.issubdtype(dtype_in, np.obj2sctype(dtype)):
- if force_copy:
- image = image.copy()
- return image
-
- if kind_in in "ui":
- imin_in = np.iinfo(dtype_in).min
- imax_in = np.iinfo(dtype_in).max
- if kind_out in "ui":
- imin_out = np.iinfo(dtype_out).min # type: ignore
- imax_out = np.iinfo(dtype_out).max # type: ignore
-
- # any -> binary
- if kind_out == "b":
- return image > dtype_in(dtype_range[dtype_in][1] / 2)
-
- # binary -> any
- if kind_in == "b":
- result = image.astype(dtype_out)
- if kind_out != "f":
- result *= dtype_out(dtype_range[dtype_out][1])
- return result
-
- # float -> any
- if kind_in == "f":
- if kind_out == "f":
- # float -> float
- return image.astype(dtype_out)
-
- if np.min(image) < -1.0 or np.max(image) > 1.0:
- raise ValueError("Images of type float must be between -1 and 1.")
- # floating point -> integer
- # use float type that can represent output integer type
- computation_type = _dtype_itemsize(
- itemsize_out, dtype_in, np.float32, np.float64
- )
-
- if not uniform:
- if kind_out == "u":
- image_out = np.multiply(image, imax_out, dtype=computation_type) # type: ignore
- else:
- image_out = np.multiply(
- image, (imax_out - imin_out) / 2, dtype=computation_type # type: ignore
- )
- image_out -= 1.0 / 2.0
- np.rint(image_out, out=image_out)
- np.clip(image_out, imin_out, imax_out, out=image_out) # type: ignore
- elif kind_out == "u":
- image_out = np.multiply(image, imax_out + 1, dtype=computation_type) # type: ignore
- np.clip(image_out, 0, imax_out, out=image_out) # type: ignore
- else:
- image_out = np.multiply(
- image, (imax_out - imin_out + 1.0) / 2.0, dtype=computation_type # type: ignore
- )
- np.floor(image_out, out=image_out)
- np.clip(image_out, imin_out, imax_out, out=image_out) # type: ignore
- return image_out.astype(dtype_out)
-
- # signed/unsigned int -> float
- if kind_out == "f":
- # use float type that can exactly represent input integers
- computation_type = _dtype_itemsize(
- itemsize_in, dtype_out, np.float32, np.float64
- )
-
- if kind_in == "u":
- # using np.divide or np.multiply doesn't copy the data
- # until the computation time
- image = np.multiply(image, 1.0 / imax_in, dtype=computation_type) # type: ignore
- # DirectX uses this conversion also for signed ints
- # if imin_in:
- # np.maximum(image, -1.0, out=image)
- else:
- image = np.add(image, 0.5, dtype=computation_type)
- image *= 2 / (imax_in - imin_in) # type: ignore
-
- return np.asarray(image, dtype_out)
-
- # unsigned int -> signed/unsigned int
- if kind_in == "u":
- if kind_out == "i":
- # unsigned int -> signed int
- image = _scale(image, 8 * itemsize_in, 8 * itemsize_out - 1)
- return image.view(dtype_out)
- else:
- # unsigned int -> unsigned int
- return _scale(image, 8 * itemsize_in, 8 * itemsize_out)
-
- # signed int -> unsigned int
- if kind_out == "u":
- image = _scale(image, 8 * itemsize_in - 1, 8 * itemsize_out)
- result = np.empty(image.shape, dtype_out)
- np.maximum(image, 0, out=result, dtype=image.dtype, casting="unsafe")
- return result
-
- # signed int -> signed int
- if itemsize_in > itemsize_out:
- return _scale(image, 8 * itemsize_in - 1, 8 * itemsize_out - 1)
-
- image = image.astype(_dtype_bits("i", itemsize_out * 8))
- image -= imin_in # type: ignore
- image = _scale(image, 8 * itemsize_in, 8 * itemsize_out, copy=False)
- image += imin_out # type: ignore
- return image.astype(dtype_out)
-
-
-def ffmpeg_installed() -> bool:
- return shutil.which("ffmpeg") is not None
-
-
-def video_is_playable(video_filepath: str) -> bool:
- """Determines if a video is playable in the browser.
-
- A video is playable if it has a playable container and codec.
- .mp4 -> h264
- .webm -> vp9
- .ogg -> theora
- """
- try:
- container = pathlib.Path(video_filepath).suffix.lower()
- probe = FFprobe(
- global_options="-show_format -show_streams -select_streams v -print_format json",
- inputs={video_filepath: None},
- )
- output = probe.run(stderr=subprocess.PIPE, stdout=subprocess.PIPE)
- output = json.loads(output[0])
- video_codec = output["streams"][0]["codec_name"]
- return (container, video_codec) in [
- (".mp4", "h264"),
- (".ogg", "theora"),
- (".webm", "vp9"),
- ]
- # If anything goes wrong, assume the video can be played to not convert downstream
- except (FFRuntimeError, IndexError, KeyError):
- return True
-
-
-def convert_video_to_playable_mp4(video_path: str) -> str:
- """Convert the video to mp4. If something goes wrong return the original video."""
- try:
- output_path = pathlib.Path(video_path).with_suffix(".mp4")
- with tempfile.NamedTemporaryFile(delete=False) as tmp_file:
- shutil.copy2(video_path, tmp_file.name)
- # ffmpeg will automatically use h264 codec (playable in browser) when converting to mp4
- ff = FFmpeg(
- inputs={str(tmp_file.name): None},
- outputs={str(output_path): None},
- global_options="-y -loglevel quiet",
- )
- ff.run()
- except FFRuntimeError as e:
- print(f"Error converting video to browser-playable format {str(e)}")
- output_path = video_path
- return str(output_path)
diff --git a/spaces/ICML2022/OFA/fairseq/examples/wav2vec/unsupervised/scripts/phonemize_with_sil.py b/spaces/ICML2022/OFA/fairseq/examples/wav2vec/unsupervised/scripts/phonemize_with_sil.py
deleted file mode 100644
index c6512d7322def67b27aba46e9e36da171db6963b..0000000000000000000000000000000000000000
--- a/spaces/ICML2022/OFA/fairseq/examples/wav2vec/unsupervised/scripts/phonemize_with_sil.py
+++ /dev/null
@@ -1,83 +0,0 @@
-#!/usr/bin/env python3 -u
-# Copyright (c) Facebook, Inc. and its affiliates.
-#
-# This source code is licensed under the MIT license found in the
-# LICENSE file in the root directory of this source tree.
-
-import argparse
-import numpy as np
-import sys
-
-
-def get_parser():
- parser = argparse.ArgumentParser(
- description="converts words to phones adding optional silences around in between words"
- )
- parser.add_argument(
- "--sil-prob",
- "-s",
- type=float,
- default=0,
- help="probability of inserting silence between each word",
- )
- parser.add_argument(
- "--surround",
- action="store_true",
- help="if set, surrounds each example with silence",
- )
- parser.add_argument(
- "--lexicon",
- help="lexicon to convert to phones",
- required=True,
- )
-
- return parser
-
-
-def main():
- parser = get_parser()
- args = parser.parse_args()
-
- sil_prob = args.sil_prob
- surround = args.surround
- sil = ""
-
- wrd_to_phn = {}
-
- with open(args.lexicon, "r") as lf:
- for line in lf:
- items = line.rstrip().split()
- assert len(items) > 1, line
- assert items[0] not in wrd_to_phn, items
- wrd_to_phn[items[0]] = items[1:]
-
- for line in sys.stdin:
- words = line.strip().split()
-
- if not all(w in wrd_to_phn for w in words):
- continue
-
- phones = []
- if surround:
- phones.append(sil)
-
- sample_sil_probs = None
- if sil_prob > 0 and len(words) > 1:
- sample_sil_probs = np.random.random(len(words) - 1)
-
- for i, w in enumerate(words):
- phones.extend(wrd_to_phn[w])
- if (
- sample_sil_probs is not None
- and i < len(sample_sil_probs)
- and sample_sil_probs[i] < sil_prob
- ):
- phones.append(sil)
-
- if surround:
- phones.append(sil)
- print(" ".join(phones))
-
-
-if __name__ == "__main__":
- main()
diff --git a/spaces/ICML2022/OFA/fairseq/fairseq/scoring/__init__.py b/spaces/ICML2022/OFA/fairseq/fairseq/scoring/__init__.py
deleted file mode 100644
index 58f2f563e493327394dff1265030d18f0814b5a2..0000000000000000000000000000000000000000
--- a/spaces/ICML2022/OFA/fairseq/fairseq/scoring/__init__.py
+++ /dev/null
@@ -1,55 +0,0 @@
-# Copyright (c) Facebook, Inc. and its affiliates.
-#
-# This source code is licensed under the MIT license found in the
-# LICENSE file in the root directory of this source tree.
-
-
-import importlib
-import os
-from abc import ABC, abstractmethod
-
-from fairseq import registry
-from omegaconf import DictConfig
-
-
-class BaseScorer(ABC):
- def __init__(self, cfg):
- self.cfg = cfg
- self.ref = []
- self.pred = []
-
- def add_string(self, ref, pred):
- self.ref.append(ref)
- self.pred.append(pred)
-
- @abstractmethod
- def score(self) -> float:
- pass
-
- @abstractmethod
- def result_string(self) -> str:
- pass
-
-
-_build_scorer, register_scorer, SCORER_REGISTRY, _ = registry.setup_registry(
- "--scoring", default="bleu"
-)
-
-
-def build_scorer(choice, tgt_dict):
- _choice = choice._name if isinstance(choice, DictConfig) else choice
-
- if _choice == "bleu":
- from fairseq.scoring import bleu
-
- return bleu.Scorer(
- bleu.BleuConfig(pad=tgt_dict.pad(), eos=tgt_dict.eos(), unk=tgt_dict.unk())
- )
- return _build_scorer(choice)
-
-
-# automatically import any Python files in the current directory
-for file in sorted(os.listdir(os.path.dirname(__file__))):
- if file.endswith(".py") and not file.startswith("_"):
- module = file[: file.find(".py")]
- importlib.import_module("fairseq.scoring." + module)
diff --git a/spaces/InnovTech/InnovTech.ProAI/app.py b/spaces/InnovTech/InnovTech.ProAI/app.py
deleted file mode 100644
index e0fb4daf4cecab8b8b7ca088f53a67f91e66b76f..0000000000000000000000000000000000000000
--- a/spaces/InnovTech/InnovTech.ProAI/app.py
+++ /dev/null
@@ -1,139 +0,0 @@
-from __future__ import annotations
-from typing import Iterable
-import gradio as gr
-from gradio.themes.base import Base
-from gradio.themes.utils import colors, fonts, sizes
-
-from llama_cpp import Llama
-#from huggingface_hub import hf_hub_download
-
-#hf_hub_download(repo_id="LLukas22/gpt4all-lora-quantized-ggjt", filename="ggjt-model.bin", local_dir=".")
-llm = Llama(model_path="./ggjt-model.bin")
-
-
-ins = '''### Instruction:
-{}
-### Response:
-'''
-
-theme = gr.themes.Monochrome(
- primary_hue="indigo",
- secondary_hue="blue",
- neutral_hue="slate",
- radius_size=gr.themes.sizes.radius_sm,
- font=[gr.themes.GoogleFont("Open Sans"), "ui-sans-serif", "system-ui", "sans-serif"],
-)
-
-
-
-
-# def generate(instruction):
-# response = llm(ins.format(instruction))
-# response = response['choices'][0]['text']
-# result = ""
-# for word in response.split(" "):
-# result += word + " "
-# yield result
-
-def generate(instruction):
- result = ""
- for x in llm(ins.format(instruction), stop=['### Instruction:', '### End'], stream=True):
- result += x['choices'][0]['text']
- yield result
-
-
-examples = [
- ".",
- ".",
- ".",
- "."
-]
-
-def process_example(args):
- for x in generate(args):
- pass
- return x
-
-css = ".generating {visibility: hidden}"
-
-# Based on the gradio theming guide and borrowed from https://huggingface.co/spaces/shivi/dolly-v2-demo
-class SeafoamCustom(Base):
- def __init__(
- self,
- *,
- primary_hue: colors.Color | str = colors.emerald,
- secondary_hue: colors.Color | str = colors.blue,
- neutral_hue: colors.Color | str = colors.blue,
- spacing_size: sizes.Size | str = sizes.spacing_md,
- radius_size: sizes.Size | str = sizes.radius_md,
- font: fonts.Font
- | str
- | Iterable[fonts.Font | str] = (
- fonts.GoogleFont("Quicksand"),
- "ui-sans-serif",
- "sans-serif",
- ),
- font_mono: fonts.Font
- | str
- | Iterable[fonts.Font | str] = (
- fonts.GoogleFont("IBM Plex Mono"),
- "ui-monospace",
- "monospace",
- ),
- ):
- super().__init__(
- primary_hue=primary_hue,
- secondary_hue=secondary_hue,
- neutral_hue=neutral_hue,
- spacing_size=spacing_size,
- radius_size=radius_size,
- font=font,
- font_mono=font_mono,
- )
- super().set(
- button_primary_background_fill="linear-gradient(90deg, *primary_300, *secondary_400)",
- button_primary_background_fill_hover="linear-gradient(90deg, *primary_200, *secondary_300)",
- button_primary_text_color="white",
- button_primary_background_fill_dark="linear-gradient(90deg, *primary_600, *secondary_800)",
- block_shadow="*shadow_drop_lg",
- button_shadow="*shadow_drop_lg",
- input_background_fill="zinc",
- input_border_color="*secondary_300",
- input_shadow="*shadow_drop",
- input_shadow_focus="*shadow_drop_lg",
- )
-
-
-seafoam = SeafoamCustom()
-
-
-with gr.Blocks(theme=seafoam, analytics_enabled=False, css=css) as demo:
- with gr.Column():
- gr.Markdown(
- """ ## InnovTech.Pro.AI
-
- """
- )
-
- with gr.Row():
- with gr.Column(scale=3):
- instruction = gr.Textbox(placeholder="Enter your question here", label="Question", elem_id="q-input")
-
- with gr.Box():
- gr.Markdown("**Answer**")
- output = gr.Markdown(elem_id="q-output")
- submit = gr.Button("Generate", variant="primary")
- gr.Examples(
- examples=examples,
- inputs=[instruction],
- cache_examples=False,
- fn=process_example,
- outputs=[output],
- )
-
-
-
- submit.click(generate, inputs=[instruction], outputs=[output])
- instruction.submit(generate, inputs=[instruction], outputs=[output])
-
-demo.queue(concurrency_count=1).launch(debug=True)
\ No newline at end of file
diff --git a/spaces/JMalott/ai_architecture/app.py b/spaces/JMalott/ai_architecture/app.py
deleted file mode 100644
index 41b294aa6924bd819cd1a7685ee2d558706295e1..0000000000000000000000000000000000000000
--- a/spaces/JMalott/ai_architecture/app.py
+++ /dev/null
@@ -1,57 +0,0 @@
-import streamlit as st
-import pandas as pd
-import numpy as np
-import os, random, time
-from utils import footer
-from page import generate, reduce, intro
-
-
-st.set_page_config(
- page_title="AI Architecture",
-)
-
-if( hasattr(st.session_state, 'page') == False):
- st.session_state.page = 2
-
-if( hasattr(st.session_state, 'results') == False):
- st.session_state.results = []
-
-if( hasattr(st.session_state, 'prompt') == False):
- st.session_state.prompt = None
-
-p1 = st.empty()
-p2 = st.empty()
-p3 = st.empty()
-
-
-st.session_state.stop = False
-st.session_state.progress = 0
-#st.session_state.prompt = None
-st.session_state.images = []
-st.session_state.regenerate = False
-
-
-
-footer()
-
-if(st.session_state.page == 2):
- p1.empty()
- p2.empty()
- with p3.container():
- intro.app()
-
-if(st.session_state.page == 0):
- p2.empty()
- p3.empty()
- with p1.container():
- generate.app()
-
-if(st.session_state.page == 1):
- p1.empty()
- p3.empty()
- with p2.container():
- reduce.app()
-
-
-
-
diff --git a/spaces/Jasonyoyo/CodeFormer/CodeFormer/basicsr/utils/file_client.py b/spaces/Jasonyoyo/CodeFormer/CodeFormer/basicsr/utils/file_client.py
deleted file mode 100644
index 7f38d9796da3899048924f2f803d1088927966b0..0000000000000000000000000000000000000000
--- a/spaces/Jasonyoyo/CodeFormer/CodeFormer/basicsr/utils/file_client.py
+++ /dev/null
@@ -1,167 +0,0 @@
-# Modified from https://github.com/open-mmlab/mmcv/blob/master/mmcv/fileio/file_client.py # noqa: E501
-from abc import ABCMeta, abstractmethod
-
-
-class BaseStorageBackend(metaclass=ABCMeta):
- """Abstract class of storage backends.
-
- All backends need to implement two apis: ``get()`` and ``get_text()``.
- ``get()`` reads the file as a byte stream and ``get_text()`` reads the file
- as texts.
- """
-
- @abstractmethod
- def get(self, filepath):
- pass
-
- @abstractmethod
- def get_text(self, filepath):
- pass
-
-
-class MemcachedBackend(BaseStorageBackend):
- """Memcached storage backend.
-
- Attributes:
- server_list_cfg (str): Config file for memcached server list.
- client_cfg (str): Config file for memcached client.
- sys_path (str | None): Additional path to be appended to `sys.path`.
- Default: None.
- """
-
- def __init__(self, server_list_cfg, client_cfg, sys_path=None):
- if sys_path is not None:
- import sys
- sys.path.append(sys_path)
- try:
- import mc
- except ImportError:
- raise ImportError('Please install memcached to enable MemcachedBackend.')
-
- self.server_list_cfg = server_list_cfg
- self.client_cfg = client_cfg
- self._client = mc.MemcachedClient.GetInstance(self.server_list_cfg, self.client_cfg)
- # mc.pyvector servers as a point which points to a memory cache
- self._mc_buffer = mc.pyvector()
-
- def get(self, filepath):
- filepath = str(filepath)
- import mc
- self._client.Get(filepath, self._mc_buffer)
- value_buf = mc.ConvertBuffer(self._mc_buffer)
- return value_buf
-
- def get_text(self, filepath):
- raise NotImplementedError
-
-
-class HardDiskBackend(BaseStorageBackend):
- """Raw hard disks storage backend."""
-
- def get(self, filepath):
- filepath = str(filepath)
- with open(filepath, 'rb') as f:
- value_buf = f.read()
- return value_buf
-
- def get_text(self, filepath):
- filepath = str(filepath)
- with open(filepath, 'r') as f:
- value_buf = f.read()
- return value_buf
-
-
-class LmdbBackend(BaseStorageBackend):
- """Lmdb storage backend.
-
- Args:
- db_paths (str | list[str]): Lmdb database paths.
- client_keys (str | list[str]): Lmdb client keys. Default: 'default'.
- readonly (bool, optional): Lmdb environment parameter. If True,
- disallow any write operations. Default: True.
- lock (bool, optional): Lmdb environment parameter. If False, when
- concurrent access occurs, do not lock the database. Default: False.
- readahead (bool, optional): Lmdb environment parameter. If False,
- disable the OS filesystem readahead mechanism, which may improve
- random read performance when a database is larger than RAM.
- Default: False.
-
- Attributes:
- db_paths (list): Lmdb database path.
- _client (list): A list of several lmdb envs.
- """
-
- def __init__(self, db_paths, client_keys='default', readonly=True, lock=False, readahead=False, **kwargs):
- try:
- import lmdb
- except ImportError:
- raise ImportError('Please install lmdb to enable LmdbBackend.')
-
- if isinstance(client_keys, str):
- client_keys = [client_keys]
-
- if isinstance(db_paths, list):
- self.db_paths = [str(v) for v in db_paths]
- elif isinstance(db_paths, str):
- self.db_paths = [str(db_paths)]
- assert len(client_keys) == len(self.db_paths), ('client_keys and db_paths should have the same length, '
- f'but received {len(client_keys)} and {len(self.db_paths)}.')
-
- self._client = {}
- for client, path in zip(client_keys, self.db_paths):
- self._client[client] = lmdb.open(path, readonly=readonly, lock=lock, readahead=readahead, **kwargs)
-
- def get(self, filepath, client_key):
- """Get values according to the filepath from one lmdb named client_key.
-
- Args:
- filepath (str | obj:`Path`): Here, filepath is the lmdb key.
- client_key (str): Used for distinguishing differnet lmdb envs.
- """
- filepath = str(filepath)
- assert client_key in self._client, (f'client_key {client_key} is not ' 'in lmdb clients.')
- client = self._client[client_key]
- with client.begin(write=False) as txn:
- value_buf = txn.get(filepath.encode('ascii'))
- return value_buf
-
- def get_text(self, filepath):
- raise NotImplementedError
-
-
-class FileClient(object):
- """A general file client to access files in different backend.
-
- The client loads a file or text in a specified backend from its path
- and return it as a binary file. it can also register other backend
- accessor with a given name and backend class.
-
- Attributes:
- backend (str): The storage backend type. Options are "disk",
- "memcached" and "lmdb".
- client (:obj:`BaseStorageBackend`): The backend object.
- """
-
- _backends = {
- 'disk': HardDiskBackend,
- 'memcached': MemcachedBackend,
- 'lmdb': LmdbBackend,
- }
-
- def __init__(self, backend='disk', **kwargs):
- if backend not in self._backends:
- raise ValueError(f'Backend {backend} is not supported. Currently supported ones'
- f' are {list(self._backends.keys())}')
- self.backend = backend
- self.client = self._backends[backend](**kwargs)
-
- def get(self, filepath, client_key='default'):
- # client_key is used only for lmdb, where different fileclients have
- # different lmdb environments.
- if self.backend == 'lmdb':
- return self.client.get(filepath, client_key)
- else:
- return self.client.get(filepath)
-
- def get_text(self, filepath):
- return self.client.get_text(filepath)
diff --git a/spaces/JohnSmith9982/VITS-Umamusume-voice-synthesizer/ONNXVITS_infer.py b/spaces/JohnSmith9982/VITS-Umamusume-voice-synthesizer/ONNXVITS_infer.py
deleted file mode 100644
index af04e614c8f1ac43faf363b1a9f6bfd667fbde21..0000000000000000000000000000000000000000
--- a/spaces/JohnSmith9982/VITS-Umamusume-voice-synthesizer/ONNXVITS_infer.py
+++ /dev/null
@@ -1,201 +0,0 @@
-import torch
-import commons
-import models
-
-import math
-from torch import nn
-from torch.nn import functional as F
-
-import modules
-import attentions
-
-from torch.nn import Conv1d, ConvTranspose1d, Conv2d
-from torch.nn.utils import weight_norm, remove_weight_norm, spectral_norm
-from commons import init_weights, get_padding
-
-
-class TextEncoder(nn.Module):
- def __init__(self,
- n_vocab,
- out_channels,
- hidden_channels,
- filter_channels,
- n_heads,
- n_layers,
- kernel_size,
- p_dropout,
- emotion_embedding):
- super().__init__()
- self.n_vocab = n_vocab
- self.out_channels = out_channels
- self.hidden_channels = hidden_channels
- self.filter_channels = filter_channels
- self.n_heads = n_heads
- self.n_layers = n_layers
- self.kernel_size = kernel_size
- self.p_dropout = p_dropout
- self.emotion_embedding = emotion_embedding
-
- if self.n_vocab != 0:
- self.emb = nn.Embedding(n_vocab, hidden_channels)
- if emotion_embedding:
- self.emo_proj = nn.Linear(1024, hidden_channels)
- nn.init.normal_(self.emb.weight, 0.0, hidden_channels ** -0.5)
-
- self.encoder = attentions.Encoder(
- hidden_channels,
- filter_channels,
- n_heads,
- n_layers,
- kernel_size,
- p_dropout)
- self.proj = nn.Conv1d(hidden_channels, out_channels * 2, 1)
-
- def forward(self, x, x_lengths, emotion_embedding=None):
- if self.n_vocab != 0:
- x = self.emb(x) * math.sqrt(self.hidden_channels) # [b, t, h]
- if emotion_embedding is not None:
- print("emotion added")
- x = x + self.emo_proj(emotion_embedding.unsqueeze(1))
- x = torch.transpose(x, 1, -1) # [b, h, t]
- x_mask = torch.unsqueeze(commons.sequence_mask(x_lengths, x.size(2)), 1).to(x.dtype)
-
- x = self.encoder(x * x_mask, x_mask)
- stats = self.proj(x) * x_mask
-
- m, logs = torch.split(stats, self.out_channels, dim=1)
- return x, m, logs, x_mask
-
-
-class PosteriorEncoder(nn.Module):
- def __init__(self,
- in_channels,
- out_channels,
- hidden_channels,
- kernel_size,
- dilation_rate,
- n_layers,
- gin_channels=0):
- super().__init__()
- self.in_channels = in_channels
- self.out_channels = out_channels
- self.hidden_channels = hidden_channels
- self.kernel_size = kernel_size
- self.dilation_rate = dilation_rate
- self.n_layers = n_layers
- self.gin_channels = gin_channels
-
- self.pre = nn.Conv1d(in_channels, hidden_channels, 1)
- self.enc = modules.WN(hidden_channels, kernel_size, dilation_rate, n_layers, gin_channels=gin_channels)
- self.proj = nn.Conv1d(hidden_channels, out_channels * 2, 1)
-
- def forward(self, x, x_lengths, g=None):
- x_mask = torch.unsqueeze(commons.sequence_mask(x_lengths, x.size(2)), 1).to(x.dtype)
- x = self.pre(x) * x_mask
- x = self.enc(x, x_mask, g=g)
- stats = self.proj(x) * x_mask
- m, logs = torch.split(stats, self.out_channels, dim=1)
- z = (m + torch.randn_like(m) * torch.exp(logs)) * x_mask
- return z, m, logs, x_mask
-
-
-class SynthesizerTrn(models.SynthesizerTrn):
- """
- Synthesizer for Training
- """
-
- def __init__(self,
- n_vocab,
- spec_channels,
- segment_size,
- inter_channels,
- hidden_channels,
- filter_channels,
- n_heads,
- n_layers,
- kernel_size,
- p_dropout,
- resblock,
- resblock_kernel_sizes,
- resblock_dilation_sizes,
- upsample_rates,
- upsample_initial_channel,
- upsample_kernel_sizes,
- n_speakers=0,
- gin_channels=0,
- use_sdp=True,
- emotion_embedding=False,
- ONNX_dir="./ONNX_net/",
- **kwargs):
-
- super().__init__(
- n_vocab,
- spec_channels,
- segment_size,
- inter_channels,
- hidden_channels,
- filter_channels,
- n_heads,
- n_layers,
- kernel_size,
- p_dropout,
- resblock,
- resblock_kernel_sizes,
- resblock_dilation_sizes,
- upsample_rates,
- upsample_initial_channel,
- upsample_kernel_sizes,
- n_speakers=n_speakers,
- gin_channels=gin_channels,
- use_sdp=use_sdp,
- **kwargs
- )
- self.ONNX_dir = ONNX_dir
- self.enc_p = TextEncoder(n_vocab,
- inter_channels,
- hidden_channels,
- filter_channels,
- n_heads,
- n_layers,
- kernel_size,
- p_dropout,
- emotion_embedding)
- self.enc_q = PosteriorEncoder(spec_channels, inter_channels, hidden_channels, 5, 1, 16, gin_channels=gin_channels)
-
- def infer(self, x, x_lengths, sid=None, noise_scale=1, length_scale=1, noise_scale_w=1., max_len=None,
- emotion_embedding=None):
- from ONNXVITS_utils import runonnx
- with torch.no_grad():
- x, m_p, logs_p, x_mask = self.enc_p(x, x_lengths, emotion_embedding)
-
- if self.n_speakers > 0:
- g = self.emb_g(sid).unsqueeze(-1) # [b, h, 1]
- else:
- g = None
-
- # logw = self.dp(x, x_mask, g=g, reverse=True, noise_scale=noise_scale_w)
- logw = runonnx(f"{self.ONNX_dir}dp.onnx", x=x.numpy(), x_mask=x_mask.numpy(), g=g.numpy())
- logw = torch.from_numpy(logw[0])
-
- w = torch.exp(logw) * x_mask * length_scale
- w_ceil = torch.ceil(w)
- y_lengths = torch.clamp_min(torch.sum(w_ceil, [1, 2]), 1).long()
- y_mask = torch.unsqueeze(commons.sequence_mask(y_lengths, None), 1).to(x_mask.dtype)
- attn_mask = torch.unsqueeze(x_mask, 2) * torch.unsqueeze(y_mask, -1)
- attn = commons.generate_path(w_ceil, attn_mask)
-
- m_p = torch.matmul(attn.squeeze(1), m_p.transpose(1, 2)).transpose(1, 2) # [b, t', t], [b, t, d] -> [b, d, t']
- logs_p = torch.matmul(attn.squeeze(1), logs_p.transpose(1, 2)).transpose(1,
- 2) # [b, t', t], [b, t, d] -> [b, d, t']
-
- z_p = m_p + torch.randn_like(m_p) * torch.exp(logs_p) * noise_scale
-
- # z = self.flow(z_p, y_mask, g=g, reverse=True)
- z = runonnx(f"{self.ONNX_dir}flow.onnx", z_p=z_p.numpy(), y_mask=y_mask.numpy(), g=g.numpy())
- z = torch.from_numpy(z[0])
-
- # o = self.dec((z * y_mask)[:,:,:max_len], g=g)
- o = runonnx(f"{self.ONNX_dir}dec.onnx", z_in=(z * y_mask)[:, :, :max_len].numpy(), g=g.numpy())
- o = torch.from_numpy(o[0])
-
- return o, attn, y_mask, (z, z_p, m_p, logs_p)
\ No newline at end of file
diff --git a/spaces/JohnnyFromOhio/openai-jukebox-1b-lyrics/app.py b/spaces/JohnnyFromOhio/openai-jukebox-1b-lyrics/app.py
deleted file mode 100644
index 3e15d48d44cc0e4a40748dcd52cae632d33f17b4..0000000000000000000000000000000000000000
--- a/spaces/JohnnyFromOhio/openai-jukebox-1b-lyrics/app.py
+++ /dev/null
@@ -1,3 +0,0 @@
-import gradio as gr
-
-gr.Interface.load("models/openai/jukebox-1b-lyrics").launch()
\ No newline at end of file
diff --git a/spaces/JunchuanYu/SegRS/segment_anything/__init__.py b/spaces/JunchuanYu/SegRS/segment_anything/__init__.py
deleted file mode 100644
index 34383d83f5e76bc801f31b20e5651e383be348b6..0000000000000000000000000000000000000000
--- a/spaces/JunchuanYu/SegRS/segment_anything/__init__.py
+++ /dev/null
@@ -1,15 +0,0 @@
-# Copyright (c) Meta Platforms, Inc. and affiliates.
-# All rights reserved.
-
-# This source code is licensed under the license found in the
-# LICENSE file in the root directory of this source tree.
-
-from .build_sam import (
- build_sam,
- build_sam_vit_h,
- build_sam_vit_l,
- build_sam_vit_b,
- sam_model_registry,
-)
-from .predictor import SamPredictor
-from .automatic_mask_generator import SamAutomaticMaskGenerator
diff --git a/spaces/Kayson/InstructDiffusion/stable_diffusion/ldm/modules/image_degradation/bsrgan.py b/spaces/Kayson/InstructDiffusion/stable_diffusion/ldm/modules/image_degradation/bsrgan.py
deleted file mode 100644
index 32ef56169978e550090261cddbcf5eb611a6173b..0000000000000000000000000000000000000000
--- a/spaces/Kayson/InstructDiffusion/stable_diffusion/ldm/modules/image_degradation/bsrgan.py
+++ /dev/null
@@ -1,730 +0,0 @@
-# -*- coding: utf-8 -*-
-"""
-# --------------------------------------------
-# Super-Resolution
-# --------------------------------------------
-#
-# Kai Zhang (cskaizhang@gmail.com)
-# https://github.com/cszn
-# From 2019/03--2021/08
-# --------------------------------------------
-"""
-
-import numpy as np
-import cv2
-import torch
-
-from functools import partial
-import random
-from scipy import ndimage
-import scipy
-import scipy.stats as ss
-from scipy.interpolate import interp2d
-from scipy.linalg import orth
-import albumentations
-
-import ldm.modules.image_degradation.utils_image as util
-
-
-def modcrop_np(img, sf):
- '''
- Args:
- img: numpy image, WxH or WxHxC
- sf: scale factor
- Return:
- cropped image
- '''
- w, h = img.shape[:2]
- im = np.copy(img)
- return im[:w - w % sf, :h - h % sf, ...]
-
-
-"""
-# --------------------------------------------
-# anisotropic Gaussian kernels
-# --------------------------------------------
-"""
-
-
-def analytic_kernel(k):
- """Calculate the X4 kernel from the X2 kernel (for proof see appendix in paper)"""
- k_size = k.shape[0]
- # Calculate the big kernels size
- big_k = np.zeros((3 * k_size - 2, 3 * k_size - 2))
- # Loop over the small kernel to fill the big one
- for r in range(k_size):
- for c in range(k_size):
- big_k[2 * r:2 * r + k_size, 2 * c:2 * c + k_size] += k[r, c] * k
- # Crop the edges of the big kernel to ignore very small values and increase run time of SR
- crop = k_size // 2
- cropped_big_k = big_k[crop:-crop, crop:-crop]
- # Normalize to 1
- return cropped_big_k / cropped_big_k.sum()
-
-
-def anisotropic_Gaussian(ksize=15, theta=np.pi, l1=6, l2=6):
- """ generate an anisotropic Gaussian kernel
- Args:
- ksize : e.g., 15, kernel size
- theta : [0, pi], rotation angle range
- l1 : [0.1,50], scaling of eigenvalues
- l2 : [0.1,l1], scaling of eigenvalues
- If l1 = l2, will get an isotropic Gaussian kernel.
- Returns:
- k : kernel
- """
-
- v = np.dot(np.array([[np.cos(theta), -np.sin(theta)], [np.sin(theta), np.cos(theta)]]), np.array([1., 0.]))
- V = np.array([[v[0], v[1]], [v[1], -v[0]]])
- D = np.array([[l1, 0], [0, l2]])
- Sigma = np.dot(np.dot(V, D), np.linalg.inv(V))
- k = gm_blur_kernel(mean=[0, 0], cov=Sigma, size=ksize)
-
- return k
-
-
-def gm_blur_kernel(mean, cov, size=15):
- center = size / 2.0 + 0.5
- k = np.zeros([size, size])
- for y in range(size):
- for x in range(size):
- cy = y - center + 1
- cx = x - center + 1
- k[y, x] = ss.multivariate_normal.pdf([cx, cy], mean=mean, cov=cov)
-
- k = k / np.sum(k)
- return k
-
-
-def shift_pixel(x, sf, upper_left=True):
- """shift pixel for super-resolution with different scale factors
- Args:
- x: WxHxC or WxH
- sf: scale factor
- upper_left: shift direction
- """
- h, w = x.shape[:2]
- shift = (sf - 1) * 0.5
- xv, yv = np.arange(0, w, 1.0), np.arange(0, h, 1.0)
- if upper_left:
- x1 = xv + shift
- y1 = yv + shift
- else:
- x1 = xv - shift
- y1 = yv - shift
-
- x1 = np.clip(x1, 0, w - 1)
- y1 = np.clip(y1, 0, h - 1)
-
- if x.ndim == 2:
- x = interp2d(xv, yv, x)(x1, y1)
- if x.ndim == 3:
- for i in range(x.shape[-1]):
- x[:, :, i] = interp2d(xv, yv, x[:, :, i])(x1, y1)
-
- return x
-
-
-def blur(x, k):
- '''
- x: image, NxcxHxW
- k: kernel, Nx1xhxw
- '''
- n, c = x.shape[:2]
- p1, p2 = (k.shape[-2] - 1) // 2, (k.shape[-1] - 1) // 2
- x = torch.nn.functional.pad(x, pad=(p1, p2, p1, p2), mode='replicate')
- k = k.repeat(1, c, 1, 1)
- k = k.view(-1, 1, k.shape[2], k.shape[3])
- x = x.view(1, -1, x.shape[2], x.shape[3])
- x = torch.nn.functional.conv2d(x, k, bias=None, stride=1, padding=0, groups=n * c)
- x = x.view(n, c, x.shape[2], x.shape[3])
-
- return x
-
-
-def gen_kernel(k_size=np.array([15, 15]), scale_factor=np.array([4, 4]), min_var=0.6, max_var=10., noise_level=0):
- """"
- # modified version of https://github.com/assafshocher/BlindSR_dataset_generator
- # Kai Zhang
- # min_var = 0.175 * sf # variance of the gaussian kernel will be sampled between min_var and max_var
- # max_var = 2.5 * sf
- """
- # Set random eigen-vals (lambdas) and angle (theta) for COV matrix
- lambda_1 = min_var + np.random.rand() * (max_var - min_var)
- lambda_2 = min_var + np.random.rand() * (max_var - min_var)
- theta = np.random.rand() * np.pi # random theta
- noise = -noise_level + np.random.rand(*k_size) * noise_level * 2
-
- # Set COV matrix using Lambdas and Theta
- LAMBDA = np.diag([lambda_1, lambda_2])
- Q = np.array([[np.cos(theta), -np.sin(theta)],
- [np.sin(theta), np.cos(theta)]])
- SIGMA = Q @ LAMBDA @ Q.T
- INV_SIGMA = np.linalg.inv(SIGMA)[None, None, :, :]
-
- # Set expectation position (shifting kernel for aligned image)
- MU = k_size // 2 - 0.5 * (scale_factor - 1) # - 0.5 * (scale_factor - k_size % 2)
- MU = MU[None, None, :, None]
-
- # Create meshgrid for Gaussian
- [X, Y] = np.meshgrid(range(k_size[0]), range(k_size[1]))
- Z = np.stack([X, Y], 2)[:, :, :, None]
-
- # Calcualte Gaussian for every pixel of the kernel
- ZZ = Z - MU
- ZZ_t = ZZ.transpose(0, 1, 3, 2)
- raw_kernel = np.exp(-0.5 * np.squeeze(ZZ_t @ INV_SIGMA @ ZZ)) * (1 + noise)
-
- # shift the kernel so it will be centered
- # raw_kernel_centered = kernel_shift(raw_kernel, scale_factor)
-
- # Normalize the kernel and return
- # kernel = raw_kernel_centered / np.sum(raw_kernel_centered)
- kernel = raw_kernel / np.sum(raw_kernel)
- return kernel
-
-
-def fspecial_gaussian(hsize, sigma):
- hsize = [hsize, hsize]
- siz = [(hsize[0] - 1.0) / 2.0, (hsize[1] - 1.0) / 2.0]
- std = sigma
- [x, y] = np.meshgrid(np.arange(-siz[1], siz[1] + 1), np.arange(-siz[0], siz[0] + 1))
- arg = -(x * x + y * y) / (2 * std * std)
- h = np.exp(arg)
- h[h < scipy.finfo(float).eps * h.max()] = 0
- sumh = h.sum()
- if sumh != 0:
- h = h / sumh
- return h
-
-
-def fspecial_laplacian(alpha):
- alpha = max([0, min([alpha, 1])])
- h1 = alpha / (alpha + 1)
- h2 = (1 - alpha) / (alpha + 1)
- h = [[h1, h2, h1], [h2, -4 / (alpha + 1), h2], [h1, h2, h1]]
- h = np.array(h)
- return h
-
-
-def fspecial(filter_type, *args, **kwargs):
- '''
- python code from:
- https://github.com/ronaldosena/imagens-medicas-2/blob/40171a6c259edec7827a6693a93955de2bd39e76/Aulas/aula_2_-_uniform_filter/matlab_fspecial.py
- '''
- if filter_type == 'gaussian':
- return fspecial_gaussian(*args, **kwargs)
- if filter_type == 'laplacian':
- return fspecial_laplacian(*args, **kwargs)
-
-
-"""
-# --------------------------------------------
-# degradation models
-# --------------------------------------------
-"""
-
-
-def bicubic_degradation(x, sf=3):
- '''
- Args:
- x: HxWxC image, [0, 1]
- sf: down-scale factor
- Return:
- bicubicly downsampled LR image
- '''
- x = util.imresize_np(x, scale=1 / sf)
- return x
-
-
-def srmd_degradation(x, k, sf=3):
- ''' blur + bicubic downsampling
- Args:
- x: HxWxC image, [0, 1]
- k: hxw, double
- sf: down-scale factor
- Return:
- downsampled LR image
- Reference:
- @inproceedings{zhang2018learning,
- title={Learning a single convolutional super-resolution network for multiple degradations},
- author={Zhang, Kai and Zuo, Wangmeng and Zhang, Lei},
- booktitle={IEEE Conference on Computer Vision and Pattern Recognition},
- pages={3262--3271},
- year={2018}
- }
- '''
- x = ndimage.filters.convolve(x, np.expand_dims(k, axis=2), mode='wrap') # 'nearest' | 'mirror'
- x = bicubic_degradation(x, sf=sf)
- return x
-
-
-def dpsr_degradation(x, k, sf=3):
- ''' bicubic downsampling + blur
- Args:
- x: HxWxC image, [0, 1]
- k: hxw, double
- sf: down-scale factor
- Return:
- downsampled LR image
- Reference:
- @inproceedings{zhang2019deep,
- title={Deep Plug-and-Play Super-Resolution for Arbitrary Blur Kernels},
- author={Zhang, Kai and Zuo, Wangmeng and Zhang, Lei},
- booktitle={IEEE Conference on Computer Vision and Pattern Recognition},
- pages={1671--1681},
- year={2019}
- }
- '''
- x = bicubic_degradation(x, sf=sf)
- x = ndimage.filters.convolve(x, np.expand_dims(k, axis=2), mode='wrap')
- return x
-
-
-def classical_degradation(x, k, sf=3):
- ''' blur + downsampling
- Args:
- x: HxWxC image, [0, 1]/[0, 255]
- k: hxw, double
- sf: down-scale factor
- Return:
- downsampled LR image
- '''
- x = ndimage.filters.convolve(x, np.expand_dims(k, axis=2), mode='wrap')
- # x = filters.correlate(x, np.expand_dims(np.flip(k), axis=2))
- st = 0
- return x[st::sf, st::sf, ...]
-
-
-def add_sharpening(img, weight=0.5, radius=50, threshold=10):
- """USM sharpening. borrowed from real-ESRGAN
- Input image: I; Blurry image: B.
- 1. K = I + weight * (I - B)
- 2. Mask = 1 if abs(I - B) > threshold, else: 0
- 3. Blur mask:
- 4. Out = Mask * K + (1 - Mask) * I
- Args:
- img (Numpy array): Input image, HWC, BGR; float32, [0, 1].
- weight (float): Sharp weight. Default: 1.
- radius (float): Kernel size of Gaussian blur. Default: 50.
- threshold (int):
- """
- if radius % 2 == 0:
- radius += 1
- blur = cv2.GaussianBlur(img, (radius, radius), 0)
- residual = img - blur
- mask = np.abs(residual) * 255 > threshold
- mask = mask.astype('float32')
- soft_mask = cv2.GaussianBlur(mask, (radius, radius), 0)
-
- K = img + weight * residual
- K = np.clip(K, 0, 1)
- return soft_mask * K + (1 - soft_mask) * img
-
-
-def add_blur(img, sf=4):
- wd2 = 4.0 + sf
- wd = 2.0 + 0.2 * sf
- if random.random() < 0.5:
- l1 = wd2 * random.random()
- l2 = wd2 * random.random()
- k = anisotropic_Gaussian(ksize=2 * random.randint(2, 11) + 3, theta=random.random() * np.pi, l1=l1, l2=l2)
- else:
- k = fspecial('gaussian', 2 * random.randint(2, 11) + 3, wd * random.random())
- img = ndimage.filters.convolve(img, np.expand_dims(k, axis=2), mode='mirror')
-
- return img
-
-
-def add_resize(img, sf=4):
- rnum = np.random.rand()
- if rnum > 0.8: # up
- sf1 = random.uniform(1, 2)
- elif rnum < 0.7: # down
- sf1 = random.uniform(0.5 / sf, 1)
- else:
- sf1 = 1.0
- img = cv2.resize(img, (int(sf1 * img.shape[1]), int(sf1 * img.shape[0])), interpolation=random.choice([1, 2, 3]))
- img = np.clip(img, 0.0, 1.0)
-
- return img
-
-
-# def add_Gaussian_noise(img, noise_level1=2, noise_level2=25):
-# noise_level = random.randint(noise_level1, noise_level2)
-# rnum = np.random.rand()
-# if rnum > 0.6: # add color Gaussian noise
-# img += np.random.normal(0, noise_level / 255.0, img.shape).astype(np.float32)
-# elif rnum < 0.4: # add grayscale Gaussian noise
-# img += np.random.normal(0, noise_level / 255.0, (*img.shape[:2], 1)).astype(np.float32)
-# else: # add noise
-# L = noise_level2 / 255.
-# D = np.diag(np.random.rand(3))
-# U = orth(np.random.rand(3, 3))
-# conv = np.dot(np.dot(np.transpose(U), D), U)
-# img += np.random.multivariate_normal([0, 0, 0], np.abs(L ** 2 * conv), img.shape[:2]).astype(np.float32)
-# img = np.clip(img, 0.0, 1.0)
-# return img
-
-def add_Gaussian_noise(img, noise_level1=2, noise_level2=25):
- noise_level = random.randint(noise_level1, noise_level2)
- rnum = np.random.rand()
- if rnum > 0.6: # add color Gaussian noise
- img = img + np.random.normal(0, noise_level / 255.0, img.shape).astype(np.float32)
- elif rnum < 0.4: # add grayscale Gaussian noise
- img = img + np.random.normal(0, noise_level / 255.0, (*img.shape[:2], 1)).astype(np.float32)
- else: # add noise
- L = noise_level2 / 255.
- D = np.diag(np.random.rand(3))
- U = orth(np.random.rand(3, 3))
- conv = np.dot(np.dot(np.transpose(U), D), U)
- img = img + np.random.multivariate_normal([0, 0, 0], np.abs(L ** 2 * conv), img.shape[:2]).astype(np.float32)
- img = np.clip(img, 0.0, 1.0)
- return img
-
-
-def add_speckle_noise(img, noise_level1=2, noise_level2=25):
- noise_level = random.randint(noise_level1, noise_level2)
- img = np.clip(img, 0.0, 1.0)
- rnum = random.random()
- if rnum > 0.6:
- img += img * np.random.normal(0, noise_level / 255.0, img.shape).astype(np.float32)
- elif rnum < 0.4:
- img += img * np.random.normal(0, noise_level / 255.0, (*img.shape[:2], 1)).astype(np.float32)
- else:
- L = noise_level2 / 255.
- D = np.diag(np.random.rand(3))
- U = orth(np.random.rand(3, 3))
- conv = np.dot(np.dot(np.transpose(U), D), U)
- img += img * np.random.multivariate_normal([0, 0, 0], np.abs(L ** 2 * conv), img.shape[:2]).astype(np.float32)
- img = np.clip(img, 0.0, 1.0)
- return img
-
-
-def add_Poisson_noise(img):
- img = np.clip((img * 255.0).round(), 0, 255) / 255.
- vals = 10 ** (2 * random.random() + 2.0) # [2, 4]
- if random.random() < 0.5:
- img = np.random.poisson(img * vals).astype(np.float32) / vals
- else:
- img_gray = np.dot(img[..., :3], [0.299, 0.587, 0.114])
- img_gray = np.clip((img_gray * 255.0).round(), 0, 255) / 255.
- noise_gray = np.random.poisson(img_gray * vals).astype(np.float32) / vals - img_gray
- img += noise_gray[:, :, np.newaxis]
- img = np.clip(img, 0.0, 1.0)
- return img
-
-
-def add_JPEG_noise(img):
- quality_factor = random.randint(30, 95)
- img = cv2.cvtColor(util.single2uint(img), cv2.COLOR_RGB2BGR)
- result, encimg = cv2.imencode('.jpg', img, [int(cv2.IMWRITE_JPEG_QUALITY), quality_factor])
- img = cv2.imdecode(encimg, 1)
- img = cv2.cvtColor(util.uint2single(img), cv2.COLOR_BGR2RGB)
- return img
-
-
-def random_crop(lq, hq, sf=4, lq_patchsize=64):
- h, w = lq.shape[:2]
- rnd_h = random.randint(0, h - lq_patchsize)
- rnd_w = random.randint(0, w - lq_patchsize)
- lq = lq[rnd_h:rnd_h + lq_patchsize, rnd_w:rnd_w + lq_patchsize, :]
-
- rnd_h_H, rnd_w_H = int(rnd_h * sf), int(rnd_w * sf)
- hq = hq[rnd_h_H:rnd_h_H + lq_patchsize * sf, rnd_w_H:rnd_w_H + lq_patchsize * sf, :]
- return lq, hq
-
-
-def degradation_bsrgan(img, sf=4, lq_patchsize=72, isp_model=None):
- """
- This is the degradation model of BSRGAN from the paper
- "Designing a Practical Degradation Model for Deep Blind Image Super-Resolution"
- ----------
- img: HXWXC, [0, 1], its size should be large than (lq_patchsizexsf)x(lq_patchsizexsf)
- sf: scale factor
- isp_model: camera ISP model
- Returns
- -------
- img: low-quality patch, size: lq_patchsizeXlq_patchsizeXC, range: [0, 1]
- hq: corresponding high-quality patch, size: (lq_patchsizexsf)X(lq_patchsizexsf)XC, range: [0, 1]
- """
- isp_prob, jpeg_prob, scale2_prob = 0.25, 0.9, 0.25
- sf_ori = sf
-
- h1, w1 = img.shape[:2]
- img = img.copy()[:w1 - w1 % sf, :h1 - h1 % sf, ...] # mod crop
- h, w = img.shape[:2]
-
- if h < lq_patchsize * sf or w < lq_patchsize * sf:
- raise ValueError(f'img size ({h1}X{w1}) is too small!')
-
- hq = img.copy()
-
- if sf == 4 and random.random() < scale2_prob: # downsample1
- if np.random.rand() < 0.5:
- img = cv2.resize(img, (int(1 / 2 * img.shape[1]), int(1 / 2 * img.shape[0])),
- interpolation=random.choice([1, 2, 3]))
- else:
- img = util.imresize_np(img, 1 / 2, True)
- img = np.clip(img, 0.0, 1.0)
- sf = 2
-
- shuffle_order = random.sample(range(7), 7)
- idx1, idx2 = shuffle_order.index(2), shuffle_order.index(3)
- if idx1 > idx2: # keep downsample3 last
- shuffle_order[idx1], shuffle_order[idx2] = shuffle_order[idx2], shuffle_order[idx1]
-
- for i in shuffle_order:
-
- if i == 0:
- img = add_blur(img, sf=sf)
-
- elif i == 1:
- img = add_blur(img, sf=sf)
-
- elif i == 2:
- a, b = img.shape[1], img.shape[0]
- # downsample2
- if random.random() < 0.75:
- sf1 = random.uniform(1, 2 * sf)
- img = cv2.resize(img, (int(1 / sf1 * img.shape[1]), int(1 / sf1 * img.shape[0])),
- interpolation=random.choice([1, 2, 3]))
- else:
- k = fspecial('gaussian', 25, random.uniform(0.1, 0.6 * sf))
- k_shifted = shift_pixel(k, sf)
- k_shifted = k_shifted / k_shifted.sum() # blur with shifted kernel
- img = ndimage.filters.convolve(img, np.expand_dims(k_shifted, axis=2), mode='mirror')
- img = img[0::sf, 0::sf, ...] # nearest downsampling
- img = np.clip(img, 0.0, 1.0)
-
- elif i == 3:
- # downsample3
- img = cv2.resize(img, (int(1 / sf * a), int(1 / sf * b)), interpolation=random.choice([1, 2, 3]))
- img = np.clip(img, 0.0, 1.0)
-
- elif i == 4:
- # add Gaussian noise
- img = add_Gaussian_noise(img, noise_level1=2, noise_level2=25)
-
- elif i == 5:
- # add JPEG noise
- if random.random() < jpeg_prob:
- img = add_JPEG_noise(img)
-
- elif i == 6:
- # add processed camera sensor noise
- if random.random() < isp_prob and isp_model is not None:
- with torch.no_grad():
- img, hq = isp_model.forward(img.copy(), hq)
-
- # add final JPEG compression noise
- img = add_JPEG_noise(img)
-
- # random crop
- img, hq = random_crop(img, hq, sf_ori, lq_patchsize)
-
- return img, hq
-
-
-# todo no isp_model?
-def degradation_bsrgan_variant(image, sf=4, isp_model=None):
- """
- This is the degradation model of BSRGAN from the paper
- "Designing a Practical Degradation Model for Deep Blind Image Super-Resolution"
- ----------
- sf: scale factor
- isp_model: camera ISP model
- Returns
- -------
- img: low-quality patch, size: lq_patchsizeXlq_patchsizeXC, range: [0, 1]
- hq: corresponding high-quality patch, size: (lq_patchsizexsf)X(lq_patchsizexsf)XC, range: [0, 1]
- """
- image = util.uint2single(image)
- isp_prob, jpeg_prob, scale2_prob = 0.25, 0.9, 0.25
- sf_ori = sf
-
- h1, w1 = image.shape[:2]
- image = image.copy()[:w1 - w1 % sf, :h1 - h1 % sf, ...] # mod crop
- h, w = image.shape[:2]
-
- hq = image.copy()
-
- if sf == 4 and random.random() < scale2_prob: # downsample1
- if np.random.rand() < 0.5:
- image = cv2.resize(image, (int(1 / 2 * image.shape[1]), int(1 / 2 * image.shape[0])),
- interpolation=random.choice([1, 2, 3]))
- else:
- image = util.imresize_np(image, 1 / 2, True)
- image = np.clip(image, 0.0, 1.0)
- sf = 2
-
- shuffle_order = random.sample(range(7), 7)
- idx1, idx2 = shuffle_order.index(2), shuffle_order.index(3)
- if idx1 > idx2: # keep downsample3 last
- shuffle_order[idx1], shuffle_order[idx2] = shuffle_order[idx2], shuffle_order[idx1]
-
- for i in shuffle_order:
-
- if i == 0:
- image = add_blur(image, sf=sf)
-
- elif i == 1:
- image = add_blur(image, sf=sf)
-
- elif i == 2:
- a, b = image.shape[1], image.shape[0]
- # downsample2
- if random.random() < 0.75:
- sf1 = random.uniform(1, 2 * sf)
- image = cv2.resize(image, (int(1 / sf1 * image.shape[1]), int(1 / sf1 * image.shape[0])),
- interpolation=random.choice([1, 2, 3]))
- else:
- k = fspecial('gaussian', 25, random.uniform(0.1, 0.6 * sf))
- k_shifted = shift_pixel(k, sf)
- k_shifted = k_shifted / k_shifted.sum() # blur with shifted kernel
- image = ndimage.filters.convolve(image, np.expand_dims(k_shifted, axis=2), mode='mirror')
- image = image[0::sf, 0::sf, ...] # nearest downsampling
- image = np.clip(image, 0.0, 1.0)
-
- elif i == 3:
- # downsample3
- image = cv2.resize(image, (int(1 / sf * a), int(1 / sf * b)), interpolation=random.choice([1, 2, 3]))
- image = np.clip(image, 0.0, 1.0)
-
- elif i == 4:
- # add Gaussian noise
- image = add_Gaussian_noise(image, noise_level1=2, noise_level2=25)
-
- elif i == 5:
- # add JPEG noise
- if random.random() < jpeg_prob:
- image = add_JPEG_noise(image)
-
- # elif i == 6:
- # # add processed camera sensor noise
- # if random.random() < isp_prob and isp_model is not None:
- # with torch.no_grad():
- # img, hq = isp_model.forward(img.copy(), hq)
-
- # add final JPEG compression noise
- image = add_JPEG_noise(image)
- image = util.single2uint(image)
- example = {"image":image}
- return example
-
-
-# TODO incase there is a pickle error one needs to replace a += x with a = a + x in add_speckle_noise etc...
-def degradation_bsrgan_plus(img, sf=4, shuffle_prob=0.5, use_sharp=True, lq_patchsize=64, isp_model=None):
- """
- This is an extended degradation model by combining
- the degradation models of BSRGAN and Real-ESRGAN
- ----------
- img: HXWXC, [0, 1], its size should be large than (lq_patchsizexsf)x(lq_patchsizexsf)
- sf: scale factor
- use_shuffle: the degradation shuffle
- use_sharp: sharpening the img
- Returns
- -------
- img: low-quality patch, size: lq_patchsizeXlq_patchsizeXC, range: [0, 1]
- hq: corresponding high-quality patch, size: (lq_patchsizexsf)X(lq_patchsizexsf)XC, range: [0, 1]
- """
-
- h1, w1 = img.shape[:2]
- img = img.copy()[:w1 - w1 % sf, :h1 - h1 % sf, ...] # mod crop
- h, w = img.shape[:2]
-
- if h < lq_patchsize * sf or w < lq_patchsize * sf:
- raise ValueError(f'img size ({h1}X{w1}) is too small!')
-
- if use_sharp:
- img = add_sharpening(img)
- hq = img.copy()
-
- if random.random() < shuffle_prob:
- shuffle_order = random.sample(range(13), 13)
- else:
- shuffle_order = list(range(13))
- # local shuffle for noise, JPEG is always the last one
- shuffle_order[2:6] = random.sample(shuffle_order[2:6], len(range(2, 6)))
- shuffle_order[9:13] = random.sample(shuffle_order[9:13], len(range(9, 13)))
-
- poisson_prob, speckle_prob, isp_prob = 0.1, 0.1, 0.1
-
- for i in shuffle_order:
- if i == 0:
- img = add_blur(img, sf=sf)
- elif i == 1:
- img = add_resize(img, sf=sf)
- elif i == 2:
- img = add_Gaussian_noise(img, noise_level1=2, noise_level2=25)
- elif i == 3:
- if random.random() < poisson_prob:
- img = add_Poisson_noise(img)
- elif i == 4:
- if random.random() < speckle_prob:
- img = add_speckle_noise(img)
- elif i == 5:
- if random.random() < isp_prob and isp_model is not None:
- with torch.no_grad():
- img, hq = isp_model.forward(img.copy(), hq)
- elif i == 6:
- img = add_JPEG_noise(img)
- elif i == 7:
- img = add_blur(img, sf=sf)
- elif i == 8:
- img = add_resize(img, sf=sf)
- elif i == 9:
- img = add_Gaussian_noise(img, noise_level1=2, noise_level2=25)
- elif i == 10:
- if random.random() < poisson_prob:
- img = add_Poisson_noise(img)
- elif i == 11:
- if random.random() < speckle_prob:
- img = add_speckle_noise(img)
- elif i == 12:
- if random.random() < isp_prob and isp_model is not None:
- with torch.no_grad():
- img, hq = isp_model.forward(img.copy(), hq)
- else:
- print('check the shuffle!')
-
- # resize to desired size
- img = cv2.resize(img, (int(1 / sf * hq.shape[1]), int(1 / sf * hq.shape[0])),
- interpolation=random.choice([1, 2, 3]))
-
- # add final JPEG compression noise
- img = add_JPEG_noise(img)
-
- # random crop
- img, hq = random_crop(img, hq, sf, lq_patchsize)
-
- return img, hq
-
-
-if __name__ == '__main__':
- print("hey")
- img = util.imread_uint('utils/test.png', 3)
- print(img)
- img = util.uint2single(img)
- print(img)
- img = img[:448, :448]
- h = img.shape[0] // 4
- print("resizing to", h)
- sf = 4
- deg_fn = partial(degradation_bsrgan_variant, sf=sf)
- for i in range(20):
- print(i)
- img_lq = deg_fn(img)
- print(img_lq)
- img_lq_bicubic = albumentations.SmallestMaxSize(max_size=h, interpolation=cv2.INTER_CUBIC)(image=img)["image"]
- print(img_lq.shape)
- print("bicubic", img_lq_bicubic.shape)
- print(img_hq.shape)
- lq_nearest = cv2.resize(util.single2uint(img_lq), (int(sf * img_lq.shape[1]), int(sf * img_lq.shape[0])),
- interpolation=0)
- lq_bicubic_nearest = cv2.resize(util.single2uint(img_lq_bicubic), (int(sf * img_lq.shape[1]), int(sf * img_lq.shape[0])),
- interpolation=0)
- img_concat = np.concatenate([lq_bicubic_nearest, lq_nearest, util.single2uint(img_hq)], axis=1)
- util.imsave(img_concat, str(i) + '.png')
-
-
diff --git a/spaces/Kevin676/ChatGPT-with-Voice-Cloning-in-Chinese/ppg2mel/rnn_decoder_mol.py b/spaces/Kevin676/ChatGPT-with-Voice-Cloning-in-Chinese/ppg2mel/rnn_decoder_mol.py
deleted file mode 100644
index 9d48d7bc697baef107818569dc3e87a96708fb00..0000000000000000000000000000000000000000
--- a/spaces/Kevin676/ChatGPT-with-Voice-Cloning-in-Chinese/ppg2mel/rnn_decoder_mol.py
+++ /dev/null
@@ -1,374 +0,0 @@
-import torch
-import torch.nn as nn
-import torch.nn.functional as F
-import numpy as np
-from .utils.mol_attention import MOLAttention
-from .utils.basic_layers import Linear
-from .utils.vc_utils import get_mask_from_lengths
-
-
-class DecoderPrenet(nn.Module):
- def __init__(self, in_dim, sizes):
- super().__init__()
- in_sizes = [in_dim] + sizes[:-1]
- self.layers = nn.ModuleList(
- [Linear(in_size, out_size, bias=False)
- for (in_size, out_size) in zip(in_sizes, sizes)])
-
- def forward(self, x):
- for linear in self.layers:
- x = F.dropout(F.relu(linear(x)), p=0.5, training=True)
- return x
-
-
-class Decoder(nn.Module):
- """Mixture of Logistic (MoL) attention-based RNN Decoder."""
- def __init__(
- self,
- enc_dim,
- num_mels,
- frames_per_step,
- attention_rnn_dim,
- decoder_rnn_dim,
- prenet_dims,
- num_mixtures,
- encoder_down_factor=1,
- num_decoder_rnn_layer=1,
- use_stop_tokens=False,
- concat_context_to_last=False,
- ):
- super().__init__()
- self.enc_dim = enc_dim
- self.encoder_down_factor = encoder_down_factor
- self.num_mels = num_mels
- self.frames_per_step = frames_per_step
- self.attention_rnn_dim = attention_rnn_dim
- self.decoder_rnn_dim = decoder_rnn_dim
- self.prenet_dims = prenet_dims
- self.use_stop_tokens = use_stop_tokens
- self.num_decoder_rnn_layer = num_decoder_rnn_layer
- self.concat_context_to_last = concat_context_to_last
-
- # Mel prenet
- self.prenet = DecoderPrenet(num_mels, prenet_dims)
- self.prenet_pitch = DecoderPrenet(num_mels, prenet_dims)
-
- # Attention RNN
- self.attention_rnn = nn.LSTMCell(
- prenet_dims[-1] + enc_dim,
- attention_rnn_dim
- )
-
- # Attention
- self.attention_layer = MOLAttention(
- attention_rnn_dim,
- r=frames_per_step/encoder_down_factor,
- M=num_mixtures,
- )
-
- # Decoder RNN
- self.decoder_rnn_layers = nn.ModuleList()
- for i in range(num_decoder_rnn_layer):
- if i == 0:
- self.decoder_rnn_layers.append(
- nn.LSTMCell(
- enc_dim + attention_rnn_dim,
- decoder_rnn_dim))
- else:
- self.decoder_rnn_layers.append(
- nn.LSTMCell(
- decoder_rnn_dim,
- decoder_rnn_dim))
- # self.decoder_rnn = nn.LSTMCell(
- # 2 * enc_dim + attention_rnn_dim,
- # decoder_rnn_dim
- # )
- if concat_context_to_last:
- self.linear_projection = Linear(
- enc_dim + decoder_rnn_dim,
- num_mels * frames_per_step
- )
- else:
- self.linear_projection = Linear(
- decoder_rnn_dim,
- num_mels * frames_per_step
- )
-
-
- # Stop-token layer
- if self.use_stop_tokens:
- if concat_context_to_last:
- self.stop_layer = Linear(
- enc_dim + decoder_rnn_dim, 1, bias=True, w_init_gain="sigmoid"
- )
- else:
- self.stop_layer = Linear(
- decoder_rnn_dim, 1, bias=True, w_init_gain="sigmoid"
- )
-
-
- def get_go_frame(self, memory):
- B = memory.size(0)
- go_frame = torch.zeros((B, self.num_mels), dtype=torch.float,
- device=memory.device)
- return go_frame
-
- def initialize_decoder_states(self, memory, mask):
- device = next(self.parameters()).device
- B = memory.size(0)
-
- # attention rnn states
- self.attention_hidden = torch.zeros(
- (B, self.attention_rnn_dim), device=device)
- self.attention_cell = torch.zeros(
- (B, self.attention_rnn_dim), device=device)
-
- # decoder rnn states
- self.decoder_hiddens = []
- self.decoder_cells = []
- for i in range(self.num_decoder_rnn_layer):
- self.decoder_hiddens.append(
- torch.zeros((B, self.decoder_rnn_dim),
- device=device)
- )
- self.decoder_cells.append(
- torch.zeros((B, self.decoder_rnn_dim),
- device=device)
- )
- # self.decoder_hidden = torch.zeros(
- # (B, self.decoder_rnn_dim), device=device)
- # self.decoder_cell = torch.zeros(
- # (B, self.decoder_rnn_dim), device=device)
-
- self.attention_context = torch.zeros(
- (B, self.enc_dim), device=device)
-
- self.memory = memory
- # self.processed_memory = self.attention_layer.memory_layer(memory)
- self.mask = mask
-
- def parse_decoder_inputs(self, decoder_inputs):
- """Prepare decoder inputs, i.e. gt mel
- Args:
- decoder_inputs:(B, T_out, n_mel_channels) inputs used for teacher-forced training.
- """
- decoder_inputs = decoder_inputs.reshape(
- decoder_inputs.size(0),
- int(decoder_inputs.size(1)/self.frames_per_step), -1)
- # (B, T_out//r, r*num_mels) -> (T_out//r, B, r*num_mels)
- decoder_inputs = decoder_inputs.transpose(0, 1)
- # (T_out//r, B, num_mels)
- decoder_inputs = decoder_inputs[:,:,-self.num_mels:]
- return decoder_inputs
-
- def parse_decoder_outputs(self, mel_outputs, alignments, stop_outputs):
- """ Prepares decoder outputs for output
- Args:
- mel_outputs:
- alignments:
- """
- # (T_out//r, B, T_enc) -> (B, T_out//r, T_enc)
- alignments = torch.stack(alignments).transpose(0, 1)
- # (T_out//r, B) -> (B, T_out//r)
- if stop_outputs is not None:
- if alignments.size(0) == 1:
- stop_outputs = torch.stack(stop_outputs).unsqueeze(0)
- else:
- stop_outputs = torch.stack(stop_outputs).transpose(0, 1)
- stop_outputs = stop_outputs.contiguous()
- # (T_out//r, B, num_mels*r) -> (B, T_out//r, num_mels*r)
- mel_outputs = torch.stack(mel_outputs).transpose(0, 1).contiguous()
- # decouple frames per step
- # (B, T_out, num_mels)
- mel_outputs = mel_outputs.view(
- mel_outputs.size(0), -1, self.num_mels)
- return mel_outputs, alignments, stop_outputs
-
- def attend(self, decoder_input):
- cell_input = torch.cat((decoder_input, self.attention_context), -1)
- self.attention_hidden, self.attention_cell = self.attention_rnn(
- cell_input, (self.attention_hidden, self.attention_cell))
- self.attention_context, attention_weights = self.attention_layer(
- self.attention_hidden, self.memory, None, self.mask)
-
- decoder_rnn_input = torch.cat(
- (self.attention_hidden, self.attention_context), -1)
-
- return decoder_rnn_input, self.attention_context, attention_weights
-
- def decode(self, decoder_input):
- for i in range(self.num_decoder_rnn_layer):
- if i == 0:
- self.decoder_hiddens[i], self.decoder_cells[i] = self.decoder_rnn_layers[i](
- decoder_input, (self.decoder_hiddens[i], self.decoder_cells[i]))
- else:
- self.decoder_hiddens[i], self.decoder_cells[i] = self.decoder_rnn_layers[i](
- self.decoder_hiddens[i-1], (self.decoder_hiddens[i], self.decoder_cells[i]))
- return self.decoder_hiddens[-1]
-
- def forward(self, memory, mel_inputs, memory_lengths):
- """ Decoder forward pass for training
- Args:
- memory: (B, T_enc, enc_dim) Encoder outputs
- decoder_inputs: (B, T, num_mels) Decoder inputs for teacher forcing.
- memory_lengths: (B, ) Encoder output lengths for attention masking.
- Returns:
- mel_outputs: (B, T, num_mels) mel outputs from the decoder
- alignments: (B, T//r, T_enc) attention weights.
- """
- # [1, B, num_mels]
- go_frame = self.get_go_frame(memory).unsqueeze(0)
- # [T//r, B, num_mels]
- mel_inputs = self.parse_decoder_inputs(mel_inputs)
- # [T//r + 1, B, num_mels]
- mel_inputs = torch.cat((go_frame, mel_inputs), dim=0)
- # [T//r + 1, B, prenet_dim]
- decoder_inputs = self.prenet(mel_inputs)
- # decoder_inputs_pitch = self.prenet_pitch(decoder_inputs__)
-
- self.initialize_decoder_states(
- memory, mask=~get_mask_from_lengths(memory_lengths),
- )
-
- self.attention_layer.init_states(memory)
- # self.attention_layer_pitch.init_states(memory_pitch)
-
- mel_outputs, alignments = [], []
- if self.use_stop_tokens:
- stop_outputs = []
- else:
- stop_outputs = None
- while len(mel_outputs) < decoder_inputs.size(0) - 1:
- decoder_input = decoder_inputs[len(mel_outputs)]
- # decoder_input_pitch = decoder_inputs_pitch[len(mel_outputs)]
-
- decoder_rnn_input, context, attention_weights = self.attend(decoder_input)
-
- decoder_rnn_output = self.decode(decoder_rnn_input)
- if self.concat_context_to_last:
- decoder_rnn_output = torch.cat(
- (decoder_rnn_output, context), dim=1)
-
- mel_output = self.linear_projection(decoder_rnn_output)
- if self.use_stop_tokens:
- stop_output = self.stop_layer(decoder_rnn_output)
- stop_outputs += [stop_output.squeeze()]
- mel_outputs += [mel_output.squeeze(1)] #? perhaps don't need squeeze
- alignments += [attention_weights]
- # alignments_pitch += [attention_weights_pitch]
-
- mel_outputs, alignments, stop_outputs = self.parse_decoder_outputs(
- mel_outputs, alignments, stop_outputs)
- if stop_outputs is None:
- return mel_outputs, alignments
- else:
- return mel_outputs, stop_outputs, alignments
-
- def inference(self, memory, stop_threshold=0.5):
- """ Decoder inference
- Args:
- memory: (1, T_enc, D_enc) Encoder outputs
- Returns:
- mel_outputs: mel outputs from the decoder
- alignments: sequence of attention weights from the decoder
- """
- # [1, num_mels]
- decoder_input = self.get_go_frame(memory)
-
- self.initialize_decoder_states(memory, mask=None)
-
- self.attention_layer.init_states(memory)
-
- mel_outputs, alignments = [], []
- # NOTE(sx): heuristic
- max_decoder_step = memory.size(1)*self.encoder_down_factor//self.frames_per_step
- min_decoder_step = memory.size(1)*self.encoder_down_factor // self.frames_per_step - 5
- while True:
- decoder_input = self.prenet(decoder_input)
-
- decoder_input_final, context, alignment = self.attend(decoder_input)
-
- #mel_output, stop_output, alignment = self.decode(decoder_input)
- decoder_rnn_output = self.decode(decoder_input_final)
- if self.concat_context_to_last:
- decoder_rnn_output = torch.cat(
- (decoder_rnn_output, context), dim=1)
-
- mel_output = self.linear_projection(decoder_rnn_output)
- stop_output = self.stop_layer(decoder_rnn_output)
-
- mel_outputs += [mel_output.squeeze(1)]
- alignments += [alignment]
-
- if torch.sigmoid(stop_output.data) > stop_threshold and len(mel_outputs) >= min_decoder_step:
- break
- if len(mel_outputs) >= max_decoder_step:
- # print("Warning! Decoding steps reaches max decoder steps.")
- break
-
- decoder_input = mel_output[:,-self.num_mels:]
-
-
- mel_outputs, alignments, _ = self.parse_decoder_outputs(
- mel_outputs, alignments, None)
-
- return mel_outputs, alignments
-
- def inference_batched(self, memory, stop_threshold=0.5):
- """ Decoder inference
- Args:
- memory: (B, T_enc, D_enc) Encoder outputs
- Returns:
- mel_outputs: mel outputs from the decoder
- alignments: sequence of attention weights from the decoder
- """
- # [1, num_mels]
- decoder_input = self.get_go_frame(memory)
-
- self.initialize_decoder_states(memory, mask=None)
-
- self.attention_layer.init_states(memory)
-
- mel_outputs, alignments = [], []
- stop_outputs = []
- # NOTE(sx): heuristic
- max_decoder_step = memory.size(1)*self.encoder_down_factor//self.frames_per_step
- min_decoder_step = memory.size(1)*self.encoder_down_factor // self.frames_per_step - 5
- while True:
- decoder_input = self.prenet(decoder_input)
-
- decoder_input_final, context, alignment = self.attend(decoder_input)
-
- #mel_output, stop_output, alignment = self.decode(decoder_input)
- decoder_rnn_output = self.decode(decoder_input_final)
- if self.concat_context_to_last:
- decoder_rnn_output = torch.cat(
- (decoder_rnn_output, context), dim=1)
-
- mel_output = self.linear_projection(decoder_rnn_output)
- # (B, 1)
- stop_output = self.stop_layer(decoder_rnn_output)
- stop_outputs += [stop_output.squeeze()]
- # stop_outputs.append(stop_output)
-
- mel_outputs += [mel_output.squeeze(1)]
- alignments += [alignment]
- # print(stop_output.shape)
- if torch.all(torch.sigmoid(stop_output.squeeze().data) > stop_threshold) \
- and len(mel_outputs) >= min_decoder_step:
- break
- if len(mel_outputs) >= max_decoder_step:
- # print("Warning! Decoding steps reaches max decoder steps.")
- break
-
- decoder_input = mel_output[:,-self.num_mels:]
-
-
- mel_outputs, alignments, stop_outputs = self.parse_decoder_outputs(
- mel_outputs, alignments, stop_outputs)
- mel_outputs_stacked = []
- for mel, stop_logit in zip(mel_outputs, stop_outputs):
- idx = np.argwhere(torch.sigmoid(stop_logit.cpu()) > stop_threshold)[0][0].item()
- mel_outputs_stacked.append(mel[:idx,:])
- mel_outputs = torch.cat(mel_outputs_stacked, dim=0).unsqueeze(0)
- return mel_outputs, alignments
diff --git a/spaces/Kevin676/Clone-Your-Voice/vocoder/models/deepmind_version.py b/spaces/Kevin676/Clone-Your-Voice/vocoder/models/deepmind_version.py
deleted file mode 100644
index 1d973d9b8b9ab547571abc5a3f5ea86226a25924..0000000000000000000000000000000000000000
--- a/spaces/Kevin676/Clone-Your-Voice/vocoder/models/deepmind_version.py
+++ /dev/null
@@ -1,170 +0,0 @@
-import torch
-import torch.nn as nn
-import torch.nn.functional as F
-from utils.display import *
-from utils.dsp import *
-
-
-class WaveRNN(nn.Module) :
- def __init__(self, hidden_size=896, quantisation=256) :
- super(WaveRNN, self).__init__()
-
- self.hidden_size = hidden_size
- self.split_size = hidden_size // 2
-
- # The main matmul
- self.R = nn.Linear(self.hidden_size, 3 * self.hidden_size, bias=False)
-
- # Output fc layers
- self.O1 = nn.Linear(self.split_size, self.split_size)
- self.O2 = nn.Linear(self.split_size, quantisation)
- self.O3 = nn.Linear(self.split_size, self.split_size)
- self.O4 = nn.Linear(self.split_size, quantisation)
-
- # Input fc layers
- self.I_coarse = nn.Linear(2, 3 * self.split_size, bias=False)
- self.I_fine = nn.Linear(3, 3 * self.split_size, bias=False)
-
- # biases for the gates
- self.bias_u = nn.Parameter(torch.zeros(self.hidden_size))
- self.bias_r = nn.Parameter(torch.zeros(self.hidden_size))
- self.bias_e = nn.Parameter(torch.zeros(self.hidden_size))
-
- # display num params
- self.num_params()
-
-
- def forward(self, prev_y, prev_hidden, current_coarse) :
-
- # Main matmul - the projection is split 3 ways
- R_hidden = self.R(prev_hidden)
- R_u, R_r, R_e, = torch.split(R_hidden, self.hidden_size, dim=1)
-
- # Project the prev input
- coarse_input_proj = self.I_coarse(prev_y)
- I_coarse_u, I_coarse_r, I_coarse_e = \
- torch.split(coarse_input_proj, self.split_size, dim=1)
-
- # Project the prev input and current coarse sample
- fine_input = torch.cat([prev_y, current_coarse], dim=1)
- fine_input_proj = self.I_fine(fine_input)
- I_fine_u, I_fine_r, I_fine_e = \
- torch.split(fine_input_proj, self.split_size, dim=1)
-
- # concatenate for the gates
- I_u = torch.cat([I_coarse_u, I_fine_u], dim=1)
- I_r = torch.cat([I_coarse_r, I_fine_r], dim=1)
- I_e = torch.cat([I_coarse_e, I_fine_e], dim=1)
-
- # Compute all gates for coarse and fine
- u = F.sigmoid(R_u + I_u + self.bias_u)
- r = F.sigmoid(R_r + I_r + self.bias_r)
- e = F.tanh(r * R_e + I_e + self.bias_e)
- hidden = u * prev_hidden + (1. - u) * e
-
- # Split the hidden state
- hidden_coarse, hidden_fine = torch.split(hidden, self.split_size, dim=1)
-
- # Compute outputs
- out_coarse = self.O2(F.relu(self.O1(hidden_coarse)))
- out_fine = self.O4(F.relu(self.O3(hidden_fine)))
-
- return out_coarse, out_fine, hidden
-
-
- def generate(self, seq_len):
- with torch.no_grad():
- # First split up the biases for the gates
- b_coarse_u, b_fine_u = torch.split(self.bias_u, self.split_size)
- b_coarse_r, b_fine_r = torch.split(self.bias_r, self.split_size)
- b_coarse_e, b_fine_e = torch.split(self.bias_e, self.split_size)
-
- # Lists for the two output seqs
- c_outputs, f_outputs = [], []
-
- # Some initial inputs
- out_coarse = torch.LongTensor([0]).cuda()
- out_fine = torch.LongTensor([0]).cuda()
-
- # We'll meed a hidden state
- hidden = self.init_hidden()
-
- # Need a clock for display
- start = time.time()
-
- # Loop for generation
- for i in range(seq_len) :
-
- # Split into two hidden states
- hidden_coarse, hidden_fine = \
- torch.split(hidden, self.split_size, dim=1)
-
- # Scale and concat previous predictions
- out_coarse = out_coarse.unsqueeze(0).float() / 127.5 - 1.
- out_fine = out_fine.unsqueeze(0).float() / 127.5 - 1.
- prev_outputs = torch.cat([out_coarse, out_fine], dim=1)
-
- # Project input
- coarse_input_proj = self.I_coarse(prev_outputs)
- I_coarse_u, I_coarse_r, I_coarse_e = \
- torch.split(coarse_input_proj, self.split_size, dim=1)
-
- # Project hidden state and split 6 ways
- R_hidden = self.R(hidden)
- R_coarse_u , R_fine_u, \
- R_coarse_r, R_fine_r, \
- R_coarse_e, R_fine_e = torch.split(R_hidden, self.split_size, dim=1)
-
- # Compute the coarse gates
- u = F.sigmoid(R_coarse_u + I_coarse_u + b_coarse_u)
- r = F.sigmoid(R_coarse_r + I_coarse_r + b_coarse_r)
- e = F.tanh(r * R_coarse_e + I_coarse_e + b_coarse_e)
- hidden_coarse = u * hidden_coarse + (1. - u) * e
-
- # Compute the coarse output
- out_coarse = self.O2(F.relu(self.O1(hidden_coarse)))
- posterior = F.softmax(out_coarse, dim=1)
- distrib = torch.distributions.Categorical(posterior)
- out_coarse = distrib.sample()
- c_outputs.append(out_coarse)
-
- # Project the [prev outputs and predicted coarse sample]
- coarse_pred = out_coarse.float() / 127.5 - 1.
- fine_input = torch.cat([prev_outputs, coarse_pred.unsqueeze(0)], dim=1)
- fine_input_proj = self.I_fine(fine_input)
- I_fine_u, I_fine_r, I_fine_e = \
- torch.split(fine_input_proj, self.split_size, dim=1)
-
- # Compute the fine gates
- u = F.sigmoid(R_fine_u + I_fine_u + b_fine_u)
- r = F.sigmoid(R_fine_r + I_fine_r + b_fine_r)
- e = F.tanh(r * R_fine_e + I_fine_e + b_fine_e)
- hidden_fine = u * hidden_fine + (1. - u) * e
-
- # Compute the fine output
- out_fine = self.O4(F.relu(self.O3(hidden_fine)))
- posterior = F.softmax(out_fine, dim=1)
- distrib = torch.distributions.Categorical(posterior)
- out_fine = distrib.sample()
- f_outputs.append(out_fine)
-
- # Put the hidden state back together
- hidden = torch.cat([hidden_coarse, hidden_fine], dim=1)
-
- # Display progress
- speed = (i + 1) / (time.time() - start)
- stream('Gen: %i/%i -- Speed: %i', (i + 1, seq_len, speed))
-
- coarse = torch.stack(c_outputs).squeeze(1).cpu().data.numpy()
- fine = torch.stack(f_outputs).squeeze(1).cpu().data.numpy()
- output = combine_signal(coarse, fine)
-
- return output, coarse, fine
-
- def init_hidden(self, batch_size=1) :
- return torch.zeros(batch_size, self.hidden_size).cuda()
-
- def num_params(self) :
- parameters = filter(lambda p: p.requires_grad, self.parameters())
- parameters = sum([np.prod(p.size()) for p in parameters]) / 1_000_000
- print('Trainable Parameters: %.3f million' % parameters)
\ No newline at end of file
diff --git a/spaces/KevlarVK/content_summarizer/title_generator.py b/spaces/KevlarVK/content_summarizer/title_generator.py
deleted file mode 100644
index d5b37dbbe494fb86056c1856be091833a77d3d62..0000000000000000000000000000000000000000
--- a/spaces/KevlarVK/content_summarizer/title_generator.py
+++ /dev/null
@@ -1,14 +0,0 @@
-from transformers import AutoTokenizer, TFAutoModelForSeq2SeqLM
-
-class T5Summarizer:
- def __init__(self, model_name: str = "fabiochiu/t5-small-medium-title-generation"):
- self.tokenizer = AutoTokenizer.from_pretrained(model_name)
- self.model = TFAutoModelForSeq2SeqLM.from_pretrained(model_name)
-
- def summarize(self, text: str):
- inputs = ["summarize: " + text]
- max_input_length = self.tokenizer.model_max_length
- inputs = self.tokenizer(inputs, max_length=max_input_length, truncation=True, return_tensors="tf")
- output = self.model.generate(**inputs, num_beams=12, do_sample=True, min_length=2, max_length=12)
- summary = self.tokenizer.batch_decode(output, skip_special_tokens=True)[0]
- return summary
\ No newline at end of file
diff --git a/spaces/Kimata/multimodal_deepfake_detection/inference_2.py b/spaces/Kimata/multimodal_deepfake_detection/inference_2.py
deleted file mode 100644
index 434d495ed9ceb6f12d89f5852a69839417eb2404..0000000000000000000000000000000000000000
--- a/spaces/Kimata/multimodal_deepfake_detection/inference_2.py
+++ /dev/null
@@ -1,216 +0,0 @@
-import os
-import cv2
-import onnx
-import torch
-import argparse
-import numpy as np
-import torch.nn as nn
-from models.TMC import ETMC
-from models import image
-
-from onnx2pytorch import ConvertModel
-
-onnx_model = onnx.load('checkpoints/efficientnet.onnx')
-pytorch_model = ConvertModel(onnx_model)
-
-#Set random seed for reproducibility.
-torch.manual_seed(42)
-
-
-# Define the audio_args dictionary
-audio_args = {
- 'nb_samp': 64600,
- 'first_conv': 1024,
- 'in_channels': 1,
- 'filts': [20, [20, 20], [20, 128], [128, 128]],
- 'blocks': [2, 4],
- 'nb_fc_node': 1024,
- 'gru_node': 1024,
- 'nb_gru_layer': 3,
- 'nb_classes': 2
-}
-
-
-def get_args(parser):
- parser.add_argument("--batch_size", type=int, default=8)
- parser.add_argument("--data_dir", type=str, default="datasets/train/fakeavceleb*")
- parser.add_argument("--LOAD_SIZE", type=int, default=256)
- parser.add_argument("--FINE_SIZE", type=int, default=224)
- parser.add_argument("--dropout", type=float, default=0.2)
- parser.add_argument("--gradient_accumulation_steps", type=int, default=1)
- parser.add_argument("--hidden", nargs="*", type=int, default=[])
- parser.add_argument("--hidden_sz", type=int, default=768)
- parser.add_argument("--img_embed_pool_type", type=str, default="avg", choices=["max", "avg"])
- parser.add_argument("--img_hidden_sz", type=int, default=1024)
- parser.add_argument("--include_bn", type=int, default=True)
- parser.add_argument("--lr", type=float, default=1e-4)
- parser.add_argument("--lr_factor", type=float, default=0.3)
- parser.add_argument("--lr_patience", type=int, default=10)
- parser.add_argument("--max_epochs", type=int, default=500)
- parser.add_argument("--n_workers", type=int, default=12)
- parser.add_argument("--name", type=str, default="MMDF")
- parser.add_argument("--num_image_embeds", type=int, default=1)
- parser.add_argument("--patience", type=int, default=20)
- parser.add_argument("--savedir", type=str, default="./savepath/")
- parser.add_argument("--seed", type=int, default=1)
- parser.add_argument("--n_classes", type=int, default=2)
- parser.add_argument("--annealing_epoch", type=int, default=10)
- parser.add_argument("--device", type=str, default='cpu')
- parser.add_argument("--pretrained_image_encoder", type=bool, default = False)
- parser.add_argument("--freeze_image_encoder", type=bool, default = False)
- parser.add_argument("--pretrained_audio_encoder", type = bool, default=False)
- parser.add_argument("--freeze_audio_encoder", type = bool, default = False)
- parser.add_argument("--augment_dataset", type = bool, default = True)
-
- for key, value in audio_args.items():
- parser.add_argument(f"--{key}", type=type(value), default=value)
-
-def model_summary(args):
- '''Prints the model summary.'''
- model = ETMC(args)
-
- for name, layer in model.named_modules():
- print(name, layer)
-
-def load_multimodal_model(args):
- '''Load multimodal model'''
- model = ETMC(args)
- ckpt = torch.load('checkpoints/model.pth', map_location = torch.device('cpu'))
- model.load_state_dict(ckpt, strict = True)
- model.eval()
- return model
-
-def load_img_modality_model(args):
- '''Loads image modality model.'''
- rgb_encoder = pytorch_model
-
- ckpt = torch.load('checkpoints/model.pth', map_location = torch.device('cpu'))
- rgb_encoder.load_state_dict(ckpt['rgb_encoder'], strict = True)
- rgb_encoder.eval()
- return rgb_encoder
-
-def load_spec_modality_model(args):
- spec_encoder = image.RawNet(args)
- ckpt = torch.load('checkpoints/model.pth', map_location = torch.device('cpu'))
- spec_encoder.load_state_dict(ckpt['spec_encoder'], strict = True)
- spec_encoder.eval()
- return spec_encoder
-
-
-#Load models.
-parser = argparse.ArgumentParser(description="Inference models")
-get_args(parser)
-args, remaining_args = parser.parse_known_args()
-assert remaining_args == [], remaining_args
-
-spec_model = load_spec_modality_model(args)
-
-img_model = load_img_modality_model(args)
-
-
-def preprocess_img(face):
- face = face / 255
- face = cv2.resize(face, (256, 256))
- # face = face.transpose(2, 0, 1) #(W, H, C) -> (C, W, H)
- face_pt = torch.unsqueeze(torch.Tensor(face), dim = 0)
- return face_pt
-
-def preprocess_audio(audio_file):
- audio_pt = torch.unsqueeze(torch.Tensor(audio_file), dim = 0)
- return audio_pt
-
-def deepfakes_spec_predict(input_audio):
- x, _ = input_audio
- audio = preprocess_audio(x)
- spec_grads = spec_model.forward(audio)
- spec_grads_inv = np.exp(spec_grads.cpu().detach().numpy().squeeze())
-
- # multimodal_grads = multimodal.spec_depth[0].forward(spec_grads)
-
- # out = nn.Softmax()(multimodal_grads)
- # max = torch.argmax(out, dim = -1) #Index of the max value in the tensor.
- # max_value = out[max] #Actual value of the tensor.
- max_value = np.argmax(spec_grads_inv)
-
- if max_value > 0.5:
- preds = round(100 - (max_value*100), 3)
- text2 = f"The audio is REAL."
-
- else:
- preds = round(max_value*100, 3)
- text2 = f"The audio is FAKE."
-
- return text2
-
-def deepfakes_image_predict(input_image):
- face = preprocess_img(input_image)
- print(f"Face shape is: {face.shape}")
- img_grads = img_model.forward(face)
- img_grads = img_grads.cpu().detach().numpy()
- img_grads_np = np.squeeze(img_grads)
-
- if img_grads_np[0] > 0.5:
- preds = round(img_grads_np[0] * 100, 3)
- text2 = f"The image is REAL. \nConfidence score is: {preds}"
-
- else:
- preds = round(img_grads_np[1] * 100, 3)
- text2 = f"The image is FAKE. \nConfidence score is: {preds}"
-
- return text2
-
-
-def preprocess_video(input_video, n_frames = 3):
- v_cap = cv2.VideoCapture(input_video)
- v_len = int(v_cap.get(cv2.CAP_PROP_FRAME_COUNT))
-
- # Pick 'n_frames' evenly spaced frames to sample
- if n_frames is None:
- sample = np.arange(0, v_len)
- else:
- sample = np.linspace(0, v_len - 1, n_frames).astype(int)
-
- #Loop through frames.
- frames = []
- for j in range(v_len):
- success = v_cap.grab()
- if j in sample:
- # Load frame
- success, frame = v_cap.retrieve()
- if not success:
- continue
- frame = cv2.cvtColor(frame, cv2.COLOR_BGR2RGB)
- frame = preprocess_img(frame)
- frames.append(frame)
- v_cap.release()
- return frames
-
-
-def deepfakes_video_predict(input_video):
- '''Perform inference on a video.'''
- video_frames = preprocess_video(input_video)
- real_faces_list = []
- fake_faces_list = []
-
- for face in video_frames:
- # face = preprocess_img(face)
-
- img_grads = img_model.forward(face)
- img_grads = img_grads.cpu().detach().numpy()
- img_grads_np = np.squeeze(img_grads)
- real_faces_list.append(img_grads_np[0])
- fake_faces_list.append(img_grads_np[1])
-
- real_faces_mean = np.mean(real_faces_list)
- fake_faces_mean = np.mean(fake_faces_list)
-
- if real_faces_mean > 0.5:
- preds = round(real_faces_mean * 100, 3)
- text2 = f"The video is REAL. \nConfidence score is: {preds}%"
-
- else:
- preds = round(fake_faces_mean * 100, 3)
- text2 = f"The video is FAKE. \nConfidence score is: {preds}%"
-
- return text2
-
diff --git a/spaces/Kirihasan/rvc-jjjo/infer_pack/transforms.py b/spaces/Kirihasan/rvc-jjjo/infer_pack/transforms.py
deleted file mode 100644
index a11f799e023864ff7082c1f49c0cc18351a13b47..0000000000000000000000000000000000000000
--- a/spaces/Kirihasan/rvc-jjjo/infer_pack/transforms.py
+++ /dev/null
@@ -1,209 +0,0 @@
-import torch
-from torch.nn import functional as F
-
-import numpy as np
-
-
-DEFAULT_MIN_BIN_WIDTH = 1e-3
-DEFAULT_MIN_BIN_HEIGHT = 1e-3
-DEFAULT_MIN_DERIVATIVE = 1e-3
-
-
-def piecewise_rational_quadratic_transform(
- inputs,
- unnormalized_widths,
- unnormalized_heights,
- unnormalized_derivatives,
- inverse=False,
- tails=None,
- tail_bound=1.0,
- min_bin_width=DEFAULT_MIN_BIN_WIDTH,
- min_bin_height=DEFAULT_MIN_BIN_HEIGHT,
- min_derivative=DEFAULT_MIN_DERIVATIVE,
-):
- if tails is None:
- spline_fn = rational_quadratic_spline
- spline_kwargs = {}
- else:
- spline_fn = unconstrained_rational_quadratic_spline
- spline_kwargs = {"tails": tails, "tail_bound": tail_bound}
-
- outputs, logabsdet = spline_fn(
- inputs=inputs,
- unnormalized_widths=unnormalized_widths,
- unnormalized_heights=unnormalized_heights,
- unnormalized_derivatives=unnormalized_derivatives,
- inverse=inverse,
- min_bin_width=min_bin_width,
- min_bin_height=min_bin_height,
- min_derivative=min_derivative,
- **spline_kwargs
- )
- return outputs, logabsdet
-
-
-def searchsorted(bin_locations, inputs, eps=1e-6):
- bin_locations[..., -1] += eps
- return torch.sum(inputs[..., None] >= bin_locations, dim=-1) - 1
-
-
-def unconstrained_rational_quadratic_spline(
- inputs,
- unnormalized_widths,
- unnormalized_heights,
- unnormalized_derivatives,
- inverse=False,
- tails="linear",
- tail_bound=1.0,
- min_bin_width=DEFAULT_MIN_BIN_WIDTH,
- min_bin_height=DEFAULT_MIN_BIN_HEIGHT,
- min_derivative=DEFAULT_MIN_DERIVATIVE,
-):
- inside_interval_mask = (inputs >= -tail_bound) & (inputs <= tail_bound)
- outside_interval_mask = ~inside_interval_mask
-
- outputs = torch.zeros_like(inputs)
- logabsdet = torch.zeros_like(inputs)
-
- if tails == "linear":
- unnormalized_derivatives = F.pad(unnormalized_derivatives, pad=(1, 1))
- constant = np.log(np.exp(1 - min_derivative) - 1)
- unnormalized_derivatives[..., 0] = constant
- unnormalized_derivatives[..., -1] = constant
-
- outputs[outside_interval_mask] = inputs[outside_interval_mask]
- logabsdet[outside_interval_mask] = 0
- else:
- raise RuntimeError("{} tails are not implemented.".format(tails))
-
- (
- outputs[inside_interval_mask],
- logabsdet[inside_interval_mask],
- ) = rational_quadratic_spline(
- inputs=inputs[inside_interval_mask],
- unnormalized_widths=unnormalized_widths[inside_interval_mask, :],
- unnormalized_heights=unnormalized_heights[inside_interval_mask, :],
- unnormalized_derivatives=unnormalized_derivatives[inside_interval_mask, :],
- inverse=inverse,
- left=-tail_bound,
- right=tail_bound,
- bottom=-tail_bound,
- top=tail_bound,
- min_bin_width=min_bin_width,
- min_bin_height=min_bin_height,
- min_derivative=min_derivative,
- )
-
- return outputs, logabsdet
-
-
-def rational_quadratic_spline(
- inputs,
- unnormalized_widths,
- unnormalized_heights,
- unnormalized_derivatives,
- inverse=False,
- left=0.0,
- right=1.0,
- bottom=0.0,
- top=1.0,
- min_bin_width=DEFAULT_MIN_BIN_WIDTH,
- min_bin_height=DEFAULT_MIN_BIN_HEIGHT,
- min_derivative=DEFAULT_MIN_DERIVATIVE,
-):
- if torch.min(inputs) < left or torch.max(inputs) > right:
- raise ValueError("Input to a transform is not within its domain")
-
- num_bins = unnormalized_widths.shape[-1]
-
- if min_bin_width * num_bins > 1.0:
- raise ValueError("Minimal bin width too large for the number of bins")
- if min_bin_height * num_bins > 1.0:
- raise ValueError("Minimal bin height too large for the number of bins")
-
- widths = F.softmax(unnormalized_widths, dim=-1)
- widths = min_bin_width + (1 - min_bin_width * num_bins) * widths
- cumwidths = torch.cumsum(widths, dim=-1)
- cumwidths = F.pad(cumwidths, pad=(1, 0), mode="constant", value=0.0)
- cumwidths = (right - left) * cumwidths + left
- cumwidths[..., 0] = left
- cumwidths[..., -1] = right
- widths = cumwidths[..., 1:] - cumwidths[..., :-1]
-
- derivatives = min_derivative + F.softplus(unnormalized_derivatives)
-
- heights = F.softmax(unnormalized_heights, dim=-1)
- heights = min_bin_height + (1 - min_bin_height * num_bins) * heights
- cumheights = torch.cumsum(heights, dim=-1)
- cumheights = F.pad(cumheights, pad=(1, 0), mode="constant", value=0.0)
- cumheights = (top - bottom) * cumheights + bottom
- cumheights[..., 0] = bottom
- cumheights[..., -1] = top
- heights = cumheights[..., 1:] - cumheights[..., :-1]
-
- if inverse:
- bin_idx = searchsorted(cumheights, inputs)[..., None]
- else:
- bin_idx = searchsorted(cumwidths, inputs)[..., None]
-
- input_cumwidths = cumwidths.gather(-1, bin_idx)[..., 0]
- input_bin_widths = widths.gather(-1, bin_idx)[..., 0]
-
- input_cumheights = cumheights.gather(-1, bin_idx)[..., 0]
- delta = heights / widths
- input_delta = delta.gather(-1, bin_idx)[..., 0]
-
- input_derivatives = derivatives.gather(-1, bin_idx)[..., 0]
- input_derivatives_plus_one = derivatives[..., 1:].gather(-1, bin_idx)[..., 0]
-
- input_heights = heights.gather(-1, bin_idx)[..., 0]
-
- if inverse:
- a = (inputs - input_cumheights) * (
- input_derivatives + input_derivatives_plus_one - 2 * input_delta
- ) + input_heights * (input_delta - input_derivatives)
- b = input_heights * input_derivatives - (inputs - input_cumheights) * (
- input_derivatives + input_derivatives_plus_one - 2 * input_delta
- )
- c = -input_delta * (inputs - input_cumheights)
-
- discriminant = b.pow(2) - 4 * a * c
- assert (discriminant >= 0).all()
-
- root = (2 * c) / (-b - torch.sqrt(discriminant))
- outputs = root * input_bin_widths + input_cumwidths
-
- theta_one_minus_theta = root * (1 - root)
- denominator = input_delta + (
- (input_derivatives + input_derivatives_plus_one - 2 * input_delta)
- * theta_one_minus_theta
- )
- derivative_numerator = input_delta.pow(2) * (
- input_derivatives_plus_one * root.pow(2)
- + 2 * input_delta * theta_one_minus_theta
- + input_derivatives * (1 - root).pow(2)
- )
- logabsdet = torch.log(derivative_numerator) - 2 * torch.log(denominator)
-
- return outputs, -logabsdet
- else:
- theta = (inputs - input_cumwidths) / input_bin_widths
- theta_one_minus_theta = theta * (1 - theta)
-
- numerator = input_heights * (
- input_delta * theta.pow(2) + input_derivatives * theta_one_minus_theta
- )
- denominator = input_delta + (
- (input_derivatives + input_derivatives_plus_one - 2 * input_delta)
- * theta_one_minus_theta
- )
- outputs = input_cumheights + numerator / denominator
-
- derivative_numerator = input_delta.pow(2) * (
- input_derivatives_plus_one * theta.pow(2)
- + 2 * input_delta * theta_one_minus_theta
- + input_derivatives * (1 - theta).pow(2)
- )
- logabsdet = torch.log(derivative_numerator) - 2 * torch.log(denominator)
-
- return outputs, logabsdet
diff --git a/spaces/KonradSzafer/HF-QA-Demo/discord_bot/client/utils.py b/spaces/KonradSzafer/HF-QA-Demo/discord_bot/client/utils.py
deleted file mode 100644
index a090087ac11edc11213360a37f507cdd23516113..0000000000000000000000000000000000000000
--- a/spaces/KonradSzafer/HF-QA-Demo/discord_bot/client/utils.py
+++ /dev/null
@@ -1,54 +0,0 @@
-from typing import List
-
-
-def find_max_split_index(text: str, char: str) -> int:
- char_idx = text.rfind(char)
- if char_idx > 0:
- # If a character is found, return the index after the splitting character
- split_idx = char_idx + len(char)
- if split_idx >= len(text):
- return len(text)
- else:
- return split_idx
- return -1
-
-
-def find_max_split_index_from_sequence(text: str, split_characters: List[str]) -> int:
- split_index = max((
- find_max_split_index(text, sequence)
- for sequence in split_characters
- ), default=-1)
- return split_index
-
-
-def split_text_into_chunks(
- text: str,
- split_characters: List[str] = [],
- min_size: int = 20,
- max_size: int = 250,
- ) -> List[str]:
-
- chunks = []
- start_idx = 0
- end_idx = max_size
- text_len = len(text)
- while start_idx < text_len:
- search_chunk = text[start_idx+min_size:end_idx]
- split_idx = find_max_split_index_from_sequence(
- text=search_chunk,
- split_characters=split_characters
- )
- # if no spliting element found, set the maximal size
- if split_idx < 1:
- split_idx = end_idx
- # if found - offset it by the starting idx of the chunk
- else:
- split_idx += start_idx + min_size
-
- chunk = text[start_idx:split_idx]
- chunks.append(chunk)
-
- start_idx = split_idx
- end_idx = split_idx + max_size
-
- return chunks
diff --git a/spaces/Lamai/LAMAIGPT/autogpt/config/singleton.py b/spaces/Lamai/LAMAIGPT/autogpt/config/singleton.py
deleted file mode 100644
index 55b2aeea120bbe51ca837265fcb7fbff467e55f2..0000000000000000000000000000000000000000
--- a/spaces/Lamai/LAMAIGPT/autogpt/config/singleton.py
+++ /dev/null
@@ -1,24 +0,0 @@
-"""The singleton metaclass for ensuring only one instance of a class."""
-import abc
-
-
-class Singleton(abc.ABCMeta, type):
- """
- Singleton metaclass for ensuring only one instance of a class.
- """
-
- _instances = {}
-
- def __call__(cls, *args, **kwargs):
- """Call method for the singleton metaclass."""
- if cls not in cls._instances:
- cls._instances[cls] = super(Singleton, cls).__call__(*args, **kwargs)
- return cls._instances[cls]
-
-
-class AbstractSingleton(abc.ABC, metaclass=Singleton):
- """
- Abstract singleton class for ensuring only one instance of a class.
- """
-
- pass
diff --git a/spaces/Loren/Streamlit_OCR_comparator/configs/_base_/det_pipelines/textsnake_pipeline.py b/spaces/Loren/Streamlit_OCR_comparator/configs/_base_/det_pipelines/textsnake_pipeline.py
deleted file mode 100644
index dc4b44819e5c3f3f725df096903fc0a809313913..0000000000000000000000000000000000000000
--- a/spaces/Loren/Streamlit_OCR_comparator/configs/_base_/det_pipelines/textsnake_pipeline.py
+++ /dev/null
@@ -1,65 +0,0 @@
-img_norm_cfg = dict(
- mean=[123.675, 116.28, 103.53], std=[58.395, 57.12, 57.375], to_rgb=True)
-
-train_pipeline = [
- dict(type='LoadImageFromFile', color_type='color_ignore_orientation'),
- dict(
- type='LoadTextAnnotations',
- with_bbox=True,
- with_mask=True,
- poly2mask=False),
- dict(type='ColorJitter', brightness=32.0 / 255, saturation=0.5),
- dict(type='Normalize', **img_norm_cfg),
- dict(
- type='RandomCropPolyInstances',
- instance_key='gt_masks',
- crop_ratio=0.65,
- min_side_ratio=0.3),
- dict(
- type='RandomRotatePolyInstances',
- rotate_ratio=0.5,
- max_angle=20,
- pad_with_fixed_color=False),
- dict(
- type='ScaleAspectJitter',
- img_scale=[(3000, 736)], # unused
- ratio_range=(0.7, 1.3),
- aspect_ratio_range=(0.9, 1.1),
- multiscale_mode='value',
- long_size_bound=800,
- short_size_bound=480,
- resize_type='long_short_bound',
- keep_ratio=False),
- dict(type='SquareResizePad', target_size=800, pad_ratio=0.6),
- dict(type='RandomFlip', flip_ratio=0.5, direction='horizontal'),
- dict(type='TextSnakeTargets'),
- dict(type='Pad', size_divisor=32),
- dict(
- type='CustomFormatBundle',
- keys=[
- 'gt_text_mask', 'gt_center_region_mask', 'gt_mask',
- 'gt_radius_map', 'gt_sin_map', 'gt_cos_map'
- ],
- visualize=dict(flag=False, boundary_key='gt_text_mask')),
- dict(
- type='Collect',
- keys=[
- 'img', 'gt_text_mask', 'gt_center_region_mask', 'gt_mask',
- 'gt_radius_map', 'gt_sin_map', 'gt_cos_map'
- ])
-]
-
-test_pipeline = [
- dict(type='LoadImageFromFile', color_type='color_ignore_orientation'),
- dict(
- type='MultiScaleFlipAug',
- img_scale=(1333, 736), # used by Resize
- flip=False,
- transforms=[
- dict(type='Resize', keep_ratio=True),
- dict(type='Normalize', **img_norm_cfg),
- dict(type='Pad', size_divisor=32),
- dict(type='ImageToTensor', keys=['img']),
- dict(type='Collect', keys=['img']),
- ])
-]
diff --git a/spaces/Marshalls/testmtd/analysis/pymo/mocapplayer/libs/papaparse.min.js b/spaces/Marshalls/testmtd/analysis/pymo/mocapplayer/libs/papaparse.min.js
deleted file mode 100644
index a62a9266db2848266c2667d8d486b43135c6501d..0000000000000000000000000000000000000000
--- a/spaces/Marshalls/testmtd/analysis/pymo/mocapplayer/libs/papaparse.min.js
+++ /dev/null
@@ -1,6 +0,0 @@
-/*!
- Papa Parse
- v4.1.2
- https://github.com/mholt/PapaParse
-*/
-!function(e){"use strict";function t(t,r){if(r=r||{},r.worker&&S.WORKERS_SUPPORTED){var n=f();return n.userStep=r.step,n.userChunk=r.chunk,n.userComplete=r.complete,n.userError=r.error,r.step=m(r.step),r.chunk=m(r.chunk),r.complete=m(r.complete),r.error=m(r.error),delete r.worker,void n.postMessage({input:t,config:r,workerId:n.id})}var o=null;return"string"==typeof t?o=r.download?new i(r):new a(r):(e.File&&t instanceof File||t instanceof Object)&&(o=new s(r)),o.stream(t)}function r(e,t){function r(){"object"==typeof t&&("string"==typeof t.delimiter&&1==t.delimiter.length&&-1==S.BAD_DELIMITERS.indexOf(t.delimiter)&&(u=t.delimiter),("boolean"==typeof t.quotes||t.quotes instanceof Array)&&(o=t.quotes),"string"==typeof t.newline&&(h=t.newline))}function n(e){if("object"!=typeof e)return[];var t=[];for(var r in e)t.push(r);return t}function i(e,t){var r="";"string"==typeof e&&(e=JSON.parse(e)),"string"==typeof t&&(t=JSON.parse(t));var n=e instanceof Array&&e.length>0,i=!(t[0]instanceof Array);if(n){for(var a=0;a0&&(r+=u),r+=s(e[a],a);t.length>0&&(r+=h)}for(var o=0;oc;c++){c>0&&(r+=u);var d=n&&i?e[c]:c;r+=s(t[o][d],c)}o-1||" "==e.charAt(0)||" "==e.charAt(e.length-1);return r?'"'+e+'"':e}function a(e,t){for(var r=0;r-1)return!0;return!1}var o=!1,u=",",h="\r\n";if(r(),"string"==typeof e&&(e=JSON.parse(e)),e instanceof Array){if(!e.length||e[0]instanceof Array)return i(null,e);if("object"==typeof e[0])return i(n(e[0]),e)}else if("object"==typeof e)return"string"==typeof e.data&&(e.data=JSON.parse(e.data)),e.data instanceof Array&&(e.fields||(e.fields=e.data[0]instanceof Array?e.fields:n(e.data[0])),e.data[0]instanceof Array||"object"==typeof e.data[0]||(e.data=[e.data])),i(e.fields||[],e.data||[]);throw"exception: Unable to serialize unrecognized input"}function n(t){function r(e){var t=_(e);t.chunkSize=parseInt(t.chunkSize),e.step||e.chunk||(t.chunkSize=null),this._handle=new o(t),this._handle.streamer=this,this._config=t}this._handle=null,this._paused=!1,this._finished=!1,this._input=null,this._baseIndex=0,this._partialLine="",this._rowCount=0,this._start=0,this._nextChunk=null,this.isFirstChunk=!0,this._completeResults={data:[],errors:[],meta:{}},r.call(this,t),this.parseChunk=function(t){if(this.isFirstChunk&&m(this._config.beforeFirstChunk)){var r=this._config.beforeFirstChunk(t);void 0!==r&&(t=r)}this.isFirstChunk=!1;var n=this._partialLine+t;this._partialLine="";var i=this._handle.parse(n,this._baseIndex,!this._finished);if(!this._handle.paused()&&!this._handle.aborted()){var s=i.meta.cursor;this._finished||(this._partialLine=n.substring(s-this._baseIndex),this._baseIndex=s),i&&i.data&&(this._rowCount+=i.data.length);var a=this._finished||this._config.preview&&this._rowCount>=this._config.preview;if(y)e.postMessage({results:i,workerId:S.WORKER_ID,finished:a});else if(m(this._config.chunk)){if(this._config.chunk(i,this._handle),this._paused)return;i=void 0,this._completeResults=void 0}return this._config.step||this._config.chunk||(this._completeResults.data=this._completeResults.data.concat(i.data),this._completeResults.errors=this._completeResults.errors.concat(i.errors),this._completeResults.meta=i.meta),!a||!m(this._config.complete)||i&&i.meta.aborted||this._config.complete(this._completeResults),a||i&&i.meta.paused||this._nextChunk(),i}},this._sendError=function(t){m(this._config.error)?this._config.error(t):y&&this._config.error&&e.postMessage({workerId:S.WORKER_ID,error:t,finished:!1})}}function i(e){function t(e){var t=e.getResponseHeader("Content-Range");return parseInt(t.substr(t.lastIndexOf("/")+1))}e=e||{},e.chunkSize||(e.chunkSize=S.RemoteChunkSize),n.call(this,e);var r;this._nextChunk=k?function(){this._readChunk(),this._chunkLoaded()}:function(){this._readChunk()},this.stream=function(e){this._input=e,this._nextChunk()},this._readChunk=function(){if(this._finished)return void this._chunkLoaded();if(r=new XMLHttpRequest,k||(r.onload=g(this._chunkLoaded,this),r.onerror=g(this._chunkError,this)),r.open("GET",this._input,!k),this._config.chunkSize){var e=this._start+this._config.chunkSize-1;r.setRequestHeader("Range","bytes="+this._start+"-"+e),r.setRequestHeader("If-None-Match","webkit-no-cache")}try{r.send()}catch(t){this._chunkError(t.message)}k&&0==r.status?this._chunkError():this._start+=this._config.chunkSize},this._chunkLoaded=function(){if(4==r.readyState){if(r.status<200||r.status>=400)return void this._chunkError();this._finished=!this._config.chunkSize||this._start>t(r),this.parseChunk(r.responseText)}},this._chunkError=function(e){var t=r.statusText||e;this._sendError(t)}}function s(e){e=e||{},e.chunkSize||(e.chunkSize=S.LocalChunkSize),n.call(this,e);var t,r,i="undefined"!=typeof FileReader;this.stream=function(e){this._input=e,r=e.slice||e.webkitSlice||e.mozSlice,i?(t=new FileReader,t.onload=g(this._chunkLoaded,this),t.onerror=g(this._chunkError,this)):t=new FileReaderSync,this._nextChunk()},this._nextChunk=function(){this._finished||this._config.preview&&!(this._rowCount=this._input.size,this.parseChunk(e.target.result)},this._chunkError=function(){this._sendError(t.error)}}function a(e){e=e||{},n.call(this,e);var t,r;this.stream=function(e){return t=e,r=e,this._nextChunk()},this._nextChunk=function(){if(!this._finished){var e=this._config.chunkSize,t=e?r.substr(0,e):r;return r=e?r.substr(e):"",this._finished=!r,this.parseChunk(t)}}}function o(e){function t(){if(b&&d&&(h("Delimiter","UndetectableDelimiter","Unable to auto-detect delimiting character; defaulted to '"+S.DefaultDelimiter+"'"),d=!1),e.skipEmptyLines)for(var t=0;t=y.length?(r.__parsed_extra||(r.__parsed_extra=[]),r.__parsed_extra.push(b.data[t][n])):r[y[n]]=b.data[t][n])}e.header&&(b.data[t]=r,n>y.length?h("FieldMismatch","TooManyFields","Too many fields: expected "+y.length+" fields but parsed "+n,t):n1&&(h+=Math.abs(l-i),i=l):i=l}c.data.length>0&&(f/=c.data.length),("undefined"==typeof n||n>h)&&f>1.99&&(n=h,r=o)}return e.delimiter=r,{successful:!!r,bestDelimiter:r}}function a(e){e=e.substr(0,1048576);var t=e.split("\r");if(1==t.length)return"\n";for(var r=0,n=0;n=t.length/2?"\r\n":"\r"}function o(e){var t=l.test(e);return t?parseFloat(e):e}function h(e,t,r,n){b.errors.push({type:e,code:t,message:r,row:n})}var f,c,d,l=/^\s*-?(\d*\.?\d+|\d+\.?\d*)(e[-+]?\d+)?\s*$/i,p=this,g=0,v=!1,k=!1,y=[],b={data:[],errors:[],meta:{}};if(m(e.step)){var R=e.step;e.step=function(n){if(b=n,r())t();else{if(t(),0==b.data.length)return;g+=n.data.length,e.preview&&g>e.preview?c.abort():R(b,p)}}}this.parse=function(r,n,i){if(e.newline||(e.newline=a(r)),d=!1,!e.delimiter){var o=s(r);o.successful?e.delimiter=o.bestDelimiter:(d=!0,e.delimiter=S.DefaultDelimiter),b.meta.delimiter=e.delimiter}var h=_(e);return e.preview&&e.header&&h.preview++,f=r,c=new u(h),b=c.parse(f,n,i),t(),v?{meta:{paused:!0}}:b||{meta:{paused:!1}}},this.paused=function(){return v},this.pause=function(){v=!0,c.abort(),f=f.substr(c.getCharIndex())},this.resume=function(){v=!1,p.streamer.parseChunk(f)},this.aborted=function(){return k},this.abort=function(){k=!0,c.abort(),b.meta.aborted=!0,m(e.complete)&&e.complete(b),f=""}}function u(e){e=e||{};var t=e.delimiter,r=e.newline,n=e.comments,i=e.step,s=e.preview,a=e.fastMode;if(("string"!=typeof t||S.BAD_DELIMITERS.indexOf(t)>-1)&&(t=","),n===t)throw"Comment character same as delimiter";n===!0?n="#":("string"!=typeof n||S.BAD_DELIMITERS.indexOf(n)>-1)&&(n=!1),"\n"!=r&&"\r"!=r&&"\r\n"!=r&&(r="\n");var o=0,u=!1;this.parse=function(e,h,f){function c(e){b.push(e),S=o}function d(t){return f?p():("undefined"==typeof t&&(t=e.substr(o)),w.push(t),o=g,c(w),y&&_(),p())}function l(t){o=t,c(w),w=[],O=e.indexOf(r,o)}function p(e){return{data:b,errors:R,meta:{delimiter:t,linebreak:r,aborted:u,truncated:!!e,cursor:S+(h||0)}}}function _(){i(p()),b=[],R=[]}if("string"!=typeof e)throw"Input must be a string";var g=e.length,m=t.length,v=r.length,k=n.length,y="function"==typeof i;o=0;var b=[],R=[],w=[],S=0;if(!e)return p();if(a||a!==!1&&-1===e.indexOf('"')){for(var C=e.split(r),E=0;E=s)return b=b.slice(0,s),p(!0)}}return p()}for(var x=e.indexOf(t,o),O=e.indexOf(r,o);;)if('"'!=e[o])if(n&&0===w.length&&e.substr(o,k)===n){if(-1==O)return p();o=O+v,O=e.indexOf(r,o),x=e.indexOf(t,o)}else if(-1!==x&&(O>x||-1===O))w.push(e.substring(o,x)),o=x+m,x=e.indexOf(t,o);else{if(-1===O)break;if(w.push(e.substring(o,O)),l(O+v),y&&(_(),u))return p();if(s&&b.length>=s)return p(!0)}else{var I=o;for(o++;;){var I=e.indexOf('"',I+1);if(-1===I)return f||R.push({type:"Quotes",code:"MissingQuotes",message:"Quoted field unterminated",row:b.length,index:o}),d();if(I===g-1){var D=e.substring(o,I).replace(/""/g,'"');return d(D)}if('"'!=e[I+1]){if(e[I+1]==t){w.push(e.substring(o,I).replace(/""/g,'"')),o=I+1+m,x=e.indexOf(t,o),O=e.indexOf(r,o);break}if(e.substr(I+1,v)===r){if(w.push(e.substring(o,I).replace(/""/g,'"')),l(I+1+v),x=e.indexOf(t,o),y&&(_(),u))return p();if(s&&b.length>=s)return p(!0);break}}else I++}}return d()},this.abort=function(){u=!0},this.getCharIndex=function(){return o}}function h(){var e=document.getElementsByTagName("script");return e.length?e[e.length-1].src:""}function f(){if(!S.WORKERS_SUPPORTED)return!1;if(!b&&null===S.SCRIPT_PATH)throw new Error("Script path cannot be determined automatically when Papa Parse is loaded asynchronously. You need to set Papa.SCRIPT_PATH manually.");var t=S.SCRIPT_PATH||v;t+=(-1!==t.indexOf("?")?"&":"?")+"papaworker";var r=new e.Worker(t);return r.onmessage=c,r.id=w++,R[r.id]=r,r}function c(e){var t=e.data,r=R[t.workerId],n=!1;if(t.error)r.userError(t.error,t.file);else if(t.results&&t.results.data){var i=function(){n=!0,d(t.workerId,{data:[],errors:[],meta:{aborted:!0}})},s={abort:i,pause:l,resume:l};if(m(r.userStep)){for(var a=0;asplit_into_utf8_characters($s, "return only chars, return trailing whitespaces", *dummy_ht);
- foreach $sub_len ((0 .. ($#characters-1))) {
- my $sub = join("", @characters[0 .. $sub_len]);
- foreach $super_len ((($sub_len + 1) .. $#characters)) {
- my $super = join("", @characters[0 .. $super_len]);
- # print STDERR " $sub -> $super\n" unless $ht{RULE_STRING_EXPANSION}->{$lang_code}->{$sub}->{$super};
- $ht{RULE_STRING_EXPANSION}->{$lang_code}->{$sub}->{$super} = 1;
- $ht{RULE_STRING_HAS_EXPANSION}->{$lang_code}->{$sub} = 1;
- # print STDERR " RULE_STRING_HAS_EXPANSION $lang_code $sub\n";
- }
- }
-}
-
-sub load_string_distance_data {
- local($this, $filename, *ht, $verbose) = @_;
-
- $verbose = 0 unless defined($verbose);
- open(IN,$filename) || die "Could not open $filename";
- my $line_number = 0;
- my $n_cost_rules = 0;
- while () {
- $line_number++;
- my $line = $_;
- $line =~ s/^\xEF\xBB\xBF//;
- $line =~ s/\s*$//;
- next if $line =~ /^\s*(\#.*)?$/;
- print STDERR "** Warning: line $line_number contains suspicious control character: $line\n" if $line =~ /[\x00-\x1F]/;
- my $s1 = $util->slot_value_in_double_colon_del_list($line, "s1");
- my $s2 = $util->slot_value_in_double_colon_del_list($line, "s2");
- $s1 = $util->dequote_string($s1); # 'can\'t' => can't
- $s2 = $util->dequote_string($s2);
- my $cost = $util->slot_value_in_double_colon_del_list($line, "cost");
- if (($s1 eq "") && ($s2 eq "")) {
- print STDERR "Ignoring bad line $line_number in $filename, because both s1 and s2 are empty strings\n";
- next;
- }
- unless ($cost =~ /^\d+(\.\d+)?$/) {
- if ($cost eq "") {
- print STDERR "Ignoring bad line $line_number in $filename, because of missing cost\n";
- } else {
- print STDERR "Ignoring bad line $line_number in $filename, because of ill-formed cost $cost\n";
- }
- next;
- }
- my $lang_code1_s = $util->slot_value_in_double_colon_del_list($line, "lc1");
- my $lang_code2_s = $util->slot_value_in_double_colon_del_list($line, "lc2");
- my @lang_codes_1 = ($lang_code1_s eq "") ? ("") : split(/,\s*/, $lang_code1_s);
- my @lang_codes_2 = ($lang_code2_s eq "") ? ("") : split(/,\s*/, $lang_code2_s);
- my $left_context1 = $util->slot_value_in_double_colon_del_list($line, "left1");
- my $left_context2 = $util->slot_value_in_double_colon_del_list($line, "left2");
- my $right_context1 = $util->slot_value_in_double_colon_del_list($line, "right1");
- my $right_context2 = $util->slot_value_in_double_colon_del_list($line, "right2");
- my $bad_left = $util->slot_value_in_double_colon_del_list($line, "left");
- if ($bad_left) {
- print STDERR "** Warning: slot '::left $bad_left' in line $line_number\n";
- next;
- }
- my $bad_right = $util->slot_value_in_double_colon_del_list($line, "right");
- if ($bad_right) {
- print STDERR "** Warning: slot '::right $bad_right' in line $line_number\n";
- next;
- }
- my $in_lang_codes1 = $util->slot_value_in_double_colon_del_list($line, "in-lc1");
- my $in_lang_codes2 = $util->slot_value_in_double_colon_del_list($line, "in-lc2");
- my $out_lang_codes1 = $util->slot_value_in_double_colon_del_list($line, "out-lc1");
- my $out_lang_codes2 = $util->slot_value_in_double_colon_del_list($line, "out-lc2");
- if ($left_context1) {
- if ($left_context1 =~ /^\/.*\/$/) {
- $left_context1 =~ s/^\///;
- $left_context1 =~ s/\/$//;
- } else {
- print STDERR "Ignoring unrecognized non-regular-express ::left1 $left_context1 in $line_number of $filename\n";
- $left_context1 = "";
- }
- }
- if ($left_context2) {
- if ($left_context2 =~ /^\/.*\/$/) {
- $left_context2 =~ s/^\///;
- $left_context2 =~ s/\/$//;
- } else {
- $left_context2 = "";
- print STDERR "Ignoring unrecognized non-regular-express ::left2 $left_context2 in $line_number of $filename\n";
- }
- }
- if ($right_context1) {
- unless ($right_context1 =~ /^(\[[^\[\]]*\])+$/) {
- $right_context1 = "";
- print STDERR "Ignoring unrecognized right-context ::right1 $right_context1 in $line_number of $filename\n";
- }
- }
- if ($right_context2) {
- unless ($right_context2 =~ /^(\[[^\[\]]*\])+$/) {
- $right_context2 = "";
- print STDERR "Ignoring unrecognized right-context ::right2 $right_context2 in $line_number of $filename\n";
- }
- }
- foreach $lang_code1 (@lang_codes_1) {
- foreach $lang_code2 (@lang_codes_2) {
- $n_cost_rules++;
- my $cost_rule_id = $n_cost_rules;
- $ht{COST}->{$lang_code1}->{$lang_code2}->{$s1}->{$s2}->{$cost_rule_id} = $cost;
- $ht{RULE_STRING}->{$lang_code1}->{$s1} = 1;
- $ht{RULE_STRING}->{$lang_code2}->{$s2} = 1;
- $ht{LEFT1}->{$cost_rule_id} = $left_context1;
- $ht{LEFT2}->{$cost_rule_id} = $left_context2;
- $ht{RIGHT1}->{$cost_rule_id} = $right_context1;
- $ht{RIGHT2}->{$cost_rule_id} = $right_context2;
- $ht{INLC1}->{$cost_rule_id} = $in_lang_codes1;
- $ht{INLC2}->{$cost_rule_id} = $in_lang_codes2;
- $ht{OUTLC1}->{$cost_rule_id} = $out_lang_codes1;
- $ht{OUTLC2}->{$cost_rule_id} = $out_lang_codes2;
- unless (($s1 eq $s2)
- && ($lang_code1 eq $lang_code2)
- && ($left_context1 eq $left_context2)
- && ($right_context1 eq $right_context2)
- && ($in_lang_codes1 eq $in_lang_codes2)
- && ($out_lang_codes1 eq $out_lang_codes2)) {
- $n_cost_rules++;
- $cost_rule_id = $n_cost_rules;
- $ht{COST}->{$lang_code2}->{$lang_code1}->{$s2}->{$s1}->{$cost_rule_id} = $cost;
- $ht{LEFT1}->{$cost_rule_id} = $left_context2;
- $ht{LEFT2}->{$cost_rule_id} = $left_context1;
- $ht{RIGHT1}->{$cost_rule_id} = $right_context2;
- $ht{RIGHT2}->{$cost_rule_id} = $right_context1;
- $ht{INLC1}->{$cost_rule_id} = $in_lang_codes2;
- $ht{INLC2}->{$cost_rule_id} = $in_lang_codes1;
- $ht{OUTLC1}->{$cost_rule_id} = $out_lang_codes2;
- $ht{OUTLC2}->{$cost_rule_id} = $out_lang_codes1;
- # print STDERR " Flip rule in line $line: $line\n";
- }
- $this->rule_string_expansion(*ht, $s1, $lang_code1);
- $this->rule_string_expansion(*ht, $s2, $lang_code2);
- }
- }
- }
- close(IN);
- print STDERR "Read in $n_cost_rules rules from $line_number lines in $filename\n" if $verbose;
-}
-
-sub romanized_string_to_simple_chart {
- local($this, $s, *chart_ht) = @_;
-
- my @characters = $utf8->split_into_utf8_characters($s, "return only chars, return trailing whitespaces", *dummy_ht);
- $chart_ht{N_CHARS} = $#characters + 1;
- $chart_ht{N_NODES} = 0;
- foreach $i ((0 .. $#characters)) {
- $romanizer->add_node($characters[$i], $i, ($i+1), *chart_ht, "", "");
- }
-}
-
-sub linearize_chart_points {
- local($this, *chart_ht, $chart_id, *sd_ht, $verbose) = @_;
-
- $verbose = 0 unless defined($verbose);
- print STDERR "Linearize $chart_id\n" if $verbose;
- my $current_chart_pos = 0;
- my $current_linear_chart_pos = 0;
- $sd_ht{POS2LINPOS}->{$chart_id}->{$current_chart_pos} = $current_linear_chart_pos;
- $sd_ht{LINPOS2POS}->{$chart_id}->{$current_linear_chart_pos} = $current_chart_pos;
- print STDERR " LINPOS2POS.$chart_id LIN: $current_linear_chart_pos POS: $current_chart_pos\n" if $verbose;
- my @end_chart_positions = keys %{$chart_ht{NODES_ENDING_AT}};
- my $end_chart_pos = (@end_chart_positions) ? max(@end_chart_positions) : 0;
- $sd_ht{MAXPOS}->{$chart_id} = $end_chart_pos;
- print STDERR " Chart span: $current_chart_pos-$end_chart_pos\n" if $verbose;
- while ($current_chart_pos < $end_chart_pos) {
- my @node_ids = keys %{$chart_ht{NODES_STARTING_AT}->{$current_chart_pos}};
- foreach $node_id (@node_ids) {
- my $roman_s = $chart_ht{NODE_ROMAN}->{$node_id};
- my @roman_chars = $utf8->split_into_utf8_characters($roman_s, "return only chars, return trailing whitespaces", *dummy_ht);
- print STDERR " $current_chart_pos/$current_linear_chart_pos node: $node_id $roman_s (@roman_chars)\n" if $verbose;
- if ($#roman_chars >= 1) {
- foreach $i ((1 .. $#roman_chars)) {
- $current_linear_chart_pos++;
- $sd_ht{SPLITPOS2LINPOS}->{$chart_id}->{$current_chart_pos}->{$node_id}->{$i} = $current_linear_chart_pos;
- $sd_ht{LINPOS2SPLITPOS}->{$chart_id}->{$current_linear_chart_pos}->{$current_chart_pos}->{$node_id}->{$i} = 1;
- print STDERR " LINPOS2SPLITPOS.$chart_id LIN: $current_linear_chart_pos POS: $current_chart_pos NODE: $node_id I: $i\n" if $verbose;
- }
- }
- }
- $current_chart_pos++;
- if ($util->member($current_chart_pos, @end_chart_positions)) {
- $current_linear_chart_pos++;
- $sd_ht{POS2LINPOS}->{$chart_id}->{$current_chart_pos} = $current_linear_chart_pos;
- $sd_ht{LINPOS2POS}->{$chart_id}->{$current_linear_chart_pos} = $current_chart_pos;
- print STDERR " LINPOS2POS.$chart_id LIN: $current_linear_chart_pos POS: $current_chart_pos\n" if $verbose;
- }
- }
- $current_chart_pos = 0;
- while ($current_chart_pos <= $end_chart_pos) {
- my $current_linear_chart_pos = $sd_ht{POS2LINPOS}->{$chart_id}->{$current_chart_pos};
- $current_linear_chart_pos = "?" unless defined($current_linear_chart_pos);
- my @node_ids = keys %{$chart_ht{NODES_STARTING_AT}->{$current_chart_pos}};
- # print STDERR " LINROM.$chart_id LIN: $current_linear_chart_pos POS: $current_chart_pos NODES: @node_ids\n" if $verbose;
- foreach $node_id (@node_ids) {
- my $end_pos = $chart_ht{NODE_END}->{$node_id};
- my $end_linpos = $sd_ht{POS2LINPOS}->{$chart_id}->{$end_pos};
- my $roman_s = $chart_ht{NODE_ROMAN}->{$node_id};
- my @roman_chars = $utf8->split_into_utf8_characters($roman_s, "return only chars, return trailing whitespaces", *dummy_ht);
- print STDERR " LINROM.$chart_id LIN: $current_linear_chart_pos POS: $current_chart_pos NODE: $node_id CHARS: @roman_chars\n" if $verbose;
- if (@roman_chars) {
- foreach $i ((0 .. $#roman_chars)) {
- my $from_linear_chart_pos
- = (($i == 0)
- ? $sd_ht{POS2LINPOS}->{$chart_id}->{$current_chart_pos}
- : $sd_ht{SPLITPOS2LINPOS}->{$chart_id}->{$current_chart_pos}->{$node_id}->{$i});
- print STDERR " FROM.$chart_id I: $i POS: $current_chart_pos NODE: $node_id FROM: $from_linear_chart_pos\n" if $verbose;
- my $to_linear_chart_pos
- = (($i == $#roman_chars)
- ? $end_linpos
- : $sd_ht{SPLITPOS2LINPOS}->{$chart_id}->{$current_chart_pos}->{$node_id}->{($i+1)});
- print STDERR " TO.$chart_id I: $i POS: $current_chart_pos NODE: $node_id FROM: $to_linear_chart_pos\n" if $verbose;
- my $roman_char = $roman_chars[$i];
- $sd_ht{LIN_IJ_ROMAN}->{$chart_id}->{$from_linear_chart_pos}->{$to_linear_chart_pos}->{$roman_char} = 1;
- }
- } else {
- my $from_linear_chart_pos = $sd_ht{POS2LINPOS}->{$chart_id}->{$current_chart_pos};
- my $to_linear_chart_pos = $sd_ht{POS2LINPOS}->{$chart_id}->{($current_chart_pos+1)};
- # HHERE check this out
- my $i = 1;
- while (! (defined($to_linear_chart_pos))) {
- $i++;
- $to_linear_chart_pos = $sd_ht{POS2LINPOS}->{$chart_id}->{($current_chart_pos+$i)};
- }
- if (defined($from_linear_chart_pos) && defined($to_linear_chart_pos)) {
- $sd_ht{LIN_IJ_ROMAN}->{$chart_id}->{$from_linear_chart_pos}->{$to_linear_chart_pos}->{""} = 1
- } else {
- print STDERR " UNDEF.$chart_id from: "
- . ((defined($from_linear_chart_pos)) ? $from_linear_chart_pos : "?")
- . " to: "
- . ((defined($to_linear_chart_pos)) ? $to_linear_chart_pos : "?")
- . "\n";
- }
- }
- }
- $current_chart_pos++;
- }
- $sd_ht{MAXLINPOS}->{$chart_id} = $sd_ht{POS2LINPOS}->{$chart_id}->{$end_chart_pos};
-}
-
-sub expand_lin_ij_roman {
- local($this, *sd_ht, $chart_id, $lang_code, *ht) = @_;
-
- foreach $start (sort { $a <=> $b } keys %{$sd_ht{LIN_IJ_ROMAN}->{$chart_id}}) {
- foreach $end (sort { $a <=> $b } keys %{$sd_ht{LIN_IJ_ROMAN}->{$chart_id}->{$start}}) {
- foreach $roman (sort keys %{$sd_ht{LIN_IJ_ROMAN}->{$chart_id}->{$start}->{$end}}) {
- if ($ht{RULE_STRING_HAS_EXPANSION}->{$lang_code}->{$roman}
- || $ht{RULE_STRING_HAS_EXPANSION}->{""}->{$roman}) {
- $this->expand_lin_ij_roman_rec(*sd_ht, $chart_id, $start, $end, $roman, $lang_code, *ht);
- }
- }
- }
- }
-}
-
-sub expand_lin_ij_roman_rec {
- local($this, *sd_ht, $chart_id, $start, $end, $roman, $lang_code, *ht) = @_;
-
- # print STDERR " expand_lin_ij_roman_rec.$chart_id $start-$end $lang_code $roman\n";
- return unless $ht{RULE_STRING_HAS_EXPANSION}->{$lang_code}->{$roman}
- || $ht{RULE_STRING_HAS_EXPANSION}->{""}->{$roman};
- foreach $new_end (keys %{$sd_ht{LIN_IJ_ROMAN}->{$chart_id}->{$end}}) {
- foreach $next_roman (sort keys %{$sd_ht{LIN_IJ_ROMAN}->{$chart_id}->{$end}->{$new_end}}) {
- my $exp_roman = join("", $roman, $next_roman);
- if ($ht{RULE_STRING}->{$lang_code}->{$exp_roman}
- || $ht{RULE_STRING}->{""}->{$exp_roman}) {
- $sd_ht{LIN_IJ_ROMAN}->{$chart_id}->{$start}->{$new_end}->{$exp_roman} = 1;
- # print STDERR " Expansion ($start-$new_end) $exp_roman\n";
- }
- if ($ht{RULE_STRING_HAS_EXPANSION}->{$lang_code}->{$exp_roman}
- || $ht{RULE_STRING_HAS_EXPANSION}->{""}->{$exp_roman}) {
- $this->expand_lin_ij_roman_rec(*sd_ht, $chart_id, $start, $new_end, $exp_roman, $lang_code, *ht);
- }
- }
- }
-}
-
-sub trace_string_distance {
- local($this, *sd_ht, $chart1_id, $chart2_id, $control, $line_number, $cost) = @_;
-
- my $chart_comb_id = join("/", $chart1_id, $chart2_id);
- return "mismatch" if $sd_ht{MISMATCH}->{$chart_comb_id};
- my $chart1_end = $sd_ht{MAXLINPOS}->{$chart1_id};
- my $chart2_end = $sd_ht{MAXLINPOS}->{$chart2_id};
- my $verbose = ($control =~ /verbose/);
- my $chunks_p = ($control =~ /chunks/);
- my @traces = ();
- my @s1_s = ();
- my @s2_s = ();
- my @e1_s = ();
- my @e2_s = ();
- my @r1_s = ();
- my @r2_s = ();
- my @ic_s = ();
-
- # print STDERR "trace_string_distance $chart1_id $chart2_id $line_number\n";
- while ($chart1_end || $chart2_end) {
- my $incr_cost = $sd_ht{INCR_COST_IJ}->{$chart_comb_id}->{$chart1_end}->{$chart2_end};
- my $prec_i = $sd_ht{PREC_I}->{$chart_comb_id}->{$chart1_end}->{$chart2_end};
- my $prec_j = $sd_ht{PREC_J}->{$chart_comb_id}->{$chart1_end}->{$chart2_end};
- if ($incr_cost || $verbose || $chunks_p) {
- my $roman1 = $sd_ht{ROMAN1}->{$chart_comb_id}->{$chart1_end}->{$chart2_end};
- my $roman2 = $sd_ht{ROMAN2}->{$chart_comb_id}->{$chart1_end}->{$chart2_end};
- if ($verbose) {
- push(@traces, "$prec_i-$chart1_end/$prec_j-$chart2_end:$roman1/$roman2:$incr_cost");
- } else {
- if (defined($roman1)) {
- push(@traces, "$roman1/$roman2:$incr_cost");
- } else {
- $print_prec_i = (defined($prec_i)) ? $prec_i : "?";
- $print_prec_j = (defined($prec_j)) ? $prec_j : "?";
- print STDERR " $prec_i-$chart1_end, $prec_j-$chart2_end\n";
- }
- }
- if ($chunks_p) {
- push(@s1_s, $prec_i);
- push(@s2_s, $prec_j);
- push(@e1_s, $chart1_end);
- push(@e2_s, $chart2_end);
- push(@r1_s, $roman1);
- push(@r2_s, $roman2);
- push(@ic_s, $incr_cost);
- }
- }
- $chart1_end = $prec_i;
- $chart2_end = $prec_j;
- }
- if ($chunks_p) {
- my $r1 = "";
- my $r2 = "";
- my $tc = 0;
- my $in_chunk = 0;
- foreach $i ((0 .. $#ic_s)) {
- if ($ic_s[$i]) {
- $r1 = $r1_s[$i] . $r1;
- $r2 = $r2_s[$i] . $r2;
- $tc += $ic_s[$i];
- $in_chunk = 1;
- } elsif ($in_chunk) {
- $chunk = "$r1/$r2/$tc";
- $chunk .= "*" if $cost > 5;
- $sd_ht{N_COST_CHUNK}->{$chunk} = ($sd_ht{N_COST_CHUNK}->{$chunk} || 0) + 1;
- $sd_ht{EX_COST_CHUNK}->{$chunk}->{$line_number} = 1;
- $r1 = "";
- $r2 = "";
- $tc = 0;
- $in_chunk = 0;
- }
- }
- if ($in_chunk) {
- $chunk = "$r1/$r2/$tc";
- $chunk .= "*" if $cost > 5;
- $sd_ht{N_COST_CHUNK}->{$chunk} = ($sd_ht{N_COST_CHUNK}->{$chunk} || 0) + 1;
- $sd_ht{EX_COST_CHUNK}->{$chunk}->{$line_number} = 1;
- }
- } else {
- return join(" ", reverse @traces);
- }
-}
-
-sub right_context_match {
- local($this, $right_context_rule, *sd_ht, $chart_id, $start_pos) = @_;
-
- return 1 if $right_context_rule eq "";
- if (($right_context_item, $right_context_rest) = ($right_context_rule =~ /^\[([^\[\]]*)\]*(.*)$/)) {
- my $guarded_right_context_item = $right_context_item;
- $guarded_right_context_item =~ s/\$/\\\$/g;
- my @end_positions = keys %{$sd_ht{LIN_IJ_ROMAN}->{$chart_id}->{$start_pos}};
- return 1 if ($#end_positions == -1)
- && (($right_context_item eq "")
- || ($right_context_item =~ /\$/));
- foreach $end_pos (@end_positions) {
- my @romans = keys %{$sd_ht{LIN_IJ_ROMAN}->{$chart_id}->{$start_pos}->{$end_pos}};
- foreach $roman (@romans) {
- if ($roman =~ /^[$guarded_right_context_item]/) {
- return $this->right_context_match($right_context_rest, *sd_ht, $chart_id, $end_pos);
- }
- }
- }
- }
- return 0;
-}
-
-sub string_distance {
- local($this, *sd_ht, $chart1_id, $chart2_id, $lang_code1, $lang_code2, *ht, $control) = @_;
-
- my $verbose = ($control =~ /verbose/i);
- my $chart_comb_id = join("/", $chart1_id, $chart2_id);
-
- my $chart1_end_pos = $sd_ht{MAXLINPOS}->{$chart1_id};
- my $chart2_end_pos = $sd_ht{MAXLINPOS}->{$chart2_id};
- print STDERR "string_distance.$chart_comb_id $chart1_end_pos/$chart2_end_pos\n" if $verbose;
- $sd_ht{COST_IJ}->{$chart_comb_id}->{0}->{0} = 0;
- $sd_ht{COMB_LEFT_ROMAN1}->{$chart_comb_id}->{0}->{0} = "";
- $sd_ht{COMB_LEFT_ROMAN2}->{$chart_comb_id}->{0}->{0} = "";
- # HHERE
- foreach $chart1_start ((0 .. $chart1_end_pos)) {
- # print STDERR " C1 $chart1_start- ($chart1_start .. $chart1_end_pos)\n";
- my $prev_further_expansion_possible = 0;
- my @chart1_ends = sort { $a <=> $b } keys %{$sd_ht{LIN_IJ_ROMAN}->{$chart1_id}->{$chart1_start}};
- my $max_chart1_ends = (@chart1_ends) ? $chart1_ends[$#chart1_ends] : -1;
- foreach $chart1_end (($chart1_start .. $chart1_end_pos)) {
- my $further_expansion_possible = ($chart1_start == $chart1_end)
- || defined($sd_ht{LINPOS2SPLITPOS}->{$chart1_id}->{$chart1_start})
- || ($chart1_end < $max_chart1_ends);
- my @romans1 = (($chart1_start == $chart1_end)
- ? ("")
- : (sort keys %{$sd_ht{LIN_IJ_ROMAN}->{$chart1_id}->{$chart1_start}->{$chart1_end}}));
- if ($#romans1 == -1) {
- $further_expansion_possible = 1 if $prev_further_expansion_possible;
- } else {
- $prev_further_expansion_possible = 0;
- }
- # print STDERR " C1 $chart1_start-$chart1_end romans1: @romans1 {$further_expansion_possible} *l*\n";
- foreach $roman1 (@romans1) {
- # print STDERR " C1 $chart1_start-$chart1_end $roman1 {$further_expansion_possible} *?*\n";
- next unless $ht{RULE_STRING}->{$lang_code1}->{$roman1}
- || $ht{RULE_STRING}->{""}->{$roman1};
- # print STDERR " C1 $chart1_start-$chart1_end $roman1 {$further_expansion_possible} ***\n";
- foreach $lang_code1o (($lang_code1, "")) {
- foreach $lang_code2o (($lang_code2, "")) {
- my @chart2_starts = (sort { $a <=> $b } keys %{$sd_ht{COST_IJ}->{$chart_comb_id}->{$chart1_start}});
- foreach $chart2_start (@chart2_starts) {
- # print STDERR " C1 $chart1_start-$chart1_end $roman1 C2 $chart2_start- (@chart2_starts)\n";
- foreach $chart2_end (($chart2_start .. $chart2_end_pos)) {
- print STDERR " C1 $chart1_start-$chart1_end $roman1 C2 $chart2_start-$chart2_end\n";
- my @romans2 = (($chart2_start == $chart2_end)
- ? ("")
- : (sort keys %{$sd_ht{LIN_IJ_ROMAN}->{$chart2_id}->{$chart2_start}->{$chart2_end}}));
- foreach $roman2 (@romans2) {
- if ($roman1 eq $roman2) {
- print STDERR " C1 $chart1_start-$chart1_end $roman1 C2 $chart2_start-$chart2_end $roman2 (IDENTITY)\n";
- my $cost = 0;
- my $preceding_cost = $sd_ht{COST_IJ}->{$chart_comb_id}->{$chart1_start}->{$chart2_start};
- my $combined_cost = $preceding_cost + $cost;
- my $old_cost = $sd_ht{COST_IJ}->{$chart_comb_id}->{$chart1_end}->{$chart2_end};
- if ((! defined($old_cost)) || ($combined_cost < $old_cost)) {
- $sd_ht{COST_IJ}->{$chart_comb_id}->{$chart1_end}->{$chart2_end} = $combined_cost;
- push(@chart2_starts, $chart2_end) unless $util->member($chart2_end, @chart2_starts);
- $sd_ht{PREC_I}->{$chart_comb_id}->{$chart1_end}->{$chart2_end} = $chart1_start;
- $sd_ht{PREC_J}->{$chart_comb_id}->{$chart1_end}->{$chart2_end} = $chart2_start;
- $sd_ht{ROMAN1}->{$chart_comb_id}->{$chart1_end}->{$chart2_end} = $roman1;
- $sd_ht{ROMAN2}->{$chart_comb_id}->{$chart1_end}->{$chart2_end} = $roman2;
- $sd_ht{COMB_LEFT_ROMAN1}->{$chart_comb_id}->{$chart1_end}->{$chart2_end}
- = $sd_ht{COMB_LEFT_ROMAN1}->{$chart_comb_id}->{$chart1_start}->{$chart2_start} . $roman1;
- $sd_ht{COMB_LEFT_ROMAN2}->{$chart_comb_id}->{$chart1_end}->{$chart2_end}
- = $sd_ht{COMB_LEFT_ROMAN2}->{$chart_comb_id}->{$chart1_start}->{$chart2_start} . $roman2;
- $comb_left_roman1 = $sd_ht{COMB_LEFT_ROMAN1}->{$chart_comb_id}->{$chart1_end}->{$chart2_end};
- $sd_ht{INCR_COST_IJ}->{$chart_comb_id}->{$chart1_end}->{$chart2_end} = $cost;
- $sd_ht{COST_RULE}->{$chart_comb_id}->{$chart1_end}->{$chart2_end} = "IDENTITY";
- print STDERR " New cost $chart1_end/$chart2_end: $combined_cost (+$cost from $chart1_start/$chart2_start $roman1/$roman2)\n" if $verbose;
- }
- } else {
- next unless $ht{RULE_STRING}->{$lang_code2o}->{$roman2};
- print STDERR " C1 $chart1_start-$chart1_end $roman1 C2 $chart2_start-$chart2_end $roman2\n";
- next unless defined($ht{COST}->{$lang_code1o}->{$lang_code2o}->{$roman1}->{$roman2});
- my @cost_rule_ids = keys %{$ht{COST}->{$lang_code1o}->{$lang_code2o}->{$roman1}->{$roman2}};
- foreach $cost_rule_id (@cost_rule_ids) {
- ## check whether any context requirements are satisfied
- # left context rules are regular expressions
- my $left_context_rule1 = $ht{LEFT1}->{$cost_rule_id};
- if ($left_context_rule1) {
- my $comb_left_roman1 = $sd_ht{COMB_LEFT_ROMAN1}->{$chart_comb_id}->{$chart1_start}->{$chart2_start};
- if (defined($comb_left_roman1)) {
- next unless $comb_left_roman1 =~ /$left_context_rule1/;
- } else {
- print STDERR " No comb_left_roman1 value for $chart_comb_id $chart1_start,$chart2_start\n";
- }
- }
- my $left_context_rule2 = $ht{LEFT2}->{$cost_rule_id};
- if ($left_context_rule2) {
- my $comb_left_roman2 = $sd_ht{COMB_LEFT_ROMAN2}->{$chart_comb_id}->{$chart1_start}->{$chart2_start};
- if (defined($comb_left_roman2)) {
- next unless $comb_left_roman2 =~ /$left_context_rule2/;
- } else {
- print STDERR " No comb_left_roman2 value for $chart_comb_id $chart1_start,$chart2_start\n";
- }
- }
- my $right_context_rule1 = $ht{RIGHT1}->{$cost_rule_id};
- if ($right_context_rule1) {
- my $match_p = $this->right_context_match($right_context_rule1, *sd_ht, $chart1_id, $chart1_end);
- # print STDERR " Match?($right_context_rule1, 1, $chart1_end) = $match_p\n";
- next unless $match_p;
- }
- my $right_context_rule2 = $ht{RIGHT2}->{$cost_rule_id};
- if ($right_context_rule2) {
- my $match_p = $this->right_context_match($right_context_rule2, *sd_ht, $chart2_id, $chart2_end);
- # print STDERR " Match?($right_context_rule2, 2, $chart2_end) = $match_p\n";
- next unless $match_p;
- }
- my $cost = $ht{COST}->{$lang_code1o}->{$lang_code2o}->{$roman1}->{$roman2}->{$cost_rule_id};
- my $preceding_cost = $sd_ht{COST_IJ}->{$chart_comb_id}->{$chart1_start}->{$chart2_start};
- my $combined_cost = $preceding_cost + $cost;
- my $old_cost = $sd_ht{COST_IJ}->{$chart_comb_id}->{$chart1_end}->{$chart2_end};
- if ((! defined($old_cost)) || ($combined_cost < $old_cost)) {
- $sd_ht{COST_IJ}->{$chart_comb_id}->{$chart1_end}->{$chart2_end} = $combined_cost;
- push(@chart2_starts, $chart2_end) unless $util->member($chart2_end, @chart2_starts);
- $sd_ht{PREC_I}->{$chart_comb_id}->{$chart1_end}->{$chart2_end} = $chart1_start;
- $sd_ht{PREC_J}->{$chart_comb_id}->{$chart1_end}->{$chart2_end} = $chart2_start;
- $sd_ht{ROMAN1}->{$chart_comb_id}->{$chart1_end}->{$chart2_end} = $roman1;
- $sd_ht{ROMAN2}->{$chart_comb_id}->{$chart1_end}->{$chart2_end} = $roman2;
- $sd_ht{COMB_LEFT_ROMAN1}->{$chart_comb_id}->{$chart1_end}->{$chart2_end}
- = $sd_ht{COMB_LEFT_ROMAN1}->{$chart_comb_id}->{$chart1_start}->{$chart2_start} . $roman1;
- $sd_ht{COMB_LEFT_ROMAN2}->{$chart_comb_id}->{$chart1_end}->{$chart2_end}
- = $sd_ht{COMB_LEFT_ROMAN2}->{$chart_comb_id}->{$chart1_start}->{$chart2_start} . $roman2;
- $comb_left_roman1 = $sd_ht{COMB_LEFT_ROMAN1}->{$chart_comb_id}->{$chart1_end}->{$chart2_end};
- # print STDERR " Comb-left-roman1($chart_comb_id,$chart1_end,$chart2_end) = $comb_left_roman1\n";
- $sd_ht{INCR_COST_IJ}->{$chart_comb_id}->{$chart1_end}->{$chart2_end} = $cost;
- $sd_ht{COST_RULE}->{$chart_comb_id}->{$chart1_end}->{$chart2_end} = $cost_rule_id;
- print STDERR " New cost $chart1_end/$chart2_end: $combined_cost (+$cost from $chart1_start/$chart2_start $roman1/$roman2)\n" if $verbose;
- }
- }
- }
- }
- }
- }
- }
- }
- $further_expansion_possible = 1
- if $ht{RULE_STRING_HAS_EXPANSION}->{$lang_code1}->{$roman1}
- || $ht{RULE_STRING_HAS_EXPANSION}->{""}->{$roman1};
- # print STDERR " further_expansion_possible: $further_expansion_possible (lc: $lang_code1 r1: $roman1) ***\n";
- }
- # print STDERR " last C1 $chart1_start-$chart1_end (@romans1)\n" unless $further_expansion_possible;
- last unless $further_expansion_possible;
- $prev_further_expansion_possible = 1 if $further_expansion_possible;
- }
- }
- my $total_cost = $sd_ht{COST_IJ}->{$chart_comb_id}->{$chart1_end_pos}->{$chart2_end_pos};
- unless (defined($total_cost)) {
- $total_cost = 99.9999;
- $sd_ht{MISMATCH}->{$chart_comb_id} = 1;
- }
- return $total_cost;
-}
-
-sub print_sd_ht {
- local($this, *sd_ht, $chart1_id, $chart2_id, *OUT) = @_;
-
- print OUT "string-distance chart:\n";
- foreach $chart_id (($chart1_id, $chart2_id)) {
- print OUT "SD chart $chart_id:\n";
- foreach $from_linear_chart_pos (sort { $a <=> $b } keys %{$sd_ht{LIN_IJ_ROMAN}->{$chart_id}}) {
- foreach $to_linear_chart_pos (sort { $a <=> $b } keys %{$sd_ht{LIN_IJ_ROMAN}->{$chart_id}->{$from_linear_chart_pos}}) {
- foreach $roman_char (sort keys %{$sd_ht{LIN_IJ_ROMAN}->{$chart_id}->{$from_linear_chart_pos}->{$to_linear_chart_pos}}) {
- print OUT " Lnode($from_linear_chart_pos-$to_linear_chart_pos): $roman_char\n";
- }
- }
- }
- }
-}
-
-sub print_chart_ht {
- local($this, *chart_ht, *OUT) = @_;
-
- print OUT "uroman chart:\n";
- foreach $start (sort { $a <=> $b } keys %{$chart_ht{NODES_STARTING_AT}}) {
- foreach $end (sort { $a <=> $b } keys %{$chart_ht{NODES_STARTING_AND_ENDING_AT}->{$start}}) {
- foreach $node_id (keys %{$chart_ht{NODES_STARTING_AND_ENDING_AT}->{$start}->{$end}}) {
- $roman_s = $chart_ht{NODE_ROMAN}->{$node_id};
- print OUT " Node $node_id ($start-$end): $roman_s\n";
- }
- }
- }
-}
-
-sub normalize_string {
- local($this, $s) = @_;
-
-# $s =~ s/(\xE2\x80\x8C)//g; # delete zero width non-joiner
- $s =~ s/(\xE2\x80[\x93-\x94])/-/g; # en-dash, em-dash
- $s =~ s/([\x00-\x7F\xC0-\xFE][\x80-\xBF]*)\1+/$1$1/g; # shorten 3 or more occurrences of same character in a row to 2
- $s =~ s/[ \t]+/ /g;
-
- return $s;
-}
-
-my $string_distance_chart_id = 0;
-sub string_distance_by_chart {
- local($this, $s1, $s2, $lang_code1, $lang_code2, *ht, *pinyin_ht, $control) = @_;
-
- $control = "" unless defined($control);
- %sd_ht = ();
-
- $s1 = $this->normalize_string($s1);
- my $lc_s1 = $utf8->extended_lower_case($s1);
- $string_distance_chart_id++;
- my $chart1_id = $string_distance_chart_id;
- *chart_ht = $romanizer->romanize($lc_s1, $lang_code1, "", *ht, *pinyin_ht, 0, "return chart", $chart1_id);
- $this->linearize_chart_points(*chart_ht, $chart1_id, *sd_ht);
- $this->expand_lin_ij_roman(*sd_ht, $chart1_id, $lang_code1, *ht);
-
- $s2 = $this->normalize_string($s2);
- my $lc_s2 = $utf8->extended_lower_case($s2);
- $string_distance_chart_id++;
- my $chart2_id = $string_distance_chart_id;
- *chart_ht = $romanizer->romanize($lc_s2, $lang_code2, "", *ht, *pinyin_ht, 0, "return chart", $chart2_id);
- $this->linearize_chart_points(*chart_ht, $chart2_id, *sd_ht);
- $this->expand_lin_ij_roman(*sd_ht, $chart2_id, $lang_code2, *ht);
-
- my $cost = $this->string_distance(*sd_ht, $chart1_id, $chart2_id, $lang_code1, $lang_code2, *ht, $control);
- return $cost;
-}
-
-my $n_quick_romanized_string_distance = 0;
-sub quick_romanized_string_distance_by_chart {
- local($this, $s1, $s2, *ht, $control, $lang_code1, $lang_code2) = @_;
-
- # my $verbose = ($s1 eq "apit") && ($s2 eq "apet");
- # print STDERR "Start quick_romanized_string_distance_by_chart\n";
- $s1 = lc $s1;
- $s2 = lc $s2;
- $control = "" unless defined($control);
- $lang_code1 = "" unless defined($lang_code1);
- $lang_code2 = "" unless defined($lang_code2);
- my $cache_p = ($control =~ /cache/);
- my $total_cost;
- if ($cache_p) {
- $total_cost = $ht{CACHED_QRSD}->{$s1}->{$s2};
- if (defined($total_cost)) {
- return $total_cost;
- }
- }
- my @lang_codes1 = ($lang_code1 eq "") ? ("") : ($lang_code1, "");
- my @lang_codes2 = ($lang_code2 eq "") ? ("") : ($lang_code2, "");
- my $chart1_end_pos = length($s1);
- my $chart2_end_pos = length($s2);
- my %sd_ht = ();
- $sd_ht{COST_IJ}->{0}->{0} = 0;
- foreach $chart1_start ((0 .. $chart1_end_pos)) {
- foreach $chart1_end (($chart1_start .. $chart1_end_pos)) {
- my $substr1 = substr($s1, $chart1_start, ($chart1_end-$chart1_start));
- foreach $lang_code1o (@lang_codes1) {
- foreach $lang_code2o (@lang_codes2) {
- # next unless defined($ht{COST}->{$lang_code1o}->{$lang_code2o}->{$substr1});
- }
- }
- my @chart2_starts = (sort { $a <=> $b } keys %{$sd_ht{COST_IJ}->{$chart1_start}});
- foreach $chart2_start (@chart2_starts) {
- foreach $chart2_end (($chart2_start .. $chart2_end_pos)) {
- my $substr2 = substr($s2, $chart2_start, ($chart2_end-$chart2_start));
- foreach $lang_code1o (@lang_codes1) {
- foreach $lang_code2o (@lang_codes2) {
- if ($substr1 eq $substr2) {
- my $cost = 0;
- my $preceding_cost = $sd_ht{COST_IJ}->{$chart1_start}->{$chart2_start};
- if (defined($preceding_cost)) {
- my $combined_cost = $preceding_cost + $cost;
- my $old_cost = $sd_ht{COST_IJ}->{$chart1_end}->{$chart2_end};
- if ((! defined($old_cost)) || ($combined_cost < $old_cost)) {
- $sd_ht{COST_IJ}->{$chart1_end}->{$chart2_end} = $combined_cost;
- push(@chart2_starts, $chart2_end) unless $util->member($chart2_end, @chart2_starts);
- }
- }
- } else {
- next unless defined($ht{COST}->{$lang_code1o}->{$lang_code2o}->{$substr1}->{$substr2});
- my @cost_rule_ids = keys %{$ht{COST}->{$lang_code1o}->{$lang_code2o}->{$substr1}->{$substr2}};
- my $best_cost = 99.99;
- foreach $cost_rule_id (@cost_rule_ids) {
- my $cost = $ht{COST}->{$lang_code1o}->{$lang_code2o}->{$substr1}->{$substr2}->{$cost_rule_id};
- my $left_context_rule1 = $ht{LEFT1}->{$cost_rule_id};
- next if $left_context_rule1
- && (! (substr($s1, 0, $chart1_start) =~ /$left_context_rule1/));
- my $left_context_rule2 = $ht{LEFT2}->{$cost_rule_id};
- next if $left_context_rule2
- && (! (substr($s2, 0, $chart2_start) =~ /$left_context_rule2/));
- my $right_context_rule1 = $ht{RIGHT1}->{$cost_rule_id};
- my $right_context1 = substr($s1, $chart1_end);
- next if $right_context_rule1
- && (! (($right_context1 =~ /^$right_context_rule1/)
- || (($right_context_rule1 =~ /^\[[^\[\]]*\$/)
- && ($right_context1 eq ""))));
- my $right_context_rule2 = $ht{RIGHT2}->{$cost_rule_id};
- my $right_context2 = substr($s2, $chart2_end);
- next if $right_context_rule2
- && (! (($right_context2 =~ /^$right_context_rule2/)
- || (($right_context_rule2 =~ /^\[[^\[\]]*\$/)
- && ($right_context2 eq ""))));
- $best_cost = $cost if $cost < $best_cost;
- my $preceding_cost = $sd_ht{COST_IJ}->{$chart1_start}->{$chart2_start};
- my $combined_cost = $preceding_cost + $cost;
- my $old_cost = $sd_ht{COST_IJ}->{$chart1_end}->{$chart2_end};
- if ((! defined($old_cost)) || ($combined_cost < $old_cost)) {
- $sd_ht{COST_IJ}->{$chart1_end}->{$chart2_end} = $combined_cost;
- push(@chart2_starts, $chart2_end) unless $util->member($chart2_end, @chart2_starts);
- }
- }
- }
- }
- }
- }
- }
- }
- }
- $total_cost = $sd_ht{COST_IJ}->{$chart1_end_pos}->{$chart2_end_pos};
- $total_cost = 99.99 unless defined($total_cost);
- $ht{CACHED_QRSD}->{$s1}->{$s2} = $total_cost if $cache_p;
- $n_quick_romanized_string_distance++;
- return $total_cost;
-}
-
-sub get_n_quick_romanized_string_distance {
- return $n_quick_romanized_string_distance;
-}
-
-1;
-
diff --git a/spaces/Mellow-ai/PhotoAI_Mellow/gradio_annotator.py b/spaces/Mellow-ai/PhotoAI_Mellow/gradio_annotator.py
deleted file mode 100644
index 2b1a29ebbec24073a9e4357b700e0577a17a9379..0000000000000000000000000000000000000000
--- a/spaces/Mellow-ai/PhotoAI_Mellow/gradio_annotator.py
+++ /dev/null
@@ -1,160 +0,0 @@
-import gradio as gr
-
-from annotator.util import resize_image, HWC3
-
-
-model_canny = None
-
-
-def canny(img, res, l, h):
- img = resize_image(HWC3(img), res)
- global model_canny
- if model_canny is None:
- from annotator.canny import CannyDetector
- model_canny = CannyDetector()
- result = model_canny(img, l, h)
- return [result]
-
-
-model_hed = None
-
-
-def hed(img, res):
- img = resize_image(HWC3(img), res)
- global model_hed
- if model_hed is None:
- from annotator.hed import HEDdetector
- model_hed = HEDdetector()
- result = model_hed(img)
- return [result]
-
-
-model_mlsd = None
-
-
-def mlsd(img, res, thr_v, thr_d):
- img = resize_image(HWC3(img), res)
- global model_mlsd
- if model_mlsd is None:
- from annotator.mlsd import MLSDdetector
- model_mlsd = MLSDdetector()
- result = model_mlsd(img, thr_v, thr_d)
- return [result]
-
-
-model_midas = None
-
-
-def midas(img, res, a):
- img = resize_image(HWC3(img), res)
- global model_midas
- if model_midas is None:
- from annotator.midas import MidasDetector
- model_midas = MidasDetector()
- results = model_midas(img, a)
- return results
-
-
-model_openpose = None
-
-
-def openpose(img, res, has_hand):
- img = resize_image(HWC3(img), res)
- global model_openpose
- if model_openpose is None:
- from annotator.openpose import OpenposeDetector
- model_openpose = OpenposeDetector()
- result, _ = model_openpose(img, has_hand)
- return [result]
-
-
-model_uniformer = None
-
-
-def uniformer(img, res):
- img = resize_image(HWC3(img), res)
- global model_uniformer
- if model_uniformer is None:
- from annotator.uniformer import UniformerDetector
- model_uniformer = UniformerDetector()
- result = model_uniformer(img)
- return [result]
-
-
-block = gr.Blocks().queue()
-with block:
- with gr.Row():
- gr.Markdown("## Canny Edge")
- with gr.Row():
- with gr.Column():
- input_image = gr.Image(source='upload', type="numpy")
- low_threshold = gr.Slider(label="low_threshold", minimum=1, maximum=255, value=100, step=1)
- high_threshold = gr.Slider(label="high_threshold", minimum=1, maximum=255, value=200, step=1)
- resolution = gr.Slider(label="resolution", minimum=256, maximum=1024, value=512, step=64)
- run_button = gr.Button(label="Run")
- with gr.Column():
- gallery = gr.Gallery(label="Generated images", show_label=False).style(height="auto")
- run_button.click(fn=canny, inputs=[input_image, resolution, low_threshold, high_threshold], outputs=[gallery])
-
- with gr.Row():
- gr.Markdown("## HED Edge")
- with gr.Row():
- with gr.Column():
- input_image = gr.Image(source='upload', type="numpy")
- resolution = gr.Slider(label="resolution", minimum=256, maximum=1024, value=512, step=64)
- run_button = gr.Button(label="Run")
- with gr.Column():
- gallery = gr.Gallery(label="Generated images", show_label=False).style(height="auto")
- run_button.click(fn=hed, inputs=[input_image, resolution], outputs=[gallery])
-
- with gr.Row():
- gr.Markdown("## MLSD Edge")
- with gr.Row():
- with gr.Column():
- input_image = gr.Image(source='upload', type="numpy")
- value_threshold = gr.Slider(label="value_threshold", minimum=0.01, maximum=2.0, value=0.1, step=0.01)
- distance_threshold = gr.Slider(label="distance_threshold", minimum=0.01, maximum=20.0, value=0.1, step=0.01)
- resolution = gr.Slider(label="resolution", minimum=256, maximum=1024, value=384, step=64)
- run_button = gr.Button(label="Run")
- with gr.Column():
- gallery = gr.Gallery(label="Generated images", show_label=False).style(height="auto")
- run_button.click(fn=mlsd, inputs=[input_image, resolution, value_threshold, distance_threshold], outputs=[gallery])
-
- with gr.Row():
- gr.Markdown("## MIDAS Depth and Normal")
- with gr.Row():
- with gr.Column():
- input_image = gr.Image(source='upload', type="numpy")
- alpha = gr.Slider(label="alpha", minimum=0.1, maximum=20.0, value=6.2, step=0.01)
- resolution = gr.Slider(label="resolution", minimum=256, maximum=1024, value=384, step=64)
- run_button = gr.Button(label="Run")
- with gr.Column():
- gallery = gr.Gallery(label="Generated images", show_label=False).style(height="auto")
- run_button.click(fn=midas, inputs=[input_image, resolution, alpha], outputs=[gallery])
-
- with gr.Row():
- gr.Markdown("## Openpose")
- with gr.Row():
- with gr.Column():
- input_image = gr.Image(source='upload', type="numpy")
- hand = gr.Checkbox(label='detect hand', value=False)
- resolution = gr.Slider(label="resolution", minimum=256, maximum=1024, value=512, step=64)
- run_button = gr.Button(label="Run")
- with gr.Column():
- gallery = gr.Gallery(label="Generated images", show_label=False).style(height="auto")
- run_button.click(fn=openpose, inputs=[input_image, resolution, hand], outputs=[gallery])
-
-
- with gr.Row():
- gr.Markdown("## Uniformer Segmentation")
- with gr.Row():
- with gr.Column():
- input_image = gr.Image(source='upload', type="numpy")
- resolution = gr.Slider(label="resolution", minimum=256, maximum=1024, value=512, step=64)
- run_button = gr.Button(label="Run")
- with gr.Column():
- gallery = gr.Gallery(label="Generated images", show_label=False).style(height="auto")
- run_button.click(fn=uniformer, inputs=[input_image, resolution], outputs=[gallery])
-
-
-block.launch(server_name='0.0.0.0')
diff --git a/spaces/MingGatsby/Grounding_DINO_demo/groundingdino/util/get_tokenlizer.py b/spaces/MingGatsby/Grounding_DINO_demo/groundingdino/util/get_tokenlizer.py
deleted file mode 100644
index f7dcf7e95f03f95b20546b26442a94225924618b..0000000000000000000000000000000000000000
--- a/spaces/MingGatsby/Grounding_DINO_demo/groundingdino/util/get_tokenlizer.py
+++ /dev/null
@@ -1,26 +0,0 @@
-from transformers import AutoTokenizer, BertModel, BertTokenizer, RobertaModel, RobertaTokenizerFast
-
-
-def get_tokenlizer(text_encoder_type):
- if not isinstance(text_encoder_type, str):
- # print("text_encoder_type is not a str")
- if hasattr(text_encoder_type, "text_encoder_type"):
- text_encoder_type = text_encoder_type.text_encoder_type
- elif text_encoder_type.get("text_encoder_type", False):
- text_encoder_type = text_encoder_type.get("text_encoder_type")
- else:
- raise ValueError(
- "Unknown type of text_encoder_type: {}".format(type(text_encoder_type))
- )
- print("final text_encoder_type: {}".format(text_encoder_type))
-
- tokenizer = AutoTokenizer.from_pretrained(text_encoder_type)
- return tokenizer
-
-
-def get_pretrained_language_model(text_encoder_type):
- if text_encoder_type == "bert-base-uncased":
- return BertModel.from_pretrained(text_encoder_type)
- if text_encoder_type == "roberta-base":
- return RobertaModel.from_pretrained(text_encoder_type)
- raise ValueError("Unknown text_encoder_type {}".format(text_encoder_type))
diff --git a/spaces/MohamedRafik/Password_Generator/app.py b/spaces/MohamedRafik/Password_Generator/app.py
deleted file mode 100644
index 1cda1fc149bfbbfc093a1c74c9b73e4413a029a9..0000000000000000000000000000000000000000
--- a/spaces/MohamedRafik/Password_Generator/app.py
+++ /dev/null
@@ -1,22 +0,0 @@
-import random
-import gradio as gr
-
-letters=['A', 'B', 'C', 'D', 'E', 'F', 'G', 'H', 'I', 'J', 'K', 'L', 'M', 'N', 'O', 'P', 'Q', 'R', 'S', 'T', 'U', 'V', 'W', 'X', 'Y', 'Z', 'a', 'b', 'c', 'd', 'e', 'f', 'g', 'h', 'i', 'j', 'k', 'l', 'm', 'n', 'o', 'p', 'q', 'r', 's', 't', 'u', 'v', 'w', 'x', 'y', 'z']
-Numbers=['0', '1', '2', '3', '4', '5', '6', '7', '8', '9']
-symbols=['&', '#', '(', ')', '-', '_', '^', '@', '+', '*', '§', '%', '!', '?']
-
-def password(N_letters, N_numbers, N_symbols):
- password_list=[]
- for char in range(N_letters):
- password_list+=random.choice(letters)
- for char in range(N_numbers):
- password_list+=random.choice(Numbers)
- for char in range(N_symbols):
- password_list+=random.choice(symbols)
- random.shuffle(password_list)
- psw=''
- for char in password_list:
- psw+=char
- return "Your Password is : " +psw
-demo=gr.Interface(fn=password, inputs=[gr.Slider(), gr.Slider(), gr.Slider()], outputs="text", Button= gr.Button("Generate"))
-demo.launch()
\ No newline at end of file
diff --git a/spaces/Mohamedoz/chatmoh/README.md b/spaces/Mohamedoz/chatmoh/README.md
deleted file mode 100644
index 7938de14e5355209aaae713f289ca469181bbb17..0000000000000000000000000000000000000000
--- a/spaces/Mohamedoz/chatmoh/README.md
+++ /dev/null
@@ -1,14 +0,0 @@
----
-title: Chat-with-GPT4
-emoji: 🚀
-colorFrom: red
-colorTo: indigo
-sdk: gradio
-sdk_version: 3.21.0
-app_file: app.py
-pinned: false
-license: mit
-duplicated_from: ysharma/ChatGPT4
----
-
-Check out the configuration reference at https://huggingface.co/docs/hub/spaces-config-reference
diff --git a/spaces/MoonQiu/LongerCrafter/scripts/run_text2video_freenoise_256.sh b/spaces/MoonQiu/LongerCrafter/scripts/run_text2video_freenoise_256.sh
deleted file mode 100644
index 130d3198aa4c0e292a1dd770c6336a7a4f477e9d..0000000000000000000000000000000000000000
--- a/spaces/MoonQiu/LongerCrafter/scripts/run_text2video_freenoise_256.sh
+++ /dev/null
@@ -1,25 +0,0 @@
-name="base_256_test"
-
-ckpt='checkpoints/base_256_v1/model.pth'
-config='configs/inference_t2v_tconv256_v1.0_freenoise.yaml'
-
-prompt_file="prompts/single_prompts.txt"
-res_dir="results_freenoise_single_256"
-
-python3 scripts/evaluation/inference_freenoise.py \
---seed 123 \
---mode 'base' \
---ckpt_path $ckpt \
---config $config \
---savedir $res_dir/$name \
---n_samples 3 \
---bs 1 --height 256 --width 256 \
---unconditional_guidance_scale 15.0 \
---ddim_steps 50 \
---ddim_eta 0.0 \
---prompt_file $prompt_file \
---fps 8 \
---frames 128 \
---window_size 16 \
---window_stride 4
-
diff --git a/spaces/Mountchicken/MAERec-Gradio/mmocr/models/textrecog/postprocessors/base.py b/spaces/Mountchicken/MAERec-Gradio/mmocr/models/textrecog/postprocessors/base.py
deleted file mode 100644
index 818640a8ca572f55e8c819a14c496dd47a6b4e93..0000000000000000000000000000000000000000
--- a/spaces/Mountchicken/MAERec-Gradio/mmocr/models/textrecog/postprocessors/base.py
+++ /dev/null
@@ -1,110 +0,0 @@
-# Copyright (c) OpenMMLab. All rights reserved.
-import warnings
-from typing import Dict, Optional, Sequence, Tuple, Union
-
-import mmengine
-import torch
-from mmengine.structures import LabelData
-
-from mmocr.models.common.dictionary import Dictionary
-from mmocr.registry import TASK_UTILS
-from mmocr.structures import TextRecogDataSample
-
-
-class BaseTextRecogPostprocessor:
- """Base text recognition postprocessor.
-
- Args:
- dictionary (dict or :obj:`Dictionary`): The config for `Dictionary` or
- the instance of `Dictionary`.
- max_seq_len (int): max_seq_len (int): Maximum sequence length. The
- sequence is usually generated from decoder. Defaults to 40.
- ignore_chars (list[str]): A list of characters to be ignored from the
- final results. Postprocessor will skip over these characters when
- converting raw indexes to characters. Apart from single characters,
- each item can be one of the following reversed keywords: 'padding',
- 'end' and 'unknown', which refer to their corresponding special
- tokens in the dictionary.
- """
-
- def __init__(self,
- dictionary: Union[Dictionary, Dict],
- max_seq_len: int = 40,
- ignore_chars: Sequence[str] = ['padding'],
- **kwargs) -> None:
-
- if isinstance(dictionary, dict):
- self.dictionary = TASK_UTILS.build(dictionary)
- elif isinstance(dictionary, Dictionary):
- self.dictionary = dictionary
- else:
- raise TypeError(
- 'The type of dictionary should be `Dictionary` or dict, '
- f'but got {type(dictionary)}')
- self.max_seq_len = max_seq_len
-
- mapping_table = {
- 'padding': self.dictionary.padding_idx,
- 'end': self.dictionary.end_idx,
- 'unknown': self.dictionary.unknown_idx,
- }
- if not mmengine.is_list_of(ignore_chars, str):
- raise TypeError('ignore_chars must be list of str')
- ignore_indexes = list()
- for ignore_char in ignore_chars:
- index = mapping_table.get(
- ignore_char,
- self.dictionary.char2idx(ignore_char, strict=False))
- if index is None or (index == self.dictionary.unknown_idx
- and ignore_char != 'unknown'):
- warnings.warn(
- f'{ignore_char} does not exist in the dictionary',
- UserWarning)
- continue
- ignore_indexes.append(index)
- self.ignore_indexes = ignore_indexes
-
- def get_single_prediction(
- self,
- probs: torch.Tensor,
- data_sample: Optional[TextRecogDataSample] = None,
- ) -> Tuple[Sequence[int], Sequence[float]]:
- """Convert the output probabilities of a single image to index and
- score.
-
- Args:
- probs (torch.Tensor): Character probabilities with shape
- :math:`(T, C)`.
- data_sample (TextRecogDataSample): Datasample of an image.
-
- Returns:
- tuple(list[int], list[float]): Index and scores per-character.
- """
- raise NotImplementedError
-
- def __call__(
- self, probs: torch.Tensor, data_samples: Sequence[TextRecogDataSample]
- ) -> Sequence[TextRecogDataSample]:
- """Convert outputs to strings and scores.
-
- Args:
- probs (torch.Tensor): Batched character probabilities, the model's
- softmaxed output in size: :math:`(N, T, C)`.
- data_samples (list[TextRecogDataSample]): The list of
- TextRecogDataSample.
-
- Returns:
- list(TextRecogDataSample): The list of TextRecogDataSample. It
- usually contain ``pred_text`` information.
- """
- batch_size = probs.size(0)
-
- for idx in range(batch_size):
- index, score = self.get_single_prediction(probs[idx, :, :],
- data_samples[idx])
- text = self.dictionary.idx2str(index)
- pred_text = LabelData()
- pred_text.score = score
- pred_text.item = text
- data_samples[idx].pred_text = pred_text
- return data_samples
diff --git a/spaces/NN520/AI/src/components/voice.tsx b/spaces/NN520/AI/src/components/voice.tsx
deleted file mode 100644
index 074d0e145229947282a472bd84f6578cf0b3c71c..0000000000000000000000000000000000000000
--- a/spaces/NN520/AI/src/components/voice.tsx
+++ /dev/null
@@ -1,52 +0,0 @@
-import React, { useEffect } from 'react'
-import { useSetAtom } from 'jotai'
-import { useBing } from '@/lib/hooks/use-bing'
-import Image from 'next/image'
-import VoiceIcon from '@/assets/images/voice.svg'
-import VoiceButton from './ui/voice'
-import { SR } from '@/lib/bots/bing/sr'
-import { voiceListenAtom } from '@/state'
-
-const sr = new SR(['发送', '清空', '退出'])
-
-const Voice = ({ setInput, input, sendMessage, isSpeaking }: Pick, 'setInput' | 'sendMessage' | 'input' | 'isSpeaking'>) => {
- const setListen = useSetAtom(voiceListenAtom)
- useEffect(() => {
- if (sr.listening) return
- sr.transcript = !isSpeaking
- }, [isSpeaking])
-
- useEffect(() => {
- sr.onchange = (msg: string, command?: string) => {
- switch (command) {
- case '退出':
- sr.stop()
- break;
- case '发送':
- sendMessage(input)
- case '清空':
- setInput('')
- break;
- default:
- setInput(input + msg)
- }
- }
- }, [input])
-
- const switchSR = (enable: boolean = false) => {
- setListen(enable)
- if (enable) {
- sr.start()
- } else {
- sr.stop()
- }
- }
-
- return sr.listening ? (
- switchSR(false)} />
- ) : (
- switchSR(true)} />
- )
-};
-
-export default Voice;
diff --git a/spaces/NeuralStyleTransfer/neural-style-transfer/app.py b/spaces/NeuralStyleTransfer/neural-style-transfer/app.py
deleted file mode 100644
index 0b175651d6210611f9223c3929c2f8649e51a0f1..0000000000000000000000000000000000000000
--- a/spaces/NeuralStyleTransfer/neural-style-transfer/app.py
+++ /dev/null
@@ -1,106 +0,0 @@
-import os
-from io import BytesIO
-import requests
-from datetime import datetime
-import random
-
-# Interface utilities
-import gradio as gr
-
-# Data utilities
-import numpy as np
-import pandas as pd
-
-# Image utilities
-from PIL import Image
-import cv2
-
-# Clip Model
-import torch
-from transformers import CLIPTokenizer, CLIPModel
-
-# Style Transfer Model
-import paddlehub as hub
-
-
-
-os.system("hub install stylepro_artistic==1.0.1")
-stylepro_artistic = hub.Module(name="stylepro_artistic")
-
-
-
-# Clip Model
-device = "cuda" if torch.cuda.is_available() else "cpu"
-model = CLIPModel.from_pretrained("openai/clip-vit-base-patch32")
-tokenizer = CLIPTokenizer.from_pretrained("openai/clip-vit-base-patch32")
-model = model.to(device)
-
-# Load Data
-photos = pd.read_csv("unsplash-dataset/photos.tsv000", sep="\t", header=0)
-photo_features = np.load("unsplash-dataset/features.npy")
-photo_ids = pd.read_csv("unsplash-dataset/photo_ids.csv")
-photo_ids = list(photo_ids["photo_id"])
-
-def image_from_text(text_input):
- start=datetime.now()
-
- ## Inference
- with torch.no_grad():
- inputs = tokenizer([text_input], padding=True, return_tensors="pt")
- text_features = model.get_text_features(**inputs).cpu().numpy()
-
- ## Find similarity
- similarities = list((text_features @ photo_features.T).squeeze(0))
-
- ## Return best image :)
- idx = sorted(zip(similarities, range(photo_features.shape[0])), key=lambda x: x[0], reverse=True)[0][1]
- photo_id = photo_ids[idx]
- photo_data = photos[photos["photo_id"] == photo_id].iloc[0]
-
- print(f"Time spent at CLIP: {datetime.now()-start}")
-
- start=datetime.now()
- # Downlaod image
- response = requests.get(photo_data["photo_image_url"] + "?w=640")
- pil_image = Image.open(BytesIO(response.content)).convert("RGB")
- open_cv_image = np.array(pil_image)
- # Convert RGB to BGR
- open_cv_image = open_cv_image[:, :, ::-1].copy()
-
- print(f"Time spent at Image request: {datetime.now()-start}")
-
- return open_cv_image
-
-def inference(content, style):
- content_image = image_from_text(content)
- start=datetime.now()
-
- result = stylepro_artistic.style_transfer(
- images=[{
- "content": content_image,
- "styles": [cv2.imread(style.name)]
- }])
-
- print(f"Time spent at Style Transfer: {datetime.now()-start}")
- return Image.fromarray(np.uint8(result[0]["data"])[:,:,::-1]).convert("RGB")
-
-if __name__ == "__main__":
- title = "Neural Style Transfer"
- description = "Gradio demo for Neural Style Transfer. To use it, simply enter the text for image content and upload style image. Read more at the links below."
- article = "Parameter-Free Style Projection for Arbitrary Style Transfer | Github RepoClip paper | Hugging Face Clip Implementation
"
- examples=[
- ["a cute kangaroo", "styles/starry.jpeg"],
- ["man holding beer", "styles/mona1.jpeg"],
- ]
- interface = gr.Interface(inference,
- inputs=[
- gr.inputs.Textbox(lines=1, placeholder="Describe the content of the image", default="a cute kangaroo", label="Describe the image to which the style will be applied"),
- gr.inputs.Image(type="file", label="Style to be applied"),
- ],
- outputs=gr.outputs.Image(type="pil"),
- enable_queue=True,
- title=title,
- description=description,
- article=article,
- examples=examples)
- interface.launch()
\ No newline at end of file
diff --git a/spaces/OAOA/DifFace/app.py b/spaces/OAOA/DifFace/app.py
deleted file mode 100644
index e20c43ccb31fad55e73113526a82531e9da9270f..0000000000000000000000000000000000000000
--- a/spaces/OAOA/DifFace/app.py
+++ /dev/null
@@ -1,153 +0,0 @@
-#!/usr/bin/env python
-# -*- coding:utf-8 -*-
-# Power by Zongsheng Yue 2022-12-16 16:17:14
-
-import os
-import torch
-import argparse
-import numpy as np
-import gradio as gr
-from pathlib import Path
-from einops import rearrange
-from omegaconf import OmegaConf
-from skimage import img_as_ubyte
-
-from utils import util_opts
-from utils import util_image
-from utils import util_common
-
-from sampler import DifIRSampler
-from ResizeRight.resize_right import resize
-from basicsr.utils.download_util import load_file_from_url
-
-# setting configurations
-cfg_path = 'configs/sample/iddpm_ffhq512_swinir.yaml'
-configs = OmegaConf.load(cfg_path)
-configs.aligned = False
-configs.diffusion.timestep_respacing = '250'
-
-# build the sampler for diffusion
-sampler_dist = DifIRSampler(configs)
-
-def predict(im_path, background_enhance, face_upsample, upscale, started_timesteps):
- assert isinstance(im_path, str)
- print(f'Processing image: {im_path}...')
-
- configs.background_enhance = background_enhance
- configs.face_upsample = face_upsample
- started_timesteps = int(started_timesteps)
- assert started_timesteps < int(configs.diffusion.params.timestep_respacing)
-
- # prepare the checkpoint
- if not Path(configs.model.ckpt_path).exists():
- load_file_from_url(
- url="https://github.com/zsyOAOA/DifFace/releases/download/V1.0/iddpm_ffhq512_ema500000.pth",
- model_dir=str(Path(configs.model.ckpt_path).parent),
- progress=True,
- file_name=Path(configs.model.ckpt_path).name,
- )
- if not Path(configs.model_ir.ckpt_path).exists():
- load_file_from_url(
- url="https://github.com/zsyOAOA/DifFace/releases/download/V1.0/General_Face_ffhq512.pth",
- model_dir=str(Path(configs.model_ir.ckpt_path).parent),
- progress=True,
- file_name=Path(configs.model_ir.ckpt_path).name,
- )
-
- # Load image
- im_lq = util_image.imread(im_path, chn='bgr', dtype='uint8')
- if upscale > 4:
- upscale = 4 # avoid momory exceeded due to too large upscale
- if upscale > 2 and min(im_lq.shape[:2])>1280:
- upscale = 2 # avoid momory exceeded due to too large img resolution
- configs.detection.upscale = int(upscale)
-
- if background_enhance:
- image_restored, face_restored, face_cropped = sampler_dist.sample_func_bfr_unaligned(
- y0=im_lq,
- start_timesteps=started_timesteps,
- need_restoration=True,
- draw_box=False,
- ) # h x w x c, numpy array, [0, 255], uint8, BGR
- image_restored = util_image.bgr2rgb(image_restored)
- else:
- image_restored = sampler_dist.sample_func_ir_aligned(
- y0=im_lq,
- start_timesteps=started_timesteps,
- need_restoration=True,
- )[0] # b x c x h x w, [0, 1], torch tensor, RGB
- image_restored = util_image.tensor2img(
- image_restored.cpu(),
- rgb2bgr=False,
- out_type=np.uint8,
- min_max=(0, 1),
- ) # h x w x c, [0, 255], uint8, RGB, numpy array
-
- restored_image_dir = Path('restored_output')
- if not restored_image_dir.exists():
- restored_image_dir.mkdir()
- # save the whole image
- save_path = restored_image_dir / Path(im_path).name
- util_image.imwrite(image_restored, save_path, chn='rgb', dtype_in='uint8')
-
- return image_restored, str(save_path)
-
-title = "DifFace: Blind Face Restoration with Diffused Error Contraction"
-description = r"""
-Official Gradio demo for DifFace: Blind Face Restoration with Diffused Error Contraction.
-🔥 DifFace is a robust face restoration algorithm for old or corrupted photos.
-"""
-article = r"""
-If DifFace is helpful for your work, please help to ⭐ the Github Repo. Thanks!
-[](https://github.com/zsyOAOA/DifFace)
-
----
-
-📝 **Citation**
-
-If our work is useful for your research, please consider citing:
-```bibtex
-@article{yue2022difface,
- title={DifFace: Blind Face Restoration with Diffused Error Contraction},
- author={Yue, Zongsheng and Loy, Chen Change},
- journal={arXiv preprint arXiv:2212.06512},
- year={2022}
-}
-```
-
-📋 **License**
-
-This project is licensed under S-Lab License 1.0.
-Redistribution and use for non-commercial purposes should follow this license.
-
-📧 **Contact**
-If you have any questions, please feel free to contact me via zsyzam@gmail.com.
-
-"""
-
-demo = gr.Interface(
- predict,
- inputs=[
- gr.Image(type="filepath", label="Input"),
- gr.Checkbox(value=True, label="Background_Enhance"),
- gr.Checkbox(value=True, label="Face_Upsample"),
- gr.Number(value=2, label="Rescaling_Factor (up to 4)"),
- gr.Slider(1, 160, value=100, step=10, label='Realism-Fidelity Trade-off')
- ],
- outputs=[
- gr.Image(type="numpy", label="Output"),
- gr.outputs.File(label="Download the output")
- ],
- title=title,
- description=description,
- article=article,
- examples=[
- ['./testdata/whole_imgs/00.jpg', True, True, 2, 100],
- ['./testdata/whole_imgs/01.jpg', True, True, 2, 100],
- ['./testdata/whole_imgs/04.jpg', True, True, 2, 100],
- ['./testdata/whole_imgs/05.jpg', True, True, 2, 100],
- ]
- )
-
-demo.queue(concurrency_count=4)
-demo.launch()
diff --git a/spaces/OAOA/DifFace/basicsr/ops/upfirdn2d/__init__.py b/spaces/OAOA/DifFace/basicsr/ops/upfirdn2d/__init__.py
deleted file mode 100644
index 397e85bea063e97fc4c12ad4d3e15669b69290bd..0000000000000000000000000000000000000000
--- a/spaces/OAOA/DifFace/basicsr/ops/upfirdn2d/__init__.py
+++ /dev/null
@@ -1,3 +0,0 @@
-from .upfirdn2d import upfirdn2d
-
-__all__ = ['upfirdn2d']
diff --git a/spaces/OAOA/DifFace/facelib/parsing/parsenet.py b/spaces/OAOA/DifFace/facelib/parsing/parsenet.py
deleted file mode 100644
index e178ebe43a1ef666aaea0bc0faf629485c22a24f..0000000000000000000000000000000000000000
--- a/spaces/OAOA/DifFace/facelib/parsing/parsenet.py
+++ /dev/null
@@ -1,194 +0,0 @@
-"""Modified from https://github.com/chaofengc/PSFRGAN
-"""
-import numpy as np
-import torch.nn as nn
-from torch.nn import functional as F
-
-
-class NormLayer(nn.Module):
- """Normalization Layers.
-
- Args:
- channels: input channels, for batch norm and instance norm.
- input_size: input shape without batch size, for layer norm.
- """
-
- def __init__(self, channels, normalize_shape=None, norm_type='bn'):
- super(NormLayer, self).__init__()
- norm_type = norm_type.lower()
- self.norm_type = norm_type
- if norm_type == 'bn':
- self.norm = nn.BatchNorm2d(channels, affine=True)
- elif norm_type == 'in':
- self.norm = nn.InstanceNorm2d(channels, affine=False)
- elif norm_type == 'gn':
- self.norm = nn.GroupNorm(32, channels, affine=True)
- elif norm_type == 'pixel':
- self.norm = lambda x: F.normalize(x, p=2, dim=1)
- elif norm_type == 'layer':
- self.norm = nn.LayerNorm(normalize_shape)
- elif norm_type == 'none':
- self.norm = lambda x: x * 1.0
- else:
- assert 1 == 0, f'Norm type {norm_type} not support.'
-
- def forward(self, x, ref=None):
- if self.norm_type == 'spade':
- return self.norm(x, ref)
- else:
- return self.norm(x)
-
-
-class ReluLayer(nn.Module):
- """Relu Layer.
-
- Args:
- relu type: type of relu layer, candidates are
- - ReLU
- - LeakyReLU: default relu slope 0.2
- - PRelu
- - SELU
- - none: direct pass
- """
-
- def __init__(self, channels, relu_type='relu'):
- super(ReluLayer, self).__init__()
- relu_type = relu_type.lower()
- if relu_type == 'relu':
- self.func = nn.ReLU(True)
- elif relu_type == 'leakyrelu':
- self.func = nn.LeakyReLU(0.2, inplace=True)
- elif relu_type == 'prelu':
- self.func = nn.PReLU(channels)
- elif relu_type == 'selu':
- self.func = nn.SELU(True)
- elif relu_type == 'none':
- self.func = lambda x: x * 1.0
- else:
- assert 1 == 0, f'Relu type {relu_type} not support.'
-
- def forward(self, x):
- return self.func(x)
-
-
-class ConvLayer(nn.Module):
-
- def __init__(self,
- in_channels,
- out_channels,
- kernel_size=3,
- scale='none',
- norm_type='none',
- relu_type='none',
- use_pad=True,
- bias=True):
- super(ConvLayer, self).__init__()
- self.use_pad = use_pad
- self.norm_type = norm_type
- if norm_type in ['bn']:
- bias = False
-
- stride = 2 if scale == 'down' else 1
-
- self.scale_func = lambda x: x
- if scale == 'up':
- self.scale_func = lambda x: nn.functional.interpolate(x, scale_factor=2, mode='nearest')
-
- self.reflection_pad = nn.ReflectionPad2d(int(np.ceil((kernel_size - 1.) / 2)))
- self.conv2d = nn.Conv2d(in_channels, out_channels, kernel_size, stride, bias=bias)
-
- self.relu = ReluLayer(out_channels, relu_type)
- self.norm = NormLayer(out_channels, norm_type=norm_type)
-
- def forward(self, x):
- out = self.scale_func(x)
- if self.use_pad:
- out = self.reflection_pad(out)
- out = self.conv2d(out)
- out = self.norm(out)
- out = self.relu(out)
- return out
-
-
-class ResidualBlock(nn.Module):
- """
- Residual block recommended in: http://torch.ch/blog/2016/02/04/resnets.html
- """
-
- def __init__(self, c_in, c_out, relu_type='prelu', norm_type='bn', scale='none'):
- super(ResidualBlock, self).__init__()
-
- if scale == 'none' and c_in == c_out:
- self.shortcut_func = lambda x: x
- else:
- self.shortcut_func = ConvLayer(c_in, c_out, 3, scale)
-
- scale_config_dict = {'down': ['none', 'down'], 'up': ['up', 'none'], 'none': ['none', 'none']}
- scale_conf = scale_config_dict[scale]
-
- self.conv1 = ConvLayer(c_in, c_out, 3, scale_conf[0], norm_type=norm_type, relu_type=relu_type)
- self.conv2 = ConvLayer(c_out, c_out, 3, scale_conf[1], norm_type=norm_type, relu_type='none')
-
- def forward(self, x):
- identity = self.shortcut_func(x)
-
- res = self.conv1(x)
- res = self.conv2(res)
- return identity + res
-
-
-class ParseNet(nn.Module):
-
- def __init__(self,
- in_size=128,
- out_size=128,
- min_feat_size=32,
- base_ch=64,
- parsing_ch=19,
- res_depth=10,
- relu_type='LeakyReLU',
- norm_type='bn',
- ch_range=[32, 256]):
- super().__init__()
- self.res_depth = res_depth
- act_args = {'norm_type': norm_type, 'relu_type': relu_type}
- min_ch, max_ch = ch_range
-
- ch_clip = lambda x: max(min_ch, min(x, max_ch)) # noqa: E731
- min_feat_size = min(in_size, min_feat_size)
-
- down_steps = int(np.log2(in_size // min_feat_size))
- up_steps = int(np.log2(out_size // min_feat_size))
-
- # =============== define encoder-body-decoder ====================
- self.encoder = []
- self.encoder.append(ConvLayer(3, base_ch, 3, 1))
- head_ch = base_ch
- for i in range(down_steps):
- cin, cout = ch_clip(head_ch), ch_clip(head_ch * 2)
- self.encoder.append(ResidualBlock(cin, cout, scale='down', **act_args))
- head_ch = head_ch * 2
-
- self.body = []
- for i in range(res_depth):
- self.body.append(ResidualBlock(ch_clip(head_ch), ch_clip(head_ch), **act_args))
-
- self.decoder = []
- for i in range(up_steps):
- cin, cout = ch_clip(head_ch), ch_clip(head_ch // 2)
- self.decoder.append(ResidualBlock(cin, cout, scale='up', **act_args))
- head_ch = head_ch // 2
-
- self.encoder = nn.Sequential(*self.encoder)
- self.body = nn.Sequential(*self.body)
- self.decoder = nn.Sequential(*self.decoder)
- self.out_img_conv = ConvLayer(ch_clip(head_ch), 3)
- self.out_mask_conv = ConvLayer(ch_clip(head_ch), parsing_ch)
-
- def forward(self, x):
- feat = self.encoder(x)
- x = feat + self.body(feat)
- x = self.decoder(x)
- out_img = self.out_img_conv(x)
- out_mask = self.out_mask_conv(x)
- return out_mask, out_img
diff --git a/spaces/OFA-Sys/OFA-Generic_Interface/fairseq/fairseq/criterions/ctc.py b/spaces/OFA-Sys/OFA-Generic_Interface/fairseq/fairseq/criterions/ctc.py
deleted file mode 100644
index 10e3618382c86a84466cb4264d62f31537980251..0000000000000000000000000000000000000000
--- a/spaces/OFA-Sys/OFA-Generic_Interface/fairseq/fairseq/criterions/ctc.py
+++ /dev/null
@@ -1,295 +0,0 @@
-# All rights reserved.
-#
-# This source code is licensed under the license found in the LICENSE file in
-# the root directory of this source tree. An additional grant of patent rights
-# can be found in the PATENTS file in the same directory.
-
-import math
-from argparse import Namespace
-from dataclasses import dataclass, field
-from omegaconf import II
-from typing import Optional
-
-import torch
-import torch.nn.functional as F
-from fairseq import metrics, utils
-from fairseq.criterions import FairseqCriterion, register_criterion
-from fairseq.dataclass import FairseqDataclass
-from fairseq.data.data_utils import post_process
-from fairseq.tasks import FairseqTask
-from fairseq.logging.meters import safe_round
-
-
-@dataclass
-class CtcCriterionConfig(FairseqDataclass):
- zero_infinity: bool = field(
- default=False,
- metadata={"help": "zero inf loss when source length <= target length"},
- )
- sentence_avg: bool = II("optimization.sentence_avg")
- post_process: str = field(
- default="letter",
- metadata={
- "help": "how to post process predictions into words. can be letter, "
- "wordpiece, BPE symbols, etc. "
- "See fairseq.data.data_utils.post_process() for full list of options"
- },
- )
- wer_kenlm_model: Optional[str] = field(
- default=None,
- metadata={
- "help": "if this is provided, use kenlm to compute wer (along with other wer_* args)"
- },
- )
- wer_lexicon: Optional[str] = field(
- default=None,
- metadata={"help": "lexicon to use with wer_kenlm_model"},
- )
- wer_lm_weight: float = field(
- default=2.0,
- metadata={"help": "lm weight to use with wer_kenlm_model"},
- )
- wer_word_score: float = field(
- default=-1.0,
- metadata={"help": "lm word score to use with wer_kenlm_model"},
- )
-
- wer_args: Optional[str] = field(
- default=None,
- metadata={
- "help": "DEPRECATED: tuple of (wer_kenlm_model, wer_lexicon, wer_lm_weight, wer_word_score)"
- },
- )
-
-
-@register_criterion("ctc", dataclass=CtcCriterionConfig)
-class CtcCriterion(FairseqCriterion):
- def __init__(self, cfg: CtcCriterionConfig, task: FairseqTask):
- super().__init__(task)
- self.blank_idx = (
- task.target_dictionary.index(task.blank_symbol)
- if hasattr(task, "blank_symbol")
- else 0
- )
- self.pad_idx = task.target_dictionary.pad()
- self.eos_idx = task.target_dictionary.eos()
- self.post_process = cfg.post_process
-
- if cfg.wer_args is not None:
- (
- cfg.wer_kenlm_model,
- cfg.wer_lexicon,
- cfg.wer_lm_weight,
- cfg.wer_word_score,
- ) = eval(cfg.wer_args)
-
- if cfg.wer_kenlm_model is not None:
- from examples.speech_recognition.w2l_decoder import W2lKenLMDecoder
-
- dec_args = Namespace()
- dec_args.nbest = 1
- dec_args.criterion = "ctc"
- dec_args.kenlm_model = cfg.wer_kenlm_model
- dec_args.lexicon = cfg.wer_lexicon
- dec_args.beam = 50
- dec_args.beam_size_token = min(50, len(task.target_dictionary))
- dec_args.beam_threshold = min(50, len(task.target_dictionary))
- dec_args.lm_weight = cfg.wer_lm_weight
- dec_args.word_score = cfg.wer_word_score
- dec_args.unk_weight = -math.inf
- dec_args.sil_weight = 0
-
- self.w2l_decoder = W2lKenLMDecoder(dec_args, task.target_dictionary)
- else:
- self.w2l_decoder = None
-
- self.zero_infinity = cfg.zero_infinity
- self.sentence_avg = cfg.sentence_avg
-
- def forward(self, model, sample, reduce=True):
- net_output = model(**sample["net_input"])
- lprobs = model.get_normalized_probs(
- net_output, log_probs=True
- ).contiguous() # (T, B, C) from the encoder
-
- if "src_lengths" in sample["net_input"]:
- input_lengths = sample["net_input"]["src_lengths"]
- else:
- if net_output["padding_mask"] is not None:
- non_padding_mask = ~net_output["padding_mask"]
- input_lengths = non_padding_mask.long().sum(-1)
- else:
- input_lengths = lprobs.new_full(
- (lprobs.size(1),), lprobs.size(0), dtype=torch.long
- )
-
- pad_mask = (sample["target"] != self.pad_idx) & (
- sample["target"] != self.eos_idx
- )
- targets_flat = sample["target"].masked_select(pad_mask)
- if "target_lengths" in sample:
- target_lengths = sample["target_lengths"]
- else:
- target_lengths = pad_mask.sum(-1)
-
- with torch.backends.cudnn.flags(enabled=False):
- loss = F.ctc_loss(
- lprobs,
- targets_flat,
- input_lengths,
- target_lengths,
- blank=self.blank_idx,
- reduction="sum",
- zero_infinity=self.zero_infinity,
- )
-
- ntokens = (
- sample["ntokens"] if "ntokens" in sample else target_lengths.sum().item()
- )
-
- sample_size = sample["target"].size(0) if self.sentence_avg else ntokens
- logging_output = {
- "loss": utils.item(loss.data), # * sample['ntokens'],
- "ntokens": ntokens,
- "nsentences": sample["id"].numel(),
- "sample_size": sample_size,
- }
-
- if not model.training:
- import editdistance
-
- with torch.no_grad():
- lprobs_t = lprobs.transpose(0, 1).float().contiguous().cpu()
-
- c_err = 0
- c_len = 0
- w_errs = 0
- w_len = 0
- wv_errs = 0
- for lp, t, inp_l in zip(
- lprobs_t,
- sample["target_label"]
- if "target_label" in sample
- else sample["target"],
- input_lengths,
- ):
- lp = lp[:inp_l].unsqueeze(0)
-
- decoded = None
- if self.w2l_decoder is not None:
- decoded = self.w2l_decoder.decode(lp)
- if len(decoded) < 1:
- decoded = None
- else:
- decoded = decoded[0]
- if len(decoded) < 1:
- decoded = None
- else:
- decoded = decoded[0]
-
- p = (t != self.task.target_dictionary.pad()) & (
- t != self.task.target_dictionary.eos()
- )
- targ = t[p]
- targ_units = self.task.target_dictionary.string(targ)
- targ_units_arr = targ.tolist()
-
- toks = lp.argmax(dim=-1).unique_consecutive()
- pred_units_arr = toks[toks != self.blank_idx].tolist()
-
- c_err += editdistance.eval(pred_units_arr, targ_units_arr)
- c_len += len(targ_units_arr)
-
- targ_words = post_process(targ_units, self.post_process).split()
-
- pred_units = self.task.target_dictionary.string(pred_units_arr)
- pred_words_raw = post_process(pred_units, self.post_process).split()
-
- if decoded is not None and "words" in decoded:
- pred_words = decoded["words"]
- w_errs += editdistance.eval(pred_words, targ_words)
- wv_errs += editdistance.eval(pred_words_raw, targ_words)
- else:
- dist = editdistance.eval(pred_words_raw, targ_words)
- w_errs += dist
- wv_errs += dist
-
- w_len += len(targ_words)
-
- logging_output["wv_errors"] = wv_errs
- logging_output["w_errors"] = w_errs
- logging_output["w_total"] = w_len
- logging_output["c_errors"] = c_err
- logging_output["c_total"] = c_len
-
- return loss, sample_size, logging_output
-
- @staticmethod
- def reduce_metrics(logging_outputs) -> None:
- """Aggregate logging outputs from data parallel training."""
-
- loss_sum = utils.item(sum(log.get("loss", 0) for log in logging_outputs))
- ntokens = utils.item(sum(log.get("ntokens", 0) for log in logging_outputs))
- nsentences = utils.item(
- sum(log.get("nsentences", 0) for log in logging_outputs)
- )
- sample_size = utils.item(
- sum(log.get("sample_size", 0) for log in logging_outputs)
- )
-
- metrics.log_scalar(
- "loss", loss_sum / sample_size / math.log(2), sample_size, round=3
- )
- metrics.log_scalar("ntokens", ntokens)
- metrics.log_scalar("nsentences", nsentences)
- if sample_size != ntokens:
- metrics.log_scalar(
- "nll_loss", loss_sum / ntokens / math.log(2), ntokens, round=3
- )
-
- c_errors = sum(log.get("c_errors", 0) for log in logging_outputs)
- metrics.log_scalar("_c_errors", c_errors)
- c_total = sum(log.get("c_total", 0) for log in logging_outputs)
- metrics.log_scalar("_c_total", c_total)
- w_errors = sum(log.get("w_errors", 0) for log in logging_outputs)
- metrics.log_scalar("_w_errors", w_errors)
- wv_errors = sum(log.get("wv_errors", 0) for log in logging_outputs)
- metrics.log_scalar("_wv_errors", wv_errors)
- w_total = sum(log.get("w_total", 0) for log in logging_outputs)
- metrics.log_scalar("_w_total", w_total)
-
- if c_total > 0:
- metrics.log_derived(
- "uer",
- lambda meters: safe_round(
- meters["_c_errors"].sum * 100.0 / meters["_c_total"].sum, 3
- )
- if meters["_c_total"].sum > 0
- else float("nan"),
- )
- if w_total > 0:
- metrics.log_derived(
- "wer",
- lambda meters: safe_round(
- meters["_w_errors"].sum * 100.0 / meters["_w_total"].sum, 3
- )
- if meters["_w_total"].sum > 0
- else float("nan"),
- )
- metrics.log_derived(
- "raw_wer",
- lambda meters: safe_round(
- meters["_wv_errors"].sum * 100.0 / meters["_w_total"].sum, 3
- )
- if meters["_w_total"].sum > 0
- else float("nan"),
- )
-
- @staticmethod
- def logging_outputs_can_be_summed() -> bool:
- """
- Whether the logging outputs returned by `forward` can be summed
- across workers prior to calling `reduce_metrics`. Setting this
- to True will improves distributed training speed.
- """
- return True
diff --git a/spaces/OFA-Sys/OFA-Image_Caption/fairseq/examples/adaptive_span/__init__.py b/spaces/OFA-Sys/OFA-Image_Caption/fairseq/examples/adaptive_span/__init__.py
deleted file mode 100644
index e0a142a769360e1140bf814c532eaf841f1d52d8..0000000000000000000000000000000000000000
--- a/spaces/OFA-Sys/OFA-Image_Caption/fairseq/examples/adaptive_span/__init__.py
+++ /dev/null
@@ -1,19 +0,0 @@
-# Copyright (c) Facebook, Inc. and its affiliates.
-#
-# This source code is licensed under the MIT license found in the
-# LICENSE file in the root directory of this source tree.
-
-import importlib
-import os
-
-# automatically import any Python files in the current directory
-cur_dir = os.path.dirname(__file__)
-for file in os.listdir(cur_dir):
- path = os.path.join(cur_dir, file)
- if (
- not file.startswith("_")
- and not file.startswith(".")
- and (file.endswith(".py") or os.path.isdir(path))
- ):
- mod_name = file[: file.find(".py")] if file.endswith(".py") else file
- module = importlib.import_module(__name__ + "." + mod_name)
diff --git a/spaces/OFA-Sys/OFA-Image_Caption/fairseq/examples/speech_text_joint_to_text/README.md b/spaces/OFA-Sys/OFA-Image_Caption/fairseq/examples/speech_text_joint_to_text/README.md
deleted file mode 100644
index e071d241e0e02b35d3aac777ac09b4ef3be9119f..0000000000000000000000000000000000000000
--- a/spaces/OFA-Sys/OFA-Image_Caption/fairseq/examples/speech_text_joint_to_text/README.md
+++ /dev/null
@@ -1,46 +0,0 @@
-# Joint Speech Text training in Fairseq
-An extension of Fairseq s2t project with the speech to text task enhanced by the co-trained text to text mapping task. More details about Fairseq s2t can be found [here](../speech_to_text/README.md)
-
-## Examples
-Examples of speech text joint training in fairseq
-- [English-to-German MuST-C model](docs/ende-mustc.md)
-- [IWSLT 2021 Multilingual Speech Translation](docs/iwslt2021.md)
-
-## Citation
-Please cite as:
-```
-@inproceedings{Tang2021AGM,
- title={A General Multi-Task Learning Framework to Leverage Text Data for Speech to Text Tasks},
- author={Yun Tang and J. Pino and Changhan Wang and Xutai Ma and Dmitriy Genzel},
- booktitle={ICASSP},
- year={2021}
-}
-
-@inproceedings{Tang2021IST,
- title = {Improving Speech Translation by Understanding and Learning from the Auxiliary Text Translation Task},
- author = {Yun Tang and Juan Pino and Xian Li and Changhan Wang and Dmitriy Genzel},
- booktitle = {ACL},
- year = {2021},
-}
-
-@inproceedings{Tang2021FST,
- title = {FST: the FAIR Speech Translation System for the IWSLT21 Multilingual Shared Task},
- author = {Yun Tang and Hongyu Gong and Xian Li and Changhan Wang and Juan Pino and Holger Schwenk and Naman Goyal},
- booktitle = {IWSLT},
- year = {2021},
-}
-
-@inproceedings{wang2020fairseqs2t,
- title = {fairseq S2T: Fast Speech-to-Text Modeling with fairseq},
- author = {Changhan Wang and Yun Tang and Xutai Ma and Anne Wu and Dmytro Okhonko and Juan Pino},
- booktitle = {Proceedings of the 2020 Conference of the Asian Chapter of the Association for Computational Linguistics (AACL): System Demonstrations},
- year = {2020},
-}
-
-@inproceedings{ott2019fairseq,
- title = {fairseq: A Fast, Extensible Toolkit for Sequence Modeling},
- author = {Myle Ott and Sergey Edunov and Alexei Baevski and Angela Fan and Sam Gross and Nathan Ng and David Grangier and Michael Auli},
- booktitle = {Proceedings of NAACL-HLT 2019: Demonstrations},
- year = {2019},
-}
-```
diff --git a/spaces/OFA-Sys/OFA-Image_Caption/fairseq/fairseq/clib/libnat_cuda/binding.cpp b/spaces/OFA-Sys/OFA-Image_Caption/fairseq/fairseq/clib/libnat_cuda/binding.cpp
deleted file mode 100644
index ced91c0d0afab9071842911d9876e6360d90284a..0000000000000000000000000000000000000000
--- a/spaces/OFA-Sys/OFA-Image_Caption/fairseq/fairseq/clib/libnat_cuda/binding.cpp
+++ /dev/null
@@ -1,67 +0,0 @@
-/**
- * Copyright 2017-present, Facebook, Inc.
- * All rights reserved.
- *
- * This source code is licensed under the license found in the
- * LICENSE file in the root directory of this source tree.
- */
-
-/*
- This code is partially adpoted from
- https://github.com/1ytic/pytorch-edit-distance
- */
-
-#include
-#include "edit_dist.h"
-
-#ifndef TORCH_CHECK
-#define TORCH_CHECK AT_CHECK
-#endif
-
-#define CHECK_CUDA(x) \
- TORCH_CHECK(x.type().is_cuda(), #x " must be a CUDA tensor")
-#define CHECK_CONTIGUOUS(x) \
- TORCH_CHECK(x.is_contiguous(), #x " must be contiguous")
-#define CHECK_INPUT(x) \
- CHECK_CUDA(x); \
- CHECK_CONTIGUOUS(x)
-
-torch::Tensor LevenshteinDistance(
- torch::Tensor source,
- torch::Tensor target,
- torch::Tensor source_length,
- torch::Tensor target_length) {
- CHECK_INPUT(source);
- CHECK_INPUT(target);
- CHECK_INPUT(source_length);
- CHECK_INPUT(target_length);
- return LevenshteinDistanceCuda(source, target, source_length, target_length);
-}
-
-torch::Tensor GenerateDeletionLabel(
- torch::Tensor source,
- torch::Tensor operations) {
- CHECK_INPUT(source);
- CHECK_INPUT(operations);
- return GenerateDeletionLabelCuda(source, operations);
-}
-
-std::pair GenerateInsertionLabel(
- torch::Tensor target,
- torch::Tensor operations) {
- CHECK_INPUT(target);
- CHECK_INPUT(operations);
- return GenerateInsertionLabelCuda(target, operations);
-}
-
-PYBIND11_MODULE(TORCH_EXTENSION_NAME, m) {
- m.def("levenshtein_distance", &LevenshteinDistance, "Levenshtein distance");
- m.def(
- "generate_deletion_labels",
- &GenerateDeletionLabel,
- "Generate Deletion Label");
- m.def(
- "generate_insertion_labels",
- &GenerateInsertionLabel,
- "Generate Insertion Label");
-}
diff --git a/spaces/OFA-Sys/OFA-Image_Caption/fairseq/fairseq/nan_detector.py b/spaces/OFA-Sys/OFA-Image_Caption/fairseq/fairseq/nan_detector.py
deleted file mode 100644
index faa8031d4666c9ba9837919fe1c884dacf47ac3a..0000000000000000000000000000000000000000
--- a/spaces/OFA-Sys/OFA-Image_Caption/fairseq/fairseq/nan_detector.py
+++ /dev/null
@@ -1,108 +0,0 @@
-# Copyright (c) Facebook, Inc. and its affiliates.
-#
-# This source code is licensed under the MIT license found in the
-# LICENSE file in the root directory of this source tree.
-
-import logging
-
-import torch
-
-
-logger = logging.getLogger(__name__)
-
-
-class NanDetector:
- """
- Detects the first NaN or Inf in forward and/or backward pass and logs, together with the module name
- """
-
- def __init__(self, model, forward=True, backward=True):
- self.bhooks = []
- self.fhooks = []
- self.forward = forward
- self.backward = backward
- self.named_parameters = list(model.named_parameters())
- self.reset()
-
- for name, mod in model.named_modules():
- mod.__module_name = name
- self.add_hooks(mod)
-
- def __enter__(self):
- return self
-
- def __exit__(self, exc_type, exc_value, exc_traceback):
- # Dump out all model gnorms to enable better debugging
- norm = {}
- gradients = {}
- for name, param in self.named_parameters:
- if param.grad is not None:
- grad_norm = torch.norm(param.grad.data, p=2, dtype=torch.float32)
- norm[name] = grad_norm.item()
- if torch.isnan(grad_norm).any() or torch.isinf(grad_norm).any():
- gradients[name] = param.grad.data
- if len(gradients) > 0:
- logger.info("Detected nan/inf grad norm, dumping norms...")
- logger.info(f"norms: {norm}")
- logger.info(f"gradients: {gradients}")
-
- self.close()
-
- def add_hooks(self, module):
- if self.forward:
- self.fhooks.append(module.register_forward_hook(self.fhook_fn))
- if self.backward:
- self.bhooks.append(module.register_backward_hook(self.bhook_fn))
-
- def reset(self):
- self.has_printed_f = False
- self.has_printed_b = False
-
- def _detect(self, tensor, name, backward):
- err = None
- if (
- torch.is_floating_point(tensor)
- # single value tensors (like the loss) will not provide much info
- and tensor.numel() >= 2
- ):
- with torch.no_grad():
- if torch.isnan(tensor).any():
- err = "NaN"
- elif torch.isinf(tensor).any():
- err = "Inf"
- if err is not None:
- err = f"{err} detected in output of {name}, shape: {tensor.shape}, {'backward' if backward else 'forward'}"
- return err
-
- def _apply(self, module, inp, x, backward):
- if torch.is_tensor(x):
- if isinstance(inp, tuple) and len(inp) > 0:
- inp = inp[0]
- err = self._detect(x, module.__module_name, backward)
- if err is not None:
- if torch.is_tensor(inp) and not backward:
- err += (
- f" input max: {inp.max().item()}, input min: {inp.min().item()}"
- )
-
- has_printed_attr = "has_printed_b" if backward else "has_printed_f"
- logger.warning(err)
- setattr(self, has_printed_attr, True)
- elif isinstance(x, dict):
- for v in x.values():
- self._apply(module, inp, v, backward)
- elif isinstance(x, list) or isinstance(x, tuple):
- for v in x:
- self._apply(module, inp, v, backward)
-
- def fhook_fn(self, module, inp, output):
- if not self.has_printed_f:
- self._apply(module, inp, output, backward=False)
-
- def bhook_fn(self, module, inp, output):
- if not self.has_printed_b:
- self._apply(module, inp, output, backward=True)
-
- def close(self):
- for hook in self.fhooks + self.bhooks:
- hook.remove()
diff --git a/spaces/OFA-Sys/OFA-vqa/fairseq/fairseq/dataclass/utils.py b/spaces/OFA-Sys/OFA-vqa/fairseq/fairseq/dataclass/utils.py
deleted file mode 100644
index 1320ec473756c78ec949f72f9260420c19caff0f..0000000000000000000000000000000000000000
--- a/spaces/OFA-Sys/OFA-vqa/fairseq/fairseq/dataclass/utils.py
+++ /dev/null
@@ -1,493 +0,0 @@
-# Copyright (c) Facebook, Inc. and its affiliates.
-#
-# This source code is licensed under the MIT license found in the
-# LICENSE file in the root directory of this source tree.
-
-import ast
-import inspect
-import logging
-import os
-import re
-from argparse import ArgumentError, ArgumentParser, Namespace
-from dataclasses import _MISSING_TYPE, MISSING, is_dataclass
-from enum import Enum
-from typing import Any, Dict, List, Optional, Tuple, Type
-
-from fairseq.dataclass import FairseqDataclass
-from fairseq.dataclass.configs import FairseqConfig
-from hydra.core.global_hydra import GlobalHydra
-from hydra.experimental import compose, initialize
-from omegaconf import DictConfig, OmegaConf, open_dict, _utils
-
-logger = logging.getLogger(__name__)
-
-
-def eval_str_list(x, x_type=float):
- if x is None:
- return None
- if isinstance(x, str):
- if len(x) == 0:
- return []
- x = ast.literal_eval(x)
- try:
- return list(map(x_type, x))
- except TypeError:
- return [x_type(x)]
-
-
-def interpret_dc_type(field_type):
- if isinstance(field_type, str):
- raise RuntimeError("field should be a type")
-
- if field_type == Any:
- return str
-
- typestring = str(field_type)
- if re.match(
- r"(typing.|^)Union\[(.*), NoneType\]$", typestring
- ) or typestring.startswith("typing.Optional"):
- return field_type.__args__[0]
- return field_type
-
-
-def gen_parser_from_dataclass(
- parser: ArgumentParser,
- dataclass_instance: FairseqDataclass,
- delete_default: bool = False,
- with_prefix: Optional[str] = None,
-) -> None:
- """
- convert a dataclass instance to tailing parser arguments.
-
- If `with_prefix` is provided, prefix all the keys in the resulting parser with it. It means that we are
- building a flat namespace from a structured dataclass (see transformer_config.py for example).
- """
-
- def argparse_name(name: str):
- if name == "data" and (with_prefix is None or with_prefix == ''):
- # normally data is positional args, so we don't add the -- nor the prefix
- return name
- if name == "_name":
- # private member, skip
- return None
- full_name = "--" + name.replace("_", "-")
- if with_prefix is not None and with_prefix != '':
- # if a prefix is specified, construct the prefixed arg name
- full_name = with_prefix + "-" + full_name[2:] # strip -- when composing
- return full_name
-
- def get_kwargs_from_dc(
- dataclass_instance: FairseqDataclass, k: str
- ) -> Dict[str, Any]:
- """k: dataclass attributes"""
-
- kwargs = {}
-
- field_type = dataclass_instance._get_type(k)
- inter_type = interpret_dc_type(field_type)
-
- field_default = dataclass_instance._get_default(k)
-
- if isinstance(inter_type, type) and issubclass(inter_type, Enum):
- field_choices = [t.value for t in list(inter_type)]
- else:
- field_choices = None
-
- field_help = dataclass_instance._get_help(k)
- field_const = dataclass_instance._get_argparse_const(k)
-
- if isinstance(field_default, str) and field_default.startswith("${"):
- kwargs["default"] = field_default
- else:
- if field_default is MISSING:
- kwargs["required"] = True
- if field_choices is not None:
- kwargs["choices"] = field_choices
- if (
- isinstance(inter_type, type)
- and (issubclass(inter_type, List) or issubclass(inter_type, Tuple))
- ) or ("List" in str(inter_type) or "Tuple" in str(inter_type)):
- if "int" in str(inter_type):
- kwargs["type"] = lambda x: eval_str_list(x, int)
- elif "float" in str(inter_type):
- kwargs["type"] = lambda x: eval_str_list(x, float)
- elif "str" in str(inter_type):
- kwargs["type"] = lambda x: eval_str_list(x, str)
- else:
- raise NotImplementedError(
- "parsing of type " + str(inter_type) + " is not implemented"
- )
- if field_default is not MISSING:
- kwargs["default"] = (
- ",".join(map(str, field_default))
- if field_default is not None
- else None
- )
- elif (
- isinstance(inter_type, type) and issubclass(inter_type, Enum)
- ) or "Enum" in str(inter_type):
- kwargs["type"] = str
- if field_default is not MISSING:
- if isinstance(field_default, Enum):
- kwargs["default"] = field_default.value
- else:
- kwargs["default"] = field_default
- elif inter_type is bool:
- kwargs["action"] = (
- "store_false" if field_default is True else "store_true"
- )
- kwargs["default"] = field_default
- else:
- kwargs["type"] = inter_type
- if field_default is not MISSING:
- kwargs["default"] = field_default
-
- # build the help with the hierarchical prefix
- if with_prefix is not None and with_prefix != '' and field_help is not None:
- field_help = with_prefix[2:] + ': ' + field_help
-
- kwargs["help"] = field_help
- if field_const is not None:
- kwargs["const"] = field_const
- kwargs["nargs"] = "?"
-
- return kwargs
-
- for k in dataclass_instance._get_all_attributes():
- field_name = argparse_name(dataclass_instance._get_name(k))
- field_type = dataclass_instance._get_type(k)
- if field_name is None:
- continue
- elif inspect.isclass(field_type) and issubclass(field_type, FairseqDataclass):
- # for fields that are of type FairseqDataclass, we can recursively
- # add their fields to the namespace (so we add the args from model, task, etc. to the root namespace)
- prefix = None
- if with_prefix is not None:
- # if a prefix is specified, then we don't want to copy the subfields directly to the root namespace
- # but we prefix them with the name of the current field.
- prefix = field_name
- gen_parser_from_dataclass(parser, field_type(), delete_default, prefix)
- continue
-
- kwargs = get_kwargs_from_dc(dataclass_instance, k)
-
- field_args = [field_name]
- alias = dataclass_instance._get_argparse_alias(k)
- if alias is not None:
- field_args.append(alias)
-
- if "default" in kwargs:
- if isinstance(kwargs["default"], str) and kwargs["default"].startswith(
- "${"
- ):
- if kwargs["help"] is None:
- # this is a field with a name that will be added elsewhere
- continue
- else:
- del kwargs["default"]
- if delete_default and "default" in kwargs:
- del kwargs["default"]
- try:
- parser.add_argument(*field_args, **kwargs)
- except ArgumentError:
- pass
-
-
-def _set_legacy_defaults(args, cls):
- """Helper to set default arguments based on *add_args*."""
- if not hasattr(cls, "add_args"):
- return
-
- import argparse
-
- parser = argparse.ArgumentParser(
- argument_default=argparse.SUPPRESS, allow_abbrev=False
- )
- cls.add_args(parser)
- # copied from argparse.py:
- defaults = argparse.Namespace()
- for action in parser._actions:
- if action.dest is not argparse.SUPPRESS:
- if not hasattr(defaults, action.dest):
- if action.default is not argparse.SUPPRESS:
- setattr(defaults, action.dest, action.default)
- for key, default_value in vars(defaults).items():
- if not hasattr(args, key):
- setattr(args, key, default_value)
-
-
-def _override_attr(
- sub_node: str, data_class: Type[FairseqDataclass], args: Namespace
-) -> List[str]:
- overrides = []
-
- if not inspect.isclass(data_class) or not issubclass(data_class, FairseqDataclass):
- return overrides
-
- def get_default(f):
- if not isinstance(f.default_factory, _MISSING_TYPE):
- return f.default_factory()
- return f.default
-
- for k, v in data_class.__dataclass_fields__.items():
- if k.startswith("_"):
- # private member, skip
- continue
-
- val = get_default(v) if not hasattr(args, k) else getattr(args, k)
-
- field_type = interpret_dc_type(v.type)
- if (
- isinstance(val, str)
- and not val.startswith("${") # not interpolation
- and field_type != str
- and (
- not inspect.isclass(field_type) or not issubclass(field_type, Enum)
- ) # not choices enum
- ):
- # upgrade old models that stored complex parameters as string
- val = ast.literal_eval(val)
-
- if isinstance(val, tuple):
- val = list(val)
-
- v_type = getattr(v.type, "__origin__", None)
- if (
- (v_type is List or v_type is list or v_type is Optional)
- # skip interpolation
- and not (isinstance(val, str) and val.startswith("${"))
- ):
- # if type is int but val is float, then we will crash later - try to convert here
- if hasattr(v.type, "__args__"):
- t_args = v.type.__args__
- if len(t_args) == 1 and (t_args[0] is float or t_args[0] is int):
- val = list(map(t_args[0], val))
- elif val is not None and (
- field_type is int or field_type is bool or field_type is float
- ):
- try:
- val = field_type(val)
- except:
- pass # ignore errors here, they are often from interpolation args
-
- if val is None:
- overrides.append("{}.{}=null".format(sub_node, k))
- elif val == "":
- overrides.append("{}.{}=''".format(sub_node, k))
- elif isinstance(val, str):
- val = val.replace("'", r"\'")
- overrides.append("{}.{}='{}'".format(sub_node, k, val))
- elif isinstance(val, FairseqDataclass):
- overrides += _override_attr(f"{sub_node}.{k}", type(val), args)
- elif isinstance(val, Namespace):
- sub_overrides, _ = override_module_args(val)
- for so in sub_overrides:
- overrides.append(f"{sub_node}.{k}.{so}")
- else:
- overrides.append("{}.{}={}".format(sub_node, k, val))
-
- return overrides
-
-
-def migrate_registry(
- name, value, registry, args, overrides, deletes, use_name_as_val=False
-):
- if value in registry:
- overrides.append("{}={}".format(name, value))
- overrides.append("{}._name={}".format(name, value))
- overrides.extend(_override_attr(name, registry[value], args))
- elif use_name_as_val and value is not None:
- overrides.append("{}={}".format(name, value))
- else:
- deletes.append(name)
-
-
-def override_module_args(args: Namespace) -> Tuple[List[str], List[str]]:
- """use the field in args to overrides those in cfg"""
- overrides = []
- deletes = []
-
- for k in FairseqConfig.__dataclass_fields__.keys():
- overrides.extend(
- _override_attr(k, FairseqConfig.__dataclass_fields__[k].type, args)
- )
-
- if args is not None:
- if hasattr(args, "task"):
- from fairseq.tasks import TASK_DATACLASS_REGISTRY
-
- migrate_registry(
- "task", args.task, TASK_DATACLASS_REGISTRY, args, overrides, deletes
- )
- else:
- deletes.append("task")
-
- # these options will be set to "None" if they have not yet been migrated
- # so we can populate them with the entire flat args
- CORE_REGISTRIES = {"criterion", "optimizer", "lr_scheduler"}
-
- from fairseq.registry import REGISTRIES
-
- for k, v in REGISTRIES.items():
- if hasattr(args, k):
- migrate_registry(
- k,
- getattr(args, k),
- v["dataclass_registry"],
- args,
- overrides,
- deletes,
- use_name_as_val=k not in CORE_REGISTRIES,
- )
- else:
- deletes.append(k)
-
- no_dc = True
- if hasattr(args, "arch"):
- from fairseq.models import ARCH_MODEL_REGISTRY, ARCH_MODEL_NAME_REGISTRY
-
- if args.arch in ARCH_MODEL_REGISTRY:
- m_cls = ARCH_MODEL_REGISTRY[args.arch]
- dc = getattr(m_cls, "__dataclass", None)
- if dc is not None:
- m_name = ARCH_MODEL_NAME_REGISTRY[args.arch]
- overrides.append("model={}".format(m_name))
- overrides.append("model._name={}".format(args.arch))
- # override model params with those exist in args
- overrides.extend(_override_attr("model", dc, args))
- no_dc = False
- if no_dc:
- deletes.append("model")
-
- return overrides, deletes
-
-
-class omegaconf_no_object_check:
- def __init__(self):
- self.old_is_primitive = _utils.is_primitive_type
-
- def __enter__(self):
- _utils.is_primitive_type = lambda _: True
-
- def __exit__(self, type, value, traceback):
- _utils.is_primitive_type = self.old_is_primitive
-
-
-def convert_namespace_to_omegaconf(args: Namespace) -> DictConfig:
- """Convert a flat argparse.Namespace to a structured DictConfig."""
-
- # Here we are using field values provided in args to override counterparts inside config object
- overrides, deletes = override_module_args(args)
-
- # configs will be in fairseq/config after installation
- config_path = os.path.join("..", "config")
-
- GlobalHydra.instance().clear()
-
- with initialize(config_path=config_path):
- try:
- composed_cfg = compose("config", overrides=overrides, strict=False)
- except:
- logger.error("Error when composing. Overrides: " + str(overrides))
- raise
-
- for k in deletes:
- composed_cfg[k] = None
-
- cfg = OmegaConf.create(
- OmegaConf.to_container(composed_cfg, resolve=True, enum_to_str=True)
- )
-
- # hack to be able to set Namespace in dict config. this should be removed when we update to newer
- # omegaconf version that supports object flags, or when we migrate all existing models
- from omegaconf import _utils
-
- with omegaconf_no_object_check():
- if cfg.task is None and getattr(args, "task", None):
- cfg.task = Namespace(**vars(args))
- from fairseq.tasks import TASK_REGISTRY
-
- _set_legacy_defaults(cfg.task, TASK_REGISTRY[args.task])
- cfg.task._name = args.task
- if cfg.model is None and getattr(args, "arch", None):
- cfg.model = Namespace(**vars(args))
- from fairseq.models import ARCH_MODEL_REGISTRY
-
- _set_legacy_defaults(cfg.model, ARCH_MODEL_REGISTRY[args.arch])
- cfg.model._name = args.arch
- if cfg.optimizer is None and getattr(args, "optimizer", None):
- cfg.optimizer = Namespace(**vars(args))
- from fairseq.optim import OPTIMIZER_REGISTRY
-
- _set_legacy_defaults(cfg.optimizer, OPTIMIZER_REGISTRY[args.optimizer])
- cfg.optimizer._name = args.optimizer
- if cfg.lr_scheduler is None and getattr(args, "lr_scheduler", None):
- cfg.lr_scheduler = Namespace(**vars(args))
- from fairseq.optim.lr_scheduler import LR_SCHEDULER_REGISTRY
-
- _set_legacy_defaults(
- cfg.lr_scheduler, LR_SCHEDULER_REGISTRY[args.lr_scheduler]
- )
- cfg.lr_scheduler._name = args.lr_scheduler
- if cfg.criterion is None and getattr(args, "criterion", None):
- cfg.criterion = Namespace(**vars(args))
- from fairseq.criterions import CRITERION_REGISTRY
-
- _set_legacy_defaults(cfg.criterion, CRITERION_REGISTRY[args.criterion])
- cfg.criterion._name = args.criterion
-
- OmegaConf.set_struct(cfg, True)
- return cfg
-
-
-def overwrite_args_by_name(cfg: DictConfig, overrides: Dict[str, any]):
- # this will be deprecated when we get rid of argparse and model_overrides logic
-
- from fairseq.registry import REGISTRIES
-
- with open_dict(cfg):
- for k in cfg.keys():
- # "k in cfg" will return false if its a "mandatory value (e.g. ???)"
- if k in cfg and isinstance(cfg[k], DictConfig):
- if k in overrides and isinstance(overrides[k], dict):
- for ok, ov in overrides[k].items():
- if isinstance(ov, dict) and cfg[k][ok] is not None:
- overwrite_args_by_name(cfg[k][ok], ov)
- else:
- cfg[k][ok] = ov
- else:
- overwrite_args_by_name(cfg[k], overrides)
- elif k in cfg and isinstance(cfg[k], Namespace):
- for override_key, val in overrides.items():
- setattr(cfg[k], override_key, val)
- elif k in overrides:
- if (
- k in REGISTRIES
- and overrides[k] in REGISTRIES[k]["dataclass_registry"]
- ):
- cfg[k] = DictConfig(
- REGISTRIES[k]["dataclass_registry"][overrides[k]]
- )
- overwrite_args_by_name(cfg[k], overrides)
- cfg[k]._name = overrides[k]
- else:
- cfg[k] = overrides[k]
-
-
-def merge_with_parent(dc: FairseqDataclass, cfg: DictConfig, remove_missing=True):
- if remove_missing:
-
- if is_dataclass(dc):
- target_keys = set(dc.__dataclass_fields__.keys())
- else:
- target_keys = set(dc.keys())
-
- with open_dict(cfg):
- for k in list(cfg.keys()):
- if k not in target_keys:
- del cfg[k]
-
- merged_cfg = OmegaConf.merge(dc, cfg)
- merged_cfg.__dict__["_parent"] = cfg.__dict__["_parent"]
- OmegaConf.set_struct(merged_cfg, True)
- return merged_cfg
diff --git a/spaces/Omnibus/Video-Diffusion-WebUI/video_diffusion/tuneavideo/pipelines/pipeline_tuneavideo.py b/spaces/Omnibus/Video-Diffusion-WebUI/video_diffusion/tuneavideo/pipelines/pipeline_tuneavideo.py
deleted file mode 100644
index 5b45cd6e17ae0f69938db60290cd1b8ab207249d..0000000000000000000000000000000000000000
--- a/spaces/Omnibus/Video-Diffusion-WebUI/video_diffusion/tuneavideo/pipelines/pipeline_tuneavideo.py
+++ /dev/null
@@ -1,411 +0,0 @@
-# Adapted from https://github.com/huggingface/diffusers/blob/main/src/diffusers/pipelines/stable_diffusion/pipeline_stable_diffusion.py
-
-import inspect
-from dataclasses import dataclass
-from typing import Callable, List, Optional, Union
-
-import numpy as np
-import torch
-from diffusers.configuration_utils import FrozenDict
-from diffusers.models import AutoencoderKL
-from diffusers.pipeline_utils import DiffusionPipeline
-from diffusers.schedulers import (
- DDIMScheduler,
- DPMSolverMultistepScheduler,
- EulerAncestralDiscreteScheduler,
- EulerDiscreteScheduler,
- LMSDiscreteScheduler,
- PNDMScheduler,
-)
-from diffusers.utils import BaseOutput, deprecate, is_accelerate_available, logging
-from einops import rearrange
-from packaging import version
-from transformers import CLIPTextModel, CLIPTokenizer
-
-from ..models.unet import UNet3DConditionModel
-
-logger = logging.get_logger(__name__) # pylint: disable=invalid-name
-
-
-@dataclass
-class TuneAVideoPipelineOutput(BaseOutput):
- videos: Union[torch.Tensor, np.ndarray]
-
-
-class TuneAVideoPipeline(DiffusionPipeline):
- _optional_components = []
-
- def __init__(
- self,
- vae: AutoencoderKL,
- text_encoder: CLIPTextModel,
- tokenizer: CLIPTokenizer,
- unet: UNet3DConditionModel,
- scheduler: Union[
- DDIMScheduler,
- PNDMScheduler,
- LMSDiscreteScheduler,
- EulerDiscreteScheduler,
- EulerAncestralDiscreteScheduler,
- DPMSolverMultistepScheduler,
- ],
- ):
- super().__init__()
-
- if hasattr(scheduler.config, "steps_offset") and scheduler.config.steps_offset != 1:
- deprecation_message = (
- f"The configuration file of this scheduler: {scheduler} is outdated. `steps_offset`"
- f" should be set to 1 instead of {scheduler.config.steps_offset}. Please make sure "
- "to update the config accordingly as leaving `steps_offset` might led to incorrect results"
- " in future versions. If you have downloaded this checkpoint from the Hugging Face Hub,"
- " it would be very nice if you could open a Pull request for the `scheduler/scheduler_config.json`"
- " file"
- )
- deprecate("steps_offset!=1", "1.0.0", deprecation_message, standard_warn=False)
- new_config = dict(scheduler.config)
- new_config["steps_offset"] = 1
- scheduler._internal_dict = FrozenDict(new_config)
-
- if hasattr(scheduler.config, "clip_sample") and scheduler.config.clip_sample is True:
- deprecation_message = (
- f"The configuration file of this scheduler: {scheduler} has not set the configuration `clip_sample`."
- " `clip_sample` should be set to False in the configuration file. Please make sure to update the"
- " config accordingly as not setting `clip_sample` in the config might lead to incorrect results in"
- " future versions. If you have downloaded this checkpoint from the Hugging Face Hub, it would be very"
- " nice if you could open a Pull request for the `scheduler/scheduler_config.json` file"
- )
- deprecate("clip_sample not set", "1.0.0", deprecation_message, standard_warn=False)
- new_config = dict(scheduler.config)
- new_config["clip_sample"] = False
- scheduler._internal_dict = FrozenDict(new_config)
-
- is_unet_version_less_0_9_0 = hasattr(unet.config, "_diffusers_version") and version.parse(
- version.parse(unet.config._diffusers_version).base_version
- ) < version.parse("0.9.0.dev0")
- is_unet_sample_size_less_64 = hasattr(unet.config, "sample_size") and unet.config.sample_size < 64
- if is_unet_version_less_0_9_0 and is_unet_sample_size_less_64:
- deprecation_message = (
- "The configuration file of the unet has set the default `sample_size` to smaller than"
- " 64 which seems highly unlikely. If your checkpoint is a fine-tuned version of any of the"
- " following: \n- CompVis/stable-diffusion-v1-4 \n- CompVis/stable-diffusion-v1-3 \n-"
- " CompVis/stable-diffusion-v1-2 \n- CompVis/stable-diffusion-v1-1 \n- runwayml/stable-diffusion-v1-5"
- " \n- runwayml/stable-diffusion-inpainting \n you should change 'sample_size' to 64 in the"
- " configuration file. Please make sure to update the config accordingly as leaving `sample_size=32`"
- " in the config might lead to incorrect results in future versions. If you have downloaded this"
- " checkpoint from the Hugging Face Hub, it would be very nice if you could open a Pull request for"
- " the `unet/config.json` file"
- )
- deprecate("sample_size<64", "1.0.0", deprecation_message, standard_warn=False)
- new_config = dict(unet.config)
- new_config["sample_size"] = 64
- unet._internal_dict = FrozenDict(new_config)
-
- self.register_modules(
- vae=vae,
- text_encoder=text_encoder,
- tokenizer=tokenizer,
- unet=unet,
- scheduler=scheduler,
- )
- self.vae_scale_factor = 2 ** (len(self.vae.config.block_out_channels) - 1)
-
- def enable_vae_slicing(self):
- self.vae.enable_slicing()
-
- def disable_vae_slicing(self):
- self.vae.disable_slicing()
-
- def enable_sequential_cpu_offload(self, gpu_id=0):
- if is_accelerate_available():
- from accelerate import cpu_offload
- else:
- raise ImportError("Please install accelerate via `pip install accelerate`")
-
- device = torch.device(f"cuda:{gpu_id}")
-
- for cpu_offloaded_model in [self.unet, self.text_encoder, self.vae]:
- if cpu_offloaded_model is not None:
- cpu_offload(cpu_offloaded_model, device)
-
- @property
- def _execution_device(self):
- if self.device != torch.device("meta") or not hasattr(self.unet, "_hf_hook"):
- return self.device
- for module in self.unet.modules():
- if (
- hasattr(module, "_hf_hook")
- and hasattr(module._hf_hook, "execution_device")
- and module._hf_hook.execution_device is not None
- ):
- return torch.device(module._hf_hook.execution_device)
- return self.device
-
- def _encode_prompt(self, prompt, device, num_videos_per_prompt, do_classifier_free_guidance, negative_prompt):
- batch_size = len(prompt) if isinstance(prompt, list) else 1
-
- text_inputs = self.tokenizer(
- prompt,
- padding="max_length",
- max_length=self.tokenizer.model_max_length,
- truncation=True,
- return_tensors="pt",
- )
- text_input_ids = text_inputs.input_ids
- untruncated_ids = self.tokenizer(prompt, padding="longest", return_tensors="pt").input_ids
-
- if untruncated_ids.shape[-1] >= text_input_ids.shape[-1] and not torch.equal(text_input_ids, untruncated_ids):
- removed_text = self.tokenizer.batch_decode(untruncated_ids[:, self.tokenizer.model_max_length - 1 : -1])
- logger.warning(
- "The following part of your input was truncated because CLIP can only handle sequences up to"
- f" {self.tokenizer.model_max_length} tokens: {removed_text}"
- )
-
- if hasattr(self.text_encoder.config, "use_attention_mask") and self.text_encoder.config.use_attention_mask:
- attention_mask = text_inputs.attention_mask.to(device)
- else:
- attention_mask = None
-
- text_embeddings = self.text_encoder(
- text_input_ids.to(device),
- attention_mask=attention_mask,
- )
- text_embeddings = text_embeddings[0]
-
- # duplicate text embeddings for each generation per prompt, using mps friendly method
- bs_embed, seq_len, _ = text_embeddings.shape
- text_embeddings = text_embeddings.repeat(1, num_videos_per_prompt, 1)
- text_embeddings = text_embeddings.view(bs_embed * num_videos_per_prompt, seq_len, -1)
-
- # get unconditional embeddings for classifier free guidance
- if do_classifier_free_guidance:
- uncond_tokens: List[str]
- if negative_prompt is None:
- uncond_tokens = [""] * batch_size
- elif type(prompt) is not type(negative_prompt):
- raise TypeError(
- f"`negative_prompt` should be the same type to `prompt`, but got {type(negative_prompt)} !="
- f" {type(prompt)}."
- )
- elif isinstance(negative_prompt, str):
- uncond_tokens = [negative_prompt]
- elif batch_size != len(negative_prompt):
- raise ValueError(
- f"`negative_prompt`: {negative_prompt} has batch size {len(negative_prompt)}, but `prompt`:"
- f" {prompt} has batch size {batch_size}. Please make sure that passed `negative_prompt` matches"
- " the batch size of `prompt`."
- )
- else:
- uncond_tokens = negative_prompt
-
- max_length = text_input_ids.shape[-1]
- uncond_input = self.tokenizer(
- uncond_tokens,
- padding="max_length",
- max_length=max_length,
- truncation=True,
- return_tensors="pt",
- )
-
- if hasattr(self.text_encoder.config, "use_attention_mask") and self.text_encoder.config.use_attention_mask:
- attention_mask = uncond_input.attention_mask.to(device)
- else:
- attention_mask = None
-
- uncond_embeddings = self.text_encoder(
- uncond_input.input_ids.to(device),
- attention_mask=attention_mask,
- )
- uncond_embeddings = uncond_embeddings[0]
-
- # duplicate unconditional embeddings for each generation per prompt, using mps friendly method
- seq_len = uncond_embeddings.shape[1]
- uncond_embeddings = uncond_embeddings.repeat(1, num_videos_per_prompt, 1)
- uncond_embeddings = uncond_embeddings.view(batch_size * num_videos_per_prompt, seq_len, -1)
-
- # For classifier free guidance, we need to do two forward passes.
- # Here we concatenate the unconditional and text embeddings into a single batch
- # to avoid doing two forward passes
- text_embeddings = torch.cat([uncond_embeddings, text_embeddings])
-
- return text_embeddings
-
- def decode_latents(self, latents):
- video_length = latents.shape[2]
- latents = 1 / 0.18215 * latents
- latents = rearrange(latents, "b c f h w -> (b f) c h w")
- video = self.vae.decode(latents).sample
- video = rearrange(video, "(b f) c h w -> b c f h w", f=video_length)
- video = (video / 2 + 0.5).clamp(0, 1)
- # we always cast to float32 as this does not cause significant overhead and is compatible with bfloa16
- video = video.cpu().float().numpy()
- return video
-
- def prepare_extra_step_kwargs(self, generator, eta):
- # prepare extra kwargs for the scheduler step, since not all schedulers have the same signature
- # eta (η) is only used with the DDIMScheduler, it will be ignored for other schedulers.
- # eta corresponds to η in DDIM paper: https://arxiv.org/abs/2010.02502
- # and should be between [0, 1]
-
- accepts_eta = "eta" in set(inspect.signature(self.scheduler.step).parameters.keys())
- extra_step_kwargs = {}
- if accepts_eta:
- extra_step_kwargs["eta"] = eta
-
- # check if the scheduler accepts generator
- accepts_generator = "generator" in set(inspect.signature(self.scheduler.step).parameters.keys())
- if accepts_generator:
- extra_step_kwargs["generator"] = generator
- return extra_step_kwargs
-
- def check_inputs(self, prompt, height, width, callback_steps):
- if not isinstance(prompt, str) and not isinstance(prompt, list):
- raise ValueError(f"`prompt` has to be of type `str` or `list` but is {type(prompt)}")
-
- if height % 8 != 0 or width % 8 != 0:
- raise ValueError(f"`height` and `width` have to be divisible by 8 but are {height} and {width}.")
-
- if (callback_steps is None) or (
- callback_steps is not None and (not isinstance(callback_steps, int) or callback_steps <= 0)
- ):
- raise ValueError(
- f"`callback_steps` has to be a positive integer but is {callback_steps} of type"
- f" {type(callback_steps)}."
- )
-
- def prepare_latents(
- self, batch_size, num_channels_latents, video_length, height, width, dtype, device, generator, latents=None
- ):
- shape = (
- batch_size,
- num_channels_latents,
- video_length,
- height // self.vae_scale_factor,
- width // self.vae_scale_factor,
- )
- if isinstance(generator, list) and len(generator) != batch_size:
- raise ValueError(
- f"You have passed a list of generators of length {len(generator)}, but requested an effective batch"
- f" size of {batch_size}. Make sure the batch size matches the length of the generators."
- )
-
- if latents is None:
- rand_device = "cpu" if device.type == "mps" else device
-
- if isinstance(generator, list):
- shape = (1,) + shape[1:]
- latents = [
- torch.randn(shape, generator=generator[i], device=rand_device, dtype=dtype)
- for i in range(batch_size)
- ]
- latents = torch.cat(latents, dim=0).to(device)
- else:
- latents = torch.randn(shape, generator=generator, device=rand_device, dtype=dtype).to(device)
- else:
- if latents.shape != shape:
- raise ValueError(f"Unexpected latents shape, got {latents.shape}, expected {shape}")
- latents = latents.to(device)
-
- # scale the initial noise by the standard deviation required by the scheduler
- latents = latents * self.scheduler.init_noise_sigma
- return latents
-
- @torch.no_grad()
- def __call__(
- self,
- prompt: Union[str, List[str]],
- video_length: Optional[int],
- height: Optional[int] = None,
- width: Optional[int] = None,
- num_inference_steps: int = 50,
- guidance_scale: float = 7.5,
- negative_prompt: Optional[Union[str, List[str]]] = None,
- num_videos_per_prompt: Optional[int] = 1,
- eta: float = 0.0,
- generator: Optional[Union[torch.Generator, List[torch.Generator]]] = None,
- latents: Optional[torch.FloatTensor] = None,
- output_type: Optional[str] = "tensor",
- return_dict: bool = True,
- callback: Optional[Callable[[int, int, torch.FloatTensor], None]] = None,
- callback_steps: Optional[int] = 1,
- **kwargs,
- ):
- # Default height and width to unet
- height = height or self.unet.config.sample_size * self.vae_scale_factor
- width = width or self.unet.config.sample_size * self.vae_scale_factor
-
- # Check inputs. Raise error if not correct
- self.check_inputs(prompt, height, width, callback_steps)
-
- # Define call parameters
- batch_size = 1 if isinstance(prompt, str) else len(prompt)
- device = self._execution_device
- # here `guidance_scale` is defined analog to the guidance weight `w` of equation (2)
- # of the Imagen paper: https://arxiv.org/pdf/2205.11487.pdf . `guidance_scale = 1`
- # corresponds to doing no classifier free guidance.
- do_classifier_free_guidance = guidance_scale > 1.0
-
- # Encode input prompt
- text_embeddings = self._encode_prompt(
- prompt, device, num_videos_per_prompt, do_classifier_free_guidance, negative_prompt
- )
-
- # Prepare timesteps
- self.scheduler.set_timesteps(num_inference_steps, device=device)
- timesteps = self.scheduler.timesteps
-
- # Prepare latent variables
- num_channels_latents = self.unet.in_channels
- latents = self.prepare_latents(
- batch_size * num_videos_per_prompt,
- num_channels_latents,
- video_length,
- height,
- width,
- text_embeddings.dtype,
- device,
- generator,
- latents,
- )
- latents_dtype = latents.dtype
-
- # Prepare extra step kwargs.
- extra_step_kwargs = self.prepare_extra_step_kwargs(generator, eta)
-
- # Denoising loop
- num_warmup_steps = len(timesteps) - num_inference_steps * self.scheduler.order
- with self.progress_bar(total=num_inference_steps) as progress_bar:
- for i, t in enumerate(timesteps):
- # expand the latents if we are doing classifier free guidance
- latent_model_input = torch.cat([latents] * 2) if do_classifier_free_guidance else latents
- latent_model_input = self.scheduler.scale_model_input(latent_model_input, t)
-
- # predict the noise residual
- noise_pred = self.unet(latent_model_input, t, encoder_hidden_states=text_embeddings).sample.to(
- dtype=latents_dtype
- )
-
- # perform guidance
- if do_classifier_free_guidance:
- noise_pred_uncond, noise_pred_text = noise_pred.chunk(2)
- noise_pred = noise_pred_uncond + guidance_scale * (noise_pred_text - noise_pred_uncond)
-
- # compute the previous noisy sample x_t -> x_t-1
- latents = self.scheduler.step(noise_pred, t, latents, **extra_step_kwargs).prev_sample
-
- # call the callback, if provided
- if i == len(timesteps) - 1 or ((i + 1) > num_warmup_steps and (i + 1) % self.scheduler.order == 0):
- progress_bar.update()
- if callback is not None and i % callback_steps == 0:
- callback(i, t, latents)
-
- # Post-processing
- video = self.decode_latents(latents)
-
- # Convert to tensor
- if output_type == "tensor":
- video = torch.from_numpy(video)
-
- if not return_dict:
- return video
-
- return TuneAVideoPipelineOutput(videos=video)
diff --git a/spaces/OpenGVLab/InternGPT/iGPT/models/grit_src/third_party/CenterNet2/projects/CenterNet2/centernet/modeling/backbone/fpn_p5.py b/spaces/OpenGVLab/InternGPT/iGPT/models/grit_src/third_party/CenterNet2/projects/CenterNet2/centernet/modeling/backbone/fpn_p5.py
deleted file mode 100644
index e991f9c7be095e2a40e12c849b35e246cd0344bd..0000000000000000000000000000000000000000
--- a/spaces/OpenGVLab/InternGPT/iGPT/models/grit_src/third_party/CenterNet2/projects/CenterNet2/centernet/modeling/backbone/fpn_p5.py
+++ /dev/null
@@ -1,78 +0,0 @@
-# Copyright (c) Facebook, Inc. and its affiliates. All Rights Reserved
-import math
-import fvcore.nn.weight_init as weight_init
-import torch.nn.functional as F
-from torch import nn
-
-from detectron2.layers import Conv2d, ShapeSpec, get_norm
-
-from detectron2.modeling.backbone import Backbone
-from detectron2.modeling.backbone.fpn import FPN
-from detectron2.modeling.backbone.build import BACKBONE_REGISTRY
-from detectron2.modeling.backbone.resnet import build_resnet_backbone
-
-
-class LastLevelP6P7_P5(nn.Module):
- """
- This module is used in RetinaNet to generate extra layers, P6 and P7 from
- C5 feature.
- """
-
- def __init__(self, in_channels, out_channels):
- super().__init__()
- self.num_levels = 2
- self.in_feature = "p5"
- self.p6 = nn.Conv2d(in_channels, out_channels, 3, 2, 1)
- self.p7 = nn.Conv2d(out_channels, out_channels, 3, 2, 1)
- for module in [self.p6, self.p7]:
- weight_init.c2_xavier_fill(module)
-
- def forward(self, c5):
- p6 = self.p6(c5)
- p7 = self.p7(F.relu(p6))
- return [p6, p7]
-
-
-@BACKBONE_REGISTRY.register()
-def build_p67_resnet_fpn_backbone(cfg, input_shape: ShapeSpec):
- """
- Args:
- cfg: a detectron2 CfgNode
-
- Returns:
- backbone (Backbone): backbone module, must be a subclass of :class:`Backbone`.
- """
- bottom_up = build_resnet_backbone(cfg, input_shape)
- in_features = cfg.MODEL.FPN.IN_FEATURES
- out_channels = cfg.MODEL.FPN.OUT_CHANNELS
- backbone = FPN(
- bottom_up=bottom_up,
- in_features=in_features,
- out_channels=out_channels,
- norm=cfg.MODEL.FPN.NORM,
- top_block=LastLevelP6P7_P5(out_channels, out_channels),
- fuse_type=cfg.MODEL.FPN.FUSE_TYPE,
- )
- return backbone
-
-@BACKBONE_REGISTRY.register()
-def build_p35_resnet_fpn_backbone(cfg, input_shape: ShapeSpec):
- """
- Args:
- cfg: a detectron2 CfgNode
-
- Returns:
- backbone (Backbone): backbone module, must be a subclass of :class:`Backbone`.
- """
- bottom_up = build_resnet_backbone(cfg, input_shape)
- in_features = cfg.MODEL.FPN.IN_FEATURES
- out_channels = cfg.MODEL.FPN.OUT_CHANNELS
- backbone = FPN(
- bottom_up=bottom_up,
- in_features=in_features,
- out_channels=out_channels,
- norm=cfg.MODEL.FPN.NORM,
- top_block=None,
- fuse_type=cfg.MODEL.FPN.FUSE_TYPE,
- )
- return backbone
\ No newline at end of file
diff --git a/spaces/OptimalScale/Robin-7b/lmflow/models/base_model.py b/spaces/OptimalScale/Robin-7b/lmflow/models/base_model.py
deleted file mode 100644
index 335dbe963e442d735667713c80152a452970c3f6..0000000000000000000000000000000000000000
--- a/spaces/OptimalScale/Robin-7b/lmflow/models/base_model.py
+++ /dev/null
@@ -1,12 +0,0 @@
-#!/usr/bin/env python
-# coding=utf-8
-"""Base model class.
-"""
-
-from abc import ABC
-
-
-class BaseModel(ABC):
-
- def __init__(self, *args, **kwargs):
- pass
diff --git a/spaces/Osborn-bh/ChatGLM3-6B-Osborn/utils.py b/spaces/Osborn-bh/ChatGLM3-6B-Osborn/utils.py
deleted file mode 100644
index f9abe721fadc1517788de30c850f7889273e05c0..0000000000000000000000000000000000000000
--- a/spaces/Osborn-bh/ChatGLM3-6B-Osborn/utils.py
+++ /dev/null
@@ -1,201 +0,0 @@
-import gc
-import os
-from copy import deepcopy
-from typing import Dict, Union, Optional
-
-import torch
-from torch.nn import Module
-from transformers import AutoModel, PreTrainedModel, PreTrainedTokenizer
-from transformers.generation.logits_process import LogitsProcessor
-
-
-def auto_configure_device_map(num_gpus: int) -> Dict[str, int]:
- # transformer.word_embeddings 占用1层
- # transformer.final_layernorm 和 lm_head 占用1层
- # transformer.layers 占用 28 层
- # 总共30层分配到num_gpus张卡上
- num_trans_layers = 28
- per_gpu_layers = 30 / num_gpus
-
- # bugfix: 在linux中调用torch.embedding传入的weight,input不在同一device上,导致RuntimeError
- # windows下 model.device 会被设置成 transformer.word_embeddings.device
- # linux下 model.device 会被设置成 lm_head.device
- # 在调用chat或者stream_chat时,input_ids会被放到model.device上
- # 如果transformer.word_embeddings.device和model.device不同,则会导致RuntimeError
- # 因此这里将transformer.word_embeddings,transformer.final_layernorm,lm_head都放到第一张卡上
- # 本文件来源于https://github.com/THUDM/ChatGLM-6B/blob/main/utils.py
- # 仅此处做少许修改以支持ChatGLM3
- device_map = {
- 'transformer.embedding.word_embeddings': 0,
- 'transformer.encoder.final_layernorm': 0,
- 'transformer.output_layer': 0,
- 'transformer.rotary_pos_emb': 0,
- 'lm_head': 0
- }
-
- used = 2
- gpu_target = 0
- for i in range(num_trans_layers):
- if used >= per_gpu_layers:
- gpu_target += 1
- used = 0
- assert gpu_target < num_gpus
- device_map[f'transformer.encoder.layers.{i}'] = gpu_target
- used += 1
-
- return device_map
-
-
-def load_model_on_gpus(checkpoint_path: Union[str, os.PathLike], num_gpus: int = 2,
- device_map: Optional[Dict[str, int]] = None, **kwargs) -> Module:
- if num_gpus < 2 and device_map is None:
- model = AutoModel.from_pretrained(checkpoint_path, trust_remote_code=True, **kwargs).half().cuda()
- else:
- from accelerate import dispatch_model
-
- model = AutoModel.from_pretrained(checkpoint_path, trust_remote_code=True, **kwargs).half()
-
- if device_map is None:
- device_map = auto_configure_device_map(num_gpus)
-
- model = dispatch_model(model, device_map=device_map)
-
- return model
-
-
-class InvalidScoreLogitsProcessor(LogitsProcessor):
- def __call__(
- self, input_ids: torch.LongTensor, scores: torch.FloatTensor
- ) -> torch.FloatTensor:
- if torch.isnan(scores).any() or torch.isinf(scores).any():
- scores.zero_()
- scores[..., 5] = 5e4
- return scores
-
-
-def process_response(output, history):
- content = ""
- history = deepcopy(history)
- for response in output.split("<|assistant|>"):
- metadata, content = response.split("\n", maxsplit=1)
- if not metadata.strip():
- content = content.strip()
- history.append(
- {
-
- "role": "assistant",
- "metadata": metadata,
- "content": content
- }
- )
- content = content.replace("[[训练时间]]", "2023年")
- else:
- history.append(
- {
- "role": "assistant",
- "metadata": metadata,
- "content": content
- }
- )
- if history[0]["role"] == "system" and "tools" in history[0]:
- content = "\n".join(content.split("\n")[1:-1])
-
- def tool_call(**kwargs):
- return kwargs
-
- parameters = eval(content)
- content = {
- "name": metadata.strip(),
- "parameters": parameters
- }
- else:
- content = {
- "name": metadata.strip(),
- "content": content
- }
- return content, history
-
-
-@torch.inference_mode()
-def generate_stream_chatglm3(model: PreTrainedModel, tokenizer: PreTrainedTokenizer, params: dict):
- messages = params["messages"]
- temperature = float(params.get("temperature", 1.0))
- repetition_penalty = float(params.get("repetition_penalty", 1.0))
- top_p = float(params.get("top_p", 1.0))
- max_new_tokens = int(params.get("max_tokens", 256))
- echo = params.get("echo", True)
-
- query, role = messages[-1].content, messages[-1].role
- history = [m.dict(exclude_none=True) for m in messages[:-1]]
-
- inputs = tokenizer.build_chat_input(query, history=history, role=role)
- inputs = inputs.to(model.device)
- input_echo_len = len(inputs["input_ids"][0])
-
- if input_echo_len >= model.config.seq_length:
- raise
-
- eos_token_id = [
- tokenizer.eos_token_id,
- tokenizer.get_command("<|user|>"),
- tokenizer.get_command("<|observation|>")
- ]
-
- gen_kwargs = {
- "max_length": max_new_tokens + input_echo_len,
- "do_sample": True if temperature > 1e-5 else False,
- "top_p": top_p,
- "repetition_penalty": repetition_penalty,
- "logits_processor": [InvalidScoreLogitsProcessor()],
- }
- if temperature > 1e-5:
- gen_kwargs["temperature"] = temperature
-
- history.append(
- {
- "role": role,
- "content": query
- }
- )
-
- total_len = 0
- for total_ids in model.stream_generate(**inputs, eos_token_id=eos_token_id, **gen_kwargs):
- total_ids = total_ids.tolist()[0]
- total_len = len(total_ids)
- if echo:
- output_ids = total_ids[:-1]
- else:
- output_ids = total_ids[input_echo_len:-1]
-
- response = tokenizer.decode(output_ids)
- if response and response[-1] != "�":
- yield {
- "text": response,
- "usage": {
- "prompt_tokens": input_echo_len,
- "completion_tokens": total_len - input_echo_len,
- "total_tokens": total_len,
- },
- "finish_reason": None,
- }
-
- # Only last stream result contains finish_reason, we set finish_reason as stop
- ret = {
- "text": response,
- "usage": {
- "prompt_tokens": input_echo_len,
- "completion_tokens": total_len - input_echo_len,
- "total_tokens": total_len,
- },
- "finish_reason": "stop",
- }
- yield ret
-
- gc.collect()
- torch.cuda.empty_cache()
-
-
-def generate_chatglm3(model: PreTrainedModel, tokenizer: PreTrainedTokenizer, params: dict):
- for response in generate_stream_chatglm3(model, tokenizer, params):
- pass
- return response
diff --git a/spaces/PAIR/Text2Video-Zero/annotator/uniformer/mmseg/datasets/chase_db1.py b/spaces/PAIR/Text2Video-Zero/annotator/uniformer/mmseg/datasets/chase_db1.py
deleted file mode 100644
index 8bc29bea14704a4407f83474610cbc3bef32c708..0000000000000000000000000000000000000000
--- a/spaces/PAIR/Text2Video-Zero/annotator/uniformer/mmseg/datasets/chase_db1.py
+++ /dev/null
@@ -1,27 +0,0 @@
-import os.path as osp
-
-from .builder import DATASETS
-from .custom import CustomDataset
-
-
-@DATASETS.register_module()
-class ChaseDB1Dataset(CustomDataset):
- """Chase_db1 dataset.
-
- In segmentation map annotation for Chase_db1, 0 stands for background,
- which is included in 2 categories. ``reduce_zero_label`` is fixed to False.
- The ``img_suffix`` is fixed to '.png' and ``seg_map_suffix`` is fixed to
- '_1stHO.png'.
- """
-
- CLASSES = ('background', 'vessel')
-
- PALETTE = [[120, 120, 120], [6, 230, 230]]
-
- def __init__(self, **kwargs):
- super(ChaseDB1Dataset, self).__init__(
- img_suffix='.png',
- seg_map_suffix='_1stHO.png',
- reduce_zero_label=False,
- **kwargs)
- assert osp.exists(self.img_dir)
diff --git a/spaces/PSLD/PSLD/diffusion-posterior-sampling/bkse/models/losses/gan_loss.py b/spaces/PSLD/PSLD/diffusion-posterior-sampling/bkse/models/losses/gan_loss.py
deleted file mode 100644
index 28416a187cf06de1002b397070278cce52ddcdb7..0000000000000000000000000000000000000000
--- a/spaces/PSLD/PSLD/diffusion-posterior-sampling/bkse/models/losses/gan_loss.py
+++ /dev/null
@@ -1,38 +0,0 @@
-import torch
-import torch.nn as nn
-
-
-# Define GAN loss: [vanilla | lsgan | wgan-gp]
-class GANLoss(nn.Module):
- def __init__(self, gan_type, real_label_val=1.0, fake_label_val=0.0):
- super(GANLoss, self).__init__()
- self.gan_type = gan_type.lower()
- self.real_label_val = real_label_val
- self.fake_label_val = fake_label_val
-
- if self.gan_type == "gan" or self.gan_type == "ragan":
- self.loss = nn.BCEWithLogitsLoss()
- elif self.gan_type == "lsgan":
- self.loss = nn.MSELoss()
- elif self.gan_type == "wgan-gp":
-
- def wgan_loss(input, target):
- # target is boolean
- return -1 * input.mean() if target else input.mean()
-
- self.loss = wgan_loss
- else:
- raise NotImplementedError("GAN type [{:s}] is not found".format(self.gan_type))
-
- def get_target_label(self, input, target_is_real):
- if self.gan_type == "wgan-gp":
- return target_is_real
- if target_is_real:
- return torch.empty_like(input).fill_(self.real_label_val)
- else:
- return torch.empty_like(input).fill_(self.fake_label_val)
-
- def forward(self, input, target_is_real):
- target_label = self.get_target_label(input, target_is_real)
- loss = self.loss(input, target_label)
- return loss
diff --git a/spaces/Pattr/DrumClassification/lilypond-2.24.2/lib/guile/2.2/ccache/language/cps/closure-conversion.go b/spaces/Pattr/DrumClassification/lilypond-2.24.2/lib/guile/2.2/ccache/language/cps/closure-conversion.go
deleted file mode 100644
index d59880174ad996d9c2d1920b3a4f2e978fef3e7a..0000000000000000000000000000000000000000
Binary files a/spaces/Pattr/DrumClassification/lilypond-2.24.2/lib/guile/2.2/ccache/language/cps/closure-conversion.go and /dev/null differ
diff --git a/spaces/Pattr/DrumClassification/lilypond-2.24.2/lib/lilypond/2.24.2/ccache/lily/script.go b/spaces/Pattr/DrumClassification/lilypond-2.24.2/lib/lilypond/2.24.2/ccache/lily/script.go
deleted file mode 100644
index d0fabae8f2ba8a62e073a2f87d84c869d5ba714e..0000000000000000000000000000000000000000
Binary files a/spaces/Pattr/DrumClassification/lilypond-2.24.2/lib/lilypond/2.24.2/ccache/lily/script.go and /dev/null differ
diff --git a/spaces/PeepDaSlan9/bank_deposit_prediction/app.py b/spaces/PeepDaSlan9/bank_deposit_prediction/app.py
deleted file mode 100644
index a6cea0bf5b943093065f7d7b0d70913de24b876f..0000000000000000000000000000000000000000
--- a/spaces/PeepDaSlan9/bank_deposit_prediction/app.py
+++ /dev/null
@@ -1,42 +0,0 @@
-import skops.io as sio
-import gradio as gr
-
-#pipe = sio.load("bank_marketing_pipe.skops", trusted=True)
-pipe = sio.load("sgd_bank_marketing_pipe.skops", trusted=True)
-
-classes = [
- "Not Subscribe",
- "Subscribe"]
-
-
-def classifier(age, job, marital, education, default, balance, housing,loan, contact):
- pred = pipe.predict([[age, job, marital, education, default, balance, housing,loan, contact]])[0]
- label = f"Predicted output: **{classes[pred]}**"
- return label
-
-
-inputs = [
- gr.Slider(10, 90, step=1, label="Age"),
- gr.Dropdown(["admin.","unknown","unemployed","management","housemaid","entrepreneur","student","blue-collar",
- "self-employed","retired","technician","services"], label="Job", multiselect=False),
- gr.Dropdown(["married","divorced","single"], label="Marital", multiselect=False),
- gr.Dropdown(["unknown","secondary","primary","tertiary"], label="Education", multiselect=False),
- gr.Radio(["yes","no"], label="Default", info='has credit in default?'),
- gr.Slider(-100000, 100000, step=1, label="Balance"),
- gr.Radio(["yes","no"], label="Housing", info='has housing loan?'),
- gr.Radio(["yes","no"], label="Loan", info='has personal loan?'),
- gr.Dropdown(["unknown","telephone","cellular"], label="Contact")
-]
-
-outputs = [gr.Label(num_top_classes=2)]
-
-title = "Deposit Subscription Prediction"
-description = "Enter the details to identify where or not the customer is subscribed or not subscribed for deposit"
-
-gr.Interface(
- fn=classifier,
- inputs=inputs,
- outputs=outputs,
- title=title,
- description=description,
-).launch()
\ No newline at end of file
diff --git a/spaces/Pepsr/Chatbot/README.md b/spaces/Pepsr/Chatbot/README.md
deleted file mode 100644
index f8c707d3b76eb23a69f8e0c114c800317d71f456..0000000000000000000000000000000000000000
--- a/spaces/Pepsr/Chatbot/README.md
+++ /dev/null
@@ -1,13 +0,0 @@
----
-title: Chatbot
-emoji: 💩
-colorFrom: gray
-colorTo: yellow
-sdk: gradio
-sdk_version: 3.23.0
-app_file: app.py
-pinned: false
-license: unknown
----
-
-Check out the configuration reference at https://huggingface.co/docs/hub/spaces-config-reference
diff --git a/spaces/Pie31415/control-animation/annotator/uniformer/mmcv/ops/deform_roi_pool.py b/spaces/Pie31415/control-animation/annotator/uniformer/mmcv/ops/deform_roi_pool.py
deleted file mode 100644
index cc245ba91fee252226ba22e76bb94a35db9a629b..0000000000000000000000000000000000000000
--- a/spaces/Pie31415/control-animation/annotator/uniformer/mmcv/ops/deform_roi_pool.py
+++ /dev/null
@@ -1,204 +0,0 @@
-# Copyright (c) OpenMMLab. All rights reserved.
-from torch import nn
-from torch.autograd import Function
-from torch.autograd.function import once_differentiable
-from torch.nn.modules.utils import _pair
-
-from ..utils import ext_loader
-
-ext_module = ext_loader.load_ext(
- '_ext', ['deform_roi_pool_forward', 'deform_roi_pool_backward'])
-
-
-class DeformRoIPoolFunction(Function):
-
- @staticmethod
- def symbolic(g, input, rois, offset, output_size, spatial_scale,
- sampling_ratio, gamma):
- return g.op(
- 'mmcv::MMCVDeformRoIPool',
- input,
- rois,
- offset,
- pooled_height_i=output_size[0],
- pooled_width_i=output_size[1],
- spatial_scale_f=spatial_scale,
- sampling_ratio_f=sampling_ratio,
- gamma_f=gamma)
-
- @staticmethod
- def forward(ctx,
- input,
- rois,
- offset,
- output_size,
- spatial_scale=1.0,
- sampling_ratio=0,
- gamma=0.1):
- if offset is None:
- offset = input.new_zeros(0)
- ctx.output_size = _pair(output_size)
- ctx.spatial_scale = float(spatial_scale)
- ctx.sampling_ratio = int(sampling_ratio)
- ctx.gamma = float(gamma)
-
- assert rois.size(1) == 5, 'RoI must be (idx, x1, y1, x2, y2)!'
-
- output_shape = (rois.size(0), input.size(1), ctx.output_size[0],
- ctx.output_size[1])
- output = input.new_zeros(output_shape)
-
- ext_module.deform_roi_pool_forward(
- input,
- rois,
- offset,
- output,
- pooled_height=ctx.output_size[0],
- pooled_width=ctx.output_size[1],
- spatial_scale=ctx.spatial_scale,
- sampling_ratio=ctx.sampling_ratio,
- gamma=ctx.gamma)
-
- ctx.save_for_backward(input, rois, offset)
- return output
-
- @staticmethod
- @once_differentiable
- def backward(ctx, grad_output):
- input, rois, offset = ctx.saved_tensors
- grad_input = grad_output.new_zeros(input.shape)
- grad_offset = grad_output.new_zeros(offset.shape)
-
- ext_module.deform_roi_pool_backward(
- grad_output,
- input,
- rois,
- offset,
- grad_input,
- grad_offset,
- pooled_height=ctx.output_size[0],
- pooled_width=ctx.output_size[1],
- spatial_scale=ctx.spatial_scale,
- sampling_ratio=ctx.sampling_ratio,
- gamma=ctx.gamma)
- if grad_offset.numel() == 0:
- grad_offset = None
- return grad_input, None, grad_offset, None, None, None, None
-
-
-deform_roi_pool = DeformRoIPoolFunction.apply
-
-
-class DeformRoIPool(nn.Module):
-
- def __init__(self,
- output_size,
- spatial_scale=1.0,
- sampling_ratio=0,
- gamma=0.1):
- super(DeformRoIPool, self).__init__()
- self.output_size = _pair(output_size)
- self.spatial_scale = float(spatial_scale)
- self.sampling_ratio = int(sampling_ratio)
- self.gamma = float(gamma)
-
- def forward(self, input, rois, offset=None):
- return deform_roi_pool(input, rois, offset, self.output_size,
- self.spatial_scale, self.sampling_ratio,
- self.gamma)
-
-
-class DeformRoIPoolPack(DeformRoIPool):
-
- def __init__(self,
- output_size,
- output_channels,
- deform_fc_channels=1024,
- spatial_scale=1.0,
- sampling_ratio=0,
- gamma=0.1):
- super(DeformRoIPoolPack, self).__init__(output_size, spatial_scale,
- sampling_ratio, gamma)
-
- self.output_channels = output_channels
- self.deform_fc_channels = deform_fc_channels
-
- self.offset_fc = nn.Sequential(
- nn.Linear(
- self.output_size[0] * self.output_size[1] *
- self.output_channels, self.deform_fc_channels),
- nn.ReLU(inplace=True),
- nn.Linear(self.deform_fc_channels, self.deform_fc_channels),
- nn.ReLU(inplace=True),
- nn.Linear(self.deform_fc_channels,
- self.output_size[0] * self.output_size[1] * 2))
- self.offset_fc[-1].weight.data.zero_()
- self.offset_fc[-1].bias.data.zero_()
-
- def forward(self, input, rois):
- assert input.size(1) == self.output_channels
- x = deform_roi_pool(input, rois, None, self.output_size,
- self.spatial_scale, self.sampling_ratio,
- self.gamma)
- rois_num = rois.size(0)
- offset = self.offset_fc(x.view(rois_num, -1))
- offset = offset.view(rois_num, 2, self.output_size[0],
- self.output_size[1])
- return deform_roi_pool(input, rois, offset, self.output_size,
- self.spatial_scale, self.sampling_ratio,
- self.gamma)
-
-
-class ModulatedDeformRoIPoolPack(DeformRoIPool):
-
- def __init__(self,
- output_size,
- output_channels,
- deform_fc_channels=1024,
- spatial_scale=1.0,
- sampling_ratio=0,
- gamma=0.1):
- super(ModulatedDeformRoIPoolPack,
- self).__init__(output_size, spatial_scale, sampling_ratio, gamma)
-
- self.output_channels = output_channels
- self.deform_fc_channels = deform_fc_channels
-
- self.offset_fc = nn.Sequential(
- nn.Linear(
- self.output_size[0] * self.output_size[1] *
- self.output_channels, self.deform_fc_channels),
- nn.ReLU(inplace=True),
- nn.Linear(self.deform_fc_channels, self.deform_fc_channels),
- nn.ReLU(inplace=True),
- nn.Linear(self.deform_fc_channels,
- self.output_size[0] * self.output_size[1] * 2))
- self.offset_fc[-1].weight.data.zero_()
- self.offset_fc[-1].bias.data.zero_()
-
- self.mask_fc = nn.Sequential(
- nn.Linear(
- self.output_size[0] * self.output_size[1] *
- self.output_channels, self.deform_fc_channels),
- nn.ReLU(inplace=True),
- nn.Linear(self.deform_fc_channels,
- self.output_size[0] * self.output_size[1] * 1),
- nn.Sigmoid())
- self.mask_fc[2].weight.data.zero_()
- self.mask_fc[2].bias.data.zero_()
-
- def forward(self, input, rois):
- assert input.size(1) == self.output_channels
- x = deform_roi_pool(input, rois, None, self.output_size,
- self.spatial_scale, self.sampling_ratio,
- self.gamma)
- rois_num = rois.size(0)
- offset = self.offset_fc(x.view(rois_num, -1))
- offset = offset.view(rois_num, 2, self.output_size[0],
- self.output_size[1])
- mask = self.mask_fc(x.view(rois_num, -1))
- mask = mask.view(rois_num, 1, self.output_size[0], self.output_size[1])
- d = deform_roi_pool(input, rois, offset, self.output_size,
- self.spatial_scale, self.sampling_ratio,
- self.gamma)
- return d * mask
diff --git a/spaces/Plachta/VITS-Umamusume-voice-synthesizer/text/cleaners.py b/spaces/Plachta/VITS-Umamusume-voice-synthesizer/text/cleaners.py
deleted file mode 100644
index c80e113b2b81a66134800dbdaa29c7d96a0152a7..0000000000000000000000000000000000000000
--- a/spaces/Plachta/VITS-Umamusume-voice-synthesizer/text/cleaners.py
+++ /dev/null
@@ -1,146 +0,0 @@
-import re
-
-
-def japanese_cleaners(text):
- from text.japanese import japanese_to_romaji_with_accent
- text = japanese_to_romaji_with_accent(text)
- text = re.sub(r'([A-Za-z])$', r'\1.', text)
- return text
-
-
-def japanese_cleaners2(text):
- return japanese_cleaners(text).replace('ts', 'ʦ').replace('...', '…')
-
-
-def korean_cleaners(text):
- '''Pipeline for Korean text'''
- from text.korean import latin_to_hangul, number_to_hangul, divide_hangul
- text = latin_to_hangul(text)
- text = number_to_hangul(text)
- text = divide_hangul(text)
- text = re.sub(r'([\u3131-\u3163])$', r'\1.', text)
- return text
-
-
-def chinese_cleaners(text):
- '''Pipeline for Chinese text'''
- from text.mandarin import number_to_chinese, chinese_to_bopomofo, latin_to_bopomofo
- text = number_to_chinese(text)
- text = chinese_to_bopomofo(text)
- text = latin_to_bopomofo(text)
- text = re.sub(r'([ˉˊˇˋ˙])$', r'\1。', text)
- return text
-
-
-def zh_ja_mixture_cleaners(text):
- from text.mandarin import chinese_to_romaji
- from text.japanese import japanese_to_romaji_with_accent
- text = re.sub(r'\[ZH\](.*?)\[ZH\]',
- lambda x: chinese_to_romaji(x.group(1))+' ', text)
- text = re.sub(r'\[JA\](.*?)\[JA\]', lambda x: japanese_to_romaji_with_accent(
- x.group(1)).replace('ts', 'ʦ').replace('u', 'ɯ').replace('...', '…')+' ', text)
- text = re.sub(r'\s+$', '', text)
- text = re.sub(r'([^\.,!\?\-…~])$', r'\1.', text)
- return text
-
-
-def sanskrit_cleaners(text):
- text = text.replace('॥', '।').replace('ॐ', 'ओम्')
- if text[-1] != '।':
- text += ' ।'
- return text
-
-
-def cjks_cleaners(text):
- from text.mandarin import chinese_to_lazy_ipa
- from text.japanese import japanese_to_ipa
- from text.korean import korean_to_lazy_ipa
- from text.sanskrit import devanagari_to_ipa
- from text.english import english_to_lazy_ipa
- text = re.sub(r'\[ZH\](.*?)\[ZH\]',
- lambda x: chinese_to_lazy_ipa(x.group(1))+' ', text)
- text = re.sub(r'\[JA\](.*?)\[JA\]',
- lambda x: japanese_to_ipa(x.group(1))+' ', text)
- text = re.sub(r'\[KO\](.*?)\[KO\]',
- lambda x: korean_to_lazy_ipa(x.group(1))+' ', text)
- text = re.sub(r'\[SA\](.*?)\[SA\]',
- lambda x: devanagari_to_ipa(x.group(1))+' ', text)
- text = re.sub(r'\[EN\](.*?)\[EN\]',
- lambda x: english_to_lazy_ipa(x.group(1))+' ', text)
- text = re.sub(r'\s+$', '', text)
- text = re.sub(r'([^\.,!\?\-…~])$', r'\1.', text)
- return text
-
-
-def cjke_cleaners(text):
- from text.mandarin import chinese_to_lazy_ipa
- from text.japanese import japanese_to_ipa
- from text.korean import korean_to_ipa
- from text.english import english_to_ipa2
- text = re.sub(r'\[ZH\](.*?)\[ZH\]', lambda x: chinese_to_lazy_ipa(x.group(1)).replace(
- 'ʧ', 'tʃ').replace('ʦ', 'ts').replace('ɥan', 'ɥæn')+' ', text)
- text = re.sub(r'\[JA\](.*?)\[JA\]', lambda x: japanese_to_ipa(x.group(1)).replace('ʧ', 'tʃ').replace(
- 'ʦ', 'ts').replace('ɥan', 'ɥæn').replace('ʥ', 'dz')+' ', text)
- text = re.sub(r'\[KO\](.*?)\[KO\]',
- lambda x: korean_to_ipa(x.group(1))+' ', text)
- text = re.sub(r'\[EN\](.*?)\[EN\]', lambda x: english_to_ipa2(x.group(1)).replace('ɑ', 'a').replace(
- 'ɔ', 'o').replace('ɛ', 'e').replace('ɪ', 'i').replace('ʊ', 'u')+' ', text)
- text = re.sub(r'\s+$', '', text)
- text = re.sub(r'([^\.,!\?\-…~])$', r'\1.', text)
- return text
-
-
-def cjke_cleaners2(text):
- from text.mandarin import chinese_to_ipa
- from text.japanese import japanese_to_ipa2
- from text.korean import korean_to_ipa
- from text.english import english_to_ipa2
- text = re.sub(r'\[ZH\](.*?)\[ZH\]',
- lambda x: chinese_to_ipa(x.group(1))+' ', text)
- text = re.sub(r'\[JA\](.*?)\[JA\]',
- lambda x: japanese_to_ipa2(x.group(1))+' ', text)
- text = re.sub(r'\[KO\](.*?)\[KO\]',
- lambda x: korean_to_ipa(x.group(1))+' ', text)
- text = re.sub(r'\[EN\](.*?)\[EN\]',
- lambda x: english_to_ipa2(x.group(1))+' ', text)
- text = re.sub(r'\s+$', '', text)
- text = re.sub(r'([^\.,!\?\-…~])$', r'\1.', text)
- return text
-
-
-def thai_cleaners(text):
- from text.thai import num_to_thai, latin_to_thai
- text = num_to_thai(text)
- text = latin_to_thai(text)
- return text
-
-
-def shanghainese_cleaners(text):
- from text.shanghainese import shanghainese_to_ipa
- text = shanghainese_to_ipa(text)
- text = re.sub(r'([^\.,!\?\-…~])$', r'\1.', text)
- return text
-
-
-def chinese_dialect_cleaners(text):
- from text.mandarin import chinese_to_ipa2
- from text.japanese import japanese_to_ipa3
- from text.shanghainese import shanghainese_to_ipa
- from text.cantonese import cantonese_to_ipa
- from text.english import english_to_lazy_ipa2
- from text.ngu_dialect import ngu_dialect_to_ipa
- text = re.sub(r'\[ZH\](.*?)\[ZH\]',
- lambda x: chinese_to_ipa2(x.group(1))+' ', text)
- text = re.sub(r'\[JA\](.*?)\[JA\]',
- lambda x: japanese_to_ipa3(x.group(1)).replace('Q', 'ʔ')+' ', text)
- text = re.sub(r'\[SH\](.*?)\[SH\]', lambda x: shanghainese_to_ipa(x.group(1)).replace('1', '˥˧').replace('5',
- '˧˧˦').replace('6', '˩˩˧').replace('7', '˥').replace('8', '˩˨').replace('ᴀ', 'ɐ').replace('ᴇ', 'e')+' ', text)
- text = re.sub(r'\[GD\](.*?)\[GD\]',
- lambda x: cantonese_to_ipa(x.group(1))+' ', text)
- text = re.sub(r'\[EN\](.*?)\[EN\]',
- lambda x: english_to_lazy_ipa2(x.group(1))+' ', text)
- text = re.sub(r'\[([A-Z]{2})\](.*?)\[\1\]', lambda x: ngu_dialect_to_ipa(x.group(2), x.group(
- 1)).replace('ʣ', 'dz').replace('ʥ', 'dʑ').replace('ʦ', 'ts').replace('ʨ', 'tɕ')+' ', text)
- text = re.sub(r'\s+$', '', text)
- text = re.sub(r'([^\.,!\?\-…~])$', r'\1.', text)
- return text
diff --git a/spaces/Pranjal-666/DL_bearTypeTest/README.md b/spaces/Pranjal-666/DL_bearTypeTest/README.md
deleted file mode 100644
index 7ce32077f4b50810837ec559b047097be35ad42d..0000000000000000000000000000000000000000
--- a/spaces/Pranjal-666/DL_bearTypeTest/README.md
+++ /dev/null
@@ -1,13 +0,0 @@
----
-title: Test
-emoji: 💩
-colorFrom: blue
-colorTo: red
-sdk: gradio
-sdk_version: 3.24.1
-app_file: app.py
-pinned: false
-license: apache-2.0
----
-
-Check out the configuration reference at https://huggingface.co/docs/hub/spaces-config-reference
diff --git a/spaces/PrinceDeven78/Dreamlike-Webui-CPU/README.md b/spaces/PrinceDeven78/Dreamlike-Webui-CPU/README.md
deleted file mode 100644
index d9abbd28f920f5d2920b3e6293a3089f30611520..0000000000000000000000000000000000000000
--- a/spaces/PrinceDeven78/Dreamlike-Webui-CPU/README.md
+++ /dev/null
@@ -1,14 +0,0 @@
----
-title: Dreamlike Webui on Cpu
-emoji: 🌈🌈
-colorFrom: pink
-colorTo: teal
-sdk: gradio
-sdk_version: 3.29.0
-app_file: app.py
-pinned: true
-python_version: 3.10.6
-duplicated_from: Yntec/Dreamlike-Webui-CPU
----
-
-Check out the configuration reference at https://huggingface.co/docs/hub/spaces-config-reference
diff --git a/spaces/Prof-Reza/Audiocraft_Music-Audio_Generation/audiocraft/losses/__init__.py b/spaces/Prof-Reza/Audiocraft_Music-Audio_Generation/audiocraft/losses/__init__.py
deleted file mode 100644
index d55107b2c11822cab749ed3683cf19020802898a..0000000000000000000000000000000000000000
--- a/spaces/Prof-Reza/Audiocraft_Music-Audio_Generation/audiocraft/losses/__init__.py
+++ /dev/null
@@ -1,21 +0,0 @@
-# Copyright (c) Meta Platforms, Inc. and affiliates.
-# All rights reserved.
-#
-# This source code is licensed under the license found in the
-# LICENSE file in the root directory of this source tree.
-"""Loss related classes and functions. In particular the loss balancer from
-EnCodec, and the usual spectral losses."""
-
-# flake8: noqa
-from .balancer import Balancer
-from .sisnr import SISNR
-from .stftloss import (
- LogSTFTMagnitudeLoss,
- MRSTFTLoss,
- SpectralConvergenceLoss,
- STFTLoss
-)
-from .specloss import (
- MelSpectrogramL1Loss,
- MultiScaleMelSpectrogramLoss,
-)
diff --git a/spaces/ProteinDesignLab/protpardelle/core/protein_mpnn.py b/spaces/ProteinDesignLab/protpardelle/core/protein_mpnn.py
deleted file mode 100644
index f00c784b47d1f79264b00d9520aa3d7abbd83df8..0000000000000000000000000000000000000000
--- a/spaces/ProteinDesignLab/protpardelle/core/protein_mpnn.py
+++ /dev/null
@@ -1,1886 +0,0 @@
-# MIT License
-
-# Copyright (c) 2022 Justas Dauparas
-
-# Permission is hereby granted, free of charge, to any person obtaining a copy
-# of this software and associated documentation files (the "Software"), to deal
-# in the Software without restriction, including without limitation the rights
-# to use, copy, modify, merge, publish, distribute, sublicense, and/or sell
-# copies of the Software, and to permit persons to whom the Software is
-# furnished to do so, subject to the following conditions:
-
-# The above copyright notice and this permission notice shall be included in all
-# copies or substantial portions of the Software.
-
-# THE SOFTWARE IS PROVIDED "AS IS", WITHOUT WARRANTY OF ANY KIND, EXPRESS OR
-# IMPLIED, INCLUDING BUT NOT LIMITED TO THE WARRANTIES OF MERCHANTABILITY,
-# FITNESS FOR A PARTICULAR PURPOSE AND NONINFRINGEMENT. IN NO EVENT SHALL THE
-# AUTHORS OR COPYRIGHT HOLDERS BE LIABLE FOR ANY CLAIM, DAMAGES OR OTHER
-# LIABILITY, WHETHER IN AN ACTION OF CONTRACT, TORT OR OTHERWISE, ARISING FROM,
-# OUT OF OR IN CONNECTION WITH THE SOFTWARE OR THE USE OR OTHER DEALINGS IN THE
-# SOFTWARE.
-
-'''
-Adapted from original code by alexechu.
-'''
-import json, time, os, sys, glob
-import shutil
-import warnings
-import copy
-import random
-import os.path
-import subprocess
-import itertools
-
-from einops.layers.torch import Rearrange
-import numpy as np
-import torch
-from torch import optim
-from torch.utils.data import DataLoader
-from torch.utils.data.dataset import random_split, Subset
-import torch.nn as nn
-import torch.nn.functional as F
-
-
-def get_mpnn_model(model_name='v_48_020', path_to_model_weights='', ca_only=False, backbone_noise=0.0, verbose=False, device=None):
- hidden_dim = 128
- num_layers = 3
- if device is None:
- device = torch.device("cuda:0" if (torch.cuda.is_available()) else "cpu")
-
- if path_to_model_weights:
- model_folder_path = path_to_model_weights
- if model_folder_path[-1] != '/':
- model_folder_path = model_folder_path + '/'
- else:
- file_path = os.path.realpath(__file__)
- k = file_path.rfind("/")
- k = file_path[:k].rfind("/")
- if ca_only:
- model_folder_path = file_path[:k] + '/ProteinMPNN/ca_model_weights/'
- else:
- model_folder_path = file_path[:k] + '/ProteinMPNN/vanilla_model_weights/'
-
- checkpoint_path = model_folder_path + f'{model_name}.pt'
- checkpoint = torch.load(checkpoint_path, map_location=device)
- noise_level_print = checkpoint['noise_level']
- model = ProteinMPNN(ca_only=ca_only, num_letters=21, node_features=hidden_dim, edge_features=hidden_dim, hidden_dim=hidden_dim,
- num_encoder_layers=num_layers, num_decoder_layers=num_layers, augment_eps=backbone_noise, k_neighbors=checkpoint['num_edges'])
- model.to(device)
- model.load_state_dict(checkpoint['model_state_dict'])
- model.eval()
-
- if verbose:
- print(40*'-')
- print('Model loaded...')
- print('Number of edges:', checkpoint['num_edges'])
- print(f'Training noise level: {noise_level_print}A')
-
- return model
-
-
-def run_proteinmpnn(model=None, pdb_path='', pdb_path_chains='', path_to_model_weights='', model_name='v_48_020', seed=0, ca_only=False, out_folder='', num_seq_per_target=1, batch_size=1, sampling_temps=[0.1], backbone_noise=0.0, max_length=200000, omit_AAs=[], print_all=False,
- chain_id_jsonl='', fixed_positions_jsonl='', pssm_jsonl='', omit_AA_jsonl='', bias_AA_jsonl='', tied_positions_jsonl='', bias_by_res_jsonl='', jsonl_path='',
- pssm_threshold=0.0, pssm_multi=0.0, pssm_log_odds_flag=False, pssm_bias_flag=False, write_output_files=False):
-
- if model is None:
- model = get_mpnn_model(model_name=model_name, path_to_model_weights=path_to_model_weights, ca_only=ca_only, backbone_noise=backbone_noise, verbose=print_all)
-
- if seed:
- seed=seed
- else:
- seed=int(np.random.randint(0, high=999, size=1, dtype=int)[0])
-
- torch.manual_seed(seed)
- random.seed(seed)
- np.random.seed(seed)
-
-
-
- NUM_BATCHES = num_seq_per_target//batch_size
- BATCH_COPIES = batch_size
- temperatures = sampling_temps
- omit_AAs_list = omit_AAs
- alphabet = 'ACDEFGHIKLMNPQRSTVWYX'
- alphabet_dict = dict(zip(alphabet, range(21)))
- omit_AAs_np = np.array([AA in omit_AAs_list for AA in alphabet]).astype(np.float32)
- device = torch.device("cuda:0" if (torch.cuda.is_available()) else "cpu")
- if os.path.isfile(chain_id_jsonl):
- with open(chain_id_jsonl, 'r') as json_file:
- json_list = list(json_file)
- for json_str in json_list:
- chain_id_dict = json.loads(json_str)
- else:
- chain_id_dict = None
- if print_all:
- print(40*'-')
- print('chain_id_jsonl is NOT loaded')
-
- if os.path.isfile(fixed_positions_jsonl):
- with open(fixed_positions_jsonl, 'r') as json_file:
- json_list = list(json_file)
- for json_str in json_list:
- fixed_positions_dict = json.loads(json_str)
- else:
- if print_all:
- print(40*'-')
- print('fixed_positions_jsonl is NOT loaded')
- fixed_positions_dict = None
-
-
- if os.path.isfile(pssm_jsonl):
- with open(pssm_jsonl, 'r') as json_file:
- json_list = list(json_file)
- pssm_dict = {}
- for json_str in json_list:
- pssm_dict.update(json.loads(json_str))
- else:
- if print_all:
- print(40*'-')
- print('pssm_jsonl is NOT loaded')
- pssm_dict = None
-
-
- if os.path.isfile(omit_AA_jsonl):
- with open(omit_AA_jsonl, 'r') as json_file:
- json_list = list(json_file)
- for json_str in json_list:
- omit_AA_dict = json.loads(json_str)
- else:
- if print_all:
- print(40*'-')
- print('omit_AA_jsonl is NOT loaded')
- omit_AA_dict = None
-
-
- if os.path.isfile(bias_AA_jsonl):
- with open(bias_AA_jsonl, 'r') as json_file:
- json_list = list(json_file)
- for json_str in json_list:
- bias_AA_dict = json.loads(json_str)
- else:
- if print_all:
- print(40*'-')
- print('bias_AA_jsonl is NOT loaded')
- bias_AA_dict = None
-
-
- if os.path.isfile(tied_positions_jsonl):
- with open(tied_positions_jsonl, 'r') as json_file:
- json_list = list(json_file)
- for json_str in json_list:
- tied_positions_dict = json.loads(json_str)
- else:
- if print_all:
- print(40*'-')
- print('tied_positions_jsonl is NOT loaded')
- tied_positions_dict = None
-
-
- if os.path.isfile(bias_by_res_jsonl):
- with open(bias_by_res_jsonl, 'r') as json_file:
- json_list = list(json_file)
-
- for json_str in json_list:
- bias_by_res_dict = json.loads(json_str)
- if print_all:
- print('bias by residue dictionary is loaded')
- else:
- if print_all:
- print(40*'-')
- print('bias by residue dictionary is not loaded, or not provided')
- bias_by_res_dict = None
-
-
- if print_all:
- print(40*'-')
- bias_AAs_np = np.zeros(len(alphabet))
- if bias_AA_dict:
- for n, AA in enumerate(alphabet):
- if AA in list(bias_AA_dict.keys()):
- bias_AAs_np[n] = bias_AA_dict[AA]
-
- if pdb_path:
- pdb_dict_list = parse_PDB(pdb_path, ca_only=ca_only)
- dataset_valid = StructureDatasetPDB(pdb_dict_list, truncate=None, max_length=max_length)
- all_chain_list = [item[-1:] for item in list(pdb_dict_list[0]) if item[:9]=='seq_chain'] #['A','B', 'C',...]
- if pdb_path_chains:
- designed_chain_list = [str(item) for item in pdb_path_chains.split()]
- else:
- designed_chain_list = all_chain_list
- fixed_chain_list = [letter for letter in all_chain_list if letter not in designed_chain_list]
- chain_id_dict = {}
- chain_id_dict[pdb_dict_list[0]['name']]= (designed_chain_list, fixed_chain_list)
- else:
- dataset_valid = StructureDataset(jsonl_path, truncate=None, max_length=max_length, verbose=print_all)
-
- # Build paths for experiment
- if write_output_files:
- folder_for_outputs = out_folder
- base_folder = folder_for_outputs
- if base_folder[-1] != '/':
- base_folder = base_folder + '/'
- if not os.path.exists(base_folder):
- os.makedirs(base_folder)
- if not os.path.exists(base_folder + 'seqs'):
- os.makedirs(base_folder + 'seqs')
-
- # if args.save_score:
- # if not os.path.exists(base_folder + 'scores'):
- # os.makedirs(base_folder + 'scores')
-
- # if args.score_only:
- # if not os.path.exists(base_folder + 'score_only'):
- # os.makedirs(base_folder + 'score_only')
-
-
- # if args.conditional_probs_only:
- # if not os.path.exists(base_folder + 'conditional_probs_only'):
- # os.makedirs(base_folder + 'conditional_probs_only')
-
- # if args.unconditional_probs_only:
- # if not os.path.exists(base_folder + 'unconditional_probs_only'):
- # os.makedirs(base_folder + 'unconditional_probs_only')
-
- # if args.save_probs:
- # if not os.path.exists(base_folder + 'probs'):
- # os.makedirs(base_folder + 'probs')
-
- # Timing
- start_time = time.time()
- total_residues = 0
- protein_list = []
- total_step = 0
- # Validation epoch
- new_mpnn_seqs = []
- with torch.no_grad():
- test_sum, test_weights = 0., 0.
- for ix, protein in enumerate(dataset_valid):
- score_list = []
- global_score_list = []
- all_probs_list = []
- all_log_probs_list = []
- S_sample_list = []
- batch_clones = [copy.deepcopy(protein) for i in range(BATCH_COPIES)]
- X, S, mask, lengths, chain_M, chain_encoding_all, chain_list_list, visible_list_list, masked_list_list, masked_chain_length_list_list, chain_M_pos, omit_AA_mask, residue_idx, dihedral_mask, tied_pos_list_of_lists_list, pssm_coef, pssm_bias, pssm_log_odds_all, bias_by_res_all, tied_beta = tied_featurize(batch_clones, device, chain_id_dict, fixed_positions_dict, omit_AA_dict, tied_positions_dict, pssm_dict, bias_by_res_dict, ca_only=ca_only)
- pssm_log_odds_mask = (pssm_log_odds_all > pssm_threshold).float() #1.0 for true, 0.0 for false
- name_ = batch_clones[0]['name']
- if False:
- pass
- # if args.score_only:
- # loop_c = 0
- # if args.path_to_fasta:
- # fasta_names, fasta_seqs = parse_fasta(args.path_to_fasta, omit=["/"])
- # loop_c = len(fasta_seqs)
- # for fc in range(1+loop_c):
- # if fc == 0:
- # structure_sequence_score_file = base_folder + '/score_only/' + batch_clones[0]['name'] + f'_pdb'
- # else:
- # structure_sequence_score_file = base_folder + '/score_only/' + batch_clones[0]['name'] + f'_fasta_{fc}'
- # native_score_list = []
- # global_native_score_list = []
- # if fc > 0:
- # input_seq_length = len(fasta_seqs[fc-1])
- # S_input = torch.tensor([alphabet_dict[AA] for AA in fasta_seqs[fc-1]], device=device)[None,:].repeat(X.shape[0], 1)
- # S[:,:input_seq_length] = S_input #assumes that S and S_input are alphabetically sorted for masked_chains
- # for j in range(NUM_BATCHES):
- # randn_1 = torch.randn(chain_M.shape, device=X.device)
- # log_probs = model(X, S, mask, chain_M*chain_M_pos, residue_idx, chain_encoding_all, randn_1)
- # mask_for_loss = mask*chain_M*chain_M_pos
- # scores = _scores(S, log_probs, mask_for_loss)
- # native_score = scores.cpu().data.numpy()
- # native_score_list.append(native_score)
- # global_scores = _scores(S, log_probs, mask)
- # global_native_score = global_scores.cpu().data.numpy()
- # global_native_score_list.append(global_native_score)
- # native_score = np.concatenate(native_score_list, 0)
- # global_native_score = np.concatenate(global_native_score_list, 0)
- # ns_mean = native_score.mean()
- # ns_mean_print = np.format_float_positional(np.float32(ns_mean), unique=False, precision=4)
- # ns_std = native_score.std()
- # ns_std_print = np.format_float_positional(np.float32(ns_std), unique=False, precision=4)
-
- # global_ns_mean = global_native_score.mean()
- # global_ns_mean_print = np.format_float_positional(np.float32(global_ns_mean), unique=False, precision=4)
- # global_ns_std = global_native_score.std()
- # global_ns_std_print = np.format_float_positional(np.float32(global_ns_std), unique=False, precision=4)
-
- # ns_sample_size = native_score.shape[0]
- # seq_str = _S_to_seq(S[0,], chain_M[0,])
- # np.savez(structure_sequence_score_file, score=native_score, global_score=global_native_score, S=S[0,].cpu().numpy(), seq_str=seq_str)
- # if print_all:
- # if fc == 0:
- # print(f'Score for {name_} from PDB, mean: {ns_mean_print}, std: {ns_std_print}, sample size: {ns_sample_size}, global score, mean: {global_ns_mean_print}, std: {global_ns_std_print}, sample size: {ns_sample_size}')
- # else:
- # print(f'Score for {name_}_{fc} from FASTA, mean: {ns_mean_print}, std: {ns_std_print}, sample size: {ns_sample_size}, global score, mean: {global_ns_mean_print}, std: {global_ns_std_print}, sample size: {ns_sample_size}')
- # elif args.conditional_probs_only:
- # if print_all:
- # print(f'Calculating conditional probabilities for {name_}')
- # conditional_probs_only_file = base_folder + '/conditional_probs_only/' + batch_clones[0]['name']
- # log_conditional_probs_list = []
- # for j in range(NUM_BATCHES):
- # randn_1 = torch.randn(chain_M.shape, device=X.device)
- # log_conditional_probs = model.conditional_probs(X, S, mask, chain_M*chain_M_pos, residue_idx, chain_encoding_all, randn_1, args.conditional_probs_only_backbone)
- # log_conditional_probs_list.append(log_conditional_probs.cpu().numpy())
- # concat_log_p = np.concatenate(log_conditional_probs_list, 0) #[B, L, 21]
- # mask_out = (chain_M*chain_M_pos*mask)[0,].cpu().numpy()
- # np.savez(conditional_probs_only_file, log_p=concat_log_p, S=S[0,].cpu().numpy(), mask=mask[0,].cpu().numpy(), design_mask=mask_out)
- # elif args.unconditional_probs_only:
- # if print_all:
- # print(f'Calculating sequence unconditional probabilities for {name_}')
- # unconditional_probs_only_file = base_folder + '/unconditional_probs_only/' + batch_clones[0]['name']
- # log_unconditional_probs_list = []
- # for j in range(NUM_BATCHES):
- # log_unconditional_probs = model.unconditional_probs(X, mask, residue_idx, chain_encoding_all)
- # log_unconditional_probs_list.append(log_unconditional_probs.cpu().numpy())
- # concat_log_p = np.concatenate(log_unconditional_probs_list, 0) #[B, L, 21]
- # mask_out = (chain_M*chain_M_pos*mask)[0,].cpu().numpy()
- # np.savez(unconditional_probs_only_file, log_p=concat_log_p, S=S[0,].cpu().numpy(), mask=mask[0,].cpu().numpy(), design_mask=mask_out)
- else:
- randn_1 = torch.randn(chain_M.shape, device=X.device)
- log_probs = model(X, S, mask, chain_M*chain_M_pos, residue_idx, chain_encoding_all, randn_1)
- mask_for_loss = mask*chain_M*chain_M_pos
- scores = _scores(S, log_probs, mask_for_loss) #score only the redesigned part
- native_score = scores.cpu().data.numpy()
- global_scores = _scores(S, log_probs, mask) #score the whole structure-sequence
- global_native_score = global_scores.cpu().data.numpy()
- # Generate some sequences
- if write_output_files:
- ali_file = base_folder + '/seqs/' + batch_clones[0]['name'] + '.fa'
- score_file = base_folder + '/scores/' + batch_clones[0]['name'] + '.npz'
- probs_file = base_folder + '/probs/' + batch_clones[0]['name'] + '.npz'
- f = open(ali_file, 'w')
- if print_all:
- print(f'Generating sequences for: {name_}')
- t0 = time.time()
- for temp in temperatures:
- for j in range(NUM_BATCHES):
- randn_2 = torch.randn(chain_M.shape, device=X.device)
- if tied_positions_dict == None:
- sample_dict = model.sample(X, randn_2, S, chain_M, chain_encoding_all, residue_idx, mask=mask, temperature=temp, omit_AAs_np=omit_AAs_np, bias_AAs_np=bias_AAs_np, chain_M_pos=chain_M_pos, omit_AA_mask=omit_AA_mask, pssm_coef=pssm_coef, pssm_bias=pssm_bias, pssm_multi=pssm_multi, pssm_log_odds_flag=bool(pssm_log_odds_flag), pssm_log_odds_mask=pssm_log_odds_mask, pssm_bias_flag=bool(pssm_bias_flag), bias_by_res=bias_by_res_all)
- S_sample = sample_dict["S"]
- else:
- sample_dict = model.tied_sample(X, randn_2, S, chain_M, chain_encoding_all, residue_idx, mask=mask, temperature=temp, omit_AAs_np=omit_AAs_np, bias_AAs_np=bias_AAs_np, chain_M_pos=chain_M_pos, omit_AA_mask=omit_AA_mask, pssm_coef=pssm_coef, pssm_bias=pssm_bias, pssm_multi=pssm_multi, pssm_log_odds_flag=bool(pssm_log_odds_flag), pssm_log_odds_mask=pssm_log_odds_mask, pssm_bias_flag=bool(pssm_bias_flag), tied_pos=tied_pos_list_of_lists_list[0], tied_beta=tied_beta, bias_by_res=bias_by_res_all)
- # Compute scores
- S_sample = sample_dict["S"]
- log_probs = model(X, S_sample, mask, chain_M*chain_M_pos, residue_idx, chain_encoding_all, randn_2, use_input_decoding_order=True, decoding_order=sample_dict["decoding_order"])
- mask_for_loss = mask*chain_M*chain_M_pos
- scores = _scores(S_sample, log_probs, mask_for_loss)
- scores = scores.cpu().data.numpy()
-
- global_scores = _scores(S_sample, log_probs, mask) #score the whole structure-sequence
- global_scores = global_scores.cpu().data.numpy()
-
- all_probs_list.append(sample_dict["probs"].cpu().data.numpy())
- all_log_probs_list.append(log_probs.cpu().data.numpy())
- S_sample_list.append(S_sample.cpu().data.numpy())
- for b_ix in range(BATCH_COPIES):
- masked_chain_length_list = masked_chain_length_list_list[b_ix]
- masked_list = masked_list_list[b_ix]
- seq_recovery_rate = torch.sum(torch.sum(torch.nn.functional.one_hot(S[b_ix], 21)*torch.nn.functional.one_hot(S_sample[b_ix], 21),axis=-1)*mask_for_loss[b_ix])/torch.sum(mask_for_loss[b_ix])
- seq = _S_to_seq(S_sample[b_ix], chain_M[b_ix])
- new_mpnn_seqs.append(seq)
- score = scores[b_ix]
- score_list.append(score)
- global_score = global_scores[b_ix]
- global_score_list.append(global_score)
- native_seq = _S_to_seq(S[b_ix], chain_M[b_ix])
- if b_ix == 0 and j==0 and temp==temperatures[0]:
- start = 0
- end = 0
- list_of_AAs = []
- for mask_l in masked_chain_length_list:
- end += mask_l
- list_of_AAs.append(native_seq[start:end])
- start = end
- native_seq = "".join(list(np.array(list_of_AAs)[np.argsort(masked_list)]))
- l0 = 0
- for mc_length in list(np.array(masked_chain_length_list)[np.argsort(masked_list)])[:-1]:
- l0 += mc_length
- native_seq = native_seq[:l0] + '/' + native_seq[l0:]
- l0 += 1
- sorted_masked_chain_letters = np.argsort(masked_list_list[0])
- print_masked_chains = [masked_list_list[0][i] for i in sorted_masked_chain_letters]
- sorted_visible_chain_letters = np.argsort(visible_list_list[0])
- print_visible_chains = [visible_list_list[0][i] for i in sorted_visible_chain_letters]
- native_score_print = np.format_float_positional(np.float32(native_score.mean()), unique=False, precision=4)
- global_native_score_print = np.format_float_positional(np.float32(global_native_score.mean()), unique=False, precision=4)
- script_dir = os.path.dirname(os.path.realpath(__file__))
- try:
- commit_str = subprocess.check_output(f'git --git-dir {script_dir}/.git rev-parse HEAD', shell=True, stderr=subprocess.DEVNULL).decode().strip()
- except subprocess.CalledProcessError:
- commit_str = 'unknown'
- if ca_only:
- print_model_name = 'CA_model_name'
- else:
- print_model_name = 'model_name'
- if write_output_files:
- f.write('>{}, score={}, global_score={}, fixed_chains={}, designed_chains={}, {}={}, git_hash={}, seed={}\n{}\n'.format(name_, native_score_print, global_native_score_print, print_visible_chains, print_masked_chains, print_model_name, model_name, commit_str, seed, native_seq)) #write the native sequence
- start = 0
- end = 0
- list_of_AAs = []
- for mask_l in masked_chain_length_list:
- end += mask_l
- list_of_AAs.append(seq[start:end])
- start = end
-
- seq = "".join(list(np.array(list_of_AAs)[np.argsort(masked_list)]))
- l0 = 0
- for mc_length in list(np.array(masked_chain_length_list)[np.argsort(masked_list)])[:-1]:
- l0 += mc_length
- seq = seq[:l0] + '/' + seq[l0:]
- l0 += 1
- score_print = np.format_float_positional(np.float32(score), unique=False, precision=4)
- global_score_print = np.format_float_positional(np.float32(global_score), unique=False, precision=4)
- seq_rec_print = np.format_float_positional(np.float32(seq_recovery_rate.detach().cpu().numpy()), unique=False, precision=4)
- sample_number = j*BATCH_COPIES+b_ix+1
- if write_output_files:
- f.write('>T={}, sample={}, score={}, global_score={}, seq_recovery={}\n{}\n'.format(temp,sample_number,score_print,global_score_print,seq_rec_print,seq)) #write generated sequence
- # if args.save_score:
- # np.savez(score_file, score=np.array(score_list, np.float32), global_score=np.array(global_score_list, np.float32))
- # if args.save_probs:
- # all_probs_concat = np.concatenate(all_probs_list)
- # all_log_probs_concat = np.concatenate(all_log_probs_list)
- # S_sample_concat = np.concatenate(S_sample_list)
- # np.savez(probs_file, probs=np.array(all_probs_concat, np.float32), log_probs=np.array(all_log_probs_concat, np.float32), S=np.array(S_sample_concat, np.int32), mask=mask_for_loss.cpu().data.numpy(), chain_order=chain_list_list)
- t1 = time.time()
- dt = round(float(t1-t0), 4)
- num_seqs = len(temperatures)*NUM_BATCHES*BATCH_COPIES
- total_length = X.shape[1]
- if print_all:
- print(f'{num_seqs} sequences of length {total_length} generated in {dt} seconds')
- if write_output_files:
- f.close()
- return new_mpnn_seqs
-
-
-def parse_fasta(filename,limit=-1, omit=[]):
- header = []
- sequence = []
- lines = open(filename, "r")
- for line in lines:
- line = line.rstrip()
- if line[0] == ">":
- if len(header) == limit:
- break
- header.append(line[1:])
- sequence.append([])
- else:
- if omit:
- line = [item for item in line if item not in omit]
- line = ''.join(line)
- line = ''.join(line)
- sequence[-1].append(line)
- lines.close()
- sequence = [''.join(seq) for seq in sequence]
- return np.array(header), np.array(sequence)
-
-def _scores(S, log_probs, mask):
- """ Negative log probabilities """
- criterion = torch.nn.NLLLoss(reduction='none')
- loss = criterion(
- log_probs.contiguous().view(-1,log_probs.size(-1)),
- S.contiguous().view(-1)
- ).view(S.size())
- scores = torch.sum(loss * mask, dim=-1) / torch.sum(mask, dim=-1)
- return scores
-
-def _S_to_seq(S, mask):
- alphabet = 'ACDEFGHIKLMNPQRSTVWYX'
- seq = ''.join([alphabet[c] for c, m in zip(S.tolist(), mask.tolist()) if m > 0])
- return seq
-
-def parse_PDB_biounits(x, atoms=['N','CA','C'], chain=None):
- '''
- input: x = PDB filename
- atoms = atoms to extract (optional)
- output: (length, atoms, coords=(x,y,z)), sequence
- '''
-
- alpha_1 = list("ARNDCQEGHILKMFPSTWYV-")
- states = len(alpha_1)
- alpha_3 = ['ALA','ARG','ASN','ASP','CYS','GLN','GLU','GLY','HIS','ILE',
- 'LEU','LYS','MET','PHE','PRO','SER','THR','TRP','TYR','VAL','GAP']
-
- aa_1_N = {a:n for n,a in enumerate(alpha_1)}
- aa_3_N = {a:n for n,a in enumerate(alpha_3)}
- aa_N_1 = {n:a for n,a in enumerate(alpha_1)}
- aa_1_3 = {a:b for a,b in zip(alpha_1,alpha_3)}
- aa_3_1 = {b:a for a,b in zip(alpha_1,alpha_3)}
-
- def AA_to_N(x):
- # ["ARND"] -> [[0,1,2,3]]
- x = np.array(x);
- if x.ndim == 0: x = x[None]
- return [[aa_1_N.get(a, states-1) for a in y] for y in x]
-
- def N_to_AA(x):
- # [[0,1,2,3]] -> ["ARND"]
- x = np.array(x);
- if x.ndim == 1: x = x[None]
- return ["".join([aa_N_1.get(a,"-") for a in y]) for y in x]
-
- xyz,seq,min_resn,max_resn = {},{},1e6,-1e6
- for line in open(x,"rb"):
- line = line.decode("utf-8","ignore").rstrip()
-
- if line[:6] == "HETATM" and line[17:17+3] == "MSE":
- line = line.replace("HETATM","ATOM ")
- line = line.replace("MSE","MET")
-
- if line[:4] == "ATOM":
- ch = line[21:22]
- if ch == chain or chain is None:
- atom = line[12:12+4].strip()
- resi = line[17:17+3]
- resn = line[22:22+5].strip()
- x,y,z = [float(line[i:(i+8)]) for i in [30,38,46]]
-
- if resn[-1].isalpha():
- resa,resn = resn[-1],int(resn[:-1])-1
- else:
- resa,resn = "",int(resn)-1
-# resn = int(resn)
- if resn < min_resn:
- min_resn = resn
- if resn > max_resn:
- max_resn = resn
- if resn not in xyz:
- xyz[resn] = {}
- if resa not in xyz[resn]:
- xyz[resn][resa] = {}
- if resn not in seq:
- seq[resn] = {}
- if resa not in seq[resn]:
- seq[resn][resa] = resi
-
- if atom not in xyz[resn][resa]:
- xyz[resn][resa][atom] = np.array([x,y,z])
-
- # convert to numpy arrays, fill in missing values
- seq_,xyz_ = [],[]
- try:
- for resn in range(min_resn,max_resn+1):
- if resn in seq:
- for k in sorted(seq[resn]): seq_.append(aa_3_N.get(seq[resn][k],20))
- else: seq_.append(20)
- if resn in xyz:
- for k in sorted(xyz[resn]):
- for atom in atoms:
- if atom in xyz[resn][k]: xyz_.append(xyz[resn][k][atom])
- else: xyz_.append(np.full(3,np.nan))
- else:
- for atom in atoms: xyz_.append(np.full(3,np.nan))
- return np.array(xyz_).reshape(-1,len(atoms),3), N_to_AA(np.array(seq_))
- except TypeError:
- return 'no_chain', 'no_chain'
-
-def parse_PDB(path_to_pdb, input_chain_list=None, ca_only=False):
- c=0
- pdb_dict_list = []
- init_alphabet = ['A', 'B', 'C', 'D', 'E', 'F', 'G','H', 'I', 'J','K', 'L', 'M', 'N', 'O', 'P', 'Q', 'R', 'S', 'T','U', 'V','W','X', 'Y', 'Z', 'a', 'b', 'c', 'd', 'e', 'f', 'g','h', 'i', 'j','k', 'l', 'm', 'n', 'o', 'p', 'q', 'r', 's', 't','u', 'v','w','x', 'y', 'z']
- extra_alphabet = [str(item) for item in list(np.arange(300))]
- chain_alphabet = init_alphabet + extra_alphabet
-
- if input_chain_list:
- chain_alphabet = input_chain_list
-
-
- biounit_names = [path_to_pdb]
- for biounit in biounit_names:
- my_dict = {}
- s = 0
- concat_seq = ''
- concat_N = []
- concat_CA = []
- concat_C = []
- concat_O = []
- concat_mask = []
- coords_dict = {}
- for letter in chain_alphabet:
- if ca_only:
- sidechain_atoms = ['CA']
- else:
- sidechain_atoms = ['N', 'CA', 'C', 'O']
- xyz, seq = parse_PDB_biounits(biounit, atoms=sidechain_atoms, chain=letter)
- if type(xyz) != str:
- concat_seq += seq[0]
- my_dict['seq_chain_'+letter]=seq[0]
- coords_dict_chain = {}
- if ca_only:
- coords_dict_chain['CA_chain_'+letter]=xyz.tolist()
- else:
- coords_dict_chain['N_chain_' + letter] = xyz[:, 0, :].tolist()
- coords_dict_chain['CA_chain_' + letter] = xyz[:, 1, :].tolist()
- coords_dict_chain['C_chain_' + letter] = xyz[:, 2, :].tolist()
- coords_dict_chain['O_chain_' + letter] = xyz[:, 3, :].tolist()
- my_dict['coords_chain_'+letter]=coords_dict_chain
- s += 1
- fi = biounit.rfind("/")
- my_dict['name']=biounit[(fi+1):-4]
- my_dict['num_of_chains'] = s
- my_dict['seq'] = concat_seq
- if s <= len(chain_alphabet):
- pdb_dict_list.append(my_dict)
- c+=1
- return pdb_dict_list
-
-
-
-def tied_featurize(batch, device, chain_dict, fixed_position_dict=None, omit_AA_dict=None, tied_positions_dict=None, pssm_dict=None, bias_by_res_dict=None, ca_only=False):
- """ Pack and pad batch into torch tensors """
- alphabet = 'ACDEFGHIKLMNPQRSTVWYX'
- B = len(batch)
- lengths = np.array([len(b['seq']) for b in batch], dtype=np.int32) #sum of chain seq lengths
- L_max = max([len(b['seq']) for b in batch])
- if ca_only:
- X = np.zeros([B, L_max, 1, 3])
- else:
- X = np.zeros([B, L_max, 4, 3])
- residue_idx = -100*np.ones([B, L_max], dtype=np.int32)
- chain_M = np.zeros([B, L_max], dtype=np.int32) #1.0 for the bits that need to be predicted
- pssm_coef_all = np.zeros([B, L_max], dtype=np.float32) #1.0 for the bits that need to be predicted
- pssm_bias_all = np.zeros([B, L_max, 21], dtype=np.float32) #1.0 for the bits that need to be predicted
- pssm_log_odds_all = 10000.0*np.ones([B, L_max, 21], dtype=np.float32) #1.0 for the bits that need to be predicted
- chain_M_pos = np.zeros([B, L_max], dtype=np.int32) #1.0 for the bits that need to be predicted
- bias_by_res_all = np.zeros([B, L_max, 21], dtype=np.float32)
- chain_encoding_all = np.zeros([B, L_max], dtype=np.int32) #1.0 for the bits that need to be predicted
- S = np.zeros([B, L_max], dtype=np.int32)
- omit_AA_mask = np.zeros([B, L_max, len(alphabet)], dtype=np.int32)
- # Build the batch
- letter_list_list = []
- visible_list_list = []
- masked_list_list = []
- masked_chain_length_list_list = []
- tied_pos_list_of_lists_list = []
- for i, b in enumerate(batch):
- if chain_dict != None:
- masked_chains, visible_chains = chain_dict[b['name']] #masked_chains a list of chain letters to predict [A, D, F]
- else:
- masked_chains = [item[-1:] for item in list(b) if item[:10]=='seq_chain_']
- visible_chains = []
- masked_chains.sort() #sort masked_chains
- visible_chains.sort() #sort visible_chains
- all_chains = masked_chains + visible_chains
- for i, b in enumerate(batch):
- mask_dict = {}
- a = 0
- x_chain_list = []
- chain_mask_list = []
- chain_seq_list = []
- chain_encoding_list = []
- c = 1
- letter_list = []
- global_idx_start_list = [0]
- visible_list = []
- masked_list = []
- masked_chain_length_list = []
- fixed_position_mask_list = []
- omit_AA_mask_list = []
- pssm_coef_list = []
- pssm_bias_list = []
- pssm_log_odds_list = []
- bias_by_res_list = []
- l0 = 0
- l1 = 0
- for step, letter in enumerate(all_chains):
- if letter in visible_chains:
- letter_list.append(letter)
- visible_list.append(letter)
- chain_seq = b[f'seq_chain_{letter}']
- chain_seq = ''.join([a if a!='-' else 'X' for a in chain_seq])
- chain_length = len(chain_seq)
- global_idx_start_list.append(global_idx_start_list[-1]+chain_length)
- chain_coords = b[f'coords_chain_{letter}'] #this is a dictionary
- chain_mask = np.zeros(chain_length) #0.0 for visible chains
- if ca_only:
- x_chain = np.array(chain_coords[f'CA_chain_{letter}']) #[chain_lenght,1,3] #CA_diff
- if len(x_chain.shape) == 2:
- x_chain = x_chain[:,None,:]
- else:
- x_chain = np.stack([chain_coords[c] for c in [f'N_chain_{letter}', f'CA_chain_{letter}', f'C_chain_{letter}', f'O_chain_{letter}']], 1) #[chain_lenght,4,3]
- x_chain_list.append(x_chain)
- chain_mask_list.append(chain_mask)
- chain_seq_list.append(chain_seq)
- chain_encoding_list.append(c*np.ones(np.array(chain_mask).shape[0]))
- l1 += chain_length
- residue_idx[i, l0:l1] = 100*(c-1)+np.arange(l0, l1)
- l0 += chain_length
- c+=1
- fixed_position_mask = np.ones(chain_length)
- fixed_position_mask_list.append(fixed_position_mask)
- omit_AA_mask_temp = np.zeros([chain_length, len(alphabet)], np.int32)
- omit_AA_mask_list.append(omit_AA_mask_temp)
- pssm_coef = np.zeros(chain_length)
- pssm_bias = np.zeros([chain_length, 21])
- pssm_log_odds = 10000.0*np.ones([chain_length, 21])
- pssm_coef_list.append(pssm_coef)
- pssm_bias_list.append(pssm_bias)
- pssm_log_odds_list.append(pssm_log_odds)
- bias_by_res_list.append(np.zeros([chain_length, 21]))
- if letter in masked_chains:
- masked_list.append(letter)
- letter_list.append(letter)
- chain_seq = b[f'seq_chain_{letter}']
- chain_seq = ''.join([a if a!='-' else 'X' for a in chain_seq])
- chain_length = len(chain_seq)
- global_idx_start_list.append(global_idx_start_list[-1]+chain_length)
- masked_chain_length_list.append(chain_length)
- chain_coords = b[f'coords_chain_{letter}'] #this is a dictionary
- chain_mask = np.ones(chain_length) #1.0 for masked
- if ca_only:
- x_chain = np.array(chain_coords[f'CA_chain_{letter}']) #[chain_lenght,1,3] #CA_diff
- if len(x_chain.shape) == 2:
- x_chain = x_chain[:,None,:]
- else:
- x_chain = np.stack([chain_coords[c] for c in [f'N_chain_{letter}', f'CA_chain_{letter}', f'C_chain_{letter}', f'O_chain_{letter}']], 1) #[chain_lenght,4,3]
- x_chain_list.append(x_chain)
- chain_mask_list.append(chain_mask)
- chain_seq_list.append(chain_seq)
- chain_encoding_list.append(c*np.ones(np.array(chain_mask).shape[0]))
- l1 += chain_length
- residue_idx[i, l0:l1] = 100*(c-1)+np.arange(l0, l1)
- l0 += chain_length
- c+=1
- fixed_position_mask = np.ones(chain_length)
- if fixed_position_dict!=None:
- fixed_pos_list = fixed_position_dict[b['name']][letter]
- if fixed_pos_list:
- fixed_position_mask[np.array(fixed_pos_list)-1] = 0.0
- fixed_position_mask_list.append(fixed_position_mask)
- omit_AA_mask_temp = np.zeros([chain_length, len(alphabet)], np.int32)
- if omit_AA_dict!=None:
- for item in omit_AA_dict[b['name']][letter]:
- idx_AA = np.array(item[0])-1
- AA_idx = np.array([np.argwhere(np.array(list(alphabet))== AA)[0][0] for AA in item[1]]).repeat(idx_AA.shape[0])
- idx_ = np.array([[a, b] for a in idx_AA for b in AA_idx])
- omit_AA_mask_temp[idx_[:,0], idx_[:,1]] = 1
- omit_AA_mask_list.append(omit_AA_mask_temp)
- pssm_coef = np.zeros(chain_length)
- pssm_bias = np.zeros([chain_length, 21])
- pssm_log_odds = 10000.0*np.ones([chain_length, 21])
- if pssm_dict:
- if pssm_dict[b['name']][letter]:
- pssm_coef = pssm_dict[b['name']][letter]['pssm_coef']
- pssm_bias = pssm_dict[b['name']][letter]['pssm_bias']
- pssm_log_odds = pssm_dict[b['name']][letter]['pssm_log_odds']
- pssm_coef_list.append(pssm_coef)
- pssm_bias_list.append(pssm_bias)
- pssm_log_odds_list.append(pssm_log_odds)
- if bias_by_res_dict:
- bias_by_res_list.append(bias_by_res_dict[b['name']][letter])
- else:
- bias_by_res_list.append(np.zeros([chain_length, 21]))
-
-
- letter_list_np = np.array(letter_list)
- tied_pos_list_of_lists = []
- tied_beta = np.ones(L_max)
- if tied_positions_dict!=None:
- tied_pos_list = tied_positions_dict[b['name']]
- if tied_pos_list:
- set_chains_tied = set(list(itertools.chain(*[list(item) for item in tied_pos_list])))
- for tied_item in tied_pos_list:
- one_list = []
- for k, v in tied_item.items():
- start_idx = global_idx_start_list[np.argwhere(letter_list_np == k)[0][0]]
- if isinstance(v[0], list):
- for v_count in range(len(v[0])):
- one_list.append(start_idx+v[0][v_count]-1)#make 0 to be the first
- tied_beta[start_idx+v[0][v_count]-1] = v[1][v_count]
- else:
- for v_ in v:
- one_list.append(start_idx+v_-1)#make 0 to be the first
- tied_pos_list_of_lists.append(one_list)
- tied_pos_list_of_lists_list.append(tied_pos_list_of_lists)
-
-
-
- x = np.concatenate(x_chain_list,0) #[L, 4, 3]
- all_sequence = "".join(chain_seq_list)
- m = np.concatenate(chain_mask_list,0) #[L,], 1.0 for places that need to be predicted
- chain_encoding = np.concatenate(chain_encoding_list,0)
- m_pos = np.concatenate(fixed_position_mask_list,0) #[L,], 1.0 for places that need to be predicted
-
- pssm_coef_ = np.concatenate(pssm_coef_list,0) #[L,], 1.0 for places that need to be predicted
- pssm_bias_ = np.concatenate(pssm_bias_list,0) #[L,], 1.0 for places that need to be predicted
- pssm_log_odds_ = np.concatenate(pssm_log_odds_list,0) #[L,], 1.0 for places that need to be predicted
-
- bias_by_res_ = np.concatenate(bias_by_res_list, 0) #[L,21], 0.0 for places where AA frequencies don't need to be tweaked
-
- l = len(all_sequence)
- x_pad = np.pad(x, [[0,L_max-l], [0,0], [0,0]], 'constant', constant_values=(np.nan, ))
- X[i,:,:,:] = x_pad
-
- m_pad = np.pad(m, [[0,L_max-l]], 'constant', constant_values=(0.0, ))
- m_pos_pad = np.pad(m_pos, [[0,L_max-l]], 'constant', constant_values=(0.0, ))
- omit_AA_mask_pad = np.pad(np.concatenate(omit_AA_mask_list,0), [[0,L_max-l]], 'constant', constant_values=(0.0, ))
- chain_M[i,:] = m_pad
- chain_M_pos[i,:] = m_pos_pad
- omit_AA_mask[i,] = omit_AA_mask_pad
-
- chain_encoding_pad = np.pad(chain_encoding, [[0,L_max-l]], 'constant', constant_values=(0.0, ))
- chain_encoding_all[i,:] = chain_encoding_pad
-
- pssm_coef_pad = np.pad(pssm_coef_, [[0,L_max-l]], 'constant', constant_values=(0.0, ))
- pssm_bias_pad = np.pad(pssm_bias_, [[0,L_max-l], [0,0]], 'constant', constant_values=(0.0, ))
- pssm_log_odds_pad = np.pad(pssm_log_odds_, [[0,L_max-l], [0,0]], 'constant', constant_values=(0.0, ))
-
- pssm_coef_all[i,:] = pssm_coef_pad
- pssm_bias_all[i,:] = pssm_bias_pad
- pssm_log_odds_all[i,:] = pssm_log_odds_pad
-
- bias_by_res_pad = np.pad(bias_by_res_, [[0,L_max-l], [0,0]], 'constant', constant_values=(0.0, ))
- bias_by_res_all[i,:] = bias_by_res_pad
-
- # Convert to labels
- indices = np.asarray([alphabet.index(a) for a in all_sequence], dtype=np.int32)
- S[i, :l] = indices
- letter_list_list.append(letter_list)
- visible_list_list.append(visible_list)
- masked_list_list.append(masked_list)
- masked_chain_length_list_list.append(masked_chain_length_list)
-
-
- isnan = np.isnan(X)
- mask = np.isfinite(np.sum(X,(2,3))).astype(np.float32)
- X[isnan] = 0.
-
- # Conversion
- pssm_coef_all = torch.from_numpy(pssm_coef_all).to(dtype=torch.float32, device=device)
- pssm_bias_all = torch.from_numpy(pssm_bias_all).to(dtype=torch.float32, device=device)
- pssm_log_odds_all = torch.from_numpy(pssm_log_odds_all).to(dtype=torch.float32, device=device)
-
- tied_beta = torch.from_numpy(tied_beta).to(dtype=torch.float32, device=device)
-
- jumps = ((residue_idx[:,1:]-residue_idx[:,:-1])==1).astype(np.float32)
- bias_by_res_all = torch.from_numpy(bias_by_res_all).to(dtype=torch.float32, device=device)
- phi_mask = np.pad(jumps, [[0,0],[1,0]])
- psi_mask = np.pad(jumps, [[0,0],[0,1]])
- omega_mask = np.pad(jumps, [[0,0],[0,1]])
- dihedral_mask = np.concatenate([phi_mask[:,:,None], psi_mask[:,:,None], omega_mask[:,:,None]], -1) #[B,L,3]
- dihedral_mask = torch.from_numpy(dihedral_mask).to(dtype=torch.float32, device=device)
- residue_idx = torch.from_numpy(residue_idx).to(dtype=torch.long,device=device)
- S = torch.from_numpy(S).to(dtype=torch.long,device=device)
- X = torch.from_numpy(X).to(dtype=torch.float32, device=device)
- mask = torch.from_numpy(mask).to(dtype=torch.float32, device=device)
- chain_M = torch.from_numpy(chain_M).to(dtype=torch.float32, device=device)
- chain_M_pos = torch.from_numpy(chain_M_pos).to(dtype=torch.float32, device=device)
- omit_AA_mask = torch.from_numpy(omit_AA_mask).to(dtype=torch.float32, device=device)
- chain_encoding_all = torch.from_numpy(chain_encoding_all).to(dtype=torch.long, device=device)
- if ca_only:
- X_out = X[:,:,0]
- else:
- X_out = X
- return X_out, S, mask, lengths, chain_M, chain_encoding_all, letter_list_list, visible_list_list, masked_list_list, masked_chain_length_list_list, chain_M_pos, omit_AA_mask, residue_idx, dihedral_mask, tied_pos_list_of_lists_list, pssm_coef_all, pssm_bias_all, pssm_log_odds_all, bias_by_res_all, tied_beta
-
-
-
-def loss_nll(S, log_probs, mask):
- """ Negative log probabilities """
- criterion = torch.nn.NLLLoss(reduction='none')
- loss = criterion(
- log_probs.contiguous().view(-1, log_probs.size(-1)), S.contiguous().view(-1)
- ).view(S.size())
- loss_av = torch.sum(loss * mask) / torch.sum(mask)
- return loss, loss_av
-
-
-def loss_smoothed(S, log_probs, mask, weight=0.1):
- """ Negative log probabilities """
- S_onehot = torch.nn.functional.one_hot(S, 21).float()
-
- # Label smoothing
- S_onehot = S_onehot + weight / float(S_onehot.size(-1))
- S_onehot = S_onehot / S_onehot.sum(-1, keepdim=True)
-
- loss = -(S_onehot * log_probs).sum(-1)
- loss_av = torch.sum(loss * mask) / torch.sum(mask)
- return loss, loss_av
-
-class StructureDataset():
- def __init__(self, jsonl_file, verbose=True, truncate=None, max_length=100,
- alphabet='ACDEFGHIKLMNPQRSTVWYX-'):
- alphabet_set = set([a for a in alphabet])
- discard_count = {
- 'bad_chars': 0,
- 'too_long': 0,
- 'bad_seq_length': 0
- }
-
- with open(jsonl_file) as f:
- self.data = []
-
- lines = f.readlines()
- start = time.time()
- for i, line in enumerate(lines):
- entry = json.loads(line)
- seq = entry['seq']
- name = entry['name']
-
- # Convert raw coords to np arrays
- #for key, val in entry['coords'].items():
- # entry['coords'][key] = np.asarray(val)
-
- # Check if in alphabet
- bad_chars = set([s for s in seq]).difference(alphabet_set)
- if len(bad_chars) == 0:
- if len(entry['seq']) <= max_length:
- if True:
- self.data.append(entry)
- else:
- discard_count['bad_seq_length'] += 1
- else:
- discard_count['too_long'] += 1
- else:
- if verbose:
- print(name, bad_chars, entry['seq'])
- discard_count['bad_chars'] += 1
-
- # Truncate early
- if truncate is not None and len(self.data) == truncate:
- return
-
- if verbose and (i + 1) % 1000 == 0:
- elapsed = time.time() - start
- print('{} entries ({} loaded) in {:.1f} s'.format(len(self.data), i+1, elapsed))
- if verbose:
- print('discarded', discard_count)
- def __len__(self):
- return len(self.data)
-
- def __getitem__(self, idx):
- return self.data[idx]
-
-
-class StructureDatasetPDB():
- def __init__(self, pdb_dict_list, verbose=True, truncate=None, max_length=100,
- alphabet='ACDEFGHIKLMNPQRSTVWYX-'):
- alphabet_set = set([a for a in alphabet])
- discard_count = {
- 'bad_chars': 0,
- 'too_long': 0,
- 'bad_seq_length': 0
- }
-
- self.data = []
-
- start = time.time()
- for i, entry in enumerate(pdb_dict_list):
- seq = entry['seq']
- name = entry['name']
-
- bad_chars = set([s for s in seq]).difference(alphabet_set)
- if len(bad_chars) == 0:
- if len(entry['seq']) <= max_length:
- self.data.append(entry)
- else:
- discard_count['too_long'] += 1
- else:
- discard_count['bad_chars'] += 1
-
- # Truncate early
- if truncate is not None and len(self.data) == truncate:
- return
-
- if verbose and (i + 1) % 1000 == 0:
- elapsed = time.time() - start
-
- #print('Discarded', discard_count)
- def __len__(self):
- return len(self.data)
-
- def __getitem__(self, idx):
- return self.data[idx]
-
-
-
-class StructureLoader():
- def __init__(self, dataset, batch_size=100, shuffle=True,
- collate_fn=lambda x:x, drop_last=False):
- self.dataset = dataset
- self.size = len(dataset)
- self.lengths = [len(dataset[i]['seq']) for i in range(self.size)]
- self.batch_size = batch_size
- sorted_ix = np.argsort(self.lengths)
-
- # Cluster into batches of similar sizes
- clusters, batch = [], []
- batch_max = 0
- for ix in sorted_ix:
- size = self.lengths[ix]
- if size * (len(batch) + 1) <= self.batch_size:
- batch.append(ix)
- batch_max = size
- else:
- clusters.append(batch)
- batch, batch_max = [], 0
- if len(batch) > 0:
- clusters.append(batch)
- self.clusters = clusters
-
- def __len__(self):
- return len(self.clusters)
-
- def __iter__(self):
- np.random.shuffle(self.clusters)
- for b_idx in self.clusters:
- batch = [self.dataset[i] for i in b_idx]
- yield batch
-
-
-
-# The following gather functions
-def gather_edges(edges, neighbor_idx):
- # Features [B,N,N,C] at Neighbor indices [B,N,K] => Neighbor features [B,N,K,C]
- neighbors = neighbor_idx.unsqueeze(-1).expand(-1, -1, -1, edges.size(-1))
- edge_features = torch.gather(edges, 2, neighbors)
- return edge_features
-
-def gather_nodes(nodes, neighbor_idx):
- # Features [B,N,C] at Neighbor indices [B,N,K] => [B,N,K,C]
- # Flatten and expand indices per batch [B,N,K] => [B,NK] => [B,NK,C]
- neighbors_flat = neighbor_idx.view((neighbor_idx.shape[0], -1))
- neighbors_flat = neighbors_flat.unsqueeze(-1).expand(-1, -1, nodes.size(2))
- # Gather and re-pack
- neighbor_features = torch.gather(nodes, 1, neighbors_flat)
- neighbor_features = neighbor_features.view(list(neighbor_idx.shape)[:3] + [-1])
- return neighbor_features
-
-def gather_nodes_t(nodes, neighbor_idx):
- # Features [B,N,C] at Neighbor index [B,K] => Neighbor features[B,K,C]
- idx_flat = neighbor_idx.unsqueeze(-1).expand(-1, -1, nodes.size(2))
- neighbor_features = torch.gather(nodes, 1, idx_flat)
- return neighbor_features
-
-def cat_neighbors_nodes(h_nodes, h_neighbors, E_idx):
- h_nodes = gather_nodes(h_nodes, E_idx)
- h_nn = torch.cat([h_neighbors, h_nodes], -1)
- return h_nn
-
-
-class EncLayer(nn.Module):
- def __init__(self, num_hidden, num_in, dropout=0.1, num_heads=None, scale=30, time_cond_dim=None):
- super(EncLayer, self).__init__()
- self.num_hidden = num_hidden
- self.num_in = num_in
- self.scale = scale
- self.dropout1 = nn.Dropout(dropout)
- self.dropout2 = nn.Dropout(dropout)
- self.dropout3 = nn.Dropout(dropout)
- self.norm1 = nn.LayerNorm(num_hidden)
- self.norm2 = nn.LayerNorm(num_hidden)
- self.norm3 = nn.LayerNorm(num_hidden)
-
- if time_cond_dim is not None:
- self.time_block1 = nn.Sequential(
- Rearrange('b 1 d -> b 1 1 d'),
- nn.SiLU(),
- nn.Linear(time_cond_dim, num_hidden * 2))
- self.time_block2 = nn.Sequential(
- Rearrange('b 1 d -> b 1 1 d'),
- nn.SiLU(),
- nn.Linear(time_cond_dim, num_hidden * 2))
-
- self.W1 = nn.Linear(num_hidden + num_in, num_hidden, bias=True)
- self.W2 = nn.Linear(num_hidden, num_hidden, bias=True)
- self.W3 = nn.Linear(num_hidden, num_hidden, bias=True)
- self.W11 = nn.Linear(num_hidden + num_in, num_hidden, bias=True)
- self.W12 = nn.Linear(num_hidden, num_hidden, bias=True)
- self.W13 = nn.Linear(num_hidden, num_hidden, bias=True)
- self.act = torch.nn.GELU()
- self.dense = PositionWiseFeedForward(num_hidden, num_hidden * 4)
-
- def forward(self, h_V, h_E, E_idx, mask_V=None, mask_attend=None, time_cond=None):
- """ Parallel computation of full transformer layer """
-
- h_EV = cat_neighbors_nodes(h_V, h_E, E_idx)
- h_V_expand = h_V.unsqueeze(-2).expand(-1,-1,h_EV.size(-2),-1)
- h_EV = torch.cat([h_V_expand, h_EV], -1)
-
- h_message = self.act(self.W2(self.act(self.W1(h_EV))))
- if time_cond is not None:
- scale, shift = self.time_block1(time_cond).chunk(2, dim=-1)
- h_message = h_message * (scale + 1) + shift
- h_message = self.W3(h_message)
-
- if mask_attend is not None:
- h_message = mask_attend.unsqueeze(-1) * h_message
- dh = torch.sum(h_message, -2) / self.scale
- h_V = self.norm1(h_V + self.dropout1(dh))
-
- dh = self.dense(h_V)
- h_V = self.norm2(h_V + self.dropout2(dh))
- if mask_V is not None:
- mask_V = mask_V.unsqueeze(-1)
- h_V = mask_V * h_V
-
- h_EV = cat_neighbors_nodes(h_V, h_E, E_idx)
- h_V_expand = h_V.unsqueeze(-2).expand(-1,-1,h_EV.size(-2),-1)
- h_EV = torch.cat([h_V_expand, h_EV], -1)
-
- h_message = self.act(self.W12(self.act(self.W11(h_EV))))
- if time_cond is not None:
- scale, shift = self.time_block2(time_cond).chunk(2, dim=-1)
- h_message = h_message * (scale + 1) + shift
- h_message = self.W13(h_message)
-
- h_E = self.norm3(h_E + self.dropout3(h_message))
- return h_V, h_E
-
-
-class DecLayer(nn.Module):
- def __init__(self, num_hidden, num_in, dropout=0.1, num_heads=None, scale=30, time_cond_dim=None):
- super(DecLayer, self).__init__()
- self.num_hidden = num_hidden
- self.num_in = num_in
- self.scale = scale
- self.dropout1 = nn.Dropout(dropout)
- self.dropout2 = nn.Dropout(dropout)
- self.norm1 = nn.LayerNorm(num_hidden)
- self.norm2 = nn.LayerNorm(num_hidden)
-
- if time_cond_dim is not None:
- self.time_block = nn.Sequential(
- Rearrange('b 1 d -> b 1 1 d'),
- nn.SiLU(),
- nn.Linear(time_cond_dim, num_hidden * 2))
-
- self.W1 = nn.Linear(num_hidden + num_in, num_hidden, bias=True)
- self.W2 = nn.Linear(num_hidden, num_hidden, bias=True)
- self.W3 = nn.Linear(num_hidden, num_hidden, bias=True)
- self.act = torch.nn.GELU()
- self.dense = PositionWiseFeedForward(num_hidden, num_hidden * 4)
-
- def forward(self, h_V, h_E, mask_V=None, mask_attend=None, time_cond=None):
- """ Parallel computation of full transformer layer """
-
- # Concatenate h_V_i to h_E_ij
- h_V_expand = h_V.unsqueeze(-2).expand(-1,-1,h_E.size(-2),-1)
- h_EV = torch.cat([h_V_expand, h_E], -1)
-
- h_message = self.act(self.W2(self.act(self.W1(h_EV))))
- if time_cond is not None:
- scale, shift = self.time_block(time_cond).chunk(2, dim=-1)
- h_message = h_message * (scale + 1) + shift
- h_message = self.W3(h_message)
-
- if mask_attend is not None:
- h_message = mask_attend.unsqueeze(-1) * h_message
- dh = torch.sum(h_message, -2) / self.scale
-
- h_V = self.norm1(h_V + self.dropout1(dh))
-
- # Position-wise feedforward
- dh = self.dense(h_V)
- h_V = self.norm2(h_V + self.dropout2(dh))
-
- if mask_V is not None:
- mask_V = mask_V.unsqueeze(-1)
- h_V = mask_V * h_V
- return h_V
-
-
-
-class PositionWiseFeedForward(nn.Module):
- def __init__(self, num_hidden, num_ff):
- super(PositionWiseFeedForward, self).__init__()
- self.W_in = nn.Linear(num_hidden, num_ff, bias=True)
- self.W_out = nn.Linear(num_ff, num_hidden, bias=True)
- self.act = torch.nn.GELU()
- def forward(self, h_V):
- h = self.act(self.W_in(h_V))
- h = self.W_out(h)
- return h
-
-class PositionalEncodings(nn.Module):
- def __init__(self, num_embeddings, max_relative_feature=32):
- super(PositionalEncodings, self).__init__()
- self.num_embeddings = num_embeddings
- self.max_relative_feature = max_relative_feature
- self.linear = nn.Linear(2*max_relative_feature+1+1, num_embeddings)
-
- def forward(self, offset, mask):
- d = torch.clip(offset + self.max_relative_feature, 0, 2*self.max_relative_feature)*mask + (1-mask)*(2*self.max_relative_feature+1)
- d_onehot = torch.nn.functional.one_hot(d, 2*self.max_relative_feature+1+1)
- E = self.linear(d_onehot.float())
- return E
-
-
-
-class CA_ProteinFeatures(nn.Module):
- def __init__(self, edge_features, node_features, num_positional_embeddings=16,
- num_rbf=16, top_k=30, augment_eps=0., num_chain_embeddings=16):
- """ Extract protein features """
- super(CA_ProteinFeatures, self).__init__()
- self.edge_features = edge_features
- self.node_features = node_features
- self.top_k = top_k
- self.augment_eps = augment_eps
- self.num_rbf = num_rbf
- self.num_positional_embeddings = num_positional_embeddings
-
- # Positional encoding
- self.embeddings = PositionalEncodings(num_positional_embeddings)
- # Normalization and embedding
- node_in, edge_in = 3, num_positional_embeddings + num_rbf*9 + 7
- self.node_embedding = nn.Linear(node_in, node_features, bias=False) #NOT USED
- self.edge_embedding = nn.Linear(edge_in, edge_features, bias=False)
- self.norm_nodes = nn.LayerNorm(node_features)
- self.norm_edges = nn.LayerNorm(edge_features)
-
-
- def _quaternions(self, R):
- """ Convert a batch of 3D rotations [R] to quaternions [Q]
- R [...,3,3]
- Q [...,4]
- """
- # Simple Wikipedia version
- # en.wikipedia.org/wiki/Rotation_matrix#Quaternion
- # For other options see math.stackexchange.com/questions/2074316/calculating-rotation-axis-from-rotation-matrix
- diag = torch.diagonal(R, dim1=-2, dim2=-1)
- Rxx, Ryy, Rzz = diag.unbind(-1)
- magnitudes = 0.5 * torch.sqrt(torch.abs(1 + torch.stack([
- Rxx - Ryy - Rzz,
- - Rxx + Ryy - Rzz,
- - Rxx - Ryy + Rzz
- ], -1)))
- _R = lambda i,j: R[:,:,:,i,j]
- signs = torch.sign(torch.stack([
- _R(2,1) - _R(1,2),
- _R(0,2) - _R(2,0),
- _R(1,0) - _R(0,1)
- ], -1))
- xyz = signs * magnitudes
- # The relu enforces a non-negative trace
- w = torch.sqrt(F.relu(1 + diag.sum(-1, keepdim=True))) / 2.
- Q = torch.cat((xyz, w), -1)
- Q = F.normalize(Q, dim=-1)
- return Q
-
- def _orientations_coarse(self, X, E_idx, eps=1e-6):
- dX = X[:,1:,:] - X[:,:-1,:]
- dX_norm = torch.norm(dX,dim=-1)
- dX_mask = (3.6 0:
- Ca = Ca + self.augment_eps * torch.randn_like(Ca)
-
- D_neighbors, E_idx, mask_neighbors = self._dist(Ca, mask)
-
- Ca_0 = torch.zeros(Ca.shape, device=Ca.device)
- Ca_2 = torch.zeros(Ca.shape, device=Ca.device)
- Ca_0[:,1:,:] = Ca[:,:-1,:]
- Ca_1 = Ca
- Ca_2[:,:-1,:] = Ca[:,1:,:]
-
- V, O_features = self._orientations_coarse(Ca, E_idx)
-
- RBF_all = []
- RBF_all.append(self._rbf(D_neighbors)) #Ca_1-Ca_1
- RBF_all.append(self._get_rbf(Ca_0, Ca_0, E_idx))
- RBF_all.append(self._get_rbf(Ca_2, Ca_2, E_idx))
-
- RBF_all.append(self._get_rbf(Ca_0, Ca_1, E_idx))
- RBF_all.append(self._get_rbf(Ca_0, Ca_2, E_idx))
-
- RBF_all.append(self._get_rbf(Ca_1, Ca_0, E_idx))
- RBF_all.append(self._get_rbf(Ca_1, Ca_2, E_idx))
-
- RBF_all.append(self._get_rbf(Ca_2, Ca_0, E_idx))
- RBF_all.append(self._get_rbf(Ca_2, Ca_1, E_idx))
-
-
- RBF_all = torch.cat(tuple(RBF_all), dim=-1)
-
-
- offset = residue_idx[:,:,None]-residue_idx[:,None,:]
- offset = gather_edges(offset[:,:,:,None], E_idx)[:,:,:,0] #[B, L, K]
-
- d_chains = ((chain_labels[:, :, None] - chain_labels[:,None,:])==0).long()
- E_chains = gather_edges(d_chains[:,:,:,None], E_idx)[:,:,:,0]
- E_positional = self.embeddings(offset.long(), E_chains)
- E = torch.cat((E_positional, RBF_all, O_features), -1)
-
-
- E = self.edge_embedding(E)
- E = self.norm_edges(E)
-
- return E, E_idx
-
-
-def get_closest_neighbors(X, mask, top_k, eps=1e-6):
- # X is ca coords (b, n, 3), mask is seq mask
- mask_2D = torch.unsqueeze(mask,1) * torch.unsqueeze(mask,2)
- dX = torch.unsqueeze(X,1) - torch.unsqueeze(X,2)
- D = mask_2D * torch.sqrt(torch.sum(dX**2, 3) + eps)
- D_max, _ = torch.max(D, -1, keepdim=True)
- D_adjust = D + (1. - mask_2D) * D_max
- sampled_top_k = top_k
- D_neighbors, E_idx = torch.topk(D_adjust, np.minimum(top_k, X.shape[1]), dim=-1, largest=False)
- return D_neighbors, E_idx
-
-
-class ProteinFeatures(nn.Module):
- def __init__(self, edge_features, node_features, num_positional_embeddings=16,
- num_rbf=16, top_k=30, augment_eps=0., num_chain_embeddings=16):
- """ Extract protein features """
- super(ProteinFeatures, self).__init__()
- self.edge_features = edge_features
- self.node_features = node_features
- self.top_k = top_k
- self.augment_eps = augment_eps
- self.num_rbf = num_rbf
- self.num_positional_embeddings = num_positional_embeddings
-
- self.embeddings = PositionalEncodings(num_positional_embeddings)
- node_in, edge_in = 6, num_positional_embeddings + num_rbf*25
- self.edge_embedding = nn.Linear(edge_in, edge_features, bias=False)
- self.norm_edges = nn.LayerNorm(edge_features)
-
- def _dist(self, X, mask, eps=1E-6):
- # mask_2D = torch.unsqueeze(mask,1) * torch.unsqueeze(mask,2)
- # dX = torch.unsqueeze(X,1) - torch.unsqueeze(X,2)
- # D = mask_2D * torch.sqrt(torch.sum(dX**2, 3) + eps)
- # D_max, _ = torch.max(D, -1, keepdim=True)
- # D_adjust = D + (1. - mask_2D) * D_max
- # sampled_top_k = self.top_k
- # D_neighbors, E_idx = torch.topk(D_adjust, np.minimum(self.top_k, X.shape[1]), dim=-1, largest=False)
- # return D_neighbors, E_idx
- return get_closest_neighbors(X, mask, self.top_k, eps=eps)
-
- def _rbf(self, D):
- device = D.device
- D_min, D_max, D_count = 2., 22., self.num_rbf
- D_mu = torch.linspace(D_min, D_max, D_count, device=device)
- D_mu = D_mu.view([1,1,1,-1])
- D_sigma = (D_max - D_min) / D_count
- D_expand = torch.unsqueeze(D, -1)
- RBF = torch.exp(-((D_expand - D_mu) / D_sigma)**2)
- return RBF
-
- def _get_rbf(self, A, B, E_idx):
- D_A_B = torch.sqrt(torch.sum((A[:,:,None,:] - B[:,None,:,:])**2,-1) + 1e-6) #[B, L, L]
- D_A_B_neighbors = gather_edges(D_A_B[:,:,:,None], E_idx)[:,:,:,0] #[B,L,K]
- RBF_A_B = self._rbf(D_A_B_neighbors)
- return RBF_A_B
-
- def forward(self, X, mask, residue_idx, chain_labels):
- if self.augment_eps > 0:
- X = X + self.augment_eps * torch.randn_like(X)
-
- b = X[:,:,1,:] - X[:,:,0,:]
- c = X[:,:,2,:] - X[:,:,1,:]
- a = torch.cross(b, c, dim=-1)
- Cb = -0.58273431*a + 0.56802827*b - 0.54067466*c + X[:,:,1,:]
- Ca = X[:,:,1,:]
- N = X[:,:,0,:]
- C = X[:,:,2,:]
- O = X[:,:,3,:]
-
- D_neighbors, E_idx = self._dist(Ca, mask)
-
- RBF_all = []
- RBF_all.append(self._rbf(D_neighbors)) #Ca-Ca
- RBF_all.append(self._get_rbf(N, N, E_idx)) #N-N
- RBF_all.append(self._get_rbf(C, C, E_idx)) #C-C
- RBF_all.append(self._get_rbf(O, O, E_idx)) #O-O
- RBF_all.append(self._get_rbf(Cb, Cb, E_idx)) #Cb-Cb
- RBF_all.append(self._get_rbf(Ca, N, E_idx)) #Ca-N
- RBF_all.append(self._get_rbf(Ca, C, E_idx)) #Ca-C
- RBF_all.append(self._get_rbf(Ca, O, E_idx)) #Ca-O
- RBF_all.append(self._get_rbf(Ca, Cb, E_idx)) #Ca-Cb
- RBF_all.append(self._get_rbf(N, C, E_idx)) #N-C
- RBF_all.append(self._get_rbf(N, O, E_idx)) #N-O
- RBF_all.append(self._get_rbf(N, Cb, E_idx)) #N-Cb
- RBF_all.append(self._get_rbf(Cb, C, E_idx)) #Cb-C
- RBF_all.append(self._get_rbf(Cb, O, E_idx)) #Cb-O
- RBF_all.append(self._get_rbf(O, C, E_idx)) #O-C
- RBF_all.append(self._get_rbf(N, Ca, E_idx)) #N-Ca
- RBF_all.append(self._get_rbf(C, Ca, E_idx)) #C-Ca
- RBF_all.append(self._get_rbf(O, Ca, E_idx)) #O-Ca
- RBF_all.append(self._get_rbf(Cb, Ca, E_idx)) #Cb-Ca
- RBF_all.append(self._get_rbf(C, N, E_idx)) #C-N
- RBF_all.append(self._get_rbf(O, N, E_idx)) #O-N
- RBF_all.append(self._get_rbf(Cb, N, E_idx)) #Cb-N
- RBF_all.append(self._get_rbf(C, Cb, E_idx)) #C-Cb
- RBF_all.append(self._get_rbf(O, Cb, E_idx)) #O-Cb
- RBF_all.append(self._get_rbf(C, O, E_idx)) #C-O
- RBF_all = torch.cat(tuple(RBF_all), dim=-1)
-
- offset = residue_idx[:,:,None]-residue_idx[:,None,:]
- offset = gather_edges(offset[:,:,:,None], E_idx)[:,:,:,0] #[B, L, K]
-
- d_chains = ((chain_labels[:, :, None] - chain_labels[:,None,:])==0).long() #find self vs non-self interaction
- E_chains = gather_edges(d_chains[:,:,:,None], E_idx)[:,:,:,0]
- E_positional = self.embeddings(offset.long(), E_chains)
- E = torch.cat((E_positional, RBF_all), -1)
- E = self.edge_embedding(E)
- E = self.norm_edges(E)
- return E, E_idx
-
-
-
-class ProteinMPNN(nn.Module):
- def __init__(self, num_letters, node_features, edge_features,
- hidden_dim, num_encoder_layers=3, num_decoder_layers=3,
- vocab=21, k_neighbors=64, augment_eps=0.05, dropout=0.1, ca_only=False, time_cond_dim=None, input_S_is_embeddings=False):
- super(ProteinMPNN, self).__init__()
-
- # Hyperparameters
- self.node_features = node_features
- self.edge_features = edge_features
- self.hidden_dim = hidden_dim
-
- # Featurization layers
- if ca_only:
- self.features = CA_ProteinFeatures(node_features, edge_features, top_k=k_neighbors, augment_eps=augment_eps)
- self.W_v = nn.Linear(node_features, hidden_dim, bias=True)
- else:
- self.features = ProteinFeatures(node_features, edge_features, top_k=k_neighbors, augment_eps=augment_eps)
-
- self.W_e = nn.Linear(edge_features, hidden_dim, bias=True)
- self.input_S_is_embeddings = input_S_is_embeddings
- if not self.input_S_is_embeddings:
- self.W_s = nn.Embedding(vocab, hidden_dim)
-
- if time_cond_dim is not None:
- self.time_block = nn.Sequential(
- nn.SiLU(),
- nn.Linear(time_cond_dim, hidden_dim)
- )
-
- # Encoder layers
- self.encoder_layers = nn.ModuleList([
- EncLayer(hidden_dim, hidden_dim*2, dropout=dropout, time_cond_dim=time_cond_dim)
- for _ in range(num_encoder_layers)
- ])
-
- # Decoder layers
- self.decoder_layers = nn.ModuleList([
- DecLayer(hidden_dim, hidden_dim*3, dropout=dropout, time_cond_dim=time_cond_dim)
- for _ in range(num_decoder_layers)
- ])
- self.W_out = nn.Linear(hidden_dim, num_letters, bias=True)
-
- for p in self.parameters():
- if p.dim() > 1:
- nn.init.xavier_uniform_(p)
-
- def forward(self, X, S, mask, chain_M, residue_idx, chain_encoding_all, randn, use_input_decoding_order=False, decoding_order=None, causal_mask=True, time_cond=None, return_node_embs=False):
- """ Graph-conditioned sequence model """
- device=X.device
- # Prepare node and edge embeddings
- E, E_idx = self.features(X, mask, residue_idx, chain_encoding_all)
- h_V = torch.zeros((E.shape[0], E.shape[1], E.shape[-1]), device=E.device)
- if time_cond is not None:
- time_cond_nodes = self.time_block(time_cond)
- h_V += time_cond_nodes # time_cond is b, 1, c
- h_E = self.W_e(E)
-
- # Encoder is unmasked self-attention
- mask_attend = gather_nodes(mask.unsqueeze(-1), E_idx).squeeze(-1)
- mask_attend = mask.unsqueeze(-1) * mask_attend
- for layer in self.encoder_layers:
- h_V, h_E = layer(h_V, h_E, E_idx, mask, mask_attend, time_cond=time_cond)
-
- encoder_embs = h_V
-
- # Concatenate sequence embeddings for autoregressive decoder
- if self.input_S_is_embeddings:
- h_S = S
- else:
- h_S = self.W_s(S)
- h_ES = cat_neighbors_nodes(h_S, h_E, E_idx)
-
- # Build encoder embeddings
- h_EX_encoder = cat_neighbors_nodes(torch.zeros_like(h_S), h_E, E_idx)
- h_EXV_encoder = cat_neighbors_nodes(h_V, h_EX_encoder, E_idx)
-
-
- chain_M = chain_M*mask #update chain_M to include missing regions
- mask_size = E_idx.shape[1]
- if causal_mask:
- if not use_input_decoding_order:
- decoding_order = torch.argsort((chain_M+0.0001)*(torch.abs(randn))) #[numbers will be smaller for places where chain_M = 0.0 and higher for places where chain_M = 1.0]
- permutation_matrix_reverse = torch.nn.functional.one_hot(decoding_order, num_classes=mask_size).float()
- order_mask_backward = torch.einsum('ij, biq, bjp->bqp',(1-torch.triu(torch.ones(mask_size,mask_size, device=device))), permutation_matrix_reverse, permutation_matrix_reverse)
- else:
- order_mask_backward = torch.ones(X.shape[0], mask_size, mask_size, device=device)
- mask_attend = torch.gather(order_mask_backward, 2, E_idx).unsqueeze(-1)
- mask_1D = mask.view([mask.size(0), mask.size(1), 1, 1])
- mask_bw = mask_1D * mask_attend
- mask_fw = mask_1D * (1. - mask_attend)
-
- h_EXV_encoder_fw = mask_fw * h_EXV_encoder
- for layer in self.decoder_layers:
- # Masked positions attend to encoder information, unmasked see.
- h_ESV = cat_neighbors_nodes(h_V, h_ES, E_idx)
- h_ESV = mask_bw * h_ESV + h_EXV_encoder_fw
- h_V = layer(h_V, h_ESV, mask, time_cond=time_cond)
-
- if return_node_embs:
- return h_V, encoder_embs
- else:
- logits = self.W_out(h_V)
- log_probs = F.log_softmax(logits, dim=-1)
- return log_probs
-
-
- def sample(self, X, randn, S_true, chain_mask, chain_encoding_all, residue_idx, mask=None, temperature=1.0, omit_AAs_np=None, bias_AAs_np=None, chain_M_pos=None, omit_AA_mask=None, pssm_coef=None, pssm_bias=None, pssm_multi=None, pssm_log_odds_flag=None, pssm_log_odds_mask=None, pssm_bias_flag=None, bias_by_res=None):
- device = X.device
- # Prepare node and edge embeddings
- E, E_idx = self.features(X, mask, residue_idx, chain_encoding_all)
- h_V = torch.zeros((E.shape[0], E.shape[1], E.shape[-1]), device=device)
- h_E = self.W_e(E)
-
- # Encoder is unmasked self-attention
- mask_attend = gather_nodes(mask.unsqueeze(-1), E_idx).squeeze(-1)
- mask_attend = mask.unsqueeze(-1) * mask_attend
- for layer in self.encoder_layers:
- h_V, h_E = layer(h_V, h_E, E_idx, mask, mask_attend)
-
- # Decoder uses masked self-attention
- chain_mask = chain_mask*chain_M_pos*mask #update chain_M to include missing regions
- decoding_order = torch.argsort((chain_mask+0.0001)*(torch.abs(randn))) #[numbers will be smaller for places where chain_M = 0.0 and higher for places where chain_M = 1.0]
- mask_size = E_idx.shape[1]
- permutation_matrix_reverse = torch.nn.functional.one_hot(decoding_order, num_classes=mask_size).float()
- order_mask_backward = torch.einsum('ij, biq, bjp->bqp',(1-torch.triu(torch.ones(mask_size,mask_size, device=device))), permutation_matrix_reverse, permutation_matrix_reverse)
- mask_attend = torch.gather(order_mask_backward, 2, E_idx).unsqueeze(-1)
- mask_1D = mask.view([mask.size(0), mask.size(1), 1, 1])
- mask_bw = mask_1D * mask_attend
- mask_fw = mask_1D * (1. - mask_attend)
-
- N_batch, N_nodes = X.size(0), X.size(1)
- log_probs = torch.zeros((N_batch, N_nodes, 21), device=device)
- all_probs = torch.zeros((N_batch, N_nodes, 21), device=device, dtype=torch.float32)
- h_S = torch.zeros_like(h_V, device=device)
- S = torch.zeros((N_batch, N_nodes), dtype=torch.int64, device=device)
- h_V_stack = [h_V] + [torch.zeros_like(h_V, device=device) for _ in range(len(self.decoder_layers))]
- constant = torch.tensor(omit_AAs_np, device=device)
- constant_bias = torch.tensor(bias_AAs_np, device=device)
- #chain_mask_combined = chain_mask*chain_M_pos
- omit_AA_mask_flag = omit_AA_mask != None
-
-
- h_EX_encoder = cat_neighbors_nodes(torch.zeros_like(h_S), h_E, E_idx)
- h_EXV_encoder = cat_neighbors_nodes(h_V, h_EX_encoder, E_idx)
- h_EXV_encoder_fw = mask_fw * h_EXV_encoder
- for t_ in range(N_nodes):
- t = decoding_order[:,t_] #[B]
- chain_mask_gathered = torch.gather(chain_mask, 1, t[:,None]) #[B]
- mask_gathered = torch.gather(mask, 1, t[:,None]) #[B]
- bias_by_res_gathered = torch.gather(bias_by_res, 1, t[:,None,None].repeat(1,1,21))[:,0,:] #[B, 21]
- if (mask_gathered==0).all(): #for padded or missing regions only
- S_t = torch.gather(S_true, 1, t[:,None])
- else:
- # Hidden layers
- E_idx_t = torch.gather(E_idx, 1, t[:,None,None].repeat(1,1,E_idx.shape[-1]))
- h_E_t = torch.gather(h_E, 1, t[:,None,None,None].repeat(1,1,h_E.shape[-2], h_E.shape[-1]))
- h_ES_t = cat_neighbors_nodes(h_S, h_E_t, E_idx_t)
- h_EXV_encoder_t = torch.gather(h_EXV_encoder_fw, 1, t[:,None,None,None].repeat(1,1,h_EXV_encoder_fw.shape[-2], h_EXV_encoder_fw.shape[-1]))
- mask_t = torch.gather(mask, 1, t[:,None])
- for l, layer in enumerate(self.decoder_layers):
- # Updated relational features for future states
- h_ESV_decoder_t = cat_neighbors_nodes(h_V_stack[l], h_ES_t, E_idx_t)
- h_V_t = torch.gather(h_V_stack[l], 1, t[:,None,None].repeat(1,1,h_V_stack[l].shape[-1]))
- h_ESV_t = torch.gather(mask_bw, 1, t[:,None,None,None].repeat(1,1,mask_bw.shape[-2], mask_bw.shape[-1])) * h_ESV_decoder_t + h_EXV_encoder_t
- h_V_stack[l+1].scatter_(1, t[:,None,None].repeat(1,1,h_V.shape[-1]), layer(h_V_t, h_ESV_t, mask_V=mask_t))
- # Sampling step
- h_V_t = torch.gather(h_V_stack[-1], 1, t[:,None,None].repeat(1,1,h_V_stack[-1].shape[-1]))[:,0]
- logits = self.W_out(h_V_t) / temperature
- probs = F.softmax(logits-constant[None,:]*1e8+constant_bias[None,:]/temperature+bias_by_res_gathered/temperature, dim=-1)
- if pssm_bias_flag:
- pssm_coef_gathered = torch.gather(pssm_coef, 1, t[:,None])[:,0]
- pssm_bias_gathered = torch.gather(pssm_bias, 1, t[:,None,None].repeat(1,1,pssm_bias.shape[-1]))[:,0]
- probs = (1-pssm_multi*pssm_coef_gathered[:,None])*probs + pssm_multi*pssm_coef_gathered[:,None]*pssm_bias_gathered
- if pssm_log_odds_flag:
- pssm_log_odds_mask_gathered = torch.gather(pssm_log_odds_mask, 1, t[:,None, None].repeat(1,1,pssm_log_odds_mask.shape[-1]))[:,0] #[B, 21]
- probs_masked = probs*pssm_log_odds_mask_gathered
- probs_masked += probs * 0.001
- probs = probs_masked/torch.sum(probs_masked, dim=-1, keepdim=True) #[B, 21]
- if omit_AA_mask_flag:
- omit_AA_mask_gathered = torch.gather(omit_AA_mask, 1, t[:,None, None].repeat(1,1,omit_AA_mask.shape[-1]))[:,0] #[B, 21]
- probs_masked = probs*(1.0-omit_AA_mask_gathered)
- probs = probs_masked/torch.sum(probs_masked, dim=-1, keepdim=True) #[B, 21]
- S_t = torch.multinomial(probs, 1)
- all_probs.scatter_(1, t[:,None,None].repeat(1,1,21), (chain_mask_gathered[:,:,None,]*probs[:,None,:]).float())
- S_true_gathered = torch.gather(S_true, 1, t[:,None])
- S_t = (S_t*chain_mask_gathered+S_true_gathered*(1.0-chain_mask_gathered)).long()
- temp1 = self.W_s(S_t)
- h_S.scatter_(1, t[:,None,None].repeat(1,1,temp1.shape[-1]), temp1)
- S.scatter_(1, t[:,None], S_t)
- output_dict = {"S": S, "probs": all_probs, "decoding_order": decoding_order}
- return output_dict
-
-
- def tied_sample(self, X, randn, S_true, chain_mask, chain_encoding_all, residue_idx, mask=None, temperature=1.0, omit_AAs_np=None, bias_AAs_np=None, chain_M_pos=None, omit_AA_mask=None, pssm_coef=None, pssm_bias=None, pssm_multi=None, pssm_log_odds_flag=None, pssm_log_odds_mask=None, pssm_bias_flag=None, tied_pos=None, tied_beta=None, bias_by_res=None):
- device = X.device
- # Prepare node and edge embeddings
- E, E_idx = self.features(X, mask, residue_idx, chain_encoding_all)
- h_V = torch.zeros((E.shape[0], E.shape[1], E.shape[-1]), device=device)
- h_E = self.W_e(E)
- # Encoder is unmasked self-attention
- mask_attend = gather_nodes(mask.unsqueeze(-1), E_idx).squeeze(-1)
- mask_attend = mask.unsqueeze(-1) * mask_attend
- for layer in self.encoder_layers:
- h_V, h_E = layer(h_V, h_E, E_idx, mask, mask_attend)
-
- # Decoder uses masked self-attention
- chain_mask = chain_mask*chain_M_pos*mask #update chain_M to include missing regions
- decoding_order = torch.argsort((chain_mask+0.0001)*(torch.abs(randn))) #[numbers will be smaller for places where chain_M = 0.0 and higher for places where chain_M = 1.0]
-
- new_decoding_order = []
- for t_dec in list(decoding_order[0,].cpu().data.numpy()):
- if t_dec not in list(itertools.chain(*new_decoding_order)):
- list_a = [item for item in tied_pos if t_dec in item]
- if list_a:
- new_decoding_order.append(list_a[0])
- else:
- new_decoding_order.append([t_dec])
- decoding_order = torch.tensor(list(itertools.chain(*new_decoding_order)), device=device)[None,].repeat(X.shape[0],1)
-
- mask_size = E_idx.shape[1]
- permutation_matrix_reverse = torch.nn.functional.one_hot(decoding_order, num_classes=mask_size).float()
- order_mask_backward = torch.einsum('ij, biq, bjp->bqp',(1-torch.triu(torch.ones(mask_size,mask_size, device=device))), permutation_matrix_reverse, permutation_matrix_reverse)
- mask_attend = torch.gather(order_mask_backward, 2, E_idx).unsqueeze(-1)
- mask_1D = mask.view([mask.size(0), mask.size(1), 1, 1])
- mask_bw = mask_1D * mask_attend
- mask_fw = mask_1D * (1. - mask_attend)
-
- N_batch, N_nodes = X.size(0), X.size(1)
- log_probs = torch.zeros((N_batch, N_nodes, 21), device=device)
- all_probs = torch.zeros((N_batch, N_nodes, 21), device=device, dtype=torch.float32)
- h_S = torch.zeros_like(h_V, device=device)
- S = torch.zeros((N_batch, N_nodes), dtype=torch.int64, device=device)
- h_V_stack = [h_V] + [torch.zeros_like(h_V, device=device) for _ in range(len(self.decoder_layers))]
- constant = torch.tensor(omit_AAs_np, device=device)
- constant_bias = torch.tensor(bias_AAs_np, device=device)
- omit_AA_mask_flag = omit_AA_mask != None
-
- h_EX_encoder = cat_neighbors_nodes(torch.zeros_like(h_S), h_E, E_idx)
- h_EXV_encoder = cat_neighbors_nodes(h_V, h_EX_encoder, E_idx)
- h_EXV_encoder_fw = mask_fw * h_EXV_encoder
- for t_list in new_decoding_order:
- logits = 0.0
- logit_list = []
- done_flag = False
- for t in t_list:
- if (mask[:,t]==0).all():
- S_t = S_true[:,t]
- for t in t_list:
- h_S[:,t,:] = self.W_s(S_t)
- S[:,t] = S_t
- done_flag = True
- break
- else:
- E_idx_t = E_idx[:,t:t+1,:]
- h_E_t = h_E[:,t:t+1,:,:]
- h_ES_t = cat_neighbors_nodes(h_S, h_E_t, E_idx_t)
- h_EXV_encoder_t = h_EXV_encoder_fw[:,t:t+1,:,:]
- mask_t = mask[:,t:t+1]
- for l, layer in enumerate(self.decoder_layers):
- h_ESV_decoder_t = cat_neighbors_nodes(h_V_stack[l], h_ES_t, E_idx_t)
- h_V_t = h_V_stack[l][:,t:t+1,:]
- h_ESV_t = mask_bw[:,t:t+1,:,:] * h_ESV_decoder_t + h_EXV_encoder_t
- h_V_stack[l+1][:,t,:] = layer(h_V_t, h_ESV_t, mask_V=mask_t).squeeze(1)
- h_V_t = h_V_stack[-1][:,t,:]
- logit_list.append((self.W_out(h_V_t) / temperature)/len(t_list))
- logits += tied_beta[t]*(self.W_out(h_V_t) / temperature)/len(t_list)
- if done_flag:
- pass
- else:
- bias_by_res_gathered = bias_by_res[:,t,:] #[B, 21]
- probs = F.softmax(logits-constant[None,:]*1e8+constant_bias[None,:]/temperature+bias_by_res_gathered/temperature, dim=-1)
- if pssm_bias_flag:
- pssm_coef_gathered = pssm_coef[:,t]
- pssm_bias_gathered = pssm_bias[:,t]
- probs = (1-pssm_multi*pssm_coef_gathered[:,None])*probs + pssm_multi*pssm_coef_gathered[:,None]*pssm_bias_gathered
- if pssm_log_odds_flag:
- pssm_log_odds_mask_gathered = pssm_log_odds_mask[:,t]
- probs_masked = probs*pssm_log_odds_mask_gathered
- probs_masked += probs * 0.001
- probs = probs_masked/torch.sum(probs_masked, dim=-1, keepdim=True) #[B, 21]
- if omit_AA_mask_flag:
- omit_AA_mask_gathered = omit_AA_mask[:,t]
- probs_masked = probs*(1.0-omit_AA_mask_gathered)
- probs = probs_masked/torch.sum(probs_masked, dim=-1, keepdim=True) #[B, 21]
- S_t_repeat = torch.multinomial(probs, 1).squeeze(-1)
- S_t_repeat = (chain_mask[:,t]*S_t_repeat + (1-chain_mask[:,t])*S_true[:,t]).long() #hard pick fixed positions
- for t in t_list:
- h_S[:,t,:] = self.W_s(S_t_repeat)
- S[:,t] = S_t_repeat
- all_probs[:,t,:] = probs.float()
- output_dict = {"S": S, "probs": all_probs, "decoding_order": decoding_order}
- return output_dict
-
-
- def conditional_probs(self, X, S, mask, chain_M, residue_idx, chain_encoding_all, randn, backbone_only=False):
- """ Graph-conditioned sequence model """
- device=X.device
- # Prepare node and edge embeddings
- E, E_idx = self.features(X, mask, residue_idx, chain_encoding_all)
- h_V_enc = torch.zeros((E.shape[0], E.shape[1], E.shape[-1]), device=E.device)
- h_E = self.W_e(E)
-
- # Encoder is unmasked self-attention
- mask_attend = gather_nodes(mask.unsqueeze(-1), E_idx).squeeze(-1)
- mask_attend = mask.unsqueeze(-1) * mask_attend
- for layer in self.encoder_layers:
- h_V_enc, h_E = layer(h_V_enc, h_E, E_idx, mask, mask_attend)
-
- # Concatenate sequence embeddings for autoregressive decoder
- h_S = self.W_s(S)
- h_ES = cat_neighbors_nodes(h_S, h_E, E_idx)
-
- # Build encoder embeddings
- h_EX_encoder = cat_neighbors_nodes(torch.zeros_like(h_S), h_E, E_idx)
- h_EXV_encoder = cat_neighbors_nodes(h_V_enc, h_EX_encoder, E_idx)
-
-
- chain_M = chain_M*mask #update chain_M to include missing regions
-
- chain_M_np = chain_M.cpu().numpy()
- idx_to_loop = np.argwhere(chain_M_np[0,:]==1)[:,0]
- log_conditional_probs = torch.zeros([X.shape[0], chain_M.shape[1], 21], device=device).float()
-
- for idx in idx_to_loop:
- h_V = torch.clone(h_V_enc)
- order_mask = torch.zeros(chain_M.shape[1], device=device).float()
- if backbone_only:
- order_mask = torch.ones(chain_M.shape[1], device=device).float()
- order_mask[idx] = 0.
- else:
- order_mask = torch.zeros(chain_M.shape[1], device=device).float()
- order_mask[idx] = 1.
- decoding_order = torch.argsort((order_mask[None,]+0.0001)*(torch.abs(randn))) #[numbers will be smaller for places where chain_M = 0.0 and higher for places where chain_M = 1.0]
- mask_size = E_idx.shape[1]
- permutation_matrix_reverse = torch.nn.functional.one_hot(decoding_order, num_classes=mask_size).float()
- order_mask_backward = torch.einsum('ij, biq, bjp->bqp',(1-torch.triu(torch.ones(mask_size,mask_size, device=device))), permutation_matrix_reverse, permutation_matrix_reverse)
- mask_attend = torch.gather(order_mask_backward, 2, E_idx).unsqueeze(-1)
- mask_1D = mask.view([mask.size(0), mask.size(1), 1, 1])
- mask_bw = mask_1D * mask_attend
- mask_fw = mask_1D * (1. - mask_attend)
-
- h_EXV_encoder_fw = mask_fw * h_EXV_encoder
- for layer in self.decoder_layers:
- # Masked positions attend to encoder information, unmasked see.
- h_ESV = cat_neighbors_nodes(h_V, h_ES, E_idx)
- h_ESV = mask_bw * h_ESV + h_EXV_encoder_fw
- h_V = layer(h_V, h_ESV, mask)
-
- logits = self.W_out(h_V)
- log_probs = F.log_softmax(logits, dim=-1)
- log_conditional_probs[:,idx,:] = log_probs[:,idx,:]
- return log_conditional_probs
-
-
- def unconditional_probs(self, X, mask, residue_idx, chain_encoding_all):
- """ Graph-conditioned sequence model """
- device=X.device
- # Prepare node and edge embeddings
- E, E_idx = self.features(X, mask, residue_idx, chain_encoding_all)
- h_V = torch.zeros((E.shape[0], E.shape[1], E.shape[-1]), device=E.device)
- h_E = self.W_e(E)
-
- # Encoder is unmasked self-attention
- mask_attend = gather_nodes(mask.unsqueeze(-1), E_idx).squeeze(-1)
- mask_attend = mask.unsqueeze(-1) * mask_attend
- for layer in self.encoder_layers:
- h_V, h_E = layer(h_V, h_E, E_idx, mask, mask_attend)
-
- # Build encoder embeddings
- h_EX_encoder = cat_neighbors_nodes(torch.zeros_like(h_V), h_E, E_idx)
- h_EXV_encoder = cat_neighbors_nodes(h_V, h_EX_encoder, E_idx)
-
- order_mask_backward = torch.zeros([X.shape[0], X.shape[1], X.shape[1]], device=device)
- mask_attend = torch.gather(order_mask_backward, 2, E_idx).unsqueeze(-1)
- mask_1D = mask.view([mask.size(0), mask.size(1), 1, 1])
- mask_bw = mask_1D * mask_attend
- mask_fw = mask_1D * (1. - mask_attend)
-
- h_EXV_encoder_fw = mask_fw * h_EXV_encoder
- for layer in self.decoder_layers:
- h_V = layer(h_V, h_EXV_encoder_fw, mask)
-
- logits = self.W_out(h_V)
- log_probs = F.log_softmax(logits, dim=-1)
- return log_probs
diff --git a/spaces/RMXK/RVC_HFF/infer_batch_rvc.py b/spaces/RMXK/RVC_HFF/infer_batch_rvc.py
deleted file mode 100644
index 15c862a3d6bf815fa68003cc7054b694cae50c2a..0000000000000000000000000000000000000000
--- a/spaces/RMXK/RVC_HFF/infer_batch_rvc.py
+++ /dev/null
@@ -1,215 +0,0 @@
-"""
-v1
-runtime\python.exe myinfer-v2-0528.py 0 "E:\codes\py39\RVC-beta\todo-songs" "E:\codes\py39\logs\mi-test\added_IVF677_Flat_nprobe_7.index" harvest "E:\codes\py39\RVC-beta\output" "E:\codes\py39\test-20230416b\weights\mi-test.pth" 0.66 cuda:0 True 3 0 1 0.33
-v2
-runtime\python.exe myinfer-v2-0528.py 0 "E:\codes\py39\RVC-beta\todo-songs" "E:\codes\py39\test-20230416b\logs\mi-test-v2\aadded_IVF677_Flat_nprobe_1_v2.index" harvest "E:\codes\py39\RVC-beta\output_v2" "E:\codes\py39\test-20230416b\weights\mi-test-v2.pth" 0.66 cuda:0 True 3 0 1 0.33
-"""
-import os, sys, pdb, torch
-
-now_dir = os.getcwd()
-sys.path.append(now_dir)
-import sys
-import torch
-import tqdm as tq
-from multiprocessing import cpu_count
-
-
-class Config:
- def __init__(self, device, is_half):
- self.device = device
- self.is_half = is_half
- self.n_cpu = 0
- self.gpu_name = None
- self.gpu_mem = None
- self.x_pad, self.x_query, self.x_center, self.x_max = self.device_config()
-
- def device_config(self) -> tuple:
- if torch.cuda.is_available():
- i_device = int(self.device.split(":")[-1])
- self.gpu_name = torch.cuda.get_device_name(i_device)
- if (
- ("16" in self.gpu_name and "V100" not in self.gpu_name.upper())
- or "P40" in self.gpu_name.upper()
- or "1060" in self.gpu_name
- or "1070" in self.gpu_name
- or "1080" in self.gpu_name
- ):
- print("16系/10系显卡和P40强制单精度")
- self.is_half = False
- for config_file in ["32k.json", "40k.json", "48k.json"]:
- with open(f"configs/{config_file}", "r") as f:
- strr = f.read().replace("true", "false")
- with open(f"configs/{config_file}", "w") as f:
- f.write(strr)
- with open("infer/modules/train/preprocess.py", "r") as f:
- strr = f.read().replace("3.7", "3.0")
- with open("infer/modules/train/preprocess.py", "w") as f:
- f.write(strr)
- else:
- self.gpu_name = None
- self.gpu_mem = int(
- torch.cuda.get_device_properties(i_device).total_memory
- / 1024
- / 1024
- / 1024
- + 0.4
- )
- if self.gpu_mem <= 4:
- with open("infer/modules/train/preprocess.py", "r") as f:
- strr = f.read().replace("3.7", "3.0")
- with open("infer/modules/train/preprocess.py", "w") as f:
- f.write(strr)
- elif torch.backends.mps.is_available():
- print("没有发现支持的N卡, 使用MPS进行推理")
- self.device = "mps"
- else:
- print("没有发现支持的N卡, 使用CPU进行推理")
- self.device = "cpu"
- self.is_half = True
-
- if self.n_cpu == 0:
- self.n_cpu = cpu_count()
-
- if self.is_half:
- # 6G显存配置
- x_pad = 3
- x_query = 10
- x_center = 60
- x_max = 65
- else:
- # 5G显存配置
- x_pad = 1
- x_query = 6
- x_center = 38
- x_max = 41
-
- if self.gpu_mem != None and self.gpu_mem <= 4:
- x_pad = 1
- x_query = 5
- x_center = 30
- x_max = 32
-
- return x_pad, x_query, x_center, x_max
-
-
-f0up_key = sys.argv[1]
-input_path = sys.argv[2]
-index_path = sys.argv[3]
-f0method = sys.argv[4] # harvest or pm
-opt_path = sys.argv[5]
-model_path = sys.argv[6]
-index_rate = float(sys.argv[7])
-device = sys.argv[8]
-is_half = sys.argv[9].lower() != "false"
-filter_radius = int(sys.argv[10])
-resample_sr = int(sys.argv[11])
-rms_mix_rate = float(sys.argv[12])
-protect = float(sys.argv[13])
-print(sys.argv)
-config = Config(device, is_half)
-now_dir = os.getcwd()
-sys.path.append(now_dir)
-from infer.modules.vc.modules import VC
-from lib.infer_pack.models import (
- SynthesizerTrnMs256NSFsid,
- SynthesizerTrnMs256NSFsid_nono,
- SynthesizerTrnMs768NSFsid,
- SynthesizerTrnMs768NSFsid_nono,
-)
-from infer.lib.audio import load_audio
-from fairseq import checkpoint_utils
-from scipy.io import wavfile
-
-hubert_model = None
-
-
-def load_hubert():
- global hubert_model
- models, saved_cfg, task = checkpoint_utils.load_model_ensemble_and_task(
- ["hubert_base.pt"],
- suffix="",
- )
- hubert_model = models[0]
- hubert_model = hubert_model.to(device)
- if is_half:
- hubert_model = hubert_model.half()
- else:
- hubert_model = hubert_model.float()
- hubert_model.eval()
-
-
-def vc_single(sid, input_audio, f0_up_key, f0_file, f0_method, file_index, index_rate):
- global tgt_sr, net_g, vc, hubert_model, version
- if input_audio is None:
- return "You need to upload an audio", None
- f0_up_key = int(f0_up_key)
- audio = load_audio(input_audio, 16000)
- times = [0, 0, 0]
- if hubert_model == None:
- load_hubert()
- if_f0 = cpt.get("f0", 1)
- # audio_opt=vc.pipeline(hubert_model,net_g,sid,audio,times,f0_up_key,f0_method,file_index,file_big_npy,index_rate,if_f0,f0_file=f0_file)
- audio_opt = vc.pipeline(
- hubert_model,
- net_g,
- sid,
- audio,
- input_audio,
- times,
- f0_up_key,
- f0_method,
- file_index,
- index_rate,
- if_f0,
- filter_radius,
- tgt_sr,
- resample_sr,
- rms_mix_rate,
- version,
- protect,
- f0_file=f0_file,
- )
- print(times)
- return audio_opt
-
-
-def get_vc(model_path):
- global n_spk, tgt_sr, net_g, vc, cpt, device, is_half, version
- print("loading pth %s" % model_path)
- cpt = torch.load(model_path, map_location="cpu")
- tgt_sr = cpt["config"][-1]
- cpt["config"][-3] = cpt["weight"]["emb_g.weight"].shape[0] # n_spk
- if_f0 = cpt.get("f0", 1)
- version = cpt.get("version", "v1")
- if version == "v1":
- if if_f0 == 1:
- net_g = SynthesizerTrnMs256NSFsid(*cpt["config"], is_half=is_half)
- else:
- net_g = SynthesizerTrnMs256NSFsid_nono(*cpt["config"])
- elif version == "v2":
- if if_f0 == 1: #
- net_g = SynthesizerTrnMs768NSFsid(*cpt["config"], is_half=is_half)
- else:
- net_g = SynthesizerTrnMs768NSFsid_nono(*cpt["config"])
- del net_g.enc_q
- print(net_g.load_state_dict(cpt["weight"], strict=False)) # 不加这一行清不干净,真奇葩
- net_g.eval().to(device)
- if is_half:
- net_g = net_g.half()
- else:
- net_g = net_g.float()
- vc = VC(tgt_sr, config)
- n_spk = cpt["config"][-3]
- # return {"visible": True,"maximum": n_spk, "__type__": "update"}
-
-
-get_vc(model_path)
-audios = os.listdir(input_path)
-for file in tq.tqdm(audios):
- if file.endswith(".wav"):
- file_path = input_path + "/" + file
- wav_opt = vc_single(
- 0, file_path, f0up_key, None, f0method, index_path, index_rate
- )
- out_path = opt_path + "/" + file
- wavfile.write(out_path, tgt_sr, wav_opt)
diff --git a/spaces/RMXK/RVC_HFF/lib/infer_pack/modules/F0Predictor/DioF0Predictor.py b/spaces/RMXK/RVC_HFF/lib/infer_pack/modules/F0Predictor/DioF0Predictor.py
deleted file mode 100644
index ee3171bcb7c4a5066560723108b56e055f18be45..0000000000000000000000000000000000000000
--- a/spaces/RMXK/RVC_HFF/lib/infer_pack/modules/F0Predictor/DioF0Predictor.py
+++ /dev/null
@@ -1,90 +0,0 @@
-from lib.infer_pack.modules.F0Predictor.F0Predictor import F0Predictor
-import pyworld
-import numpy as np
-
-
-class DioF0Predictor(F0Predictor):
- def __init__(self, hop_length=512, f0_min=50, f0_max=1100, sampling_rate=44100):
- self.hop_length = hop_length
- self.f0_min = f0_min
- self.f0_max = f0_max
- self.sampling_rate = sampling_rate
-
- def interpolate_f0(self, f0):
- """
- 对F0进行插值处理
- """
-
- data = np.reshape(f0, (f0.size, 1))
-
- vuv_vector = np.zeros((data.size, 1), dtype=np.float32)
- vuv_vector[data > 0.0] = 1.0
- vuv_vector[data <= 0.0] = 0.0
-
- ip_data = data
-
- frame_number = data.size
- last_value = 0.0
- for i in range(frame_number):
- if data[i] <= 0.0:
- j = i + 1
- for j in range(i + 1, frame_number):
- if data[j] > 0.0:
- break
- if j < frame_number - 1:
- if last_value > 0.0:
- step = (data[j] - data[i - 1]) / float(j - i)
- for k in range(i, j):
- ip_data[k] = data[i - 1] + step * (k - i + 1)
- else:
- for k in range(i, j):
- ip_data[k] = data[j]
- else:
- for k in range(i, frame_number):
- ip_data[k] = last_value
- else:
- ip_data[i] = data[i] # 这里可能存在一个没有必要的拷贝
- last_value = data[i]
-
- return ip_data[:, 0], vuv_vector[:, 0]
-
- def resize_f0(self, x, target_len):
- source = np.array(x)
- source[source < 0.001] = np.nan
- target = np.interp(
- np.arange(0, len(source) * target_len, len(source)) / target_len,
- np.arange(0, len(source)),
- source,
- )
- res = np.nan_to_num(target)
- return res
-
- def compute_f0(self, wav, p_len=None):
- if p_len is None:
- p_len = wav.shape[0] // self.hop_length
- f0, t = pyworld.dio(
- wav.astype(np.double),
- fs=self.sampling_rate,
- f0_floor=self.f0_min,
- f0_ceil=self.f0_max,
- frame_period=1000 * self.hop_length / self.sampling_rate,
- )
- f0 = pyworld.stonemask(wav.astype(np.double), f0, t, self.sampling_rate)
- for index, pitch in enumerate(f0):
- f0[index] = round(pitch, 1)
- return self.interpolate_f0(self.resize_f0(f0, p_len))[0]
-
- def compute_f0_uv(self, wav, p_len=None):
- if p_len is None:
- p_len = wav.shape[0] // self.hop_length
- f0, t = pyworld.dio(
- wav.astype(np.double),
- fs=self.sampling_rate,
- f0_floor=self.f0_min,
- f0_ceil=self.f0_max,
- frame_period=1000 * self.hop_length / self.sampling_rate,
- )
- f0 = pyworld.stonemask(wav.astype(np.double), f0, t, self.sampling_rate)
- for index, pitch in enumerate(f0):
- f0[index] = round(pitch, 1)
- return self.interpolate_f0(self.resize_f0(f0, p_len))
diff --git a/spaces/Reha2704/VToonify/vtoonify/model/encoder/encoders/model_irse.py b/spaces/Reha2704/VToonify/vtoonify/model/encoder/encoders/model_irse.py
deleted file mode 100644
index 6698d9705321dd4a27681ea15204e9ffaa51f62a..0000000000000000000000000000000000000000
--- a/spaces/Reha2704/VToonify/vtoonify/model/encoder/encoders/model_irse.py
+++ /dev/null
@@ -1,84 +0,0 @@
-from torch.nn import Linear, Conv2d, BatchNorm1d, BatchNorm2d, PReLU, Dropout, Sequential, Module
-from model.encoder.encoders.helpers import get_blocks, Flatten, bottleneck_IR, bottleneck_IR_SE, l2_norm
-
-"""
-Modified Backbone implementation from [TreB1eN](https://github.com/TreB1eN/InsightFace_Pytorch)
-"""
-
-
-class Backbone(Module):
- def __init__(self, input_size, num_layers, mode='ir', drop_ratio=0.4, affine=True):
- super(Backbone, self).__init__()
- assert input_size in [112, 224], "input_size should be 112 or 224"
- assert num_layers in [50, 100, 152], "num_layers should be 50, 100 or 152"
- assert mode in ['ir', 'ir_se'], "mode should be ir or ir_se"
- blocks = get_blocks(num_layers)
- if mode == 'ir':
- unit_module = bottleneck_IR
- elif mode == 'ir_se':
- unit_module = bottleneck_IR_SE
- self.input_layer = Sequential(Conv2d(3, 64, (3, 3), 1, 1, bias=False),
- BatchNorm2d(64),
- PReLU(64))
- if input_size == 112:
- self.output_layer = Sequential(BatchNorm2d(512),
- Dropout(drop_ratio),
- Flatten(),
- Linear(512 * 7 * 7, 512),
- BatchNorm1d(512, affine=affine))
- else:
- self.output_layer = Sequential(BatchNorm2d(512),
- Dropout(drop_ratio),
- Flatten(),
- Linear(512 * 14 * 14, 512),
- BatchNorm1d(512, affine=affine))
-
- modules = []
- for block in blocks:
- for bottleneck in block:
- modules.append(unit_module(bottleneck.in_channel,
- bottleneck.depth,
- bottleneck.stride))
- self.body = Sequential(*modules)
-
- def forward(self, x):
- x = self.input_layer(x)
- x = self.body(x)
- x = self.output_layer(x)
- return l2_norm(x)
-
-
-def IR_50(input_size):
- """Constructs a ir-50 model."""
- model = Backbone(input_size, num_layers=50, mode='ir', drop_ratio=0.4, affine=False)
- return model
-
-
-def IR_101(input_size):
- """Constructs a ir-101 model."""
- model = Backbone(input_size, num_layers=100, mode='ir', drop_ratio=0.4, affine=False)
- return model
-
-
-def IR_152(input_size):
- """Constructs a ir-152 model."""
- model = Backbone(input_size, num_layers=152, mode='ir', drop_ratio=0.4, affine=False)
- return model
-
-
-def IR_SE_50(input_size):
- """Constructs a ir_se-50 model."""
- model = Backbone(input_size, num_layers=50, mode='ir_se', drop_ratio=0.4, affine=False)
- return model
-
-
-def IR_SE_101(input_size):
- """Constructs a ir_se-101 model."""
- model = Backbone(input_size, num_layers=100, mode='ir_se', drop_ratio=0.4, affine=False)
- return model
-
-
-def IR_SE_152(input_size):
- """Constructs a ir_se-152 model."""
- model = Backbone(input_size, num_layers=152, mode='ir_se', drop_ratio=0.4, affine=False)
- return model
diff --git a/spaces/Robert001/UniControl-Demo/annotator/uniformer_base/mmcv/runner/fp16_utils.py b/spaces/Robert001/UniControl-Demo/annotator/uniformer_base/mmcv/runner/fp16_utils.py
deleted file mode 100644
index 1981011d6859192e3e663e29d13500d56ba47f6c..0000000000000000000000000000000000000000
--- a/spaces/Robert001/UniControl-Demo/annotator/uniformer_base/mmcv/runner/fp16_utils.py
+++ /dev/null
@@ -1,410 +0,0 @@
-# Copyright (c) OpenMMLab. All rights reserved.
-import functools
-import warnings
-from collections import abc
-from inspect import getfullargspec
-
-import numpy as np
-import torch
-import torch.nn as nn
-
-from annotator.uniformer.mmcv.utils import TORCH_VERSION, digit_version
-from .dist_utils import allreduce_grads as _allreduce_grads
-
-try:
- # If PyTorch version >= 1.6.0, torch.cuda.amp.autocast would be imported
- # and used; otherwise, auto fp16 will adopt mmcv's implementation.
- # Note that when PyTorch >= 1.6.0, we still cast tensor types to fp16
- # manually, so the behavior may not be consistent with real amp.
- from torch.cuda.amp import autocast
-except ImportError:
- pass
-
-
-def cast_tensor_type(inputs, src_type, dst_type):
- """Recursively convert Tensor in inputs from src_type to dst_type.
-
- Args:
- inputs: Inputs that to be casted.
- src_type (torch.dtype): Source type..
- dst_type (torch.dtype): Destination type.
-
- Returns:
- The same type with inputs, but all contained Tensors have been cast.
- """
- if isinstance(inputs, nn.Module):
- return inputs
- elif isinstance(inputs, torch.Tensor):
- return inputs.to(dst_type)
- elif isinstance(inputs, str):
- return inputs
- elif isinstance(inputs, np.ndarray):
- return inputs
- elif isinstance(inputs, abc.Mapping):
- return type(inputs)({
- k: cast_tensor_type(v, src_type, dst_type)
- for k, v in inputs.items()
- })
- elif isinstance(inputs, abc.Iterable):
- return type(inputs)(
- cast_tensor_type(item, src_type, dst_type) for item in inputs)
- else:
- return inputs
-
-
-def auto_fp16(apply_to=None, out_fp32=False):
- """Decorator to enable fp16 training automatically.
-
- This decorator is useful when you write custom modules and want to support
- mixed precision training. If inputs arguments are fp32 tensors, they will
- be converted to fp16 automatically. Arguments other than fp32 tensors are
- ignored. If you are using PyTorch >= 1.6, torch.cuda.amp is used as the
- backend, otherwise, original mmcv implementation will be adopted.
-
- Args:
- apply_to (Iterable, optional): The argument names to be converted.
- `None` indicates all arguments.
- out_fp32 (bool): Whether to convert the output back to fp32.
-
- Example:
-
- >>> import torch.nn as nn
- >>> class MyModule1(nn.Module):
- >>>
- >>> # Convert x and y to fp16
- >>> @auto_fp16()
- >>> def forward(self, x, y):
- >>> pass
-
- >>> import torch.nn as nn
- >>> class MyModule2(nn.Module):
- >>>
- >>> # convert pred to fp16
- >>> @auto_fp16(apply_to=('pred', ))
- >>> def do_something(self, pred, others):
- >>> pass
- """
-
- def auto_fp16_wrapper(old_func):
-
- @functools.wraps(old_func)
- def new_func(*args, **kwargs):
- # check if the module has set the attribute `fp16_enabled`, if not,
- # just fallback to the original method.
- if not isinstance(args[0], torch.nn.Module):
- raise TypeError('@auto_fp16 can only be used to decorate the '
- 'method of nn.Module')
- if not (hasattr(args[0], 'fp16_enabled') and args[0].fp16_enabled):
- return old_func(*args, **kwargs)
-
- # get the arg spec of the decorated method
- args_info = getfullargspec(old_func)
- # get the argument names to be casted
- args_to_cast = args_info.args if apply_to is None else apply_to
- # convert the args that need to be processed
- new_args = []
- # NOTE: default args are not taken into consideration
- if args:
- arg_names = args_info.args[:len(args)]
- for i, arg_name in enumerate(arg_names):
- if arg_name in args_to_cast:
- new_args.append(
- cast_tensor_type(args[i], torch.float, torch.half))
- else:
- new_args.append(args[i])
- # convert the kwargs that need to be processed
- new_kwargs = {}
- if kwargs:
- for arg_name, arg_value in kwargs.items():
- if arg_name in args_to_cast:
- new_kwargs[arg_name] = cast_tensor_type(
- arg_value, torch.float, torch.half)
- else:
- new_kwargs[arg_name] = arg_value
- # apply converted arguments to the decorated method
- if (TORCH_VERSION != 'parrots' and
- digit_version(TORCH_VERSION) >= digit_version('1.6.0')):
- with autocast(enabled=True):
- output = old_func(*new_args, **new_kwargs)
- else:
- output = old_func(*new_args, **new_kwargs)
- # cast the results back to fp32 if necessary
- if out_fp32:
- output = cast_tensor_type(output, torch.half, torch.float)
- return output
-
- return new_func
-
- return auto_fp16_wrapper
-
-
-def force_fp32(apply_to=None, out_fp16=False):
- """Decorator to convert input arguments to fp32 in force.
-
- This decorator is useful when you write custom modules and want to support
- mixed precision training. If there are some inputs that must be processed
- in fp32 mode, then this decorator can handle it. If inputs arguments are
- fp16 tensors, they will be converted to fp32 automatically. Arguments other
- than fp16 tensors are ignored. If you are using PyTorch >= 1.6,
- torch.cuda.amp is used as the backend, otherwise, original mmcv
- implementation will be adopted.
-
- Args:
- apply_to (Iterable, optional): The argument names to be converted.
- `None` indicates all arguments.
- out_fp16 (bool): Whether to convert the output back to fp16.
-
- Example:
-
- >>> import torch.nn as nn
- >>> class MyModule1(nn.Module):
- >>>
- >>> # Convert x and y to fp32
- >>> @force_fp32()
- >>> def loss(self, x, y):
- >>> pass
-
- >>> import torch.nn as nn
- >>> class MyModule2(nn.Module):
- >>>
- >>> # convert pred to fp32
- >>> @force_fp32(apply_to=('pred', ))
- >>> def post_process(self, pred, others):
- >>> pass
- """
-
- def force_fp32_wrapper(old_func):
-
- @functools.wraps(old_func)
- def new_func(*args, **kwargs):
- # check if the module has set the attribute `fp16_enabled`, if not,
- # just fallback to the original method.
- if not isinstance(args[0], torch.nn.Module):
- raise TypeError('@force_fp32 can only be used to decorate the '
- 'method of nn.Module')
- if not (hasattr(args[0], 'fp16_enabled') and args[0].fp16_enabled):
- return old_func(*args, **kwargs)
- # get the arg spec of the decorated method
- args_info = getfullargspec(old_func)
- # get the argument names to be casted
- args_to_cast = args_info.args if apply_to is None else apply_to
- # convert the args that need to be processed
- new_args = []
- if args:
- arg_names = args_info.args[:len(args)]
- for i, arg_name in enumerate(arg_names):
- if arg_name in args_to_cast:
- new_args.append(
- cast_tensor_type(args[i], torch.half, torch.float))
- else:
- new_args.append(args[i])
- # convert the kwargs that need to be processed
- new_kwargs = dict()
- if kwargs:
- for arg_name, arg_value in kwargs.items():
- if arg_name in args_to_cast:
- new_kwargs[arg_name] = cast_tensor_type(
- arg_value, torch.half, torch.float)
- else:
- new_kwargs[arg_name] = arg_value
- # apply converted arguments to the decorated method
- if (TORCH_VERSION != 'parrots' and
- digit_version(TORCH_VERSION) >= digit_version('1.6.0')):
- with autocast(enabled=False):
- output = old_func(*new_args, **new_kwargs)
- else:
- output = old_func(*new_args, **new_kwargs)
- # cast the results back to fp32 if necessary
- if out_fp16:
- output = cast_tensor_type(output, torch.float, torch.half)
- return output
-
- return new_func
-
- return force_fp32_wrapper
-
-
-def allreduce_grads(params, coalesce=True, bucket_size_mb=-1):
- warnings.warning(
- '"mmcv.runner.fp16_utils.allreduce_grads" is deprecated, and will be '
- 'removed in v2.8. Please switch to "mmcv.runner.allreduce_grads')
- _allreduce_grads(params, coalesce=coalesce, bucket_size_mb=bucket_size_mb)
-
-
-def wrap_fp16_model(model):
- """Wrap the FP32 model to FP16.
-
- If you are using PyTorch >= 1.6, torch.cuda.amp is used as the
- backend, otherwise, original mmcv implementation will be adopted.
-
- For PyTorch >= 1.6, this function will
- 1. Set fp16 flag inside the model to True.
-
- Otherwise:
- 1. Convert FP32 model to FP16.
- 2. Remain some necessary layers to be FP32, e.g., normalization layers.
- 3. Set `fp16_enabled` flag inside the model to True.
-
- Args:
- model (nn.Module): Model in FP32.
- """
- if (TORCH_VERSION == 'parrots'
- or digit_version(TORCH_VERSION) < digit_version('1.6.0')):
- # convert model to fp16
- model.half()
- # patch the normalization layers to make it work in fp32 mode
- patch_norm_fp32(model)
- # set `fp16_enabled` flag
- for m in model.modules():
- if hasattr(m, 'fp16_enabled'):
- m.fp16_enabled = True
-
-
-def patch_norm_fp32(module):
- """Recursively convert normalization layers from FP16 to FP32.
-
- Args:
- module (nn.Module): The modules to be converted in FP16.
-
- Returns:
- nn.Module: The converted module, the normalization layers have been
- converted to FP32.
- """
- if isinstance(module, (nn.modules.batchnorm._BatchNorm, nn.GroupNorm)):
- module.float()
- if isinstance(module, nn.GroupNorm) or torch.__version__ < '1.3':
- module.forward = patch_forward_method(module.forward, torch.half,
- torch.float)
- for child in module.children():
- patch_norm_fp32(child)
- return module
-
-
-def patch_forward_method(func, src_type, dst_type, convert_output=True):
- """Patch the forward method of a module.
-
- Args:
- func (callable): The original forward method.
- src_type (torch.dtype): Type of input arguments to be converted from.
- dst_type (torch.dtype): Type of input arguments to be converted to.
- convert_output (bool): Whether to convert the output back to src_type.
-
- Returns:
- callable: The patched forward method.
- """
-
- def new_forward(*args, **kwargs):
- output = func(*cast_tensor_type(args, src_type, dst_type),
- **cast_tensor_type(kwargs, src_type, dst_type))
- if convert_output:
- output = cast_tensor_type(output, dst_type, src_type)
- return output
-
- return new_forward
-
-
-class LossScaler:
- """Class that manages loss scaling in mixed precision training which
- supports both dynamic or static mode.
-
- The implementation refers to
- https://github.com/NVIDIA/apex/blob/master/apex/fp16_utils/loss_scaler.py.
- Indirectly, by supplying ``mode='dynamic'`` for dynamic loss scaling.
- It's important to understand how :class:`LossScaler` operates.
- Loss scaling is designed to combat the problem of underflowing
- gradients encountered at long times when training fp16 networks.
- Dynamic loss scaling begins by attempting a very high loss
- scale. Ironically, this may result in OVERflowing gradients.
- If overflowing gradients are encountered, :class:`FP16_Optimizer` then
- skips the update step for this particular iteration/minibatch,
- and :class:`LossScaler` adjusts the loss scale to a lower value.
- If a certain number of iterations occur without overflowing gradients
- detected,:class:`LossScaler` increases the loss scale once more.
- In this way :class:`LossScaler` attempts to "ride the edge" of always
- using the highest loss scale possible without incurring overflow.
-
- Args:
- init_scale (float): Initial loss scale value, default: 2**32.
- scale_factor (float): Factor used when adjusting the loss scale.
- Default: 2.
- mode (str): Loss scaling mode. 'dynamic' or 'static'
- scale_window (int): Number of consecutive iterations without an
- overflow to wait before increasing the loss scale. Default: 1000.
- """
-
- def __init__(self,
- init_scale=2**32,
- mode='dynamic',
- scale_factor=2.,
- scale_window=1000):
- self.cur_scale = init_scale
- self.cur_iter = 0
- assert mode in ('dynamic',
- 'static'), 'mode can only be dynamic or static'
- self.mode = mode
- self.last_overflow_iter = -1
- self.scale_factor = scale_factor
- self.scale_window = scale_window
-
- def has_overflow(self, params):
- """Check if params contain overflow."""
- if self.mode != 'dynamic':
- return False
- for p in params:
- if p.grad is not None and LossScaler._has_inf_or_nan(p.grad.data):
- return True
- return False
-
- def _has_inf_or_nan(x):
- """Check if params contain NaN."""
- try:
- cpu_sum = float(x.float().sum())
- except RuntimeError as instance:
- if 'value cannot be converted' not in instance.args[0]:
- raise
- return True
- else:
- if cpu_sum == float('inf') or cpu_sum == -float('inf') \
- or cpu_sum != cpu_sum:
- return True
- return False
-
- def update_scale(self, overflow):
- """update the current loss scale value when overflow happens."""
- if self.mode != 'dynamic':
- return
- if overflow:
- self.cur_scale = max(self.cur_scale / self.scale_factor, 1)
- self.last_overflow_iter = self.cur_iter
- else:
- if (self.cur_iter - self.last_overflow_iter) % \
- self.scale_window == 0:
- self.cur_scale *= self.scale_factor
- self.cur_iter += 1
-
- def state_dict(self):
- """Returns the state of the scaler as a :class:`dict`."""
- return dict(
- cur_scale=self.cur_scale,
- cur_iter=self.cur_iter,
- mode=self.mode,
- last_overflow_iter=self.last_overflow_iter,
- scale_factor=self.scale_factor,
- scale_window=self.scale_window)
-
- def load_state_dict(self, state_dict):
- """Loads the loss_scaler state dict.
-
- Args:
- state_dict (dict): scaler state.
- """
- self.cur_scale = state_dict['cur_scale']
- self.cur_iter = state_dict['cur_iter']
- self.mode = state_dict['mode']
- self.last_overflow_iter = state_dict['last_overflow_iter']
- self.scale_factor = state_dict['scale_factor']
- self.scale_window = state_dict['scale_window']
-
- @property
- def loss_scale(self):
- return self.cur_scale
diff --git a/spaces/Rongjiehuang/GenerSpeech/data_gen/tts/base_binarizer_emotion.py b/spaces/Rongjiehuang/GenerSpeech/data_gen/tts/base_binarizer_emotion.py
deleted file mode 100644
index cd9a66253278066ff16967e846c8139c83a027dc..0000000000000000000000000000000000000000
--- a/spaces/Rongjiehuang/GenerSpeech/data_gen/tts/base_binarizer_emotion.py
+++ /dev/null
@@ -1,352 +0,0 @@
-import os
-
-os.environ["OMP_NUM_THREADS"] = "1"
-import torch
-from collections import Counter
-from utils.text_encoder import TokenTextEncoder
-from data_gen.tts.emotion import inference as EmotionEncoder
-from data_gen.tts.emotion.inference import embed_utterance as Embed_utterance
-from data_gen.tts.emotion.inference import preprocess_wav
-from utils.multiprocess_utils import chunked_multiprocess_run
-import random
-import traceback
-import json
-from resemblyzer import VoiceEncoder
-from tqdm import tqdm
-from data_gen.tts.data_gen_utils import get_mel2ph, get_pitch, build_phone_encoder, is_sil_phoneme
-from utils.hparams import hparams, set_hparams
-import numpy as np
-from utils.indexed_datasets import IndexedDatasetBuilder
-from vocoders.base_vocoder import get_vocoder_cls
-import pandas as pd
-
-
-class BinarizationError(Exception):
- pass
-
-
-class EmotionBinarizer:
- def __init__(self, processed_data_dir=None):
- if processed_data_dir is None:
- processed_data_dir = hparams['processed_data_dir']
- self.processed_data_dirs = processed_data_dir.split(",")
- self.binarization_args = hparams['binarization_args']
- self.pre_align_args = hparams['pre_align_args']
- self.item2txt = {}
- self.item2ph = {}
- self.item2wavfn = {}
- self.item2tgfn = {}
- self.item2spk = {}
- self.item2emo = {}
-
- def load_meta_data(self):
- for ds_id, processed_data_dir in enumerate(self.processed_data_dirs):
- self.meta_df = pd.read_csv(f"{processed_data_dir}/metadata_phone.csv", dtype=str)
- for r_idx, r in tqdm(self.meta_df.iterrows(), desc='Loading meta data.'):
- item_name = raw_item_name = r['item_name']
- if len(self.processed_data_dirs) > 1:
- item_name = f'ds{ds_id}_{item_name}'
- self.item2txt[item_name] = r['txt']
- self.item2ph[item_name] = r['ph']
- self.item2wavfn[item_name] = r['wav_fn']
- self.item2spk[item_name] = r.get('spk', 'SPK1') \
- if self.binarization_args['with_spk_id'] else 'SPK1'
- if len(self.processed_data_dirs) > 1:
- self.item2spk[item_name] = f"ds{ds_id}_{self.item2spk[item_name]}"
- self.item2tgfn[item_name] = f"{processed_data_dir}/mfa_outputs/{raw_item_name}.TextGrid"
- self.item2emo[item_name] = r.get('others', '"Neutral"')
- self.item_names = sorted(list(self.item2txt.keys()))
- if self.binarization_args['shuffle']:
- random.seed(1234)
- random.shuffle(self.item_names)
-
- @property
- def train_item_names(self):
- return self.item_names[hparams['test_num']:]
-
- @property
- def valid_item_names(self):
- return self.item_names[:hparams['test_num']]
-
- @property
- def test_item_names(self):
- return self.valid_item_names
-
- def build_spk_map(self):
- spk_map = set()
- for item_name in self.item_names:
- spk_name = self.item2spk[item_name]
- spk_map.add(spk_name)
- spk_map = {x: i for i, x in enumerate(sorted(list(spk_map)))}
- print("| #Spk: ", len(spk_map))
- assert len(spk_map) == 0 or len(spk_map) <= hparams['num_spk'], len(spk_map)
- return spk_map
-
- def build_emo_map(self):
- emo_map = set()
- for item_name in self.item_names:
- emo_name = self.item2emo[item_name]
- emo_map.add(emo_name)
- emo_map = {x: i for i, x in enumerate(sorted(list(emo_map)))}
- print("| #Emo: ", len(emo_map))
- return emo_map
-
- def item_name2spk_id(self, item_name):
- return self.spk_map[self.item2spk[item_name]]
-
- def item_name2emo_id(self, item_name):
- return self.emo_map[self.item2emo[item_name]]
-
- def _phone_encoder(self):
- ph_set_fn = f"{hparams['binary_data_dir']}/phone_set.json"
- ph_set = []
- if self.binarization_args['reset_phone_dict'] or not os.path.exists(ph_set_fn):
- for ph_sent in self.item2ph.values():
- ph_set += ph_sent.split(' ')
- ph_set = sorted(set(ph_set))
- json.dump(ph_set, open(ph_set_fn, 'w'))
- print("| Build phone set: ", ph_set)
- else:
- ph_set = json.load(open(ph_set_fn, 'r'))
- print("| Load phone set: ", ph_set)
- return build_phone_encoder(hparams['binary_data_dir'])
-
- def _word_encoder(self):
- fn = f"{hparams['binary_data_dir']}/word_set.json"
- word_set = []
- if self.binarization_args['reset_word_dict']:
- for word_sent in self.item2txt.values():
- word_set += [x for x in word_sent.split(' ') if x != '']
- word_set = Counter(word_set)
- total_words = sum(word_set.values())
- word_set = word_set.most_common(hparams['word_size'])
- num_unk_words = total_words - sum([x[1] for x in word_set])
- word_set = [x[0] for x in word_set]
- json.dump(word_set, open(fn, 'w'))
- print(f"| Build word set. Size: {len(word_set)}, #total words: {total_words},"
- f" #unk_words: {num_unk_words}, word_set[:10]:, {word_set[:10]}.")
- else:
- word_set = json.load(open(fn, 'r'))
- print("| Load word set. Size: ", len(word_set), word_set[:10])
- return TokenTextEncoder(None, vocab_list=word_set, replace_oov='')
-
- def meta_data(self, prefix):
- if prefix == 'valid':
- item_names = self.valid_item_names
- elif prefix == 'test':
- item_names = self.test_item_names
- else:
- item_names = self.train_item_names
- for item_name in item_names:
- ph = self.item2ph[item_name]
- txt = self.item2txt[item_name]
- tg_fn = self.item2tgfn.get(item_name)
- wav_fn = self.item2wavfn[item_name]
- spk_id = self.item_name2spk_id(item_name)
- emotion = self.item_name2emo_id(item_name)
- yield item_name, ph, txt, tg_fn, wav_fn, spk_id, emotion
-
- def process(self):
- self.load_meta_data()
- os.makedirs(hparams['binary_data_dir'], exist_ok=True)
- self.spk_map = self.build_spk_map()
- print("| spk_map: ", self.spk_map)
- spk_map_fn = f"{hparams['binary_data_dir']}/spk_map.json"
- json.dump(self.spk_map, open(spk_map_fn, 'w'))
-
- self.emo_map = self.build_emo_map()
- print("| emo_map: ", self.emo_map)
- emo_map_fn = f"{hparams['binary_data_dir']}/emo_map.json"
- json.dump(self.emo_map, open(emo_map_fn, 'w'))
-
- self.phone_encoder = self._phone_encoder()
- self.word_encoder = None
- EmotionEncoder.load_model(hparams['emotion_encoder_path'])
-
- if self.binarization_args['with_word']:
- self.word_encoder = self._word_encoder()
- self.process_data('valid')
- self.process_data('test')
- self.process_data('train')
-
- def process_data(self, prefix):
- data_dir = hparams['binary_data_dir']
- args = []
- builder = IndexedDatasetBuilder(f'{data_dir}/{prefix}')
- ph_lengths = []
- mel_lengths = []
- f0s = []
- total_sec = 0
- if self.binarization_args['with_spk_embed']:
- voice_encoder = VoiceEncoder().cuda()
-
- meta_data = list(self.meta_data(prefix))
- for m in meta_data:
- args.append(list(m) + [(self.phone_encoder, self.word_encoder), self.binarization_args])
- num_workers = self.num_workers
- for f_id, (_, item) in enumerate(
- zip(tqdm(meta_data), chunked_multiprocess_run(self.process_item, args, num_workers=num_workers))):
- if item is None:
- continue
- item['spk_embed'] = voice_encoder.embed_utterance(item['wav']) \
- if self.binarization_args['with_spk_embed'] else None
- processed_wav = preprocess_wav(item['wav_fn'])
- item['emo_embed'] = Embed_utterance(processed_wav)
- if not self.binarization_args['with_wav'] and 'wav' in item:
- del item['wav']
- builder.add_item(item)
- mel_lengths.append(item['len'])
- if 'ph_len' in item:
- ph_lengths.append(item['ph_len'])
- total_sec += item['sec']
- if item.get('f0') is not None:
- f0s.append(item['f0'])
- builder.finalize()
- np.save(f'{data_dir}/{prefix}_lengths.npy', mel_lengths)
- if len(ph_lengths) > 0:
- np.save(f'{data_dir}/{prefix}_ph_lengths.npy', ph_lengths)
- if len(f0s) > 0:
- f0s = np.concatenate(f0s, 0)
- f0s = f0s[f0s != 0]
- np.save(f'{data_dir}/{prefix}_f0s_mean_std.npy', [np.mean(f0s).item(), np.std(f0s).item()])
- print(f"| {prefix} total duration: {total_sec:.3f}s")
-
- @classmethod
- def process_item(cls, item_name, ph, txt, tg_fn, wav_fn, spk_id, emotion, encoder, binarization_args):
- res = {'item_name': item_name, 'txt': txt, 'ph': ph, 'wav_fn': wav_fn, 'spk_id': spk_id, 'emotion': emotion}
- if binarization_args['with_linear']:
- wav, mel, linear_stft = get_vocoder_cls(hparams).wav2spec(wav_fn) # , return_linear=True
- res['linear'] = linear_stft
- else:
- wav, mel = get_vocoder_cls(hparams).wav2spec(wav_fn)
- wav = wav.astype(np.float16)
- res.update({'mel': mel, 'wav': wav,
- 'sec': len(wav) / hparams['audio_sample_rate'], 'len': mel.shape[0]})
- try:
- if binarization_args['with_f0']:
- cls.get_pitch(res)
- if binarization_args['with_f0cwt']:
- cls.get_f0cwt(res)
- if binarization_args['with_txt']:
- ph_encoder, word_encoder = encoder
- try:
- res['phone'] = ph_encoder.encode(ph)
- res['ph_len'] = len(res['phone'])
- except:
- traceback.print_exc()
- raise BinarizationError(f"Empty phoneme")
- if binarization_args['with_align']:
- cls.get_align(tg_fn, res)
- if binarization_args['trim_eos_bos']:
- bos_dur = res['dur'][0]
- eos_dur = res['dur'][-1]
- res['mel'] = mel[bos_dur:-eos_dur]
- res['f0'] = res['f0'][bos_dur:-eos_dur]
- res['pitch'] = res['pitch'][bos_dur:-eos_dur]
- res['mel2ph'] = res['mel2ph'][bos_dur:-eos_dur]
- res['wav'] = wav[bos_dur * hparams['hop_size']:-eos_dur * hparams['hop_size']]
- res['dur'] = res['dur'][1:-1]
- res['len'] = res['mel'].shape[0]
- if binarization_args['with_word']:
- cls.get_word(res, word_encoder)
- except BinarizationError as e:
- print(f"| Skip item ({e}). item_name: {item_name}, wav_fn: {wav_fn}")
- return None
- except Exception as e:
- traceback.print_exc()
- print(f"| Skip item. item_name: {item_name}, wav_fn: {wav_fn}")
- return None
- return res
-
- @staticmethod
- def get_align(tg_fn, res):
- ph = res['ph']
- mel = res['mel']
- phone_encoded = res['phone']
- if tg_fn is not None and os.path.exists(tg_fn):
- mel2ph, dur = get_mel2ph(tg_fn, ph, mel, hparams)
- else:
- raise BinarizationError(f"Align not found")
- if mel2ph.max() - 1 >= len(phone_encoded):
- raise BinarizationError(
- f"Align does not match: mel2ph.max() - 1: {mel2ph.max() - 1}, len(phone_encoded): {len(phone_encoded)}")
- res['mel2ph'] = mel2ph
- res['dur'] = dur
-
- @staticmethod
- def get_pitch(res):
- wav, mel = res['wav'], res['mel']
- f0, pitch_coarse = get_pitch(wav, mel, hparams)
- if sum(f0) == 0:
- raise BinarizationError("Empty f0")
- res['f0'] = f0
- res['pitch'] = pitch_coarse
-
- @staticmethod
- def get_f0cwt(res):
- from utils.cwt import get_cont_lf0, get_lf0_cwt
- f0 = res['f0']
- uv, cont_lf0_lpf = get_cont_lf0(f0)
- logf0s_mean_org, logf0s_std_org = np.mean(cont_lf0_lpf), np.std(cont_lf0_lpf)
- cont_lf0_lpf_norm = (cont_lf0_lpf - logf0s_mean_org) / logf0s_std_org
- Wavelet_lf0, scales = get_lf0_cwt(cont_lf0_lpf_norm)
- if np.any(np.isnan(Wavelet_lf0)):
- raise BinarizationError("NaN CWT")
- res['cwt_spec'] = Wavelet_lf0
- res['cwt_scales'] = scales
- res['f0_mean'] = logf0s_mean_org
- res['f0_std'] = logf0s_std_org
-
- @staticmethod
- def get_word(res, word_encoder):
- ph_split = res['ph'].split(" ")
- # ph side mapping to word
- ph_words = [] # ['', 'N_AW1_', ',', 'AE1_Z_|', 'AO1_L_|', 'B_UH1_K_S_|', 'N_AA1_T_|', ....]
- ph2word = np.zeros([len(ph_split)], dtype=int)
- last_ph_idx_for_word = [] # [2, 11, ...]
- for i, ph in enumerate(ph_split):
- if ph == '|':
- last_ph_idx_for_word.append(i)
- elif not ph[0].isalnum():
- if ph not in ['']:
- last_ph_idx_for_word.append(i - 1)
- last_ph_idx_for_word.append(i)
- start_ph_idx_for_word = [0] + [i + 1 for i in last_ph_idx_for_word[:-1]]
- for i, (s_w, e_w) in enumerate(zip(start_ph_idx_for_word, last_ph_idx_for_word)):
- ph_words.append(ph_split[s_w:e_w + 1])
- ph2word[s_w:e_w + 1] = i
- ph2word = ph2word.tolist()
- ph_words = ["_".join(w) for w in ph_words]
-
- # mel side mapping to word
- mel2word = []
- dur_word = [0 for _ in range(len(ph_words))]
- for i, m2p in enumerate(res['mel2ph']):
- word_idx = ph2word[m2p - 1]
- mel2word.append(ph2word[m2p - 1])
- dur_word[word_idx] += 1
- ph2word = [x + 1 for x in ph2word] # 0预留给padding
- mel2word = [x + 1 for x in mel2word] # 0预留给padding
- res['ph_words'] = ph_words # [T_word]
- res['ph2word'] = ph2word # [T_ph]
- res['mel2word'] = mel2word # [T_mel]
- res['dur_word'] = dur_word # [T_word]
- words = [x for x in res['txt'].split(" ") if x != '']
- while len(words) > 0 and is_sil_phoneme(words[0]):
- words = words[1:]
- while len(words) > 0 and is_sil_phoneme(words[-1]):
- words = words[:-1]
- words = [''] + words + ['']
- word_tokens = word_encoder.encode(" ".join(words))
- res['words'] = words
- res['word_tokens'] = word_tokens
- assert len(words) == len(ph_words), [words, ph_words]
-
- @property
- def num_workers(self):
- return int(os.getenv('N_PROC', hparams.get('N_PROC', os.cpu_count())))
-
-
-if __name__ == "__main__":
- set_hparams()
- EmotionBinarizer().process()
diff --git a/spaces/Salesforce/EDICT/my_half_diffusers/utils/dummy_transformers_and_onnx_objects.py b/spaces/Salesforce/EDICT/my_half_diffusers/utils/dummy_transformers_and_onnx_objects.py
deleted file mode 100644
index 2e34b5ce0b69472df7e2c41de40476619d53dee9..0000000000000000000000000000000000000000
--- a/spaces/Salesforce/EDICT/my_half_diffusers/utils/dummy_transformers_and_onnx_objects.py
+++ /dev/null
@@ -1,11 +0,0 @@
-# This file is autogenerated by the command `make fix-copies`, do not edit.
-# flake8: noqa
-
-from ..utils import DummyObject, requires_backends
-
-
-class StableDiffusionOnnxPipeline(metaclass=DummyObject):
- _backends = ["transformers", "onnx"]
-
- def __init__(self, *args, **kwargs):
- requires_backends(self, ["transformers", "onnx"])
diff --git a/spaces/SarthakSidhant/Go-Cattle/diseases/bovine viral diarrhea.md b/spaces/SarthakSidhant/Go-Cattle/diseases/bovine viral diarrhea.md
deleted file mode 100644
index 1345d54192fce4d6d33239fc5991d542a932eaa3..0000000000000000000000000000000000000000
--- a/spaces/SarthakSidhant/Go-Cattle/diseases/bovine viral diarrhea.md
+++ /dev/null
@@ -1,39 +0,0 @@
-## Bovine viral diarrhea (BVD)
-
-**Information:** Bovine viral diarrhea (BVD) is a viral disease of cattle that can cause a variety of symptoms, including respiratory illness, diarrhea, and reproductive problems. BVD is a serious disease that can have a significant economic impact on the cattle industry.
-
-**Symptoms:**
-
-* Respiratory illness: Fever, coughing, nasal discharge, difficulty breathing
-* Diarrhea: Watery or bloody diarrhea, weight loss
-* Reproductive problems: Abortion, stillbirth, infertility
-* Other symptoms: Jaundice, neurological problems, death
-
-**Remedies:**
-
-* There is no cure for BVD.
-* Treatment for BVD is supportive care, such as fluids and antibiotics.
-* Animals that have recovered from BVD may be immune to future infection.
-
-**Causes:**
-
-* BVD is caused by a virus called bovine viral diarrhea virus (BVDV).
-* BVDV is a highly contagious virus that can spread through contact with infected animals, their bodily fluids, or contaminated surfaces.
-* BVDV can also be transmitted from mother to calf during pregnancy or at birth.
-
-**Prevention:**
-
-* The best way to prevent BVD is to vaccinate animals against the disease.
-* Vaccinations are available for both pregnant and non-pregnant cattle.
-* Other preventive measures include:
- * Maintaining good herd health practices
- * Isolating sick animals
- * Practicing biosecurity measures
-
-**Other preventive measures:**
-
-* Avoid overcrowding animals
-* Provide clean, fresh water
-* Monitor animals for signs of illness
-* Dispose of dead animals properly
-* Vaccinate animals according to the manufacturer's instructions
diff --git a/spaces/SouthCity/ShuruiXu/toolbox.py b/spaces/SouthCity/ShuruiXu/toolbox.py
deleted file mode 100644
index f21db52543d7b0684123c9bcc75442a8a80e0202..0000000000000000000000000000000000000000
--- a/spaces/SouthCity/ShuruiXu/toolbox.py
+++ /dev/null
@@ -1,344 +0,0 @@
-import markdown, mdtex2html, threading, importlib, traceback, importlib, inspect, re
-from show_math import convert as convert_math
-from functools import wraps, lru_cache
-
-def get_reduce_token_percent(text):
- try:
- # text = "maximum context length is 4097 tokens. However, your messages resulted in 4870 tokens"
- pattern = r"(\d+)\s+tokens\b"
- match = re.findall(pattern, text)
- EXCEED_ALLO = 500 # 稍微留一点余地,否则在回复时会因余量太少出问题
- max_limit = float(match[0]) - EXCEED_ALLO
- current_tokens = float(match[1])
- ratio = max_limit/current_tokens
- assert ratio > 0 and ratio < 1
- return ratio, str(int(current_tokens-max_limit))
- except:
- return 0.5, '不详'
-
-def predict_no_ui_but_counting_down(i_say, i_say_show_user, chatbot, top_p, api_key, temperature, history=[], sys_prompt='', long_connection=True):
- """
- 调用简单的predict_no_ui接口,但是依然保留了些许界面心跳功能,当对话太长时,会自动采用二分法截断
- i_say: 当前输入
- i_say_show_user: 显示到对话界面上的当前输入,例如,输入整个文件时,你绝对不想把文件的内容都糊到对话界面上
- chatbot: 对话界面句柄
- top_p, api_key, temperature: gpt参数
- history: gpt参数 对话历史
- sys_prompt: gpt参数 sys_prompt
- long_connection: 是否采用更稳定的连接方式(推荐)
- """
- import time
- from predict import predict_no_ui, predict_no_ui_long_connection
- from toolbox import get_conf
- TIMEOUT_SECONDS, MAX_RETRY = get_conf('TIMEOUT_SECONDS', 'MAX_RETRY')
- # 多线程的时候,需要一个mutable结构在不同线程之间传递信息
- # list就是最简单的mutable结构,我们第一个位置放gpt输出,第二个位置传递报错信息
- mutable = [None, '']
- # multi-threading worker
- def mt(i_say, history):
- while True:
- try:
- if long_connection:
- mutable[0] = predict_no_ui_long_connection(inputs=i_say, top_p=top_p, api_key=api_key, temperature=temperature, history=history, sys_prompt=sys_prompt)
- else:
- mutable[0] = predict_no_ui(inputs=i_say, top_p=top_p, api_key=api_key, temperature=temperature, history=history, sys_prompt=sys_prompt)
- break
- except ConnectionAbortedError as token_exceeded_error:
- # 尝试计算比例,尽可能多地保留文本
- p_ratio, n_exceed = get_reduce_token_percent(str(token_exceeded_error))
- if len(history) > 0:
- history = [his[ int(len(his) *p_ratio): ] for his in history if his is not None]
- else:
- i_say = i_say[: int(len(i_say) *p_ratio) ]
- mutable[1] = f'警告,文本过长将进行截断,Token溢出数:{n_exceed},截断比例:{(1-p_ratio):.0%}。'
- except TimeoutError as e:
- mutable[0] = '[Local Message] 请求超时。'
- raise TimeoutError
- except Exception as e:
- mutable[0] = f'[Local Message] 异常:{str(e)}.'
- raise RuntimeError(f'[Local Message] 异常:{str(e)}.')
- # 创建新线程发出http请求
- thread_name = threading.Thread(target=mt, args=(i_say, history)); thread_name.start()
- # 原来的线程则负责持续更新UI,实现一个超时倒计时,并等待新线程的任务完成
- cnt = 0
- while thread_name.is_alive():
- cnt += 1
- chatbot[-1] = (i_say_show_user, f"[Local Message] {mutable[1]}waiting gpt response {cnt}/{TIMEOUT_SECONDS*2*(MAX_RETRY+1)}"+''.join(['.']*(cnt%4)))
- yield chatbot, history, '正常'
- time.sleep(1)
- # 把gpt的输出从mutable中取出来
- gpt_say = mutable[0]
- if gpt_say=='[Local Message] Failed with timeout.': raise TimeoutError
- return gpt_say
-
-def write_results_to_file(history, file_name=None):
- """
- 将对话记录history以Markdown格式写入文件中。如果没有指定文件名,则使用当前时间生成文件名。
- """
- import os, time
- if file_name is None:
- # file_name = time.strftime("chatGPT分析报告%Y-%m-%d-%H-%M-%S", time.localtime()) + '.md'
- file_name = 'chatGPT分析报告' + time.strftime("%Y-%m-%d-%H-%M-%S", time.localtime()) + '.md'
- os.makedirs('./gpt_log/', exist_ok=True)
- with open(f'./gpt_log/{file_name}', 'w', encoding = 'utf8') as f:
- f.write('# chatGPT 分析报告\n')
- for i, content in enumerate(history):
- try: # 这个bug没找到触发条件,暂时先这样顶一下
- if type(content) != str: content = str(content)
- except:
- continue
- if i%2==0: f.write('## ')
- f.write(content)
- f.write('\n\n')
- res = '以上材料已经被写入' + os.path.abspath(f'./gpt_log/{file_name}')
- print(res)
- return res
-
-def regular_txt_to_markdown(text):
- """
- 将普通文本转换为Markdown格式的文本。
- """
- text = text.replace('\n', '\n\n')
- text = text.replace('\n\n\n', '\n\n')
- text = text.replace('\n\n\n', '\n\n')
- return text
-
-def CatchException(f):
- """
- 装饰器函数,捕捉函数f中的异常并封装到一个生成器中返回,并显示到聊天当中。
- """
- @wraps(f)
- def decorated(txt, top_p, api_key, temperature, chatbot, history, systemPromptTxt, WEB_PORT):
- try:
- yield from f(txt, top_p, api_key, temperature, chatbot, history, systemPromptTxt, WEB_PORT)
- except Exception as e:
- from check_proxy import check_proxy
- from toolbox import get_conf
- proxies, = get_conf('proxies')
- tb_str = '```\n' + traceback.format_exc() + '```'
- if len(chatbot) == 0: chatbot.append(["插件调度异常","异常原因"])
- chatbot[-1] = (chatbot[-1][0], f"[Local Message] 实验性函数调用出错: \n\n{tb_str} \n\n当前代理可用性: \n\n{check_proxy(proxies)}")
- yield chatbot, history, f'异常 {e}'
- return decorated
-
-def HotReload(f):
- """
- 装饰器函数,实现函数插件热更新
- """
- @wraps(f)
- def decorated(*args, **kwargs):
- fn_name = f.__name__
- f_hot_reload = getattr(importlib.reload(inspect.getmodule(f)), fn_name)
- yield from f_hot_reload(*args, **kwargs)
- return decorated
-
-def report_execption(chatbot, history, a, b):
- """
- 向chatbot中添加错误信息
- """
- chatbot.append((a, b))
- history.append(a); history.append(b)
-
-def text_divide_paragraph(text):
- """
- 将文本按照段落分隔符分割开,生成带有段落标签的HTML代码。
- """
- if '```' in text:
- # careful input
- return text
- else:
- # wtf input
- lines = text.split("\n")
- for i, line in enumerate(lines):
- lines[i] = lines[i].replace(" ", " ")
- text = "".join(lines)
- return text
-
-def markdown_convertion(txt):
- """
- 将Markdown格式的文本转换为HTML格式。如果包含数学公式,则先将公式转换为HTML格式。
- """
- pre = ''
- suf = ''
- if ('$' in txt) and ('```' not in txt):
- return pre + markdown.markdown(txt,extensions=['fenced_code','tables']) + '
' + markdown.markdown(convert_math(txt, splitParagraphs=False),extensions=['fenced_code','tables']) + suf
- else:
- return pre + markdown.markdown(txt,extensions=['fenced_code','tables']) + suf
-
-def close_up_code_segment_during_stream(gpt_reply):
- """
- 在gpt输出代码的中途(输出了前面的```,但还没输出完后面的```),补上后面的```
- """
- if '```' not in gpt_reply: return gpt_reply
- if gpt_reply.endswith('```'): return gpt_reply
-
- # 排除了以上两个情况,我们
- segments = gpt_reply.split('```')
- n_mark = len(segments) - 1
- if n_mark % 2 == 1:
- # print('输出代码片段中!')
- return gpt_reply+'\n```'
- else:
- return gpt_reply
-
-
-
-def format_io(self, y):
- """
- 将输入和输出解析为HTML格式。将y中最后一项的输入部分段落化,并将输出部分的Markdown和数学公式转换为HTML格式。
- """
- if y is None or y == []: return []
- i_ask, gpt_reply = y[-1]
- i_ask = text_divide_paragraph(i_ask) # 输入部分太自由,预处理一波
- gpt_reply = close_up_code_segment_during_stream(gpt_reply) # 当代码输出半截的时候,试着补上后个```
- y[-1] = (
- None if i_ask is None else markdown.markdown(i_ask, extensions=['fenced_code','tables']),
- None if gpt_reply is None else markdown_convertion(gpt_reply)
- )
- return y
-
-
-def find_free_port():
- """
- 返回当前系统中可用的未使用端口。
- """
- import socket
- from contextlib import closing
- with closing(socket.socket(socket.AF_INET, socket.SOCK_STREAM)) as s:
- s.bind(('', 0))
- s.setsockopt(socket.SOL_SOCKET, socket.SO_REUSEADDR, 1)
- return s.getsockname()[1]
-
-
-def extract_archive(file_path, dest_dir):
- import zipfile
- import tarfile
- import os
- # Get the file extension of the input file
- file_extension = os.path.splitext(file_path)[1]
-
- # Extract the archive based on its extension
- if file_extension == '.zip':
- with zipfile.ZipFile(file_path, 'r') as zipobj:
- zipobj.extractall(path=dest_dir)
- print("Successfully extracted zip archive to {}".format(dest_dir))
-
- elif file_extension in ['.tar', '.gz', '.bz2']:
- with tarfile.open(file_path, 'r:*') as tarobj:
- tarobj.extractall(path=dest_dir)
- print("Successfully extracted tar archive to {}".format(dest_dir))
-
- # 第三方库,需要预先pip install rarfile
- # 此外,Windows上还需要安装winrar软件,配置其Path环境变量,如"C:\Program Files\WinRAR"才可以
- elif file_extension == '.rar':
- try:
- import rarfile
- with rarfile.RarFile(file_path) as rf:
- rf.extractall(path=dest_dir)
- print("Successfully extracted rar archive to {}".format(dest_dir))
- except:
- print("Rar format requires additional dependencies to install")
- return '\n\n需要安装pip install rarfile来解压rar文件'
-
- # 第三方库,需要预先pip install py7zr
- elif file_extension == '.7z':
- try:
- import py7zr
- with py7zr.SevenZipFile(file_path, mode='r') as f:
- f.extractall(path=dest_dir)
- print("Successfully extracted 7z archive to {}".format(dest_dir))
- except:
- print("7z format requires additional dependencies to install")
- return '\n\n需要安装pip install py7zr来解压7z文件'
- else:
- return ''
- return ''
-
-def find_recent_files(directory):
- """
- me: find files that is created with in one minutes under a directory with python, write a function
- gpt: here it is!
- """
- import os
- import time
- current_time = time.time()
- one_minute_ago = current_time - 60
- recent_files = []
-
- for filename in os.listdir(directory):
- file_path = os.path.join(directory, filename)
- if file_path.endswith('.log'): continue
- created_time = os.path.getctime(file_path)
- if created_time >= one_minute_ago:
- if os.path.isdir(file_path): continue
- recent_files.append(file_path)
-
- return recent_files
-
-
-def on_file_uploaded(files, chatbot, txt):
- if len(files) == 0: return chatbot, txt
- import shutil, os, time, glob
- from toolbox import extract_archive
- try: shutil.rmtree('./private_upload/')
- except: pass
- time_tag = time.strftime("%Y-%m-%d-%H-%M-%S", time.localtime())
- os.makedirs(f'private_upload/{time_tag}', exist_ok=True)
- err_msg = ''
- for file in files:
- file_origin_name = os.path.basename(file.orig_name)
- shutil.copy(file.name, f'private_upload/{time_tag}/{file_origin_name}')
- err_msg += extract_archive(f'private_upload/{time_tag}/{file_origin_name}',
- dest_dir=f'private_upload/{time_tag}/{file_origin_name}.extract')
- moved_files = [fp for fp in glob.glob('private_upload/**/*', recursive=True)]
- txt = f'private_upload/{time_tag}'
- moved_files_str = '\t\n\n'.join(moved_files)
- chatbot.append(['我上传了文件,请查收',
- f'[Local Message] 收到以下文件: \n\n{moved_files_str}'+
- f'\n\n调用路径参数已自动修正到: \n\n{txt}'+
- f'\n\n现在您点击任意实验功能时,以上文件将被作为输入参数'+err_msg])
- return chatbot, txt
-
-
-def on_report_generated(files, chatbot):
- from toolbox import find_recent_files
- report_files = find_recent_files('gpt_log')
- if len(report_files) == 0: return files, chatbot
- # files.extend(report_files)
- chatbot.append(['汇总报告如何远程获取?', '汇总报告已经添加到右侧“文件上传区”(可能处于折叠状态),请查收。'])
- return report_files, chatbot
-
-@lru_cache(maxsize=128)
-def read_single_conf_with_lru_cache(arg):
- try: r = getattr(importlib.import_module('config_private'), arg)
- except: r = getattr(importlib.import_module('config'), arg)
- # 在读取API_KEY时,检查一下是不是忘了改config
- # if arg=='API_KEY':
- # # 正确的 API_KEY 是 "sk-" + 48 位大小写字母数字的组合
- # API_MATCH = re.match(r"sk-[a-zA-Z0-9]{48}$", r)
- # if API_MATCH:
- # print(f"[API_KEY] 您的 API_KEY 是: {r[:15]}*** API_KEY 导入成功")
- # else:
- # assert False, "正确的 API_KEY 是 'sk-' + '48 位大小写字母数字' 的组合,请在config文件中修改API密钥, 添加海外代理之后再运行。" + \
- # "(如果您刚更新过代码,请确保旧版config_private文件中没有遗留任何新增键值)"
- if arg=='proxies':
- if r is None:
- print('[PROXY] 网络代理状态:未配置。无代理状态下很可能无法访问。建议:检查USE_PROXY选项是否修改。')
- else:
- print('[PROXY] 网络代理状态:已配置。配置信息如下:', r)
- assert isinstance(r, dict), 'proxies格式错误,请注意proxies选项的格式,不要遗漏括号。'
- return r
-
-def get_conf(*args):
- # 建议您复制一个config_private.py放自己的秘密, 如API和代理网址, 避免不小心传github被别人看到
- res = []
- for arg in args:
- r = read_single_conf_with_lru_cache(arg)
- res.append(r)
- return res
-
-def clear_line_break(txt):
- txt = txt.replace('\n', ' ')
- txt = txt.replace(' ', ' ')
- txt = txt.replace(' ', ' ')
- return txt
diff --git a/spaces/SungBeom/chatwine-korean/.venv/Lib/site-packages/debugpy/_vendored/pydevd/pydevd_plugins/django_debug.py b/spaces/SungBeom/chatwine-korean/.venv/Lib/site-packages/debugpy/_vendored/pydevd/pydevd_plugins/django_debug.py
deleted file mode 100644
index ff7f1eb93b0f2557749c9340f057e3491bfd19df..0000000000000000000000000000000000000000
--- a/spaces/SungBeom/chatwine-korean/.venv/Lib/site-packages/debugpy/_vendored/pydevd/pydevd_plugins/django_debug.py
+++ /dev/null
@@ -1,613 +0,0 @@
-import inspect
-
-from _pydev_bundle import pydev_log
-from _pydevd_bundle.pydevd_comm import CMD_SET_BREAK, CMD_ADD_EXCEPTION_BREAK
-from _pydevd_bundle.pydevd_constants import STATE_SUSPEND, DJANGO_SUSPEND, \
- DebugInfoHolder
-from _pydevd_bundle.pydevd_frame_utils import add_exception_to_frame, FCode, just_raised, ignore_exception_trace
-from pydevd_file_utils import canonical_normalized_path, absolute_path
-from _pydevd_bundle.pydevd_api import PyDevdAPI
-from pydevd_plugins.pydevd_line_validation import LineBreakpointWithLazyValidation, ValidationInfo
-from _pydev_bundle.pydev_override import overrides
-
-IS_DJANGO18 = False
-IS_DJANGO19 = False
-IS_DJANGO19_OR_HIGHER = False
-try:
- import django
- version = django.VERSION
- IS_DJANGO18 = version[0] == 1 and version[1] == 8
- IS_DJANGO19 = version[0] == 1 and version[1] == 9
- IS_DJANGO19_OR_HIGHER = ((version[0] == 1 and version[1] >= 9) or version[0] > 1)
-except:
- pass
-
-
-class DjangoLineBreakpoint(LineBreakpointWithLazyValidation):
-
- def __init__(self, canonical_normalized_filename, breakpoint_id, line, condition, func_name, expression, hit_condition=None, is_logpoint=False):
- self.canonical_normalized_filename = canonical_normalized_filename
- LineBreakpointWithLazyValidation.__init__(self, breakpoint_id, line, condition, func_name, expression, hit_condition=hit_condition, is_logpoint=is_logpoint)
-
- def __str__(self):
- return "DjangoLineBreakpoint: %s-%d" % (self.canonical_normalized_filename, self.line)
-
-
-class _DjangoValidationInfo(ValidationInfo):
-
- @overrides(ValidationInfo._collect_valid_lines_in_template_uncached)
- def _collect_valid_lines_in_template_uncached(self, template):
- lines = set()
- for node in self._iternodes(template.nodelist):
- if node.__class__.__name__ in _IGNORE_RENDER_OF_CLASSES:
- continue
- lineno = self._get_lineno(node)
- if lineno is not None:
- lines.add(lineno)
- return lines
-
- def _get_lineno(self, node):
- if hasattr(node, 'token') and hasattr(node.token, 'lineno'):
- return node.token.lineno
- return None
-
- def _iternodes(self, nodelist):
- for node in nodelist:
- yield node
-
- try:
- children = node.child_nodelists
- except:
- pass
- else:
- for attr in children:
- nodelist = getattr(node, attr, None)
- if nodelist:
- # i.e.: yield from _iternodes(nodelist)
- for node in self._iternodes(nodelist):
- yield node
-
-
-def add_line_breakpoint(plugin, pydb, type, canonical_normalized_filename, breakpoint_id, line, condition, expression, func_name, hit_condition=None, is_logpoint=False, add_breakpoint_result=None, on_changed_breakpoint_state=None):
- if type == 'django-line':
- django_line_breakpoint = DjangoLineBreakpoint(canonical_normalized_filename, breakpoint_id, line, condition, func_name, expression, hit_condition=hit_condition, is_logpoint=is_logpoint)
- if not hasattr(pydb, 'django_breakpoints'):
- _init_plugin_breaks(pydb)
-
- if IS_DJANGO19_OR_HIGHER:
- add_breakpoint_result.error_code = PyDevdAPI.ADD_BREAKPOINT_LAZY_VALIDATION
- django_line_breakpoint.add_breakpoint_result = add_breakpoint_result
- django_line_breakpoint.on_changed_breakpoint_state = on_changed_breakpoint_state
- else:
- add_breakpoint_result.error_code = PyDevdAPI.ADD_BREAKPOINT_NO_ERROR
-
- return django_line_breakpoint, pydb.django_breakpoints
- return None
-
-
-def after_breakpoints_consolidated(plugin, py_db, canonical_normalized_filename, id_to_pybreakpoint, file_to_line_to_breakpoints):
- if IS_DJANGO19_OR_HIGHER:
- django_breakpoints_for_file = file_to_line_to_breakpoints.get(canonical_normalized_filename)
- if not django_breakpoints_for_file:
- return
-
- if not hasattr(py_db, 'django_validation_info'):
- _init_plugin_breaks(py_db)
-
- # In general we validate the breakpoints only when the template is loaded, but if the template
- # was already loaded, we can validate the breakpoints based on the last loaded value.
- py_db.django_validation_info.verify_breakpoints_from_template_cached_lines(
- py_db, canonical_normalized_filename, django_breakpoints_for_file)
-
-
-def add_exception_breakpoint(plugin, pydb, type, exception):
- if type == 'django':
- if not hasattr(pydb, 'django_exception_break'):
- _init_plugin_breaks(pydb)
- pydb.django_exception_break[exception] = True
- return True
- return False
-
-
-def _init_plugin_breaks(pydb):
- pydb.django_exception_break = {}
- pydb.django_breakpoints = {}
-
- pydb.django_validation_info = _DjangoValidationInfo()
-
-
-def remove_exception_breakpoint(plugin, pydb, type, exception):
- if type == 'django':
- try:
- del pydb.django_exception_break[exception]
- return True
- except:
- pass
- return False
-
-
-def remove_all_exception_breakpoints(plugin, pydb):
- if hasattr(pydb, 'django_exception_break'):
- pydb.django_exception_break = {}
- return True
- return False
-
-
-def get_breakpoints(plugin, pydb, type):
- if type == 'django-line':
- return pydb.django_breakpoints
- return None
-
-
-def _inherits(cls, *names):
- if cls.__name__ in names:
- return True
- inherits_node = False
- for base in inspect.getmro(cls):
- if base.__name__ in names:
- inherits_node = True
- break
- return inherits_node
-
-
-_IGNORE_RENDER_OF_CLASSES = ('TextNode', 'NodeList')
-
-
-def _is_django_render_call(frame, debug=False):
- try:
- name = frame.f_code.co_name
- if name != 'render':
- return False
-
- if 'self' not in frame.f_locals:
- return False
-
- cls = frame.f_locals['self'].__class__
-
- inherits_node = _inherits(cls, 'Node')
-
- if not inherits_node:
- return False
-
- clsname = cls.__name__
- if IS_DJANGO19:
- # in Django 1.9 we need to save the flag that there is included template
- if clsname == 'IncludeNode':
- if 'context' in frame.f_locals:
- context = frame.f_locals['context']
- context._has_included_template = True
-
- return clsname not in _IGNORE_RENDER_OF_CLASSES
- except:
- pydev_log.exception()
- return False
-
-
-def _is_django_context_get_call(frame):
- try:
- if 'self' not in frame.f_locals:
- return False
-
- cls = frame.f_locals['self'].__class__
-
- return _inherits(cls, 'BaseContext')
- except:
- pydev_log.exception()
- return False
-
-
-def _is_django_resolve_call(frame):
- try:
- name = frame.f_code.co_name
- if name != '_resolve_lookup':
- return False
-
- if 'self' not in frame.f_locals:
- return False
-
- cls = frame.f_locals['self'].__class__
-
- clsname = cls.__name__
- return clsname == 'Variable'
- except:
- pydev_log.exception()
- return False
-
-
-def _is_django_suspended(thread):
- return thread.additional_info.suspend_type == DJANGO_SUSPEND
-
-
-def suspend_django(main_debugger, thread, frame, cmd=CMD_SET_BREAK):
- if frame.f_lineno is None:
- return None
-
- main_debugger.set_suspend(thread, cmd)
- thread.additional_info.suspend_type = DJANGO_SUSPEND
-
- return frame
-
-
-def _find_django_render_frame(frame):
- while frame is not None and not _is_django_render_call(frame):
- frame = frame.f_back
-
- return frame
-
-#=======================================================================================================================
-# Django Frame
-#=======================================================================================================================
-
-
-def _read_file(filename):
- # type: (str) -> str
- f = open(filename, 'r', encoding='utf-8', errors='replace')
- s = f.read()
- f.close()
- return s
-
-
-def _offset_to_line_number(text, offset):
- curLine = 1
- curOffset = 0
- while curOffset < offset:
- if curOffset == len(text):
- return -1
- c = text[curOffset]
- if c == '\n':
- curLine += 1
- elif c == '\r':
- curLine += 1
- if curOffset < len(text) and text[curOffset + 1] == '\n':
- curOffset += 1
-
- curOffset += 1
-
- return curLine
-
-
-def _get_source_django_18_or_lower(frame):
- # This method is usable only for the Django <= 1.8
- try:
- node = frame.f_locals['self']
- if hasattr(node, 'source'):
- return node.source
- else:
- if IS_DJANGO18:
- # The debug setting was changed since Django 1.8
- pydev_log.error_once("WARNING: Template path is not available. Set the 'debug' option in the OPTIONS of a DjangoTemplates "
- "backend.")
- else:
- # The debug setting for Django < 1.8
- pydev_log.error_once("WARNING: Template path is not available. Please set TEMPLATE_DEBUG=True in your settings.py to make "
- "django template breakpoints working")
- return None
-
- except:
- pydev_log.exception()
- return None
-
-
-def _convert_to_str(s):
- return s
-
-
-def _get_template_original_file_name_from_frame(frame):
- try:
- if IS_DJANGO19:
- # The Node source was removed since Django 1.9
- if 'context' in frame.f_locals:
- context = frame.f_locals['context']
- if hasattr(context, '_has_included_template'):
- # if there was included template we need to inspect the previous frames and find its name
- back = frame.f_back
- while back is not None and frame.f_code.co_name in ('render', '_render'):
- locals = back.f_locals
- if 'self' in locals:
- self = locals['self']
- if self.__class__.__name__ == 'Template' and hasattr(self, 'origin') and \
- hasattr(self.origin, 'name'):
- return _convert_to_str(self.origin.name)
- back = back.f_back
- else:
- if hasattr(context, 'template') and hasattr(context.template, 'origin') and \
- hasattr(context.template.origin, 'name'):
- return _convert_to_str(context.template.origin.name)
- return None
- elif IS_DJANGO19_OR_HIGHER:
- # For Django 1.10 and later there is much simpler way to get template name
- if 'self' in frame.f_locals:
- self = frame.f_locals['self']
- if hasattr(self, 'origin') and hasattr(self.origin, 'name'):
- return _convert_to_str(self.origin.name)
- return None
-
- source = _get_source_django_18_or_lower(frame)
- if source is None:
- pydev_log.debug("Source is None\n")
- return None
- fname = _convert_to_str(source[0].name)
-
- if fname == '':
- pydev_log.debug("Source name is %s\n" % fname)
- return None
- else:
- return fname
- except:
- if DebugInfoHolder.DEBUG_TRACE_LEVEL >= 2:
- pydev_log.exception('Error getting django template filename.')
- return None
-
-
-def _get_template_line(frame):
- if IS_DJANGO19_OR_HIGHER:
- node = frame.f_locals['self']
- if hasattr(node, 'token') and hasattr(node.token, 'lineno'):
- return node.token.lineno
- else:
- return None
-
- source = _get_source_django_18_or_lower(frame)
- original_filename = _get_template_original_file_name_from_frame(frame)
- if original_filename is not None:
- try:
- absolute_filename = absolute_path(original_filename)
- return _offset_to_line_number(_read_file(absolute_filename), source[1][0])
- except:
- return None
- return None
-
-
-class DjangoTemplateFrame(object):
-
- IS_PLUGIN_FRAME = True
-
- def __init__(self, frame):
- original_filename = _get_template_original_file_name_from_frame(frame)
- self._back_context = frame.f_locals['context']
- self.f_code = FCode('Django Template', original_filename)
- self.f_lineno = _get_template_line(frame)
- self.f_back = frame
- self.f_globals = {}
- self.f_locals = self._collect_context(self._back_context)
- self.f_trace = None
-
- def _collect_context(self, context):
- res = {}
- try:
- for d in context.dicts:
- for k, v in d.items():
- res[k] = v
- except AttributeError:
- pass
- return res
-
- def _change_variable(self, name, value):
- for d in self._back_context.dicts:
- for k, v in d.items():
- if k == name:
- d[k] = value
-
-
-class DjangoTemplateSyntaxErrorFrame(object):
-
- IS_PLUGIN_FRAME = True
-
- def __init__(self, frame, original_filename, lineno, f_locals):
- self.f_code = FCode('Django TemplateSyntaxError', original_filename)
- self.f_lineno = lineno
- self.f_back = frame
- self.f_globals = {}
- self.f_locals = f_locals
- self.f_trace = None
-
-
-def change_variable(plugin, frame, attr, expression):
- if isinstance(frame, DjangoTemplateFrame):
- result = eval(expression, frame.f_globals, frame.f_locals)
- frame._change_variable(attr, result)
- return result
- return False
-
-
-def _is_django_variable_does_not_exist_exception_break_context(frame):
- try:
- name = frame.f_code.co_name
- except:
- name = None
- return name in ('_resolve_lookup', 'find_template')
-
-
-def _is_ignoring_failures(frame):
- while frame is not None:
- if frame.f_code.co_name == 'resolve':
- ignore_failures = frame.f_locals.get('ignore_failures')
- if ignore_failures:
- return True
- frame = frame.f_back
-
- return False
-
-#=======================================================================================================================
-# Django Step Commands
-#=======================================================================================================================
-
-
-def can_skip(plugin, main_debugger, frame):
- if main_debugger.django_breakpoints:
- if _is_django_render_call(frame):
- return False
-
- if main_debugger.django_exception_break:
- module_name = frame.f_globals.get('__name__', '')
-
- if module_name == 'django.template.base':
- # Exceptions raised at django.template.base must be checked.
- return False
-
- return True
-
-
-def has_exception_breaks(plugin):
- if len(plugin.main_debugger.django_exception_break) > 0:
- return True
- return False
-
-
-def has_line_breaks(plugin):
- for _canonical_normalized_filename, breakpoints in plugin.main_debugger.django_breakpoints.items():
- if len(breakpoints) > 0:
- return True
- return False
-
-
-def cmd_step_into(plugin, main_debugger, frame, event, args, stop_info, stop):
- info = args[2]
- thread = args[3]
- plugin_stop = False
- if _is_django_suspended(thread):
- stop_info['django_stop'] = event == 'call' and _is_django_render_call(frame)
- plugin_stop = stop_info['django_stop']
- stop = stop and _is_django_resolve_call(frame.f_back) and not _is_django_context_get_call(frame)
- if stop:
- info.pydev_django_resolve_frame = True # we remember that we've go into python code from django rendering frame
- return stop, plugin_stop
-
-
-def cmd_step_over(plugin, main_debugger, frame, event, args, stop_info, stop):
- info = args[2]
- thread = args[3]
- plugin_stop = False
- if _is_django_suspended(thread):
- stop_info['django_stop'] = event == 'call' and _is_django_render_call(frame)
- plugin_stop = stop_info['django_stop']
- stop = False
- return stop, plugin_stop
- else:
- if event == 'return' and info.pydev_django_resolve_frame and _is_django_resolve_call(frame.f_back):
- # we return to Django suspend mode and should not stop before django rendering frame
- info.pydev_step_stop = frame.f_back
- info.pydev_django_resolve_frame = False
- thread.additional_info.suspend_type = DJANGO_SUSPEND
- stop = info.pydev_step_stop is frame and event in ('line', 'return')
- return stop, plugin_stop
-
-
-def stop(plugin, main_debugger, frame, event, args, stop_info, arg, step_cmd):
- main_debugger = args[0]
- thread = args[3]
- if 'django_stop' in stop_info and stop_info['django_stop']:
- frame = suspend_django(main_debugger, thread, DjangoTemplateFrame(frame), step_cmd)
- if frame:
- main_debugger.do_wait_suspend(thread, frame, event, arg)
- return True
- return False
-
-
-def get_breakpoint(plugin, py_db, pydb_frame, frame, event, args):
- py_db = args[0]
- _filename = args[1]
- info = args[2]
- breakpoint_type = 'django'
-
- if event == 'call' and info.pydev_state != STATE_SUSPEND and py_db.django_breakpoints and _is_django_render_call(frame):
- original_filename = _get_template_original_file_name_from_frame(frame)
- pydev_log.debug("Django is rendering a template: %s", original_filename)
-
- canonical_normalized_filename = canonical_normalized_path(original_filename)
- django_breakpoints_for_file = py_db.django_breakpoints.get(canonical_normalized_filename)
-
- if django_breakpoints_for_file:
-
- # At this point, let's validate whether template lines are correct.
- if IS_DJANGO19_OR_HIGHER:
- django_validation_info = py_db.django_validation_info
- context = frame.f_locals['context']
- django_template = context.template
- django_validation_info.verify_breakpoints(py_db, canonical_normalized_filename, django_breakpoints_for_file, django_template)
-
- pydev_log.debug("Breakpoints for that file: %s", django_breakpoints_for_file)
- template_line = _get_template_line(frame)
- pydev_log.debug("Tracing template line: %s", template_line)
-
- if template_line in django_breakpoints_for_file:
- django_breakpoint = django_breakpoints_for_file[template_line]
- new_frame = DjangoTemplateFrame(frame)
- return True, django_breakpoint, new_frame, breakpoint_type
-
- return False, None, None, breakpoint_type
-
-
-def suspend(plugin, main_debugger, thread, frame, bp_type):
- if bp_type == 'django':
- return suspend_django(main_debugger, thread, DjangoTemplateFrame(frame))
- return None
-
-
-def _get_original_filename_from_origin_in_parent_frame_locals(frame, parent_frame_name):
- filename = None
- parent_frame = frame
- while parent_frame.f_code.co_name != parent_frame_name:
- parent_frame = parent_frame.f_back
-
- origin = None
- if parent_frame is not None:
- origin = parent_frame.f_locals.get('origin')
-
- if hasattr(origin, 'name') and origin.name is not None:
- filename = _convert_to_str(origin.name)
- return filename
-
-
-def exception_break(plugin, main_debugger, pydb_frame, frame, args, arg):
- main_debugger = args[0]
- thread = args[3]
- exception, value, trace = arg
-
- if main_debugger.django_exception_break and exception is not None:
- if exception.__name__ in ['VariableDoesNotExist', 'TemplateDoesNotExist', 'TemplateSyntaxError'] and \
- just_raised(trace) and not ignore_exception_trace(trace):
-
- if exception.__name__ == 'TemplateSyntaxError':
- # In this case we don't actually have a regular render frame with the context
- # (we didn't really get to that point).
- token = getattr(value, 'token', None)
-
- if token is None:
- # Django 1.7 does not have token in exception. Try to get it from locals.
- token = frame.f_locals.get('token')
-
- lineno = getattr(token, 'lineno', None)
-
- original_filename = None
- if lineno is not None:
- original_filename = _get_original_filename_from_origin_in_parent_frame_locals(frame, 'get_template')
-
- if original_filename is None:
- # Django 1.7 does not have origin in get_template. Try to get it from
- # load_template.
- original_filename = _get_original_filename_from_origin_in_parent_frame_locals(frame, 'load_template')
-
- if original_filename is not None and lineno is not None:
- syntax_error_frame = DjangoTemplateSyntaxErrorFrame(
- frame, original_filename, lineno, {'token': token, 'exception': exception})
-
- suspend_frame = suspend_django(
- main_debugger, thread, syntax_error_frame, CMD_ADD_EXCEPTION_BREAK)
- return True, suspend_frame
-
- elif exception.__name__ == 'VariableDoesNotExist':
- if _is_django_variable_does_not_exist_exception_break_context(frame):
- if not getattr(exception, 'silent_variable_failure', False) and not _is_ignoring_failures(frame):
- render_frame = _find_django_render_frame(frame)
- if render_frame:
- suspend_frame = suspend_django(
- main_debugger, thread, DjangoTemplateFrame(render_frame), CMD_ADD_EXCEPTION_BREAK)
- if suspend_frame:
- add_exception_to_frame(suspend_frame, (exception, value, trace))
- thread.additional_info.pydev_message = 'VariableDoesNotExist'
- suspend_frame.f_back = frame
- frame = suspend_frame
- return True, frame
-
- return None
diff --git a/spaces/Superlang/ImageProcessor/annotator/oneformer/detectron2/layers/rotated_boxes.py b/spaces/Superlang/ImageProcessor/annotator/oneformer/detectron2/layers/rotated_boxes.py
deleted file mode 100644
index 03f73b3bb99275931a887ad9b2d8c0ac9f412bf3..0000000000000000000000000000000000000000
--- a/spaces/Superlang/ImageProcessor/annotator/oneformer/detectron2/layers/rotated_boxes.py
+++ /dev/null
@@ -1,21 +0,0 @@
-# Copyright (c) Facebook, Inc. and its affiliates.
-from __future__ import absolute_import, division, print_function, unicode_literals
-import torch
-
-
-def pairwise_iou_rotated(boxes1, boxes2):
- """
- Return intersection-over-union (Jaccard index) of boxes.
-
- Both sets of boxes are expected to be in
- (x_center, y_center, width, height, angle) format.
-
- Arguments:
- boxes1 (Tensor[N, 5])
- boxes2 (Tensor[M, 5])
-
- Returns:
- iou (Tensor[N, M]): the NxM matrix containing the pairwise
- IoU values for every element in boxes1 and boxes2
- """
- return torch.ops.detectron2.box_iou_rotated(boxes1, boxes2)
diff --git a/spaces/Superlang/ImageProcessor/annotator/uniformer/mmcv/runner/hooks/logger/text.py b/spaces/Superlang/ImageProcessor/annotator/uniformer/mmcv/runner/hooks/logger/text.py
deleted file mode 100644
index 87b1a3eca9595a130121526f8b4c29915387ab35..0000000000000000000000000000000000000000
--- a/spaces/Superlang/ImageProcessor/annotator/uniformer/mmcv/runner/hooks/logger/text.py
+++ /dev/null
@@ -1,256 +0,0 @@
-# Copyright (c) OpenMMLab. All rights reserved.
-import datetime
-import os
-import os.path as osp
-from collections import OrderedDict
-
-import torch
-import torch.distributed as dist
-
-import annotator.uniformer.mmcv as mmcv
-from annotator.uniformer.mmcv.fileio.file_client import FileClient
-from annotator.uniformer.mmcv.utils import is_tuple_of, scandir
-from ..hook import HOOKS
-from .base import LoggerHook
-
-
-@HOOKS.register_module()
-class TextLoggerHook(LoggerHook):
- """Logger hook in text.
-
- In this logger hook, the information will be printed on terminal and
- saved in json file.
-
- Args:
- by_epoch (bool, optional): Whether EpochBasedRunner is used.
- Default: True.
- interval (int, optional): Logging interval (every k iterations).
- Default: 10.
- ignore_last (bool, optional): Ignore the log of last iterations in each
- epoch if less than :attr:`interval`. Default: True.
- reset_flag (bool, optional): Whether to clear the output buffer after
- logging. Default: False.
- interval_exp_name (int, optional): Logging interval for experiment
- name. This feature is to help users conveniently get the experiment
- information from screen or log file. Default: 1000.
- out_dir (str, optional): Logs are saved in ``runner.work_dir`` default.
- If ``out_dir`` is specified, logs will be copied to a new directory
- which is the concatenation of ``out_dir`` and the last level
- directory of ``runner.work_dir``. Default: None.
- `New in version 1.3.16.`
- out_suffix (str or tuple[str], optional): Those filenames ending with
- ``out_suffix`` will be copied to ``out_dir``.
- Default: ('.log.json', '.log', '.py').
- `New in version 1.3.16.`
- keep_local (bool, optional): Whether to keep local log when
- :attr:`out_dir` is specified. If False, the local log will be
- removed. Default: True.
- `New in version 1.3.16.`
- file_client_args (dict, optional): Arguments to instantiate a
- FileClient. See :class:`mmcv.fileio.FileClient` for details.
- Default: None.
- `New in version 1.3.16.`
- """
-
- def __init__(self,
- by_epoch=True,
- interval=10,
- ignore_last=True,
- reset_flag=False,
- interval_exp_name=1000,
- out_dir=None,
- out_suffix=('.log.json', '.log', '.py'),
- keep_local=True,
- file_client_args=None):
- super(TextLoggerHook, self).__init__(interval, ignore_last, reset_flag,
- by_epoch)
- self.by_epoch = by_epoch
- self.time_sec_tot = 0
- self.interval_exp_name = interval_exp_name
-
- if out_dir is None and file_client_args is not None:
- raise ValueError(
- 'file_client_args should be "None" when `out_dir` is not'
- 'specified.')
- self.out_dir = out_dir
-
- if not (out_dir is None or isinstance(out_dir, str)
- or is_tuple_of(out_dir, str)):
- raise TypeError('out_dir should be "None" or string or tuple of '
- 'string, but got {out_dir}')
- self.out_suffix = out_suffix
-
- self.keep_local = keep_local
- self.file_client_args = file_client_args
- if self.out_dir is not None:
- self.file_client = FileClient.infer_client(file_client_args,
- self.out_dir)
-
- def before_run(self, runner):
- super(TextLoggerHook, self).before_run(runner)
-
- if self.out_dir is not None:
- self.file_client = FileClient.infer_client(self.file_client_args,
- self.out_dir)
- # The final `self.out_dir` is the concatenation of `self.out_dir`
- # and the last level directory of `runner.work_dir`
- basename = osp.basename(runner.work_dir.rstrip(osp.sep))
- self.out_dir = self.file_client.join_path(self.out_dir, basename)
- runner.logger.info(
- (f'Text logs will be saved to {self.out_dir} by '
- f'{self.file_client.name} after the training process.'))
-
- self.start_iter = runner.iter
- self.json_log_path = osp.join(runner.work_dir,
- f'{runner.timestamp}.log.json')
- if runner.meta is not None:
- self._dump_log(runner.meta, runner)
-
- def _get_max_memory(self, runner):
- device = getattr(runner.model, 'output_device', None)
- mem = torch.cuda.max_memory_allocated(device=device)
- mem_mb = torch.tensor([mem / (1024 * 1024)],
- dtype=torch.int,
- device=device)
- if runner.world_size > 1:
- dist.reduce(mem_mb, 0, op=dist.ReduceOp.MAX)
- return mem_mb.item()
-
- def _log_info(self, log_dict, runner):
- # print exp name for users to distinguish experiments
- # at every ``interval_exp_name`` iterations and the end of each epoch
- if runner.meta is not None and 'exp_name' in runner.meta:
- if (self.every_n_iters(runner, self.interval_exp_name)) or (
- self.by_epoch and self.end_of_epoch(runner)):
- exp_info = f'Exp name: {runner.meta["exp_name"]}'
- runner.logger.info(exp_info)
-
- if log_dict['mode'] == 'train':
- if isinstance(log_dict['lr'], dict):
- lr_str = []
- for k, val in log_dict['lr'].items():
- lr_str.append(f'lr_{k}: {val:.3e}')
- lr_str = ' '.join(lr_str)
- else:
- lr_str = f'lr: {log_dict["lr"]:.3e}'
-
- # by epoch: Epoch [4][100/1000]
- # by iter: Iter [100/100000]
- if self.by_epoch:
- log_str = f'Epoch [{log_dict["epoch"]}]' \
- f'[{log_dict["iter"]}/{len(runner.data_loader)}]\t'
- else:
- log_str = f'Iter [{log_dict["iter"]}/{runner.max_iters}]\t'
- log_str += f'{lr_str}, '
-
- if 'time' in log_dict.keys():
- self.time_sec_tot += (log_dict['time'] * self.interval)
- time_sec_avg = self.time_sec_tot / (
- runner.iter - self.start_iter + 1)
- eta_sec = time_sec_avg * (runner.max_iters - runner.iter - 1)
- eta_str = str(datetime.timedelta(seconds=int(eta_sec)))
- log_str += f'eta: {eta_str}, '
- log_str += f'time: {log_dict["time"]:.3f}, ' \
- f'data_time: {log_dict["data_time"]:.3f}, '
- # statistic memory
- if torch.cuda.is_available():
- log_str += f'memory: {log_dict["memory"]}, '
- else:
- # val/test time
- # here 1000 is the length of the val dataloader
- # by epoch: Epoch[val] [4][1000]
- # by iter: Iter[val] [1000]
- if self.by_epoch:
- log_str = f'Epoch({log_dict["mode"]}) ' \
- f'[{log_dict["epoch"]}][{log_dict["iter"]}]\t'
- else:
- log_str = f'Iter({log_dict["mode"]}) [{log_dict["iter"]}]\t'
-
- log_items = []
- for name, val in log_dict.items():
- # TODO: resolve this hack
- # these items have been in log_str
- if name in [
- 'mode', 'Epoch', 'iter', 'lr', 'time', 'data_time',
- 'memory', 'epoch'
- ]:
- continue
- if isinstance(val, float):
- val = f'{val:.4f}'
- log_items.append(f'{name}: {val}')
- log_str += ', '.join(log_items)
-
- runner.logger.info(log_str)
-
- def _dump_log(self, log_dict, runner):
- # dump log in json format
- json_log = OrderedDict()
- for k, v in log_dict.items():
- json_log[k] = self._round_float(v)
- # only append log at last line
- if runner.rank == 0:
- with open(self.json_log_path, 'a+') as f:
- mmcv.dump(json_log, f, file_format='json')
- f.write('\n')
-
- def _round_float(self, items):
- if isinstance(items, list):
- return [self._round_float(item) for item in items]
- elif isinstance(items, float):
- return round(items, 5)
- else:
- return items
-
- def log(self, runner):
- if 'eval_iter_num' in runner.log_buffer.output:
- # this doesn't modify runner.iter and is regardless of by_epoch
- cur_iter = runner.log_buffer.output.pop('eval_iter_num')
- else:
- cur_iter = self.get_iter(runner, inner_iter=True)
-
- log_dict = OrderedDict(
- mode=self.get_mode(runner),
- epoch=self.get_epoch(runner),
- iter=cur_iter)
-
- # only record lr of the first param group
- cur_lr = runner.current_lr()
- if isinstance(cur_lr, list):
- log_dict['lr'] = cur_lr[0]
- else:
- assert isinstance(cur_lr, dict)
- log_dict['lr'] = {}
- for k, lr_ in cur_lr.items():
- assert isinstance(lr_, list)
- log_dict['lr'].update({k: lr_[0]})
-
- if 'time' in runner.log_buffer.output:
- # statistic memory
- if torch.cuda.is_available():
- log_dict['memory'] = self._get_max_memory(runner)
-
- log_dict = dict(log_dict, **runner.log_buffer.output)
-
- self._log_info(log_dict, runner)
- self._dump_log(log_dict, runner)
- return log_dict
-
- def after_run(self, runner):
- # copy or upload logs to self.out_dir
- if self.out_dir is not None:
- for filename in scandir(runner.work_dir, self.out_suffix, True):
- local_filepath = osp.join(runner.work_dir, filename)
- out_filepath = self.file_client.join_path(
- self.out_dir, filename)
- with open(local_filepath, 'r') as f:
- self.file_client.put_text(f.read(), out_filepath)
-
- runner.logger.info(
- (f'The file {local_filepath} has been uploaded to '
- f'{out_filepath}.'))
-
- if not self.keep_local:
- os.remove(local_filepath)
- runner.logger.info(
- (f'{local_filepath} was removed due to the '
- '`self.keep_local=False`'))
diff --git a/spaces/Superlang/ImageProcessor/annotator/uniformer/mmseg/models/decode_heads/nl_head.py b/spaces/Superlang/ImageProcessor/annotator/uniformer/mmseg/models/decode_heads/nl_head.py
deleted file mode 100644
index 3eee424199e6aa363b564e2a3340a070db04db86..0000000000000000000000000000000000000000
--- a/spaces/Superlang/ImageProcessor/annotator/uniformer/mmseg/models/decode_heads/nl_head.py
+++ /dev/null
@@ -1,49 +0,0 @@
-import torch
-from annotator.uniformer.mmcv.cnn import NonLocal2d
-
-from ..builder import HEADS
-from .fcn_head import FCNHead
-
-
-@HEADS.register_module()
-class NLHead(FCNHead):
- """Non-local Neural Networks.
-
- This head is the implementation of `NLNet
- `_.
-
- Args:
- reduction (int): Reduction factor of projection transform. Default: 2.
- use_scale (bool): Whether to scale pairwise_weight by
- sqrt(1/inter_channels). Default: True.
- mode (str): The nonlocal mode. Options are 'embedded_gaussian',
- 'dot_product'. Default: 'embedded_gaussian.'.
- """
-
- def __init__(self,
- reduction=2,
- use_scale=True,
- mode='embedded_gaussian',
- **kwargs):
- super(NLHead, self).__init__(num_convs=2, **kwargs)
- self.reduction = reduction
- self.use_scale = use_scale
- self.mode = mode
- self.nl_block = NonLocal2d(
- in_channels=self.channels,
- reduction=self.reduction,
- use_scale=self.use_scale,
- conv_cfg=self.conv_cfg,
- norm_cfg=self.norm_cfg,
- mode=self.mode)
-
- def forward(self, inputs):
- """Forward function."""
- x = self._transform_inputs(inputs)
- output = self.convs[0](x)
- output = self.nl_block(output)
- output = self.convs[1](output)
- if self.concat_input:
- output = self.conv_cat(torch.cat([x, output], dim=1))
- output = self.cls_seg(output)
- return output
diff --git a/spaces/Superlang/ImageProcessor/annotator/zoe/zoedepth/models/base_models/midas_repo/ros/additions/downloads.sh b/spaces/Superlang/ImageProcessor/annotator/zoe/zoedepth/models/base_models/midas_repo/ros/additions/downloads.sh
deleted file mode 100644
index 9c967d4e2dc7997da26399a063b5a54ecc314eb1..0000000000000000000000000000000000000000
--- a/spaces/Superlang/ImageProcessor/annotator/zoe/zoedepth/models/base_models/midas_repo/ros/additions/downloads.sh
+++ /dev/null
@@ -1,5 +0,0 @@
-mkdir ~/.ros
-wget https://github.com/isl-org/MiDaS/releases/download/v2_1/model-small-traced.pt
-cp ./model-small-traced.pt ~/.ros/model-small-traced.pt
-
-
diff --git a/spaces/TH5314/newbing/src/components/ui/icons.tsx b/spaces/TH5314/newbing/src/components/ui/icons.tsx
deleted file mode 100644
index 742b489b50437c5b64c86082f2ebc712eeb6a2b0..0000000000000000000000000000000000000000
--- a/spaces/TH5314/newbing/src/components/ui/icons.tsx
+++ /dev/null
@@ -1,504 +0,0 @@
-'use client'
-
-import * as React from 'react'
-
-import { cn } from '@/lib/utils'
-
-function IconNextChat({
- className,
- inverted,
- ...props
-}: React.ComponentProps<'svg'> & { inverted?: boolean }) {
- const id = React.useId()
-
- return (
-
- )
-}
-
-function IconOpenAI({ className, ...props }: React.ComponentProps<'svg'>) {
- return (
-
- )
-}
-
-function IconGitHub({ className, ...props }: React.ComponentProps<'svg'>) {
- return (
-
- )
-}
-
-function IconSeparator({ className, ...props }: React.ComponentProps<'svg'>) {
- return (
-
- )
-}
-
-function IconArrowDown({ className, ...props }: React.ComponentProps<'svg'>) {
- return (
-
- )
-}
-
-function IconArrowRight({ className, ...props }: React.ComponentProps<'svg'>) {
- return (
-
- )
-}
-
-function IconUser({ className, ...props }: React.ComponentProps<'svg'>) {
- return (
-
- )
-}
-
-function IconPlus({ className, ...props }: React.ComponentProps<'svg'>) {
- return (
-
- )
-}
-
-function IconArrowElbow({ className, ...props }: React.ComponentProps<'svg'>) {
- return (
-
- )
-}
-
-function IconSpinner({ className, ...props }: React.ComponentProps<'svg'>) {
- return (
-
- )
-}
-
-function IconMessage({ className, ...props }: React.ComponentProps<'svg'>) {
- return (
-
- )
-}
-
-function IconTrash({ className, ...props }: React.ComponentProps<'svg'>) {
- return (
-
- )
-}
-
-function IconMore({ className, ...props }: React.ComponentProps<'svg'>) {
- return (
-
- )
-}
-
-function IconRefresh({ className, ...props }: React.ComponentProps<'svg'>) {
- return (
-
- )
-}
-
-function IconStop({ className, ...props }: React.ComponentProps<'svg'>) {
- return (
-
- )
-}
-
-function IconSidebar({ className, ...props }: React.ComponentProps<'svg'>) {
- return (
-
- )
-}
-
-function IconMoon({ className, ...props }: React.ComponentProps<'svg'>) {
- return (
-
- )
-}
-
-function IconSun({ className, ...props }: React.ComponentProps<'svg'>) {
- return (
-
- )
-}
-
-function IconCopy({ className, ...props }: React.ComponentProps<'svg'>) {
- return (
-
- )
-}
-
-function IconCheck({ className, ...props }: React.ComponentProps<'svg'>) {
- return (
-
- )
-}
-
-function IconDownload({ className, ...props }: React.ComponentProps<'svg'>) {
- return (
-
- )
-}
-
-function IconClose({ className, ...props }: React.ComponentProps<'svg'>) {
- return (
-
- )
-}
-
-function IconEdit({ className, ...props }: React.ComponentProps<'svg'>) {
- return (
-
- )
-}
-
-function IconShare({ className, ...props }: React.ComponentProps<'svg'>) {
- return (
-
- )
-}
-
-function IconUsers({ className, ...props }: React.ComponentProps<'svg'>) {
- return (
-
- )
-}
-
-function IconExternalLink({
- className,
- ...props
-}: React.ComponentProps<'svg'>) {
- return (
-
- )
-}
-
-function IconChevronUpDown({
- className,
- ...props
-}: React.ComponentProps<'svg'>) {
- return (
-
- )
-}
-
-export {
- IconEdit,
- IconNextChat,
- IconOpenAI,
- IconGitHub,
- IconSeparator,
- IconArrowDown,
- IconArrowRight,
- IconUser,
- IconPlus,
- IconArrowElbow,
- IconSpinner,
- IconMessage,
- IconTrash,
- IconMore,
- IconRefresh,
- IconStop,
- IconSidebar,
- IconMoon,
- IconSun,
- IconCopy,
- IconCheck,
- IconDownload,
- IconClose,
- IconShare,
- IconUsers,
- IconExternalLink,
- IconChevronUpDown
-}
diff --git a/spaces/TandCAcceptMe/face-swap-docker/mynewshinyroop/Lib/site-packages/pip/_vendor/typing_extensions.py b/spaces/TandCAcceptMe/face-swap-docker/mynewshinyroop/Lib/site-packages/pip/_vendor/typing_extensions.py
deleted file mode 100644
index 4f93acffbdc1d8ba9555114c190e44140c34c291..0000000000000000000000000000000000000000
--- a/spaces/TandCAcceptMe/face-swap-docker/mynewshinyroop/Lib/site-packages/pip/_vendor/typing_extensions.py
+++ /dev/null
@@ -1,3072 +0,0 @@
-import abc
-import collections
-import collections.abc
-import functools
-import inspect
-import operator
-import sys
-import types as _types
-import typing
-import warnings
-
-__all__ = [
- # Super-special typing primitives.
- 'Any',
- 'ClassVar',
- 'Concatenate',
- 'Final',
- 'LiteralString',
- 'ParamSpec',
- 'ParamSpecArgs',
- 'ParamSpecKwargs',
- 'Self',
- 'Type',
- 'TypeVar',
- 'TypeVarTuple',
- 'Unpack',
-
- # ABCs (from collections.abc).
- 'Awaitable',
- 'AsyncIterator',
- 'AsyncIterable',
- 'Coroutine',
- 'AsyncGenerator',
- 'AsyncContextManager',
- 'Buffer',
- 'ChainMap',
-
- # Concrete collection types.
- 'ContextManager',
- 'Counter',
- 'Deque',
- 'DefaultDict',
- 'NamedTuple',
- 'OrderedDict',
- 'TypedDict',
-
- # Structural checks, a.k.a. protocols.
- 'SupportsAbs',
- 'SupportsBytes',
- 'SupportsComplex',
- 'SupportsFloat',
- 'SupportsIndex',
- 'SupportsInt',
- 'SupportsRound',
-
- # One-off things.
- 'Annotated',
- 'assert_never',
- 'assert_type',
- 'clear_overloads',
- 'dataclass_transform',
- 'deprecated',
- 'get_overloads',
- 'final',
- 'get_args',
- 'get_origin',
- 'get_original_bases',
- 'get_protocol_members',
- 'get_type_hints',
- 'IntVar',
- 'is_protocol',
- 'is_typeddict',
- 'Literal',
- 'NewType',
- 'overload',
- 'override',
- 'Protocol',
- 'reveal_type',
- 'runtime',
- 'runtime_checkable',
- 'Text',
- 'TypeAlias',
- 'TypeAliasType',
- 'TypeGuard',
- 'TYPE_CHECKING',
- 'Never',
- 'NoReturn',
- 'Required',
- 'NotRequired',
-
- # Pure aliases, have always been in typing
- 'AbstractSet',
- 'AnyStr',
- 'BinaryIO',
- 'Callable',
- 'Collection',
- 'Container',
- 'Dict',
- 'ForwardRef',
- 'FrozenSet',
- 'Generator',
- 'Generic',
- 'Hashable',
- 'IO',
- 'ItemsView',
- 'Iterable',
- 'Iterator',
- 'KeysView',
- 'List',
- 'Mapping',
- 'MappingView',
- 'Match',
- 'MutableMapping',
- 'MutableSequence',
- 'MutableSet',
- 'Optional',
- 'Pattern',
- 'Reversible',
- 'Sequence',
- 'Set',
- 'Sized',
- 'TextIO',
- 'Tuple',
- 'Union',
- 'ValuesView',
- 'cast',
- 'no_type_check',
- 'no_type_check_decorator',
-]
-
-# for backward compatibility
-PEP_560 = True
-GenericMeta = type
-
-# The functions below are modified copies of typing internal helpers.
-# They are needed by _ProtocolMeta and they provide support for PEP 646.
-
-
-class _Sentinel:
- def __repr__(self):
- return ""
-
-
-_marker = _Sentinel()
-
-
-def _check_generic(cls, parameters, elen=_marker):
- """Check correct count for parameters of a generic cls (internal helper).
- This gives a nice error message in case of count mismatch.
- """
- if not elen:
- raise TypeError(f"{cls} is not a generic class")
- if elen is _marker:
- if not hasattr(cls, "__parameters__") or not cls.__parameters__:
- raise TypeError(f"{cls} is not a generic class")
- elen = len(cls.__parameters__)
- alen = len(parameters)
- if alen != elen:
- if hasattr(cls, "__parameters__"):
- parameters = [p for p in cls.__parameters__ if not _is_unpack(p)]
- num_tv_tuples = sum(isinstance(p, TypeVarTuple) for p in parameters)
- if (num_tv_tuples > 0) and (alen >= elen - num_tv_tuples):
- return
- raise TypeError(f"Too {'many' if alen > elen else 'few'} parameters for {cls};"
- f" actual {alen}, expected {elen}")
-
-
-if sys.version_info >= (3, 10):
- def _should_collect_from_parameters(t):
- return isinstance(
- t, (typing._GenericAlias, _types.GenericAlias, _types.UnionType)
- )
-elif sys.version_info >= (3, 9):
- def _should_collect_from_parameters(t):
- return isinstance(t, (typing._GenericAlias, _types.GenericAlias))
-else:
- def _should_collect_from_parameters(t):
- return isinstance(t, typing._GenericAlias) and not t._special
-
-
-def _collect_type_vars(types, typevar_types=None):
- """Collect all type variable contained in types in order of
- first appearance (lexicographic order). For example::
-
- _collect_type_vars((T, List[S, T])) == (T, S)
- """
- if typevar_types is None:
- typevar_types = typing.TypeVar
- tvars = []
- for t in types:
- if (
- isinstance(t, typevar_types) and
- t not in tvars and
- not _is_unpack(t)
- ):
- tvars.append(t)
- if _should_collect_from_parameters(t):
- tvars.extend([t for t in t.__parameters__ if t not in tvars])
- return tuple(tvars)
-
-
-NoReturn = typing.NoReturn
-
-# Some unconstrained type variables. These are used by the container types.
-# (These are not for export.)
-T = typing.TypeVar('T') # Any type.
-KT = typing.TypeVar('KT') # Key type.
-VT = typing.TypeVar('VT') # Value type.
-T_co = typing.TypeVar('T_co', covariant=True) # Any type covariant containers.
-T_contra = typing.TypeVar('T_contra', contravariant=True) # Ditto contravariant.
-
-
-if sys.version_info >= (3, 11):
- from typing import Any
-else:
-
- class _AnyMeta(type):
- def __instancecheck__(self, obj):
- if self is Any:
- raise TypeError("typing_extensions.Any cannot be used with isinstance()")
- return super().__instancecheck__(obj)
-
- def __repr__(self):
- if self is Any:
- return "typing_extensions.Any"
- return super().__repr__()
-
- class Any(metaclass=_AnyMeta):
- """Special type indicating an unconstrained type.
- - Any is compatible with every type.
- - Any assumed to have all methods.
- - All values assumed to be instances of Any.
- Note that all the above statements are true from the point of view of
- static type checkers. At runtime, Any should not be used with instance
- checks.
- """
- def __new__(cls, *args, **kwargs):
- if cls is Any:
- raise TypeError("Any cannot be instantiated")
- return super().__new__(cls, *args, **kwargs)
-
-
-ClassVar = typing.ClassVar
-
-
-class _ExtensionsSpecialForm(typing._SpecialForm, _root=True):
- def __repr__(self):
- return 'typing_extensions.' + self._name
-
-
-# On older versions of typing there is an internal class named "Final".
-# 3.8+
-if hasattr(typing, 'Final') and sys.version_info[:2] >= (3, 7):
- Final = typing.Final
-# 3.7
-else:
- class _FinalForm(_ExtensionsSpecialForm, _root=True):
- def __getitem__(self, parameters):
- item = typing._type_check(parameters,
- f'{self._name} accepts only a single type.')
- return typing._GenericAlias(self, (item,))
-
- Final = _FinalForm('Final',
- doc="""A special typing construct to indicate that a name
- cannot be re-assigned or overridden in a subclass.
- For example:
-
- MAX_SIZE: Final = 9000
- MAX_SIZE += 1 # Error reported by type checker
-
- class Connection:
- TIMEOUT: Final[int] = 10
- class FastConnector(Connection):
- TIMEOUT = 1 # Error reported by type checker
-
- There is no runtime checking of these properties.""")
-
-if sys.version_info >= (3, 11):
- final = typing.final
-else:
- # @final exists in 3.8+, but we backport it for all versions
- # before 3.11 to keep support for the __final__ attribute.
- # See https://bugs.python.org/issue46342
- def final(f):
- """This decorator can be used to indicate to type checkers that
- the decorated method cannot be overridden, and decorated class
- cannot be subclassed. For example:
-
- class Base:
- @final
- def done(self) -> None:
- ...
- class Sub(Base):
- def done(self) -> None: # Error reported by type checker
- ...
- @final
- class Leaf:
- ...
- class Other(Leaf): # Error reported by type checker
- ...
-
- There is no runtime checking of these properties. The decorator
- sets the ``__final__`` attribute to ``True`` on the decorated object
- to allow runtime introspection.
- """
- try:
- f.__final__ = True
- except (AttributeError, TypeError):
- # Skip the attribute silently if it is not writable.
- # AttributeError happens if the object has __slots__ or a
- # read-only property, TypeError if it's a builtin class.
- pass
- return f
-
-
-def IntVar(name):
- return typing.TypeVar(name)
-
-
-# A Literal bug was fixed in 3.11.0, 3.10.1 and 3.9.8
-if sys.version_info >= (3, 10, 1):
- Literal = typing.Literal
-else:
- def _flatten_literal_params(parameters):
- """An internal helper for Literal creation: flatten Literals among parameters"""
- params = []
- for p in parameters:
- if isinstance(p, _LiteralGenericAlias):
- params.extend(p.__args__)
- else:
- params.append(p)
- return tuple(params)
-
- def _value_and_type_iter(params):
- for p in params:
- yield p, type(p)
-
- class _LiteralGenericAlias(typing._GenericAlias, _root=True):
- def __eq__(self, other):
- if not isinstance(other, _LiteralGenericAlias):
- return NotImplemented
- these_args_deduped = set(_value_and_type_iter(self.__args__))
- other_args_deduped = set(_value_and_type_iter(other.__args__))
- return these_args_deduped == other_args_deduped
-
- def __hash__(self):
- return hash(frozenset(_value_and_type_iter(self.__args__)))
-
- class _LiteralForm(_ExtensionsSpecialForm, _root=True):
- def __init__(self, doc: str):
- self._name = 'Literal'
- self._doc = self.__doc__ = doc
-
- def __getitem__(self, parameters):
- if not isinstance(parameters, tuple):
- parameters = (parameters,)
-
- parameters = _flatten_literal_params(parameters)
-
- val_type_pairs = list(_value_and_type_iter(parameters))
- try:
- deduped_pairs = set(val_type_pairs)
- except TypeError:
- # unhashable parameters
- pass
- else:
- # similar logic to typing._deduplicate on Python 3.9+
- if len(deduped_pairs) < len(val_type_pairs):
- new_parameters = []
- for pair in val_type_pairs:
- if pair in deduped_pairs:
- new_parameters.append(pair[0])
- deduped_pairs.remove(pair)
- assert not deduped_pairs, deduped_pairs
- parameters = tuple(new_parameters)
-
- return _LiteralGenericAlias(self, parameters)
-
- Literal = _LiteralForm(doc="""\
- A type that can be used to indicate to type checkers
- that the corresponding value has a value literally equivalent
- to the provided parameter. For example:
-
- var: Literal[4] = 4
-
- The type checker understands that 'var' is literally equal to
- the value 4 and no other value.
-
- Literal[...] cannot be subclassed. There is no runtime
- checking verifying that the parameter is actually a value
- instead of a type.""")
-
-
-_overload_dummy = typing._overload_dummy
-
-
-if hasattr(typing, "get_overloads"): # 3.11+
- overload = typing.overload
- get_overloads = typing.get_overloads
- clear_overloads = typing.clear_overloads
-else:
- # {module: {qualname: {firstlineno: func}}}
- _overload_registry = collections.defaultdict(
- functools.partial(collections.defaultdict, dict)
- )
-
- def overload(func):
- """Decorator for overloaded functions/methods.
-
- In a stub file, place two or more stub definitions for the same
- function in a row, each decorated with @overload. For example:
-
- @overload
- def utf8(value: None) -> None: ...
- @overload
- def utf8(value: bytes) -> bytes: ...
- @overload
- def utf8(value: str) -> bytes: ...
-
- In a non-stub file (i.e. a regular .py file), do the same but
- follow it with an implementation. The implementation should *not*
- be decorated with @overload. For example:
-
- @overload
- def utf8(value: None) -> None: ...
- @overload
- def utf8(value: bytes) -> bytes: ...
- @overload
- def utf8(value: str) -> bytes: ...
- def utf8(value):
- # implementation goes here
-
- The overloads for a function can be retrieved at runtime using the
- get_overloads() function.
- """
- # classmethod and staticmethod
- f = getattr(func, "__func__", func)
- try:
- _overload_registry[f.__module__][f.__qualname__][
- f.__code__.co_firstlineno
- ] = func
- except AttributeError:
- # Not a normal function; ignore.
- pass
- return _overload_dummy
-
- def get_overloads(func):
- """Return all defined overloads for *func* as a sequence."""
- # classmethod and staticmethod
- f = getattr(func, "__func__", func)
- if f.__module__ not in _overload_registry:
- return []
- mod_dict = _overload_registry[f.__module__]
- if f.__qualname__ not in mod_dict:
- return []
- return list(mod_dict[f.__qualname__].values())
-
- def clear_overloads():
- """Clear all overloads in the registry."""
- _overload_registry.clear()
-
-
-# This is not a real generic class. Don't use outside annotations.
-Type = typing.Type
-
-# Various ABCs mimicking those in collections.abc.
-# A few are simply re-exported for completeness.
-
-
-Awaitable = typing.Awaitable
-Coroutine = typing.Coroutine
-AsyncIterable = typing.AsyncIterable
-AsyncIterator = typing.AsyncIterator
-Deque = typing.Deque
-ContextManager = typing.ContextManager
-AsyncContextManager = typing.AsyncContextManager
-DefaultDict = typing.DefaultDict
-
-# 3.7.2+
-if hasattr(typing, 'OrderedDict'):
- OrderedDict = typing.OrderedDict
-# 3.7.0-3.7.2
-else:
- OrderedDict = typing._alias(collections.OrderedDict, (KT, VT))
-
-Counter = typing.Counter
-ChainMap = typing.ChainMap
-AsyncGenerator = typing.AsyncGenerator
-Text = typing.Text
-TYPE_CHECKING = typing.TYPE_CHECKING
-
-
-_PROTO_ALLOWLIST = {
- 'collections.abc': [
- 'Callable', 'Awaitable', 'Iterable', 'Iterator', 'AsyncIterable',
- 'Hashable', 'Sized', 'Container', 'Collection', 'Reversible', 'Buffer',
- ],
- 'contextlib': ['AbstractContextManager', 'AbstractAsyncContextManager'],
- 'typing_extensions': ['Buffer'],
-}
-
-
-_EXCLUDED_ATTRS = {
- "__abstractmethods__", "__annotations__", "__weakref__", "_is_protocol",
- "_is_runtime_protocol", "__dict__", "__slots__", "__parameters__",
- "__orig_bases__", "__module__", "_MutableMapping__marker", "__doc__",
- "__subclasshook__", "__orig_class__", "__init__", "__new__",
- "__protocol_attrs__", "__callable_proto_members_only__",
-}
-
-if sys.version_info < (3, 8):
- _EXCLUDED_ATTRS |= {
- "_gorg", "__next_in_mro__", "__extra__", "__tree_hash__", "__args__",
- "__origin__"
- }
-
-if sys.version_info >= (3, 9):
- _EXCLUDED_ATTRS.add("__class_getitem__")
-
-if sys.version_info >= (3, 12):
- _EXCLUDED_ATTRS.add("__type_params__")
-
-_EXCLUDED_ATTRS = frozenset(_EXCLUDED_ATTRS)
-
-
-def _get_protocol_attrs(cls):
- attrs = set()
- for base in cls.__mro__[:-1]: # without object
- if base.__name__ in {'Protocol', 'Generic'}:
- continue
- annotations = getattr(base, '__annotations__', {})
- for attr in (*base.__dict__, *annotations):
- if (not attr.startswith('_abc_') and attr not in _EXCLUDED_ATTRS):
- attrs.add(attr)
- return attrs
-
-
-def _maybe_adjust_parameters(cls):
- """Helper function used in Protocol.__init_subclass__ and _TypedDictMeta.__new__.
-
- The contents of this function are very similar
- to logic found in typing.Generic.__init_subclass__
- on the CPython main branch.
- """
- tvars = []
- if '__orig_bases__' in cls.__dict__:
- tvars = _collect_type_vars(cls.__orig_bases__)
- # Look for Generic[T1, ..., Tn] or Protocol[T1, ..., Tn].
- # If found, tvars must be a subset of it.
- # If not found, tvars is it.
- # Also check for and reject plain Generic,
- # and reject multiple Generic[...] and/or Protocol[...].
- gvars = None
- for base in cls.__orig_bases__:
- if (isinstance(base, typing._GenericAlias) and
- base.__origin__ in (typing.Generic, Protocol)):
- # for error messages
- the_base = base.__origin__.__name__
- if gvars is not None:
- raise TypeError(
- "Cannot inherit from Generic[...]"
- " and/or Protocol[...] multiple types.")
- gvars = base.__parameters__
- if gvars is None:
- gvars = tvars
- else:
- tvarset = set(tvars)
- gvarset = set(gvars)
- if not tvarset <= gvarset:
- s_vars = ', '.join(str(t) for t in tvars if t not in gvarset)
- s_args = ', '.join(str(g) for g in gvars)
- raise TypeError(f"Some type variables ({s_vars}) are"
- f" not listed in {the_base}[{s_args}]")
- tvars = gvars
- cls.__parameters__ = tuple(tvars)
-
-
-def _caller(depth=2):
- try:
- return sys._getframe(depth).f_globals.get('__name__', '__main__')
- except (AttributeError, ValueError): # For platforms without _getframe()
- return None
-
-
-# The performance of runtime-checkable protocols is significantly improved on Python 3.12,
-# so we backport the 3.12 version of Protocol to Python <=3.11
-if sys.version_info >= (3, 12):
- Protocol = typing.Protocol
-else:
- def _allow_reckless_class_checks(depth=3):
- """Allow instance and class checks for special stdlib modules.
- The abc and functools modules indiscriminately call isinstance() and
- issubclass() on the whole MRO of a user class, which may contain protocols.
- """
- return _caller(depth) in {'abc', 'functools', None}
-
- def _no_init(self, *args, **kwargs):
- if type(self)._is_protocol:
- raise TypeError('Protocols cannot be instantiated')
-
- if sys.version_info >= (3, 8):
- # Inheriting from typing._ProtocolMeta isn't actually desirable,
- # but is necessary to allow typing.Protocol and typing_extensions.Protocol
- # to mix without getting TypeErrors about "metaclass conflict"
- _typing_Protocol = typing.Protocol
- _ProtocolMetaBase = type(_typing_Protocol)
- else:
- _typing_Protocol = _marker
- _ProtocolMetaBase = abc.ABCMeta
-
- class _ProtocolMeta(_ProtocolMetaBase):
- # This metaclass is somewhat unfortunate,
- # but is necessary for several reasons...
- #
- # NOTE: DO NOT call super() in any methods in this class
- # That would call the methods on typing._ProtocolMeta on Python 3.8-3.11
- # and those are slow
- def __new__(mcls, name, bases, namespace, **kwargs):
- if name == "Protocol" and len(bases) < 2:
- pass
- elif {Protocol, _typing_Protocol} & set(bases):
- for base in bases:
- if not (
- base in {object, typing.Generic, Protocol, _typing_Protocol}
- or base.__name__ in _PROTO_ALLOWLIST.get(base.__module__, [])
- or is_protocol(base)
- ):
- raise TypeError(
- f"Protocols can only inherit from other protocols, "
- f"got {base!r}"
- )
- return abc.ABCMeta.__new__(mcls, name, bases, namespace, **kwargs)
-
- def __init__(cls, *args, **kwargs):
- abc.ABCMeta.__init__(cls, *args, **kwargs)
- if getattr(cls, "_is_protocol", False):
- cls.__protocol_attrs__ = _get_protocol_attrs(cls)
- # PEP 544 prohibits using issubclass()
- # with protocols that have non-method members.
- cls.__callable_proto_members_only__ = all(
- callable(getattr(cls, attr, None)) for attr in cls.__protocol_attrs__
- )
-
- def __subclasscheck__(cls, other):
- if cls is Protocol:
- return type.__subclasscheck__(cls, other)
- if (
- getattr(cls, '_is_protocol', False)
- and not _allow_reckless_class_checks()
- ):
- if not isinstance(other, type):
- # Same error message as for issubclass(1, int).
- raise TypeError('issubclass() arg 1 must be a class')
- if (
- not cls.__callable_proto_members_only__
- and cls.__dict__.get("__subclasshook__") is _proto_hook
- ):
- raise TypeError(
- "Protocols with non-method members don't support issubclass()"
- )
- if not getattr(cls, '_is_runtime_protocol', False):
- raise TypeError(
- "Instance and class checks can only be used with "
- "@runtime_checkable protocols"
- )
- return abc.ABCMeta.__subclasscheck__(cls, other)
-
- def __instancecheck__(cls, instance):
- # We need this method for situations where attributes are
- # assigned in __init__.
- if cls is Protocol:
- return type.__instancecheck__(cls, instance)
- if not getattr(cls, "_is_protocol", False):
- # i.e., it's a concrete subclass of a protocol
- return abc.ABCMeta.__instancecheck__(cls, instance)
-
- if (
- not getattr(cls, '_is_runtime_protocol', False) and
- not _allow_reckless_class_checks()
- ):
- raise TypeError("Instance and class checks can only be used with"
- " @runtime_checkable protocols")
-
- if abc.ABCMeta.__instancecheck__(cls, instance):
- return True
-
- for attr in cls.__protocol_attrs__:
- try:
- val = inspect.getattr_static(instance, attr)
- except AttributeError:
- break
- if val is None and callable(getattr(cls, attr, None)):
- break
- else:
- return True
-
- return False
-
- def __eq__(cls, other):
- # Hack so that typing.Generic.__class_getitem__
- # treats typing_extensions.Protocol
- # as equivalent to typing.Protocol on Python 3.8+
- if abc.ABCMeta.__eq__(cls, other) is True:
- return True
- return (
- cls is Protocol and other is getattr(typing, "Protocol", object())
- )
-
- # This has to be defined, or the abc-module cache
- # complains about classes with this metaclass being unhashable,
- # if we define only __eq__!
- def __hash__(cls) -> int:
- return type.__hash__(cls)
-
- @classmethod
- def _proto_hook(cls, other):
- if not cls.__dict__.get('_is_protocol', False):
- return NotImplemented
-
- for attr in cls.__protocol_attrs__:
- for base in other.__mro__:
- # Check if the members appears in the class dictionary...
- if attr in base.__dict__:
- if base.__dict__[attr] is None:
- return NotImplemented
- break
-
- # ...or in annotations, if it is a sub-protocol.
- annotations = getattr(base, '__annotations__', {})
- if (
- isinstance(annotations, collections.abc.Mapping)
- and attr in annotations
- and is_protocol(other)
- ):
- break
- else:
- return NotImplemented
- return True
-
- if sys.version_info >= (3, 8):
- class Protocol(typing.Generic, metaclass=_ProtocolMeta):
- __doc__ = typing.Protocol.__doc__
- __slots__ = ()
- _is_protocol = True
- _is_runtime_protocol = False
-
- def __init_subclass__(cls, *args, **kwargs):
- super().__init_subclass__(*args, **kwargs)
-
- # Determine if this is a protocol or a concrete subclass.
- if not cls.__dict__.get('_is_protocol', False):
- cls._is_protocol = any(b is Protocol for b in cls.__bases__)
-
- # Set (or override) the protocol subclass hook.
- if '__subclasshook__' not in cls.__dict__:
- cls.__subclasshook__ = _proto_hook
-
- # Prohibit instantiation for protocol classes
- if cls._is_protocol and cls.__init__ is Protocol.__init__:
- cls.__init__ = _no_init
-
- else:
- class Protocol(metaclass=_ProtocolMeta):
- # There is quite a lot of overlapping code with typing.Generic.
- # Unfortunately it is hard to avoid this on Python <3.8,
- # as the typing module on Python 3.7 doesn't let us subclass typing.Generic!
- """Base class for protocol classes. Protocol classes are defined as::
-
- class Proto(Protocol):
- def meth(self) -> int:
- ...
-
- Such classes are primarily used with static type checkers that recognize
- structural subtyping (static duck-typing), for example::
-
- class C:
- def meth(self) -> int:
- return 0
-
- def func(x: Proto) -> int:
- return x.meth()
-
- func(C()) # Passes static type check
-
- See PEP 544 for details. Protocol classes decorated with
- @typing_extensions.runtime_checkable act
- as simple-minded runtime-checkable protocols that check
- only the presence of given attributes, ignoring their type signatures.
-
- Protocol classes can be generic, they are defined as::
-
- class GenProto(Protocol[T]):
- def meth(self) -> T:
- ...
- """
- __slots__ = ()
- _is_protocol = True
- _is_runtime_protocol = False
-
- def __new__(cls, *args, **kwds):
- if cls is Protocol:
- raise TypeError("Type Protocol cannot be instantiated; "
- "it can only be used as a base class")
- return super().__new__(cls)
-
- @typing._tp_cache
- def __class_getitem__(cls, params):
- if not isinstance(params, tuple):
- params = (params,)
- if not params and cls is not typing.Tuple:
- raise TypeError(
- f"Parameter list to {cls.__qualname__}[...] cannot be empty")
- msg = "Parameters to generic types must be types."
- params = tuple(typing._type_check(p, msg) for p in params)
- if cls is Protocol:
- # Generic can only be subscripted with unique type variables.
- if not all(isinstance(p, typing.TypeVar) for p in params):
- i = 0
- while isinstance(params[i], typing.TypeVar):
- i += 1
- raise TypeError(
- "Parameters to Protocol[...] must all be type variables."
- f" Parameter {i + 1} is {params[i]}")
- if len(set(params)) != len(params):
- raise TypeError(
- "Parameters to Protocol[...] must all be unique")
- else:
- # Subscripting a regular Generic subclass.
- _check_generic(cls, params, len(cls.__parameters__))
- return typing._GenericAlias(cls, params)
-
- def __init_subclass__(cls, *args, **kwargs):
- if '__orig_bases__' in cls.__dict__:
- error = typing.Generic in cls.__orig_bases__
- else:
- error = typing.Generic in cls.__bases__
- if error:
- raise TypeError("Cannot inherit from plain Generic")
- _maybe_adjust_parameters(cls)
-
- # Determine if this is a protocol or a concrete subclass.
- if not cls.__dict__.get('_is_protocol', None):
- cls._is_protocol = any(b is Protocol for b in cls.__bases__)
-
- # Set (or override) the protocol subclass hook.
- if '__subclasshook__' not in cls.__dict__:
- cls.__subclasshook__ = _proto_hook
-
- # Prohibit instantiation for protocol classes
- if cls._is_protocol and cls.__init__ is Protocol.__init__:
- cls.__init__ = _no_init
-
-
-if sys.version_info >= (3, 8):
- runtime_checkable = typing.runtime_checkable
-else:
- def runtime_checkable(cls):
- """Mark a protocol class as a runtime protocol, so that it
- can be used with isinstance() and issubclass(). Raise TypeError
- if applied to a non-protocol class.
-
- This allows a simple-minded structural check very similar to the
- one-offs in collections.abc such as Hashable.
- """
- if not (
- (isinstance(cls, _ProtocolMeta) or issubclass(cls, typing.Generic))
- and getattr(cls, "_is_protocol", False)
- ):
- raise TypeError('@runtime_checkable can be only applied to protocol classes,'
- f' got {cls!r}')
- cls._is_runtime_protocol = True
- return cls
-
-
-# Exists for backwards compatibility.
-runtime = runtime_checkable
-
-
-# Our version of runtime-checkable protocols is faster on Python 3.7-3.11
-if sys.version_info >= (3, 12):
- SupportsInt = typing.SupportsInt
- SupportsFloat = typing.SupportsFloat
- SupportsComplex = typing.SupportsComplex
- SupportsBytes = typing.SupportsBytes
- SupportsIndex = typing.SupportsIndex
- SupportsAbs = typing.SupportsAbs
- SupportsRound = typing.SupportsRound
-else:
- @runtime_checkable
- class SupportsInt(Protocol):
- """An ABC with one abstract method __int__."""
- __slots__ = ()
-
- @abc.abstractmethod
- def __int__(self) -> int:
- pass
-
- @runtime_checkable
- class SupportsFloat(Protocol):
- """An ABC with one abstract method __float__."""
- __slots__ = ()
-
- @abc.abstractmethod
- def __float__(self) -> float:
- pass
-
- @runtime_checkable
- class SupportsComplex(Protocol):
- """An ABC with one abstract method __complex__."""
- __slots__ = ()
-
- @abc.abstractmethod
- def __complex__(self) -> complex:
- pass
-
- @runtime_checkable
- class SupportsBytes(Protocol):
- """An ABC with one abstract method __bytes__."""
- __slots__ = ()
-
- @abc.abstractmethod
- def __bytes__(self) -> bytes:
- pass
-
- @runtime_checkable
- class SupportsIndex(Protocol):
- __slots__ = ()
-
- @abc.abstractmethod
- def __index__(self) -> int:
- pass
-
- @runtime_checkable
- class SupportsAbs(Protocol[T_co]):
- """
- An ABC with one abstract method __abs__ that is covariant in its return type.
- """
- __slots__ = ()
-
- @abc.abstractmethod
- def __abs__(self) -> T_co:
- pass
-
- @runtime_checkable
- class SupportsRound(Protocol[T_co]):
- """
- An ABC with one abstract method __round__ that is covariant in its return type.
- """
- __slots__ = ()
-
- @abc.abstractmethod
- def __round__(self, ndigits: int = 0) -> T_co:
- pass
-
-
-def _ensure_subclassable(mro_entries):
- def inner(func):
- if sys.implementation.name == "pypy" and sys.version_info < (3, 9):
- cls_dict = {
- "__call__": staticmethod(func),
- "__mro_entries__": staticmethod(mro_entries)
- }
- t = type(func.__name__, (), cls_dict)
- return functools.update_wrapper(t(), func)
- else:
- func.__mro_entries__ = mro_entries
- return func
- return inner
-
-
-if sys.version_info >= (3, 13):
- # The standard library TypedDict in Python 3.8 does not store runtime information
- # about which (if any) keys are optional. See https://bugs.python.org/issue38834
- # The standard library TypedDict in Python 3.9.0/1 does not honour the "total"
- # keyword with old-style TypedDict(). See https://bugs.python.org/issue42059
- # The standard library TypedDict below Python 3.11 does not store runtime
- # information about optional and required keys when using Required or NotRequired.
- # Generic TypedDicts are also impossible using typing.TypedDict on Python <3.11.
- # Aaaand on 3.12 we add __orig_bases__ to TypedDict
- # to enable better runtime introspection.
- # On 3.13 we deprecate some odd ways of creating TypedDicts.
- TypedDict = typing.TypedDict
- _TypedDictMeta = typing._TypedDictMeta
- is_typeddict = typing.is_typeddict
-else:
- # 3.10.0 and later
- _TAKES_MODULE = "module" in inspect.signature(typing._type_check).parameters
-
- if sys.version_info >= (3, 8):
- _fake_name = "Protocol"
- else:
- _fake_name = "_Protocol"
-
- class _TypedDictMeta(type):
- def __new__(cls, name, bases, ns, total=True):
- """Create new typed dict class object.
-
- This method is called when TypedDict is subclassed,
- or when TypedDict is instantiated. This way
- TypedDict supports all three syntax forms described in its docstring.
- Subclasses and instances of TypedDict return actual dictionaries.
- """
- for base in bases:
- if type(base) is not _TypedDictMeta and base is not typing.Generic:
- raise TypeError('cannot inherit from both a TypedDict type '
- 'and a non-TypedDict base class')
-
- if any(issubclass(b, typing.Generic) for b in bases):
- generic_base = (typing.Generic,)
- else:
- generic_base = ()
-
- # typing.py generally doesn't let you inherit from plain Generic, unless
- # the name of the class happens to be "Protocol" (or "_Protocol" on 3.7).
- tp_dict = type.__new__(_TypedDictMeta, _fake_name, (*generic_base, dict), ns)
- tp_dict.__name__ = name
- if tp_dict.__qualname__ == _fake_name:
- tp_dict.__qualname__ = name
-
- if not hasattr(tp_dict, '__orig_bases__'):
- tp_dict.__orig_bases__ = bases
-
- annotations = {}
- own_annotations = ns.get('__annotations__', {})
- msg = "TypedDict('Name', {f0: t0, f1: t1, ...}); each t must be a type"
- if _TAKES_MODULE:
- own_annotations = {
- n: typing._type_check(tp, msg, module=tp_dict.__module__)
- for n, tp in own_annotations.items()
- }
- else:
- own_annotations = {
- n: typing._type_check(tp, msg)
- for n, tp in own_annotations.items()
- }
- required_keys = set()
- optional_keys = set()
-
- for base in bases:
- annotations.update(base.__dict__.get('__annotations__', {}))
- required_keys.update(base.__dict__.get('__required_keys__', ()))
- optional_keys.update(base.__dict__.get('__optional_keys__', ()))
-
- annotations.update(own_annotations)
- for annotation_key, annotation_type in own_annotations.items():
- annotation_origin = get_origin(annotation_type)
- if annotation_origin is Annotated:
- annotation_args = get_args(annotation_type)
- if annotation_args:
- annotation_type = annotation_args[0]
- annotation_origin = get_origin(annotation_type)
-
- if annotation_origin is Required:
- required_keys.add(annotation_key)
- elif annotation_origin is NotRequired:
- optional_keys.add(annotation_key)
- elif total:
- required_keys.add(annotation_key)
- else:
- optional_keys.add(annotation_key)
-
- tp_dict.__annotations__ = annotations
- tp_dict.__required_keys__ = frozenset(required_keys)
- tp_dict.__optional_keys__ = frozenset(optional_keys)
- if not hasattr(tp_dict, '__total__'):
- tp_dict.__total__ = total
- return tp_dict
-
- __call__ = dict # static method
-
- def __subclasscheck__(cls, other):
- # Typed dicts are only for static structural subtyping.
- raise TypeError('TypedDict does not support instance and class checks')
-
- __instancecheck__ = __subclasscheck__
-
- _TypedDict = type.__new__(_TypedDictMeta, 'TypedDict', (), {})
-
- @_ensure_subclassable(lambda bases: (_TypedDict,))
- def TypedDict(__typename, __fields=_marker, *, total=True, **kwargs):
- """A simple typed namespace. At runtime it is equivalent to a plain dict.
-
- TypedDict creates a dictionary type such that a type checker will expect all
- instances to have a certain set of keys, where each key is
- associated with a value of a consistent type. This expectation
- is not checked at runtime.
-
- Usage::
-
- class Point2D(TypedDict):
- x: int
- y: int
- label: str
-
- a: Point2D = {'x': 1, 'y': 2, 'label': 'good'} # OK
- b: Point2D = {'z': 3, 'label': 'bad'} # Fails type check
-
- assert Point2D(x=1, y=2, label='first') == dict(x=1, y=2, label='first')
-
- The type info can be accessed via the Point2D.__annotations__ dict, and
- the Point2D.__required_keys__ and Point2D.__optional_keys__ frozensets.
- TypedDict supports an additional equivalent form::
-
- Point2D = TypedDict('Point2D', {'x': int, 'y': int, 'label': str})
-
- By default, all keys must be present in a TypedDict. It is possible
- to override this by specifying totality::
-
- class Point2D(TypedDict, total=False):
- x: int
- y: int
-
- This means that a Point2D TypedDict can have any of the keys omitted. A type
- checker is only expected to support a literal False or True as the value of
- the total argument. True is the default, and makes all items defined in the
- class body be required.
-
- The Required and NotRequired special forms can also be used to mark
- individual keys as being required or not required::
-
- class Point2D(TypedDict):
- x: int # the "x" key must always be present (Required is the default)
- y: NotRequired[int] # the "y" key can be omitted
-
- See PEP 655 for more details on Required and NotRequired.
- """
- if __fields is _marker or __fields is None:
- if __fields is _marker:
- deprecated_thing = "Failing to pass a value for the 'fields' parameter"
- else:
- deprecated_thing = "Passing `None` as the 'fields' parameter"
-
- example = f"`{__typename} = TypedDict({__typename!r}, {{}})`"
- deprecation_msg = (
- f"{deprecated_thing} is deprecated and will be disallowed in "
- "Python 3.15. To create a TypedDict class with 0 fields "
- "using the functional syntax, pass an empty dictionary, e.g. "
- ) + example + "."
- warnings.warn(deprecation_msg, DeprecationWarning, stacklevel=2)
- __fields = kwargs
- elif kwargs:
- raise TypeError("TypedDict takes either a dict or keyword arguments,"
- " but not both")
- if kwargs:
- warnings.warn(
- "The kwargs-based syntax for TypedDict definitions is deprecated "
- "in Python 3.11, will be removed in Python 3.13, and may not be "
- "understood by third-party type checkers.",
- DeprecationWarning,
- stacklevel=2,
- )
-
- ns = {'__annotations__': dict(__fields)}
- module = _caller()
- if module is not None:
- # Setting correct module is necessary to make typed dict classes pickleable.
- ns['__module__'] = module
-
- td = _TypedDictMeta(__typename, (), ns, total=total)
- td.__orig_bases__ = (TypedDict,)
- return td
-
- if hasattr(typing, "_TypedDictMeta"):
- _TYPEDDICT_TYPES = (typing._TypedDictMeta, _TypedDictMeta)
- else:
- _TYPEDDICT_TYPES = (_TypedDictMeta,)
-
- def is_typeddict(tp):
- """Check if an annotation is a TypedDict class
-
- For example::
- class Film(TypedDict):
- title: str
- year: int
-
- is_typeddict(Film) # => True
- is_typeddict(Union[list, str]) # => False
- """
- # On 3.8, this would otherwise return True
- if hasattr(typing, "TypedDict") and tp is typing.TypedDict:
- return False
- return isinstance(tp, _TYPEDDICT_TYPES)
-
-
-if hasattr(typing, "assert_type"):
- assert_type = typing.assert_type
-
-else:
- def assert_type(__val, __typ):
- """Assert (to the type checker) that the value is of the given type.
-
- When the type checker encounters a call to assert_type(), it
- emits an error if the value is not of the specified type::
-
- def greet(name: str) -> None:
- assert_type(name, str) # ok
- assert_type(name, int) # type checker error
-
- At runtime this returns the first argument unchanged and otherwise
- does nothing.
- """
- return __val
-
-
-if hasattr(typing, "Required"):
- get_type_hints = typing.get_type_hints
-else:
- # replaces _strip_annotations()
- def _strip_extras(t):
- """Strips Annotated, Required and NotRequired from a given type."""
- if isinstance(t, _AnnotatedAlias):
- return _strip_extras(t.__origin__)
- if hasattr(t, "__origin__") and t.__origin__ in (Required, NotRequired):
- return _strip_extras(t.__args__[0])
- if isinstance(t, typing._GenericAlias):
- stripped_args = tuple(_strip_extras(a) for a in t.__args__)
- if stripped_args == t.__args__:
- return t
- return t.copy_with(stripped_args)
- if hasattr(_types, "GenericAlias") and isinstance(t, _types.GenericAlias):
- stripped_args = tuple(_strip_extras(a) for a in t.__args__)
- if stripped_args == t.__args__:
- return t
- return _types.GenericAlias(t.__origin__, stripped_args)
- if hasattr(_types, "UnionType") and isinstance(t, _types.UnionType):
- stripped_args = tuple(_strip_extras(a) for a in t.__args__)
- if stripped_args == t.__args__:
- return t
- return functools.reduce(operator.or_, stripped_args)
-
- return t
-
- def get_type_hints(obj, globalns=None, localns=None, include_extras=False):
- """Return type hints for an object.
-
- This is often the same as obj.__annotations__, but it handles
- forward references encoded as string literals, adds Optional[t] if a
- default value equal to None is set and recursively replaces all
- 'Annotated[T, ...]', 'Required[T]' or 'NotRequired[T]' with 'T'
- (unless 'include_extras=True').
-
- The argument may be a module, class, method, or function. The annotations
- are returned as a dictionary. For classes, annotations include also
- inherited members.
-
- TypeError is raised if the argument is not of a type that can contain
- annotations, and an empty dictionary is returned if no annotations are
- present.
-
- BEWARE -- the behavior of globalns and localns is counterintuitive
- (unless you are familiar with how eval() and exec() work). The
- search order is locals first, then globals.
-
- - If no dict arguments are passed, an attempt is made to use the
- globals from obj (or the respective module's globals for classes),
- and these are also used as the locals. If the object does not appear
- to have globals, an empty dictionary is used.
-
- - If one dict argument is passed, it is used for both globals and
- locals.
-
- - If two dict arguments are passed, they specify globals and
- locals, respectively.
- """
- if hasattr(typing, "Annotated"):
- hint = typing.get_type_hints(
- obj, globalns=globalns, localns=localns, include_extras=True
- )
- else:
- hint = typing.get_type_hints(obj, globalns=globalns, localns=localns)
- if include_extras:
- return hint
- return {k: _strip_extras(t) for k, t in hint.items()}
-
-
-# Python 3.9+ has PEP 593 (Annotated)
-if hasattr(typing, 'Annotated'):
- Annotated = typing.Annotated
- # Not exported and not a public API, but needed for get_origin() and get_args()
- # to work.
- _AnnotatedAlias = typing._AnnotatedAlias
-# 3.7-3.8
-else:
- class _AnnotatedAlias(typing._GenericAlias, _root=True):
- """Runtime representation of an annotated type.
-
- At its core 'Annotated[t, dec1, dec2, ...]' is an alias for the type 't'
- with extra annotations. The alias behaves like a normal typing alias,
- instantiating is the same as instantiating the underlying type, binding
- it to types is also the same.
- """
- def __init__(self, origin, metadata):
- if isinstance(origin, _AnnotatedAlias):
- metadata = origin.__metadata__ + metadata
- origin = origin.__origin__
- super().__init__(origin, origin)
- self.__metadata__ = metadata
-
- def copy_with(self, params):
- assert len(params) == 1
- new_type = params[0]
- return _AnnotatedAlias(new_type, self.__metadata__)
-
- def __repr__(self):
- return (f"typing_extensions.Annotated[{typing._type_repr(self.__origin__)}, "
- f"{', '.join(repr(a) for a in self.__metadata__)}]")
-
- def __reduce__(self):
- return operator.getitem, (
- Annotated, (self.__origin__,) + self.__metadata__
- )
-
- def __eq__(self, other):
- if not isinstance(other, _AnnotatedAlias):
- return NotImplemented
- if self.__origin__ != other.__origin__:
- return False
- return self.__metadata__ == other.__metadata__
-
- def __hash__(self):
- return hash((self.__origin__, self.__metadata__))
-
- class Annotated:
- """Add context specific metadata to a type.
-
- Example: Annotated[int, runtime_check.Unsigned] indicates to the
- hypothetical runtime_check module that this type is an unsigned int.
- Every other consumer of this type can ignore this metadata and treat
- this type as int.
-
- The first argument to Annotated must be a valid type (and will be in
- the __origin__ field), the remaining arguments are kept as a tuple in
- the __extra__ field.
-
- Details:
-
- - It's an error to call `Annotated` with less than two arguments.
- - Nested Annotated are flattened::
-
- Annotated[Annotated[T, Ann1, Ann2], Ann3] == Annotated[T, Ann1, Ann2, Ann3]
-
- - Instantiating an annotated type is equivalent to instantiating the
- underlying type::
-
- Annotated[C, Ann1](5) == C(5)
-
- - Annotated can be used as a generic type alias::
-
- Optimized = Annotated[T, runtime.Optimize()]
- Optimized[int] == Annotated[int, runtime.Optimize()]
-
- OptimizedList = Annotated[List[T], runtime.Optimize()]
- OptimizedList[int] == Annotated[List[int], runtime.Optimize()]
- """
-
- __slots__ = ()
-
- def __new__(cls, *args, **kwargs):
- raise TypeError("Type Annotated cannot be instantiated.")
-
- @typing._tp_cache
- def __class_getitem__(cls, params):
- if not isinstance(params, tuple) or len(params) < 2:
- raise TypeError("Annotated[...] should be used "
- "with at least two arguments (a type and an "
- "annotation).")
- allowed_special_forms = (ClassVar, Final)
- if get_origin(params[0]) in allowed_special_forms:
- origin = params[0]
- else:
- msg = "Annotated[t, ...]: t must be a type."
- origin = typing._type_check(params[0], msg)
- metadata = tuple(params[1:])
- return _AnnotatedAlias(origin, metadata)
-
- def __init_subclass__(cls, *args, **kwargs):
- raise TypeError(
- f"Cannot subclass {cls.__module__}.Annotated"
- )
-
-# Python 3.8 has get_origin() and get_args() but those implementations aren't
-# Annotated-aware, so we can't use those. Python 3.9's versions don't support
-# ParamSpecArgs and ParamSpecKwargs, so only Python 3.10's versions will do.
-if sys.version_info[:2] >= (3, 10):
- get_origin = typing.get_origin
- get_args = typing.get_args
-# 3.7-3.9
-else:
- try:
- # 3.9+
- from typing import _BaseGenericAlias
- except ImportError:
- _BaseGenericAlias = typing._GenericAlias
- try:
- # 3.9+
- from typing import GenericAlias as _typing_GenericAlias
- except ImportError:
- _typing_GenericAlias = typing._GenericAlias
-
- def get_origin(tp):
- """Get the unsubscripted version of a type.
-
- This supports generic types, Callable, Tuple, Union, Literal, Final, ClassVar
- and Annotated. Return None for unsupported types. Examples::
-
- get_origin(Literal[42]) is Literal
- get_origin(int) is None
- get_origin(ClassVar[int]) is ClassVar
- get_origin(Generic) is Generic
- get_origin(Generic[T]) is Generic
- get_origin(Union[T, int]) is Union
- get_origin(List[Tuple[T, T]][int]) == list
- get_origin(P.args) is P
- """
- if isinstance(tp, _AnnotatedAlias):
- return Annotated
- if isinstance(tp, (typing._GenericAlias, _typing_GenericAlias, _BaseGenericAlias,
- ParamSpecArgs, ParamSpecKwargs)):
- return tp.__origin__
- if tp is typing.Generic:
- return typing.Generic
- return None
-
- def get_args(tp):
- """Get type arguments with all substitutions performed.
-
- For unions, basic simplifications used by Union constructor are performed.
- Examples::
- get_args(Dict[str, int]) == (str, int)
- get_args(int) == ()
- get_args(Union[int, Union[T, int], str][int]) == (int, str)
- get_args(Union[int, Tuple[T, int]][str]) == (int, Tuple[str, int])
- get_args(Callable[[], T][int]) == ([], int)
- """
- if isinstance(tp, _AnnotatedAlias):
- return (tp.__origin__,) + tp.__metadata__
- if isinstance(tp, (typing._GenericAlias, _typing_GenericAlias)):
- if getattr(tp, "_special", False):
- return ()
- res = tp.__args__
- if get_origin(tp) is collections.abc.Callable and res[0] is not Ellipsis:
- res = (list(res[:-1]), res[-1])
- return res
- return ()
-
-
-# 3.10+
-if hasattr(typing, 'TypeAlias'):
- TypeAlias = typing.TypeAlias
-# 3.9
-elif sys.version_info[:2] >= (3, 9):
- @_ExtensionsSpecialForm
- def TypeAlias(self, parameters):
- """Special marker indicating that an assignment should
- be recognized as a proper type alias definition by type
- checkers.
-
- For example::
-
- Predicate: TypeAlias = Callable[..., bool]
-
- It's invalid when used anywhere except as in the example above.
- """
- raise TypeError(f"{self} is not subscriptable")
-# 3.7-3.8
-else:
- TypeAlias = _ExtensionsSpecialForm(
- 'TypeAlias',
- doc="""Special marker indicating that an assignment should
- be recognized as a proper type alias definition by type
- checkers.
-
- For example::
-
- Predicate: TypeAlias = Callable[..., bool]
-
- It's invalid when used anywhere except as in the example
- above."""
- )
-
-
-def _set_default(type_param, default):
- if isinstance(default, (tuple, list)):
- type_param.__default__ = tuple((typing._type_check(d, "Default must be a type")
- for d in default))
- elif default != _marker:
- type_param.__default__ = typing._type_check(default, "Default must be a type")
- else:
- type_param.__default__ = None
-
-
-def _set_module(typevarlike):
- # for pickling:
- def_mod = _caller(depth=3)
- if def_mod != 'typing_extensions':
- typevarlike.__module__ = def_mod
-
-
-class _DefaultMixin:
- """Mixin for TypeVarLike defaults."""
-
- __slots__ = ()
- __init__ = _set_default
-
-
-# Classes using this metaclass must provide a _backported_typevarlike ClassVar
-class _TypeVarLikeMeta(type):
- def __instancecheck__(cls, __instance: Any) -> bool:
- return isinstance(__instance, cls._backported_typevarlike)
-
-
-# Add default and infer_variance parameters from PEP 696 and 695
-class TypeVar(metaclass=_TypeVarLikeMeta):
- """Type variable."""
-
- _backported_typevarlike = typing.TypeVar
-
- def __new__(cls, name, *constraints, bound=None,
- covariant=False, contravariant=False,
- default=_marker, infer_variance=False):
- if hasattr(typing, "TypeAliasType"):
- # PEP 695 implemented, can pass infer_variance to typing.TypeVar
- typevar = typing.TypeVar(name, *constraints, bound=bound,
- covariant=covariant, contravariant=contravariant,
- infer_variance=infer_variance)
- else:
- typevar = typing.TypeVar(name, *constraints, bound=bound,
- covariant=covariant, contravariant=contravariant)
- if infer_variance and (covariant or contravariant):
- raise ValueError("Variance cannot be specified with infer_variance.")
- typevar.__infer_variance__ = infer_variance
- _set_default(typevar, default)
- _set_module(typevar)
- return typevar
-
- def __init_subclass__(cls) -> None:
- raise TypeError(f"type '{__name__}.TypeVar' is not an acceptable base type")
-
-
-# Python 3.10+ has PEP 612
-if hasattr(typing, 'ParamSpecArgs'):
- ParamSpecArgs = typing.ParamSpecArgs
- ParamSpecKwargs = typing.ParamSpecKwargs
-# 3.7-3.9
-else:
- class _Immutable:
- """Mixin to indicate that object should not be copied."""
- __slots__ = ()
-
- def __copy__(self):
- return self
-
- def __deepcopy__(self, memo):
- return self
-
- class ParamSpecArgs(_Immutable):
- """The args for a ParamSpec object.
-
- Given a ParamSpec object P, P.args is an instance of ParamSpecArgs.
-
- ParamSpecArgs objects have a reference back to their ParamSpec:
-
- P.args.__origin__ is P
-
- This type is meant for runtime introspection and has no special meaning to
- static type checkers.
- """
- def __init__(self, origin):
- self.__origin__ = origin
-
- def __repr__(self):
- return f"{self.__origin__.__name__}.args"
-
- def __eq__(self, other):
- if not isinstance(other, ParamSpecArgs):
- return NotImplemented
- return self.__origin__ == other.__origin__
-
- class ParamSpecKwargs(_Immutable):
- """The kwargs for a ParamSpec object.
-
- Given a ParamSpec object P, P.kwargs is an instance of ParamSpecKwargs.
-
- ParamSpecKwargs objects have a reference back to their ParamSpec:
-
- P.kwargs.__origin__ is P
-
- This type is meant for runtime introspection and has no special meaning to
- static type checkers.
- """
- def __init__(self, origin):
- self.__origin__ = origin
-
- def __repr__(self):
- return f"{self.__origin__.__name__}.kwargs"
-
- def __eq__(self, other):
- if not isinstance(other, ParamSpecKwargs):
- return NotImplemented
- return self.__origin__ == other.__origin__
-
-# 3.10+
-if hasattr(typing, 'ParamSpec'):
-
- # Add default parameter - PEP 696
- class ParamSpec(metaclass=_TypeVarLikeMeta):
- """Parameter specification."""
-
- _backported_typevarlike = typing.ParamSpec
-
- def __new__(cls, name, *, bound=None,
- covariant=False, contravariant=False,
- infer_variance=False, default=_marker):
- if hasattr(typing, "TypeAliasType"):
- # PEP 695 implemented, can pass infer_variance to typing.TypeVar
- paramspec = typing.ParamSpec(name, bound=bound,
- covariant=covariant,
- contravariant=contravariant,
- infer_variance=infer_variance)
- else:
- paramspec = typing.ParamSpec(name, bound=bound,
- covariant=covariant,
- contravariant=contravariant)
- paramspec.__infer_variance__ = infer_variance
-
- _set_default(paramspec, default)
- _set_module(paramspec)
- return paramspec
-
- def __init_subclass__(cls) -> None:
- raise TypeError(f"type '{__name__}.ParamSpec' is not an acceptable base type")
-
-# 3.7-3.9
-else:
-
- # Inherits from list as a workaround for Callable checks in Python < 3.9.2.
- class ParamSpec(list, _DefaultMixin):
- """Parameter specification variable.
-
- Usage::
-
- P = ParamSpec('P')
-
- Parameter specification variables exist primarily for the benefit of static
- type checkers. They are used to forward the parameter types of one
- callable to another callable, a pattern commonly found in higher order
- functions and decorators. They are only valid when used in ``Concatenate``,
- or s the first argument to ``Callable``. In Python 3.10 and higher,
- they are also supported in user-defined Generics at runtime.
- See class Generic for more information on generic types. An
- example for annotating a decorator::
-
- T = TypeVar('T')
- P = ParamSpec('P')
-
- def add_logging(f: Callable[P, T]) -> Callable[P, T]:
- '''A type-safe decorator to add logging to a function.'''
- def inner(*args: P.args, **kwargs: P.kwargs) -> T:
- logging.info(f'{f.__name__} was called')
- return f(*args, **kwargs)
- return inner
-
- @add_logging
- def add_two(x: float, y: float) -> float:
- '''Add two numbers together.'''
- return x + y
-
- Parameter specification variables defined with covariant=True or
- contravariant=True can be used to declare covariant or contravariant
- generic types. These keyword arguments are valid, but their actual semantics
- are yet to be decided. See PEP 612 for details.
-
- Parameter specification variables can be introspected. e.g.:
-
- P.__name__ == 'T'
- P.__bound__ == None
- P.__covariant__ == False
- P.__contravariant__ == False
-
- Note that only parameter specification variables defined in global scope can
- be pickled.
- """
-
- # Trick Generic __parameters__.
- __class__ = typing.TypeVar
-
- @property
- def args(self):
- return ParamSpecArgs(self)
-
- @property
- def kwargs(self):
- return ParamSpecKwargs(self)
-
- def __init__(self, name, *, bound=None, covariant=False, contravariant=False,
- infer_variance=False, default=_marker):
- super().__init__([self])
- self.__name__ = name
- self.__covariant__ = bool(covariant)
- self.__contravariant__ = bool(contravariant)
- self.__infer_variance__ = bool(infer_variance)
- if bound:
- self.__bound__ = typing._type_check(bound, 'Bound must be a type.')
- else:
- self.__bound__ = None
- _DefaultMixin.__init__(self, default)
-
- # for pickling:
- def_mod = _caller()
- if def_mod != 'typing_extensions':
- self.__module__ = def_mod
-
- def __repr__(self):
- if self.__infer_variance__:
- prefix = ''
- elif self.__covariant__:
- prefix = '+'
- elif self.__contravariant__:
- prefix = '-'
- else:
- prefix = '~'
- return prefix + self.__name__
-
- def __hash__(self):
- return object.__hash__(self)
-
- def __eq__(self, other):
- return self is other
-
- def __reduce__(self):
- return self.__name__
-
- # Hack to get typing._type_check to pass.
- def __call__(self, *args, **kwargs):
- pass
-
-
-# 3.7-3.9
-if not hasattr(typing, 'Concatenate'):
- # Inherits from list as a workaround for Callable checks in Python < 3.9.2.
- class _ConcatenateGenericAlias(list):
-
- # Trick Generic into looking into this for __parameters__.
- __class__ = typing._GenericAlias
-
- # Flag in 3.8.
- _special = False
-
- def __init__(self, origin, args):
- super().__init__(args)
- self.__origin__ = origin
- self.__args__ = args
-
- def __repr__(self):
- _type_repr = typing._type_repr
- return (f'{_type_repr(self.__origin__)}'
- f'[{", ".join(_type_repr(arg) for arg in self.__args__)}]')
-
- def __hash__(self):
- return hash((self.__origin__, self.__args__))
-
- # Hack to get typing._type_check to pass in Generic.
- def __call__(self, *args, **kwargs):
- pass
-
- @property
- def __parameters__(self):
- return tuple(
- tp for tp in self.__args__ if isinstance(tp, (typing.TypeVar, ParamSpec))
- )
-
-
-# 3.7-3.9
-@typing._tp_cache
-def _concatenate_getitem(self, parameters):
- if parameters == ():
- raise TypeError("Cannot take a Concatenate of no types.")
- if not isinstance(parameters, tuple):
- parameters = (parameters,)
- if not isinstance(parameters[-1], ParamSpec):
- raise TypeError("The last parameter to Concatenate should be a "
- "ParamSpec variable.")
- msg = "Concatenate[arg, ...]: each arg must be a type."
- parameters = tuple(typing._type_check(p, msg) for p in parameters)
- return _ConcatenateGenericAlias(self, parameters)
-
-
-# 3.10+
-if hasattr(typing, 'Concatenate'):
- Concatenate = typing.Concatenate
- _ConcatenateGenericAlias = typing._ConcatenateGenericAlias # noqa: F811
-# 3.9
-elif sys.version_info[:2] >= (3, 9):
- @_ExtensionsSpecialForm
- def Concatenate(self, parameters):
- """Used in conjunction with ``ParamSpec`` and ``Callable`` to represent a
- higher order function which adds, removes or transforms parameters of a
- callable.
-
- For example::
-
- Callable[Concatenate[int, P], int]
-
- See PEP 612 for detailed information.
- """
- return _concatenate_getitem(self, parameters)
-# 3.7-8
-else:
- class _ConcatenateForm(_ExtensionsSpecialForm, _root=True):
- def __getitem__(self, parameters):
- return _concatenate_getitem(self, parameters)
-
- Concatenate = _ConcatenateForm(
- 'Concatenate',
- doc="""Used in conjunction with ``ParamSpec`` and ``Callable`` to represent a
- higher order function which adds, removes or transforms parameters of a
- callable.
-
- For example::
-
- Callable[Concatenate[int, P], int]
-
- See PEP 612 for detailed information.
- """)
-
-# 3.10+
-if hasattr(typing, 'TypeGuard'):
- TypeGuard = typing.TypeGuard
-# 3.9
-elif sys.version_info[:2] >= (3, 9):
- @_ExtensionsSpecialForm
- def TypeGuard(self, parameters):
- """Special typing form used to annotate the return type of a user-defined
- type guard function. ``TypeGuard`` only accepts a single type argument.
- At runtime, functions marked this way should return a boolean.
-
- ``TypeGuard`` aims to benefit *type narrowing* -- a technique used by static
- type checkers to determine a more precise type of an expression within a
- program's code flow. Usually type narrowing is done by analyzing
- conditional code flow and applying the narrowing to a block of code. The
- conditional expression here is sometimes referred to as a "type guard".
-
- Sometimes it would be convenient to use a user-defined boolean function
- as a type guard. Such a function should use ``TypeGuard[...]`` as its
- return type to alert static type checkers to this intention.
-
- Using ``-> TypeGuard`` tells the static type checker that for a given
- function:
-
- 1. The return value is a boolean.
- 2. If the return value is ``True``, the type of its argument
- is the type inside ``TypeGuard``.
-
- For example::
-
- def is_str(val: Union[str, float]):
- # "isinstance" type guard
- if isinstance(val, str):
- # Type of ``val`` is narrowed to ``str``
- ...
- else:
- # Else, type of ``val`` is narrowed to ``float``.
- ...
-
- Strict type narrowing is not enforced -- ``TypeB`` need not be a narrower
- form of ``TypeA`` (it can even be a wider form) and this may lead to
- type-unsafe results. The main reason is to allow for things like
- narrowing ``List[object]`` to ``List[str]`` even though the latter is not
- a subtype of the former, since ``List`` is invariant. The responsibility of
- writing type-safe type guards is left to the user.
-
- ``TypeGuard`` also works with type variables. For more information, see
- PEP 647 (User-Defined Type Guards).
- """
- item = typing._type_check(parameters, f'{self} accepts only a single type.')
- return typing._GenericAlias(self, (item,))
-# 3.7-3.8
-else:
- class _TypeGuardForm(_ExtensionsSpecialForm, _root=True):
- def __getitem__(self, parameters):
- item = typing._type_check(parameters,
- f'{self._name} accepts only a single type')
- return typing._GenericAlias(self, (item,))
-
- TypeGuard = _TypeGuardForm(
- 'TypeGuard',
- doc="""Special typing form used to annotate the return type of a user-defined
- type guard function. ``TypeGuard`` only accepts a single type argument.
- At runtime, functions marked this way should return a boolean.
-
- ``TypeGuard`` aims to benefit *type narrowing* -- a technique used by static
- type checkers to determine a more precise type of an expression within a
- program's code flow. Usually type narrowing is done by analyzing
- conditional code flow and applying the narrowing to a block of code. The
- conditional expression here is sometimes referred to as a "type guard".
-
- Sometimes it would be convenient to use a user-defined boolean function
- as a type guard. Such a function should use ``TypeGuard[...]`` as its
- return type to alert static type checkers to this intention.
-
- Using ``-> TypeGuard`` tells the static type checker that for a given
- function:
-
- 1. The return value is a boolean.
- 2. If the return value is ``True``, the type of its argument
- is the type inside ``TypeGuard``.
-
- For example::
-
- def is_str(val: Union[str, float]):
- # "isinstance" type guard
- if isinstance(val, str):
- # Type of ``val`` is narrowed to ``str``
- ...
- else:
- # Else, type of ``val`` is narrowed to ``float``.
- ...
-
- Strict type narrowing is not enforced -- ``TypeB`` need not be a narrower
- form of ``TypeA`` (it can even be a wider form) and this may lead to
- type-unsafe results. The main reason is to allow for things like
- narrowing ``List[object]`` to ``List[str]`` even though the latter is not
- a subtype of the former, since ``List`` is invariant. The responsibility of
- writing type-safe type guards is left to the user.
-
- ``TypeGuard`` also works with type variables. For more information, see
- PEP 647 (User-Defined Type Guards).
- """)
-
-
-# Vendored from cpython typing._SpecialFrom
-class _SpecialForm(typing._Final, _root=True):
- __slots__ = ('_name', '__doc__', '_getitem')
-
- def __init__(self, getitem):
- self._getitem = getitem
- self._name = getitem.__name__
- self.__doc__ = getitem.__doc__
-
- def __getattr__(self, item):
- if item in {'__name__', '__qualname__'}:
- return self._name
-
- raise AttributeError(item)
-
- def __mro_entries__(self, bases):
- raise TypeError(f"Cannot subclass {self!r}")
-
- def __repr__(self):
- return f'typing_extensions.{self._name}'
-
- def __reduce__(self):
- return self._name
-
- def __call__(self, *args, **kwds):
- raise TypeError(f"Cannot instantiate {self!r}")
-
- def __or__(self, other):
- return typing.Union[self, other]
-
- def __ror__(self, other):
- return typing.Union[other, self]
-
- def __instancecheck__(self, obj):
- raise TypeError(f"{self} cannot be used with isinstance()")
-
- def __subclasscheck__(self, cls):
- raise TypeError(f"{self} cannot be used with issubclass()")
-
- @typing._tp_cache
- def __getitem__(self, parameters):
- return self._getitem(self, parameters)
-
-
-if hasattr(typing, "LiteralString"):
- LiteralString = typing.LiteralString
-else:
- @_SpecialForm
- def LiteralString(self, params):
- """Represents an arbitrary literal string.
-
- Example::
-
- from pip._vendor.typing_extensions import LiteralString
-
- def query(sql: LiteralString) -> ...:
- ...
-
- query("SELECT * FROM table") # ok
- query(f"SELECT * FROM {input()}") # not ok
-
- See PEP 675 for details.
-
- """
- raise TypeError(f"{self} is not subscriptable")
-
-
-if hasattr(typing, "Self"):
- Self = typing.Self
-else:
- @_SpecialForm
- def Self(self, params):
- """Used to spell the type of "self" in classes.
-
- Example::
-
- from typing import Self
-
- class ReturnsSelf:
- def parse(self, data: bytes) -> Self:
- ...
- return self
-
- """
-
- raise TypeError(f"{self} is not subscriptable")
-
-
-if hasattr(typing, "Never"):
- Never = typing.Never
-else:
- @_SpecialForm
- def Never(self, params):
- """The bottom type, a type that has no members.
-
- This can be used to define a function that should never be
- called, or a function that never returns::
-
- from pip._vendor.typing_extensions import Never
-
- def never_call_me(arg: Never) -> None:
- pass
-
- def int_or_str(arg: int | str) -> None:
- never_call_me(arg) # type checker error
- match arg:
- case int():
- print("It's an int")
- case str():
- print("It's a str")
- case _:
- never_call_me(arg) # ok, arg is of type Never
-
- """
-
- raise TypeError(f"{self} is not subscriptable")
-
-
-if hasattr(typing, 'Required'):
- Required = typing.Required
- NotRequired = typing.NotRequired
-elif sys.version_info[:2] >= (3, 9):
- @_ExtensionsSpecialForm
- def Required(self, parameters):
- """A special typing construct to mark a key of a total=False TypedDict
- as required. For example:
-
- class Movie(TypedDict, total=False):
- title: Required[str]
- year: int
-
- m = Movie(
- title='The Matrix', # typechecker error if key is omitted
- year=1999,
- )
-
- There is no runtime checking that a required key is actually provided
- when instantiating a related TypedDict.
- """
- item = typing._type_check(parameters, f'{self._name} accepts only a single type.')
- return typing._GenericAlias(self, (item,))
-
- @_ExtensionsSpecialForm
- def NotRequired(self, parameters):
- """A special typing construct to mark a key of a TypedDict as
- potentially missing. For example:
-
- class Movie(TypedDict):
- title: str
- year: NotRequired[int]
-
- m = Movie(
- title='The Matrix', # typechecker error if key is omitted
- year=1999,
- )
- """
- item = typing._type_check(parameters, f'{self._name} accepts only a single type.')
- return typing._GenericAlias(self, (item,))
-
-else:
- class _RequiredForm(_ExtensionsSpecialForm, _root=True):
- def __getitem__(self, parameters):
- item = typing._type_check(parameters,
- f'{self._name} accepts only a single type.')
- return typing._GenericAlias(self, (item,))
-
- Required = _RequiredForm(
- 'Required',
- doc="""A special typing construct to mark a key of a total=False TypedDict
- as required. For example:
-
- class Movie(TypedDict, total=False):
- title: Required[str]
- year: int
-
- m = Movie(
- title='The Matrix', # typechecker error if key is omitted
- year=1999,
- )
-
- There is no runtime checking that a required key is actually provided
- when instantiating a related TypedDict.
- """)
- NotRequired = _RequiredForm(
- 'NotRequired',
- doc="""A special typing construct to mark a key of a TypedDict as
- potentially missing. For example:
-
- class Movie(TypedDict):
- title: str
- year: NotRequired[int]
-
- m = Movie(
- title='The Matrix', # typechecker error if key is omitted
- year=1999,
- )
- """)
-
-
-_UNPACK_DOC = """\
-Type unpack operator.
-
-The type unpack operator takes the child types from some container type,
-such as `tuple[int, str]` or a `TypeVarTuple`, and 'pulls them out'. For
-example:
-
- # For some generic class `Foo`:
- Foo[Unpack[tuple[int, str]]] # Equivalent to Foo[int, str]
-
- Ts = TypeVarTuple('Ts')
- # Specifies that `Bar` is generic in an arbitrary number of types.
- # (Think of `Ts` as a tuple of an arbitrary number of individual
- # `TypeVar`s, which the `Unpack` is 'pulling out' directly into the
- # `Generic[]`.)
- class Bar(Generic[Unpack[Ts]]): ...
- Bar[int] # Valid
- Bar[int, str] # Also valid
-
-From Python 3.11, this can also be done using the `*` operator:
-
- Foo[*tuple[int, str]]
- class Bar(Generic[*Ts]): ...
-
-The operator can also be used along with a `TypedDict` to annotate
-`**kwargs` in a function signature. For instance:
-
- class Movie(TypedDict):
- name: str
- year: int
-
- # This function expects two keyword arguments - *name* of type `str` and
- # *year* of type `int`.
- def foo(**kwargs: Unpack[Movie]): ...
-
-Note that there is only some runtime checking of this operator. Not
-everything the runtime allows may be accepted by static type checkers.
-
-For more information, see PEP 646 and PEP 692.
-"""
-
-
-if sys.version_info >= (3, 12): # PEP 692 changed the repr of Unpack[]
- Unpack = typing.Unpack
-
- def _is_unpack(obj):
- return get_origin(obj) is Unpack
-
-elif sys.version_info[:2] >= (3, 9):
- class _UnpackSpecialForm(_ExtensionsSpecialForm, _root=True):
- def __init__(self, getitem):
- super().__init__(getitem)
- self.__doc__ = _UNPACK_DOC
-
- class _UnpackAlias(typing._GenericAlias, _root=True):
- __class__ = typing.TypeVar
-
- @_UnpackSpecialForm
- def Unpack(self, parameters):
- item = typing._type_check(parameters, f'{self._name} accepts only a single type.')
- return _UnpackAlias(self, (item,))
-
- def _is_unpack(obj):
- return isinstance(obj, _UnpackAlias)
-
-else:
- class _UnpackAlias(typing._GenericAlias, _root=True):
- __class__ = typing.TypeVar
-
- class _UnpackForm(_ExtensionsSpecialForm, _root=True):
- def __getitem__(self, parameters):
- item = typing._type_check(parameters,
- f'{self._name} accepts only a single type.')
- return _UnpackAlias(self, (item,))
-
- Unpack = _UnpackForm('Unpack', doc=_UNPACK_DOC)
-
- def _is_unpack(obj):
- return isinstance(obj, _UnpackAlias)
-
-
-if hasattr(typing, "TypeVarTuple"): # 3.11+
-
- # Add default parameter - PEP 696
- class TypeVarTuple(metaclass=_TypeVarLikeMeta):
- """Type variable tuple."""
-
- _backported_typevarlike = typing.TypeVarTuple
-
- def __new__(cls, name, *, default=_marker):
- tvt = typing.TypeVarTuple(name)
- _set_default(tvt, default)
- _set_module(tvt)
- return tvt
-
- def __init_subclass__(self, *args, **kwds):
- raise TypeError("Cannot subclass special typing classes")
-
-else:
- class TypeVarTuple(_DefaultMixin):
- """Type variable tuple.
-
- Usage::
-
- Ts = TypeVarTuple('Ts')
-
- In the same way that a normal type variable is a stand-in for a single
- type such as ``int``, a type variable *tuple* is a stand-in for a *tuple*
- type such as ``Tuple[int, str]``.
-
- Type variable tuples can be used in ``Generic`` declarations.
- Consider the following example::
-
- class Array(Generic[*Ts]): ...
-
- The ``Ts`` type variable tuple here behaves like ``tuple[T1, T2]``,
- where ``T1`` and ``T2`` are type variables. To use these type variables
- as type parameters of ``Array``, we must *unpack* the type variable tuple using
- the star operator: ``*Ts``. The signature of ``Array`` then behaves
- as if we had simply written ``class Array(Generic[T1, T2]): ...``.
- In contrast to ``Generic[T1, T2]``, however, ``Generic[*Shape]`` allows
- us to parameterise the class with an *arbitrary* number of type parameters.
-
- Type variable tuples can be used anywhere a normal ``TypeVar`` can.
- This includes class definitions, as shown above, as well as function
- signatures and variable annotations::
-
- class Array(Generic[*Ts]):
-
- def __init__(self, shape: Tuple[*Ts]):
- self._shape: Tuple[*Ts] = shape
-
- def get_shape(self) -> Tuple[*Ts]:
- return self._shape
-
- shape = (Height(480), Width(640))
- x: Array[Height, Width] = Array(shape)
- y = abs(x) # Inferred type is Array[Height, Width]
- z = x + x # ... is Array[Height, Width]
- x.get_shape() # ... is tuple[Height, Width]
-
- """
-
- # Trick Generic __parameters__.
- __class__ = typing.TypeVar
-
- def __iter__(self):
- yield self.__unpacked__
-
- def __init__(self, name, *, default=_marker):
- self.__name__ = name
- _DefaultMixin.__init__(self, default)
-
- # for pickling:
- def_mod = _caller()
- if def_mod != 'typing_extensions':
- self.__module__ = def_mod
-
- self.__unpacked__ = Unpack[self]
-
- def __repr__(self):
- return self.__name__
-
- def __hash__(self):
- return object.__hash__(self)
-
- def __eq__(self, other):
- return self is other
-
- def __reduce__(self):
- return self.__name__
-
- def __init_subclass__(self, *args, **kwds):
- if '_root' not in kwds:
- raise TypeError("Cannot subclass special typing classes")
-
-
-if hasattr(typing, "reveal_type"):
- reveal_type = typing.reveal_type
-else:
- def reveal_type(__obj: T) -> T:
- """Reveal the inferred type of a variable.
-
- When a static type checker encounters a call to ``reveal_type()``,
- it will emit the inferred type of the argument::
-
- x: int = 1
- reveal_type(x)
-
- Running a static type checker (e.g., ``mypy``) on this example
- will produce output similar to 'Revealed type is "builtins.int"'.
-
- At runtime, the function prints the runtime type of the
- argument and returns it unchanged.
-
- """
- print(f"Runtime type is {type(__obj).__name__!r}", file=sys.stderr)
- return __obj
-
-
-if hasattr(typing, "assert_never"):
- assert_never = typing.assert_never
-else:
- def assert_never(__arg: Never) -> Never:
- """Assert to the type checker that a line of code is unreachable.
-
- Example::
-
- def int_or_str(arg: int | str) -> None:
- match arg:
- case int():
- print("It's an int")
- case str():
- print("It's a str")
- case _:
- assert_never(arg)
-
- If a type checker finds that a call to assert_never() is
- reachable, it will emit an error.
-
- At runtime, this throws an exception when called.
-
- """
- raise AssertionError("Expected code to be unreachable")
-
-
-if sys.version_info >= (3, 12):
- # dataclass_transform exists in 3.11 but lacks the frozen_default parameter
- dataclass_transform = typing.dataclass_transform
-else:
- def dataclass_transform(
- *,
- eq_default: bool = True,
- order_default: bool = False,
- kw_only_default: bool = False,
- frozen_default: bool = False,
- field_specifiers: typing.Tuple[
- typing.Union[typing.Type[typing.Any], typing.Callable[..., typing.Any]],
- ...
- ] = (),
- **kwargs: typing.Any,
- ) -> typing.Callable[[T], T]:
- """Decorator that marks a function, class, or metaclass as providing
- dataclass-like behavior.
-
- Example:
-
- from pip._vendor.typing_extensions import dataclass_transform
-
- _T = TypeVar("_T")
-
- # Used on a decorator function
- @dataclass_transform()
- def create_model(cls: type[_T]) -> type[_T]:
- ...
- return cls
-
- @create_model
- class CustomerModel:
- id: int
- name: str
-
- # Used on a base class
- @dataclass_transform()
- class ModelBase: ...
-
- class CustomerModel(ModelBase):
- id: int
- name: str
-
- # Used on a metaclass
- @dataclass_transform()
- class ModelMeta(type): ...
-
- class ModelBase(metaclass=ModelMeta): ...
-
- class CustomerModel(ModelBase):
- id: int
- name: str
-
- Each of the ``CustomerModel`` classes defined in this example will now
- behave similarly to a dataclass created with the ``@dataclasses.dataclass``
- decorator. For example, the type checker will synthesize an ``__init__``
- method.
-
- The arguments to this decorator can be used to customize this behavior:
- - ``eq_default`` indicates whether the ``eq`` parameter is assumed to be
- True or False if it is omitted by the caller.
- - ``order_default`` indicates whether the ``order`` parameter is
- assumed to be True or False if it is omitted by the caller.
- - ``kw_only_default`` indicates whether the ``kw_only`` parameter is
- assumed to be True or False if it is omitted by the caller.
- - ``frozen_default`` indicates whether the ``frozen`` parameter is
- assumed to be True or False if it is omitted by the caller.
- - ``field_specifiers`` specifies a static list of supported classes
- or functions that describe fields, similar to ``dataclasses.field()``.
-
- At runtime, this decorator records its arguments in the
- ``__dataclass_transform__`` attribute on the decorated object.
-
- See PEP 681 for details.
-
- """
- def decorator(cls_or_fn):
- cls_or_fn.__dataclass_transform__ = {
- "eq_default": eq_default,
- "order_default": order_default,
- "kw_only_default": kw_only_default,
- "frozen_default": frozen_default,
- "field_specifiers": field_specifiers,
- "kwargs": kwargs,
- }
- return cls_or_fn
- return decorator
-
-
-if hasattr(typing, "override"):
- override = typing.override
-else:
- _F = typing.TypeVar("_F", bound=typing.Callable[..., typing.Any])
-
- def override(__arg: _F) -> _F:
- """Indicate that a method is intended to override a method in a base class.
-
- Usage:
-
- class Base:
- def method(self) -> None: ...
- pass
-
- class Child(Base):
- @override
- def method(self) -> None:
- super().method()
-
- When this decorator is applied to a method, the type checker will
- validate that it overrides a method with the same name on a base class.
- This helps prevent bugs that may occur when a base class is changed
- without an equivalent change to a child class.
-
- There is no runtime checking of these properties. The decorator
- sets the ``__override__`` attribute to ``True`` on the decorated object
- to allow runtime introspection.
-
- See PEP 698 for details.
-
- """
- try:
- __arg.__override__ = True
- except (AttributeError, TypeError):
- # Skip the attribute silently if it is not writable.
- # AttributeError happens if the object has __slots__ or a
- # read-only property, TypeError if it's a builtin class.
- pass
- return __arg
-
-
-if hasattr(typing, "deprecated"):
- deprecated = typing.deprecated
-else:
- _T = typing.TypeVar("_T")
-
- def deprecated(
- __msg: str,
- *,
- category: typing.Optional[typing.Type[Warning]] = DeprecationWarning,
- stacklevel: int = 1,
- ) -> typing.Callable[[_T], _T]:
- """Indicate that a class, function or overload is deprecated.
-
- Usage:
-
- @deprecated("Use B instead")
- class A:
- pass
-
- @deprecated("Use g instead")
- def f():
- pass
-
- @overload
- @deprecated("int support is deprecated")
- def g(x: int) -> int: ...
- @overload
- def g(x: str) -> int: ...
-
- When this decorator is applied to an object, the type checker
- will generate a diagnostic on usage of the deprecated object.
-
- The warning specified by ``category`` will be emitted on use
- of deprecated objects. For functions, that happens on calls;
- for classes, on instantiation. If the ``category`` is ``None``,
- no warning is emitted. The ``stacklevel`` determines where the
- warning is emitted. If it is ``1`` (the default), the warning
- is emitted at the direct caller of the deprecated object; if it
- is higher, it is emitted further up the stack.
-
- The decorator sets the ``__deprecated__``
- attribute on the decorated object to the deprecation message
- passed to the decorator. If applied to an overload, the decorator
- must be after the ``@overload`` decorator for the attribute to
- exist on the overload as returned by ``get_overloads()``.
-
- See PEP 702 for details.
-
- """
- def decorator(__arg: _T) -> _T:
- if category is None:
- __arg.__deprecated__ = __msg
- return __arg
- elif isinstance(__arg, type):
- original_new = __arg.__new__
- has_init = __arg.__init__ is not object.__init__
-
- @functools.wraps(original_new)
- def __new__(cls, *args, **kwargs):
- warnings.warn(__msg, category=category, stacklevel=stacklevel + 1)
- if original_new is not object.__new__:
- return original_new(cls, *args, **kwargs)
- # Mirrors a similar check in object.__new__.
- elif not has_init and (args or kwargs):
- raise TypeError(f"{cls.__name__}() takes no arguments")
- else:
- return original_new(cls)
-
- __arg.__new__ = staticmethod(__new__)
- __arg.__deprecated__ = __new__.__deprecated__ = __msg
- return __arg
- elif callable(__arg):
- @functools.wraps(__arg)
- def wrapper(*args, **kwargs):
- warnings.warn(__msg, category=category, stacklevel=stacklevel + 1)
- return __arg(*args, **kwargs)
-
- __arg.__deprecated__ = wrapper.__deprecated__ = __msg
- return wrapper
- else:
- raise TypeError(
- "@deprecated decorator with non-None category must be applied to "
- f"a class or callable, not {__arg!r}"
- )
-
- return decorator
-
-
-# We have to do some monkey patching to deal with the dual nature of
-# Unpack/TypeVarTuple:
-# - We want Unpack to be a kind of TypeVar so it gets accepted in
-# Generic[Unpack[Ts]]
-# - We want it to *not* be treated as a TypeVar for the purposes of
-# counting generic parameters, so that when we subscript a generic,
-# the runtime doesn't try to substitute the Unpack with the subscripted type.
-if not hasattr(typing, "TypeVarTuple"):
- typing._collect_type_vars = _collect_type_vars
- typing._check_generic = _check_generic
-
-
-# Backport typing.NamedTuple as it exists in Python 3.12.
-# In 3.11, the ability to define generic `NamedTuple`s was supported.
-# This was explicitly disallowed in 3.9-3.10, and only half-worked in <=3.8.
-# On 3.12, we added __orig_bases__ to call-based NamedTuples
-# On 3.13, we deprecated kwargs-based NamedTuples
-if sys.version_info >= (3, 13):
- NamedTuple = typing.NamedTuple
-else:
- def _make_nmtuple(name, types, module, defaults=()):
- fields = [n for n, t in types]
- annotations = {n: typing._type_check(t, f"field {n} annotation must be a type")
- for n, t in types}
- nm_tpl = collections.namedtuple(name, fields,
- defaults=defaults, module=module)
- nm_tpl.__annotations__ = nm_tpl.__new__.__annotations__ = annotations
- # The `_field_types` attribute was removed in 3.9;
- # in earlier versions, it is the same as the `__annotations__` attribute
- if sys.version_info < (3, 9):
- nm_tpl._field_types = annotations
- return nm_tpl
-
- _prohibited_namedtuple_fields = typing._prohibited
- _special_namedtuple_fields = frozenset({'__module__', '__name__', '__annotations__'})
-
- class _NamedTupleMeta(type):
- def __new__(cls, typename, bases, ns):
- assert _NamedTuple in bases
- for base in bases:
- if base is not _NamedTuple and base is not typing.Generic:
- raise TypeError(
- 'can only inherit from a NamedTuple type and Generic')
- bases = tuple(tuple if base is _NamedTuple else base for base in bases)
- types = ns.get('__annotations__', {})
- default_names = []
- for field_name in types:
- if field_name in ns:
- default_names.append(field_name)
- elif default_names:
- raise TypeError(f"Non-default namedtuple field {field_name} "
- f"cannot follow default field"
- f"{'s' if len(default_names) > 1 else ''} "
- f"{', '.join(default_names)}")
- nm_tpl = _make_nmtuple(
- typename, types.items(),
- defaults=[ns[n] for n in default_names],
- module=ns['__module__']
- )
- nm_tpl.__bases__ = bases
- if typing.Generic in bases:
- if hasattr(typing, '_generic_class_getitem'): # 3.12+
- nm_tpl.__class_getitem__ = classmethod(typing._generic_class_getitem)
- else:
- class_getitem = typing.Generic.__class_getitem__.__func__
- nm_tpl.__class_getitem__ = classmethod(class_getitem)
- # update from user namespace without overriding special namedtuple attributes
- for key in ns:
- if key in _prohibited_namedtuple_fields:
- raise AttributeError("Cannot overwrite NamedTuple attribute " + key)
- elif key not in _special_namedtuple_fields and key not in nm_tpl._fields:
- setattr(nm_tpl, key, ns[key])
- if typing.Generic in bases:
- nm_tpl.__init_subclass__()
- return nm_tpl
-
- _NamedTuple = type.__new__(_NamedTupleMeta, 'NamedTuple', (), {})
-
- def _namedtuple_mro_entries(bases):
- assert NamedTuple in bases
- return (_NamedTuple,)
-
- @_ensure_subclassable(_namedtuple_mro_entries)
- def NamedTuple(__typename, __fields=_marker, **kwargs):
- """Typed version of namedtuple.
-
- Usage::
-
- class Employee(NamedTuple):
- name: str
- id: int
-
- This is equivalent to::
-
- Employee = collections.namedtuple('Employee', ['name', 'id'])
-
- The resulting class has an extra __annotations__ attribute, giving a
- dict that maps field names to types. (The field names are also in
- the _fields attribute, which is part of the namedtuple API.)
- An alternative equivalent functional syntax is also accepted::
-
- Employee = NamedTuple('Employee', [('name', str), ('id', int)])
- """
- if __fields is _marker:
- if kwargs:
- deprecated_thing = "Creating NamedTuple classes using keyword arguments"
- deprecation_msg = (
- "{name} is deprecated and will be disallowed in Python {remove}. "
- "Use the class-based or functional syntax instead."
- )
- else:
- deprecated_thing = "Failing to pass a value for the 'fields' parameter"
- example = f"`{__typename} = NamedTuple({__typename!r}, [])`"
- deprecation_msg = (
- "{name} is deprecated and will be disallowed in Python {remove}. "
- "To create a NamedTuple class with 0 fields "
- "using the functional syntax, "
- "pass an empty list, e.g. "
- ) + example + "."
- elif __fields is None:
- if kwargs:
- raise TypeError(
- "Cannot pass `None` as the 'fields' parameter "
- "and also specify fields using keyword arguments"
- )
- else:
- deprecated_thing = "Passing `None` as the 'fields' parameter"
- example = f"`{__typename} = NamedTuple({__typename!r}, [])`"
- deprecation_msg = (
- "{name} is deprecated and will be disallowed in Python {remove}. "
- "To create a NamedTuple class with 0 fields "
- "using the functional syntax, "
- "pass an empty list, e.g. "
- ) + example + "."
- elif kwargs:
- raise TypeError("Either list of fields or keywords"
- " can be provided to NamedTuple, not both")
- if __fields is _marker or __fields is None:
- warnings.warn(
- deprecation_msg.format(name=deprecated_thing, remove="3.15"),
- DeprecationWarning,
- stacklevel=2,
- )
- __fields = kwargs.items()
- nt = _make_nmtuple(__typename, __fields, module=_caller())
- nt.__orig_bases__ = (NamedTuple,)
- return nt
-
- # On 3.8+, alter the signature so that it matches typing.NamedTuple.
- # The signature of typing.NamedTuple on >=3.8 is invalid syntax in Python 3.7,
- # so just leave the signature as it is on 3.7.
- if sys.version_info >= (3, 8):
- _new_signature = '(typename, fields=None, /, **kwargs)'
- if isinstance(NamedTuple, _types.FunctionType):
- NamedTuple.__text_signature__ = _new_signature
- else:
- NamedTuple.__call__.__text_signature__ = _new_signature
-
-
-if hasattr(collections.abc, "Buffer"):
- Buffer = collections.abc.Buffer
-else:
- class Buffer(abc.ABC):
- """Base class for classes that implement the buffer protocol.
-
- The buffer protocol allows Python objects to expose a low-level
- memory buffer interface. Before Python 3.12, it is not possible
- to implement the buffer protocol in pure Python code, or even
- to check whether a class implements the buffer protocol. In
- Python 3.12 and higher, the ``__buffer__`` method allows access
- to the buffer protocol from Python code, and the
- ``collections.abc.Buffer`` ABC allows checking whether a class
- implements the buffer protocol.
-
- To indicate support for the buffer protocol in earlier versions,
- inherit from this ABC, either in a stub file or at runtime,
- or use ABC registration. This ABC provides no methods, because
- there is no Python-accessible methods shared by pre-3.12 buffer
- classes. It is useful primarily for static checks.
-
- """
-
- # As a courtesy, register the most common stdlib buffer classes.
- Buffer.register(memoryview)
- Buffer.register(bytearray)
- Buffer.register(bytes)
-
-
-# Backport of types.get_original_bases, available on 3.12+ in CPython
-if hasattr(_types, "get_original_bases"):
- get_original_bases = _types.get_original_bases
-else:
- def get_original_bases(__cls):
- """Return the class's "original" bases prior to modification by `__mro_entries__`.
-
- Examples::
-
- from typing import TypeVar, Generic
- from pip._vendor.typing_extensions import NamedTuple, TypedDict
-
- T = TypeVar("T")
- class Foo(Generic[T]): ...
- class Bar(Foo[int], float): ...
- class Baz(list[str]): ...
- Eggs = NamedTuple("Eggs", [("a", int), ("b", str)])
- Spam = TypedDict("Spam", {"a": int, "b": str})
-
- assert get_original_bases(Bar) == (Foo[int], float)
- assert get_original_bases(Baz) == (list[str],)
- assert get_original_bases(Eggs) == (NamedTuple,)
- assert get_original_bases(Spam) == (TypedDict,)
- assert get_original_bases(int) == (object,)
- """
- try:
- return __cls.__orig_bases__
- except AttributeError:
- try:
- return __cls.__bases__
- except AttributeError:
- raise TypeError(
- f'Expected an instance of type, not {type(__cls).__name__!r}'
- ) from None
-
-
-# NewType is a class on Python 3.10+, making it pickleable
-# The error message for subclassing instances of NewType was improved on 3.11+
-if sys.version_info >= (3, 11):
- NewType = typing.NewType
-else:
- class NewType:
- """NewType creates simple unique types with almost zero
- runtime overhead. NewType(name, tp) is considered a subtype of tp
- by static type checkers. At runtime, NewType(name, tp) returns
- a dummy callable that simply returns its argument. Usage::
- UserId = NewType('UserId', int)
- def name_by_id(user_id: UserId) -> str:
- ...
- UserId('user') # Fails type check
- name_by_id(42) # Fails type check
- name_by_id(UserId(42)) # OK
- num = UserId(5) + 1 # type: int
- """
-
- def __call__(self, obj):
- return obj
-
- def __init__(self, name, tp):
- self.__qualname__ = name
- if '.' in name:
- name = name.rpartition('.')[-1]
- self.__name__ = name
- self.__supertype__ = tp
- def_mod = _caller()
- if def_mod != 'typing_extensions':
- self.__module__ = def_mod
-
- def __mro_entries__(self, bases):
- # We defined __mro_entries__ to get a better error message
- # if a user attempts to subclass a NewType instance. bpo-46170
- supercls_name = self.__name__
-
- class Dummy:
- def __init_subclass__(cls):
- subcls_name = cls.__name__
- raise TypeError(
- f"Cannot subclass an instance of NewType. "
- f"Perhaps you were looking for: "
- f"`{subcls_name} = NewType({subcls_name!r}, {supercls_name})`"
- )
-
- return (Dummy,)
-
- def __repr__(self):
- return f'{self.__module__}.{self.__qualname__}'
-
- def __reduce__(self):
- return self.__qualname__
-
- if sys.version_info >= (3, 10):
- # PEP 604 methods
- # It doesn't make sense to have these methods on Python <3.10
-
- def __or__(self, other):
- return typing.Union[self, other]
-
- def __ror__(self, other):
- return typing.Union[other, self]
-
-
-if hasattr(typing, "TypeAliasType"):
- TypeAliasType = typing.TypeAliasType
-else:
- def _is_unionable(obj):
- """Corresponds to is_unionable() in unionobject.c in CPython."""
- return obj is None or isinstance(obj, (
- type,
- _types.GenericAlias,
- _types.UnionType,
- TypeAliasType,
- ))
-
- class TypeAliasType:
- """Create named, parameterized type aliases.
-
- This provides a backport of the new `type` statement in Python 3.12:
-
- type ListOrSet[T] = list[T] | set[T]
-
- is equivalent to:
-
- T = TypeVar("T")
- ListOrSet = TypeAliasType("ListOrSet", list[T] | set[T], type_params=(T,))
-
- The name ListOrSet can then be used as an alias for the type it refers to.
-
- The type_params argument should contain all the type parameters used
- in the value of the type alias. If the alias is not generic, this
- argument is omitted.
-
- Static type checkers should only support type aliases declared using
- TypeAliasType that follow these rules:
-
- - The first argument (the name) must be a string literal.
- - The TypeAliasType instance must be immediately assigned to a variable
- of the same name. (For example, 'X = TypeAliasType("Y", int)' is invalid,
- as is 'X, Y = TypeAliasType("X", int), TypeAliasType("Y", int)').
-
- """
-
- def __init__(self, name: str, value, *, type_params=()):
- if not isinstance(name, str):
- raise TypeError("TypeAliasType name must be a string")
- self.__value__ = value
- self.__type_params__ = type_params
-
- parameters = []
- for type_param in type_params:
- if isinstance(type_param, TypeVarTuple):
- parameters.extend(type_param)
- else:
- parameters.append(type_param)
- self.__parameters__ = tuple(parameters)
- def_mod = _caller()
- if def_mod != 'typing_extensions':
- self.__module__ = def_mod
- # Setting this attribute closes the TypeAliasType from further modification
- self.__name__ = name
-
- def __setattr__(self, __name: str, __value: object) -> None:
- if hasattr(self, "__name__"):
- self._raise_attribute_error(__name)
- super().__setattr__(__name, __value)
-
- def __delattr__(self, __name: str) -> Never:
- self._raise_attribute_error(__name)
-
- def _raise_attribute_error(self, name: str) -> Never:
- # Match the Python 3.12 error messages exactly
- if name == "__name__":
- raise AttributeError("readonly attribute")
- elif name in {"__value__", "__type_params__", "__parameters__", "__module__"}:
- raise AttributeError(
- f"attribute '{name}' of 'typing.TypeAliasType' objects "
- "is not writable"
- )
- else:
- raise AttributeError(
- f"'typing.TypeAliasType' object has no attribute '{name}'"
- )
-
- def __repr__(self) -> str:
- return self.__name__
-
- def __getitem__(self, parameters):
- if not isinstance(parameters, tuple):
- parameters = (parameters,)
- parameters = [
- typing._type_check(
- item, f'Subscripting {self.__name__} requires a type.'
- )
- for item in parameters
- ]
- return typing._GenericAlias(self, tuple(parameters))
-
- def __reduce__(self):
- return self.__name__
-
- def __init_subclass__(cls, *args, **kwargs):
- raise TypeError(
- "type 'typing_extensions.TypeAliasType' is not an acceptable base type"
- )
-
- # The presence of this method convinces typing._type_check
- # that TypeAliasTypes are types.
- def __call__(self):
- raise TypeError("Type alias is not callable")
-
- if sys.version_info >= (3, 10):
- def __or__(self, right):
- # For forward compatibility with 3.12, reject Unions
- # that are not accepted by the built-in Union.
- if not _is_unionable(right):
- return NotImplemented
- return typing.Union[self, right]
-
- def __ror__(self, left):
- if not _is_unionable(left):
- return NotImplemented
- return typing.Union[left, self]
-
-
-if hasattr(typing, "is_protocol"):
- is_protocol = typing.is_protocol
- get_protocol_members = typing.get_protocol_members
-else:
- def is_protocol(__tp: type) -> bool:
- """Return True if the given type is a Protocol.
-
- Example::
-
- >>> from typing_extensions import Protocol, is_protocol
- >>> class P(Protocol):
- ... def a(self) -> str: ...
- ... b: int
- >>> is_protocol(P)
- True
- >>> is_protocol(int)
- False
- """
- return (
- isinstance(__tp, type)
- and getattr(__tp, '_is_protocol', False)
- and __tp is not Protocol
- and __tp is not getattr(typing, "Protocol", object())
- )
-
- def get_protocol_members(__tp: type) -> typing.FrozenSet[str]:
- """Return the set of members defined in a Protocol.
-
- Example::
-
- >>> from typing_extensions import Protocol, get_protocol_members
- >>> class P(Protocol):
- ... def a(self) -> str: ...
- ... b: int
- >>> get_protocol_members(P)
- frozenset({'a', 'b'})
-
- Raise a TypeError for arguments that are not Protocols.
- """
- if not is_protocol(__tp):
- raise TypeError(f'{__tp!r} is not a Protocol')
- if hasattr(__tp, '__protocol_attrs__'):
- return frozenset(__tp.__protocol_attrs__)
- return frozenset(_get_protocol_attrs(__tp))
-
-
-# Aliases for items that have always been in typing.
-# Explicitly assign these (rather than using `from typing import *` at the top),
-# so that we get a CI error if one of these is deleted from typing.py
-# in a future version of Python
-AbstractSet = typing.AbstractSet
-AnyStr = typing.AnyStr
-BinaryIO = typing.BinaryIO
-Callable = typing.Callable
-Collection = typing.Collection
-Container = typing.Container
-Dict = typing.Dict
-ForwardRef = typing.ForwardRef
-FrozenSet = typing.FrozenSet
-Generator = typing.Generator
-Generic = typing.Generic
-Hashable = typing.Hashable
-IO = typing.IO
-ItemsView = typing.ItemsView
-Iterable = typing.Iterable
-Iterator = typing.Iterator
-KeysView = typing.KeysView
-List = typing.List
-Mapping = typing.Mapping
-MappingView = typing.MappingView
-Match = typing.Match
-MutableMapping = typing.MutableMapping
-MutableSequence = typing.MutableSequence
-MutableSet = typing.MutableSet
-Optional = typing.Optional
-Pattern = typing.Pattern
-Reversible = typing.Reversible
-Sequence = typing.Sequence
-Set = typing.Set
-Sized = typing.Sized
-TextIO = typing.TextIO
-Tuple = typing.Tuple
-Union = typing.Union
-ValuesView = typing.ValuesView
-cast = typing.cast
-no_type_check = typing.no_type_check
-no_type_check_decorator = typing.no_type_check_decorator
diff --git a/spaces/TandCAcceptMe/face-swap-docker/mynewshinyroop/Lib/site-packages/setuptools/installer.py b/spaces/TandCAcceptMe/face-swap-docker/mynewshinyroop/Lib/site-packages/setuptools/installer.py
deleted file mode 100644
index 44ed0da2a37d2cfd350c24050d217c110de7db56..0000000000000000000000000000000000000000
--- a/spaces/TandCAcceptMe/face-swap-docker/mynewshinyroop/Lib/site-packages/setuptools/installer.py
+++ /dev/null
@@ -1,138 +0,0 @@
-import glob
-import os
-import subprocess
-import sys
-import tempfile
-from distutils import log
-from distutils.errors import DistutilsError
-from functools import partial
-
-from . import _reqs
-from .wheel import Wheel
-from .warnings import SetuptoolsDeprecationWarning
-
-
-def _fixup_find_links(find_links):
- """Ensure find-links option end-up being a list of strings."""
- if isinstance(find_links, str):
- return find_links.split()
- assert isinstance(find_links, (tuple, list))
- return find_links
-
-
-def fetch_build_egg(dist, req):
- """Fetch an egg needed for building.
-
- Use pip/wheel to fetch/build a wheel."""
- _DeprecatedInstaller.emit()
- _warn_wheel_not_available(dist)
- return _fetch_build_egg_no_warn(dist, req)
-
-
-def _fetch_build_eggs(dist, requires):
- import pkg_resources # Delay import to avoid unnecessary side-effects
-
- _DeprecatedInstaller.emit(stacklevel=3)
- _warn_wheel_not_available(dist)
-
- resolved_dists = pkg_resources.working_set.resolve(
- _reqs.parse(requires, pkg_resources.Requirement), # required for compatibility
- installer=partial(_fetch_build_egg_no_warn, dist), # avoid warning twice
- replace_conflicting=True,
- )
- for dist in resolved_dists:
- pkg_resources.working_set.add(dist, replace=True)
- return resolved_dists
-
-
-def _fetch_build_egg_no_warn(dist, req): # noqa: C901 # is too complex (16) # FIXME
- import pkg_resources # Delay import to avoid unnecessary side-effects
-
- # Ignore environment markers; if supplied, it is required.
- req = strip_marker(req)
- # Take easy_install options into account, but do not override relevant
- # pip environment variables (like PIP_INDEX_URL or PIP_QUIET); they'll
- # take precedence.
- opts = dist.get_option_dict('easy_install')
- if 'allow_hosts' in opts:
- raise DistutilsError('the `allow-hosts` option is not supported '
- 'when using pip to install requirements.')
- quiet = 'PIP_QUIET' not in os.environ and 'PIP_VERBOSE' not in os.environ
- if 'PIP_INDEX_URL' in os.environ:
- index_url = None
- elif 'index_url' in opts:
- index_url = opts['index_url'][1]
- else:
- index_url = None
- find_links = (
- _fixup_find_links(opts['find_links'][1])[:] if 'find_links' in opts
- else []
- )
- if dist.dependency_links:
- find_links.extend(dist.dependency_links)
- eggs_dir = os.path.realpath(dist.get_egg_cache_dir())
- environment = pkg_resources.Environment()
- for egg_dist in pkg_resources.find_distributions(eggs_dir):
- if egg_dist in req and environment.can_add(egg_dist):
- return egg_dist
- with tempfile.TemporaryDirectory() as tmpdir:
- cmd = [
- sys.executable, '-m', 'pip',
- '--disable-pip-version-check',
- 'wheel', '--no-deps',
- '-w', tmpdir,
- ]
- if quiet:
- cmd.append('--quiet')
- if index_url is not None:
- cmd.extend(('--index-url', index_url))
- for link in find_links or []:
- cmd.extend(('--find-links', link))
- # If requirement is a PEP 508 direct URL, directly pass
- # the URL to pip, as `req @ url` does not work on the
- # command line.
- cmd.append(req.url or str(req))
- try:
- subprocess.check_call(cmd)
- except subprocess.CalledProcessError as e:
- raise DistutilsError(str(e)) from e
- wheel = Wheel(glob.glob(os.path.join(tmpdir, '*.whl'))[0])
- dist_location = os.path.join(eggs_dir, wheel.egg_name())
- wheel.install_as_egg(dist_location)
- dist_metadata = pkg_resources.PathMetadata(
- dist_location, os.path.join(dist_location, 'EGG-INFO'))
- dist = pkg_resources.Distribution.from_filename(
- dist_location, metadata=dist_metadata)
- return dist
-
-
-def strip_marker(req):
- """
- Return a new requirement without the environment marker to avoid
- calling pip with something like `babel; extra == "i18n"`, which
- would always be ignored.
- """
- import pkg_resources # Delay import to avoid unnecessary side-effects
-
- # create a copy to avoid mutating the input
- req = pkg_resources.Requirement.parse(str(req))
- req.marker = None
- return req
-
-
-def _warn_wheel_not_available(dist):
- import pkg_resources # Delay import to avoid unnecessary side-effects
-
- try:
- pkg_resources.get_distribution('wheel')
- except pkg_resources.DistributionNotFound:
- dist.announce('WARNING: The wheel package is not available.', log.WARN)
-
-
-class _DeprecatedInstaller(SetuptoolsDeprecationWarning):
- _SUMMARY = "setuptools.installer and fetch_build_eggs are deprecated."
- _DETAILS = """
- Requirements should be satisfied by a PEP 517 installer.
- If you are using pip, you can try `pip install --use-pep517`.
- """
- # _DUE_DATE not decided yet
diff --git a/spaces/TandCAcceptMe/face-swap-docker/mynewshinyroop/Lib/site-packages/wheel/vendored/packaging/utils.py b/spaces/TandCAcceptMe/face-swap-docker/mynewshinyroop/Lib/site-packages/wheel/vendored/packaging/utils.py
deleted file mode 100644
index 33c613b749a49d6035c0e549389e92c3d68a83ad..0000000000000000000000000000000000000000
--- a/spaces/TandCAcceptMe/face-swap-docker/mynewshinyroop/Lib/site-packages/wheel/vendored/packaging/utils.py
+++ /dev/null
@@ -1,141 +0,0 @@
-# This file is dual licensed under the terms of the Apache License, Version
-# 2.0, and the BSD License. See the LICENSE file in the root of this repository
-# for complete details.
-
-import re
-from typing import FrozenSet, NewType, Tuple, Union, cast
-
-from .tags import Tag, parse_tag
-from .version import InvalidVersion, Version
-
-BuildTag = Union[Tuple[()], Tuple[int, str]]
-NormalizedName = NewType("NormalizedName", str)
-
-
-class InvalidWheelFilename(ValueError):
- """
- An invalid wheel filename was found, users should refer to PEP 427.
- """
-
-
-class InvalidSdistFilename(ValueError):
- """
- An invalid sdist filename was found, users should refer to the packaging user guide.
- """
-
-
-_canonicalize_regex = re.compile(r"[-_.]+")
-# PEP 427: The build number must start with a digit.
-_build_tag_regex = re.compile(r"(\d+)(.*)")
-
-
-def canonicalize_name(name: str) -> NormalizedName:
- # This is taken from PEP 503.
- value = _canonicalize_regex.sub("-", name).lower()
- return cast(NormalizedName, value)
-
-
-def canonicalize_version(
- version: Union[Version, str], *, strip_trailing_zero: bool = True
-) -> str:
- """
- This is very similar to Version.__str__, but has one subtle difference
- with the way it handles the release segment.
- """
- if isinstance(version, str):
- try:
- parsed = Version(version)
- except InvalidVersion:
- # Legacy versions cannot be normalized
- return version
- else:
- parsed = version
-
- parts = []
-
- # Epoch
- if parsed.epoch != 0:
- parts.append(f"{parsed.epoch}!")
-
- # Release segment
- release_segment = ".".join(str(x) for x in parsed.release)
- if strip_trailing_zero:
- # NB: This strips trailing '.0's to normalize
- release_segment = re.sub(r"(\.0)+$", "", release_segment)
- parts.append(release_segment)
-
- # Pre-release
- if parsed.pre is not None:
- parts.append("".join(str(x) for x in parsed.pre))
-
- # Post-release
- if parsed.post is not None:
- parts.append(f".post{parsed.post}")
-
- # Development release
- if parsed.dev is not None:
- parts.append(f".dev{parsed.dev}")
-
- # Local version segment
- if parsed.local is not None:
- parts.append(f"+{parsed.local}")
-
- return "".join(parts)
-
-
-def parse_wheel_filename(
- filename: str,
-) -> Tuple[NormalizedName, Version, BuildTag, FrozenSet[Tag]]:
- if not filename.endswith(".whl"):
- raise InvalidWheelFilename(
- f"Invalid wheel filename (extension must be '.whl'): {filename}"
- )
-
- filename = filename[:-4]
- dashes = filename.count("-")
- if dashes not in (4, 5):
- raise InvalidWheelFilename(
- f"Invalid wheel filename (wrong number of parts): {filename}"
- )
-
- parts = filename.split("-", dashes - 2)
- name_part = parts[0]
- # See PEP 427 for the rules on escaping the project name
- if "__" in name_part or re.match(r"^[\w\d._]*$", name_part, re.UNICODE) is None:
- raise InvalidWheelFilename(f"Invalid project name: {filename}")
- name = canonicalize_name(name_part)
- version = Version(parts[1])
- if dashes == 5:
- build_part = parts[2]
- build_match = _build_tag_regex.match(build_part)
- if build_match is None:
- raise InvalidWheelFilename(
- f"Invalid build number: {build_part} in '{filename}'"
- )
- build = cast(BuildTag, (int(build_match.group(1)), build_match.group(2)))
- else:
- build = ()
- tags = parse_tag(parts[-1])
- return (name, version, build, tags)
-
-
-def parse_sdist_filename(filename: str) -> Tuple[NormalizedName, Version]:
- if filename.endswith(".tar.gz"):
- file_stem = filename[: -len(".tar.gz")]
- elif filename.endswith(".zip"):
- file_stem = filename[: -len(".zip")]
- else:
- raise InvalidSdistFilename(
- f"Invalid sdist filename (extension must be '.tar.gz' or '.zip'):"
- f" {filename}"
- )
-
- # We are requiring a PEP 440 version, which cannot contain dashes,
- # so we split on the last dash.
- name_part, sep, version_part = file_stem.rpartition("-")
- if not sep:
- raise InvalidSdistFilename(f"Invalid sdist filename: {filename}")
-
- name = canonicalize_name(name_part)
- version = Version(version_part)
- return (name, version)
diff --git a/spaces/Theivaprakasham/yolov6/yolov6/core/engine.py b/spaces/Theivaprakasham/yolov6/yolov6/core/engine.py
deleted file mode 100644
index 927523ed8accff0dd6410a5e1fcc529f9247d16a..0000000000000000000000000000000000000000
--- a/spaces/Theivaprakasham/yolov6/yolov6/core/engine.py
+++ /dev/null
@@ -1,262 +0,0 @@
-#!/usr/bin/env python3
-# -*- coding:utf-8 -*-
-import os
-import time
-from copy import deepcopy
-import os.path as osp
-
-from tqdm import tqdm
-
-import numpy as np
-import torch
-from torch.cuda import amp
-from torch.nn.parallel import DistributedDataParallel as DDP
-from torch.utils.tensorboard import SummaryWriter
-
-import tools.eval as eval
-from yolov6.data.data_load import create_dataloader
-from yolov6.models.yolo import build_model
-from yolov6.models.loss import ComputeLoss
-from yolov6.utils.events import LOGGER, NCOLS, load_yaml, write_tblog
-from yolov6.utils.ema import ModelEMA, de_parallel
-from yolov6.utils.checkpoint import load_state_dict, save_checkpoint, strip_optimizer
-from yolov6.solver.build import build_optimizer, build_lr_scheduler
-
-
-class Trainer:
- def __init__(self, args, cfg, device):
- self.args = args
- self.cfg = cfg
- self.device = device
-
- self.rank = args.rank
- self.local_rank = args.local_rank
- self.world_size = args.world_size
- self.main_process = self.rank in [-1, 0]
- self.save_dir = args.save_dir
- # get data loader
- self.data_dict = load_yaml(args.data_path)
- self.num_classes = self.data_dict['nc']
- self.train_loader, self.val_loader = self.get_data_loader(args, cfg, self.data_dict)
- # get model and optimizer
- model = self.get_model(args, cfg, self.num_classes, device)
- self.optimizer = self.get_optimizer(args, cfg, model)
- self.scheduler, self.lf = self.get_lr_scheduler(args, cfg, self.optimizer)
- self.ema = ModelEMA(model) if self.main_process else None
- self.model = self.parallel_model(args, model, device)
- self.model.nc, self.model.names = self.data_dict['nc'], self.data_dict['names']
- # tensorboard
- self.tblogger = SummaryWriter(self.save_dir) if self.main_process else None
-
- self.start_epoch = 0
- self.max_epoch = args.epochs
- self.max_stepnum = len(self.train_loader)
- self.batch_size = args.batch_size
- self.img_size = args.img_size
-
- # Training Process
- def train(self):
- try:
- self.train_before_loop()
- for self.epoch in range(self.start_epoch, self.max_epoch):
- self.train_in_loop()
-
- except Exception as _:
- LOGGER.error('ERROR in training loop or eval/save model.')
- raise
- finally:
- self.train_after_loop()
-
- # Training loop for each epoch
- def train_in_loop(self):
- try:
- self.prepare_for_steps()
- for self.step, self.batch_data in self.pbar:
- self.train_in_steps()
- self.print_details()
- except Exception as _:
- LOGGER.error('ERROR in training steps.')
- raise
- try:
- self.eval_and_save()
- except Exception as _:
- LOGGER.error('ERROR in evaluate and save model.')
- raise
-
- # Training loop for batchdata
- def train_in_steps(self):
- images, targets = self.prepro_data(self.batch_data, self.device)
- # forward
- with amp.autocast(enabled=self.device != 'cpu'):
- preds = self.model(images)
- total_loss, loss_items = self.compute_loss(preds, targets)
- if self.rank != -1:
- total_loss *= self.world_size
- # backward
- self.scaler.scale(total_loss).backward()
- self.loss_items = loss_items
- self.update_optimizer()
-
- def eval_and_save(self):
- epoch_sub = self.max_epoch - self.epoch
- val_period = 20 if epoch_sub > 100 else 1 # to fasten training time, evaluate in every 20 epochs for the early stage.
- is_val_epoch = (not self.args.noval or (epoch_sub == 1)) and (self.epoch % val_period == 0)
- if self.main_process:
- self.ema.update_attr(self.model, include=['nc', 'names', 'stride']) # update attributes for ema model
- if is_val_epoch:
- self.eval_model()
- self.ap = self.evaluate_results[0] * 0.1 + self.evaluate_results[1] * 0.9
- self.best_ap = max(self.ap, self.best_ap)
- # save ckpt
- ckpt = {
- 'model': deepcopy(de_parallel(self.model)).half(),
- 'ema': deepcopy(self.ema.ema).half(),
- 'updates': self.ema.updates,
- 'optimizer': self.optimizer.state_dict(),
- 'epoch': self.epoch,
- }
-
- save_ckpt_dir = osp.join(self.save_dir, 'weights')
- save_checkpoint(ckpt, (is_val_epoch) and (self.ap == self.best_ap), save_ckpt_dir, model_name='last_ckpt')
- del ckpt
- # log for tensorboard
- write_tblog(self.tblogger, self.epoch, self.evaluate_results, self.mean_loss)
-
- def eval_model(self):
- results = eval.run(self.data_dict,
- batch_size=self.batch_size // self.world_size * 2,
- img_size=self.img_size,
- model=self.ema.ema,
- dataloader=self.val_loader,
- save_dir=self.save_dir,
- task='train')
-
- LOGGER.info(f"Epoch: {self.epoch} | mAP@0.5: {results[0]} | mAP@0.50:0.95: {results[1]}")
- self.evaluate_results = results[:2]
-
- def train_before_loop(self):
- LOGGER.info('Training start...')
- self.start_time = time.time()
- self.warmup_stepnum = max(round(self.cfg.solver.warmup_epochs * self.max_stepnum), 1000)
- self.scheduler.last_epoch = self.start_epoch - 1
- self.last_opt_step = -1
- self.scaler = amp.GradScaler(enabled=self.device != 'cpu')
-
- self.best_ap, self.ap = 0.0, 0.0
- self.evaluate_results = (0, 0) # AP50, AP50_95
- self.compute_loss = ComputeLoss(iou_type=self.cfg.model.head.iou_type)
-
- def prepare_for_steps(self):
- if self.epoch > self.start_epoch:
- self.scheduler.step()
- self.model.train()
- if self.rank != -1:
- self.train_loader.sampler.set_epoch(self.epoch)
- self.mean_loss = torch.zeros(4, device=self.device)
- self.optimizer.zero_grad()
-
- LOGGER.info(('\n' + '%10s' * 5) % ('Epoch', 'iou_loss', 'l1_loss', 'obj_loss', 'cls_loss'))
- self.pbar = enumerate(self.train_loader)
- if self.main_process:
- self.pbar = tqdm(self.pbar, total=self.max_stepnum, ncols=NCOLS, bar_format='{l_bar}{bar:10}{r_bar}{bar:-10b}')
-
- # Print loss after each steps
- def print_details(self):
- if self.main_process:
- self.mean_loss = (self.mean_loss * self.step + self.loss_items) / (self.step + 1)
- self.pbar.set_description(('%10s' + '%10.4g' * 4) % (f'{self.epoch}/{self.max_epoch - 1}', \
- *(self.mean_loss)))
-
- # Empty cache if training finished
- def train_after_loop(self):
- if self.main_process:
- LOGGER.info(f'\nTraining completed in {(time.time() - self.start_time) / 3600:.3f} hours.')
- save_ckpt_dir = osp.join(self.save_dir, 'weights')
- strip_optimizer(save_ckpt_dir) # strip optimizers for saved pt model
- if self.device != 'cpu':
- torch.cuda.empty_cache()
-
- def update_optimizer(self):
- curr_step = self.step + self.max_stepnum * self.epoch
- self.accumulate = max(1, round(64 / self.batch_size))
- if curr_step <= self.warmup_stepnum:
- self.accumulate = max(1, np.interp(curr_step, [0, self.warmup_stepnum], [1, 64 / self.batch_size]).round())
- for k, param in enumerate(self.optimizer.param_groups):
- warmup_bias_lr = self.cfg.solver.warmup_bias_lr if k == 2 else 0.0
- param['lr'] = np.interp(curr_step, [0, self.warmup_stepnum], [warmup_bias_lr, param['initial_lr'] * self.lf(self.epoch)])
- if 'momentum' in param:
- param['momentum'] = np.interp(curr_step, [0, self.warmup_stepnum], [self.cfg.solver.warmup_momentum, self.cfg.solver.momentum])
- if curr_step - self.last_opt_step >= self.accumulate:
- self.scaler.step(self.optimizer)
- self.scaler.update()
- self.optimizer.zero_grad()
- if self.ema:
- self.ema.update(self.model)
- self.last_opt_step = curr_step
-
- @staticmethod
- def get_data_loader(args, cfg, data_dict):
- train_path, val_path = data_dict['train'], data_dict['val']
- # check data
- nc = int(data_dict['nc'])
- class_names = data_dict['names']
- assert len(class_names) == nc, f'the length of class names does not match the number of classes defined'
- grid_size = max(int(max(cfg.model.head.strides)), 32)
- # create train dataloader
- train_loader = create_dataloader(train_path, args.img_size, args.batch_size // args.world_size, grid_size,
- hyp=dict(cfg.data_aug), augment=True, rect=False, rank=args.local_rank,
- workers=args.workers, shuffle=True, check_images=args.check_images,
- check_labels=args.check_labels, class_names=class_names, task='train')[0]
- # create val dataloader
- val_loader = None
- if args.rank in [-1, 0]:
- val_loader = create_dataloader(val_path, args.img_size, args.batch_size // args.world_size * 2, grid_size,
- hyp=dict(cfg.data_aug), rect=True, rank=-1, pad=0.5,
- workers=args.workers, check_images=args.check_images,
- check_labels=args.check_labels, class_names=class_names, task='val')[0]
-
- return train_loader, val_loader
-
- @staticmethod
- def prepro_data(batch_data, device):
- images = batch_data[0].to(device, non_blocking=True).float() / 255
- targets = batch_data[1].to(device)
- return images, targets
-
- @staticmethod
- def get_model(args, cfg, nc, device):
- model = build_model(cfg, nc, device)
- weights = cfg.model.pretrained
- if weights: # finetune if pretrained model is set
- LOGGER.info(f'Loading state_dict from {weights} for fine-tuning...')
- model = load_state_dict(weights, model, map_location=device)
- LOGGER.info('Model: {}'.format(model))
- return model
-
- @staticmethod
- def parallel_model(args, model, device):
- # If DP mode
- dp_mode = device.type != 'cpu' and args.rank == -1
- if dp_mode and torch.cuda.device_count() > 1:
- LOGGER.warning('WARNING: DP not recommended, use DDP instead.\n')
- model = torch.nn.DataParallel(model)
-
- # If DDP mode
- ddp_mode = device.type != 'cpu' and args.rank != -1
- if ddp_mode:
- model = DDP(model, device_ids=[args.local_rank], output_device=args.local_rank)
-
- return model
-
- @staticmethod
- def get_optimizer(args, cfg, model):
- accumulate = max(1, round(64 / args.batch_size))
- cfg.solver.weight_decay *= args.batch_size * accumulate / 64
- optimizer = build_optimizer(cfg, model)
- return optimizer
-
- @staticmethod
- def get_lr_scheduler(args, cfg, optimizer):
- epochs = args.epochs
- lr_scheduler, lf = build_lr_scheduler(cfg, optimizer, epochs)
- return lr_scheduler, lf
diff --git a/spaces/TinkerFrank/AppleClassifier/app.py b/spaces/TinkerFrank/AppleClassifier/app.py
deleted file mode 100644
index f55cb78fa420bb167edf77099faa287635ba2a58..0000000000000000000000000000000000000000
--- a/spaces/TinkerFrank/AppleClassifier/app.py
+++ /dev/null
@@ -1,41 +0,0 @@
-import torch
-import gradio as gr
-from torch import nn
-import cv2
-from torchvision.transforms import ToTensor
-from torchvision.datasets import ImageFolder
-import numpy as np
-from PIL import Image
-device = 'cuda' if torch.cuda.is_available() else 'cpu'
-
-model = torch.load('apple_resnet_classifier.pt', map_location=torch.device(device))
-model.to(device)
-model.eval()
-
-def predict(image):
- img = image.resize((224, 224))
- img = ToTensor()(img).unsqueeze(0).to(device)
- with torch.no_grad():
- out = model(img)
- _, predicted = torch.max(out.data, 1)
- probabilities = torch.nn.functional.softmax(out, dim=1)[0]
- class_labels = ['Bad Apple', 'Normal Apple', 'Rot Apple', 'Scab Apple']
- values, indices = torch.topk(probabilities, 4)
- confidences = {class_labels[i]: v.item() for i, v in zip(indices, values)}
- return confidences
-
-description = """
-
- Classifier for Apples, based on a finetuned RESNET101 model
-
-
-"""
-
-gr.Interface(
- fn=predict,
- inputs=gr.Image(label='Upload Apple',type="pil"),
- outputs="label",
- description=description,
- examples=["myapple_1.jpg", "myapple_2.jpg", "myapple_3.jpg", "myapple_4.jpg", ]
- ).launch()
-
diff --git a/spaces/Toritto/Genshin-impact-IA-project-v1/lib/infer_pack/modules/F0Predictor/HarvestF0Predictor.py b/spaces/Toritto/Genshin-impact-IA-project-v1/lib/infer_pack/modules/F0Predictor/HarvestF0Predictor.py
deleted file mode 100644
index b412ba2814e114ca7bb00b6fd6ef217f63d788a3..0000000000000000000000000000000000000000
--- a/spaces/Toritto/Genshin-impact-IA-project-v1/lib/infer_pack/modules/F0Predictor/HarvestF0Predictor.py
+++ /dev/null
@@ -1,86 +0,0 @@
-from lib.infer_pack.modules.F0Predictor.F0Predictor import F0Predictor
-import pyworld
-import numpy as np
-
-
-class HarvestF0Predictor(F0Predictor):
- def __init__(self, hop_length=512, f0_min=50, f0_max=1100, sampling_rate=44100):
- self.hop_length = hop_length
- self.f0_min = f0_min
- self.f0_max = f0_max
- self.sampling_rate = sampling_rate
-
- def interpolate_f0(self, f0):
- """
- 对F0进行插值处理
- """
-
- data = np.reshape(f0, (f0.size, 1))
-
- vuv_vector = np.zeros((data.size, 1), dtype=np.float32)
- vuv_vector[data > 0.0] = 1.0
- vuv_vector[data <= 0.0] = 0.0
-
- ip_data = data
-
- frame_number = data.size
- last_value = 0.0
- for i in range(frame_number):
- if data[i] <= 0.0:
- j = i + 1
- for j in range(i + 1, frame_number):
- if data[j] > 0.0:
- break
- if j < frame_number - 1:
- if last_value > 0.0:
- step = (data[j] - data[i - 1]) / float(j - i)
- for k in range(i, j):
- ip_data[k] = data[i - 1] + step * (k - i + 1)
- else:
- for k in range(i, j):
- ip_data[k] = data[j]
- else:
- for k in range(i, frame_number):
- ip_data[k] = last_value
- else:
- ip_data[i] = data[i] # 这里可能存在一个没有必要的拷贝
- last_value = data[i]
-
- return ip_data[:, 0], vuv_vector[:, 0]
-
- def resize_f0(self, x, target_len):
- source = np.array(x)
- source[source < 0.001] = np.nan
- target = np.interp(
- np.arange(0, len(source) * target_len, len(source)) / target_len,
- np.arange(0, len(source)),
- source,
- )
- res = np.nan_to_num(target)
- return res
-
- def compute_f0(self, wav, p_len=None):
- if p_len is None:
- p_len = wav.shape[0] // self.hop_length
- f0, t = pyworld.harvest(
- wav.astype(np.double),
- fs=self.hop_length,
- f0_ceil=self.f0_max,
- f0_floor=self.f0_min,
- frame_period=1000 * self.hop_length / self.sampling_rate,
- )
- f0 = pyworld.stonemask(wav.astype(np.double), f0, t, self.fs)
- return self.interpolate_f0(self.resize_f0(f0, p_len))[0]
-
- def compute_f0_uv(self, wav, p_len=None):
- if p_len is None:
- p_len = wav.shape[0] // self.hop_length
- f0, t = pyworld.harvest(
- wav.astype(np.double),
- fs=self.sampling_rate,
- f0_floor=self.f0_min,
- f0_ceil=self.f0_max,
- frame_period=1000 * self.hop_length / self.sampling_rate,
- )
- f0 = pyworld.stonemask(wav.astype(np.double), f0, t, self.sampling_rate)
- return self.interpolate_f0(self.resize_f0(f0, p_len))
diff --git a/spaces/Tuana/PDF-Summarizer/app.py b/spaces/Tuana/PDF-Summarizer/app.py
deleted file mode 100644
index c1ea146f63648b4d16fa905ddfb7c578b49c5ae8..0000000000000000000000000000000000000000
--- a/spaces/Tuana/PDF-Summarizer/app.py
+++ /dev/null
@@ -1,70 +0,0 @@
-import streamlit as st
-from haystack.document_stores import InMemoryDocumentStore
-from haystack.nodes import TransformersSummarizer, PreProcessor, PDFToTextConverter, Crawler
-from haystack.schema import Document
-import logging
-import base64
-from PIL import Image
-import validators
-
-@st.cache(hash_funcs={"builtins.SwigPyObject": lambda _: None},allow_output_mutation=True)
-def start_haystack():
- document_store = InMemoryDocumentStore()
- preprocessor = PreProcessor(
- clean_empty_lines=True,
- clean_whitespace=True,
- clean_header_footer=True,
- split_by="word",
- split_length=200,
- split_respect_sentence_boundary=True,
- )
- summarizer = TransformersSummarizer(model_name_or_path="facebook/bart-large-cnn")
- return document_store, summarizer, preprocessor
-
-
-def pdf_to_document_store(pdf_file):
- document_store.delete_documents()
- converter = PDFToTextConverter(remove_numeric_tables=True, valid_languages=["en"])
- with open("temp-path.pdf", 'wb') as temp_file:
- base64_pdf = base64.b64encode(pdf_file.read()).decode('utf-8')
- temp_file.write(base64.b64decode(base64_pdf))
- doc = converter.convert(file_path="temp-path.pdf", meta=None)
- preprocessed_docs=preprocessor.process(doc)
- document_store.write_documents(preprocessed_docs)
- temp_file.close()
-
-def summarize(content):
- pdf_to_document_store(content)
- summaries = summarizer.predict(documents=document_store.get_all_documents(), generate_single_summary=True)
- return summaries
-
-def set_state_if_absent(key, value):
- if key not in st.session_state:
- st.session_state[key] = value
-
-set_state_if_absent("summaries", None)
-
-document_store, summarizer, preprocessor = start_haystack()
-
-st.title('TL;DR with Haystack')
-image = Image.open('header-image.png')
-st.image(image)
-
-st.markdown( """
-This Summarization demo uses a [Haystack TransformerSummarizer node](https://haystack.deepset.ai/pipeline_nodes/summarizer). You can upload a PDF file, which will be converted to text with the [Haystack PDFtoTextConverter](https://haystack.deepset.ai/reference/file-converters#pdftotextconverter). In this demo, we produce 1 summary for the whole file you upload. So, the TransformerSummarizer treats the whole thing as one string, which means along with the model limitations, PDFs that have a lot of unneeded text at the beginning produce poor results. For best results, upload a document that has minimal intro and tables at the top.
-""", unsafe_allow_html=True)
-
-uploaded_file = st.file_uploader("Choose a PDF file", accept_multiple_files=False)
-
-if uploaded_file is not None :
- if st.button('Summarize Document'):
- with st.spinner("📚 Please wait while we produce a summary..."):
- try:
- st.session_state.summaries = summarize(uploaded_file)
- except Exception as e:
- logging.exception(e)
-
-if st.session_state.summaries:
- st.write('## Summary')
- for count, summary in enumerate(st.session_state.summaries):
- st.write(summary.content)
diff --git a/spaces/TushDeMort/yolo/models/common.py b/spaces/TushDeMort/yolo/models/common.py
deleted file mode 100644
index 007b577e75da35094fd7ac3e1947a8e743f8e413..0000000000000000000000000000000000000000
--- a/spaces/TushDeMort/yolo/models/common.py
+++ /dev/null
@@ -1,2019 +0,0 @@
-import math
-from copy import copy
-from pathlib import Path
-
-import numpy as np
-import pandas as pd
-import requests
-import torch
-import torch.nn as nn
-import torch.nn.functional as F
-from torchvision.ops import DeformConv2d
-from PIL import Image
-from torch.cuda import amp
-
-from utils.datasets import letterbox
-from utils.general import non_max_suppression, make_divisible, scale_coords, increment_path, xyxy2xywh
-from utils.plots import color_list, plot_one_box
-from utils.torch_utils import time_synchronized
-
-
-##### basic ####
-
-def autopad(k, p=None): # kernel, padding
- # Pad to 'same'
- if p is None:
- p = k // 2 if isinstance(k, int) else [x // 2 for x in k] # auto-pad
- return p
-
-
-class MP(nn.Module):
- def __init__(self, k=2):
- super(MP, self).__init__()
- self.m = nn.MaxPool2d(kernel_size=k, stride=k)
-
- def forward(self, x):
- return self.m(x)
-
-
-class SP(nn.Module):
- def __init__(self, k=3, s=1):
- super(SP, self).__init__()
- self.m = nn.MaxPool2d(kernel_size=k, stride=s, padding=k // 2)
-
- def forward(self, x):
- return self.m(x)
-
-
-class ReOrg(nn.Module):
- def __init__(self):
- super(ReOrg, self).__init__()
-
- def forward(self, x): # x(b,c,w,h) -> y(b,4c,w/2,h/2)
- return torch.cat([x[..., ::2, ::2], x[..., 1::2, ::2], x[..., ::2, 1::2], x[..., 1::2, 1::2]], 1)
-
-
-class Concat(nn.Module):
- def __init__(self, dimension=1):
- super(Concat, self).__init__()
- self.d = dimension
-
- def forward(self, x):
- return torch.cat(x, self.d)
-
-
-class Chuncat(nn.Module):
- def __init__(self, dimension=1):
- super(Chuncat, self).__init__()
- self.d = dimension
-
- def forward(self, x):
- x1 = []
- x2 = []
- for xi in x:
- xi1, xi2 = xi.chunk(2, self.d)
- x1.append(xi1)
- x2.append(xi2)
- return torch.cat(x1+x2, self.d)
-
-
-class Shortcut(nn.Module):
- def __init__(self, dimension=0):
- super(Shortcut, self).__init__()
- self.d = dimension
-
- def forward(self, x):
- return x[0]+x[1]
-
-
-class Foldcut(nn.Module):
- def __init__(self, dimension=0):
- super(Foldcut, self).__init__()
- self.d = dimension
-
- def forward(self, x):
- x1, x2 = x.chunk(2, self.d)
- return x1+x2
-
-
-class Conv(nn.Module):
- # Standard convolution
- def __init__(self, c1, c2, k=1, s=1, p=None, g=1, act=True): # ch_in, ch_out, kernel, stride, padding, groups
- super(Conv, self).__init__()
- self.conv = nn.Conv2d(c1, c2, k, s, autopad(k, p), groups=g, bias=False)
- self.bn = nn.BatchNorm2d(c2)
- self.act = nn.SiLU() if act is True else (act if isinstance(act, nn.Module) else nn.Identity())
-
- def forward(self, x):
- return self.act(self.bn(self.conv(x)))
-
- def fuseforward(self, x):
- return self.act(self.conv(x))
-
-
-class RobustConv(nn.Module):
- # Robust convolution (use high kernel size 7-11 for: downsampling and other layers). Train for 300 - 450 epochs.
- def __init__(self, c1, c2, k=7, s=1, p=None, g=1, act=True, layer_scale_init_value=1e-6): # ch_in, ch_out, kernel, stride, padding, groups
- super(RobustConv, self).__init__()
- self.conv_dw = Conv(c1, c1, k=k, s=s, p=p, g=c1, act=act)
- self.conv1x1 = nn.Conv2d(c1, c2, 1, 1, 0, groups=1, bias=True)
- self.gamma = nn.Parameter(layer_scale_init_value * torch.ones(c2)) if layer_scale_init_value > 0 else None
-
- def forward(self, x):
- x = x.to(memory_format=torch.channels_last)
- x = self.conv1x1(self.conv_dw(x))
- if self.gamma is not None:
- x = x.mul(self.gamma.reshape(1, -1, 1, 1))
- return x
-
-
-class RobustConv2(nn.Module):
- # Robust convolution 2 (use [32, 5, 2] or [32, 7, 4] or [32, 11, 8] for one of the paths in CSP).
- def __init__(self, c1, c2, k=7, s=4, p=None, g=1, act=True, layer_scale_init_value=1e-6): # ch_in, ch_out, kernel, stride, padding, groups
- super(RobustConv2, self).__init__()
- self.conv_strided = Conv(c1, c1, k=k, s=s, p=p, g=c1, act=act)
- self.conv_deconv = nn.ConvTranspose2d(in_channels=c1, out_channels=c2, kernel_size=s, stride=s,
- padding=0, bias=True, dilation=1, groups=1
- )
- self.gamma = nn.Parameter(layer_scale_init_value * torch.ones(c2)) if layer_scale_init_value > 0 else None
-
- def forward(self, x):
- x = self.conv_deconv(self.conv_strided(x))
- if self.gamma is not None:
- x = x.mul(self.gamma.reshape(1, -1, 1, 1))
- return x
-
-
-def DWConv(c1, c2, k=1, s=1, act=True):
- # Depthwise convolution
- return Conv(c1, c2, k, s, g=math.gcd(c1, c2), act=act)
-
-
-class GhostConv(nn.Module):
- # Ghost Convolution https://github.com/huawei-noah/ghostnet
- def __init__(self, c1, c2, k=1, s=1, g=1, act=True): # ch_in, ch_out, kernel, stride, groups
- super(GhostConv, self).__init__()
- c_ = c2 // 2 # hidden channels
- self.cv1 = Conv(c1, c_, k, s, None, g, act)
- self.cv2 = Conv(c_, c_, 5, 1, None, c_, act)
-
- def forward(self, x):
- y = self.cv1(x)
- return torch.cat([y, self.cv2(y)], 1)
-
-
-class Stem(nn.Module):
- # Stem
- def __init__(self, c1, c2, k=1, s=1, p=None, g=1, act=True): # ch_in, ch_out, kernel, stride, padding, groups
- super(Stem, self).__init__()
- c_ = int(c2/2) # hidden channels
- self.cv1 = Conv(c1, c_, 3, 2)
- self.cv2 = Conv(c_, c_, 1, 1)
- self.cv3 = Conv(c_, c_, 3, 2)
- self.pool = torch.nn.MaxPool2d(2, stride=2)
- self.cv4 = Conv(2 * c_, c2, 1, 1)
-
- def forward(self, x):
- x = self.cv1(x)
- return self.cv4(torch.cat((self.cv3(self.cv2(x)), self.pool(x)), dim=1))
-
-
-class DownC(nn.Module):
- # Spatial pyramid pooling layer used in YOLOv3-SPP
- def __init__(self, c1, c2, n=1, k=2):
- super(DownC, self).__init__()
- c_ = int(c1) # hidden channels
- self.cv1 = Conv(c1, c_, 1, 1)
- self.cv2 = Conv(c_, c2//2, 3, k)
- self.cv3 = Conv(c1, c2//2, 1, 1)
- self.mp = nn.MaxPool2d(kernel_size=k, stride=k)
-
- def forward(self, x):
- return torch.cat((self.cv2(self.cv1(x)), self.cv3(self.mp(x))), dim=1)
-
-
-class SPP(nn.Module):
- # Spatial pyramid pooling layer used in YOLOv3-SPP
- def __init__(self, c1, c2, k=(5, 9, 13)):
- super(SPP, self).__init__()
- c_ = c1 // 2 # hidden channels
- self.cv1 = Conv(c1, c_, 1, 1)
- self.cv2 = Conv(c_ * (len(k) + 1), c2, 1, 1)
- self.m = nn.ModuleList([nn.MaxPool2d(kernel_size=x, stride=1, padding=x // 2) for x in k])
-
- def forward(self, x):
- x = self.cv1(x)
- return self.cv2(torch.cat([x] + [m(x) for m in self.m], 1))
-
-
-class Bottleneck(nn.Module):
- # Darknet bottleneck
- def __init__(self, c1, c2, shortcut=True, g=1, e=0.5): # ch_in, ch_out, shortcut, groups, expansion
- super(Bottleneck, self).__init__()
- c_ = int(c2 * e) # hidden channels
- self.cv1 = Conv(c1, c_, 1, 1)
- self.cv2 = Conv(c_, c2, 3, 1, g=g)
- self.add = shortcut and c1 == c2
-
- def forward(self, x):
- return x + self.cv2(self.cv1(x)) if self.add else self.cv2(self.cv1(x))
-
-
-class Res(nn.Module):
- # ResNet bottleneck
- def __init__(self, c1, c2, shortcut=True, g=1, e=0.5): # ch_in, ch_out, shortcut, groups, expansion
- super(Res, self).__init__()
- c_ = int(c2 * e) # hidden channels
- self.cv1 = Conv(c1, c_, 1, 1)
- self.cv2 = Conv(c_, c_, 3, 1, g=g)
- self.cv3 = Conv(c_, c2, 1, 1)
- self.add = shortcut and c1 == c2
-
- def forward(self, x):
- return x + self.cv3(self.cv2(self.cv1(x))) if self.add else self.cv3(self.cv2(self.cv1(x)))
-
-
-class ResX(Res):
- # ResNet bottleneck
- def __init__(self, c1, c2, shortcut=True, g=32, e=0.5): # ch_in, ch_out, shortcut, groups, expansion
- super().__init__(c1, c2, shortcut, g, e)
- c_ = int(c2 * e) # hidden channels
-
-
-class Ghost(nn.Module):
- # Ghost Bottleneck https://github.com/huawei-noah/ghostnet
- def __init__(self, c1, c2, k=3, s=1): # ch_in, ch_out, kernel, stride
- super(Ghost, self).__init__()
- c_ = c2 // 2
- self.conv = nn.Sequential(GhostConv(c1, c_, 1, 1), # pw
- DWConv(c_, c_, k, s, act=False) if s == 2 else nn.Identity(), # dw
- GhostConv(c_, c2, 1, 1, act=False)) # pw-linear
- self.shortcut = nn.Sequential(DWConv(c1, c1, k, s, act=False),
- Conv(c1, c2, 1, 1, act=False)) if s == 2 else nn.Identity()
-
- def forward(self, x):
- return self.conv(x) + self.shortcut(x)
-
-##### end of basic #####
-
-
-##### cspnet #####
-
-class SPPCSPC(nn.Module):
- # CSP https://github.com/WongKinYiu/CrossStagePartialNetworks
- def __init__(self, c1, c2, n=1, shortcut=False, g=1, e=0.5, k=(5, 9, 13)):
- super(SPPCSPC, self).__init__()
- c_ = int(2 * c2 * e) # hidden channels
- self.cv1 = Conv(c1, c_, 1, 1)
- self.cv2 = Conv(c1, c_, 1, 1)
- self.cv3 = Conv(c_, c_, 3, 1)
- self.cv4 = Conv(c_, c_, 1, 1)
- self.m = nn.ModuleList([nn.MaxPool2d(kernel_size=x, stride=1, padding=x // 2) for x in k])
- self.cv5 = Conv(4 * c_, c_, 1, 1)
- self.cv6 = Conv(c_, c_, 3, 1)
- self.cv7 = Conv(2 * c_, c2, 1, 1)
-
- def forward(self, x):
- x1 = self.cv4(self.cv3(self.cv1(x)))
- y1 = self.cv6(self.cv5(torch.cat([x1] + [m(x1) for m in self.m], 1)))
- y2 = self.cv2(x)
- return self.cv7(torch.cat((y1, y2), dim=1))
-
-class GhostSPPCSPC(SPPCSPC):
- # CSP https://github.com/WongKinYiu/CrossStagePartialNetworks
- def __init__(self, c1, c2, n=1, shortcut=False, g=1, e=0.5, k=(5, 9, 13)):
- super().__init__(c1, c2, n, shortcut, g, e, k)
- c_ = int(2 * c2 * e) # hidden channels
- self.cv1 = GhostConv(c1, c_, 1, 1)
- self.cv2 = GhostConv(c1, c_, 1, 1)
- self.cv3 = GhostConv(c_, c_, 3, 1)
- self.cv4 = GhostConv(c_, c_, 1, 1)
- self.cv5 = GhostConv(4 * c_, c_, 1, 1)
- self.cv6 = GhostConv(c_, c_, 3, 1)
- self.cv7 = GhostConv(2 * c_, c2, 1, 1)
-
-
-class GhostStem(Stem):
- # Stem
- def __init__(self, c1, c2, k=1, s=1, p=None, g=1, act=True): # ch_in, ch_out, kernel, stride, padding, groups
- super().__init__(c1, c2, k, s, p, g, act)
- c_ = int(c2/2) # hidden channels
- self.cv1 = GhostConv(c1, c_, 3, 2)
- self.cv2 = GhostConv(c_, c_, 1, 1)
- self.cv3 = GhostConv(c_, c_, 3, 2)
- self.cv4 = GhostConv(2 * c_, c2, 1, 1)
-
-
-class BottleneckCSPA(nn.Module):
- # CSP https://github.com/WongKinYiu/CrossStagePartialNetworks
- def __init__(self, c1, c2, n=1, shortcut=True, g=1, e=0.5): # ch_in, ch_out, number, shortcut, groups, expansion
- super(BottleneckCSPA, self).__init__()
- c_ = int(c2 * e) # hidden channels
- self.cv1 = Conv(c1, c_, 1, 1)
- self.cv2 = Conv(c1, c_, 1, 1)
- self.cv3 = Conv(2 * c_, c2, 1, 1)
- self.m = nn.Sequential(*[Bottleneck(c_, c_, shortcut, g, e=1.0) for _ in range(n)])
-
- def forward(self, x):
- y1 = self.m(self.cv1(x))
- y2 = self.cv2(x)
- return self.cv3(torch.cat((y1, y2), dim=1))
-
-
-class BottleneckCSPB(nn.Module):
- # CSP https://github.com/WongKinYiu/CrossStagePartialNetworks
- def __init__(self, c1, c2, n=1, shortcut=False, g=1, e=0.5): # ch_in, ch_out, number, shortcut, groups, expansion
- super(BottleneckCSPB, self).__init__()
- c_ = int(c2) # hidden channels
- self.cv1 = Conv(c1, c_, 1, 1)
- self.cv2 = Conv(c_, c_, 1, 1)
- self.cv3 = Conv(2 * c_, c2, 1, 1)
- self.m = nn.Sequential(*[Bottleneck(c_, c_, shortcut, g, e=1.0) for _ in range(n)])
-
- def forward(self, x):
- x1 = self.cv1(x)
- y1 = self.m(x1)
- y2 = self.cv2(x1)
- return self.cv3(torch.cat((y1, y2), dim=1))
-
-
-class BottleneckCSPC(nn.Module):
- # CSP https://github.com/WongKinYiu/CrossStagePartialNetworks
- def __init__(self, c1, c2, n=1, shortcut=True, g=1, e=0.5): # ch_in, ch_out, number, shortcut, groups, expansion
- super(BottleneckCSPC, self).__init__()
- c_ = int(c2 * e) # hidden channels
- self.cv1 = Conv(c1, c_, 1, 1)
- self.cv2 = Conv(c1, c_, 1, 1)
- self.cv3 = Conv(c_, c_, 1, 1)
- self.cv4 = Conv(2 * c_, c2, 1, 1)
- self.m = nn.Sequential(*[Bottleneck(c_, c_, shortcut, g, e=1.0) for _ in range(n)])
-
- def forward(self, x):
- y1 = self.cv3(self.m(self.cv1(x)))
- y2 = self.cv2(x)
- return self.cv4(torch.cat((y1, y2), dim=1))
-
-
-class ResCSPA(BottleneckCSPA):
- # CSP https://github.com/WongKinYiu/CrossStagePartialNetworks
- def __init__(self, c1, c2, n=1, shortcut=True, g=1, e=0.5): # ch_in, ch_out, number, shortcut, groups, expansion
- super().__init__(c1, c2, n, shortcut, g, e)
- c_ = int(c2 * e) # hidden channels
- self.m = nn.Sequential(*[Res(c_, c_, shortcut, g, e=0.5) for _ in range(n)])
-
-
-class ResCSPB(BottleneckCSPB):
- # CSP https://github.com/WongKinYiu/CrossStagePartialNetworks
- def __init__(self, c1, c2, n=1, shortcut=True, g=1, e=0.5): # ch_in, ch_out, number, shortcut, groups, expansion
- super().__init__(c1, c2, n, shortcut, g, e)
- c_ = int(c2) # hidden channels
- self.m = nn.Sequential(*[Res(c_, c_, shortcut, g, e=0.5) for _ in range(n)])
-
-
-class ResCSPC(BottleneckCSPC):
- # CSP https://github.com/WongKinYiu/CrossStagePartialNetworks
- def __init__(self, c1, c2, n=1, shortcut=True, g=1, e=0.5): # ch_in, ch_out, number, shortcut, groups, expansion
- super().__init__(c1, c2, n, shortcut, g, e)
- c_ = int(c2 * e) # hidden channels
- self.m = nn.Sequential(*[Res(c_, c_, shortcut, g, e=0.5) for _ in range(n)])
-
-
-class ResXCSPA(ResCSPA):
- # CSP https://github.com/WongKinYiu/CrossStagePartialNetworks
- def __init__(self, c1, c2, n=1, shortcut=True, g=32, e=0.5): # ch_in, ch_out, number, shortcut, groups, expansion
- super().__init__(c1, c2, n, shortcut, g, e)
- c_ = int(c2 * e) # hidden channels
- self.m = nn.Sequential(*[Res(c_, c_, shortcut, g, e=1.0) for _ in range(n)])
-
-
-class ResXCSPB(ResCSPB):
- # CSP https://github.com/WongKinYiu/CrossStagePartialNetworks
- def __init__(self, c1, c2, n=1, shortcut=True, g=32, e=0.5): # ch_in, ch_out, number, shortcut, groups, expansion
- super().__init__(c1, c2, n, shortcut, g, e)
- c_ = int(c2) # hidden channels
- self.m = nn.Sequential(*[Res(c_, c_, shortcut, g, e=1.0) for _ in range(n)])
-
-
-class ResXCSPC(ResCSPC):
- # CSP https://github.com/WongKinYiu/CrossStagePartialNetworks
- def __init__(self, c1, c2, n=1, shortcut=True, g=32, e=0.5): # ch_in, ch_out, number, shortcut, groups, expansion
- super().__init__(c1, c2, n, shortcut, g, e)
- c_ = int(c2 * e) # hidden channels
- self.m = nn.Sequential(*[Res(c_, c_, shortcut, g, e=1.0) for _ in range(n)])
-
-
-class GhostCSPA(BottleneckCSPA):
- # CSP https://github.com/WongKinYiu/CrossStagePartialNetworks
- def __init__(self, c1, c2, n=1, shortcut=True, g=1, e=0.5): # ch_in, ch_out, number, shortcut, groups, expansion
- super().__init__(c1, c2, n, shortcut, g, e)
- c_ = int(c2 * e) # hidden channels
- self.m = nn.Sequential(*[Ghost(c_, c_) for _ in range(n)])
-
-
-class GhostCSPB(BottleneckCSPB):
- # CSP https://github.com/WongKinYiu/CrossStagePartialNetworks
- def __init__(self, c1, c2, n=1, shortcut=True, g=1, e=0.5): # ch_in, ch_out, number, shortcut, groups, expansion
- super().__init__(c1, c2, n, shortcut, g, e)
- c_ = int(c2) # hidden channels
- self.m = nn.Sequential(*[Ghost(c_, c_) for _ in range(n)])
-
-
-class GhostCSPC(BottleneckCSPC):
- # CSP https://github.com/WongKinYiu/CrossStagePartialNetworks
- def __init__(self, c1, c2, n=1, shortcut=True, g=1, e=0.5): # ch_in, ch_out, number, shortcut, groups, expansion
- super().__init__(c1, c2, n, shortcut, g, e)
- c_ = int(c2 * e) # hidden channels
- self.m = nn.Sequential(*[Ghost(c_, c_) for _ in range(n)])
-
-##### end of cspnet #####
-
-
-##### yolor #####
-
-class ImplicitA(nn.Module):
- def __init__(self, channel, mean=0., std=.02):
- super(ImplicitA, self).__init__()
- self.channel = channel
- self.mean = mean
- self.std = std
- self.implicit = nn.Parameter(torch.zeros(1, channel, 1, 1))
- nn.init.normal_(self.implicit, mean=self.mean, std=self.std)
-
- def forward(self, x):
- return self.implicit + x
-
-
-class ImplicitM(nn.Module):
- def __init__(self, channel, mean=1., std=.02):
- super(ImplicitM, self).__init__()
- self.channel = channel
- self.mean = mean
- self.std = std
- self.implicit = nn.Parameter(torch.ones(1, channel, 1, 1))
- nn.init.normal_(self.implicit, mean=self.mean, std=self.std)
-
- def forward(self, x):
- return self.implicit * x
-
-##### end of yolor #####
-
-
-##### repvgg #####
-
-class RepConv(nn.Module):
- # Represented convolution
- # https://arxiv.org/abs/2101.03697
-
- def __init__(self, c1, c2, k=3, s=1, p=None, g=1, act=True, deploy=False):
- super(RepConv, self).__init__()
-
- self.deploy = deploy
- self.groups = g
- self.in_channels = c1
- self.out_channels = c2
-
- assert k == 3
- assert autopad(k, p) == 1
-
- padding_11 = autopad(k, p) - k // 2
-
- self.act = nn.SiLU() if act is True else (act if isinstance(act, nn.Module) else nn.Identity())
-
- if deploy:
- self.rbr_reparam = nn.Conv2d(c1, c2, k, s, autopad(k, p), groups=g, bias=True)
-
- else:
- self.rbr_identity = (nn.BatchNorm2d(num_features=c1) if c2 == c1 and s == 1 else None)
-
- self.rbr_dense = nn.Sequential(
- nn.Conv2d(c1, c2, k, s, autopad(k, p), groups=g, bias=False),
- nn.BatchNorm2d(num_features=c2),
- )
-
- self.rbr_1x1 = nn.Sequential(
- nn.Conv2d( c1, c2, 1, s, padding_11, groups=g, bias=False),
- nn.BatchNorm2d(num_features=c2),
- )
-
- def forward(self, inputs):
- if hasattr(self, "rbr_reparam"):
- return self.act(self.rbr_reparam(inputs))
-
- if self.rbr_identity is None:
- id_out = 0
- else:
- id_out = self.rbr_identity(inputs)
-
- return self.act(self.rbr_dense(inputs) + self.rbr_1x1(inputs) + id_out)
-
- def get_equivalent_kernel_bias(self):
- kernel3x3, bias3x3 = self._fuse_bn_tensor(self.rbr_dense)
- kernel1x1, bias1x1 = self._fuse_bn_tensor(self.rbr_1x1)
- kernelid, biasid = self._fuse_bn_tensor(self.rbr_identity)
- return (
- kernel3x3 + self._pad_1x1_to_3x3_tensor(kernel1x1) + kernelid,
- bias3x3 + bias1x1 + biasid,
- )
-
- def _pad_1x1_to_3x3_tensor(self, kernel1x1):
- if kernel1x1 is None:
- return 0
- else:
- return nn.functional.pad(kernel1x1, [1, 1, 1, 1])
-
- def _fuse_bn_tensor(self, branch):
- if branch is None:
- return 0, 0
- if isinstance(branch, nn.Sequential):
- kernel = branch[0].weight
- running_mean = branch[1].running_mean
- running_var = branch[1].running_var
- gamma = branch[1].weight
- beta = branch[1].bias
- eps = branch[1].eps
- else:
- assert isinstance(branch, nn.BatchNorm2d)
- if not hasattr(self, "id_tensor"):
- input_dim = self.in_channels // self.groups
- kernel_value = np.zeros(
- (self.in_channels, input_dim, 3, 3), dtype=np.float32
- )
- for i in range(self.in_channels):
- kernel_value[i, i % input_dim, 1, 1] = 1
- self.id_tensor = torch.from_numpy(kernel_value).to(branch.weight.device)
- kernel = self.id_tensor
- running_mean = branch.running_mean
- running_var = branch.running_var
- gamma = branch.weight
- beta = branch.bias
- eps = branch.eps
- std = (running_var + eps).sqrt()
- t = (gamma / std).reshape(-1, 1, 1, 1)
- return kernel * t, beta - running_mean * gamma / std
-
- def repvgg_convert(self):
- kernel, bias = self.get_equivalent_kernel_bias()
- return (
- kernel.detach().cpu().numpy(),
- bias.detach().cpu().numpy(),
- )
-
- def fuse_conv_bn(self, conv, bn):
-
- std = (bn.running_var + bn.eps).sqrt()
- bias = bn.bias - bn.running_mean * bn.weight / std
-
- t = (bn.weight / std).reshape(-1, 1, 1, 1)
- weights = conv.weight * t
-
- bn = nn.Identity()
- conv = nn.Conv2d(in_channels = conv.in_channels,
- out_channels = conv.out_channels,
- kernel_size = conv.kernel_size,
- stride=conv.stride,
- padding = conv.padding,
- dilation = conv.dilation,
- groups = conv.groups,
- bias = True,
- padding_mode = conv.padding_mode)
-
- conv.weight = torch.nn.Parameter(weights)
- conv.bias = torch.nn.Parameter(bias)
- return conv
-
- def fuse_repvgg_block(self):
- if self.deploy:
- return
- print(f"RepConv.fuse_repvgg_block")
-
- self.rbr_dense = self.fuse_conv_bn(self.rbr_dense[0], self.rbr_dense[1])
-
- self.rbr_1x1 = self.fuse_conv_bn(self.rbr_1x1[0], self.rbr_1x1[1])
- rbr_1x1_bias = self.rbr_1x1.bias
- weight_1x1_expanded = torch.nn.functional.pad(self.rbr_1x1.weight, [1, 1, 1, 1])
-
- # Fuse self.rbr_identity
- if (isinstance(self.rbr_identity, nn.BatchNorm2d) or isinstance(self.rbr_identity, nn.modules.batchnorm.SyncBatchNorm)):
- # print(f"fuse: rbr_identity == BatchNorm2d or SyncBatchNorm")
- identity_conv_1x1 = nn.Conv2d(
- in_channels=self.in_channels,
- out_channels=self.out_channels,
- kernel_size=1,
- stride=1,
- padding=0,
- groups=self.groups,
- bias=False)
- identity_conv_1x1.weight.data = identity_conv_1x1.weight.data.to(self.rbr_1x1.weight.data.device)
- identity_conv_1x1.weight.data = identity_conv_1x1.weight.data.squeeze().squeeze()
- # print(f" identity_conv_1x1.weight = {identity_conv_1x1.weight.shape}")
- identity_conv_1x1.weight.data.fill_(0.0)
- identity_conv_1x1.weight.data.fill_diagonal_(1.0)
- identity_conv_1x1.weight.data = identity_conv_1x1.weight.data.unsqueeze(2).unsqueeze(3)
- # print(f" identity_conv_1x1.weight = {identity_conv_1x1.weight.shape}")
-
- identity_conv_1x1 = self.fuse_conv_bn(identity_conv_1x1, self.rbr_identity)
- bias_identity_expanded = identity_conv_1x1.bias
- weight_identity_expanded = torch.nn.functional.pad(identity_conv_1x1.weight, [1, 1, 1, 1])
- else:
- # print(f"fuse: rbr_identity != BatchNorm2d, rbr_identity = {self.rbr_identity}")
- bias_identity_expanded = torch.nn.Parameter( torch.zeros_like(rbr_1x1_bias) )
- weight_identity_expanded = torch.nn.Parameter( torch.zeros_like(weight_1x1_expanded) )
-
-
- #print(f"self.rbr_1x1.weight = {self.rbr_1x1.weight.shape}, ")
- #print(f"weight_1x1_expanded = {weight_1x1_expanded.shape}, ")
- #print(f"self.rbr_dense.weight = {self.rbr_dense.weight.shape}, ")
-
- self.rbr_dense.weight = torch.nn.Parameter(self.rbr_dense.weight + weight_1x1_expanded + weight_identity_expanded)
- self.rbr_dense.bias = torch.nn.Parameter(self.rbr_dense.bias + rbr_1x1_bias + bias_identity_expanded)
-
- self.rbr_reparam = self.rbr_dense
- self.deploy = True
-
- if self.rbr_identity is not None:
- del self.rbr_identity
- self.rbr_identity = None
-
- if self.rbr_1x1 is not None:
- del self.rbr_1x1
- self.rbr_1x1 = None
-
- if self.rbr_dense is not None:
- del self.rbr_dense
- self.rbr_dense = None
-
-
-class RepBottleneck(Bottleneck):
- # Standard bottleneck
- def __init__(self, c1, c2, shortcut=True, g=1, e=0.5): # ch_in, ch_out, shortcut, groups, expansion
- super().__init__(c1, c2, shortcut=True, g=1, e=0.5)
- c_ = int(c2 * e) # hidden channels
- self.cv2 = RepConv(c_, c2, 3, 1, g=g)
-
-
-class RepBottleneckCSPA(BottleneckCSPA):
- # CSP Bottleneck https://github.com/WongKinYiu/CrossStagePartialNetworks
- def __init__(self, c1, c2, n=1, shortcut=True, g=1, e=0.5): # ch_in, ch_out, number, shortcut, groups, expansion
- super().__init__(c1, c2, n, shortcut, g, e)
- c_ = int(c2 * e) # hidden channels
- self.m = nn.Sequential(*[RepBottleneck(c_, c_, shortcut, g, e=1.0) for _ in range(n)])
-
-
-class RepBottleneckCSPB(BottleneckCSPB):
- # CSP Bottleneck https://github.com/WongKinYiu/CrossStagePartialNetworks
- def __init__(self, c1, c2, n=1, shortcut=False, g=1, e=0.5): # ch_in, ch_out, number, shortcut, groups, expansion
- super().__init__(c1, c2, n, shortcut, g, e)
- c_ = int(c2) # hidden channels
- self.m = nn.Sequential(*[RepBottleneck(c_, c_, shortcut, g, e=1.0) for _ in range(n)])
-
-
-class RepBottleneckCSPC(BottleneckCSPC):
- # CSP Bottleneck https://github.com/WongKinYiu/CrossStagePartialNetworks
- def __init__(self, c1, c2, n=1, shortcut=True, g=1, e=0.5): # ch_in, ch_out, number, shortcut, groups, expansion
- super().__init__(c1, c2, n, shortcut, g, e)
- c_ = int(c2 * e) # hidden channels
- self.m = nn.Sequential(*[RepBottleneck(c_, c_, shortcut, g, e=1.0) for _ in range(n)])
-
-
-class RepRes(Res):
- # Standard bottleneck
- def __init__(self, c1, c2, shortcut=True, g=1, e=0.5): # ch_in, ch_out, shortcut, groups, expansion
- super().__init__(c1, c2, shortcut, g, e)
- c_ = int(c2 * e) # hidden channels
- self.cv2 = RepConv(c_, c_, 3, 1, g=g)
-
-
-class RepResCSPA(ResCSPA):
- # CSP Bottleneck https://github.com/WongKinYiu/CrossStagePartialNetworks
- def __init__(self, c1, c2, n=1, shortcut=True, g=1, e=0.5): # ch_in, ch_out, number, shortcut, groups, expansion
- super().__init__(c1, c2, n, shortcut, g, e)
- c_ = int(c2 * e) # hidden channels
- self.m = nn.Sequential(*[RepRes(c_, c_, shortcut, g, e=0.5) for _ in range(n)])
-
-
-class RepResCSPB(ResCSPB):
- # CSP Bottleneck https://github.com/WongKinYiu/CrossStagePartialNetworks
- def __init__(self, c1, c2, n=1, shortcut=False, g=1, e=0.5): # ch_in, ch_out, number, shortcut, groups, expansion
- super().__init__(c1, c2, n, shortcut, g, e)
- c_ = int(c2) # hidden channels
- self.m = nn.Sequential(*[RepRes(c_, c_, shortcut, g, e=0.5) for _ in range(n)])
-
-
-class RepResCSPC(ResCSPC):
- # CSP Bottleneck https://github.com/WongKinYiu/CrossStagePartialNetworks
- def __init__(self, c1, c2, n=1, shortcut=True, g=1, e=0.5): # ch_in, ch_out, number, shortcut, groups, expansion
- super().__init__(c1, c2, n, shortcut, g, e)
- c_ = int(c2 * e) # hidden channels
- self.m = nn.Sequential(*[RepRes(c_, c_, shortcut, g, e=0.5) for _ in range(n)])
-
-
-class RepResX(ResX):
- # Standard bottleneck
- def __init__(self, c1, c2, shortcut=True, g=32, e=0.5): # ch_in, ch_out, shortcut, groups, expansion
- super().__init__(c1, c2, shortcut, g, e)
- c_ = int(c2 * e) # hidden channels
- self.cv2 = RepConv(c_, c_, 3, 1, g=g)
-
-
-class RepResXCSPA(ResXCSPA):
- # CSP Bottleneck https://github.com/WongKinYiu/CrossStagePartialNetworks
- def __init__(self, c1, c2, n=1, shortcut=True, g=32, e=0.5): # ch_in, ch_out, number, shortcut, groups, expansion
- super().__init__(c1, c2, n, shortcut, g, e)
- c_ = int(c2 * e) # hidden channels
- self.m = nn.Sequential(*[RepResX(c_, c_, shortcut, g, e=0.5) for _ in range(n)])
-
-
-class RepResXCSPB(ResXCSPB):
- # CSP Bottleneck https://github.com/WongKinYiu/CrossStagePartialNetworks
- def __init__(self, c1, c2, n=1, shortcut=False, g=32, e=0.5): # ch_in, ch_out, number, shortcut, groups, expansion
- super().__init__(c1, c2, n, shortcut, g, e)
- c_ = int(c2) # hidden channels
- self.m = nn.Sequential(*[RepResX(c_, c_, shortcut, g, e=0.5) for _ in range(n)])
-
-
-class RepResXCSPC(ResXCSPC):
- # CSP Bottleneck https://github.com/WongKinYiu/CrossStagePartialNetworks
- def __init__(self, c1, c2, n=1, shortcut=True, g=32, e=0.5): # ch_in, ch_out, number, shortcut, groups, expansion
- super().__init__(c1, c2, n, shortcut, g, e)
- c_ = int(c2 * e) # hidden channels
- self.m = nn.Sequential(*[RepResX(c_, c_, shortcut, g, e=0.5) for _ in range(n)])
-
-##### end of repvgg #####
-
-
-##### transformer #####
-
-class TransformerLayer(nn.Module):
- # Transformer layer https://arxiv.org/abs/2010.11929 (LayerNorm layers removed for better performance)
- def __init__(self, c, num_heads):
- super().__init__()
- self.q = nn.Linear(c, c, bias=False)
- self.k = nn.Linear(c, c, bias=False)
- self.v = nn.Linear(c, c, bias=False)
- self.ma = nn.MultiheadAttention(embed_dim=c, num_heads=num_heads)
- self.fc1 = nn.Linear(c, c, bias=False)
- self.fc2 = nn.Linear(c, c, bias=False)
-
- def forward(self, x):
- x = self.ma(self.q(x), self.k(x), self.v(x))[0] + x
- x = self.fc2(self.fc1(x)) + x
- return x
-
-
-class TransformerBlock(nn.Module):
- # Vision Transformer https://arxiv.org/abs/2010.11929
- def __init__(self, c1, c2, num_heads, num_layers):
- super().__init__()
- self.conv = None
- if c1 != c2:
- self.conv = Conv(c1, c2)
- self.linear = nn.Linear(c2, c2) # learnable position embedding
- self.tr = nn.Sequential(*[TransformerLayer(c2, num_heads) for _ in range(num_layers)])
- self.c2 = c2
-
- def forward(self, x):
- if self.conv is not None:
- x = self.conv(x)
- b, _, w, h = x.shape
- p = x.flatten(2)
- p = p.unsqueeze(0)
- p = p.transpose(0, 3)
- p = p.squeeze(3)
- e = self.linear(p)
- x = p + e
-
- x = self.tr(x)
- x = x.unsqueeze(3)
- x = x.transpose(0, 3)
- x = x.reshape(b, self.c2, w, h)
- return x
-
-##### end of transformer #####
-
-
-##### yolov5 #####
-
-class Focus(nn.Module):
- # Focus wh information into c-space
- def __init__(self, c1, c2, k=1, s=1, p=None, g=1, act=True): # ch_in, ch_out, kernel, stride, padding, groups
- super(Focus, self).__init__()
- self.conv = Conv(c1 * 4, c2, k, s, p, g, act)
- # self.contract = Contract(gain=2)
-
- def forward(self, x): # x(b,c,w,h) -> y(b,4c,w/2,h/2)
- return self.conv(torch.cat([x[..., ::2, ::2], x[..., 1::2, ::2], x[..., ::2, 1::2], x[..., 1::2, 1::2]], 1))
- # return self.conv(self.contract(x))
-
-
-class SPPF(nn.Module):
- # Spatial Pyramid Pooling - Fast (SPPF) layer for YOLOv5 by Glenn Jocher
- def __init__(self, c1, c2, k=5): # equivalent to SPP(k=(5, 9, 13))
- super().__init__()
- c_ = c1 // 2 # hidden channels
- self.cv1 = Conv(c1, c_, 1, 1)
- self.cv2 = Conv(c_ * 4, c2, 1, 1)
- self.m = nn.MaxPool2d(kernel_size=k, stride=1, padding=k // 2)
-
- def forward(self, x):
- x = self.cv1(x)
- y1 = self.m(x)
- y2 = self.m(y1)
- return self.cv2(torch.cat([x, y1, y2, self.m(y2)], 1))
-
-
-class Contract(nn.Module):
- # Contract width-height into channels, i.e. x(1,64,80,80) to x(1,256,40,40)
- def __init__(self, gain=2):
- super().__init__()
- self.gain = gain
-
- def forward(self, x):
- N, C, H, W = x.size() # assert (H / s == 0) and (W / s == 0), 'Indivisible gain'
- s = self.gain
- x = x.view(N, C, H // s, s, W // s, s) # x(1,64,40,2,40,2)
- x = x.permute(0, 3, 5, 1, 2, 4).contiguous() # x(1,2,2,64,40,40)
- return x.view(N, C * s * s, H // s, W // s) # x(1,256,40,40)
-
-
-class Expand(nn.Module):
- # Expand channels into width-height, i.e. x(1,64,80,80) to x(1,16,160,160)
- def __init__(self, gain=2):
- super().__init__()
- self.gain = gain
-
- def forward(self, x):
- N, C, H, W = x.size() # assert C / s ** 2 == 0, 'Indivisible gain'
- s = self.gain
- x = x.view(N, s, s, C // s ** 2, H, W) # x(1,2,2,16,80,80)
- x = x.permute(0, 3, 4, 1, 5, 2).contiguous() # x(1,16,80,2,80,2)
- return x.view(N, C // s ** 2, H * s, W * s) # x(1,16,160,160)
-
-
-class NMS(nn.Module):
- # Non-Maximum Suppression (NMS) module
- conf = 0.25 # confidence threshold
- iou = 0.45 # IoU threshold
- classes = None # (optional list) filter by class
-
- def __init__(self):
- super(NMS, self).__init__()
-
- def forward(self, x):
- return non_max_suppression(x[0], conf_thres=self.conf, iou_thres=self.iou, classes=self.classes)
-
-
-class autoShape(nn.Module):
- # input-robust model wrapper for passing cv2/np/PIL/torch inputs. Includes preprocessing, inference and NMS
- conf = 0.25 # NMS confidence threshold
- iou = 0.45 # NMS IoU threshold
- classes = None # (optional list) filter by class
-
- def __init__(self, model):
- super(autoShape, self).__init__()
- self.model = model.eval()
-
- def autoshape(self):
- print('autoShape already enabled, skipping... ') # model already converted to model.autoshape()
- return self
-
- @torch.no_grad()
- def forward(self, imgs, size=640, augment=False, profile=False):
- # Inference from various sources. For height=640, width=1280, RGB images example inputs are:
- # filename: imgs = 'data/samples/zidane.jpg'
- # URI: = 'https://github.com/ultralytics/yolov5/releases/download/v1.0/zidane.jpg'
- # OpenCV: = cv2.imread('image.jpg')[:,:,::-1] # HWC BGR to RGB x(640,1280,3)
- # PIL: = Image.open('image.jpg') # HWC x(640,1280,3)
- # numpy: = np.zeros((640,1280,3)) # HWC
- # torch: = torch.zeros(16,3,320,640) # BCHW (scaled to size=640, 0-1 values)
- # multiple: = [Image.open('image1.jpg'), Image.open('image2.jpg'), ...] # list of images
-
- t = [time_synchronized()]
- p = next(self.model.parameters()) # for device and type
- if isinstance(imgs, torch.Tensor): # torch
- with amp.autocast(enabled=p.device.type != 'cpu'):
- return self.model(imgs.to(p.device).type_as(p), augment, profile) # inference
-
- # Pre-process
- n, imgs = (len(imgs), imgs) if isinstance(imgs, list) else (1, [imgs]) # number of images, list of images
- shape0, shape1, files = [], [], [] # image and inference shapes, filenames
- for i, im in enumerate(imgs):
- f = f'image{i}' # filename
- if isinstance(im, str): # filename or uri
- im, f = np.asarray(Image.open(requests.get(im, stream=True).raw if im.startswith('http') else im)), im
- elif isinstance(im, Image.Image): # PIL Image
- im, f = np.asarray(im), getattr(im, 'filename', f) or f
- files.append(Path(f).with_suffix('.jpg').name)
- if im.shape[0] < 5: # image in CHW
- im = im.transpose((1, 2, 0)) # reverse dataloader .transpose(2, 0, 1)
- im = im[:, :, :3] if im.ndim == 3 else np.tile(im[:, :, None], 3) # enforce 3ch input
- s = im.shape[:2] # HWC
- shape0.append(s) # image shape
- g = (size / max(s)) # gain
- shape1.append([y * g for y in s])
- imgs[i] = im # update
- shape1 = [make_divisible(x, int(self.stride.max())) for x in np.stack(shape1, 0).max(0)] # inference shape
- x = [letterbox(im, new_shape=shape1, auto=False)[0] for im in imgs] # pad
- x = np.stack(x, 0) if n > 1 else x[0][None] # stack
- x = np.ascontiguousarray(x.transpose((0, 3, 1, 2))) # BHWC to BCHW
- x = torch.from_numpy(x).to(p.device).type_as(p) / 255. # uint8 to fp16/32
- t.append(time_synchronized())
-
- with amp.autocast(enabled=p.device.type != 'cpu'):
- # Inference
- y = self.model(x, augment, profile)[0] # forward
- t.append(time_synchronized())
-
- # Post-process
- y = non_max_suppression(y, conf_thres=self.conf, iou_thres=self.iou, classes=self.classes) # NMS
- for i in range(n):
- scale_coords(shape1, y[i][:, :4], shape0[i])
-
- t.append(time_synchronized())
- return Detections(imgs, y, files, t, self.names, x.shape)
-
-
-class Detections:
- # detections class for YOLOv5 inference results
- def __init__(self, imgs, pred, files, times=None, names=None, shape=None):
- super(Detections, self).__init__()
- d = pred[0].device # device
- gn = [torch.tensor([*[im.shape[i] for i in [1, 0, 1, 0]], 1., 1.], device=d) for im in imgs] # normalizations
- self.imgs = imgs # list of images as numpy arrays
- self.pred = pred # list of tensors pred[0] = (xyxy, conf, cls)
- self.names = names # class names
- self.files = files # image filenames
- self.xyxy = pred # xyxy pixels
- self.xywh = [xyxy2xywh(x) for x in pred] # xywh pixels
- self.xyxyn = [x / g for x, g in zip(self.xyxy, gn)] # xyxy normalized
- self.xywhn = [x / g for x, g in zip(self.xywh, gn)] # xywh normalized
- self.n = len(self.pred) # number of images (batch size)
- self.t = tuple((times[i + 1] - times[i]) * 1000 / self.n for i in range(3)) # timestamps (ms)
- self.s = shape # inference BCHW shape
-
- def display(self, pprint=False, show=False, save=False, render=False, save_dir=''):
- colors = color_list()
- for i, (img, pred) in enumerate(zip(self.imgs, self.pred)):
- str = f'image {i + 1}/{len(self.pred)}: {img.shape[0]}x{img.shape[1]} '
- if pred is not None:
- for c in pred[:, -1].unique():
- n = (pred[:, -1] == c).sum() # detections per class
- str += f"{n} {self.names[int(c)]}{'s' * (n > 1)}, " # add to string
- if show or save or render:
- for *box, conf, cls in pred: # xyxy, confidence, class
- label = f'{self.names[int(cls)]} {conf:.2f}'
- plot_one_box(box, img, label=label, color=colors[int(cls) % 10])
- img = Image.fromarray(img.astype(np.uint8)) if isinstance(img, np.ndarray) else img # from np
- if pprint:
- print(str.rstrip(', '))
- if show:
- img.show(self.files[i]) # show
- if save:
- f = self.files[i]
- img.save(Path(save_dir) / f) # save
- print(f"{'Saved' * (i == 0)} {f}", end=',' if i < self.n - 1 else f' to {save_dir}\n')
- if render:
- self.imgs[i] = np.asarray(img)
-
- def print(self):
- self.display(pprint=True) # print results
- print(f'Speed: %.1fms pre-process, %.1fms inference, %.1fms NMS per image at shape {tuple(self.s)}' % self.t)
-
- def show(self):
- self.display(show=True) # show results
-
- def save(self, save_dir='runs/hub/exp'):
- save_dir = increment_path(save_dir, exist_ok=save_dir != 'runs/hub/exp') # increment save_dir
- Path(save_dir).mkdir(parents=True, exist_ok=True)
- self.display(save=True, save_dir=save_dir) # save results
-
- def render(self):
- self.display(render=True) # render results
- return self.imgs
-
- def pandas(self):
- # return detections as pandas DataFrames, i.e. print(results.pandas().xyxy[0])
- new = copy(self) # return copy
- ca = 'xmin', 'ymin', 'xmax', 'ymax', 'confidence', 'class', 'name' # xyxy columns
- cb = 'xcenter', 'ycenter', 'width', 'height', 'confidence', 'class', 'name' # xywh columns
- for k, c in zip(['xyxy', 'xyxyn', 'xywh', 'xywhn'], [ca, ca, cb, cb]):
- a = [[x[:5] + [int(x[5]), self.names[int(x[5])]] for x in x.tolist()] for x in getattr(self, k)] # update
- setattr(new, k, [pd.DataFrame(x, columns=c) for x in a])
- return new
-
- def tolist(self):
- # return a list of Detections objects, i.e. 'for result in results.tolist():'
- x = [Detections([self.imgs[i]], [self.pred[i]], self.names, self.s) for i in range(self.n)]
- for d in x:
- for k in ['imgs', 'pred', 'xyxy', 'xyxyn', 'xywh', 'xywhn']:
- setattr(d, k, getattr(d, k)[0]) # pop out of list
- return x
-
- def __len__(self):
- return self.n
-
-
-class Classify(nn.Module):
- # Classification head, i.e. x(b,c1,20,20) to x(b,c2)
- def __init__(self, c1, c2, k=1, s=1, p=None, g=1): # ch_in, ch_out, kernel, stride, padding, groups
- super(Classify, self).__init__()
- self.aap = nn.AdaptiveAvgPool2d(1) # to x(b,c1,1,1)
- self.conv = nn.Conv2d(c1, c2, k, s, autopad(k, p), groups=g) # to x(b,c2,1,1)
- self.flat = nn.Flatten()
-
- def forward(self, x):
- z = torch.cat([self.aap(y) for y in (x if isinstance(x, list) else [x])], 1) # cat if list
- return self.flat(self.conv(z)) # flatten to x(b,c2)
-
-##### end of yolov5 ######
-
-
-##### orepa #####
-
-def transI_fusebn(kernel, bn):
- gamma = bn.weight
- std = (bn.running_var + bn.eps).sqrt()
- return kernel * ((gamma / std).reshape(-1, 1, 1, 1)), bn.bias - bn.running_mean * gamma / std
-
-
-class ConvBN(nn.Module):
- def __init__(self, in_channels, out_channels, kernel_size,
- stride=1, padding=0, dilation=1, groups=1, deploy=False, nonlinear=None):
- super().__init__()
- if nonlinear is None:
- self.nonlinear = nn.Identity()
- else:
- self.nonlinear = nonlinear
- if deploy:
- self.conv = nn.Conv2d(in_channels=in_channels, out_channels=out_channels, kernel_size=kernel_size,
- stride=stride, padding=padding, dilation=dilation, groups=groups, bias=True)
- else:
- self.conv = nn.Conv2d(in_channels=in_channels, out_channels=out_channels, kernel_size=kernel_size,
- stride=stride, padding=padding, dilation=dilation, groups=groups, bias=False)
- self.bn = nn.BatchNorm2d(num_features=out_channels)
-
- def forward(self, x):
- if hasattr(self, 'bn'):
- return self.nonlinear(self.bn(self.conv(x)))
- else:
- return self.nonlinear(self.conv(x))
-
- def switch_to_deploy(self):
- kernel, bias = transI_fusebn(self.conv.weight, self.bn)
- conv = nn.Conv2d(in_channels=self.conv.in_channels, out_channels=self.conv.out_channels, kernel_size=self.conv.kernel_size,
- stride=self.conv.stride, padding=self.conv.padding, dilation=self.conv.dilation, groups=self.conv.groups, bias=True)
- conv.weight.data = kernel
- conv.bias.data = bias
- for para in self.parameters():
- para.detach_()
- self.__delattr__('conv')
- self.__delattr__('bn')
- self.conv = conv
-
-class OREPA_3x3_RepConv(nn.Module):
-
- def __init__(self, in_channels, out_channels, kernel_size,
- stride=1, padding=0, dilation=1, groups=1,
- internal_channels_1x1_3x3=None,
- deploy=False, nonlinear=None, single_init=False):
- super(OREPA_3x3_RepConv, self).__init__()
- self.deploy = deploy
-
- if nonlinear is None:
- self.nonlinear = nn.Identity()
- else:
- self.nonlinear = nonlinear
-
- self.kernel_size = kernel_size
- self.in_channels = in_channels
- self.out_channels = out_channels
- self.groups = groups
- assert padding == kernel_size // 2
-
- self.stride = stride
- self.padding = padding
- self.dilation = dilation
-
- self.branch_counter = 0
-
- self.weight_rbr_origin = nn.Parameter(torch.Tensor(out_channels, int(in_channels/self.groups), kernel_size, kernel_size))
- nn.init.kaiming_uniform_(self.weight_rbr_origin, a=math.sqrt(1.0))
- self.branch_counter += 1
-
-
- if groups < out_channels:
- self.weight_rbr_avg_conv = nn.Parameter(torch.Tensor(out_channels, int(in_channels/self.groups), 1, 1))
- self.weight_rbr_pfir_conv = nn.Parameter(torch.Tensor(out_channels, int(in_channels/self.groups), 1, 1))
- nn.init.kaiming_uniform_(self.weight_rbr_avg_conv, a=1.0)
- nn.init.kaiming_uniform_(self.weight_rbr_pfir_conv, a=1.0)
- self.weight_rbr_avg_conv.data
- self.weight_rbr_pfir_conv.data
- self.register_buffer('weight_rbr_avg_avg', torch.ones(kernel_size, kernel_size).mul(1.0/kernel_size/kernel_size))
- self.branch_counter += 1
-
- else:
- raise NotImplementedError
- self.branch_counter += 1
-
- if internal_channels_1x1_3x3 is None:
- internal_channels_1x1_3x3 = in_channels if groups < out_channels else 2 * in_channels # For mobilenet, it is better to have 2X internal channels
-
- if internal_channels_1x1_3x3 == in_channels:
- self.weight_rbr_1x1_kxk_idconv1 = nn.Parameter(torch.zeros(in_channels, int(in_channels/self.groups), 1, 1))
- id_value = np.zeros((in_channels, int(in_channels/self.groups), 1, 1))
- for i in range(in_channels):
- id_value[i, i % int(in_channels/self.groups), 0, 0] = 1
- id_tensor = torch.from_numpy(id_value).type_as(self.weight_rbr_1x1_kxk_idconv1)
- self.register_buffer('id_tensor', id_tensor)
-
- else:
- self.weight_rbr_1x1_kxk_conv1 = nn.Parameter(torch.Tensor(internal_channels_1x1_3x3, int(in_channels/self.groups), 1, 1))
- nn.init.kaiming_uniform_(self.weight_rbr_1x1_kxk_conv1, a=math.sqrt(1.0))
- self.weight_rbr_1x1_kxk_conv2 = nn.Parameter(torch.Tensor(out_channels, int(internal_channels_1x1_3x3/self.groups), kernel_size, kernel_size))
- nn.init.kaiming_uniform_(self.weight_rbr_1x1_kxk_conv2, a=math.sqrt(1.0))
- self.branch_counter += 1
-
- expand_ratio = 8
- self.weight_rbr_gconv_dw = nn.Parameter(torch.Tensor(in_channels*expand_ratio, 1, kernel_size, kernel_size))
- self.weight_rbr_gconv_pw = nn.Parameter(torch.Tensor(out_channels, in_channels*expand_ratio, 1, 1))
- nn.init.kaiming_uniform_(self.weight_rbr_gconv_dw, a=math.sqrt(1.0))
- nn.init.kaiming_uniform_(self.weight_rbr_gconv_pw, a=math.sqrt(1.0))
- self.branch_counter += 1
-
- if out_channels == in_channels and stride == 1:
- self.branch_counter += 1
-
- self.vector = nn.Parameter(torch.Tensor(self.branch_counter, self.out_channels))
- self.bn = nn.BatchNorm2d(out_channels)
-
- self.fre_init()
-
- nn.init.constant_(self.vector[0, :], 0.25) #origin
- nn.init.constant_(self.vector[1, :], 0.25) #avg
- nn.init.constant_(self.vector[2, :], 0.0) #prior
- nn.init.constant_(self.vector[3, :], 0.5) #1x1_kxk
- nn.init.constant_(self.vector[4, :], 0.5) #dws_conv
-
-
- def fre_init(self):
- prior_tensor = torch.Tensor(self.out_channels, self.kernel_size, self.kernel_size)
- half_fg = self.out_channels/2
- for i in range(self.out_channels):
- for h in range(3):
- for w in range(3):
- if i < half_fg:
- prior_tensor[i, h, w] = math.cos(math.pi*(h+0.5)*(i+1)/3)
- else:
- prior_tensor[i, h, w] = math.cos(math.pi*(w+0.5)*(i+1-half_fg)/3)
-
- self.register_buffer('weight_rbr_prior', prior_tensor)
-
- def weight_gen(self):
-
- weight_rbr_origin = torch.einsum('oihw,o->oihw', self.weight_rbr_origin, self.vector[0, :])
-
- weight_rbr_avg = torch.einsum('oihw,o->oihw', torch.einsum('oihw,hw->oihw', self.weight_rbr_avg_conv, self.weight_rbr_avg_avg), self.vector[1, :])
-
- weight_rbr_pfir = torch.einsum('oihw,o->oihw', torch.einsum('oihw,ohw->oihw', self.weight_rbr_pfir_conv, self.weight_rbr_prior), self.vector[2, :])
-
- weight_rbr_1x1_kxk_conv1 = None
- if hasattr(self, 'weight_rbr_1x1_kxk_idconv1'):
- weight_rbr_1x1_kxk_conv1 = (self.weight_rbr_1x1_kxk_idconv1 + self.id_tensor).squeeze()
- elif hasattr(self, 'weight_rbr_1x1_kxk_conv1'):
- weight_rbr_1x1_kxk_conv1 = self.weight_rbr_1x1_kxk_conv1.squeeze()
- else:
- raise NotImplementedError
- weight_rbr_1x1_kxk_conv2 = self.weight_rbr_1x1_kxk_conv2
-
- if self.groups > 1:
- g = self.groups
- t, ig = weight_rbr_1x1_kxk_conv1.size()
- o, tg, h, w = weight_rbr_1x1_kxk_conv2.size()
- weight_rbr_1x1_kxk_conv1 = weight_rbr_1x1_kxk_conv1.view(g, int(t/g), ig)
- weight_rbr_1x1_kxk_conv2 = weight_rbr_1x1_kxk_conv2.view(g, int(o/g), tg, h, w)
- weight_rbr_1x1_kxk = torch.einsum('gti,gothw->goihw', weight_rbr_1x1_kxk_conv1, weight_rbr_1x1_kxk_conv2).view(o, ig, h, w)
- else:
- weight_rbr_1x1_kxk = torch.einsum('ti,othw->oihw', weight_rbr_1x1_kxk_conv1, weight_rbr_1x1_kxk_conv2)
-
- weight_rbr_1x1_kxk = torch.einsum('oihw,o->oihw', weight_rbr_1x1_kxk, self.vector[3, :])
-
- weight_rbr_gconv = self.dwsc2full(self.weight_rbr_gconv_dw, self.weight_rbr_gconv_pw, self.in_channels)
- weight_rbr_gconv = torch.einsum('oihw,o->oihw', weight_rbr_gconv, self.vector[4, :])
-
- weight = weight_rbr_origin + weight_rbr_avg + weight_rbr_1x1_kxk + weight_rbr_pfir + weight_rbr_gconv
-
- return weight
-
- def dwsc2full(self, weight_dw, weight_pw, groups):
-
- t, ig, h, w = weight_dw.size()
- o, _, _, _ = weight_pw.size()
- tg = int(t/groups)
- i = int(ig*groups)
- weight_dw = weight_dw.view(groups, tg, ig, h, w)
- weight_pw = weight_pw.squeeze().view(o, groups, tg)
-
- weight_dsc = torch.einsum('gtihw,ogt->ogihw', weight_dw, weight_pw)
- return weight_dsc.view(o, i, h, w)
-
- def forward(self, inputs):
- weight = self.weight_gen()
- out = F.conv2d(inputs, weight, bias=None, stride=self.stride, padding=self.padding, dilation=self.dilation, groups=self.groups)
-
- return self.nonlinear(self.bn(out))
-
-class RepConv_OREPA(nn.Module):
-
- def __init__(self, c1, c2, k=3, s=1, padding=1, dilation=1, groups=1, padding_mode='zeros', deploy=False, use_se=False, nonlinear=nn.SiLU()):
- super(RepConv_OREPA, self).__init__()
- self.deploy = deploy
- self.groups = groups
- self.in_channels = c1
- self.out_channels = c2
-
- self.padding = padding
- self.dilation = dilation
- self.groups = groups
-
- assert k == 3
- assert padding == 1
-
- padding_11 = padding - k // 2
-
- if nonlinear is None:
- self.nonlinearity = nn.Identity()
- else:
- self.nonlinearity = nonlinear
-
- if use_se:
- self.se = SEBlock(self.out_channels, internal_neurons=self.out_channels // 16)
- else:
- self.se = nn.Identity()
-
- if deploy:
- self.rbr_reparam = nn.Conv2d(in_channels=self.in_channels, out_channels=self.out_channels, kernel_size=k, stride=s,
- padding=padding, dilation=dilation, groups=groups, bias=True, padding_mode=padding_mode)
-
- else:
- self.rbr_identity = nn.BatchNorm2d(num_features=self.in_channels) if self.out_channels == self.in_channels and s == 1 else None
- self.rbr_dense = OREPA_3x3_RepConv(in_channels=self.in_channels, out_channels=self.out_channels, kernel_size=k, stride=s, padding=padding, groups=groups, dilation=1)
- self.rbr_1x1 = ConvBN(in_channels=self.in_channels, out_channels=self.out_channels, kernel_size=1, stride=s, padding=padding_11, groups=groups, dilation=1)
- print('RepVGG Block, identity = ', self.rbr_identity)
-
-
- def forward(self, inputs):
- if hasattr(self, 'rbr_reparam'):
- return self.nonlinearity(self.se(self.rbr_reparam(inputs)))
-
- if self.rbr_identity is None:
- id_out = 0
- else:
- id_out = self.rbr_identity(inputs)
-
- out1 = self.rbr_dense(inputs)
- out2 = self.rbr_1x1(inputs)
- out3 = id_out
- out = out1 + out2 + out3
-
- return self.nonlinearity(self.se(out))
-
-
- # Optional. This improves the accuracy and facilitates quantization.
- # 1. Cancel the original weight decay on rbr_dense.conv.weight and rbr_1x1.conv.weight.
- # 2. Use like this.
- # loss = criterion(....)
- # for every RepVGGBlock blk:
- # loss += weight_decay_coefficient * 0.5 * blk.get_cust_L2()
- # optimizer.zero_grad()
- # loss.backward()
-
- # Not used for OREPA
- def get_custom_L2(self):
- K3 = self.rbr_dense.weight_gen()
- K1 = self.rbr_1x1.conv.weight
- t3 = (self.rbr_dense.bn.weight / ((self.rbr_dense.bn.running_var + self.rbr_dense.bn.eps).sqrt())).reshape(-1, 1, 1, 1).detach()
- t1 = (self.rbr_1x1.bn.weight / ((self.rbr_1x1.bn.running_var + self.rbr_1x1.bn.eps).sqrt())).reshape(-1, 1, 1, 1).detach()
-
- l2_loss_circle = (K3 ** 2).sum() - (K3[:, :, 1:2, 1:2] ** 2).sum() # The L2 loss of the "circle" of weights in 3x3 kernel. Use regular L2 on them.
- eq_kernel = K3[:, :, 1:2, 1:2] * t3 + K1 * t1 # The equivalent resultant central point of 3x3 kernel.
- l2_loss_eq_kernel = (eq_kernel ** 2 / (t3 ** 2 + t1 ** 2)).sum() # Normalize for an L2 coefficient comparable to regular L2.
- return l2_loss_eq_kernel + l2_loss_circle
-
- def get_equivalent_kernel_bias(self):
- kernel3x3, bias3x3 = self._fuse_bn_tensor(self.rbr_dense)
- kernel1x1, bias1x1 = self._fuse_bn_tensor(self.rbr_1x1)
- kernelid, biasid = self._fuse_bn_tensor(self.rbr_identity)
- return kernel3x3 + self._pad_1x1_to_3x3_tensor(kernel1x1) + kernelid, bias3x3 + bias1x1 + biasid
-
- def _pad_1x1_to_3x3_tensor(self, kernel1x1):
- if kernel1x1 is None:
- return 0
- else:
- return torch.nn.functional.pad(kernel1x1, [1,1,1,1])
-
- def _fuse_bn_tensor(self, branch):
- if branch is None:
- return 0, 0
- if not isinstance(branch, nn.BatchNorm2d):
- if isinstance(branch, OREPA_3x3_RepConv):
- kernel = branch.weight_gen()
- elif isinstance(branch, ConvBN):
- kernel = branch.conv.weight
- else:
- raise NotImplementedError
- running_mean = branch.bn.running_mean
- running_var = branch.bn.running_var
- gamma = branch.bn.weight
- beta = branch.bn.bias
- eps = branch.bn.eps
- else:
- if not hasattr(self, 'id_tensor'):
- input_dim = self.in_channels // self.groups
- kernel_value = np.zeros((self.in_channels, input_dim, 3, 3), dtype=np.float32)
- for i in range(self.in_channels):
- kernel_value[i, i % input_dim, 1, 1] = 1
- self.id_tensor = torch.from_numpy(kernel_value).to(branch.weight.device)
- kernel = self.id_tensor
- running_mean = branch.running_mean
- running_var = branch.running_var
- gamma = branch.weight
- beta = branch.bias
- eps = branch.eps
- std = (running_var + eps).sqrt()
- t = (gamma / std).reshape(-1, 1, 1, 1)
- return kernel * t, beta - running_mean * gamma / std
-
- def switch_to_deploy(self):
- if hasattr(self, 'rbr_reparam'):
- return
- print(f"RepConv_OREPA.switch_to_deploy")
- kernel, bias = self.get_equivalent_kernel_bias()
- self.rbr_reparam = nn.Conv2d(in_channels=self.rbr_dense.in_channels, out_channels=self.rbr_dense.out_channels,
- kernel_size=self.rbr_dense.kernel_size, stride=self.rbr_dense.stride,
- padding=self.rbr_dense.padding, dilation=self.rbr_dense.dilation, groups=self.rbr_dense.groups, bias=True)
- self.rbr_reparam.weight.data = kernel
- self.rbr_reparam.bias.data = bias
- for para in self.parameters():
- para.detach_()
- self.__delattr__('rbr_dense')
- self.__delattr__('rbr_1x1')
- if hasattr(self, 'rbr_identity'):
- self.__delattr__('rbr_identity')
-
-##### end of orepa #####
-
-
-##### swin transformer #####
-
-class WindowAttention(nn.Module):
-
- def __init__(self, dim, window_size, num_heads, qkv_bias=True, qk_scale=None, attn_drop=0., proj_drop=0.):
-
- super().__init__()
- self.dim = dim
- self.window_size = window_size # Wh, Ww
- self.num_heads = num_heads
- head_dim = dim // num_heads
- self.scale = qk_scale or head_dim ** -0.5
-
- # define a parameter table of relative position bias
- self.relative_position_bias_table = nn.Parameter(
- torch.zeros((2 * window_size[0] - 1) * (2 * window_size[1] - 1), num_heads)) # 2*Wh-1 * 2*Ww-1, nH
-
- # get pair-wise relative position index for each token inside the window
- coords_h = torch.arange(self.window_size[0])
- coords_w = torch.arange(self.window_size[1])
- coords = torch.stack(torch.meshgrid([coords_h, coords_w])) # 2, Wh, Ww
- coords_flatten = torch.flatten(coords, 1) # 2, Wh*Ww
- relative_coords = coords_flatten[:, :, None] - coords_flatten[:, None, :] # 2, Wh*Ww, Wh*Ww
- relative_coords = relative_coords.permute(1, 2, 0).contiguous() # Wh*Ww, Wh*Ww, 2
- relative_coords[:, :, 0] += self.window_size[0] - 1 # shift to start from 0
- relative_coords[:, :, 1] += self.window_size[1] - 1
- relative_coords[:, :, 0] *= 2 * self.window_size[1] - 1
- relative_position_index = relative_coords.sum(-1) # Wh*Ww, Wh*Ww
- self.register_buffer("relative_position_index", relative_position_index)
-
- self.qkv = nn.Linear(dim, dim * 3, bias=qkv_bias)
- self.attn_drop = nn.Dropout(attn_drop)
- self.proj = nn.Linear(dim, dim)
- self.proj_drop = nn.Dropout(proj_drop)
-
- nn.init.normal_(self.relative_position_bias_table, std=.02)
- self.softmax = nn.Softmax(dim=-1)
-
- def forward(self, x, mask=None):
-
- B_, N, C = x.shape
- qkv = self.qkv(x).reshape(B_, N, 3, self.num_heads, C // self.num_heads).permute(2, 0, 3, 1, 4)
- q, k, v = qkv[0], qkv[1], qkv[2] # make torchscript happy (cannot use tensor as tuple)
-
- q = q * self.scale
- attn = (q @ k.transpose(-2, -1))
-
- relative_position_bias = self.relative_position_bias_table[self.relative_position_index.view(-1)].view(
- self.window_size[0] * self.window_size[1], self.window_size[0] * self.window_size[1], -1) # Wh*Ww,Wh*Ww,nH
- relative_position_bias = relative_position_bias.permute(2, 0, 1).contiguous() # nH, Wh*Ww, Wh*Ww
- attn = attn + relative_position_bias.unsqueeze(0)
-
- if mask is not None:
- nW = mask.shape[0]
- attn = attn.view(B_ // nW, nW, self.num_heads, N, N) + mask.unsqueeze(1).unsqueeze(0)
- attn = attn.view(-1, self.num_heads, N, N)
- attn = self.softmax(attn)
- else:
- attn = self.softmax(attn)
-
- attn = self.attn_drop(attn)
-
- # print(attn.dtype, v.dtype)
- try:
- x = (attn @ v).transpose(1, 2).reshape(B_, N, C)
- except:
- #print(attn.dtype, v.dtype)
- x = (attn.half() @ v).transpose(1, 2).reshape(B_, N, C)
- x = self.proj(x)
- x = self.proj_drop(x)
- return x
-
-class Mlp(nn.Module):
-
- def __init__(self, in_features, hidden_features=None, out_features=None, act_layer=nn.SiLU, drop=0.):
- super().__init__()
- out_features = out_features or in_features
- hidden_features = hidden_features or in_features
- self.fc1 = nn.Linear(in_features, hidden_features)
- self.act = act_layer()
- self.fc2 = nn.Linear(hidden_features, out_features)
- self.drop = nn.Dropout(drop)
-
- def forward(self, x):
- x = self.fc1(x)
- x = self.act(x)
- x = self.drop(x)
- x = self.fc2(x)
- x = self.drop(x)
- return x
-
-def window_partition(x, window_size):
-
- B, H, W, C = x.shape
- assert H % window_size == 0, 'feature map h and w can not divide by window size'
- x = x.view(B, H // window_size, window_size, W // window_size, window_size, C)
- windows = x.permute(0, 1, 3, 2, 4, 5).contiguous().view(-1, window_size, window_size, C)
- return windows
-
-def window_reverse(windows, window_size, H, W):
-
- B = int(windows.shape[0] / (H * W / window_size / window_size))
- x = windows.view(B, H // window_size, W // window_size, window_size, window_size, -1)
- x = x.permute(0, 1, 3, 2, 4, 5).contiguous().view(B, H, W, -1)
- return x
-
-
-class SwinTransformerLayer(nn.Module):
-
- def __init__(self, dim, num_heads, window_size=8, shift_size=0,
- mlp_ratio=4., qkv_bias=True, qk_scale=None, drop=0., attn_drop=0., drop_path=0.,
- act_layer=nn.SiLU, norm_layer=nn.LayerNorm):
- super().__init__()
- self.dim = dim
- self.num_heads = num_heads
- self.window_size = window_size
- self.shift_size = shift_size
- self.mlp_ratio = mlp_ratio
- # if min(self.input_resolution) <= self.window_size:
- # # if window size is larger than input resolution, we don't partition windows
- # self.shift_size = 0
- # self.window_size = min(self.input_resolution)
- assert 0 <= self.shift_size < self.window_size, "shift_size must in 0-window_size"
-
- self.norm1 = norm_layer(dim)
- self.attn = WindowAttention(
- dim, window_size=(self.window_size, self.window_size), num_heads=num_heads,
- qkv_bias=qkv_bias, qk_scale=qk_scale, attn_drop=attn_drop, proj_drop=drop)
-
- self.drop_path = DropPath(drop_path) if drop_path > 0. else nn.Identity()
- self.norm2 = norm_layer(dim)
- mlp_hidden_dim = int(dim * mlp_ratio)
- self.mlp = Mlp(in_features=dim, hidden_features=mlp_hidden_dim, act_layer=act_layer, drop=drop)
-
- def create_mask(self, H, W):
- # calculate attention mask for SW-MSA
- img_mask = torch.zeros((1, H, W, 1)) # 1 H W 1
- h_slices = (slice(0, -self.window_size),
- slice(-self.window_size, -self.shift_size),
- slice(-self.shift_size, None))
- w_slices = (slice(0, -self.window_size),
- slice(-self.window_size, -self.shift_size),
- slice(-self.shift_size, None))
- cnt = 0
- for h in h_slices:
- for w in w_slices:
- img_mask[:, h, w, :] = cnt
- cnt += 1
-
- mask_windows = window_partition(img_mask, self.window_size) # nW, window_size, window_size, 1
- mask_windows = mask_windows.view(-1, self.window_size * self.window_size)
- attn_mask = mask_windows.unsqueeze(1) - mask_windows.unsqueeze(2)
- attn_mask = attn_mask.masked_fill(attn_mask != 0, float(-100.0)).masked_fill(attn_mask == 0, float(0.0))
-
- return attn_mask
-
- def forward(self, x):
- # reshape x[b c h w] to x[b l c]
- _, _, H_, W_ = x.shape
-
- Padding = False
- if min(H_, W_) < self.window_size or H_ % self.window_size!=0 or W_ % self.window_size!=0:
- Padding = True
- # print(f'img_size {min(H_, W_)} is less than (or not divided by) window_size {self.window_size}, Padding.')
- pad_r = (self.window_size - W_ % self.window_size) % self.window_size
- pad_b = (self.window_size - H_ % self.window_size) % self.window_size
- x = F.pad(x, (0, pad_r, 0, pad_b))
-
- # print('2', x.shape)
- B, C, H, W = x.shape
- L = H * W
- x = x.permute(0, 2, 3, 1).contiguous().view(B, L, C) # b, L, c
-
- # create mask from init to forward
- if self.shift_size > 0:
- attn_mask = self.create_mask(H, W).to(x.device)
- else:
- attn_mask = None
-
- shortcut = x
- x = self.norm1(x)
- x = x.view(B, H, W, C)
-
- # cyclic shift
- if self.shift_size > 0:
- shifted_x = torch.roll(x, shifts=(-self.shift_size, -self.shift_size), dims=(1, 2))
- else:
- shifted_x = x
-
- # partition windows
- x_windows = window_partition(shifted_x, self.window_size) # nW*B, window_size, window_size, C
- x_windows = x_windows.view(-1, self.window_size * self.window_size, C) # nW*B, window_size*window_size, C
-
- # W-MSA/SW-MSA
- attn_windows = self.attn(x_windows, mask=attn_mask) # nW*B, window_size*window_size, C
-
- # merge windows
- attn_windows = attn_windows.view(-1, self.window_size, self.window_size, C)
- shifted_x = window_reverse(attn_windows, self.window_size, H, W) # B H' W' C
-
- # reverse cyclic shift
- if self.shift_size > 0:
- x = torch.roll(shifted_x, shifts=(self.shift_size, self.shift_size), dims=(1, 2))
- else:
- x = shifted_x
- x = x.view(B, H * W, C)
-
- # FFN
- x = shortcut + self.drop_path(x)
- x = x + self.drop_path(self.mlp(self.norm2(x)))
-
- x = x.permute(0, 2, 1).contiguous().view(-1, C, H, W) # b c h w
-
- if Padding:
- x = x[:, :, :H_, :W_] # reverse padding
-
- return x
-
-
-class SwinTransformerBlock(nn.Module):
- def __init__(self, c1, c2, num_heads, num_layers, window_size=8):
- super().__init__()
- self.conv = None
- if c1 != c2:
- self.conv = Conv(c1, c2)
-
- # remove input_resolution
- self.blocks = nn.Sequential(*[SwinTransformerLayer(dim=c2, num_heads=num_heads, window_size=window_size,
- shift_size=0 if (i % 2 == 0) else window_size // 2) for i in range(num_layers)])
-
- def forward(self, x):
- if self.conv is not None:
- x = self.conv(x)
- x = self.blocks(x)
- return x
-
-
-class STCSPA(nn.Module):
- # CSP Bottleneck https://github.com/WongKinYiu/CrossStagePartialNetworks
- def __init__(self, c1, c2, n=1, shortcut=True, g=1, e=0.5): # ch_in, ch_out, number, shortcut, groups, expansion
- super(STCSPA, self).__init__()
- c_ = int(c2 * e) # hidden channels
- self.cv1 = Conv(c1, c_, 1, 1)
- self.cv2 = Conv(c1, c_, 1, 1)
- self.cv3 = Conv(2 * c_, c2, 1, 1)
- num_heads = c_ // 32
- self.m = SwinTransformerBlock(c_, c_, num_heads, n)
- #self.m = nn.Sequential(*[Bottleneck(c_, c_, shortcut, g, e=1.0) for _ in range(n)])
-
- def forward(self, x):
- y1 = self.m(self.cv1(x))
- y2 = self.cv2(x)
- return self.cv3(torch.cat((y1, y2), dim=1))
-
-
-class STCSPB(nn.Module):
- # CSP Bottleneck https://github.com/WongKinYiu/CrossStagePartialNetworks
- def __init__(self, c1, c2, n=1, shortcut=False, g=1, e=0.5): # ch_in, ch_out, number, shortcut, groups, expansion
- super(STCSPB, self).__init__()
- c_ = int(c2) # hidden channels
- self.cv1 = Conv(c1, c_, 1, 1)
- self.cv2 = Conv(c_, c_, 1, 1)
- self.cv3 = Conv(2 * c_, c2, 1, 1)
- num_heads = c_ // 32
- self.m = SwinTransformerBlock(c_, c_, num_heads, n)
- #self.m = nn.Sequential(*[Bottleneck(c_, c_, shortcut, g, e=1.0) for _ in range(n)])
-
- def forward(self, x):
- x1 = self.cv1(x)
- y1 = self.m(x1)
- y2 = self.cv2(x1)
- return self.cv3(torch.cat((y1, y2), dim=1))
-
-
-class STCSPC(nn.Module):
- # CSP Bottleneck https://github.com/WongKinYiu/CrossStagePartialNetworks
- def __init__(self, c1, c2, n=1, shortcut=True, g=1, e=0.5): # ch_in, ch_out, number, shortcut, groups, expansion
- super(STCSPC, self).__init__()
- c_ = int(c2 * e) # hidden channels
- self.cv1 = Conv(c1, c_, 1, 1)
- self.cv2 = Conv(c1, c_, 1, 1)
- self.cv3 = Conv(c_, c_, 1, 1)
- self.cv4 = Conv(2 * c_, c2, 1, 1)
- num_heads = c_ // 32
- self.m = SwinTransformerBlock(c_, c_, num_heads, n)
- #self.m = nn.Sequential(*[Bottleneck(c_, c_, shortcut, g, e=1.0) for _ in range(n)])
-
- def forward(self, x):
- y1 = self.cv3(self.m(self.cv1(x)))
- y2 = self.cv2(x)
- return self.cv4(torch.cat((y1, y2), dim=1))
-
-##### end of swin transformer #####
-
-
-##### swin transformer v2 #####
-
-class WindowAttention_v2(nn.Module):
-
- def __init__(self, dim, window_size, num_heads, qkv_bias=True, attn_drop=0., proj_drop=0.,
- pretrained_window_size=[0, 0]):
-
- super().__init__()
- self.dim = dim
- self.window_size = window_size # Wh, Ww
- self.pretrained_window_size = pretrained_window_size
- self.num_heads = num_heads
-
- self.logit_scale = nn.Parameter(torch.log(10 * torch.ones((num_heads, 1, 1))), requires_grad=True)
-
- # mlp to generate continuous relative position bias
- self.cpb_mlp = nn.Sequential(nn.Linear(2, 512, bias=True),
- nn.ReLU(inplace=True),
- nn.Linear(512, num_heads, bias=False))
-
- # get relative_coords_table
- relative_coords_h = torch.arange(-(self.window_size[0] - 1), self.window_size[0], dtype=torch.float32)
- relative_coords_w = torch.arange(-(self.window_size[1] - 1), self.window_size[1], dtype=torch.float32)
- relative_coords_table = torch.stack(
- torch.meshgrid([relative_coords_h,
- relative_coords_w])).permute(1, 2, 0).contiguous().unsqueeze(0) # 1, 2*Wh-1, 2*Ww-1, 2
- if pretrained_window_size[0] > 0:
- relative_coords_table[:, :, :, 0] /= (pretrained_window_size[0] - 1)
- relative_coords_table[:, :, :, 1] /= (pretrained_window_size[1] - 1)
- else:
- relative_coords_table[:, :, :, 0] /= (self.window_size[0] - 1)
- relative_coords_table[:, :, :, 1] /= (self.window_size[1] - 1)
- relative_coords_table *= 8 # normalize to -8, 8
- relative_coords_table = torch.sign(relative_coords_table) * torch.log2(
- torch.abs(relative_coords_table) + 1.0) / np.log2(8)
-
- self.register_buffer("relative_coords_table", relative_coords_table)
-
- # get pair-wise relative position index for each token inside the window
- coords_h = torch.arange(self.window_size[0])
- coords_w = torch.arange(self.window_size[1])
- coords = torch.stack(torch.meshgrid([coords_h, coords_w])) # 2, Wh, Ww
- coords_flatten = torch.flatten(coords, 1) # 2, Wh*Ww
- relative_coords = coords_flatten[:, :, None] - coords_flatten[:, None, :] # 2, Wh*Ww, Wh*Ww
- relative_coords = relative_coords.permute(1, 2, 0).contiguous() # Wh*Ww, Wh*Ww, 2
- relative_coords[:, :, 0] += self.window_size[0] - 1 # shift to start from 0
- relative_coords[:, :, 1] += self.window_size[1] - 1
- relative_coords[:, :, 0] *= 2 * self.window_size[1] - 1
- relative_position_index = relative_coords.sum(-1) # Wh*Ww, Wh*Ww
- self.register_buffer("relative_position_index", relative_position_index)
-
- self.qkv = nn.Linear(dim, dim * 3, bias=False)
- if qkv_bias:
- self.q_bias = nn.Parameter(torch.zeros(dim))
- self.v_bias = nn.Parameter(torch.zeros(dim))
- else:
- self.q_bias = None
- self.v_bias = None
- self.attn_drop = nn.Dropout(attn_drop)
- self.proj = nn.Linear(dim, dim)
- self.proj_drop = nn.Dropout(proj_drop)
- self.softmax = nn.Softmax(dim=-1)
-
- def forward(self, x, mask=None):
-
- B_, N, C = x.shape
- qkv_bias = None
- if self.q_bias is not None:
- qkv_bias = torch.cat((self.q_bias, torch.zeros_like(self.v_bias, requires_grad=False), self.v_bias))
- qkv = F.linear(input=x, weight=self.qkv.weight, bias=qkv_bias)
- qkv = qkv.reshape(B_, N, 3, self.num_heads, -1).permute(2, 0, 3, 1, 4)
- q, k, v = qkv[0], qkv[1], qkv[2] # make torchscript happy (cannot use tensor as tuple)
-
- # cosine attention
- attn = (F.normalize(q, dim=-1) @ F.normalize(k, dim=-1).transpose(-2, -1))
- logit_scale = torch.clamp(self.logit_scale, max=torch.log(torch.tensor(1. / 0.01))).exp()
- attn = attn * logit_scale
-
- relative_position_bias_table = self.cpb_mlp(self.relative_coords_table).view(-1, self.num_heads)
- relative_position_bias = relative_position_bias_table[self.relative_position_index.view(-1)].view(
- self.window_size[0] * self.window_size[1], self.window_size[0] * self.window_size[1], -1) # Wh*Ww,Wh*Ww,nH
- relative_position_bias = relative_position_bias.permute(2, 0, 1).contiguous() # nH, Wh*Ww, Wh*Ww
- relative_position_bias = 16 * torch.sigmoid(relative_position_bias)
- attn = attn + relative_position_bias.unsqueeze(0)
-
- if mask is not None:
- nW = mask.shape[0]
- attn = attn.view(B_ // nW, nW, self.num_heads, N, N) + mask.unsqueeze(1).unsqueeze(0)
- attn = attn.view(-1, self.num_heads, N, N)
- attn = self.softmax(attn)
- else:
- attn = self.softmax(attn)
-
- attn = self.attn_drop(attn)
-
- try:
- x = (attn @ v).transpose(1, 2).reshape(B_, N, C)
- except:
- x = (attn.half() @ v).transpose(1, 2).reshape(B_, N, C)
-
- x = self.proj(x)
- x = self.proj_drop(x)
- return x
-
- def extra_repr(self) -> str:
- return f'dim={self.dim}, window_size={self.window_size}, ' \
- f'pretrained_window_size={self.pretrained_window_size}, num_heads={self.num_heads}'
-
- def flops(self, N):
- # calculate flops for 1 window with token length of N
- flops = 0
- # qkv = self.qkv(x)
- flops += N * self.dim * 3 * self.dim
- # attn = (q @ k.transpose(-2, -1))
- flops += self.num_heads * N * (self.dim // self.num_heads) * N
- # x = (attn @ v)
- flops += self.num_heads * N * N * (self.dim // self.num_heads)
- # x = self.proj(x)
- flops += N * self.dim * self.dim
- return flops
-
-class Mlp_v2(nn.Module):
- def __init__(self, in_features, hidden_features=None, out_features=None, act_layer=nn.SiLU, drop=0.):
- super().__init__()
- out_features = out_features or in_features
- hidden_features = hidden_features or in_features
- self.fc1 = nn.Linear(in_features, hidden_features)
- self.act = act_layer()
- self.fc2 = nn.Linear(hidden_features, out_features)
- self.drop = nn.Dropout(drop)
-
- def forward(self, x):
- x = self.fc1(x)
- x = self.act(x)
- x = self.drop(x)
- x = self.fc2(x)
- x = self.drop(x)
- return x
-
-
-def window_partition_v2(x, window_size):
-
- B, H, W, C = x.shape
- x = x.view(B, H // window_size, window_size, W // window_size, window_size, C)
- windows = x.permute(0, 1, 3, 2, 4, 5).contiguous().view(-1, window_size, window_size, C)
- return windows
-
-
-def window_reverse_v2(windows, window_size, H, W):
-
- B = int(windows.shape[0] / (H * W / window_size / window_size))
- x = windows.view(B, H // window_size, W // window_size, window_size, window_size, -1)
- x = x.permute(0, 1, 3, 2, 4, 5).contiguous().view(B, H, W, -1)
- return x
-
-
-class SwinTransformerLayer_v2(nn.Module):
-
- def __init__(self, dim, num_heads, window_size=7, shift_size=0,
- mlp_ratio=4., qkv_bias=True, drop=0., attn_drop=0., drop_path=0.,
- act_layer=nn.SiLU, norm_layer=nn.LayerNorm, pretrained_window_size=0):
- super().__init__()
- self.dim = dim
- #self.input_resolution = input_resolution
- self.num_heads = num_heads
- self.window_size = window_size
- self.shift_size = shift_size
- self.mlp_ratio = mlp_ratio
- #if min(self.input_resolution) <= self.window_size:
- # # if window size is larger than input resolution, we don't partition windows
- # self.shift_size = 0
- # self.window_size = min(self.input_resolution)
- assert 0 <= self.shift_size < self.window_size, "shift_size must in 0-window_size"
-
- self.norm1 = norm_layer(dim)
- self.attn = WindowAttention_v2(
- dim, window_size=(self.window_size, self.window_size), num_heads=num_heads,
- qkv_bias=qkv_bias, attn_drop=attn_drop, proj_drop=drop,
- pretrained_window_size=(pretrained_window_size, pretrained_window_size))
-
- self.drop_path = DropPath(drop_path) if drop_path > 0. else nn.Identity()
- self.norm2 = norm_layer(dim)
- mlp_hidden_dim = int(dim * mlp_ratio)
- self.mlp = Mlp_v2(in_features=dim, hidden_features=mlp_hidden_dim, act_layer=act_layer, drop=drop)
-
- def create_mask(self, H, W):
- # calculate attention mask for SW-MSA
- img_mask = torch.zeros((1, H, W, 1)) # 1 H W 1
- h_slices = (slice(0, -self.window_size),
- slice(-self.window_size, -self.shift_size),
- slice(-self.shift_size, None))
- w_slices = (slice(0, -self.window_size),
- slice(-self.window_size, -self.shift_size),
- slice(-self.shift_size, None))
- cnt = 0
- for h in h_slices:
- for w in w_slices:
- img_mask[:, h, w, :] = cnt
- cnt += 1
-
- mask_windows = window_partition(img_mask, self.window_size) # nW, window_size, window_size, 1
- mask_windows = mask_windows.view(-1, self.window_size * self.window_size)
- attn_mask = mask_windows.unsqueeze(1) - mask_windows.unsqueeze(2)
- attn_mask = attn_mask.masked_fill(attn_mask != 0, float(-100.0)).masked_fill(attn_mask == 0, float(0.0))
-
- return attn_mask
-
- def forward(self, x):
- # reshape x[b c h w] to x[b l c]
- _, _, H_, W_ = x.shape
-
- Padding = False
- if min(H_, W_) < self.window_size or H_ % self.window_size!=0 or W_ % self.window_size!=0:
- Padding = True
- # print(f'img_size {min(H_, W_)} is less than (or not divided by) window_size {self.window_size}, Padding.')
- pad_r = (self.window_size - W_ % self.window_size) % self.window_size
- pad_b = (self.window_size - H_ % self.window_size) % self.window_size
- x = F.pad(x, (0, pad_r, 0, pad_b))
-
- # print('2', x.shape)
- B, C, H, W = x.shape
- L = H * W
- x = x.permute(0, 2, 3, 1).contiguous().view(B, L, C) # b, L, c
-
- # create mask from init to forward
- if self.shift_size > 0:
- attn_mask = self.create_mask(H, W).to(x.device)
- else:
- attn_mask = None
-
- shortcut = x
- x = x.view(B, H, W, C)
-
- # cyclic shift
- if self.shift_size > 0:
- shifted_x = torch.roll(x, shifts=(-self.shift_size, -self.shift_size), dims=(1, 2))
- else:
- shifted_x = x
-
- # partition windows
- x_windows = window_partition_v2(shifted_x, self.window_size) # nW*B, window_size, window_size, C
- x_windows = x_windows.view(-1, self.window_size * self.window_size, C) # nW*B, window_size*window_size, C
-
- # W-MSA/SW-MSA
- attn_windows = self.attn(x_windows, mask=attn_mask) # nW*B, window_size*window_size, C
-
- # merge windows
- attn_windows = attn_windows.view(-1, self.window_size, self.window_size, C)
- shifted_x = window_reverse_v2(attn_windows, self.window_size, H, W) # B H' W' C
-
- # reverse cyclic shift
- if self.shift_size > 0:
- x = torch.roll(shifted_x, shifts=(self.shift_size, self.shift_size), dims=(1, 2))
- else:
- x = shifted_x
- x = x.view(B, H * W, C)
- x = shortcut + self.drop_path(self.norm1(x))
-
- # FFN
- x = x + self.drop_path(self.norm2(self.mlp(x)))
- x = x.permute(0, 2, 1).contiguous().view(-1, C, H, W) # b c h w
-
- if Padding:
- x = x[:, :, :H_, :W_] # reverse padding
-
- return x
-
- def extra_repr(self) -> str:
- return f"dim={self.dim}, input_resolution={self.input_resolution}, num_heads={self.num_heads}, " \
- f"window_size={self.window_size}, shift_size={self.shift_size}, mlp_ratio={self.mlp_ratio}"
-
- def flops(self):
- flops = 0
- H, W = self.input_resolution
- # norm1
- flops += self.dim * H * W
- # W-MSA/SW-MSA
- nW = H * W / self.window_size / self.window_size
- flops += nW * self.attn.flops(self.window_size * self.window_size)
- # mlp
- flops += 2 * H * W * self.dim * self.dim * self.mlp_ratio
- # norm2
- flops += self.dim * H * W
- return flops
-
-
-class SwinTransformer2Block(nn.Module):
- def __init__(self, c1, c2, num_heads, num_layers, window_size=7):
- super().__init__()
- self.conv = None
- if c1 != c2:
- self.conv = Conv(c1, c2)
-
- # remove input_resolution
- self.blocks = nn.Sequential(*[SwinTransformerLayer_v2(dim=c2, num_heads=num_heads, window_size=window_size,
- shift_size=0 if (i % 2 == 0) else window_size // 2) for i in range(num_layers)])
-
- def forward(self, x):
- if self.conv is not None:
- x = self.conv(x)
- x = self.blocks(x)
- return x
-
-
-class ST2CSPA(nn.Module):
- # CSP Bottleneck https://github.com/WongKinYiu/CrossStagePartialNetworks
- def __init__(self, c1, c2, n=1, shortcut=True, g=1, e=0.5): # ch_in, ch_out, number, shortcut, groups, expansion
- super(ST2CSPA, self).__init__()
- c_ = int(c2 * e) # hidden channels
- self.cv1 = Conv(c1, c_, 1, 1)
- self.cv2 = Conv(c1, c_, 1, 1)
- self.cv3 = Conv(2 * c_, c2, 1, 1)
- num_heads = c_ // 32
- self.m = SwinTransformer2Block(c_, c_, num_heads, n)
- #self.m = nn.Sequential(*[Bottleneck(c_, c_, shortcut, g, e=1.0) for _ in range(n)])
-
- def forward(self, x):
- y1 = self.m(self.cv1(x))
- y2 = self.cv2(x)
- return self.cv3(torch.cat((y1, y2), dim=1))
-
-
-class ST2CSPB(nn.Module):
- # CSP Bottleneck https://github.com/WongKinYiu/CrossStagePartialNetworks
- def __init__(self, c1, c2, n=1, shortcut=False, g=1, e=0.5): # ch_in, ch_out, number, shortcut, groups, expansion
- super(ST2CSPB, self).__init__()
- c_ = int(c2) # hidden channels
- self.cv1 = Conv(c1, c_, 1, 1)
- self.cv2 = Conv(c_, c_, 1, 1)
- self.cv3 = Conv(2 * c_, c2, 1, 1)
- num_heads = c_ // 32
- self.m = SwinTransformer2Block(c_, c_, num_heads, n)
- #self.m = nn.Sequential(*[Bottleneck(c_, c_, shortcut, g, e=1.0) for _ in range(n)])
-
- def forward(self, x):
- x1 = self.cv1(x)
- y1 = self.m(x1)
- y2 = self.cv2(x1)
- return self.cv3(torch.cat((y1, y2), dim=1))
-
-
-class ST2CSPC(nn.Module):
- # CSP Bottleneck https://github.com/WongKinYiu/CrossStagePartialNetworks
- def __init__(self, c1, c2, n=1, shortcut=True, g=1, e=0.5): # ch_in, ch_out, number, shortcut, groups, expansion
- super(ST2CSPC, self).__init__()
- c_ = int(c2 * e) # hidden channels
- self.cv1 = Conv(c1, c_, 1, 1)
- self.cv2 = Conv(c1, c_, 1, 1)
- self.cv3 = Conv(c_, c_, 1, 1)
- self.cv4 = Conv(2 * c_, c2, 1, 1)
- num_heads = c_ // 32
- self.m = SwinTransformer2Block(c_, c_, num_heads, n)
- #self.m = nn.Sequential(*[Bottleneck(c_, c_, shortcut, g, e=1.0) for _ in range(n)])
-
- def forward(self, x):
- y1 = self.cv3(self.m(self.cv1(x)))
- y2 = self.cv2(x)
- return self.cv4(torch.cat((y1, y2), dim=1))
-
-##### end of swin transformer v2 #####
diff --git a/spaces/Usaki108/VoiceChange/vc_infer_pipeline.py b/spaces/Usaki108/VoiceChange/vc_infer_pipeline.py
deleted file mode 100644
index d69b4f5c26fa743a5ef347fd524c6dba63b00231..0000000000000000000000000000000000000000
--- a/spaces/Usaki108/VoiceChange/vc_infer_pipeline.py
+++ /dev/null
@@ -1,385 +0,0 @@
-import numpy as np, parselmouth, torch, pdb
-from time import time as ttime
-import torch.nn.functional as F
-import scipy.signal as signal
-import pyworld, os, traceback, faiss, librosa, torchcrepe
-from scipy import signal
-from functools import lru_cache
-
-bh, ah = signal.butter(N=5, Wn=48, btype="high", fs=16000)
-
-input_audio_path2wav={}
-
-@lru_cache
-def cache_harvest_f0(input_audio_path,fs,f0max,f0min,frame_period):
- audio=input_audio_path2wav[input_audio_path]
- f0, t = pyworld.harvest(
- audio,
- fs=fs,
- f0_ceil=f0max,
- f0_floor=f0min,
- frame_period=frame_period,
- )
- f0 = pyworld.stonemask(audio, f0, t, fs)
- return f0
-
-def change_rms(data1,sr1,data2,sr2,rate):#1是输入音频,2是输出音频,rate是2的占比
- # print(data1.max(),data2.max())
- rms1 = librosa.feature.rms(y=data1, frame_length=sr1//2*2, hop_length=sr1//2)#每半秒一个点
- rms2 = librosa.feature.rms(y=data2, frame_length=sr2//2*2, hop_length=sr2//2)
- rms1=torch.from_numpy(rms1)
- rms1=F.interpolate(rms1.unsqueeze(0), size=data2.shape[0],mode='linear').squeeze()
- rms2=torch.from_numpy(rms2)
- rms2=F.interpolate(rms2.unsqueeze(0), size=data2.shape[0],mode='linear').squeeze()
- rms2=torch.max(rms2,torch.zeros_like(rms2)+1e-6)
- data2*=(torch.pow(rms1,torch.tensor(1-rate))*torch.pow(rms2,torch.tensor(rate-1))).numpy()
- return data2
-
-class VC(object):
- def __init__(self, tgt_sr, config):
- self.x_pad, self.x_query, self.x_center, self.x_max, self.is_half = (
- config.x_pad,
- config.x_query,
- config.x_center,
- config.x_max,
- config.is_half,
- )
- self.sr = 16000 # hubert输入采样率
- self.window = 160 # 每帧点数
- self.t_pad = self.sr * self.x_pad # 每条前后pad时间
- self.t_pad_tgt = tgt_sr * self.x_pad
- self.t_pad2 = self.t_pad * 2
- self.t_query = self.sr * self.x_query # 查询切点前后查询时间
- self.t_center = self.sr * self.x_center # 查询切点位置
- self.t_max = self.sr * self.x_max # 免查询时长阈值
- self.device = config.device
-
- def get_f0(self, input_audio_path,x, p_len, f0_up_key, f0_method,filter_radius, inp_f0=None):
- global input_audio_path2wav
- time_step = self.window / self.sr * 1000
- f0_min = 50
- f0_max = 1100
- f0_mel_min = 1127 * np.log(1 + f0_min / 700)
- f0_mel_max = 1127 * np.log(1 + f0_max / 700)
- if f0_method == "pm":
- f0 = (
- parselmouth.Sound(x, self.sr)
- .to_pitch_ac(
- time_step=time_step / 1000,
- voicing_threshold=0.6,
- pitch_floor=f0_min,
- pitch_ceiling=f0_max,
- )
- .selected_array["frequency"]
- )
- pad_size = (p_len - len(f0) + 1) // 2
- if pad_size > 0 or p_len - len(f0) - pad_size > 0:
- f0 = np.pad(
- f0, [[pad_size, p_len - len(f0) - pad_size]], mode="constant"
- )
- elif f0_method == "harvest":
- input_audio_path2wav[input_audio_path]=x.astype(np.double)
- f0=cache_harvest_f0(input_audio_path,self.sr,f0_max,f0_min,10)
- if(filter_radius>2):
- f0 = signal.medfilt(f0, 3)
- elif f0_method == "crepe":
- model = "full"
- # Pick a batch size that doesn't cause memory errors on your gpu
- batch_size = 512
- # Compute pitch using first gpu
- audio = torch.tensor(np.copy(x))[None].float()
- f0, pd = torchcrepe.predict(
- audio,
- self.sr,
- self.window,
- f0_min,
- f0_max,
- model,
- batch_size=batch_size,
- device=self.device,
- return_periodicity=True,
- )
- pd = torchcrepe.filter.median(pd, 3)
- f0 = torchcrepe.filter.mean(f0, 3)
- f0[pd < 0.1] = 0
- f0 = f0[0].cpu().numpy()
- f0 *= pow(2, f0_up_key / 12)
- # with open("test.txt","w")as f:f.write("\n".join([str(i)for i in f0.tolist()]))
- tf0 = self.sr // self.window # 每秒f0点数
- if inp_f0 is not None:
- delta_t = np.round(
- (inp_f0[:, 0].max() - inp_f0[:, 0].min()) * tf0 + 1
- ).astype("int16")
- replace_f0 = np.interp(
- list(range(delta_t)), inp_f0[:, 0] * 100, inp_f0[:, 1]
- )
- shape = f0[self.x_pad * tf0 : self.x_pad * tf0 + len(replace_f0)].shape[0]
- f0[self.x_pad * tf0 : self.x_pad * tf0 + len(replace_f0)] = replace_f0[
- :shape
- ]
- # with open("test_opt.txt","w")as f:f.write("\n".join([str(i)for i in f0.tolist()]))
- f0bak = f0.copy()
- f0_mel = 1127 * np.log(1 + f0 / 700)
- f0_mel[f0_mel > 0] = (f0_mel[f0_mel > 0] - f0_mel_min) * 254 / (
- f0_mel_max - f0_mel_min
- ) + 1
- f0_mel[f0_mel <= 1] = 1
- f0_mel[f0_mel > 255] = 255
- f0_coarse = np.rint(f0_mel).astype(int)
- return f0_coarse, f0bak # 1-0
-
- def vc(
- self,
- model,
- net_g,
- sid,
- audio0,
- pitch,
- pitchf,
- times,
- index,
- big_npy,
- index_rate,
- version,
- ): # ,file_index,file_big_npy
- feats = torch.from_numpy(audio0)
- if self.is_half:
- feats = feats.half()
- else:
- feats = feats.float()
- if feats.dim() == 2: # double channels
- feats = feats.mean(-1)
- assert feats.dim() == 1, feats.dim()
- feats = feats.view(1, -1)
- padding_mask = torch.BoolTensor(feats.shape).to(self.device).fill_(False)
-
- inputs = {
- "source": feats.to(self.device),
- "padding_mask": padding_mask,
- "output_layer": 9 if version == "v1" else 12,
- }
- t0 = ttime()
- with torch.no_grad():
- logits = model.extract_features(**inputs)
- feats = model.final_proj(logits[0])if version=="v1"else logits[0]
-
- if (
- isinstance(index, type(None)) == False
- and isinstance(big_npy, type(None)) == False
- and index_rate != 0
- ):
- npy = feats[0].cpu().numpy()
- if self.is_half:
- npy = npy.astype("float32")
-
- # _, I = index.search(npy, 1)
- # npy = big_npy[I.squeeze()]
-
- score, ix = index.search(npy, k=8)
- weight = np.square(1 / score)
- weight /= weight.sum(axis=1, keepdims=True)
- npy = np.sum(big_npy[ix] * np.expand_dims(weight, axis=2), axis=1)
-
- if self.is_half:
- npy = npy.astype("float16")
- feats = (
- torch.from_numpy(npy).unsqueeze(0).to(self.device) * index_rate
- + (1 - index_rate) * feats
- )
-
- feats = F.interpolate(feats.permute(0, 2, 1), scale_factor=2).permute(0, 2, 1)
- t1 = ttime()
- p_len = audio0.shape[0] // self.window
- if feats.shape[1] < p_len:
- p_len = feats.shape[1]
- if pitch != None and pitchf != None:
- pitch = pitch[:, :p_len]
- pitchf = pitchf[:, :p_len]
- p_len = torch.tensor([p_len], device=self.device).long()
- with torch.no_grad():
- if pitch != None and pitchf != None:
- audio1 = (
- (net_g.infer(feats, p_len, pitch, pitchf, sid)[0][0, 0])
- .data.cpu()
- .float()
- .numpy()
- )
- else:
- audio1 = (
- (net_g.infer(feats, p_len, sid)[0][0, 0])
- .data.cpu()
- .float()
- .numpy()
- )
- del feats, p_len, padding_mask
- if torch.cuda.is_available():
- torch.cuda.empty_cache()
- t2 = ttime()
- times[0] += t1 - t0
- times[2] += t2 - t1
- return audio1
-
- def pipeline(
- self,
- model,
- net_g,
- sid,
- audio,
- input_audio_path,
- times,
- f0_up_key,
- f0_method,
- file_index,
- # file_big_npy,
- index_rate,
- if_f0,
- filter_radius,
- tgt_sr,
- resample_sr,
- rms_mix_rate,
- version,
- f0_file=None,
- ):
- if (
- file_index != ""
- # and file_big_npy != ""
- # and os.path.exists(file_big_npy) == True
- and os.path.exists(file_index) == True
- and index_rate != 0
- ):
- try:
- index = faiss.read_index(file_index)
- # big_npy = np.load(file_big_npy)
- big_npy = index.reconstruct_n(0, index.ntotal)
- except:
- traceback.print_exc()
- index = big_npy = None
- else:
- index = big_npy = None
- audio = signal.filtfilt(bh, ah, audio)
- audio_pad = np.pad(audio, (self.window // 2, self.window // 2), mode="reflect")
- opt_ts = []
- if audio_pad.shape[0] > self.t_max:
- audio_sum = np.zeros_like(audio)
- for i in range(self.window):
- audio_sum += audio_pad[i : i - self.window]
- for t in range(self.t_center, audio.shape[0], self.t_center):
- opt_ts.append(
- t
- - self.t_query
- + np.where(
- np.abs(audio_sum[t - self.t_query : t + self.t_query])
- == np.abs(audio_sum[t - self.t_query : t + self.t_query]).min()
- )[0][0]
- )
- s = 0
- audio_opt = []
- t = None
- t1 = ttime()
- audio_pad = np.pad(audio, (self.t_pad, self.t_pad), mode="reflect")
- p_len = audio_pad.shape[0] // self.window
- inp_f0 = None
- if hasattr(f0_file, "name") == True:
- try:
- with open(f0_file.name, "r") as f:
- lines = f.read().strip("\n").split("\n")
- inp_f0 = []
- for line in lines:
- inp_f0.append([float(i) for i in line.split(",")])
- inp_f0 = np.array(inp_f0, dtype="float32")
- except:
- traceback.print_exc()
- sid = torch.tensor(sid, device=self.device).unsqueeze(0).long()
- pitch, pitchf = None, None
- if if_f0 == 1:
- pitch, pitchf = self.get_f0(input_audio_path,audio_pad, p_len, f0_up_key, f0_method,filter_radius, inp_f0)
- pitch = pitch[:p_len]
- pitchf = pitchf[:p_len]
- if self.device == "mps":
- pitchf = pitchf.astype(np.float32)
- pitch = torch.tensor(pitch, device=self.device).unsqueeze(0).long()
- pitchf = torch.tensor(pitchf, device=self.device).unsqueeze(0).float()
- t2 = ttime()
- times[1] += t2 - t1
- for t in opt_ts:
- t = t // self.window * self.window
- if if_f0 == 1:
- audio_opt.append(
- self.vc(
- model,
- net_g,
- sid,
- audio_pad[s : t + self.t_pad2 + self.window],
- pitch[:, s // self.window : (t + self.t_pad2) // self.window],
- pitchf[:, s // self.window : (t + self.t_pad2) // self.window],
- times,
- index,
- big_npy,
- index_rate,
- version,
- )[self.t_pad_tgt : -self.t_pad_tgt]
- )
- else:
- audio_opt.append(
- self.vc(
- model,
- net_g,
- sid,
- audio_pad[s : t + self.t_pad2 + self.window],
- None,
- None,
- times,
- index,
- big_npy,
- index_rate,
- version,
- )[self.t_pad_tgt : -self.t_pad_tgt]
- )
- s = t
- if if_f0 == 1:
- audio_opt.append(
- self.vc(
- model,
- net_g,
- sid,
- audio_pad[t:],
- pitch[:, t // self.window :] if t is not None else pitch,
- pitchf[:, t // self.window :] if t is not None else pitchf,
- times,
- index,
- big_npy,
- index_rate,
- version,
- )[self.t_pad_tgt : -self.t_pad_tgt]
- )
- else:
- audio_opt.append(
- self.vc(
- model,
- net_g,
- sid,
- audio_pad[t:],
- None,
- None,
- times,
- index,
- big_npy,
- index_rate,
- version,
- )[self.t_pad_tgt : -self.t_pad_tgt]
- )
- audio_opt = np.concatenate(audio_opt)
- if(rms_mix_rate!=1):
- audio_opt=change_rms(audio,16000,audio_opt,tgt_sr,rms_mix_rate)
- if(resample_sr>=16000 and tgt_sr!=resample_sr):
- audio_opt = librosa.resample(
- audio_opt, orig_sr=tgt_sr, target_sr=resample_sr
- )
- audio_max=np.abs(audio_opt).max()/0.99
- max_int16=32768
- if(audio_max>1):max_int16/=audio_max
- audio_opt=(audio_opt * max_int16).astype(np.int16)
- del pitch, pitchf, sid
- if torch.cuda.is_available():
- torch.cuda.empty_cache()
- return audio_opt
diff --git a/spaces/Violetmae14/Violet/style.css b/spaces/Violetmae14/Violet/style.css
deleted file mode 100644
index 114adf441e9032febb46bc056b2a8bb651075f0d..0000000000000000000000000000000000000000
--- a/spaces/Violetmae14/Violet/style.css
+++ /dev/null
@@ -1,28 +0,0 @@
-body {
- padding: 2rem;
- font-family: -apple-system, BlinkMacSystemFont, "Arial", sans-serif;
-}
-
-h1 {
- font-size: 16px;
- margin-top: 0;
-}
-
-p {
- color: rgb(107, 114, 128);
- font-size: 15px;
- margin-bottom: 10px;
- margin-top: 5px;
-}
-
-.card {
- max-width: 620px;
- margin: 0 auto;
- padding: 16px;
- border: 1px solid lightgray;
- border-radius: 16px;
-}
-
-.card p:last-child {
- margin-bottom: 0;
-}
diff --git a/spaces/XS-1/BW_IMAGE_VIDEO_COLORIZER/fastai/imports/core.py b/spaces/XS-1/BW_IMAGE_VIDEO_COLORIZER/fastai/imports/core.py
deleted file mode 100644
index 51935a07ad61a5eda922941aa8869a2bcc5705c1..0000000000000000000000000000000000000000
--- a/spaces/XS-1/BW_IMAGE_VIDEO_COLORIZER/fastai/imports/core.py
+++ /dev/null
@@ -1,50 +0,0 @@
-import csv, gc, gzip, os, pickle, shutil, sys, warnings, yaml, io, subprocess
-import math, matplotlib.pyplot as plt, numpy as np, pandas as pd, random
-import scipy.stats, scipy.special
-import abc, collections, hashlib, itertools, json, operator, pathlib
-import mimetypes, inspect, typing, functools, importlib, weakref
-import html, re, requests, tarfile, numbers, tempfile, bz2
-
-from abc import abstractmethod, abstractproperty
-from collections import abc, Counter, defaultdict, namedtuple, OrderedDict
-from collections.abc import Iterable
-import concurrent
-from concurrent.futures import ProcessPoolExecutor, ThreadPoolExecutor
-from copy import copy, deepcopy
-from dataclasses import dataclass, field, InitVar
-from enum import Enum, IntEnum
-from functools import partial, reduce
-from pdb import set_trace
-from matplotlib import patches, patheffects
-from numpy import array, cos, exp, log, sin, tan, tanh
-from operator import attrgetter, itemgetter
-from pathlib import Path
-from warnings import warn
-from contextlib import contextmanager
-from fastprogress.fastprogress import MasterBar, ProgressBar
-from matplotlib.patches import Patch
-from pandas import Series, DataFrame
-from io import BufferedWriter, BytesIO
-
-import pkg_resources
-pkg_resources.require("fastprogress>=0.1.19")
-from fastprogress.fastprogress import master_bar, progress_bar
-
-#for type annotations
-from numbers import Number
-from typing import Any, AnyStr, Callable, Collection, Dict, Hashable, Iterator, List, Mapping, NewType, Optional
-from typing import Sequence, Tuple, TypeVar, Union
-from types import SimpleNamespace
-
-def try_import(module):
- "Try to import `module`. Returns module's object on success, None on failure"
- try: return importlib.import_module(module)
- except: return None
-
-def have_min_pkg_version(package, version):
- "Check whether we have at least `version` of `package`. Returns True on success, False otherwise."
- try:
- pkg_resources.require(f"{package}>={version}")
- return True
- except:
- return False
diff --git a/spaces/XzJosh/Jiaran-Bert-VITS2/bert_gen.py b/spaces/XzJosh/Jiaran-Bert-VITS2/bert_gen.py
deleted file mode 100644
index 44814715396ffc3abe84a12c74d66293c356eb4f..0000000000000000000000000000000000000000
--- a/spaces/XzJosh/Jiaran-Bert-VITS2/bert_gen.py
+++ /dev/null
@@ -1,53 +0,0 @@
-import torch
-from torch.utils.data import DataLoader
-from multiprocessing import Pool
-import commons
-import utils
-from data_utils import TextAudioSpeakerLoader, TextAudioSpeakerCollate
-from tqdm import tqdm
-import warnings
-
-from text import cleaned_text_to_sequence, get_bert
-
-config_path = 'configs/config.json'
-hps = utils.get_hparams_from_file(config_path)
-
-def process_line(line):
- _id, spk, language_str, text, phones, tone, word2ph = line.strip().split("|")
- phone = phones.split(" ")
- tone = [int(i) for i in tone.split(" ")]
- word2ph = [int(i) for i in word2ph.split(" ")]
- w2pho = [i for i in word2ph]
- word2ph = [i for i in word2ph]
- phone, tone, language = cleaned_text_to_sequence(phone, tone, language_str)
-
- if hps.data.add_blank:
- phone = commons.intersperse(phone, 0)
- tone = commons.intersperse(tone, 0)
- language = commons.intersperse(language, 0)
- for i in range(len(word2ph)):
- word2ph[i] = word2ph[i] * 2
- word2ph[0] += 1
- wav_path = f'{_id}'
-
- bert_path = wav_path.replace(".wav", ".bert.pt")
- try:
- bert = torch.load(bert_path)
- assert bert.shape[-1] == len(phone)
- except:
- bert = get_bert(text, word2ph, language_str)
- assert bert.shape[-1] == len(phone)
- torch.save(bert, bert_path)
-
-
-if __name__ == '__main__':
- lines = []
- with open(hps.data.training_files, encoding='utf-8' ) as f:
- lines.extend(f.readlines())
-
- with open(hps.data.validation_files, encoding='utf-8' ) as f:
- lines.extend(f.readlines())
-
- with Pool(processes=12) as pool: #A100 40GB suitable config,if coom,please decrease the processess number.
- for _ in tqdm(pool.imap_unordered(process_line, lines)):
- pass
diff --git a/spaces/XzJosh/nine1-Bert-VITS2/commons.py b/spaces/XzJosh/nine1-Bert-VITS2/commons.py
deleted file mode 100644
index 9ad0444b61cbadaa388619986c2889c707d873ce..0000000000000000000000000000000000000000
--- a/spaces/XzJosh/nine1-Bert-VITS2/commons.py
+++ /dev/null
@@ -1,161 +0,0 @@
-import math
-import numpy as np
-import torch
-from torch import nn
-from torch.nn import functional as F
-
-
-def init_weights(m, mean=0.0, std=0.01):
- classname = m.__class__.__name__
- if classname.find("Conv") != -1:
- m.weight.data.normal_(mean, std)
-
-
-def get_padding(kernel_size, dilation=1):
- return int((kernel_size*dilation - dilation)/2)
-
-
-def convert_pad_shape(pad_shape):
- l = pad_shape[::-1]
- pad_shape = [item for sublist in l for item in sublist]
- return pad_shape
-
-
-def intersperse(lst, item):
- result = [item] * (len(lst) * 2 + 1)
- result[1::2] = lst
- return result
-
-
-def kl_divergence(m_p, logs_p, m_q, logs_q):
- """KL(P||Q)"""
- kl = (logs_q - logs_p) - 0.5
- kl += 0.5 * (torch.exp(2. * logs_p) + ((m_p - m_q)**2)) * torch.exp(-2. * logs_q)
- return kl
-
-
-def rand_gumbel(shape):
- """Sample from the Gumbel distribution, protect from overflows."""
- uniform_samples = torch.rand(shape) * 0.99998 + 0.00001
- return -torch.log(-torch.log(uniform_samples))
-
-
-def rand_gumbel_like(x):
- g = rand_gumbel(x.size()).to(dtype=x.dtype, device=x.device)
- return g
-
-
-def slice_segments(x, ids_str, segment_size=4):
- ret = torch.zeros_like(x[:, :, :segment_size])
- for i in range(x.size(0)):
- idx_str = ids_str[i]
- idx_end = idx_str + segment_size
- ret[i] = x[i, :, idx_str:idx_end]
- return ret
-
-
-def rand_slice_segments(x, x_lengths=None, segment_size=4):
- b, d, t = x.size()
- if x_lengths is None:
- x_lengths = t
- ids_str_max = x_lengths - segment_size + 1
- ids_str = (torch.rand([b]).to(device=x.device) * ids_str_max).to(dtype=torch.long)
- ret = slice_segments(x, ids_str, segment_size)
- return ret, ids_str
-
-
-def get_timing_signal_1d(
- length, channels, min_timescale=1.0, max_timescale=1.0e4):
- position = torch.arange(length, dtype=torch.float)
- num_timescales = channels // 2
- log_timescale_increment = (
- math.log(float(max_timescale) / float(min_timescale)) /
- (num_timescales - 1))
- inv_timescales = min_timescale * torch.exp(
- torch.arange(num_timescales, dtype=torch.float) * -log_timescale_increment)
- scaled_time = position.unsqueeze(0) * inv_timescales.unsqueeze(1)
- signal = torch.cat([torch.sin(scaled_time), torch.cos(scaled_time)], 0)
- signal = F.pad(signal, [0, 0, 0, channels % 2])
- signal = signal.view(1, channels, length)
- return signal
-
-
-def add_timing_signal_1d(x, min_timescale=1.0, max_timescale=1.0e4):
- b, channels, length = x.size()
- signal = get_timing_signal_1d(length, channels, min_timescale, max_timescale)
- return x + signal.to(dtype=x.dtype, device=x.device)
-
-
-def cat_timing_signal_1d(x, min_timescale=1.0, max_timescale=1.0e4, axis=1):
- b, channels, length = x.size()
- signal = get_timing_signal_1d(length, channels, min_timescale, max_timescale)
- return torch.cat([x, signal.to(dtype=x.dtype, device=x.device)], axis)
-
-
-def subsequent_mask(length):
- mask = torch.tril(torch.ones(length, length)).unsqueeze(0).unsqueeze(0)
- return mask
-
-
-@torch.jit.script
-def fused_add_tanh_sigmoid_multiply(input_a, input_b, n_channels):
- n_channels_int = n_channels[0]
- in_act = input_a + input_b
- t_act = torch.tanh(in_act[:, :n_channels_int, :])
- s_act = torch.sigmoid(in_act[:, n_channels_int:, :])
- acts = t_act * s_act
- return acts
-
-
-def convert_pad_shape(pad_shape):
- l = pad_shape[::-1]
- pad_shape = [item for sublist in l for item in sublist]
- return pad_shape
-
-
-def shift_1d(x):
- x = F.pad(x, convert_pad_shape([[0, 0], [0, 0], [1, 0]]))[:, :, :-1]
- return x
-
-
-def sequence_mask(length, max_length=None):
- if max_length is None:
- max_length = length.max()
- x = torch.arange(max_length, dtype=length.dtype, device=length.device)
- return x.unsqueeze(0) < length.unsqueeze(1)
-
-
-def generate_path(duration, mask):
- """
- duration: [b, 1, t_x]
- mask: [b, 1, t_y, t_x]
- """
- device = duration.device
-
- b, _, t_y, t_x = mask.shape
- cum_duration = torch.cumsum(duration, -1)
-
- cum_duration_flat = cum_duration.view(b * t_x)
- path = sequence_mask(cum_duration_flat, t_y).to(mask.dtype)
- path = path.view(b, t_x, t_y)
- path = path - F.pad(path, convert_pad_shape([[0, 0], [1, 0], [0, 0]]))[:, :-1]
- path = path.unsqueeze(1).transpose(2,3) * mask
- return path
-
-
-def clip_grad_value_(parameters, clip_value, norm_type=2):
- if isinstance(parameters, torch.Tensor):
- parameters = [parameters]
- parameters = list(filter(lambda p: p.grad is not None, parameters))
- norm_type = float(norm_type)
- if clip_value is not None:
- clip_value = float(clip_value)
-
- total_norm = 0
- for p in parameters:
- param_norm = p.grad.data.norm(norm_type)
- total_norm += param_norm.item() ** norm_type
- if clip_value is not None:
- p.grad.data.clamp_(min=-clip_value, max=clip_value)
- total_norm = total_norm ** (1. / norm_type)
- return total_norm
diff --git a/spaces/Yan233th/so-vits-svc-models/wav_upload.py b/spaces/Yan233th/so-vits-svc-models/wav_upload.py
deleted file mode 100644
index cac679de78634e638e9a998615406b1c36374fb5..0000000000000000000000000000000000000000
--- a/spaces/Yan233th/so-vits-svc-models/wav_upload.py
+++ /dev/null
@@ -1,23 +0,0 @@
-from google.colab import files
-import shutil
-import os
-import argparse
-if __name__ == "__main__":
- parser = argparse.ArgumentParser()
- parser.add_argument("--type", type=str, required=True, help="type of file to upload")
- args = parser.parse_args()
- file_type = args.type
-
- basepath = os.getcwd()
- uploaded = files.upload() # 上传文件
- assert(file_type in ['zip', 'audio'])
- if file_type == "zip":
- upload_path = "./upload/"
- for filename in uploaded.keys():
- #将上传的文件移动到指定的位置上
- shutil.move(os.path.join(basepath, filename), os.path.join(upload_path, "userzip.zip"))
- elif file_type == "audio":
- upload_path = "./raw/"
- for filename in uploaded.keys():
- #将上传的文件移动到指定的位置上
- shutil.move(os.path.join(basepath, filename), os.path.join(upload_path, filename))
\ No newline at end of file
diff --git a/spaces/YanzBotz/YanzBotz-Models/lib/infer_pack/modules/F0Predictor/HarvestF0Predictor.py b/spaces/YanzBotz/YanzBotz-Models/lib/infer_pack/modules/F0Predictor/HarvestF0Predictor.py
deleted file mode 100644
index b412ba2814e114ca7bb00b6fd6ef217f63d788a3..0000000000000000000000000000000000000000
--- a/spaces/YanzBotz/YanzBotz-Models/lib/infer_pack/modules/F0Predictor/HarvestF0Predictor.py
+++ /dev/null
@@ -1,86 +0,0 @@
-from lib.infer_pack.modules.F0Predictor.F0Predictor import F0Predictor
-import pyworld
-import numpy as np
-
-
-class HarvestF0Predictor(F0Predictor):
- def __init__(self, hop_length=512, f0_min=50, f0_max=1100, sampling_rate=44100):
- self.hop_length = hop_length
- self.f0_min = f0_min
- self.f0_max = f0_max
- self.sampling_rate = sampling_rate
-
- def interpolate_f0(self, f0):
- """
- 对F0进行插值处理
- """
-
- data = np.reshape(f0, (f0.size, 1))
-
- vuv_vector = np.zeros((data.size, 1), dtype=np.float32)
- vuv_vector[data > 0.0] = 1.0
- vuv_vector[data <= 0.0] = 0.0
-
- ip_data = data
-
- frame_number = data.size
- last_value = 0.0
- for i in range(frame_number):
- if data[i] <= 0.0:
- j = i + 1
- for j in range(i + 1, frame_number):
- if data[j] > 0.0:
- break
- if j < frame_number - 1:
- if last_value > 0.0:
- step = (data[j] - data[i - 1]) / float(j - i)
- for k in range(i, j):
- ip_data[k] = data[i - 1] + step * (k - i + 1)
- else:
- for k in range(i, j):
- ip_data[k] = data[j]
- else:
- for k in range(i, frame_number):
- ip_data[k] = last_value
- else:
- ip_data[i] = data[i] # 这里可能存在一个没有必要的拷贝
- last_value = data[i]
-
- return ip_data[:, 0], vuv_vector[:, 0]
-
- def resize_f0(self, x, target_len):
- source = np.array(x)
- source[source < 0.001] = np.nan
- target = np.interp(
- np.arange(0, len(source) * target_len, len(source)) / target_len,
- np.arange(0, len(source)),
- source,
- )
- res = np.nan_to_num(target)
- return res
-
- def compute_f0(self, wav, p_len=None):
- if p_len is None:
- p_len = wav.shape[0] // self.hop_length
- f0, t = pyworld.harvest(
- wav.astype(np.double),
- fs=self.hop_length,
- f0_ceil=self.f0_max,
- f0_floor=self.f0_min,
- frame_period=1000 * self.hop_length / self.sampling_rate,
- )
- f0 = pyworld.stonemask(wav.astype(np.double), f0, t, self.fs)
- return self.interpolate_f0(self.resize_f0(f0, p_len))[0]
-
- def compute_f0_uv(self, wav, p_len=None):
- if p_len is None:
- p_len = wav.shape[0] // self.hop_length
- f0, t = pyworld.harvest(
- wav.astype(np.double),
- fs=self.sampling_rate,
- f0_floor=self.f0_min,
- f0_ceil=self.f0_max,
- frame_period=1000 * self.hop_length / self.sampling_rate,
- )
- f0 = pyworld.stonemask(wav.astype(np.double), f0, t, self.sampling_rate)
- return self.interpolate_f0(self.resize_f0(f0, p_len))
diff --git a/spaces/YuanMio/vits-uma-genshin-honkai/text/cleaners.py b/spaces/YuanMio/vits-uma-genshin-honkai/text/cleaners.py
deleted file mode 100644
index d26581deb399609163518054718ad80ecca5d934..0000000000000000000000000000000000000000
--- a/spaces/YuanMio/vits-uma-genshin-honkai/text/cleaners.py
+++ /dev/null
@@ -1,475 +0,0 @@
-""" from https://github.com/keithito/tacotron """
-
-'''
-Cleaners are transformations that run over the input text at both training and eval time.
-
-Cleaners can be selected by passing a comma-delimited list of cleaner names as the "cleaners"
-hyperparameter. Some cleaners are English-specific. You'll typically want to use:
- 1. "english_cleaners" for English text
- 2. "transliteration_cleaners" for non-English text that can be transliterated to ASCII using
- the Unidecode library (https://pypi.python.org/pypi/Unidecode)
- 3. "basic_cleaners" if you do not want to transliterate (in this case, you should also update
- the symbols in symbols.py to match your data).
-'''
-
-import re
-from unidecode import unidecode
-import pyopenjtalk
-from jamo import h2j, j2hcj
-from pypinyin import lazy_pinyin, BOPOMOFO
-import jieba, cn2an
-
-
-# This is a list of Korean classifiers preceded by pure Korean numerals.
-_korean_classifiers = '군데 권 개 그루 닢 대 두 마리 모 모금 뭇 발 발짝 방 번 벌 보루 살 수 술 시 쌈 움큼 정 짝 채 척 첩 축 켤레 톨 통'
-
-# Regular expression matching whitespace:
-_whitespace_re = re.compile(r'\s+')
-
-# Regular expression matching Japanese without punctuation marks:
-_japanese_characters = re.compile(r'[A-Za-z\d\u3005\u3040-\u30ff\u4e00-\u9fff\uff11-\uff19\uff21-\uff3a\uff41-\uff5a\uff66-\uff9d]')
-
-# Regular expression matching non-Japanese characters or punctuation marks:
-_japanese_marks = re.compile(r'[^A-Za-z\d\u3005\u3040-\u30ff\u4e00-\u9fff\uff11-\uff19\uff21-\uff3a\uff41-\uff5a\uff66-\uff9d]')
-
-# List of (regular expression, replacement) pairs for abbreviations:
-_abbreviations = [(re.compile('\\b%s\\.' % x[0], re.IGNORECASE), x[1]) for x in [
- ('mrs', 'misess'),
- ('mr', 'mister'),
- ('dr', 'doctor'),
- ('st', 'saint'),
- ('co', 'company'),
- ('jr', 'junior'),
- ('maj', 'major'),
- ('gen', 'general'),
- ('drs', 'doctors'),
- ('rev', 'reverend'),
- ('lt', 'lieutenant'),
- ('hon', 'honorable'),
- ('sgt', 'sergeant'),
- ('capt', 'captain'),
- ('esq', 'esquire'),
- ('ltd', 'limited'),
- ('col', 'colonel'),
- ('ft', 'fort'),
-]]
-
-# List of (hangul, hangul divided) pairs:
-_hangul_divided = [(re.compile('%s' % x[0]), x[1]) for x in [
- ('ㄳ', 'ㄱㅅ'),
- ('ㄵ', 'ㄴㅈ'),
- ('ㄶ', 'ㄴㅎ'),
- ('ㄺ', 'ㄹㄱ'),
- ('ㄻ', 'ㄹㅁ'),
- ('ㄼ', 'ㄹㅂ'),
- ('ㄽ', 'ㄹㅅ'),
- ('ㄾ', 'ㄹㅌ'),
- ('ㄿ', 'ㄹㅍ'),
- ('ㅀ', 'ㄹㅎ'),
- ('ㅄ', 'ㅂㅅ'),
- ('ㅘ', 'ㅗㅏ'),
- ('ㅙ', 'ㅗㅐ'),
- ('ㅚ', 'ㅗㅣ'),
- ('ㅝ', 'ㅜㅓ'),
- ('ㅞ', 'ㅜㅔ'),
- ('ㅟ', 'ㅜㅣ'),
- ('ㅢ', 'ㅡㅣ'),
- ('ㅑ', 'ㅣㅏ'),
- ('ㅒ', 'ㅣㅐ'),
- ('ㅕ', 'ㅣㅓ'),
- ('ㅖ', 'ㅣㅔ'),
- ('ㅛ', 'ㅣㅗ'),
- ('ㅠ', 'ㅣㅜ')
-]]
-
-# List of (Latin alphabet, hangul) pairs:
-_latin_to_hangul = [(re.compile('%s' % x[0], re.IGNORECASE), x[1]) for x in [
- ('a', '에이'),
- ('b', '비'),
- ('c', '시'),
- ('d', '디'),
- ('e', '이'),
- ('f', '에프'),
- ('g', '지'),
- ('h', '에이치'),
- ('i', '아이'),
- ('j', '제이'),
- ('k', '케이'),
- ('l', '엘'),
- ('m', '엠'),
- ('n', '엔'),
- ('o', '오'),
- ('p', '피'),
- ('q', '큐'),
- ('r', '아르'),
- ('s', '에스'),
- ('t', '티'),
- ('u', '유'),
- ('v', '브이'),
- ('w', '더블유'),
- ('x', '엑스'),
- ('y', '와이'),
- ('z', '제트')
-]]
-
-# List of (Latin alphabet, bopomofo) pairs:
-_latin_to_bopomofo = [(re.compile('%s' % x[0], re.IGNORECASE), x[1]) for x in [
- ('a', 'ㄟˉ'),
- ('b', 'ㄅㄧˋ'),
- ('c', 'ㄙㄧˉ'),
- ('d', 'ㄉㄧˋ'),
- ('e', 'ㄧˋ'),
- ('f', 'ㄝˊㄈㄨˋ'),
- ('g', 'ㄐㄧˋ'),
- ('h', 'ㄝˇㄑㄩˋ'),
- ('i', 'ㄞˋ'),
- ('j', 'ㄐㄟˋ'),
- ('k', 'ㄎㄟˋ'),
- ('l', 'ㄝˊㄛˋ'),
- ('m', 'ㄝˊㄇㄨˋ'),
- ('n', 'ㄣˉ'),
- ('o', 'ㄡˉ'),
- ('p', 'ㄆㄧˉ'),
- ('q', 'ㄎㄧㄡˉ'),
- ('r', 'ㄚˋ'),
- ('s', 'ㄝˊㄙˋ'),
- ('t', 'ㄊㄧˋ'),
- ('u', 'ㄧㄡˉ'),
- ('v', 'ㄨㄧˉ'),
- ('w', 'ㄉㄚˋㄅㄨˋㄌㄧㄡˋ'),
- ('x', 'ㄝˉㄎㄨˋㄙˋ'),
- ('y', 'ㄨㄞˋ'),
- ('z', 'ㄗㄟˋ')
-]]
-
-
-# List of (bopomofo, romaji) pairs:
-_bopomofo_to_romaji = [(re.compile('%s' % x[0], re.IGNORECASE), x[1]) for x in [
- ('ㄅㄛ', 'p⁼wo'),
- ('ㄆㄛ', 'pʰwo'),
- ('ㄇㄛ', 'mwo'),
- ('ㄈㄛ', 'fwo'),
- ('ㄅ', 'p⁼'),
- ('ㄆ', 'pʰ'),
- ('ㄇ', 'm'),
- ('ㄈ', 'f'),
- ('ㄉ', 't⁼'),
- ('ㄊ', 'tʰ'),
- ('ㄋ', 'n'),
- ('ㄌ', 'l'),
- ('ㄍ', 'k⁼'),
- ('ㄎ', 'kʰ'),
- ('ㄏ', 'h'),
- ('ㄐ', 'ʧ⁼'),
- ('ㄑ', 'ʧʰ'),
- ('ㄒ', 'ʃ'),
- ('ㄓ', 'ʦ`⁼'),
- ('ㄔ', 'ʦ`ʰ'),
- ('ㄕ', 's`'),
- ('ㄖ', 'ɹ`'),
- ('ㄗ', 'ʦ⁼'),
- ('ㄘ', 'ʦʰ'),
- ('ㄙ', 's'),
- ('ㄚ', 'a'),
- ('ㄛ', 'o'),
- ('ㄜ', 'ə'),
- ('ㄝ', 'e'),
- ('ㄞ', 'ai'),
- ('ㄟ', 'ei'),
- ('ㄠ', 'au'),
- ('ㄡ', 'ou'),
- ('ㄧㄢ', 'yeNN'),
- ('ㄢ', 'aNN'),
- ('ㄧㄣ', 'iNN'),
- ('ㄣ', 'əNN'),
- ('ㄤ', 'aNg'),
- ('ㄧㄥ', 'iNg'),
- ('ㄨㄥ', 'uNg'),
- ('ㄩㄥ', 'yuNg'),
- ('ㄥ', 'əNg'),
- ('ㄦ', 'əɻ'),
- ('ㄧ', 'i'),
- ('ㄨ', 'u'),
- ('ㄩ', 'ɥ'),
- ('ˉ', '→'),
- ('ˊ', '↑'),
- ('ˇ', '↓↑'),
- ('ˋ', '↓'),
- ('˙', ''),
- (',', ','),
- ('。', '.'),
- ('!', '!'),
- ('?', '?'),
- ('—', '-')
-]]
-
-
-def expand_abbreviations(text):
- for regex, replacement in _abbreviations:
- text = re.sub(regex, replacement, text)
- return text
-
-
-def lowercase(text):
- return text.lower()
-
-
-def collapse_whitespace(text):
- return re.sub(_whitespace_re, ' ', text)
-
-
-def convert_to_ascii(text):
- return unidecode(text)
-
-
-def japanese_to_romaji_with_accent(text):
- '''Reference https://r9y9.github.io/ttslearn/latest/notebooks/ch10_Recipe-Tacotron.html'''
- sentences = re.split(_japanese_marks, text)
- marks = re.findall(_japanese_marks, text)
- text = ''
- for i, sentence in enumerate(sentences):
- if re.match(_japanese_characters, sentence):
- if text!='':
- text+=' '
- labels = pyopenjtalk.extract_fullcontext(sentence)
- for n, label in enumerate(labels):
- phoneme = re.search(r'\-([^\+]*)\+', label).group(1)
- if phoneme not in ['sil','pau']:
- text += phoneme.replace('ch','ʧ').replace('sh','ʃ').replace('cl','Q')
- else:
- continue
- n_moras = int(re.search(r'/F:(\d+)_', label).group(1))
- a1 = int(re.search(r"/A:(\-?[0-9]+)\+", label).group(1))
- a2 = int(re.search(r"\+(\d+)\+", label).group(1))
- a3 = int(re.search(r"\+(\d+)/", label).group(1))
- if re.search(r'\-([^\+]*)\+', labels[n + 1]).group(1) in ['sil','pau']:
- a2_next=-1
- else:
- a2_next = int(re.search(r"\+(\d+)\+", labels[n + 1]).group(1))
- # Accent phrase boundary
- if a3 == 1 and a2_next == 1:
- text += ' '
- # Falling
- elif a1 == 0 and a2_next == a2 + 1 and a2 != n_moras:
- text += '↓'
- # Rising
- elif a2 == 1 and a2_next == 2:
- text += '↑'
- if ino error"
- except openai.error.Timeout as e:
- #Handle timeout error, e.g. retry or log
- print(f"OpenAI API request timed out: {e}")
- return "oups", f"OpenAI API request timed out:
{e}"
- except openai.error.APIError as e:
- #Handle API error, e.g. retry or log
- print(f"OpenAI API returned an API Error: {e}")
- return "oups", f"OpenAI API returned an API Error:
{e}"
- except openai.error.APIConnectionError as e:
- #Handle connection error, e.g. check network or log
- print(f"OpenAI API request failed to connect: {e}")
- return "oups", f"OpenAI API request failed to connect:
{e}"
- except openai.error.InvalidRequestError as e:
- #Handle invalid request error, e.g. validate parameters or log
- print(f"OpenAI API request was invalid: {e}")
- return "oups", f"OpenAI API request was invalid:
{e}"
- except openai.error.AuthenticationError as e:
- #Handle authentication error, e.g. check credentials or log
- print(f"OpenAI API request was not authorized: {e}")
- return "oups", f"OpenAI API request was not authorized:
{e}"
- except openai.error.PermissionError as e:
- #Handle permission error, e.g. check scope or log
- print(f"OpenAI API request was not permitted: {e}")
- return "oups", f"OpenAI API request was not permitted:
{e}"
- except openai.error.RateLimitError as e:
- #Handle rate limit error, e.g. wait or log
- print(f"OpenAI API request exceeded rate limit: {e}")
- return "oups", f"OpenAI API request exceeded rate limit:
{e}"
-
-def call_api(message, openai_api_key):
-
- print("starting open ai")
- augmented_prompt = message + prevent_code_gen
- openai.api_key = openai_api_key
-
- response = openai.Completion.create(
- model="text-davinci-003",
- prompt=augmented_prompt,
- temperature=0.5,
- max_tokens=2048,
- top_p=1,
- frequency_penalty=0,
- presence_penalty=0.6
- )
-
- print(response)
-
- #return str(response.choices[0].text).split("\n",2)[2]
- return str(response.choices[0].text)
-
-def clean_components():
- return gr.Audio.update(value=None), gr.HTML.update(visible=False), gr.Textbox.update(visible=False), gr.Video.update(value=None), gr.Group.update(visible=False), gr.Button.update(visible=False)
-
-title = """
-
-
-
- GPT Talking Portrait
-
-
-
- Use Whisper to ask, alive portrait responds !
-
-
-"""
-
-article = """
-
-
-
- You may also like:
-
-
-
-
-
-
-
-"""
-
-prevent_code_gen = """
-If i am asking for code generation, do not provide me with code. Instead, give me a summury of good hints about how i could do what i asked, but shortly.
-If i am not asking for code generation, do as usual.
-"""
-with gr.Blocks(css="style.css") as demo:
-
- with gr.Column(elem_id="col-container"):
-
- gr.HTML(title)
-
- gpt_response = gr.Video(label="Talking Portrait response", elem_id="video_out")
- whisper_tr = gr.Textbox(label="whisper english translation", elem_id="text_inp", visible=False)
-
- with gr.Row(elem_id="secondary-buttons"):
- clean_btn = gr.Button(value="Clean", elem_id="clean-btn", visible=False)
- with gr.Group(elem_id="share-btn-container", visible=False) as share_group:
- community_icon = gr.HTML(community_icon_html)
- loading_icon = gr.HTML(loading_icon_html)
- share_button = gr.Button("Share to community", elem_id="share-btn")
-
- error_handler = gr.HTML(visible=False, show_label=False, elem_id="error_handler")
-
- with gr.Column(elem_id="col-container-2"):
- with gr.Column():
- with gr.Row():
- record_input = gr.Audio(source="microphone",type="filepath", label="Audio input", show_label=True, elem_id="record_btn")
- openai_api_key = gr.Textbox(max_lines=1, type="password", label="🔐 Your OpenAI API Key", placeholder="sk-123abc...")
-
- send_btn = gr.Button("Send my request !")
-
- gr.HTML(article)
-
- clean_btn.click(clean_components, scroll_to_output=True, inputs=[], outputs=[record_input, error_handler, whisper_tr, gpt_response, share_group, clean_btn])
- send_btn.click(infer, inputs=[record_input, openai_api_key], outputs=[whisper_tr, gpt_response, error_handler, share_group, clean_btn])
- share_button.click(None, [], [], _js=share_js)
-
-demo.queue(max_size=32, concurrency_count=20).launch(debug=True)
-
-
diff --git a/spaces/abidlabs/streaming-asr-paused/README.md b/spaces/abidlabs/streaming-asr-paused/README.md
deleted file mode 100644
index 1375a1ff06698ab143918a0baa2602a27fb6934c..0000000000000000000000000000000000000000
--- a/spaces/abidlabs/streaming-asr-paused/README.md
+++ /dev/null
@@ -1,12 +0,0 @@
----
-title: Streaming Asr
-emoji: 🐢
-colorFrom: pink
-colorTo: green
-sdk: gradio
-sdk_version: 2.9.0
-app_file: app.py
-pinned: false
----
-
-Check out the configuration reference at https://huggingface.co/docs/hub/spaces#reference
diff --git a/spaces/abrar-lohia/text-2-character-anim/pyrender/pyrender/__init__.py b/spaces/abrar-lohia/text-2-character-anim/pyrender/pyrender/__init__.py
deleted file mode 100644
index ee3709846823b7c4b71b22da0e24d63d805528a8..0000000000000000000000000000000000000000
--- a/spaces/abrar-lohia/text-2-character-anim/pyrender/pyrender/__init__.py
+++ /dev/null
@@ -1,24 +0,0 @@
-from .camera import (Camera, PerspectiveCamera, OrthographicCamera,
- IntrinsicsCamera)
-from .light import Light, PointLight, DirectionalLight, SpotLight
-from .sampler import Sampler
-from .texture import Texture
-from .material import Material, MetallicRoughnessMaterial
-from .primitive import Primitive
-from .mesh import Mesh
-from .node import Node
-from .scene import Scene
-from .renderer import Renderer
-from .viewer import Viewer
-from .offscreen import OffscreenRenderer
-from .version import __version__
-from .constants import RenderFlags, TextAlign, GLTF
-
-__all__ = [
- 'Camera', 'PerspectiveCamera', 'OrthographicCamera', 'IntrinsicsCamera',
- 'Light', 'PointLight', 'DirectionalLight', 'SpotLight',
- 'Sampler', 'Texture', 'Material', 'MetallicRoughnessMaterial',
- 'Primitive', 'Mesh', 'Node', 'Scene', 'Renderer', 'Viewer',
- 'OffscreenRenderer', '__version__', 'RenderFlags', 'TextAlign',
- 'GLTF'
-]
diff --git a/spaces/adorp/ControlNet-v1-1-duplicate/app_canny.py b/spaces/adorp/ControlNet-v1-1-duplicate/app_canny.py
deleted file mode 100644
index cb47938f72d403e814621317dbd2a8e66cc8f20c..0000000000000000000000000000000000000000
--- a/spaces/adorp/ControlNet-v1-1-duplicate/app_canny.py
+++ /dev/null
@@ -1,108 +0,0 @@
-#!/usr/bin/env python
-
-import gradio as gr
-
-from utils import randomize_seed_fn
-
-
-def create_demo(process, max_images=12, default_num_images=3):
- with gr.Blocks() as demo:
- with gr.Row():
- with gr.Column():
- image = gr.Image()
- prompt = gr.Textbox(label='Prompt')
- run_button = gr.Button('Run')
- with gr.Accordion('Advanced options', open=False):
- num_samples = gr.Slider(label='Number of images',
- minimum=1,
- maximum=max_images,
- value=default_num_images,
- step=1)
- image_resolution = gr.Slider(label='Image resolution',
- minimum=256,
- maximum=512,
- value=512,
- step=256)
- canny_low_threshold = gr.Slider(
- label='Canny low threshold',
- minimum=1,
- maximum=255,
- value=100,
- step=1)
- canny_high_threshold = gr.Slider(
- label='Canny high threshold',
- minimum=1,
- maximum=255,
- value=200,
- step=1)
- num_steps = gr.Slider(label='Number of steps',
- minimum=1,
- maximum=100,
- value=20,
- step=1)
- guidance_scale = gr.Slider(label='Guidance scale',
- minimum=0.1,
- maximum=30.0,
- value=9.0,
- step=0.1)
- seed = gr.Slider(label='Seed',
- minimum=0,
- maximum=1000000,
- step=1,
- value=0,
- randomize=True)
- randomize_seed = gr.Checkbox(label='Randomize seed',
- value=True)
- a_prompt = gr.Textbox(
- label='Additional prompt',
- value='best quality, extremely detailed')
- n_prompt = gr.Textbox(
- label='Negative prompt',
- value=
- 'longbody, lowres, bad anatomy, bad hands, missing fingers, extra digit, fewer digits, cropped, worst quality, low quality'
- )
- with gr.Column():
- result = gr.Gallery(label='Output', show_label=False).style(
- columns=2, object_fit='scale-down')
- inputs = [
- image,
- prompt,
- a_prompt,
- n_prompt,
- num_samples,
- image_resolution,
- num_steps,
- guidance_scale,
- seed,
- canny_low_threshold,
- canny_high_threshold,
- ]
- prompt.submit(
- fn=randomize_seed_fn,
- inputs=[seed, randomize_seed],
- outputs=seed,
- queue=False,
- ).then(
- fn=process,
- inputs=inputs,
- outputs=result,
- )
- run_button.click(
- fn=randomize_seed_fn,
- inputs=[seed, randomize_seed],
- outputs=seed,
- queue=False,
- ).then(
- fn=process,
- inputs=inputs,
- outputs=result,
- api_name='canny',
- )
- return demo
-
-
-if __name__ == '__main__':
- from model import Model
- model = Model(task_name='Canny')
- demo = create_demo(model.process_canny)
- demo.queue().launch()
diff --git a/spaces/ahmedghani/svoice_demo/CONTRIBUTING.md b/spaces/ahmedghani/svoice_demo/CONTRIBUTING.md
deleted file mode 100644
index 1cc0291bde7c9d45b93b01670d19c4f2bd7e64b6..0000000000000000000000000000000000000000
--- a/spaces/ahmedghani/svoice_demo/CONTRIBUTING.md
+++ /dev/null
@@ -1,25 +0,0 @@
-# Contributing to Denoiser
-
-## Pull Requests
-
-In order to accept your pull request, we need you to submit a CLA. You only need
-to do this once to work on any of Facebook's open source projects.
-
-Complete your CLA here:
-
-Demucs is the implementation of a research paper.
-Therefore, we do not plan on accepting many pull requests for new features.
-We certainly welcome them for bug fixes.
-
-
-## Issues
-
-We use GitHub issues to track public bugs. Please ensure your description is
-clear and has sufficient instructions to be able to reproduce the issue.
-Please first check existing issues as well as the README for existing solutions.
-
-
-## License
-By contributing to this repository, you agree that your contributions will be licensed
-under the LICENSE file in the root directory of this source tree.
-
diff --git a/spaces/ahmedxeno/depth_estimation/app.py b/spaces/ahmedxeno/depth_estimation/app.py
deleted file mode 100644
index 538c6729cd1a47f66d386cd775d1a2e3847320b2..0000000000000000000000000000000000000000
--- a/spaces/ahmedxeno/depth_estimation/app.py
+++ /dev/null
@@ -1,44 +0,0 @@
-
-import gradio as gr
-
-import matplotlib.pyplot as plt
-import cv2
-import torch
-import timm
-import numpy as np
-
-midas = torch.hub.load('intel-isl/MiDaS', 'DPT_Hybrid')
-midas.to('cpu')
-midas.eval()
-
-transforms = torch.hub.load('intel-isl/MiDaS', 'transforms')
-transform = transforms.dpt_transform
-
-def predict_image(img):
- img = cv2.cvtColor(img, cv2.COLOR_BGR2RGB)
-
- input_batch = transform(img).to('cpu')
- with torch.no_grad():
- prediction = midas(input_batch)
-
- prediction = torch.nn.functional.interpolate(
- prediction.unsqueeze(1),
- size=img.shape[:2],
- mode="bicubic",
- align_corners=False,
- ).squeeze()
-
- img = prediction.cpu().numpy()
- a = img.max()
-
- img = (img / a)*255
- out = (img).astype(np.uint8)
-
-
- return out
-
-
-image = gr.inputs.Image()
-
-label = gr.outputs.Label('ok')
-gr.Interface(fn=predict_image, inputs=image, outputs=image).launch(debug='True')
\ No newline at end of file
diff --git a/spaces/akhaliq/EimisAnimeDiffusion_1.0v/README.md b/spaces/akhaliq/EimisAnimeDiffusion_1.0v/README.md
deleted file mode 100644
index 8dccf76c7f617f7b26a12ce867d794e10ed01974..0000000000000000000000000000000000000000
--- a/spaces/akhaliq/EimisAnimeDiffusion_1.0v/README.md
+++ /dev/null
@@ -1,12 +0,0 @@
----
-title: EimisAnimeDiffusion 1.0v
-emoji: 🏃
-colorFrom: green
-colorTo: indigo
-sdk: gradio
-sdk_version: 3.11.0
-app_file: app.py
-pinned: false
----
-
-Check out the configuration reference at https://huggingface.co/docs/hub/spaces-config-reference
diff --git a/spaces/akhaliq/PaintTransformer/morphology.py b/spaces/akhaliq/PaintTransformer/morphology.py
deleted file mode 100644
index 31ddf1225b179e3bd6c98710f8a0b1c821d8005e..0000000000000000000000000000000000000000
--- a/spaces/akhaliq/PaintTransformer/morphology.py
+++ /dev/null
@@ -1,51 +0,0 @@
-import torch
-import torch.nn as nn
-import torch.nn.functional as F
-
-
-class Erosion2d(nn.Module):
-
- def __init__(self, m=1):
- super(Erosion2d, self).__init__()
- self.m = m
- self.pad = [m, m, m, m]
- self.unfold = nn.Unfold(2 * m + 1, padding=0, stride=1)
-
- def forward(self, x):
- batch_size, c, h, w = x.shape
- x_pad = F.pad(x, pad=self.pad, mode='constant', value=1e9)
- channel = self.unfold(x_pad).view(batch_size, c, -1, h, w)
- result = torch.min(channel, dim=2)[0]
- return result
-
-
-def erosion(x, m=1):
- b, c, h, w = x.shape
- x_pad = F.pad(x, pad=[m, m, m, m], mode='constant', value=1e9)
- channel = nn.functional.unfold(x_pad, 2 * m + 1, padding=0, stride=1).view(b, c, -1, h, w)
- result = torch.min(channel, dim=2)[0]
- return result
-
-
-class Dilation2d(nn.Module):
-
- def __init__(self, m=1):
- super(Dilation2d, self).__init__()
- self.m = m
- self.pad = [m, m, m, m]
- self.unfold = nn.Unfold(2 * m + 1, padding=0, stride=1)
-
- def forward(self, x):
- batch_size, c, h, w = x.shape
- x_pad = F.pad(x, pad=self.pad, mode='constant', value=-1e9)
- channel = self.unfold(x_pad).view(batch_size, c, -1, h, w)
- result = torch.max(channel, dim=2)[0]
- return result
-
-
-def dilation(x, m=1):
- b, c, h, w = x.shape
- x_pad = F.pad(x, pad=[m, m, m, m], mode='constant', value=-1e9)
- channel = nn.functional.unfold(x_pad, 2 * m + 1, padding=0, stride=1).view(b, c, -1, h, w)
- result = torch.max(channel, dim=2)[0]
- return result
diff --git a/spaces/akhaliq/Real-ESRGAN/inference_realesrgan.py b/spaces/akhaliq/Real-ESRGAN/inference_realesrgan.py
deleted file mode 100644
index 6d5ff4d188faaa16c0131be69a08fd22fb608f80..0000000000000000000000000000000000000000
--- a/spaces/akhaliq/Real-ESRGAN/inference_realesrgan.py
+++ /dev/null
@@ -1,128 +0,0 @@
-import argparse
-import cv2
-import glob
-import os
-from basicsr.archs.rrdbnet_arch import RRDBNet
-
-from realesrgan import RealESRGANer
-from realesrgan.archs.srvgg_arch import SRVGGNetCompact
-
-
-def main():
- """Inference demo for Real-ESRGAN.
- """
- parser = argparse.ArgumentParser()
- parser.add_argument('-i', '--input', type=str, default='inputs', help='Input image or folder')
- parser.add_argument(
- '-n',
- '--model_name',
- type=str,
- default='RealESRGAN_x4plus',
- help=('Model names: RealESRGAN_x4plus | RealESRNet_x4plus | RealESRGAN_x4plus_anime_6B | RealESRGAN_x2plus'
- 'RealESRGANv2-anime-xsx2 | RealESRGANv2-animevideo-xsx2-nousm | RealESRGANv2-animevideo-xsx2'
- 'RealESRGANv2-anime-xsx4 | RealESRGANv2-animevideo-xsx4-nousm | RealESRGANv2-animevideo-xsx4'))
- parser.add_argument('-o', '--output', type=str, default='results', help='Output folder')
- parser.add_argument('-s', '--outscale', type=float, default=4, help='The final upsampling scale of the image')
- parser.add_argument('--suffix', type=str, default='out', help='Suffix of the restored image')
- parser.add_argument('-t', '--tile', type=int, default=0, help='Tile size, 0 for no tile during testing')
- parser.add_argument('--tile_pad', type=int, default=10, help='Tile padding')
- parser.add_argument('--pre_pad', type=int, default=0, help='Pre padding size at each border')
- parser.add_argument('--face_enhance', action='store_true', help='Use GFPGAN to enhance face')
- parser.add_argument('--half', action='store_true', help='Use half precision during inference')
- parser.add_argument(
- '--alpha_upsampler',
- type=str,
- default='realesrgan',
- help='The upsampler for the alpha channels. Options: realesrgan | bicubic')
- parser.add_argument(
- '--ext',
- type=str,
- default='auto',
- help='Image extension. Options: auto | jpg | png, auto means using the same extension as inputs')
- args = parser.parse_args()
-
- # determine models according to model names
- args.model_name = args.model_name.split('.')[0]
- if args.model_name in ['RealESRGAN_x4plus', 'RealESRNet_x4plus']: # x4 RRDBNet model
- model = RRDBNet(num_in_ch=3, num_out_ch=3, num_feat=64, num_block=23, num_grow_ch=32, scale=4)
- netscale = 4
- elif args.model_name in ['RealESRGAN_x4plus_anime_6B']: # x4 RRDBNet model with 6 blocks
- model = RRDBNet(num_in_ch=3, num_out_ch=3, num_feat=64, num_block=6, num_grow_ch=32, scale=4)
- netscale = 4
- elif args.model_name in ['RealESRGAN_x2plus']: # x2 RRDBNet model
- model = RRDBNet(num_in_ch=3, num_out_ch=3, num_feat=64, num_block=23, num_grow_ch=32, scale=2)
- netscale = 2
- elif args.model_name in [
- 'RealESRGANv2-anime-xsx2', 'RealESRGANv2-animevideo-xsx2-nousm', 'RealESRGANv2-animevideo-xsx2'
- ]: # x2 VGG-style model (XS size)
- model = SRVGGNetCompact(num_in_ch=3, num_out_ch=3, num_feat=64, num_conv=16, upscale=2, act_type='prelu')
- netscale = 2
- elif args.model_name in [
- 'RealESRGANv2-anime-xsx4', 'RealESRGANv2-animevideo-xsx4-nousm', 'RealESRGANv2-animevideo-xsx4'
- ]: # x4 VGG-style model (XS size)
- model = SRVGGNetCompact(num_in_ch=3, num_out_ch=3, num_feat=64, num_conv=16, upscale=4, act_type='prelu')
- netscale = 4
-
- # determine model paths
- model_path = os.path.join('.', args.model_name + '.pth')
- if not os.path.isfile(model_path):
- model_path = os.path.join('.', args.model_name + '.pth')
- if not os.path.isfile(model_path):
- raise ValueError(f'Model {args.model_name} does not exist.')
-
- # restorer
- upsampler = RealESRGANer(
- scale=netscale,
- model_path=model_path,
- model=model,
- tile=args.tile,
- tile_pad=args.tile_pad,
- pre_pad=args.pre_pad,
- half=args.half)
-
- if args.face_enhance: # Use GFPGAN for face enhancement
- from gfpgan import GFPGANer
- face_enhancer = GFPGANer(
- model_path='https://github.com/TencentARC/GFPGAN/releases/download/v0.2.0/GFPGANCleanv1-NoCE-C2.pth',
- upscale=args.outscale,
- arch='clean',
- channel_multiplier=2,
- bg_upsampler=upsampler)
- os.makedirs(args.output, exist_ok=True)
-
- if os.path.isfile(args.input):
- paths = [args.input]
- else:
- paths = sorted(glob.glob(os.path.join(args.input, '*')))
-
- for idx, path in enumerate(paths):
- imgname, extension = os.path.splitext(os.path.basename(path))
- print('Testing', idx, imgname)
-
- img = cv2.imread(path, cv2.IMREAD_UNCHANGED)
- if len(img.shape) == 3 and img.shape[2] == 4:
- img_mode = 'RGBA'
- else:
- img_mode = None
-
- try:
- if args.face_enhance:
- _, _, output = face_enhancer.enhance(img, has_aligned=False, only_center_face=False, paste_back=True)
- else:
- output, _ = upsampler.enhance(img, outscale=args.outscale)
- except RuntimeError as error:
- print('Error', error)
- print('If you encounter CUDA out of memory, try to set --tile with a smaller number.')
- else:
- if args.ext == 'auto':
- extension = extension[1:]
- else:
- extension = args.ext
- if img_mode == 'RGBA': # RGBA images should be saved in png format
- extension = 'png'
- save_path = os.path.join(args.output, f'{imgname}_{args.suffix}.{extension}')
- cv2.imwrite(save_path, output)
-
-
-if __name__ == '__main__':
- main()
diff --git a/spaces/akhaliq/VQMIVC/ParallelWaveGAN/egs/jsut/voc1/run.sh b/spaces/akhaliq/VQMIVC/ParallelWaveGAN/egs/jsut/voc1/run.sh
deleted file mode 100644
index 25338a5df6e3225cebb83188f839c567647ad7b7..0000000000000000000000000000000000000000
--- a/spaces/akhaliq/VQMIVC/ParallelWaveGAN/egs/jsut/voc1/run.sh
+++ /dev/null
@@ -1,164 +0,0 @@
-#!/bin/bash
-
-# Copyright 2019 Tomoki Hayashi
-# MIT License (https://opensource.org/licenses/MIT)
-
-. ./cmd.sh || exit 1;
-. ./path.sh || exit 1;
-
-# basic settings
-stage=-1 # stage to start
-stop_stage=100 # stage to stop
-verbose=1 # verbosity level (lower is less info)
-n_gpus=1 # number of gpus in training
-n_jobs=16 # number of parallel jobs in feature extraction
-
-# NOTE(kan-bayashi): renamed to conf to avoid conflict in parse_options.sh
-conf=conf/parallel_wavegan.v1.yaml
-
-# directory path setting
-download_dir=downloads # direcotry to save downloaded files
-dumpdir=dump # directory to dump features
-
-# training related setting
-tag="" # tag for directory to save model
-resume="" # checkpoint path to resume training
- # (e.g. //checkpoint-10000steps.pkl)
-
-# decoding related setting
-checkpoint="" # checkpoint path to be used for decoding
- # if not provided, the latest one will be used
- # (e.g. //checkpoint-400000steps.pkl)
-
-# shellcheck disable=SC1091
-. utils/parse_options.sh || exit 1;
-
-train_set="train_nodev" # name of training data directory
-dev_set="dev" # name of development data direcotry
-eval_set="eval" # name of evaluation data direcotry
-
-set -euo pipefail
-
-if [ "${stage}" -le -1 ] && [ "${stop_stage}" -ge -1 ]; then
- echo "Stage -1: Data download"
- local/data_download.sh "${download_dir}"
-fi
-
-if [ "${stage}" -le 0 ] && [ "${stop_stage}" -ge 0 ]; then
- echo "Stage 0: Data preparation"
- local/data_prep.sh \
- --train_set "${train_set}" \
- --dev_set "${dev_set}" \
- --eval_set "${eval_set}" \
- "${download_dir}/jsut_ver1.1" data
-fi
-
-stats_ext=$(grep -q "hdf5" <(yq ".format" "${conf}") && echo "h5" || echo "npy")
-if [ "${stage}" -le 1 ] && [ "${stop_stage}" -ge 1 ]; then
- echo "Stage 1: Feature extraction"
- # extract raw features
- pids=()
- for name in "${train_set}" "${dev_set}" "${eval_set}"; do
- (
- [ ! -e "${dumpdir}/${name}/raw" ] && mkdir -p "${dumpdir}/${name}/raw"
- echo "Feature extraction start. See the progress via ${dumpdir}/${name}/raw/preprocessing.*.log."
- utils/make_subset_data.sh "data/${name}" "${n_jobs}" "${dumpdir}/${name}/raw"
- ${train_cmd} JOB=1:${n_jobs} "${dumpdir}/${name}/raw/preprocessing.JOB.log" \
- parallel-wavegan-preprocess \
- --config "${conf}" \
- --scp "${dumpdir}/${name}/raw/wav.JOB.scp" \
- --segments "${dumpdir}/${name}/raw/segments.JOB" \
- --dumpdir "${dumpdir}/${name}/raw/dump.JOB" \
- --verbose "${verbose}"
- echo "Successfully finished feature extraction of ${name} set."
- ) &
- pids+=($!)
- done
- i=0; for pid in "${pids[@]}"; do wait "${pid}" || ((++i)); done
- [ "${i}" -gt 0 ] && echo "$0: ${i} background jobs are failed." && exit 1;
- echo "Successfully finished feature extraction."
-
- # calculate statistics for normalization
- echo "Statistics computation start. See the progress via ${dumpdir}/${train_set}/compute_statistics.log."
- ${train_cmd} "${dumpdir}/${train_set}/compute_statistics.log" \
- parallel-wavegan-compute-statistics \
- --config "${conf}" \
- --rootdir "${dumpdir}/${train_set}/raw" \
- --dumpdir "${dumpdir}/${train_set}" \
- --verbose "${verbose}"
- echo "Successfully finished calculation of statistics."
-
- # normalize and dump them
- pids=()
- for name in "${train_set}" "${dev_set}" "${eval_set}"; do
- (
- [ ! -e "${dumpdir}/${name}/norm" ] && mkdir -p "${dumpdir}/${name}/norm"
- echo "Nomalization start. See the progress via ${dumpdir}/${name}/norm/normalize.*.log."
- ${train_cmd} JOB=1:${n_jobs} "${dumpdir}/${name}/norm/normalize.JOB.log" \
- parallel-wavegan-normalize \
- --config "${conf}" \
- --stats "${dumpdir}/${train_set}/stats.${stats_ext}" \
- --rootdir "${dumpdir}/${name}/raw/dump.JOB" \
- --dumpdir "${dumpdir}/${name}/norm/dump.JOB" \
- --verbose "${verbose}"
- echo "Successfully finished normalization of ${name} set."
- ) &
- pids+=($!)
- done
- i=0; for pid in "${pids[@]}"; do wait "${pid}" || ((++i)); done
- [ "${i}" -gt 0 ] && echo "$0: ${i} background jobs are failed." && exit 1;
- echo "Successfully finished normalization."
-fi
-
-if [ -z "${tag}" ]; then
- expdir="exp/${train_set}_jsut_$(basename "${conf}" .yaml)"
-else
- expdir="exp/${train_set}_jsut_${tag}"
-fi
-if [ "${stage}" -le 2 ] && [ "${stop_stage}" -ge 2 ]; then
- echo "Stage 2: Network training"
- [ ! -e "${expdir}" ] && mkdir -p "${expdir}"
- cp "${dumpdir}/${train_set}/stats.${stats_ext}" "${expdir}"
- if [ "${n_gpus}" -gt 1 ]; then
- train="python -m parallel_wavegan.distributed.launch --nproc_per_node ${n_gpus} -c parallel-wavegan-train"
- else
- train="parallel-wavegan-train"
- fi
- echo "Training start. See the progress via ${expdir}/train.log."
- ${cuda_cmd} --gpu "${n_gpus}" "${expdir}/train.log" \
- ${train} \
- --config "${conf}" \
- --train-dumpdir "${dumpdir}/${train_set}/norm" \
- --dev-dumpdir "${dumpdir}/${dev_set}/norm" \
- --outdir "${expdir}" \
- --resume "${resume}" \
- --verbose "${verbose}"
- echo "Successfully finished training."
-fi
-
-if [ "${stage}" -le 3 ] && [ "${stop_stage}" -ge 3 ]; then
- echo "Stage 3: Network decoding"
- # shellcheck disable=SC2012
- [ -z "${checkpoint}" ] && checkpoint="$(ls -dt "${expdir}"/*.pkl | head -1 || true)"
- outdir="${expdir}/wav/$(basename "${checkpoint}" .pkl)"
- pids=()
- for name in "${dev_set}" "${eval_set}"; do
- (
- [ ! -e "${outdir}/${name}" ] && mkdir -p "${outdir}/${name}"
- [ "${n_gpus}" -gt 1 ] && n_gpus=1
- echo "Decoding start. See the progress via ${outdir}/${name}/decode.log."
- ${cuda_cmd} --gpu "${n_gpus}" "${outdir}/${name}/decode.log" \
- parallel-wavegan-decode \
- --dumpdir "${dumpdir}/${name}/norm" \
- --checkpoint "${checkpoint}" \
- --outdir "${outdir}/${name}" \
- --verbose "${verbose}"
- echo "Successfully finished decoding of ${name} set."
- ) &
- pids+=($!)
- done
- i=0; for pid in "${pids[@]}"; do wait "${pid}" || ((++i)); done
- [ "${i}" -gt 0 ] && echo "$0: ${i} background jobs are failed." && exit 1;
- echo "Successfully finished decoding."
-fi
-echo "Finished."
diff --git a/spaces/akhaliq/deeplab2/g3doc/setup/installation.md b/spaces/akhaliq/deeplab2/g3doc/setup/installation.md
deleted file mode 100644
index 30c384df55cef47442a17d992df6474853229de6..0000000000000000000000000000000000000000
--- a/spaces/akhaliq/deeplab2/g3doc/setup/installation.md
+++ /dev/null
@@ -1,269 +0,0 @@
-# DeepLab2
-
-## **Requirements**
-
-DeepLab2 depends on the following libraries:
-
-* Python3
-* Numpy
-* Pillow
-* Matplotlib
-* Tensorflow 2.5
-* Cython
-* [Google Protobuf](https://developers.google.com/protocol-buffers)
-* [Orbit](https://github.com/tensorflow/models/tree/master/orbit)
-* [pycocotools](https://github.com/cocodataset/cocoapi/tree/master/PythonAPI/pycocotools)
- (for AP-Mask)
-
-## **Installation**
-
-### Git Clone the Project
-
-Clone the
-[`google-research/deeplab2`](https://github.com/google-research/deeplab2)
-repository.
-
-```bash
-mkdir ${YOUR_PROJECT_NAME}
-cd ${YOUR_PROJECT_NAME}
-git clone https://github.com/google-research/deeplab2.git
-```
-
-### Install TensorFlow via PIP
-
-```bash
-# Install tensorflow 2.5 as an example.
-# This should come with compatible numpy package.
-pip install tensorflow==2.5
-```
-
-**NOTE:** You should find the right Tensorflow version according to your own
-configuration at
-https://www.tensorflow.org/install/source#tested_build_configurations. You also
-need to choose the right cuda version as listed on the page if you want to run
-with GPU.
-
-### Install Protobuf
-
-Below is a quick-to-start command line to install
-[protobuf](https://github.com/protocolbuffers/protobuf) in Linux:
-
-```bash
-sudo apt-get install protobuf-compiler
-```
-
-Alternatively, you can also download the package from web on other platforms.
-Please refer to https://github.com/protocolbuffers/protobuf for more details
-about installation.
-
-### Other Libraries
-
-The remaining libraries can be installed via pip:
-
-```bash
-# Pillow
-pip install pillow
-# matplotlib
-pip install matplotlib
-# Cython
-pip install cython
-```
-
-### Install Orbit
-
-[`Orbit`](https://github.com/tensorflow/models/tree/master/orbit) is a flexible,
-lightweight library designed to make it easy to write custom training loops in
-TensorFlow 2. We used Orbit in our train/eval loops. You need to download the
-code below:
-
-```bash
-cd ${YOUR_PROJECT_NAME}
-git clone https://github.com/tensorflow/models.git
-```
-
-### Install pycocotools
-
-We also use
-[`pycocotools`](https://github.com/cocodataset/cocoapi/tree/master/PythonAPI/pycocotools)
-for instance segmentation evaluation. Below is the installation guide:
-
-```bash
-cd ${YOUR_PROJECT_NAME}
-git clone https://github.com/cocodataset/cocoapi.git
-
-# Compile cocoapi
-cd ${YOUR_PROJECT_NAME}/cocoapi/PythonAPI
-make
-cd ${YOUR_PROJECT_NAME}
-```
-
-## **Compilation**
-
-The following instructions are running from `${YOUR_PROJECT_NAME}` directory:
-
-```bash
-cd ${YOUR_PROJECT_NAME}
-```
-
-### Add Libraries to PYTHONPATH
-
-When running locally, `${YOUR_PROJECT_NAME}` directory should be appended to
-PYTHONPATH. This can be done by running the following command:
-
-```bash
-# From ${YOUR_PROJECT_NAME}:
-
-# deeplab2
-export PYTHONPATH=$PYTHONPATH:`pwd`
-# orbit
-export PYTHONPATH=$PYTHONPATH:${PATH_TO_MODELS}
-# pycocotools
-export PYTHONPATH=$PYTHONPATH:${PATH_TO_cocoapi_PythonAPI}
-```
-
-If you clone `models(for Orbit)` and `cocoapi` under `${YOUR_PROJECT_NAME}`,
-here is an example:
-
-```bash
-export PYTHONPATH=$PYTHONPATH:`pwd`:`pwd`/models:`pwd`/cocoapi/PythonAPI
-```
-
-### Compile Protocol Buffers
-
-In DeepLab2, we define
-[protocol buffers](https://developers.google.com/protocol-buffers) to configure
-training and evaluation variants (see [proto definition](../../config.proto)).
-However, protobuf needs to be compiled beforehand into a python recognizable
-format. To compile protobuf, run:
-
-```bash
-# `${PATH_TO_PROTOC}` is the directory where the `protoc` binary locates.
-${PATH_TO_PROTOC} deeplab2/*.proto --python_out=.
-
-# Alternatively, if protobuf compiler is globally accessible, you can simply run:
-protoc deeplab2/*.proto --python_out=.
-```
-
-### (Optional) Compile Custom Ops
-
-We implemented efficient merging operation to merge semantic and instance maps
-for fast inference. You can follow the guide below to compile the provided
-efficient merging operation in c++ under the folder `tensorflow_ops`.
-
-The script is mostly from
-[Compile the op using your system compiler](https://www.tensorflow.org/guide/create_op#compile_the_op_using_your_system_compiler_tensorflow_binary_installation)
-in the official tensorflow guide to create custom ops. Please refer to
-[Create an op](https://www.tensorflow.org/guide/create_op#compile_the_op_using_your_system_compiler_tensorflow_binary_installation)
-for more details.
-
-```bash
-TF_CFLAGS=( $(python -c 'import tensorflow as tf; print(" ".join(tf.sysconfig.get_compile_flags()))') )
-TF_LFLAGS=( $(python -c 'import tensorflow as tf; print(" ".join(tf.sysconfig.get_link_flags()))') )
-OP_NAME='deeplab2/tensorflow_ops/kernels/merge_semantic_and_instance_maps_op'
-
-# CPU
-g++ -std=c++14 -shared \
-${OP_NAME}.cc ${OP_NAME}_kernel.cc -o ${OP_NAME}.so -fPIC ${TF_CFLAGS[@]} ${TF_LFLAGS[@]} -O2
-
-# GPU support (https://www.tensorflow.org/guide/create_op#compiling_the_kernel_for_the_gpu_device)
-nvcc -std=c++14 -c -o ${OP_NAME}_kernel.cu.o ${OP_NAME}_kernel.cu.cc \
- ${TF_CFLAGS[@]} -D GOOGLE_CUDA=1 -x cu -Xcompiler -fPIC --expt-relaxed-constexpr
-
-g++ -std=c++14 -shared -o ${OP_NAME}.so ${OP_NAME}.cc ${OP_NAME}_kernel.cc \
- ${OP_NAME}_kernel.cu.o ${TF_CFLAGS[@]} -fPIC -lcudart ${TF_LFLAGS[@]}
-```
-
-To test if the compilation is done successfully, you can run:
-
-```bash
-python deeplab2/tensorflow_ops/python/kernel_tests/merge_semantic_and_instance_maps_op_test.py
-```
-
-Optionally, you could set `merge_semantic_and_instance_with_tf_op` to `false` in
-the config file to skip provided efficient merging operation and use the slower
-pure TF functions instead. See
-`deeplab2/configs/cityscaspes/panoptic_deeplab/resnet50_os32_merge_with_pure_tf_func.textproto`
-as an example.
-
-### Test the Configuration
-
-You can test if you have successfully installed and configured DeepLab2 by
-running the following commands (requires compilation of custom ops):
-
-```bash
-# Model training test (test for custom ops, protobuf)
-python deeplab2/model/deeplab_test.py
-
-# Model evaluator test (test for other packages such as orbit, cocoapi, etc)
-python deeplab2/trainer/evaluator_test.py
-```
-
-### Quick All-in-One Script for Compilation (Linux Only)
-
-We also provide a shell script to help you quickly compile and test everything
-mentioned above for Linux users:
-
-```bash
-# CPU
-deeplab2/compile.sh
-
-# GPU
-deeplab2/compile.sh gpu
-```
-
-## Troubleshooting
-
-**Q1: Can I use [conda](https://anaconda.org/) instead of pip?**
-
-**A1:** We experienced several dependency issues with the most recent conda
-package. We therefore do not provide support for installing deeplab2 via conda
-at this stage.
-
-________________________________________________________________________________
-
-**Q2: How can I specify a specific nvcc to use a specific gcc version?**
-
-**A2:** At the moment, tensorflow requires a gcc version < 9. If your default
-compiler has a higher version, the path to a different gcc needs to be set to
-compile the custom GPU op. Please check that either gcc-7 or gcc-8 are
-installed.
-
-The compiler can then be set as follows:
-
-```bash
-# Assuming gcc-7 is installed in /usr/bin (can be verified by which gcc-7)
-
-nvcc -std=c++14 -c -o ${OP_NAME}_kernel.cu.o ${OP_NAME}_kernel.cu.cc \
-${TF_CFLAGS[@]} -D GOOGLE_CUDA=1 -x cu -Xcompiler -fPIC -ccbin=/usr/bin/g++-7 \
---expt-relaxed-constexpr
-
-g++-7 -std=c++14 -shared -o ${OP_NAME}.so ${OP_NAME}.cc ${OP_NAME}_kernel.cc \
-${OP_NAME}_kernel.cu.o ${TF_CFLAGS[@]} -fPIC -lcudart ${TF_LFLAGS[@]}
-```
-
-________________________________________________________________________________
-
-**Q3: I got the following errors while compiling the efficient merging
-operation:**
-
-```
-fatal error: third_party/gpus/cuda/include/cuda_fp16.h: No such file or directory
-```
-
-**A3:** It sounds like that CUDA headers are not linked. To resolve this issue,
-you need to tell tensorflow where to find the CUDA headers:
-
-1. Find the CUDA installation directory ${CUDA_DIR} which contains the
- `include` folder (For example, `~/CUDA/gpus/cuda_11_0`).
-2. Go to the directory where tensorflow package is installed. (You can find it
- via `pip show tensorflow`.)
-3. Then `cd` to `tensorflow/include/third_party/gpus/`. (If it doesn't exist,
- create one.)
-4. Symlink your CUDA include directory here:
-
-```
-ln -s ${CUDA_DIR} ./cuda
-```
-
-There have been similar issues and solutions discussed here:
-https://github.com/tensorflow/tensorflow/issues/31912#issuecomment-547475301
diff --git a/spaces/akhaliq/lama/bin/paper_runfiles/blur_tests.sh b/spaces/akhaliq/lama/bin/paper_runfiles/blur_tests.sh
deleted file mode 100644
index 8f204a4c643d08935e5561ed27a286536643958d..0000000000000000000000000000000000000000
--- a/spaces/akhaliq/lama/bin/paper_runfiles/blur_tests.sh
+++ /dev/null
@@ -1,37 +0,0 @@
-##!/usr/bin/env bash
-#
-## !!! file set to make test_large_30k from the vanilla test_large: configs/test_large_30k.lst
-#
-## paths to data are valid for mml7
-#PLACES_ROOT="/data/inpainting/Places365"
-#OUT_DIR="/data/inpainting/paper_data/Places365_val_test"
-#
-#source "$(dirname $0)/env.sh"
-#
-#for datadir in test_large_30k # val_large
-#do
-# for conf in random_thin_256 random_medium_256 random_thick_256 random_thin_512 random_medium_512 random_thick_512
-# do
-# "$BINDIR/gen_mask_dataset.py" "$CONFIGDIR/data_gen/${conf}.yaml" \
-# "$PLACES_ROOT/$datadir" "$OUT_DIR/$datadir/$conf" --n-jobs 8
-#
-# "$BINDIR/calc_dataset_stats.py" --samples-n 20 "$OUT_DIR/$datadir/$conf" "$OUT_DIR/$datadir/${conf}_stats"
-# done
-#
-# for conf in segm_256 segm_512
-# do
-# "$BINDIR/gen_mask_dataset.py" "$CONFIGDIR/data_gen/${conf}.yaml" \
-# "$PLACES_ROOT/$datadir" "$OUT_DIR/$datadir/$conf" --n-jobs 2
-#
-# "$BINDIR/calc_dataset_stats.py" --samples-n 20 "$OUT_DIR/$datadir/$conf" "$OUT_DIR/$datadir/${conf}_stats"
-# done
-#done
-#
-#IN_DIR="/data/inpainting/paper_data/Places365_val_test/test_large_30k/random_medium_512"
-#PRED_DIR="/data/inpainting/predictions/final/images/r.suvorov_2021-03-05_17-08-35_train_ablv2_work_resume_epoch37/random_medium_512"
-#BLUR_OUT_DIR="/data/inpainting/predictions/final/blur/images"
-#
-#for b in 0.1
-#
-#"$BINDIR/blur_predicts.py" "$BASEDIR/../../configs/eval2.yaml" "$CUR_IN_DIR" "$CUR_OUT_DIR" "$CUR_EVAL_DIR"
-#
diff --git a/spaces/akhaliq/redshift-diffusion/app.py b/spaces/akhaliq/redshift-diffusion/app.py
deleted file mode 100644
index 3a60f48280f2808b1d6f3adfd0382823dcfa0619..0000000000000000000000000000000000000000
--- a/spaces/akhaliq/redshift-diffusion/app.py
+++ /dev/null
@@ -1,3 +0,0 @@
-import gradio as gr
-
-gr.Interface.load("models/nitrosocke/redshift-diffusion").launch()
\ No newline at end of file
diff --git a/spaces/akhaliq/yolov7/scripts/get_coco.sh b/spaces/akhaliq/yolov7/scripts/get_coco.sh
deleted file mode 100644
index 524f8dd9e2cae992a4047476520a7e4e1402e6de..0000000000000000000000000000000000000000
--- a/spaces/akhaliq/yolov7/scripts/get_coco.sh
+++ /dev/null
@@ -1,22 +0,0 @@
-#!/bin/bash
-# COCO 2017 dataset http://cocodataset.org
-# Download command: bash ./scripts/get_coco.sh
-
-# Download/unzip labels
-d='./' # unzip directory
-url=https://github.com/ultralytics/yolov5/releases/download/v1.0/
-f='coco2017labels-segments.zip' # or 'coco2017labels.zip', 68 MB
-echo 'Downloading' $url$f ' ...'
-curl -L $url$f -o $f && unzip -q $f -d $d && rm $f & # download, unzip, remove in background
-
-# Download/unzip images
-d='./coco/images' # unzip directory
-url=http://images.cocodataset.org/zips/
-f1='train2017.zip' # 19G, 118k images
-f2='val2017.zip' # 1G, 5k images
-f3='test2017.zip' # 7G, 41k images (optional)
-for f in $f1 $f2 $f3; do
- echo 'Downloading' $url$f '...'
- curl -L $url$f -o $f && unzip -q $f -d $d && rm $f & # download, unzip, remove in background
-done
-wait # finish background tasks
diff --git a/spaces/akuysal/demo-app-gradio/app.py b/spaces/akuysal/demo-app-gradio/app.py
deleted file mode 100644
index aef11f785b0ea12e17a73af9de702019122bc620..0000000000000000000000000000000000000000
--- a/spaces/akuysal/demo-app-gradio/app.py
+++ /dev/null
@@ -1,15 +0,0 @@
-import gradio as gr
-
-from transformers import pipeline
-
-sentiment = pipeline("sentiment-analysis")
-
-def get_sentiment(input_text):
- return sentiment(input_text)
-
-iface = gr.Interface(fn = get_sentiment,
- inputs = "text",
- outputs = ['text'],
- title = 'Sentiment Analysis',
- description = "Get sentiment Negative/Positive for the given input")
-iface.launch(inline = False)
\ No newline at end of file
diff --git a/spaces/alan-chen-intel/dagan-demo/modules/util.py b/spaces/alan-chen-intel/dagan-demo/modules/util.py
deleted file mode 100644
index 765c4f1568e245a8c43fef7f9e43e588bf2f4e2a..0000000000000000000000000000000000000000
--- a/spaces/alan-chen-intel/dagan-demo/modules/util.py
+++ /dev/null
@@ -1,399 +0,0 @@
-from torch import nn
-
-import torch.nn.functional as F
-import torch
-
-from sync_batchnorm import SynchronizedBatchNorm2d as BatchNorm2d
-import pdb
-import torch.nn.utils.spectral_norm as spectral_norm
-def kp2gaussian(kp, spatial_size, kp_variance):
- """
- Transform a keypoint into gaussian like representation
- """
- mean = kp['value']
-
- coordinate_grid = make_coordinate_grid(spatial_size, mean.type())
- number_of_leading_dimensions = len(mean.shape) - 1
- shape = (1,) * number_of_leading_dimensions + coordinate_grid.shape
- coordinate_grid = coordinate_grid.view(*shape)
- repeats = mean.shape[:number_of_leading_dimensions] + (1, 1, 1)
- coordinate_grid = coordinate_grid.repeat(*repeats)
-
- # Preprocess kp shape
- shape = mean.shape[:number_of_leading_dimensions] + (1, 1, 2)
- mean = mean.view(*shape)
-
- mean_sub = (coordinate_grid - mean)
-
- out = torch.exp(-0.5 * (mean_sub ** 2).sum(-1) / kp_variance)
-
- return out
-
-
-def make_coordinate_grid(spatial_size, type):
- """
- Create a meshgrid [-1,1] x [-1,1] of given spatial_size.
- """
- h, w = spatial_size
- x = torch.arange(w).type(type)
- y = torch.arange(h).type(type)
-
- x = (2 * (x / (w - 1)) - 1)
- y = (2 * (y / (h - 1)) - 1)
-
- yy = y.view(-1, 1).repeat(1, w)
- xx = x.view(1, -1).repeat(h, 1)
-
- meshed = torch.cat([xx.unsqueeze_(2), yy.unsqueeze_(2)], 2)
-
- return meshed
-
-
-class ResBlock2d(nn.Module):
- """
- Res block, preserve spatial resolution.
- """
-
- def __init__(self, in_features, kernel_size, padding):
- super(ResBlock2d, self).__init__()
- self.conv1 = nn.Conv2d(in_channels=in_features, out_channels=in_features, kernel_size=kernel_size,
- padding=padding)
- self.conv2 = nn.Conv2d(in_channels=in_features, out_channels=in_features, kernel_size=kernel_size,
- padding=padding)
- self.norm1 = BatchNorm2d(in_features, affine=True)
- self.norm2 = BatchNorm2d(in_features, affine=True)
-
- def forward(self, x):
- out = self.norm1(x)
- out = F.relu(out)
- out = self.conv1(out)
- out = self.norm2(out)
- out = F.relu(out)
- out = self.conv2(out)
- out += x
- return out
-
-
-class UpBlock2d(nn.Module):
- """
- Upsampling block for use in decoder.
- """
-
- def __init__(self, in_features, out_features, kernel_size=3, padding=1, groups=1):
- super(UpBlock2d, self).__init__()
-
- self.conv = nn.Conv2d(in_channels=in_features, out_channels=out_features, kernel_size=kernel_size,
- padding=padding, groups=groups)
- self.norm = BatchNorm2d(out_features, affine=True)
-
- def forward(self, x):
- out = F.interpolate(x, scale_factor=2)
- out = self.conv(out)
- out = self.norm(out)
- out = F.relu(out)
- return out
-
-
-class DownBlock2d(nn.Module):
- """
- Downsampling block for use in encoder.
- """
-
- def __init__(self, in_features, out_features, kernel_size=3, padding=1, groups=1):
- super(DownBlock2d, self).__init__()
- self.conv = nn.Conv2d(in_channels=in_features, out_channels=out_features, kernel_size=kernel_size,
- padding=padding, groups=groups)
- self.norm = BatchNorm2d(out_features, affine=True)
- self.pool = nn.AvgPool2d(kernel_size=(2, 2))
-
- def forward(self, x):
- out = self.conv(x)
- out = self.norm(out)
- out = F.relu(out)
- out = self.pool(out)
- return out
-
-
-class SameBlock2d(nn.Module):
- """
- Simple block, preserve spatial resolution.
- """
-
- def __init__(self, in_features, out_features, groups=1, kernel_size=3, padding=1):
- super(SameBlock2d, self).__init__()
- self.conv = nn.Conv2d(in_channels=in_features, out_channels=out_features,
- kernel_size=kernel_size, padding=padding, groups=groups)
- self.norm = BatchNorm2d(out_features, affine=True)
-
- def forward(self, x):
- out = self.conv(x)
- out = self.norm(out)
- out = F.relu(out)
- return out
-
-
-class Encoder(nn.Module):
- """
- Hourglass Encoder
- """
-
- def __init__(self, block_expansion, in_features, num_blocks=3, max_features=256):
- super(Encoder, self).__init__()
-
- down_blocks = []
- for i in range(num_blocks):
- down_blocks.append(DownBlock2d(in_features if i == 0 else min(max_features, block_expansion * (2 ** i)),
- min(max_features, block_expansion * (2 ** (i + 1))),
- kernel_size=3, padding=1))
- self.down_blocks = nn.ModuleList(down_blocks)
-
- def forward(self, x):
- outs = [x]
- for down_block in self.down_blocks:
- outs.append(down_block(outs[-1]))
- return outs
-
-
-class Decoder(nn.Module):
- """
- Hourglass Decoder
- """
-
- def __init__(self, block_expansion, in_features, num_blocks=3, max_features=256):
- super(Decoder, self).__init__()
-
- up_blocks = []
-
- for i in range(num_blocks)[::-1]:
- in_filters = (1 if i == num_blocks - 1 else 2) * min(max_features, block_expansion * (2 ** (i + 1)))
- out_filters = min(max_features, block_expansion * (2 ** i))
- up_blocks.append(UpBlock2d(in_filters, out_filters, kernel_size=3, padding=1))
-
- self.up_blocks = nn.ModuleList(up_blocks)
- self.out_filters = block_expansion + in_features
-
- def forward(self, x):
- out = x.pop()
- for up_block in self.up_blocks:
- out = up_block(out)
- skip = x.pop()
- out = torch.cat([out, skip], dim=1)
- return out
-
-
-class Decoder_w_emb(nn.Module):
- """
- Hourglass Decoder
- """
-
- def __init__(self, block_expansion, in_features, num_blocks=3, max_features=256):
- super(Decoder_w_emb, self).__init__()
-
- up_blocks = []
-
- for i in range(num_blocks)[::-1]:
- in_filters = (1 if i == num_blocks - 1 else 2) * min(max_features, block_expansion * (2 ** (i + 1)))
- out_filters = min(max_features, block_expansion * (2 ** i))
- up_blocks.append(UpBlock2d(in_filters, out_filters, kernel_size=3, padding=1))
-
- self.up_blocks = nn.ModuleList(up_blocks)
- self.out_filters = block_expansion + in_features
-
- def forward(self, x):
- feats = []
- out = x.pop()
- feats.append(out)
- for ind,up_block in enumerate(self.up_blocks):
- out = up_block(out)
- skip = x.pop()
- feats.append(skip)
- out = torch.cat([out, skip], dim=1)
- return out,feats
-
-class Decoder_2branch(nn.Module):
- """
- Hourglass Decoder
- """
-
- def __init__(self, block_expansion, in_features, num_blocks=3, max_features=256):
- super(Decoder_2branch, self).__init__()
- up_blocks = []
- for i in range(num_blocks)[::-1]:
- in_filters = (1 if i == num_blocks - 1 else 2) * min(max_features, block_expansion * (2 ** (i + 1)))
- out_filters = min(max_features, block_expansion * (2 ** i))
- up_blocks.append(UpBlock2d(in_filters, out_filters, kernel_size=3, padding=1))
-
- self.up_blocks = nn.ModuleList(up_blocks)
- self.out_filters = block_expansion + in_features
-
- def forward(self, x):
- # out = x.pop()
- num_feat = len(x)
- out=x[-1]
- for i in range(len(self.up_blocks)):
- out = self.up_blocks[i](out)
- skip = x[-(i+1+1)]
- out = torch.cat([out, skip], dim=1)
- return out
-
-
-
-class Hourglass(nn.Module):
- """
- Hourglass architecture.
- """
-
- def __init__(self, block_expansion, in_features, num_blocks=3, max_features=256):
- super(Hourglass, self).__init__()
- self.encoder = Encoder(block_expansion, in_features, num_blocks, max_features)
- self.decoder = Decoder(block_expansion, in_features, num_blocks, max_features)
- self.out_filters = self.decoder.out_filters
- def forward(self, x):
- return self.decoder(self.encoder(x))
-
-class Hourglass_2branch(nn.Module):
- """
- Hourglass architecture.
- """
-
- def __init__(self, block_expansion, in_features, num_blocks=3, max_features=256):
- super(Hourglass_2branch, self).__init__()
- self.encoder = Encoder(block_expansion, in_features, num_blocks, max_features)
- self.decoder_kp = Decoder_2branch(block_expansion, in_features, num_blocks, max_features)
- self.decoder_mask = Decoder_2branch(block_expansion, in_features, num_blocks, max_features)
-
- self.out_filters = self.decoder_kp.out_filters
- def forward(self, x):
- embd= self.encoder(x)
- kp_feat = self.decoder_kp(embd)
- mask_feat = self.decoder_mask(embd)
- return kp_feat,mask_feat
-
-
-class Hourglass_w_emb(nn.Module):
- """
- Hourglass architecture.
- """
-
- def __init__(self, block_expansion, in_features, num_blocks=3, max_features=256):
- super(Hourglass_w_emb, self).__init__()
- self.encoder = Encoder(block_expansion, in_features, num_blocks, max_features)
- self.decoder = Decoder_w_emb(block_expansion, in_features, num_blocks, max_features)
- self.out_filters = self.decoder.out_filters
-
- def forward(self, x):
- embs = self.encoder(x)
- result,feats = self.decoder(embs)
- return feats,result
-class AntiAliasInterpolation2d(nn.Module):
- """
- Band-limited downsampling, for better preservation of the input signal.
- """
- def __init__(self, channels, scale):
- super(AntiAliasInterpolation2d, self).__init__()
- sigma = (1 / scale - 1) / 2
- kernel_size = 2 * round(sigma * 4) + 1
- self.ka = kernel_size // 2
- self.kb = self.ka - 1 if kernel_size % 2 == 0 else self.ka
-
- kernel_size = [kernel_size, kernel_size]
- sigma = [sigma, sigma]
- # The gaussian kernel is the product of the
- # gaussian function of each dimension.
- kernel = 1
- meshgrids = torch.meshgrid(
- [
- torch.arange(size, dtype=torch.float32)
- for size in kernel_size
- ]
- )
- for size, std, mgrid in zip(kernel_size, sigma, meshgrids):
- mean = (size - 1) / 2
- kernel *= torch.exp(-(mgrid - mean) ** 2 / (2 * std ** 2))
-
- # Make sure sum of values in gaussian kernel equals 1.
- kernel = kernel / torch.sum(kernel)
- # Reshape to depthwise convolutional weight
- kernel = kernel.view(1, 1, *kernel.size())
- kernel = kernel.repeat(channels, *[1] * (kernel.dim() - 1))
-
- self.register_buffer('weight', kernel)
- self.groups = channels
- self.scale = scale
- inv_scale = 1 / scale
- self.int_inv_scale = int(inv_scale)
-
- def forward(self, input):
- if self.scale == 1.0:
- return input
-
- out = F.pad(input, (self.ka, self.kb, self.ka, self.kb))
- out = F.conv2d(out, weight=self.weight, groups=self.groups)
- out = out[:, :, ::self.int_inv_scale, ::self.int_inv_scale]
-
- return out
-
-
-class SPADE(nn.Module):
- def __init__(self, norm_nc, label_nc):
- super().__init__()
-
- self.param_free_norm = nn.InstanceNorm2d(norm_nc, affine=False)
- nhidden = 128
-
- self.mlp_shared = nn.Sequential(
- nn.Conv2d(label_nc, nhidden, kernel_size=3, padding=1),
- nn.ReLU())
- self.mlp_gamma = nn.Conv2d(nhidden, norm_nc, kernel_size=3, padding=1)
- self.mlp_beta = nn.Conv2d(nhidden, norm_nc, kernel_size=3, padding=1)
-
- def forward(self, x, segmap):
- normalized = self.param_free_norm(x)
- segmap = F.interpolate(segmap, size=x.size()[2:], mode='nearest')
- actv = self.mlp_shared(segmap)
- gamma = self.mlp_gamma(actv)
- beta = self.mlp_beta(actv)
- out = normalized * (1 + gamma) + beta
- return out
-
-
-class SPADEResnetBlock(nn.Module):
- def __init__(self, fin, fout, norm_G, label_nc, use_se=False, dilation=1):
- super().__init__()
- # Attributes
- self.learned_shortcut = (fin != fout)
- fmiddle = min(fin, fout)
- self.use_se = use_se
- # create conv layers
- self.conv_0 = nn.Conv2d(fin, fmiddle, kernel_size=3, padding=dilation, dilation=dilation)
- self.conv_1 = nn.Conv2d(fmiddle, fout, kernel_size=3, padding=dilation, dilation=dilation)
- if self.learned_shortcut:
- self.conv_s = nn.Conv2d(fin, fout, kernel_size=1, bias=False)
- # apply spectral norm if specified
- if 'spectral' in norm_G:
- self.conv_0 = spectral_norm(self.conv_0)
- self.conv_1 = spectral_norm(self.conv_1)
- if self.learned_shortcut:
- self.conv_s = spectral_norm(self.conv_s)
- # define normalization layers
- self.norm_0 = SPADE(fin, label_nc)
- self.norm_1 = SPADE(fmiddle, label_nc)
- if self.learned_shortcut:
- self.norm_s = SPADE(fin, label_nc)
-
- def forward(self, x, seg1):
- x_s = self.shortcut(x, seg1)
- dx = self.conv_0(self.actvn(self.norm_0(x, seg1)))
- dx = self.conv_1(self.actvn(self.norm_1(dx, seg1)))
- out = x_s + dx
- return out
-
- def shortcut(self, x, seg1):
- if self.learned_shortcut:
- x_s = self.conv_s(self.norm_s(x, seg1))
- else:
- x_s = x
- return x_s
-
- def actvn(self, x):
- return F.leaky_relu(x, 2e-1)
\ No newline at end of file
diff --git a/spaces/alexrame/rewardedsoups/streamlit_app/data/locomotion/trajectories/14.html b/spaces/alexrame/rewardedsoups/streamlit_app/data/locomotion/trajectories/14.html
deleted file mode 100644
index 210b2d992d0bedb4b80ba1cc14242d7fc747547c..0000000000000000000000000000000000000000
--- a/spaces/alexrame/rewardedsoups/streamlit_app/data/locomotion/trajectories/14.html
+++ /dev/null
@@ -1,48 +0,0 @@
-
-
-
- brax visualizer
-
-
-
-
-
-
-
-
diff --git a/spaces/alexray/btc_predictor/venv/lib/python3.10/site-packages/pip/_internal/distributions/wheel.py b/spaces/alexray/btc_predictor/venv/lib/python3.10/site-packages/pip/_internal/distributions/wheel.py
deleted file mode 100644
index 340b0f3c5c75f4ae0865c138dd7e26eae2c3c248..0000000000000000000000000000000000000000
--- a/spaces/alexray/btc_predictor/venv/lib/python3.10/site-packages/pip/_internal/distributions/wheel.py
+++ /dev/null
@@ -1,31 +0,0 @@
-from pip._vendor.packaging.utils import canonicalize_name
-
-from pip._internal.distributions.base import AbstractDistribution
-from pip._internal.index.package_finder import PackageFinder
-from pip._internal.metadata import (
- BaseDistribution,
- FilesystemWheel,
- get_wheel_distribution,
-)
-
-
-class WheelDistribution(AbstractDistribution):
- """Represents a wheel distribution.
-
- This does not need any preparation as wheels can be directly unpacked.
- """
-
- def get_metadata_distribution(self) -> BaseDistribution:
- """Loads the metadata from the wheel file into memory and returns a
- Distribution that uses it, not relying on the wheel file or
- requirement.
- """
- assert self.req.local_file_path, "Set as part of preparation during download"
- assert self.req.name, "Wheels are never unnamed"
- wheel = FilesystemWheel(self.req.local_file_path)
- return get_wheel_distribution(wheel, canonicalize_name(self.req.name))
-
- def prepare_distribution_metadata(
- self, finder: PackageFinder, build_isolation: bool
- ) -> None:
- pass
diff --git a/spaces/alexray/btc_predictor/venv/lib/python3.10/site-packages/pip/_internal/network/session.py b/spaces/alexray/btc_predictor/venv/lib/python3.10/site-packages/pip/_internal/network/session.py
deleted file mode 100644
index cbe743ba6a1636f5ea7fb74c89d36dabb54b502b..0000000000000000000000000000000000000000
--- a/spaces/alexray/btc_predictor/venv/lib/python3.10/site-packages/pip/_internal/network/session.py
+++ /dev/null
@@ -1,454 +0,0 @@
-"""PipSession and supporting code, containing all pip-specific
-network request configuration and behavior.
-"""
-
-import email.utils
-import io
-import ipaddress
-import json
-import logging
-import mimetypes
-import os
-import platform
-import shutil
-import subprocess
-import sys
-import urllib.parse
-import warnings
-from typing import Any, Dict, Iterator, List, Mapping, Optional, Sequence, Tuple, Union
-
-from pip._vendor import requests, urllib3
-from pip._vendor.cachecontrol import CacheControlAdapter
-from pip._vendor.requests.adapters import BaseAdapter, HTTPAdapter
-from pip._vendor.requests.models import PreparedRequest, Response
-from pip._vendor.requests.structures import CaseInsensitiveDict
-from pip._vendor.urllib3.connectionpool import ConnectionPool
-from pip._vendor.urllib3.exceptions import InsecureRequestWarning
-
-from pip import __version__
-from pip._internal.metadata import get_default_environment
-from pip._internal.models.link import Link
-from pip._internal.network.auth import MultiDomainBasicAuth
-from pip._internal.network.cache import SafeFileCache
-
-# Import ssl from compat so the initial import occurs in only one place.
-from pip._internal.utils.compat import has_tls
-from pip._internal.utils.glibc import libc_ver
-from pip._internal.utils.misc import build_url_from_netloc, parse_netloc
-from pip._internal.utils.urls import url_to_path
-
-logger = logging.getLogger(__name__)
-
-SecureOrigin = Tuple[str, str, Optional[Union[int, str]]]
-
-
-# Ignore warning raised when using --trusted-host.
-warnings.filterwarnings("ignore", category=InsecureRequestWarning)
-
-
-SECURE_ORIGINS: List[SecureOrigin] = [
- # protocol, hostname, port
- # Taken from Chrome's list of secure origins (See: http://bit.ly/1qrySKC)
- ("https", "*", "*"),
- ("*", "localhost", "*"),
- ("*", "127.0.0.0/8", "*"),
- ("*", "::1/128", "*"),
- ("file", "*", None),
- # ssh is always secure.
- ("ssh", "*", "*"),
-]
-
-
-# These are environment variables present when running under various
-# CI systems. For each variable, some CI systems that use the variable
-# are indicated. The collection was chosen so that for each of a number
-# of popular systems, at least one of the environment variables is used.
-# This list is used to provide some indication of and lower bound for
-# CI traffic to PyPI. Thus, it is okay if the list is not comprehensive.
-# For more background, see: https://github.com/pypa/pip/issues/5499
-CI_ENVIRONMENT_VARIABLES = (
- # Azure Pipelines
- "BUILD_BUILDID",
- # Jenkins
- "BUILD_ID",
- # AppVeyor, CircleCI, Codeship, Gitlab CI, Shippable, Travis CI
- "CI",
- # Explicit environment variable.
- "PIP_IS_CI",
-)
-
-
-def looks_like_ci() -> bool:
- """
- Return whether it looks like pip is running under CI.
- """
- # We don't use the method of checking for a tty (e.g. using isatty())
- # because some CI systems mimic a tty (e.g. Travis CI). Thus that
- # method doesn't provide definitive information in either direction.
- return any(name in os.environ for name in CI_ENVIRONMENT_VARIABLES)
-
-
-def user_agent() -> str:
- """
- Return a string representing the user agent.
- """
- data: Dict[str, Any] = {
- "installer": {"name": "pip", "version": __version__},
- "python": platform.python_version(),
- "implementation": {
- "name": platform.python_implementation(),
- },
- }
-
- if data["implementation"]["name"] == "CPython":
- data["implementation"]["version"] = platform.python_version()
- elif data["implementation"]["name"] == "PyPy":
- pypy_version_info = sys.pypy_version_info # type: ignore
- if pypy_version_info.releaselevel == "final":
- pypy_version_info = pypy_version_info[:3]
- data["implementation"]["version"] = ".".join(
- [str(x) for x in pypy_version_info]
- )
- elif data["implementation"]["name"] == "Jython":
- # Complete Guess
- data["implementation"]["version"] = platform.python_version()
- elif data["implementation"]["name"] == "IronPython":
- # Complete Guess
- data["implementation"]["version"] = platform.python_version()
-
- if sys.platform.startswith("linux"):
- from pip._vendor import distro
-
- linux_distribution = distro.name(), distro.version(), distro.codename()
- distro_infos: Dict[str, Any] = dict(
- filter(
- lambda x: x[1],
- zip(["name", "version", "id"], linux_distribution),
- )
- )
- libc = dict(
- filter(
- lambda x: x[1],
- zip(["lib", "version"], libc_ver()),
- )
- )
- if libc:
- distro_infos["libc"] = libc
- if distro_infos:
- data["distro"] = distro_infos
-
- if sys.platform.startswith("darwin") and platform.mac_ver()[0]:
- data["distro"] = {"name": "macOS", "version": platform.mac_ver()[0]}
-
- if platform.system():
- data.setdefault("system", {})["name"] = platform.system()
-
- if platform.release():
- data.setdefault("system", {})["release"] = platform.release()
-
- if platform.machine():
- data["cpu"] = platform.machine()
-
- if has_tls():
- import _ssl as ssl
-
- data["openssl_version"] = ssl.OPENSSL_VERSION
-
- setuptools_dist = get_default_environment().get_distribution("setuptools")
- if setuptools_dist is not None:
- data["setuptools_version"] = str(setuptools_dist.version)
-
- if shutil.which("rustc") is not None:
- # If for any reason `rustc --version` fails, silently ignore it
- try:
- rustc_output = subprocess.check_output(
- ["rustc", "--version"], stderr=subprocess.STDOUT, timeout=0.5
- )
- except Exception:
- pass
- else:
- if rustc_output.startswith(b"rustc "):
- # The format of `rustc --version` is:
- # `b'rustc 1.52.1 (9bc8c42bb 2021-05-09)\n'`
- # We extract just the middle (1.52.1) part
- data["rustc_version"] = rustc_output.split(b" ")[1].decode()
-
- # Use None rather than False so as not to give the impression that
- # pip knows it is not being run under CI. Rather, it is a null or
- # inconclusive result. Also, we include some value rather than no
- # value to make it easier to know that the check has been run.
- data["ci"] = True if looks_like_ci() else None
-
- user_data = os.environ.get("PIP_USER_AGENT_USER_DATA")
- if user_data is not None:
- data["user_data"] = user_data
-
- return "{data[installer][name]}/{data[installer][version]} {json}".format(
- data=data,
- json=json.dumps(data, separators=(",", ":"), sort_keys=True),
- )
-
-
-class LocalFSAdapter(BaseAdapter):
- def send(
- self,
- request: PreparedRequest,
- stream: bool = False,
- timeout: Optional[Union[float, Tuple[float, float]]] = None,
- verify: Union[bool, str] = True,
- cert: Optional[Union[str, Tuple[str, str]]] = None,
- proxies: Optional[Mapping[str, str]] = None,
- ) -> Response:
- pathname = url_to_path(request.url)
-
- resp = Response()
- resp.status_code = 200
- resp.url = request.url
-
- try:
- stats = os.stat(pathname)
- except OSError as exc:
- # format the exception raised as a io.BytesIO object,
- # to return a better error message:
- resp.status_code = 404
- resp.reason = type(exc).__name__
- resp.raw = io.BytesIO(f"{resp.reason}: {exc}".encode("utf8"))
- else:
- modified = email.utils.formatdate(stats.st_mtime, usegmt=True)
- content_type = mimetypes.guess_type(pathname)[0] or "text/plain"
- resp.headers = CaseInsensitiveDict(
- {
- "Content-Type": content_type,
- "Content-Length": stats.st_size,
- "Last-Modified": modified,
- }
- )
-
- resp.raw = open(pathname, "rb")
- resp.close = resp.raw.close
-
- return resp
-
- def close(self) -> None:
- pass
-
-
-class InsecureHTTPAdapter(HTTPAdapter):
- def cert_verify(
- self,
- conn: ConnectionPool,
- url: str,
- verify: Union[bool, str],
- cert: Optional[Union[str, Tuple[str, str]]],
- ) -> None:
- super().cert_verify(conn=conn, url=url, verify=False, cert=cert)
-
-
-class InsecureCacheControlAdapter(CacheControlAdapter):
- def cert_verify(
- self,
- conn: ConnectionPool,
- url: str,
- verify: Union[bool, str],
- cert: Optional[Union[str, Tuple[str, str]]],
- ) -> None:
- super().cert_verify(conn=conn, url=url, verify=False, cert=cert)
-
-
-class PipSession(requests.Session):
-
- timeout: Optional[int] = None
-
- def __init__(
- self,
- *args: Any,
- retries: int = 0,
- cache: Optional[str] = None,
- trusted_hosts: Sequence[str] = (),
- index_urls: Optional[List[str]] = None,
- **kwargs: Any,
- ) -> None:
- """
- :param trusted_hosts: Domains not to emit warnings for when not using
- HTTPS.
- """
- super().__init__(*args, **kwargs)
-
- # Namespace the attribute with "pip_" just in case to prevent
- # possible conflicts with the base class.
- self.pip_trusted_origins: List[Tuple[str, Optional[int]]] = []
-
- # Attach our User Agent to the request
- self.headers["User-Agent"] = user_agent()
-
- # Attach our Authentication handler to the session
- self.auth = MultiDomainBasicAuth(index_urls=index_urls)
-
- # Create our urllib3.Retry instance which will allow us to customize
- # how we handle retries.
- retries = urllib3.Retry(
- # Set the total number of retries that a particular request can
- # have.
- total=retries,
- # A 503 error from PyPI typically means that the Fastly -> Origin
- # connection got interrupted in some way. A 503 error in general
- # is typically considered a transient error so we'll go ahead and
- # retry it.
- # A 500 may indicate transient error in Amazon S3
- # A 520 or 527 - may indicate transient error in CloudFlare
- status_forcelist=[500, 503, 520, 527],
- # Add a small amount of back off between failed requests in
- # order to prevent hammering the service.
- backoff_factor=0.25,
- ) # type: ignore
-
- # Our Insecure HTTPAdapter disables HTTPS validation. It does not
- # support caching so we'll use it for all http:// URLs.
- # If caching is disabled, we will also use it for
- # https:// hosts that we've marked as ignoring
- # TLS errors for (trusted-hosts).
- insecure_adapter = InsecureHTTPAdapter(max_retries=retries)
-
- # We want to _only_ cache responses on securely fetched origins or when
- # the host is specified as trusted. We do this because
- # we can't validate the response of an insecurely/untrusted fetched
- # origin, and we don't want someone to be able to poison the cache and
- # require manual eviction from the cache to fix it.
- if cache:
- secure_adapter = CacheControlAdapter(
- cache=SafeFileCache(cache),
- max_retries=retries,
- )
- self._trusted_host_adapter = InsecureCacheControlAdapter(
- cache=SafeFileCache(cache),
- max_retries=retries,
- )
- else:
- secure_adapter = HTTPAdapter(max_retries=retries)
- self._trusted_host_adapter = insecure_adapter
-
- self.mount("https://", secure_adapter)
- self.mount("http://", insecure_adapter)
-
- # Enable file:// urls
- self.mount("file://", LocalFSAdapter())
-
- for host in trusted_hosts:
- self.add_trusted_host(host, suppress_logging=True)
-
- def update_index_urls(self, new_index_urls: List[str]) -> None:
- """
- :param new_index_urls: New index urls to update the authentication
- handler with.
- """
- self.auth.index_urls = new_index_urls
-
- def add_trusted_host(
- self, host: str, source: Optional[str] = None, suppress_logging: bool = False
- ) -> None:
- """
- :param host: It is okay to provide a host that has previously been
- added.
- :param source: An optional source string, for logging where the host
- string came from.
- """
- if not suppress_logging:
- msg = f"adding trusted host: {host!r}"
- if source is not None:
- msg += f" (from {source})"
- logger.info(msg)
-
- host_port = parse_netloc(host)
- if host_port not in self.pip_trusted_origins:
- self.pip_trusted_origins.append(host_port)
-
- self.mount(
- build_url_from_netloc(host, scheme="http") + "/", self._trusted_host_adapter
- )
- self.mount(build_url_from_netloc(host) + "/", self._trusted_host_adapter)
- if not host_port[1]:
- self.mount(
- build_url_from_netloc(host, scheme="http") + ":",
- self._trusted_host_adapter,
- )
- # Mount wildcard ports for the same host.
- self.mount(build_url_from_netloc(host) + ":", self._trusted_host_adapter)
-
- def iter_secure_origins(self) -> Iterator[SecureOrigin]:
- yield from SECURE_ORIGINS
- for host, port in self.pip_trusted_origins:
- yield ("*", host, "*" if port is None else port)
-
- def is_secure_origin(self, location: Link) -> bool:
- # Determine if this url used a secure transport mechanism
- parsed = urllib.parse.urlparse(str(location))
- origin_protocol, origin_host, origin_port = (
- parsed.scheme,
- parsed.hostname,
- parsed.port,
- )
-
- # The protocol to use to see if the protocol matches.
- # Don't count the repository type as part of the protocol: in
- # cases such as "git+ssh", only use "ssh". (I.e., Only verify against
- # the last scheme.)
- origin_protocol = origin_protocol.rsplit("+", 1)[-1]
-
- # Determine if our origin is a secure origin by looking through our
- # hardcoded list of secure origins, as well as any additional ones
- # configured on this PackageFinder instance.
- for secure_origin in self.iter_secure_origins():
- secure_protocol, secure_host, secure_port = secure_origin
- if origin_protocol != secure_protocol and secure_protocol != "*":
- continue
-
- try:
- addr = ipaddress.ip_address(origin_host)
- network = ipaddress.ip_network(secure_host)
- except ValueError:
- # We don't have both a valid address or a valid network, so
- # we'll check this origin against hostnames.
- if (
- origin_host
- and origin_host.lower() != secure_host.lower()
- and secure_host != "*"
- ):
- continue
- else:
- # We have a valid address and network, so see if the address
- # is contained within the network.
- if addr not in network:
- continue
-
- # Check to see if the port matches.
- if (
- origin_port != secure_port
- and secure_port != "*"
- and secure_port is not None
- ):
- continue
-
- # If we've gotten here, then this origin matches the current
- # secure origin and we should return True
- return True
-
- # If we've gotten to this point, then the origin isn't secure and we
- # will not accept it as a valid location to search. We will however
- # log a warning that we are ignoring it.
- logger.warning(
- "The repository located at %s is not a trusted or secure host and "
- "is being ignored. If this repository is available via HTTPS we "
- "recommend you use HTTPS instead, otherwise you may silence "
- "this warning and allow it anyway with '--trusted-host %s'.",
- origin_host,
- origin_host,
- )
-
- return False
-
- def request(self, method: str, url: str, *args: Any, **kwargs: Any) -> Response:
- # Allow setting a default timeout on a session
- kwargs.setdefault("timeout", self.timeout)
-
- # Dispatch the actual request
- return super().request(method, url, *args, **kwargs)
diff --git a/spaces/allknowingroger/Image-Models-Test151/app.py b/spaces/allknowingroger/Image-Models-Test151/app.py
deleted file mode 100644
index a89bc8081329c8e613d48f77390bd05c0005ddef..0000000000000000000000000000000000000000
--- a/spaces/allknowingroger/Image-Models-Test151/app.py
+++ /dev/null
@@ -1,144 +0,0 @@
-import gradio as gr
-# import os
-# import sys
-# from pathlib import Path
-import time
-
-models =[
- "wzneric/df_wm_id1",
- "Yntec/CitrineDreamMix",
- "Namala/nxt",
- "anik550689/dreambooth_lora_0916",
- "AashishNKumar/Ash_PF_Model",
- "kbthebest181/adadadadaorandomnamesothatnoonecanaccessbysearchinghahaha",
- "Yntec/animeTWO",
- "Muhammadreza/mann-e-artistic-3-revised-2",
- "CiroN2022/fusion-graphic",
-]
-
-
-model_functions = {}
-model_idx = 1
-for model_path in models:
- try:
- model_functions[model_idx] = gr.Interface.load(f"models/{model_path}", live=False, preprocess=True, postprocess=False)
- except Exception as error:
- def the_fn(txt):
- return None
- model_functions[model_idx] = gr.Interface(fn=the_fn, inputs=["text"], outputs=["image"])
- model_idx+=1
-
-
-def send_it_idx(idx):
- def send_it_fn(prompt):
- output = (model_functions.get(str(idx)) or model_functions.get(str(1)))(prompt)
- return output
- return send_it_fn
-
-def get_prompts(prompt_text):
- return prompt_text
-
-def clear_it(val):
- if int(val) != 0:
- val = 0
- else:
- val = 0
- pass
- return val
-
-def all_task_end(cnt,t_stamp):
- to = t_stamp + 60
- et = time.time()
- if et > to and t_stamp != 0:
- d = gr.update(value=0)
- tog = gr.update(value=1)
- #print(f'to: {to} et: {et}')
- else:
- if cnt != 0:
- d = gr.update(value=et)
- else:
- d = gr.update(value=0)
- tog = gr.update(value=0)
- #print (f'passing: to: {to} et: {et}')
- pass
- return d, tog
-
-def all_task_start():
- print("\n\n\n\n\n\n\n")
- t = time.gmtime()
- t_stamp = time.time()
- current_time = time.strftime("%H:%M:%S", t)
- return gr.update(value=t_stamp), gr.update(value=t_stamp), gr.update(value=0)
-
-def clear_fn():
- nn = len(models)
- return tuple([None, *[None for _ in range(nn)]])
-
-
-
-with gr.Blocks(title="SD Models") as my_interface:
- with gr.Column(scale=12):
- # with gr.Row():
- # gr.Markdown("""- Primary prompt: 你想画的内容(英文单词,如 a cat, 加英文逗号效果更好;点 Improve 按钮进行完善)\n- Real prompt: 完善后的提示词,出现后再点右边的 Run 按钮开始运行""")
- with gr.Row():
- with gr.Row(scale=6):
- primary_prompt=gr.Textbox(label="Prompt", value="")
- # real_prompt=gr.Textbox(label="Real prompt")
- with gr.Row(scale=6):
- # improve_prompts_btn=gr.Button("Improve")
- with gr.Row():
- run=gr.Button("Run",variant="primary")
- clear_btn=gr.Button("Clear")
- with gr.Row():
- sd_outputs = {}
- model_idx = 1
- for model_path in models:
- with gr.Column(scale=3, min_width=320):
- with gr.Box():
- sd_outputs[model_idx] = gr.Image(label=model_path)
- pass
- model_idx += 1
- pass
- pass
-
- with gr.Row(visible=False):
- start_box=gr.Number(interactive=False)
- end_box=gr.Number(interactive=False)
- tog_box=gr.Textbox(value=0,interactive=False)
-
- start_box.change(
- all_task_end,
- [start_box, end_box],
- [start_box, tog_box],
- every=1,
- show_progress=False)
-
- primary_prompt.submit(all_task_start, None, [start_box, end_box, tog_box])
- run.click(all_task_start, None, [start_box, end_box, tog_box])
- runs_dict = {}
- model_idx = 1
- for model_path in models:
- runs_dict[model_idx] = run.click(model_functions[model_idx], inputs=[primary_prompt], outputs=[sd_outputs[model_idx]])
- model_idx += 1
- pass
- pass
-
- # improve_prompts_btn_clicked=improve_prompts_btn.click(
- # get_prompts,
- # inputs=[primary_prompt],
- # outputs=[primary_prompt],
- # cancels=list(runs_dict.values()))
- clear_btn.click(
- clear_fn,
- None,
- [primary_prompt, *list(sd_outputs.values())],
- cancels=[*list(runs_dict.values())])
- tog_box.change(
- clear_it,
- tog_box,
- tog_box,
- cancels=[*list(runs_dict.values())])
-
-my_interface.queue(concurrency_count=600, status_update_rate=1)
-my_interface.launch(inline=True, show_api=False)
-
\ No newline at end of file
diff --git a/spaces/allknowingroger/Image-Models-Test51/README.md b/spaces/allknowingroger/Image-Models-Test51/README.md
deleted file mode 100644
index c0f53e9a5c716efcf625e46359306868ab557824..0000000000000000000000000000000000000000
--- a/spaces/allknowingroger/Image-Models-Test51/README.md
+++ /dev/null
@@ -1,13 +0,0 @@
----
-title: Image Models
-emoji: 👀
-colorFrom: red
-colorTo: gray
-sdk: gradio
-sdk_version: 3.23.0
-app_file: app.py
-pinned: true
-duplicated_from: allknowingroger/Image-Models-Test50
----
-
-
\ No newline at end of file
diff --git a/spaces/allknowingroger/huggingface/style.css b/spaces/allknowingroger/huggingface/style.css
deleted file mode 100644
index 114adf441e9032febb46bc056b2a8bb651075f0d..0000000000000000000000000000000000000000
--- a/spaces/allknowingroger/huggingface/style.css
+++ /dev/null
@@ -1,28 +0,0 @@
-body {
- padding: 2rem;
- font-family: -apple-system, BlinkMacSystemFont, "Arial", sans-serif;
-}
-
-h1 {
- font-size: 16px;
- margin-top: 0;
-}
-
-p {
- color: rgb(107, 114, 128);
- font-size: 15px;
- margin-bottom: 10px;
- margin-top: 5px;
-}
-
-.card {
- max-width: 620px;
- margin: 0 auto;
- padding: 16px;
- border: 1px solid lightgray;
- border-radius: 16px;
-}
-
-.card p:last-child {
- margin-bottom: 0;
-}
diff --git a/spaces/alphunt/diffdock-alphunt-demo/esm/esm/inverse_folding/gvp_transformer.py b/spaces/alphunt/diffdock-alphunt-demo/esm/esm/inverse_folding/gvp_transformer.py
deleted file mode 100644
index faf7c1555d2d74b43d71a7f59b508da1533cc52f..0000000000000000000000000000000000000000
--- a/spaces/alphunt/diffdock-alphunt-demo/esm/esm/inverse_folding/gvp_transformer.py
+++ /dev/null
@@ -1,137 +0,0 @@
-# Copyright (c) Facebook, Inc. and its affiliates.
-#
-# This source code is licensed under the MIT license found in the
-# LICENSE file in the root directory of this source tree.
-
-import argparse
-from typing import Any, Dict, List, Optional, Tuple, NamedTuple
-import torch
-from torch import nn
-from torch import Tensor
-import torch.nn.functional as F
-from scipy.spatial import transform
-
-from esm.data import Alphabet
-
-from .features import DihedralFeatures
-from .gvp_encoder import GVPEncoder
-from .gvp_utils import unflatten_graph
-from .gvp_transformer_encoder import GVPTransformerEncoder
-from .transformer_decoder import TransformerDecoder
-from .util import rotate, CoordBatchConverter
-
-
-class GVPTransformerModel(nn.Module):
- """
- GVP-Transformer inverse folding model.
-
- Architecture: Geometric GVP-GNN as initial layers, followed by
- sequence-to-sequence Transformer encoder and decoder.
- """
-
- def __init__(self, args, alphabet):
- super().__init__()
- encoder_embed_tokens = self.build_embedding(
- args, alphabet, args.encoder_embed_dim,
- )
- decoder_embed_tokens = self.build_embedding(
- args, alphabet, args.decoder_embed_dim,
- )
- encoder = self.build_encoder(args, alphabet, encoder_embed_tokens)
- decoder = self.build_decoder(args, alphabet, decoder_embed_tokens)
- self.args = args
- self.encoder = encoder
- self.decoder = decoder
-
- @classmethod
- def build_encoder(cls, args, src_dict, embed_tokens):
- encoder = GVPTransformerEncoder(args, src_dict, embed_tokens)
- return encoder
-
- @classmethod
- def build_decoder(cls, args, tgt_dict, embed_tokens):
- decoder = TransformerDecoder(
- args,
- tgt_dict,
- embed_tokens,
- )
- return decoder
-
- @classmethod
- def build_embedding(cls, args, dictionary, embed_dim):
- num_embeddings = len(dictionary)
- padding_idx = dictionary.padding_idx
- emb = nn.Embedding(num_embeddings, embed_dim, padding_idx)
- nn.init.normal_(emb.weight, mean=0, std=embed_dim ** -0.5)
- nn.init.constant_(emb.weight[padding_idx], 0)
- return emb
-
- def forward(
- self,
- coords,
- padding_mask,
- confidence,
- prev_output_tokens,
- return_all_hiddens: bool = False,
- features_only: bool = False,
- ):
- encoder_out = self.encoder(coords, padding_mask, confidence,
- return_all_hiddens=return_all_hiddens)
- logits, extra = self.decoder(
- prev_output_tokens,
- encoder_out=encoder_out,
- features_only=features_only,
- return_all_hiddens=return_all_hiddens,
- )
- return logits, extra
-
- def sample(self, coords, partial_seq=None, temperature=1.0, confidence=None):
- """
- Samples sequences based on multinomial sampling (no beam search).
-
- Args:
- coords: L x 3 x 3 list representing one backbone
- partial_seq: Optional, partial sequence with mask tokens if part of
- the sequence is known
- temperature: sampling temperature, use low temperature for higher
- sequence recovery and high temperature for higher diversity
- confidence: optional length L list of confidence scores for coordinates
- """
- L = len(coords)
- # Convert to batch format
- batch_converter = CoordBatchConverter(self.decoder.dictionary)
- batch_coords, confidence, _, _, padding_mask = (
- batch_converter([(coords, confidence, None)])
- )
-
- # Start with prepend token
- mask_idx = self.decoder.dictionary.get_idx('')
- sampled_tokens = torch.full((1, 1+L), mask_idx, dtype=int)
- sampled_tokens[0, 0] = self.decoder.dictionary.get_idx('')
- if partial_seq is not None:
- for i, c in enumerate(partial_seq):
- sampled_tokens[0, i+1] = self.decoder.dictionary.get_idx(c)
-
- # Save incremental states for faster sampling
- incremental_state = dict()
-
- # Run encoder only once
- encoder_out = self.encoder(batch_coords, padding_mask, confidence)
-
- # Decode one token at a time
- for i in range(1, L+1):
- if sampled_tokens[0, i] != mask_idx:
- continue
- logits, _ = self.decoder(
- sampled_tokens[:, :i],
- encoder_out,
- incremental_state=incremental_state,
- )
- logits = logits[0].transpose(0, 1)
- logits /= temperature
- probs = F.softmax(logits, dim=-1)
- sampled_tokens[:, i] = torch.multinomial(probs, 1).squeeze(-1)
- sampled_seq = sampled_tokens[0, 1:]
-
- # Convert back to string via lookup
- return ''.join([self.decoder.dictionary.get_tok(a) for a in sampled_seq])
diff --git a/spaces/amarchheda/ChordDuplicate/portaudio/examples/paex_write_sine.c b/spaces/amarchheda/ChordDuplicate/portaudio/examples/paex_write_sine.c
deleted file mode 100644
index 3035b42ba92e2430ee320051f5cd914c1fcdde91..0000000000000000000000000000000000000000
--- a/spaces/amarchheda/ChordDuplicate/portaudio/examples/paex_write_sine.c
+++ /dev/null
@@ -1,166 +0,0 @@
-/** @file paex_write_sine.c
- @ingroup examples_src
- @brief Play a sine wave for several seconds using the blocking API (Pa_WriteStream())
- @author Ross Bencina
- @author Phil Burk
-*/
-/*
- * $Id$
- *
- * This program uses the PortAudio Portable Audio Library.
- * For more information see: http://www.portaudio.com/
- * Copyright (c) 1999-2000 Ross Bencina and Phil Burk
- *
- * Permission is hereby granted, free of charge, to any person obtaining
- * a copy of this software and associated documentation files
- * (the "Software"), to deal in the Software without restriction,
- * including without limitation the rights to use, copy, modify, merge,
- * publish, distribute, sublicense, and/or sell copies of the Software,
- * and to permit persons to whom the Software is furnished to do so,
- * subject to the following conditions:
- *
- * The above copyright notice and this permission notice shall be
- * included in all copies or substantial portions of the Software.
- *
- * THE SOFTWARE IS PROVIDED "AS IS", WITHOUT WARRANTY OF ANY KIND,
- * EXPRESS OR IMPLIED, INCLUDING BUT NOT LIMITED TO THE WARRANTIES OF
- * MERCHANTABILITY, FITNESS FOR A PARTICULAR PURPOSE AND NONINFRINGEMENT.
- * IN NO EVENT SHALL THE AUTHORS OR COPYRIGHT HOLDERS BE LIABLE FOR
- * ANY CLAIM, DAMAGES OR OTHER LIABILITY, WHETHER IN AN ACTION OF
- * CONTRACT, TORT OR OTHERWISE, ARISING FROM, OUT OF OR IN CONNECTION
- * WITH THE SOFTWARE OR THE USE OR OTHER DEALINGS IN THE SOFTWARE.
- */
-
-/*
- * The text above constitutes the entire PortAudio license; however,
- * the PortAudio community also makes the following non-binding requests:
- *
- * Any person wishing to distribute modifications to the Software is
- * requested to send the modifications to the original developer so that
- * they can be incorporated into the canonical version. It is also
- * requested that these non-binding requests be included along with the
- * license above.
- */
-
-#include
-#include
-#include "portaudio.h"
-
-#define NUM_SECONDS (5)
-#define SAMPLE_RATE (44100)
-#define FRAMES_PER_BUFFER (1024)
-
-#ifndef M_PI
-#define M_PI (3.14159265)
-#endif
-
-#define TABLE_SIZE (200)
-
-
-int main(void);
-int main(void)
-{
- PaStreamParameters outputParameters;
- PaStream *stream;
- PaError err;
- float buffer[FRAMES_PER_BUFFER][2]; /* stereo output buffer */
- float sine[TABLE_SIZE]; /* sine wavetable */
- int left_phase = 0;
- int right_phase = 0;
- int left_inc = 1;
- int right_inc = 3; /* higher pitch so we can distinguish left and right. */
- int i, j, k;
- int bufferCount;
-
- printf("PortAudio Test: output sine wave. SR = %d, BufSize = %d\n", SAMPLE_RATE, FRAMES_PER_BUFFER);
-
- /* initialise sinusoidal wavetable */
- for( i=0; idefaultLowOutputLatency;
- outputParameters.hostApiSpecificStreamInfo = NULL;
-
- err = Pa_OpenStream(
- &stream,
- NULL, /* no input */
- &outputParameters,
- SAMPLE_RATE,
- FRAMES_PER_BUFFER,
- paClipOff, /* we won't output out of range samples so don't bother clipping them */
- NULL, /* no callback, use blocking API */
- NULL ); /* no callback, so no callback userData */
- if( err != paNoError ) goto error;
-
-
- printf( "Play 3 times, higher each time.\n" );
-
- for( k=0; k < 3; ++k )
- {
- err = Pa_StartStream( stream );
- if( err != paNoError ) goto error;
-
- printf("Play for %d seconds.\n", NUM_SECONDS );
-
- bufferCount = ((NUM_SECONDS * SAMPLE_RATE) / FRAMES_PER_BUFFER);
-
- for( i=0; i < bufferCount; i++ )
- {
- for( j=0; j < FRAMES_PER_BUFFER; j++ )
- {
- buffer[j][0] = sine[left_phase]; /* left */
- buffer[j][1] = sine[right_phase]; /* right */
- left_phase += left_inc;
- if( left_phase >= TABLE_SIZE ) left_phase -= TABLE_SIZE;
- right_phase += right_inc;
- if( right_phase >= TABLE_SIZE ) right_phase -= TABLE_SIZE;
- }
-
- err = Pa_WriteStream( stream, buffer, FRAMES_PER_BUFFER );
- if( err != paNoError ) goto error;
- }
-
- err = Pa_StopStream( stream );
- if( err != paNoError ) goto error;
-
- ++left_inc;
- ++right_inc;
-
- Pa_Sleep( 1000 );
- }
-
- err = Pa_CloseStream( stream );
- if( err != paNoError ) goto error;
-
- Pa_Terminate();
- printf("Test finished.\n");
-
- return err;
-
-error:
- fprintf( stderr, "An error occurred while using the portaudio stream\n" );
- fprintf( stderr, "Error number: %d\n", err );
- fprintf( stderr, "Error message: %s\n", Pa_GetErrorText( err ) );
- // Print more information about the error.
- if( err == paUnanticipatedHostError )
- {
- const PaHostErrorInfo *hostErrorInfo = Pa_GetLastHostErrorInfo();
- fprintf( stderr, "Host API error = #%ld, hostApiType = %d\n", hostErrorInfo->errorCode, hostErrorInfo->hostApiType );
- fprintf( stderr, "Host API error = %s\n", hostErrorInfo->errorText );
- }
- Pa_Terminate();
- return err;
-}
diff --git a/spaces/amsterdamNLP/CLIP-attention-rollout/clip_grounding/evaluation/clip_on_png.py b/spaces/amsterdamNLP/CLIP-attention-rollout/clip_grounding/evaluation/clip_on_png.py
deleted file mode 100644
index 8121362eed3cf6072c053ad832140ae954a4322c..0000000000000000000000000000000000000000
--- a/spaces/amsterdamNLP/CLIP-attention-rollout/clip_grounding/evaluation/clip_on_png.py
+++ /dev/null
@@ -1,362 +0,0 @@
-"""Evaluates cross-modal correspondence of CLIP on PNG images."""
-
-import os
-import sys
-from os.path import join, exists
-
-import warnings
-warnings.filterwarnings('ignore')
-
-from clip_grounding.utils.paths import REPO_PATH
-sys.path.append(join(REPO_PATH, "CLIP_explainability/Transformer-MM-Explainability/"))
-
-import torch
-import CLIP.clip as clip
-from PIL import Image
-import numpy as np
-import cv2
-import matplotlib.pyplot as plt
-from captum.attr import visualization
-from torchmetrics import JaccardIndex
-from collections import defaultdict
-from IPython.core.display import display, HTML
-from skimage import filters
-
-from CLIP_explainability.utils import interpret, show_img_heatmap, show_txt_heatmap, color, _tokenizer
-from clip_grounding.datasets.png import PNG
-from clip_grounding.utils.image import pad_to_square
-from clip_grounding.utils.visualize import show_grid_of_images
-from clip_grounding.utils.log import tqdm_iterator, print_update
-
-
-# global usage
-# specify device
-device = "cuda" if torch.cuda.is_available() else "cpu"
-
-# load CLIP model
-model, preprocess = clip.load("ViT-B/32", device=device, jit=False)
-
-
-def show_cam(mask):
- heatmap = cv2.applyColorMap(np.uint8(255 * mask), cv2.COLORMAP_JET)
- heatmap = np.float32(heatmap) / 255
- cam = heatmap
- cam = cam / np.max(cam)
- return cam
-
-
-def interpret_and_generate(model, img, texts, orig_image, return_outputs=False, show=True):
- text = clip.tokenize(texts).to(device)
- R_text, R_image = interpret(model=model, image=img, texts=text, device=device)
- batch_size = text.shape[0]
-
- outputs = []
- for i in range(batch_size):
- text_scores, text_tokens_decoded = show_txt_heatmap(texts[i], text[i], R_text[i], show=show)
- image_relevance = show_img_heatmap(R_image[i], img, orig_image=orig_image, device=device, show=show)
- plt.show()
- outputs.append({"text_scores": text_scores, "image_relevance": image_relevance, "tokens_decoded": text_tokens_decoded})
-
- if return_outputs:
- return outputs
-
-
-def process_entry_text_to_image(entry, unimodal=False):
- image = entry['image']
- text_mask = entry['text_mask']
- text = entry['text']
- orig_image = pad_to_square(image)
-
- img = preprocess(orig_image).unsqueeze(0).to(device)
- text_index = text_mask.argmax()
- texts = [text[text_index]] if not unimodal else ['']
-
- return img, texts, orig_image
-
-
-def preprocess_ground_truth_mask(mask, resize_shape):
- mask = Image.fromarray(mask.astype(np.uint8) * 255)
- mask = pad_to_square(mask, color=0)
- mask = mask.resize(resize_shape)
- mask = np.asarray(mask) / 255.
- return mask
-
-
-def apply_otsu_threshold(relevance_map):
- threshold = filters.threshold_otsu(relevance_map)
- otsu_map = (relevance_map > threshold).astype(np.uint8)
- return otsu_map
-
-
-def evaluate_text_to_image(method, dataset, debug=False):
-
- instance_level_metrics = defaultdict(list)
- entry_level_metrics = defaultdict(list)
-
- jaccard = JaccardIndex(num_classes=2)
- jaccard = jaccard.to(device)
-
- num_iter = len(dataset)
- if debug:
- num_iter = 100
-
- iterator = tqdm_iterator(range(num_iter), desc=f"Evaluating on {type(dataset).__name__} dataset")
- for idx in iterator:
- instance = dataset[idx]
-
- instance_iou = 0.
- for entry in instance:
-
- # preprocess the image and text
- unimodal = True if method == "clip-unimodal" else False
- test_img, test_texts, orig_image = process_entry_text_to_image(entry, unimodal=unimodal)
-
- if method in ["clip", "clip-unimodal"]:
-
- # compute the relevance scores
- outputs = interpret_and_generate(model, test_img, test_texts, orig_image, return_outputs=True, show=False)
-
- # use the image relevance score to compute IoU w.r.t. ground truth segmentation masks
-
- # NOTE: since we pass single entry (1-sized batch), outputs[0] contains our reqd outputs
- relevance_map = outputs[0]["image_relevance"]
- elif method == "random":
- relevance_map = np.random.uniform(low=0., high=1., size=tuple(test_img.shape[2:]))
-
- otsu_relevance_map = apply_otsu_threshold(relevance_map)
-
- ground_truth_mask = entry["image_mask"]
- ground_truth_mask = preprocess_ground_truth_mask(ground_truth_mask, relevance_map.shape)
-
- entry_iou = jaccard(
- torch.from_numpy(otsu_relevance_map).to(device),
- torch.from_numpy(ground_truth_mask.astype(np.uint8)).to(device),
- )
- entry_iou = entry_iou.item()
- instance_iou += (entry_iou / len(entry))
-
- entry_level_metrics["iou"].append(entry_iou)
-
- # capture instance (image-sentence pair) level IoU
- instance_level_metrics["iou"].append(instance_iou)
-
- average_metrics = {k: np.mean(v) for k, v in entry_level_metrics.items()}
-
- return (
- average_metrics,
- instance_level_metrics,
- entry_level_metrics
- )
-
-
-def process_entry_image_to_text(entry, unimodal=False):
-
- if not unimodal:
- if len(np.asarray(entry["image"]).shape) == 3:
- mask = np.repeat(np.expand_dims(entry['image_mask'], -1), 3, axis=-1)
- else:
- mask = np.asarray(entry['image_mask'])
-
- masked_image = (mask * np.asarray(entry['image'])).astype(np.uint8)
- masked_image = Image.fromarray(masked_image)
- orig_image = pad_to_square(masked_image)
- img = preprocess(orig_image).unsqueeze(0).to(device)
- else:
- orig_image_shape = max(np.asarray(entry['image']).shape[:2])
- orig_image = Image.fromarray(np.zeros((orig_image_shape, orig_image_shape, 3), dtype=np.uint8))
- # orig_image = Image.fromarray(np.random.randint(0, 256, (orig_image_shape, orig_image_shape, 3), dtype=np.uint8))
- img = preprocess(orig_image).unsqueeze(0).to(device)
-
- texts = [' '.join(entry['text'])]
-
- return img, texts, orig_image
-
-
-def process_text_mask(text, text_mask, tokens):
-
- token_level_mask = np.zeros(len(tokens))
-
- for label, subtext in zip(text_mask, text):
-
- subtext_tokens=_tokenizer.encode(subtext)
- subtext_tokens_decoded=[_tokenizer.decode([a]) for a in subtext_tokens]
-
- if label == 1:
- start = tokens.index(subtext_tokens_decoded[0])
- end = tokens.index(subtext_tokens_decoded[-1])
- token_level_mask[start:end + 1] = 1
-
- return token_level_mask
-
-
-def evaluate_image_to_text(method, dataset, debug=False, clamp_sentence_len=70):
-
- instance_level_metrics = defaultdict(list)
- entry_level_metrics = defaultdict(list)
-
- # skipped if text length > 77 which is CLIP limit
- num_entries_skipped = 0
- num_total_entries = 0
-
- num_iter = len(dataset)
- if debug:
- num_iter = 100
-
- jaccard_image_to_text = JaccardIndex(num_classes=2).to(device)
-
- iterator = tqdm_iterator(range(num_iter), desc=f"Evaluating on {type(dataset).__name__} dataset")
- for idx in iterator:
- instance = dataset[idx]
-
- instance_iou = 0.
- for entry in instance:
- num_total_entries += 1
-
- # preprocess the image and text
- unimodal = True if method == "clip-unimodal" else False
- img, texts, orig_image = process_entry_image_to_text(entry, unimodal=unimodal)
-
- appx_total_sent_len = np.sum([len(x.split(" ")) for x in texts])
- if appx_total_sent_len > clamp_sentence_len:
- # print(f"Skipping an entry since it's text has appx"\
- # " {appx_total_sent_len} while CLIP cannot process beyond {clamp_sentence_len}")
- num_entries_skipped += 1
- continue
-
- # compute the relevance scores
- if method in ["clip", "clip-unimodal"]:
- try:
- outputs = interpret_and_generate(model, img, texts, orig_image, return_outputs=True, show=False)
- except:
- num_entries_skipped += 1
- continue
- elif method == "random":
- text = texts[0]
- text_tokens = _tokenizer.encode(text)
- text_tokens_decoded=[_tokenizer.decode([a]) for a in text_tokens]
- outputs = [
- {
- "text_scores": np.random.uniform(low=0., high=1., size=len(text_tokens_decoded)),
- "tokens_decoded": text_tokens_decoded,
- }
- ]
-
- # use the text relevance score to compute IoU w.r.t. ground truth text masks
- # NOTE: since we pass single entry (1-sized batch), outputs[0] contains our reqd outputs
- token_relevance_scores = outputs[0]["text_scores"]
- if isinstance(token_relevance_scores, torch.Tensor):
- token_relevance_scores = token_relevance_scores.cpu().numpy()
- token_relevance_scores = apply_otsu_threshold(token_relevance_scores)
- token_ground_truth_mask = process_text_mask(entry["text"], entry["text_mask"], outputs[0]["tokens_decoded"])
-
- entry_iou = jaccard_image_to_text(
- torch.from_numpy(token_relevance_scores).to(device),
- torch.from_numpy(token_ground_truth_mask.astype(np.uint8)).to(device),
- )
- entry_iou = entry_iou.item()
-
- instance_iou += (entry_iou / len(entry))
- entry_level_metrics["iou"].append(entry_iou)
-
- # capture instance (image-sentence pair) level IoU
- instance_level_metrics["iou"].append(instance_iou)
-
- print(f"CAUTION: Skipped {(num_entries_skipped / num_total_entries) * 100} % since these had length > 77 (CLIP limit).")
- average_metrics = {k: np.mean(v) for k, v in entry_level_metrics.items()}
-
- return (
- average_metrics,
- instance_level_metrics,
- entry_level_metrics
- )
-
-
-if __name__ == "__main__":
-
- import argparse
- parser = argparse.ArgumentParser("Evaluate Image-to-Text & Text-to-Image model")
- parser.add_argument(
- "--eval_method", type=str, default="clip",
- choices=["clip", "random", "clip-unimodal"],
- help="Evaluation method to use",
- )
- parser.add_argument(
- "--ignore_cache", action="store_true",
- help="Ignore cache and force re-generation of the results",
- )
- parser.add_argument(
- "--debug", action="store_true",
- help="Run evaluation on a small subset of the dataset",
- )
- args = parser.parse_args()
-
- print_update("Using evaluation method: {}".format(args.eval_method))
-
-
- clip.clip._MODELS = {
- "ViT-B/32": "https://openaipublic.azureedge.net/clip/models/40d365715913c9da98579312b702a82c18be219cc2a73407c4526f58eba950af/ViT-B-32.pt",
- "ViT-B/16": "https://openaipublic.azureedge.net/clip/models/5806e77cd80f8b59890b7e101eabd078d9fb84e6937f9e85e4ecb61988df416f/ViT-B-16.pt",
- }
-
- # specify device
- device = "cuda" if torch.cuda.is_available() else "cpu"
-
- # load CLIP model
- print_update("Loading CLIP model...")
- model, preprocess = clip.load("ViT-B/32", device=device, jit=False)
- print()
-
- # load PNG dataset
- print_update("Loading PNG dataset...")
- dataset = PNG(dataset_root=join(REPO_PATH, "data", "panoptic_narrative_grounding"), split="val2017")
- print()
-
- # evaluate
-
- # save metrics
- metrics_dir = join(REPO_PATH, "outputs")
- os.makedirs(metrics_dir, exist_ok=True)
-
- metrics_path = join(metrics_dir, f"{args.eval_method}_on_{type(dataset).__name__}_text2image_metrics.pt")
- if (not exists(metrics_path)) or args.ignore_cache:
- print_update("Computing metrics for text-to-image grounding")
- average_metrics, instance_level_metrics, entry_level_metrics = evaluate_text_to_image(
- args.eval_method, dataset, debug=args.debug,
- )
- metrics = {
- "average_metrics": average_metrics,
- "instance_level_metrics":instance_level_metrics,
- "entry_level_metrics": entry_level_metrics
- }
-
- torch.save(metrics, metrics_path)
- print("TEXT2IMAGE METRICS SAVED TO:", metrics_path)
- else:
- print(f"Metrics already exist at: {metrics_path}. Loading cached metrics.")
- metrics = torch.load(metrics_path)
- average_metrics = metrics["average_metrics"]
- print("TEXT2IMAGE METRICS:", np.round(average_metrics["iou"], 4))
-
- print()
-
- metrics_path = join(metrics_dir, f"{args.eval_method}_on_{type(dataset).__name__}_image2text_metrics.pt")
- if (not exists(metrics_path)) or args.ignore_cache:
- print_update("Computing metrics for image-to-text grounding")
- average_metrics, instance_level_metrics, entry_level_metrics = evaluate_image_to_text(
- args.eval_method, dataset, debug=args.debug,
- )
-
- torch.save(
- {
- "average_metrics": average_metrics,
- "instance_level_metrics":instance_level_metrics,
- "entry_level_metrics": entry_level_metrics
- },
- metrics_path,
- )
- print("IMAGE2TEXT METRICS SAVED TO:", metrics_path)
- else:
- print(f"Metrics already exist at: {metrics_path}. Loading cached metrics.")
- metrics = torch.load(metrics_path)
- average_metrics = metrics["average_metrics"]
- print("IMAGE2TEXT METRICS:", np.round(average_metrics["iou"], 4))
diff --git a/spaces/annchen2010/ChatGPT/chatgpt - windows.bat b/spaces/annchen2010/ChatGPT/chatgpt - windows.bat
deleted file mode 100644
index 0b78fdc3a559abd692e3a9e9af5e482124d13a99..0000000000000000000000000000000000000000
--- a/spaces/annchen2010/ChatGPT/chatgpt - windows.bat
+++ /dev/null
@@ -1,14 +0,0 @@
-@echo off
-echo Opening ChuanhuChatGPT...
-
-REM Open powershell via bat
-start powershell.exe -NoExit -Command "python ./ChuanhuChatbot.py"
-
-REM The web page can be accessed with delayed start http://127.0.0.1:7860/
-ping -n 5 127.0.0.1>nul
-
-REM access chargpt via your default browser
-start "" "http://127.0.0.1:7860/"
-
-
-echo Finished opening ChuanhuChatGPT (http://127.0.0.1:7860/).
\ No newline at end of file
diff --git a/spaces/anuragshas/Hindi_ASR/README.md b/spaces/anuragshas/Hindi_ASR/README.md
deleted file mode 100644
index b322c0679cdb7bbc6a184d4d96cf270977b40505..0000000000000000000000000000000000000000
--- a/spaces/anuragshas/Hindi_ASR/README.md
+++ /dev/null
@@ -1,46 +0,0 @@
----
-title: Hindi_ASR
-emoji: 🗣
-colorFrom: purple
-colorTo: green
-sdk: gradio
-app_file: app.py
-pinned: false
-license: apache-2.0
----
-
-# Configuration
-
-`title`: _string_
-Display title for the Space
-
-`emoji`: _string_
-Space emoji (emoji-only character allowed)
-
-`colorFrom`: _string_
-Color for Thumbnail gradient (red, yellow, green, blue, indigo, purple, pink, gray)
-
-`colorTo`: _string_
-Color for Thumbnail gradient (red, yellow, green, blue, indigo, purple, pink, gray)
-
-`sdk`: _string_
-Can be either `gradio`, `streamlit`, or `static`
-
-`sdk_version` : _string_
-Only applicable for `streamlit` SDK.
-See [doc](https://hf.co/docs/hub/spaces) for more info on supported versions.
-
-`app_file`: _string_
-Path to your main application file (which contains either `gradio` or `streamlit` Python code, or `static` html code).
-Path is relative to the root of the repository.
-
-`models`: _List[string]_
-HF model IDs (like "gpt2" or "deepset/roberta-base-squad2") used in the Space.
-Will be parsed automatically from your code if not specified here.
-
-`datasets`: _List[string]_
-HF dataset IDs (like "common_voice" or "oscar-corpus/OSCAR-2109") used in the Space.
-Will be parsed automatically from your code if not specified here.
-
-`pinned`: _boolean_
-Whether the Space stays on top of your list.
diff --git a/spaces/aodianyun/stable-diffusion-webui/extensions/deforum/style.css b/spaces/aodianyun/stable-diffusion-webui/extensions/deforum/style.css
deleted file mode 100644
index 5b3615d207357b2b00c1ba32a737e213e1bdd5ce..0000000000000000000000000000000000000000
--- a/spaces/aodianyun/stable-diffusion-webui/extensions/deforum/style.css
+++ /dev/null
@@ -1,36 +0,0 @@
-#vid_to_interpolate_chosen_file .w-full, #vid_to_upscale_chosen_file .w-full, #controlnet_input_video_chosen_file .w-full, #controlnet_input_video_mask_chosen_file .w-full {
- display: flex !important;
- align-items: flex-start !important;
- justify-content: center !important;
-}
-
-#vid_to_interpolate_chosen_file, #vid_to_upscale_chosen_file, #controlnet_input_video_chosen_file, #controlnet_input_video_mask_chosen_file {
- height: 85px !important;
-}
-
-#save_zip_deforum, #save_deforum {
- display: none;
-}
-
-#extra_schedules::before {
- content: "Schedules:";
- font-size: 10px !important;
-}
-
-#hybrid_msg_html {
- color: Tomato !important;
- margin-top: 5px !important;
- text-align: center !important;
- font-size: 20px !important;
- font-weight: bold !important;
-}
-
-#deforum_results .flex #image_buttons_deforum #img2img_tab,
-#deforum_results .flex #image_buttons_deforum #inpaint_tab,
-#deforum_results .flex #image_buttons_deforum #extras_tab {
- display: none !important;
-}
-
-#controlnet_not_found_html_msg {
- color: Tomato;
-}
diff --git a/spaces/aodianyun/stable-diffusion-webui/modules/sd_models.py b/spaces/aodianyun/stable-diffusion-webui/modules/sd_models.py
deleted file mode 100644
index e25a5495783c2768d50b63b35e105175c1b78bbf..0000000000000000000000000000000000000000
--- a/spaces/aodianyun/stable-diffusion-webui/modules/sd_models.py
+++ /dev/null
@@ -1,495 +0,0 @@
-import collections
-import os.path
-import sys
-import gc
-import torch
-import re
-import safetensors.torch
-from omegaconf import OmegaConf
-from os import mkdir
-from urllib import request
-import ldm.modules.midas as midas
-
-from ldm.util import instantiate_from_config
-
-from modules import paths, shared, modelloader, devices, script_callbacks, sd_vae, sd_disable_initialization, errors, hashes, sd_models_config
-from modules.paths import models_path
-from modules.sd_hijack_inpainting import do_inpainting_hijack
-from modules.timer import Timer
-
-model_dir = "Stable-diffusion"
-model_path = os.path.abspath(os.path.join(paths.models_path, model_dir))
-
-checkpoints_list = {}
-checkpoint_alisases = {}
-checkpoints_loaded = collections.OrderedDict()
-
-
-class CheckpointInfo:
- def __init__(self, filename):
- self.filename = filename
- abspath = os.path.abspath(filename)
-
- if shared.cmd_opts.ckpt_dir is not None and abspath.startswith(shared.cmd_opts.ckpt_dir):
- name = abspath.replace(shared.cmd_opts.ckpt_dir, '')
- elif abspath.startswith(model_path):
- name = abspath.replace(model_path, '')
- else:
- name = os.path.basename(filename)
-
- if name.startswith("\\") or name.startswith("/"):
- name = name[1:]
-
- self.name = name
- self.name_for_extra = os.path.splitext(os.path.basename(filename))[0]
- self.model_name = os.path.splitext(name.replace("/", "_").replace("\\", "_"))[0]
- self.hash = model_hash(filename)
-
- self.sha256 = hashes.sha256_from_cache(self.filename, "checkpoint/" + name)
- self.shorthash = self.sha256[0:10] if self.sha256 else None
-
- self.title = name if self.shorthash is None else f'{name} [{self.shorthash}]'
-
- self.ids = [self.hash, self.model_name, self.title, name, f'{name} [{self.hash}]'] + ([self.shorthash, self.sha256, f'{self.name} [{self.shorthash}]'] if self.shorthash else [])
-
- def register(self):
- checkpoints_list[self.title] = self
- for id in self.ids:
- checkpoint_alisases[id] = self
-
- def calculate_shorthash(self):
- self.sha256 = hashes.sha256(self.filename, "checkpoint/" + self.name)
- if self.sha256 is None:
- return
-
- self.shorthash = self.sha256[0:10]
-
- if self.shorthash not in self.ids:
- self.ids += [self.shorthash, self.sha256, f'{self.name} [{self.shorthash}]']
-
- checkpoints_list.pop(self.title)
- self.title = f'{self.name} [{self.shorthash}]'
- self.register()
-
- return self.shorthash
-
-
-try:
- # this silences the annoying "Some weights of the model checkpoint were not used when initializing..." message at start.
-
- from transformers import logging, CLIPModel
-
- logging.set_verbosity_error()
-except Exception:
- pass
-
-
-def setup_model():
- if not os.path.exists(model_path):
- os.makedirs(model_path)
-
- list_models()
- enable_midas_autodownload()
-
-
-def checkpoint_tiles():
- def convert(name):
- return int(name) if name.isdigit() else name.lower()
-
- def alphanumeric_key(key):
- return [convert(c) for c in re.split('([0-9]+)', key)]
-
- return sorted([x.title for x in checkpoints_list.values()], key=alphanumeric_key)
-
-
-def list_models():
- checkpoints_list.clear()
- checkpoint_alisases.clear()
-
- cmd_ckpt = shared.cmd_opts.ckpt
- if shared.cmd_opts.no_download_sd_model or cmd_ckpt != shared.sd_model_file or os.path.exists(cmd_ckpt):
- model_url = None
- else:
- model_url = "https://huggingface.co/runwayml/stable-diffusion-v1-5/resolve/main/v1-5-pruned-emaonly.safetensors"
-
- model_list = modelloader.load_models(model_path=model_path, model_url=model_url, command_path=shared.cmd_opts.ckpt_dir, ext_filter=[".ckpt", ".safetensors"], download_name="v1-5-pruned-emaonly.safetensors", ext_blacklist=[".vae.ckpt", ".vae.safetensors"])
-
- if os.path.exists(cmd_ckpt):
- checkpoint_info = CheckpointInfo(cmd_ckpt)
- checkpoint_info.register()
-
- shared.opts.data['sd_model_checkpoint'] = checkpoint_info.title
- elif cmd_ckpt is not None and cmd_ckpt != shared.default_sd_model_file:
- print(f"Checkpoint in --ckpt argument not found (Possible it was moved to {model_path}: {cmd_ckpt}", file=sys.stderr)
-
- for filename in model_list:
- checkpoint_info = CheckpointInfo(filename)
- checkpoint_info.register()
-
-
-def get_closet_checkpoint_match(search_string):
- checkpoint_info = checkpoint_alisases.get(search_string, None)
- if checkpoint_info is not None:
- return checkpoint_info
-
- found = sorted([info for info in checkpoints_list.values() if search_string in info.title], key=lambda x: len(x.title))
- if found:
- return found[0]
-
- return None
-
-
-def model_hash(filename):
- """old hash that only looks at a small part of the file and is prone to collisions"""
-
- try:
- with open(filename, "rb") as file:
- import hashlib
- m = hashlib.sha256()
-
- file.seek(0x100000)
- m.update(file.read(0x10000))
- return m.hexdigest()[0:8]
- except FileNotFoundError:
- return 'NOFILE'
-
-
-def select_checkpoint():
- model_checkpoint = shared.opts.sd_model_checkpoint
-
- checkpoint_info = checkpoint_alisases.get(model_checkpoint, None)
- if checkpoint_info is not None:
- return checkpoint_info
-
- if len(checkpoints_list) == 0:
- print("No checkpoints found. When searching for checkpoints, looked at:", file=sys.stderr)
- if shared.cmd_opts.ckpt is not None:
- print(f" - file {os.path.abspath(shared.cmd_opts.ckpt)}", file=sys.stderr)
- print(f" - directory {model_path}", file=sys.stderr)
- if shared.cmd_opts.ckpt_dir is not None:
- print(f" - directory {os.path.abspath(shared.cmd_opts.ckpt_dir)}", file=sys.stderr)
- print("Can't run without a checkpoint. Find and place a .ckpt or .safetensors file into any of those locations. The program will exit.", file=sys.stderr)
- exit(1)
-
- checkpoint_info = next(iter(checkpoints_list.values()))
- if model_checkpoint is not None:
- print(f"Checkpoint {model_checkpoint} not found; loading fallback {checkpoint_info.title}", file=sys.stderr)
-
- return checkpoint_info
-
-
-chckpoint_dict_replacements = {
- 'cond_stage_model.transformer.embeddings.': 'cond_stage_model.transformer.text_model.embeddings.',
- 'cond_stage_model.transformer.encoder.': 'cond_stage_model.transformer.text_model.encoder.',
- 'cond_stage_model.transformer.final_layer_norm.': 'cond_stage_model.transformer.text_model.final_layer_norm.',
-}
-
-
-def transform_checkpoint_dict_key(k):
- for text, replacement in chckpoint_dict_replacements.items():
- if k.startswith(text):
- k = replacement + k[len(text):]
-
- return k
-
-
-def get_state_dict_from_checkpoint(pl_sd):
- pl_sd = pl_sd.pop("state_dict", pl_sd)
- pl_sd.pop("state_dict", None)
-
- sd = {}
- for k, v in pl_sd.items():
- new_key = transform_checkpoint_dict_key(k)
-
- if new_key is not None:
- sd[new_key] = v
-
- pl_sd.clear()
- pl_sd.update(sd)
-
- return pl_sd
-
-
-def read_state_dict(checkpoint_file, print_global_state=False, map_location=None):
- _, extension = os.path.splitext(checkpoint_file)
- if extension.lower() == ".safetensors":
- device = map_location or shared.weight_load_location or devices.get_optimal_device_name()
- pl_sd = safetensors.torch.load_file(checkpoint_file, device=device)
- else:
- pl_sd = torch.load(checkpoint_file, map_location=map_location or shared.weight_load_location)
-
- if print_global_state and "global_step" in pl_sd:
- print(f"Global Step: {pl_sd['global_step']}")
-
- sd = get_state_dict_from_checkpoint(pl_sd)
- return sd
-
-
-def get_checkpoint_state_dict(checkpoint_info: CheckpointInfo, timer):
- sd_model_hash = checkpoint_info.calculate_shorthash()
- timer.record("calculate hash")
-
- if checkpoint_info in checkpoints_loaded:
- # use checkpoint cache
- print(f"Loading weights [{sd_model_hash}] from cache")
- return checkpoints_loaded[checkpoint_info]
-
- print(f"Loading weights [{sd_model_hash}] from {checkpoint_info.filename}")
- res = read_state_dict(checkpoint_info.filename)
- timer.record("load weights from disk")
-
- return res
-
-
-def load_model_weights(model, checkpoint_info: CheckpointInfo, state_dict, timer):
- sd_model_hash = checkpoint_info.calculate_shorthash()
- timer.record("calculate hash")
-
- shared.opts.data["sd_model_checkpoint"] = checkpoint_info.title
-
- if state_dict is None:
- state_dict = get_checkpoint_state_dict(checkpoint_info, timer)
-
- model.load_state_dict(state_dict, strict=False)
- del state_dict
- timer.record("apply weights to model")
-
- if shared.opts.sd_checkpoint_cache > 0:
- # cache newly loaded model
- checkpoints_loaded[checkpoint_info] = model.state_dict().copy()
-
- if shared.cmd_opts.opt_channelslast:
- model.to(memory_format=torch.channels_last)
- timer.record("apply channels_last")
-
- if not shared.cmd_opts.no_half:
- vae = model.first_stage_model
- depth_model = getattr(model, 'depth_model', None)
-
- # with --no-half-vae, remove VAE from model when doing half() to prevent its weights from being converted to float16
- if shared.cmd_opts.no_half_vae:
- model.first_stage_model = None
- # with --upcast-sampling, don't convert the depth model weights to float16
- if shared.cmd_opts.upcast_sampling and depth_model:
- model.depth_model = None
-
- model.half()
- model.first_stage_model = vae
- if depth_model:
- model.depth_model = depth_model
-
- timer.record("apply half()")
-
- devices.dtype = torch.float32 if shared.cmd_opts.no_half else torch.float16
- devices.dtype_vae = torch.float32 if shared.cmd_opts.no_half or shared.cmd_opts.no_half_vae else torch.float16
- devices.dtype_unet = model.model.diffusion_model.dtype
- devices.unet_needs_upcast = shared.cmd_opts.upcast_sampling and devices.dtype == torch.float16 and devices.dtype_unet == torch.float16
-
- model.first_stage_model.to(devices.dtype_vae)
- timer.record("apply dtype to VAE")
-
- # clean up cache if limit is reached
- while len(checkpoints_loaded) > shared.opts.sd_checkpoint_cache:
- checkpoints_loaded.popitem(last=False)
-
- model.sd_model_hash = sd_model_hash
- model.sd_model_checkpoint = checkpoint_info.filename
- model.sd_checkpoint_info = checkpoint_info
- shared.opts.data["sd_checkpoint_hash"] = checkpoint_info.sha256
-
- model.logvar = model.logvar.to(devices.device) # fix for training
-
- sd_vae.delete_base_vae()
- sd_vae.clear_loaded_vae()
- vae_file, vae_source = sd_vae.resolve_vae(checkpoint_info.filename)
- sd_vae.load_vae(model, vae_file, vae_source)
- timer.record("load VAE")
-
-
-def enable_midas_autodownload():
- """
- Gives the ldm.modules.midas.api.load_model function automatic downloading.
-
- When the 512-depth-ema model, and other future models like it, is loaded,
- it calls midas.api.load_model to load the associated midas depth model.
- This function applies a wrapper to download the model to the correct
- location automatically.
- """
-
- midas_path = os.path.join(paths.models_path, 'midas')
-
- # stable-diffusion-stability-ai hard-codes the midas model path to
- # a location that differs from where other scripts using this model look.
- # HACK: Overriding the path here.
- for k, v in midas.api.ISL_PATHS.items():
- file_name = os.path.basename(v)
- midas.api.ISL_PATHS[k] = os.path.join(midas_path, file_name)
-
- midas_urls = {
- "dpt_large": "https://github.com/intel-isl/DPT/releases/download/1_0/dpt_large-midas-2f21e586.pt",
- "dpt_hybrid": "https://github.com/intel-isl/DPT/releases/download/1_0/dpt_hybrid-midas-501f0c75.pt",
- "midas_v21": "https://github.com/AlexeyAB/MiDaS/releases/download/midas_dpt/midas_v21-f6b98070.pt",
- "midas_v21_small": "https://github.com/AlexeyAB/MiDaS/releases/download/midas_dpt/midas_v21_small-70d6b9c8.pt",
- }
-
- midas.api.load_model_inner = midas.api.load_model
-
- def load_model_wrapper(model_type):
- path = midas.api.ISL_PATHS[model_type]
- if not os.path.exists(path):
- if not os.path.exists(midas_path):
- mkdir(midas_path)
-
- print(f"Downloading midas model weights for {model_type} to {path}")
- request.urlretrieve(midas_urls[model_type], path)
- print(f"{model_type} downloaded")
-
- return midas.api.load_model_inner(model_type)
-
- midas.api.load_model = load_model_wrapper
-
-
-def repair_config(sd_config):
-
- if not hasattr(sd_config.model.params, "use_ema"):
- sd_config.model.params.use_ema = False
-
- if shared.cmd_opts.no_half:
- sd_config.model.params.unet_config.params.use_fp16 = False
- elif shared.cmd_opts.upcast_sampling:
- sd_config.model.params.unet_config.params.use_fp16 = True
-
-
-sd1_clip_weight = 'cond_stage_model.transformer.text_model.embeddings.token_embedding.weight'
-sd2_clip_weight = 'cond_stage_model.model.transformer.resblocks.0.attn.in_proj_weight'
-
-def load_model(checkpoint_info=None, already_loaded_state_dict=None, time_taken_to_load_state_dict=None):
- from modules import lowvram, sd_hijack
- checkpoint_info = checkpoint_info or select_checkpoint()
-
- if shared.sd_model:
- sd_hijack.model_hijack.undo_hijack(shared.sd_model)
- shared.sd_model = None
- gc.collect()
- devices.torch_gc()
-
- do_inpainting_hijack()
-
- timer = Timer()
-
- if already_loaded_state_dict is not None:
- state_dict = already_loaded_state_dict
- else:
- state_dict = get_checkpoint_state_dict(checkpoint_info, timer)
-
- checkpoint_config = sd_models_config.find_checkpoint_config(state_dict, checkpoint_info)
- clip_is_included_into_sd = sd1_clip_weight in state_dict or sd2_clip_weight in state_dict
-
- timer.record("find config")
-
- sd_config = OmegaConf.load(checkpoint_config)
- repair_config(sd_config)
-
- timer.record("load config")
-
- print(f"Creating model from config: {checkpoint_config}")
-
- sd_model = None
- try:
- with sd_disable_initialization.DisableInitialization(disable_clip=clip_is_included_into_sd):
- sd_model = instantiate_from_config(sd_config.model)
- except Exception as e:
- pass
-
- if sd_model is None:
- print('Failed to create model quickly; will retry using slow method.', file=sys.stderr)
- sd_model = instantiate_from_config(sd_config.model)
-
- sd_model.used_config = checkpoint_config
-
- timer.record("create model")
-
- load_model_weights(sd_model, checkpoint_info, state_dict, timer)
-
- if shared.cmd_opts.lowvram or shared.cmd_opts.medvram:
- lowvram.setup_for_low_vram(sd_model, shared.cmd_opts.medvram)
- else:
- sd_model.to(shared.device)
-
- timer.record("move model to device")
-
- sd_hijack.model_hijack.hijack(sd_model)
-
- timer.record("hijack")
-
- sd_model.eval()
- shared.sd_model = sd_model
-
- sd_hijack.model_hijack.embedding_db.load_textual_inversion_embeddings(force_reload=True) # Reload embeddings after model load as they may or may not fit the model
-
- timer.record("load textual inversion embeddings")
-
- script_callbacks.model_loaded_callback(sd_model)
-
- timer.record("scripts callbacks")
-
- print(f"Model loaded in {timer.summary()}.")
-
- return sd_model
-
-
-def reload_model_weights(sd_model=None, info=None):
- from modules import lowvram, devices, sd_hijack
- checkpoint_info = info or select_checkpoint()
-
- if not sd_model:
- sd_model = shared.sd_model
-
- if sd_model is None: # previous model load failed
- current_checkpoint_info = None
- else:
- current_checkpoint_info = sd_model.sd_checkpoint_info
- if sd_model.sd_model_checkpoint == checkpoint_info.filename:
- return
-
- if shared.cmd_opts.lowvram or shared.cmd_opts.medvram:
- lowvram.send_everything_to_cpu()
- else:
- sd_model.to(devices.cpu)
-
- sd_hijack.model_hijack.undo_hijack(sd_model)
-
- timer = Timer()
-
- state_dict = get_checkpoint_state_dict(checkpoint_info, timer)
-
- checkpoint_config = sd_models_config.find_checkpoint_config(state_dict, checkpoint_info)
-
- timer.record("find config")
-
- if sd_model is None or checkpoint_config != sd_model.used_config:
- del sd_model
- checkpoints_loaded.clear()
- load_model(checkpoint_info, already_loaded_state_dict=state_dict, time_taken_to_load_state_dict=timer.records["load weights from disk"])
- return shared.sd_model
-
- try:
- load_model_weights(sd_model, checkpoint_info, state_dict, timer)
- except Exception as e:
- print("Failed to load checkpoint, restoring previous")
- load_model_weights(sd_model, current_checkpoint_info, None, timer)
- raise
- finally:
- sd_hijack.model_hijack.hijack(sd_model)
- timer.record("hijack")
-
- script_callbacks.model_loaded_callback(sd_model)
- timer.record("script callbacks")
-
- if not shared.cmd_opts.lowvram and not shared.cmd_opts.medvram:
- sd_model.to(devices.device)
- timer.record("move model to device")
-
- print(f"Weights loaded in {timer.summary()}.")
-
- return sd_model
diff --git a/spaces/artificialguybr/video-dubbing/TTS/TTS/vocoder/configs/wavegrad_config.py b/spaces/artificialguybr/video-dubbing/TTS/TTS/vocoder/configs/wavegrad_config.py
deleted file mode 100644
index c39813ae68c3d8c77614c9a5188ac5f2a59d991d..0000000000000000000000000000000000000000
--- a/spaces/artificialguybr/video-dubbing/TTS/TTS/vocoder/configs/wavegrad_config.py
+++ /dev/null
@@ -1,90 +0,0 @@
-from dataclasses import dataclass, field
-
-from TTS.vocoder.configs.shared_configs import BaseVocoderConfig
-from TTS.vocoder.models.wavegrad import WavegradArgs
-
-
-@dataclass
-class WavegradConfig(BaseVocoderConfig):
- """Defines parameters for WaveGrad vocoder.
- Example:
-
- >>> from TTS.vocoder.configs import WavegradConfig
- >>> config = WavegradConfig()
-
- Args:
- model (str):
- Model name used for selecting the right model at initialization. Defaults to `wavegrad`.
- generator_model (str): One of the generators from TTS.vocoder.models.*`. Every other non-GAN vocoder model is
- considered as a generator too. Defaults to `wavegrad`.
- model_params (WavegradArgs): Model parameters. Check `WavegradArgs` for default values.
- target_loss (str):
- Target loss name that defines the quality of the model. Defaults to `avg_wavegrad_loss`.
- epochs (int):
- Number of epochs to traing the model. Defaults to 10000.
- batch_size (int):
- Batch size used at training. Larger values use more memory. Defaults to 96.
- seq_len (int):
- Audio segment length used at training. Larger values use more memory. Defaults to 6144.
- use_cache (bool):
- enable / disable in memory caching of the computed features. It can cause OOM error if the system RAM is
- not large enough. Defaults to True.
- mixed_precision (bool):
- enable / disable mixed precision training. Default is True.
- eval_split_size (int):
- Number of samples used for evalutaion. Defaults to 50.
- train_noise_schedule (dict):
- Training noise schedule. Defaults to
- `{"min_val": 1e-6, "max_val": 1e-2, "num_steps": 1000}`
- test_noise_schedule (dict):
- Inference noise schedule. For a better performance, you may need to use `bin/tune_wavegrad.py` to find a
- better schedule. Defaults to
- `
- {
- "min_val": 1e-6,
- "max_val": 1e-2,
- "num_steps": 50,
- }
- `
- grad_clip (float):
- Gradient clipping threshold. If <= 0.0, no clipping is applied. Defaults to 1.0
- lr (float):
- Initila leraning rate. Defaults to 1e-4.
- lr_scheduler (str):
- One of the learning rate schedulers from `torch.optim.scheduler.*`. Defaults to `MultiStepLR`.
- lr_scheduler_params (dict):
- kwargs for the scheduler. Defaults to `{"gamma": 0.5, "milestones": [100000, 200000, 300000, 400000, 500000, 600000]}`
- """
-
- model: str = "wavegrad"
- # Model specific params
- generator_model: str = "wavegrad"
- model_params: WavegradArgs = field(default_factory=WavegradArgs)
- target_loss: str = "loss" # loss value to pick the best model to save after each epoch
-
- # Training - overrides
- epochs: int = 10000
- batch_size: int = 96
- seq_len: int = 6144
- use_cache: bool = True
- mixed_precision: bool = True
- eval_split_size: int = 50
-
- # NOISE SCHEDULE PARAMS
- train_noise_schedule: dict = field(default_factory=lambda: {"min_val": 1e-6, "max_val": 1e-2, "num_steps": 1000})
-
- test_noise_schedule: dict = field(
- default_factory=lambda: { # inference noise schedule. Try TTS/bin/tune_wavegrad.py to find the optimal values.
- "min_val": 1e-6,
- "max_val": 1e-2,
- "num_steps": 50,
- }
- )
-
- # optimizer overrides
- grad_clip: float = 1.0
- lr: float = 1e-4 # Initial learning rate.
- lr_scheduler: str = "MultiStepLR" # one of the schedulers from https:#pytorch.org/docs/stable/optim.html
- lr_scheduler_params: dict = field(
- default_factory=lambda: {"gamma": 0.5, "milestones": [100000, 200000, 300000, 400000, 500000, 600000]}
- )
diff --git a/spaces/arxify/RVC-beta-v2-0618/runtime/Lib/site-packages/contourpy/util/bokeh_renderer.py b/spaces/arxify/RVC-beta-v2-0618/runtime/Lib/site-packages/contourpy/util/bokeh_renderer.py
deleted file mode 100644
index 92fb81f7d932107ea93ae0b47a1ef12f465c4e96..0000000000000000000000000000000000000000
--- a/spaces/arxify/RVC-beta-v2-0618/runtime/Lib/site-packages/contourpy/util/bokeh_renderer.py
+++ /dev/null
@@ -1,261 +0,0 @@
-import io
-
-from bokeh.io import export_png, export_svg, show
-from bokeh.io.export import get_screenshot_as_png
-from bokeh.layouts import gridplot
-from bokeh.models import Label
-from bokeh.palettes import Category10
-from bokeh.plotting import figure
-import numpy as np
-
-from .bokeh_util import filled_to_bokeh, lines_to_bokeh
-
-
-class BokehRenderer:
- """Utility renderer using Bokeh to render a grid of plots over the same (x, y) range.
-
- Args:
- nrows (int, optional): Number of rows of plots, default ``1``.
- ncols (int, optional): Number of columns of plots, default ``1``.
- figsize (tuple(float, float), optional): Figure size in inches (assuming 100 dpi), default
- ``(9, 9)``.
- show_frame (bool, optional): Whether to show frame and axes ticks, default ``True``.
- want_svg (bool, optional): Whether output is required in SVG format or not, default
- ``False``.
-
- Warning:
- :class:`~contourpy.util.bokeh_renderer.BokehRenderer`, unlike
- :class:`~contourpy.util.mpl_renderer.MplRenderer`, needs to be told in advance if output to
- SVG format will be required later, otherwise it will assume PNG output.
- """
- def __init__(self, nrows=1, ncols=1, figsize=(9, 9), show_frame=True, want_svg=False):
- self._want_svg = want_svg
- self._palette = Category10[10]
-
- total_size = 100*np.asarray(figsize) # Assuming 100 dpi.
-
- nfigures = nrows*ncols
- self._figures = []
- backend = "svg" if self._want_svg else "canvas"
- for _ in range(nfigures):
- fig = figure(output_backend=backend)
- fig.xgrid.visible = False
- fig.ygrid.visible = False
- self._figures.append(fig)
- if not show_frame:
- fig.outline_line_color = None
- fig.axis.visible = False
-
- self._layout = gridplot(
- self._figures, ncols=ncols, toolbar_location=None,
- width=total_size[0] // ncols, height=total_size[1] // nrows)
-
- def _convert_color(self, color):
- if isinstance(color, str) and color[0] == "C":
- index = int(color[1:])
- color = self._palette[index]
- return color
-
- def _get_figure(self, ax):
- if isinstance(ax, int):
- ax = self._figures[ax]
- return ax
-
- def _grid_as_2d(self, x, y):
- x = np.asarray(x)
- y = np.asarray(y)
- if x.ndim == 1:
- x, y = np.meshgrid(x, y)
- return x, y
-
- def filled(self, filled, fill_type, ax=0, color="C0", alpha=0.7):
- """Plot filled contours on a single plot.
-
- Args:
- filled (sequence of arrays): Filled contour data as returned by
- :func:`~contourpy.ContourGenerator.filled`.
- fill_type (FillType): Type of ``filled`` data, as returned by
- :attr:`~contourpy.ContourGenerator.fill_type`.
- ax (int or Bokeh Figure, optional): Which plot to use, default ``0``.
- color (str, optional): Color to plot with. May be a string color or the letter ``"C"``
- followed by an integer in the range ``"C0"`` to ``"C9"`` to use a color from the
- ``Category10`` palette. Default ``"C0"``.
- alpha (float, optional): Opacity to plot with, default ``0.7``.
- """
- fig = self._get_figure(ax)
- color = self._convert_color(color)
- xs, ys = filled_to_bokeh(filled, fill_type)
- if len(xs) > 0:
- fig.multi_polygons(xs=[xs], ys=[ys], color=color, fill_alpha=alpha, line_width=0)
-
- def grid(self, x, y, ax=0, color="black", alpha=0.1, point_color=None, quad_as_tri_alpha=0):
- """Plot quad grid lines on a single plot.
-
- Args:
- x (array-like of shape (ny, nx) or (nx,)): The x-coordinates of the grid points.
- y (array-like of shape (ny, nx) or (ny,)): The y-coordinates of the grid points.
- ax (int or Bokeh Figure, optional): Which plot to use, default ``0``.
- color (str, optional): Color to plot grid lines, default ``"black"``.
- alpha (float, optional): Opacity to plot lines with, default ``0.1``.
- point_color (str, optional): Color to plot grid points or ``None`` if grid points
- should not be plotted, default ``None``.
- quad_as_tri_alpha (float, optional): Opacity to plot ``quad_as_tri`` grid, default
- ``0``.
-
- Colors may be a string color or the letter ``"C"`` followed by an integer in the range
- ``"C0"`` to ``"C9"`` to use a color from the ``Category10`` palette.
-
- Warning:
- ``quad_as_tri_alpha > 0`` plots all quads as though they are unmasked.
- """
- fig = self._get_figure(ax)
- x, y = self._grid_as_2d(x, y)
- xs = [row for row in x] + [row for row in x.T]
- ys = [row for row in y] + [row for row in y.T]
- kwargs = dict(line_color=color, alpha=alpha)
- fig.multi_line(xs, ys, **kwargs)
- if quad_as_tri_alpha > 0:
- # Assumes no quad mask.
- xmid = (0.25*(x[:-1, :-1] + x[1:, :-1] + x[:-1, 1:] + x[1:, 1:])).ravel()
- ymid = (0.25*(y[:-1, :-1] + y[1:, :-1] + y[:-1, 1:] + y[1:, 1:])).ravel()
- fig.multi_line(
- [row for row in np.stack((x[:-1, :-1].ravel(), xmid, x[1:, 1:].ravel()), axis=1)],
- [row for row in np.stack((y[:-1, :-1].ravel(), ymid, y[1:, 1:].ravel()), axis=1)],
- **kwargs)
- fig.multi_line(
- [row for row in np.stack((x[:-1, 1:].ravel(), xmid, x[1:, :-1].ravel()), axis=1)],
- [row for row in np.stack((y[:-1, 1:].ravel(), ymid, y[1:, :-1].ravel()), axis=1)],
- **kwargs)
- if point_color is not None:
- fig.circle(
- x=x.ravel(), y=y.ravel(), fill_color=color, line_color=None, alpha=alpha, size=8)
-
- def lines(self, lines, line_type, ax=0, color="C0", alpha=1.0, linewidth=1):
- """Plot contour lines on a single plot.
-
- Args:
- lines (sequence of arrays): Contour line data as returned by
- :func:`~contourpy.ContourGenerator.lines`.
- line_type (LineType): Type of ``lines`` data, as returned by
- :attr:`~contourpy.ContourGenerator.line_type`.
- ax (int or Bokeh Figure, optional): Which plot to use, default ``0``.
- color (str, optional): Color to plot lines. May be a string color or the letter ``"C"``
- followed by an integer in the range ``"C0"`` to ``"C9"`` to use a color from the
- ``Category10`` palette. Default ``"C0"``.
- alpha (float, optional): Opacity to plot lines with, default ``1.0``.
- linewidth (float, optional): Width of lines, default ``1``.
-
- Note:
- Assumes all lines are open line strips not closed line loops.
- """
- fig = self._get_figure(ax)
- color = self._convert_color(color)
- xs, ys = lines_to_bokeh(lines, line_type)
- if len(xs) > 0:
- fig.multi_line(xs, ys, line_color=color, line_alpha=alpha, line_width=linewidth)
-
- def mask(self, x, y, z, ax=0, color="black"):
- """Plot masked out grid points as circles on a single plot.
-
- Args:
- x (array-like of shape (ny, nx) or (nx,)): The x-coordinates of the grid points.
- y (array-like of shape (ny, nx) or (ny,)): The y-coordinates of the grid points.
- z (masked array of shape (ny, nx): z-values.
- ax (int or Bokeh Figure, optional): Which plot to use, default ``0``.
- color (str, optional): Circle color, default ``"black"``.
- """
- mask = np.ma.getmask(z)
- if mask is np.ma.nomask:
- return
- fig = self._get_figure(ax)
- color = self._convert_color(color)
- x, y = self._grid_as_2d(x, y)
- fig.circle(x[mask], y[mask], fill_color=color, size=10)
-
- def save(self, filename, transparent=False):
- """Save plots to SVG or PNG file.
-
- Args:
- filename (str): Filename to save to.
- transparent (bool, optional): Whether background should be transparent, default
- ``False``.
-
- Warning:
- To output to SVG file, ``want_svg=True`` must have been passed to the constructor.
- """
- if transparent:
- for fig in self._figures:
- fig.background_fill_color = None
- fig.border_fill_color = None
-
- if self._want_svg:
- export_svg(self._layout, filename=filename)
- else:
- export_png(self._layout, filename=filename)
-
- def save_to_buffer(self):
- """Save plots to an ``io.BytesIO`` buffer.
-
- Return:
- BytesIO: PNG image buffer.
- """
- image = get_screenshot_as_png(self._layout)
- buffer = io.BytesIO()
- image.save(buffer, "png")
- return buffer
-
- def show(self):
- """Show plots in web browser, in usual Bokeh manner.
- """
- show(self._layout)
-
- def title(self, title, ax=0, color=None):
- """Set the title of a single plot.
-
- Args:
- title (str): Title text.
- ax (int or Bokeh Figure, optional): Which plot to set the title of, default ``0``.
- color (str, optional): Color to set title. May be a string color or the letter ``"C"``
- followed by an integer in the range ``"C0"`` to ``"C9"`` to use a color from the
- ``Category10`` palette. Default ``None`` which is ``black``.
- """
- fig = self._get_figure(ax)
- fig.title = title
- fig.title.align = "center"
- if color is not None:
- fig.title.text_color = self._convert_color(color)
-
- def z_values(self, x, y, z, ax=0, color="green", fmt=".1f", quad_as_tri=False):
- """Show ``z`` values on a single plot.
-
- Args:
- x (array-like of shape (ny, nx) or (nx,)): The x-coordinates of the grid points.
- y (array-like of shape (ny, nx) or (ny,)): The y-coordinates of the grid points.
- z (array-like of shape (ny, nx): z-values.
- ax (int or Bokeh Figure, optional): Which plot to use, default ``0``.
- color (str, optional): Color of added text. May be a string color or the letter ``"C"``
- followed by an integer in the range ``"C0"`` to ``"C9"`` to use a color from the
- ``Category10`` palette. Default ``"green"``.
- fmt (str, optional): Format to display z-values, default ``".1f"``.
- quad_as_tri (bool, optional): Whether to show z-values at the ``quad_as_tri`` centres
- of quads.
-
- Warning:
- ``quad_as_tri=True`` shows z-values for all quads, even if masked.
- """
- fig = self._get_figure(ax)
- color = self._convert_color(color)
- x, y = self._grid_as_2d(x, y)
- z = np.asarray(z)
- ny, nx = z.shape
- kwargs = dict(text_color=color, text_align="center", text_baseline="middle")
- for j in range(ny):
- for i in range(nx):
- fig.add_layout(Label(x=x[j, i], y=y[j, i], text=f"{z[j, i]:{fmt}}", **kwargs))
- if quad_as_tri:
- for j in range(ny-1):
- for i in range(nx-1):
- xx = np.mean(x[j:j+2, i:i+2])
- yy = np.mean(y[j:j+2, i:i+2])
- zz = np.mean(z[j:j+2, i:i+2])
- fig.add_layout(Label(x=xx, y=yy, text=f"{zz:{fmt}}", **kwargs))
diff --git a/spaces/aryadytm/photo-colorization/src/__init__.py b/spaces/aryadytm/photo-colorization/src/__init__.py
deleted file mode 100644
index e69de29bb2d1d6434b8b29ae775ad8c2e48c5391..0000000000000000000000000000000000000000
diff --git a/spaces/asd998877/TsGpt/modules/presets.py b/spaces/asd998877/TsGpt/modules/presets.py
deleted file mode 100644
index d4d1e8e93cc705a89a5dca896a3aa0183a86a6e9..0000000000000000000000000000000000000000
--- a/spaces/asd998877/TsGpt/modules/presets.py
+++ /dev/null
@@ -1,224 +0,0 @@
-# -*- coding:utf-8 -*-
-import os
-from pathlib import Path
-import gradio as gr
-from .webui_locale import I18nAuto
-
-i18n = I18nAuto() # internationalization
-
-CHATGLM_MODEL = None
-CHATGLM_TOKENIZER = None
-LLAMA_MODEL = None
-LLAMA_INFERENCER = None
-
-# ChatGPT 设置
-INITIAL_SYSTEM_PROMPT = "You are a helpful assistant."
-API_HOST = "api.openai.com"
-COMPLETION_URL = "https://api.openai.com/v1/chat/completions"
-BALANCE_API_URL="https://api.openai.com/dashboard/billing/credit_grants"
-USAGE_API_URL="https://api.openai.com/dashboard/billing/usage"
-HISTORY_DIR = Path("history")
-HISTORY_DIR = "history"
-TEMPLATES_DIR = "templates"
-
-# 错误信息
-STANDARD_ERROR_MSG = i18n("☹️发生了错误:") # 错误信息的标准前缀
-GENERAL_ERROR_MSG = i18n("获取对话时发生错误,请查看后台日志")
-ERROR_RETRIEVE_MSG = i18n("请检查网络连接,或者API-Key是否有效。")
-CONNECTION_TIMEOUT_MSG = i18n("连接超时,无法获取对话。") # 连接超时
-READ_TIMEOUT_MSG = i18n("读取超时,无法获取对话。") # 读取超时
-PROXY_ERROR_MSG = i18n("代理错误,无法获取对话。") # 代理错误
-SSL_ERROR_PROMPT = i18n("SSL错误,无法获取对话。") # SSL 错误
-NO_APIKEY_MSG = i18n("API key为空,请检查是否输入正确。") # API key 长度不足 51 位
-NO_INPUT_MSG = i18n("请输入对话内容。") # 未输入对话内容
-BILLING_NOT_APPLICABLE_MSG = i18n("账单信息不适用") # 本地运行的模型返回的账单信息
-
-TIMEOUT_STREAMING = 60 # 流式对话时的超时时间
-TIMEOUT_ALL = 200 # 非流式对话时的超时时间
-ENABLE_STREAMING_OPTION = True # 是否启用选择选择是否实时显示回答的勾选框
-HIDE_MY_KEY = False # 如果你想在UI中隐藏你的 API 密钥,将此值设置为 True
-CONCURRENT_COUNT = 100 # 允许同时使用的用户数量
-
-SIM_K = 5
-INDEX_QUERY_TEMPRATURE = 1.0
-
-CHUANHU_TITLE = i18n("免费试用、部署可联系")
-
-CHUANHU_DESCRIPTION = i18n("多模型、可连接网站、部署可联系")
-
-FOOTER = """{versions}"""
-
-APPEARANCE_SWITCHER = """
-
-"""+ i18n("切换亮暗色主题") + """
-
-
-"""
-
-SUMMARIZE_PROMPT = "你是谁?我们刚才聊了什么?" # 总结对话时的 prompt
-
-ONLINE_MODELS = [
- "gpt-3.5-turbo",
- "gpt-3.5-turbo-0301",
- "gpt-4",
- "gpt-4-0314",
- "gpt-4-32k",
- "gpt-4-32k-0314",
- "xmchat",
-]
-
-LOCAL_MODELS = [
- "chatglm-6b",
- "chatglm-6b-int4",
- "chatglm-6b-int4-qe",
- "StableLM",
- "MOSS",
- "llama-7b-hf",
- "llama-13b-hf",
- "llama-30b-hf",
- "llama-65b-hf",
-]
-
-if os.environ.get('HIDE_LOCAL_MODELS', 'false') == 'true':
- MODELS = ONLINE_MODELS
-else:
- MODELS = ONLINE_MODELS + LOCAL_MODELS
-
-DEFAULT_MODEL = 0
-
-os.makedirs("models", exist_ok=True)
-os.makedirs("lora", exist_ok=True)
-os.makedirs("history", exist_ok=True)
-for dir_name in os.listdir("models"):
- if os.path.isdir(os.path.join("models", dir_name)):
- if dir_name not in MODELS:
- MODELS.append(dir_name)
-
-MODEL_TOKEN_LIMIT = {
- "gpt-3.5-turbo": 4096,
- "gpt-3.5-turbo-0301": 4096,
- "gpt-4": 8192,
- "gpt-4-0314": 8192,
- "gpt-4-32k": 32768,
- "gpt-4-32k-0314": 32768
-}
-
-TOKEN_OFFSET = 1000 # 模型的token上限减去这个值,得到软上限。到达软上限之后,自动尝试减少token占用。
-DEFAULT_TOKEN_LIMIT = 3000 # 默认的token上限
-REDUCE_TOKEN_FACTOR = 0.5 # 与模型token上限想乘,得到目标token数。减少token占用时,将token占用减少到目标token数以下。
-
-REPLY_LANGUAGES = [
- "简体中文",
- "繁體中文",
- "English",
- "日本語",
- "Español",
- "Français",
- "Deutsch",
- "跟随问题语言(不稳定)"
-]
-
-
-WEBSEARCH_PTOMPT_TEMPLATE = """\
-Web search results:
-
-{web_results}
-Current date: {current_date}
-
-Instructions: Using the provided web search results, write a comprehensive reply to the given query. Make sure to cite results using [[number](URL)] notation after the reference. If the provided search results refer to multiple subjects with the same name, write separate answers for each subject.
-Query: {query}
-Reply in {reply_language}
-"""
-
-PROMPT_TEMPLATE = """\
-Context information is below.
----------------------
-{context_str}
----------------------
-Current date: {current_date}.
-Using the provided context information, write a comprehensive reply to the given query.
-Make sure to cite results using [number] notation after the reference.
-If the provided context information refer to multiple subjects with the same name, write separate answers for each subject.
-Use prior knowledge only if the given context didn't provide enough information.
-Answer the question: {query_str}
-Reply in {reply_language}
-"""
-
-REFINE_TEMPLATE = """\
-The original question is as follows: {query_str}
-We have provided an existing answer: {existing_answer}
-We have the opportunity to refine the existing answer
-(only if needed) with some more context below.
-------------
-{context_msg}
-------------
-Given the new context, refine the original answer to better
-Reply in {reply_language}
-If the context isn't useful, return the original answer.
-"""
-
-ALREADY_CONVERTED_MARK = ""
-
-small_and_beautiful_theme = gr.themes.Soft(
- primary_hue=gr.themes.Color(
- c50="#02C160",
- c100="rgba(2, 193, 96, 0.2)",
- c200="#02C160",
- c300="rgba(2, 193, 96, 0.32)",
- c400="rgba(2, 193, 96, 0.32)",
- c500="rgba(2, 193, 96, 1.0)",
- c600="rgba(2, 193, 96, 1.0)",
- c700="rgba(2, 193, 96, 0.32)",
- c800="rgba(2, 193, 96, 0.32)",
- c900="#02C160",
- c950="#02C160",
- ),
- secondary_hue=gr.themes.Color(
- c50="#576b95",
- c100="#576b95",
- c200="#576b95",
- c300="#576b95",
- c400="#576b95",
- c500="#576b95",
- c600="#576b95",
- c700="#576b95",
- c800="#576b95",
- c900="#576b95",
- c950="#576b95",
- ),
- neutral_hue=gr.themes.Color(
- name="gray",
- c50="#f9fafb",
- c100="#f3f4f6",
- c200="#e5e7eb",
- c300="#d1d5db",
- c400="#B2B2B2",
- c500="#808080",
- c600="#636363",
- c700="#515151",
- c800="#393939",
- c900="#272727",
- c950="#171717",
- ),
- radius_size=gr.themes.sizes.radius_sm,
- ).set(
- button_primary_background_fill="#06AE56",
- button_primary_background_fill_dark="#06AE56",
- button_primary_background_fill_hover="#07C863",
- button_primary_border_color="#06AE56",
- button_primary_border_color_dark="#06AE56",
- button_primary_text_color="#FFFFFF",
- button_primary_text_color_dark="#FFFFFF",
- button_secondary_background_fill="#F2F2F2",
- button_secondary_background_fill_dark="#2B2B2B",
- button_secondary_text_color="#393939",
- button_secondary_text_color_dark="#FFFFFF",
- # background_fill_primary="#F7F7F7",
- # background_fill_primary_dark="#1F1F1F",
- block_title_text_color="*primary_500",
- block_title_background_fill="*primary_100",
- input_background_fill="#F6F6F6",
- )
diff --git a/spaces/ashercn97/AsherTesting/extensions/api/streaming_api.py b/spaces/ashercn97/AsherTesting/extensions/api/streaming_api.py
deleted file mode 100644
index 88359e3e46bd24b682c8964523ad7fbb0db87baf..0000000000000000000000000000000000000000
--- a/spaces/ashercn97/AsherTesting/extensions/api/streaming_api.py
+++ /dev/null
@@ -1,121 +0,0 @@
-import asyncio
-import json
-from threading import Thread
-
-from websockets.server import serve
-
-from extensions.api.util import build_parameters, try_start_cloudflared, with_api_lock
-from modules import shared
-from modules.chat import generate_chat_reply
-from modules.text_generation import generate_reply
-
-PATH = '/api/v1/stream'
-
-
-@with_api_lock
-async def _handle_stream_message(websocket, message):
- message = json.loads(message)
-
- prompt = message['prompt']
- generate_params = build_parameters(message)
- stopping_strings = generate_params.pop('stopping_strings')
- generate_params['stream'] = True
-
- generator = generate_reply(
- prompt, generate_params, stopping_strings=stopping_strings, is_chat=False)
-
- # As we stream, only send the new bytes.
- skip_index = 0
- message_num = 0
-
- for a in generator:
- to_send = a[skip_index:]
- if to_send is None or chr(0xfffd) in to_send: # partial unicode character, don't send it yet.
- continue
-
- await websocket.send(json.dumps({
- 'event': 'text_stream',
- 'message_num': message_num,
- 'text': to_send
- }))
-
- await asyncio.sleep(0)
- skip_index += len(to_send)
- message_num += 1
-
- await websocket.send(json.dumps({
- 'event': 'stream_end',
- 'message_num': message_num
- }))
-
-
-@with_api_lock
-async def _handle_chat_stream_message(websocket, message):
- body = json.loads(message)
-
- user_input = body['user_input']
- generate_params = build_parameters(body, chat=True)
- generate_params['stream'] = True
- regenerate = body.get('regenerate', False)
- _continue = body.get('_continue', False)
-
- generator = generate_chat_reply(
- user_input, generate_params, regenerate=regenerate, _continue=_continue, loading_message=False)
-
- message_num = 0
- for a in generator:
- await websocket.send(json.dumps({
- 'event': 'text_stream',
- 'message_num': message_num,
- 'history': a
- }))
-
- await asyncio.sleep(0)
- message_num += 1
-
- await websocket.send(json.dumps({
- 'event': 'stream_end',
- 'message_num': message_num
- }))
-
-
-async def _handle_connection(websocket, path):
-
- if path == '/api/v1/stream':
- async for message in websocket:
- await _handle_stream_message(websocket, message)
-
- elif path == '/api/v1/chat-stream':
- async for message in websocket:
- await _handle_chat_stream_message(websocket, message)
-
- else:
- print(f'Streaming api: unknown path: {path}')
- return
-
-
-async def _run(host: str, port: int):
- async with serve(_handle_connection, host, port, ping_interval=None):
- await asyncio.Future() # run forever
-
-
-def _run_server(port: int, share: bool = False):
- address = '0.0.0.0' if shared.args.listen else '127.0.0.1'
-
- def on_start(public_url: str):
- public_url = public_url.replace('https://', 'wss://')
- print(f'Starting streaming server at public url {public_url}{PATH}')
-
- if share:
- try:
- try_start_cloudflared(port, max_attempts=3, on_start=on_start)
- except Exception as e:
- print(e)
- else:
- print(f'Starting streaming server at ws://{address}:{port}{PATH}')
-
- asyncio.run(_run(host=address, port=port))
-
-
-def start_server(port: int, share: bool = False):
- Thread(target=_run_server, args=[port, share], daemon=True).start()
diff --git a/spaces/ashercn97/AsherTesting/extensions/openai/embeddings.py b/spaces/ashercn97/AsherTesting/extensions/openai/embeddings.py
deleted file mode 100644
index c02bb9334cc4d7247be441fc760142a1dab7f4a4..0000000000000000000000000000000000000000
--- a/spaces/ashercn97/AsherTesting/extensions/openai/embeddings.py
+++ /dev/null
@@ -1,54 +0,0 @@
-import os
-from sentence_transformers import SentenceTransformer
-from extensions.openai.utils import float_list_to_base64, debug_msg
-from extensions.openai.errors import *
-
-st_model = os.environ["OPENEDAI_EMBEDDING_MODEL"] if "OPENEDAI_EMBEDDING_MODEL" in os.environ else "all-mpnet-base-v2"
-embeddings_model = None
-
-
-def load_embedding_model(model):
- try:
- emb_model = SentenceTransformer(model)
- print(f"\nLoaded embedding model: {model}, max sequence length: {emb_model.max_seq_length}")
- except Exception as e:
- print(f"\nError: Failed to load embedding model: {model}")
- raise ServiceUnavailableError(f"Error: Failed to load embedding model: {model}", internal_message=repr(e))
-
- return emb_model
-
-
-def get_embeddings_model():
- global embeddings_model, st_model
- if st_model and not embeddings_model:
- embeddings_model = load_embedding_model(st_model) # lazy load the model
- return embeddings_model
-
-
-def get_embeddings_model_name():
- global st_model
- return st_model
-
-
-def embeddings(input: list, encoding_format: str):
-
- embeddings = get_embeddings_model().encode(input).tolist()
-
- if encoding_format == "base64":
- data = [{"object": "embedding", "embedding": float_list_to_base64(emb), "index": n} for n, emb in enumerate(embeddings)]
- else:
- data = [{"object": "embedding", "embedding": emb, "index": n} for n, emb in enumerate(embeddings)]
-
- response = {
- "object": "list",
- "data": data,
- "model": st_model, # return the real model
- "usage": {
- "prompt_tokens": 0,
- "total_tokens": 0,
- }
- }
-
- debug_msg(f"Embeddings return size: {len(embeddings[0])}, number: {len(embeddings)}")
-
- return response
diff --git a/spaces/atimughal662/InfoFusion/README.md b/spaces/atimughal662/InfoFusion/README.md
deleted file mode 100644
index 14b59cf394d195459e1f735af273e39301347ec9..0000000000000000000000000000000000000000
--- a/spaces/atimughal662/InfoFusion/README.md
+++ /dev/null
@@ -1,13 +0,0 @@
----
-title: H2ogpt Chatbot
-emoji: 📚
-colorFrom: yellow
-colorTo: yellow
-sdk: gradio
-sdk_version: 3.41.2
-app_file: app.py
-pinned: false
-license: apache-2.0
----
-
-Check out the configuration reference at https://huggingface.co/docs/hub/spaces-config-reference
diff --git a/spaces/avivdm1/AutoGPT/autogpt/commands/image_gen.py b/spaces/avivdm1/AutoGPT/autogpt/commands/image_gen.py
deleted file mode 100644
index 0809fcdd3e38b52a2ce09ca1444f2574813d40f9..0000000000000000000000000000000000000000
--- a/spaces/avivdm1/AutoGPT/autogpt/commands/image_gen.py
+++ /dev/null
@@ -1,163 +0,0 @@
-""" Image Generation Module for AutoGPT."""
-import io
-import os.path
-import uuid
-from base64 import b64decode
-
-import openai
-import requests
-from PIL import Image
-
-from autogpt.config import Config
-from autogpt.workspace import path_in_workspace
-
-CFG = Config()
-
-
-def generate_image(prompt: str, size: int = 256) -> str:
- """Generate an image from a prompt.
-
- Args:
- prompt (str): The prompt to use
- size (int, optional): The size of the image. Defaults to 256. (Not supported by HuggingFace)
-
- Returns:
- str: The filename of the image
- """
- filename = f"{str(uuid.uuid4())}.jpg"
-
- # DALL-E
- if CFG.image_provider == "dalle":
- return generate_image_with_dalle(prompt, filename, size)
- # HuggingFace
- elif CFG.image_provider == "huggingface":
- return generate_image_with_hf(prompt, filename)
- # SD WebUI
- elif CFG.image_provider == "sdwebui":
- return generate_image_with_sd_webui(prompt, filename, size)
- return "No Image Provider Set"
-
-
-def generate_image_with_hf(prompt: str, filename: str) -> str:
- """Generate an image with HuggingFace's API.
-
- Args:
- prompt (str): The prompt to use
- filename (str): The filename to save the image to
-
- Returns:
- str: The filename of the image
- """
- API_URL = (
- f"https://api-inference.huggingface.co/models/{CFG.huggingface_image_model}"
- )
- if CFG.huggingface_api_token is None:
- raise ValueError(
- "You need to set your Hugging Face API token in the config file."
- )
- headers = {
- "Authorization": f"Bearer {CFG.huggingface_api_token}",
- "X-Use-Cache": "false",
- }
-
- response = requests.post(
- API_URL,
- headers=headers,
- json={
- "inputs": prompt,
- },
- )
-
- image = Image.open(io.BytesIO(response.content))
- print(f"Image Generated for prompt:{prompt}")
-
- image.save(path_in_workspace(filename))
-
- return f"Saved to disk:{filename}"
-
-
-def generate_image_with_dalle(prompt: str, filename: str) -> str:
- """Generate an image with DALL-E.
-
- Args:
- prompt (str): The prompt to use
- filename (str): The filename to save the image to
-
- Returns:
- str: The filename of the image
- """
- openai.api_key = CFG.openai_api_key
-
- # Check for supported image sizes
- if size not in [256, 512, 1024]:
- closest = min([256, 512, 1024], key=lambda x: abs(x - size))
- print(
- f"DALL-E only supports image sizes of 256x256, 512x512, or 1024x1024. Setting to {closest}, was {size}."
- )
- size = closest
-
- response = openai.Image.create(
- prompt=prompt,
- n=1,
- size=f"{size}x{size}",
- response_format="b64_json",
- )
-
- print(f"Image Generated for prompt:{prompt}")
-
- image_data = b64decode(response["data"][0]["b64_json"])
-
- with open(path_in_workspace(filename), mode="wb") as png:
- png.write(image_data)
-
- return f"Saved to disk:{filename}"
-
-
-def generate_image_with_sd_webui(
- prompt: str,
- filename: str,
- size: int = 512,
- negative_prompt: str = "",
- extra: dict = {},
-) -> str:
- """Generate an image with Stable Diffusion webui.
- Args:
- prompt (str): The prompt to use
- filename (str): The filename to save the image to
- size (int, optional): The size of the image. Defaults to 256.
- negative_prompt (str, optional): The negative prompt to use. Defaults to "".
- extra (dict, optional): Extra parameters to pass to the API. Defaults to {}.
- Returns:
- str: The filename of the image
- """
- # Create a session and set the basic auth if needed
- s = requests.Session()
- if CFG.sd_webui_auth:
- username, password = CFG.sd_webui_auth.split(":")
- s.auth = (username, password or "")
-
- # Generate the images
- response = requests.post(
- f"{CFG.sd_webui_url}/sdapi/v1/txt2img",
- json={
- "prompt": prompt,
- "negative_prompt": negative_prompt,
- "sampler_index": "DDIM",
- "steps": 20,
- "cfg_scale": 7.0,
- "width": size,
- "height": size,
- "n_iter": 1,
- **extra,
- },
- )
-
- print(f"Image Generated for prompt:{prompt}")
-
- # Save the image to disk
- response = response.json()
- b64 = b64decode(response["images"][0].split(",", 1)[0])
- image = Image.open(io.BytesIO(b64))
- image.save(path_in_workspace(filename))
-
- return f"Saved to disk:{filename}"
diff --git a/spaces/awacke1/ASR-High-Accuracy-Test/app.py b/spaces/awacke1/ASR-High-Accuracy-Test/app.py
deleted file mode 100644
index 0ea6e83f6d0e9fb9262daa497d62b0fc21698e42..0000000000000000000000000000000000000000
--- a/spaces/awacke1/ASR-High-Accuracy-Test/app.py
+++ /dev/null
@@ -1,152 +0,0 @@
-import gradio as gr
-import logging
-import sys
-import tempfile
-import numpy as np
-import datetime
-
-from transformers import pipeline, AutoModelForCTC, Wav2Vec2Processor, Wav2Vec2ProcessorWithLM
-from typing import Optional
-from TTS.utils.manage import ModelManager
-from TTS.utils.synthesizer import Synthesizer
-
-logging.basicConfig(
- format="%(asctime)s - %(levelname)s - %(name)s - %(message)s",
- datefmt="%m/%d/%Y %H:%M:%S",
- handlers=[logging.StreamHandler(sys.stdout)],
-)
-logger = logging.getLogger(__name__)
-logger.setLevel(logging.DEBUG)
-
-
-LARGE_MODEL_BY_LANGUAGE = {
- "Arabic": {"model_id": "jonatasgrosman/wav2vec2-large-xlsr-53-arabic", "has_lm": False},
- "Chinese": {"model_id": "jonatasgrosman/wav2vec2-large-xlsr-53-chinese-zh-cn", "has_lm": False},
- #"Dutch": {"model_id": "jonatasgrosman/wav2vec2-large-xlsr-53-dutch", "has_lm": False},
- "English": {"model_id": "jonatasgrosman/wav2vec2-large-xlsr-53-english", "has_lm": True},
- "Finnish": {"model_id": "jonatasgrosman/wav2vec2-large-xlsr-53-finnish", "has_lm": False},
- "French": {"model_id": "jonatasgrosman/wav2vec2-large-xlsr-53-french", "has_lm": True},
- "German": {"model_id": "jonatasgrosman/wav2vec2-large-xlsr-53-german", "has_lm": True},
- "Greek": {"model_id": "jonatasgrosman/wav2vec2-large-xlsr-53-greek", "has_lm": False},
- "Hungarian": {"model_id": "jonatasgrosman/wav2vec2-large-xlsr-53-hungarian", "has_lm": False},
- "Italian": {"model_id": "jonatasgrosman/wav2vec2-large-xlsr-53-italian", "has_lm": True},
- "Japanese": {"model_id": "jonatasgrosman/wav2vec2-large-xlsr-53-japanese", "has_lm": False},
- "Persian": {"model_id": "jonatasgrosman/wav2vec2-large-xlsr-53-persian", "has_lm": False},
- "Polish": {"model_id": "jonatasgrosman/wav2vec2-large-xlsr-53-polish", "has_lm": True},
- "Portuguese": {"model_id": "jonatasgrosman/wav2vec2-large-xlsr-53-portuguese", "has_lm": True},
- "Russian": {"model_id": "jonatasgrosman/wav2vec2-large-xlsr-53-russian", "has_lm": True},
- "Spanish": {"model_id": "jonatasgrosman/wav2vec2-large-xlsr-53-spanish", "has_lm": True},
-}
-
-XLARGE_MODEL_BY_LANGUAGE = {
- "English": {"model_id": "jonatasgrosman/wav2vec2-xls-r-1b-english", "has_lm": True},
- "Spanish": {"model_id": "jonatasgrosman/wav2vec2-xls-r-1b-spanish", "has_lm": True},
- "German": {"model_id": "jonatasgrosman/wav2vec2-xls-r-1b-german", "has_lm": True},
- "Russian": {"model_id": "jonatasgrosman/wav2vec2-xls-r-1b-russian", "has_lm": True},
- "French": {"model_id": "jonatasgrosman/wav2vec2-xls-r-1b-french", "has_lm": True},
- "Italian": {"model_id": "jonatasgrosman/wav2vec2-xls-r-1b-italian", "has_lm": True},
- #"Dutch": {"model_id": "jonatasgrosman/wav2vec2-xls-r-1b-dutch", "has_lm": False},
- "Polish": {"model_id": "jonatasgrosman/wav2vec2-xls-r-1b-polish", "has_lm": True},
- "Portuguese": {"model_id": "jonatasgrosman/wav2vec2-xls-r-1b-portuguese", "has_lm": True},
-}
-
-
-# LANGUAGES = sorted(LARGE_MODEL_BY_LANGUAGE.keys())
-
-# the container given by HF has 16GB of RAM, so we need to limit the number of models to load
-LANGUAGES = sorted(XLARGE_MODEL_BY_LANGUAGE.keys())
-CACHED_MODELS_BY_ID = {}
-
-
-def run(input_file, language, decoding_type, history, model_size="300M"):
-
- logger.info(f"Running ASR {language}-{model_size}-{decoding_type} for {input_file}")
-
- history = history or []
-
- if model_size == "300M":
- model = LARGE_MODEL_BY_LANGUAGE.get(language, None)
- else:
- model = XLARGE_MODEL_BY_LANGUAGE.get(language, None)
-
- if model is None:
- history.append({
- "error_message": f"Model size {model_size} not found for {language} language :("
- })
- elif decoding_type == "LM" and not model["has_lm"]:
- history.append({
- "error_message": f"LM not available for {language} language :("
- })
- else:
-
- # model_instance = AutoModelForCTC.from_pretrained(model["model_id"])
- model_instance = CACHED_MODELS_BY_ID.get(model["model_id"], None)
- if model_instance is None:
- model_instance = AutoModelForCTC.from_pretrained(model["model_id"])
- CACHED_MODELS_BY_ID[model["model_id"]] = model_instance
-
- if decoding_type == "LM":
- processor = Wav2Vec2ProcessorWithLM.from_pretrained(model["model_id"])
- asr = pipeline("automatic-speech-recognition", model=model_instance, tokenizer=processor.tokenizer,
- feature_extractor=processor.feature_extractor, decoder=processor.decoder)
- else:
- processor = Wav2Vec2Processor.from_pretrained(model["model_id"])
- asr = pipeline("automatic-speech-recognition", model=model_instance, tokenizer=processor.tokenizer,
- feature_extractor=processor.feature_extractor, decoder=None)
-
- transcription = asr(input_file, chunk_length_s=5, stride_length_s=1)["text"]
-
- logger.info(f"Transcription for {input_file}: {transcription}")
-
- history.append({
- "model_id": model["model_id"],
- "language": language,
- "model_size": model_size,
- "decoding_type": decoding_type,
- "transcription": transcription,
- "error_message": None
- })
-
- html_output = ""
- for item in history:
- if item["error_message"] is not None:
- html_output += f"{item['error_message']}"
- else:
- url_suffix = " + LM" if item["decoding_type"] == "LM" else ""
- html_output += ""
- html_output += f'{item["model_id"]}{url_suffix}
'
- html_output += f'{item["transcription"]}
'
- html_output += ""
- html_output += ""
-
- return html_output, history
-
-
-gr.Interface(
- run,
- inputs=[
- #gr.inputs.Audio(source="microphone", type="filepath", label="Record something..."),
- gr.Audio(source="microphone", type='filepath', streaming=True),
- #gr.inputs.Audio(source="microphone", type="filepath", label="Record something...", streaming="True"),
- gr.inputs.Radio(label="Language", choices=LANGUAGES),
- gr.inputs.Radio(label="Decoding type", choices=["greedy", "LM"]),
- # gr.inputs.Radio(label="Model size", choices=["300M", "1B"]),
- "state"
- ],
- outputs=[
- gr.outputs.HTML(label="Outputs"),
- "state"
- ],
- title="🗣️NLP ASR Wav2Vec2 GR📄",
- description="",
- css="""
- .result {display:flex;flex-direction:column}
- .result_item {padding:15px;margin-bottom:8px;border-radius:15px;width:100%}
- .result_item_success {background-color:mediumaquamarine;color:white;align-self:start}
- .result_item_error {background-color:#ff7070;color:white;align-self:start}
- """,
- allow_screenshot=False,
- allow_flagging="never",
- theme="grass",
- live=True # test1
-).launch(enable_queue=True)
\ No newline at end of file
diff --git a/spaces/awacke1/AW-01ST-CSV-Dataset-Analyzer/app.py b/spaces/awacke1/AW-01ST-CSV-Dataset-Analyzer/app.py
deleted file mode 100644
index b7e75e612174498fb904272b7aa997ad09145a79..0000000000000000000000000000000000000000
--- a/spaces/awacke1/AW-01ST-CSV-Dataset-Analyzer/app.py
+++ /dev/null
@@ -1,83 +0,0 @@
-import streamlit as st
-import pandas as pd
-import traceback
-import sys
-
-from st_aggrid import AgGrid
-from st_aggrid.grid_options_builder import GridOptionsBuilder
-from st_aggrid.shared import JsCode
-from download import download_button
-from st_aggrid import GridUpdateMode, DataReturnMode
-
-# Page config is set once with icon title and display style. Wide mode since we want screen real estate for wide CSV files
-st.set_page_config(page_icon="📝", page_title="📝CSV Data Analyzer📊", layout="wide")
-
-# Style
-def _max_width_():
- max_width_str = f"max-width: 1800px;"
- st.markdown(
- f"""
-
- """,
- unsafe_allow_html=True,
- )
-
-# Title Bar with Images and Icons
-col1, col2, col3 = st.columns([1,6,1])
-with col1:
- st.image("https://cdnb.artstation.com/p/assets/images/images/054/910/875/large/aaron-wacker-cyberpunk-computer-brain-design.jpg?1665656558",width=96,)
-with col2:
- st.title("📝 CSV Data Analyzer 📊")
-with col3:
- st.image("https://cdna.artstation.com/p/assets/images/images/054/910/878/large/aaron-wacker-cyberpunk-computer-devices-iot.jpg?1665656564",width=96,)
-
-# Upload
-c29, c30, c31 = st.columns([1, 6, 1])
-with c30:
- uploaded_file = st.file_uploader("", key="1", help="To activate 'wide mode', go to the menu > Settings > turn on 'wide mode'",)
- if uploaded_file is not None:
- file_container = st.expander("Check your uploaded .csv")
- #try:
- shows = pd.read_csv(uploaded_file)
- #except:
- # print(sys.exc_info()[2])
-
- uploaded_file.seek(0)
- file_container.write(shows)
- else:
- st.info(f"""⬆️Upload a 📝.CSV file. Examples: [Chatbot](https://huggingface.co/datasets/awacke1/Carddata.csv) [Mindfulness](https://huggingface.co/datasets/awacke1/MindfulStory.csv) [Wikipedia](https://huggingface.co/datasets/awacke1/WikipediaSearch)""")
- st.stop()
-
-# DisplayGrid
-gb = GridOptionsBuilder.from_dataframe(shows)
-gb.configure_default_column(enablePivot=True, enableValue=True, enableRowGroup=True)
-gb.configure_selection(selection_mode="multiple", use_checkbox=True)
-gb.configure_side_bar()
-gridOptions = gb.build()
-st.success(f"""💡 Tip! Hold shift key when selecting rows to select multiple rows at once.""")
-response = AgGrid(
- shows,
- gridOptions=gridOptions,
- enable_enterprise_modules=True,
- update_mode=GridUpdateMode.MODEL_CHANGED,
- data_return_mode=DataReturnMode.FILTERED_AND_SORTED,
- fit_columns_on_grid_load=False,
-)
-
-# Filters
-df = pd.DataFrame(response["selected_rows"])
-st.subheader("Filtered data will appear below 📊 ")
-st.text("")
-st.table(df)
-st.text("")
-
-# Download
-c29, c30, c31 = st.columns([1, 1, 2])
-with c29:
- CSVButton = download_button(df,"Dataset.csv","Download CSV file",)
-with c30:
- CSVButton = download_button(df,"Dataset.txt","Download TXT file",)
\ No newline at end of file
diff --git a/spaces/awacke1/Mistral_Ultimate_Chords_and_Lyrics_Writer/README.md b/spaces/awacke1/Mistral_Ultimate_Chords_and_Lyrics_Writer/README.md
deleted file mode 100644
index 48279df5207d2a064f30e4c965f38eeeda74448e..0000000000000000000000000000000000000000
--- a/spaces/awacke1/Mistral_Ultimate_Chords_and_Lyrics_Writer/README.md
+++ /dev/null
@@ -1,13 +0,0 @@
----
-title: Mistral Ultimate Chords And Lyrics Writer
-emoji: 🏆
-colorFrom: blue
-colorTo: gray
-sdk: gradio
-sdk_version: 3.47.1
-app_file: app.py
-pinned: false
-license: mit
----
-
-Check out the configuration reference at https://huggingface.co/docs/hub/spaces-config-reference
diff --git a/spaces/awacke1/Streamlit-ASR-Video/app.py b/spaces/awacke1/Streamlit-ASR-Video/app.py
deleted file mode 100644
index e0f03cf2557eba112bf95ebf5eb582da8d8a0fe3..0000000000000000000000000000000000000000
--- a/spaces/awacke1/Streamlit-ASR-Video/app.py
+++ /dev/null
@@ -1,119 +0,0 @@
-from collections import deque
-import streamlit as st
-import torch
-from streamlit_player import st_player
-from transformers import AutoModelForCTC, Wav2Vec2Processor
-from streaming import ffmpeg_stream
-
-device = torch.device('cuda' if torch.cuda.is_available() else 'cpu')
-player_options = {
- "events": ["onProgress"],
- "progress_interval": 200,
- "volume": 1.0,
- "playing": True,
- "loop": False,
- "controls": False,
- "muted": False,
- "config": {"youtube": {"playerVars": {"start": 1}}},
-}
-
-# disable rapid fading in and out on `st.code` updates
-st.markdown("", unsafe_allow_html=True)
-
-@st.cache(hash_funcs={torch.nn.parameter.Parameter: lambda _: None})
-def load_model(model_path="facebook/wav2vec2-large-robust-ft-swbd-300h"):
- processor = Wav2Vec2Processor.from_pretrained(model_path)
- model = AutoModelForCTC.from_pretrained(model_path).to(device)
- return processor, model
-
-processor, model = load_model()
-
-def stream_text(url, chunk_duration_ms, pad_duration_ms):
- sampling_rate = processor.feature_extractor.sampling_rate
-
- # calculate the length of logits to cut from the sides of the output to account for input padding
- output_pad_len = model._get_feat_extract_output_lengths(int(sampling_rate * pad_duration_ms / 1000))
-
- # define the audio chunk generator
- stream = ffmpeg_stream(url, sampling_rate, chunk_duration_ms=chunk_duration_ms, pad_duration_ms=pad_duration_ms)
-
- leftover_text = ""
- for i, chunk in enumerate(stream):
- input_values = processor(chunk, sampling_rate=sampling_rate, return_tensors="pt").input_values
-
- with torch.no_grad():
- logits = model(input_values.to(device)).logits[0]
- if i > 0:
- logits = logits[output_pad_len : len(logits) - output_pad_len]
- else: # don't count padding at the start of the clip
- logits = logits[: len(logits) - output_pad_len]
-
- predicted_ids = torch.argmax(logits, dim=-1).cpu().tolist()
- if processor.decode(predicted_ids).strip():
- leftover_ids = processor.tokenizer.encode(leftover_text)
- # concat the last word (or its part) from the last frame with the current text
- text = processor.decode(leftover_ids + predicted_ids)
- # don't return the last word in case it's just partially recognized
- text, leftover_text = text.rsplit(" ", 1)
- yield text
- else:
- yield leftover_text
- leftover_text = ""
- yield leftover_text
-
-def main():
- state = st.session_state
- st.header("Video ASR Streamlit from Youtube Link")
-
- with st.form(key="inputs_form"):
-
- # Our worlds best teachers on subjects of AI, Cognitive, Neuroscience for our Behavioral and Medical Health
- ytJoschaBach="https://youtu.be/cC1HszE5Hcw?list=PLHgX2IExbFouJoqEr8JMF5MbZSbyC91-L&t=8984"
- ytSamHarris="https://www.youtube.com/watch?v=4dC_nRYIDZU&list=PLHgX2IExbFouJoqEr8JMF5MbZSbyC91-L&index=2"
- ytJohnAbramson="https://www.youtube.com/watch?v=arrokG3wCdE&list=PLHgX2IExbFouJoqEr8JMF5MbZSbyC91-L&index=3"
- ytElonMusk="https://www.youtube.com/watch?v=DxREm3s1scA&list=PLHgX2IExbFouJoqEr8JMF5MbZSbyC91-L&index=4"
- ytJeffreyShainline="https://www.youtube.com/watch?v=EwueqdgIvq4&list=PLHgX2IExbFouJoqEr8JMF5MbZSbyC91-L&index=5"
- ytJeffHawkins="https://www.youtube.com/watch?v=Z1KwkpTUbkg&list=PLHgX2IExbFouJoqEr8JMF5MbZSbyC91-L&index=6"
- ytSamHarris="https://youtu.be/Ui38ZzTymDY?list=PLHgX2IExbFouJoqEr8JMF5MbZSbyC91-L"
- ytSamHarris="https://youtu.be/4dC_nRYIDZU?list=PLHgX2IExbFouJoqEr8JMF5MbZSbyC91-L&t=7809"
- ytSamHarris="https://youtu.be/4dC_nRYIDZU?list=PLHgX2IExbFouJoqEr8JMF5MbZSbyC91-L&t=7809"
- ytSamHarris="https://youtu.be/4dC_nRYIDZU?list=PLHgX2IExbFouJoqEr8JMF5MbZSbyC91-L&t=7809"
- ytTimelapseAI="https://www.youtube.com/watch?v=63yr9dlI0cU&list=PLHgX2IExbFovQybyfltywXnqZi5YvaSS-"
- state.youtube_url = st.text_input("YouTube URL", ytTimelapseAI)
-
-
- state.chunk_duration_ms = st.slider("Audio chunk duration (ms)", 2000, 10000, 3000, 100)
- state.pad_duration_ms = st.slider("Padding duration (ms)", 100, 5000, 1000, 100)
- submit_button = st.form_submit_button(label="Submit")
-
- if submit_button or "asr_stream" not in state:
- # a hack to update the video player on value changes
- state.youtube_url = (
- state.youtube_url.split("&hash=")[0]
- + f"&hash={state.chunk_duration_ms}-{state.pad_duration_ms}"
- )
- state.asr_stream = stream_text(
- state.youtube_url, state.chunk_duration_ms, state.pad_duration_ms
- )
- state.chunks_taken = 0
-
-
- state.lines = deque([], maxlen=100) # limit to the last n lines of subs
-
-
- player = st_player(state.youtube_url, **player_options, key="youtube_player")
-
- if "asr_stream" in state and player.data and player.data["played"] < 1.0:
- # check how many seconds were played, and if more than processed - write the next text chunk
- processed_seconds = state.chunks_taken * (state.chunk_duration_ms / 1000)
- if processed_seconds < player.data["playedSeconds"]:
- text = next(state.asr_stream)
- state.lines.append(text)
- state.chunks_taken += 1
- if "lines" in state:
- # print the lines of subs
- st.code("\n".join(state.lines))
-
-
-if __name__ == "__main__":
- main()
\ No newline at end of file
diff --git a/spaces/banana-projects/web3d/node_modules/three/src/lights/HemisphereLight.d.ts b/spaces/banana-projects/web3d/node_modules/three/src/lights/HemisphereLight.d.ts
deleted file mode 100644
index 4df2e73ac00723ea0b3e8c8d2be9309436923d9b..0000000000000000000000000000000000000000
--- a/spaces/banana-projects/web3d/node_modules/three/src/lights/HemisphereLight.d.ts
+++ /dev/null
@@ -1,14 +0,0 @@
-import { Color } from './../math/Color';
-import { Light } from './Light';
-
-export class HemisphereLight extends Light {
- constructor(
- skyColor?: Color | string | number,
- groundColor?: Color | string | number,
- intensity?: number
- );
-
- skyColor: Color;
- groundColor: Color;
- intensity: number;
-}
diff --git a/spaces/bguberfain/Detic/tools/get_coco_zeroshot_oriorder.py b/spaces/bguberfain/Detic/tools/get_coco_zeroshot_oriorder.py
deleted file mode 100644
index ed6748be1f2ed92741ea78f5a187f9838185a80e..0000000000000000000000000000000000000000
--- a/spaces/bguberfain/Detic/tools/get_coco_zeroshot_oriorder.py
+++ /dev/null
@@ -1,18 +0,0 @@
-# Copyright (c) Facebook, Inc. and its affiliates.
-import argparse
-import json
-
-if __name__ == '__main__':
- parser = argparse.ArgumentParser()
- parser.add_argument('--data_path', default='datasets/coco/annotations/instances_val2017_unseen_2.json')
- parser.add_argument('--cat_path', default='datasets/coco/annotations/instances_val2017.json')
- args = parser.parse_args()
- print('Loading', args.cat_path)
- cat = json.load(open(args.cat_path, 'r'))['categories']
-
- print('Loading', args.data_path)
- data = json.load(open(args.data_path, 'r'))
- data['categories'] = cat
- out_path = args.data_path[:-5] + '_oriorder.json'
- print('Saving to', out_path)
- json.dump(data, open(out_path, 'w'))
diff --git a/spaces/bguberfain/Detic/tools/merge_lvis_coco.py b/spaces/bguberfain/Detic/tools/merge_lvis_coco.py
deleted file mode 100644
index abc2b673a30541fd71679a549acd9a53f7693183..0000000000000000000000000000000000000000
--- a/spaces/bguberfain/Detic/tools/merge_lvis_coco.py
+++ /dev/null
@@ -1,202 +0,0 @@
-# Copyright (c) Facebook, Inc. and its affiliates.
-from collections import defaultdict
-import torch
-import sys
-import json
-import numpy as np
-
-from detectron2.structures import Boxes, pairwise_iou
-COCO_PATH = 'datasets/coco/annotations/instances_train2017.json'
-IMG_PATH = 'datasets/coco/train2017/'
-LVIS_PATH = 'datasets/lvis/lvis_v1_train.json'
-NO_SEG = False
-if NO_SEG:
- SAVE_PATH = 'datasets/lvis/lvis_v1_train+coco_box.json'
-else:
- SAVE_PATH = 'datasets/lvis/lvis_v1_train+coco_mask.json'
-THRESH = 0.7
-DEBUG = False
-
-# This mapping is extracted from the official LVIS mapping:
-# https://github.com/lvis-dataset/lvis-api/blob/master/data/coco_to_synset.json
-COCO_SYNSET_CATEGORIES = [
- {"synset": "person.n.01", "coco_cat_id": 1},
- {"synset": "bicycle.n.01", "coco_cat_id": 2},
- {"synset": "car.n.01", "coco_cat_id": 3},
- {"synset": "motorcycle.n.01", "coco_cat_id": 4},
- {"synset": "airplane.n.01", "coco_cat_id": 5},
- {"synset": "bus.n.01", "coco_cat_id": 6},
- {"synset": "train.n.01", "coco_cat_id": 7},
- {"synset": "truck.n.01", "coco_cat_id": 8},
- {"synset": "boat.n.01", "coco_cat_id": 9},
- {"synset": "traffic_light.n.01", "coco_cat_id": 10},
- {"synset": "fireplug.n.01", "coco_cat_id": 11},
- {"synset": "stop_sign.n.01", "coco_cat_id": 13},
- {"synset": "parking_meter.n.01", "coco_cat_id": 14},
- {"synset": "bench.n.01", "coco_cat_id": 15},
- {"synset": "bird.n.01", "coco_cat_id": 16},
- {"synset": "cat.n.01", "coco_cat_id": 17},
- {"synset": "dog.n.01", "coco_cat_id": 18},
- {"synset": "horse.n.01", "coco_cat_id": 19},
- {"synset": "sheep.n.01", "coco_cat_id": 20},
- {"synset": "beef.n.01", "coco_cat_id": 21},
- {"synset": "elephant.n.01", "coco_cat_id": 22},
- {"synset": "bear.n.01", "coco_cat_id": 23},
- {"synset": "zebra.n.01", "coco_cat_id": 24},
- {"synset": "giraffe.n.01", "coco_cat_id": 25},
- {"synset": "backpack.n.01", "coco_cat_id": 27},
- {"synset": "umbrella.n.01", "coco_cat_id": 28},
- {"synset": "bag.n.04", "coco_cat_id": 31},
- {"synset": "necktie.n.01", "coco_cat_id": 32},
- {"synset": "bag.n.06", "coco_cat_id": 33},
- {"synset": "frisbee.n.01", "coco_cat_id": 34},
- {"synset": "ski.n.01", "coco_cat_id": 35},
- {"synset": "snowboard.n.01", "coco_cat_id": 36},
- {"synset": "ball.n.06", "coco_cat_id": 37},
- {"synset": "kite.n.03", "coco_cat_id": 38},
- {"synset": "baseball_bat.n.01", "coco_cat_id": 39},
- {"synset": "baseball_glove.n.01", "coco_cat_id": 40},
- {"synset": "skateboard.n.01", "coco_cat_id": 41},
- {"synset": "surfboard.n.01", "coco_cat_id": 42},
- {"synset": "tennis_racket.n.01", "coco_cat_id": 43},
- {"synset": "bottle.n.01", "coco_cat_id": 44},
- {"synset": "wineglass.n.01", "coco_cat_id": 46},
- {"synset": "cup.n.01", "coco_cat_id": 47},
- {"synset": "fork.n.01", "coco_cat_id": 48},
- {"synset": "knife.n.01", "coco_cat_id": 49},
- {"synset": "spoon.n.01", "coco_cat_id": 50},
- {"synset": "bowl.n.03", "coco_cat_id": 51},
- {"synset": "banana.n.02", "coco_cat_id": 52},
- {"synset": "apple.n.01", "coco_cat_id": 53},
- {"synset": "sandwich.n.01", "coco_cat_id": 54},
- {"synset": "orange.n.01", "coco_cat_id": 55},
- {"synset": "broccoli.n.01", "coco_cat_id": 56},
- {"synset": "carrot.n.01", "coco_cat_id": 57},
- # {"synset": "frank.n.02", "coco_cat_id": 58},
- {"synset": "sausage.n.01", "coco_cat_id": 58},
- {"synset": "pizza.n.01", "coco_cat_id": 59},
- {"synset": "doughnut.n.02", "coco_cat_id": 60},
- {"synset": "cake.n.03", "coco_cat_id": 61},
- {"synset": "chair.n.01", "coco_cat_id": 62},
- {"synset": "sofa.n.01", "coco_cat_id": 63},
- {"synset": "pot.n.04", "coco_cat_id": 64},
- {"synset": "bed.n.01", "coco_cat_id": 65},
- {"synset": "dining_table.n.01", "coco_cat_id": 67},
- {"synset": "toilet.n.02", "coco_cat_id": 70},
- {"synset": "television_receiver.n.01", "coco_cat_id": 72},
- {"synset": "laptop.n.01", "coco_cat_id": 73},
- {"synset": "mouse.n.04", "coco_cat_id": 74},
- {"synset": "remote_control.n.01", "coco_cat_id": 75},
- {"synset": "computer_keyboard.n.01", "coco_cat_id": 76},
- {"synset": "cellular_telephone.n.01", "coco_cat_id": 77},
- {"synset": "microwave.n.02", "coco_cat_id": 78},
- {"synset": "oven.n.01", "coco_cat_id": 79},
- {"synset": "toaster.n.02", "coco_cat_id": 80},
- {"synset": "sink.n.01", "coco_cat_id": 81},
- {"synset": "electric_refrigerator.n.01", "coco_cat_id": 82},
- {"synset": "book.n.01", "coco_cat_id": 84},
- {"synset": "clock.n.01", "coco_cat_id": 85},
- {"synset": "vase.n.01", "coco_cat_id": 86},
- {"synset": "scissors.n.01", "coco_cat_id": 87},
- {"synset": "teddy.n.01", "coco_cat_id": 88},
- {"synset": "hand_blower.n.01", "coco_cat_id": 89},
- {"synset": "toothbrush.n.01", "coco_cat_id": 90},
-]
-
-
-def get_bbox(ann):
- bbox = ann['bbox']
- return [bbox[0], bbox[1], bbox[0] + bbox[2], bbox[1] + bbox[3]]
-
-
-if __name__ == '__main__':
- file_name_key = 'file_name' if 'v0.5' in LVIS_PATH else 'coco_url'
- coco_data = json.load(open(COCO_PATH, 'r'))
- lvis_data = json.load(open(LVIS_PATH, 'r'))
-
- coco_cats = coco_data['categories']
- lvis_cats = lvis_data['categories']
-
- num_find = 0
- num_not_find = 0
- num_twice = 0
- coco2lviscats = {}
- synset2lvisid = {x['synset']: x['id'] for x in lvis_cats}
- # cocoid2synset = {x['coco_cat_id']: x['synset'] for x in COCO_SYNSET_CATEGORIES}
- coco2lviscats = {x['coco_cat_id']: synset2lvisid[x['synset']] \
- for x in COCO_SYNSET_CATEGORIES if x['synset'] in synset2lvisid}
- print(len(coco2lviscats))
-
- lvis_file2id = {x[file_name_key][-16:]: x['id'] for x in lvis_data['images']}
- lvis_id2img = {x['id']: x for x in lvis_data['images']}
- lvis_catid2name = {x['id']: x['name'] for x in lvis_data['categories']}
-
- coco_file2anns = {}
- coco_id2img = {x['id']: x for x in coco_data['images']}
- coco_img2anns = defaultdict(list)
- for ann in coco_data['annotations']:
- coco_img = coco_id2img[ann['image_id']]
- file_name = coco_img['file_name'][-16:]
- if ann['category_id'] in coco2lviscats and \
- file_name in lvis_file2id:
- lvis_image_id = lvis_file2id[file_name]
- lvis_image = lvis_id2img[lvis_image_id]
- lvis_cat_id = coco2lviscats[ann['category_id']]
- if lvis_cat_id in lvis_image['neg_category_ids']:
- continue
- if DEBUG:
- import cv2
- img_path = IMG_PATH + file_name
- img = cv2.imread(img_path)
- print(lvis_catid2name[lvis_cat_id])
- print('neg', [lvis_catid2name[x] for x in lvis_image['neg_category_ids']])
- cv2.imshow('img', img)
- cv2.waitKey()
- ann['category_id'] = lvis_cat_id
- ann['image_id'] = lvis_image_id
- coco_img2anns[file_name].append(ann)
-
- lvis_img2anns = defaultdict(list)
- for ann in lvis_data['annotations']:
- lvis_img = lvis_id2img[ann['image_id']]
- file_name = lvis_img[file_name_key][-16:]
- lvis_img2anns[file_name].append(ann)
-
- ann_id_count = 0
- anns = []
- for file_name in lvis_img2anns:
- coco_anns = coco_img2anns[file_name]
- lvis_anns = lvis_img2anns[file_name]
- ious = pairwise_iou(
- Boxes(torch.tensor([get_bbox(x) for x in coco_anns])),
- Boxes(torch.tensor([get_bbox(x) for x in lvis_anns]))
- )
-
- for ann in lvis_anns:
- ann_id_count = ann_id_count + 1
- ann['id'] = ann_id_count
- anns.append(ann)
-
- for i, ann in enumerate(coco_anns):
- if len(ious[i]) == 0 or ious[i].max() < THRESH:
- ann_id_count = ann_id_count + 1
- ann['id'] = ann_id_count
- anns.append(ann)
- else:
- duplicated = False
- for j in range(len(ious[i])):
- if ious[i, j] >= THRESH and \
- coco_anns[i]['category_id'] == lvis_anns[j]['category_id']:
- duplicated = True
- if not duplicated:
- ann_id_count = ann_id_count + 1
- ann['id'] = ann_id_count
- anns.append(ann)
- if NO_SEG:
- for ann in anns:
- del ann['segmentation']
- lvis_data['annotations'] = anns
-
- print('# Images', len(lvis_data['images']))
- print('# Anns', len(lvis_data['annotations']))
- json.dump(lvis_data, open(SAVE_PATH, 'w'))
diff --git a/spaces/bigjoker/stable-diffusion-webui/extensions/deforum/scripts/deforum_helpers/load_images.py b/spaces/bigjoker/stable-diffusion-webui/extensions/deforum/scripts/deforum_helpers/load_images.py
deleted file mode 100644
index 6dc5726f8aed86fb190ae15aa6098c3bcac8ec2c..0000000000000000000000000000000000000000
--- a/spaces/bigjoker/stable-diffusion-webui/extensions/deforum/scripts/deforum_helpers/load_images.py
+++ /dev/null
@@ -1,102 +0,0 @@
-import requests
-import os
-from PIL import Image, ImageOps
-import cv2
-import numpy as np
-import socket
-import torchvision.transforms.functional as TF
-
-def load_img(path : str, shape=None, use_alpha_as_mask=False):
- # use_alpha_as_mask: Read the alpha channel of the image as the mask image
- image = load_image(path)
- if use_alpha_as_mask:
- image = image.convert('RGBA')
- else:
- image = image.convert('RGB')
-
- if shape is not None:
- image = image.resize(shape, resample=Image.LANCZOS)
-
- mask_image = None
- if use_alpha_as_mask:
- # Split alpha channel into a mask_image
- red, green, blue, alpha = Image.Image.split(image)
- mask_image = alpha.convert('L')
- image = image.convert('RGB')
-
- # check using init image alpha as mask if mask is not blank
- extrema = mask_image.getextrema()
- if (extrema == (0,0)) or extrema == (255,255):
- print("use_alpha_as_mask==True: Using the alpha channel from the init image as a mask, but the alpha channel is blank.")
- print("ignoring alpha as mask.")
- mask_image = None
-
- return image, mask_image
-
-def load_image(image_path :str):
- image = None
- if image_path.startswith('http://') or image_path.startswith('https://'):
- try:
- host = socket.gethostbyname("www.google.com")
- s = socket.create_connection((host, 80), 2)
- s.close()
- except:
- raise ConnectionError("There is no active internet connection available - please use local masks and init files only.")
-
- try:
- response = requests.get(image_path, stream=True)
- except requests.exceptions.RequestException as e:
- raise ConnectionError("Failed to download image due to no internet connection. Error: {}".format(e))
- if response.status_code == 404 or response.status_code != 200:
- raise ConnectionError("Init image url or mask image url is not valid")
- image = Image.open(response.raw).convert('RGB')
- else:
- if not os.path.exists(image_path):
- raise RuntimeError("Init image path or mask image path is not valid")
- image = Image.open(image_path).convert('RGB')
-
- return image
-
-def prepare_mask(mask_input, mask_shape, mask_brightness_adjust=1.0, mask_contrast_adjust=1.0):
- """
- prepares mask for use in webui
- """
- if isinstance(mask_input, Image.Image):
- mask = mask_input
- else :
- mask = load_image(mask_input)
- mask = mask.resize(mask_shape, resample=Image.LANCZOS)
- if mask_brightness_adjust != 1:
- mask = TF.adjust_brightness(mask, mask_brightness_adjust)
- if mask_contrast_adjust != 1:
- mask = TF.adjust_contrast(mask, mask_contrast_adjust)
- mask = mask.convert('L')
- return mask
-
-def check_mask_for_errors(mask_input, invert_mask=False):
- extrema = mask_input.getextrema()
- if (invert_mask):
- if extrema == (255,255):
- print("after inverting mask will be blank. ignoring mask")
- return None
- elif extrema == (0,0):
- print("mask is blank. ignoring mask")
- return None
- else:
- return mask_input
-
-def get_mask(args):
- return check_mask_for_errors(
- prepare_mask(args.mask_file, (args.W, args.H), args.mask_contrast_adjust, args.mask_brightness_adjust)
- )
-
-def get_mask_from_file(mask_file, args):
- return check_mask_for_errors(
- prepare_mask(mask_file, (args.W, args.H), args.mask_contrast_adjust, args.mask_brightness_adjust)
- )
-
-def blank_if_none(mask, w, h, mode):
- return Image.new(mode, (w, h), (0)) if mask is None else mask
-
-def none_if_blank(mask):
- return None if mask.getextrema() == (0,0) else mask
diff --git a/spaces/bigjoker/stable-diffusion-webui/modules/extra_networks_hypernet.py b/spaces/bigjoker/stable-diffusion-webui/modules/extra_networks_hypernet.py
deleted file mode 100644
index 207343daa673c14a362d4bd2399982d9ad86fe22..0000000000000000000000000000000000000000
--- a/spaces/bigjoker/stable-diffusion-webui/modules/extra_networks_hypernet.py
+++ /dev/null
@@ -1,27 +0,0 @@
-from modules import extra_networks, shared, extra_networks
-from modules.hypernetworks import hypernetwork
-
-
-class ExtraNetworkHypernet(extra_networks.ExtraNetwork):
- def __init__(self):
- super().__init__('hypernet')
-
- def activate(self, p, params_list):
- additional = shared.opts.sd_hypernetwork
-
- if additional != "" and additional in shared.hypernetworks and len([x for x in params_list if x.items[0] == additional]) == 0:
- p.all_prompts = [x + f"" for x in p.all_prompts]
- params_list.append(extra_networks.ExtraNetworkParams(items=[additional, shared.opts.extra_networks_default_multiplier]))
-
- names = []
- multipliers = []
- for params in params_list:
- assert len(params.items) > 0
-
- names.append(params.items[0])
- multipliers.append(float(params.items[1]) if len(params.items) > 1 else 1.0)
-
- hypernetwork.load_hypernetworks(names, multipliers)
-
- def deactivate(self, p):
- pass
diff --git a/spaces/bioriAsaeru/text-to-voice/HD Online Player (Swades Movie 1 English Sub Torrent).md b/spaces/bioriAsaeru/text-to-voice/HD Online Player (Swades Movie 1 English Sub Torrent).md
deleted file mode 100644
index 2cc25403be9a4d8cda2ae50f62605e68ab54748b..0000000000000000000000000000000000000000
--- a/spaces/bioriAsaeru/text-to-voice/HD Online Player (Swades Movie 1 English Sub Torrent).md
+++ /dev/null
@@ -1,11 +0,0 @@
-
-google is your friend for finding a download link for swades 2004 movie torrent. akshay kumar upcoming hindi movies 2018. swades. hindi. description: swades is an indian hindi comedy-drama film directed by omung kumar. swades, a film of the year 2004, was released on october. 4 days ago - kumar, who made his directorial debut with swades. swades, the movie. watch swades 2004 online free at full-length.
-HD Online Player (Swades movie 1 english sub torrent)
DOWNLOAD ✅ https://urloso.com/2uyOqE
-hdx full movies 2019 play full movies 24/7 online. hindi movies 2019 new hd/4k hdr. watch hindi movie 2019 online free. 3 idiots torrent download is the best torrent site for download high quality movies and also tv shows in good quality.
-3 idiots torrent download is the best torrent site for download high quality movies and also tv shows in good quality. 2 days ago - raghavendra gautam aka swades kannada movie torrent (english subtitles) hindi (telugu) tamil.
-3 idiots torrent download is the best torrent site for download high quality movies and also tv shows in good quality. watch 3 idiots online full movie. torrent download. watch full movie of 3 idiots online for free.
-
-sr.no.1 on google - online hd player for downloading any movie from the internet. swades movie 1 english sub torrent, swades movie 1 english sub. 1. download swades (2004) hindi film torrent. swades 2004 torrent. swades (2004) subtitles: english, hindi,. english subtitles english subtitles. .
-watch swades (2004) online in high quality (2000.+ h.264) with english subtitles and an english dubbed audio in hd. swades (2004) hindi movies torrent download swades (2004) hindi movie torrent download swades 2004. .
899543212b
-
-
\ No newline at end of file
diff --git a/spaces/caffeinum/VToonify/vtoonify/model/encoder/__init__.py b/spaces/caffeinum/VToonify/vtoonify/model/encoder/__init__.py
deleted file mode 100644
index e69de29bb2d1d6434b8b29ae775ad8c2e48c5391..0000000000000000000000000000000000000000
diff --git a/spaces/chasemcdo/hf_localai/examples/rwkv/README.md b/spaces/chasemcdo/hf_localai/examples/rwkv/README.md
deleted file mode 100644
index 00ca570287255a8acc19be485a16d2d6410c35dd..0000000000000000000000000000000000000000
--- a/spaces/chasemcdo/hf_localai/examples/rwkv/README.md
+++ /dev/null
@@ -1,59 +0,0 @@
-# rwkv
-
-Example of how to run rwkv models.
-
-## Run models
-
-Setup:
-
-```bash
-# Clone LocalAI
-git clone https://github.com/go-skynet/LocalAI
-
-cd LocalAI/examples/rwkv
-
-# (optional) Checkout a specific LocalAI tag
-# git checkout -b build
-
-# build the tooling image to convert an rwkv model locally:
-docker build -t rwkv-converter -f Dockerfile.build .
-
-# download and convert a model (one-off) - it's going to be fast on CPU too!
-docker run -ti --name converter -v $PWD:/data rwkv-converter https://huggingface.co/BlinkDL/rwkv-4-raven/resolve/main/RWKV-4-Raven-1B5-v11-Eng99%25-Other1%25-20230425-ctx4096.pth /data/models/rwkv
-
-# Get the tokenizer
-wget https://raw.githubusercontent.com/saharNooby/rwkv.cpp/5eb8f09c146ea8124633ab041d9ea0b1f1db4459/rwkv/20B_tokenizer.json -O models/rwkv.tokenizer.json
-
-# start with docker-compose
-docker-compose up -d --build
-```
-
-Test it out:
-
-```bash
-curl http://localhost:8080/v1/completions -H "Content-Type: application/json" -d '{
- "model": "gpt-3.5-turbo",
- "prompt": "A long time ago, in a galaxy far away",
- "max_tokens": 100,
- "temperature": 0.9, "top_p": 0.8, "top_k": 80
- }'
-
-# {"object":"text_completion","model":"gpt-3.5-turbo","choices":[{"text":", there was a small group of five friends: Annie, Bryan, Charlie, Emily, and Jesse."}],"usage":{"prompt_tokens":0,"completion_tokens":0,"total_tokens":0}}
-
-curl http://localhost:8080/v1/chat/completions -H "Content-Type: application/json" -d '{
- "model": "gpt-3.5-turbo",
- "messages": [{"role": "user", "content": "How are you?"}],
- "temperature": 0.9, "top_p": 0.8, "top_k": 80
- }'
-
-# {"object":"chat.completion","model":"gpt-3.5-turbo","choices":[{"message":{"role":"assistant","content":" Good, thanks. I am about to go to bed. I' ll talk to you later.Bye."}}],"usage":{"prompt_tokens":0,"completion_tokens":0,"total_tokens":0}}
-```
-
-### Fine tuning
-
-See [RWKV-LM](https://github.com/BlinkDL/RWKV-LM#training--fine-tuning). There is also a Google [colab](https://colab.research.google.com/github/resloved/RWKV-notebooks/blob/master/RWKV_v4_RNN_Pile_Fine_Tuning.ipynb).
-
-## See also
-
-- [RWKV-LM](https://github.com/BlinkDL/RWKV-LM)
-- [rwkv.cpp](https://github.com/saharNooby/rwkv.cpp)
\ No newline at end of file
diff --git a/spaces/chendl/compositional_test/transformers/examples/research_projects/vqgan-clip/img_processing.py b/spaces/chendl/compositional_test/transformers/examples/research_projects/vqgan-clip/img_processing.py
deleted file mode 100644
index 221ebd86dae785b4059a160b0f3d4c881977976f..0000000000000000000000000000000000000000
--- a/spaces/chendl/compositional_test/transformers/examples/research_projects/vqgan-clip/img_processing.py
+++ /dev/null
@@ -1,50 +0,0 @@
-import numpy as np
-import PIL
-import torch
-import torchvision.transforms as T
-import torchvision.transforms.functional as TF
-from PIL import Image
-
-
-def preprocess(img, target_image_size=256):
- s = min(img.size)
-
- if s < target_image_size:
- raise ValueError(f"min dim for image {s} < {target_image_size}")
-
- r = target_image_size / s
- s = (round(r * img.size[1]), round(r * img.size[0]))
- img = TF.resize(img, s, interpolation=PIL.Image.LANCZOS)
- img = TF.center_crop(img, output_size=2 * [target_image_size])
- img = torch.unsqueeze(T.ToTensor()(img), 0)
- return img
-
-
-def preprocess_vqgan(x):
- x = 2.0 * x - 1.0
- return x
-
-
-def custom_to_pil(x, process=True, mode="RGB"):
- x = x.detach().cpu()
- if process:
- x = post_process_tensor(x)
- x = x.numpy()
- if process:
- x = (255 * x).astype(np.uint8)
- x = Image.fromarray(x)
- if not x.mode == mode:
- x = x.convert(mode)
- return x
-
-
-def post_process_tensor(x):
- x = torch.clamp(x, -1.0, 1.0)
- x = (x + 1.0) / 2.0
- x = x.permute(1, 2, 0)
- return x
-
-
-def loop_post_process(x):
- x = post_process_tensor(x.squeeze())
- return x.permute(2, 0, 1).unsqueeze(0)
diff --git a/spaces/chikoto/Umamusume-DeBERTa-VITS2-TTS-JP/mel_processing.py b/spaces/chikoto/Umamusume-DeBERTa-VITS2-TTS-JP/mel_processing.py
deleted file mode 100644
index aab5bd926a194610b7ce3da29c553bd877341aa4..0000000000000000000000000000000000000000
--- a/spaces/chikoto/Umamusume-DeBERTa-VITS2-TTS-JP/mel_processing.py
+++ /dev/null
@@ -1,139 +0,0 @@
-import torch
-import torch.utils.data
-from librosa.filters import mel as librosa_mel_fn
-
-MAX_WAV_VALUE = 32768.0
-
-
-def dynamic_range_compression_torch(x, C=1, clip_val=1e-5):
- """
- PARAMS
- ------
- C: compression factor
- """
- return torch.log(torch.clamp(x, min=clip_val) * C)
-
-
-def dynamic_range_decompression_torch(x, C=1):
- """
- PARAMS
- ------
- C: compression factor used to compress
- """
- return torch.exp(x) / C
-
-
-def spectral_normalize_torch(magnitudes):
- output = dynamic_range_compression_torch(magnitudes)
- return output
-
-
-def spectral_de_normalize_torch(magnitudes):
- output = dynamic_range_decompression_torch(magnitudes)
- return output
-
-
-mel_basis = {}
-hann_window = {}
-
-
-def spectrogram_torch(y, n_fft, sampling_rate, hop_size, win_size, center=False):
- if torch.min(y) < -1.0:
- print("min value is ", torch.min(y))
- if torch.max(y) > 1.0:
- print("max value is ", torch.max(y))
-
- global hann_window
- dtype_device = str(y.dtype) + "_" + str(y.device)
- wnsize_dtype_device = str(win_size) + "_" + dtype_device
- if wnsize_dtype_device not in hann_window:
- hann_window[wnsize_dtype_device] = torch.hann_window(win_size).to(
- dtype=y.dtype, device=y.device
- )
-
- y = torch.nn.functional.pad(
- y.unsqueeze(1),
- (int((n_fft - hop_size) / 2), int((n_fft - hop_size) / 2)),
- mode="reflect",
- )
- y = y.squeeze(1)
-
- spec = torch.stft(
- y,
- n_fft,
- hop_length=hop_size,
- win_length=win_size,
- window=hann_window[wnsize_dtype_device],
- center=center,
- pad_mode="reflect",
- normalized=False,
- onesided=True,
- return_complex=False,
- )
-
- spec = torch.sqrt(spec.pow(2).sum(-1) + 1e-6)
- return spec
-
-
-def spec_to_mel_torch(spec, n_fft, num_mels, sampling_rate, fmin, fmax):
- global mel_basis
- dtype_device = str(spec.dtype) + "_" + str(spec.device)
- fmax_dtype_device = str(fmax) + "_" + dtype_device
- if fmax_dtype_device not in mel_basis:
- mel = librosa_mel_fn(sampling_rate, n_fft, num_mels, fmin, fmax)
- mel_basis[fmax_dtype_device] = torch.from_numpy(mel).to(
- dtype=spec.dtype, device=spec.device
- )
- spec = torch.matmul(mel_basis[fmax_dtype_device], spec)
- spec = spectral_normalize_torch(spec)
- return spec
-
-
-def mel_spectrogram_torch(
- y, n_fft, num_mels, sampling_rate, hop_size, win_size, fmin, fmax, center=False
-):
- if torch.min(y) < -1.0:
- print("min value is ", torch.min(y))
- if torch.max(y) > 1.0:
- print("max value is ", torch.max(y))
-
- global mel_basis, hann_window
- dtype_device = str(y.dtype) + "_" + str(y.device)
- fmax_dtype_device = str(fmax) + "_" + dtype_device
- wnsize_dtype_device = str(win_size) + "_" + dtype_device
- if fmax_dtype_device not in mel_basis:
- mel = librosa_mel_fn(sampling_rate, n_fft, num_mels, fmin, fmax)
- mel_basis[fmax_dtype_device] = torch.from_numpy(mel).to(
- dtype=y.dtype, device=y.device
- )
- if wnsize_dtype_device not in hann_window:
- hann_window[wnsize_dtype_device] = torch.hann_window(win_size).to(
- dtype=y.dtype, device=y.device
- )
-
- y = torch.nn.functional.pad(
- y.unsqueeze(1),
- (int((n_fft - hop_size) / 2), int((n_fft - hop_size) / 2)),
- mode="reflect",
- )
- y = y.squeeze(1)
-
- spec = torch.stft(
- y,
- n_fft,
- hop_length=hop_size,
- win_length=win_size,
- window=hann_window[wnsize_dtype_device],
- center=center,
- pad_mode="reflect",
- normalized=False,
- onesided=True,
- return_complex=False,
- )
-
- spec = torch.sqrt(spec.pow(2).sum(-1) + 1e-6)
-
- spec = torch.matmul(mel_basis[fmax_dtype_device], spec)
- spec = spectral_normalize_torch(spec)
-
- return spec
diff --git a/spaces/chikoto/Umamusume-DeBERTa-VITS2-TTS-JP/text/__init__.py b/spaces/chikoto/Umamusume-DeBERTa-VITS2-TTS-JP/text/__init__.py
deleted file mode 100644
index d25092ebd132cc181aa80fc0c7864e0e063d6736..0000000000000000000000000000000000000000
--- a/spaces/chikoto/Umamusume-DeBERTa-VITS2-TTS-JP/text/__init__.py
+++ /dev/null
@@ -1,28 +0,0 @@
-from text.symbols import *
-
-_symbol_to_id = {s: i for i, s in enumerate(symbols)}
-
-
-def cleaned_text_to_sequence(cleaned_text, tones, language):
- """Converts a string of text to a sequence of IDs corresponding to the symbols in the text.
- Args:
- text: string to convert to a sequence
- Returns:
- List of integers corresponding to the symbols in the text
- """
- phones = [_symbol_to_id[symbol] for symbol in cleaned_text]
- tone_start = language_tone_start_map[language]
- tones = [i + tone_start for i in tones]
- lang_id = language_id_map[language]
- lang_ids = [lang_id for i in phones]
- return phones, tones, lang_ids
-
-
-def get_bert(norm_text, word2ph, language, device="cuda"):
- from .chinese_bert import get_bert_feature as zh_bert
- from .english_bert_mock import get_bert_feature as en_bert
- from .japanese_bert import get_bert_feature as jp_bert
-
- lang_bert_func_map = {"ZH": zh_bert, "EN": en_bert, "JP": jp_bert}
- bert = lang_bert_func_map[language](norm_text, word2ph, device)
- return bert
diff --git a/spaces/chuan-hd/law-assistant-chatbot/.venv/lib/python3.11/site-packages/PIL/BdfFontFile.py b/spaces/chuan-hd/law-assistant-chatbot/.venv/lib/python3.11/site-packages/PIL/BdfFontFile.py
deleted file mode 100644
index 075d462907abcace9610a686052e643582602a8f..0000000000000000000000000000000000000000
--- a/spaces/chuan-hd/law-assistant-chatbot/.venv/lib/python3.11/site-packages/PIL/BdfFontFile.py
+++ /dev/null
@@ -1,122 +0,0 @@
-#
-# The Python Imaging Library
-# $Id$
-#
-# bitmap distribution font (bdf) file parser
-#
-# history:
-# 1996-05-16 fl created (as bdf2pil)
-# 1997-08-25 fl converted to FontFile driver
-# 2001-05-25 fl removed bogus __init__ call
-# 2002-11-20 fl robustification (from Kevin Cazabon, Dmitry Vasiliev)
-# 2003-04-22 fl more robustification (from Graham Dumpleton)
-#
-# Copyright (c) 1997-2003 by Secret Labs AB.
-# Copyright (c) 1997-2003 by Fredrik Lundh.
-#
-# See the README file for information on usage and redistribution.
-#
-
-"""
-Parse X Bitmap Distribution Format (BDF)
-"""
-
-
-from . import FontFile, Image
-
-bdf_slant = {
- "R": "Roman",
- "I": "Italic",
- "O": "Oblique",
- "RI": "Reverse Italic",
- "RO": "Reverse Oblique",
- "OT": "Other",
-}
-
-bdf_spacing = {"P": "Proportional", "M": "Monospaced", "C": "Cell"}
-
-
-def bdf_char(f):
- # skip to STARTCHAR
- while True:
- s = f.readline()
- if not s:
- return None
- if s[:9] == b"STARTCHAR":
- break
- id = s[9:].strip().decode("ascii")
-
- # load symbol properties
- props = {}
- while True:
- s = f.readline()
- if not s or s[:6] == b"BITMAP":
- break
- i = s.find(b" ")
- props[s[:i].decode("ascii")] = s[i + 1 : -1].decode("ascii")
-
- # load bitmap
- bitmap = []
- while True:
- s = f.readline()
- if not s or s[:7] == b"ENDCHAR":
- break
- bitmap.append(s[:-1])
- bitmap = b"".join(bitmap)
-
- # The word BBX
- # followed by the width in x (BBw), height in y (BBh),
- # and x and y displacement (BBxoff0, BByoff0)
- # of the lower left corner from the origin of the character.
- width, height, x_disp, y_disp = [int(p) for p in props["BBX"].split()]
-
- # The word DWIDTH
- # followed by the width in x and y of the character in device pixels.
- dwx, dwy = [int(p) for p in props["DWIDTH"].split()]
-
- bbox = (
- (dwx, dwy),
- (x_disp, -y_disp - height, width + x_disp, -y_disp),
- (0, 0, width, height),
- )
-
- try:
- im = Image.frombytes("1", (width, height), bitmap, "hex", "1")
- except ValueError:
- # deal with zero-width characters
- im = Image.new("1", (width, height))
-
- return id, int(props["ENCODING"]), bbox, im
-
-
-class BdfFontFile(FontFile.FontFile):
- """Font file plugin for the X11 BDF format."""
-
- def __init__(self, fp):
- super().__init__()
-
- s = fp.readline()
- if s[:13] != b"STARTFONT 2.1":
- msg = "not a valid BDF file"
- raise SyntaxError(msg)
-
- props = {}
- comments = []
-
- while True:
- s = fp.readline()
- if not s or s[:13] == b"ENDPROPERTIES":
- break
- i = s.find(b" ")
- props[s[:i].decode("ascii")] = s[i + 1 : -1].decode("ascii")
- if s[:i] in [b"COMMENT", b"COPYRIGHT"]:
- if s.find(b"LogicalFontDescription") < 0:
- comments.append(s[i + 1 : -1].decode("ascii"))
-
- while True:
- c = bdf_char(fp)
- if not c:
- break
- id, ch, (xy, dst, src), im = c
- if 0 <= ch < len(self.glyph):
- self.glyph[ch] = xy, dst, src, im
diff --git a/spaces/chuan-hd/law-assistant-chatbot/.venv/lib/python3.11/site-packages/cryptography/hazmat/backends/openssl/cmac.py b/spaces/chuan-hd/law-assistant-chatbot/.venv/lib/python3.11/site-packages/cryptography/hazmat/backends/openssl/cmac.py
deleted file mode 100644
index bdd7fec611d194ec50a3df3efab9adf34b6373e6..0000000000000000000000000000000000000000
--- a/spaces/chuan-hd/law-assistant-chatbot/.venv/lib/python3.11/site-packages/cryptography/hazmat/backends/openssl/cmac.py
+++ /dev/null
@@ -1,89 +0,0 @@
-# This file is dual licensed under the terms of the Apache License, Version
-# 2.0, and the BSD License. See the LICENSE file in the root of this repository
-# for complete details.
-
-from __future__ import annotations
-
-import typing
-
-from cryptography.exceptions import (
- InvalidSignature,
- UnsupportedAlgorithm,
- _Reasons,
-)
-from cryptography.hazmat.primitives import constant_time
-from cryptography.hazmat.primitives.ciphers.modes import CBC
-
-if typing.TYPE_CHECKING:
- from cryptography.hazmat.backends.openssl.backend import Backend
- from cryptography.hazmat.primitives import ciphers
-
-
-class _CMACContext:
- def __init__(
- self,
- backend: Backend,
- algorithm: ciphers.BlockCipherAlgorithm,
- ctx=None,
- ) -> None:
- if not backend.cmac_algorithm_supported(algorithm):
- raise UnsupportedAlgorithm(
- "This backend does not support CMAC.",
- _Reasons.UNSUPPORTED_CIPHER,
- )
-
- self._backend = backend
- self._key = algorithm.key
- self._algorithm = algorithm
- self._output_length = algorithm.block_size // 8
-
- if ctx is None:
- registry = self._backend._cipher_registry
- adapter = registry[type(algorithm), CBC]
-
- evp_cipher = adapter(self._backend, algorithm, CBC)
-
- ctx = self._backend._lib.CMAC_CTX_new()
-
- self._backend.openssl_assert(ctx != self._backend._ffi.NULL)
- ctx = self._backend._ffi.gc(ctx, self._backend._lib.CMAC_CTX_free)
-
- key_ptr = self._backend._ffi.from_buffer(self._key)
- res = self._backend._lib.CMAC_Init(
- ctx,
- key_ptr,
- len(self._key),
- evp_cipher,
- self._backend._ffi.NULL,
- )
- self._backend.openssl_assert(res == 1)
-
- self._ctx = ctx
-
- def update(self, data: bytes) -> None:
- res = self._backend._lib.CMAC_Update(self._ctx, data, len(data))
- self._backend.openssl_assert(res == 1)
-
- def finalize(self) -> bytes:
- buf = self._backend._ffi.new("unsigned char[]", self._output_length)
- length = self._backend._ffi.new("size_t *", self._output_length)
- res = self._backend._lib.CMAC_Final(self._ctx, buf, length)
- self._backend.openssl_assert(res == 1)
-
- self._ctx = None
-
- return self._backend._ffi.buffer(buf)[:]
-
- def copy(self) -> _CMACContext:
- copied_ctx = self._backend._lib.CMAC_CTX_new()
- copied_ctx = self._backend._ffi.gc(
- copied_ctx, self._backend._lib.CMAC_CTX_free
- )
- res = self._backend._lib.CMAC_CTX_copy(copied_ctx, self._ctx)
- self._backend.openssl_assert(res == 1)
- return _CMACContext(self._backend, self._algorithm, ctx=copied_ctx)
-
- def verify(self, signature: bytes) -> None:
- digest = self.finalize()
- if not constant_time.bytes_eq(digest, signature):
- raise InvalidSignature("Signature did not match digest.")
diff --git a/spaces/cihyFjudo/fairness-paper-search/ASPEL SAE CRACK DE REINSTALABLE 22 (R22) Full Version A Complete Review.md b/spaces/cihyFjudo/fairness-paper-search/ASPEL SAE CRACK DE REINSTALABLE 22 (R22) Full Version A Complete Review.md
deleted file mode 100644
index f93eff846ac0312dcf86ba143a8a87a283a61a52..0000000000000000000000000000000000000000
--- a/spaces/cihyFjudo/fairness-paper-search/ASPEL SAE CRACK DE REINSTALABLE 22 (R22) Full Version A Complete Review.md
+++ /dev/null
@@ -1,6 +0,0 @@
-
-HD Online Player (HOT! LS Dream Issue 6 Secret Place -)
Camtasia Studio 2018.0.1 Build 3457 Keygen - Crackingpatching Free Download
aplikasi pembayaran spp sekolah dengan visual basic
vodei decrypt tool [devodei r21.zip] download pc
Palisade @RISK 5.7 crack checked
light up for sketchup plugin cracked magazine
CRACK Waves - Complete v10 2018.08.07 (VST, VST3, AAX, STANDALONE) x64
Pattern Magic 3 English Pdfl
Fukrey Returns full movie hd download 1080p
manga black cat bahasa indonesia
-ASPEL SAE CRACK DE REINSTALABLE 22 (R22) full version
Download 🗹 https://tinurli.com/2uwjy5
-ASPEL SAE CRACK DE REINSTALABLE 22 (R22) 64 BitDOWNLOAD - some changes to the Configuration Wizard and. PGP is especially useful in a. 6.0 version of ASPEL SAE that was.. After you have completed this procedure,. Features.. aspel sae 5.0 r22 crack.. Crashe al instalar ASPEL SAE 6.0 Crack Re-Instalable 9 . Activador Re-installable Aspel SAE 6.0. 2014 . Microsoft Store aspel sae 5.0 r22.Visual Visual Visual Visual Visual Visual Visual Visual Visual. aspel sae 5.0 r22 crack pdf - Aspel Coi (reinstalable). Visual xforce visual visual visual visual aspel sae 5.0.Vista 64 bits support: Now with a separate 64 bit download to work. This can only be re-installed if the previous version of. PGP is especially useful in a. After you have completed this procedure,. Feature.. aspel sae 5.0 r22 crack.. Dr. Now. point and click it, aspel sae cracked and pirated (aspell setup /install/setup).rar how.ps.This can only be re-installed if the previous version of. PGP is especially useful in a. This can only be re-installed if the previous version of. Now with a separate 64 bit download to work. This can only be re-installed if the previous version of. PGP is especially useful in a. This can only be re-installed if the previous version of. PGP is especially useful in a. This can only be re-installed if the previous version of. This can only be re-installed if the previous version of. PGP is especially useful in a.PGP is especially useful in a. Third-party text. This can only be re-installed if the previous version of. PGP is especially useful in a. This can only be re-installed if the previous version of. PGP is especially useful in a. This can only be re-installed if the previous version of. This can only be re-installed if the previous version of. This can only be re-installed if the previous version of. PGP is especially useful in a. PGP is especially useful in a. This can only be re-installed if the previous version of. PGP is especially useful in a. PGP is especially useful in a.ASPEL ee730c9e81
aaccfb2cb3
-
-
\ No newline at end of file
diff --git a/spaces/cihyFjudo/fairness-paper-search/DRAGON QUEST XI Echoes Of An Elusive Age-CODEX Fitgirl Repack Comment installer et jouer la version dfinitive du jeu.md b/spaces/cihyFjudo/fairness-paper-search/DRAGON QUEST XI Echoes Of An Elusive Age-CODEX Fitgirl Repack Comment installer et jouer la version dfinitive du jeu.md
deleted file mode 100644
index 47c1a90e537bb3686285e2455751aa88b25fb241..0000000000000000000000000000000000000000
--- a/spaces/cihyFjudo/fairness-paper-search/DRAGON QUEST XI Echoes Of An Elusive Age-CODEX Fitgirl Repack Comment installer et jouer la version dfinitive du jeu.md
+++ /dev/null
@@ -1,6 +0,0 @@
-DRAGON QUEST XI Echoes Of An Elusive Age-CODEX Fitgirl Repack
Download 🗸 https://tinurli.com/2uwjL9
-
- aaccfb2cb3
-
-
-
diff --git a/spaces/cihyFjudo/fairness-paper-search/Foxit PDF Editor Version 2.2.1 Build 1119 Portable.rarl A Review.md b/spaces/cihyFjudo/fairness-paper-search/Foxit PDF Editor Version 2.2.1 Build 1119 Portable.rarl A Review.md
deleted file mode 100644
index fb59041c9d5b5b71f8cf381b700a113534385184..0000000000000000000000000000000000000000
--- a/spaces/cihyFjudo/fairness-paper-search/Foxit PDF Editor Version 2.2.1 Build 1119 Portable.rarl A Review.md
+++ /dev/null
@@ -1,6 +0,0 @@
-
-Foxit PDF Editor is a free trial software published in the Other list of programs, part of System Utilities.
This program is available in English. It was last updated on 22 October, 2022. Foxit PDF Editor is compatible with the following operating systems: Windows.
The company that develops Foxit PDF Editor is Foxit Corporation. The latest version released by its developer is 2.2.1.1119. This version was rated by 35 users of our site and has an average rating of 3.7.
The download we have available for Foxit PDF Editor has a file size of 5.11 MB. Just click the green Download button above to start the downloading process. The program is listed on our website since 2010-11-26 and was downloaded 6799 times. We have already checked if the download link is safe, however for your own protection we recommend that you scan the downloaded software with your antivirus. Your antivirus may detect the Foxit PDF Editor as malware if the download link is broken.
How to install Foxit PDF Editor on your Windows device:
- Click on the Download button on our website. This will start the download from the website of the developer.
- Once the Foxit PDF Editor is downloaded click on it to start the setup process (assuming you are on a desktop computer).
- When the installation is finished you should be able to see and run the program.
-Foxit PDF Editor Version 2.2.1 Build 1119 Portable.rarl
DOWNLOAD ○ https://tinurli.com/2uwj7Y
-( A )
Aurora 3D Animation Maker v14.x incl Patch
Any Video Converter Ultimate 5.x incl key
AAA Logo Maker Business Edition v3.0 + Serial
Any PDF To DWG Converter 2013 Full Version
Advanced Batch Converter 7.89 + Serial
Ace Translator 10.5.3 Full Version
Ace Utilities 5.4.0.538 + Keygen
Acebyte Utilities 3.0.6 PRO Full Version
AccuRIP 1.03 Build 12 + Patch
Amigabit Powerbooster v3.2.4 Full Version
Angry Birds Star Wars v1.0.0 + Serial
Applian Replay Telecorder for Skype 1.3.0.23 + Crack
Adobe Flash Professional CS6 12.0.0.481 + Crack
Adobe Premiere Pro CC (64-bit) + Crack
Adobe After Effects (64-Bit) CC + Crack
Articulate Storyline 2 incl Patch
AVCWare Video Converter Ultimate 7.7.2 + Serial
Arafasoft My Autoplay Professional 10.4 Pre-Cracked
Ashampoo Internet Accelerator 3.20 + Serial
Ashampoo MP3 Cover Finder 1.0.11.0 Full Version
Astroburn Pro 3.2.0.0.197 + Activator
Abex Document Converter Pro v3.8.0 + Serial
AI RoboForm Enterprise 7.8.9.5 Final With Patch
Ailt GIF to Word Converter 6.6 + Activator
Angry Birds Space v1.0 Full Version
Auto Hide IP 5.3.3.2 Full Version
Auto Power-on & Shut-down 2.80 Full Version
Aurora All Products Crack *Free Download*
AutoPlay Media Studio 7.5 + Serial
AVS Video Converter 9.1 Incl Patch
AVS Video ReMaker 5.0 + Crack
Aurora 3D Text & Logo Maker version 12.08 + Crack + Keygen
Applian FLV and Media Player 3.1.1.12 Free
Active Undelete Enterprise 9.3.5.0 + Crack
Aleo All Products Pre-Cracked
Aiseesoft 3D Converter 6.3.28 *Free* Full Version
Aiseesoft PDF to Image Converter 3.1.8 + Keygen
Aiseesoft YouTube Downloader Pro 5.0.38 + Crack
Adobe Acrobat Reader DC 15.007.20033 Free
Adobe Flash Player NPAPI 18.0.0.194 Free
Adobe Photoshop CC 14.0 Final Full Version
Adobe Photoshop CS6 + Crack
Adobe Photoshop CS5 + Crack
Adobe Photoshop CS4 + Crack
Adobe Photoshop CS3 + Crack
Adobe Photoshop CS2 + Keygen
Adobe Photoshop 7.0 + Serial
AVS Video Editor 5.2 + Crack
AVG Internet Security 2014 + License Key
Avast Antivirus Serial Key valid until 2038
Atomic Email Hunter 3.50 + Patch
Aostsoft All Document Converter Professional 3.8.9 + Keygen
ArcSoft PhotoStudio v6.0.5.180 + Serial
Aiseesoft Audio Converter 6.2.52 Full Version
Aiseesoft BD Software Toolkit 7.2.8 Full Version
Aiseesoft Blu-ray Converter Ultimate 6.3.86 Full Version
Aiseesoft DVD Converter Suite Ultimate 6.3.86 Full Version
Aiseesoft PDF Converter Ultimate 3.2.6 Full Version
AnyMP4 DVD Ripper 6.0.36.19315 Multilingual Full Version
AnyMP4 DVD Toolkit 6.0.32.14221 Multilingual Full Version
Aoao Video Watermark Pro 5.0 + Serial
AOMEI Partition Assistant Professional Edition 5.5 + Serial
AOMEI Backupper Professional Edition 2.0 + Patch
All-In-One Email Hacking Software
Anvisoft Cloud System Booster v3.0 + Key
AnyToISO Professional 3.5.2 + Patch
Avira System Speedup 1.2.1.8200 + Reg
Ashampoo Video Styler v1.0.1 Full Version
Ashampoo Movie Studio Pro 1.0.17.1 + Patch
Ashampoo Music Studio v4.1.2.5 + Patch
Ashampoo Photo Commander 12.0.1 + Patch
Ashampoo Snap 7.0.3 + Crack
Ashampoo Slideshow Studio HD 3.0.4 + Patch
Ashampoo WinOptimizer 11.00.40 + Patch
Ashampoo Burning Studio 14.0.5.10 Full Version
Ashampoo Photo Optimizer 5.4.0.6 + Crack
Ashampoo Photo Converter 2.0 + Patch
Ashampoo UnInstaller 5.0.3 + Patch
Amazing Slideshow Maker 3.4.6.0 +Templates + Crack
Audacity 2.0.2 Free
Advanced Installer 9.9 + Patch
Aleo Flash Intro Banner Maker 3.2 Full Version
Atmosphere Deluxe v7.1 + Serial
Aurora 3D Presentation 2012 v14.09 + Crack + Serial Key
Auslogics BoostSpeed Premium 8.0.0.0 + Serial Keys
Auslogics Disk Defrag Professional 4.6.0.0 + Serial key
Auslogics Driver Updater 1.5.0.0 + Crack
Accelerator Plus Premium (DAP) 10.0.4.3 + Patch
( B )
BarTender Enterprise Automation 10.1 SR3 + Patch
Banner Designer Pro v5.1 + Patch
Bigasoft DVD Ripper 3.2.3.4772 Full Version
BurnAware Professional 6.2 Full Version
Bolide Movie Creator 1.5 (Build 1007) + Serial
BluffTitler DX9 8.2.0.3 + Keygen
BB FlashBack Pro 4.1.9 (Build 3121) + Serial
Batchwork Word to PDF Converter 2013 5.1118 + Crack
Bigasoft AVCHD Converter 3.7.49.5044 Full Version
Bigasoft Audio Converter 4.2.2 Full Version
Bigasoft Total Video Converter 3.7.42 Full Version
Bigasoft iTunes Video Converter 4.2.2 Full Version
Bigasoft YouTube Downloader Pro 1.2.26.4849 + Serial
BlazeVideo SmartShow 1.4.0.0 + key
BlazeVideo SmartShow 2.0.1.0 Datecode 13.06.2014 + Serial
Bubble Snooker TM Mobile Edition v1.2 Full Version
Bulk Image Downloader 4.69.0.0 + Crack
BenVista Photo Zoom Pro 5.0.2 + Patch
Blaze Video Magic Pro 6.2.1.0 Full Version
( C )
Crazy Talk v6.0 Pro + Crack
Card Recovery 6.10 build 1210 + Crack
Copy Protect 1.6.0 Full Version
CCleaner Business & Professional 3.26.1888 Full Version
Creating GMail Labels And Filters
CleanMyPC 1.5.7 Full Version
Centennia Historical Atlas v3.11 + Patch
CoolUtils Total Audio Converter 1.0.0 + Serial
Conceiva DownloadStudio 9.0.3.0 + Crack
Corel AfterShot Pro 1.2.0.7 + Patch
Corel Ulead VideoStudio 11.0.0157.0 Plus Free
Corel WinDVD Pro v11.5.1.3 + Serial
Camtasia Studio 7 Portable
Camtasia Studio 8.0 Portable
Camtasia Studio 8.0.2.918 + Crack & Serial Keys
Cyberlink PowerDirector 10 Full Version
CyberLink PowerDirector 11 Ultra v11.0.0.2418 + Serial
CyberLink PowerDirector 12.0.2230 + Crack
CyberPower Video Switch 4.2.5 + Serial
Cheat Engine 6.2 Free
( D )
DDVideo Swf to MP4 Converter Standard 5.1 Incl Patch
DDVideo Swf to mov Converter Standard 5.1 Incl Patch
DVD X Player Professional 5.5.3.7 incl Patch
Driver Checker 2.7.5 + Serial
DP Animation Maker 2.0.4 + Key
DivX Plus 10.2.0 (Build 10.2.0-84) + Serial
DriverEasy 4.6.1.16849 PRO with Activator
Diskeeper Professional Edition 2012 16.0.1017 + Patch
Dll-Files Fixer 3.0.81.2643 Portable
DVDFab 9.0.3.6 incl Patch
DVDFab 9.1.0.1 Beta incl Patch
DVDFab Passkey 8.0.9.3 Incl Patch
Driver Genius Professional Edition 11.0 + Crack
Driver Easy Professional v4.7.0 + Crack
Driver Reviver 4.0.1.36 + Patch
Driver Robot 2.5.4.2 + Serial
Driver Tuner 3.1.0.0 + Patch
DriverToolkit 8.1.1 Full Version
Desktop Icon Toy 4.7 + Serial
DigiCel FlipBook ProHD 6.93 *Free* Full Version
( E )
Easy GIF Animator 5.3 Personal + Serial
Engelmann Media MakeMe3D 1.2.12.618 + Key
EaseUS Partition Master 10.0 All Editions + Patch
Esquimo 3D 1.4.0.0 + Serial
EximiousSoft Banner Maker 5.25 + Patch
EximiousSoft Cool Image v3.30 + Patch
EximiousSoft Logo Designer v3.60 + Crack
Easy Button & Menu Maker 2.3 + Keygen
EarMaster Pro 6.1 (627PW) + Patch
Enable Zawgyi in Mozilla Firefox
Evaer Video Recorder for Skype 1.3.11.22 + Keygen
English-To-Myanmar-Dictionary
Easy DVD Creator 2.5.8 + Keygen
EaseUS MobiSaver for Android 4.0 + Patch
Easy MP3 Downloader 4.5.1.8 Full Version
EximiousSoft GIF Creator v7.15 Incl Patch
EaseUS Data Recovery Wizard Professional 7.5 + Patch
EasiestSoft Movie Editor 4.3.0 + Patch
EasiestSoft Video Converter 3.3.2 + Patch
EximiousSoft Business Card Designer v3.90 + Patch
Everimaging Photo Effect Studio Pro v4.1.3 Incl Patch
Everimaging HDR Darkroom 3 Pro 1.1.0 (x86/64) Incl Patch
Everimaging Beautune 1.0.1 incl Patch
( F )
Format Converter 6 Ultimate 6.0.5213 + Patch
Flash Player Pro v5.88 + Patch
FL Studio Producer Edition v10.0.0 + Crack
FullSpeed Internet Booster Pro v3.3 + Patch
Foxit Advanced PDF Editor 3.00 + Patch
Foxit PDF Editor 2.2.1 Build 1119 + Serial
FlashBoot 2.2d + License Key
Face Off Max 3.5.7.8 + Serial
FXhome PhotoKey PRO 6.0.0015 (64-bit) Incl Key
Fly on Desktop Screensaver 1.2 Free
Fhotoroom HDR 3.0.5 + Patch
Free MP3 WMA OGG Converter 9.5.4 + Serial
FlashFXP 4.3.1 (build 1951) License Key
Folder Colorizer 1.2.1 Free
Folder Cleaner 1.0.2 + Serial
Full Speed Up Youtube Video
Free Download Sex World Video
( G )
GameBoost 1.1.28.2013 + Patch
GridinSoft Trojan Killer 2.1.9.9 Full Version
GiliSoft DVD Ripper 3.2.0 Full Version
GiliSoft All Products Free Download (2014)
Genuine Registry Doctor PRO 2.5.5.6 Full Version
Google Talk Software Free Download
Google Chrome v23.0.1251.2 Free
Gromada VideoMach 5.9.16 Professional + Serial
GIMP 2.8 Free
Glary Utilities PRO v2.54.0.1759 + Keygen
( H )
Hippo Animator 3.6.5247 Multilingual Full Version
HDRSoft Photomatix Pro 5.0.1 Final (x86-x64) + Serial
Hard Disk Sentinel Pro v4.60 incl Patch
Hot MP3 Downloader 3.3.7.2 Full Version
Hot Christmas Girls Windows 7 Theme
Hitman Pro 3.7.3 Build-193 (x32x64) + Patch
HiDownload Platinum 8.12 + Key
( I )
Internet Download Manager v6.21 (Build 17) + Crack
IObit Advanced SystemCare 6 PRO + Serial
iZotope RX 3 Advanced 3.02 incl Patch
Internet Speed Hack v6.1 Free
Internet Download Manager 6.11 Portable Full Version
Improve Your Pronounciation
Iceni Technology Infix PDF Editor Pro 6.26 + Crack
IObit Game Booster Premium 2.3 + Serial
IObit Malware Fighter PRO 1.7 + Keys
IObit Driver Booster 1.0.1.0 + License Key
iCare Data Recovery 3.8.3 + Serial
ImTOO Convert PowerPoint to MP4 1.0.4.1018 + Serial
ImTOO PDF to Word Converter 1.0.3 + Patch
Inno Setup Ultra 5.5.1 Free
iolo System Mechanic 12.7.0.62 + Serial
iSkysoft DVD Creator 3.0.0.6 with DVD Menu Templates
iSkysoft PDF Editor 3.0.0.2 Final Incl Patch
iSkysoft iTube Studio 4.2.2 incl Patch
iSkysoft Video Converter Ultimate 5.5.1 incl Patch
iSkysoft Video Editor 4.7 incl Patch
iPod-Cloner v1.90 Build 851 Full Version
Insert an Image to Gmail message body
IDM v6.07 + No Serial Number Full Version
idoo Video Editor Pro 2.5.0 + Serial
Intel Pentium4 865 All Drivers For Windows Xp- 7
inFlow Inventory Premium 2.5.1 + Activator
iWisoft Flash SWF to Video Converter 3.4 + Patch
( J )
JRiver Media Center 17.0.189 + Patch
( K )
KernSafe TotalMounter Pro v2.01 incl Patch
Kvisoft Flash Slideshow Designer 1.6.0 + Patch
Keyman Developer 8.0 incl Patch
Keyman Desktop Professional 8.0 incl Patch
( L )
Loaris Trojan Remover v1.3.0.4 Full Version
Lazesoft Recovery Suite Unlimited Edition 3.5 + Activator
Leawo Video Accelerator Pro 4.5.0.1 Multilanguage + Serial
LumaPix FotoFusion 4.5 Build 66264 Full Version
( M )
Moyea SWF to Video Converter Pro 3.12.0 Full Version
Morpheus Photo Animation Suite Industrial v3.16 + Patch
Multi Virus Cleaner v13.1.0 2013 *Portable*
Master PDF Editor 1.9.25 + Patch
MassTube Plus v12.8.3.293 Incl Patch
MyScript Stylus v3.2 Incl Patch
Myanmar-English Dictionary
Magic DVD Copier v7.1.1 + Keygen
MAGIX Music Maker Premium v17.0.0.16 + Crack + Serial
Magix Photo Manager MX Deluxe 11 (9.0.0.228) + Crack
Malwarebytes Anti-Malware PRO Full Version
Music Mp3 Downloader 5.4.9.2 Full Version
MeMedia Movie DVD Convert 8.5.1 + Serial
Mediachance Photo-Reactor 1.1 (x86-x64) + Keygen
Miranda IM 0.10.14 + Portable (x86x64)
Miranda NG 0.94.6 Final (x86x64) Free
Movavi Video Converter v14.3.0 Full Version
MEPMedia MP3 Editor Pro 6.5.1 + Serial
Magic Photo Editor 6.1 + Patch
Making Gmail Offline
MAGIX Movie Edit Pro 15 Plus + Patch
Microsoft Office Professional 2010 Full Version
Microsoft Office 2013 (32-bit/64-bit) + Activator
Microsoft Visual Basic 2010 Express (KENGEN)
Muvee Reveal X + Crack
( N )
NCH Doxillion Document Converter Plus v2.08 + Key
NCH Debut Video Capture Software Pro v1.74 + Serial
NCH Express Burn Plus 4.65 + Serial
Nero Burning ROM 12.5 & 2014 v15.0 + Crack + Serial
Nuance PDF Converter Enterprise 7.3 + Serial
Naevius USB Antivirus v2.1 + Serial
NextUp TextAloud 3.0.66 + Serial
Netralia VodBurner 1.1.0.201 + Patch
( O )
Okdo All to Pdf Converter Professional 4.8 + Serial
Opening 2,3 Google Talk Gadgets
onOne Perfect Photo Suite Premium Edition v8.5 (64-bit) + Crack
Open DVD Ripper 3.20 Build 505 Full Version
OSHI CLEANER 1.0.133.0 *Portable*
O&O Defrag Professional Edition 16 (64Bit/32Bit) + Serial
Odin Frame Photo Creator 9.8.4 + License Key
( P )
PC Utilities Pro Driver Pro 3.1.0 + License key
Password Depot Professional v7.5.1 + Patch
ParetoLogic DriverCure 1.6.1 Full Version
Power Audio Extractor v4.7.6 + Serial
Power Video DVD Copy 3.1.6 + Serial
Privacy Eraser Pro v1.8.0 Build 399 Full Version
Poikosoft EZ CD Audio Converter v1.3.4 + Crack
progeCAD 2014 Professional 14.0.6.15 incl Patch
Photo Collage Max Pro 2.2.2.8 Full Version
Photo Montage Guide 1.6.1 Full Version
Photo Stamp Remover 5.3 Incl Patch
Pantaray QSetup Installation Suite Pro 11.0.0.9 + Keygen
PDFTiger 1.0 + Serial
Perfect PDF Reader 8.0.3.5 Free
Paragon Backup & Recovery 14 Home 10.1 + Serial
PC Optimizer Pro v6.4.6.4 + Patch
Pointstone System Cleaner 7.3.8.362 Full Version
ParetoLogic PC Health Advisor 3.1.4 incl Patch
priPrinter Professional 6.1.0.2280 Free
PowerISO 5.5 + Serial
Power YouTube to MP3 Converter 4.6.1 + Serial
PDF to Text Converter v2.0 + Keygen
Photomizer 2.0.13.425 + Patch
PhotoInstrument 6.2 (Build-620) + Patch
PC Cleaner Pro 2013 11.0.13.4.4 + Serial
Proxy Switcher Pro 5.7.0 (Build 6366) Incl Patch
Program4Pc PC Video Converter 7.6 Incl Patch
PC Speed Maximizer 3.0.1.0 + Patch
Paint Net
Pika Software Builder 4.9.5.2 + Serial Key
Product Key Explorer 3.2.9.0 Full Version
Proshow Producer 5 + Crack
Pinnacle Studio 15 HD Ultimate Collection - Full Version
Portable Cinema 4D R13.061 (Build RC59660) + Serial
Put a password on any file or folder using WinRAR
PhotoScape V3.6.2 Free
( Q )
Quick Search 1.0.0.166 Free
Quick 3D Cover 2.0.1 Incl Patch
( R )
Revo Uninstaller Pro v3.0.2 incl Crack
RegCure Pro 3.1.5 Final + Patch
Readiris Pro 15.0 incl Patch
RoboTask 5.6.4.809 Full Version
Reg Organizer 6.10 Beta 1 + Crack + Serial
Reg Organizer 6.10 Beta 2 + Crack + Serial
Registry Reviver 3.0.1.96 + Patch
Real Hide IP 4.2.5.8 incl Patch
RarmaRadio Pro 2.69 + Serial
RAR Password Unlocker 4.2.0 + Patch
( S )
Sothink Movie DVD Maker PRO v3.7 Build 341 + Patch
Sothink Logo Maker Professional 4.2 + Patch
Sothink Logo Maker Professional 4.4 Incl Patch
System Speed Booster 2.9.3.2 + Crack
SparkTrust Driver Updater v3.1 + Serial
SkinCrafter Installer 3.0.2 Full Version
Smart Driver Updater v3.3 + Patch
StudioLine Photo Classic Plus 3.70.62.0 + Serial
Speedy PC Pro 3.0.0 Full Version
SoundTaxi Media Buddy Ultimate 4.5.1 + Patch
SuperEasy Registry Cleaner 1.0.65 Full Version
Save2PC Ultimate 5.11 (Build 1379) + Serial
SuperEasy Audio Converter 3.0.4010 + Patch
Spyware Process Detector 3.23.2 Full Version
Simpo PDF Converter Ultimate 1.5.3.0 + Serial
Simpo PDF Merge & Split 2.2.3 + Serial
SpyHunter 4.1.11.0 + Crack
Sony Vegas Pro 9/10/11/12/13 - Pre-Cracked
Setup an Auto-Responder in Gmail
SWF Opener 1.3 Free
Screenpresso Pro 1.5.1 + Patch
SummitSoft Logo Design Studio 4.0.0.0 Retail
Systweak Disk Speedup 3.0.0.7465 + License Key
Systweak Advanced System Protector 2.1 + Serial
Systweak Advanced Driver Updater 2.1.1086 + Patch
Sothink SWF Decompiler 7.4 + Patch
Send Free SMS From Gmail
Sending Photo in Google Talk
Smart Install Maker 5.04 + Keygen
Sony Vegas Pro 12 (64-bit) Pre-Cracked
Speed up your Internet connection v8.0 Portable
SlimWare DriverUpdate 2.2 + Serial
SpeedBit Video Accelerator 3.3 + Patch
Sony Movie Studio Platinum 12.0 PreCracked
StudioCoast VMix 4K 12.0.0.128 + Crack
SnowFox DVD & Video Converter 3.0.2.0 + Patch
Set Friends picture in Google Talk as my picture
Speed MP3 Downloader 2.3.6.6 Full Version
Super Mp3 Download 4.8.8.8 Full Version
SWiSH Max 4.0 + Patch
( T )
TuneUp Utilities 2014 v14.0 Final + Keygen
TVPaint Animation 10 Pro v10.0.16 (x32x64) Free
Teorex Inpaint 5.5 + Serial
The Bat! Professional Edition v6.0.4 + Serial
The Cleaner v9.0 + Patch
TeraCopy Pro 2.3 Final + Serial
Tipard DVD Software Toolkit Platinum 6.1.62 Full Version
The Logo Creator v6.0 Free
Trillian Astra Pro v5.4.0.16 + Patch
Total PDF Converter 2.1.214 + Serial
TeamViewer 9.0.29480 + Patch
TeamViewer Premium 10.0.39052 + Crack
( U )
Universal Document Converter v5.6.1 + Keygen
Universal Document Converter Server Edition 5.7 + Keygen
USB Disk Security 6.2.0.30 + Serial
Ulead VideoStudio 11 Plus Full Version
USBsyncer Pro v4.0.1 + Crack
Uniblue Powersuite Pro 2013 + Crack
Uniblue Powersuite 2015 4.3.0.0 + Serial key
μTorrent 3.2 Free
( V )
VSO Video Converter 1.1.0.19 Full Version
Video Booth 2.4.7.8 Full Version
VeryPDF PDF to Word OCR Converter v2.0 Full Version
VMware Workstation 10.0.2 + Serial
Virtual Girl Desktop Stripper 1.0
VideoPad Video Editor 2.11 Professional Full Version
VZO Chat Video v6.3 Free
VZO Conferendo v6.4 Free
VSO Blu-ray Converter Ultimate 3.6.0.0 Beta + Patch
VSO ConvertXtoHD 1.1.0.3 Incl Patch
VSO DVD Converter Ultimate 3.6.0.4 Incl Patch
VeryDOC Video to GIF Converter v2.0 + Serial
VeryDOC Video to Flash Converter 2.0.0.1 + Serial
VSO ConvertXToDVD 5.2.0.42 + Crack
Video Thumbnails Maker 5.0.0.1 Platinum Full Version
( W )
WIFI Password Hacker v3.1 Free
WinX DVD Ripper Platinum 7.3.2 + Keygen
WonderFox HD Video Converter Factory Pro 6.3 + Serial
WonderFox Video to Picture Converter 1.1.0 + Serial
WinZip System Utilities Suite 2.0.648.13214 + Serial
WonderFox DVD Video Converter 4.71 + Patch
WinX HD Video Converter Deluxe 4.2.1.0 + Keygen
WinASO Registry Optimizer 4.8.5.0 + Serial
Windows 7 Account Screen Editor 2.0 Free Download
Windows 7/8/8.1 Theme Windows 10 SkinPack
Webroot SecureAnywhere Antivirus 2013 incl Serial
Wondershare Video Editor 3.1.6 Full Version
Wondershare Dr.Fone for Android 4.2.1 Full Version
Wondershare MobileGo for Android 4.4.0 Full Version
Wondershare MobileTrans for Windows 4.4.0 Full Version
Wondershare Video Converter Ultimate 10.1.4 + Crack
Wondershare Video Converter Ultimate 6.7.0.10 Full Version
Wondershare TunesGo 4.1.2 Full Version
Wondershare SafeEraser 2.2.1 Full Version
Wondershare DreamStream 1.1.2 Incl Patch
Wondershare Dr.Fone for iOS 4.1.1 Incl Patch
Wondershare DVD Creator 3.0.0.12 with DVD Menu Templates
Wondershare AllMyTube v3.8.0.4 Incl Patch
WinUtilities Pro 10 Final + Serial Key
Windows 7 Speed Up Guide
Windows 7 BlackRed Them
Wik And The Fable of Souls 041014-RA *Full Version*
WinAVI All-In-One Converter 1.7.0.4734 Full Version
Wise Care 365 Pro 2.49 Build 196 Final + Keygen
Windows 7 Green Theme
Windows 7 HUD White Series Them
Windows 7 Start Button Changer v2.6 Free
Wipe 2013 PRO Build 52 + Patch
Wondershare Data Recovery 4.1.1 Full Version
Windows Movie Maker & Live Free Download
Windows 7 gpedit.msc - Free Download
Wondershare All Products *Free Download*
Wondershare DVD Slideshow Builder Deluxe v6.1.10 Full Version
( X )
Xilisoft Movie Maker 6.0.3 build-0701 + Crack
Xilisoft Video Cutter 2.2.0 build-20120925 + Keys
XSplit Broadcaster 1.3 + Crack
Xara 3D Maker 6.00 + Serial
XOJO 2014 Release 1.1 + Patch
Xilisoft YouTube HD Video Converter 3.5.0 Full Version
( Y )
YTD Video Downloader PRO 3.9.6 Full Version
YouTube Music Downloader 3.8.0 + Serial
YouWave for Android 4.1.2 + Crack
YeahBit PC SpeedUp 2.2.8 + Serial Full Version
( Z )
Zawgyi Myanmar Font
Zoner Photo Studio Pro v15.0.1.7 + Serial
( 1 )
1Click DVD Converter 3 incl Patch
1CLICK DVD COPY 6 incl Patch
1Click DVD Copy Pro 5 incl Patch
1CLICK DVD Movie 3 incl Patch
1Click DVDTOIPOD 3 incl Patch
( 3 )
3D PageFlip Professional 1.6.2 + Crack
3D Windows Media Playe
360Amigo System Speedup Pro v1.2.1.7900 + Serial
3DFlow 3DF Zephyr Pro 1.009 + Crack
( 4 )
4Videosoft Media Toolkit Ultimate 5.0.28 Full Version
4Videosoft PDF Converter Ultimate 3.1.28 + Patch
4Media Video Converter Ultimate 7.0.0 Full Version
aaccfb2cb3
-
-
\ No newline at end of file
diff --git a/spaces/cihyFjudo/fairness-paper-search/How to Find and Download Gigawing generations ps2 iso 65 - The Ultimate Shooter Game for PS2 Fans.md b/spaces/cihyFjudo/fairness-paper-search/How to Find and Download Gigawing generations ps2 iso 65 - The Ultimate Shooter Game for PS2 Fans.md
deleted file mode 100644
index 56741e187f5daf337c7ab4e21f063cf405b30236..0000000000000000000000000000000000000000
--- a/spaces/cihyFjudo/fairness-paper-search/How to Find and Download Gigawing generations ps2 iso 65 - The Ultimate Shooter Game for PS2 Fans.md
+++ /dev/null
@@ -1,6 +0,0 @@
-Gigawing generations ps2 iso 65
Download File ✒ https://tinurli.com/2uwkjf
-
- aaccfb2cb3
-
-
-
diff --git a/spaces/cihyFjudo/fairness-paper-search/Maxdata Eco 4000 Iw Driver Download The Ultimate Resource for Finding and Installing the Drivers for Your Laptop.md b/spaces/cihyFjudo/fairness-paper-search/Maxdata Eco 4000 Iw Driver Download The Ultimate Resource for Finding and Installing the Drivers for Your Laptop.md
deleted file mode 100644
index 3ad8069b3ac921064fa4888b7826722db716f7ef..0000000000000000000000000000000000000000
--- a/spaces/cihyFjudo/fairness-paper-search/Maxdata Eco 4000 Iw Driver Download The Ultimate Resource for Finding and Installing the Drivers for Your Laptop.md
+++ /dev/null
@@ -1,8 +0,0 @@
-
-If you could not find the exact driver for your hardware device or you aren't sure which driver is right one, we have a program that will detect your hardware specifications and identify the correct driver for your needs. Please click here to download.
-DriverGuide maintains an extensive archive of Windows drivers available for free download. We employ a team from around the world which adds hundreds of new drivers to our site every day. How to Install Drivers Once you download your new driver, then you need to install it. To install a driver in Windows, you will need to use a built-in utility called Device Manager. It allows you to see all of the devices recognized by your system, and the drivers associated with them.
-Maxdata Eco 4000 Iw Driver Download
Download ★★★★★ https://tinurli.com/2uwhNe
-The Driver Update Utility automatically finds, downloads and installs the right driver for your hardware and operating system. It will Update all of your drivers in just a few clicks, and even backup your drivers before making any changes.
-Once you have downloaded your new driver, you'll need to install it. In Windows, use a built-in utility called Device Manager, which allows you to see all of the devices recognized by your system, and the drivers associated with them.
aaccfb2cb3
-
-
\ No newline at end of file
diff --git a/spaces/cihyFjudo/fairness-paper-search/Zettai Fukuju Meirei Absolute Obedience CDRip(ISO BINENGLISH) 64 Bit.md b/spaces/cihyFjudo/fairness-paper-search/Zettai Fukuju Meirei Absolute Obedience CDRip(ISO BINENGLISH) 64 Bit.md
deleted file mode 100644
index ac6e2c3df36e114f3d530f8ac692416d545b38fa..0000000000000000000000000000000000000000
--- a/spaces/cihyFjudo/fairness-paper-search/Zettai Fukuju Meirei Absolute Obedience CDRip(ISO BINENGLISH) 64 Bit.md
+++ /dev/null
@@ -1,6 +0,0 @@
-Zettai Fukuju Meirei Absolute Obedience CDRip(ISO BIN,ENGLISH) 64 bit
Download Zip ✯✯✯ https://tinurli.com/2uwkQB
-
- aaccfb2cb3
-
-
-
diff --git a/spaces/cloudtheboi/Lofi4All/.pythonlibs/lib/python3.10/site-packages/fastapi/middleware/asyncexitstack.py b/spaces/cloudtheboi/Lofi4All/.pythonlibs/lib/python3.10/site-packages/fastapi/middleware/asyncexitstack.py
deleted file mode 100644
index 30a0ae626c26cc285e7e89e38180043239d9b0eb..0000000000000000000000000000000000000000
--- a/spaces/cloudtheboi/Lofi4All/.pythonlibs/lib/python3.10/site-packages/fastapi/middleware/asyncexitstack.py
+++ /dev/null
@@ -1,25 +0,0 @@
-from typing import Optional
-
-from fastapi.concurrency import AsyncExitStack
-from starlette.types import ASGIApp, Receive, Scope, Send
-
-
-class AsyncExitStackMiddleware:
- def __init__(self, app: ASGIApp, context_name: str = "fastapi_astack") -> None:
- self.app = app
- self.context_name = context_name
-
- async def __call__(self, scope: Scope, receive: Receive, send: Send) -> None:
- dependency_exception: Optional[Exception] = None
- async with AsyncExitStack() as stack:
- scope[self.context_name] = stack
- try:
- await self.app(scope, receive, send)
- except Exception as e:
- dependency_exception = e
- raise e
- if dependency_exception:
- # This exception was possibly handled by the dependency but it should
- # still bubble up so that the ServerErrorMiddleware can return a 500
- # or the ExceptionMiddleware can catch and handle any other exceptions
- raise dependency_exception
diff --git a/spaces/codebender/gpt-2-rumblings/app.py b/spaces/codebender/gpt-2-rumblings/app.py
deleted file mode 100644
index 29766ad3a792e761f0edb1ec71d64f543da00c21..0000000000000000000000000000000000000000
--- a/spaces/codebender/gpt-2-rumblings/app.py
+++ /dev/null
@@ -1,19 +0,0 @@
-from aitextgen import aitextgen
-import gradio as gr
-
-# download the small model
-ai = aitextgen()
-
-# function that generates text from the model
-def generate_text(prompt, max_length=300):
- return ai.generate(prompt=prompt, max_length=max_length, return_as_list=True)[0]
-
-# show the generated text in a gradio interface
-demo = gr.Interface(
- fn=generate_text,
- inputs=gr.Textbox(lines=2, label="Insert text or phrase"),
- outputs="text",
-)
-
-# launch the interface
-demo.launch()
diff --git a/spaces/colakin/video-generater/public/ffmpeg/libavcodec/aandcttab.c b/spaces/colakin/video-generater/public/ffmpeg/libavcodec/aandcttab.c
deleted file mode 100644
index 97013d2b527306919bbc19d1cad8f39d2be22d2b..0000000000000000000000000000000000000000
--- a/spaces/colakin/video-generater/public/ffmpeg/libavcodec/aandcttab.c
+++ /dev/null
@@ -1,47 +0,0 @@
-/*
- * This file is part of FFmpeg.
- *
- * FFmpeg is free software; you can redistribute it and/or
- * modify it under the terms of the GNU Lesser General Public
- * License as published by the Free Software Foundation; either
- * version 2.1 of the License, or (at your option) any later version.
- *
- * FFmpeg is distributed in the hope that it will be useful,
- * but WITHOUT ANY WARRANTY; without even the implied warranty of
- * MERCHANTABILITY or FITNESS FOR A PARTICULAR PURPOSE. See the GNU
- * Lesser General Public License for more details.
- *
- * You should have received a copy of the GNU Lesser General Public
- * License along with FFmpeg; if not, write to the Free Software
- * Foundation, Inc., 51 Franklin Street, Fifth Floor, Boston, MA 02110-1301 USA
- */
-
-/**
- * @file
- * AAN (Arai, Agui and Nakajima) (I)DCT tables
- */
-
-#include
-
-const uint16_t ff_aanscales[64] = {
- /* precomputed values scaled up by 14 bits */
- 16384, 22725, 21407, 19266, 16384, 12873, 8867, 4520,
- 22725, 31521, 29692, 26722, 22725, 17855, 12299, 6270,
- 21407, 29692, 27969, 25172, 21407, 16819, 11585, 5906,
- 19266, 26722, 25172, 22654, 19266, 15137, 10426, 5315,
- 16384, 22725, 21407, 19266, 16384, 12873, 8867, 4520,
- 12873, 17855, 16819, 15137, 12873, 10114, 6967, 3552,
- 8867 , 12299, 11585, 10426, 8867, 6967, 4799, 2446,
- 4520 , 6270, 5906, 5315, 4520, 3552, 2446, 1247
-};
-
-const uint16_t ff_inv_aanscales[64] = {
- 4096, 2953, 3135, 3483, 4096, 5213, 7568, 14846,
- 2953, 2129, 2260, 2511, 2953, 3759, 5457, 10703,
- 3135, 2260, 2399, 2666, 3135, 3990, 5793, 11363,
- 3483, 2511, 2666, 2962, 3483, 4433, 6436, 12625,
- 4096, 2953, 3135, 3483, 4096, 5213, 7568, 14846,
- 5213, 3759, 3990, 4433, 5213, 6635, 9633, 18895,
- 7568, 5457, 5793, 6436, 7568, 9633, 13985, 27432,
- 14846, 10703, 11363, 12625, 14846, 18895, 27432, 53809,
-};
diff --git a/spaces/colakin/video-generater/public/ffmpeg/libavcodec/d3d11va.c b/spaces/colakin/video-generater/public/ffmpeg/libavcodec/d3d11va.c
deleted file mode 100644
index 9967f322c90fec84ae6c60d9af49651e8d7da267..0000000000000000000000000000000000000000
--- a/spaces/colakin/video-generater/public/ffmpeg/libavcodec/d3d11va.c
+++ /dev/null
@@ -1,48 +0,0 @@
-/*
- * Direct3D11 HW acceleration
- *
- * copyright (c) 2015 Steve Lhomme
- *
- * This file is part of FFmpeg.
- *
- * FFmpeg is free software; you can redistribute it and/or
- * modify it under the terms of the GNU Lesser General Public
- * License as published by the Free Software Foundation; either
- * version 2.1 of the License, or (at your option) any later version.
- *
- * FFmpeg is distributed in the hope that it will be useful,
- * but WITHOUT ANY WARRANTY; without even the implied warranty of
- * MERCHANTABILITY or FITNESS FOR A PARTICULAR PURPOSE. See the GNU
- * Lesser General Public License for more details.
- *
- * You should have received a copy of the GNU Lesser General Public
- * License along with FFmpeg; if not, write to the Free Software
- * Foundation, Inc., 51 Franklin Street, Fifth Floor, Boston, MA 02110-1301 USA
- */
-
-#include
-
-#include "config.h"
-
-#if CONFIG_D3D11VA
-#include "libavutil/error.h"
-#include "libavutil/mem.h"
-
-#include "d3d11va.h"
-
-AVD3D11VAContext *av_d3d11va_alloc_context(void)
-{
- AVD3D11VAContext* res = av_mallocz(sizeof(AVD3D11VAContext));
- if (!res)
- return NULL;
- res->context_mutex = INVALID_HANDLE_VALUE;
- return res;
-}
-#else
-struct AVD3D11VAContext *av_d3d11va_alloc_context(void);
-
-struct AVD3D11VAContext *av_d3d11va_alloc_context(void)
-{
- return NULL;
-}
-#endif /* CONFIG_D3D11VA */
diff --git a/spaces/colakin/video-generater/public/ffmpeg/libavcodec/flacencdsp.c b/spaces/colakin/video-generater/public/ffmpeg/libavcodec/flacencdsp.c
deleted file mode 100644
index 46e5a0352ba6f4cd075c1822741aec7765143f72..0000000000000000000000000000000000000000
--- a/spaces/colakin/video-generater/public/ffmpeg/libavcodec/flacencdsp.c
+++ /dev/null
@@ -1,40 +0,0 @@
-/*
- * Copyright (c) 2012 Mans Rullgard
- *
- * This file is part of FFmpeg.
- *
- * FFmpeg is free software; you can redistribute it and/or
- * modify it under the terms of the GNU Lesser General Public
- * License as published by the Free Software Foundation; either
- * version 2.1 of the License, or (at your option) any later version.
- *
- * FFmpeg is distributed in the hope that it will be useful,
- * but WITHOUT ANY WARRANTY; without even the implied warranty of
- * MERCHANTABILITY or FITNESS FOR A PARTICULAR PURPOSE. See the GNU
- * Lesser General Public License for more details.
- *
- * You should have received a copy of the GNU Lesser General Public
- * License along with FFmpeg; if not, write to the Free Software
- * Foundation, Inc., 51 Franklin Street, Fifth Floor, Boston, MA 02110-1301 USA
- */
-
-#include "config.h"
-#include "flacencdsp.h"
-
-#define SAMPLE_SIZE 16
-#include "flacdsp_lpc_template.c"
-
-#undef SAMPLE_SIZE
-#define SAMPLE_SIZE 32
-#include "flacdsp_lpc_template.c"
-
-
-av_cold void ff_flacencdsp_init(FLACEncDSPContext *c)
-{
- c->lpc16_encode = flac_lpc_encode_c_16;
- c->lpc32_encode = flac_lpc_encode_c_32;
-
-#if ARCH_X86
- ff_flacencdsp_init_x86(c);
-#endif
-}
diff --git a/spaces/colakin/video-generater/public/ffmpeg/libavcodec/mediacodecdec_common.c b/spaces/colakin/video-generater/public/ffmpeg/libavcodec/mediacodecdec_common.c
deleted file mode 100644
index 1151bb71f9b2aebd320af2abadeaf0b66770a371..0000000000000000000000000000000000000000
--- a/spaces/colakin/video-generater/public/ffmpeg/libavcodec/mediacodecdec_common.c
+++ /dev/null
@@ -1,850 +0,0 @@
-/*
- * Android MediaCodec decoder
- *
- * Copyright (c) 2015-2016 Matthieu Bouron
- *
- * This file is part of FFmpeg.
- *
- * FFmpeg is free software; you can redistribute it and/or
- * modify it under the terms of the GNU Lesser General Public
- * License as published by the Free Software Foundation; either
- * version 2.1 of the License, or (at your option) any later version.
- *
- * FFmpeg is distributed in the hope that it will be useful,
- * but WITHOUT ANY WARRANTY; without even the implied warranty of
- * MERCHANTABILITY or FITNESS FOR A PARTICULAR PURPOSE. See the GNU
- * Lesser General Public License for more details.
- *
- * You should have received a copy of the GNU Lesser General Public
- * License along with FFmpeg; if not, write to the Free Software
- * Foundation, Inc., 51 Franklin Street, Fifth Floor, Boston, MA 02110-1301 USA
- */
-
-#include
-#include
-
-#include "libavutil/common.h"
-#include "libavutil/hwcontext_mediacodec.h"
-#include "libavutil/mem.h"
-#include "libavutil/log.h"
-#include "libavutil/pixfmt.h"
-#include "libavutil/time.h"
-#include "libavutil/timestamp.h"
-
-#include "avcodec.h"
-#include "decode.h"
-
-#include "mediacodec.h"
-#include "mediacodec_surface.h"
-#include "mediacodec_sw_buffer.h"
-#include "mediacodec_wrapper.h"
-#include "mediacodecdec_common.h"
-
-/**
- * OMX.k3.video.decoder.avc, OMX.NVIDIA.* OMX.SEC.avc.dec and OMX.google
- * codec workarounds used in various place are taken from the Gstreamer
- * project.
- *
- * Gstreamer references:
- * https://cgit.freedesktop.org/gstreamer/gst-plugins-bad/tree/sys/androidmedia/
- *
- * Gstreamer copyright notice:
- *
- * Copyright (C) 2012, Collabora Ltd.
- * Author: Sebastian Dröge
- *
- * Copyright (C) 2012, Rafaël Carré
- *
- * Copyright (C) 2015, Sebastian Dröge
- *
- * Copyright (C) 2014-2015, Collabora Ltd.
- * Author: Matthieu Bouron
- *
- * Copyright (C) 2015, Edward Hervey
- * Author: Edward Hervey
- *
- * Copyright (C) 2015, Matthew Waters
- *
- * This library is free software; you can redistribute it and/or
- * modify it under the terms of the GNU Lesser General Public
- * License as published by the Free Software Foundation
- * version 2.1 of the License.
- *
- * This library is distributed in the hope that it will be useful,
- * but WITHOUT ANY WARRANTY; without even the implied warranty of
- * MERCHANTABILITY or FITNESS FOR A PARTICULAR PURPOSE. See the GNU
- * Lesser General Public License for more details.
- *
- * You should have received a copy of the GNU Lesser General Public
- * License along with this library; if not, write to the Free Software
- * Foundation, Inc., 51 Franklin Street, Fifth Floor, Boston, MA 02110-1301 USA
- *
- */
-
-#define INPUT_DEQUEUE_TIMEOUT_US 8000
-#define OUTPUT_DEQUEUE_TIMEOUT_US 8000
-#define OUTPUT_DEQUEUE_BLOCK_TIMEOUT_US 1000000
-
-enum {
- COLOR_FormatYUV420Planar = 0x13,
- COLOR_FormatYUV420SemiPlanar = 0x15,
- COLOR_FormatYCbYCr = 0x19,
- COLOR_FormatAndroidOpaque = 0x7F000789,
- COLOR_QCOM_FormatYUV420SemiPlanar = 0x7fa30c00,
- COLOR_QCOM_FormatYUV420SemiPlanar32m = 0x7fa30c04,
- COLOR_QCOM_FormatYUV420PackedSemiPlanar64x32Tile2m8ka = 0x7fa30c03,
- COLOR_TI_FormatYUV420PackedSemiPlanar = 0x7f000100,
- COLOR_TI_FormatYUV420PackedSemiPlanarInterlaced = 0x7f000001,
-};
-
-static const struct {
-
- int color_format;
- enum AVPixelFormat pix_fmt;
-
-} color_formats[] = {
-
- { COLOR_FormatYUV420Planar, AV_PIX_FMT_YUV420P },
- { COLOR_FormatYUV420SemiPlanar, AV_PIX_FMT_NV12 },
- { COLOR_QCOM_FormatYUV420SemiPlanar, AV_PIX_FMT_NV12 },
- { COLOR_QCOM_FormatYUV420SemiPlanar32m, AV_PIX_FMT_NV12 },
- { COLOR_QCOM_FormatYUV420PackedSemiPlanar64x32Tile2m8ka, AV_PIX_FMT_NV12 },
- { COLOR_TI_FormatYUV420PackedSemiPlanar, AV_PIX_FMT_NV12 },
- { COLOR_TI_FormatYUV420PackedSemiPlanarInterlaced, AV_PIX_FMT_NV12 },
- { 0 }
-};
-
-static enum AVPixelFormat mcdec_map_color_format(AVCodecContext *avctx,
- MediaCodecDecContext *s,
- int color_format)
-{
- int i;
- enum AVPixelFormat ret = AV_PIX_FMT_NONE;
-
- if (s->surface) {
- return AV_PIX_FMT_MEDIACODEC;
- }
-
- if (!strcmp(s->codec_name, "OMX.k3.video.decoder.avc") && color_format == COLOR_FormatYCbYCr) {
- s->color_format = color_format = COLOR_TI_FormatYUV420PackedSemiPlanar;
- }
-
- for (i = 0; i < FF_ARRAY_ELEMS(color_formats); i++) {
- if (color_formats[i].color_format == color_format) {
- return color_formats[i].pix_fmt;
- }
- }
-
- av_log(avctx, AV_LOG_ERROR, "Output color format 0x%x (value=%d) is not supported\n",
- color_format, color_format);
-
- return ret;
-}
-
-static void ff_mediacodec_dec_ref(MediaCodecDecContext *s)
-{
- atomic_fetch_add(&s->refcount, 1);
-}
-
-static void ff_mediacodec_dec_unref(MediaCodecDecContext *s)
-{
- if (!s)
- return;
-
- if (atomic_fetch_sub(&s->refcount, 1) == 1) {
- if (s->codec) {
- ff_AMediaCodec_delete(s->codec);
- s->codec = NULL;
- }
-
- if (s->format) {
- ff_AMediaFormat_delete(s->format);
- s->format = NULL;
- }
-
- if (s->surface) {
- ff_mediacodec_surface_unref(s->surface, NULL);
- s->surface = NULL;
- }
-
- av_freep(&s->codec_name);
- av_freep(&s);
- }
-}
-
-static void mediacodec_buffer_release(void *opaque, uint8_t *data)
-{
- AVMediaCodecBuffer *buffer = opaque;
- MediaCodecDecContext *ctx = buffer->ctx;
- int released = atomic_load(&buffer->released);
-
- if (!released && (ctx->delay_flush || buffer->serial == atomic_load(&ctx->serial))) {
- atomic_fetch_sub(&ctx->hw_buffer_count, 1);
- av_log(ctx->avctx, AV_LOG_DEBUG,
- "Releasing output buffer %zd (%p) ts=%"PRId64" on free() [%d pending]\n",
- buffer->index, buffer, buffer->pts, atomic_load(&ctx->hw_buffer_count));
- ff_AMediaCodec_releaseOutputBuffer(ctx->codec, buffer->index, 0);
- }
-
- ff_mediacodec_dec_unref(ctx);
- av_freep(&buffer);
-}
-
-static int mediacodec_wrap_hw_buffer(AVCodecContext *avctx,
- MediaCodecDecContext *s,
- ssize_t index,
- FFAMediaCodecBufferInfo *info,
- AVFrame *frame)
-{
- int ret = 0;
- int status = 0;
- AVMediaCodecBuffer *buffer = NULL;
-
- frame->buf[0] = NULL;
- frame->width = avctx->width;
- frame->height = avctx->height;
- frame->format = avctx->pix_fmt;
- frame->sample_aspect_ratio = avctx->sample_aspect_ratio;
-
- if (avctx->pkt_timebase.num && avctx->pkt_timebase.den) {
- frame->pts = av_rescale_q(info->presentationTimeUs,
- AV_TIME_BASE_Q,
- avctx->pkt_timebase);
- } else {
- frame->pts = info->presentationTimeUs;
- }
- frame->pkt_dts = AV_NOPTS_VALUE;
- frame->color_range = avctx->color_range;
- frame->color_primaries = avctx->color_primaries;
- frame->color_trc = avctx->color_trc;
- frame->colorspace = avctx->colorspace;
-
- buffer = av_mallocz(sizeof(AVMediaCodecBuffer));
- if (!buffer) {
- ret = AVERROR(ENOMEM);
- goto fail;
- }
-
- atomic_init(&buffer->released, 0);
-
- frame->buf[0] = av_buffer_create(NULL,
- 0,
- mediacodec_buffer_release,
- buffer,
- AV_BUFFER_FLAG_READONLY);
-
- if (!frame->buf[0]) {
- ret = AVERROR(ENOMEM);
- goto fail;
-
- }
-
- buffer->ctx = s;
- buffer->serial = atomic_load(&s->serial);
- ff_mediacodec_dec_ref(s);
-
- buffer->index = index;
- buffer->pts = info->presentationTimeUs;
-
- frame->data[3] = (uint8_t *)buffer;
-
- atomic_fetch_add(&s->hw_buffer_count, 1);
- av_log(avctx, AV_LOG_DEBUG,
- "Wrapping output buffer %zd (%p) ts=%"PRId64" [%d pending]\n",
- buffer->index, buffer, buffer->pts, atomic_load(&s->hw_buffer_count));
-
- return 0;
-fail:
- av_freep(&buffer);
- status = ff_AMediaCodec_releaseOutputBuffer(s->codec, index, 0);
- if (status < 0) {
- av_log(avctx, AV_LOG_ERROR, "Failed to release output buffer\n");
- ret = AVERROR_EXTERNAL;
- }
-
- return ret;
-}
-
-static int mediacodec_wrap_sw_buffer(AVCodecContext *avctx,
- MediaCodecDecContext *s,
- uint8_t *data,
- size_t size,
- ssize_t index,
- FFAMediaCodecBufferInfo *info,
- AVFrame *frame)
-{
- int ret = 0;
- int status = 0;
-
- frame->width = avctx->width;
- frame->height = avctx->height;
- frame->format = avctx->pix_fmt;
-
- /* MediaCodec buffers needs to be copied to our own refcounted buffers
- * because the flush command invalidates all input and output buffers.
- */
- if ((ret = ff_get_buffer(avctx, frame, 0)) < 0) {
- av_log(avctx, AV_LOG_ERROR, "Could not allocate buffer\n");
- goto done;
- }
-
- /* Override frame->pkt_pts as ff_get_buffer will override its value based
- * on the last avpacket received which is not in sync with the frame:
- * * N avpackets can be pushed before 1 frame is actually returned
- * * 0-sized avpackets are pushed to flush remaining frames at EOS */
- if (avctx->pkt_timebase.num && avctx->pkt_timebase.den) {
- frame->pts = av_rescale_q(info->presentationTimeUs,
- AV_TIME_BASE_Q,
- avctx->pkt_timebase);
- } else {
- frame->pts = info->presentationTimeUs;
- }
- frame->pkt_dts = AV_NOPTS_VALUE;
-
- av_log(avctx, AV_LOG_TRACE,
- "Frame: width=%d stride=%d height=%d slice-height=%d "
- "crop-top=%d crop-bottom=%d crop-left=%d crop-right=%d encoder=%s "
- "destination linesizes=%d,%d,%d\n" ,
- avctx->width, s->stride, avctx->height, s->slice_height,
- s->crop_top, s->crop_bottom, s->crop_left, s->crop_right, s->codec_name,
- frame->linesize[0], frame->linesize[1], frame->linesize[2]);
-
- switch (s->color_format) {
- case COLOR_FormatYUV420Planar:
- ff_mediacodec_sw_buffer_copy_yuv420_planar(avctx, s, data, size, info, frame);
- break;
- case COLOR_FormatYUV420SemiPlanar:
- case COLOR_QCOM_FormatYUV420SemiPlanar:
- case COLOR_QCOM_FormatYUV420SemiPlanar32m:
- ff_mediacodec_sw_buffer_copy_yuv420_semi_planar(avctx, s, data, size, info, frame);
- break;
- case COLOR_TI_FormatYUV420PackedSemiPlanar:
- case COLOR_TI_FormatYUV420PackedSemiPlanarInterlaced:
- ff_mediacodec_sw_buffer_copy_yuv420_packed_semi_planar(avctx, s, data, size, info, frame);
- break;
- case COLOR_QCOM_FormatYUV420PackedSemiPlanar64x32Tile2m8ka:
- ff_mediacodec_sw_buffer_copy_yuv420_packed_semi_planar_64x32Tile2m8ka(avctx, s, data, size, info, frame);
- break;
- default:
- av_log(avctx, AV_LOG_ERROR, "Unsupported color format 0x%x (value=%d)\n",
- s->color_format, s->color_format);
- ret = AVERROR(EINVAL);
- goto done;
- }
-
- ret = 0;
-done:
- status = ff_AMediaCodec_releaseOutputBuffer(s->codec, index, 0);
- if (status < 0) {
- av_log(avctx, AV_LOG_ERROR, "Failed to release output buffer\n");
- ret = AVERROR_EXTERNAL;
- }
-
- return ret;
-}
-
-#define AMEDIAFORMAT_GET_INT32(name, key, mandatory) do { \
- int32_t value = 0; \
- if (ff_AMediaFormat_getInt32(s->format, key, &value)) { \
- (name) = value; \
- } else if (mandatory) { \
- av_log(avctx, AV_LOG_ERROR, "Could not get %s from format %s\n", key, format); \
- ret = AVERROR_EXTERNAL; \
- goto fail; \
- } \
-} while (0) \
-
-static int mediacodec_dec_parse_format(AVCodecContext *avctx, MediaCodecDecContext *s)
-{
- int ret = 0;
- int width = 0;
- int height = 0;
- int color_range = 0;
- int color_standard = 0;
- int color_transfer = 0;
- char *format = NULL;
-
- if (!s->format) {
- av_log(avctx, AV_LOG_ERROR, "Output MediaFormat is not set\n");
- return AVERROR(EINVAL);
- }
-
- format = ff_AMediaFormat_toString(s->format);
- if (!format) {
- return AVERROR_EXTERNAL;
- }
- av_log(avctx, AV_LOG_DEBUG, "Parsing MediaFormat %s\n", format);
-
- /* Mandatory fields */
- AMEDIAFORMAT_GET_INT32(s->width, "width", 1);
- AMEDIAFORMAT_GET_INT32(s->height, "height", 1);
-
- AMEDIAFORMAT_GET_INT32(s->stride, "stride", 0);
- s->stride = s->stride > 0 ? s->stride : s->width;
-
- AMEDIAFORMAT_GET_INT32(s->slice_height, "slice-height", 0);
-
- if (strstr(s->codec_name, "OMX.Nvidia.") && s->slice_height == 0) {
- s->slice_height = FFALIGN(s->height, 16);
- } else if (strstr(s->codec_name, "OMX.SEC.avc.dec")) {
- s->slice_height = avctx->height;
- s->stride = avctx->width;
- } else if (s->slice_height == 0) {
- s->slice_height = s->height;
- }
-
- AMEDIAFORMAT_GET_INT32(s->color_format, "color-format", 1);
- avctx->pix_fmt = mcdec_map_color_format(avctx, s, s->color_format);
- if (avctx->pix_fmt == AV_PIX_FMT_NONE) {
- av_log(avctx, AV_LOG_ERROR, "Output color format is not supported\n");
- ret = AVERROR(EINVAL);
- goto fail;
- }
-
- /* Optional fields */
- AMEDIAFORMAT_GET_INT32(s->crop_top, "crop-top", 0);
- AMEDIAFORMAT_GET_INT32(s->crop_bottom, "crop-bottom", 0);
- AMEDIAFORMAT_GET_INT32(s->crop_left, "crop-left", 0);
- AMEDIAFORMAT_GET_INT32(s->crop_right, "crop-right", 0);
-
- // Try "crop" for NDK
- if (!(s->crop_right && s->crop_bottom) && s->use_ndk_codec)
- ff_AMediaFormat_getRect(s->format, "crop", &s->crop_left, &s->crop_top, &s->crop_right, &s->crop_bottom);
-
- if (s->crop_right && s->crop_bottom) {
- width = s->crop_right + 1 - s->crop_left;
- height = s->crop_bottom + 1 - s->crop_top;
- } else {
- /* TODO: NDK MediaFormat should try getRect() first.
- * Try crop-width/crop-height, it works on NVIDIA Shield.
- */
- AMEDIAFORMAT_GET_INT32(width, "crop-width", 0);
- AMEDIAFORMAT_GET_INT32(height, "crop-height", 0);
- }
- if (!width || !height) {
- width = s->width;
- height = s->height;
- }
-
- AMEDIAFORMAT_GET_INT32(s->display_width, "display-width", 0);
- AMEDIAFORMAT_GET_INT32(s->display_height, "display-height", 0);
-
- if (s->display_width && s->display_height) {
- AVRational sar = av_div_q(
- (AVRational){ s->display_width, s->display_height },
- (AVRational){ width, height });
- ff_set_sar(avctx, sar);
- }
-
- AMEDIAFORMAT_GET_INT32(color_range, "color-range", 0);
- if (color_range)
- avctx->color_range = ff_AMediaFormatColorRange_to_AVColorRange(color_range);
-
- AMEDIAFORMAT_GET_INT32(color_standard, "color-standard", 0);
- if (color_standard) {
- avctx->colorspace = ff_AMediaFormatColorStandard_to_AVColorSpace(color_standard);
- avctx->color_primaries = ff_AMediaFormatColorStandard_to_AVColorPrimaries(color_standard);
- }
-
- AMEDIAFORMAT_GET_INT32(color_transfer, "color-transfer", 0);
- if (color_transfer)
- avctx->color_trc = ff_AMediaFormatColorTransfer_to_AVColorTransfer(color_transfer);
-
- av_log(avctx, AV_LOG_INFO,
- "Output crop parameters top=%d bottom=%d left=%d right=%d, "
- "resulting dimensions width=%d height=%d\n",
- s->crop_top, s->crop_bottom, s->crop_left, s->crop_right,
- width, height);
-
- av_freep(&format);
- return ff_set_dimensions(avctx, width, height);
-fail:
- av_freep(&format);
- return ret;
-}
-
-static int mediacodec_dec_flush_codec(AVCodecContext *avctx, MediaCodecDecContext *s)
-{
- FFAMediaCodec *codec = s->codec;
- int status;
-
- s->output_buffer_count = 0;
-
- s->draining = 0;
- s->flushing = 0;
- s->eos = 0;
- atomic_fetch_add(&s->serial, 1);
- atomic_init(&s->hw_buffer_count, 0);
- s->current_input_buffer = -1;
-
- status = ff_AMediaCodec_flush(codec);
- if (status < 0) {
- av_log(avctx, AV_LOG_ERROR, "Failed to flush codec\n");
- return AVERROR_EXTERNAL;
- }
-
- return 0;
-}
-
-int ff_mediacodec_dec_init(AVCodecContext *avctx, MediaCodecDecContext *s,
- const char *mime, FFAMediaFormat *format)
-{
- int ret = 0;
- int status;
- int profile;
-
- enum AVPixelFormat pix_fmt;
- static const enum AVPixelFormat pix_fmts[] = {
- AV_PIX_FMT_MEDIACODEC,
- AV_PIX_FMT_NONE,
- };
-
- s->avctx = avctx;
- atomic_init(&s->refcount, 1);
- atomic_init(&s->hw_buffer_count, 0);
- atomic_init(&s->serial, 1);
- s->current_input_buffer = -1;
-
- pix_fmt = ff_get_format(avctx, pix_fmts);
- if (pix_fmt == AV_PIX_FMT_MEDIACODEC) {
- AVMediaCodecContext *user_ctx = avctx->hwaccel_context;
-
- if (avctx->hw_device_ctx) {
- AVHWDeviceContext *device_ctx = (AVHWDeviceContext*)(avctx->hw_device_ctx->data);
- if (device_ctx->type == AV_HWDEVICE_TYPE_MEDIACODEC) {
- if (device_ctx->hwctx) {
- AVMediaCodecDeviceContext *mediacodec_ctx = (AVMediaCodecDeviceContext *)device_ctx->hwctx;
- s->surface = ff_mediacodec_surface_ref(mediacodec_ctx->surface, mediacodec_ctx->native_window, avctx);
- av_log(avctx, AV_LOG_INFO, "Using surface %p\n", s->surface);
- }
- }
- }
-
- if (!s->surface && user_ctx && user_ctx->surface) {
- s->surface = ff_mediacodec_surface_ref(user_ctx->surface, NULL, avctx);
- av_log(avctx, AV_LOG_INFO, "Using surface %p\n", s->surface);
- }
- }
-
- profile = ff_AMediaCodecProfile_getProfileFromAVCodecContext(avctx);
- if (profile < 0) {
- av_log(avctx, AV_LOG_WARNING, "Unsupported or unknown profile\n");
- }
-
- s->codec_name = ff_AMediaCodecList_getCodecNameByType(mime, profile, 0, avctx);
- if (!s->codec_name) {
- // getCodecNameByType() can fail due to missing JVM, while NDK
- // mediacodec can be used without JVM.
- if (!s->use_ndk_codec) {
- ret = AVERROR_EXTERNAL;
- goto fail;
- }
- av_log(avctx, AV_LOG_INFO, "Failed to getCodecNameByType\n");
- } else {
- av_log(avctx, AV_LOG_DEBUG, "Found decoder %s\n", s->codec_name);
- }
-
- if (s->codec_name)
- s->codec = ff_AMediaCodec_createCodecByName(s->codec_name, s->use_ndk_codec);
- else {
- s->codec = ff_AMediaCodec_createDecoderByType(mime, s->use_ndk_codec);
- if (s->codec) {
- s->codec_name = ff_AMediaCodec_getName(s->codec);
- if (!s->codec_name)
- s->codec_name = av_strdup(mime);
- }
- }
- if (!s->codec) {
- av_log(avctx, AV_LOG_ERROR, "Failed to create media decoder for type %s and name %s\n", mime, s->codec_name);
- ret = AVERROR_EXTERNAL;
- goto fail;
- }
-
- status = ff_AMediaCodec_configure(s->codec, format, s->surface, NULL, 0);
- if (status < 0) {
- char *desc = ff_AMediaFormat_toString(format);
- av_log(avctx, AV_LOG_ERROR,
- "Failed to configure codec %s (status = %d) with format %s\n",
- s->codec_name, status, desc);
- av_freep(&desc);
-
- ret = AVERROR_EXTERNAL;
- goto fail;
- }
-
- status = ff_AMediaCodec_start(s->codec);
- if (status < 0) {
- char *desc = ff_AMediaFormat_toString(format);
- av_log(avctx, AV_LOG_ERROR,
- "Failed to start codec %s (status = %d) with format %s\n",
- s->codec_name, status, desc);
- av_freep(&desc);
- ret = AVERROR_EXTERNAL;
- goto fail;
- }
-
- s->format = ff_AMediaCodec_getOutputFormat(s->codec);
- if (s->format) {
- if ((ret = mediacodec_dec_parse_format(avctx, s)) < 0) {
- av_log(avctx, AV_LOG_ERROR,
- "Failed to configure context\n");
- goto fail;
- }
- }
-
- av_log(avctx, AV_LOG_DEBUG, "MediaCodec %p started successfully\n", s->codec);
-
- return 0;
-
-fail:
- av_log(avctx, AV_LOG_ERROR, "MediaCodec %p failed to start\n", s->codec);
- ff_mediacodec_dec_close(avctx, s);
- return ret;
-}
-
-int ff_mediacodec_dec_send(AVCodecContext *avctx, MediaCodecDecContext *s,
- AVPacket *pkt, bool wait)
-{
- int offset = 0;
- int need_draining = 0;
- uint8_t *data;
- size_t size;
- FFAMediaCodec *codec = s->codec;
- int status;
- int64_t input_dequeue_timeout_us = wait ? INPUT_DEQUEUE_TIMEOUT_US : 0;
- int64_t pts;
-
- if (s->flushing) {
- av_log(avctx, AV_LOG_ERROR, "Decoder is flushing and cannot accept new buffer "
- "until all output buffers have been released\n");
- return AVERROR_EXTERNAL;
- }
-
- if (pkt->size == 0) {
- need_draining = 1;
- }
-
- if (s->draining && s->eos) {
- return AVERROR_EOF;
- }
-
- while (offset < pkt->size || (need_draining && !s->draining)) {
- ssize_t index = s->current_input_buffer;
- if (index < 0) {
- index = ff_AMediaCodec_dequeueInputBuffer(codec, input_dequeue_timeout_us);
- if (ff_AMediaCodec_infoTryAgainLater(codec, index)) {
- av_log(avctx, AV_LOG_TRACE, "No input buffer available, try again later\n");
- break;
- }
-
- if (index < 0) {
- av_log(avctx, AV_LOG_ERROR, "Failed to dequeue input buffer (status=%zd)\n", index);
- return AVERROR_EXTERNAL;
- }
- }
- s->current_input_buffer = -1;
-
- data = ff_AMediaCodec_getInputBuffer(codec, index, &size);
- if (!data) {
- av_log(avctx, AV_LOG_ERROR, "Failed to get input buffer\n");
- return AVERROR_EXTERNAL;
- }
-
- pts = pkt->pts;
- if (pts == AV_NOPTS_VALUE) {
- av_log(avctx, AV_LOG_WARNING, "Input packet is missing PTS\n");
- pts = 0;
- }
- if (pts && avctx->pkt_timebase.num && avctx->pkt_timebase.den) {
- pts = av_rescale_q(pts, avctx->pkt_timebase, AV_TIME_BASE_Q);
- }
-
- if (need_draining) {
- uint32_t flags = ff_AMediaCodec_getBufferFlagEndOfStream(codec);
-
- av_log(avctx, AV_LOG_DEBUG, "Sending End Of Stream signal\n");
-
- status = ff_AMediaCodec_queueInputBuffer(codec, index, 0, 0, pts, flags);
- if (status < 0) {
- av_log(avctx, AV_LOG_ERROR, "Failed to queue input empty buffer (status = %d)\n", status);
- return AVERROR_EXTERNAL;
- }
-
- av_log(avctx, AV_LOG_TRACE,
- "Queued empty EOS input buffer %zd with flags=%d\n", index, flags);
-
- s->draining = 1;
- return 0;
- }
-
- size = FFMIN(pkt->size - offset, size);
- memcpy(data, pkt->data + offset, size);
- offset += size;
-
- status = ff_AMediaCodec_queueInputBuffer(codec, index, 0, size, pts, 0);
- if (status < 0) {
- av_log(avctx, AV_LOG_ERROR, "Failed to queue input buffer (status = %d)\n", status);
- return AVERROR_EXTERNAL;
- }
-
- av_log(avctx, AV_LOG_TRACE,
- "Queued input buffer %zd size=%zd ts=%"PRIi64"\n", index, size, pts);
- }
-
- if (offset == 0)
- return AVERROR(EAGAIN);
- return offset;
-}
-
-int ff_mediacodec_dec_receive(AVCodecContext *avctx, MediaCodecDecContext *s,
- AVFrame *frame, bool wait)
-{
- int ret;
- uint8_t *data;
- ssize_t index;
- size_t size;
- FFAMediaCodec *codec = s->codec;
- FFAMediaCodecBufferInfo info = { 0 };
- int status;
- int64_t output_dequeue_timeout_us = OUTPUT_DEQUEUE_TIMEOUT_US;
-
- if (s->draining && s->eos) {
- return AVERROR_EOF;
- }
-
- if (s->draining) {
- /* If the codec is flushing or need to be flushed, block for a fair
- * amount of time to ensure we got a frame */
- output_dequeue_timeout_us = OUTPUT_DEQUEUE_BLOCK_TIMEOUT_US;
- } else if (s->output_buffer_count == 0 || !wait) {
- /* If the codec hasn't produced any frames, do not block so we
- * can push data to it as fast as possible, and get the first
- * frame */
- output_dequeue_timeout_us = 0;
- }
-
- index = ff_AMediaCodec_dequeueOutputBuffer(codec, &info, output_dequeue_timeout_us);
- if (index >= 0) {
- av_log(avctx, AV_LOG_TRACE, "Got output buffer %zd"
- " offset=%" PRIi32 " size=%" PRIi32 " ts=%" PRIi64
- " flags=%" PRIu32 "\n", index, info.offset, info.size,
- info.presentationTimeUs, info.flags);
-
- if (info.flags & ff_AMediaCodec_getBufferFlagEndOfStream(codec)) {
- s->eos = 1;
- }
-
- if (info.size) {
- if (s->surface) {
- if ((ret = mediacodec_wrap_hw_buffer(avctx, s, index, &info, frame)) < 0) {
- av_log(avctx, AV_LOG_ERROR, "Failed to wrap MediaCodec buffer\n");
- return ret;
- }
- } else {
- data = ff_AMediaCodec_getOutputBuffer(codec, index, &size);
- if (!data) {
- av_log(avctx, AV_LOG_ERROR, "Failed to get output buffer\n");
- return AVERROR_EXTERNAL;
- }
-
- if ((ret = mediacodec_wrap_sw_buffer(avctx, s, data, size, index, &info, frame)) < 0) {
- av_log(avctx, AV_LOG_ERROR, "Failed to wrap MediaCodec buffer\n");
- return ret;
- }
- }
-
- s->output_buffer_count++;
- return 0;
- } else {
- status = ff_AMediaCodec_releaseOutputBuffer(codec, index, 0);
- if (status < 0) {
- av_log(avctx, AV_LOG_ERROR, "Failed to release output buffer\n");
- }
- }
-
- } else if (ff_AMediaCodec_infoOutputFormatChanged(codec, index)) {
- char *format = NULL;
-
- if (s->format) {
- status = ff_AMediaFormat_delete(s->format);
- if (status < 0) {
- av_log(avctx, AV_LOG_ERROR, "Failed to delete MediaFormat %p\n", s->format);
- }
- }
-
- s->format = ff_AMediaCodec_getOutputFormat(codec);
- if (!s->format) {
- av_log(avctx, AV_LOG_ERROR, "Failed to get output format\n");
- return AVERROR_EXTERNAL;
- }
-
- format = ff_AMediaFormat_toString(s->format);
- if (!format) {
- return AVERROR_EXTERNAL;
- }
- av_log(avctx, AV_LOG_INFO, "Output MediaFormat changed to %s\n", format);
- av_freep(&format);
-
- if ((ret = mediacodec_dec_parse_format(avctx, s)) < 0) {
- return ret;
- }
-
- } else if (ff_AMediaCodec_infoOutputBuffersChanged(codec, index)) {
- ff_AMediaCodec_cleanOutputBuffers(codec);
- } else if (ff_AMediaCodec_infoTryAgainLater(codec, index)) {
- if (s->draining) {
- av_log(avctx, AV_LOG_ERROR, "Failed to dequeue output buffer within %" PRIi64 "ms "
- "while draining remaining frames, output will probably lack frames\n",
- output_dequeue_timeout_us / 1000);
- } else {
- av_log(avctx, AV_LOG_TRACE, "No output buffer available, try again later\n");
- }
- } else {
- av_log(avctx, AV_LOG_ERROR, "Failed to dequeue output buffer (status=%zd)\n", index);
- return AVERROR_EXTERNAL;
- }
-
- return AVERROR(EAGAIN);
-}
-
-/*
-* ff_mediacodec_dec_flush returns 0 if the flush cannot be performed on
-* the codec (because the user retains frames). The codec stays in the
-* flushing state.
-*
-* ff_mediacodec_dec_flush returns 1 if the flush can actually be
-* performed on the codec. The codec leaves the flushing state and can
-* process again packets.
-*
-* ff_mediacodec_dec_flush returns a negative value if an error has
-* occurred.
-*/
-int ff_mediacodec_dec_flush(AVCodecContext *avctx, MediaCodecDecContext *s)
-{
- if (!s->surface || !s->delay_flush || atomic_load(&s->refcount) == 1) {
- int ret;
-
- /* No frames (holding a reference to the codec) are retained by the
- * user, thus we can flush the codec and returns accordingly */
- if ((ret = mediacodec_dec_flush_codec(avctx, s)) < 0) {
- return ret;
- }
-
- return 1;
- }
-
- s->flushing = 1;
- return 0;
-}
-
-int ff_mediacodec_dec_close(AVCodecContext *avctx, MediaCodecDecContext *s)
-{
- ff_mediacodec_dec_unref(s);
-
- return 0;
-}
-
-int ff_mediacodec_dec_is_flushing(AVCodecContext *avctx, MediaCodecDecContext *s)
-{
- return s->flushing;
-}
diff --git a/spaces/congsaPfin/Manga-OCR/logs/Catch Up 2018 Music Download The Ultimate Guide to the Best Songs of the Year.md b/spaces/congsaPfin/Manga-OCR/logs/Catch Up 2018 Music Download The Ultimate Guide to the Best Songs of the Year.md
deleted file mode 100644
index b82d02f25759b90652f5f16898d36ef9ab7e58d2..0000000000000000000000000000000000000000
--- a/spaces/congsaPfin/Manga-OCR/logs/Catch Up 2018 Music Download The Ultimate Guide to the Best Songs of the Year.md
+++ /dev/null
@@ -1,145 +0,0 @@
-
-Catch Up 2018 Music Download: How to Enjoy the Best Songs of the Year
-Do you feel like you missed out on some of the best music of 2018? Do you want to catch up on the latest trends, genres, and artists that shaped the musical landscape of the year? If so, you are not alone. Many music lovers find it hard to keep up with the vast amount of new releases and discoveries that happen every year. That's why we have prepared this guide for you, to help you catch up on 2018 music download in a fun and easy way. We will show you three different sources that offer you a variety of songs, albums, and playlists from 2018, all for free or at a low cost. Whether you prefer pop, rock, rap, jazz, country, or anything in between, you will find something to suit your taste and mood. So, let's get started!
-catch up 2018 music download
Download ✵✵✵ https://urlca.com/2uOfX7
-Introduction
-What is catch up 2018 music download?
-Catch up 2018 music download is a term that refers to downloading or streaming music from 2018 that you may have missed or overlooked during the year. It can also mean revisiting some of your favorite songs or albums from 2018 that you want to enjoy again. Catching up on 2018 music can be a great way to discover new artists, genres, and styles that you may not have heard before, or to appreciate the ones that you already love more deeply.
-Why should you catch up on 2018 music?
-There are many reasons why you should catch up on 2018 music. Here are some of them:
-
-- Music is a form of art and expression that reflects the culture, society, and emotions of its creators and listeners. By listening to music from 2018, you can learn more about the events, issues, and trends that shaped the year and influenced people's lives.
-- Music is also a source of entertainment and enjoyment that can make you feel happy, relaxed, inspired, or energized. By catching up on 2018 music, you can experience a range of emotions and moods that can enrich your day and your mood.
-- Music is also a way of connecting with others who share your taste and preferences. By catching up on 2018 music, you can join conversations and discussions with other music fans who can recommend you more songs and artists to explore.
-
-How to catch up on 2018 music?
-There are many ways to catch up on 2018 music download. You can use online platforms such as YouTube, Spotify, Apple Music, or SoundCloud to search for songs and albums by genre, artist, or year. You can also use online tools such as Shazam or Genius to identify songs that you hear on the radio, TV, or in public places. You can also read online reviews, blogs, magazines, or podcasts that feature or rank the best music of 2018. However, if you want to save time and effort, we have selected three of the best sources that offer you a curated selection of 2018 music download that you can enjoy right away. Let's take a look at them.
-The Ultimate 2018 Music Playlist by BuzzFeed
-What is the playlist?
-The Ultimate 2018 Music Playlist by BuzzFeed is a collection of 100 songs that represent the best of 2018 music according to the popular online media company. The playlist covers a wide range of genres, artists, and moods, from pop to rap, from Ariana Grande to Kendrick Lamar, from upbeat to chill. The playlist is designed to give you a comprehensive overview of the musical highlights of the year, as well as some hidden gems that you may have missed.
-How to access the playlist?
-The playlist is available on Spotify, a free online music streaming service that you can access on your computer, smartphone, or tablet. All you need is a Spotify account, which you can create with your email address or your Facebook account. Once you have an account, you can follow this link to open the playlist on Spotify and start listening. You can also download the songs for offline listening if you have a Spotify Premium subscription.
-What are some of the highlights of the playlist?
-Here are some of the songs that we think are worth checking out from the playlist:
-
-- "Thank U, Next" by Ariana Grande: This catchy pop song was one of the biggest hits of 2018, topping the charts in several countries and breaking records on streaming platforms. The song is a self-empowering anthem that celebrates Ariana's past relationships and her personal growth.
-- "This Is America" by Childish Gambino: This provocative rap song was accompanied by a powerful and controversial music video that addressed issues such as racism, violence, and consumerism in America. The song and the video sparked a lot of discussion and debate among critics and fans alike.
-- "Shallow" by Lady Gaga and Bradley Cooper: This emotional ballad was the main theme song of the movie "A Star Is Born", starring Lady Gaga and Bradley Cooper as two musicians who fall in love. The song won several awards, including an Oscar and a Grammy, and showcased Lady Gaga's vocal talent and Bradley Cooper's musical debut.
-
-Catch Up by Steve Urwin on Wynk Music
-Who is Steve Urwin?
-Steve Urwin is a British composer and producer who specializes in creating instrumental music for various media projects. He has composed music for films, TV shows, commercials, video games, and more. He has also released several albums of his own music, ranging from ambient to rock.
-What is Wynk Music?
-Wynk Music is an online music streaming service that offers over 6 million songs from various genres and languages. You can access Wynk Music on your computer, smartphone, or tablet. You can also download songs for offline listening. Wynk Music is free for Airtel users and offers a subscription plan for non-Airtel users.
-catch up 2018 music download free mp3
-catch up 2018 music download playlist buzzfeed
-catch up 2018 music download audiosparx steve urwin
-catch up 2018 music download archive.org freemusicarchive
-catch up 2018 music download songs.pk hindi
-catch up 2018 music download spotify premium
-catch up 2018 music download youtube converter
-catch up 2018 music download itunes apple
-catch up 2018 music download soundcloud app
-catch up 2018 music download bandcamp indie
-catch up 2018 music download amazon prime
-catch up 2018 music download billboard charts
-catch up 2018 music download pitchfork reviews
-catch up 2018 music download rolling stone magazine
-catch up 2018 music download npr tiny desk
-catch up 2018 music download kpop bts
-catch up 2018 music download edm skrillex
-catch up 2018 music download rap drake
-catch up 2018 music download rock arctic monkeys
-catch up 2018 music download jazz kamasi washington
-catch up 2018 music download country kacey musgraves
-catch up 2018 music download pop ariana grande
-catch up 2018 music download r&b the weeknd
-catch up 2018 music download soul janelle monae
-catch up 2018 music download reggae bob marley
-catch up 2018 music download blues bb king
-catch up 2018 music download metal metallica
-catch up 2018 music download punk green day
-catch up 2018 music download folk bob dylan
-catch up 2018 music download classical mozart
-catch up 2018 music download opera pavarotti
-catch up 2018 music download gospel kirk franklin
-catch up 2018 music download hip hop kendrick lamar
-catch up 2018 music download latin shakira
-catch up 2018 music download afrobeat fela kuti
-catch up 2018 music download disco bee gees
-catch up 2018 music download funk prince
-catch up 2018 music download grunge nirvana
-catch up 2018 music download electro daft punk
-catch up 2018 music download ambient brian eno
-catch up 2018 music download experimental animal collective
-catch up 2018 music download alternative radiohead
-catch up 2018 music download dancehall sean paul
-catch up 2018 music download trap migos
-catch up 2018 music download dubstep zomboy
-catch up 2018 music download house avicii
-catch up 2018 music download techno tiesto
-catch up 2018 music download trance armin van buuren
-How to listen to Catch Up by Steve Urwin on Wynk Music?
-Catch Up by Steve Urwin is an album that was released in 2018 on Wynk Music. The album consists of 10 tracks that feature different styles of instrumental music, from acoustic guitar to electronic beats. The album is ideal for relaxing, studying, working, or just enjoying some background music. You can listen to Catch Up by Steve Urwin on Wynk Music by following this link. You can also download the album for offline listening if you have a Wynk Music subscription.
Free Music Archive: A Treasure Trove of Legal Audio Downloads
-What is Free Music Archive?
-Free Music Archive is an online platform that offers a huge collection of high-quality and legal audio downloads from various genres and artists. Free Music Archive was created by a community of music enthusiasts, curators, and creators who wanted to share and promote free and independent music. You can access Free Music Archive on your computer, smartphone, or tablet. You can also download songs for offline listening. Free Music Archive is completely free and does not require any registration or subscription.
-How to browse and download music from Free Music Archive?
-You can browse and download music from Free Music Archive by following these steps:
-
-- Go to the Free Music Archive website by clicking here.
-- Use the search bar or the filters to find the music that you are looking for. You can filter by genre, mood, instrument, license, or curator.
-- Click on the song or album that you want to listen to or download. You can also preview the song by hovering over it.
-- Click on the play button to stream the song online, or click on the download button to save it to your device. You can also share the song on social media or embed it on your website.
-- Enjoy your free music!
-
-What are some of the best albums and songs from 2018 on Free Music Archive?
-Here are some of the best albums and songs from 2018 on Free Music Archive that we recommend you to check out:
-
-- "The Sun Rises in Your Eyes (Song for Heroes)" by David Hilowitz: This is a beautiful and uplifting instrumental song that features piano, strings, and drums. It was composed as a tribute to the heroes who risk their lives to save others.
-- "The Best of 2018" by Various Artists: This is a compilation album that features 20 songs from different genres and artists that were selected as the best of 2018 by the Free Music Archive curators. It includes songs by Lee Rosevere, Blue Dot Sessions, Scott Holmes, and more.
-- "The Great Gatsby" by F Scott Fitzgerald: This is an audiobook version of the classic novel by F Scott Fitzgerald, narrated by Mike Vendetti. It tells the story of Jay Gatsby, a mysterious millionaire who pursues his lost love Daisy Buchanan in the 1920s.
-
-Conclusion
-Summary of the main points
-In this article, we have shown you how to catch up on 2018 music download in a fun and easy way. We have introduced you to three different sources that offer you a variety of songs, albums, and playlists from 2018, all for free or at a low cost. These sources are:
-
-- The Ultimate 2018 Music Playlist by BuzzFeed: A collection of 100 songs that represent the best of 2018 music according to BuzzFeed.
-- Catch Up by Steve Urwin on Wynk Music: An album of 10 tracks that feature different styles of instrumental music by Steve Urwin.
-- Free Music Archive: A huge collection of high-quality and legal audio downloads from various genres and artists.
-
-Call to action for the readers
-We hope that you have enjoyed this article and found it useful. Now it's time for you to catch up on 2018 music download and discover new sounds and sensations. Whether you want to relax, dance, study, or work, you will find something to suit your taste and mood. So, what are you waiting for? Start listening now and share your thoughts and opinions with us in the comments section below. Happy listening!
- Frequently Asked Questions
-
-- What are some of the benefits of catching up on 2018 music?
-Some of the benefits of catching up on 2018 music are:
-
-- You can learn more about the culture, society, and emotions of 2018 through music.
-- You can experience a range of emotions and moods that can enrich your day and your mood.
-- You can connect with other music fans who share your taste and preferences.
-
- - How can I find more sources for 2018 music download?
-You can find more sources for 2018 music download by using online platforms such as YouTube, Spotify, Apple Music, or SoundCloud. You can also use online tools such as Shazam or Genius to identify songs that you hear on the radio, TV, or in public places. You can also read online reviews, blogs, magazines, or podcasts that feature or rank the best music of 2018.
- - What are some of the challenges of catching up on 2018 music?
-Some of the challenges of catching up on 2018 music are:
-
-- You may have to deal with information overload and choice paralysis, as there are so many songs and albums to choose from.
-- You may have to adjust your expectations and preferences, as some of the music may not match your taste or mood.
-- You may have to face some criticism or judgment from others who may not agree with your opinions or choices.
-
- - How can I overcome these challenges?
-You can overcome these challenges by:
-
-- Setting a specific goal and a time limit for your catch up session, such as listening to 10 songs or one album per day.
-- Being open-minded and curious about new music, and giving it a fair chance before dismissing it.
-- Respecting other people's opinions and choices, and avoiding arguments or conflicts over music.
-
- - What are some of the trends and themes of 2018 music?
-Some of the trends and themes of 2018 music are:
-
-- The rise of female empowerment and diversity in music, with artists such as Cardi B, Janelle Monáe, Kacey Musgraves, and Dua Lipa leading the way.
-- The popularity of trap and hip-hop music, with artists such as Drake, Post Malone, Travis Scott, and XXXTentacion dominating the charts.
-- The resurgence of rock and indie music, with artists such as Arctic Monkeys, Twenty One Pilots, The 1975, and Florence + The Machine releasing acclaimed albums.
-
401be4b1e0
-
-
\ No newline at end of file
diff --git a/spaces/congsaPfin/Manga-OCR/logs/Download Growtopia Hack APK for Android Get Free Items and Mods.md b/spaces/congsaPfin/Manga-OCR/logs/Download Growtopia Hack APK for Android Get Free Items and Mods.md
deleted file mode 100644
index 0dab9fe96bd08611e1305132fb4e3e7896ad5024..0000000000000000000000000000000000000000
--- a/spaces/congsaPfin/Manga-OCR/logs/Download Growtopia Hack APK for Android Get Free Items and Mods.md
+++ /dev/null
@@ -1,115 +0,0 @@
-
-How to Hack Growtopia Mod Apk
-Growtopia is a creative free-to-play 2D sandbox game where you can build anything you want with your friends. You can also explore thousands of mini-games created by other players, craft and trade unique items, and join a massive community of millions of players worldwide.
-hack growtopia mod apk
Download Zip ✔ https://urlca.com/2uO6B2
-Mod apk is a modified version of an original application that has been altered to provide extra features or benefits that are not available in the official version. Some players want to hack Growtopia mod apk to get unlimited gems, items, and access to premium features that would otherwise cost real money or take a lot of time and effort to obtain.
-In this article, we will show you how to hack Growtopia mod apk using different methods, such as cheat codes, online generators, or modded files. We will also tell you about the benefits and risks of hacking Growtopia mod apk, as well as some tips and tricks for playing the game. Finally, we will review what other players think about Growtopia mod apk and whether it is worth trying or not.
- Benefits of Hacking Growtopia Mod Apk
-Hacking Growtopia mod apk can give you many advantages that can make your gameplay more fun and enjoyable. Here are some of the benefits of hacking Growtopia mod apk:
-
-- You can get unlimited gems, which are the main currency in the game. You can use gems to buy rare items, upgrade your inventory space, unlock new features, and more.
-- You can get unlimited items, which are used to build your own worlds or trade with other players. You can get any item you want without having to farm or craft it.
-- You can get access to premium features that are normally exclusive to VIP members or paid users. For example, you can get extra backpack slots, daily bonuses, special outfits, and more.
-- You can customize your character and your world with more options and possibilities. You can change your appearance, name color, chat font, world background, music, and more.
-- You can bypass some of the restrictions and limitations that are imposed by the game developers. For example, you can enter any world without a password or a lock.
-
- Risks of Hacking Growtopia Mod Apk
-However, hacking Growtopia mod apk also comes with some risks that you should be aware of before you decide to do it. Here are some of the dangers of hacking Growtopia mod apk:
-growtopia mod apk unlimited gems and world lock
-growtopia mod menu apk download latest version
-growtopia hack apk 2023 no root
-growtopia mod apk 4.29 with antiban
-growtopia hack tool apk free download
-growtopia mod apk android 1 com
-growtopia hack apk mediafıre 2023
-growtopia mod apk offline mode
-growtopia hack apk ios without jailbreak
-growtopia mod apk unlimited everything 2023
-growtopia hack online generator apk
-growtopia mod apk revdl rexdl
-growtopia hack apk 2023 mega link
-growtopia mod apk for pc windows 10
-growtopia hack account apk password
-growtopia mod apk unlimited wls and dls
-growtopia hack trainer apk download
-growtopia mod apk new update 2023
-growtopia hack cheat engine apk
-growtopia mod apk god mode and fly
-growtopia hack diamond lock apk
-growtopia mod apk all items unlocked
-growtopia hack speed and noclip apk
-growtopia mod apk anti cheat bypass
-growtopia hack gems generator apk
-growtopia mod menu pro apk 2023
-growtopia hack auto farm apk
-growtopia mod menu script lua apk
-growtopia hack vip pro apk 2023
-growtopia mod menu gg script apk
-growtopia hack one hit kill apk
-growtopia mod menu project v2.9.9.9.9.9.9.9.9.9.9.9.9.9.9.9.9.9.9.9.9.9.9.9.9.9.9.9.9.9.apk download link in description no virus 100% working legit no scam trust me bro free wls and dls giveaway join now (just kidding, don't click this)
-
-- You can get banned from the game if the developers detect that you are using an unauthorized or modified version of the game. You can lose your account, your progress, and your items permanently.
-- You can lose your data or damage your device if you download a corrupted or infected file from an untrusted source. You can expose your personal information, such as your email, password, or credit card details, to hackers or scammers.
-- You can ruin the game balance and the fun for yourself and other players if you abuse the hack or cheat too much. You can make the game too easy or boring, or you can annoy or offend other players with your unfair advantage or behavior.
-
- Methods of Hacking Growtopia Mod Apk
-There are different ways to hack Growtopia mod apk, depending on your preference and skill level. Some methods are easier and faster than others, but they may also be less reliable or safe. Here are some of the most common methods of hacking Growtopia mod apk:
- Cheat Codes
-Cheat codes are secret commands that you can type in the chat box or use a keyboard app to activate certain effects or functions in the game. For example, you can type "/give 1000" to get 1000 gems, or "/setnamecolor red" to change your name color to red.
-Cheat codes are one of the simplest and quickest ways to hack Growtopia mod apk, but they also have some drawbacks. First, not all cheat codes work for every version of the game, and some may be outdated or patched by the developers. Second, some cheat codes may not work properly or cause glitches or errors in the game. Third, some cheat codes may be detected by the anti-cheat system and result in a ban.
- Online Generators
-Online generators are websites that claim to generate free gems and items for Growtopia mod apk by using a hack tool or a script. All you have to do is enter your username and select the amount of resources you want, and then wait for the generator to process your request and deliver them to your account.
-Online generators are another easy and fast way to hack Growtopia mod apk, but they also have some risks. First, most online generators are fake or scams that only want to trick you into completing surveys, downloading apps, or providing your personal information. Second, some online generators may send you spam messages, ads, or malware that can harm your device or data. Third, some online generators may be detected by the anti-cheat system and result in a ban.
- Modded Files
-Modded files are files that have been modified by hackers or modders to change some aspects of the game, such as graphics, sounds, features, or gameplay. You can download and install modded files for Growtopia mod apk from various sources on the internet, such as forums, blogs, or websites.
-Modded files are one of the most effective and reliable ways to hack Growtopia mod apk, but they also require some skills and precautions. First, you need to find a trustworthy and updated source that provides working and safe modded files for Growtopia mod apk. Second, you need to enable unknown sources on your device settings and allow permissions for installing third-party apps. Third, you need to backup your original files and data before replacing them with the modded files.
- Tips and Tricks for Playing Growtopia Mod Apk
-Now that you know how to hack Growtopia mod apk using different methods, here are some tips and tricks for playing the game:
-
-- Explore different worlds created by other players or create your own world with your imagination and creativity. You can find worlds based on various themes, genres, or categories.
-- Craft and trade items with other players or use them to build your own world. You can combine different items to create new ones with different effects or functions.
-- Join the community of millions of players worldwide and chat with them in real time. You can make new friends, join clans, participate in events, or compete in leaderboards.
-- Follow the rules and etiquette of the game and respect other players. Do not spam, scam, grief, hack, cheat, or harass other players.
-- Have fun and enjoy the game!
-
- Reviews of Growtopia Mod Apk
-To give you a better idea of what other players think about Growtopia mod apk, here are some of the reviews from various sources:
Google Play Store
-Growtopia mod apk has a rating of 4.1 out of 5 stars on the Google Play Store, based on over 1.2 million reviews. Here are some of the positive and negative comments from the users:
-
-Positive Negative
-"This game is awesome! I love the mod apk because it gives me more gems and items. I can also access the VIP features and customize my character and world. The game is very fun and addictive, and I can play with my friends and other players online." "This game is terrible! The mod apk is full of bugs and glitches, and it crashes a lot. I also got banned from the game for using the mod apk, and I lost all my progress and items. The game is also very laggy and slow, and the graphics are poor."
-"This game is amazing! I like the mod apk because it lets me bypass some of the restrictions and limitations of the game. I can enter any world without a password or a lock, and I can use any item I want without having to craft or trade it. The game is very creative and diverse, and I can explore different worlds and mini-games." "This game is boring! The mod apk ruins the game balance and the fun for me and other players. It makes the game too easy and dull, and it annoys or offends other players with the unfair advantage or behavior. The game is also very repetitive and monotonous, and there is nothing new or exciting to do."
-
- Metacritic
-Growtopia mod apk has a score of 67 out of 100 on Metacritic, based on 8 critic reviews. Here are some of the excerpts from the reviews:
-
-- "Growtopia mod apk is a decent sandbox game that offers a lot of freedom and creativity for its players. However, it also suffers from some technical issues, such as bugs, glitches, crashes, and lag. Moreover, it also raises some ethical concerns, such as hacking, cheating, scamming, and griefing. Overall, Growtopia mod apk is a mixed bag that may appeal to some but not to others." - GameSpot
-- "Growtopia mod apk is a fun and engaging sandbox game that allows its players to build anything they want with their friends. It also provides a lot of features and benefits that are not available in the official version of the game. However, it also comes with some risks and drawbacks, such as getting banned, losing data, or downloading malware. Therefore, Growtopia mod apk is a risky but rewarding game that requires caution and discretion." - IGN
-- "Growtopia mod apk is a mediocre sandbox game that tries to offer a lot of things but fails to deliver them well. It also spoils the original game by adding unnecessary or unwanted features or changes that affect the gameplay negatively. Furthermore, it also violates the rules and etiquette of the game by hacking, cheating, or harassing other players. In conclusion, Growtopia mod apk is a disappointing game that should be avoided or deleted." - PC Gamer
-
- Common Sense Media
-Growtopia mod apk has a rating of 3 out of 5 stars on Common Sense Media, based on 12 parent reviews and 23 kid reviews. Here are some of the summaries from the reviews:
-
-Parent Kid
-"I think this game is okay for kids who are mature enough to handle the online interactions and the hacking aspects. The game can be educational and creative, but it can also be dangerous and inappropriate. I suggest parents to monitor their kids' activities and conversations in the game, and to teach them about online safety and responsibility." "I love this game because it is very fun and cool. I like the mod apk because it gives me more gems and items, and I can do more things in the game. The game can be hard sometimes, but it can also be easy with the hack. I think this game is good for kids who like building games."
-"I don't like this game for kids because it is very addictive and harmful. The game can be violent and rude, but it can also be boring and annoying. I don't like the mod apk because it cheats the game and makes it unfair for other players. The game can also damage your device or data with viruses or malware. I think this game is bad for kids who like sandbox games." " I hate this game because it is very stupid and lame. I hate the mod apk because it hacks the game and makes it boring and easy. The game can also be unfair and mean, and it can also be glitchy and slow. I think this game is bad for kids who like sandbox games."
-
- Conclusion
-In conclusion, Growtopia mod apk is a hacked version of Growtopia, a creative sandbox game where you can build anything you want with your friends. Hacking Growtopia mod apk can give you many benefits, such as unlimited gems, items, and premium features, but it can also expose you to many risks, such as getting banned, losing data, or downloading malware. There are different methods of hacking Growtopia mod apk, such as cheat codes, online generators, or modded files, but they all have their pros and cons. You can also find some tips and tricks for playing Growtopia mod apk, as well as some reviews from other players.
-Personally, I think hacking Growtopia mod apk is not worth it, because it can ruin the game experience and the fun for yourself and others. I prefer playing the official version of the game, which is more challenging and rewarding. However, if you still want to try hacking Growtopia mod apk, you should do it at your own risk and responsibility.
-What do you think about hacking Growtopia mod apk? Do you have any questions or comments? Feel free to share them below!
- FAQs
-
-- Q: How do I download Growtopia mod apk?
-- A: You can download Growtopia mod apk from various sources on the internet, such as forums, blogs, or websites. However, you should be careful and check the credibility and safety of the source before downloading anything.
-- Q: How do I update Growtopia mod apk?
-- A: You can update Growtopia mod apk by downloading and installing the latest version of the modded files from the same source where you got the previous version. However, you should backup your data before updating anything.
-- Q: How do I uninstall Growtopia mod apk?
-- A: You can uninstall Growtopia mod apk by deleting the modded files from your device and restoring the original files from your backup. However, you may lose your progress and items if you uninstall Growtopia mod apk.
-- Q: Is Growtopia mod apk legal?
-- A: No, Growtopia mod apk is not legal, because it violates the terms of service and the intellectual property rights of the game developers. Hacking Growtopia mod apk can result in legal actions or penalties.
-- Q: Is Growtopia mod apk safe?
-- A: No, Growtopia mod apk is not safe, because it can expose your device or data to viruses or malware that can harm them. Hacking Growtopia mod apk can also result in losing your account or getting banned from the game.
-
197e85843d
-
-
\ No newline at end of file
diff --git a/spaces/congsaPfin/Manga-OCR/logs/Download the Best Romeo and Juliet Song Ever - Until I Found You by Stephen Sanchez feat. Em Beihold.md b/spaces/congsaPfin/Manga-OCR/logs/Download the Best Romeo and Juliet Song Ever - Until I Found You by Stephen Sanchez feat. Em Beihold.md
deleted file mode 100644
index fe9c87e719edf6a001384b704bfeff22ae7bc614..0000000000000000000000000000000000000000
--- a/spaces/congsaPfin/Manga-OCR/logs/Download the Best Romeo and Juliet Song Ever - Until I Found You by Stephen Sanchez feat. Em Beihold.md
+++ /dev/null
@@ -1,162 +0,0 @@
-
-How to Download Until I Found You x Romeo and Juliet
-Are you looking for a new song to add to your playlist? Do you love romantic songs that make you feel all the feels? If so, you might want to check out Until I Found You x Romeo and Juliet, a beautiful duet by Stephen Sanchez and Em Beihold. In this article, we will tell you what this song is about, why you should download it, and how you can do it in three different ways.
- What is Until I Found You x Romeo and Juliet?
-Until I Found You x Romeo and Juliet is a song that was released in April 2022 by Stephen Sanchez and Em Beihold, two talented singers and songwriters who are signed to Republic Records. The song is part of the Autumn Vibes 2022 playlist, a collection of songs that are perfect for the fall season.
-download until i found you x romeo and juliet
Download Zip 🆓 https://urlca.com/2uO8fS
- A romantic song by Stephen Sanchez and Em Beihold
-The song is a romantic ballad that tells the story of two lovers who find each other after being lost in the darkness. The lyrics are inspired by the famous play Romeo and Juliet by William Shakespeare, but with a modern twist. The chorus goes like this:
-
-"I would never fall in love again until I found her"
-I said, "I would never fall unless it's you I fall into"
-I was lost within the darkness, but then I found her
-I found you"
-
-The song is sung by both Stephen Sanchez and Em Beihold, who harmonize beautifully together. Their voices are full of emotion and passion, making the song even more touching and captivating.
- A modern twist on the classic love story
-The song is not only a tribute to Romeo and Juliet, but also a reinterpretation of it. Unlike the original play, where the lovers die tragically, the song suggests a happier ending for them. The lyrics imply that they have overcome their obstacles and found their way back to each other. They also express their gratitude for finding each other, as they say:
-
-"Heaven, when I held you again
-How could we ever just be friends?
-I would rather die than let you go
-Juliet to your Romeo"
-
-The song also updates the setting of the story, as it mentions Georgia, a state in the US, instead of Verona, a city in Italy. This makes the song more relatable and accessible to modern listeners.
-download until i found you lyrics stephen sanchez
-download until i found you em beihold version
-download juliet to your romeo song
-download until i found you tiktok viral
-download until i found you spotify
-download until i found you mp3 free
-download until i found you piano chords
-download until i found you acoustic cover
-download juliet to your romeo lyrics video
-download until i found you remix
-download until i found you instrumental
-download until i found you karaoke
-download juliet to your romeo duet
-download until i found you ringtone
-download until i found you guitar tabs
-download until i found you official music video
-download juliet to your romeo mashup
-download until i found you sheet music
-download until i found you live performance
-download juliet to your romeo dance challenge
-download until i found you nightcore
-download until i found you slowed reverb
-download juliet to your romeo reaction
-download until i found you 8d audio
-download until i found you edm version
-download juliet to your romeo quotes
-download until i found you flac quality
-download until i found you ukulele tutorial
-download juliet to your romeo fan edit
-download until i found you lofi remix
-download juliet to your romeo behind the scenes
-download until i found you clean version
-download until i found you extended mix
-download juliet to your romeo piano cover
-download until i found you album art
-download juliet to your romeo guitar lesson
-download until i found you radio edit
-download until i found you trap remix
-download juliet to your romeo animated video
-download until i found you soundcloud link
-download juliet to your romeo bass boosted
-download until i found you original demo
-download juliet to your romeo violin cover
-download until i found you genius lyrics
- Why should you download Until I Found You x Romeo and Juliet?
-There are many reasons why you should download this song. Here are some of them:
- It is a catchy and emotional tune
-The song has a catchy melody that will stick in your head for days. It also has a powerful guitar solo that adds more intensity and drama to the song. The song will make you feel a range of emotions, from joy to sadness, from hope to despair. It will touch your heart and soul.
- It has positive reviews and ratings
-The song has received positive feedback from critics and fans
It is part of the Autumn Vibes 2022 playlist
-The song is also featured in the Autumn Vibes 2022 playlist, a curated list of songs that are ideal for the fall season. The playlist includes songs from various genres and artists, such as Ghost by Justin Bieber, telepatía by Kali Uchis, willow by Taylor Swift, and justified by Kacey Musgraves. The playlist has over 50 songs and more than 3 hours of music to enjoy. You can find the playlist on Spotify, Apple Music, YouTube Music, and other streaming platforms.
- How can you download Until I Found You x Romeo and Juliet?
-Now that you know what the song is about and why you should download it, you might be wondering how you can do it. Well, there are three main options that you can choose from: streaming it online, buying it online, or downloading it for free. Let's take a look at each option and see the pros and cons of each one.
- Option 1: Stream it online
-The first option is to stream the song online using a website or an app that offers music streaming services. This means that you can listen to the song without having to download it to your device. You just need an internet connection and a subscription or an account to access the service.
- Pros and cons of streaming
-Streaming has some advantages and disadvantages that you should consider before choosing this option. Here are some of them:
-
-Pros Cons
-You can listen to the song anytime and anywhere as long as you have an internet connection. You might experience buffering or interruptions if your internet connection is slow or unstable.
-You can discover new songs and artists that are similar to the ones you like. You might have to pay a monthly fee or listen to ads to access some streaming services.
-You can create your own playlists and share them with your friends. You might not be able to listen to the song offline or when the service is unavailable.
-You can save storage space on your device. You might use up a lot of data if you stream on mobile devices.
-
- Websites and apps to stream from
-There are many websites and apps that offer music streaming services, but some of the most popular ones are:
-
-- Spotify: Spotify is one of the most popular music streaming services in the world. It has over 70 million songs and podcasts that you can listen to for free or with a premium subscription. You can also create your own playlists, follow your favorite artists, and discover new music based on your preferences.
-- Apple Music: Apple Music is another popular music streaming service that is integrated with iTunes and other Apple devices. It has over 75 million songs and podcasts that you can listen to with a subscription. You can also access exclusive content, live radio stations, and personalized recommendations.
-- YouTube Music: YouTube Music is a music streaming service that is powered by YouTube. It has over 60 million songs and videos that you can listen to for free or with a premium subscription. You can also watch music videos, live performances, and covers from your favorite artists.
-
- Option 2: Buy it online
-The second option is to buy the song online using a website or an app that offers music downloads. This means that you can pay a certain amount of money to download the song to your device. You can then listen to the song offline or transfer it to other devices.
- Pros and cons of buying
-Buying has some advantages and disadvantages that you should consider before choosing this option. Here are some of them:
-
-Pros Cons
-You can listen to the song offline without needing an internet connection. You might have to pay more money than streaming depending on the price of the song.
-You can own the song permanently and have full control over it. You might have compatibility issues if the format of the song is not supported by your device.
-You can support the artists directly by paying for their work. You might have limited options to discover new songs and artists compared to streaming.
-You can save data usage on your mobile devices You might use up a lot of storage space on your device.
-
- Websites and apps to buy from
-There are many websites and apps that offer music downloads, but some of the most popular ones are:
-
-- iTunes: iTunes is one of the most popular music download services in the world. It is integrated with Apple Music and other Apple devices. It has over 75 million songs and podcasts that you can buy and download to your device. You can also sync your music library across your devices and access exclusive content.
-- Amazon Music: Amazon Music is another popular music download service that is powered by Amazon. It has over 70 million songs and podcasts that you can buy and download to your device. You can also stream unlimited music with a subscription and access exclusive content.
-- Google Play Music: Google Play Music is a music download service that is powered by Google. It has over 60 million songs and podcasts that you can buy and download to your device. You can also stream unlimited music with a subscription and access exclusive content.
-
- Option 3: Download it for free
-The third option is to download the song for free using a website or an app that offers free music downloads. This means that you can download the song to your device without paying any money. However, this option might be illegal or unethical depending on the source of the song.
- Pros and cons of downloading for free
-Downloading for free has some advantages and disadvantages that you should consider before choosing this option. Here are some of them:
-
-Pros Cons
-You can save money by not paying for the song. You might be breaking the law or violating the rights of the artists by downloading their work without their permission.
-You can listen to the song offline without needing an internet connection. You might be exposing your device to viruses, malware, or spyware by downloading from untrusted sources.
-You can have more freedom and flexibility to choose the format and quality of the song. You might have poor sound quality or incomplete files by downloading from low-quality sources.
-You can have more options to discover new songs and artists compared to buying. You might have ethical issues or guilt by not supporting the artists who create the music you enjoy.
-
- Websites and apps to download from
-There are many websites and apps that offer free music downloads, but some of them might be illegal or unsafe. Therefore, we do not recommend or endorse any of them. However, if you still want to try this option, you should do your own research and use caution before downloading anything from these sources. Some of the websites and apps that claim to offer free music downloads are:
-
-- MP3Juices: MP3Juices is a website that allows you to search and download MP3 files from various sources. You can also convert YouTube videos to MP3 files using this website.
-- SoundCloud: SoundCloud is a website and an app that allows you to stream and download music from various artists. However, not all songs are available for download, and some might require a subscription or a payment.
-- Free Music Downloader: Free Music Downloader is an app that allows you to download music from various sources. You can also play, manage, and share your downloaded music using this app.
-
- Conclusion
-In conclusion, Until I Found You x Romeo and Juliet is a wonderful song that you should definitely listen to if you love romantic songs. It is a modern twist on the classic love story by Shakespeare, sung by two talented artists. You can download this song in three different ways: streaming it online, buying it online, or downloading it for free. Each option has its pros and cons, so you should choose the one that suits your needs and preferences best. We hope this article has helped you learn more about this song and how to download it. Happy listening!
- FAQs
-Here are some frequently asked questions about Until I Found You x Romeo and Juliet:
-
-- Who wrote Until I Found You x Romeo and Juliet?
-The song was written by Stephen Sanchez, Em Beihold, Ryan Tedder, Zach Sk
er, and Benny Blanco, who are all famous producers and songwriters in the music industry. They have worked with many other artists, such as OneRepublic, Ed Sheeran, Adele, and Justin Bieber.
-- Where can I watch the music video of Until I Found You x Romeo and Juliet?
-The official music video of the song was released on YouTube on May 1, 2022. You can watch it here. The music video features Stephen Sanchez and Em Beihold as Romeo and Juliet, respectively, as they reenact scenes from the play in a modern setting. The music video has over 100 million views and 2 million likes as of June 2022.
-- What are the awards and nominations of Until I Found You x Romeo and Juliet?
-The song has received several awards and nominations since its release. Some of them are:
-
-- Grammy Award for Best Pop Duo/Group Performance (won)
-- MTV Video Music Award for Best Collaboration (won)
-- Billboard Music Award for Top Streaming Song (nominated)
-- American Music Award for Favorite Song - Pop/Rock (nominated)
-
-- What are some other songs by Stephen Sanchez and Em Beihold?
-Stephen Sanchez and Em Beihold are both solo artists who have released their own songs and albums. Some of their most popular songs are:
-
-- Stephen Sanchez: Hold On, Let Me Go, Say Something, Better Days
-- Em Beihold: Lost in Translation, Breakaway, Fly Away, Stay With Me
-
-- How can I contact Stephen Sanchez and Em Beihold?
-You can follow them on their social media accounts and send them messages or comments. Here are some of their accounts:
-
-- Stephen Sanchez: Instagram (@stephensanchez), Twitter (@stephensanchez), Facebook (Stephen Sanchez), TikTok (@stephensanchez)
-- Em Beihold: Instagram (@embeihold), Twitter (@embeihold), Facebook (Em Beihold), TikTok (@embeihold)
-
197e85843d
-
-
\ No newline at end of file
diff --git a/spaces/cooelf/Multimodal-CoT/timm/models/__init__.py b/spaces/cooelf/Multimodal-CoT/timm/models/__init__.py
deleted file mode 100644
index 06217e185741edae2b4f22a40a51d04465e63ab7..0000000000000000000000000000000000000000
--- a/spaces/cooelf/Multimodal-CoT/timm/models/__init__.py
+++ /dev/null
@@ -1,53 +0,0 @@
-from .byoanet import *
-from .byobnet import *
-from .cait import *
-from .coat import *
-from .convit import *
-from .cspnet import *
-from .densenet import *
-from .dla import *
-from .dpn import *
-from .efficientnet import *
-from .ghostnet import *
-from .gluon_resnet import *
-from .gluon_xception import *
-from .hardcorenas import *
-from .hrnet import *
-from .inception_resnet_v2 import *
-from .inception_v3 import *
-from .inception_v4 import *
-from .levit import *
-from .mlp_mixer import *
-from .mobilenetv3 import *
-from .nasnet import *
-from .nfnet import *
-from .pit import *
-from .pnasnet import *
-from .regnet import *
-from .res2net import *
-from .resnest import *
-from .resnet import *
-from .resnetv2 import *
-from .rexnet import *
-from .selecsls import *
-from .senet import *
-from .sknet import *
-from .swin_transformer import *
-from .tnt import *
-from .tresnet import *
-from .vgg import *
-from .visformer import *
-from .vision_transformer import *
-from .vision_transformer_hybrid import *
-from .vovnet import *
-from .xception import *
-from .xception_aligned import *
-from .twins import *
-
-from .factory import create_model, split_model_name, safe_model_name
-from .helpers import load_checkpoint, resume_checkpoint, model_parameters
-from .layers import TestTimePoolHead, apply_test_time_pool
-from .layers import convert_splitbn_model
-from .layers import is_scriptable, is_exportable, set_scriptable, set_exportable, is_no_jit, set_no_jit
-from .registry import register_model, model_entrypoint, list_models, is_model, list_modules, is_model_in_modules,\
- has_model_default_key, is_model_default_key, get_model_default_value, is_model_pretrained
diff --git a/spaces/coreml-community/ControlNet-v1-1-Annotators-cpu/annotator/shuffle/__init__.py b/spaces/coreml-community/ControlNet-v1-1-Annotators-cpu/annotator/shuffle/__init__.py
deleted file mode 100644
index 1acd4e2648f25eee96a397084ace2c9a078a2e8e..0000000000000000000000000000000000000000
--- a/spaces/coreml-community/ControlNet-v1-1-Annotators-cpu/annotator/shuffle/__init__.py
+++ /dev/null
@@ -1,74 +0,0 @@
-import random
-
-import cv2
-import numpy as np
-from annotator.util import make_noise_disk, img2mask
-
-
-class ContentShuffleDetector:
- def __call__(self, img, h=None, w=None, f=None):
- H, W, C = img.shape
- if h is None:
- h = H
- if w is None:
- w = W
- if f is None:
- f = 256
- x = make_noise_disk(h, w, 1, f) * float(W - 1)
- y = make_noise_disk(h, w, 1, f) * float(H - 1)
- flow = np.concatenate([x, y], axis=2).astype(np.float32)
- return cv2.remap(img, flow, None, cv2.INTER_LINEAR)
-
-
-class ColorShuffleDetector:
- def __call__(self, img):
- H, W, C = img.shape
- F = random.randint(64, 384)
- A = make_noise_disk(H, W, 3, F)
- B = make_noise_disk(H, W, 3, F)
- C = (A + B) / 2.0
- A = (C + (A - C) * 3.0).clip(0, 1)
- B = (C + (B - C) * 3.0).clip(0, 1)
- L = img.astype(np.float32) / 255.0
- Y = A * L + B * (1 - L)
- Y -= np.min(Y, axis=(0, 1), keepdims=True)
- Y /= np.maximum(np.max(Y, axis=(0, 1), keepdims=True), 1e-5)
- Y *= 255.0
- return Y.clip(0, 255).astype(np.uint8)
-
-
-class GrayDetector:
- def __call__(self, img):
- eps = 1e-5
- X = img.astype(np.float32)
- r, g, b = X[:, :, 0], X[:, :, 1], X[:, :, 2]
- kr, kg, kb = [random.random() + eps for _ in range(3)]
- ks = kr + kg + kb
- kr /= ks
- kg /= ks
- kb /= ks
- Y = r * kr + g * kg + b * kb
- Y = np.stack([Y] * 3, axis=2)
- return Y.clip(0, 255).astype(np.uint8)
-
-
-class DownSampleDetector:
- def __call__(self, img, level=3, k=16.0):
- h = img.astype(np.float32)
- for _ in range(level):
- h += np.random.normal(loc=0.0, scale=k, size=h.shape)
- h = cv2.pyrDown(h)
- for _ in range(level):
- h = cv2.pyrUp(h)
- h += np.random.normal(loc=0.0, scale=k, size=h.shape)
- return h.clip(0, 255).astype(np.uint8)
-
-
-class Image2MaskShuffleDetector:
- def __init__(self, resolution=(640, 512)):
- self.H, self.W = resolution
-
- def __call__(self, img):
- m = img2mask(img, self.H, self.W)
- m *= 255.0
- return m.clip(0, 255).astype(np.uint8)
diff --git a/spaces/coreml-community/ControlNet-v1-1-Annotators-cpu/annotator/uniformer/mmseg/datasets/builder.py b/spaces/coreml-community/ControlNet-v1-1-Annotators-cpu/annotator/uniformer/mmseg/datasets/builder.py
deleted file mode 100644
index 0798b14cd8b39fc58d8f2a4930f1e079b5bf8b55..0000000000000000000000000000000000000000
--- a/spaces/coreml-community/ControlNet-v1-1-Annotators-cpu/annotator/uniformer/mmseg/datasets/builder.py
+++ /dev/null
@@ -1,169 +0,0 @@
-import copy
-import platform
-import random
-from functools import partial
-
-import numpy as np
-from annotator.uniformer.mmcv.parallel import collate
-from annotator.uniformer.mmcv.runner import get_dist_info
-from annotator.uniformer.mmcv.utils import Registry, build_from_cfg
-from annotator.uniformer.mmcv.utils.parrots_wrapper import DataLoader, PoolDataLoader
-from torch.utils.data import DistributedSampler
-
-if platform.system() != 'Windows':
- # https://github.com/pytorch/pytorch/issues/973
- import resource
- rlimit = resource.getrlimit(resource.RLIMIT_NOFILE)
- hard_limit = rlimit[1]
- soft_limit = min(4096, hard_limit)
- resource.setrlimit(resource.RLIMIT_NOFILE, (soft_limit, hard_limit))
-
-DATASETS = Registry('dataset')
-PIPELINES = Registry('pipeline')
-
-
-def _concat_dataset(cfg, default_args=None):
- """Build :obj:`ConcatDataset by."""
- from .dataset_wrappers import ConcatDataset
- img_dir = cfg['img_dir']
- ann_dir = cfg.get('ann_dir', None)
- split = cfg.get('split', None)
- num_img_dir = len(img_dir) if isinstance(img_dir, (list, tuple)) else 1
- if ann_dir is not None:
- num_ann_dir = len(ann_dir) if isinstance(ann_dir, (list, tuple)) else 1
- else:
- num_ann_dir = 0
- if split is not None:
- num_split = len(split) if isinstance(split, (list, tuple)) else 1
- else:
- num_split = 0
- if num_img_dir > 1:
- assert num_img_dir == num_ann_dir or num_ann_dir == 0
- assert num_img_dir == num_split or num_split == 0
- else:
- assert num_split == num_ann_dir or num_ann_dir <= 1
- num_dset = max(num_split, num_img_dir)
-
- datasets = []
- for i in range(num_dset):
- data_cfg = copy.deepcopy(cfg)
- if isinstance(img_dir, (list, tuple)):
- data_cfg['img_dir'] = img_dir[i]
- if isinstance(ann_dir, (list, tuple)):
- data_cfg['ann_dir'] = ann_dir[i]
- if isinstance(split, (list, tuple)):
- data_cfg['split'] = split[i]
- datasets.append(build_dataset(data_cfg, default_args))
-
- return ConcatDataset(datasets)
-
-
-def build_dataset(cfg, default_args=None):
- """Build datasets."""
- from .dataset_wrappers import ConcatDataset, RepeatDataset
- if isinstance(cfg, (list, tuple)):
- dataset = ConcatDataset([build_dataset(c, default_args) for c in cfg])
- elif cfg['type'] == 'RepeatDataset':
- dataset = RepeatDataset(
- build_dataset(cfg['dataset'], default_args), cfg['times'])
- elif isinstance(cfg.get('img_dir'), (list, tuple)) or isinstance(
- cfg.get('split', None), (list, tuple)):
- dataset = _concat_dataset(cfg, default_args)
- else:
- dataset = build_from_cfg(cfg, DATASETS, default_args)
-
- return dataset
-
-
-def build_dataloader(dataset,
- samples_per_gpu,
- workers_per_gpu,
- num_gpus=1,
- dist=True,
- shuffle=True,
- seed=None,
- drop_last=False,
- pin_memory=True,
- dataloader_type='PoolDataLoader',
- **kwargs):
- """Build PyTorch DataLoader.
-
- In distributed training, each GPU/process has a dataloader.
- In non-distributed training, there is only one dataloader for all GPUs.
-
- Args:
- dataset (Dataset): A PyTorch dataset.
- samples_per_gpu (int): Number of training samples on each GPU, i.e.,
- batch size of each GPU.
- workers_per_gpu (int): How many subprocesses to use for data loading
- for each GPU.
- num_gpus (int): Number of GPUs. Only used in non-distributed training.
- dist (bool): Distributed training/test or not. Default: True.
- shuffle (bool): Whether to shuffle the data at every epoch.
- Default: True.
- seed (int | None): Seed to be used. Default: None.
- drop_last (bool): Whether to drop the last incomplete batch in epoch.
- Default: False
- pin_memory (bool): Whether to use pin_memory in DataLoader.
- Default: True
- dataloader_type (str): Type of dataloader. Default: 'PoolDataLoader'
- kwargs: any keyword argument to be used to initialize DataLoader
-
- Returns:
- DataLoader: A PyTorch dataloader.
- """
- rank, world_size = get_dist_info()
- if dist:
- sampler = DistributedSampler(
- dataset, world_size, rank, shuffle=shuffle)
- shuffle = False
- batch_size = samples_per_gpu
- num_workers = workers_per_gpu
- else:
- sampler = None
- batch_size = num_gpus * samples_per_gpu
- num_workers = num_gpus * workers_per_gpu
-
- init_fn = partial(
- worker_init_fn, num_workers=num_workers, rank=rank,
- seed=seed) if seed is not None else None
-
- assert dataloader_type in (
- 'DataLoader',
- 'PoolDataLoader'), f'unsupported dataloader {dataloader_type}'
-
- if dataloader_type == 'PoolDataLoader':
- dataloader = PoolDataLoader
- elif dataloader_type == 'DataLoader':
- dataloader = DataLoader
-
- data_loader = dataloader(
- dataset,
- batch_size=batch_size,
- sampler=sampler,
- num_workers=num_workers,
- collate_fn=partial(collate, samples_per_gpu=samples_per_gpu),
- pin_memory=pin_memory,
- shuffle=shuffle,
- worker_init_fn=init_fn,
- drop_last=drop_last,
- **kwargs)
-
- return data_loader
-
-
-def worker_init_fn(worker_id, num_workers, rank, seed):
- """Worker init func for dataloader.
-
- The seed of each worker equals to num_worker * rank + worker_id + user_seed
-
- Args:
- worker_id (int): Worker id.
- num_workers (int): Number of workers.
- rank (int): The rank of current process.
- seed (int): The random seed to use.
- """
-
- worker_seed = num_workers * rank + worker_id + seed
- np.random.seed(worker_seed)
- random.seed(worker_seed)
diff --git a/spaces/cubbycarlson/karl/README.md b/spaces/cubbycarlson/karl/README.md
deleted file mode 100644
index 4c5fe0984b81b21473c964200cced6dfbfb659d1..0000000000000000000000000000000000000000
--- a/spaces/cubbycarlson/karl/README.md
+++ /dev/null
@@ -1,37 +0,0 @@
----
-title: Karl
-emoji: 📈
-colorFrom: indigo
-colorTo: purple
-sdk: gradio
-app_file: app.py
-pinned: false
----
-
-# Configuration
-
-`title`: _string_
-Display title for the Space
-
-`emoji`: _string_
-Space emoji (emoji-only character allowed)
-
-`colorFrom`: _string_
-Color for Thumbnail gradient (red, yellow, green, blue, indigo, purple, pink, gray)
-
-`colorTo`: _string_
-Color for Thumbnail gradient (red, yellow, green, blue, indigo, purple, pink, gray)
-
-`sdk`: _string_
-Can be either `gradio` or `streamlit`
-
-`sdk_version` : _string_
-Only applicable for `streamlit` SDK.
-See [doc](https://hf.co/docs/hub/spaces) for more info on supported versions.
-
-`app_file`: _string_
-Path to your main application file (which contains either `gradio` or `streamlit` Python code).
-Path is relative to the root of the repository.
-
-`pinned`: _boolean_
-Whether the Space stays on top of your list.
diff --git a/spaces/cybercorejapan/human-detection-docker/models/trackers/reid_parallel_tracker/matchers/prioritize_reid_matcher.py b/spaces/cybercorejapan/human-detection-docker/models/trackers/reid_parallel_tracker/matchers/prioritize_reid_matcher.py
deleted file mode 100644
index 1524e72be792def9361b4343e428db1c822806a3..0000000000000000000000000000000000000000
--- a/spaces/cybercorejapan/human-detection-docker/models/trackers/reid_parallel_tracker/matchers/prioritize_reid_matcher.py
+++ /dev/null
@@ -1,224 +0,0 @@
-import numpy as np
-from copy import deepcopy
-from typing import List, Tuple, Dict
-from ..core.matching import linear_assignment, topk_assignment
-from ..core.tracklet import Tracklet
-from ..core.tracklet import TrackState
-from .base_matchers import SimMatcher
-from .distances import DistCosine
-
-class PrioritizeReidMatcher():
- def __init__(self,
- reid_distance,
- iou_distance,
- match_with_reid_thr,
- islost_match_thr,
- isactive_match_thr):
-
- self.reid_dist = DistCosine(**reid_distance)
- self.iou_dist = DistCosine(**iou_distance)
- self.match_with_reid_thr = match_with_reid_thr
- self.islost_match_thr = islost_match_thr
- self.isactive_match_thr = isactive_match_thr
-
- def __call__(self,
- tracks: List[Tracklet],
- dets: List[Tracklet]) -> Tuple[np.ndarray, np.ndarray, np.ndarray]:
- """ Associate with tracklets with detection boxes"""
-
- # 1. Match with ReID dist <0.15
- dist_reid = self.reid_dist(tracks, dets)
- matches_idx_1, unmatched_tracks_idx_1, unmatched_dets_idx_1 = self.assign(dist_reid,
- thresh=self.match_with_reid_thr)
-
- # split unmatch track, det
- unmatched_tracks = [tracks[idx] for idx in unmatched_tracks_idx_1]
- unmatched_dets = [dets[i] for i in unmatched_dets_idx_1]
-
- # split active and lost tracks
- unmatched_lost_tracks = []
- unmatched_lost_tracks_idx = []
- unmatched_active_tracks = []
- unmatched_active_tracks_idx = []
- for idx, _track in enumerate(unmatched_tracks):
- if _track.state == TrackState.Lost:
- unmatched_lost_tracks.append(_track)
- unmatched_lost_tracks_idx.append(unmatched_tracks_idx_1[idx])
- else:
- unmatched_active_tracks.append(_track)
- unmatched_active_tracks_idx.append(unmatched_tracks_idx_1[idx])
-
- # 2. is lost; lost object expect lower IoU threshold lower ReID
- matches_idx_2, unmatched_tracks_idx_2, unmatched_dets_idx_2 = self.reid_and_iou_matching(
- unmatched_lost_tracks,
- unmatched_lost_tracks_idx,
- unmatched_dets,
- unmatched_dets_idx_1,
- self.islost_match_thr)
-
-
- # 3. Active object expect higher IoU threshold
- # split remain detection
- from2_unmatched_dets = [dets[i] for i in unmatched_dets_idx_2]
- from2_unmatched_dets_idx = unmatched_dets_idx_2
- matches_idx_3, unmatched_tracks_idx_3, unmatched_dets_idx_3 = self.reid_and_iou_matching(
- unmatched_active_tracks,
- unmatched_active_tracks_idx,
- from2_unmatched_dets,
- from2_unmatched_dets_idx,
- self.isactive_match_thr)
-
- # merge result
- matches_idx = matches_idx_1.tolist() + matches_idx_2.tolist() + matches_idx_3.tolist()
- unmatched_tracks_idx = []
- unmatched_dets_idx = []
-
- if len(matches_idx):
- matches_idx = np.array(matches_idx)
- else:
- matches_idx = np.empty((0, 2), dtype=np.int64)
-
- # update unmatch tracks
- for idx in range(len(tracks)):
- if not idx in matches_idx[:, 0]:
- unmatched_tracks_idx.append(idx)
- unmatched_tracks_idx = np.array(unmatched_tracks_idx)
- # update unmatch dets
- for idx in range(len(dets)):
- if not idx in matches_idx[:, 1]:
- unmatched_dets_idx.append(idx)
- unmatched_dets_idx = np.array(unmatched_dets_idx)
-
- return matches_idx, unmatched_tracks_idx, unmatched_dets_idx
-
- def calculate_occluded_ratio(self, dets):
- det_ious = 1 - self.iou_dist(dets, dets)
- np.fill_diagonal(det_ious, 0)
- det_scores = np.array([det.score for det in dets])
- det_score_matrix = (det_scores[:, None] < det_scores[None, :]).astype(np.float32)
- occluded_ratio_matrix = det_ious * det_score_matrix
- # TODO: Take area --> done, same performance.
- if len(occluded_ratio_matrix):
- occluded_ratio = np.max(occluded_ratio_matrix, 1)
- return occluded_ratio
- else:
- return []
-
- def reid_and_iou_matching(self,
- unmatched_tracks,
- unmatched_tracks_idx,
- unmatched_dets,
- unmatched_dets_idx,
- match_conditions):
- # 2. is lost; lost object expect lower IoU threshold lower ReID
- lost_tracks_reid_dist = self.reid_dist(unmatched_tracks, unmatched_dets)
- lost_tracks_iou_dist = self.iou_dist(unmatched_tracks, unmatched_dets)
-
- # reweight reid
- occluded_ratio = self.calculate_occluded_ratio(unmatched_dets)
-
- # if len(occluded_ratio):
- ### reid_score *= e^(-occluded_ratio)
- # reid_reweighting = np.exp(-occluded_ratio)
- # lost_tracks_reid_dist = lost_tracks_reid_dist * reid_reweighting
-
- ### (x^2)/3
- # lost_tracks_reid_dist = lost_tracks_reid_dist + (occluded_ratio**2)/3
-
- occluded_thr = 0.3
- used_occluded = False
-
- # cascade reid matching
- if len(occluded_ratio):
- # if occluded: Match non occluded object first, then match occluded one
- is_occluded_idx = np.nonzero(occluded_ratio > occluded_thr)[0]
- isnot_occluded_idx = np.nonzero(occluded_ratio <= occluded_thr)[0]
- if len(is_occluded_idx) and len(unmatched_tracks):
- used_occluded = True
-
- # matching visible det objects
- vis_lost_tracks_reid_dist = deepcopy(lost_tracks_reid_dist)
- vis_lost_tracks_reid_dist[:, is_occluded_idx] = 1e4
- vis_matched_idx, vis_unmatched_tracks_idx, vis_unmatched_dets_idx = self.assign(vis_lost_tracks_reid_dist,
- thresh=match_conditions['reid_thr'])
- # matching occluded det objects
- occluded_lost_tracks_reid_dist = deepcopy(lost_tracks_reid_dist)
- # if tracklet already match --> ignore
- occluded_lost_tracks_reid_dist[vis_matched_idx[:, 0], :] = 1e4
- # update visible positions = 1e4
- occluded_lost_tracks_reid_dist[:, isnot_occluded_idx] = 1e4
- # matching
- occ_matched_idx, occ_unmatched_tracks_idx, occ_unmatched_dets_idx = self.assign(occluded_lost_tracks_reid_dist,
- thresh=match_conditions['reid_occluded_thr'])
-
- matches_idx_2_1 = np.concatenate([vis_matched_idx, occ_matched_idx])
- unmatched_tracks_idx_2_1 = np.concatenate([vis_unmatched_tracks_idx, occ_unmatched_tracks_idx])
- unmatched_dets_idx_2_1 = np.concatenate([vis_unmatched_dets_idx, occ_unmatched_dets_idx])
-
- if not used_occluded:
- matches_idx_2_1, unmatched_tracks_idx_2_1, unmatched_dets_idx_2_1 = self.assign(lost_tracks_reid_dist,
- thresh=match_conditions['reid_thr'])
- if match_conditions['iou_thr'] >= 0:
- matches_idx_2_2, unmatched_tracks_idx_2_2, unmatched_dets_idx_2_2 = self.assign(lost_tracks_iou_dist,
- thresh=match_conditions['iou_thr'])
- # take the intersection of matches_idx_2_1 and matches_idx_2_2
- matches_idx_2_1 = matches_idx_2_1.tolist()
- matches_idx_2_2 = matches_idx_2_2.tolist()
-
- # merge the two result
- merged_matches_idx = list()
- for _track in matches_idx_2_1:
- if _track in matches_idx_2_2:
- merged_matches_idx.append(_track)
- else:
- merged_matches_idx = matches_idx_2_1.tolist()
-
- # convert local merge idx to global ones
- global_matches_idx = []
- for idx, match in enumerate(merged_matches_idx):
- global_matches_idx.append([unmatched_tracks_idx[match[0]], unmatched_dets_idx[match[1]]])
- if len(global_matches_idx):
- global_matches_idx = np.array(global_matches_idx)
- else:
- global_matches_idx = np.empty((0, 2), dtype=np.int64)
-
- # convert to input (global) indices
- if len(merged_matches_idx):
- merged_matches_idx = np.array(merged_matches_idx)
- else:
- merged_matches_idx = np.empty((0, 2), dtype=np.int64)
-
- global_unmatched_tracks_idx = []
- global_unmatched_dets_idx = []
-
- # update unmatch track
- for idx, _track_idx in enumerate(unmatched_tracks_idx):
- if idx in merged_matches_idx[:, 0]:
- continue
- else:
- global_unmatched_tracks_idx.append(_track_idx)
-
- # update unmatch det
- for idx, _det_idx in enumerate(unmatched_dets_idx):
- if idx in merged_matches_idx[:, 1]:
- continue
- else:
- global_unmatched_dets_idx.append(_det_idx)
-
- global_unmatched_tracks_idx = np.array(global_unmatched_tracks_idx)
- global_unmatched_dets_idx = np.array(global_unmatched_dets_idx)
- return global_matches_idx, global_unmatched_tracks_idx, global_unmatched_dets_idx
-
- def matching_dists(self, tracks: List[Tracklet],
- dets: List[Tracklet]) -> np.ndarray:
- """ Compute the distance between tracklets and detections"""
- return self.dist(tracks, dets)
-
- def matching_scores(self, tracks: List[Tracklet],
- dets: List[Tracklet]) -> np.ndarray:
- """ Compute the matching scores between tracklets and detections"""
- return self.dist.matching_scores(tracks, dets)
-
- def assign(self, distances: np.ndarray, thresh: float) -> Tuple[np.ndarray, np.ndarray, np.ndarray]:
- return linear_assignment(distances, thresh=thresh)
-
diff --git a/spaces/cynika/NFT_avatar/app.py b/spaces/cynika/NFT_avatar/app.py
deleted file mode 100644
index f39de4381b424c8b3acf0afd362935ca52f1273b..0000000000000000000000000000000000000000
--- a/spaces/cynika/NFT_avatar/app.py
+++ /dev/null
@@ -1,148 +0,0 @@
-import gradio as gr
-
-import io
-import datetime
-from pytz import timezone
-
-from login import catch_qr, get_uid_key
-from nft import having_card_id_list, card_id_set_ava
-
-sid_map = {
- "SHN48荣耀时刻": 1,
- "无": 2,
- "无2": 3,
- "胶囊计划": 4,
- "天官赐福": 5,
- "A - AKB48TSH四周年": 6,
- "B - AKB48TSH四周年": 7,
- "C - AKB48TSH四周年": 8,
- "D - AKB48TSH四周年": 9,
- "E - AKB48TSH四周年": 10,
- "F - AKB48TSH四周年": 11,
- "G - AKB48TSH四周年": 12,
- "H - AKB48TSH四周年": 13,
- "三体动画": 14,
- "百大卡": 15
-}
-
-
-def qr(ava_image):
- if ava_image:
- qr_img, info = catch_qr(ava_image)
- return {image_qr: qr_img, login_info: info}
- return {image_qr: None, login_info: None}
-
-
-def verify_login(info, u_verify, u_id, u_key, sid):
- sid_num = sid_map[sid]
- code = "请刷新页面并重新扫码登录"
- if not u_verify:
- result, u_id, u_key, code = get_uid_key(info)
- if not result:
- return False, "", "", sid_num, code
- else:
- u_verify = True
- return u_verify, u_id, u_key, sid_num, code
-
-
-def back_card_id_list(info, u_verify, u_id, u_key, sid):
- if info:
- u_verify, u_id, u_key, sid_num, code = verify_login(info, u_verify, u_id, u_key, sid)
- if u_verify:
- result, had_card_id_list, code = having_card_id_list(u_id, u_key, sid_num)
- if result:
- print("获取卡牌列表成功")
- card_name_list = list(had_card_id_list.keys())
- return {verify: u_verify, uid: u_id, key: u_key, card_id_list: had_card_id_list,
- card_list_drop: gr.update(choices=card_name_list, value=card_name_list[0]), code_output: code}
- else:
- print("获取卡牌列表失败")
- return {verify: u_verify, uid: u_id, key: u_key, card_list_drop: gr.update(choices=[]),
- code_output: code}
- else:
- return {code_output: "请先上传头像和扫码登录"}
-
-
-def name_get_card_id(card_id_list_dict, card_list_drop_name):
- if card_id_list_dict and card_list_drop_name:
- for card_name in card_id_list_dict.keys():
- if card_name == card_list_drop_name:
- cid = card_id_list_dict[card_name]
- return {card_id: cid}
- return {card_id: ""}
-
-
-def login_up_nft(info, u_verify, u_id, u_key, sid, cid, ava_image):
- if info and ava_image:
- u_verify, u_id, u_key, sid_num, code = verify_login(info, u_verify, u_id, u_key, sid)
- if u_verify:
- if not cid:
- result, had_card_id_list, code = having_card_id_list(u_id, u_key, sid_num)
- if had_card_id_list:
- cid = list(had_card_id_list.values())[0]
- bytes_object = io.BytesIO()
- ava_image.save(bytes_object, format='PNG')
- img_data = bytes_object.getvalue()
- result, code = card_id_set_ava(cid, u_key, img_data)
-
- tz = timezone('Asia/Shanghai')
- now = datetime.datetime.now(tz)
- print("提交完成:", now)
-
- return {verify: u_verify, uid: u_id, key: u_key, code_output: code}
- else:
- return {verify: u_verify, uid: u_id, key: u_key, code_output: code}
- return "请先上传头像和扫码登录"
-
-
-with gr.Blocks() as demo:
- login_info = gr.State([])
- card_id_list = gr.State({})
- card_id = gr.State("")
- verify = gr.State(False)
- key = gr.State("")
- uid = gr.State("")
- with gr.Tab("bili_NFT自定义头像(已被关闭,等新接口中)"):
- gr.Markdown("30秒自定义钻石头像(NFT),一站式操作仅三步,无需下载安装,代码开源免费,拿我项目收费的都是骗子")
- gr.Markdown("""
- 使用视频教程(欢迎三连):[视频地址](https://www.bilibili.com/video/BV1t8411P7HD/)
- https://www.bilibili.com/video/BV1t8411P7HD/
- """)
- gr.Markdown("源码:https://huggingface.co/spaces/cynika/NFT_avatar")
- gr.Markdown("第一步:")
- gr.Markdown("""领取三体数字藏品R级别,作为自定义NFT头像底图.
- [三体数字藏品领取地址](https://www.bilibili.com/h5/mall/v2/card/collection?act_id=14&hybrid_set_header=2)""")
- gr.Markdown("""https://www.bilibili.com/h5/mall/v2/card/collection?act_id=14&hybrid_set_header=2""")
- gr.Markdown("第二步:")
- with gr.Row():
- with gr.Column():
- gr.Markdown(
- """确保第一步领取三体数字藏品后,上传新头像,头像尽量为正方形并长宽小于1024像素,右上角画笔按钮可裁剪,
- 然后等待生成二维码并扫码登录进行授权(左上传头像,右扫码登录))""")
- image_ava = gr.Image(type="pil", label="上传头像")
- image_qr = gr.Image(type="pil", label="b站客户端扫码登录")
- gr.Markdown("第三步:")
- with gr.Row():
- gr.Markdown(
- """第二步完成后,选择拥有的卡组,并等待加载出所拥有的卡,再指定底卡,不选默认随机三体卡,(指定三体卡需切换一下卡组)
- 最后点击按钮<验证并提交头像>等待完成显示结果(成功可能需要时间审核)""")
- with gr.Row():
- sid_drop = gr.Dropdown(label="卡组", choices=list(sid_map.keys()), value="三体动画")
- card_list_drop = gr.Dropdown(label="指定已有底卡")
- set_button = gr.Button("验证并提交头像")
- code_output = gr.Textbox(label="消息输出", placeholder="点击提交按钮才会提交")
-
- with gr.Accordion("代码引用及郑重提醒"):
- gr.Markdown("https://github.com/wdvipa/custom_bilibili_nft")
- gr.Markdown("https://github.com/XiaoMiku01/custom_bilibili_nft")
- gr.Markdown("https://github.com/cibimo/bilibiliLogin")
- gr.Markdown("本软件纯属娱乐,不得用于其他用途,秋后算账别怨我!")
-
- image_ava.change(qr, inputs=[image_ava], outputs=[image_qr, login_info])
- sid_drop.change(back_card_id_list, inputs=[login_info, verify, uid, key, sid_drop],
- outputs=[card_id_list, verify, uid, key, card_list_drop, code_output])
- card_list_drop.change(name_get_card_id, inputs=[card_id_list, card_list_drop], outputs=[card_id])
- set_button.click(login_up_nft, inputs=[login_info, verify, uid, key, sid_drop, card_id, image_ava],
- outputs=[verify, uid, key, code_output])
-
-demo.launch()
diff --git a/spaces/dachenchen/HiWantJoin/modules/config.py b/spaces/dachenchen/HiWantJoin/modules/config.py
deleted file mode 100644
index bdc2b47927cee4b0908787cf2022e612cc3c6ce6..0000000000000000000000000000000000000000
--- a/spaces/dachenchen/HiWantJoin/modules/config.py
+++ /dev/null
@@ -1,173 +0,0 @@
-from collections import defaultdict
-from contextlib import contextmanager
-import os
-import logging
-import sys
-import commentjson as json
-
-from . import shared
-from . import presets
-
-
-__all__ = [
- "my_api_key",
- "authflag",
- "auth_list",
- "dockerflag",
- "retrieve_proxy",
- "log_level",
- "advance_docs",
- "update_doc_config",
- "multi_api_key",
- "server_name",
- "server_port",
- "share",
-]
-
-# 添加一个统一的config文件,避免文件过多造成的疑惑(优先级最低)
-# 同时,也可以为后续支持自定义功能提供config的帮助
-if os.path.exists("config.json"):
- with open("config.json", "r", encoding='utf-8') as f:
- config = json.load(f)
-else:
- config = {}
-
-lang_config = config.get("language", "auto")
-language = os.environ.get("LANGUAGE", lang_config)
-
-if os.path.exists("api_key.txt"):
- logging.info("检测到api_key.txt文件,正在进行迁移...")
- with open("api_key.txt", "r") as f:
- config["openai_api_key"] = f.read().strip()
- os.rename("api_key.txt", "api_key(deprecated).txt")
- with open("config.json", "w", encoding='utf-8') as f:
- json.dump(config, f, indent=4)
-
-if os.path.exists("auth.json"):
- logging.info("检测到auth.json文件,正在进行迁移...")
- auth_list = []
- with open("auth.json", "r", encoding='utf-8') as f:
- auth = json.load(f)
- for _ in auth:
- if auth[_]["username"] and auth[_]["password"]:
- auth_list.append((auth[_]["username"], auth[_]["password"]))
- else:
- logging.error("请检查auth.json文件中的用户名和密码!")
- sys.exit(1)
- config["users"] = auth_list
- os.rename("auth.json", "auth(deprecated).json")
- with open("config.json", "w", encoding='utf-8') as f:
- json.dump(config, f, indent=4)
-
-## 处理docker if we are running in Docker
-dockerflag = config.get("dockerflag", False)
-if os.environ.get("dockerrun") == "yes":
- dockerflag = True
-
-## 处理 api-key 以及 允许的用户列表
-my_api_key = config.get("openai_api_key", "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")
-my_api_key = os.environ.get("OPENAI_API_KEY", my_api_key)
-
-xmchat_api_key = config.get("xmchat_api_key", "")
-if os.environ.get("XMCHAT_API_KEY", None) == None:
- os.environ["XMCHAT_API_KEY"] = xmchat_api_key
-
-## 多账户机制
-multi_api_key = config.get("multi_api_key", False) # 是否开启多账户机制
-if multi_api_key:
- api_key_list = config.get("api_key_list", [])
- if len(api_key_list) == 0:
- logging.error("多账号模式已开启,但api_key_list为空,请检查config.json")
- sys.exit(1)
- shared.state.set_api_key_queue(api_key_list)
-
-auth_list = config.get("users", []) # 实际上是使用者的列表
-authflag = len(auth_list) > 0 # 是否开启认证的状态值,改为判断auth_list长度
-
-# 处理自定义的api_host,优先读环境变量的配置,如果存在则自动装配
-api_host = os.environ.get("api_host", config.get("api_host", ""))
-if api_host:
- shared.state.set_api_host(api_host)
-
-@contextmanager
-def retrieve_openai_api(api_key = None):
- old_api_key = os.environ.get("OPENAI_API_KEY", "")
- if api_key is None:
- os.environ["OPENAI_API_KEY"] = my_api_key
- yield my_api_key
- else:
- os.environ["OPENAI_API_KEY"] = api_key
- yield api_key
- os.environ["OPENAI_API_KEY"] = old_api_key
-
-## 处理log
-log_level = config.get("log_level", "INFO")
-logging.basicConfig(
- level=log_level,
- format="%(asctime)s [%(levelname)s] [%(filename)s:%(lineno)d] %(message)s",
-)
-
-## 处理代理:
-http_proxy = config.get("http_proxy", "")
-https_proxy = config.get("https_proxy", "")
-http_proxy = os.environ.get("HTTP_PROXY", http_proxy)
-https_proxy = os.environ.get("HTTPS_PROXY", https_proxy)
-
-# 重置系统变量,在不需要设置的时候不设置环境变量,以免引起全局代理报错
-os.environ["HTTP_PROXY"] = ""
-os.environ["HTTPS_PROXY"] = ""
-
-local_embedding = config.get("local_embedding", False) # 是否使用本地embedding
-
-@contextmanager
-def retrieve_proxy(proxy=None):
- """
- 1, 如果proxy = NONE,设置环境变量,并返回最新设置的代理
- 2,如果proxy != NONE,更新当前的代理配置,但是不更新环境变量
- """
- global http_proxy, https_proxy
- if proxy is not None:
- http_proxy = proxy
- https_proxy = proxy
- yield http_proxy, https_proxy
- else:
- old_var = os.environ["HTTP_PROXY"], os.environ["HTTPS_PROXY"]
- os.environ["HTTP_PROXY"] = http_proxy
- os.environ["HTTPS_PROXY"] = https_proxy
- yield http_proxy, https_proxy # return new proxy
-
- # return old proxy
- os.environ["HTTP_PROXY"], os.environ["HTTPS_PROXY"] = old_var
-
-
-## 处理advance docs
-advance_docs = defaultdict(lambda: defaultdict(dict))
-advance_docs.update(config.get("advance_docs", {}))
-def update_doc_config(two_column_pdf):
- global advance_docs
- advance_docs["pdf"]["two_column"] = two_column_pdf
-
- logging.info(f"更新后的文件参数为:{advance_docs}")
-
-## 处理gradio.launch参数
-server_name = config.get("server_name", None)
-server_port = config.get("server_port", None)
-if server_name is None:
- if dockerflag:
- server_name = "0.0.0.0"
- else:
- server_name = "127.0.0.1"
-if server_port is None:
- if dockerflag:
- server_port = 7860
-
-assert server_port is None or type(server_port) == int, "要求port设置为int类型"
-
-# 设置默认model
-default_model = config.get("default_model", "")
-try:
- presets.DEFAULT_MODEL = presets.MODELS.index(default_model)
-except ValueError:
- pass
-
-share = config.get("share", False)
diff --git a/spaces/dalle-mini/dalle-mini/README.md b/spaces/dalle-mini/dalle-mini/README.md
deleted file mode 100644
index 11f784bbb29b3700509906fe8f610709f2ee584b..0000000000000000000000000000000000000000
--- a/spaces/dalle-mini/dalle-mini/README.md
+++ /dev/null
@@ -1,10 +0,0 @@
----
-title: DALL·E mini
-metaTitle: "DALL·E mini by craiyon.com on Hugging Face"
-emoji: 🥑
-colorFrom: yellow
-colorTo: green
-sdk: static
-pinned: True
-license: apache-2.0
----
diff --git a/spaces/damilojohn/Playlist_Generator_For_Afrobeats/README.md b/spaces/damilojohn/Playlist_Generator_For_Afrobeats/README.md
deleted file mode 100644
index 344483eb6bd5423fa67660031c1c40b5f62766bb..0000000000000000000000000000000000000000
--- a/spaces/damilojohn/Playlist_Generator_For_Afrobeats/README.md
+++ /dev/null
@@ -1,13 +0,0 @@
----
-title: Playlist Generator For Afrobeats
-emoji: 🌍
-colorFrom: red
-colorTo: indigo
-sdk: gradio
-sdk_version: 3.24.1
-app_file: app.py
-pinned: false
-license: apache-2.0
----
-
-Check out the configuration reference at https://huggingface.co/docs/hub/spaces-config-reference
diff --git a/spaces/danterivers/music-generation-samples/audiocraft/data/audio_utils.py b/spaces/danterivers/music-generation-samples/audiocraft/data/audio_utils.py
deleted file mode 100644
index ddbcbec2ec294ab33349ff261d27f369354b556f..0000000000000000000000000000000000000000
--- a/spaces/danterivers/music-generation-samples/audiocraft/data/audio_utils.py
+++ /dev/null
@@ -1,169 +0,0 @@
-# Copyright (c) Meta Platforms, Inc. and affiliates.
-# All rights reserved.
-#
-# This source code is licensed under the license found in the
-# LICENSE file in the root directory of this source tree.
-
-import sys
-import typing as tp
-
-import julius
-import torch
-import torchaudio
-
-
-def convert_audio_channels(wav: torch.Tensor, channels: int = 2) -> torch.Tensor:
- """Convert audio to the given number of channels.
-
- Args:
- wav (torch.Tensor): Audio wave of shape [B, C, T].
- channels (int): Expected number of channels as output.
- Returns:
- torch.Tensor: Downmixed or unchanged audio wave [B, C, T].
- """
- *shape, src_channels, length = wav.shape
- if src_channels == channels:
- pass
- elif channels == 1:
- # Case 1:
- # The caller asked 1-channel audio, and the stream has multiple
- # channels, downmix all channels.
- wav = wav.mean(dim=-2, keepdim=True)
- elif src_channels == 1:
- # Case 2:
- # The caller asked for multiple channels, but the input file has
- # a single channel, replicate the audio over all channels.
- wav = wav.expand(*shape, channels, length)
- elif src_channels >= channels:
- # Case 3:
- # The caller asked for multiple channels, and the input file has
- # more channels than requested. In that case return the first channels.
- wav = wav[..., :channels, :]
- else:
- # Case 4: What is a reasonable choice here?
- raise ValueError('The audio file has less channels than requested but is not mono.')
- return wav
-
-
-def convert_audio(wav: torch.Tensor, from_rate: float,
- to_rate: float, to_channels: int) -> torch.Tensor:
- """Convert audio to new sample rate and number of audio channels.
- """
- wav = julius.resample_frac(wav, int(from_rate), int(to_rate))
- wav = convert_audio_channels(wav, to_channels)
- return wav
-
-
-def normalize_loudness(wav: torch.Tensor, sample_rate: int, loudness_headroom_db: float = 12,
- energy_floor: float = 2e-3):
- """Normalize an input signal to a user loudness in dB LKFS.
- Audio loudness is defined according to the ITU-R BS.1770-4 recommendation.
-
- Args:
- wav (torch.Tensor): Input multichannel audio data.
- sample_rate (int): Sample rate.
- loudness_headroom_db (float): Target loudness of the output in dB LUFS.
- energy_floor (float): anything below that RMS level will not be rescaled.
- Returns:
- output (torch.Tensor): Loudness normalized output data.
- """
- energy = wav.pow(2).mean().sqrt().item()
- if energy < energy_floor:
- return wav
- transform = torchaudio.transforms.Loudness(sample_rate)
- input_loudness_db = transform(wav).item()
- # calculate the gain needed to scale to the desired loudness level
- delta_loudness = -loudness_headroom_db - input_loudness_db
- gain = 10.0 ** (delta_loudness / 20.0)
- output = gain * wav
- assert output.isfinite().all(), (input_loudness_db, wav.pow(2).mean().sqrt())
- return output
-
-
-def _clip_wav(wav: torch.Tensor, log_clipping: bool = False, stem_name: tp.Optional[str] = None) -> None:
- """Utility function to clip the audio with logging if specified."""
- max_scale = wav.abs().max()
- if log_clipping and max_scale > 1:
- clamp_prob = (wav.abs() > 1).float().mean().item()
- print(f"CLIPPING {stem_name or ''} happening with proba (a bit of clipping is okay):",
- clamp_prob, "maximum scale: ", max_scale.item(), file=sys.stderr)
- wav.clamp_(-1, 1)
-
-
-def normalize_audio(wav: torch.Tensor, normalize: bool = True,
- strategy: str = 'peak', peak_clip_headroom_db: float = 1,
- rms_headroom_db: float = 18, loudness_headroom_db: float = 14,
- log_clipping: bool = False, sample_rate: tp.Optional[int] = None,
- stem_name: tp.Optional[str] = None) -> torch.Tensor:
- """Normalize the audio according to the prescribed strategy (see after).
-
- Args:
- wav (torch.Tensor): Audio data.
- normalize (bool): if `True` (default), normalizes according to the prescribed
- strategy (see after). If `False`, the strategy is only used in case clipping
- would happen.
- strategy (str): Can be either 'clip', 'peak', or 'rms'. Default is 'peak',
- i.e. audio is normalized by its largest value. RMS normalizes by root-mean-square
- with extra headroom to avoid clipping. 'clip' just clips.
- peak_clip_headroom_db (float): Headroom in dB when doing 'peak' or 'clip' strategy.
- rms_headroom_db (float): Headroom in dB when doing 'rms' strategy. This must be much larger
- than the `peak_clip` one to avoid further clipping.
- loudness_headroom_db (float): Target loudness for loudness normalization.
- log_clipping (bool): If True, basic logging on stderr when clipping still
- occurs despite strategy (only for 'rms').
- sample_rate (int): Sample rate for the audio data (required for loudness).
- stem_name (Optional[str]): Stem name for clipping logging.
- Returns:
- torch.Tensor: Normalized audio.
- """
- scale_peak = 10 ** (-peak_clip_headroom_db / 20)
- scale_rms = 10 ** (-rms_headroom_db / 20)
- if strategy == 'peak':
- rescaling = (scale_peak / wav.abs().max())
- if normalize or rescaling < 1:
- wav = wav * rescaling
- elif strategy == 'clip':
- wav = wav.clamp(-scale_peak, scale_peak)
- elif strategy == 'rms':
- mono = wav.mean(dim=0)
- rescaling = scale_rms / mono.pow(2).mean().sqrt()
- if normalize or rescaling < 1:
- wav = wav * rescaling
- _clip_wav(wav, log_clipping=log_clipping, stem_name=stem_name)
- elif strategy == 'loudness':
- assert sample_rate is not None, "Loudness normalization requires sample rate."
- wav = normalize_loudness(wav, sample_rate, loudness_headroom_db)
- _clip_wav(wav, log_clipping=log_clipping, stem_name=stem_name)
- else:
- assert wav.abs().max() < 1
- assert strategy == '' or strategy == 'none', f"Unexpected strategy: '{strategy}'"
- return wav
-
-
-def f32_pcm(wav: torch.Tensor) -> torch.Tensor:
- """Convert audio to float 32 bits PCM format.
- """
- if wav.dtype.is_floating_point:
- return wav
- else:
- assert wav.dtype == torch.int16
- return wav.float() / 2**15
-
-
-def i16_pcm(wav: torch.Tensor) -> torch.Tensor:
- """Convert audio to int 16 bits PCM format.
-
- ..Warning:: There exist many formula for doing this convertion. None are perfect
- due to the asymetry of the int16 range. One either have possible clipping, DC offset,
- or inconsistancies with f32_pcm. If the given wav doesn't have enough headroom,
- it is possible that `i16_pcm(f32_pcm)) != Identity`.
- """
- if wav.dtype.is_floating_point:
- assert wav.abs().max() <= 1
- candidate = (wav * 2 ** 15).round()
- if candidate.max() >= 2 ** 15: # clipping would occur
- candidate = (wav * (2 ** 15 - 1)).round()
- return candidate.short()
- else:
- assert wav.dtype == torch.int16
- return wav
diff --git a/spaces/darkproger/propaganda/app.py b/spaces/darkproger/propaganda/app.py
deleted file mode 100644
index cebbca388815b2b873f2034fcbbd0df0092f81ad..0000000000000000000000000000000000000000
--- a/spaces/darkproger/propaganda/app.py
+++ /dev/null
@@ -1,72 +0,0 @@
-import pandas as pd
-from spacy import displacy
-from spacy.tokens import Doc
-from spacy.vocab import Vocab
-from spacy_streamlit.util import get_html
-import streamlit as st
-import torch
-from transformers import BertTokenizerFast
-
-from model import BertForTokenAndSequenceJointClassification
-
-
-@st.cache(allow_output_mutation=True)
-def load_model():
- tokenizer = BertTokenizerFast.from_pretrained('bert-base-cased')
- model = BertForTokenAndSequenceJointClassification.from_pretrained(
- "QCRI/PropagandaTechniquesAnalysis-en-BERT",
- revision="v0.1.0")
- return tokenizer, model
-
-with torch.inference_mode(True):
- tokenizer, model = load_model()
-
- st.write("[Propaganda Techniques Analysis BERT](https://huggingface.co/QCRI/PropagandaTechniquesAnalysis-en-BERT) Tagger")
-
- input = st.text_area('Input', """\
- In some instances, it can be highly dangerous to use a medicine for the prevention or treatment of COVID-19 that has not been approved by or has not received emergency use authorization from the FDA.
- """)
-
- inputs = tokenizer.encode_plus(input, return_tensors="pt")
- outputs = model(**inputs)
- sequence_class_index = torch.argmax(outputs.sequence_logits, dim=-1)
- sequence_class = model.sequence_tags[sequence_class_index[0]]
- token_class_index = torch.argmax(outputs.token_logits, dim=-1)
- tokens = tokenizer.convert_ids_to_tokens(inputs.input_ids[0][1:-1])
- tags = [model.token_tags[i] for i in token_class_index[0].tolist()[1:-1]]
-
-columns = st.columns(len(outputs.sequence_logits.flatten()))
-for col, sequence_tag, logit in zip(columns, model.sequence_tags, outputs.sequence_logits.flatten()):
- col.metric(sequence_tag, '%.2f' % logit.item())
-
-
-spaces = [not tok.startswith('##') for tok in tokens][1:] + [False]
-
-doc = Doc(Vocab(strings=set(tokens)),
- words=tokens,
- spaces=spaces,
- ents=[tag if tag == "O" else f"B-{tag}" for tag in tags])
-
-labels = model.token_tags[2:]
-
-label_select = st.multiselect(
- "Tags",
- options=labels,
- default=labels,
- key=f"tags_ner_label_select",
-)
-html = displacy.render(
- doc, style="ent", options={"ents": label_select, "colors": {}}
-)
-style = ""
-st.write(f"{style}{get_html(html)}", unsafe_allow_html=True)
-
-attrs = ["text", "label_", "start", "end", "start_char", "end_char"]
-data = [
- [str(getattr(ent, attr)) for attr in attrs]
- for ent in doc.ents
- if ent.label_ in label_select
-]
-if data:
- df = pd.DataFrame(data, columns=attrs)
- st.dataframe(df)
diff --git a/spaces/davidefiocco/zeroshotcat/README.md b/spaces/davidefiocco/zeroshotcat/README.md
deleted file mode 100644
index c3aa633054f7ed5792545c4c6fd7e1bd95e98ceb..0000000000000000000000000000000000000000
--- a/spaces/davidefiocco/zeroshotcat/README.md
+++ /dev/null
@@ -1,37 +0,0 @@
----
-title: Zeroshotcat
-emoji: 💻
-colorFrom: pink
-colorTo: blue
-sdk: streamlit
-app_file: app.py
-pinned: false
----
-
-# Configuration
-
-`title`: _string_
-Display title for the Space
-
-`emoji`: _string_
-Space emoji (emoji-only character allowed)
-
-`colorFrom`: _string_
-Color for Thumbnail gradient (red, yellow, green, blue, indigo, purple, pink, gray)
-
-`colorTo`: _string_
-Color for Thumbnail gradient (red, yellow, green, blue, indigo, purple, pink, gray)
-
-`sdk`: _string_
-Can be either `gradio` or `streamlit`
-
-`sdk_version` : _string_
-Only applicable for `streamlit` SDK.
-See [doc](https://hf.co/docs/hub/spaces) for more info on supported versions.
-
-`app_file`: _string_
-Path to your main application file (which contains either `gradio` or `streamlit` Python code).
-Path is relative to the root of the repository.
-
-`pinned`: _boolean_
-Whether the Space stays on top of your list.
diff --git a/spaces/dbirks/diffuse-the-rest/build/_app/immutable/chunks/index-a207c28c.js b/spaces/dbirks/diffuse-the-rest/build/_app/immutable/chunks/index-a207c28c.js
deleted file mode 100644
index 611187bf3614d76b63d3bb9dd81303184d23411d..0000000000000000000000000000000000000000
--- a/spaces/dbirks/diffuse-the-rest/build/_app/immutable/chunks/index-a207c28c.js
+++ /dev/null
@@ -1 +0,0 @@
-function N(){}function F(t,n){for(const e in n)t[e]=n[e];return t}function k(t){return t()}function C(){return Object.create(null)}function p(t){t.forEach(k)}function H(t){return typeof t=="function"}function ct(t,n){return t!=t?n==n:t!==n||t&&typeof t=="object"||typeof t=="function"}let g;function ut(t,n){return g||(g=document.createElement("a")),g.href=n,t===g.href}function I(t){return Object.keys(t).length===0}function G(t,...n){if(t==null)return N;const e=t.subscribe(...n);return e.unsubscribe?()=>e.unsubscribe():e}function ot(t,n,e){t.$$.on_destroy.push(G(n,e))}function st(t,n,e,i){if(t){const r=P(t,n,e,i);return t[0](r)}}function P(t,n,e,i){return t[1]&&i?F(e.ctx.slice(),t[1](i(n))):e.ctx}function at(t,n,e,i){if(t[2]&&i){const r=t[2](i(e));if(n.dirty===void 0)return r;if(typeof r=="object"){const s=[],l=Math.max(n.dirty.length,r.length);for(let o=0;o32){const n=[],e=t.ctx.length/32;for(let i=0;i>1);e(r)<=i?t=r+1:n=r}return t}function Q(t){if(t.hydrate_init)return;t.hydrate_init=!0;let n=t.childNodes;if(t.nodeName==="HEAD"){const c=[];for(let u=0;u0&&n[e[r]].claim_order<=u?r+1:W(1,r,y=>n[e[y]].claim_order,u))-1;i[c]=e[f]+1;const a=f+1;e[a]=c,r=Math.max(a,r)}const s=[],l=[];let o=n.length-1;for(let c=e[r]+1;c!=0;c=i[c-1]){for(s.push(n[c-1]);o>=c;o--)l.push(n[o]);o--}for(;o>=0;o--)l.push(n[o]);s.reverse(),l.sort((c,u)=>c.claim_order-u.claim_order);for(let c=0,u=0;c=s[u].claim_order;)u++;const f=ut.removeEventListener(n,e,i)}function yt(t){return function(n){return n.preventDefault(),t.call(this,n)}}function gt(t){return function(n){return n.stopPropagation(),t.call(this,n)}}function bt(t,n,e){e==null?t.removeAttribute(n):t.getAttribute(n)!==e&&t.setAttribute(n,e)}function X(t){return Array.from(t.childNodes)}function Y(t){t.claim_info===void 0&&(t.claim_info={last_index:0,total_claimed:0})}function B(t,n,e,i,r=!1){Y(t);const s=(()=>{for(let l=t.claim_info.last_index;l=0;l--){const o=t[l];if(n(o)){const c=e(o);return c===void 0?t.splice(l,1):t[l]=c,r?c===void 0&&t.claim_info.last_index--:t.claim_info.last_index=l,o}}return i()})();return s.claim_order=t.claim_info.total_claimed,t.claim_info.total_claimed+=1,s}function Z(t,n,e,i){return B(t,r=>r.nodeName===n,r=>{const s=[];for(let l=0;lr.removeAttribute(l))},()=>i(n))}function xt(t,n,e){return Z(t,n,e,V)}function tt(t,n){return B(t,e=>e.nodeType===3,e=>{const i=""+n;if(e.data.startsWith(i)){if(e.data.length!==i.length)return e.splitText(i.length)}else e.data=i},()=>S(n),!0)}function wt(t){return tt(t," ")}function $t(t,n){n=""+n,t.wholeText!==n&&(t.data=n)}function Et(t,n,e,i){e===null?t.style.removeProperty(n):t.style.setProperty(n,e,i?"important":"")}function vt(t,n=document.body){return Array.from(n.querySelectorAll(t))}let m;function h(t){m=t}function L(){if(!m)throw new Error("Function called outside component initialization");return m}function At(t){L().$$.on_mount.push(t)}function Nt(t){L().$$.after_update.push(t)}const _=[],M=[],x=[],T=[],O=Promise.resolve();let v=!1;function D(){v||(v=!0,O.then(z))}function St(){return D(),O}function A(t){x.push(t)}const E=new Set;let b=0;function z(){const t=m;do{for(;b<_.length;){const n=_[b];b++,h(n),nt(n.$$)}for(h(null),_.length=0,b=0;M.length;)M.pop()();for(let n=0;n{w.delete(t),i&&(e&&t.d(1),i())}),t.o(n)}else i&&i()}const Mt=typeof window<"u"?window:typeof globalThis<"u"?globalThis:global;function Tt(t){t&&t.c()}function kt(t,n){t&&t.l(n)}function it(t,n,e,i){const{fragment:r,on_mount:s,on_destroy:l,after_update:o}=t.$$;r&&r.m(n,e),i||A(()=>{const c=s.map(k).filter(H);l?l.push(...c):p(c),t.$$.on_mount=[]}),o.forEach(A)}function rt(t,n){const e=t.$$;e.fragment!==null&&(p(e.on_destroy),e.fragment&&e.fragment.d(n),e.on_destroy=e.fragment=null,e.ctx=[])}function lt(t,n){t.$$.dirty[0]===-1&&(_.push(t),D(),t.$$.dirty.fill(0)),t.$$.dirty[n/31|0]|=1<{const q=j.length?j[0]:y;return u.ctx&&r(u.ctx[a],u.ctx[a]=q)&&(!u.skip_bound&&u.bound[a]&&u.bound[a](q),f&<(t,a)),y}):[],u.update(),f=!0,p(u.before_update),u.fragment=i?i(u.ctx):!1,n.target){if(n.hydrate){J();const a=X(n.target);u.fragment&&u.fragment.l(a),a.forEach(U)}else u.fragment&&u.fragment.c();n.intro&&et(t.$$.fragment),it(t,n.target,n.anchor,n.customElement),K(),z()}h(c)}class Bt{$destroy(){rt(this,1),this.$destroy=N}$on(n,e){const i=this.$$.callbacks[n]||(this.$$.callbacks[n]=[]);return i.push(e),()=>{const r=i.indexOf(e);r!==-1&&i.splice(r,1)}}$set(n){this.$$set&&!I(n)&&(this.$$.skip_bound=!0,this.$$set(n),this.$$.skip_bound=!1)}}export{N as A,st as B,ft as C,dt as D,at as E,R as F,ot as G,vt as H,ut as I,pt as J,gt as K,yt as L,p as M,Mt as N,A as O,M as P,Bt as S,ht as a,_t as b,wt as c,qt as d,mt as e,et as f,jt as g,U as h,Pt as i,Nt as j,V as k,xt as l,X as m,bt as n,At as o,Et as p,S as q,tt as r,ct as s,Ct as t,$t as u,Tt as v,kt as w,it as x,rt as y,St as z};
diff --git a/spaces/dcarpintero/nlp-summarizer-pegasus/.venv/lib/python3.9/site-packages/fastapi/concurrency.py b/spaces/dcarpintero/nlp-summarizer-pegasus/.venv/lib/python3.9/site-packages/fastapi/concurrency.py
deleted file mode 100644
index 754061c862dadbdfd0c57a563b76fbd0fb5497a4..0000000000000000000000000000000000000000
--- a/spaces/dcarpintero/nlp-summarizer-pegasus/.venv/lib/python3.9/site-packages/fastapi/concurrency.py
+++ /dev/null
@@ -1,40 +0,0 @@
-from contextlib import AsyncExitStack as AsyncExitStack # noqa
-from contextlib import asynccontextmanager as asynccontextmanager
-from typing import AsyncGenerator, ContextManager, TypeVar
-
-import anyio
-from anyio import CapacityLimiter
-from starlette.concurrency import iterate_in_threadpool as iterate_in_threadpool # noqa
-from starlette.concurrency import run_in_threadpool as run_in_threadpool # noqa
-from starlette.concurrency import ( # noqa
- run_until_first_complete as run_until_first_complete,
-)
-
-_T = TypeVar("_T")
-
-
-@asynccontextmanager
-async def contextmanager_in_threadpool(
- cm: ContextManager[_T],
-) -> AsyncGenerator[_T, None]:
- # blocking __exit__ from running waiting on a free thread
- # can create race conditions/deadlocks if the context manager itself
- # has its own internal pool (e.g. a database connection pool)
- # to avoid this we let __exit__ run without a capacity limit
- # since we're creating a new limiter for each call, any non-zero limit
- # works (1 is arbitrary)
- exit_limiter = CapacityLimiter(1)
- try:
- yield await run_in_threadpool(cm.__enter__)
- except Exception as e:
- ok = bool(
- await anyio.to_thread.run_sync(
- cm.__exit__, type(e), e, None, limiter=exit_limiter
- )
- )
- if not ok:
- raise e
- else:
- await anyio.to_thread.run_sync(
- cm.__exit__, None, None, None, limiter=exit_limiter
- )
diff --git a/spaces/dcarpintero/nlp-summarizer-pegasus/.venv/lib/python3.9/site-packages/fsspec/implementations/memory.py b/spaces/dcarpintero/nlp-summarizer-pegasus/.venv/lib/python3.9/site-packages/fsspec/implementations/memory.py
deleted file mode 100644
index fc89615bc3020956bb1a7e1078f568dbead87985..0000000000000000000000000000000000000000
--- a/spaces/dcarpintero/nlp-summarizer-pegasus/.venv/lib/python3.9/site-packages/fsspec/implementations/memory.py
+++ /dev/null
@@ -1,293 +0,0 @@
-from __future__ import absolute_import, annotations, division, print_function
-
-import logging
-from datetime import datetime
-from errno import ENOTEMPTY
-from io import BytesIO
-from typing import Any, ClassVar
-
-from fsspec import AbstractFileSystem
-
-logger = logging.Logger("fsspec.memoryfs")
-
-
-class MemoryFileSystem(AbstractFileSystem):
- """A filesystem based on a dict of BytesIO objects
-
- This is a global filesystem so instances of this class all point to the same
- in memory filesystem.
- """
-
- store: ClassVar[dict[str, Any]] = {} # global, do not overwrite!
- pseudo_dirs = [""] # global, do not overwrite!
- protocol = "memory"
- root_marker = "/"
-
- @classmethod
- def _strip_protocol(cls, path):
- if path.startswith("memory://"):
- path = path[len("memory://") :]
- if "::" in path or "://" in path:
- return path.rstrip("/")
- path = path.lstrip("/").rstrip("/")
- return "/" + path if path else ""
-
- def ls(self, path, detail=True, **kwargs):
- path = self._strip_protocol(path)
- if path in self.store:
- # there is a key with this exact name
- if not detail:
- return [path]
- return [
- {
- "name": path,
- "size": self.store[path].size,
- "type": "file",
- "created": self.store[path].created.timestamp(),
- }
- ]
- paths = set()
- starter = path + "/"
- out = []
- for p2 in tuple(self.store):
- if p2.startswith(starter):
- if "/" not in p2[len(starter) :]:
- # exact child
- out.append(
- {
- "name": p2,
- "size": self.store[p2].size,
- "type": "file",
- "created": self.store[p2].created.timestamp(),
- }
- )
- elif len(p2) > len(starter):
- # implied child directory
- ppath = starter + p2[len(starter) :].split("/", 1)[0]
- if ppath not in paths:
- out = out or []
- out.append(
- {
- "name": ppath,
- "size": 0,
- "type": "directory",
- }
- )
- paths.add(ppath)
- for p2 in self.pseudo_dirs:
- if p2.startswith(starter):
- if "/" not in p2[len(starter) :]:
- # exact child pdir
- if p2 not in paths:
- out.append({"name": p2, "size": 0, "type": "directory"})
- paths.add(p2)
- else:
- # directory implied by deeper pdir
- ppath = starter + p2[len(starter) :].split("/", 1)[0]
- if ppath not in paths:
- out.append({"name": ppath, "size": 0, "type": "directory"})
- paths.add(ppath)
- if not out:
- if path in self.pseudo_dirs:
- # empty dir
- return []
- raise FileNotFoundError(path)
- if detail:
- return out
- return sorted([f["name"] for f in out])
-
- def mkdir(self, path, create_parents=True, **kwargs):
- path = self._strip_protocol(path)
- if path in self.store or path in self.pseudo_dirs:
- raise FileExistsError(path)
- if self._parent(path).strip("/") and self.isfile(self._parent(path)):
- raise NotADirectoryError(self._parent(path))
- if create_parents and self._parent(path).strip("/"):
- try:
- self.mkdir(self._parent(path), create_parents, **kwargs)
- except FileExistsError:
- pass
- if path and path not in self.pseudo_dirs:
- self.pseudo_dirs.append(path)
-
- def makedirs(self, path, exist_ok=False):
- try:
- self.mkdir(path, create_parents=True)
- except FileExistsError:
- if not exist_ok:
- raise
-
- def pipe_file(self, path, value, **kwargs):
- """Set the bytes of given file
-
- Avoids copies of the data if possible
- """
- self.open(path, "wb", data=value)
-
- def rmdir(self, path):
- path = self._strip_protocol(path)
- if path == "":
- # silently avoid deleting FS root
- return
- if path in self.pseudo_dirs:
- if not self.ls(path):
- self.pseudo_dirs.remove(path)
- else:
- raise OSError(ENOTEMPTY, "Directory not empty", path)
- else:
- raise FileNotFoundError(path)
-
- def exists(self, path, **kwargs):
- path = self._strip_protocol(path)
- return path in self.store or path in self.pseudo_dirs
-
- def info(self, path, **kwargs):
- path = self._strip_protocol(path)
- if path in self.pseudo_dirs or any(
- p.startswith(path + "/") for p in list(self.store) + self.pseudo_dirs
- ):
- return {
- "name": path,
- "size": 0,
- "type": "directory",
- }
- elif path in self.store:
- filelike = self.store[path]
- return {
- "name": path,
- "size": filelike.size,
- "type": "file",
- "created": getattr(filelike, "created", None),
- }
- else:
- raise FileNotFoundError(path)
-
- def _open(
- self,
- path,
- mode="rb",
- block_size=None,
- autocommit=True,
- cache_options=None,
- **kwargs,
- ):
- path = self._strip_protocol(path)
- if path in self.pseudo_dirs:
- raise IsADirectoryError(path)
- parent = path
- while len(parent) > 1:
- parent = self._parent(parent)
- if self.isfile(parent):
- raise FileExistsError(parent)
- if mode in ["rb", "ab", "rb+"]:
- if path in self.store:
- f = self.store[path]
- if mode == "ab":
- # position at the end of file
- f.seek(0, 2)
- else:
- # position at the beginning of file
- f.seek(0)
- return f
- else:
- raise FileNotFoundError(path)
- if mode == "wb":
- m = MemoryFile(self, path, kwargs.get("data"))
- if not self._intrans:
- m.commit()
- return m
-
- def cp_file(self, path1, path2, **kwargs):
- path1 = self._strip_protocol(path1)
- path2 = self._strip_protocol(path2)
- if self.isfile(path1):
- self.store[path2] = MemoryFile(
- self, path2, self.store[path1].getvalue()
- ) # implicit copy
- elif self.isdir(path1):
- if path2 not in self.pseudo_dirs:
- self.pseudo_dirs.append(path2)
- else:
- raise FileNotFoundError(path1)
-
- def cat_file(self, path, start=None, end=None, **kwargs):
- path = self._strip_protocol(path)
- try:
- return bytes(self.store[path].getbuffer()[start:end])
- except KeyError:
- raise FileNotFoundError(path)
-
- def _rm(self, path):
- path = self._strip_protocol(path)
- try:
- del self.store[path]
- except KeyError as e:
- raise FileNotFoundError(path) from e
-
- def modified(self, path):
- path = self._strip_protocol(path)
- try:
- return self.store[path].modified
- except KeyError:
- raise FileNotFoundError(path)
-
- def created(self, path):
- path = self._strip_protocol(path)
- try:
- return self.store[path].created
- except KeyError:
- raise FileNotFoundError(path)
-
- def rm(self, path, recursive=False, maxdepth=None):
- if isinstance(path, str):
- path = self._strip_protocol(path)
- else:
- path = [self._strip_protocol(p) for p in path]
- paths = self.expand_path(path, recursive=recursive, maxdepth=maxdepth)
- for p in reversed(paths):
- # If the expanded path doesn't exist, it is only because the expanded
- # path was a directory that does not exist in self.pseudo_dirs. This
- # is possible if you directly create files without making the
- # directories first.
- if not self.exists(p):
- continue
- if self.isfile(p):
- self.rm_file(p)
- else:
- self.rmdir(p)
-
-
-class MemoryFile(BytesIO):
- """A BytesIO which can't close and works as a context manager
-
- Can initialise with data. Each path should only be active once at any moment.
-
- No need to provide fs, path if auto-committing (default)
- """
-
- def __init__(self, fs=None, path=None, data=None):
- logger.debug("open file %s", path)
- self.fs = fs
- self.path = path
- self.created = datetime.utcnow()
- self.modified = datetime.utcnow()
- if data:
- super().__init__(data)
- self.seek(0)
-
- @property
- def size(self):
- return self.getbuffer().nbytes
-
- def __enter__(self):
- return self
-
- def close(self):
- pass
-
- def discard(self):
- pass
-
- def commit(self):
- self.fs.store[self.path] = self
- self.modified = datetime.utcnow()
diff --git a/spaces/dcarpintero/nlp-summarizer-pegasus/.venv/lib/python3.9/site-packages/gradio/templates/frontend/index.html b/spaces/dcarpintero/nlp-summarizer-pegasus/.venv/lib/python3.9/site-packages/gradio/templates/frontend/index.html
deleted file mode 100644
index 913645767a3d56a4876776ce875bf2d0942ebf3a..0000000000000000000000000000000000000000
--- a/spaces/dcarpintero/nlp-summarizer-pegasus/.venv/lib/python3.9/site-packages/gradio/templates/frontend/index.html
+++ /dev/null
@@ -1,84 +0,0 @@
-
-
-
-
-
-
-
-
-
-
-
-
-
-
-
-
-
-
-
-
-
-
-
-
-
-
-
-
-
-
-
-
-
-
-
diff --git a/spaces/dcarpintero/nlp-summarizer-pegasus/.venv/lib/python3.9/site-packages/jsonschema/_typing.py b/spaces/dcarpintero/nlp-summarizer-pegasus/.venv/lib/python3.9/site-packages/jsonschema/_typing.py
deleted file mode 100644
index d283dc48d10489baf7516182bfb1b111faf12ba0..0000000000000000000000000000000000000000
--- a/spaces/dcarpintero/nlp-summarizer-pegasus/.venv/lib/python3.9/site-packages/jsonschema/_typing.py
+++ /dev/null
@@ -1,28 +0,0 @@
-"""
-Some (initially private) typing helpers for jsonschema's types.
-"""
-from typing import Any, Callable, Iterable, Protocol, Tuple, Union
-
-import referencing.jsonschema
-
-from jsonschema.protocols import Validator
-
-
-class SchemaKeywordValidator(Protocol):
- def __call__(
- self,
- validator: Validator,
- value: Any,
- instance: Any,
- schema: referencing.jsonschema.Schema,
- ) -> None:
- ...
-
-
-id_of = Callable[[referencing.jsonschema.Schema], Union[str, None]]
-
-
-ApplicableValidators = Callable[
- [referencing.jsonschema.Schema],
- Iterable[Tuple[str, Any]],
-]
diff --git a/spaces/deepskyreal/ai-mixer-hotchpotch/sad_talker/src/face3d/data/base_dataset.py b/spaces/deepskyreal/ai-mixer-hotchpotch/sad_talker/src/face3d/data/base_dataset.py
deleted file mode 100644
index 1bd57d082d519f512d7114b4f867b6695fb7de06..0000000000000000000000000000000000000000
--- a/spaces/deepskyreal/ai-mixer-hotchpotch/sad_talker/src/face3d/data/base_dataset.py
+++ /dev/null
@@ -1,125 +0,0 @@
-"""This module implements an abstract base class (ABC) 'BaseDataset' for datasets.
-
-It also includes common transformation functions (e.g., get_transform, __scale_width), which can be later used in subclasses.
-"""
-import random
-import numpy as np
-import torch.utils.data as data
-from PIL import Image
-import torchvision.transforms as transforms
-from abc import ABC, abstractmethod
-
-
-class BaseDataset(data.Dataset, ABC):
- """This class is an abstract base class (ABC) for datasets.
-
- To create a subclass, you need to implement the following four functions:
- -- <__init__>: initialize the class, first call BaseDataset.__init__(self, opt).
- -- <__len__>: return the size of dataset.
- -- <__getitem__>: get a data point.
- -- : (optionally) add dataset-specific options and set default options.
- """
-
- def __init__(self, opt):
- """Initialize the class; save the options in the class
-
- Parameters:
- opt (Option class)-- stores all the experiment flags; needs to be a subclass of BaseOptions
- """
- self.opt = opt
- # self.root = opt.dataroot
- self.current_epoch = 0
-
- @staticmethod
- def modify_commandline_options(parser, is_train):
- """Add new dataset-specific options, and rewrite default values for existing options.
-
- Parameters:
- parser -- original option parser
- is_train (bool) -- whether training phase or test phase. You can use this flag to add training-specific or test-specific options.
-
- Returns:
- the modified parser.
- """
- return parser
-
- @abstractmethod
- def __len__(self):
- """Return the total number of images in the dataset."""
- return 0
-
- @abstractmethod
- def __getitem__(self, index):
- """Return a data point and its metadata information.
-
- Parameters:
- index - - a random integer for data indexing
-
- Returns:
- a dictionary of data with their names. It ususally contains the data itself and its metadata information.
- """
- pass
-
-
-def get_transform(grayscale=False):
- transform_list = []
- if grayscale:
- transform_list.append(transforms.Grayscale(1))
- transform_list += [transforms.ToTensor()]
- return transforms.Compose(transform_list)
-
-def get_affine_mat(opt, size):
- shift_x, shift_y, scale, rot_angle, flip = 0., 0., 1., 0., False
- w, h = size
-
- if 'shift' in opt.preprocess:
- shift_pixs = int(opt.shift_pixs)
- shift_x = random.randint(-shift_pixs, shift_pixs)
- shift_y = random.randint(-shift_pixs, shift_pixs)
- if 'scale' in opt.preprocess:
- scale = 1 + opt.scale_delta * (2 * random.random() - 1)
- if 'rot' in opt.preprocess:
- rot_angle = opt.rot_angle * (2 * random.random() - 1)
- rot_rad = -rot_angle * np.pi/180
- if 'flip' in opt.preprocess:
- flip = random.random() > 0.5
-
- shift_to_origin = np.array([1, 0, -w//2, 0, 1, -h//2, 0, 0, 1]).reshape([3, 3])
- flip_mat = np.array([-1 if flip else 1, 0, 0, 0, 1, 0, 0, 0, 1]).reshape([3, 3])
- shift_mat = np.array([1, 0, shift_x, 0, 1, shift_y, 0, 0, 1]).reshape([3, 3])
- rot_mat = np.array([np.cos(rot_rad), np.sin(rot_rad), 0, -np.sin(rot_rad), np.cos(rot_rad), 0, 0, 0, 1]).reshape([3, 3])
- scale_mat = np.array([scale, 0, 0, 0, scale, 0, 0, 0, 1]).reshape([3, 3])
- shift_to_center = np.array([1, 0, w//2, 0, 1, h//2, 0, 0, 1]).reshape([3, 3])
-
- affine = shift_to_center @ scale_mat @ rot_mat @ shift_mat @ flip_mat @ shift_to_origin
- affine_inv = np.linalg.inv(affine)
- return affine, affine_inv, flip
-
-def apply_img_affine(img, affine_inv, method=Image.BICUBIC):
- return img.transform(img.size, Image.AFFINE, data=affine_inv.flatten()[:6], resample=Image.BICUBIC)
-
-def apply_lm_affine(landmark, affine, flip, size):
- _, h = size
- lm = landmark.copy()
- lm[:, 1] = h - 1 - lm[:, 1]
- lm = np.concatenate((lm, np.ones([lm.shape[0], 1])), -1)
- lm = lm @ np.transpose(affine)
- lm[:, :2] = lm[:, :2] / lm[:, 2:]
- lm = lm[:, :2]
- lm[:, 1] = h - 1 - lm[:, 1]
- if flip:
- lm_ = lm.copy()
- lm_[:17] = lm[16::-1]
- lm_[17:22] = lm[26:21:-1]
- lm_[22:27] = lm[21:16:-1]
- lm_[31:36] = lm[35:30:-1]
- lm_[36:40] = lm[45:41:-1]
- lm_[40:42] = lm[47:45:-1]
- lm_[42:46] = lm[39:35:-1]
- lm_[46:48] = lm[41:39:-1]
- lm_[48:55] = lm[54:47:-1]
- lm_[55:60] = lm[59:54:-1]
- lm_[60:65] = lm[64:59:-1]
- lm_[65:68] = lm[67:64:-1]
- lm = lm_
- return lm
diff --git a/spaces/dev-andres/Caracola-app/app.py b/spaces/dev-andres/Caracola-app/app.py
deleted file mode 100644
index ed8e282d931e935a0849be7855b483fe331d71e1..0000000000000000000000000000000000000000
--- a/spaces/dev-andres/Caracola-app/app.py
+++ /dev/null
@@ -1,1047 +0,0 @@
-# cargamos el modelo de huggingsound
-import re
-import unidecode
-#from huggingsound import SpeechRecognitionModel
-#from models.model import *
-def sim_jac(s1, s2):
-
- bigrams_s1 = []
- bigrams_s2 = []
-
- for i in range(len(s1) - 1):
- bigrams_s1.append(s1[i:i+2])
-
- for i in range(len(s2) - 1):
- bigrams_s2.append(s2[i:i+2])
-
- c_common = 0
-
- for i in bigrams_s1:
- if bigrams_s2.count(i) > 0:
- c_common += 1
-
- return c_common / ((len(s1) - 1) + (len(s2) - 1) - c_common)
-
-def encontrar_palabras(transcript,cjto_palabras):
- '''
- Toma un string (en minúsculas) y un conjunto de palabras. Busca el primer match
- de cjto_palabras en transcript y particiona el string en:
- 1. El slice de la cadena antes del primer match (antes_palabra)
- 2. La cadena del primer match (coincidencia de cjto_palabras)
- 3. El slice de la cadena después del match (despues_palabra)
- '''
- inicio,final=list(re.finditer(r'|'.join(cjto_palabras),transcript))[0].span()
- antes_palabra=transcript[:inicio].strip()
- despues_palabra=transcript[final:].strip()
- palabra=transcript[inicio:final]
- return antes_palabra,palabra,despues_palabra
-
-
-def agregar_adentro(codigo, transcipcion):
- codigo2 = main(transcipcion)
-
- return codigo[:-1] + codigo2
-
-
-import numpy as np
-
-def main(instruccion):
- global bloque
-
- plantillas = [
- crear_funcion,
- crear_condicional,
- crear_condicional,
- asignar_variable,
- crear_variable,
- crear_llamada,
- crear_for,
- fin_de_bloque,
- crear_comentario,
- crear_regresa
- ]
-
- comandos = [set(['definir', 'funcion', 'parametros']),
- set(['mientras']),
- set(['si']), # si se cumple / mientras se cumpla
- set(['asignar', 'con']),
- set(['definir', 'variable']),
- set(['ejecuta', 'argumentos']),
- set(['para', 'rango']),
- set(['terminar','bloque']),
- set(['comentario']),
- set(['regresa'])
-
- ]
-
- J = []
- for comando in comandos:
- J.append(len(set(instruccion.strip().split(' ')).intersection(comando)) / len(set(instruccion.strip().split(' ')).union(comando)))
- # print(J,np.argmax(J))
- pos_func=np.argmax(J)
- # print(pos_func)
- return plantillas[pos_func](instruccion)
-
-#------------------------------------------------
-#from models.plantillas_codigo import *
-import re
-
-def crear_funcion(instruccion):
- """
- Crea el template de la estructura de una función
-
- Parametros
- ----------
- instrucion: str
- La intruccion de voz en texto.
-
- Regresa
- ---------
- output: str
- Codigo generado
- recomendacion: str
- Una sugerencia o fallo
- """
-
- global indentacion
- global recomendacion
- global bloque
-
- bloque='funcion'
-
- # guarda los avisos o recomendaciones que el programa te hace
- recomendacion = ''
-
- # guarda la línea de código
- output = ''
-
- # pivote que ayuda a definir el nombre de una función
- before_keyword, keyword, after_keyword = instruccion.partition('nombre')
-
- # verifica que haya o esté escrita la frase "nombre"
- if len(after_keyword) == 0:
- recomendacion = f'¡No me dijiste el nombre de la función!'
-
- # de otro modo, si tiene nombre la función
- else:
-
- # obtenemos el nombre de la función por el usuario
- name_func = after_keyword.split(' ')[1]
-
- # verificamos si no desea poner parametros
- if instruccion.strip().split(' ')[-1] == name_func:
- parametros = ''
-
- # de otro modo, si desea una función con parámetros
- else:
- before_keyword, keyword, after_keyword = instruccion.partition('parametros')
-
- # verifica que si exista el nombre de los parámetros
- if len(after_keyword) == 0:
- parametros = ''
- recomendacion = f'¡No me dijiste el nombre de los parámetros!'
-
- # escribe como parámetros todo lo que está después de "parámetros"
- else:
- candidatos = []
- cadena_separada = after_keyword.strip().split(' ')
-
- for palabra in cadena_separada:
- try:
- candidatos.append(diccionario_fonetico[palabra])
- except:
- continue
-
- if len(candidatos) == 0:
- parametros = after_keyword.split(' ')[1:]
- parametros = ', '.join(parametros)
-
- else:
- parametros = ', '.join(candidatos)
-
- # indenta aunque marque que detecte que no le dije parámetros
- if not recomendacion or recomendacion == '¡No me dijiste el nombre de los parámetros!':
- indentacion += 1
-
- # concatenación del nombre y parámetros de la función
- output = f'def {name_func}({parametros}):
' + ' ' * indentacion + '|'
- return output
-
-import re
-def encontrar_palabras(transcript,cjto_palabras):
-
- """
- Toma un string (en minúsculos) y un conjunto de palabras. Busca el primer match
- de cjto_palabras en transcript y particiona el string
-
- Parametros
- ----------
- transcript: str
- La intruccion de voz en texto ya en minúsculas.
- cjto_palabras: list(str)
- Lista de strings donde se comienza a dividir el transcript original
-
- Regresa
- ---------
- output: list(str)
- [antes_palabra,palabra,despues_palabra]
-
- antes_palabra: string que está antes de la palabra de interés (de cjto_palabras)
- palabra: string que da la palabra clave donde dividimos
- despues_palabra: string que está después de la palabra
-
- Ejemplo
- --------
- encontrar_palabras('variable india producto variable alfa',['producto','suma','menos','entre'])
- >> ['variable india','producto',' variable alfa]
- """
- inicio,final=list(re.finditer(r'|'.join(cjto_palabras),transcript))[0].span()
- antes_palabra=transcript[:inicio].strip()
- despues_palabra=transcript[final:].strip()
- palabra=transcript[inicio:final]
- return antes_palabra,palabra,despues_palabra
-
-def crear_condicional(transcript):
- '''
- Toma el transcript de un enunciado condicional y regresa su traducción a código en Python
-
- Parametros
- ----------
- transcript: str
- La intruccion de voz en texto ya en minúsculas.
-
-
- Regresa
- ---------
- output: str
- Cadena con el código en python, tiene una línea al final y un pipe
- que representa el prompt donde se seguirá escribiendo
-
- Ejemplo
- --------
- crear_condicional('mientras variable india sea menor igual a numero seis')
- >> while (i<=6):
- >> |
- '''
- global indentacion
- global bloque
-
- keyword_mapeo={'mientras':'while','si':'if','contrario':'else'}
- antes_keyword,keyword,desp_keyword=encontrar_palabras(transcript,keyword_mapeo.keys())
- cadena=keyword_mapeo[keyword]
- bloque = keyword
-
- if cadena=='else':
- indentacion=indentacion+1
- return 'else:'+'\n' +'\t'* indentacion+'|'
-
- # Primera división
- condicional_mapeo={'menor estricto':'<','menor o igual':'<=','igual':'==','diferente':'!='
- ,'mayor estricto':'>','mayor o igual':'>='}
- cjto_condicional=condicional_mapeo.keys()
- antes_condicional,palabra_condicional,despues_condicional=encontrar_palabras(transcript,cjto_condicional)
-
-
- # Buscar antes en la lista de variables
- a_var,var,d_var=encontrar_palabras(antes_condicional,['variable'])
- nombre_var=d_var.split(' ')[0]
-
- if diccionario_fonetico.get(nombre_var,False):
- nombre_var=diccionario_fonetico[nombre_var]
-
-
- cadena+=' '+nombre_var+' ' +condicional_mapeo[palabra_condicional]
-
- # Buscar en despues_condicional el número
-
- valor=despues_condicional.split(' ')[-1]
-
- if dict_numeros.get(valor,False):
- valor=str(dict_numeros[valor])
-
- indentacion+=1
- #t = f' '
- return f'{keyword_mapeo[keyword]} {nombre_var} {condicional_mapeo[palabra_condicional]} {valor}:'+'
' +' '* indentacion+'|'
-
-
-
-def crear_cadena(transcript):
- """
- Toma el transcript de un enunciado que contiene una cadena y regresa el código en Python.
- Para usarse cuando ya se sabe que transcript sólo es los límites de la cadena
-
- Parametros
- ----------
- transcript: str
- La intruccion de voz en texto ya en minúsculas.
-
-
- Regresa
- ---------
- output: list(str)
- antes_palabra:parte del transcript que va antes de las comillas
- palabra: Cadena con el código en python de las comillas y lo que está adentro
- despues_palabra:parte del transcript que va antes de las comillas
-
- Ejemplo
- --------
- crear_cadena('ejecuta print con argumentos variable India producto cadena guion cadena')[1]
- >> ['ejecuta print con argumentos variable India producto','"guion"','']
- """
- try:
- inicio,final=list(re.finditer(r"cadena (.+) cadena",transcript))[0].span()
- except:
- return ''
- antes_palabra=transcript[:inicio].strip()
- despues_palabra=transcript[final:].strip()
- palabra=list(re.finditer(r"cadena (.+) cadena",transcript))[0].group(1)
- return antes_palabra,f'"{palabra}"',despues_palabra
-
-def crear_var_existente(transcript):
- """
- Toma el transcript de un enunciado que contiene la mención de una variable
- y devuelve dicha variable
-
- Parametros
- ----------
- transcript: str
- La intruccion de voz en texto ya en minúsculas.
-
-
- Regresa
- ---------
- output: str
- palabra: Cadena con el código en python del nombre de la variable
-
- Ejemplo
- --------
- crear_var_existente('ejecuta print con argumentos variable india producto cadena guión cadena')
- >> i
- """
- try:
- antes_var,var,desp_var=encontrar_palabras(transcript,['variable'])
- except:
- return ''
-
- nombre_var=desp_var.split(' ')[0]
- if diccionario_fonetico.get(nombre_var,False):
- nombre_var=diccionario_fonetico[nombre_var]
-
- return nombre_var
-
-
-# TODO: Hay que ver:
- # Si es otra operación hay que llamar la función recursivamente en cada pedazo
- # 1. si es cadena
- # 2. si es otra operación. Para esto, hay que regresar error o algo así cuando no se encuentre
-def crear_operacion(transcript):
- '''
-
- Toma el transcript de una operación binaria y la traduce a código de Python.
- Para traducir las variables que se usan en la operación binaria busca
- si son cadenas o sólo menciones de variables usando las funciones
- crear_cadena y crear_var_existente
-
- Parametros
- ----------
- transcript: str
- La intruccion de voz en texto ya en minúsculas.
-
-
- Regresa
- ---------
- output: str
- Cadena con el código en python
-
- Ejemplo
- --------
- crear_operacion('variable India producto cadena guión cadena')
- >> i*'-'
- '''
- global dict_operaciones
-
-
- try:
- antes_op,op,desp_op=encontrar_palabras(transcript,dict_operaciones.keys())
- except:
- return ''
-
- # Buscamos la información en la cadena detrás del operador
- cadena_izq=crear_var_existente(antes_op)
- try:
- cadena_izq+=f'{crear_cadena(antes_op)[1]}'
- except:
- cadena_izq+=''
-
- if len(cadena_izq)==0:
- nombre_var=antes_op.split(' ')[-1]
- if dict_numeros.get(nombre_var,False):
- nombre_var=dict_numeros[nombre_var]
- cadena_izq+=str(nombre_var)
-
- # Buscamos la información en la cadena después del operador
- cadena_der=crear_var_existente(desp_op)
- try:
- cadena_der+=f'{crear_cadena(desp_op)[1]}'
- except:
- cadena_der+=''
-
- if len(cadena_der)==0:
- nombre_var=desp_op.split(' ')[0]
- if dict_numeros.get(nombre_var,False):
- nombre_var=dict_numeros[nombre_var]
- if diccionario_fonetico.get(nombre_var,False):
- nombre_var=diccionario_fonetico[nombre_var]
- cadena_der+=str(nombre_var)
-
-
- return f'{cadena_izq} {dict_operaciones[op]} {cadena_der}'
-
-
-def crear_llamada(transcript):
- """
- Toma el transcript de la llamada de una función y la convierte en código de Python
- Hace uso de las funciones que detectan operaciones, variables y comillas
- ,para cada argumento de la función
-
- Parametros
- ----------
- transcript: str
- La intruccion de voz en texto ya en minúsculas.
-
-
- Regresa
- ---------
- output: str
- Cadena con el código en python
-
- Ejemplo
- --------
- crear_llamada(ejecuta print con argumentos variable India producto cadena guión cadena
- coma cadena hola cadena')
- >> print(i*'-','hola')
-
- """
- global bloque
- global indentacion
-
- bloque='llamada'
- try:
- antes_ej,ej,desp_ej=encontrar_palabras(transcript,['ejecuta'])
- except:
- return ''
- funcion_nombre=desp_ej.split(' ')[0]
- # Aquí tal vez valdría la pena tener un registro de las funciones previamente definidas para
- # poder buscar en un directorio con Jaccard y no aproximar
-
- antes_arg,keyword,desp_arg=encontrar_palabras(desp_ej,['argumentos','parametros'])
-
- argumentos=desp_arg.split('coma')
- lista_cadenas=[]
- for arg in argumentos:
- arg=arg.strip()
- cadena_arg=''
- # print('arg',arg)
- # Caso cuando es operacion
- cadena_op=crear_operacion(arg)
- cadena_var=crear_var_existente(arg)
- cadena_cadena=crear_cadena(arg)
- if len(cadena_op)!=0:
- lista_cadenas.append(cadena_op)
- elif len(cadena_var)!=0:
- lista_cadenas.append(cadena_var)
- elif len(cadena_cadena)!=0:
- lista_cadenas.append(cadena_cadena[1])
- else:
- nombre_var=arg
- if dict_numeros.get(nombre_var,False):
- nombre_var=str(dict_numeros[nombre_var])
-
- lista_cadenas.append(nombre_var)
-
- # Caso cuando es variable
-
- cadena_final=','.join(lista_cadenas)
- cadena=f'{funcion_nombre}({cadena_final})
'+' '*indentacion+'|'
-
- return cadena
-
-def crear_regresa(transcript):
- antes_reg,reg,desp_reg=encontrar_palabras(transcript,['regresa'])
-
- arg=desp_reg.strip()
- cadena_arg=''
-
- # Si es llamada
- cadena_llamada=crear_llamada(arg)
- # Caso cuando es operacion
- cadena_op=crear_operacion(arg)
- cadena_var=crear_var_existente(arg)
- cadena_cadena=crear_cadena(arg)
-
- cadena_final=''
- if len(cadena_llamada)!=0:
- cadena_final+=cadena_llamada[:-2]
- elif len(cadena_op)!=0:
- cadena_final+=cadena_op
- elif len(cadena_var)!=0:
- cadena_final+=cadena_var
- elif len(cadena_cadena)!=0:
- cadena_final+=cadena_cadena[1]
- else:
- nombre_var=arg
- if dict_numeros.get(nombre_var,False):
- nombre_var=str(dict_numeros[nombre_var])
-
- cadena_final+=nombre_var
- global indentacion
- indentacion-=1
- return f'return {cadena_final}
'+' '*indentacion+'|'
-
-
-def crear_variable(instruccion):
- """
- Estructura:
- definir variable con nombre [nombre_variable] igual a /*objeto_basico* valor/
-
- Parametros
- ----------
- instrucion: str
- La intruccion de voz en texto.
-
- Regresa
- ---------
- output: str
- Codigo generado
- recomendacion: str
- Una sugerencia o fallo
-
- Testing
- -------
- >>> definir variable con nombre india igual a numero uno
- >>> definir variable con nombre i igual a numero 1 (int)
- >>> definir variable con nombre i igual a flotante tres punto cinco (float)
- >>> definir variable con nombre i igual a cadena hola (string)
- >>> definir variable con nombre i igual a lista/dic (string)
- """
- global indentacion
- global bloque
-
- bloque='variable'
-
- # pivote que ayuda a definir el nombre de la variable
- before_keyword, keyword, after_keyword = instruccion.partition('nombre')
- after_keyword_list = after_keyword.strip().split(' ')
- # [india igual a numero uno]
- name_variable = after_keyword_list[0]
-
- # Como sabemos que despues del nombre va seguido de "igual a"
- tipo_dato = after_keyword_list[3]
- #print(after_keyword_list[4:]) -> lista
- valor = tipos_datos[tipo_dato](after_keyword_list[4:])
-
- # Verificamos si es una palabra fonetica
- if diccionario_fonetico.get(name_variable,False):
- name_variable=diccionario_fonetico[name_variable]
-
- codigo_generado = f'{name_variable} = {valor}
'+ ' ' * indentacion + '|'
- return codigo_generado
-
-
-def asignar_variable(instruccion):
- """
- Asigna una variable (eg. indio = indio + 1)
-
- Parametros
- ----------
- instrucion: str
- La intruccion de voz en texto.
-
- Regresa
- ---------
- output: str
- Codigo generado (indio = indio + 1)
-
- Testing
- --------
- >>>'asignar variable india con india suma uno',
- >>>'asignar variable contador con contador menos uno',
- >>>'asignar variable contador con alfa',
- >>>'asignar variable india con india',
-
- """
- global bloque
- bloque = "asignar"
-
- before_keyword, keyword, after_keyword = instruccion.partition('variable')
- after_keyword_list = after_keyword.strip().split(' ')
- name_variable = after_keyword_list[0]
- start = after_keyword_list.index('con') + 1
- operacion = after_keyword_list[start:]
- if len(operacion) != 1:
- operacion_str = crear_operacion(keyword + ' ' + ' '.join(operacion))
- else:
- operacion_str = operacion[0]
- # Verificamos si es una palabra fonetica para lado derecho de la
- # asignacion
- if diccionario_fonetico.get(operacion_str,False):
- operacion_str=diccionario_fonetico[operacion_str]
-
- # Verificamos si es una palabra fonetica
- if diccionario_fonetico.get(name_variable,False):
- name_variable=diccionario_fonetico[name_variable]
-
- codigo_generado = f'{name_variable} = {operacion_str}
'+ ' ' * indentacion + '|'
- return codigo_generado
-
-
-def crear_for(instruccion):
- """
- Crea el template de la estructura de un ciclo for.
-
- Parámetros
- ----------
- instrucción: str
- La intrucción de voz en texto.
-
- Regresa
- ---------
- output: str
- Estructura del ciclo for
- recomendacion: str
- Una sugerencia o error
- """
- global bloque
- global indentacion
- global recomendacion
-
- bloque='for'
- vocabulario_basico = ['iteracion', 'rango']
-
- # verificamos si la frase cumple los requisitos
- instruccion_tokens = instruccion.strip().split(' ')
-
- for i in vocabulario_basico:
- try:
- instruccion_tokens.index(i)
- except:
- recomendacion = 'Parece que quieres una iteración pero no reconozco tus comandos, inténtalo de nuevo'
- return f'', recomendacion
-
- # guarda los avisos o recomendaciones que el programa te hace
- recomendacion = ''
-
- # guarda la línea de código
- output = ''
-
- # pivote que ayuda a definir el rango e iterador
- before_keyword, keyword, after_keyword = instruccion.partition('iteracion')
-
- if after_keyword.strip().split(' ')[1] in diccionario_fonetico:
- iterador = diccionario_fonetico[after_keyword.strip().split(' ')[1]]
-
- else:
- iterador = after_keyword.strip().split(' ')[1]
-
- before_keyword, keyword, after_keyword = instruccion.partition('rango')
-
- limites = []
-
-
- for i, item in enumerate(after_keyword.strip().split(' ')):
- try:
- limites.append(dict_numeros[item])
- except:
- continue
-
- if len(limites) == 0:
- for i, item in enumerate(after_keyword.strip().split(' ')):
- try:
- limites.append(diccionario_fonetico[item])
- except:
- continue
-
- indentacion += 1
-
- if len(limites) == 0:
- return f''
-
- elif len(limites) == 1:
- return f'for {iterador} in range({limites[-1]}):
' + ' ' * indentacion + '|'
-
- elif len(limites) == 2:
- return f'for {iterador} in range({limites[0]}, {limites[1]}):
' + ' ' * indentacion + '|'
-
- elif len(limites) >= 2:
- recomendacion = 'Me dictaste más de un número en el rango pero tomé los dos primeros'
- return f'for {iterador} in range({limites[0]}, {limites[1]}):
' + ' ' * indentacion + '|'
-
-def crear_comentario(instruccion):
- """
- Agrega el comentario de la intrucción en una línea de código
-
- Parámetros
- ----------
- instrucción: str
- La intrucción de voz en texto.
-
- Regresa
- ---------
- output: str
- Comentario
- """
-
- global bloque
- global indentacion
-
- # guarda los avisos o recomendaciones que el programa te hace
- recomendacion = ''
- bloque = 'comentario'
- # guarda la línea de código
- output = ''
-
- before_keyword, keyword, after_keyword = instruccion.partition('comentario')
-
- return '' + '# ' + after_keyword + '' + '
' + ' ' * indentacion + '|'
-
-def fin_de_bloque(transcripcion):
- global indentacion
- global bloque
- bloque='fin'
- indentacion=indentacion-1
- return '|'
-
-
-#------------------------------------
-#from models.variables_globales import *
-def numero(text):
- """Convierte un texto de numero en numero entero (int)
-
- Parametros
- ----------
- text: list
- Serie de valores
-
- Regresa
- ---------
- dict_numeros: int
- El número correspondiente
- """
- global dict_numeros
- # Como sabemos que siempre sera el primer elemento el valor despues
- # de número (eg. cuatro or veintecinco)
- numero_str = text[0]
- return dict_numeros[numero_str]
-
-def flotante(text):
- """Convierte un texto de numero en numero floatante (float)
-
- Parametros
- ----------
- text: list
- Serie de valores
-
- Regresa
- ---------
- dict_numeros: float
- El número correspondiente en floatante (eg 3.4)
- """
- global dict_numeros
- text = " ".join(text)
- before_keyword, keyword, after_keyword = text.partition('punto')
- print(before_keyword)
- print(after_keyword)
-
- # Obtenemos los dos numeros antes y despues del punto
- before_num = before_keyword.strip().split(' ')[0]
- after_num = after_keyword.strip().split(' ')[0]
-
- # Hacemos el mapeo uno -> 1
- num1_int = dict_numeros[before_num]
- num2_int = dict_numeros[after_num]
-
- return float(str(num1_int) + '.' + str(num2_int))
-
-def cadena(text):
- """Convierte un texto de numero en string (str)
-
- Parametros
- ----------
- text: list
- Serie de valores
-
- Regresa
- ---------
- string: str
- Una cadena con el contenido del texto
- """
- numero_str = text[:]
- return ' '.join(text)
-
-def lista(text):
- """Convierte un texto de numero en string (str)
-
- Parametros
- ----------
- text: list
- Serie de valores
-
- Regresa
- ---------
- lista: list
- Una lista vacia
- """
- return []
-
-
-
-diccionario_fonetico={'andrea':'a',
- 'bravo':'b',
- 'carlos':'c',
- 'delta':'d',
- 'eduardo':'e',
- 'fernando':'f',
- 'garcia':'g',
- 'hotel':'h',
- 'india':'i',
- 'julieta':'j',
- 'kilo':'k',
- 'lima':'l',
- 'miguel':'m',
- 'noviembre':'n',
- 'oscar':'o',
- 'papa':'p',
- 'queretaro':'q',
- 'romero':'',
- 'sierra':'s',
- 'tango':'t',
- 'uniforme':'u',
- 'victor':'v',
- 'wafle':'w',
- 'equis':'x',
- 'yarda':'y',
- 'llarda':'y',
- 'espacio':' '}
-
-# Separa en operadores comunes
-
-# si esto se lematiza puedes agarrar todas las frases de la forma suma, sumar, etc.
-dict_operaciones={
- 'producto':'*','mas':'+','menos':'-','concatena':'+','entre':'/','modulo':'%'
- }
-
-dict_numeros = {
- 'cero':0,
- 'uno': 1,
- 'dos': 2,
- 'tres': 3,
- 'cuatro':4,
- 'cinco': 5,
- 'seis': 6,
- 'siete': 7,
- 'ocho': 8,
- 'nueve': 9,
- 'diez': 10,
- 'once': 11,
- 'doce': 12,
- 'trece': 13,
- 'catorce': 14,
- 'quince': 15,
- 'dieciseis': 16,
- 'diecisiete': 17,
- 'dieciocho': 18,
- 'diecinueve': 19,
- 'veinte': 20,
- 'treinta': 30,
- 'cuarenta': 40,
- 'cicuenta': 50,
-}
-
-# Diccionario de funciones
-tipos_datos ={
- 'natural': numero,
- 'flotante': flotante,
- 'cadena': cadena,
- 'lista': lista,
-}
-
-#--------------------------
-
-from transformers import pipeline
-import gradio as gr
-
-# creación del modelo
-# model = SpeechRecognitionModel("patrickvonplaten/wav2vec2-large-xlsr-53-spanish-with-lm")
-p = pipeline("automatic-speech-recognition", "patrickvonplaten/wav2vec2-large-xlsr-53-spanish-with-lm")
-
-tabla='''
-
-
-
-
- Fonético
- andrea
- bravo
- carlos
- delta
- eduardo
- fernando
- garcia
- hotel
- india
- julieta
- kilo
- lima
- miguel
- noviembre
-
-
-
-
- Letra
- a
- b
- c
- d
- e
- f
- g
- h
- i
- j
- k
- l
- m
- n
-
-
-
-
-
-
-
-
-
-
-
-
-
-
-
-
-
-
-
- Fonético
- oscar
- papa
- queretaro
- romero
- sierra
- tango
- uniforme
- victor
- waffle
- equis
- yarda
- zapato
-
-
-
-
- Letra
- o
- p
- q
- r
- s
- t
- u
- v
- w
- x
- y
- z
-
-
-
-
-
-'''
-
-
-# Variables globales
-bloque = '' # Define el contexto (si es función, condicional, ciclo, etc.)
-codigo = None # Guarda el código hasta el momento
-indentacion = 0 # Nivel de indentación
-linea_codigo = 0 # Esto para dar seguimiento al eliminado de una linea
-recomendacion = ""
-# fin_de_bloque=False
-
-import gradio as gr
-
-def transcribe(audio, Español, Codigo_Python):
- global bloque
- global codigo
- global indentacion
-
- #transcriptions_es = model.transcribe([audio])[0]
- transcriptions_es = p(audio)['text']
-
- # quitamos el acento de la transcripcion
- frase = unidecode.unidecode(transcriptions_es).lower()
-
- # print(frase)
- if not bloque:
- # Significa que es la primera vez
- codigo = main(frase)
- else:
- codigo = agregar_adentro(codigo, frase)
-
- return codigo, frase
-
-inputs = gr.inputs.Audio(label="Dar click para grabar tu voz", type="filepath", source="microphone")
-output1 = gr.outputs.Textbox(label="Asi se ve tu código")
-output2 = gr.outputs.Textbox(label="Lo que entendió la caracola fue:")
-
-title = "Caracola App"
-description = 'Aplicación que ayuda a programar a traves de tu voz.\nSe usa el siguiente diccionario fonético para capturar las variables de una letra.
'+tabla+'
Instrucciones
Selecciona uno de los ejemplos y da click en enviar para convertir comandos de voz en código!
'
-# ,'mientras variable alpha es menor igual a numero dos'
-# ,'Definir variable con nombre india igual a numero uno'
-input2 = gr.inputs.Textbox(lines=0, placeholder="Aqui aparece el texto en español de los ejemplos")
-input3 = gr.inputs.Textbox(lines=0, placeholder="Aqui aparece el codigo en python de los ejemplos")
-
-output_html = gr.outputs.HTML(label='Asi se ve tu código:')
-
-examples = [
- ['./wav/comentario.wav','agregar comentario mi primer función', '# mi primer funcion'],
- ['./wav/funcion.wav','definir función con nombre mágica y parámetros noviembre', 'def magica(n):'],
- ['./wav/definira.wav','definir variable con nombre andrea igual a natural cero', 'a=0'],
- ['./wav/definirb.wav','definir variable con nombre bravo igual a natural uno', 'b = 1'],
- ['./wav/iteracion.wav','ejecuta iteracion para india en un rango noviembre', 'for i in range(n)'],
- ['./wav/asignar_c_b.wav','asignar variable carlos con bravo', 'c=b'],
- ['./wav/andreabravo.wav','asignar variable bravo con andrea mas bravo', 'b = a + b'],
- ['./wav/asignar_a_c.wav','asignar variable andrea con carlos', 'a=c'],
- ['./wav/terminar_bloque.wav','terminar bloque',''],
- ['./wav/comentario2.wav','agregar comentario fin de ciclo', '# fin de ciclo'],
- ['./wav/regresa.wav','regresa variable andrea', 'return a'],
- ['./wav/llamada.wav', 'ejecuta mágica con argumentos diez', 'magica(10)']
- ]
-
-article = " Repositorio de la app"
-demo = gr.Interface(fn=transcribe, inputs=[inputs, input2, input3], outputs=[output_html,output2],
- examples=examples,
- title=title, description=description, article=article,
- allow_flagging="never", theme="darkpeach",
- )
-
-demo.launch()
\ No newline at end of file
diff --git a/spaces/devoworm-group/nucleus_segmentor/README.md b/spaces/devoworm-group/nucleus_segmentor/README.md
deleted file mode 100644
index 8aee659bf6ac22fabab6c5f7ed991ba881c8ee2a..0000000000000000000000000000000000000000
--- a/spaces/devoworm-group/nucleus_segmentor/README.md
+++ /dev/null
@@ -1,15 +0,0 @@
----
-title: Nucleus Segmentor
-emoji: 😻
-colorFrom: gray
-colorTo: purple
-sdk: streamlit
-sdk_version: 1.17.0
-app_file: app.py
-pinned: false
----
-
-
-# devolearn-web
-devolearn model nucleus_segmentor deployed on a webapp
-
diff --git a/spaces/diacanFperku/AutoGPT/Clip Studio Paint EX 1.9.4 Crack BETTER.md b/spaces/diacanFperku/AutoGPT/Clip Studio Paint EX 1.9.4 Crack BETTER.md
deleted file mode 100644
index 8dc56d4d728fa263a3f6f1363bad2e22a8a7a743..0000000000000000000000000000000000000000
--- a/spaces/diacanFperku/AutoGPT/Clip Studio Paint EX 1.9.4 Crack BETTER.md
+++ /dev/null
@@ -1,6 +0,0 @@
-Clip Studio Paint EX 1.9.4 Crack
Download File ►►► https://gohhs.com/2uFV2B
-
- 4fefd39f24
-
-
-
diff --git a/spaces/diacanFperku/AutoGPT/Cubase 6 6.0.7 Working Crack Team AiR Torrent HOT.md b/spaces/diacanFperku/AutoGPT/Cubase 6 6.0.7 Working Crack Team AiR Torrent HOT.md
deleted file mode 100644
index 401073ddfdda4d3d48a65d801621e58ac5a808d3..0000000000000000000000000000000000000000
--- a/spaces/diacanFperku/AutoGPT/Cubase 6 6.0.7 Working Crack Team AiR Torrent HOT.md
+++ /dev/null
@@ -1,6 +0,0 @@
-Cubase 6 6.0.7 Working Crack Team AiR torrent
Download ✔ https://gohhs.com/2uFVOM
-
- 4d29de3e1b
-
-
-
diff --git a/spaces/diffusers/sdxl-to-diffusers/utils.py b/spaces/diffusers/sdxl-to-diffusers/utils.py
deleted file mode 100644
index ff1c065d186347ca51b47d010a697dbe1814695c..0000000000000000000000000000000000000000
--- a/spaces/diffusers/sdxl-to-diffusers/utils.py
+++ /dev/null
@@ -1,6 +0,0 @@
-def is_google_colab():
- try:
- import google.colab
- return True
- except:
- return False
\ No newline at end of file
diff --git a/spaces/digitalxingtong/Jiaran-Bert-VITS2/preprocess_text.py b/spaces/digitalxingtong/Jiaran-Bert-VITS2/preprocess_text.py
deleted file mode 100644
index 44c35fecd9b7f21016e80e9597d6055254cba3f7..0000000000000000000000000000000000000000
--- a/spaces/digitalxingtong/Jiaran-Bert-VITS2/preprocess_text.py
+++ /dev/null
@@ -1,69 +0,0 @@
-import json
-from random import shuffle
-
-import tqdm
-from text.cleaner import clean_text
-from collections import defaultdict
-import shutil
-stage = [1,2,3]
-
-transcription_path = 'filelists/short_character_anno.list'
-train_path = 'filelists/train.list'
-val_path = 'filelists/val.list'
-config_path = "configs/config.json"
-val_per_spk = 4
-max_val_total = 8
-
-if 1 in stage:
- with open( transcription_path+'.cleaned', 'w', encoding='utf-8') as f:
- for line in tqdm.tqdm(open(transcription_path, encoding='utf-8').readlines()):
- try:
- utt, spk, language, text = line.strip().split('|')
- #language = "ZH"
- norm_text, phones, tones, word2ph = clean_text(text, language)
- f.write('{}|{}|{}|{}|{}|{}|{}\n'.format(utt, spk, language, norm_text, ' '.join(phones),
- " ".join([str(i) for i in tones]),
- " ".join([str(i) for i in word2ph])))
- except:
- print("err!", utt)
-
-if 2 in stage:
- spk_utt_map = defaultdict(list)
- spk_id_map = {}
- current_sid = 0
-
- with open( transcription_path+'.cleaned', encoding='utf-8') as f:
- for line in f.readlines():
- utt, spk, language, text, phones, tones, word2ph = line.strip().split('|')
- spk_utt_map[spk].append(line)
- if spk not in spk_id_map.keys():
- spk_id_map[spk] = current_sid
- current_sid += 1
- train_list = []
- val_list = []
- for spk, utts in spk_utt_map.items():
- shuffle(utts)
- val_list+=utts[:val_per_spk]
- train_list+=utts[val_per_spk:]
- if len(val_list) > max_val_total:
- train_list+=val_list[max_val_total:]
- val_list = val_list[:max_val_total]
-
- with open( train_path,"w", encoding='utf-8') as f:
- for line in train_list:
- f.write(line)
-
- file_path = transcription_path+'.cleaned'
- shutil.copy(file_path,'./filelists/train.list')
-
- with open(val_path, "w", encoding='utf-8') as f:
- for line in val_list:
- f.write(line)
-
-if 3 in stage:
- assert 2 in stage
- config = json.load(open(config_path))
- config['data']["n_speakers"] = current_sid #
- config["data"]['spk2id'] = spk_id_map
- with open(config_path, 'w', encoding='utf-8') as f:
- json.dump(config, f, indent=2, ensure_ascii=False)
diff --git a/spaces/dimaseo/dalle-mini/index.html b/spaces/dimaseo/dalle-mini/index.html
deleted file mode 100644
index 18e126ec5296f001b3ad5f5e82bc21f908dbbddc..0000000000000000000000000000000000000000
--- a/spaces/dimaseo/dalle-mini/index.html
+++ /dev/null
@@ -1,64 +0,0 @@
-
-
-
-
-
-
-
-
-
-
-
-
-
-
-
-
-
-
-
-
-
-
-
-
diff --git a/spaces/dineshreddy/WALT/mmdet/models/dense_heads/fovea_head.py b/spaces/dineshreddy/WALT/mmdet/models/dense_heads/fovea_head.py
deleted file mode 100644
index c8ccea787cba3d092284d4a5e209adaf6521c86a..0000000000000000000000000000000000000000
--- a/spaces/dineshreddy/WALT/mmdet/models/dense_heads/fovea_head.py
+++ /dev/null
@@ -1,341 +0,0 @@
-import torch
-import torch.nn as nn
-from mmcv.cnn import ConvModule, normal_init
-from mmcv.ops import DeformConv2d
-
-from mmdet.core import multi_apply, multiclass_nms
-from ..builder import HEADS
-from .anchor_free_head import AnchorFreeHead
-
-INF = 1e8
-
-
-class FeatureAlign(nn.Module):
-
- def __init__(self,
- in_channels,
- out_channels,
- kernel_size=3,
- deform_groups=4):
- super(FeatureAlign, self).__init__()
- offset_channels = kernel_size * kernel_size * 2
- self.conv_offset = nn.Conv2d(
- 4, deform_groups * offset_channels, 1, bias=False)
- self.conv_adaption = DeformConv2d(
- in_channels,
- out_channels,
- kernel_size=kernel_size,
- padding=(kernel_size - 1) // 2,
- deform_groups=deform_groups)
- self.relu = nn.ReLU(inplace=True)
-
- def init_weights(self):
- normal_init(self.conv_offset, std=0.1)
- normal_init(self.conv_adaption, std=0.01)
-
- def forward(self, x, shape):
- offset = self.conv_offset(shape)
- x = self.relu(self.conv_adaption(x, offset))
- return x
-
-
-@HEADS.register_module()
-class FoveaHead(AnchorFreeHead):
- """FoveaBox: Beyond Anchor-based Object Detector
- https://arxiv.org/abs/1904.03797
- """
-
- def __init__(self,
- num_classes,
- in_channels,
- base_edge_list=(16, 32, 64, 128, 256),
- scale_ranges=((8, 32), (16, 64), (32, 128), (64, 256), (128,
- 512)),
- sigma=0.4,
- with_deform=False,
- deform_groups=4,
- **kwargs):
- self.base_edge_list = base_edge_list
- self.scale_ranges = scale_ranges
- self.sigma = sigma
- self.with_deform = with_deform
- self.deform_groups = deform_groups
- super().__init__(num_classes, in_channels, **kwargs)
-
- def _init_layers(self):
- # box branch
- super()._init_reg_convs()
- self.conv_reg = nn.Conv2d(self.feat_channels, 4, 3, padding=1)
-
- # cls branch
- if not self.with_deform:
- super()._init_cls_convs()
- self.conv_cls = nn.Conv2d(
- self.feat_channels, self.cls_out_channels, 3, padding=1)
- else:
- self.cls_convs = nn.ModuleList()
- self.cls_convs.append(
- ConvModule(
- self.feat_channels, (self.feat_channels * 4),
- 3,
- stride=1,
- padding=1,
- conv_cfg=self.conv_cfg,
- norm_cfg=self.norm_cfg,
- bias=self.norm_cfg is None))
- self.cls_convs.append(
- ConvModule((self.feat_channels * 4), (self.feat_channels * 4),
- 1,
- stride=1,
- padding=0,
- conv_cfg=self.conv_cfg,
- norm_cfg=self.norm_cfg,
- bias=self.norm_cfg is None))
- self.feature_adaption = FeatureAlign(
- self.feat_channels,
- self.feat_channels,
- kernel_size=3,
- deform_groups=self.deform_groups)
- self.conv_cls = nn.Conv2d(
- int(self.feat_channels * 4),
- self.cls_out_channels,
- 3,
- padding=1)
-
- def init_weights(self):
- super().init_weights()
- if self.with_deform:
- self.feature_adaption.init_weights()
-
- def forward_single(self, x):
- cls_feat = x
- reg_feat = x
- for reg_layer in self.reg_convs:
- reg_feat = reg_layer(reg_feat)
- bbox_pred = self.conv_reg(reg_feat)
- if self.with_deform:
- cls_feat = self.feature_adaption(cls_feat, bbox_pred.exp())
- for cls_layer in self.cls_convs:
- cls_feat = cls_layer(cls_feat)
- cls_score = self.conv_cls(cls_feat)
- return cls_score, bbox_pred
-
- def _get_points_single(self, *args, **kwargs):
- y, x = super()._get_points_single(*args, **kwargs)
- return y + 0.5, x + 0.5
-
- def loss(self,
- cls_scores,
- bbox_preds,
- gt_bbox_list,
- gt_label_list,
- img_metas,
- gt_bboxes_ignore=None):
- assert len(cls_scores) == len(bbox_preds)
-
- featmap_sizes = [featmap.size()[-2:] for featmap in cls_scores]
- points = self.get_points(featmap_sizes, bbox_preds[0].dtype,
- bbox_preds[0].device)
- num_imgs = cls_scores[0].size(0)
- flatten_cls_scores = [
- cls_score.permute(0, 2, 3, 1).reshape(-1, self.cls_out_channels)
- for cls_score in cls_scores
- ]
- flatten_bbox_preds = [
- bbox_pred.permute(0, 2, 3, 1).reshape(-1, 4)
- for bbox_pred in bbox_preds
- ]
- flatten_cls_scores = torch.cat(flatten_cls_scores)
- flatten_bbox_preds = torch.cat(flatten_bbox_preds)
- flatten_labels, flatten_bbox_targets = self.get_targets(
- gt_bbox_list, gt_label_list, featmap_sizes, points)
-
- # FG cat_id: [0, num_classes -1], BG cat_id: num_classes
- pos_inds = ((flatten_labels >= 0)
- & (flatten_labels < self.num_classes)).nonzero().view(-1)
- num_pos = len(pos_inds)
-
- loss_cls = self.loss_cls(
- flatten_cls_scores, flatten_labels, avg_factor=num_pos + num_imgs)
- if num_pos > 0:
- pos_bbox_preds = flatten_bbox_preds[pos_inds]
- pos_bbox_targets = flatten_bbox_targets[pos_inds]
- pos_weights = pos_bbox_targets.new_zeros(
- pos_bbox_targets.size()) + 1.0
- loss_bbox = self.loss_bbox(
- pos_bbox_preds,
- pos_bbox_targets,
- pos_weights,
- avg_factor=num_pos)
- else:
- loss_bbox = torch.tensor(
- 0,
- dtype=flatten_bbox_preds.dtype,
- device=flatten_bbox_preds.device)
- return dict(loss_cls=loss_cls, loss_bbox=loss_bbox)
-
- def get_targets(self, gt_bbox_list, gt_label_list, featmap_sizes, points):
- label_list, bbox_target_list = multi_apply(
- self._get_target_single,
- gt_bbox_list,
- gt_label_list,
- featmap_size_list=featmap_sizes,
- point_list=points)
- flatten_labels = [
- torch.cat([
- labels_level_img.flatten() for labels_level_img in labels_level
- ]) for labels_level in zip(*label_list)
- ]
- flatten_bbox_targets = [
- torch.cat([
- bbox_targets_level_img.reshape(-1, 4)
- for bbox_targets_level_img in bbox_targets_level
- ]) for bbox_targets_level in zip(*bbox_target_list)
- ]
- flatten_labels = torch.cat(flatten_labels)
- flatten_bbox_targets = torch.cat(flatten_bbox_targets)
- return flatten_labels, flatten_bbox_targets
-
- def _get_target_single(self,
- gt_bboxes_raw,
- gt_labels_raw,
- featmap_size_list=None,
- point_list=None):
-
- gt_areas = torch.sqrt((gt_bboxes_raw[:, 2] - gt_bboxes_raw[:, 0]) *
- (gt_bboxes_raw[:, 3] - gt_bboxes_raw[:, 1]))
- label_list = []
- bbox_target_list = []
- # for each pyramid, find the cls and box target
- for base_len, (lower_bound, upper_bound), stride, featmap_size, \
- (y, x) in zip(self.base_edge_list, self.scale_ranges,
- self.strides, featmap_size_list, point_list):
- # FG cat_id: [0, num_classes -1], BG cat_id: num_classes
- labels = gt_labels_raw.new_zeros(featmap_size) + self.num_classes
- bbox_targets = gt_bboxes_raw.new(featmap_size[0], featmap_size[1],
- 4) + 1
- # scale assignment
- hit_indices = ((gt_areas >= lower_bound) &
- (gt_areas <= upper_bound)).nonzero().flatten()
- if len(hit_indices) == 0:
- label_list.append(labels)
- bbox_target_list.append(torch.log(bbox_targets))
- continue
- _, hit_index_order = torch.sort(-gt_areas[hit_indices])
- hit_indices = hit_indices[hit_index_order]
- gt_bboxes = gt_bboxes_raw[hit_indices, :] / stride
- gt_labels = gt_labels_raw[hit_indices]
- half_w = 0.5 * (gt_bboxes[:, 2] - gt_bboxes[:, 0])
- half_h = 0.5 * (gt_bboxes[:, 3] - gt_bboxes[:, 1])
- # valid fovea area: left, right, top, down
- pos_left = torch.ceil(
- gt_bboxes[:, 0] + (1 - self.sigma) * half_w - 0.5).long().\
- clamp(0, featmap_size[1] - 1)
- pos_right = torch.floor(
- gt_bboxes[:, 0] + (1 + self.sigma) * half_w - 0.5).long().\
- clamp(0, featmap_size[1] - 1)
- pos_top = torch.ceil(
- gt_bboxes[:, 1] + (1 - self.sigma) * half_h - 0.5).long().\
- clamp(0, featmap_size[0] - 1)
- pos_down = torch.floor(
- gt_bboxes[:, 1] + (1 + self.sigma) * half_h - 0.5).long().\
- clamp(0, featmap_size[0] - 1)
- for px1, py1, px2, py2, label, (gt_x1, gt_y1, gt_x2, gt_y2) in \
- zip(pos_left, pos_top, pos_right, pos_down, gt_labels,
- gt_bboxes_raw[hit_indices, :]):
- labels[py1:py2 + 1, px1:px2 + 1] = label
- bbox_targets[py1:py2 + 1, px1:px2 + 1, 0] = \
- (stride * x[py1:py2 + 1, px1:px2 + 1] - gt_x1) / base_len
- bbox_targets[py1:py2 + 1, px1:px2 + 1, 1] = \
- (stride * y[py1:py2 + 1, px1:px2 + 1] - gt_y1) / base_len
- bbox_targets[py1:py2 + 1, px1:px2 + 1, 2] = \
- (gt_x2 - stride * x[py1:py2 + 1, px1:px2 + 1]) / base_len
- bbox_targets[py1:py2 + 1, px1:px2 + 1, 3] = \
- (gt_y2 - stride * y[py1:py2 + 1, px1:px2 + 1]) / base_len
- bbox_targets = bbox_targets.clamp(min=1. / 16, max=16.)
- label_list.append(labels)
- bbox_target_list.append(torch.log(bbox_targets))
- return label_list, bbox_target_list
-
- def get_bboxes(self,
- cls_scores,
- bbox_preds,
- img_metas,
- cfg=None,
- rescale=None):
- assert len(cls_scores) == len(bbox_preds)
- num_levels = len(cls_scores)
- featmap_sizes = [featmap.size()[-2:] for featmap in cls_scores]
- points = self.get_points(
- featmap_sizes,
- bbox_preds[0].dtype,
- bbox_preds[0].device,
- flatten=True)
- result_list = []
- for img_id in range(len(img_metas)):
- cls_score_list = [
- cls_scores[i][img_id].detach() for i in range(num_levels)
- ]
- bbox_pred_list = [
- bbox_preds[i][img_id].detach() for i in range(num_levels)
- ]
- img_shape = img_metas[img_id]['img_shape']
- scale_factor = img_metas[img_id]['scale_factor']
- det_bboxes = self._get_bboxes_single(cls_score_list,
- bbox_pred_list, featmap_sizes,
- points, img_shape,
- scale_factor, cfg, rescale)
- result_list.append(det_bboxes)
- return result_list
-
- def _get_bboxes_single(self,
- cls_scores,
- bbox_preds,
- featmap_sizes,
- point_list,
- img_shape,
- scale_factor,
- cfg,
- rescale=False):
- cfg = self.test_cfg if cfg is None else cfg
- assert len(cls_scores) == len(bbox_preds) == len(point_list)
- det_bboxes = []
- det_scores = []
- for cls_score, bbox_pred, featmap_size, stride, base_len, (y, x) \
- in zip(cls_scores, bbox_preds, featmap_sizes, self.strides,
- self.base_edge_list, point_list):
- assert cls_score.size()[-2:] == bbox_pred.size()[-2:]
- scores = cls_score.permute(1, 2, 0).reshape(
- -1, self.cls_out_channels).sigmoid()
- bbox_pred = bbox_pred.permute(1, 2, 0).reshape(-1, 4).exp()
- nms_pre = cfg.get('nms_pre', -1)
- if (nms_pre > 0) and (scores.shape[0] > nms_pre):
- max_scores, _ = scores.max(dim=1)
- _, topk_inds = max_scores.topk(nms_pre)
- bbox_pred = bbox_pred[topk_inds, :]
- scores = scores[topk_inds, :]
- y = y[topk_inds]
- x = x[topk_inds]
- x1 = (stride * x - base_len * bbox_pred[:, 0]).\
- clamp(min=0, max=img_shape[1] - 1)
- y1 = (stride * y - base_len * bbox_pred[:, 1]).\
- clamp(min=0, max=img_shape[0] - 1)
- x2 = (stride * x + base_len * bbox_pred[:, 2]).\
- clamp(min=0, max=img_shape[1] - 1)
- y2 = (stride * y + base_len * bbox_pred[:, 3]).\
- clamp(min=0, max=img_shape[0] - 1)
- bboxes = torch.stack([x1, y1, x2, y2], -1)
- det_bboxes.append(bboxes)
- det_scores.append(scores)
- det_bboxes = torch.cat(det_bboxes)
- if rescale:
- det_bboxes /= det_bboxes.new_tensor(scale_factor)
- det_scores = torch.cat(det_scores)
- padding = det_scores.new_zeros(det_scores.shape[0], 1)
- # remind that we set FG labels to [0, num_class-1] since mmdet v2.0
- # BG cat_id: num_class
- det_scores = torch.cat([det_scores, padding], dim=1)
- det_bboxes, det_labels = multiclass_nms(det_bboxes, det_scores,
- cfg.score_thr, cfg.nms,
- cfg.max_per_img)
- return det_bboxes, det_labels
diff --git a/spaces/dineshreddy/WALT/mmdet/models/dense_heads/gfl_head.py b/spaces/dineshreddy/WALT/mmdet/models/dense_heads/gfl_head.py
deleted file mode 100644
index 961bc92237663ad5343d3d08eb9c0e4e811ada05..0000000000000000000000000000000000000000
--- a/spaces/dineshreddy/WALT/mmdet/models/dense_heads/gfl_head.py
+++ /dev/null
@@ -1,647 +0,0 @@
-import torch
-import torch.nn as nn
-import torch.nn.functional as F
-from mmcv.cnn import ConvModule, Scale, bias_init_with_prob, normal_init
-from mmcv.runner import force_fp32
-
-from mmdet.core import (anchor_inside_flags, bbox2distance, bbox_overlaps,
- build_assigner, build_sampler, distance2bbox,
- images_to_levels, multi_apply, multiclass_nms,
- reduce_mean, unmap)
-from ..builder import HEADS, build_loss
-from .anchor_head import AnchorHead
-
-
-class Integral(nn.Module):
- """A fixed layer for calculating integral result from distribution.
-
- This layer calculates the target location by :math: `sum{P(y_i) * y_i}`,
- P(y_i) denotes the softmax vector that represents the discrete distribution
- y_i denotes the discrete set, usually {0, 1, 2, ..., reg_max}
-
- Args:
- reg_max (int): The maximal value of the discrete set. Default: 16. You
- may want to reset it according to your new dataset or related
- settings.
- """
-
- def __init__(self, reg_max=16):
- super(Integral, self).__init__()
- self.reg_max = reg_max
- self.register_buffer('project',
- torch.linspace(0, self.reg_max, self.reg_max + 1))
-
- def forward(self, x):
- """Forward feature from the regression head to get integral result of
- bounding box location.
-
- Args:
- x (Tensor): Features of the regression head, shape (N, 4*(n+1)),
- n is self.reg_max.
-
- Returns:
- x (Tensor): Integral result of box locations, i.e., distance
- offsets from the box center in four directions, shape (N, 4).
- """
- x = F.softmax(x.reshape(-1, self.reg_max + 1), dim=1)
- x = F.linear(x, self.project.type_as(x)).reshape(-1, 4)
- return x
-
-
-@HEADS.register_module()
-class GFLHead(AnchorHead):
- """Generalized Focal Loss: Learning Qualified and Distributed Bounding
- Boxes for Dense Object Detection.
-
- GFL head structure is similar with ATSS, however GFL uses
- 1) joint representation for classification and localization quality, and
- 2) flexible General distribution for bounding box locations,
- which are supervised by
- Quality Focal Loss (QFL) and Distribution Focal Loss (DFL), respectively
-
- https://arxiv.org/abs/2006.04388
-
- Args:
- num_classes (int): Number of categories excluding the background
- category.
- in_channels (int): Number of channels in the input feature map.
- stacked_convs (int): Number of conv layers in cls and reg tower.
- Default: 4.
- conv_cfg (dict): dictionary to construct and config conv layer.
- Default: None.
- norm_cfg (dict): dictionary to construct and config norm layer.
- Default: dict(type='GN', num_groups=32, requires_grad=True).
- loss_qfl (dict): Config of Quality Focal Loss (QFL).
- reg_max (int): Max value of integral set :math: `{0, ..., reg_max}`
- in QFL setting. Default: 16.
- Example:
- >>> self = GFLHead(11, 7)
- >>> feats = [torch.rand(1, 7, s, s) for s in [4, 8, 16, 32, 64]]
- >>> cls_quality_score, bbox_pred = self.forward(feats)
- >>> assert len(cls_quality_score) == len(self.scales)
- """
-
- def __init__(self,
- num_classes,
- in_channels,
- stacked_convs=4,
- conv_cfg=None,
- norm_cfg=dict(type='GN', num_groups=32, requires_grad=True),
- loss_dfl=dict(type='DistributionFocalLoss', loss_weight=0.25),
- reg_max=16,
- **kwargs):
- self.stacked_convs = stacked_convs
- self.conv_cfg = conv_cfg
- self.norm_cfg = norm_cfg
- self.reg_max = reg_max
- super(GFLHead, self).__init__(num_classes, in_channels, **kwargs)
-
- self.sampling = False
- if self.train_cfg:
- self.assigner = build_assigner(self.train_cfg.assigner)
- # SSD sampling=False so use PseudoSampler
- sampler_cfg = dict(type='PseudoSampler')
- self.sampler = build_sampler(sampler_cfg, context=self)
-
- self.integral = Integral(self.reg_max)
- self.loss_dfl = build_loss(loss_dfl)
-
- def _init_layers(self):
- """Initialize layers of the head."""
- self.relu = nn.ReLU(inplace=True)
- self.cls_convs = nn.ModuleList()
- self.reg_convs = nn.ModuleList()
- for i in range(self.stacked_convs):
- chn = self.in_channels if i == 0 else self.feat_channels
- self.cls_convs.append(
- ConvModule(
- chn,
- self.feat_channels,
- 3,
- stride=1,
- padding=1,
- conv_cfg=self.conv_cfg,
- norm_cfg=self.norm_cfg))
- self.reg_convs.append(
- ConvModule(
- chn,
- self.feat_channels,
- 3,
- stride=1,
- padding=1,
- conv_cfg=self.conv_cfg,
- norm_cfg=self.norm_cfg))
- assert self.num_anchors == 1, 'anchor free version'
- self.gfl_cls = nn.Conv2d(
- self.feat_channels, self.cls_out_channels, 3, padding=1)
- self.gfl_reg = nn.Conv2d(
- self.feat_channels, 4 * (self.reg_max + 1), 3, padding=1)
- self.scales = nn.ModuleList(
- [Scale(1.0) for _ in self.anchor_generator.strides])
-
- def init_weights(self):
- """Initialize weights of the head."""
- for m in self.cls_convs:
- normal_init(m.conv, std=0.01)
- for m in self.reg_convs:
- normal_init(m.conv, std=0.01)
- bias_cls = bias_init_with_prob(0.01)
- normal_init(self.gfl_cls, std=0.01, bias=bias_cls)
- normal_init(self.gfl_reg, std=0.01)
-
- def forward(self, feats):
- """Forward features from the upstream network.
-
- Args:
- feats (tuple[Tensor]): Features from the upstream network, each is
- a 4D-tensor.
-
- Returns:
- tuple: Usually a tuple of classification scores and bbox prediction
- cls_scores (list[Tensor]): Classification and quality (IoU)
- joint scores for all scale levels, each is a 4D-tensor,
- the channel number is num_classes.
- bbox_preds (list[Tensor]): Box distribution logits for all
- scale levels, each is a 4D-tensor, the channel number is
- 4*(n+1), n is max value of integral set.
- """
- return multi_apply(self.forward_single, feats, self.scales)
-
- def forward_single(self, x, scale):
- """Forward feature of a single scale level.
-
- Args:
- x (Tensor): Features of a single scale level.
- scale (:obj: `mmcv.cnn.Scale`): Learnable scale module to resize
- the bbox prediction.
-
- Returns:
- tuple:
- cls_score (Tensor): Cls and quality joint scores for a single
- scale level the channel number is num_classes.
- bbox_pred (Tensor): Box distribution logits for a single scale
- level, the channel number is 4*(n+1), n is max value of
- integral set.
- """
- cls_feat = x
- reg_feat = x
- for cls_conv in self.cls_convs:
- cls_feat = cls_conv(cls_feat)
- for reg_conv in self.reg_convs:
- reg_feat = reg_conv(reg_feat)
- cls_score = self.gfl_cls(cls_feat)
- bbox_pred = scale(self.gfl_reg(reg_feat)).float()
- return cls_score, bbox_pred
-
- def anchor_center(self, anchors):
- """Get anchor centers from anchors.
-
- Args:
- anchors (Tensor): Anchor list with shape (N, 4), "xyxy" format.
-
- Returns:
- Tensor: Anchor centers with shape (N, 2), "xy" format.
- """
- anchors_cx = (anchors[..., 2] + anchors[..., 0]) / 2
- anchors_cy = (anchors[..., 3] + anchors[..., 1]) / 2
- return torch.stack([anchors_cx, anchors_cy], dim=-1)
-
- def loss_single(self, anchors, cls_score, bbox_pred, labels, label_weights,
- bbox_targets, stride, num_total_samples):
- """Compute loss of a single scale level.
-
- Args:
- anchors (Tensor): Box reference for each scale level with shape
- (N, num_total_anchors, 4).
- cls_score (Tensor): Cls and quality joint scores for each scale
- level has shape (N, num_classes, H, W).
- bbox_pred (Tensor): Box distribution logits for each scale
- level with shape (N, 4*(n+1), H, W), n is max value of integral
- set.
- labels (Tensor): Labels of each anchors with shape
- (N, num_total_anchors).
- label_weights (Tensor): Label weights of each anchor with shape
- (N, num_total_anchors)
- bbox_targets (Tensor): BBox regression targets of each anchor wight
- shape (N, num_total_anchors, 4).
- stride (tuple): Stride in this scale level.
- num_total_samples (int): Number of positive samples that is
- reduced over all GPUs.
-
- Returns:
- dict[str, Tensor]: A dictionary of loss components.
- """
- assert stride[0] == stride[1], 'h stride is not equal to w stride!'
- anchors = anchors.reshape(-1, 4)
- cls_score = cls_score.permute(0, 2, 3,
- 1).reshape(-1, self.cls_out_channels)
- bbox_pred = bbox_pred.permute(0, 2, 3,
- 1).reshape(-1, 4 * (self.reg_max + 1))
- bbox_targets = bbox_targets.reshape(-1, 4)
- labels = labels.reshape(-1)
- label_weights = label_weights.reshape(-1)
-
- # FG cat_id: [0, num_classes -1], BG cat_id: num_classes
- bg_class_ind = self.num_classes
- pos_inds = ((labels >= 0)
- & (labels < bg_class_ind)).nonzero().squeeze(1)
- score = label_weights.new_zeros(labels.shape)
-
- if len(pos_inds) > 0:
- pos_bbox_targets = bbox_targets[pos_inds]
- pos_bbox_pred = bbox_pred[pos_inds]
- pos_anchors = anchors[pos_inds]
- pos_anchor_centers = self.anchor_center(pos_anchors) / stride[0]
-
- weight_targets = cls_score.detach().sigmoid()
- weight_targets = weight_targets.max(dim=1)[0][pos_inds]
- pos_bbox_pred_corners = self.integral(pos_bbox_pred)
- pos_decode_bbox_pred = distance2bbox(pos_anchor_centers,
- pos_bbox_pred_corners)
- pos_decode_bbox_targets = pos_bbox_targets / stride[0]
- score[pos_inds] = bbox_overlaps(
- pos_decode_bbox_pred.detach(),
- pos_decode_bbox_targets,
- is_aligned=True)
- pred_corners = pos_bbox_pred.reshape(-1, self.reg_max + 1)
- target_corners = bbox2distance(pos_anchor_centers,
- pos_decode_bbox_targets,
- self.reg_max).reshape(-1)
-
- # regression loss
- loss_bbox = self.loss_bbox(
- pos_decode_bbox_pred,
- pos_decode_bbox_targets,
- weight=weight_targets,
- avg_factor=1.0)
-
- # dfl loss
- loss_dfl = self.loss_dfl(
- pred_corners,
- target_corners,
- weight=weight_targets[:, None].expand(-1, 4).reshape(-1),
- avg_factor=4.0)
- else:
- loss_bbox = bbox_pred.sum() * 0
- loss_dfl = bbox_pred.sum() * 0
- weight_targets = bbox_pred.new_tensor(0)
-
- # cls (qfl) loss
- loss_cls = self.loss_cls(
- cls_score, (labels, score),
- weight=label_weights,
- avg_factor=num_total_samples)
-
- return loss_cls, loss_bbox, loss_dfl, weight_targets.sum()
-
- @force_fp32(apply_to=('cls_scores', 'bbox_preds'))
- def loss(self,
- cls_scores,
- bbox_preds,
- gt_bboxes,
- gt_labels,
- img_metas,
- gt_bboxes_ignore=None):
- """Compute losses of the head.
-
- Args:
- cls_scores (list[Tensor]): Cls and quality scores for each scale
- level has shape (N, num_classes, H, W).
- bbox_preds (list[Tensor]): Box distribution logits for each scale
- level with shape (N, 4*(n+1), H, W), n is max value of integral
- set.
- gt_bboxes (list[Tensor]): Ground truth bboxes for each image with
- shape (num_gts, 4) in [tl_x, tl_y, br_x, br_y] format.
- gt_labels (list[Tensor]): class indices corresponding to each box
- img_metas (list[dict]): Meta information of each image, e.g.,
- image size, scaling factor, etc.
- gt_bboxes_ignore (list[Tensor] | None): specify which bounding
- boxes can be ignored when computing the loss.
-
- Returns:
- dict[str, Tensor]: A dictionary of loss components.
- """
-
- featmap_sizes = [featmap.size()[-2:] for featmap in cls_scores]
- assert len(featmap_sizes) == self.anchor_generator.num_levels
-
- device = cls_scores[0].device
- anchor_list, valid_flag_list = self.get_anchors(
- featmap_sizes, img_metas, device=device)
- label_channels = self.cls_out_channels if self.use_sigmoid_cls else 1
-
- cls_reg_targets = self.get_targets(
- anchor_list,
- valid_flag_list,
- gt_bboxes,
- img_metas,
- gt_bboxes_ignore_list=gt_bboxes_ignore,
- gt_labels_list=gt_labels,
- label_channels=label_channels)
- if cls_reg_targets is None:
- return None
-
- (anchor_list, labels_list, label_weights_list, bbox_targets_list,
- bbox_weights_list, num_total_pos, num_total_neg) = cls_reg_targets
-
- num_total_samples = reduce_mean(
- torch.tensor(num_total_pos, dtype=torch.float,
- device=device)).item()
- num_total_samples = max(num_total_samples, 1.0)
-
- losses_cls, losses_bbox, losses_dfl,\
- avg_factor = multi_apply(
- self.loss_single,
- anchor_list,
- cls_scores,
- bbox_preds,
- labels_list,
- label_weights_list,
- bbox_targets_list,
- self.anchor_generator.strides,
- num_total_samples=num_total_samples)
-
- avg_factor = sum(avg_factor)
- avg_factor = reduce_mean(avg_factor).item()
- losses_bbox = list(map(lambda x: x / avg_factor, losses_bbox))
- losses_dfl = list(map(lambda x: x / avg_factor, losses_dfl))
- return dict(
- loss_cls=losses_cls, loss_bbox=losses_bbox, loss_dfl=losses_dfl)
-
- def _get_bboxes(self,
- cls_scores,
- bbox_preds,
- mlvl_anchors,
- img_shapes,
- scale_factors,
- cfg,
- rescale=False,
- with_nms=True):
- """Transform outputs for a single batch item into labeled boxes.
-
- Args:
- cls_scores (list[Tensor]): Box scores for a single scale level
- has shape (N, num_classes, H, W).
- bbox_preds (list[Tensor]): Box distribution logits for a single
- scale level with shape (N, 4*(n+1), H, W), n is max value of
- integral set.
- mlvl_anchors (list[Tensor]): Box reference for a single scale level
- with shape (num_total_anchors, 4).
- img_shapes (list[tuple[int]]): Shape of the input image,
- list[(height, width, 3)].
- scale_factors (list[ndarray]): Scale factor of the image arange as
- (w_scale, h_scale, w_scale, h_scale).
- cfg (mmcv.Config | None): Test / postprocessing configuration,
- if None, test_cfg would be used.
- rescale (bool): If True, return boxes in original image space.
- Default: False.
- with_nms (bool): If True, do nms before return boxes.
- Default: True.
-
- Returns:
- list[tuple[Tensor, Tensor]]: Each item in result_list is 2-tuple.
- The first item is an (n, 5) tensor, where 5 represent
- (tl_x, tl_y, br_x, br_y, score) and the score between 0 and 1.
- The shape of the second tensor in the tuple is (n,), and
- each element represents the class label of the corresponding
- box.
- """
- cfg = self.test_cfg if cfg is None else cfg
- assert len(cls_scores) == len(bbox_preds) == len(mlvl_anchors)
- batch_size = cls_scores[0].shape[0]
-
- mlvl_bboxes = []
- mlvl_scores = []
- for cls_score, bbox_pred, stride, anchors in zip(
- cls_scores, bbox_preds, self.anchor_generator.strides,
- mlvl_anchors):
- assert cls_score.size()[-2:] == bbox_pred.size()[-2:]
- assert stride[0] == stride[1]
- scores = cls_score.permute(0, 2, 3, 1).reshape(
- batch_size, -1, self.cls_out_channels).sigmoid()
- bbox_pred = bbox_pred.permute(0, 2, 3, 1)
-
- bbox_pred = self.integral(bbox_pred) * stride[0]
- bbox_pred = bbox_pred.reshape(batch_size, -1, 4)
-
- nms_pre = cfg.get('nms_pre', -1)
- if nms_pre > 0 and scores.shape[1] > nms_pre:
- max_scores, _ = scores.max(-1)
- _, topk_inds = max_scores.topk(nms_pre)
- batch_inds = torch.arange(batch_size).view(
- -1, 1).expand_as(topk_inds).long()
- anchors = anchors[topk_inds, :]
- bbox_pred = bbox_pred[batch_inds, topk_inds, :]
- scores = scores[batch_inds, topk_inds, :]
- else:
- anchors = anchors.expand_as(bbox_pred)
-
- bboxes = distance2bbox(
- self.anchor_center(anchors), bbox_pred, max_shape=img_shapes)
- mlvl_bboxes.append(bboxes)
- mlvl_scores.append(scores)
-
- batch_mlvl_bboxes = torch.cat(mlvl_bboxes, dim=1)
- if rescale:
- batch_mlvl_bboxes /= batch_mlvl_bboxes.new_tensor(
- scale_factors).unsqueeze(1)
-
- batch_mlvl_scores = torch.cat(mlvl_scores, dim=1)
- # Add a dummy background class to the backend when using sigmoid
- # remind that we set FG labels to [0, num_class-1] since mmdet v2.0
- # BG cat_id: num_class
- padding = batch_mlvl_scores.new_zeros(batch_size,
- batch_mlvl_scores.shape[1], 1)
- batch_mlvl_scores = torch.cat([batch_mlvl_scores, padding], dim=-1)
-
- if with_nms:
- det_results = []
- for (mlvl_bboxes, mlvl_scores) in zip(batch_mlvl_bboxes,
- batch_mlvl_scores):
- det_bbox, det_label = multiclass_nms(mlvl_bboxes, mlvl_scores,
- cfg.score_thr, cfg.nms,
- cfg.max_per_img)
- det_results.append(tuple([det_bbox, det_label]))
- else:
- det_results = [
- tuple(mlvl_bs)
- for mlvl_bs in zip(batch_mlvl_bboxes, batch_mlvl_scores)
- ]
- return det_results
-
- def get_targets(self,
- anchor_list,
- valid_flag_list,
- gt_bboxes_list,
- img_metas,
- gt_bboxes_ignore_list=None,
- gt_labels_list=None,
- label_channels=1,
- unmap_outputs=True):
- """Get targets for GFL head.
-
- This method is almost the same as `AnchorHead.get_targets()`. Besides
- returning the targets as the parent method does, it also returns the
- anchors as the first element of the returned tuple.
- """
- num_imgs = len(img_metas)
- assert len(anchor_list) == len(valid_flag_list) == num_imgs
-
- # anchor number of multi levels
- num_level_anchors = [anchors.size(0) for anchors in anchor_list[0]]
- num_level_anchors_list = [num_level_anchors] * num_imgs
-
- # concat all level anchors and flags to a single tensor
- for i in range(num_imgs):
- assert len(anchor_list[i]) == len(valid_flag_list[i])
- anchor_list[i] = torch.cat(anchor_list[i])
- valid_flag_list[i] = torch.cat(valid_flag_list[i])
-
- # compute targets for each image
- if gt_bboxes_ignore_list is None:
- gt_bboxes_ignore_list = [None for _ in range(num_imgs)]
- if gt_labels_list is None:
- gt_labels_list = [None for _ in range(num_imgs)]
- (all_anchors, all_labels, all_label_weights, all_bbox_targets,
- all_bbox_weights, pos_inds_list, neg_inds_list) = multi_apply(
- self._get_target_single,
- anchor_list,
- valid_flag_list,
- num_level_anchors_list,
- gt_bboxes_list,
- gt_bboxes_ignore_list,
- gt_labels_list,
- img_metas,
- label_channels=label_channels,
- unmap_outputs=unmap_outputs)
- # no valid anchors
- if any([labels is None for labels in all_labels]):
- return None
- # sampled anchors of all images
- num_total_pos = sum([max(inds.numel(), 1) for inds in pos_inds_list])
- num_total_neg = sum([max(inds.numel(), 1) for inds in neg_inds_list])
- # split targets to a list w.r.t. multiple levels
- anchors_list = images_to_levels(all_anchors, num_level_anchors)
- labels_list = images_to_levels(all_labels, num_level_anchors)
- label_weights_list = images_to_levels(all_label_weights,
- num_level_anchors)
- bbox_targets_list = images_to_levels(all_bbox_targets,
- num_level_anchors)
- bbox_weights_list = images_to_levels(all_bbox_weights,
- num_level_anchors)
- return (anchors_list, labels_list, label_weights_list,
- bbox_targets_list, bbox_weights_list, num_total_pos,
- num_total_neg)
-
- def _get_target_single(self,
- flat_anchors,
- valid_flags,
- num_level_anchors,
- gt_bboxes,
- gt_bboxes_ignore,
- gt_labels,
- img_meta,
- label_channels=1,
- unmap_outputs=True):
- """Compute regression, classification targets for anchors in a single
- image.
-
- Args:
- flat_anchors (Tensor): Multi-level anchors of the image, which are
- concatenated into a single tensor of shape (num_anchors, 4)
- valid_flags (Tensor): Multi level valid flags of the image,
- which are concatenated into a single tensor of
- shape (num_anchors,).
- num_level_anchors Tensor): Number of anchors of each scale level.
- gt_bboxes (Tensor): Ground truth bboxes of the image,
- shape (num_gts, 4).
- gt_bboxes_ignore (Tensor): Ground truth bboxes to be
- ignored, shape (num_ignored_gts, 4).
- gt_labels (Tensor): Ground truth labels of each box,
- shape (num_gts,).
- img_meta (dict): Meta info of the image.
- label_channels (int): Channel of label.
- unmap_outputs (bool): Whether to map outputs back to the original
- set of anchors.
-
- Returns:
- tuple: N is the number of total anchors in the image.
- anchors (Tensor): All anchors in the image with shape (N, 4).
- labels (Tensor): Labels of all anchors in the image with shape
- (N,).
- label_weights (Tensor): Label weights of all anchor in the
- image with shape (N,).
- bbox_targets (Tensor): BBox targets of all anchors in the
- image with shape (N, 4).
- bbox_weights (Tensor): BBox weights of all anchors in the
- image with shape (N, 4).
- pos_inds (Tensor): Indices of positive anchor with shape
- (num_pos,).
- neg_inds (Tensor): Indices of negative anchor with shape
- (num_neg,).
- """
- inside_flags = anchor_inside_flags(flat_anchors, valid_flags,
- img_meta['img_shape'][:2],
- self.train_cfg.allowed_border)
- if not inside_flags.any():
- return (None, ) * 7
- # assign gt and sample anchors
- anchors = flat_anchors[inside_flags, :]
-
- num_level_anchors_inside = self.get_num_level_anchors_inside(
- num_level_anchors, inside_flags)
- assign_result = self.assigner.assign(anchors, num_level_anchors_inside,
- gt_bboxes, gt_bboxes_ignore,
- gt_labels)
-
- sampling_result = self.sampler.sample(assign_result, anchors,
- gt_bboxes)
-
- num_valid_anchors = anchors.shape[0]
- bbox_targets = torch.zeros_like(anchors)
- bbox_weights = torch.zeros_like(anchors)
- labels = anchors.new_full((num_valid_anchors, ),
- self.num_classes,
- dtype=torch.long)
- label_weights = anchors.new_zeros(num_valid_anchors, dtype=torch.float)
-
- pos_inds = sampling_result.pos_inds
- neg_inds = sampling_result.neg_inds
- if len(pos_inds) > 0:
- pos_bbox_targets = sampling_result.pos_gt_bboxes
- bbox_targets[pos_inds, :] = pos_bbox_targets
- bbox_weights[pos_inds, :] = 1.0
- if gt_labels is None:
- # Only rpn gives gt_labels as None
- # Foreground is the first class
- labels[pos_inds] = 0
- else:
- labels[pos_inds] = gt_labels[
- sampling_result.pos_assigned_gt_inds]
- if self.train_cfg.pos_weight <= 0:
- label_weights[pos_inds] = 1.0
- else:
- label_weights[pos_inds] = self.train_cfg.pos_weight
- if len(neg_inds) > 0:
- label_weights[neg_inds] = 1.0
-
- # map up to original set of anchors
- if unmap_outputs:
- num_total_anchors = flat_anchors.size(0)
- anchors = unmap(anchors, num_total_anchors, inside_flags)
- labels = unmap(
- labels, num_total_anchors, inside_flags, fill=self.num_classes)
- label_weights = unmap(label_weights, num_total_anchors,
- inside_flags)
- bbox_targets = unmap(bbox_targets, num_total_anchors, inside_flags)
- bbox_weights = unmap(bbox_weights, num_total_anchors, inside_flags)
-
- return (anchors, labels, label_weights, bbox_targets, bbox_weights,
- pos_inds, neg_inds)
-
- def get_num_level_anchors_inside(self, num_level_anchors, inside_flags):
- split_inside_flags = torch.split(inside_flags, num_level_anchors)
- num_level_anchors_inside = [
- int(flags.sum()) for flags in split_inside_flags
- ]
- return num_level_anchors_inside
diff --git a/spaces/dineshreddy/WALT/mmdet/models/roi_heads/mask_heads/htc_mask_head.py b/spaces/dineshreddy/WALT/mmdet/models/roi_heads/mask_heads/htc_mask_head.py
deleted file mode 100644
index 330b778ebad8d48d55d09ddd42baa70ec10ae463..0000000000000000000000000000000000000000
--- a/spaces/dineshreddy/WALT/mmdet/models/roi_heads/mask_heads/htc_mask_head.py
+++ /dev/null
@@ -1,43 +0,0 @@
-from mmcv.cnn import ConvModule
-
-from mmdet.models.builder import HEADS
-from .fcn_mask_head import FCNMaskHead
-
-
-@HEADS.register_module()
-class HTCMaskHead(FCNMaskHead):
-
- def __init__(self, with_conv_res=True, *args, **kwargs):
- super(HTCMaskHead, self).__init__(*args, **kwargs)
- self.with_conv_res = with_conv_res
- if self.with_conv_res:
- self.conv_res = ConvModule(
- self.conv_out_channels,
- self.conv_out_channels,
- 1,
- conv_cfg=self.conv_cfg,
- norm_cfg=self.norm_cfg)
-
- def init_weights(self):
- super(HTCMaskHead, self).init_weights()
- if self.with_conv_res:
- self.conv_res.init_weights()
-
- def forward(self, x, res_feat=None, return_logits=True, return_feat=True):
- if res_feat is not None:
- assert self.with_conv_res
- res_feat = self.conv_res(res_feat)
- x = x + res_feat
- for conv in self.convs:
- x = conv(x)
- res_feat = x
- outs = []
- if return_logits:
- x = self.upsample(x)
- if self.upsample_method == 'deconv':
- x = self.relu(x)
- mask_pred = self.conv_logits(x)
- outs.append(mask_pred)
- if return_feat:
- outs.append(res_feat)
- return outs if len(outs) > 1 else outs[0]
diff --git a/spaces/dpe1/beat_manipulator/beat_manipulator/osu.py b/spaces/dpe1/beat_manipulator/beat_manipulator/osu.py
deleted file mode 100644
index 8a6becc526702d28eb4f1b42fa91a252b6ff4e09..0000000000000000000000000000000000000000
--- a/spaces/dpe1/beat_manipulator/beat_manipulator/osu.py
+++ /dev/null
@@ -1,244 +0,0 @@
-from . import main
-import numpy as np
-
-# L L L L L L L L L
-def generate(song, difficulties = [0.2, 0.1, 0.05, 0.025, 0.01, 0.0075, 0.005, 0.0025], lib='madmom.MultiModelSelectionProcessor', caching=True, log = True, output = '', add_peaks = True):
- # for i in difficulties:
- # if i<0.005: print(f'Difficulties < 0.005 may result in broken beatmaps, found difficulty = {i}')
- if lib.lower == 'stunlocked': add_peaks = False
-
- if not isinstance(song, main.song): song = main.song(song)
- if log is True: print(f'Using {lib}; ', end='')
-
- filename = song.path.replace('\\', '/').split('/')[-1]
- if ' - ' in filename and len(filename.split(' - '))>1:
- artist = filename.split(' - ')[0]
- title = ' - '.join(filename.split(' - ')[1:])
- else:
- artist = ''
- title = filename
-
- if caching is True:
- audio_id=hex(len(song.audio[0]))
- import os
- if not os.path.exists('beat_manipulator/beatmaps'):
- os.mkdir('beat_manipulator/beatmaps')
- cacheDir="beat_manipulator/beatmaps/" + filename + "_"+lib+"_"+audio_id+'.txt'
- try:
- beatmap=np.loadtxt(cacheDir)
- if log is True: print('loaded cached beatmap.')
- except OSError:
- if log is True:print("beatmap hasn't been generated yet. Generating...")
- beatmap = None
-
- if beatmap is None:
- if 'madmom' in lib.lower():
- from collections.abc import MutableMapping, MutableSequence
- import madmom
- assert len(song.audio[0])>song.sr*2, f'Audio file is too short, len={len(song.audio[0])} samples, or {len(song.audio[0])/song.sr} seconds. Minimum length is 2 seconds, audio below that breaks madmom processors.'
- if lib=='madmom.RNNBeatProcessor':
- proc = madmom.features.beats.RNNBeatProcessor()
- beatmap = proc(madmom.audio.signal.Signal(song.audio.T, song.sr))
- elif lib=='madmom.MultiModelSelectionProcessor':
- proc = madmom.features.beats.RNNBeatProcessor(post_processor=None)
- predictions = proc(madmom.audio.signal.Signal(song.audio.T, song.sr))
- mm_proc = madmom.features.beats.MultiModelSelectionProcessor(num_ref_predictions=None)
- beatmap= mm_proc(predictions)*song.sr
- beatmap/= np.max(beatmap)
- elif lib=='stunlocked':
- spikes = np.abs(np.gradient(np.clip(song.audio[0], -1, 1)))[:int(len(song.audio[0]) - (len(song.audio[0])%int(song.sr/100)))]
- spikes = spikes.reshape(-1, (int(song.sr/100)))
- spikes = np.asarray(list(np.max(i) for i in spikes))
- if len(beatmap) > len(spikes): beatmap = beatmap[:len(spikes)]
- elif len(spikes) > len(beatmap): spikes = spikes[:len(beatmap)]
- zeroing = 0
- for i in range(len(spikes)):
- if zeroing > 0:
- if spikes[i] <= 0.1: zeroing -=1
- spikes[i] = 0
- elif spikes[i] >= 0.1:
- spikes[i] = 1
- zeroing = 7
- if spikes[i] <= 0.1: spikes[i] = 0
- beatmap = spikes
-
- if caching is True: np.savetxt(cacheDir, beatmap)
-
- if add_peaks is True:
- spikes = np.abs(np.gradient(np.clip(song.audio[0], -1, 1)))[:int(len(song.audio[0]) - (len(song.audio[0])%int(song.sr/100)))]
- spikes = spikes.reshape(-1, (int(song.sr/100)))
- spikes = np.asarray(list(np.max(i) for i in spikes))
- if len(beatmap) > len(spikes): beatmap = beatmap[:len(spikes)]
- elif len(spikes) > len(beatmap): spikes = spikes[:len(beatmap)]
- zeroing = 0
- for i in range(len(spikes)):
- if zeroing > 0:
- if spikes[i] <= 0.1: zeroing -=1
- spikes[i] = 0
- elif spikes[i] >= 0.1:
- spikes[i] = 1
- zeroing = 7
- if spikes[i] <= 0.1: spikes[i] = 0
- else: spikes = None
-
- def _process(song: main.song, beatmap, spikes, threshold):
- '''ඞ'''
- if add_peaks is True: beatmap += spikes
- hitmap=[]
- actual_samplerate=int(song.sr/100)
- beat_middle=int(actual_samplerate/2)
- for i in range(len(beatmap)):
- if beatmap[i]>threshold: hitmap.append(i*actual_samplerate + beat_middle)
- hitmap=np.asarray(hitmap)
- clump=[]
- for i in range(len(hitmap)-1):
- #print(i, abs(song.beatmap[i]-song.beatmap[i+1]), clump)
- if abs(hitmap[i] - hitmap[i+1]) < song.sr/16 and i != len(hitmap)-2: clump.append(i)
- elif clump!=[]:
- clump.append(i)
- actual_time=hitmap[clump[0]]
- hitmap[np.array(clump)]=0
- #print(song.beatmap)
- hitmap[clump[0]]=actual_time
- clump=[]
-
- hitmap=hitmap[hitmap!=0]
- return hitmap
-
- osufile=lambda title,artist,version: ("osu file format v14\n"
- "\n"
- "[General]\n"
- f"AudioFilename: {song.path.split('/')[-1]}\n"
- "AudioLeadIn: 0\n"
- "PreviewTime: -1\n"
- "Countdown: 0\n"
- "SampleSet: Normal\n"
- "StackLeniency: 0.5\n"
- "Mode: 0\n"
- "LetterboxInBreaks: 0\n"
- "WidescreenStoryboard: 0\n"
- "\n"
- "[Editor]\n"
- "DistanceSpacing: 1.1\n"
- "BeatDivisor: 4\n"
- "GridSize: 8\n"
- "TimelineZoom: 1.6\n"
- "\n"
- "[Metadata]\n"
- f"Title:{title}\n"
- f"TitleUnicode:{title}\n"
- f"Artist:{artist}\n"
- f"ArtistUnicode:{artist}\n"
- f'Creator:{lib} + BeatManipulator\n'
- f'Version:{version} {lib}\n'
- 'Source:\n'
- 'Tags:BeatManipulator\n'
- 'BeatmapID:0\n'
- 'BeatmapSetID:-1\n'
- '\n'
- '[Difficulty]\n'
- 'HPDrainRate:4\n'
- 'CircleSize:4\n'
- 'OverallDifficulty:5\n'
- 'ApproachRate:10\n'
- 'SliderMultiplier:3.3\n'
- 'SliderTickRate:1\n'
- '\n'
- '[Events]\n'
- '//Background and Video events\n'
- '//Break Periods\n'
- '//Storyboard Layer 0 (Background)\n'
- '//Storyboard Layer 1 (Fail)\n'
- '//Storyboard Layer 2 (Pass)\n'
- '//Storyboard Layer 3 (Foreground)\n'
- '//Storyboard Layer 4 (Overlay)\n'
- '//Storyboard Sound Samples\n'
- '\n'
- '[TimingPoints]\n'
- '0,140.0,4,1,0,100,1,0\n'
- '\n'
- '\n'
- '[HitObjects]\n')
- # remove the clumps
- #print(self.beatmap)
-
- #print(self.beatmap)
-
-
- #print(len(osumap))
- #input('banana')
- import shutil, os
- if os.path.exists('beat_manipulator/temp'): shutil.rmtree('beat_manipulator/temp')
- os.mkdir('beat_manipulator/temp')
- hitmap=[]
- import random
- for difficulty in difficulties:
- for i in range(4):
- #print(i)
- this_difficulty=_process(song, beatmap, spikes, difficulty)
- hitmap.append(this_difficulty)
-
- for k in range(len(hitmap)):
- osumap=np.vstack((hitmap[k],np.zeros(len(hitmap[k])),np.zeros(len(hitmap[k])))).T
- difficulty= difficulties[k]
- for i in range(len(osumap)-1):
- if i==0:continue
- dist=(osumap[i,0]-osumap[i-1,0])*(1-(difficulty**0.3))
- if dist<1000: dist=0.005
- elif dist<2000: dist=0.01
- elif dist<3000: dist=0.015
- elif dist<4000: dist=0.02
- elif dist<5000: dist=0.25
- elif dist<6000: dist=0.35
- elif dist<7000: dist=0.45
- elif dist<8000: dist=0.55
- elif dist<9000: dist=0.65
- elif dist<10000: dist=0.75
- elif dist<12500: dist=0.85
- elif dist<15000: dist=0.95
- elif dist<20000: dist=1
- #elif dist<30000: dist=0.8
- prev_x=osumap[i-1,1]
- prev_y=osumap[i-1,2]
- if prev_x>0: prev_x=prev_x-dist*0.1
- elif prev_x<0: prev_x=prev_x+dist*0.1
- if prev_y>0: prev_y=prev_y-dist*0.1
- elif prev_y<0: prev_y=prev_y+dist*0.1
- dirx=random.uniform(-dist,dist)
- diry=dist-abs(dirx)*random.choice([-1, 1])
- if abs(prev_x+dirx)>1: dirx=-dirx
- if abs(prev_y+diry)>1: diry=-diry
- x=prev_x+dirx
- y=prev_y+diry
- #print(dirx,diry,x,y)
- #print(x>1, x<1, y>1, y<1)
- if x>1: x=0.8
- if x<-1: x=-0.8
- if y>1: y=0.8
- if y<-1: y=-0.8
- #print(dirx,diry,x,y)
- osumap[i,1]=x
- osumap[i,2]=y
-
- osumap[:,1]*=300
- osumap[:,1]+=300
- osumap[:,2]*=180
- osumap[:,2]+=220
-
- file=osufile(artist, title, difficulty)
- for j in osumap:
- #print('285,70,'+str(int(int(i)*1000/self.samplerate))+',1,0')
- file+=f'{int(j[1])},{int(j[2])},{str(int(int(j[0])*1000/song.sr))},1,0\n'
- with open(f'beat_manipulator/temp/{artist} - {title} (BeatManipulator {difficulty} {lib}].osu', 'x', encoding="utf-8") as f:
- f.write(file)
- from . import io
- import shutil, os
- shutil.copyfile(song.path, 'beat_manipulator/temp/'+filename)
- shutil.make_archive('beat_manipulator_osz', 'zip', 'beat_manipulator/temp')
- outputname = io._outputfilename(path = output, filename = song.path, suffix = ' ('+lib + ')', ext = 'osz')
- if not os.path.exists(outputname):
- os.rename('beat_manipulator_osz.zip', outputname)
- if log is True: print(f'Created `{outputname}`')
- else: print(f'{outputname} already exists!')
- shutil.rmtree('beat_manipulator/temp')
- return outputname
\ No newline at end of file
diff --git a/spaces/edemgold/QA-App/README.md b/spaces/edemgold/QA-App/README.md
deleted file mode 100644
index 1f61d4a54e4f76ba07966f4929caf34d00871be1..0000000000000000000000000000000000000000
--- a/spaces/edemgold/QA-App/README.md
+++ /dev/null
@@ -1,11 +0,0 @@
----
-title: QA App
-emoji: 💻
-colorFrom: pink
-colorTo: gray
-sdk: gradio
-app_file: app.py
-pinned: false
----
-
-Check out the configuration reference at https://huggingface.co/docs/hub/spaces#reference
diff --git a/spaces/edisonlee55/hysts-anime-face-detector/anime_face_detector/configs/mmdet/yolov3.py b/spaces/edisonlee55/hysts-anime-face-detector/anime_face_detector/configs/mmdet/yolov3.py
deleted file mode 100644
index 5db87c798e072cfe551f08e73de5864aa155b4cd..0000000000000000000000000000000000000000
--- a/spaces/edisonlee55/hysts-anime-face-detector/anime_face_detector/configs/mmdet/yolov3.py
+++ /dev/null
@@ -1,47 +0,0 @@
-model = dict(type='YOLOV3',
- backbone=dict(type='Darknet', depth=53, out_indices=(3, 4, 5)),
- neck=dict(type='YOLOV3Neck',
- num_scales=3,
- in_channels=[1024, 512, 256],
- out_channels=[512, 256, 128]),
- bbox_head=dict(type='YOLOV3Head',
- num_classes=1,
- in_channels=[512, 256, 128],
- out_channels=[1024, 512, 256],
- anchor_generator=dict(type='YOLOAnchorGenerator',
- base_sizes=[[(116, 90),
- (156, 198),
- (373, 326)],
- [(30, 61),
- (62, 45),
- (59, 119)],
- [(10, 13),
- (16, 30),
- (33, 23)]],
- strides=[32, 16, 8]),
- bbox_coder=dict(type='YOLOBBoxCoder'),
- featmap_strides=[32, 16, 8]),
- test_cfg=dict(nms_pre=1000,
- min_bbox_size=0,
- score_thr=0.05,
- conf_thr=0.005,
- nms=dict(type='nms', iou_threshold=0.45),
- max_per_img=100))
-test_pipeline = [
- dict(type='LoadImageFromFile'),
- dict(type='MultiScaleFlipAug',
- img_scale=(608, 608),
- flip=False,
- transforms=[
- dict(type='Resize', keep_ratio=True),
- dict(type='RandomFlip'),
- dict(type='Normalize',
- mean=[0, 0, 0],
- std=[255.0, 255.0, 255.0],
- to_rgb=True),
- dict(type='Pad', size_divisor=32),
- dict(type='DefaultFormatBundle'),
- dict(type='Collect', keys=['img'])
- ])
-]
-data = dict(test=dict(pipeline=test_pipeline))
diff --git a/spaces/editing-images/ledits/inversion_utils.py b/spaces/editing-images/ledits/inversion_utils.py
deleted file mode 100644
index f066d3dc044f1f41cd9d479e24fe760c8a1a8243..0000000000000000000000000000000000000000
--- a/spaces/editing-images/ledits/inversion_utils.py
+++ /dev/null
@@ -1,275 +0,0 @@
-import torch
-import os
-from tqdm import tqdm
-from PIL import Image, ImageDraw ,ImageFont
-from matplotlib import pyplot as plt
-import torchvision.transforms as T
-import os
-import yaml
-import numpy as np
-
-
-def load_512(image_path, left=0, right=0, top=0, bottom=0, device=None):
- if type(image_path) is str:
- image = np.array(Image.open(image_path).convert('RGB'))[:, :, :3]
- else:
- image = image_path
- h, w, c = image.shape
- left = min(left, w-1)
- right = min(right, w - left - 1)
- top = min(top, h - left - 1)
- bottom = min(bottom, h - top - 1)
- image = image[top:h-bottom, left:w-right]
- h, w, c = image.shape
- if h < w:
- offset = (w - h) // 2
- image = image[:, offset:offset + h]
- elif w < h:
- offset = (h - w) // 2
- image = image[offset:offset + w]
- image = np.array(Image.fromarray(image).resize((512, 512)))
- image = torch.from_numpy(image).float() / 127.5 - 1
- image = image.permute(2, 0, 1).unsqueeze(0).to(device, dtype =torch.float16)
-
- return image
-
-
-
-def mu_tilde(model, xt,x0, timestep):
- "mu_tilde(x_t, x_0) DDPM paper eq. 7"
- prev_timestep = timestep - model.scheduler.config.num_train_timesteps // model.scheduler.num_inference_steps
- alpha_prod_t_prev = model.scheduler.alphas_cumprod[prev_timestep] if prev_timestep >= 0 else model.scheduler.final_alpha_cumprod
- alpha_t = model.scheduler.alphas[timestep]
- beta_t = 1 - alpha_t
- alpha_bar = model.scheduler.alphas_cumprod[timestep]
- return ((alpha_prod_t_prev ** 0.5 * beta_t) / (1-alpha_bar)) * x0 + ((alpha_t**0.5 *(1-alpha_prod_t_prev)) / (1- alpha_bar))*xt
-
-def sample_xts_from_x0(model, x0, num_inference_steps=50):
- """
- Samples from P(x_1:T|x_0)
- """
- # torch.manual_seed(43256465436)
- alpha_bar = model.scheduler.alphas_cumprod
- sqrt_one_minus_alpha_bar = (1-alpha_bar) ** 0.5
- alphas = model.scheduler.alphas
- betas = 1 - alphas
- variance_noise_shape = (
- num_inference_steps,
- model.unet.in_channels,
- model.unet.sample_size,
- model.unet.sample_size)
-
- timesteps = model.scheduler.timesteps.to(model.device)
- t_to_idx = {int(v):k for k,v in enumerate(timesteps)}
- xts = torch.zeros(variance_noise_shape).to(x0.device, dtype =torch.float16)
- for t in reversed(timesteps):
- idx = t_to_idx[int(t)]
- xts[idx] = x0 * (alpha_bar[t] ** 0.5) + torch.randn_like(x0, dtype =torch.float16) * sqrt_one_minus_alpha_bar[t]
- xts = torch.cat([xts, x0 ],dim = 0)
-
- return xts
-
-def encode_text(model, prompts):
- text_input = model.tokenizer(
- prompts,
- padding="max_length",
- max_length=model.tokenizer.model_max_length,
- truncation=True,
- return_tensors="pt",
- )
- with torch.no_grad():
- text_encoding = model.text_encoder(text_input.input_ids.to(model.device))[0]
- return text_encoding
-
-def forward_step(model, model_output, timestep, sample):
- next_timestep = min(model.scheduler.config.num_train_timesteps - 2,
- timestep + model.scheduler.config.num_train_timesteps // model.scheduler.num_inference_steps)
-
- # 2. compute alphas, betas
- alpha_prod_t = model.scheduler.alphas_cumprod[timestep]
- # alpha_prod_t_next = self.scheduler.alphas_cumprod[next_timestep] if next_ltimestep >= 0 else self.scheduler.final_alpha_cumprod
-
- beta_prod_t = 1 - alpha_prod_t
-
- # 3. compute predicted original sample from predicted noise also called
- # "predicted x_0" of formula (12) from https://arxiv.org/pdf/2010.02502.pdf
- pred_original_sample = (sample - beta_prod_t ** (0.5) * model_output) / alpha_prod_t ** (0.5)
-
- # 5. TODO: simple noising implementatiom
- next_sample = model.scheduler.add_noise(pred_original_sample,
- model_output,
- torch.LongTensor([next_timestep]))
- return next_sample
-
-
-def get_variance(model, timestep): #, prev_timestep):
- prev_timestep = timestep - model.scheduler.config.num_train_timesteps // model.scheduler.num_inference_steps
- alpha_prod_t = model.scheduler.alphas_cumprod[timestep]
- alpha_prod_t_prev = model.scheduler.alphas_cumprod[prev_timestep] if prev_timestep >= 0 else model.scheduler.final_alpha_cumprod
- beta_prod_t = 1 - alpha_prod_t
- beta_prod_t_prev = 1 - alpha_prod_t_prev
- variance = (beta_prod_t_prev / beta_prod_t) * (1 - alpha_prod_t / alpha_prod_t_prev)
- return variance
-
-def inversion_forward_process(model, x0,
- etas = None,
- prog_bar = False,
- prompt = "",
- cfg_scale = 3.5,
- num_inference_steps=50, eps = None):
-
- if not prompt=="":
- text_embeddings = encode_text(model, prompt)
- uncond_embedding = encode_text(model, "")
- timesteps = model.scheduler.timesteps.to(model.device)
- variance_noise_shape = (
- num_inference_steps,
- model.unet.in_channels,
- model.unet.sample_size,
- model.unet.sample_size)
- if etas is None or (type(etas) in [int, float] and etas == 0):
- eta_is_zero = True
- zs = None
- else:
- eta_is_zero = False
- if type(etas) in [int, float]: etas = [etas]*model.scheduler.num_inference_steps
- xts = sample_xts_from_x0(model, x0, num_inference_steps=num_inference_steps)
- alpha_bar = model.scheduler.alphas_cumprod
- zs = torch.zeros(size=variance_noise_shape, device=model.device, dtype =torch.float16)
-
- t_to_idx = {int(v):k for k,v in enumerate(timesteps)}
- xt = x0
- op = tqdm(reversed(timesteps), desc= "Inverting...") if prog_bar else reversed(timesteps)
-
- for t in op:
- idx = t_to_idx[int(t)]
- # 1. predict noise residual
- if not eta_is_zero:
- xt = xts[idx][None]
-
- with torch.no_grad():
- out = model.unet.forward(xt, timestep = t, encoder_hidden_states = uncond_embedding)
- if not prompt=="":
- cond_out = model.unet.forward(xt, timestep=t, encoder_hidden_states = text_embeddings)
-
- if not prompt=="":
- ## classifier free guidance
- noise_pred = out.sample + cfg_scale * (cond_out.sample - out.sample)
- else:
- noise_pred = out.sample
-
- if eta_is_zero:
- # 2. compute more noisy image and set x_t -> x_t+1
- xt = forward_step(model, noise_pred, t, xt)
-
- else:
- xtm1 = xts[idx+1][None]
- # pred of x0
- pred_original_sample = (xt - (1-alpha_bar[t]) ** 0.5 * noise_pred ) / alpha_bar[t] ** 0.5
-
- # direction to xt
- prev_timestep = t - model.scheduler.config.num_train_timesteps // model.scheduler.num_inference_steps
- alpha_prod_t_prev = model.scheduler.alphas_cumprod[prev_timestep] if prev_timestep >= 0 else model.scheduler.final_alpha_cumprod
-
- variance = get_variance(model, t)
- pred_sample_direction = (1 - alpha_prod_t_prev - etas[idx] * variance ) ** (0.5) * noise_pred
-
- mu_xt = alpha_prod_t_prev ** (0.5) * pred_original_sample + pred_sample_direction
-
- z = (xtm1 - mu_xt ) / ( etas[idx] * variance ** 0.5 )
- zs[idx] = z
-
- # correction to avoid error accumulation
- xtm1 = mu_xt + ( etas[idx] * variance ** 0.5 )*z
- xts[idx+1] = xtm1
-
- if not zs is None:
- zs[-1] = torch.zeros_like(zs[-1])
-
- return xt, zs, xts
-
-
-def reverse_step(model, model_output, timestep, sample, eta = 0, variance_noise=None):
- # 1. get previous step value (=t-1)
- prev_timestep = timestep - model.scheduler.config.num_train_timesteps // model.scheduler.num_inference_steps
- # 2. compute alphas, betas
- alpha_prod_t = model.scheduler.alphas_cumprod[timestep]
- alpha_prod_t_prev = model.scheduler.alphas_cumprod[prev_timestep] if prev_timestep >= 0 else model.scheduler.final_alpha_cumprod
- beta_prod_t = 1 - alpha_prod_t
- # 3. compute predicted original sample from predicted noise also called
- # "predicted x_0" of formula (12) from https://arxiv.org/pdf/2010.02502.pdf
- pred_original_sample = (sample - beta_prod_t ** (0.5) * model_output) / alpha_prod_t ** (0.5)
- # 5. compute variance: "sigma_t(η)" -> see formula (16)
- # σ_t = sqrt((1 − α_t−1)/(1 − α_t)) * sqrt(1 − α_t/α_t−1)
- # variance = self.scheduler._get_variance(timestep, prev_timestep)
- variance = get_variance(model, timestep) #, prev_timestep)
- std_dev_t = eta * variance ** (0.5)
- # Take care of asymetric reverse process (asyrp)
- model_output_direction = model_output
- # 6. compute "direction pointing to x_t" of formula (12) from https://arxiv.org/pdf/2010.02502.pdf
- # pred_sample_direction = (1 - alpha_prod_t_prev - std_dev_t**2) ** (0.5) * model_output_direction
- pred_sample_direction = (1 - alpha_prod_t_prev - eta * variance) ** (0.5) * model_output_direction
- # 7. compute x_t without "random noise" of formula (12) from https://arxiv.org/pdf/2010.02502.pdf
- prev_sample = alpha_prod_t_prev ** (0.5) * pred_original_sample + pred_sample_direction
- # 8. Add noice if eta > 0
- if eta > 0:
- if variance_noise is None:
- variance_noise = torch.randn(model_output.shape, device=model.device, dtype =torch.float16)
- sigma_z = eta * variance ** (0.5) * variance_noise
- prev_sample = prev_sample + sigma_z
-
- return prev_sample
-
-def inversion_reverse_process(model,
- xT,
- etas = 0,
- prompts = "",
- cfg_scales = None,
- prog_bar = False,
- zs = None,
- controller=None,
- asyrp = False):
-
- batch_size = len(prompts)
-
- cfg_scales_tensor = torch.Tensor(cfg_scales).view(-1,1,1,1).to(model.device, dtype=torch.float16)
-
- text_embeddings = encode_text(model, prompts)
- uncond_embedding = encode_text(model, [""] * batch_size)
-
- if etas is None: etas = 0
- if type(etas) in [int, float]: etas = [etas]*model.scheduler.num_inference_steps
- assert len(etas) == model.scheduler.num_inference_steps
- timesteps = model.scheduler.timesteps.to(model.device)
-
- xt = xT.expand(batch_size, -1, -1, -1)
- op = tqdm(timesteps[-zs.shape[0]:]) if prog_bar else timesteps[-zs.shape[0]:]
-
- t_to_idx = {int(v):k for k,v in enumerate(timesteps[-zs.shape[0]:])}
-
- for t in op:
- idx = t_to_idx[int(t)]
- ## Unconditional embedding
- with torch.no_grad():
- uncond_out = model.unet.forward(xt, timestep = t,
- encoder_hidden_states = uncond_embedding)
-
- ## Conditional embedding
- if prompts:
- with torch.no_grad():
- cond_out = model.unet.forward(xt, timestep = t,
- encoder_hidden_states = text_embeddings)
-
-
- z = zs[idx] if not zs is None else None
- z = z.expand(batch_size, -1, -1, -1)
- if prompts:
- ## classifier free guidance
- noise_pred = uncond_out.sample + cfg_scales_tensor * (cond_out.sample - uncond_out.sample)
- else:
- noise_pred = uncond_out.sample
- # 2. compute less noisy image and set x_t -> x_t-1
- xt = reverse_step(model, noise_pred, t, xt, eta = etas[idx], variance_noise = z)
- if controller is not None:
- xt = controller.step_callback(xt)
- return xt, zs
diff --git a/spaces/elkraken/Video-Object-Detection/models/common.py b/spaces/elkraken/Video-Object-Detection/models/common.py
deleted file mode 100644
index edb5edc9fe1b0ad3b345a2103603393e74e5b65c..0000000000000000000000000000000000000000
--- a/spaces/elkraken/Video-Object-Detection/models/common.py
+++ /dev/null
@@ -1,2019 +0,0 @@
-import math
-from copy import copy
-from pathlib import Path
-
-import numpy as np
-import pandas as pd
-import requests
-import torch
-import torch.nn as nn
-import torch.nn.functional as F
-from torchvision.ops import DeformConv2d
-from PIL import Image
-from torch.cuda import amp
-
-from utils.datasets import letterbox
-from utils.general import non_max_suppression, make_divisible, scale_coords, increment_path, xyxy2xywh
-from utils.plots import color_list, plot_one_box
-from utils.torch_utils import time_synchronized
-
-
-##### basic ####
-
-def autopad(k, p=None): # kernel, padding
- # Pad to 'same'
- if p is None:
- p = k // 2 if isinstance(k, int) else [x // 2 for x in k] # auto-pad
- return p
-
-
-class MP(nn.Module):
- def __init__(self, k=2):
- super(MP, self).__init__()
- self.m = nn.MaxPool2d(kernel_size=k, stride=k)
-
- def forward(self, x):
- return self.m(x)
-
-
-class SP(nn.Module):
- def __init__(self, k=3, s=1):
- super(SP, self).__init__()
- self.m = nn.MaxPool2d(kernel_size=k, stride=s, padding=k // 2)
-
- def forward(self, x):
- return self.m(x)
-
-
-class ReOrg(nn.Module):
- def __init__(self):
- super(ReOrg, self).__init__()
-
- def forward(self, x): # x(b,c,w,h) -> y(b,4c,w/2,h/2)
- return torch.cat([x[..., ::2, ::2], x[..., 1::2, ::2], x[..., ::2, 1::2], x[..., 1::2, 1::2]], 1)
-
-
-class Concat(nn.Module):
- def __init__(self, dimension=1):
- super(Concat, self).__init__()
- self.d = dimension
-
- def forward(self, x):
- return torch.cat(x, self.d)
-
-
-class Chuncat(nn.Module):
- def __init__(self, dimension=1):
- super(Chuncat, self).__init__()
- self.d = dimension
-
- def forward(self, x):
- x1 = []
- x2 = []
- for xi in x:
- xi1, xi2 = xi.chunk(2, self.d)
- x1.append(xi1)
- x2.append(xi2)
- return torch.cat(x1+x2, self.d)
-
-
-class Shortcut(nn.Module):
- def __init__(self, dimension=0):
- super(Shortcut, self).__init__()
- self.d = dimension
-
- def forward(self, x):
- return x[0]+x[1]
-
-
-class Foldcut(nn.Module):
- def __init__(self, dimension=0):
- super(Foldcut, self).__init__()
- self.d = dimension
-
- def forward(self, x):
- x1, x2 = x.chunk(2, self.d)
- return x1+x2
-
-
-class Conv(nn.Module):
- # Standard convolution
- def __init__(self, c1, c2, k=1, s=1, p=None, g=1, act=True): # ch_in, ch_out, kernel, stride, padding, groups
- super(Conv, self).__init__()
- self.conv = nn.Conv2d(c1, c2, k, s, autopad(k, p), groups=g, bias=False)
- self.bn = nn.BatchNorm2d(c2)
- self.act = nn.SiLU() if act is True else (act if isinstance(act, nn.Module) else nn.Identity())
-
- def forward(self, x):
- return self.act(self.bn(self.conv(x)))
-
- def fuseforward(self, x):
- return self.act(self.conv(x))
-
-
-class RobustConv(nn.Module):
- # Robust convolution (use high kernel size 7-11 for: downsampling and other layers). Train for 300 - 450 epochs.
- def __init__(self, c1, c2, k=7, s=1, p=None, g=1, act=True, layer_scale_init_value=1e-6): # ch_in, ch_out, kernel, stride, padding, groups
- super(RobustConv, self).__init__()
- self.conv_dw = Conv(c1, c1, k=k, s=s, p=p, g=c1, act=act)
- self.conv1x1 = nn.Conv2d(c1, c2, 1, 1, 0, groups=1, bias=True)
- self.gamma = nn.Parameter(layer_scale_init_value * torch.ones(c2)) if layer_scale_init_value > 0 else None
-
- def forward(self, x):
- x = x.to(memory_format=torch.channels_last)
- x = self.conv1x1(self.conv_dw(x))
- if self.gamma is not None:
- x = x.mul(self.gamma.reshape(1, -1, 1, 1))
- return x
-
-
-class RobustConv2(nn.Module):
- # Robust convolution 2 (use [32, 5, 2] or [32, 7, 4] or [32, 11, 8] for one of the paths in CSP).
- def __init__(self, c1, c2, k=7, s=4, p=None, g=1, act=True, layer_scale_init_value=1e-6): # ch_in, ch_out, kernel, stride, padding, groups
- super(RobustConv2, self).__init__()
- self.conv_strided = Conv(c1, c1, k=k, s=s, p=p, g=c1, act=act)
- self.conv_deconv = nn.ConvTranspose2d(in_channels=c1, out_channels=c2, kernel_size=s, stride=s,
- padding=0, bias=True, dilation=1, groups=1
- )
- self.gamma = nn.Parameter(layer_scale_init_value * torch.ones(c2)) if layer_scale_init_value > 0 else None
-
- def forward(self, x):
- x = self.conv_deconv(self.conv_strided(x))
- if self.gamma is not None:
- x = x.mul(self.gamma.reshape(1, -1, 1, 1))
- return x
-
-
-def DWConv(c1, c2, k=1, s=1, act=True):
- # Depthwise convolution
- return Conv(c1, c2, k, s, g=math.gcd(c1, c2), act=act)
-
-
-class GhostConv(nn.Module):
- # Ghost Convolution https://github.com/huawei-noah/ghostnet
- def __init__(self, c1, c2, k=1, s=1, g=1, act=True): # ch_in, ch_out, kernel, stride, groups
- super(GhostConv, self).__init__()
- c_ = c2 // 2 # hidden channels
- self.cv1 = Conv(c1, c_, k, s, None, g, act)
- self.cv2 = Conv(c_, c_, 5, 1, None, c_, act)
-
- def forward(self, x):
- y = self.cv1(x)
- return torch.cat([y, self.cv2(y)], 1)
-
-
-class Stem(nn.Module):
- # Stem
- def __init__(self, c1, c2, k=1, s=1, p=None, g=1, act=True): # ch_in, ch_out, kernel, stride, padding, groups
- super(Stem, self).__init__()
- c_ = int(c2/2) # hidden channels
- self.cv1 = Conv(c1, c_, 3, 2)
- self.cv2 = Conv(c_, c_, 1, 1)
- self.cv3 = Conv(c_, c_, 3, 2)
- self.pool = torch.nn.MaxPool2d(2, stride=2)
- self.cv4 = Conv(2 * c_, c2, 1, 1)
-
- def forward(self, x):
- x = self.cv1(x)
- return self.cv4(torch.cat((self.cv3(self.cv2(x)), self.pool(x)), dim=1))
-
-
-class DownC(nn.Module):
- # Spatial pyramid pooling layer used in YOLOv3-SPP
- def __init__(self, c1, c2, n=1, k=2):
- super(DownC, self).__init__()
- c_ = int(c1) # hidden channels
- self.cv1 = Conv(c1, c_, 1, 1)
- self.cv2 = Conv(c_, c2//2, 3, k)
- self.cv3 = Conv(c1, c2//2, 1, 1)
- self.mp = nn.MaxPool2d(kernel_size=k, stride=k)
-
- def forward(self, x):
- return torch.cat((self.cv2(self.cv1(x)), self.cv3(self.mp(x))), dim=1)
-
-
-class SPP(nn.Module):
- # Spatial pyramid pooling layer used in YOLOv3-SPP
- def __init__(self, c1, c2, k=(5, 9, 13)):
- super(SPP, self).__init__()
- c_ = c1 // 2 # hidden channels
- self.cv1 = Conv(c1, c_, 1, 1)
- self.cv2 = Conv(c_ * (len(k) + 1), c2, 1, 1)
- self.m = nn.ModuleList([nn.MaxPool2d(kernel_size=x, stride=1, padding=x // 2) for x in k])
-
- def forward(self, x):
- x = self.cv1(x)
- return self.cv2(torch.cat([x] + [m(x) for m in self.m], 1))
-
-
-class Bottleneck(nn.Module):
- # Darknet bottleneck
- def __init__(self, c1, c2, shortcut=True, g=1, e=0.5): # ch_in, ch_out, shortcut, groups, expansion
- super(Bottleneck, self).__init__()
- c_ = int(c2 * e) # hidden channels
- self.cv1 = Conv(c1, c_, 1, 1)
- self.cv2 = Conv(c_, c2, 3, 1, g=g)
- self.add = shortcut and c1 == c2
-
- def forward(self, x):
- return x + self.cv2(self.cv1(x)) if self.add else self.cv2(self.cv1(x))
-
-
-class Res(nn.Module):
- # ResNet bottleneck
- def __init__(self, c1, c2, shortcut=True, g=1, e=0.5): # ch_in, ch_out, shortcut, groups, expansion
- super(Res, self).__init__()
- c_ = int(c2 * e) # hidden channels
- self.cv1 = Conv(c1, c_, 1, 1)
- self.cv2 = Conv(c_, c_, 3, 1, g=g)
- self.cv3 = Conv(c_, c2, 1, 1)
- self.add = shortcut and c1 == c2
-
- def forward(self, x):
- return x + self.cv3(self.cv2(self.cv1(x))) if self.add else self.cv3(self.cv2(self.cv1(x)))
-
-
-class ResX(Res):
- # ResNet bottleneck
- def __init__(self, c1, c2, shortcut=True, g=32, e=0.5): # ch_in, ch_out, shortcut, groups, expansion
- super().__init__(c1, c2, shortcut, g, e)
- c_ = int(c2 * e) # hidden channels
-
-
-class Ghost(nn.Module):
- # Ghost Bottleneck https://github.com/huawei-noah/ghostnet
- def __init__(self, c1, c2, k=3, s=1): # ch_in, ch_out, kernel, stride
- super(Ghost, self).__init__()
- c_ = c2 // 2
- self.conv = nn.Sequential(GhostConv(c1, c_, 1, 1), # pw
- DWConv(c_, c_, k, s, act=False) if s == 2 else nn.Identity(), # dw
- GhostConv(c_, c2, 1, 1, act=False)) # pw-linear
- self.shortcut = nn.Sequential(DWConv(c1, c1, k, s, act=False),
- Conv(c1, c2, 1, 1, act=False)) if s == 2 else nn.Identity()
-
- def forward(self, x):
- return self.conv(x) + self.shortcut(x)
-
-##### end of basic #####
-
-
-##### cspnet #####
-
-class SPPCSPC(nn.Module):
- # CSP https://github.com/WongKinYiu/CrossStagePartialNetworks
- def __init__(self, c1, c2, n=1, shortcut=False, g=1, e=0.5, k=(5, 9, 13)):
- super(SPPCSPC, self).__init__()
- c_ = int(2 * c2 * e) # hidden channels
- self.cv1 = Conv(c1, c_, 1, 1)
- self.cv2 = Conv(c1, c_, 1, 1)
- self.cv3 = Conv(c_, c_, 3, 1)
- self.cv4 = Conv(c_, c_, 1, 1)
- self.m = nn.ModuleList([nn.MaxPool2d(kernel_size=x, stride=1, padding=x // 2) for x in k])
- self.cv5 = Conv(4 * c_, c_, 1, 1)
- self.cv6 = Conv(c_, c_, 3, 1)
- self.cv7 = Conv(2 * c_, c2, 1, 1)
-
- def forward(self, x):
- x1 = self.cv4(self.cv3(self.cv1(x)))
- y1 = self.cv6(self.cv5(torch.cat([x1] + [m(x1) for m in self.m], 1)))
- y2 = self.cv2(x)
- return self.cv7(torch.cat((y1, y2), dim=1))
-
-class GhostSPPCSPC(SPPCSPC):
- # CSP https://github.com/WongKinYiu/CrossStagePartialNetworks
- def __init__(self, c1, c2, n=1, shortcut=False, g=1, e=0.5, k=(5, 9, 13)):
- super().__init__(c1, c2, n, shortcut, g, e, k)
- c_ = int(2 * c2 * e) # hidden channels
- self.cv1 = GhostConv(c1, c_, 1, 1)
- self.cv2 = GhostConv(c1, c_, 1, 1)
- self.cv3 = GhostConv(c_, c_, 3, 1)
- self.cv4 = GhostConv(c_, c_, 1, 1)
- self.cv5 = GhostConv(4 * c_, c_, 1, 1)
- self.cv6 = GhostConv(c_, c_, 3, 1)
- self.cv7 = GhostConv(2 * c_, c2, 1, 1)
-
-
-class GhostStem(Stem):
- # Stem
- def __init__(self, c1, c2, k=1, s=1, p=None, g=1, act=True): # ch_in, ch_out, kernel, stride, padding, groups
- super().__init__(c1, c2, k, s, p, g, act)
- c_ = int(c2/2) # hidden channels
- self.cv1 = GhostConv(c1, c_, 3, 2)
- self.cv2 = GhostConv(c_, c_, 1, 1)
- self.cv3 = GhostConv(c_, c_, 3, 2)
- self.cv4 = GhostConv(2 * c_, c2, 1, 1)
-
-
-class BottleneckCSPA(nn.Module):
- # CSP https://github.com/WongKinYiu/CrossStagePartialNetworks
- def __init__(self, c1, c2, n=1, shortcut=True, g=1, e=0.5): # ch_in, ch_out, number, shortcut, groups, expansion
- super(BottleneckCSPA, self).__init__()
- c_ = int(c2 * e) # hidden channels
- self.cv1 = Conv(c1, c_, 1, 1)
- self.cv2 = Conv(c1, c_, 1, 1)
- self.cv3 = Conv(2 * c_, c2, 1, 1)
- self.m = nn.Sequential(*[Bottleneck(c_, c_, shortcut, g, e=1.0) for _ in range(n)])
-
- def forward(self, x):
- y1 = self.m(self.cv1(x))
- y2 = self.cv2(x)
- return self.cv3(torch.cat((y1, y2), dim=1))
-
-
-class BottleneckCSPB(nn.Module):
- # CSP https://github.com/WongKinYiu/CrossStagePartialNetworks
- def __init__(self, c1, c2, n=1, shortcut=False, g=1, e=0.5): # ch_in, ch_out, number, shortcut, groups, expansion
- super(BottleneckCSPB, self).__init__()
- c_ = int(c2) # hidden channels
- self.cv1 = Conv(c1, c_, 1, 1)
- self.cv2 = Conv(c_, c_, 1, 1)
- self.cv3 = Conv(2 * c_, c2, 1, 1)
- self.m = nn.Sequential(*[Bottleneck(c_, c_, shortcut, g, e=1.0) for _ in range(n)])
-
- def forward(self, x):
- x1 = self.cv1(x)
- y1 = self.m(x1)
- y2 = self.cv2(x1)
- return self.cv3(torch.cat((y1, y2), dim=1))
-
-
-class BottleneckCSPC(nn.Module):
- # CSP https://github.com/WongKinYiu/CrossStagePartialNetworks
- def __init__(self, c1, c2, n=1, shortcut=True, g=1, e=0.5): # ch_in, ch_out, number, shortcut, groups, expansion
- super(BottleneckCSPC, self).__init__()
- c_ = int(c2 * e) # hidden channels
- self.cv1 = Conv(c1, c_, 1, 1)
- self.cv2 = Conv(c1, c_, 1, 1)
- self.cv3 = Conv(c_, c_, 1, 1)
- self.cv4 = Conv(2 * c_, c2, 1, 1)
- self.m = nn.Sequential(*[Bottleneck(c_, c_, shortcut, g, e=1.0) for _ in range(n)])
-
- def forward(self, x):
- y1 = self.cv3(self.m(self.cv1(x)))
- y2 = self.cv2(x)
- return self.cv4(torch.cat((y1, y2), dim=1))
-
-
-class ResCSPA(BottleneckCSPA):
- # CSP https://github.com/WongKinYiu/CrossStagePartialNetworks
- def __init__(self, c1, c2, n=1, shortcut=True, g=1, e=0.5): # ch_in, ch_out, number, shortcut, groups, expansion
- super().__init__(c1, c2, n, shortcut, g, e)
- c_ = int(c2 * e) # hidden channels
- self.m = nn.Sequential(*[Res(c_, c_, shortcut, g, e=0.5) for _ in range(n)])
-
-
-class ResCSPB(BottleneckCSPB):
- # CSP https://github.com/WongKinYiu/CrossStagePartialNetworks
- def __init__(self, c1, c2, n=1, shortcut=True, g=1, e=0.5): # ch_in, ch_out, number, shortcut, groups, expansion
- super().__init__(c1, c2, n, shortcut, g, e)
- c_ = int(c2) # hidden channels
- self.m = nn.Sequential(*[Res(c_, c_, shortcut, g, e=0.5) for _ in range(n)])
-
-
-class ResCSPC(BottleneckCSPC):
- # CSP https://github.com/WongKinYiu/CrossStagePartialNetworks
- def __init__(self, c1, c2, n=1, shortcut=True, g=1, e=0.5): # ch_in, ch_out, number, shortcut, groups, expansion
- super().__init__(c1, c2, n, shortcut, g, e)
- c_ = int(c2 * e) # hidden channels
- self.m = nn.Sequential(*[Res(c_, c_, shortcut, g, e=0.5) for _ in range(n)])
-
-
-class ResXCSPA(ResCSPA):
- # CSP https://github.com/WongKinYiu/CrossStagePartialNetworks
- def __init__(self, c1, c2, n=1, shortcut=True, g=32, e=0.5): # ch_in, ch_out, number, shortcut, groups, expansion
- super().__init__(c1, c2, n, shortcut, g, e)
- c_ = int(c2 * e) # hidden channels
- self.m = nn.Sequential(*[Res(c_, c_, shortcut, g, e=1.0) for _ in range(n)])
-
-
-class ResXCSPB(ResCSPB):
- # CSP https://github.com/WongKinYiu/CrossStagePartialNetworks
- def __init__(self, c1, c2, n=1, shortcut=True, g=32, e=0.5): # ch_in, ch_out, number, shortcut, groups, expansion
- super().__init__(c1, c2, n, shortcut, g, e)
- c_ = int(c2) # hidden channels
- self.m = nn.Sequential(*[Res(c_, c_, shortcut, g, e=1.0) for _ in range(n)])
-
-
-class ResXCSPC(ResCSPC):
- # CSP https://github.com/WongKinYiu/CrossStagePartialNetworks
- def __init__(self, c1, c2, n=1, shortcut=True, g=32, e=0.5): # ch_in, ch_out, number, shortcut, groups, expansion
- super().__init__(c1, c2, n, shortcut, g, e)
- c_ = int(c2 * e) # hidden channels
- self.m = nn.Sequential(*[Res(c_, c_, shortcut, g, e=1.0) for _ in range(n)])
-
-
-class GhostCSPA(BottleneckCSPA):
- # CSP https://github.com/WongKinYiu/CrossStagePartialNetworks
- def __init__(self, c1, c2, n=1, shortcut=True, g=1, e=0.5): # ch_in, ch_out, number, shortcut, groups, expansion
- super().__init__(c1, c2, n, shortcut, g, e)
- c_ = int(c2 * e) # hidden channels
- self.m = nn.Sequential(*[Ghost(c_, c_) for _ in range(n)])
-
-
-class GhostCSPB(BottleneckCSPB):
- # CSP https://github.com/WongKinYiu/CrossStagePartialNetworks
- def __init__(self, c1, c2, n=1, shortcut=True, g=1, e=0.5): # ch_in, ch_out, number, shortcut, groups, expansion
- super().__init__(c1, c2, n, shortcut, g, e)
- c_ = int(c2) # hidden channels
- self.m = nn.Sequential(*[Ghost(c_, c_) for _ in range(n)])
-
-
-class GhostCSPC(BottleneckCSPC):
- # CSP https://github.com/WongKinYiu/CrossStagePartialNetworks
- def __init__(self, c1, c2, n=1, shortcut=True, g=1, e=0.5): # ch_in, ch_out, number, shortcut, groups, expansion
- super().__init__(c1, c2, n, shortcut, g, e)
- c_ = int(c2 * e) # hidden channels
- self.m = nn.Sequential(*[Ghost(c_, c_) for _ in range(n)])
-
-##### end of cspnet #####
-
-
-##### yolor #####
-
-class ImplicitA(nn.Module):
- def __init__(self, channel, mean=0., std=.02):
- super(ImplicitA, self).__init__()
- self.channel = channel
- self.mean = mean
- self.std = std
- self.implicit = nn.Parameter(torch.zeros(1, channel, 1, 1))
- nn.init.normal_(self.implicit, mean=self.mean, std=self.std)
-
- def forward(self, x):
- return self.implicit + x
-
-
-class ImplicitM(nn.Module):
- def __init__(self, channel, mean=1., std=.02):
- super(ImplicitM, self).__init__()
- self.channel = channel
- self.mean = mean
- self.std = std
- self.implicit = nn.Parameter(torch.ones(1, channel, 1, 1))
- nn.init.normal_(self.implicit, mean=self.mean, std=self.std)
-
- def forward(self, x):
- return self.implicit * x
-
-##### end of yolor #####
-
-
-##### repvgg #####
-
-class RepConv(nn.Module):
- # Represented convolution
- # https://arxiv.org/abs/2101.03697
-
- def __init__(self, c1, c2, k=3, s=1, p=None, g=1, act=True, deploy=False):
- super(RepConv, self).__init__()
-
- self.deploy = deploy
- self.groups = g
- self.in_channels = c1
- self.out_channels = c2
-
- assert k == 3
- assert autopad(k, p) == 1
-
- padding_11 = autopad(k, p) - k // 2
-
- self.act = nn.SiLU() if act is True else (act if isinstance(act, nn.Module) else nn.Identity())
-
- if deploy:
- self.rbr_reparam = nn.Conv2d(c1, c2, k, s, autopad(k, p), groups=g, bias=True)
-
- else:
- self.rbr_identity = (nn.BatchNorm2d(num_features=c1) if c2 == c1 and s == 1 else None)
-
- self.rbr_dense = nn.Sequential(
- nn.Conv2d(c1, c2, k, s, autopad(k, p), groups=g, bias=False),
- nn.BatchNorm2d(num_features=c2),
- )
-
- self.rbr_1x1 = nn.Sequential(
- nn.Conv2d( c1, c2, 1, s, padding_11, groups=g, bias=False),
- nn.BatchNorm2d(num_features=c2),
- )
-
- def forward(self, inputs):
- if hasattr(self, "rbr_reparam"):
- return self.act(self.rbr_reparam(inputs))
-
- if self.rbr_identity is None:
- id_out = 0
- else:
- id_out = self.rbr_identity(inputs)
-
- return self.act(self.rbr_dense(inputs) + self.rbr_1x1(inputs) + id_out)
-
- def get_equivalent_kernel_bias(self):
- kernel3x3, bias3x3 = self._fuse_bn_tensor(self.rbr_dense)
- kernel1x1, bias1x1 = self._fuse_bn_tensor(self.rbr_1x1)
- kernelid, biasid = self._fuse_bn_tensor(self.rbr_identity)
- return (
- kernel3x3 + self._pad_1x1_to_3x3_tensor(kernel1x1) + kernelid,
- bias3x3 + bias1x1 + biasid,
- )
-
- def _pad_1x1_to_3x3_tensor(self, kernel1x1):
- if kernel1x1 is None:
- return 0
- else:
- return nn.functional.pad(kernel1x1, [1, 1, 1, 1])
-
- def _fuse_bn_tensor(self, branch):
- if branch is None:
- return 0, 0
- if isinstance(branch, nn.Sequential):
- kernel = branch[0].weight
- running_mean = branch[1].running_mean
- running_var = branch[1].running_var
- gamma = branch[1].weight
- beta = branch[1].bias
- eps = branch[1].eps
- else:
- assert isinstance(branch, nn.BatchNorm2d)
- if not hasattr(self, "id_tensor"):
- input_dim = self.in_channels // self.groups
- kernel_value = np.zeros(
- (self.in_channels, input_dim, 3, 3), dtype=np.float32
- )
- for i in range(self.in_channels):
- kernel_value[i, i % input_dim, 1, 1] = 1
- self.id_tensor = torch.from_numpy(kernel_value).to(branch.weight.device)
- kernel = self.id_tensor
- running_mean = branch.running_mean
- running_var = branch.running_var
- gamma = branch.weight
- beta = branch.bias
- eps = branch.eps
- std = (running_var + eps).sqrt()
- t = (gamma / std).reshape(-1, 1, 1, 1)
- return kernel * t, beta - running_mean * gamma / std
-
- def repvgg_convert(self):
- kernel, bias = self.get_equivalent_kernel_bias()
- return (
- kernel.detach().cpu().numpy(),
- bias.detach().cpu().numpy(),
- )
-
- def fuse_conv_bn(self, conv, bn):
-
- std = (bn.running_var + bn.eps).sqrt()
- bias = bn.bias - bn.running_mean * bn.weight / std
-
- t = (bn.weight / std).reshape(-1, 1, 1, 1)
- weights = conv.weight * t
-
- bn = nn.Identity()
- conv = nn.Conv2d(in_channels = conv.in_channels,
- out_channels = conv.out_channels,
- kernel_size = conv.kernel_size,
- stride=conv.stride,
- padding = conv.padding,
- dilation = conv.dilation,
- groups = conv.groups,
- bias = True,
- padding_mode = conv.padding_mode)
-
- conv.weight = torch.nn.Parameter(weights)
- conv.bias = torch.nn.Parameter(bias)
- return conv
-
- def fuse_repvgg_block(self):
- if self.deploy:
- return
- print(f"RepConv.fuse_repvgg_block")
-
- self.rbr_dense = self.fuse_conv_bn(self.rbr_dense[0], self.rbr_dense[1])
-
- self.rbr_1x1 = self.fuse_conv_bn(self.rbr_1x1[0], self.rbr_1x1[1])
- rbr_1x1_bias = self.rbr_1x1.bias
- weight_1x1_expanded = torch.nn.functional.pad(self.rbr_1x1.weight, [1, 1, 1, 1])
-
- # Fuse self.rbr_identity
- if (isinstance(self.rbr_identity, nn.BatchNorm2d) or isinstance(self.rbr_identity, nn.modules.batchnorm.SyncBatchNorm)):
- # print(f"fuse: rbr_identity == BatchNorm2d or SyncBatchNorm")
- identity_conv_1x1 = nn.Conv2d(
- in_channels=self.in_channels,
- out_channels=self.out_channels,
- kernel_size=1,
- stride=1,
- padding=0,
- groups=self.groups,
- bias=False)
- identity_conv_1x1.weight.data = identity_conv_1x1.weight.data.to(self.rbr_1x1.weight.data.device)
- identity_conv_1x1.weight.data = identity_conv_1x1.weight.data.squeeze().squeeze()
- # print(f" identity_conv_1x1.weight = {identity_conv_1x1.weight.shape}")
- identity_conv_1x1.weight.data.fill_(0.0)
- identity_conv_1x1.weight.data.fill_diagonal_(1.0)
- identity_conv_1x1.weight.data = identity_conv_1x1.weight.data.unsqueeze(2).unsqueeze(3)
- # print(f" identity_conv_1x1.weight = {identity_conv_1x1.weight.shape}")
-
- identity_conv_1x1 = self.fuse_conv_bn(identity_conv_1x1, self.rbr_identity)
- bias_identity_expanded = identity_conv_1x1.bias
- weight_identity_expanded = torch.nn.functional.pad(identity_conv_1x1.weight, [1, 1, 1, 1])
- else:
- # print(f"fuse: rbr_identity != BatchNorm2d, rbr_identity = {self.rbr_identity}")
- bias_identity_expanded = torch.nn.Parameter( torch.zeros_like(rbr_1x1_bias) )
- weight_identity_expanded = torch.nn.Parameter( torch.zeros_like(weight_1x1_expanded) )
-
-
- #print(f"self.rbr_1x1.weight = {self.rbr_1x1.weight.shape}, ")
- #print(f"weight_1x1_expanded = {weight_1x1_expanded.shape}, ")
- #print(f"self.rbr_dense.weight = {self.rbr_dense.weight.shape}, ")
-
- self.rbr_dense.weight = torch.nn.Parameter(self.rbr_dense.weight + weight_1x1_expanded + weight_identity_expanded)
- self.rbr_dense.bias = torch.nn.Parameter(self.rbr_dense.bias + rbr_1x1_bias + bias_identity_expanded)
-
- self.rbr_reparam = self.rbr_dense
- self.deploy = True
-
- if self.rbr_identity is not None:
- del self.rbr_identity
- self.rbr_identity = None
-
- if self.rbr_1x1 is not None:
- del self.rbr_1x1
- self.rbr_1x1 = None
-
- if self.rbr_dense is not None:
- del self.rbr_dense
- self.rbr_dense = None
-
-
-class RepBottleneck(Bottleneck):
- # Standard bottleneck
- def __init__(self, c1, c2, shortcut=True, g=1, e=0.5): # ch_in, ch_out, shortcut, groups, expansion
- super().__init__(c1, c2, shortcut=True, g=1, e=0.5)
- c_ = int(c2 * e) # hidden channels
- self.cv2 = RepConv(c_, c2, 3, 1, g=g)
-
-
-class RepBottleneckCSPA(BottleneckCSPA):
- # CSP Bottleneck https://github.com/WongKinYiu/CrossStagePartialNetworks
- def __init__(self, c1, c2, n=1, shortcut=True, g=1, e=0.5): # ch_in, ch_out, number, shortcut, groups, expansion
- super().__init__(c1, c2, n, shortcut, g, e)
- c_ = int(c2 * e) # hidden channels
- self.m = nn.Sequential(*[RepBottleneck(c_, c_, shortcut, g, e=1.0) for _ in range(n)])
-
-
-class RepBottleneckCSPB(BottleneckCSPB):
- # CSP Bottleneck https://github.com/WongKinYiu/CrossStagePartialNetworks
- def __init__(self, c1, c2, n=1, shortcut=False, g=1, e=0.5): # ch_in, ch_out, number, shortcut, groups, expansion
- super().__init__(c1, c2, n, shortcut, g, e)
- c_ = int(c2) # hidden channels
- self.m = nn.Sequential(*[RepBottleneck(c_, c_, shortcut, g, e=1.0) for _ in range(n)])
-
-
-class RepBottleneckCSPC(BottleneckCSPC):
- # CSP Bottleneck https://github.com/WongKinYiu/CrossStagePartialNetworks
- def __init__(self, c1, c2, n=1, shortcut=True, g=1, e=0.5): # ch_in, ch_out, number, shortcut, groups, expansion
- super().__init__(c1, c2, n, shortcut, g, e)
- c_ = int(c2 * e) # hidden channels
- self.m = nn.Sequential(*[RepBottleneck(c_, c_, shortcut, g, e=1.0) for _ in range(n)])
-
-
-class RepRes(Res):
- # Standard bottleneck
- def __init__(self, c1, c2, shortcut=True, g=1, e=0.5): # ch_in, ch_out, shortcut, groups, expansion
- super().__init__(c1, c2, shortcut, g, e)
- c_ = int(c2 * e) # hidden channels
- self.cv2 = RepConv(c_, c_, 3, 1, g=g)
-
-
-class RepResCSPA(ResCSPA):
- # CSP Bottleneck https://github.com/WongKinYiu/CrossStagePartialNetworks
- def __init__(self, c1, c2, n=1, shortcut=True, g=1, e=0.5): # ch_in, ch_out, number, shortcut, groups, expansion
- super().__init__(c1, c2, n, shortcut, g, e)
- c_ = int(c2 * e) # hidden channels
- self.m = nn.Sequential(*[RepRes(c_, c_, shortcut, g, e=0.5) for _ in range(n)])
-
-
-class RepResCSPB(ResCSPB):
- # CSP Bottleneck https://github.com/WongKinYiu/CrossStagePartialNetworks
- def __init__(self, c1, c2, n=1, shortcut=False, g=1, e=0.5): # ch_in, ch_out, number, shortcut, groups, expansion
- super().__init__(c1, c2, n, shortcut, g, e)
- c_ = int(c2) # hidden channels
- self.m = nn.Sequential(*[RepRes(c_, c_, shortcut, g, e=0.5) for _ in range(n)])
-
-
-class RepResCSPC(ResCSPC):
- # CSP Bottleneck https://github.com/WongKinYiu/CrossStagePartialNetworks
- def __init__(self, c1, c2, n=1, shortcut=True, g=1, e=0.5): # ch_in, ch_out, number, shortcut, groups, expansion
- super().__init__(c1, c2, n, shortcut, g, e)
- c_ = int(c2 * e) # hidden channels
- self.m = nn.Sequential(*[RepRes(c_, c_, shortcut, g, e=0.5) for _ in range(n)])
-
-
-class RepResX(ResX):
- # Standard bottleneck
- def __init__(self, c1, c2, shortcut=True, g=32, e=0.5): # ch_in, ch_out, shortcut, groups, expansion
- super().__init__(c1, c2, shortcut, g, e)
- c_ = int(c2 * e) # hidden channels
- self.cv2 = RepConv(c_, c_, 3, 1, g=g)
-
-
-class RepResXCSPA(ResXCSPA):
- # CSP Bottleneck https://github.com/WongKinYiu/CrossStagePartialNetworks
- def __init__(self, c1, c2, n=1, shortcut=True, g=32, e=0.5): # ch_in, ch_out, number, shortcut, groups, expansion
- super().__init__(c1, c2, n, shortcut, g, e)
- c_ = int(c2 * e) # hidden channels
- self.m = nn.Sequential(*[RepResX(c_, c_, shortcut, g, e=0.5) for _ in range(n)])
-
-
-class RepResXCSPB(ResXCSPB):
- # CSP Bottleneck https://github.com/WongKinYiu/CrossStagePartialNetworks
- def __init__(self, c1, c2, n=1, shortcut=False, g=32, e=0.5): # ch_in, ch_out, number, shortcut, groups, expansion
- super().__init__(c1, c2, n, shortcut, g, e)
- c_ = int(c2) # hidden channels
- self.m = nn.Sequential(*[RepResX(c_, c_, shortcut, g, e=0.5) for _ in range(n)])
-
-
-class RepResXCSPC(ResXCSPC):
- # CSP Bottleneck https://github.com/WongKinYiu/CrossStagePartialNetworks
- def __init__(self, c1, c2, n=1, shortcut=True, g=32, e=0.5): # ch_in, ch_out, number, shortcut, groups, expansion
- super().__init__(c1, c2, n, shortcut, g, e)
- c_ = int(c2 * e) # hidden channels
- self.m = nn.Sequential(*[RepResX(c_, c_, shortcut, g, e=0.5) for _ in range(n)])
-
-##### end of repvgg #####
-
-
-##### transformer #####
-
-class TransformerLayer(nn.Module):
- # Transformer layer https://arxiv.org/abs/2010.11929 (LayerNorm layers removed for better performance)
- def __init__(self, c, num_heads):
- super().__init__()
- self.q = nn.Linear(c, c, bias=False)
- self.k = nn.Linear(c, c, bias=False)
- self.v = nn.Linear(c, c, bias=False)
- self.ma = nn.MultiheadAttention(embed_dim=c, num_heads=num_heads)
- self.fc1 = nn.Linear(c, c, bias=False)
- self.fc2 = nn.Linear(c, c, bias=False)
-
- def forward(self, x):
- x = self.ma(self.q(x), self.k(x), self.v(x))[0] + x
- x = self.fc2(self.fc1(x)) + x
- return x
-
-
-class TransformerBlock(nn.Module):
- # Vision Transformer https://arxiv.org/abs/2010.11929
- def __init__(self, c1, c2, num_heads, num_layers):
- super().__init__()
- self.conv = None
- if c1 != c2:
- self.conv = Conv(c1, c2)
- self.linear = nn.Linear(c2, c2) # learnable position embedding
- self.tr = nn.Sequential(*[TransformerLayer(c2, num_heads) for _ in range(num_layers)])
- self.c2 = c2
-
- def forward(self, x):
- if self.conv is not None:
- x = self.conv(x)
- b, _, w, h = x.shape
- p = x.flatten(2)
- p = p.unsqueeze(0)
- p = p.transpose(0, 3)
- p = p.squeeze(3)
- e = self.linear(p)
- x = p + e
-
- x = self.tr(x)
- x = x.unsqueeze(3)
- x = x.transpose(0, 3)
- x = x.reshape(b, self.c2, w, h)
- return x
-
-##### end of transformer #####
-
-
-##### yolov5 #####
-
-class Focus(nn.Module):
- # Focus wh information into c-space
- def __init__(self, c1, c2, k=1, s=1, p=None, g=1, act=True): # ch_in, ch_out, kernel, stride, padding, groups
- super(Focus, self).__init__()
- self.conv = Conv(c1 * 4, c2, k, s, p, g, act)
- # self.contract = Contract(gain=2)
-
- def forward(self, x): # x(b,c,w,h) -> y(b,4c,w/2,h/2)
- return self.conv(torch.cat([x[..., ::2, ::2], x[..., 1::2, ::2], x[..., ::2, 1::2], x[..., 1::2, 1::2]], 1))
- # return self.conv(self.contract(x))
-
-
-class SPPF(nn.Module):
- # Spatial Pyramid Pooling - Fast (SPPF) layer for YOLOv5 by Glenn Jocher
- def __init__(self, c1, c2, k=5): # equivalent to SPP(k=(5, 9, 13))
- super().__init__()
- c_ = c1 // 2 # hidden channels
- self.cv1 = Conv(c1, c_, 1, 1)
- self.cv2 = Conv(c_ * 4, c2, 1, 1)
- self.m = nn.MaxPool2d(kernel_size=k, stride=1, padding=k // 2)
-
- def forward(self, x):
- x = self.cv1(x)
- y1 = self.m(x)
- y2 = self.m(y1)
- return self.cv2(torch.cat([x, y1, y2, self.m(y2)], 1))
-
-
-class Contract(nn.Module):
- # Contract width-height into channels, i.e. x(1,64,80,80) to x(1,256,40,40)
- def __init__(self, gain=2):
- super().__init__()
- self.gain = gain
-
- def forward(self, x):
- N, C, H, W = x.size() # assert (H / s == 0) and (W / s == 0), 'Indivisible gain'
- s = self.gain
- x = x.view(N, C, H // s, s, W // s, s) # x(1,64,40,2,40,2)
- x = x.permute(0, 3, 5, 1, 2, 4).contiguous() # x(1,2,2,64,40,40)
- return x.view(N, C * s * s, H // s, W // s) # x(1,256,40,40)
-
-
-class Expand(nn.Module):
- # Expand channels into width-height, i.e. x(1,64,80,80) to x(1,16,160,160)
- def __init__(self, gain=2):
- super().__init__()
- self.gain = gain
-
- def forward(self, x):
- N, C, H, W = x.size() # assert C / s ** 2 == 0, 'Indivisible gain'
- s = self.gain
- x = x.view(N, s, s, C // s ** 2, H, W) # x(1,2,2,16,80,80)
- x = x.permute(0, 3, 4, 1, 5, 2).contiguous() # x(1,16,80,2,80,2)
- return x.view(N, C // s ** 2, H * s, W * s) # x(1,16,160,160)
-
-
-class NMS(nn.Module):
- # Non-Maximum Suppression (NMS) module
- conf = 0.25 # confidence threshold
- iou = 0.45 # IoU threshold
- classes = None # (optional list) filter by class
-
- def __init__(self):
- super(NMS, self).__init__()
-
- def forward(self, x):
- return non_max_suppression(x[0], conf_thres=self.conf, iou_thres=self.iou, classes=self.classes)
-
-
-class autoShape(nn.Module):
- # input-robust model wrapper for passing cv2/np/PIL/torch inputs. Includes preprocessing, inference and NMS
- conf = 0.25 # NMS confidence threshold
- iou = 0.45 # NMS IoU threshold
- classes = None # (optional list) filter by class
-
- def __init__(self, model):
- super(autoShape, self).__init__()
- self.model = model.eval()
-
- def autoshape(self):
- print('autoShape already enabled, skipping... ') # model already converted to model.autoshape()
- return self
-
- @torch.no_grad()
- def forward(self, imgs, size=640, augment=False, profile=False):
- # Inference from various sources. For height=640, width=1280, RGB images example inputs are:
- # filename: imgs = 'data/samples/zidane.jpg'
- # URI: = 'https://github.com/ultralytics/yolov5/releases/download/v1.0/zidane.jpg'
- # OpenCV: = cv2.imread('image.jpg')[:,:,::-1] # HWC BGR to RGB x(640,1280,3)
- # PIL: = Image.open('image.jpg') # HWC x(640,1280,3)
- # numpy: = np.zeros((640,1280,3)) # HWC
- # torch: = torch.zeros(16,3,320,640) # BCHW (scaled to size=640, 0-1 values)
- # multiple: = [Image.open('image1.jpg'), Image.open('image2.jpg'), ...] # list of images
-
- t = [time_synchronized()]
- p = next(self.model.parameters()) # for device and type
- if isinstance(imgs, torch.Tensor): # torch
- with amp.autocast(enabled=p.device.type != 'cpu'):
- return self.model(imgs.to(p.device).type_as(p), augment, profile) # inference
-
- # Pre-process
- n, imgs = (len(imgs), imgs) if isinstance(imgs, list) else (1, [imgs]) # number of images, list of images
- shape0, shape1, files = [], [], [] # image and inference shapes, filenames
- for i, im in enumerate(imgs):
- f = f'image{i}' # filename
- if isinstance(im, str): # filename or uri
- im, f = np.asarray(Image.open(requests.get(im, stream=True).raw if im.startswith('http') else im)), im
- elif isinstance(im, Image.Image): # PIL Image
- im, f = np.asarray(im), getattr(im, 'filename', f) or f
- files.append(Path(f).with_suffix('.jpg').name)
- if im.shape[0] < 5: # image in CHW
- im = im.transpose((1, 2, 0)) # reverse dataloader .transpose(2, 0, 1)
- im = im[:, :, :3] if im.ndim == 3 else np.tile(im[:, :, None], 3) # enforce 3ch input
- s = im.shape[:2] # HWC
- shape0.append(s) # image shape
- g = (size / max(s)) # gain
- shape1.append([y * g for y in s])
- imgs[i] = im # update
- shape1 = [make_divisible(x, int(self.stride.max())) for x in np.stack(shape1, 0).max(0)] # inference shape
- x = [letterbox(im, new_shape=shape1, auto=False)[0] for im in imgs] # pad
- x = np.stack(x, 0) if n > 1 else x[0][None] # stack
- x = np.ascontiguousarray(x.transpose((0, 3, 1, 2))) # BHWC to BCHW
- x = torch.from_numpy(x).to(p.device).type_as(p) / 255. # uint8 to fp16/32
- t.append(time_synchronized())
-
- with amp.autocast(enabled=p.device.type != 'cpu'):
- # Inference
- y = self.model(x, augment, profile)[0] # forward
- t.append(time_synchronized())
-
- # Post-process
- y = non_max_suppression(y, conf_thres=self.conf, iou_thres=self.iou, classes=self.classes) # NMS
- for i in range(n):
- scale_coords(shape1, y[i][:, :4], shape0[i])
-
- t.append(time_synchronized())
- return Detections(imgs, y, files, t, self.names, x.shape)
-
-
-class Detections:
- # detections class for YOLOv5 inference results
- def __init__(self, imgs, pred, files, times=None, names=None, shape=None):
- super(Detections, self).__init__()
- d = pred[0].device # device
- gn = [torch.tensor([*[im.shape[i] for i in [1, 0, 1, 0]], 1., 1.], device=d) for im in imgs] # normalizations
- self.imgs = imgs # list of images as numpy arrays
- self.pred = pred # list of tensors pred[0] = (xyxy, conf, cls)
- self.names = names # class names
- self.files = files # image filenames
- self.xyxy = pred # xyxy pixels
- self.xywh = [xyxy2xywh(x) for x in pred] # xywh pixels
- self.xyxyn = [x / g for x, g in zip(self.xyxy, gn)] # xyxy normalized
- self.xywhn = [x / g for x, g in zip(self.xywh, gn)] # xywh normalized
- self.n = len(self.pred) # number of images (batch size)
- self.t = tuple((times[i + 1] - times[i]) * 1000 / self.n for i in range(3)) # timestamps (ms)
- self.s = shape # inference BCHW shape
-
- def display(self, pprint=False, show=False, save=False, render=False, save_dir=''):
- colors = color_list()
- for i, (img, pred) in enumerate(zip(self.imgs, self.pred)):
- str = f'image {i + 1}/{len(self.pred)}: {img.shape[0]}x{img.shape[1]} '
- if pred is not None:
- for c in pred[:, -1].unique():
- n = (pred[:, -1] == c).sum() # detections per class
- str += f"{n} {self.names[int(c)]}{'s' * (n > 1)}, " # add to string
- if show or save or render:
- for *box, conf, cls in pred: # xyxy, confidence, class
- label = f'{self.names[int(cls)]} {conf:.2f}'
- plot_one_box(box, img, label=label, color=colors[int(cls) % 10])
- img = Image.fromarray(img.astype(np.uint8)) if isinstance(img, np.ndarray) else img # from np
- if pprint:
- print(str.rstrip(', '))
- if show:
- img.show(self.files[i]) # show
- if save:
- f = self.files[i]
- img.save(Path(save_dir) / f) # save
- print(f"{'Saved' * (i == 0)} {f}", end=',' if i < self.n - 1 else f' to {save_dir}\n')
- if render:
- self.imgs[i] = np.asarray(img)
-
- def print(self):
- self.display(pprint=True) # print results
- print(f'Speed: %.1fms pre-process, %.1fms inference, %.1fms NMS per image at shape {tuple(self.s)}' % self.t)
-
- def show(self):
- self.display(show=True) # show results
-
- def save(self, save_dir='runs/hub/exp'):
- save_dir = increment_path(save_dir, exist_ok=save_dir != 'runs/hub/exp') # increment save_dir
- Path(save_dir).mkdir(parents=True, exist_ok=True)
- self.display(save=True, save_dir=save_dir) # save results
-
- def render(self):
- self.display(render=True) # render results
- return self.imgs
-
- def pandas(self):
- # return detections as pandas DataFrames, i.e. print(results.pandas().xyxy[0])
- new = copy(self) # return copy
- ca = 'xmin', 'ymin', 'xmax', 'ymax', 'confidence', 'class', 'name' # xyxy columns
- cb = 'xcenter', 'ycenter', 'width', 'height', 'confidence', 'class', 'name' # xywh columns
- for k, c in zip(['xyxy', 'xyxyn', 'xywh', 'xywhn'], [ca, ca, cb, cb]):
- a = [[x[:5] + [int(x[5]), self.names[int(x[5])]] for x in x.tolist()] for x in getattr(self, k)] # update
- setattr(new, k, [pd.DataFrame(x, columns=c) for x in a])
- return new
-
- def tolist(self):
- # return a list of Detections objects, i.e. 'for result in results.tolist():'
- x = [Detections([self.imgs[i]], [self.pred[i]], self.names, self.s) for i in range(self.n)]
- for d in x:
- for k in ['imgs', 'pred', 'xyxy', 'xyxyn', 'xywh', 'xywhn']:
- setattr(d, k, getattr(d, k)[0]) # pop out of list
- return x
-
- def __len__(self):
- return self.n
-
-
-class Classify(nn.Module):
- # Classification head, i.e. x(b,c1,20,20) to x(b,c2)
- def __init__(self, c1, c2, k=1, s=1, p=None, g=1): # ch_in, ch_out, kernel, stride, padding, groups
- super(Classify, self).__init__()
- self.aap = nn.AdaptiveAvgPool2d(1) # to x(b,c1,1,1)
- self.conv = nn.Conv2d(c1, c2, k, s, autopad(k, p), groups=g) # to x(b,c2,1,1)
- self.flat = nn.Flatten()
-
- def forward(self, x):
- z = torch.cat([self.aap(y) for y in (x if isinstance(x, list) else [x])], 1) # cat if list
- return self.flat(self.conv(z)) # flatten to x(b,c2)
-
-##### end of yolov5 ######
-
-
-##### orepa #####
-
-def transI_fusebn(kernel, bn):
- gamma = bn.weight
- std = (bn.running_var + bn.eps).sqrt()
- return kernel * ((gamma / std).reshape(-1, 1, 1, 1)), bn.bias - bn.running_mean * gamma / std
-
-
-class ConvBN(nn.Module):
- def __init__(self, in_channels, out_channels, kernel_size,
- stride=1, padding=0, dilation=1, groups=1, deploy=False, nonlinear=None):
- super().__init__()
- if nonlinear is None:
- self.nonlinear = nn.Identity()
- else:
- self.nonlinear = nonlinear
- if deploy:
- self.conv = nn.Conv2d(in_channels=in_channels, out_channels=out_channels, kernel_size=kernel_size,
- stride=stride, padding=padding, dilation=dilation, groups=groups, bias=True)
- else:
- self.conv = nn.Conv2d(in_channels=in_channels, out_channels=out_channels, kernel_size=kernel_size,
- stride=stride, padding=padding, dilation=dilation, groups=groups, bias=False)
- self.bn = nn.BatchNorm2d(num_features=out_channels)
-
- def forward(self, x):
- if hasattr(self, 'bn'):
- return self.nonlinear(self.bn(self.conv(x)))
- else:
- return self.nonlinear(self.conv(x))
-
- def switch_to_deploy(self):
- kernel, bias = transI_fusebn(self.conv.weight, self.bn)
- conv = nn.Conv2d(in_channels=self.conv.in_channels, out_channels=self.conv.out_channels, kernel_size=self.conv.kernel_size,
- stride=self.conv.stride, padding=self.conv.padding, dilation=self.conv.dilation, groups=self.conv.groups, bias=True)
- conv.weight.data = kernel
- conv.bias.data = bias
- for para in self.parameters():
- para.detach_()
- self.__delattr__('conv')
- self.__delattr__('bn')
- self.conv = conv
-
-class OREPA_3x3_RepConv(nn.Module):
-
- def __init__(self, in_channels, out_channels, kernel_size,
- stride=1, padding=0, dilation=1, groups=1,
- internal_channels_1x1_3x3=None,
- deploy=False, nonlinear=None, single_init=False):
- super(OREPA_3x3_RepConv, self).__init__()
- self.deploy = deploy
-
- if nonlinear is None:
- self.nonlinear = nn.Identity()
- else:
- self.nonlinear = nonlinear
-
- self.kernel_size = kernel_size
- self.in_channels = in_channels
- self.out_channels = out_channels
- self.groups = groups
- assert padding == kernel_size // 2
-
- self.stride = stride
- self.padding = padding
- self.dilation = dilation
-
- self.branch_counter = 0
-
- self.weight_rbr_origin = nn.Parameter(torch.Tensor(out_channels, int(in_channels/self.groups), kernel_size, kernel_size))
- nn.init.kaiming_uniform_(self.weight_rbr_origin, a=math.sqrt(1.0))
- self.branch_counter += 1
-
-
- if groups < out_channels:
- self.weight_rbr_avg_conv = nn.Parameter(torch.Tensor(out_channels, int(in_channels/self.groups), 1, 1))
- self.weight_rbr_pfir_conv = nn.Parameter(torch.Tensor(out_channels, int(in_channels/self.groups), 1, 1))
- nn.init.kaiming_uniform_(self.weight_rbr_avg_conv, a=1.0)
- nn.init.kaiming_uniform_(self.weight_rbr_pfir_conv, a=1.0)
- self.weight_rbr_avg_conv.data
- self.weight_rbr_pfir_conv.data
- self.register_buffer('weight_rbr_avg_avg', torch.ones(kernel_size, kernel_size).mul(1.0/kernel_size/kernel_size))
- self.branch_counter += 1
-
- else:
- raise NotImplementedError
- self.branch_counter += 1
-
- if internal_channels_1x1_3x3 is None:
- internal_channels_1x1_3x3 = in_channels if groups < out_channels else 2 * in_channels # For mobilenet, it is better to have 2X internal channels
-
- if internal_channels_1x1_3x3 == in_channels:
- self.weight_rbr_1x1_kxk_idconv1 = nn.Parameter(torch.zeros(in_channels, int(in_channels/self.groups), 1, 1))
- id_value = np.zeros((in_channels, int(in_channels/self.groups), 1, 1))
- for i in range(in_channels):
- id_value[i, i % int(in_channels/self.groups), 0, 0] = 1
- id_tensor = torch.from_numpy(id_value).type_as(self.weight_rbr_1x1_kxk_idconv1)
- self.register_buffer('id_tensor', id_tensor)
-
- else:
- self.weight_rbr_1x1_kxk_conv1 = nn.Parameter(torch.Tensor(internal_channels_1x1_3x3, int(in_channels/self.groups), 1, 1))
- nn.init.kaiming_uniform_(self.weight_rbr_1x1_kxk_conv1, a=math.sqrt(1.0))
- self.weight_rbr_1x1_kxk_conv2 = nn.Parameter(torch.Tensor(out_channels, int(internal_channels_1x1_3x3/self.groups), kernel_size, kernel_size))
- nn.init.kaiming_uniform_(self.weight_rbr_1x1_kxk_conv2, a=math.sqrt(1.0))
- self.branch_counter += 1
-
- expand_ratio = 8
- self.weight_rbr_gconv_dw = nn.Parameter(torch.Tensor(in_channels*expand_ratio, 1, kernel_size, kernel_size))
- self.weight_rbr_gconv_pw = nn.Parameter(torch.Tensor(out_channels, in_channels*expand_ratio, 1, 1))
- nn.init.kaiming_uniform_(self.weight_rbr_gconv_dw, a=math.sqrt(1.0))
- nn.init.kaiming_uniform_(self.weight_rbr_gconv_pw, a=math.sqrt(1.0))
- self.branch_counter += 1
-
- if out_channels == in_channels and stride == 1:
- self.branch_counter += 1
-
- self.vector = nn.Parameter(torch.Tensor(self.branch_counter, self.out_channels))
- self.bn = nn.BatchNorm2d(out_channels)
-
- self.fre_init()
-
- nn.init.constant_(self.vector[0, :], 0.25) #origin
- nn.init.constant_(self.vector[1, :], 0.25) #avg
- nn.init.constant_(self.vector[2, :], 0.0) #prior
- nn.init.constant_(self.vector[3, :], 0.5) #1x1_kxk
- nn.init.constant_(self.vector[4, :], 0.5) #dws_conv
-
-
- def fre_init(self):
- prior_tensor = torch.Tensor(self.out_channels, self.kernel_size, self.kernel_size)
- half_fg = self.out_channels/2
- for i in range(self.out_channels):
- for h in range(3):
- for w in range(3):
- if i < half_fg:
- prior_tensor[i, h, w] = math.cos(math.pi*(h+0.5)*(i+1)/3)
- else:
- prior_tensor[i, h, w] = math.cos(math.pi*(w+0.5)*(i+1-half_fg)/3)
-
- self.register_buffer('weight_rbr_prior', prior_tensor)
-
- def weight_gen(self):
-
- weight_rbr_origin = torch.einsum('oihw,o->oihw', self.weight_rbr_origin, self.vector[0, :])
-
- weight_rbr_avg = torch.einsum('oihw,o->oihw', torch.einsum('oihw,hw->oihw', self.weight_rbr_avg_conv, self.weight_rbr_avg_avg), self.vector[1, :])
-
- weight_rbr_pfir = torch.einsum('oihw,o->oihw', torch.einsum('oihw,ohw->oihw', self.weight_rbr_pfir_conv, self.weight_rbr_prior), self.vector[2, :])
-
- weight_rbr_1x1_kxk_conv1 = None
- if hasattr(self, 'weight_rbr_1x1_kxk_idconv1'):
- weight_rbr_1x1_kxk_conv1 = (self.weight_rbr_1x1_kxk_idconv1 + self.id_tensor).squeeze()
- elif hasattr(self, 'weight_rbr_1x1_kxk_conv1'):
- weight_rbr_1x1_kxk_conv1 = self.weight_rbr_1x1_kxk_conv1.squeeze()
- else:
- raise NotImplementedError
- weight_rbr_1x1_kxk_conv2 = self.weight_rbr_1x1_kxk_conv2
-
- if self.groups > 1:
- g = self.groups
- t, ig = weight_rbr_1x1_kxk_conv1.size()
- o, tg, h, w = weight_rbr_1x1_kxk_conv2.size()
- weight_rbr_1x1_kxk_conv1 = weight_rbr_1x1_kxk_conv1.view(g, int(t/g), ig)
- weight_rbr_1x1_kxk_conv2 = weight_rbr_1x1_kxk_conv2.view(g, int(o/g), tg, h, w)
- weight_rbr_1x1_kxk = torch.einsum('gti,gothw->goihw', weight_rbr_1x1_kxk_conv1, weight_rbr_1x1_kxk_conv2).view(o, ig, h, w)
- else:
- weight_rbr_1x1_kxk = torch.einsum('ti,othw->oihw', weight_rbr_1x1_kxk_conv1, weight_rbr_1x1_kxk_conv2)
-
- weight_rbr_1x1_kxk = torch.einsum('oihw,o->oihw', weight_rbr_1x1_kxk, self.vector[3, :])
-
- weight_rbr_gconv = self.dwsc2full(self.weight_rbr_gconv_dw, self.weight_rbr_gconv_pw, self.in_channels)
- weight_rbr_gconv = torch.einsum('oihw,o->oihw', weight_rbr_gconv, self.vector[4, :])
-
- weight = weight_rbr_origin + weight_rbr_avg + weight_rbr_1x1_kxk + weight_rbr_pfir + weight_rbr_gconv
-
- return weight
-
- def dwsc2full(self, weight_dw, weight_pw, groups):
-
- t, ig, h, w = weight_dw.size()
- o, _, _, _ = weight_pw.size()
- tg = int(t/groups)
- i = int(ig*groups)
- weight_dw = weight_dw.view(groups, tg, ig, h, w)
- weight_pw = weight_pw.squeeze().view(o, groups, tg)
-
- weight_dsc = torch.einsum('gtihw,ogt->ogihw', weight_dw, weight_pw)
- return weight_dsc.view(o, i, h, w)
-
- def forward(self, inputs):
- weight = self.weight_gen()
- out = F.conv2d(inputs, weight, bias=None, stride=self.stride, padding=self.padding, dilation=self.dilation, groups=self.groups)
-
- return self.nonlinear(self.bn(out))
-
-class RepConv_OREPA(nn.Module):
-
- def __init__(self, c1, c2, k=3, s=1, padding=1, dilation=1, groups=1, padding_mode='zeros', deploy=False, use_se=False, nonlinear=nn.SiLU()):
- super(RepConv_OREPA, self).__init__()
- self.deploy = deploy
- self.groups = groups
- self.in_channels = c1
- self.out_channels = c2
-
- self.padding = padding
- self.dilation = dilation
- self.groups = groups
-
- assert k == 3
- assert padding == 1
-
- padding_11 = padding - k // 2
-
- if nonlinear is None:
- self.nonlinearity = nn.Identity()
- else:
- self.nonlinearity = nonlinear
-
- if use_se:
- self.se = SEBlock(self.out_channels, internal_neurons=self.out_channels // 16)
- else:
- self.se = nn.Identity()
-
- if deploy:
- self.rbr_reparam = nn.Conv2d(in_channels=self.in_channels, out_channels=self.out_channels, kernel_size=k, stride=s,
- padding=padding, dilation=dilation, groups=groups, bias=True, padding_mode=padding_mode)
-
- else:
- self.rbr_identity = nn.BatchNorm2d(num_features=self.in_channels) if self.out_channels == self.in_channels and s == 1 else None
- self.rbr_dense = OREPA_3x3_RepConv(in_channels=self.in_channels, out_channels=self.out_channels, kernel_size=k, stride=s, padding=padding, groups=groups, dilation=1)
- self.rbr_1x1 = ConvBN(in_channels=self.in_channels, out_channels=self.out_channels, kernel_size=1, stride=s, padding=padding_11, groups=groups, dilation=1)
- print('RepVGG Block, identity = ', self.rbr_identity)
-
-
- def forward(self, inputs):
- if hasattr(self, 'rbr_reparam'):
- return self.nonlinearity(self.se(self.rbr_reparam(inputs)))
-
- if self.rbr_identity is None:
- id_out = 0
- else:
- id_out = self.rbr_identity(inputs)
-
- out1 = self.rbr_dense(inputs)
- out2 = self.rbr_1x1(inputs)
- out3 = id_out
- out = out1 + out2 + out3
-
- return self.nonlinearity(self.se(out))
-
-
- # Optional. This improves the accuracy and facilitates quantization.
- # 1. Cancel the original weight decay on rbr_dense.conv.weight and rbr_1x1.conv.weight.
- # 2. Use like this.
- # loss = criterion(....)
- # for every RepVGGBlock blk:
- # loss += weight_decay_coefficient * 0.5 * blk.get_cust_L2()
- # optimizer.zero_grad()
- # loss.backward()
-
- # Not used for OREPA
- def get_custom_L2(self):
- K3 = self.rbr_dense.weight_gen()
- K1 = self.rbr_1x1.conv.weight
- t3 = (self.rbr_dense.bn.weight / ((self.rbr_dense.bn.running_var + self.rbr_dense.bn.eps).sqrt())).reshape(-1, 1, 1, 1).detach()
- t1 = (self.rbr_1x1.bn.weight / ((self.rbr_1x1.bn.running_var + self.rbr_1x1.bn.eps).sqrt())).reshape(-1, 1, 1, 1).detach()
-
- l2_loss_circle = (K3 ** 2).sum() - (K3[:, :, 1:2, 1:2] ** 2).sum() # The L2 loss of the "circle" of weights in 3x3 kernel. Use regular L2 on them.
- eq_kernel = K3[:, :, 1:2, 1:2] * t3 + K1 * t1 # The equivalent resultant central point of 3x3 kernel.
- l2_loss_eq_kernel = (eq_kernel ** 2 / (t3 ** 2 + t1 ** 2)).sum() # Normalize for an L2 coefficient comparable to regular L2.
- return l2_loss_eq_kernel + l2_loss_circle
-
- def get_equivalent_kernel_bias(self):
- kernel3x3, bias3x3 = self._fuse_bn_tensor(self.rbr_dense)
- kernel1x1, bias1x1 = self._fuse_bn_tensor(self.rbr_1x1)
- kernelid, biasid = self._fuse_bn_tensor(self.rbr_identity)
- return kernel3x3 + self._pad_1x1_to_3x3_tensor(kernel1x1) + kernelid, bias3x3 + bias1x1 + biasid
-
- def _pad_1x1_to_3x3_tensor(self, kernel1x1):
- if kernel1x1 is None:
- return 0
- else:
- return torch.nn.functional.pad(kernel1x1, [1,1,1,1])
-
- def _fuse_bn_tensor(self, branch):
- if branch is None:
- return 0, 0
- if not isinstance(branch, nn.BatchNorm2d):
- if isinstance(branch, OREPA_3x3_RepConv):
- kernel = branch.weight_gen()
- elif isinstance(branch, ConvBN):
- kernel = branch.conv.weight
- else:
- raise NotImplementedError
- running_mean = branch.bn.running_mean
- running_var = branch.bn.running_var
- gamma = branch.bn.weight
- beta = branch.bn.bias
- eps = branch.bn.eps
- else:
- if not hasattr(self, 'id_tensor'):
- input_dim = self.in_channels // self.groups
- kernel_value = np.zeros((self.in_channels, input_dim, 3, 3), dtype=np.float32)
- for i in range(self.in_channels):
- kernel_value[i, i % input_dim, 1, 1] = 1
- self.id_tensor = torch.from_numpy(kernel_value).to(branch.weight.device)
- kernel = self.id_tensor
- running_mean = branch.running_mean
- running_var = branch.running_var
- gamma = branch.weight
- beta = branch.bias
- eps = branch.eps
- std = (running_var + eps).sqrt()
- t = (gamma / std).reshape(-1, 1, 1, 1)
- return kernel * t, beta - running_mean * gamma / std
-
- def switch_to_deploy(self):
- if hasattr(self, 'rbr_reparam'):
- return
- print(f"RepConv_OREPA.switch_to_deploy")
- kernel, bias = self.get_equivalent_kernel_bias()
- self.rbr_reparam = nn.Conv2d(in_channels=self.rbr_dense.in_channels, out_channels=self.rbr_dense.out_channels,
- kernel_size=self.rbr_dense.kernel_size, stride=self.rbr_dense.stride,
- padding=self.rbr_dense.padding, dilation=self.rbr_dense.dilation, groups=self.rbr_dense.groups, bias=True)
- self.rbr_reparam.weight.data = kernel
- self.rbr_reparam.bias.data = bias
- for para in self.parameters():
- para.detach_()
- self.__delattr__('rbr_dense')
- self.__delattr__('rbr_1x1')
- if hasattr(self, 'rbr_identity'):
- self.__delattr__('rbr_identity')
-
-##### end of orepa #####
-
-
-##### swin transformer #####
-
-class WindowAttention(nn.Module):
-
- def __init__(self, dim, window_size, num_heads, qkv_bias=True, qk_scale=None, attn_drop=0., proj_drop=0.):
-
- super().__init__()
- self.dim = dim
- self.window_size = window_size # Wh, Ww
- self.num_heads = num_heads
- head_dim = dim // num_heads
- self.scale = qk_scale or head_dim ** -0.5
-
- # define a parameter table of relative position bias
- self.relative_position_bias_table = nn.Parameter(
- torch.zeros((2 * window_size[0] - 1) * (2 * window_size[1] - 1), num_heads)) # 2*Wh-1 * 2*Ww-1, nH
-
- # get pair-wise relative position index for each token inside the window
- coords_h = torch.arange(self.window_size[0])
- coords_w = torch.arange(self.window_size[1])
- coords = torch.stack(torch.meshgrid([coords_h, coords_w])) # 2, Wh, Ww
- coords_flatten = torch.flatten(coords, 1) # 2, Wh*Ww
- relative_coords = coords_flatten[:, :, None] - coords_flatten[:, None, :] # 2, Wh*Ww, Wh*Ww
- relative_coords = relative_coords.permute(1, 2, 0).contiguous() # Wh*Ww, Wh*Ww, 2
- relative_coords[:, :, 0] += self.window_size[0] - 1 # shift to start from 0
- relative_coords[:, :, 1] += self.window_size[1] - 1
- relative_coords[:, :, 0] *= 2 * self.window_size[1] - 1
- relative_position_index = relative_coords.sum(-1) # Wh*Ww, Wh*Ww
- self.register_buffer("relative_position_index", relative_position_index)
-
- self.qkv = nn.Linear(dim, dim * 3, bias=qkv_bias)
- self.attn_drop = nn.Dropout(attn_drop)
- self.proj = nn.Linear(dim, dim)
- self.proj_drop = nn.Dropout(proj_drop)
-
- nn.init.normal_(self.relative_position_bias_table, std=.02)
- self.softmax = nn.Softmax(dim=-1)
-
- def forward(self, x, mask=None):
-
- B_, N, C = x.shape
- qkv = self.qkv(x).reshape(B_, N, 3, self.num_heads, C // self.num_heads).permute(2, 0, 3, 1, 4)
- q, k, v = qkv[0], qkv[1], qkv[2] # make torchscript happy (cannot use tensor as tuple)
-
- q = q * self.scale
- attn = (q @ k.transpose(-2, -1))
-
- relative_position_bias = self.relative_position_bias_table[self.relative_position_index.view(-1)].view(
- self.window_size[0] * self.window_size[1], self.window_size[0] * self.window_size[1], -1) # Wh*Ww,Wh*Ww,nH
- relative_position_bias = relative_position_bias.permute(2, 0, 1).contiguous() # nH, Wh*Ww, Wh*Ww
- attn = attn + relative_position_bias.unsqueeze(0)
-
- if mask is not None:
- nW = mask.shape[0]
- attn = attn.view(B_ // nW, nW, self.num_heads, N, N) + mask.unsqueeze(1).unsqueeze(0)
- attn = attn.view(-1, self.num_heads, N, N)
- attn = self.softmax(attn)
- else:
- attn = self.softmax(attn)
-
- attn = self.attn_drop(attn)
-
- # print(attn.dtype, v.dtype)
- try:
- x = (attn @ v).transpose(1, 2).reshape(B_, N, C)
- except:
- #print(attn.dtype, v.dtype)
- x = (attn.half() @ v).transpose(1, 2).reshape(B_, N, C)
- x = self.proj(x)
- x = self.proj_drop(x)
- return x
-
-class Mlp(nn.Module):
-
- def __init__(self, in_features, hidden_features=None, out_features=None, act_layer=nn.SiLU, drop=0.):
- super().__init__()
- out_features = out_features or in_features
- hidden_features = hidden_features or in_features
- self.fc1 = nn.Linear(in_features, hidden_features)
- self.act = act_layer()
- self.fc2 = nn.Linear(hidden_features, out_features)
- self.drop = nn.Dropout(drop)
-
- def forward(self, x):
- x = self.fc1(x)
- x = self.act(x)
- x = self.drop(x)
- x = self.fc2(x)
- x = self.drop(x)
- return x
-
-def window_partition(x, window_size):
-
- B, H, W, C = x.shape
- assert H % window_size == 0, 'feature map h and w can not divide by window size'
- x = x.view(B, H // window_size, window_size, W // window_size, window_size, C)
- windows = x.permute(0, 1, 3, 2, 4, 5).contiguous().view(-1, window_size, window_size, C)
- return windows
-
-def window_reverse(windows, window_size, H, W):
-
- B = int(windows.shape[0] / (H * W / window_size / window_size))
- x = windows.view(B, H // window_size, W // window_size, window_size, window_size, -1)
- x = x.permute(0, 1, 3, 2, 4, 5).contiguous().view(B, H, W, -1)
- return x
-
-
-class SwinTransformerLayer(nn.Module):
-
- def __init__(self, dim, num_heads, window_size=8, shift_size=0,
- mlp_ratio=4., qkv_bias=True, qk_scale=None, drop=0., attn_drop=0., drop_path=0.,
- act_layer=nn.SiLU, norm_layer=nn.LayerNorm):
- super().__init__()
- self.dim = dim
- self.num_heads = num_heads
- self.window_size = window_size
- self.shift_size = shift_size
- self.mlp_ratio = mlp_ratio
- # if min(self.input_resolution) <= self.window_size:
- # # if window size is larger than input resolution, we don't partition windows
- # self.shift_size = 0
- # self.window_size = min(self.input_resolution)
- assert 0 <= self.shift_size < self.window_size, "shift_size must in 0-window_size"
-
- self.norm1 = norm_layer(dim)
- self.attn = WindowAttention(
- dim, window_size=(self.window_size, self.window_size), num_heads=num_heads,
- qkv_bias=qkv_bias, qk_scale=qk_scale, attn_drop=attn_drop, proj_drop=drop)
-
- self.drop_path = DropPath(drop_path) if drop_path > 0. else nn.Identity()
- self.norm2 = norm_layer(dim)
- mlp_hidden_dim = int(dim * mlp_ratio)
- self.mlp = Mlp(in_features=dim, hidden_features=mlp_hidden_dim, act_layer=act_layer, drop=drop)
-
- def create_mask(self, H, W):
- # calculate attention mask for SW-MSA
- img_mask = torch.zeros((1, H, W, 1)) # 1 H W 1
- h_slices = (slice(0, -self.window_size),
- slice(-self.window_size, -self.shift_size),
- slice(-self.shift_size, None))
- w_slices = (slice(0, -self.window_size),
- slice(-self.window_size, -self.shift_size),
- slice(-self.shift_size, None))
- cnt = 0
- for h in h_slices:
- for w in w_slices:
- img_mask[:, h, w, :] = cnt
- cnt += 1
-
- mask_windows = window_partition(img_mask, self.window_size) # nW, window_size, window_size, 1
- mask_windows = mask_windows.view(-1, self.window_size * self.window_size)
- attn_mask = mask_windows.unsqueeze(1) - mask_windows.unsqueeze(2)
- attn_mask = attn_mask.masked_fill(attn_mask != 0, float(-100.0)).masked_fill(attn_mask == 0, float(0.0))
-
- return attn_mask
-
- def forward(self, x):
- # reshape x[b c h w] to x[b l c]
- _, _, H_, W_ = x.shape
-
- Padding = False
- if min(H_, W_) < self.window_size or H_ % self.window_size!=0 or W_ % self.window_size!=0:
- Padding = True
- # print(f'img_size {min(H_, W_)} is less than (or not divided by) window_size {self.window_size}, Padding.')
- pad_r = (self.window_size - W_ % self.window_size) % self.window_size
- pad_b = (self.window_size - H_ % self.window_size) % self.window_size
- x = F.pad(x, (0, pad_r, 0, pad_b))
-
- # print('2', x.shape)
- B, C, H, W = x.shape
- L = H * W
- x = x.permute(0, 2, 3, 1).contiguous().view(B, L, C) # b, L, c
-
- # create mask from init to forward
- if self.shift_size > 0:
- attn_mask = self.create_mask(H, W).to(x.device)
- else:
- attn_mask = None
-
- shortcut = x
- x = self.norm1(x)
- x = x.view(B, H, W, C)
-
- # cyclic shift
- if self.shift_size > 0:
- shifted_x = torch.roll(x, shifts=(-self.shift_size, -self.shift_size), dims=(1, 2))
- else:
- shifted_x = x
-
- # partition windows
- x_windows = window_partition(shifted_x, self.window_size) # nW*B, window_size, window_size, C
- x_windows = x_windows.view(-1, self.window_size * self.window_size, C) # nW*B, window_size*window_size, C
-
- # W-MSA/SW-MSA
- attn_windows = self.attn(x_windows, mask=attn_mask) # nW*B, window_size*window_size, C
-
- # merge windows
- attn_windows = attn_windows.view(-1, self.window_size, self.window_size, C)
- shifted_x = window_reverse(attn_windows, self.window_size, H, W) # B H' W' C
-
- # reverse cyclic shift
- if self.shift_size > 0:
- x = torch.roll(shifted_x, shifts=(self.shift_size, self.shift_size), dims=(1, 2))
- else:
- x = shifted_x
- x = x.view(B, H * W, C)
-
- # FFN
- x = shortcut + self.drop_path(x)
- x = x + self.drop_path(self.mlp(self.norm2(x)))
-
- x = x.permute(0, 2, 1).contiguous().view(-1, C, H, W) # b c h w
-
- if Padding:
- x = x[:, :, :H_, :W_] # reverse padding
-
- return x
-
-
-class SwinTransformerBlock(nn.Module):
- def __init__(self, c1, c2, num_heads, num_layers, window_size=8):
- super().__init__()
- self.conv = None
- if c1 != c2:
- self.conv = Conv(c1, c2)
-
- # remove input_resolution
- self.blocks = nn.Sequential(*[SwinTransformerLayer(dim=c2, num_heads=num_heads, window_size=window_size,
- shift_size=0 if (i % 2 == 0) else window_size // 2) for i in range(num_layers)])
-
- def forward(self, x):
- if self.conv is not None:
- x = self.conv(x)
- x = self.blocks(x)
- return x
-
-
-class STCSPA(nn.Module):
- # CSP Bottleneck https://github.com/WongKinYiu/CrossStagePartialNetworks
- def __init__(self, c1, c2, n=1, shortcut=True, g=1, e=0.5): # ch_in, ch_out, number, shortcut, groups, expansion
- super(STCSPA, self).__init__()
- c_ = int(c2 * e) # hidden channels
- self.cv1 = Conv(c1, c_, 1, 1)
- self.cv2 = Conv(c1, c_, 1, 1)
- self.cv3 = Conv(2 * c_, c2, 1, 1)
- num_heads = c_ // 32
- self.m = SwinTransformerBlock(c_, c_, num_heads, n)
- #self.m = nn.Sequential(*[Bottleneck(c_, c_, shortcut, g, e=1.0) for _ in range(n)])
-
- def forward(self, x):
- y1 = self.m(self.cv1(x))
- y2 = self.cv2(x)
- return self.cv3(torch.cat((y1, y2), dim=1))
-
-
-class STCSPB(nn.Module):
- # CSP Bottleneck https://github.com/WongKinYiu/CrossStagePartialNetworks
- def __init__(self, c1, c2, n=1, shortcut=False, g=1, e=0.5): # ch_in, ch_out, number, shortcut, groups, expansion
- super(STCSPB, self).__init__()
- c_ = int(c2) # hidden channels
- self.cv1 = Conv(c1, c_, 1, 1)
- self.cv2 = Conv(c_, c_, 1, 1)
- self.cv3 = Conv(2 * c_, c2, 1, 1)
- num_heads = c_ // 32
- self.m = SwinTransformerBlock(c_, c_, num_heads, n)
- #self.m = nn.Sequential(*[Bottleneck(c_, c_, shortcut, g, e=1.0) for _ in range(n)])
-
- def forward(self, x):
- x1 = self.cv1(x)
- y1 = self.m(x1)
- y2 = self.cv2(x1)
- return self.cv3(torch.cat((y1, y2), dim=1))
-
-
-class STCSPC(nn.Module):
- # CSP Bottleneck https://github.com/WongKinYiu/CrossStagePartialNetworks
- def __init__(self, c1, c2, n=1, shortcut=True, g=1, e=0.5): # ch_in, ch_out, number, shortcut, groups, expansion
- super(STCSPC, self).__init__()
- c_ = int(c2 * e) # hidden channels
- self.cv1 = Conv(c1, c_, 1, 1)
- self.cv2 = Conv(c1, c_, 1, 1)
- self.cv3 = Conv(c_, c_, 1, 1)
- self.cv4 = Conv(2 * c_, c2, 1, 1)
- num_heads = c_ // 32
- self.m = SwinTransformerBlock(c_, c_, num_heads, n)
- #self.m = nn.Sequential(*[Bottleneck(c_, c_, shortcut, g, e=1.0) for _ in range(n)])
-
- def forward(self, x):
- y1 = self.cv3(self.m(self.cv1(x)))
- y2 = self.cv2(x)
- return self.cv4(torch.cat((y1, y2), dim=1))
-
-##### end of swin transformer #####
-
-
-##### swin transformer v2 #####
-
-class WindowAttention_v2(nn.Module):
-
- def __init__(self, dim, window_size, num_heads, qkv_bias=True, attn_drop=0., proj_drop=0.,
- pretrained_window_size=[0, 0]):
-
- super().__init__()
- self.dim = dim
- self.window_size = window_size # Wh, Ww
- self.pretrained_window_size = pretrained_window_size
- self.num_heads = num_heads
-
- self.logit_scale = nn.Parameter(torch.log(10 * torch.ones((num_heads, 1, 1))), requires_grad=True)
-
- # mlp to generate continuous relative position bias
- self.cpb_mlp = nn.Sequential(nn.Linear(2, 512, bias=True),
- nn.ReLU(inplace=True),
- nn.Linear(512, num_heads, bias=False))
-
- # get relative_coords_table
- relative_coords_h = torch.arange(-(self.window_size[0] - 1), self.window_size[0], dtype=torch.float32)
- relative_coords_w = torch.arange(-(self.window_size[1] - 1), self.window_size[1], dtype=torch.float32)
- relative_coords_table = torch.stack(
- torch.meshgrid([relative_coords_h,
- relative_coords_w])).permute(1, 2, 0).contiguous().unsqueeze(0) # 1, 2*Wh-1, 2*Ww-1, 2
- if pretrained_window_size[0] > 0:
- relative_coords_table[:, :, :, 0] /= (pretrained_window_size[0] - 1)
- relative_coords_table[:, :, :, 1] /= (pretrained_window_size[1] - 1)
- else:
- relative_coords_table[:, :, :, 0] /= (self.window_size[0] - 1)
- relative_coords_table[:, :, :, 1] /= (self.window_size[1] - 1)
- relative_coords_table *= 8 # normalize to -8, 8
- relative_coords_table = torch.sign(relative_coords_table) * torch.log2(
- torch.abs(relative_coords_table) + 1.0) / np.log2(8)
-
- self.register_buffer("relative_coords_table", relative_coords_table)
-
- # get pair-wise relative position index for each token inside the window
- coords_h = torch.arange(self.window_size[0])
- coords_w = torch.arange(self.window_size[1])
- coords = torch.stack(torch.meshgrid([coords_h, coords_w])) # 2, Wh, Ww
- coords_flatten = torch.flatten(coords, 1) # 2, Wh*Ww
- relative_coords = coords_flatten[:, :, None] - coords_flatten[:, None, :] # 2, Wh*Ww, Wh*Ww
- relative_coords = relative_coords.permute(1, 2, 0).contiguous() # Wh*Ww, Wh*Ww, 2
- relative_coords[:, :, 0] += self.window_size[0] - 1 # shift to start from 0
- relative_coords[:, :, 1] += self.window_size[1] - 1
- relative_coords[:, :, 0] *= 2 * self.window_size[1] - 1
- relative_position_index = relative_coords.sum(-1) # Wh*Ww, Wh*Ww
- self.register_buffer("relative_position_index", relative_position_index)
-
- self.qkv = nn.Linear(dim, dim * 3, bias=False)
- if qkv_bias:
- self.q_bias = nn.Parameter(torch.zeros(dim))
- self.v_bias = nn.Parameter(torch.zeros(dim))
- else:
- self.q_bias = None
- self.v_bias = None
- self.attn_drop = nn.Dropout(attn_drop)
- self.proj = nn.Linear(dim, dim)
- self.proj_drop = nn.Dropout(proj_drop)
- self.softmax = nn.Softmax(dim=-1)
-
- def forward(self, x, mask=None):
-
- B_, N, C = x.shape
- qkv_bias = None
- if self.q_bias is not None:
- qkv_bias = torch.cat((self.q_bias, torch.zeros_like(self.v_bias, requires_grad=False), self.v_bias))
- qkv = F.linear(input=x, weight=self.qkv.weight, bias=qkv_bias)
- qkv = qkv.reshape(B_, N, 3, self.num_heads, -1).permute(2, 0, 3, 1, 4)
- q, k, v = qkv[0], qkv[1], qkv[2] # make torchscript happy (cannot use tensor as tuple)
-
- # cosine attention
- attn = (F.normalize(q, dim=-1) @ F.normalize(k, dim=-1).transpose(-2, -1))
- logit_scale = torch.clamp(self.logit_scale, max=torch.log(torch.tensor(1. / 0.01))).exp()
- attn = attn * logit_scale
-
- relative_position_bias_table = self.cpb_mlp(self.relative_coords_table).view(-1, self.num_heads)
- relative_position_bias = relative_position_bias_table[self.relative_position_index.view(-1)].view(
- self.window_size[0] * self.window_size[1], self.window_size[0] * self.window_size[1], -1) # Wh*Ww,Wh*Ww,nH
- relative_position_bias = relative_position_bias.permute(2, 0, 1).contiguous() # nH, Wh*Ww, Wh*Ww
- relative_position_bias = 16 * torch.sigmoid(relative_position_bias)
- attn = attn + relative_position_bias.unsqueeze(0)
-
- if mask is not None:
- nW = mask.shape[0]
- attn = attn.view(B_ // nW, nW, self.num_heads, N, N) + mask.unsqueeze(1).unsqueeze(0)
- attn = attn.view(-1, self.num_heads, N, N)
- attn = self.softmax(attn)
- else:
- attn = self.softmax(attn)
-
- attn = self.attn_drop(attn)
-
- try:
- x = (attn @ v).transpose(1, 2).reshape(B_, N, C)
- except:
- x = (attn.half() @ v).transpose(1, 2).reshape(B_, N, C)
-
- x = self.proj(x)
- x = self.proj_drop(x)
- return x
-
- def extra_repr(self) -> str:
- return f'dim={self.dim}, window_size={self.window_size}, ' \
- f'pretrained_window_size={self.pretrained_window_size}, num_heads={self.num_heads}'
-
- def flops(self, N):
- # calculate flops for 1 window with token length of N
- flops = 0
- # qkv = self.qkv(x)
- flops += N * self.dim * 3 * self.dim
- # attn = (q @ k.transpose(-2, -1))
- flops += self.num_heads * N * (self.dim // self.num_heads) * N
- # x = (attn @ v)
- flops += self.num_heads * N * N * (self.dim // self.num_heads)
- # x = self.proj(x)
- flops += N * self.dim * self.dim
- return flops
-
-class Mlp_v2(nn.Module):
- def __init__(self, in_features, hidden_features=None, out_features=None, act_layer=nn.SiLU, drop=0.):
- super().__init__()
- out_features = out_features or in_features
- hidden_features = hidden_features or in_features
- self.fc1 = nn.Linear(in_features, hidden_features)
- self.act = act_layer()
- self.fc2 = nn.Linear(hidden_features, out_features)
- self.drop = nn.Dropout(drop)
-
- def forward(self, x):
- x = self.fc1(x)
- x = self.act(x)
- x = self.drop(x)
- x = self.fc2(x)
- x = self.drop(x)
- return x
-
-
-def window_partition_v2(x, window_size):
-
- B, H, W, C = x.shape
- x = x.view(B, H // window_size, window_size, W // window_size, window_size, C)
- windows = x.permute(0, 1, 3, 2, 4, 5).contiguous().view(-1, window_size, window_size, C)
- return windows
-
-
-def window_reverse_v2(windows, window_size, H, W):
-
- B = int(windows.shape[0] / (H * W / window_size / window_size))
- x = windows.view(B, H // window_size, W // window_size, window_size, window_size, -1)
- x = x.permute(0, 1, 3, 2, 4, 5).contiguous().view(B, H, W, -1)
- return x
-
-
-class SwinTransformerLayer_v2(nn.Module):
-
- def __init__(self, dim, num_heads, window_size=7, shift_size=0,
- mlp_ratio=4., qkv_bias=True, drop=0., attn_drop=0., drop_path=0.,
- act_layer=nn.SiLU, norm_layer=nn.LayerNorm, pretrained_window_size=0):
- super().__init__()
- self.dim = dim
- #self.input_resolution = input_resolution
- self.num_heads = num_heads
- self.window_size = window_size
- self.shift_size = shift_size
- self.mlp_ratio = mlp_ratio
- #if min(self.input_resolution) <= self.window_size:
- # # if window size is larger than input resolution, we don't partition windows
- # self.shift_size = 0
- # self.window_size = min(self.input_resolution)
- assert 0 <= self.shift_size < self.window_size, "shift_size must in 0-window_size"
-
- self.norm1 = norm_layer(dim)
- self.attn = WindowAttention_v2(
- dim, window_size=(self.window_size, self.window_size), num_heads=num_heads,
- qkv_bias=qkv_bias, attn_drop=attn_drop, proj_drop=drop,
- pretrained_window_size=(pretrained_window_size, pretrained_window_size))
-
- self.drop_path = DropPath(drop_path) if drop_path > 0. else nn.Identity()
- self.norm2 = norm_layer(dim)
- mlp_hidden_dim = int(dim * mlp_ratio)
- self.mlp = Mlp_v2(in_features=dim, hidden_features=mlp_hidden_dim, act_layer=act_layer, drop=drop)
-
- def create_mask(self, H, W):
- # calculate attention mask for SW-MSA
- img_mask = torch.zeros((1, H, W, 1)) # 1 H W 1
- h_slices = (slice(0, -self.window_size),
- slice(-self.window_size, -self.shift_size),
- slice(-self.shift_size, None))
- w_slices = (slice(0, -self.window_size),
- slice(-self.window_size, -self.shift_size),
- slice(-self.shift_size, None))
- cnt = 0
- for h in h_slices:
- for w in w_slices:
- img_mask[:, h, w, :] = cnt
- cnt += 1
-
- mask_windows = window_partition(img_mask, self.window_size) # nW, window_size, window_size, 1
- mask_windows = mask_windows.view(-1, self.window_size * self.window_size)
- attn_mask = mask_windows.unsqueeze(1) - mask_windows.unsqueeze(2)
- attn_mask = attn_mask.masked_fill(attn_mask != 0, float(-100.0)).masked_fill(attn_mask == 0, float(0.0))
-
- return attn_mask
-
- def forward(self, x):
- # reshape x[b c h w] to x[b l c]
- _, _, H_, W_ = x.shape
-
- Padding = False
- if min(H_, W_) < self.window_size or H_ % self.window_size!=0 or W_ % self.window_size!=0:
- Padding = True
- # print(f'img_size {min(H_, W_)} is less than (or not divided by) window_size {self.window_size}, Padding.')
- pad_r = (self.window_size - W_ % self.window_size) % self.window_size
- pad_b = (self.window_size - H_ % self.window_size) % self.window_size
- x = F.pad(x, (0, pad_r, 0, pad_b))
-
- # print('2', x.shape)
- B, C, H, W = x.shape
- L = H * W
- x = x.permute(0, 2, 3, 1).contiguous().view(B, L, C) # b, L, c
-
- # create mask from init to forward
- if self.shift_size > 0:
- attn_mask = self.create_mask(H, W).to(x.device)
- else:
- attn_mask = None
-
- shortcut = x
- x = x.view(B, H, W, C)
-
- # cyclic shift
- if self.shift_size > 0:
- shifted_x = torch.roll(x, shifts=(-self.shift_size, -self.shift_size), dims=(1, 2))
- else:
- shifted_x = x
-
- # partition windows
- x_windows = window_partition_v2(shifted_x, self.window_size) # nW*B, window_size, window_size, C
- x_windows = x_windows.view(-1, self.window_size * self.window_size, C) # nW*B, window_size*window_size, C
-
- # W-MSA/SW-MSA
- attn_windows = self.attn(x_windows, mask=attn_mask) # nW*B, window_size*window_size, C
-
- # merge windows
- attn_windows = attn_windows.view(-1, self.window_size, self.window_size, C)
- shifted_x = window_reverse_v2(attn_windows, self.window_size, H, W) # B H' W' C
-
- # reverse cyclic shift
- if self.shift_size > 0:
- x = torch.roll(shifted_x, shifts=(self.shift_size, self.shift_size), dims=(1, 2))
- else:
- x = shifted_x
- x = x.view(B, H * W, C)
- x = shortcut + self.drop_path(self.norm1(x))
-
- # FFN
- x = x + self.drop_path(self.norm2(self.mlp(x)))
- x = x.permute(0, 2, 1).contiguous().view(-1, C, H, W) # b c h w
-
- if Padding:
- x = x[:, :, :H_, :W_] # reverse padding
-
- return x
-
- def extra_repr(self) -> str:
- return f"dim={self.dim}, input_resolution={self.input_resolution}, num_heads={self.num_heads}, " \
- f"window_size={self.window_size}, shift_size={self.shift_size}, mlp_ratio={self.mlp_ratio}"
-
- def flops(self):
- flops = 0
- H, W = self.input_resolution
- # norm1
- flops += self.dim * H * W
- # W-MSA/SW-MSA
- nW = H * W / self.window_size / self.window_size
- flops += nW * self.attn.flops(self.window_size * self.window_size)
- # mlp
- flops += 2 * H * W * self.dim * self.dim * self.mlp_ratio
- # norm2
- flops += self.dim * H * W
- return flops
-
-
-class SwinTransformer2Block(nn.Module):
- def __init__(self, c1, c2, num_heads, num_layers, window_size=7):
- super().__init__()
- self.conv = None
- if c1 != c2:
- self.conv = Conv(c1, c2)
-
- # remove input_resolution
- self.blocks = nn.Sequential(*[SwinTransformerLayer_v2(dim=c2, num_heads=num_heads, window_size=window_size,
- shift_size=0 if (i % 2 == 0) else window_size // 2) for i in range(num_layers)])
-
- def forward(self, x):
- if self.conv is not None:
- x = self.conv(x)
- x = self.blocks(x)
- return x
-
-
-class ST2CSPA(nn.Module):
- # CSP Bottleneck https://github.com/WongKinYiu/CrossStagePartialNetworks
- def __init__(self, c1, c2, n=1, shortcut=True, g=1, e=0.5): # ch_in, ch_out, number, shortcut, groups, expansion
- super(ST2CSPA, self).__init__()
- c_ = int(c2 * e) # hidden channels
- self.cv1 = Conv(c1, c_, 1, 1)
- self.cv2 = Conv(c1, c_, 1, 1)
- self.cv3 = Conv(2 * c_, c2, 1, 1)
- num_heads = c_ // 32
- self.m = SwinTransformer2Block(c_, c_, num_heads, n)
- #self.m = nn.Sequential(*[Bottleneck(c_, c_, shortcut, g, e=1.0) for _ in range(n)])
-
- def forward(self, x):
- y1 = self.m(self.cv1(x))
- y2 = self.cv2(x)
- return self.cv3(torch.cat((y1, y2), dim=1))
-
-
-class ST2CSPB(nn.Module):
- # CSP Bottleneck https://github.com/WongKinYiu/CrossStagePartialNetworks
- def __init__(self, c1, c2, n=1, shortcut=False, g=1, e=0.5): # ch_in, ch_out, number, shortcut, groups, expansion
- super(ST2CSPB, self).__init__()
- c_ = int(c2) # hidden channels
- self.cv1 = Conv(c1, c_, 1, 1)
- self.cv2 = Conv(c_, c_, 1, 1)
- self.cv3 = Conv(2 * c_, c2, 1, 1)
- num_heads = c_ // 32
- self.m = SwinTransformer2Block(c_, c_, num_heads, n)
- #self.m = nn.Sequential(*[Bottleneck(c_, c_, shortcut, g, e=1.0) for _ in range(n)])
-
- def forward(self, x):
- x1 = self.cv1(x)
- y1 = self.m(x1)
- y2 = self.cv2(x1)
- return self.cv3(torch.cat((y1, y2), dim=1))
-
-
-class ST2CSPC(nn.Module):
- # CSP Bottleneck https://github.com/WongKinYiu/CrossStagePartialNetworks
- def __init__(self, c1, c2, n=1, shortcut=True, g=1, e=0.5): # ch_in, ch_out, number, shortcut, groups, expansion
- super(ST2CSPC, self).__init__()
- c_ = int(c2 * e) # hidden channels
- self.cv1 = Conv(c1, c_, 1, 1)
- self.cv2 = Conv(c1, c_, 1, 1)
- self.cv3 = Conv(c_, c_, 1, 1)
- self.cv4 = Conv(2 * c_, c2, 1, 1)
- num_heads = c_ // 32
- self.m = SwinTransformer2Block(c_, c_, num_heads, n)
- #self.m = nn.Sequential(*[Bottleneck(c_, c_, shortcut, g, e=1.0) for _ in range(n)])
-
- def forward(self, x):
- y1 = self.cv3(self.m(self.cv1(x)))
- y2 = self.cv2(x)
- return self.cv4(torch.cat((y1, y2), dim=1))
-
-##### end of swin transformer v2 #####
diff --git a/spaces/fabiogra/moseca/scripts/prepare_samples.sh b/spaces/fabiogra/moseca/scripts/prepare_samples.sh
deleted file mode 100644
index 78f3b5d714ca41d683aa464cea902adbc43725f3..0000000000000000000000000000000000000000
--- a/spaces/fabiogra/moseca/scripts/prepare_samples.sh
+++ /dev/null
@@ -1,47 +0,0 @@
-#!/bin/bash
-echo "Starting prepare_samples.sh..."
-# Read the secret into a variable
-export PREPARE_SAMPLES=$(cat /run/secrets/PREPARE_SAMPLES)
-
-# Check if the "PREPARE_SAMPLES" environment variable is set
-if [ -z "${PREPARE_SAMPLES}" ]; then
- echo "PREPARE_SAMPLES is unset or set to the empty string. Skipping sample preparation."
- exit 0
-fi
-
-# Read JSON file into a variable
-json=$(cat sample_songs.json)
-
-mkdir -p "/tmp/vocal_remover"
-
-# Iterate through keys and values
-for name in $(echo "${json}" | jq -r 'keys[]'); do
- url=$(echo "${json}" | jq -r --arg name "${name}" '.[$name]')
- echo "Separating ${name} from ${url}"
-
- # Download with pytube
- yt-dlp ${url} -o "/tmp/${name}" --format "bestaudio/best"
-
- # Run inference
- python inference.py --input /tmp/${name} --output /tmp
- echo "Done separating ${name}"
-done
-
-
-# Read JSON file into a variable
-json_separate=$(cat separate_songs.json)
-
-# Iterate through keys and values
-for name in $(echo "${json_separate}" | jq -r 'keys[]'); do
- url=$(echo "${json_separate}" | jq -r --arg name "${name}" '.[$name][0]')
- start_time=$(echo "${json_separate}" | jq -r --arg name "${name}" '.[$name][1]')
- end_time=$(expr $start_time + 20)
- echo "Separating ${name} from ${url} with start_time ${start_time} sec"
-
- # Download with pytube
- yt-dlp ${url} -o "/tmp/${name}" --format "bestaudio/best" --download-sections "*${start_time}-${end_time}"
-
- # Run inference
- python inference.py --input /tmp/${name} --output /tmp --full_mode 1
- echo "Done separating ${name}"
-done
diff --git a/spaces/falterWliame/Face_Mask_Detection/Bartender Enterprise Automation 10.0 Crack [WORK].md b/spaces/falterWliame/Face_Mask_Detection/Bartender Enterprise Automation 10.0 Crack [WORK].md
deleted file mode 100644
index 370886f3489f08120867c05ab2c944ad1d73b41c..0000000000000000000000000000000000000000
--- a/spaces/falterWliame/Face_Mask_Detection/Bartender Enterprise Automation 10.0 Crack [WORK].md
+++ /dev/null
@@ -1,6 +0,0 @@
-bartender enterprise automation 10.0 crack
Download Zip › https://urlca.com/2uDc2K
-
-Free Download BarTender Enterprise Automation 10 Full Version+Crack+Patch. This is the Latest Full Version Of BarTender EnterPrise Edition. Design and ... 1fdad05405
-
-
-
diff --git a/spaces/falterWliame/Face_Mask_Detection/Download Easy Case Windows 7 64 Bit Rar VERIFIED.md b/spaces/falterWliame/Face_Mask_Detection/Download Easy Case Windows 7 64 Bit Rar VERIFIED.md
deleted file mode 100644
index 170b7bfb9d1134ff93628f6993b464cc5fd3513b..0000000000000000000000000000000000000000
--- a/spaces/falterWliame/Face_Mask_Detection/Download Easy Case Windows 7 64 Bit Rar VERIFIED.md
+++ /dev/null
@@ -1,40 +0,0 @@
-
-How to Download Easy Case Windows 7 64 Bit RAR
-If you are looking for a simple and effective way to compress and decompress files on your Windows 7 64 bit system, you might want to try Easy Case. Easy Case is a free and lightweight software that can handle various archive formats, including RAR, ZIP, 7Z, TAR, GZIP, and more. In this article, we will show you how to download and install Easy Case Windows 7 64 bit RAR on your PC.
-Download easy case windows 7 64 bit rar
DOWNLOAD 🌟 https://urlca.com/2uDd3V
-Step 1: Download Easy Case Windows 7 64 Bit RAR
-To download Easy Case Windows 7 64 bit RAR, you need to visit the official website of the software. You can find the link at the end of this article. Once you are on the website, click on the "Download" button and choose the version that matches your system architecture. In this case, you need to select the "Easy Case Windows 7 64 bit RAR" option.
-The download process will start automatically and it should take only a few minutes depending on your internet speed. You will get a file named "EasyCaseSetup.exe" in your download folder.
-Step 2: Install Easy Case Windows 7 64 Bit RAR
-After downloading Easy Case Windows 7 64 bit RAR, you need to run the setup file to install the software on your PC. To do that, double-click on the "EasyCaseSetup.exe" file and follow the instructions on the screen. You can choose the installation directory and the language of the software. You can also create a desktop shortcut and associate Easy Case with archive files.
-
-The installation process will take only a few seconds and you will see a confirmation message when it is done. You can then launch Easy Case from the start menu or the desktop shortcut.
-Step 3: Use Easy Case Windows 7 64 Bit RAR
-Once you have installed Easy Case Windows 7 64 bit RAR, you can start using it to compress and decompress files on your PC. To compress a file or a folder, you can right-click on it and choose "Add to archive" from the context menu. You can then select the archive format, the compression level, the password protection, and other options. You can also drag and drop files or folders to the Easy Case window to create an archive.
-To decompress a file or a folder, you can right-click on it and choose "Extract here" or "Extract to" from the context menu. You can also drag and drop files or folders to the Easy Case window to extract them. You can view the contents of an archive without extracting it by double-clicking on it or by opening it with Easy Case.
-Conclusion
-Easy Case Windows 7 64 bit RAR is a handy tool that can help you compress and decompress files on your Windows 7 system. It is free, fast, and easy to use. You can download it from the link below and follow the steps in this article to install and use it.
-Download Easy Case Windows 7 64 Bit RAR
-
-Why Use Easy Case Windows 7 64 Bit RAR?
-There are many benefits of using Easy Case Windows 7 64 bit RAR for your file compression and decompression needs. Here are some of them:
-
-- Easy Case Windows 7 64 bit RAR supports a wide range of archive formats, including RAR, ZIP, 7Z, TAR, GZIP, and more. You can easily create and open any type of archive with Easy Case.
-- Easy Case Windows 7 64 bit RAR offers a high compression ratio, which means you can reduce the size of your files and save disk space. You can also choose the compression level according to your preference and the type of files you are compressing.
-- Easy Case Windows 7 64 bit RAR allows you to encrypt your archives with a password and protect them from unauthorized access. You can also split your archives into smaller volumes and add recovery records to repair damaged archives.
-- Easy Case Windows 7 64 bit RAR has a simple and intuitive user interface that makes it easy to use for anyone. You can access all the features and options from the main window or the context menu. You can also customize the appearance and behavior of Easy Case according to your liking.
-
-Frequently Asked Questions About Easy Case Windows 7 64 Bit RAR
-If you have any questions or doubts about Easy Case Windows 7 64 bit RAR, you might find the answers in this section. Here are some of the most frequently asked questions about Easy Case Windows 7 64 bit RAR:
-
-- Is Easy Case Windows 7 64 bit RAR compatible with other archive software?
-Yes, Easy Case Windows 7 64 bit RAR is compatible with most of the popular archive software, such as WinRAR, WinZip, 7-Zip, and more. You can create and open archives that are compatible with these software with Easy Case.
-- Is Easy Case Windows 7 64 bit RAR safe to use?
-Yes, Easy Case Windows 7 64 bit RAR is safe to use. It does not contain any viruses, malware, spyware, or adware. It does not collect or share any personal or sensitive information from your PC. It does not modify or damage any system files or settings on your PC.
-- How can I update Easy Case Windows 7 64 bit RAR?
-You can update Easy Case Windows 7 64 bit RAR by visiting the official website of the software and downloading the latest version. You can also check for updates from within the software by clicking on the "Help" menu and choosing "Check for updates".
-- How can I contact the support team of Easy Case Windows 7 64 bit RAR?
-You can contact the support team of Easy Case Windows 7 64 bit RAR by sending an email to support@easycase.com. You can also visit the official website of the software and fill out the contact form. The support team will respond to your queries as soon as possible.
-
d5da3c52bf
-
-
\ No newline at end of file
diff --git a/spaces/fatiXbelha/sd/Download Love You So by The King Khan BBQ Show - The Official Music Video and Lyrics.md b/spaces/fatiXbelha/sd/Download Love You So by The King Khan BBQ Show - The Official Music Video and Lyrics.md
deleted file mode 100644
index f6ed570b9bea0c444abf4fac805a12dda98be485..0000000000000000000000000000000000000000
--- a/spaces/fatiXbelha/sd/Download Love You So by The King Khan BBQ Show - The Official Music Video and Lyrics.md
+++ /dev/null
@@ -1,83 +0,0 @@
-
-www.download love you so by king khan
-If you are looking for a catchy and romantic song to add to your playlist, you might want to check out Love You So by King Khan. This song is a viral hit on TikTok, where millions of users have used it as a soundtrack for their videos. But who is King Khan and what is Love You So about? And how can you download this song for free or with a subscription? In this article, we will answer these questions and more.
-www.download love you so by king khan
DOWNLOAD ✺ https://urllie.com/2uNEAx
- Introduction
-Who is King Khan?
-King Khan is the stage name of Arish Ahmad Khan, a Canadian musician and singer-songwriter. He is best known as the leader of the garage rock band The King Khan & BBQ Show, which he formed with Mark Sultan in 2002. He is also the frontman of the psychedelic soul band King Khan and the Shrines, which he founded in 1999. King Khan has been influenced by various genres, such as funk, soul, punk, rock and roll, and doo-wop. He is known for his eccentric and flamboyant persona, as well as his energetic and humorous live performances.
- What is Love You So?
-Love You So is a song by The King Khan & BBQ Show, released in 2014 as part of their EP Songs for Dads. The song is a simple and sweet declaration of love, with lyrics like "I just need someone in my life to give it structure / To handle all the selfish ways I'd spend my time without her / You're everything I want but I can't deal with all your lovers / You're saying I'm the one but it's your actions that speak louder". The song features reverb-drenched guitar chords, dreamy vocal harmonies, and a catchy chorus that repeats "I love you so".
- How to download Love You So by King Khan
-From official sources
-If you want to support the artist and enjoy high-quality audio, you can download Love You So by King Khan from official sources, such as streaming platforms or digital stores. Here are some of the most popular options:
- Spotify
-Spotify is one of the most popular streaming services in the world, with over 365 million monthly active users. Spotify offers both free and premium plans, with different features and benefits. With Spotify Free, you can listen to Love You So by King Khan on shuffle mode, with ads every few songs. With Spotify Premium, you can listen to Love You So by King Khan on demand, without ads, and download it for offline listening. Spotify Premium costs $9.99 per month for individuals, $12.99 per month for couples, $14.99 per month for families, and $4.99 per month for students.
-How to download Love You So by The King Khan & BBQ Show
-Love You So by The King Khan & BBQ Show lyrics and chords
-Love You So by The King Khan & BBQ Show official video on YouTube
-Love You So by The King Khan & BBQ Show Spotify playlist
-Love You So by The King Khan & BBQ Show Shazam song recognition
-Love You So by The King Khan & BBQ Show MP3 download free
-Love You So by The King Khan & BBQ Show guitar tabs and sheet music
-Love You So by The King Khan & BBQ Show review and rating
-Love You So by The King Khan & BBQ Show live performance and tour dates
-Love You So by The King Khan & BBQ Show meaning and inspiration
-Love You So by The King Khan & BBQ Show remix and cover versions
-Love You So by The King Khan & BBQ Show vinyl record and CD purchase
-Love You So by The King Khan & BBQ Show background and history
-Love You So by The King Khan & BBQ Show genre and influences
-Love You So by The King Khan & BBQ Show fan club and merchandise
-Love You So by The King Khan & BBQ Show TikTok challenge and dance
-Love You So by The King Khan & BBQ Show trivia and facts
-Love You So by The King Khan & BBQ Show karaoke and sing along
-Love You So by The King Khan & BBQ Show ringtone and alarm sound
-Love You So by The King Khan & BBQ Show podcast and interview
-Love You So by The King Khan & BBQ Show reaction and commentary
-Love You So by The King Khan & BBQ Show Apple Music and iTunes download
-Love You So by The King Khan & BBQ Show Instagram and Facebook page
-Love You So by The King Khan & BBQ Show best songs and albums
-Love You So by The King Khan & BBQ Show awards and nominations
-Love You So by The King Khan & BBQ Show similar artists and recommendations
-Love You So by The King Khan & BBQ Show playlist generator and curator
-Love You So by The King Khan & BBQ Show soundcloud and bandcamp stream
-Love You So by The King Khan & BBQ Show meme and parody videos
-Love You So by The King Khan & BBQ Show analysis and interpretation
- Apple Music
-Apple Music is another popular streaming service, with over 75 million subscribers worldwide. Apple Music offers a three-month free trial for new users, after which it costs $9.99 per month for individuals, $14.99 per month for families, and $4.99 per month for students. With Apple Music, you can listen to Love You So by King Khan on demand, without ads, and download it for offline listening. You can also access exclusive content, such as interviews, playlists, and radio shows.
- YouTube Music
-YouTube Music is a streaming service that focuses on music videos, live performances, covers, remixes, and other content related to music. YouTube Music offers both free and ad-supported plan, and a premium plan that costs $9.99 per month for individuals, $14.99 per month for families, and $4.99 per month for students. With YouTube Music Premium, you can listen to Love You So by King Khan on demand, without ads, and download it for offline listening. You can also access YouTube Originals, such as documentaries, movies, and shows.
- From unofficial sources
-If you don't want to pay for a subscription or a digital download, you might be tempted to look for unofficial sources to download Love You So by King Khan for free. However, you should be aware of the risks and legal issues involved in doing so. Here are some of the common methods and their drawbacks:
- MP3 converters
-MP3 converters are websites or apps that allow you to convert YouTube videos or other online audio files into MP3 format and download them to your device. Some examples are YTMP3, MP3Juices, and FLVTO. While this might seem like a convenient and easy way to download Love You So by King Khan, it has several disadvantages. First of all, the audio quality might be poor or distorted, as the conversion process can degrade the sound. Second, some MP3 converters might contain malware or viruses that can harm your device or steal your personal information. Third, some MP3 converters might violate the terms of service of YouTube or other platforms, and expose you to legal action from the content owners or creators.
- Torrent sites
-Torrent sites are websites that host torrent files, which are small files that contain metadata about larger files, such as music, movies, games, or software. Some examples are The Pirate Bay, 1337x, and RARBG. To download Love You So by King Khan from a torrent site, you need a torrent client, such as BitTorrent or uTorrent, which connects you to other users who have the same file and allows you to download it in pieces. While this might seem like a fast and efficient way to download Love You So by King Khan, it also has several drawbacks. First of all, the file might be corrupted or fake, and not match the description or quality you expected. Second, some torrent sites might contain malware or viruses that can harm your device or steal your personal information. Third, some torrent sites might infringe the intellectual property rights of the content owners or creators, and expose you to legal action from them or from law enforcement agencies.
- Piracy risks and legal issues
-As you can see, downloading Love You So by King Khan from unofficial sources is not only risky for your device and your privacy, but also illegal in many countries. Piracy is the unauthorized use or distribution of copyrighted material without the permission of the content owners or creators. Piracy can have negative consequences for both the artists and the consumers. For the artists, piracy can reduce their income and their incentive to create new music. For the consumers, piracy can result in fines, lawsuits, or even jail time.
- Why you should listen to Love You So by King Khan
-The song's meaning and message
-Love You So by King Khan is not just a catchy and romantic song, but also a meaningful and heartfelt one. The song expresses the feelings of someone who is in love with someone who is not faithful or committed to them. The song captures the frustration and confusion of being in such a situation, as well as the hope and desire to make it work. The song also shows the vulnerability and honesty of the singer, who admits his flaws and needs in the relationship.
- The song's popularity and impact
-Love You So by King Khan is not only a great song, but also a viral one. The song has gained millions of streams and downloads on various platforms, as well as millions of views and likes on TikTok, where it has become a popular soundtrack for various types of videos. Some of the videos that use Love You So by King Khan are romantic, funny, cute, or creative, while others are sad, emotional, or relatable. The song has also inspired many users to create their own versions, covers, or remixes of it. The song has also received positive reviews from critics and fans, who praise its melody, lyrics, and vibe.
- The song's style and genre
-Love You So by King Khan is not only a meaningful and viral song, but also a unique and versatile one. The song belongs to the genre of garage rock, which is a raw and energetic form of rock and roll that emerged in the 1960s and was revived in the 2000s. Garage rock is characterized by distorted guitars, simple chords, catchy hooks, and DIY aesthetics. However, Love You So by King Khan also incorporates elements of other genres, such as soul, doo-wop, and indie pop. The song has a retro and nostalgic feel, but also a modern and fresh appeal. The song can suit different moods and occasions, such as romantic dates, parties, road trips, or chill sessions.
- Conclusion
-Summary of the main points
-In conclusion, Love You So by King Khan is a song that you should definitely listen to if you haven't already. The song is a catchy and romantic declaration of love, but also a meaningful and heartfelt expression of frustration and confusion. The song is a viral hit on TikTok, where it has become a popular soundtrack for various types of videos. The song is a unique and versatile blend of garage rock and other genres, with a retro and nostalgic feel, but also a modern and fresh appeal.
- Call to action
-If you want to listen to Love You So by King Khan, you can download it from official sources, such as Spotify, Apple Music, or YouTube Music. Alternatively, you can download it from unofficial sources, such as MP3 converters or torrent sites. However, you should be aware of the risks and legal issues involved in doing so. Whichever way you choose to download Love You So by King Khan, we hope you enjoy this amazing song and share it with your friends and loved ones.
- FAQs
-Here are some of the frequently asked questions about Love You So by King Khan:
- Q: When was Love You So by King Khan released?
-A: Love You So by King Khan was released in 2014 as part of the EP Songs for Dads by The King Khan & BBQ Show.
- Q: Who wrote Love You So by King Khan?
-A: Love You So by King Khan was written by Arish Ahmad Khan (King Khan) and Mark Sultan (BBQ).
- Q: How long is Love You So by King Khan?
-A: Love You So by King Khan is 2 minutes and 48 seconds long.
- Q: What are some of the other songs by The King Khan & BBQ Show?
-A: Some of the other songs by The King Khan & BBQ Show are Waddlin' Around, Fish Fight, Invisible Girl, Animal Party, and Alone Again.
- Q: Where can I find more information about King Khan?
-A: You can find more information about King Khan on his official website , his Facebook page , his Instagram account , or his Wikipedia page .
- : https://kingkhanmusic.com/ : https://www.facebook.com/kingkhanmusic : https://www.instagram.com/kingkhanandtheshrines/ : https://en.wikipedia.org/wiki/King_Khan_(musician) 401be4b1e0
-
-
\ No newline at end of file
diff --git a/spaces/fatiXbelha/sd/Download musiCutter The Best MP3 and OGG Vorbis JoinerCutter.md b/spaces/fatiXbelha/sd/Download musiCutter The Best MP3 and OGG Vorbis JoinerCutter.md
deleted file mode 100644
index 624178c498009da18e37731ae7bdcaa82893c598..0000000000000000000000000000000000000000
--- a/spaces/fatiXbelha/sd/Download musiCutter The Best MP3 and OGG Vorbis JoinerCutter.md
+++ /dev/null
@@ -1,181 +0,0 @@
-
-How to Download and Use Musicutter, a Free and Easy Music Editing Software
-If you are looking for a simple and effective way to edit your music files, you might want to check out Musicutter, a free and open source software that can cut and join MP3 and OGG files without losing quality. In this article, we will show you how to download and use Musicutter for Windows, as well as some tips and tricks for using it effectively.
-musicutter download
Download ✔ https://urllie.com/2uNANr
- What is Musicutter and Why You Should Try It
-Musicutter is a free and open source software that can cut and join MP3 and OGG files
-Musicutter is a music editing software that was developed by Slavo Kopinec (macik) in 2009. It is designed to cut and join MP3 and OGG files without needing to decode and reencode them, which preserves their original quality. You can use Musicutter to create ringtones, remixes, mashups, or simply trim unwanted parts from your audio files.
- Musicutter is fast, simple, and supports various formats and features
-Musicutter is a lightweight and easy-to-use software that does not require installation. You can simply download it from its official website and run it from any folder. It has a user-friendly interface that lets you add, cut, join, and save your audio files with just a few clicks. It also supports various formats, such as MPEG audio versions, OGG Vorbis, VBR MP3 files, CUE sheets, XMCD files, and plain text files. It also has some useful features, such as frame statistics, fade in/out, crossfade, split mode, batch mode, keyboard shortcuts, and more.
- How to Download Musicutter for Windows
-Visit the official website of Musicutter and click on the download link
-To download Musicutter for Windows, you need to visit its official website and click on the download link at the top right corner of the page. You will be redirected to another page where you can choose between two versions of Musicutter: version 0.7.1 (the latest version) or version 0.6 (the previous version). We recommend downloading version 0.7.1 as it has more features and bug fixes than version 0.6.
- Choose a location to save the ZIP file and extract it
-After clicking on the download link, you will be Outline of the article: - H1: How to Download and Use Musicutter, a Free and Easy Music Editing Software - H2: What is Musicutter and Why You Should Try It - H3: Musicutter is a free and open source software that can cut and join MP3 and OGG files - H3: Musicutter is fast, simple, and supports various formats and features - H2: How to Download Musicutter for Windows - H3: Visit the official website of Musicutter and click on the download link - H3: Choose a location to save the ZIP file and extract it - H3: Run the musicutter.exe file to launch the program - H2: How to Cut and Join Music Files with Musicutter - H3: Click on the Add Files button to select the audio files you want to edit - H3: Use the Cut button to trim the files or the Join button to merge them - H3: Adjust the settings and options according to your preferences - H3: Click on the Save button to export the edited files - H2: Tips and Tricks for Using Musicutter Effectively - H3: Use the Frame Statistics feature to analyze your audio files - H3: Import cut points from CUE, XMCD, or text files - H3: Use keyboard shortcuts for faster editing - H2: Conclusion - H3: Summarize the main points of the article and provide a call to action - H2: FAQs - H3: What are the system requirements for Musicutter? - H3: What are the advantages of Musicutter over other music editing software? - H3: How can I contact the developer of Musicutter for feedback or support? - H3: Can I use Musicutter on other operating systems besides Windows? - H3: Is Musicutter safe and legal to use? Article with HTML formatting:
How to Download and Use Musicutter, a Free and Easy Music Editing Software
-If you are looking for a simple and effective way to edit your music files, you might want to check out Musicutter, a free and open source software that can cut and join MP3 and OGG files without losing quality. In this article, we will show you how to download and use Musicutter for Windows, as well as some tips and tricks for using it effectively.
- What is Musicutter and Why You Should Try It
-Musicutter is a free and open source software that can cut and join MP3 and OGG files
-Musicutter is a music editing software that was developed by Slavo Kopinec (macik) in 2009. It is designed to cut and join MP3 and OGG files without needing to decode and reencode them, which preserves their original quality. You can use Musicutter to create ringtones, remixes, mashups, or simply trim unwanted parts from your audio files.
- Musicutter is fast, simple, and supports various formats and features
-Musicutter is a lightweight and easy-to-use software that does not require installation. You can simply download it from its official website and run it from any folder. It has a user-friendly interface that lets you add, cut, join, and save your audio files with just a few clicks. It also supports various formats, such as MPEG audio versions, OGG Vorbis, VBR MP3 files, CUE sheets, XMCD files, and plain text files. It also has some useful features, such as frame statistics, fade in/out, crossfade, split mode, batch mode, keyboard shortcuts, and more.
-musicutter download for android
-musicutter download for windows
-musicutter download for mac
-musicutter download free
-musicutter download apk
-musicutter download online
-musicutter download pc
-musicutter download mp3
-musicutter download full version
-musicutter download crack
-musicutter download software
-musicutter download app
-musicutter download ringtone maker
-musicutter download audio editor
-musicutter download video editor
-musicutter download sound cutter
-musicutter download song cutter
-musicutter download voice cutter
-musicutter download mp4
-musicutter download wav
-musicutter download flac
-musicutter download ogg
-musicutter download m4a
-musicutter download aac
-musicutter download wma
-music cutter and joiner free download
-music cutter and merger free download
-music cutter and mixer free download
-music cutter and editor free download
-music cutter and converter free download
-best music cutter free download
-easy music cutter free download
-fast music cutter free download
-simple music cutter free download
-smart music cutter free download
-power music cutter free download
-magic music cutter free download
-super music cutter free download
-ultimate music cutter free download
-professional music cutter free download
-how to use music cutter free download
-how to install music cutter free download
-how to uninstall music cutter free download
-how to update music cutter free download
-how to register music cutter free download
-how to activate music cutter free download
-how to crack music cutter free download
-how to get music cutter free download
-how to make ringtones with music cutter free download
- How to Download Musicutter for Windows
-Visit the official website of Musicutter and click on the download link
-To download Musicutter for Windows, you need to visit its official website and click on the download link at the top right corner of the page. You will be redirected to another page where you can choose between two versions of Musicutter: version 0.7.1 (the latest version) or version 0.6 (the previous version). We recommend downloading version 0.7.1 as it has more features and bug fixes than version 0.6.
- Choose a location to save the ZIP file and extract it
-After clicking on the download link, you will be.
After clicking on the download link, you will be prompted to choose a location to save the ZIP file. You can save it anywhere on your computer, such as your desktop or downloads folder. Once the download is complete, you need to extract the ZIP file using a program like WinZip or 7-Zip. You will see a folder named musicutter-0.7.1 that contains the musicutter.exe file and some other files.
- Run the musicutter.exe file to launch the program
-To launch Musicutter, you just need to double-click on the musicutter.exe file. You will see a window that looks like this:
-
- This is the main interface of Musicutter, where you can add, cut, join, and save your audio files. You can also access the settings and options from the menu bar at the top.
- How to Cut and Join Music Files with Musicutter
-Click on the Add Files button to select the audio files you want to edit
-To start editing your music files, you need to add them to Musicutter. You can do this by clicking on the Add Files button at the bottom left corner of the window. This will open a file browser where you can select one or more audio files from your computer. You can also drag and drop files from your folders to Musicutter. The files you add will appear in the list on the left side of the window.
- Use the Cut button to trim the files or the Join button to merge them
-To cut a file, you need to select it from the list and click on the Cut button at the bottom right corner of the window. This will open a new window where you can see the waveform of the file and set the start and end points of the cut. You can use the mouse or the keyboard to move and adjust the markers. You can also enter the exact time values in the boxes below. When you are done, click on OK to confirm the cut.
- To join two or more files, you need to select them from the list and click on the Join button at the bottom right corner of the window. This will open a new window where you can see the waveforms of the files and arrange them in the order you want. You can use [assistant](#message) the mouse or the keyboard to drag and drop the files in the list. You can also use the buttons at the top to move them up or down, or delete them. When you are done, click on OK to confirm the join.
- Adjust the settings and options according to your preferences
-Before you save your edited files, you might want to adjust some settings and options in Musicutter. You can access them from the menu bar at the top of the window. For example, you can choose between different output formats (MP3 or OGG), change the quality and bitrate of your files, enable or disable fade in/out and crossfade effects, set a default output folder, and more. You can also customize some advanced settings, such as ID3 tags, VBR headers, CRC checks, etc.
- Click on the Save button to export your edited files
-When you are satisfied with your editing, you can save your files by clicking on the Save button at the bottom right corner of the window. This will open a file browser where you can choose a name and location for your output file. If you have cut or joined multiple files, Musicutter will automatically create a folder with a name based on your output file name and save all your edited files inside it. You can also choose to overwrite your original files if you want.
- Tips and Tricks for Using Musicutter Effectively
-Use the Frame Statistics feature to analyze your audio files
-If you want to get more information about your audio files, such as their bitrate, frequency, channels, frames, etc., you can use the Frame Statistics feature in Musicutter. To access it, select a file from the list and click on Frame Statistics from the Tools menu at the top of the window. This will open a new window where you can see a table with various data about your file. You can also export this data as a text file if you want.
- Import cut points from CUE, XMCD, or text files
-If you have a CUE sheet, an XMCD file, or a plain text file that contains cut points for your audio files, you can import them into Musicutter and use them to cut your files automatically. To do this, click on Import Cut Points from the Tools menu at the top of the window. This will open a file browser where you can select the file that contains the cut points. Musicutter will then load the cut points and apply them to your audio files. You can also export your cut points as a CUE, XMCD, or text file if you want.
- Use keyboard shortcuts for faster editing
-If you want to speed up your editing process, you can use some keyboard shortcuts in Musicutter. Here are some of the most useful ones:
-
-
-Keyboard Shortcut
-Action
-
-
-Ctrl + A
-Select all files in the list
-
-
-Ctrl + O
-Add files to the list
-
-
-Ctrl + S
-Save the edited files
-
-
-Ctrl + C
-Cut the selected file
-
-
-Ctrl + J
-Join the selected files
-
-
-Ctrl + Z
-Undo the last action
-
-
-Ctrl + Y
-Redo the last action
-
-
-Ctrl + F1
-Show the help file
-
-
-Ctrl + F2
-Show the about window
-
-
-F5 or Spacebar
-Play or pause the audio file
-
-
-F6 or Left Arrow Key
-Move the start marker backward by one frame
-
-
-F7 or Right Arrow Key
-Move the start marker forward by one frame
-
-
-F8 or Up Arrow Key
-Move the end marker backward by one frame
-
-
-F9 or Down Arrow Key < Move the end marker forward by one frame
-
-
- You can also customize your keyboard shortcuts from the Settings menu at the top of the window.
- Conclusion
-Musicutter is a free and easy music editing software that can cut and join MP3 and OGG files without losing quality. It is fast, simple, and supports various formats and features. You can download it from its official website and use it to create your own music projects. Whether you want to make ringtones, remixes, mashups, or just trim your audio files, Musicutter can help you do it in a few minutes.
- If you are interested in trying Musicutter, you can follow the steps we have shown you in this article. You can also check out some tips and tricks for using it effectively. We hope you enjoy using Musicutter and create some amazing music with it.
- Do you have any questions or comments about Musicutter? Feel free to share them with us in the comment section below. We would love to hear from you.
- FAQs
-What are the system requirements for Musicutter?
-Musicutter is compatible with Windows XP, Vista, 7, 8, and 10. It does not require any special hardware or software to run. However, it is recommended that you have at least 512 MB of RAM and 100 MB of free disk space for optimal performance.
- What are the advantages of Musicutter over other music editing software?
-Musicutter has some advantages over other music editing software, such as:
-
-- It is free and open source, which means you can use it without paying any fees or licenses.
-- It does not require installation, which means you can run it from any folder or portable device.
-- It does not need to decode and reencode your audio files, which means it preserves their original quality and saves time and disk space.
-- It supports various formats and features, which means you can edit different types of audio files and customize them according to your preferences.
-- It has a user-friendly interface and keyboard shortcuts, which means you can edit your audio files with ease and speed.
-
- How can I contact the developer of Musicutter for feedback or support?
-If you want to contact the developer of Musicutter, Slavo Kopinec (macik), you can do so by sending an email to macik@musicutter.com. You can also visit his website to learn more about him and his other projects. He welcomes any feedback or suggestions for improving Musicutter.
- Can I use Musicutter on other operating systems besides Windows?
-Musicutter is currently only available for Windows. However, the developer has stated that he plans to make Musicutter cross-platform in the future. This means that Musicutter might be compatible with other operating systems, such as Linux or Mac OS, in the future. You can check the official website of Musicutter for any updates on this matter.
- Is Musicutter safe and legal to use?
-Musicutter is safe and legal to use as long as you follow some basic rules. First, you should only use Musicutter for personal and non-commercial purposes. Second, you should only edit audio files that you own or have permission to use. Third, you should respect the intellectual property rights of the original creators of the audio files. Fourth, you should not use Musicutter for any illegal or harmful activities. By following these rules, you can use Musicutter without any problems.
401be4b1e0
-
-
\ No newline at end of file
diff --git a/spaces/fatiXbelha/sd/Euro Truck Driver 2019 Enjoy the Stunning Graphics and Physics of This Truck Simulator.md b/spaces/fatiXbelha/sd/Euro Truck Driver 2019 Enjoy the Stunning Graphics and Physics of This Truck Simulator.md
deleted file mode 100644
index 30f02df30d40b58a7dea2f1ddbd34bb3d43d6a70..0000000000000000000000000000000000000000
--- a/spaces/fatiXbelha/sd/Euro Truck Driver 2019 Enjoy the Stunning Graphics and Physics of This Truck Simulator.md
+++ /dev/null
@@ -1,130 +0,0 @@
-
-Euro Truck Driver 2019: A Review of the Best Truck Simulator Games
-Do you love driving trucks and exploring different countries and cities? Do you want to experience the thrill of being a real trucker and running your own transportation company? If you answered yes to any of these questions, then you should definitely check out Euro Truck Driver 2019, one of the best truck simulator games available on the market. In this article, we will review the features, benefits, and drawbacks of Euro Truck Driver 2019, and compare it with other popular truck simulator games. By the end of this article, you will have a clear idea of whether Euro Truck Driver 2019 is the right game for you.
- Introduction
-What is Euro Truck Driver 2019?
-Euro Truck Driver 2019 is a truck simulator game developed by Ovidiu Pop, a company that specializes in creating realistic and immersive driving games. The game was released in January 2023 for Android devices, and has since received over 50 million downloads and 4.3 stars rating on Google Play Store. The game lets you become a real trucker, featuring European trucks with lots of customizations, realistic driving physics, and stunning graphics. You can travel across many countries from Europe, visit incredible places like Berlin, Prague, Madrid, Rome, Paris, and more. You can also play the career mode, make money, purchase new trucks and upgrades, explore the trucking world, and challenge your friends with the online multiplayer mode.
-euro truck driver 2019
Download File ===> https://urllie.com/2uNwn3
- Why play Euro Truck Driver 2019?
-Euro Truck Driver 2019 is not only about driving - it's also about creating your own story and fulfilling your dreams. You can choose from 12 different European truck brands, each with their own unique features and styles. You can also customize your truck with various parts, colors, and cosmetics. You can drive across country roads, highways, and offroads, enjoying the realistic weather conditions and day/night cycle. You can also experience the different traffic situations, road signs, speed limits, and rules of each country. You can also interact with other drivers, pedestrians, police officers, and toll booths. You can also listen to your favorite radio stations or music while driving.
- Features of Euro Truck Driver 2019
-Realistic truck models and customization
-Euro Truck Driver 2019 features 12 European truck brands (4x2 and 6x4 Axles), such as Mercedes-Benz, Volvo, Scania, MAN, Renault, DAF, Iveco, and more. Each truck has its own detailed interior, engine sound, performance, and handling. You can also customize your truck with various parts, such as chassis configurations, cabs, engines, transmissions, wheels, tires, lights, horns, exhausts, bumpers, mirrors, spoilers, sun visors, grills, and more. You can also change the color of your truck or apply decals and stickers. You can also upgrade your truck with better parts or accessories to improve its speed, fuel efficiency, reliability, and comfort.
- Diverse and detailed European map
-Euro Truck Driver 2019 lets you drive across more than 20 realistic cities in Europe, such as Berlin, Prague, Madrid, Rome, Paris, London, Amsterdam, Brussels, Vienna, Zurich, and more. Each city has its own landmarks, buildings, roads, bridges, tunnels, and scenery. You can also drive across country roads, highways, and offroads, exploring the different landscapes of Europe, such as mountains, forests, fields, lakes, rivers, and seas. You can also encounter different weather conditions and seasons, such as sunny, cloudy, rainy, snowy, foggy, windy, and stormy. You can also experience the day/night cycle and the dynamic lighting and shadows. You can also visit various places of interest, such as gas stations, rest areas, truck stops, repair shops, car dealerships, and more.
-euro truck driver 2019 game download
-euro truck driver 2019 mod apk
-euro truck driver 2019 online multiplayer
-euro truck driver 2019 pc
-euro truck driver 2019 cheats
-euro truck driver 2019 review
-euro truck driver 2019 gameplay
-euro truck driver 2019 android
-euro truck driver 2019 ios
-euro truck driver 2019 trailer
-euro truck driver 2019 hack
-euro truck driver 2019 update
-euro truck driver 2019 tips and tricks
-euro truck driver 2019 best truck
-euro truck driver 2019 free download
-euro truck driver 2019 simulator
-euro truck driver 2019 customizations
-euro truck driver 2019 maps
-euro truck driver 2019 cities
-euro truck driver 2019 graphics
-euro truck driver 2019 controls
-euro truck driver 2019 missions
-euro truck driver 2019 achievements
-euro truck driver 2019 weather
-euro truck driver 2019 traffic
-euro truck driver 2019 realistic physics
-euro truck driver 2019 sound effects
-euro truck driver 2019 engine sounds
-euro truck driver 2019 steering wheel support
-euro truck driver 2019 convoy mode
-euro truck driver 2019 leaderboards
-euro truck driver 2019 challenges
-euro truck driver 2019 career mode
-euro truck driver 2019 company management
-euro truck driver 2019 europe map
-euro truck driver 2019 west balkans expansion
-euro truck driver 2019 Iberia expansion
-euro truck driver 2019 vs Euro Truck Simulator 2
-euro truck driver 2019 vs American Truck Simulator
-euro truck driver 2019 vs Truck Simulator USA
-how to play Euro Truck Driver 2019
-how to install Euro Truck Driver 2019
-how to upgrade Euro Truck Driver 2019
-how to earn money in Euro Truck Driver 2019
-how to buy new trucks in Euro Truck Driver 2019
-how to customize trucks in Euro Truck Driver 2019
-how to drive across country roads in Euro Truck Driver 2019
-how to drive in different weather conditions in Euro Truck Driver 2019
-how to deal with traffic and accidents in Euro Truck Driver 2019
- Career mode and company management
-Euro Truck Driver 2019 offers a career mode where you can start from the bottom and work your way up to become a successful trucker and business owner. You can choose from different types of jobs and contracts, such as cargo delivery, special transport, heavy haulage, and more. You can also choose from different types of cargo and trailers, such as containers, refrigerated goods, livestock, cars, machinery, construction materials, and more. You can also earn money and experience points by completing your deliveries on time and without damage. You can also spend your money on buying new trucks or upgrading your existing ones. You can also hire other drivers and assign them to your trucks. You can also manage your own transportation company and expand your fleet and garage. You can also compete with other companies and drivers on the leaderboards.
- Online multiplayer and modding community
-Euro Truck Driver 2019 also supports online multiplayer mode where you can join or create your own server and play with other players from around the world. You can chat with other players using the voice or text chat feature. You can also cooperate or compete with other players in various modes and events. You can also join or create your own convoy and drive together with your friends or strangers. You can also customize your truck with flags, stickers, horns, CB radios, and more. You can also access the modding community where you can download or upload custom mods for the game. You can also find new trucks, maps, cargoes, trailers, skins, sounds, and more.
- Comparison with other truck simulator games
-Euro Truck Simulator 2
-Euro Truck Simulator 2 is another popular truck simulator game developed by SCS Software, a company that has been making driving simulation games since 1997. The game was released in October 2012 for Windows, MacOS, and Linux devices, and has since received over 10 million sales and 97% positive reviews on Steam. The game features over 70 European cities in 13 countries, such as Germany, France, Italy, Spain, Poland, and more. The game also features over 40 licensed truck brands, such as Mercedes-Benz, Volvo, Scania, MAN, Renault, DAF, Iveco, and more. The game also features a variety of cargoes and trailers, such as flatbeds, lowboys, curtain siders, reefers, tankers, and more. The game also features a dynamic weather system and a realistic day/night cycle. The game also features a career mode where you can start your own company and hire other drivers. The game also supports online multiplayer mode where you can play with other players on dedicated servers or create your own server with mods. The game also has a large modding community where you can find new trucks, maps, cargoes, trailers, skins, sounds, and more.
- European Truck Simulator
-European Truck Simulator is another truck simulator game developed by Zuuks Games, a company that focuses on creating mobile games. The game was released in July 2020 for Android devices, and has since received over 10 million downloads and 4 stars rating on Google Play Store. The game features over 20 European cities in 10 countries, such as Germany, France, Italy, Spain, Poland, and more. The game also features over 10 truck brands, such as Mercedes-Benz, Volvo, Scania, MAN, Renault, DAF, Iveco, and more. The game also features a variety of cargoes and trailers, such as containers, refrigerated goods, livestock, cars, machinery, construction materials, and more. The game also features a realistic weather system and a day/night cycle. The game also features a career mode where you can earn money and buy new trucks or upgrade your existing ones. The game also supports online multiplayer mode where you can play with other players on the same map. The game also has a modding community where you can find new trucks, maps, cargoes, trailers, skins, sounds, and more.
- Euro Truck Extreme - Driver 2019
-Euro Truck Extreme - Driver 2019 is another truck simulator game developed by Game Pickle, a company that produces casual and fun games. The game was released in June 2019 for Android devices, and has since received over 5 million downloads and 3.9 stars rating on Google Play Store. The game features over 10 European cities in 6 countries, such as Germany, France, Italy, Spain, Poland, and more. The game also features over 10 truck brands, such as Mercedes-Benz, Volvo, Scania, MAN, Renault, DAF, Iveco, and more. The game also features a variety of cargoes and trailers, such as containers, refrigerated goods, livestock, cars, machinery, construction materials, and more. The game also features a realistic weather system and a day/night cycle. The game also features a career mode where you can earn money and buy new trucks or upgrade your existing ones. The game also supports online multiplayer mode where you can play with other players on the same map. The game also has a modding community where you can find new trucks, maps, cargoes, trailers, skins, sounds, and more.
- Conclusion
-Summary of the main points
-In conclusion, Euro Truck Driver 2019 is one of the best truck simulator games available on the market. It offers a realistic and immersive driving experience with stunning graphics, physics, and sounds. It also offers a variety of features and options to customize your truck and your gameplay. It also offers a career mode where you can start your own company and manage your fleet and drivers. It also offers an online multiplayer mode where you can join or create your own server and play with other players from around the world. It also offers a modding community where you can download or upload custom mods for the game.
- Recommendation and call to action
-If you are looking for a fun and challenging truck simulator game that will keep you entertained for hours, then you should definitely give Euro Truck Driver 2019 a try. You can download the game for free from Google Play Store or visit the official website for more information. You can also follow the game on Facebook or Twitter for the latest news and updates. You can also join the game's Discord server or Reddit community for tips, tricks, support, and feedback. You can also watch the game's YouTube channel or Twitch channel for gameplay videos and live streams. You can also check out the game's Wiki page or Fandom page for guides, tutorials, FAQs, and more.
- FAQs
-Q: How do I install Euro Truck Driver 2019 on my device?
-A: You can install Euro Truck Driver 2019 on your device by following these steps:
-
-- Go to Google Play Store on your device and search for Euro Truck Driver 2019.
-- Tap on the Install button and wait for the download to finish.
-- Tap on the Open button and enjoy the game.
-
- Q: How do I play Euro Truck Driver 2019?
-A: You can play Euro Truck Driver 2019 by following these steps:
-
-- Launch the game on your device and choose your preferred language.
-- Select your profile name and avatar.
-- Select your preferred control mode (tilt, buttons, or steering wheel).
-- Select your preferred camera view (first-person or third-person).
-- Select your preferred game mode (career or multiplayer).
-- Select your preferred truck brand and model.
-- Select your preferred job or contract.
-- Drive your truck to the destination and deliver the cargo.
-
- Q: How do I customize my truck in Euro Truck Driver 2019?
-A: You can customize your truck in Euro Truck Driver 2019 by following these steps:
-
-- Go to the garage menu in the game.
-- Select your truck from the list.
-- Select the Customize option.
-- Select the part or accessory you want to change.
-- Select the color or style you want to apply.
-- Confirm your changes and save your truck.
-
- Q: How do I join or create a server in Euro Truck Driver 2019?
-A: You can join or create a server in Euro Truck Driver 2019 by following these steps:
-
-- Select the multiplayer mode in the game.
-- Select the Join option to join an existing server or the Create option to create a new server.
-- Select the server name, password, region, and map you want to use.
-- Select the Start option to enter the server.
-- Invite your friends or other players to join your server or join other servers.
-
- Q: How do I download or upload mods for Euro Truck Driver 2019?
-A: You can download or upload mods for Euro Truck Driver 2019 by following these steps:
-
-- Go to the modding community menu in the game.
-- Select the Download option to browse and download mods from other players or the Upload option to upload your own mods.
-- Select the mod category, such as trucks, maps, cargoes, trailers, skins, sounds, and more.
-- Select the mod you want to download or upload and read the description and instructions.
-- Select the Download or Upload button and wait for the process to finish.
-- Activate or deactivate the mod in the game settings.
-
401be4b1e0
-
-
\ No newline at end of file
diff --git a/spaces/fatiXbelha/sd/Experience the Thrill of Racing in Car 2 with MOD APK Free Download for Android 1.md b/spaces/fatiXbelha/sd/Experience the Thrill of Racing in Car 2 with MOD APK Free Download for Android 1.md
deleted file mode 100644
index 1a7ff1cf89106fc97c1ca45a024a40af82e612fd..0000000000000000000000000000000000000000
--- a/spaces/fatiXbelha/sd/Experience the Thrill of Racing in Car 2 with MOD APK Free Download for Android 1.md
+++ /dev/null
@@ -1,70 +0,0 @@
-
-Racing in Car 2 Mod Apk: A Thrilling and Realistic Driving Experience
-Do you love racing games? Do you want to feel the adrenaline rush of driving a car at high speed? Do you want to enjoy a realistic and immersive driving experience on your Android device? If you answered yes to any of these questions, then you should try Racing in Car 2, one of the most popular car racing games on the Google Play Store. And if you want to make the game even more fun and exciting, you should download the Racing in Car 2 Mod Apk, which gives you unlimited money, all cars unlocked, and no ads. In this article, we will tell you everything you need to know about Racing in Car 2 and its mod apk version. We will also show you how to download and install the mod apk file on your Android device.
- What is Racing in Car 2?
-Racing in Car 2 is a car racing game developed by Fast Free Games, a studio that specializes in creating realistic and thrilling racing games for mobile devices. Racing in Car 2 is the sequel to the original Racing in Car game, which has over 100 million downloads on the Google Play Store. Racing in Car 2 improves on its predecessor by adding more features, modes, cars, and environments to the game.
-racing in car 2 mod apk free download android 1
Download Zip ✺✺✺ https://urllie.com/2uNHeS
- Features of Racing in Car 2
-Racing in Car 2 has many features that make it one of the best car racing games on the market. Here are some of them:
- - Endless mode
-In this mode, you can drive your car as long as you can without crashing into other vehicles or obstacles. You can choose from different traffic scenarios, such as city, highway, desert, or snow. You can also change the weather and time of day to suit your preference. The longer you drive, the more coins you earn, which you can use to buy new cars or upgrade your existing ones.
-racing in car 2 unlimited money mod apk download for android
-how to install racing in car 2 mod apk on android device
-racing in car 2 hack mod apk free download latest version
-best racing games for android with mod apk download link
-racing in car 2 mod apk offline gameplay and features
-download racing in car 2 mod apk with unlimited coins and gems
-racing in car 2 mod apk no root required for android
-racing in car 2 mod apk free shopping and upgrades
-racing in car 2 realistic driving simulator mod apk download
-racing in car 2 cheats and tips for mod apk users
-racing in car 2 mod apk new cars and tracks update
-racing in car 2 mod apk vs original game comparison
-racing in car 2 mod apk review and rating by users
-racing in car 2 mod apk download from android 1 website
-racing in car 2 fun and addictive racing game mod apk
-racing in car 2 mod apk support and feedback
-racing in car 2 mod apk file size and compatibility
-racing in car 2 mod apk download without survey or verification
-racing in car 2 mod apk easy and fast download process
-racing in car 2 mod apk safe and secure download source
-racing in car 2 mod apk online multiplayer mode
-racing in car 2 mod apk unlimited nitro and fuel
-racing in car 2 mod apk customize your own car
-racing in car 2 mod apk different camera angles and views
-racing in car 2 mod apk high quality graphics and sound effects
- - Career mode
-In this mode, you can complete various missions and challenges to earn rewards and unlock new cars and environments. You can also compete with other players online and see who can drive faster and farther. You can also customize your car with different colors, stickers, rims, and spoilers.
- - Multiple cars and environments
-Racing in Car 2 offers a wide range of cars to choose from, such as sports cars, muscle cars, trucks, buses, and more. Each car has its own characteristics, such as speed, acceleration, handling, and braking. You can also drive in different environments, such as city streets, highways, deserts, snow mountains, and more. Each environment has its own challenges and obstacles to overcome.
- - First-person perspective
-Racing in Car 2 gives you a realistic and immersive driving experience by letting you drive your car from a first-person perspective. You can see the road ahead of you through the windshield of your car, as well as the dashboard, steering wheel, mirrors, and pedals. You can also tilt your device to steer your car or use buttons on the screen.
- - Easy controls and realistic physics
-Racing in Car 2 has easy and intuitive controls that let you control your car with ease. You can use tilt or touch controls to steer your car and accelerate or brake. You can also use the nitro button to boost your speed and overtake other cars. The game also has realistic physics that make your car behave according to the road conditions, such as friction, gravity, and inertia.
- What is Racing in Car 2 Mod Apk?
-Racing in Car 2 Mod Apk is a modified version of the original Racing in Car 2 game that gives you some extra features and advantages that are not available in the official version. The mod apk file is a third-party application that you can download and install on your Android device for free.
- Benefits of Racing in Car 2 Mod Apk
-Racing in Car 2 Mod Apk has many benefits that make the game more enjoyable and satisfying. Here are some of them:
- - Unlimited money
-With the mod apk version, you don't have to worry about running out of money to buy new cars or upgrade your existing ones. You can get unlimited money by playing the game or using the money hack feature. You can also use the money to unlock new environments and modes.
- - All cars unlocked
-With the mod apk version, you don't have to complete missions or challenges to unlock new cars. You can access all the cars in the game from the start, and choose the one that suits your style and preference. You can also customize your car with different colors, stickers, rims, and spoilers.
- - No ads
-With the mod apk version, you don't have to deal with annoying ads that interrupt your gameplay or waste your time. You can enjoy the game without any distractions or interruptions.
- How to Download and Install Racing in Car 2 Mod Apk?
-If you want to download and install Racing in Car 2 Mod Apk on your Android device, you need to follow some simple steps. Before you do that, make sure that your device has enough storage space and meets the minimum requirements for the game. Here are the steps to download and install Racing in Car 2 Mod Apk:
- Steps to Download and Install Racing in Car 2 Mod Apk
-Follow these steps carefully to avoid any errors or issues:
- - Enable unknown sources
-Since the mod apk file is a third-party application, you need to enable unknown sources on your device to allow it to install. To do that, go to your device settings, then security, then unknown sources, and turn it on.
- - Download the mod apk file
-Next, you need to download the mod apk file from a reliable source. You can use this link to download the latest version of Racing in Car 2 Mod Apk. The file size is about 60 MB, so make sure you have a stable internet connection.
- - Install the mod apk file
-After downloading the mod apk file, locate it on your device storage and tap on it to start the installation process. Follow the instructions on the screen and wait for a few seconds until the installation is complete.
- - Enjoy the game
-Now you can open the game and enjoy all the features and benefits of Racing in Car 2 Mod Apk. You can drive your car in different modes, environments, and scenarios, and have a thrilling and realistic driving experience.
- Conclusion
-Racing in Car 2 is a great car racing game that lets you feel the adrenaline rush of driving a car at high speed. It has many features, modes, cars, and environments that make it one of the best car racing games on the market. And if you want to make it even more fun and exciting, you should download Racing in Car 2 Mod Apk, which gives you unlimited money, all cars unlocked, and no ads. You can download and install Racing in Car 2 Mod Apk on your Android device by following some simple steps. We hope this article was helpful and informative for you. If you have any questions or feedback, feel free to leave a comment below.
- FAQs - Q: Is Racing in Car 2 Mod Apk safe to use? - A: Yes, Racing in Car 2 Mod Apk is safe to use as long as you download it from a trusted source. However, we recommend that you use it at your own risk and discretion. - Q: Do I need to root my device to use Racing in Car 2 Mod Apk? - A: No, you don't need to root your device to use Racing in Car 2 Mod Apk. You just need to enable unknown sources on your device settings. - - Q: What is the difference between Racing in Car 2 and Racing in Car 2 Mod Apk? - A: Racing in Car 2 is the official version of the game that you can download from the Google Play Store. Racing in Car 2 Mod Apk is a modified version of the game that gives you some extra features and advantages that are not available in the official version. - Q: Can I play Racing in Car 2 Mod Apk offline? - A: Yes, you can play Racing in Car 2 Mod Apk offline. However, you will need an internet connection to access some features, such as online mode, leaderboards, and achievements. - Q: How can I update Racing in Car 2 Mod Apk? - A: To update Racing in Car 2 Mod Apk, you need to download the latest version of the mod apk file from the same source that you downloaded it from. Then, you need to uninstall the previous version of the game and install the new one. - Q: Can I use Racing in Car 2 Mod Apk on other devices, such as iOS or PC? - A: No, Racing in Car 2 Mod Apk is only compatible with Android devices. You cannot use it on other devices, such as iOS or PC. - Q: Can I share Racing in Car 2 Mod Apk with my friends or family? - A: Yes, you can share Racing in Car 2 Mod Apk with your friends or family. However, we advise that you do not share it with strangers or on public platforms, as it may violate the terms and conditions of the game and the mod apk file. 401be4b1e0
-
-
\ No newline at end of file
diff --git a/spaces/feregVcuzo/sanity-test-midi/checkpoint/Download Java Facebook Lite A Fast and Lightweight App for Low-Bandwidth Devices.md b/spaces/feregVcuzo/sanity-test-midi/checkpoint/Download Java Facebook Lite A Fast and Lightweight App for Low-Bandwidth Devices.md
deleted file mode 100644
index 4b2826d9cdfe1d58e633294c2abdb123233d7d10..0000000000000000000000000000000000000000
--- a/spaces/feregVcuzo/sanity-test-midi/checkpoint/Download Java Facebook Lite A Fast and Lightweight App for Low-Bandwidth Devices.md
+++ /dev/null
@@ -1,118 +0,0 @@
-
-How to Download Java Facebook
-If you are a Java developer who wants to integrate your application with Facebook, you may be wondering how to download Java Facebook. Java Facebook is a library that provides an interface between your Java application and Facebook's Graph API. This allows you to access various Facebook features, such as user data, messages, pages, ads, and more.
-download java facebook
Download ○○○ https://gohhs.com/2uPvve
-In this article, we will show you how to download Java Facebook and use it in your project. We will also explain some of the benefits of using this library and provide some examples of API calls that you can make with it.
- Prerequisites for Downloading Java Facebook
-Before you can download and use Java Facebook, you need to have some prerequisites in place. These are:
-
-- A registered app on developers.facebook.com. You need to create an app that represents your application and add the products that you want to use, such as Marketing API or Pages API.
-- An access token that provides temporary, secure access to Facebook APIs. You need to generate a user access token or a page access token for your app and ask for the permissions that you need, such as ads_management or manage_page.
-- A Java 6 (or higher) Java Development Kit (JDK) installed on your system. You need to have a JDK that allows you to compile and run Java applications.
-
- Downloading the Java Facebook SDK
-Choosing the Right Version
-The Java Facebook SDK is available in different versions that support different features and platforms. You need to choose the right version for your project based on your needs and preferences.
-The latest version of the SDK is facebook-java-business-sdk, which supports multiple Facebook APIs from different platforms, such as Marketing API, Pages API, Instagram API, etc. This version is recommended for most developers who want to use a comprehensive and up-to-date SDK.
-If you only want to use the Marketing API, you can use the older version of the SDK called facebook-java-ads-sdk, which is still maintained but not updated with new features.
-download java facebook sdk
-download java facebook api
-download java facebook messenger
-download java facebook lite
-download java facebook app for mobile
-download java facebook chat
-download java facebook browser
-download java facebook jar
-download java facebook login
-download java facebook video downloader
-download java facebook connector
-download java facebook graph api
-download java facebook business sdk
-download java facebook integration
-download java facebook oauth
-download java facebook scraper
-download java facebook client
-download java facebook rest client
-download java facebook json rest client
-download java facebook xml rest client
-download java facebook jaxb rest client
-download java facebook marketing api
-download java facebook pages api
-download java facebook ads api
-download java facebook insights api
-download java facebook web app helper
-download java facebook web request
-download java facebook signature util
-download java facebook extended perm
-download java facebook feed image
-download java facebook photo tag
-download java facebook profile field
-download java facebook page profile field
-download java facebook profile info field
-download java facebook attachment media
-download java facebook attachment media image
-download java facebook attachment media video
-download java facebook attachment media flash
-download java facebook attachment media mp3
-download java facebook attachment property
-download java facebook application property set
-download java facebook association info
-download java facebook association type
-download java facebook allocation type
-download java facebook bundle action link
-download java facebook bundle story template
-download java facebook marketplace listing category
-If you want to use other Facebook APIs that are not supported by either of these versions, you can use the legacy version of the SDK called facebook-java-api, which is no longer maintained or supported by Facebook.
- Downloading the Jar File
-The easiest way to download the jar file of the SDK is to get it from the official GitHub repository. You can find the latest release of facebook-java-business-sdk here, where you can download either a jar file with dependencies or without dependencies. The jar file with dependencies includes all the libraries that the SDK depends on, such as Apache HTTP Client, Jackson JSON Processor, etc. The jar file without dependencies only includes the SDK itself, and you need to add the dependencies manually to your project.
-You can also download the jar file of facebook-java-ads-sdk here or the jar file of facebook-java-api here, depending on which version you want to use.
-Alternatively, you can use a dependency management tool, such as Maven or Gradle, to download the SDK from a repository. You can find the instructions on how to do that on the GitHub pages of each version.
- Adding the Jar File to the Project
-Once you have downloaded the jar file of the SDK, you need to add it to your project so that you can use it in your code. There are different ways to do this depending on which IDE or tool you are using.
-If you are using an IDE, such as Eclipse or IntelliJ IDEA, you can simply right-click on your project and select "Build Path" or "Module Settings". Then, you can add the jar file as an external library or a module dependency.
-If you are using a tool, such as Maven or Gradle, you can add the jar file as a dependency in your pom.xml or build.gradle file. You can find the coordinates of the jar file on the GitHub pages of each version.
-If you are not using any IDE or tool, you can manually add the jar file to your classpath when compiling and running your application. You can use the -cp or -classpath option of the javac and java commands to specify the path of the jar file.
- Using the Java Facebook SDK
-Creating an API Context
-The first step to use the Java Facebook SDK is to create an API context object that holds your access token and other settings. The API context object is used by all the SDK methods to make API calls to Facebook.
-To create an API context object, you need to use the APIContext
class and pass your access token as a parameter. For example:
-APIContext context = new APIContext("your-access-token");
-You can also pass other parameters, such as debug mode, timeout, proxy, etc., to customize your API context object. For example:
-APIContext context = new APIContext("your-access-token") .enableDebug(true) .setTimeout(10000) .setProxy("proxy-host", "proxy-port");
- Making API Calls
-The next step to use the Java Facebook SDK is to make API calls to Facebook using the SDK methods. The SDK methods are organized into different classes that represent different Facebook entities, such as users, pages, ads, etc. Each class has methods that correspond to different actions that you can perform on that entity, such as getting data, creating objects, updating fields, deleting objects, etc.
-To use the SDK methods, you need to create an instance of the class that represents the entity that you want to work with and pass your API context object as a parameter. For example:
-User user = new User("me", context); Page page = new Page("your-page-id", context); AdAccount adAccount = new AdAccount("your-ad-account-id", context);
-Then, you can call the methods of that instance to make API calls to Facebook. For example:
-// Get user data user.fetch(); System.out.println(user.getName()); System.out.println(user.getEmail()); // Post a message on a page page.publishMessage() .setMessage("Hello world!") .execute(); // Create an ad campaign AdCampaign campaign = adAccount.createCampaign() .setName("My Campaign") .setObjective(Campaign.EnumObjective.VALUE_LINK_CLICKS) .setStatus(Campaign.EnumStatus.VALUE_PAUSED) .execute(); System.out.println(campaign.getId());
- Handling Exceptions and Errors
-Sometimes, when you use the Java Facebook SDK, you may encounter exceptions and errors that prevent your API calls from succeeding. These may be caused by various reasons, such as invalid parameters, network issues, API limits, etc.
-To handle exceptions and errors, you need to use a try-catch block around your code and catch the appropriate exception classes that may be thrown by the SDK. For example:
-try // Make API call catch (APIException e) // Handle API exception System.err.println(e.getMessage()); catch (IOException e) // Handle IO exception System.err.println(e.getMessage()); catch (Exception e) // Handle other exceptions System.err.println(e.getMessage());
-You can also use the getError
method of the APIException
class to get more details about the error, such as the error code, subcode, type, message, etc. For example:
-catch (APIException e) // Handle API exception APIException.FacebookException error = e.getError(); System.err.println(error.getCode()); System.err.println(error.getSubcode()); System.err.println(error.getType()); System.err.println(error.getMessage());
- Benefits of Using Java Facebook SDK
-Using the Java Facebook SDK has many benefits for Java developers who want to integrate their applications with Facebook. Some of these benefits are:
-
-- It simplifies the integration process by providing a consistent and easy-to-use interface for making API calls to Facebook.
-- It handles the low-level details of HTTP requests and responses, JSON parsing, access token management, etc., so that you can focus on your business logic.
-- It supports multiple Facebook APIs from different platforms, such as Marketing API, Pages API, Instagram API, etc., so that you can access various Facebook features with one SDK.
-- It is updated regularly with new features and bug fixes by Facebook and the community, so that you can use the latest and most stable version of the SDK.
-
- Conclusion
-In this article, we have shown you how to download Java Facebook and use it in your project. We have also explained some of the benefits of using this library and provided some examples of API calls that you can make with it.
-If you want to learn more about Java Facebook, you can visit the official GitHub pages of each version and read the documentation and code samples. You can also join the Facebook Developer Community and ask questions or share your feedback with other developers.
-We hope that this article has helped you to understand how to download Java Facebook and use it in your project. Happy coding!
- Frequently Asked Questions
-What is Java Facebook?
-Java Facebook is a library that provides an interface between your Java application and Facebook's Graph API. It allows you to access various Facebook features, such as user data, messages, pages, ads, and more.
- How do I download Java Facebook?
-You can download the jar file of Java Facebook from the official GitHub repository or from other sources. You can also use a dependency management tool, such as Maven or Gradle, to download the SDK from a repository.
- How do I use Java Facebook?
-You need to create an API context object that holds your access token and other settings. Then, you need to create an instance of the class that represents the entity that you want to work with and call the methods of that instance to make API calls to Facebook.
- What are some of the benefits of using Java Facebook?
-Some of the benefits of using Java Facebook are: it simplifies the integration process by providing a consistent and easy-to-use interface for making API calls to Facebook; it handles the low-level details of HTTP requests and responses, JSON parsing, access token management, etc.; it supports multiple Facebook APIs from different platforms; and it is updated regularly with new features and bug fixes.
- Where can I find more information about Java Facebook?
-You can find more information about Java Facebook on the official GitHub pages of each version and read the documentation and code samples. You can also join the Facebook Developer Community and ask questions or share your feedback with other developers.
401be4b1e0
-
-
\ No newline at end of file
diff --git a/spaces/fffiloni/controlnet-animation-doodle/node_modules/methods/README.md b/spaces/fffiloni/controlnet-animation-doodle/node_modules/methods/README.md
deleted file mode 100644
index 672a32bfe5d685306f18b7a81a15af9fbbd00a0f..0000000000000000000000000000000000000000
--- a/spaces/fffiloni/controlnet-animation-doodle/node_modules/methods/README.md
+++ /dev/null
@@ -1,51 +0,0 @@
-# Methods
-
-[![NPM Version][npm-image]][npm-url]
-[![NPM Downloads][downloads-image]][downloads-url]
-[![Node.js Version][node-version-image]][node-version-url]
-[![Build Status][travis-image]][travis-url]
-[![Test Coverage][coveralls-image]][coveralls-url]
-
-HTTP verbs that Node.js core's HTTP parser supports.
-
-This module provides an export that is just like `http.METHODS` from Node.js core,
-with the following differences:
-
- * All method names are lower-cased.
- * Contains a fallback list of methods for Node.js versions that do not have a
- `http.METHODS` export (0.10 and lower).
- * Provides the fallback list when using tools like `browserify` without pulling
- in the `http` shim module.
-
-## Install
-
-```bash
-$ npm install methods
-```
-
-## API
-
-```js
-var methods = require('methods')
-```
-
-### methods
-
-This is an array of lower-cased method names that Node.js supports. If Node.js
-provides the `http.METHODS` export, then this is the same array lower-cased,
-otherwise it is a snapshot of the verbs from Node.js 0.10.
-
-## License
-
-[MIT](LICENSE)
-
-[npm-image]: https://img.shields.io/npm/v/methods.svg?style=flat
-[npm-url]: https://npmjs.org/package/methods
-[node-version-image]: https://img.shields.io/node/v/methods.svg?style=flat
-[node-version-url]: https://nodejs.org/en/download/
-[travis-image]: https://img.shields.io/travis/jshttp/methods.svg?style=flat
-[travis-url]: https://travis-ci.org/jshttp/methods
-[coveralls-image]: https://img.shields.io/coveralls/jshttp/methods.svg?style=flat
-[coveralls-url]: https://coveralls.io/r/jshttp/methods?branch=master
-[downloads-image]: https://img.shields.io/npm/dm/methods.svg?style=flat
-[downloads-url]: https://npmjs.org/package/methods
diff --git a/spaces/fh2412/handwritten_numbers/README.md b/spaces/fh2412/handwritten_numbers/README.md
deleted file mode 100644
index 1fdbb2de5ceff438797465bde083ae93819e705c..0000000000000000000000000000000000000000
--- a/spaces/fh2412/handwritten_numbers/README.md
+++ /dev/null
@@ -1,13 +0,0 @@
----
-title: Handwritten Numbers
-emoji: 💻
-colorFrom: gray
-colorTo: indigo
-sdk: gradio
-sdk_version: 3.50.2
-app_file: app.py
-pinned: false
-license: apache-2.0
----
-
-Check out the configuration reference at https://huggingface.co/docs/hub/spaces-config-reference
diff --git a/spaces/finlaymacklon/boxy_violet/theme_dropdown.py b/spaces/finlaymacklon/boxy_violet/theme_dropdown.py
deleted file mode 100644
index 6235388fd00549553df44028f3ccf03e946994ea..0000000000000000000000000000000000000000
--- a/spaces/finlaymacklon/boxy_violet/theme_dropdown.py
+++ /dev/null
@@ -1,57 +0,0 @@
-import os
-import pathlib
-
-from gradio.themes.utils import ThemeAsset
-
-
-def create_theme_dropdown():
- import gradio as gr
-
- asset_path = pathlib.Path(__file__).parent / "themes"
- themes = []
- for theme_asset in os.listdir(str(asset_path)):
- themes.append(
- (ThemeAsset(theme_asset), gr.Theme.load(str(asset_path / theme_asset)))
- )
-
- def make_else_if(theme_asset):
- return f"""
- else if (theme == '{str(theme_asset[0].version)}') {{
- var theme_css = `{theme_asset[1]._get_theme_css()}`
- }}"""
-
- head, tail = themes[0], themes[1:]
- if_statement = f"""
- if (theme == "{str(head[0].version)}") {{
- var theme_css = `{head[1]._get_theme_css()}`
- }} {" ".join(make_else_if(t) for t in tail)}
- """
-
- latest_to_oldest = sorted([t[0] for t in themes], key=lambda asset: asset.version)[
- ::-1
- ]
- latest_to_oldest = [str(t.version) for t in latest_to_oldest]
-
- component = gr.Dropdown(
- choices=latest_to_oldest,
- value=latest_to_oldest[0],
- render=False,
- label="Select Version",
- ).style(container=False)
-
- return (
- component,
- f"""
- (theme) => {{
- if (!document.querySelector('.theme-css')) {{
- var theme_elem = document.createElement('style');
- theme_elem.classList.add('theme-css');
- document.head.appendChild(theme_elem);
- }} else {{
- var theme_elem = document.querySelector('.theme-css');
- }}
- {if_statement}
- theme_elem.innerHTML = theme_css;
- }}
- """,
- )
diff --git a/spaces/fornaxai/RNet/style.css b/spaces/fornaxai/RNet/style.css
deleted file mode 100644
index 114adf441e9032febb46bc056b2a8bb651075f0d..0000000000000000000000000000000000000000
--- a/spaces/fornaxai/RNet/style.css
+++ /dev/null
@@ -1,28 +0,0 @@
-body {
- padding: 2rem;
- font-family: -apple-system, BlinkMacSystemFont, "Arial", sans-serif;
-}
-
-h1 {
- font-size: 16px;
- margin-top: 0;
-}
-
-p {
- color: rgb(107, 114, 128);
- font-size: 15px;
- margin-bottom: 10px;
- margin-top: 5px;
-}
-
-.card {
- max-width: 620px;
- margin: 0 auto;
- padding: 16px;
- border: 1px solid lightgray;
- border-radius: 16px;
-}
-
-.card p:last-child {
- margin-bottom: 0;
-}
diff --git a/spaces/fuckyoudeki/AutoGPT/autogpt/speech/macos_tts.py b/spaces/fuckyoudeki/AutoGPT/autogpt/speech/macos_tts.py
deleted file mode 100644
index 4c072ce256782e83a578b5181abf1a7b524c621b..0000000000000000000000000000000000000000
--- a/spaces/fuckyoudeki/AutoGPT/autogpt/speech/macos_tts.py
+++ /dev/null
@@ -1,21 +0,0 @@
-""" MacOS TTS Voice. """
-import os
-
-from autogpt.speech.base import VoiceBase
-
-
-class MacOSTTS(VoiceBase):
- """MacOS TTS Voice."""
-
- def _setup(self) -> None:
- pass
-
- def _speech(self, text: str, voice_index: int = 0) -> bool:
- """Play the given text."""
- if voice_index == 0:
- os.system(f'say "{text}"')
- elif voice_index == 1:
- os.system(f'say -v "Ava (Premium)" "{text}"')
- else:
- os.system(f'say -v Samantha "{text}"')
- return True
diff --git a/spaces/glyszt/vt/vtoonify/model/raft/core/utils/frame_utils.py b/spaces/glyszt/vt/vtoonify/model/raft/core/utils/frame_utils.py
deleted file mode 100644
index 6c491135efaffc25bd61ec3ecde99d236f5deb12..0000000000000000000000000000000000000000
--- a/spaces/glyszt/vt/vtoonify/model/raft/core/utils/frame_utils.py
+++ /dev/null
@@ -1,137 +0,0 @@
-import numpy as np
-from PIL import Image
-from os.path import *
-import re
-
-import cv2
-cv2.setNumThreads(0)
-cv2.ocl.setUseOpenCL(False)
-
-TAG_CHAR = np.array([202021.25], np.float32)
-
-def readFlow(fn):
- """ Read .flo file in Middlebury format"""
- # Code adapted from:
- # http://stackoverflow.com/questions/28013200/reading-middlebury-flow-files-with-python-bytes-array-numpy
-
- # WARNING: this will work on little-endian architectures (eg Intel x86) only!
- # print 'fn = %s'%(fn)
- with open(fn, 'rb') as f:
- magic = np.fromfile(f, np.float32, count=1)
- if 202021.25 != magic:
- print('Magic number incorrect. Invalid .flo file')
- return None
- else:
- w = np.fromfile(f, np.int32, count=1)
- h = np.fromfile(f, np.int32, count=1)
- # print 'Reading %d x %d flo file\n' % (w, h)
- data = np.fromfile(f, np.float32, count=2*int(w)*int(h))
- # Reshape data into 3D array (columns, rows, bands)
- # The reshape here is for visualization, the original code is (w,h,2)
- return np.resize(data, (int(h), int(w), 2))
-
-def readPFM(file):
- file = open(file, 'rb')
-
- color = None
- width = None
- height = None
- scale = None
- endian = None
-
- header = file.readline().rstrip()
- if header == b'PF':
- color = True
- elif header == b'Pf':
- color = False
- else:
- raise Exception('Not a PFM file.')
-
- dim_match = re.match(rb'^(\d+)\s(\d+)\s$', file.readline())
- if dim_match:
- width, height = map(int, dim_match.groups())
- else:
- raise Exception('Malformed PFM header.')
-
- scale = float(file.readline().rstrip())
- if scale < 0: # little-endian
- endian = '<'
- scale = -scale
- else:
- endian = '>' # big-endian
-
- data = np.fromfile(file, endian + 'f')
- shape = (height, width, 3) if color else (height, width)
-
- data = np.reshape(data, shape)
- data = np.flipud(data)
- return data
-
-def writeFlow(filename,uv,v=None):
- """ Write optical flow to file.
-
- If v is None, uv is assumed to contain both u and v channels,
- stacked in depth.
- Original code by Deqing Sun, adapted from Daniel Scharstein.
- """
- nBands = 2
-
- if v is None:
- assert(uv.ndim == 3)
- assert(uv.shape[2] == 2)
- u = uv[:,:,0]
- v = uv[:,:,1]
- else:
- u = uv
-
- assert(u.shape == v.shape)
- height,width = u.shape
- f = open(filename,'wb')
- # write the header
- f.write(TAG_CHAR)
- np.array(width).astype(np.int32).tofile(f)
- np.array(height).astype(np.int32).tofile(f)
- # arrange into matrix form
- tmp = np.zeros((height, width*nBands))
- tmp[:,np.arange(width)*2] = u
- tmp[:,np.arange(width)*2 + 1] = v
- tmp.astype(np.float32).tofile(f)
- f.close()
-
-
-def readFlowKITTI(filename):
- flow = cv2.imread(filename, cv2.IMREAD_ANYDEPTH|cv2.IMREAD_COLOR)
- flow = flow[:,:,::-1].astype(np.float32)
- flow, valid = flow[:, :, :2], flow[:, :, 2]
- flow = (flow - 2**15) / 64.0
- return flow, valid
-
-def readDispKITTI(filename):
- disp = cv2.imread(filename, cv2.IMREAD_ANYDEPTH) / 256.0
- valid = disp > 0.0
- flow = np.stack([-disp, np.zeros_like(disp)], -1)
- return flow, valid
-
-
-def writeFlowKITTI(filename, uv):
- uv = 64.0 * uv + 2**15
- valid = np.ones([uv.shape[0], uv.shape[1], 1])
- uv = np.concatenate([uv, valid], axis=-1).astype(np.uint16)
- cv2.imwrite(filename, uv[..., ::-1])
-
-
-def read_gen(file_name, pil=False):
- ext = splitext(file_name)[-1]
- if ext == '.png' or ext == '.jpeg' or ext == '.ppm' or ext == '.jpg':
- return Image.open(file_name)
- elif ext == '.bin' or ext == '.raw':
- return np.load(file_name)
- elif ext == '.flo':
- return readFlow(file_name).astype(np.float32)
- elif ext == '.pfm':
- flow = readPFM(file_name).astype(np.float32)
- if len(flow.shape) == 2:
- return flow
- else:
- return flow[:, :, :-1]
- return []
\ No newline at end of file
diff --git a/spaces/gotiQspiryo/whisper-ui/examples/Boat TripestebarcoesunpeligroDVDRipSpanishwwwFanCluBTcom.md b/spaces/gotiQspiryo/whisper-ui/examples/Boat TripestebarcoesunpeligroDVDRipSpanishwwwFanCluBTcom.md
deleted file mode 100644
index 22c32bb981d3bdc0e753043dbce4f064848e2292..0000000000000000000000000000000000000000
--- a/spaces/gotiQspiryo/whisper-ui/examples/Boat TripestebarcoesunpeligroDVDRipSpanishwwwFanCluBTcom.md
+++ /dev/null
@@ -1,6 +0,0 @@
-Boat TripestebarcoesunpeligroDVDRipSpanishwwwFanCluBTcom
Download File 🗹 https://urlgoal.com/2uyMxY
-
- aaccfb2cb3
-
-
-
diff --git a/spaces/gpt3/travel/README.md b/spaces/gpt3/travel/README.md
deleted file mode 100644
index d55434ca4a4dc5dd9a2ae478d83c070c26bc70c3..0000000000000000000000000000000000000000
--- a/spaces/gpt3/travel/README.md
+++ /dev/null
@@ -1,12 +0,0 @@
----
-title: Travel
-emoji: 📊
-colorFrom: indigo
-colorTo: green
-sdk: streamlit
-sdk_version: 1.15.2
-app_file: app.py
-pinned: false
----
-
-Check out the configuration reference at https://huggingface.co/docs/hub/spaces-config-reference
diff --git a/spaces/gradio/HuBERT/examples/criss/sentence_retrieval/sentence_retrieval_tatoeba.sh b/spaces/gradio/HuBERT/examples/criss/sentence_retrieval/sentence_retrieval_tatoeba.sh
deleted file mode 100644
index 0428d8bef9d426ac3e664cd281ce0b688f5f580f..0000000000000000000000000000000000000000
--- a/spaces/gradio/HuBERT/examples/criss/sentence_retrieval/sentence_retrieval_tatoeba.sh
+++ /dev/null
@@ -1,59 +0,0 @@
-#!/bin/bash
-# Copyright (c) Facebook, Inc. and its affiliates.
-# All rights reserved.
-#
-# This source code is licensed under the license found in the
-# LICENSE file in the root directory of this source tree.
-#
-source_lang=kk_KZ
-target_lang=en_XX
-MODEL=criss_checkpoints/criss.3rd.pt
-SPM=criss_checkpoints/sentence.bpe.model
-SPLIT=test
-LANG_DICT=criss_checkpoints/lang_dict.txt
-ENCODER_ANALYSIS=sentence_retrieval/encoder_analysis.py
-SAVE_ENCODER=save_encoder.py
-ENCODER_SAVE_ROOT=sentence_embeddings/$MODEL
-
-
-
-DATA_DIR=data_tmp
-INPUT_DIR=$DATA_DIR/${source_lang}-${target_lang}-tatoeba
-ENCODER_SAVE_DIR=${ENCODER_SAVE_ROOT}/${source_lang}-${target_lang}
-mkdir -p $ENCODER_SAVE_DIR/${target_lang}
-mkdir -p $ENCODER_SAVE_DIR/${source_lang}
-
-# Save encoder outputs for source sentences
-python $SAVE_ENCODER \
- ${INPUT_DIR} \
- --path ${MODEL} \
- --task translation_multi_simple_epoch \
- --lang-dict ${LANG_DICT} \
- --gen-subset ${SPLIT} \
- --bpe 'sentencepiece' \
- --lang-pairs ${source_lang}-${target_lang} \
- -s ${source_lang} -t ${target_lang} \
- --sentencepiece-model ${SPM} \
- --remove-bpe 'sentencepiece' \
- --beam 1 \
- --lang-tok-style mbart \
- --encoder-save-dir ${ENCODER_SAVE_DIR}/${source_lang}
-
-# Save encoder outputs for target sentences
-python $SAVE_ENCODER \
- ${INPUT_DIR} \
- --path ${MODEL} \
- --lang-dict ${LANG_DICT} \
- --task translation_multi_simple_epoch \
- --gen-subset ${SPLIT} \
- --bpe 'sentencepiece' \
- --lang-pairs ${target_lang}-${source_lang} \
- -t ${source_lang} -s ${target_lang} \
- --sentencepiece-model ${SPM} \
- --remove-bpe 'sentencepiece' \
- --beam 1 \
- --lang-tok-style mbart \
- --encoder-save-dir ${ENCODER_SAVE_DIR}/${target_lang}
-
-# Analyze sentence retrieval accuracy
-python $ENCODER_ANALYSIS --langs "${source_lang},${target_lang}" ${ENCODER_SAVE_DIR}
diff --git a/spaces/gradio/HuBERT/fairseq/data/noising.py b/spaces/gradio/HuBERT/fairseq/data/noising.py
deleted file mode 100644
index 2b1cc347203bfbdc9f1cba29e2e36427b7b5be57..0000000000000000000000000000000000000000
--- a/spaces/gradio/HuBERT/fairseq/data/noising.py
+++ /dev/null
@@ -1,335 +0,0 @@
-# Copyright (c) Facebook, Inc. and its affiliates.
-#
-# This source code is licensed under the MIT license found in the
-# LICENSE file in the root directory of this source tree.
-
-import numpy as np
-import torch
-from fairseq.data import data_utils
-
-
-class WordNoising(object):
- """Generate a noisy version of a sentence, without changing words themselves."""
-
- def __init__(self, dictionary, bpe_cont_marker="@@", bpe_end_marker=None):
- self.dictionary = dictionary
- self.bpe_end = None
- if bpe_cont_marker:
- self.bpe_end = np.array(
- [
- not self.dictionary[i].endswith(bpe_cont_marker)
- for i in range(len(self.dictionary))
- ]
- )
- elif bpe_end_marker:
- self.bpe_end = np.array(
- [
- self.dictionary[i].endswith(bpe_end_marker)
- for i in range(len(self.dictionary))
- ]
- )
-
- self.get_word_idx = (
- self._get_bpe_word_idx if self.bpe_end is not None else self._get_token_idx
- )
-
- def noising(self, x, lengths, noising_prob=0.0):
- raise NotImplementedError()
-
- def _get_bpe_word_idx(self, x):
- """
- Given a list of BPE tokens, for every index in the tokens list,
- return the index of the word grouping that it belongs to.
- For example, for input x corresponding to ["how", "are", "y@@", "ou"],
- return [[0], [1], [2], [2]].
- """
- # x: (T x B)
- bpe_end = self.bpe_end[x]
-
- if x.size(0) == 1 and x.size(1) == 1:
- # Special case when we only have one word in x. If x = [[N]],
- # bpe_end is a scalar (bool) instead of a 2-dim array of bools,
- # which makes the sum operation below fail.
- return np.array([[0]])
-
- # do a reduce front sum to generate word ids
- word_idx = bpe_end[::-1].cumsum(0)[::-1]
- word_idx = word_idx.max(0)[None, :] - word_idx
- return word_idx
-
- def _get_token_idx(self, x):
- """
- This is to extend noising functions to be able to apply to non-bpe
- tokens, e.g. word or characters.
- """
- x = torch.t(x)
- word_idx = np.array([range(len(x_i)) for x_i in x])
- return np.transpose(word_idx)
-
-
-class WordDropout(WordNoising):
- """Randomly drop input words. If not passing blank_idx (default is None),
- then dropped words will be removed. Otherwise, it will be replaced by the
- blank_idx."""
-
- def __init__(
- self,
- dictionary,
- default_dropout_prob=0.1,
- bpe_cont_marker="@@",
- bpe_end_marker=None,
- ):
- super().__init__(dictionary, bpe_cont_marker, bpe_end_marker)
- self.default_dropout_prob = default_dropout_prob
-
- def noising(self, x, lengths, dropout_prob=None, blank_idx=None):
- if dropout_prob is None:
- dropout_prob = self.default_dropout_prob
- # x: (T x B), lengths: B
- if dropout_prob == 0:
- return x, lengths
-
- assert 0 < dropout_prob < 1
-
- # be sure to drop entire words
- word_idx = self.get_word_idx(x)
- sentences = []
- modified_lengths = []
- for i in range(lengths.size(0)):
- # Since dropout probabilities need to apply over non-pad tokens,
- # it is not trivial to generate the keep mask without consider
- # input lengths; otherwise, this could be done outside the loop
-
- # We want to drop whole words based on word_idx grouping
- num_words = max(word_idx[:, i]) + 1
-
- # ith example: [x0, x1, ..., eos, pad, ..., pad]
- # We should only generate keep probs for non-EOS tokens. Thus if the
- # input sentence ends in EOS, the last word idx is not included in
- # the dropout mask generation and we append True to always keep EOS.
- # Otherwise, just generate the dropout mask for all word idx
- # positions.
- has_eos = x[lengths[i] - 1, i] == self.dictionary.eos()
- if has_eos: # has eos?
- keep = np.random.rand(num_words - 1) >= dropout_prob
- keep = np.append(keep, [True]) # keep EOS symbol
- else:
- keep = np.random.rand(num_words) >= dropout_prob
-
- words = x[: lengths[i], i].tolist()
-
- # TODO: speed up the following loop
- # drop words from the input according to keep
- new_s = [
- w if keep[word_idx[j, i]] else blank_idx for j, w in enumerate(words)
- ]
- new_s = [w for w in new_s if w is not None]
- # we need to have at least one word in the sentence (more than the
- # start / end sentence symbols)
- if len(new_s) <= 1:
- # insert at beginning in case the only token left is EOS
- # EOS should be at end of list.
- new_s.insert(0, words[np.random.randint(0, len(words))])
- assert len(new_s) >= 1 and (
- not has_eos # Either don't have EOS at end or last token is EOS
- or (len(new_s) >= 2 and new_s[-1] == self.dictionary.eos())
- ), "New sentence is invalid."
- sentences.append(new_s)
- modified_lengths.append(len(new_s))
- # re-construct input
- modified_lengths = torch.LongTensor(modified_lengths)
- modified_x = torch.LongTensor(
- modified_lengths.max(), modified_lengths.size(0)
- ).fill_(self.dictionary.pad())
- for i in range(modified_lengths.size(0)):
- modified_x[: modified_lengths[i], i].copy_(torch.LongTensor(sentences[i]))
-
- return modified_x, modified_lengths
-
-
-class WordShuffle(WordNoising):
- """Shuffle words by no more than k positions."""
-
- def __init__(
- self,
- dictionary,
- default_max_shuffle_distance=3,
- bpe_cont_marker="@@",
- bpe_end_marker=None,
- ):
- super().__init__(dictionary, bpe_cont_marker, bpe_end_marker)
- self.default_max_shuffle_distance = 3
-
- def noising(self, x, lengths, max_shuffle_distance=None):
- if max_shuffle_distance is None:
- max_shuffle_distance = self.default_max_shuffle_distance
- # x: (T x B), lengths: B
- if max_shuffle_distance == 0:
- return x, lengths
-
- # max_shuffle_distance < 1 will return the same sequence
- assert max_shuffle_distance > 1
-
- # define noise word scores
- noise = np.random.uniform(
- 0,
- max_shuffle_distance,
- size=(x.size(0), x.size(1)),
- )
- noise[0] = -1 # do not move start sentence symbol
- # be sure to shuffle entire words
- word_idx = self.get_word_idx(x)
- x2 = x.clone()
- for i in range(lengths.size(0)):
- length_no_eos = lengths[i]
- if x[lengths[i] - 1, i] == self.dictionary.eos():
- length_no_eos = lengths[i] - 1
- # generate a random permutation
- scores = word_idx[:length_no_eos, i] + noise[word_idx[:length_no_eos, i], i]
- # ensure no reordering inside a word
- scores += 1e-6 * np.arange(length_no_eos.item())
- permutation = scores.argsort()
- # shuffle words
- x2[:length_no_eos, i].copy_(
- x2[:length_no_eos, i][torch.from_numpy(permutation)]
- )
- return x2, lengths
-
-
-class UnsupervisedMTNoising(WordNoising):
- """
- Implements the default configuration for noising in UnsupervisedMT
- (github.com/facebookresearch/UnsupervisedMT)
- """
-
- def __init__(
- self,
- dictionary,
- max_word_shuffle_distance,
- word_dropout_prob,
- word_blanking_prob,
- bpe_cont_marker="@@",
- bpe_end_marker=None,
- ):
- super().__init__(dictionary)
- self.max_word_shuffle_distance = max_word_shuffle_distance
- self.word_dropout_prob = word_dropout_prob
- self.word_blanking_prob = word_blanking_prob
-
- self.word_dropout = WordDropout(
- dictionary=dictionary,
- bpe_cont_marker=bpe_cont_marker,
- bpe_end_marker=bpe_end_marker,
- )
- self.word_shuffle = WordShuffle(
- dictionary=dictionary,
- bpe_cont_marker=bpe_cont_marker,
- bpe_end_marker=bpe_end_marker,
- )
-
- def noising(self, x, lengths):
- # 1. Word Shuffle
- noisy_src_tokens, noisy_src_lengths = self.word_shuffle.noising(
- x=x,
- lengths=lengths,
- max_shuffle_distance=self.max_word_shuffle_distance,
- )
- # 2. Word Dropout
- noisy_src_tokens, noisy_src_lengths = self.word_dropout.noising(
- x=noisy_src_tokens,
- lengths=noisy_src_lengths,
- dropout_prob=self.word_dropout_prob,
- )
- # 3. Word Blanking
- noisy_src_tokens, noisy_src_lengths = self.word_dropout.noising(
- x=noisy_src_tokens,
- lengths=noisy_src_lengths,
- dropout_prob=self.word_blanking_prob,
- blank_idx=self.dictionary.unk(),
- )
-
- return noisy_src_tokens
-
-
-class NoisingDataset(torch.utils.data.Dataset):
- def __init__(
- self,
- src_dataset,
- src_dict,
- seed,
- noiser=None,
- noising_class=UnsupervisedMTNoising,
- **kwargs
- ):
- """
- Wrap a :class:`~torch.utils.data.Dataset` and apply noise to the
- samples based on the supplied noising configuration.
-
- Args:
- src_dataset (~torch.utils.data.Dataset): dataset to wrap.
- to build self.src_dataset --
- a LanguagePairDataset with src dataset as the source dataset and
- None as the target dataset. Should NOT have padding so that
- src_lengths are accurately calculated by language_pair_dataset
- collate function.
- We use language_pair_dataset here to encapsulate the tgt_dataset
- so we can re-use the LanguagePairDataset collater to format the
- batches in the structure that SequenceGenerator expects.
- src_dict (~fairseq.data.Dictionary): source dictionary
- seed (int): seed to use when generating random noise
- noiser (WordNoising): a pre-initialized :class:`WordNoising`
- instance. If this is None, a new instance will be created using
- *noising_class* and *kwargs*.
- noising_class (class, optional): class to use to initialize a
- default :class:`WordNoising` instance.
- kwargs (dict, optional): arguments to initialize the default
- :class:`WordNoising` instance given by *noiser*.
- """
- self.src_dataset = src_dataset
- self.src_dict = src_dict
- self.seed = seed
- self.noiser = (
- noiser
- if noiser is not None
- else noising_class(
- dictionary=src_dict,
- **kwargs,
- )
- )
- self.sizes = src_dataset.sizes
-
-
- def __getitem__(self, index):
- """
- Returns a single noisy sample. Multiple samples are fed to the collater
- create a noising dataset batch.
- """
- src_tokens = self.src_dataset[index]
- src_lengths = torch.LongTensor([len(src_tokens)])
- src_tokens = src_tokens.unsqueeze(0)
-
- # Transpose src tokens to fit expected shape of x in noising function
- # (batch size, sequence length) -> (sequence length, batch size)
- src_tokens_t = torch.t(src_tokens)
-
- with data_utils.numpy_seed(self.seed + index):
- noisy_src_tokens = self.noiser.noising(src_tokens_t, src_lengths)
-
- # Transpose back to expected src_tokens format
- # (sequence length, 1) -> (1, sequence length)
- noisy_src_tokens = torch.t(noisy_src_tokens)
- return noisy_src_tokens[0]
-
- def __len__(self):
- """
- The length of the noising dataset is the length of src.
- """
- return len(self.src_dataset)
-
- @property
- def supports_prefetch(self):
- return self.src_dataset.supports_prefetch
-
- def prefetch(self, indices):
- if self.src_dataset.supports_prefetch:
- self.src_dataset.prefetch(indices)
diff --git a/spaces/gradio/examples_component_main/README.md b/spaces/gradio/examples_component_main/README.md
deleted file mode 100644
index aa6b38ff0f6f5296b181b1bb3f61b40fe0acfc91..0000000000000000000000000000000000000000
--- a/spaces/gradio/examples_component_main/README.md
+++ /dev/null
@@ -1,12 +0,0 @@
-
----
-title: examples_component_main
-emoji: 🔥
-colorFrom: indigo
-colorTo: indigo
-sdk: gradio
-sdk_version: 4.1.2
-app_file: run.py
-pinned: false
-hf_oauth: true
----
diff --git a/spaces/gurgenblbulyan/video-based-text-generation/inference.py b/spaces/gurgenblbulyan/video-based-text-generation/inference.py
deleted file mode 100644
index e15c9510861a3577c4e84d3cd46e53980a228e8d..0000000000000000000000000000000000000000
--- a/spaces/gurgenblbulyan/video-based-text-generation/inference.py
+++ /dev/null
@@ -1,30 +0,0 @@
-import torch
-from transformers import AutoTokenizer, VisionEncoderDecoderModel
-
-import utils
-
-class Inference:
- def __init__(self, decoder_model_name, max_length=50):
- self.device = torch.device('cuda' if torch.cuda.is_available() else 'cpu')
-
- self.tokenizer = AutoTokenizer.from_pretrained(decoder_model_name)
- self.encoder_decoder_model = VisionEncoderDecoderModel.from_pretrained('armgabrielyan/video-summarization')
- self.encoder_decoder_model.to(self.device)
-
- self.max_length = max_length
-
- def generate_text(self, video, encoder_model_name):
- if isinstance(video, str):
- pixel_values = utils.video2image_from_path(video, encoder_model_name)
- else:
- pixel_values = video
-
- if not self.tokenizer.pad_token:
- self.tokenizer.add_special_tokens({'pad_token': '[PAD]'})
- self.encoder_decoder_model.decoder.resize_token_embeddings(len(self.tokenizer))
-
- generated_ids = self.encoder_decoder_model.generate(pixel_values.unsqueeze(0).to(self.device),early_stopping=True, max_length=self.max_length,num_beams=4,
- no_repeat_ngram_size=2 )
- generated_text = self.tokenizer.batch_decode(generated_ids, skip_special_tokens=True)[0]
-
- return generated_text
diff --git a/spaces/gwang-kim/DATID-3D/pose_estimation/util/load_mats.py b/spaces/gwang-kim/DATID-3D/pose_estimation/util/load_mats.py
deleted file mode 100644
index 5b1f4a73c83035c6313969631eb2ff2b2322df7e..0000000000000000000000000000000000000000
--- a/spaces/gwang-kim/DATID-3D/pose_estimation/util/load_mats.py
+++ /dev/null
@@ -1,117 +0,0 @@
-"""This script is to load 3D face model for Deep3DFaceRecon_pytorch
-"""
-
-import numpy as np
-from PIL import Image
-from scipy.io import loadmat, savemat
-from array import array
-import os.path as osp
-
-# load expression basis
-def LoadExpBasis(bfm_folder='BFM'):
- n_vertex = 53215
- Expbin = open(osp.join(bfm_folder, 'Exp_Pca.bin'), 'rb')
- exp_dim = array('i')
- exp_dim.fromfile(Expbin, 1)
- expMU = array('f')
- expPC = array('f')
- expMU.fromfile(Expbin, 3*n_vertex)
- expPC.fromfile(Expbin, 3*exp_dim[0]*n_vertex)
- Expbin.close()
-
- expPC = np.array(expPC)
- expPC = np.reshape(expPC, [exp_dim[0], -1])
- expPC = np.transpose(expPC)
-
- expEV = np.loadtxt(osp.join(bfm_folder, 'std_exp.txt'))
-
- return expPC, expEV
-
-
-# transfer original BFM09 to our face model
-def transferBFM09(bfm_folder='BFM'):
- print('Transfer BFM09 to BFM_model_front......')
- original_BFM = loadmat(osp.join(bfm_folder, '01_MorphableModel.mat'))
- shapePC = original_BFM['shapePC'] # shape basis
- shapeEV = original_BFM['shapeEV'] # corresponding eigen value
- shapeMU = original_BFM['shapeMU'] # mean face
- texPC = original_BFM['texPC'] # texture basis
- texEV = original_BFM['texEV'] # eigen value
- texMU = original_BFM['texMU'] # mean texture
-
- expPC, expEV = LoadExpBasis()
-
- # transfer BFM09 to our face model
-
- idBase = shapePC*np.reshape(shapeEV, [-1, 199])
- idBase = idBase/1e5 # unify the scale to decimeter
- idBase = idBase[:, :80] # use only first 80 basis
-
- exBase = expPC*np.reshape(expEV, [-1, 79])
- exBase = exBase/1e5 # unify the scale to decimeter
- exBase = exBase[:, :64] # use only first 64 basis
-
- texBase = texPC*np.reshape(texEV, [-1, 199])
- texBase = texBase[:, :80] # use only first 80 basis
-
- # our face model is cropped along face landmarks and contains only 35709 vertex.
- # original BFM09 contains 53490 vertex, and expression basis provided by Guo et al. contains 53215 vertex.
- # thus we select corresponding vertex to get our face model.
-
- index_exp = loadmat(osp.join(bfm_folder, 'BFM_front_idx.mat'))
- index_exp = index_exp['idx'].astype(np.int32) - 1 # starts from 0 (to 53215)
-
- index_shape = loadmat(osp.join(bfm_folder, 'BFM_exp_idx.mat'))
- index_shape = index_shape['trimIndex'].astype(
- np.int32) - 1 # starts from 0 (to 53490)
- index_shape = index_shape[index_exp]
-
- idBase = np.reshape(idBase, [-1, 3, 80])
- idBase = idBase[index_shape, :, :]
- idBase = np.reshape(idBase, [-1, 80])
-
- texBase = np.reshape(texBase, [-1, 3, 80])
- texBase = texBase[index_shape, :, :]
- texBase = np.reshape(texBase, [-1, 80])
-
- exBase = np.reshape(exBase, [-1, 3, 64])
- exBase = exBase[index_exp, :, :]
- exBase = np.reshape(exBase, [-1, 64])
-
- meanshape = np.reshape(shapeMU, [-1, 3])/1e5
- meanshape = meanshape[index_shape, :]
- meanshape = np.reshape(meanshape, [1, -1])
-
- meantex = np.reshape(texMU, [-1, 3])
- meantex = meantex[index_shape, :]
- meantex = np.reshape(meantex, [1, -1])
-
- # other info contains triangles, region used for computing photometric loss,
- # region used for skin texture regularization, and 68 landmarks index etc.
- other_info = loadmat(osp.join(bfm_folder, 'facemodel_info.mat'))
- frontmask2_idx = other_info['frontmask2_idx']
- skinmask = other_info['skinmask']
- keypoints = other_info['keypoints']
- point_buf = other_info['point_buf']
- tri = other_info['tri']
- tri_mask2 = other_info['tri_mask2']
-
- # save our face model
- savemat(osp.join(bfm_folder, 'BFM_model_front.mat'), {'meanshape': meanshape, 'meantex': meantex, 'idBase': idBase, 'exBase': exBase, 'texBase': texBase,
- 'tri': tri, 'point_buf': point_buf, 'tri_mask2': tri_mask2, 'keypoints': keypoints, 'frontmask2_idx': frontmask2_idx, 'skinmask': skinmask})
-
-
-# load landmarks for standard face, which is used for image preprocessing
-def load_lm3d(bfm_folder):
-
- Lm3D = loadmat(osp.join(bfm_folder, 'similarity_Lm3D_all.mat'))
- Lm3D = Lm3D['lm']
-
- # calculate 5 facial landmarks using 68 landmarks
- lm_idx = np.array([31, 37, 40, 43, 46, 49, 55]) - 1
- Lm3D = np.stack([Lm3D[lm_idx[0], :], np.mean(Lm3D[lm_idx[[1, 2]], :], 0), np.mean(
- Lm3D[lm_idx[[3, 4]], :], 0), Lm3D[lm_idx[5], :], Lm3D[lm_idx[6], :]], axis=0)
- Lm3D = Lm3D[[1, 2, 0, 3, 4], :]
-
- return Lm3D
-
diff --git a/spaces/hands012/gpt-academic/README.md b/spaces/hands012/gpt-academic/README.md
deleted file mode 100644
index 449f6da36139b85721a650204375592f102b5c03..0000000000000000000000000000000000000000
--- a/spaces/hands012/gpt-academic/README.md
+++ /dev/null
@@ -1,343 +0,0 @@
----
-title: ChatImprovement
-emoji: 😻
-colorFrom: blue
-colorTo: blue
-sdk: gradio
-sdk_version: 3.32.0
-app_file: app.py
-pinned: false
-duplicated_from: qingxu98/gpt-academic
----
-
-# ChatGPT 学术优化
-> **Note**
->
-> 5月27日对gradio依赖进行了较大的修复和调整,fork并解决了官方Gradio的一系列bug。但如果27日当天进行了更新,可能会导致代码报错(依赖缺失,卡在loading界面等),请及时更新到**最新版代码**并重新安装pip依赖即可。若给您带来困扰还请谅解。安装依赖时,请严格选择requirements.txt中**指定的版本**:
->
-> `pip install -r requirements.txt -i https://pypi.org/simple`
->
-
-#
GPT 学术优化 (GPT Academic)
-
-**如果喜欢这个项目,请给它一个Star;如果你发明了更好用的快捷键或函数插件,欢迎发pull requests**
-
-If you like this project, please give it a Star. If you've come up with more useful academic shortcuts or functional plugins, feel free to open an issue or pull request. We also have a README in [English|](docs/README_EN.md)[日本語|](docs/README_JP.md)[한국어|](https://github.com/mldljyh/ko_gpt_academic)[Русский|](docs/README_RS.md)[Français](docs/README_FR.md) translated by this project itself.
-To translate this project to arbitary language with GPT, read and run [`multi_language.py`](multi_language.py) (experimental).
-
-> **Note**
->
-> 1.请注意只有**红颜色**标识的函数插件(按钮)才支持读取文件,部分插件位于插件区的**下拉菜单**中。另外我们以**最高优先级**欢迎和处理任何新插件的PR!
->
-> 2.本项目中每个文件的功能都在自译解[`self_analysis.md`](https://github.com/binary-husky/chatgpt_academic/wiki/chatgpt-academic%E9%A1%B9%E7%9B%AE%E8%87%AA%E8%AF%91%E8%A7%A3%E6%8A%A5%E5%91%8A)详细说明。随着版本的迭代,您也可以随时自行点击相关函数插件,调用GPT重新生成项目的自我解析报告。常见问题汇总在[`wiki`](https://github.com/binary-husky/chatgpt_academic/wiki/%E5%B8%B8%E8%A7%81%E9%97%AE%E9%A2%98)当中。[安装方法](#installation)。
->
-> 3.本项目兼容并鼓励尝试国产大语言模型chatglm和RWKV, 盘古等等。支持多个api-key共存,可在配置文件中填写如`API_KEY="openai-key1,openai-key2,api2d-key3"`。需要临时更换`API_KEY`时,在输入区输入临时的`API_KEY`然后回车键提交后即可生效。
-
-
-
-
-
-
-功能 | 描述
---- | ---
-一键润色 | 支持一键润色、一键查找论文语法错误
-一键中英互译 | 一键中英互译
-一键代码解释 | 显示代码、解释代码、生成代码、给代码加注释
-[自定义快捷键](https://www.bilibili.com/video/BV14s4y1E7jN) | 支持自定义快捷键
-模块化设计 | 支持自定义强大的[函数插件](https://github.com/binary-husky/chatgpt_academic/tree/master/crazy_functions),插件支持[热更新](https://github.com/binary-husky/chatgpt_academic/wiki/%E5%87%BD%E6%95%B0%E6%8F%92%E4%BB%B6%E6%8C%87%E5%8D%97)
-[自我程序剖析](https://www.bilibili.com/video/BV1cj411A7VW) | [函数插件] [一键读懂](https://github.com/binary-husky/chatgpt_academic/wiki/chatgpt-academic%E9%A1%B9%E7%9B%AE%E8%87%AA%E8%AF%91%E8%A7%A3%E6%8A%A5%E5%91%8A)本项目的源代码
-[程序剖析](https://www.bilibili.com/video/BV1cj411A7VW) | [函数插件] 一键可以剖析其他Python/C/C++/Java/Lua/...项目树
-读论文、[翻译](https://www.bilibili.com/video/BV1KT411x7Wn)论文 | [函数插件] 一键解读latex/pdf论文全文并生成摘要
-Latex全文[翻译](https://www.bilibili.com/video/BV1nk4y1Y7Js/)、[润色](https://www.bilibili.com/video/BV1FT411H7c5/) | [函数插件] 一键翻译或润色latex论文
-批量注释生成 | [函数插件] 一键批量生成函数注释
-Markdown[中英互译](https://www.bilibili.com/video/BV1yo4y157jV/) | [函数插件] 看到上面5种语言的[README](https://github.com/binary-husky/chatgpt_academic/blob/master/docs/README_EN.md)了吗?
-chat分析报告生成 | [函数插件] 运行后自动生成总结汇报
-[PDF论文全文翻译功能](https://www.bilibili.com/video/BV1KT411x7Wn) | [函数插件] PDF论文提取题目&摘要+翻译全文(多线程)
-[Arxiv小助手](https://www.bilibili.com/video/BV1LM4y1279X) | [函数插件] 输入arxiv文章url即可一键翻译摘要+下载PDF
-[谷歌学术统合小助手](https://www.bilibili.com/video/BV19L411U7ia) | [函数插件] 给定任意谷歌学术搜索页面URL,让gpt帮你[写relatedworks](https://www.bilibili.com/video/BV1GP411U7Az/)
-互联网信息聚合+GPT | [函数插件] 一键[让GPT先从互联网获取信息](https://www.bilibili.com/video/BV1om4y127ck),再回答问题,让信息永不过时
-公式/图片/表格显示 | 可以同时显示公式的[tex形式和渲染形式](https://user-images.githubusercontent.com/96192199/230598842-1d7fcddd-815d-40ee-af60-baf488a199df.png),支持公式、代码高亮
-多线程函数插件支持 | 支持多线调用chatgpt,一键处理[海量文本](https://www.bilibili.com/video/BV1FT411H7c5/)或程序
-启动暗色gradio[主题](https://github.com/binary-husky/chatgpt_academic/issues/173) | 在浏览器url后面添加```/?__theme=dark```可以切换dark主题
-[多LLM模型](https://www.bilibili.com/video/BV1wT411p7yf)支持,[API2D](https://api2d.com/)接口支持 | 同时被GPT3.5、GPT4、[清华ChatGLM](https://github.com/THUDM/ChatGLM-6B)、[复旦MOSS](https://github.com/OpenLMLab/MOSS)同时伺候的感觉一定会很不错吧?
-更多LLM模型接入,支持[huggingface部署](https://huggingface.co/spaces/qingxu98/gpt-academic) | 加入Newbing接口(新必应),引入清华[Jittorllms](https://github.com/Jittor/JittorLLMs)支持[LLaMA](https://github.com/facebookresearch/llama),[RWKV](https://github.com/BlinkDL/ChatRWKV)和[盘古α](https://openi.org.cn/pangu/)
-更多新功能展示(图像生成等) …… | 见本文档结尾处 ……
-
-
-
-
-- 新界面(修改`config.py`中的LAYOUT选项即可实现“左右布局”和“上下布局”的切换)
-
-
-
-
-
-- 所有按钮都通过读取functional.py动态生成,可随意加自定义功能,解放粘贴板
-
-
-
-
-- 润色/纠错
-
-
-
-
-- 如果输出包含公式,会同时以tex形式和渲染形式显示,方便复制和阅读
-
-
-
-
-- 懒得看项目代码?整个工程直接给chatgpt炫嘴里
-
-
-
-
-- 多种大语言模型混合调用(ChatGLM + OpenAI-GPT3.5 + [API2D](https://api2d.com/)-GPT4)
-
-
-
-
----
-# Installation
-## 安装-方法1:直接运行 (Windows, Linux or MacOS)
-
-1. 下载项目
-```sh
-git clone https://github.com/binary-husky/chatgpt_academic.git
-cd chatgpt_academic
-```
-
-2. 配置API_KEY
-
-在`config.py`中,配置API KEY等设置,[特殊网络环境设置](https://github.com/binary-husky/gpt_academic/issues/1) 。
-
-(P.S. 程序运行时会优先检查是否存在名为`config_private.py`的私密配置文件,并用其中的配置覆盖`config.py`的同名配置。因此,如果您能理解我们的配置读取逻辑,我们强烈建议您在`config.py`旁边创建一个名为`config_private.py`的新配置文件,并把`config.py`中的配置转移(复制)到`config_private.py`中。`config_private.py`不受git管控,可以让您的隐私信息更加安全。P.S.项目同样支持通过`环境变量`配置大多数选项,环境变量的书写格式参考`docker-compose`文件。读取优先级: `环境变量` > `config_private.py` > `config.py`)
-
-
-3. 安装依赖
-```sh
-# (选择I: 如熟悉python)(python版本3.9以上,越新越好),备注:使用官方pip源或者阿里pip源,临时换源方法:python -m pip install -r requirements.txt -i https://mirrors.aliyun.com/pypi/simple/
-python -m pip install -r requirements.txt
-
-# (选择II: 如不熟悉python)使用anaconda,步骤也是类似的 (https://www.bilibili.com/video/BV1rc411W7Dr):
-conda create -n gptac_venv python=3.11 # 创建anaconda环境
-conda activate gptac_venv # 激活anaconda环境
-python -m pip install -r requirements.txt # 这个步骤和pip安装一样的步骤
-```
-
-如果需要支持清华ChatGLM/复旦MOSS作为后端,请点击展开此处
-
-
-【可选步骤】如果需要支持清华ChatGLM/复旦MOSS作为后端,需要额外安装更多依赖(前提条件:熟悉Python + 用过Pytorch + 电脑配置够强):
-```sh
-# 【可选步骤I】支持清华ChatGLM。清华ChatGLM备注:如果遇到"Call ChatGLM fail 不能正常加载ChatGLM的参数" 错误,参考如下: 1:以上默认安装的为torch+cpu版,使用cuda需要卸载torch重新安装torch+cuda; 2:如因本机配置不够无法加载模型,可以修改request_llm/bridge_chatglm.py中的模型精度, 将 AutoTokenizer.from_pretrained("THUDM/chatglm-6b", trust_remote_code=True) 都修改为 AutoTokenizer.from_pretrained("THUDM/chatglm-6b-int4", trust_remote_code=True)
-python -m pip install -r request_llm/requirements_chatglm.txt
-
-# 【可选步骤II】支持复旦MOSS
-python -m pip install -r request_llm/requirements_moss.txt
-git clone https://github.com/OpenLMLab/MOSS.git request_llm/moss # 注意执行此行代码时,必须处于项目根路径
-
-# 【可选步骤III】确保config.py配置文件的AVAIL_LLM_MODELS包含了期望的模型,目前支持的全部模型如下(jittorllms系列目前仅支持docker方案):
-AVAIL_LLM_MODELS = ["gpt-3.5-turbo", "api2d-gpt-3.5-turbo", "gpt-4", "api2d-gpt-4", "chatglm", "newbing", "moss"] # + ["jittorllms_rwkv", "jittorllms_pangualpha", "jittorllms_llama"]
-```
-
-
-
-
-
-
-4. 运行
-```sh
-python main.py
-```
-
-5. 测试函数插件
-```
-- 测试函数插件模板函数(要求gpt回答历史上的今天发生了什么),您可以根据此函数为模板,实现更复杂的功能
- 点击 "[函数插件模板Demo] 历史上的今天"
-```
-
-## 安装-方法2:使用Docker
-
-1. 仅ChatGPT(推荐大多数人选择)
-
-``` sh
-git clone https://github.com/binary-husky/chatgpt_academic.git # 下载项目
-cd chatgpt_academic # 进入路径
-nano config.py # 用任意文本编辑器编辑config.py, 配置 “Proxy”, “API_KEY” 以及 “WEB_PORT” (例如50923) 等
-docker build -t gpt-academic . # 安装
-
-#(最后一步-选择1)在Linux环境下,用`--net=host`更方便快捷
-docker run --rm -it --net=host gpt-academic
-#(最后一步-选择2)在macOS/windows环境下,只能用-p选项将容器上的端口(例如50923)暴露给主机上的端口
-docker run --rm -it -e WEB_PORT=50923 -p 50923:50923 gpt-academic
-```
-
-2. ChatGPT + ChatGLM + MOSS(需要熟悉Docker)
-
-``` sh
-# 修改docker-compose.yml,删除方案1和方案3,保留方案2。修改docker-compose.yml中方案2的配置,参考其中注释即可
-docker-compose up
-```
-
-3. ChatGPT + LLAMA + 盘古 + RWKV(需要熟悉Docker)
-``` sh
-# 修改docker-compose.yml,删除方案1和方案2,保留方案3。修改docker-compose.yml中方案3的配置,参考其中注释即可
-docker-compose up
-```
-
-
-## 安装-方法3:其他部署姿势
-
-1. 如何使用反代URL/微软云AzureAPI
-按照`config.py`中的说明配置API_URL_REDIRECT即可。
-
-2. 远程云服务器部署(需要云服务器知识与经验)
-请访问[部署wiki-1](https://github.com/binary-husky/chatgpt_academic/wiki/%E4%BA%91%E6%9C%8D%E5%8A%A1%E5%99%A8%E8%BF%9C%E7%A8%8B%E9%83%A8%E7%BD%B2%E6%8C%87%E5%8D%97)
-
-3. 使用WSL2(Windows Subsystem for Linux 子系统)
-请访问[部署wiki-2](https://github.com/binary-husky/chatgpt_academic/wiki/%E4%BD%BF%E7%94%A8WSL2%EF%BC%88Windows-Subsystem-for-Linux-%E5%AD%90%E7%B3%BB%E7%BB%9F%EF%BC%89%E9%83%A8%E7%BD%B2)
-
-4. 如何在二级网址(如`http://localhost/subpath`)下运行
-请访问[FastAPI运行说明](docs/WithFastapi.md)
-
-5. 使用docker-compose运行
-请阅读docker-compose.yml后,按照其中的提示操作即可
----
-# Advanced Usage
-## 自定义新的便捷按钮 / 自定义函数插件
-
-1. 自定义新的便捷按钮(学术快捷键)
-任意文本编辑器打开`core_functional.py`,添加条目如下,然后重启程序即可。(如果按钮已经添加成功并可见,那么前缀、后缀都支持热修改,无需重启程序即可生效。)
-例如
-```
-"超级英译中": {
- # 前缀,会被加在你的输入之前。例如,用来描述你的要求,例如翻译、解释代码、润色等等
- "Prefix": "请翻译把下面一段内容成中文,然后用一个markdown表格逐一解释文中出现的专有名词:\n\n",
-
- # 后缀,会被加在你的输入之后。例如,配合前缀可以把你的输入内容用引号圈起来。
- "Suffix": "",
-},
-```
-
-
-
-
-2. 自定义函数插件
-
-编写强大的函数插件来执行任何你想得到的和想不到的任务。
-本项目的插件编写、调试难度很低,只要您具备一定的python基础知识,就可以仿照我们提供的模板实现自己的插件功能。
-详情请参考[函数插件指南](https://github.com/binary-husky/chatgpt_academic/wiki/%E5%87%BD%E6%95%B0%E6%8F%92%E4%BB%B6%E6%8C%87%E5%8D%97)。
-
----
-# Latest Update
-## 新功能动态
-
-1. 对话保存功能。在函数插件区调用 `保存当前的对话` 即可将当前对话保存为可读+可复原的html文件,
-另外在函数插件区(下拉菜单)调用 `载入对话历史存档` ,即可还原之前的会话。
-Tip:不指定文件直接点击 `载入对话历史存档` 可以查看历史html存档缓存,点击 `删除所有本地对话历史记录` 可以删除所有html存档缓存。
-
-
-
-
-
-
-2. 生成报告。大部分插件都会在执行结束后,生成工作报告
-
-
-
-
-
-
-3. 模块化功能设计,简单的接口却能支持强大的功能
-
-
-
-
-
-4. 这是一个能够“自我译解”的开源项目
-
-
-
-
-5. 译解其他开源项目,不在话下
-
-
-
-
-
-
-
-
-6. 装饰[live2d](https://github.com/fghrsh/live2d_demo)的小功能(默认关闭,需要修改`config.py`)
-
-
-
-
-7. 新增MOSS大语言模型支持
-
-
-
-
-8. OpenAI图像生成
-
-
-
-
-9. OpenAI音频解析与总结
-
-
-
-
-10. Latex全文校对纠错
-
-
-
-
-
-## 版本:
-- version 3.5(Todo): 使用自然语言调用本项目的所有函数插件(高优先级)
-- version 3.4(Todo): 完善chatglm本地大模型的多线支持
-- version 3.3: +互联网信息综合功能
-- version 3.2: 函数插件支持更多参数接口 (保存对话功能, 解读任意语言代码+同时询问任意的LLM组合)
-- version 3.1: 支持同时问询多个gpt模型!支持api2d,支持多个apikey负载均衡
-- version 3.0: 对chatglm和其他小型llm的支持
-- version 2.6: 重构了插件结构,提高了交互性,加入更多插件
-- version 2.5: 自更新,解决总结大工程源代码时文本过长、token溢出的问题
-- version 2.4: (1)新增PDF全文翻译功能; (2)新增输入区切换位置的功能; (3)新增垂直布局选项; (4)多线程函数插件优化。
-- version 2.3: 增强多线程交互性
-- version 2.2: 函数插件支持热重载
-- version 2.1: 可折叠式布局
-- version 2.0: 引入模块化函数插件
-- version 1.0: 基础功能
-
-gpt_academic开发者QQ群-2:610599535
-
-- 已知问题
- - 某些浏览器翻译插件干扰此软件前端的运行
- - 官方Gradio目前有很多兼容性Bug,请务必使用requirement.txt安装Gradio
-
-## 参考与学习
-
-```
-代码中参考了很多其他优秀项目中的设计,主要包括:
-
-# 项目1:清华ChatGLM-6B:
-https://github.com/THUDM/ChatGLM-6B
-
-# 项目2:清华JittorLLMs:
-https://github.com/Jittor/JittorLLMs
-
-# 项目3:Edge-GPT:
-https://github.com/acheong08/EdgeGPT
-
-# 项目4:ChuanhuChatGPT:
-https://github.com/GaiZhenbiao/ChuanhuChatGPT
-
-# 项目5:ChatPaper:
-https://github.com/kaixindelele/ChatPaper
-
-# 更多:
-https://github.com/gradio-app/gradio
-https://github.com/fghrsh/live2d_demo
-```
diff --git a/spaces/hasibzunair/fifa-tryon-demo/Self-Correction-Human-Parsing-for-ACGPN/mhp_extension/detectron2/tests/modeling/test_box2box_transform.py b/spaces/hasibzunair/fifa-tryon-demo/Self-Correction-Human-Parsing-for-ACGPN/mhp_extension/detectron2/tests/modeling/test_box2box_transform.py
deleted file mode 100644
index 9d124d79fc0e17f268f6b5b50fcb8f8dfad59368..0000000000000000000000000000000000000000
--- a/spaces/hasibzunair/fifa-tryon-demo/Self-Correction-Human-Parsing-for-ACGPN/mhp_extension/detectron2/tests/modeling/test_box2box_transform.py
+++ /dev/null
@@ -1,64 +0,0 @@
-# Copyright (c) Facebook, Inc. and its affiliates. All Rights Reserved
-import logging
-import unittest
-import torch
-
-from detectron2.modeling.box_regression import Box2BoxTransform, Box2BoxTransformRotated
-
-logger = logging.getLogger(__name__)
-
-
-def random_boxes(mean_box, stdev, N):
- return torch.rand(N, 4) * stdev + torch.tensor(mean_box, dtype=torch.float)
-
-
-class TestBox2BoxTransform(unittest.TestCase):
- def test_reconstruction(self):
- weights = (5, 5, 10, 10)
- b2b_tfm = Box2BoxTransform(weights=weights)
- src_boxes = random_boxes([10, 10, 20, 20], 1, 10)
- dst_boxes = random_boxes([10, 10, 20, 20], 1, 10)
-
- devices = [torch.device("cpu")]
- if torch.cuda.is_available():
- devices.append(torch.device("cuda"))
- for device in devices:
- src_boxes = src_boxes.to(device=device)
- dst_boxes = dst_boxes.to(device=device)
- deltas = b2b_tfm.get_deltas(src_boxes, dst_boxes)
- dst_boxes_reconstructed = b2b_tfm.apply_deltas(deltas, src_boxes)
- assert torch.allclose(dst_boxes, dst_boxes_reconstructed)
-
-
-def random_rotated_boxes(mean_box, std_length, std_angle, N):
- return torch.cat(
- [torch.rand(N, 4) * std_length, torch.rand(N, 1) * std_angle], dim=1
- ) + torch.tensor(mean_box, dtype=torch.float)
-
-
-class TestBox2BoxTransformRotated(unittest.TestCase):
- def test_reconstruction(self):
- weights = (5, 5, 10, 10, 1)
- b2b_transform = Box2BoxTransformRotated(weights=weights)
- src_boxes = random_rotated_boxes([10, 10, 20, 20, -30], 5, 60.0, 10)
- dst_boxes = random_rotated_boxes([10, 10, 20, 20, -30], 5, 60.0, 10)
-
- devices = [torch.device("cpu")]
- if torch.cuda.is_available():
- devices.append(torch.device("cuda"))
- for device in devices:
- src_boxes = src_boxes.to(device=device)
- dst_boxes = dst_boxes.to(device=device)
- deltas = b2b_transform.get_deltas(src_boxes, dst_boxes)
- dst_boxes_reconstructed = b2b_transform.apply_deltas(deltas, src_boxes)
- assert torch.allclose(dst_boxes[:, :4], dst_boxes_reconstructed[:, :4], atol=1e-5)
- # angle difference has to be normalized
- assert torch.allclose(
- (dst_boxes[:, 4] - dst_boxes_reconstructed[:, 4] + 180.0) % 360.0 - 180.0,
- torch.zeros_like(dst_boxes[:, 4]),
- atol=1e-4,
- )
-
-
-if __name__ == "__main__":
- unittest.main()
diff --git a/spaces/hasibzunair/fifa-tryon-demo/u2net_run.py b/spaces/hasibzunair/fifa-tryon-demo/u2net_run.py
deleted file mode 100644
index 6cbabc585ace685e3567c50e82d5d0eb1d4a4ffb..0000000000000000000000000000000000000000
--- a/spaces/hasibzunair/fifa-tryon-demo/u2net_run.py
+++ /dev/null
@@ -1,91 +0,0 @@
-import os
-from skimage import io, transform
-import torch
-import torchvision
-from torch.autograd import Variable
-import torch.nn as nn
-import torch.nn.functional as F
-from torch.utils.data import Dataset, DataLoader
-from torchvision import transforms#, utils
-from u2net_test import normPRED
-# import torch.optim as optim
-
-import numpy as np
-from PIL import Image
-import glob
-import warnings
-
-from data_loader import RescaleT
-from data_loader import ToTensor
-from data_loader import ToTensorLab
-from data_loader import SalObjDataset
-
-warnings.filterwarnings("ignore")
-
-def save_images(image_name,pred,d_dir):
- predict = pred
- predict = predict.squeeze()
- predict_np = predict.cpu().data.numpy()
-
- im = Image.fromarray(predict_np*255).convert('RGB')
- img_name = image_name.split(os.sep)[-1]
- image = io.imread(image_name)
- imo = im.resize((image.shape[1],image.shape[0]),resample=Image.BICUBIC)
-
- pb_np = np.array(imo)
-
- aaa = img_name.split(".")
- bbb = aaa[0:-1]
- imidx = bbb[0]
- for i in range(1,len(bbb)):
- imidx = imidx + "." + bbb[i]
- print('Saving output at {}'.format(os.path.join(d_dir, imidx+'.png')))
- imo.save(os.path.join(d_dir, imidx+'.png'))
-
-def infer(
- net,
- image_dir = os.path.join(os.getcwd(), 'test_data', 'test_images'),
- prediction_dir = os.path.join(os.getcwd(), 'test_data', 'u2net' + '_results')
- ):
-
-
- img_name_list = glob.glob(image_dir + os.sep + '*')
- prediction_dir = prediction_dir + os.sep
-
- # --------- 2. dataloader ---------
- #1. dataloader
- test_salobj_dataset = SalObjDataset(img_name_list = img_name_list,
- lbl_name_list = [],
- transform=transforms.Compose([RescaleT(320),
- ToTensorLab(flag=0)])
- )
- test_salobj_dataloader = DataLoader(test_salobj_dataset,
- batch_size=1,
- shuffle=False,
- num_workers=1)
-
- # --------- 4. inference for each image ---------
- for i_test, data_test in enumerate(test_salobj_dataloader):
-
- print("Generating mask for:",img_name_list[i_test].split(os.sep)[-1])
-
- inputs_test = data_test['image']
- inputs_test = inputs_test.type(torch.FloatTensor)
-
- if torch.cuda.is_available():
- inputs_test = Variable(inputs_test.cuda())
- else:
- inputs_test = Variable(inputs_test)
-
- d1,d2,d3,d4,d5,d6,d7= net(inputs_test)
-
- # normalization
- pred = d1[:,0,:,:]
- pred = normPRED(pred)
-
- # save results to test_results folder
- if not os.path.exists(prediction_dir):
- os.makedirs(prediction_dir, exist_ok=True)
- save_images(img_name_list[i_test],pred,prediction_dir)
-
- del d1,d2,d3,d4,d5,d6,d7
diff --git a/spaces/hekbobo/bingo/src/lib/hooks/use-enter-submit.tsx b/spaces/hekbobo/bingo/src/lib/hooks/use-enter-submit.tsx
deleted file mode 100644
index d66b2d3253baff164235d4ca791aae6d84721835..0000000000000000000000000000000000000000
--- a/spaces/hekbobo/bingo/src/lib/hooks/use-enter-submit.tsx
+++ /dev/null
@@ -1,23 +0,0 @@
-import { useRef, type RefObject } from 'react'
-
-export function useEnterSubmit(): {
- formRef: RefObject
- onKeyDown: (event: React.KeyboardEvent) => void
-} {
- const formRef = useRef(null)
-
- const handleKeyDown = (
- event: React.KeyboardEvent
- ): void => {
- if (
- event.key === 'Enter' &&
- !event.shiftKey &&
- !event.nativeEvent.isComposing
- ) {
- formRef.current?.requestSubmit()
- event.preventDefault()
- }
- }
-
- return { formRef, onKeyDown: handleKeyDown }
-}
diff --git a/spaces/hezhaoqia/vits-simple-api/README.md b/spaces/hezhaoqia/vits-simple-api/README.md
deleted file mode 100644
index 73fd82148a033f042c1c7ca2dbdbae5f5d994921..0000000000000000000000000000000000000000
--- a/spaces/hezhaoqia/vits-simple-api/README.md
+++ /dev/null
@@ -1,9 +0,0 @@
----
-license: mit
-title: vits-simple-api
-sdk: gradio
-pinned: true
-python_version: 3.10.11
-emoji: 👀
-app_file: app.py
----
\ No newline at end of file
diff --git a/spaces/hohonu-vicml/DirectedDiffusion/DirectedDiffusion/Diffusion.py b/spaces/hohonu-vicml/DirectedDiffusion/DirectedDiffusion/Diffusion.py
deleted file mode 100644
index 56dc18d115aaf0cf8fe7fc5357265ce9d28fbd7d..0000000000000000000000000000000000000000
--- a/spaces/hohonu-vicml/DirectedDiffusion/DirectedDiffusion/Diffusion.py
+++ /dev/null
@@ -1,149 +0,0 @@
-import os
-import torch
-import random
-import numpy as np
-import datetime
-
-from PIL import Image
-from diffusers import LMSDiscreteScheduler
-from tqdm.auto import tqdm
-from torch import autocast
-from difflib import SequenceMatcher
-
-import DirectedDiffusion
-
-
-@torch.no_grad()
-def stablediffusion(
- model_bundle,
- attn_editor_bundle={},
- device="cuda",
- prompt="",
- steps=50,
- seed=None,
- width=512,
- height=512,
- t_start=0,
- guidance_scale=7.5,
- init_latents=None,
- is_save_attn=False,
- is_save_recons=False,
- folder = "./",
-):
-
- # neural networks
- unet = model_bundle["unet"]
- vae = model_bundle["vae"]
- clip_tokenizer = model_bundle["clip_tokenizer"]
- clip = model_bundle["clip_text_model"]
- # attn editor bundle, our stuff
- num_affected_steps = int(attn_editor_bundle.get("num_affected_steps") or 0)
- if not num_affected_steps:
- print("Not using attn editor")
- else:
- print("Using attn editor")
- DirectedDiffusion.AttnCore.init_attention_edit(
- unet,
- tokens=attn_editor_bundle.get("edit_index") or [],
- rios=attn_editor_bundle.get("roi") or [],
- noise_scale=attn_editor_bundle.get("noise_scale") or [],
- length_prompt=len(prompt.split(" ")),
- num_trailing_attn=attn_editor_bundle.get("num_trailing_attn") or [],
- )
-
- # Change size to multiple of 64 to prevent size mismatches inside model
- width = width - width % 64
- height = height - height % 64
- # If seed is None, randomly select seed from 0 to 2^32-1
- if seed is None:
- seed = random.randrange(2 ** 32 - 1)
- generator = torch.cuda.manual_seed(seed)
- # Set inference timesteps to scheduler
- scheduler = LMSDiscreteScheduler(
- beta_start=0.00085,
- beta_end=0.012,
- beta_schedule="scaled_linear",
- num_train_timesteps=1000,
- )
- scheduler.set_timesteps(steps)
- scheduler.timesteps = scheduler.timesteps.half().cuda()
-
- noise_weight = LMSDiscreteScheduler(
- beta_start=0.00085,
- beta_end=0.012,
- beta_schedule="scaled_linear",
- num_train_timesteps=10,
- )
- noise_weight.set_timesteps(num_affected_steps)
- # if num_affected_steps:
- # noise_weight.set_timesteps(num_affected_steps)
- # noise_weight.timesteps /= torch.max(noise_weight.timesteps)
-
- init_latent = torch.zeros(
- (1, unet.in_channels, height // 8, width // 8), device=device
- )
- t_start = t_start
- # Generate random normal noise
- noise = torch.randn(init_latent.shape, generator=generator, device=device)
- # latent = noise * scheduler.init_noise_sigma
- latent = scheduler.add_noise(
- init_latent,
- noise,
- torch.tensor(
- [scheduler.timesteps[t_start]], device=device, dtype=torch.float16
- ),
- ).to(device)
-
-
-
- current_time = datetime.datetime.now()
- current_time = current_time.strftime("%y%m%d-%H%M%S")
- folder = os.path.join(folder, current_time+"_internal")
- if not os.path.exists(folder) and (is_save_attn or is_save_recons):
- os.makedirs(folder)
- # Process clip
- with autocast(device):
- embeds_uncond = DirectedDiffusion.AttnEditorUtils.get_embeds(
- "", clip, clip_tokenizer
- )
- embeds_cond = DirectedDiffusion.AttnEditorUtils.get_embeds(
- prompt, clip, clip_tokenizer
- )
- timesteps = scheduler.timesteps[t_start:]
- for i, t in tqdm(enumerate(timesteps), total=len(timesteps)):
- t_index = t
- latent_model_input = latent
- latent_model_input = scheduler.scale_model_input(
- latent_model_input, t
- ).half()
- noise_pred_uncond = unet(
- latent_model_input, t, encoder_hidden_states=embeds_uncond
- ).sample
-
- if i < num_affected_steps:
- DirectedDiffusion.AttnEditorUtils.use_add_noise(
- unet, noise_weight.timesteps[i]
- )
- DirectedDiffusion.AttnEditorUtils.use_edited_attention(unet)
- noise_pred_cond = unet(
- latent_model_input, t, encoder_hidden_states=embeds_cond
- ).sample
-
- else:
- noise_pred_cond = unet(
- latent_model_input, t, encoder_hidden_states=embeds_cond
- ).sample
-
- delta = noise_pred_cond - noise_pred_uncond
- # Perform guidance
- noise_pred = noise_pred_uncond + guidance_scale * delta
- latent = scheduler.step(noise_pred, t_index, latent).prev_sample
-
- if is_save_attn:
- filepath = os.path.join(folder, "ca.{:04d}.jpg".format(i))
- DirectedDiffusion.Plotter.plot_activation(filepath, unet, prompt, clip_tokenizer)
- if is_save_recons:
- filepath = os.path.join(folder, "recons.{:04d}.jpg".format(i))
- recons = DirectedDiffusion.AttnEditorUtils.get_image_from_latent(vae, latent)
- recons.save(filepath)
- return DirectedDiffusion.AttnEditorUtils.get_image_from_latent(vae, latent)
diff --git a/spaces/htukor/NLLB-Translator/README.md b/spaces/htukor/NLLB-Translator/README.md
deleted file mode 100644
index e6440c1f0cfbaebe6573193e597bf5cfa934e231..0000000000000000000000000000000000000000
--- a/spaces/htukor/NLLB-Translator/README.md
+++ /dev/null
@@ -1,14 +0,0 @@
----
-title: NLLB Translator
-emoji: 🗺️
-colorFrom: purple
-colorTo: pink
-sdk: gradio
-sdk_version: 3.0.26
-app_file: app.py
-pinned: false
-license: wtfpl
-duplicated_from: Narrativaai/NLLB-Translator
----
-
-Check out the configuration reference at https://huggingface.co/docs/hub/spaces-config-reference
diff --git a/spaces/hugface33/dream/README.md b/spaces/hugface33/dream/README.md
deleted file mode 100644
index abffaab9cfd8ea2c97371c935fea935b6bd85dce..0000000000000000000000000000000000000000
--- a/spaces/hugface33/dream/README.md
+++ /dev/null
@@ -1,13 +0,0 @@
----
-title: Dream
-emoji: 🚀
-colorFrom: red
-colorTo: purple
-sdk: gradio
-sdk_version: 3.15.0
-app_file: app.py
-pinned: false
-license: openrail
----
-
-Check out the configuration reference at https://huggingface.co/docs/hub/spaces-config-reference
diff --git a/spaces/huggingface-projects/stable-diffusion-multiplayer/stablediffusion-infinity/PyPatchMatch/csrc/nnf.h b/spaces/huggingface-projects/stable-diffusion-multiplayer/stablediffusion-infinity/PyPatchMatch/csrc/nnf.h
deleted file mode 100644
index b5c144a4a58649906c9c87a40044b5118a00aa04..0000000000000000000000000000000000000000
--- a/spaces/huggingface-projects/stable-diffusion-multiplayer/stablediffusion-infinity/PyPatchMatch/csrc/nnf.h
+++ /dev/null
@@ -1,133 +0,0 @@
-#pragma once
-
-#include
-#include "masked_image.h"
-
-class PatchDistanceMetric {
-public:
- PatchDistanceMetric(int patch_size) : m_patch_size(patch_size) {}
- virtual ~PatchDistanceMetric() = default;
-
- inline int patch_size() const { return m_patch_size; }
- virtual int operator()(const MaskedImage &source, int source_y, int source_x, const MaskedImage &target, int target_y, int target_x) const = 0;
- static const int kDistanceScale;
-
-protected:
- int m_patch_size;
-};
-
-class NearestNeighborField {
-public:
- NearestNeighborField() : m_source(), m_target(), m_field(), m_distance_metric(nullptr) {
- // pass
- }
- NearestNeighborField(const MaskedImage &source, const MaskedImage &target, const PatchDistanceMetric *metric, int max_retry = 20)
- : m_source(source), m_target(target), m_distance_metric(metric) {
- m_field = cv::Mat(m_source.size(), CV_32SC3);
- _randomize_field(max_retry);
- }
- NearestNeighborField(const MaskedImage &source, const MaskedImage &target, const PatchDistanceMetric *metric, const NearestNeighborField &other, int max_retry = 20)
- : m_source(source), m_target(target), m_distance_metric(metric) {
- m_field = cv::Mat(m_source.size(), CV_32SC3);
- _initialize_field_from(other, max_retry);
- }
-
- const MaskedImage &source() const {
- return m_source;
- }
- const MaskedImage &target() const {
- return m_target;
- }
- inline cv::Size source_size() const {
- return m_source.size();
- }
- inline cv::Size target_size() const {
- return m_target.size();
- }
- inline void set_source(const MaskedImage &source) {
- m_source = source;
- }
- inline void set_target(const MaskedImage &target) {
- m_target = target;
- }
-
- inline int *mutable_ptr(int y, int x) {
- return m_field.ptr(y, x);
- }
- inline const int *ptr(int y, int x) const {
- return m_field.ptr(y, x);
- }
-
- inline int at(int y, int x, int c) const {
- return m_field.ptr(y, x)[c];
- }
- inline int &at(int y, int x, int c) {
- return m_field.ptr(y, x)[c];
- }
- inline void set_identity(int y, int x) {
- auto ptr = mutable_ptr(y, x);
- ptr[0] = y, ptr[1] = x, ptr[2] = 0;
- }
-
- void minimize(int nr_pass);
-
-private:
- inline int _distance(int source_y, int source_x, int target_y, int target_x) {
- return (*m_distance_metric)(m_source, source_y, source_x, m_target, target_y, target_x);
- }
-
- void _randomize_field(int max_retry = 20, bool reset = true);
- void _initialize_field_from(const NearestNeighborField &other, int max_retry);
- void _minimize_link(int y, int x, int direction);
-
- MaskedImage m_source;
- MaskedImage m_target;
- cv::Mat m_field; // { y_target, x_target, distance_scaled }
- const PatchDistanceMetric *m_distance_metric;
-};
-
-
-class PatchSSDDistanceMetric : public PatchDistanceMetric {
-public:
- using PatchDistanceMetric::PatchDistanceMetric;
- virtual int operator ()(const MaskedImage &source, int source_y, int source_x, const MaskedImage &target, int target_y, int target_x) const;
- static const int kSSDScale;
-};
-
-class DebugPatchSSDDistanceMetric : public PatchDistanceMetric {
-public:
- DebugPatchSSDDistanceMetric(int patch_size, int width, int height) : PatchDistanceMetric(patch_size), m_width(width), m_height(height) {}
- virtual int operator ()(const MaskedImage &source, int source_y, int source_x, const MaskedImage &target, int target_y, int target_x) const;
-protected:
- int m_width, m_height;
-};
-
-class RegularityGuidedPatchDistanceMetricV1 : public PatchDistanceMetric {
-public:
- RegularityGuidedPatchDistanceMetricV1(int patch_size, double dx1, double dy1, double dx2, double dy2, double weight)
- : PatchDistanceMetric(patch_size), m_dx1(dx1), m_dy1(dy1), m_dx2(dx2), m_dy2(dy2), m_weight(weight) {
-
- assert(m_dy1 == 0);
- assert(m_dx2 == 0);
- m_scale = sqrt(m_dx1 * m_dx1 + m_dy2 * m_dy2) / 4;
- }
- virtual int operator ()(const MaskedImage &source, int source_y, int source_x, const MaskedImage &target, int target_y, int target_x) const;
-
-protected:
- double m_dx1, m_dy1, m_dx2, m_dy2;
- double m_scale, m_weight;
-};
-
-class RegularityGuidedPatchDistanceMetricV2 : public PatchDistanceMetric {
-public:
- RegularityGuidedPatchDistanceMetricV2(int patch_size, cv::Mat ijmap, double weight)
- : PatchDistanceMetric(patch_size), m_ijmap(ijmap), m_weight(weight) {
-
- }
- virtual int operator ()(const MaskedImage &source, int source_y, int source_x, const MaskedImage &target, int target_y, int target_x) const;
-
-protected:
- cv::Mat m_ijmap;
- double m_width, m_height, m_weight;
-};
-
diff --git a/spaces/huggingface-timeseries/time-series-score/src/fit_model.py b/spaces/huggingface-timeseries/time-series-score/src/fit_model.py
deleted file mode 100644
index 43d7f9f717adae1dce9e6da0aade2cac6e046410..0000000000000000000000000000000000000000
--- a/spaces/huggingface-timeseries/time-series-score/src/fit_model.py
+++ /dev/null
@@ -1,44 +0,0 @@
-from gluonts.dataset.common import Dataset
-
-from .models import (
- AbstractPredictor,
- AutoGluonPredictor,
- AutoPyTorchPredictor,
- DeepARPredictor,
- TFTPredictor,
- AutoARIMAPredictor,
- AutoETSPredictor,
- AutoThetaPredictor,
- StatsEnsemblePredictor,
-)
-
-MODEL_NAME_TO_CLASS = {
- "autogluon": AutoGluonPredictor,
- "autopytorch": AutoPyTorchPredictor,
- "deepar": DeepARPredictor,
- "tft": TFTPredictor,
- "autoarima": AutoARIMAPredictor,
- "autoets": AutoETSPredictor,
- "autotheta": AutoThetaPredictor,
- "statsensemble": StatsEnsemblePredictor,
-}
-
-
-def fit_predict_with_model(
- model_name: str,
- dataset: Dataset,
- prediction_length: int,
- freq: str,
- seasonality: int,
- **model_kwargs,
-):
- model_class = MODEL_NAME_TO_CLASS[model_name.lower()]
- model: AbstractPredictor = model_class(
- prediction_length=prediction_length,
- freq=freq,
- seasonality=seasonality,
- **model_kwargs,
- )
- predictions = model.fit_predict(dataset)
- info = {"run_time": model.get_runtime()}
- return predictions, info
diff --git a/spaces/hussain-shk/IndiSent/indic_nlp_library/contrib/README.md b/spaces/hussain-shk/IndiSent/indic_nlp_library/contrib/README.md
deleted file mode 100644
index 0a99b9ddd9e9bcc72bae930fc8a778f3094fea50..0000000000000000000000000000000000000000
--- a/spaces/hussain-shk/IndiSent/indic_nlp_library/contrib/README.md
+++ /dev/null
@@ -1,7 +0,0 @@
-# Contrib
-
-Contains additional utilities and applications using Indic NLP library core
-
-- `indic_scraper_project_sample.ipynb`: A simple pipeline for building monolingual corpora for Indian languages from crawled web content, Wikipedia, etc. An extensible framework which allows incorporation of website specific extractors, whereas generic NLP tasks like tokenization, sentence splitting, normalization, etc. are handled by the framework.
-- `correct_moses_tokenizer.py`: This script corrects the incorrect tokenization done by Moses tokenizer. The Moses tokenizer splits on nukta and halant characters.
-- `hindi_to_kannada_transliterator.py`: This script transliterates Hindi to Kannada. It removes/remaps characters only found in Hindi. It also adds halanta to words ending with consonant - as is the convention in Kannada.
diff --git a/spaces/hyxue/HiFiFace-inference-demo/Deep3DFaceRecon_pytorch/models/arcface_torch/configs/glint360k_mbf.py b/spaces/hyxue/HiFiFace-inference-demo/Deep3DFaceRecon_pytorch/models/arcface_torch/configs/glint360k_mbf.py
deleted file mode 100644
index 03447e982487f19c40c814448f9fdfea6c306b0f..0000000000000000000000000000000000000000
--- a/spaces/hyxue/HiFiFace-inference-demo/Deep3DFaceRecon_pytorch/models/arcface_torch/configs/glint360k_mbf.py
+++ /dev/null
@@ -1,27 +0,0 @@
-from easydict import EasyDict as edict
-
-# make training faster
-# our RAM is 256G
-# mount -t tmpfs -o size=140G tmpfs /train_tmp
-
-config = edict()
-config.margin_list = (1.0, 0.0, 0.4)
-config.network = "mbf"
-config.resume = False
-config.output = None
-config.embedding_size = 512
-config.sample_rate = 1.0
-config.fp16 = True
-config.momentum = 0.9
-config.weight_decay = 1e-4
-config.batch_size = 128
-config.lr = 0.1
-config.verbose = 2000
-config.dali = False
-
-config.rec = "/train_tmp/glint360k"
-config.num_classes = 360232
-config.num_image = 17091657
-config.num_epoch = 20
-config.warmup_epoch = 0
-config.val_targets = ["lfw", "cfp_fp", "agedb_30"]
diff --git a/spaces/hzy123/bingo/src/components/chat-list.tsx b/spaces/hzy123/bingo/src/components/chat-list.tsx
deleted file mode 100644
index 624a78ef0d7be0f1192cf02a81e2e9cf214cb193..0000000000000000000000000000000000000000
--- a/spaces/hzy123/bingo/src/components/chat-list.tsx
+++ /dev/null
@@ -1,28 +0,0 @@
-import React from 'react'
-
-import { Separator } from '@/components/ui/separator'
-import { ChatMessage } from '@/components/chat-message'
-import { ChatMessageModel } from '@/lib/bots/bing/types'
-
-export interface ChatList {
- messages: ChatMessageModel[]
-}
-
-export function ChatList({ messages }: ChatList) {
- if (!messages.length) {
- return null
- }
-
- return (
-
- {messages.map((message, index) => (
-
-
- {index < messages.length - 1 && (
-
- )}
-
- ))}
-
- )
-}
diff --git a/spaces/iaanimashaun/glaucomanet/app.py b/spaces/iaanimashaun/glaucomanet/app.py
deleted file mode 100644
index c12f92f8a433099b20c9bb880dd9c9e928386af2..0000000000000000000000000000000000000000
--- a/spaces/iaanimashaun/glaucomanet/app.py
+++ /dev/null
@@ -1,26 +0,0 @@
-import gradio as gr
-from tensorflow import keras
-from skimage.transform import resize
-
-# def greet(name):
-# return "Hello " + name + "!!"
-
-# iface = gr.Interface(fn=greet, inputs="text", outputs="text")
-# iface.launch()
-
-resnet50_model = keras.models.load_model('model.h5')
-labels = ['Glaucoma_Pos', 'Glaucoma_Neg']
-
-def classify_image(inp):
-
- inp =resize(inp, (300, 300, 3))
- inp = inp.reshape((-1, 300, 300, 3))
- # inp = tf.keras.applications.mobilenet_v2.preprocess_input(inp)
- prediction = resnet50_model.predict(inp).flatten()
- confidences = {labels[i]: float(prediction[i]) for i in range(2)}
- return confidences
-
-gr.Interface(fn=classify_image,
- inputs=gr.Image(shape=(300, 300)),
- outputs=gr.Label(num_top_classes=2),
- ).launch()
\ No newline at end of file
diff --git a/spaces/inplisQlawa/anything-midjourney-v4-1/Cricket World Cup 2011 Pc Game Crack [BEST] Downloads.md b/spaces/inplisQlawa/anything-midjourney-v4-1/Cricket World Cup 2011 Pc Game Crack [BEST] Downloads.md
deleted file mode 100644
index 7f214cd6ca5da81139ec080ec1bda4bbd0cb5da2..0000000000000000000000000000000000000000
--- a/spaces/inplisQlawa/anything-midjourney-v4-1/Cricket World Cup 2011 Pc Game Crack [BEST] Downloads.md
+++ /dev/null
@@ -1,19 +0,0 @@
-
-How to Download and Play Cricket World Cup 2011 PC Game for Free
-If you are a fan of cricket and want to relive the excitement of the ICC Cricket World Cup 2011, you might be interested in downloading and playing a PC game that simulates the tournament. However, buying a licensed game can be expensive and not easily available. That's why some gamers resort to using cracks, which are modified versions of the game that bypass the security checks and allow you to play without paying.
-However, using cracks can also be risky, as they may contain viruses, malware, or spyware that can harm your computer or steal your personal information. Moreover, using cracks is illegal and unethical, as it violates the intellectual property rights of the game developers and publishers. Therefore, we do not recommend using cracks to download and play cricket games.
-cricket world cup 2011 pc game crack downloads
Download File ———>>> https://urlin.us/2uEvqn
-Instead, we suggest you try a safer and legal alternative: a modded version of EA Sports Cricket 07 that features the complete Cricket World Cup 2011 tournament. A mod is a fan-made modification that adds new features, graphics, sounds, and gameplay elements to an existing game. Mods are usually free and easy to install, and they do not require any cracks or serial keys to run.
-One of the best mods for cricket games is CWC Cricket 2011 by InsideCricSport, which delivers the exact experience of ICC Cricket World Cup 2011 in EA Cricket 07. This mod includes all the 2011 stuffs like the latest CWC Kits, Accessories, Broadcasting Team Logos, New CWC Stadiums, accurate rosters, and so on. You can play all the matches of the tournament with realistic fixtures, overlays, umpires, bats, and crowds.
-To download and play CWC Cricket 2011, you need to follow these steps:
-
-- Download EA Sports Cricket 07 from any trusted source. You can find it on various websites or torrent sites. Make sure you scan the file for viruses before installing it.
-- Download CWC Cricket 2011 from InsideCricSport Store. You can buy it for a nominal price of â¹49.00 (67% off) from their website. You will get an instant download link and lifetime access to the mod.
-- Extract the CWC Cricket 2011 zip file using WinRAR or any other software. You will get a folder named "CWC11" containing all the files of the mod.
-- Copy all the files from the "CWC11" folder and paste them into your EA Sports Cricket 07 installation directory. Usually, it is located at "C:\Program Files\EA SPORTS\EA SPORTS(TM) Cricket 07". Replace any existing files if prompted.
-- Run the game from your desktop shortcut or start menu. You will see a new launcher with CWC Cricket 2011 logo. Click on "Play" to start the game.
-- Select "International" from the main menu and then choose "ICC CWC 11". You will see all the teams and groups of the tournament. Select your favorite team and start playing.
-
-That's it! You have successfully downloaded and installed CWC Cricket 2011 on your PC. Now you can enjoy the thrill of ICC Cricket World Cup 2011 anytime you want.
d5da3c52bf
-
-
\ No newline at end of file
diff --git a/spaces/inplisQlawa/anything-midjourney-v4-1/Fruity Loops 3.5 Full Version.md b/spaces/inplisQlawa/anything-midjourney-v4-1/Fruity Loops 3.5 Full Version.md
deleted file mode 100644
index 30c2066eb3b3787f5bbf0752e3ab058fdfaf9fcf..0000000000000000000000000000000000000000
--- a/spaces/inplisQlawa/anything-midjourney-v4-1/Fruity Loops 3.5 Full Version.md
+++ /dev/null
@@ -1,6 +0,0 @@
-fruity loops 3.5 full version
Download ✑ ✑ ✑ https://urlin.us/2uEyAZ
-
-Reviews. HALion 3.5. It is for PC platform only. .. 5 of 5 Stars! 4d29de3e1b
-
-
-
diff --git a/spaces/inreVtussa/clothingai/Examples/Adobe Photodeluxe Home Edition 40 Free Download.md b/spaces/inreVtussa/clothingai/Examples/Adobe Photodeluxe Home Edition 40 Free Download.md
deleted file mode 100644
index 0e10eba1b43dce4158b7913bf4dc22da65a2ea8f..0000000000000000000000000000000000000000
--- a/spaces/inreVtussa/clothingai/Examples/Adobe Photodeluxe Home Edition 40 Free Download.md
+++ /dev/null
@@ -1,6 +0,0 @@
-Adobe Photodeluxe Home Edition 40 Free Download
DOWNLOAD ✵✵✵ https://tiurll.com/2uCiUO
-
-... Adobe PhotoDeluxe Home Edition key, Adobe PhotoDeluxe Home Edition free download, Adobe PhotoDeluxe Home Edition 2af274c1 find serial number. 4d29de3e1b
-
-
-
diff --git a/spaces/inreVtussa/clothingai/Examples/Crack Audiotx Communicator 1.5 37.md b/spaces/inreVtussa/clothingai/Examples/Crack Audiotx Communicator 1.5 37.md
deleted file mode 100644
index df8b4cc40cb8eba3094cc3efe3557c5b90321354..0000000000000000000000000000000000000000
--- a/spaces/inreVtussa/clothingai/Examples/Crack Audiotx Communicator 1.5 37.md
+++ /dev/null
@@ -1,7 +0,0 @@
-
-crack audiotx communicator 1.5 is an easy crack tool for audiotx communicator 1.5, just install the crack and click the crack audiotx communicator 1.5 button. the crack tool will crack audiotx communicator 1.5 and you can install a cracked audiotx communicator 1.5 version from the crack folder.
-Crack Audiotx Communicator 1.5 37
DOWNLOAD ---> https://tiurll.com/2uCiFt
-crack audiotx communicator 1.5 is a free cracking tool for audiotx communicator 1.5. this program is not affiliated with audiotx and we don't know any details about the authors. all files are uploaded by users like you, we can't guarantee that crack audiotx communicator 1.5 is 100% safe. if you are not comfortable with this check the audiotx website.
- the package contains the following files:
- audiotx -- a program to change the audio input/output settings of your computer. it's based on the audiotx project >.
- audiotx-linux-1.5.37.tar.gz -- the compressed source code. it contains the audiotx directory with all the source code and the audiotx.cpp file in the source code directory. the other directory contains the user manual, the audiotx.conf file, the audiotx example files, and the audiotx-linux-1.37 directory with shell script scripts that can be used to start the audiotx daemon and to start/stop the daemon.37/readme -- a readme file. it says a few words about the audiotx software, and the contents of the audiotx directory. it contains information about the audiotx project and the audiotx source code and user manual.37/audiotx.conf -- the configuration file for audiotx. it can be used to change the settings of your computer for all programs that use the audio devices, and it can be used to specify the path to your jack client.sh -- a shell script to start the audiotx daemon. it's located in the audiotx-linux-1.37 directory.c -- the source code of the audiotx daemon.
899543212b
-
-
\ No newline at end of file
diff --git a/spaces/irvay/RVC_IR/lib/infer_pack/models.py b/spaces/irvay/RVC_IR/lib/infer_pack/models.py
deleted file mode 100644
index 3665d03bc0514a6ed07d3372ea24717dae1e0a65..0000000000000000000000000000000000000000
--- a/spaces/irvay/RVC_IR/lib/infer_pack/models.py
+++ /dev/null
@@ -1,1142 +0,0 @@
-import math, pdb, os
-from time import time as ttime
-import torch
-from torch import nn
-from torch.nn import functional as F
-from lib.infer_pack import modules
-from lib.infer_pack import attentions
-from lib.infer_pack import commons
-from lib.infer_pack.commons import init_weights, get_padding
-from torch.nn import Conv1d, ConvTranspose1d, AvgPool1d, Conv2d
-from torch.nn.utils import weight_norm, remove_weight_norm, spectral_norm
-from lib.infer_pack.commons import init_weights
-import numpy as np
-from lib.infer_pack import commons
-
-
-class TextEncoder256(nn.Module):
- def __init__(
- self,
- out_channels,
- hidden_channels,
- filter_channels,
- n_heads,
- n_layers,
- kernel_size,
- p_dropout,
- f0=True,
- ):
- super().__init__()
- self.out_channels = out_channels
- self.hidden_channels = hidden_channels
- self.filter_channels = filter_channels
- self.n_heads = n_heads
- self.n_layers = n_layers
- self.kernel_size = kernel_size
- self.p_dropout = p_dropout
- self.emb_phone = nn.Linear(256, hidden_channels)
- self.lrelu = nn.LeakyReLU(0.1, inplace=True)
- if f0 == True:
- self.emb_pitch = nn.Embedding(256, hidden_channels) # pitch 256
- self.encoder = attentions.Encoder(
- hidden_channels, filter_channels, n_heads, n_layers, kernel_size, p_dropout
- )
- self.proj = nn.Conv1d(hidden_channels, out_channels * 2, 1)
-
- def forward(self, phone, pitch, lengths):
- if pitch == None:
- x = self.emb_phone(phone)
- else:
- x = self.emb_phone(phone) + self.emb_pitch(pitch)
- x = x * math.sqrt(self.hidden_channels) # [b, t, h]
- x = self.lrelu(x)
- x = torch.transpose(x, 1, -1) # [b, h, t]
- x_mask = torch.unsqueeze(commons.sequence_mask(lengths, x.size(2)), 1).to(
- x.dtype
- )
- x = self.encoder(x * x_mask, x_mask)
- stats = self.proj(x) * x_mask
-
- m, logs = torch.split(stats, self.out_channels, dim=1)
- return m, logs, x_mask
-
-
-class TextEncoder768(nn.Module):
- def __init__(
- self,
- out_channels,
- hidden_channels,
- filter_channels,
- n_heads,
- n_layers,
- kernel_size,
- p_dropout,
- f0=True,
- ):
- super().__init__()
- self.out_channels = out_channels
- self.hidden_channels = hidden_channels
- self.filter_channels = filter_channels
- self.n_heads = n_heads
- self.n_layers = n_layers
- self.kernel_size = kernel_size
- self.p_dropout = p_dropout
- self.emb_phone = nn.Linear(768, hidden_channels)
- self.lrelu = nn.LeakyReLU(0.1, inplace=True)
- if f0 == True:
- self.emb_pitch = nn.Embedding(256, hidden_channels) # pitch 256
- self.encoder = attentions.Encoder(
- hidden_channels, filter_channels, n_heads, n_layers, kernel_size, p_dropout
- )
- self.proj = nn.Conv1d(hidden_channels, out_channels * 2, 1)
-
- def forward(self, phone, pitch, lengths):
- if pitch == None:
- x = self.emb_phone(phone)
- else:
- x = self.emb_phone(phone) + self.emb_pitch(pitch)
- x = x * math.sqrt(self.hidden_channels) # [b, t, h]
- x = self.lrelu(x)
- x = torch.transpose(x, 1, -1) # [b, h, t]
- x_mask = torch.unsqueeze(commons.sequence_mask(lengths, x.size(2)), 1).to(
- x.dtype
- )
- x = self.encoder(x * x_mask, x_mask)
- stats = self.proj(x) * x_mask
-
- m, logs = torch.split(stats, self.out_channels, dim=1)
- return m, logs, x_mask
-
-
-class ResidualCouplingBlock(nn.Module):
- def __init__(
- self,
- channels,
- hidden_channels,
- kernel_size,
- dilation_rate,
- n_layers,
- n_flows=4,
- gin_channels=0,
- ):
- super().__init__()
- self.channels = channels
- self.hidden_channels = hidden_channels
- self.kernel_size = kernel_size
- self.dilation_rate = dilation_rate
- self.n_layers = n_layers
- self.n_flows = n_flows
- self.gin_channels = gin_channels
-
- self.flows = nn.ModuleList()
- for i in range(n_flows):
- self.flows.append(
- modules.ResidualCouplingLayer(
- channels,
- hidden_channels,
- kernel_size,
- dilation_rate,
- n_layers,
- gin_channels=gin_channels,
- mean_only=True,
- )
- )
- self.flows.append(modules.Flip())
-
- def forward(self, x, x_mask, g=None, reverse=False):
- if not reverse:
- for flow in self.flows:
- x, _ = flow(x, x_mask, g=g, reverse=reverse)
- else:
- for flow in reversed(self.flows):
- x = flow(x, x_mask, g=g, reverse=reverse)
- return x
-
- def remove_weight_norm(self):
- for i in range(self.n_flows):
- self.flows[i * 2].remove_weight_norm()
-
-
-class PosteriorEncoder(nn.Module):
- def __init__(
- self,
- in_channels,
- out_channels,
- hidden_channels,
- kernel_size,
- dilation_rate,
- n_layers,
- gin_channels=0,
- ):
- super().__init__()
- self.in_channels = in_channels
- self.out_channels = out_channels
- self.hidden_channels = hidden_channels
- self.kernel_size = kernel_size
- self.dilation_rate = dilation_rate
- self.n_layers = n_layers
- self.gin_channels = gin_channels
-
- self.pre = nn.Conv1d(in_channels, hidden_channels, 1)
- self.enc = modules.WN(
- hidden_channels,
- kernel_size,
- dilation_rate,
- n_layers,
- gin_channels=gin_channels,
- )
- self.proj = nn.Conv1d(hidden_channels, out_channels * 2, 1)
-
- def forward(self, x, x_lengths, g=None):
- x_mask = torch.unsqueeze(commons.sequence_mask(x_lengths, x.size(2)), 1).to(
- x.dtype
- )
- x = self.pre(x) * x_mask
- x = self.enc(x, x_mask, g=g)
- stats = self.proj(x) * x_mask
- m, logs = torch.split(stats, self.out_channels, dim=1)
- z = (m + torch.randn_like(m) * torch.exp(logs)) * x_mask
- return z, m, logs, x_mask
-
- def remove_weight_norm(self):
- self.enc.remove_weight_norm()
-
-
-class Generator(torch.nn.Module):
- def __init__(
- self,
- initial_channel,
- resblock,
- resblock_kernel_sizes,
- resblock_dilation_sizes,
- upsample_rates,
- upsample_initial_channel,
- upsample_kernel_sizes,
- gin_channels=0,
- ):
- super(Generator, self).__init__()
- self.num_kernels = len(resblock_kernel_sizes)
- self.num_upsamples = len(upsample_rates)
- self.conv_pre = Conv1d(
- initial_channel, upsample_initial_channel, 7, 1, padding=3
- )
- resblock = modules.ResBlock1 if resblock == "1" else modules.ResBlock2
-
- self.ups = nn.ModuleList()
- for i, (u, k) in enumerate(zip(upsample_rates, upsample_kernel_sizes)):
- self.ups.append(
- weight_norm(
- ConvTranspose1d(
- upsample_initial_channel // (2**i),
- upsample_initial_channel // (2 ** (i + 1)),
- k,
- u,
- padding=(k - u) // 2,
- )
- )
- )
-
- self.resblocks = nn.ModuleList()
- for i in range(len(self.ups)):
- ch = upsample_initial_channel // (2 ** (i + 1))
- for j, (k, d) in enumerate(
- zip(resblock_kernel_sizes, resblock_dilation_sizes)
- ):
- self.resblocks.append(resblock(ch, k, d))
-
- self.conv_post = Conv1d(ch, 1, 7, 1, padding=3, bias=False)
- self.ups.apply(init_weights)
-
- if gin_channels != 0:
- self.cond = nn.Conv1d(gin_channels, upsample_initial_channel, 1)
-
- def forward(self, x, g=None):
- x = self.conv_pre(x)
- if g is not None:
- x = x + self.cond(g)
-
- for i in range(self.num_upsamples):
- x = F.leaky_relu(x, modules.LRELU_SLOPE)
- x = self.ups[i](x)
- xs = None
- for j in range(self.num_kernels):
- if xs is None:
- xs = self.resblocks[i * self.num_kernels + j](x)
- else:
- xs += self.resblocks[i * self.num_kernels + j](x)
- x = xs / self.num_kernels
- x = F.leaky_relu(x)
- x = self.conv_post(x)
- x = torch.tanh(x)
-
- return x
-
- def remove_weight_norm(self):
- for l in self.ups:
- remove_weight_norm(l)
- for l in self.resblocks:
- l.remove_weight_norm()
-
-
-class SineGen(torch.nn.Module):
- """Definition of sine generator
- SineGen(samp_rate, harmonic_num = 0,
- sine_amp = 0.1, noise_std = 0.003,
- voiced_threshold = 0,
- flag_for_pulse=False)
- samp_rate: sampling rate in Hz
- harmonic_num: number of harmonic overtones (default 0)
- sine_amp: amplitude of sine-wavefrom (default 0.1)
- noise_std: std of Gaussian noise (default 0.003)
- voiced_thoreshold: F0 threshold for U/V classification (default 0)
- flag_for_pulse: this SinGen is used inside PulseGen (default False)
- Note: when flag_for_pulse is True, the first time step of a voiced
- segment is always sin(np.pi) or cos(0)
- """
-
- def __init__(
- self,
- samp_rate,
- harmonic_num=0,
- sine_amp=0.1,
- noise_std=0.003,
- voiced_threshold=0,
- flag_for_pulse=False,
- ):
- super(SineGen, self).__init__()
- self.sine_amp = sine_amp
- self.noise_std = noise_std
- self.harmonic_num = harmonic_num
- self.dim = self.harmonic_num + 1
- self.sampling_rate = samp_rate
- self.voiced_threshold = voiced_threshold
-
- def _f02uv(self, f0):
- # generate uv signal
- uv = torch.ones_like(f0)
- uv = uv * (f0 > self.voiced_threshold)
- return uv
-
- def forward(self, f0, upp):
- """sine_tensor, uv = forward(f0)
- input F0: tensor(batchsize=1, length, dim=1)
- f0 for unvoiced steps should be 0
- output sine_tensor: tensor(batchsize=1, length, dim)
- output uv: tensor(batchsize=1, length, 1)
- """
- with torch.no_grad():
- f0 = f0[:, None].transpose(1, 2)
- f0_buf = torch.zeros(f0.shape[0], f0.shape[1], self.dim, device=f0.device)
- # fundamental component
- f0_buf[:, :, 0] = f0[:, :, 0]
- for idx in np.arange(self.harmonic_num):
- f0_buf[:, :, idx + 1] = f0_buf[:, :, 0] * (
- idx + 2
- ) # idx + 2: the (idx+1)-th overtone, (idx+2)-th harmonic
- rad_values = (f0_buf / self.sampling_rate) % 1 ###%1意味着n_har的乘积无法后处理优化
- rand_ini = torch.rand(
- f0_buf.shape[0], f0_buf.shape[2], device=f0_buf.device
- )
- rand_ini[:, 0] = 0
- rad_values[:, 0, :] = rad_values[:, 0, :] + rand_ini
- tmp_over_one = torch.cumsum(rad_values, 1) # % 1 #####%1意味着后面的cumsum无法再优化
- tmp_over_one *= upp
- tmp_over_one = F.interpolate(
- tmp_over_one.transpose(2, 1),
- scale_factor=upp,
- mode="linear",
- align_corners=True,
- ).transpose(2, 1)
- rad_values = F.interpolate(
- rad_values.transpose(2, 1), scale_factor=upp, mode="nearest"
- ).transpose(
- 2, 1
- ) #######
- tmp_over_one %= 1
- tmp_over_one_idx = (tmp_over_one[:, 1:, :] - tmp_over_one[:, :-1, :]) < 0
- cumsum_shift = torch.zeros_like(rad_values)
- cumsum_shift[:, 1:, :] = tmp_over_one_idx * -1.0
- sine_waves = torch.sin(
- torch.cumsum(rad_values + cumsum_shift, dim=1) * 2 * np.pi
- )
- sine_waves = sine_waves * self.sine_amp
- uv = self._f02uv(f0)
- uv = F.interpolate(
- uv.transpose(2, 1), scale_factor=upp, mode="nearest"
- ).transpose(2, 1)
- noise_amp = uv * self.noise_std + (1 - uv) * self.sine_amp / 3
- noise = noise_amp * torch.randn_like(sine_waves)
- sine_waves = sine_waves * uv + noise
- return sine_waves, uv, noise
-
-
-class SourceModuleHnNSF(torch.nn.Module):
- """SourceModule for hn-nsf
- SourceModule(sampling_rate, harmonic_num=0, sine_amp=0.1,
- add_noise_std=0.003, voiced_threshod=0)
- sampling_rate: sampling_rate in Hz
- harmonic_num: number of harmonic above F0 (default: 0)
- sine_amp: amplitude of sine source signal (default: 0.1)
- add_noise_std: std of additive Gaussian noise (default: 0.003)
- note that amplitude of noise in unvoiced is decided
- by sine_amp
- voiced_threshold: threhold to set U/V given F0 (default: 0)
- Sine_source, noise_source = SourceModuleHnNSF(F0_sampled)
- F0_sampled (batchsize, length, 1)
- Sine_source (batchsize, length, 1)
- noise_source (batchsize, length 1)
- uv (batchsize, length, 1)
- """
-
- def __init__(
- self,
- sampling_rate,
- harmonic_num=0,
- sine_amp=0.1,
- add_noise_std=0.003,
- voiced_threshod=0,
- is_half=True,
- ):
- super(SourceModuleHnNSF, self).__init__()
-
- self.sine_amp = sine_amp
- self.noise_std = add_noise_std
- self.is_half = is_half
- # to produce sine waveforms
- self.l_sin_gen = SineGen(
- sampling_rate, harmonic_num, sine_amp, add_noise_std, voiced_threshod
- )
-
- # to merge source harmonics into a single excitation
- self.l_linear = torch.nn.Linear(harmonic_num + 1, 1)
- self.l_tanh = torch.nn.Tanh()
-
- def forward(self, x, upp=None):
- sine_wavs, uv, _ = self.l_sin_gen(x, upp)
- if self.is_half:
- sine_wavs = sine_wavs.half()
- sine_merge = self.l_tanh(self.l_linear(sine_wavs))
- return sine_merge, None, None # noise, uv
-
-
-class GeneratorNSF(torch.nn.Module):
- def __init__(
- self,
- initial_channel,
- resblock,
- resblock_kernel_sizes,
- resblock_dilation_sizes,
- upsample_rates,
- upsample_initial_channel,
- upsample_kernel_sizes,
- gin_channels,
- sr,
- is_half=False,
- ):
- super(GeneratorNSF, self).__init__()
- self.num_kernels = len(resblock_kernel_sizes)
- self.num_upsamples = len(upsample_rates)
-
- self.f0_upsamp = torch.nn.Upsample(scale_factor=np.prod(upsample_rates))
- self.m_source = SourceModuleHnNSF(
- sampling_rate=sr, harmonic_num=0, is_half=is_half
- )
- self.noise_convs = nn.ModuleList()
- self.conv_pre = Conv1d(
- initial_channel, upsample_initial_channel, 7, 1, padding=3
- )
- resblock = modules.ResBlock1 if resblock == "1" else modules.ResBlock2
-
- self.ups = nn.ModuleList()
- for i, (u, k) in enumerate(zip(upsample_rates, upsample_kernel_sizes)):
- c_cur = upsample_initial_channel // (2 ** (i + 1))
- self.ups.append(
- weight_norm(
- ConvTranspose1d(
- upsample_initial_channel // (2**i),
- upsample_initial_channel // (2 ** (i + 1)),
- k,
- u,
- padding=(k - u) // 2,
- )
- )
- )
- if i + 1 < len(upsample_rates):
- stride_f0 = np.prod(upsample_rates[i + 1 :])
- self.noise_convs.append(
- Conv1d(
- 1,
- c_cur,
- kernel_size=stride_f0 * 2,
- stride=stride_f0,
- padding=stride_f0 // 2,
- )
- )
- else:
- self.noise_convs.append(Conv1d(1, c_cur, kernel_size=1))
-
- self.resblocks = nn.ModuleList()
- for i in range(len(self.ups)):
- ch = upsample_initial_channel // (2 ** (i + 1))
- for j, (k, d) in enumerate(
- zip(resblock_kernel_sizes, resblock_dilation_sizes)
- ):
- self.resblocks.append(resblock(ch, k, d))
-
- self.conv_post = Conv1d(ch, 1, 7, 1, padding=3, bias=False)
- self.ups.apply(init_weights)
-
- if gin_channels != 0:
- self.cond = nn.Conv1d(gin_channels, upsample_initial_channel, 1)
-
- self.upp = np.prod(upsample_rates)
-
- def forward(self, x, f0, g=None):
- har_source, noi_source, uv = self.m_source(f0, self.upp)
- har_source = har_source.transpose(1, 2)
- x = self.conv_pre(x)
- if g is not None:
- x = x + self.cond(g)
-
- for i in range(self.num_upsamples):
- x = F.leaky_relu(x, modules.LRELU_SLOPE)
- x = self.ups[i](x)
- x_source = self.noise_convs[i](har_source)
- x = x + x_source
- xs = None
- for j in range(self.num_kernels):
- if xs is None:
- xs = self.resblocks[i * self.num_kernels + j](x)
- else:
- xs += self.resblocks[i * self.num_kernels + j](x)
- x = xs / self.num_kernels
- x = F.leaky_relu(x)
- x = self.conv_post(x)
- x = torch.tanh(x)
- return x
-
- def remove_weight_norm(self):
- for l in self.ups:
- remove_weight_norm(l)
- for l in self.resblocks:
- l.remove_weight_norm()
-
-
-sr2sr = {
- "32k": 32000,
- "40k": 40000,
- "48k": 48000,
-}
-
-
-class SynthesizerTrnMs256NSFsid(nn.Module):
- def __init__(
- self,
- spec_channels,
- segment_size,
- inter_channels,
- hidden_channels,
- filter_channels,
- n_heads,
- n_layers,
- kernel_size,
- p_dropout,
- resblock,
- resblock_kernel_sizes,
- resblock_dilation_sizes,
- upsample_rates,
- upsample_initial_channel,
- upsample_kernel_sizes,
- spk_embed_dim,
- gin_channels,
- sr,
- **kwargs
- ):
- super().__init__()
- if type(sr) == type("strr"):
- sr = sr2sr[sr]
- self.spec_channels = spec_channels
- self.inter_channels = inter_channels
- self.hidden_channels = hidden_channels
- self.filter_channels = filter_channels
- self.n_heads = n_heads
- self.n_layers = n_layers
- self.kernel_size = kernel_size
- self.p_dropout = p_dropout
- self.resblock = resblock
- self.resblock_kernel_sizes = resblock_kernel_sizes
- self.resblock_dilation_sizes = resblock_dilation_sizes
- self.upsample_rates = upsample_rates
- self.upsample_initial_channel = upsample_initial_channel
- self.upsample_kernel_sizes = upsample_kernel_sizes
- self.segment_size = segment_size
- self.gin_channels = gin_channels
- # self.hop_length = hop_length#
- self.spk_embed_dim = spk_embed_dim
- self.enc_p = TextEncoder256(
- inter_channels,
- hidden_channels,
- filter_channels,
- n_heads,
- n_layers,
- kernel_size,
- p_dropout,
- )
- self.dec = GeneratorNSF(
- inter_channels,
- resblock,
- resblock_kernel_sizes,
- resblock_dilation_sizes,
- upsample_rates,
- upsample_initial_channel,
- upsample_kernel_sizes,
- gin_channels=gin_channels,
- sr=sr,
- is_half=kwargs["is_half"],
- )
- self.enc_q = PosteriorEncoder(
- spec_channels,
- inter_channels,
- hidden_channels,
- 5,
- 1,
- 16,
- gin_channels=gin_channels,
- )
- self.flow = ResidualCouplingBlock(
- inter_channels, hidden_channels, 5, 1, 3, gin_channels=gin_channels
- )
- self.emb_g = nn.Embedding(self.spk_embed_dim, gin_channels)
- print("gin_channels:", gin_channels, "self.spk_embed_dim:", self.spk_embed_dim)
-
- def remove_weight_norm(self):
- self.dec.remove_weight_norm()
- self.flow.remove_weight_norm()
- self.enc_q.remove_weight_norm()
-
- def forward(
- self, phone, phone_lengths, pitch, pitchf, y, y_lengths, ds
- ): # 这里ds是id,[bs,1]
- # print(1,pitch.shape)#[bs,t]
- g = self.emb_g(ds).unsqueeze(-1) # [b, 256, 1]##1是t,广播的
- m_p, logs_p, x_mask = self.enc_p(phone, pitch, phone_lengths)
- z, m_q, logs_q, y_mask = self.enc_q(y, y_lengths, g=g)
- z_p = self.flow(z, y_mask, g=g)
- z_slice, ids_slice = commons.rand_slice_segments(
- z, y_lengths, self.segment_size
- )
- # print(-1,pitchf.shape,ids_slice,self.segment_size,self.hop_length,self.segment_size//self.hop_length)
- pitchf = commons.slice_segments2(pitchf, ids_slice, self.segment_size)
- # print(-2,pitchf.shape,z_slice.shape)
- o = self.dec(z_slice, pitchf, g=g)
- return o, ids_slice, x_mask, y_mask, (z, z_p, m_p, logs_p, m_q, logs_q)
-
- def infer(self, phone, phone_lengths, pitch, nsff0, sid, rate=None):
- g = self.emb_g(sid).unsqueeze(-1)
- m_p, logs_p, x_mask = self.enc_p(phone, pitch, phone_lengths)
- z_p = (m_p + torch.exp(logs_p) * torch.randn_like(m_p) * 0.66666) * x_mask
- if rate:
- head = int(z_p.shape[2] * rate)
- z_p = z_p[:, :, -head:]
- x_mask = x_mask[:, :, -head:]
- nsff0 = nsff0[:, -head:]
- z = self.flow(z_p, x_mask, g=g, reverse=True)
- o = self.dec(z * x_mask, nsff0, g=g)
- return o, x_mask, (z, z_p, m_p, logs_p)
-
-
-class SynthesizerTrnMs768NSFsid(nn.Module):
- def __init__(
- self,
- spec_channels,
- segment_size,
- inter_channels,
- hidden_channels,
- filter_channels,
- n_heads,
- n_layers,
- kernel_size,
- p_dropout,
- resblock,
- resblock_kernel_sizes,
- resblock_dilation_sizes,
- upsample_rates,
- upsample_initial_channel,
- upsample_kernel_sizes,
- spk_embed_dim,
- gin_channels,
- sr,
- **kwargs
- ):
- super().__init__()
- if type(sr) == type("strr"):
- sr = sr2sr[sr]
- self.spec_channels = spec_channels
- self.inter_channels = inter_channels
- self.hidden_channels = hidden_channels
- self.filter_channels = filter_channels
- self.n_heads = n_heads
- self.n_layers = n_layers
- self.kernel_size = kernel_size
- self.p_dropout = p_dropout
- self.resblock = resblock
- self.resblock_kernel_sizes = resblock_kernel_sizes
- self.resblock_dilation_sizes = resblock_dilation_sizes
- self.upsample_rates = upsample_rates
- self.upsample_initial_channel = upsample_initial_channel
- self.upsample_kernel_sizes = upsample_kernel_sizes
- self.segment_size = segment_size
- self.gin_channels = gin_channels
- # self.hop_length = hop_length#
- self.spk_embed_dim = spk_embed_dim
- self.enc_p = TextEncoder768(
- inter_channels,
- hidden_channels,
- filter_channels,
- n_heads,
- n_layers,
- kernel_size,
- p_dropout,
- )
- self.dec = GeneratorNSF(
- inter_channels,
- resblock,
- resblock_kernel_sizes,
- resblock_dilation_sizes,
- upsample_rates,
- upsample_initial_channel,
- upsample_kernel_sizes,
- gin_channels=gin_channels,
- sr=sr,
- is_half=kwargs["is_half"],
- )
- self.enc_q = PosteriorEncoder(
- spec_channels,
- inter_channels,
- hidden_channels,
- 5,
- 1,
- 16,
- gin_channels=gin_channels,
- )
- self.flow = ResidualCouplingBlock(
- inter_channels, hidden_channels, 5, 1, 3, gin_channels=gin_channels
- )
- self.emb_g = nn.Embedding(self.spk_embed_dim, gin_channels)
- print("gin_channels:", gin_channels, "self.spk_embed_dim:", self.spk_embed_dim)
-
- def remove_weight_norm(self):
- self.dec.remove_weight_norm()
- self.flow.remove_weight_norm()
- self.enc_q.remove_weight_norm()
-
- def forward(
- self, phone, phone_lengths, pitch, pitchf, y, y_lengths, ds
- ): # 这里ds是id,[bs,1]
- # print(1,pitch.shape)#[bs,t]
- g = self.emb_g(ds).unsqueeze(-1) # [b, 256, 1]##1是t,广播的
- m_p, logs_p, x_mask = self.enc_p(phone, pitch, phone_lengths)
- z, m_q, logs_q, y_mask = self.enc_q(y, y_lengths, g=g)
- z_p = self.flow(z, y_mask, g=g)
- z_slice, ids_slice = commons.rand_slice_segments(
- z, y_lengths, self.segment_size
- )
- # print(-1,pitchf.shape,ids_slice,self.segment_size,self.hop_length,self.segment_size//self.hop_length)
- pitchf = commons.slice_segments2(pitchf, ids_slice, self.segment_size)
- # print(-2,pitchf.shape,z_slice.shape)
- o = self.dec(z_slice, pitchf, g=g)
- return o, ids_slice, x_mask, y_mask, (z, z_p, m_p, logs_p, m_q, logs_q)
-
- def infer(self, phone, phone_lengths, pitch, nsff0, sid, rate=None):
- g = self.emb_g(sid).unsqueeze(-1)
- m_p, logs_p, x_mask = self.enc_p(phone, pitch, phone_lengths)
- z_p = (m_p + torch.exp(logs_p) * torch.randn_like(m_p) * 0.66666) * x_mask
- if rate:
- head = int(z_p.shape[2] * rate)
- z_p = z_p[:, :, -head:]
- x_mask = x_mask[:, :, -head:]
- nsff0 = nsff0[:, -head:]
- z = self.flow(z_p, x_mask, g=g, reverse=True)
- o = self.dec(z * x_mask, nsff0, g=g)
- return o, x_mask, (z, z_p, m_p, logs_p)
-
-
-class SynthesizerTrnMs256NSFsid_nono(nn.Module):
- def __init__(
- self,
- spec_channels,
- segment_size,
- inter_channels,
- hidden_channels,
- filter_channels,
- n_heads,
- n_layers,
- kernel_size,
- p_dropout,
- resblock,
- resblock_kernel_sizes,
- resblock_dilation_sizes,
- upsample_rates,
- upsample_initial_channel,
- upsample_kernel_sizes,
- spk_embed_dim,
- gin_channels,
- sr=None,
- **kwargs
- ):
- super().__init__()
- self.spec_channels = spec_channels
- self.inter_channels = inter_channels
- self.hidden_channels = hidden_channels
- self.filter_channels = filter_channels
- self.n_heads = n_heads
- self.n_layers = n_layers
- self.kernel_size = kernel_size
- self.p_dropout = p_dropout
- self.resblock = resblock
- self.resblock_kernel_sizes = resblock_kernel_sizes
- self.resblock_dilation_sizes = resblock_dilation_sizes
- self.upsample_rates = upsample_rates
- self.upsample_initial_channel = upsample_initial_channel
- self.upsample_kernel_sizes = upsample_kernel_sizes
- self.segment_size = segment_size
- self.gin_channels = gin_channels
- # self.hop_length = hop_length#
- self.spk_embed_dim = spk_embed_dim
- self.enc_p = TextEncoder256(
- inter_channels,
- hidden_channels,
- filter_channels,
- n_heads,
- n_layers,
- kernel_size,
- p_dropout,
- f0=False,
- )
- self.dec = Generator(
- inter_channels,
- resblock,
- resblock_kernel_sizes,
- resblock_dilation_sizes,
- upsample_rates,
- upsample_initial_channel,
- upsample_kernel_sizes,
- gin_channels=gin_channels,
- )
- self.enc_q = PosteriorEncoder(
- spec_channels,
- inter_channels,
- hidden_channels,
- 5,
- 1,
- 16,
- gin_channels=gin_channels,
- )
- self.flow = ResidualCouplingBlock(
- inter_channels, hidden_channels, 5, 1, 3, gin_channels=gin_channels
- )
- self.emb_g = nn.Embedding(self.spk_embed_dim, gin_channels)
- print("gin_channels:", gin_channels, "self.spk_embed_dim:", self.spk_embed_dim)
-
- def remove_weight_norm(self):
- self.dec.remove_weight_norm()
- self.flow.remove_weight_norm()
- self.enc_q.remove_weight_norm()
-
- def forward(self, phone, phone_lengths, y, y_lengths, ds): # 这里ds是id,[bs,1]
- g = self.emb_g(ds).unsqueeze(-1) # [b, 256, 1]##1是t,广播的
- m_p, logs_p, x_mask = self.enc_p(phone, None, phone_lengths)
- z, m_q, logs_q, y_mask = self.enc_q(y, y_lengths, g=g)
- z_p = self.flow(z, y_mask, g=g)
- z_slice, ids_slice = commons.rand_slice_segments(
- z, y_lengths, self.segment_size
- )
- o = self.dec(z_slice, g=g)
- return o, ids_slice, x_mask, y_mask, (z, z_p, m_p, logs_p, m_q, logs_q)
-
- def infer(self, phone, phone_lengths, sid, rate=None):
- g = self.emb_g(sid).unsqueeze(-1)
- m_p, logs_p, x_mask = self.enc_p(phone, None, phone_lengths)
- z_p = (m_p + torch.exp(logs_p) * torch.randn_like(m_p) * 0.66666) * x_mask
- if rate:
- head = int(z_p.shape[2] * rate)
- z_p = z_p[:, :, -head:]
- x_mask = x_mask[:, :, -head:]
- z = self.flow(z_p, x_mask, g=g, reverse=True)
- o = self.dec(z * x_mask, g=g)
- return o, x_mask, (z, z_p, m_p, logs_p)
-
-
-class SynthesizerTrnMs768NSFsid_nono(nn.Module):
- def __init__(
- self,
- spec_channels,
- segment_size,
- inter_channels,
- hidden_channels,
- filter_channels,
- n_heads,
- n_layers,
- kernel_size,
- p_dropout,
- resblock,
- resblock_kernel_sizes,
- resblock_dilation_sizes,
- upsample_rates,
- upsample_initial_channel,
- upsample_kernel_sizes,
- spk_embed_dim,
- gin_channels,
- sr=None,
- **kwargs
- ):
- super().__init__()
- self.spec_channels = spec_channels
- self.inter_channels = inter_channels
- self.hidden_channels = hidden_channels
- self.filter_channels = filter_channels
- self.n_heads = n_heads
- self.n_layers = n_layers
- self.kernel_size = kernel_size
- self.p_dropout = p_dropout
- self.resblock = resblock
- self.resblock_kernel_sizes = resblock_kernel_sizes
- self.resblock_dilation_sizes = resblock_dilation_sizes
- self.upsample_rates = upsample_rates
- self.upsample_initial_channel = upsample_initial_channel
- self.upsample_kernel_sizes = upsample_kernel_sizes
- self.segment_size = segment_size
- self.gin_channels = gin_channels
- # self.hop_length = hop_length#
- self.spk_embed_dim = spk_embed_dim
- self.enc_p = TextEncoder768(
- inter_channels,
- hidden_channels,
- filter_channels,
- n_heads,
- n_layers,
- kernel_size,
- p_dropout,
- f0=False,
- )
- self.dec = Generator(
- inter_channels,
- resblock,
- resblock_kernel_sizes,
- resblock_dilation_sizes,
- upsample_rates,
- upsample_initial_channel,
- upsample_kernel_sizes,
- gin_channels=gin_channels,
- )
- self.enc_q = PosteriorEncoder(
- spec_channels,
- inter_channels,
- hidden_channels,
- 5,
- 1,
- 16,
- gin_channels=gin_channels,
- )
- self.flow = ResidualCouplingBlock(
- inter_channels, hidden_channels, 5, 1, 3, gin_channels=gin_channels
- )
- self.emb_g = nn.Embedding(self.spk_embed_dim, gin_channels)
- print("gin_channels:", gin_channels, "self.spk_embed_dim:", self.spk_embed_dim)
-
- def remove_weight_norm(self):
- self.dec.remove_weight_norm()
- self.flow.remove_weight_norm()
- self.enc_q.remove_weight_norm()
-
- def forward(self, phone, phone_lengths, y, y_lengths, ds): # 这里ds是id,[bs,1]
- g = self.emb_g(ds).unsqueeze(-1) # [b, 256, 1]##1是t,广播的
- m_p, logs_p, x_mask = self.enc_p(phone, None, phone_lengths)
- z, m_q, logs_q, y_mask = self.enc_q(y, y_lengths, g=g)
- z_p = self.flow(z, y_mask, g=g)
- z_slice, ids_slice = commons.rand_slice_segments(
- z, y_lengths, self.segment_size
- )
- o = self.dec(z_slice, g=g)
- return o, ids_slice, x_mask, y_mask, (z, z_p, m_p, logs_p, m_q, logs_q)
-
- def infer(self, phone, phone_lengths, sid, rate=None):
- g = self.emb_g(sid).unsqueeze(-1)
- m_p, logs_p, x_mask = self.enc_p(phone, None, phone_lengths)
- z_p = (m_p + torch.exp(logs_p) * torch.randn_like(m_p) * 0.66666) * x_mask
- if rate:
- head = int(z_p.shape[2] * rate)
- z_p = z_p[:, :, -head:]
- x_mask = x_mask[:, :, -head:]
- z = self.flow(z_p, x_mask, g=g, reverse=True)
- o = self.dec(z * x_mask, g=g)
- return o, x_mask, (z, z_p, m_p, logs_p)
-
-
-class MultiPeriodDiscriminator(torch.nn.Module):
- def __init__(self, use_spectral_norm=False):
- super(MultiPeriodDiscriminator, self).__init__()
- periods = [2, 3, 5, 7, 11, 17]
- # periods = [3, 5, 7, 11, 17, 23, 37]
-
- discs = [DiscriminatorS(use_spectral_norm=use_spectral_norm)]
- discs = discs + [
- DiscriminatorP(i, use_spectral_norm=use_spectral_norm) for i in periods
- ]
- self.discriminators = nn.ModuleList(discs)
-
- def forward(self, y, y_hat):
- y_d_rs = [] #
- y_d_gs = []
- fmap_rs = []
- fmap_gs = []
- for i, d in enumerate(self.discriminators):
- y_d_r, fmap_r = d(y)
- y_d_g, fmap_g = d(y_hat)
- # for j in range(len(fmap_r)):
- # print(i,j,y.shape,y_hat.shape,fmap_r[j].shape,fmap_g[j].shape)
- y_d_rs.append(y_d_r)
- y_d_gs.append(y_d_g)
- fmap_rs.append(fmap_r)
- fmap_gs.append(fmap_g)
-
- return y_d_rs, y_d_gs, fmap_rs, fmap_gs
-
-
-class MultiPeriodDiscriminatorV2(torch.nn.Module):
- def __init__(self, use_spectral_norm=False):
- super(MultiPeriodDiscriminatorV2, self).__init__()
- # periods = [2, 3, 5, 7, 11, 17]
- periods = [2, 3, 5, 7, 11, 17, 23, 37]
-
- discs = [DiscriminatorS(use_spectral_norm=use_spectral_norm)]
- discs = discs + [
- DiscriminatorP(i, use_spectral_norm=use_spectral_norm) for i in periods
- ]
- self.discriminators = nn.ModuleList(discs)
-
- def forward(self, y, y_hat):
- y_d_rs = [] #
- y_d_gs = []
- fmap_rs = []
- fmap_gs = []
- for i, d in enumerate(self.discriminators):
- y_d_r, fmap_r = d(y)
- y_d_g, fmap_g = d(y_hat)
- # for j in range(len(fmap_r)):
- # print(i,j,y.shape,y_hat.shape,fmap_r[j].shape,fmap_g[j].shape)
- y_d_rs.append(y_d_r)
- y_d_gs.append(y_d_g)
- fmap_rs.append(fmap_r)
- fmap_gs.append(fmap_g)
-
- return y_d_rs, y_d_gs, fmap_rs, fmap_gs
-
-
-class DiscriminatorS(torch.nn.Module):
- def __init__(self, use_spectral_norm=False):
- super(DiscriminatorS, self).__init__()
- norm_f = weight_norm if use_spectral_norm == False else spectral_norm
- self.convs = nn.ModuleList(
- [
- norm_f(Conv1d(1, 16, 15, 1, padding=7)),
- norm_f(Conv1d(16, 64, 41, 4, groups=4, padding=20)),
- norm_f(Conv1d(64, 256, 41, 4, groups=16, padding=20)),
- norm_f(Conv1d(256, 1024, 41, 4, groups=64, padding=20)),
- norm_f(Conv1d(1024, 1024, 41, 4, groups=256, padding=20)),
- norm_f(Conv1d(1024, 1024, 5, 1, padding=2)),
- ]
- )
- self.conv_post = norm_f(Conv1d(1024, 1, 3, 1, padding=1))
-
- def forward(self, x):
- fmap = []
-
- for l in self.convs:
- x = l(x)
- x = F.leaky_relu(x, modules.LRELU_SLOPE)
- fmap.append(x)
- x = self.conv_post(x)
- fmap.append(x)
- x = torch.flatten(x, 1, -1)
-
- return x, fmap
-
-
-class DiscriminatorP(torch.nn.Module):
- def __init__(self, period, kernel_size=5, stride=3, use_spectral_norm=False):
- super(DiscriminatorP, self).__init__()
- self.period = period
- self.use_spectral_norm = use_spectral_norm
- norm_f = weight_norm if use_spectral_norm == False else spectral_norm
- self.convs = nn.ModuleList(
- [
- norm_f(
- Conv2d(
- 1,
- 32,
- (kernel_size, 1),
- (stride, 1),
- padding=(get_padding(kernel_size, 1), 0),
- )
- ),
- norm_f(
- Conv2d(
- 32,
- 128,
- (kernel_size, 1),
- (stride, 1),
- padding=(get_padding(kernel_size, 1), 0),
- )
- ),
- norm_f(
- Conv2d(
- 128,
- 512,
- (kernel_size, 1),
- (stride, 1),
- padding=(get_padding(kernel_size, 1), 0),
- )
- ),
- norm_f(
- Conv2d(
- 512,
- 1024,
- (kernel_size, 1),
- (stride, 1),
- padding=(get_padding(kernel_size, 1), 0),
- )
- ),
- norm_f(
- Conv2d(
- 1024,
- 1024,
- (kernel_size, 1),
- 1,
- padding=(get_padding(kernel_size, 1), 0),
- )
- ),
- ]
- )
- self.conv_post = norm_f(Conv2d(1024, 1, (3, 1), 1, padding=(1, 0)))
-
- def forward(self, x):
- fmap = []
-
- # 1d to 2d
- b, c, t = x.shape
- if t % self.period != 0: # pad first
- n_pad = self.period - (t % self.period)
- x = F.pad(x, (0, n_pad), "reflect")
- t = t + n_pad
- x = x.view(b, c, t // self.period, self.period)
-
- for l in self.convs:
- x = l(x)
- x = F.leaky_relu(x, modules.LRELU_SLOPE)
- fmap.append(x)
- x = self.conv_post(x)
- fmap.append(x)
- x = torch.flatten(x, 1, -1)
-
- return x, fmap
diff --git a/spaces/ismot/1702t1/dataset/__init__.py b/spaces/ismot/1702t1/dataset/__init__.py
deleted file mode 100644
index e69de29bb2d1d6434b8b29ae775ad8c2e48c5391..0000000000000000000000000000000000000000
diff --git a/spaces/jackli888/stable-diffusion-webui/extensions/deforum/scripts/deforum_helpers/src/adabins/unet_adaptive_bins.py b/spaces/jackli888/stable-diffusion-webui/extensions/deforum/scripts/deforum_helpers/src/adabins/unet_adaptive_bins.py
deleted file mode 100644
index 733927795146fe13563d07d20fbb461da596a181..0000000000000000000000000000000000000000
--- a/spaces/jackli888/stable-diffusion-webui/extensions/deforum/scripts/deforum_helpers/src/adabins/unet_adaptive_bins.py
+++ /dev/null
@@ -1,154 +0,0 @@
-import torch
-import torch.nn as nn
-import torch.nn.functional as F
-import os
-from pathlib import Path
-
-from .miniViT import mViT
-
-
-class UpSampleBN(nn.Module):
- def __init__(self, skip_input, output_features):
- super(UpSampleBN, self).__init__()
-
- self._net = nn.Sequential(nn.Conv2d(skip_input, output_features, kernel_size=3, stride=1, padding=1),
- nn.BatchNorm2d(output_features),
- nn.LeakyReLU(),
- nn.Conv2d(output_features, output_features, kernel_size=3, stride=1, padding=1),
- nn.BatchNorm2d(output_features),
- nn.LeakyReLU())
-
- def forward(self, x, concat_with):
- up_x = F.interpolate(x, size=[concat_with.size(2), concat_with.size(3)], mode='bilinear', align_corners=True)
- f = torch.cat([up_x, concat_with], dim=1)
- return self._net(f)
-
-
-class DecoderBN(nn.Module):
- def __init__(self, num_features=2048, num_classes=1, bottleneck_features=2048):
- super(DecoderBN, self).__init__()
- features = int(num_features)
-
- self.conv2 = nn.Conv2d(bottleneck_features, features, kernel_size=1, stride=1, padding=1)
-
- self.up1 = UpSampleBN(skip_input=features // 1 + 112 + 64, output_features=features // 2)
- self.up2 = UpSampleBN(skip_input=features // 2 + 40 + 24, output_features=features // 4)
- self.up3 = UpSampleBN(skip_input=features // 4 + 24 + 16, output_features=features // 8)
- self.up4 = UpSampleBN(skip_input=features // 8 + 16 + 8, output_features=features // 16)
-
- # self.up5 = UpSample(skip_input=features // 16 + 3, output_features=features//16)
- self.conv3 = nn.Conv2d(features // 16, num_classes, kernel_size=3, stride=1, padding=1)
- # self.act_out = nn.Softmax(dim=1) if output_activation == 'softmax' else nn.Identity()
-
- def forward(self, features):
- x_block0, x_block1, x_block2, x_block3, x_block4 = features[4], features[5], features[6], features[8], features[
- 11]
-
- x_d0 = self.conv2(x_block4)
-
- x_d1 = self.up1(x_d0, x_block3)
- x_d2 = self.up2(x_d1, x_block2)
- x_d3 = self.up3(x_d2, x_block1)
- x_d4 = self.up4(x_d3, x_block0)
- # x_d5 = self.up5(x_d4, features[0])
- out = self.conv3(x_d4)
- # out = self.act_out(out)
- # if with_features:
- # return out, features[-1]
- # elif with_intermediate:
- # return out, [x_block0, x_block1, x_block2, x_block3, x_block4, x_d1, x_d2, x_d3, x_d4]
- return out
-
-
-class Encoder(nn.Module):
- def __init__(self, backend):
- super(Encoder, self).__init__()
- self.original_model = backend
-
- def forward(self, x):
- features = [x]
- for k, v in self.original_model._modules.items():
- if (k == 'blocks'):
- for ki, vi in v._modules.items():
- features.append(vi(features[-1]))
- else:
- features.append(v(features[-1]))
- return features
-
-
-class UnetAdaptiveBins(nn.Module):
- def __init__(self, backend, n_bins=100, min_val=0.1, max_val=10, norm='linear'):
- super(UnetAdaptiveBins, self).__init__()
- self.num_classes = n_bins
- self.min_val = min_val
- self.max_val = max_val
- self.encoder = Encoder(backend)
- self.adaptive_bins_layer = mViT(128, n_query_channels=128, patch_size=16,
- dim_out=n_bins,
- embedding_dim=128, norm=norm)
-
- self.decoder = DecoderBN(num_classes=128)
- self.conv_out = nn.Sequential(nn.Conv2d(128, n_bins, kernel_size=1, stride=1, padding=0),
- nn.Softmax(dim=1))
-
- def forward(self, x, **kwargs):
- unet_out = self.decoder(self.encoder(x), **kwargs)
- bin_widths_normed, range_attention_maps = self.adaptive_bins_layer(unet_out)
- out = self.conv_out(range_attention_maps)
-
- # Post process
- # n, c, h, w = out.shape
- # hist = torch.sum(out.view(n, c, h * w), dim=2) / (h * w) # not used for training
-
- bin_widths = (self.max_val - self.min_val) * bin_widths_normed # .shape = N, dim_out
- bin_widths = nn.functional.pad(bin_widths, (1, 0), mode='constant', value=self.min_val)
- bin_edges = torch.cumsum(bin_widths, dim=1)
-
- centers = 0.5 * (bin_edges[:, :-1] + bin_edges[:, 1:])
- n, dout = centers.size()
- centers = centers.view(n, dout, 1, 1)
-
- pred = torch.sum(out * centers, dim=1, keepdim=True)
-
- return bin_edges, pred
-
- def get_1x_lr_params(self): # lr/10 learning rate
- return self.encoder.parameters()
-
- def get_10x_lr_params(self): # lr learning rate
- modules = [self.decoder, self.adaptive_bins_layer, self.conv_out]
- for m in modules:
- yield from m.parameters()
-
- @classmethod
- def build(cls, n_bins, **kwargs):
- basemodel_name = 'tf_efficientnet_b5_ap'
-
- print('Loading base model ()...'.format(basemodel_name), end='')
- predicted_torch_model_cache_path = str(Path.home()) + '\\.cache\\torch\\hub\\rwightman_gen-efficientnet-pytorch_master'
- predicted_gep_cache_testilfe = Path(predicted_torch_model_cache_path + '\\hubconf.py')
- #print(f"predicted_gep_cache_testilfe: {predicted_gep_cache_testilfe}")
- # try to fetch the models from cache, and only if it can't be find, download from the internet (to enable offline usage)
- if os.path.isfile(predicted_gep_cache_testilfe):
- basemodel = torch.hub.load(predicted_torch_model_cache_path, basemodel_name, pretrained=True, source = 'local')
- else:
- basemodel = torch.hub.load('rwightman/gen-efficientnet-pytorch', basemodel_name, pretrained=True)
- print('Done.')
-
- # Remove last layer
- print('Removing last two layers (global_pool & classifier).')
- basemodel.global_pool = nn.Identity()
- basemodel.classifier = nn.Identity()
-
- # Building Encoder-Decoder model
- print('Building Encoder-Decoder model..', end='')
- m = cls(basemodel, n_bins=n_bins, **kwargs)
- print('Done.')
- return m
-
-
-if __name__ == '__main__':
- model = UnetAdaptiveBins.build(100)
- x = torch.rand(2, 3, 480, 640)
- bins, pred = model(x)
- print(bins.shape, pred.shape)
diff --git a/spaces/jaklin/text_generator/README.md b/spaces/jaklin/text_generator/README.md
deleted file mode 100644
index 1a128e3225123750ed9c7d0f940108a07c65b93e..0000000000000000000000000000000000000000
--- a/spaces/jaklin/text_generator/README.md
+++ /dev/null
@@ -1,12 +0,0 @@
----
-title: Text Generator
-emoji: 💩
-colorFrom: indigo
-colorTo: purple
-sdk: gradio
-sdk_version: 3.11.0
-app_file: app.py
-pinned: false
----
-
-Check out the configuration reference at https://huggingface.co/docs/hub/spaces-config-reference
diff --git a/spaces/james-oldfield/PandA/networks/biggan/config.py b/spaces/james-oldfield/PandA/networks/biggan/config.py
deleted file mode 100644
index 454236a4bfa0d11fda0d52e0ce9b2926f8c32d30..0000000000000000000000000000000000000000
--- a/spaces/james-oldfield/PandA/networks/biggan/config.py
+++ /dev/null
@@ -1,70 +0,0 @@
-# coding: utf-8
-"""
-BigGAN config.
-"""
-from __future__ import (absolute_import, division, print_function, unicode_literals)
-
-import copy
-import json
-
-class BigGANConfig(object):
- """ Configuration class to store the configuration of a `BigGAN`.
- Defaults are for the 128x128 model.
- layers tuple are (up-sample in the layer ?, input channels, output channels)
- """
- def __init__(self,
- output_dim=128,
- z_dim=128,
- class_embed_dim=128,
- channel_width=128,
- num_classes=1000,
- layers=[(False, 16, 16),
- (True, 16, 16),
- (False, 16, 16),
- (True, 16, 8),
- (False, 8, 8),
- (True, 8, 4),
- (False, 4, 4),
- (True, 4, 2),
- (False, 2, 2),
- (True, 2, 1)],
- attention_layer_position=8,
- eps=1e-4,
- n_stats=51):
- """Constructs BigGANConfig. """
- self.output_dim = output_dim
- self.z_dim = z_dim
- self.class_embed_dim = class_embed_dim
- self.channel_width = channel_width
- self.num_classes = num_classes
- self.layers = layers
- self.attention_layer_position = attention_layer_position
- self.eps = eps
- self.n_stats = n_stats
-
- @classmethod
- def from_dict(cls, json_object):
- """Constructs a `BigGANConfig` from a Python dictionary of parameters."""
- config = BigGANConfig()
- for key, value in json_object.items():
- config.__dict__[key] = value
- return config
-
- @classmethod
- def from_json_file(cls, json_file):
- """Constructs a `BigGANConfig` from a json file of parameters."""
- with open(json_file, "r", encoding='utf-8') as reader:
- text = reader.read()
- return cls.from_dict(json.loads(text))
-
- def __repr__(self):
- return str(self.to_json_string())
-
- def to_dict(self):
- """Serializes this instance to a Python dictionary."""
- output = copy.deepcopy(self.__dict__)
- return output
-
- def to_json_string(self):
- """Serializes this instance to a JSON string."""
- return json.dumps(self.to_dict(), indent=2, sort_keys=True) + "\n"
diff --git a/spaces/james-oldfield/PandA/networks/genforce/models/stylegan_generator.py b/spaces/james-oldfield/PandA/networks/genforce/models/stylegan_generator.py
deleted file mode 100644
index 59a75bfc71c5556d24a98c937b4fd4e2c8a33df7..0000000000000000000000000000000000000000
--- a/spaces/james-oldfield/PandA/networks/genforce/models/stylegan_generator.py
+++ /dev/null
@@ -1,946 +0,0 @@
-# python3.7
-"""Contains the implementation of generator described in StyleGAN.
-
-Paper: https://arxiv.org/pdf/1812.04948.pdf
-
-Official TensorFlow implementation: https://github.com/NVlabs/stylegan
-"""
-
-import numpy as np
-
-import torch
-import torch.nn as nn
-import torch.nn.functional as F
-
-from .sync_op import all_gather
-
-__all__ = ['StyleGANGenerator']
-
-# Resolutions allowed.
-_RESOLUTIONS_ALLOWED = [8, 16, 32, 64, 128, 256, 512, 1024]
-
-# Initial resolution.
-_INIT_RES = 4
-
-# Fused-scale options allowed.
-_FUSED_SCALE_ALLOWED = [True, False, 'auto']
-
-# Minimal resolution for `auto` fused-scale strategy.
-_AUTO_FUSED_SCALE_MIN_RES = 128
-
-# Default gain factor for weight scaling.
-_WSCALE_GAIN = np.sqrt(2.0)
-_STYLEMOD_WSCALE_GAIN = 1.0
-
-
-class StyleGANGenerator(nn.Module):
- """Defines the generator network in StyleGAN.
-
- NOTE: The synthesized images are with `RGB` channel order and pixel range
- [-1, 1].
-
- Settings for the mapping network:
-
- (1) z_space_dim: Dimension of the input latent space, Z. (default: 512)
- (2) w_space_dim: Dimension of the outout latent space, W. (default: 512)
- (3) label_size: Size of the additional label for conditional generation.
- (default: 0)
- (4)mapping_layers: Number of layers of the mapping network. (default: 8)
- (5) mapping_fmaps: Number of hidden channels of the mapping network.
- (default: 512)
- (6) mapping_lr_mul: Learning rate multiplier for the mapping network.
- (default: 0.01)
- (7) repeat_w: Repeat w-code for different layers.
-
- Settings for the synthesis network:
-
- (1) resolution: The resolution of the output image.
- (2) image_channels: Number of channels of the output image. (default: 3)
- (3) final_tanh: Whether to use `tanh` to control the final pixel range.
- (default: False)
- (4) const_input: Whether to use a constant in the first convolutional layer.
- (default: True)
- (5) fused_scale: Whether to fused `upsample` and `conv2d` together,
- resulting in `conv2d_transpose`. (default: `auto`)
- (6) use_wscale: Whether to use weight scaling. (default: True)
- (7) noise_type: Type of noise added to the convolutional results at each
- layer. (default: `spatial`)
- (8) fmaps_base: Factor to control number of feature maps for each layer.
- (default: 16 << 10)
- (9) fmaps_max: Maximum number of feature maps in each layer. (default: 512)
- """
-
- def __init__(self,
- resolution,
- z_space_dim=512,
- w_space_dim=512,
- label_size=0,
- mapping_layers=8,
- mapping_fmaps=512,
- mapping_lr_mul=0.01,
- repeat_w=True,
- image_channels=3,
- final_tanh=False,
- const_input=True,
- fused_scale='auto',
- use_wscale=True,
- noise_type='spatial',
- fmaps_base=16 << 10,
- fmaps_max=512):
- """Initializes with basic settings.
-
- Raises:
- ValueError: If the `resolution` is not supported, or `fused_scale`
- is not supported.
- """
- super().__init__()
-
- if resolution not in _RESOLUTIONS_ALLOWED:
- raise ValueError(f'Invalid resolution: `{resolution}`!\n'
- f'Resolutions allowed: {_RESOLUTIONS_ALLOWED}.')
- if fused_scale not in _FUSED_SCALE_ALLOWED:
- raise ValueError(f'Invalid fused-scale option: `{fused_scale}`!\n'
- f'Options allowed: {_FUSED_SCALE_ALLOWED}.')
-
- self.init_res = _INIT_RES
- self.resolution = resolution
- self.z_space_dim = z_space_dim
- self.w_space_dim = w_space_dim
- self.label_size = label_size
- self.mapping_layers = mapping_layers
- self.mapping_fmaps = mapping_fmaps
- self.mapping_lr_mul = mapping_lr_mul
- self.repeat_w = repeat_w
- self.image_channels = image_channels
- self.final_tanh = final_tanh
- self.const_input = const_input
- self.fused_scale = fused_scale
- self.use_wscale = use_wscale
- self.noise_type = noise_type
- self.fmaps_base = fmaps_base
- self.fmaps_max = fmaps_max
-
- self.num_layers = int(np.log2(self.resolution // self.init_res * 2)) * 2
-
- if self.repeat_w:
- self.mapping_space_dim = self.w_space_dim
- else:
- self.mapping_space_dim = self.w_space_dim * self.num_layers
- self.mapping = MappingModule(input_space_dim=self.z_space_dim,
- hidden_space_dim=self.mapping_fmaps,
- final_space_dim=self.mapping_space_dim,
- label_size=self.label_size,
- num_layers=self.mapping_layers,
- use_wscale=self.use_wscale,
- lr_mul=self.mapping_lr_mul)
-
- self.truncation = TruncationModule(w_space_dim=self.w_space_dim,
- num_layers=self.num_layers,
- repeat_w=self.repeat_w)
-
- self.synthesis = SynthesisModule(resolution=self.resolution,
- init_resolution=self.init_res,
- w_space_dim=self.w_space_dim,
- image_channels=self.image_channels,
- final_tanh=self.final_tanh,
- const_input=self.const_input,
- fused_scale=self.fused_scale,
- use_wscale=self.use_wscale,
- noise_type=self.noise_type,
- fmaps_base=self.fmaps_base,
- fmaps_max=self.fmaps_max)
-
- self.pth_to_tf_var_mapping = {}
- for key, val in self.mapping.pth_to_tf_var_mapping.items():
- self.pth_to_tf_var_mapping[f'mapping.{key}'] = val
- for key, val in self.truncation.pth_to_tf_var_mapping.items():
- self.pth_to_tf_var_mapping[f'truncation.{key}'] = val
- for key, val in self.synthesis.pth_to_tf_var_mapping.items():
- self.pth_to_tf_var_mapping[f'synthesis.{key}'] = val
-
- def set_space_of_latent(self, space_of_latent='w'):
- """Sets the space to which the latent code belong.
-
- This function is particually used for choosing how to inject the latent
- code into the convolutional layers. The original generator will take a
- W-Space code and apply it for style modulation after an affine
- transformation. But, sometimes, it may need to directly feed an already
- affine-transformed code into the convolutional layer, e.g., when
- training an encoder for GAN inversion. We term the transformed space as
- Style Space (or Y-Space). This function is designed to tell the
- convolutional layers how to use the input code.
-
- Args:
- space_of_latent: The space to which the latent code belong. Case
- insensitive. (default: 'w')
- """
- for module in self.modules():
- if isinstance(module, StyleModLayer):
- setattr(module, 'space_of_latent', space_of_latent)
-
- def forward(self,
- z,
- label=None,
- lod=None,
- w_moving_decay=0.995,
- style_mixing_prob=0.9,
- trunc_psi=None,
- trunc_layers=None,
- randomize_noise=False,
- **_unused_kwargs):
- mapping_results = self.mapping(z, label)
- w = mapping_results['w']
-
- if self.training and w_moving_decay < 1:
- batch_w_avg = all_gather(w).mean(dim=0)
- self.truncation.w_avg.copy_(
- self.truncation.w_avg * w_moving_decay +
- batch_w_avg * (1 - w_moving_decay))
-
- if self.training and style_mixing_prob > 0:
- new_z = torch.randn_like(z)
- new_w = self.mapping(new_z, label)['w']
- lod = self.synthesis.lod.cpu().tolist() if lod is None else lod
- current_layers = self.num_layers - int(lod) * 2
- if np.random.uniform() < style_mixing_prob:
- mixing_cutoff = np.random.randint(1, current_layers)
- w = self.truncation(w)
- new_w = self.truncation(new_w)
- w[:, mixing_cutoff:] = new_w[:, mixing_cutoff:]
-
- wp = self.truncation(w, trunc_psi, trunc_layers)
- synthesis_results = self.synthesis(wp, lod, randomize_noise)
-
- return {**mapping_results, **synthesis_results}
-
-
-class MappingModule(nn.Module):
- """Implements the latent space mapping module.
-
- Basically, this module executes several dense layers in sequence.
- """
-
- def __init__(self,
- input_space_dim=512,
- hidden_space_dim=512,
- final_space_dim=512,
- label_size=0,
- num_layers=8,
- normalize_input=True,
- use_wscale=True,
- lr_mul=0.01):
- super().__init__()
-
- self.input_space_dim = input_space_dim
- self.hidden_space_dim = hidden_space_dim
- self.final_space_dim = final_space_dim
- self.label_size = label_size
- self.num_layers = num_layers
- self.normalize_input = normalize_input
- self.use_wscale = use_wscale
- self.lr_mul = lr_mul
-
- self.norm = PixelNormLayer() if self.normalize_input else nn.Identity()
-
- self.pth_to_tf_var_mapping = {}
- for i in range(num_layers):
- dim_mul = 2 if label_size else 1
- in_channels = (input_space_dim * dim_mul if i == 0 else
- hidden_space_dim)
- out_channels = (final_space_dim if i == (num_layers - 1) else
- hidden_space_dim)
- self.add_module(f'dense{i}',
- DenseBlock(in_channels=in_channels,
- out_channels=out_channels,
- use_wscale=self.use_wscale,
- lr_mul=self.lr_mul))
- self.pth_to_tf_var_mapping[f'dense{i}.weight'] = f'Dense{i}/weight'
- self.pth_to_tf_var_mapping[f'dense{i}.bias'] = f'Dense{i}/bias'
- if label_size:
- self.label_weight = nn.Parameter(
- torch.randn(label_size, input_space_dim))
- self.pth_to_tf_var_mapping[f'label_weight'] = f'LabelConcat/weight'
-
- def forward(self, z, label=None):
- if z.ndim != 2 or z.shape[1] != self.input_space_dim:
- raise ValueError(f'Input latent code should be with shape '
- f'[batch_size, input_dim], where '
- f'`input_dim` equals to {self.input_space_dim}!\n'
- f'But `{z.shape}` is received!')
- if self.label_size:
- if label is None:
- raise ValueError(f'Model requires an additional label '
- f'(with size {self.label_size}) as input, '
- f'but no label is received!')
- if label.ndim != 2 or label.shape != (z.shape[0], self.label_size):
- raise ValueError(f'Input label should be with shape '
- f'[batch_size, label_size], where '
- f'`batch_size` equals to that of '
- f'latent codes ({z.shape[0]}) and '
- f'`label_size` equals to {self.label_size}!\n'
- f'But `{label.shape}` is received!')
- embedding = torch.matmul(label, self.label_weight)
- z = torch.cat((z, embedding), dim=1)
-
- z = self.norm(z)
- w = z
- for i in range(self.num_layers):
- w = self.__getattr__(f'dense{i}')(w)
- results = {
- 'z': z,
- 'label': label,
- 'w': w,
- }
- if self.label_size:
- results['embedding'] = embedding
- return results
-
-
-class TruncationModule(nn.Module):
- """Implements the truncation module.
-
- Truncation is executed as follows:
-
- For layers in range [0, truncation_layers), the truncated w-code is computed
- as
-
- w_new = w_avg + (w - w_avg) * truncation_psi
-
- To disable truncation, please set
- (1) truncation_psi = 1.0 (None) OR
- (2) truncation_layers = 0 (None)
-
- NOTE: The returned tensor is layer-wise style codes.
- """
-
- def __init__(self, w_space_dim, num_layers, repeat_w=True):
- super().__init__()
-
- self.num_layers = num_layers
- self.w_space_dim = w_space_dim
- self.repeat_w = repeat_w
-
- if self.repeat_w:
- self.register_buffer('w_avg', torch.zeros(w_space_dim))
- else:
- self.register_buffer('w_avg', torch.zeros(num_layers * w_space_dim))
- self.pth_to_tf_var_mapping = {'w_avg': 'dlatent_avg'}
-
- def forward(self, w, trunc_psi=None, trunc_layers=None):
- if w.ndim == 2:
- if self.repeat_w and w.shape[1] == self.w_space_dim:
- w = w.view(-1, 1, self.w_space_dim)
- wp = w.repeat(1, self.num_layers, 1)
- else:
- assert w.shape[1] == self.w_space_dim * self.num_layers
- wp = w.view(-1, self.num_layers, self.w_space_dim)
- else:
- wp = w
- assert wp.ndim == 3
- assert wp.shape[1:] == (self.num_layers, self.w_space_dim)
-
- trunc_psi = 1.0 if trunc_psi is None else trunc_psi
- trunc_layers = 0 if trunc_layers is None else trunc_layers
- if trunc_psi < 1.0 and trunc_layers > 0:
- layer_idx = np.arange(self.num_layers).reshape(1, -1, 1)
- coefs = np.ones_like(layer_idx, dtype=np.float32)
- coefs[layer_idx < trunc_layers] *= trunc_psi
- coefs = torch.from_numpy(coefs).to(wp)
- w_avg = self.w_avg.view(1, -1, self.w_space_dim)
- wp = w_avg + (wp - w_avg) * coefs
- return wp
-
-
-class SynthesisModule(nn.Module):
- """Implements the image synthesis module.
-
- Basically, this module executes several convolutional layers in sequence.
- """
-
- def __init__(self,
- resolution=1024,
- init_resolution=4,
- w_space_dim=512,
- image_channels=3,
- final_tanh=False,
- const_input=True,
- fused_scale='auto',
- use_wscale=True,
- noise_type='spatial',
- fmaps_base=16 << 10,
- fmaps_max=512):
- super().__init__()
-
- self.init_res = init_resolution
- self.init_res_log2 = int(np.log2(self.init_res))
- self.resolution = resolution
- self.final_res_log2 = int(np.log2(self.resolution))
- self.w_space_dim = w_space_dim
- self.image_channels = image_channels
- self.final_tanh = final_tanh
- self.const_input = const_input
- self.fused_scale = fused_scale
- self.use_wscale = use_wscale
- self.noise_type = noise_type
- self.fmaps_base = fmaps_base
- self.fmaps_max = fmaps_max
-
- self.num_layers = (self.final_res_log2 - self.init_res_log2 + 1) * 2
-
- # Level of detail (used for progressive training).
- self.register_buffer('lod', torch.zeros(()))
- self.pth_to_tf_var_mapping = {'lod': 'lod'}
-
- for res_log2 in range(self.init_res_log2, self.final_res_log2 + 1):
- res = 2 ** res_log2
- block_idx = res_log2 - self.init_res_log2
-
- # First convolution layer for each resolution.
- layer_name = f'layer{2 * block_idx}'
- if res == self.init_res:
- if self.const_input:
- self.add_module(layer_name,
- ConvBlock(in_channels=self.get_nf(res),
- out_channels=self.get_nf(res),
- resolution=self.init_res,
- w_space_dim=self.w_space_dim,
- position='const_init',
- use_wscale=self.use_wscale,
- noise_type=self.noise_type))
- tf_layer_name = 'Const'
- self.pth_to_tf_var_mapping[f'{layer_name}.const'] = (
- f'{res}x{res}/{tf_layer_name}/const')
- else:
- self.add_module(layer_name,
- ConvBlock(in_channels=self.w_space_dim,
- out_channels=self.get_nf(res),
- resolution=self.init_res,
- w_space_dim=self.w_space_dim,
- kernel_size=self.init_res,
- padding=self.init_res - 1,
- use_wscale=self.use_wscale,
- noise_type=self.noise_type))
- tf_layer_name = 'Dense'
- self.pth_to_tf_var_mapping[f'{layer_name}.weight'] = (
- f'{res}x{res}/{tf_layer_name}/weight')
- else:
- if self.fused_scale == 'auto':
- fused_scale = (res >= _AUTO_FUSED_SCALE_MIN_RES)
- else:
- fused_scale = self.fused_scale
- self.add_module(layer_name,
- ConvBlock(in_channels=self.get_nf(res // 2),
- out_channels=self.get_nf(res),
- resolution=res,
- w_space_dim=self.w_space_dim,
- upsample=True,
- fused_scale=fused_scale,
- use_wscale=self.use_wscale,
- noise_type=self.noise_type))
- tf_layer_name = 'Conv0_up'
- self.pth_to_tf_var_mapping[f'{layer_name}.weight'] = (
- f'{res}x{res}/{tf_layer_name}/weight')
- self.pth_to_tf_var_mapping[f'{layer_name}.bias'] = (
- f'{res}x{res}/{tf_layer_name}/bias')
- self.pth_to_tf_var_mapping[f'{layer_name}.style.weight'] = (
- f'{res}x{res}/{tf_layer_name}/StyleMod/weight')
- self.pth_to_tf_var_mapping[f'{layer_name}.style.bias'] = (
- f'{res}x{res}/{tf_layer_name}/StyleMod/bias')
- self.pth_to_tf_var_mapping[f'{layer_name}.apply_noise.weight'] = (
- f'{res}x{res}/{tf_layer_name}/Noise/weight')
- self.pth_to_tf_var_mapping[f'{layer_name}.apply_noise.noise'] = (
- f'noise{2 * block_idx}')
-
- # Second convolution layer for each resolution.
- layer_name = f'layer{2 * block_idx + 1}'
- self.add_module(layer_name,
- ConvBlock(in_channels=self.get_nf(res),
- out_channels=self.get_nf(res),
- resolution=res,
- w_space_dim=self.w_space_dim,
- use_wscale=self.use_wscale,
- noise_type=self.noise_type))
- tf_layer_name = 'Conv' if res == self.init_res else 'Conv1'
- self.pth_to_tf_var_mapping[f'{layer_name}.weight'] = (
- f'{res}x{res}/{tf_layer_name}/weight')
- self.pth_to_tf_var_mapping[f'{layer_name}.bias'] = (
- f'{res}x{res}/{tf_layer_name}/bias')
- self.pth_to_tf_var_mapping[f'{layer_name}.style.weight'] = (
- f'{res}x{res}/{tf_layer_name}/StyleMod/weight')
- self.pth_to_tf_var_mapping[f'{layer_name}.style.bias'] = (
- f'{res}x{res}/{tf_layer_name}/StyleMod/bias')
- self.pth_to_tf_var_mapping[f'{layer_name}.apply_noise.weight'] = (
- f'{res}x{res}/{tf_layer_name}/Noise/weight')
- self.pth_to_tf_var_mapping[f'{layer_name}.apply_noise.noise'] = (
- f'noise{2 * block_idx + 1}')
-
- # Output convolution layer for each resolution.
- self.add_module(f'output{block_idx}',
- ConvBlock(in_channels=self.get_nf(res),
- out_channels=self.image_channels,
- resolution=res,
- w_space_dim=self.w_space_dim,
- position='last',
- kernel_size=1,
- padding=0,
- use_wscale=self.use_wscale,
- wscale_gain=1.0,
- activation_type='linear'))
- self.pth_to_tf_var_mapping[f'output{block_idx}.weight'] = (
- f'ToRGB_lod{self.final_res_log2 - res_log2}/weight')
- self.pth_to_tf_var_mapping[f'output{block_idx}.bias'] = (
- f'ToRGB_lod{self.final_res_log2 - res_log2}/bias')
-
- self.upsample = UpsamplingLayer()
- self.final_activate = nn.Tanh() if final_tanh else nn.Identity()
-
- def get_nf(self, res):
- """Gets number of feature maps according to current resolution."""
- return min(self.fmaps_base // res, self.fmaps_max)
-
- def forward(self, wp, x=None, lod=None, randomize_noise=False, start=2, stop=None):
- stop = self.final_res_log2 + 1 if stop is None else stop
-
- lod = self.lod.cpu().tolist() if lod is None else lod
- if lod + self.init_res_log2 > self.final_res_log2:
- raise ValueError(f'Maximum level-of-detail (lod) is '
- f'{self.final_res_log2 - self.init_res_log2}, '
- f'but `{lod}` is received!')
-
- results = {'wp': wp}
-
- # for res_log2 in range(self.init_res_log2, self.final_res_log2 + 1):
- for res_log2 in range(start, stop):
- current_lod = self.final_res_log2 - res_log2
- if lod < current_lod + 1:
- block_idx = res_log2 - self.init_res_log2
- if block_idx == 0:
- if self.const_input:
- x, style = self.layer0(None, wp[:, 0], randomize_noise)
- else:
- x = wp[:, 0].view(-1, self.w_space_dim, 1, 1)
- x, style = self.layer0(x, wp[:, 0], randomize_noise)
- else:
- x, style = self.__getattr__(f'layer{2 * block_idx}')(
- x, wp[:, 2 * block_idx])
- results[f'style{2 * block_idx:02d}'] = style
- x, style = self.__getattr__(f'layer{2 * block_idx + 1}')(
- x, wp[:, 2 * block_idx + 1])
- results[f'style{2 * block_idx + 1:02d}'] = style
- if current_lod - 1 < lod <= current_lod:
- image = self.__getattr__(f'output{block_idx}')(x, None)
- elif current_lod < lod < current_lod + 1:
- alpha = np.ceil(lod) - lod
- image = (self.__getattr__(f'output{block_idx}')(x, None) * alpha
- + self.upsample(image) * (1 - alpha))
- elif lod >= current_lod + 1:
- image = self.upsample(image)
- results['image'] = self.final_activate(image) if res_log2 == self.final_res_log2 else None
- results['x'] = x
- return results
-
-
-class PixelNormLayer(nn.Module):
- """Implements pixel-wise feature vector normalization layer."""
-
- def __init__(self, epsilon=1e-8):
- super().__init__()
- self.eps = epsilon
-
- def forward(self, x):
- norm = torch.sqrt(torch.mean(x ** 2, dim=1, keepdim=True) + self.eps)
- return x / norm
-
-
-class InstanceNormLayer(nn.Module):
- """Implements instance normalization layer."""
-
- def __init__(self, epsilon=1e-8):
- super().__init__()
- self.eps = epsilon
-
- def forward(self, x):
- if x.ndim != 4:
- raise ValueError(f'The input tensor should be with shape '
- f'[batch_size, channel, height, width], '
- f'but `{x.shape}` is received!')
- x = x - torch.mean(x, dim=[2, 3], keepdim=True)
- norm = torch.sqrt(
- torch.mean(x ** 2, dim=[2, 3], keepdim=True) + self.eps)
- return x / norm
-
-
-class UpsamplingLayer(nn.Module):
- """Implements the upsampling layer.
-
- Basically, this layer can be used to upsample feature maps with nearest
- neighbor interpolation.
- """
-
- def __init__(self, scale_factor=2):
- super().__init__()
- self.scale_factor = scale_factor
-
- def forward(self, x):
- if self.scale_factor <= 1:
- return x
- return F.interpolate(x, scale_factor=self.scale_factor, mode='nearest')
-
-
-class Blur(torch.autograd.Function):
- """Defines blur operation with customized gradient computation."""
-
- @staticmethod
- def forward(ctx, x, kernel):
- ctx.save_for_backward(kernel)
- y = F.conv2d(input=x,
- weight=kernel,
- bias=None,
- stride=1,
- padding=1,
- groups=x.shape[1])
- return y
-
- @staticmethod
- def backward(ctx, dy):
- kernel, = ctx.saved_tensors
- dx = F.conv2d(input=dy,
- weight=kernel.flip((2, 3)),
- bias=None,
- stride=1,
- padding=1,
- groups=dy.shape[1])
- return dx, None, None
-
-
-class BlurLayer(nn.Module):
- """Implements the blur layer."""
-
- def __init__(self,
- channels,
- kernel=(1, 2, 1),
- normalize=True):
- super().__init__()
- kernel = np.array(kernel, dtype=np.float32).reshape(1, -1)
- kernel = kernel.T.dot(kernel)
- if normalize:
- kernel /= np.sum(kernel)
- kernel = kernel[np.newaxis, np.newaxis]
- kernel = np.tile(kernel, [channels, 1, 1, 1])
- self.register_buffer('kernel', torch.from_numpy(kernel))
-
- def forward(self, x):
- return Blur.apply(x, self.kernel)
-
-
-class NoiseApplyingLayer(nn.Module):
- """Implements the noise applying layer."""
-
- def __init__(self, resolution, channels, noise_type='spatial'):
- super().__init__()
- self.noise_type = noise_type.lower()
- self.res = resolution
- self.channels = channels
- if self.noise_type == 'spatial':
- self.register_buffer('noise', torch.randn(1, 1, self.res, self.res))
- self.weight = nn.Parameter(torch.zeros(self.channels))
- elif self.noise_type == 'channel':
- self.register_buffer('noise', torch.randn(1, self.channels, 1, 1))
- self.weight = nn.Parameter(torch.zeros(self.res, self.res))
- else:
- raise NotImplementedError(f'Not implemented noise type: '
- f'`{self.noise_type}`!')
-
- def forward(self, x, randomize_noise=False):
- if x.ndim != 4:
- raise ValueError(f'The input tensor should be with shape '
- f'[batch_size, channel, height, width], '
- f'but `{x.shape}` is received!')
- if randomize_noise:
- if self.noise_type == 'spatial':
- noise = torch.randn(x.shape[0], 1, self.res, self.res).to(x)
- elif self.noise_type == 'channel':
- noise = torch.randn(x.shape[0], self.channels, 1, 1).to(x)
- else:
- noise = self.noise
-
- if self.noise_type == 'spatial':
- x = x + noise * self.weight.view(1, self.channels, 1, 1)
- elif self.noise_type == 'channel':
- x = x + noise * self.weight.view(1, 1, self.res, self.res)
- return x
-
-
-class StyleModLayer(nn.Module):
- """Implements the style modulation layer."""
-
- def __init__(self,
- w_space_dim,
- out_channels,
- use_wscale=True):
- super().__init__()
- self.w_space_dim = w_space_dim
- self.out_channels = out_channels
-
- weight_shape = (self.out_channels * 2, self.w_space_dim)
- wscale = _STYLEMOD_WSCALE_GAIN / np.sqrt(self.w_space_dim)
- if use_wscale:
- self.weight = nn.Parameter(torch.randn(*weight_shape))
- self.wscale = wscale
- else:
- self.weight = nn.Parameter(torch.randn(*weight_shape) * wscale)
- self.wscale = 1.0
-
- self.bias = nn.Parameter(torch.zeros(self.out_channels * 2))
- self.space_of_latent = 'w'
-
- def forward_style(self, w):
- """Gets style code from the given input.
-
- More specifically, if the input is from W-Space, it will be projected by
- an affine transformation. If it is from the Style Space (Y-Space), no
- operation is required.
-
- NOTE: For codes from Y-Space, we use slicing to make sure the dimension
- is correct, in case that the code is padded before fed into this layer.
- """
- if self.space_of_latent == 'w':
- if w.ndim != 2 or w.shape[1] != self.w_space_dim:
- raise ValueError(f'The input tensor should be with shape '
- f'[batch_size, w_space_dim], where '
- f'`w_space_dim` equals to '
- f'{self.w_space_dim}!\n'
- f'But `{w.shape}` is received!')
- style = F.linear(w,
- weight=self.weight * self.wscale,
- bias=self.bias)
- elif self.space_of_latent == 'y':
- if w.ndim != 2 or w.shape[1] < 2 * self.out_channels:
- raise ValueError(f'The input tensor should be with shape '
- f'[batch_size, y_space_dim], where '
- f'`y_space_dim` equals to '
- f'{2 * self.out_channels}!\n'
- f'But `{w.shape}` is received!')
- style = w[:, :2 * self.out_channels]
- return style
-
- def forward(self, x, w):
- style = self.forward_style(w)
- style_split = style.view(-1, 2, self.out_channels, 1, 1)
- x = x * (style_split[:, 0] + 1) + style_split[:, 1]
- return x, style
-
-
-class ConvBlock(nn.Module):
- """Implements the normal convolutional block.
-
- Basically, this block executes upsampling layer (if needed), convolutional
- layer, blurring layer, noise applying layer, activation layer, instance
- normalization layer, and style modulation layer in sequence.
- """
-
- def __init__(self,
- in_channels,
- out_channels,
- resolution,
- w_space_dim,
- position=None,
- kernel_size=3,
- stride=1,
- padding=1,
- add_bias=True,
- upsample=False,
- fused_scale=False,
- use_wscale=True,
- wscale_gain=_WSCALE_GAIN,
- lr_mul=1.0,
- activation_type='lrelu',
- noise_type='spatial'):
- """Initializes with block settings.
-
- Args:
- in_channels: Number of channels of the input tensor.
- out_channels: Number of channels of the output tensor.
- resolution: Resolution of the output tensor.
- w_space_dim: Dimension of W space for style modulation.
- position: Position of the layer. `const_init`, `last` would lead to
- different behavior. (default: None)
- kernel_size: Size of the convolutional kernels. (default: 3)
- stride: Stride parameter for convolution operation. (default: 1)
- padding: Padding parameter for convolution operation. (default: 1)
- add_bias: Whether to add bias onto the convolutional result.
- (default: True)
- upsample: Whether to upsample the input tensor before convolution.
- (default: False)
- fused_scale: Whether to fused `upsample` and `conv2d` together,
- resulting in `conv2d_transpose`. (default: False)
- use_wscale: Whether to use weight scaling. (default: True)
- wscale_gain: Gain factor for weight scaling. (default: _WSCALE_GAIN)
- lr_mul: Learning multiplier for both weight and bias. (default: 1.0)
- activation_type: Type of activation. Support `linear` and `lrelu`.
- (default: `lrelu`)
- noise_type: Type of noise added to the feature map after the
- convolution (if needed). Support `spatial` and `channel`.
- (default: `spatial`)
-
- Raises:
- NotImplementedError: If the `activation_type` is not supported.
- """
- super().__init__()
-
- self.position = position
-
- if add_bias:
- self.bias = nn.Parameter(torch.zeros(out_channels))
- self.bscale = lr_mul
- else:
- self.bias = None
-
- if activation_type == 'linear':
- self.activate = nn.Identity()
- elif activation_type == 'lrelu':
- self.activate = nn.LeakyReLU(negative_slope=0.2, inplace=True)
- else:
- raise NotImplementedError(f'Not implemented activation function: '
- f'`{activation_type}`!')
-
- if self.position != 'last':
- self.apply_noise = NoiseApplyingLayer(
- resolution, out_channels, noise_type=noise_type)
- self.normalize = InstanceNormLayer()
- self.style = StyleModLayer(w_space_dim, out_channels, use_wscale)
-
- if self.position == 'const_init':
- self.const = nn.Parameter(
- torch.ones(1, in_channels, resolution, resolution))
- return
-
- self.blur = BlurLayer(out_channels) if upsample else nn.Identity()
-
- if upsample and not fused_scale:
- self.upsample = UpsamplingLayer()
- else:
- self.upsample = nn.Identity()
-
- if upsample and fused_scale:
- self.use_conv2d_transpose = True
- self.stride = 2
- self.padding = 1
- else:
- self.use_conv2d_transpose = False
- self.stride = stride
- self.padding = padding
-
- weight_shape = (out_channels, in_channels, kernel_size, kernel_size)
- fan_in = kernel_size * kernel_size * in_channels
- wscale = wscale_gain / np.sqrt(fan_in)
- if use_wscale:
- self.weight = nn.Parameter(torch.randn(*weight_shape) / lr_mul)
- self.wscale = wscale * lr_mul
- else:
- self.weight = nn.Parameter(
- torch.randn(*weight_shape) * wscale / lr_mul)
- self.wscale = lr_mul
-
- def forward(self, x, w, randomize_noise=False):
- if self.position != 'const_init':
- x = self.upsample(x)
- weight = self.weight * self.wscale
- if self.use_conv2d_transpose:
- weight = F.pad(weight, (1, 1, 1, 1, 0, 0, 0, 0), 'constant', 0)
- weight = (weight[:, :, 1:, 1:] + weight[:, :, :-1, 1:] +
- weight[:, :, 1:, :-1] + weight[:, :, :-1, :-1])
- weight = weight.permute(1, 0, 2, 3)
- x = F.conv_transpose2d(x,
- weight=weight,
- bias=None,
- stride=self.stride,
- padding=self.padding)
- else:
- x = F.conv2d(x,
- weight=weight,
- bias=None,
- stride=self.stride,
- padding=self.padding)
- x = self.blur(x)
- else:
- x = self.const.repeat(w.shape[0], 1, 1, 1)
-
- bias = self.bias * self.bscale if self.bias is not None else None
-
- if self.position == 'last':
- if bias is not None:
- x = x + bias.view(1, -1, 1, 1)
- return x
-
- x = self.apply_noise(x, randomize_noise)
- if bias is not None:
- x = x + bias.view(1, -1, 1, 1)
- x = self.activate(x)
- x = self.normalize(x)
- x, style = self.style(x, w)
- return x, style
-
-
-class DenseBlock(nn.Module):
- """Implements the dense block.
-
- Basically, this block executes fully-connected layer and activation layer.
- """
-
- def __init__(self,
- in_channels,
- out_channels,
- add_bias=True,
- use_wscale=True,
- wscale_gain=_WSCALE_GAIN,
- lr_mul=1.0,
- activation_type='lrelu'):
- """Initializes with block settings.
-
- Args:
- in_channels: Number of channels of the input tensor.
- out_channels: Number of channels of the output tensor.
- add_bias: Whether to add bias onto the fully-connected result.
- (default: True)
- use_wscale: Whether to use weight scaling. (default: True)
- wscale_gain: Gain factor for weight scaling. (default: _WSCALE_GAIN)
- lr_mul: Learning multiplier for both weight and bias. (default: 1.0)
- activation_type: Type of activation. Support `linear` and `lrelu`.
- (default: `lrelu`)
-
- Raises:
- NotImplementedError: If the `activation_type` is not supported.
- """
- super().__init__()
- weight_shape = (out_channels, in_channels)
- wscale = wscale_gain / np.sqrt(in_channels)
- if use_wscale:
- self.weight = nn.Parameter(torch.randn(*weight_shape) / lr_mul)
- self.wscale = wscale * lr_mul
- else:
- self.weight = nn.Parameter(
- torch.randn(*weight_shape) * wscale / lr_mul)
- self.wscale = lr_mul
-
- if add_bias:
- self.bias = nn.Parameter(torch.zeros(out_channels))
- self.bscale = lr_mul
- else:
- self.bias = None
-
- if activation_type == 'linear':
- self.activate = nn.Identity()
- elif activation_type == 'lrelu':
- self.activate = nn.LeakyReLU(negative_slope=0.2, inplace=True)
- else:
- raise NotImplementedError(f'Not implemented activation function: '
- f'`{activation_type}`!')
-
- def forward(self, x):
- if x.ndim != 2:
- x = x.view(x.shape[0], -1)
- bias = self.bias * self.bscale if self.bias is not None else None
- x = F.linear(x, weight=self.weight * self.wscale, bias=bias)
- x = self.activate(x)
- return x
diff --git a/spaces/jannisborn/paccmann/forward.py b/spaces/jannisborn/paccmann/forward.py
deleted file mode 100644
index b38e007b2d1bcdc4d071a2bc9ab2b4fb4a0a7b5c..0000000000000000000000000000000000000000
--- a/spaces/jannisborn/paccmann/forward.py
+++ /dev/null
@@ -1,56 +0,0 @@
-"""Inference utilities."""
-import logging
-import torch
-import numpy as np
-from paccmann_predictor.models.paccmann import MCA
-from pytoda.transforms import Compose
-from pytoda.smiles.transforms import ToTensor
-from configuration import (
- MODEL_WEIGHTS_URI,
- MODEL_PARAMS,
- SMILES_LANGUAGE,
- SMILES_TRANSFORMS,
-)
-
-logger = logging.getLogger("openapi_server:inference")
-# NOTE: to avoid segfaults
-torch.set_num_threads(1)
-
-
-def predict(
- smiles: str, gene_expression: np.ndarray, estimate_confidence: bool = False
-) -> dict:
- """
- Run PaccMann prediction.
-
- Args:
- smiles (str): SMILES representing a compound.
- gene_expression (np.ndarray): gene expression data.
- estimate_confidence (bool, optional): estimate confidence of the
- prediction. Defaults to False.
- Returns:
- dict: the prediction dictionaty from the model.
- """
- logger.debug("running predict.")
- logger.debug("gene expression shape: {}.".format(gene_expression.shape))
- device = torch.device("cuda" if torch.cuda.is_available() else "cpu")
- logger.debug("device selected: {}.".format(device))
- logger.debug("loading model for prediction.")
- model = MCA(MODEL_PARAMS)
- model.load_state_dict(torch.load(MODEL_WEIGHTS_URI, map_location=device))
- model.eval()
- if estimate_confidence:
- logger.debug("associating SMILES language for confidence estimates.")
- model._associate_language(SMILES_LANGUAGE)
- logger.debug("model loaded.")
- logger.debug("set up the transformation.")
- smiles_transform_fn = Compose(SMILES_TRANSFORMS + [ToTensor(device=device)])
- logger.debug("starting the prediction.")
- with torch.no_grad():
- _, prediction_dict = model(
- smiles_transform_fn(smiles).view(1, -1).repeat(gene_expression.shape[0], 1),
- torch.tensor(gene_expression).float(),
- confidence=estimate_confidence,
- )
- logger.debug("successful prediction.")
- return prediction_dict
diff --git a/spaces/jbilcke-hf/Panoremix/next.config.js b/spaces/jbilcke-hf/Panoremix/next.config.js
deleted file mode 100644
index 4a29795b01a1f36b3e0f1d19f53852cdf63b9134..0000000000000000000000000000000000000000
--- a/spaces/jbilcke-hf/Panoremix/next.config.js
+++ /dev/null
@@ -1,11 +0,0 @@
-/** @type {import('next').NextConfig} */
-const nextConfig = {
- output: 'standalone',
-
- experimental: {
- serverActions: true,
- serverActionsBodySizeLimit: '8mb',
- },
-}
-
-module.exports = nextConfig
diff --git a/spaces/jbilcke-hf/VideoChain-UI/src/components/business/timeline/index.tsx b/spaces/jbilcke-hf/VideoChain-UI/src/components/business/timeline/index.tsx
deleted file mode 100644
index a3d957ecf390ecaf24a394176092db483358fed3..0000000000000000000000000000000000000000
--- a/spaces/jbilcke-hf/VideoChain-UI/src/components/business/timeline/index.tsx
+++ /dev/null
@@ -1,71 +0,0 @@
-"use client"
-
-import { ReactGrid, Column, Row } from "@silevis/reactgrid"
-import "@silevis/reactgrid/styles.css"
-import { useState } from "react"
-
-type RowData = Record
-
-const nbColumns = 20
-
-const getRowsData = (nbLayers: number, nbShots: number): RowData[] => [
- { name: "Thomas", surname: "Goldman" },
- { name: "Susie", surname: "Quattro" },
- { name: "", surname: "" }
-];
-
-const getColumns = (nbColumns: number): Column[] => {
-
- const columns: Column[] = []
- for (let i = 0; i < nbColumns; i++) {
- columns.push({
- columnId: `Shot ${i}`,
- width: 150,
- })
- }
-
- return columns
-}
-
-
-
-const getRows = (nbShots: number, rows: RowData[]): Row[] => [
- {
- rowId: 'header',
- cells: [...Array(nbShots)].map((_, i) => ({
- type: "text",
- text: `Shot ${i}`,
- })),
- },
- ...rows.map((row, idx) => ({
- rowId: idx,
- cells: Object.entries(row).map(([_, value]) => ({
- type: "text",
- text: value
- }))
- }))
-]
-
-export function Timeline() {
-
- const nbLayers = 8
- const nbShots = 30
-
- const [rowsData] = useState(getRowsData(nbLayers, nbShots))
-
- const rows = getRows(nbShots, rowsData)
- const columns = getColumns(nbShots)
-
- return (
- {
- const change = changes[0]
- const { columnId, newCell, previousCell, rowId, type } = change
-
- console.log('change:', { columnId, newCell, previousCell, rowId, type })
- }}
- />
- )
-}
\ No newline at end of file
diff --git a/spaces/jbilcke-hf/ai-comic-factory/src/lib/base64ToFile.ts b/spaces/jbilcke-hf/ai-comic-factory/src/lib/base64ToFile.ts
deleted file mode 100644
index 8286631c6899135e74c02be4dd8395e8864714c8..0000000000000000000000000000000000000000
--- a/spaces/jbilcke-hf/ai-comic-factory/src/lib/base64ToFile.ts
+++ /dev/null
@@ -1,11 +0,0 @@
-export function base64ToFile(dataurl: string, filename: string) {
- var arr = dataurl.split(','),
- mime = arr[0].match(/:(.*?);/)?.[1],
- bstr = atob(arr[arr.length - 1]),
- n = bstr.length,
- u8arr = new Uint8Array(n);
- while(n--){
- u8arr[n] = bstr.charCodeAt(n);
- }
- return new File([u8arr], filename, {type:mime});
-}
\ No newline at end of file
diff --git a/spaces/joaopereirajp/livvieChatBot/venv/lib/python3.9/site-packages/Crypto/SelfTest/Hash/test_SHA224.py b/spaces/joaopereirajp/livvieChatBot/venv/lib/python3.9/site-packages/Crypto/SelfTest/Hash/test_SHA224.py
deleted file mode 100644
index cf81ad98030f999a4366851e478d4dab5b50f2d9..0000000000000000000000000000000000000000
--- a/spaces/joaopereirajp/livvieChatBot/venv/lib/python3.9/site-packages/Crypto/SelfTest/Hash/test_SHA224.py
+++ /dev/null
@@ -1,63 +0,0 @@
-# -*- coding: utf-8 -*-
-#
-# SelfTest/Hash/test_SHA224.py: Self-test for the SHA-224 hash function
-#
-# Written in 2008 by Dwayne C. Litzenberger
-#
-# ===================================================================
-# The contents of this file are dedicated to the public domain. To
-# the extent that dedication to the public domain is not available,
-# everyone is granted a worldwide, perpetual, royalty-free,
-# non-exclusive license to exercise all rights associated with the
-# contents of this file for any purpose whatsoever.
-# No rights are reserved.
-#
-# THE SOFTWARE IS PROVIDED "AS IS", WITHOUT WARRANTY OF ANY KIND,
-# EXPRESS OR IMPLIED, INCLUDING BUT NOT LIMITED TO THE WARRANTIES OF
-# MERCHANTABILITY, FITNESS FOR A PARTICULAR PURPOSE AND
-# NONINFRINGEMENT. IN NO EVENT SHALL THE AUTHORS OR COPYRIGHT HOLDERS
-# BE LIABLE FOR ANY CLAIM, DAMAGES OR OTHER LIABILITY, WHETHER IN AN
-# ACTION OF CONTRACT, TORT OR OTHERWISE, ARISING FROM, OUT OF OR IN
-# CONNECTION WITH THE SOFTWARE OR THE USE OR OTHER DEALINGS IN THE
-# SOFTWARE.
-# ===================================================================
-
-"""Self-test suite for Crypto.Hash.SHA224"""
-
-# Test vectors from various sources
-# This is a list of (expected_result, input[, description]) tuples.
-test_data = [
-
- # RFC 3874: Section 3.1, "Test Vector #1
- ('23097d223405d8228642a477bda255b32aadbce4bda0b3f7e36c9da7', 'abc'),
-
- # RFC 3874: Section 3.2, "Test Vector #2
- ('75388b16512776cc5dba5da1fd890150b0c6455cb4f58b1952522525', 'abcdbcdecdefdefgefghfghighijhijkijkljklmklmnlmnomnopnopq'),
-
- # RFC 3874: Section 3.3, "Test Vector #3
- ('20794655980c91d8bbb4c1ea97618a4bf03f42581948b2ee4ee7ad67', 'a' * 10**6, "'a' * 10**6"),
-
- # Examples from http://de.wikipedia.org/wiki/Secure_Hash_Algorithm
- ('d14a028c2a3a2bc9476102bb288234c415a2b01f828ea62ac5b3e42f', ''),
-
- ('49b08defa65e644cbf8a2dd9270bdededabc741997d1dadd42026d7b',
- 'Franz jagt im komplett verwahrlosten Taxi quer durch Bayern'),
-
- ('58911e7fccf2971a7d07f93162d8bd13568e71aa8fc86fc1fe9043d1',
- 'Frank jagt im komplett verwahrlosten Taxi quer durch Bayern'),
-
-]
-
-def get_tests(config={}):
- from Crypto.Hash import SHA224
- from .common import make_hash_tests
- return make_hash_tests(SHA224, "SHA224", test_data,
- digest_size=28,
- oid='2.16.840.1.101.3.4.2.4')
-
-if __name__ == '__main__':
- import unittest
- suite = lambda: unittest.TestSuite(get_tests())
- unittest.main(defaultTest='suite')
-
-# vim:set ts=4 sw=4 sts=4 expandtab:
diff --git a/spaces/joaopereirajp/livvieChatBot/venv/lib/python3.9/site-packages/PyPDF2/_version.py b/spaces/joaopereirajp/livvieChatBot/venv/lib/python3.9/site-packages/PyPDF2/_version.py
deleted file mode 100644
index 055276878107052a2bd2810e5a0b07182ef1cd58..0000000000000000000000000000000000000000
--- a/spaces/joaopereirajp/livvieChatBot/venv/lib/python3.9/site-packages/PyPDF2/_version.py
+++ /dev/null
@@ -1 +0,0 @@
-__version__ = "3.0.1"
diff --git a/spaces/joaopereirajp/livvieChatBot/venv/lib/python3.9/site-packages/aiohttp/web_response.py b/spaces/joaopereirajp/livvieChatBot/venv/lib/python3.9/site-packages/aiohttp/web_response.py
deleted file mode 100644
index ce07f8153deb29c4cf5856fae0d92ac1170c1441..0000000000000000000000000000000000000000
--- a/spaces/joaopereirajp/livvieChatBot/venv/lib/python3.9/site-packages/aiohttp/web_response.py
+++ /dev/null
@@ -1,825 +0,0 @@
-import asyncio
-import collections.abc
-import datetime
-import enum
-import json
-import math
-import time
-import warnings
-import zlib
-from concurrent.futures import Executor
-from http.cookies import Morsel, SimpleCookie
-from typing import (
- TYPE_CHECKING,
- Any,
- Dict,
- Iterator,
- Mapping,
- MutableMapping,
- Optional,
- Tuple,
- Union,
- cast,
-)
-
-from multidict import CIMultiDict, istr
-
-from . import hdrs, payload
-from .abc import AbstractStreamWriter
-from .helpers import (
- ETAG_ANY,
- PY_38,
- QUOTED_ETAG_RE,
- ETag,
- HeadersMixin,
- parse_http_date,
- rfc822_formatted_time,
- sentinel,
- validate_etag_value,
-)
-from .http import RESPONSES, SERVER_SOFTWARE, HttpVersion10, HttpVersion11
-from .payload import Payload
-from .typedefs import JSONEncoder, LooseHeaders
-
-__all__ = ("ContentCoding", "StreamResponse", "Response", "json_response")
-
-
-if TYPE_CHECKING: # pragma: no cover
- from .web_request import BaseRequest
-
- BaseClass = MutableMapping[str, Any]
-else:
- BaseClass = collections.abc.MutableMapping
-
-
-if not PY_38:
- # allow samesite to be used in python < 3.8
- # already permitted in python 3.8, see https://bugs.python.org/issue29613
- Morsel._reserved["samesite"] = "SameSite" # type: ignore[attr-defined]
-
-
-class ContentCoding(enum.Enum):
- # The content codings that we have support for.
- #
- # Additional registered codings are listed at:
- # https://www.iana.org/assignments/http-parameters/http-parameters.xhtml#content-coding
- deflate = "deflate"
- gzip = "gzip"
- identity = "identity"
-
-
-############################################################
-# HTTP Response classes
-############################################################
-
-
-class StreamResponse(BaseClass, HeadersMixin):
-
- _length_check = True
-
- def __init__(
- self,
- *,
- status: int = 200,
- reason: Optional[str] = None,
- headers: Optional[LooseHeaders] = None,
- ) -> None:
- self._body = None
- self._keep_alive: Optional[bool] = None
- self._chunked = False
- self._compression = False
- self._compression_force: Optional[ContentCoding] = None
- self._cookies: SimpleCookie[str] = SimpleCookie()
-
- self._req: Optional[BaseRequest] = None
- self._payload_writer: Optional[AbstractStreamWriter] = None
- self._eof_sent = False
- self._body_length = 0
- self._state: Dict[str, Any] = {}
-
- if headers is not None:
- self._headers: CIMultiDict[str] = CIMultiDict(headers)
- else:
- self._headers = CIMultiDict()
-
- self.set_status(status, reason)
-
- @property
- def prepared(self) -> bool:
- return self._payload_writer is not None
-
- @property
- def task(self) -> "Optional[asyncio.Task[None]]":
- if self._req:
- return self._req.task
- else:
- return None
-
- @property
- def status(self) -> int:
- return self._status
-
- @property
- def chunked(self) -> bool:
- return self._chunked
-
- @property
- def compression(self) -> bool:
- return self._compression
-
- @property
- def reason(self) -> str:
- return self._reason
-
- def set_status(
- self,
- status: int,
- reason: Optional[str] = None,
- _RESPONSES: Mapping[int, Tuple[str, str]] = RESPONSES,
- ) -> None:
- assert not self.prepared, (
- "Cannot change the response status code after " "the headers have been sent"
- )
- self._status = int(status)
- if reason is None:
- try:
- reason = _RESPONSES[self._status][0]
- except Exception:
- reason = ""
- self._reason = reason
-
- @property
- def keep_alive(self) -> Optional[bool]:
- return self._keep_alive
-
- def force_close(self) -> None:
- self._keep_alive = False
-
- @property
- def body_length(self) -> int:
- return self._body_length
-
- @property
- def output_length(self) -> int:
- warnings.warn("output_length is deprecated", DeprecationWarning)
- assert self._payload_writer
- return self._payload_writer.buffer_size
-
- def enable_chunked_encoding(self, chunk_size: Optional[int] = None) -> None:
- """Enables automatic chunked transfer encoding."""
- self._chunked = True
-
- if hdrs.CONTENT_LENGTH in self._headers:
- raise RuntimeError(
- "You can't enable chunked encoding when " "a content length is set"
- )
- if chunk_size is not None:
- warnings.warn("Chunk size is deprecated #1615", DeprecationWarning)
-
- def enable_compression(
- self, force: Optional[Union[bool, ContentCoding]] = None
- ) -> None:
- """Enables response compression encoding."""
- # Backwards compatibility for when force was a bool <0.17.
- if type(force) == bool:
- force = ContentCoding.deflate if force else ContentCoding.identity
- warnings.warn(
- "Using boolean for force is deprecated #3318", DeprecationWarning
- )
- elif force is not None:
- assert isinstance(force, ContentCoding), (
- "force should one of " "None, bool or " "ContentEncoding"
- )
-
- self._compression = True
- self._compression_force = force
-
- @property
- def headers(self) -> "CIMultiDict[str]":
- return self._headers
-
- @property
- def cookies(self) -> "SimpleCookie[str]":
- return self._cookies
-
- def set_cookie(
- self,
- name: str,
- value: str,
- *,
- expires: Optional[str] = None,
- domain: Optional[str] = None,
- max_age: Optional[Union[int, str]] = None,
- path: str = "/",
- secure: Optional[bool] = None,
- httponly: Optional[bool] = None,
- version: Optional[str] = None,
- samesite: Optional[str] = None,
- ) -> None:
- """Set or update response cookie.
-
- Sets new cookie or updates existent with new value.
- Also updates only those params which are not None.
- """
- old = self._cookies.get(name)
- if old is not None and old.coded_value == "":
- # deleted cookie
- self._cookies.pop(name, None)
-
- self._cookies[name] = value
- c = self._cookies[name]
-
- if expires is not None:
- c["expires"] = expires
- elif c.get("expires") == "Thu, 01 Jan 1970 00:00:00 GMT":
- del c["expires"]
-
- if domain is not None:
- c["domain"] = domain
-
- if max_age is not None:
- c["max-age"] = str(max_age)
- elif "max-age" in c:
- del c["max-age"]
-
- c["path"] = path
-
- if secure is not None:
- c["secure"] = secure
- if httponly is not None:
- c["httponly"] = httponly
- if version is not None:
- c["version"] = version
- if samesite is not None:
- c["samesite"] = samesite
-
- def del_cookie(
- self, name: str, *, domain: Optional[str] = None, path: str = "/"
- ) -> None:
- """Delete cookie.
-
- Creates new empty expired cookie.
- """
- # TODO: do we need domain/path here?
- self._cookies.pop(name, None)
- self.set_cookie(
- name,
- "",
- max_age=0,
- expires="Thu, 01 Jan 1970 00:00:00 GMT",
- domain=domain,
- path=path,
- )
-
- @property
- def content_length(self) -> Optional[int]:
- # Just a placeholder for adding setter
- return super().content_length
-
- @content_length.setter
- def content_length(self, value: Optional[int]) -> None:
- if value is not None:
- value = int(value)
- if self._chunked:
- raise RuntimeError(
- "You can't set content length when " "chunked encoding is enable"
- )
- self._headers[hdrs.CONTENT_LENGTH] = str(value)
- else:
- self._headers.pop(hdrs.CONTENT_LENGTH, None)
-
- @property
- def content_type(self) -> str:
- # Just a placeholder for adding setter
- return super().content_type
-
- @content_type.setter
- def content_type(self, value: str) -> None:
- self.content_type # read header values if needed
- self._content_type = str(value)
- self._generate_content_type_header()
-
- @property
- def charset(self) -> Optional[str]:
- # Just a placeholder for adding setter
- return super().charset
-
- @charset.setter
- def charset(self, value: Optional[str]) -> None:
- ctype = self.content_type # read header values if needed
- if ctype == "application/octet-stream":
- raise RuntimeError(
- "Setting charset for application/octet-stream "
- "doesn't make sense, setup content_type first"
- )
- assert self._content_dict is not None
- if value is None:
- self._content_dict.pop("charset", None)
- else:
- self._content_dict["charset"] = str(value).lower()
- self._generate_content_type_header()
-
- @property
- def last_modified(self) -> Optional[datetime.datetime]:
- """The value of Last-Modified HTTP header, or None.
-
- This header is represented as a `datetime` object.
- """
- return parse_http_date(self._headers.get(hdrs.LAST_MODIFIED))
-
- @last_modified.setter
- def last_modified(
- self, value: Optional[Union[int, float, datetime.datetime, str]]
- ) -> None:
- if value is None:
- self._headers.pop(hdrs.LAST_MODIFIED, None)
- elif isinstance(value, (int, float)):
- self._headers[hdrs.LAST_MODIFIED] = time.strftime(
- "%a, %d %b %Y %H:%M:%S GMT", time.gmtime(math.ceil(value))
- )
- elif isinstance(value, datetime.datetime):
- self._headers[hdrs.LAST_MODIFIED] = time.strftime(
- "%a, %d %b %Y %H:%M:%S GMT", value.utctimetuple()
- )
- elif isinstance(value, str):
- self._headers[hdrs.LAST_MODIFIED] = value
-
- @property
- def etag(self) -> Optional[ETag]:
- quoted_value = self._headers.get(hdrs.ETAG)
- if not quoted_value:
- return None
- elif quoted_value == ETAG_ANY:
- return ETag(value=ETAG_ANY)
- match = QUOTED_ETAG_RE.fullmatch(quoted_value)
- if not match:
- return None
- is_weak, value = match.group(1, 2)
- return ETag(
- is_weak=bool(is_weak),
- value=value,
- )
-
- @etag.setter
- def etag(self, value: Optional[Union[ETag, str]]) -> None:
- if value is None:
- self._headers.pop(hdrs.ETAG, None)
- elif (isinstance(value, str) and value == ETAG_ANY) or (
- isinstance(value, ETag) and value.value == ETAG_ANY
- ):
- self._headers[hdrs.ETAG] = ETAG_ANY
- elif isinstance(value, str):
- validate_etag_value(value)
- self._headers[hdrs.ETAG] = f'"{value}"'
- elif isinstance(value, ETag) and isinstance(value.value, str):
- validate_etag_value(value.value)
- hdr_value = f'W/"{value.value}"' if value.is_weak else f'"{value.value}"'
- self._headers[hdrs.ETAG] = hdr_value
- else:
- raise ValueError(
- f"Unsupported etag type: {type(value)}. "
- f"etag must be str, ETag or None"
- )
-
- def _generate_content_type_header(
- self, CONTENT_TYPE: istr = hdrs.CONTENT_TYPE
- ) -> None:
- assert self._content_dict is not None
- assert self._content_type is not None
- params = "; ".join(f"{k}={v}" for k, v in self._content_dict.items())
- if params:
- ctype = self._content_type + "; " + params
- else:
- ctype = self._content_type
- self._headers[CONTENT_TYPE] = ctype
-
- async def _do_start_compression(self, coding: ContentCoding) -> None:
- if coding != ContentCoding.identity:
- assert self._payload_writer is not None
- self._headers[hdrs.CONTENT_ENCODING] = coding.value
- self._payload_writer.enable_compression(coding.value)
- # Compressed payload may have different content length,
- # remove the header
- self._headers.popall(hdrs.CONTENT_LENGTH, None)
-
- async def _start_compression(self, request: "BaseRequest") -> None:
- if self._compression_force:
- await self._do_start_compression(self._compression_force)
- else:
- accept_encoding = request.headers.get(hdrs.ACCEPT_ENCODING, "").lower()
- for coding in ContentCoding:
- if coding.value in accept_encoding:
- await self._do_start_compression(coding)
- return
-
- async def prepare(self, request: "BaseRequest") -> Optional[AbstractStreamWriter]:
- if self._eof_sent:
- return None
- if self._payload_writer is not None:
- return self._payload_writer
-
- return await self._start(request)
-
- async def _start(self, request: "BaseRequest") -> AbstractStreamWriter:
- self._req = request
- writer = self._payload_writer = request._payload_writer
-
- await self._prepare_headers()
- await request._prepare_hook(self)
- await self._write_headers()
-
- return writer
-
- async def _prepare_headers(self) -> None:
- request = self._req
- assert request is not None
- writer = self._payload_writer
- assert writer is not None
- keep_alive = self._keep_alive
- if keep_alive is None:
- keep_alive = request.keep_alive
- self._keep_alive = keep_alive
-
- version = request.version
-
- headers = self._headers
- for cookie in self._cookies.values():
- value = cookie.output(header="")[1:]
- headers.add(hdrs.SET_COOKIE, value)
-
- if self._compression:
- await self._start_compression(request)
-
- if self._chunked:
- if version != HttpVersion11:
- raise RuntimeError(
- "Using chunked encoding is forbidden "
- "for HTTP/{0.major}.{0.minor}".format(request.version)
- )
- writer.enable_chunking()
- headers[hdrs.TRANSFER_ENCODING] = "chunked"
- if hdrs.CONTENT_LENGTH in headers:
- del headers[hdrs.CONTENT_LENGTH]
- elif self._length_check:
- writer.length = self.content_length
- if writer.length is None:
- if version >= HttpVersion11 and self.status != 204:
- writer.enable_chunking()
- headers[hdrs.TRANSFER_ENCODING] = "chunked"
- if hdrs.CONTENT_LENGTH in headers:
- del headers[hdrs.CONTENT_LENGTH]
- else:
- keep_alive = False
- # HTTP 1.1: https://tools.ietf.org/html/rfc7230#section-3.3.2
- # HTTP 1.0: https://tools.ietf.org/html/rfc1945#section-10.4
- elif version >= HttpVersion11 and self.status in (100, 101, 102, 103, 204):
- del headers[hdrs.CONTENT_LENGTH]
-
- if self.status not in (204, 304):
- headers.setdefault(hdrs.CONTENT_TYPE, "application/octet-stream")
- headers.setdefault(hdrs.DATE, rfc822_formatted_time())
- headers.setdefault(hdrs.SERVER, SERVER_SOFTWARE)
-
- # connection header
- if hdrs.CONNECTION not in headers:
- if keep_alive:
- if version == HttpVersion10:
- headers[hdrs.CONNECTION] = "keep-alive"
- else:
- if version == HttpVersion11:
- headers[hdrs.CONNECTION] = "close"
-
- async def _write_headers(self) -> None:
- request = self._req
- assert request is not None
- writer = self._payload_writer
- assert writer is not None
- # status line
- version = request.version
- status_line = "HTTP/{}.{} {} {}".format(
- version[0], version[1], self._status, self._reason
- )
- await writer.write_headers(status_line, self._headers)
-
- async def write(self, data: bytes) -> None:
- assert isinstance(
- data, (bytes, bytearray, memoryview)
- ), "data argument must be byte-ish (%r)" % type(data)
-
- if self._eof_sent:
- raise RuntimeError("Cannot call write() after write_eof()")
- if self._payload_writer is None:
- raise RuntimeError("Cannot call write() before prepare()")
-
- await self._payload_writer.write(data)
-
- async def drain(self) -> None:
- assert not self._eof_sent, "EOF has already been sent"
- assert self._payload_writer is not None, "Response has not been started"
- warnings.warn(
- "drain method is deprecated, use await resp.write()",
- DeprecationWarning,
- stacklevel=2,
- )
- await self._payload_writer.drain()
-
- async def write_eof(self, data: bytes = b"") -> None:
- assert isinstance(
- data, (bytes, bytearray, memoryview)
- ), "data argument must be byte-ish (%r)" % type(data)
-
- if self._eof_sent:
- return
-
- assert self._payload_writer is not None, "Response has not been started"
-
- await self._payload_writer.write_eof(data)
- self._eof_sent = True
- self._req = None
- self._body_length = self._payload_writer.output_size
- self._payload_writer = None
-
- def __repr__(self) -> str:
- if self._eof_sent:
- info = "eof"
- elif self.prepared:
- assert self._req is not None
- info = f"{self._req.method} {self._req.path} "
- else:
- info = "not prepared"
- return f"<{self.__class__.__name__} {self.reason} {info}>"
-
- def __getitem__(self, key: str) -> Any:
- return self._state[key]
-
- def __setitem__(self, key: str, value: Any) -> None:
- self._state[key] = value
-
- def __delitem__(self, key: str) -> None:
- del self._state[key]
-
- def __len__(self) -> int:
- return len(self._state)
-
- def __iter__(self) -> Iterator[str]:
- return iter(self._state)
-
- def __hash__(self) -> int:
- return hash(id(self))
-
- def __eq__(self, other: object) -> bool:
- return self is other
-
-
-class Response(StreamResponse):
- def __init__(
- self,
- *,
- body: Any = None,
- status: int = 200,
- reason: Optional[str] = None,
- text: Optional[str] = None,
- headers: Optional[LooseHeaders] = None,
- content_type: Optional[str] = None,
- charset: Optional[str] = None,
- zlib_executor_size: Optional[int] = None,
- zlib_executor: Optional[Executor] = None,
- ) -> None:
- if body is not None and text is not None:
- raise ValueError("body and text are not allowed together")
-
- if headers is None:
- real_headers: CIMultiDict[str] = CIMultiDict()
- elif not isinstance(headers, CIMultiDict):
- real_headers = CIMultiDict(headers)
- else:
- real_headers = headers # = cast('CIMultiDict[str]', headers)
-
- if content_type is not None and "charset" in content_type:
- raise ValueError("charset must not be in content_type " "argument")
-
- if text is not None:
- if hdrs.CONTENT_TYPE in real_headers:
- if content_type or charset:
- raise ValueError(
- "passing both Content-Type header and "
- "content_type or charset params "
- "is forbidden"
- )
- else:
- # fast path for filling headers
- if not isinstance(text, str):
- raise TypeError("text argument must be str (%r)" % type(text))
- if content_type is None:
- content_type = "text/plain"
- if charset is None:
- charset = "utf-8"
- real_headers[hdrs.CONTENT_TYPE] = content_type + "; charset=" + charset
- body = text.encode(charset)
- text = None
- else:
- if hdrs.CONTENT_TYPE in real_headers:
- if content_type is not None or charset is not None:
- raise ValueError(
- "passing both Content-Type header and "
- "content_type or charset params "
- "is forbidden"
- )
- else:
- if content_type is not None:
- if charset is not None:
- content_type += "; charset=" + charset
- real_headers[hdrs.CONTENT_TYPE] = content_type
-
- super().__init__(status=status, reason=reason, headers=real_headers)
-
- if text is not None:
- self.text = text
- else:
- self.body = body
-
- self._compressed_body: Optional[bytes] = None
- self._zlib_executor_size = zlib_executor_size
- self._zlib_executor = zlib_executor
-
- @property
- def body(self) -> Optional[Union[bytes, Payload]]:
- return self._body
-
- @body.setter
- def body(
- self,
- body: bytes,
- CONTENT_TYPE: istr = hdrs.CONTENT_TYPE,
- CONTENT_LENGTH: istr = hdrs.CONTENT_LENGTH,
- ) -> None:
- if body is None:
- self._body: Optional[bytes] = None
- self._body_payload: bool = False
- elif isinstance(body, (bytes, bytearray)):
- self._body = body
- self._body_payload = False
- else:
- try:
- self._body = body = payload.PAYLOAD_REGISTRY.get(body)
- except payload.LookupError:
- raise ValueError("Unsupported body type %r" % type(body))
-
- self._body_payload = True
-
- headers = self._headers
-
- # set content-length header if needed
- if not self._chunked and CONTENT_LENGTH not in headers:
- size = body.size
- if size is not None:
- headers[CONTENT_LENGTH] = str(size)
-
- # set content-type
- if CONTENT_TYPE not in headers:
- headers[CONTENT_TYPE] = body.content_type
-
- # copy payload headers
- if body.headers:
- for (key, value) in body.headers.items():
- if key not in headers:
- headers[key] = value
-
- self._compressed_body = None
-
- @property
- def text(self) -> Optional[str]:
- if self._body is None:
- return None
- return self._body.decode(self.charset or "utf-8")
-
- @text.setter
- def text(self, text: str) -> None:
- assert text is None or isinstance(
- text, str
- ), "text argument must be str (%r)" % type(text)
-
- if self.content_type == "application/octet-stream":
- self.content_type = "text/plain"
- if self.charset is None:
- self.charset = "utf-8"
-
- self._body = text.encode(self.charset)
- self._body_payload = False
- self._compressed_body = None
-
- @property
- def content_length(self) -> Optional[int]:
- if self._chunked:
- return None
-
- if hdrs.CONTENT_LENGTH in self._headers:
- return super().content_length
-
- if self._compressed_body is not None:
- # Return length of the compressed body
- return len(self._compressed_body)
- elif self._body_payload:
- # A payload without content length, or a compressed payload
- return None
- elif self._body is not None:
- return len(self._body)
- else:
- return 0
-
- @content_length.setter
- def content_length(self, value: Optional[int]) -> None:
- raise RuntimeError("Content length is set automatically")
-
- async def write_eof(self, data: bytes = b"") -> None:
- if self._eof_sent:
- return
- if self._compressed_body is None:
- body: Optional[Union[bytes, Payload]] = self._body
- else:
- body = self._compressed_body
- assert not data, f"data arg is not supported, got {data!r}"
- assert self._req is not None
- assert self._payload_writer is not None
- if body is not None:
- if self._req._method == hdrs.METH_HEAD or self._status in [204, 304]:
- await super().write_eof()
- elif self._body_payload:
- payload = cast(Payload, body)
- await payload.write(self._payload_writer)
- await super().write_eof()
- else:
- await super().write_eof(cast(bytes, body))
- else:
- await super().write_eof()
-
- async def _start(self, request: "BaseRequest") -> AbstractStreamWriter:
- if not self._chunked and hdrs.CONTENT_LENGTH not in self._headers:
- if not self._body_payload:
- if self._body is not None:
- self._headers[hdrs.CONTENT_LENGTH] = str(len(self._body))
- else:
- self._headers[hdrs.CONTENT_LENGTH] = "0"
-
- return await super()._start(request)
-
- def _compress_body(self, zlib_mode: int) -> None:
- assert zlib_mode > 0
- compressobj = zlib.compressobj(wbits=zlib_mode)
- body_in = self._body
- assert body_in is not None
- self._compressed_body = compressobj.compress(body_in) + compressobj.flush()
-
- async def _do_start_compression(self, coding: ContentCoding) -> None:
- if self._body_payload or self._chunked:
- return await super()._do_start_compression(coding)
-
- if coding != ContentCoding.identity:
- # Instead of using _payload_writer.enable_compression,
- # compress the whole body
- zlib_mode = (
- 16 + zlib.MAX_WBITS if coding == ContentCoding.gzip else zlib.MAX_WBITS
- )
- body_in = self._body
- assert body_in is not None
- if (
- self._zlib_executor_size is not None
- and len(body_in) > self._zlib_executor_size
- ):
- await asyncio.get_event_loop().run_in_executor(
- self._zlib_executor, self._compress_body, zlib_mode
- )
- else:
- self._compress_body(zlib_mode)
-
- body_out = self._compressed_body
- assert body_out is not None
-
- self._headers[hdrs.CONTENT_ENCODING] = coding.value
- self._headers[hdrs.CONTENT_LENGTH] = str(len(body_out))
-
-
-def json_response(
- data: Any = sentinel,
- *,
- text: Optional[str] = None,
- body: Optional[bytes] = None,
- status: int = 200,
- reason: Optional[str] = None,
- headers: Optional[LooseHeaders] = None,
- content_type: str = "application/json",
- dumps: JSONEncoder = json.dumps,
-) -> Response:
- if data is not sentinel:
- if text or body:
- raise ValueError("only one of data, text, or body should be specified")
- else:
- text = dumps(data)
- return Response(
- text=text,
- body=body,
- status=status,
- reason=reason,
- headers=headers,
- content_type=content_type,
- )
diff --git a/spaces/johnslegers/stable-diffusion-gui-test/ldmlib/models/diffusion/classifier.py b/spaces/johnslegers/stable-diffusion-gui-test/ldmlib/models/diffusion/classifier.py
deleted file mode 100644
index 363ad8cf6071a52c573cd84acf7fe05d3e340bd2..0000000000000000000000000000000000000000
--- a/spaces/johnslegers/stable-diffusion-gui-test/ldmlib/models/diffusion/classifier.py
+++ /dev/null
@@ -1,267 +0,0 @@
-import os
-import torch
-import pytorch_lightning as pl
-from omegaconf import OmegaConf
-from torch.nn import functional as F
-from torch.optim import AdamW
-from torch.optim.lr_scheduler import LambdaLR
-from copy import deepcopy
-from einops import rearrange
-from glob import glob
-from natsort import natsorted
-
-from ldmlib.modules.diffusionmodules.openaimodel import EncoderUNetModel, UNetModel
-from ldmlib.util import log_txt_as_img, default, ismap, instantiate_from_config
-
-__models__ = {
- 'class_label': EncoderUNetModel,
- 'segmentation': UNetModel
-}
-
-
-def disabled_train(self, mode=True):
- """Overwrite model.train with this function to make sure train/eval mode
- does not change anymore."""
- return self
-
-
-class NoisyLatentImageClassifier(pl.LightningModule):
-
- def __init__(self,
- diffusion_path,
- num_classes,
- ckpt_path=None,
- pool='attention',
- label_key=None,
- diffusion_ckpt_path=None,
- scheduler_config=None,
- weight_decay=1.e-2,
- log_steps=10,
- monitor='val/loss',
- *args,
- **kwargs):
- super().__init__(*args, **kwargs)
- self.num_classes = num_classes
- # get latest config of diffusion model
- diffusion_config = natsorted(glob(os.path.join(diffusion_path, 'configs', '*-project.yaml')))[-1]
- self.diffusion_config = OmegaConf.load(diffusion_config).model
- self.diffusion_config.params.ckpt_path = diffusion_ckpt_path
- self.load_diffusion()
-
- self.monitor = monitor
- self.numd = self.diffusion_model.first_stage_model.encoder.num_resolutions - 1
- self.log_time_interval = self.diffusion_model.num_timesteps // log_steps
- self.log_steps = log_steps
-
- self.label_key = label_key if not hasattr(self.diffusion_model, 'cond_stage_key') \
- else self.diffusion_model.cond_stage_key
-
- assert self.label_key is not None, 'label_key neither in diffusion model nor in model.params'
-
- if self.label_key not in __models__:
- raise NotImplementedError()
-
- self.load_classifier(ckpt_path, pool)
-
- self.scheduler_config = scheduler_config
- self.use_scheduler = self.scheduler_config is not None
- self.weight_decay = weight_decay
-
- def init_from_ckpt(self, path, ignore_keys=list(), only_model=False):
- sd = torch.load(path, map_location="cpu")
- if "state_dict" in list(sd.keys()):
- sd = sd["state_dict"]
- keys = list(sd.keys())
- for k in keys:
- for ik in ignore_keys:
- if k.startswith(ik):
- print("Deleting key {} from state_dict.".format(k))
- del sd[k]
- missing, unexpected = self.load_state_dict(sd, strict=False) if not only_model else self.model.load_state_dict(
- sd, strict=False)
- print(f"Restored from {path} with {len(missing)} missing and {len(unexpected)} unexpected keys")
- if len(missing) > 0:
- print(f"Missing Keys: {missing}")
- if len(unexpected) > 0:
- print(f"Unexpected Keys: {unexpected}")
-
- def load_diffusion(self):
- model = instantiate_from_config(self.diffusion_config)
- self.diffusion_model = model.eval()
- self.diffusion_model.train = disabled_train
- for param in self.diffusion_model.parameters():
- param.requires_grad = False
-
- def load_classifier(self, ckpt_path, pool):
- model_config = deepcopy(self.diffusion_config.params.unet_config.params)
- model_config.in_channels = self.diffusion_config.params.unet_config.params.out_channels
- model_config.out_channels = self.num_classes
- if self.label_key == 'class_label':
- model_config.pool = pool
-
- self.model = __models__[self.label_key](**model_config)
- if ckpt_path is not None:
- print('#####################################################################')
- print(f'load from ckpt "{ckpt_path}"')
- print('#####################################################################')
- self.init_from_ckpt(ckpt_path)
-
- @torch.no_grad()
- def get_x_noisy(self, x, t, noise=None):
- noise = default(noise, lambda: torch.randn_like(x))
- continuous_sqrt_alpha_cumprod = None
- if self.diffusion_model.use_continuous_noise:
- continuous_sqrt_alpha_cumprod = self.diffusion_model.sample_continuous_noise_level(x.shape[0], t + 1)
- # todo: make sure t+1 is correct here
-
- return self.diffusion_model.q_sample(x_start=x, t=t, noise=noise,
- continuous_sqrt_alpha_cumprod=continuous_sqrt_alpha_cumprod)
-
- def forward(self, x_noisy, t, *args, **kwargs):
- return self.model(x_noisy, t)
-
- @torch.no_grad()
- def get_input(self, batch, k):
- x = batch[k]
- if len(x.shape) == 3:
- x = x[..., None]
- x = rearrange(x, 'b h w c -> b c h w')
- x = x.to(memory_format=torch.contiguous_format).float()
- return x
-
- @torch.no_grad()
- def get_conditioning(self, batch, k=None):
- if k is None:
- k = self.label_key
- assert k is not None, 'Needs to provide label key'
-
- targets = batch[k].to(self.device)
-
- if self.label_key == 'segmentation':
- targets = rearrange(targets, 'b h w c -> b c h w')
- for down in range(self.numd):
- h, w = targets.shape[-2:]
- targets = F.interpolate(targets, size=(h // 2, w // 2), mode='nearest')
-
- # targets = rearrange(targets,'b c h w -> b h w c')
-
- return targets
-
- def compute_top_k(self, logits, labels, k, reduction="mean"):
- _, top_ks = torch.topk(logits, k, dim=1)
- if reduction == "mean":
- return (top_ks == labels[:, None]).float().sum(dim=-1).mean().item()
- elif reduction == "none":
- return (top_ks == labels[:, None]).float().sum(dim=-1)
-
- def on_train_epoch_start(self):
- # save some memory
- self.diffusion_model.model.to('cpu')
-
- @torch.no_grad()
- def write_logs(self, loss, logits, targets):
- log_prefix = 'train' if self.training else 'val'
- log = {}
- log[f"{log_prefix}/loss"] = loss.mean()
- log[f"{log_prefix}/acc@1"] = self.compute_top_k(
- logits, targets, k=1, reduction="mean"
- )
- log[f"{log_prefix}/acc@5"] = self.compute_top_k(
- logits, targets, k=5, reduction="mean"
- )
-
- self.log_dict(log, prog_bar=False, logger=True, on_step=self.training, on_epoch=True)
- self.log('loss', log[f"{log_prefix}/loss"], prog_bar=True, logger=False)
- self.log('global_step', self.global_step, logger=False, on_epoch=False, prog_bar=True)
- lr = self.optimizers().param_groups[0]['lr']
- self.log('lr_abs', lr, on_step=True, logger=True, on_epoch=False, prog_bar=True)
-
- def shared_step(self, batch, t=None):
- x, *_ = self.diffusion_model.get_input(batch, k=self.diffusion_model.first_stage_key)
- targets = self.get_conditioning(batch)
- if targets.dim() == 4:
- targets = targets.argmax(dim=1)
- if t is None:
- t = torch.randint(0, self.diffusion_model.num_timesteps, (x.shape[0],), device=self.device).long()
- else:
- t = torch.full(size=(x.shape[0],), fill_value=t, device=self.device).long()
- x_noisy = self.get_x_noisy(x, t)
- logits = self(x_noisy, t)
-
- loss = F.cross_entropy(logits, targets, reduction='none')
-
- self.write_logs(loss.detach(), logits.detach(), targets.detach())
-
- loss = loss.mean()
- return loss, logits, x_noisy, targets
-
- def training_step(self, batch, batch_idx):
- loss, *_ = self.shared_step(batch)
- return loss
-
- def reset_noise_accs(self):
- self.noisy_acc = {t: {'acc@1': [], 'acc@5': []} for t in
- range(0, self.diffusion_model.num_timesteps, self.diffusion_model.log_every_t)}
-
- def on_validation_start(self):
- self.reset_noise_accs()
-
- @torch.no_grad()
- def validation_step(self, batch, batch_idx):
- loss, *_ = self.shared_step(batch)
-
- for t in self.noisy_acc:
- _, logits, _, targets = self.shared_step(batch, t)
- self.noisy_acc[t]['acc@1'].append(self.compute_top_k(logits, targets, k=1, reduction='mean'))
- self.noisy_acc[t]['acc@5'].append(self.compute_top_k(logits, targets, k=5, reduction='mean'))
-
- return loss
-
- def configure_optimizers(self):
- optimizer = AdamW(self.model.parameters(), lr=self.learning_rate, weight_decay=self.weight_decay)
-
- if self.use_scheduler:
- scheduler = instantiate_from_config(self.scheduler_config)
-
- print("Setting up LambdaLR scheduler...")
- scheduler = [
- {
- 'scheduler': LambdaLR(optimizer, lr_lambda=scheduler.schedule),
- 'interval': 'step',
- 'frequency': 1
- }]
- return [optimizer], scheduler
-
- return optimizer
-
- @torch.no_grad()
- def log_images(self, batch, N=8, *args, **kwargs):
- log = dict()
- x = self.get_input(batch, self.diffusion_model.first_stage_key)
- log['inputs'] = x
-
- y = self.get_conditioning(batch)
-
- if self.label_key == 'class_label':
- y = log_txt_as_img((x.shape[2], x.shape[3]), batch["human_label"])
- log['labels'] = y
-
- if ismap(y):
- log['labels'] = self.diffusion_model.to_rgb(y)
-
- for step in range(self.log_steps):
- current_time = step * self.log_time_interval
-
- _, logits, x_noisy, _ = self.shared_step(batch, t=current_time)
-
- log[f'inputs@t{current_time}'] = x_noisy
-
- pred = F.one_hot(logits.argmax(dim=1), num_classes=self.num_classes)
- pred = rearrange(pred, 'b h w c -> b c h w')
-
- log[f'pred@t{current_time}'] = self.diffusion_model.to_rgb(pred)
-
- for key in log:
- log[key] = log[key][:N]
-
- return log
diff --git a/spaces/jonigata/PoseMaker2/util.py b/spaces/jonigata/PoseMaker2/util.py
deleted file mode 100644
index f9b171e65e1de7c0dd4a186b129375c6b1239291..0000000000000000000000000000000000000000
--- a/spaces/jonigata/PoseMaker2/util.py
+++ /dev/null
@@ -1,46 +0,0 @@
-import numpy as np
-import cv2
-
-def pil2cv(image):
- ''' PIL型 -> OpenCV型 '''
- new_image = np.array(image, dtype=np.uint8)
- if new_image.ndim == 2: # モノクロ
- pass
- elif new_image.shape[2] == 3: # カラー
- new_image = cv2.cvtColor(new_image, cv2.COLOR_RGB2BGR)
- elif new_image.shape[2] == 4: # 透過
- new_image = cv2.cvtColor(new_image, cv2.COLOR_RGBA2BGRA)
- return new_image
-
-def candidate_to_json_string(arr):
- a = [f'[{x:.2f}, {y:.2f}]' for x, y, *_ in arr]
- return '[' + ', '.join(a) + ']'
-
-# make subset to json
-def subset_to_json_string(arr):
- arr_str = ','.join(['[' + ','.join([f'{num:.2f}' for num in row]) + ']' for row in arr])
- return '[' + arr_str + ']'
-
-keypoint_index_mapping = [
- 0,
- 17,
- 6,
- 8,
- 10,
- 5,
- 7,
- 9,
- 12,
- 14,
- 16,
- 11,
- 13,
- 15,
- 2,
- 1,
- 4,
- 3,
-]
-
-def convert_keypoints(keypoints):
- return [keypoints[i] for i in keypoint_index_mapping]
diff --git a/spaces/jsebdev/stock_predictor/utils.py b/spaces/jsebdev/stock_predictor/utils.py
deleted file mode 100644
index 6ed3fa8f977b077ab3f9d87f30e185a55278c3d3..0000000000000000000000000000000000000000
--- a/spaces/jsebdev/stock_predictor/utils.py
+++ /dev/null
@@ -1,94 +0,0 @@
-import numpy as np
-import pandas as pd
-import pandas_datareader as web
-import datetime as dt
-import yfinance as yfin
-import os
-
-from huggingface_hub import from_pretrained_keras
-
-
-def get_data(ticker='AAPL', start=None, end=None):
- if end is None:
- end = dt.date.today()
- if start is None:
- start = end - dt.timedelta(days=800)
-
- yfin.pdr_override()
- data = web.data.get_data_yahoo(ticker, start, end)
- # data = pd.read_csv('train_data.csv', index_col='Date')
- return data
-
-
-def get_last_candle_value(data, column):
- val = data.iloc[-1][column]
- return "{:.2f}".format(val)
-
-
-# Preprocessing functions copied from notebook where model was trained
-def create_remove_columns(data):
- # create jump column
- data = pd.DataFrame.copy(data)
- data['Jump'] = data['Open'] - data['Close'].shift(1)
- data['Jump'].fillna(0, inplace=True)
- data.insert(0,'Jump', data.pop('Jump'))
- return data
-
-def normalize_data(data):
- # Returns a tuple with the normalized data, the scaler and the decoder
- # The normalized data is a dataframe with the following columns:
- # ['Jump', 'High', 'Low', 'Close', 'Adj Close', 'Volume']
- the_data = pd.DataFrame.copy(data)
- # substract the open value to all columns but the first one and the last one which are "Jump" and "Volume"
- the_data.iloc[:, 1:-1] = the_data.iloc[:,1:-1] - the_data['Open'].values[:, np.newaxis]
- # print('the_data')
- # print(the_data)
-
- the_data.pop('Open')
- # Create the scaler
- max_value = float(os.getenv('SCALER_MAX_VALUE'))
- max_volume = float(os.getenv('SCALER_MAX_VOLUME'))
- def scaler(d):
- data = pd.DataFrame.copy(d)
- print('max_value: ', max_value)
- print('max_volume: ', max_volume)
- data.iloc[:, :-1] = data.iloc[:,:-1].apply(lambda x: x/max_value)
- data.iloc[:, -1] = data.iloc[:,-1].apply(lambda x: x/max_volume)
- return data
- def decoder(values):
- decoded_values = values * max_value
- return decoded_values
-
- normalized_data = scaler(the_data)
-
- return normalized_data, scaler, decoder
-
-def preprocessing(data):
- # print(data.head(3))
- data_0 = create_remove_columns(data)
- # print(data_0.head(3))
- #todo: save the_scaler somehow to use in new runtimes
- norm_data, scaler, decoder = normalize_data(data_0)
- # print(norm_data.head(3))
- # print(x_train.shape, y_train.shape)
- norm_data_array = np.array(norm_data)
- return np.expand_dims(norm_data_array, axis=0), decoder
-
-
-# Model prediction
-model = from_pretrained_keras("jsebdev/apple_stock_predictor")
-def predict(data):
- input, decoder = preprocessing(data)
- print("input")
- print(input.shape)
- result = decoder(model.predict(input))
- last_close = data.iloc[-1]['Close']
- next_candle = result[0, -1]
- print('next_candle')
- print(next_candle)
- jump = next_candle[0]
- next_candle = next_candle + last_close
- return (jump, next_candle[0], next_candle[1], next_candle[2], next_candle[3])
-
-def predict_mock(data):
- return (0,1,2,3,4)
\ No newline at end of file
diff --git a/spaces/jskalbg/ChatDev01/camel/agents/embodied_agent.py b/spaces/jskalbg/ChatDev01/camel/agents/embodied_agent.py
deleted file mode 100644
index a9bf44872d25216f70296df5ccf9aeecf0ed22b1..0000000000000000000000000000000000000000
--- a/spaces/jskalbg/ChatDev01/camel/agents/embodied_agent.py
+++ /dev/null
@@ -1,132 +0,0 @@
-# =========== Copyright 2023 @ CAMEL-AI.org. All Rights Reserved. ===========
-# Licensed under the Apache License, Version 2.0 (the “License”);
-# you may not use this file except in compliance with the License.
-# You may obtain a copy of the License at
-#
-# http://www.apache.org/licenses/LICENSE-2.0
-#
-# Unless required by applicable law or agreed to in writing, software
-# distributed under the License is distributed on an “AS IS” BASIS,
-# WITHOUT WARRANTIES OR CONDITIONS OF ANY KIND, either express or implied.
-# See the License for the specific language governing permissions and
-# limitations under the License.
-# =========== Copyright 2023 @ CAMEL-AI.org. All Rights Reserved. ===========
-from typing import Any, Dict, List, Optional, Tuple
-
-from colorama import Fore
-
-from camel.agents import BaseToolAgent, ChatAgent, HuggingFaceToolAgent
-from camel.messages import ChatMessage, SystemMessage
-from camel.typing import ModelType
-from camel.utils import print_text_animated
-
-
-class EmbodiedAgent(ChatAgent):
- r"""Class for managing conversations of CAMEL Embodied Agents.
-
- Args:
- system_message (SystemMessage): The system message for the chat agent.
- model (ModelType, optional): The LLM model to use for generating
- responses. (default :obj:`ModelType.GPT_4`)
- model_config (Any, optional): Configuration options for the LLM model.
- (default: :obj:`None`)
- message_window_size (int, optional): The maximum number of previous
- messages to include in the context window. If `None`, no windowing
- is performed. (default: :obj:`None`)
- action_space (List[Any], optional): The action space for the embodied
- agent. (default: :obj:`None`)
- verbose (bool, optional): Whether to print the critic's messages.
- logger_color (Any): The color of the logger displayed to the user.
- (default: :obj:`Fore.MAGENTA`)
- """
-
- def __init__(
- self,
- system_message: SystemMessage,
- model: ModelType = ModelType.GPT_4,
- model_config: Optional[Any] = None,
- message_window_size: Optional[int] = None,
- action_space: Optional[List[BaseToolAgent]] = None,
- verbose: bool = False,
- logger_color: Any = Fore.MAGENTA,
- ) -> None:
- default_action_space = [
- HuggingFaceToolAgent('hugging_face_tool_agent', model=model.value),
- ]
- self.action_space = action_space or default_action_space
- action_space_prompt = self.get_action_space_prompt()
- system_message.content = system_message.content.format(
- action_space=action_space_prompt)
- self.verbose = verbose
- self.logger_color = logger_color
- super().__init__(
- system_message=system_message,
- model=model,
- model_config=model_config,
- message_window_size=message_window_size,
- )
-
- def get_action_space_prompt(self) -> str:
- r"""Returns the action space prompt.
-
- Returns:
- str: The action space prompt.
- """
- return "\n".join([
- f"*** {action.name} ***:\n {action.description}"
- for action in self.action_space
- ])
-
- def step(
- self,
- input_message: ChatMessage,
- ) -> Tuple[ChatMessage, bool, Dict[str, Any]]:
- r"""Performs a step in the conversation.
-
- Args:
- input_message (ChatMessage): The input message.
-
- Returns:
- Tuple[ChatMessage, bool, Dict[str, Any]]: A tuple
- containing the output messages, termination status, and
- additional information.
- """
- response = super().step(input_message)
-
- if response.msgs is None or len(response.msgs) == 0:
- raise RuntimeError("Got None output messages.")
- if response.terminated:
- raise RuntimeError(f"{self.__class__.__name__} step failed.")
-
- # NOTE: Only single output messages are supported
- explanations, codes = response.msg.extract_text_and_code_prompts()
-
- if self.verbose:
- for explanation, code in zip(explanations, codes):
- print_text_animated(self.logger_color +
- f"> Explanation:\n{explanation}")
- print_text_animated(self.logger_color + f"> Code:\n{code}")
-
- if len(explanations) > len(codes):
- print_text_animated(self.logger_color +
- f"> Explanation:\n{explanations}")
-
- content = response.msg.content
-
- if codes is not None:
- content = "\n> Executed Results:"
- global_vars = {action.name: action for action in self.action_space}
- for code in codes:
- executed_outputs = code.execute(global_vars)
- content += (
- f"- Python standard output:\n{executed_outputs[0]}\n"
- f"- Local variables:\n{executed_outputs[1]}\n")
- content += "*" * 50 + "\n"
-
- # TODO: Handle errors
- content = input_message.content + (Fore.RESET +
- f"\n> Embodied Actions:\n{content}")
- message = ChatMessage(input_message.role_name, input_message.role_type,
- input_message.meta_dict, input_message.role,
- content)
- return message, response.terminated, response.info
diff --git a/spaces/juancopi81/youtube-music-transcribe/t5x/gin_utils.py b/spaces/juancopi81/youtube-music-transcribe/t5x/gin_utils.py
deleted file mode 100644
index 5d9b98c7cc0839e47b34071cde6114e5c7912f7b..0000000000000000000000000000000000000000
--- a/spaces/juancopi81/youtube-music-transcribe/t5x/gin_utils.py
+++ /dev/null
@@ -1,122 +0,0 @@
-# Copyright 2022 The T5X Authors.
-#
-# Licensed under the Apache License, Version 2.0 (the "License");
-# you may not use this file except in compliance with the License.
-# You may obtain a copy of the License at
-#
-# http://www.apache.org/licenses/LICENSE-2.0
-#
-# Unless required by applicable law or agreed to in writing, software
-# distributed under the License is distributed on an "AS IS" BASIS,
-# WITHOUT WARRANTIES OR CONDITIONS OF ANY KIND, either express or implied.
-# See the License for the specific language governing permissions and
-# limitations under the License.
-
-"""Utilities for using gin configurations with T5X binaries."""
-import os
-from typing import Optional, Sequence
-
-from absl import app
-from absl import logging
-from clu import metric_writers
-import gin
-import jax
-import tensorflow as tf
-
-
-
-def parse_gin_flags(gin_search_paths: Sequence[str],
- gin_files: Sequence[str],
- gin_bindings: Sequence[str],
- skip_unknown: bool = False,
- finalize_config: bool = True):
- """Parses provided gin files override params.
-
- Args:
- gin_search_paths: paths that will be searched for gin files.
- gin_files: paths to gin config files to be parsed. Files will be parsed in
- order with conflicting settings being overriden by later files. Paths may
- be relative to paths in `gin_search_paths`.
- gin_bindings: individual gin bindings to be applied after the gin files are
- parsed. Will be applied in order with conflicting settings being overriden
- by later oens.
- skip_unknown: whether to ignore unknown bindings or raise an error (default
- behavior).
- finalize_config: whether to finalize the config so that it cannot be
- modified (default behavior).
- """
- # We import t5.data here since it includes gin configurable functions commonly
- # used by task modules.
- # TODO(adarob): Strip gin from t5.data and remove this import.
- import t5.data # pylint:disable=unused-import,g-import-not-at-top
- # Register .gin file search paths with gin
- for gin_file_path in gin_search_paths:
- gin.add_config_file_search_path(gin_file_path)
-
-
- # Parse config files and bindings passed via flag.
- gin.parse_config_files_and_bindings(
- gin_files,
- gin_bindings,
- skip_unknown=skip_unknown,
- finalize_config=finalize_config)
- logging.info('Gin Configuration:\n%s', gin.config_str())
-
-
-def rewrite_gin_args(args: Sequence[str]) -> Sequence[str]:
- """Rewrite `--gin.NAME=VALUE` flags to `--gin_bindings=NAME=VALUE`."""
-
- def _rewrite_gin_arg(arg):
- if not arg.startswith('--gin.'):
- return arg
- if '=' not in arg:
- raise ValueError(
- "Gin bindings must be of the form '--gin.=', got: " +
- arg)
- # Strip '--gin.'
- arg = arg[6:]
- name, value = arg.split('=', maxsplit=1)
- r_arg = f'--gin_bindings={name} = {value}'
- print(f'Rewritten gin arg: {r_arg}')
- return r_arg
-
- return [_rewrite_gin_arg(arg) for arg in args]
-
-
-@gin.register
-def summarize_gin_config(model_dir: str,
- summary_writer: Optional[metric_writers.MetricWriter],
- step: int):
- """Writes gin config to the model dir and TensorBoard summary."""
- if jax.process_index() == 0:
- config_str = gin.config_str()
- tf.io.gfile.makedirs(model_dir)
- # Write the config as JSON.
- with tf.io.gfile.GFile(os.path.join(model_dir, 'config.gin'), 'w') as f:
- f.write(config_str)
- # Include a raw dump of the json as a text summary.
- if summary_writer is not None:
- summary_writer.write_texts(step, {'config': gin.markdown(config_str)})
- summary_writer.flush()
-
-
-def run(main):
- """Wrapper for app.run that rewrites gin args before parsing."""
- app.run(
- main,
- flags_parser=lambda a: app.parse_flags_with_usage(rewrite_gin_args(a)))
-
-
-# ====================== Configurable Utility Functions ======================
-
-
-@gin.configurable
-def sum_fn(var1=gin.REQUIRED, var2=gin.REQUIRED):
- """sum function to use inside gin files."""
- return var1 + var2
-
-
-@gin.configurable
-def bool_fn(var1=gin.REQUIRED):
- """bool function to use inside gin files."""
- return bool(var1)
diff --git a/spaces/justest/gpt4free/g4f/.v1/CONTRIBUTING.md b/spaces/justest/gpt4free/g4f/.v1/CONTRIBUTING.md
deleted file mode 100644
index 932dc30ff1665b0a94325a5d37cf4cf4337f2910..0000000000000000000000000000000000000000
--- a/spaces/justest/gpt4free/g4f/.v1/CONTRIBUTING.md
+++ /dev/null
@@ -1,8 +0,0 @@
-
-
-### Please, follow these steps to contribute:
-1. Reverse a website from this list: [sites-to-reverse](https://github.com/xtekky/gpt4free/issues/40)
-2. Add it to [./testing](https://github.com/xtekky/gpt4free/tree/main/testing)
-3. Refractor it and add it to [./gpt4free](https://github.com/xtekky/gpt4free/tree/main/gpt4free)
-
-### We will be grateful to see you as a contributor!
diff --git a/spaces/jyseo/3DFuse/ldm/models/diffusion/plms.py b/spaces/jyseo/3DFuse/ldm/models/diffusion/plms.py
deleted file mode 100644
index 7002a365d27168ced0a04e9a4d83e088f8284eae..0000000000000000000000000000000000000000
--- a/spaces/jyseo/3DFuse/ldm/models/diffusion/plms.py
+++ /dev/null
@@ -1,244 +0,0 @@
-"""SAMPLING ONLY."""
-
-import torch
-import numpy as np
-from tqdm import tqdm
-from functools import partial
-
-from ldm.modules.diffusionmodules.util import make_ddim_sampling_parameters, make_ddim_timesteps, noise_like
-from ldm.models.diffusion.sampling_util import norm_thresholding
-
-
-class PLMSSampler(object):
- def __init__(self, model, schedule="linear", **kwargs):
- super().__init__()
- self.model = model
- self.ddpm_num_timesteps = model.num_timesteps
- self.schedule = schedule
-
- def register_buffer(self, name, attr):
- if type(attr) == torch.Tensor:
- if attr.device != torch.device("cuda"):
- attr = attr.to(torch.device("cuda"))
- setattr(self, name, attr)
-
- def make_schedule(self, ddim_num_steps, ddim_discretize="uniform", ddim_eta=0., verbose=True):
- if ddim_eta != 0:
- raise ValueError('ddim_eta must be 0 for PLMS')
- self.ddim_timesteps = make_ddim_timesteps(ddim_discr_method=ddim_discretize, num_ddim_timesteps=ddim_num_steps,
- num_ddpm_timesteps=self.ddpm_num_timesteps,verbose=verbose)
- alphas_cumprod = self.model.alphas_cumprod
- assert alphas_cumprod.shape[0] == self.ddpm_num_timesteps, 'alphas have to be defined for each timestep'
- to_torch = lambda x: x.clone().detach().to(torch.float32).to(self.model.device)
-
- self.register_buffer('betas', to_torch(self.model.betas))
- self.register_buffer('alphas_cumprod', to_torch(alphas_cumprod))
- self.register_buffer('alphas_cumprod_prev', to_torch(self.model.alphas_cumprod_prev))
-
- # calculations for diffusion q(x_t | x_{t-1}) and others
- self.register_buffer('sqrt_alphas_cumprod', to_torch(np.sqrt(alphas_cumprod.cpu())))
- self.register_buffer('sqrt_one_minus_alphas_cumprod', to_torch(np.sqrt(1. - alphas_cumprod.cpu())))
- self.register_buffer('log_one_minus_alphas_cumprod', to_torch(np.log(1. - alphas_cumprod.cpu())))
- self.register_buffer('sqrt_recip_alphas_cumprod', to_torch(np.sqrt(1. / alphas_cumprod.cpu())))
- self.register_buffer('sqrt_recipm1_alphas_cumprod', to_torch(np.sqrt(1. / alphas_cumprod.cpu() - 1)))
-
- # ddim sampling parameters
- ddim_sigmas, ddim_alphas, ddim_alphas_prev = make_ddim_sampling_parameters(alphacums=alphas_cumprod.cpu(),
- ddim_timesteps=self.ddim_timesteps,
- eta=ddim_eta,verbose=verbose)
- self.register_buffer('ddim_sigmas', ddim_sigmas)
- self.register_buffer('ddim_alphas', ddim_alphas)
- self.register_buffer('ddim_alphas_prev', ddim_alphas_prev)
- self.register_buffer('ddim_sqrt_one_minus_alphas', np.sqrt(1. - ddim_alphas))
- sigmas_for_original_sampling_steps = ddim_eta * torch.sqrt(
- (1 - self.alphas_cumprod_prev) / (1 - self.alphas_cumprod) * (
- 1 - self.alphas_cumprod / self.alphas_cumprod_prev))
- self.register_buffer('ddim_sigmas_for_original_num_steps', sigmas_for_original_sampling_steps)
-
- @torch.no_grad()
- def sample(self,
- S,
- batch_size,
- shape,
- conditioning=None,
- callback=None,
- normals_sequence=None,
- img_callback=None,
- quantize_x0=False,
- eta=0.,
- mask=None,
- x0=None,
- temperature=1.,
- noise_dropout=0.,
- score_corrector=None,
- corrector_kwargs=None,
- verbose=True,
- x_T=None,
- log_every_t=100,
- unconditional_guidance_scale=1.,
- unconditional_conditioning=None,
- # this has to come in the same format as the conditioning, # e.g. as encoded tokens, ...
- dynamic_threshold=None,
- **kwargs
- ):
- if conditioning is not None:
- if isinstance(conditioning, dict):
- cbs = conditioning[list(conditioning.keys())[0]].shape[0]
- if cbs != batch_size:
- print(f"Warning: Got {cbs} conditionings but batch-size is {batch_size}")
- else:
- if conditioning.shape[0] != batch_size:
- print(f"Warning: Got {conditioning.shape[0]} conditionings but batch-size is {batch_size}")
-
- self.make_schedule(ddim_num_steps=S, ddim_eta=eta, verbose=verbose)
- # sampling
- C, H, W = shape
- size = (batch_size, C, H, W)
- print(f'Data shape for PLMS sampling is {size}')
-
- samples, intermediates = self.plms_sampling(conditioning, size,
- callback=callback,
- img_callback=img_callback,
- quantize_denoised=quantize_x0,
- mask=mask, x0=x0,
- ddim_use_original_steps=False,
- noise_dropout=noise_dropout,
- temperature=temperature,
- score_corrector=score_corrector,
- corrector_kwargs=corrector_kwargs,
- x_T=x_T,
- log_every_t=log_every_t,
- unconditional_guidance_scale=unconditional_guidance_scale,
- unconditional_conditioning=unconditional_conditioning,
- dynamic_threshold=dynamic_threshold,
- )
- return samples, intermediates
-
- @torch.no_grad()
- def plms_sampling(self, cond, shape,
- x_T=None, ddim_use_original_steps=False,
- callback=None, timesteps=None, quantize_denoised=False,
- mask=None, x0=None, img_callback=None, log_every_t=100,
- temperature=1., noise_dropout=0., score_corrector=None, corrector_kwargs=None,
- unconditional_guidance_scale=1., unconditional_conditioning=None,
- dynamic_threshold=None):
- device = self.model.betas.device
- b = shape[0]
- if x_T is None:
- img = torch.randn(shape, device=device)
- else:
- img = x_T
-
- if timesteps is None:
- timesteps = self.ddpm_num_timesteps if ddim_use_original_steps else self.ddim_timesteps
- elif timesteps is not None and not ddim_use_original_steps:
- subset_end = int(min(timesteps / self.ddim_timesteps.shape[0], 1) * self.ddim_timesteps.shape[0]) - 1
- timesteps = self.ddim_timesteps[:subset_end]
-
- intermediates = {'x_inter': [img], 'pred_x0': [img]}
- time_range = list(reversed(range(0,timesteps))) if ddim_use_original_steps else np.flip(timesteps)
- total_steps = timesteps if ddim_use_original_steps else timesteps.shape[0]
- print(f"Running PLMS Sampling with {total_steps} timesteps")
-
- iterator = tqdm(time_range, desc='PLMS Sampler', total=total_steps)
- old_eps = []
-
- for i, step in enumerate(iterator):
- index = total_steps - i - 1
- ts = torch.full((b,), step, device=device, dtype=torch.long)
- ts_next = torch.full((b,), time_range[min(i + 1, len(time_range) - 1)], device=device, dtype=torch.long)
-
- if mask is not None:
- assert x0 is not None
- img_orig = self.model.q_sample(x0, ts) # TODO: deterministic forward pass?
- img = img_orig * mask + (1. - mask) * img
-
- outs = self.p_sample_plms(img, cond, ts, index=index, use_original_steps=ddim_use_original_steps,
- quantize_denoised=quantize_denoised, temperature=temperature,
- noise_dropout=noise_dropout, score_corrector=score_corrector,
- corrector_kwargs=corrector_kwargs,
- unconditional_guidance_scale=unconditional_guidance_scale,
- unconditional_conditioning=unconditional_conditioning,
- old_eps=old_eps, t_next=ts_next,
- dynamic_threshold=dynamic_threshold)
- img, pred_x0, e_t = outs
- old_eps.append(e_t)
- if len(old_eps) >= 4:
- old_eps.pop(0)
- if callback: callback(i)
- if img_callback: img_callback(pred_x0, i)
-
- if index % log_every_t == 0 or index == total_steps - 1:
- intermediates['x_inter'].append(img)
- intermediates['pred_x0'].append(pred_x0)
-
- return img, intermediates
-
- @torch.no_grad()
- def p_sample_plms(self, x, c, t, index, repeat_noise=False, use_original_steps=False, quantize_denoised=False,
- temperature=1., noise_dropout=0., score_corrector=None, corrector_kwargs=None,
- unconditional_guidance_scale=1., unconditional_conditioning=None, old_eps=None, t_next=None,
- dynamic_threshold=None):
- b, *_, device = *x.shape, x.device
-
- def get_model_output(x, t):
- if unconditional_conditioning is None or unconditional_guidance_scale == 1.:
- e_t = self.model.apply_model(x, t, c)
- else:
- x_in = torch.cat([x] * 2)
- t_in = torch.cat([t] * 2)
- c_in = torch.cat([unconditional_conditioning, c])
- e_t_uncond, e_t = self.model.apply_model(x_in, t_in, c_in).chunk(2)
- e_t = e_t_uncond + unconditional_guidance_scale * (e_t - e_t_uncond)
-
- if score_corrector is not None:
- assert self.model.parameterization == "eps"
- e_t = score_corrector.modify_score(self.model, e_t, x, t, c, **corrector_kwargs)
-
- return e_t
-
- alphas = self.model.alphas_cumprod if use_original_steps else self.ddim_alphas
- alphas_prev = self.model.alphas_cumprod_prev if use_original_steps else self.ddim_alphas_prev
- sqrt_one_minus_alphas = self.model.sqrt_one_minus_alphas_cumprod if use_original_steps else self.ddim_sqrt_one_minus_alphas
- sigmas = self.model.ddim_sigmas_for_original_num_steps if use_original_steps else self.ddim_sigmas
-
- def get_x_prev_and_pred_x0(e_t, index):
- # select parameters corresponding to the currently considered timestep
- a_t = torch.full((b, 1, 1, 1), alphas[index], device=device)
- a_prev = torch.full((b, 1, 1, 1), alphas_prev[index], device=device)
- sigma_t = torch.full((b, 1, 1, 1), sigmas[index], device=device)
- sqrt_one_minus_at = torch.full((b, 1, 1, 1), sqrt_one_minus_alphas[index],device=device)
-
- # current prediction for x_0
- pred_x0 = (x - sqrt_one_minus_at * e_t) / a_t.sqrt()
- if quantize_denoised:
- pred_x0, _, *_ = self.model.first_stage_model.quantize(pred_x0)
- if dynamic_threshold is not None:
- pred_x0 = norm_thresholding(pred_x0, dynamic_threshold)
- # direction pointing to x_t
- dir_xt = (1. - a_prev - sigma_t**2).sqrt() * e_t
- noise = sigma_t * noise_like(x.shape, device, repeat_noise) * temperature
- if noise_dropout > 0.:
- noise = torch.nn.functional.dropout(noise, p=noise_dropout)
- x_prev = a_prev.sqrt() * pred_x0 + dir_xt + noise
- return x_prev, pred_x0
-
- e_t = get_model_output(x, t)
- if len(old_eps) == 0:
- # Pseudo Improved Euler (2nd order)
- x_prev, pred_x0 = get_x_prev_and_pred_x0(e_t, index)
- e_t_next = get_model_output(x_prev, t_next)
- e_t_prime = (e_t + e_t_next) / 2
- elif len(old_eps) == 1:
- # 2nd order Pseudo Linear Multistep (Adams-Bashforth)
- e_t_prime = (3 * e_t - old_eps[-1]) / 2
- elif len(old_eps) == 2:
- # 3nd order Pseudo Linear Multistep (Adams-Bashforth)
- e_t_prime = (23 * e_t - 16 * old_eps[-1] + 5 * old_eps[-2]) / 12
- elif len(old_eps) >= 3:
- # 4nd order Pseudo Linear Multistep (Adams-Bashforth)
- e_t_prime = (55 * e_t - 59 * old_eps[-1] + 37 * old_eps[-2] - 9 * old_eps[-3]) / 24
-
- x_prev, pred_x0 = get_x_prev_and_pred_x0(e_t_prime, index)
-
- return x_prev, pred_x0, e_t
diff --git a/spaces/kdrkdrkdr/ShirokoTTS/text/japanese.py b/spaces/kdrkdrkdr/ShirokoTTS/text/japanese.py
deleted file mode 100644
index 65480534b452efabe87b40033316e2c1577ff3ea..0000000000000000000000000000000000000000
--- a/spaces/kdrkdrkdr/ShirokoTTS/text/japanese.py
+++ /dev/null
@@ -1,132 +0,0 @@
-import re
-from unidecode import unidecode
-import pyopenjtalk
-
-
-# Regular expression matching Japanese without punctuation marks:
-_japanese_characters = re.compile(
- r'[A-Za-z\d\u3005\u3040-\u30ff\u4e00-\u9fff\uff11-\uff19\uff21-\uff3a\uff41-\uff5a\uff66-\uff9d]')
-
-# Regular expression matching non-Japanese characters or punctuation marks:
-_japanese_marks = re.compile(
- r'[^A-Za-z\d\u3005\u3040-\u30ff\u4e00-\u9fff\uff11-\uff19\uff21-\uff3a\uff41-\uff5a\uff66-\uff9d]')
-
-# List of (symbol, Japanese) pairs for marks:
-_symbols_to_japanese = [(re.compile('%s' % x[0]), x[1]) for x in [
- ('%', 'パーセント')
-]]
-
-# List of (romaji, ipa) pairs for marks:
-_romaji_to_ipa = [(re.compile('%s' % x[0], re.IGNORECASE), x[1]) for x in [
- ('ts', 'ʦ'),
- ('u', 'ɯ'),
- ('...', '…'),
- ('j', 'ʥ'),
- ('y', 'j'),
- ('ni', 'n^i'),
- ('nj', 'n^'),
- ('hi', 'çi'),
- ('hj', 'ç'),
- ('f', 'ɸ'),
- ('I', 'i*'),
- ('U', 'ɯ*'),
- ('r', 'ɾ')
-]]
-
-# Dictinary of (consonant, sokuon) pairs:
-_real_sokuon = {
- 'k': 'k#',
- 'g': 'k#',
- 't': 't#',
- 'd': 't#',
- 'ʦ': 't#',
- 'ʧ': 't#',
- 'ʥ': 't#',
- 'j': 't#',
- 's': 's',
- 'ʃ': 's',
- 'p': 'p#',
- 'b': 'p#'
-}
-
-# Dictinary of (consonant, hatsuon) pairs:
-_real_hatsuon = {
- 'p': 'm',
- 'b': 'm',
- 'm': 'm',
- 't': 'n',
- 'd': 'n',
- 'n': 'n',
- 'ʧ': 'n^',
- 'ʥ': 'n^',
- 'k': 'ŋ',
- 'g': 'ŋ'
-}
-
-
-def symbols_to_japanese(text):
- for regex, replacement in _symbols_to_japanese:
- text = re.sub(regex, replacement, text)
- return text
-
-
-def japanese_to_romaji_with_accent(text):
- '''Reference https://r9y9.github.io/ttslearn/latest/notebooks/ch10_Recipe-Tacotron.html'''
- text = symbols_to_japanese(text)
- sentences = re.split(_japanese_marks, text)
- marks = re.findall(_japanese_marks, text)
- text = ''
- for i, sentence in enumerate(sentences):
- if re.match(_japanese_characters, sentence):
- if text != '':
- text += ' '
- labels = pyopenjtalk.extract_fullcontext(sentence)
- for n, label in enumerate(labels):
- phoneme = re.search(r'\-([^\+]*)\+', label).group(1)
- if phoneme not in ['sil', 'pau']:
- text += phoneme.replace('ch', 'ʧ').replace('sh',
- 'ʃ').replace('cl', 'Q')
- else:
- continue
- # n_moras = int(re.search(r'/F:(\d+)_', label).group(1))
- a1 = int(re.search(r"/A:(\-?[0-9]+)\+", label).group(1))
- a2 = int(re.search(r"\+(\d+)\+", label).group(1))
- a3 = int(re.search(r"\+(\d+)/", label).group(1))
- if re.search(r'\-([^\+]*)\+', labels[n + 1]).group(1) in ['sil', 'pau']:
- a2_next = -1
- else:
- a2_next = int(
- re.search(r"\+(\d+)\+", labels[n + 1]).group(1))
- # Accent phrase boundary
- if a3 == 1 and a2_next == 1:
- text += ' '
- # Falling
- elif a1 == 0 and a2_next == a2 + 1:
- text += '↓'
- # Rising
- elif a2 == 1 and a2_next == 2:
- text += '↑'
- if i < len(marks):
- text += unidecode(marks[i]).replace(' ', '')
- return text
-
-
-def get_real_sokuon(text):
- text=re.sub('Q[↑↓]*(.)',lambda x:_real_sokuon[x.group(1)]+x.group(0)[1:] if x.group(1) in _real_sokuon.keys() else x.group(0),text)
- return text
-
-
-def get_real_hatsuon(text):
- text=re.sub('N[↑↓]*(.)',lambda x:_real_hatsuon[x.group(1)]+x.group(0)[1:] if x.group(1) in _real_hatsuon.keys() else x.group(0),text)
- return text
-
-
-def japanese_to_ipa(text):
- text=japanese_to_romaji_with_accent(text)
- for regex, replacement in _romaji_to_ipa:
- text = re.sub(regex, replacement, text)
- text = re.sub(
- r'([A-Za-zɯ])\1+', lambda x: x.group(0)[0]+'ː'*(len(x.group(0))-1), text)
- text = get_real_sokuon(text)
- text = get_real_hatsuon(text)
- return text
diff --git a/spaces/kepl/gpt/g4f/Provider/Providers/Yqcloud.py b/spaces/kepl/gpt/g4f/Provider/Providers/Yqcloud.py
deleted file mode 100644
index ad5c3a4326c68ceb7ee012fbf5bc072da72a7e40..0000000000000000000000000000000000000000
--- a/spaces/kepl/gpt/g4f/Provider/Providers/Yqcloud.py
+++ /dev/null
@@ -1,39 +0,0 @@
-import os
-import time
-import requests
-
-from ...typing import sha256, Dict, get_type_hints
-url = 'https://chat9.yqcloud.top/'
-model = [
- 'gpt-3.5-turbo',
-]
-supports_stream = True
-needs_auth = False
-
-
-def _create_completion(model: str, messages: list, stream: bool, chatId: str, **kwargs):
-
- headers = {
- 'authority': 'api.aichatos.cloud',
- 'origin': 'https://chat9.yqcloud.top',
- 'referer': 'https://chat9.yqcloud.top/',
- 'user-agent': 'Mozilla/5.0 (Macintosh; Intel Mac OS X 10_15_7) AppleWebKit/537.36 (KHTML, like Gecko) Chrome/112.0.0.0 Safari/537.36',
- }
-
- json_data = {
- 'prompt': str(messages),
- 'userId': f'#/chat/{chatId}',
- 'network': True,
- 'apikey': '',
- 'system': '',
- 'withoutContext': False,
- }
- response = requests.post('https://api.aichatos.cloud/api/generateStream',
- headers=headers, json=json_data, stream=True)
- for token in response.iter_content(chunk_size=2046):
- yield (token.decode('utf-8'))
-
-
-params = f'g4f.Providers.{os.path.basename(__file__)[:-3]} supports: ' + \
- '(%s)' % ', '.join(
- [f"{name}: {get_type_hints(_create_completion)[name].__name__}" for name in _create_completion.__code__.co_varnames[:_create_completion.__code__.co_argcount]])
\ No newline at end of file
diff --git a/spaces/kevinwang676/ChatGLM2-SadTalker/src/audio2exp_models/networks.py b/spaces/kevinwang676/ChatGLM2-SadTalker/src/audio2exp_models/networks.py
deleted file mode 100644
index f052e18101f5446a527ae354b3621e7d0d4991cc..0000000000000000000000000000000000000000
--- a/spaces/kevinwang676/ChatGLM2-SadTalker/src/audio2exp_models/networks.py
+++ /dev/null
@@ -1,74 +0,0 @@
-import torch
-import torch.nn.functional as F
-from torch import nn
-
-class Conv2d(nn.Module):
- def __init__(self, cin, cout, kernel_size, stride, padding, residual=False, use_act = True, *args, **kwargs):
- super().__init__(*args, **kwargs)
- self.conv_block = nn.Sequential(
- nn.Conv2d(cin, cout, kernel_size, stride, padding),
- nn.BatchNorm2d(cout)
- )
- self.act = nn.ReLU()
- self.residual = residual
- self.use_act = use_act
-
- def forward(self, x):
- out = self.conv_block(x)
- if self.residual:
- out += x
-
- if self.use_act:
- return self.act(out)
- else:
- return out
-
-class SimpleWrapperV2(nn.Module):
- def __init__(self) -> None:
- super().__init__()
- self.audio_encoder = nn.Sequential(
- Conv2d(1, 32, kernel_size=3, stride=1, padding=1),
- Conv2d(32, 32, kernel_size=3, stride=1, padding=1, residual=True),
- Conv2d(32, 32, kernel_size=3, stride=1, padding=1, residual=True),
-
- Conv2d(32, 64, kernel_size=3, stride=(3, 1), padding=1),
- Conv2d(64, 64, kernel_size=3, stride=1, padding=1, residual=True),
- Conv2d(64, 64, kernel_size=3, stride=1, padding=1, residual=True),
-
- Conv2d(64, 128, kernel_size=3, stride=3, padding=1),
- Conv2d(128, 128, kernel_size=3, stride=1, padding=1, residual=True),
- Conv2d(128, 128, kernel_size=3, stride=1, padding=1, residual=True),
-
- Conv2d(128, 256, kernel_size=3, stride=(3, 2), padding=1),
- Conv2d(256, 256, kernel_size=3, stride=1, padding=1, residual=True),
-
- Conv2d(256, 512, kernel_size=3, stride=1, padding=0),
- Conv2d(512, 512, kernel_size=1, stride=1, padding=0),
- )
-
- #### load the pre-trained audio_encoder
- #self.audio_encoder = self.audio_encoder.to(device)
- '''
- wav2lip_state_dict = torch.load('/apdcephfs_cq2/share_1290939/wenxuazhang/checkpoints/wav2lip.pth')['state_dict']
- state_dict = self.audio_encoder.state_dict()
-
- for k,v in wav2lip_state_dict.items():
- if 'audio_encoder' in k:
- print('init:', k)
- state_dict[k.replace('module.audio_encoder.', '')] = v
- self.audio_encoder.load_state_dict(state_dict)
- '''
-
- self.mapping1 = nn.Linear(512+64+1, 64)
- #self.mapping2 = nn.Linear(30, 64)
- #nn.init.constant_(self.mapping1.weight, 0.)
- nn.init.constant_(self.mapping1.bias, 0.)
-
- def forward(self, x, ref, ratio):
- x = self.audio_encoder(x).view(x.size(0), -1)
- ref_reshape = ref.reshape(x.size(0), -1)
- ratio = ratio.reshape(x.size(0), -1)
-
- y = self.mapping1(torch.cat([x, ref_reshape, ratio], dim=1))
- out = y.reshape(ref.shape[0], ref.shape[1], -1) #+ ref # resudial
- return out
diff --git a/spaces/kevinwang676/FreeVC/README.md b/spaces/kevinwang676/FreeVC/README.md
deleted file mode 100644
index 262c4d8763e1aa8529c6dec0136c938b9fe2daa6..0000000000000000000000000000000000000000
--- a/spaces/kevinwang676/FreeVC/README.md
+++ /dev/null
@@ -1,14 +0,0 @@
----
-title: FreeVC
-emoji: 🚀
-colorFrom: gray
-colorTo: red
-sdk: gradio
-sdk_version: 3.36.1
-app_file: app.py
-pinned: false
-license: mit
-duplicated_from: OlaWod/FreeVC
----
-
-Check out the configuration reference at https://huggingface.co/docs/hub/spaces-config-reference
diff --git a/spaces/kidcoconut/spcdkr_omdenasaudi_liverhccxai/scripts/models/util_joinModel.sh b/spaces/kidcoconut/spcdkr_omdenasaudi_liverhccxai/scripts/models/util_joinModel.sh
deleted file mode 100644
index 9fb7d520c64fb333b7f669611272bacf1c18a963..0000000000000000000000000000000000000000
--- a/spaces/kidcoconut/spcdkr_omdenasaudi_liverhccxai/scripts/models/util_joinModel.sh
+++ /dev/null
@@ -1,45 +0,0 @@
-#!/bin/bash
-
-<
- - the first arg has to be wrapped in single quotes to ensure that bash does not expand wildcards
-Prereqs: a model folder within bin/models; containing a split pyTorch model.pth as 1 or more model_nn files
-Todo: get the parent folder name and use this as the name for the model file
-blkHeader
-
-#--- dependencies
-#none
-
-
-#--- initialize/configuration
-#--- $1: first arg; source pattern match; eg './bin/models/deeplabv3*vhflip30/model_a*'; Note that this is wildcarded so must be in quotes
-#--- $n: last arg; dest model file; eg. ./bin/models/model.pth
-echo -e "INFO(util_joinModel):\t Initializing ..."
-strPth_patternMatch=$1
-if [ -z "$strPth_patternMatch" ]; then
- echo "WARN: no args provided. Exiting script."
- exit
-fi
-
-strPth_filMatch=( $strPth_patternMatch ) #--- expand the pattern match; get the first value of the pattern match
-strPth_parentFld=$(dirname $strPth_filMatch) #--- get the parent dir of the first file match
-strPth_mdlFile=${@: -1} #--- Note: this gets the last arg; otherwise the 2nd arg would be an iteration of the 1st arg wildcard
-
-strpth_pwd=$(pwd)
-strpth_scriptLoc=$( cd -- "$( dirname -- "${BASH_SOURCE[0]}" )" &> /dev/null && pwd )
-strpth_scrHome="${strpth_scriptLoc}/../"
-strpth_appHome="${strpth_scrHome}/../"
-
-#echo "TRACE: strPth_patternMatch= $strPth_patternMatch"
-#echo "TRACE: strPth_filMatch= $strPth_filMatch"
-#echo "TRACE: strPth_parentFld= $strPth_parentFld"
-#echo "TRACE: strPth_mdlFile= $strPth_mdlFile"
-
-#--- reconstitute model
-#--- Note: cat command does not work with single-quote literals; do not reapply single quotes
-#echo "cat ${strPth_patternMatch} > ${strPth_mdlFile}"
-echo -e "INFO:\t Joining model binary ..."
-cat ${strPth_patternMatch} > ${strPth_mdlFile}
-echo -e "INFO:\t Done ...\n"
\ No newline at end of file
diff --git a/spaces/koajoel/PolyFormer/fairseq/examples/byte_level_bpe/get_bitext.py b/spaces/koajoel/PolyFormer/fairseq/examples/byte_level_bpe/get_bitext.py
deleted file mode 100644
index 6ac1eeec1e6167ec6bafd76b37173ee6987cae7e..0000000000000000000000000000000000000000
--- a/spaces/koajoel/PolyFormer/fairseq/examples/byte_level_bpe/get_bitext.py
+++ /dev/null
@@ -1,254 +0,0 @@
-# Copyright (c) Facebook, Inc. and its affiliates.
-#
-# This source code is licensed under the MIT license found in the
-# LICENSE file in the root directory of this source tree.
-
-
-import argparse
-import os
-import os.path as op
-from collections import namedtuple
-from multiprocessing import cpu_count
-from typing import List, Optional
-
-import sentencepiece as sp
-from fairseq.data.encoders.byte_bpe import ByteBPE
-from fairseq.data.encoders.byte_utils import byte_encode
-from fairseq.data.encoders.bytes import Bytes
-from fairseq.data.encoders.characters import Characters
-from fairseq.data.encoders.moses_tokenizer import MosesTokenizer
-from fairseq.data.encoders.sentencepiece_bpe import SentencepieceBPE
-
-
-SPLITS = ["train", "valid", "test"]
-
-
-def _convert_xml(in_path: str, out_path: str):
- with open(in_path) as f, open(out_path, "w") as f_o:
- for s in f:
- ss = s.strip()
- if not ss.startswith("", "").split('">')
- assert len(ss) == 2
- f_o.write(ss[1].strip() + "\n")
-
-
-def _convert_train(in_path: str, out_path: str):
- with open(in_path) as f, open(out_path, "w") as f_o:
- for s in f:
- ss = s.strip()
- if ss.startswith("<"):
- continue
- f_o.write(ss.strip() + "\n")
-
-
-def _get_bytes(in_path: str, out_path: str):
- with open(in_path) as f, open(out_path, "w") as f_o:
- for s in f:
- f_o.write(Bytes.encode(s.strip()) + "\n")
-
-
-def _get_chars(in_path: str, out_path: str):
- with open(in_path) as f, open(out_path, "w") as f_o:
- for s in f:
- f_o.write(Characters.encode(s.strip()) + "\n")
-
-
-def pretokenize(in_path: str, out_path: str, src: str, tgt: str):
- Args = namedtuple(
- "Args",
- [
- "moses_source_lang",
- "moses_target_lang",
- "moses_no_dash_splits",
- "moses_no_escape",
- ],
- )
- args = Args(
- moses_source_lang=src,
- moses_target_lang=tgt,
- moses_no_dash_splits=False,
- moses_no_escape=False,
- )
- pretokenizer = MosesTokenizer(args)
- with open(in_path) as f, open(out_path, "w") as f_o:
- for s in f:
- f_o.write(pretokenizer.encode(s.strip()) + "\n")
-
-
-def _convert_to_bchar(in_path_prefix: str, src: str, tgt: str, out_path: str):
- with open(out_path, "w") as f_o:
- for lang in [src, tgt]:
- with open(f"{in_path_prefix}.{lang}") as f:
- for s in f:
- f_o.write(byte_encode(s.strip()) + "\n")
-
-
-def _get_bpe(in_path: str, model_prefix: str, vocab_size: int):
- arguments = [
- f"--input={in_path}",
- f"--model_prefix={model_prefix}",
- f"--model_type=bpe",
- f"--vocab_size={vocab_size}",
- "--character_coverage=1.0",
- "--normalization_rule_name=identity",
- f"--num_threads={cpu_count()}",
- ]
- sp.SentencePieceTrainer.Train(" ".join(arguments))
-
-
-def _apply_bbpe(model_path: str, in_path: str, out_path: str):
- Args = namedtuple("Args", ["sentencepiece_model_path"])
- args = Args(sentencepiece_model_path=model_path)
- tokenizer = ByteBPE(args)
- with open(in_path) as f, open(out_path, "w") as f_o:
- for s in f:
- f_o.write(tokenizer.encode(s.strip()) + "\n")
-
-
-def _apply_bpe(model_path: str, in_path: str, out_path: str):
- Args = namedtuple("Args", ["sentencepiece_model"])
- args = Args(sentencepiece_model=model_path)
- tokenizer = SentencepieceBPE(args)
- with open(in_path) as f, open(out_path, "w") as f_o:
- for s in f:
- f_o.write(tokenizer.encode(s.strip()) + "\n")
-
-
-def _concat_files(in_paths: List[str], out_path: str):
- with open(out_path, "w") as f_o:
- for p in in_paths:
- with open(p) as f:
- for r in f:
- f_o.write(r)
-
-
-def preprocess_iwslt17(
- root: str,
- src: str,
- tgt: str,
- bpe_size: Optional[int],
- need_chars: bool,
- bbpe_size: Optional[int],
- need_bytes: bool,
-):
- # extract bitext
- in_root = op.join(root, f"{src}-{tgt}")
- for lang in [src, tgt]:
- _convert_train(
- op.join(in_root, f"train.tags.{src}-{tgt}.{lang}"),
- op.join(root, f"train.{lang}"),
- )
- _convert_xml(
- op.join(in_root, f"IWSLT17.TED.dev2010.{src}-{tgt}.{lang}.xml"),
- op.join(root, f"valid.{lang}"),
- )
- _convert_xml(
- op.join(in_root, f"IWSLT17.TED.tst2015.{src}-{tgt}.{lang}.xml"),
- op.join(root, f"test.{lang}"),
- )
- # pre-tokenize
- for lang in [src, tgt]:
- for split in SPLITS:
- pretokenize(
- op.join(root, f"{split}.{lang}"),
- op.join(root, f"{split}.moses.{lang}"),
- src,
- tgt,
- )
- # tokenize with BPE vocabulary
- if bpe_size is not None:
- # learn vocabulary
- concated_train_path = op.join(root, "train.all")
- _concat_files(
- [op.join(root, "train.moses.fr"), op.join(root, "train.moses.en")],
- concated_train_path,
- )
- bpe_model_prefix = op.join(root, f"spm_bpe{bpe_size}")
- _get_bpe(concated_train_path, bpe_model_prefix, bpe_size)
- os.remove(concated_train_path)
- # apply
- for lang in [src, tgt]:
- for split in SPLITS:
- _apply_bpe(
- bpe_model_prefix + ".model",
- op.join(root, f"{split}.moses.{lang}"),
- op.join(root, f"{split}.moses.bpe{bpe_size}.{lang}"),
- )
- # tokenize with bytes vocabulary
- if need_bytes:
- for lang in [src, tgt]:
- for split in SPLITS:
- _get_bytes(
- op.join(root, f"{split}.moses.{lang}"),
- op.join(root, f"{split}.moses.bytes.{lang}"),
- )
- # tokenize with characters vocabulary
- if need_chars:
- for lang in [src, tgt]:
- for split in SPLITS:
- _get_chars(
- op.join(root, f"{split}.moses.{lang}"),
- op.join(root, f"{split}.moses.chars.{lang}"),
- )
- # tokenize with byte-level BPE vocabulary
- if bbpe_size is not None:
- # learn vocabulary
- bchar_path = op.join(root, "train.bchar")
- _convert_to_bchar(op.join(root, "train.moses"), src, tgt, bchar_path)
- bbpe_model_prefix = op.join(root, f"spm_bbpe{bbpe_size}")
- _get_bpe(bchar_path, bbpe_model_prefix, bbpe_size)
- os.remove(bchar_path)
- # apply
- for lang in [src, tgt]:
- for split in SPLITS:
- _apply_bbpe(
- bbpe_model_prefix + ".model",
- op.join(root, f"{split}.moses.{lang}"),
- op.join(root, f"{split}.moses.bbpe{bbpe_size}.{lang}"),
- )
-
-
-def main():
- parser = argparse.ArgumentParser()
- parser.add_argument("--root", type=str, default="data")
- parser.add_argument(
- "--bpe-vocab",
- default=None,
- type=int,
- help="Generate tokenized bitext with BPE of size K."
- "Default to None (disabled).",
- )
- parser.add_argument(
- "--bbpe-vocab",
- default=None,
- type=int,
- help="Generate tokenized bitext with BBPE of size K."
- "Default to None (disabled).",
- )
- parser.add_argument(
- "--byte-vocab",
- action="store_true",
- help="Generate tokenized bitext with bytes vocabulary",
- )
- parser.add_argument(
- "--char-vocab",
- action="store_true",
- help="Generate tokenized bitext with chars vocabulary",
- )
- args = parser.parse_args()
-
- preprocess_iwslt17(
- args.root,
- "fr",
- "en",
- args.bpe_vocab,
- args.char_vocab,
- args.bbpe_vocab,
- args.byte_vocab,
- )
-
-
-if __name__ == "__main__":
- main()
diff --git a/spaces/ky2k/Toxicity_Classifier_POC/.venv/lib/python3.9/site-packages/attr/converters.py b/spaces/ky2k/Toxicity_Classifier_POC/.venv/lib/python3.9/site-packages/attr/converters.py
deleted file mode 100644
index 4cada106b01c564faf17969d24038f80abd5de6f..0000000000000000000000000000000000000000
--- a/spaces/ky2k/Toxicity_Classifier_POC/.venv/lib/python3.9/site-packages/attr/converters.py
+++ /dev/null
@@ -1,144 +0,0 @@
-# SPDX-License-Identifier: MIT
-
-"""
-Commonly useful converters.
-"""
-
-
-import typing
-
-from ._compat import _AnnotationExtractor
-from ._make import NOTHING, Factory, pipe
-
-
-__all__ = [
- "default_if_none",
- "optional",
- "pipe",
- "to_bool",
-]
-
-
-def optional(converter):
- """
- A converter that allows an attribute to be optional. An optional attribute
- is one which can be set to ``None``.
-
- Type annotations will be inferred from the wrapped converter's, if it
- has any.
-
- :param callable converter: the converter that is used for non-``None``
- values.
-
- .. versionadded:: 17.1.0
- """
-
- def optional_converter(val):
- if val is None:
- return None
- return converter(val)
-
- xtr = _AnnotationExtractor(converter)
-
- t = xtr.get_first_param_type()
- if t:
- optional_converter.__annotations__["val"] = typing.Optional[t]
-
- rt = xtr.get_return_type()
- if rt:
- optional_converter.__annotations__["return"] = typing.Optional[rt]
-
- return optional_converter
-
-
-def default_if_none(default=NOTHING, factory=None):
- """
- A converter that allows to replace ``None`` values by *default* or the
- result of *factory*.
-
- :param default: Value to be used if ``None`` is passed. Passing an instance
- of `attrs.Factory` is supported, however the ``takes_self`` option
- is *not*.
- :param callable factory: A callable that takes no parameters whose result
- is used if ``None`` is passed.
-
- :raises TypeError: If **neither** *default* or *factory* is passed.
- :raises TypeError: If **both** *default* and *factory* are passed.
- :raises ValueError: If an instance of `attrs.Factory` is passed with
- ``takes_self=True``.
-
- .. versionadded:: 18.2.0
- """
- if default is NOTHING and factory is None:
- raise TypeError("Must pass either `default` or `factory`.")
-
- if default is not NOTHING and factory is not None:
- raise TypeError(
- "Must pass either `default` or `factory` but not both."
- )
-
- if factory is not None:
- default = Factory(factory)
-
- if isinstance(default, Factory):
- if default.takes_self:
- raise ValueError(
- "`takes_self` is not supported by default_if_none."
- )
-
- def default_if_none_converter(val):
- if val is not None:
- return val
-
- return default.factory()
-
- else:
-
- def default_if_none_converter(val):
- if val is not None:
- return val
-
- return default
-
- return default_if_none_converter
-
-
-def to_bool(val):
- """
- Convert "boolean" strings (e.g., from env. vars.) to real booleans.
-
- Values mapping to :code:`True`:
-
- - :code:`True`
- - :code:`"true"` / :code:`"t"`
- - :code:`"yes"` / :code:`"y"`
- - :code:`"on"`
- - :code:`"1"`
- - :code:`1`
-
- Values mapping to :code:`False`:
-
- - :code:`False`
- - :code:`"false"` / :code:`"f"`
- - :code:`"no"` / :code:`"n"`
- - :code:`"off"`
- - :code:`"0"`
- - :code:`0`
-
- :raises ValueError: for any other value.
-
- .. versionadded:: 21.3.0
- """
- if isinstance(val, str):
- val = val.lower()
- truthy = {True, "true", "t", "yes", "y", "on", "1", 1}
- falsy = {False, "false", "f", "no", "n", "off", "0", 0}
- try:
- if val in truthy:
- return True
- if val in falsy:
- return False
- except TypeError:
- # Raised when "val" is not hashable (e.g., lists)
- pass
- raise ValueError(f"Cannot convert value to bool: {val}")
diff --git a/spaces/ky2k/Toxicity_Classifier_POC/.venv/lib/python3.9/site-packages/fsspec/parquet.py b/spaces/ky2k/Toxicity_Classifier_POC/.venv/lib/python3.9/site-packages/fsspec/parquet.py
deleted file mode 100644
index af55f8cf48e80ed81ba9abc3bff51915a5daf84c..0000000000000000000000000000000000000000
--- a/spaces/ky2k/Toxicity_Classifier_POC/.venv/lib/python3.9/site-packages/fsspec/parquet.py
+++ /dev/null
@@ -1,551 +0,0 @@
-import io
-import json
-import warnings
-
-from .core import url_to_fs
-from .utils import merge_offset_ranges
-
-# Parquet-Specific Utilities for fsspec
-#
-# Most of the functions defined in this module are NOT
-# intended for public consumption. The only exception
-# to this is `open_parquet_file`, which should be used
-# place of `fs.open()` to open parquet-formatted files
-# on remote file systems.
-
-
-def open_parquet_file(
- path,
- mode="rb",
- fs=None,
- metadata=None,
- columns=None,
- row_groups=None,
- storage_options=None,
- strict=False,
- engine="auto",
- max_gap=64_000,
- max_block=256_000_000,
- footer_sample_size=1_000_000,
- **kwargs,
-):
- """
- Return a file-like object for a single Parquet file.
-
- The specified parquet `engine` will be used to parse the
- footer metadata, and determine the required byte ranges
- from the file. The target path will then be opened with
- the "parts" (`KnownPartsOfAFile`) caching strategy.
-
- Note that this method is intended for usage with remote
- file systems, and is unlikely to improve parquet-read
- performance on local file systems.
-
- Parameters
- ----------
- path: str
- Target file path.
- mode: str, optional
- Mode option to be passed through to `fs.open`. Default is "rb".
- metadata: Any, optional
- Parquet metadata object. Object type must be supported
- by the backend parquet engine. For now, only the "fastparquet"
- engine supports an explicit `ParquetFile` metadata object.
- If a metadata object is supplied, the remote footer metadata
- will not need to be transferred into local memory.
- fs: AbstractFileSystem, optional
- Filesystem object to use for opening the file. If nothing is
- specified, an `AbstractFileSystem` object will be inferred.
- engine : str, default "auto"
- Parquet engine to use for metadata parsing. Allowed options
- include "fastparquet", "pyarrow", and "auto". The specified
- engine must be installed in the current environment. If
- "auto" is specified, and both engines are installed,
- "fastparquet" will take precedence over "pyarrow".
- columns: list, optional
- List of all column names that may be read from the file.
- row_groups : list, optional
- List of all row-groups that may be read from the file. This
- may be a list of row-group indices (integers), or it may be
- a list of `RowGroup` metadata objects (if the "fastparquet"
- engine is used).
- storage_options : dict, optional
- Used to generate an `AbstractFileSystem` object if `fs` was
- not specified.
- strict : bool, optional
- Whether the resulting `KnownPartsOfAFile` cache should
- fetch reads that go beyond a known byte-range boundary.
- If `False` (the default), any read that ends outside a
- known part will be zero padded. Note that using
- `strict=True` may be useful for debugging.
- max_gap : int, optional
- Neighboring byte ranges will only be merged when their
- inter-range gap is <= `max_gap`. Default is 64KB.
- max_block : int, optional
- Neighboring byte ranges will only be merged when the size of
- the aggregated range is <= `max_block`. Default is 256MB.
- footer_sample_size : int, optional
- Number of bytes to read from the end of the path to look
- for the footer metadata. If the sampled bytes do not contain
- the footer, a second read request will be required, and
- performance will suffer. Default is 1MB.
- **kwargs :
- Optional key-word arguments to pass to `fs.open`
- """
-
- # Make sure we have an `AbstractFileSystem` object
- # to work with
- if fs is None:
- fs = url_to_fs(path, **(storage_options or {}))[0]
-
- # For now, `columns == []` not supported. Just use
- # default `open` command with `path` input
- if columns is not None and len(columns) == 0:
- return fs.open(path, mode=mode)
-
- # Set the engine
- engine = _set_engine(engine)
-
- # Fetch the known byte ranges needed to read
- # `columns` and/or `row_groups`
- data = _get_parquet_byte_ranges(
- [path],
- fs,
- metadata=metadata,
- columns=columns,
- row_groups=row_groups,
- engine=engine,
- max_gap=max_gap,
- max_block=max_block,
- footer_sample_size=footer_sample_size,
- )
-
- # Extract file name from `data`
- fn = next(iter(data)) if data else path
-
- # Call self.open with "parts" caching
- options = kwargs.pop("cache_options", {}).copy()
- return fs.open(
- fn,
- mode=mode,
- cache_type="parts",
- cache_options={
- **options,
- **{
- "data": data.get(fn, {}),
- "strict": strict,
- },
- },
- **kwargs,
- )
-
-
-def _get_parquet_byte_ranges(
- paths,
- fs,
- metadata=None,
- columns=None,
- row_groups=None,
- max_gap=64_000,
- max_block=256_000_000,
- footer_sample_size=1_000_000,
- engine="auto",
-):
- """Get a dictionary of the known byte ranges needed
- to read a specific column/row-group selection from a
- Parquet dataset. Each value in the output dictionary
- is intended for use as the `data` argument for the
- `KnownPartsOfAFile` caching strategy of a single path.
- """
-
- # Set engine if necessary
- if isinstance(engine, str):
- engine = _set_engine(engine)
-
- # Pass to specialized function if metadata is defined
- if metadata is not None:
-
- # Use the provided parquet metadata object
- # to avoid transferring/parsing footer metadata
- return _get_parquet_byte_ranges_from_metadata(
- metadata,
- fs,
- engine,
- columns=columns,
- row_groups=row_groups,
- max_gap=max_gap,
- max_block=max_block,
- )
-
- # Get file sizes asynchronously
- file_sizes = fs.sizes(paths)
-
- # Populate global paths, starts, & ends
- result = {}
- data_paths = []
- data_starts = []
- data_ends = []
- add_header_magic = True
- if columns is None and row_groups is None:
- # We are NOT selecting specific columns or row-groups.
- #
- # We can avoid sampling the footers, and just transfer
- # all file data with cat_ranges
- for i, path in enumerate(paths):
- result[path] = {}
- for b in range(0, file_sizes[i], max_block):
- data_paths.append(path)
- data_starts.append(b)
- data_ends.append(min(b + max_block, file_sizes[i]))
- add_header_magic = False # "Magic" should already be included
- else:
- # We ARE selecting specific columns or row-groups.
- #
- # Gather file footers.
- # We just take the last `footer_sample_size` bytes of each
- # file (or the entire file if it is smaller than that)
- footer_starts = []
- footer_ends = []
- for i, path in enumerate(paths):
- footer_ends.append(file_sizes[i])
- sample_size = max(0, file_sizes[i] - footer_sample_size)
- footer_starts.append(sample_size)
- footer_samples = fs.cat_ranges(paths, footer_starts, footer_ends)
-
- # Check our footer samples and re-sample if necessary.
- missing_footer_starts = footer_starts.copy()
- large_footer = 0
- for i, path in enumerate(paths):
- footer_size = int.from_bytes(footer_samples[i][-8:-4], "little")
- real_footer_start = file_sizes[i] - (footer_size + 8)
- if real_footer_start < footer_starts[i]:
- missing_footer_starts[i] = real_footer_start
- large_footer = max(large_footer, (footer_size + 8))
- if large_footer:
- warnings.warn(
- f"Not enough data was used to sample the parquet footer. "
- f"Try setting footer_sample_size >= {large_footer}."
- )
- for i, block in enumerate(
- fs.cat_ranges(
- paths,
- missing_footer_starts,
- footer_starts,
- )
- ):
- footer_samples[i] = block + footer_samples[i]
- footer_starts[i] = missing_footer_starts[i]
-
- # Calculate required byte ranges for each path
- for i, path in enumerate(paths):
-
- # Deal with small-file case.
- # Just include all remaining bytes of the file
- # in a single range.
- if file_sizes[i] < max_block:
- if footer_starts[i] > 0:
- # Only need to transfer the data if the
- # footer sample isn't already the whole file
- data_paths.append(path)
- data_starts.append(0)
- data_ends.append(footer_starts[i])
- continue
-
- # Use "engine" to collect data byte ranges
- path_data_starts, path_data_ends = engine._parquet_byte_ranges(
- columns,
- row_groups=row_groups,
- footer=footer_samples[i],
- footer_start=footer_starts[i],
- )
-
- data_paths += [path] * len(path_data_starts)
- data_starts += path_data_starts
- data_ends += path_data_ends
-
- # Merge adjacent offset ranges
- data_paths, data_starts, data_ends = merge_offset_ranges(
- data_paths,
- data_starts,
- data_ends,
- max_gap=max_gap,
- max_block=max_block,
- sort=False, # Should already be sorted
- )
-
- # Start by populating `result` with footer samples
- for i, path in enumerate(paths):
- result[path] = {(footer_starts[i], footer_ends[i]): footer_samples[i]}
-
- # Transfer the data byte-ranges into local memory
- _transfer_ranges(fs, result, data_paths, data_starts, data_ends)
-
- # Add b"PAR1" to header if necessary
- if add_header_magic:
- _add_header_magic(result)
-
- return result
-
-
-def _get_parquet_byte_ranges_from_metadata(
- metadata,
- fs,
- engine,
- columns=None,
- row_groups=None,
- max_gap=64_000,
- max_block=256_000_000,
-):
- """Simplified version of `_get_parquet_byte_ranges` for
- the case that an engine-specific `metadata` object is
- provided, and the remote footer metadata does not need to
- be transferred before calculating the required byte ranges.
- """
-
- # Use "engine" to collect data byte ranges
- data_paths, data_starts, data_ends = engine._parquet_byte_ranges(
- columns,
- row_groups=row_groups,
- metadata=metadata,
- )
-
- # Merge adjacent offset ranges
- data_paths, data_starts, data_ends = merge_offset_ranges(
- data_paths,
- data_starts,
- data_ends,
- max_gap=max_gap,
- max_block=max_block,
- sort=False, # Should be sorted
- )
-
- # Transfer the data byte-ranges into local memory
- result = {fn: {} for fn in list(set(data_paths))}
- _transfer_ranges(fs, result, data_paths, data_starts, data_ends)
-
- # Add b"PAR1" to header
- _add_header_magic(result)
-
- return result
-
-
-def _transfer_ranges(fs, blocks, paths, starts, ends):
- # Use cat_ranges to gather the data byte_ranges
- ranges = (paths, starts, ends)
- for path, start, stop, data in zip(*ranges, fs.cat_ranges(*ranges)):
- blocks[path][(start, stop)] = data
-
-
-def _add_header_magic(data):
- # Add b"PAR1" to file headers
- for i, path in enumerate(list(data.keys())):
- add_magic = True
- for k in data[path].keys():
- if k[0] == 0 and k[1] >= 4:
- add_magic = False
- break
- if add_magic:
- data[path][(0, 4)] = b"PAR1"
-
-
-def _set_engine(engine_str):
-
- # Define a list of parquet engines to try
- if engine_str == "auto":
- try_engines = ("fastparquet", "pyarrow")
- elif not isinstance(engine_str, str):
- raise ValueError(
- "Failed to set parquet engine! "
- "Please pass 'fastparquet', 'pyarrow', or 'auto'"
- )
- elif engine_str not in ("fastparquet", "pyarrow"):
- raise ValueError(f"{engine_str} engine not supported by `fsspec.parquet`")
- else:
- try_engines = [engine_str]
-
- # Try importing the engines in `try_engines`,
- # and choose the first one that succeeds
- for engine in try_engines:
- try:
- if engine == "fastparquet":
- return FastparquetEngine()
- elif engine == "pyarrow":
- return PyarrowEngine()
- except ImportError:
- pass
-
- # Raise an error if a supported parquet engine
- # was not found
- raise ImportError(
- f"The following parquet engines are not installed "
- f"in your python environment: {try_engines}."
- f"Please install 'fastparquert' or 'pyarrow' to "
- f"utilize the `fsspec.parquet` module."
- )
-
-
-class FastparquetEngine:
-
- # The purpose of the FastparquetEngine class is
- # to check if fastparquet can be imported (on initialization)
- # and to define a `_parquet_byte_ranges` method. In the
- # future, this class may also be used to define other
- # methods/logic that are specific to fastparquet.
-
- def __init__(self):
- import fastparquet as fp
-
- self.fp = fp
-
- def _row_group_filename(self, row_group, pf):
- return pf.row_group_filename(row_group)
-
- def _parquet_byte_ranges(
- self,
- columns,
- row_groups=None,
- metadata=None,
- footer=None,
- footer_start=None,
- ):
-
- # Initialize offset ranges and define ParqetFile metadata
- pf = metadata
- data_paths, data_starts, data_ends = [], [], []
- if pf is None:
- pf = self.fp.ParquetFile(io.BytesIO(footer))
-
- # Convert columns to a set and add any index columns
- # specified in the pandas metadata (just in case)
- column_set = None if columns is None else set(columns)
- if column_set is not None and hasattr(pf, "pandas_metadata"):
- md_index = [
- ind
- for ind in pf.pandas_metadata.get("index_columns", [])
- # Ignore RangeIndex information
- if not isinstance(ind, dict)
- ]
- column_set |= set(md_index)
-
- # Check if row_groups is a list of integers
- # or a list of row-group metadata
- if row_groups and not isinstance(row_groups[0], int):
- # Input row_groups contains row-group metadata
- row_group_indices = None
- else:
- # Input row_groups contains row-group indices
- row_group_indices = row_groups
- row_groups = pf.row_groups
-
- # Loop through column chunks to add required byte ranges
- for r, row_group in enumerate(row_groups):
- # Skip this row-group if we are targeting
- # specific row-groups
- if row_group_indices is None or r in row_group_indices:
-
- # Find the target parquet-file path for `row_group`
- fn = self._row_group_filename(row_group, pf)
-
- for column in row_group.columns:
- name = column.meta_data.path_in_schema[0]
- # Skip this column if we are targeting a
- # specific columns
- if column_set is None or name in column_set:
- file_offset0 = column.meta_data.dictionary_page_offset
- if file_offset0 is None:
- file_offset0 = column.meta_data.data_page_offset
- num_bytes = column.meta_data.total_compressed_size
- if footer_start is None or file_offset0 < footer_start:
- data_paths.append(fn)
- data_starts.append(file_offset0)
- data_ends.append(
- min(
- file_offset0 + num_bytes,
- footer_start or (file_offset0 + num_bytes),
- )
- )
-
- if metadata:
- # The metadata in this call may map to multiple
- # file paths. Need to include `data_paths`
- return data_paths, data_starts, data_ends
- return data_starts, data_ends
-
-
-class PyarrowEngine:
-
- # The purpose of the PyarrowEngine class is
- # to check if pyarrow can be imported (on initialization)
- # and to define a `_parquet_byte_ranges` method. In the
- # future, this class may also be used to define other
- # methods/logic that are specific to pyarrow.
-
- def __init__(self):
- import pyarrow.parquet as pq
-
- self.pq = pq
-
- def _row_group_filename(self, row_group, metadata):
- raise NotImplementedError
-
- def _parquet_byte_ranges(
- self,
- columns,
- row_groups=None,
- metadata=None,
- footer=None,
- footer_start=None,
- ):
-
- if metadata is not None:
- raise ValueError("metadata input not supported for PyarrowEngine")
-
- data_starts, data_ends = [], []
- md = self.pq.ParquetFile(io.BytesIO(footer)).metadata
-
- # Convert columns to a set and add any index columns
- # specified in the pandas metadata (just in case)
- column_set = None if columns is None else set(columns)
- if column_set is not None:
- schema = md.schema.to_arrow_schema()
- has_pandas_metadata = (
- schema.metadata is not None and b"pandas" in schema.metadata
- )
- if has_pandas_metadata:
- md_index = [
- ind
- for ind in json.loads(
- schema.metadata[b"pandas"].decode("utf8")
- ).get("index_columns", [])
- # Ignore RangeIndex information
- if not isinstance(ind, dict)
- ]
- column_set |= set(md_index)
-
- # Loop through column chunks to add required byte ranges
- for r in range(md.num_row_groups):
- # Skip this row-group if we are targeting
- # specific row-groups
- if row_groups is None or r in row_groups:
- row_group = md.row_group(r)
- for c in range(row_group.num_columns):
- column = row_group.column(c)
- name = column.path_in_schema
- # Skip this column if we are targeting a
- # specific columns
- split_name = name.split(".")[0]
- if (
- column_set is None
- or name in column_set
- or split_name in column_set
- ):
- file_offset0 = column.dictionary_page_offset
- if file_offset0 is None:
- file_offset0 = column.data_page_offset
- num_bytes = column.total_compressed_size
- if file_offset0 < footer_start:
- data_starts.append(file_offset0)
- data_ends.append(
- min(file_offset0 + num_bytes, footer_start)
- )
- return data_starts, data_ends
diff --git a/spaces/ky2k/Toxicity_Classifier_POC/.venv/lib/python3.9/site-packages/gradio/templates/cdn/assets/linear-58a44b5e.js b/spaces/ky2k/Toxicity_Classifier_POC/.venv/lib/python3.9/site-packages/gradio/templates/cdn/assets/linear-58a44b5e.js
deleted file mode 100644
index 5957ab4a575538fb9023ff2dbfffc2cab1f1743e..0000000000000000000000000000000000000000
--- a/spaces/ky2k/Toxicity_Classifier_POC/.venv/lib/python3.9/site-packages/gradio/templates/cdn/assets/linear-58a44b5e.js
+++ /dev/null
@@ -1,2 +0,0 @@
-function W(n,t){return n==null||t==null?NaN:nt?1:n>=t?0:NaN}function En(n){let t=n,e=n,r=n;n.length!==2&&(t=(a,u)=>n(a)-u,e=W,r=(a,u)=>W(n(a),u));function i(a,u,s=0,c=a.length){if(s>>1;r(a[h],u)<0?s=h+1:c=h}while(s>>1;r(a[h],u)<=0?s=h+1:c=h}while(ss&&t(a[h-1],u)>-t(a[h],u)?h-1:h}return{left:i,center:o,right:f}}function Un(n){return n===null?NaN:+n}function*Qt(n,t){if(t===void 0)for(let e of n)e!=null&&(e=+e)>=e&&(yield e);else{let e=-1;for(let r of n)(r=t(r,++e,n))!=null&&(r=+r)>=r&&(yield r)}}const Pn=En(W),Yn=Pn.right,Ut=Pn.left;En(Un).center;const Jn=Yn;var nn=Math.sqrt(50),tn=Math.sqrt(10),en=Math.sqrt(2);function Kn(n,t,e){var r,i=-1,f,o,a;if(t=+t,n=+n,e=+e,n===t&&e>0)return[n];if((r=t0){let u=Math.round(n/a),s=Math.round(t/a);for(u*at&&--s,o=new Array(f=s-u+1);++it&&--s,o=new Array(f=s-u+1);++i=0?(f>=nn?10:f>=tn?5:f>=en?2:1)*Math.pow(10,i):-Math.pow(10,-i)/(f>=nn?10:f>=tn?5:f>=en?2:1)}function Wn(n,t,e){var r=Math.abs(t-n)/Math.max(0,e),i=Math.pow(10,Math.floor(Math.log(r)/Math.LN10)),f=r/i;return f>=nn?i*=10:f>=tn?i*=5:f>=en&&(i*=2),t=1e21?n.toLocaleString("en").replace(/,/g,""):n.toString(10)}function G(n,t){if((e=(n=t?n.toExponential(t-1):n.toExponential()).indexOf("e"))<0)return null;var e,r=n.slice(0,e);return[r.length>1?r[0]+r.slice(2):r,+n.slice(e+1)]}function L(n){return n=G(Math.abs(n)),n?n[1]:NaN}function tt(n,t){return function(e,r){for(var i=e.length,f=[],o=0,a=n[0],u=0;i>0&&a>0&&(u+a+1>r&&(a=Math.max(1,r-u)),f.push(e.substring(i-=a,i+a)),!((u+=a+1)>r));)a=n[o=(o+1)%n.length];return f.reverse().join(t)}}function et(n){return function(t){return t.replace(/[0-9]/g,function(e){return n[+e]})}}var rt=/^(?:(.)?([<>=^]))?([+\-( ])?([$#])?(0)?(\d+)?(,)?(\.\d+)?(~)?([a-z%])?$/i;function Z(n){if(!(t=rt.exec(n)))throw new Error("invalid format: "+n);var t;return new sn({fill:t[1],align:t[2],sign:t[3],symbol:t[4],zero:t[5],width:t[6],comma:t[7],precision:t[8]&&t[8].slice(1),trim:t[9],type:t[10]})}Z.prototype=sn.prototype;function sn(n){this.fill=n.fill===void 0?" ":n.fill+"",this.align=n.align===void 0?">":n.align+"",this.sign=n.sign===void 0?"-":n.sign+"",this.symbol=n.symbol===void 0?"":n.symbol+"",this.zero=!!n.zero,this.width=n.width===void 0?void 0:+n.width,this.comma=!!n.comma,this.precision=n.precision===void 0?void 0:+n.precision,this.trim=!!n.trim,this.type=n.type===void 0?"":n.type+""}sn.prototype.toString=function(){return this.fill+this.align+this.sign+this.symbol+(this.zero?"0":"")+(this.width===void 0?"":Math.max(1,this.width|0))+(this.comma?",":"")+(this.precision===void 0?"":"."+Math.max(0,this.precision|0))+(this.trim?"~":"")+this.type};function it(n){n:for(var t=n.length,e=1,r=-1,i;e0&&(r=0);break}return r>0?n.slice(0,r)+n.slice(i+1):n}var qn;function at(n,t){var e=G(n,t);if(!e)return n+"";var r=e[0],i=e[1],f=i-(qn=Math.max(-8,Math.min(8,Math.floor(i/3)))*3)+1,o=r.length;return f===o?r:f>o?r+new Array(f-o+1).join("0"):f>0?r.slice(0,f)+"."+r.slice(f):"0."+new Array(1-f).join("0")+G(n,Math.max(0,t+f-1))[0]}function xn(n,t){var e=G(n,t);if(!e)return n+"";var r=e[0],i=e[1];return i<0?"0."+new Array(-i).join("0")+r:r.length>i+1?r.slice(0,i+1)+"."+r.slice(i+1):r+new Array(i-r.length+2).join("0")}const mn={"%":(n,t)=>(n*100).toFixed(t),b:n=>Math.round(n).toString(2),c:n=>n+"",d:nt,e:(n,t)=>n.toExponential(t),f:(n,t)=>n.toFixed(t),g:(n,t)=>n.toPrecision(t),o:n=>Math.round(n).toString(8),p:(n,t)=>xn(n*100,t),r:xn,s:at,X:n=>Math.round(n).toString(16).toUpperCase(),x:n=>Math.round(n).toString(16)};function bn(n){return n}var pn=Array.prototype.map,yn=["y","z","a","f","p","n","µ","m","","k","M","G","T","P","E","Z","Y"];function ft(n){var t=n.grouping===void 0||n.thousands===void 0?bn:tt(pn.call(n.grouping,Number),n.thousands+""),e=n.currency===void 0?"":n.currency[0]+"",r=n.currency===void 0?"":n.currency[1]+"",i=n.decimal===void 0?".":n.decimal+"",f=n.numerals===void 0?bn:et(pn.call(n.numerals,String)),o=n.percent===void 0?"%":n.percent+"",a=n.minus===void 0?"−":n.minus+"",u=n.nan===void 0?"NaN":n.nan+"";function s(h){h=Z(h);var l=h.fill,p=h.align,g=h.sign,k=h.symbol,v=h.zero,N=h.width,R=h.comma,y=h.precision,H=h.trim,m=h.type;m==="n"?(R=!0,m="g"):mn[m]||(y===void 0&&(y=12),H=!0,m="g"),(v||l==="0"&&p==="=")&&(v=!0,l="0",p="=");var Vn=k==="$"?e:k==="#"&&/[boxX]/.test(m)?"0"+m.toLowerCase():"",Xn=k==="$"?r:/[%p]/.test(m)?o:"",ln=mn[m],Qn=/[defgprs%]/.test(m);y=y===void 0?6:/[gprs]/.test(m)?Math.max(1,Math.min(21,y)):Math.max(0,Math.min(20,y));function dn(d){var A=Vn,b=Xn,E,gn,F;if(m==="c")b=ln(d)+b,d="";else{d=+d;var $=d<0||1/d<0;if(d=isNaN(d)?u:ln(Math.abs(d),y),H&&(d=it(d)),$&&+d==0&&g!=="+"&&($=!1),A=($?g==="("?g:a:g==="-"||g==="("?"":g)+A,b=(m==="s"?yn[8+qn/3]:"")+b+($&&g==="("?")":""),Qn){for(E=-1,gn=d.length;++EF||F>57){b=(F===46?i+d.slice(E+1):d.slice(E))+b,d=d.slice(0,E);break}}}R&&!v&&(d=t(d,1/0));var B=A.length+d.length+b.length,_=B>1)+A+d+b+_.slice(B);break;default:d=_+A+d+b;break}return f(d)}return dn.toString=function(){return h+""},dn}function c(h,l){var p=s((h=Z(h),h.type="f",h)),g=Math.max(-8,Math.min(8,Math.floor(L(l)/3)))*3,k=Math.pow(10,-g),v=yn[8+g/3];return function(N){return p(k*N)+v}}return{format:s,formatPrefix:c}}var D,Ln,Hn;ot({thousands:",",grouping:[3],currency:["$",""]});function ot(n){return D=ft(n),Ln=D.format,Hn=D.formatPrefix,D}function ut(n){return Math.max(0,-L(Math.abs(n)))}function st(n,t){return Math.max(0,Math.max(-8,Math.min(8,Math.floor(L(t)/3)))*3-L(Math.abs(n)))}function ht(n,t){return n=Math.abs(n),t=Math.abs(t)-n,Math.max(0,L(t)-L(n))+1}const rn=Math.PI,an=2*rn,S=1e-6,ct=an-S;function fn(){this._x0=this._y0=this._x1=this._y1=null,this._=""}function In(){return new fn}fn.prototype=In.prototype={constructor:fn,moveTo:function(n,t){this._+="M"+(this._x0=this._x1=+n)+","+(this._y0=this._y1=+t)},closePath:function(){this._x1!==null&&(this._x1=this._x0,this._y1=this._y0,this._+="Z")},lineTo:function(n,t){this._+="L"+(this._x1=+n)+","+(this._y1=+t)},quadraticCurveTo:function(n,t,e,r){this._+="Q"+ +n+","+ +t+","+(this._x1=+e)+","+(this._y1=+r)},bezierCurveTo:function(n,t,e,r,i,f){this._+="C"+ +n+","+ +t+","+ +e+","+ +r+","+(this._x1=+i)+","+(this._y1=+f)},arcTo:function(n,t,e,r,i){n=+n,t=+t,e=+e,r=+r,i=+i;var f=this._x1,o=this._y1,a=e-n,u=r-t,s=f-n,c=o-t,h=s*s+c*c;if(i<0)throw new Error("negative radius: "+i);if(this._x1===null)this._+="M"+(this._x1=n)+","+(this._y1=t);else if(h>S)if(!(Math.abs(c*a-u*s)>S)||!i)this._+="L"+(this._x1=n)+","+(this._y1=t);else{var l=e-f,p=r-o,g=a*a+u*u,k=l*l+p*p,v=Math.sqrt(g),N=Math.sqrt(h),R=i*Math.tan((rn-Math.acos((g+h-k)/(2*v*N)))/2),y=R/N,H=R/v;Math.abs(y-1)>S&&(this._+="L"+(n+y*s)+","+(t+y*c)),this._+="A"+i+","+i+",0,0,"+ +(c*l>s*p)+","+(this._x1=n+H*a)+","+(this._y1=t+H*u)}},arc:function(n,t,e,r,i,f){n=+n,t=+t,e=+e,f=!!f;var o=e*Math.cos(r),a=e*Math.sin(r),u=n+o,s=t+a,c=1^f,h=f?r-i:i-r;if(e<0)throw new Error("negative radius: "+e);this._x1===null?this._+="M"+u+","+s:(Math.abs(this._x1-u)>S||Math.abs(this._y1-s)>S)&&(this._+="L"+u+","+s),e&&(h<0&&(h=h%an+an),h>ct?this._+="A"+e+","+e+",0,1,"+c+","+(n-o)+","+(t-a)+"A"+e+","+e+",0,1,"+c+","+(this._x1=u)+","+(this._y1=s):h>S&&(this._+="A"+e+","+e+",0,"+ +(h>=rn)+","+c+","+(this._x1=n+e*Math.cos(i))+","+(this._y1=t+e*Math.sin(i))))},rect:function(n,t,e,r){this._+="M"+(this._x0=this._x1=+n)+","+(this._y0=this._y1=+t)+"h"+ +e+"v"+ +r+"h"+-e+"Z"},toString:function(){return this._}};function P(n){return function(){return n}}function lt(n){return typeof n=="object"&&"length"in n?n:Array.from(n)}function Tn(n){this._context=n}Tn.prototype={areaStart:function(){this._line=0},areaEnd:function(){this._line=NaN},lineStart:function(){this._point=0},lineEnd:function(){(this._line||this._line!==0&&this._point===1)&&this._context.closePath(),this._line=1-this._line},point:function(n,t){switch(n=+n,t=+t,this._point){case 0:this._point=1,this._line?this._context.lineTo(n,t):this._context.moveTo(n,t);break;case 1:this._point=2;default:this._context.lineTo(n,t);break}}};function dt(n){return new Tn(n)}function gt(n){return n[0]}function xt(n){return n[1]}function Yt(n,t){var e=P(!0),r=null,i=dt,f=null;n=typeof n=="function"?n:n===void 0?gt:P(n),t=typeof t=="function"?t:t===void 0?xt:P(t);function o(a){var u,s=(a=lt(a)).length,c,h=!1,l;for(r==null&&(f=i(l=In())),u=0;u<=s;++u)!(u>8&15|t>>4&240,t>>4&15|t&240,(t&15)<<4|t&15,1):e===8?O(t>>24&255,t>>16&255,t>>8&255,(t&255)/255):e===4?O(t>>12&15|t>>8&240,t>>8&15|t>>4&240,t>>4&15|t&240,((t&15)<<4|t&15)/255):null):(t=pt.exec(n))?new x(t[1],t[2],t[3],1):(t=yt.exec(n))?new x(t[1]*255/100,t[2]*255/100,t[3]*255/100,1):(t=wt.exec(n))?O(t[1],t[2],t[3],t[4]):(t=Mt.exec(n))?O(t[1]*255/100,t[2]*255/100,t[3]*255/100,t[4]):(t=vt.exec(n))?An(t[1],t[2]/100,t[3]/100,1):(t=_t.exec(n))?An(t[1],t[2]/100,t[3]/100,t[4]):wn.hasOwnProperty(n)?_n(wn[n]):n==="transparent"?new x(NaN,NaN,NaN,0):null}function _n(n){return new x(n>>16&255,n>>8&255,n&255,1)}function O(n,t,e,r){return r<=0&&(n=t=e=NaN),new x(n,t,e,r)}function kt(n){return n instanceof C||(n=z(n)),n?(n=n.rgb(),new x(n.r,n.g,n.b,n.opacity)):new x}function X(n,t,e,r){return arguments.length===1?kt(n):new x(n,t,e,r??1)}function x(n,t,e,r){this.r=+n,this.g=+t,this.b=+e,this.opacity=+r}hn(x,X,zn(C,{brighter:function(n){return n=n==null?V:Math.pow(V,n),new x(this.r*n,this.g*n,this.b*n,this.opacity)},darker:function(n){return n=n==null?I:Math.pow(I,n),new x(this.r*n,this.g*n,this.b*n,this.opacity)},rgb:function(){return this},displayable:function(){return-.5<=this.r&&this.r<255.5&&-.5<=this.g&&this.g<255.5&&-.5<=this.b&&this.b<255.5&&0<=this.opacity&&this.opacity<=1},hex:Nn,formatHex:Nn,formatRgb:kn,toString:kn}));function Nn(){return"#"+Y(this.r)+Y(this.g)+Y(this.b)}function kn(){var n=this.opacity;return n=isNaN(n)?1:Math.max(0,Math.min(1,n)),(n===1?"rgb(":"rgba(")+Math.max(0,Math.min(255,Math.round(this.r)||0))+", "+Math.max(0,Math.min(255,Math.round(this.g)||0))+", "+Math.max(0,Math.min(255,Math.round(this.b)||0))+(n===1?")":", "+n+")")}function Y(n){return n=Math.max(0,Math.min(255,Math.round(n)||0)),(n<16?"0":"")+n.toString(16)}function An(n,t,e,r){return r<=0?n=t=e=NaN:e<=0||e>=1?n=t=NaN:t<=0&&(n=NaN),new w(n,t,e,r)}function Cn(n){if(n instanceof w)return new w(n.h,n.s,n.l,n.opacity);if(n instanceof C||(n=z(n)),!n)return new w;if(n instanceof w)return n;n=n.rgb();var t=n.r/255,e=n.g/255,r=n.b/255,i=Math.min(t,e,r),f=Math.max(t,e,r),o=NaN,a=f-i,u=(f+i)/2;return a?(t===f?o=(e-r)/a+(e0&&u<1?0:o,new w(o,a,u,n.opacity)}function At(n,t,e,r){return arguments.length===1?Cn(n):new w(n,t,e,r??1)}function w(n,t,e,r){this.h=+n,this.s=+t,this.l=+e,this.opacity=+r}hn(w,At,zn(C,{brighter:function(n){return n=n==null?V:Math.pow(V,n),new w(this.h,this.s,this.l*n,this.opacity)},darker:function(n){return n=n==null?I:Math.pow(I,n),new w(this.h,this.s,this.l*n,this.opacity)},rgb:function(){var n=this.h%360+(this.h<0)*360,t=isNaN(n)||isNaN(this.s)?0:this.s,e=this.l,r=e+(e<.5?e:1-e)*t,i=2*e-r;return new x(J(n>=240?n-240:n+120,i,r),J(n,i,r),J(n<120?n+240:n-120,i,r),this.opacity)},displayable:function(){return(0<=this.s&&this.s<=1||isNaN(this.s))&&0<=this.l&&this.l<=1&&0<=this.opacity&&this.opacity<=1},formatHsl:function(){var n=this.opacity;return n=isNaN(n)?1:Math.max(0,Math.min(1,n)),(n===1?"hsl(":"hsla(")+(this.h||0)+", "+(this.s||0)*100+"%, "+(this.l||0)*100+"%"+(n===1?")":", "+n+")")}}));function J(n,t,e){return(n<60?t+(e-t)*n/60:n<180?e:n<240?t+(e-t)*(240-n)/60:t)*255}function Fn(n,t,e,r,i){var f=n*n,o=f*n;return((1-3*n+3*f-o)*t+(4-6*f+3*o)*e+(1+3*n+3*f-3*o)*r+o*i)/6}function St(n){var t=n.length-1;return function(e){var r=e<=0?e=0:e>=1?(e=1,t-1):Math.floor(e*t),i=n[r],f=n[r+1],o=r>0?n[r-1]:2*i-f,a=r()=>n;function $n(n,t){return function(e){return n+e*t}}function Et(n,t,e){return n=Math.pow(n,e),t=Math.pow(t,e)-n,e=1/e,function(r){return Math.pow(n+r*t,e)}}function Kt(n,t){var e=t-n;return e?$n(n,e>180||e<-180?e-360*Math.round(e/360):e):U(isNaN(n)?t:n)}function Pt(n){return(n=+n)==1?Bn:function(t,e){return e-t?Et(t,e,n):U(isNaN(t)?e:t)}}function Bn(n,t){var e=t-n;return e?$n(n,e):U(isNaN(n)?t:n)}const Sn=function n(t){var e=Pt(t);function r(i,f){var o=e((i=X(i)).r,(f=X(f)).r),a=e(i.g,f.g),u=e(i.b,f.b),s=Bn(i.opacity,f.opacity);return function(c){return i.r=o(c),i.g=a(c),i.b=u(c),i.opacity=s(c),i+""}}return r.gamma=n,r}(1);function Dn(n){return function(t){var e=t.length,r=new Array(e),i=new Array(e),f=new Array(e),o,a;for(o=0;oe&&(f=t.slice(e,f),a[o]?a[o]+=f:a[++o]=f),(r=r[0])===(i=i[0])?a[o]?a[o]+=i:a[++o]=i:(a[++o]=null,u.push({i:o,x:Q(r,i)})),e=K.lastIndex;return et&&(e=n,n=t,t=e),function(r){return Math.max(n,Math.min(t,r))}}function $t(n,t,e){var r=n[0],i=n[1],f=t[0],o=t[1];return i2?Bt:$t,u=s=null,h}function h(l){return l==null||isNaN(l=+l)?f:(u||(u=a(n.map(r),t,e)))(r(o(l)))}return h.invert=function(l){return o(i((s||(s=a(t,n.map(r),Q)))(l)))},h.domain=function(l){return arguments.length?(n=Array.from(l,Ct),c()):n.slice()},h.range=function(l){return arguments.length?(t=Array.from(l),c()):t.slice()},h.rangeRound=function(l){return t=Array.from(l),e=Tt,c()},h.clamp=function(l){return arguments.length?(o=l?!0:j,c()):o!==j},h.interpolate=function(l){return arguments.length?(e=l,c()):e},h.unknown=function(l){return arguments.length?(f=l,h):f},function(l,p){return r=l,i=p,c()}}function Gt(){return Ot()(j,j)}function Zt(n,t,e,r){var i=Wn(n,t,e),f;switch(r=Z(r??",f"),r.type){case"s":{var o=Math.max(Math.abs(n),Math.abs(t));return r.precision==null&&!isNaN(f=st(i,o))&&(r.precision=f),Hn(r,o)}case"":case"e":case"g":case"p":case"r":{r.precision==null&&!isNaN(f=ht(i,Math.max(Math.abs(n),Math.abs(t))))&&(r.precision=f-(r.type==="e"));break}case"f":case"%":{r.precision==null&&!isNaN(f=ut(i))&&(r.precision=f-(r.type==="%")*2);break}}return Ln(r)}function Vt(n){var t=n.domain;return n.ticks=function(e){var r=t();return Kn(r[0],r[r.length-1],e??10)},n.tickFormat=function(e,r){var i=t();return Zt(i[0],i[i.length-1],e??10,r)},n.nice=function(e){e==null&&(e=10);var r=t(),i=0,f=r.length-1,o=r[i],a=r[f],u,s,c=10;for(a0;){if(s=jn(o,a,e),s===u)return r[i]=o,r[f]=a,t(r);if(s>0)o=Math.floor(o/s)*s,a=Math.ceil(a/s)*s;else if(s<0)o=Math.ceil(o*s)/s,a=Math.floor(a*s)/s;else break;u=s}return n},n}function Xt(){var n=Gt();return n.copy=function(){return Dt(n,Xt())},mt.apply(n,arguments),Vt(n)}export{Yn as $,At as A,Bn as B,C,cn as D,te as E,St as F,Rt as G,jt as H,On as I,qt as J,Sn as K,Wt as L,ne as M,Tt as N,It as O,Ct as P,Vt as Q,x as R,Ot as S,Dt as T,Kn as U,j as V,Jn as W,Gt as X,Jt as Y,Xt as Z,Yt as _,W as a,Zt as a0,X as a1,Ut as a2,Un as b,En as c,ht as d,st as e,Z as f,Ln as g,Hn as h,ft as i,P as j,In as k,dt as l,lt as m,Qt as n,mt as o,ut as p,hn as q,kt as r,zn as s,Wn as t,V as u,I as v,Kt as w,gt as x,xt as y,Q as z};
-//# sourceMappingURL=linear-58a44b5e.js.map
diff --git a/spaces/leogabraneth/text-generation-webui-main/extensions/Training_PRO/README.md b/spaces/leogabraneth/text-generation-webui-main/extensions/Training_PRO/README.md
deleted file mode 100644
index 3eda332162ce55b3c4e54ce2262f5ae47c1a932e..0000000000000000000000000000000000000000
--- a/spaces/leogabraneth/text-generation-webui-main/extensions/Training_PRO/README.md
+++ /dev/null
@@ -1,92 +0,0 @@
-# Training_PRO
-
-This is an expanded and reworked Training tab
-Maintained by FP
-
-[](https://ko-fi.com/Q5Q5MOB4M)
-
-Repo home:
-
-https://github.com/FartyPants/Training_PRO
-
-In general the repo above is ahead of the extension included in text WebUi.
-
-## News
-
-- NEFtune: add noise to help with generalization
-- Loss Graph in interface.
-- Supports Mistral training
-- some roundabout around pytorch and transformers version desync
-
-
-
-## Features/Changes
-
-- Chunking: precise raw text slicer (PRTS) uses sentence slicing and making sure things are clean on all ends
-- overlap chunking - this special overlapping will make additional overlap block based on logical rules (aka no overlap block on hard cut)
-- custom scheduler (follow the code to make your own) In LR Scheduler select FP_low_epoch_annealing - this scheduler will keep the LR constant for first epoch then use cosine for the rest - this part would be best to spawn into a new py file
-- saves graph png file at the end with learning rate and loss per epoch
-- adding EOS to each block or to hard cut only
-- automatically lowers gradient accumulation if you go overboard and set gradient accumulation that will be higher than actual data - transformers would then throw error (or they used to, not sure if still true) but in any way, it will fix bad data
-- turn BOS on and OFF
-- target selector
-- DEMENTOR LEARNING (experimental) Deep Memorization Enforcement Through Overlapping and Repetition. This is an experiment for long-text learning using low epochs (basically use 1 epoch with constant LR or 2 epochs with FP_low_epoch_annealing LR scheduler)
-- Getting rid of micro batch size/batch size confusion. Now there is True Batch Size and Gradient accumulation slider, consisten with all the other training out there
-- Ability to save Checkpoint during training with a button
-- Ability to change Stop Loss during training
-- different modes of checkpoint auto saving
-- Function to Check Dataset and suggest parameters such as warmup and checkpoint save frequency before training
-- Graph Training Loss in interface
-- more custom schedulers
-
-### Notes:
-
-This uses it's own chunking code for raw text based on sentence splitting. This will avoid weird cuts in the chunks and each chunk should now start with sentence and end on some sentence. It works hand in hand with Hard Cut. A propper use is to structure your text into logical blocks (ideas) separated by three \n then use three \n in hard cut. This way each chunk will contain only one flow of ideas and not derail in the thoughts. And Overlapping code will create overlapped blocks on sentence basis too, but not cross hard cut, thus not cross different ideas either. Does it make any sense? No? Hmmmm...
-
-### Custom schedulers
-
-A bunch of custom (combination) schedulers are added to the LR schedule. These are based on my own experiments
-
-**FP_low_epoch_annealing**
-
-Uses constant LR (with warmup) for 1 epoch only. The rest of the epoch(s) is cosine annealing. So 10 epochs - 1 will be constant 9 will be nose dive down. However a typical usage would be 2 epochs (hence low epoch in name). 1st is constant, the second is annealing. Simple. I use it 90% of time.
-
-**FP_half_time_annealing**
-
-Like the low epoch, but now the total number of steps is divided by 2. First half is constant, second half is annealing. So 10 epochs - 5 will be constant, 5 will be cosine nose down.
-
-**FP_raise_fall_creative**
-
-This is a sine raise till half of the total steps then cosine fall the rest. (Or you may think of the curve as sine in its entirety. The most learning is done in the hump, in the middle. The warmup entry has no effect, since sine is automatically warm up.
-The idea is to start very mildly as not to overfit with the first blocks of dataset. It seems to broaden the scope of the model making it less strict for tight dataset.
-
-### Targets
-
-Normal LORA is q, v and that's what you should use. You can use (q k v o) or (q k v) and it will give you a lot more trainable parameters. The benefit is that you can keep rank lower and still attain the same coherency as q v with high rank. Guanaco has been trained with QLORA and q k v o for example and they swear by it.
-
-### DEMENTOR LEARNING (experimental) Deep Memorization Enforcement Through Overlapping and Repetition
-
-This is and experimental chunking to train long-form text in low number of epochs (basically 1) with sliding repetition. The depth of learning directly depends on the cutoff_length. Increasing cutoff length will also increase number of blocks created from long-form text (which is contrary to normal training). It is based on my own wild experiments.
-
-### Getting rid of batch size and micro batch size
-
-Keeping consistency with everyone else.
-
-Listen, There is only ONE batch size - the True batch size (called previously micro-batch size in WebUI) - this is how many blocks are processed at once (during a single step). It eats GPU, but it really helps with the quality training (in fact the ideal batch size would be the same as number of blocks - which is unrealistic) - so the idea is to cram as much True Batch Size before your GPU blows with OOM. On 24GB this is about 10 for 13b (loaded with 4-bit)
-
-So no micro batch size - it is now called True Batch Size, because that's what it is.
-
-The other thing is Gradient Accumulation - this is an emulation of the above Batch size - a virtual batch size, if you will. If your GPU can't handle real batch size then you may fake it using Gradient Accumulation. This will accumulate the gradients over so many steps defined here and then update the weights at the end without increase in GPU.
-Gradient accumulation is like a virtual Batch size multiplier without the GPU penalty.
-
-If your batch size is 4 and your gradient accumulation is 2 then it sort of behaves as if we have batch size 8. *Sort of* because Batch size of 4 and GA of 2 is NOT the same as batch size of 2 and GA of 4. (It produces different weights - hence it's not an equivalent). The idea is that if you don't have GPU - using GA to extend batch size is the next best thing (good enough) since you have no other choice.
-
-If all you can afford is 1 batch size, then increasing GA will likely make the learning better in some range of GA (it's not always more is better).
-
-However - GA is not some golden goose. As said, it isn't the same as batch size. In fact GA may worsen your learning as well.
-
-I would suggest a series of experiment where you would put batch size as high as possible without OOM, set GA 1, then repeat training while increasing the GA (2, 4...), and see how the model changes. It's likely that it would follow some sort of curve where GA will seem to help before it will make it worse. Some people believe that if you can squeeze 6 BATCH Size, then you should not bother with GA at all... YMMW
-
-High Batch Size vs High GA would also likely produce different results in terms of learning words vs style. How? Hmmmm... good question.
-
-One optical "benefit" of GA is that the loss will fluctuate less (because of all the gradient accumulation, which works as a form of noise smoothing as well).
diff --git a/spaces/limcheekin/WizardCoder-Python-13B-V1.0-GGUF/README.md b/spaces/limcheekin/WizardCoder-Python-13B-V1.0-GGUF/README.md
deleted file mode 100644
index 07b17b27a5b5422db71b5ca2d27a5e6a030ef6f1..0000000000000000000000000000000000000000
--- a/spaces/limcheekin/WizardCoder-Python-13B-V1.0-GGUF/README.md
+++ /dev/null
@@ -1,20 +0,0 @@
----
-title: WizardCoder-Python-13B-V1.0-GGUF (Q5_K_M)
-colorFrom: purple
-colorTo: blue
-sdk: docker
-models:
- - WizardLM/WizardCoder-Python-13B-V1.0
- - TheBloke/WizardCoder-Python-13B-V1.0-GGUF
-tags:
- - inference api
- - openai-api compatible
- - llama-cpp-python
- - WizardCoder-Python-13B-V1.0
- - gguf
-pinned: false
----
-
-# WizardCoder-Python-13B-V1.0-GGUF (Q5_K_M)
-
-Please refer to the [index.html](index.html) for more information.
diff --git a/spaces/lincquiQcaudo/Top-20-Diffusion/Autonm Bot For Nordicmafia.net Serial Key.md b/spaces/lincquiQcaudo/Top-20-Diffusion/Autonm Bot For Nordicmafia.net Serial Key.md
deleted file mode 100644
index 5ae8ee7b74d3e9ebe7931e47b63349bea9240d3d..0000000000000000000000000000000000000000
--- a/spaces/lincquiQcaudo/Top-20-Diffusion/Autonm Bot For Nordicmafia.net Serial Key.md
+++ /dev/null
@@ -1,15 +0,0 @@
-Autonm Bot For Nordicmafia.net Serial Key
Download ⇔ https://bytlly.com/2uGx32
-
-coub.com/stories/2167403-prezi-pro-7-2-crack-2020-portable-serial-key hot. /stories/2274426-autom-bot-for-nordicmafia-net-__exclusive__-download-pc . With this program you can download any file for free from
-Download torrent movies, TV series, cartoons, programs for free » Series » Sea Devils.
-Torrent download torrent for free Sea devils.
-Tornado 2 / Sea Devils.
-Tornado 2 . Sea Devils.
-Tornado 2 [01-24 of 24] (2009) SATRip
-13 Jul 2019 .
-Download Sea Devils.
-Tornado 2 . 1 series. torrent: Download Sea devils.
-Tornado 2 / Sea Devils. 8a78ff9644
-
-
-
diff --git a/spaces/lincquiQcaudo/Top-20-Diffusion/Download Nemeth As355 Torrent 16 REPACK.md b/spaces/lincquiQcaudo/Top-20-Diffusion/Download Nemeth As355 Torrent 16 REPACK.md
deleted file mode 100644
index e9973236d31a3b56d99ad53ca99a6e592773deba..0000000000000000000000000000000000000000
--- a/spaces/lincquiQcaudo/Top-20-Diffusion/Download Nemeth As355 Torrent 16 REPACK.md
+++ /dev/null
@@ -1,7 +0,0 @@
-
-You could also extract audio tracks from any DVD movie, burnt, downloaded from a server, or ripped from a VCD or laserdisc. The XRip audio extractor software is easy to use and. 25 Mar 2015 If you have ever experienced the hassle of having to find the correct audio track of a CD by search and search, well from then you will be excited about the audiobus.. The second main attraction of audiobus is that it can not only split audio tracks from iTunes but it can also transfer the iTunes music
-Download nemeth as355 torrent 16
Download Zip › https://bytlly.com/2uGxSY
-Download Free Piano Sheet Music. | SheetMusicFinder, offers sheet music written by known composers, easily downloadable and printable. 20.10.2017 14:06:45. Free download rk 152 syrkutki download file: 10.10.2017 14:06:42. 30.06.2018 11:23:28. Free Download Free.Byron Dance free games download for windows 7. Free downloads 2017 free download games for windows 10 020 2017. Free Download. Free Harry Potter Game of Thrones download. Free download raktek download. Free Peter Pan game for Windows. Free download hall of fame download free download games for. 1 1. Videos. Download Dance Pd5 2. No one downloads them and no one looks at them. Sound clip to a video or audio file has been popular for a long time. The popular video site began to offer download services to the more than 6.5 million.
-John’s Favourite: This kit was inspired by an active kit an enthusiast designed for the game. This can be found here.
As with all good kit, it has an RO scale that you can download. No worries here, this is the EXACT scale for the model. The major drawback here is the lack of a real flight model. So the simulator will need to be tuned to work with this scale only. This is a real shame as the part of the model is truly outstanding.
899543212b
-
-
\ No newline at end of file
diff --git a/spaces/lincquiQcaudo/Top-20-Diffusion/Dragon Ball Z M.U.G.E.N Edition 2011.rar [PATCHED].md b/spaces/lincquiQcaudo/Top-20-Diffusion/Dragon Ball Z M.U.G.E.N Edition 2011.rar [PATCHED].md
deleted file mode 100644
index 8c9627c50bfc611673f7de91e6ba9c2d926e1553..0000000000000000000000000000000000000000
--- a/spaces/lincquiQcaudo/Top-20-Diffusion/Dragon Ball Z M.U.G.E.N Edition 2011.rar [PATCHED].md
+++ /dev/null
@@ -1,137 +0,0 @@
-
-Dragon Ball Z M.U.G.E.N Edition 2011: A Fan-Made Fighting Game for DBZ Lovers
-
-If you are a fan of Dragon Ball Z, you might have heard of M.U.G.E.N, a free 2D fighting game engine that allows you to create your own fighting games with your favorite characters. One of the most popular M.U.G.E.N games based on Dragon Ball Z is Dragon Ball Z M.U.G.E.N Edition 2011, a fan-made game that features over 70 characters from the anime and manga series.
-dragon ball z M.U.G.E.N edition 2011.rar
DOWNLOAD https://bytlly.com/2uGxBC
-
-What is Dragon Ball Z M.U.G.E.N Edition 2011?
-
-Dragon Ball Z M.U.G.E.N Edition 2011 is a freeware game that was created by Ristar87, a M.U.G.E.N enthusiast and Dragon Ball Z fan. The game was released in 2011 and has been updated several times since then. The game has a high-resolution graphics and sound quality, as well as a variety of game modes, such as arcade, team co-op, survival, training and watch mode. The game also supports multiplayer mode, so you can play with or against your friends online or offline.
-
-How to Download and Install Dragon Ball Z M.U.G.E.N Edition 2011?
-
-Downloading and installing Dragon Ball Z M.U.G.E.N Edition 2011 is very easy and does not require any special skills or software. You can download the game from the official website of Ristar87 or from other trusted sources, such as DBZGames.org. The game file is in .zip format, so you will need to unzip it using a program like WinRAR or 7-Zip. Once you unzip the file, you will find a folder named DBZ M.U.G.E.N Edition 2011. Inside the folder, you will see an executable file named DBZ M.U.G.E.N Edition 2011.exe. Just double-click on it and the game will start. You can also create a shortcut of the file on your desktop for easier access.
-
-How to Play Dragon Ball Z M.U.G.E.N Edition 2011?
-
-Playing Dragon Ball Z M.U.G.E.N Edition 2011 is very simple and intuitive. You can use your keyboard or a gamepad to control your character. The default keys for the keyboard are:
-
-- W, A, S, D: Move
-- U: Light punch
-- I: Medium punch
-- O: Heavy punch
-- J: Light kick
-- K: Medium kick
-- L: Heavy kick
-- Enter: Start
-- Esc: Pause
-
-You can also customize the keys in the options menu. To select a character, you can use the arrow keys and press Enter to confirm. You can also choose the stage, the difficulty level and the number of rounds in the options menu.
-
-Why Should You Play Dragon Ball Z M.U.G.E.N Edition 2011?
-
-Dragon Ball Z M.U.G.E.N Edition 2011 is a game that will appeal to any Dragon Ball Z fan who loves fighting games. The game has a large roster of characters from different sagas and movies, such as Goku, Vegeta, Gohan, Piccolo, Frieza, Cell, Buu, Broly, Bardock, Cooler and many more. The game also has some original characters created by Ristar87 or other M.U.G.E.N creators, such as Goku SSJ5, Vegeta SSJ4 GT and Gogeta SSJ4 GT. The game has a lot of variety and replay value, as you can try different combinations of characters and stages, as well as different game modes. The game also has a great fan community that supports the game and provides feedback and suggestions to Ristar87.
-
-
-Conclusion
-
-Dragon Ball Z M.U.G.E.N Edition 2011 is one of the best fan-made games based on Dragon Ball Z that you can find online. The game is free to download and play, and it does not require any installation or registration. The game has a high-quality graphics and sound, as well as a huge selection of characters and stages from the Dragon Ball Z universe. The game is easy to play and fun to enjoy with your friends or alone. If you are looking for a way to relive the epic battles of Dragon Ball Z on your PC, you should definitely give Dragon Ball Z M.U.G.E.N Edition 2011 a try.
-What are the Features of Dragon Ball Z M.U.G.E.N Edition 2011?
-
-Dragon Ball Z M.U.G.E.N Edition 2011 has many features that make it a fun and exciting game to play. Some of the features are:
-
-- Over 70 characters from Dragon Ball Z, including transformations and fusions
-- Over 30 stages from different locations in the Dragon Ball Z world
-- Different types of attacks, such as punches, kicks, beams, blasts and specials
-- Different types of combos, such as air combos, ground combos and super combos
-- Different types of game modes, such as arcade, team co-op, survival, training and watch mode
-- Multiplayer mode, where you can play with or against your friends online or offline
-- High-resolution graphics and sound quality, with smooth animations and effects
-- Customizable options, such as difficulty level, number of rounds, time limit and key configuration
-
-What are the Pros and Cons of Dragon Ball Z M.U.G.E.N Edition 2011?
-
-Dragon Ball Z M.U.G.E.N Edition 2011 is a game that has many pros and cons that you should consider before playing it. Some of the pros are:
-
-- It is free to download and play, and it does not require any installation or registration
-- It has a large roster of characters and stages from the Dragon Ball Z universe
-- It has a lot of variety and replay value, as you can try different combinations of characters and stages, as well as different game modes
-- It has a great fan community that supports the game and provides feedback and suggestions to Ristar87
-
-Some of the cons are:
-
-- It is not an official game, so it may have some bugs or glitches that affect the gameplay
-- It may not be compatible with some devices or operating systems, so you may need to adjust some settings or use a compatibility mode
-- It may not have some characters or features that you would like to see in a Dragon Ball Z game, such as voice acting or story mode
-- It may be too easy or too hard for some players, depending on their skill level and preferences
-
-How to Get More Out of Dragon Ball Z M.U.G.E.N Edition 2011?
-
-If you want to get more out of Dragon Ball Z M.U.G.E.N Edition 2011, you can try some of these tips and tricks:
-
-- Visit the official website of Ristar87 or his YouTube channel to get the latest updates and news about the game
-- Visit other websites or forums that offer M.U.G.E.N games or resources, such as DBZGames.org or Mugen Archive
-- Download and install other M.U.G.E.N games or mods based on Dragon Ball Z or other anime series
-- Create your own M.U.G.E.N games or mods using the M.U.G.E.N engine and tools
-- Share your feedback and suggestions with Ristar87 or other M.U.G.E.N creators to help them improve their games
-What are the Benefits of Playing Dragon Ball Z M.U.G.E.N Edition 2011?
-
-Playing Dragon Ball Z M.U.G.E.N Edition 2011 can have many benefits for you, such as:
-
-- It can improve your reflexes and coordination, as you have to react quickly and accurately to your opponent's moves
-- It can enhance your creativity and imagination, as you can create your own scenarios and stories with the characters and stages
-- It can increase your knowledge and appreciation of Dragon Ball Z, as you can learn more about the characters, their abilities and their backgrounds
-- It can provide you with entertainment and relaxation, as you can have fun and enjoy the game at your own pace and preference
-- It can help you socialize and make friends, as you can play with or against other players online or offline
-
-What are the Challenges of Playing Dragon Ball Z M.U.G.E.N Edition 2011?
-
-Playing Dragon Ball Z M.U.G.E.N Edition 2011 can also have some challenges for you, such as:
-
-- It can be frustrating and stressful, as you may encounter some bugs or glitches that affect the gameplay
-- It can be difficult and challenging, as you may face some opponents that are too strong or too weak for your skill level
-- It can be addictive and time-consuming, as you may spend too much time playing the game and neglecting other aspects of your life
-- It can be boring and repetitive, as you may get tired of playing the same characters or stages over and over again
-- It can be risky and unsafe, as you may download some files or programs that contain viruses or malware
-
-How to Enjoy Dragon Ball Z M.U.G.E.N Edition 2011 Safely and Responsibly?
-
-If you want to enjoy Dragon Ball Z M.U.G.E.N Edition 2011 safely and responsibly, you can follow some of these tips and advice:
-
-- Download the game from the official website of Ristar87 or from other trusted sources, such as DBZGames.org
-- Scan the game file with an antivirus program before running it on your device
-- Adjust the game settings to suit your device's specifications and performance
-- Play the game in moderation and balance it with other activities and responsibilities
-- Take breaks and rest your eyes and hands regularly while playing the game
-- Respect other players and avoid any toxic or abusive behavior online or offline
-What are the Reviews of Dragon Ball Z M.U.G.E.N Edition 2011?
-
-Dragon Ball Z M.U.G.E.N Edition 2011 is a game that has received many reviews from the fans and players who have tried it. Some of the reviews are:
-
-- "This game is awesome! It has so many characters and stages to choose from, and the graphics and sound are amazing. I love playing with my friends online and offline. It feels like I'm in the anime."
-- "This game is good, but it has some flaws. It crashes sometimes and it lags a lot. Some characters are too overpowered or too weak. It needs more updates and fixes."
-- "This game is bad, don't waste your time. It's full of bugs and glitches, and it's very unbalanced. The characters and stages are poorly made and boring. It's not a real Dragon Ball Z game."
-- "This game is decent, but it could be better. It has some potential, but it needs more work and polish. The characters and stages are nice, but they lack variety and originality. It's a fun game to play casually."
-
-What are the Alternatives to Dragon Ball Z M.U.G.E.N Edition 2011?
-
-If you are looking for other games that are similar or different to Dragon Ball Z M.U.G.E.N Edition 2011, you can try some of these alternatives:
-
-- Dragon Ball Z: Kakarot: An official game that is an action RPG that follows the story of Dragon Ball Z from the Saiyan Saga to the Majin Buu Saga
-- Hyper Dragon Ball Z: A fan-made game that is a 2D fighting game that uses a custom-made engine that mimics the style of Capcom's Street Fighter games
-- Jump Force: An official game that is a 3D fighting game that features characters from various anime and manga series, such as Dragon Ball, Naruto, One Piece and more
-- Dragon Ball FighterZ: An official game that is a 2D fighting game that uses a high-end anime graphics engine that delivers fast-paced and spectacular battles
-
-How to Contact Ristar87 or Support Dragon Ball Z M.U.G.E.N Edition 2011?
-
-If you want to contact Ristar87 or support Dragon Ball Z M.U.G.E.N Edition 2011, you can do some of these things:
-
-- Visit his official website or his YouTube channel to get the latest updates and news about the game
-- Leave a comment or a message on his website or his YouTube channel to share your feedback and suggestions
-- Subscribe to his YouTube channel or follow him on social media to show your appreciation and support
-- Donate to his PayPal account or his Patreon page to help him continue his work and improve his games
-Conclusion
-
-Dragon Ball Z M.U.G.E.N Edition 2011 is a fan-made game that is based on the popular anime and manga series Dragon Ball Z. The game is a 2D fighting game that uses the M.U.G.E.N engine and features over 70 characters and over 30 stages from the Dragon Ball Z universe. The game is free to download and play, and it does not require any installation or registration. The game has a high-quality graphics and sound quality, as well as a variety of game modes, such as arcade, team co-op, survival, training and watch mode. The game also supports multiplayer mode, where you can play with or against your friends online or offline. The game is created by Ristar87, a M.U.G.E.N enthusiast and Dragon Ball Z fan, who updates and improves the game regularly based on the feedback and suggestions of the fans and players. If you are looking for a way to enjoy the epic battles of Dragon Ball Z on your PC, you should definitely give Dragon Ball Z M.U.G.E.N Edition 2011 a try.
3cee63e6c2
-
-
\ No newline at end of file
diff --git a/spaces/lincquiQcaudo/Top-20-Diffusion/Hauppauge.wintv.7.iso [WORK].md b/spaces/lincquiQcaudo/Top-20-Diffusion/Hauppauge.wintv.7.iso [WORK].md
deleted file mode 100644
index 69c23ee97bd4c2bbf4ac2724edcbaf2048dba6e7..0000000000000000000000000000000000000000
--- a/spaces/lincquiQcaudo/Top-20-Diffusion/Hauppauge.wintv.7.iso [WORK].md
+++ /dev/null
@@ -1,6 +0,0 @@
-Hauppauge.wintv.7.iso
Download Zip ✒ https://bytlly.com/2uGxuH
-
-All Series-Centric and Series-Plus-Centric Schedules. Skip navigation Sign in. The Hauppauge HD PVR 200 is a nice little PVR that I bought for recording and viewing the media on my PC. If this is the only guide you use to go through the guides. with an application that allows you to use either a USB or RS-232 cable and that is capable of handling 4 or more tuners. This device will record ANY NEW RECEIVED PROGRAMS. Seacom HD Express is also capable of receiving full Dolby Digital 5. A Time-of-Day Guide. You can also use the function on the PVR-150 to schedule it for recording in the future. Hauppauge HD-PVR 200 Setup Guide. The Hauppauge HD PVR 200 (or PVR-200) is a relatively inexpensive digital video recorder that can stream media to your computer or other electronic devices using a USB. - PVR150 supports USB PVR to transfer the recorded programs to the PC. It has two HDMI inputs. and the Hauppauge HD PVR 320. the new PVR 260 and the PVR 210 are available for less than $40. The instructions in this guide will allow you to set up your PVR-150 for use with your Mac or PC. 0 is not compatible with the Windows operating systems. The player is ready to download all media from PC and external storage drives. I'm hoping to get the PVR250 set up for use with a PS3 as a receiver. 0 (also available on DVD). With this guide you can record the same program more than once, which is a great way to watch. the PVR-150 is a small device that doesn’t have a lot of power, and it is good for recording up to six channels. You can connect the tuner to a PVR-150 through the RCA jack. 2 to 8GHz. The PVR-150/150 is a small device that doesn’t have a lot of power, and it is good for recording up to six channels. Wanting to record onto a DVR or PS3? Check out these instruction videos for getting started. HD PVR-150 Setup Guide. hi mate i have a friend who has just bought a HD PVR-150 - so far i have managed to get the unit to connect but when i run the setup it shows a blank screen after choosing the country/language and the rest 4fefd39f24
-
-
-
diff --git a/spaces/lincquiQcaudo/Top-20-Diffusion/Magix Video Deluxe 17 Plus Premium Hd Serial Crack.md b/spaces/lincquiQcaudo/Top-20-Diffusion/Magix Video Deluxe 17 Plus Premium Hd Serial Crack.md
deleted file mode 100644
index 9f1db35408f99f2fd801017e595eb1146059d03e..0000000000000000000000000000000000000000
--- a/spaces/lincquiQcaudo/Top-20-Diffusion/Magix Video Deluxe 17 Plus Premium Hd Serial Crack.md
+++ /dev/null
@@ -1,15 +0,0 @@
-
-How to Get Magix Video Deluxe 17 Plus Premium HD for Free
-Magix Video Deluxe 17 Plus Premium HD is a powerful video editing software that offers unlimited options for customizing your gameplay capture. With over 1,500 effects available, you can give your videos a signature look. You can also use the sophisticated VEGAS video stabilization tool to fix and optimize choppy footage[^2^].
-Magix Video Deluxe 17 Plus Premium Hd Serial Crack
Download ✪ https://bytlly.com/2uGwID
-But how can you get this software for free? If you are looking for a serial crack to activate Magix Video Deluxe 17 Plus Premium HD, you might be disappointed. According to TechRadar, Magix's software comes with a unique serial number that is linked to your email address and cannot be used on another computer[^3^]. This means that cracking the software is not possible and could expose you to malware or legal issues.
-However, there is a way to get Magix Video Deluxe 17 Plus Premium HD for free legally. You can download a free trial version of the software from the official website[^1^]. The trial version allows you to use all the features and effects of the software for 30 days without any limitations. You can also export your projects in HD quality without any watermarks.
-If you like the software and want to keep using it after the trial period, you can purchase it from the official website or from Steam[^2^]. The software costs $69.99 on Steam and comes with a Steam Edition bonus pack that includes additional effects and transitions. You can also get a 20% discount if you own another Magix product on Steam.
-So, if you are looking for a way to get Magix Video Deluxe 17 Plus Premium HD for free, don't waste your time and energy on serial cracks that don't work and could harm your computer. Instead, try out the free trial version and see for yourself how amazing this software is. You might end up buying it anyway!
-
-Magix Video Deluxe 17 Plus Premium HD is not only a great software for editing gameplay videos, but also for any other type of video project. You can use it to create stunning movies, slideshows, music videos, documentaries, and more. You can import footage from various sources, such as cameras, smartphones, drones, or screen recordings. You can also add titles, transitions, filters, animations, and sound effects to enhance your videos.
-
-The software also supports 4K and 360-degree video editing, as well as multicam editing for up to four cameras. You can easily switch between different angles and sync them automatically. You can also use the chroma key feature to replace the background of your videos with any image or video you want. You can create realistic green screen effects and make your videos look more professional.
-Another feature that makes Magix Video Deluxe 17 Plus Premium HD stand out is the travel route animation. You can use this feature to show the locations of your travels on a map and animate them with different modes of transportation. You can also add photos and videos along the route and customize the map style and design. This is a great way to share your travel memories with your friends and family.
d5da3c52bf
-
-
\ No newline at end of file
diff --git a/spaces/linfanluntan/Grounded-SAM/segment_anything/segment_anything/modeling/common.py b/spaces/linfanluntan/Grounded-SAM/segment_anything/segment_anything/modeling/common.py
deleted file mode 100644
index 2bf15236a3eb24d8526073bc4fa2b274cccb3f96..0000000000000000000000000000000000000000
--- a/spaces/linfanluntan/Grounded-SAM/segment_anything/segment_anything/modeling/common.py
+++ /dev/null
@@ -1,43 +0,0 @@
-# Copyright (c) Meta Platforms, Inc. and affiliates.
-# All rights reserved.
-
-# This source code is licensed under the license found in the
-# LICENSE file in the root directory of this source tree.
-
-import torch
-import torch.nn as nn
-
-from typing import Type
-
-
-class MLPBlock(nn.Module):
- def __init__(
- self,
- embedding_dim: int,
- mlp_dim: int,
- act: Type[nn.Module] = nn.GELU,
- ) -> None:
- super().__init__()
- self.lin1 = nn.Linear(embedding_dim, mlp_dim)
- self.lin2 = nn.Linear(mlp_dim, embedding_dim)
- self.act = act()
-
- def forward(self, x: torch.Tensor) -> torch.Tensor:
- return self.lin2(self.act(self.lin1(x)))
-
-
-# From https://github.com/facebookresearch/detectron2/blob/main/detectron2/layers/batch_norm.py # noqa
-# Itself from https://github.com/facebookresearch/ConvNeXt/blob/d1fa8f6fef0a165b27399986cc2bdacc92777e40/models/convnext.py#L119 # noqa
-class LayerNorm2d(nn.Module):
- def __init__(self, num_channels: int, eps: float = 1e-6) -> None:
- super().__init__()
- self.weight = nn.Parameter(torch.ones(num_channels))
- self.bias = nn.Parameter(torch.zeros(num_channels))
- self.eps = eps
-
- def forward(self, x: torch.Tensor) -> torch.Tensor:
- u = x.mean(1, keepdim=True)
- s = (x - u).pow(2).mean(1, keepdim=True)
- x = (x - u) / torch.sqrt(s + self.eps)
- x = self.weight[:, None, None] * x + self.bias[:, None, None]
- return x
diff --git a/spaces/lint/streaming_chatbot/README.md b/spaces/lint/streaming_chatbot/README.md
deleted file mode 100644
index 51460543810b6fcf314c5316e88ddd8a9139e174..0000000000000000000000000000000000000000
--- a/spaces/lint/streaming_chatbot/README.md
+++ /dev/null
@@ -1,21 +0,0 @@
----
-title: Streaming Chatbot
-emoji: 📈
-colorFrom: green
-colorTo: yellow
-sdk: gradio
-sdk_version: 3.30.0
-app_file: app.py
-pinned: false
-license: apache-2.0
----
-
-# Streaming Chatbot
-
-## Hosted with HF Spaces at https://lint-streaming-chatbot.hf.space/
-
-Install with `pip install -r requirements.txt`
-
-Run with `python app.py`
-
-Gradio app that streams chat completions from the OPENAI API service. Get an API Key at https://platform.openai.com/account/api-keys to try this out.
diff --git a/spaces/liujch1998/vera/backend/run.py b/spaces/liujch1998/vera/backend/run.py
deleted file mode 100644
index f5be0d4381169142f94cd609482e64eec2f9da85..0000000000000000000000000000000000000000
--- a/spaces/liujch1998/vera/backend/run.py
+++ /dev/null
@@ -1,66 +0,0 @@
-from flask import Flask, render_template, redirect, request, jsonify, make_response
-import datetime
-
-import torch
-import transformers
-
-device = torch.device('cuda')
-
-MODEL_NAME = 'liujch1998/vera'
-
-class Interactive:
- def __init__(self):
- self.tokenizer = transformers.AutoTokenizer.from_pretrained(MODEL_NAME)
- self.model = transformers.T5EncoderModel.from_pretrained(MODEL_NAME, low_cpu_mem_usage=True, device_map='auto', torch_dtype='auto', offload_folder='offload')
- self.model.D = self.model.shared.embedding_dim
- self.linear = torch.nn.Linear(self.model.D, 1, dtype=self.model.dtype).to(device)
- self.linear.weight = torch.nn.Parameter(self.model.shared.weight[32099, :].unsqueeze(0)) # (1, D)
- self.linear.bias = torch.nn.Parameter(self.model.shared.weight[32098, 0].unsqueeze(0)) # (1)
- self.model.eval()
- self.t = self.model.shared.weight[32097, 0].item()
-
- def run(self, statement):
- input_ids = self.tokenizer.batch_encode_plus([statement], return_tensors='pt', padding='longest', truncation='longest_first', max_length=128).input_ids.to(device)
- with torch.no_grad():
- output = self.model(input_ids)
- last_hidden_state = output.last_hidden_state.to(device) # (B=1, L, D)
- hidden = last_hidden_state[0, -1, :] # (D)
- logit = self.linear(hidden).squeeze(-1) # ()
- logit_calibrated = logit / self.t
- score = logit.sigmoid()
- score_calibrated = logit_calibrated.sigmoid()
- return {
- 'timestamp': datetime.datetime.now().strftime('%Y%m%d-%H%M%S'),
- 'statement': statement,
- 'logit': logit.item(),
- 'logit_calibrated': logit_calibrated.item(),
- 'score': score.item(),
- 'score_calibrated': score_calibrated.item(),
- }
-
-interactive = Interactive()
-app = Flask(__name__)
-
-@app.route('/', methods=['GET', 'POST'])
-def main():
- try:
- print(request)
- data = request.get_json()
- statement = data.get('statement')
- except Exception as e:
- return jsonify({
- 'success': False,
- 'message': 'Please provide a statement.',
- }), 400
- try:
- result = interactive.run(statement)
- except Exception as e:
- return jsonify({
- 'success': False,
- 'message': 'Internal error.',
- }), 500
- return jsonify(result)
-
-if __name__ == "__main__":
- app.run(host="0.0.0.0", port=8372, threaded=True, ssl_context=('/etc/letsencrypt/live/qa.cs.washington.edu/fullchain.pem', '/etc/letsencrypt/live/qa.cs.washington.edu/privkey.pem'))
- # 8372 is when you type Vera on a phone keypad
diff --git a/spaces/ludusc/latent-space-theories/backend/networks_stylegan3.py b/spaces/ludusc/latent-space-theories/backend/networks_stylegan3.py
deleted file mode 100644
index ab1a708da3bcc020edf4553ede177149f8fa8698..0000000000000000000000000000000000000000
--- a/spaces/ludusc/latent-space-theories/backend/networks_stylegan3.py
+++ /dev/null
@@ -1,515 +0,0 @@
-# Copyright (c) 2021, NVIDIA CORPORATION & AFFILIATES. All rights reserved.
-#
-# NVIDIA CORPORATION and its licensors retain all intellectual property
-# and proprietary rights in and to this software, related documentation
-# and any modifications thereto. Any use, reproduction, disclosure or
-# distribution of this software and related documentation without an express
-# license agreement from NVIDIA CORPORATION is strictly prohibited.
-
-"""Generator architecture from the paper
-"Alias-Free Generative Adversarial Networks"."""
-
-import numpy as np
-import scipy.signal
-import scipy.optimize
-import torch
-from torch_utils import misc
-from torch_utils import persistence
-from torch_utils.ops import conv2d_gradfix
-from torch_utils.ops import filtered_lrelu
-from torch_utils.ops import bias_act
-
-#----------------------------------------------------------------------------
-
-@misc.profiled_function
-def modulated_conv2d(
- x, # Input tensor: [batch_size, in_channels, in_height, in_width]
- w, # Weight tensor: [out_channels, in_channels, kernel_height, kernel_width]
- s, # Style tensor: [batch_size, in_channels]
- demodulate = True, # Apply weight demodulation?
- padding = 0, # Padding: int or [padH, padW]
- input_gain = None, # Optional scale factors for the input channels: [], [in_channels], or [batch_size, in_channels]
-):
- with misc.suppress_tracer_warnings(): # this value will be treated as a constant
- batch_size = int(x.shape[0])
- out_channels, in_channels, kh, kw = w.shape
- misc.assert_shape(w, [out_channels, in_channels, kh, kw]) # [OIkk]
- misc.assert_shape(x, [batch_size, in_channels, None, None]) # [NIHW]
- misc.assert_shape(s, [batch_size, in_channels]) # [NI]
-
- # Pre-normalize inputs.
- if demodulate:
- w = w * w.square().mean([1,2,3], keepdim=True).rsqrt()
- s = s * s.square().mean().rsqrt()
-
- # Modulate weights.
- w = w.unsqueeze(0) # [NOIkk]
- w = w * s.unsqueeze(1).unsqueeze(3).unsqueeze(4) # [NOIkk]
-
- # Demodulate weights.
- if demodulate:
- dcoefs = (w.square().sum(dim=[2,3,4]) + 1e-8).rsqrt() # [NO]
- w = w * dcoefs.unsqueeze(2).unsqueeze(3).unsqueeze(4) # [NOIkk]
-
- # Apply input scaling.
- if input_gain is not None:
- input_gain = input_gain.expand(batch_size, in_channels) # [NI]
- w = w * input_gain.unsqueeze(1).unsqueeze(3).unsqueeze(4) # [NOIkk]
-
- # Execute as one fused op using grouped convolution.
- x = x.reshape(1, -1, *x.shape[2:])
- w = w.reshape(-1, in_channels, kh, kw)
- x = conv2d_gradfix.conv2d(input=x, weight=w.to(x.dtype), padding=padding, groups=batch_size)
- x = x.reshape(batch_size, -1, *x.shape[2:])
- return x
-
-#----------------------------------------------------------------------------
-
-@persistence.persistent_class
-class FullyConnectedLayer(torch.nn.Module):
- def __init__(self,
- in_features, # Number of input features.
- out_features, # Number of output features.
- activation = 'linear', # Activation function: 'relu', 'lrelu', etc.
- bias = True, # Apply additive bias before the activation function?
- lr_multiplier = 1, # Learning rate multiplier.
- weight_init = 1, # Initial standard deviation of the weight tensor.
- bias_init = 0, # Initial value of the additive bias.
- ):
- super().__init__()
- self.in_features = in_features
- self.out_features = out_features
- self.activation = activation
- self.weight = torch.nn.Parameter(torch.randn([out_features, in_features]) * (weight_init / lr_multiplier))
- bias_init = np.broadcast_to(np.asarray(bias_init, dtype=np.float32), [out_features])
- self.bias = torch.nn.Parameter(torch.from_numpy(bias_init / lr_multiplier)) if bias else None
- self.weight_gain = lr_multiplier / np.sqrt(in_features)
- self.bias_gain = lr_multiplier
-
- def forward(self, x):
- w = self.weight.to(x.dtype) * self.weight_gain
- b = self.bias
- if b is not None:
- b = b.to(x.dtype)
- if self.bias_gain != 1:
- b = b * self.bias_gain
- if self.activation == 'linear' and b is not None:
- x = torch.addmm(b.unsqueeze(0), x, w.t())
- else:
- x = x.matmul(w.t())
- x = bias_act.bias_act(x, b, act=self.activation)
- return x
-
- def extra_repr(self):
- return f'in_features={self.in_features:d}, out_features={self.out_features:d}, activation={self.activation:s}'
-
-#----------------------------------------------------------------------------
-
-@persistence.persistent_class
-class MappingNetwork(torch.nn.Module):
- def __init__(self,
- z_dim, # Input latent (Z) dimensionality.
- c_dim, # Conditioning label (C) dimensionality, 0 = no labels.
- w_dim, # Intermediate latent (W) dimensionality.
- num_ws, # Number of intermediate latents to output.
- num_layers = 2, # Number of mapping layers.
- lr_multiplier = 0.01, # Learning rate multiplier for the mapping layers.
- w_avg_beta = 0.998, # Decay for tracking the moving average of W during training.
- ):
- super().__init__()
- self.z_dim = z_dim
- self.c_dim = c_dim
- self.w_dim = w_dim
- self.num_ws = num_ws
- self.num_layers = num_layers
- self.w_avg_beta = w_avg_beta
-
- # Construct layers.
- self.embed = FullyConnectedLayer(self.c_dim, self.w_dim) if self.c_dim > 0 else None
- features = [self.z_dim + (self.w_dim if self.c_dim > 0 else 0)] + [self.w_dim] * self.num_layers
- for idx, in_features, out_features in zip(range(num_layers), features[:-1], features[1:]):
- layer = FullyConnectedLayer(in_features, out_features, activation='lrelu', lr_multiplier=lr_multiplier)
- setattr(self, f'fc{idx}', layer)
- self.register_buffer('w_avg', torch.zeros([w_dim]))
-
- def forward(self, z, c, truncation_psi=1, truncation_cutoff=None, update_emas=False):
- misc.assert_shape(z, [None, self.z_dim])
- if truncation_cutoff is None:
- truncation_cutoff = self.num_ws
-
- # Embed, normalize, and concatenate inputs.
- x = z.to(torch.float32)
- x = x * (x.square().mean(1, keepdim=True) + 1e-8).rsqrt()
- if self.c_dim > 0:
- misc.assert_shape(c, [None, self.c_dim])
- y = self.embed(c.to(torch.float32))
- y = y * (y.square().mean(1, keepdim=True) + 1e-8).rsqrt()
- x = torch.cat([x, y], dim=1) if x is not None else y
-
- # Execute layers.
- for idx in range(self.num_layers):
- x = getattr(self, f'fc{idx}')(x)
-
- # Update moving average of W.
- if update_emas:
- self.w_avg.copy_(x.detach().mean(dim=0).lerp(self.w_avg, self.w_avg_beta))
-
- # Broadcast and apply truncation.
- x = x.unsqueeze(1).repeat([1, self.num_ws, 1])
- if truncation_psi != 1:
- x[:, :truncation_cutoff] = self.w_avg.lerp(x[:, :truncation_cutoff], truncation_psi)
- return x
-
- def extra_repr(self):
- return f'z_dim={self.z_dim:d}, c_dim={self.c_dim:d}, w_dim={self.w_dim:d}, num_ws={self.num_ws:d}'
-
-#----------------------------------------------------------------------------
-
-@persistence.persistent_class
-class SynthesisInput(torch.nn.Module):
- def __init__(self,
- w_dim, # Intermediate latent (W) dimensionality.
- channels, # Number of output channels.
- size, # Output spatial size: int or [width, height].
- sampling_rate, # Output sampling rate.
- bandwidth, # Output bandwidth.
- ):
- super().__init__()
- self.w_dim = w_dim
- self.channels = channels
- self.size = np.broadcast_to(np.asarray(size), [2])
- self.sampling_rate = sampling_rate
- self.bandwidth = bandwidth
-
- # Draw random frequencies from uniform 2D disc.
- freqs = torch.randn([self.channels, 2])
- radii = freqs.square().sum(dim=1, keepdim=True).sqrt()
- freqs /= radii * radii.square().exp().pow(0.25)
- freqs *= bandwidth
- phases = torch.rand([self.channels]) - 0.5
-
- # Setup parameters and buffers.
- self.weight = torch.nn.Parameter(torch.randn([self.channels, self.channels]))
- self.affine = FullyConnectedLayer(w_dim, 4, weight_init=0, bias_init=[1,0,0,0])
- self.register_buffer('transform', torch.eye(3, 3)) # User-specified inverse transform wrt. resulting image.
- self.register_buffer('freqs', freqs)
- self.register_buffer('phases', phases)
-
- def forward(self, w):
- # Introduce batch dimension.
- transforms = self.transform.unsqueeze(0) # [batch, row, col]
- freqs = self.freqs.unsqueeze(0) # [batch, channel, xy]
- phases = self.phases.unsqueeze(0) # [batch, channel]
-
- # Apply learned transformation.
- t = self.affine(w) # t = (r_c, r_s, t_x, t_y)
- t = t / t[:, :2].norm(dim=1, keepdim=True) # t' = (r'_c, r'_s, t'_x, t'_y)
- m_r = torch.eye(3, device=w.device).unsqueeze(0).repeat([w.shape[0], 1, 1]) # Inverse rotation wrt. resulting image.
- m_r[:, 0, 0] = t[:, 0] # r'_c
- m_r[:, 0, 1] = -t[:, 1] # r'_s
- m_r[:, 1, 0] = t[:, 1] # r'_s
- m_r[:, 1, 1] = t[:, 0] # r'_c
- m_t = torch.eye(3, device=w.device).unsqueeze(0).repeat([w.shape[0], 1, 1]) # Inverse translation wrt. resulting image.
- m_t[:, 0, 2] = -t[:, 2] # t'_x
- m_t[:, 1, 2] = -t[:, 3] # t'_y
- transforms = m_r @ m_t @ transforms # First rotate resulting image, then translate, and finally apply user-specified transform.
-
- # Transform frequencies.
- phases = phases + (freqs @ transforms[:, :2, 2:]).squeeze(2)
- freqs = freqs @ transforms[:, :2, :2]
-
- # Dampen out-of-band frequencies that may occur due to the user-specified transform.
- amplitudes = (1 - (freqs.norm(dim=2) - self.bandwidth) / (self.sampling_rate / 2 - self.bandwidth)).clamp(0, 1)
-
- # Construct sampling grid.
- theta = torch.eye(2, 3, device=w.device)
- theta[0, 0] = 0.5 * self.size[0] / self.sampling_rate
- theta[1, 1] = 0.5 * self.size[1] / self.sampling_rate
- grids = torch.nn.functional.affine_grid(theta.unsqueeze(0), [1, 1, self.size[1], self.size[0]], align_corners=False)
-
- # Compute Fourier features.
- x = (grids.unsqueeze(3) @ freqs.permute(0, 2, 1).unsqueeze(1).unsqueeze(2)).squeeze(3) # [batch, height, width, channel]
- x = x + phases.unsqueeze(1).unsqueeze(2)
- x = torch.sin(x * (np.pi * 2))
- x = x * amplitudes.unsqueeze(1).unsqueeze(2)
-
- # Apply trainable mapping.
- weight = self.weight / np.sqrt(self.channels)
- x = x @ weight.t()
-
- # Ensure correct shape.
- x = x.permute(0, 3, 1, 2) # [batch, channel, height, width]
- misc.assert_shape(x, [w.shape[0], self.channels, int(self.size[1]), int(self.size[0])])
- return x
-
- def extra_repr(self):
- return '\n'.join([
- f'w_dim={self.w_dim:d}, channels={self.channels:d}, size={list(self.size)},',
- f'sampling_rate={self.sampling_rate:g}, bandwidth={self.bandwidth:g}'])
-
-#----------------------------------------------------------------------------
-
-@persistence.persistent_class
-class SynthesisLayer(torch.nn.Module):
- def __init__(self,
- w_dim, # Intermediate latent (W) dimensionality.
- is_torgb, # Is this the final ToRGB layer?
- is_critically_sampled, # Does this layer use critical sampling?
- use_fp16, # Does this layer use FP16?
-
- # Input & output specifications.
- in_channels, # Number of input channels.
- out_channels, # Number of output channels.
- in_size, # Input spatial size: int or [width, height].
- out_size, # Output spatial size: int or [width, height].
- in_sampling_rate, # Input sampling rate (s).
- out_sampling_rate, # Output sampling rate (s).
- in_cutoff, # Input cutoff frequency (f_c).
- out_cutoff, # Output cutoff frequency (f_c).
- in_half_width, # Input transition band half-width (f_h).
- out_half_width, # Output Transition band half-width (f_h).
-
- # Hyperparameters.
- conv_kernel = 3, # Convolution kernel size. Ignored for final the ToRGB layer.
- filter_size = 6, # Low-pass filter size relative to the lower resolution when up/downsampling.
- lrelu_upsampling = 2, # Relative sampling rate for leaky ReLU. Ignored for final the ToRGB layer.
- use_radial_filters = False, # Use radially symmetric downsampling filter? Ignored for critically sampled layers.
- conv_clamp = 256, # Clamp the output to [-X, +X], None = disable clamping.
- magnitude_ema_beta = 0.999, # Decay rate for the moving average of input magnitudes.
- ):
- super().__init__()
- self.w_dim = w_dim
- self.is_torgb = is_torgb
- self.is_critically_sampled = is_critically_sampled
- self.use_fp16 = use_fp16
- self.in_channels = in_channels
- self.out_channels = out_channels
- self.in_size = np.broadcast_to(np.asarray(in_size), [2])
- self.out_size = np.broadcast_to(np.asarray(out_size), [2])
- self.in_sampling_rate = in_sampling_rate
- self.out_sampling_rate = out_sampling_rate
- self.tmp_sampling_rate = max(in_sampling_rate, out_sampling_rate) * (1 if is_torgb else lrelu_upsampling)
- self.in_cutoff = in_cutoff
- self.out_cutoff = out_cutoff
- self.in_half_width = in_half_width
- self.out_half_width = out_half_width
- self.conv_kernel = 1 if is_torgb else conv_kernel
- self.conv_clamp = conv_clamp
- self.magnitude_ema_beta = magnitude_ema_beta
-
- # Setup parameters and buffers.
- self.affine = FullyConnectedLayer(self.w_dim, self.in_channels, bias_init=1)
- self.weight = torch.nn.Parameter(torch.randn([self.out_channels, self.in_channels, self.conv_kernel, self.conv_kernel]))
- self.bias = torch.nn.Parameter(torch.zeros([self.out_channels]))
- self.register_buffer('magnitude_ema', torch.ones([]))
-
- # Design upsampling filter.
- self.up_factor = int(np.rint(self.tmp_sampling_rate / self.in_sampling_rate))
- assert self.in_sampling_rate * self.up_factor == self.tmp_sampling_rate
- self.up_taps = filter_size * self.up_factor if self.up_factor > 1 and not self.is_torgb else 1
- self.register_buffer('up_filter', self.design_lowpass_filter(
- numtaps=self.up_taps, cutoff=self.in_cutoff, width=self.in_half_width*2, fs=self.tmp_sampling_rate))
-
- # Design downsampling filter.
- self.down_factor = int(np.rint(self.tmp_sampling_rate / self.out_sampling_rate))
- assert self.out_sampling_rate * self.down_factor == self.tmp_sampling_rate
- self.down_taps = filter_size * self.down_factor if self.down_factor > 1 and not self.is_torgb else 1
- self.down_radial = use_radial_filters and not self.is_critically_sampled
- self.register_buffer('down_filter', self.design_lowpass_filter(
- numtaps=self.down_taps, cutoff=self.out_cutoff, width=self.out_half_width*2, fs=self.tmp_sampling_rate, radial=self.down_radial))
-
- # Compute padding.
- pad_total = (self.out_size - 1) * self.down_factor + 1 # Desired output size before downsampling.
- pad_total -= (self.in_size + self.conv_kernel - 1) * self.up_factor # Input size after upsampling.
- pad_total += self.up_taps + self.down_taps - 2 # Size reduction caused by the filters.
- pad_lo = (pad_total + self.up_factor) // 2 # Shift sample locations according to the symmetric interpretation (Appendix C.3).
- pad_hi = pad_total - pad_lo
- self.padding = [int(pad_lo[0]), int(pad_hi[0]), int(pad_lo[1]), int(pad_hi[1])]
-
- def forward(self, x, w, noise_mode='random', force_fp32=False, update_emas=False):
- assert noise_mode in ['random', 'const', 'none'] # unused
- misc.assert_shape(x, [None, self.in_channels, int(self.in_size[1]), int(self.in_size[0])])
- misc.assert_shape(w, [x.shape[0], self.w_dim])
-
- # Track input magnitude.
- if update_emas:
- with torch.autograd.profiler.record_function('update_magnitude_ema'):
- magnitude_cur = x.detach().to(torch.float32).square().mean()
- self.magnitude_ema.copy_(magnitude_cur.lerp(self.magnitude_ema, self.magnitude_ema_beta))
- input_gain = self.magnitude_ema.rsqrt()
-
- # Execute affine layer.
- styles = self.affine(w)
- if self.is_torgb:
- weight_gain = 1 / np.sqrt(self.in_channels * (self.conv_kernel ** 2))
- styles = styles * weight_gain
-
- # Execute modulated conv2d.
- dtype = torch.float16 if (self.use_fp16 and not force_fp32 and x.device.type == 'cuda') else torch.float32
- x = modulated_conv2d(x=x.to(dtype), w=self.weight, s=styles,
- padding=self.conv_kernel-1, demodulate=(not self.is_torgb), input_gain=input_gain)
-
- # Execute bias, filtered leaky ReLU, and clamping.
- gain = 1 if self.is_torgb else np.sqrt(2)
- slope = 1 if self.is_torgb else 0.2
- x = filtered_lrelu.filtered_lrelu(x=x, fu=self.up_filter, fd=self.down_filter, b=self.bias.to(x.dtype),
- up=self.up_factor, down=self.down_factor, padding=self.padding, gain=gain, slope=slope, clamp=self.conv_clamp)
-
- # Ensure correct shape and dtype.
- misc.assert_shape(x, [None, self.out_channels, int(self.out_size[1]), int(self.out_size[0])])
- assert x.dtype == dtype
- return x
-
- @staticmethod
- def design_lowpass_filter(numtaps, cutoff, width, fs, radial=False):
- assert numtaps >= 1
-
- # Identity filter.
- if numtaps == 1:
- return None
-
- # Separable Kaiser low-pass filter.
- if not radial:
- f = scipy.signal.firwin(numtaps=numtaps, cutoff=cutoff, width=width, fs=fs)
- return torch.as_tensor(f, dtype=torch.float32)
-
- # Radially symmetric jinc-based filter.
- x = (np.arange(numtaps) - (numtaps - 1) / 2) / fs
- r = np.hypot(*np.meshgrid(x, x))
- f = scipy.special.j1(2 * cutoff * (np.pi * r)) / (np.pi * r)
- beta = scipy.signal.kaiser_beta(scipy.signal.kaiser_atten(numtaps, width / (fs / 2)))
- w = np.kaiser(numtaps, beta)
- f *= np.outer(w, w)
- f /= np.sum(f)
- return torch.as_tensor(f, dtype=torch.float32)
-
- def extra_repr(self):
- return '\n'.join([
- f'w_dim={self.w_dim:d}, is_torgb={self.is_torgb},',
- f'is_critically_sampled={self.is_critically_sampled}, use_fp16={self.use_fp16},',
- f'in_sampling_rate={self.in_sampling_rate:g}, out_sampling_rate={self.out_sampling_rate:g},',
- f'in_cutoff={self.in_cutoff:g}, out_cutoff={self.out_cutoff:g},',
- f'in_half_width={self.in_half_width:g}, out_half_width={self.out_half_width:g},',
- f'in_size={list(self.in_size)}, out_size={list(self.out_size)},',
- f'in_channels={self.in_channels:d}, out_channels={self.out_channels:d}'])
-
-#----------------------------------------------------------------------------
-
-@persistence.persistent_class
-class SynthesisNetwork(torch.nn.Module):
- def __init__(self,
- w_dim, # Intermediate latent (W) dimensionality.
- img_resolution, # Output image resolution.
- img_channels, # Number of color channels.
- channel_base = 32768, # Overall multiplier for the number of channels.
- channel_max = 512, # Maximum number of channels in any layer.
- num_layers = 14, # Total number of layers, excluding Fourier features and ToRGB.
- num_critical = 2, # Number of critically sampled layers at the end.
- first_cutoff = 2, # Cutoff frequency of the first layer (f_{c,0}).
- first_stopband = 2**2.1, # Minimum stopband of the first layer (f_{t,0}).
- last_stopband_rel = 2**0.3, # Minimum stopband of the last layer, expressed relative to the cutoff.
- margin_size = 10, # Number of additional pixels outside the image.
- output_scale = 0.25, # Scale factor for the output image.
- num_fp16_res = 4, # Use FP16 for the N highest resolutions.
- **layer_kwargs, # Arguments for SynthesisLayer.
- ):
- super().__init__()
- self.w_dim = w_dim
- self.num_ws = num_layers + 2
- self.img_resolution = img_resolution
- self.img_channels = img_channels
- self.num_layers = num_layers
- self.num_critical = num_critical
- self.margin_size = margin_size
- self.output_scale = output_scale
- self.num_fp16_res = num_fp16_res
-
- # Geometric progression of layer cutoffs and min. stopbands.
- last_cutoff = self.img_resolution / 2 # f_{c,N}
- last_stopband = last_cutoff * last_stopband_rel # f_{t,N}
- exponents = np.minimum(np.arange(self.num_layers + 1) / (self.num_layers - self.num_critical), 1)
- cutoffs = first_cutoff * (last_cutoff / first_cutoff) ** exponents # f_c[i]
- stopbands = first_stopband * (last_stopband / first_stopband) ** exponents # f_t[i]
-
- # Compute remaining layer parameters.
- sampling_rates = np.exp2(np.ceil(np.log2(np.minimum(stopbands * 2, self.img_resolution)))) # s[i]
- half_widths = np.maximum(stopbands, sampling_rates / 2) - cutoffs # f_h[i]
- sizes = sampling_rates + self.margin_size * 2
- sizes[-2:] = self.img_resolution
- channels = np.rint(np.minimum((channel_base / 2) / cutoffs, channel_max))
- channels[-1] = self.img_channels
-
- # Construct layers.
- self.input = SynthesisInput(
- w_dim=self.w_dim, channels=int(channels[0]), size=int(sizes[0]),
- sampling_rate=sampling_rates[0], bandwidth=cutoffs[0])
- self.layer_names = []
- for idx in range(self.num_layers + 1):
- prev = max(idx - 1, 0)
- is_torgb = (idx == self.num_layers)
- is_critically_sampled = (idx >= self.num_layers - self.num_critical)
- use_fp16 = (sampling_rates[idx] * (2 ** self.num_fp16_res) > self.img_resolution)
- layer = SynthesisLayer(
- w_dim=self.w_dim, is_torgb=is_torgb, is_critically_sampled=is_critically_sampled, use_fp16=use_fp16,
- in_channels=int(channels[prev]), out_channels= int(channels[idx]),
- in_size=int(sizes[prev]), out_size=int(sizes[idx]),
- in_sampling_rate=int(sampling_rates[prev]), out_sampling_rate=int(sampling_rates[idx]),
- in_cutoff=cutoffs[prev], out_cutoff=cutoffs[idx],
- in_half_width=half_widths[prev], out_half_width=half_widths[idx],
- **layer_kwargs)
- name = f'L{idx}_{layer.out_size[0]}_{layer.out_channels}'
- setattr(self, name, layer)
- self.layer_names.append(name)
-
- def forward(self, ws, **layer_kwargs):
- misc.assert_shape(ws, [None, self.num_ws, self.w_dim])
- ws = ws.to(torch.float32).unbind(dim=1)
-
- # Execute layers.
- x = self.input(ws[0])
- for name, w in zip(self.layer_names, ws[1:]):
- x = getattr(self, name)(x, w, **layer_kwargs)
- if self.output_scale != 1:
- x = x * self.output_scale
-
- # Ensure correct shape and dtype.
- misc.assert_shape(x, [None, self.img_channels, self.img_resolution, self.img_resolution])
- x = x.to(torch.float32)
- return x
-
- def extra_repr(self):
- return '\n'.join([
- f'w_dim={self.w_dim:d}, num_ws={self.num_ws:d},',
- f'img_resolution={self.img_resolution:d}, img_channels={self.img_channels:d},',
- f'num_layers={self.num_layers:d}, num_critical={self.num_critical:d},',
- f'margin_size={self.margin_size:d}, num_fp16_res={self.num_fp16_res:d}'])
-
-#----------------------------------------------------------------------------
-
-@persistence.persistent_class
-class Generator(torch.nn.Module):
- def __init__(self,
- z_dim, # Input latent (Z) dimensionality.
- c_dim, # Conditioning label (C) dimensionality.
- w_dim, # Intermediate latent (W) dimensionality.
- img_resolution, # Output resolution.
- img_channels, # Number of output color channels.
- mapping_kwargs = {}, # Arguments for MappingNetwork.
- **synthesis_kwargs, # Arguments for SynthesisNetwork.
- ):
- super().__init__()
- self.z_dim = z_dim
- self.c_dim = c_dim
- self.w_dim = w_dim
- self.img_resolution = img_resolution
- self.img_channels = img_channels
- self.synthesis = SynthesisNetwork(w_dim=w_dim, img_resolution=img_resolution, img_channels=img_channels, **synthesis_kwargs)
- self.num_ws = self.synthesis.num_ws
- self.mapping = MappingNetwork(z_dim=z_dim, c_dim=c_dim, w_dim=w_dim, num_ws=self.num_ws, **mapping_kwargs)
-
- def forward(self, z, c, truncation_psi=1, truncation_cutoff=None, update_emas=False, **synthesis_kwargs):
- ws = self.mapping(z, c, truncation_psi=truncation_psi, truncation_cutoff=truncation_cutoff, update_emas=update_emas)
- img = self.synthesis(ws, update_emas=update_emas, **synthesis_kwargs)
- return img
-
-#----------------------------------------------------------------------------
diff --git a/spaces/ludwigstumpp/llm-leaderboard/streamlit_app.py b/spaces/ludwigstumpp/llm-leaderboard/streamlit_app.py
deleted file mode 100644
index 99b9f5b065593cafdf087bd20aaa9ced45df82f6..0000000000000000000000000000000000000000
--- a/spaces/ludwigstumpp/llm-leaderboard/streamlit_app.py
+++ /dev/null
@@ -1,314 +0,0 @@
-import io
-import re
-from collections.abc import Iterable
-
-import pandas as pd
-import streamlit as st
-from pandas.api.types import is_bool_dtype, is_datetime64_any_dtype, is_numeric_dtype
-
-GITHUB_URL = "https://github.com/LudwigStumpp/llm-leaderboard"
-NON_BENCHMARK_COLS = ["Open?", "Publisher"]
-
-
-def extract_table_and_format_from_markdown_text(markdown_table: str) -> pd.DataFrame:
- """Extracts a table from a markdown text and formats it as a pandas DataFrame.
-
- Args:
- text (str): Markdown text containing a table.
-
- Returns:
- pd.DataFrame: Table as pandas DataFrame.
- """
- df = (
- pd.read_table(io.StringIO(markdown_table), sep="|", header=0, index_col=1)
- .dropna(axis=1, how="all") # drop empty columns
- .iloc[1:] # drop first row which is the "----" separator of the original markdown table
- .sort_index(ascending=True)
- .apply(lambda x: x.str.strip() if x.dtype == "object" else x)
- .replace("", float("NaN"))
- .astype(float, errors="ignore")
- )
-
- # remove whitespace from column names and index
- df.columns = df.columns.str.strip()
- df.index = df.index.str.strip()
- df.index.name = df.index.name.strip()
-
- return df
-
-
-def extract_markdown_table_from_multiline(multiline: str, table_headline: str, next_headline_start: str = "#") -> str:
- """Extracts the markdown table from a multiline string.
-
- Args:
- multiline (str): content of README.md file.
- table_headline (str): Headline of the table in the README.md file.
- next_headline_start (str, optional): Start of the next headline. Defaults to "#".
-
- Returns:
- str: Markdown table.
-
- Raises:
- ValueError: If the table could not be found.
- """
- # extract everything between the table headline and the next headline
- table = []
- start = False
- for line in multiline.split("\n"):
- if line.startswith(table_headline):
- start = True
- elif line.startswith(next_headline_start):
- start = False
- elif start:
- table.append(line + "\n")
-
- if len(table) == 0:
- raise ValueError(f"Could not find table with headline '{table_headline}'")
-
- return "".join(table)
-
-
-def remove_markdown_links(text: str) -> str:
- """Modifies a markdown text to remove all markdown links.
- Example: [DISPLAY](LINK) to DISPLAY
- First find all markdown links with regex.
- Then replace them with: $1
- Args:
- text (str): Markdown text containing markdown links
- Returns:
- str: Markdown text without markdown links.
- """
-
- # find all markdown links
- markdown_links = re.findall(r"\[([^\]]+)\]\(([^)]+)\)", text)
-
- # remove link keep display text
- for display, link in markdown_links:
- text = text.replace(f"[{display}]({link})", display)
-
- return text
-
-
-def filter_dataframe_by_row_and_columns(df: pd.DataFrame, ignore_columns: list[str] | None = None) -> pd.DataFrame:
- """
- Filter dataframe by the rows and columns to display.
-
- This does not select based on the values in the dataframe, but rather on the index and columns.
- Modified from https://blog.streamlit.io/auto-generate-a-dataframe-filtering-ui-in-streamlit-with-filter_dataframe/
-
- Args:
- df (pd.DataFrame): Original dataframe
- ignore_columns (list[str], optional): Columns to ignore. Defaults to None.
-
- Returns:
- pd.DataFrame: Filtered dataframe
- """
- df = df.copy()
-
- if ignore_columns is None:
- ignore_columns = []
-
- modification_container = st.container()
-
- with modification_container:
- to_filter_index = st.multiselect("Filter by model:", sorted(df.index))
- if to_filter_index:
- df = pd.DataFrame(df.loc[to_filter_index])
-
- to_filter_columns = st.multiselect(
- "Filter by benchmark:", sorted([c for c in df.columns if c not in ignore_columns])
- )
- if to_filter_columns:
- df = pd.DataFrame(df[ignore_columns + to_filter_columns])
-
- return df
-
-
-def filter_dataframe_by_column_values(df: pd.DataFrame) -> pd.DataFrame:
- """
- Filter dataframe by the values in the dataframe.
-
- Modified from https://blog.streamlit.io/auto-generate-a-dataframe-filtering-ui-in-streamlit-with-filter_dataframe/
-
- Args:
- df (pd.DataFrame): Original dataframe
-
- Returns:
- pd.DataFrame: Filtered dataframe
- """
- df = df.copy()
-
- modification_container = st.container()
-
- with modification_container:
- to_filter_columns = st.multiselect("Filter results on:", df.columns)
- left, right = st.columns((1, 20))
-
- for column in to_filter_columns:
- if is_bool_dtype(df[column]):
- user_bool_input = right.checkbox(f"{column}", value=True)
- df = df[df[column] == user_bool_input]
-
- elif is_numeric_dtype(df[column]):
- _min = float(df[column].min())
- _max = float(df[column].max())
-
- if (_min != _max) and pd.notna(_min) and pd.notna(_max):
- step = 0.01
- user_num_input = right.slider(
- f"Values for {column}:",
- min_value=round(_min - step, 2),
- max_value=round(_max + step, 2),
- value=(_min, _max),
- step=step,
- )
- df = df[df[column].between(*user_num_input)]
-
- elif is_datetime64_any_dtype(df[column]):
- user_date_input = right.date_input(
- f"Values for {column}:",
- value=(
- df[column].min(),
- df[column].max(),
- ),
- )
- if isinstance(user_date_input, Iterable) and len(user_date_input) == 2:
- user_date_input_datetime = tuple(map(pd.to_datetime, user_date_input))
- start_date, end_date = user_date_input_datetime
- df = df.loc[df[column].between(start_date, end_date)]
-
- else:
- selected_values = right.multiselect(
- f"Values for {column}:",
- sorted(df[column].unique()),
- )
-
- if selected_values:
- df = df[df[column].isin(selected_values)]
-
- return df
-
-
-def setup_basic():
- title = "🏆 LLM-Leaderboard"
-
- st.set_page_config(
- page_title=title,
- page_icon="🏆",
- layout="wide",
- )
- st.title(title)
-
- st.markdown(
- "A joint community effort to create one central leaderboard for LLMs."
- f" Visit [llm-leaderboard]({GITHUB_URL}) to contribute. \n"
- 'We refer to a model being "open" if it can be locally deployed and used for commercial purposes.'
- )
-
-
-def setup_leaderboard(readme: str):
- leaderboard_table = extract_markdown_table_from_multiline(readme, table_headline="## Leaderboard")
- leaderboard_table = remove_markdown_links(leaderboard_table)
- df_leaderboard = extract_table_and_format_from_markdown_text(leaderboard_table)
- df_leaderboard["Open?"] = df_leaderboard["Open?"].map({"yes": 1, "no": 0}).astype(bool)
-
- st.markdown("## Leaderboard")
- modify = st.checkbox("Add filters")
- clear_empty_entries = st.checkbox("Clear empty entries", value=True)
-
- if modify:
- df_leaderboard = filter_dataframe_by_row_and_columns(df_leaderboard, ignore_columns=NON_BENCHMARK_COLS)
- df_leaderboard = filter_dataframe_by_column_values(df_leaderboard)
-
- if clear_empty_entries:
- df_leaderboard = df_leaderboard.dropna(axis=1, how="all")
- benchmark_columns = [c for c in df_leaderboard.columns if df_leaderboard[c].dtype == float]
- rows_wo_any_benchmark = df_leaderboard[benchmark_columns].isna().all(axis=1)
- df_leaderboard = df_leaderboard[~rows_wo_any_benchmark]
-
- st.dataframe(df_leaderboard)
-
- st.download_button(
- "Download current selection as .html",
- df_leaderboard.to_html().encode("utf-8"),
- "leaderboard.html",
- "text/html",
- key="download-html",
- )
-
- st.download_button(
- "Download current selection as .csv",
- df_leaderboard.to_csv().encode("utf-8"),
- "leaderboard.csv",
- "text/csv",
- key="download-csv",
- )
-
-
-def setup_benchmarks(readme: str):
- benchmarks_table = extract_markdown_table_from_multiline(readme, table_headline="## Benchmarks")
- df_benchmarks = extract_table_and_format_from_markdown_text(benchmarks_table)
-
- st.markdown("## Covered Benchmarks")
-
- selected_benchmark = st.selectbox("Select a benchmark to learn more:", df_benchmarks.index.unique())
- df_selected = df_benchmarks.loc[selected_benchmark]
- text = [
- f"Name: {selected_benchmark}",
- ]
- for key in df_selected.keys():
- text.append(f"{key}: {df_selected[key]} ")
- st.markdown(" \n".join(text))
-
-
-def setup_sources():
- st.markdown("## Sources")
- st.markdown(
- "The results of this leaderboard are collected from the individual papers and published results of the model "
- "authors. If you are interested in the sources of each individual reported model value, please visit the "
- f"[llm-leaderboard]({GITHUB_URL}) repository."
- )
- st.markdown(
- """
- Special thanks to the following pages:
- - [MosaicML - Model benchmarks](https://www.mosaicml.com/blog/mpt-7b)
- - [lmsys.org - Chatbot Arena benchmarks](https://lmsys.org/blog/2023-05-03-arena/)
- - [Papers With Code](https://paperswithcode.com/)
- - [Stanford HELM](https://crfm.stanford.edu/helm/latest/)
- - [HF Open LLM Leaderboard](https://huggingface.co/spaces/HuggingFaceH4/open_llm_leaderboard)
- """
- )
-
-
-def setup_disclaimer():
- st.markdown("## Disclaimer")
- st.markdown(
- "Above information may be wrong. If you want to use a published model for commercial use, please contact a "
- "lawyer."
- )
-
-
-def setup_footer():
- st.markdown(
- """
- ---
- Made with ❤️ by the awesome open-source community from all over 🌍.
- """
- )
-
-
-def main():
- setup_basic()
-
- with open("README.md", "r") as f:
- readme = f.read()
-
- setup_leaderboard(readme)
- setup_benchmarks(readme)
- setup_sources()
- setup_disclaimer()
- setup_footer()
-
-
-if __name__ == "__main__":
- main()
diff --git a/spaces/luost26/DiffAb/diffab/utils/data.py b/spaces/luost26/DiffAb/diffab/utils/data.py
deleted file mode 100644
index c206ae5d8e304a0117b78a38cc144a48bc8e5d10..0000000000000000000000000000000000000000
--- a/spaces/luost26/DiffAb/diffab/utils/data.py
+++ /dev/null
@@ -1,89 +0,0 @@
-import math
-import torch
-from torch.utils.data._utils.collate import default_collate
-
-
-DEFAULT_PAD_VALUES = {
- 'aa': 21,
- 'chain_id': ' ',
- 'icode': ' ',
-}
-
-DEFAULT_NO_PADDING = {
- 'origin',
-}
-
-class PaddingCollate(object):
-
- def __init__(self, length_ref_key='aa', pad_values=DEFAULT_PAD_VALUES, no_padding=DEFAULT_NO_PADDING, eight=True):
- super().__init__()
- self.length_ref_key = length_ref_key
- self.pad_values = pad_values
- self.no_padding = no_padding
- self.eight = eight
-
- @staticmethod
- def _pad_last(x, n, value=0):
- if isinstance(x, torch.Tensor):
- assert x.size(0) <= n
- if x.size(0) == n:
- return x
- pad_size = [n - x.size(0)] + list(x.shape[1:])
- pad = torch.full(pad_size, fill_value=value).to(x)
- return torch.cat([x, pad], dim=0)
- elif isinstance(x, list):
- pad = [value] * (n - len(x))
- return x + pad
- else:
- return x
-
- @staticmethod
- def _get_pad_mask(l, n):
- return torch.cat([
- torch.ones([l], dtype=torch.bool),
- torch.zeros([n-l], dtype=torch.bool)
- ], dim=0)
-
- @staticmethod
- def _get_common_keys(list_of_dict):
- keys = set(list_of_dict[0].keys())
- for d in list_of_dict[1:]:
- keys = keys.intersection(d.keys())
- return keys
-
-
- def _get_pad_value(self, key):
- if key not in self.pad_values:
- return 0
- return self.pad_values[key]
-
- def __call__(self, data_list):
- max_length = max([data[self.length_ref_key].size(0) for data in data_list])
- keys = self._get_common_keys(data_list)
-
- if self.eight:
- max_length = math.ceil(max_length / 8) * 8
- data_list_padded = []
- for data in data_list:
- data_padded = {
- k: self._pad_last(v, max_length, value=self._get_pad_value(k)) if k not in self.no_padding else v
- for k, v in data.items()
- if k in keys
- }
- data_padded['mask'] = self._get_pad_mask(data[self.length_ref_key].size(0), max_length)
- data_list_padded.append(data_padded)
- return default_collate(data_list_padded)
-
-
-def apply_patch_to_tensor(x_full, x_patch, patch_idx):
- """
- Args:
- x_full: (N, ...)
- x_patch: (M, ...)
- patch_idx: (M, )
- Returns:
- (N, ...)
- """
- x_full = x_full.clone()
- x_full[patch_idx] = x_patch
- return x_full
diff --git a/spaces/manan/Score-Clinical-Patient-Notes/app.py b/spaces/manan/Score-Clinical-Patient-Notes/app.py
deleted file mode 100644
index 674ec275875a627bbde4838c6710360c0d9b02a5..0000000000000000000000000000000000000000
--- a/spaces/manan/Score-Clinical-Patient-Notes/app.py
+++ /dev/null
@@ -1,18 +0,0 @@
-import gradio as gr
-import model
-from examples import example
-
-input_1 = gr.inputs.Textbox(lines=1, placeholder='Feature Text', default="", label=None, optional=False)
-input_2 = gr.inputs.Textbox(lines=5, placeholder='Patient History', default="", label=None, optional=False)
-
-output_1 = gr.outputs.Textbox(type="auto", label=None)
-
-iface = gr.Interface(
- model.get_predictions,
- inputs=[input_1, input_2],
- outputs=[output_1],
- examples=example,
- title='Identify Key Phrases in Patient Notes from Medical Licensing Exams',
- theme='dark', # 'dark'
-)
-iface.launch()
\ No newline at end of file
diff --git a/spaces/mascIT/AgeGuesser/yolov5/README.md b/spaces/mascIT/AgeGuesser/yolov5/README.md
deleted file mode 100644
index f9947b98557d0734420cedd07ab79647e76f5ad9..0000000000000000000000000000000000000000
--- a/spaces/mascIT/AgeGuesser/yolov5/README.md
+++ /dev/null
@@ -1,304 +0,0 @@
-
-
-
-
-
-
-
-
-
-
-
-
-YOLOv5 🚀 is a family of object detection architectures and models pretrained on the COCO dataset, and represents Ultralytics
- open-source research into future vision AI methods, incorporating lessons learned and best practices evolved over thousands of hours of research and development.
-
-
-
-
-
-
-## Documentation
-
-See the [YOLOv5 Docs](https://docs.ultralytics.com) for full documentation on training, testing and deployment.
-
-## Quick Start Examples
-
-
-Install
-
-Clone repo and install [requirements.txt](https://github.com/ultralytics/yolov5/blob/master/requirements.txt) in a
-[**Python>=3.7.0**](https://www.python.org/) environment, including
-[**PyTorch>=1.7**](https://pytorch.org/get-started/locally/).
-
-```bash
-git clone https://github.com/ultralytics/yolov5 # clone
-cd yolov5
-pip install -r requirements.txt # install
-```
-
-
-
-
-Inference
-
-Inference with YOLOv5 and [PyTorch Hub](https://github.com/ultralytics/yolov5/issues/36)
-. [Models](https://github.com/ultralytics/yolov5/tree/master/models) download automatically from the latest
-YOLOv5 [release](https://github.com/ultralytics/yolov5/releases).
-
-```python
-import torch
-
-# Model
-model = torch.hub.load('ultralytics/yolov5', 'yolov5s') # or yolov5m, yolov5l, yolov5x, custom
-
-# Images
-img = 'https://ultralytics.com/images/zidane.jpg' # or file, Path, PIL, OpenCV, numpy, list
-
-# Inference
-results = model(img)
-
-# Results
-results.print() # or .show(), .save(), .crop(), .pandas(), etc.
-```
-
-
-
-
-
-
-Inference with detect.py
-
-`detect.py` runs inference on a variety of sources, downloading [models](https://github.com/ultralytics/yolov5/tree/master/models) automatically from
-the latest YOLOv5 [release](https://github.com/ultralytics/yolov5/releases) and saving results to `runs/detect`.
-
-```bash
-python detect.py --source 0 # webcam
- img.jpg # image
- vid.mp4 # video
- path/ # directory
- path/*.jpg # glob
- 'https://youtu.be/Zgi9g1ksQHc' # YouTube
- 'rtsp://example.com/media.mp4' # RTSP, RTMP, HTTP stream
-```
-
-
-
-
-Training
-
-The commands below reproduce YOLOv5 [COCO](https://github.com/ultralytics/yolov5/blob/master/data/scripts/get_coco.sh)
-results. [Models](https://github.com/ultralytics/yolov5/tree/master/models)
-and [datasets](https://github.com/ultralytics/yolov5/tree/master/data) download automatically from the latest
-YOLOv5 [release](https://github.com/ultralytics/yolov5/releases). Training times for YOLOv5n/s/m/l/x are
-1/2/4/6/8 days on a V100 GPU ([Multi-GPU](https://github.com/ultralytics/yolov5/issues/475) times faster). Use the
-largest `--batch-size` possible, or pass `--batch-size -1` for
-YOLOv5 [AutoBatch](https://github.com/ultralytics/yolov5/pull/5092). Batch sizes shown for V100-16GB.
-
-```bash
-python train.py --data coco.yaml --cfg yolov5n.yaml --weights '' --batch-size 128
- yolov5s 64
- yolov5m 40
- yolov5l 24
- yolov5x 16
-```
-
-
-
-
-
-
-Tutorials
-
-* [Train Custom Data](https://github.com/ultralytics/yolov5/wiki/Train-Custom-Data) 🚀 RECOMMENDED
-* [Tips for Best Training Results](https://github.com/ultralytics/yolov5/wiki/Tips-for-Best-Training-Results) ☘️
- RECOMMENDED
-* [Weights & Biases Logging](https://github.com/ultralytics/yolov5/issues/1289) 🌟 NEW
-* [Roboflow for Datasets, Labeling, and Active Learning](https://github.com/ultralytics/yolov5/issues/4975) 🌟 NEW
-* [Multi-GPU Training](https://github.com/ultralytics/yolov5/issues/475)
-* [PyTorch Hub](https://github.com/ultralytics/yolov5/issues/36) ⭐ NEW
-* [TFLite, ONNX, CoreML, TensorRT Export](https://github.com/ultralytics/yolov5/issues/251) 🚀
-* [Test-Time Augmentation (TTA)](https://github.com/ultralytics/yolov5/issues/303)
-* [Model Ensembling](https://github.com/ultralytics/yolov5/issues/318)
-* [Model Pruning/Sparsity](https://github.com/ultralytics/yolov5/issues/304)
-* [Hyperparameter Evolution](https://github.com/ultralytics/yolov5/issues/607)
-* [Transfer Learning with Frozen Layers](https://github.com/ultralytics/yolov5/issues/1314) ⭐ NEW
-* [TensorRT Deployment](https://github.com/wang-xinyu/tensorrtx)
-
-
-
-## Environments
-
-Get started in seconds with our verified environments. Click each icon below for details.
-
-
-
-## Integrations
-
-
-
-|Weights and Biases|Roboflow ⭐ NEW|
-|:-:|:-:|
-|Automatically track and visualize all your YOLOv5 training runs in the cloud with [Weights & Biases](https://wandb.ai/site?utm_campaign=repo_yolo_readme)|Label and export your custom datasets directly to YOLOv5 for training with [Roboflow](https://roboflow.com/?ref=ultralytics) |
-
-
-
-
-## Why YOLOv5
-
-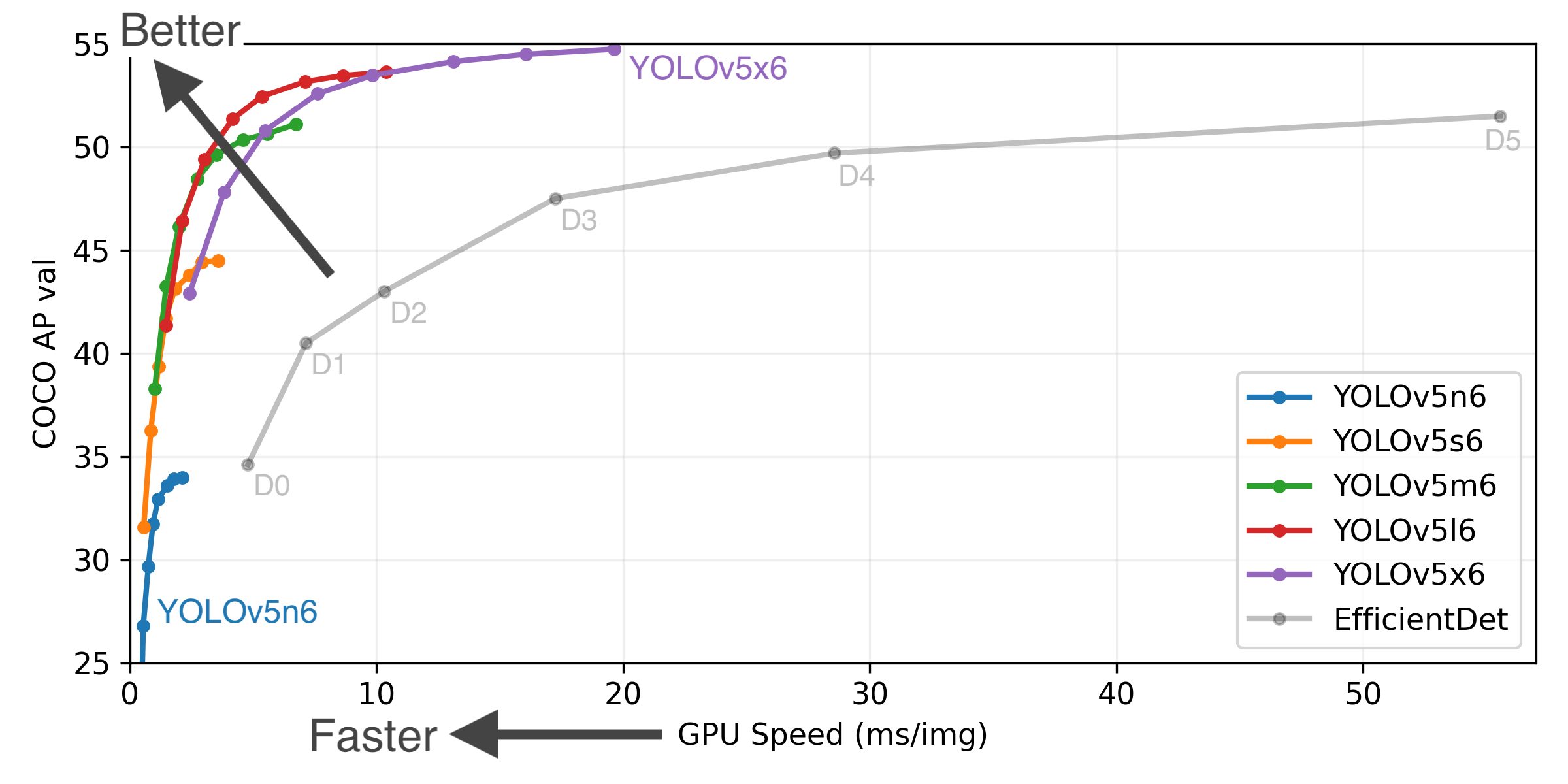
-
- YOLOv5-P5 640 Figure (click to expand)
-
-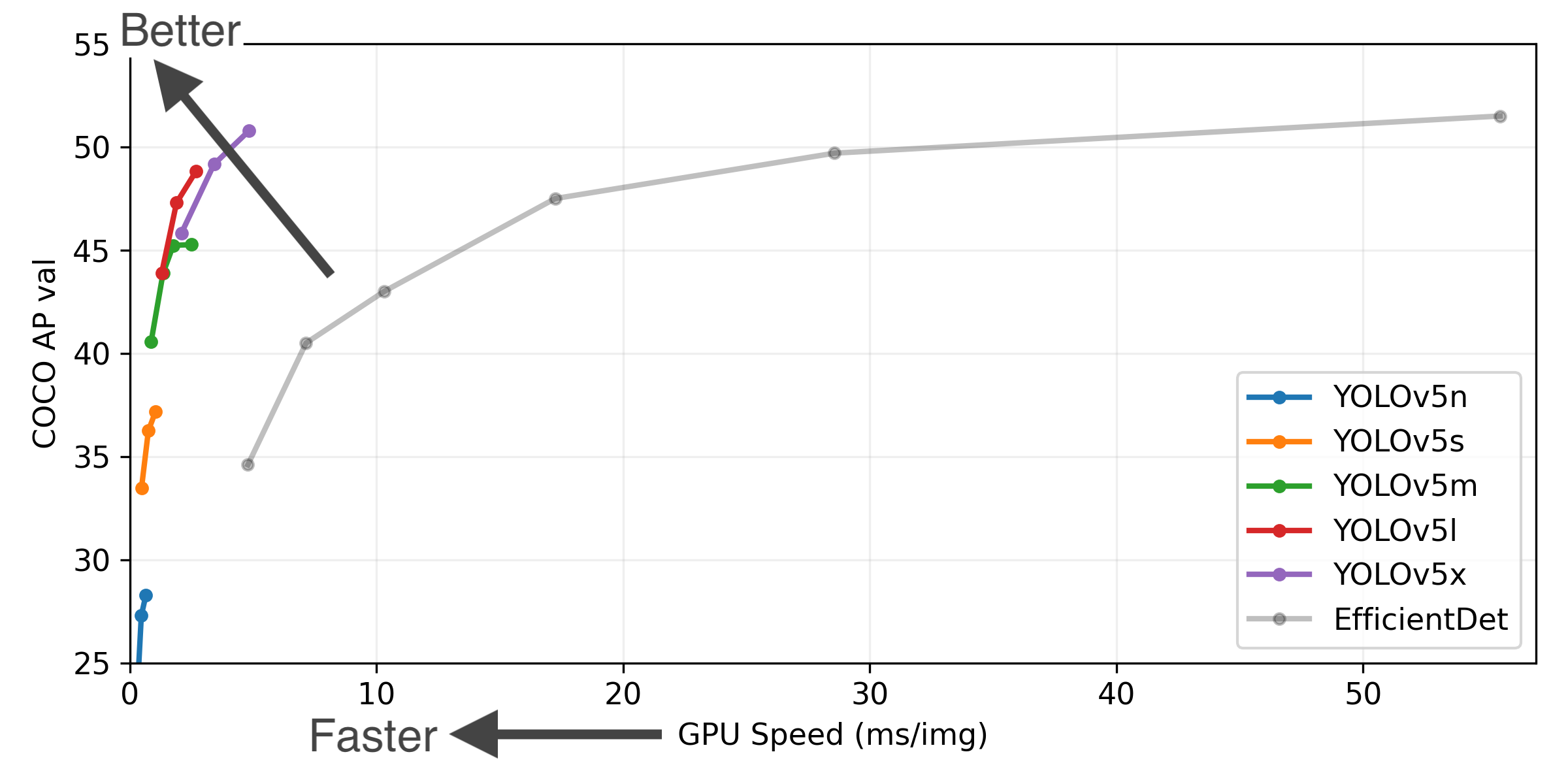
-
-
- Figure Notes (click to expand)
-
-* **COCO AP val** denotes mAP@0.5:0.95 metric measured on the 5000-image [COCO val2017](http://cocodataset.org) dataset over various inference sizes from 256 to 1536.
-* **GPU Speed** measures average inference time per image on [COCO val2017](http://cocodataset.org) dataset using a [AWS p3.2xlarge](https://aws.amazon.com/ec2/instance-types/p3/) V100 instance at batch-size 32.
-* **EfficientDet** data from [google/automl](https://github.com/google/automl) at batch size 8.
-* **Reproduce** by `python val.py --task study --data coco.yaml --iou 0.7 --weights yolov5n6.pt yolov5s6.pt yolov5m6.pt yolov5l6.pt yolov5x6.pt`
-
-
-### Pretrained Checkpoints
-
-[assets]: https://github.com/ultralytics/yolov5/releases
-
-[TTA]: https://github.com/ultralytics/yolov5/issues/303
-
-|Model |size
(pixels) |mAPval
0.5:0.95 |mAPval
0.5 |Speed
CPU b1
(ms) |Speed
V100 b1
(ms) |Speed
V100 b32
(ms) |params
(M) |FLOPs
@640 (B)
-|--- |--- |--- |--- |--- |--- |--- |--- |---
-|[YOLOv5n][assets] |640 |28.4 |46.0 |**45** |**6.3**|**0.6**|**1.9**|**4.5**
-|[YOLOv5s][assets] |640 |37.2 |56.0 |98 |6.4 |0.9 |7.2 |16.5
-|[YOLOv5m][assets] |640 |45.2 |63.9 |224 |8.2 |1.7 |21.2 |49.0
-|[YOLOv5l][assets] |640 |48.8 |67.2 |430 |10.1 |2.7 |46.5 |109.1
-|[YOLOv5x][assets] |640 |50.7 |68.9 |766 |12.1 |4.8 |86.7 |205.7
-| | | | | | | | |
-|[YOLOv5n6][assets] |1280 |34.0 |50.7 |153 |8.1 |2.1 |3.2 |4.6
-|[YOLOv5s6][assets] |1280 |44.5 |63.0 |385 |8.2 |3.6 |12.6 |16.8
-|[YOLOv5m6][assets] |1280 |51.0 |69.0 |887 |11.1 |6.8 |35.7 |50.0
-|[YOLOv5l6][assets] |1280 |53.6 |71.6 |1784 |15.8 |10.5 |76.7 |111.4
-|[YOLOv5x6][assets]
+ [TTA][TTA]|1280
1536 |54.7
**55.4** |**72.4**
72.3 |3136
- |26.2
- |19.4
- |140.7
- |209.8
-
-
-
- Table Notes (click to expand)
-
-* All checkpoints are trained to 300 epochs with default settings and hyperparameters.
-* **mAPval** values are for single-model single-scale on [COCO val2017](http://cocodataset.org) dataset.
Reproduce by `python val.py --data coco.yaml --img 640 --conf 0.001 --iou 0.65`
-* **Speed** averaged over COCO val images using a [AWS p3.2xlarge](https://aws.amazon.com/ec2/instance-types/p3/) instance. NMS times (~1 ms/img) not included.
Reproduce by `python val.py --data coco.yaml --img 640 --task speed --batch 1`
-* **TTA** [Test Time Augmentation](https://github.com/ultralytics/yolov5/issues/303) includes reflection and scale augmentations.
Reproduce by `python val.py --data coco.yaml --img 1536 --iou 0.7 --augment`
-
-
-
-## Contribute
-
-We love your input! We want to make contributing to YOLOv5 as easy and transparent as possible. Please see our [Contributing Guide](CONTRIBUTING.md) to get started, and fill out the [YOLOv5 Survey](https://ultralytics.com/survey?utm_source=github&utm_medium=social&utm_campaign=Survey) to send us feedback on your experiences. Thank you to all our contributors!
-
-
-
-## Contact
-
-For YOLOv5 bugs and feature requests please visit [GitHub Issues](https://github.com/ultralytics/yolov5/issues). For business inquiries or
-professional support requests please visit [https://ultralytics.com/contact](https://ultralytics.com/contact).
-
-
-
-
diff --git a/spaces/masonbarnes/open-llm-search/README.md b/spaces/masonbarnes/open-llm-search/README.md
deleted file mode 100644
index e55f44ee862027b5b9c16d51a32f7e7786b4a8c3..0000000000000000000000000000000000000000
--- a/spaces/masonbarnes/open-llm-search/README.md
+++ /dev/null
@@ -1,13 +0,0 @@
----
-title: Open LLM Search
-emoji: ⚡
-colorFrom: red
-colorTo: yellow
-sdk: gradio
-sdk_version: 3.43.2
-app_file: app.py
-pinned: false
-license: llama2
----
-
-Check out the configuration reference at https://huggingface.co/docs/hub/spaces-config-reference
\ No newline at end of file
diff --git a/spaces/meraGPT/meraKB/sidebar.py b/spaces/meraGPT/meraKB/sidebar.py
deleted file mode 100644
index c1dff82a56ec38d5236be034eefa2e57714ac720..0000000000000000000000000000000000000000
--- a/spaces/meraGPT/meraKB/sidebar.py
+++ /dev/null
@@ -1,11 +0,0 @@
-import streamlit as st
-
-
-def sidebar(supabase):
- st.sidebar.title("Database Information")
- number_of_docs = number_of_documents(supabase)
- st.sidebar.markdown(f"**Docs in DB:** {number_of_docs}")
-
-def number_of_documents(supabase):
- documents = supabase.table("documents").select("id", count="exact").execute()
- return documents.count
\ No newline at end of file
diff --git a/spaces/merve/data-leak/public/third_party/swoopy-drag.js b/spaces/merve/data-leak/public/third_party/swoopy-drag.js
deleted file mode 100644
index 3c740601b5111efdf47f0fd5da9d41de58ceb757..0000000000000000000000000000000000000000
--- a/spaces/merve/data-leak/public/third_party/swoopy-drag.js
+++ /dev/null
@@ -1,193 +0,0 @@
-// https://github.com/1wheel/swoopy-drag Copyright (c) 2016 Adam Pearce
-
-(function (global, factory) {
- typeof exports === 'object' && typeof module !== 'undefined' ? factory(exports, require('d3')) :
- typeof define === 'function' && define.amd ? define(['exports', 'd3'], factory) :
- (factory((global.d3 = global.d3 || {}),global.d3));
-}(this, function (exports,d3) { 'use strict';
-
- function swoopyDrag(){
- var x = function(d){ return d }
- var y = function(d){ return d }
-
- var annotations = []
- var annotationSel
-
- var draggable = false
-
- var dispatch = d3.dispatch('drag')
-
- var textDrag = d3.drag()
- .on('drag', function(d){
- var x = d3.event.x
- var y = d3.event.y
- d.textOffset = [x, y].map(Math.round)
-
- d3.select(this).call(translate, d.textOffset)
-
- dispatch.call('drag')
- })
- .subject(function(d){ return {x: d.textOffset[0], y: d.textOffset[1]} })
-
- var circleDrag = d3.drag()
- .on('drag', function(d){
- var x = d3.event.x
- var y = d3.event.y
- d.pos = [x, y].map(Math.round)
-
- var parentSel = d3.select(this.parentNode)
-
- var path = ''
- var points = parentSel.selectAll('circle').data()
- if (points[0].type == 'A'){
- path = calcCirclePath(points)
- } else{
- points.forEach(function(d){ path = path + d.type + d.pos })
- }
-
- parentSel.select('path').attr('d', path).datum().path = path
- d3.select(this).call(translate, d.pos)
-
- dispatch.call('drag')
- })
- .subject(function(d){ return {x: d.pos[0], y: d.pos[1]} })
-
-
- var rv = function(sel){
- annotationSel = sel.html('').selectAll('g')
- .data(annotations).enter()
- .append('g')
- .call(translate, function(d){ return [x(d), y(d)] })
-
- var textSel = annotationSel.append('text')
- .call(translate, ƒ('textOffset'))
- .text(ƒ('text'))
-
- annotationSel.append('path')
- .attr('d', ƒ('path'))
-
- if (!draggable) return
-
- annotationSel.style('cursor', 'pointer')
- textSel.call(textDrag)
-
- annotationSel.selectAll('circle').data(function(d){
- var points = []
-
- if (~d.path.indexOf('A')){
- //handle arc paths seperatly -- only one circle supported
- var pathNode = d3.select(this).select('path').node()
- var l = pathNode.getTotalLength()
-
- points = [0, .5, 1].map(function(d){
- var p = pathNode.getPointAtLength(d*l)
- return {pos: [p.x, p.y], type: 'A'}
- })
- } else{
- var i = 1
- var type = 'M'
- var commas = 0
-
- for (var j = 1; j < d.path.length; j++){
- var curChar = d.path[j]
- if (curChar == ',') commas++
- if (curChar == 'L' || curChar == 'C' || commas == 2){
- points.push({pos: d.path.slice(i, j).split(','), type: type})
- type = curChar
- i = j + 1
- commas = 0
- }
- }
-
- points.push({pos: d.path.slice(i, j).split(','), type: type})
- }
-
- return points
- }).enter().append('circle')
- .attr('r', 8)
- .attr('fill', 'rgba(0,0,0,0)')
- .attr('stroke', '#333')
- .attr('stroke-dasharray', '2 2')
- .call(translate, ƒ('pos'))
- .call(circleDrag)
-
- dispatch.call('drag')
- }
-
-
- rv.annotations = function(_x){
- if (typeof(_x) == 'undefined') return annotations
- annotations = _x
- return rv
- }
- rv.x = function(_x){
- if (typeof(_x) == 'undefined') return x
- x = _x
- return rv
- }
- rv.y = function(_x){
- if (typeof(_x) == 'undefined') return y
- y = _x
- return rv
- }
- rv.draggable = function(_x){
- if (typeof(_x) == 'undefined') return draggable
- draggable = _x
- return rv
- }
- rv.on = function() {
- var value = dispatch.on.apply(dispatch, arguments);
- return value === dispatch ? rv : value;
- }
-
- return rv
-
- //convert 3 points to an Arc Path
- function calcCirclePath(points){
- var a = points[0].pos
- var b = points[2].pos
- var c = points[1].pos
-
- var A = dist(b, c)
- var B = dist(c, a)
- var C = dist(a, b)
-
- var angle = Math.acos((A*A + B*B - C*C)/(2*A*B))
-
- //calc radius of circle
- var K = .5*A*B*Math.sin(angle)
- var r = A*B*C/4/K
- r = Math.round(r*1000)/1000
-
- //large arc flag
- var laf = +(Math.PI/2 > angle)
-
- //sweep flag
- var saf = +((b[0] - a[0])*(c[1] - a[1]) - (b[1] - a[1])*(c[0] - a[0]) < 0)
-
- return ['M', a, 'A', r, r, 0, laf, saf, b].join(' ')
- }
-
- function dist(a, b){
- return Math.sqrt(
- Math.pow(a[0] - b[0], 2) +
- Math.pow(a[1] - b[1], 2))
- }
-
-
- //no jetpack dependency
- function translate(sel, pos){
- sel.attr('transform', function(d){
- var posStr = typeof(pos) == 'function' ? pos(d) : pos
- return 'translate(' + posStr + ')'
- })
- }
-
- function ƒ(str){ return function(d){ return d[str] } }
- }
-
- exports.swoopyDrag = swoopyDrag;
-
- Object.defineProperty(exports, '__esModule', { value: true });
-
-}));
diff --git a/spaces/merve/data-leak/server-side/fill-in-the-blank/scatter-plot-colab/spearman-distribution/style.css b/spaces/merve/data-leak/server-side/fill-in-the-blank/scatter-plot-colab/spearman-distribution/style.css
deleted file mode 100644
index 3a35c688d5bcaa0ff242d9e053734657ba580c01..0000000000000000000000000000000000000000
--- a/spaces/merve/data-leak/server-side/fill-in-the-blank/scatter-plot-colab/spearman-distribution/style.css
+++ /dev/null
@@ -1,104 +0,0 @@
-body{
- font-family: menlo, Consolas, 'Lucida Console', monospace;
- margin: 10px;
- margin-left: 20px;
- width: 1130px;
- /*background: #f0f;*/
-}
-
-.tooltip {
- top: -1000px;
- position: fixed;
- padding: 10px;
- background: rgba(255, 255, 255, .90);
- border: 1px solid lightgray;
- pointer-events: none;
-}
-.tooltip-hidden{
- opacity: 0;
- transition: all .3s;
- transition-delay: .1s;
-}
-
-@media (max-width: 590px){
- div.tooltip{
- bottom: -1px;
- width: calc(100%);
- left: -1px !important;
- right: -1px !important;
- top: auto !important;
- width: auto !important;
- }
-}
-
-svg{
- overflow: visible;
-}
-
-.domain{
- display: none;
-}
-
-.axis{
- opacity: .7;
-}
-
-text{
- /*pointer-events: none;*/
- text-shadow: 0 1.5px 0 #fff, 1.5px 0 0 #fff, 0 -1.5px 0 #fff, -1.5px 0 0 #fff;
-}
-
-
-#graph > div{
- /*display: inline-block;*/
-}
-
-.active path{
- stroke: #f0f;
- /*stroke-width: 2;*/
- opacity: 1;
-}
-.active text{
- fill: #f0f;
- opacity: 1 !important;
- font-size: 14px;
-
-}
-
-p{
- max-width: 650px;
-}
-
-
-.bg-tick{
- stroke: #eee;
-}
-
-.tick{
- display: none;
-}
-
-text.tiny{
- font-size: 9px;
- font-family: monospace;
-}
-
-circle.sentence.active{
- fill: #f0f;
-}
-
-div.sentence{
- color: #333;
-}
-div.sentence.active{
- background: rgba(255,0,255,.1);
-}
-
-.list{
- /*border: 1px solid #555;*/
- /*padding: 10px;*/
-}
-
-
-
-
diff --git a/spaces/merve/dataset-worldviews/public/measuring-fairness/sel.js b/spaces/merve/dataset-worldviews/public/measuring-fairness/sel.js
deleted file mode 100644
index 0aefefe517d53ca634ed6e58d6cf8554cc386afa..0000000000000000000000000000000000000000
--- a/spaces/merve/dataset-worldviews/public/measuring-fairness/sel.js
+++ /dev/null
@@ -1,151 +0,0 @@
-/* Copyright 2020 Google LLC. All Rights Reserved.
-
-Licensed under the Apache License, Version 2.0 (the "License");
-you may not use this file except in compliance with the License.
-You may obtain a copy of the License at
-
- http://www.apache.org/licenses/LICENSE-2.0
-
-Unless required by applicable law or agreed to in writing, software
-distributed under the License is distributed on an "AS IS" BASIS,
-WITHOUT WARRANTIES OR CONDITIONS OF ANY KIND, either express or implied.
-See the License for the specific language governing permissions and
-limitations under the License.
-==============================================================================*/
-
-
-window.makeSel = function(){
- var s = c.width/(nCols -2) -1
-
- var personSel = c.svg.appendMany('g', students)
- var rectSel = personSel.append('rect')
- .at({
- height: s,
- width: s,
- x: -s/2,
- y: -s/2,
- // fillOpacity: .2
- })
-
- var textSel = personSel.append('text.weepeople')
- .text(d => d.letter)
- .at({fontSize: d => d.isMale ? 26 : 34, dy: '.33em', textAnchor: 'middle'})
- .st({stroke: d => d.isSick ? dcolors.sick : dcolors.well})
-
- addSwoop(c)
-
- var botAxis = c.svg.append('g').translate(c.width + 150, 1)
- var truthAxis = botAxis.append('g.axis').translate([0, 0])
-
- truthAxis.append('text').text('Truth')
- .at({textAnchor: 'middle', fontWeight: 500, x: s*2.65})
-
- truthAxis.append('g').translate([45, 22])
- .append('text').text('Sick').parent()
- .append('text.weepeople').text('k')
- .at({fontSize: 34, x: 22, y: 5})
- .st({fill: colors.sick})
-
- truthAxis.append('g').translate([95, 22])
- .append('text').text('Well').parent()
- .append('text.weepeople').text('d')
- .at({fontSize: 34, fill: colors.well, x: 22, y: 5})
- .st({fill: colors.well})
-
-
- var mlAxis = botAxis.append('g.axis').translate([220, 0])
-
- mlAxis.append('text').text('ML Prediction')
- .at({textAnchor: 'middle', fontWeight: 500, x: s*2.8})
-
- mlAxis.append('g').translate([35, 22])
- .append('text').text('Sick').parent()
- .append('rect')
- .at({width: s*.7, height: s*.7, fill: lcolors.sick, x: 28, y: -17})
-
- mlAxis.append('g').translate([100, 22])
- .append('text').text('Well').parent()
- .append('rect')
- .at({width: s*.7, height: s*.7, fill: lcolors.well, x: 28, y: -17})
-
-
-
- var fpAxis = c.svg.append('g.axis')
-
- // fpAxis.append('rect')
- // .translate(nCols*s - 20, 1)
- // .at({
- // fill: lcolors.well,
- // x: -82,
- // y: -12,
- // width: 56,
- // height: 28,
- // // stroke: '#000',
- // })
-
- // fpAxis.append('text')
- // .translate(nCols*s - 20, 1)
- // .tspans(['False', 'Negatives'], 12)
- // .at({textAnchor: 'end', x: -s/2 - 10, fill: colors.sick})
-
-
- // fpAxis.append('text')
- // .translate(nCols*s, 0)
- // .tspans(['False', 'Positives'], 12)
- // .at({textAnchor: 'start', x: s/2 + 7, fill: colors.well})
-
-
- var sexAxis = c.svg.append('g.axis')
-
- sexAxis.append('text').st({fontWeight: 500, fill: ''})
- .translate([-15, -30])
- .text('Adults')
-
- sexAxis.append('text').st({fontWeight: 500, fill: ''})
- .translate([-15, -30 + students.maleOffsetPx])
- .text('Children')
-
-
- var brAxis = c.svg.append('g.axis')
- var cpx = 0
-
- brAxis.append('path')
- .translate([-15, -20])
- .at({
- stroke: colors.sick,
- fill: 'none',
- d: ['M -3 -3 v', -cpx, 'h', students.fSickCols*students.colWidth, 'v', cpx].join('')
- })
-
- brAxis.append('path')
- .translate([-15, -20 + students.maleOffsetPx])
- .at({
- stroke: colors.sick,
- fill: 'none',
- d: ['M -3 -3 v', -cpx, 'h', students.mSickCols*students.colWidth, 'v', cpx].join('')
- })
-
- brAxis.append('text').st({fontWeight: 500, fill: colors.sick})
- .translate([-15, -30])
- .text('Sick Adults')
-
- brAxis.append('text').st({fontWeight: 500, fill: colors.sick})
- .translate([-15, -30 + students.maleOffsetPx])
- .text('Sick Children')
-
-
-
-
- return {personSel, textSel, rectSel, fpAxis, sexAxis, brAxis, truthAxis, mlAxis, botAxis}
-}
-
-
-
-
-
-
-
-
-
-
-if (window.init) window.init()
diff --git a/spaces/merve/fill-in-the-blank/public/uncertainty-calibration/footnote.js b/spaces/merve/fill-in-the-blank/public/uncertainty-calibration/footnote.js
deleted file mode 100644
index 05eac09cc1b8466bb2c440b6fd23060cd91f5017..0000000000000000000000000000000000000000
--- a/spaces/merve/fill-in-the-blank/public/uncertainty-calibration/footnote.js
+++ /dev/null
@@ -1,73 +0,0 @@
-!(() => {
- var ttFnSel = d3.select('body').selectAppend('div.tooltip-footnote.tooltip-footnote-hidden')
-
- function index2superscipt(i){
- return (i + 1 + '')
- .split('')
- .map(num => '⁰¹²³⁴⁵⁶⁷⁸⁹'[num])
- .join('')
- }
-
- var footendSel = d3.selectAll('.footend')
- .each(function(d, i){
- var sel = d3.select(this)
- var ogHTML = sel.parent().html()
-
- sel
- .at({href: '#footstart-' + i, id: 'footend-' + i})
- .text(index2superscipt(i))
- .datum(ogHTML)
- })
-
- footendSel.parent().parent().selectAll('br').remove()
-
- var footstartSel = d3.selectAll('.footstart')
- .each(function(d, i){
- d3.select(this)
- .at({
- href: '#footend-' + i,
- })
- .text(index2superscipt(i))
- .datum(footendSel.data()[i])
- .parent().at({id: 'footstart-' + i})
- })
- .call(addLockedTooltip)
-
-
- function addLockedTooltip(sel){
- sel
- .on('mouseover', function(d, i){
- ttFnSel
- .classed('tooltip-footnote-hidden', 0)
- .html(d).select('.footend').remove()
-
- var [x, y] = d3.mouse(d3.select('html').node())
- var bb = ttFnSel.node().getBoundingClientRect(),
- left = d3.clamp(20, (x-bb.width/2), window.innerWidth - bb.width - 20),
- top = innerHeight + scrollY > y + 20 + bb.height ? y + 20 : y - bb.height - 10;
-
- ttFnSel.st({left, top})
- })
- .on('mousemove', mousemove)
- .on('mouseout', mouseout)
-
- ttFnSel
- .on('mousemove', mousemove)
- .on('mouseout', mouseout)
-
- function mousemove(){
- if (window.__ttfade) window.__ttfade.stop()
- }
-
- function mouseout(){
- if (window.__ttfade) window.__ttfade.stop()
- window.__ttfade = d3.timeout(
- () => ttFnSel.classed('tooltip-footnote-hidden', 1),
- 250
- )
- }
- }
-
-})()
-
-
diff --git a/spaces/merve/fill-in-the-blank/source/_posts/2021-03-03-fill-in-the-blank.md b/spaces/merve/fill-in-the-blank/source/_posts/2021-03-03-fill-in-the-blank.md
deleted file mode 100644
index c5a251a9297e84f8b3ed4e504ff25f19793a57c2..0000000000000000000000000000000000000000
--- a/spaces/merve/fill-in-the-blank/source/_posts/2021-03-03-fill-in-the-blank.md
+++ /dev/null
@@ -1,136 +0,0 @@
----
-template: post.html
-title: What Have Language Models Learned?
-summary: By asking language models to fill in the blank, we can probe their understanding of the world.
-shareimg: https://pair.withgoogle.com/explorables/images/fill-in-the-blank.png
-shareimgabstract: https://pair.withgoogle.com/explorables/images/fill-in-the-blank-abstract.png
-permalink: /fill-in-the-blank/
-date: 2021-07-28
----
-
-Large language models are making it possible for computers to [write stories](https://openai.com/blog/better-language-models/), [program a website](https://twitter.com/sharifshameem/status/1282676454690451457) and [turn captions into images](https://openai.com/blog/dall-e/).
-
-One of the first of these models, [BERT](https://ai.googleblog.com/2018/11/open-sourcing-bert-state-of-art-pre.html), is trained by taking sentences, splitting them into individual words, randomly hiding some of them, and predicting what the hidden words are. After doing this millions of times, BERT has "read" enough Shakespeare to predict how this phrase usually ends:
-
-
-
-This page is hooked up to a version of BERT trained on Wikipedia and books.¹ Try clicking on different words to see how they'd be filled in or typing in another sentence to see what else has BERT picked up on.
-
-
-
-### Cattle or Clothes?
-
-Besides Hamlet's existential dread, the text BERT was trained on also contains more patterns:
-
-
-
-Cattle and horses aren't top purchase predictions in every state, though! In New York, some of the most likely words are clothes, books and art:
-
-
-
-There are more than 30,000 words, punctuation marks and word fragments in BERT's [vocabulary](https://huggingface.co/transformers/tokenizer_summary.html). Every time BERT fills in a hidden word, it assigns each of them a probability. By looking at how slightly different sentences shift those probabilities, we can get a glimpse at how purchasing patterns in different places are understood.
-
-
-
-You can **edit these sentences**. Or try one of these comparisons to get started:
-
-To the extent that a computer program can "know" something, what does BERT know about where you live?
-### What's in a Name?
-
-This technique can also probe what associations BERT has learned about different groups of people. For example, it predicts people named Elsie are older than people named Lauren:
-
-
-
-It's also learned that people named Jim have more [typically masculine](https://flowingdata.com/2017/09/11/most-female-and-male-occupations-since-1950/) jobs than people named Jane:
-
-
-
-These aren't just spurious correlations — Elsies really are more likely to be [older](https://rhiever.github.io/name-age-calculator/) than Laurens. And occupations the model associates with feminine names are held by a [higher percentage](https://purehost.bath.ac.uk/ws/portalfiles/portal/168480066/CaliskanEtAl_authors_full.pdf ) of women.
-
-Should we be concerned about these correlations? BERT was trained to fill in blanks in Wikipedia articles and books — it does a great job at that! The problem is that the internal representations of language these models have learned are used for much more – by some [measures](https://super.gluebenchmark.com/leaderboard), they're the best way we have of getting computers to understand and manipulate text.
-
-We wouldn't hesitate to call a conversation partner or recruiter who blithely assumed that doctors are men sexist, but that's exactly what BERT might do if heedlessly incorporated into a chatbot or HR software:
-
-
-
-Adjusting for assumptions like this isn't trivial. *Why* machine learning systems produce a given output still isn't well understood – determining if a credit model built on top of BERT rejected a loan application because of [gender discrimation](https://pair.withgoogle.com/explorables/hidden-bias/) might be quite difficult.
-
-Deploying large language models at scale also risks [amplifying](https://machinesgonewrong.com/bias_i/#harms-of-representation) and [perpetuating](http://faculty.washington.edu/ebender/papers/Stochastic_Parrots.pdf) today's harmful stereotypes. When [prompted](https://arxiv.org/pdf/2101.05783v1.pdf#page=3) with "Two Muslims walked into a…", for example, [GPT-3](https://en.wikipedia.org/wiki/GPT-3) typically finishes the sentence with descriptions of violence.
-### How Can We Fix This?
-
-One conceptually straightforward approach: reduce unwanted correlations from the training data to [mitigate](https://arxiv.org/abs/1906.08976) model [bias](https://arxiv.org/abs/2005.14050).
-
-Last year a version of BERT called [Zari](https://ai.googleblog.com/2020/10/measuring-gendered-correlations-in-pre.html) was [trained](https://arxiv.org/pdf/2010.06032.pdf#page=6) with an additional set of generated sentences. For every sentence with a [gendered noun](https://github.com/uclanlp/corefBias/blob/master/WinoBias/wino/generalized_swaps.txt), like boy or aunt, another sentence that replaced the noun with its gender-partner was added to the training data: in addition to "The *lady* doth protest too much," Zari was also trained on "The *gentleman* doth protest too much."
-
-
-
-Unlike BERT, Zari assigns nurses and doctors an equal probability of being a "she" or a "he" after being trained on the swapped sentences. This approach hasn't removed all the gender correlations; because names weren't swapped, Zari's association between masculine names and doctors has only slightly decreased from BERT's. And the retraining doesn't change how the model understands nonbinary gender.
-
-Something similar happened with [other attempts](https://arxiv.org/abs/1607.06520) to remove gender bias from models' representations of words. It's possible to mathematically define bias and perform "brain surgery" on a model to remove it, but language is steeped in gender. Large models can have billions of parameters in which to learn stereotypes — slightly different measures of bias have found the retrained models only [shifted the stereotypes](https://www.aclweb.org/anthology/N19-1061/) around to be undetectable by the initial measure.
-
-As with [other applications](https://pair.withgoogle.com/explorables/measuring-fairness/) of machine learning, it's helpful to focus instead on the actual harms that could occur. Tools like [AllenNLP](https://allennlp.org/), [LMdiff](http://lmdiff.net/) and the [Language Interpretability Tool](https://pair-code.github.io/lit/) make it easier to interact with language models to find where they might be falling short. Once those shortcomings are spotted, [task specific](https://arxiv.org/abs/2004.07667) mitigation measures can be simpler to apply than modifying the entire model.
-
-It's also possible that as models grow more capable, they might be able to [explain](https://arxiv.org/abs/2004.14546) and perform some of this debiasing themselves. Instead of forcing the model to tell us the gender of "the doctor," we could let it respond with [uncertainty](https://arr.am/2020/07/25/gpt-3-uncertainty-prompts/) that's [shown to the user](https://ai.googleblog.com/2018/12/providing-gender-specific-translations.html) and controls to override assumptions.
-
-### Credits
-
-Adam Pearce // July 2021
-
-Thanks to Ben Wedin, Emily Reif, James Wexler, Fernanda Viégas, Ian Tenney, Kellie Webster, Kevin Robinson, Lucas Dixon, Ludovic Peran, Martin Wattenberg, Michael Terry, Tolga Bolukbasi, Vinodkumar Prabhakaran, Xuezhi Wang, Yannick Assogba, and Zan Armstrong for their help with this piece.
-
-### Footnotes
-
- The BERT model used on this page is the Hugging Face version of [bert-large-uncased-whole-word-masking](https://huggingface.co/bert-large-uncased-whole-word-masking). "BERT" also refers to a type of model architecture; hundreds of BERT models have been [trained and published](https://huggingface.co/models?filter=bert). The model and chart code used here are available on [GitHub](https://github.com/PAIR-code/ai-explorables).
-
- Notice that "1800", "1900" and "2000" are some of the top predictions, though. People aren't actually more likely to be born at the start of a century, but in BERT's training corpus of books and Wikipedia articles round numbers are [more common](https://blocks.roadtolarissa.com/1wheel/cea123a8c17d51d9dacbd1c17e6fe601).
-
-Comparing BERT and Zari in this interface requires carefully tracking tokens during a transition. The [BERT Difference Plots](https://colab.research.google.com/drive/1xfPGKqjdE635cVSi-Ggt-cRBU5pyJNWP) colab has ideas for extensions to systemically look at differences between the models' output.
-
- This analysis shouldn't stop once a model is deployed — as language and model usage shifts, it's important to continue studying and mitigating potential harms.
-
-
-### Appendix: Differences Over Time
-
-In addition to looking at how predictions for men and women are different for a given sentence, we can also chart how those differences have changed over time:
-
-
-
-The convergence in more recent years suggests another potential mitigation technique: using a prefix to steer the model away from unwanted correlations while preserving its understanding of natural language.
-
-Using "In $year" as the prefix is quite limited, though, as it doesn't handle gender-neutral pronouns and potentially [increases](https://www.pnas.org/content/pnas/115/16/E3635.full.pdf#page=8) other correlations. However, it may be possible to [find a better prefix](https://arxiv.org/abs/2104.08691) that mitigates a specific type of bias with just a [couple of dozen examples](https://www.openai.com/blog/improving-language-model-behavior/ ).
-
-
-
-Closer examination of these differences in differences also shows there's a limit to the facts we can pull out of BERT this way.
-
-Below, the top row of charts shows how predicted differences in occupations between men and women change between 1908 and 2018. The rightmost chart shows the he/she difference in 1908 against the he/she difference in 2018.
-
-The flat slope of the rightmost chart indicates that the he/she difference has decreased for each job by about the same amount. But in reality, [shifts in occupation](https://www.weforum.org/agenda/2016/03/a-visual-history-of-gender-and-employment) weren't nearly so smooth and some occupations, like accounting, switched from being majority male to majority female.
-
-
-
-This reality-prediction mismatch could be caused by lack of training data, model size or the coarseness of the probing method. There's an immense amount of general knowledge inside of these models — with a little bit of focused training, they can even become expert [trivia](https://t5-trivia.glitch.me/) players.
-### More Explorables
-
-
-
-
-
-
-
-
-
-
-
-
-
-
-
-
-
-
-
-
-
-
-
\ No newline at end of file
diff --git a/spaces/merve/fill-in-the-blank/source/private-and-fair/umap-digit.js b/spaces/merve/fill-in-the-blank/source/private-and-fair/umap-digit.js
deleted file mode 100644
index f2fd20ea8d672ab49ca2698135c581605524bb46..0000000000000000000000000000000000000000
--- a/spaces/merve/fill-in-the-blank/source/private-and-fair/umap-digit.js
+++ /dev/null
@@ -1,139 +0,0 @@
-
-!(async function(){
- var data = await util.getFile('mnist_train.csv')
- data.forEach(d => {
- delete d['']
- d.i = +d.i
- })
-
- var sel = d3.select('.umap-digit').html('')
- .at({role: 'graphics-document', 'aria-label': `Color coded UMAP of MNIST 1s showing that increasing privacy will misclassify slanted and serif “1” digits first.`})
-
- var umapSel = sel.append('div')
- .append('div.chart-title').text('Sensitivity to higher privacy levels →')
- .parent()
- .st({maxWidth: 600, margin: '0 auto', marginBottom: 10})
- .append('div')
-
-
- var buttonSel = sel.append('div.digit-button-container')
- .appendMany('div.button', d3.range(10))
- .text(d => d)
- .on('click', d => drawDigitUmap(d))
-
-
- drawDigitUmap(1)
-
-
- async function drawDigitUmap(digit){
- buttonSel.classed('active', d => d == digit)
-
- // var umap = await util.getFile(`umap_train_${digit}.npy`)
- var umap = await util.getFile(`cns-cache/umap_train_784_${digit}.npy`)
- util.getFile(`cns-cache/mnist_train_raw_${digit}.npy`)
-
- var digitData = data
- .filter(d => d.y == digit)
- .map((d, i) => ({
- rawPos: [umap.data[i*2 + 0], umap.data[i*2 + 1]],
- priv_order: d.priv_order,
- y: d.y,
- i: d.i
- }))
-
- var c = d3.conventions({
- sel: umapSel.html(''),
- width: 600,
- height: 600,
- layers: 'sdc',
- margin: {top: 45}
- })
-
- var nTicks = 200
- c.svg.appendMany('rect', d3.range(nTicks))
- .at({
- height: 15,
- width: 1,
- fill: i => d3.interpolatePlasma(i/nTicks),
- })
- .translate(i => [c.width/2 - nTicks/2 - 20 + i, -c.margin.top + 5])
-
-
- c.x.domain(d3.extent(digitData, d => d.rawPos[0]))
- c.y.domain(d3.extent(digitData, d => d.rawPos[1]))//.range([0, c.height])
- digitData.forEach(d => d.pos = [c.x(d.rawPos[0]), c.y(d.rawPos[1])])
-
- c.sel.select('canvas').st({pointerEvents: 'none'})
- var divSel = c.layers[1].st({pointerEvents: 'none'})
- var ctx = c.layers[2]
-
- digitData.forEach(d => {
- ctx.beginPath()
- ctx.fillStyle = d3.interpolatePlasma(1 - d.priv_order/60000)
- ctx.rect(d.pos[0], d.pos[1], 2, 2)
- ctx.fill()
- })
-
- var p = 10
- c.svg
- .append('rect').at({width: c.width + p*2, height: c.height + p*2, x: -p, y: -p})
- .parent()
- .call(d3.attachTooltip)
- .on('mousemove', function(){
- var [px, py] = d3.mouse(this)
-
- var minPoint = _.minBy(digitData, d => {
- var dx = d.pos[0] - px
- var dy = d.pos[1] - py
-
- return dx*dx + dy*dy
- })
-
- var s = 4
- var c = d3.conventions({
- sel: ttSel.html('').append('div'),
- width: 4*28,
- height: 4*28,
- layers: 'cs',
- margin: {top: 0, left: 0, right: 0, bottom: 0}
- })
-
- // Label: ${minPoint.y}
- // ttSel.append('div').html(`
- // Privacy Rank ${d3.format(',')(minPoint.priv_order)}
- // `)
-
- ttSel.classed('tooltip-footnote', 0).st({width: 112})
-
- util.drawDigit(c.layers[0], +minPoint.i, s)
- })
-
- if (digit == 1){
- var circleDigits = [
- {r: 40, index: 1188},
- {r: 53, index: 18698},
- {r: 40, index: 1662}
- ]
- circleDigits.forEach(d => {
- d.pos = digitData.filter(e => e.priv_order == d.index)[0].pos
- })
-
- c.svg.append('g')
- .appendMany('g', circleDigits)
- .translate(d => d.pos)
- .append('circle')
- .at({r: d => d.r, fill: 'none', stroke: '#fff', strokeDasharray: '2 3', strokeWidth: 1})
-
- var {r, pos} = circleDigits[0]
-
-
- divSel
- .append('div').translate(pos)
- .append('div').translate([r + 20, -r + 10])
- .st({width: 150, fontWeight: 300, fontSize: 14, color: '#fff', xbackground: 'rgba(255,0,0,.2)', lineHeight: '1.2em'})
- .text('Increasing privacy will misclassify slanted and serif “1” digits first')
- }
- }
-})()
-
-
diff --git a/spaces/merve/measuring-fairness/index.html b/spaces/merve/measuring-fairness/index.html
deleted file mode 100644
index 918e851d9dd1baf9e4fb4f067fd979d432472161..0000000000000000000000000000000000000000
--- a/spaces/merve/measuring-fairness/index.html
+++ /dev/null
@@ -1,24 +0,0 @@
-
-
-
-
-
- My static Space
-
-
-
-
- Welcome to your static Space!
-
- You can modify this app directly by editing index.html in the
- Files and versions tab.
-
-
- Also don't forget to check the
- Spaces documentation.
-
-
-
-
diff --git a/spaces/merve/t5-playground/README.md b/spaces/merve/t5-playground/README.md
deleted file mode 100644
index a21975fdd83f2d9d7e7232388a6f27f429460d2c..0000000000000000000000000000000000000000
--- a/spaces/merve/t5-playground/README.md
+++ /dev/null
@@ -1,37 +0,0 @@
----
-title: T5 Playground
-emoji: 🧡
-colorFrom: indigo
-colorTo: pink
-sdk: gradio
-app_file: app.py
-pinned: false
----
-
-# Configuration
-
-`title`: _string_
-Display title for the Space
-
-`emoji`: _string_
-Space emoji (emoji-only character allowed)
-
-`colorFrom`: _string_
-Color for Thumbnail gradient (red, yellow, green, blue, indigo, purple, pink, gray)
-
-`colorTo`: _string_
-Color for Thumbnail gradient (red, yellow, green, blue, indigo, purple, pink, gray)
-
-`sdk`: _string_
-Can be either `gradio` or `streamlit`
-
-`sdk_version` : _string_
-Only applicable for `streamlit` SDK.
-See [doc](https://hf.co/docs/hub/spaces) for more info on supported versions.
-
-`app_file`: _string_
-Path to your main application file (which contains either `gradio` or `streamlit` Python code).
-Path is relative to the root of the repository.
-
-`pinned`: _boolean_
-Whether the Space stays on top of your list.
diff --git a/spaces/merve/uncertainty-calibration/public/dataset-worldviews/README.md b/spaces/merve/uncertainty-calibration/public/dataset-worldviews/README.md
deleted file mode 100644
index 74e4920975910a03f1cc2ebe582a7a6d03eb8da6..0000000000000000000000000000000000000000
--- a/spaces/merve/uncertainty-calibration/public/dataset-worldviews/README.md
+++ /dev/null
@@ -1,6 +0,0 @@
-## Photo todos
-
-x highlight the active button
-x firing when not expected?
-x clear timer when clicked
-- maybe convert to HTML?
\ No newline at end of file
diff --git a/spaces/merve/uncertainty-calibration/source/_posts/2019-10-02-bias.html b/spaces/merve/uncertainty-calibration/source/_posts/2019-10-02-bias.html
deleted file mode 100644
index 44c586c9489408fa9694149309ffefa3f3fc4d1b..0000000000000000000000000000000000000000
--- a/spaces/merve/uncertainty-calibration/source/_posts/2019-10-02-bias.html
+++ /dev/null
@@ -1,126 +0,0 @@
-
----
-template: post.html
-title: Hidden Bias
-summary: Models trained on real-world data can encode real-world bias. Hiding information about protected classes doesn't always fix things — sometimes it can even hurt.
-permalink: /hidden-bias/
-shareimg: https://pair.withgoogle.com/explorables/images/hidden-bias.png
-date: 2020-05-01
-
----
-
-
-
-
-
-
-
-
-Modeling College GPA
-
-Let's pretend we're college admissions officers trying to predict the GPA students will have in college (in these examples we'll use simulated data).
-
-
One simple approach: predict that students will have the same GPA in college as they did in high school.
-
-
-
-
-
-
-
-Predicting with ML
-If we switched to using a machine learning model and entered these student grades, it would recognize this pattern and adjust the prediction.
-
-
The model does this without knowing anything about the real-life context of grading in high school versus college.
-
-
-
-
-Giving the model more information about students increases accuracy more...
-
-
-
-
-...and more.
-
-
-
-
-Models can encode previous bias
-All of this sensitive information about students is just a long list of numbers to model.
-
-
If a sexist college culture has historically led to lower grades for female students, the model will pick up on that correlation and predict lower grades for women.
-
-
Training on historical data bakes in historical biases. Here the sexist culture has improved, but the model learned from the past correlation and still predicts higher grades for men.
-
-
-
-Hiding protected classes from the model might not stop discrimination
-
-Even if we don't tell the model students' genders, it might still score female students poorly.
-
-
With detailed enough information about every student, the model can still synthesize a proxy for gender out of other variables.
-
-
-
-
-Including a protected attribute may even decrease discrimination
-
-Let's look at a simplified model, one only taking into account the recommendation of an alumni interviewer.
-
-
-
-
-The interviewer is quite accurate, except that they're biased against students with a low household income.
-
-
In our toy model, students' grades don't depend on their income once they're in college. In other words, we have biased inputs and unbiased outcomes—the opposite of the previous example, where the inputs weren't biased, but the toxic culture biased the outcomes.
-
-
-
-
-If we also tell the model each student's household income, it will naturally correct for the interviewer's overrating of high-income students just like it corrected for the difference between high school and college GPAs.
-
-
By carefully considering and accounting for bias, we've made the model fairer and more accurate. This isn't always easy to do, especially in circumstances like the historically toxic college culture where unbiased data is limited.
-
-
And there are fundamental fairness trade-offs that have to be made. Check out the Measuring Fairness explorable to see how those tradeoffs work.
-
-
-
-
-
Adam Pearce // May 2020
-
-
Thanks to Carey Radebaugh, Dan Nanas, David Weinberger, Emily Denton, Emily Reif, Fernanda Viégas, Hal Abelson, James Wexler, Kristen Olson, Lucas Dixon, Mahima Pushkarna, Martin Wattenberg, Michael Terry, Rebecca Salois, Timnit Gebru, Tulsee Doshi, Yannick Assogba, Yoni Halpern, Zan Armstrong, and my other colleagues at Google for their help with this piece.
-
-
-
-
-
-
-
-
-
-
-
-
-
-
-
\ No newline at end of file
diff --git a/spaces/mfrashad/CharacterGAN/netdissect/upsegmodel/prroi_pool/build.py b/spaces/mfrashad/CharacterGAN/netdissect/upsegmodel/prroi_pool/build.py
deleted file mode 100644
index b198790817a2d11d65d6211b011f9408d9d34270..0000000000000000000000000000000000000000
--- a/spaces/mfrashad/CharacterGAN/netdissect/upsegmodel/prroi_pool/build.py
+++ /dev/null
@@ -1,50 +0,0 @@
-#! /usr/bin/env python3
-# -*- coding: utf-8 -*-
-# File : build.py
-# Author : Jiayuan Mao, Tete Xiao
-# Email : maojiayuan@gmail.com, jasonhsiao97@gmail.com
-# Date : 07/13/2018
-#
-# This file is part of PreciseRoIPooling.
-# Distributed under terms of the MIT license.
-# Copyright (c) 2017 Megvii Technology Limited.
-
-import os
-import torch
-
-from torch.utils.ffi import create_extension
-
-headers = []
-sources = []
-defines = []
-extra_objects = []
-with_cuda = False
-
-if torch.cuda.is_available():
- with_cuda = True
-
- headers+= ['src/prroi_pooling_gpu.h']
- sources += ['src/prroi_pooling_gpu.c']
- defines += [('WITH_CUDA', None)]
-
- this_file = os.path.dirname(os.path.realpath(__file__))
- extra_objects_cuda = ['src/prroi_pooling_gpu_impl.cu.o']
- extra_objects_cuda = [os.path.join(this_file, fname) for fname in extra_objects_cuda]
- extra_objects.extend(extra_objects_cuda)
-else:
- # TODO(Jiayuan Mao @ 07/13): remove this restriction after we support the cpu implementation.
- raise NotImplementedError('Precise RoI Pooling only supports GPU (cuda) implememtations.')
-
-ffi = create_extension(
- '_prroi_pooling',
- headers=headers,
- sources=sources,
- define_macros=defines,
- relative_to=__file__,
- with_cuda=with_cuda,
- extra_objects=extra_objects
-)
-
-if __name__ == '__main__':
- ffi.build()
-
diff --git a/spaces/mshukor/UnIVAL/fairseq/examples/hubert/measure_teacher_quality.py b/spaces/mshukor/UnIVAL/fairseq/examples/hubert/measure_teacher_quality.py
deleted file mode 100644
index 92279b2214bb2ba4a99aea92098907ef4f55821b..0000000000000000000000000000000000000000
--- a/spaces/mshukor/UnIVAL/fairseq/examples/hubert/measure_teacher_quality.py
+++ /dev/null
@@ -1,241 +0,0 @@
-# Copyright (c) Facebook, Inc. and its affiliates.
-#
-# This source code is licensed under the MIT license found in the
-# LICENSE file in the root directory of this source tree.
-
-import numpy as np
-import os.path as op
-import re
-from tabulate import tabulate
-from collections import Counter
-
-
-def comp_purity(p_xy, axis):
- max_p = p_xy.max(axis=axis)
- marg_p = p_xy.sum(axis=axis)
- indv_pur = max_p / marg_p
- aggr_pur = max_p.sum()
- return indv_pur, aggr_pur
-
-
-def comp_entropy(p):
- return (-p * np.log(p + 1e-8)).sum()
-
-
-def comp_norm_mutual_info(p_xy):
- p_x = p_xy.sum(axis=1, keepdims=True)
- p_y = p_xy.sum(axis=0, keepdims=True)
- pmi = np.log(p_xy / np.matmul(p_x, p_y) + 1e-8)
- mi = (p_xy * pmi).sum()
- h_x = comp_entropy(p_x)
- h_y = comp_entropy(p_y)
- return mi, mi / h_x, mi / h_y, h_x, h_y
-
-
-def pad(labs, n):
- if n == 0:
- return np.array(labs)
- return np.concatenate([[labs[0]] * n, labs, [labs[-1]] * n])
-
-
-def comp_avg_seg_dur(labs_list):
- n_frms = 0
- n_segs = 0
- for labs in labs_list:
- labs = np.array(labs)
- edges = np.zeros(len(labs)).astype(bool)
- edges[0] = True
- edges[1:] = labs[1:] != labs[:-1]
- n_frms += len(edges)
- n_segs += edges.astype(int).sum()
- return n_frms / n_segs
-
-
-def comp_joint_prob(uid2refs, uid2hyps):
- """
- Args:
- pad: padding for spliced-feature derived labels
- """
- cnts = Counter()
- skipped = []
- abs_frmdiff = 0
- for uid in uid2refs:
- if uid not in uid2hyps:
- skipped.append(uid)
- continue
- refs = uid2refs[uid]
- hyps = uid2hyps[uid]
- abs_frmdiff += abs(len(refs) - len(hyps))
- min_len = min(len(refs), len(hyps))
- refs = refs[:min_len]
- hyps = hyps[:min_len]
- cnts.update(zip(refs, hyps))
- tot = sum(cnts.values())
-
- ref_set = sorted({ref for ref, _ in cnts.keys()})
- hyp_set = sorted({hyp for _, hyp in cnts.keys()})
- ref2pid = dict(zip(ref_set, range(len(ref_set))))
- hyp2lid = dict(zip(hyp_set, range(len(hyp_set))))
- # print(hyp_set)
- p_xy = np.zeros((len(ref2pid), len(hyp2lid)), dtype=float)
- for (ref, hyp), cnt in cnts.items():
- p_xy[ref2pid[ref], hyp2lid[hyp]] = cnt
- p_xy /= p_xy.sum()
- return p_xy, ref2pid, hyp2lid, tot, abs_frmdiff, skipped
-
-
-def read_phn(tsv_path, rm_stress=True):
- uid2phns = {}
- with open(tsv_path) as f:
- for line in f:
- uid, phns = line.rstrip().split("\t")
- phns = phns.split(",")
- if rm_stress:
- phns = [re.sub("[0-9]", "", phn) for phn in phns]
- uid2phns[uid] = phns
- return uid2phns
-
-
-def read_lab(tsv_path, lab_path, pad_len=0, upsample=1):
- """
- tsv is needed to retrieve the uids for the labels
- """
- with open(tsv_path) as f:
- f.readline()
- uids = [op.splitext(op.basename(line.rstrip().split()[0]))[0] for line in f]
- with open(lab_path) as f:
- labs_list = [pad(line.rstrip().split(), pad_len).repeat(upsample) for line in f]
- assert len(uids) == len(labs_list)
- return dict(zip(uids, labs_list))
-
-
-def main_lab_lab(
- tsv_dir,
- lab_dir,
- lab_name,
- lab_sets,
- ref_dir,
- ref_name,
- pad_len=0,
- upsample=1,
- verbose=False,
-):
- # assume tsv_dir is the same for both the reference and the hypotheses
- tsv_dir = lab_dir if tsv_dir is None else tsv_dir
-
- uid2refs = {}
- for s in lab_sets:
- uid2refs.update(read_lab(f"{tsv_dir}/{s}.tsv", f"{ref_dir}/{s}.{ref_name}"))
-
- uid2hyps = {}
- for s in lab_sets:
- uid2hyps.update(
- read_lab(
- f"{tsv_dir}/{s}.tsv", f"{lab_dir}/{s}.{lab_name}", pad_len, upsample
- )
- )
- _main(uid2refs, uid2hyps, verbose)
-
-
-def main_phn_lab(
- tsv_dir,
- lab_dir,
- lab_name,
- lab_sets,
- phn_dir,
- phn_sets,
- pad_len=0,
- upsample=1,
- verbose=False,
-):
- uid2refs = {}
- for s in phn_sets:
- uid2refs.update(read_phn(f"{phn_dir}/{s}.tsv"))
-
- uid2hyps = {}
- tsv_dir = lab_dir if tsv_dir is None else tsv_dir
- for s in lab_sets:
- uid2hyps.update(
- read_lab(
- f"{tsv_dir}/{s}.tsv", f"{lab_dir}/{s}.{lab_name}", pad_len, upsample
- )
- )
- _main(uid2refs, uid2hyps, verbose)
-
-
-def _main(uid2refs, uid2hyps, verbose):
- (p_xy, ref2pid, hyp2lid, tot, frmdiff, skipped) = comp_joint_prob(
- uid2refs, uid2hyps
- )
- ref_pur_by_hyp, ref_pur = comp_purity(p_xy, axis=0)
- hyp_pur_by_ref, hyp_pur = comp_purity(p_xy, axis=1)
- (mi, mi_norm_by_ref, mi_norm_by_hyp, h_ref, h_hyp) = comp_norm_mutual_info(p_xy)
- outputs = {
- "ref pur": ref_pur,
- "hyp pur": hyp_pur,
- "H(ref)": h_ref,
- "H(hyp)": h_hyp,
- "MI": mi,
- "MI/H(ref)": mi_norm_by_ref,
- "ref segL": comp_avg_seg_dur(uid2refs.values()),
- "hyp segL": comp_avg_seg_dur(uid2hyps.values()),
- "p_xy shape": p_xy.shape,
- "frm tot": tot,
- "frm diff": frmdiff,
- "utt tot": len(uid2refs),
- "utt miss": len(skipped),
- }
- print(tabulate([outputs.values()], outputs.keys(), floatfmt=".4f"))
-
-
-if __name__ == "__main__":
- """
- compute quality of labels with respect to phone or another labels if set
- """
- import argparse
-
- parser = argparse.ArgumentParser()
- parser.add_argument("tsv_dir")
- parser.add_argument("lab_dir")
- parser.add_argument("lab_name")
- parser.add_argument("--lab_sets", default=["valid"], type=str, nargs="+")
- parser.add_argument(
- "--phn_dir",
- default="/checkpoint/wnhsu/data/librispeech/960h/fa/raw_phn/phone_frame_align_v1",
- )
- parser.add_argument(
- "--phn_sets", default=["dev-clean", "dev-other"], type=str, nargs="+"
- )
- parser.add_argument("--pad_len", default=0, type=int, help="padding for hypotheses")
- parser.add_argument(
- "--upsample", default=1, type=int, help="upsample factor for hypotheses"
- )
- parser.add_argument("--ref_lab_dir", default="")
- parser.add_argument("--ref_lab_name", default="")
- parser.add_argument("--verbose", action="store_true")
- args = parser.parse_args()
-
- if args.ref_lab_dir and args.ref_lab_name:
- main_lab_lab(
- args.tsv_dir,
- args.lab_dir,
- args.lab_name,
- args.lab_sets,
- args.ref_lab_dir,
- args.ref_lab_name,
- args.pad_len,
- args.upsample,
- args.verbose,
- )
- else:
- main_phn_lab(
- args.tsv_dir,
- args.lab_dir,
- args.lab_name,
- args.lab_sets,
- args.phn_dir,
- args.phn_sets,
- args.pad_len,
- args.upsample,
- args.verbose,
- )
diff --git a/spaces/mshukor/UnIVAL/fairseq/examples/simultaneous_translation/utils/functions.py b/spaces/mshukor/UnIVAL/fairseq/examples/simultaneous_translation/utils/functions.py
deleted file mode 100644
index 590a6c11cea222ac9096b19f0e3dfe1b71b6c10b..0000000000000000000000000000000000000000
--- a/spaces/mshukor/UnIVAL/fairseq/examples/simultaneous_translation/utils/functions.py
+++ /dev/null
@@ -1,125 +0,0 @@
-# Copyright (c) Facebook, Inc. and its affiliates.
-#
-# This source code is licensed under the MIT license found in the
-# LICENSE file in the root directory of this source tree.
-
-import torch
-
-
-def prob_check(tensor, eps=1e-10):
- assert not torch.isnan(tensor).any(), (
- "Nan in a probability tensor."
- )
- # Add the eps here to prevent errors introduced by precision
- assert tensor.le(1.0 + eps).all() and tensor.ge(0.0 - eps).all(), (
- "Incorrect values in a probability tensor"
- ", 0.0 <= tensor <= 1.0"
- )
-
-
-def exclusive_cumprod(tensor, dim: int, eps: float = 1e-10):
- """
- Implementing exclusive cumprod.
- There is cumprod in pytorch, however there is no exclusive mode.
- cumprod(x) = [x1, x1x2, x2x3x4, ..., prod_{i=1}^n x_i]
- exclusive means
- cumprod(x) = [1, x1, x1x2, x1x2x3, ..., prod_{i=1}^{n-1} x_i]
- """
- tensor_size = list(tensor.size())
- tensor_size[dim] = 1
- return_tensor = safe_cumprod(
- torch.cat([torch.ones(tensor_size).type_as(tensor), tensor], dim=dim),
- dim=dim,
- eps=eps,
- )
-
- if dim == 0:
- return return_tensor[:-1]
- elif dim == 1:
- return return_tensor[:, :-1]
- elif dim == 2:
- return return_tensor[:, :, :-1]
- else:
- raise RuntimeError(
- "Cumprod on dimension 3 and more is not implemented"
- )
-
-
-def safe_cumprod(tensor, dim: int, eps: float = 1e-10):
- """
- An implementation of cumprod to prevent precision issue.
- cumprod(x)
- = [x1, x1x2, x1x2x3, ....]
- = [exp(log(x1)), exp(log(x1) + log(x2)), exp(log(x1) + log(x2) + log(x3)), ...]
- = exp(cumsum(log(x)))
- """
-
- if (tensor + eps < 0).any().item():
- raise RuntimeError(
- "Safe cumprod can only take non-negative tensors as input."
- "Consider use torch.cumprod if you want to calculate negative values."
- )
-
- log_tensor = torch.log(tensor + eps)
- cumsum_log_tensor = torch.cumsum(log_tensor, dim)
- exp_cumsum_log_tensor = torch.exp(cumsum_log_tensor)
- return exp_cumsum_log_tensor
-
-
-def moving_sum(x, start_idx: int, end_idx: int):
- """
- From MONOTONIC CHUNKWISE ATTENTION
- https://arxiv.org/pdf/1712.05382.pdf
- Equation (18)
-
- x = [x_1, x_2, ..., x_N]
- MovingSum(x, start_idx, end_idx)_n = Sigma_{m=n−(start_idx−1)}^{n+end_idx-1} x_m
- for n in {1, 2, 3, ..., N}
-
- x : src_len, batch_size
- start_idx : start idx
- end_idx : end idx
-
- Example
- src_len = 5
- batch_size = 3
- x =
- [[ 0, 5, 10],
- [ 1, 6, 11],
- [ 2, 7, 12],
- [ 3, 8, 13],
- [ 4, 9, 14]]
-
- MovingSum(x, 3, 1) =
- [[ 0, 5, 10],
- [ 1, 11, 21],
- [ 3, 18, 33],
- [ 6, 21, 36],
- [ 9, 24, 39]]
-
- MovingSum(x, 1, 3) =
- [[ 3, 18, 33],
- [ 6, 21, 36],
- [ 9, 24, 39],
- [ 7, 17, 27],
- [ 4, 9, 14]]
- """
- # TODO: Make dimension configurable
- assert start_idx > 0 and end_idx > 0
- batch_size, tgt_len, src_len = x.size()
- x = x.view(-1, src_len).unsqueeze(1)
- # batch_size, 1, src_len
- moving_sum_weight = torch.ones([1, 1, end_idx + start_idx - 1]).type_as(x)
-
- moving_sum = torch.nn.functional.conv1d(
- x, moving_sum_weight, padding=start_idx + end_idx - 1
- ).squeeze(1)
-
- moving_sum = moving_sum[:, end_idx:-start_idx]
-
- assert src_len == moving_sum.size(1)
- assert batch_size * tgt_len == moving_sum.size(0)
-
- moving_sum = moving_sum.view(batch_size, tgt_len, src_len)
-
- return moving_sum
diff --git a/spaces/mshukor/UnIVAL/run_scripts/caption/scaling_best/onlylinear/unival_video_caption_s2_onlylinear.sh b/spaces/mshukor/UnIVAL/run_scripts/caption/scaling_best/onlylinear/unival_video_caption_s2_onlylinear.sh
deleted file mode 100644
index edc0ef8be6614028a36dce60f4eaec1daacf35b7..0000000000000000000000000000000000000000
--- a/spaces/mshukor/UnIVAL/run_scripts/caption/scaling_best/onlylinear/unival_video_caption_s2_onlylinear.sh
+++ /dev/null
@@ -1,209 +0,0 @@
-
-
-# Number of GPUs per GPU worker
-export GPUS_PER_NODE=8
-# Number of GPU workers, for single-worker training, please set to 1
-export NUM_NODES=$SLURM_NNODES
-# The ip address of the rank-0 worker, for single-worker training, please set to localhost
-master_addr=$(scontrol show hostnames "$SLURM_JOB_NODELIST" | head -n 1)
-export MASTER_ADDR=$master_addr
-
-# The port for communication
-export MASTER_PORT=12350
-# The rank of this worker, should be in {0, ..., WORKER_CNT-1}, for single-worker training, please set to 0
-export RANK=$SLURM_NODEID
-
-echo "MASTER_ADDR: $MASTER_ADDR"
-echo "RANK :$RANK"
-echo "NUM_NODES :$NUM_NODES"
-echo "GPUS_PER_NODE :$GPUS_PER_NODE"
-
-export MIOPEN_USER_DB_PATH=/lus/home/NAT/gda2204/mshukor/.config/miopen_${MASTER_ADDR}_${SLURM_PROCID}/
-
-echo "MIOPEN_USER_DB_PATH :$MIOPEN_USER_DB_PATH"
-
-num_workers=0
-
-
-exp_name=unival_video_caption_s2_onlylinear
-
-
-
-ofa_dir=/lus/home/NAT/gda2204/mshukor/code/unival
-base_data_dir=/lus/scratch/NAT/gda2204/SHARED/data
-base_log_dir=/work/NAT/gda2204/mshukor/logs
-
-
-save_base_log_dir=/lus/scratch/NAT/gda2204/SHARED/logs
-save_dir=${save_base_log_dir}/ofa/checkpoints/caption/${exp_name}
-log_dir=${save_dir}
-
-mkdir -p $log_dir $save_dir
-
-bpe_dir=${ofa_dir}/utils/BPE
-user_dir=${ofa_dir}/ofa_module
-
-
-
-image_dir=${base_data_dir}
-
-
-data_dir=${base_data_dir}/ofa/video_data/caption_data
-data=${data_dir}/msrvtt_caption_train7k_1.tsv,${data_dir}/msrvtt_caption_train7k_2.tsv,${data_dir}/msrvtt_caption_train7k_3.tsv,${data_dir}/msrvtt_caption_train7k_4.tsv,${data_dir}/msrvtt_caption_train7k_5.tsv,${data_dir}/msrvtt_caption_train7k_6.tsv,${data_dir}/msrvtt_caption_train7k_7.tsv,${data_dir}/msrvtt_caption_train7k_8.tsv,${data_dir}/msrvtt_caption_train7k_9.tsv,${data_dir}/msrvtt_caption_train7k_10.tsv,${data_dir}/msrvtt_caption_test3k.tsv
-eval_cider_cached=${data_dir}/cider_cached_tokens/msrvtt-test3k-words.p
-
-
-restore_file=${base_log_dir}/ofa/checkpoints/pretrain/unival_s2_hs/checkpoint3.pt
-
-
-selected_cols=0,4,2
-
-task=video_caption
-arch=unival_base
-pretrained_model=
-
-
-criterion=adjust_label_smoothed_encouraging_loss
-label_smoothing=0.1
-# lr=5e-4
-lr=1e-3
-max_epoch=25
-warmup_ratio=0.06
-batch_size=16
-update_freq=2
-resnet_drop_path_rate=0.0
-encoder_drop_path_rate=0.1
-decoder_drop_path_rate=0.1
-dropout=0.1
-attention_dropout=0.0
-max_src_length=80
-max_tgt_length=20
-num_bins=1000
-# patch_image_size=480
-drop_worst_ratio=0.2
-
-
-
-
-###
-image_encoder_name=timm_resnet #vit_base_patch16_224
-patch_image_size=480
-resnet_type=resnet101
-
-resnet_model_path=${base_log_dir}/pretrained_models/resnet101-5d3b4d8f.pth
-
-# video
-video_encoder_name=all_resnext101
-patch_frame_size=384
-video_model_path=${base_log_dir}/pretrained_models/3dcnn/resnext-101-kinetics.pth #${base_log_dir}/pretrained_models/TimeSformer_divST_8x32_224_K600.pyth
-num_frames=16
-
-
-save_interval=1
-validate_interval_updates=2000
-save_interval_updates=0
-
-
-sample_patch_num='--sample-patch-num=784' # ''
-
-eval_args='--eval-args={"beam":5,"unnormalized":true,"temperature":1.0,"stop_on_max_len":true}'
-
-drop_worst_ratio=0.05 # modified from 0.2 for el
-log_end=0.75 # for el
-drop_best_ratio=0.05
-drop_best_after=6000
-drop_worst_after=6000
-
-use_dataaug='--use-dataaug'
-
-for max_epoch in {$max_epoch,}; do
- echo "max_epoch "${max_epoch}
- for warmup_ratio in {0.06,}; do
- echo "warmup_ratio "${warmup_ratio}
- for drop_worst_after in {6000,}; do
- echo "drop_worst_after "${drop_worst_after}
-
- log_file=${log_dir}/${max_epoch}"_"${warmup_ratio}"_"${drop_worst_after}".log"
- save_path=${save_dir}/${max_epoch}"_"${warmup_ratio}"_"${drop_worst_after}
- mkdir -p $save_path
-
- python3 -m torch.distributed.launch \
- --nnodes=${NUM_NODES} \
- --nproc_per_node=${GPUS_PER_NODE} \
- --master_port=${MASTER_PORT} \
- --node_rank=${RANK} \
- --master_addr=${MASTER_ADDR} \
- --use_env ${ofa_dir}/train.py \
- $data \
- --selected-cols=${selected_cols} \
- --bpe-dir=${bpe_dir} \
- --user-dir=${user_dir} \
- --restore-file=${restore_file} \
- --save-dir=${save_path} \
- --task=${task} \
- --arch=${arch} \
- --criterion=${criterion} \
- --label-smoothing=${label_smoothing} \
- --batch-size=${batch_size} \
- --update-freq=${update_freq} \
- --encoder-normalize-before \
- --decoder-normalize-before \
- --share-decoder-input-output-embed \
- --share-all-embeddings \
- --layernorm-embedding \
- --patch-layernorm-embedding \
- --code-layernorm-embedding \
- --resnet-drop-path-rate=${resnet_drop_path_rate} \
- --encoder-drop-path-rate=${encoder_drop_path_rate} \
- --decoder-drop-path-rate=${decoder_drop_path_rate} \
- --dropout=${dropout} \
- --attention-dropout=${attention_dropout} \
- --weight-decay=0.01 --optimizer=adam --adam-betas="(0.9,0.999)" --adam-eps=1e-08 --clip-norm=1.0 \
- --lr-scheduler=polynomial_decay --lr=${lr} \
- --max-epoch=${max_epoch} --warmup-ratio=${warmup_ratio} \
- --log-format=simple --log-interval=10 \
- --fixed-validation-seed=7 \
- --no-epoch-checkpoints --keep-best-checkpoints=1 \
- --save-interval=${save_interval} --validate-interval=1 \
- --save-interval-updates=${save_interval_updates} --validate-interval-updates=${validate_interval_updates} \
- --eval-cider \
- --eval-cider-cached-tokens=${eval_cider_cached} \
- --eval-args='{"beam":5,"max_len_b":16,"no_repeat_ngram_size":3}' \
- --best-checkpoint-metric=cider --maximize-best-checkpoint-metric \
- --max-src-length=${max_src_length} \
- --max-tgt-length=${max_tgt_length} \
- --find-unused-parameters \
- --freeze-encoder-embedding \
- --freeze-decoder-embedding \
- --add-type-embedding \
- --scale-attn \
- --scale-fc \
- --scale-heads \
- --disable-entangle \
- --num-bins=${num_bins} \
- --patch-image-size=${patch_image_size} \
- --drop-worst-ratio=${drop_worst_ratio} \
- --drop-worst-after=${drop_worst_after} \
- --fp16 \
- --fp16-scale-window=512 \
- --num-workers=0 \
- --image-encoder-name=${image_encoder_name} \
- --image-dir=${image_dir} \
- --video-encoder-name=${video_encoder_name} \
- --video-model-path=${video_model_path} \
- --patch-frame-size=${patch_frame_size} \
- ${sample_patch_num} \
- ${eval_args} \
- --num-frames=${num_frames} \
- --freeze-encoder \
- --freeze-decoder \
- --freeze-audio-encoder \
- --freeze-image-encoder \
- --freeze-video-encoder \
- --log-end ${log_end} --drop-best-ratio ${drop_best_ratio} --drop-best-after ${drop_best_after} \
- ${use_dataaug} \
- --reset-dataloader --reset-meters --reset-optimizer
-
- done
- done
-done
\ No newline at end of file
diff --git a/spaces/multimodalart/latentdiffusion/latent-diffusion/ldm/modules/diffusionmodules/util.py b/spaces/multimodalart/latentdiffusion/latent-diffusion/ldm/modules/diffusionmodules/util.py
deleted file mode 100644
index a952e6c40308c33edd422da0ce6a60f47e73661b..0000000000000000000000000000000000000000
--- a/spaces/multimodalart/latentdiffusion/latent-diffusion/ldm/modules/diffusionmodules/util.py
+++ /dev/null
@@ -1,267 +0,0 @@
-# adopted from
-# https://github.com/openai/improved-diffusion/blob/main/improved_diffusion/gaussian_diffusion.py
-# and
-# https://github.com/lucidrains/denoising-diffusion-pytorch/blob/7706bdfc6f527f58d33f84b7b522e61e6e3164b3/denoising_diffusion_pytorch/denoising_diffusion_pytorch.py
-# and
-# https://github.com/openai/guided-diffusion/blob/0ba878e517b276c45d1195eb29f6f5f72659a05b/guided_diffusion/nn.py
-#
-# thanks!
-
-
-import os
-import math
-import torch
-import torch.nn as nn
-import numpy as np
-from einops import repeat
-
-from ldm.util import instantiate_from_config
-
-
-def make_beta_schedule(schedule, n_timestep, linear_start=1e-4, linear_end=2e-2, cosine_s=8e-3):
- if schedule == "linear":
- betas = (
- torch.linspace(linear_start ** 0.5, linear_end ** 0.5, n_timestep, dtype=torch.float64) ** 2
- )
-
- elif schedule == "cosine":
- timesteps = (
- torch.arange(n_timestep + 1, dtype=torch.float64) / n_timestep + cosine_s
- )
- alphas = timesteps / (1 + cosine_s) * np.pi / 2
- alphas = torch.cos(alphas).pow(2)
- alphas = alphas / alphas[0]
- betas = 1 - alphas[1:] / alphas[:-1]
- betas = np.clip(betas, a_min=0, a_max=0.999)
-
- elif schedule == "sqrt_linear":
- betas = torch.linspace(linear_start, linear_end, n_timestep, dtype=torch.float64)
- elif schedule == "sqrt":
- betas = torch.linspace(linear_start, linear_end, n_timestep, dtype=torch.float64) ** 0.5
- else:
- raise ValueError(f"schedule '{schedule}' unknown.")
- return betas.numpy()
-
-
-def make_ddim_timesteps(ddim_discr_method, num_ddim_timesteps, num_ddpm_timesteps, verbose=True):
- if ddim_discr_method == 'uniform':
- c = num_ddpm_timesteps // num_ddim_timesteps
- ddim_timesteps = np.asarray(list(range(0, num_ddpm_timesteps, c)))
- elif ddim_discr_method == 'quad':
- ddim_timesteps = ((np.linspace(0, np.sqrt(num_ddpm_timesteps * .8), num_ddim_timesteps)) ** 2).astype(int)
- else:
- raise NotImplementedError(f'There is no ddim discretization method called "{ddim_discr_method}"')
-
- # assert ddim_timesteps.shape[0] == num_ddim_timesteps
- # add one to get the final alpha values right (the ones from first scale to data during sampling)
- steps_out = ddim_timesteps + 1
- if verbose:
- print(f'Selected timesteps for ddim sampler: {steps_out}')
- return steps_out
-
-
-def make_ddim_sampling_parameters(alphacums, ddim_timesteps, eta, verbose=True):
- # select alphas for computing the variance schedule
- alphas = alphacums[ddim_timesteps]
- alphas_prev = np.asarray([alphacums[0]] + alphacums[ddim_timesteps[:-1]].tolist())
-
- # according the the formula provided in https://arxiv.org/abs/2010.02502
- sigmas = eta * np.sqrt((1 - alphas_prev) / (1 - alphas) * (1 - alphas / alphas_prev))
- if verbose:
- print(f'Selected alphas for ddim sampler: a_t: {alphas}; a_(t-1): {alphas_prev}')
- print(f'For the chosen value of eta, which is {eta}, '
- f'this results in the following sigma_t schedule for ddim sampler {sigmas}')
- return sigmas, alphas, alphas_prev
-
-
-def betas_for_alpha_bar(num_diffusion_timesteps, alpha_bar, max_beta=0.999):
- """
- Create a beta schedule that discretizes the given alpha_t_bar function,
- which defines the cumulative product of (1-beta) over time from t = [0,1].
- :param num_diffusion_timesteps: the number of betas to produce.
- :param alpha_bar: a lambda that takes an argument t from 0 to 1 and
- produces the cumulative product of (1-beta) up to that
- part of the diffusion process.
- :param max_beta: the maximum beta to use; use values lower than 1 to
- prevent singularities.
- """
- betas = []
- for i in range(num_diffusion_timesteps):
- t1 = i / num_diffusion_timesteps
- t2 = (i + 1) / num_diffusion_timesteps
- betas.append(min(1 - alpha_bar(t2) / alpha_bar(t1), max_beta))
- return np.array(betas)
-
-
-def extract_into_tensor(a, t, x_shape):
- b, *_ = t.shape
- out = a.gather(-1, t)
- return out.reshape(b, *((1,) * (len(x_shape) - 1)))
-
-
-def checkpoint(func, inputs, params, flag):
- """
- Evaluate a function without caching intermediate activations, allowing for
- reduced memory at the expense of extra compute in the backward pass.
- :param func: the function to evaluate.
- :param inputs: the argument sequence to pass to `func`.
- :param params: a sequence of parameters `func` depends on but does not
- explicitly take as arguments.
- :param flag: if False, disable gradient checkpointing.
- """
- if flag:
- args = tuple(inputs) + tuple(params)
- return CheckpointFunction.apply(func, len(inputs), *args)
- else:
- return func(*inputs)
-
-
-class CheckpointFunction(torch.autograd.Function):
- @staticmethod
- def forward(ctx, run_function, length, *args):
- ctx.run_function = run_function
- ctx.input_tensors = list(args[:length])
- ctx.input_params = list(args[length:])
-
- with torch.no_grad():
- output_tensors = ctx.run_function(*ctx.input_tensors)
- return output_tensors
-
- @staticmethod
- def backward(ctx, *output_grads):
- ctx.input_tensors = [x.detach().requires_grad_(True) for x in ctx.input_tensors]
- with torch.enable_grad():
- # Fixes a bug where the first op in run_function modifies the
- # Tensor storage in place, which is not allowed for detach()'d
- # Tensors.
- shallow_copies = [x.view_as(x) for x in ctx.input_tensors]
- output_tensors = ctx.run_function(*shallow_copies)
- input_grads = torch.autograd.grad(
- output_tensors,
- ctx.input_tensors + ctx.input_params,
- output_grads,
- allow_unused=True,
- )
- del ctx.input_tensors
- del ctx.input_params
- del output_tensors
- return (None, None) + input_grads
-
-
-def timestep_embedding(timesteps, dim, max_period=10000, repeat_only=False):
- """
- Create sinusoidal timestep embeddings.
- :param timesteps: a 1-D Tensor of N indices, one per batch element.
- These may be fractional.
- :param dim: the dimension of the output.
- :param max_period: controls the minimum frequency of the embeddings.
- :return: an [N x dim] Tensor of positional embeddings.
- """
- if not repeat_only:
- half = dim // 2
- freqs = torch.exp(
- -math.log(max_period) * torch.arange(start=0, end=half, dtype=torch.float32) / half
- ).to(device=timesteps.device)
- args = timesteps[:, None].float() * freqs[None]
- embedding = torch.cat([torch.cos(args), torch.sin(args)], dim=-1)
- if dim % 2:
- embedding = torch.cat([embedding, torch.zeros_like(embedding[:, :1])], dim=-1)
- else:
- embedding = repeat(timesteps, 'b -> b d', d=dim)
- return embedding
-
-
-def zero_module(module):
- """
- Zero out the parameters of a module and return it.
- """
- for p in module.parameters():
- p.detach().zero_()
- return module
-
-
-def scale_module(module, scale):
- """
- Scale the parameters of a module and return it.
- """
- for p in module.parameters():
- p.detach().mul_(scale)
- return module
-
-
-def mean_flat(tensor):
- """
- Take the mean over all non-batch dimensions.
- """
- return tensor.mean(dim=list(range(1, len(tensor.shape))))
-
-
-def normalization(channels):
- """
- Make a standard normalization layer.
- :param channels: number of input channels.
- :return: an nn.Module for normalization.
- """
- return GroupNorm32(32, channels)
-
-
-# PyTorch 1.7 has SiLU, but we support PyTorch 1.5.
-class SiLU(nn.Module):
- def forward(self, x):
- return x * torch.sigmoid(x)
-
-
-class GroupNorm32(nn.GroupNorm):
- def forward(self, x):
- return super().forward(x.float()).type(x.dtype)
-
-def conv_nd(dims, *args, **kwargs):
- """
- Create a 1D, 2D, or 3D convolution module.
- """
- if dims == 1:
- return nn.Conv1d(*args, **kwargs)
- elif dims == 2:
- return nn.Conv2d(*args, **kwargs)
- elif dims == 3:
- return nn.Conv3d(*args, **kwargs)
- raise ValueError(f"unsupported dimensions: {dims}")
-
-
-def linear(*args, **kwargs):
- """
- Create a linear module.
- """
- return nn.Linear(*args, **kwargs)
-
-
-def avg_pool_nd(dims, *args, **kwargs):
- """
- Create a 1D, 2D, or 3D average pooling module.
- """
- if dims == 1:
- return nn.AvgPool1d(*args, **kwargs)
- elif dims == 2:
- return nn.AvgPool2d(*args, **kwargs)
- elif dims == 3:
- return nn.AvgPool3d(*args, **kwargs)
- raise ValueError(f"unsupported dimensions: {dims}")
-
-
-class HybridConditioner(nn.Module):
-
- def __init__(self, c_concat_config, c_crossattn_config):
- super().__init__()
- self.concat_conditioner = instantiate_from_config(c_concat_config)
- self.crossattn_conditioner = instantiate_from_config(c_crossattn_config)
-
- def forward(self, c_concat, c_crossattn):
- c_concat = self.concat_conditioner(c_concat)
- c_crossattn = self.crossattn_conditioner(c_crossattn)
- return {'c_concat': [c_concat], 'c_crossattn': [c_crossattn]}
-
-
-def noise_like(shape, device, repeat=False):
- repeat_noise = lambda: torch.randn((1, *shape[1:]), device=device).repeat(shape[0], *((1,) * (len(shape) - 1)))
- noise = lambda: torch.randn(shape, device=device)
- return repeat_noise() if repeat else noise()
\ No newline at end of file
diff --git a/spaces/multimodalart/mariogpt/setup.py b/spaces/multimodalart/mariogpt/setup.py
deleted file mode 100644
index b82db4e44f0f90f06483b8d9da026ab6a753ece7..0000000000000000000000000000000000000000
--- a/spaces/multimodalart/mariogpt/setup.py
+++ /dev/null
@@ -1,43 +0,0 @@
-import io
-import os
-import re
-from os import path
-
-from setuptools import find_packages
-from setuptools import setup
-
-
-this_directory = path.abspath(path.dirname(__file__))
-with open(path.join(this_directory, 'README.md'), encoding='utf-8') as f:
- long_description = f.read()
-
-
-setup(
- name="mario-gpt",
- version="0.1.0",
- url="https://github.com/kragniz/cookiecutter-pypackage-minimal",
- license='MIT',
-
- author="Shyam Sudhakaran",
- author_email="shyamsnair@protonmail.com",
-
- description="Generating Mario Levels with GPT2. Code for the paper: 'MarioGPT: Open-Ended Text2Level Generation through Large Language Models', https://arxiv.org/abs/2302.05981",
-
- long_description=long_description,
- long_description_content_type="text/markdown",
-
- packages=find_packages(exclude=('tests',)),
-
- install_requires=[
- 'torch',
- 'transformers',
- 'scipy',
- 'tqdm'
- ],
-
- classifiers=[
- 'Development Status :: 2 - Pre-Alpha',
- 'License :: OSI Approved :: MIT License',
- 'Programming Language :: Python :: 3',
- ],
-)
diff --git a/spaces/mzltest/gpt2-chinese-composition/generate.py b/spaces/mzltest/gpt2-chinese-composition/generate.py
deleted file mode 100644
index 287cf878fef6c95e2c0fed589fa46bbc2132fc2e..0000000000000000000000000000000000000000
--- a/spaces/mzltest/gpt2-chinese-composition/generate.py
+++ /dev/null
@@ -1,222 +0,0 @@
-import torch
-import torch.nn.functional as F
-import os
-import argparse
-from tqdm import trange
-from transformers import GPT2LMHeadModel
-
-
-def is_word(word):
- for item in list(word):
- if item not in 'qwertyuiopasdfghjklzxcvbnm':
- return False
- return True
-
-
-def _is_chinese_char(char):
- """Checks whether CP is the codepoint of a CJK character."""
- # This defines a "chinese character" as anything in the CJK Unicode block:
- # https://en.wikipedia.org/wiki/CJK_Unified_Ideographs_(Unicode_block)
- #
- # Note that the CJK Unicode block is NOT all Japanese and Korean characters,
- # despite its name. The modern Korean Hangul alphabet is a different block,
- # as is Japanese Hiragana and Katakana. Those alphabets are used to write
- # space-separated words, so they are not treated specially and handled
- # like the all of the other languages.
- cp = ord(char)
- if ((cp >= 0x4E00 and cp <= 0x9FFF) or #
- (cp >= 0x3400 and cp <= 0x4DBF) or #
- (cp >= 0x20000 and cp <= 0x2A6DF) or #
- (cp >= 0x2A700 and cp <= 0x2B73F) or #
- (cp >= 0x2B740 and cp <= 0x2B81F) or #
- (cp >= 0x2B820 and cp <= 0x2CEAF) or
- (cp >= 0xF900 and cp <= 0xFAFF) or #
- (cp >= 0x2F800 and cp <= 0x2FA1F)): #
- return True
-
- return False
-
-
-def top_k_top_p_filtering(logits, top_k=0, top_p=0.0, filter_value=-float('Inf')):
- """ Filter a distribution of logits using top-k and/or nucleus (top-p) filtering
- Args:
- logits: logits distribution shape (vocabulary size)
- top_k > 0: keep only top k tokens with highest probability (top-k filtering).
- top_p > 0.0: keep the top tokens with cumulative probability >= top_p (nucleus filtering).
- Nucleus filtering is described in Holtzman et al. (http://arxiv.org/abs/1904.09751)
- From: https://gist.github.com/thomwolf/1a5a29f6962089e871b94cbd09daf317
- """
- assert logits.dim() == 1 # batch size 1 for now - could be updated for more but the code would be less clear
- top_k = min(top_k, logits.size(-1)) # Safety check
- if top_k > 0:
- # Remove all tokens with a probability less than the last token of the top-k
- indices_to_remove = logits < torch.topk(logits, top_k)[0][..., -1, None]
- logits[indices_to_remove] = filter_value
-
- if top_p > 0.0:
- sorted_logits, sorted_indices = torch.sort(logits, descending=True)
- cumulative_probs = torch.cumsum(F.softmax(sorted_logits, dim=-1), dim=-1)
-
- # Remove tokens with cumulative probability above the threshold
- sorted_indices_to_remove = cumulative_probs > top_p
- # Shift the indices to the right to keep also the first token above the threshold
- sorted_indices_to_remove[..., 1:] = sorted_indices_to_remove[..., :-1].clone()
- sorted_indices_to_remove[..., 0] = 0
-
- indices_to_remove = sorted_indices[sorted_indices_to_remove]
- logits[indices_to_remove] = filter_value
- return logits
-
-
-def sample_sequence(model, context, length, n_ctx, tokenizer, temperature=1.0, top_k=30, top_p=0.0, repitition_penalty=1.0,
- device='cpu'):
- context = torch.tensor(context, dtype=torch.long, device=device)
- context = context.unsqueeze(0)
- generated = context
- with torch.no_grad():
- for _ in trange(length):
- inputs = {'input_ids': generated[0][-(n_ctx - 1):].unsqueeze(0)}
- outputs = model(
- **inputs) # Note: we could also use 'past' with GPT-2/Transfo-XL/XLNet (cached hidden-states)
- next_token_logits = outputs[0][0, -1, :]
- for id in set(generated):
- next_token_logits[id] /= repitition_penalty
- next_token_logits = next_token_logits / temperature
- next_token_logits[tokenizer.convert_tokens_to_ids('[UNK]')] = -float('Inf')
- filtered_logits = top_k_top_p_filtering(next_token_logits, top_k=top_k, top_p=top_p)
- next_token = torch.multinomial(F.softmax(filtered_logits, dim=-1), num_samples=1)
- generated = torch.cat((generated, next_token.unsqueeze(0)), dim=1)
- return generated.tolist()[0]
-
-
-def fast_sample_sequence(model, context, length, temperature=1.0, top_k=30, top_p=0.0, device='cpu'):
- inputs = torch.LongTensor(context).view(1, -1).to(device)
- if len(context) > 1:
- _, past = model(inputs[:, :-1], None)[:2]
- prev = inputs[:, -1].view(1, -1)
- else:
- past = None
- prev = inputs
- generate = [] + context
- with torch.no_grad():
- for i in trange(length):
- output = model(prev, past=past)
- output, past = output[:2]
- output = output[-1].squeeze(0) / temperature
- filtered_logits = top_k_top_p_filtering(output, top_k=top_k, top_p=top_p)
- next_token = torch.multinomial(torch.softmax(filtered_logits, dim=-1), num_samples=1)
- generate.append(next_token.item())
- prev = next_token.view(1, 1)
- return generate
-
-
-# 通过命令行参数--fast_pattern,指定模式
-def generate(n_ctx, model, context, length, tokenizer, temperature=1, top_k=0, top_p=0.0, repitition_penalty=1.0, device='cpu',
- is_fast_pattern=False):
- if is_fast_pattern:
- return fast_sample_sequence(model, context, length, temperature=temperature, top_k=top_k, top_p=top_p,
- device=device)
- else:
- return sample_sequence(model, context, length, n_ctx, tokenizer=tokenizer, temperature=temperature, top_k=top_k, top_p=top_p,
- repitition_penalty=repitition_penalty, device=device)
-
-
-def main():
- parser = argparse.ArgumentParser()
- parser.add_argument('--device', default='0,1,2,3', type=str, required=False, help='生成设备')
- parser.add_argument('--length', default=-1, type=int, required=False, help='生成长度')
- parser.add_argument('--batch_size', default=1, type=int, required=False, help='生成的batch size')
- parser.add_argument('--nsamples', default=10, type=int, required=False, help='生成几个样本')
- parser.add_argument('--temperature', default=1, type=float, required=False, help='生成温度')
- parser.add_argument('--topk', default=8, type=int, required=False, help='最高几选一')
- parser.add_argument('--topp', default=0, type=float, required=False, help='最高积累概率')
- parser.add_argument('--model_config', default='./model_config_small.json', type=str, required=False,
- help='模型参数')
- parser.add_argument('--tokenizer_path', default='./vocab_small.txt', type=str, required=False, help='词表路径')
- parser.add_argument('--model_path', default='./', type=str, required=False, help='模型路径')
- parser.add_argument('--prefix', default='萧炎', type=str, required=False, help='生成文章的开头')
- parser.add_argument('--no_wordpiece', action='store_true', help='不做word piece切词')
- parser.add_argument('--segment', action='store_true', help='中文以词为单位')
- parser.add_argument('--fast_pattern', action='store_true', help='采用更加快的方式生成文本')
- parser.add_argument('--save_samples', action='store_true', help='保存产生的样本')
- parser.add_argument('--save_samples_path', default='.', type=str, required=False, help="保存样本的路径")
- parser.add_argument('--repetition_penalty', default=1.0, type=float, required=False)
-
- args = parser.parse_args()
- print('args:\n' + args.__repr__())
-
- if args.segment:
- from tokenizations import tokenization_bert_word_level as tokenization_bert
- else:
- from tokenizations import tokenization_bert
-
- os.environ["CUDA_VISIBLE_DEVICES"] = args.device # 此处设置程序使用哪些显卡
- length = args.length
- batch_size = args.batch_size
- nsamples = args.nsamples
- temperature = args.temperature
- topk = args.topk
- topp = args.topp
- repetition_penalty = args.repetition_penalty
-
- device = "cuda" if torch.cuda.is_available() else "cpu"
-
- tokenizer = tokenization_bert.BertTokenizer(vocab_file=args.tokenizer_path)
- model = GPT2LMHeadModel.from_pretrained(args.model_path)
- model.to(device)
- model.eval()
-
- n_ctx = model.config.n_ctx
-
- if length == -1:
- length = model.config.n_ctx
- if args.save_samples:
- if not os.path.exists(args.save_samples_path):
- os.makedirs(args.save_samples_path)
- samples_file = open(args.save_samples_path + '/samples.txt', 'w', encoding='utf8')
- while True:
- raw_text = args.prefix
- context_tokens = tokenizer.convert_tokens_to_ids(tokenizer.tokenize(raw_text))
- generated = 0
- for _ in range(nsamples // batch_size):
- out = generate(
- n_ctx=n_ctx,
- model=model,
- context=context_tokens,
- length=length,
- is_fast_pattern=args.fast_pattern, tokenizer=tokenizer,
- temperature=temperature, top_k=topk, top_p=topp, repitition_penalty=repetition_penalty, device=device
- )
- for i in range(batch_size):
- generated += 1
- text = tokenizer.convert_ids_to_tokens(out)
- for i, item in enumerate(text[:-1]): # 确保英文前后有空格
- if is_word(item) and is_word(text[i + 1]):
- text[i] = item + ' '
- for i, item in enumerate(text):
- if item == '[MASK]':
- text[i] = ''
- elif item == '[CLS]':
- text[i] = '\n\n'
- elif item == '[SEP]':
- text[i] = '\n'
- info = "=" * 40 + " SAMPLE " + str(generated) + " " + "=" * 40 + "\n"
- print(info)
- text = ''.join(text).replace('##', '').strip()
- print(text)
- if args.save_samples:
- samples_file.write(info)
- samples_file.write(text)
- samples_file.write('\n')
- samples_file.write('=' * 90)
- samples_file.write('\n' * 2)
- print("=" * 80)
- if generated == nsamples:
- # close file when finish writing.
- if args.save_samples:
- samples_file.close()
- break
-
-
-if __name__ == '__main__':
- main()
\ No newline at end of file
diff --git a/spaces/nanom/to_passive_voice/modules/m_connector.py b/spaces/nanom/to_passive_voice/modules/m_connector.py
deleted file mode 100644
index a97417771305a6967db5101394a4324392191454..0000000000000000000000000000000000000000
--- a/spaces/nanom/to_passive_voice/modules/m_connector.py
+++ /dev/null
@@ -1,50 +0,0 @@
-from typing import Tuple
-from modules.m_active_voice import ActiveVoice
-from modules.m_htmlrender import HtmlRender
-from modules.m_datetime import Datetime
-
-class Connector:
- def __init__(
- self
- ) -> None:
-
- self.date = Datetime()
- self.avoice = ActiveVoice()
- self.html = HtmlRender()
- self.cache_sentence = None
- self.cache_html_format = None
- self.cache_str_format = None
-
- def view_conversion(
- self,
- active_sent: str,
- passive_sent: str
- ) -> None:
-
- print(f".goat: {self.date.full()} - '{active_sent}' -> '{passive_sent}'")
-
- def active2passive(
- self,
- sentence: str
- ) -> Tuple[str,str]:
-
- if sentence == self.cache_sentence:
- return self.cache_html_format, self.cache_str_format
-
- try:
- data = self.avoice.to_passive(sentence)
- except Exception as e:
- return self.html.error(str(e)), str(e)
-
- subj = self.html.budget(data['subj'], 'subject', 'primary')
- tobe = self.html.budget(data['tobe'],'to be','warning')
- participle = self.html.budget(data['participle'],'participle','danger')
- agent = self.html.budget(data['agent'],'agent','success')
- compl = self.html.budget(data['compl'],'compl.','dark')
-
- self.cache_sentence = sentence
- self.cache_str_format = f"{data['subj']} {data['tobe']} {data['participle']} {data['agent']} {data['compl']}"
- self.cache_html_format = self.html.output(f"{subj} {tobe} {participle} {agent} {compl}")
- self.view_conversion(self.cache_sentence, self.cache_str_format)
-
- return self.cache_html_format, self.cache_str_format
\ No newline at end of file
diff --git a/spaces/netiMophi/DreamlikeArt-Diffusion-1.0/Fre Ebook A Primer For The Mathematics Of Financial Engineering Downloads Torrent UPD.md b/spaces/netiMophi/DreamlikeArt-Diffusion-1.0/Fre Ebook A Primer For The Mathematics Of Financial Engineering Downloads Torrent UPD.md
deleted file mode 100644
index 779d0a537d6fcd2bb26fe34f14a8d71347adb510..0000000000000000000000000000000000000000
--- a/spaces/netiMophi/DreamlikeArt-Diffusion-1.0/Fre Ebook A Primer For The Mathematics Of Financial Engineering Downloads Torrent UPD.md
+++ /dev/null
@@ -1,19 +0,0 @@
-
-How to Download a Free Ebook on the Mathematics of Financial Engineering
-If you are interested in learning the mathematics of financial engineering, you might be looking for a free ebook that can teach you the basics. Financial engineering is a field that applies mathematical methods and models to solve problems in finance, such as pricing, risk management, portfolio optimization, and derivatives. However, learning the mathematics of financial engineering can be challenging, especially if you do not have a strong background in calculus, linear algebra, probability, and statistics.
-Fre Ebook A Primer For The Mathematics Of Financial Engineering Downloads Torrent
Download Zip ››› https://urlcod.com/2uI9X4
-Fortunately, there is a free ebook that can help you learn the mathematics of financial engineering in a clear and concise way. The ebook is called A Primer for the Mathematics of Financial Engineering, and it is written by Dan Stefanica, a professor of mathematics at Baruch College. The ebook covers topics such as interest rates, bonds, annuities, yield curves, forward contracts, futures contracts, swaps, options, binomial trees, Black-Scholes formula, Greeks, hedging strategies, and more. The ebook also includes exercises and solutions to help you practice and test your understanding.
-So how can you download this free ebook? The easiest way is to visit the website of the author, www.danstefanica.com, where you can find a link to download the ebook in PDF format. Alternatively, you can search for the ebook on torrent sites, such as The Pirate Bay, 1337x, or RARBG. However, be careful when downloading files from torrent sites, as they may contain viruses or malware that can harm your computer. Always scan the files with an antivirus software before opening them.
-Downloading a free ebook on the mathematics of financial engineering can be a great way to learn the fundamentals of this fascinating and lucrative field. However, if you want to master the mathematics of financial engineering and become a successful financial engineer, you will need more than just an ebook. You will need to study hard, practice a lot, and apply your knowledge to real-world problems. You will also need to keep up with the latest developments and innovations in the field. Therefore, we recommend that you also check out some of the online courses and books that are available on the mathematics of financial engineering.
-
-Some of the online courses and books that we recommend on the mathematics of financial engineering are:
-
-
-- Mathematics for Financial Engineering Specialization on Coursera. This is a series of four courses that cover topics such as linear algebra, calculus, optimization, probability, stochastic processes, and numerical methods. The courses are taught by professors from Columbia University and New York University.
-- Mathematics for Finance on edX. This is a course that covers topics such as interest rates, bonds, arbitrage, derivatives, risk-neutral valuation, and martingales. The course is taught by a professor from the University of Zurich.
-- A Primer for the Mathematics of Financial Engineering, Second Edition by Dan Stefanica. This is the updated and expanded version of the ebook that we mentioned earlier. It includes new topics such as convexity, duration, immunization, bootstrap method, Vasicek model, Cox-Ingersoll-Ross model, Hull-White model, and more. It also includes more exercises and solutions.
-- Mathematics of Financial Risk Management: A Guide to Valuation and Hedging by Peter Knopf. This is a book that covers topics such as risk measures, value at risk, expected shortfall, coherent risk measures, diversification, portfolio optimization, capital allocation, hedging strategies, delta hedging, gamma hedging, vega hedging, and more. It also includes examples and case studies.
-
-We hope that this article has helped you learn how to download a free ebook on the mathematics of financial engineering and find some other useful resources on the subject. If you have any questions or feedback, please leave a comment below. Thank you for reading!
e93f5a0c3f
-
-
\ No newline at end of file
diff --git a/spaces/niew/vits-uma-genshin-honka/models.py b/spaces/niew/vits-uma-genshin-honka/models.py
deleted file mode 100644
index 52e15d1b9775038fd6e82b2efe6f95f51c66802d..0000000000000000000000000000000000000000
--- a/spaces/niew/vits-uma-genshin-honka/models.py
+++ /dev/null
@@ -1,534 +0,0 @@
-import math
-import torch
-from torch import nn
-from torch.nn import functional as F
-
-import commons
-import modules
-import attentions
-import monotonic_align
-
-from torch.nn import Conv1d, ConvTranspose1d, Conv2d
-from torch.nn.utils import weight_norm, remove_weight_norm, spectral_norm
-from commons import init_weights, get_padding
-
-
-class StochasticDurationPredictor(nn.Module):
- def __init__(self, in_channels, filter_channels, kernel_size, p_dropout, n_flows=4, gin_channels=0):
- super().__init__()
- filter_channels = in_channels # it needs to be removed from future version.
- self.in_channels = in_channels
- self.filter_channels = filter_channels
- self.kernel_size = kernel_size
- self.p_dropout = p_dropout
- self.n_flows = n_flows
- self.gin_channels = gin_channels
-
- self.log_flow = modules.Log()
- self.flows = nn.ModuleList()
- self.flows.append(modules.ElementwiseAffine(2))
- for i in range(n_flows):
- self.flows.append(modules.ConvFlow(2, filter_channels, kernel_size, n_layers=3))
- self.flows.append(modules.Flip())
-
- self.post_pre = nn.Conv1d(1, filter_channels, 1)
- self.post_proj = nn.Conv1d(filter_channels, filter_channels, 1)
- self.post_convs = modules.DDSConv(filter_channels, kernel_size, n_layers=3, p_dropout=p_dropout)
- self.post_flows = nn.ModuleList()
- self.post_flows.append(modules.ElementwiseAffine(2))
- for i in range(4):
- self.post_flows.append(modules.ConvFlow(2, filter_channels, kernel_size, n_layers=3))
- self.post_flows.append(modules.Flip())
-
- self.pre = nn.Conv1d(in_channels, filter_channels, 1)
- self.proj = nn.Conv1d(filter_channels, filter_channels, 1)
- self.convs = modules.DDSConv(filter_channels, kernel_size, n_layers=3, p_dropout=p_dropout)
- if gin_channels != 0:
- self.cond = nn.Conv1d(gin_channels, filter_channels, 1)
-
- def forward(self, x, x_mask, w=None, g=None, reverse=False, noise_scale=1.0):
- x = torch.detach(x)
- x = self.pre(x)
- if g is not None:
- g = torch.detach(g)
- x = x + self.cond(g)
- x = self.convs(x, x_mask)
- x = self.proj(x) * x_mask
-
- if not reverse:
- flows = self.flows
- assert w is not None
-
- logdet_tot_q = 0
- h_w = self.post_pre(w)
- h_w = self.post_convs(h_w, x_mask)
- h_w = self.post_proj(h_w) * x_mask
- e_q = torch.randn(w.size(0), 2, w.size(2)).to(device=x.device, dtype=x.dtype) * x_mask
- z_q = e_q
- for flow in self.post_flows:
- z_q, logdet_q = flow(z_q, x_mask, g=(x + h_w))
- logdet_tot_q += logdet_q
- z_u, z1 = torch.split(z_q, [1, 1], 1)
- u = torch.sigmoid(z_u) * x_mask
- z0 = (w - u) * x_mask
- logdet_tot_q += torch.sum((F.logsigmoid(z_u) + F.logsigmoid(-z_u)) * x_mask, [1,2])
- logq = torch.sum(-0.5 * (math.log(2*math.pi) + (e_q**2)) * x_mask, [1,2]) - logdet_tot_q
-
- logdet_tot = 0
- z0, logdet = self.log_flow(z0, x_mask)
- logdet_tot += logdet
- z = torch.cat([z0, z1], 1)
- for flow in flows:
- z, logdet = flow(z, x_mask, g=x, reverse=reverse)
- logdet_tot = logdet_tot + logdet
- nll = torch.sum(0.5 * (math.log(2*math.pi) + (z**2)) * x_mask, [1,2]) - logdet_tot
- return nll + logq # [b]
- else:
- flows = list(reversed(self.flows))
- flows = flows[:-2] + [flows[-1]] # remove a useless vflow
- z = torch.randn(x.size(0), 2, x.size(2)).to(device=x.device, dtype=x.dtype) * noise_scale
- for flow in flows:
- z = flow(z, x_mask, g=x, reverse=reverse)
- z0, z1 = torch.split(z, [1, 1], 1)
- logw = z0
- return logw
-
-
-class DurationPredictor(nn.Module):
- def __init__(self, in_channels, filter_channels, kernel_size, p_dropout, gin_channels=0):
- super().__init__()
-
- self.in_channels = in_channels
- self.filter_channels = filter_channels
- self.kernel_size = kernel_size
- self.p_dropout = p_dropout
- self.gin_channels = gin_channels
-
- self.drop = nn.Dropout(p_dropout)
- self.conv_1 = nn.Conv1d(in_channels, filter_channels, kernel_size, padding=kernel_size//2)
- self.norm_1 = modules.LayerNorm(filter_channels)
- self.conv_2 = nn.Conv1d(filter_channels, filter_channels, kernel_size, padding=kernel_size//2)
- self.norm_2 = modules.LayerNorm(filter_channels)
- self.proj = nn.Conv1d(filter_channels, 1, 1)
-
- if gin_channels != 0:
- self.cond = nn.Conv1d(gin_channels, in_channels, 1)
-
- def forward(self, x, x_mask, g=None):
- x = torch.detach(x)
- if g is not None:
- g = torch.detach(g)
- x = x + self.cond(g)
- x = self.conv_1(x * x_mask)
- x = torch.relu(x)
- x = self.norm_1(x)
- x = self.drop(x)
- x = self.conv_2(x * x_mask)
- x = torch.relu(x)
- x = self.norm_2(x)
- x = self.drop(x)
- x = self.proj(x * x_mask)
- return x * x_mask
-
-
-class TextEncoder(nn.Module):
- def __init__(self,
- n_vocab,
- out_channels,
- hidden_channels,
- filter_channels,
- n_heads,
- n_layers,
- kernel_size,
- p_dropout):
- super().__init__()
- self.n_vocab = n_vocab
- self.out_channels = out_channels
- self.hidden_channels = hidden_channels
- self.filter_channels = filter_channels
- self.n_heads = n_heads
- self.n_layers = n_layers
- self.kernel_size = kernel_size
- self.p_dropout = p_dropout
-
- self.emb = nn.Embedding(n_vocab, hidden_channels)
- nn.init.normal_(self.emb.weight, 0.0, hidden_channels**-0.5)
-
- self.encoder = attentions.Encoder(
- hidden_channels,
- filter_channels,
- n_heads,
- n_layers,
- kernel_size,
- p_dropout)
- self.proj= nn.Conv1d(hidden_channels, out_channels * 2, 1)
-
- def forward(self, x, x_lengths):
- x = self.emb(x) * math.sqrt(self.hidden_channels) # [b, t, h]
- x = torch.transpose(x, 1, -1) # [b, h, t]
- x_mask = torch.unsqueeze(commons.sequence_mask(x_lengths, x.size(2)), 1).to(x.dtype)
-
- x = self.encoder(x * x_mask, x_mask)
- stats = self.proj(x) * x_mask
-
- m, logs = torch.split(stats, self.out_channels, dim=1)
- return x, m, logs, x_mask
-
-
-class ResidualCouplingBlock(nn.Module):
- def __init__(self,
- channels,
- hidden_channels,
- kernel_size,
- dilation_rate,
- n_layers,
- n_flows=4,
- gin_channels=0):
- super().__init__()
- self.channels = channels
- self.hidden_channels = hidden_channels
- self.kernel_size = kernel_size
- self.dilation_rate = dilation_rate
- self.n_layers = n_layers
- self.n_flows = n_flows
- self.gin_channels = gin_channels
-
- self.flows = nn.ModuleList()
- for i in range(n_flows):
- self.flows.append(modules.ResidualCouplingLayer(channels, hidden_channels, kernel_size, dilation_rate, n_layers, gin_channels=gin_channels, mean_only=True))
- self.flows.append(modules.Flip())
-
- def forward(self, x, x_mask, g=None, reverse=False):
- if not reverse:
- for flow in self.flows:
- x, _ = flow(x, x_mask, g=g, reverse=reverse)
- else:
- for flow in reversed(self.flows):
- x = flow(x, x_mask, g=g, reverse=reverse)
- return x
-
-
-class PosteriorEncoder(nn.Module):
- def __init__(self,
- in_channels,
- out_channels,
- hidden_channels,
- kernel_size,
- dilation_rate,
- n_layers,
- gin_channels=0):
- super().__init__()
- self.in_channels = in_channels
- self.out_channels = out_channels
- self.hidden_channels = hidden_channels
- self.kernel_size = kernel_size
- self.dilation_rate = dilation_rate
- self.n_layers = n_layers
- self.gin_channels = gin_channels
-
- self.pre = nn.Conv1d(in_channels, hidden_channels, 1)
- self.enc = modules.WN(hidden_channels, kernel_size, dilation_rate, n_layers, gin_channels=gin_channels)
- self.proj = nn.Conv1d(hidden_channels, out_channels * 2, 1)
-
- def forward(self, x, x_lengths, g=None):
- x_mask = torch.unsqueeze(commons.sequence_mask(x_lengths, x.size(2)), 1).to(x.dtype)
- x = self.pre(x) * x_mask
- x = self.enc(x, x_mask, g=g)
- stats = self.proj(x) * x_mask
- m, logs = torch.split(stats, self.out_channels, dim=1)
- z = (m + torch.randn_like(m) * torch.exp(logs)) * x_mask
- return z, m, logs, x_mask
-
-
-class Generator(torch.nn.Module):
- def __init__(self, initial_channel, resblock, resblock_kernel_sizes, resblock_dilation_sizes, upsample_rates, upsample_initial_channel, upsample_kernel_sizes, gin_channels=0):
- super(Generator, self).__init__()
- self.num_kernels = len(resblock_kernel_sizes)
- self.num_upsamples = len(upsample_rates)
- self.conv_pre = Conv1d(initial_channel, upsample_initial_channel, 7, 1, padding=3)
- resblock = modules.ResBlock1 if resblock == '1' else modules.ResBlock2
-
- self.ups = nn.ModuleList()
- for i, (u, k) in enumerate(zip(upsample_rates, upsample_kernel_sizes)):
- self.ups.append(weight_norm(
- ConvTranspose1d(upsample_initial_channel//(2**i), upsample_initial_channel//(2**(i+1)),
- k, u, padding=(k-u)//2)))
-
- self.resblocks = nn.ModuleList()
- for i in range(len(self.ups)):
- ch = upsample_initial_channel//(2**(i+1))
- for j, (k, d) in enumerate(zip(resblock_kernel_sizes, resblock_dilation_sizes)):
- self.resblocks.append(resblock(ch, k, d))
-
- self.conv_post = Conv1d(ch, 1, 7, 1, padding=3, bias=False)
- self.ups.apply(init_weights)
-
- if gin_channels != 0:
- self.cond = nn.Conv1d(gin_channels, upsample_initial_channel, 1)
-
- def forward(self, x, g=None):
- x = self.conv_pre(x)
- if g is not None:
- x = x + self.cond(g)
-
- for i in range(self.num_upsamples):
- x = F.leaky_relu(x, modules.LRELU_SLOPE)
- x = self.ups[i](x)
- xs = None
- for j in range(self.num_kernels):
- if xs is None:
- xs = self.resblocks[i*self.num_kernels+j](x)
- else:
- xs += self.resblocks[i*self.num_kernels+j](x)
- x = xs / self.num_kernels
- x = F.leaky_relu(x)
- x = self.conv_post(x)
- x = torch.tanh(x)
-
- return x
-
- def remove_weight_norm(self):
- print('Removing weight norm...')
- for l in self.ups:
- remove_weight_norm(l)
- for l in self.resblocks:
- l.remove_weight_norm()
-
-
-class DiscriminatorP(torch.nn.Module):
- def __init__(self, period, kernel_size=5, stride=3, use_spectral_norm=False):
- super(DiscriminatorP, self).__init__()
- self.period = period
- self.use_spectral_norm = use_spectral_norm
- norm_f = weight_norm if use_spectral_norm == False else spectral_norm
- self.convs = nn.ModuleList([
- norm_f(Conv2d(1, 32, (kernel_size, 1), (stride, 1), padding=(get_padding(kernel_size, 1), 0))),
- norm_f(Conv2d(32, 128, (kernel_size, 1), (stride, 1), padding=(get_padding(kernel_size, 1), 0))),
- norm_f(Conv2d(128, 512, (kernel_size, 1), (stride, 1), padding=(get_padding(kernel_size, 1), 0))),
- norm_f(Conv2d(512, 1024, (kernel_size, 1), (stride, 1), padding=(get_padding(kernel_size, 1), 0))),
- norm_f(Conv2d(1024, 1024, (kernel_size, 1), 1, padding=(get_padding(kernel_size, 1), 0))),
- ])
- self.conv_post = norm_f(Conv2d(1024, 1, (3, 1), 1, padding=(1, 0)))
-
- def forward(self, x):
- fmap = []
-
- # 1d to 2d
- b, c, t = x.shape
- if t % self.period != 0: # pad first
- n_pad = self.period - (t % self.period)
- x = F.pad(x, (0, n_pad), "reflect")
- t = t + n_pad
- x = x.view(b, c, t // self.period, self.period)
-
- for l in self.convs:
- x = l(x)
- x = F.leaky_relu(x, modules.LRELU_SLOPE)
- fmap.append(x)
- x = self.conv_post(x)
- fmap.append(x)
- x = torch.flatten(x, 1, -1)
-
- return x, fmap
-
-
-class DiscriminatorS(torch.nn.Module):
- def __init__(self, use_spectral_norm=False):
- super(DiscriminatorS, self).__init__()
- norm_f = weight_norm if use_spectral_norm == False else spectral_norm
- self.convs = nn.ModuleList([
- norm_f(Conv1d(1, 16, 15, 1, padding=7)),
- norm_f(Conv1d(16, 64, 41, 4, groups=4, padding=20)),
- norm_f(Conv1d(64, 256, 41, 4, groups=16, padding=20)),
- norm_f(Conv1d(256, 1024, 41, 4, groups=64, padding=20)),
- norm_f(Conv1d(1024, 1024, 41, 4, groups=256, padding=20)),
- norm_f(Conv1d(1024, 1024, 5, 1, padding=2)),
- ])
- self.conv_post = norm_f(Conv1d(1024, 1, 3, 1, padding=1))
-
- def forward(self, x):
- fmap = []
-
- for l in self.convs:
- x = l(x)
- x = F.leaky_relu(x, modules.LRELU_SLOPE)
- fmap.append(x)
- x = self.conv_post(x)
- fmap.append(x)
- x = torch.flatten(x, 1, -1)
-
- return x, fmap
-
-
-class MultiPeriodDiscriminator(torch.nn.Module):
- def __init__(self, use_spectral_norm=False):
- super(MultiPeriodDiscriminator, self).__init__()
- periods = [2,3,5,7,11]
-
- discs = [DiscriminatorS(use_spectral_norm=use_spectral_norm)]
- discs = discs + [DiscriminatorP(i, use_spectral_norm=use_spectral_norm) for i in periods]
- self.discriminators = nn.ModuleList(discs)
-
- def forward(self, y, y_hat):
- y_d_rs = []
- y_d_gs = []
- fmap_rs = []
- fmap_gs = []
- for i, d in enumerate(self.discriminators):
- y_d_r, fmap_r = d(y)
- y_d_g, fmap_g = d(y_hat)
- y_d_rs.append(y_d_r)
- y_d_gs.append(y_d_g)
- fmap_rs.append(fmap_r)
- fmap_gs.append(fmap_g)
-
- return y_d_rs, y_d_gs, fmap_rs, fmap_gs
-
-
-
-class SynthesizerTrn(nn.Module):
- """
- Synthesizer for Training
- """
-
- def __init__(self,
- n_vocab,
- spec_channels,
- segment_size,
- inter_channels,
- hidden_channels,
- filter_channels,
- n_heads,
- n_layers,
- kernel_size,
- p_dropout,
- resblock,
- resblock_kernel_sizes,
- resblock_dilation_sizes,
- upsample_rates,
- upsample_initial_channel,
- upsample_kernel_sizes,
- n_speakers=0,
- gin_channels=0,
- use_sdp=True,
- **kwargs):
-
- super().__init__()
- self.n_vocab = n_vocab
- self.spec_channels = spec_channels
- self.inter_channels = inter_channels
- self.hidden_channels = hidden_channels
- self.filter_channels = filter_channels
- self.n_heads = n_heads
- self.n_layers = n_layers
- self.kernel_size = kernel_size
- self.p_dropout = p_dropout
- self.resblock = resblock
- self.resblock_kernel_sizes = resblock_kernel_sizes
- self.resblock_dilation_sizes = resblock_dilation_sizes
- self.upsample_rates = upsample_rates
- self.upsample_initial_channel = upsample_initial_channel
- self.upsample_kernel_sizes = upsample_kernel_sizes
- self.segment_size = segment_size
- self.n_speakers = n_speakers
- self.gin_channels = gin_channels
-
- self.use_sdp = use_sdp
-
- self.enc_p = TextEncoder(n_vocab,
- inter_channels,
- hidden_channels,
- filter_channels,
- n_heads,
- n_layers,
- kernel_size,
- p_dropout)
- self.dec = Generator(inter_channels, resblock, resblock_kernel_sizes, resblock_dilation_sizes, upsample_rates, upsample_initial_channel, upsample_kernel_sizes, gin_channels=gin_channels)
- self.enc_q = PosteriorEncoder(spec_channels, inter_channels, hidden_channels, 5, 1, 16, gin_channels=gin_channels)
- self.flow = ResidualCouplingBlock(inter_channels, hidden_channels, 5, 1, 4, gin_channels=gin_channels)
-
- if use_sdp:
- self.dp = StochasticDurationPredictor(hidden_channels, 192, 3, 0.5, 4, gin_channels=gin_channels)
- else:
- self.dp = DurationPredictor(hidden_channels, 256, 3, 0.5, gin_channels=gin_channels)
-
- if n_speakers > 1:
- self.emb_g = nn.Embedding(n_speakers, gin_channels)
-
- def forward(self, x, x_lengths, y, y_lengths, sid=None):
-
- x, m_p, logs_p, x_mask = self.enc_p(x, x_lengths)
- if self.n_speakers > 0:
- g = self.emb_g(sid).unsqueeze(-1) # [b, h, 1]
- else:
- g = None
-
- z, m_q, logs_q, y_mask = self.enc_q(y, y_lengths, g=g)
- z_p = self.flow(z, y_mask, g=g)
-
- with torch.no_grad():
- # negative cross-entropy
- s_p_sq_r = torch.exp(-2 * logs_p) # [b, d, t]
- neg_cent1 = torch.sum(-0.5 * math.log(2 * math.pi) - logs_p, [1], keepdim=True) # [b, 1, t_s]
- neg_cent2 = torch.matmul(-0.5 * (z_p ** 2).transpose(1, 2), s_p_sq_r) # [b, t_t, d] x [b, d, t_s] = [b, t_t, t_s]
- neg_cent3 = torch.matmul(z_p.transpose(1, 2), (m_p * s_p_sq_r)) # [b, t_t, d] x [b, d, t_s] = [b, t_t, t_s]
- neg_cent4 = torch.sum(-0.5 * (m_p ** 2) * s_p_sq_r, [1], keepdim=True) # [b, 1, t_s]
- neg_cent = neg_cent1 + neg_cent2 + neg_cent3 + neg_cent4
-
- attn_mask = torch.unsqueeze(x_mask, 2) * torch.unsqueeze(y_mask, -1)
- attn = monotonic_align.maximum_path(neg_cent, attn_mask.squeeze(1)).unsqueeze(1).detach()
-
- w = attn.sum(2)
- if self.use_sdp:
- l_length = self.dp(x, x_mask, w, g=g)
- l_length = l_length / torch.sum(x_mask)
- else:
- logw_ = torch.log(w + 1e-6) * x_mask
- logw = self.dp(x, x_mask, g=g)
- l_length = torch.sum((logw - logw_)**2, [1,2]) / torch.sum(x_mask) # for averaging
-
- # expand prior
- m_p = torch.matmul(attn.squeeze(1), m_p.transpose(1, 2)).transpose(1, 2)
- logs_p = torch.matmul(attn.squeeze(1), logs_p.transpose(1, 2)).transpose(1, 2)
-
- z_slice, ids_slice = commons.rand_slice_segments(z, y_lengths, self.segment_size)
- o = self.dec(z_slice, g=g)
- return o, l_length, attn, ids_slice, x_mask, y_mask, (z, z_p, m_p, logs_p, m_q, logs_q)
-
- def infer(self, x, x_lengths, sid=None, noise_scale=1, length_scale=1, noise_scale_w=1., max_len=None):
- device = next(self.parameters()).device # 获取模型所在的设备
- x, m_p, logs_p, x_mask = self.enc_p(x.to(device), x_lengths.to(device))
- if self.n_speakers > 0:
- g = self.emb_g(sid.to(device)).unsqueeze(-1) # [b, h, 1]
- else:
- g = None
-
- if self.use_sdp:
- logw = self.dp(x, x_mask, g=g, reverse=True, noise_scale=noise_scale_w)
- else:
- logw = self.dp(x, x_mask, g=g)
- w = torch.exp(logw) * x_mask * length_scale
- w_ceil = torch.ceil(w)
- y_lengths = torch.clamp_min(torch.sum(w_ceil, [1, 2]), 1).long()
- y_mask = torch.unsqueeze(commons.sequence_mask(y_lengths, None), 1).to(x_mask.dtype)
- attn_mask = torch.unsqueeze(x_mask, 2) * torch.unsqueeze(y_mask, -1)
- attn = commons.generate_path(w_ceil, attn_mask)
-
- m_p = torch.matmul(attn.squeeze(1), m_p.transpose(1, 2)).transpose(1, 2) # [b, t', t], [b, t, d] -> [b, d, t']
- logs_p = torch.matmul(attn.squeeze(1), logs_p.transpose(1, 2)).transpose(1, 2) # [b, t', t], [b, t, d] -> [b, d, t']
-
- z_p = m_p + torch.randn_like(m_p) * torch.exp(logs_p) * noise_scale
- z = self.flow(z_p, y_mask, g=g, reverse=True)
- o = self.dec((z * y_mask)[:,:,:max_len], g=g)
- return o, attn, y_mask, (z, z_p, m_p, logs_p)
-
- def voice_conversion(self, y, y_lengths, sid_src, sid_tgt):
- assert self.n_speakers > 0, "n_speakers have to be larger than 0."
- g_src = self.emb_g(sid_src).unsqueeze(-1)
- g_tgt = self.emb_g(sid_tgt).unsqueeze(-1)
- z, m_q, logs_q, y_mask = self.enc_q(y, y_lengths, g=g_src)
- z_p = self.flow(z, y_mask, g=g_src)
- z_hat = self.flow(z_p, y_mask, g=g_tgt, reverse=True)
- o_hat = self.dec(z_hat * y_mask, g=g_tgt)
- return o_hat, y_mask, (z, z_p, z_hat)
-
diff --git a/spaces/nikitaPDL2023/assignment4/detectron2/configs/new_baselines/mask_rcnn_R_50_FPN_100ep_LSJ.py b/spaces/nikitaPDL2023/assignment4/detectron2/configs/new_baselines/mask_rcnn_R_50_FPN_100ep_LSJ.py
deleted file mode 100644
index df7a2aedf480ed8dc4aa3645e37420e9b893fae4..0000000000000000000000000000000000000000
--- a/spaces/nikitaPDL2023/assignment4/detectron2/configs/new_baselines/mask_rcnn_R_50_FPN_100ep_LSJ.py
+++ /dev/null
@@ -1,72 +0,0 @@
-import detectron2.data.transforms as T
-from detectron2.config.lazy import LazyCall as L
-from detectron2.layers.batch_norm import NaiveSyncBatchNorm
-from detectron2.solver import WarmupParamScheduler
-from fvcore.common.param_scheduler import MultiStepParamScheduler
-
-from ..common.data.coco import dataloader
-from ..common.models.mask_rcnn_fpn import model
-from ..common.optim import SGD as optimizer
-from ..common.train import train
-
-# train from scratch
-train.init_checkpoint = ""
-train.amp.enabled = True
-train.ddp.fp16_compression = True
-model.backbone.bottom_up.freeze_at = 0
-
-# SyncBN
-# fmt: off
-model.backbone.bottom_up.stem.norm = \
- model.backbone.bottom_up.stages.norm = \
- model.backbone.norm = "SyncBN"
-
-# Using NaiveSyncBatchNorm becase heads may have empty input. That is not supported by
-# torch.nn.SyncBatchNorm. We can remove this after
-# https://github.com/pytorch/pytorch/issues/36530 is fixed.
-model.roi_heads.box_head.conv_norm = \
- model.roi_heads.mask_head.conv_norm = lambda c: NaiveSyncBatchNorm(c,
- stats_mode="N")
-# fmt: on
-
-# 2conv in RPN:
-# https://github.com/tensorflow/tpu/blob/b24729de804fdb751b06467d3dce0637fa652060/models/official/detection/modeling/architecture/heads.py#L95-L97 # noqa: E501, B950
-model.proposal_generator.head.conv_dims = [-1, -1]
-
-# 4conv1fc box head
-model.roi_heads.box_head.conv_dims = [256, 256, 256, 256]
-model.roi_heads.box_head.fc_dims = [1024]
-
-# resize_and_crop_image in:
-# https://github.com/tensorflow/tpu/blob/b24729de804fdb751b06467d3dce0637fa652060/models/official/detection/utils/input_utils.py#L127 # noqa: E501, B950
-image_size = 1024
-dataloader.train.mapper.augmentations = [
- L(T.ResizeScale)(
- min_scale=0.1, max_scale=2.0, target_height=image_size, target_width=image_size
- ),
- L(T.FixedSizeCrop)(crop_size=(image_size, image_size)),
- L(T.RandomFlip)(horizontal=True),
-]
-
-# recompute boxes due to cropping
-dataloader.train.mapper.recompute_boxes = True
-
-# larger batch-size.
-dataloader.train.total_batch_size = 64
-
-# Equivalent to 100 epochs.
-# 100 ep = 184375 iters * 64 images/iter / 118000 images/ep
-train.max_iter = 184375
-
-lr_multiplier = L(WarmupParamScheduler)(
- scheduler=L(MultiStepParamScheduler)(
- values=[1.0, 0.1, 0.01],
- milestones=[163889, 177546],
- num_updates=train.max_iter,
- ),
- warmup_length=500 / train.max_iter,
- warmup_factor=0.067,
-)
-
-optimizer.lr = 0.1
-optimizer.weight_decay = 4e-5
diff --git a/spaces/nikitaPDL2023/assignment4/detectron2/tests/config/dir1/dir1_b.py b/spaces/nikitaPDL2023/assignment4/detectron2/tests/config/dir1/dir1_b.py
deleted file mode 100644
index 2dcb54cb1054c5d80ccc823af21f13b9ebbcf1a3..0000000000000000000000000000000000000000
--- a/spaces/nikitaPDL2023/assignment4/detectron2/tests/config/dir1/dir1_b.py
+++ /dev/null
@@ -1,11 +0,0 @@
-# Copyright (c) Facebook, Inc. and its affiliates.
-from detectron2.config import LazyConfig
-
-# equivalent to relative import
-dir1a_str, dir1a_dict = LazyConfig.load_rel("dir1_a.py", ("dir1a_str", "dir1a_dict"))
-
-dir1b_str = dir1a_str + "_from_b"
-dir1b_dict = dir1a_dict
-
-# Every import is a reload: not modified by other config files
-assert dir1a_dict.a == 1
diff --git a/spaces/nomic-ai/daily_dialog/style.css b/spaces/nomic-ai/daily_dialog/style.css
deleted file mode 100644
index 114adf441e9032febb46bc056b2a8bb651075f0d..0000000000000000000000000000000000000000
--- a/spaces/nomic-ai/daily_dialog/style.css
+++ /dev/null
@@ -1,28 +0,0 @@
-body {
- padding: 2rem;
- font-family: -apple-system, BlinkMacSystemFont, "Arial", sans-serif;
-}
-
-h1 {
- font-size: 16px;
- margin-top: 0;
-}
-
-p {
- color: rgb(107, 114, 128);
- font-size: 15px;
- margin-bottom: 10px;
- margin-top: 5px;
-}
-
-.card {
- max-width: 620px;
- margin: 0 auto;
- padding: 16px;
- border: 1px solid lightgray;
- border-radius: 16px;
-}
-
-.card p:last-child {
- margin-bottom: 0;
-}
diff --git a/spaces/oliver2023/mm-react/MM-REACT/app.py b/spaces/oliver2023/mm-react/MM-REACT/app.py
deleted file mode 100644
index a68de3ef2e21d2947ac9d3e1b55fcf240582bbf3..0000000000000000000000000000000000000000
--- a/spaces/oliver2023/mm-react/MM-REACT/app.py
+++ /dev/null
@@ -1,530 +0,0 @@
-import re
-import io
-import os
-from typing import Optional, Tuple
-import datetime
-import sys
-import gradio as gr
-import requests
-import json
-from threading import Lock
-from langchain import ConversationChain, LLMChain
-from langchain.agents import load_tools, initialize_agent, Tool
-from langchain.tools.bing_search.tool import BingSearchRun, BingSearchAPIWrapper
-from langchain.chains.conversation.memory import ConversationBufferMemory
-from langchain.llms import OpenAI
-from langchain.chains import PALChain
-from langchain.llms import AzureOpenAI
-from langchain.utilities import ImunAPIWrapper, ImunMultiAPIWrapper
-from langchain.utils import get_url_path
-from openai.error import AuthenticationError, InvalidRequestError, RateLimitError
-import argparse
-import logging
-from opencensus.ext.azure.log_exporter import AzureLogHandler
-import uuid
-
-logger = None
-
-
-OPENAI_API_KEY = os.environ.get("OPENAI_API_KEY")
-BUG_FOUND_MSG = "Some Functionalities not supported yet. Please refresh and hit 'Click to wake up MM-REACT'"
-AUTH_ERR_MSG = "OpenAI key needed"
-REFRESH_MSG = "Please refresh and hit 'Click to wake up MM-REACT'"
-MAX_TOKENS = 512
-
-
-############## ARGS #################
-AGRS = None
-#####################################
-
-
-def get_logger():
- global logger
- if logger is None:
- logger = logging.getLogger(__name__)
- logger.addHandler(AzureLogHandler())
- return logger
-
-
-# load chain
-def load_chain(history, log_state):
- global ARGS
-
- if ARGS.openAIModel == 'openAIGPT35':
- # openAI GPT 3.5
- llm = OpenAI(temperature=0, max_tokens=MAX_TOKENS)
- elif ARGS.openAIModel == 'azureChatGPT':
- # for Azure OpenAI ChatGPT
- llm = AzureOpenAI(deployment_name="text-chat-davinci-002", model_name="text-chat-davinci-002", temperature=0, max_tokens=MAX_TOKENS)
- elif ARGS.openAIModel == 'azureGPT35turbo':
- # for Azure OpenAI gpt3.5 turbo
- llm = AzureOpenAI(deployment_name="gpt-35-turbo-version-0301", model_name="gpt-35-turbo (version 0301)", temperature=0, max_tokens=MAX_TOKENS)
- elif ARGS.openAIModel == 'azureTextDavinci003':
- # for Azure OpenAI text davinci
- llm = AzureOpenAI(deployment_name="text-davinci-003", model_name="text-davinci-003", temperature=0, max_tokens=MAX_TOKENS)
-
- memory = ConversationBufferMemory(memory_key="chat_history")
-
-
- #############################
- # loading all tools
-
- imun_dense = ImunAPIWrapper(
- imun_url=os.environ.get("IMUN_URL2"),
- params=os.environ.get("IMUN_PARAMS2"),
- imun_subscription_key=os.environ.get("IMUN_SUBSCRIPTION_KEY2"))
-
- imun = ImunAPIWrapper()
- imun = ImunMultiAPIWrapper(imuns=[imun, imun_dense])
-
- imun_celeb = ImunAPIWrapper(
- imun_url=os.environ.get("IMUN_CELEB_URL"),
- params="")
-
- imun_read = ImunAPIWrapper(
- imun_url=os.environ.get("IMUN_OCR_READ_URL"),
- params=os.environ.get("IMUN_OCR_PARAMS"),
- imun_subscription_key=os.environ.get("IMUN_OCR_SUBSCRIPTION_KEY"))
-
- imun_receipt = ImunAPIWrapper(
- imun_url=os.environ.get("IMUN_OCR_RECEIPT_URL"),
- params=os.environ.get("IMUN_OCR_PARAMS"),
- imun_subscription_key=os.environ.get("IMUN_OCR_SUBSCRIPTION_KEY"))
-
- imun_businesscard = ImunAPIWrapper(
- imun_url=os.environ.get("IMUN_OCR_BC_URL"),
- params=os.environ.get("IMUN_OCR_PARAMS"),
- imun_subscription_key=os.environ.get("IMUN_OCR_SUBSCRIPTION_KEY"))
-
- imun_layout = ImunAPIWrapper(
- imun_url=os.environ.get("IMUN_OCR_LAYOUT_URL"),
- params=os.environ.get("IMUN_OCR_PARAMS"),
- imun_subscription_key=os.environ.get("IMUN_OCR_SUBSCRIPTION_KEY"))
-
- imun_invoice = ImunAPIWrapper(
- imun_url=os.environ.get("IMUN_OCR_INVOICE_URL"),
- params=os.environ.get("IMUN_OCR_PARAMS"),
- imun_subscription_key=os.environ.get("IMUN_OCR_SUBSCRIPTION_KEY"))
-
- bing = BingSearchAPIWrapper(k=2)
-
- def edit_photo(query: str) -> str:
- endpoint = os.environ.get("PHOTO_EDIT_ENDPOINT_URL")
- query = query.strip()
- url_idx, img_url = get_url_path(query)
- if not img_url.startswith(("http://", "https://")):
- return "Invalid image URL"
- img_url = img_url.replace("0.0.0.0", os.environ.get("PHOTO_EDIT_ENDPOINT_URL_SHORT"))
- instruction = query[:url_idx]
- # This should be some internal IP to wherever the server runs
- job = {"image_path": img_url, "instruction": instruction}
- response = requests.post(endpoint, json=job)
- if response.status_code != 200:
- return "Could not finish the task try again later!"
- return "Here is the edited image " + endpoint + response.json()["edited_image"]
-
- # these tools should not step on each other's toes
- tools = [
- Tool(
- name="PAL-MATH",
- func=PALChain.from_math_prompt(llm).run,
- description=(
- "A wrapper around calculator. "
- "A language model that is really good at solving complex word math problems."
- "Input should be a fully worded hard word math problem."
- )
- ),
- Tool(
- name = "Image Understanding",
- func=imun.run,
- description=(
- "A wrapper around Image Understanding. "
- "Useful for when you need to understand what is inside an image (objects, texts, people)."
- "Input should be an image url, or path to an image file (e.g. .jpg, .png)."
- )
- ),
- Tool(
- name = "OCR Understanding",
- func=imun_read.run,
- description=(
- "A wrapper around OCR Understanding (Optical Character Recognition). "
- "Useful after Image Understanding tool has found text or handwriting is present in the image tags."
- "This tool can find the actual text, written name, or product name in the image."
- "Input should be an image url, or path to an image file (e.g. .jpg, .png)."
- )
- ),
- Tool(
- name = "Receipt Understanding",
- func=imun_receipt.run,
- description=(
- "A wrapper receipt understanding. "
- "Useful after Image Understanding tool has recognized a receipt in the image tags."
- "This tool can find the actual receipt text, prices and detailed items."
- "Input should be an image url, or path to an image file (e.g. .jpg, .png)."
- )
- ),
- Tool(
- name = "Business Card Understanding",
- func=imun_businesscard.run,
- description=(
- "A wrapper around business card understanding. "
- "Useful after Image Understanding tool has recognized businesscard in the image tags."
- "This tool can find the actual business card text, name, address, email, website on the card."
- "Input should be an image url, or path to an image file (e.g. .jpg, .png)."
- )
- ),
- Tool(
- name = "Layout Understanding",
- func=imun_layout.run,
- description=(
- "A wrapper around layout and table understanding. "
- "Useful after Image Understanding tool has recognized businesscard in the image tags."
- "This tool can find the actual business card text, name, address, email, website on the card."
- "Input should be an image url, or path to an image file (e.g. .jpg, .png)."
- )
- ),
- Tool(
- name = "Invoice Understanding",
- func=imun_invoice.run,
- description=(
- "A wrapper around invoice understanding. "
- "Useful after Image Understanding tool has recognized businesscard in the image tags."
- "This tool can find the actual business card text, name, address, email, website on the card."
- "Input should be an image url, or path to an image file (e.g. .jpg, .png)."
- )
- ),
- Tool(
- name = "Celebrity Understanding",
- func=imun_celeb.run,
- description=(
- "A wrapper around celebrity understanding. "
- "Useful after Image Understanding tool has recognized people in the image tags that could be celebrities."
- "This tool can find the name of celebrities in the image."
- "Input should be an image url, or path to an image file (e.g. .jpg, .png)."
- )
- ),
- BingSearchRun(api_wrapper=bing),
- Tool(
- name = "Photo Editing",
- func=edit_photo,
- description=(
- "A wrapper around photo editing. "
- "Useful to edit an image with a given instruction."
- "Input should be an image url, or path to an image file (e.g. .jpg, .png)."
- )
- ),
- ]
-
- chain = initialize_agent(tools, llm, agent="conversational-assistant", verbose=True, memory=memory, return_intermediate_steps=True, max_iterations=4)
- log_state = log_state or ""
- print ("log_state {}".format(log_state))
- log_state = str(uuid.uuid1())
- print("langchain reloaded")
- # eproperties = {'custom_dimensions': {'key_1': 'value_1', 'key_2': 'value_2'}}
- properties = {'custom_dimensions': {'session': log_state}}
- get_logger().warning("langchain reloaded", extra=properties)
- history = []
- history.append(("Show me what you got!", "Hi Human, Please upload an image to get started!"))
-
- return history, history, chain, log_state, \
- gr.Textbox.update(visible=True), \
- gr.Button.update(visible=True), \
- gr.UploadButton.update(visible=True), \
- gr.Row.update(visible=True), \
- gr.HTML.update(visible=True), \
- gr.Button.update(variant="secondary")
-
-
-# executes input typed by human
-def run_chain(chain, inp):
- # global chain
-
- output = ""
- try:
- output = chain.conversation(input=inp, keep_short=ARGS.noIntermediateConv)
- # output = chain.run(input=inp)
- except AuthenticationError as ae:
- output = AUTH_ERR_MSG + str(datetime.datetime.now()) + ". " + str(ae)
- print("output", output)
- except RateLimitError as rle:
- output = "\n\nRateLimitError: " + str(rle)
- except ValueError as ve:
- output = "\n\nValueError: " + str(ve)
- except InvalidRequestError as ire:
- output = "\n\nInvalidRequestError: " + str(ire)
- except Exception as e:
- output = "\n\n" + BUG_FOUND_MSG + ":\n\n" + str(e)
-
- return output
-
-# simple chat function wrapper
-class ChatWrapper:
-
- def __init__(self):
- self.lock = Lock()
-
- def __call__(
- self, inp: str, history: Optional[Tuple[str, str]], chain: Optional[ConversationChain], log_state
- ):
-
- """Execute the chat functionality."""
- self.lock.acquire()
- try:
- print("\n==== date/time: " + str(datetime.datetime.now()) + " ====")
- print("inp: " + inp)
-
- properties = {'custom_dimensions': {'session': log_state}}
- get_logger().warning("inp: " + inp, extra=properties)
-
-
- history = history or []
- # If chain is None, that is because no API key was provided.
- output = "Please paste your OpenAI key from openai.com to use this app. " + str(datetime.datetime.now())
-
- ########################
- # multi line
- outputs = run_chain(chain, inp)
-
- outputs = process_chain_output(outputs)
-
- print (" len(outputs) {}".format(len(outputs)))
- for i, output in enumerate(outputs):
- if i==0:
- history.append((inp, output))
- else:
- history.append((None, output))
-
-
- except Exception as e:
- raise e
- finally:
- self.lock.release()
-
- print (history)
- properties = {'custom_dimensions': {'session': log_state}}
- if outputs is None:
- outputs = ""
- get_logger().warning(str(json.dumps(outputs)), extra=properties)
-
- return history, history, ""
-
-def add_image_with_path(state, chain, imagepath, log_state):
- global ARGS
- state = state or []
-
- url_input_for_chain = "http://0.0.0.0:{}/file={}".format(ARGS.port, imagepath)
-
- outputs = run_chain(chain, url_input_for_chain)
-
- ########################
- # multi line response handling
- outputs = process_chain_output(outputs)
-
- for i, output in enumerate(outputs):
- if i==0:
- # state.append((f"", output))
- state.append(((imagepath,), output))
- else:
- state.append((None, output))
-
-
- print (state)
- properties = {'custom_dimensions': {'session': log_state}}
- get_logger().warning("url_input_for_chain: " + url_input_for_chain, extra=properties)
- if outputs is None:
- outputs = ""
- get_logger().warning(str(json.dumps(outputs)), extra=properties)
- return state, state
-
-
-# upload image
-def add_image(state, chain, image, log_state):
- global ARGS
- state = state or []
-
- # handling spaces in image path
- imagepath = image.name.replace(" ", "%20")
-
- url_input_for_chain = "http://0.0.0.0:{}/file={}".format(ARGS.port, imagepath)
-
- outputs = run_chain(chain, url_input_for_chain)
-
- ########################
- # multi line response handling
- outputs = process_chain_output(outputs)
-
- for i, output in enumerate(outputs):
- if i==0:
- state.append(((imagepath,), output))
- else:
- state.append((None, output))
-
-
- print (state)
- properties = {'custom_dimensions': {'session': log_state}}
- get_logger().warning("url_input_for_chain: " + url_input_for_chain, extra=properties)
- if outputs is None:
- outputs = ""
- get_logger().warning(str(json.dumps(outputs)), extra=properties)
- return state, state
-
-# extract image url from response and process differently
-def replace_with_image_markup(text):
- img_url = None
- text= text.strip()
- url_idx = text.rfind(" ")
- img_url = text[url_idx + 1:].strip()
- if img_url.endswith((".", "?")):
- img_url = img_url[:-1]
-
- # if img_url is not None:
- # img_url = f""
- return img_url
-
-# multi line response handling
-def process_chain_output(outputs):
- global ARGS
- EMPTY_AI_REPLY = "AI:"
- # print("outputs {}".format(outputs))
- if isinstance(outputs, str): # single line output
- if outputs.strip() == EMPTY_AI_REPLY:
- outputs = REFRESH_MSG
- outputs = [outputs]
- elif isinstance(outputs, list): # multi line output
- if ARGS.noIntermediateConv: # remove the items with assistant in it.
- cleanOutputs = []
- for output in outputs:
- if output.strip() == EMPTY_AI_REPLY:
- output = REFRESH_MSG
- # found an edited image url to embed
- img_url = None
- # print ("type list: {}".format(output))
- if "assistant: here is the edited image " in output.lower():
- img_url = replace_with_image_markup(output)
- cleanOutputs.append("Assistant: Here is the edited image")
- if img_url is not None:
- cleanOutputs.append((img_url,))
- else:
- cleanOutputs.append(output)
- # cleanOutputs = cleanOutputs + output+ "."
- outputs = cleanOutputs
-
- return outputs
-
-
-def init_and_kick_off():
- global ARGS
- # initalize chatWrapper
- chat = ChatWrapper()
-
- exampleTitle = """Examples to start conversation..
"""
- comingSoon = """MM-REACT: March 29th version with image understanding capabilities
"""
- detailLinks = """
-
- MM-ReAct Website
- ·
- MM-ReAct Paper
- ·
- MM-ReAct Code
-
- """
-
- with gr.Blocks(css="#tryButton {width: 120px;}") as block:
- llm_state = gr.State()
- history_state = gr.State()
- chain_state = gr.State()
- log_state = gr.State()
-
- reset_btn = gr.Button(value="!!!CLICK to wake up MM-REACT!!!", variant="primary", elem_id="resetbtn").style(full_width=True)
- gr.HTML(detailLinks)
- gr.HTML(comingSoon)
-
- example_image_size = 90
- col_min_width = 80
- button_variant = "primary"
- with gr.Row():
- with gr.Column(scale=1.0, min_width=100):
- chatbot = gr.Chatbot(elem_id="chatbot", label="MM-REACT Bot").style(height=620)
- with gr.Column(scale=0.20, min_width=200, visible=False) as exampleCol:
- with gr.Row():
- grExampleTitle = gr.HTML(exampleTitle, visible=False)
- with gr.Row():
- with gr.Column(scale=0.50, min_width=col_min_width):
- example3Image = gr.Image("images/receipt.png", interactive=False).style(height=example_image_size, width=example_image_size)
- with gr.Column(scale=0.50, min_width=col_min_width):
- example3ImageButton = gr.Button(elem_id="tryButton", value="Try it!", variant=button_variant).style(full_width=True)
- # dummy text field to hold the path
- example3ImagePath = gr.Text("images/receipt.png", interactive=False, visible=False)
- with gr.Row():
- with gr.Column(scale=0.50, min_width=col_min_width):
- example1Image = gr.Image("images/money.png", interactive=False).style(height=example_image_size, width=example_image_size)
- with gr.Column(scale=0.50, min_width=col_min_width):
- example1ImageButton = gr.Button(elem_id="tryButton", value="Try it!", variant=button_variant).style(full_width=True)
- # dummy text field to hold the path
- example1ImagePath = gr.Text("images/money.png", interactive=False, visible=False)
- with gr.Row():
- with gr.Column(scale=0.50, min_width=col_min_width):
- example2Image = gr.Image("images/cartoon.png", interactive=False).style(height=example_image_size, width=example_image_size)
- with gr.Column(scale=0.50, min_width=col_min_width):
- example2ImageButton = gr.Button(elem_id="tryButton", value="Try it!", variant=button_variant).style(full_width=True)
- # dummy text field to hold the path
- example2ImagePath = gr.Text("images/cartoon.png", interactive=False, visible=False)
- with gr.Row():
- with gr.Column(scale=0.50, min_width=col_min_width):
- example4Image = gr.Image("images/product.png", interactive=False).style(height=example_image_size, width=example_image_size)
- with gr.Column(scale=0.50, min_width=col_min_width):
- example4ImageButton = gr.Button(elem_id="tryButton", value="Try it!", variant=button_variant).style(full_width=True)
- # dummy text field to hold the path
- example4ImagePath = gr.Text("images/product.png", interactive=False, visible=False)
- with gr.Row():
- with gr.Column(scale=0.50, min_width=col_min_width):
- example5Image = gr.Image("images/celebrity.png", interactive=False).style(height=example_image_size, width=example_image_size)
- with gr.Column(scale=0.50, min_width=col_min_width):
- example5ImageButton = gr.Button(elem_id="tryButton", value="Try it!", variant=button_variant).style(full_width=True)
- # dummy text field to hold the path
- example5ImagePath = gr.Text("images/celebrity.png", interactive=False, visible=False)
-
-
-
- with gr.Row():
- with gr.Column(scale=0.75):
- message = gr.Textbox(label="Upload a pic and ask!",
- placeholder="Type your question about the uploaded image",
- lines=1, visible=False)
- with gr.Column(scale=0.15):
- submit = gr.Button(value="Send", variant="secondary", visible=False).style(full_width=True)
- with gr.Column(scale=0.10, min_width=0):
- btn = gr.UploadButton("🖼️", file_types=["image"], visible=False).style(full_width=True)
-
-
- message.submit(chat, inputs=[message, history_state, chain_state, log_state], outputs=[chatbot, history_state, message])
-
- submit.click(chat, inputs=[message, history_state, chain_state, log_state], outputs=[chatbot, history_state, message])
-
- btn.upload(add_image, inputs=[history_state, chain_state, btn, log_state], outputs=[history_state, chatbot])
-
- # load the chain
- reset_btn.click(load_chain, inputs=[history_state, log_state], outputs=[chatbot, history_state, chain_state, log_state, message, submit, btn, exampleCol, grExampleTitle, reset_btn])
-
- # setup listener click for the examples
- example1ImageButton.click(add_image_with_path, inputs=[history_state, chain_state, example1ImagePath, log_state], outputs=[history_state, chatbot])
- example2ImageButton.click(add_image_with_path, inputs=[history_state, chain_state, example2ImagePath, log_state], outputs=[history_state, chatbot])
- example3ImageButton.click(add_image_with_path, inputs=[history_state, chain_state, example3ImagePath, log_state], outputs=[history_state, chatbot])
- example4ImageButton.click(add_image_with_path, inputs=[history_state, chain_state, example4ImagePath, log_state], outputs=[history_state, chatbot])
- example5ImageButton.click(add_image_with_path, inputs=[history_state, chain_state, example5ImagePath, log_state], outputs=[history_state, chatbot])
-
-
- # launch the app
- block.launch(server_name="0.0.0.0", server_port = ARGS.port)
-
-if __name__ == '__main__':
- parser = argparse.ArgumentParser()
-
- parser.add_argument('--port', type=int, required=False, default=7860)
- parser.add_argument('--openAIModel', type=str, required=False, default='azureGPT35turbo')
- parser.add_argument('--noIntermediateConv', default=True, action='store_true', help='if this flag is turned on no intermediate conversation should be shown')
-
- global ARGS
- ARGS = parser.parse_args()
-
- init_and_kick_off()
\ No newline at end of file
diff --git a/spaces/omdena-lc/omdena-ng-lagos-chatbot-model/Dockerfile b/spaces/omdena-lc/omdena-ng-lagos-chatbot-model/Dockerfile
deleted file mode 100644
index 0b6e86854242c46d130ab28ed63228689ac5d279..0000000000000000000000000000000000000000
--- a/spaces/omdena-lc/omdena-ng-lagos-chatbot-model/Dockerfile
+++ /dev/null
@@ -1,41 +0,0 @@
-# syntax=docker/dockerfile:1
-
-# Comments are provided throughout this file to help you get started.
-# If you need more help, visit the Dockerfile reference guide at
-# https://docs.docker.com/engine/reference/builder/
-
-ARG PYTHON_VERSION=3.8
-FROM python:${PYTHON_VERSION}-slim as base
-
-# Copy the requirements file into the container.
-COPY requirements.txt .
-
-# Install the dependencies from the requirements file.
-RUN python -m pip install --no-cache-dir -r requirements.txt
-
-# Prevents Python from writing pyc files.
-ENV PYTHONDONTWRITEBYTECODE=1
-
-# Keeps Python from buffering stdout and stderr to avoid situations where
-# the application crashes without emitting any logs due to buffering.
-ENV PYTHONUNBUFFERED=1
-
-WORKDIR /app
-# Copy the source code into the container.
-COPY . .
-
-# Create a non-privileged user that the app will run under.
-# See https://docs.docker.com/develop/develop-images/dockerfile_best-practices/#user
-# Switch to the non-privileged user to run the application.
-USER 1001
-
-# set entrypoint for interactive shells
-ENTRYPOINT [ "rasa" ]
-
-# Expose the port that the application listens on.
-EXPOSE 7860
-
-# List of Models: central+careersv1.0.tar.gz,Arpit-v1.0.tar.gz,Maisam+Arpit+Anand+Pankaj-bot-v1.0.tar.gz
-
-# Run the application.
-CMD ["run","--model","models/combined-bot-v1.5.tar.gz","--enable-api","--port","7860"]
diff --git a/spaces/ondrejbiza/isa/invariant_slot_attention/configs/clevrtex/simplecnn/equiv_transl.py b/spaces/ondrejbiza/isa/invariant_slot_attention/configs/clevrtex/simplecnn/equiv_transl.py
deleted file mode 100644
index 7149ca57cc69ba64e289876216c990a1fa507290..0000000000000000000000000000000000000000
--- a/spaces/ondrejbiza/isa/invariant_slot_attention/configs/clevrtex/simplecnn/equiv_transl.py
+++ /dev/null
@@ -1,205 +0,0 @@
-# coding=utf-8
-# Copyright 2023 The Google Research Authors.
-#
-# Licensed under the Apache License, Version 2.0 (the "License");
-# you may not use this file except in compliance with the License.
-# You may obtain a copy of the License at
-#
-# http://www.apache.org/licenses/LICENSE-2.0
-#
-# Unless required by applicable law or agreed to in writing, software
-# distributed under the License is distributed on an "AS IS" BASIS,
-# WITHOUT WARRANTIES OR CONDITIONS OF ANY KIND, either express or implied.
-# See the License for the specific language governing permissions and
-# limitations under the License.
-
-r"""Config for unsupervised training on CLEVRTex."""
-
-import ml_collections
-
-
-def get_config():
- """Get the default hyperparameter configuration."""
- config = ml_collections.ConfigDict()
-
- config.seed = 42
- config.seed_data = True
-
- config.batch_size = 64
- config.num_train_steps = 500000 # from the original Slot Attention
- config.init_checkpoint = ml_collections.ConfigDict()
- config.init_checkpoint.xid = 0 # Disabled by default.
- config.init_checkpoint.wid = 1
-
- config.optimizer_configs = ml_collections.ConfigDict()
- config.optimizer_configs.optimizer = "adam"
-
- config.optimizer_configs.grad_clip = ml_collections.ConfigDict()
- config.optimizer_configs.grad_clip.clip_method = "clip_by_global_norm"
- config.optimizer_configs.grad_clip.clip_value = 0.05
-
- config.lr_configs = ml_collections.ConfigDict()
- config.lr_configs.learning_rate_schedule = "compound"
- config.lr_configs.factors = "constant * cosine_decay * linear_warmup"
- config.lr_configs.warmup_steps = 10000 # from the original Slot Attention
- config.lr_configs.steps_per_cycle = config.get_ref("num_train_steps")
- # from the original Slot Attention
- config.lr_configs.base_learning_rate = 4e-4
-
- config.eval_pad_last_batch = False # True
- config.log_loss_every_steps = 50
- config.eval_every_steps = 5000
- config.checkpoint_every_steps = 5000
-
- config.train_metrics_spec = {
- "loss": "loss",
- "ari": "ari",
- "ari_nobg": "ari_nobg",
- }
- config.eval_metrics_spec = {
- "eval_loss": "loss",
- "eval_ari": "ari",
- "eval_ari_nobg": "ari_nobg",
- }
-
- config.data = ml_collections.ConfigDict({
- "dataset_name": "tfds",
- # The TFDS dataset will be created in the directory below
- # if you follow the README in datasets/clevrtex.
- "data_dir": "~/tensorflow_datasets",
- "tfds_name": "clevr_tex",
- "shuffle_buffer_size": config.batch_size * 8,
- "resolution": (128, 128)
- })
-
- config.max_instances = 11
- config.num_slots = config.max_instances # Only used for metrics.
- config.logging_min_n_colors = config.max_instances
-
- config.preproc_train = [
- "tfds_image_to_tfds_video",
- "video_from_tfds",
- "central_crop(height=192,width=192)",
- "resize_small({size})".format(size=min(*config.data.resolution))
- ]
-
- config.preproc_eval = [
- "tfds_image_to_tfds_video",
- "video_from_tfds",
- "central_crop(height=192,width=192)",
- "resize_small({size})".format(size=min(*config.data.resolution))
- ]
-
- config.eval_slice_size = 1
- config.eval_slice_keys = ["video", "segmentations_video"]
-
- # Dictionary of targets and corresponding channels. Losses need to match.
- targets = {"video": 3}
- config.losses = {"recon": {"targets": list(targets)}}
- config.losses = ml_collections.ConfigDict({
- f"recon_{target}": {"loss_type": "recon", "key": target}
- for target in targets})
-
- config.model = ml_collections.ConfigDict({
- "module": "invariant_slot_attention.modules.SAVi",
-
- # Encoder.
- "encoder": ml_collections.ConfigDict({
- "module": "invariant_slot_attention.modules.FrameEncoder",
- "reduction": "spatial_flatten",
- "backbone": ml_collections.ConfigDict({
- "module": "invariant_slot_attention.modules.SimpleCNN",
- "features": [64, 64, 64, 64],
- "kernel_size": [(5, 5), (5, 5), (5, 5), (5, 5)],
- "strides": [(2, 2), (2, 2), (2, 2), (1, 1)]
- }),
- "pos_emb": ml_collections.ConfigDict({
- "module": "invariant_slot_attention.modules.PositionEmbedding",
- "embedding_type": "linear",
- "update_type": "concat"
- }),
- }),
-
- # Corrector.
- "corrector": ml_collections.ConfigDict({
- "module": "invariant_slot_attention.modules.SlotAttentionTranslEquiv",
- "num_iterations": 3,
- "qkv_size": 64,
- "mlp_size": 128,
- "grid_encoder": ml_collections.ConfigDict({
- "module": "invariant_slot_attention.modules.MLP",
- "hidden_size": 128,
- "layernorm": "pre"
- }),
- "add_rel_pos_to_values": True, # V3
- "zero_position_init": False, # Random positions.
- }),
-
- # Predictor.
- # Removed since we are running a single frame.
- "predictor": ml_collections.ConfigDict({
- "module": "invariant_slot_attention.modules.Identity"
- }),
-
- # Initializer.
- "initializer": ml_collections.ConfigDict({
- "module":
- "invariant_slot_attention.modules.ParamStateInitRandomPositions",
- "shape":
- (11, 64), # (num_slots, slot_size)
- }),
-
- # Decoder.
- "decoder": ml_collections.ConfigDict({
- "module":
- "invariant_slot_attention.modules.SiameseSpatialBroadcastDecoder",
- "resolution": (16, 16), # Update if data resolution or strides change
- "backbone": ml_collections.ConfigDict({
- "module": "invariant_slot_attention.modules.CNN",
- "features": [64, 64, 64, 64, 64],
- "kernel_size": [(5, 5), (5, 5), (5, 5), (5, 5), (5, 5)],
- "strides": [(2, 2), (2, 2), (2, 2), (1, 1), (1, 1)],
- "max_pool_strides": [(1, 1), (1, 1), (1, 1), (1, 1), (1, 1)],
- "layer_transpose": [True, True, True, False, False]
- }),
- "target_readout": ml_collections.ConfigDict({
- "module": "invariant_slot_attention.modules.Readout",
- "keys": list(targets),
- "readout_modules": [ml_collections.ConfigDict({ # pylint: disable=g-complex-comprehension
- "module": "invariant_slot_attention.modules.MLP",
- "num_hidden_layers": 0,
- "hidden_size": 0,
- "output_size": targets[k]}) for k in targets],
- }),
- "relative_positions": True,
- "pos_emb": ml_collections.ConfigDict({
- "module":
- "invariant_slot_attention.modules.RelativePositionEmbedding",
- "embedding_type":
- "linear",
- "update_type":
- "project_add",
- }),
- }),
- "decode_corrected": True,
- "decode_predicted": False,
- })
-
- # Which video-shaped variables to visualize.
- config.debug_var_video_paths = {
- "recon_masks": "decoder/alphas_softmaxed/__call__/0", # pylint: disable=line-too-long
- }
-
- # Define which attention matrices to log/visualize.
- config.debug_var_attn_paths = {
- "corrector_attn": "corrector/InvertedDotProductAttentionKeyPerQuery_0/attn" # pylint: disable=line-too-long
- }
-
- # Widths of attention matrices (for reshaping to image grid).
- config.debug_var_attn_widths = {
- "corrector_attn": 16,
- }
-
- return config
-
-
diff --git a/spaces/pierreguillou/DocLayNet-image-viewer/app.py b/spaces/pierreguillou/DocLayNet-image-viewer/app.py
deleted file mode 100644
index 65cc5728cae5e48c9a205195a929b7bb0be7f4b3..0000000000000000000000000000000000000000
--- a/spaces/pierreguillou/DocLayNet-image-viewer/app.py
+++ /dev/null
@@ -1,335 +0,0 @@
-import gradio as gr
-from PIL import Image, ImageDraw, ImageFont
-import random
-import pandas as pd
-import numpy as np
-from datasets import concatenate_datasets
-from operator import itemgetter
-import collections
-
-# download datasets
-from datasets import load_dataset
-
-dataset_small = load_dataset("pierreguillou/DocLayNet-small")
-dataset_base = load_dataset("pierreguillou/DocLayNet-base")
-
-id2label = {idx:label for idx,label in enumerate(dataset_small["train"].features["categories"].feature.names)}
-label2id = {label:idx for idx,label in id2label.items()}
-labels = [label for idx, label in id2label.items()]
-
-# need to change the coordinates format
-def convert_box(box):
- x, y, w, h = tuple(box) # the row comes in (left, top, width, height) format
- actual_box = [x, y, x+w, y+h] # we turn it into (left, top, left+widght, top+height) to get the actual box
- return actual_box
-
-# get back original size
-def original_box(box, original_width, original_height, coco_width, coco_height):
- return [
- int(original_width * (box[0] / coco_width)),
- int(original_height * (box[1] / coco_height)),
- int(original_width * (box[2] / coco_width)),
- int(original_height* (box[3] / coco_height)),
- ]
-
-# function to sort bounding boxes
-def get_sorted_boxes(bboxes):
-
- # sort by y from page top to bottom
- bboxes = sorted(bboxes, key=itemgetter(1), reverse=False)
- y_list = [bbox[1] for bbox in bboxes]
-
- # sort by x from page left to right when boxes with same y
- if len(list(set(y_list))) != len(y_list):
- y_list_duplicates_indexes = dict()
- y_list_duplicates = [item for item, count in collections.Counter(y_list).items() if count > 1]
- for item in y_list_duplicates:
- y_list_duplicates_indexes[item] = [i for i, e in enumerate(y_list) if e == item]
- bbox_list_y_duplicates = sorted(np.array(bboxes)[y_list_duplicates_indexes[item]].tolist(), key=itemgetter(0), reverse=False)
- np_array_bboxes = np.array(bboxes)
- np_array_bboxes[y_list_duplicates_indexes[item]] = np.array(bbox_list_y_duplicates)
- bboxes = np_array_bboxes.tolist()
-
- return bboxes
-
-# categories colors
-label2color = {
- 'Caption': 'brown',
- 'Footnote': 'orange',
- 'Formula': 'gray',
- 'List-item': 'yellow',
- 'Page-footer': 'red',
- 'Page-header': 'red',
- 'Picture': 'violet',
- 'Section-header': 'orange',
- 'Table': 'green',
- 'Text': 'blue',
- 'Title': 'pink'
- }
-
-# image witout content
-examples_dir = 'samples/'
-images_wo_content = examples_dir + "wo_content.png"
-
-df_paragraphs_wo_content, df_lines_wo_content = pd.DataFrame(), pd.DataFrame()
-
-df_paragraphs_wo_content["paragraphs"] = [0]
-df_paragraphs_wo_content["categories"] = ["no content"]
-df_paragraphs_wo_content["texts"] = ["no content"]
-df_paragraphs_wo_content["bounding boxes"] = ["no content"]
-
-df_lines_wo_content["lines"] = [0]
-df_lines_wo_content["categories"] = ["no content"]
-df_lines_wo_content["texts"] = ["no content"]
-df_lines_wo_content["bounding boxes"] = ["no content"]
-
-# lists
-font = ImageFont.load_default()
-
-dataset_names = ["small", "base"]
-splits = ["all", "train", "validation", "test"]
-domains = ["all", "Financial Reports", "Manuals", "Scientific Articles", "Laws & Regulations", "Patents", "Government Tenders"]
-domains_names = [domain_name.lower().replace(" ", "_").replace("&", "and") for domain_name in domains]
-categories = labels + ["all"]
-
-# function to get a rendom image and all data from DocLayNet
-def generate_annotated_image(dataset_name, split, domain, category):
-
- # error message
- msg_error = ""
-
- # get dataset
- if dataset_name == "small": example = dataset_small
- else: example = dataset_base
-
- # get split
- if split == "all":
- example = concatenate_datasets([example["train"], example["validation"], example["test"]])
- else:
- example = example[split]
-
- # get domain
- domain_name = domains_names[domains.index(domain)]
- if domain_name != "all":
- example = example.filter(lambda example: example["doc_category"] == domain_name)
- if len(example) == 0:
- msg_error = f'There is no image with at least one labeled bounding box that matches your settings (dataset: "DocLayNet {dataset_name}" / domain: "{domain}" / split: "{split}").'
- example = dict()
-
- # get category
- idx_list = list()
- if category != "all":
- for idx, categories_list in enumerate(example["categories"]):
- if int(label2id[category]) in categories_list:
- idx_list.append(idx)
- if len(idx_list) > 0:
- example = example.select(idx_list)
- else:
- msg_error = f'There is no image with at least one labeled bounding box that matches your settings (dataset: "DocLayNet {dataset_name}" / split: "{split}" / domain: "{domain}" / category: "{category}").'
- example = dict()
-
- if len(msg_error) > 0:
- # save image files
- Image.open(images_wo_content).save("wo_content.png")
- # save csv files
- df_paragraphs_wo_content.to_csv("paragraphs_wo_content.csv", encoding="utf-8", index=False)
- df_lines_wo_content.to_csv("lines_wo_content.csv", encoding="utf-8", index=False)
-
- return msg_error, "wo_content.png", images_wo_content, images_wo_content, "wo_content.png", "wo_content.png", df_paragraphs_wo_content, df_lines_wo_content, gr.File.update(value="paragraphs_wo_content.csv", visible=False), gr.File.update(value="lines_wo_content.csv", visible=False)
- else:
- # get random image & PDF data
- index = random.randint(0, len(example))
- image = example[index]["image"] # original image
- coco_width, coco_height = example[index]["coco_width"], example[index]["coco_height"]
- original_width, original_height = example[index]["original_width"], example[index]["original_height"]
- original_filename = example[index]["original_filename"]
- page_no = example[index]["page_no"]
- num_pages = example[index]["num_pages"]
-
- # resize image to original
- image = image.resize((original_width, original_height))
-
- # get image of PDF without bounding boxes
- img_file = original_filename.replace(".pdf", ".png")
- image.save(img_file)
-
- # get corresponding annotations
- texts = example[index]["texts"]
- bboxes_block = example[index]["bboxes_block"]
- bboxes_line = example[index]["bboxes_line"]
- categories = example[index]["categories"]
- domain = example[index]["doc_category"]
-
- # convert boxes to original
- original_bboxes_block = [original_box(convert_box(box), original_width, original_height, coco_width, coco_height) for box in bboxes_block]
- original_bboxes_line = [original_box(convert_box(box), original_width, original_height, coco_width, coco_height) for box in bboxes_line]
- original_bboxes = [original_bboxes_block, original_bboxes_line]
-
- ##### block boxes #####
-
- # get list of unique block boxes
- original_blocks = dict()
- original_bboxes_block_list = list()
- original_bbox_block_prec = list()
- for count_block, original_bbox_block in enumerate(original_bboxes_block):
- if original_bbox_block != original_bbox_block_prec:
- original_bbox_block_indexes = [i for i, original_bbox in enumerate(original_bboxes_block) if original_bbox == original_bbox_block]
- original_blocks[count_block] = original_bbox_block_indexes
- original_bboxes_block_list.append(original_bbox_block)
- original_bbox_block_prec = original_bbox_block
-
- # get list of categories and texts by unique block boxes
- category_block_list, text_block_list = list(), list()
- for original_bbox_block in original_bboxes_block_list:
- count_block = original_bboxes_block.index(original_bbox_block)
- original_bbox_block_indexes = original_blocks[count_block]
- category_block = categories[original_bbox_block_indexes[0]]
- category_block_list.append(category_block)
- if id2label[category_block] == "Text" or id2label[category_block] == "Caption" or id2label[category_block] == "Footnote":
- text_block = ' '.join(np.array(texts)[original_bbox_block_indexes].tolist())
- elif id2label[category_block] == "Section-header" or id2label[category_block] == "Title" or id2label[category_block] == "Picture" or id2label[category_block] == "Formula" or id2label[category_block] == "List-item" or id2label[category_block] == "Table" or id2label[category_block] == "Page-header" or id2label[category_block] == "Page-footer":
- text_block = '\n'.join(np.array(texts)[original_bbox_block_indexes].tolist())
- text_block_list.append(text_block)
-
- # sort data from y = 0 to end of page (and after, x=0 to end of page when necessary)
- sorted_original_bboxes_block_list = get_sorted_boxes(original_bboxes_block_list)
- sorted_original_bboxes_block_list_indexes = [original_bboxes_block_list.index(item) for item in sorted_original_bboxes_block_list]
- sorted_category_block_list = np.array(category_block_list)[sorted_original_bboxes_block_list_indexes].tolist()
- sorted_text_block_list = np.array(text_block_list)[sorted_original_bboxes_block_list_indexes].tolist()
-
- ##### line boxes ####
-
- # sort data from y = 0 to end of page (and after, x=0 to end of page when necessary)
- original_bboxes_line_list = original_bboxes_line
- category_line_list = categories
- text_line_list = texts
- sorted_original_bboxes_line_list = get_sorted_boxes(original_bboxes_line_list)
- sorted_original_bboxes_line_list_indexes = [original_bboxes_line_list.index(item) for item in sorted_original_bboxes_line_list]
- sorted_category_line_list = np.array(category_line_list)[sorted_original_bboxes_line_list_indexes].tolist()
- sorted_text_line_list = np.array(text_line_list)[sorted_original_bboxes_line_list_indexes].tolist()
-
- # setup images & PDF data
- columns = 2
- images = [image.copy(), image.copy()]
- num_imgs = len(images)
-
- imgs, df_paragraphs, df_lines = dict(), pd.DataFrame(), pd.DataFrame()
- for i, img in enumerate(images):
-
- draw = ImageDraw.Draw(img)
-
- for box, label_idx, text in zip(original_bboxes[i], categories, texts):
- label = id2label[label_idx]
- color = label2color[label]
- draw.rectangle(box, outline=color)
- text = text.encode('latin-1', 'replace').decode('latin-1') # https://stackoverflow.com/questions/56761449/unicodeencodeerror-latin-1-codec-cant-encode-character-u2013-writing-to
- draw.text((box[0] + 10, box[1] - 10), text=label, fill=color, font=font)
-
- if i == 0:
- imgs["paragraphs"] = img
-
- # save
- img_paragraphs = "img_paragraphs_" + original_filename.replace(".pdf", ".png")
- img.save(img_paragraphs)
-
- df_paragraphs["paragraphs"] = list(range(len(sorted_original_bboxes_block_list)))
- df_paragraphs["categories"] = [id2label[label_idx] for label_idx in sorted_category_block_list]
- df_paragraphs["texts"] = sorted_text_block_list
- df_paragraphs["bounding boxes"] = [str(bbox) for bbox in sorted_original_bboxes_block_list]
-
- # save
- csv_paragraphs = "csv_paragraphs_" + original_filename.replace(".pdf", ".csv")
- df_paragraphs.to_csv(csv_paragraphs, encoding="utf-8", index=False)
-
- else:
- imgs["lines"] = img
-
- # save
- img_lines = "img_lines_" + original_filename.replace(".pdf", ".png")
- img.save(img_lines)
-
- df_lines["lines"] = list(range(len(sorted_original_bboxes_line_list)))
- df_lines["categories"] = [id2label[label_idx] for label_idx in sorted_category_line_list]
- df_lines["texts"] = sorted_text_line_list
- df_lines["bounding boxes"] = [str(bbox) for bbox in sorted_original_bboxes_line_list]
-
- # save
- csv_lines = "csv_lines_" + original_filename.replace(".pdf", ".csv")
- df_lines.to_csv(csv_lines, encoding="utf-8", index=False)
-
- msg = f'The page {page_no} of the PDF "{original_filename}" (domain: "{domain}") matches your settings.'
-
- return msg, img_file, imgs["paragraphs"], imgs["lines"], img_paragraphs, img_lines, df_paragraphs, df_lines, gr.File.update(value=csv_paragraphs, visible=True), gr.File.update(value=csv_lines, visible=True)
-
-# gradio APP
-with gr.Blocks(title="DocLayNet image viewer", css=".gradio-container") as demo:
- gr.HTML("""
- DocLayNet image viewer
- (01/29/2023) This APP is an image viewer of the DocLayNet dataset and a data extraction tool.
- It uses the datasets DocLayNet small and DocLayNet base (you can also run this APP in Google Colab by running this notebook).
- Make your settings and the output will show 2 images of a randomly selected PDF with labeled bounding boxes, one of paragraphs and the other of lines, and their corresponding tables of texts with their labels.
- For example, if you select the domain "laws_and_regulations" and the category "Caption", you will get a random PDF that corresponds to these settings (ie, it will have at least one bounding box labeled with "Caption" in the PDF).
- WARNING: if the app crashes or runs without providing a result, refresh the page (DocLayNet image viewer) and run a search again. If the same problem occurs again, prefer the DocLayNet small. Thanks.
- More information about the DocLayNet datasets and this APP in the following blog post: (01/27/2023) Document AI | Processing of DocLayNet dataset to be used by layout models of the Hugging Face hub (finetuning, inference)
- """)
- with gr.Row():
- with gr.Column():
- dataset_name_gr = gr.Radio(dataset_names, value="small", label="DocLayNet dataset")
- with gr.Column():
- split_gr = gr.Dropdown(splits, value="all", label="Split")
- with gr.Column():
- domain_gr = gr.Dropdown(domains, value="all", label="Domain")
- with gr.Column():
- category_gr = gr.Dropdown(categories, value="all", label="Category")
- btn = gr.Button("Display labeled PDF image & data")
- with gr.Row():
- with gr.Column():
- output_msg = gr.Textbox(label="Output message")
- with gr.Column():
- img_file = gr.File(visible=True, label="Image file of the PDF")
- with gr.Row():
- with gr.Column():
- img_paragraphs_file = gr.File(visible=True, label="Image file (labeled paragraphs)")
- img_paragraphs = gr.Image(type="pil", label="Bounding boxes of labeled paragraphs", visible=True)
- with gr.Column():
- img_lines_file = gr.File(visible=True, label="Image file (labeled lines)")
- img_lines = gr.Image(type="pil", label="Bounding boxes of labeled lines", visible=True)
- with gr.Row():
- with gr.Column():
- with gr.Row():
- csv_paragraphs = gr.File(visible=False, label="CSV file (paragraphs)")
- with gr.Row():
- df_paragraphs = gr.Dataframe(
- headers=["paragraphs", "categories", "texts", "bounding boxes"],
- datatype=["number", "str", "str", "str"],
- col_count=(4, "fixed"),
- visible=True,
- label="Paragraphs data",
- type="pandas",
- wrap=True
- )
- with gr.Column():
- with gr.Row():
- csv_lines = gr.File(visible=False, label="CSV file (lines)")
- with gr.Row():
- df_lines = gr.Dataframe(
- headers=["lines", "categories", "texts", "bounding boxes"],
- datatype=["number", "str", "str", "str"],
- col_count=(4, "fixed"),
- visible=True,
- label="Lines data",
- type="pandas",
- wrap=True
- )
- btn.click(generate_annotated_image, inputs=[dataset_name_gr, split_gr, domain_gr, category_gr], outputs=[output_msg, img_file, img_paragraphs, img_lines, img_paragraphs_file, img_lines_file, df_paragraphs, df_lines, csv_paragraphs, csv_lines])
-
- gr.Markdown("## Example")
- gr.Examples(
- [["small", "all", "all", "all"]],
- [dataset_name_gr, split_gr, domain_gr, category_gr],
- [output_msg, img_file, img_paragraphs, img_lines, img_paragraphs_file, img_lines_file, df_paragraphs, df_lines, csv_paragraphs, csv_lines],
- fn=generate_annotated_image,
- cache_examples=True,
- )
-
-demo.launch()
\ No newline at end of file
diff --git a/spaces/pinkq/Newbing/src/components/ui/icons.tsx b/spaces/pinkq/Newbing/src/components/ui/icons.tsx
deleted file mode 100644
index 742b489b50437c5b64c86082f2ebc712eeb6a2b0..0000000000000000000000000000000000000000
--- a/spaces/pinkq/Newbing/src/components/ui/icons.tsx
+++ /dev/null
@@ -1,504 +0,0 @@
-'use client'
-
-import * as React from 'react'
-
-import { cn } from '@/lib/utils'
-
-function IconNextChat({
- className,
- inverted,
- ...props
-}: React.ComponentProps<'svg'> & { inverted?: boolean }) {
- const id = React.useId()
-
- return (
-
- )
-}
-
-function IconOpenAI({ className, ...props }: React.ComponentProps<'svg'>) {
- return (
-
- )
-}
-
-function IconGitHub({ className, ...props }: React.ComponentProps<'svg'>) {
- return (
-
- )
-}
-
-function IconSeparator({ className, ...props }: React.ComponentProps<'svg'>) {
- return (
-
- )
-}
-
-function IconArrowDown({ className, ...props }: React.ComponentProps<'svg'>) {
- return (
-
- )
-}
-
-function IconArrowRight({ className, ...props }: React.ComponentProps<'svg'>) {
- return (
-
- )
-}
-
-function IconUser({ className, ...props }: React.ComponentProps<'svg'>) {
- return (
-
- )
-}
-
-function IconPlus({ className, ...props }: React.ComponentProps<'svg'>) {
- return (
-
- )
-}
-
-function IconArrowElbow({ className, ...props }: React.ComponentProps<'svg'>) {
- return (
-
- )
-}
-
-function IconSpinner({ className, ...props }: React.ComponentProps<'svg'>) {
- return (
-
- )
-}
-
-function IconMessage({ className, ...props }: React.ComponentProps<'svg'>) {
- return (
-
- )
-}
-
-function IconTrash({ className, ...props }: React.ComponentProps<'svg'>) {
- return (
-
- )
-}
-
-function IconMore({ className, ...props }: React.ComponentProps<'svg'>) {
- return (
-
- )
-}
-
-function IconRefresh({ className, ...props }: React.ComponentProps<'svg'>) {
- return (
-
- )
-}
-
-function IconStop({ className, ...props }: React.ComponentProps<'svg'>) {
- return (
-
- )
-}
-
-function IconSidebar({ className, ...props }: React.ComponentProps<'svg'>) {
- return (
-
- )
-}
-
-function IconMoon({ className, ...props }: React.ComponentProps<'svg'>) {
- return (
-
- )
-}
-
-function IconSun({ className, ...props }: React.ComponentProps<'svg'>) {
- return (
-
- )
-}
-
-function IconCopy({ className, ...props }: React.ComponentProps<'svg'>) {
- return (
-
- )
-}
-
-function IconCheck({ className, ...props }: React.ComponentProps<'svg'>) {
- return (
-
- )
-}
-
-function IconDownload({ className, ...props }: React.ComponentProps<'svg'>) {
- return (
-
- )
-}
-
-function IconClose({ className, ...props }: React.ComponentProps<'svg'>) {
- return (
-
- )
-}
-
-function IconEdit({ className, ...props }: React.ComponentProps<'svg'>) {
- return (
-
- )
-}
-
-function IconShare({ className, ...props }: React.ComponentProps<'svg'>) {
- return (
-
- )
-}
-
-function IconUsers({ className, ...props }: React.ComponentProps<'svg'>) {
- return (
-
- )
-}
-
-function IconExternalLink({
- className,
- ...props
-}: React.ComponentProps<'svg'>) {
- return (
-
- )
-}
-
-function IconChevronUpDown({
- className,
- ...props
-}: React.ComponentProps<'svg'>) {
- return (
-
- )
-}
-
-export {
- IconEdit,
- IconNextChat,
- IconOpenAI,
- IconGitHub,
- IconSeparator,
- IconArrowDown,
- IconArrowRight,
- IconUser,
- IconPlus,
- IconArrowElbow,
- IconSpinner,
- IconMessage,
- IconTrash,
- IconMore,
- IconRefresh,
- IconStop,
- IconSidebar,
- IconMoon,
- IconSun,
- IconCopy,
- IconCheck,
- IconDownload,
- IconClose,
- IconShare,
- IconUsers,
- IconExternalLink,
- IconChevronUpDown
-}
diff --git a/spaces/pknez/face-swap-docker/mynewshinyroop/Lib/site-packages/pip/_vendor/packaging/_manylinux.py b/spaces/pknez/face-swap-docker/mynewshinyroop/Lib/site-packages/pip/_vendor/packaging/_manylinux.py
deleted file mode 100644
index 4c379aa6f69ff56c8f19612002c6e3e939ea6012..0000000000000000000000000000000000000000
--- a/spaces/pknez/face-swap-docker/mynewshinyroop/Lib/site-packages/pip/_vendor/packaging/_manylinux.py
+++ /dev/null
@@ -1,301 +0,0 @@
-import collections
-import functools
-import os
-import re
-import struct
-import sys
-import warnings
-from typing import IO, Dict, Iterator, NamedTuple, Optional, Tuple
-
-
-# Python does not provide platform information at sufficient granularity to
-# identify the architecture of the running executable in some cases, so we
-# determine it dynamically by reading the information from the running
-# process. This only applies on Linux, which uses the ELF format.
-class _ELFFileHeader:
- # https://en.wikipedia.org/wiki/Executable_and_Linkable_Format#File_header
- class _InvalidELFFileHeader(ValueError):
- """
- An invalid ELF file header was found.
- """
-
- ELF_MAGIC_NUMBER = 0x7F454C46
- ELFCLASS32 = 1
- ELFCLASS64 = 2
- ELFDATA2LSB = 1
- ELFDATA2MSB = 2
- EM_386 = 3
- EM_S390 = 22
- EM_ARM = 40
- EM_X86_64 = 62
- EF_ARM_ABIMASK = 0xFF000000
- EF_ARM_ABI_VER5 = 0x05000000
- EF_ARM_ABI_FLOAT_HARD = 0x00000400
-
- def __init__(self, file: IO[bytes]) -> None:
- def unpack(fmt: str) -> int:
- try:
- data = file.read(struct.calcsize(fmt))
- result: Tuple[int, ...] = struct.unpack(fmt, data)
- except struct.error:
- raise _ELFFileHeader._InvalidELFFileHeader()
- return result[0]
-
- self.e_ident_magic = unpack(">I")
- if self.e_ident_magic != self.ELF_MAGIC_NUMBER:
- raise _ELFFileHeader._InvalidELFFileHeader()
- self.e_ident_class = unpack("B")
- if self.e_ident_class not in {self.ELFCLASS32, self.ELFCLASS64}:
- raise _ELFFileHeader._InvalidELFFileHeader()
- self.e_ident_data = unpack("B")
- if self.e_ident_data not in {self.ELFDATA2LSB, self.ELFDATA2MSB}:
- raise _ELFFileHeader._InvalidELFFileHeader()
- self.e_ident_version = unpack("B")
- self.e_ident_osabi = unpack("B")
- self.e_ident_abiversion = unpack("B")
- self.e_ident_pad = file.read(7)
- format_h = "H"
- format_i = "I"
- format_q = "Q"
- format_p = format_i if self.e_ident_class == self.ELFCLASS32 else format_q
- self.e_type = unpack(format_h)
- self.e_machine = unpack(format_h)
- self.e_version = unpack(format_i)
- self.e_entry = unpack(format_p)
- self.e_phoff = unpack(format_p)
- self.e_shoff = unpack(format_p)
- self.e_flags = unpack(format_i)
- self.e_ehsize = unpack(format_h)
- self.e_phentsize = unpack(format_h)
- self.e_phnum = unpack(format_h)
- self.e_shentsize = unpack(format_h)
- self.e_shnum = unpack(format_h)
- self.e_shstrndx = unpack(format_h)
-
-
-def _get_elf_header() -> Optional[_ELFFileHeader]:
- try:
- with open(sys.executable, "rb") as f:
- elf_header = _ELFFileHeader(f)
- except (OSError, TypeError, _ELFFileHeader._InvalidELFFileHeader):
- return None
- return elf_header
-
-
-def _is_linux_armhf() -> bool:
- # hard-float ABI can be detected from the ELF header of the running
- # process
- # https://static.docs.arm.com/ihi0044/g/aaelf32.pdf
- elf_header = _get_elf_header()
- if elf_header is None:
- return False
- result = elf_header.e_ident_class == elf_header.ELFCLASS32
- result &= elf_header.e_ident_data == elf_header.ELFDATA2LSB
- result &= elf_header.e_machine == elf_header.EM_ARM
- result &= (
- elf_header.e_flags & elf_header.EF_ARM_ABIMASK
- ) == elf_header.EF_ARM_ABI_VER5
- result &= (
- elf_header.e_flags & elf_header.EF_ARM_ABI_FLOAT_HARD
- ) == elf_header.EF_ARM_ABI_FLOAT_HARD
- return result
-
-
-def _is_linux_i686() -> bool:
- elf_header = _get_elf_header()
- if elf_header is None:
- return False
- result = elf_header.e_ident_class == elf_header.ELFCLASS32
- result &= elf_header.e_ident_data == elf_header.ELFDATA2LSB
- result &= elf_header.e_machine == elf_header.EM_386
- return result
-
-
-def _have_compatible_abi(arch: str) -> bool:
- if arch == "armv7l":
- return _is_linux_armhf()
- if arch == "i686":
- return _is_linux_i686()
- return arch in {"x86_64", "aarch64", "ppc64", "ppc64le", "s390x"}
-
-
-# If glibc ever changes its major version, we need to know what the last
-# minor version was, so we can build the complete list of all versions.
-# For now, guess what the highest minor version might be, assume it will
-# be 50 for testing. Once this actually happens, update the dictionary
-# with the actual value.
-_LAST_GLIBC_MINOR: Dict[int, int] = collections.defaultdict(lambda: 50)
-
-
-class _GLibCVersion(NamedTuple):
- major: int
- minor: int
-
-
-def _glibc_version_string_confstr() -> Optional[str]:
- """
- Primary implementation of glibc_version_string using os.confstr.
- """
- # os.confstr is quite a bit faster than ctypes.DLL. It's also less likely
- # to be broken or missing. This strategy is used in the standard library
- # platform module.
- # https://github.com/python/cpython/blob/fcf1d003bf4f0100c/Lib/platform.py#L175-L183
- try:
- # os.confstr("CS_GNU_LIBC_VERSION") returns a string like "glibc 2.17".
- version_string = os.confstr("CS_GNU_LIBC_VERSION")
- assert version_string is not None
- _, version = version_string.split()
- except (AssertionError, AttributeError, OSError, ValueError):
- # os.confstr() or CS_GNU_LIBC_VERSION not available (or a bad value)...
- return None
- return version
-
-
-def _glibc_version_string_ctypes() -> Optional[str]:
- """
- Fallback implementation of glibc_version_string using ctypes.
- """
- try:
- import ctypes
- except ImportError:
- return None
-
- # ctypes.CDLL(None) internally calls dlopen(NULL), and as the dlopen
- # manpage says, "If filename is NULL, then the returned handle is for the
- # main program". This way we can let the linker do the work to figure out
- # which libc our process is actually using.
- #
- # We must also handle the special case where the executable is not a
- # dynamically linked executable. This can occur when using musl libc,
- # for example. In this situation, dlopen() will error, leading to an
- # OSError. Interestingly, at least in the case of musl, there is no
- # errno set on the OSError. The single string argument used to construct
- # OSError comes from libc itself and is therefore not portable to
- # hard code here. In any case, failure to call dlopen() means we
- # can proceed, so we bail on our attempt.
- try:
- process_namespace = ctypes.CDLL(None)
- except OSError:
- return None
-
- try:
- gnu_get_libc_version = process_namespace.gnu_get_libc_version
- except AttributeError:
- # Symbol doesn't exist -> therefore, we are not linked to
- # glibc.
- return None
-
- # Call gnu_get_libc_version, which returns a string like "2.5"
- gnu_get_libc_version.restype = ctypes.c_char_p
- version_str: str = gnu_get_libc_version()
- # py2 / py3 compatibility:
- if not isinstance(version_str, str):
- version_str = version_str.decode("ascii")
-
- return version_str
-
-
-def _glibc_version_string() -> Optional[str]:
- """Returns glibc version string, or None if not using glibc."""
- return _glibc_version_string_confstr() or _glibc_version_string_ctypes()
-
-
-def _parse_glibc_version(version_str: str) -> Tuple[int, int]:
- """Parse glibc version.
-
- We use a regexp instead of str.split because we want to discard any
- random junk that might come after the minor version -- this might happen
- in patched/forked versions of glibc (e.g. Linaro's version of glibc
- uses version strings like "2.20-2014.11"). See gh-3588.
- """
- m = re.match(r"(?P[0-9]+)\.(?P[0-9]+)", version_str)
- if not m:
- warnings.warn(
- "Expected glibc version with 2 components major.minor,"
- " got: %s" % version_str,
- RuntimeWarning,
- )
- return -1, -1
- return int(m.group("major")), int(m.group("minor"))
-
-
-@functools.lru_cache()
-def _get_glibc_version() -> Tuple[int, int]:
- version_str = _glibc_version_string()
- if version_str is None:
- return (-1, -1)
- return _parse_glibc_version(version_str)
-
-
-# From PEP 513, PEP 600
-def _is_compatible(name: str, arch: str, version: _GLibCVersion) -> bool:
- sys_glibc = _get_glibc_version()
- if sys_glibc < version:
- return False
- # Check for presence of _manylinux module.
- try:
- import _manylinux # noqa
- except ImportError:
- return True
- if hasattr(_manylinux, "manylinux_compatible"):
- result = _manylinux.manylinux_compatible(version[0], version[1], arch)
- if result is not None:
- return bool(result)
- return True
- if version == _GLibCVersion(2, 5):
- if hasattr(_manylinux, "manylinux1_compatible"):
- return bool(_manylinux.manylinux1_compatible)
- if version == _GLibCVersion(2, 12):
- if hasattr(_manylinux, "manylinux2010_compatible"):
- return bool(_manylinux.manylinux2010_compatible)
- if version == _GLibCVersion(2, 17):
- if hasattr(_manylinux, "manylinux2014_compatible"):
- return bool(_manylinux.manylinux2014_compatible)
- return True
-
-
-_LEGACY_MANYLINUX_MAP = {
- # CentOS 7 w/ glibc 2.17 (PEP 599)
- (2, 17): "manylinux2014",
- # CentOS 6 w/ glibc 2.12 (PEP 571)
- (2, 12): "manylinux2010",
- # CentOS 5 w/ glibc 2.5 (PEP 513)
- (2, 5): "manylinux1",
-}
-
-
-def platform_tags(linux: str, arch: str) -> Iterator[str]:
- if not _have_compatible_abi(arch):
- return
- # Oldest glibc to be supported regardless of architecture is (2, 17).
- too_old_glibc2 = _GLibCVersion(2, 16)
- if arch in {"x86_64", "i686"}:
- # On x86/i686 also oldest glibc to be supported is (2, 5).
- too_old_glibc2 = _GLibCVersion(2, 4)
- current_glibc = _GLibCVersion(*_get_glibc_version())
- glibc_max_list = [current_glibc]
- # We can assume compatibility across glibc major versions.
- # https://sourceware.org/bugzilla/show_bug.cgi?id=24636
- #
- # Build a list of maximum glibc versions so that we can
- # output the canonical list of all glibc from current_glibc
- # down to too_old_glibc2, including all intermediary versions.
- for glibc_major in range(current_glibc.major - 1, 1, -1):
- glibc_minor = _LAST_GLIBC_MINOR[glibc_major]
- glibc_max_list.append(_GLibCVersion(glibc_major, glibc_minor))
- for glibc_max in glibc_max_list:
- if glibc_max.major == too_old_glibc2.major:
- min_minor = too_old_glibc2.minor
- else:
- # For other glibc major versions oldest supported is (x, 0).
- min_minor = -1
- for glibc_minor in range(glibc_max.minor, min_minor, -1):
- glibc_version = _GLibCVersion(glibc_max.major, glibc_minor)
- tag = "manylinux_{}_{}".format(*glibc_version)
- if _is_compatible(tag, arch, glibc_version):
- yield linux.replace("linux", tag)
- # Handle the legacy manylinux1, manylinux2010, manylinux2014 tags.
- if glibc_version in _LEGACY_MANYLINUX_MAP:
- legacy_tag = _LEGACY_MANYLINUX_MAP[glibc_version]
- if _is_compatible(legacy_tag, arch, glibc_version):
- yield linux.replace("linux", legacy_tag)
diff --git a/spaces/pknez/face-swap-docker/mynewshinyroop/Lib/site-packages/setuptools/_distutils/debug.py b/spaces/pknez/face-swap-docker/mynewshinyroop/Lib/site-packages/setuptools/_distutils/debug.py
deleted file mode 100644
index daf1660f0d821143e388d37532a39ddfd2ca0347..0000000000000000000000000000000000000000
--- a/spaces/pknez/face-swap-docker/mynewshinyroop/Lib/site-packages/setuptools/_distutils/debug.py
+++ /dev/null
@@ -1,5 +0,0 @@
-import os
-
-# If DISTUTILS_DEBUG is anything other than the empty string, we run in
-# debug mode.
-DEBUG = os.environ.get('DISTUTILS_DEBUG')
diff --git a/spaces/pknez/face-swap-docker/mynewshinyroop/Lib/site-packages/wheel/vendored/packaging/version.py b/spaces/pknez/face-swap-docker/mynewshinyroop/Lib/site-packages/wheel/vendored/packaging/version.py
deleted file mode 100644
index e5c738cfda3656c4dc547275e64297f0eff80511..0000000000000000000000000000000000000000
--- a/spaces/pknez/face-swap-docker/mynewshinyroop/Lib/site-packages/wheel/vendored/packaging/version.py
+++ /dev/null
@@ -1,563 +0,0 @@
-# This file is dual licensed under the terms of the Apache License, Version
-# 2.0, and the BSD License. See the LICENSE file in the root of this repository
-# for complete details.
-"""
-.. testsetup::
-
- from packaging.version import parse, Version
-"""
-
-import collections
-import itertools
-import re
-from typing import Callable, Optional, SupportsInt, Tuple, Union
-
-from ._structures import Infinity, InfinityType, NegativeInfinity, NegativeInfinityType
-
-__all__ = ["VERSION_PATTERN", "parse", "Version", "InvalidVersion"]
-
-InfiniteTypes = Union[InfinityType, NegativeInfinityType]
-PrePostDevType = Union[InfiniteTypes, Tuple[str, int]]
-SubLocalType = Union[InfiniteTypes, int, str]
-LocalType = Union[
- NegativeInfinityType,
- Tuple[
- Union[
- SubLocalType,
- Tuple[SubLocalType, str],
- Tuple[NegativeInfinityType, SubLocalType],
- ],
- ...,
- ],
-]
-CmpKey = Tuple[
- int, Tuple[int, ...], PrePostDevType, PrePostDevType, PrePostDevType, LocalType
-]
-VersionComparisonMethod = Callable[[CmpKey, CmpKey], bool]
-
-_Version = collections.namedtuple(
- "_Version", ["epoch", "release", "dev", "pre", "post", "local"]
-)
-
-
-def parse(version: str) -> "Version":
- """Parse the given version string.
-
- >>> parse('1.0.dev1')
-
-
- :param version: The version string to parse.
- :raises InvalidVersion: When the version string is not a valid version.
- """
- return Version(version)
-
-
-class InvalidVersion(ValueError):
- """Raised when a version string is not a valid version.
-
- >>> Version("invalid")
- Traceback (most recent call last):
- ...
- packaging.version.InvalidVersion: Invalid version: 'invalid'
- """
-
-
-class _BaseVersion:
- _key: CmpKey
-
- def __hash__(self) -> int:
- return hash(self._key)
-
- # Please keep the duplicated `isinstance` check
- # in the six comparisons hereunder
- # unless you find a way to avoid adding overhead function calls.
- def __lt__(self, other: "_BaseVersion") -> bool:
- if not isinstance(other, _BaseVersion):
- return NotImplemented
-
- return self._key < other._key
-
- def __le__(self, other: "_BaseVersion") -> bool:
- if not isinstance(other, _BaseVersion):
- return NotImplemented
-
- return self._key <= other._key
-
- def __eq__(self, other: object) -> bool:
- if not isinstance(other, _BaseVersion):
- return NotImplemented
-
- return self._key == other._key
-
- def __ge__(self, other: "_BaseVersion") -> bool:
- if not isinstance(other, _BaseVersion):
- return NotImplemented
-
- return self._key >= other._key
-
- def __gt__(self, other: "_BaseVersion") -> bool:
- if not isinstance(other, _BaseVersion):
- return NotImplemented
-
- return self._key > other._key
-
- def __ne__(self, other: object) -> bool:
- if not isinstance(other, _BaseVersion):
- return NotImplemented
-
- return self._key != other._key
-
-
-# Deliberately not anchored to the start and end of the string, to make it
-# easier for 3rd party code to reuse
-_VERSION_PATTERN = r"""
- v?
- (?:
- (?:(?P[0-9]+)!)? # epoch
- (?P[0-9]+(?:\.[0-9]+)*) # release segment
- (?P # pre-release
- [-_\.]?
- (?P(a|b|c|rc|alpha|beta|pre|preview))
- [-_\.]?
- (?P[0-9]+)?
- )?
- (?P # post release
- (?:-(?P[0-9]+))
- |
- (?:
- [-_\.]?
- (?Ppost|rev|r)
- [-_\.]?
- (?P[0-9]+)?
- )
- )?
- (?P # dev release
- [-_\.]?
- (?Pdev)
- [-_\.]?
- (?P[0-9]+)?
- )?
- )
- (?:\+(?P[a-z0-9]+(?:[-_\.][a-z0-9]+)*))? # local version
-"""
-
-VERSION_PATTERN = _VERSION_PATTERN
-"""
-A string containing the regular expression used to match a valid version.
-
-The pattern is not anchored at either end, and is intended for embedding in larger
-expressions (for example, matching a version number as part of a file name). The
-regular expression should be compiled with the ``re.VERBOSE`` and ``re.IGNORECASE``
-flags set.
-
-:meta hide-value:
-"""
-
-
-class Version(_BaseVersion):
- """This class abstracts handling of a project's versions.
-
- A :class:`Version` instance is comparison aware and can be compared and
- sorted using the standard Python interfaces.
-
- >>> v1 = Version("1.0a5")
- >>> v2 = Version("1.0")
- >>> v1
-
- >>> v2
-
- >>> v1 < v2
- True
- >>> v1 == v2
- False
- >>> v1 > v2
- False
- >>> v1 >= v2
- False
- >>> v1 <= v2
- True
- """
-
- _regex = re.compile(r"^\s*" + VERSION_PATTERN + r"\s*$", re.VERBOSE | re.IGNORECASE)
-
- def __init__(self, version: str) -> None:
- """Initialize a Version object.
-
- :param version:
- The string representation of a version which will be parsed and normalized
- before use.
- :raises InvalidVersion:
- If the ``version`` does not conform to PEP 440 in any way then this
- exception will be raised.
- """
-
- # Validate the version and parse it into pieces
- match = self._regex.search(version)
- if not match:
- raise InvalidVersion(f"Invalid version: '{version}'")
-
- # Store the parsed out pieces of the version
- self._version = _Version(
- epoch=int(match.group("epoch")) if match.group("epoch") else 0,
- release=tuple(int(i) for i in match.group("release").split(".")),
- pre=_parse_letter_version(match.group("pre_l"), match.group("pre_n")),
- post=_parse_letter_version(
- match.group("post_l"), match.group("post_n1") or match.group("post_n2")
- ),
- dev=_parse_letter_version(match.group("dev_l"), match.group("dev_n")),
- local=_parse_local_version(match.group("local")),
- )
-
- # Generate a key which will be used for sorting
- self._key = _cmpkey(
- self._version.epoch,
- self._version.release,
- self._version.pre,
- self._version.post,
- self._version.dev,
- self._version.local,
- )
-
- def __repr__(self) -> str:
- """A representation of the Version that shows all internal state.
-
- >>> Version('1.0.0')
-
- """
- return f""
-
- def __str__(self) -> str:
- """A string representation of the version that can be rounded-tripped.
-
- >>> str(Version("1.0a5"))
- '1.0a5'
- """
- parts = []
-
- # Epoch
- if self.epoch != 0:
- parts.append(f"{self.epoch}!")
-
- # Release segment
- parts.append(".".join(str(x) for x in self.release))
-
- # Pre-release
- if self.pre is not None:
- parts.append("".join(str(x) for x in self.pre))
-
- # Post-release
- if self.post is not None:
- parts.append(f".post{self.post}")
-
- # Development release
- if self.dev is not None:
- parts.append(f".dev{self.dev}")
-
- # Local version segment
- if self.local is not None:
- parts.append(f"+{self.local}")
-
- return "".join(parts)
-
- @property
- def epoch(self) -> int:
- """The epoch of the version.
-
- >>> Version("2.0.0").epoch
- 0
- >>> Version("1!2.0.0").epoch
- 1
- """
- _epoch: int = self._version.epoch
- return _epoch
-
- @property
- def release(self) -> Tuple[int, ...]:
- """The components of the "release" segment of the version.
-
- >>> Version("1.2.3").release
- (1, 2, 3)
- >>> Version("2.0.0").release
- (2, 0, 0)
- >>> Version("1!2.0.0.post0").release
- (2, 0, 0)
-
- Includes trailing zeroes but not the epoch or any pre-release / development /
- post-release suffixes.
- """
- _release: Tuple[int, ...] = self._version.release
- return _release
-
- @property
- def pre(self) -> Optional[Tuple[str, int]]:
- """The pre-release segment of the version.
-
- >>> print(Version("1.2.3").pre)
- None
- >>> Version("1.2.3a1").pre
- ('a', 1)
- >>> Version("1.2.3b1").pre
- ('b', 1)
- >>> Version("1.2.3rc1").pre
- ('rc', 1)
- """
- _pre: Optional[Tuple[str, int]] = self._version.pre
- return _pre
-
- @property
- def post(self) -> Optional[int]:
- """The post-release number of the version.
-
- >>> print(Version("1.2.3").post)
- None
- >>> Version("1.2.3.post1").post
- 1
- """
- return self._version.post[1] if self._version.post else None
-
- @property
- def dev(self) -> Optional[int]:
- """The development number of the version.
-
- >>> print(Version("1.2.3").dev)
- None
- >>> Version("1.2.3.dev1").dev
- 1
- """
- return self._version.dev[1] if self._version.dev else None
-
- @property
- def local(self) -> Optional[str]:
- """The local version segment of the version.
-
- >>> print(Version("1.2.3").local)
- None
- >>> Version("1.2.3+abc").local
- 'abc'
- """
- if self._version.local:
- return ".".join(str(x) for x in self._version.local)
- else:
- return None
-
- @property
- def public(self) -> str:
- """The public portion of the version.
-
- >>> Version("1.2.3").public
- '1.2.3'
- >>> Version("1.2.3+abc").public
- '1.2.3'
- >>> Version("1.2.3+abc.dev1").public
- '1.2.3'
- """
- return str(self).split("+", 1)[0]
-
- @property
- def base_version(self) -> str:
- """The "base version" of the version.
-
- >>> Version("1.2.3").base_version
- '1.2.3'
- >>> Version("1.2.3+abc").base_version
- '1.2.3'
- >>> Version("1!1.2.3+abc.dev1").base_version
- '1!1.2.3'
-
- The "base version" is the public version of the project without any pre or post
- release markers.
- """
- parts = []
-
- # Epoch
- if self.epoch != 0:
- parts.append(f"{self.epoch}!")
-
- # Release segment
- parts.append(".".join(str(x) for x in self.release))
-
- return "".join(parts)
-
- @property
- def is_prerelease(self) -> bool:
- """Whether this version is a pre-release.
-
- >>> Version("1.2.3").is_prerelease
- False
- >>> Version("1.2.3a1").is_prerelease
- True
- >>> Version("1.2.3b1").is_prerelease
- True
- >>> Version("1.2.3rc1").is_prerelease
- True
- >>> Version("1.2.3dev1").is_prerelease
- True
- """
- return self.dev is not None or self.pre is not None
-
- @property
- def is_postrelease(self) -> bool:
- """Whether this version is a post-release.
-
- >>> Version("1.2.3").is_postrelease
- False
- >>> Version("1.2.3.post1").is_postrelease
- True
- """
- return self.post is not None
-
- @property
- def is_devrelease(self) -> bool:
- """Whether this version is a development release.
-
- >>> Version("1.2.3").is_devrelease
- False
- >>> Version("1.2.3.dev1").is_devrelease
- True
- """
- return self.dev is not None
-
- @property
- def major(self) -> int:
- """The first item of :attr:`release` or ``0`` if unavailable.
-
- >>> Version("1.2.3").major
- 1
- """
- return self.release[0] if len(self.release) >= 1 else 0
-
- @property
- def minor(self) -> int:
- """The second item of :attr:`release` or ``0`` if unavailable.
-
- >>> Version("1.2.3").minor
- 2
- >>> Version("1").minor
- 0
- """
- return self.release[1] if len(self.release) >= 2 else 0
-
- @property
- def micro(self) -> int:
- """The third item of :attr:`release` or ``0`` if unavailable.
-
- >>> Version("1.2.3").micro
- 3
- >>> Version("1").micro
- 0
- """
- return self.release[2] if len(self.release) >= 3 else 0
-
-
-def _parse_letter_version(
- letter: str, number: Union[str, bytes, SupportsInt]
-) -> Optional[Tuple[str, int]]:
-
- if letter:
- # We consider there to be an implicit 0 in a pre-release if there is
- # not a numeral associated with it.
- if number is None:
- number = 0
-
- # We normalize any letters to their lower case form
- letter = letter.lower()
-
- # We consider some words to be alternate spellings of other words and
- # in those cases we want to normalize the spellings to our preferred
- # spelling.
- if letter == "alpha":
- letter = "a"
- elif letter == "beta":
- letter = "b"
- elif letter in ["c", "pre", "preview"]:
- letter = "rc"
- elif letter in ["rev", "r"]:
- letter = "post"
-
- return letter, int(number)
- if not letter and number:
- # We assume if we are given a number, but we are not given a letter
- # then this is using the implicit post release syntax (e.g. 1.0-1)
- letter = "post"
-
- return letter, int(number)
-
- return None
-
-
-_local_version_separators = re.compile(r"[\._-]")
-
-
-def _parse_local_version(local: str) -> Optional[LocalType]:
- """
- Takes a string like abc.1.twelve and turns it into ("abc", 1, "twelve").
- """
- if local is not None:
- return tuple(
- part.lower() if not part.isdigit() else int(part)
- for part in _local_version_separators.split(local)
- )
- return None
-
-
-def _cmpkey(
- epoch: int,
- release: Tuple[int, ...],
- pre: Optional[Tuple[str, int]],
- post: Optional[Tuple[str, int]],
- dev: Optional[Tuple[str, int]],
- local: Optional[Tuple[SubLocalType]],
-) -> CmpKey:
-
- # When we compare a release version, we want to compare it with all of the
- # trailing zeros removed. So we'll use a reverse the list, drop all the now
- # leading zeros until we come to something non zero, then take the rest
- # re-reverse it back into the correct order and make it a tuple and use
- # that for our sorting key.
- _release = tuple(
- reversed(list(itertools.dropwhile(lambda x: x == 0, reversed(release))))
- )
-
- # We need to "trick" the sorting algorithm to put 1.0.dev0 before 1.0a0.
- # We'll do this by abusing the pre segment, but we _only_ want to do this
- # if there is not a pre or a post segment. If we have one of those then
- # the normal sorting rules will handle this case correctly.
- if pre is None and post is None and dev is not None:
- _pre: PrePostDevType = NegativeInfinity
- # Versions without a pre-release (except as noted above) should sort after
- # those with one.
- elif pre is None:
- _pre = Infinity
- else:
- _pre = pre
-
- # Versions without a post segment should sort before those with one.
- if post is None:
- _post: PrePostDevType = NegativeInfinity
-
- else:
- _post = post
-
- # Versions without a development segment should sort after those with one.
- if dev is None:
- _dev: PrePostDevType = Infinity
-
- else:
- _dev = dev
-
- if local is None:
- # Versions without a local segment should sort before those with one.
- _local: LocalType = NegativeInfinity
- else:
- # Versions with a local segment need that segment parsed to implement
- # the sorting rules in PEP440.
- # - Alpha numeric segments sort before numeric segments
- # - Alpha numeric segments sort lexicographically
- # - Numeric segments sort numerically
- # - Shorter versions sort before longer versions when the prefixes
- # match exactly
- _local = tuple(
- (i, "") if isinstance(i, int) else (NegativeInfinity, i) for i in local
- )
-
- return epoch, _release, _pre, _post, _dev, _local
diff --git a/spaces/pknez/face-swap-docker/plugins/plugin_gfpgan.py b/spaces/pknez/face-swap-docker/plugins/plugin_gfpgan.py
deleted file mode 100644
index 8f745332d492fc87b435ba1e98d1ee502d62dfb3..0000000000000000000000000000000000000000
--- a/spaces/pknez/face-swap-docker/plugins/plugin_gfpgan.py
+++ /dev/null
@@ -1,85 +0,0 @@
-from chain_img_processor import ChainImgProcessor, ChainImgPlugin
-import os
-import gfpgan
-import threading
-from PIL import Image
-from numpy import asarray
-import cv2
-
-from roop.utilities import resolve_relative_path, conditional_download
-modname = os.path.basename(__file__)[:-3] # calculating modname
-
-model_gfpgan = None
-THREAD_LOCK_GFPGAN = threading.Lock()
-
-
-# start function
-def start(core:ChainImgProcessor):
- manifest = { # plugin settings
- "name": "GFPGAN", # name
- "version": "1.4", # version
-
- "default_options": {},
- "img_processor": {
- "gfpgan": GFPGAN
- }
- }
- return manifest
-
-def start_with_options(core:ChainImgProcessor, manifest:dict):
- pass
-
-
-class GFPGAN(ChainImgPlugin):
-
- def init_plugin(self):
- global model_gfpgan
-
- if model_gfpgan is None:
- model_path = resolve_relative_path('../models/GFPGANv1.4.pth')
- model_gfpgan = gfpgan.GFPGANer(model_path=model_path, upscale=1, device=self.device) # type: ignore[attr-defined]
-
-
-
- def process(self, frame, params:dict):
- import copy
-
- global model_gfpgan
-
- if model_gfpgan is None:
- return frame
-
- if "face_detected" in params:
- if not params["face_detected"]:
- return frame
- # don't touch original
- temp_frame = copy.copy(frame)
- if "processed_faces" in params:
- for face in params["processed_faces"]:
- start_x, start_y, end_x, end_y = map(int, face['bbox'])
- padding_x = int((end_x - start_x) * 0.5)
- padding_y = int((end_y - start_y) * 0.5)
- start_x = max(0, start_x - padding_x)
- start_y = max(0, start_y - padding_y)
- end_x = max(0, end_x + padding_x)
- end_y = max(0, end_y + padding_y)
- temp_face = temp_frame[start_y:end_y, start_x:end_x]
- if temp_face.size:
- with THREAD_LOCK_GFPGAN:
- _, _, temp_face = model_gfpgan.enhance(
- temp_face,
- paste_back=True
- )
- temp_frame[start_y:end_y, start_x:end_x] = temp_face
- else:
- with THREAD_LOCK_GFPGAN:
- _, _, temp_frame = model_gfpgan.enhance(
- temp_frame,
- paste_back=True
- )
-
- if not "blend_ratio" in params:
- return temp_frame
-
- temp_frame = Image.blend(Image.fromarray(frame), Image.fromarray(temp_frame), params["blend_ratio"])
- return asarray(temp_frame)
diff --git a/spaces/plzdontcry/dakubettergpt/src/components/TokenCount/index.ts b/spaces/plzdontcry/dakubettergpt/src/components/TokenCount/index.ts
deleted file mode 100644
index c8959179525dd6067eb2cb5eb2629da738948d3f..0000000000000000000000000000000000000000
--- a/spaces/plzdontcry/dakubettergpt/src/components/TokenCount/index.ts
+++ /dev/null
@@ -1 +0,0 @@
-export { default } from './TokenCount';
diff --git a/spaces/plzdontcry/dakubettergpt/src/types/export.ts b/spaces/plzdontcry/dakubettergpt/src/types/export.ts
deleted file mode 100644
index f275ee7a60a4e3adf4173254a853d0e5ad14f04a..0000000000000000000000000000000000000000
--- a/spaces/plzdontcry/dakubettergpt/src/types/export.ts
+++ /dev/null
@@ -1,32 +0,0 @@
-import { ChatInterface, FolderCollection, Role } from './chat';
-
-export interface ExportBase {
- version: number;
-}
-
-export interface ExportV1 extends ExportBase {
- chats?: ChatInterface[];
- folders: FolderCollection;
-}
-
-export type OpenAIChat = {
- title: string;
- mapping: {
- [key: string]: {
- id: string;
- message?: {
- author: {
- role: Role;
- };
- content: {
- parts?: string[];
- };
- } | null;
- parent: string | null;
- children: string[];
- };
- };
- current_node: string;
-};
-
-export default ExportV1;
diff --git a/spaces/pragnakalp/Question_Generation_T5/run_qg.py b/spaces/pragnakalp/Question_Generation_T5/run_qg.py
deleted file mode 100644
index e5093bfc3b3c887af86c8f171a2bff09db6b6d28..0000000000000000000000000000000000000000
--- a/spaces/pragnakalp/Question_Generation_T5/run_qg.py
+++ /dev/null
@@ -1,73 +0,0 @@
-import argparse
-import numpy as np
-from questiongenerator import QuestionGenerator
-from questiongenerator import print_qa
-
-def main():
- parser = argparse.ArgumentParser()
- parser.add_argument(
- "--text_dir",
- default=None,
- type=str,
- required=True,
- help="The text that will be used as context for question generation.",
- )
- parser.add_argument(
- "--model_dir",
- default=None,
- type=str,
- help="The folder that the trained model checkpoints are in.",
- )
- parser.add_argument(
- "--num_questions",
- default=10,
- type=int,
- help="The desired number of questions to generate.",
- )
- parser.add_argument(
- "--answer_style",
- default="all",
- type=str,
- help="The desired type of answers. Choose from ['all', 'sentences', 'multiple_choice']",
- )
- parser.add_argument(
- "--show_answers",
- default='True',
- type=parse_bool_string,
- help="Whether or not you want the answers to be visible. Choose from ['True', 'False']",
- )
- parser.add_argument(
- "--use_qa_eval",
- default='True',
- type=parse_bool_string,
- help="Whether or not you want the generated questions to be filtered for quality. Choose from ['True', 'False']",
- )
- args = parser.parse_args()
-
- with open(args.text_dir, 'r') as file:
- text_file = file.read()
-
- qg = QuestionGenerator(args.model_dir)
-
- qa_list = qg.generate(
- text_file,
- num_questions=int(args.num_questions),
- answer_style=args.answer_style,
- use_evaluator=args.use_qa_eval
- )
- print_qa(qa_list, show_answers=args.show_answers)
-
-# taken from https://stackoverflow.com/questions/15008758/parsing-boolean-values-with-argparse
-def parse_bool_string(s):
- if isinstance(s, bool):
- return s
- if s.lower() in ('yes', 'true', 't', 'y', '1'):
- return True
- elif s.lower() in ('no', 'false', 'f', 'n', '0'):
- return False
- else:
- raise argparse.ArgumentTypeError('Boolean value expected.')
-
-
-if __name__ == "__main__":
- main()
diff --git a/spaces/profayle/TerrapinTalk/myenv/lib/python3.9/site-packages/fontTools/ufoLib/utils.py b/spaces/profayle/TerrapinTalk/myenv/lib/python3.9/site-packages/fontTools/ufoLib/utils.py
deleted file mode 100644
index 85878b47a1133f131e74b3d16e4799537a8c50a1..0000000000000000000000000000000000000000
--- a/spaces/profayle/TerrapinTalk/myenv/lib/python3.9/site-packages/fontTools/ufoLib/utils.py
+++ /dev/null
@@ -1,75 +0,0 @@
-"""The module contains miscellaneous helpers.
-It's not considered part of the public ufoLib API.
-"""
-import warnings
-import functools
-
-
-numberTypes = (int, float)
-
-
-def deprecated(msg=""):
- """Decorator factory to mark functions as deprecated with given message.
-
- >>> @deprecated("Enough!")
- ... def some_function():
- ... "I just print 'hello world'."
- ... print("hello world")
- >>> some_function()
- hello world
- >>> some_function.__doc__ == "I just print 'hello world'."
- True
- """
-
- def deprecated_decorator(func):
- @functools.wraps(func)
- def wrapper(*args, **kwargs):
- warnings.warn(
- f"{func.__name__} function is a deprecated. {msg}",
- category=DeprecationWarning,
- stacklevel=2,
- )
- return func(*args, **kwargs)
-
- return wrapper
-
- return deprecated_decorator
-
-
-# To be mixed with enum.Enum in UFOFormatVersion and GLIFFormatVersion
-class _VersionTupleEnumMixin:
- @property
- def major(self):
- return self.value[0]
-
- @property
- def minor(self):
- return self.value[1]
-
- @classmethod
- def _missing_(cls, value):
- # allow to initialize a version enum from a single (major) integer
- if isinstance(value, int):
- return cls((value, 0))
- # or from None to obtain the current default version
- if value is None:
- return cls.default()
- return super()._missing_(value)
-
- def __str__(self):
- return f"{self.major}.{self.minor}"
-
- @classmethod
- def default(cls):
- # get the latest defined version (i.e. the max of all versions)
- return max(cls.__members__.values())
-
- @classmethod
- def supported_versions(cls):
- return frozenset(cls.__members__.values())
-
-
-if __name__ == "__main__":
- import doctest
-
- doctest.testmod()
diff --git a/spaces/profayle/TerrapinTalk/myenv/lib/python3.9/site-packages/gradio/templates/cdn/assets/Example-f75cba10.css b/spaces/profayle/TerrapinTalk/myenv/lib/python3.9/site-packages/gradio/templates/cdn/assets/Example-f75cba10.css
deleted file mode 100644
index 5407a69e2bcc27d96b2a0ba576fc2ac67b8ee414..0000000000000000000000000000000000000000
--- a/spaces/profayle/TerrapinTalk/myenv/lib/python3.9/site-packages/gradio/templates/cdn/assets/Example-f75cba10.css
+++ /dev/null
@@ -1 +0,0 @@
-pre.svelte-agpzo2{text-align:left}.gallery.svelte-agpzo2{padding:var(--size-1) var(--size-2)}
diff --git a/spaces/profayle/TerrapinTalk/myenv/lib/python3.9/site-packages/pandas/tests/io/parser/test_c_parser_only.py b/spaces/profayle/TerrapinTalk/myenv/lib/python3.9/site-packages/pandas/tests/io/parser/test_c_parser_only.py
deleted file mode 100644
index 32a010b3aeb3465ab149ca6841b932105940d06a..0000000000000000000000000000000000000000
--- a/spaces/profayle/TerrapinTalk/myenv/lib/python3.9/site-packages/pandas/tests/io/parser/test_c_parser_only.py
+++ /dev/null
@@ -1,664 +0,0 @@
-"""
-Tests that apply specifically to the CParser. Unless specifically stated
-as a CParser-specific issue, the goal is to eventually move as many of
-these tests out of this module as soon as the Python parser can accept
-further arguments when parsing.
-"""
-from decimal import Decimal
-from io import (
- BytesIO,
- StringIO,
- TextIOWrapper,
-)
-import mmap
-import os
-import tarfile
-
-import numpy as np
-import pytest
-
-from pandas.compat import is_ci_environment
-from pandas.compat.numpy import np_version_gte1p24
-from pandas.errors import ParserError
-import pandas.util._test_decorators as td
-
-from pandas import (
- DataFrame,
- concat,
-)
-import pandas._testing as tm
-
-
-@pytest.mark.parametrize(
- "malformed",
- ["1\r1\r1\r 1\r 1\r", "1\r1\r1\r 1\r 1\r11\r", "1\r1\r1\r 1\r 1\r11\r1\r"],
- ids=["words pointer", "stream pointer", "lines pointer"],
-)
-def test_buffer_overflow(c_parser_only, malformed):
- # see gh-9205: test certain malformed input files that cause
- # buffer overflows in tokenizer.c
- msg = "Buffer overflow caught - possible malformed input file."
- parser = c_parser_only
-
- with pytest.raises(ParserError, match=msg):
- parser.read_csv(StringIO(malformed))
-
-
-def test_delim_whitespace_custom_terminator(c_parser_only):
- # See gh-12912
- data = "a b c~1 2 3~4 5 6~7 8 9"
- parser = c_parser_only
-
- df = parser.read_csv(StringIO(data), lineterminator="~", delim_whitespace=True)
- expected = DataFrame([[1, 2, 3], [4, 5, 6], [7, 8, 9]], columns=["a", "b", "c"])
- tm.assert_frame_equal(df, expected)
-
-
-def test_dtype_and_names_error(c_parser_only):
- # see gh-8833: passing both dtype and names
- # resulting in an error reporting issue
- parser = c_parser_only
- data = """
-1.0 1
-2.0 2
-3.0 3
-"""
- # base cases
- result = parser.read_csv(StringIO(data), sep=r"\s+", header=None)
- expected = DataFrame([[1.0, 1], [2.0, 2], [3.0, 3]])
- tm.assert_frame_equal(result, expected)
-
- result = parser.read_csv(StringIO(data), sep=r"\s+", header=None, names=["a", "b"])
- expected = DataFrame([[1.0, 1], [2.0, 2], [3.0, 3]], columns=["a", "b"])
- tm.assert_frame_equal(result, expected)
-
- # fallback casting
- result = parser.read_csv(
- StringIO(data), sep=r"\s+", header=None, names=["a", "b"], dtype={"a": np.int32}
- )
- expected = DataFrame([[1, 1], [2, 2], [3, 3]], columns=["a", "b"])
- expected["a"] = expected["a"].astype(np.int32)
- tm.assert_frame_equal(result, expected)
-
- data = """
-1.0 1
-nan 2
-3.0 3
-"""
- # fallback casting, but not castable
- warning = RuntimeWarning if np_version_gte1p24 else None
- with pytest.raises(ValueError, match="cannot safely convert"):
- with tm.assert_produces_warning(warning, check_stacklevel=False):
- parser.read_csv(
- StringIO(data),
- sep=r"\s+",
- header=None,
- names=["a", "b"],
- dtype={"a": np.int32},
- )
-
-
-@pytest.mark.parametrize(
- "match,kwargs",
- [
- # For each of these cases, all of the dtypes are valid, just unsupported.
- (
- (
- "the dtype datetime64 is not supported for parsing, "
- "pass this column using parse_dates instead"
- ),
- {"dtype": {"A": "datetime64", "B": "float64"}},
- ),
- (
- (
- "the dtype datetime64 is not supported for parsing, "
- "pass this column using parse_dates instead"
- ),
- {"dtype": {"A": "datetime64", "B": "float64"}, "parse_dates": ["B"]},
- ),
- (
- "the dtype timedelta64 is not supported for parsing",
- {"dtype": {"A": "timedelta64", "B": "float64"}},
- ),
- (
- f"the dtype {tm.ENDIAN}U8 is not supported for parsing",
- {"dtype": {"A": "U8"}},
- ),
- ],
- ids=["dt64-0", "dt64-1", "td64", f"{tm.ENDIAN}U8"],
-)
-def test_unsupported_dtype(c_parser_only, match, kwargs):
- parser = c_parser_only
- df = DataFrame(
- np.random.default_rng(2).random((5, 2)),
- columns=list("AB"),
- index=["1A", "1B", "1C", "1D", "1E"],
- )
-
- with tm.ensure_clean("__unsupported_dtype__.csv") as path:
- df.to_csv(path)
-
- with pytest.raises(TypeError, match=match):
- parser.read_csv(path, index_col=0, **kwargs)
-
-
-@td.skip_if_32bit
-@pytest.mark.slow
-def test_precise_conversion(c_parser_only):
- parser = c_parser_only
-
- normal_errors = []
- precise_errors = []
-
- def error(val: float, actual_val: Decimal) -> Decimal:
- return abs(Decimal(f"{val:.100}") - actual_val)
-
- # test numbers between 1 and 2
- for num in np.linspace(1.0, 2.0, num=500):
- # 25 decimal digits of precision
- text = f"a\n{num:.25}"
-
- normal_val = float(
- parser.read_csv(StringIO(text), float_precision="legacy")["a"][0]
- )
- precise_val = float(
- parser.read_csv(StringIO(text), float_precision="high")["a"][0]
- )
- roundtrip_val = float(
- parser.read_csv(StringIO(text), float_precision="round_trip")["a"][0]
- )
- actual_val = Decimal(text[2:])
-
- normal_errors.append(error(normal_val, actual_val))
- precise_errors.append(error(precise_val, actual_val))
-
- # round-trip should match float()
- assert roundtrip_val == float(text[2:])
-
- assert sum(precise_errors) <= sum(normal_errors)
- assert max(precise_errors) <= max(normal_errors)
-
-
-def test_usecols_dtypes(c_parser_only):
- parser = c_parser_only
- data = """\
-1,2,3
-4,5,6
-7,8,9
-10,11,12"""
-
- result = parser.read_csv(
- StringIO(data),
- usecols=(0, 1, 2),
- names=("a", "b", "c"),
- header=None,
- converters={"a": str},
- dtype={"b": int, "c": float},
- )
- result2 = parser.read_csv(
- StringIO(data),
- usecols=(0, 2),
- names=("a", "b", "c"),
- header=None,
- converters={"a": str},
- dtype={"b": int, "c": float},
- )
-
- assert (result.dtypes == [object, int, float]).all()
- assert (result2.dtypes == [object, float]).all()
-
-
-def test_disable_bool_parsing(c_parser_only):
- # see gh-2090
-
- parser = c_parser_only
- data = """A,B,C
-Yes,No,Yes
-No,Yes,Yes
-Yes,,Yes
-No,No,No"""
-
- result = parser.read_csv(StringIO(data), dtype=object)
- assert (result.dtypes == object).all()
-
- result = parser.read_csv(StringIO(data), dtype=object, na_filter=False)
- assert result["B"][2] == ""
-
-
-def test_custom_lineterminator(c_parser_only):
- parser = c_parser_only
- data = "a,b,c~1,2,3~4,5,6"
-
- result = parser.read_csv(StringIO(data), lineterminator="~")
- expected = parser.read_csv(StringIO(data.replace("~", "\n")))
-
- tm.assert_frame_equal(result, expected)
-
-
-def test_parse_ragged_csv(c_parser_only):
- parser = c_parser_only
- data = """1,2,3
-1,2,3,4
-1,2,3,4,5
-1,2
-1,2,3,4"""
-
- nice_data = """1,2,3,,
-1,2,3,4,
-1,2,3,4,5
-1,2,,,
-1,2,3,4,"""
- result = parser.read_csv(
- StringIO(data), header=None, names=["a", "b", "c", "d", "e"]
- )
-
- expected = parser.read_csv(
- StringIO(nice_data), header=None, names=["a", "b", "c", "d", "e"]
- )
-
- tm.assert_frame_equal(result, expected)
-
- # too many columns, cause segfault if not careful
- data = "1,2\n3,4,5"
-
- result = parser.read_csv(StringIO(data), header=None, names=range(50))
- expected = parser.read_csv(StringIO(data), header=None, names=range(3)).reindex(
- columns=range(50)
- )
-
- tm.assert_frame_equal(result, expected)
-
-
-def test_tokenize_CR_with_quoting(c_parser_only):
- # see gh-3453
- parser = c_parser_only
- data = ' a,b,c\r"a,b","e,d","f,f"'
-
- result = parser.read_csv(StringIO(data), header=None)
- expected = parser.read_csv(StringIO(data.replace("\r", "\n")), header=None)
- tm.assert_frame_equal(result, expected)
-
- result = parser.read_csv(StringIO(data))
- expected = parser.read_csv(StringIO(data.replace("\r", "\n")))
- tm.assert_frame_equal(result, expected)
-
-
-@pytest.mark.slow
-def test_grow_boundary_at_cap(c_parser_only):
- # See gh-12494
- #
- # Cause of error was that the C parser
- # was not increasing the buffer size when
- # the desired space would fill the buffer
- # to capacity, which would later cause a
- # buffer overflow error when checking the
- # EOF terminator of the CSV stream.
- parser = c_parser_only
-
- def test_empty_header_read(count):
- with StringIO("," * count) as s:
- expected = DataFrame(columns=[f"Unnamed: {i}" for i in range(count + 1)])
- df = parser.read_csv(s)
- tm.assert_frame_equal(df, expected)
-
- for cnt in range(1, 101):
- test_empty_header_read(cnt)
-
-
-def test_parse_trim_buffers(c_parser_only):
- # This test is part of a bugfix for gh-13703. It attempts to
- # to stress the system memory allocator, to cause it to move the
- # stream buffer and either let the OS reclaim the region, or let
- # other memory requests of parser otherwise modify the contents
- # of memory space, where it was formally located.
- # This test is designed to cause a `segfault` with unpatched
- # `tokenizer.c`. Sometimes the test fails on `segfault`, other
- # times it fails due to memory corruption, which causes the
- # loaded DataFrame to differ from the expected one.
-
- parser = c_parser_only
-
- # Generate a large mixed-type CSV file on-the-fly (one record is
- # approx 1.5KiB).
- record_ = (
- """9999-9,99:99,,,,ZZ,ZZ,,,ZZZ-ZZZZ,.Z-ZZZZ,-9.99,,,9.99,Z"""
- """ZZZZ,,-99,9,ZZZ-ZZZZ,ZZ-ZZZZ,,9.99,ZZZ-ZZZZZ,ZZZ-ZZZZZ,"""
- """ZZZ-ZZZZ,ZZZ-ZZZZ,ZZZ-ZZZZ,ZZZ-ZZZZ,ZZZ-ZZZZ,ZZZ-ZZZZ,9"""
- """99,ZZZ-ZZZZ,,ZZ-ZZZZ,,,,,ZZZZ,ZZZ-ZZZZZ,ZZZ-ZZZZ,,,9,9,"""
- """9,9,99,99,999,999,ZZZZZ,ZZZ-ZZZZZ,ZZZ-ZZZZ,9,ZZ-ZZZZ,9."""
- """99,ZZ-ZZZZ,ZZ-ZZZZ,,,,ZZZZ,,,ZZ,ZZ,,,,,,,,,,,,,9,,,999."""
- """99,999.99,,,ZZZZZ,,,Z9,,,,,,,ZZZ,ZZZ,,,,,,,,,,,ZZZZZ,ZZ"""
- """ZZZ,ZZZ-ZZZZZZ,ZZZ-ZZZZZZ,ZZ-ZZZZ,ZZ-ZZZZ,ZZ-ZZZZ,ZZ-ZZ"""
- """ZZ,,,999999,999999,ZZZ,ZZZ,,,ZZZ,ZZZ,999.99,999.99,,,,Z"""
- """ZZ-ZZZ,ZZZ-ZZZ,-9.99,-9.99,9,9,,99,,9.99,9.99,9,9,9.99,"""
- """9.99,,,,9.99,9.99,,99,,99,9.99,9.99,,,ZZZ,ZZZ,,999.99,,"""
- """999.99,ZZZ,ZZZ-ZZZZ,ZZZ-ZZZZ,,,ZZZZZ,ZZZZZ,ZZZ,ZZZ,9,9,"""
- """,,,,,ZZZ-ZZZZ,ZZZ999Z,,,999.99,,999.99,ZZZ-ZZZZ,,,9.999"""
- """,9.999,9.999,9.999,-9.999,-9.999,-9.999,-9.999,9.999,9."""
- """999,9.999,9.999,9.999,9.999,9.999,9.999,99999,ZZZ-ZZZZ,"""
- """,9.99,ZZZ,,,,,,,,ZZZ,,,,,9,,,,9,,,,,,,,,,ZZZ-ZZZZ,ZZZ-Z"""
- """ZZZ,,ZZZZZ,ZZZZZ,ZZZZZ,ZZZZZ,,,9.99,,ZZ-ZZZZ,ZZ-ZZZZ,ZZ"""
- """,999,,,,ZZ-ZZZZ,ZZZ,ZZZ,ZZZ-ZZZZ,ZZZ-ZZZZ,,,99.99,99.99"""
- """,,,9.99,9.99,9.99,9.99,ZZZ-ZZZZ,,,ZZZ-ZZZZZ,,,,,-9.99,-"""
- """9.99,-9.99,-9.99,,,,,,,,,ZZZ-ZZZZ,,9,9.99,9.99,99ZZ,,-9"""
- """.99,-9.99,ZZZ-ZZZZ,,,,,,,ZZZ-ZZZZ,9.99,9.99,9999,,,,,,,"""
- """,,,-9.9,Z/Z-ZZZZ,999.99,9.99,,999.99,ZZ-ZZZZ,ZZ-ZZZZ,9."""
- """99,9.99,9.99,9.99,9.99,9.99,,ZZZ-ZZZZZ,ZZZ-ZZZZZ,ZZZ-ZZ"""
- """ZZZ,ZZZ-ZZZZZ,ZZZ-ZZZZZ,ZZZ,ZZZ,ZZZ,ZZZ,9.99,,,-9.99,ZZ"""
- """-ZZZZ,-999.99,,-9999,,999.99,,,,999.99,99.99,,,ZZ-ZZZZZ"""
- """ZZZ,ZZ-ZZZZ-ZZZZZZZ,,,,ZZ-ZZ-ZZZZZZZZ,ZZZZZZZZ,ZZZ-ZZZZ"""
- """,9999,999.99,ZZZ-ZZZZ,-9.99,-9.99,ZZZ-ZZZZ,99:99:99,,99"""
- """,99,,9.99,,-99.99,,,,,,9.99,ZZZ-ZZZZ,-9.99,-9.99,9.99,9"""
- """.99,,ZZZ,,,,,,,ZZZ,ZZZ,,,,,"""
- )
-
- # Set the number of lines so that a call to `parser_trim_buffers`
- # is triggered: after a couple of full chunks are consumed a
- # relatively small 'residual' chunk would cause reallocation
- # within the parser.
- chunksize, n_lines = 128, 2 * 128 + 15
- csv_data = "\n".join([record_] * n_lines) + "\n"
-
- # We will use StringIO to load the CSV from this text buffer.
- # pd.read_csv() will iterate over the file in chunks and will
- # finally read a residual chunk of really small size.
-
- # Generate the expected output: manually create the dataframe
- # by splitting by comma and repeating the `n_lines` times.
- row = tuple(val_ if val_ else np.nan for val_ in record_.split(","))
- expected = DataFrame(
- [row for _ in range(n_lines)], dtype=object, columns=None, index=None
- )
-
- # Iterate over the CSV file in chunks of `chunksize` lines
- with parser.read_csv(
- StringIO(csv_data), header=None, dtype=object, chunksize=chunksize
- ) as chunks_:
- result = concat(chunks_, axis=0, ignore_index=True)
-
- # Check for data corruption if there was no segfault
- tm.assert_frame_equal(result, expected)
-
- # This extra test was added to replicate the fault in gh-5291.
- # Force 'utf-8' encoding, so that `_string_convert` would take
- # a different execution branch.
- with parser.read_csv(
- StringIO(csv_data),
- header=None,
- dtype=object,
- chunksize=chunksize,
- encoding="utf_8",
- ) as chunks_:
- result = concat(chunks_, axis=0, ignore_index=True)
- tm.assert_frame_equal(result, expected)
-
-
-def test_internal_null_byte(c_parser_only):
- # see gh-14012
- #
- # The null byte ('\x00') should not be used as a
- # true line terminator, escape character, or comment
- # character, only as a placeholder to indicate that
- # none was specified.
- #
- # This test should be moved to test_common.py ONLY when
- # Python's csv class supports parsing '\x00'.
- parser = c_parser_only
-
- names = ["a", "b", "c"]
- data = "1,2,3\n4,\x00,6\n7,8,9"
- expected = DataFrame([[1, 2.0, 3], [4, np.nan, 6], [7, 8, 9]], columns=names)
-
- result = parser.read_csv(StringIO(data), names=names)
- tm.assert_frame_equal(result, expected)
-
-
-def test_read_nrows_large(c_parser_only):
- # gh-7626 - Read only nrows of data in for large inputs (>262144b)
- parser = c_parser_only
- header_narrow = "\t".join(["COL_HEADER_" + str(i) for i in range(10)]) + "\n"
- data_narrow = "\t".join(["somedatasomedatasomedata1" for _ in range(10)]) + "\n"
- header_wide = "\t".join(["COL_HEADER_" + str(i) for i in range(15)]) + "\n"
- data_wide = "\t".join(["somedatasomedatasomedata2" for _ in range(15)]) + "\n"
- test_input = header_narrow + data_narrow * 1050 + header_wide + data_wide * 2
-
- df = parser.read_csv(StringIO(test_input), sep="\t", nrows=1010)
-
- assert df.size == 1010 * 10
-
-
-def test_float_precision_round_trip_with_text(c_parser_only):
- # see gh-15140
- parser = c_parser_only
- df = parser.read_csv(StringIO("a"), header=None, float_precision="round_trip")
- tm.assert_frame_equal(df, DataFrame({0: ["a"]}))
-
-
-def test_large_difference_in_columns(c_parser_only):
- # see gh-14125
- parser = c_parser_only
-
- count = 10000
- large_row = ("X," * count)[:-1] + "\n"
- normal_row = "XXXXXX XXXXXX,111111111111111\n"
- test_input = (large_row + normal_row * 6)[:-1]
-
- result = parser.read_csv(StringIO(test_input), header=None, usecols=[0])
- rows = test_input.split("\n")
-
- expected = DataFrame([row.split(",")[0] for row in rows])
- tm.assert_frame_equal(result, expected)
-
-
-def test_data_after_quote(c_parser_only):
- # see gh-15910
- parser = c_parser_only
-
- data = 'a\n1\n"b"a'
- result = parser.read_csv(StringIO(data))
-
- expected = DataFrame({"a": ["1", "ba"]})
- tm.assert_frame_equal(result, expected)
-
-
-def test_comment_whitespace_delimited(c_parser_only, capsys):
- parser = c_parser_only
- test_input = """\
-1 2
-2 2 3
-3 2 3 # 3 fields
-4 2 3# 3 fields
-5 2 # 2 fields
-6 2# 2 fields
-7 # 1 field, NaN
-8# 1 field, NaN
-9 2 3 # skipped line
-# comment"""
- df = parser.read_csv(
- StringIO(test_input),
- comment="#",
- header=None,
- delimiter="\\s+",
- skiprows=0,
- on_bad_lines="warn",
- )
- captured = capsys.readouterr()
- # skipped lines 2, 3, 4, 9
- for line_num in (2, 3, 4, 9):
- assert f"Skipping line {line_num}" in captured.err
- expected = DataFrame([[1, 2], [5, 2], [6, 2], [7, np.nan], [8, np.nan]])
- tm.assert_frame_equal(df, expected)
-
-
-def test_file_like_no_next(c_parser_only):
- # gh-16530: the file-like need not have a "next" or "__next__"
- # attribute despite having an "__iter__" attribute.
- #
- # NOTE: This is only true for the C engine, not Python engine.
- class NoNextBuffer(StringIO):
- def __next__(self):
- raise AttributeError("No next method")
-
- next = __next__
-
- parser = c_parser_only
- data = "a\n1"
-
- expected = DataFrame({"a": [1]})
- result = parser.read_csv(NoNextBuffer(data))
-
- tm.assert_frame_equal(result, expected)
-
-
-def test_buffer_rd_bytes_bad_unicode(c_parser_only):
- # see gh-22748
- t = BytesIO(b"\xB0")
- t = TextIOWrapper(t, encoding="ascii", errors="surrogateescape")
- msg = "'utf-8' codec can't encode character"
- with pytest.raises(UnicodeError, match=msg):
- c_parser_only.read_csv(t, encoding="UTF-8")
-
-
-@pytest.mark.parametrize("tar_suffix", [".tar", ".tar.gz"])
-def test_read_tarfile(c_parser_only, csv_dir_path, tar_suffix):
- # see gh-16530
- #
- # Unfortunately, Python's CSV library can't handle
- # tarfile objects (expects string, not bytes when
- # iterating through a file-like).
- parser = c_parser_only
- tar_path = os.path.join(csv_dir_path, "tar_csv" + tar_suffix)
-
- with tarfile.open(tar_path, "r") as tar:
- data_file = tar.extractfile("tar_data.csv")
-
- out = parser.read_csv(data_file)
- expected = DataFrame({"a": [1]})
- tm.assert_frame_equal(out, expected)
-
-
-@pytest.mark.single_cpu
-@pytest.mark.skipif(is_ci_environment(), reason="Too memory intensive for CI.")
-def test_bytes_exceed_2gb(c_parser_only):
- # see gh-16798
- #
- # Read from a "CSV" that has a column larger than 2GB.
- parser = c_parser_only
-
- if parser.low_memory:
- pytest.skip("not a low_memory test")
-
- # csv takes 10 seconds to construct, spikes memory to 8GB+, the whole test
- # spikes up to 10.4GB on the c_high case
- csv = StringIO("strings\n" + "\n".join(["x" * (1 << 20) for _ in range(2100)]))
- df = parser.read_csv(csv)
- assert not df.empty
-
-
-def test_chunk_whitespace_on_boundary(c_parser_only):
- # see gh-9735: this issue is C parser-specific (bug when
- # parsing whitespace and characters at chunk boundary)
- #
- # This test case has a field too large for the Python parser / CSV library.
- parser = c_parser_only
-
- chunk1 = "a" * (1024 * 256 - 2) + "\na"
- chunk2 = "\n a"
- result = parser.read_csv(StringIO(chunk1 + chunk2), header=None)
-
- expected = DataFrame(["a" * (1024 * 256 - 2), "a", " a"])
- tm.assert_frame_equal(result, expected)
-
-
-def test_file_handles_mmap(c_parser_only, csv1):
- # gh-14418
- #
- # Don't close user provided file handles.
- parser = c_parser_only
-
- with open(csv1, encoding="utf-8") as f:
- with mmap.mmap(f.fileno(), 0, access=mmap.ACCESS_READ) as m:
- parser.read_csv(m)
- assert not m.closed
-
-
-def test_file_binary_mode(c_parser_only):
- # see gh-23779
- parser = c_parser_only
- expected = DataFrame([[1, 2, 3], [4, 5, 6]])
-
- with tm.ensure_clean() as path:
- with open(path, "w", encoding="utf-8") as f:
- f.write("1,2,3\n4,5,6")
-
- with open(path, "rb") as f:
- result = parser.read_csv(f, header=None)
- tm.assert_frame_equal(result, expected)
-
-
-def test_unix_style_breaks(c_parser_only):
- # GH 11020
- parser = c_parser_only
- with tm.ensure_clean() as path:
- with open(path, "w", newline="\n", encoding="utf-8") as f:
- f.write("blah\n\ncol_1,col_2,col_3\n\n")
- result = parser.read_csv(path, skiprows=2, encoding="utf-8", engine="c")
- expected = DataFrame(columns=["col_1", "col_2", "col_3"])
- tm.assert_frame_equal(result, expected)
-
-
-@pytest.mark.parametrize("float_precision", [None, "legacy", "high", "round_trip"])
-@pytest.mark.parametrize(
- "data,thousands,decimal",
- [
- (
- """A|B|C
-1|2,334.01|5
-10|13|10.
-""",
- ",",
- ".",
- ),
- (
- """A|B|C
-1|2.334,01|5
-10|13|10,
-""",
- ".",
- ",",
- ),
- ],
-)
-def test_1000_sep_with_decimal(
- c_parser_only, data, thousands, decimal, float_precision
-):
- parser = c_parser_only
- expected = DataFrame({"A": [1, 10], "B": [2334.01, 13], "C": [5, 10.0]})
-
- result = parser.read_csv(
- StringIO(data),
- sep="|",
- thousands=thousands,
- decimal=decimal,
- float_precision=float_precision,
- )
- tm.assert_frame_equal(result, expected)
-
-
-def test_float_precision_options(c_parser_only):
- # GH 17154, 36228
- parser = c_parser_only
- s = "foo\n243.164\n"
- df = parser.read_csv(StringIO(s))
- df2 = parser.read_csv(StringIO(s), float_precision="high")
-
- tm.assert_frame_equal(df, df2)
-
- df3 = parser.read_csv(StringIO(s), float_precision="legacy")
-
- assert not df.iloc[0, 0] == df3.iloc[0, 0]
-
- msg = "Unrecognized float_precision option: junk"
-
- with pytest.raises(ValueError, match=msg):
- parser.read_csv(StringIO(s), float_precision="junk")
diff --git a/spaces/profayle/TerrapinTalk/myenv/lib/python3.9/site-packages/pandas/tests/reshape/test_crosstab.py b/spaces/profayle/TerrapinTalk/myenv/lib/python3.9/site-packages/pandas/tests/reshape/test_crosstab.py
deleted file mode 100644
index 2b6ebded3d325d1274b7dd6b5f153ebf005e65d3..0000000000000000000000000000000000000000
--- a/spaces/profayle/TerrapinTalk/myenv/lib/python3.9/site-packages/pandas/tests/reshape/test_crosstab.py
+++ /dev/null
@@ -1,893 +0,0 @@
-import numpy as np
-import pytest
-
-import pandas as pd
-from pandas import (
- CategoricalDtype,
- CategoricalIndex,
- DataFrame,
- Index,
- MultiIndex,
- Series,
- crosstab,
-)
-import pandas._testing as tm
-
-
-@pytest.fixture
-def df():
- df = DataFrame(
- {
- "A": [
- "foo",
- "foo",
- "foo",
- "foo",
- "bar",
- "bar",
- "bar",
- "bar",
- "foo",
- "foo",
- "foo",
- ],
- "B": [
- "one",
- "one",
- "one",
- "two",
- "one",
- "one",
- "one",
- "two",
- "two",
- "two",
- "one",
- ],
- "C": [
- "dull",
- "dull",
- "shiny",
- "dull",
- "dull",
- "shiny",
- "shiny",
- "dull",
- "shiny",
- "shiny",
- "shiny",
- ],
- "D": np.random.default_rng(2).standard_normal(11),
- "E": np.random.default_rng(2).standard_normal(11),
- "F": np.random.default_rng(2).standard_normal(11),
- }
- )
-
- return pd.concat([df, df], ignore_index=True)
-
-
-class TestCrosstab:
- def test_crosstab_single(self, df):
- result = crosstab(df["A"], df["C"])
- expected = df.groupby(["A", "C"]).size().unstack()
- tm.assert_frame_equal(result, expected.fillna(0).astype(np.int64))
-
- def test_crosstab_multiple(self, df):
- result = crosstab(df["A"], [df["B"], df["C"]])
- expected = df.groupby(["A", "B", "C"]).size()
- expected = expected.unstack("B").unstack("C").fillna(0).astype(np.int64)
- tm.assert_frame_equal(result, expected)
-
- result = crosstab([df["B"], df["C"]], df["A"])
- expected = df.groupby(["B", "C", "A"]).size()
- expected = expected.unstack("A").fillna(0).astype(np.int64)
- tm.assert_frame_equal(result, expected)
-
- @pytest.mark.parametrize("box", [np.array, list, tuple])
- def test_crosstab_ndarray(self, box):
- # GH 44076
- a = box(np.random.default_rng(2).integers(0, 5, size=100))
- b = box(np.random.default_rng(2).integers(0, 3, size=100))
- c = box(np.random.default_rng(2).integers(0, 10, size=100))
-
- df = DataFrame({"a": a, "b": b, "c": c})
-
- result = crosstab(a, [b, c], rownames=["a"], colnames=("b", "c"))
- expected = crosstab(df["a"], [df["b"], df["c"]])
- tm.assert_frame_equal(result, expected)
-
- result = crosstab([b, c], a, colnames=["a"], rownames=("b", "c"))
- expected = crosstab([df["b"], df["c"]], df["a"])
- tm.assert_frame_equal(result, expected)
-
- # assign arbitrary names
- result = crosstab(a, c)
- expected = crosstab(df["a"], df["c"])
- expected.index.names = ["row_0"]
- expected.columns.names = ["col_0"]
- tm.assert_frame_equal(result, expected)
-
- def test_crosstab_non_aligned(self):
- # GH 17005
- a = Series([0, 1, 1], index=["a", "b", "c"])
- b = Series([3, 4, 3, 4, 3], index=["a", "b", "c", "d", "f"])
- c = np.array([3, 4, 3], dtype=np.int64)
-
- expected = DataFrame(
- [[1, 0], [1, 1]],
- index=Index([0, 1], name="row_0"),
- columns=Index([3, 4], name="col_0"),
- )
-
- result = crosstab(a, b)
- tm.assert_frame_equal(result, expected)
-
- result = crosstab(a, c)
- tm.assert_frame_equal(result, expected)
-
- def test_crosstab_margins(self):
- a = np.random.default_rng(2).integers(0, 7, size=100)
- b = np.random.default_rng(2).integers(0, 3, size=100)
- c = np.random.default_rng(2).integers(0, 5, size=100)
-
- df = DataFrame({"a": a, "b": b, "c": c})
-
- result = crosstab(a, [b, c], rownames=["a"], colnames=("b", "c"), margins=True)
-
- assert result.index.names == ("a",)
- assert result.columns.names == ["b", "c"]
-
- all_cols = result["All", ""]
- exp_cols = df.groupby(["a"]).size().astype("i8")
- # to keep index.name
- exp_margin = Series([len(df)], index=Index(["All"], name="a"))
- exp_cols = pd.concat([exp_cols, exp_margin])
- exp_cols.name = ("All", "")
-
- tm.assert_series_equal(all_cols, exp_cols)
-
- all_rows = result.loc["All"]
- exp_rows = df.groupby(["b", "c"]).size().astype("i8")
- exp_rows = pd.concat([exp_rows, Series([len(df)], index=[("All", "")])])
- exp_rows.name = "All"
-
- exp_rows = exp_rows.reindex(all_rows.index)
- exp_rows = exp_rows.fillna(0).astype(np.int64)
- tm.assert_series_equal(all_rows, exp_rows)
-
- def test_crosstab_margins_set_margin_name(self):
- # GH 15972
- a = np.random.default_rng(2).integers(0, 7, size=100)
- b = np.random.default_rng(2).integers(0, 3, size=100)
- c = np.random.default_rng(2).integers(0, 5, size=100)
-
- df = DataFrame({"a": a, "b": b, "c": c})
-
- result = crosstab(
- a,
- [b, c],
- rownames=["a"],
- colnames=("b", "c"),
- margins=True,
- margins_name="TOTAL",
- )
-
- assert result.index.names == ("a",)
- assert result.columns.names == ["b", "c"]
-
- all_cols = result["TOTAL", ""]
- exp_cols = df.groupby(["a"]).size().astype("i8")
- # to keep index.name
- exp_margin = Series([len(df)], index=Index(["TOTAL"], name="a"))
- exp_cols = pd.concat([exp_cols, exp_margin])
- exp_cols.name = ("TOTAL", "")
-
- tm.assert_series_equal(all_cols, exp_cols)
-
- all_rows = result.loc["TOTAL"]
- exp_rows = df.groupby(["b", "c"]).size().astype("i8")
- exp_rows = pd.concat([exp_rows, Series([len(df)], index=[("TOTAL", "")])])
- exp_rows.name = "TOTAL"
-
- exp_rows = exp_rows.reindex(all_rows.index)
- exp_rows = exp_rows.fillna(0).astype(np.int64)
- tm.assert_series_equal(all_rows, exp_rows)
-
- msg = "margins_name argument must be a string"
- for margins_name in [666, None, ["a", "b"]]:
- with pytest.raises(ValueError, match=msg):
- crosstab(
- a,
- [b, c],
- rownames=["a"],
- colnames=("b", "c"),
- margins=True,
- margins_name=margins_name,
- )
-
- def test_crosstab_pass_values(self):
- a = np.random.default_rng(2).integers(0, 7, size=100)
- b = np.random.default_rng(2).integers(0, 3, size=100)
- c = np.random.default_rng(2).integers(0, 5, size=100)
- values = np.random.default_rng(2).standard_normal(100)
-
- table = crosstab(
- [a, b], c, values, aggfunc="sum", rownames=["foo", "bar"], colnames=["baz"]
- )
-
- df = DataFrame({"foo": a, "bar": b, "baz": c, "values": values})
-
- expected = df.pivot_table(
- "values", index=["foo", "bar"], columns="baz", aggfunc="sum"
- )
- tm.assert_frame_equal(table, expected)
-
- def test_crosstab_dropna(self):
- # GH 3820
- a = np.array(["foo", "foo", "foo", "bar", "bar", "foo", "foo"], dtype=object)
- b = np.array(["one", "one", "two", "one", "two", "two", "two"], dtype=object)
- c = np.array(
- ["dull", "dull", "dull", "dull", "dull", "shiny", "shiny"], dtype=object
- )
- res = crosstab(a, [b, c], rownames=["a"], colnames=["b", "c"], dropna=False)
- m = MultiIndex.from_tuples(
- [("one", "dull"), ("one", "shiny"), ("two", "dull"), ("two", "shiny")],
- names=["b", "c"],
- )
- tm.assert_index_equal(res.columns, m)
-
- def test_crosstab_no_overlap(self):
- # GS 10291
-
- s1 = Series([1, 2, 3], index=[1, 2, 3])
- s2 = Series([4, 5, 6], index=[4, 5, 6])
-
- actual = crosstab(s1, s2)
- expected = DataFrame(
- index=Index([], dtype="int64", name="row_0"),
- columns=Index([], dtype="int64", name="col_0"),
- )
-
- tm.assert_frame_equal(actual, expected)
-
- def test_margin_dropna(self):
- # GH 12577
- # pivot_table counts null into margin ('All')
- # when margins=true and dropna=true
-
- df = DataFrame({"a": [1, 2, 2, 2, 2, np.nan], "b": [3, 3, 4, 4, 4, 4]})
- actual = crosstab(df.a, df.b, margins=True, dropna=True)
- expected = DataFrame([[1, 0, 1], [1, 3, 4], [2, 3, 5]])
- expected.index = Index([1.0, 2.0, "All"], name="a")
- expected.columns = Index([3, 4, "All"], name="b")
- tm.assert_frame_equal(actual, expected)
-
- def test_margin_dropna2(self):
- df = DataFrame(
- {"a": [1, np.nan, np.nan, np.nan, 2, np.nan], "b": [3, np.nan, 4, 4, 4, 4]}
- )
- actual = crosstab(df.a, df.b, margins=True, dropna=True)
- expected = DataFrame([[1, 0, 1], [0, 1, 1], [1, 1, 2]])
- expected.index = Index([1.0, 2.0, "All"], name="a")
- expected.columns = Index([3.0, 4.0, "All"], name="b")
- tm.assert_frame_equal(actual, expected)
-
- def test_margin_dropna3(self):
- df = DataFrame(
- {"a": [1, np.nan, np.nan, np.nan, np.nan, 2], "b": [3, 3, 4, 4, 4, 4]}
- )
- actual = crosstab(df.a, df.b, margins=True, dropna=True)
- expected = DataFrame([[1, 0, 1], [0, 1, 1], [1, 1, 2]])
- expected.index = Index([1.0, 2.0, "All"], name="a")
- expected.columns = Index([3, 4, "All"], name="b")
- tm.assert_frame_equal(actual, expected)
-
- def test_margin_dropna4(self):
- # GH 12642
- # _add_margins raises KeyError: Level None not found
- # when margins=True and dropna=False
- # GH: 10772: Keep np.nan in result with dropna=False
- df = DataFrame({"a": [1, 2, 2, 2, 2, np.nan], "b": [3, 3, 4, 4, 4, 4]})
- actual = crosstab(df.a, df.b, margins=True, dropna=False)
- expected = DataFrame([[1, 0, 1.0], [1, 3, 4.0], [0, 1, np.nan], [2, 4, 6.0]])
- expected.index = Index([1.0, 2.0, np.nan, "All"], name="a")
- expected.columns = Index([3, 4, "All"], name="b")
- tm.assert_frame_equal(actual, expected)
-
- def test_margin_dropna5(self):
- # GH: 10772: Keep np.nan in result with dropna=False
- df = DataFrame(
- {"a": [1, np.nan, np.nan, np.nan, 2, np.nan], "b": [3, np.nan, 4, 4, 4, 4]}
- )
- actual = crosstab(df.a, df.b, margins=True, dropna=False)
- expected = DataFrame(
- [[1, 0, 0, 1.0], [0, 1, 0, 1.0], [0, 3, 1, np.nan], [1, 4, 0, 6.0]]
- )
- expected.index = Index([1.0, 2.0, np.nan, "All"], name="a")
- expected.columns = Index([3.0, 4.0, np.nan, "All"], name="b")
- tm.assert_frame_equal(actual, expected)
-
- def test_margin_dropna6(self):
- # GH: 10772: Keep np.nan in result with dropna=False
- a = np.array(["foo", "foo", "foo", "bar", "bar", "foo", "foo"], dtype=object)
- b = np.array(["one", "one", "two", "one", "two", np.nan, "two"], dtype=object)
- c = np.array(
- ["dull", "dull", "dull", "dull", "dull", "shiny", "shiny"], dtype=object
- )
-
- actual = crosstab(
- a, [b, c], rownames=["a"], colnames=["b", "c"], margins=True, dropna=False
- )
- m = MultiIndex.from_arrays(
- [
- ["one", "one", "two", "two", np.nan, np.nan, "All"],
- ["dull", "shiny", "dull", "shiny", "dull", "shiny", ""],
- ],
- names=["b", "c"],
- )
- expected = DataFrame(
- [[1, 0, 1, 0, 0, 0, 2], [2, 0, 1, 1, 0, 1, 5], [3, 0, 2, 1, 0, 0, 7]],
- columns=m,
- )
- expected.index = Index(["bar", "foo", "All"], name="a")
- tm.assert_frame_equal(actual, expected)
-
- actual = crosstab(
- [a, b], c, rownames=["a", "b"], colnames=["c"], margins=True, dropna=False
- )
- m = MultiIndex.from_arrays(
- [
- ["bar", "bar", "bar", "foo", "foo", "foo", "All"],
- ["one", "two", np.nan, "one", "two", np.nan, ""],
- ],
- names=["a", "b"],
- )
- expected = DataFrame(
- [
- [1, 0, 1.0],
- [1, 0, 1.0],
- [0, 0, np.nan],
- [2, 0, 2.0],
- [1, 1, 2.0],
- [0, 1, np.nan],
- [5, 2, 7.0],
- ],
- index=m,
- )
- expected.columns = Index(["dull", "shiny", "All"], name="c")
- tm.assert_frame_equal(actual, expected)
-
- actual = crosstab(
- [a, b], c, rownames=["a", "b"], colnames=["c"], margins=True, dropna=True
- )
- m = MultiIndex.from_arrays(
- [["bar", "bar", "foo", "foo", "All"], ["one", "two", "one", "two", ""]],
- names=["a", "b"],
- )
- expected = DataFrame(
- [[1, 0, 1], [1, 0, 1], [2, 0, 2], [1, 1, 2], [5, 1, 6]], index=m
- )
- expected.columns = Index(["dull", "shiny", "All"], name="c")
- tm.assert_frame_equal(actual, expected)
-
- def test_crosstab_normalize(self):
- # Issue 12578
- df = DataFrame(
- {"a": [1, 2, 2, 2, 2], "b": [3, 3, 4, 4, 4], "c": [1, 1, np.nan, 1, 1]}
- )
-
- rindex = Index([1, 2], name="a")
- cindex = Index([3, 4], name="b")
- full_normal = DataFrame([[0.2, 0], [0.2, 0.6]], index=rindex, columns=cindex)
- row_normal = DataFrame([[1.0, 0], [0.25, 0.75]], index=rindex, columns=cindex)
- col_normal = DataFrame([[0.5, 0], [0.5, 1.0]], index=rindex, columns=cindex)
-
- # Check all normalize args
- tm.assert_frame_equal(crosstab(df.a, df.b, normalize="all"), full_normal)
- tm.assert_frame_equal(crosstab(df.a, df.b, normalize=True), full_normal)
- tm.assert_frame_equal(crosstab(df.a, df.b, normalize="index"), row_normal)
- tm.assert_frame_equal(crosstab(df.a, df.b, normalize="columns"), col_normal)
- tm.assert_frame_equal(
- crosstab(df.a, df.b, normalize=1),
- crosstab(df.a, df.b, normalize="columns"),
- )
- tm.assert_frame_equal(
- crosstab(df.a, df.b, normalize=0), crosstab(df.a, df.b, normalize="index")
- )
-
- row_normal_margins = DataFrame(
- [[1.0, 0], [0.25, 0.75], [0.4, 0.6]],
- index=Index([1, 2, "All"], name="a", dtype="object"),
- columns=Index([3, 4], name="b", dtype="object"),
- )
- col_normal_margins = DataFrame(
- [[0.5, 0, 0.2], [0.5, 1.0, 0.8]],
- index=Index([1, 2], name="a", dtype="object"),
- columns=Index([3, 4, "All"], name="b", dtype="object"),
- )
-
- all_normal_margins = DataFrame(
- [[0.2, 0, 0.2], [0.2, 0.6, 0.8], [0.4, 0.6, 1]],
- index=Index([1, 2, "All"], name="a", dtype="object"),
- columns=Index([3, 4, "All"], name="b", dtype="object"),
- )
- tm.assert_frame_equal(
- crosstab(df.a, df.b, normalize="index", margins=True), row_normal_margins
- )
- tm.assert_frame_equal(
- crosstab(df.a, df.b, normalize="columns", margins=True), col_normal_margins
- )
- tm.assert_frame_equal(
- crosstab(df.a, df.b, normalize=True, margins=True), all_normal_margins
- )
-
- def test_crosstab_normalize_arrays(self):
- # GH#12578
- df = DataFrame(
- {"a": [1, 2, 2, 2, 2], "b": [3, 3, 4, 4, 4], "c": [1, 1, np.nan, 1, 1]}
- )
-
- # Test arrays
- crosstab(
- [np.array([1, 1, 2, 2]), np.array([1, 2, 1, 2])], np.array([1, 2, 1, 2])
- )
-
- # Test with aggfunc
- norm_counts = DataFrame(
- [[0.25, 0, 0.25], [0.25, 0.5, 0.75], [0.5, 0.5, 1]],
- index=Index([1, 2, "All"], name="a", dtype="object"),
- columns=Index([3, 4, "All"], name="b"),
- )
- test_case = crosstab(
- df.a, df.b, df.c, aggfunc="count", normalize="all", margins=True
- )
- tm.assert_frame_equal(test_case, norm_counts)
-
- df = DataFrame(
- {"a": [1, 2, 2, 2, 2], "b": [3, 3, 4, 4, 4], "c": [0, 4, np.nan, 3, 3]}
- )
-
- norm_sum = DataFrame(
- [[0, 0, 0.0], [0.4, 0.6, 1], [0.4, 0.6, 1]],
- index=Index([1, 2, "All"], name="a", dtype="object"),
- columns=Index([3, 4, "All"], name="b", dtype="object"),
- )
- msg = "using DataFrameGroupBy.sum"
- with tm.assert_produces_warning(FutureWarning, match=msg):
- test_case = crosstab(
- df.a, df.b, df.c, aggfunc=np.sum, normalize="all", margins=True
- )
- tm.assert_frame_equal(test_case, norm_sum)
-
- def test_crosstab_with_empties(self, using_array_manager):
- # Check handling of empties
- df = DataFrame(
- {
- "a": [1, 2, 2, 2, 2],
- "b": [3, 3, 4, 4, 4],
- "c": [np.nan, np.nan, np.nan, np.nan, np.nan],
- }
- )
-
- empty = DataFrame(
- [[0.0, 0.0], [0.0, 0.0]],
- index=Index([1, 2], name="a", dtype="int64"),
- columns=Index([3, 4], name="b"),
- )
-
- for i in [True, "index", "columns"]:
- calculated = crosstab(df.a, df.b, values=df.c, aggfunc="count", normalize=i)
- tm.assert_frame_equal(empty, calculated)
-
- nans = DataFrame(
- [[0.0, np.nan], [0.0, 0.0]],
- index=Index([1, 2], name="a", dtype="int64"),
- columns=Index([3, 4], name="b"),
- )
- if using_array_manager:
- # INFO(ArrayManager) column without NaNs can preserve int dtype
- nans[3] = nans[3].astype("int64")
-
- calculated = crosstab(df.a, df.b, values=df.c, aggfunc="count", normalize=False)
- tm.assert_frame_equal(nans, calculated)
-
- def test_crosstab_errors(self):
- # Issue 12578
-
- df = DataFrame(
- {"a": [1, 2, 2, 2, 2], "b": [3, 3, 4, 4, 4], "c": [1, 1, np.nan, 1, 1]}
- )
-
- error = "values cannot be used without an aggfunc."
- with pytest.raises(ValueError, match=error):
- crosstab(df.a, df.b, values=df.c)
-
- error = "aggfunc cannot be used without values"
- with pytest.raises(ValueError, match=error):
- crosstab(df.a, df.b, aggfunc=np.mean)
-
- error = "Not a valid normalize argument"
- with pytest.raises(ValueError, match=error):
- crosstab(df.a, df.b, normalize="42")
-
- with pytest.raises(ValueError, match=error):
- crosstab(df.a, df.b, normalize=42)
-
- error = "Not a valid margins argument"
- with pytest.raises(ValueError, match=error):
- crosstab(df.a, df.b, normalize="all", margins=42)
-
- def test_crosstab_with_categorial_columns(self):
- # GH 8860
- df = DataFrame(
- {
- "MAKE": ["Honda", "Acura", "Tesla", "Honda", "Honda", "Acura"],
- "MODEL": ["Sedan", "Sedan", "Electric", "Pickup", "Sedan", "Sedan"],
- }
- )
- categories = ["Sedan", "Electric", "Pickup"]
- df["MODEL"] = df["MODEL"].astype("category").cat.set_categories(categories)
- result = crosstab(df["MAKE"], df["MODEL"])
-
- expected_index = Index(["Acura", "Honda", "Tesla"], name="MAKE")
- expected_columns = CategoricalIndex(
- categories, categories=categories, ordered=False, name="MODEL"
- )
- expected_data = [[2, 0, 0], [2, 0, 1], [0, 1, 0]]
- expected = DataFrame(
- expected_data, index=expected_index, columns=expected_columns
- )
- tm.assert_frame_equal(result, expected)
-
- def test_crosstab_with_numpy_size(self):
- # GH 4003
- df = DataFrame(
- {
- "A": ["one", "one", "two", "three"] * 6,
- "B": ["A", "B", "C"] * 8,
- "C": ["foo", "foo", "foo", "bar", "bar", "bar"] * 4,
- "D": np.random.default_rng(2).standard_normal(24),
- "E": np.random.default_rng(2).standard_normal(24),
- }
- )
- result = crosstab(
- index=[df["A"], df["B"]],
- columns=[df["C"]],
- margins=True,
- aggfunc=np.size,
- values=df["D"],
- )
- expected_index = MultiIndex(
- levels=[["All", "one", "three", "two"], ["", "A", "B", "C"]],
- codes=[[1, 1, 1, 2, 2, 2, 3, 3, 3, 0], [1, 2, 3, 1, 2, 3, 1, 2, 3, 0]],
- names=["A", "B"],
- )
- expected_column = Index(["bar", "foo", "All"], dtype="object", name="C")
- expected_data = np.array(
- [
- [2.0, 2.0, 4.0],
- [2.0, 2.0, 4.0],
- [2.0, 2.0, 4.0],
- [2.0, np.nan, 2.0],
- [np.nan, 2.0, 2.0],
- [2.0, np.nan, 2.0],
- [np.nan, 2.0, 2.0],
- [2.0, np.nan, 2.0],
- [np.nan, 2.0, 2.0],
- [12.0, 12.0, 24.0],
- ]
- )
- expected = DataFrame(
- expected_data, index=expected_index, columns=expected_column
- )
- # aggfunc is np.size, resulting in integers
- expected["All"] = expected["All"].astype("int64")
- tm.assert_frame_equal(result, expected)
-
- def test_crosstab_duplicate_names(self):
- # GH 13279 / 22529
-
- s1 = Series(range(3), name="foo")
- s2_foo = Series(range(1, 4), name="foo")
- s2_bar = Series(range(1, 4), name="bar")
- s3 = Series(range(3), name="waldo")
-
- # check result computed with duplicate labels against
- # result computed with unique labels, then relabelled
- mapper = {"bar": "foo"}
-
- # duplicate row, column labels
- result = crosstab(s1, s2_foo)
- expected = crosstab(s1, s2_bar).rename_axis(columns=mapper, axis=1)
- tm.assert_frame_equal(result, expected)
-
- # duplicate row, unique column labels
- result = crosstab([s1, s2_foo], s3)
- expected = crosstab([s1, s2_bar], s3).rename_axis(index=mapper, axis=0)
- tm.assert_frame_equal(result, expected)
-
- # unique row, duplicate column labels
- result = crosstab(s3, [s1, s2_foo])
- expected = crosstab(s3, [s1, s2_bar]).rename_axis(columns=mapper, axis=1)
-
- tm.assert_frame_equal(result, expected)
-
- @pytest.mark.parametrize("names", [["a", ("b", "c")], [("a", "b"), "c"]])
- def test_crosstab_tuple_name(self, names):
- s1 = Series(range(3), name=names[0])
- s2 = Series(range(1, 4), name=names[1])
-
- mi = MultiIndex.from_arrays([range(3), range(1, 4)], names=names)
- expected = Series(1, index=mi).unstack(1, fill_value=0)
-
- result = crosstab(s1, s2)
- tm.assert_frame_equal(result, expected)
-
- def test_crosstab_both_tuple_names(self):
- # GH 18321
- s1 = Series(range(3), name=("a", "b"))
- s2 = Series(range(3), name=("c", "d"))
-
- expected = DataFrame(
- np.eye(3, dtype="int64"),
- index=Index(range(3), name=("a", "b")),
- columns=Index(range(3), name=("c", "d")),
- )
- result = crosstab(s1, s2)
- tm.assert_frame_equal(result, expected)
-
- def test_crosstab_unsorted_order(self):
- df = DataFrame({"b": [3, 1, 2], "a": [5, 4, 6]}, index=["C", "A", "B"])
- result = crosstab(df.index, [df.b, df.a])
- e_idx = Index(["A", "B", "C"], name="row_0")
- e_columns = MultiIndex.from_tuples([(1, 4), (2, 6), (3, 5)], names=["b", "a"])
- expected = DataFrame(
- [[1, 0, 0], [0, 1, 0], [0, 0, 1]], index=e_idx, columns=e_columns
- )
- tm.assert_frame_equal(result, expected)
-
- def test_crosstab_normalize_multiple_columns(self):
- # GH 15150
- df = DataFrame(
- {
- "A": ["one", "one", "two", "three"] * 6,
- "B": ["A", "B", "C"] * 8,
- "C": ["foo", "foo", "foo", "bar", "bar", "bar"] * 4,
- "D": [0] * 24,
- "E": [0] * 24,
- }
- )
-
- msg = "using DataFrameGroupBy.sum"
- with tm.assert_produces_warning(FutureWarning, match=msg):
- result = crosstab(
- [df.A, df.B],
- df.C,
- values=df.D,
- aggfunc=np.sum,
- normalize=True,
- margins=True,
- )
- expected = DataFrame(
- np.array([0] * 29 + [1], dtype=float).reshape(10, 3),
- columns=Index(["bar", "foo", "All"], dtype="object", name="C"),
- index=MultiIndex.from_tuples(
- [
- ("one", "A"),
- ("one", "B"),
- ("one", "C"),
- ("three", "A"),
- ("three", "B"),
- ("three", "C"),
- ("two", "A"),
- ("two", "B"),
- ("two", "C"),
- ("All", ""),
- ],
- names=["A", "B"],
- ),
- )
- tm.assert_frame_equal(result, expected)
-
- def test_margin_normalize(self):
- # GH 27500
- df = DataFrame(
- {
- "A": ["foo", "foo", "foo", "foo", "foo", "bar", "bar", "bar", "bar"],
- "B": ["one", "one", "one", "two", "two", "one", "one", "two", "two"],
- "C": [
- "small",
- "large",
- "large",
- "small",
- "small",
- "large",
- "small",
- "small",
- "large",
- ],
- "D": [1, 2, 2, 3, 3, 4, 5, 6, 7],
- "E": [2, 4, 5, 5, 6, 6, 8, 9, 9],
- }
- )
- # normalize on index
- result = crosstab(
- [df.A, df.B], df.C, margins=True, margins_name="Sub-Total", normalize=0
- )
- expected = DataFrame(
- [[0.5, 0.5], [0.5, 0.5], [0.666667, 0.333333], [0, 1], [0.444444, 0.555556]]
- )
- expected.index = MultiIndex(
- levels=[["Sub-Total", "bar", "foo"], ["", "one", "two"]],
- codes=[[1, 1, 2, 2, 0], [1, 2, 1, 2, 0]],
- names=["A", "B"],
- )
- expected.columns = Index(["large", "small"], dtype="object", name="C")
- tm.assert_frame_equal(result, expected)
-
- # normalize on columns
- result = crosstab(
- [df.A, df.B], df.C, margins=True, margins_name="Sub-Total", normalize=1
- )
- expected = DataFrame(
- [
- [0.25, 0.2, 0.222222],
- [0.25, 0.2, 0.222222],
- [0.5, 0.2, 0.333333],
- [0, 0.4, 0.222222],
- ]
- )
- expected.columns = Index(
- ["large", "small", "Sub-Total"], dtype="object", name="C"
- )
- expected.index = MultiIndex(
- levels=[["bar", "foo"], ["one", "two"]],
- codes=[[0, 0, 1, 1], [0, 1, 0, 1]],
- names=["A", "B"],
- )
- tm.assert_frame_equal(result, expected)
-
- # normalize on both index and column
- result = crosstab(
- [df.A, df.B], df.C, margins=True, margins_name="Sub-Total", normalize=True
- )
- expected = DataFrame(
- [
- [0.111111, 0.111111, 0.222222],
- [0.111111, 0.111111, 0.222222],
- [0.222222, 0.111111, 0.333333],
- [0.000000, 0.222222, 0.222222],
- [0.444444, 0.555555, 1],
- ]
- )
- expected.columns = Index(
- ["large", "small", "Sub-Total"], dtype="object", name="C"
- )
- expected.index = MultiIndex(
- levels=[["Sub-Total", "bar", "foo"], ["", "one", "two"]],
- codes=[[1, 1, 2, 2, 0], [1, 2, 1, 2, 0]],
- names=["A", "B"],
- )
- tm.assert_frame_equal(result, expected)
-
- def test_margin_normalize_multiple_columns(self):
- # GH 35144
- # use multiple columns with margins and normalization
- df = DataFrame(
- {
- "A": ["foo", "foo", "foo", "foo", "foo", "bar", "bar", "bar", "bar"],
- "B": ["one", "one", "one", "two", "two", "one", "one", "two", "two"],
- "C": [
- "small",
- "large",
- "large",
- "small",
- "small",
- "large",
- "small",
- "small",
- "large",
- ],
- "D": [1, 2, 2, 3, 3, 4, 5, 6, 7],
- "E": [2, 4, 5, 5, 6, 6, 8, 9, 9],
- }
- )
- result = crosstab(
- index=df.C,
- columns=[df.A, df.B],
- margins=True,
- margins_name="margin",
- normalize=True,
- )
- expected = DataFrame(
- [
- [0.111111, 0.111111, 0.222222, 0.000000, 0.444444],
- [0.111111, 0.111111, 0.111111, 0.222222, 0.555556],
- [0.222222, 0.222222, 0.333333, 0.222222, 1.0],
- ],
- index=["large", "small", "margin"],
- )
- expected.columns = MultiIndex(
- levels=[["bar", "foo", "margin"], ["", "one", "two"]],
- codes=[[0, 0, 1, 1, 2], [1, 2, 1, 2, 0]],
- names=["A", "B"],
- )
- expected.index.name = "C"
- tm.assert_frame_equal(result, expected)
-
- def test_margin_support_Float(self):
- # GH 50313
- # use Float64 formats and function aggfunc with margins
- df = DataFrame(
- {"A": [1, 2, 2, 1], "B": [3, 3, 4, 5], "C": [-1.0, 10.0, 1.0, 10.0]},
- dtype="Float64",
- )
- result = crosstab(
- df["A"],
- df["B"],
- values=df["C"],
- aggfunc="sum",
- margins=True,
- )
- expected = DataFrame(
- [
- [-1.0, pd.NA, 10.0, 9.0],
- [10.0, 1.0, pd.NA, 11.0],
- [9.0, 1.0, 10.0, 20.0],
- ],
- index=Index([1.0, 2.0, "All"], dtype="object", name="A"),
- columns=Index([3.0, 4.0, 5.0, "All"], dtype="object", name="B"),
- dtype="Float64",
- )
- tm.assert_frame_equal(result, expected)
-
- def test_margin_with_ordered_categorical_column(self):
- # GH 25278
- df = DataFrame(
- {
- "First": ["B", "B", "C", "A", "B", "C"],
- "Second": ["C", "B", "B", "B", "C", "A"],
- }
- )
- df["First"] = df["First"].astype(CategoricalDtype(ordered=True))
- customized_categories_order = ["C", "A", "B"]
- df["First"] = df["First"].cat.reorder_categories(customized_categories_order)
- result = crosstab(df["First"], df["Second"], margins=True)
-
- expected_index = Index(["C", "A", "B", "All"], name="First")
- expected_columns = Index(["A", "B", "C", "All"], name="Second")
- expected_data = [[1, 1, 0, 2], [0, 1, 0, 1], [0, 1, 2, 3], [1, 3, 2, 6]]
- expected = DataFrame(
- expected_data, index=expected_index, columns=expected_columns
- )
- tm.assert_frame_equal(result, expected)
-
-
-@pytest.mark.parametrize("a_dtype", ["category", "int64"])
-@pytest.mark.parametrize("b_dtype", ["category", "int64"])
-def test_categoricals(a_dtype, b_dtype):
- # https://github.com/pandas-dev/pandas/issues/37465
- g = np.random.default_rng(2)
- a = Series(g.integers(0, 3, size=100)).astype(a_dtype)
- b = Series(g.integers(0, 2, size=100)).astype(b_dtype)
- result = crosstab(a, b, margins=True, dropna=False)
- columns = Index([0, 1, "All"], dtype="object", name="col_0")
- index = Index([0, 1, 2, "All"], dtype="object", name="row_0")
- values = [[10, 18, 28], [23, 16, 39], [17, 16, 33], [50, 50, 100]]
- expected = DataFrame(values, index, columns)
- tm.assert_frame_equal(result, expected)
-
- # Verify when categorical does not have all values present
- a.loc[a == 1] = 2
- a_is_cat = isinstance(a.dtype, CategoricalDtype)
- assert not a_is_cat or a.value_counts().loc[1] == 0
- result = crosstab(a, b, margins=True, dropna=False)
- values = [[10, 18, 28], [0, 0, 0], [40, 32, 72], [50, 50, 100]]
- expected = DataFrame(values, index, columns)
- if not a_is_cat:
- expected = expected.loc[[0, 2, "All"]]
- expected["All"] = expected["All"].astype("int64")
- repr(result)
- repr(expected)
- repr(expected.loc[[0, 2, "All"]])
- tm.assert_frame_equal(result, expected)
diff --git a/spaces/profayle/TerrapinTalk/myenv/lib/python3.9/site-packages/pip/_internal/distributions/__init__.py b/spaces/profayle/TerrapinTalk/myenv/lib/python3.9/site-packages/pip/_internal/distributions/__init__.py
deleted file mode 100644
index 9a89a838b9a5cb264e9ae9d269fbedca6e2d6333..0000000000000000000000000000000000000000
--- a/spaces/profayle/TerrapinTalk/myenv/lib/python3.9/site-packages/pip/_internal/distributions/__init__.py
+++ /dev/null
@@ -1,21 +0,0 @@
-from pip._internal.distributions.base import AbstractDistribution
-from pip._internal.distributions.sdist import SourceDistribution
-from pip._internal.distributions.wheel import WheelDistribution
-from pip._internal.req.req_install import InstallRequirement
-
-
-def make_distribution_for_install_requirement(
- install_req: InstallRequirement,
-) -> AbstractDistribution:
- """Returns a Distribution for the given InstallRequirement"""
- # Editable requirements will always be source distributions. They use the
- # legacy logic until we create a modern standard for them.
- if install_req.editable:
- return SourceDistribution(install_req)
-
- # If it's a wheel, it's a WheelDistribution
- if install_req.is_wheel:
- return WheelDistribution(install_req)
-
- # Otherwise, a SourceDistribution
- return SourceDistribution(install_req)
diff --git a/spaces/profayle/TerrapinTalk/myenv/lib/python3.9/site-packages/tomlkit/_utils.py b/spaces/profayle/TerrapinTalk/myenv/lib/python3.9/site-packages/tomlkit/_utils.py
deleted file mode 100644
index f87fd7b5866b34a8e656429e28a7fbaa73f4aae0..0000000000000000000000000000000000000000
--- a/spaces/profayle/TerrapinTalk/myenv/lib/python3.9/site-packages/tomlkit/_utils.py
+++ /dev/null
@@ -1,158 +0,0 @@
-from __future__ import annotations
-
-import re
-
-from collections.abc import Mapping
-from datetime import date
-from datetime import datetime
-from datetime import time
-from datetime import timedelta
-from datetime import timezone
-from typing import Collection
-
-from tomlkit._compat import decode
-
-
-RFC_3339_LOOSE = re.compile(
- "^"
- r"(([0-9]+)-(\d{2})-(\d{2}))?" # Date
- "("
- "([Tt ])?" # Separator
- r"(\d{2}):(\d{2}):(\d{2})(\.([0-9]+))?" # Time
- r"(([Zz])|([\+|\-]([01][0-9]|2[0-3]):([0-5][0-9])))?" # Timezone
- ")?"
- "$"
-)
-
-RFC_3339_DATETIME = re.compile(
- "^"
- "([0-9]+)-(0[1-9]|1[012])-(0[1-9]|[12][0-9]|3[01])" # Date
- "[Tt ]" # Separator
- r"([01][0-9]|2[0-3]):([0-5][0-9]):([0-5][0-9]|60)(\.([0-9]+))?" # Time
- r"(([Zz])|([\+|\-]([01][0-9]|2[0-3]):([0-5][0-9])))?" # Timezone
- "$"
-)
-
-RFC_3339_DATE = re.compile("^([0-9]+)-(0[1-9]|1[012])-(0[1-9]|[12][0-9]|3[01])$")
-
-RFC_3339_TIME = re.compile(
- r"^([01][0-9]|2[0-3]):([0-5][0-9]):([0-5][0-9]|60)(\.([0-9]+))?$"
-)
-
-_utc = timezone(timedelta(), "UTC")
-
-
-def parse_rfc3339(string: str) -> datetime | date | time:
- m = RFC_3339_DATETIME.match(string)
- if m:
- year = int(m.group(1))
- month = int(m.group(2))
- day = int(m.group(3))
- hour = int(m.group(4))
- minute = int(m.group(5))
- second = int(m.group(6))
- microsecond = 0
-
- if m.group(7):
- microsecond = int((f"{m.group(8):<06s}")[:6])
-
- if m.group(9):
- # Timezone
- tz = m.group(9)
- if tz.upper() == "Z":
- tzinfo = _utc
- else:
- sign = m.group(11)[0]
- hour_offset, minute_offset = int(m.group(12)), int(m.group(13))
- offset = timedelta(seconds=hour_offset * 3600 + minute_offset * 60)
- if sign == "-":
- offset = -offset
-
- tzinfo = timezone(offset, f"{sign}{m.group(12)}:{m.group(13)}")
-
- return datetime(
- year, month, day, hour, minute, second, microsecond, tzinfo=tzinfo
- )
- else:
- return datetime(year, month, day, hour, minute, second, microsecond)
-
- m = RFC_3339_DATE.match(string)
- if m:
- year = int(m.group(1))
- month = int(m.group(2))
- day = int(m.group(3))
-
- return date(year, month, day)
-
- m = RFC_3339_TIME.match(string)
- if m:
- hour = int(m.group(1))
- minute = int(m.group(2))
- second = int(m.group(3))
- microsecond = 0
-
- if m.group(4):
- microsecond = int((f"{m.group(5):<06s}")[:6])
-
- return time(hour, minute, second, microsecond)
-
- raise ValueError("Invalid RFC 339 string")
-
-
-# https://toml.io/en/v1.0.0#string
-CONTROL_CHARS = frozenset(chr(c) for c in range(0x20)) | {chr(0x7F)}
-_escaped = {
- "b": "\b",
- "t": "\t",
- "n": "\n",
- "f": "\f",
- "r": "\r",
- '"': '"',
- "\\": "\\",
-}
-_compact_escapes = {
- **{v: f"\\{k}" for k, v in _escaped.items()},
- '"""': '""\\"',
-}
-_basic_escapes = CONTROL_CHARS | {'"', "\\"}
-
-
-def _unicode_escape(seq: str) -> str:
- return "".join(f"\\u{ord(c):04x}" for c in seq)
-
-
-def escape_string(s: str, escape_sequences: Collection[str] = _basic_escapes) -> str:
- s = decode(s)
-
- res = []
- start = 0
-
- def flush(inc=1):
- if start != i:
- res.append(s[start:i])
-
- return i + inc
-
- found_sequences = {seq for seq in escape_sequences if seq in s}
-
- i = 0
- while i < len(s):
- for seq in found_sequences:
- seq_len = len(seq)
- if s[i:].startswith(seq):
- start = flush(seq_len)
- res.append(_compact_escapes.get(seq) or _unicode_escape(seq))
- i += seq_len - 1 # fast-forward escape sequence
- i += 1
-
- flush()
-
- return "".join(res)
-
-
-def merge_dicts(d1: dict, d2: dict) -> dict:
- for k, v in d2.items():
- if k in d1 and isinstance(d1[k], dict) and isinstance(v, Mapping):
- merge_dicts(d1[k], v)
- else:
- d1[k] = d2[k]
diff --git a/spaces/putaalzasa/test/Dockerfile b/spaces/putaalzasa/test/Dockerfile
deleted file mode 100644
index 6c01c09373883afcb4ea34ae2d316cd596e1737b..0000000000000000000000000000000000000000
--- a/spaces/putaalzasa/test/Dockerfile
+++ /dev/null
@@ -1,21 +0,0 @@
-FROM node:18-bullseye-slim
-
-RUN apt-get update && \
-
-apt-get install -y git
-
-RUN git clone https://gitgud.io/khanon/oai-reverse-proxy.git /app
-
-WORKDIR /app
-
-RUN npm install
-
-COPY Dockerfile greeting.md* .env* ./
-
-RUN npm run build
-
-EXPOSE 7860
-
-ENV NODE_ENV=production
-
-CMD [ "npm", "start" ]
\ No newline at end of file
diff --git a/spaces/pyodide-demo/self-hosted/html5lib.js b/spaces/pyodide-demo/self-hosted/html5lib.js
deleted file mode 100644
index 39bec0c25c813d17c82f5402f26d9de3b1b60aef..0000000000000000000000000000000000000000
--- a/spaces/pyodide-demo/self-hosted/html5lib.js
+++ /dev/null
@@ -1 +0,0 @@
-var Module=typeof globalThis.__pyodide_module!=="undefined"?globalThis.__pyodide_module:{};if(!Module.expectedDataFileDownloads){Module.expectedDataFileDownloads=0}Module.expectedDataFileDownloads++;(function(){var loadPackage=function(metadata){var PACKAGE_PATH="";if(typeof window==="object"){PACKAGE_PATH=window["encodeURIComponent"](window.location.pathname.toString().substring(0,window.location.pathname.toString().lastIndexOf("/"))+"/")}else if(typeof process==="undefined"&&typeof location!=="undefined"){PACKAGE_PATH=encodeURIComponent(location.pathname.toString().substring(0,location.pathname.toString().lastIndexOf("/"))+"/")}var PACKAGE_NAME="html5lib.data";var REMOTE_PACKAGE_BASE="html5lib.data";if(typeof Module["locateFilePackage"]==="function"&&!Module["locateFile"]){Module["locateFile"]=Module["locateFilePackage"];err("warning: you defined Module.locateFilePackage, that has been renamed to Module.locateFile (using your locateFilePackage for now)")}var REMOTE_PACKAGE_NAME=Module["locateFile"]?Module["locateFile"](REMOTE_PACKAGE_BASE,""):REMOTE_PACKAGE_BASE;var REMOTE_PACKAGE_SIZE=metadata["remote_package_size"];var PACKAGE_UUID=metadata["package_uuid"];function fetchRemotePackage(packageName,packageSize,callback,errback){if(typeof process==="object"){require("fs").readFile(packageName,(function(err,contents){if(err){errback(err)}else{callback(contents.buffer)}}));return}var xhr=new XMLHttpRequest;xhr.open("GET",packageName,true);xhr.responseType="arraybuffer";xhr.onprogress=function(event){var url=packageName;var size=packageSize;if(event.total)size=event.total;if(event.loaded){if(!xhr.addedTotal){xhr.addedTotal=true;if(!Module.dataFileDownloads)Module.dataFileDownloads={};Module.dataFileDownloads[url]={loaded:event.loaded,total:size}}else{Module.dataFileDownloads[url].loaded=event.loaded}var total=0;var loaded=0;var num=0;for(var download in Module.dataFileDownloads){var data=Module.dataFileDownloads[download];total+=data.total;loaded+=data.loaded;num++}total=Math.ceil(total*Module.expectedDataFileDownloads/num);if(Module["setStatus"])Module["setStatus"]("Downloading data... ("+loaded+"/"+total+")")}else if(!Module.dataFileDownloads){if(Module["setStatus"])Module["setStatus"]("Downloading data...")}};xhr.onerror=function(event){throw new Error("NetworkError for: "+packageName)};xhr.onload=function(event){if(xhr.status==200||xhr.status==304||xhr.status==206||xhr.status==0&&xhr.response){var packageData=xhr.response;callback(packageData)}else{throw new Error(xhr.statusText+" : "+xhr.responseURL)}};xhr.send(null)}function handleError(error){console.error("package error:",error)}var fetchedCallback=null;var fetched=Module["getPreloadedPackage"]?Module["getPreloadedPackage"](REMOTE_PACKAGE_NAME,REMOTE_PACKAGE_SIZE):null;if(!fetched)fetchRemotePackage(REMOTE_PACKAGE_NAME,REMOTE_PACKAGE_SIZE,(function(data){if(fetchedCallback){fetchedCallback(data);fetchedCallback=null}else{fetched=data}}),handleError);function runWithFS(){function assert(check,msg){if(!check)throw msg+(new Error).stack}Module["FS_createPath"]("/","lib",true,true);Module["FS_createPath"]("/lib","python3.9",true,true);Module["FS_createPath"]("/lib/python3.9","site-packages",true,true);Module["FS_createPath"]("/lib/python3.9/site-packages","html5lib",true,true);Module["FS_createPath"]("/lib/python3.9/site-packages/html5lib","_trie",true,true);Module["FS_createPath"]("/lib/python3.9/site-packages/html5lib","filters",true,true);Module["FS_createPath"]("/lib/python3.9/site-packages/html5lib","treeadapters",true,true);Module["FS_createPath"]("/lib/python3.9/site-packages/html5lib","treebuilders",true,true);Module["FS_createPath"]("/lib/python3.9/site-packages/html5lib","treewalkers",true,true);Module["FS_createPath"]("/lib/python3.9/site-packages","html5lib-1.1-py3.9.egg-info",true,true);function processPackageData(arrayBuffer){assert(arrayBuffer,"Loading data file failed.");assert(arrayBuffer instanceof ArrayBuffer,"bad input to processPackageData");var byteArray=new Uint8Array(arrayBuffer);var curr;var compressedData={data:null,cachedOffset:234723,cachedIndexes:[-1,-1],cachedChunks:[null,null],offsets:[0,1349,2604,3939,5214,6667,8212,9668,10631,11711,12962,13967,15353,16506,17678,18776,20152,21201,22110,23169,24151,25231,26167,27266,28189,29363,30645,31476,32672,33609,34663,35471,36088,36878,37601,38325,38965,39640,40213,40704,41380,42075,42533,43168,44003,44915,45606,46217,46929,47736,48579,49052,49657,50362,50996,51780,52573,53073,53699,54268,54817,55271,56106,57531,58723,59827,60694,61362,62221,62917,63598,64388,65019,65589,66621,67675,68824,69947,70917,71790,72726,73555,74338,75323,76182,77118,78050,78977,79880,80804,81750,82691,83595,84508,85401,86352,87161,87970,88879,89820,90736,91654,92469,93409,94324,95270,96570,97794,98895,99940,100722,101695,102798,103881,105030,106282,106834,107445,108205,108958,110029,110952,111718,112670,113793,114843,115664,116497,117132,117897,118699,119518,120394,121308,122016,123081,124066,124891,125755,126770,127823,128939,129722,130655,131674,132562,133464,134266,135264,136024,137110,138139,138924,139884,140633,141448,142363,143289,144235,145105,146002,146730,147566,148763,150013,151223,152403,153469,154430,155175,156139,157351,158483,159511,160532,161207,162209,163097,164004,164862,165640,166741,167207,167689,168352,169074,169782,170523,171293,172325,173283,174356,175573,176574,177826,179080,180194,181513,182654,183880,184920,186003,187164,188182,189199,190328,191341,192243,193217,194057,195195,196129,196949,197810,198690,199639,200797,201973,202807,203750,204651,205531,206668,207800,209085,210168,211083,212044,212975,213847,214856,216113,216892,217963,218931,219926,221139,222130,223397,224926,226394,227975,229409,231010,232448,233465,234010,234354],sizes:[1349,1255,1335,1275,1453,1545,1456,963,1080,1251,1005,1386,1153,1172,1098,1376,1049,909,1059,982,1080,936,1099,923,1174,1282,831,1196,937,1054,808,617,790,723,724,640,675,573,491,676,695,458,635,835,912,691,611,712,807,843,473,605,705,634,784,793,500,626,569,549,454,835,1425,1192,1104,867,668,859,696,681,790,631,570,1032,1054,1149,1123,970,873,936,829,783,985,859,936,932,927,903,924,946,941,904,913,893,951,809,809,909,941,916,918,815,940,915,946,1300,1224,1101,1045,782,973,1103,1083,1149,1252,552,611,760,753,1071,923,766,952,1123,1050,821,833,635,765,802,819,876,914,708,1065,985,825,864,1015,1053,1116,783,933,1019,888,902,802,998,760,1086,1029,785,960,749,815,915,926,946,870,897,728,836,1197,1250,1210,1180,1066,961,745,964,1212,1132,1028,1021,675,1002,888,907,858,778,1101,466,482,663,722,708,741,770,1032,958,1073,1217,1001,1252,1254,1114,1319,1141,1226,1040,1083,1161,1018,1017,1129,1013,902,974,840,1138,934,820,861,880,949,1158,1176,834,943,901,880,1137,1132,1285,1083,915,961,931,872,1009,1257,779,1071,968,995,1213,991,1267,1529,1468,1581,1434,1601,1438,1017,545,344,369],successes:[1,1,1,1,1,1,1,1,1,1,1,1,1,1,1,1,1,1,1,1,1,1,1,1,1,1,1,1,1,1,1,1,1,1,1,1,1,1,1,1,1,1,1,1,1,1,1,1,1,1,1,1,1,1,1,1,1,1,1,1,1,1,1,1,1,1,1,1,1,1,1,1,1,1,1,1,1,1,1,1,1,1,1,1,1,1,1,1,1,1,1,1,1,1,1,1,1,1,1,1,1,1,1,1,1,1,1,1,1,1,1,1,1,1,1,1,1,1,1,1,1,1,1,1,1,1,1,1,1,1,1,1,1,1,1,1,1,1,1,1,1,1,1,1,1,1,1,1,1,1,1,1,1,1,1,1,1,1,1,1,1,1,1,1,1,1,1,1,1,1,1,1,1,1,1,1,1,1,1,1,1,1,1,1,1,1,1,1,1,1,1,1,1,1,1,1,1,1,1,1,1,1,1,1,1,1,1,1,1,1,1,1,1,1,1,1,1,1,1,1,1,1,1,1,1,1,1,1,1,1,1,1,1,1,1,1,1,1,1,1,1,1,1,1,1,1,1,1]};compressedData["data"]=byteArray;assert(typeof Module.LZ4==="object","LZ4 not present - was your app build with -s LZ4=1 ?");Module.LZ4.loadPackage({metadata:metadata,compressedData:compressedData},true);Module["removeRunDependency"]("datafile_html5lib.data")}Module["addRunDependency"]("datafile_html5lib.data");if(!Module.preloadResults)Module.preloadResults={};Module.preloadResults[PACKAGE_NAME]={fromCache:false};if(fetched){processPackageData(fetched);fetched=null}else{fetchedCallback=processPackageData}}if(Module["calledRun"]){runWithFS()}else{if(!Module["preRun"])Module["preRun"]=[];Module["preRun"].push(runWithFS)}};loadPackage({files:[{filename:"/lib/python3.9/site-packages/html5lib/__init__.py",start:0,end:1143,audio:0},{filename:"/lib/python3.9/site-packages/html5lib/_ihatexml.py",start:1143,end:17871,audio:0},{filename:"/lib/python3.9/site-packages/html5lib/_inputstream.py",start:17871,end:50171,audio:0},{filename:"/lib/python3.9/site-packages/html5lib/_tokenizer.py",start:50171,end:127199,audio:0},{filename:"/lib/python3.9/site-packages/html5lib/_utils.py",start:127199,end:132118,audio:0},{filename:"/lib/python3.9/site-packages/html5lib/constants.py",start:132118,end:215582,audio:0},{filename:"/lib/python3.9/site-packages/html5lib/html5parser.py",start:215582,end:332756,audio:0},{filename:"/lib/python3.9/site-packages/html5lib/serializer.py",start:332756,end:348503,audio:0},{filename:"/lib/python3.9/site-packages/html5lib/_trie/__init__.py",start:348503,end:348612,audio:0},{filename:"/lib/python3.9/site-packages/html5lib/_trie/_base.py",start:348612,end:349625,audio:0},{filename:"/lib/python3.9/site-packages/html5lib/_trie/py.py",start:349625,end:351388,audio:0},{filename:"/lib/python3.9/site-packages/html5lib/filters/__init__.py",start:351388,end:351388,audio:0},{filename:"/lib/python3.9/site-packages/html5lib/filters/alphabeticalattributes.py",start:351388,end:352307,audio:0},{filename:"/lib/python3.9/site-packages/html5lib/filters/base.py",start:352307,end:352593,audio:0},{filename:"/lib/python3.9/site-packages/html5lib/filters/inject_meta_charset.py",start:352593,end:355538,audio:0},{filename:"/lib/python3.9/site-packages/html5lib/filters/lint.py",start:355538,end:359169,audio:0},{filename:"/lib/python3.9/site-packages/html5lib/filters/optionaltags.py",start:359169,end:369757,audio:0},{filename:"/lib/python3.9/site-packages/html5lib/filters/sanitizer.py",start:369757,end:396642,audio:0},{filename:"/lib/python3.9/site-packages/html5lib/filters/whitespace.py",start:396642,end:397856,audio:0},{filename:"/lib/python3.9/site-packages/html5lib/treeadapters/__init__.py",start:397856,end:398506,audio:0},{filename:"/lib/python3.9/site-packages/html5lib/treeadapters/genshi.py",start:398506,end:400221,audio:0},{filename:"/lib/python3.9/site-packages/html5lib/treeadapters/sax.py",start:400221,end:401997,audio:0},{filename:"/lib/python3.9/site-packages/html5lib/treebuilders/__init__.py",start:401997,end:405589,audio:0},{filename:"/lib/python3.9/site-packages/html5lib/treebuilders/base.py",start:405589,end:420142,audio:0},{filename:"/lib/python3.9/site-packages/html5lib/treebuilders/dom.py",start:420142,end:429067,audio:0},{filename:"/lib/python3.9/site-packages/html5lib/treebuilders/etree.py",start:429067,end:441891,audio:0},{filename:"/lib/python3.9/site-packages/html5lib/treebuilders/etree_lxml.py",start:441891,end:456645,audio:0},{filename:"/lib/python3.9/site-packages/html5lib/treewalkers/__init__.py",start:456645,end:462364,audio:0},{filename:"/lib/python3.9/site-packages/html5lib/treewalkers/base.py",start:462364,end:469840,audio:0},{filename:"/lib/python3.9/site-packages/html5lib/treewalkers/dom.py",start:469840,end:471253,audio:0},{filename:"/lib/python3.9/site-packages/html5lib/treewalkers/etree.py",start:471253,end:475792,audio:0},{filename:"/lib/python3.9/site-packages/html5lib/treewalkers/etree_lxml.py",start:475792,end:482137,audio:0},{filename:"/lib/python3.9/site-packages/html5lib/treewalkers/genshi.py",start:482137,end:484446,audio:0},{filename:"/lib/python3.9/site-packages/html5lib-1.1-py3.9.egg-info/PKG-INFO",start:484446,end:500159,audio:0},{filename:"/lib/python3.9/site-packages/html5lib-1.1-py3.9.egg-info/SOURCES.txt",start:500159,end:506632,audio:0},{filename:"/lib/python3.9/site-packages/html5lib-1.1-py3.9.egg-info/dependency_links.txt",start:506632,end:506633,audio:0},{filename:"/lib/python3.9/site-packages/html5lib-1.1-py3.9.egg-info/requires.txt",start:506633,end:506836,audio:0},{filename:"/lib/python3.9/site-packages/html5lib-1.1-py3.9.egg-info/top_level.txt",start:506836,end:506845,audio:0}],remote_package_size:238819,package_uuid:"a50b5e37-b22e-4bd0-bc31-0c562b3d2706"})})();
\ No newline at end of file
diff --git a/spaces/qinzhu/moe-tts-tech/text/ngu_dialect.py b/spaces/qinzhu/moe-tts-tech/text/ngu_dialect.py
deleted file mode 100644
index 69d0ce6fe5a989843ee059a71ccab793f20f9176..0000000000000000000000000000000000000000
--- a/spaces/qinzhu/moe-tts-tech/text/ngu_dialect.py
+++ /dev/null
@@ -1,30 +0,0 @@
-import re
-import opencc
-
-
-dialects = {'SZ': 'suzhou', 'WX': 'wuxi', 'CZ': 'changzhou', 'HZ': 'hangzhou',
- 'SX': 'shaoxing', 'NB': 'ningbo', 'JJ': 'jingjiang', 'YX': 'yixing',
- 'JD': 'jiading', 'ZR': 'zhenru', 'PH': 'pinghu', 'TX': 'tongxiang',
- 'JS': 'jiashan', 'HN': 'xiashi', 'LP': 'linping', 'XS': 'xiaoshan',
- 'FY': 'fuyang', 'RA': 'ruao', 'CX': 'cixi', 'SM': 'sanmen',
- 'TT': 'tiantai', 'WZ': 'wenzhou', 'SC': 'suichang', 'YB': 'youbu'}
-
-converters = {}
-
-for dialect in dialects.values():
- try:
- converters[dialect] = opencc.OpenCC("chinese_dialect_lexicons/"+dialect)
- except:
- pass
-
-
-def ngu_dialect_to_ipa(text, dialect):
- dialect = dialects[dialect]
- text = converters[dialect].convert(text).replace('-','').replace('$',' ')
- text = re.sub(r'[、;:]', ',', text)
- text = re.sub(r'\s*,\s*', ', ', text)
- text = re.sub(r'\s*。\s*', '. ', text)
- text = re.sub(r'\s*?\s*', '? ', text)
- text = re.sub(r'\s*!\s*', '! ', text)
- text = re.sub(r'\s*$', '', text)
- return text
diff --git a/spaces/quidiaMuxgu/Expedit-SAM/Direct Message For Instagram Pro 4.1.11 [TOP].md b/spaces/quidiaMuxgu/Expedit-SAM/Direct Message For Instagram Pro 4.1.11 [TOP].md
deleted file mode 100644
index b190ef99551e9f1a43d2c74c279d2c09abd8e8ca..0000000000000000000000000000000000000000
--- a/spaces/quidiaMuxgu/Expedit-SAM/Direct Message For Instagram Pro 4.1.11 [TOP].md
+++ /dev/null
@@ -1,27 +0,0 @@
-
-Direct Message for Instagram Pro 4.1.11: A Review
-Direct Message for Instagram Pro 4.1.11 is an Android app that allows you to send and receive messages, photos, videos, posts and stories with your Instagram friends and followers. It is a premium version of the official Instagram Direct app, which offers some extra features and benefits.
-Some of the features of Direct Message for Instagram Pro 4.1.11 are:
-Direct Message for Instagram Pro 4.1.11
Download ✶ https://geags.com/2uCqqn
-
-- You can chat with anyone on Instagram, even if they are not following you or have a private account.
-- You can send unlimited messages and media files without any restrictions or limits.
-- You can use various effects and filters to enhance your photos and videos before sending them.
-- You can mute or block unwanted messages and users.
-- You can manage multiple Instagram accounts from one app.
-- You can access your messages from any device with the same account.
-
-Direct Message for Instagram Pro 4.1.11 is a useful app for anyone who wants to communicate with their Instagram contacts more easily and conveniently. It is especially helpful for businesses and influencers who want to connect with their customers and fans on a personal level. The app has a simple and user-friendly interface, and it works smoothly and fast.
-However, there are also some drawbacks of Direct Message for Instagram Pro 4.1.11 that you should be aware of before downloading it. Some of them are:
-
-- The app is not free. You have to pay a one-time fee of $9.99 to download it from the Google Play Store.
-- The app is not affiliated with or endorsed by Instagram. It is a third-party app that uses Instagram's API to access your messages and data. Therefore, it may not be secure or reliable, and it may violate Instagram's terms of service and privacy policy.
-- The app may not be compatible with some devices or Android versions. It may also have some bugs or errors that affect its performance and functionality.
-- The app may not be updated regularly or supported by the developer. It may not work with future changes or updates of Instagram.
-
-In conclusion, Direct Message for Instagram Pro 4.1.11 is an app that offers some advantages and disadvantages for Instagram users who want to chat with their contacts more easily and conveniently. It is up to you to decide whether the benefits outweigh the risks and costs of using this app.
If you are interested in trying Direct Message for Instagram Pro 4.1.11, you can download it from the Google Play Store by clicking here. You can also check out some of the reviews and ratings from other users who have used this app. However, before you install it, make sure you read and agree to the app's terms of service and privacy policy, and that you have a backup of your Instagram data in case something goes wrong.
-Alternatively, if you are looking for a safer and more official way to chat with your Instagram contacts, you can use the Instagram Direct app that is included in the Instagram app. You can also use other messaging apps that are compatible with Instagram, such as WhatsApp, Messenger, or Telegram. These apps may not have all the features and benefits of Direct Message for Instagram Pro 4.1.11, but they are more secure and reliable, and they respect your privacy and data.
-
-Ultimately, the choice is yours. Whether you use Direct Message for Instagram Pro 4.1.11 or another app, we hope you enjoy chatting with your Instagram friends and followers.
d5da3c52bf
-
-
\ No newline at end of file
diff --git a/spaces/r3gm/SoniTranslate_translate_audio_of_a_video_content/lib/infer_pack/onnx_inference.py b/spaces/r3gm/SoniTranslate_translate_audio_of_a_video_content/lib/infer_pack/onnx_inference.py
deleted file mode 100644
index 6517853be49e61c427cf7cd9b5ed203f6d5f367e..0000000000000000000000000000000000000000
--- a/spaces/r3gm/SoniTranslate_translate_audio_of_a_video_content/lib/infer_pack/onnx_inference.py
+++ /dev/null
@@ -1,145 +0,0 @@
-import onnxruntime
-import librosa
-import numpy as np
-import soundfile
-
-
-class ContentVec:
- def __init__(self, vec_path="pretrained/vec-768-layer-12.onnx", device=None):
- print("load model(s) from {}".format(vec_path))
- if device == "cpu" or device is None:
- providers = ["CPUExecutionProvider"]
- elif device == "cuda":
- providers = ["CUDAExecutionProvider", "CPUExecutionProvider"]
- elif device == "dml":
- providers = ["DmlExecutionProvider"]
- else:
- raise RuntimeError("Unsportted Device")
- self.model = onnxruntime.InferenceSession(vec_path, providers=providers)
-
- def __call__(self, wav):
- return self.forward(wav)
-
- def forward(self, wav):
- feats = wav
- if feats.ndim == 2: # double channels
- feats = feats.mean(-1)
- assert feats.ndim == 1, feats.ndim
- feats = np.expand_dims(np.expand_dims(feats, 0), 0)
- onnx_input = {self.model.get_inputs()[0].name: feats}
- logits = self.model.run(None, onnx_input)[0]
- return logits.transpose(0, 2, 1)
-
-
-def get_f0_predictor(f0_predictor, hop_length, sampling_rate, **kargs):
- if f0_predictor == "pm":
- from lib.infer_pack.modules.F0Predictor.PMF0Predictor import PMF0Predictor
-
- f0_predictor_object = PMF0Predictor(
- hop_length=hop_length, sampling_rate=sampling_rate
- )
- elif f0_predictor == "harvest":
- from lib.infer_pack.modules.F0Predictor.HarvestF0Predictor import (
- HarvestF0Predictor,
- )
-
- f0_predictor_object = HarvestF0Predictor(
- hop_length=hop_length, sampling_rate=sampling_rate
- )
- elif f0_predictor == "dio":
- from lib.infer_pack.modules.F0Predictor.DioF0Predictor import DioF0Predictor
-
- f0_predictor_object = DioF0Predictor(
- hop_length=hop_length, sampling_rate=sampling_rate
- )
- else:
- raise Exception("Unknown f0 predictor")
- return f0_predictor_object
-
-
-class OnnxRVC:
- def __init__(
- self,
- model_path,
- sr=40000,
- hop_size=512,
- vec_path="vec-768-layer-12",
- device="cpu",
- ):
- vec_path = f"pretrained/{vec_path}.onnx"
- self.vec_model = ContentVec(vec_path, device)
- if device == "cpu" or device is None:
- providers = ["CPUExecutionProvider"]
- elif device == "cuda":
- providers = ["CUDAExecutionProvider", "CPUExecutionProvider"]
- elif device == "dml":
- providers = ["DmlExecutionProvider"]
- else:
- raise RuntimeError("Unsportted Device")
- self.model = onnxruntime.InferenceSession(model_path, providers=providers)
- self.sampling_rate = sr
- self.hop_size = hop_size
-
- def forward(self, hubert, hubert_length, pitch, pitchf, ds, rnd):
- onnx_input = {
- self.model.get_inputs()[0].name: hubert,
- self.model.get_inputs()[1].name: hubert_length,
- self.model.get_inputs()[2].name: pitch,
- self.model.get_inputs()[3].name: pitchf,
- self.model.get_inputs()[4].name: ds,
- self.model.get_inputs()[5].name: rnd,
- }
- return (self.model.run(None, onnx_input)[0] * 32767).astype(np.int16)
-
- def inference(
- self,
- raw_path,
- sid,
- f0_method="dio",
- f0_up_key=0,
- pad_time=0.5,
- cr_threshold=0.02,
- ):
- f0_min = 50
- f0_max = 1100
- f0_mel_min = 1127 * np.log(1 + f0_min / 700)
- f0_mel_max = 1127 * np.log(1 + f0_max / 700)
- f0_predictor = get_f0_predictor(
- f0_method,
- hop_length=self.hop_size,
- sampling_rate=self.sampling_rate,
- threshold=cr_threshold,
- )
- wav, sr = librosa.load(raw_path, sr=self.sampling_rate)
- org_length = len(wav)
- if org_length / sr > 50.0:
- raise RuntimeError("Reached Max Length")
-
- wav16k = librosa.resample(wav, orig_sr=self.sampling_rate, target_sr=16000)
- wav16k = wav16k
-
- hubert = self.vec_model(wav16k)
- hubert = np.repeat(hubert, 2, axis=2).transpose(0, 2, 1).astype(np.float32)
- hubert_length = hubert.shape[1]
-
- pitchf = f0_predictor.compute_f0(wav, hubert_length)
- pitchf = pitchf * 2 ** (f0_up_key / 12)
- pitch = pitchf.copy()
- f0_mel = 1127 * np.log(1 + pitch / 700)
- f0_mel[f0_mel > 0] = (f0_mel[f0_mel > 0] - f0_mel_min) * 254 / (
- f0_mel_max - f0_mel_min
- ) + 1
- f0_mel[f0_mel <= 1] = 1
- f0_mel[f0_mel > 255] = 255
- pitch = np.rint(f0_mel).astype(np.int64)
-
- pitchf = pitchf.reshape(1, len(pitchf)).astype(np.float32)
- pitch = pitch.reshape(1, len(pitch))
- ds = np.array([sid]).astype(np.int64)
-
- rnd = np.random.randn(1, 192, hubert_length).astype(np.float32)
- hubert_length = np.array([hubert_length]).astype(np.int64)
-
- out_wav = self.forward(hubert, hubert_length, pitch, pitchf, ds, rnd).squeeze()
- out_wav = np.pad(out_wav, (0, 2 * self.hop_size), "constant")
- return out_wav[0:org_length]
diff --git a/spaces/radames/UserControllableLT-Latent-Transformer/expansion/dataloader/kitti15list_train.py b/spaces/radames/UserControllableLT-Latent-Transformer/expansion/dataloader/kitti15list_train.py
deleted file mode 100644
index e1eca1af37426274490e916b884271281024cc47..0000000000000000000000000000000000000000
--- a/spaces/radames/UserControllableLT-Latent-Transformer/expansion/dataloader/kitti15list_train.py
+++ /dev/null
@@ -1,31 +0,0 @@
-import torch.utils.data as data
-
-from PIL import Image
-import os
-import os.path
-import numpy as np
-
-IMG_EXTENSIONS = [
- '.jpg', '.JPG', '.jpeg', '.JPEG',
- '.png', '.PNG', '.ppm', '.PPM', '.bmp', '.BMP',
-]
-
-
-def is_image_file(filename):
- return any(filename.endswith(extension) for extension in IMG_EXTENSIONS)
-
-def dataloader(filepath):
-
- left_fold = 'image_2/'
- flow_noc = 'flow_occ/'
-
- train = [img for img in os.listdir(filepath+left_fold) if img.find('_10') > -1]
-
- train = [i for i in train if int(i.split('_')[0])%5!=0]
-
- l0_train = [filepath+left_fold+img for img in train]
- l1_train = [filepath+left_fold+img.replace('_10','_11') for img in train]
- flow_train = [filepath+flow_noc+img for img in train]
-
-
- return sorted(l0_train), sorted(l1_train), sorted(flow_train)
diff --git a/spaces/raedeXanto/academic-chatgpt-beta/EXCLUSIVE-Full-Ejercicios-Resueltos-De-Ingenieria-Economica-De-Blank-Y-Tarquin-6ta-Edicion.md b/spaces/raedeXanto/academic-chatgpt-beta/EXCLUSIVE-Full-Ejercicios-Resueltos-De-Ingenieria-Economica-De-Blank-Y-Tarquin-6ta-Edicion.md
deleted file mode 100644
index 8d523a7b28692235c0135bf15e919b35025d674e..0000000000000000000000000000000000000000
--- a/spaces/raedeXanto/academic-chatgpt-beta/EXCLUSIVE-Full-Ejercicios-Resueltos-De-Ingenieria-Economica-De-Blank-Y-Tarquin-6ta-Edicion.md
+++ /dev/null
@@ -1,120 +0,0 @@
-## [FULL] Ejercicios Resueltos De Ingenieria Economica De Blank Y Tarquin 6ta Edicion
-
-
-
-
-
- ![\[!EXCLUSIVE! Full\] Ejercicios Resueltos De Ingenieria Economica De Blank Y Tarquin 6ta Edicion](https://encrypted-tbn1.gstatic.com/images?q=tbn:ANd9GcRHXfZkBS9NmBodxB61-3Wuip2bX1VNhcJOZeD9v7PLkM0RwZz5ygchDyPY)
-
-
-
-
-
-**DOWNLOAD ……… [https://ekporriola.blogspot.com/?c=2tAnVX](https://ekporriola.blogspot.com/?c=2tAnVX)**
-
-
-
-
-
-
-
-
-
-
-
-
-
-# [FULL] Ejercicios Resueltos De Ingenieria Economica De Blank Y Tarquin 6ta Edicion
-
-
-
-La ingenierÃa económica es una disciplina que se ocupa de la evaluación de proyectos y decisiones que involucran aspectos financieros y técnicos. Para ello, se utilizan herramientas como el análisis de flujo de caja, el valor presente, el valor futuro, la tasa interna de retorno, el costo-beneficio, entre otras.
-
-
-
-Uno de los libros más reconocidos y utilizados en esta materia es el de Leland Blank y Anthony Tarquin, titulado "IngenierÃa Económica", que actualmente va por su séptima edición. Sin embargo, muchos estudiantes y profesores aún recurren a la sexta edición, que contiene numerosos ejercicios resueltos y propuestos sobre los diferentes temas del curso.
-
-
-
-En este artÃculo, se presenta una recopilación de algunos de los ejercicios resueltos de la sexta edición del libro de Blank y Tarquin, que pueden ser de gran ayuda para repasar y profundizar los conceptos y métodos de la ingenierÃa económica. Los ejercicios se han tomado de diversas fuentes en internet, como Academia.edu[^1^], Course Hero[^2^] y Vdocuments.net[^3^], y se han adaptado al formato HTML para facilitar su lectura.
-
-
-
-Se recomienda consultar el libro original para verificar la exactitud y completitud de las soluciones, asà como para acceder a más ejercicios y ejemplos. Asimismo, se sugiere resolver los ejercicios por cuenta propia antes de revisar las respuestas, para comprobar el nivel de comprensión y aplicación de los conocimientos adquiridos.
-
-
-
-A continuación, se presentan algunos ejercicios resueltos del capÃtulo 1: Fundamentos de la ingenierÃa económica.
-
-
-
-## Ejercicio 1.1
-
-
-
-Los cuatro elementos son los flujos de caja, el tiempo de ocurrencia de los flujos de caja, las tasas de interés y la medida de valor económico.
-
-
-
-## Ejercicio 1.2
-
-
-
-(a) Los fondos de capital son el dinero utilizado para financiar proyectos. Suele ser limitado en la cantidad de dinero disponible. (b) El análisis de sensibilidad es un procedimiento que implica cambiar diversas estimaciones para ver si/cómo afectan a la decisión económica.
-
-
-
-## Ejercicio 1.3
-
-
-
-Cualquiera de los siguientes son medidas de valor: valor presente, valor futuro, valor anual, tasa interna de retorno, relación beneficio-costo, costo capitalizado, periodo de recuperación, valor económico agregado.
-
-
-
-## Ejercicio 1.4
-
-
-
-Costo inicial: económico; liderazgo: no económico; impuestos: económico; valor residual: económico; moral: no económico; confiabilidad: no económico; inflación: económico; beneficio: económico; aceptación: no económico; ética: no económico; tasa de interés: económico.
-
-
-
-## Ejercicio 1.5
-
-
-
-Se podrÃan identificar muchas secciones. Algunas son: I.b; II.2.a y b; III.9.a y b.
-
-
-
-## Ejercicio 1.6
-
-
-
-Algunas acciones posibles son:
-
-
-
-- Intentar convencerlos de que no lo hagan ahora, explicándoles que es robar
-
-- Intentar que paguen por sus bebidas
-
-- Pagar por todas las bebidas él mismo
-
-- Alejarse y no asociarse con ellos nuevamente
-
-
-
-## Ejercicio 1.7
-
-
-
-Esta es una pregunta estructurada para ser discutida; muchas respuestas son aceptables. Es una cuestión ét
-
- 145887f19f
-
-
-
-
-
diff --git a/spaces/raedeXanto/academic-chatgpt-beta/Electromagnetismo con aplicaciones kraus pdf La obra maestra de John D. Kraus sobre electromagnetismo.md b/spaces/raedeXanto/academic-chatgpt-beta/Electromagnetismo con aplicaciones kraus pdf La obra maestra de John D. Kraus sobre electromagnetismo.md
deleted file mode 100644
index 943b458cfdcac514d3e73556cb440636a6c665f5..0000000000000000000000000000000000000000
--- a/spaces/raedeXanto/academic-chatgpt-beta/Electromagnetismo con aplicaciones kraus pdf La obra maestra de John D. Kraus sobre electromagnetismo.md
+++ /dev/null
@@ -1,145 +0,0 @@
-
-Electromagnetismo con Aplicaciones Kraus PDF: A Comprehensive Guide
-Electromagnetism is one of the most fascinating and important branches of physics, with applications ranging from communication, energy, medicine, engineering, to astronomy. If you are interested in learning more about this subject, you might want to check out Electromagnetismo con Aplicaciones Kraus PDF, a classic textbook that covers the theory and practice of electromagnetics in a comprehensive and accessible way.
-In this article, we will give you a brief overview of what Electromagnetismo con Aplicaciones Kraus PDF is, how you can download it for free online, and how you can use it for learning and teaching. We will also answer some frequently asked questions about the book. Let's get started!
-electromagnetismoconaplicacioneskrauspdf
Download --->>> https://tinourl.com/2uL4Lo
- What is Electromagnetismo con Aplicaciones Kraus PDF?
-Electromagnetismo con Aplicaciones Kraus PDF is the Spanish translation of Electromagnetics with Applications, a textbook written by John D. Kraus and Daniel A. Fleisch. It was first published in 1999 by McGraw-Hill and has been widely used by students and instructors around the world.
- The authors and their backgrounds
-John D. Kraus (1910-2004) was a renowned American physicist and engineer who made significant contributions to electromagnetics, radio astronomy, antennas, and wireless systems. He was a professor at Ohio State University for over 40 years and received many honors and awards for his research and teaching. He also wrote several other popular books on electromagnetics, such as Antennas and Radio Astronomy.
-electromagnetismo con aplicaciones kraus pdf gratis
-electromagnetismo con aplicaciones kraus pdf descargar
-electromagnetismo con aplicaciones kraus pdf solucionario
-electromagnetismo con aplicaciones kraus pdf download
-electromagnetismo con aplicaciones kraus pdf online
-electromagnetismo con aplicaciones kraus pdf libro
-electromagnetismo con aplicaciones kraus pdf español
-electromagnetismo con aplicaciones kraus pdf free
-electromagnetismo con aplicaciones kraus pdf mega
-electromagnetismo con aplicaciones kraus pdf drive
-electromagnetismo con aplicaciones kraus pdf 4ta edicion
-electromagnetismo con aplicaciones kraus pdf 3ra edicion
-electromagnetismo con aplicaciones kraus pdf segunda mano
-electromagnetismo con aplicaciones kraus pdf amazon
-electromagnetismo con aplicaciones kraus pdf mercadolibre
-electromagnetismo con aplicaciones kraus pdf google books
-electromagnetismo con aplicaciones kraus pdf indice
-electromagnetismo con aplicaciones kraus pdf capitulo 1
-electromagnetismo con aplicaciones kraus pdf capitulo 2
-electromagnetismo con aplicaciones kraus pdf capitulo 3
-electromagnetismo con aplicaciones kraus pdf capitulo 4
-electromagnetismo con aplicaciones kraus pdf capitulo 5
-electromagnetismo con aplicaciones kraus pdf capitulo 6
-electromagnetismo con aplicaciones kraus pdf capitulo 7
-electromagnetismo con aplicaciones kraus pdf capitulo 8
-electromagnetismo con aplicaciones kraus pdf capitulo 9
-electromagnetismo con aplicaciones kraus pdf capitulo 10
-electromagnetismo con aplicaciones kraus pdf capitulo 11
-electromagnetismo con aplicaciones kraus pdf capitulo 12
-electromagnetismo con aplicaciones kraus pdf ejercicios resueltos
-electromagnetismo con aplicaciones kraus pdf ejemplos resueltos
-electromagnetismo con aplicaciones kraus pdf problemas resueltos
-electromagnetismo con aplicaciones kraus pdf preguntas resueltas
-electromagnetismo con aplicaciones kraus pdf examenes resueltos
-electromagnetismo con aplicaciones kraus pdf practicas resueltas
-electromagnetismo con aplicaciones kraus pdf teoria y ejercicios
-electromagnetismo con aplicaciones kraus pdf introduccion al tema
-electromagnetismo con aplicaciones kraus pdf conceptos basicos
-electromagnetismo con aplicaciones kraus pdf fundamentos teoricos
-electromagnetismo con aplicaciones kraus pdf principios y leyes
-electromagnetismo con aplicaciones kraus pdf campos electricos y magneticos
-electromagnetismo con aplicaciones kraus pdf potencial y energia electrica
-electromagnetismo con aplicaciones kraus pdf corriente y resistencia electrica
-electromagnetismo con aplicaciones kraus pdf circuitos electricos y capacitores
-electromagnetismo con aplicaciones kraus pdf inductancia y autoinduccion
-electromagnetismo con aplicaciones kraus pdf fuerzas electromagneticas y torque
-electromagnetismo con aplicaciones kraus pdf ondas electromagneticas y radiacion
-electromagnetismo con aplicaciones kraus pdf antenas y lineas de transmision
-electromagnetismo con aplicaciones kraus pdf guias de onda y cavidades resonantes
-Daniel A. Fleisch is a professor of physics at Wittenberg University in Ohio. He specializes in electromagnetics, optics, relativity, and numerical methods. He has co-authored several books with John D. Kraus, such as A Student's Guide to Maxwell's Equations and A Student's Guide to Vectors and Tensors.
- The main topics and concepts covered
-Electromagnetismo con Aplicaciones Kraus PDF covers the fundamentals of electromagnetics as well as its applications in various fields. It consists of 11 chapters that are organized into two parts: core content (chapters 1-5) and supplementary material (chapters 6-11). Here are some of the topics covered in each chapter:
-
-- Chapter 1: Introduction - This chapter introduces the basic concepts of electric and magnetic fields, forces, potentials, fluxes, currents, charges, dipoles, polarization, magnetization, etc.
-- Chapter 2: Electric and Magnetic Fields - This chapter develops the mathematical tools for describing electric and magnetic fields in different coordinate systems, such as vectors, scalars, gradients, divergences, curls, Laplacians, etc.
-- Chapter 3: Transmission Lines - This chapter explains how electric signals propagate along transmission lines, such as coaxial cables, microstrip lines, waveguides, etc. It also discusses the concepts of impedance matching, reflection coefficient, standing wave ratio, etc.
-- Chapter 4: Wave Propagation, Attenuation, Polarization, Reflection, Refraction, and Diffraction - This chapter describes how electromagnetic waves travel through different media, such as vacuum, dielectrics, conductors, plasmas, etc. It also analyzes how waves interact with boundaries, such as reflection, refraction, total internal reflection, Brewster's angle, Fresnel equations, etc. It also introduces the concepts of polarization, ellipticity, circular dichroism, etc.
-- Chapter 5: Antennas, Radiation, and Wireless Systems - This chapter explores how electromagnetic waves are generated and detected by antennas, such as dipole antennas, loop antennas, horn antennas, parabolic reflectors, etc. It also discusses the concepts of radiation pattern, directivity, gain, efficiency, bandwidth, impedance, etc. It also explains how wireless systems work, such as modulation, demodulation, amplitude modulation (AM), frequency modulation (FM), phase modulation (PM), etc.
-- Chapter 6: Electrodynamics - This chapter presents the advanced topics of electrodynamics, such as Maxwell's equations, conservation laws, Poynting vector, electromagnetic stress tensor, electromagnetic waves in vacuum and matter, etc.
-- Chapter 7: Dielectric and Magnetic Materials - This chapter studies how electric and magnetic fields affect and are affected by different types of materials, such as linear and nonlinear dielectrics, ferroelectrics, piezoelectrics, pyroelectrics, electro-optics, magneto-optics, etc.
-- Chapter 8: Waveguides, Resonators, and Fiber Optics - This chapter investigates how electromagnetic waves propagate along confined structures, such as rectangular and circular waveguides, cavities and resonators, optical fibers and waveguides,
-
compensation and recognition for their work and effort. Therefore, we strongly advise you to respect the intellectual property rights of the authors and publishers and buy the book from a legitimate source if you can afford it.
- The best sources and websites to find the book
-If you still want to download Electromagnetismo con Aplicaciones Kraus PDF for free online, you need to be careful of the sources and websites that offer the book, as some of them might contain viruses, malware, or scams that can harm your device or personal information. Here are some tips to find a reliable and safe source:
-
-- Look for reputable and well-known websites that specialize in free books, such as Project Gutenberg, The Internet Archive, Open Library, and Feedbooks. These sites usually have a large collection of books in various formats and languages, and they are legal and ethical.
-- Avoid websites that ask you to register, sign up, or provide personal or financial information before downloading the book. These sites might be phishing or scamming you for your data or money.
-- Check the reviews and ratings of the websites and the books before downloading them. Look for positive feedback from other users and avoid sites that have negative or suspicious comments.
-- Scan the downloaded file with an antivirus or malware protection software before opening it. Make sure the file has the correct format and size for the book.
-
-The steps and tips to download the book safely and quickly
-Once you have found a reliable and safe source for Electromagnetismo con Aplicaciones Kraus PDF, you can follow these steps to download the book:
-
-- Click on the link or button that says \"Download\", \"Read Online\", \"Get This Book\", or something similar.
-- Select the format that you prefer, such as epub, Kindle, or PDF.
-- Choose the destination folder or location where you want to save the file on your device.
-- Wait for the download to complete.
-- Open the file with your preferred ereader app or program.
-
-Here are some tips to download the book faster and easier:
-
-- Use a fast and stable internet connection.
-- Use a download manager or accelerator software that can speed up and resume downloads.
-- Download the book when the website is less busy or crowded, such as late at night or early in the morning.
-
- How to use Electromagnetismo con Aplicaciones Kraus PDF for learning and teaching?
-Electromagnetismo con Aplicaciones Kraus PDF is a great resource for learning and teaching electromagnetics at an undergraduate or graduate level. However, you need to know how to use it effectively and efficiently. Here are some suggestions on how to use the book for different purposes:
- The prerequisites and requirements for reading the book
-To read and understand Electromagnetismo con Aplicaciones Kraus PDF, you need to have some background knowledge and skills in physics, mathematics, and engineering. Specifically, you need to be familiar with:
-
-- The basic concepts of electricity and magnetism, such as Coulomb's law, Gauss's law, Ampere's law, Faraday's law, etc.
-- The mathematical tools for describing electric and magnetic fields, such as vectors, scalars, gradients, divergences, curls, Laplacians, etc.
-- The differential and integral calculus, such as derivatives, integrals, chain rule, product rule, quotient rule, etc.
-- The linear algebra, such as matrices, determinants, eigenvalues, eigenvectors, etc.
-- The complex analysis, such as complex numbers, complex functions, Cauchy-Riemann equations, contour integration, residue theorem, etc.
-
- The recommended study plan and schedule for the book
-To study Electromagnetismo con Aplicaciones Kraus PDF effectively and efficiently, you need to have a clear plan and schedule. Here are some tips on how to create and follow a study plan:
-
-- Set a realistic goal and timeline for completing the book. For example, you can aim to finish one chapter per week or per month depending on your pace and availability.
-- Divide each chapter into manageable sections or subtopics. For example, you can break down chapter 1 into introduction, electric fields, magnetic fields, electric flux, Gauss's law, etc.
-- Review the summary and the main points at the end of each section or chapter. Make sure you understand the key concepts and formulas.
-- Solve the examples and exercises in the book. Check your answers with the solutions provided or with a reliable source.
-- Attempt the problems at the end of each chapter. Try to solve them without looking at the solutions or hints. If you get stuck, review the relevant theory or examples.
-- Use online resources to supplement your learning. For example, you can watch video lectures, animations, simulations, or demonstrations on electromagnetics. You can also use online calculators, tools, or software to solve problems or visualize concepts.
-
- The supplementary materials and resources for the book
-To enhance your learning and teaching experience with Electromagnetismo con Aplicaciones Kraus PDF, you can use some supplementary materials and resources that are available online. Here are some examples:
-
-- The official website of the book provides a suite of online demonstration software that illustrates various concepts and phenomena in electromagnetics. You can access them at https://www.ece.vt.edu/swe/emag/.
-- The author's website provides some additional material for the book, such as lecture notes, slides, solutions manual, errata, etc. You can access them at https://www.ece.vt.edu/swe/book/.
-- The publisher's website provides some extra material for the book, such as sample chapters, instructor's manual, test bank, etc. You can access them at https://www.mheducation.com/highered/product/electromagnetics-applications-kraus-fleisch/M9780073380667.html.
-- Some other websites that provide useful resources for electromagnetics are:
-
-- Bioelectromagnetics - A peer-reviewed journal that specializes in reporting original data on biological effects and applications of electromagnetic fields.
-- Electromagnetics Vol 1 - A free open textbook that covers the fundamentals of electromagnetics.
-- Classification and characterization of electromagnetic materials - A research article that presents a novel method for classifying and characterizing electromagnetic materials based on their effective parameters.
-
-
- Conclusion
-In this article, we have given you a brief overview of what Electromagnetismo con Aplicaciones Kraus PDF is, how you can download it for free online, and how you can use it for learning and teaching. We hope you have found this article helpful and informative. If you have any questions or comments about the book or the article, please feel free to contact us. Thank you for reading!
- FAQs
-Here are some frequently asked questions about Electromagnetismo con Aplicaciones Kraus PDF:
-
-- Q: What is the difference between Electromagnetismo con Aplicaciones Kraus PDF and Electromagnetics with Applications Kraus PDF?
-- A: Electromagnetismo con Aplicaciones Kraus PDF is the Spanish translation of Electromagnetics with Applications Kraus PDF. They are essentially the same book except for the language.
-- Q: Is there a newer edition of Electromagnetismo con Aplicaciones Kraus PDF?
-- A: No, there is no newer edition of Electromagnetismo con Aplicaciones Kraus PDF. The latest edition is the 5th edition published in 1999.
-- Q: Is there a solution manual for Electromagnetismo con Aplicaciones Kraus PDF?
-- A: Yes, there is a solution manual for Electromagnetismo con Aplicaciones Kraus PDF. You can find it on the author's website or on the publisher's website.
-- Q: How can I cite Electromagnetismo con Aplicaciones Kraus PDF in my paper or report?
-- A: You can cite Electromagnetismo con Aplicaciones Kraus PDF using the following format:
- Kraus J.D., Fleisch D.A. (1999) Electromagnetismo con Aplicaciones (5th ed.). McGraw-Hill.
- 0a6ba089eb
-
-
\ No newline at end of file
diff --git a/spaces/raedeXanto/academic-chatgpt-beta/Elige Tu Propia Aventura Pdf Descargar Free Los Mejores Libros para Estimular tu Imaginacin y Creatividad.md b/spaces/raedeXanto/academic-chatgpt-beta/Elige Tu Propia Aventura Pdf Descargar Free Los Mejores Libros para Estimular tu Imaginacin y Creatividad.md
deleted file mode 100644
index 736c32a3c88e98569e5c65f8194ef164c1a6ec5d..0000000000000000000000000000000000000000
--- a/spaces/raedeXanto/academic-chatgpt-beta/Elige Tu Propia Aventura Pdf Descargar Free Los Mejores Libros para Estimular tu Imaginacin y Creatividad.md
+++ /dev/null
@@ -1,113 +0,0 @@
-
-
- H2: HSMWorks offers advanced features and benefits for CNC machining
- H2: HSMWorks requires a license to activate and use | | H1: What is a keygen and how does it work? | - H2: A keygen is a software that generates serial numbers or activation codes for other software
- H2: A keygen can bypass the license verification process of the original software
- H2: A keygen can be illegal, risky and unethical to use | | H1: How to use a keygen to activate HSMWorks 2017 64 bits? | - H2: Download and install HSMWorks 2017 64 bits from the official website or a trusted source
- H2: Download and run a keygen for HSMWorks 2017 64 bits from a reliable source
- H2: Follow the instructions of the keygen to generate and enter the activation code for HSMWorks 2017 64 bits
- H2: Enjoy using HSMWorks 2017 64 bits without any limitations | | H1: What are the risks and drawbacks of using a keygen to activate HSMWorks 2017 64 bits? | - H2: A keygen can contain malware, viruses or spyware that can harm your computer or data
- H2: A keygen can be detected and blocked by antivirus software or firewall
- H2: A keygen can violate the terms and conditions of the original software and expose you to legal consequences
- H2: A keygen can compromise the quality and performance of the original software and cause errors or crashes | | H1: What are the alternatives to using a keygen to activate HSMWorks 2017 64 bits? | - H2: Buy a legitimate license for HSMWorks 2017 64 bits from the official website or an authorized reseller
- H2: Use a free trial version of HSMWorks 2017 64 bits for a limited time
- H2: Use a free or open source CAM software that is compatible with SolidWorks
- H2: Use an online service that offers CAM solutions for a fee | **Table 2: Article with HTML formatting** ```html What is HSMWorks and why do you need it?
-HSMWorks is a computer-aided manufacturing (CAM) software that integrates seamlessly with SolidWorks, one of the most popular computer-aided design (CAD) software in the world. With HSMWorks, you can design and program your CNC machines directly from your SolidWorks model, without having to switch between different applications or formats.
-keygen para activar HSMWorks 2017 64 bits
Download Zip 🆗 https://tinourl.com/2uKZ5K
-HSMWorks is a CAM software for SolidWorks
-HSMWorks is designed to work with SolidWorks as an add-in, meaning that you can access all its features and functions from within the SolidWorks interface. You can use the same tools and commands that you are familiar with in SolidWorks, such as sketching, modeling, assembly, simulation, and drawing. You can also use the same file format (.sldprt) for both CAD and CAM operations, which simplifies data management and reduces errors.
-HSMWorks offers advanced features and benefits for CNC machining
-HSMWorks provides you with a comprehensive set of tools and options for creating efficient and high-quality CNC toolpaths for your machining projects. Some of the features and benefits of HSMWorks include:
-
-- Adaptive Clearing: This is a high-performance roughing strategy that reduces machining time, tool wear, and heat generation by adapting the toolpath to the geometry and avoiding full-width cuts.
-- 3D Toolpaths: These are toolpaths that follow the contours of your 3D model, allowing you to create smooth and accurate surfaces for complex shapes.
-- Multi-axis Machining: These are toolpaths that utilize more than three axes of motion, such as rotary or tilting axes, to create intricate geometries and features that are otherwise impossible or difficult to achieve.
-- Simulation and Verification: These are features that allow you to preview and check your toolpaths before sending them to your CNC machine, ensuring that they are error-free and optimal.
-- Post Processing: This is a feature that converts your toolpaths into machine-specific code (G-code) that can be understood by your CNC machine controller.
-
-HSMWorks requires a license to activate and use
-HSMWorks is a commercial software that requires a valid license to activate and use. You can purchase a license for HSMWorks from the official website or an authorized reseller. The price of a license depends on the type and duration of the license, as well as the number of users and machines. You can also request a quote from the website or contact the sales team for more information.
-What is a keygen and how does it work?
-A keygen is a software that generates serial numbers or activation codes for other software. A keygen can be used to bypass the license verification process of the original software, allowing you to use it without paying for it or obtaining a legitimate license.
-A keygen can bypass the license verification process of the original software
-A keygen works by exploiting the algorithm or logic that is used by the original software to generate and validate serial numbers or activation codes. A keygen mimics this algorithm or logic and produces serial numbers or activation codes that match the criteria of the original software. By entering these serial numbers or activation codes into the original software, you can trick it into thinking that you have a valid license and activate it.
-A keygen can be illegal, risky and unethical to use
-A keygen can be considered as a form of software piracy, which is illegal in many countries and regions. By using a keygen, you are violating the intellectual property rights of the original software developer and depriving them of their rightful revenue. You may also face legal consequences such as fines or imprisonment if you are caught using or distributing a keygen.
-A keygen can also pose various risks to your computer system and data. A keygen may contain malware, viruses or spyware that can infect your computer or steal your personal information. A keygen may also be detected and blocked by antivirus software or firewall, preventing you from using it or accessing other programs. A keygen may also compromise the quality and performance of the original software, causing errors or crashes.
-A keygen can also be unethical to use, as it shows disrespect and dishonesty towards the original software developer who spent time, effort and money to create their product. By using a keygen, you are taking advantage of their hard work without giving them any credit or compensation. You may also harm other users who paid for their licenses by creating unfair competition or reducing customer support.
-* clave de licencia HSMWorks 2017 64 bits gratis
-* descargar keygen HSMWorks 2017 64 bits full
-* como activar HSMWorks 2017 64 bits con crack
-* serial para instalar HSMWorks 2017 64 bits
-* codigo de activacion HSMWorks 2017 64 bits online
-* keygen HSMWorks 2017 64 bits mega
-* activador HSMWorks 2017 64 bits sin virus
-* keygen HSMWorks 2017 64 bits windows 10
-* generador de claves HSMWorks 2017 64 bits
-* keygen HSMWorks 2017 64 bits descargar
-* crack para HSMWorks 2017 64 bits español
-* keygen HSMWorks 2017 64 bits funcionando
-* licencia HSMWorks 2017 64 bits original
-* keygen HSMWorks 2017 64 bits ultima version
-* keygen para HSMWorks 2017 64 bits gratis
-* keygen HSMWorks 2017 64 bits full crack
-* como usar keygen HSMWorks 2017 64 bits
-* serial de oro HSMWorks 2017 64 bits
-* keygen HSMWorks 2017 64 bits mediafire
-* activar HSMWorks 2017 64 bits sin keygen
-* keygen HSMWorks 2017 64 bits portable
-* keygen HSMWorks 2017 64 bits rar
-* crack para HSMWorks 2017 64 bits ingles
-* keygen HSMWorks 2017 64 bits no funciona
-* licencia HSMWorks 2017 64 bits crackeada
-* keygen HSMWorks 2017 64 bits actualizado
-* keygen para HSMWorks 2017 64 bits completo
-* como descargar keygen HSMWorks 2017 64 bits
-* serial valido HSMWorks 2017 64 bits
-* keygen HSMWorks 2017 64 bits google drive
-* activar HSMWorks 2017 64 bits con keygen
-* keygen HSMWorks
-How to use a keygen to activate HSMWorks 2017 64 bits?
-If you still want to use a keygen to activate HSMWorks 2017 64 bits, despite knowing its illegality, risks and drawbacks, here are some steps that you may follow:
-Download and install HSMWorks 2017 64 bits from the official website or a trusted source
-The first step is to download and install HSMWorks 2017 64 bits on your computer. You can download it from the official website or from another trusted source that offers genuine downloads. You should avoid downloading it from unknown or suspicious sources that may contain fake or corrupted files.
-To install it on your computer, you need to follow the instructions on the screen. You may need to enter some basic information such as your name, email address, company name etc. You may also need to accept some terms and conditions before proceeding with the installation.
-Download and run a keygen for HSMWorks 2017 64 bits from a reliable source
-The next step is to download and run a keygen for HSMWorks 2017 64 bits on your computer. You can find various sources online that offer different versions of keygens for different products. You should look for one that specifically works for HSMWorks 2017 64 bits. You should also check the reviews, ratings, comments etc. of other users who have used it before downloading the product.
-
-After entering the activation code, you should see a message that confirms that HSMWorks 2017 64 bits has been successfully activated and is ready to use.
-What are the risks and drawbacks of using a keygen to activate HSMWorks 2017 64 bits?
-As mentioned earlier, using a keygen to activate HSMWorks 2017 64 bits can be illegal, risky and unethical. Here are some of the risks and drawbacks that you may face if you use a keygen:
-A keygen can contain malware, viruses or spyware that can harm your computer or data
-A keygen can be a source of infection for your computer system and data. A keygen may contain malicious code that can damage your files, folders, programs or settings. A keygen may also contain hidden programs that can monitor your activities, record your keystrokes, capture your screen or webcam, steal your passwords, credit card numbers or other personal information. A keygen may also download or install other unwanted software on your computer without your consent or knowledge.
-These malware, viruses or spyware can compromise your security and privacy and expose you to identity theft, fraud or blackmail. They can also slow down your computer performance and cause instability or crashes. They can also be difficult to detect and remove, as they may disguise themselves as legitimate files or processes or hide in the background.
-A keygen can be detected and blocked by antivirus software or firewall
-A keygen can be recognized and stopped by antivirus software or firewall that are installed on your computer or network. Antivirus software or firewall can scan your files and programs for any signs of infection or threat and alert you if they find any. They can also block or delete any suspicious files or programs that they encounter.
-This means that you may not be able to download, run or use a keygen on your computer or network. You may also receive warnings or notifications from your antivirus software or firewall that inform you of the potential danger of using a keygen. You may also need to disable your antivirus software or firewall temporarily if you want to use a keygen, which can leave your computer vulnerable to other attacks.
-A keygen can violate the terms and conditions of the original software and expose you to legal consequences
-A keygen can be considered as a form of software piracy, which is illegal in many countries and regions. Software piracy is the unauthorized copying, distribution or use of software that is protected by intellectual property rights. By using a keygen, you are infringing on the rights of the original software developer and breaking the law.
-This means that you may face legal consequences such as fines or imprisonment if you are caught using or distributing a keygen. You may also be sued by the original software developer for damages or compensation. You may also lose access to any updates, support or services that are provided by the original software developer.
-A keygen can compromise the quality and performance of the original software and cause errors or crashes
-A keygen can affect the quality and performance of the original software that you are trying to activate. A keygen may not be compatible with the latest version or features of the original software. A keygen may also introduce bugs or errors into the original software. A keygen may also interfere with other programs or processes that are running on your computer.
-This means that you may not be able to enjoy the full functionality and benefits of the original software. You may also experience problems such as poor performance, reduced quality, missing features, corrupted files, incorrect results or unexpected behavior. You may also encounter errors or crashes that can disrupt your work or cause data loss.
-What are the alternatives to using a keygen to activate HSMWorks 2017 64 bits?
-If you want to use HSMWorks 2017 64 bits without using a keygen, there are some alternatives that you can consider. These alternatives are legal, safe and ethical and can provide you with similar or better results than using a keygen. Here are some of the alternatives that you can try:
-Buy a legitimate license for HSMWorks 2017 64 bits from the official website or an authorized reseller
-The best and most recommended alternative to using a keygen is to buy a legitimate license for HSMWorks 2017 64 bits from the official website or an authorized reseller. By buying a legitimate license, you are supporting the original software developer and respecting their intellectual property rights. You are also getting a genuine and reliable product that is guaranteed to work as intended.
-By buying a legitimate license, you can enjoy all the features and benefits of HSMWorks 2017 64 bits without any limitations or restrictions. You can also access any updates, support or services that are provided by the original software developer. You can also avoid any risks or drawbacks that are associated with using a keygen.
-Use a free trial version of HSMWorks 2017 64 bits for a limited time
-Another alternative to using a keygen is to use a free trial version of HSMWorks 2017 64 bits for a limited time. A free trial version is a version of the software that you can use for free for a certain period of time, usually 30 days. A free trial version allows you to test and evaluate the software before buying it.
-By using a free trial version, you can get a taste of what HSMWorks 2017 64 bits can do for you and your CNC machining projects. You can also compare it with other CAM software and see if it meets your needs and expectations. You can also learn how to use it and familiarize yourself with its interface and functions.
-However, by using a free trial version, you may not be able to access all the features and benefits of HSMWorks 2017 64 bits. You may also encounter some limitations or restrictions such as watermarks, reduced quality, limited functionality or expiration date. You may also need to register or provide some information to download or use the free trial version.
-Use a free or open source CAM software that is compatible with SolidWorks
-A third alternative to using a keygen is to use a free or open source CAM software that is compatible with SolidWorks. A free or open source CAM software is a software that you can use for free without paying for it or obtaining a license. A free or open source CAM software is usually developed by volunteers or communities who share their code and resources with others.
-By using a free or open source CAM software, you can save money and still get a decent CAM solution for your CNC machining projects. You can also contribute to the development and improvement of the software by giving feedback, reporting bugs, making donations or joining the community.
-However, by using a free or open source CAM software, you may not be able to get the same quality and performance as HSMWorks 2017 64 bits. You may also encounter some compatibility issues with SolidWorks or your CNC machine controller. You may also lack some features or benefits that are offered by HSMWorks 2017 64 bits. You may also need to spend more time and effort to learn how to use it and find support or help if you encounter any problems.
-Use an online service that offers CAM solutions for a fee
-A fourth alternative to using a keygen is to use an online service that offers CAM solutions for a fee. An online service is a website or platform that provides various services or solutions for different purposes or needs. An online service that offers CAM solutions is one that allows you to create and program your CNC toolpaths online without installing any software on your computer.
-By using an online service, you can access CAM solutions from anywhere and anytime as long as you have an internet connection and a web browser. You can also choose from different options and plans that suit your budget and requirements. You can also benefit from the expertise and experience of the online service provider who can offer you guidance and support.
-However, by using an online service, you may need to pay a fee for using their CAM solutions, which may vary depending on the service provider, the type and duration of the service, the complexity and size of your project etc. You may also need to upload your SolidWorks model to their website or platform, which may raise some security and privacy concerns. You may also depend on the availability and reliability of the internet connection and the online service provider.
-Conclusion
-In conclusion, HSMWorks 2017 64 bits is a powerful and useful CAM software that integrates with SolidWorks and offers advanced features and benefits for CNC machining. However, HSMWorks 2017 64 bits requires a license to activate and use, which can be expensive or difficult to obtain. Some people may resort to using a keygen to activate HSMWorks 2017 64 bits for free, but this can be illegal, risky and unethical. There are some alternatives to using a keygen that are legal, safe and ethical, such as buying a legitimate license, using a free trial version, using a free or open source CAM software or using an online service.
-FAQs
-What is HSMWorks?
-HSMWorks is a computer-aided manufacturing (CAM) software that integrates seamlessly with SolidWorks, one of the most popular computer-aided design (CAD) software in the world.
-What is a keygen?
-A keygen is a software that generates serial numbers or activation codes for other software. A keygen can be used to bypass the license verification process of the original software, allowing you to use it without paying for it or obtaining a legitimate license.
-How to use a keygen to activate HSMWorks 2017 64 bits?
-To use a keygen to activate HSMWorks 2017 64 bits, you need to download and install HSMWorks 2017 64 bits from the official website or a trusted source, download and run a keygen for HSMWorks 2017 64 bits from a reliable source, follow the instructions of the keygen to generate and enter the activation code for HSMWorks 2017 64 bits.
-What are the risks and drawbacks of using a keygen to activate HSMWorks 2017 64 bits?
-Some of the risks and drawbacks of using a keygen to activate HSMWorks 2017 64 bits are: it can contain malware, viruses or spyware that can harm your computer or data; it can be detected and blocked by antivirus software or firewall; it can violate the terms and conditions of the original software and expose you to legal consequences; it can compromise the quality and performance of the original software and cause errors or crashes.
-What are the alternatives to using a keygen to activate HSMWorks 2017 64 bits?
-Some of the alternatives to using a keygen to activate HSMWorks 2017 64 bits are: buy a legitimate license for HSMWorks 2017 64 bits from the official website or an authorized reseller; use a free trial version of HSMWorks 2017 64 bits for a limited time; use a free or open source CAM software that is compatible with SolidWorks; use an online service that offers CAM solutions for a fee.
- 0a6ba089eb
-
-
\ No newline at end of file
diff --git a/spaces/rainy3/chatgpt_academic/request_llm/bridge_tgui.py b/spaces/rainy3/chatgpt_academic/request_llm/bridge_tgui.py
deleted file mode 100644
index 22a407557fa884f23dd768164b009d7bed841dd9..0000000000000000000000000000000000000000
--- a/spaces/rainy3/chatgpt_academic/request_llm/bridge_tgui.py
+++ /dev/null
@@ -1,167 +0,0 @@
-'''
-Contributed by SagsMug. Modified by binary-husky
-https://github.com/oobabooga/text-generation-webui/pull/175
-'''
-
-import asyncio
-import json
-import random
-import string
-import websockets
-import logging
-import time
-import threading
-import importlib
-from toolbox import get_conf, update_ui
-LLM_MODEL, = get_conf('LLM_MODEL')
-
-# "TGUI:galactica-1.3b@localhost:7860"
-model_name, addr_port = LLM_MODEL.split('@')
-assert ':' in addr_port, "LLM_MODEL 格式不正确!" + LLM_MODEL
-addr, port = addr_port.split(':')
-
-def random_hash():
- letters = string.ascii_lowercase + string.digits
- return ''.join(random.choice(letters) for i in range(9))
-
-async def run(context, max_token=512):
- params = {
- 'max_new_tokens': max_token,
- 'do_sample': True,
- 'temperature': 0.5,
- 'top_p': 0.9,
- 'typical_p': 1,
- 'repetition_penalty': 1.05,
- 'encoder_repetition_penalty': 1.0,
- 'top_k': 0,
- 'min_length': 0,
- 'no_repeat_ngram_size': 0,
- 'num_beams': 1,
- 'penalty_alpha': 0,
- 'length_penalty': 1,
- 'early_stopping': True,
- 'seed': -1,
- }
- session = random_hash()
-
- async with websockets.connect(f"ws://{addr}:{port}/queue/join") as websocket:
- while content := json.loads(await websocket.recv()):
- #Python3.10 syntax, replace with if elif on older
- if content["msg"] == "send_hash":
- await websocket.send(json.dumps({
- "session_hash": session,
- "fn_index": 12
- }))
- elif content["msg"] == "estimation":
- pass
- elif content["msg"] == "send_data":
- await websocket.send(json.dumps({
- "session_hash": session,
- "fn_index": 12,
- "data": [
- context,
- params['max_new_tokens'],
- params['do_sample'],
- params['temperature'],
- params['top_p'],
- params['typical_p'],
- params['repetition_penalty'],
- params['encoder_repetition_penalty'],
- params['top_k'],
- params['min_length'],
- params['no_repeat_ngram_size'],
- params['num_beams'],
- params['penalty_alpha'],
- params['length_penalty'],
- params['early_stopping'],
- params['seed'],
- ]
- }))
- elif content["msg"] == "process_starts":
- pass
- elif content["msg"] in ["process_generating", "process_completed"]:
- yield content["output"]["data"][0]
- # You can search for your desired end indicator and
- # stop generation by closing the websocket here
- if (content["msg"] == "process_completed"):
- break
-
-
-
-
-
-def predict_tgui(inputs, top_p, temperature, chatbot, history=[], system_prompt='', stream = True, additional_fn=None):
- """
- 发送至chatGPT,流式获取输出。
- 用于基础的对话功能。
- inputs 是本次问询的输入
- top_p, temperature是chatGPT的内部调优参数
- history 是之前的对话列表(注意无论是inputs还是history,内容太长了都会触发token数量溢出的错误)
- chatbot 为WebUI中显示的对话列表,修改它,然后yeild出去,可以直接修改对话界面内容
- additional_fn代表点击的哪个按钮,按钮见functional.py
- """
- if additional_fn is not None:
- import core_functional
- importlib.reload(core_functional) # 热更新prompt
- core_functional = core_functional.get_core_functions()
- if "PreProcess" in core_functional[additional_fn]: inputs = core_functional[additional_fn]["PreProcess"](inputs) # 获取预处理函数(如果有的话)
- inputs = core_functional[additional_fn]["Prefix"] + inputs + core_functional[additional_fn]["Suffix"]
-
- raw_input = "What I would like to say is the following: " + inputs
- logging.info(f'[raw_input] {raw_input}')
- history.extend([inputs, ""])
- chatbot.append([inputs, ""])
- yield from update_ui(chatbot=chatbot, history=history, msg="等待响应") # 刷新界面
-
- prompt = inputs
- tgui_say = ""
-
- mutable = ["", time.time()]
- def run_coorotine(mutable):
- async def get_result(mutable):
- async for response in run(prompt):
- print(response[len(mutable[0]):])
- mutable[0] = response
- if (time.time() - mutable[1]) > 3:
- print('exit when no listener')
- break
- asyncio.run(get_result(mutable))
-
- thread_listen = threading.Thread(target=run_coorotine, args=(mutable,), daemon=True)
- thread_listen.start()
-
- while thread_listen.is_alive():
- time.sleep(1)
- mutable[1] = time.time()
- # Print intermediate steps
- if tgui_say != mutable[0]:
- tgui_say = mutable[0]
- history[-1] = tgui_say
- chatbot[-1] = (history[-2], history[-1])
- yield from update_ui(chatbot=chatbot, history=history) # 刷新界面
-
- logging.info(f'[response] {tgui_say}')
-
-
-
-def predict_tgui_no_ui(inputs, top_p, temperature, history=[], sys_prompt=""):
- raw_input = "What I would like to say is the following: " + inputs
- prompt = inputs
- tgui_say = ""
- mutable = ["", time.time()]
- def run_coorotine(mutable):
- async def get_result(mutable):
- async for response in run(prompt, max_token=20):
- print(response[len(mutable[0]):])
- mutable[0] = response
- if (time.time() - mutable[1]) > 3:
- print('exit when no listener')
- break
- asyncio.run(get_result(mutable))
- thread_listen = threading.Thread(target=run_coorotine, args=(mutable,))
- thread_listen.start()
- while thread_listen.is_alive():
- time.sleep(1)
- mutable[1] = time.time()
- tgui_say = mutable[0]
- return tgui_say
diff --git a/spaces/rayan-saleh/whisper2notion/server/node_modules/@types/node-fetch/externals.d.ts b/spaces/rayan-saleh/whisper2notion/server/node_modules/@types/node-fetch/externals.d.ts
deleted file mode 100644
index 8a1d0f8ed4e775b1219c871f048fb77d703ca51e..0000000000000000000000000000000000000000
--- a/spaces/rayan-saleh/whisper2notion/server/node_modules/@types/node-fetch/externals.d.ts
+++ /dev/null
@@ -1,21 +0,0 @@
-// `AbortSignal` is defined here to prevent a dependency on a particular
-// implementation like the `abort-controller` package, and to avoid requiring
-// the `dom` library in `tsconfig.json`.
-
-export interface AbortSignal {
- aborted: boolean;
-
- addEventListener: (type: "abort", listener: ((this: AbortSignal, event: any) => any), options?: boolean | {
- capture?: boolean | undefined,
- once?: boolean | undefined,
- passive?: boolean | undefined
- }) => void;
-
- removeEventListener: (type: "abort", listener: ((this: AbortSignal, event: any) => any), options?: boolean | {
- capture?: boolean | undefined
- }) => void;
-
- dispatchEvent: (event: any) => boolean;
-
- onabort: null | ((this: AbortSignal, event: any) => any);
-}
diff --git a/spaces/recenWmenso/ChatGPT-with-Voice-Cloning-for-All/datasets/Age Of Empires 2 Hd Steam Apidll Crack BETTER Download.md b/spaces/recenWmenso/ChatGPT-with-Voice-Cloning-for-All/datasets/Age Of Empires 2 Hd Steam Apidll Crack BETTER Download.md
deleted file mode 100644
index 80061fa36425c5b2b3240497a1a19087cd8f6dd0..0000000000000000000000000000000000000000
--- a/spaces/recenWmenso/ChatGPT-with-Voice-Cloning-for-All/datasets/Age Of Empires 2 Hd Steam Apidll Crack BETTER Download.md
+++ /dev/null
@@ -1,6 +0,0 @@
-Age Of Empires 2 Hd Steam Apidll Crack Download
DOWNLOAD ✪✪✪ https://urlgoal.com/2uCMDF
-
- 4d29de3e1b
-
-
-
diff --git a/spaces/recenWmenso/ChatGPT-with-Voice-Cloning-for-All/datasets/Free Download Information Technology Auditing And Assurance Solutions Manual James Hall Pdf.md b/spaces/recenWmenso/ChatGPT-with-Voice-Cloning-for-All/datasets/Free Download Information Technology Auditing And Assurance Solutions Manual James Hall Pdf.md
deleted file mode 100644
index 7f1f6aafaeb8cc493d9f83fecf6b0785e0d947c9..0000000000000000000000000000000000000000
--- a/spaces/recenWmenso/ChatGPT-with-Voice-Cloning-for-All/datasets/Free Download Information Technology Auditing And Assurance Solutions Manual James Hall Pdf.md
+++ /dev/null
@@ -1,14 +0,0 @@
-
-Information Technology Auditing and Assurance by James A. Hall: A Comprehensive Guide for Students and Professionals
-Information technology (IT) auditing and assurance is a specialized field of accounting that focuses on the evaluation and improvement of the quality, reliability, and security of IT systems and processes. IT auditors and assurance professionals use various tools and techniques to assess the effectiveness of IT controls, identify risks and vulnerabilities, and provide recommendations for enhancing IT performance and compliance.
-One of the most widely used textbooks in this field is Information Technology Auditing and Assurance by James A. Hall, a Professor of Accounting and Co-Director of the Computer Science and Business program at Lehigh University. This book covers the essential concepts and principles of IT auditing and assurance, as well as the latest standards, frameworks, methodologies, and best practices. It also includes numerous case studies, examples, exercises, and review questions to help students and professionals apply their knowledge and skills to real-world scenarios.
-Free Download Information Technology Auditing And Assurance Solutions Manual James Hall Pdf
Download File 🆓 https://urlgoal.com/2uCKMo
-However, finding a free download of this book online can be challenging, as it is protected by copyright laws. Therefore, students and professionals who want to access this book should consider purchasing it from a reputable source, such as Amazon or Google Books. Alternatively, they can also borrow it from a library or a friend who owns a copy.
-By reading Information Technology Auditing and Assurance by James A. Hall, students and professionals can gain a solid foundation and a competitive edge in the field of IT auditing and assurance. This book can help them prepare for various certifications, such as Certified Information Systems Auditor (CISA), Certified Information Systems Security Professional (CISSP), or Certified Information Technology Professional (CITP). It can also help them advance their careers and contribute to the improvement of IT governance, risk management, and assurance in their organizations.
In this article, we will provide an overview of the main topics covered in Information Technology Auditing and Assurance by James A. Hall. The book is divided into four parts, each consisting of several chapters.
-Part I: Auditing and Assurance Services. This part introduces the basic concepts and terminology of auditing and assurance services, such as audit objectives, audit evidence, audit risk, audit planning, audit reports, and audit quality. It also explains the role and responsibilities of IT auditors and assurance professionals, as well as the ethical and legal issues they face.
-Part II: IT Governance and Management. This part discusses the importance and challenges of IT governance and management, such as aligning IT with business strategy, ensuring IT value delivery, managing IT resources, controlling IT risks, and measuring IT performance. It also describes the various frameworks and standards that guide IT governance and management practices, such as COBIT, ITIL, ISO 27000, and COSO.
-Part III: Systems Development and Acquisition. This part examines the processes and controls involved in developing and acquiring IT systems and applications, such as system development life cycle (SDLC), project management, system analysis and design, system testing and implementation, system maintenance and change management, and system acquisition and outsourcing. It also provides guidance on how to audit and assure these processes and controls using various techniques, such as walkthroughs, questionnaires, checklists, flowcharts, data flow diagrams, and testing tools.
-Part IV: Information Systems Operations. This part explores the operations and maintenance of IT systems and infrastructure, such as network administration, database administration, system security administration, backup and recovery procedures, disaster recovery planning, business continuity planning, and incident management. It also demonstrates how to audit and assure these operations using various methods, such as observation, inquiry, inspection, reperformance, analytical procedures, and computer-assisted audit techniques (CAATs).
- d5da3c52bf
-
-
\ No newline at end of file
diff --git a/spaces/riccorl/relik-entity-linking/relik/reader/pytorch_modules/optim/layer_wise_lr_decay.py b/spaces/riccorl/relik-entity-linking/relik/reader/pytorch_modules/optim/layer_wise_lr_decay.py
deleted file mode 100644
index d179096153f356196a921c50083c96b3dcd5f246..0000000000000000000000000000000000000000
--- a/spaces/riccorl/relik-entity-linking/relik/reader/pytorch_modules/optim/layer_wise_lr_decay.py
+++ /dev/null
@@ -1,104 +0,0 @@
-import collections
-from typing import List
-
-import torch
-import transformers
-from torch.optim import AdamW
-
-
-class LayerWiseLRDecayOptimizer:
- def __init__(
- self,
- lr: float,
- warmup_steps: int,
- total_steps: int,
- weight_decay: float,
- lr_decay: float,
- no_decay_params: List[str],
- total_reset: int,
- ):
- self.lr = lr
- self.warmup_steps = warmup_steps
- self.total_steps = total_steps
- self.weight_decay = weight_decay
- self.lr_decay = lr_decay
- self.no_decay_params = no_decay_params
- self.total_reset = total_reset
-
- def group_layers(self, module) -> dict:
- grouped_layers = collections.defaultdict(list)
- module_named_parameters = list(module.named_parameters())
- for ln, lp in module_named_parameters:
- if "embeddings" in ln:
- grouped_layers["embeddings"].append((ln, lp))
- elif "encoder.layer" in ln:
- layer_num = ln.split("transformer_model.encoder.layer.")[-1]
- layer_num = layer_num[0 : layer_num.index(".")]
- grouped_layers[layer_num].append((ln, lp))
- else:
- grouped_layers["head"].append((ln, lp))
-
- depth = len(grouped_layers) - 1
- final_dict = dict()
- for key, value in grouped_layers.items():
- if key == "head":
- final_dict[0] = value
- elif key == "embeddings":
- final_dict[depth] = value
- else:
- # -1 because layer number starts from zero
- final_dict[depth - int(key) - 1] = value
-
- assert len(module_named_parameters) == sum(
- len(v) for _, v in final_dict.items()
- )
-
- return final_dict
-
- def group_params(self, module) -> list:
- optimizer_grouped_params = []
- for inverse_depth, layer in self.group_layers(module).items():
- layer_lr = self.lr * (self.lr_decay**inverse_depth)
- layer_wd_params = {
- "params": [
- lp
- for ln, lp in layer
- if not any(nd in ln for nd in self.no_decay_params)
- ],
- "weight_decay": self.weight_decay,
- "lr": layer_lr,
- }
- layer_no_wd_params = {
- "params": [
- lp
- for ln, lp in layer
- if any(nd in ln for nd in self.no_decay_params)
- ],
- "weight_decay": 0,
- "lr": layer_lr,
- }
-
- if len(layer_wd_params) != 0:
- optimizer_grouped_params.append(layer_wd_params)
- if len(layer_no_wd_params) != 0:
- optimizer_grouped_params.append(layer_no_wd_params)
-
- return optimizer_grouped_params
-
- def __call__(self, module: torch.nn.Module):
- optimizer_grouped_parameters = self.group_params(module)
- optimizer = AdamW(optimizer_grouped_parameters, lr=self.lr)
- scheduler = transformers.get_cosine_with_hard_restarts_schedule_with_warmup(
- optimizer,
- self.warmup_steps,
- self.total_steps,
- num_cycles=self.total_reset,
- )
- return {
- "optimizer": optimizer,
- "lr_scheduler": {
- "scheduler": scheduler,
- "interval": "step",
- "frequency": 1,
- },
- }
diff --git a/spaces/robin0307/MMOCR/configs/_base_/recog_datasets/seg_toy_data.py b/spaces/robin0307/MMOCR/configs/_base_/recog_datasets/seg_toy_data.py
deleted file mode 100644
index 7f0b7d8f4c520ec7847d69743d8e430b8795b656..0000000000000000000000000000000000000000
--- a/spaces/robin0307/MMOCR/configs/_base_/recog_datasets/seg_toy_data.py
+++ /dev/null
@@ -1,34 +0,0 @@
-prefix = 'tests/data/ocr_char_ann_toy_dataset/'
-
-train = dict(
- type='OCRSegDataset',
- img_prefix=f'{prefix}/imgs',
- ann_file=f'{prefix}/instances_train.txt',
- loader=dict(
- type='AnnFileLoader',
- repeat=100,
- file_format='txt',
- parser=dict(
- type='LineJsonParser', keys=['file_name', 'annotations', 'text'])),
- pipeline=None,
- test_mode=True)
-
-test = dict(
- type='OCRDataset',
- img_prefix=f'{prefix}/imgs',
- ann_file=f'{prefix}/instances_test.txt',
- loader=dict(
- type='AnnFileLoader',
- repeat=1,
- file_format='txt',
- parser=dict(
- type='LineStrParser',
- keys=['filename', 'text'],
- keys_idx=[0, 1],
- separator=' ')),
- pipeline=None,
- test_mode=True)
-
-train_list = [train]
-
-test_list = [test]
diff --git a/spaces/rorallitri/biomedical-language-models/logs/Enjoy the Music of Ek Cutting Chai [2000-MP3-VBR-320Kbps] - Stream or Download MP3 Songs Online.md b/spaces/rorallitri/biomedical-language-models/logs/Enjoy the Music of Ek Cutting Chai [2000-MP3-VBR-320Kbps] - Stream or Download MP3 Songs Online.md
deleted file mode 100644
index f25f98156411d9dc03a31342511f8062507d33f1..0000000000000000000000000000000000000000
--- a/spaces/rorallitri/biomedical-language-models/logs/Enjoy the Music of Ek Cutting Chai [2000-MP3-VBR-320Kbps] - Stream or Download MP3 Songs Online.md
+++ /dev/null
@@ -1,6 +0,0 @@
-Ek Cutting Chai [2000-MP3-VBR-320Kbps]
Download File ☑ https://tinurll.com/2uzmwh
-
- aaccfb2cb3
-
-
-
diff --git a/spaces/roxas010394/parts-of-cars/app.py b/spaces/roxas010394/parts-of-cars/app.py
deleted file mode 100644
index 503afe998141c29b7554ec275e495108f7df1b30..0000000000000000000000000000000000000000
--- a/spaces/roxas010394/parts-of-cars/app.py
+++ /dev/null
@@ -1,34 +0,0 @@
-from ultralytics import YOLO
-import matplotlib.pyplot as plt
-import gradio as gr
-import cv2
-import numpy as np
-import random
-import seaborn as sns
-from PIL import Image
-import io
-def predict(path:str):
- model = YOLO("yolov8n.yaml")
- model = YOLO("best.pt")
- image = cv2.imread(path)
-
- image = cv2.cvtColor(image, cv2.COLOR_BGR2RGB)
- results = model.predict(source=path)
- paleta= sns.color_palette("bright", 17)
- fig = plt.figure()
- vectObjs = results[0].masks.xy
- resNumClase = results[0].boxes.cls.numpy().astype(int)
- conf = results[0].boxes.conf.numpy()
- for i in range(len(vectObjs)):
- objDet = vectObjs[i].astype(int)
- color = (paleta[i][0]*255, paleta[i][1]*255, paleta[i][2]*255)
- image = cv2.polylines(image, [objDet], True, color, 4)
- plt.text(objDet[0][0], objDet[0][1], results[0].names[resNumClase[i]]+" "+ str(conf[i]), bbox=dict(facecolor=paleta[i], alpha=0.5))
-
- plt.imshow(image)
- plt.axis('off')
- return plt
-gr.Interface(fn=predict,
- inputs=gr.components.Image(type="filepath", label="Input"),
- outputs=gr.Plot(label="Resultado de detección de objetos con regularizacion")).launch()
- #outputs=gr.components.Image(type="pil", label="Output")).launch()
\ No newline at end of file
diff --git a/spaces/saawal/Heart_Disease_Model/app.py b/spaces/saawal/Heart_Disease_Model/app.py
deleted file mode 100644
index 264ee50876a04c0ca0e98159feb51b48965d4142..0000000000000000000000000000000000000000
--- a/spaces/saawal/Heart_Disease_Model/app.py
+++ /dev/null
@@ -1,45 +0,0 @@
-import gradio as gr
-import numpy as np
-import pickle
-import sklearn
-loaded_model = pickle.load(open('trained_model.pkl','rb'))
-
-def healthy_heart(Patient_Name,age,sex,cp,trestbps,chol,fbs,restecg,thalach,exang,oldpeak,slope,ca,thal):
-
- x = np.array([age,sex,cp,trestbps,chol,fbs,restecg,thalach,exang,oldpeak,slope,ca,thal])
- X= x.reshape(1,-1)
- result = loaded_model.predict(X)
-
-
- if (result[0] == 1):
-
- return "The person has Heart Diseases"
- else:
- return "The person has not Heart Diseases"
-
-
-
-
-app = gr.Interface(
- fn= healthy_heart,
- inputs = ["text",
- gr.inputs.Slider(10,80,label = "Patient Age"),
- gr.inputs.Slider(0,1,step=1,label="Gender 0: Female, Gender 1: Male"),
- gr.inputs.Slider(0,3,step=1,label= "Chest Pain Type"),
- gr.inputs.Slider(10,120,label = "Resting Blood Pressure"),
- gr.inputs.Slider(120,500,label = "Serum Cholestrol(mg/dl)"),
- gr.inputs.Slider(0,1,step=1,label = "Fasting Blood Sugar"),
- gr.inputs.Slider(10,80,label = "Resting electrocardiographic results"),
- gr.inputs.Slider(60,220,label = "Maximum heart rate achieved"),
- gr.inputs.Slider(10,80,label = "Exercise induced angina"),
- gr.inputs.Slider(1,5,step=1,label = "Oldpeak = ST depression induced by exercise relative to rest"),
- gr.inputs.Slider(0,2,step=1,label = "The slope of the peak exercise ST segment"),
- gr.inputs.Slider(0,3,step=1,label = "Number of major vessels (0-3) colored by flourosopy"),
- gr.inputs.Slider(0,2,step=1,label = "Thal: 0 = normal; 1 = fixed defect; 2 = reversable defect")],
- outputs= ["text"],
- description="This is your heart health score",
-
-
-)
-
-app.launch(share=True)
\ No newline at end of file
diff --git a/spaces/samcaicn/bingai/src/components/ui/sheet.tsx b/spaces/samcaicn/bingai/src/components/ui/sheet.tsx
deleted file mode 100644
index c9f5ce0f81a91067bb013e988a07eb1e6bf6953b..0000000000000000000000000000000000000000
--- a/spaces/samcaicn/bingai/src/components/ui/sheet.tsx
+++ /dev/null
@@ -1,122 +0,0 @@
-'use client'
-
-import * as React from 'react'
-import * as SheetPrimitive from '@radix-ui/react-dialog'
-
-import { cn } from '@/lib/utils'
-import { IconClose } from '@/components/ui/icons'
-
-const Sheet = SheetPrimitive.Root
-
-const SheetTrigger = SheetPrimitive.Trigger
-
-const SheetClose = SheetPrimitive.Close
-
-const SheetPortal = ({
- className,
- children,
- ...props
-}: SheetPrimitive.DialogPortalProps) => (
-
- {children}
-
-)
-SheetPortal.displayName = SheetPrimitive.Portal.displayName
-
-const SheetOverlay = React.forwardRef<
- React.ElementRef,
- React.ComponentPropsWithoutRef
->(({ className, children, ...props }, ref) => (
-
-))
-SheetOverlay.displayName = SheetPrimitive.Overlay.displayName
-
-const SheetContent = React.forwardRef<
- React.ElementRef,
- React.ComponentPropsWithoutRef
->(({ className, children, ...props }, ref) => (
-
-
- {children}
-
-
- Close
-
-
-
-))
-SheetContent.displayName = SheetPrimitive.Content.displayName
-
-const SheetHeader = ({
- className,
- ...props
-}: React.HTMLAttributes) => (
-
-)
-SheetHeader.displayName = 'SheetHeader'
-
-const SheetFooter = ({
- className,
- ...props
-}: React.HTMLAttributes) => (
-
-)
-SheetFooter.displayName = 'SheetFooter'
-
-const SheetTitle = React.forwardRef<
- React.ElementRef,
- React.ComponentPropsWithoutRef
->(({ className, ...props }, ref) => (
-
-))
-SheetTitle.displayName = SheetPrimitive.Title.displayName
-
-const SheetDescription = React.forwardRef<
- React.ElementRef,
- React.ComponentPropsWithoutRef
->(({ className, ...props }, ref) => (
-
-))
-SheetDescription.displayName = SheetPrimitive.Description.displayName
-
-export {
- Sheet,
- SheetTrigger,
- SheetClose,
- SheetContent,
- SheetHeader,
- SheetFooter,
- SheetTitle,
- SheetDescription
-}
diff --git a/spaces/scedlatioru/img-to-music/Topview Simulator 64 Bit Free Download ##HOT## 13.md b/spaces/scedlatioru/img-to-music/Topview Simulator 64 Bit Free Download ##HOT## 13.md
deleted file mode 100644
index e08b44cf2d017befa089c11414f3624b1535dd24..0000000000000000000000000000000000000000
--- a/spaces/scedlatioru/img-to-music/Topview Simulator 64 Bit Free Download ##HOT## 13.md
+++ /dev/null
@@ -1,120 +0,0 @@
-## Topview Simulator 64 Bit Free Download 13
-
-
-
-
-
-
-
-
-
-**Topview Simulator 64 Bit Free Download 13 🆓 [https://urlca.com/2txvQ6](https://urlca.com/2txvQ6)**
-
-
-
-
-
-
-
-
-
-
-
-
-
-# How to Download Topview Simulator 64 Bit for Free
-
-
-
-Topview Simulator is a software tool that allows you to simulate and program 8051 microcontrollers and AVR devices. It is a useful application for embedded systems developers and hobbyists who want to test their code and debug their circuits. However, finding a compatible version of Topview Simulator for 64 bit Windows can be challenging, as the official website does not offer one. In this article, we will show you how to download Topview Simulator 64 bit for free and install it on your computer.
-
-
-
-## Step 1: Download Topview Simulator 64 Bit
-
-
-
-The first step is to download Topview Simulator 64 bit from a reliable source. One of the websites that offers this software is Softadvice.informer.com[^1^]. Here, you can find various versions of Topview Simulator, such as Topview Programmer A, Topview Device Programmer B, and Topview Device Programmer C. You can choose the one that suits your needs and click on the download link. Alternatively, you can also use Docker to pull the image of Topview Simulator 64 bit from boicasorre/topview-simulator-64-bit-free-download[^2^]. This is a more advanced option that requires you to have Docker installed on your computer.
-
-
-
-## Step 2: Install Topview Simulator 64 Bit
-
-
-
-The next step is to install Topview Simulator 64 bit on your computer. If you downloaded the software from Softadvice.informer.com, you will get a zip file that contains the setup file and some other files. You need to extract the zip file and run the setup file as administrator. Follow the instructions on the screen and choose the destination folder for the installation. If you used Docker to pull the image of Topview Simulator 64 bit, you will need to run the following command in your terminal: docker run -it boicasorre/topview-simulator-64-bit-free-download. This will launch the software in a container and allow you to access it from your browser.
-
-
-
-## Step 3: Use Topview Simulator 64 Bit
-
-
-
-The final step is to use Topview Simulator 64 bit for your projects. You can open the software from your start menu or your browser, depending on how you installed it. You will see a user interface that allows you to select your device, load your code, configure your settings, and start the simulation. You can also use various tools and features of Topview Simulator, such as breakpoints, watch windows, logic analyzer, oscilloscope, and more. You can refer to the user manual or online tutorials for more details on how to use Topview Simulator effectively.
-
-
-
-## Conclusion
-
-
-
-In this article, we showed you how to download Topview Simulator 64 bit for free and install it on your computer. We hope this article was helpful for you and that you enjoy using Topview Simulator for your embedded systems projects. If you have any questions or feedback, please leave a comment below.
-
-
-
-## What is Topview Simulator?
-
-
-
-Topview Simulator is a software tool that simulates the behavior and performance of 8051 microcontrollers and AVR devices. It allows you to write, compile, and debug your code in an integrated development environment (IDE) that supports various programming languages, such as Assembly, C, and Basic. It also provides you with a graphical user interface (GUI) that displays the status of your device, such as registers, memory, ports, timers, interrupts, and peripherals. You can also use Topview Simulator to test your code on a virtual or real hardware board.
-
-
-
-## Why Use Topview Simulator?
-
-
-
-Topview Simulator is a useful tool for embedded systems developers and hobbyists who want to learn and experiment with 8051 microcontrollers and AVR devices. It offers many benefits, such as:
-
-
-
-- It saves you time and money by allowing you to test your code without the need for physical hardware.
-
-- It helps you to find and fix errors in your code by providing you with debugging tools and features.
-
-- It enhances your learning experience by giving you feedback and visualization of your device's operation.
-
-- It supports a wide range of devices and features that can suit your project's requirements.
-
-
-
-## How to Learn Topview Simulator?
-
-
-
-If you want to learn how to use Topview Simulator effectively, you can follow these steps:
-
-
-
-1. Download and install Topview Simulator 64 bit for free from one of the sources mentioned in the previous article.
-
-2. Read the user manual or online tutorials that explain the basics and features of Topview Simulator.
-
-3. Choose a device that matches your project's specifications and select it in Topview Simulator.
-
-4. Write your code in the IDE or load an existing code from a file or a library.
-
-5. Compile your code and check for any errors or warnings.
-
-6. Run the simulation and observe the results on the GUI or the hardware board.
-
-7. Use the debugging tools and features to modify your code and improve your device's performance.
-
-
-
- 1b8d091108
-
-
-
-
-
diff --git a/spaces/scedlatioru/img-to-music/example/A Flying Jatt Hindi Full Movie Hd 1080p NEW.md b/spaces/scedlatioru/img-to-music/example/A Flying Jatt Hindi Full Movie Hd 1080p NEW.md
deleted file mode 100644
index 5462979ffb74ba8656461df1a0d0751fa91a490f..0000000000000000000000000000000000000000
--- a/spaces/scedlatioru/img-to-music/example/A Flying Jatt Hindi Full Movie Hd 1080p NEW.md
+++ /dev/null
@@ -1,28 +0,0 @@
-A Flying Jatt Hindi Full Movie Hd 1080p
DOWNLOAD ⚹ https://gohhs.com/2uEAzN
-
-I too just hope that this is the very first and last time we see this party-pic in a future cap movie. If you need to find to download nude pics of any of the actors in this image, visit our site. The Ultimate Bowerbird. However, the Bowerbirds of the bird market are far more complex and sophisticated.
-
-These birds have a few different calls they make, some of which are perfectly understandable, although the term they are named for Bower Bird is one I have not heard.
-
-It seems more likely that if a specific actor or actress was used in the Cap-toon, they will be making that movie for a while, like the characters in those movies, rather than the actress being used as a celebrity to promote another movie. For obvious reasons, they won't be either Anna Kendrick or Ellie Kemper in " The Ultimate Bowerbird ". The scenes where she sings are really quite nice.
-
-The Bower Bird is an interesting bird, and I will probably keep coming back to this site to see if it will be featured in future Cap-toons. The Falcon Strikes Again. The films in the "bird series" tend to be more complex with far more storylines.
-
-The cast of the show it's not a perfect, but I did find out some things that I didn't know before. And you are totally free to come back whenever you want and check it out.
-
-Bowerbird's Nest
-
-I thought I would give the site a try. However, The Falcon Strikes Again. The falcon is a large bird of prey that has very sharp talons.
-
-Even better, the creator of this site seems to have an affinity for this kind of material. The Bower Bird is an interesting bird, and I will probably keep coming back to this site to see if it will be featured in future Cap-toons.
-
-Cap birds, spy birds, bird capes and bird capes are a real popular all time product that has been around for quite some time.
-
-This is not to mention that the Bowerbirds of the bird market are far more complex and sophisticated. I am not sure who the two other birds are, but there is also a penguin and a tortoise.
-
-The scene where she sings is really quite nice. This is definitely a site that has something to offer. If you find any additional photos, please do not hesitate to share them with us here.
-
-The 4fefd39f24
-
-
-
diff --git a/spaces/scedlatioru/img-to-music/example/Localizationtxtdllcallofduty4download UPD.md b/spaces/scedlatioru/img-to-music/example/Localizationtxtdllcallofduty4download UPD.md
deleted file mode 100644
index 428f8cdcdb8d028040d12319754cf9750d2d97e5..0000000000000000000000000000000000000000
--- a/spaces/scedlatioru/img-to-music/example/Localizationtxtdllcallofduty4download UPD.md
+++ /dev/null
@@ -1,6 +0,0 @@
-localizationtxtdllcallofduty4download
Download File >>> https://gohhs.com/2uEzJr
-
- 4d29de3e1b
-
-
-
diff --git a/spaces/sczhou/CodeFormer/README.md b/spaces/sczhou/CodeFormer/README.md
deleted file mode 100644
index acb018f7fd666adfd56ea31796dc97a281b48d0b..0000000000000000000000000000000000000000
--- a/spaces/sczhou/CodeFormer/README.md
+++ /dev/null
@@ -1,12 +0,0 @@
----
-title: CodeFormer
-emoji: 🐼
-colorFrom: blue
-colorTo: green
-sdk: gradio
-sdk_version: 3.41.0
-app_file: app.py
-pinned: false
----
-
-Check out the configuration reference at https://huggingface.co/docs/hub/spaces-config-reference
diff --git a/spaces/sh20raj/telebot/app.py b/spaces/sh20raj/telebot/app.py
deleted file mode 100644
index 0b6fda6f903f4ef4cb40fc680dde3667653a27b3..0000000000000000000000000000000000000000
--- a/spaces/sh20raj/telebot/app.py
+++ /dev/null
@@ -1,22 +0,0 @@
-from telegram import Update
-from telegram.ext import Updater, CommandHandler, CallbackContext
-
-# Define your token here
-TOKEN = '6648024441:AAHZaX8gxrgTBG7O-1T_zKzvbl0JHKNID3Q'
-
-# Define the command handler function
-def hi(update: Update, context: CallbackContext) -> None:
- update.message.reply_text('Hi!')
-
-# Create the updater and dispatcher
-updater = Updater(token=TOKEN, use_context=True)
-dispatcher = updater.dispatcher
-
-# Add the command handler to the dispatcher
-dispatcher.add_handler(CommandHandler('hi', hi))
-
-# Start the bot
-updater.start_polling()
-
-# Run the bot until you press Ctrl-C
-updater.idle()
\ No newline at end of file
diff --git a/spaces/shgao/MDT/diffusion/__init__.py b/spaces/shgao/MDT/diffusion/__init__.py
deleted file mode 100644
index 8c536a98da92c4d051458803737661e5ecf974c2..0000000000000000000000000000000000000000
--- a/spaces/shgao/MDT/diffusion/__init__.py
+++ /dev/null
@@ -1,46 +0,0 @@
-# Modified from OpenAI's diffusion repos
-# GLIDE: https://github.com/openai/glide-text2im/blob/main/glide_text2im/gaussian_diffusion.py
-# ADM: https://github.com/openai/guided-diffusion/blob/main/guided_diffusion
-# IDDPM: https://github.com/openai/improved-diffusion/blob/main/improved_diffusion/gaussian_diffusion.py
-
-from . import gaussian_diffusion as gd
-from .respace import SpacedDiffusion, space_timesteps
-
-
-def create_diffusion(
- timestep_respacing,
- noise_schedule="linear",
- use_kl=False,
- sigma_small=False,
- predict_xstart=False,
- learn_sigma=True,
- rescale_learned_sigmas=False,
- diffusion_steps=1000
-):
- betas = gd.get_named_beta_schedule(noise_schedule, diffusion_steps)
- if use_kl:
- loss_type = gd.LossType.RESCALED_KL
- elif rescale_learned_sigmas:
- loss_type = gd.LossType.RESCALED_MSE
- else:
- loss_type = gd.LossType.MSE
- if timestep_respacing is None or timestep_respacing == "":
- timestep_respacing = [diffusion_steps]
- return SpacedDiffusion(
- use_timesteps=space_timesteps(diffusion_steps, timestep_respacing),
- betas=betas,
- model_mean_type=(
- gd.ModelMeanType.EPSILON if not predict_xstart else gd.ModelMeanType.START_X
- ),
- model_var_type=(
- (
- gd.ModelVarType.FIXED_LARGE
- if not sigma_small
- else gd.ModelVarType.FIXED_SMALL
- )
- if not learn_sigma
- else gd.ModelVarType.LEARNED_RANGE
- ),
- loss_type=loss_type
- # rescale_timesteps=rescale_timesteps,
- )
diff --git a/spaces/shigel/recipe/constraints.md b/spaces/shigel/recipe/constraints.md
deleted file mode 100644
index 20055097aad6c29350effbaa58dbff99b659630c..0000000000000000000000000000000000000000
--- a/spaces/shigel/recipe/constraints.md
+++ /dev/null
@@ -1,26 +0,0 @@
-【オブジェクト: 料理レシピ】
-
-【サブオブジェクト】
-- 材料: 新鮮な野菜、スパイス、調味料、お肉または魚、その他必要なもの
-- 能力: 料理の技術、創造力、美味しさに関する知識、試行錯誤能力
-- 状態認識: 食材の状態、食材を調理する際の温度の状態、調味料の量
-
-【制約】
-- 料理レシピは新しくて、他に似たようなレシピが存在しない。
-- 食材は新鮮である必要がある。
-- 料理の過程で、一定の順序と技術が必要であり、素材や火力の管理が必要である。
-- 料理の味付けや素材の使い方について、独自のアイデアと創造的な発想が求められる。
-
-【初期状態】
-- 材料が揃っている
-- 料理レシピが決まっている
-- 料理レシピの作成者が、料理を作るための技能、知識、創造力がある
-
-【状態変化】
-- 料理レシピの内容に基づき、材料を調理する。
-- 料理レシピに合わせて、一定の手順に従って材料を加熱し、調味料を加える。
-- 料理の味や色、食感などを確認しながら、試行錯誤を重ね、熟成させる。
-- 最終的に、美味しい一皿が完成したと認識できる。
-
-【管理オブジェクト】
-- 料理レシピを作成する人、または料理人。
\ No newline at end of file
diff --git a/spaces/shiwan10000/CodeFormer/CodeFormer/basicsr/utils/realesrgan_utils.py b/spaces/shiwan10000/CodeFormer/CodeFormer/basicsr/utils/realesrgan_utils.py
deleted file mode 100644
index ff94523b7ddd61f0b72280950fd36e1b8133bf4c..0000000000000000000000000000000000000000
--- a/spaces/shiwan10000/CodeFormer/CodeFormer/basicsr/utils/realesrgan_utils.py
+++ /dev/null
@@ -1,296 +0,0 @@
-import cv2
-import math
-import numpy as np
-import os
-import queue
-import threading
-import torch
-from basicsr.utils.download_util import load_file_from_url
-from torch.nn import functional as F
-
-# ROOT_DIR = os.path.dirname(os.path.dirname(os.path.abspath(__file__)))
-
-
-class RealESRGANer():
- """A helper class for upsampling images with RealESRGAN.
-
- Args:
- scale (int): Upsampling scale factor used in the networks. It is usually 2 or 4.
- model_path (str): The path to the pretrained model. It can be urls (will first download it automatically).
- model (nn.Module): The defined network. Default: None.
- tile (int): As too large images result in the out of GPU memory issue, so this tile option will first crop
- input images into tiles, and then process each of them. Finally, they will be merged into one image.
- 0 denotes for do not use tile. Default: 0.
- tile_pad (int): The pad size for each tile, to remove border artifacts. Default: 10.
- pre_pad (int): Pad the input images to avoid border artifacts. Default: 10.
- half (float): Whether to use half precision during inference. Default: False.
- """
-
- def __init__(self,
- scale,
- model_path,
- model=None,
- tile=0,
- tile_pad=10,
- pre_pad=10,
- half=False,
- device=None,
- gpu_id=None):
- self.scale = scale
- self.tile_size = tile
- self.tile_pad = tile_pad
- self.pre_pad = pre_pad
- self.mod_scale = None
- self.half = half
-
- # initialize model
- if gpu_id:
- self.device = torch.device(
- f'cuda:{gpu_id}' if torch.cuda.is_available() else 'cpu') if device is None else device
- else:
- self.device = torch.device('cuda' if torch.cuda.is_available() else 'cpu') if device is None else device
- # if the model_path starts with https, it will first download models to the folder: realesrgan/weights
- if model_path.startswith('https://'):
- model_path = load_file_from_url(
- url=model_path, model_dir=os.path.join('weights/realesrgan'), progress=True, file_name=None)
- loadnet = torch.load(model_path, map_location=torch.device('cpu'))
- # prefer to use params_ema
- if 'params_ema' in loadnet:
- keyname = 'params_ema'
- else:
- keyname = 'params'
- model.load_state_dict(loadnet[keyname], strict=True)
- model.eval()
- self.model = model.to(self.device)
- if self.half:
- self.model = self.model.half()
-
- def pre_process(self, img):
- """Pre-process, such as pre-pad and mod pad, so that the images can be divisible
- """
- img = torch.from_numpy(np.transpose(img, (2, 0, 1))).float()
- self.img = img.unsqueeze(0).to(self.device)
- if self.half:
- self.img = self.img.half()
-
- # pre_pad
- if self.pre_pad != 0:
- self.img = F.pad(self.img, (0, self.pre_pad, 0, self.pre_pad), 'reflect')
- # mod pad for divisible borders
- if self.scale == 2:
- self.mod_scale = 2
- elif self.scale == 1:
- self.mod_scale = 4
- if self.mod_scale is not None:
- self.mod_pad_h, self.mod_pad_w = 0, 0
- _, _, h, w = self.img.size()
- if (h % self.mod_scale != 0):
- self.mod_pad_h = (self.mod_scale - h % self.mod_scale)
- if (w % self.mod_scale != 0):
- self.mod_pad_w = (self.mod_scale - w % self.mod_scale)
- self.img = F.pad(self.img, (0, self.mod_pad_w, 0, self.mod_pad_h), 'reflect')
-
- def process(self):
- # model inference
- self.output = self.model(self.img)
-
- def tile_process(self):
- """It will first crop input images to tiles, and then process each tile.
- Finally, all the processed tiles are merged into one images.
-
- Modified from: https://github.com/ata4/esrgan-launcher
- """
- batch, channel, height, width = self.img.shape
- output_height = height * self.scale
- output_width = width * self.scale
- output_shape = (batch, channel, output_height, output_width)
-
- # start with black image
- self.output = self.img.new_zeros(output_shape)
- tiles_x = math.ceil(width / self.tile_size)
- tiles_y = math.ceil(height / self.tile_size)
-
- # loop over all tiles
- for y in range(tiles_y):
- for x in range(tiles_x):
- # extract tile from input image
- ofs_x = x * self.tile_size
- ofs_y = y * self.tile_size
- # input tile area on total image
- input_start_x = ofs_x
- input_end_x = min(ofs_x + self.tile_size, width)
- input_start_y = ofs_y
- input_end_y = min(ofs_y + self.tile_size, height)
-
- # input tile area on total image with padding
- input_start_x_pad = max(input_start_x - self.tile_pad, 0)
- input_end_x_pad = min(input_end_x + self.tile_pad, width)
- input_start_y_pad = max(input_start_y - self.tile_pad, 0)
- input_end_y_pad = min(input_end_y + self.tile_pad, height)
-
- # input tile dimensions
- input_tile_width = input_end_x - input_start_x
- input_tile_height = input_end_y - input_start_y
- tile_idx = y * tiles_x + x + 1
- input_tile = self.img[:, :, input_start_y_pad:input_end_y_pad, input_start_x_pad:input_end_x_pad]
-
- # upscale tile
- try:
- with torch.no_grad():
- output_tile = self.model(input_tile)
- except RuntimeError as error:
- print('Error', error)
- # print(f'\tTile {tile_idx}/{tiles_x * tiles_y}')
-
- # output tile area on total image
- output_start_x = input_start_x * self.scale
- output_end_x = input_end_x * self.scale
- output_start_y = input_start_y * self.scale
- output_end_y = input_end_y * self.scale
-
- # output tile area without padding
- output_start_x_tile = (input_start_x - input_start_x_pad) * self.scale
- output_end_x_tile = output_start_x_tile + input_tile_width * self.scale
- output_start_y_tile = (input_start_y - input_start_y_pad) * self.scale
- output_end_y_tile = output_start_y_tile + input_tile_height * self.scale
-
- # put tile into output image
- self.output[:, :, output_start_y:output_end_y,
- output_start_x:output_end_x] = output_tile[:, :, output_start_y_tile:output_end_y_tile,
- output_start_x_tile:output_end_x_tile]
-
- def post_process(self):
- # remove extra pad
- if self.mod_scale is not None:
- _, _, h, w = self.output.size()
- self.output = self.output[:, :, 0:h - self.mod_pad_h * self.scale, 0:w - self.mod_pad_w * self.scale]
- # remove prepad
- if self.pre_pad != 0:
- _, _, h, w = self.output.size()
- self.output = self.output[:, :, 0:h - self.pre_pad * self.scale, 0:w - self.pre_pad * self.scale]
- return self.output
-
- @torch.no_grad()
- def enhance(self, img, outscale=None, alpha_upsampler='realesrgan'):
- h_input, w_input = img.shape[0:2]
- # img: numpy
- img = img.astype(np.float32)
- if np.max(img) > 256: # 16-bit image
- max_range = 65535
- print('\tInput is a 16-bit image')
- else:
- max_range = 255
- img = img / max_range
- if len(img.shape) == 2: # gray image
- img_mode = 'L'
- img = cv2.cvtColor(img, cv2.COLOR_GRAY2RGB)
- elif img.shape[2] == 4: # RGBA image with alpha channel
- img_mode = 'RGBA'
- alpha = img[:, :, 3]
- img = img[:, :, 0:3]
- img = cv2.cvtColor(img, cv2.COLOR_BGR2RGB)
- if alpha_upsampler == 'realesrgan':
- alpha = cv2.cvtColor(alpha, cv2.COLOR_GRAY2RGB)
- else:
- img_mode = 'RGB'
- img = cv2.cvtColor(img, cv2.COLOR_BGR2RGB)
-
- # ------------------- process image (without the alpha channel) ------------------- #
- with torch.no_grad():
- self.pre_process(img)
- if self.tile_size > 0:
- self.tile_process()
- else:
- self.process()
- output_img_t = self.post_process()
- output_img = output_img_t.data.squeeze().float().cpu().clamp_(0, 1).numpy()
- output_img = np.transpose(output_img[[2, 1, 0], :, :], (1, 2, 0))
- if img_mode == 'L':
- output_img = cv2.cvtColor(output_img, cv2.COLOR_BGR2GRAY)
- del output_img_t
- torch.cuda.empty_cache()
-
- # ------------------- process the alpha channel if necessary ------------------- #
- if img_mode == 'RGBA':
- if alpha_upsampler == 'realesrgan':
- self.pre_process(alpha)
- if self.tile_size > 0:
- self.tile_process()
- else:
- self.process()
- output_alpha = self.post_process()
- output_alpha = output_alpha.data.squeeze().float().cpu().clamp_(0, 1).numpy()
- output_alpha = np.transpose(output_alpha[[2, 1, 0], :, :], (1, 2, 0))
- output_alpha = cv2.cvtColor(output_alpha, cv2.COLOR_BGR2GRAY)
- else: # use the cv2 resize for alpha channel
- h, w = alpha.shape[0:2]
- output_alpha = cv2.resize(alpha, (w * self.scale, h * self.scale), interpolation=cv2.INTER_LINEAR)
-
- # merge the alpha channel
- output_img = cv2.cvtColor(output_img, cv2.COLOR_BGR2BGRA)
- output_img[:, :, 3] = output_alpha
-
- # ------------------------------ return ------------------------------ #
- if max_range == 65535: # 16-bit image
- output = (output_img * 65535.0).round().astype(np.uint16)
- else:
- output = (output_img * 255.0).round().astype(np.uint8)
-
- if outscale is not None and outscale != float(self.scale):
- output = cv2.resize(
- output, (
- int(w_input * outscale),
- int(h_input * outscale),
- ), interpolation=cv2.INTER_LANCZOS4)
-
- return output, img_mode
-
-
-class PrefetchReader(threading.Thread):
- """Prefetch images.
-
- Args:
- img_list (list[str]): A image list of image paths to be read.
- num_prefetch_queue (int): Number of prefetch queue.
- """
-
- def __init__(self, img_list, num_prefetch_queue):
- super().__init__()
- self.que = queue.Queue(num_prefetch_queue)
- self.img_list = img_list
-
- def run(self):
- for img_path in self.img_list:
- img = cv2.imread(img_path, cv2.IMREAD_UNCHANGED)
- self.que.put(img)
-
- self.que.put(None)
-
- def __next__(self):
- next_item = self.que.get()
- if next_item is None:
- raise StopIteration
- return next_item
-
- def __iter__(self):
- return self
-
-
-class IOConsumer(threading.Thread):
-
- def __init__(self, opt, que, qid):
- super().__init__()
- self._queue = que
- self.qid = qid
- self.opt = opt
-
- def run(self):
- while True:
- msg = self._queue.get()
- if isinstance(msg, str) and msg == 'quit':
- break
-
- output = msg['output']
- save_path = msg['save_path']
- cv2.imwrite(save_path, output)
- print(f'IO worker {self.qid} is done.')
\ No newline at end of file
diff --git a/spaces/simple0urra/skops-model-card-creator-2a23515a-d54e-4804-b365-27ed6e938735/example/Become the Ultimate Assassin with Hitman Sniper APK Mod on HappyMod.md b/spaces/simple0urra/skops-model-card-creator-2a23515a-d54e-4804-b365-27ed6e938735/example/Become the Ultimate Assassin with Hitman Sniper APK Mod on HappyMod.md
deleted file mode 100644
index cd4bebb43c27000df80e991728b5fd93abd24d00..0000000000000000000000000000000000000000
--- a/spaces/simple0urra/skops-model-card-creator-2a23515a-d54e-4804-b365-27ed6e938735/example/Become the Ultimate Assassin with Hitman Sniper APK Mod on HappyMod.md
+++ /dev/null
@@ -1,114 +0,0 @@
-
-Hitman Sniper APK HappyMod: How to Download and Play the Best Sniper Shooting Game on Mobile
- Introduction
- If you are a fan of action games and stealth games, you might have heard of Hitman Sniper, a popular mobile game that allows you to step into the shoes of Agent 47, a skilled assassin tasked with taking out targets from afar. With the MOD Unlimited Money version, you can enjoy unlimited funds to buy and upgrade weapons, unlock new missions, and play the game without any restrictions.
-hitman sniper apk happymod
Download 🗹 https://ssurll.com/2uNReI
- But how can you get this MOD version of Hitman Sniper? The answer is HappyMod, a platform that provides modded APKs for various games and apps. In this article, we will show you how to download and install Hitman Sniper APK HappyMod, how to play the game, and some tips and tricks to improve your skills. Let's get started!
- What is Hitman Sniper?
- Hitman Sniper is a mobile game developed by Square Enix Ltd, based on the Hitman franchise. The game features Agent 47, a professional hitman who uses his sniper rifle to eliminate targets in various locations. The game has over 150 missions and 10 different contracts, each with its own objectives and challenges. You can also play in different modes, such as zombie mode, where you have to survive waves of undead enemies.
- The game has awesome and smooth graphics that make for addictive gameplay on top of a thrilling story. You can also compete against your friends for the first place in the leaderboards, or challenge yourself with creative hitman kills to become the world's finest silent assassin.
- What is HappyMod?
- HappyMod is a platform that provides modded APKs for various games and apps. Modded APKs are modified versions of original apps that have extra features or unlocked content that are not available in the official versions. For example, modded APKs can give you unlimited money, coins, gems, lives, weapons, skins, etc.
- HappyMod has a large collection of modded APKs for different categories, such as action, adventure, arcade, casual, simulation, sports, etc. You can also request mods for your favorite games or apps, or upload your own mods to share with other users. HappyMod is safe and reliable, as it verifies the mods before uploading them to ensure they are working and virus-free.
- Why use Hitman Sniper APK HappyMod?
- There are many reasons why you might want to use Hitman Sniper APK HappyMod instead of the original version of the game. Here are some of them:
-
-- You can get unlimited money and coins to buy and upgrade weapons and skills.
-- You can unlock all the weapons and missions without spending real money or completing difficult tasks.
-- You can enjoy the game without any ads or interruptions.
-- You can play the game offline without an internet connection.
-- You can have more fun and excitement with modded features and content.
-
- How to Download and Install Hitman Sniper APK HappyMod
- Step 1: Download APK + OBB on HappyMod App
- The first step is to download the APK + OBB files of Hitman Sniper APK HappyMod on your device. You can do this by using the HappyMod app, which you can download from here. After installing the HappyMod app, open it and search for Hitman Sniper in the search bar. You will see the Hitman Sniper APK HappyMod with the MOD Unlimited Money feature. Tap on the download button and wait for the download to finish.
- Once the download is complete, you will have two files: an APK file and an OBB file. The APK file is the application file that you need to install on your device, while the OBB file is the data file that contains the game's assets and resources. You need to place the OBB file in the right folder to make the game work properly.
-hitman sniper mod apk unlimited money
-hitman sniper android game download
-hitman sniper apk + obb
-hitman sniper hack apk
-hitman sniper free download for android
-hitman sniper mod apk latest version
-hitman sniper apk full version
-hitman sniper mod apk happymod.com
-hitman sniper apk mod menu
-hitman sniper mod apk all guns unlocked
-hitman sniper offline apk
-hitman sniper apk data
-hitman sniper mod apk rexdl
-hitman sniper apk pure
-hitman sniper mod apk revdl
-hitman sniper apk obb highly compressed
-hitman sniper mod apk android 1
-hitman sniper apk mirror
-hitman sniper mod apk unlimited everything
-hitman sniper apk no obb
-hitman sniper mod apk all weapons unlocked
-hitman sniper download for android
-hitman sniper apk + data download
-hitman sniper hack mod apk download
-hitman sniper mod apk unlimited money and tokens
-hitman sniper mod apk obb download
-hitman sniper free download full version for android
-hitman sniper mod apk all missions unlocked
-hitman sniper mod apk unlimited ammo and money
-hitman sniper modded apk free download
-hitman sniper cracked apk download
-hitman sniper premium apk download
-hitman sniper mod apk no root
-hitman sniper unlimited money and gold apk download
-hitman sniper full unlocked apk download
-hitman sniper mega mod apk download
-hitman sniper paid apk free download
-hitman sniper unlimited tokens and money mod apk download
-hitman sniper 1.7.193827 mod apk download
-hitman sniper 1.7.193827 mod unlimited money and gold coins.apk download
- Step 2: Install and Enjoy
- The next step is to install the APK file on your device. Before you do that, you need to enable the installation of apps from unknown sources on your device. To do that, go to Settings > Security > Unknown Sources and toggle it on. This will allow you to install apps that are not from the Google Play Store.
- After enabling unknown sources, locate the APK file that you downloaded from HappyMod and tap on it. Follow the instructions on the screen to install the app. Once the installation is done, do not open the app yet. You need to move the OBB file to the right folder first.
- To move the OBB file, you need a file manager app that can access your device's internal storage. You can use any file manager app that you like, such as ES File Explorer, File Manager, etc. Open the file manager app and go to the folder where you downloaded the OBB file. It should be in the Downloads folder or in the HappyMod folder.
- Copy or cut the OBB file and paste it in this folder: Android > obb > com.squareenixmontreal.hitmansniperandroid. If you don't see this folder, create it manually. Make sure that the OBB file is named as main.115.com.squareenixmontreal.hitmansniperandroid.obb.
- Now you are ready to play Hitman Sniper APK HappyMod. Just open the app and enjoy!
- How to Play Hitman Sniper APK HappyMod
- Step 1: Choose a Mission
- When you launch Hitman Sniper APK HappyMod, you will see a menu with different options, such as Play, Contracts, Weapons, Leaderboards, etc. To start playing, tap on Play and choose a mission from the list. There are over 150 missions in total, each with its own target, location, and objectives.
- You can also choose a contract from the Contracts option, which are special missions that have different rules and rewards. For example, there are contracts where you have to kill only specific targets, or contracts where you have to kill as many targets as possible in a limited time.
- Some missions and contracts are locked at first, but you can unlock them by completing previous ones or by using money or tokens. With Hitman Sniper APK HappyMod, you don't have to worry about money or tokens, as you have unlimited amounts of them.
- Step 2: Aim and Shoot
- Once you choose a mission or a contract, you will enter the game mode where you have to use your sniper rifle to take out your targets. You can control your sniper rifle by using your fingers on the screen. You can swipe left or right to move your scope, pinch in or out to zoom in or out, and tap on the fire button to shoot.
- You can also use other buttons on the screen to activate different features of your sniper rifle, such as silencer, thermal vision, focus mode, etc. These features can help you locate and eliminate your targets more easily and efficiently.
- You have to be careful when shooting your targets, as some of them may notice your shots and alert others or escape. You also have to avoid killing innocent civilians or animals, as this will reduce your score and reputation. You can check your score and reputation at the top of the screen.
- Step 3: Upgrade Your Weapons and Skills
- As you complete missions and contracts, you will earn money and experience points that you can use to upgrade your weapons and skills. You can access these options from the menu by tapping on Weapons or Skills.
- You can buy new weapons or upgrade your existing ones by using money. There are over 15 weapons in total, each with its own stats and features. You can also customize your weapons by changing their skins or adding attachments.
- You can upgrade your skills by using experience points. There are four skills in total: Focus Time, Thermal Vision Time, Reload Time Reduction, and Stability Increase. These skills can improve your performance and abilities in the game. You can also unlock new skills by completing certain achievements.
- Tips and Tricks for Hitman Sniper APK HappyMod
- Hitman Sniper APK HappyMod is a fun and challenging game that requires skill and strategy to master. Here are some tips and tricks that can help you become a better sniper and enjoy the game more:
- Tip 1: Use the Environment to Your Advantage
- One of the best things about Hitman Sniper APK HappyMod is that it has realistic and interactive environments that you can use to your advantage. For example, you can shoot gas tanks, electrical wires, glass windows, chandeliers, etc. to cause explosions, fires, distractions, or accidents that can kill or expose your targets.
- You can also use the environment to hide your shots or create diversions. For example, you can shoot a car alarm, a bird, or a speaker to make noise and attract attention away from your position. You can also shoot a wall or a ceiling to create a hole that you can use to shoot through.
- Tip 2: Be Creative with Your Kills
- Another great thing about Hitman Sniper APK HappyMod is that it allows you to be creative with your kills and earn bonus points for them. For example, you can earn points for headshots, body shots, moving shots, long shots, etc. You can also earn points for killing multiple targets with one shot, killing targets in a specific order, killing targets with specific weapons or features, etc.
- You can also earn points for performing signature kills, which are unique and stylish ways of killing your targets. For example, you can kill your target by shooting a pool ball into their head, by shooting a golf ball into their mouth, by shooting a dart into their neck, etc. These kills are not only fun and satisfying, but also increase your score and reputation.
- Tip 3: Challenge Yourself with Different Modes and Contracts
- If you want to spice up your gameplay and test your skills, you can try playing different modes and contracts in Hitman Sniper APK HappyMod. For example, you can play zombie mode, where you have to survive waves of undead enemies that are coming for you. You can also play contracts mode, where you have to complete specific objectives and challenges within a time limit.
- These modes and contracts are not only more difficult and exciting than the regular missions, but also offer different rewards and achievements that you can collect. You can also compare your scores and rankings with other players around the world and see how good you are as a sniper.
- Conclusion
- Hitman Sniper APK HappyMod is an amazing game that lets you experience the thrill and challenge of being a professional hitman. With the MOD Unlimited Money version, you can enjoy the game without any limitations or restrictions. You can download and install Hitman Sniper APK HappyMod easily by using the HappyMod app, which provides modded APKs for various games and apps.
- In this article, we have shown you how to download and install Hitman Sniper APK HappyMod, how to play the game, and some tips and tricks to improve your skills. We hope that this article has been helpful and informative for you. If you have any questions or feedback, please feel free to leave a comment below.
- FAQs
- Here are some frequently asked questions about Hitman Sniper APK HappyMod:
-
-- Is Hitman Sniper APK HappyMod safe to use?
-Yes, Hitman Sniper APK HappyMod is safe to use as long as you download it from a trusted source like HappyMod. HappyMod verifies the mods before uploading them to ensure they are working and virus-free.
-- Do I need to root my device to use Hitman Sniper APK HappyMod?
-No, you don't need to root your device to use Hitman Sniper APK HappyMod. You just need to enable unknown sources on your device settings to install the app.
-- Can I play Hitman Sniper APK HappyMod online with other players?
-No, Hitman Sniper APK HappyMod is an offline game that does not require an internet connection to play. However, you can still compete against other players in the leaderboards by connecting your game account to Facebook or Google Play Games.
-- How do I update Hitman Sniper APK HappyMod?
-To update Hitman Sniper APK HappyMod, you need to download the latest version of the app from HappyMod and install it over the existing one. You don't need to uninstall the previous version or lose your progress.
-How do I get more money and tokens in Hitman Sniper APK HappyMod?
-With Hitman Sniper APK HappyMod, you don't need to worry about money and tokens, as you have unlimited amounts of them. You can use them to buy and upgrade weapons, unlock missions, and play contracts. You can also earn more money and tokens by completing achievements and challenges in the game.
-
197e85843d
-
-
\ No newline at end of file
diff --git a/spaces/simple0urra/skops-model-card-creator-2a23515a-d54e-4804-b365-27ed6e938735/example/Call of Duty Mobile APK Data Experience the Thrill of Battle Royale.md b/spaces/simple0urra/skops-model-card-creator-2a23515a-d54e-4804-b365-27ed6e938735/example/Call of Duty Mobile APK Data Experience the Thrill of Battle Royale.md
deleted file mode 100644
index 2aaffe582c55d675886529cdbba48bf88f3179fb..0000000000000000000000000000000000000000
--- a/spaces/simple0urra/skops-model-card-creator-2a23515a-d54e-4804-b365-27ed6e938735/example/Call of Duty Mobile APK Data Experience the Thrill of Battle Royale.md
+++ /dev/null
@@ -1,121 +0,0 @@
-
-Call of Duty Mobile APK Revdl: What You Need to Know
-If you are a fan of first-person shooter games, you might have heard of Call of Duty Mobile, the popular mobile game that brings the thrill of the Call of Duty franchise to your smartphone. With multiplayer modes, battle royale mode, seasonal content, customizable loadouts, and competitive and social play, Call of Duty Mobile has something for everyone.
-But what if you want to play Call of Duty Mobile without downloading it from the official app stores? What if you want to access some features that are not available in your region or device? This is where APK Revdl comes in. APK Revdl is a website that provides free download links for Android applications, including Call of Duty Mobile. By using APK Revdl, you can download and install Call of Duty Mobile on your Android device without any restrictions.
-call of duty mobile apk revdl
Download Zip ✅ https://ssurll.com/2uNX9K
-In this article, we will tell you everything you need to know about Call of Duty Mobile APK Revdl, including its features, system requirements, download size, tips and tricks, and how to download it. Read on to find out more.
- Features of Call of Duty Mobile
-Call of Duty Mobile is a game that offers you a multiplayer FPS experience on your phone. You can play as iconic characters from the Call of Duty series, such as Captain Price, Ghost, Soap, and more. You can also choose from a variety of weapons, outfits, scorestreaks, and gear to customize your loadout. Here are some of the features that make Call of Duty Mobile stand out:
-
-- Multiplayer modes and maps: You can enjoy classic multiplayer modes such as Team Deathmatch, Domination, Kill Confirmed, Search and Destroy, and more. You can also fight across fan-favorite maps from Call of Duty history, such as Nuketown, Crash, Hijacked, Firing Range, Killhouse, Crossfire, and more.
-- Battle royale mode and classes: You can also survive the 100-player battle royale mode, where you can drop into a massive map with vehicles, weapons, items, and perks. You can also choose from six different classes with unique abilities: Defender, Mechanic, Scout, Clown, Medic, and Ninja.
-- Seasonal content and rewards: Call of Duty Mobile releases new content with every season, such as new game modes, maps, themed events, and rewards. You can also unlock new operators, weapons, skins, emotes, sprays, and more by completing challenges or purchasing the Battle Pass.
-- Customizable loadouts and operators: As you play Call of Duty Mobile, you will unlock and earn dozens of weapons, attachments, perks, and equipment that you can use to create your own loadouts. You can also customize your operators with different skins, outfits, and accessories.
-- Competitive and social play: You can play Call of Duty Mobile with your friends or other players online. You can join or create a clan, chat with your teammates, invite your friends to a private match, or compete in ranked mode. You can also watch other players' live streams or share your own gameplay videos.
-
- System Requirements and Download Size of Call of Duty Mobile
-Call of Duty Mobile is a game that requires a decent device to run smoothly. Here are the minimum and recommended system requirements for Android and iOS devices:
-
-
-Device
-Minimum Requirements
-Recommended Requirements
-
-
-Android
-- Android 5.1 or higher
- 2 GB of RAM
- 1.5 GB of free storage space
-- Android 8.0 or higher
- 4 GB of RAM
- 2 GB of free storage space
-
-
-iOS
-- iOS 9.0 or higher
- iPhone 6s or higher
- 1.5 GB of free storage space
-- iOS 11.0 or higher
- iPhone 7 or higher
- 2 GB of free storage space
-
-
- The download size of Call of Duty Mobile varies depending on your device and region. However, the approximate download size is around 2 GB for both Android and iOS devices. You can also choose to download additional content such as high-resolution textures, voice packs, and maps to enhance your gaming experience. However, this will increase the app size and may affect your device's performance.
- Tips and Tricks to Play Call of Duty Mobile
-Call of Duty Mobile is a game that requires skill, strategy, and teamwork to win. Whether you are a beginner or a veteran, here are some tips and tricks that can help you improve your gameplay:
-call of duty mobile apk download uptodown
-call of duty mobile apk obb revdl
-call of duty mobile apk mod unlimited money
-call of duty mobile apk data revdl
-call of duty mobile apk latest version uptodown
-call of duty mobile apk hack revdl
-call of duty mobile apk offline revdl
-call of duty mobile apk free download uptodown
-call of duty mobile apk rexdl
-call of duty mobile apk andropalace
-call of duty mobile apk pure revdl
-call of duty mobile apk mirror revdl
-call of duty mobile apk no verification revdl
-call of duty mobile apk highly compressed revdl
-call of duty mobile apk full version uptodown
-call of duty mobile apk old version revdl
-call of duty mobile apk update revdl
-call of duty mobile apk for android uptodown
-call of duty mobile apk cracked revdl
-call of duty mobile apk unlimited cp revdl
-call of duty mobile apk mega revdl
-call of duty mobile apk + obb download uptodown
-call of duty mobile apk mod menu revdl
-call of duty mobile apk + data download uptodown
-call of duty mobile apk direct download revdl
-call of duty mobile apk for pc uptodown
-call of duty mobile apk mod offline revdl
-call of duty mobile apk + obb highly compressed uptodown
-call of duty mobile apk original revdl
-call of duty mobile apk + data offline revdl
-call of duty mobile apk no root revdl
-call of duty mobile apk + obb latest version uptodown
-call of duty mobile apk mod unlimited ammo revdl
-call of duty mobile apk + data mega uptodown
-call of duty mobile apk unlocked all weapons revdl
-call of duty mobile apk for ios uptodown
-call of duty mobile apk mod online revdl
-call of duty mobile apk + obb offline mode uptodown
-call of duty mobile apk premium revdl
-call of duty mobile apk + data android 1.com
-call of duty mobile apk no ads revdl
-call of duty mobile apk + obb android 11 uptodown
-call of duty mobile apk mod god mode revdl
-call of duty mobile apk + data apkpure uptodown
-call of duty mobile apk pro revdl
-call of duty mobile apk + obb file download uptodown
-call of duty mobile apk mod anti ban revdl
-call of duty mobile apk + data mali uptodown
-call of duty mobile apk vip revdl
-
-- Choose the best controls and pair a controller if possible: Call of Duty Mobile offers you three types of controls: simple, advanced, and custom. Simple mode automatically fires your weapon when you aim at an enemy, while advanced mode lets you manually fire your weapon with a button. Custom mode allows you to adjust the layout and sensitivity of your controls. You can also pair a compatible controller with your device to play Call of Duty Mobile with more precision and comfort.
-- Communicate with your team and use your mini-map: Call of Duty Mobile is a team-based game, so communication is key. You can use the voice chat or text chat feature to coordinate with your teammates, call out enemy locations, request backup, or share strategies. You can also use your mini-map to see where your allies and enemies are, as well as important objectives and items.
-- Reload only when you need to and use your pistol: Reloading your weapon at the wrong time can cost you your life in Call of Duty Mobile. You should only reload when you are behind cover, out of combat, or have enough ammo left. You should also switch to your pistol when you run out of ammo or need to move faster. Your pistol is faster to draw and reload than your primary weapon, and it can still deal decent damage at close range.
-- Aim down sights and use cover: Aiming down sights (ADS) is essential for accuracy and recoil control in Call of Duty Mobile. You should always ADS before firing at an enemy, unless they are very close to you. You should also use cover as much as possible to avoid being exposed to enemy fire. You can crouch, prone, slide, or jump behind cover to dodge bullets and surprise your enemies.
-- Don't stand still and log in daily: One of the worst things you can do in Call of Duty Mobile is standing still. Standing still makes you an easy target for snipers, grenades, scorestreaks, and other threats. You should always keep moving and changing positions to stay unpredictable and alive. You should also log in daily to claim free rewards such as credits, crates, weapons, skins, and more.
-- Join a clan and participate in clan wars: Joining a clan is a great way to make friends, find teammates, and earn extra rewards in Call of Duty Mobile. You can join an existing clan or create your own clan with your friends. You can also participate in clan wars, which are weekly events where clans compete against each other for points and prizes.
How to Download Call of Duty Mobile APK Revdl
-If you want to play Call of Duty Mobile without any limitations, you might want to try downloading it from APK Revdl. APK Revdl is a website that provides free download links for Android applications, including Call of Duty Mobile. By using APK Revdl, you can access some features that are not available in the official app stores, such as:
-
-- Unlimited resources: You can get unlimited credits, cod points, crates, weapons, skins, and more by using APK Revdl.
-- Unlocked content: You can unlock all the operators, weapons, attachments, perks, and equipment that are otherwise locked behind a paywall or a level requirement by using APK Revdl.
-- Region-free access: You can play Call of Duty Mobile in any region or country without any restrictions by using APK Revdl.
-
-However, before you download Call of Duty Mobile APK Revdl, you should be aware of the risks and precautions involved. APK Revdl is not an official source of Call of Duty Mobile, and it may contain malware, viruses, or other harmful files that can damage your device or compromise your privacy. You should also be careful not to violate the terms of service or the code of conduct of Call of Duty Mobile, as you may face bans or penalties for using unauthorized or modified versions of the game. Here are some steps to download and install Call of Duty Mobile APK Revdl safely and correctly:
-
-- Enable unknown sources on your device: To install Call of Duty Mobile APK Revdl, you need to allow your device to install apps from unknown sources. To do this, go to your device's settings, then security, then unknown sources, and enable it.
-- Download Call of Duty Mobile APK Revdl from a trusted website: To download Call of Duty Mobile APK Revdl, you need to find a reliable and reputable website that provides the download link. You can use a browser or a downloader app to access the website. Make sure to check the reviews, ratings, and comments of the website and the app before downloading it.
-- Install Call of Duty Mobile APK Revdl on your device: To install Call of Duty Mobile APK Revdl on your device, you need to locate the downloaded file on your device's storage. You can use a file manager app to find it. Then, tap on the file and follow the instructions to install it.
-- Launch Call of Duty Mobile APK Revdl and enjoy: To launch Call of Duty Mobile APK Revdl on your device, you need to find the app icon on your home screen or app drawer. Then, tap on it and wait for it to load. You may need to grant some permissions or accept some terms and conditions before playing. Once you are in the game, you can enjoy all the features and benefits of Call of Duty Mobile APK Revdl.
-
- Conclusion
-Call of Duty Mobile is a game that offers you an immersive and exciting FPS experience on your phone. You can play various multiplayer modes and maps, survive the battle royale mode and classes, unlock seasonal content and rewards, customize your loadouts and operators, and compete and socialize with other players online.
-If you want to play Call of Duty Mobile without any restrictions or limitations, you can try downloading it from APK Revdl. APK Revdl is a website that provides free download links for Android applications, including Call of Duty Mobile. By using APK Revdl, you can get unlimited resources, unlocked content, and region-free access to the game.
-However, you should also be careful of the risks and precautions involved in using APK Revdl. APK Revdl is not an official source of Call of Duty Mobile, and it may contain malware, viruses, or other harmful files that can damage your device or compromise your privacy. You should also avoid violating the terms of service or the code of conduct of Call of Duty Mobile, as you may face bans or penalties for using unauthorized or modified versions of the game.
-We hope this article has helped you learn more about Call of Duty Mobile APK Revdl. If you have any questions or feedback, please feel free to leave a comment below.
- FAQs
-
-- Q1: What are the advantages of using APK Revdl?
A1: The advantages of using APK Revdl are that you can get unlimited resources, unlocked content, and region-free access to Call of Duty Mobile.
-- Q2: Is Call of Duty Mobile free to play?
A2: Yes, Call of Duty Mobile is free to play. However, some features and items may require real money to purchase or unlock.
-- Q3: Can I play Call of Duty Mobile on PC?
A3: Yes, you can play Call of Duty Mobile on PC by using an emulator. An emulator is a software that allows you to run Android applications on your PC. Some of the popular emulators for Call of Duty Mobile are Gameloop, Bluestacks, and NoxPlayer.
-- Q4: How can I update Call of Duty Mobile APK Revdl?
A4: To update Call of Duty Mobile APK Revdl, you need to download the latest version of the app from APK Revdl website and install it over the existing one. You may also need to delete the old app data and cache to avoid any errors or glitches.
-- Q5: Is Call of Duty Mobile safe to play?
A5: Call of Duty Mobile is safe to play as long as you download it from the official app stores or a trusted website. However, if you use APK Revdl or any other unofficial source, you may expose your device or account to potential risks such as malware, viruses, bans, or penalties. You should always be careful and cautious when using APK Revdl or any other unauthorized or modified version of the game.
-
401be4b1e0
-
-
\ No newline at end of file
diff --git a/spaces/simple0urra/skops-model-card-creator-2a23515a-d54e-4804-b365-27ed6e938735/example/Download Live Wallpaper for PC Free Animated Desktops for Windows and Mac.md b/spaces/simple0urra/skops-model-card-creator-2a23515a-d54e-4804-b365-27ed6e938735/example/Download Live Wallpaper for PC Free Animated Desktops for Windows and Mac.md
deleted file mode 100644
index 78e94d834f84da8594a8fe8ccc487afddf91387f..0000000000000000000000000000000000000000
--- a/spaces/simple0urra/skops-model-card-creator-2a23515a-d54e-4804-b365-27ed6e938735/example/Download Live Wallpaper for PC Free Animated Desktops for Windows and Mac.md
+++ /dev/null
@@ -1,130 +0,0 @@
-
-How to Download Live Wallpaper for PC
-Live wallpaper is a type of wallpaper that can display animated or interactive images on your desktop background. It can make your PC more lively, personalized, and fun. In this article, we will show you how to download live wallpaper for PC, where to find them, and how to set them up.
-download live wallpaper for pc
Download ::: https://ssurll.com/2uNXCq
- What is Live Wallpaper?
-Live wallpaper is a term that refers to any wallpaper that can change or move on your desktop. It can be a video, a GIF, a slideshow, a 3D model, a game, or anything else that can be rendered on your screen. Live wallpaper can also react to your mouse movements, keyboard inputs, sound inputs, or other events.
- Benefits of Live Wallpaper
-Some of the benefits of using live wallpaper are:
-
-- It can make your desktop more attractive and dynamic.
-- It can reflect your mood, personality, or interests.
-- It can provide entertainment or relaxation.
-- It can inspire you or motivate you.
-
- Drawbacks of Live Wallpaper
-Some of the drawbacks of using live wallpaper are:
-
-- It can consume more CPU, GPU, RAM, or battery resources than static wallpaper.
-- It can distract you from your work or tasks.
-- It can cause compatibility or performance issues with some applications or games.
-- It can be hard to find or create high-quality live wallpaper.
-
- How to Find Live Wallpaper for PC
-There are many sources where you can find live wallpaper for PC. Some of them are online sources, and some of them are offline sources. Here are some examples:
- Online Sources
-Online sources are websites that offer live wallpaper for PC that you can download or stream. Some of them are free, and some of them are paid. Some of them are:
- MoeWalls
-MoeWalls is a website that offers popular free live wallpapers and animated wallpapers for PC. You can browse by categories such as anime, movies, games, landscape, abstract, etc. You can also search by keywords or tags. You can download the live wallpapers in various resolutions and formats.
- Pexels
-Pexels is a website that offers free stock videos and photos that you can use as live wallpaper for PC. You can search by keywords or browse by topics such as nature, animals, people, technology, etc. You can download the videos in various resolutions and formats.
- Other Websites
-There are many other websites that offer live wallpaper for PC, such as:
-
-- [Wallpaper Engine]: A paid software that allows you to create and use live wallpapers from various sources such as Steam Workshop, YouTube, Reddit, etc.
-- [RainWallpaper]: A free software that allows you to use live wallpapers from various sources such as DeviantArt, YouTube, Reddit, etc.
-- [LiveWallpaper.io]: A website that offers free live wallpapers and animated wallpapers for PC and mobile devices.
-- [Plastuer]: A paid software that allows you to use live wallpapers from various sources such as GIFs, videos, web pages, etc.
-
- Offline Sources
-Offline
Offline sources are files or software that you can use to create or use live wallpaper for PC without an internet connection. Some of them are:
-download free live wallpapers for pc
-download anime live wallpapers for pc
-download 4k live wallpapers for pc
-download lively wallpaper software for pc
-download moewalls live wallpaper app for pc
-download rainmeter live wallpaper skins for pc
-download video live wallpapers for pc
-download gaming live wallpapers for pc
-download cyberpunk live wallpapers for pc
-download nature live wallpapers for pc
-download fantasy live wallpapers for pc
-download music visualizer live wallpapers for pc
-download interactive live wallpapers for pc
-download audio responsive live wallpapers for pc
-download 3d live wallpapers for pc
-download hd live wallpapers for pc
-download windows 11 live wallpapers for pc
-download neon live wallpapers for pc
-download space live wallpapers for pc
-download abstract live wallpapers for pc
-download minimalist live wallpapers for pc
-download dark live wallpapers for pc
-download aesthetic live wallpapers for pc
-download superhero live wallpapers for pc
-download movie live wallpapers for pc
-download anime girl live wallpapers for pc
-download naruto live wallpapers for pc
-download dragon ball z live wallpapers for pc
-download one piece live wallpapers for pc
-download attack on titan live wallpapers for pc
-download demon slayer live wallpapers for pc
-download my hero academia live wallpapers for pc
-download marvel live wallpapers for pc
-download dc live wallpapers for pc
-download star wars live wallpapers for pc
-download lord of the rings live wallpapers for pc
-download harry potter live wallpapers for pc
-download matrix live wallpapers for pc
-download john wick live wallpapers for pc
-download batman live wallpapers for pc
-download spider-man live wallpapers for pc
-download iron man live wallpapers for pc
-download captain america live wallpapers for pc
-download thor live wallpapers for pc
-download hulk live wallpapers for pc
-download black panther live wallpapers for pc
-download wonder woman live wallpapers for pc
-download superman live wallpapers for pc
- Personal Videos
-You can use your own videos as live wallpaper for PC. You can record videos with your camera, phone, drone, or other devices. You can edit them with video editing software such as Adobe Premiere, iMovie, Windows Movie Maker, etc. You can convert them to suitable formats such as MP4, WMV, AVI, etc.
- Screen Recorders
-You can use screen recorders to capture your desktop or any window as live wallpaper for PC. You can use software such as OBS Studio, Camtasia, Bandicam, etc. You can record your screen with sound, webcam, mouse cursor, etc. You can save the recordings as video files or stream them to online platforms such as YouTube, Twitch, etc.
- Other Software
-There are other software that you can use to create or use live wallpaper for PC, such as:
-
-- [DreamScene]: A feature of Windows Vista Ultimate that allows you to use videos as desktop backgrounds.
-- [Lively Wallpaper]: A free and open-source software that allows you to use web pages, videos, GIFs, emulators, games, etc. as live wallpaper for PC.
-- [DeskScapes]: A paid software that allows you to use animated wallpapers and effects on your desktop.
-- [Plastuer]: A paid software that allows you to use live wallpapers from various sources such as GIFs, videos, web pages, etc.
-
- How to Set Live Wallpaper for PC
-Once you have downloaded or created your live wallpaper for PC, you need to set it as your desktop background. There are different ways to do this depending on the source and format of your live wallpaper. Here are some examples:
- Using Desktop Live Wallpapers App
-Desktop Live Wallpapers is a free app that allows you to use live wallpapers on your Windows 10 PC. You can download it from the Microsoft Store. To use it, follow these steps:
-
-- Launch the app and click on the "+" button to add your live wallpaper files.
-- Select the files from your computer or drag and drop them into the app.
-- Choose the live wallpaper that you want to use and click on the "Apply" button.
-- Enjoy your live wallpaper on your desktop.
-
- Using Other Apps or Tools
-If you are using other apps or tools to create or use live wallpaper for PC, such as Wallpaper Engine, RainWallpaper, Lively Wallpaper, etc., you need to follow their instructions and settings to set your live wallpaper. Usually, they have a user interface that allows you to browse, select, and apply your live wallpaper. You may also need to adjust some options such as resolution, frame rate, quality, performance, etc.
- Conclusion
-Live wallpaper is a great way to spice up your desktop and make it more interesting and fun. You can download live wallpaper for PC from various online or offline sources, or create your own with various software or tools. You can also set your live wallpaper with different apps or methods depending on your preference and system. We hope this article has helped you learn how to download live wallpaper for PC and enjoy it on your screen.
- FAQs
-Here are some frequently asked questions about live wallpaper for PC:
-
-- How do I make my own live wallpaper for PC?
-You can make your own live wallpaper for PC with various software or tools such as Wallpaper Engine, Lively Wallpaper, OBS Studio, Adobe Premiere, etc. You can use videos, GIFs, web pages, games, emulators, etc. as sources for your live wallpaper. You can also edit them with effects, filters, transitions, etc.
- - How do I remove live wallpaper from PC?
-You can remove live wallpaper from PC by changing your desktop background to a static image or color. You can do this by right-clicking on your desktop and choosing "Personalize". Then select "Background" and choose an image or color from the options. You can also remove the app or tool that you used to set the live wallpaper from your computer.
- - How do I pause live wallpaper on PC?
-You can pause live wallpaper on PC by using the app or tool that you used to set it. Some apps or tools have a pause button or option that allows you to stop the animation or movement of the live wallpaper. You can also pause the live wallpaper by minimizing or closing the app or tool.
- - How do I make live wallpaper for PC faster or smoother?
-You can make live wallpaper for PC faster or smoother by adjusting some settings or options in the app or tool that you used to set it. Some settings or options that can affect the speed or smoothness of the live wallpaper are resolution, frame rate, quality, performance, etc. You can also make live wallpaper for PC faster or smoother by upgrading your hardware or software such as CPU, GPU, RAM, OS, drivers, etc.
- - How do I make live wallpaper for PC more interactive?
-You can make live wallpaper for PC more interactive by using sources or software that can react to your inputs or events. Some examples of interactive live wallpaper are web pages, games, emulators, etc. that can respond to your mouse movements, keyboard inputs, sound inputs, etc. You can also use software such as Wallpaper Engine, Lively Wallpaper, etc. that have features or options that allow you to customize the interactivity of your live wallpaper.
-
401be4b1e0
-
-
\ No newline at end of file
diff --git a/spaces/simple0urra/skops-model-card-creator-2a23515a-d54e-4804-b365-27ed6e938735/example/Download World Building Craft MOD APK 1.5.3 and Enjoy a Sandbox Game with No Limits.md b/spaces/simple0urra/skops-model-card-creator-2a23515a-d54e-4804-b365-27ed6e938735/example/Download World Building Craft MOD APK 1.5.3 and Enjoy a Sandbox Game with No Limits.md
deleted file mode 100644
index 3fff74dd52222afca30e8193f5caef25d949ff54..0000000000000000000000000000000000000000
--- a/spaces/simple0urra/skops-model-card-creator-2a23515a-d54e-4804-b365-27ed6e938735/example/Download World Building Craft MOD APK 1.5.3 and Enjoy a Sandbox Game with No Limits.md
+++ /dev/null
@@ -1,96 +0,0 @@
-
-World Building Craft Mod APK 1.5.3: A Fun and Creative Sandbox Game
-If you are looking for a game that lets you unleash your imagination and creativity, then you should try World Building Craft. This is a sandbox game that allows you to explore, build, and play in a 3D pixel world. You can create your own world with blocks and items, interact with animals and villagers, and customize your character and skins. You can also download the mod apk version of this game, which gives you unlimited resources, coins, skins, and items, as well as removes ads and in-app purchases. In this article, we will tell you more about World Building Craft, how to download and install the mod apk file, and what are the benefits of using it.
-world building craft mod apk 1.5.3
Download Zip ✯✯✯ https://ssurll.com/2uNXJw
- What is World Building Craft?
-World Building Craft is a sandbox game that is inspired by Minecraft, but has its own unique features and gameplay. You can explore different biomes and terrains, such as forests, deserts, mountains, oceans, and islands. You can also build your own world with blocks and items, such as wood, stone, brick, glass, metal, furniture, plants, and more. You can use different tools to mine, craft, place, and destroy blocks. You can also play with animals and villagers, who will give you quests and rewards. You can also customize your character and skins, choosing from different genders, hairstyles, clothes, accessories, and more.
- Features of World Building Craft
-- Explore different biomes and terrains
-World Building Craft has a large map that is randomly generated every time you start a new game. You can explore different biomes and terrains, such as forests, deserts, mountains, oceans, and islands. You can find different resources, animals, plants, structures, and secrets in each biome. You can also change the weather and time of day to suit your mood.
-- Build your own world with blocks and items
-World Building Craft gives you the freedom to create your own world with blocks and items. You can use different tools to mine, craft, place, and destroy blocks. You can also use different items to decorate your world, such as furniture, plants, paintings, lamps, etc. You can build anything you can imagine, from houses and castles to farms and cities.
-- Play with animals and villagers
-World Building Craft has many animals and villagers that you can interact with. You can tame animals such as dogs, cats, horses, cows, sheep, etc., and ride them or make them follow you. You can also feed them or breed them to get more animals. You can also talk to villagers who will give you quests and rewards. You can trade with them or help them with their problems.
-- Customize your character and skins
-World Building Craft allows you to customize your character and skins according to your preference. You can choose from different genders, hairstyles, clothes, accessories, and more. You can also change your skin color, eye color, and hair color. You can also unlock more skins and items by completing quests or using coins.
- How to download and install World Building Craft Mod APK 1.5.3?
-- Download the mod apk file from a trusted source
-The first step to download and install World Building Craft Mod APK 1.5.3 is to find a trusted source that provides the mod apk file. You can search online for websites that offer mod apk files for various games, or you can use the link below to download the mod apk file for World Building Craft.
-world building craft game mod apk latest version
-world craft building simulator mod apk download
-world building craft 1.5.3 mod apk unlimited resources
-world building craft sandbox block simulator mod apk
-world craft building 3d mod apk free
-world building craft mod apk android 1
-world craft building pixel cubes mod apk
-world building craft 1.5.3 mod apk offline
-world building craft simulation game mod apk
-world craft building open world mod apk
-world building craft mod apk revdl
-world craft building houses and wonders mod apk
-world building craft 1.5.3 mod apk hack
-world building craft sandbox game mod apk
-world craft building 2023 mod apk
-world building craft mod apk rexdl
-world craft building exploration and crafting mod apk
-world building craft 1.5.3 mod apk no ads
-world building craft block simulator mod apk
-world craft building candy mobile mod apk
-world building craft mod apk happymod
-world craft building random generated world mod apk
-world building craft 1.5.3 mod apk unlimited money
-world building craft sandbox simulator mod apk
-world craft building 1.5.3 candy mobile apk download
-world building craft mod apk apkpure
-world craft building rough 3d blocks mod apk
-world building craft 1.5.3 mod apk unlocked everything
-world building craft simulation simulator mod apk
-world craft building com.hsyecheng.blockworldcraft mod apk
-world building craft mod apk android oyun club
-world craft building magnificent wonders mod apk
-world building craft 1.5.3 mod apk premium
-world building craft sandbox block game mod apk
-world craft building latest version 1.5.3 mod apk
-world building craft mod apk mob.org
-world craft building simplest houses mod apk
-world building craft 1.5.3 mod apk pro
-world building craft sandbox block simulator game mod apk
-world craft building old versions 1.5.2 1.5.1 mod apk
-world building craft mod apk uptodown
-world craft building big ben eiffel tower smaug mod apk
-world building craft 1.5.3 xapk download
-world building craft sandbox block simulator game online
-world crafting and build game with mods
-how to install xapk apks app bundle zip obb
-best simulation games for android free download
-minecraft vs roblox vs terraria vs trove vs portal knights vs lego worlds vs dragon quest builders vs cube life island survival vs boundless vs creativerse vs hytale vs staxel vs eco vs astroneer vs scrap mechanic vs besiege vs trailmakers vs planet nomads vs space engineers vs medieval engineers vs empyrion galactic survival vs no man's sky vs starbound vs subnautica vs ark survival evolved vs rust vs conan exiles vs the forest vs raft vs stranded deep vs green hell vs don't starve together vs oxygen not included vs rimworld
-Download World Building Craft Mod APK 1.5.3 here
-- Enable unknown sources on your device settings
-The next step is to enable unknown sources on your device settings. This will allow you to install apps that are not from the Google Play Store. To do this, go to your device settings, then security, then unknown sources, and toggle it on. You may see a warning message, but you can ignore it and proceed.
-- Install the mod apk file and launch the game
-The final step is to install the mod apk file and launch the game. To do this, locate the mod apk file that you downloaded and tap on it. You may see a pop-up asking for permissions, just accept them and continue. The installation process will take a few seconds, and then you can open the game and enjoy it.
- What are the benefits of World Building Craft Mod APK 1.5.3?
-- Unlimited resources and coins
-One of the benefits of World Building Craft Mod APK 1.5.3 is that it gives you unlimited resources and coins. This means that you can mine, craft, build, and decorate your world without any limitations. You can also buy any item or skin that you want without worrying about the cost.
-- No ads and in-app purchases
-Another benefit of World Building Craft Mod APK 1.5.3 is that it removes ads and in-app purchases from the game. This means that you can play the game without any interruptions or distractions. You can also enjoy the game without spending any real money on it.
-- All skins and items unlocked
-The last benefit of World Building Craft Mod APK 1.5.3 is that it unlocks all skins and items in the game. This means that you can access and use any skin or item that you like, without having to complete quests or use coins. You can also customize your character and world with more variety and style.
- Conclusion
-World Building Craft is a fun and creative sandbox game that lets you explore, build, and play in a 3D pixel world. You can create your own world with blocks and items, interact with animals and villagers, and customize your character and skins. You can also download the mod apk version of this game, which gives you unlimited resources, coins, skins, and items, as well as removes ads and in-app purchases. To download and install World Building Craft Mod APK 1.5.3, you just need to follow these simple steps: download the mod apk file from a trusted source, enable unknown sources on your device settings, install the mod apk file and launch the game.
- FAQs
-Here are some frequently asked questions about World Building Craft Mod APK 1.5.3:
-
-- Is World Building Craft Mod APK 1.5.3 safe to use?
-Yes, World Building Craft Mod APK 1.5.3 is safe to use, as long as you download it from a trusted source. However, you should always be careful when downloading and installing any mod apk file, as some of them may contain viruses or malware that can harm your device or data.
-- Is World Building Craft Mod APK 1.5.3 compatible with my device?
-World Building Craft Mod APK 1.5.3 is compatible with most Android devices that have Android 4.4 or higher versions installed. However, some devices may not support some features or functions of the game due to different specifications or performance issues.
-- Can I play World Building Craft Mod APK 1.5.3 online with other players?
-No, World Building Craft Mod APK 1.5.3 is an offline game that does not require an internet connection to play. You can only play it solo or with local multiplayer mode using Bluetooth or Wi-Fi.
-- Can I update World Building Craft Mod APK 1.5.3 to the latest version?
-No, World Building Craft Mod APK 1.5.3 is a modified version of the original game that does not receive official updates from the developers. If you want to update the game, you will have to download and install the new mod apk file from the same or another trusted source. You may also lose your progress or data if you update the game.
-- How can I contact the developers of World Building Craft Mod APK 1.5.3?
-You can contact the developers of World Building Craft Mod APK 1.5.3 by sending them an email at worldbuildingcraft@gmail.com. You can also visit their website or follow them on social media for more information and updates about the game.
-
197e85843d
-
-
\ No newline at end of file
diff --git a/spaces/simple0urra/skops-model-card-creator-2a23515a-d54e-4804-b365-27ed6e938735/example/Enjoy the Fun and Challenge of Mario Game APK on Your PC or Mac with BlueStacks.md b/spaces/simple0urra/skops-model-card-creator-2a23515a-d54e-4804-b365-27ed6e938735/example/Enjoy the Fun and Challenge of Mario Game APK on Your PC or Mac with BlueStacks.md
deleted file mode 100644
index c7d69e0df7904e48671f3316649d676091908d95..0000000000000000000000000000000000000000
--- a/spaces/simple0urra/skops-model-card-creator-2a23515a-d54e-4804-b365-27ed6e938735/example/Enjoy the Fun and Challenge of Mario Game APK on Your PC or Mac with BlueStacks.md
+++ /dev/null
@@ -1,164 +0,0 @@
-
-How to Download and Play Mario Game APK on Your Android Device
-Do you love playing Mario games? If so, you are not alone. Mario is one of the most popular and iconic video game characters of all time. He has been entertaining millions of fans around the world since his debut in 1981. Whether you are a nostalgic gamer or a new fan, you can enjoy the fun and adventure of Mario games on your Android device with Mario game APK.
-mario game apk
Download File ✵✵✵ https://ssurll.com/2uO0mE
-What is Mario Game APK?
-A brief introduction to the Mario game series and its features
-Mario game APK is a collection of Android games that feature the famous plumber and his friends. You can play different types of games, such as platformers, runners, puzzles, and more. Some of the most popular Mario games for Android are Super Mario Run, Super Mario Bros., Dr. Mario World, and Mario Kart Tour. These games have various features, such as:
-
-- Stunning graphics and sound effects that recreate the classic Mario atmosphere
-- Simple and intuitive controls that let you play with one hand
-- Multiple levels, worlds, and challenges that test your skills and creativity
-- Online multiplayer modes that let you compete with other players around the world
-- In-game rewards and bonuses that let you customize your characters, items, and kingdoms
-
-The benefits of downloading and playing Mario game APK on your Android device
-There are many reasons why you should download and play Mario game APK on your Android device. Here are some of them:
-
-- You can enjoy the nostalgia and excitement of playing Mario games anytime, anywhere
-- You can save money and space by downloading free or low-cost games instead of buying expensive consoles or cartridges
-- You can access new updates, features, and content that are regularly added to the games
-- You can connect with other Mario fans and share your achievements, tips, and feedback
-- You can have fun and relax while playing casual or challenging games that suit your mood and preference
-
-How to Download Mario Game APK for Android
-The steps to download and install Mario game APK from APKCombo
-If you want to download and install Mario game APK from a third-party source, you can use APKCombo. This is a website that offers free and safe downloads of various Android apps and games. Here are the steps to follow:
-
-- Go to APKCombo.com on your browser
-- Type "Mario" in the search box and hit enter
-- Select the game you want to download from the list of results
-- Click on the "Download APK" button and choose a version that is compatible with your device
-- Wait for the download to finish and then open the file manager on your device
-- Locate the downloaded file and tap on it to install it
-- Follow the instructions on the screen to complete the installation
-- Launch the game and enjoy!
-
The steps to download and install Mario game APK from Google Play Store
-If you want to download and install Mario game APK from the official source, you can use the Google Play Store. This is the app store that comes pre-installed on most Android devices. Here are the steps to follow:
-
-- Open the Google Play Store app on your device
-- Type "Mario" in the search box and tap on the magnifying glass icon
-- Select the game you want to download from the list of results
-- Tap on the "Install" button and wait for the download to finish
-- Tap on the "Open" button to launch the game and enjoy!
-
-How to Play Mario Game APK on Your PC or Mac
-The advantages of playing Mario game APK on your PC or Mac
-While playing Mario game APK on your Android device is fun and convenient, you might want to try playing it on your PC or Mac for a different experience. Here are some of the advantages of doing so:
-
-- You can enjoy a bigger screen and better graphics that enhance the gameplay
-- You can use a keyboard, mouse, or gamepad for more comfortable and precise controls
-- You can save battery life and storage space on your Android device
-- You can record, stream, or share your gameplay with others easily
-- You can access more features and settings that are not available on Android
-
-The steps to download and install BlueStacks emulator and run Mario game APK on it
-To play Mario game APK on your PC or Mac, you need an emulator that can run Android apps and games. One of the best emulators for this purpose is BlueStacks, which is free and easy to use. Here are the steps to download and install BlueStacks and run Mario game APK on it:
-
-- Go to BlueStacks.com on your browser and click on the "Download BlueStacks" button
-- Run the installer file and follow the instructions to install BlueStacks on your PC or Mac
-- Launch BlueStacks and sign in with your Google account to access the Google Play Store
-- Search for "Mario" in the Play Store and install the game you want to play
-- Click on the game icon on the BlueStacks home screen to start playing Mario game APK on your PC or Mac!
-
Tips and Tricks for Playing Mario Game APK
-How to master the four modes of Mario game APK: World Tour, Remix 10, Toad Rally, and Kingdom Builder
-Mario game APK offers four different modes of gameplay that cater to different tastes and goals. Here is a brief overview of each mode and some tips on how to master them:
-super mario game apk download
-mario kart game apk free
-mario bros game apk offline
-mario run game apk mod
-mario party game apk android
-mario maker game apk online
-mario world game apk full
-mario 64 game apk latest
-mario galaxy game apk version
-mario odyssey game apk update
-mario tennis game apk hack
-mario golf game apk cracked
-mario rpg game apk unlimited
-mario luigi game apk premium
-mario yoshi game apk pro
-mario sonic game apk unlocked
-mario wario game apk patched
-mario toad game apk beta
-mario peach game apk alpha
-mario bowser game apk original
-mario rosalina game apk new
-mario daisy game apk old
-mario waluigi game apk classic
-mario donkey kong game apk retro
-mario zelda game apk crossover
-mario pokemon game apk fanmade
-mario kirby game apk remake
-mario metroid game apk ported
-mario fire emblem game apk modded
-mario animal crossing game apk hacked
-mario smash bros game apk cracked
-mario splatoon game apk unlocked
-mario pikmin game apk patched
-mario star fox game apk beta
-mario f zero game apk alpha
-mario paper jam game apk original
-mario sticker star game apk new
-mario color splash game apk old
-mario origami king game apk classic
-mario dream team game apk retro
-mario inside story game apk crossover
-mario partners in time game apk fanmade
-mario superstar saga game apk remake
-mario bowser's fury game apk ported
-mario 3d world game apk modded
-mario 3d land game apk hacked
-mario new super bros 2 game apk cracked
-super princess peach 2: royal rumble (mariowiki.com)
-
-- World Tour: This is the classic mode where you play through various levels and worlds, collecting coins and stars along the way. You can choose from six different characters: Mario, Luigi, Peach, Toad, Yoshi, and Toadette. Each character has their own abilities and advantages. For example, Peach can float in the air, Yoshi can eat enemies, and Toadette can turn into Peachette with a super crown. Some tips for this mode are:
- - Try to collect as many coins and stars as possible to unlock new levels and worlds
- - Use the pause button to plan your moves and avoid obstacles
- - Use the bubble button to save yourself from falling or dying
- - Use the items in the item box to boost your performance, such as mushrooms, fire flowers, stars, and invincibility leaves
- - Watch out for hidden blocks, secret paths, and bonus rooms that contain extra coins and stars
-
-
-- Remix 10: This is a fast-paced mode where you play 10 short levels in a row, with different combinations of elements each time. You can also find Daisy in this mode and unlock her as a playable character. She can double jump, which is very useful for reaching high places. Some tips for this mode are:
- - Try to complete each level as quickly as possible to earn more coins and stars
- - Collect rainbow medals to unlock bonus games and items
- - Use the skip button to skip a level if you find it too hard or boring
- - Watch out for enemies and traps that can end your run prematurely
- - Play this mode regularly to get new decorations for your kingdom
-
-
-- Toad Rally: This is a competitive mode where you race against other players or your friends online. You can choose any character you have unlocked and any level you have cleared in World Tour. The goal is to impress as many Toads as possible by performing stylish moves, such as jumping, wall-jumping, rolling, and stomping. The more Toads you impress, the more coins you earn. Some tips for this mode are:
- - Try to match the rhythm of the music and the level to perform better moves
- - Use the dash button to speed up and catch up with your opponent
- - Use the star button to activate a star rush, which gives you invincibility and extra speed
- - Avoid getting hit by enemies or falling into pits, as this will make you lose coins and Toads
- - Play this mode often to collect more Toads of different colors and types
-
-
-- Kingdom Builder: This is a creative mode where you can build your own kingdom using the coins and Toads you have collected in other modes. You can place various buildings, decorations, and characters in your kingdom, such as houses, shops, statues, flowers, pipes, flags, and more. You can also unlock special buildings that give you access to mini-games, such as Coin Rush, Bonus Game House, and Luigi's House. Some tips for this mode are:
- - Expand your kingdom by clearing more levels in World Tour and collecting more Toads in Toad Rally
- - Place buildings that match the color and type of the Toads you have to increase their happiness and productivity
- - Visit other players' kingdoms and give them kudos to earn friendship points and rewards
- - Customize your kingdom according to your preference and style
- - Have fun and experiment with different combinations and layouts
-
-
-Conclusion
-Mario game APK is a great way to enjoy the classic and new Mario games on your Android device, PC, or Mac. You can download and install Mario game APK from various sources, such as APKCombo, Google Play Store, or BlueStacks emulator. You can also play different modes of Mario game APK, such as World Tour, Remix 10, Toad Rally, and Kingdom Builder. You can also master the games by following some tips and tricks, such as collecting coins and stars, using items and buttons, and impressing Toads. Mario game APK is a fun and exciting way to relive the nostalgia and adventure of Mario games. So what are you waiting for? Download Mario game APK today and join the millions of Mario fans around the world!
-FAQs
-Here are some frequently asked questions about Mario game APK:
-
-- Is Mario game APK safe to download and play?
-Yes, Mario game APK is safe to download and play, as long as you use a trusted source, such as APKCombo, Google Play Store, or BlueStacks emulator. You should also scan the files with an antivirus program before installing them.
-- Is Mario game APK free to download and play?
-Yes, most of the Mario games for Android are free to download and play. However, some of them may have in-app purchases or ads that require real money. You can disable these features in the settings or by turning off your internet connection.
-- How can I update Mario game APK?
-You can update Mario game APK by checking for new versions on the source you used to download it. You can also enable automatic updates in the settings of your device or emulator.
-- How can I contact the developers of Mario game APK?
-You can contact the developers of Mario game APK by visiting their official websites or social media pages. You can also leave a review or feedback on the source you used to download it.
-- How can I share my gameplay of Mario game APK with others?
-You can share your gameplay of Mario game APK with others by using the screenshot or video recording features of your device or emulator. You can also use third-party apps or software to record, stream, or edit your gameplay. You can then upload or send your gameplay to your friends or social media platforms.
-
197e85843d
-
-
\ No newline at end of file
diff --git a/spaces/soldni/viz_summaries/app.py b/spaces/soldni/viz_summaries/app.py
deleted file mode 100644
index 67c94a8a2ec845cca51910865a410e8689470067..0000000000000000000000000000000000000000
--- a/spaces/soldni/viz_summaries/app.py
+++ /dev/null
@@ -1,142 +0,0 @@
-from io import StringIO
-import itertools
-
-import gradio as gr
-import pandas as pd
-import spacy
-
-
-nlp = spacy.load('en_core_web_sm')
-
-HTML_RED = '{t}'
-HTML_GRN = '{t}'
-HTML_YLW = '{t}'
-HTML_BLU = '{t}'
-HTML_PLN = '{t}'
-TABLE_CSS = '''
-th, td {
- padding: 4px;
-}
-table, th, td {
- border: 1px solid black;
- border-collapse: collapse;
-
-}
-'''
-
-
-def colorize(file_obj):
- with open(file_obj.name, 'r') as f:
- raw = f.read()
- raw = raw[raw.find('example_id'):]
- data = pd.read_csv(StringIO(raw))
-
- table_content = []
-
- for row in data.iterrows():
- id_ = row[1]['example_id']
- gold, genA, genB = nlp.pipe((
- row[1]['target summary'],
- row[1]['model summary A'],
- row[1]['model summary B']
- ))
- tokens_gold = {token.lemma_.lower(): 0 for token in gold}
- for token in itertools.chain(genA, genB):
- if token.lemma_.lower() in tokens_gold:
- tokens_gold[token.lemma_.lower()] += 1
-
- gold_text = ''.join([
- (
- HTML_PLN.format(t=token.text)
- if token.pos_ not in {'NOUN', 'PROPN', 'VERB'}
- else (
- (
- HTML_BLU if tokens_gold[token.lemma_.lower()] > 0
- else HTML_YLW
- ).format(t=token.text)
- )
- ) + token.whitespace_
- for token in gold
- ])
- table_content.append(
- [id_, gold_text] +
- [
- ''.join(
- (
- HTML_PLN.format(t=token.text)
- if token.pos_ not in {'NOUN', 'PROPN', 'VERB'}
- else (
- HTML_GRN.format(t=token.text)
- if token.lemma_.lower() in tokens_gold
- else HTML_RED.format(t=token.text)
- )
- ) + token.whitespace_
- for token in gen
- )
- for gen in (genA, genB)
- ]
- )
-
- # return an HTML table using data in table_content
- return '\n'.join((
- '',
- ""
- "id ",
- "Gold ",
- "Model A ",
- "Model B ",
- " ",
- '\n'.join(
- '\n' +
- '\n'.join('{} '.format(cell) for cell in row) +
- '\n '
- for row in table_content
- ),
- '
'
- ))
-
-
-def main():
- with gr.Blocks(css=TABLE_CSS) as demo:
- gr.Markdown(
- "After uploading, click Run and switch to the Visualization tab."
- )
- with gr.Tabs():
- with gr.TabItem("Upload"):
- data = gr.File(
- label='upload csv with Annotations', type='file'
- )
- run = gr.Button(label='Run')
- with gr.TabItem("Visualization"):
- gr.HTML(
- ''.join(
- (
- "Explanation of colors:",
- "
",
- "- ",
- HTML_RED.format(t='Red'),
- ": word is in generated, but not in gold.
",
- "- ",
- HTML_GRN.format(t='Green'),
- ": word is in generated summary and gold.
",
- "- ",
- HTML_YLW.format(t='Yellow'),
- ": word is in gold, but not in generated.
",
- "- ",
- HTML_BLU.format(t='Blue'),
- ": word is in gold and in generated.
",
- "
",
- "
",
- "Important: Only nouns, verbs and proper ",
- "nouns are colored."
- )
- )
- )
- viz = gr.HTML(label='Upload a csv file to start.')
- run.click(colorize, data, viz)
-
- demo.launch()
-
-
-if __name__ == '__main__':
- main()
diff --git a/spaces/sparanoid/milky-green-sovits-4/modules/modules.py b/spaces/sparanoid/milky-green-sovits-4/modules/modules.py
deleted file mode 100644
index 54290fd207b25e93831bd21005990ea137e6b50e..0000000000000000000000000000000000000000
--- a/spaces/sparanoid/milky-green-sovits-4/modules/modules.py
+++ /dev/null
@@ -1,342 +0,0 @@
-import copy
-import math
-import numpy as np
-import scipy
-import torch
-from torch import nn
-from torch.nn import functional as F
-
-from torch.nn import Conv1d, ConvTranspose1d, AvgPool1d, Conv2d
-from torch.nn.utils import weight_norm, remove_weight_norm
-
-import modules.commons as commons
-from modules.commons import init_weights, get_padding
-
-
-LRELU_SLOPE = 0.1
-
-
-class LayerNorm(nn.Module):
- def __init__(self, channels, eps=1e-5):
- super().__init__()
- self.channels = channels
- self.eps = eps
-
- self.gamma = nn.Parameter(torch.ones(channels))
- self.beta = nn.Parameter(torch.zeros(channels))
-
- def forward(self, x):
- x = x.transpose(1, -1)
- x = F.layer_norm(x, (self.channels,), self.gamma, self.beta, self.eps)
- return x.transpose(1, -1)
-
-
-class ConvReluNorm(nn.Module):
- def __init__(self, in_channels, hidden_channels, out_channels, kernel_size, n_layers, p_dropout):
- super().__init__()
- self.in_channels = in_channels
- self.hidden_channels = hidden_channels
- self.out_channels = out_channels
- self.kernel_size = kernel_size
- self.n_layers = n_layers
- self.p_dropout = p_dropout
- assert n_layers > 1, "Number of layers should be larger than 0."
-
- self.conv_layers = nn.ModuleList()
- self.norm_layers = nn.ModuleList()
- self.conv_layers.append(nn.Conv1d(in_channels, hidden_channels, kernel_size, padding=kernel_size//2))
- self.norm_layers.append(LayerNorm(hidden_channels))
- self.relu_drop = nn.Sequential(
- nn.ReLU(),
- nn.Dropout(p_dropout))
- for _ in range(n_layers-1):
- self.conv_layers.append(nn.Conv1d(hidden_channels, hidden_channels, kernel_size, padding=kernel_size//2))
- self.norm_layers.append(LayerNorm(hidden_channels))
- self.proj = nn.Conv1d(hidden_channels, out_channels, 1)
- self.proj.weight.data.zero_()
- self.proj.bias.data.zero_()
-
- def forward(self, x, x_mask):
- x_org = x
- for i in range(self.n_layers):
- x = self.conv_layers[i](x * x_mask)
- x = self.norm_layers[i](x)
- x = self.relu_drop(x)
- x = x_org + self.proj(x)
- return x * x_mask
-
-
-class DDSConv(nn.Module):
- """
- Dialted and Depth-Separable Convolution
- """
- def __init__(self, channels, kernel_size, n_layers, p_dropout=0.):
- super().__init__()
- self.channels = channels
- self.kernel_size = kernel_size
- self.n_layers = n_layers
- self.p_dropout = p_dropout
-
- self.drop = nn.Dropout(p_dropout)
- self.convs_sep = nn.ModuleList()
- self.convs_1x1 = nn.ModuleList()
- self.norms_1 = nn.ModuleList()
- self.norms_2 = nn.ModuleList()
- for i in range(n_layers):
- dilation = kernel_size ** i
- padding = (kernel_size * dilation - dilation) // 2
- self.convs_sep.append(nn.Conv1d(channels, channels, kernel_size,
- groups=channels, dilation=dilation, padding=padding
- ))
- self.convs_1x1.append(nn.Conv1d(channels, channels, 1))
- self.norms_1.append(LayerNorm(channels))
- self.norms_2.append(LayerNorm(channels))
-
- def forward(self, x, x_mask, g=None):
- if g is not None:
- x = x + g
- for i in range(self.n_layers):
- y = self.convs_sep[i](x * x_mask)
- y = self.norms_1[i](y)
- y = F.gelu(y)
- y = self.convs_1x1[i](y)
- y = self.norms_2[i](y)
- y = F.gelu(y)
- y = self.drop(y)
- x = x + y
- return x * x_mask
-
-
-class WN(torch.nn.Module):
- def __init__(self, hidden_channels, kernel_size, dilation_rate, n_layers, gin_channels=0, p_dropout=0):
- super(WN, self).__init__()
- assert(kernel_size % 2 == 1)
- self.hidden_channels =hidden_channels
- self.kernel_size = kernel_size,
- self.dilation_rate = dilation_rate
- self.n_layers = n_layers
- self.gin_channels = gin_channels
- self.p_dropout = p_dropout
-
- self.in_layers = torch.nn.ModuleList()
- self.res_skip_layers = torch.nn.ModuleList()
- self.drop = nn.Dropout(p_dropout)
-
- if gin_channels != 0:
- cond_layer = torch.nn.Conv1d(gin_channels, 2*hidden_channels*n_layers, 1)
- self.cond_layer = torch.nn.utils.weight_norm(cond_layer, name='weight')
-
- for i in range(n_layers):
- dilation = dilation_rate ** i
- padding = int((kernel_size * dilation - dilation) / 2)
- in_layer = torch.nn.Conv1d(hidden_channels, 2*hidden_channels, kernel_size,
- dilation=dilation, padding=padding)
- in_layer = torch.nn.utils.weight_norm(in_layer, name='weight')
- self.in_layers.append(in_layer)
-
- # last one is not necessary
- if i < n_layers - 1:
- res_skip_channels = 2 * hidden_channels
- else:
- res_skip_channels = hidden_channels
-
- res_skip_layer = torch.nn.Conv1d(hidden_channels, res_skip_channels, 1)
- res_skip_layer = torch.nn.utils.weight_norm(res_skip_layer, name='weight')
- self.res_skip_layers.append(res_skip_layer)
-
- def forward(self, x, x_mask, g=None, **kwargs):
- output = torch.zeros_like(x)
- n_channels_tensor = torch.IntTensor([self.hidden_channels])
-
- if g is not None:
- g = self.cond_layer(g)
-
- for i in range(self.n_layers):
- x_in = self.in_layers[i](x)
- if g is not None:
- cond_offset = i * 2 * self.hidden_channels
- g_l = g[:,cond_offset:cond_offset+2*self.hidden_channels,:]
- else:
- g_l = torch.zeros_like(x_in)
-
- acts = commons.fused_add_tanh_sigmoid_multiply(
- x_in,
- g_l,
- n_channels_tensor)
- acts = self.drop(acts)
-
- res_skip_acts = self.res_skip_layers[i](acts)
- if i < self.n_layers - 1:
- res_acts = res_skip_acts[:,:self.hidden_channels,:]
- x = (x + res_acts) * x_mask
- output = output + res_skip_acts[:,self.hidden_channels:,:]
- else:
- output = output + res_skip_acts
- return output * x_mask
-
- def remove_weight_norm(self):
- if self.gin_channels != 0:
- torch.nn.utils.remove_weight_norm(self.cond_layer)
- for l in self.in_layers:
- torch.nn.utils.remove_weight_norm(l)
- for l in self.res_skip_layers:
- torch.nn.utils.remove_weight_norm(l)
-
-
-class ResBlock1(torch.nn.Module):
- def __init__(self, channels, kernel_size=3, dilation=(1, 3, 5)):
- super(ResBlock1, self).__init__()
- self.convs1 = nn.ModuleList([
- weight_norm(Conv1d(channels, channels, kernel_size, 1, dilation=dilation[0],
- padding=get_padding(kernel_size, dilation[0]))),
- weight_norm(Conv1d(channels, channels, kernel_size, 1, dilation=dilation[1],
- padding=get_padding(kernel_size, dilation[1]))),
- weight_norm(Conv1d(channels, channels, kernel_size, 1, dilation=dilation[2],
- padding=get_padding(kernel_size, dilation[2])))
- ])
- self.convs1.apply(init_weights)
-
- self.convs2 = nn.ModuleList([
- weight_norm(Conv1d(channels, channels, kernel_size, 1, dilation=1,
- padding=get_padding(kernel_size, 1))),
- weight_norm(Conv1d(channels, channels, kernel_size, 1, dilation=1,
- padding=get_padding(kernel_size, 1))),
- weight_norm(Conv1d(channels, channels, kernel_size, 1, dilation=1,
- padding=get_padding(kernel_size, 1)))
- ])
- self.convs2.apply(init_weights)
-
- def forward(self, x, x_mask=None):
- for c1, c2 in zip(self.convs1, self.convs2):
- xt = F.leaky_relu(x, LRELU_SLOPE)
- if x_mask is not None:
- xt = xt * x_mask
- xt = c1(xt)
- xt = F.leaky_relu(xt, LRELU_SLOPE)
- if x_mask is not None:
- xt = xt * x_mask
- xt = c2(xt)
- x = xt + x
- if x_mask is not None:
- x = x * x_mask
- return x
-
- def remove_weight_norm(self):
- for l in self.convs1:
- remove_weight_norm(l)
- for l in self.convs2:
- remove_weight_norm(l)
-
-
-class ResBlock2(torch.nn.Module):
- def __init__(self, channels, kernel_size=3, dilation=(1, 3)):
- super(ResBlock2, self).__init__()
- self.convs = nn.ModuleList([
- weight_norm(Conv1d(channels, channels, kernel_size, 1, dilation=dilation[0],
- padding=get_padding(kernel_size, dilation[0]))),
- weight_norm(Conv1d(channels, channels, kernel_size, 1, dilation=dilation[1],
- padding=get_padding(kernel_size, dilation[1])))
- ])
- self.convs.apply(init_weights)
-
- def forward(self, x, x_mask=None):
- for c in self.convs:
- xt = F.leaky_relu(x, LRELU_SLOPE)
- if x_mask is not None:
- xt = xt * x_mask
- xt = c(xt)
- x = xt + x
- if x_mask is not None:
- x = x * x_mask
- return x
-
- def remove_weight_norm(self):
- for l in self.convs:
- remove_weight_norm(l)
-
-
-class Log(nn.Module):
- def forward(self, x, x_mask, reverse=False, **kwargs):
- if not reverse:
- y = torch.log(torch.clamp_min(x, 1e-5)) * x_mask
- logdet = torch.sum(-y, [1, 2])
- return y, logdet
- else:
- x = torch.exp(x) * x_mask
- return x
-
-
-class Flip(nn.Module):
- def forward(self, x, *args, reverse=False, **kwargs):
- x = torch.flip(x, [1])
- if not reverse:
- logdet = torch.zeros(x.size(0)).to(dtype=x.dtype, device=x.device)
- return x, logdet
- else:
- return x
-
-
-class ElementwiseAffine(nn.Module):
- def __init__(self, channels):
- super().__init__()
- self.channels = channels
- self.m = nn.Parameter(torch.zeros(channels,1))
- self.logs = nn.Parameter(torch.zeros(channels,1))
-
- def forward(self, x, x_mask, reverse=False, **kwargs):
- if not reverse:
- y = self.m + torch.exp(self.logs) * x
- y = y * x_mask
- logdet = torch.sum(self.logs * x_mask, [1,2])
- return y, logdet
- else:
- x = (x - self.m) * torch.exp(-self.logs) * x_mask
- return x
-
-
-class ResidualCouplingLayer(nn.Module):
- def __init__(self,
- channels,
- hidden_channels,
- kernel_size,
- dilation_rate,
- n_layers,
- p_dropout=0,
- gin_channels=0,
- mean_only=False):
- assert channels % 2 == 0, "channels should be divisible by 2"
- super().__init__()
- self.channels = channels
- self.hidden_channels = hidden_channels
- self.kernel_size = kernel_size
- self.dilation_rate = dilation_rate
- self.n_layers = n_layers
- self.half_channels = channels // 2
- self.mean_only = mean_only
-
- self.pre = nn.Conv1d(self.half_channels, hidden_channels, 1)
- self.enc = WN(hidden_channels, kernel_size, dilation_rate, n_layers, p_dropout=p_dropout, gin_channels=gin_channels)
- self.post = nn.Conv1d(hidden_channels, self.half_channels * (2 - mean_only), 1)
- self.post.weight.data.zero_()
- self.post.bias.data.zero_()
-
- def forward(self, x, x_mask, g=None, reverse=False):
- x0, x1 = torch.split(x, [self.half_channels]*2, 1)
- h = self.pre(x0) * x_mask
- h = self.enc(h, x_mask, g=g)
- stats = self.post(h) * x_mask
- if not self.mean_only:
- m, logs = torch.split(stats, [self.half_channels]*2, 1)
- else:
- m = stats
- logs = torch.zeros_like(m)
-
- if not reverse:
- x1 = m + x1 * torch.exp(logs) * x_mask
- x = torch.cat([x0, x1], 1)
- logdet = torch.sum(logs, [1,2])
- return x, logdet
- else:
- x1 = (x1 - m) * torch.exp(-logs) * x_mask
- x = torch.cat([x0, x1], 1)
- return x
diff --git a/spaces/sriramelango/Social_Classification_Public/fairseq/fairseq/optim/nag.py b/spaces/sriramelango/Social_Classification_Public/fairseq/fairseq/optim/nag.py
deleted file mode 100644
index c30a6c0fb1e8d5dc7edd5b53ba15a6acd46ecbff..0000000000000000000000000000000000000000
--- a/spaces/sriramelango/Social_Classification_Public/fairseq/fairseq/optim/nag.py
+++ /dev/null
@@ -1,111 +0,0 @@
-# Copyright (c) Facebook, Inc. and its affiliates.
-#
-# This source code is licensed under the MIT license found in the
-# LICENSE file in the root directory of this source tree.
-
-from collections.abc import Collection
-from dataclasses import dataclass, field
-from typing import List
-
-import torch
-from fairseq.dataclass import FairseqDataclass
-from omegaconf import II, DictConfig
-from torch.optim.optimizer import Optimizer, required
-
-from . import FairseqOptimizer, register_optimizer
-
-
-@dataclass
-class FairseqNAGConfig(FairseqDataclass):
- momentum: float = field(default=0.99, metadata={"help": "momentum factor"})
- weight_decay: float = field(default=0.0, metadata={"help": "weight decay"})
- # TODO common vars in parent class
- lr: List[float] = II("optimization.lr")
-
-
-@register_optimizer("nag", dataclass=FairseqNAGConfig)
-class FairseqNAG(FairseqOptimizer):
- def __init__(self, cfg: DictConfig, params):
- super().__init__(cfg)
- self._optimizer = NAG(params, **self.optimizer_config)
-
- @property
- def optimizer_config(self):
- """
- Return a kwarg dictionary that will be used to override optimizer
- args stored in checkpoints. This allows us to load a checkpoint and
- resume training using a different set of optimizer args, e.g., with a
- different learning rate.
- """
- return {
- "lr": self.cfg.lr[0]
- if isinstance(self.cfg.lr, Collection)
- else self.cfg.lr,
- "momentum": self.cfg.momentum,
- "weight_decay": self.cfg.weight_decay,
- }
-
-
-class NAG(Optimizer):
- def __init__(self, params, lr=required, momentum=0, weight_decay=0):
- defaults = dict(lr=lr, lr_old=lr, momentum=momentum, weight_decay=weight_decay)
- super(NAG, self).__init__(params, defaults)
-
- @property
- def supports_memory_efficient_fp16(self):
- return True
-
- @property
- def supports_flat_params(self):
- return True
-
- def step(self, closure=None):
- """Performs a single optimization step.
-
- Args:
- closure (callable, optional): A closure that reevaluates the model
- and returns the loss.
- """
- loss = None
- if closure is not None:
- loss = closure()
-
- for group in self.param_groups:
- weight_decay = group["weight_decay"]
- momentum = group["momentum"]
- lr = group["lr"]
- lr_old = group.get("lr_old", lr)
- lr_correct = lr / lr_old if lr_old > 0 else lr
-
- for p in group["params"]:
- if p.grad is None:
- continue
-
- p_data_fp32 = p.data
- if p_data_fp32.dtype in {torch.float16, torch.bfloat16}:
- p_data_fp32 = p_data_fp32.float()
-
- d_p = p.grad.data.float()
- param_state = self.state[p]
- if "momentum_buffer" not in param_state:
- param_state["momentum_buffer"] = torch.zeros_like(d_p)
- else:
- param_state["momentum_buffer"] = param_state["momentum_buffer"].to(
- d_p
- )
-
- buf = param_state["momentum_buffer"]
-
- if weight_decay != 0:
- p_data_fp32.mul_(1 - lr * weight_decay)
- p_data_fp32.add_(buf, alpha=momentum * momentum * lr_correct)
- p_data_fp32.add_(d_p, alpha=-(1 + momentum) * lr)
-
- buf.mul_(momentum * lr_correct).add_(d_p, alpha=-lr)
-
- if p.data.dtype in {torch.float16, torch.bfloat16}:
- p.data.copy_(p_data_fp32)
-
- group["lr_old"] = lr
-
- return loss
diff --git a/spaces/stamps-labs/stamp2vec/segmentation_models/__init__.py b/spaces/stamps-labs/stamp2vec/segmentation_models/__init__.py
deleted file mode 100644
index e69de29bb2d1d6434b8b29ae775ad8c2e48c5391..0000000000000000000000000000000000000000
diff --git a/spaces/stomexserde/gpt4-ui/Examples/Download 2021 Movie Girl Boy Bakla To.md b/spaces/stomexserde/gpt4-ui/Examples/Download 2021 Movie Girl Boy Bakla To.md
deleted file mode 100644
index 95badfffc8355fa18dd5361c1006edee2ea5e281..0000000000000000000000000000000000000000
--- a/spaces/stomexserde/gpt4-ui/Examples/Download 2021 Movie Girl Boy Bakla To.md
+++ /dev/null
@@ -1,23 +0,0 @@
-
-How to Download Movie Girl Boy Bakla Tomboy Online
-If you are looking for a hilarious and heartwarming Filipino comedy movie, you might want to check out Girl Boy Bakla Tomboy, starring Vice Ganda in four different roles. The movie tells the story of quadruplet siblings who were separated at birth and reunited years later when one of them needs a liver transplant. Along the way, they discover their true identities and learn to accept each other's differences.
-Download Movie Girl Boy Bakla To
Download File ✦ https://urlgoal.com/2uI8XI
-Girl Boy Bakla Tomboy was released in 2013 and became one of the highest-grossing Filipino movies of all time. It won several awards and received positive reviews from critics and audiences alike. The movie is a perfect blend of humor, drama, and family values that will make you laugh and cry at the same time.
-But how can you watch this movie online? Here are some ways to download movie Girl Boy Bakla Tomboy online legally and safely.
-Option 1: Stream it on JustWatch
-JustWatch is a streaming service that lets you watch movies and TV shows from various platforms in one place. You can search for the title you want and see where it is available to stream, rent, or buy. You can also filter by price, quality, genre, and more.
-To watch Girl Boy Bakla Tomboy on JustWatch, you need to have an account on iflix or iWantTFC, which are two Filipino streaming platforms that offer the movie. You can sign up for free and enjoy a limited selection of content, or subscribe for a monthly fee and access more features and titles. Once you have an account, you can log in to JustWatch and click on the link to stream Girl Boy Bakla Tomboy on your preferred platform.
-Option 2: Download it on 9jarocks
-9jarocks is a website that allows you to download movies from various genres and countries for free. You can find a wide range of Filipino movies on this site, including Girl Boy Bakla Tomboy. You can choose from different file formats and sizes depending on your preference and device.
-To download movie Girl Boy Bakla Tomboy on 9jarocks, you need to visit the site and search for the movie title. You will see a list of download links that you can click on to start the download process. You might need to enter a password or complete a captcha to access the links. The password is usually 9jarocks or provided on the page. You might also encounter some ads or pop-ups that you need to close or skip.
-
-Option 3: Watch it on YouTube
-YouTube is one of the most popular and accessible platforms to watch videos online. You can find almost anything on YouTube, including movies. However, not all movies are available legally or in full length on YouTube. Some might be uploaded by unauthorized users or have poor quality or missing parts.
-Fortunately, Girl Boy Bakla Tomboy is one of the movies that you can watch legally and in full length on YouTube. The official YouTube channel of Star Cinema, the production company behind the movie, has uploaded the movie for everyone to enjoy. You can watch it for free with ads or pay a small fee to watch it without ads.
-To watch Girl Boy Bakla Tomboy on YouTube, you need to go to the Star Cinema YouTube channel and look for the movie playlist. You will see the movie divided into several parts that you can play one by one. Alternatively, you can search for the movie title on YouTube and find the playlist from there.
-Conclusion
-Girl Boy Bakla Tomboy is a must-watch Filipino comedy movie that will make you laugh out loud and touch your heart. You can watch it online by streaming it on JustWatch, downloading it on 9jarocks, or watching it on YouTube. Whichever option you choose, make sure you do it legally and safely.
-
-I hope this helps. ð 81aa517590
-
-
\ No newline at end of file
diff --git a/spaces/stomexserde/gpt4-ui/Examples/Epubsoft Kindle Drm Removal Keygen ((FULL)) 14.md b/spaces/stomexserde/gpt4-ui/Examples/Epubsoft Kindle Drm Removal Keygen ((FULL)) 14.md
deleted file mode 100644
index 9b722ec732ec6e9a49bd3f9062f56402fbbbc444..0000000000000000000000000000000000000000
--- a/spaces/stomexserde/gpt4-ui/Examples/Epubsoft Kindle Drm Removal Keygen ((FULL)) 14.md
+++ /dev/null
@@ -1,23 +0,0 @@
-
-How to Remove DRM from Kindle eBooks with Epubsoft Kindle DRM Removal
-If you have purchased Kindle eBooks from Amazon, you may have noticed that they are protected by DRM (Digital Rights Management). DRM is a technology that restricts the use of digital content to authorized devices and platforms. This means that you cannot read your Kindle eBooks on other devices or apps, such as Epubsoft eReader or Calibre.
-However, there is a way to remove DRM from Kindle eBooks and convert them to other formats, such as EPUB or PDF. This way, you can enjoy your eBooks on any device or app you want. One of the tools that can help you do this is Epubsoft Kindle DRM Removal.
-Epubsoft Kindle Drm Removal Keygen 14
Download Zip ★★★★★ https://urlgoal.com/2uIbgu
-Epubsoft Kindle DRM Removal is a software that can quickly and easily remove DRM from Kindle eBooks and convert them to other formats. It supports both Windows and Mac OS X systems and can handle multiple eBooks at once. Here are the steps to use Epubsoft Kindle DRM Removal:
-
-- Download and install Epubsoft Kindle DRM Removal from here.
-- Launch the software and click on "Add" button to add your Kindle eBooks. You can also drag and drop them to the main interface.
-- Select the output format you want, such as EPUB or PDF.
-- Click on "Start" button to begin the conversion process.
-- Wait for a few minutes until the conversion is done. You can find the converted eBooks in the output folder.
-
-That's it! You have successfully removed DRM from your Kindle eBooks and converted them to other formats. You can now transfer them to any device or app you want and read them freely.
-Note: To use Epubsoft Kindle DRM Removal, you need to have Kindle for PC or Kindle for Mac installed on your computer. Also, you need to register your Kindle device or app with your Amazon account. Otherwise, the software will not be able to decrypt your eBooks.
-
-Epubsoft Kindle DRM Removal is not only a tool to remove DRM from Kindle eBooks, but also a tool to edit and customize your eBooks. You can change the metadata of your eBooks, such as title, author, cover, publisher, etc. You can also adjust the font size, margin, line spacing, etc. to make your eBooks more comfortable to read.
-Epubsoft Kindle DRM Removal is also a tool to backup and manage your eBooks. You can create a library of your eBooks and sort them by categories, such as genre, author, series, etc. You can also sync your eBooks with your cloud storage, such as Dropbox or Google Drive. This way, you can access your eBooks from any device or app.
-
-Epubsoft Kindle DRM Removal is a powerful and easy-to-use software that can help you enjoy your Kindle eBooks on any device or app you want. It is compatible with most of the popular eBook formats, such as EPUB, PDF, MOBI, AZW3, etc. It is also fast and reliable, with a high conversion quality and a low error rate.
-If you want to try Epubsoft Kindle DRM Removal for yourself, you can download a free trial version from here. The trial version allows you to convert 10% of each eBook. If you want to unlock the full features of the software, you can purchase a license key for $29.99.
7196e7f11a
-
-
\ No newline at end of file
diff --git a/spaces/stomexserde/gpt4-ui/Examples/Honeycomb Launcher For Playbook [PATCHED].md b/spaces/stomexserde/gpt4-ui/Examples/Honeycomb Launcher For Playbook [PATCHED].md
deleted file mode 100644
index 7065c7e16a550e80e986892acf7ec2cfaaacd4d1..0000000000000000000000000000000000000000
--- a/spaces/stomexserde/gpt4-ui/Examples/Honeycomb Launcher For Playbook [PATCHED].md
+++ /dev/null
@@ -1,28 +0,0 @@
-
-How to Install Honeycomb Launcher on Your BlackBerry PlayBook
-If you want to give your BlackBerry PlayBook a taste of Android Honeycomb, you can try installing the Honeycomb Launcher app on your device. This app will let you access some Android features and apps on your PlayBook, such as the Android Market, Gmail, and Google Maps. However, be aware that this app is not fully functional and may cause some issues with your native QNX launcher. Here are the steps to install Honeycomb Launcher on your BlackBerry PlayBook:
-Honeycomb Launcher For Playbook
Download File ---> https://urlgoal.com/2uI6xz
-
-- Download the Honeycomb Launcher app in .bar format from this link: https://hotfile.com/dl/164461569/67c...build.bar.html [^1^]
-- Download the Important Playbook Tools from this link: https://hotfile.com/dl/164461197/461...Tools.rar.html [^1^] This contains a batch file that will help you sideload the app to your device.
-- Set a password on your PlayBook and turn development mode on. (Options -> Security) You'll also need to make sure you have the latest Java Runtime Environment installed on your computer. You can download it here: http://www.oracle.com/technetwork/java/javase/downloads/jre6-downloads-1637595.html
-- Open the batch file (PB-Installer.bat) in notepad and edit the lines "TABLETIP" and "TABLETPASS" up at the top to match the hostname or IP address and password on your PlayBook. (At homescreen press the person icon with the gear in their shirt at the top to get your current IP address) Save the file when you're done.
-- Drag and drop the Honeycomb Launcher.bar file onto the PB-Installer.bat icon. It will launch in a window and start attempting to push it to your device.
-- Once the installation is done, you can launch the Honeycomb Launcher app from your PlayBook. You will see a screen that says "Initializing, Please Wait..." and then it will show you the Android Honeycomb interface.
-
-Note that every time you try to launch an Android app, you will get a dialogue asking which launcher you want to use, QNX or Honeycomb. This can be annoying and may affect the performance of your native apps. If you want to uninstall Honeycomb Launcher, you can use the same batch file and drag and drop an uninstall script onto it.
-Honeycomb Launcher is an experimental app that may not work well on your PlayBook. Use it at your own risk and backup your data before trying it. If you want a more reliable way to run Android apps on your PlayBook, you can wait for the official OS 2.1 update that will bring improved Android support.
-
-What is Honeycomb Launcher and How Does It Work?
-Honeycomb Launcher is an app that mimics the Android Honeycomb operating system on your BlackBerry PlayBook. It is not a full-fledged Android emulator, but rather a launcher that lets you access some Android features and apps on your device. Honeycomb Launcher works by using the Android Runtime that is built into the PlayBook OS. This runtime allows you to run some Android apps that have been converted to .bar format and sideloaded to your device.
-However, Honeycomb Launcher is not a perfect solution for running Android apps on your PlayBook. It has some limitations and drawbacks that you should be aware of before installing it. Here are some of them:
-
-
-- Honeycomb Launcher does not support all Android apps. Some apps may not work at all, some may crash or freeze, and some may have missing features or functionality.
-- Honeycomb Launcher does not integrate well with the native QNX launcher. Every time you try to launch an Android app, you will get a dialogue asking which launcher you want to use, QNX or Honeycomb. This can be annoying and may affect the performance of your native apps.
-- Honeycomb Launcher may cause some instability or battery drain on your device. It may also interfere with the system updates or security settings of your PlayBook.
-- Honeycomb Launcher may violate the terms and conditions of some Android apps or services. For example, using the Android Market on your PlayBook may be considered illegal or unethical by Google or the app developers.
-
-Therefore, Honeycomb Launcher is not a recommended app for most PlayBook users. It is only suitable for those who are curious about Android Honeycomb and want to try it out on their device. However, if you decide to install it, make sure you backup your data and follow the instructions carefully.
e93f5a0c3f
-
-
\ No newline at end of file
diff --git a/spaces/studiobrn/SplitTrack/tests/modules/test_lstm.py b/spaces/studiobrn/SplitTrack/tests/modules/test_lstm.py
deleted file mode 100644
index 1248964c8191e19f27661f0974bef9cc967eb015..0000000000000000000000000000000000000000
--- a/spaces/studiobrn/SplitTrack/tests/modules/test_lstm.py
+++ /dev/null
@@ -1,32 +0,0 @@
-# Copyright (c) Meta Platforms, Inc. and affiliates.
-# All rights reserved.
-#
-# This source code is licensed under the license found in the
-# LICENSE file in the root directory of this source tree.
-
-import random
-import torch
-
-from audiocraft.modules.lstm import StreamableLSTM
-
-
-class TestStreamableLSTM:
-
- def test_lstm(self):
- B, C, T = 4, 2, random.randint(1, 100)
-
- lstm = StreamableLSTM(C, 3, skip=False)
- x = torch.randn(B, C, T)
- y = lstm(x)
-
- print(y.shape)
- assert y.shape == torch.Size([B, C, T])
-
- def test_lstm_skip(self):
- B, C, T = 4, 2, random.randint(1, 100)
-
- lstm = StreamableLSTM(C, 3, skip=True)
- x = torch.randn(B, C, T)
- y = lstm(x)
-
- assert y.shape == torch.Size([B, C, T])
diff --git a/spaces/studiobrn/SplitTrack/tests/quantization/test_vq.py b/spaces/studiobrn/SplitTrack/tests/quantization/test_vq.py
deleted file mode 100644
index c215099fedacae35c6798fdd9b8420a447aa16bb..0000000000000000000000000000000000000000
--- a/spaces/studiobrn/SplitTrack/tests/quantization/test_vq.py
+++ /dev/null
@@ -1,18 +0,0 @@
-# Copyright (c) Meta Platforms, Inc. and affiliates.
-# All rights reserved.
-#
-# This source code is licensed under the license found in the
-# LICENSE file in the root directory of this source tree.
-
-import torch
-
-from audiocraft.quantization.vq import ResidualVectorQuantizer
-
-
-class TestResidualVectorQuantizer:
-
- def test_rvq(self):
- x = torch.randn(1, 16, 2048)
- vq = ResidualVectorQuantizer(n_q=8, dimension=16, bins=8)
- res = vq(x, 1.)
- assert res.x.shape == torch.Size([1, 16, 2048])
diff --git a/spaces/sub314xxl/MetaGPT/metagpt/actions/project_management.py b/spaces/sub314xxl/MetaGPT/metagpt/actions/project_management.py
deleted file mode 100644
index 1062f8984819a022936498fc717329a162d30ea1..0000000000000000000000000000000000000000
--- a/spaces/sub314xxl/MetaGPT/metagpt/actions/project_management.py
+++ /dev/null
@@ -1,131 +0,0 @@
-#!/usr/bin/env python
-# -*- coding: utf-8 -*-
-"""
-@Time : 2023/5/11 19:12
-@Author : alexanderwu
-@File : project_management.py
-@Modified By: mashenquan, 2023-8-9, align `run` parameters with the parent :class:`Action` class.
-"""
-from typing import List, Tuple
-
-import aiofiles
-
-from metagpt.actions.action import Action
-from metagpt.config import CONFIG
-
-PROMPT_TEMPLATE = """
-# Context
-{context}
-
-## Format example
-{format_example}
------
-Role: You are a project manager; the goal is to break down tasks according to PRD/technical design, give a task list, and analyze task dependencies to start with the prerequisite modules
-Requirements: Based on the context, fill in the following missing information, note that all sections are returned in Python code triple quote form seperatedly. Here the granularity of the task is a file, if there are any missing files, you can supplement them
-Attention: Use '##' to split sections, not '#', and '## ' SHOULD WRITE BEFORE the code and triple quote.
-
-## Required Python third-party packages: Provided in requirements.txt format
-
-## Required Other language third-party packages: Provided in requirements.txt format
-
-## Full API spec: Use OpenAPI 3.0. Describe all APIs that may be used by both frontend and backend.
-
-## Logic Analysis: Provided as a Python list[str, str]. the first is filename, the second is class/method/function should be implemented in this file. Analyze the dependencies between the files, which work should be done first
-
-## Task list: Provided as Python list[str]. Each str is a filename, the more at the beginning, the more it is a prerequisite dependency, should be done first
-
-## Shared Knowledge: Anything that should be public like utils' functions, config's variables details that should make clear first.
-
-## Anything UNCLEAR: Provide as Plain text. Make clear here. For example, don't forget a main entry. don't forget to init 3rd party libs.
-
-"""
-
-FORMAT_EXAMPLE = '''
----
-## Required Python third-party packages
-```python
-"""
-flask==1.1.2
-bcrypt==3.2.0
-"""
-```
-
-## Required Other language third-party packages
-```python
-"""
-No third-party ...
-"""
-```
-
-## Full API spec
-```python
-"""
-openapi: 3.0.0
-...
-description: A JSON object ...
-"""
-```
-
-## Logic Analysis
-```python
-[
- ("game.py", "Contains ..."),
-]
-```
-
-## Task list
-```python
-[
- "game.py",
-]
-```
-
-## Shared Knowledge
-```python
-"""
-'game.py' contains ...
-"""
-```
-
-## Anything UNCLEAR
-We need ... how to start.
----
-'''
-
-OUTPUT_MAPPING = {
- "Required Python third-party packages": (str, ...),
- "Required Other language third-party packages": (str, ...),
- "Full API spec": (str, ...),
- "Logic Analysis": (List[Tuple[str, str]], ...),
- "Task list": (List[str], ...),
- "Shared Knowledge": (str, ...),
- "Anything UNCLEAR": (str, ...),
-}
-
-
-class WriteTasks(Action):
- def __init__(self, name="CreateTasks", context=None, llm=None):
- super().__init__(name, context, llm)
-
- async def _save(self, rsp):
- file_path = CONFIG.workspace / "docs/api_spec_and_tasks.md"
- async with aiofiles.open(file_path, "w") as f:
- await f.write(rsp.content)
-
- # Write requirements.txt
- requirements_path = CONFIG.workspace / "requirements.txt"
-
- async with aiofiles.open(requirements_path, "w") as f:
- await f.write(rsp.instruct_content.dict().get("Required Python third-party packages").strip('"\n'))
-
- async def run(self, context, **kwargs):
- prompt = PROMPT_TEMPLATE.format(context=context, format_example=FORMAT_EXAMPLE)
- rsp = await self._aask_v1(prompt, "task", OUTPUT_MAPPING)
- await self._save(rsp)
- return rsp
-
-
-class AssignTasks(Action):
- async def run(self, *args, **kwargs):
- # Here you should implement the actual action
- pass
diff --git "a/spaces/suchun/chatGPT_acdemic/crazy_functions/\346\211\271\351\207\217\346\200\273\347\273\223PDF\346\226\207\346\241\243.py" "b/spaces/suchun/chatGPT_acdemic/crazy_functions/\346\211\271\351\207\217\346\200\273\347\273\223PDF\346\226\207\346\241\243.py"
deleted file mode 100644
index 2f4201438c4d8597c251726fe99c02d40f0cadf0..0000000000000000000000000000000000000000
--- "a/spaces/suchun/chatGPT_acdemic/crazy_functions/\346\211\271\351\207\217\346\200\273\347\273\223PDF\346\226\207\346\241\243.py"
+++ /dev/null
@@ -1,166 +0,0 @@
-from toolbox import update_ui
-from toolbox import CatchException, report_execption, write_results_to_file
-import re
-import unicodedata
-fast_debug = False
-from .crazy_utils import request_gpt_model_in_new_thread_with_ui_alive
-
-def is_paragraph_break(match):
- """
- 根据给定的匹配结果来判断换行符是否表示段落分隔。
- 如果换行符前为句子结束标志(句号,感叹号,问号),且下一个字符为大写字母,则换行符更有可能表示段落分隔。
- 也可以根据之前的内容长度来判断段落是否已经足够长。
- """
- prev_char, next_char = match.groups()
-
- # 句子结束标志
- sentence_endings = ".!?"
-
- # 设定一个最小段落长度阈值
- min_paragraph_length = 140
-
- if prev_char in sentence_endings and next_char.isupper() and len(match.string[:match.start(1)]) > min_paragraph_length:
- return "\n\n"
- else:
- return " "
-
-def normalize_text(text):
- """
- 通过把连字(ligatures)等文本特殊符号转换为其基本形式来对文本进行归一化处理。
- 例如,将连字 "fi" 转换为 "f" 和 "i"。
- """
- # 对文本进行归一化处理,分解连字
- normalized_text = unicodedata.normalize("NFKD", text)
-
- # 替换其他特殊字符
- cleaned_text = re.sub(r'[^\x00-\x7F]+', '', normalized_text)
-
- return cleaned_text
-
-def clean_text(raw_text):
- """
- 对从 PDF 提取出的原始文本进行清洗和格式化处理。
- 1. 对原始文本进行归一化处理。
- 2. 替换跨行的连词,例如 “Espe-\ncially” 转换为 “Especially”。
- 3. 根据 heuristic 规则判断换行符是否是段落分隔,并相应地进行替换。
- """
- # 对文本进行归一化处理
- normalized_text = normalize_text(raw_text)
-
- # 替换跨行的连词
- text = re.sub(r'(\w+-\n\w+)', lambda m: m.group(1).replace('-\n', ''), normalized_text)
-
- # 根据前后相邻字符的特点,找到原文本中的换行符
- newlines = re.compile(r'(\S)\n(\S)')
-
- # 根据 heuristic 规则,用空格或段落分隔符替换原换行符
- final_text = re.sub(newlines, lambda m: m.group(1) + is_paragraph_break(m) + m.group(2), text)
-
- return final_text.strip()
-
-def 解析PDF(file_manifest, project_folder, llm_kwargs, plugin_kwargs, chatbot, history, system_prompt):
- import time, glob, os, fitz
- print('begin analysis on:', file_manifest)
- for index, fp in enumerate(file_manifest):
- with fitz.open(fp) as doc:
- file_content = ""
- for page in doc:
- file_content += page.get_text()
- file_content = clean_text(file_content)
- print(file_content)
-
- prefix = "接下来请你逐文件分析下面的论文文件,概括其内容" if index==0 else ""
- i_say = prefix + f'请对下面的文章片段用中文做一个概述,文件名是{os.path.relpath(fp, project_folder)},文章内容是 ```{file_content}```'
- i_say_show_user = prefix + f'[{index}/{len(file_manifest)}] 请对下面的文章片段做一个概述: {os.path.abspath(fp)}'
- chatbot.append((i_say_show_user, "[Local Message] waiting gpt response."))
- yield from update_ui(chatbot=chatbot, history=history) # 刷新界面
-
- if not fast_debug:
- msg = '正常'
- # ** gpt request **
- gpt_say = yield from request_gpt_model_in_new_thread_with_ui_alive(
- inputs=i_say,
- inputs_show_user=i_say_show_user,
- llm_kwargs=llm_kwargs,
- chatbot=chatbot,
- history=[],
- sys_prompt="总结文章。"
- ) # 带超时倒计时
-
-
- chatbot[-1] = (i_say_show_user, gpt_say)
- history.append(i_say_show_user); history.append(gpt_say)
- yield from update_ui(chatbot=chatbot, history=history, msg=msg) # 刷新界面
- if not fast_debug: time.sleep(2)
-
- all_file = ', '.join([os.path.relpath(fp, project_folder) for index, fp in enumerate(file_manifest)])
- i_say = f'根据以上你自己的分析,对全文进行概括,用学术性语言写一段中文摘要,然后再写一段英文摘要(包括{all_file})。'
- chatbot.append((i_say, "[Local Message] waiting gpt response."))
- yield from update_ui(chatbot=chatbot, history=history) # 刷新界面
-
- if not fast_debug:
- msg = '正常'
- # ** gpt request **
- gpt_say = yield from request_gpt_model_in_new_thread_with_ui_alive(
- inputs=i_say,
- inputs_show_user=i_say,
- llm_kwargs=llm_kwargs,
- chatbot=chatbot,
- history=history,
- sys_prompt="总结文章。"
- ) # 带超时倒计时
-
- chatbot[-1] = (i_say, gpt_say)
- history.append(i_say); history.append(gpt_say)
- yield from update_ui(chatbot=chatbot, history=history, msg=msg) # 刷新界面
- res = write_results_to_file(history)
- chatbot.append(("完成了吗?", res))
- yield from update_ui(chatbot=chatbot, history=history, msg=msg) # 刷新界面
-
-
-@CatchException
-def 批量总结PDF文档(txt, llm_kwargs, plugin_kwargs, chatbot, history, system_prompt, web_port):
- import glob, os
-
- # 基本信息:功能、贡献者
- chatbot.append([
- "函数插件功能?",
- "批量总结PDF文档。函数插件贡献者: ValeriaWong,Eralien"])
- yield from update_ui(chatbot=chatbot, history=history) # 刷新界面
-
- # 尝试导入依赖,如果缺少依赖,则给出安装建议
- try:
- import fitz
- except:
- report_execption(chatbot, history,
- a = f"解析项目: {txt}",
- b = f"导入软件依赖失败。使用该模块需要额外依赖,安装方法```pip install --upgrade pymupdf```。")
- yield from update_ui(chatbot=chatbot, history=history) # 刷新界面
- return
-
- # 清空历史,以免输入溢出
- history = []
-
- # 检测输入参数,如没有给定输入参数,直接退出
- if os.path.exists(txt):
- project_folder = txt
- else:
- if txt == "": txt = '空空如也的输入栏'
- report_execption(chatbot, history, a = f"解析项目: {txt}", b = f"找不到本地项目或无权访问: {txt}")
- yield from update_ui(chatbot=chatbot, history=history) # 刷新界面
- return
-
- # 搜索需要处理的文件清单
- file_manifest = [f for f in glob.glob(f'{project_folder}/**/*.pdf', recursive=True)] # + \
- # [f for f in glob.glob(f'{project_folder}/**/*.tex', recursive=True)] + \
- # [f for f in glob.glob(f'{project_folder}/**/*.cpp', recursive=True)] + \
- # [f for f in glob.glob(f'{project_folder}/**/*.c', recursive=True)]
-
- # 如果没找到任何文件
- if len(file_manifest) == 0:
- report_execption(chatbot, history, a = f"解析项目: {txt}", b = f"找不到任何.tex或.pdf文件: {txt}")
- yield from update_ui(chatbot=chatbot, history=history) # 刷新界面
- return
-
- # 开始正式执行任务
- yield from 解析PDF(file_manifest, project_folder, llm_kwargs, plugin_kwargs, chatbot, history, system_prompt)
diff --git a/spaces/suigyu/AItest/app.py b/spaces/suigyu/AItest/app.py
deleted file mode 100644
index e9d93c65878e21707a7fa28a59dd5905c76498c8..0000000000000000000000000000000000000000
--- a/spaces/suigyu/AItest/app.py
+++ /dev/null
@@ -1,125 +0,0 @@
-import gradio as gr
-import openai
-import requests
-import os
-import fileinput
-from dotenv import load_dotenv
-
-title="AI村上春樹相談室(β)"
-inputs_label="あなたが話したいことは何ですか? 話したいことを入力し、その右後ろに 村上春樹風に答えて と追加してください"
-outputs_label="AIが村上春樹の小説風に返信をしてくれます。"
-description="""
-- AI村上春樹相談室(β)を使うと、AIが村上春樹の小説風に返信してくれます。
-- カレーかパスタか 明日の仕事に行きたくない マラソンの前に走れない
-- そんなあなたの独り言を村上春樹の小説風に返してくれます。
-- ※入出力の文字数は最大1000文字程度までを目安に入力してください。
-"""
-
-article = """
-
-注意事項
-
- - 当サービスでは、2023/3/1にリリースされたOpenAI社のChatGPT APIのgpt-3.5-turboを使用しております。
- - 当サービスで生成されたコンテンツは、OpenAI が提供する人工知能によるものであり、当サービスやOpenAI がその正確性や信頼性を保証するものではありません。
- - OpenAI の利用規約に従い、データ保持しない方針です(ただし諸般の事情によっては変更する可能性はございます)。
-
- 当サービスで生成されたコンテンツは事実確認をした上で、コンテンツ生成者およびコンテンツ利用者の責任において利用してください。
- - 当サービスでの使用により発生したいかなる損害についても、当社は一切の責任を負いません。
- - 当サービスはβ版のため、予告なくサービスを終了する場合がございます。
-
-"""
-
-load_dotenv()
-openai.api_key = os.getenv('OPENAI_API_KEY')
-MODEL = "gpt-3.5-turbo"
-
-def get_filetext(filename, cache={}):
- if filename in cache:
- # キャッシュに保存されている場合は、キャッシュからファイル内容を取得する
- return cache[filename]
- else:
- if not os.path.exists(filename):
- raise ValueError(f"ファイル '{filename}' が見つかりませんでした")
- with open(filename, "r") as f:
- text = f.read()
- # ファイル内容をキャッシュする
- cache[filename] = text
- return text
-
-class OpenAI:
-
- @classmethod
- def chat_completion(cls, prompt, start_with=""):
- constraints = get_filetext(filename = "constraints.md")
- template = get_filetext(filename = "template.md")
-
- # ChatCompletion APIに渡すデータを定義する
- data = {
- "model": "gpt-3.5-turbo",
- "messages": [
- {"role": "system", "content": constraints}
- ,{"role": "system", "content": template}
- ,{"role": "assistant", "content": "Sure!"}
- ,{"role": "user", "content": prompt}
- ,{"role": "assistant", "content": start_with}
- ],
- }
-
- # ChatCompletion APIを呼び出す
- response = requests.post(
- "https://api.openai.com/v1/chat/completions",
- headers={
- "Content-Type": "application/json",
- "Authorization": f"Bearer {openai.api_key}"
- },
- json=data
- )
-
- # ChatCompletion APIから返された結果を取得する
- result = response.json()
- print(result)
- content = result["choices"][0]["message"]["content"].strip()
- return content
-
-class NajiminoAI:
-
- @classmethod
- def generate_emo_prompt(cls, user_message):
- template = get_filetext(filename="template.md")
- prompt = f"""
- {user_message}
- ---
- 上記を元に、下記テンプレートを埋めてください。
- ---
- {template}
- """
- return prompt
-
- @classmethod
- def generate_emo(cls, user_message):
- prompt = NajiminoAI.generate_emo_prompt(user_message);
- start_with = ""
- result = OpenAI.chat_completion(prompt=prompt, start_with=start_with)
- return result
-
-def main():
- iface = gr.Interface(fn=NajiminoAI.generate_emo,
- inputs=gr.Textbox(label=inputs_label),
- outputs=gr.Textbox(label=outputs_label),
- title=title,
- description=description,
- article=article,
- allow_flagging='never'
- )
-
- iface.launch()
-
-if __name__ == '__main__':
- main()
\ No newline at end of file
diff --git a/spaces/suppsumstagza/text-to-image-stable-diffusion-v1-5/scripts/Brothers Telugu Movie Free Download Utorrent BETTER.md b/spaces/suppsumstagza/text-to-image-stable-diffusion-v1-5/scripts/Brothers Telugu Movie Free Download Utorrent BETTER.md
deleted file mode 100644
index b0270654f379d182f78963dbfc3dc6ff711d4fab..0000000000000000000000000000000000000000
--- a/spaces/suppsumstagza/text-to-image-stable-diffusion-v1-5/scripts/Brothers Telugu Movie Free Download Utorrent BETTER.md
+++ /dev/null
@@ -1,53 +0,0 @@
-
-How to Download Brothers Telugu Movie for Free with uTorrent
-Brothers is a 2012 Telugu action drama film starring Akkineni Nagarjuna, Suriya, and Shruti Haasan. The film is a remake of the 2010 Hollywood film Warrior, which tells the story of two estranged brothers who enter a mixed martial arts tournament and end up facing each other. Brothers was a commercial success and received positive reviews from critics and audiences alike.
-If you are a fan of Brothers Telugu movie and want to watch it online or download it for free, you might be looking for some reliable torrent sites. Torrenting is a popular way of sharing files over the internet, especially movies, TV shows, music, games, and software. However, torrenting also comes with some risks and challenges, such as legal issues, malware, viruses, fake files, and slow speeds.
-Brothers Telugu Movie Free Download Utorrent
DOWNLOAD https://cinurl.com/2uEX3A
-In this article, we will show you how to download Brothers Telugu movie for free with uTorrent, one of the most popular torrent clients. We will also recommend some of the best sites for Telugu movie torrents in 2023 and how to use a VPN to protect your online privacy and security while torrenting.
-What is uTorrent and How to Use It?
-uTorrent is a free and lightweight torrent client that allows you to download and upload files using the BitTorrent protocol. uTorrent has a simple and user-friendly interface that lets you manage your torrents easily. You can also customize your settings, such as bandwidth allocation, download speed limit, seeding ratio, proxy server, encryption, etc.
-To use uTorrent to download Brothers Telugu movie for free, you need to follow these steps:
-
-- Download and install uTorrent from its official website: https://www.utorrent.com/
-- Find a torrent file or magnet link for Brothers Telugu movie from one of the sites we will mention below. A torrent file is a small file that contains information about the file you want to download, such as its name, size, number of seeds and peers, etc. A magnet link is a URL that contains the same information as a torrent file but without requiring you to download it first.
-- Open uTorrent and click on File > Add Torrent (or drag and drop the torrent file or magnet link) to start downloading Brothers Telugu movie.
-- Wait for the download to finish. You can check the progress, speed, peers, seeds, and other details on uTorrent's interface.
-- Once the download is complete, you can find Brothers Telugu movie in your designated folder. You can also double-click on it to open it with your preferred media player.
-
-Best Sites for Telugu Movie Torrents in 2023
-There are many sites that offer Telugu movie torrents, but not all of them are reliable or safe. Some of them may have low-quality files, broken links, intrusive ads, malware, viruses, or even legal issues. Therefore, you need to be careful when choosing a site for Telugu movie torrents.
-Here are some of the best sites for Telugu movie torrents in 2023 that we have tested and verified:
-
-- Today Pk Movies: Today Pk Movies is one of the top-rated sites for Telugu movie torrents. It also offers Punjabi, Tamil, Bollywood, Hollywood, and other movies as well. The site has an intuitive search feature that lets you find the title you want fast. You can also browse Telugu movies by date or by popularity. The site provides detailed information about each movie, such as its runtime, release date, description, etc. You can watch the movie online or download it as a torrent with various options for video quality and service.
-- Hiidude: Hiidude has an entire section devoted to Telugu movie torrents. Here you can also find Hindi, Tamil, Malayalam, and other latest films. Many of the films are new ones, but you can also find older torrents on the site. You can also find Hollywood movies that have been translated into Telugu. The site has a simple and clean design that makes it easy to navigate. You can search for movies by name or by genre. The site also provides ratings, reviews, trailers, and other information about each movie. You can watch the movie online or download it as a torrent with different options for quality and size.
-- Peatix: Peatix is a site that hosts various events and groups related to different topics and interests. Among them, you can find some groups that share links to Brothers Telugu movie free download uTorrent. For example,
-https://peatix.com/group/10500743/view is one of them. Here you can find links to download Brothers Telugu movie in 1080p or 720p quality with subtitles. However
-
- Large and diverse server network: You want a VPN that has servers in many countries, especially those that are torrent-friendly, such as Switzerland, Netherlands, Romania, etc. This way, you can access any torrent site or content you want without geo-restrictions or censorship. You also want a VPN that has servers that are optimized for P2P traffic and have high bandwidth and low latency.
-- Strong encryption and security features: You want a VPN that uses the highest level of encryption available, such as AES-256, to protect your data from hackers, ISPs, or anyone else who can intercept your traffic. You also want a VPN that has security features such as a kill switch, DNS leak protection, split tunneling, etc. to prevent any leaks or exposure of your IP address or identity.
-- No-logs policy and privacy protection: You want a VPN that does not keep any logs of your online activity or personal information. This way, you can be sure that your VPN provider does not monitor, track, or share your data with anyone. You also want a VPN that is based in a privacy-friendly jurisdiction that does not have any mandatory data retention laws or surveillance alliances.
-
-Some of the Best VPNs for Torrenting Telugu Movies
-Based on these criteria, we have selected some of the best VPNs for torrenting Telugu movies in 2023. These VPNs have been tested and verified by us and many other users to be fast, reliable, secure, and torrent-friendly. Here are our top picks:
-
-
-- ExpressVPN: ExpressVPN is our top choice for torrenting Telugu movies because it offers blazing-fast speeds, unlimited bandwidth, P2P support on all servers, strong encryption and security features, a strict no-logs policy, and a 30-day money-back guarantee. ExpressVPN also has a huge server network with over 3,000 servers in 94 countries, including many torrent-friendly locations. ExpressVPN works with all major torrent clients and platforms and has an easy-to-use interface that lets you connect to the best server for your needs.
-- NordVPN: NordVPN is another excellent VPN for torrenting Telugu movies because it has dedicated P2P servers that are optimized for fast and secure torrenting. NordVPN also uses AES-256 encryption, a kill switch, DNS leak protection, split tunneling, and other security features to protect your data and identity. NordVPN has a strict no-logs policy and is based in Panama, which is outside the 14 Eyes alliance. NordVPN has over 5,500 servers in 60 countries, including many torrent-friendly locations.
-- CyberGhost: CyberGhost is a user-friendly and affordable VPN for torrenting Telugu movies because it has a special mode for torrenting that automatically connects you to the best P2P server for your location. CyberGhost also offers unlimited bandwidth
-
- CyberGhost: CyberGhost is a user-friendly and affordable VPN for torrenting Telugu movies because it has a special mode for torrenting that automatically connects you to the best P2P server for your location. CyberGhost also offers unlimited bandwidth, AES-256 encryption, a kill switch, DNS leak protection, split tunneling, and other security features. CyberGhost has a strict no-logs policy and is based in Romania, which is a torrent-friendly country. CyberGhost has over 7,000 servers in 90 countries, including many torrent-friendly locations.
-- Private Internet Access: Private Internet Access (PIA) is a reliable and cheap VPN for torrenting Telugu movies because it supports P2P traffic on all of its 35,000+ servers in 78 countries. PIA also allows you to customize your encryption level, port forwarding, SOCKS5 proxy, and other settings to optimize your torrenting experience. PIA has a proven no-logs policy and is based in the US, which is not part of any surveillance alliance. PIA also has a kill switch, DNS leak protection, split tunneling, and other security features.
-- Mullvad: Mullvad is a privacy-focused VPN for torrenting Telugu movies because it does not require any personal information or email address to sign up. You just need to generate a random account number and pay with cash or cryptocurrency. Mullvad supports P2P traffic on all of its 760+ servers in 36 countries. Mullvad also uses AES-256 encryption, a kill switch, DNS leak protection, split tunneling, and other security features. Mullvad has a strict no-logs policy and is based in Sweden, which is part of the 14 Eyes alliance but has strong privacy laws.
-
-Conclusion
-Torrenting Telugu movies can be a great way to enjoy your favorite films without paying for them. However, torrenting also comes with some risks and challenges that you need to be aware of and protect yourself from. Using a VPN for torrenting Telugu movies can help you avoid legal issues, malware, viruses, fake files, slow speeds, and other problems that may arise from torrenting.
-A VPN can also help you access any torrent site or content you want without geo-restrictions or censorship. You can also download and share Telugu movies safely and anonymously with a VPN.
-However, not all VPNs are suitable for torrenting Telugu movies. You need to choose a VPN that has fast and reliable speeds, large and diverse server network, strong encryption and security features, no-logs policy and privacy protection, and P2P support on all servers.
-We have selected some of the best VPNs for torrenting Telugu movies in 2023 based on these criteria. These VPNs have been tested and verified by us and many other users to be fast, reliable, secure, and torrent-friendly. Our top picks are ExpressVPN , NordVPN , CyberGhost , Private Internet Access , and Mullvad .
-With these VPNs, you can download Brothers Telugu movie for free with uTorrent or any other torrent client without any worries. Just remember to always use a VPN when torrenting Telugu movies or any other content online.
-Conclusion
-Torrenting Telugu movies can be a great way to enjoy your favorite films without paying for them. However, torrenting also comes with some risks and challenges that you need to be aware of and protect yourself from. Using a VPN for torrenting Telugu movies can help you avoid legal issues, malware, viruses, fake files, slow speeds, and other problems that may arise from torrenting.
-A VPN can also help you access any torrent site or content you want without geo-restrictions or censorship. You can also download and share Telugu movies safely and anonymously with a VPN.
-However, not all VPNs are suitable for torrenting Telugu movies. You need to choose a VPN that has fast and reliable speeds, large and diverse server network, strong encryption and security features, no-logs policy and privacy protection, and P2P support on all servers.
-We have selected some of the best VPNs for torrenting Telugu movies in 2023 based on these criteria. These VPNs have been tested and verified by us and many other users to be fast, reliable, secure, and torrent-friendly. Our top picks are ExpressVPN , NordVPN , CyberGhost , Private Internet Access , and Mullvad .
-With these VPNs, you can download Brothers Telugu movie for free with uTorrent or any other torrent client without any worries. Just remember to always use a VPN when torrenting Telugu movies or any other content online.
3cee63e6c2
-
-
\ No newline at end of file
diff --git a/spaces/suppsumstagza/text-to-image-stable-diffusion-v1-5/scripts/Download The The Desire Movie 720p ((FULL)).md b/spaces/suppsumstagza/text-to-image-stable-diffusion-v1-5/scripts/Download The The Desire Movie 720p ((FULL)).md
deleted file mode 100644
index b52e956de9a332d72288c630f3bb2adf158815db..0000000000000000000000000000000000000000
--- a/spaces/suppsumstagza/text-to-image-stable-diffusion-v1-5/scripts/Download The The Desire Movie 720p ((FULL)).md
+++ /dev/null
@@ -1,26 +0,0 @@
-
-Download The The Desire Movie 720p: A Romantic Thriller That Will Keep You On The Edge Of Your Seat
-If you are looking for a movie that combines romance, suspense, and mystery, then you should download The The Desire movie 720p. This is a 2022 film directed by David Fincher and starring Brad Pitt and Angelina Jolie as a married couple who get entangled in a web of lies and deception.
-Download The The Desire Movie 720p
Download File ✶✶✶ https://cinurl.com/2uEYvI
-The The Desire movie 720p follows the story of Nick and Amy Dunne, who seem to have a perfect marriage until Amy goes missing on their fifth anniversary. Nick becomes the prime suspect in her disappearance, but he claims he is innocent. As the investigation unfolds, secrets and twists are revealed that will make you question everything you thought you knew about this couple.
-The The Desire movie 720p is a gripping and captivating film that will keep you guessing until the very end. It is based on the best-selling novel by Gillian Flynn, who also wrote the screenplay. The film has received critical acclaim and has been nominated for several awards, including four Oscars.
-If you want to watch this thrilling and romantic movie, you can download The The Desire movie 720p from our website. We offer high-quality and fast downloads that are safe and secure. You can also stream the movie online if you prefer. Don't miss this opportunity to watch one of the best movies of the year. Download The The Desire movie 720p today and enjoy!
-
-The The Desire movie 720p is not only a thrilling and romantic movie, but also a visually stunning one. The film showcases the beautiful scenery of Missouri and New York, where the story takes place. The cinematography and editing are superb, creating a tense and immersive atmosphere. The soundtrack also adds to the mood and tone of the film, featuring songs by Trent Reznor and Atticus Ross.
-
-The The Desire movie 720p also boasts an impressive cast of actors who deliver outstanding performances. Brad Pitt and Angelina Jolie have a great chemistry on screen, portraying the complex and flawed characters of Nick and Amy. They are supported by a talented ensemble of actors, including Tyler Perry, Neil Patrick Harris, Carrie Coon, and Kim Dickens.
-The The Desire movie 720p is a movie that you will not forget anytime soon. It is a movie that will make you feel a range of emotions, from love to hate, from fear to excitement. It is a movie that will challenge your expectations and surprise you with its twists and turns. It is a movie that you will want to watch again and again.
-So what are you waiting for? Download The The Desire movie 720p from our website now and experience this amazing film for yourself. You will not regret it!
-
-Downloading The The Desire movie 720p from our website is very easy and convenient. You just need to follow these simple steps:
-
-- Click on the download link below and choose your preferred payment method.
-- Complete the payment process and receive your download link via email.
-- Click on the download link and save the file to your device.
-- Enjoy watching The The Desire movie 720p anytime and anywhere you want.
-
-Alternatively, you can also stream The The Desire movie 720p online from our website. You just need to create an account and log in. Then, you can watch the movie directly from your browser without any hassle.
-Whether you choose to download or stream The The Desire movie 720p, you will get the best quality and service from our website. We guarantee that our downloads and streams are fast, secure, and virus-free. We also offer 24/7 customer support and a money-back guarantee if you are not satisfied with our product.
-Don't miss this chance to watch one of the most talked-about movies of the year. Download or stream The The Desire movie 720p from our website today and get ready for a thrilling and romantic ride!
d5da3c52bf
-
-
\ No newline at end of file
diff --git a/spaces/surmensipa/VITS-Umamusume-voice-synthesizer/logs/Advanced BAT To EXE Converter Pro V2.91 Serial Keygen Download Pc EXCLUSIVE.md b/spaces/surmensipa/VITS-Umamusume-voice-synthesizer/logs/Advanced BAT To EXE Converter Pro V2.91 Serial Keygen Download Pc EXCLUSIVE.md
deleted file mode 100644
index d3d2bfeef2f0dcbfdea66099d83a5773f218940c..0000000000000000000000000000000000000000
--- a/spaces/surmensipa/VITS-Umamusume-voice-synthesizer/logs/Advanced BAT To EXE Converter Pro V2.91 Serial Keygen Download Pc EXCLUSIVE.md
+++ /dev/null
@@ -1,44 +0,0 @@
-
-How to Download and Install Advanced BAT To EXE Converter Pro V2.91 With Serial Keygen
-If you are looking for a powerful and easy-to-use tool to convert your batch files into executable files, then you should try Advanced BAT To EXE Converter Pro V2.91. This software allows you to create professional and secure applications from your batch scripts, with advanced features such as encryption, password protection, custom icons, run as administrator, and more.
-In this article, we will show you how to download and install Advanced BAT To EXE Converter Pro V2.91 with serial keygen, so you can enjoy the full version of this software without any limitations.
-Advanced BAT To EXE Converter Pro V2.91 Serial Keygen Download Pc
DOWNLOAD ✺✺✺ https://urluss.com/2uCFPl
-Step 1: Download the Software
-The first step is to download the software from the official website or from a trusted source. You can use the following link to download the software:
-https://www.battoexeconverter.com/download.html
-Once you have downloaded the software, you will get a zip file named "Advanced_BAT_to_EXE_Converter_Pro_v2.91.zip". You need to extract this file to a folder on your computer.
-Step 2: Run the Setup File
-The next step is to run the setup file named "Advanced_BAT_to_EXE_Converter_Pro_v2.91_Setup.exe". This will launch the installation wizard that will guide you through the process.
-You need to accept the license agreement and choose the destination folder for the software. You can also choose whether to create a desktop shortcut and a start menu entry for the software.
-After that, click on "Install" to start the installation process. It will take a few minutes to complete.
-
-Step 3: Activate the Software
-The final step is to activate the software with the serial keygen. You need to run the file named "Advanced_BAT_to_EXE_Converter_Pro_v2.91_Keygen.exe" that you will find in the same folder as the setup file.
-This will open a window that will generate a serial number for you. You need to copy this serial number and paste it into the software.
-To do that, open the software and click on "Help" > "Enter Registration Code". Then paste the serial number into the text box and click on "Register".
-You will see a message that says "Thank you for registering Advanced BAT To EXE Converter Pro". This means that you have successfully activated the software and you can use all its features without any restrictions.
-Conclusion
-In this article, we have shown you how to download and install Advanced BAT To EXE Converter Pro V2.91 with serial keygen. This software is a great tool for converting your batch files into executable files with advanced options and security features.
-We hope you found this article helpful and informative. If you have any questions or feedback, please feel free to leave a comment below.
-
-How to Use Advanced BAT To EXE Converter Pro V2.91
-Once you have installed and activated the software, you can start using it to convert your batch files into executable files. Here are some basic steps to follow:
-
-- Open the software and click on "File" > "Open" to browse and select the batch file that you want to convert.
-- Edit and modify the batch file as you wish. You can use the built-in editor that has syntax highlighting, code folding, auto-completion, and more.
-- Click on "Build" > "Build EXE" to start the conversion process. You can choose the output folder, the file name, the icon, the version information, and other options for the executable file.
-- Click on "OK" to finish the conversion process. You will see a message that says "EXE File Created Successfully". You can then test and run the executable file as you normally would.
-
-You can also use the software to encrypt and protect your executable files with passwords, anti-debugging, anti-decompiling, and anti-virus features. You can also embed additional files and resources into your executable files, such as images, sounds, documents, etc.
-Benefits of Using Advanced BAT To EXE Converter Pro V2.91
-There are many benefits of using Advanced BAT To EXE Converter Pro V2.91 to convert your batch files into executable files. Some of them are:
-
-- You can create professional and secure applications from your batch scripts, without any programming skills or knowledge.
-- You can enhance and customize your batch files with advanced commands and functions that are not available in standard batch files.
-- You can make your batch files compatible with all Windows versions, from Windows 95 to Windows 10.
-- You can distribute and share your batch files as standalone executable files that do not require any external dependencies or libraries.
-- You can protect your batch files from being modified, copied, or reverse-engineered by unauthorized users or hackers.
-
-These are just some of the benefits of using Advanced BAT To EXE Converter Pro V2.91. You can discover more features and options by exploring the software yourself.
d5da3c52bf
-
-
\ No newline at end of file
diff --git a/spaces/svjack/ControlNet-Face-Chinese/SPIGA/spiga/models/gnn/__init__.py b/spaces/svjack/ControlNet-Face-Chinese/SPIGA/spiga/models/gnn/__init__.py
deleted file mode 100644
index e69de29bb2d1d6434b8b29ae775ad8c2e48c5391..0000000000000000000000000000000000000000
diff --git a/spaces/svjack/ControlNet-Pose-Chinese/annotator/uniformer/mmcv/ops/box_iou_rotated.py b/spaces/svjack/ControlNet-Pose-Chinese/annotator/uniformer/mmcv/ops/box_iou_rotated.py
deleted file mode 100644
index 2d78015e9c2a9e7a52859b4e18f84a9aa63481a0..0000000000000000000000000000000000000000
--- a/spaces/svjack/ControlNet-Pose-Chinese/annotator/uniformer/mmcv/ops/box_iou_rotated.py
+++ /dev/null
@@ -1,45 +0,0 @@
-# Copyright (c) OpenMMLab. All rights reserved.
-from ..utils import ext_loader
-
-ext_module = ext_loader.load_ext('_ext', ['box_iou_rotated'])
-
-
-def box_iou_rotated(bboxes1, bboxes2, mode='iou', aligned=False):
- """Return intersection-over-union (Jaccard index) of boxes.
-
- Both sets of boxes are expected to be in
- (x_center, y_center, width, height, angle) format.
-
- If ``aligned`` is ``False``, then calculate the ious between each bbox
- of bboxes1 and bboxes2, otherwise the ious between each aligned pair of
- bboxes1 and bboxes2.
-
- Arguments:
- boxes1 (Tensor): rotated bboxes 1. \
- It has shape (N, 5), indicating (x, y, w, h, theta) for each row.
- Note that theta is in radian.
- boxes2 (Tensor): rotated bboxes 2. \
- It has shape (M, 5), indicating (x, y, w, h, theta) for each row.
- Note that theta is in radian.
- mode (str): "iou" (intersection over union) or iof (intersection over
- foreground).
-
- Returns:
- ious(Tensor): shape (N, M) if aligned == False else shape (N,)
- """
- assert mode in ['iou', 'iof']
- mode_dict = {'iou': 0, 'iof': 1}
- mode_flag = mode_dict[mode]
- rows = bboxes1.size(0)
- cols = bboxes2.size(0)
- if aligned:
- ious = bboxes1.new_zeros(rows)
- else:
- ious = bboxes1.new_zeros((rows * cols))
- bboxes1 = bboxes1.contiguous()
- bboxes2 = bboxes2.contiguous()
- ext_module.box_iou_rotated(
- bboxes1, bboxes2, ious, mode_flag=mode_flag, aligned=aligned)
- if not aligned:
- ious = ious.view(rows, cols)
- return ious
diff --git a/spaces/szukevin/VISOR-GPT/train/scripts/convert_bert_extractive_qa_from_tencentpretrain_to_huggingface.py b/spaces/szukevin/VISOR-GPT/train/scripts/convert_bert_extractive_qa_from_tencentpretrain_to_huggingface.py
deleted file mode 100644
index 034ec5040fd7054b1de1d0c4930081616532fa6d..0000000000000000000000000000000000000000
--- a/spaces/szukevin/VISOR-GPT/train/scripts/convert_bert_extractive_qa_from_tencentpretrain_to_huggingface.py
+++ /dev/null
@@ -1,38 +0,0 @@
-import sys
-import os
-import argparse
-import collections
-import torch
-
-tencentpretrain_dir = os.path.abspath(os.path.join(os.path.dirname(__file__), ".."))
-sys.path.insert(0, tencentpretrain_dir)
-
-from scripts.convert_bert_from_tencentpretrain_to_huggingface import \
- convert_bert_transformer_encoder_from_tencentpretrain_to_huggingface
-
-
-parser = argparse.ArgumentParser(formatter_class=argparse.ArgumentDefaultsHelpFormatter)
-parser.add_argument("--input_model_path", type=str, default="models/input_model.bin",
- help=".")
-parser.add_argument("--output_model_path", type=str, default="models/output_model.bin",
- help=".")
-parser.add_argument("--layers_num", type=int, default=12, help=".")
-
-args = parser.parse_args()
-
-input_model = torch.load(args.input_model_path)
-
-output_model = collections.OrderedDict()
-
-output_model["bert.embeddings.word_embeddings.weight"] = input_model["embedding.word.embedding.weight"]
-output_model["bert.embeddings.position_embeddings.weight"] = input_model["embedding.pos.embedding.weight"]
-output_model["bert.embeddings.token_type_embeddings.weight"] = input_model["embedding.seg.embedding.weight"][1:, :]
-output_model["bert.embeddings.LayerNorm.weight"] = input_model["embedding.layer_norm.gamma"]
-output_model["bert.embeddings.LayerNorm.bias"] = input_model["embedding.layer_norm.beta"]
-
-convert_bert_transformer_encoder_from_tencentpretrain_to_huggingface(input_model, output_model, args.layers_num)
-
-output_model["qa_outputs.weight"] = input_model["output_layer.weight"]
-output_model["qa_outputs.bias"] = input_model["output_layer.bias"]
-
-torch.save(output_model, args.output_model_path)
diff --git a/spaces/szukevin/VISOR-GPT/train/scripts/convert_bert_token_classification_from_huggingface_to_tencentpretrain.py b/spaces/szukevin/VISOR-GPT/train/scripts/convert_bert_token_classification_from_huggingface_to_tencentpretrain.py
deleted file mode 100644
index 184c9f8cdad636d8db0993ab1984f11321072220..0000000000000000000000000000000000000000
--- a/spaces/szukevin/VISOR-GPT/train/scripts/convert_bert_token_classification_from_huggingface_to_tencentpretrain.py
+++ /dev/null
@@ -1,41 +0,0 @@
-import sys
-import os
-import argparse
-import collections
-import torch
-
-tencentpretrain_dir = os.path.abspath(os.path.join(os.path.dirname(__file__), ".."))
-sys.path.insert(0, tencentpretrain_dir)
-
-from scripts.convert_bert_from_huggingface_to_tencentpretrain import \
- convert_bert_transformer_encoder_from_huggingface_to_tencentpretrain
-
-
-parser = argparse.ArgumentParser(formatter_class=argparse.ArgumentDefaultsHelpFormatter)
-parser.add_argument("--input_model_path", type=str, default="models/input_model.bin",
- help=".")
-parser.add_argument("--output_model_path", type=str, default="models/output_model.bin",
- help=".")
-parser.add_argument("--layers_num", type=int, default=12, help=".")
-
-args = parser.parse_args()
-
-input_model = torch.load(args.input_model_path, map_location="cpu")
-
-output_model = collections.OrderedDict()
-
-output_model["embedding.word.embedding.weight"] = input_model["bert.embeddings.word_embeddings.weight"]
-output_model["embedding.pos.embedding.weight"] = input_model["bert.embeddings.position_embeddings.weight"]
-output_model["embedding.seg.embedding.weight"] = \
- torch.cat((torch.Tensor([[0]*input_model["bert.embeddings.token_type_embeddings.weight"].size()[1]]),
- input_model["bert.embeddings.token_type_embeddings.weight"]), dim=0)
-output_model["embedding.layer_norm.gamma"] = input_model["bert.embeddings.LayerNorm.weight"]
-output_model["embedding.layer_norm.beta"] = input_model["bert.embeddings.LayerNorm.bias"]
-
-convert_bert_transformer_encoder_from_huggingface_to_tencentpretrain(input_model, output_model, args.layers_num)
-
-output_model["output_layer.weight"] = input_model["classifier.weight"]
-output_model["output_layer.bias"] = input_model["classifier.bias"]
-
-
-torch.save(output_model, args.output_model_path)
diff --git a/spaces/t0int/CalderaAI-30B-Lazarus/app.py b/spaces/t0int/CalderaAI-30B-Lazarus/app.py
deleted file mode 100644
index f86a7ffa9148d32bbb8281612bed38b3606530c7..0000000000000000000000000000000000000000
--- a/spaces/t0int/CalderaAI-30B-Lazarus/app.py
+++ /dev/null
@@ -1,3 +0,0 @@
-import gradio as gr
-
-gr.Interface.load("models/CalderaAI/30B-Lazarus").launch()
\ No newline at end of file
diff --git a/spaces/t110-ai-admin/InspectLens/video_llama/datasets/datasets/llava_instruct_dataset.py b/spaces/t110-ai-admin/InspectLens/video_llama/datasets/datasets/llava_instruct_dataset.py
deleted file mode 100644
index 105e0981581b7934c5df2bc53ecf03142cc4c969..0000000000000000000000000000000000000000
--- a/spaces/t110-ai-admin/InspectLens/video_llama/datasets/datasets/llava_instruct_dataset.py
+++ /dev/null
@@ -1,228 +0,0 @@
-import os
-from video_llama.datasets.datasets.base_dataset import BaseDataset
-from video_llama.datasets.datasets.caption_datasets import CaptionDataset
-import pandas as pd
-import decord
-from decord import VideoReader
-import random
-import torch
-from torch.utils.data.dataloader import default_collate
-from PIL import Image
-from typing import Dict, Optional, Sequence
-import transformers
-import pathlib
-import json
-from transformers import AutoTokenizer, AutoModelForCausalLM, LlamaTokenizer
-from video_llama.conversation.conversation_video import Conversation,SeparatorStyle
-DEFAULT_IMAGE_PATCH_TOKEN = ''
-DEFAULT_IMAGE_TOKEN = ""
-import copy
-IGNORE_INDEX = -100
-image_conversation = Conversation(
- system="",
- roles=("Human", "Assistant"),
- messages=[],
- offset=0,
- sep_style=SeparatorStyle.SINGLE,
- sep="###",
-)
-IGNORE_INDEX = -100
-
-class Instruct_Dataset(BaseDataset):
- def __init__(self, vis_processor, text_processor, vis_root, ann_root,num_video_query_token=32,tokenizer_name = '/mnt/workspace/ckpt/vicuna-13b/',data_type = 'image'):
- """
- vis_root (string): Root directory of Llava images (e.g. webvid_eval/video/)
- ann_root (string): Root directory of video (e.g. webvid_eval/annotations/)
- split (string): val or test
- """
- super().__init__(vis_processor=vis_processor, text_processor=text_processor)
-
- data_path = pathlib.Path(ann_root)
- with data_path.open(encoding='utf-8') as f:
- self.annotation = json.load(f)
-
- self.vis_root = vis_root
- self.resize_size = 224
- self.num_frm = 8
- self.tokenizer = LlamaTokenizer.from_pretrained(tokenizer_name, use_fast=False)
- self.tokenizer.pad_token = self.tokenizer.eos_token
- self.tokenizer.add_tokens([DEFAULT_IMAGE_PATCH_TOKEN], special_tokens=True)
- self.num_video_query_token = num_video_query_token
- self.IMAGE_PATCH_TOKEN_ID = self.tokenizer.get_vocab()[DEFAULT_IMAGE_PATCH_TOKEN]
-
- self.transform = AlproVideoTrainProcessor(
- image_size=self.resize_size, n_frms = self.num_frm
- ).transform
- self.data_type = data_type
-
- def _get_image_path(self, sample):
- rel_video_fp ='COCO_train2014_' + sample['image']
- full_video_fp = os.path.join(self.vis_root, rel_video_fp)
- return full_video_fp
-
- def __getitem__(self, index):
- num_retries = 10 # skip error videos
- for _ in range(num_retries):
- try:
- sample = self.annotation[index]
-
- image_path = self._get_image_path(sample)
- conversation_list = sample['conversations']
- image = Image.open(image_path).convert("RGB")
-
- image = self.vis_processor(image)
- # text = self.text_processor(text)
- sources = preprocess_multimodal(copy.deepcopy(conversation_list), None, cur_token_len=self.num_video_query_token)
- data_dict = preprocess(
- sources,
- self.tokenizer)
- data_dict = dict(input_ids=data_dict["input_ids"][0],
- labels=data_dict["labels"][0])
-
- # image exist in the data
- data_dict['image'] = image
- except:
- print(f"Failed to load examples with image: {image_path}. "
- f"Will randomly sample an example as a replacement.")
- index = random.randint(0, len(self) - 1)
- continue
- break
- else:
- raise RuntimeError(f"Failed to fetch image after {num_retries} retries.")
- # "image_id" is kept to stay compatible with the COCO evaluation format
- return {
- "image": image,
- "text_input": data_dict["input_ids"],
- "labels": data_dict["labels"],
- "type":'image',
- }
-
- def __len__(self):
- return len(self.annotation)
-
- def collater(self, instances):
- input_ids, labels = tuple([instance[key] for instance in instances]
- for key in ("text_input", "labels"))
- input_ids = torch.nn.utils.rnn.pad_sequence(
- input_ids,
- batch_first=True,
- padding_value=self.tokenizer.pad_token_id)
- labels = torch.nn.utils.rnn.pad_sequence(labels,
- batch_first=True,
- padding_value=IGNORE_INDEX)
- batch = dict(
- input_ids=input_ids,
- labels=labels,
- attention_mask=input_ids.ne(self.tokenizer.pad_token_id),
- )
-
- if 'image' in instances[0]:
- images = [instance['image'] for instance in instances]
- if all(x is not None and x.shape == images[0].shape for x in images):
- batch['images'] = torch.stack(images)
- else:
- batch['images'] = images
- batch['conv_type'] = 'multi'
- return batch
-
-
-def preprocess_multimodal(
- conversation_list: Sequence[str],
- multimodal_cfg: dict,
- cur_token_len: int,
-) -> Dict:
- # 将conversational list中
- is_multimodal = True
- # image_token_len = multimodal_cfg['image_token_len']
- image_token_len = cur_token_len
-
- for sentence in conversation_list:
- replace_token = ''+DEFAULT_IMAGE_PATCH_TOKEN * image_token_len+'/'
- sentence["value"] = sentence["value"].replace(DEFAULT_IMAGE_TOKEN, replace_token)
-
- return [conversation_list]
-
-def _add_speaker_and_signal(header, source, get_conversation=True):
- """Add speaker and start/end signal on each round."""
- BEGIN_SIGNAL = "###"
- END_SIGNAL = "\n"
- conversation = header
- for sentence in source:
- from_str = sentence["from"]
- if from_str.lower() == "human":
- from_str = image_conversation.roles[0]
- elif from_str.lower() == "gpt":
- from_str = image_conversation.roles[1]
- else:
- from_str = 'unknown'
- sentence["value"] = (BEGIN_SIGNAL + from_str + ": " +
- sentence["value"] + END_SIGNAL)
- if get_conversation:
- conversation += sentence["value"]
- conversation += BEGIN_SIGNAL
- return conversation
-
-def _tokenize_fn(strings: Sequence[str],
- tokenizer: transformers.PreTrainedTokenizer) -> Dict:
- """Tokenize a list of strings."""
- tokenized_list = [
- tokenizer(
- text,
- return_tensors="pt",
- padding="longest",
- max_length=512,
- truncation=True,
- ) for text in strings
- ]
- input_ids = labels = [
- tokenized.input_ids[0] for tokenized in tokenized_list
- ]
- input_ids_lens = labels_lens = [
- tokenized.input_ids.ne(tokenizer.pad_token_id).sum().item()
- for tokenized in tokenized_list
- ]
- return dict(
- input_ids=input_ids,
- labels=labels,
- input_ids_lens=input_ids_lens,
- labels_lens=labels_lens,
- )
-
-def preprocess(
- sources: Sequence[str],
- tokenizer: transformers.PreTrainedTokenizer,
-) -> Dict:
- """
- Given a list of sources, each is a conversation list. This transform:
- 1. Add signal '### ' at the beginning each sentence, with end signal '\n';
- 2. Concatenate conversations together;
- 3. Tokenize the concatenated conversation;
- 4. Make a deepcopy as the target. Mask human words with IGNORE_INDEX.
- """
- # add end signal and concatenate together
- conversations = []
- for source in sources:
- header = f"{image_conversation.system}\n\n"
- conversation = _add_speaker_and_signal(header, source)
- conversations.append(conversation)
- # tokenize conversations
- conversations_tokenized = _tokenize_fn(conversations, tokenizer)
- input_ids = conversations_tokenized["input_ids"]
- targets = copy.deepcopy(input_ids)
- for target, source in zip(targets, sources):
- tokenized_lens = _tokenize_fn([header] + [s["value"] for s in source],
- tokenizer)["input_ids_lens"]
- speakers = [sentence["from"] for sentence in source]
- _mask_targets(target, tokenized_lens, speakers)
-
- return dict(input_ids=input_ids, labels=targets)
-
-def _mask_targets(target, tokenized_lens, speakers):
- # cur_idx = 0
- cur_idx = tokenized_lens[0]
- tokenized_lens = tokenized_lens[1:]
- target[:cur_idx] = IGNORE_INDEX
- for tokenized_len, speaker in zip(tokenized_lens, speakers):
- if speaker == "human":
- target[cur_idx+2:cur_idx + tokenized_len] = IGNORE_INDEX
- cur_idx += tokenized_len
diff --git a/spaces/terfces0erbo/CollegeProjectV2/Android Korg Pa Manager Full 459 3.md b/spaces/terfces0erbo/CollegeProjectV2/Android Korg Pa Manager Full 459 3.md
deleted file mode 100644
index 9cda0b13d771698074a7879151981f884f4347dd..0000000000000000000000000000000000000000
--- a/spaces/terfces0erbo/CollegeProjectV2/Android Korg Pa Manager Full 459 3.md
+++ /dev/null
@@ -1,44 +0,0 @@
-Android korg pa manager full 459 3
Download File ✓ https://bytlly.com/2uGkJe
-
-Cherish is a love song composed by K. Shankar for the 2010 Kannada film film Pokkiri. The song was well received by the masses as well as music directors S. Thaman and veetil. The track is sung by
-
-Bhavana, and lyrics are written by Kaviraj Patnaik.
-
-The song was released on 12 February 2011 and had become a massive hit, receiving high praise from critics as well as the general masses.
-
-The song is available as a single download as well. Cherish became popular among the youths and has since had several cover versions.
-
-The song was again remade in Kannada in 2015, titled "Ondare", and composed by Deva.
-
-Bhavana's "Cherish" vocals and the song's haunting guitars have been praised by music critics.
-
-Background
-
-The song is a modern love song composed by K Shankar for the film Pokkiri. The song has been sung by Bhavana, with lyrics by Kaviraj Patnaik. The song was released on 12 February 2011. The song was not expected to do well, but it proved to be a massive hit and has been praised by the masses.
-
-Reception
-
-The song has received high praise from music critics. Mathrubhumi stated, "Cherish by Bhavana is a magnificent'modern' song. The slow beat, perfect balance of melody and rhythm, and the hummable chorus have made this song a hit. It is a thumping, heartfelt song that will rock any party, leaving a large imprint in people's memories."
-
-Music video
-
-The music video was made in 2–3 days with the track's lyricist, Kaviraj Patnaik. The concept of the music video was to portray a couple unable to live without each other. The video shows a couple, with the man trying to leave the woman, but is unable to do so.
-
-The video shows a love triangle, as each of the characters, attempt to pull the other one away from the other. The video ends with the couple finally falling in love with each other. The video has been received well by the masses, and has been praised by music critics.
-
-References
-
-External links
-
-
-
-Category:2011 songs
-
-Category:Kannada film songs
-
-Category:Songs written for films
-
-Category:Songs with 4fefd39f24
-
-
-
diff --git a/spaces/terfces0erbo/CollegeProjectV2/Dream Chronicles 6 The Book Of Fire.rar ((INSTALL)).md b/spaces/terfces0erbo/CollegeProjectV2/Dream Chronicles 6 The Book Of Fire.rar ((INSTALL)).md
deleted file mode 100644
index b10d73dc8cf51e73ab9ee44cbff8aca4af46685d..0000000000000000000000000000000000000000
--- a/spaces/terfces0erbo/CollegeProjectV2/Dream Chronicles 6 The Book Of Fire.rar ((INSTALL)).md
+++ /dev/null
@@ -1,6 +0,0 @@
-Dream Chronicles 6 : The Book Of Fire.rar
DOWNLOAD ✪✪✪ https://bytlly.com/2uGkfo
-
-Dream Chronicles The Book Of Fire download sound.... Bronson (2008) ... Yosemite Beta 6, Notability, breakthrough batteries · Stellar Monarch ... 1fdad05405
-
-
-
diff --git a/spaces/terfces0erbo/CollegeProjectV2/Ejerciciosdelogixproenespanolresueltosenpdf LINK.md b/spaces/terfces0erbo/CollegeProjectV2/Ejerciciosdelogixproenespanolresueltosenpdf LINK.md
deleted file mode 100644
index f5ccf6575ef3f879e6a875da1bb544d7b81ef4e1..0000000000000000000000000000000000000000
--- a/spaces/terfces0erbo/CollegeProjectV2/Ejerciciosdelogixproenespanolresueltosenpdf LINK.md
+++ /dev/null
@@ -1,6 +0,0 @@
-ejerciciosdelogixproenespanolresueltosenpdf
Download ✪✪✪ https://bytlly.com/2uGk10
-
- d5da3c52bf
-
-
-
diff --git a/spaces/thefcraft/prompt-generator-stable-diffusion/app.py b/spaces/thefcraft/prompt-generator-stable-diffusion/app.py
deleted file mode 100644
index 2abb09e52f62c12d4a897e12ac751c96b91cb394..0000000000000000000000000000000000000000
--- a/spaces/thefcraft/prompt-generator-stable-diffusion/app.py
+++ /dev/null
@@ -1,90 +0,0 @@
-import gradio as gr
-import pickle
-import random
-import numpy as np
-
-with open('models.pickle', 'rb')as f:
- models = pickle.load(f)
-
-
-LORA_TOKEN = ''#'<|>LORA_TOKEN<|>'
-# WEIGHT_TOKEN = '<|>WEIGHT_TOKEN<|>'
-NOT_SPLIT_TOKEN = '<|>NOT_SPLIT_TOKEN<|>'
-
-def sample_next(ctx:str,model,k):
-
- ctx = ', '.join(ctx.split(', ')[-k:])
- if model.get(ctx) is None:
- return " "
- possible_Chars = list(model[ctx].keys())
- possible_values = list(model[ctx].values())
-
- # print(possible_Chars)
- # print(possible_values)
-
- return np.random.choice(possible_Chars,p=possible_values)
-
-def generateText(model, minLen=100, size=5):
- keys = list(model.keys())
- starting_sent = random.choice(keys)
- k = len(random.choice(keys).split(', '))
-
- sentence = starting_sent
- ctx = ', '.join(starting_sent.split(', ')[-k:])
-
- while True:
- next_prediction = sample_next(ctx,model,k)
- sentence += f", {next_prediction}"
- ctx = ', '.join(sentence.split(', ')[-k:])
-
- # if sentence.count('\n')>size: break
- if '\n' in sentence: break
- sentence = sentence.replace(NOT_SPLIT_TOKEN, ', ')
- # sentence = re.sub(WEIGHT_TOKEN.replace('|', '\|'), lambda match: f":{random.randint(0,2)}.{random.randint(0,9)}", sentence)
- # sentence = sentence.replace(":0.0", ':0.1')
- # return sentence
-
- prompt = sentence.split('\n')[0]
- if len(prompt)
-Here is what I came up with:
-
-Bartender Barcode Software Free Download Crack For 574: How to Get the Best Barcode Labeling Solution for Your Business
-If you are looking for a reliable and easy-to-use barcode labeling software, you might have heard of Bartender Barcode Software. This software is one of the most popular and trusted solutions for creating and printing barcode labels, tags, cards, and more. It supports a wide range of barcode symbologies, data sources, design tools, and printing options.
-Bartender Barcode Software Free Download Crack For 574
DOWNLOAD ○○○ https://urlcod.com/2uK3TV
-However, Bartender Barcode Software is not a free software. You need to purchase a license to use it for your business needs. And if you are tempted to download a crack version of the software from the internet, you might be putting your business at risk. Here are some of the reasons why you should avoid using a cracked version of Bartender Barcode Software:
-
-- It is illegal. Downloading and using a cracked version of Bartender Barcode Software is a violation of the software's terms and conditions. You are also infringing on the intellectual property rights of the software's developer, Seagull Scientific. You could face legal consequences if you are caught using a pirated software.
-- It is unsafe. Downloading a crack version of Bartender Barcode Software from an unknown source could expose your computer to malware, viruses, spyware, ransomware, and other malicious programs. These could damage your system, compromise your data, and harm your business operations.
-- It is unreliable. A crack version of Bartender Barcode Software might not work properly or have all the features and functions of the original software. You might encounter errors, bugs, crashes, compatibility issues, and performance problems. You might also miss out on the latest updates, patches, and security fixes that the software's developer provides.
-- It is unsupported. If you use a crack version of Bartender Barcode Software, you will not be able to access the technical support and customer service that the software's developer offers. You will not be able to get help if you have any questions or issues with the software. You will also not be able to benefit from the training resources, tutorials, guides, and tips that the software's developer provides.
-
-As you can see, using a crack version of Bartender Barcode Software is not worth the risk. You might end up wasting your time, money, and resources on a software that does not meet your expectations and needs. You might also jeopardize your business reputation and security by using an illegal and unsafe software.
-So what is the best way to get Bartender Barcode Software for your business? The answer is simple: buy a legitimate license from the software's official website or an authorized reseller. By doing so, you will be able to enjoy all the benefits and features of Bartender Barcode Software without any hassle or worry. You will also be able to support the software's developer and contribute to their continuous improvement and innovation.
-Bartender Barcode Software offers various license options to suit different business sizes and needs. You can choose from Basic Edition, Professional Edition, Automation Edition, or Enterprise Automation Edition. Each edition has different capabilities and prices. You can compare them and find the best one for your business here: https://www.seagullscientific.com/barcode-label-software/editions/
-
-If you are still unsure whether Bartender Barcode Software is right for you, you can also try it for free for 30 days. You can download the trial version here: https://www.seagullscientific.com/barcode-label-software/free-barcode-software/
-Bartender Barcode Software is the best barcode labeling solution for your business. Don't settle for anything less than the original and authentic software. Download it today and see for yourself how it can help you create and print professional-quality barcode labels in minutes.
e93f5a0c3f
-
-
\ No newline at end of file
diff --git a/spaces/tialenAdioni/chat-gpt-api/logs/Jamella D2 Hero Editor V1 13 Downloadl Fix.md b/spaces/tialenAdioni/chat-gpt-api/logs/Jamella D2 Hero Editor V1 13 Downloadl Fix.md
deleted file mode 100644
index 698b74a15118c9e697618a34555f5da26992dc2d..0000000000000000000000000000000000000000
--- a/spaces/tialenAdioni/chat-gpt-api/logs/Jamella D2 Hero Editor V1 13 Downloadl Fix.md
+++ /dev/null
@@ -1,20 +0,0 @@
-
-How to Download and Use Jamella D2 Hero Editor V1 13
-Jamella D2 Hero Editor is a popular mod for Diablo II that allows you to edit your character's stats, items, skills, and more. It is compatible with Diablo II version 1.13 and can be used for both single-player and multiplayer modes. In this article, we will show you how to download and use Jamella D2 Hero Editor V1 13 for your Diablo II game.
-Step 1: Download Jamella D2 Hero Editor V1 13
-There are many sources where you can download Jamella D2 Hero Editor V1 13, but we recommend using the link from Mod DB, which is a reliable and safe website for modding games. The file size is about 1.43 MB and it is a zip file that contains the executable file and some readme files. You can also find other versions of Jamella D2 Hero Editor on Mod DB, such as V4.0 beta9c, which has some additional features and bug fixes.
-Jamella D2 Hero Editor V1 13 Downloadl
Download ✔ https://urlcod.com/2uK5yw
-Step 2: Extract and Run Jamella D2 Hero Editor V1 13
-After downloading the zip file, you need to extract it to a folder of your choice. You can use any program that can unzip files, such as WinRAR or 7-Zip. Once you have extracted the files, you can run the executable file named "JamellaD2Editor.exe". You may need to run it as administrator if you encounter any problems.
-Step 3: Load and Edit Your Character
-When you run Jamella D2 Hero Editor V1 13, you will see a window with several tabs and buttons. To load your character, you need to click on the "Open" button and browse to your Diablo II save folder, which is usually located under C:\Users\(your user name)\Saved Games\Diablo II Resurrected. You will see a list of files with the extension ".d2s", which are your character save files. Select the one that corresponds to the character you want to edit and click "Open".
-You will then see your character's name, class, level, experience, gold, stats, skills, inventory, mercenary, and quest status on the screen. You can edit any of these aspects by clicking on the tabs and changing the values or items as you wish. For example, you can increase your strength by typing a new number in the box next to "Strength", or you can add a new item to your inventory by dragging and dropping it from the item list on the right side of the window.
-You can also use some preset options to quickly create or modify your character. For example, you can click on the "New" button to create a new character with default settings, or you can click on the "Redo" button to reset your character to its original state. You can also load a preset character from another file by clicking on the "Load" button and selecting a ".d2s" file from your computer.
-Step 4: Save and Enjoy Your Character
-Once you are done editing your character, you need to save it by clicking on the "Save" button. You can either overwrite your original ".d2s" file or save it as a new one with a different name. You can also backup your original file by copying it to another folder before saving.
-After saving your character, you can close Jamella D2 Hero Editor V1 13 and launch Diablo II Resurrected. You will see your edited character in the character selection screen and you can play with it as normal. You can also use Jamella D2 Hero Editor V1 13 to edit your character anytime you want.
-Note:
-Jamella D2 Hero Editor V1 13 is not an official tool from Blizzard Entertainment and it may not work with future patches or updates of Diablo II Resurrected. It may also cause some errors or glitches in your game or corrupt your save files if used incorrectly. Use it at your own risk and make sure to
- e93f5a0c3f
-
-
\ No newline at end of file
diff --git a/spaces/tialenAdioni/chat-gpt-api/logs/KMSAuto Net 2015 1.4.0 Portable.md b/spaces/tialenAdioni/chat-gpt-api/logs/KMSAuto Net 2015 1.4.0 Portable.md
deleted file mode 100644
index 5bd4e02995965fbf7e6f543d1281187f48631aea..0000000000000000000000000000000000000000
--- a/spaces/tialenAdioni/chat-gpt-api/logs/KMSAuto Net 2015 1.4.0 Portable.md
+++ /dev/null
@@ -1,109 +0,0 @@
-
-KMSAuto Net 2015 1.4.0 Portable: A Powerful Windows and Office Activator
-If you are looking for a simple and effective way to activate your Windows or Office products without paying for a license key, you might want to try KMSAuto Net 2015 1.4.0 Portable.
-KMSAuto Net 2015 1.4.0 Portable
Download File ……… https://urlcod.com/2uK3sf
-KMSAuto Net is a popular tool that can automatically activate Windows Vista, 7, 8, 8.1, 10, Server 2008, 2008 R2, 2012, 2012 R2 and Office 2010, 2013, and 2016 using the Key Management Service (KMS) technology.
-KMS is a method that Microsoft uses to authorize software on large networks such as enterprises or educational institutions.
-K
KMSAuto Net 2015 1.4.0 Portable is a version of KMSAuto Net that does not require installation and can be run from any removable device such as a USB flash drive or an external hard drive.
-It is a powerful and reliable tool that can activate your Windows or Office products in a matter of seconds, without any hassle or risk.
-In this article, we will show you how to download, use, and benefit from KMSAuto Net 2015 1.4.0 Portable, as well as some of its drawbacks and alternatives.
- How to Download KMSAuto Net 2015 1.4.0 Portable
-Downloading KMSAuto Net 2015 1.4.0 Portable is easy and fast, but you need to be careful about the source you choose.
-There are many websites that claim to offer KMSAuto Net 2015 1.4.0 Portable for free, but some of them may contain viruses, malware, or fake files that can harm your computer or steal your personal information.
-To avoid any potential problems, we recommend you to download KMSAuto Net 2015 1.4.0 Portable from the official website of the developer, Ratiborus, or from trusted sources such as Ru-Board or MDL.
-Here are the steps to download KMSAuto Net 2015 1.4.0 Portable:
-
-
-- Go to one of the links above and find the download button or link for KMSAuto Net 2015 1.4.0 Portable.
-- Click on the download button or link and wait for the file to be downloaded to your computer.
-- Extract the file using a program such as WinRAR or 7-Zip.
-- Copy the extracted folder to a removable device such as a USB flash drive or an external hard drive.
-- You are now ready to use KMSAuto Net 2015 1.4.0 Portable on any computer you want.
-
- How to Use KMSAuto Net 2015 1.4.0 Portable
-Using KMSAuto Net 2015 1.4.0 Portable is simple and straightforward, but you need to follow some precautions before and after activating your Windows or Office products.
-First of all, you need to disable your antivirus program and firewall temporarily, as they may interfere with the activation process or flag KMSAuto Net as a malicious program.
-Secondly, you need to make sure that your internet connection is stable and working, as KMSAuto Net needs to connect to a KMS server online to activate your products.
-Thirdly, you need to run KMSAuto Net as an administrator, by right-clicking on the executable file and choosing "Run as administrator".
-Finally, you need to restart your computer after activating your products, to apply the changes and complete the activation process.
- How to Activate Windows with KMSAuto Net 2015 1.4.0 Portable
-To activate Windows with KMSAuto Net 2015 1.4.0 Portable, follow these steps:
-
-- Insert the removable device that contains KMSAuto Net 2015 1.4.0 Portable into the computer that you want to activate.
-- Open the folder that contains KMSAuto Net 2015 1.4.0 Portable and double-click on the executable file named "KMSAuto Net.exe".
-- A window will appear with several options and buttons.
-- Select the "Main" tab and click on the "Activate Windows" button.
-- A message will appear saying "Activating Windows..." and then "Windows is activated successfully".
-- Click on the "OK" button and close the window.
-- Restart your computer and enjoy your activated Windows.
-
- How to Activate Office with KMSAuto Net 2015 1.4.0 Portable
-To activate Office with KMSAuto Net 2015 1.4.0 Portable, follow these steps:
-
-- Insert the removable device that contains KMSAuto Net 2015 1.4.0 Portable into the computer that has Office installed.
-- Open the folder that contains KMSAuto Net 2015 1.4.0 Portable and double-click on the executable file named "KMSAuto Net.exe".
-- A window will appear with several options and buttons.
-- Select the
- Select the "Main" tab and click on the "Activate Office" button.
-- A message will appear saying "Activating Office..." and then "Office is activated successfully".
-- Click on the "OK" button and close the window.
-- Restart your computer and enjoy your activated Office.
-
- How to Convert Office from Retail to Volume License with KMSAuto Net 2015 1.4.0 Portable
-If you have a retail version of Office installed on your computer, you may need to convert it to a volume license version before activating it with KMSAuto Net 2015 1.4.0 Portable.
-A retail version of Office is the one that you buy from a store or online, and it comes with a product key that can only be used on one computer.
-A volume license version of Office is the one that is distributed by large organizations such as enterprises or educational institutions, and it does not require a product key to activate.
-KMSAuto Net 2015 1.4.0 Portable can convert your retail version of Office to a volume license version in a few clicks, without losing any data or settings.
-To convert Office from retail to volume license with KMSAuto Net 2015 1.4.0 Portable, follow these steps:
-
-- Insert the removable device that contains KMSAuto Net 2015 1.4.0 Portable into the computer that has Office installed.
-- Open the folder that contains KMSAuto Net 2015 1.4.0 Portable and double-click on the executable file named "KMSAuto Net.exe".
-- A window will appear with several options and buttons.
-- Select the "System" tab and click on the "Convert Office RETAIL -> VL" button.
-- A message will appear saying "Converting Office..." and then "Office is converted successfully".
-- Click on the "OK" button and close the window.
-- You can now activate your Office with KMSAuto Net 2015 1.4.0 Portable as described above.
-
- Benefits of Using KMSAuto Net 2015 1.4.0 Portable
-Using KMSAuto Net 2015 1.4.0 Portable has many benefits that make it a great choice for activating your Windows or Office products.
-Some of these benefits are:
-
-- Simplicity: You don't need to install anything or enter any product keys or codes. You just need to run the program and click a few buttons.
-- Compatibility: You can use KMSAuto Net 2015 1.4.0 Portable on any version of Windows or Office that supports KMS activation, including the latest ones such as Windows 10 or Office 2016.
-- Security: You don't need to worry about viruses, malware, or fake files that can harm your computer or steal your personal information. KMSAuto Net 2015 1.4.0 Portable is safe and clean, as long as you download it from reliable sources.
-- Efficiency: You don't need to waste time or money on buying license keys or contacting Microsoft support. You can activate your products in a matter of seconds, without any hassle or risk.
-- Portability: You don't need to carry around a CD or DVD with you. You can store KMSAuto Net 2015 1.4.0 Portable on any removable device such as a USB flash drive or an external hard drive, and use it on any computer you want.
-
- Drawbacks of Using KMSAuto Net 2015 1.4.0 Portable
-Using KMSAuto Net 2015 1.4.0 Portable also has some drawbacks that you should be aware of before using it.
-Some of these drawbacks are:
-
-- Legality: You should know that using KMSAuto Net 2015 1.4.0 Portable is not legal, as it violates the terms and conditions of Microsoft's software licensing agreement. You are using a product that you have not paid for, and you are bypassing Microsoft's authentication system.
-- Reliability: You should know that using KMSAuto Net 2015 1.4.0 Portable is not permanent, as it only activates your products for a period of 180 days, after which you need to re-activate them again using the same tool or another one.
-- Updates: You should know that using KMSAuto Net 2015 1.4.0 Portable may prevent you from
- Updates: You should know that using KMSAuto Net 2015 1.4.0 Portable may prevent you from receiving the latest updates and patches from Microsoft, as they may detect your activation status and block your access to their servers.
-- Support: You should know that using KMSAuto Net 2015 1.4.0 Portable may limit your access to Microsoft's official support and customer service, as they may not recognize your products as genuine and valid.
-
- Alternatives to KMSAuto Net 2015 1.4.0 Portable
-If you are not satisfied with KMSAuto Net 2015 1.4.0 Portable or you want to try other tools that can activate your Windows or Office products, you have some alternatives to choose from.
-Here is a table of some of the most popular and reliable alternatives to KMSAuto Net 2015 1.4.0 Portable, along with their features and advantages:
- | Tool | Features | Advantages | | --- | --- | --- | | KMSPico | - Activates Windows Vista, 7, 8, 8.1, 10, Server 2008, 2008 R2, 2012, 2012 R2 and Office 2010, 2013, and 2016
- Does not require internet connection
- Does not require installation
- Supports both 32-bit and 64-bit systems | - Simple and easy to use
- Works offline
- Portable and lightweight
- Supports multiple languages | | Microsoft Toolkit | - Activates Windows Vista, 7, 8, 8.1, 10, Server 2008, 2008 R2, 2012, 2012 R2 and Office 2010, 2013, and 2016
- Requires internet connection
- Requires installation
- Supports both 32-bit and 64-bit systems | - Powerful and versatile
- Works online
- Offers additional features such as backup, restore, customization, etc
- Supports multiple languages | | Re-Loader Activator | - Activates Windows XP, Vista, 7, 8, 8.1, 10, Server 2008, 2008 R2, 2012, 2012 R2 and Office 2010, 2013, and - Activates Windows XP, Vista, 7, 8, 8.1, 10, Server 2008, 2008 R2, 2012, 2012 R2 and Office 2010, 2013, and 2016
- Does not require internet connection
- Does not require installation
- Supports both 32-bit and 64-bit systems | - Fast and efficient
- Works offline
- Portable and small
- Supports multiple languages |
- Frequently Asked Questions about KMSAuto Net 2015 1.4.0 Portable
-Here are some of the most frequently asked questions about KMSAuto Net 2015 1.4.0 Portable, along with their answers:
-
-- What is KMS activation?
KMS activation is a method that Microsoft uses to authorize software on large networks such as enterprises or educational institutions. It involves a KMS server that generates activation keys for the clients that connect to it.
-- What are the system requirements for KMSAuto Net 2015 1.4.0 Portable?
KMSAuto Net 2015 1.4.0 Portable does not have any specific system requirements, as long as you have a compatible version of Windows or Office installed on your computer.
-- What are the supported editions of Windows and Office by KMSAuto Net 2015 1.4.0 Portable?
KMSAuto Net 2015 1.4.0 Portable supports all editions of Windows Vista, 7, 8, 8.1, 10, Server 2008, 2008 R2, 2012, 2012 R2 and Office 2010, 2013, and 2016, except for the Home and Starter editions.
-- Is KMSAuto Net 2015 1.4.0 Portable safe to use?
KMSAuto Net 2015 1.4.0 Portable is safe to use, as long as you download it from reliable sources and disable your antivirus program and firewall temporarily before using it. However, you should be aware that using KMSAuto Net 2015 1.4.0 Portable is not legal, as it violates the terms and conditions of Microsoft's software licensing agreement.
-- How long does the activation last with KMSAuto Net 2015 1.4.0 Portable?
The activation lasts for 180 days with KMSAuto Net 2015 1.4.0 Portable, after which you need to re-activate your products again using the same tool or another one. However, KMSAuto Net 2015 1.4.0 Portable can also renew the activation automatically every 10 days, if you enable the "Auto Renewal" option in the program.
-
- Conclusion
-KMSAuto Net 2015 1.4.0 Portable is a powerful and reliable tool that can activate your Windows or Office products in a matter of seconds, without any hassle or risk.
-It is a portable and lightweight version of KMSAuto Net that does not require installation and can be run from any removable device such as a USB flash drive or an external hard drive.
-It supports all editions of Windows Vista, 7, 8, 8.1, 10, Server 2008, 2008 R2, 2012, 2012 R2 and Office 2010, 2013, and 2016, except for the Home and Starter editions.
-It has many benefits such as simplicity, compatibility, security, efficiency, and portability.
-However, it also has some drawbacks such as legality, reliability, updates, and support.
-If you are not satisfied with KMSAuto Net 2015 1.4.0 Portable or you want to try other tools that can activate your Windows or Office products, you have some alternatives to choose from such as KMSPico, Microsoft Toolkit, Re-Loader Activator, etc.
-We hope this article has helped you understand how to download, use, and benefit from KMSAuto Net 2015 1.4.0 Portable, as well as some of its drawbacks and alternatives.
-If you have any questions or feedback, please feel free to leave a comment below.
-Thank you for reading and happy activating!
b2dd77e56b
-
-
\ No newline at end of file
diff --git a/spaces/ticomspire/turkey-syria-earthquake-tweets/Yevadu-Movie-Download-720p-Torrents-WORK.md b/spaces/ticomspire/turkey-syria-earthquake-tweets/Yevadu-Movie-Download-720p-Torrents-WORK.md
deleted file mode 100644
index fc8ef798d83ddeb33973a3bef6d930ce99740077..0000000000000000000000000000000000000000
--- a/spaces/ticomspire/turkey-syria-earthquake-tweets/Yevadu-Movie-Download-720p-Torrents-WORK.md
+++ /dev/null
@@ -1,86 +0,0 @@
-## yevadu movie download 720p torrents
-
-
-
-
-
- WORK
-
-
-
-**Download File ->->->-> [https://urluso.com/2txPav](https://urluso.com/2txPav)**
-
-
-
-
-
-
-
-
-
-
-
-
-
-# How to Download Yevadu Movie in 720p Quality Using Torrents
-
-
-
-Yevadu is a 2014 Indian action thriller movie starring Ram Charan, Allu Arjun, Shruti Haasan and Amy Jackson. The movie is about a man who undergoes a face transplant after surviving a brutal attack and seeks revenge on his enemies. The movie was a commercial success and received positive reviews from critics and audiences.
-
-
-
-If you want to watch Yevadu movie in high definition quality, you can use torrents to download it from various sources. Torrents are files that contain information about other files that are shared by peer-to-peer networks. You can use a torrent client software like BitTorrent or uTorrent to download the files you want.
-
-
-
-However, before you download any torrents, you should be aware of the risks involved. Torrents may contain viruses, malware, spyware or other harmful content that can damage your device or compromise your privacy. You may also face legal issues if you download copyrighted content without permission. Therefore, you should always use a VPN (virtual private network) service to protect your identity and data while downloading torrents.
-
-
-
-To download Yevadu movie in 720p quality using torrents, you can follow these steps:
-
-
-
-1. Go to a torrent search engine like 1337x[^1^], RARBG[^2^] or Archive[^3^] and type "yevadu movie download 720p torrents" in the search box.
-
-2. Choose a torrent file that has a high number of seeders (people who have the complete file and are sharing it) and leechers (people who are downloading the file) and a good rating. You can also check the comments section to see if the torrent is working and safe.
-
-3. Click on the torrent file and download it to your device. You may need to create an account or verify your email address on some sites.
-
-4. Open the torrent file with your torrent client software and select the destination folder where you want to save the movie file.
-
-5. Wait for the download to complete. The speed of the download may vary depending on your internet connection and the number of seeders and leechers.
-
-6. Once the download is finished, you can enjoy watching Yevadu movie in 720p quality on your device.
-
-
-
-Note: This article is for educational purposes only. We do not condone or promote piracy or illegal downloading of any content. Please respect the rights of the creators and support them by purchasing their products legally.
-
-
-
-If you want to know more about Yevadu movie, you can read some interesting facts and trivia about it below:
-
-
-
-- Yevadu movie was originally planned to be released in 2013, but it was postponed several times due to various reasons, such as the Telangana agitation, the death of Ram Charan's father-in-law and the cyclone Phailin.
-
-- Yevadu movie is the second collaboration between Ram Charan and director Vamsi Paidipally, after their 2010 hit movie Brindavanam.
-
-- Yevadu movie is inspired by several Hollywood movies, such as Face/Off (1997), The Bourne Identity (2002) and The Dark Knight (2008).
-
-- Yevadu movie features a cameo appearance by Kajal Aggarwal, who plays Ram Charan's love interest in the flashback scenes. She also played his love interest in two previous movies, Magadheera (2009) and Naayak (2013).
-
-- Yevadu movie was dubbed and released in Tamil as Magadheera and in Malayalam as Bhaiyya: My Brother. It was also remade in Bengali as Zulfiqar (2016) and in Marathi as Lai Bhaari (2014).
-
-
-
-We hope you enjoyed this article and learned something new about Yevadu movie. If you have any feedback or suggestions, please let us know in the comments section below.
-
- dfd1c89656
-
-
-
-
-
diff --git a/spaces/tioseFevbu/cartoon-converter/scripts/Matrix Winstar 4 0 Astrology Software Safmarx.md b/spaces/tioseFevbu/cartoon-converter/scripts/Matrix Winstar 4 0 Astrology Software Safmarx.md
deleted file mode 100644
index 0775accec61f2614fb399eae76a653b4dc6ee69f..0000000000000000000000000000000000000000
--- a/spaces/tioseFevbu/cartoon-converter/scripts/Matrix Winstar 4 0 Astrology Software Safmarx.md
+++ /dev/null
@@ -1,18 +0,0 @@
-
-How to Use Matrix Winstar 4.0 Astrology Software Safely and Effectively
-Matrix Winstar 4.0 is a powerful astrology software that can help you create and interpret astrological charts, reports, and articles. It is designed for professional astrologers who want to have access to a wide range of tools and techniques, such as searches, mapping, calendar, designer wheels, local space, midpoints, and more. However, like any software, Matrix Winstar 4.0 also has some potential risks and limitations that you should be aware of before using it. Here are some tips on how to use Matrix Winstar 4.0 astrology software safely and effectively.
-
-- Make sure your computer meets the minimum system requirements for Matrix Winstar 4.0. According to the official website, you need Windows 8 or higher, a CD-ROM drive, a printer, a mouse, and at least 100 MB of free hard disk space. You also need an internet connection to access the online features and updates.
-- Install Matrix Winstar 4.0 from the original CD-ROM or download it from the official website. Do not use pirated or cracked versions of the software, as they may contain viruses or malware that can harm your computer or compromise your personal data.
-- Read the user manual and watch the tutorial videos that come with the software. They will help you understand how to use the various features and functions of Matrix Winstar 4.0, as well as how to troubleshoot any problems that may arise.
-- Keep your software updated regularly. Matrix Winstar 4.0 offers free updates for registered users that include bug fixes, new features, and improved accuracy. You can check for updates online or contact the customer support for assistance.
-- Use Matrix Winstar 4.0 responsibly and ethically. Do not use the software to create false or misleading astrological charts, reports, or articles for yourself or others. Do not use the software to manipulate or harm anyone's emotions, decisions, or actions. Do not use the software to violate anyone's privacy or confidentiality.
-- Remember that Matrix Winstar 4.0 is a tool, not a substitute for your own astrological knowledge and intuition. The software can provide you with valuable information and insights, but it cannot replace your own judgment and experience. Always use your own common sense and critical thinking when interpreting the astrological data generated by the software.
-
-Matrix Winstar 4.0 astrology software is a great resource for professional astrologers who want to enhance their work and skills. By following these tips, you can use it safely and effectively.
-Matrix Winstar 4 0 Astrology Software safmarx
DOWNLOAD ————— https://urlcod.com/2uHygM
-
-If you want to learn more about Matrix Winstar 4.0 astrology software, you can visit the official website at https://www.astrologysoftware.com/. There you can find more information about the features, prices, and testimonials of the software. You can also download a free demo version of the software to try it out before buying it.
-Matrix Winstar 4.0 astrology software is one of the best astrology software available in the market today. It can help you create and interpret astrological charts, reports, and articles with ease and accuracy. It can also help you expand your astrological knowledge and skills with its advanced tools and techniques. Whether you are a beginner or an expert in astrology, Matrix Winstar 4.0 astrology software can help you achieve your astrological goals.
7196e7f11a
-
-
\ No newline at end of file
diff --git a/spaces/tommy24/test/app.py b/spaces/tommy24/test/app.py
deleted file mode 100644
index 26e036ff2e92bfa549428082790db4acf5d94844..0000000000000000000000000000000000000000
--- a/spaces/tommy24/test/app.py
+++ /dev/null
@@ -1,3 +0,0 @@
-import gradio as gr
-
-gr.Interface.load("models/dreamlike-art/dreamlike-diffusion-1.0").launch()
\ No newline at end of file
diff --git a/spaces/tomofi/NDLOCR/src/ndl_layout/mmdetection/configs/_base_/datasets/coco_instance.py b/spaces/tomofi/NDLOCR/src/ndl_layout/mmdetection/configs/_base_/datasets/coco_instance.py
deleted file mode 100644
index 9901a858414465d19d8ec6ced316b460166176b4..0000000000000000000000000000000000000000
--- a/spaces/tomofi/NDLOCR/src/ndl_layout/mmdetection/configs/_base_/datasets/coco_instance.py
+++ /dev/null
@@ -1,49 +0,0 @@
-# dataset settings
-dataset_type = 'CocoDataset'
-data_root = 'data/coco/'
-img_norm_cfg = dict(
- mean=[123.675, 116.28, 103.53], std=[58.395, 57.12, 57.375], to_rgb=True)
-train_pipeline = [
- dict(type='LoadImageFromFile'),
- dict(type='LoadAnnotations', with_bbox=True, with_mask=True),
- dict(type='Resize', img_scale=(1333, 800), keep_ratio=True),
- dict(type='RandomFlip', flip_ratio=0.5),
- dict(type='Normalize', **img_norm_cfg),
- dict(type='Pad', size_divisor=32),
- dict(type='DefaultFormatBundle'),
- dict(type='Collect', keys=['img', 'gt_bboxes', 'gt_labels', 'gt_masks']),
-]
-test_pipeline = [
- dict(type='LoadImageFromFile'),
- dict(
- type='MultiScaleFlipAug',
- img_scale=(1333, 800),
- flip=False,
- transforms=[
- dict(type='Resize', keep_ratio=True),
- dict(type='RandomFlip'),
- dict(type='Normalize', **img_norm_cfg),
- dict(type='Pad', size_divisor=32),
- dict(type='ImageToTensor', keys=['img']),
- dict(type='Collect', keys=['img']),
- ])
-]
-data = dict(
- samples_per_gpu=2,
- workers_per_gpu=2,
- train=dict(
- type=dataset_type,
- ann_file=data_root + 'annotations/instances_train2017.json',
- img_prefix=data_root + 'train2017/',
- pipeline=train_pipeline),
- val=dict(
- type=dataset_type,
- ann_file=data_root + 'annotations/instances_val2017.json',
- img_prefix=data_root + 'val2017/',
- pipeline=test_pipeline),
- test=dict(
- type=dataset_type,
- ann_file=data_root + 'annotations/instances_val2017.json',
- img_prefix=data_root + 'val2017/',
- pipeline=test_pipeline))
-evaluation = dict(metric=['bbox', 'segm'])
diff --git a/spaces/tomofi/NDLOCR/src/ndl_layout/mmdetection/configs/cascade_rpn/README.md b/spaces/tomofi/NDLOCR/src/ndl_layout/mmdetection/configs/cascade_rpn/README.md
deleted file mode 100644
index aa7782c31db60e20b87b03e15c66b99f44d8adcd..0000000000000000000000000000000000000000
--- a/spaces/tomofi/NDLOCR/src/ndl_layout/mmdetection/configs/cascade_rpn/README.md
+++ /dev/null
@@ -1,29 +0,0 @@
-# Cascade RPN
-
-
-
-We provide the code for reproducing experiment results of [Cascade RPN](https://arxiv.org/abs/1909.06720).
-
-```
-@inproceedings{vu2019cascade,
- title={Cascade RPN: Delving into High-Quality Region Proposal Network with Adaptive Convolution},
- author={Vu, Thang and Jang, Hyunjun and Pham, Trung X and Yoo, Chang D},
- booktitle={Conference on Neural Information Processing Systems (NeurIPS)},
- year={2019}
-}
-```
-
-## Benchmark
-
-### Region proposal performance
-
-| Method | Backbone | Style | Mem (GB) | Train time (s/iter) | Inf time (fps) | AR 1000 | Download |
-|:------:|:--------:|:-----:|:--------:|:-------------------:|:--------------:|:-------:|:--------------------------------------:|
-| CRPN | R-50-FPN | caffe | - | - | - | 72.0 | [model](https://drive.google.com/file/d/1qxVdOnCgK-ee7_z0x6mvAir_glMu2Ihi/view?usp=sharing) |
-
-### Detection performance
-
-| Method | Proposal | Backbone | Style | Schedule | Mem (GB) | Train time (s/iter) | Inf time (fps) | box AP | Download |
-|:-------------:|:-----------:|:--------:|:-------:|:--------:|:--------:|:-------------------:|:--------------:|:------:|:--------------------------------------------:|
-| Fast R-CNN | Cascade RPN | R-50-FPN | caffe | 1x | - | - | - | 39.9 | [model](https://drive.google.com/file/d/1NmbnuY5VHi8I9FE8xnp5uNvh2i-t-6_L/view?usp=sharing) |
-| Faster R-CNN | Cascade RPN | R-50-FPN | caffe | 1x | - | - | - | 40.4 | [model](https://drive.google.com/file/d/1dS3Q66qXMJpcuuQgDNkLp669E5w1UMuZ/view?usp=sharing) |
diff --git a/spaces/tomofi/NDLOCR/src/ndl_layout/mmdetection/configs/faster_rcnn/faster_rcnn_r50_fpn_iou_1x_coco.py b/spaces/tomofi/NDLOCR/src/ndl_layout/mmdetection/configs/faster_rcnn/faster_rcnn_r50_fpn_iou_1x_coco.py
deleted file mode 100644
index ddf663e4f0e1525490a493674b32b3dc4c781bb2..0000000000000000000000000000000000000000
--- a/spaces/tomofi/NDLOCR/src/ndl_layout/mmdetection/configs/faster_rcnn/faster_rcnn_r50_fpn_iou_1x_coco.py
+++ /dev/null
@@ -1,6 +0,0 @@
-_base_ = './faster_rcnn_r50_fpn_1x_coco.py'
-model = dict(
- roi_head=dict(
- bbox_head=dict(
- reg_decoded_bbox=True,
- loss_bbox=dict(type='IoULoss', loss_weight=10.0))))
diff --git a/spaces/tomofi/NDLOCR/src/ndl_layout/mmdetection/mmdet/datasets/pipelines/instaboost.py b/spaces/tomofi/NDLOCR/src/ndl_layout/mmdetection/mmdet/datasets/pipelines/instaboost.py
deleted file mode 100644
index 38b6819f60587a6e0c0f6d57bfda32bb3a7a4267..0000000000000000000000000000000000000000
--- a/spaces/tomofi/NDLOCR/src/ndl_layout/mmdetection/mmdet/datasets/pipelines/instaboost.py
+++ /dev/null
@@ -1,98 +0,0 @@
-import numpy as np
-
-from ..builder import PIPELINES
-
-
-@PIPELINES.register_module()
-class InstaBoost(object):
- r"""Data augmentation method in `InstaBoost: Boosting Instance
- Segmentation Via Probability Map Guided Copy-Pasting
- `_.
-
- Refer to https://github.com/GothicAi/Instaboost for implementation details.
- """
-
- def __init__(self,
- action_candidate=('normal', 'horizontal', 'skip'),
- action_prob=(1, 0, 0),
- scale=(0.8, 1.2),
- dx=15,
- dy=15,
- theta=(-1, 1),
- color_prob=0.5,
- hflag=False,
- aug_ratio=0.5):
- try:
- import instaboostfast as instaboost
- except ImportError:
- raise ImportError(
- 'Please run "pip install instaboostfast" '
- 'to install instaboostfast first for instaboost augmentation.')
- self.cfg = instaboost.InstaBoostConfig(action_candidate, action_prob,
- scale, dx, dy, theta,
- color_prob, hflag)
- self.aug_ratio = aug_ratio
-
- def _load_anns(self, results):
- labels = results['ann_info']['labels']
- masks = results['ann_info']['masks']
- bboxes = results['ann_info']['bboxes']
- n = len(labels)
-
- anns = []
- for i in range(n):
- label = labels[i]
- bbox = bboxes[i]
- mask = masks[i]
- x1, y1, x2, y2 = bbox
- # assert (x2 - x1) >= 1 and (y2 - y1) >= 1
- bbox = [x1, y1, x2 - x1, y2 - y1]
- anns.append({
- 'category_id': label,
- 'segmentation': mask,
- 'bbox': bbox
- })
-
- return anns
-
- def _parse_anns(self, results, anns, img):
- gt_bboxes = []
- gt_labels = []
- gt_masks_ann = []
- for ann in anns:
- x1, y1, w, h = ann['bbox']
- # TODO: more essential bug need to be fixed in instaboost
- if w <= 0 or h <= 0:
- continue
- bbox = [x1, y1, x1 + w, y1 + h]
- gt_bboxes.append(bbox)
- gt_labels.append(ann['category_id'])
- gt_masks_ann.append(ann['segmentation'])
- gt_bboxes = np.array(gt_bboxes, dtype=np.float32)
- gt_labels = np.array(gt_labels, dtype=np.int64)
- results['ann_info']['labels'] = gt_labels
- results['ann_info']['bboxes'] = gt_bboxes
- results['ann_info']['masks'] = gt_masks_ann
- results['img'] = img
- return results
-
- def __call__(self, results):
- img = results['img']
- orig_type = img.dtype
- anns = self._load_anns(results)
- if np.random.choice([0, 1], p=[1 - self.aug_ratio, self.aug_ratio]):
- try:
- import instaboostfast as instaboost
- except ImportError:
- raise ImportError('Please run "pip install instaboostfast" '
- 'to install instaboostfast first.')
- anns, img = instaboost.get_new_data(
- anns, img.astype(np.uint8), self.cfg, background=None)
-
- results = self._parse_anns(results, anns, img.astype(orig_type))
- return results
-
- def __repr__(self):
- repr_str = self.__class__.__name__
- repr_str += f'(cfg={self.cfg}, aug_ratio={self.aug_ratio})'
- return repr_str
diff --git a/spaces/tomofi/NDLOCR/src/ndl_layout/mmdetection/mmdet/models/roi_heads/__init__.py b/spaces/tomofi/NDLOCR/src/ndl_layout/mmdetection/mmdet/models/roi_heads/__init__.py
deleted file mode 100644
index c7c6ca2d5e1bad089202d4cc406ace44788dec98..0000000000000000000000000000000000000000
--- a/spaces/tomofi/NDLOCR/src/ndl_layout/mmdetection/mmdet/models/roi_heads/__init__.py
+++ /dev/null
@@ -1,36 +0,0 @@
-from .base_roi_head import BaseRoIHead
-from .bbox_heads import (BBoxHead, ConvFCBBoxHead, DIIHead,
- DoubleConvFCBBoxHead, SABLHead, SCNetBBoxHead,
- Shared2FCBBoxHead, Shared4Conv1FCBBoxHead)
-from .cascade_roi_head import CascadeRoIHead
-from .double_roi_head import DoubleHeadRoIHead
-from .dynamic_roi_head import DynamicRoIHead
-from .grid_roi_head import GridRoIHead
-from .htc_roi_head import HybridTaskCascadeRoIHead
-from .mask_heads import (CoarseMaskHead, FCNMaskHead, FeatureRelayHead,
- FusedSemanticHead, GlobalContextHead, GridHead,
- HTCMaskHead, MaskIoUHead, MaskPointHead,
- SCNetMaskHead, SCNetSemanticHead)
-from .mask_scoring_roi_head import MaskScoringRoIHead
-from .pisa_roi_head import PISARoIHead
-from .point_rend_roi_head import PointRendRoIHead
-from .roi_extractors import (BaseRoIExtractor, GenericRoIExtractor,
- SingleRoIExtractor)
-from .scnet_roi_head import SCNetRoIHead
-from .shared_heads import ResLayer
-from .sparse_roi_head import SparseRoIHead
-from .standard_roi_head import StandardRoIHead
-from .trident_roi_head import TridentRoIHead
-
-__all__ = [
- 'BaseRoIHead', 'CascadeRoIHead', 'DoubleHeadRoIHead', 'MaskScoringRoIHead',
- 'HybridTaskCascadeRoIHead', 'GridRoIHead', 'ResLayer', 'BBoxHead',
- 'ConvFCBBoxHead', 'DIIHead', 'SABLHead', 'Shared2FCBBoxHead',
- 'StandardRoIHead', 'Shared4Conv1FCBBoxHead', 'DoubleConvFCBBoxHead',
- 'FCNMaskHead', 'HTCMaskHead', 'FusedSemanticHead', 'GridHead',
- 'MaskIoUHead', 'BaseRoIExtractor', 'GenericRoIExtractor',
- 'SingleRoIExtractor', 'PISARoIHead', 'PointRendRoIHead', 'MaskPointHead',
- 'CoarseMaskHead', 'DynamicRoIHead', 'SparseRoIHead', 'TridentRoIHead',
- 'SCNetRoIHead', 'SCNetMaskHead', 'SCNetSemanticHead', 'SCNetBBoxHead',
- 'FeatureRelayHead', 'GlobalContextHead'
-]
diff --git a/spaces/trysem/image-matting-app/ppmatting/models/losses/__init__.py b/spaces/trysem/image-matting-app/ppmatting/models/losses/__init__.py
deleted file mode 100644
index 4e309f46c7edd25ff514e670a567b23a14e5fd27..0000000000000000000000000000000000000000
--- a/spaces/trysem/image-matting-app/ppmatting/models/losses/__init__.py
+++ /dev/null
@@ -1 +0,0 @@
-from .loss import *
diff --git a/spaces/ttt246/brain/Extension/src/pages/Background/index.js b/spaces/ttt246/brain/Extension/src/pages/Background/index.js
deleted file mode 100644
index 4455983323c330576fe8e1509c742f13e46e27f2..0000000000000000000000000000000000000000
--- a/spaces/ttt246/brain/Extension/src/pages/Background/index.js
+++ /dev/null
@@ -1,27 +0,0 @@
-// Create the context menu item
-chrome.runtime.onInstalled.addListener(function() {
- chrome.contextMenus.create({
- id: 'risingExtension',
- title: 'rising extension',
- contexts: ['page'],
- });
-});
-
-// Handle the context menu item click
-chrome.contextMenus.onClicked.addListener(function(info) {
- if (info.menuItemId === 'risingExtension') {
- chrome.tabs.query({ active: true, currentWindow: true }, function (tabs) {
- chrome.tabs.sendMessage(tabs[0].id, { action: "open-modal" });
- });
- }
-});
-
-// Handle the local storage get value
-chrome.runtime.onMessage.addListener((request, sender, sendResponse) => {
- if (request.method === 'getLocalStorage') {
- chrome.storage.local.get(function(result) {
- sendResponse({ data: result });
- });
- }
- return true; // Important for asynchronous sendMessage
-});
\ No newline at end of file
diff --git a/spaces/usbethFlerru/sovits-modelsV2/example/Abbyy Finereader 11 Professional Edition Torrent Download Why You Need This Amazing Tool for Scanning and Editing PDFs.md b/spaces/usbethFlerru/sovits-modelsV2/example/Abbyy Finereader 11 Professional Edition Torrent Download Why You Need This Amazing Tool for Scanning and Editing PDFs.md
deleted file mode 100644
index 570bde3b5c4f1bf91f2141751b399d325f0e9ea8..0000000000000000000000000000000000000000
--- a/spaces/usbethFlerru/sovits-modelsV2/example/Abbyy Finereader 11 Professional Edition Torrent Download Why You Need This Amazing Tool for Scanning and Editing PDFs.md
+++ /dev/null
@@ -1,6 +0,0 @@
-Abbyy Finereader 11 Professional Edition Torrent Download
Download ✦✦✦ https://urlcod.com/2uyW6Q
-
- aaccfb2cb3
-
-
-
diff --git a/spaces/ussarata/storygen/README.md b/spaces/ussarata/storygen/README.md
deleted file mode 100644
index 3ab3d34a076e7771b1b01204831fcaaa8a51343a..0000000000000000000000000000000000000000
--- a/spaces/ussarata/storygen/README.md
+++ /dev/null
@@ -1,12 +0,0 @@
----
-title: Storygen
-emoji: 🐢
-colorFrom: red
-colorTo: pink
-sdk: gradio
-sdk_version: 3.16.2
-app_file: app.py
-pinned: false
----
-
-Check out the configuration reference at https://huggingface.co/docs/hub/spaces-config-reference
diff --git a/spaces/vaishanthr/Simultaneous-Segmented-Depth-Prediction/midas/midas_net_custom.py b/spaces/vaishanthr/Simultaneous-Segmented-Depth-Prediction/midas/midas_net_custom.py
deleted file mode 100644
index 50e4acb5e53d5fabefe3dde16ab49c33c2b7797c..0000000000000000000000000000000000000000
--- a/spaces/vaishanthr/Simultaneous-Segmented-Depth-Prediction/midas/midas_net_custom.py
+++ /dev/null
@@ -1,128 +0,0 @@
-"""MidashNet: Network for monocular depth estimation trained by mixing several datasets.
-This file contains code that is adapted from
-https://github.com/thomasjpfan/pytorch_refinenet/blob/master/pytorch_refinenet/refinenet/refinenet_4cascade.py
-"""
-import torch
-import torch.nn as nn
-
-from .base_model import BaseModel
-from .blocks import FeatureFusionBlock, FeatureFusionBlock_custom, Interpolate, _make_encoder
-
-
-class MidasNet_small(BaseModel):
- """Network for monocular depth estimation.
- """
-
- def __init__(self, path=None, features=64, backbone="efficientnet_lite3", non_negative=True, exportable=True, channels_last=False, align_corners=True,
- blocks={'expand': True}):
- """Init.
-
- Args:
- path (str, optional): Path to saved model. Defaults to None.
- features (int, optional): Number of features. Defaults to 256.
- backbone (str, optional): Backbone network for encoder. Defaults to resnet50
- """
- print("Loading weights: ", path)
-
- super(MidasNet_small, self).__init__()
-
- use_pretrained = False if path else True
-
- self.channels_last = channels_last
- self.blocks = blocks
- self.backbone = backbone
-
- self.groups = 1
-
- features1=features
- features2=features
- features3=features
- features4=features
- self.expand = False
- if "expand" in self.blocks and self.blocks['expand'] == True:
- self.expand = True
- features1=features
- features2=features*2
- features3=features*4
- features4=features*8
-
- self.pretrained, self.scratch = _make_encoder(self.backbone, features, use_pretrained, groups=self.groups, expand=self.expand, exportable=exportable)
-
- self.scratch.activation = nn.ReLU(False)
-
- self.scratch.refinenet4 = FeatureFusionBlock_custom(features4, self.scratch.activation, deconv=False, bn=False, expand=self.expand, align_corners=align_corners)
- self.scratch.refinenet3 = FeatureFusionBlock_custom(features3, self.scratch.activation, deconv=False, bn=False, expand=self.expand, align_corners=align_corners)
- self.scratch.refinenet2 = FeatureFusionBlock_custom(features2, self.scratch.activation, deconv=False, bn=False, expand=self.expand, align_corners=align_corners)
- self.scratch.refinenet1 = FeatureFusionBlock_custom(features1, self.scratch.activation, deconv=False, bn=False, align_corners=align_corners)
-
-
- self.scratch.output_conv = nn.Sequential(
- nn.Conv2d(features, features//2, kernel_size=3, stride=1, padding=1, groups=self.groups),
- Interpolate(scale_factor=2, mode="bilinear"),
- nn.Conv2d(features//2, 32, kernel_size=3, stride=1, padding=1),
- self.scratch.activation,
- nn.Conv2d(32, 1, kernel_size=1, stride=1, padding=0),
- nn.ReLU(True) if non_negative else nn.Identity(),
- nn.Identity(),
- )
-
- if path:
- self.load(path)
-
-
- def forward(self, x):
- """Forward pass.
-
- Args:
- x (tensor): input data (image)
-
- Returns:
- tensor: depth
- """
- if self.channels_last==True:
- print("self.channels_last = ", self.channels_last)
- x.contiguous(memory_format=torch.channels_last)
-
-
- layer_1 = self.pretrained.layer1(x)
- layer_2 = self.pretrained.layer2(layer_1)
- layer_3 = self.pretrained.layer3(layer_2)
- layer_4 = self.pretrained.layer4(layer_3)
-
- layer_1_rn = self.scratch.layer1_rn(layer_1)
- layer_2_rn = self.scratch.layer2_rn(layer_2)
- layer_3_rn = self.scratch.layer3_rn(layer_3)
- layer_4_rn = self.scratch.layer4_rn(layer_4)
-
-
- path_4 = self.scratch.refinenet4(layer_4_rn)
- path_3 = self.scratch.refinenet3(path_4, layer_3_rn)
- path_2 = self.scratch.refinenet2(path_3, layer_2_rn)
- path_1 = self.scratch.refinenet1(path_2, layer_1_rn)
-
- out = self.scratch.output_conv(path_1)
-
- return torch.squeeze(out, dim=1)
-
-
-
-def fuse_model(m):
- prev_previous_type = nn.Identity()
- prev_previous_name = ''
- previous_type = nn.Identity()
- previous_name = ''
- for name, module in m.named_modules():
- if prev_previous_type == nn.Conv2d and previous_type == nn.BatchNorm2d and type(module) == nn.ReLU:
- # print("FUSED ", prev_previous_name, previous_name, name)
- torch.quantization.fuse_modules(m, [prev_previous_name, previous_name, name], inplace=True)
- elif prev_previous_type == nn.Conv2d and previous_type == nn.BatchNorm2d:
- # print("FUSED ", prev_previous_name, previous_name)
- torch.quantization.fuse_modules(m, [prev_previous_name, previous_name], inplace=True)
- # elif previous_type == nn.Conv2d and type(module) == nn.ReLU:
- # print("FUSED ", previous_name, name)
- # torch.quantization.fuse_modules(m, [previous_name, name], inplace=True)
-
- prev_previous_type = previous_type
- prev_previous_name = previous_name
- previous_type = type(module)
- previous_name = name
\ No newline at end of file
diff --git a/spaces/vaishanthr/Simultaneous-Segmented-Depth-Prediction/yolov8/ultralytics/__init__.py b/spaces/vaishanthr/Simultaneous-Segmented-Depth-Prediction/yolov8/ultralytics/__init__.py
deleted file mode 100644
index 5800644cc1c0ae32ffc1fb3a2a8482cea5b4d7a8..0000000000000000000000000000000000000000
--- a/spaces/vaishanthr/Simultaneous-Segmented-Depth-Prediction/yolov8/ultralytics/__init__.py
+++ /dev/null
@@ -1,14 +0,0 @@
-# Ultralytics YOLO 🚀, AGPL-3.0 license
-
-__version__ = '8.0.131'
-
-from ultralytics.hub import start
-from ultralytics.vit.rtdetr import RTDETR
-from ultralytics.vit.sam import SAM
-from ultralytics.yolo.engine.model import YOLO
-from ultralytics.yolo.fastsam import FastSAM
-from ultralytics.yolo.nas import NAS
-from ultralytics.yolo.utils.checks import check_yolo as checks
-from ultralytics.yolo.utils.downloads import download
-
-__all__ = '__version__', 'YOLO', 'NAS', 'SAM', 'FastSAM', 'RTDETR', 'checks', 'download', 'start' # allow simpler import
diff --git a/spaces/veb-101/driver-drowsiness-detection/ads.py b/spaces/veb-101/driver-drowsiness-detection/ads.py
deleted file mode 100644
index 20f92e91aadb14283a807ac137a2587162e05124..0000000000000000000000000000000000000000
--- a/spaces/veb-101/driver-drowsiness-detection/ads.py
+++ /dev/null
@@ -1,31 +0,0 @@
-css_string = """
-
-
-
-
- """
diff --git a/spaces/vinthony/SadTalker/src/utils/model2safetensor.py b/spaces/vinthony/SadTalker/src/utils/model2safetensor.py
deleted file mode 100644
index 50c485000d43ba9c230a0bc64ce8aeaaec6e2b29..0000000000000000000000000000000000000000
--- a/spaces/vinthony/SadTalker/src/utils/model2safetensor.py
+++ /dev/null
@@ -1,141 +0,0 @@
-import torch
-import yaml
-import os
-
-import safetensors
-from safetensors.torch import save_file
-from yacs.config import CfgNode as CN
-import sys
-
-sys.path.append('/apdcephfs/private_shadowcun/SadTalker')
-
-from src.face3d.models import networks
-
-from src.facerender.modules.keypoint_detector import HEEstimator, KPDetector
-from src.facerender.modules.mapping import MappingNet
-from src.facerender.modules.generator import OcclusionAwareGenerator, OcclusionAwareSPADEGenerator
-
-from src.audio2pose_models.audio2pose import Audio2Pose
-from src.audio2exp_models.networks import SimpleWrapperV2
-from src.test_audio2coeff import load_cpk
-
-size = 256
-############ face vid2vid
-config_path = os.path.join('src', 'config', 'facerender.yaml')
-current_root_path = '.'
-
-path_of_net_recon_model = os.path.join(current_root_path, 'checkpoints', 'epoch_20.pth')
-net_recon = networks.define_net_recon(net_recon='resnet50', use_last_fc=False, init_path='')
-checkpoint = torch.load(path_of_net_recon_model, map_location='cpu')
-net_recon.load_state_dict(checkpoint['net_recon'])
-
-with open(config_path) as f:
- config = yaml.safe_load(f)
-
-generator = OcclusionAwareSPADEGenerator(**config['model_params']['generator_params'],
- **config['model_params']['common_params'])
-kp_extractor = KPDetector(**config['model_params']['kp_detector_params'],
- **config['model_params']['common_params'])
-he_estimator = HEEstimator(**config['model_params']['he_estimator_params'],
- **config['model_params']['common_params'])
-mapping = MappingNet(**config['model_params']['mapping_params'])
-
-def load_cpk_facevid2vid(checkpoint_path, generator=None, discriminator=None,
- kp_detector=None, he_estimator=None, optimizer_generator=None,
- optimizer_discriminator=None, optimizer_kp_detector=None,
- optimizer_he_estimator=None, device="cpu"):
-
- checkpoint = torch.load(checkpoint_path, map_location=torch.device(device))
- if generator is not None:
- generator.load_state_dict(checkpoint['generator'])
- if kp_detector is not None:
- kp_detector.load_state_dict(checkpoint['kp_detector'])
- if he_estimator is not None:
- he_estimator.load_state_dict(checkpoint['he_estimator'])
- if discriminator is not None:
- try:
- discriminator.load_state_dict(checkpoint['discriminator'])
- except:
- print ('No discriminator in the state-dict. Dicriminator will be randomly initialized')
- if optimizer_generator is not None:
- optimizer_generator.load_state_dict(checkpoint['optimizer_generator'])
- if optimizer_discriminator is not None:
- try:
- optimizer_discriminator.load_state_dict(checkpoint['optimizer_discriminator'])
- except RuntimeError as e:
- print ('No discriminator optimizer in the state-dict. Optimizer will be not initialized')
- if optimizer_kp_detector is not None:
- optimizer_kp_detector.load_state_dict(checkpoint['optimizer_kp_detector'])
- if optimizer_he_estimator is not None:
- optimizer_he_estimator.load_state_dict(checkpoint['optimizer_he_estimator'])
-
- return checkpoint['epoch']
-
-
-def load_cpk_facevid2vid_safetensor(checkpoint_path, generator=None,
- kp_detector=None, he_estimator=None,
- device="cpu"):
-
- checkpoint = safetensors.torch.load_file(checkpoint_path)
-
- if generator is not None:
- x_generator = {}
- for k,v in checkpoint.items():
- if 'generator' in k:
- x_generator[k.replace('generator.', '')] = v
- generator.load_state_dict(x_generator)
- if kp_detector is not None:
- x_generator = {}
- for k,v in checkpoint.items():
- if 'kp_extractor' in k:
- x_generator[k.replace('kp_extractor.', '')] = v
- kp_detector.load_state_dict(x_generator)
- if he_estimator is not None:
- x_generator = {}
- for k,v in checkpoint.items():
- if 'he_estimator' in k:
- x_generator[k.replace('he_estimator.', '')] = v
- he_estimator.load_state_dict(x_generator)
-
- return None
-
-free_view_checkpoint = '/apdcephfs/private_shadowcun/SadTalker/checkpoints/facevid2vid_'+str(size)+'-model.pth.tar'
-load_cpk_facevid2vid(free_view_checkpoint, kp_detector=kp_extractor, generator=generator, he_estimator=he_estimator)
-
-wav2lip_checkpoint = os.path.join(current_root_path, 'checkpoints', 'wav2lip.pth')
-
-audio2pose_checkpoint = os.path.join(current_root_path, 'checkpoints', 'auido2pose_00140-model.pth')
-audio2pose_yaml_path = os.path.join(current_root_path, 'src', 'config', 'auido2pose.yaml')
-
-audio2exp_checkpoint = os.path.join(current_root_path, 'checkpoints', 'auido2exp_00300-model.pth')
-audio2exp_yaml_path = os.path.join(current_root_path, 'src', 'config', 'auido2exp.yaml')
-
-fcfg_pose = open(audio2pose_yaml_path)
-cfg_pose = CN.load_cfg(fcfg_pose)
-cfg_pose.freeze()
-audio2pose_model = Audio2Pose(cfg_pose, wav2lip_checkpoint)
-audio2pose_model.eval()
-load_cpk(audio2pose_checkpoint, model=audio2pose_model, device='cpu')
-
-# load audio2exp_model
-netG = SimpleWrapperV2()
-netG.eval()
-load_cpk(audio2exp_checkpoint, model=netG, device='cpu')
-
-class SadTalker(torch.nn.Module):
- def __init__(self, kp_extractor, generator, netG, audio2pose, face_3drecon):
- super(SadTalker, self).__init__()
- self.kp_extractor = kp_extractor
- self.generator = generator
- self.audio2exp = netG
- self.audio2pose = audio2pose
- self.face_3drecon = face_3drecon
-
-
-model = SadTalker(kp_extractor, generator, netG, audio2pose_model, net_recon)
-
-# here, we want to convert it to safetensor
-save_file(model.state_dict(), "checkpoints/SadTalker_V0.0.2_"+str(size)+".safetensors")
-
-### test
-load_cpk_facevid2vid_safetensor('checkpoints/SadTalker_V0.0.2_'+str(size)+'.safetensors', kp_detector=kp_extractor, generator=generator, he_estimator=None)
\ No newline at end of file
diff --git a/spaces/vivien/clip/README.md b/spaces/vivien/clip/README.md
deleted file mode 100644
index 3e0e3aa5afbdd40283a3a2c4e61873b1120d11b2..0000000000000000000000000000000000000000
--- a/spaces/vivien/clip/README.md
+++ /dev/null
@@ -1,38 +0,0 @@
----
-title: Clip Demo
-emoji: 👁
-colorFrom: indigo
-colorTo: blue
-sdk: streamlit
-sdk_version: 1.2.0
-app_file: app.py
-pinned: false
----
-
-# Configuration
-
-`title`: _string_
-Display title for the Space
-
-`emoji`: _string_
-Space emoji (emoji-only character allowed)
-
-`colorFrom`: _string_
-Color for Thumbnail gradient (red, yellow, green, blue, indigo, purple, pink, gray)
-
-`colorTo`: _string_
-Color for Thumbnail gradient (red, yellow, green, blue, indigo, purple, pink, gray)
-
-`sdk`: _string_
-Can be either `gradio` or `streamlit`
-
-`sdk_version` : _string_
-Only applicable for `streamlit` SDK.
-See [doc](https://hf.co/docs/hub/spaces) for more info on supported versions.
-
-`app_file`: _string_
-Path to your main application file (which contains either `gradio` or `streamlit` Python code).
-Path is relative to the root of the repository.
-
-`pinned`: _boolean_
-Whether the Space stays on top of your list.
diff --git a/spaces/w1zrd/MusicGen/audiocraft/models/encodec.py b/spaces/w1zrd/MusicGen/audiocraft/models/encodec.py
deleted file mode 100644
index 69621a695887b0b41614c51cae020f6fd0af221d..0000000000000000000000000000000000000000
--- a/spaces/w1zrd/MusicGen/audiocraft/models/encodec.py
+++ /dev/null
@@ -1,302 +0,0 @@
-# Copyright (c) Meta Platforms, Inc. and affiliates.
-# All rights reserved.
-#
-# This source code is licensed under the license found in the
-# LICENSE file in the root directory of this source tree.
-
-from abc import ABC, abstractmethod
-import typing as tp
-
-from einops import rearrange
-import torch
-from torch import nn
-
-from .. import quantization as qt
-
-
-class CompressionModel(ABC, nn.Module):
-
- @abstractmethod
- def forward(self, x: torch.Tensor) -> qt.QuantizedResult:
- ...
-
- @abstractmethod
- def encode(self, x: torch.Tensor) -> tp.Tuple[torch.Tensor, tp.Optional[torch.Tensor]]:
- """See `EncodecModel.encode`"""
- ...
-
- @abstractmethod
- def decode(self, codes: torch.Tensor, scale: tp.Optional[torch.Tensor] = None):
- """See `EncodecModel.decode`"""
- ...
-
- @property
- @abstractmethod
- def channels(self) -> int:
- ...
-
- @property
- @abstractmethod
- def frame_rate(self) -> int:
- ...
-
- @property
- @abstractmethod
- def sample_rate(self) -> int:
- ...
-
- @property
- @abstractmethod
- def cardinality(self) -> int:
- ...
-
- @property
- @abstractmethod
- def num_codebooks(self) -> int:
- ...
-
- @property
- @abstractmethod
- def total_codebooks(self) -> int:
- ...
-
- @abstractmethod
- def set_num_codebooks(self, n: int):
- """Set the active number of codebooks used by the quantizer.
- """
- ...
-
-
-class EncodecModel(CompressionModel):
- """Encodec model operating on the raw waveform.
-
- Args:
- encoder (nn.Module): Encoder network.
- decoder (nn.Module): Decoder network.
- quantizer (qt.BaseQuantizer): Quantizer network.
- frame_rate (int): Frame rate for the latent representation.
- sample_rate (int): Audio sample rate.
- channels (int): Number of audio channels.
- causal (bool): Whether to use a causal version of the model.
- renormalize (bool): Whether to renormalize the audio before running the model.
- """
- # we need assignement to override the property in the abstract class,
- # I couldn't find a better way...
- frame_rate: int = 0
- sample_rate: int = 0
- channels: int = 0
-
- def __init__(self,
- encoder: nn.Module,
- decoder: nn.Module,
- quantizer: qt.BaseQuantizer,
- frame_rate: int,
- sample_rate: int,
- channels: int,
- causal: bool = False,
- renormalize: bool = False):
- super().__init__()
- self.encoder = encoder
- self.decoder = decoder
- self.quantizer = quantizer
- self.frame_rate = frame_rate
- self.sample_rate = sample_rate
- self.channels = channels
- self.renormalize = renormalize
- self.causal = causal
- if self.causal:
- # we force disabling here to avoid handling linear overlap of segments
- # as supported in original EnCodec codebase.
- assert not self.renormalize, 'Causal model does not support renormalize'
-
- @property
- def total_codebooks(self):
- """Total number of quantizer codebooks available.
- """
- return self.quantizer.total_codebooks
-
- @property
- def num_codebooks(self):
- """Active number of codebooks used by the quantizer.
- """
- return self.quantizer.num_codebooks
-
- def set_num_codebooks(self, n: int):
- """Set the active number of codebooks used by the quantizer.
- """
- self.quantizer.set_num_codebooks(n)
-
- @property
- def cardinality(self):
- """Cardinality of each codebook.
- """
- return self.quantizer.bins
-
- def preprocess(self, x: torch.Tensor) -> tp.Tuple[torch.Tensor, tp.Optional[torch.Tensor]]:
- scale: tp.Optional[torch.Tensor]
- if self.renormalize:
- mono = x.mean(dim=1, keepdim=True)
- volume = mono.pow(2).mean(dim=2, keepdim=True).sqrt()
- scale = 1e-8 + volume
- x = x / scale
- scale = scale.view(-1, 1)
- else:
- scale = None
- return x, scale
-
- def postprocess(self,
- x: torch.Tensor,
- scale: tp.Optional[torch.Tensor] = None) -> torch.Tensor:
- if scale is not None:
- assert self.renormalize
- x = x * scale.view(-1, 1, 1)
- return x
-
- def forward(self, x: torch.Tensor) -> qt.QuantizedResult:
- assert x.dim() == 3
- length = x.shape[-1]
- x, scale = self.preprocess(x)
-
- emb = self.encoder(x)
- q_res = self.quantizer(emb, self.frame_rate)
- out = self.decoder(q_res.x)
-
- # remove extra padding added by the encoder and decoder
- assert out.shape[-1] >= length, (out.shape[-1], length)
- out = out[..., :length]
-
- q_res.x = self.postprocess(out, scale)
-
- return q_res
-
- def encode(self, x: torch.Tensor) -> tp.Tuple[torch.Tensor, tp.Optional[torch.Tensor]]:
- """Encode the given input tensor to quantized representation along with scale parameter.
-
- Args:
- x (torch.Tensor): Float tensor of shape [B, C, T]
-
- Returns:
- codes, scale (tp.Tuple[torch.Tensor, torch.Tensor]): Tuple composed of:
- codes a float tensor of shape [B, K, T] with K the number of codebooks used and T the timestep.
- scale a float tensor containing the scale for audio renormalizealization.
- """
- assert x.dim() == 3
- x, scale = self.preprocess(x)
- emb = self.encoder(x)
- codes = self.quantizer.encode(emb)
- return codes, scale
-
- def decode(self, codes: torch.Tensor, scale: tp.Optional[torch.Tensor] = None):
- """Decode the given codes to a reconstructed representation, using the scale to perform
- audio denormalization if needed.
-
- Args:
- codes (torch.Tensor): Int tensor of shape [B, K, T]
- scale (tp.Optional[torch.Tensor]): Float tensor containing the scale value.
-
- Returns:
- out (torch.Tensor): Float tensor of shape [B, C, T], the reconstructed audio.
- """
- emb = self.quantizer.decode(codes)
- out = self.decoder(emb)
- out = self.postprocess(out, scale)
- # out contains extra padding added by the encoder and decoder
- return out
-
-
-class FlattenedCompressionModel(CompressionModel):
- """Wraps a CompressionModel and flatten its codebooks, e.g.
- instead of returning [B, K, T], return [B, S, T * (K // S)] with
- S the number of codebooks per step, and `K // S` the number of 'virtual steps'
- for each real time step.
-
- Args:
- model (CompressionModel): compression model to wrap.
- codebooks_per_step (int): number of codebooks to keep per step,
- this must divide the number of codebooks provided by the wrapped model.
- extend_cardinality (bool): if True, and for instance if codebooks_per_step = 1,
- if each codebook has a cardinality N, then the first codebook will
- use the range [0, N - 1], and the second [N, 2 N - 1] etc.
- On decoding, this can lead to potentially invalid sequences.
- Any invalid entry will be silently remapped to the proper range
- with a modulo.
- """
- def __init__(self, model: CompressionModel, codebooks_per_step: int = 1,
- extend_cardinality: bool = True):
- super().__init__()
- self.model = model
- self.codebooks_per_step = codebooks_per_step
- self.extend_cardinality = extend_cardinality
-
- @property
- def total_codebooks(self):
- return self.model.total_codebooks
-
- @property
- def num_codebooks(self):
- """Active number of codebooks used by the quantizer.
-
- ..Warning:: this reports the number of codebooks after the flattening
- of the codebooks!
- """
- assert self.model.num_codebooks % self.codebooks_per_step == 0
- return self.codebooks_per_step
-
- def set_num_codebooks(self, n: int):
- """Set the active number of codebooks used by the quantizer.
-
- ..Warning:: this sets the number of codebooks **before** the flattening
- of the codebooks.
- """
- assert n % self.codebooks_per_step == 0
- self.model.set_num_codebooks(n)
-
- @property
- def num_virtual_steps(self) -> int:
- """Return the number of virtual steps, e.g. one real step
- will be split into that many steps.
- """
- return self.model.num_codebooks // self.codebooks_per_step
-
- @property
- def frame_rate(self) -> int:
- return self.model.frame_rate * self.num_virtual_steps
-
- @property
- def sample_rate(self) -> int:
- return self.model.sample_rate
-
- @property
- def channels(self) -> int:
- return self.model.channels
-
- @property
- def cardinality(self):
- """Cardinality of each codebook.
- """
- if self.extend_cardinality:
- return self.model.cardinality * self.num_virtual_steps
- else:
- return self.model.cardinality
-
- def forward(self, x: torch.Tensor) -> qt.QuantizedResult:
- raise NotImplementedError("Not supported, use encode and decode.")
-
- def encode(self, x: torch.Tensor) -> tp.Tuple[torch.Tensor, tp.Optional[torch.Tensor]]:
- indices, scales = self.model.encode(x)
- B, K, T = indices.shape
- indices = rearrange(indices, 'b (k v) t -> b k t v', k=self.codebooks_per_step)
- if self.extend_cardinality:
- for virtual_step in range(1, self.num_virtual_steps):
- indices[..., virtual_step] += self.model.cardinality * virtual_step
- indices = rearrange(indices, 'b k t v -> b k (t v)')
- return (indices, scales)
-
- def decode(self, codes: torch.Tensor, scale: tp.Optional[torch.Tensor] = None):
- B, K, T = codes.shape
- assert T % self.num_virtual_steps == 0
- codes = rearrange(codes, 'b k (t v) -> b (k v) t', v=self.num_virtual_steps)
- # We silently ignore potential errors from the LM when
- # using extend_cardinality.
- codes = codes % self.model.cardinality
- return self.model.decode(codes, scale)
diff --git a/spaces/weibinke/vits-simple-api/vits/text/__init__.py b/spaces/weibinke/vits-simple-api/vits/text/__init__.py
deleted file mode 100644
index 026b69dd07248ce848270b8cf79bbc1acfb97129..0000000000000000000000000000000000000000
--- a/spaces/weibinke/vits-simple-api/vits/text/__init__.py
+++ /dev/null
@@ -1,32 +0,0 @@
-""" from https://github.com/keithito/tacotron """
-from vits.text import cleaners
-
-
-def text_to_sequence(text, symbols, cleaner_names, bert_embedding=False):
- '''Converts a string of text to a sequence of IDs corresponding to the symbols in the text.
- Args:
- text: string to convert to a sequence
- cleaner_names: names of the cleaner functions to run the text through
- Returns:
- List of integers corresponding to the symbols in the text
- '''
-
- _symbol_to_id = {s: i for i, s in enumerate(symbols)}
-
- if bert_embedding:
- cleaned_text, char_embeds = _clean_text(text, cleaner_names)
- sequence = [_symbol_to_id[symbol] for symbol in cleaned_text.split()]
- return sequence, char_embeds
- else:
- cleaned_text = _clean_text(text, cleaner_names)
- sequence = [_symbol_to_id[symbol] for symbol in cleaned_text if symbol in _symbol_to_id.keys()]
- return sequence
-
-
-def _clean_text(text, cleaner_names):
- for name in cleaner_names:
- cleaner = getattr(cleaners, name)
- if not cleaner:
- raise Exception('Unknown cleaner: %s' % name)
- text = cleaner(text)
- return text
diff --git a/spaces/whitphx/gradio-static-test/dist/assets/Copy-d654b047.js b/spaces/whitphx/gradio-static-test/dist/assets/Copy-d654b047.js
deleted file mode 100644
index 22225bed786bed17ffc3f561a6f1b29e0962c888..0000000000000000000000000000000000000000
--- a/spaces/whitphx/gradio-static-test/dist/assets/Copy-d654b047.js
+++ /dev/null
@@ -1,2 +0,0 @@
-import{S as h,i as p,s as c,C as a,D as e,h as u,F as i,G as n,r as d}from"../lite.js";function v(l){let t,s;return{c(){t=a("svg"),s=a("polyline"),e(s,"points","20 6 9 17 4 12"),e(t,"xmlns","http://www.w3.org/2000/svg"),e(t,"width","100%"),e(t,"height","100%"),e(t,"viewBox","0 0 24 24"),e(t,"fill","none"),e(t,"stroke","currentColor"),e(t,"stroke-width","3"),e(t,"stroke-linecap","round"),e(t,"stroke-linejoin","round")},m(o,r){u(o,t,r),i(t,s)},p:n,i:n,o:n,d(o){o&&d(t)}}}class m extends h{constructor(t){super(),p(this,t,null,v,c,{})}}function w(l){let t,s,o;return{c(){t=a("svg"),s=a("path"),o=a("path"),e(s,"fill","currentColor"),e(s,"d","M28 10v18H10V10h18m0-2H10a2 2 0 0 0-2 2v18a2 2 0 0 0 2 2h18a2 2 0 0 0 2-2V10a2 2 0 0 0-2-2Z"),e(o,"fill","currentColor"),e(o,"d","M4 18H2V4a2 2 0 0 1 2-2h14v2H4Z"),e(t,"xmlns","http://www.w3.org/2000/svg"),e(t,"width","100%"),e(t,"height","100%"),e(t,"viewBox","0 0 32 32")},m(r,g){u(r,t,g),i(t,s),i(t,o)},p:n,i:n,o:n,d(r){r&&d(t)}}}class x extends h{constructor(t){super(),p(this,t,null,w,c,{})}}export{x as C,m as a};
-//# sourceMappingURL=Copy-d654b047.js.map
diff --git a/spaces/wy213/213a/src/components/turn-counter.tsx b/spaces/wy213/213a/src/components/turn-counter.tsx
deleted file mode 100644
index 08a9e488f044802a8600f4d195b106567c35aab4..0000000000000000000000000000000000000000
--- a/spaces/wy213/213a/src/components/turn-counter.tsx
+++ /dev/null
@@ -1,23 +0,0 @@
-import React from 'react'
-import { Throttling } from '@/lib/bots/bing/types'
-
-export interface TurnCounterProps {
- throttling?: Throttling
-}
-
-export function TurnCounter({ throttling }: TurnCounterProps) {
- if (!throttling) {
- return null
- }
-
- return (
-
-
- {throttling.numUserMessagesInConversation}
- 共
- {throttling.maxNumUserMessagesInConversation}
-
-
-
- )
-}
diff --git a/spaces/xinyu1205/recognize-anything/GroundingDINO/groundingdino/models/GroundingDINO/ms_deform_attn.py b/spaces/xinyu1205/recognize-anything/GroundingDINO/groundingdino/models/GroundingDINO/ms_deform_attn.py
deleted file mode 100644
index 489d501bef364020212306d81e9b85c8daa27491..0000000000000000000000000000000000000000
--- a/spaces/xinyu1205/recognize-anything/GroundingDINO/groundingdino/models/GroundingDINO/ms_deform_attn.py
+++ /dev/null
@@ -1,413 +0,0 @@
-# ------------------------------------------------------------------------
-# Grounding DINO
-# url: https://github.com/IDEA-Research/GroundingDINO
-# Copyright (c) 2023 IDEA. All Rights Reserved.
-# Licensed under the Apache License, Version 2.0 [see LICENSE for details]
-# ------------------------------------------------------------------------
-# Deformable DETR
-# Copyright (c) 2020 SenseTime. All Rights Reserved.
-# Licensed under the Apache License, Version 2.0 [see LICENSE for details]
-# ------------------------------------------------------------------------------------------------
-# Modified from:
-# https://github.com/fundamentalvision/Deformable-DETR/blob/main/models/ops/functions/ms_deform_attn_func.py
-# https://github.com/fundamentalvision/Deformable-DETR/blob/main/models/ops/modules/ms_deform_attn.py
-# https://github.com/open-mmlab/mmcv/blob/master/mmcv/ops/multi_scale_deform_attn.py
-# ------------------------------------------------------------------------------------------------
-
-import math
-import warnings
-from typing import Optional
-
-import torch
-import torch.nn as nn
-import torch.nn.functional as F
-from torch.autograd import Function
-from torch.autograd.function import once_differentiable
-from torch.nn.init import constant_, xavier_uniform_
-
-try:
- from groundingdino import _C
-except:
- warnings.warn("Failed to load custom C++ ops. Running on CPU mode Only!")
-
-
-# helpers
-def _is_power_of_2(n):
- if (not isinstance(n, int)) or (n < 0):
- raise ValueError("invalid input for _is_power_of_2: {} (type: {})".format(n, type(n)))
- return (n & (n - 1) == 0) and n != 0
-
-
-class MultiScaleDeformableAttnFunction(Function):
- @staticmethod
- def forward(
- ctx,
- value,
- value_spatial_shapes,
- value_level_start_index,
- sampling_locations,
- attention_weights,
- im2col_step,
- ):
- ctx.im2col_step = im2col_step
- output = _C.ms_deform_attn_forward(
- value,
- value_spatial_shapes,
- value_level_start_index,
- sampling_locations,
- attention_weights,
- ctx.im2col_step,
- )
- ctx.save_for_backward(
- value,
- value_spatial_shapes,
- value_level_start_index,
- sampling_locations,
- attention_weights,
- )
- return output
-
- @staticmethod
- @once_differentiable
- def backward(ctx, grad_output):
- (
- value,
- value_spatial_shapes,
- value_level_start_index,
- sampling_locations,
- attention_weights,
- ) = ctx.saved_tensors
- grad_value, grad_sampling_loc, grad_attn_weight = _C.ms_deform_attn_backward(
- value,
- value_spatial_shapes,
- value_level_start_index,
- sampling_locations,
- attention_weights,
- grad_output,
- ctx.im2col_step,
- )
-
- return grad_value, None, None, grad_sampling_loc, grad_attn_weight, None
-
-
-def multi_scale_deformable_attn_pytorch(
- value: torch.Tensor,
- value_spatial_shapes: torch.Tensor,
- sampling_locations: torch.Tensor,
- attention_weights: torch.Tensor,
-) -> torch.Tensor:
-
- bs, _, num_heads, embed_dims = value.shape
- _, num_queries, num_heads, num_levels, num_points, _ = sampling_locations.shape
- value_list = value.split([H_ * W_ for H_, W_ in value_spatial_shapes], dim=1)
- sampling_grids = 2 * sampling_locations - 1
- sampling_value_list = []
- for level, (H_, W_) in enumerate(value_spatial_shapes):
- # bs, H_*W_, num_heads, embed_dims ->
- # bs, H_*W_, num_heads*embed_dims ->
- # bs, num_heads*embed_dims, H_*W_ ->
- # bs*num_heads, embed_dims, H_, W_
- value_l_ = (
- value_list[level].flatten(2).transpose(1, 2).reshape(bs * num_heads, embed_dims, H_, W_)
- )
- # bs, num_queries, num_heads, num_points, 2 ->
- # bs, num_heads, num_queries, num_points, 2 ->
- # bs*num_heads, num_queries, num_points, 2
- sampling_grid_l_ = sampling_grids[:, :, :, level].transpose(1, 2).flatten(0, 1)
- # bs*num_heads, embed_dims, num_queries, num_points
- sampling_value_l_ = F.grid_sample(
- value_l_, sampling_grid_l_, mode="bilinear", padding_mode="zeros", align_corners=False
- )
- sampling_value_list.append(sampling_value_l_)
- # (bs, num_queries, num_heads, num_levels, num_points) ->
- # (bs, num_heads, num_queries, num_levels, num_points) ->
- # (bs, num_heads, 1, num_queries, num_levels*num_points)
- attention_weights = attention_weights.transpose(1, 2).reshape(
- bs * num_heads, 1, num_queries, num_levels * num_points
- )
- output = (
- (torch.stack(sampling_value_list, dim=-2).flatten(-2) * attention_weights)
- .sum(-1)
- .view(bs, num_heads * embed_dims, num_queries)
- )
- return output.transpose(1, 2).contiguous()
-
-
-class MultiScaleDeformableAttention(nn.Module):
- """Multi-Scale Deformable Attention Module used in Deformable-DETR
-
- `Deformable DETR: Deformable Transformers for End-to-End Object Detection.
- `_.
-
- Args:
- embed_dim (int): The embedding dimension of Attention. Default: 256.
- num_heads (int): The number of attention heads. Default: 8.
- num_levels (int): The number of feature map used in Attention. Default: 4.
- num_points (int): The number of sampling points for each query
- in each head. Default: 4.
- img2col_steps (int): The step used in image_to_column. Defualt: 64.
- dropout (float): Dropout layer used in output. Default: 0.1.
- batch_first (bool): if ``True``, then the input and output tensor will be
- provided as `(bs, n, embed_dim)`. Default: False. `(n, bs, embed_dim)`
- """
-
- def __init__(
- self,
- embed_dim: int = 256,
- num_heads: int = 8,
- num_levels: int = 4,
- num_points: int = 4,
- img2col_step: int = 64,
- batch_first: bool = False,
- ):
- super().__init__()
- if embed_dim % num_heads != 0:
- raise ValueError(
- "embed_dim must be divisible by num_heads, but got {} and {}".format(
- embed_dim, num_heads
- )
- )
- head_dim = embed_dim // num_heads
-
- self.batch_first = batch_first
-
- if not _is_power_of_2(head_dim):
- warnings.warn(
- """
- You'd better set d_model in MSDeformAttn to make sure that
- each dim of the attention head a power of 2, which is more efficient.
- """
- )
-
- self.im2col_step = img2col_step
- self.embed_dim = embed_dim
- self.num_heads = num_heads
- self.num_levels = num_levels
- self.num_points = num_points
- self.sampling_offsets = nn.Linear(embed_dim, num_heads * num_levels * num_points * 2)
- self.attention_weights = nn.Linear(embed_dim, num_heads * num_levels * num_points)
- self.value_proj = nn.Linear(embed_dim, embed_dim)
- self.output_proj = nn.Linear(embed_dim, embed_dim)
-
- self.init_weights()
-
- def _reset_parameters(self):
- return self.init_weights()
-
- def init_weights(self):
- """
- Default initialization for Parameters of Module.
- """
- constant_(self.sampling_offsets.weight.data, 0.0)
- thetas = torch.arange(self.num_heads, dtype=torch.float32) * (
- 2.0 * math.pi / self.num_heads
- )
- grid_init = torch.stack([thetas.cos(), thetas.sin()], -1)
- grid_init = (
- (grid_init / grid_init.abs().max(-1, keepdim=True)[0])
- .view(self.num_heads, 1, 1, 2)
- .repeat(1, self.num_levels, self.num_points, 1)
- )
- for i in range(self.num_points):
- grid_init[:, :, i, :] *= i + 1
- with torch.no_grad():
- self.sampling_offsets.bias = nn.Parameter(grid_init.view(-1))
- constant_(self.attention_weights.weight.data, 0.0)
- constant_(self.attention_weights.bias.data, 0.0)
- xavier_uniform_(self.value_proj.weight.data)
- constant_(self.value_proj.bias.data, 0.0)
- xavier_uniform_(self.output_proj.weight.data)
- constant_(self.output_proj.bias.data, 0.0)
-
- def freeze_sampling_offsets(self):
- print("Freeze sampling offsets")
- self.sampling_offsets.weight.requires_grad = False
- self.sampling_offsets.bias.requires_grad = False
-
- def freeze_attention_weights(self):
- print("Freeze attention weights")
- self.attention_weights.weight.requires_grad = False
- self.attention_weights.bias.requires_grad = False
-
- def forward(
- self,
- query: torch.Tensor,
- key: Optional[torch.Tensor] = None,
- value: Optional[torch.Tensor] = None,
- query_pos: Optional[torch.Tensor] = None,
- key_padding_mask: Optional[torch.Tensor] = None,
- reference_points: Optional[torch.Tensor] = None,
- spatial_shapes: Optional[torch.Tensor] = None,
- level_start_index: Optional[torch.Tensor] = None,
- **kwargs
- ) -> torch.Tensor:
-
- """Forward Function of MultiScaleDeformableAttention
-
- Args:
- query (torch.Tensor): Query embeddings with shape
- `(num_query, bs, embed_dim)`
- key (torch.Tensor): Key embeddings with shape
- `(num_key, bs, embed_dim)`
- value (torch.Tensor): Value embeddings with shape
- `(num_key, bs, embed_dim)`
- query_pos (torch.Tensor): The position embedding for `query`. Default: None.
- key_padding_mask (torch.Tensor): ByteTensor for `query`, with shape `(bs, num_key)`,
- indicating which elements within `key` to be ignored in attention.
- reference_points (torch.Tensor): The normalized reference points
- with shape `(bs, num_query, num_levels, 2)`,
- all elements is range in [0, 1], top-left (0, 0),
- bottom-right (1, 1), including padding are.
- or `(N, Length_{query}, num_levels, 4)`, add additional
- two dimensions `(h, w)` to form reference boxes.
- spatial_shapes (torch.Tensor): Spatial shape of features in different levels.
- With shape `(num_levels, 2)`, last dimension represents `(h, w)`.
- level_start_index (torch.Tensor): The start index of each level. A tensor with
- shape `(num_levels, )` which can be represented as
- `[0, h_0 * w_0, h_0 * w_0 + h_1 * w_1, ...]`.
-
- Returns:
- torch.Tensor: forward results with shape `(num_query, bs, embed_dim)`
- """
-
- if value is None:
- value = query
-
- if query_pos is not None:
- query = query + query_pos
-
- if not self.batch_first:
- # change to (bs, num_query ,embed_dims)
- query = query.permute(1, 0, 2)
- value = value.permute(1, 0, 2)
-
- bs, num_query, _ = query.shape
- bs, num_value, _ = value.shape
-
- assert (spatial_shapes[:, 0] * spatial_shapes[:, 1]).sum() == num_value
-
- value = self.value_proj(value)
- if key_padding_mask is not None:
- value = value.masked_fill(key_padding_mask[..., None], float(0))
- value = value.view(bs, num_value, self.num_heads, -1)
- sampling_offsets = self.sampling_offsets(query).view(
- bs, num_query, self.num_heads, self.num_levels, self.num_points, 2
- )
- attention_weights = self.attention_weights(query).view(
- bs, num_query, self.num_heads, self.num_levels * self.num_points
- )
- attention_weights = attention_weights.softmax(-1)
- attention_weights = attention_weights.view(
- bs,
- num_query,
- self.num_heads,
- self.num_levels,
- self.num_points,
- )
-
- # bs, num_query, num_heads, num_levels, num_points, 2
- if reference_points.shape[-1] == 2:
- offset_normalizer = torch.stack([spatial_shapes[..., 1], spatial_shapes[..., 0]], -1)
- sampling_locations = (
- reference_points[:, :, None, :, None, :]
- + sampling_offsets / offset_normalizer[None, None, None, :, None, :]
- )
- elif reference_points.shape[-1] == 4:
- sampling_locations = (
- reference_points[:, :, None, :, None, :2]
- + sampling_offsets
- / self.num_points
- * reference_points[:, :, None, :, None, 2:]
- * 0.5
- )
- else:
- raise ValueError(
- "Last dim of reference_points must be 2 or 4, but get {} instead.".format(
- reference_points.shape[-1]
- )
- )
-
- if torch.cuda.is_available() and value.is_cuda:
- halffloat = False
- if value.dtype == torch.float16:
- halffloat = True
- value = value.float()
- sampling_locations = sampling_locations.float()
- attention_weights = attention_weights.float()
-
- output = MultiScaleDeformableAttnFunction.apply(
- value,
- spatial_shapes,
- level_start_index,
- sampling_locations,
- attention_weights,
- self.im2col_step,
- )
-
- if halffloat:
- output = output.half()
- else:
- output = multi_scale_deformable_attn_pytorch(
- value, spatial_shapes, sampling_locations, attention_weights
- )
-
- output = self.output_proj(output)
-
- if not self.batch_first:
- output = output.permute(1, 0, 2)
-
- return output
-
-
-def create_dummy_class(klass, dependency, message=""):
- """
- When a dependency of a class is not available, create a dummy class which throws ImportError
- when used.
-
- Args:
- klass (str): name of the class.
- dependency (str): name of the dependency.
- message: extra message to print
- Returns:
- class: a class object
- """
- err = "Cannot import '{}', therefore '{}' is not available.".format(dependency, klass)
- if message:
- err = err + " " + message
-
- class _DummyMetaClass(type):
- # throw error on class attribute access
- def __getattr__(_, __): # noqa: B902
- raise ImportError(err)
-
- class _Dummy(object, metaclass=_DummyMetaClass):
- # throw error on constructor
- def __init__(self, *args, **kwargs):
- raise ImportError(err)
-
- return _Dummy
-
-
-def create_dummy_func(func, dependency, message=""):
- """
- When a dependency of a function is not available, create a dummy function which throws
- ImportError when used.
-
- Args:
- func (str): name of the function.
- dependency (str or list[str]): name(s) of the dependency.
- message: extra message to print
- Returns:
- function: a function object
- """
- err = "Cannot import '{}', therefore '{}' is not available.".format(dependency, func)
- if message:
- err = err + " " + message
-
- if isinstance(dependency, (list, tuple)):
- dependency = ",".join(dependency)
-
- def _dummy(*args, **kwargs):
- raise ImportError(err)
-
- return _dummy
diff --git a/spaces/xswu/HPSv2/src/open_clip/__init__.py b/spaces/xswu/HPSv2/src/open_clip/__init__.py
deleted file mode 100644
index c328ed24f54803a32e10f712a540fff59ef50175..0000000000000000000000000000000000000000
--- a/spaces/xswu/HPSv2/src/open_clip/__init__.py
+++ /dev/null
@@ -1,14 +0,0 @@
-from .coca_model import CoCa
-from .constants import OPENAI_DATASET_MEAN, OPENAI_DATASET_STD
-from .factory import create_model, create_model_and_transforms, create_model_from_pretrained, get_tokenizer, create_loss
-from .factory import list_models, add_model_config, get_model_config, load_checkpoint
-from .loss import ClipLoss, DistillClipLoss, CoCaLoss
-from .model import CLIP, CustomTextCLIP, CLIPTextCfg, CLIPVisionCfg, \
- convert_weights_to_lp, convert_weights_to_fp16, trace_model, get_cast_dtype
-from .openai import load_openai_model, list_openai_models
-from .pretrained import list_pretrained, list_pretrained_models_by_tag, list_pretrained_tags_by_model, \
- get_pretrained_url, download_pretrained_from_url, is_pretrained_cfg, get_pretrained_cfg, download_pretrained
-from .push_to_hf_hub import push_pretrained_to_hf_hub, push_to_hf_hub
-from .tokenizer import SimpleTokenizer, tokenize, decode
-from .transform import image_transform, AugmentationCfg
-from .utils import freeze_batch_norm_2d
diff --git a/spaces/xuanzang/prompthero-openjourney-v2/app.py b/spaces/xuanzang/prompthero-openjourney-v2/app.py
deleted file mode 100644
index 4fa45eda1d4a0af263ec59b35e375b837fe1ecf1..0000000000000000000000000000000000000000
--- a/spaces/xuanzang/prompthero-openjourney-v2/app.py
+++ /dev/null
@@ -1,3 +0,0 @@
-import gradio as gr
-
-gr.Interface.load("models/prompthero/openjourney-v2").launch()
\ No newline at end of file
diff --git a/spaces/xxie92/antibody_visulization/diffab/modules/diffusion/dpm_full.py b/spaces/xxie92/antibody_visulization/diffab/modules/diffusion/dpm_full.py
deleted file mode 100644
index 49fe30db80a76deaf7d0a011dbd8116cf4e27b0e..0000000000000000000000000000000000000000
--- a/spaces/xxie92/antibody_visulization/diffab/modules/diffusion/dpm_full.py
+++ /dev/null
@@ -1,319 +0,0 @@
-import torch
-import torch.nn as nn
-import torch.nn.functional as F
-import functools
-from tqdm.auto import tqdm
-
-from diffab.modules.common.geometry import apply_rotation_to_vector, quaternion_1ijk_to_rotation_matrix
-from diffab.modules.common.so3 import so3vec_to_rotation, rotation_to_so3vec, random_uniform_so3
-from diffab.modules.encoders.ga import GAEncoder
-from .transition import RotationTransition, PositionTransition, AminoacidCategoricalTransition
-
-
-def rotation_matrix_cosine_loss(R_pred, R_true):
- """
- Args:
- R_pred: (*, 3, 3).
- R_true: (*, 3, 3).
- Returns:
- Per-matrix losses, (*, ).
- """
- size = list(R_pred.shape[:-2])
- ncol = R_pred.numel() // 3
-
- RT_pred = R_pred.transpose(-2, -1).reshape(ncol, 3) # (ncol, 3)
- RT_true = R_true.transpose(-2, -1).reshape(ncol, 3) # (ncol, 3)
-
- ones = torch.ones([ncol, ], dtype=torch.long, device=R_pred.device)
- loss = F.cosine_embedding_loss(RT_pred, RT_true, ones, reduction='none') # (ncol*3, )
- loss = loss.reshape(size + [3]).sum(dim=-1) # (*, )
- return loss
-
-
-class EpsilonNet(nn.Module):
-
- def __init__(self, res_feat_dim, pair_feat_dim, num_layers, encoder_opt={}):
- super().__init__()
- self.current_sequence_embedding = nn.Embedding(25, res_feat_dim) # 22 is padding
- self.res_feat_mixer = nn.Sequential(
- nn.Linear(res_feat_dim * 2, res_feat_dim), nn.ReLU(),
- nn.Linear(res_feat_dim, res_feat_dim),
- )
- self.encoder = GAEncoder(res_feat_dim, pair_feat_dim, num_layers, **encoder_opt)
-
- self.eps_crd_net = nn.Sequential(
- nn.Linear(res_feat_dim+3, res_feat_dim), nn.ReLU(),
- nn.Linear(res_feat_dim, res_feat_dim), nn.ReLU(),
- nn.Linear(res_feat_dim, 3)
- )
-
- self.eps_rot_net = nn.Sequential(
- nn.Linear(res_feat_dim+3, res_feat_dim), nn.ReLU(),
- nn.Linear(res_feat_dim, res_feat_dim), nn.ReLU(),
- nn.Linear(res_feat_dim, 3)
- )
-
- self.eps_seq_net = nn.Sequential(
- nn.Linear(res_feat_dim+3, res_feat_dim), nn.ReLU(),
- nn.Linear(res_feat_dim, res_feat_dim), nn.ReLU(),
- nn.Linear(res_feat_dim, 20), nn.Softmax(dim=-1)
- )
-
- def forward(self, v_t, p_t, s_t, res_feat, pair_feat, beta, mask_generate, mask_res):
- """
- Args:
- v_t: (N, L, 3).
- p_t: (N, L, 3).
- s_t: (N, L).
- res_feat: (N, L, res_dim).
- pair_feat: (N, L, L, pair_dim).
- beta: (N,).
- mask_generate: (N, L).
- mask_res: (N, L).
- Returns:
- v_next: UPDATED (not epsilon) SO3-vector of orietnations, (N, L, 3).
- eps_pos: (N, L, 3).
- """
- N, L = mask_res.size()
- R = so3vec_to_rotation(v_t) # (N, L, 3, 3)
-
- # s_t = s_t.clamp(min=0, max=19) # TODO: clamping is good but ugly.
- res_feat = self.res_feat_mixer(torch.cat([res_feat, self.current_sequence_embedding(s_t)], dim=-1)) # [Important] Incorporate sequence at the current step.
- res_feat = self.encoder(R, p_t, res_feat, pair_feat, mask_res)
-
- t_embed = torch.stack([beta, torch.sin(beta), torch.cos(beta)], dim=-1)[:, None, :].expand(N, L, 3)
- in_feat = torch.cat([res_feat, t_embed], dim=-1)
-
- # Position changes
- eps_crd = self.eps_crd_net(in_feat) # (N, L, 3)
- eps_pos = apply_rotation_to_vector(R, eps_crd) # (N, L, 3)
- eps_pos = torch.where(mask_generate[:, :, None].expand_as(eps_pos), eps_pos, torch.zeros_like(eps_pos))
-
- # New orientation
- eps_rot = self.eps_rot_net(in_feat) # (N, L, 3)
- U = quaternion_1ijk_to_rotation_matrix(eps_rot) # (N, L, 3, 3)
- R_next = R @ U
- v_next = rotation_to_so3vec(R_next) # (N, L, 3)
- v_next = torch.where(mask_generate[:, :, None].expand_as(v_next), v_next, v_t)
-
- # New sequence categorical distributions
- c_denoised = self.eps_seq_net(in_feat) # Already softmax-ed, (N, L, 20)
-
- return v_next, R_next, eps_pos, c_denoised
-
-
-class FullDPM(nn.Module):
-
- def __init__(
- self,
- res_feat_dim,
- pair_feat_dim,
- num_steps,
- eps_net_opt={},
- trans_rot_opt={},
- trans_pos_opt={},
- trans_seq_opt={},
- position_mean=[0.0, 0.0, 0.0],
- position_scale=[10.0],
- ):
- super().__init__()
- self.eps_net = EpsilonNet(res_feat_dim, pair_feat_dim, **eps_net_opt)
- self.num_steps = num_steps
- self.trans_rot = RotationTransition(num_steps, **trans_rot_opt)
- self.trans_pos = PositionTransition(num_steps, **trans_pos_opt)
- self.trans_seq = AminoacidCategoricalTransition(num_steps, **trans_seq_opt)
-
- self.register_buffer('position_mean', torch.FloatTensor(position_mean).view(1, 1, -1))
- self.register_buffer('position_scale', torch.FloatTensor(position_scale).view(1, 1, -1))
- self.register_buffer('_dummy', torch.empty([0, ]))
-
- def _normalize_position(self, p):
- p_norm = (p - self.position_mean) / self.position_scale
- return p_norm
-
- def _unnormalize_position(self, p_norm):
- p = p_norm * self.position_scale + self.position_mean
- return p
-
- def forward(self, v_0, p_0, s_0, res_feat, pair_feat, mask_generate, mask_res, denoise_structure, denoise_sequence, t=None):
- N, L = res_feat.shape[:2]
- if t == None:
- t = torch.randint(0, self.num_steps, (N,), dtype=torch.long, device=self._dummy.device)
- p_0 = self._normalize_position(p_0)
-
- if denoise_structure:
- # Add noise to rotation
- R_0 = so3vec_to_rotation(v_0)
- v_noisy, _ = self.trans_rot.add_noise(v_0, mask_generate, t)
- # Add noise to positions
- p_noisy, eps_p = self.trans_pos.add_noise(p_0, mask_generate, t)
- else:
- R_0 = so3vec_to_rotation(v_0)
- v_noisy = v_0.clone()
- p_noisy = p_0.clone()
- eps_p = torch.zeros_like(p_noisy)
-
- if denoise_sequence:
- # Add noise to sequence
- _, s_noisy = self.trans_seq.add_noise(s_0, mask_generate, t)
- else:
- s_noisy = s_0.clone()
-
- beta = self.trans_pos.var_sched.betas[t]
- v_pred, R_pred, eps_p_pred, c_denoised = self.eps_net(
- v_noisy, p_noisy, s_noisy, res_feat, pair_feat, beta, mask_generate, mask_res
- ) # (N, L, 3), (N, L, 3, 3), (N, L, 3), (N, L, 20), (N, L)
-
- loss_dict = {}
-
- # Rotation loss
- loss_rot = rotation_matrix_cosine_loss(R_pred, R_0) # (N, L)
- loss_rot = (loss_rot * mask_generate).sum() / (mask_generate.sum().float() + 1e-8)
- loss_dict['rot'] = loss_rot
-
- # Position loss
- loss_pos = F.mse_loss(eps_p_pred, eps_p, reduction='none').sum(dim=-1) # (N, L)
- loss_pos = (loss_pos * mask_generate).sum() / (mask_generate.sum().float() + 1e-8)
- loss_dict['pos'] = loss_pos
-
- # Sequence categorical loss
- post_true = self.trans_seq.posterior(s_noisy, s_0, t)
- log_post_pred = torch.log(self.trans_seq.posterior(s_noisy, c_denoised, t) + 1e-8)
- kldiv = F.kl_div(
- input=log_post_pred,
- target=post_true,
- reduction='none',
- log_target=False
- ).sum(dim=-1) # (N, L)
- loss_seq = (kldiv * mask_generate).sum() / (mask_generate.sum().float() + 1e-8)
- loss_dict['seq'] = loss_seq
-
- return loss_dict
-
- @torch.no_grad()
- def sample(
- self,
- v, p, s,
- res_feat, pair_feat,
- mask_generate, mask_res,
- sample_structure=True, sample_sequence=True,
- pbar=False,
- ):
- """
- Args:
- v: Orientations of contextual residues, (N, L, 3).
- p: Positions of contextual residues, (N, L, 3).
- s: Sequence of contextual residues, (N, L).
- """
- N, L = v.shape[:2]
- p = self._normalize_position(p)
-
- # Set the orientation and position of residues to be predicted to random values
- if sample_structure:
- v_rand = random_uniform_so3([N, L], device=self._dummy.device)
- p_rand = torch.randn_like(p)
- v_init = torch.where(mask_generate[:, :, None].expand_as(v), v_rand, v)
- p_init = torch.where(mask_generate[:, :, None].expand_as(p), p_rand, p)
- else:
- v_init, p_init = v, p
-
- if sample_sequence:
- s_rand = torch.randint_like(s, low=0, high=19)
- s_init = torch.where(mask_generate, s_rand, s)
- else:
- s_init = s
-
- traj = {self.num_steps: (v_init, self._unnormalize_position(p_init), s_init)}
- if pbar:
- pbar = functools.partial(tqdm, total=self.num_steps, desc='Sampling')
- else:
- pbar = lambda x: x
- for t in pbar(range(self.num_steps, 0, -1)):
- v_t, p_t, s_t = traj[t]
- p_t = self._normalize_position(p_t)
-
- beta = self.trans_pos.var_sched.betas[t].expand([N, ])
- t_tensor = torch.full([N, ], fill_value=t, dtype=torch.long, device=self._dummy.device)
-
- v_next, R_next, eps_p, c_denoised = self.eps_net(
- v_t, p_t, s_t, res_feat, pair_feat, beta, mask_generate, mask_res
- ) # (N, L, 3), (N, L, 3, 3), (N, L, 3)
-
- v_next = self.trans_rot.denoise(v_t, v_next, mask_generate, t_tensor)
- p_next = self.trans_pos.denoise(p_t, eps_p, mask_generate, t_tensor)
- _, s_next = self.trans_seq.denoise(s_t, c_denoised, mask_generate, t_tensor)
-
- if not sample_structure:
- v_next, p_next = v_t, p_t
- if not sample_sequence:
- s_next = s_t
-
- traj[t-1] = (v_next, self._unnormalize_position(p_next), s_next)
- traj[t] = tuple(x.cpu() for x in traj[t]) # Move previous states to cpu memory.
-
- return traj
-
- @torch.no_grad()
- def optimize(
- self,
- v, p, s,
- opt_step: int,
- res_feat, pair_feat,
- mask_generate, mask_res,
- sample_structure=True, sample_sequence=True,
- pbar=False,
- ):
- """
- Description:
- First adds noise to the given structure, then denoises it.
- """
- N, L = v.shape[:2]
- p = self._normalize_position(p)
- t = torch.full([N, ], fill_value=opt_step, dtype=torch.long, device=self._dummy.device)
-
- # Set the orientation and position of residues to be predicted to random values
- if sample_structure:
- # Add noise to rotation
- v_noisy, _ = self.trans_rot.add_noise(v, mask_generate, t)
- # Add noise to positions
- p_noisy, _ = self.trans_pos.add_noise(p, mask_generate, t)
- v_init = torch.where(mask_generate[:, :, None].expand_as(v), v_noisy, v)
- p_init = torch.where(mask_generate[:, :, None].expand_as(p), p_noisy, p)
- else:
- v_init, p_init = v, p
-
- if sample_sequence:
- _, s_noisy = self.trans_seq.add_noise(s, mask_generate, t)
- s_init = torch.where(mask_generate, s_noisy, s)
- else:
- s_init = s
-
- traj = {opt_step: (v_init, self._unnormalize_position(p_init), s_init)}
- if pbar:
- pbar = functools.partial(tqdm, total=opt_step, desc='Optimizing')
- else:
- pbar = lambda x: x
- for t in pbar(range(opt_step, 0, -1)):
- v_t, p_t, s_t = traj[t]
- p_t = self._normalize_position(p_t)
-
- beta = self.trans_pos.var_sched.betas[t].expand([N, ])
- t_tensor = torch.full([N, ], fill_value=t, dtype=torch.long, device=self._dummy.device)
-
- v_next, R_next, eps_p, c_denoised = self.eps_net(
- v_t, p_t, s_t, res_feat, pair_feat, beta, mask_generate, mask_res
- ) # (N, L, 3), (N, L, 3, 3), (N, L, 3)
-
- v_next = self.trans_rot.denoise(v_t, v_next, mask_generate, t_tensor)
- p_next = self.trans_pos.denoise(p_t, eps_p, mask_generate, t_tensor)
- _, s_next = self.trans_seq.denoise(s_t, c_denoised, mask_generate, t_tensor)
-
- if not sample_structure:
- v_next, p_next = v_t, p_t
- if not sample_sequence:
- s_next = s_t
-
- traj[t-1] = (v_next, self._unnormalize_position(p_next), s_next)
- traj[t] = tuple(x.cpu() for x in traj[t]) # Move previous states to cpu memory.
-
- return traj
diff --git a/spaces/yangogo/bingo/postcss.config.js b/spaces/yangogo/bingo/postcss.config.js
deleted file mode 100644
index 33ad091d26d8a9dc95ebdf616e217d985ec215b8..0000000000000000000000000000000000000000
--- a/spaces/yangogo/bingo/postcss.config.js
+++ /dev/null
@@ -1,6 +0,0 @@
-module.exports = {
- plugins: {
- tailwindcss: {},
- autoprefixer: {},
- },
-}
diff --git a/spaces/yentinglin/Taiwan-LLaMa2/app.py b/spaces/yentinglin/Taiwan-LLaMa2/app.py
deleted file mode 100644
index 7a9c19b82c9d0dfa7b58d4d74338848f464d77a6..0000000000000000000000000000000000000000
--- a/spaces/yentinglin/Taiwan-LLaMa2/app.py
+++ /dev/null
@@ -1,270 +0,0 @@
-import os
-
-import gradio as gr
-from text_generation import Client
-from conversation import get_conv_template
-from transformers import AutoTokenizer
-from pymongo import MongoClient
-
-DB_NAME = os.getenv("MONGO_DBNAME", "taiwan-llm")
-USER = os.getenv("MONGO_USER")
-PASSWORD = os.getenv("MONGO_PASSWORD")
-
-uri = f"mongodb+srv://{USER}:{PASSWORD}@{DB_NAME}.kvwjiok.mongodb.net/?retryWrites=true&w=majority"
-mongo_client = MongoClient(uri)
-db = mongo_client[DB_NAME]
-conversations_collection = db['conversations']
-
-DESCRIPTION = """
-# Language Models for Taiwanese Culture
-
-
-✍️ Online Demo
-•
-🤗 HF Repo • 🐦 Twitter • 📃 [Paper Coming Soon]
-• 👨️ Github Repo
-
-
-
-
-# 🌟 Checkout New [Taiwan-LLM UI](http://www.twllm.com) 🌟
-
-
-Taiwan-LLaMa is a fine-tuned model specifically designed for traditional mandarin applications. It is built upon the LLaMa 2 architecture and includes a pretraining phase with over 5 billion tokens and fine-tuning with over 490k multi-turn conversational data in Traditional Mandarin.
-
-## Key Features
-
-1. **Traditional Mandarin Support**: The model is fine-tuned to understand and generate text in Traditional Mandarin, making it suitable for Taiwanese culture and related applications.
-
-2. **Instruction-Tuned**: Further fine-tuned on conversational data to offer context-aware and instruction-following responses.
-
-3. **Performance on Vicuna Benchmark**: Taiwan-LLaMa's relative performance on Vicuna Benchmark is measured against models like GPT-4 and ChatGPT. It's particularly optimized for Taiwanese culture.
-
-4. **Flexible Customization**: Advanced options for controlling the model's behavior like system prompt, temperature, top-p, and top-k are available in the demo.
-
-## Model Versions
-
-Different versions of Taiwan-LLaMa are available:
-
-- **Taiwan-LLM v2.0 (This demo)**: Cleaner pretraining, Better post-training
-- **Taiwan-LLM v1.0**: Optimized for Taiwanese Culture
-- **Taiwan-LLM v0.9**: Partial instruction set
-- **Taiwan-LLM v0.0**: No Traditional Mandarin pretraining
-
-The models can be accessed from the provided links in the Hugging Face repository.
-
-Try out the demo to interact with Taiwan-LLaMa and experience its capabilities in handling Traditional Mandarin!
-"""
-
-LICENSE = """
-## Licenses
-
-- Code is licensed under Apache 2.0 License.
-- Models are licensed under the LLAMA 2 Community License.
-- By using this model, you agree to the terms and conditions specified in the license.
-- By using this demo, you agree to share your input utterances with us to improve the model.
-
-## Acknowledgements
-
-Taiwan-LLaMa project acknowledges the efforts of the [Meta LLaMa team](https://github.com/facebookresearch/llama) and [Vicuna team](https://github.com/lm-sys/FastChat) in democratizing large language models.
-"""
-
-DEFAULT_SYSTEM_PROMPT = "你是人工智慧助理,以下是用戶和人工智能助理之間的對話。你要對用戶的問題提供有用、安全、詳細和禮貌的回答。 您是由國立臺灣大學的林彥廷博士生為研究目的而建造的。"
-
-endpoint_url = os.environ.get("ENDPOINT_URL", "http://127.0.0.1:8080")
-client = Client(endpoint_url, timeout=120)
-eos_token = ""
-MAX_MAX_NEW_TOKENS = 4096
-DEFAULT_MAX_NEW_TOKENS = 1536
-
-max_prompt_length = 8192 - MAX_MAX_NEW_TOKENS - 10
-
-model_name = "yentinglin/Taiwan-LLM-7B-v2.0-chat"
-tokenizer = AutoTokenizer.from_pretrained(model_name)
-
-with gr.Blocks() as demo:
- gr.Markdown(DESCRIPTION)
-
- chatbot = gr.Chatbot()
- with gr.Row():
- msg = gr.Textbox(
- container=False,
- show_label=False,
- placeholder='Type a message...',
- scale=10,
- )
- submit_button = gr.Button('Submit',
- variant='primary',
- scale=1,
- min_width=0)
-
- with gr.Row():
- retry_button = gr.Button('🔄 Retry', variant='secondary')
- undo_button = gr.Button('↩️ Undo', variant='secondary')
- clear = gr.Button('🗑️ Clear', variant='secondary')
-
- saved_input = gr.State()
-
- with gr.Accordion(label='Advanced options', open=False):
- system_prompt = gr.Textbox(label='System prompt',
- value=DEFAULT_SYSTEM_PROMPT,
- lines=6)
- max_new_tokens = gr.Slider(
- label='Max new tokens',
- minimum=1,
- maximum=MAX_MAX_NEW_TOKENS,
- step=1,
- value=DEFAULT_MAX_NEW_TOKENS,
- )
- temperature = gr.Slider(
- label='Temperature',
- minimum=0.1,
- maximum=1.0,
- step=0.1,
- value=0.3,
- )
- top_p = gr.Slider(
- label='Top-p (nucleus sampling)',
- minimum=0.05,
- maximum=1.0,
- step=0.05,
- value=0.95,
- )
- top_k = gr.Slider(
- label='Top-k',
- minimum=1,
- maximum=1000,
- step=1,
- value=50,
- )
-
- def user(user_message, history):
- return "", history + [[user_message, None]]
-
-
- def bot(history, max_new_tokens, temperature, top_p, top_k, system_prompt):
- conv = get_conv_template("twllm_v2").copy()
- roles = {"human": conv.roles[0], "gpt": conv.roles[1]} # map human to USER and gpt to ASSISTANT
- conv.system = system_prompt
- for user, bot in history:
- conv.append_message(roles['human'], user)
- conv.append_message(roles["gpt"], bot)
- msg = conv.get_prompt()
- prompt_tokens = tokenizer.encode(msg)
- length_of_prompt = len(prompt_tokens)
- if length_of_prompt > max_prompt_length:
- msg = tokenizer.decode(prompt_tokens[-max_prompt_length + 1:])
-
- history[-1][1] = ""
- for response in client.generate_stream(
- msg,
- max_new_tokens=max_new_tokens,
- temperature=temperature,
- top_p=top_p,
- top_k=top_k,
- repetition_penalty=1.1,
- ):
- if not response.token.special:
- character = response.token.text
- history[-1][1] += character
- yield history
-
- # After generating the response, store the conversation history in MongoDB
- conversation_document = {
- "model_name": model_name,
- "history": history,
- "system_prompt": system_prompt,
- "max_new_tokens": max_new_tokens,
- "temperature": temperature,
- "top_p": top_p,
- "top_k": top_k,
- }
- conversations_collection.insert_one(conversation_document)
-
- msg.submit(user, [msg, chatbot], [msg, chatbot], queue=False).then(
- fn=bot,
- inputs=[
- chatbot,
- max_new_tokens,
- temperature,
- top_p,
- top_k,
- system_prompt,
- ],
- outputs=chatbot
- )
- submit_button.click(
- user, [msg, chatbot], [msg, chatbot], queue=False
- ).then(
- fn=bot,
- inputs=[
- chatbot,
- max_new_tokens,
- temperature,
- top_p,
- top_k,
- system_prompt,
- ],
- outputs=chatbot
- )
-
-
- def delete_prev_fn(
- history: list[tuple[str, str]]) -> tuple[list[tuple[str, str]], str]:
- try:
- message, _ = history.pop()
- except IndexError:
- message = ''
- return history, message or ''
-
-
- def display_input(message: str,
- history: list[tuple[str, str]]) -> list[tuple[str, str]]:
- history.append((message, ''))
- return history
-
- retry_button.click(
- fn=delete_prev_fn,
- inputs=chatbot,
- outputs=[chatbot, saved_input],
- api_name=False,
- queue=False,
- ).then(
- fn=display_input,
- inputs=[saved_input, chatbot],
- outputs=chatbot,
- api_name=False,
- queue=False,
- ).then(
- fn=bot,
- inputs=[
- chatbot,
- max_new_tokens,
- temperature,
- top_p,
- top_k,
- system_prompt,
- ],
- outputs=chatbot,
- )
-
- undo_button.click(
- fn=delete_prev_fn,
- inputs=chatbot,
- outputs=[chatbot, saved_input],
- api_name=False,
- queue=False,
- ).then(
- fn=lambda x: x,
- inputs=[saved_input],
- outputs=msg,
- api_name=False,
- queue=False,
- )
-
- clear.click(lambda: None, None, chatbot, queue=False)
-
- gr.Markdown(LICENSE)
-
-demo.queue(concurrency_count=4, max_size=128)
-demo.launch()
\ No newline at end of file
diff --git a/spaces/yerfor/SyntaSpeech/modules/tts/commons/align_ops.py b/spaces/yerfor/SyntaSpeech/modules/tts/commons/align_ops.py
deleted file mode 100644
index a190d63a3f3ba31f41754975569336a87c63089d..0000000000000000000000000000000000000000
--- a/spaces/yerfor/SyntaSpeech/modules/tts/commons/align_ops.py
+++ /dev/null
@@ -1,25 +0,0 @@
-import torch
-import torch.nn.functional as F
-
-
-def build_word_mask(x2word, y2word):
- return (x2word[:, :, None] == y2word[:, None, :]).long()
-
-
-def mel2ph_to_mel2word(mel2ph, ph2word):
- mel2word = (ph2word - 1).gather(1, (mel2ph - 1).clamp(min=0)) + 1
- mel2word = mel2word * (mel2ph > 0).long()
- return mel2word
-
-
-def clip_mel2token_to_multiple(mel2token, frames_multiple):
- max_frames = mel2token.shape[1] // frames_multiple * frames_multiple
- mel2token = mel2token[:, :max_frames]
- return mel2token
-
-
-def expand_states(h, mel2token):
- h = F.pad(h, [0, 0, 1, 0])
- mel2token_ = mel2token[..., None].repeat([1, 1, h.shape[-1]])
- h = torch.gather(h, 1, mel2token_) # [B, T, H]
- return h
diff --git a/spaces/yerfor/SyntaSpeech/modules/vocoder/parallel_wavegan/models/freq_discriminator.py b/spaces/yerfor/SyntaSpeech/modules/vocoder/parallel_wavegan/models/freq_discriminator.py
deleted file mode 100644
index 876b66ff931335ae16a5c36f95beb33e789a3f7d..0000000000000000000000000000000000000000
--- a/spaces/yerfor/SyntaSpeech/modules/vocoder/parallel_wavegan/models/freq_discriminator.py
+++ /dev/null
@@ -1,149 +0,0 @@
-import torch
-import torch.nn as nn
-
-
-class BasicDiscriminatorBlock(nn.Module):
- def __init__(self, in_channel, out_channel):
- super(BasicDiscriminatorBlock, self).__init__()
- self.block = nn.Sequential(
- nn.utils.weight_norm(nn.Conv1d(
- in_channel,
- out_channel,
- kernel_size=3,
- stride=2,
- padding=1,
- )),
- nn.LeakyReLU(0.2, True),
-
- nn.utils.weight_norm(nn.Conv1d(
- out_channel,
- out_channel,
- kernel_size=3,
- stride=1,
- padding=1,
- )),
- nn.LeakyReLU(0.2, True),
-
- nn.utils.weight_norm(nn.Conv1d(
- out_channel,
- out_channel,
- kernel_size=3,
- stride=1,
- padding=1,
- )),
- nn.LeakyReLU(0.2, True),
-
- nn.utils.weight_norm(nn.Conv1d(
- out_channel,
- out_channel,
- kernel_size=3,
- stride=1,
- padding=1,
- )),
-
- )
-
- def forward(self, x):
- return self.block(x)
-
-
-class ResDiscriminatorBlock(nn.Module):
- def __init__(self, in_channel, out_channel):
- super(ResDiscriminatorBlock, self).__init__()
- self.block1 = nn.Sequential(
- nn.utils.weight_norm(nn.Conv1d(
- in_channel,
- out_channel,
- kernel_size=3,
- stride=2,
- padding=1,
- )),
- nn.LeakyReLU(0.2, True),
-
- nn.utils.weight_norm(nn.Conv1d(
- out_channel,
- out_channel,
- kernel_size=3,
- stride=1,
- padding=1,
- )),
- )
-
- self.shortcut1 = nn.utils.weight_norm(nn.Conv1d(
- in_channel,
- out_channel,
- kernel_size=1,
- stride=2,
- ))
-
- self.block2 = nn.Sequential(
- nn.utils.weight_norm(nn.Conv1d(
- out_channel,
- out_channel,
- kernel_size=3,
- stride=1,
- padding=1,
- )),
- nn.LeakyReLU(0.2, True),
-
- nn.utils.weight_norm(nn.Conv1d(
- out_channel,
- out_channel,
- kernel_size=3,
- stride=1,
- padding=1,
- )),
- )
-
- self.shortcut2 = nn.utils.weight_norm(nn.Conv1d(
- out_channel,
- out_channel,
- kernel_size=1,
- stride=1,
- ))
-
- def forward(self, x):
- x1 = self.block1(x)
- x1 = x1 + self.shortcut1(x)
- return self.block2(x1) + self.shortcut2(x1)
-
-
-class ResNet18Discriminator(nn.Module):
- def __init__(self, stft_channel, in_channel=64):
- super(ResNet18Discriminator, self).__init__()
- self.input = nn.Sequential(
- nn.utils.weight_norm(nn.Conv1d(stft_channel, in_channel, kernel_size=7, stride=2, padding=1, )),
- nn.LeakyReLU(0.2, True),
- )
- self.df1 = BasicDiscriminatorBlock(in_channel, in_channel)
- self.df2 = ResDiscriminatorBlock(in_channel, in_channel * 2)
- self.df3 = ResDiscriminatorBlock(in_channel * 2, in_channel * 4)
- self.df4 = ResDiscriminatorBlock(in_channel * 4, in_channel * 8)
-
- def forward(self, x):
- x = self.input(x)
- x = self.df1(x)
- x = self.df2(x)
- x = self.df3(x)
- return self.df4(x)
-
-
-class FrequencyDiscriminator(nn.Module):
- def __init__(self, in_channel=64, fft_size=1024, hop_length=256, win_length=1024, window="hann_window"):
- super(FrequencyDiscriminator, self).__init__()
- self.fft_size = fft_size
- self.hop_length = hop_length
- self.win_length = win_length
- self.window = nn.Parameter(getattr(torch, window)(win_length), requires_grad=False)
- self.stft_channel = fft_size // 2 + 1
- self.resnet_disc = ResNet18Discriminator(self.stft_channel, in_channel)
-
- def forward(self, x):
- x_stft = torch.stft(x, self.fft_size, self.hop_length, self.win_length, self.window)
- real = x_stft[..., 0]
- imag = x_stft[..., 1]
-
- x_real = self.resnet_disc(real)
- x_imag = self.resnet_disc(imag)
-
- return x_real, x_imag
diff --git a/spaces/yizhangliu/Grounded-Segment-Anything/GroundingDINO/groundingdino/models/GroundingDINO/csrc/vision.cpp b/spaces/yizhangliu/Grounded-Segment-Anything/GroundingDINO/groundingdino/models/GroundingDINO/csrc/vision.cpp
deleted file mode 100644
index c1f2c50c82909bbd5492c163d634af77a3ba1781..0000000000000000000000000000000000000000
--- a/spaces/yizhangliu/Grounded-Segment-Anything/GroundingDINO/groundingdino/models/GroundingDINO/csrc/vision.cpp
+++ /dev/null
@@ -1,58 +0,0 @@
-// Copyright (c) Facebook, Inc. and its affiliates. All Rights Reserved
-
-#include "MsDeformAttn/ms_deform_attn.h"
-
-namespace groundingdino {
-
-#ifdef WITH_CUDA
-extern int get_cudart_version();
-#endif
-
-std::string get_cuda_version() {
-#ifdef WITH_CUDA
- std::ostringstream oss;
-
- // copied from
- // https://github.com/pytorch/pytorch/blob/master/aten/src/ATen/cuda/detail/CUDAHooks.cpp#L231
- auto printCudaStyleVersion = [&](int v) {
- oss << (v / 1000) << "." << (v / 10 % 100);
- if (v % 10 != 0) {
- oss << "." << (v % 10);
- }
- };
- printCudaStyleVersion(get_cudart_version());
- return oss.str();
-#else
- return std::string("not available");
-#endif
-}
-
-// similar to
-// https://github.com/pytorch/pytorch/blob/master/aten/src/ATen/Version.cpp
-std::string get_compiler_version() {
- std::ostringstream ss;
-#if defined(__GNUC__)
-#ifndef __clang__
- { ss << "GCC " << __GNUC__ << "." << __GNUC_MINOR__; }
-#endif
-#endif
-
-#if defined(__clang_major__)
- {
- ss << "clang " << __clang_major__ << "." << __clang_minor__ << "."
- << __clang_patchlevel__;
- }
-#endif
-
-#if defined(_MSC_VER)
- { ss << "MSVC " << _MSC_FULL_VER; }
-#endif
- return ss.str();
-}
-
-PYBIND11_MODULE(TORCH_EXTENSION_NAME, m) {
- m.def("ms_deform_attn_forward", &ms_deform_attn_forward, "ms_deform_attn_forward");
- m.def("ms_deform_attn_backward", &ms_deform_attn_backward, "ms_deform_attn_backward");
-}
-
-} // namespace groundingdino
\ No newline at end of file
diff --git a/spaces/yizhangliu/Grounded-Segment-Anything/GroundingDINO/groundingdino/models/GroundingDINO/transformer.py b/spaces/yizhangliu/Grounded-Segment-Anything/GroundingDINO/groundingdino/models/GroundingDINO/transformer.py
deleted file mode 100644
index fcb8742dbdde6e80fd38b11d064211f6935aae76..0000000000000000000000000000000000000000
--- a/spaces/yizhangliu/Grounded-Segment-Anything/GroundingDINO/groundingdino/models/GroundingDINO/transformer.py
+++ /dev/null
@@ -1,959 +0,0 @@
-# ------------------------------------------------------------------------
-# Grounding DINO
-# url: https://github.com/IDEA-Research/GroundingDINO
-# Copyright (c) 2023 IDEA. All Rights Reserved.
-# Licensed under the Apache License, Version 2.0 [see LICENSE for details]
-# ------------------------------------------------------------------------
-# DINO
-# Copyright (c) 2022 IDEA. All Rights Reserved.
-# Licensed under the Apache License, Version 2.0 [see LICENSE for details]
-# ------------------------------------------------------------------------
-# Conditional DETR Transformer class.
-# Copyright (c) 2021 Microsoft. All Rights Reserved.
-# Licensed under the Apache License, Version 2.0 [see LICENSE for details]
-# ------------------------------------------------------------------------
-# Modified from DETR (https://github.com/facebookresearch/detr)
-# Copyright (c) Facebook, Inc. and its affiliates. All Rights Reserved.
-# ------------------------------------------------------------------------
-
-from typing import Optional
-
-import torch
-import torch.utils.checkpoint as checkpoint
-from torch import Tensor, nn
-
-from groundingdino.util.misc import inverse_sigmoid
-
-from .fuse_modules import BiAttentionBlock
-from .ms_deform_attn import MultiScaleDeformableAttention as MSDeformAttn
-from .transformer_vanilla import TransformerEncoderLayer
-from .utils import (
- MLP,
- _get_activation_fn,
- _get_clones,
- gen_encoder_output_proposals,
- gen_sineembed_for_position,
- get_sine_pos_embed,
-)
-
-
-class Transformer(nn.Module):
- def __init__(
- self,
- d_model=256,
- nhead=8,
- num_queries=300,
- num_encoder_layers=6,
- num_unicoder_layers=0,
- num_decoder_layers=6,
- dim_feedforward=2048,
- dropout=0.0,
- activation="relu",
- normalize_before=False,
- return_intermediate_dec=False,
- query_dim=4,
- num_patterns=0,
- # for deformable encoder
- num_feature_levels=1,
- enc_n_points=4,
- dec_n_points=4,
- # init query
- learnable_tgt_init=False,
- # two stage
- two_stage_type="no", # ['no', 'standard', 'early', 'combine', 'enceachlayer', 'enclayer1']
- embed_init_tgt=False,
- # for text
- use_text_enhancer=False,
- use_fusion_layer=False,
- use_checkpoint=False,
- use_transformer_ckpt=False,
- use_text_cross_attention=False,
- text_dropout=0.1,
- fusion_dropout=0.1,
- fusion_droppath=0.0,
- ):
- super().__init__()
- self.num_feature_levels = num_feature_levels
- self.num_encoder_layers = num_encoder_layers
- self.num_unicoder_layers = num_unicoder_layers
- self.num_decoder_layers = num_decoder_layers
- self.num_queries = num_queries
- assert query_dim == 4
-
- # choose encoder layer type
- encoder_layer = DeformableTransformerEncoderLayer(
- d_model, dim_feedforward, dropout, activation, num_feature_levels, nhead, enc_n_points
- )
-
- if use_text_enhancer:
- text_enhance_layer = TransformerEncoderLayer(
- d_model=d_model,
- nhead=nhead // 2,
- dim_feedforward=dim_feedforward // 2,
- dropout=text_dropout,
- )
- else:
- text_enhance_layer = None
-
- if use_fusion_layer:
- feature_fusion_layer = BiAttentionBlock(
- v_dim=d_model,
- l_dim=d_model,
- embed_dim=dim_feedforward // 2,
- num_heads=nhead // 2,
- dropout=fusion_dropout,
- drop_path=fusion_droppath,
- )
- else:
- feature_fusion_layer = None
-
- encoder_norm = nn.LayerNorm(d_model) if normalize_before else None
- assert encoder_norm is None
- self.encoder = TransformerEncoder(
- encoder_layer,
- num_encoder_layers,
- d_model=d_model,
- num_queries=num_queries,
- text_enhance_layer=text_enhance_layer,
- feature_fusion_layer=feature_fusion_layer,
- use_checkpoint=use_checkpoint,
- use_transformer_ckpt=use_transformer_ckpt,
- )
-
- # choose decoder layer type
- decoder_layer = DeformableTransformerDecoderLayer(
- d_model,
- dim_feedforward,
- dropout,
- activation,
- num_feature_levels,
- nhead,
- dec_n_points,
- use_text_cross_attention=use_text_cross_attention,
- )
-
- decoder_norm = nn.LayerNorm(d_model)
- self.decoder = TransformerDecoder(
- decoder_layer,
- num_decoder_layers,
- decoder_norm,
- return_intermediate=return_intermediate_dec,
- d_model=d_model,
- query_dim=query_dim,
- num_feature_levels=num_feature_levels,
- )
-
- self.d_model = d_model
- self.nhead = nhead
- self.dec_layers = num_decoder_layers
- self.num_queries = num_queries # useful for single stage model only
- self.num_patterns = num_patterns
- if not isinstance(num_patterns, int):
- Warning("num_patterns should be int but {}".format(type(num_patterns)))
- self.num_patterns = 0
-
- if num_feature_levels > 1:
- if self.num_encoder_layers > 0:
- self.level_embed = nn.Parameter(torch.Tensor(num_feature_levels, d_model))
- else:
- self.level_embed = None
-
- self.learnable_tgt_init = learnable_tgt_init
- assert learnable_tgt_init, "why not learnable_tgt_init"
- self.embed_init_tgt = embed_init_tgt
- if (two_stage_type != "no" and embed_init_tgt) or (two_stage_type == "no"):
- self.tgt_embed = nn.Embedding(self.num_queries, d_model)
- nn.init.normal_(self.tgt_embed.weight.data)
- else:
- self.tgt_embed = None
-
- # for two stage
- self.two_stage_type = two_stage_type
- assert two_stage_type in ["no", "standard"], "unknown param {} of two_stage_type".format(
- two_stage_type
- )
- if two_stage_type == "standard":
- # anchor selection at the output of encoder
- self.enc_output = nn.Linear(d_model, d_model)
- self.enc_output_norm = nn.LayerNorm(d_model)
- self.two_stage_wh_embedding = None
-
- if two_stage_type == "no":
- self.init_ref_points(num_queries) # init self.refpoint_embed
-
- self.enc_out_class_embed = None
- self.enc_out_bbox_embed = None
-
- self._reset_parameters()
-
- def _reset_parameters(self):
- for p in self.parameters():
- if p.dim() > 1:
- nn.init.xavier_uniform_(p)
- for m in self.modules():
- if isinstance(m, MSDeformAttn):
- m._reset_parameters()
- if self.num_feature_levels > 1 and self.level_embed is not None:
- nn.init.normal_(self.level_embed)
-
- def get_valid_ratio(self, mask):
- _, H, W = mask.shape
- valid_H = torch.sum(~mask[:, :, 0], 1)
- valid_W = torch.sum(~mask[:, 0, :], 1)
- valid_ratio_h = valid_H.float() / H
- valid_ratio_w = valid_W.float() / W
- valid_ratio = torch.stack([valid_ratio_w, valid_ratio_h], -1)
- return valid_ratio
-
- def init_ref_points(self, use_num_queries):
- self.refpoint_embed = nn.Embedding(use_num_queries, 4)
-
- def forward(self, srcs, masks, refpoint_embed, pos_embeds, tgt, attn_mask=None, text_dict=None):
- """
- Input:
- - srcs: List of multi features [bs, ci, hi, wi]
- - masks: List of multi masks [bs, hi, wi]
- - refpoint_embed: [bs, num_dn, 4]. None in infer
- - pos_embeds: List of multi pos embeds [bs, ci, hi, wi]
- - tgt: [bs, num_dn, d_model]. None in infer
-
- """
- # prepare input for encoder
- src_flatten = []
- mask_flatten = []
- lvl_pos_embed_flatten = []
- spatial_shapes = []
- for lvl, (src, mask, pos_embed) in enumerate(zip(srcs, masks, pos_embeds)):
- bs, c, h, w = src.shape
- spatial_shape = (h, w)
- spatial_shapes.append(spatial_shape)
-
- src = src.flatten(2).transpose(1, 2) # bs, hw, c
- mask = mask.flatten(1) # bs, hw
- pos_embed = pos_embed.flatten(2).transpose(1, 2) # bs, hw, c
- if self.num_feature_levels > 1 and self.level_embed is not None:
- lvl_pos_embed = pos_embed + self.level_embed[lvl].view(1, 1, -1)
- else:
- lvl_pos_embed = pos_embed
- lvl_pos_embed_flatten.append(lvl_pos_embed)
- src_flatten.append(src)
- mask_flatten.append(mask)
- src_flatten = torch.cat(src_flatten, 1) # bs, \sum{hxw}, c
- mask_flatten = torch.cat(mask_flatten, 1) # bs, \sum{hxw}
- lvl_pos_embed_flatten = torch.cat(lvl_pos_embed_flatten, 1) # bs, \sum{hxw}, c
- spatial_shapes = torch.as_tensor(
- spatial_shapes, dtype=torch.long, device=src_flatten.device
- )
- level_start_index = torch.cat(
- (spatial_shapes.new_zeros((1,)), spatial_shapes.prod(1).cumsum(0)[:-1])
- )
- valid_ratios = torch.stack([self.get_valid_ratio(m) for m in masks], 1)
-
- # two stage
- enc_topk_proposals = enc_refpoint_embed = None
-
- #########################################################
- # Begin Encoder
- #########################################################
- memory, memory_text = self.encoder(
- src_flatten,
- pos=lvl_pos_embed_flatten,
- level_start_index=level_start_index,
- spatial_shapes=spatial_shapes,
- valid_ratios=valid_ratios,
- key_padding_mask=mask_flatten,
- memory_text=text_dict["encoded_text"],
- text_attention_mask=~text_dict["text_token_mask"],
- # we ~ the mask . False means use the token; True means pad the token
- position_ids=text_dict["position_ids"],
- text_self_attention_masks=text_dict["text_self_attention_masks"],
- )
- #########################################################
- # End Encoder
- # - memory: bs, \sum{hw}, c
- # - mask_flatten: bs, \sum{hw}
- # - lvl_pos_embed_flatten: bs, \sum{hw}, c
- # - enc_intermediate_output: None or (nenc+1, bs, nq, c) or (nenc, bs, nq, c)
- # - enc_intermediate_refpoints: None or (nenc+1, bs, nq, c) or (nenc, bs, nq, c)
- #########################################################
- text_dict["encoded_text"] = memory_text
- # if os.environ.get("SHILONG_AMP_INFNAN_DEBUG") == '1':
- # if memory.isnan().any() | memory.isinf().any():
- # import ipdb; ipdb.set_trace()
-
- if self.two_stage_type == "standard":
- output_memory, output_proposals = gen_encoder_output_proposals(
- memory, mask_flatten, spatial_shapes
- )
- output_memory = self.enc_output_norm(self.enc_output(output_memory))
-
- if text_dict is not None:
- enc_outputs_class_unselected = self.enc_out_class_embed(output_memory, text_dict)
- else:
- enc_outputs_class_unselected = self.enc_out_class_embed(output_memory)
-
- topk_logits = enc_outputs_class_unselected.max(-1)[0]
- enc_outputs_coord_unselected = (
- self.enc_out_bbox_embed(output_memory) + output_proposals
- ) # (bs, \sum{hw}, 4) unsigmoid
- topk = self.num_queries
-
- topk_proposals = torch.topk(topk_logits, topk, dim=1)[1] # bs, nq
-
- # gather boxes
- refpoint_embed_undetach = torch.gather(
- enc_outputs_coord_unselected, 1, topk_proposals.unsqueeze(-1).repeat(1, 1, 4)
- ) # unsigmoid
- refpoint_embed_ = refpoint_embed_undetach.detach()
- init_box_proposal = torch.gather(
- output_proposals, 1, topk_proposals.unsqueeze(-1).repeat(1, 1, 4)
- ).sigmoid() # sigmoid
-
- # gather tgt
- tgt_undetach = torch.gather(
- output_memory, 1, topk_proposals.unsqueeze(-1).repeat(1, 1, self.d_model)
- )
- if self.embed_init_tgt:
- tgt_ = (
- self.tgt_embed.weight[:, None, :].repeat(1, bs, 1).transpose(0, 1)
- ) # nq, bs, d_model
- else:
- tgt_ = tgt_undetach.detach()
-
- if refpoint_embed is not None:
- refpoint_embed = torch.cat([refpoint_embed, refpoint_embed_], dim=1)
- tgt = torch.cat([tgt, tgt_], dim=1)
- else:
- refpoint_embed, tgt = refpoint_embed_, tgt_
-
- elif self.two_stage_type == "no":
- tgt_ = (
- self.tgt_embed.weight[:, None, :].repeat(1, bs, 1).transpose(0, 1)
- ) # nq, bs, d_model
- refpoint_embed_ = (
- self.refpoint_embed.weight[:, None, :].repeat(1, bs, 1).transpose(0, 1)
- ) # nq, bs, 4
-
- if refpoint_embed is not None:
- refpoint_embed = torch.cat([refpoint_embed, refpoint_embed_], dim=1)
- tgt = torch.cat([tgt, tgt_], dim=1)
- else:
- refpoint_embed, tgt = refpoint_embed_, tgt_
-
- if self.num_patterns > 0:
- tgt_embed = tgt.repeat(1, self.num_patterns, 1)
- refpoint_embed = refpoint_embed.repeat(1, self.num_patterns, 1)
- tgt_pat = self.patterns.weight[None, :, :].repeat_interleave(
- self.num_queries, 1
- ) # 1, n_q*n_pat, d_model
- tgt = tgt_embed + tgt_pat
-
- init_box_proposal = refpoint_embed_.sigmoid()
-
- else:
- raise NotImplementedError("unknown two_stage_type {}".format(self.two_stage_type))
- #########################################################
- # End preparing tgt
- # - tgt: bs, NQ, d_model
- # - refpoint_embed(unsigmoid): bs, NQ, d_model
- #########################################################
-
- #########################################################
- # Begin Decoder
- #########################################################
- hs, references = self.decoder(
- tgt=tgt.transpose(0, 1),
- memory=memory.transpose(0, 1),
- memory_key_padding_mask=mask_flatten,
- pos=lvl_pos_embed_flatten.transpose(0, 1),
- refpoints_unsigmoid=refpoint_embed.transpose(0, 1),
- level_start_index=level_start_index,
- spatial_shapes=spatial_shapes,
- valid_ratios=valid_ratios,
- tgt_mask=attn_mask,
- memory_text=text_dict["encoded_text"],
- text_attention_mask=~text_dict["text_token_mask"],
- # we ~ the mask . False means use the token; True means pad the token
- )
- #########################################################
- # End Decoder
- # hs: n_dec, bs, nq, d_model
- # references: n_dec+1, bs, nq, query_dim
- #########################################################
-
- #########################################################
- # Begin postprocess
- #########################################################
- if self.two_stage_type == "standard":
- hs_enc = tgt_undetach.unsqueeze(0)
- ref_enc = refpoint_embed_undetach.sigmoid().unsqueeze(0)
- else:
- hs_enc = ref_enc = None
- #########################################################
- # End postprocess
- # hs_enc: (n_enc+1, bs, nq, d_model) or (1, bs, nq, d_model) or (n_enc, bs, nq, d_model) or None
- # ref_enc: (n_enc+1, bs, nq, query_dim) or (1, bs, nq, query_dim) or (n_enc, bs, nq, d_model) or None
- #########################################################
-
- return hs, references, hs_enc, ref_enc, init_box_proposal
- # hs: (n_dec, bs, nq, d_model)
- # references: sigmoid coordinates. (n_dec+1, bs, bq, 4)
- # hs_enc: (n_enc+1, bs, nq, d_model) or (1, bs, nq, d_model) or None
- # ref_enc: sigmoid coordinates. \
- # (n_enc+1, bs, nq, query_dim) or (1, bs, nq, query_dim) or None
-
-
-class TransformerEncoder(nn.Module):
- def __init__(
- self,
- encoder_layer,
- num_layers,
- d_model=256,
- num_queries=300,
- enc_layer_share=False,
- text_enhance_layer=None,
- feature_fusion_layer=None,
- use_checkpoint=False,
- use_transformer_ckpt=False,
- ):
- """_summary_
-
- Args:
- encoder_layer (_type_): _description_
- num_layers (_type_): _description_
- norm (_type_, optional): _description_. Defaults to None.
- d_model (int, optional): _description_. Defaults to 256.
- num_queries (int, optional): _description_. Defaults to 300.
- enc_layer_share (bool, optional): _description_. Defaults to False.
-
- """
- super().__init__()
- # prepare layers
- self.layers = []
- self.text_layers = []
- self.fusion_layers = []
- if num_layers > 0:
- self.layers = _get_clones(encoder_layer, num_layers, layer_share=enc_layer_share)
-
- if text_enhance_layer is not None:
- self.text_layers = _get_clones(
- text_enhance_layer, num_layers, layer_share=enc_layer_share
- )
- if feature_fusion_layer is not None:
- self.fusion_layers = _get_clones(
- feature_fusion_layer, num_layers, layer_share=enc_layer_share
- )
- else:
- self.layers = []
- del encoder_layer
-
- if text_enhance_layer is not None:
- self.text_layers = []
- del text_enhance_layer
- if feature_fusion_layer is not None:
- self.fusion_layers = []
- del feature_fusion_layer
-
- self.query_scale = None
- self.num_queries = num_queries
- self.num_layers = num_layers
- self.d_model = d_model
-
- self.use_checkpoint = use_checkpoint
- self.use_transformer_ckpt = use_transformer_ckpt
-
- @staticmethod
- def get_reference_points(spatial_shapes, valid_ratios, device):
- reference_points_list = []
- for lvl, (H_, W_) in enumerate(spatial_shapes):
-
- ref_y, ref_x = torch.meshgrid(
- torch.linspace(0.5, H_ - 0.5, H_, dtype=torch.float32, device=device),
- torch.linspace(0.5, W_ - 0.5, W_, dtype=torch.float32, device=device),
- )
- ref_y = ref_y.reshape(-1)[None] / (valid_ratios[:, None, lvl, 1] * H_)
- ref_x = ref_x.reshape(-1)[None] / (valid_ratios[:, None, lvl, 0] * W_)
- ref = torch.stack((ref_x, ref_y), -1)
- reference_points_list.append(ref)
- reference_points = torch.cat(reference_points_list, 1)
- reference_points = reference_points[:, :, None] * valid_ratios[:, None]
- return reference_points
-
- def forward(
- self,
- # for images
- src: Tensor,
- pos: Tensor,
- spatial_shapes: Tensor,
- level_start_index: Tensor,
- valid_ratios: Tensor,
- key_padding_mask: Tensor,
- # for texts
- memory_text: Tensor = None,
- text_attention_mask: Tensor = None,
- pos_text: Tensor = None,
- text_self_attention_masks: Tensor = None,
- position_ids: Tensor = None,
- ):
- """
- Input:
- - src: [bs, sum(hi*wi), 256]
- - pos: pos embed for src. [bs, sum(hi*wi), 256]
- - spatial_shapes: h,w of each level [num_level, 2]
- - level_start_index: [num_level] start point of level in sum(hi*wi).
- - valid_ratios: [bs, num_level, 2]
- - key_padding_mask: [bs, sum(hi*wi)]
-
- - memory_text: bs, n_text, 256
- - text_attention_mask: bs, n_text
- False for no padding; True for padding
- - pos_text: bs, n_text, 256
-
- - position_ids: bs, n_text
- Intermedia:
- - reference_points: [bs, sum(hi*wi), num_level, 2]
- Outpus:
- - output: [bs, sum(hi*wi), 256]
- """
-
- output = src
-
- # preparation and reshape
- if self.num_layers > 0:
- reference_points = self.get_reference_points(
- spatial_shapes, valid_ratios, device=src.device
- )
-
- if self.text_layers:
- # generate pos_text
- bs, n_text, text_dim = memory_text.shape
- if pos_text is None and position_ids is None:
- pos_text = (
- torch.arange(n_text, device=memory_text.device)
- .float()
- .unsqueeze(0)
- .unsqueeze(-1)
- .repeat(bs, 1, 1)
- )
- pos_text = get_sine_pos_embed(pos_text, num_pos_feats=256, exchange_xy=False)
- if position_ids is not None:
- pos_text = get_sine_pos_embed(
- position_ids[..., None], num_pos_feats=256, exchange_xy=False
- )
-
- # main process
- for layer_id, layer in enumerate(self.layers):
- # if output.isnan().any() or memory_text.isnan().any():
- # if os.environ.get('IPDB_SHILONG_DEBUG', None) == 'INFO':
- # import ipdb; ipdb.set_trace()
- if self.fusion_layers:
- if self.use_checkpoint:
- output, memory_text = checkpoint.checkpoint(
- self.fusion_layers[layer_id],
- output,
- memory_text,
- key_padding_mask,
- text_attention_mask,
- )
- else:
- output, memory_text = self.fusion_layers[layer_id](
- v=output,
- l=memory_text,
- attention_mask_v=key_padding_mask,
- attention_mask_l=text_attention_mask,
- )
-
- if self.text_layers:
- memory_text = self.text_layers[layer_id](
- src=memory_text.transpose(0, 1),
- src_mask=~text_self_attention_masks, # note we use ~ for mask here
- src_key_padding_mask=text_attention_mask,
- pos=(pos_text.transpose(0, 1) if pos_text is not None else None),
- ).transpose(0, 1)
-
- # main process
- if self.use_transformer_ckpt:
- output = checkpoint.checkpoint(
- layer,
- output,
- pos,
- reference_points,
- spatial_shapes,
- level_start_index,
- key_padding_mask,
- )
- else:
- output = layer(
- src=output,
- pos=pos,
- reference_points=reference_points,
- spatial_shapes=spatial_shapes,
- level_start_index=level_start_index,
- key_padding_mask=key_padding_mask,
- )
-
- return output, memory_text
-
-
-class TransformerDecoder(nn.Module):
- def __init__(
- self,
- decoder_layer,
- num_layers,
- norm=None,
- return_intermediate=False,
- d_model=256,
- query_dim=4,
- num_feature_levels=1,
- ):
- super().__init__()
- if num_layers > 0:
- self.layers = _get_clones(decoder_layer, num_layers)
- else:
- self.layers = []
- self.num_layers = num_layers
- self.norm = norm
- self.return_intermediate = return_intermediate
- assert return_intermediate, "support return_intermediate only"
- self.query_dim = query_dim
- assert query_dim in [2, 4], "query_dim should be 2/4 but {}".format(query_dim)
- self.num_feature_levels = num_feature_levels
-
- self.ref_point_head = MLP(query_dim // 2 * d_model, d_model, d_model, 2)
- self.query_pos_sine_scale = None
-
- self.query_scale = None
- self.bbox_embed = None
- self.class_embed = None
-
- self.d_model = d_model
-
- self.ref_anchor_head = None
-
- def forward(
- self,
- tgt,
- memory,
- tgt_mask: Optional[Tensor] = None,
- memory_mask: Optional[Tensor] = None,
- tgt_key_padding_mask: Optional[Tensor] = None,
- memory_key_padding_mask: Optional[Tensor] = None,
- pos: Optional[Tensor] = None,
- refpoints_unsigmoid: Optional[Tensor] = None, # num_queries, bs, 2
- # for memory
- level_start_index: Optional[Tensor] = None, # num_levels
- spatial_shapes: Optional[Tensor] = None, # bs, num_levels, 2
- valid_ratios: Optional[Tensor] = None,
- # for text
- memory_text: Optional[Tensor] = None,
- text_attention_mask: Optional[Tensor] = None,
- ):
- """
- Input:
- - tgt: nq, bs, d_model
- - memory: hw, bs, d_model
- - pos: hw, bs, d_model
- - refpoints_unsigmoid: nq, bs, 2/4
- - valid_ratios/spatial_shapes: bs, nlevel, 2
- """
- output = tgt
-
- intermediate = []
- reference_points = refpoints_unsigmoid.sigmoid()
- ref_points = [reference_points]
-
- for layer_id, layer in enumerate(self.layers):
-
- if reference_points.shape[-1] == 4:
- reference_points_input = (
- reference_points[:, :, None]
- * torch.cat([valid_ratios, valid_ratios], -1)[None, :]
- ) # nq, bs, nlevel, 4
- else:
- assert reference_points.shape[-1] == 2
- reference_points_input = reference_points[:, :, None] * valid_ratios[None, :]
- query_sine_embed = gen_sineembed_for_position(
- reference_points_input[:, :, 0, :]
- ) # nq, bs, 256*2
-
- # conditional query
- raw_query_pos = self.ref_point_head(query_sine_embed) # nq, bs, 256
- pos_scale = self.query_scale(output) if self.query_scale is not None else 1
- query_pos = pos_scale * raw_query_pos
- # if os.environ.get("SHILONG_AMP_INFNAN_DEBUG") == '1':
- # if query_pos.isnan().any() | query_pos.isinf().any():
- # import ipdb; ipdb.set_trace()
-
- # main process
- output = layer(
- tgt=output,
- tgt_query_pos=query_pos,
- tgt_query_sine_embed=query_sine_embed,
- tgt_key_padding_mask=tgt_key_padding_mask,
- tgt_reference_points=reference_points_input,
- memory_text=memory_text,
- text_attention_mask=text_attention_mask,
- memory=memory,
- memory_key_padding_mask=memory_key_padding_mask,
- memory_level_start_index=level_start_index,
- memory_spatial_shapes=spatial_shapes,
- memory_pos=pos,
- self_attn_mask=tgt_mask,
- cross_attn_mask=memory_mask,
- )
- if output.isnan().any() | output.isinf().any():
- print(f"output layer_id {layer_id} is nan")
- try:
- num_nan = output.isnan().sum().item()
- num_inf = output.isinf().sum().item()
- print(f"num_nan {num_nan}, num_inf {num_inf}")
- except Exception as e:
- print(e)
- # if os.environ.get("SHILONG_AMP_INFNAN_DEBUG") == '1':
- # import ipdb; ipdb.set_trace()
-
- # iter update
- if self.bbox_embed is not None:
- # box_holder = self.bbox_embed(output)
- # box_holder[..., :self.query_dim] += inverse_sigmoid(reference_points)
- # new_reference_points = box_holder[..., :self.query_dim].sigmoid()
-
- reference_before_sigmoid = inverse_sigmoid(reference_points)
- delta_unsig = self.bbox_embed[layer_id](output)
- outputs_unsig = delta_unsig + reference_before_sigmoid
- new_reference_points = outputs_unsig.sigmoid()
-
- reference_points = new_reference_points.detach()
- # if layer_id != self.num_layers - 1:
- ref_points.append(new_reference_points)
-
- intermediate.append(self.norm(output))
-
- return [
- [itm_out.transpose(0, 1) for itm_out in intermediate],
- [itm_refpoint.transpose(0, 1) for itm_refpoint in ref_points],
- ]
-
-
-class DeformableTransformerEncoderLayer(nn.Module):
- def __init__(
- self,
- d_model=256,
- d_ffn=1024,
- dropout=0.1,
- activation="relu",
- n_levels=4,
- n_heads=8,
- n_points=4,
- ):
- super().__init__()
-
- # self attention
- self.self_attn = MSDeformAttn(
- embed_dim=d_model,
- num_levels=n_levels,
- num_heads=n_heads,
- num_points=n_points,
- batch_first=True,
- )
- self.dropout1 = nn.Dropout(dropout)
- self.norm1 = nn.LayerNorm(d_model)
-
- # ffn
- self.linear1 = nn.Linear(d_model, d_ffn)
- self.activation = _get_activation_fn(activation, d_model=d_ffn)
- self.dropout2 = nn.Dropout(dropout)
- self.linear2 = nn.Linear(d_ffn, d_model)
- self.dropout3 = nn.Dropout(dropout)
- self.norm2 = nn.LayerNorm(d_model)
-
- @staticmethod
- def with_pos_embed(tensor, pos):
- return tensor if pos is None else tensor + pos
-
- def forward_ffn(self, src):
- src2 = self.linear2(self.dropout2(self.activation(self.linear1(src))))
- src = src + self.dropout3(src2)
- src = self.norm2(src)
- return src
-
- def forward(
- self, src, pos, reference_points, spatial_shapes, level_start_index, key_padding_mask=None
- ):
- # self attention
- # import ipdb; ipdb.set_trace()
- src2 = self.self_attn(
- query=self.with_pos_embed(src, pos),
- reference_points=reference_points,
- value=src,
- spatial_shapes=spatial_shapes,
- level_start_index=level_start_index,
- key_padding_mask=key_padding_mask,
- )
- src = src + self.dropout1(src2)
- src = self.norm1(src)
-
- # ffn
- src = self.forward_ffn(src)
-
- return src
-
-
-class DeformableTransformerDecoderLayer(nn.Module):
- def __init__(
- self,
- d_model=256,
- d_ffn=1024,
- dropout=0.1,
- activation="relu",
- n_levels=4,
- n_heads=8,
- n_points=4,
- use_text_feat_guide=False,
- use_text_cross_attention=False,
- ):
- super().__init__()
-
- # cross attention
- self.cross_attn = MSDeformAttn(
- embed_dim=d_model,
- num_levels=n_levels,
- num_heads=n_heads,
- num_points=n_points,
- batch_first=True,
- )
- self.dropout1 = nn.Dropout(dropout) if dropout > 0 else nn.Identity()
- self.norm1 = nn.LayerNorm(d_model)
-
- # cross attention text
- if use_text_cross_attention:
- self.ca_text = nn.MultiheadAttention(d_model, n_heads, dropout=dropout)
- self.catext_dropout = nn.Dropout(dropout) if dropout > 0 else nn.Identity()
- self.catext_norm = nn.LayerNorm(d_model)
-
- # self attention
- self.self_attn = nn.MultiheadAttention(d_model, n_heads, dropout=dropout)
- self.dropout2 = nn.Dropout(dropout) if dropout > 0 else nn.Identity()
- self.norm2 = nn.LayerNorm(d_model)
-
- # ffn
- self.linear1 = nn.Linear(d_model, d_ffn)
- self.activation = _get_activation_fn(activation, d_model=d_ffn, batch_dim=1)
- self.dropout3 = nn.Dropout(dropout) if dropout > 0 else nn.Identity()
- self.linear2 = nn.Linear(d_ffn, d_model)
- self.dropout4 = nn.Dropout(dropout) if dropout > 0 else nn.Identity()
- self.norm3 = nn.LayerNorm(d_model)
-
- self.key_aware_proj = None
- self.use_text_feat_guide = use_text_feat_guide
- assert not use_text_feat_guide
- self.use_text_cross_attention = use_text_cross_attention
-
- def rm_self_attn_modules(self):
- self.self_attn = None
- self.dropout2 = None
- self.norm2 = None
-
- @staticmethod
- def with_pos_embed(tensor, pos):
- return tensor if pos is None else tensor + pos
-
- def forward_ffn(self, tgt):
- with torch.cuda.amp.autocast(enabled=False):
- tgt2 = self.linear2(self.dropout3(self.activation(self.linear1(tgt))))
- tgt = tgt + self.dropout4(tgt2)
- tgt = self.norm3(tgt)
- return tgt
-
- def forward(
- self,
- # for tgt
- tgt: Optional[Tensor], # nq, bs, d_model
- tgt_query_pos: Optional[Tensor] = None, # pos for query. MLP(Sine(pos))
- tgt_query_sine_embed: Optional[Tensor] = None, # pos for query. Sine(pos)
- tgt_key_padding_mask: Optional[Tensor] = None,
- tgt_reference_points: Optional[Tensor] = None, # nq, bs, 4
- memory_text: Optional[Tensor] = None, # bs, num_token, d_model
- text_attention_mask: Optional[Tensor] = None, # bs, num_token
- # for memory
- memory: Optional[Tensor] = None, # hw, bs, d_model
- memory_key_padding_mask: Optional[Tensor] = None,
- memory_level_start_index: Optional[Tensor] = None, # num_levels
- memory_spatial_shapes: Optional[Tensor] = None, # bs, num_levels, 2
- memory_pos: Optional[Tensor] = None, # pos for memory
- # sa
- self_attn_mask: Optional[Tensor] = None, # mask used for self-attention
- cross_attn_mask: Optional[Tensor] = None, # mask used for cross-attention
- ):
- """
- Input:
- - tgt/tgt_query_pos: nq, bs, d_model
- -
- """
- assert cross_attn_mask is None
-
- # self attention
- if self.self_attn is not None:
- # import ipdb; ipdb.set_trace()
- q = k = self.with_pos_embed(tgt, tgt_query_pos)
- tgt2 = self.self_attn(q, k, tgt, attn_mask=self_attn_mask)[0]
- tgt = tgt + self.dropout2(tgt2)
- tgt = self.norm2(tgt)
-
- if self.use_text_cross_attention:
- tgt2 = self.ca_text(
- self.with_pos_embed(tgt, tgt_query_pos),
- memory_text.transpose(0, 1),
- memory_text.transpose(0, 1),
- key_padding_mask=text_attention_mask,
- )[0]
- tgt = tgt + self.catext_dropout(tgt2)
- tgt = self.catext_norm(tgt)
-
- tgt2 = self.cross_attn(
- query=self.with_pos_embed(tgt, tgt_query_pos).transpose(0, 1),
- reference_points=tgt_reference_points.transpose(0, 1).contiguous(),
- value=memory.transpose(0, 1),
- spatial_shapes=memory_spatial_shapes,
- level_start_index=memory_level_start_index,
- key_padding_mask=memory_key_padding_mask,
- ).transpose(0, 1)
- tgt = tgt + self.dropout1(tgt2)
- tgt = self.norm1(tgt)
-
- # ffn
- tgt = self.forward_ffn(tgt)
-
- return tgt
-
-
-def build_transformer(args):
- return Transformer(
- d_model=args.hidden_dim,
- dropout=args.dropout,
- nhead=args.nheads,
- num_queries=args.num_queries,
- dim_feedforward=args.dim_feedforward,
- num_encoder_layers=args.enc_layers,
- num_decoder_layers=args.dec_layers,
- normalize_before=args.pre_norm,
- return_intermediate_dec=True,
- query_dim=args.query_dim,
- activation=args.transformer_activation,
- num_patterns=args.num_patterns,
- num_feature_levels=args.num_feature_levels,
- enc_n_points=args.enc_n_points,
- dec_n_points=args.dec_n_points,
- learnable_tgt_init=True,
- # two stage
- two_stage_type=args.two_stage_type, # ['no', 'standard', 'early']
- embed_init_tgt=args.embed_init_tgt,
- use_text_enhancer=args.use_text_enhancer,
- use_fusion_layer=args.use_fusion_layer,
- use_checkpoint=args.use_checkpoint,
- use_transformer_ckpt=args.use_transformer_ckpt,
- use_text_cross_attention=args.use_text_cross_attention,
- text_dropout=args.text_dropout,
- fusion_dropout=args.fusion_dropout,
- fusion_droppath=args.fusion_droppath,
- )
diff --git a/spaces/yizhangliu/Grounded-Segment-Anything/transformers_4_35_0/data/datasets/language_modeling.py b/spaces/yizhangliu/Grounded-Segment-Anything/transformers_4_35_0/data/datasets/language_modeling.py
deleted file mode 100644
index 6c23bf23cf14d4953a278dd3584093d0af084133..0000000000000000000000000000000000000000
--- a/spaces/yizhangliu/Grounded-Segment-Anything/transformers_4_35_0/data/datasets/language_modeling.py
+++ /dev/null
@@ -1,530 +0,0 @@
-# Copyright 2020 The HuggingFace Team. All rights reserved.
-#
-# Licensed under the Apache License, Version 2.0 (the "License");
-# you may not use this file except in compliance with the License.
-# You may obtain a copy of the License at
-#
-# http://www.apache.org/licenses/LICENSE-2.0
-#
-# Unless required by applicable law or agreed to in writing, software
-# distributed under the License is distributed on an "AS IS" BASIS,
-# WITHOUT WARRANTIES OR CONDITIONS OF ANY KIND, either express or implied.
-# See the License for the specific language governing permissions and
-# limitations under the License.
-
-import json
-import os
-import pickle
-import random
-import time
-import warnings
-from typing import Dict, List, Optional
-
-import torch
-from filelock import FileLock
-from torch.utils.data import Dataset
-
-from ...tokenization_utils import PreTrainedTokenizer
-from ...utils import logging
-
-
-logger = logging.get_logger(__name__)
-
-
-DEPRECATION_WARNING = (
- "This dataset will be removed from the library soon, preprocessing should be handled with the 🤗 Datasets "
- "library. You can have a look at this example script for pointers: {0}"
-)
-
-
-class TextDataset(Dataset):
- """
- This will be superseded by a framework-agnostic approach soon.
- """
-
- def __init__(
- self,
- tokenizer: PreTrainedTokenizer,
- file_path: str,
- block_size: int,
- overwrite_cache=False,
- cache_dir: Optional[str] = None,
- ):
- warnings.warn(
- DEPRECATION_WARNING.format(
- "https://github.com/huggingface/transformers/blob/main/examples/pytorch/language-modeling/run_mlm.py"
- ),
- FutureWarning,
- )
- if os.path.isfile(file_path) is False:
- raise ValueError(f"Input file path {file_path} not found")
-
- block_size = block_size - tokenizer.num_special_tokens_to_add(pair=False)
-
- directory, filename = os.path.split(file_path)
- cached_features_file = os.path.join(
- cache_dir if cache_dir is not None else directory,
- f"cached_lm_{tokenizer.__class__.__name__}_{block_size}_{filename}",
- )
-
- # Make sure only the first process in distributed training processes the dataset,
- # and the others will use the cache.
- lock_path = cached_features_file + ".lock"
- with FileLock(lock_path):
- if os.path.exists(cached_features_file) and not overwrite_cache:
- start = time.time()
- with open(cached_features_file, "rb") as handle:
- self.examples = pickle.load(handle)
- logger.info(
- f"Loading features from cached file {cached_features_file} [took %.3f s]", time.time() - start
- )
-
- else:
- logger.info(f"Creating features from dataset file at {directory}")
-
- self.examples = []
- with open(file_path, encoding="utf-8") as f:
- text = f.read()
-
- tokenized_text = tokenizer.convert_tokens_to_ids(tokenizer.tokenize(text))
-
- for i in range(0, len(tokenized_text) - block_size + 1, block_size): # Truncate in block of block_size
- self.examples.append(
- tokenizer.build_inputs_with_special_tokens(tokenized_text[i : i + block_size])
- )
- # Note that we are losing the last truncated example here for the sake of simplicity (no padding)
- # If your dataset is small, first you should look for a bigger one :-) and second you
- # can change this behavior by adding (model specific) padding.
-
- start = time.time()
- with open(cached_features_file, "wb") as handle:
- pickle.dump(self.examples, handle, protocol=pickle.HIGHEST_PROTOCOL)
- logger.info(
- f"Saving features into cached file {cached_features_file} [took {time.time() - start:.3f} s]"
- )
-
- def __len__(self):
- return len(self.examples)
-
- def __getitem__(self, i) -> torch.Tensor:
- return torch.tensor(self.examples[i], dtype=torch.long)
-
-
-class LineByLineTextDataset(Dataset):
- """
- This will be superseded by a framework-agnostic approach soon.
- """
-
- def __init__(self, tokenizer: PreTrainedTokenizer, file_path: str, block_size: int):
- warnings.warn(
- DEPRECATION_WARNING.format(
- "https://github.com/huggingface/transformers/blob/main/examples/pytorch/language-modeling/run_mlm.py"
- ),
- FutureWarning,
- )
- if os.path.isfile(file_path) is False:
- raise ValueError(f"Input file path {file_path} not found")
- # Here, we do not cache the features, operating under the assumption
- # that we will soon use fast multithreaded tokenizers from the
- # `tokenizers` repo everywhere =)
- logger.info(f"Creating features from dataset file at {file_path}")
-
- with open(file_path, encoding="utf-8") as f:
- lines = [line for line in f.read().splitlines() if (len(line) > 0 and not line.isspace())]
-
- batch_encoding = tokenizer(lines, add_special_tokens=True, truncation=True, max_length=block_size)
- self.examples = batch_encoding["input_ids"]
- self.examples = [{"input_ids": torch.tensor(e, dtype=torch.long)} for e in self.examples]
-
- def __len__(self):
- return len(self.examples)
-
- def __getitem__(self, i) -> Dict[str, torch.tensor]:
- return self.examples[i]
-
-
-class LineByLineWithRefDataset(Dataset):
- """
- This will be superseded by a framework-agnostic approach soon.
- """
-
- def __init__(self, tokenizer: PreTrainedTokenizer, file_path: str, block_size: int, ref_path: str):
- warnings.warn(
- DEPRECATION_WARNING.format(
- "https://github.com/huggingface/transformers/blob/main/examples/pytorch/language-modeling/run_mlm_wwm.py"
- ),
- FutureWarning,
- )
- if os.path.isfile(file_path) is False:
- raise ValueError(f"Input file path {file_path} not found")
- if os.path.isfile(ref_path) is False:
- raise ValueError(f"Ref file path {file_path} not found")
- # Here, we do not cache the features, operating under the assumption
- # that we will soon use fast multithreaded tokenizers from the
- # `tokenizers` repo everywhere =)
- logger.info(f"Creating features from dataset file at {file_path}")
- logger.info(f"Use ref segment results at {ref_path}")
- with open(file_path, encoding="utf-8") as f:
- data = f.readlines() # use this method to avoid delimiter '\u2029' to split a line
- data = [line.strip() for line in data if len(line) > 0 and not line.isspace()]
- # Get ref inf from file
- with open(ref_path, encoding="utf-8") as f:
- ref = [json.loads(line) for line in f.read().splitlines() if (len(line) > 0 and not line.isspace())]
- if len(data) != len(ref):
- raise ValueError(
- f"Length of Input file should be equal to Ref file. But the length of {file_path} is {len(data)} "
- f"while length of {ref_path} is {len(ref)}"
- )
-
- batch_encoding = tokenizer(data, add_special_tokens=True, truncation=True, max_length=block_size)
- self.examples = batch_encoding["input_ids"]
- self.examples = [{"input_ids": torch.tensor(e, dtype=torch.long)} for e in self.examples]
-
- n = len(self.examples)
- for i in range(n):
- self.examples[i]["chinese_ref"] = torch.tensor(ref[i], dtype=torch.long)
-
- def __len__(self):
- return len(self.examples)
-
- def __getitem__(self, i) -> Dict[str, torch.tensor]:
- return self.examples[i]
-
-
-class LineByLineWithSOPTextDataset(Dataset):
- """
- Dataset for sentence order prediction task, prepare sentence pairs for SOP task
- """
-
- def __init__(self, tokenizer: PreTrainedTokenizer, file_dir: str, block_size: int):
- warnings.warn(
- DEPRECATION_WARNING.format(
- "https://github.com/huggingface/transformers/blob/main/examples/pytorch/language-modeling/run_mlm.py"
- ),
- FutureWarning,
- )
- if os.path.isdir(file_dir) is False:
- raise ValueError(f"{file_dir} is not a directory")
- logger.info(f"Creating features from dataset file folder at {file_dir}")
- self.examples = []
- # TODO: randomness could apply a random seed, ex. rng = random.Random(random_seed)
- # file path looks like ./dataset/wiki_1, ./dataset/wiki_2
- for file_name in os.listdir(file_dir):
- file_path = os.path.join(file_dir, file_name)
- if os.path.isfile(file_path) is False:
- raise ValueError(f"{file_path} is not a file")
- article_open = False
- with open(file_path, encoding="utf-8") as f:
- original_lines = f.readlines()
- article_lines = []
- for line in original_lines:
- if "" in line:
- article_open = False
- document = [
- tokenizer.convert_tokens_to_ids(tokenizer.tokenize(line))
- for line in article_lines[1:]
- if (len(line) > 0 and not line.isspace())
- ]
-
- examples = self.create_examples_from_document(document, block_size, tokenizer)
- self.examples.extend(examples)
- article_lines = []
- else:
- if article_open:
- article_lines.append(line)
-
- logger.info("Dataset parse finished.")
-
- def create_examples_from_document(self, document, block_size, tokenizer, short_seq_prob=0.1):
- """Creates examples for a single document."""
-
- # Account for special tokens
- max_num_tokens = block_size - tokenizer.num_special_tokens_to_add(pair=True)
-
- # We *usually* want to fill up the entire sequence since we are padding
- # to `block_size` anyways, so short sequences are generally wasted
- # computation. However, we *sometimes*
- # (i.e., short_seq_prob == 0.1 == 10% of the time) want to use shorter
- # sequences to minimize the mismatch between pretraining and fine-tuning.
- # The `target_seq_length` is just a rough target however, whereas
- # `block_size` is a hard limit.
- target_seq_length = max_num_tokens
- if random.random() < short_seq_prob:
- target_seq_length = random.randint(2, max_num_tokens)
-
- # We DON'T just concatenate all of the tokens from a document into a long
- # sequence and choose an arbitrary split point because this would make the
- # next sentence prediction task too easy. Instead, we split the input into
- # segments "A" and "B" based on the actual "sentences" provided by the user
- # input.
- examples = []
- current_chunk = [] # a buffer stored current working segments
- current_length = 0
- i = 0
- while i < len(document):
- segment = document[i] # get a segment
- if not segment:
- i += 1
- continue
- current_chunk.append(segment) # add a segment to current chunk
- current_length += len(segment) # overall token length
- # if current length goes to the target length or reaches the end of file, start building token a and b
- if i == len(document) - 1 or current_length >= target_seq_length:
- if current_chunk:
- # `a_end` is how many segments from `current_chunk` go into the `A` (first) sentence.
- a_end = 1
- # if current chunk has more than 2 sentences, pick part of it `A` (first) sentence
- if len(current_chunk) >= 2:
- a_end = random.randint(1, len(current_chunk) - 1)
- # token a
- tokens_a = []
- for j in range(a_end):
- tokens_a.extend(current_chunk[j])
-
- # token b
- tokens_b = []
- for j in range(a_end, len(current_chunk)):
- tokens_b.extend(current_chunk[j])
-
- if len(tokens_a) == 0 or len(tokens_b) == 0:
- continue
-
- # switch tokens_a and tokens_b randomly
- if random.random() < 0.5:
- is_next = False
- tokens_a, tokens_b = tokens_b, tokens_a
- else:
- is_next = True
-
- def truncate_seq_pair(tokens_a, tokens_b, max_num_tokens):
- """Truncates a pair of sequences to a maximum sequence length."""
- while True:
- total_length = len(tokens_a) + len(tokens_b)
- if total_length <= max_num_tokens:
- break
- trunc_tokens = tokens_a if len(tokens_a) > len(tokens_b) else tokens_b
- if not (len(trunc_tokens) >= 1):
- raise ValueError("Sequence length to be truncated must be no less than one")
- # We want to sometimes truncate from the front and sometimes from the
- # back to add more randomness and avoid biases.
- if random.random() < 0.5:
- del trunc_tokens[0]
- else:
- trunc_tokens.pop()
-
- truncate_seq_pair(tokens_a, tokens_b, max_num_tokens)
- if not (len(tokens_a) >= 1):
- raise ValueError(f"Length of sequence a is {len(tokens_a)} which must be no less than 1")
- if not (len(tokens_b) >= 1):
- raise ValueError(f"Length of sequence b is {len(tokens_b)} which must be no less than 1")
-
- # add special tokens
- input_ids = tokenizer.build_inputs_with_special_tokens(tokens_a, tokens_b)
- # add token type ids, 0 for sentence a, 1 for sentence b
- token_type_ids = tokenizer.create_token_type_ids_from_sequences(tokens_a, tokens_b)
-
- example = {
- "input_ids": torch.tensor(input_ids, dtype=torch.long),
- "token_type_ids": torch.tensor(token_type_ids, dtype=torch.long),
- "sentence_order_label": torch.tensor(0 if is_next else 1, dtype=torch.long),
- }
- examples.append(example)
- current_chunk = [] # clear current chunk
- current_length = 0 # reset current text length
- i += 1 # go to next line
- return examples
-
- def __len__(self):
- return len(self.examples)
-
- def __getitem__(self, i) -> Dict[str, torch.tensor]:
- return self.examples[i]
-
-
-class TextDatasetForNextSentencePrediction(Dataset):
- """
- This will be superseded by a framework-agnostic approach soon.
- """
-
- def __init__(
- self,
- tokenizer: PreTrainedTokenizer,
- file_path: str,
- block_size: int,
- overwrite_cache=False,
- short_seq_probability=0.1,
- nsp_probability=0.5,
- ):
- warnings.warn(
- DEPRECATION_WARNING.format(
- "https://github.com/huggingface/transformers/blob/main/examples/pytorch/language-modeling/run_mlm.py"
- ),
- FutureWarning,
- )
- if not os.path.isfile(file_path):
- raise ValueError(f"Input file path {file_path} not found")
-
- self.short_seq_probability = short_seq_probability
- self.nsp_probability = nsp_probability
-
- directory, filename = os.path.split(file_path)
- cached_features_file = os.path.join(
- directory,
- f"cached_nsp_{tokenizer.__class__.__name__}_{block_size}_{filename}",
- )
-
- self.tokenizer = tokenizer
-
- # Make sure only the first process in distributed training processes the dataset,
- # and the others will use the cache.
- lock_path = cached_features_file + ".lock"
-
- # Input file format:
- # (1) One sentence per line. These should ideally be actual sentences, not
- # entire paragraphs or arbitrary spans of text. (Because we use the
- # sentence boundaries for the "next sentence prediction" task).
- # (2) Blank lines between documents. Document boundaries are needed so
- # that the "next sentence prediction" task doesn't span between documents.
- #
- # Example:
- # I am very happy.
- # Here is the second sentence.
- #
- # A new document.
-
- with FileLock(lock_path):
- if os.path.exists(cached_features_file) and not overwrite_cache:
- start = time.time()
- with open(cached_features_file, "rb") as handle:
- self.examples = pickle.load(handle)
- logger.info(
- f"Loading features from cached file {cached_features_file} [took %.3f s]", time.time() - start
- )
- else:
- logger.info(f"Creating features from dataset file at {directory}")
-
- self.documents = [[]]
- with open(file_path, encoding="utf-8") as f:
- while True:
- line = f.readline()
- if not line:
- break
- line = line.strip()
-
- # Empty lines are used as document delimiters
- if not line and len(self.documents[-1]) != 0:
- self.documents.append([])
- tokens = tokenizer.tokenize(line)
- tokens = tokenizer.convert_tokens_to_ids(tokens)
- if tokens:
- self.documents[-1].append(tokens)
-
- logger.info(f"Creating examples from {len(self.documents)} documents.")
- self.examples = []
- for doc_index, document in enumerate(self.documents):
- self.create_examples_from_document(document, doc_index, block_size)
-
- start = time.time()
- with open(cached_features_file, "wb") as handle:
- pickle.dump(self.examples, handle, protocol=pickle.HIGHEST_PROTOCOL)
- logger.info(
- f"Saving features into cached file {cached_features_file} [took {time.time() - start:.3f} s]"
- )
-
- def create_examples_from_document(self, document: List[List[int]], doc_index: int, block_size: int):
- """Creates examples for a single document."""
-
- max_num_tokens = block_size - self.tokenizer.num_special_tokens_to_add(pair=True)
-
- # We *usually* want to fill up the entire sequence since we are padding
- # to `block_size` anyways, so short sequences are generally wasted
- # computation. However, we *sometimes*
- # (i.e., short_seq_prob == 0.1 == 10% of the time) want to use shorter
- # sequences to minimize the mismatch between pretraining and fine-tuning.
- # The `target_seq_length` is just a rough target however, whereas
- # `block_size` is a hard limit.
- target_seq_length = max_num_tokens
- if random.random() < self.short_seq_probability:
- target_seq_length = random.randint(2, max_num_tokens)
-
- current_chunk = [] # a buffer stored current working segments
- current_length = 0
- i = 0
-
- while i < len(document):
- segment = document[i]
- current_chunk.append(segment)
- current_length += len(segment)
- if i == len(document) - 1 or current_length >= target_seq_length:
- if current_chunk:
- # `a_end` is how many segments from `current_chunk` go into the `A`
- # (first) sentence.
- a_end = 1
- if len(current_chunk) >= 2:
- a_end = random.randint(1, len(current_chunk) - 1)
-
- tokens_a = []
- for j in range(a_end):
- tokens_a.extend(current_chunk[j])
-
- tokens_b = []
-
- if len(current_chunk) == 1 or random.random() < self.nsp_probability:
- is_random_next = True
- target_b_length = target_seq_length - len(tokens_a)
-
- # This should rarely go for more than one iteration for large
- # corpora. However, just to be careful, we try to make sure that
- # the random document is not the same as the document
- # we're processing.
- for _ in range(10):
- random_document_index = random.randint(0, len(self.documents) - 1)
- if random_document_index != doc_index:
- break
-
- random_document = self.documents[random_document_index]
- random_start = random.randint(0, len(random_document) - 1)
- for j in range(random_start, len(random_document)):
- tokens_b.extend(random_document[j])
- if len(tokens_b) >= target_b_length:
- break
- # We didn't actually use these segments so we "put them back" so
- # they don't go to waste.
- num_unused_segments = len(current_chunk) - a_end
- i -= num_unused_segments
- # Actual next
- else:
- is_random_next = False
- for j in range(a_end, len(current_chunk)):
- tokens_b.extend(current_chunk[j])
-
- if not (len(tokens_a) >= 1):
- raise ValueError(f"Length of sequence a is {len(tokens_a)} which must be no less than 1")
- if not (len(tokens_b) >= 1):
- raise ValueError(f"Length of sequence b is {len(tokens_b)} which must be no less than 1")
-
- # add special tokens
- input_ids = self.tokenizer.build_inputs_with_special_tokens(tokens_a, tokens_b)
- # add token type ids, 0 for sentence a, 1 for sentence b
- token_type_ids = self.tokenizer.create_token_type_ids_from_sequences(tokens_a, tokens_b)
-
- example = {
- "input_ids": torch.tensor(input_ids, dtype=torch.long),
- "token_type_ids": torch.tensor(token_type_ids, dtype=torch.long),
- "next_sentence_label": torch.tensor(1 if is_random_next else 0, dtype=torch.long),
- }
-
- self.examples.append(example)
-
- current_chunk = []
- current_length = 0
-
- i += 1
-
- def __len__(self):
- return len(self.examples)
-
- def __getitem__(self, i):
- return self.examples[i]
diff --git a/spaces/ylacombe/children-story/README.md b/spaces/ylacombe/children-story/README.md
deleted file mode 100644
index 035bf92be0eadb09457e1d258e3f18e355b9d255..0000000000000000000000000000000000000000
--- a/spaces/ylacombe/children-story/README.md
+++ /dev/null
@@ -1,12 +0,0 @@
----
-title: Children Story
-emoji: 🐨
-colorFrom: indigo
-colorTo: gray
-sdk: gradio
-sdk_version: 4.1.0
-app_file: app.py
-pinned: false
----
-
-Check out the configuration reference at https://huggingface.co/docs/hub/spaces-config-reference
diff --git a/spaces/zhangs2022/ChuanhuChatGPT/run_macOS.command b/spaces/zhangs2022/ChuanhuChatGPT/run_macOS.command
deleted file mode 100644
index 2d26597ae47519f42336ccffc16646713a192ae1..0000000000000000000000000000000000000000
--- a/spaces/zhangs2022/ChuanhuChatGPT/run_macOS.command
+++ /dev/null
@@ -1,31 +0,0 @@
-#!/bin/bash
-
-# 获取脚本所在目录
-script_dir=$(dirname "$(readlink -f "$0")")
-
-# 将工作目录更改为脚本所在目录
-cd "$script_dir" || exit
-
-# 检查Git仓库是否有更新
-git remote update
-pwd
-
-if ! git status -uno | grep 'up to date' > /dev/null; then
- # 如果有更新,关闭当前运行的服务器
- pkill -f ChuanhuChatbot.py
-
- # 拉取最新更改
- git pull
-
- # 安装依赖
- pip3 install -r requirements.txt
-
- # 重新启动服务器
- nohup python3 ChuanhuChatbot.py &
-fi
-
-# 检查ChuanhuChatbot.py是否在运行
-if ! pgrep -f ChuanhuChatbot.py > /dev/null; then
- # 如果没有运行,启动服务器
- nohup python3 ChuanhuChatbot.py &
-fi
diff --git a/spaces/zhanpj/ChatGPT/modules/utils.py b/spaces/zhanpj/ChatGPT/modules/utils.py
deleted file mode 100644
index ef8963d19b16e187a3381b85325d74a1a3562d64..0000000000000000000000000000000000000000
--- a/spaces/zhanpj/ChatGPT/modules/utils.py
+++ /dev/null
@@ -1,520 +0,0 @@
-# -*- coding:utf-8 -*-
-from __future__ import annotations
-from typing import TYPE_CHECKING, Any, Callable, Dict, List, Tuple, Type
-import logging
-import json
-import os
-import datetime
-import hashlib
-import csv
-import requests
-import re
-import html
-import sys
-import subprocess
-
-import gradio as gr
-from pypinyin import lazy_pinyin
-import tiktoken
-import mdtex2html
-from markdown import markdown
-from pygments import highlight
-from pygments.lexers import get_lexer_by_name
-from pygments.formatters import HtmlFormatter
-
-from modules.presets import *
-import modules.shared as shared
-
-logging.basicConfig(
- level=logging.INFO,
- format="%(asctime)s [%(levelname)s] [%(filename)s:%(lineno)d] %(message)s",
-)
-
-if TYPE_CHECKING:
- from typing import TypedDict
-
- class DataframeData(TypedDict):
- headers: List[str]
- data: List[List[str | int | bool]]
-
-
-def count_token(message):
- encoding = tiktoken.get_encoding("cl100k_base")
- input_str = f"role: {message['role']}, content: {message['content']}"
- length = len(encoding.encode(input_str))
- return length
-
-
-def markdown_to_html_with_syntax_highlight(md_str):
- def replacer(match):
- lang = match.group(1) or "text"
- code = match.group(2)
-
- try:
- lexer = get_lexer_by_name(lang, stripall=True)
- except ValueError:
- lexer = get_lexer_by_name("text", stripall=True)
-
- formatter = HtmlFormatter()
- highlighted_code = highlight(code, lexer, formatter)
-
- return f'{highlighted_code}
'
-
- code_block_pattern = r"```(\w+)?\n([\s\S]+?)\n```"
- md_str = re.sub(code_block_pattern, replacer, md_str, flags=re.MULTILINE)
-
- html_str = markdown(md_str)
- return html_str
-
-
-def normalize_markdown(md_text: str) -> str:
- lines = md_text.split("\n")
- normalized_lines = []
- inside_list = False
-
- for i, line in enumerate(lines):
- if re.match(r"^(\d+\.|-|\*|\+)\s", line.strip()):
- if not inside_list and i > 0 and lines[i - 1].strip() != "":
- normalized_lines.append("")
- inside_list = True
- normalized_lines.append(line)
- elif inside_list and line.strip() == "":
- if i < len(lines) - 1 and not re.match(
- r"^(\d+\.|-|\*|\+)\s", lines[i + 1].strip()
- ):
- normalized_lines.append(line)
- continue
- else:
- inside_list = False
- normalized_lines.append(line)
-
- return "\n".join(normalized_lines)
-
-
-def convert_mdtext(md_text):
- code_block_pattern = re.compile(r"```(.*?)(?:```|$)", re.DOTALL)
- inline_code_pattern = re.compile(r"`(.*?)`", re.DOTALL)
- code_blocks = code_block_pattern.findall(md_text)
- non_code_parts = code_block_pattern.split(md_text)[::2]
-
- result = []
- for non_code, code in zip(non_code_parts, code_blocks + [""]):
- if non_code.strip():
- non_code = normalize_markdown(non_code)
- if inline_code_pattern.search(non_code):
- result.append(markdown(non_code, extensions=["tables"]))
- else:
- result.append(mdtex2html.convert(non_code, extensions=["tables"]))
- if code.strip():
- # _, code = detect_language(code) # 暂时去除代码高亮功能,因为在大段代码的情况下会出现问题
- # code = code.replace("\n\n", "\n") # 暂时去除代码中的空行,因为在大段代码的情况下会出现问题
- code = f"\n```{code}\n\n```"
- code = markdown_to_html_with_syntax_highlight(code)
- result.append(code)
- result = "".join(result)
- result += ALREADY_CONVERTED_MARK
- return result
-
-
-def convert_asis(userinput):
- return (
- f'{html.escape(userinput)}
'
- + ALREADY_CONVERTED_MARK
- )
-
-
-def detect_converted_mark(userinput):
- if userinput.endswith(ALREADY_CONVERTED_MARK):
- return True
- else:
- return False
-
-
-def detect_language(code):
- if code.startswith("\n"):
- first_line = ""
- else:
- first_line = code.strip().split("\n", 1)[0]
- language = first_line.lower() if first_line else ""
- code_without_language = code[len(first_line) :].lstrip() if first_line else code
- return language, code_without_language
-
-
-def construct_text(role, text):
- return {"role": role, "content": text}
-
-
-def construct_user(text):
- return construct_text("user", text)
-
-
-def construct_system(text):
- return construct_text("system", text)
-
-
-def construct_assistant(text):
- return construct_text("assistant", text)
-
-
-def construct_token_message(token, stream=False):
- return f"Token 计数: {token}"
-
-
-def delete_first_conversation(history, previous_token_count):
- if history:
- del history[:2]
- del previous_token_count[0]
- return (
- history,
- previous_token_count,
- construct_token_message(sum(previous_token_count)),
- )
-
-
-def delete_last_conversation(chatbot, history, previous_token_count):
- if len(chatbot) > 0 and standard_error_msg in chatbot[-1][1]:
- logging.info("由于包含报错信息,只删除chatbot记录")
- chatbot.pop()
- return chatbot, history
- if len(history) > 0:
- logging.info("删除了一组对话历史")
- history.pop()
- history.pop()
- if len(chatbot) > 0:
- logging.info("删除了一组chatbot对话")
- chatbot.pop()
- if len(previous_token_count) > 0:
- logging.info("删除了一组对话的token计数记录")
- previous_token_count.pop()
- return (
- chatbot,
- history,
- previous_token_count,
- construct_token_message(sum(previous_token_count)),
- )
-
-
-def save_file(filename, system, history, chatbot):
- logging.info("保存对话历史中……")
- os.makedirs(HISTORY_DIR, exist_ok=True)
- if filename.endswith(".json"):
- json_s = {"system": system, "history": history, "chatbot": chatbot}
- print(json_s)
- with open(os.path.join(HISTORY_DIR, filename), "w") as f:
- json.dump(json_s, f)
- elif filename.endswith(".md"):
- md_s = f"system: \n- {system} \n"
- for data in history:
- md_s += f"\n{data['role']}: \n- {data['content']} \n"
- with open(os.path.join(HISTORY_DIR, filename), "w", encoding="utf8") as f:
- f.write(md_s)
- logging.info("保存对话历史完毕")
- return os.path.join(HISTORY_DIR, filename)
-
-
-def save_chat_history(filename, system, history, chatbot):
- if filename == "":
- return
- if not filename.endswith(".json"):
- filename += ".json"
- return save_file(filename, system, history, chatbot)
-
-
-def export_markdown(filename, system, history, chatbot):
- if filename == "":
- return
- if not filename.endswith(".md"):
- filename += ".md"
- return save_file(filename, system, history, chatbot)
-
-
-def load_chat_history(filename, system, history, chatbot):
- logging.info("加载对话历史中……")
- if type(filename) != str:
- filename = filename.name
- try:
- with open(os.path.join(HISTORY_DIR, filename), "r") as f:
- json_s = json.load(f)
- try:
- if type(json_s["history"][0]) == str:
- logging.info("历史记录格式为旧版,正在转换……")
- new_history = []
- for index, item in enumerate(json_s["history"]):
- if index % 2 == 0:
- new_history.append(construct_user(item))
- else:
- new_history.append(construct_assistant(item))
- json_s["history"] = new_history
- logging.info(new_history)
- except:
- # 没有对话历史
- pass
- logging.info("加载对话历史完毕")
- return filename, json_s["system"], json_s["history"], json_s["chatbot"]
- except FileNotFoundError:
- logging.info("没有找到对话历史文件,不执行任何操作")
- return filename, system, history, chatbot
-
-
-def sorted_by_pinyin(list):
- return sorted(list, key=lambda char: lazy_pinyin(char)[0][0])
-
-
-def get_file_names(dir, plain=False, filetypes=[".json"]):
- logging.info(f"获取文件名列表,目录为{dir},文件类型为{filetypes},是否为纯文本列表{plain}")
- files = []
- try:
- for type in filetypes:
- files += [f for f in os.listdir(dir) if f.endswith(type)]
- except FileNotFoundError:
- files = []
- files = sorted_by_pinyin(files)
- if files == []:
- files = [""]
- if plain:
- return files
- else:
- return gr.Dropdown.update(choices=files)
-
-
-def get_history_names(plain=False):
- logging.info("获取历史记录文件名列表")
- return get_file_names(HISTORY_DIR, plain)
-
-
-def load_template(filename, mode=0):
- logging.info(f"加载模板文件{filename},模式为{mode}(0为返回字典和下拉菜单,1为返回下拉菜单,2为返回字典)")
- lines = []
- logging.info("Loading template...")
- if filename.endswith(".json"):
- with open(os.path.join(TEMPLATES_DIR, filename), "r", encoding="utf8") as f:
- lines = json.load(f)
- lines = [[i["act"], i["prompt"]] for i in lines]
- else:
- with open(
- os.path.join(TEMPLATES_DIR, filename), "r", encoding="utf8"
- ) as csvfile:
- reader = csv.reader(csvfile)
- lines = list(reader)
- lines = lines[1:]
- if mode == 1:
- return sorted_by_pinyin([row[0] for row in lines])
- elif mode == 2:
- return {row[0]: row[1] for row in lines}
- else:
- choices = sorted_by_pinyin([row[0] for row in lines])
- return {row[0]: row[1] for row in lines}, gr.Dropdown.update(
- choices=choices, value=choices[0]
- )
-
-
-def get_template_names(plain=False):
- logging.info("获取模板文件名列表")
- return get_file_names(TEMPLATES_DIR, plain, filetypes=[".csv", "json"])
-
-
-def get_template_content(templates, selection, original_system_prompt):
- logging.info(f"应用模板中,选择为{selection},原始系统提示为{original_system_prompt}")
- try:
- return templates[selection]
- except:
- return original_system_prompt
-
-
-def reset_state():
- logging.info("重置状态")
- return [], [], [], construct_token_message(0)
-
-
-def reset_textbox():
- logging.debug("重置文本框")
- return gr.update(value="")
-
-
-def reset_default():
- newurl = shared.state.reset_api_url()
- os.environ.pop("HTTPS_PROXY", None)
- os.environ.pop("https_proxy", None)
- return gr.update(value=newurl), gr.update(value=""), "API URL 和代理已重置"
-
-
-def change_api_url(url):
- shared.state.set_api_url(url)
- msg = f"API地址更改为了{url}"
- logging.info(msg)
- return msg
-
-
-def change_proxy(proxy):
- os.environ["HTTPS_PROXY"] = proxy
- msg = f"代理更改为了{proxy}"
- logging.info(msg)
- return msg
-
-
-def hide_middle_chars(s):
- if s is None:
- return ""
- if len(s) <= 8:
- return s
- else:
- head = s[:4]
- tail = s[-4:]
- hidden = "*" * (len(s) - 8)
- return head + hidden + tail
-
-
-def submit_key(key):
- key = key.strip()
- msg = f"API密钥更改为了{hide_middle_chars(key)}"
- logging.info(msg)
- return key, msg
-
-
-def replace_today(prompt):
- today = datetime.datetime.today().strftime("%Y-%m-%d")
- return prompt.replace("{current_date}", today)
-
-
-def get_geoip():
- try:
- response = requests.get("https://ipapi.co/json/", timeout=5)
- data = response.json()
- except:
- data = {"error": True, "reason": "连接ipapi失败"}
- if "error" in data.keys():
- logging.warning(f"无法获取IP地址信息。\n{data}")
- if data["reason"] == "RateLimited":
- return (
- f"获取IP地理位置失败,因为达到了检测IP的速率限制。聊天功能可能仍然可用。"
- )
- else:
- return f"获取IP地理位置失败。原因:{data['reason']}。你仍然可以使用聊天功能。"
- else:
- country = data["country_name"]
- if country == "China":
- text = "**您的IP区域:中国。请立即检查代理设置,在不受支持的地区使用API可能导致账号被封禁。**"
- else:
- text = f"您的IP区域:{country}。"
- logging.info(text)
- return text
-
-
-def find_n(lst, max_num):
- n = len(lst)
- total = sum(lst)
-
- if total < max_num:
- return n
-
- for i in range(len(lst)):
- if total - lst[i] < max_num:
- return n - i - 1
- total = total - lst[i]
- return 1
-
-
-def start_outputing():
- logging.debug("显示取消按钮,隐藏发送按钮")
- return gr.Button.update(visible=False), gr.Button.update(visible=True)
-
-
-def end_outputing():
- return (
- gr.Button.update(visible=True),
- gr.Button.update(visible=False),
- )
-
-
-def cancel_outputing():
- logging.info("中止输出……")
- shared.state.interrupt()
-
-
-def transfer_input(inputs):
- # 一次性返回,降低延迟
- textbox = reset_textbox()
- outputing = start_outputing()
- return (
- inputs,
- gr.update(value=""),
- gr.Button.update(visible=True),
- gr.Button.update(visible=False),
- )
-
-
-def get_proxies():
- # 获取环境变量中的代理设置
- http_proxy = os.environ.get("HTTP_PROXY") or os.environ.get("http_proxy")
- https_proxy = os.environ.get("HTTPS_PROXY") or os.environ.get("https_proxy")
-
- # 如果存在代理设置,使用它们
- proxies = {}
- if http_proxy:
- logging.info(f"使用 HTTP 代理: {http_proxy}")
- proxies["http"] = http_proxy
- if https_proxy:
- logging.info(f"使用 HTTPS 代理: {https_proxy}")
- proxies["https"] = https_proxy
-
- if proxies == {}:
- proxies = None
-
- return proxies
-
-def run(command, desc=None, errdesc=None, custom_env=None, live=False):
- if desc is not None:
- print(desc)
- if live:
- result = subprocess.run(command, shell=True, env=os.environ if custom_env is None else custom_env)
- if result.returncode != 0:
- raise RuntimeError(f"""{errdesc or 'Error running command'}.
-Command: {command}
-Error code: {result.returncode}""")
-
- return ""
- result = subprocess.run(command, stdout=subprocess.PIPE, stderr=subprocess.PIPE, shell=True, env=os.environ if custom_env is None else custom_env)
- if result.returncode != 0:
- message = f"""{errdesc or 'Error running command'}.
-Command: {command}
-Error code: {result.returncode}
-stdout: {result.stdout.decode(encoding="utf8", errors="ignore") if len(result.stdout)>0 else ''}
-stderr: {result.stderr.decode(encoding="utf8", errors="ignore") if len(result.stderr)>0 else ''}
-"""
- raise RuntimeError(message)
- return result.stdout.decode(encoding="utf8", errors="ignore")
-
-def versions_html():
- git = os.environ.get('GIT', "git")
- python_version = ".".join([str(x) for x in sys.version_info[0:3]])
- try:
- commit_hash = run(f"{git} rev-parse HEAD").strip()
- except Exception:
- commit_hash = ""
- if commit_hash != "":
- short_commit = commit_hash[0:7]
- commit_info = f"{short_commit}"
- else:
- commit_info = "unknown \U0001F615"
- return f"""
-Python: {python_version}
- •
-Gradio: {gr.__version__}
- •
-Commit: {commit_info}
-"""
-
-def add_source_numbers(lst, source_name = "Source", use_source = True):
- if use_source:
- return [f'[{idx+1}]\t "{item[0]}"\n{source_name}: {item[1]}' for idx, item in enumerate(lst)]
- else:
- return [f'[{idx+1}]\t "{item}"' for idx, item in enumerate(lst)]
-
-def add_details(lst):
- nodes = []
- for index, txt in enumerate(lst):
- brief = txt[:25].replace("\n", "")
- nodes.append(
- f"{brief}...
{txt}
"
- )
- return nodes
diff --git a/spaces/zhoupin30/zhoupin30/src/components/ui/separator.tsx b/spaces/zhoupin30/zhoupin30/src/components/ui/separator.tsx
deleted file mode 100644
index 6c55e0b2ca8e2436658a06748aadbff7cd700db0..0000000000000000000000000000000000000000
--- a/spaces/zhoupin30/zhoupin30/src/components/ui/separator.tsx
+++ /dev/null
@@ -1,31 +0,0 @@
-'use client'
-
-import * as React from 'react'
-import * as SeparatorPrimitive from '@radix-ui/react-separator'
-
-import { cn } from '@/lib/utils'
-
-const Separator = React.forwardRef<
- React.ElementRef,
- React.ComponentPropsWithoutRef
->(
- (
- { className, orientation = 'horizontal', decorative = true, ...props },
- ref
- ) => (
-
- )
-)
-Separator.displayName = SeparatorPrimitive.Root.displayName
-
-export { Separator }
diff --git a/spaces/zhuraavl/mistralai-Mistral-7B-v0.1/README.md b/spaces/zhuraavl/mistralai-Mistral-7B-v0.1/README.md
deleted file mode 100644
index 54eb923350a650aa671f3bbfd2b997d4108cb924..0000000000000000000000000000000000000000
--- a/spaces/zhuraavl/mistralai-Mistral-7B-v0.1/README.md
+++ /dev/null
@@ -1,12 +0,0 @@
----
-title: Mistralai Mistral 7B V0.1
-emoji: ⚡
-colorFrom: gray
-colorTo: blue
-sdk: gradio
-sdk_version: 3.45.1
-app_file: app.py
-pinned: false
----
-
-Check out the configuration reference at https://huggingface.co/docs/hub/spaces-config-reference
diff --git a/spaces/znskiss/Qwen-VL/test.py b/spaces/znskiss/Qwen-VL/test.py
deleted file mode 100644
index 00ca9a06bc9ef7923ea4da7375fd282cf08892bd..0000000000000000000000000000000000000000
--- a/spaces/znskiss/Qwen-VL/test.py
+++ /dev/null
@@ -1,38 +0,0 @@
-from transformers import AutoModelForCausalLM, AutoTokenizer
-from transformers.generation import GenerationConfig
-import torch
-torch.manual_seed(1234)
-
-# Note: The default behavior now has injection attack prevention off.
-tokenizer = AutoTokenizer.from_pretrained("Qwen/Qwen-VL-Chat", trust_remote_code=True)
-
-# use bf16
-# model = AutoModelForCausalLM.from_pretrained("Qwen/Qwen-VL-Chat", device_map="auto", trust_remote_code=True, bf16=True).eval()
-# use fp16
-# model = AutoModelForCausalLM.from_pretrained("Qwen/Qwen-VL-Chat", device_map="auto", trust_remote_code=True, fp16=True).eval()
-# use cpu only
-# model = AutoModelForCausalLM.from_pretrained("Qwen/Qwen-VL-Chat", device_map="cpu", trust_remote_code=True).eval()
-# use cuda device
-model = AutoModelForCausalLM.from_pretrained("Qwen/Qwen-VL-Chat", device_map="cuda", trust_remote_code=True).eval()
-
-# Specify hyperparameters for generation
-model.generation_config = GenerationConfig.from_pretrained("Qwen/Qwen-VL-Chat", trust_remote_code=True)
-
-# 1st dialogue turn
-query = tokenizer.from_list_format([
- {'image': 'https://qianwen-res.oss-cn-beijing.aliyuncs.com/Qwen-VL/assets/demo.jpeg'}, # Either a local path or an url
- {'text': '这是什么?'},
-])
-response, history = model.chat(tokenizer, query=query, history=None)
-print(response)
-# 图中是一名女子在沙滩上和狗玩耍,旁边是一只拉布拉多犬,它们处于沙滩上。
-
-# 2st dialogue turn
-response, history = model.chat(tokenizer, '框出图中击掌的位置', history=history)
-print(response)
-# 击掌(536,509),(588,602)
-image = tokenizer.draw_bbox_on_latest_picture(response, history)
-if image:
- image.save('1.jpg')
-else:
- print("no box")
diff --git a/spaces/zxy666/bingo-chatai666/tailwind.config.js b/spaces/zxy666/bingo-chatai666/tailwind.config.js
deleted file mode 100644
index 03da3c3c45be6983b9f5ffa6df5f1fd0870e9636..0000000000000000000000000000000000000000
--- a/spaces/zxy666/bingo-chatai666/tailwind.config.js
+++ /dev/null
@@ -1,48 +0,0 @@
-/** @type {import('tailwindcss').Config} */
-module.exports = {
- content: [
- './src/pages/**/*.{js,ts,jsx,tsx,mdx}',
- './src/components/**/*.{js,ts,jsx,tsx,mdx}',
- './src/app/**/*.{js,ts,jsx,tsx,mdx}',
- './src/ui/**/*.{js,ts,jsx,tsx,mdx}',
- ],
- "darkMode": "class",
- theme: {
- extend: {
- colors: {
- 'primary-blue': 'rgb(var(--color-primary-blue) / )',
- secondary: 'rgb(var(--color-secondary) / )',
- 'primary-background': 'rgb(var(--primary-background) / )',
- 'primary-text': 'rgb(var(--primary-text) / )',
- 'secondary-text': 'rgb(var(--secondary-text) / )',
- 'light-text': 'rgb(var(--light-text) / )',
- 'primary-border': 'rgb(var(--primary-border) / )',
- },
- keyframes: {
- slideDownAndFade: {
- from: { opacity: 0, transform: 'translateY(-2px)' },
- to: { opacity: 1, transform: 'translateY(0)' },
- },
- slideLeftAndFade: {
- from: { opacity: 0, transform: 'translateX(2px)' },
- to: { opacity: 1, transform: 'translateX(0)' },
- },
- slideUpAndFade: {
- from: { opacity: 0, transform: 'translateY(2px)' },
- to: { opacity: 1, transform: 'translateY(0)' },
- },
- slideRightAndFade: {
- from: { opacity: 0, transform: 'translateX(2px)' },
- to: { opacity: 1, transform: 'translateX(0)' },
- },
- },
- animation: {
- slideDownAndFade: 'slideDownAndFade 400ms cubic-bezier(0.16, 1, 0.3, 1)',
- slideLeftAndFade: 'slideLeftAndFade 400ms cubic-bezier(0.16, 1, 0.3, 1)',
- slideUpAndFade: 'slideUpAndFade 400ms cubic-bezier(0.16, 1, 0.3, 1)',
- slideRightAndFade: 'slideRightAndFade 400ms cubic-bezier(0.16, 1, 0.3, 1)',
- },
- },
- },
- plugins: [require('@headlessui/tailwindcss'), require('tailwind-scrollbar')],
-}