diff --git a/spaces/0x90e/ESRGAN-MANGA/ESRGAN_plus/architecture.py b/spaces/0x90e/ESRGAN-MANGA/ESRGAN_plus/architecture.py
deleted file mode 100644
index 6ac437cfa8c1713eb060d1caa7ca70e1d2e9bb00..0000000000000000000000000000000000000000
--- a/spaces/0x90e/ESRGAN-MANGA/ESRGAN_plus/architecture.py
+++ /dev/null
@@ -1,38 +0,0 @@
-import math
-import torch
-import torch.nn as nn
-import ESRGAN_plus.block as B
-
-
-class RRDB_Net(nn.Module):
- def __init__(self, in_nc, out_nc, nf, nb, gc=32, upscale=4, norm_type=None, act_type='leakyrelu', \
- mode='CNA', res_scale=1, upsample_mode='upconv'):
- super(RRDB_Net, self).__init__()
- n_upscale = int(math.log(upscale, 2))
- if upscale == 3:
- n_upscale = 1
-
- fea_conv = B.conv_block(in_nc, nf, kernel_size=3, norm_type=None, act_type=None)
- rb_blocks = [B.RRDB(nf, kernel_size=3, gc=32, stride=1, bias=True, pad_type='zero', \
- norm_type=norm_type, act_type=act_type, mode='CNA') for _ in range(nb)]
- LR_conv = B.conv_block(nf, nf, kernel_size=3, norm_type=norm_type, act_type=None, mode=mode)
-
- if upsample_mode == 'upconv':
- upsample_block = B.upconv_blcok
- elif upsample_mode == 'pixelshuffle':
- upsample_block = B.pixelshuffle_block
- else:
- raise NotImplementedError('upsample mode [%s] is not found' % upsample_mode)
- if upscale == 3:
- upsampler = upsample_block(nf, nf, 3, act_type=act_type)
- else:
- upsampler = [upsample_block(nf, nf, act_type=act_type) for _ in range(n_upscale)]
- HR_conv0 = B.conv_block(nf, nf, kernel_size=3, norm_type=None, act_type=act_type)
- HR_conv1 = B.conv_block(nf, out_nc, kernel_size=3, norm_type=None, act_type=None)
-
- self.model = B.sequential(fea_conv, B.ShortcutBlock(B.sequential(*rb_blocks, LR_conv)),\
- *upsampler, HR_conv0, HR_conv1)
-
- def forward(self, x):
- x = self.model(x)
- return x
diff --git a/spaces/101-5/gpt4free/g4f/.v1/gui/streamlit_chat_app.py b/spaces/101-5/gpt4free/g4f/.v1/gui/streamlit_chat_app.py
deleted file mode 100644
index af3969e6fa682b0b237b0a7b8c228de83e7209ae..0000000000000000000000000000000000000000
--- a/spaces/101-5/gpt4free/g4f/.v1/gui/streamlit_chat_app.py
+++ /dev/null
@@ -1,156 +0,0 @@
-import atexit
-import Levenshtein
-import os
-import sys
-
-sys.path.append(os.path.join(os.path.dirname(__file__), os.path.pardir))
-
-import streamlit as st
-from streamlit_chat import message
-from query_methods import query, avail_query_methods
-import pickle
-
-conversations_file = "conversations.pkl"
-
-def load_conversations():
- try:
- with open(conversations_file, "rb") as f:
- return pickle.load(f)
- except FileNotFoundError:
- return []
- except EOFError:
- return []
-
-
-def save_conversations(conversations, current_conversation):
- updated = False
- for idx, conversation in enumerate(conversations):
- if conversation == current_conversation:
- conversations[idx] = current_conversation
- updated = True
- break
- if not updated:
- conversations.append(current_conversation)
-
- temp_conversations_file = "temp_" + conversations_file
- with open(temp_conversations_file, "wb") as f:
- pickle.dump(conversations, f)
-
- os.replace(temp_conversations_file, conversations_file)
-
-def delete_conversation(conversations, current_conversation):
- for idx, conversation in enumerate(conversations):
- conversations[idx] = current_conversation
- break
- conversations.remove(current_conversation)
-
- temp_conversations_file = "temp_" + conversations_file
- with open(temp_conversations_file, "wb") as f:
- pickle.dump(conversations, f)
-
- os.replace(temp_conversations_file, conversations_file)
-
-def exit_handler():
- print("Exiting, saving data...")
- # Perform cleanup operations here, like saving data or closing open files.
- save_conversations(st.session_state.conversations, st.session_state.current_conversation)
-
-
-# Register the exit_handler function to be called when the program is closing.
-atexit.register(exit_handler)
-
-st.header("Chat Placeholder")
-
-if 'conversations' not in st.session_state:
- st.session_state['conversations'] = load_conversations()
-
-if 'input_text' not in st.session_state:
- st.session_state['input_text'] = ''
-
-if 'selected_conversation' not in st.session_state:
- st.session_state['selected_conversation'] = None
-
-if 'input_field_key' not in st.session_state:
- st.session_state['input_field_key'] = 0
-
-if 'query_method' not in st.session_state:
- st.session_state['query_method'] = query
-
-if 'search_query' not in st.session_state:
- st.session_state['search_query'] = ''
-
-# Initialize new conversation
-if 'current_conversation' not in st.session_state or st.session_state['current_conversation'] is None:
- st.session_state['current_conversation'] = {'user_inputs': [], 'generated_responses': []}
-
-input_placeholder = st.empty()
-user_input = input_placeholder.text_input(
- 'You:', value=st.session_state['input_text'], key=f'input_text_-1'#{st.session_state["input_field_key"]}
-)
-submit_button = st.button("Submit")
-
-if (user_input and user_input != st.session_state['input_text']) or submit_button:
- output = query(user_input, st.session_state['query_method'])
-
- escaped_output = output.encode('utf-8').decode('unicode-escape')
-
- st.session_state['current_conversation']['user_inputs'].append(user_input)
- st.session_state.current_conversation['generated_responses'].append(escaped_output)
- save_conversations(st.session_state.conversations, st.session_state.current_conversation)
- st.session_state['input_text'] = ''
- st.session_state['input_field_key'] += 1 # Increment key value for new widget
- user_input = input_placeholder.text_input(
- 'You:', value=st.session_state['input_text'], key=f'input_text_{st.session_state["input_field_key"]}'
- ) # Clear the input field
-
-# Add a button to create a new conversation
-if st.sidebar.button("New Conversation"):
- st.session_state['selected_conversation'] = None
- st.session_state['current_conversation'] = {'user_inputs': [], 'generated_responses': []}
- st.session_state['input_field_key'] += 1 # Increment key value for new widget
- st.session_state['query_method'] = st.sidebar.selectbox("Select API:", options=avail_query_methods, index=0)
-
-# Proxy
-st.session_state['proxy'] = st.sidebar.text_input("Proxy: ")
-
-# Searchbar
-search_query = st.sidebar.text_input("Search Conversations:", value=st.session_state.get('search_query', ''), key='search')
-
-if search_query:
- filtered_conversations = []
- indices = []
- for idx, conversation in enumerate(st.session_state.conversations):
- if search_query in conversation['user_inputs'][0]:
- filtered_conversations.append(conversation)
- indices.append(idx)
-
- filtered_conversations = list(zip(indices, filtered_conversations))
- conversations = sorted(filtered_conversations, key=lambda x: Levenshtein.distance(search_query, x[1]['user_inputs'][0]))
-
- sidebar_header = f"Search Results ({len(conversations)})"
-else:
- conversations = st.session_state.conversations
- sidebar_header = "Conversation History"
-
-# Sidebar
-st.sidebar.header(sidebar_header)
-sidebar_col1, sidebar_col2 = st.sidebar.columns([5,1])
-for idx, conversation in enumerate(conversations):
- if sidebar_col1.button(f"Conversation {idx + 1}: {conversation['user_inputs'][0]}", key=f"sidebar_btn_{idx}"):
- st.session_state['selected_conversation'] = idx
- st.session_state['current_conversation'] = conversation
- if sidebar_col2.button('🗑️', key=f"sidebar_btn_delete_{idx}"):
- if st.session_state['selected_conversation'] == idx:
- st.session_state['selected_conversation'] = None
- st.session_state['current_conversation'] = {'user_inputs': [], 'generated_responses': []}
- delete_conversation(conversations, conversation)
- st.experimental_rerun()
-if st.session_state['selected_conversation'] is not None:
- conversation_to_display = conversations[st.session_state['selected_conversation']]
-else:
- conversation_to_display = st.session_state.current_conversation
-
-if conversation_to_display['generated_responses']:
- for i in range(len(conversation_to_display['generated_responses']) - 1, -1, -1):
- message(conversation_to_display["generated_responses"][i], key=f"display_generated_{i}")
- message(conversation_to_display['user_inputs'][i], is_user=True, key=f"display_user_{i}")
\ No newline at end of file
diff --git a/spaces/1acneusushi/gradio-2dmoleculeeditor/data/Fusion 360 2008 32 Bit __EXCLUSIVE__ Xforce Keygen.md b/spaces/1acneusushi/gradio-2dmoleculeeditor/data/Fusion 360 2008 32 Bit __EXCLUSIVE__ Xforce Keygen.md
deleted file mode 100644
index eedc826a9493fcf65c062f6b97cca98922c7195f..0000000000000000000000000000000000000000
--- a/spaces/1acneusushi/gradio-2dmoleculeeditor/data/Fusion 360 2008 32 Bit __EXCLUSIVE__ Xforce Keygen.md
+++ /dev/null
@@ -1,40 +0,0 @@
-
-
Fusion 360 2008 32 Bit Xforce Keygen: How to Activate Autodesk Products for Free
- If you are looking for a powerful and versatile software for designing, modeling, and engineering, you might have heard of Fusion 360. Fusion 360 is a cloud-based CAD/CAM/CAE software that allows you to create, edit, and share your designs in various formats. It also integrates with other Autodesk products and services, such as AutoCAD, Inventor, Revit, Maya, and more.
-Fusion 360 2008 32 Bit Xforce Keygen
Download File →→→ https://byltly.com/2uKxYI
- However, Fusion 360 is not a cheap software. It requires a monthly or yearly subscription fee that can range from $25 to $500 depending on your plan. If you want to use Fusion 360 without paying anything, you might be tempted to use a Xforce keygen. Xforce keygen is a crack tool that can generate activation codes for any Autodesk product. By using Xforce keygen, you can bypass the license verification process and activate Fusion 360 for free.
- But is it worth it? In this article, we will show you how to use Xforce keygen for Fusion 360 2008 32 bit, as well as the benefits and risks of doing so. We will also answer some frequently asked questions about Fusion 360 and Xforce keygen. Read on to find out more.
- How to Download and Install Fusion 360 2008 32 Bit
- Before you can use Xforce keygen for Fusion 360, you need to download and install the software first. Here are the steps to do so:
- Step 1: Download Fusion 360 2008 32 bit from the official website or a trusted source. You can find the download link here: . Make sure you choose the correct version for your operating system and system requirements. - Step 2: Run the setup file and follow the instructions. You may need to accept the terms and conditions, choose the installation location, and select the components you want to install. The installation process may take some time depending on your internet speed and computer performance. - Step 3: Choose the trial version or enter a serial number. If you have a valid serial number, you can enter it and activate Fusion 360. If not, you can choose the trial version and use it for 30 days. You can also sign in with your Autodesk account if you have one. How to Download and Use Xforce Keygen for Fusion 360 2008 32 Bit
- Once you have installed Fusion 360, you can use Xforce keygen to activate it for free. Here are the steps to do so:
- - Step 1: Download Xforce keygen from a reliable source. You can find many websites that offer Xforce keygen for various Autodesk products, but be careful of malware and viruses. One of the most popular sources is , but use it at your own risk. - Step 2: Disable your antivirus and internet connection. This is important because Xforce keygen is detected as a malicious program by most antivirus software and may be blocked or deleted. Also, disconnecting from the internet will prevent Autodesk from verifying your license online. - Step 3: Run Xforce keygen as administrator and click on "Patch". You will see a window that asks you to locate the file "xf-adsk2018_x86.exe" in your Fusion 360 installation folder. Usually, it is located in "C:\Program Files\Autodesk\Fusion 360\bin". Select the file and click on "Open". - Step 4: Copy the request code from Fusion 360 and paste it into Xforce keygen. To get the request code, open Fusion 360 and go to the "Help" menu. Click on "Register" and then on "Activate". You will see a screen that shows your serial number and a request code. Copy the request code and paste it into Xforce keygen where it says "Request". - Step 5: Click on "Generate" and copy the activation code from Xforce keygen. You will see a long string of letters and numbers that is your activation code. Copy it and go back to Fusion 360. - Step 6: Paste the activation code into Fusion 360 and click on "Next". You will see a message that says "Activation successful". Click on "Finish" and enjoy using Fusion 360 for free. How to Verify that Fusion 360 is Activated
- To make sure that Fusion 360 is activated, you can do the following:
- - Step 1: Open Fusion 360 and go to the "Help" menu. - Step 2: Click on "About Autodesk Fusion 360". - Step 3: Check the license status and expiration date. You should see that your license is valid until January 1, 2060, which means that you have activated Fusion 360 with Xforce keygen. Benefits of Using Fusion 360 with Xforce Keygen
- By using Xforce keygen for Fusion 360, you can enjoy some benefits, such as:
-
- - Access to all features and updates of Fusion 360. You can use all the tools and functions of Fusion 360 without any limitations or restrictions. You can also download and install any updates or patches that are released by Autodesk. - No need to pay for a subscription or license. You can save money by not having to pay for a monthly or yearly fee to use Fusion 360. You can also avoid any renewal or cancellation issues that may arise with a subscription plan. - Ability to create, edit, and share designs in various formats. You can work on any type of design project with Fusion 360, from sketching to rendering to simulation. You can also export and import your designs in different formats, such as DWG, DXF, STL, OBJ, IGES, STEP, etc. - Compatibility with other Autodesk products and services. You can integrate your designs with other Autodesk software, such as AutoCAD, Inventor, Revit, Maya, etc. You can also access other Autodesk services, such as A360 cloud storage, Autodesk Gallery, Autodesk Education Community, etc. Risks and Drawbacks of Using Fusion 360 with Xforce Keygen
- However, using Xforce keygen for Fusion 360 also comes with some risks and drawbacks, such as:
- - Legal and ethical issues of using - Legal and ethical issues of using pirated software. By using Xforce keygen, you are violating the terms and conditions of Autodesk and infringing their intellectual property rights. You are also depriving them of their revenue and supporting software piracy. This can lead to legal consequences, such as fines, lawsuits, or even jail time. You are also acting unethically, as you are not respecting the work and effort of the software developers and creators. - Potential malware and virus infection from untrusted sources. Xforce keygen is not an official or authorized tool from Autodesk. It is created by hackers and crackers who may have malicious intentions. They may embed malware or viruses into the Xforce keygen file or the websites that offer it. This can compromise your computer security and privacy, as well as damage your files and data. - Possible errors and glitches in the software performance. Xforce keygen may not work properly with Fusion 360, especially if there are updates or patches from Autodesk. It may cause errors, crashes, or glitches in the software functionality or stability. It may also interfere with other programs or applications on your computer. - No technical support or customer service from Autodesk. If you encounter any problems or issues with Fusion 360, you cannot contact Autodesk for help or assistance. You are on your own, as Autodesk does not provide any support or service for pirated software. You may also miss out on some features or benefits that are only available for licensed users, such as online forums, tutorials, feedback, etc. Conclusion
- Fusion 360 is a great software for design, modeling, and engineering. However, it is not a cheap software, and you may want to use Xforce keygen to activate it for free. Xforce keygen is a crack tool that can generate activation codes for any Autodesk product, including Fusion 360.
- In this article, we have shown you how to use Xforce keygen for Fusion 360 2008 32 bit, as well as the benefits and risks of doing so. We have also answered some frequently asked questions about Fusion 360 and Xforce keygen.
- Using Xforce keygen for Fusion 360 can give you access to all features and updates of the software without paying anything. However, it can also expose you to legal and ethical issues, malware and virus infection, errors and glitches, and no technical support or customer service.
- Ultimately, the decision is yours to make. We do not recommend or endorse using Xforce keygen for Fusion 360 or any other Autodesk product. We advise you to use the official website or a trusted source to download and install Fusion 360, and to pay for a subscription or license if you want to use it legally and ethically.
- If you found this article helpful, please share it with your friends and colleagues who may be interested in using Fusion 360 with Xforce keygen. If you have any questions or feedback, please leave a comment below. Thank you for reading.
- FAQs
- Here are some of the most common questions that people ask about Fusion 360 and Xforce keygen:
- What is the difference between Fusion 360 and AutoCAD?
- Fusion 360 and AutoCAD are both products from Autodesk that are used for design and engineering. However, they have some differences in their features and functions.
- AutoCAD is a 2D and 3D CAD software that is mainly used for drafting, documentation, and detailing. It has a wide range of tools and commands that allow you to create precise and accurate drawings and models.
- Fusion 360 is a cloud-based CAD/CAM/CAE software that is mainly used for design, modeling, and simulation. It has a more intuitive and user-friendly interface that allows you to create complex and organic shapes and forms. It also integrates with other Autodesk products and services, such as Inventor, Revit, Maya, etc.
- Is Fusion 360 free for students and educators?
- Yes, Fusion 360 is free for students and educators who are enrolled in or employed by a qualified educational institution. You can apply for a free educational license that will allow you to use Fusion 360 for non-commercial purposes for up to three years.
- To get a free educational license for Fusion 360, you need to create an Autodesk account with your educational email address. Then, you need to verify your eligibility by providing proof of your enrollment or employment status. After that, you can download and install Fusion 360 from the Autodesk Education Community website.
- How can I update Fusion 360 after using Xforce keygen?
- If you have used Xforce keygen to activate Fusion 360, you may not be able to update it automatically from the software itself. This is because Autodesk may detect - your license as invalid or expired and prevent you from updating. You may also lose your activation if you update Fusion 360 with Xforce keygen. - To update Fusion 360 after using Xforce keygen, you need to download the latest version of the software from the official website or a trusted source. Then, you need to uninstall the previous version of Fusion 360 and install the new one. After that, you need to use Xforce keygen again to activate the new version of Fusion 360. - Alternatively, you can use a patcher tool that can update Fusion 360 without affecting your activation. One of the most popular patchers is , but use it at your own risk.
What are some alternatives to Xforce keygen for activating Autodesk products?
- Xforce keygen is not the only crack tool that can activate Autodesk products. There are some other tools that can do the same thing, such as:
- - : This is a universal keygen that can generate serial numbers and product keys for any Autodesk product. It also has a patch function that can modify the software files and bypass the license verification process. - : This is a patcher tool that can activate any Autodesk product by modifying the registry entries and the hosts file. It also has a backup and restore function that can save and restore your activation data. - : This is a loader tool that can activate any Autodesk product by injecting a DLL file into the software process. It also has a stealth mode that can hide the loader from detection. However, these tools are also illegal and unethical, and they may have the same risks and drawbacks as Xforce keygen. We do not recommend or endorse using these tools for activating Autodesk products.
- Where can I find more tutorials and resources for Fusion 360?
- If you want to learn more about Fusion 360 and how to use it effectively, you can find many tutorials and resources online, such as:
- - : This is the official website of Fusion 360, where you can find information, documentation, downloads, updates, forums, blogs, videos, webinars, events, and more. - : This is the official YouTube channel of Fusion 360, where you can find tutorials, tips, tricks, demos, showcases, live streams, and more. - : This is an online learning platform that offers courses and lessons on Fusion 360 and other Autodesk products. You can learn from experts and professionals at your own pace and level. - : This is an online community of Fusion 360 users, where you can share your designs, projects, questions, feedback, ideas, and more. You can also join groups, challenges, contests, and events. These are some of the best sources for learning and improving your skills in Fusion 360. You can also search for other websites, blogs, podcasts, books, magazines, etc. that offer Fusion 360 tutorials and resources.
b2dd77e56b
-
-
\ No newline at end of file
diff --git a/spaces/1gistliPinn/ChatGPT4/Examples/Cossacks Back To War __EXCLUSIVE__ Full Game Download.md b/spaces/1gistliPinn/ChatGPT4/Examples/Cossacks Back To War __EXCLUSIVE__ Full Game Download.md
deleted file mode 100644
index 00ece12c7fb7edd72ead4f01599b2189051d1711..0000000000000000000000000000000000000000
--- a/spaces/1gistliPinn/ChatGPT4/Examples/Cossacks Back To War __EXCLUSIVE__ Full Game Download.md
+++ /dev/null
@@ -1,32 +0,0 @@
-cossacks back to war full game download
Download ►►► https://imgfil.com/2uy1Yf
-
-ZOMBIE ATTACK (2014)
-
-NOVOLAND
-
-Jakob Keseberg
-
-As zombie hordes gather in occupied Europe, a powerful armada of airborne attack vehicles emerge to fight them. Heroes have to fight the undead from the skies or else face the devastating consequences.
-
-Jakob Keseberg grew up in Germany as a creative hobbyist with a passion for game development. He was already making video games at the age of 12, inspired by old arcade titles. Later he founded a company called Geek Bytes Studio, and to this day he continues to develop games.
-
-After finishing school he moved to Hamburg to work as a web developer. In 2011 Jakob Keseberg started studying to be a game developer and took his first steps in the game industry by creating the text adventure game "The Vampyricium" and the social game "Heroes of Shadow".
-
-ZOMBIE ATTACK (2014) (click the thumbnail for the official trailer)
-
-REAL-TIME STRATEGY WITH A HISTORICAL BACKGROUND
-
-The game puts the player in the shoes of a powerful airborne commander. The player takes control of ground and air forces to lead military operations on the continent of Europe. The player creates a strategic combination of military forces to defeat the invading zombie hordes.
-
-You have the opportunity to operate military operations at the strategic level of European politics, and the challenge of making history. The year is 1530, and Europe is about to plunge into chaos. Religiously motivated wars are spreading like wildfire across the continent, while great powers like Spain, France, and England are preparing to fight for the control of the new world. During this time, new kinds of weapons become available. It is your mission to determine who will survive, and to rule the world in their place.
-
-ZOMBIE ATTACK (2014) is a strategy game inspired by the German popular saying "Zombie attack!"
-
-TO THE ROOF OF EUROPE
-
-Europe has been invaded by hordes of undead, and the continent is experiencing its worst war. Now, armed with sword, pistol, and shotgun, you will lead the only force of its kind to make history. At the helm of your military force you will shape the destiny of the continent.
-
-In ZOMBIE ATTACK (2014) you will lead your forces to the roof of Europe. Through 4fefd39f24
-
-
-
diff --git a/spaces/1gistliPinn/ChatGPT4/Examples/Fallout 3 Crack [BEST]ed Launcher 1.7.md b/spaces/1gistliPinn/ChatGPT4/Examples/Fallout 3 Crack [BEST]ed Launcher 1.7.md
deleted file mode 100644
index 8b4910fd04168bb9f87e165632d461578a937577..0000000000000000000000000000000000000000
--- a/spaces/1gistliPinn/ChatGPT4/Examples/Fallout 3 Crack [BEST]ed Launcher 1.7.md
+++ /dev/null
@@ -1,6 +0,0 @@
-
-bethesda's fallout 4 is a completely overhauled version of the fallout engine. the title is based on the "true" fallout universe established by fallout 3 and fallout new vegas, and the setting takes place in boston, which has been the center of civilization in the present and future. fallout 4 will include a full open world, with large towns, a government to rule, factions to choose from, and a dynamic main quest. the game's creators say it will also be the first entry in the series to feature a linear narrative.
-fallout 3 cracked launcher 1.7
Download ---> https://imgfil.com/2uy19L
-bethesda softworks also announced a new version of the game would be released on march 27, 2011. it was available on steam on march 29, 2011, and costs $15 for a pc edition or $40 for a game plus the director's cut. the director's cut consists of six new expansion packs, including fallout: new california, fallout: new vegas, fallout: brotherhood of steel, fallout: brotherhood of steel - the pitt, fallout: brotherhood of steel - point lookout and fallout: brotherhood of steel - mothership zeta, all free of drm and released on a weekly basis. [3] bethesda softworks also announced a new version of the game would be released on march 27, 2011. [4] the game also includes an enhanced version of the companion app, which allows players to access the in-game radio, map and quick-travel to an area with the iphone or ipod touch. the game was also re-released as a part of a bundle pack with the first two expansion packs, which included the game and the first two expansion packs, which included the game and the first two expansion packs.
899543212b
-
-
\ No newline at end of file
diff --git a/spaces/1phancelerku/anime-remove-background/Download Free Games for MacBook Air and Experience the Thrill of Gaming.md b/spaces/1phancelerku/anime-remove-background/Download Free Games for MacBook Air and Experience the Thrill of Gaming.md
deleted file mode 100644
index 9fba39c1d1846127f65fab6b66a12b65421dd1c4..0000000000000000000000000000000000000000
--- a/spaces/1phancelerku/anime-remove-background/Download Free Games for MacBook Air and Experience the Thrill of Gaming.md
+++ /dev/null
@@ -1,140 +0,0 @@
-
-How to Download Free Games for MacBook Air
-If you are a MacBook Air user, you might be wondering how to download free games for your device. After all, gaming is not only fun but also a great way to relax and unwind. However, finding free games for Mac can be challenging, as not all games are compatible with macOS or optimized for the MacBook Air's performance. In this article, we will show you how to download free games for MacBook Air using different methods, such as Steam, the App Store, and iPad games. We will also recommend some of the best free games that you can enjoy on your MacBook Air.
- Install Steam on Mac
-Steam is one of the most popular online gaming platforms for PC and Mac users. It offers thousands of games across various genres, many of which are free to play or have free demos. To use Steam on your Mac, you need to install the Steam app first. Here's how:
-download free games for macbook air
DOWNLOAD ✫✫✫ https://jinyurl.com/2uNNPs
-
-- Go to steampowered.com in your browser.
-- Click Install Steam at the top right of the webpage.
-- Choose Install Steam.
-- Next, click the show downloads button.
-- Double-click on steam.dmg to launch the installer.
-- Drag Steam into the Applications folder.
-- Exit the window.
-
- Download a Steam game on Mac
-Once you have installed Steam on your Mac, you can browse and download games from its library. To download a Steam game on your Mac, follow these steps:
-
-- Launch Steam from the Applications folder.
-- Choose Open, if applicable.
-- Wait for Steam to update.
-- Create a new account or log in into an existing account if you have one.
-- Go to Store.
-- Browse or search for the game that you want to download. Make sure it has an Apple logo next to it, which means it is compatible with Mac.
-- Click on the title you want to download.
-- Choose Add to Cart.
-- Click Purchase for myself.
-- Select your payment method. You can also use your Steam Wallet balance if you have any.
-- Fill out your payment information.
-- Click Continue.
-- Click the checkbox to agree to the terms.
-- Click Purchase.
-
-From here, your game will begin to download, and you can start playing as soon as it's done. You can also manage your downloads and library from the Library tab in Steam.
- Download apps from the App Store on Mac
-If you prefer not to use Steam or want to find more games that are designed for macOS, you can use the App Store on your Mac. The App Store has a wide selection of apps and games for your Mac, some of which are free or have free trials. To download apps from the App Store on your Mac, follow these steps:
-
-- Open the App Store from the Dock or the Applications folder.
-- Sign in with your Apple ID and password if you haven't already.
-- Go to Games.
-- Browse or search for the game that you want to download. You can also filter by categories, ratings, prices, and more.
-- Click on the game that you want to download.
-- Click Get if the game is free, or click the price if it is paid.
-- Enter your Apple ID password or use Touch ID if prompted.
-- Wait for the game to download and install on your Mac.
-
-You can find your downloaded games in the Launchpad or the Applications folder. You can also manage your purchases and updates from the App Store.
- Download iPad games on Mac with Apple Silicon
-If you have a MacBook Air with an Apple Silicon processor, such as the M1 chip, you can also download and play iPad games on your Mac. This is because Apple Silicon Macs can run iOS apps natively, without any emulation or compatibility issues. However, not all iPad games are available for Mac, as some developers may choose to opt out of this feature. To download iPad games on your Mac with Apple Silicon, follow these steps:
-download free games for macbook air 2023
-download free games for macbook air offline
-download free games for macbook air without app store
-download free games for macbook air full version
-download free games for macbook air no internet
-download free games for macbook air from steam
-download free games for macbook air adventure
-download free games for macbook air racing
-download free games for macbook air puzzle
-download free games for macbook air strategy
-download free games for macbook air simulation
-download free games for macbook air action
-download free games for macbook air horror
-download free games for macbook air rpg
-download free games for macbook air multiplayer
-download free games for macbook air shooting
-download free games for macbook air sports
-download free games for macbook air educational
-download free games for macbook air casual
-download free games for macbook air hidden object
-download free games for macbook air arcade
-download free games for macbook air platformer
-download free games for macbook air sandbox
-download free games for macbook air survival
-download free games for macbook air tycoon
-download free games for macbook air card
-download free games for macbook air board
-download free games for macbook air trivia
-download free games for macbook air word
-download free games for macbook air match 3
-download free games for macbook air solitaire
-download free games for macbook air mahjong
-download free games for macbook air sudoku
-download free games for macbook air crossword
-download free games for macbook air chess
-download free games for macbook air checkers
-download free games for macbook air backgammon
-download free games for macbook air minesweeper
-download free games for macbook air snake
-download free games for macbook air tetris
-download free games for macbook air pacman
-download free games for macbook air pinball
-download free games for macbook air breakout
-download free games for macbook air space invaders
-download free games for macbook air frogger
-download free games for macbook air asteroids
-download free games for macbook air pong
-download free games for macbook air galaga
-download free games for macbook air donkey kong
-
-- Open the App Store from the Dock or the Applications folder.
-- Sign in with your Apple ID and password if you haven't already.
-- Go to Games.
-- Click on iPhone & iPad Apps in the sidebar.
-- Browse or search for the game that you want to download. You can also filter by categories, ratings, prices, and more.
-- Click on the game that you want to download.
-- Click Get if the game is free, or click the price if it is paid.
-- Enter your Apple ID password or use Touch ID if prompted.
-- Wait for the game to download and install on your Mac.
-
-You can find your downloaded iPad games in the Launchpad or the Applications folder. You can also manage your purchases and updates from the App Store.
- Best free games for MacBook Air
-Now that you know how to download free games for MacBook Air using different methods, you might be wondering what are some of the best free games that you can play on your device. Here are some of our recommendations:
-
-- Dota 2: Dota 2 is one of the most popular and competitive multiplayer online battle arena (MOBA) games in the world. It features hundreds of heroes, each with their own unique abilities and playstyles, and a variety of game modes and maps. You can team up with friends or strangers and fight against other players in matches that can last from 20 minutes to over an hour. Dota 2 is free to play on Steam, but you can also buy cosmetic items and battle passes to enhance your experience. Download Dota 2 here.
-- Among Us: Among Us is a social deduction game that has taken the internet by storm. It is set in a spaceship where one or more impostors are trying to sabotage and kill the crewmates, while the crewmates are trying to complete tasks and find out who the impostors are. You can play online with up to 10 players, or locally with up to 4 players. Among Us is free to play on iOS devices, but you can also buy it for $4.99 on Steam or $6.99 on the App Store for Mac. Download Among Us here.
-- Fallout Shelter: Fallout Shelter is a simulation game based on the Fallout series. It puts you in charge of a vault, where you have to build rooms, assign dwellers, manage resources, and protect your vault from disasters and enemies. You can also explore the wasteland, send dwellers on quests, and collect weapons and outfits. Fallout Shelter is free to play on iOS devices, but you can also buy it for $9.99 on Steam or $14.99 on the App Store for Mac. Download Fallout Shelter here.
-
- Conclusion
-In this article, we have shown you how to download free games for MacBook Air using different methods, such as Steam, the App Store, and iPad games. We have also recommended some of the best free games that you can play on your MacBook Air, such as Dota 2, Among Us, and Fallout Shelter. We hope you have found this article helpful and informative, and that you have fun playing these games on your MacBook Air. Here are some FAQs that you might have about downloading free games for MacBook Air.
- FAQs
-
-- How do I uninstall a game from my MacBook Air?
-- To uninstall a game from your MacBook Air, you can either drag it to the Trash from the Applications folder, or use an uninstaller app that can remove all the associated files and folders. You can also delete a game from Steam or the App Store by right-clicking on it and choosing Delete or Move to Trash.
-- How do I update a game on my MacBook Air?
-- To update a game on your MacBook Air, you can either check for updates manually from the game's menu or settings, or enable automatic updates from Steam or the App Store. You can also check for updates from the Updates tab in the App Store, or from the Downloads tab in Steam.
-- How do I optimize a game's performance on my MacBook Air?
-- To optimize a game's performance on your MacBook Air, you can try some of the following tips:
-- Close any unnecessary apps or tabs that are running in the background.
-- Adjust the game's graphics settings to lower the resolution, quality, or effects.
-- Use an external monitor, keyboard, and mouse for better gaming experience.
-- Keep your MacBook Air cool and ventilated by using a cooling pad or fan.
-- Update your macOS and drivers to the latest versions.
-
-- How do I play online games on my MacBook Air?
-- To play online games on your MacBook Air, you need to have a stable and fast internet connection, preferably wired or Wi-Fi. You also need to have an account for the online gaming platform or service that you are using, such as Steam, Origin, Epic Games, etc. You may also need to pay a subscription fee or buy in-game currency or items for some online games.
-- How do I play Windows games on my MacBook Air?
-- To play Windows games on your MacBook Air, you need to use a software that can run Windows applications on Mac, such as Boot Camp, Parallels Desktop, Wine, or Crossover. However, not all Windows games are compatible with Mac, and some may have performance issues or bugs. You also need to have a valid license for Windows and enough disk space and memory for the installation.
-
197e85843d
-
-
\ No newline at end of file
diff --git a/spaces/1toTree/lora_test/ppdiffusers/pipelines/stable_diffusion/pipeline_cycle_diffusion.py b/spaces/1toTree/lora_test/ppdiffusers/pipelines/stable_diffusion/pipeline_cycle_diffusion.py
deleted file mode 100644
index a352efdc98e0aad330fca4f490a0616e5175c873..0000000000000000000000000000000000000000
--- a/spaces/1toTree/lora_test/ppdiffusers/pipelines/stable_diffusion/pipeline_cycle_diffusion.py
+++ /dev/null
@@ -1,631 +0,0 @@
-# Copyright (c) 2022 PaddlePaddle Authors. All Rights Reserved.
-# Copyright 2022 The HuggingFace Team. All rights reserved.
-#
-# Licensed under the Apache License, Version 2.0 (the "License");
-# you may not use this file except in compliance with the License.
-# You may obtain a copy of the License at
-#
-# http://www.apache.org/licenses/LICENSE-2.0
-#
-# Unless required by applicable law or agreed to in writing, software
-# distributed under the License is distributed on an "AS IS" BASIS,
-# WITHOUT WARRANTIES OR CONDITIONS OF ANY KIND, either express or implied.
-# See the License for the specific language governing permissions and
-# limitations under the License.
-
-import inspect
-from typing import Callable, List, Optional, Union
-
-import numpy as np
-import paddle
-import PIL
-from packaging import version
-
-from paddlenlp.transformers import CLIPFeatureExtractor, CLIPTextModel, CLIPTokenizer
-
-from ...configuration_utils import FrozenDict
-from ...models import AutoencoderKL, UNet2DConditionModel
-from ...pipeline_utils import DiffusionPipeline
-from ...schedulers import DDIMScheduler
-from ...utils import PIL_INTERPOLATION, deprecate, logging
-from . import StableDiffusionPipelineOutput
-from .safety_checker import StableDiffusionSafetyChecker
-
-logger = logging.get_logger(__name__) # pylint: disable=invalid-name
-
-
-# Copied from diffusers.pipelines.stable_diffusion.pipeline_stable_diffusion_img2img.preprocess
-def preprocess(image):
- if isinstance(image, paddle.Tensor):
- return image
- elif isinstance(image, PIL.Image.Image):
- image = [image]
-
- if isinstance(image[0], PIL.Image.Image):
- w, h = image[0].size
- w, h = map(lambda x: x - x % 32, (w, h)) # resize to integer multiple of 32
-
- image = [np.array(i.resize((w, h), resample=PIL_INTERPOLATION["lanczos"]))[None, :] for i in image]
- image = np.concatenate(image, axis=0)
- image = np.array(image).astype(np.float32) / 255.0
- image = image.transpose(0, 3, 1, 2)
- image = 2.0 * image - 1.0
- image = paddle.to_tensor(image)
- elif isinstance(image[0], paddle.Tensor):
- image = paddle.concat(image, axis=0)
- return image
-
-
-def posterior_sample(scheduler, latents, timestep, clean_latents, generator, eta):
- # 1. get previous step value (=t-1)
- prev_timestep = timestep - scheduler.config.num_train_timesteps // scheduler.num_inference_steps
-
- if prev_timestep <= 0:
- return clean_latents
-
- # 2. compute alphas, betas
- alpha_prod_t = scheduler.alphas_cumprod[timestep]
- alpha_prod_t_prev = (
- scheduler.alphas_cumprod[prev_timestep] if prev_timestep >= 0 else scheduler.final_alpha_cumprod
- )
-
- variance = scheduler._get_variance(timestep, prev_timestep)
- std_dev_t = eta * variance ** (0.5)
-
- # direction pointing to x_t
- e_t = (latents - alpha_prod_t ** (0.5) * clean_latents) / (1 - alpha_prod_t) ** (0.5)
- dir_xt = (1.0 - alpha_prod_t_prev - std_dev_t**2) ** (0.5) * e_t
- noise = std_dev_t * paddle.randn(clean_latents.shape, dtype=clean_latents.dtype, generator=generator)
- prev_latents = alpha_prod_t_prev ** (0.5) * clean_latents + dir_xt + noise
-
- return prev_latents
-
-
-def compute_noise(scheduler, prev_latents, latents, timestep, noise_pred, eta):
- # 1. get previous step value (=t-1)
- prev_timestep = timestep - scheduler.config.num_train_timesteps // scheduler.num_inference_steps
-
- # 2. compute alphas, betas
- alpha_prod_t = scheduler.alphas_cumprod[timestep]
- alpha_prod_t_prev = (
- scheduler.alphas_cumprod[prev_timestep] if prev_timestep >= 0 else scheduler.final_alpha_cumprod
- )
-
- beta_prod_t = 1 - alpha_prod_t
-
- # 3. compute predicted original sample from predicted noise also called
- # "predicted x_0" of formula (12) from https://arxiv.org/pdf/2010.02502.pdf
- pred_original_sample = (latents - beta_prod_t ** (0.5) * noise_pred) / alpha_prod_t ** (0.5)
-
- # 4. Clip "predicted x_0"
- if scheduler.config.clip_sample:
- pred_original_sample = pred_original_sample.clip(-1, 1)
-
- # 5. compute variance: "sigma_t(η)" -> see formula (16)
- # σ_t = sqrt((1 − α_t−1)/(1 − α_t)) * sqrt(1 − α_t/α_t−1)
- variance = scheduler._get_variance(timestep, prev_timestep)
- std_dev_t = eta * variance ** (0.5)
-
- # 6. compute "direction pointing to x_t" of formula (12) from https://arxiv.org/pdf/2010.02502.pdf
- pred_sample_direction = (1 - alpha_prod_t_prev - std_dev_t**2) ** (0.5) * noise_pred
-
- noise = (prev_latents - (alpha_prod_t_prev ** (0.5) * pred_original_sample + pred_sample_direction)) / (
- variance ** (0.5) * eta
- )
- return noise
-
-
-class CycleDiffusionPipeline(DiffusionPipeline):
- r"""
- Pipeline for text-guided image to image generation using Stable Diffusion.
-
- This model inherits from [`DiffusionPipeline`]. Check the superclass documentation for the generic methods the
- library implements for all the pipelines (such as downloading or saving, running on a particular xxxx, etc.)
-
- Args:
- vae ([`AutoencoderKL`]):
- Variational Auto-Encoder (VAE) Model to encode and decode images to and from latent representations.
- text_encoder ([`CLIPTextModel`]):
- Frozen text-encoder. Stable Diffusion uses the text portion of
- [CLIP](https://huggingface.co/docs/transformers/model_doc/clip#transformers.CLIPTextModel), specifically
- the [clip-vit-large-patch14](https://huggingface.co/openai/clip-vit-large-patch14) variant.
- tokenizer (`CLIPTokenizer`):
- Tokenizer of class
- [CLIPTokenizer](https://huggingface.co/docs/transformers/v4.21.0/en/model_doc/clip#transformers.CLIPTokenizer).
- unet ([`UNet2DConditionModel`]): Conditional U-Net architecture to denoise the encoded image latents.
- scheduler ([`SchedulerMixin`]):
- A scheduler to be used in combination with `unet` to denoise the encoded image latents. Can be one of
- [`DDIMScheduler`], [`LMSDiscreteScheduler`], or [`PNDMScheduler`].
- safety_checker ([`StableDiffusionSafetyChecker`]):
- Classification module that estimates whether generated images could be considered offensive or harmful.
- Please, refer to the [model card](https://huggingface.co/CompVis/stable-diffusion-v1-4) for details.
- feature_extractor ([`CLIPFeatureExtractor`]):
- Model that extracts features from generated images to be used as inputs for the `safety_checker`.
- """
- _optional_components = ["safety_checker", "feature_extractor"]
-
- def __init__(
- self,
- vae: AutoencoderKL,
- text_encoder: CLIPTextModel,
- tokenizer: CLIPTokenizer,
- unet: UNet2DConditionModel,
- scheduler: DDIMScheduler,
- safety_checker: StableDiffusionSafetyChecker,
- feature_extractor: CLIPFeatureExtractor,
- requires_safety_checker: bool = True,
- ):
- super().__init__()
-
- if hasattr(scheduler.config, "steps_offset") and scheduler.config.steps_offset != 1:
- deprecation_message = (
- f"The configuration file of this scheduler: {scheduler} is outdated. `steps_offset`"
- f" should be set to 1 instead of {scheduler.config.steps_offset}. Please make sure "
- "to update the config accordingly as leaving `steps_offset` might led to incorrect results"
- " in future versions. If you have downloaded this checkpoint from the Hugging Face Hub,"
- " it would be very nice if you could open a Pull request for the `scheduler/scheduler_config.json`"
- " file"
- )
- deprecate("steps_offset!=1", "1.0.0", deprecation_message, standard_warn=False)
- new_config = dict(scheduler.config)
- new_config["steps_offset"] = 1
- scheduler._internal_dict = FrozenDict(new_config)
-
- if safety_checker is None and requires_safety_checker:
- logger.warning(
- f"You have disabled the safety checker for {self.__class__} by passing `safety_checker=None`. Ensure"
- " that you abide to the conditions of the Stable Diffusion license and do not expose unfiltered"
- " results in services or applications open to the public. PaddleNLP team, diffusers team and Hugging Face"
- " strongly recommend to keep the safety filter enabled in all public facing circumstances, disabling"
- " it only for use-cases that involve analyzing network behavior or auditing its results. For more"
- " information, please have a look at https://github.com/huggingface/diffusers/pull/254 ."
- )
- if safety_checker is not None and feature_extractor is None:
- raise ValueError(
- "Make sure to define a feature extractor when loading {self.__class__} if you want to use the safety"
- " checker. If you do not want to use the safety checker, you can pass `'safety_checker=None'` instead."
- )
- is_unet_version_less_0_9_0 = hasattr(unet.config, "_ppdiffusers_version") and version.parse(
- version.parse(unet.config._ppdiffusers_version).base_version
- ) < version.parse("0.9.0.dev0")
- is_unet_sample_size_less_64 = hasattr(unet.config, "sample_size") and unet.config.sample_size < 64
- if is_unet_version_less_0_9_0 and is_unet_sample_size_less_64:
- deprecation_message = (
- "The configuration file of the unet has set the default `sample_size` to smaller than"
- " 64 which seems highly unlikely. If your checkpoint is a fine-tuned version of any of the"
- " following: \n- CompVis/stable-diffusion-v1-4 \n- CompVis/stable-diffusion-v1-3 \n-"
- " CompVis/stable-diffusion-v1-2 \n- CompVis/stable-diffusion-v1-1 \n- runwayml/stable-diffusion-v1-5"
- " \n- runwayml/stable-diffusion-inpainting \n you should change 'sample_size' to 64 in the"
- " configuration file. Please make sure to update the config accordingly as leaving `sample_size=32`"
- " in the config might lead to incorrect results in future versions. If you have downloaded this"
- " checkpoint from the Hugging Face Hub, it would be very nice if you could open a Pull request for"
- " the `unet/config.json` file"
- )
- deprecate("sample_size<64", "1.0.0", deprecation_message, standard_warn=False)
- new_config = dict(unet.config)
- new_config["sample_size"] = 64
- unet._internal_dict = FrozenDict(new_config)
-
- self.register_modules(
- vae=vae,
- text_encoder=text_encoder,
- tokenizer=tokenizer,
- unet=unet,
- scheduler=scheduler,
- safety_checker=safety_checker,
- feature_extractor=feature_extractor,
- )
-
- self.register_to_config(requires_safety_checker=requires_safety_checker)
-
- # Copied from diffusers.pipelines.stable_diffusion.pipeline_stable_diffusion.StableDiffusionPipeline._encode_prompt
- def _encode_prompt(self, prompt, num_images_per_prompt, do_classifier_free_guidance, negative_prompt):
- r"""
- Encodes the prompt into text encoder hidden states.
-
- Args:
- prompt (`str` or `list(int)`):
- prompt to be encoded
- num_images_per_prompt (`int`):
- number of images that should be generated per prompt
- do_classifier_free_guidance (`bool`):
- whether to use classifier free guidance or not
- negative_prompt (`str` or `List[str]`):
- The prompt or prompts not to guide the image generation. Ignored when not using guidance (i.e., ignored
- if `guidance_scale` is less than `1`).
- """
- batch_size = len(prompt) if isinstance(prompt, list) else 1
-
- text_inputs = self.tokenizer(
- prompt,
- padding="max_length",
- max_length=self.tokenizer.model_max_length,
- truncation=True,
- return_tensors="pd",
- )
- text_input_ids = text_inputs.input_ids
- untruncated_ids = self.tokenizer(prompt, padding="longest", return_tensors="pd").input_ids
-
- if untruncated_ids.shape[-1] >= text_input_ids.shape[-1] and not paddle.equal_all(
- text_input_ids, untruncated_ids
- ):
- removed_text = self.tokenizer.batch_decode(untruncated_ids[:, self.tokenizer.model_max_length - 1 : -1])
- logger.warning(
- "The following part of your input was truncated because CLIP can only handle sequences up to"
- f" {self.tokenizer.model_max_length} tokens: {removed_text}"
- )
-
- if hasattr(self.text_encoder.config, "use_attention_mask") and self.text_encoder.config.use_attention_mask:
- attention_mask = text_inputs.attention_mask
- else:
- attention_mask = None
-
- text_embeddings = self.text_encoder(
- text_input_ids,
- attention_mask=attention_mask,
- )
- text_embeddings = text_embeddings[0]
-
- # duplicate text embeddings for each generation per prompt, using mps friendly method
- bs_embed, seq_len, _ = text_embeddings.shape
- text_embeddings = text_embeddings.tile([1, num_images_per_prompt, 1])
- text_embeddings = text_embeddings.reshape([bs_embed * num_images_per_prompt, seq_len, -1])
-
- # get unconditional embeddings for classifier free guidance
- if do_classifier_free_guidance:
- uncond_tokens: List[str]
- if negative_prompt is None:
- uncond_tokens = [""] * batch_size
- elif type(prompt) is not type(negative_prompt):
- raise TypeError(
- f"`negative_prompt` should be the same type to `prompt`, but got {type(negative_prompt)} !="
- f" {type(prompt)}."
- )
- elif isinstance(negative_prompt, str):
- uncond_tokens = [negative_prompt]
- elif batch_size != len(negative_prompt):
- raise ValueError(
- f"`negative_prompt`: {negative_prompt} has batch size {len(negative_prompt)}, but `prompt`:"
- f" {prompt} has batch size {batch_size}. Please make sure that passed `negative_prompt` matches"
- " the batch size of `prompt`."
- )
- else:
- uncond_tokens = negative_prompt
-
- max_length = text_input_ids.shape[-1]
- uncond_input = self.tokenizer(
- uncond_tokens,
- padding="max_length",
- max_length=max_length,
- truncation=True,
- return_tensors="pd",
- )
-
- if hasattr(self.text_encoder.config, "use_attention_mask") and self.text_encoder.config.use_attention_mask:
- attention_mask = uncond_input.attention_mask
- else:
- attention_mask = None
-
- uncond_embeddings = self.text_encoder(
- uncond_input.input_ids,
- attention_mask=attention_mask,
- )
- uncond_embeddings = uncond_embeddings[0]
-
- # duplicate unconditional embeddings for each generation per prompt, using mps friendly method
- seq_len = uncond_embeddings.shape[1]
- uncond_embeddings = uncond_embeddings.tile([1, num_images_per_prompt, 1])
- uncond_embeddings = uncond_embeddings.reshape([batch_size * num_images_per_prompt, seq_len, -1])
-
- # For classifier free guidance, we need to do two forward passes.
- # Here we concatenate the unconditional and text embeddings into a single batch
- # to avoid doing two forward passes
- text_embeddings = paddle.concat([uncond_embeddings, text_embeddings])
-
- return text_embeddings
-
- # Copied from diffusers.pipelines.stable_diffusion.pipeline_stable_diffusion_img2img.StableDiffusionImg2ImgPipeline.check_inputs
- def check_inputs(self, prompt, strength, callback_steps):
- if not isinstance(prompt, str) and not isinstance(prompt, list):
- raise ValueError(f"`prompt` has to be of type `str` or `list` but is {type(prompt)}")
-
- if strength < 0 or strength > 1:
- raise ValueError(f"The value of strength should in [1.0, 1.0] but is {strength}")
-
- if (callback_steps is None) or (
- callback_steps is not None and (not isinstance(callback_steps, int) or callback_steps <= 0)
- ):
- raise ValueError(
- f"`callback_steps` has to be a positive integer but is {callback_steps} of type"
- f" {type(callback_steps)}."
- )
-
- # Copied from diffusers.pipelines.stable_diffusion.pipeline_stable_diffusion.StableDiffusionPipeline.prepare_extra_step_kwargs
- def prepare_extra_step_kwargs(self, generator, eta):
- # prepare extra kwargs for the scheduler step, since not all schedulers have the same signature
- # eta (η) is only used with the DDIMScheduler, it will be ignored for other schedulers.
- # eta corresponds to η in DDIM paper: https://arxiv.org/abs/2010.02502
- # and should be between [0, 1]
-
- accepts_eta = "eta" in set(inspect.signature(self.scheduler.step).parameters.keys())
- extra_step_kwargs = {}
- if accepts_eta:
- extra_step_kwargs["eta"] = eta
-
- # check if the scheduler accepts generator
- accepts_generator = "generator" in set(inspect.signature(self.scheduler.step).parameters.keys())
- if accepts_generator:
- extra_step_kwargs["generator"] = generator
- return extra_step_kwargs
-
- # Copied from diffusers.pipelines.stable_diffusion.pipeline_stable_diffusion.StableDiffusionPipeline.run_safety_checker
- def run_safety_checker(self, image, dtype):
- if self.safety_checker is not None:
- safety_checker_input = self.feature_extractor(self.numpy_to_pil(image), return_tensors="pd")
- image, has_nsfw_concept = self.safety_checker(
- images=image, clip_input=safety_checker_input.pixel_values.cast(dtype)
- )
- else:
- has_nsfw_concept = None
- return image, has_nsfw_concept
-
- # Copied from diffusers.pipelines.stable_diffusion.pipeline_stable_diffusion.StableDiffusionPipeline.decode_latents
- def decode_latents(self, latents):
- latents = 1 / 0.18215 * latents
- image = self.vae.decode(latents).sample
- image = (image / 2 + 0.5).clip(0, 1)
- # we always cast to float32 as this does not cause significant overhead and is compatible with bfloa16
- image = image.transpose([0, 2, 3, 1]).cast("float32").numpy()
- return image
-
- # Copied from diffusers.pipelines.stable_diffusion.pipeline_stable_diffusion_img2img.StableDiffusionImg2ImgPipeline.get_timesteps
- def get_timesteps(self, num_inference_steps, strength):
- # get the original timestep using init_timestep
- init_timestep = min(int(num_inference_steps * strength), num_inference_steps)
-
- t_start = max(num_inference_steps - init_timestep, 0)
- timesteps = self.scheduler.timesteps[t_start:]
-
- return timesteps, num_inference_steps - t_start
-
- def prepare_latents(self, image, timestep, batch_size, num_images_per_prompt, dtype, generator=None):
- image = image.cast(dtype=dtype)
-
- batch_size = image.shape[0]
- if isinstance(generator, list) and len(generator) != batch_size:
- raise ValueError(
- f"You have passed a list of generators of length {len(generator)}, but requested an effective batch"
- f" size of {batch_size}. Make sure the batch size matches the length of the generators."
- )
-
- if isinstance(generator, list):
- init_latents = [
- self.vae.encode(image[i : i + 1]).latent_dist.sample(generator[i]) for i in range(batch_size)
- ]
- init_latents = paddle.concat(init_latents, axis=0)
- else:
- init_latents = self.vae.encode(image).latent_dist.sample(generator)
- init_latents = 0.18215 * init_latents
-
- if batch_size > init_latents.shape[0] and batch_size % init_latents.shape[0] == 0:
- # expand init_latents for batch_size
- deprecation_message = (
- f"You have passed {batch_size} text prompts (`prompt`), but only {init_latents.shape[0]} initial"
- " images (`image`). Initial images are now duplicating to match the number of text prompts. Note"
- " that this behavior is deprecated and will be removed in a version 1.0.0. Please make sure to update"
- " your script to pass as many initial images as text prompts to suppress this warning."
- )
- deprecate("len(prompt) != len(image)", "1.0.0", deprecation_message, standard_warn=False)
- additional_image_per_prompt = batch_size // init_latents.shape[0]
- init_latents = paddle.concat([init_latents] * additional_image_per_prompt * num_images_per_prompt, axis=0)
- elif batch_size > init_latents.shape[0] and batch_size % init_latents.shape[0] != 0:
- raise ValueError(
- f"Cannot duplicate `image` of batch size {init_latents.shape[0]} to {batch_size} text prompts."
- )
- else:
- init_latents = paddle.concat([init_latents] * num_images_per_prompt, axis=0)
-
- # add noise to latents using the timestep
- shape = init_latents.shape
- if isinstance(generator, list):
- shape = [
- 1,
- ] + shape[1:]
- noise = [paddle.randn(shape, generator=generator[i], dtype=dtype) for i in range(batch_size)]
- noise = paddle.concat(noise, axis=0)
- else:
- noise = paddle.randn(shape, generator=generator, dtype=dtype)
-
- # get latents
- clean_latents = init_latents
- init_latents = self.scheduler.add_noise(init_latents, noise, timestep)
- latents = init_latents
-
- return latents, clean_latents
-
- @paddle.no_grad()
- def __call__(
- self,
- prompt: Union[str, List[str]],
- source_prompt: Union[str, List[str]],
- image: Union[paddle.Tensor, PIL.Image.Image] = None,
- strength: float = 0.8,
- num_inference_steps: Optional[int] = 50,
- guidance_scale: Optional[float] = 7.5,
- source_guidance_scale: Optional[float] = 1,
- num_images_per_prompt: Optional[int] = 1,
- eta: Optional[float] = 0.1,
- generator: Optional[Union[paddle.Generator, List[paddle.Generator]]] = None,
- output_type: Optional[str] = "pil",
- return_dict: bool = True,
- callback: Optional[Callable[[int, int, paddle.Tensor], None]] = None,
- callback_steps: Optional[int] = 1,
- ):
- r"""
- Function invoked when calling the pipeline for generation.
-
- Args:
- prompt (`str` or `List[str]`):
- The prompt or prompts to guide the image generation.
- image (`paddle.Tensor` or `PIL.Image.Image`):
- `Image`, or tensor representing an image batch, that will be used as the starting point for the
- process.
- strength (`float`, *optional*, defaults to 0.8):
- Conceptually, indicates how much to transform the reference `image`. Must be between 0 and 1.
- `image` will be used as a starting point, adding more noise to it the larger the `strength`. The
- number of denoising steps depends on the amount of noise initially added. When `strength` is 1, added
- noise will be maximum and the denoising process will run for the full number of iterations specified in
- `num_inference_steps`. A value of 1, therefore, essentially ignores `image`.
- num_inference_steps (`int`, *optional*, defaults to 50):
- The number of denoising steps. More denoising steps usually lead to a higher quality image at the
- expense of slower inference. This parameter will be modulated by `strength`.
- guidance_scale (`float`, *optional*, defaults to 7.5):
- Guidance scale as defined in [Classifier-Free Diffusion Guidance](https://arxiv.org/abs/2207.12598).
- `guidance_scale` is defined as `w` of equation 2. of [Imagen
- Paper](https://arxiv.org/pdf/2205.11487.pdf). Guidance scale is enabled by setting `guidance_scale >
- 1`. Higher guidance scale encourages to generate images that are closely linked to the text `prompt`,
- usually at the expense of lower image quality.
- source_guidance_scale (`float`, *optional*, defaults to 1):
- Guidance scale for the source prompt. This is useful to control the amount of influence the source
- prompt for encoding.
- num_images_per_prompt (`int`, *optional*, defaults to 1):
- The number of images to generate per prompt.
- eta (`float`, *optional*, defaults to 0.1):
- Corresponds to parameter eta (η) in the DDIM paper: https://arxiv.org/abs/2010.02502. Only applies to
- [`schedulers.DDIMScheduler`], will be ignored for others.
- generator (`paddle.Generator`, *optional*):
- One or a list of paddle generator(s) to make generation deterministic.
- output_type (`str`, *optional*, defaults to `"pil"`):
- The output format of the generate image. Choose between
- [PIL](https://pillow.readthedocs.io/en/stable/): `PIL.Image.Image` or `np.array`.
- return_dict (`bool`, *optional*, defaults to `True`):
- Whether or not to return a [`~pipelines.stable_diffusion.StableDiffusionPipelineOutput`] instead of a
- plain tuple.
- callback (`Callable`, *optional*):
- A function that will be called every `callback_steps` steps during inference. The function will be
- called with the following arguments: `callback(step: int, timestep: int, latents: paddle.Tensor)`.
- callback_steps (`int`, *optional*, defaults to 1):
- The frequency at which the `callback` function will be called. If not specified, the callback will be
- called at every step.
-
- Returns:
- [`~pipelines.stable_diffusion.StableDiffusionPipelineOutput`] or `tuple`:
- [`~pipelines.stable_diffusion.StableDiffusionPipelineOutput`] if `return_dict` is True, otherwise a `tuple.
- When returning a tuple, the first element is a list with the generated images, and the second element is a
- list of `bool`s denoting whether the corresponding generated image likely represents "not-safe-for-work"
- (nsfw) content, according to the `safety_checker`.
- """
- # 1. Check inputs
- self.check_inputs(prompt, strength, callback_steps)
-
- # 2. Define call parameters
- batch_size = 1 if isinstance(prompt, str) else len(prompt)
- # here `guidance_scale` is defined analog to the guidance weight `w` of equation (2)
- # of the Imagen paper: https://arxiv.org/pdf/2205.11487.pdf . `guidance_scale = 1`
- # corresponds to doing no classifier free guidance.
- do_classifier_free_guidance = guidance_scale > 1.0
-
- # 3. Encode input prompt
- text_embeddings = self._encode_prompt(prompt, num_images_per_prompt, do_classifier_free_guidance, None)
- source_text_embeddings = self._encode_prompt(
- source_prompt, num_images_per_prompt, do_classifier_free_guidance, None
- )
-
- # 4. Preprocess image
- image = preprocess(image)
-
- # 5. Prepare timesteps
- self.scheduler.set_timesteps(num_inference_steps)
- timesteps, num_inference_steps = self.get_timesteps(num_inference_steps, strength)
- latent_timestep = timesteps[:1].tile([batch_size * num_images_per_prompt])
-
- # 6. Prepare latent variables
- latents, clean_latents = self.prepare_latents(
- image, latent_timestep, batch_size, num_images_per_prompt, text_embeddings.dtype, generator
- )
- source_latents = latents
-
- # 7. Prepare extra step kwargs. TODO: Logic should ideally just be moved out of the pipeline
- extra_step_kwargs = self.prepare_extra_step_kwargs(generator, eta)
- generator = extra_step_kwargs.pop("generator", None)
-
- # 8. Denoising loop
- num_warmup_steps = len(timesteps) - num_inference_steps * self.scheduler.order
- with self.progress_bar(total=num_inference_steps) as progress_bar:
- for i, t in enumerate(timesteps):
- # expand the latents if we are doing classifier free guidance
- latent_model_input = paddle.concat([latents] * 2)
- source_latent_model_input = paddle.concat([source_latents] * 2)
- latent_model_input = self.scheduler.scale_model_input(latent_model_input, t)
- source_latent_model_input = self.scheduler.scale_model_input(source_latent_model_input, t)
-
- # predict the noise residual
- concat_latent_model_input = paddle.stack(
- [
- source_latent_model_input[0],
- latent_model_input[0],
- source_latent_model_input[1],
- latent_model_input[1],
- ],
- axis=0,
- )
- concat_text_embeddings = paddle.stack(
- [
- source_text_embeddings[0],
- text_embeddings[0],
- source_text_embeddings[1],
- text_embeddings[1],
- ],
- axis=0,
- )
- concat_noise_pred = self.unet(
- concat_latent_model_input, t, encoder_hidden_states=concat_text_embeddings
- ).sample
-
- # perform guidance
- (
- source_noise_pred_uncond,
- noise_pred_uncond,
- source_noise_pred_text,
- noise_pred_text,
- ) = concat_noise_pred.chunk(4, axis=0)
- noise_pred = noise_pred_uncond + guidance_scale * (noise_pred_text - noise_pred_uncond)
- source_noise_pred = source_noise_pred_uncond + source_guidance_scale * (
- source_noise_pred_text - source_noise_pred_uncond
- )
-
- # Sample source_latents from the posterior distribution.
- prev_source_latents = posterior_sample(
- self.scheduler, source_latents, t, clean_latents, generator=generator, **extra_step_kwargs
- )
- # Compute noise.
- noise = compute_noise(
- self.scheduler, prev_source_latents, source_latents, t, source_noise_pred, **extra_step_kwargs
- )
- source_latents = prev_source_latents
-
- # compute the previous noisy sample x_t -> x_t-1
- latents = self.scheduler.step(
- noise_pred, t, latents, variance_noise=noise, **extra_step_kwargs
- ).prev_sample
-
- # call the callback, if provided
- if i == len(timesteps) - 1 or ((i + 1) > num_warmup_steps and (i + 1) % self.scheduler.order == 0):
- progress_bar.update()
- if callback is not None and i % callback_steps == 0:
- callback(i, t, latents)
-
- # 9. Post-processing
- image = self.decode_latents(latents)
-
- # 10. Run safety checker
- image, has_nsfw_concept = self.run_safety_checker(image, text_embeddings.dtype)
-
- # 11. Convert to PIL
- if output_type == "pil":
- image = self.numpy_to_pil(image)
-
- if not return_dict:
- return (image, has_nsfw_concept)
-
- return StableDiffusionPipelineOutput(images=image, nsfw_content_detected=has_nsfw_concept)
diff --git a/spaces/7thHeaven/ochyai_food/README.md b/spaces/7thHeaven/ochyai_food/README.md
deleted file mode 100644
index f16c0c5cc9994b0e5e8cc517ed40c523be62278c..0000000000000000000000000000000000000000
--- a/spaces/7thHeaven/ochyai_food/README.md
+++ /dev/null
@@ -1,13 +0,0 @@
----
-title: ochyai_food
-emoji: 🍛
-colorFrom: yellow
-colorTo: red
-sdk: gradio
-sdk_version: 3.19.1
-app_file: app.py
-pinned: false
-duplicated_from: ochyai/ochyai_food
----
-
-Check out the configuration reference at https://huggingface.co/docs/hub/spaces-config-reference
\ No newline at end of file
diff --git a/spaces/801artistry/RVC801/infer/lib/infer_pack/onnx_inference.py b/spaces/801artistry/RVC801/infer/lib/infer_pack/onnx_inference.py
deleted file mode 100644
index 6633659fc83b19d82611d3c9cc840e9c547734d0..0000000000000000000000000000000000000000
--- a/spaces/801artistry/RVC801/infer/lib/infer_pack/onnx_inference.py
+++ /dev/null
@@ -1,149 +0,0 @@
-import librosa
-import numpy as np
-import onnxruntime
-import soundfile
-
-import logging
-
-logger = logging.getLogger(__name__)
-
-
-class ContentVec:
- def __init__(self, vec_path="pretrained/vec-768-layer-12.onnx", device=None):
- logger.info("Load model(s) from {}".format(vec_path))
- if device == "cpu" or device is None:
- providers = ["CPUExecutionProvider"]
- elif device == "cuda":
- providers = ["CUDAExecutionProvider", "CPUExecutionProvider"]
- elif device == "dml":
- providers = ["DmlExecutionProvider"]
- else:
- raise RuntimeError("Unsportted Device")
- self.model = onnxruntime.InferenceSession(vec_path, providers=providers)
-
- def __call__(self, wav):
- return self.forward(wav)
-
- def forward(self, wav):
- feats = wav
- if feats.ndim == 2: # double channels
- feats = feats.mean(-1)
- assert feats.ndim == 1, feats.ndim
- feats = np.expand_dims(np.expand_dims(feats, 0), 0)
- onnx_input = {self.model.get_inputs()[0].name: feats}
- logits = self.model.run(None, onnx_input)[0]
- return logits.transpose(0, 2, 1)
-
-
-def get_f0_predictor(f0_predictor, hop_length, sampling_rate, **kargs):
- if f0_predictor == "pm":
- from lib.infer_pack.modules.F0Predictor.PMF0Predictor import PMF0Predictor
-
- f0_predictor_object = PMF0Predictor(
- hop_length=hop_length, sampling_rate=sampling_rate
- )
- elif f0_predictor == "harvest":
- from lib.infer_pack.modules.F0Predictor.HarvestF0Predictor import (
- HarvestF0Predictor,
- )
-
- f0_predictor_object = HarvestF0Predictor(
- hop_length=hop_length, sampling_rate=sampling_rate
- )
- elif f0_predictor == "dio":
- from lib.infer_pack.modules.F0Predictor.DioF0Predictor import DioF0Predictor
-
- f0_predictor_object = DioF0Predictor(
- hop_length=hop_length, sampling_rate=sampling_rate
- )
- else:
- raise Exception("Unknown f0 predictor")
- return f0_predictor_object
-
-
-class OnnxRVC:
- def __init__(
- self,
- model_path,
- sr=40000,
- hop_size=512,
- vec_path="vec-768-layer-12",
- device="cpu",
- ):
- vec_path = f"pretrained/{vec_path}.onnx"
- self.vec_model = ContentVec(vec_path, device)
- if device == "cpu" or device is None:
- providers = ["CPUExecutionProvider"]
- elif device == "cuda":
- providers = ["CUDAExecutionProvider", "CPUExecutionProvider"]
- elif device == "dml":
- providers = ["DmlExecutionProvider"]
- else:
- raise RuntimeError("Unsportted Device")
- self.model = onnxruntime.InferenceSession(model_path, providers=providers)
- self.sampling_rate = sr
- self.hop_size = hop_size
-
- def forward(self, hubert, hubert_length, pitch, pitchf, ds, rnd):
- onnx_input = {
- self.model.get_inputs()[0].name: hubert,
- self.model.get_inputs()[1].name: hubert_length,
- self.model.get_inputs()[2].name: pitch,
- self.model.get_inputs()[3].name: pitchf,
- self.model.get_inputs()[4].name: ds,
- self.model.get_inputs()[5].name: rnd,
- }
- return (self.model.run(None, onnx_input)[0] * 32767).astype(np.int16)
-
- def inference(
- self,
- raw_path,
- sid,
- f0_method="dio",
- f0_up_key=0,
- pad_time=0.5,
- cr_threshold=0.02,
- ):
- f0_min = 50
- f0_max = 1100
- f0_mel_min = 1127 * np.log(1 + f0_min / 700)
- f0_mel_max = 1127 * np.log(1 + f0_max / 700)
- f0_predictor = get_f0_predictor(
- f0_method,
- hop_length=self.hop_size,
- sampling_rate=self.sampling_rate,
- threshold=cr_threshold,
- )
- wav, sr = librosa.load(raw_path, sr=self.sampling_rate)
- org_length = len(wav)
- if org_length / sr > 50.0:
- raise RuntimeError("Reached Max Length")
-
- wav16k = librosa.resample(wav, orig_sr=self.sampling_rate, target_sr=16000)
- wav16k = wav16k
-
- hubert = self.vec_model(wav16k)
- hubert = np.repeat(hubert, 2, axis=2).transpose(0, 2, 1).astype(np.float32)
- hubert_length = hubert.shape[1]
-
- pitchf = f0_predictor.compute_f0(wav, hubert_length)
- pitchf = pitchf * 2 ** (f0_up_key / 12)
- pitch = pitchf.copy()
- f0_mel = 1127 * np.log(1 + pitch / 700)
- f0_mel[f0_mel > 0] = (f0_mel[f0_mel > 0] - f0_mel_min) * 254 / (
- f0_mel_max - f0_mel_min
- ) + 1
- f0_mel[f0_mel <= 1] = 1
- f0_mel[f0_mel > 255] = 255
- pitch = np.rint(f0_mel).astype(np.int64)
-
- pitchf = pitchf.reshape(1, len(pitchf)).astype(np.float32)
- pitch = pitch.reshape(1, len(pitch))
- ds = np.array([sid]).astype(np.int64)
-
- rnd = np.random.randn(1, 192, hubert_length).astype(np.float32)
- hubert_length = np.array([hubert_length]).astype(np.int64)
-
- out_wav = self.forward(hubert, hubert_length, pitch, pitchf, ds, rnd).squeeze()
- out_wav = np.pad(out_wav, (0, 2 * self.hop_size), "constant")
- return out_wav[0:org_length]
diff --git a/spaces/AIFILMS/StyleGANEX/models/stylegan2/op_ori/upfirdn2d.cpp b/spaces/AIFILMS/StyleGANEX/models/stylegan2/op_ori/upfirdn2d.cpp
deleted file mode 100644
index d2e633dc896433c205e18bc3e455539192ff968e..0000000000000000000000000000000000000000
--- a/spaces/AIFILMS/StyleGANEX/models/stylegan2/op_ori/upfirdn2d.cpp
+++ /dev/null
@@ -1,23 +0,0 @@
-#include
-
-
-torch::Tensor upfirdn2d_op(const torch::Tensor& input, const torch::Tensor& kernel,
- int up_x, int up_y, int down_x, int down_y,
- int pad_x0, int pad_x1, int pad_y0, int pad_y1);
-
-#define CHECK_CUDA(x) TORCH_CHECK(x.type().is_cuda(), #x " must be a CUDA tensor")
-#define CHECK_CONTIGUOUS(x) TORCH_CHECK(x.is_contiguous(), #x " must be contiguous")
-#define CHECK_INPUT(x) CHECK_CUDA(x); CHECK_CONTIGUOUS(x)
-
-torch::Tensor upfirdn2d(const torch::Tensor& input, const torch::Tensor& kernel,
- int up_x, int up_y, int down_x, int down_y,
- int pad_x0, int pad_x1, int pad_y0, int pad_y1) {
- CHECK_CUDA(input);
- CHECK_CUDA(kernel);
-
- return upfirdn2d_op(input, kernel, up_x, up_y, down_x, down_y, pad_x0, pad_x1, pad_y0, pad_y1);
-}
-
-PYBIND11_MODULE(TORCH_EXTENSION_NAME, m) {
- m.def("upfirdn2d", &upfirdn2d, "upfirdn2d (CUDA)");
-}
\ No newline at end of file
diff --git a/spaces/AIGC-Audio/Make_An_Audio/ldm/modules/diffusionmodules/__init__.py b/spaces/AIGC-Audio/Make_An_Audio/ldm/modules/diffusionmodules/__init__.py
deleted file mode 100644
index e69de29bb2d1d6434b8b29ae775ad8c2e48c5391..0000000000000000000000000000000000000000
diff --git a/spaces/AIGC-Audio/Make_An_Audio_inpaint/ldm/modules/losses_audio/vggishish/predict.py b/spaces/AIGC-Audio/Make_An_Audio_inpaint/ldm/modules/losses_audio/vggishish/predict.py
deleted file mode 100644
index e9d13f30153cd43a4a8bcfe2da4b9a53846bf1eb..0000000000000000000000000000000000000000
--- a/spaces/AIGC-Audio/Make_An_Audio_inpaint/ldm/modules/losses_audio/vggishish/predict.py
+++ /dev/null
@@ -1,90 +0,0 @@
-import os
-from torch.utils.data import DataLoader
-import torchvision
-from tqdm import tqdm
-from dataset import VGGSound
-import torch
-import torch.nn as nn
-from metrics import metrics
-from omegaconf import OmegaConf
-from model import VGGishish
-from transforms import Crop, StandardNormalizeAudio, ToTensor
-
-
-if __name__ == '__main__':
- cfg_cli = OmegaConf.from_cli()
- print(cfg_cli.config)
- cfg_yml = OmegaConf.load(cfg_cli.config)
- # the latter arguments are prioritized
- cfg = OmegaConf.merge(cfg_yml, cfg_cli)
- OmegaConf.set_readonly(cfg, True)
- print(OmegaConf.to_yaml(cfg))
-
- # logger = LoggerWithTBoard(cfg)
- transforms = [
- StandardNormalizeAudio(cfg.mels_path),
- ToTensor(),
- ]
- if cfg.cropped_size not in [None, 'None', 'none']:
- transforms.append(Crop(cfg.cropped_size))
- transforms = torchvision.transforms.transforms.Compose(transforms)
-
- datasets = {
- 'test': VGGSound('test', cfg.mels_path, transforms),
- }
-
- loaders = {
- 'test': DataLoader(datasets['test'], batch_size=cfg.batch_size,
- num_workers=cfg.num_workers, pin_memory=True)
- }
-
- device = torch.device(cfg.device if torch.cuda.is_available() else 'cpu')
- model = VGGishish(cfg.conv_layers, cfg.use_bn, num_classes=len(datasets['test'].target2label))
- model = model.to(device)
-
- optimizer = torch.optim.Adam(model.parameters(), lr=cfg.learning_rate)
- criterion = nn.CrossEntropyLoss()
-
- # loading the best model
- folder_name = os.path.split(cfg.config)[0].split('/')[-1]
- print(folder_name)
- ckpt = torch.load(f'./logs/{folder_name}/vggishish-{folder_name}.pt', map_location='cpu')
- model.load_state_dict(ckpt['model'])
- print((f'The model was trained for {ckpt["epoch"]} epochs. Loss: {ckpt["loss"]:.4f}'))
-
- # Testing the model
- model.eval()
- running_loss = 0
- preds_from_each_batch = []
- targets_from_each_batch = []
-
- for i, batch in enumerate(tqdm(loaders['test'])):
- inputs = batch['input'].to(device)
- targets = batch['target'].to(device)
-
- # zero the parameter gradients
- optimizer.zero_grad()
-
- # forward + backward + optimize
- with torch.set_grad_enabled(False):
- outputs = model(inputs)
- loss = criterion(outputs, targets)
-
- # loss
- running_loss += loss.item()
-
- # for metrics calculation later on
- preds_from_each_batch += [outputs.detach().cpu()]
- targets_from_each_batch += [targets.cpu()]
-
- # logging metrics
- preds_from_each_batch = torch.cat(preds_from_each_batch)
- targets_from_each_batch = torch.cat(targets_from_each_batch)
- test_metrics_dict = metrics(targets_from_each_batch, preds_from_each_batch)
- test_metrics_dict['avg_loss'] = running_loss / len(loaders['test'])
- test_metrics_dict['param_num'] = sum(p.numel() for p in model.parameters() if p.requires_grad)
-
- # TODO: I have no idea why tboard doesn't keep metrics (hparams) in a tensorboard when
- # I run this experiment from cli: `python main.py config=./configs/vggish.yaml`
- # while when I run it in vscode debugger the metrics are present in the tboard (weird)
- print(test_metrics_dict)
diff --git a/spaces/ATang0729/Forecast4Muses/Model/Model6/Model6_2_ProfileRecogition/mmpretrain/configs/resnet/resnet50_8xb16_cifar100.py b/spaces/ATang0729/Forecast4Muses/Model/Model6/Model6_2_ProfileRecogition/mmpretrain/configs/resnet/resnet50_8xb16_cifar100.py
deleted file mode 100644
index ebde6c76ecca6d23b58edfb85ebc3b72ce15a2b2..0000000000000000000000000000000000000000
--- a/spaces/ATang0729/Forecast4Muses/Model/Model6/Model6_2_ProfileRecogition/mmpretrain/configs/resnet/resnet50_8xb16_cifar100.py
+++ /dev/null
@@ -1,19 +0,0 @@
-_base_ = [
- '../_base_/models/resnet50_cifar.py',
- '../_base_/datasets/cifar100_bs16.py',
- '../_base_/schedules/cifar10_bs128.py',
- '../_base_/default_runtime.py',
-]
-
-# model settings
-model = dict(head=dict(num_classes=100))
-
-# schedule settings
-optim_wrapper = dict(optimizer=dict(weight_decay=0.0005))
-
-param_scheduler = dict(
- type='MultiStepLR',
- by_epoch=True,
- milestones=[60, 120, 160],
- gamma=0.2,
-)
diff --git a/spaces/Adapter/CoAdapter/ldm/models/autoencoder.py b/spaces/Adapter/CoAdapter/ldm/models/autoencoder.py
deleted file mode 100644
index e3ff5fe3ed0f70de8b31f1af27e107b93fbb94ca..0000000000000000000000000000000000000000
--- a/spaces/Adapter/CoAdapter/ldm/models/autoencoder.py
+++ /dev/null
@@ -1,211 +0,0 @@
-import torch
-import pytorch_lightning as pl
-import torch.nn.functional as F
-import torch.nn as nn
-from contextlib import contextmanager
-
-from ldm.modules.diffusionmodules.model import Encoder, Decoder
-from ldm.modules.distributions.distributions import DiagonalGaussianDistribution
-
-from ldm.util import instantiate_from_config
-from ldm.modules.ema import LitEma
-
-
-class AutoencoderKL(pl.LightningModule):
- def __init__(self,
- ddconfig,
- lossconfig,
- embed_dim,
- ckpt_path=None,
- ignore_keys=[],
- image_key="image",
- colorize_nlabels=None,
- monitor=None,
- ema_decay=None,
- learn_logvar=False
- ):
- super().__init__()
- self.learn_logvar = learn_logvar
- self.image_key = image_key
- self.encoder = Encoder(**ddconfig)
- self.decoder = Decoder(**ddconfig)
- self.loss = instantiate_from_config(lossconfig)
- assert ddconfig["double_z"]
- self.quant_conv = torch.nn.Conv2d(2*ddconfig["z_channels"], 2*embed_dim, 1)
- self.post_quant_conv = torch.nn.Conv2d(embed_dim, ddconfig["z_channels"], 1)
- self.embed_dim = embed_dim
- if colorize_nlabels is not None:
- assert type(colorize_nlabels)==int
- self.register_buffer("colorize", torch.randn(3, colorize_nlabels, 1, 1))
- if monitor is not None:
- self.monitor = monitor
-
- self.use_ema = ema_decay is not None
- if self.use_ema:
- self.ema_decay = ema_decay
- assert 0. < ema_decay < 1.
- self.model_ema = LitEma(self, decay=ema_decay)
- print(f"Keeping EMAs of {len(list(self.model_ema.buffers()))}.")
-
- if ckpt_path is not None:
- self.init_from_ckpt(ckpt_path, ignore_keys=ignore_keys)
-
- def init_from_ckpt(self, path, ignore_keys=list()):
- sd = torch.load(path, map_location="cpu")["state_dict"]
- keys = list(sd.keys())
- for k in keys:
- for ik in ignore_keys:
- if k.startswith(ik):
- print("Deleting key {} from state_dict.".format(k))
- del sd[k]
- self.load_state_dict(sd, strict=False)
- print(f"Restored from {path}")
-
- @contextmanager
- def ema_scope(self, context=None):
- if self.use_ema:
- self.model_ema.store(self.parameters())
- self.model_ema.copy_to(self)
- if context is not None:
- print(f"{context}: Switched to EMA weights")
- try:
- yield None
- finally:
- if self.use_ema:
- self.model_ema.restore(self.parameters())
- if context is not None:
- print(f"{context}: Restored training weights")
-
- def on_train_batch_end(self, *args, **kwargs):
- if self.use_ema:
- self.model_ema(self)
-
- def encode(self, x):
- h = self.encoder(x)
- moments = self.quant_conv(h)
- posterior = DiagonalGaussianDistribution(moments)
- return posterior
-
- def decode(self, z):
- z = self.post_quant_conv(z)
- dec = self.decoder(z)
- return dec
-
- def forward(self, input, sample_posterior=True):
- posterior = self.encode(input)
- if sample_posterior:
- z = posterior.sample()
- else:
- z = posterior.mode()
- dec = self.decode(z)
- return dec, posterior
-
- def get_input(self, batch, k):
- x = batch[k]
- if len(x.shape) == 3:
- x = x[..., None]
- x = x.permute(0, 3, 1, 2).to(memory_format=torch.contiguous_format).float()
- return x
-
- def training_step(self, batch, batch_idx, optimizer_idx):
- inputs = self.get_input(batch, self.image_key)
- reconstructions, posterior = self(inputs)
-
- if optimizer_idx == 0:
- # train encoder+decoder+logvar
- aeloss, log_dict_ae = self.loss(inputs, reconstructions, posterior, optimizer_idx, self.global_step,
- last_layer=self.get_last_layer(), split="train")
- self.log("aeloss", aeloss, prog_bar=True, logger=True, on_step=True, on_epoch=True)
- self.log_dict(log_dict_ae, prog_bar=False, logger=True, on_step=True, on_epoch=False)
- return aeloss
-
- if optimizer_idx == 1:
- # train the discriminator
- discloss, log_dict_disc = self.loss(inputs, reconstructions, posterior, optimizer_idx, self.global_step,
- last_layer=self.get_last_layer(), split="train")
-
- self.log("discloss", discloss, prog_bar=True, logger=True, on_step=True, on_epoch=True)
- self.log_dict(log_dict_disc, prog_bar=False, logger=True, on_step=True, on_epoch=False)
- return discloss
-
- def validation_step(self, batch, batch_idx):
- log_dict = self._validation_step(batch, batch_idx)
- with self.ema_scope():
- log_dict_ema = self._validation_step(batch, batch_idx, postfix="_ema")
- return log_dict
-
- def _validation_step(self, batch, batch_idx, postfix=""):
- inputs = self.get_input(batch, self.image_key)
- reconstructions, posterior = self(inputs)
- aeloss, log_dict_ae = self.loss(inputs, reconstructions, posterior, 0, self.global_step,
- last_layer=self.get_last_layer(), split="val"+postfix)
-
- discloss, log_dict_disc = self.loss(inputs, reconstructions, posterior, 1, self.global_step,
- last_layer=self.get_last_layer(), split="val"+postfix)
-
- self.log(f"val{postfix}/rec_loss", log_dict_ae[f"val{postfix}/rec_loss"])
- self.log_dict(log_dict_ae)
- self.log_dict(log_dict_disc)
- return self.log_dict
-
- def configure_optimizers(self):
- lr = self.learning_rate
- ae_params_list = list(self.encoder.parameters()) + list(self.decoder.parameters()) + list(
- self.quant_conv.parameters()) + list(self.post_quant_conv.parameters())
- if self.learn_logvar:
- print(f"{self.__class__.__name__}: Learning logvar")
- ae_params_list.append(self.loss.logvar)
- opt_ae = torch.optim.Adam(ae_params_list,
- lr=lr, betas=(0.5, 0.9))
- opt_disc = torch.optim.Adam(self.loss.discriminator.parameters(),
- lr=lr, betas=(0.5, 0.9))
- return [opt_ae, opt_disc], []
-
- def get_last_layer(self):
- return self.decoder.conv_out.weight
-
- @torch.no_grad()
- def log_images(self, batch, only_inputs=False, log_ema=False, **kwargs):
- log = dict()
- x = self.get_input(batch, self.image_key)
- x = x.to(self.device)
- if not only_inputs:
- xrec, posterior = self(x)
- if x.shape[1] > 3:
- # colorize with random projection
- assert xrec.shape[1] > 3
- x = self.to_rgb(x)
- xrec = self.to_rgb(xrec)
- log["samples"] = self.decode(torch.randn_like(posterior.sample()))
- log["reconstructions"] = xrec
- log["inputs"] = x
- return log
-
- def to_rgb(self, x):
- assert self.image_key == "segmentation"
- if not hasattr(self, "colorize"):
- self.register_buffer("colorize", torch.randn(3, x.shape[1], 1, 1).to(x))
- x = F.conv2d(x, weight=self.colorize)
- x = 2.*(x-x.min())/(x.max()-x.min()) - 1.
- return x
-
-
-class IdentityFirstStage(nn.Module):
- def __init__(self, *args, vq_interface=False, **kwargs):
- self.vq_interface = vq_interface
- super().__init__()
-
- def encode(self, x, *args, **kwargs):
- return x
-
- def decode(self, x, *args, **kwargs):
- return x
-
- def quantize(self, x, *args, **kwargs):
- if self.vq_interface:
- return x, None, [None, None, None]
- return x
-
- def forward(self, x, *args, **kwargs):
- return x
-
diff --git a/spaces/Adapter/CoAdapter/ldm/models/diffusion/dpm_solver/sampler.py b/spaces/Adapter/CoAdapter/ldm/models/diffusion/dpm_solver/sampler.py
deleted file mode 100644
index fc2c96baf2bf5f8de3684c198bcd1b0df5b51149..0000000000000000000000000000000000000000
--- a/spaces/Adapter/CoAdapter/ldm/models/diffusion/dpm_solver/sampler.py
+++ /dev/null
@@ -1,87 +0,0 @@
-"""SAMPLING ONLY."""
-import torch
-
-from .dpm_solver import NoiseScheduleVP, model_wrapper, DPM_Solver
-
-
-MODEL_TYPES = {
- "eps": "noise",
- "v": "v"
-}
-
-
-class DPMSolverSampler(object):
- def __init__(self, model, **kwargs):
- super().__init__()
- self.model = model
- to_torch = lambda x: x.clone().detach().to(torch.float32).to(model.device)
- self.register_buffer('alphas_cumprod', to_torch(model.alphas_cumprod))
-
- def register_buffer(self, name, attr):
- if type(attr) == torch.Tensor:
- if attr.device != torch.device("cuda"):
- attr = attr.to(torch.device("cuda"))
- setattr(self, name, attr)
-
- @torch.no_grad()
- def sample(self,
- S,
- batch_size,
- shape,
- conditioning=None,
- callback=None,
- normals_sequence=None,
- img_callback=None,
- quantize_x0=False,
- eta=0.,
- mask=None,
- x0=None,
- temperature=1.,
- noise_dropout=0.,
- score_corrector=None,
- corrector_kwargs=None,
- verbose=True,
- x_T=None,
- log_every_t=100,
- unconditional_guidance_scale=1.,
- unconditional_conditioning=None,
- # this has to come in the same format as the conditioning, # e.g. as encoded tokens, ...
- **kwargs
- ):
- if conditioning is not None:
- if isinstance(conditioning, dict):
- cbs = conditioning[list(conditioning.keys())[0]].shape[0]
- if cbs != batch_size:
- print(f"Warning: Got {cbs} conditionings but batch-size is {batch_size}")
- else:
- if conditioning.shape[0] != batch_size:
- print(f"Warning: Got {conditioning.shape[0]} conditionings but batch-size is {batch_size}")
-
- # sampling
- C, H, W = shape
- size = (batch_size, C, H, W)
-
- print(f'Data shape for DPM-Solver sampling is {size}, sampling steps {S}')
-
- device = self.model.betas.device
- if x_T is None:
- img = torch.randn(size, device=device)
- else:
- img = x_T
-
- ns = NoiseScheduleVP('discrete', alphas_cumprod=self.alphas_cumprod)
-
- model_fn = model_wrapper(
- lambda x, t, c: self.model.apply_model(x, t, c),
- ns,
- model_type=MODEL_TYPES[self.model.parameterization],
- guidance_type="classifier-free",
- condition=conditioning,
- unconditional_condition=unconditional_conditioning,
- guidance_scale=unconditional_guidance_scale,
- )
-
- dpm_solver = DPM_Solver(model_fn, ns, predict_x0=True, thresholding=False)
- x = dpm_solver.sample(img, steps=S, skip_type="time_uniform", method="multistep", order=2, lower_order_final=True)
-
- return x.to(device), None
diff --git a/spaces/Adapter/CoAdapter/ldm/modules/diffusionmodules/openaimodel.py b/spaces/Adapter/CoAdapter/ldm/modules/diffusionmodules/openaimodel.py
deleted file mode 100644
index 09972d58e1a65b88909dfe35c12c9126851da5cf..0000000000000000000000000000000000000000
--- a/spaces/Adapter/CoAdapter/ldm/modules/diffusionmodules/openaimodel.py
+++ /dev/null
@@ -1,798 +0,0 @@
-from abc import abstractmethod
-import math
-import torch
-
-import numpy as np
-import torch as th
-import torch.nn as nn
-import torch.nn.functional as F
-
-from ldm.modules.diffusionmodules.util import (
- checkpoint,
- conv_nd,
- linear,
- avg_pool_nd,
- zero_module,
- normalization,
- timestep_embedding,
-)
-from ldm.modules.attention import SpatialTransformer
-from ldm.util import exists
-
-
-# dummy replace
-def convert_module_to_f16(x):
- pass
-
-def convert_module_to_f32(x):
- pass
-
-
-## go
-class AttentionPool2d(nn.Module):
- """
- Adapted from CLIP: https://github.com/openai/CLIP/blob/main/clip/model.py
- """
-
- def __init__(
- self,
- spacial_dim: int,
- embed_dim: int,
- num_heads_channels: int,
- output_dim: int = None,
- ):
- super().__init__()
- self.positional_embedding = nn.Parameter(th.randn(embed_dim, spacial_dim ** 2 + 1) / embed_dim ** 0.5)
- self.qkv_proj = conv_nd(1, embed_dim, 3 * embed_dim, 1)
- self.c_proj = conv_nd(1, embed_dim, output_dim or embed_dim, 1)
- self.num_heads = embed_dim // num_heads_channels
- self.attention = QKVAttention(self.num_heads)
-
- def forward(self, x):
- b, c, *_spatial = x.shape
- x = x.reshape(b, c, -1) # NC(HW)
- x = th.cat([x.mean(dim=-1, keepdim=True), x], dim=-1) # NC(HW+1)
- x = x + self.positional_embedding[None, :, :].to(x.dtype) # NC(HW+1)
- x = self.qkv_proj(x)
- x = self.attention(x)
- x = self.c_proj(x)
- return x[:, :, 0]
-
-
-class TimestepBlock(nn.Module):
- """
- Any module where forward() takes timestep embeddings as a second argument.
- """
-
- @abstractmethod
- def forward(self, x, emb):
- """
- Apply the module to `x` given `emb` timestep embeddings.
- """
-
-
-class TimestepEmbedSequential(nn.Sequential, TimestepBlock):
- """
- A sequential module that passes timestep embeddings to the children that
- support it as an extra input.
- """
-
- def forward(self, x, emb, context=None):
- for layer in self:
- if isinstance(layer, TimestepBlock):
- x = layer(x, emb)
- elif isinstance(layer, SpatialTransformer):
- x = layer(x, context)
- else:
- x = layer(x)
- return x
-
-
-class Upsample(nn.Module):
- """
- An upsampling layer with an optional convolution.
- :param channels: channels in the inputs and outputs.
- :param use_conv: a bool determining if a convolution is applied.
- :param dims: determines if the signal is 1D, 2D, or 3D. If 3D, then
- upsampling occurs in the inner-two dimensions.
- """
-
- def __init__(self, channels, use_conv, dims=2, out_channels=None, padding=1):
- super().__init__()
- self.channels = channels
- self.out_channels = out_channels or channels
- self.use_conv = use_conv
- self.dims = dims
- if use_conv:
- self.conv = conv_nd(dims, self.channels, self.out_channels, 3, padding=padding)
-
- def forward(self, x):
- assert x.shape[1] == self.channels
- if self.dims == 3:
- x = F.interpolate(
- x, (x.shape[2], x.shape[3] * 2, x.shape[4] * 2), mode="nearest"
- )
- else:
- x = F.interpolate(x, scale_factor=2, mode="nearest")
- if self.use_conv:
- x = self.conv(x)
- return x
-
-class TransposedUpsample(nn.Module):
- 'Learned 2x upsampling without padding'
- def __init__(self, channels, out_channels=None, ks=5):
- super().__init__()
- self.channels = channels
- self.out_channels = out_channels or channels
-
- self.up = nn.ConvTranspose2d(self.channels,self.out_channels,kernel_size=ks,stride=2)
-
- def forward(self,x):
- return self.up(x)
-
-
-class Downsample(nn.Module):
- """
- A downsampling layer with an optional convolution.
- :param channels: channels in the inputs and outputs.
- :param use_conv: a bool determining if a convolution is applied.
- :param dims: determines if the signal is 1D, 2D, or 3D. If 3D, then
- downsampling occurs in the inner-two dimensions.
- """
-
- def __init__(self, channels, use_conv, dims=2, out_channels=None,padding=1):
- super().__init__()
- self.channels = channels
- self.out_channels = out_channels or channels
- self.use_conv = use_conv
- self.dims = dims
- stride = 2 if dims != 3 else (1, 2, 2)
- if use_conv:
- self.op = conv_nd(
- dims, self.channels, self.out_channels, 3, stride=stride, padding=padding
- )
- else:
- assert self.channels == self.out_channels
- self.op = avg_pool_nd(dims, kernel_size=stride, stride=stride)
-
- def forward(self, x):
- assert x.shape[1] == self.channels
- return self.op(x)
-
-
-class ResBlock(TimestepBlock):
- """
- A residual block that can optionally change the number of channels.
- :param channels: the number of input channels.
- :param emb_channels: the number of timestep embedding channels.
- :param dropout: the rate of dropout.
- :param out_channels: if specified, the number of out channels.
- :param use_conv: if True and out_channels is specified, use a spatial
- convolution instead of a smaller 1x1 convolution to change the
- channels in the skip connection.
- :param dims: determines if the signal is 1D, 2D, or 3D.
- :param use_checkpoint: if True, use gradient checkpointing on this module.
- :param up: if True, use this block for upsampling.
- :param down: if True, use this block for downsampling.
- """
-
- def __init__(
- self,
- channels,
- emb_channels,
- dropout,
- out_channels=None,
- use_conv=False,
- use_scale_shift_norm=False,
- dims=2,
- use_checkpoint=False,
- up=False,
- down=False,
- ):
- super().__init__()
- self.channels = channels
- self.emb_channels = emb_channels
- self.dropout = dropout
- self.out_channels = out_channels or channels
- self.use_conv = use_conv
- self.use_checkpoint = use_checkpoint
- self.use_scale_shift_norm = use_scale_shift_norm
-
- self.in_layers = nn.Sequential(
- normalization(channels),
- nn.SiLU(),
- conv_nd(dims, channels, self.out_channels, 3, padding=1),
- )
-
- self.updown = up or down
-
- if up:
- self.h_upd = Upsample(channels, False, dims)
- self.x_upd = Upsample(channels, False, dims)
- elif down:
- self.h_upd = Downsample(channels, False, dims)
- self.x_upd = Downsample(channels, False, dims)
- else:
- self.h_upd = self.x_upd = nn.Identity()
-
- self.emb_layers = nn.Sequential(
- nn.SiLU(),
- linear(
- emb_channels,
- 2 * self.out_channels if use_scale_shift_norm else self.out_channels,
- ),
- )
- self.out_layers = nn.Sequential(
- normalization(self.out_channels),
- nn.SiLU(),
- nn.Dropout(p=dropout),
- zero_module(
- conv_nd(dims, self.out_channels, self.out_channels, 3, padding=1)
- ),
- )
-
- if self.out_channels == channels:
- self.skip_connection = nn.Identity()
- elif use_conv:
- self.skip_connection = conv_nd(
- dims, channels, self.out_channels, 3, padding=1
- )
- else:
- self.skip_connection = conv_nd(dims, channels, self.out_channels, 1)
-
- def forward(self, x, emb):
- """
- Apply the block to a Tensor, conditioned on a timestep embedding.
- :param x: an [N x C x ...] Tensor of features.
- :param emb: an [N x emb_channels] Tensor of timestep embeddings.
- :return: an [N x C x ...] Tensor of outputs.
- """
- return checkpoint(
- self._forward, (x, emb), self.parameters(), self.use_checkpoint
- )
-
-
- def _forward(self, x, emb):
- if self.updown:
- in_rest, in_conv = self.in_layers[:-1], self.in_layers[-1]
- h = in_rest(x)
- h = self.h_upd(h)
- x = self.x_upd(x)
- h = in_conv(h)
- else:
- h = self.in_layers(x)
- emb_out = self.emb_layers(emb).type(h.dtype)
- while len(emb_out.shape) < len(h.shape):
- emb_out = emb_out[..., None]
- if self.use_scale_shift_norm:
- out_norm, out_rest = self.out_layers[0], self.out_layers[1:]
- scale, shift = th.chunk(emb_out, 2, dim=1)
- h = out_norm(h) * (1 + scale) + shift
- h = out_rest(h)
- else:
- h = h + emb_out
- h = self.out_layers(h)
- return self.skip_connection(x) + h
-
-
-class AttentionBlock(nn.Module):
- """
- An attention block that allows spatial positions to attend to each other.
- Originally ported from here, but adapted to the N-d case.
- https://github.com/hojonathanho/diffusion/blob/1e0dceb3b3495bbe19116a5e1b3596cd0706c543/diffusion_tf/models/unet.py#L66.
- """
-
- def __init__(
- self,
- channels,
- num_heads=1,
- num_head_channels=-1,
- use_checkpoint=False,
- use_new_attention_order=False,
- ):
- super().__init__()
- self.channels = channels
- if num_head_channels == -1:
- self.num_heads = num_heads
- else:
- assert (
- channels % num_head_channels == 0
- ), f"q,k,v channels {channels} is not divisible by num_head_channels {num_head_channels}"
- self.num_heads = channels // num_head_channels
- self.use_checkpoint = use_checkpoint
- self.norm = normalization(channels)
- self.qkv = conv_nd(1, channels, channels * 3, 1)
- if use_new_attention_order:
- # split qkv before split heads
- self.attention = QKVAttention(self.num_heads)
- else:
- # split heads before split qkv
- self.attention = QKVAttentionLegacy(self.num_heads)
-
- self.proj_out = zero_module(conv_nd(1, channels, channels, 1))
-
- def forward(self, x):
- return checkpoint(self._forward, (x,), self.parameters(), True) # TODO: check checkpoint usage, is True # TODO: fix the .half call!!!
- #return pt_checkpoint(self._forward, x) # pytorch
-
- def _forward(self, x):
- b, c, *spatial = x.shape
- x = x.reshape(b, c, -1)
- qkv = self.qkv(self.norm(x))
- h = self.attention(qkv)
- h = self.proj_out(h)
- return (x + h).reshape(b, c, *spatial)
-
-
-def count_flops_attn(model, _x, y):
- """
- A counter for the `thop` package to count the operations in an
- attention operation.
- Meant to be used like:
- macs, params = thop.profile(
- model,
- inputs=(inputs, timestamps),
- custom_ops={QKVAttention: QKVAttention.count_flops},
- )
- """
- b, c, *spatial = y[0].shape
- num_spatial = int(np.prod(spatial))
- # We perform two matmuls with the same number of ops.
- # The first computes the weight matrix, the second computes
- # the combination of the value vectors.
- matmul_ops = 2 * b * (num_spatial ** 2) * c
- model.total_ops += th.DoubleTensor([matmul_ops])
-
-
-class QKVAttentionLegacy(nn.Module):
- """
- A module which performs QKV attention. Matches legacy QKVAttention + input/ouput heads shaping
- """
-
- def __init__(self, n_heads):
- super().__init__()
- self.n_heads = n_heads
-
- def forward(self, qkv):
- """
- Apply QKV attention.
- :param qkv: an [N x (H * 3 * C) x T] tensor of Qs, Ks, and Vs.
- :return: an [N x (H * C) x T] tensor after attention.
- """
- bs, width, length = qkv.shape
- assert width % (3 * self.n_heads) == 0
- ch = width // (3 * self.n_heads)
- q, k, v = qkv.reshape(bs * self.n_heads, ch * 3, length).split(ch, dim=1)
- scale = 1 / math.sqrt(math.sqrt(ch))
- weight = th.einsum(
- "bct,bcs->bts", q * scale, k * scale
- ) # More stable with f16 than dividing afterwards
- weight = th.softmax(weight.float(), dim=-1).type(weight.dtype)
- a = th.einsum("bts,bcs->bct", weight, v)
- return a.reshape(bs, -1, length)
-
- @staticmethod
- def count_flops(model, _x, y):
- return count_flops_attn(model, _x, y)
-
-
-class QKVAttention(nn.Module):
- """
- A module which performs QKV attention and splits in a different order.
- """
-
- def __init__(self, n_heads):
- super().__init__()
- self.n_heads = n_heads
-
- def forward(self, qkv):
- """
- Apply QKV attention.
- :param qkv: an [N x (3 * H * C) x T] tensor of Qs, Ks, and Vs.
- :return: an [N x (H * C) x T] tensor after attention.
- """
- bs, width, length = qkv.shape
- assert width % (3 * self.n_heads) == 0
- ch = width // (3 * self.n_heads)
- q, k, v = qkv.chunk(3, dim=1)
- scale = 1 / math.sqrt(math.sqrt(ch))
- weight = th.einsum(
- "bct,bcs->bts",
- (q * scale).view(bs * self.n_heads, ch, length),
- (k * scale).view(bs * self.n_heads, ch, length),
- ) # More stable with f16 than dividing afterwards
- weight = th.softmax(weight.float(), dim=-1).type(weight.dtype)
- a = th.einsum("bts,bcs->bct", weight, v.reshape(bs * self.n_heads, ch, length))
- return a.reshape(bs, -1, length)
-
- @staticmethod
- def count_flops(model, _x, y):
- return count_flops_attn(model, _x, y)
-
-
-class UNetModel(nn.Module):
- """
- The full UNet model with attention and timestep embedding.
- :param in_channels: channels in the input Tensor.
- :param model_channels: base channel count for the model.
- :param out_channels: channels in the output Tensor.
- :param num_res_blocks: number of residual blocks per downsample.
- :param attention_resolutions: a collection of downsample rates at which
- attention will take place. May be a set, list, or tuple.
- For example, if this contains 4, then at 4x downsampling, attention
- will be used.
- :param dropout: the dropout probability.
- :param channel_mult: channel multiplier for each level of the UNet.
- :param conv_resample: if True, use learned convolutions for upsampling and
- downsampling.
- :param dims: determines if the signal is 1D, 2D, or 3D.
- :param num_classes: if specified (as an int), then this model will be
- class-conditional with `num_classes` classes.
- :param use_checkpoint: use gradient checkpointing to reduce memory usage.
- :param num_heads: the number of attention heads in each attention layer.
- :param num_heads_channels: if specified, ignore num_heads and instead use
- a fixed channel width per attention head.
- :param num_heads_upsample: works with num_heads to set a different number
- of heads for upsampling. Deprecated.
- :param use_scale_shift_norm: use a FiLM-like conditioning mechanism.
- :param resblock_updown: use residual blocks for up/downsampling.
- :param use_new_attention_order: use a different attention pattern for potentially
- increased efficiency.
- """
-
- def __init__(
- self,
- image_size,
- in_channels,
- model_channels,
- out_channels,
- num_res_blocks,
- attention_resolutions,
- dropout=0,
- channel_mult=(1, 2, 4, 8),
- conv_resample=True,
- dims=2,
- num_classes=None,
- use_checkpoint=False,
- use_fp16=False,
- num_heads=-1,
- num_head_channels=-1,
- num_heads_upsample=-1,
- use_scale_shift_norm=False,
- resblock_updown=False,
- use_new_attention_order=False,
- use_spatial_transformer=False, # custom transformer support
- transformer_depth=1, # custom transformer support
- context_dim=None, # custom transformer support
- n_embed=None, # custom support for prediction of discrete ids into codebook of first stage vq model
- legacy=True,
- disable_self_attentions=None,
- num_attention_blocks=None,
- disable_middle_self_attn=False,
- use_linear_in_transformer=False,
- ):
- super().__init__()
- if use_spatial_transformer:
- assert context_dim is not None, 'Fool!! You forgot to include the dimension of your cross-attention conditioning...'
-
- if context_dim is not None:
- assert use_spatial_transformer, 'Fool!! You forgot to use the spatial transformer for your cross-attention conditioning...'
- from omegaconf.listconfig import ListConfig
- if type(context_dim) == ListConfig:
- context_dim = list(context_dim)
-
- if num_heads_upsample == -1:
- num_heads_upsample = num_heads
-
- if num_heads == -1:
- assert num_head_channels != -1, 'Either num_heads or num_head_channels has to be set'
-
- if num_head_channels == -1:
- assert num_heads != -1, 'Either num_heads or num_head_channels has to be set'
-
- self.image_size = image_size
- self.in_channels = in_channels
- self.model_channels = model_channels
- self.out_channels = out_channels
- if isinstance(num_res_blocks, int):
- self.num_res_blocks = len(channel_mult) * [num_res_blocks]
- else:
- if len(num_res_blocks) != len(channel_mult):
- raise ValueError("provide num_res_blocks either as an int (globally constant) or "
- "as a list/tuple (per-level) with the same length as channel_mult")
- self.num_res_blocks = num_res_blocks
- if disable_self_attentions is not None:
- # should be a list of booleans, indicating whether to disable self-attention in TransformerBlocks or not
- assert len(disable_self_attentions) == len(channel_mult)
- if num_attention_blocks is not None:
- assert len(num_attention_blocks) == len(self.num_res_blocks)
- assert all(map(lambda i: self.num_res_blocks[i] >= num_attention_blocks[i], range(len(num_attention_blocks))))
- print(f"Constructor of UNetModel received num_attention_blocks={num_attention_blocks}. "
- f"This option has LESS priority than attention_resolutions {attention_resolutions}, "
- f"i.e., in cases where num_attention_blocks[i] > 0 but 2**i not in attention_resolutions, "
- f"attention will still not be set.")
-
- self.attention_resolutions = attention_resolutions
- self.dropout = dropout
- self.channel_mult = channel_mult
- self.conv_resample = conv_resample
- self.num_classes = num_classes
- self.use_checkpoint = use_checkpoint
- self.dtype = th.float16 if use_fp16 else th.float32
- self.num_heads = num_heads
- self.num_head_channels = num_head_channels
- self.num_heads_upsample = num_heads_upsample
- self.predict_codebook_ids = n_embed is not None
-
- time_embed_dim = model_channels * 4
- self.time_embed = nn.Sequential(
- linear(model_channels, time_embed_dim),
- nn.SiLU(),
- linear(time_embed_dim, time_embed_dim),
- )
-
- if self.num_classes is not None:
- if isinstance(self.num_classes, int):
- self.label_emb = nn.Embedding(num_classes, time_embed_dim)
- elif self.num_classes == "continuous":
- print("setting up linear c_adm embedding layer")
- self.label_emb = nn.Linear(1, time_embed_dim)
- else:
- raise ValueError()
-
- self.input_blocks = nn.ModuleList(
- [
- TimestepEmbedSequential(
- conv_nd(dims, in_channels, model_channels, 3, padding=1)
- )
- ]
- )
- self._feature_size = model_channels
- input_block_chans = [model_channels]
- ch = model_channels
- ds = 1
- for level, mult in enumerate(channel_mult):
- for nr in range(self.num_res_blocks[level]):
- layers = [
- ResBlock(
- ch,
- time_embed_dim,
- dropout,
- out_channels=mult * model_channels,
- dims=dims,
- use_checkpoint=use_checkpoint,
- use_scale_shift_norm=use_scale_shift_norm,
- )
- ]
- ch = mult * model_channels
- if ds in attention_resolutions:
- if num_head_channels == -1:
- dim_head = ch // num_heads
- else:
- num_heads = ch // num_head_channels
- dim_head = num_head_channels
- if legacy:
- #num_heads = 1
- dim_head = ch // num_heads if use_spatial_transformer else num_head_channels
- if exists(disable_self_attentions):
- disabled_sa = disable_self_attentions[level]
- else:
- disabled_sa = False
-
- if not exists(num_attention_blocks) or nr < num_attention_blocks[level]:
- layers.append(
- AttentionBlock(
- ch,
- use_checkpoint=use_checkpoint,
- num_heads=num_heads,
- num_head_channels=dim_head,
- use_new_attention_order=use_new_attention_order,
- ) if not use_spatial_transformer else SpatialTransformer(
- ch, num_heads, dim_head, depth=transformer_depth, context_dim=context_dim,
- disable_self_attn=disabled_sa, use_linear=use_linear_in_transformer,
- use_checkpoint=use_checkpoint
- )
- )
- self.input_blocks.append(TimestepEmbedSequential(*layers))
- self._feature_size += ch
- input_block_chans.append(ch)
- if level != len(channel_mult) - 1:
- out_ch = ch
- self.input_blocks.append(
- TimestepEmbedSequential(
- ResBlock(
- ch,
- time_embed_dim,
- dropout,
- out_channels=out_ch,
- dims=dims,
- use_checkpoint=use_checkpoint,
- use_scale_shift_norm=use_scale_shift_norm,
- down=True,
- )
- if resblock_updown
- else Downsample(
- ch, conv_resample, dims=dims, out_channels=out_ch
- )
- )
- )
- ch = out_ch
- input_block_chans.append(ch)
- ds *= 2
- self._feature_size += ch
-
- if num_head_channels == -1:
- dim_head = ch // num_heads
- else:
- num_heads = ch // num_head_channels
- dim_head = num_head_channels
- if legacy:
- #num_heads = 1
- dim_head = ch // num_heads if use_spatial_transformer else num_head_channels
- self.middle_block = TimestepEmbedSequential(
- ResBlock(
- ch,
- time_embed_dim,
- dropout,
- dims=dims,
- use_checkpoint=use_checkpoint,
- use_scale_shift_norm=use_scale_shift_norm,
- ),
- AttentionBlock(
- ch,
- use_checkpoint=use_checkpoint,
- num_heads=num_heads,
- num_head_channels=dim_head,
- use_new_attention_order=use_new_attention_order,
- ) if not use_spatial_transformer else SpatialTransformer( # always uses a self-attn
- ch, num_heads, dim_head, depth=transformer_depth, context_dim=context_dim,
- disable_self_attn=disable_middle_self_attn, use_linear=use_linear_in_transformer,
- use_checkpoint=use_checkpoint
- ),
- ResBlock(
- ch,
- time_embed_dim,
- dropout,
- dims=dims,
- use_checkpoint=use_checkpoint,
- use_scale_shift_norm=use_scale_shift_norm,
- ),
- )
- self._feature_size += ch
-
- self.output_blocks = nn.ModuleList([])
- for level, mult in list(enumerate(channel_mult))[::-1]:
- for i in range(self.num_res_blocks[level] + 1):
- ich = input_block_chans.pop()
- layers = [
- ResBlock(
- ch + ich,
- time_embed_dim,
- dropout,
- out_channels=model_channels * mult,
- dims=dims,
- use_checkpoint=use_checkpoint,
- use_scale_shift_norm=use_scale_shift_norm,
- )
- ]
- ch = model_channels * mult
- if ds in attention_resolutions:
- if num_head_channels == -1:
- dim_head = ch // num_heads
- else:
- num_heads = ch // num_head_channels
- dim_head = num_head_channels
- if legacy:
- #num_heads = 1
- dim_head = ch // num_heads if use_spatial_transformer else num_head_channels
- if exists(disable_self_attentions):
- disabled_sa = disable_self_attentions[level]
- else:
- disabled_sa = False
-
- if not exists(num_attention_blocks) or i < num_attention_blocks[level]:
- layers.append(
- AttentionBlock(
- ch,
- use_checkpoint=use_checkpoint,
- num_heads=num_heads_upsample,
- num_head_channels=dim_head,
- use_new_attention_order=use_new_attention_order,
- ) if not use_spatial_transformer else SpatialTransformer(
- ch, num_heads, dim_head, depth=transformer_depth, context_dim=context_dim,
- disable_self_attn=disabled_sa, use_linear=use_linear_in_transformer,
- use_checkpoint=use_checkpoint
- )
- )
- if level and i == self.num_res_blocks[level]:
- out_ch = ch
- layers.append(
- ResBlock(
- ch,
- time_embed_dim,
- dropout,
- out_channels=out_ch,
- dims=dims,
- use_checkpoint=use_checkpoint,
- use_scale_shift_norm=use_scale_shift_norm,
- up=True,
- )
- if resblock_updown
- else Upsample(ch, conv_resample, dims=dims, out_channels=out_ch)
- )
- ds //= 2
- self.output_blocks.append(TimestepEmbedSequential(*layers))
- self._feature_size += ch
-
- self.out = nn.Sequential(
- normalization(ch),
- nn.SiLU(),
- zero_module(conv_nd(dims, model_channels, out_channels, 3, padding=1)),
- )
- if self.predict_codebook_ids:
- self.id_predictor = nn.Sequential(
- normalization(ch),
- conv_nd(dims, model_channels, n_embed, 1),
- #nn.LogSoftmax(dim=1) # change to cross_entropy and produce non-normalized logits
- )
-
- def convert_to_fp16(self):
- """
- Convert the torso of the model to float16.
- """
- self.input_blocks.apply(convert_module_to_f16)
- self.middle_block.apply(convert_module_to_f16)
- self.output_blocks.apply(convert_module_to_f16)
-
- def convert_to_fp32(self):
- """
- Convert the torso of the model to float32.
- """
- self.input_blocks.apply(convert_module_to_f32)
- self.middle_block.apply(convert_module_to_f32)
- self.output_blocks.apply(convert_module_to_f32)
-
- def forward(self, x, timesteps=None, context=None, y=None, features_adapter=None, append_to_context=None, **kwargs):
- """
- Apply the model to an input batch.
- :param x: an [N x C x ...] Tensor of inputs.
- :param timesteps: a 1-D batch of timesteps.
- :param context: conditioning plugged in via crossattn
- :param y: an [N] Tensor of labels, if class-conditional.
- :return: an [N x C x ...] Tensor of outputs.
- """
- assert (y is not None) == (
- self.num_classes is not None
- ), "must specify y if and only if the model is class-conditional"
- hs = []
- t_emb = timestep_embedding(timesteps, self.model_channels, repeat_only=False)
- emb = self.time_embed(t_emb)
-
- if self.num_classes is not None:
- assert y.shape[0] == x.shape[0]
- emb = emb + self.label_emb(y)
-
- h = x.type(self.dtype)
-
- if append_to_context is not None:
- context = torch.cat([context, append_to_context], dim=1)
-
- adapter_idx = 0
- for id, module in enumerate(self.input_blocks):
- h = module(h, emb, context)
- if ((id+1)%3 == 0) and features_adapter is not None:
- h = h + features_adapter[adapter_idx]
- adapter_idx += 1
- hs.append(h)
- if features_adapter is not None:
- assert len(features_adapter)==adapter_idx, 'Wrong features_adapter'
-
- h = self.middle_block(h, emb, context)
- for module in self.output_blocks:
- h = th.cat([h, hs.pop()], dim=1)
- h = module(h, emb, context)
- h = h.type(x.dtype)
- if self.predict_codebook_ids:
- return self.id_predictor(h)
- else:
- return self.out(h)
diff --git a/spaces/Aditya9790/yolo7-object-tracking/utils/aws/userdata.sh b/spaces/Aditya9790/yolo7-object-tracking/utils/aws/userdata.sh
deleted file mode 100644
index 5a99d4bec7400a08069ce40e8b02928d4b4e06ee..0000000000000000000000000000000000000000
--- a/spaces/Aditya9790/yolo7-object-tracking/utils/aws/userdata.sh
+++ /dev/null
@@ -1,27 +0,0 @@
-#!/bin/bash
-# AWS EC2 instance startup script https://docs.aws.amazon.com/AWSEC2/latest/UserGuide/user-data.html
-# This script will run only once on first instance start (for a re-start script see mime.sh)
-# /home/ubuntu (ubuntu) or /home/ec2-user (amazon-linux) is working dir
-# Use >300 GB SSD
-
-cd home/ubuntu
-if [ ! -d yolor ]; then
- echo "Running first-time script." # install dependencies, download COCO, pull Docker
- git clone -b main https://github.com/WongKinYiu/yolov7 && sudo chmod -R 777 yolov7
- cd yolov7
- bash data/scripts/get_coco.sh && echo "Data done." &
- sudo docker pull nvcr.io/nvidia/pytorch:21.08-py3 && echo "Docker done." &
- python -m pip install --upgrade pip && pip install -r requirements.txt && python detect.py && echo "Requirements done." &
- wait && echo "All tasks done." # finish background tasks
-else
- echo "Running re-start script." # resume interrupted runs
- i=0
- list=$(sudo docker ps -qa) # container list i.e. $'one\ntwo\nthree\nfour'
- while IFS= read -r id; do
- ((i++))
- echo "restarting container $i: $id"
- sudo docker start $id
- # sudo docker exec -it $id python train.py --resume # single-GPU
- sudo docker exec -d $id python utils/aws/resume.py # multi-scenario
- done <<<"$list"
-fi
diff --git a/spaces/Al-Chan/Vits_League_of_Legends_Yuumi_TTS/monotonic_align/core.c b/spaces/Al-Chan/Vits_League_of_Legends_Yuumi_TTS/monotonic_align/core.c
deleted file mode 100644
index 5631d20a9a00db29e143a6e8e4e5c378d6bb850a..0000000000000000000000000000000000000000
--- a/spaces/Al-Chan/Vits_League_of_Legends_Yuumi_TTS/monotonic_align/core.c
+++ /dev/null
@@ -1,21299 +0,0 @@
-/* Generated by Cython 0.29.21 */
-
-/* BEGIN: Cython Metadata
-{
- "distutils": {
- "name": "monotonic_align.core",
- "sources": [
- "core.pyx"
- ]
- },
- "module_name": "monotonic_align.core"
-}
-END: Cython Metadata */
-
-#define PY_SSIZE_T_CLEAN
-#include "Python.h"
-#ifndef Py_PYTHON_H
- #error Python headers needed to compile C extensions, please install development version of Python.
-#elif PY_VERSION_HEX < 0x02060000 || (0x03000000 <= PY_VERSION_HEX && PY_VERSION_HEX < 0x03030000)
- #error Cython requires Python 2.6+ or Python 3.3+.
-#else
-#define CYTHON_ABI "0_29_21"
-#define CYTHON_HEX_VERSION 0x001D15F0
-#define CYTHON_FUTURE_DIVISION 0
-#include
-#ifndef offsetof
- #define offsetof(type, member) ( (size_t) & ((type*)0) -> member )
-#endif
-#if !defined(WIN32) && !defined(MS_WINDOWS)
- #ifndef __stdcall
- #define __stdcall
- #endif
- #ifndef __cdecl
- #define __cdecl
- #endif
- #ifndef __fastcall
- #define __fastcall
- #endif
-#endif
-#ifndef DL_IMPORT
- #define DL_IMPORT(t) t
-#endif
-#ifndef DL_EXPORT
- #define DL_EXPORT(t) t
-#endif
-#define __PYX_COMMA ,
-#ifndef HAVE_LONG_LONG
- #if PY_VERSION_HEX >= 0x02070000
- #define HAVE_LONG_LONG
- #endif
-#endif
-#ifndef PY_LONG_LONG
- #define PY_LONG_LONG LONG_LONG
-#endif
-#ifndef Py_HUGE_VAL
- #define Py_HUGE_VAL HUGE_VAL
-#endif
-#ifdef PYPY_VERSION
- #define CYTHON_COMPILING_IN_PYPY 1
- #define CYTHON_COMPILING_IN_PYSTON 0
- #define CYTHON_COMPILING_IN_CPYTHON 0
- #undef CYTHON_USE_TYPE_SLOTS
- #define CYTHON_USE_TYPE_SLOTS 0
- #undef CYTHON_USE_PYTYPE_LOOKUP
- #define CYTHON_USE_PYTYPE_LOOKUP 0
- #if PY_VERSION_HEX < 0x03050000
- #undef CYTHON_USE_ASYNC_SLOTS
- #define CYTHON_USE_ASYNC_SLOTS 0
- #elif !defined(CYTHON_USE_ASYNC_SLOTS)
- #define CYTHON_USE_ASYNC_SLOTS 1
- #endif
- #undef CYTHON_USE_PYLIST_INTERNALS
- #define CYTHON_USE_PYLIST_INTERNALS 0
- #undef CYTHON_USE_UNICODE_INTERNALS
- #define CYTHON_USE_UNICODE_INTERNALS 0
- #undef CYTHON_USE_UNICODE_WRITER
- #define CYTHON_USE_UNICODE_WRITER 0
- #undef CYTHON_USE_PYLONG_INTERNALS
- #define CYTHON_USE_PYLONG_INTERNALS 0
- #undef CYTHON_AVOID_BORROWED_REFS
- #define CYTHON_AVOID_BORROWED_REFS 1
- #undef CYTHON_ASSUME_SAFE_MACROS
- #define CYTHON_ASSUME_SAFE_MACROS 0
- #undef CYTHON_UNPACK_METHODS
- #define CYTHON_UNPACK_METHODS 0
- #undef CYTHON_FAST_THREAD_STATE
- #define CYTHON_FAST_THREAD_STATE 0
- #undef CYTHON_FAST_PYCALL
- #define CYTHON_FAST_PYCALL 0
- #undef CYTHON_PEP489_MULTI_PHASE_INIT
- #define CYTHON_PEP489_MULTI_PHASE_INIT 0
- #undef CYTHON_USE_TP_FINALIZE
- #define CYTHON_USE_TP_FINALIZE 0
- #undef CYTHON_USE_DICT_VERSIONS
- #define CYTHON_USE_DICT_VERSIONS 0
- #undef CYTHON_USE_EXC_INFO_STACK
- #define CYTHON_USE_EXC_INFO_STACK 0
-#elif defined(PYSTON_VERSION)
- #define CYTHON_COMPILING_IN_PYPY 0
- #define CYTHON_COMPILING_IN_PYSTON 1
- #define CYTHON_COMPILING_IN_CPYTHON 0
- #ifndef CYTHON_USE_TYPE_SLOTS
- #define CYTHON_USE_TYPE_SLOTS 1
- #endif
- #undef CYTHON_USE_PYTYPE_LOOKUP
- #define CYTHON_USE_PYTYPE_LOOKUP 0
- #undef CYTHON_USE_ASYNC_SLOTS
- #define CYTHON_USE_ASYNC_SLOTS 0
- #undef CYTHON_USE_PYLIST_INTERNALS
- #define CYTHON_USE_PYLIST_INTERNALS 0
- #ifndef CYTHON_USE_UNICODE_INTERNALS
- #define CYTHON_USE_UNICODE_INTERNALS 1
- #endif
- #undef CYTHON_USE_UNICODE_WRITER
- #define CYTHON_USE_UNICODE_WRITER 0
- #undef CYTHON_USE_PYLONG_INTERNALS
- #define CYTHON_USE_PYLONG_INTERNALS 0
- #ifndef CYTHON_AVOID_BORROWED_REFS
- #define CYTHON_AVOID_BORROWED_REFS 0
- #endif
- #ifndef CYTHON_ASSUME_SAFE_MACROS
- #define CYTHON_ASSUME_SAFE_MACROS 1
- #endif
- #ifndef CYTHON_UNPACK_METHODS
- #define CYTHON_UNPACK_METHODS 1
- #endif
- #undef CYTHON_FAST_THREAD_STATE
- #define CYTHON_FAST_THREAD_STATE 0
- #undef CYTHON_FAST_PYCALL
- #define CYTHON_FAST_PYCALL 0
- #undef CYTHON_PEP489_MULTI_PHASE_INIT
- #define CYTHON_PEP489_MULTI_PHASE_INIT 0
- #undef CYTHON_USE_TP_FINALIZE
- #define CYTHON_USE_TP_FINALIZE 0
- #undef CYTHON_USE_DICT_VERSIONS
- #define CYTHON_USE_DICT_VERSIONS 0
- #undef CYTHON_USE_EXC_INFO_STACK
- #define CYTHON_USE_EXC_INFO_STACK 0
-#else
- #define CYTHON_COMPILING_IN_PYPY 0
- #define CYTHON_COMPILING_IN_PYSTON 0
- #define CYTHON_COMPILING_IN_CPYTHON 1
- #ifndef CYTHON_USE_TYPE_SLOTS
- #define CYTHON_USE_TYPE_SLOTS 1
- #endif
- #if PY_VERSION_HEX < 0x02070000
- #undef CYTHON_USE_PYTYPE_LOOKUP
- #define CYTHON_USE_PYTYPE_LOOKUP 0
- #elif !defined(CYTHON_USE_PYTYPE_LOOKUP)
- #define CYTHON_USE_PYTYPE_LOOKUP 1
- #endif
- #if PY_MAJOR_VERSION < 3
- #undef CYTHON_USE_ASYNC_SLOTS
- #define CYTHON_USE_ASYNC_SLOTS 0
- #elif !defined(CYTHON_USE_ASYNC_SLOTS)
- #define CYTHON_USE_ASYNC_SLOTS 1
- #endif
- #if PY_VERSION_HEX < 0x02070000
- #undef CYTHON_USE_PYLONG_INTERNALS
- #define CYTHON_USE_PYLONG_INTERNALS 0
- #elif !defined(CYTHON_USE_PYLONG_INTERNALS)
- #define CYTHON_USE_PYLONG_INTERNALS 1
- #endif
- #ifndef CYTHON_USE_PYLIST_INTERNALS
- #define CYTHON_USE_PYLIST_INTERNALS 1
- #endif
- #ifndef CYTHON_USE_UNICODE_INTERNALS
- #define CYTHON_USE_UNICODE_INTERNALS 1
- #endif
- #if PY_VERSION_HEX < 0x030300F0
- #undef CYTHON_USE_UNICODE_WRITER
- #define CYTHON_USE_UNICODE_WRITER 0
- #elif !defined(CYTHON_USE_UNICODE_WRITER)
- #define CYTHON_USE_UNICODE_WRITER 1
- #endif
- #ifndef CYTHON_AVOID_BORROWED_REFS
- #define CYTHON_AVOID_BORROWED_REFS 0
- #endif
- #ifndef CYTHON_ASSUME_SAFE_MACROS
- #define CYTHON_ASSUME_SAFE_MACROS 1
- #endif
- #ifndef CYTHON_UNPACK_METHODS
- #define CYTHON_UNPACK_METHODS 1
- #endif
- #ifndef CYTHON_FAST_THREAD_STATE
- #define CYTHON_FAST_THREAD_STATE 1
- #endif
- #ifndef CYTHON_FAST_PYCALL
- #define CYTHON_FAST_PYCALL 1
- #endif
- #ifndef CYTHON_PEP489_MULTI_PHASE_INIT
- #define CYTHON_PEP489_MULTI_PHASE_INIT (PY_VERSION_HEX >= 0x03050000)
- #endif
- #ifndef CYTHON_USE_TP_FINALIZE
- #define CYTHON_USE_TP_FINALIZE (PY_VERSION_HEX >= 0x030400a1)
- #endif
- #ifndef CYTHON_USE_DICT_VERSIONS
- #define CYTHON_USE_DICT_VERSIONS (PY_VERSION_HEX >= 0x030600B1)
- #endif
- #ifndef CYTHON_USE_EXC_INFO_STACK
- #define CYTHON_USE_EXC_INFO_STACK (PY_VERSION_HEX >= 0x030700A3)
- #endif
-#endif
-#if !defined(CYTHON_FAST_PYCCALL)
-#define CYTHON_FAST_PYCCALL (CYTHON_FAST_PYCALL && PY_VERSION_HEX >= 0x030600B1)
-#endif
-#if CYTHON_USE_PYLONG_INTERNALS
- #include "longintrepr.h"
- #undef SHIFT
- #undef BASE
- #undef MASK
- #ifdef SIZEOF_VOID_P
- enum { __pyx_check_sizeof_voidp = 1 / (int)(SIZEOF_VOID_P == sizeof(void*)) };
- #endif
-#endif
-#ifndef __has_attribute
- #define __has_attribute(x) 0
-#endif
-#ifndef __has_cpp_attribute
- #define __has_cpp_attribute(x) 0
-#endif
-#ifndef CYTHON_RESTRICT
- #if defined(__GNUC__)
- #define CYTHON_RESTRICT __restrict__
- #elif defined(_MSC_VER) && _MSC_VER >= 1400
- #define CYTHON_RESTRICT __restrict
- #elif defined (__STDC_VERSION__) && __STDC_VERSION__ >= 199901L
- #define CYTHON_RESTRICT restrict
- #else
- #define CYTHON_RESTRICT
- #endif
-#endif
-#ifndef CYTHON_UNUSED
-# if defined(__GNUC__)
-# if !(defined(__cplusplus)) || (__GNUC__ > 3 || (__GNUC__ == 3 && __GNUC_MINOR__ >= 4))
-# define CYTHON_UNUSED __attribute__ ((__unused__))
-# else
-# define CYTHON_UNUSED
-# endif
-# elif defined(__ICC) || (defined(__INTEL_COMPILER) && !defined(_MSC_VER))
-# define CYTHON_UNUSED __attribute__ ((__unused__))
-# else
-# define CYTHON_UNUSED
-# endif
-#endif
-#ifndef CYTHON_MAYBE_UNUSED_VAR
-# if defined(__cplusplus)
- template void CYTHON_MAYBE_UNUSED_VAR( const T& ) { }
-# else
-# define CYTHON_MAYBE_UNUSED_VAR(x) (void)(x)
-# endif
-#endif
-#ifndef CYTHON_NCP_UNUSED
-# if CYTHON_COMPILING_IN_CPYTHON
-# define CYTHON_NCP_UNUSED
-# else
-# define CYTHON_NCP_UNUSED CYTHON_UNUSED
-# endif
-#endif
-#define __Pyx_void_to_None(void_result) ((void)(void_result), Py_INCREF(Py_None), Py_None)
-#ifdef _MSC_VER
- #ifndef _MSC_STDINT_H_
- #if _MSC_VER < 1300
- typedef unsigned char uint8_t;
- typedef unsigned int uint32_t;
- #else
- typedef unsigned __int8 uint8_t;
- typedef unsigned __int32 uint32_t;
- #endif
- #endif
-#else
- #include
-#endif
-#ifndef CYTHON_FALLTHROUGH
- #if defined(__cplusplus) && __cplusplus >= 201103L
- #if __has_cpp_attribute(fallthrough)
- #define CYTHON_FALLTHROUGH [[fallthrough]]
- #elif __has_cpp_attribute(clang::fallthrough)
- #define CYTHON_FALLTHROUGH [[clang::fallthrough]]
- #elif __has_cpp_attribute(gnu::fallthrough)
- #define CYTHON_FALLTHROUGH [[gnu::fallthrough]]
- #endif
- #endif
- #ifndef CYTHON_FALLTHROUGH
- #if __has_attribute(fallthrough)
- #define CYTHON_FALLTHROUGH __attribute__((fallthrough))
- #else
- #define CYTHON_FALLTHROUGH
- #endif
- #endif
- #if defined(__clang__ ) && defined(__apple_build_version__)
- #if __apple_build_version__ < 7000000
- #undef CYTHON_FALLTHROUGH
- #define CYTHON_FALLTHROUGH
- #endif
- #endif
-#endif
-
-#ifndef CYTHON_INLINE
- #if defined(__clang__)
- #define CYTHON_INLINE __inline__ __attribute__ ((__unused__))
- #elif defined(__GNUC__)
- #define CYTHON_INLINE __inline__
- #elif defined(_MSC_VER)
- #define CYTHON_INLINE __inline
- #elif defined (__STDC_VERSION__) && __STDC_VERSION__ >= 199901L
- #define CYTHON_INLINE inline
- #else
- #define CYTHON_INLINE
- #endif
-#endif
-
-#if CYTHON_COMPILING_IN_PYPY && PY_VERSION_HEX < 0x02070600 && !defined(Py_OptimizeFlag)
- #define Py_OptimizeFlag 0
-#endif
-#define __PYX_BUILD_PY_SSIZE_T "n"
-#define CYTHON_FORMAT_SSIZE_T "z"
-#if PY_MAJOR_VERSION < 3
- #define __Pyx_BUILTIN_MODULE_NAME "__builtin__"
- #define __Pyx_PyCode_New(a, k, l, s, f, code, c, n, v, fv, cell, fn, name, fline, lnos)\
- PyCode_New(a+k, l, s, f, code, c, n, v, fv, cell, fn, name, fline, lnos)
- #define __Pyx_DefaultClassType PyClass_Type
-#else
- #define __Pyx_BUILTIN_MODULE_NAME "builtins"
-#if PY_VERSION_HEX >= 0x030800A4 && PY_VERSION_HEX < 0x030800B2
- #define __Pyx_PyCode_New(a, k, l, s, f, code, c, n, v, fv, cell, fn, name, fline, lnos)\
- PyCode_New(a, 0, k, l, s, f, code, c, n, v, fv, cell, fn, name, fline, lnos)
-#else
- #define __Pyx_PyCode_New(a, k, l, s, f, code, c, n, v, fv, cell, fn, name, fline, lnos)\
- PyCode_New(a, k, l, s, f, code, c, n, v, fv, cell, fn, name, fline, lnos)
-#endif
- #define __Pyx_DefaultClassType PyType_Type
-#endif
-#ifndef Py_TPFLAGS_CHECKTYPES
- #define Py_TPFLAGS_CHECKTYPES 0
-#endif
-#ifndef Py_TPFLAGS_HAVE_INDEX
- #define Py_TPFLAGS_HAVE_INDEX 0
-#endif
-#ifndef Py_TPFLAGS_HAVE_NEWBUFFER
- #define Py_TPFLAGS_HAVE_NEWBUFFER 0
-#endif
-#ifndef Py_TPFLAGS_HAVE_FINALIZE
- #define Py_TPFLAGS_HAVE_FINALIZE 0
-#endif
-#ifndef METH_STACKLESS
- #define METH_STACKLESS 0
-#endif
-#if PY_VERSION_HEX <= 0x030700A3 || !defined(METH_FASTCALL)
- #ifndef METH_FASTCALL
- #define METH_FASTCALL 0x80
- #endif
- typedef PyObject *(*__Pyx_PyCFunctionFast) (PyObject *self, PyObject *const *args, Py_ssize_t nargs);
- typedef PyObject *(*__Pyx_PyCFunctionFastWithKeywords) (PyObject *self, PyObject *const *args,
- Py_ssize_t nargs, PyObject *kwnames);
-#else
- #define __Pyx_PyCFunctionFast _PyCFunctionFast
- #define __Pyx_PyCFunctionFastWithKeywords _PyCFunctionFastWithKeywords
-#endif
-#if CYTHON_FAST_PYCCALL
-#define __Pyx_PyFastCFunction_Check(func)\
- ((PyCFunction_Check(func) && (METH_FASTCALL == (PyCFunction_GET_FLAGS(func) & ~(METH_CLASS | METH_STATIC | METH_COEXIST | METH_KEYWORDS | METH_STACKLESS)))))
-#else
-#define __Pyx_PyFastCFunction_Check(func) 0
-#endif
-#if CYTHON_COMPILING_IN_PYPY && !defined(PyObject_Malloc)
- #define PyObject_Malloc(s) PyMem_Malloc(s)
- #define PyObject_Free(p) PyMem_Free(p)
- #define PyObject_Realloc(p) PyMem_Realloc(p)
-#endif
-#if CYTHON_COMPILING_IN_CPYTHON && PY_VERSION_HEX < 0x030400A1
- #define PyMem_RawMalloc(n) PyMem_Malloc(n)
- #define PyMem_RawRealloc(p, n) PyMem_Realloc(p, n)
- #define PyMem_RawFree(p) PyMem_Free(p)
-#endif
-#if CYTHON_COMPILING_IN_PYSTON
- #define __Pyx_PyCode_HasFreeVars(co) PyCode_HasFreeVars(co)
- #define __Pyx_PyFrame_SetLineNumber(frame, lineno) PyFrame_SetLineNumber(frame, lineno)
-#else
- #define __Pyx_PyCode_HasFreeVars(co) (PyCode_GetNumFree(co) > 0)
- #define __Pyx_PyFrame_SetLineNumber(frame, lineno) (frame)->f_lineno = (lineno)
-#endif
-#if !CYTHON_FAST_THREAD_STATE || PY_VERSION_HEX < 0x02070000
- #define __Pyx_PyThreadState_Current PyThreadState_GET()
-#elif PY_VERSION_HEX >= 0x03060000
- #define __Pyx_PyThreadState_Current _PyThreadState_UncheckedGet()
-#elif PY_VERSION_HEX >= 0x03000000
- #define __Pyx_PyThreadState_Current PyThreadState_GET()
-#else
- #define __Pyx_PyThreadState_Current _PyThreadState_Current
-#endif
-#if PY_VERSION_HEX < 0x030700A2 && !defined(PyThread_tss_create) && !defined(Py_tss_NEEDS_INIT)
-#include "pythread.h"
-#define Py_tss_NEEDS_INIT 0
-typedef int Py_tss_t;
-static CYTHON_INLINE int PyThread_tss_create(Py_tss_t *key) {
- *key = PyThread_create_key();
- return 0;
-}
-static CYTHON_INLINE Py_tss_t * PyThread_tss_alloc(void) {
- Py_tss_t *key = (Py_tss_t *)PyObject_Malloc(sizeof(Py_tss_t));
- *key = Py_tss_NEEDS_INIT;
- return key;
-}
-static CYTHON_INLINE void PyThread_tss_free(Py_tss_t *key) {
- PyObject_Free(key);
-}
-static CYTHON_INLINE int PyThread_tss_is_created(Py_tss_t *key) {
- return *key != Py_tss_NEEDS_INIT;
-}
-static CYTHON_INLINE void PyThread_tss_delete(Py_tss_t *key) {
- PyThread_delete_key(*key);
- *key = Py_tss_NEEDS_INIT;
-}
-static CYTHON_INLINE int PyThread_tss_set(Py_tss_t *key, void *value) {
- return PyThread_set_key_value(*key, value);
-}
-static CYTHON_INLINE void * PyThread_tss_get(Py_tss_t *key) {
- return PyThread_get_key_value(*key);
-}
-#endif
-#if CYTHON_COMPILING_IN_CPYTHON || defined(_PyDict_NewPresized)
-#define __Pyx_PyDict_NewPresized(n) ((n <= 8) ? PyDict_New() : _PyDict_NewPresized(n))
-#else
-#define __Pyx_PyDict_NewPresized(n) PyDict_New()
-#endif
-#if PY_MAJOR_VERSION >= 3 || CYTHON_FUTURE_DIVISION
- #define __Pyx_PyNumber_Divide(x,y) PyNumber_TrueDivide(x,y)
- #define __Pyx_PyNumber_InPlaceDivide(x,y) PyNumber_InPlaceTrueDivide(x,y)
-#else
- #define __Pyx_PyNumber_Divide(x,y) PyNumber_Divide(x,y)
- #define __Pyx_PyNumber_InPlaceDivide(x,y) PyNumber_InPlaceDivide(x,y)
-#endif
-#if CYTHON_COMPILING_IN_CPYTHON && PY_VERSION_HEX >= 0x030500A1 && CYTHON_USE_UNICODE_INTERNALS
-#define __Pyx_PyDict_GetItemStr(dict, name) _PyDict_GetItem_KnownHash(dict, name, ((PyASCIIObject *) name)->hash)
-#else
-#define __Pyx_PyDict_GetItemStr(dict, name) PyDict_GetItem(dict, name)
-#endif
-#if PY_VERSION_HEX > 0x03030000 && defined(PyUnicode_KIND)
- #define CYTHON_PEP393_ENABLED 1
- #define __Pyx_PyUnicode_READY(op) (likely(PyUnicode_IS_READY(op)) ?\
- 0 : _PyUnicode_Ready((PyObject *)(op)))
- #define __Pyx_PyUnicode_GET_LENGTH(u) PyUnicode_GET_LENGTH(u)
- #define __Pyx_PyUnicode_READ_CHAR(u, i) PyUnicode_READ_CHAR(u, i)
- #define __Pyx_PyUnicode_MAX_CHAR_VALUE(u) PyUnicode_MAX_CHAR_VALUE(u)
- #define __Pyx_PyUnicode_KIND(u) PyUnicode_KIND(u)
- #define __Pyx_PyUnicode_DATA(u) PyUnicode_DATA(u)
- #define __Pyx_PyUnicode_READ(k, d, i) PyUnicode_READ(k, d, i)
- #define __Pyx_PyUnicode_WRITE(k, d, i, ch) PyUnicode_WRITE(k, d, i, ch)
- #if defined(PyUnicode_IS_READY) && defined(PyUnicode_GET_SIZE)
- #define __Pyx_PyUnicode_IS_TRUE(u) (0 != (likely(PyUnicode_IS_READY(u)) ? PyUnicode_GET_LENGTH(u) : PyUnicode_GET_SIZE(u)))
- #else
- #define __Pyx_PyUnicode_IS_TRUE(u) (0 != PyUnicode_GET_LENGTH(u))
- #endif
-#else
- #define CYTHON_PEP393_ENABLED 0
- #define PyUnicode_1BYTE_KIND 1
- #define PyUnicode_2BYTE_KIND 2
- #define PyUnicode_4BYTE_KIND 4
- #define __Pyx_PyUnicode_READY(op) (0)
- #define __Pyx_PyUnicode_GET_LENGTH(u) PyUnicode_GET_SIZE(u)
- #define __Pyx_PyUnicode_READ_CHAR(u, i) ((Py_UCS4)(PyUnicode_AS_UNICODE(u)[i]))
- #define __Pyx_PyUnicode_MAX_CHAR_VALUE(u) ((sizeof(Py_UNICODE) == 2) ? 65535 : 1114111)
- #define __Pyx_PyUnicode_KIND(u) (sizeof(Py_UNICODE))
- #define __Pyx_PyUnicode_DATA(u) ((void*)PyUnicode_AS_UNICODE(u))
- #define __Pyx_PyUnicode_READ(k, d, i) ((void)(k), (Py_UCS4)(((Py_UNICODE*)d)[i]))
- #define __Pyx_PyUnicode_WRITE(k, d, i, ch) (((void)(k)), ((Py_UNICODE*)d)[i] = ch)
- #define __Pyx_PyUnicode_IS_TRUE(u) (0 != PyUnicode_GET_SIZE(u))
-#endif
-#if CYTHON_COMPILING_IN_PYPY
- #define __Pyx_PyUnicode_Concat(a, b) PyNumber_Add(a, b)
- #define __Pyx_PyUnicode_ConcatSafe(a, b) PyNumber_Add(a, b)
-#else
- #define __Pyx_PyUnicode_Concat(a, b) PyUnicode_Concat(a, b)
- #define __Pyx_PyUnicode_ConcatSafe(a, b) ((unlikely((a) == Py_None) || unlikely((b) == Py_None)) ?\
- PyNumber_Add(a, b) : __Pyx_PyUnicode_Concat(a, b))
-#endif
-#if CYTHON_COMPILING_IN_PYPY && !defined(PyUnicode_Contains)
- #define PyUnicode_Contains(u, s) PySequence_Contains(u, s)
-#endif
-#if CYTHON_COMPILING_IN_PYPY && !defined(PyByteArray_Check)
- #define PyByteArray_Check(obj) PyObject_TypeCheck(obj, &PyByteArray_Type)
-#endif
-#if CYTHON_COMPILING_IN_PYPY && !defined(PyObject_Format)
- #define PyObject_Format(obj, fmt) PyObject_CallMethod(obj, "__format__", "O", fmt)
-#endif
-#define __Pyx_PyString_FormatSafe(a, b) ((unlikely((a) == Py_None || (PyString_Check(b) && !PyString_CheckExact(b)))) ? PyNumber_Remainder(a, b) : __Pyx_PyString_Format(a, b))
-#define __Pyx_PyUnicode_FormatSafe(a, b) ((unlikely((a) == Py_None || (PyUnicode_Check(b) && !PyUnicode_CheckExact(b)))) ? PyNumber_Remainder(a, b) : PyUnicode_Format(a, b))
-#if PY_MAJOR_VERSION >= 3
- #define __Pyx_PyString_Format(a, b) PyUnicode_Format(a, b)
-#else
- #define __Pyx_PyString_Format(a, b) PyString_Format(a, b)
-#endif
-#if PY_MAJOR_VERSION < 3 && !defined(PyObject_ASCII)
- #define PyObject_ASCII(o) PyObject_Repr(o)
-#endif
-#if PY_MAJOR_VERSION >= 3
- #define PyBaseString_Type PyUnicode_Type
- #define PyStringObject PyUnicodeObject
- #define PyString_Type PyUnicode_Type
- #define PyString_Check PyUnicode_Check
- #define PyString_CheckExact PyUnicode_CheckExact
-#ifndef PyObject_Unicode
- #define PyObject_Unicode PyObject_Str
-#endif
-#endif
-#if PY_MAJOR_VERSION >= 3
- #define __Pyx_PyBaseString_Check(obj) PyUnicode_Check(obj)
- #define __Pyx_PyBaseString_CheckExact(obj) PyUnicode_CheckExact(obj)
-#else
- #define __Pyx_PyBaseString_Check(obj) (PyString_Check(obj) || PyUnicode_Check(obj))
- #define __Pyx_PyBaseString_CheckExact(obj) (PyString_CheckExact(obj) || PyUnicode_CheckExact(obj))
-#endif
-#ifndef PySet_CheckExact
- #define PySet_CheckExact(obj) (Py_TYPE(obj) == &PySet_Type)
-#endif
-#if PY_VERSION_HEX >= 0x030900A4
- #define __Pyx_SET_REFCNT(obj, refcnt) Py_SET_REFCNT(obj, refcnt)
- #define __Pyx_SET_SIZE(obj, size) Py_SET_SIZE(obj, size)
-#else
- #define __Pyx_SET_REFCNT(obj, refcnt) Py_REFCNT(obj) = (refcnt)
- #define __Pyx_SET_SIZE(obj, size) Py_SIZE(obj) = (size)
-#endif
-#if CYTHON_ASSUME_SAFE_MACROS
- #define __Pyx_PySequence_SIZE(seq) Py_SIZE(seq)
-#else
- #define __Pyx_PySequence_SIZE(seq) PySequence_Size(seq)
-#endif
-#if PY_MAJOR_VERSION >= 3
- #define PyIntObject PyLongObject
- #define PyInt_Type PyLong_Type
- #define PyInt_Check(op) PyLong_Check(op)
- #define PyInt_CheckExact(op) PyLong_CheckExact(op)
- #define PyInt_FromString PyLong_FromString
- #define PyInt_FromUnicode PyLong_FromUnicode
- #define PyInt_FromLong PyLong_FromLong
- #define PyInt_FromSize_t PyLong_FromSize_t
- #define PyInt_FromSsize_t PyLong_FromSsize_t
- #define PyInt_AsLong PyLong_AsLong
- #define PyInt_AS_LONG PyLong_AS_LONG
- #define PyInt_AsSsize_t PyLong_AsSsize_t
- #define PyInt_AsUnsignedLongMask PyLong_AsUnsignedLongMask
- #define PyInt_AsUnsignedLongLongMask PyLong_AsUnsignedLongLongMask
- #define PyNumber_Int PyNumber_Long
-#endif
-#if PY_MAJOR_VERSION >= 3
- #define PyBoolObject PyLongObject
-#endif
-#if PY_MAJOR_VERSION >= 3 && CYTHON_COMPILING_IN_PYPY
- #ifndef PyUnicode_InternFromString
- #define PyUnicode_InternFromString(s) PyUnicode_FromString(s)
- #endif
-#endif
-#if PY_VERSION_HEX < 0x030200A4
- typedef long Py_hash_t;
- #define __Pyx_PyInt_FromHash_t PyInt_FromLong
- #define __Pyx_PyInt_AsHash_t PyInt_AsLong
-#else
- #define __Pyx_PyInt_FromHash_t PyInt_FromSsize_t
- #define __Pyx_PyInt_AsHash_t PyInt_AsSsize_t
-#endif
-#if PY_MAJOR_VERSION >= 3
- #define __Pyx_PyMethod_New(func, self, klass) ((self) ? ((void)(klass), PyMethod_New(func, self)) : __Pyx_NewRef(func))
-#else
- #define __Pyx_PyMethod_New(func, self, klass) PyMethod_New(func, self, klass)
-#endif
-#if CYTHON_USE_ASYNC_SLOTS
- #if PY_VERSION_HEX >= 0x030500B1
- #define __Pyx_PyAsyncMethodsStruct PyAsyncMethods
- #define __Pyx_PyType_AsAsync(obj) (Py_TYPE(obj)->tp_as_async)
- #else
- #define __Pyx_PyType_AsAsync(obj) ((__Pyx_PyAsyncMethodsStruct*) (Py_TYPE(obj)->tp_reserved))
- #endif
-#else
- #define __Pyx_PyType_AsAsync(obj) NULL
-#endif
-#ifndef __Pyx_PyAsyncMethodsStruct
- typedef struct {
- unaryfunc am_await;
- unaryfunc am_aiter;
- unaryfunc am_anext;
- } __Pyx_PyAsyncMethodsStruct;
-#endif
-
-#if defined(WIN32) || defined(MS_WINDOWS)
- #define _USE_MATH_DEFINES
-#endif
-#include
-#ifdef NAN
-#define __PYX_NAN() ((float) NAN)
-#else
-static CYTHON_INLINE float __PYX_NAN() {
- float value;
- memset(&value, 0xFF, sizeof(value));
- return value;
-}
-#endif
-#if defined(__CYGWIN__) && defined(_LDBL_EQ_DBL)
-#define __Pyx_truncl trunc
-#else
-#define __Pyx_truncl truncl
-#endif
-
-#define __PYX_MARK_ERR_POS(f_index, lineno) \
- { __pyx_filename = __pyx_f[f_index]; (void)__pyx_filename; __pyx_lineno = lineno; (void)__pyx_lineno; __pyx_clineno = __LINE__; (void)__pyx_clineno; }
-#define __PYX_ERR(f_index, lineno, Ln_error) \
- { __PYX_MARK_ERR_POS(f_index, lineno) goto Ln_error; }
-
-#ifndef __PYX_EXTERN_C
- #ifdef __cplusplus
- #define __PYX_EXTERN_C extern "C"
- #else
- #define __PYX_EXTERN_C extern
- #endif
-#endif
-
-#define __PYX_HAVE__monotonic_align__core
-#define __PYX_HAVE_API__monotonic_align__core
-/* Early includes */
-#include "pythread.h"
-#include
-#include
-#include
-#include "pystate.h"
-#ifdef _OPENMP
-#include
-#endif /* _OPENMP */
-
-#if defined(PYREX_WITHOUT_ASSERTIONS) && !defined(CYTHON_WITHOUT_ASSERTIONS)
-#define CYTHON_WITHOUT_ASSERTIONS
-#endif
-
-typedef struct {PyObject **p; const char *s; const Py_ssize_t n; const char* encoding;
- const char is_unicode; const char is_str; const char intern; } __Pyx_StringTabEntry;
-
-#define __PYX_DEFAULT_STRING_ENCODING_IS_ASCII 0
-#define __PYX_DEFAULT_STRING_ENCODING_IS_UTF8 0
-#define __PYX_DEFAULT_STRING_ENCODING_IS_DEFAULT (PY_MAJOR_VERSION >= 3 && __PYX_DEFAULT_STRING_ENCODING_IS_UTF8)
-#define __PYX_DEFAULT_STRING_ENCODING ""
-#define __Pyx_PyObject_FromString __Pyx_PyBytes_FromString
-#define __Pyx_PyObject_FromStringAndSize __Pyx_PyBytes_FromStringAndSize
-#define __Pyx_uchar_cast(c) ((unsigned char)c)
-#define __Pyx_long_cast(x) ((long)x)
-#define __Pyx_fits_Py_ssize_t(v, type, is_signed) (\
- (sizeof(type) < sizeof(Py_ssize_t)) ||\
- (sizeof(type) > sizeof(Py_ssize_t) &&\
- likely(v < (type)PY_SSIZE_T_MAX ||\
- v == (type)PY_SSIZE_T_MAX) &&\
- (!is_signed || likely(v > (type)PY_SSIZE_T_MIN ||\
- v == (type)PY_SSIZE_T_MIN))) ||\
- (sizeof(type) == sizeof(Py_ssize_t) &&\
- (is_signed || likely(v < (type)PY_SSIZE_T_MAX ||\
- v == (type)PY_SSIZE_T_MAX))) )
-static CYTHON_INLINE int __Pyx_is_valid_index(Py_ssize_t i, Py_ssize_t limit) {
- return (size_t) i < (size_t) limit;
-}
-#if defined (__cplusplus) && __cplusplus >= 201103L
- #include
- #define __Pyx_sst_abs(value) std::abs(value)
-#elif SIZEOF_INT >= SIZEOF_SIZE_T
- #define __Pyx_sst_abs(value) abs(value)
-#elif SIZEOF_LONG >= SIZEOF_SIZE_T
- #define __Pyx_sst_abs(value) labs(value)
-#elif defined (_MSC_VER)
- #define __Pyx_sst_abs(value) ((Py_ssize_t)_abs64(value))
-#elif defined (__STDC_VERSION__) && __STDC_VERSION__ >= 199901L
- #define __Pyx_sst_abs(value) llabs(value)
-#elif defined (__GNUC__)
- #define __Pyx_sst_abs(value) __builtin_llabs(value)
-#else
- #define __Pyx_sst_abs(value) ((value<0) ? -value : value)
-#endif
-static CYTHON_INLINE const char* __Pyx_PyObject_AsString(PyObject*);
-static CYTHON_INLINE const char* __Pyx_PyObject_AsStringAndSize(PyObject*, Py_ssize_t* length);
-#define __Pyx_PyByteArray_FromString(s) PyByteArray_FromStringAndSize((const char*)s, strlen((const char*)s))
-#define __Pyx_PyByteArray_FromStringAndSize(s, l) PyByteArray_FromStringAndSize((const char*)s, l)
-#define __Pyx_PyBytes_FromString PyBytes_FromString
-#define __Pyx_PyBytes_FromStringAndSize PyBytes_FromStringAndSize
-static CYTHON_INLINE PyObject* __Pyx_PyUnicode_FromString(const char*);
-#if PY_MAJOR_VERSION < 3
- #define __Pyx_PyStr_FromString __Pyx_PyBytes_FromString
- #define __Pyx_PyStr_FromStringAndSize __Pyx_PyBytes_FromStringAndSize
-#else
- #define __Pyx_PyStr_FromString __Pyx_PyUnicode_FromString
- #define __Pyx_PyStr_FromStringAndSize __Pyx_PyUnicode_FromStringAndSize
-#endif
-#define __Pyx_PyBytes_AsWritableString(s) ((char*) PyBytes_AS_STRING(s))
-#define __Pyx_PyBytes_AsWritableSString(s) ((signed char*) PyBytes_AS_STRING(s))
-#define __Pyx_PyBytes_AsWritableUString(s) ((unsigned char*) PyBytes_AS_STRING(s))
-#define __Pyx_PyBytes_AsString(s) ((const char*) PyBytes_AS_STRING(s))
-#define __Pyx_PyBytes_AsSString(s) ((const signed char*) PyBytes_AS_STRING(s))
-#define __Pyx_PyBytes_AsUString(s) ((const unsigned char*) PyBytes_AS_STRING(s))
-#define __Pyx_PyObject_AsWritableString(s) ((char*) __Pyx_PyObject_AsString(s))
-#define __Pyx_PyObject_AsWritableSString(s) ((signed char*) __Pyx_PyObject_AsString(s))
-#define __Pyx_PyObject_AsWritableUString(s) ((unsigned char*) __Pyx_PyObject_AsString(s))
-#define __Pyx_PyObject_AsSString(s) ((const signed char*) __Pyx_PyObject_AsString(s))
-#define __Pyx_PyObject_AsUString(s) ((const unsigned char*) __Pyx_PyObject_AsString(s))
-#define __Pyx_PyObject_FromCString(s) __Pyx_PyObject_FromString((const char*)s)
-#define __Pyx_PyBytes_FromCString(s) __Pyx_PyBytes_FromString((const char*)s)
-#define __Pyx_PyByteArray_FromCString(s) __Pyx_PyByteArray_FromString((const char*)s)
-#define __Pyx_PyStr_FromCString(s) __Pyx_PyStr_FromString((const char*)s)
-#define __Pyx_PyUnicode_FromCString(s) __Pyx_PyUnicode_FromString((const char*)s)
-static CYTHON_INLINE size_t __Pyx_Py_UNICODE_strlen(const Py_UNICODE *u) {
- const Py_UNICODE *u_end = u;
- while (*u_end++) ;
- return (size_t)(u_end - u - 1);
-}
-#define __Pyx_PyUnicode_FromUnicode(u) PyUnicode_FromUnicode(u, __Pyx_Py_UNICODE_strlen(u))
-#define __Pyx_PyUnicode_FromUnicodeAndLength PyUnicode_FromUnicode
-#define __Pyx_PyUnicode_AsUnicode PyUnicode_AsUnicode
-#define __Pyx_NewRef(obj) (Py_INCREF(obj), obj)
-#define __Pyx_Owned_Py_None(b) __Pyx_NewRef(Py_None)
-static CYTHON_INLINE PyObject * __Pyx_PyBool_FromLong(long b);
-static CYTHON_INLINE int __Pyx_PyObject_IsTrue(PyObject*);
-static CYTHON_INLINE int __Pyx_PyObject_IsTrueAndDecref(PyObject*);
-static CYTHON_INLINE PyObject* __Pyx_PyNumber_IntOrLong(PyObject* x);
-#define __Pyx_PySequence_Tuple(obj)\
- (likely(PyTuple_CheckExact(obj)) ? __Pyx_NewRef(obj) : PySequence_Tuple(obj))
-static CYTHON_INLINE Py_ssize_t __Pyx_PyIndex_AsSsize_t(PyObject*);
-static CYTHON_INLINE PyObject * __Pyx_PyInt_FromSize_t(size_t);
-#if CYTHON_ASSUME_SAFE_MACROS
-#define __pyx_PyFloat_AsDouble(x) (PyFloat_CheckExact(x) ? PyFloat_AS_DOUBLE(x) : PyFloat_AsDouble(x))
-#else
-#define __pyx_PyFloat_AsDouble(x) PyFloat_AsDouble(x)
-#endif
-#define __pyx_PyFloat_AsFloat(x) ((float) __pyx_PyFloat_AsDouble(x))
-#if PY_MAJOR_VERSION >= 3
-#define __Pyx_PyNumber_Int(x) (PyLong_CheckExact(x) ? __Pyx_NewRef(x) : PyNumber_Long(x))
-#else
-#define __Pyx_PyNumber_Int(x) (PyInt_CheckExact(x) ? __Pyx_NewRef(x) : PyNumber_Int(x))
-#endif
-#define __Pyx_PyNumber_Float(x) (PyFloat_CheckExact(x) ? __Pyx_NewRef(x) : PyNumber_Float(x))
-#if PY_MAJOR_VERSION < 3 && __PYX_DEFAULT_STRING_ENCODING_IS_ASCII
-static int __Pyx_sys_getdefaultencoding_not_ascii;
-static int __Pyx_init_sys_getdefaultencoding_params(void) {
- PyObject* sys;
- PyObject* default_encoding = NULL;
- PyObject* ascii_chars_u = NULL;
- PyObject* ascii_chars_b = NULL;
- const char* default_encoding_c;
- sys = PyImport_ImportModule("sys");
- if (!sys) goto bad;
- default_encoding = PyObject_CallMethod(sys, (char*) "getdefaultencoding", NULL);
- Py_DECREF(sys);
- if (!default_encoding) goto bad;
- default_encoding_c = PyBytes_AsString(default_encoding);
- if (!default_encoding_c) goto bad;
- if (strcmp(default_encoding_c, "ascii") == 0) {
- __Pyx_sys_getdefaultencoding_not_ascii = 0;
- } else {
- char ascii_chars[128];
- int c;
- for (c = 0; c < 128; c++) {
- ascii_chars[c] = c;
- }
- __Pyx_sys_getdefaultencoding_not_ascii = 1;
- ascii_chars_u = PyUnicode_DecodeASCII(ascii_chars, 128, NULL);
- if (!ascii_chars_u) goto bad;
- ascii_chars_b = PyUnicode_AsEncodedString(ascii_chars_u, default_encoding_c, NULL);
- if (!ascii_chars_b || !PyBytes_Check(ascii_chars_b) || memcmp(ascii_chars, PyBytes_AS_STRING(ascii_chars_b), 128) != 0) {
- PyErr_Format(
- PyExc_ValueError,
- "This module compiled with c_string_encoding=ascii, but default encoding '%.200s' is not a superset of ascii.",
- default_encoding_c);
- goto bad;
- }
- Py_DECREF(ascii_chars_u);
- Py_DECREF(ascii_chars_b);
- }
- Py_DECREF(default_encoding);
- return 0;
-bad:
- Py_XDECREF(default_encoding);
- Py_XDECREF(ascii_chars_u);
- Py_XDECREF(ascii_chars_b);
- return -1;
-}
-#endif
-#if __PYX_DEFAULT_STRING_ENCODING_IS_DEFAULT && PY_MAJOR_VERSION >= 3
-#define __Pyx_PyUnicode_FromStringAndSize(c_str, size) PyUnicode_DecodeUTF8(c_str, size, NULL)
-#else
-#define __Pyx_PyUnicode_FromStringAndSize(c_str, size) PyUnicode_Decode(c_str, size, __PYX_DEFAULT_STRING_ENCODING, NULL)
-#if __PYX_DEFAULT_STRING_ENCODING_IS_DEFAULT
-static char* __PYX_DEFAULT_STRING_ENCODING;
-static int __Pyx_init_sys_getdefaultencoding_params(void) {
- PyObject* sys;
- PyObject* default_encoding = NULL;
- char* default_encoding_c;
- sys = PyImport_ImportModule("sys");
- if (!sys) goto bad;
- default_encoding = PyObject_CallMethod(sys, (char*) (const char*) "getdefaultencoding", NULL);
- Py_DECREF(sys);
- if (!default_encoding) goto bad;
- default_encoding_c = PyBytes_AsString(default_encoding);
- if (!default_encoding_c) goto bad;
- __PYX_DEFAULT_STRING_ENCODING = (char*) malloc(strlen(default_encoding_c) + 1);
- if (!__PYX_DEFAULT_STRING_ENCODING) goto bad;
- strcpy(__PYX_DEFAULT_STRING_ENCODING, default_encoding_c);
- Py_DECREF(default_encoding);
- return 0;
-bad:
- Py_XDECREF(default_encoding);
- return -1;
-}
-#endif
-#endif
-
-
-/* Test for GCC > 2.95 */
-#if defined(__GNUC__) && (__GNUC__ > 2 || (__GNUC__ == 2 && (__GNUC_MINOR__ > 95)))
- #define likely(x) __builtin_expect(!!(x), 1)
- #define unlikely(x) __builtin_expect(!!(x), 0)
-#else /* !__GNUC__ or GCC < 2.95 */
- #define likely(x) (x)
- #define unlikely(x) (x)
-#endif /* __GNUC__ */
-static CYTHON_INLINE void __Pyx_pretend_to_initialize(void* ptr) { (void)ptr; }
-
-static PyObject *__pyx_m = NULL;
-static PyObject *__pyx_d;
-static PyObject *__pyx_b;
-static PyObject *__pyx_cython_runtime = NULL;
-static PyObject *__pyx_empty_tuple;
-static PyObject *__pyx_empty_bytes;
-static PyObject *__pyx_empty_unicode;
-static int __pyx_lineno;
-static int __pyx_clineno = 0;
-static const char * __pyx_cfilenm= __FILE__;
-static const char *__pyx_filename;
-
-
-static const char *__pyx_f[] = {
- "core.pyx",
- "stringsource",
-};
-/* NoFastGil.proto */
-#define __Pyx_PyGILState_Ensure PyGILState_Ensure
-#define __Pyx_PyGILState_Release PyGILState_Release
-#define __Pyx_FastGIL_Remember()
-#define __Pyx_FastGIL_Forget()
-#define __Pyx_FastGilFuncInit()
-
-/* MemviewSliceStruct.proto */
-struct __pyx_memoryview_obj;
-typedef struct {
- struct __pyx_memoryview_obj *memview;
- char *data;
- Py_ssize_t shape[8];
- Py_ssize_t strides[8];
- Py_ssize_t suboffsets[8];
-} __Pyx_memviewslice;
-#define __Pyx_MemoryView_Len(m) (m.shape[0])
-
-/* Atomics.proto */
-#include
-#ifndef CYTHON_ATOMICS
- #define CYTHON_ATOMICS 1
-#endif
-#define __pyx_atomic_int_type int
-#if CYTHON_ATOMICS && __GNUC__ >= 4 && (__GNUC_MINOR__ > 1 ||\
- (__GNUC_MINOR__ == 1 && __GNUC_PATCHLEVEL >= 2)) &&\
- !defined(__i386__)
- #define __pyx_atomic_incr_aligned(value, lock) __sync_fetch_and_add(value, 1)
- #define __pyx_atomic_decr_aligned(value, lock) __sync_fetch_and_sub(value, 1)
- #ifdef __PYX_DEBUG_ATOMICS
- #warning "Using GNU atomics"
- #endif
-#elif CYTHON_ATOMICS && defined(_MSC_VER) && 0
- #include
- #undef __pyx_atomic_int_type
- #define __pyx_atomic_int_type LONG
- #define __pyx_atomic_incr_aligned(value, lock) InterlockedIncrement(value)
- #define __pyx_atomic_decr_aligned(value, lock) InterlockedDecrement(value)
- #ifdef __PYX_DEBUG_ATOMICS
- #pragma message ("Using MSVC atomics")
- #endif
-#elif CYTHON_ATOMICS && (defined(__ICC) || defined(__INTEL_COMPILER)) && 0
- #define __pyx_atomic_incr_aligned(value, lock) _InterlockedIncrement(value)
- #define __pyx_atomic_decr_aligned(value, lock) _InterlockedDecrement(value)
- #ifdef __PYX_DEBUG_ATOMICS
- #warning "Using Intel atomics"
- #endif
-#else
- #undef CYTHON_ATOMICS
- #define CYTHON_ATOMICS 0
- #ifdef __PYX_DEBUG_ATOMICS
- #warning "Not using atomics"
- #endif
-#endif
-typedef volatile __pyx_atomic_int_type __pyx_atomic_int;
-#if CYTHON_ATOMICS
- #define __pyx_add_acquisition_count(memview)\
- __pyx_atomic_incr_aligned(__pyx_get_slice_count_pointer(memview), memview->lock)
- #define __pyx_sub_acquisition_count(memview)\
- __pyx_atomic_decr_aligned(__pyx_get_slice_count_pointer(memview), memview->lock)
-#else
- #define __pyx_add_acquisition_count(memview)\
- __pyx_add_acquisition_count_locked(__pyx_get_slice_count_pointer(memview), memview->lock)
- #define __pyx_sub_acquisition_count(memview)\
- __pyx_sub_acquisition_count_locked(__pyx_get_slice_count_pointer(memview), memview->lock)
-#endif
-
-/* ForceInitThreads.proto */
-#ifndef __PYX_FORCE_INIT_THREADS
- #define __PYX_FORCE_INIT_THREADS 0
-#endif
-
-/* BufferFormatStructs.proto */
-#define IS_UNSIGNED(type) (((type) -1) > 0)
-struct __Pyx_StructField_;
-#define __PYX_BUF_FLAGS_PACKED_STRUCT (1 << 0)
-typedef struct {
- const char* name;
- struct __Pyx_StructField_* fields;
- size_t size;
- size_t arraysize[8];
- int ndim;
- char typegroup;
- char is_unsigned;
- int flags;
-} __Pyx_TypeInfo;
-typedef struct __Pyx_StructField_ {
- __Pyx_TypeInfo* type;
- const char* name;
- size_t offset;
-} __Pyx_StructField;
-typedef struct {
- __Pyx_StructField* field;
- size_t parent_offset;
-} __Pyx_BufFmt_StackElem;
-typedef struct {
- __Pyx_StructField root;
- __Pyx_BufFmt_StackElem* head;
- size_t fmt_offset;
- size_t new_count, enc_count;
- size_t struct_alignment;
- int is_complex;
- char enc_type;
- char new_packmode;
- char enc_packmode;
- char is_valid_array;
-} __Pyx_BufFmt_Context;
-
-
-/*--- Type declarations ---*/
-struct __pyx_array_obj;
-struct __pyx_MemviewEnum_obj;
-struct __pyx_memoryview_obj;
-struct __pyx_memoryviewslice_obj;
-struct __pyx_opt_args_15monotonic_align_4core_maximum_path_each;
-
-/* "monotonic_align/core.pyx":7
- * @cython.boundscheck(False)
- * @cython.wraparound(False)
- * cdef void maximum_path_each(int[:,::1] path, float[:,::1] value, int t_y, int t_x, float max_neg_val=-1e9) nogil: # <<<<<<<<<<<<<<
- * cdef int x
- * cdef int y
- */
-struct __pyx_opt_args_15monotonic_align_4core_maximum_path_each {
- int __pyx_n;
- float max_neg_val;
-};
-
-/* "View.MemoryView":105
- *
- * @cname("__pyx_array")
- * cdef class array: # <<<<<<<<<<<<<<
- *
- * cdef:
- */
-struct __pyx_array_obj {
- PyObject_HEAD
- struct __pyx_vtabstruct_array *__pyx_vtab;
- char *data;
- Py_ssize_t len;
- char *format;
- int ndim;
- Py_ssize_t *_shape;
- Py_ssize_t *_strides;
- Py_ssize_t itemsize;
- PyObject *mode;
- PyObject *_format;
- void (*callback_free_data)(void *);
- int free_data;
- int dtype_is_object;
-};
-
-
-/* "View.MemoryView":279
- *
- * @cname('__pyx_MemviewEnum')
- * cdef class Enum(object): # <<<<<<<<<<<<<<
- * cdef object name
- * def __init__(self, name):
- */
-struct __pyx_MemviewEnum_obj {
- PyObject_HEAD
- PyObject *name;
-};
-
-
-/* "View.MemoryView":330
- *
- * @cname('__pyx_memoryview')
- * cdef class memoryview(object): # <<<<<<<<<<<<<<
- *
- * cdef object obj
- */
-struct __pyx_memoryview_obj {
- PyObject_HEAD
- struct __pyx_vtabstruct_memoryview *__pyx_vtab;
- PyObject *obj;
- PyObject *_size;
- PyObject *_array_interface;
- PyThread_type_lock lock;
- __pyx_atomic_int acquisition_count[2];
- __pyx_atomic_int *acquisition_count_aligned_p;
- Py_buffer view;
- int flags;
- int dtype_is_object;
- __Pyx_TypeInfo *typeinfo;
-};
-
-
-/* "View.MemoryView":965
- *
- * @cname('__pyx_memoryviewslice')
- * cdef class _memoryviewslice(memoryview): # <<<<<<<<<<<<<<
- * "Internal class for passing memoryview slices to Python"
- *
- */
-struct __pyx_memoryviewslice_obj {
- struct __pyx_memoryview_obj __pyx_base;
- __Pyx_memviewslice from_slice;
- PyObject *from_object;
- PyObject *(*to_object_func)(char *);
- int (*to_dtype_func)(char *, PyObject *);
-};
-
-
-
-/* "View.MemoryView":105
- *
- * @cname("__pyx_array")
- * cdef class array: # <<<<<<<<<<<<<<
- *
- * cdef:
- */
-
-struct __pyx_vtabstruct_array {
- PyObject *(*get_memview)(struct __pyx_array_obj *);
-};
-static struct __pyx_vtabstruct_array *__pyx_vtabptr_array;
-
-
-/* "View.MemoryView":330
- *
- * @cname('__pyx_memoryview')
- * cdef class memoryview(object): # <<<<<<<<<<<<<<
- *
- * cdef object obj
- */
-
-struct __pyx_vtabstruct_memoryview {
- char *(*get_item_pointer)(struct __pyx_memoryview_obj *, PyObject *);
- PyObject *(*is_slice)(struct __pyx_memoryview_obj *, PyObject *);
- PyObject *(*setitem_slice_assignment)(struct __pyx_memoryview_obj *, PyObject *, PyObject *);
- PyObject *(*setitem_slice_assign_scalar)(struct __pyx_memoryview_obj *, struct __pyx_memoryview_obj *, PyObject *);
- PyObject *(*setitem_indexed)(struct __pyx_memoryview_obj *, PyObject *, PyObject *);
- PyObject *(*convert_item_to_object)(struct __pyx_memoryview_obj *, char *);
- PyObject *(*assign_item_from_object)(struct __pyx_memoryview_obj *, char *, PyObject *);
-};
-static struct __pyx_vtabstruct_memoryview *__pyx_vtabptr_memoryview;
-
-
-/* "View.MemoryView":965
- *
- * @cname('__pyx_memoryviewslice')
- * cdef class _memoryviewslice(memoryview): # <<<<<<<<<<<<<<
- * "Internal class for passing memoryview slices to Python"
- *
- */
-
-struct __pyx_vtabstruct__memoryviewslice {
- struct __pyx_vtabstruct_memoryview __pyx_base;
-};
-static struct __pyx_vtabstruct__memoryviewslice *__pyx_vtabptr__memoryviewslice;
-
-/* --- Runtime support code (head) --- */
-/* Refnanny.proto */
-#ifndef CYTHON_REFNANNY
- #define CYTHON_REFNANNY 0
-#endif
-#if CYTHON_REFNANNY
- typedef struct {
- void (*INCREF)(void*, PyObject*, int);
- void (*DECREF)(void*, PyObject*, int);
- void (*GOTREF)(void*, PyObject*, int);
- void (*GIVEREF)(void*, PyObject*, int);
- void* (*SetupContext)(const char*, int, const char*);
- void (*FinishContext)(void**);
- } __Pyx_RefNannyAPIStruct;
- static __Pyx_RefNannyAPIStruct *__Pyx_RefNanny = NULL;
- static __Pyx_RefNannyAPIStruct *__Pyx_RefNannyImportAPI(const char *modname);
- #define __Pyx_RefNannyDeclarations void *__pyx_refnanny = NULL;
-#ifdef WITH_THREAD
- #define __Pyx_RefNannySetupContext(name, acquire_gil)\
- if (acquire_gil) {\
- PyGILState_STATE __pyx_gilstate_save = PyGILState_Ensure();\
- __pyx_refnanny = __Pyx_RefNanny->SetupContext((name), __LINE__, __FILE__);\
- PyGILState_Release(__pyx_gilstate_save);\
- } else {\
- __pyx_refnanny = __Pyx_RefNanny->SetupContext((name), __LINE__, __FILE__);\
- }
-#else
- #define __Pyx_RefNannySetupContext(name, acquire_gil)\
- __pyx_refnanny = __Pyx_RefNanny->SetupContext((name), __LINE__, __FILE__)
-#endif
- #define __Pyx_RefNannyFinishContext()\
- __Pyx_RefNanny->FinishContext(&__pyx_refnanny)
- #define __Pyx_INCREF(r) __Pyx_RefNanny->INCREF(__pyx_refnanny, (PyObject *)(r), __LINE__)
- #define __Pyx_DECREF(r) __Pyx_RefNanny->DECREF(__pyx_refnanny, (PyObject *)(r), __LINE__)
- #define __Pyx_GOTREF(r) __Pyx_RefNanny->GOTREF(__pyx_refnanny, (PyObject *)(r), __LINE__)
- #define __Pyx_GIVEREF(r) __Pyx_RefNanny->GIVEREF(__pyx_refnanny, (PyObject *)(r), __LINE__)
- #define __Pyx_XINCREF(r) do { if((r) != NULL) {__Pyx_INCREF(r); }} while(0)
- #define __Pyx_XDECREF(r) do { if((r) != NULL) {__Pyx_DECREF(r); }} while(0)
- #define __Pyx_XGOTREF(r) do { if((r) != NULL) {__Pyx_GOTREF(r); }} while(0)
- #define __Pyx_XGIVEREF(r) do { if((r) != NULL) {__Pyx_GIVEREF(r);}} while(0)
-#else
- #define __Pyx_RefNannyDeclarations
- #define __Pyx_RefNannySetupContext(name, acquire_gil)
- #define __Pyx_RefNannyFinishContext()
- #define __Pyx_INCREF(r) Py_INCREF(r)
- #define __Pyx_DECREF(r) Py_DECREF(r)
- #define __Pyx_GOTREF(r)
- #define __Pyx_GIVEREF(r)
- #define __Pyx_XINCREF(r) Py_XINCREF(r)
- #define __Pyx_XDECREF(r) Py_XDECREF(r)
- #define __Pyx_XGOTREF(r)
- #define __Pyx_XGIVEREF(r)
-#endif
-#define __Pyx_XDECREF_SET(r, v) do {\
- PyObject *tmp = (PyObject *) r;\
- r = v; __Pyx_XDECREF(tmp);\
- } while (0)
-#define __Pyx_DECREF_SET(r, v) do {\
- PyObject *tmp = (PyObject *) r;\
- r = v; __Pyx_DECREF(tmp);\
- } while (0)
-#define __Pyx_CLEAR(r) do { PyObject* tmp = ((PyObject*)(r)); r = NULL; __Pyx_DECREF(tmp);} while(0)
-#define __Pyx_XCLEAR(r) do { if((r) != NULL) {PyObject* tmp = ((PyObject*)(r)); r = NULL; __Pyx_DECREF(tmp);}} while(0)
-
-/* PyObjectGetAttrStr.proto */
-#if CYTHON_USE_TYPE_SLOTS
-static CYTHON_INLINE PyObject* __Pyx_PyObject_GetAttrStr(PyObject* obj, PyObject* attr_name);
-#else
-#define __Pyx_PyObject_GetAttrStr(o,n) PyObject_GetAttr(o,n)
-#endif
-
-/* GetBuiltinName.proto */
-static PyObject *__Pyx_GetBuiltinName(PyObject *name);
-
-/* MemviewSliceInit.proto */
-#define __Pyx_BUF_MAX_NDIMS %(BUF_MAX_NDIMS)d
-#define __Pyx_MEMVIEW_DIRECT 1
-#define __Pyx_MEMVIEW_PTR 2
-#define __Pyx_MEMVIEW_FULL 4
-#define __Pyx_MEMVIEW_CONTIG 8
-#define __Pyx_MEMVIEW_STRIDED 16
-#define __Pyx_MEMVIEW_FOLLOW 32
-#define __Pyx_IS_C_CONTIG 1
-#define __Pyx_IS_F_CONTIG 2
-static int __Pyx_init_memviewslice(
- struct __pyx_memoryview_obj *memview,
- int ndim,
- __Pyx_memviewslice *memviewslice,
- int memview_is_new_reference);
-static CYTHON_INLINE int __pyx_add_acquisition_count_locked(
- __pyx_atomic_int *acquisition_count, PyThread_type_lock lock);
-static CYTHON_INLINE int __pyx_sub_acquisition_count_locked(
- __pyx_atomic_int *acquisition_count, PyThread_type_lock lock);
-#define __pyx_get_slice_count_pointer(memview) (memview->acquisition_count_aligned_p)
-#define __pyx_get_slice_count(memview) (*__pyx_get_slice_count_pointer(memview))
-#define __PYX_INC_MEMVIEW(slice, have_gil) __Pyx_INC_MEMVIEW(slice, have_gil, __LINE__)
-#define __PYX_XDEC_MEMVIEW(slice, have_gil) __Pyx_XDEC_MEMVIEW(slice, have_gil, __LINE__)
-static CYTHON_INLINE void __Pyx_INC_MEMVIEW(__Pyx_memviewslice *, int, int);
-static CYTHON_INLINE void __Pyx_XDEC_MEMVIEW(__Pyx_memviewslice *, int, int);
-
-/* RaiseArgTupleInvalid.proto */
-static void __Pyx_RaiseArgtupleInvalid(const char* func_name, int exact,
- Py_ssize_t num_min, Py_ssize_t num_max, Py_ssize_t num_found);
-
-/* RaiseDoubleKeywords.proto */
-static void __Pyx_RaiseDoubleKeywordsError(const char* func_name, PyObject* kw_name);
-
-/* ParseKeywords.proto */
-static int __Pyx_ParseOptionalKeywords(PyObject *kwds, PyObject **argnames[],\
- PyObject *kwds2, PyObject *values[], Py_ssize_t num_pos_args,\
- const char* function_name);
-
-/* None.proto */
-static CYTHON_INLINE void __Pyx_RaiseUnboundLocalError(const char *varname);
-
-/* ArgTypeTest.proto */
-#define __Pyx_ArgTypeTest(obj, type, none_allowed, name, exact)\
- ((likely((Py_TYPE(obj) == type) | (none_allowed && (obj == Py_None)))) ? 1 :\
- __Pyx__ArgTypeTest(obj, type, name, exact))
-static int __Pyx__ArgTypeTest(PyObject *obj, PyTypeObject *type, const char *name, int exact);
-
-/* PyObjectCall.proto */
-#if CYTHON_COMPILING_IN_CPYTHON
-static CYTHON_INLINE PyObject* __Pyx_PyObject_Call(PyObject *func, PyObject *arg, PyObject *kw);
-#else
-#define __Pyx_PyObject_Call(func, arg, kw) PyObject_Call(func, arg, kw)
-#endif
-
-/* PyThreadStateGet.proto */
-#if CYTHON_FAST_THREAD_STATE
-#define __Pyx_PyThreadState_declare PyThreadState *__pyx_tstate;
-#define __Pyx_PyThreadState_assign __pyx_tstate = __Pyx_PyThreadState_Current;
-#define __Pyx_PyErr_Occurred() __pyx_tstate->curexc_type
-#else
-#define __Pyx_PyThreadState_declare
-#define __Pyx_PyThreadState_assign
-#define __Pyx_PyErr_Occurred() PyErr_Occurred()
-#endif
-
-/* PyErrFetchRestore.proto */
-#if CYTHON_FAST_THREAD_STATE
-#define __Pyx_PyErr_Clear() __Pyx_ErrRestore(NULL, NULL, NULL)
-#define __Pyx_ErrRestoreWithState(type, value, tb) __Pyx_ErrRestoreInState(PyThreadState_GET(), type, value, tb)
-#define __Pyx_ErrFetchWithState(type, value, tb) __Pyx_ErrFetchInState(PyThreadState_GET(), type, value, tb)
-#define __Pyx_ErrRestore(type, value, tb) __Pyx_ErrRestoreInState(__pyx_tstate, type, value, tb)
-#define __Pyx_ErrFetch(type, value, tb) __Pyx_ErrFetchInState(__pyx_tstate, type, value, tb)
-static CYTHON_INLINE void __Pyx_ErrRestoreInState(PyThreadState *tstate, PyObject *type, PyObject *value, PyObject *tb);
-static CYTHON_INLINE void __Pyx_ErrFetchInState(PyThreadState *tstate, PyObject **type, PyObject **value, PyObject **tb);
-#if CYTHON_COMPILING_IN_CPYTHON
-#define __Pyx_PyErr_SetNone(exc) (Py_INCREF(exc), __Pyx_ErrRestore((exc), NULL, NULL))
-#else
-#define __Pyx_PyErr_SetNone(exc) PyErr_SetNone(exc)
-#endif
-#else
-#define __Pyx_PyErr_Clear() PyErr_Clear()
-#define __Pyx_PyErr_SetNone(exc) PyErr_SetNone(exc)
-#define __Pyx_ErrRestoreWithState(type, value, tb) PyErr_Restore(type, value, tb)
-#define __Pyx_ErrFetchWithState(type, value, tb) PyErr_Fetch(type, value, tb)
-#define __Pyx_ErrRestoreInState(tstate, type, value, tb) PyErr_Restore(type, value, tb)
-#define __Pyx_ErrFetchInState(tstate, type, value, tb) PyErr_Fetch(type, value, tb)
-#define __Pyx_ErrRestore(type, value, tb) PyErr_Restore(type, value, tb)
-#define __Pyx_ErrFetch(type, value, tb) PyErr_Fetch(type, value, tb)
-#endif
-
-/* RaiseException.proto */
-static void __Pyx_Raise(PyObject *type, PyObject *value, PyObject *tb, PyObject *cause);
-
-/* PyCFunctionFastCall.proto */
-#if CYTHON_FAST_PYCCALL
-static CYTHON_INLINE PyObject *__Pyx_PyCFunction_FastCall(PyObject *func, PyObject **args, Py_ssize_t nargs);
-#else
-#define __Pyx_PyCFunction_FastCall(func, args, nargs) (assert(0), NULL)
-#endif
-
-/* PyFunctionFastCall.proto */
-#if CYTHON_FAST_PYCALL
-#define __Pyx_PyFunction_FastCall(func, args, nargs)\
- __Pyx_PyFunction_FastCallDict((func), (args), (nargs), NULL)
-#if 1 || PY_VERSION_HEX < 0x030600B1
-static PyObject *__Pyx_PyFunction_FastCallDict(PyObject *func, PyObject **args, Py_ssize_t nargs, PyObject *kwargs);
-#else
-#define __Pyx_PyFunction_FastCallDict(func, args, nargs, kwargs) _PyFunction_FastCallDict(func, args, nargs, kwargs)
-#endif
-#define __Pyx_BUILD_ASSERT_EXPR(cond)\
- (sizeof(char [1 - 2*!(cond)]) - 1)
-#ifndef Py_MEMBER_SIZE
-#define Py_MEMBER_SIZE(type, member) sizeof(((type *)0)->member)
-#endif
- static size_t __pyx_pyframe_localsplus_offset = 0;
- #include "frameobject.h"
- #define __Pxy_PyFrame_Initialize_Offsets()\
- ((void)__Pyx_BUILD_ASSERT_EXPR(sizeof(PyFrameObject) == offsetof(PyFrameObject, f_localsplus) + Py_MEMBER_SIZE(PyFrameObject, f_localsplus)),\
- (void)(__pyx_pyframe_localsplus_offset = ((size_t)PyFrame_Type.tp_basicsize) - Py_MEMBER_SIZE(PyFrameObject, f_localsplus)))
- #define __Pyx_PyFrame_GetLocalsplus(frame)\
- (assert(__pyx_pyframe_localsplus_offset), (PyObject **)(((char *)(frame)) + __pyx_pyframe_localsplus_offset))
-#endif
-
-/* PyObjectCall2Args.proto */
-static CYTHON_UNUSED PyObject* __Pyx_PyObject_Call2Args(PyObject* function, PyObject* arg1, PyObject* arg2);
-
-/* PyObjectCallMethO.proto */
-#if CYTHON_COMPILING_IN_CPYTHON
-static CYTHON_INLINE PyObject* __Pyx_PyObject_CallMethO(PyObject *func, PyObject *arg);
-#endif
-
-/* PyObjectCallOneArg.proto */
-static CYTHON_INLINE PyObject* __Pyx_PyObject_CallOneArg(PyObject *func, PyObject *arg);
-
-/* IncludeStringH.proto */
-#include
-
-/* BytesEquals.proto */
-static CYTHON_INLINE int __Pyx_PyBytes_Equals(PyObject* s1, PyObject* s2, int equals);
-
-/* UnicodeEquals.proto */
-static CYTHON_INLINE int __Pyx_PyUnicode_Equals(PyObject* s1, PyObject* s2, int equals);
-
-/* StrEquals.proto */
-#if PY_MAJOR_VERSION >= 3
-#define __Pyx_PyString_Equals __Pyx_PyUnicode_Equals
-#else
-#define __Pyx_PyString_Equals __Pyx_PyBytes_Equals
-#endif
-
-/* None.proto */
-static CYTHON_INLINE Py_ssize_t __Pyx_div_Py_ssize_t(Py_ssize_t, Py_ssize_t);
-
-/* UnaryNegOverflows.proto */
-#define UNARY_NEG_WOULD_OVERFLOW(x)\
- (((x) < 0) & ((unsigned long)(x) == 0-(unsigned long)(x)))
-
-static CYTHON_UNUSED int __pyx_array_getbuffer(PyObject *__pyx_v_self, Py_buffer *__pyx_v_info, int __pyx_v_flags); /*proto*/
-static PyObject *__pyx_array_get_memview(struct __pyx_array_obj *); /*proto*/
-/* GetAttr.proto */
-static CYTHON_INLINE PyObject *__Pyx_GetAttr(PyObject *, PyObject *);
-
-/* GetItemInt.proto */
-#define __Pyx_GetItemInt(o, i, type, is_signed, to_py_func, is_list, wraparound, boundscheck)\
- (__Pyx_fits_Py_ssize_t(i, type, is_signed) ?\
- __Pyx_GetItemInt_Fast(o, (Py_ssize_t)i, is_list, wraparound, boundscheck) :\
- (is_list ? (PyErr_SetString(PyExc_IndexError, "list index out of range"), (PyObject*)NULL) :\
- __Pyx_GetItemInt_Generic(o, to_py_func(i))))
-#define __Pyx_GetItemInt_List(o, i, type, is_signed, to_py_func, is_list, wraparound, boundscheck)\
- (__Pyx_fits_Py_ssize_t(i, type, is_signed) ?\
- __Pyx_GetItemInt_List_Fast(o, (Py_ssize_t)i, wraparound, boundscheck) :\
- (PyErr_SetString(PyExc_IndexError, "list index out of range"), (PyObject*)NULL))
-static CYTHON_INLINE PyObject *__Pyx_GetItemInt_List_Fast(PyObject *o, Py_ssize_t i,
- int wraparound, int boundscheck);
-#define __Pyx_GetItemInt_Tuple(o, i, type, is_signed, to_py_func, is_list, wraparound, boundscheck)\
- (__Pyx_fits_Py_ssize_t(i, type, is_signed) ?\
- __Pyx_GetItemInt_Tuple_Fast(o, (Py_ssize_t)i, wraparound, boundscheck) :\
- (PyErr_SetString(PyExc_IndexError, "tuple index out of range"), (PyObject*)NULL))
-static CYTHON_INLINE PyObject *__Pyx_GetItemInt_Tuple_Fast(PyObject *o, Py_ssize_t i,
- int wraparound, int boundscheck);
-static PyObject *__Pyx_GetItemInt_Generic(PyObject *o, PyObject* j);
-static CYTHON_INLINE PyObject *__Pyx_GetItemInt_Fast(PyObject *o, Py_ssize_t i,
- int is_list, int wraparound, int boundscheck);
-
-/* ObjectGetItem.proto */
-#if CYTHON_USE_TYPE_SLOTS
-static CYTHON_INLINE PyObject *__Pyx_PyObject_GetItem(PyObject *obj, PyObject* key);
-#else
-#define __Pyx_PyObject_GetItem(obj, key) PyObject_GetItem(obj, key)
-#endif
-
-/* decode_c_string_utf16.proto */
-static CYTHON_INLINE PyObject *__Pyx_PyUnicode_DecodeUTF16(const char *s, Py_ssize_t size, const char *errors) {
- int byteorder = 0;
- return PyUnicode_DecodeUTF16(s, size, errors, &byteorder);
-}
-static CYTHON_INLINE PyObject *__Pyx_PyUnicode_DecodeUTF16LE(const char *s, Py_ssize_t size, const char *errors) {
- int byteorder = -1;
- return PyUnicode_DecodeUTF16(s, size, errors, &byteorder);
-}
-static CYTHON_INLINE PyObject *__Pyx_PyUnicode_DecodeUTF16BE(const char *s, Py_ssize_t size, const char *errors) {
- int byteorder = 1;
- return PyUnicode_DecodeUTF16(s, size, errors, &byteorder);
-}
-
-/* decode_c_string.proto */
-static CYTHON_INLINE PyObject* __Pyx_decode_c_string(
- const char* cstring, Py_ssize_t start, Py_ssize_t stop,
- const char* encoding, const char* errors,
- PyObject* (*decode_func)(const char *s, Py_ssize_t size, const char *errors));
-
-/* PyErrExceptionMatches.proto */
-#if CYTHON_FAST_THREAD_STATE
-#define __Pyx_PyErr_ExceptionMatches(err) __Pyx_PyErr_ExceptionMatchesInState(__pyx_tstate, err)
-static CYTHON_INLINE int __Pyx_PyErr_ExceptionMatchesInState(PyThreadState* tstate, PyObject* err);
-#else
-#define __Pyx_PyErr_ExceptionMatches(err) PyErr_ExceptionMatches(err)
-#endif
-
-/* GetAttr3.proto */
-static CYTHON_INLINE PyObject *__Pyx_GetAttr3(PyObject *, PyObject *, PyObject *);
-
-/* PyDictVersioning.proto */
-#if CYTHON_USE_DICT_VERSIONS && CYTHON_USE_TYPE_SLOTS
-#define __PYX_DICT_VERSION_INIT ((PY_UINT64_T) -1)
-#define __PYX_GET_DICT_VERSION(dict) (((PyDictObject*)(dict))->ma_version_tag)
-#define __PYX_UPDATE_DICT_CACHE(dict, value, cache_var, version_var)\
- (version_var) = __PYX_GET_DICT_VERSION(dict);\
- (cache_var) = (value);
-#define __PYX_PY_DICT_LOOKUP_IF_MODIFIED(VAR, DICT, LOOKUP) {\
- static PY_UINT64_T __pyx_dict_version = 0;\
- static PyObject *__pyx_dict_cached_value = NULL;\
- if (likely(__PYX_GET_DICT_VERSION(DICT) == __pyx_dict_version)) {\
- (VAR) = __pyx_dict_cached_value;\
- } else {\
- (VAR) = __pyx_dict_cached_value = (LOOKUP);\
- __pyx_dict_version = __PYX_GET_DICT_VERSION(DICT);\
- }\
-}
-static CYTHON_INLINE PY_UINT64_T __Pyx_get_tp_dict_version(PyObject *obj);
-static CYTHON_INLINE PY_UINT64_T __Pyx_get_object_dict_version(PyObject *obj);
-static CYTHON_INLINE int __Pyx_object_dict_version_matches(PyObject* obj, PY_UINT64_T tp_dict_version, PY_UINT64_T obj_dict_version);
-#else
-#define __PYX_GET_DICT_VERSION(dict) (0)
-#define __PYX_UPDATE_DICT_CACHE(dict, value, cache_var, version_var)
-#define __PYX_PY_DICT_LOOKUP_IF_MODIFIED(VAR, DICT, LOOKUP) (VAR) = (LOOKUP);
-#endif
-
-/* GetModuleGlobalName.proto */
-#if CYTHON_USE_DICT_VERSIONS
-#define __Pyx_GetModuleGlobalName(var, name) {\
- static PY_UINT64_T __pyx_dict_version = 0;\
- static PyObject *__pyx_dict_cached_value = NULL;\
- (var) = (likely(__pyx_dict_version == __PYX_GET_DICT_VERSION(__pyx_d))) ?\
- (likely(__pyx_dict_cached_value) ? __Pyx_NewRef(__pyx_dict_cached_value) : __Pyx_GetBuiltinName(name)) :\
- __Pyx__GetModuleGlobalName(name, &__pyx_dict_version, &__pyx_dict_cached_value);\
-}
-#define __Pyx_GetModuleGlobalNameUncached(var, name) {\
- PY_UINT64_T __pyx_dict_version;\
- PyObject *__pyx_dict_cached_value;\
- (var) = __Pyx__GetModuleGlobalName(name, &__pyx_dict_version, &__pyx_dict_cached_value);\
-}
-static PyObject *__Pyx__GetModuleGlobalName(PyObject *name, PY_UINT64_T *dict_version, PyObject **dict_cached_value);
-#else
-#define __Pyx_GetModuleGlobalName(var, name) (var) = __Pyx__GetModuleGlobalName(name)
-#define __Pyx_GetModuleGlobalNameUncached(var, name) (var) = __Pyx__GetModuleGlobalName(name)
-static CYTHON_INLINE PyObject *__Pyx__GetModuleGlobalName(PyObject *name);
-#endif
-
-/* RaiseTooManyValuesToUnpack.proto */
-static CYTHON_INLINE void __Pyx_RaiseTooManyValuesError(Py_ssize_t expected);
-
-/* RaiseNeedMoreValuesToUnpack.proto */
-static CYTHON_INLINE void __Pyx_RaiseNeedMoreValuesError(Py_ssize_t index);
-
-/* RaiseNoneIterError.proto */
-static CYTHON_INLINE void __Pyx_RaiseNoneNotIterableError(void);
-
-/* ExtTypeTest.proto */
-static CYTHON_INLINE int __Pyx_TypeTest(PyObject *obj, PyTypeObject *type);
-
-/* GetTopmostException.proto */
-#if CYTHON_USE_EXC_INFO_STACK
-static _PyErr_StackItem * __Pyx_PyErr_GetTopmostException(PyThreadState *tstate);
-#endif
-
-/* SaveResetException.proto */
-#if CYTHON_FAST_THREAD_STATE
-#define __Pyx_ExceptionSave(type, value, tb) __Pyx__ExceptionSave(__pyx_tstate, type, value, tb)
-static CYTHON_INLINE void __Pyx__ExceptionSave(PyThreadState *tstate, PyObject **type, PyObject **value, PyObject **tb);
-#define __Pyx_ExceptionReset(type, value, tb) __Pyx__ExceptionReset(__pyx_tstate, type, value, tb)
-static CYTHON_INLINE void __Pyx__ExceptionReset(PyThreadState *tstate, PyObject *type, PyObject *value, PyObject *tb);
-#else
-#define __Pyx_ExceptionSave(type, value, tb) PyErr_GetExcInfo(type, value, tb)
-#define __Pyx_ExceptionReset(type, value, tb) PyErr_SetExcInfo(type, value, tb)
-#endif
-
-/* GetException.proto */
-#if CYTHON_FAST_THREAD_STATE
-#define __Pyx_GetException(type, value, tb) __Pyx__GetException(__pyx_tstate, type, value, tb)
-static int __Pyx__GetException(PyThreadState *tstate, PyObject **type, PyObject **value, PyObject **tb);
-#else
-static int __Pyx_GetException(PyObject **type, PyObject **value, PyObject **tb);
-#endif
-
-/* SwapException.proto */
-#if CYTHON_FAST_THREAD_STATE
-#define __Pyx_ExceptionSwap(type, value, tb) __Pyx__ExceptionSwap(__pyx_tstate, type, value, tb)
-static CYTHON_INLINE void __Pyx__ExceptionSwap(PyThreadState *tstate, PyObject **type, PyObject **value, PyObject **tb);
-#else
-static CYTHON_INLINE void __Pyx_ExceptionSwap(PyObject **type, PyObject **value, PyObject **tb);
-#endif
-
-/* Import.proto */
-static PyObject *__Pyx_Import(PyObject *name, PyObject *from_list, int level);
-
-/* FastTypeChecks.proto */
-#if CYTHON_COMPILING_IN_CPYTHON
-#define __Pyx_TypeCheck(obj, type) __Pyx_IsSubtype(Py_TYPE(obj), (PyTypeObject *)type)
-static CYTHON_INLINE int __Pyx_IsSubtype(PyTypeObject *a, PyTypeObject *b);
-static CYTHON_INLINE int __Pyx_PyErr_GivenExceptionMatches(PyObject *err, PyObject *type);
-static CYTHON_INLINE int __Pyx_PyErr_GivenExceptionMatches2(PyObject *err, PyObject *type1, PyObject *type2);
-#else
-#define __Pyx_TypeCheck(obj, type) PyObject_TypeCheck(obj, (PyTypeObject *)type)
-#define __Pyx_PyErr_GivenExceptionMatches(err, type) PyErr_GivenExceptionMatches(err, type)
-#define __Pyx_PyErr_GivenExceptionMatches2(err, type1, type2) (PyErr_GivenExceptionMatches(err, type1) || PyErr_GivenExceptionMatches(err, type2))
-#endif
-#define __Pyx_PyException_Check(obj) __Pyx_TypeCheck(obj, PyExc_Exception)
-
-static CYTHON_UNUSED int __pyx_memoryview_getbuffer(PyObject *__pyx_v_self, Py_buffer *__pyx_v_info, int __pyx_v_flags); /*proto*/
-/* ListCompAppend.proto */
-#if CYTHON_USE_PYLIST_INTERNALS && CYTHON_ASSUME_SAFE_MACROS
-static CYTHON_INLINE int __Pyx_ListComp_Append(PyObject* list, PyObject* x) {
- PyListObject* L = (PyListObject*) list;
- Py_ssize_t len = Py_SIZE(list);
- if (likely(L->allocated > len)) {
- Py_INCREF(x);
- PyList_SET_ITEM(list, len, x);
- __Pyx_SET_SIZE(list, len + 1);
- return 0;
- }
- return PyList_Append(list, x);
-}
-#else
-#define __Pyx_ListComp_Append(L,x) PyList_Append(L,x)
-#endif
-
-/* PyIntBinop.proto */
-#if !CYTHON_COMPILING_IN_PYPY
-static PyObject* __Pyx_PyInt_AddObjC(PyObject *op1, PyObject *op2, long intval, int inplace, int zerodivision_check);
-#else
-#define __Pyx_PyInt_AddObjC(op1, op2, intval, inplace, zerodivision_check)\
- (inplace ? PyNumber_InPlaceAdd(op1, op2) : PyNumber_Add(op1, op2))
-#endif
-
-/* ListExtend.proto */
-static CYTHON_INLINE int __Pyx_PyList_Extend(PyObject* L, PyObject* v) {
-#if CYTHON_COMPILING_IN_CPYTHON
- PyObject* none = _PyList_Extend((PyListObject*)L, v);
- if (unlikely(!none))
- return -1;
- Py_DECREF(none);
- return 0;
-#else
- return PyList_SetSlice(L, PY_SSIZE_T_MAX, PY_SSIZE_T_MAX, v);
-#endif
-}
-
-/* ListAppend.proto */
-#if CYTHON_USE_PYLIST_INTERNALS && CYTHON_ASSUME_SAFE_MACROS
-static CYTHON_INLINE int __Pyx_PyList_Append(PyObject* list, PyObject* x) {
- PyListObject* L = (PyListObject*) list;
- Py_ssize_t len = Py_SIZE(list);
- if (likely(L->allocated > len) & likely(len > (L->allocated >> 1))) {
- Py_INCREF(x);
- PyList_SET_ITEM(list, len, x);
- __Pyx_SET_SIZE(list, len + 1);
- return 0;
- }
- return PyList_Append(list, x);
-}
-#else
-#define __Pyx_PyList_Append(L,x) PyList_Append(L,x)
-#endif
-
-/* None.proto */
-static CYTHON_INLINE long __Pyx_div_long(long, long);
-
-/* ImportFrom.proto */
-static PyObject* __Pyx_ImportFrom(PyObject* module, PyObject* name);
-
-/* HasAttr.proto */
-static CYTHON_INLINE int __Pyx_HasAttr(PyObject *, PyObject *);
-
-/* PyObject_GenericGetAttrNoDict.proto */
-#if CYTHON_USE_TYPE_SLOTS && CYTHON_USE_PYTYPE_LOOKUP && PY_VERSION_HEX < 0x03070000
-static CYTHON_INLINE PyObject* __Pyx_PyObject_GenericGetAttrNoDict(PyObject* obj, PyObject* attr_name);
-#else
-#define __Pyx_PyObject_GenericGetAttrNoDict PyObject_GenericGetAttr
-#endif
-
-/* PyObject_GenericGetAttr.proto */
-#if CYTHON_USE_TYPE_SLOTS && CYTHON_USE_PYTYPE_LOOKUP && PY_VERSION_HEX < 0x03070000
-static PyObject* __Pyx_PyObject_GenericGetAttr(PyObject* obj, PyObject* attr_name);
-#else
-#define __Pyx_PyObject_GenericGetAttr PyObject_GenericGetAttr
-#endif
-
-/* SetVTable.proto */
-static int __Pyx_SetVtable(PyObject *dict, void *vtable);
-
-/* PyObjectGetAttrStrNoError.proto */
-static CYTHON_INLINE PyObject* __Pyx_PyObject_GetAttrStrNoError(PyObject* obj, PyObject* attr_name);
-
-/* SetupReduce.proto */
-static int __Pyx_setup_reduce(PyObject* type_obj);
-
-/* CLineInTraceback.proto */
-#ifdef CYTHON_CLINE_IN_TRACEBACK
-#define __Pyx_CLineForTraceback(tstate, c_line) (((CYTHON_CLINE_IN_TRACEBACK)) ? c_line : 0)
-#else
-static int __Pyx_CLineForTraceback(PyThreadState *tstate, int c_line);
-#endif
-
-/* CodeObjectCache.proto */
-typedef struct {
- PyCodeObject* code_object;
- int code_line;
-} __Pyx_CodeObjectCacheEntry;
-struct __Pyx_CodeObjectCache {
- int count;
- int max_count;
- __Pyx_CodeObjectCacheEntry* entries;
-};
-static struct __Pyx_CodeObjectCache __pyx_code_cache = {0,0,NULL};
-static int __pyx_bisect_code_objects(__Pyx_CodeObjectCacheEntry* entries, int count, int code_line);
-static PyCodeObject *__pyx_find_code_object(int code_line);
-static void __pyx_insert_code_object(int code_line, PyCodeObject* code_object);
-
-/* AddTraceback.proto */
-static void __Pyx_AddTraceback(const char *funcname, int c_line,
- int py_line, const char *filename);
-
-#if PY_MAJOR_VERSION < 3
- static int __Pyx_GetBuffer(PyObject *obj, Py_buffer *view, int flags);
- static void __Pyx_ReleaseBuffer(Py_buffer *view);
-#else
- #define __Pyx_GetBuffer PyObject_GetBuffer
- #define __Pyx_ReleaseBuffer PyBuffer_Release
-#endif
-
-
-/* BufferStructDeclare.proto */
-typedef struct {
- Py_ssize_t shape, strides, suboffsets;
-} __Pyx_Buf_DimInfo;
-typedef struct {
- size_t refcount;
- Py_buffer pybuffer;
-} __Pyx_Buffer;
-typedef struct {
- __Pyx_Buffer *rcbuffer;
- char *data;
- __Pyx_Buf_DimInfo diminfo[8];
-} __Pyx_LocalBuf_ND;
-
-/* MemviewSliceIsContig.proto */
-static int __pyx_memviewslice_is_contig(const __Pyx_memviewslice mvs, char order, int ndim);
-
-/* OverlappingSlices.proto */
-static int __pyx_slices_overlap(__Pyx_memviewslice *slice1,
- __Pyx_memviewslice *slice2,
- int ndim, size_t itemsize);
-
-/* Capsule.proto */
-static CYTHON_INLINE PyObject *__pyx_capsule_create(void *p, const char *sig);
-
-/* IsLittleEndian.proto */
-static CYTHON_INLINE int __Pyx_Is_Little_Endian(void);
-
-/* BufferFormatCheck.proto */
-static const char* __Pyx_BufFmt_CheckString(__Pyx_BufFmt_Context* ctx, const char* ts);
-static void __Pyx_BufFmt_Init(__Pyx_BufFmt_Context* ctx,
- __Pyx_BufFmt_StackElem* stack,
- __Pyx_TypeInfo* type);
-
-/* TypeInfoCompare.proto */
-static int __pyx_typeinfo_cmp(__Pyx_TypeInfo *a, __Pyx_TypeInfo *b);
-
-/* MemviewSliceValidateAndInit.proto */
-static int __Pyx_ValidateAndInit_memviewslice(
- int *axes_specs,
- int c_or_f_flag,
- int buf_flags,
- int ndim,
- __Pyx_TypeInfo *dtype,
- __Pyx_BufFmt_StackElem stack[],
- __Pyx_memviewslice *memviewslice,
- PyObject *original_obj);
-
-/* ObjectToMemviewSlice.proto */
-static CYTHON_INLINE __Pyx_memviewslice __Pyx_PyObject_to_MemoryviewSlice_d_d_dc_int(PyObject *, int writable_flag);
-
-/* ObjectToMemviewSlice.proto */
-static CYTHON_INLINE __Pyx_memviewslice __Pyx_PyObject_to_MemoryviewSlice_d_d_dc_float(PyObject *, int writable_flag);
-
-/* ObjectToMemviewSlice.proto */
-static CYTHON_INLINE __Pyx_memviewslice __Pyx_PyObject_to_MemoryviewSlice_dc_int(PyObject *, int writable_flag);
-
-/* CIntToPy.proto */
-static CYTHON_INLINE PyObject* __Pyx_PyInt_From_int(int value);
-
-/* CIntToPy.proto */
-static CYTHON_INLINE PyObject* __Pyx_PyInt_From_long(long value);
-
-/* MemviewSliceCopyTemplate.proto */
-static __Pyx_memviewslice
-__pyx_memoryview_copy_new_contig(const __Pyx_memviewslice *from_mvs,
- const char *mode, int ndim,
- size_t sizeof_dtype, int contig_flag,
- int dtype_is_object);
-
-/* CIntFromPy.proto */
-static CYTHON_INLINE int __Pyx_PyInt_As_int(PyObject *);
-
-/* CIntFromPy.proto */
-static CYTHON_INLINE long __Pyx_PyInt_As_long(PyObject *);
-
-/* CIntFromPy.proto */
-static CYTHON_INLINE char __Pyx_PyInt_As_char(PyObject *);
-
-/* CheckBinaryVersion.proto */
-static int __Pyx_check_binary_version(void);
-
-/* InitStrings.proto */
-static int __Pyx_InitStrings(__Pyx_StringTabEntry *t);
-
-static PyObject *__pyx_array_get_memview(struct __pyx_array_obj *__pyx_v_self); /* proto*/
-static char *__pyx_memoryview_get_item_pointer(struct __pyx_memoryview_obj *__pyx_v_self, PyObject *__pyx_v_index); /* proto*/
-static PyObject *__pyx_memoryview_is_slice(struct __pyx_memoryview_obj *__pyx_v_self, PyObject *__pyx_v_obj); /* proto*/
-static PyObject *__pyx_memoryview_setitem_slice_assignment(struct __pyx_memoryview_obj *__pyx_v_self, PyObject *__pyx_v_dst, PyObject *__pyx_v_src); /* proto*/
-static PyObject *__pyx_memoryview_setitem_slice_assign_scalar(struct __pyx_memoryview_obj *__pyx_v_self, struct __pyx_memoryview_obj *__pyx_v_dst, PyObject *__pyx_v_value); /* proto*/
-static PyObject *__pyx_memoryview_setitem_indexed(struct __pyx_memoryview_obj *__pyx_v_self, PyObject *__pyx_v_index, PyObject *__pyx_v_value); /* proto*/
-static PyObject *__pyx_memoryview_convert_item_to_object(struct __pyx_memoryview_obj *__pyx_v_self, char *__pyx_v_itemp); /* proto*/
-static PyObject *__pyx_memoryview_assign_item_from_object(struct __pyx_memoryview_obj *__pyx_v_self, char *__pyx_v_itemp, PyObject *__pyx_v_value); /* proto*/
-static PyObject *__pyx_memoryviewslice_convert_item_to_object(struct __pyx_memoryviewslice_obj *__pyx_v_self, char *__pyx_v_itemp); /* proto*/
-static PyObject *__pyx_memoryviewslice_assign_item_from_object(struct __pyx_memoryviewslice_obj *__pyx_v_self, char *__pyx_v_itemp, PyObject *__pyx_v_value); /* proto*/
-
-/* Module declarations from 'cython.view' */
-
-/* Module declarations from 'cython' */
-
-/* Module declarations from 'monotonic_align.core' */
-static PyTypeObject *__pyx_array_type = 0;
-static PyTypeObject *__pyx_MemviewEnum_type = 0;
-static PyTypeObject *__pyx_memoryview_type = 0;
-static PyTypeObject *__pyx_memoryviewslice_type = 0;
-static PyObject *generic = 0;
-static PyObject *strided = 0;
-static PyObject *indirect = 0;
-static PyObject *contiguous = 0;
-static PyObject *indirect_contiguous = 0;
-static int __pyx_memoryview_thread_locks_used;
-static PyThread_type_lock __pyx_memoryview_thread_locks[8];
-static void __pyx_f_15monotonic_align_4core_maximum_path_each(__Pyx_memviewslice, __Pyx_memviewslice, int, int, struct __pyx_opt_args_15monotonic_align_4core_maximum_path_each *__pyx_optional_args); /*proto*/
-static void __pyx_f_15monotonic_align_4core_maximum_path_c(__Pyx_memviewslice, __Pyx_memviewslice, __Pyx_memviewslice, __Pyx_memviewslice, int __pyx_skip_dispatch); /*proto*/
-static struct __pyx_array_obj *__pyx_array_new(PyObject *, Py_ssize_t, char *, char *, char *); /*proto*/
-static void *__pyx_align_pointer(void *, size_t); /*proto*/
-static PyObject *__pyx_memoryview_new(PyObject *, int, int, __Pyx_TypeInfo *); /*proto*/
-static CYTHON_INLINE int __pyx_memoryview_check(PyObject *); /*proto*/
-static PyObject *_unellipsify(PyObject *, int); /*proto*/
-static PyObject *assert_direct_dimensions(Py_ssize_t *, int); /*proto*/
-static struct __pyx_memoryview_obj *__pyx_memview_slice(struct __pyx_memoryview_obj *, PyObject *); /*proto*/
-static int __pyx_memoryview_slice_memviewslice(__Pyx_memviewslice *, Py_ssize_t, Py_ssize_t, Py_ssize_t, int, int, int *, Py_ssize_t, Py_ssize_t, Py_ssize_t, int, int, int, int); /*proto*/
-static char *__pyx_pybuffer_index(Py_buffer *, char *, Py_ssize_t, Py_ssize_t); /*proto*/
-static int __pyx_memslice_transpose(__Pyx_memviewslice *); /*proto*/
-static PyObject *__pyx_memoryview_fromslice(__Pyx_memviewslice, int, PyObject *(*)(char *), int (*)(char *, PyObject *), int); /*proto*/
-static __Pyx_memviewslice *__pyx_memoryview_get_slice_from_memoryview(struct __pyx_memoryview_obj *, __Pyx_memviewslice *); /*proto*/
-static void __pyx_memoryview_slice_copy(struct __pyx_memoryview_obj *, __Pyx_memviewslice *); /*proto*/
-static PyObject *__pyx_memoryview_copy_object(struct __pyx_memoryview_obj *); /*proto*/
-static PyObject *__pyx_memoryview_copy_object_from_slice(struct __pyx_memoryview_obj *, __Pyx_memviewslice *); /*proto*/
-static Py_ssize_t abs_py_ssize_t(Py_ssize_t); /*proto*/
-static char __pyx_get_best_slice_order(__Pyx_memviewslice *, int); /*proto*/
-static void _copy_strided_to_strided(char *, Py_ssize_t *, char *, Py_ssize_t *, Py_ssize_t *, Py_ssize_t *, int, size_t); /*proto*/
-static void copy_strided_to_strided(__Pyx_memviewslice *, __Pyx_memviewslice *, int, size_t); /*proto*/
-static Py_ssize_t __pyx_memoryview_slice_get_size(__Pyx_memviewslice *, int); /*proto*/
-static Py_ssize_t __pyx_fill_contig_strides_array(Py_ssize_t *, Py_ssize_t *, Py_ssize_t, int, char); /*proto*/
-static void *__pyx_memoryview_copy_data_to_temp(__Pyx_memviewslice *, __Pyx_memviewslice *, char, int); /*proto*/
-static int __pyx_memoryview_err_extents(int, Py_ssize_t, Py_ssize_t); /*proto*/
-static int __pyx_memoryview_err_dim(PyObject *, char *, int); /*proto*/
-static int __pyx_memoryview_err(PyObject *, char *); /*proto*/
-static int __pyx_memoryview_copy_contents(__Pyx_memviewslice, __Pyx_memviewslice, int, int, int); /*proto*/
-static void __pyx_memoryview_broadcast_leading(__Pyx_memviewslice *, int, int); /*proto*/
-static void __pyx_memoryview_refcount_copying(__Pyx_memviewslice *, int, int, int); /*proto*/
-static void __pyx_memoryview_refcount_objects_in_slice_with_gil(char *, Py_ssize_t *, Py_ssize_t *, int, int); /*proto*/
-static void __pyx_memoryview_refcount_objects_in_slice(char *, Py_ssize_t *, Py_ssize_t *, int, int); /*proto*/
-static void __pyx_memoryview_slice_assign_scalar(__Pyx_memviewslice *, int, size_t, void *, int); /*proto*/
-static void __pyx_memoryview__slice_assign_scalar(char *, Py_ssize_t *, Py_ssize_t *, int, size_t, void *); /*proto*/
-static PyObject *__pyx_unpickle_Enum__set_state(struct __pyx_MemviewEnum_obj *, PyObject *); /*proto*/
-static __Pyx_TypeInfo __Pyx_TypeInfo_int = { "int", NULL, sizeof(int), { 0 }, 0, IS_UNSIGNED(int) ? 'U' : 'I', IS_UNSIGNED(int), 0 };
-static __Pyx_TypeInfo __Pyx_TypeInfo_float = { "float", NULL, sizeof(float), { 0 }, 0, 'R', 0, 0 };
-#define __Pyx_MODULE_NAME "monotonic_align.core"
-extern int __pyx_module_is_main_monotonic_align__core;
-int __pyx_module_is_main_monotonic_align__core = 0;
-
-/* Implementation of 'monotonic_align.core' */
-static PyObject *__pyx_builtin_range;
-static PyObject *__pyx_builtin_ValueError;
-static PyObject *__pyx_builtin_MemoryError;
-static PyObject *__pyx_builtin_enumerate;
-static PyObject *__pyx_builtin_TypeError;
-static PyObject *__pyx_builtin_Ellipsis;
-static PyObject *__pyx_builtin_id;
-static PyObject *__pyx_builtin_IndexError;
-static const char __pyx_k_O[] = "O";
-static const char __pyx_k_c[] = "c";
-static const char __pyx_k_id[] = "id";
-static const char __pyx_k_new[] = "__new__";
-static const char __pyx_k_obj[] = "obj";
-static const char __pyx_k_base[] = "base";
-static const char __pyx_k_dict[] = "__dict__";
-static const char __pyx_k_main[] = "__main__";
-static const char __pyx_k_mode[] = "mode";
-static const char __pyx_k_name[] = "name";
-static const char __pyx_k_ndim[] = "ndim";
-static const char __pyx_k_pack[] = "pack";
-static const char __pyx_k_size[] = "size";
-static const char __pyx_k_step[] = "step";
-static const char __pyx_k_stop[] = "stop";
-static const char __pyx_k_t_xs[] = "t_xs";
-static const char __pyx_k_t_ys[] = "t_ys";
-static const char __pyx_k_test[] = "__test__";
-static const char __pyx_k_ASCII[] = "ASCII";
-static const char __pyx_k_class[] = "__class__";
-static const char __pyx_k_error[] = "error";
-static const char __pyx_k_flags[] = "flags";
-static const char __pyx_k_paths[] = "paths";
-static const char __pyx_k_range[] = "range";
-static const char __pyx_k_shape[] = "shape";
-static const char __pyx_k_start[] = "start";
-static const char __pyx_k_encode[] = "encode";
-static const char __pyx_k_format[] = "format";
-static const char __pyx_k_import[] = "__import__";
-static const char __pyx_k_name_2[] = "__name__";
-static const char __pyx_k_pickle[] = "pickle";
-static const char __pyx_k_reduce[] = "__reduce__";
-static const char __pyx_k_struct[] = "struct";
-static const char __pyx_k_unpack[] = "unpack";
-static const char __pyx_k_update[] = "update";
-static const char __pyx_k_values[] = "values";
-static const char __pyx_k_fortran[] = "fortran";
-static const char __pyx_k_memview[] = "memview";
-static const char __pyx_k_Ellipsis[] = "Ellipsis";
-static const char __pyx_k_getstate[] = "__getstate__";
-static const char __pyx_k_itemsize[] = "itemsize";
-static const char __pyx_k_pyx_type[] = "__pyx_type";
-static const char __pyx_k_setstate[] = "__setstate__";
-static const char __pyx_k_TypeError[] = "TypeError";
-static const char __pyx_k_enumerate[] = "enumerate";
-static const char __pyx_k_pyx_state[] = "__pyx_state";
-static const char __pyx_k_reduce_ex[] = "__reduce_ex__";
-static const char __pyx_k_IndexError[] = "IndexError";
-static const char __pyx_k_ValueError[] = "ValueError";
-static const char __pyx_k_pyx_result[] = "__pyx_result";
-static const char __pyx_k_pyx_vtable[] = "__pyx_vtable__";
-static const char __pyx_k_MemoryError[] = "MemoryError";
-static const char __pyx_k_PickleError[] = "PickleError";
-static const char __pyx_k_pyx_checksum[] = "__pyx_checksum";
-static const char __pyx_k_stringsource[] = "stringsource";
-static const char __pyx_k_pyx_getbuffer[] = "__pyx_getbuffer";
-static const char __pyx_k_reduce_cython[] = "__reduce_cython__";
-static const char __pyx_k_View_MemoryView[] = "View.MemoryView";
-static const char __pyx_k_allocate_buffer[] = "allocate_buffer";
-static const char __pyx_k_dtype_is_object[] = "dtype_is_object";
-static const char __pyx_k_pyx_PickleError[] = "__pyx_PickleError";
-static const char __pyx_k_setstate_cython[] = "__setstate_cython__";
-static const char __pyx_k_pyx_unpickle_Enum[] = "__pyx_unpickle_Enum";
-static const char __pyx_k_cline_in_traceback[] = "cline_in_traceback";
-static const char __pyx_k_strided_and_direct[] = "";
-static const char __pyx_k_strided_and_indirect[] = "";
-static const char __pyx_k_contiguous_and_direct[] = "";
-static const char __pyx_k_MemoryView_of_r_object[] = "";
-static const char __pyx_k_MemoryView_of_r_at_0x_x[] = "";
-static const char __pyx_k_contiguous_and_indirect[] = "";
-static const char __pyx_k_Cannot_index_with_type_s[] = "Cannot index with type '%s'";
-static const char __pyx_k_Invalid_shape_in_axis_d_d[] = "Invalid shape in axis %d: %d.";
-static const char __pyx_k_itemsize_0_for_cython_array[] = "itemsize <= 0 for cython.array";
-static const char __pyx_k_unable_to_allocate_array_data[] = "unable to allocate array data.";
-static const char __pyx_k_strided_and_direct_or_indirect[] = "";
-static const char __pyx_k_Buffer_view_does_not_expose_stri[] = "Buffer view does not expose strides";
-static const char __pyx_k_Can_only_create_a_buffer_that_is[] = "Can only create a buffer that is contiguous in memory.";
-static const char __pyx_k_Cannot_assign_to_read_only_memor[] = "Cannot assign to read-only memoryview";
-static const char __pyx_k_Cannot_create_writable_memory_vi[] = "Cannot create writable memory view from read-only memoryview";
-static const char __pyx_k_Empty_shape_tuple_for_cython_arr[] = "Empty shape tuple for cython.array";
-static const char __pyx_k_Incompatible_checksums_s_vs_0xb0[] = "Incompatible checksums (%s vs 0xb068931 = (name))";
-static const char __pyx_k_Indirect_dimensions_not_supporte[] = "Indirect dimensions not supported";
-static const char __pyx_k_Invalid_mode_expected_c_or_fortr[] = "Invalid mode, expected 'c' or 'fortran', got %s";
-static const char __pyx_k_Out_of_bounds_on_buffer_access_a[] = "Out of bounds on buffer access (axis %d)";
-static const char __pyx_k_Unable_to_convert_item_to_object[] = "Unable to convert item to object";
-static const char __pyx_k_got_differing_extents_in_dimensi[] = "got differing extents in dimension %d (got %d and %d)";
-static const char __pyx_k_no_default___reduce___due_to_non[] = "no default __reduce__ due to non-trivial __cinit__";
-static const char __pyx_k_unable_to_allocate_shape_and_str[] = "unable to allocate shape and strides.";
-static PyObject *__pyx_n_s_ASCII;
-static PyObject *__pyx_kp_s_Buffer_view_does_not_expose_stri;
-static PyObject *__pyx_kp_s_Can_only_create_a_buffer_that_is;
-static PyObject *__pyx_kp_s_Cannot_assign_to_read_only_memor;
-static PyObject *__pyx_kp_s_Cannot_create_writable_memory_vi;
-static PyObject *__pyx_kp_s_Cannot_index_with_type_s;
-static PyObject *__pyx_n_s_Ellipsis;
-static PyObject *__pyx_kp_s_Empty_shape_tuple_for_cython_arr;
-static PyObject *__pyx_kp_s_Incompatible_checksums_s_vs_0xb0;
-static PyObject *__pyx_n_s_IndexError;
-static PyObject *__pyx_kp_s_Indirect_dimensions_not_supporte;
-static PyObject *__pyx_kp_s_Invalid_mode_expected_c_or_fortr;
-static PyObject *__pyx_kp_s_Invalid_shape_in_axis_d_d;
-static PyObject *__pyx_n_s_MemoryError;
-static PyObject *__pyx_kp_s_MemoryView_of_r_at_0x_x;
-static PyObject *__pyx_kp_s_MemoryView_of_r_object;
-static PyObject *__pyx_n_b_O;
-static PyObject *__pyx_kp_s_Out_of_bounds_on_buffer_access_a;
-static PyObject *__pyx_n_s_PickleError;
-static PyObject *__pyx_n_s_TypeError;
-static PyObject *__pyx_kp_s_Unable_to_convert_item_to_object;
-static PyObject *__pyx_n_s_ValueError;
-static PyObject *__pyx_n_s_View_MemoryView;
-static PyObject *__pyx_n_s_allocate_buffer;
-static PyObject *__pyx_n_s_base;
-static PyObject *__pyx_n_s_c;
-static PyObject *__pyx_n_u_c;
-static PyObject *__pyx_n_s_class;
-static PyObject *__pyx_n_s_cline_in_traceback;
-static PyObject *__pyx_kp_s_contiguous_and_direct;
-static PyObject *__pyx_kp_s_contiguous_and_indirect;
-static PyObject *__pyx_n_s_dict;
-static PyObject *__pyx_n_s_dtype_is_object;
-static PyObject *__pyx_n_s_encode;
-static PyObject *__pyx_n_s_enumerate;
-static PyObject *__pyx_n_s_error;
-static PyObject *__pyx_n_s_flags;
-static PyObject *__pyx_n_s_format;
-static PyObject *__pyx_n_s_fortran;
-static PyObject *__pyx_n_u_fortran;
-static PyObject *__pyx_n_s_getstate;
-static PyObject *__pyx_kp_s_got_differing_extents_in_dimensi;
-static PyObject *__pyx_n_s_id;
-static PyObject *__pyx_n_s_import;
-static PyObject *__pyx_n_s_itemsize;
-static PyObject *__pyx_kp_s_itemsize_0_for_cython_array;
-static PyObject *__pyx_n_s_main;
-static PyObject *__pyx_n_s_memview;
-static PyObject *__pyx_n_s_mode;
-static PyObject *__pyx_n_s_name;
-static PyObject *__pyx_n_s_name_2;
-static PyObject *__pyx_n_s_ndim;
-static PyObject *__pyx_n_s_new;
-static PyObject *__pyx_kp_s_no_default___reduce___due_to_non;
-static PyObject *__pyx_n_s_obj;
-static PyObject *__pyx_n_s_pack;
-static PyObject *__pyx_n_s_paths;
-static PyObject *__pyx_n_s_pickle;
-static PyObject *__pyx_n_s_pyx_PickleError;
-static PyObject *__pyx_n_s_pyx_checksum;
-static PyObject *__pyx_n_s_pyx_getbuffer;
-static PyObject *__pyx_n_s_pyx_result;
-static PyObject *__pyx_n_s_pyx_state;
-static PyObject *__pyx_n_s_pyx_type;
-static PyObject *__pyx_n_s_pyx_unpickle_Enum;
-static PyObject *__pyx_n_s_pyx_vtable;
-static PyObject *__pyx_n_s_range;
-static PyObject *__pyx_n_s_reduce;
-static PyObject *__pyx_n_s_reduce_cython;
-static PyObject *__pyx_n_s_reduce_ex;
-static PyObject *__pyx_n_s_setstate;
-static PyObject *__pyx_n_s_setstate_cython;
-static PyObject *__pyx_n_s_shape;
-static PyObject *__pyx_n_s_size;
-static PyObject *__pyx_n_s_start;
-static PyObject *__pyx_n_s_step;
-static PyObject *__pyx_n_s_stop;
-static PyObject *__pyx_kp_s_strided_and_direct;
-static PyObject *__pyx_kp_s_strided_and_direct_or_indirect;
-static PyObject *__pyx_kp_s_strided_and_indirect;
-static PyObject *__pyx_kp_s_stringsource;
-static PyObject *__pyx_n_s_struct;
-static PyObject *__pyx_n_s_t_xs;
-static PyObject *__pyx_n_s_t_ys;
-static PyObject *__pyx_n_s_test;
-static PyObject *__pyx_kp_s_unable_to_allocate_array_data;
-static PyObject *__pyx_kp_s_unable_to_allocate_shape_and_str;
-static PyObject *__pyx_n_s_unpack;
-static PyObject *__pyx_n_s_update;
-static PyObject *__pyx_n_s_values;
-static PyObject *__pyx_pf_15monotonic_align_4core_maximum_path_c(CYTHON_UNUSED PyObject *__pyx_self, __Pyx_memviewslice __pyx_v_paths, __Pyx_memviewslice __pyx_v_values, __Pyx_memviewslice __pyx_v_t_ys, __Pyx_memviewslice __pyx_v_t_xs); /* proto */
-static int __pyx_array___pyx_pf_15View_dot_MemoryView_5array___cinit__(struct __pyx_array_obj *__pyx_v_self, PyObject *__pyx_v_shape, Py_ssize_t __pyx_v_itemsize, PyObject *__pyx_v_format, PyObject *__pyx_v_mode, int __pyx_v_allocate_buffer); /* proto */
-static int __pyx_array___pyx_pf_15View_dot_MemoryView_5array_2__getbuffer__(struct __pyx_array_obj *__pyx_v_self, Py_buffer *__pyx_v_info, int __pyx_v_flags); /* proto */
-static void __pyx_array___pyx_pf_15View_dot_MemoryView_5array_4__dealloc__(struct __pyx_array_obj *__pyx_v_self); /* proto */
-static PyObject *__pyx_pf_15View_dot_MemoryView_5array_7memview___get__(struct __pyx_array_obj *__pyx_v_self); /* proto */
-static Py_ssize_t __pyx_array___pyx_pf_15View_dot_MemoryView_5array_6__len__(struct __pyx_array_obj *__pyx_v_self); /* proto */
-static PyObject *__pyx_array___pyx_pf_15View_dot_MemoryView_5array_8__getattr__(struct __pyx_array_obj *__pyx_v_self, PyObject *__pyx_v_attr); /* proto */
-static PyObject *__pyx_array___pyx_pf_15View_dot_MemoryView_5array_10__getitem__(struct __pyx_array_obj *__pyx_v_self, PyObject *__pyx_v_item); /* proto */
-static int __pyx_array___pyx_pf_15View_dot_MemoryView_5array_12__setitem__(struct __pyx_array_obj *__pyx_v_self, PyObject *__pyx_v_item, PyObject *__pyx_v_value); /* proto */
-static PyObject *__pyx_pf___pyx_array___reduce_cython__(CYTHON_UNUSED struct __pyx_array_obj *__pyx_v_self); /* proto */
-static PyObject *__pyx_pf___pyx_array_2__setstate_cython__(CYTHON_UNUSED struct __pyx_array_obj *__pyx_v_self, CYTHON_UNUSED PyObject *__pyx_v___pyx_state); /* proto */
-static int __pyx_MemviewEnum___pyx_pf_15View_dot_MemoryView_4Enum___init__(struct __pyx_MemviewEnum_obj *__pyx_v_self, PyObject *__pyx_v_name); /* proto */
-static PyObject *__pyx_MemviewEnum___pyx_pf_15View_dot_MemoryView_4Enum_2__repr__(struct __pyx_MemviewEnum_obj *__pyx_v_self); /* proto */
-static PyObject *__pyx_pf___pyx_MemviewEnum___reduce_cython__(struct __pyx_MemviewEnum_obj *__pyx_v_self); /* proto */
-static PyObject *__pyx_pf___pyx_MemviewEnum_2__setstate_cython__(struct __pyx_MemviewEnum_obj *__pyx_v_self, PyObject *__pyx_v___pyx_state); /* proto */
-static int __pyx_memoryview___pyx_pf_15View_dot_MemoryView_10memoryview___cinit__(struct __pyx_memoryview_obj *__pyx_v_self, PyObject *__pyx_v_obj, int __pyx_v_flags, int __pyx_v_dtype_is_object); /* proto */
-static void __pyx_memoryview___pyx_pf_15View_dot_MemoryView_10memoryview_2__dealloc__(struct __pyx_memoryview_obj *__pyx_v_self); /* proto */
-static PyObject *__pyx_memoryview___pyx_pf_15View_dot_MemoryView_10memoryview_4__getitem__(struct __pyx_memoryview_obj *__pyx_v_self, PyObject *__pyx_v_index); /* proto */
-static int __pyx_memoryview___pyx_pf_15View_dot_MemoryView_10memoryview_6__setitem__(struct __pyx_memoryview_obj *__pyx_v_self, PyObject *__pyx_v_index, PyObject *__pyx_v_value); /* proto */
-static int __pyx_memoryview___pyx_pf_15View_dot_MemoryView_10memoryview_8__getbuffer__(struct __pyx_memoryview_obj *__pyx_v_self, Py_buffer *__pyx_v_info, int __pyx_v_flags); /* proto */
-static PyObject *__pyx_pf_15View_dot_MemoryView_10memoryview_1T___get__(struct __pyx_memoryview_obj *__pyx_v_self); /* proto */
-static PyObject *__pyx_pf_15View_dot_MemoryView_10memoryview_4base___get__(struct __pyx_memoryview_obj *__pyx_v_self); /* proto */
-static PyObject *__pyx_pf_15View_dot_MemoryView_10memoryview_5shape___get__(struct __pyx_memoryview_obj *__pyx_v_self); /* proto */
-static PyObject *__pyx_pf_15View_dot_MemoryView_10memoryview_7strides___get__(struct __pyx_memoryview_obj *__pyx_v_self); /* proto */
-static PyObject *__pyx_pf_15View_dot_MemoryView_10memoryview_10suboffsets___get__(struct __pyx_memoryview_obj *__pyx_v_self); /* proto */
-static PyObject *__pyx_pf_15View_dot_MemoryView_10memoryview_4ndim___get__(struct __pyx_memoryview_obj *__pyx_v_self); /* proto */
-static PyObject *__pyx_pf_15View_dot_MemoryView_10memoryview_8itemsize___get__(struct __pyx_memoryview_obj *__pyx_v_self); /* proto */
-static PyObject *__pyx_pf_15View_dot_MemoryView_10memoryview_6nbytes___get__(struct __pyx_memoryview_obj *__pyx_v_self); /* proto */
-static PyObject *__pyx_pf_15View_dot_MemoryView_10memoryview_4size___get__(struct __pyx_memoryview_obj *__pyx_v_self); /* proto */
-static Py_ssize_t __pyx_memoryview___pyx_pf_15View_dot_MemoryView_10memoryview_10__len__(struct __pyx_memoryview_obj *__pyx_v_self); /* proto */
-static PyObject *__pyx_memoryview___pyx_pf_15View_dot_MemoryView_10memoryview_12__repr__(struct __pyx_memoryview_obj *__pyx_v_self); /* proto */
-static PyObject *__pyx_memoryview___pyx_pf_15View_dot_MemoryView_10memoryview_14__str__(struct __pyx_memoryview_obj *__pyx_v_self); /* proto */
-static PyObject *__pyx_memoryview___pyx_pf_15View_dot_MemoryView_10memoryview_16is_c_contig(struct __pyx_memoryview_obj *__pyx_v_self); /* proto */
-static PyObject *__pyx_memoryview___pyx_pf_15View_dot_MemoryView_10memoryview_18is_f_contig(struct __pyx_memoryview_obj *__pyx_v_self); /* proto */
-static PyObject *__pyx_memoryview___pyx_pf_15View_dot_MemoryView_10memoryview_20copy(struct __pyx_memoryview_obj *__pyx_v_self); /* proto */
-static PyObject *__pyx_memoryview___pyx_pf_15View_dot_MemoryView_10memoryview_22copy_fortran(struct __pyx_memoryview_obj *__pyx_v_self); /* proto */
-static PyObject *__pyx_pf___pyx_memoryview___reduce_cython__(CYTHON_UNUSED struct __pyx_memoryview_obj *__pyx_v_self); /* proto */
-static PyObject *__pyx_pf___pyx_memoryview_2__setstate_cython__(CYTHON_UNUSED struct __pyx_memoryview_obj *__pyx_v_self, CYTHON_UNUSED PyObject *__pyx_v___pyx_state); /* proto */
-static void __pyx_memoryviewslice___pyx_pf_15View_dot_MemoryView_16_memoryviewslice___dealloc__(struct __pyx_memoryviewslice_obj *__pyx_v_self); /* proto */
-static PyObject *__pyx_pf_15View_dot_MemoryView_16_memoryviewslice_4base___get__(struct __pyx_memoryviewslice_obj *__pyx_v_self); /* proto */
-static PyObject *__pyx_pf___pyx_memoryviewslice___reduce_cython__(CYTHON_UNUSED struct __pyx_memoryviewslice_obj *__pyx_v_self); /* proto */
-static PyObject *__pyx_pf___pyx_memoryviewslice_2__setstate_cython__(CYTHON_UNUSED struct __pyx_memoryviewslice_obj *__pyx_v_self, CYTHON_UNUSED PyObject *__pyx_v___pyx_state); /* proto */
-static PyObject *__pyx_pf_15View_dot_MemoryView___pyx_unpickle_Enum(CYTHON_UNUSED PyObject *__pyx_self, PyObject *__pyx_v___pyx_type, long __pyx_v___pyx_checksum, PyObject *__pyx_v___pyx_state); /* proto */
-static PyObject *__pyx_tp_new_array(PyTypeObject *t, PyObject *a, PyObject *k); /*proto*/
-static PyObject *__pyx_tp_new_Enum(PyTypeObject *t, PyObject *a, PyObject *k); /*proto*/
-static PyObject *__pyx_tp_new_memoryview(PyTypeObject *t, PyObject *a, PyObject *k); /*proto*/
-static PyObject *__pyx_tp_new__memoryviewslice(PyTypeObject *t, PyObject *a, PyObject *k); /*proto*/
-static PyObject *__pyx_int_0;
-static PyObject *__pyx_int_1;
-static PyObject *__pyx_int_184977713;
-static PyObject *__pyx_int_neg_1;
-static float __pyx_k_;
-static PyObject *__pyx_tuple__2;
-static PyObject *__pyx_tuple__3;
-static PyObject *__pyx_tuple__4;
-static PyObject *__pyx_tuple__5;
-static PyObject *__pyx_tuple__6;
-static PyObject *__pyx_tuple__7;
-static PyObject *__pyx_tuple__8;
-static PyObject *__pyx_tuple__9;
-static PyObject *__pyx_slice__16;
-static PyObject *__pyx_tuple__10;
-static PyObject *__pyx_tuple__11;
-static PyObject *__pyx_tuple__12;
-static PyObject *__pyx_tuple__13;
-static PyObject *__pyx_tuple__14;
-static PyObject *__pyx_tuple__15;
-static PyObject *__pyx_tuple__17;
-static PyObject *__pyx_tuple__18;
-static PyObject *__pyx_tuple__19;
-static PyObject *__pyx_tuple__20;
-static PyObject *__pyx_tuple__21;
-static PyObject *__pyx_tuple__22;
-static PyObject *__pyx_tuple__23;
-static PyObject *__pyx_tuple__24;
-static PyObject *__pyx_tuple__25;
-static PyObject *__pyx_codeobj__26;
-/* Late includes */
-
-/* "monotonic_align/core.pyx":7
- * @cython.boundscheck(False)
- * @cython.wraparound(False)
- * cdef void maximum_path_each(int[:,::1] path, float[:,::1] value, int t_y, int t_x, float max_neg_val=-1e9) nogil: # <<<<<<<<<<<<<<
- * cdef int x
- * cdef int y
- */
-
-static void __pyx_f_15monotonic_align_4core_maximum_path_each(__Pyx_memviewslice __pyx_v_path, __Pyx_memviewslice __pyx_v_value, int __pyx_v_t_y, int __pyx_v_t_x, struct __pyx_opt_args_15monotonic_align_4core_maximum_path_each *__pyx_optional_args) {
- float __pyx_v_max_neg_val = __pyx_k_;
- int __pyx_v_x;
- int __pyx_v_y;
- float __pyx_v_v_prev;
- float __pyx_v_v_cur;
- int __pyx_v_index;
- int __pyx_t_1;
- int __pyx_t_2;
- int __pyx_t_3;
- long __pyx_t_4;
- int __pyx_t_5;
- long __pyx_t_6;
- long __pyx_t_7;
- int __pyx_t_8;
- Py_ssize_t __pyx_t_9;
- Py_ssize_t __pyx_t_10;
- float __pyx_t_11;
- float __pyx_t_12;
- float __pyx_t_13;
- int __pyx_t_14;
- Py_ssize_t __pyx_t_15;
- Py_ssize_t __pyx_t_16;
- if (__pyx_optional_args) {
- if (__pyx_optional_args->__pyx_n > 0) {
- __pyx_v_max_neg_val = __pyx_optional_args->max_neg_val;
- }
- }
-
- /* "monotonic_align/core.pyx":13
- * cdef float v_cur
- * cdef float tmp
- * cdef int index = t_x - 1 # <<<<<<<<<<<<<<
- *
- * for y in range(t_y):
- */
- __pyx_v_index = (__pyx_v_t_x - 1);
-
- /* "monotonic_align/core.pyx":15
- * cdef int index = t_x - 1
- *
- * for y in range(t_y): # <<<<<<<<<<<<<<
- * for x in range(max(0, t_x + y - t_y), min(t_x, y + 1)):
- * if x == y:
- */
- __pyx_t_1 = __pyx_v_t_y;
- __pyx_t_2 = __pyx_t_1;
- for (__pyx_t_3 = 0; __pyx_t_3 < __pyx_t_2; __pyx_t_3+=1) {
- __pyx_v_y = __pyx_t_3;
-
- /* "monotonic_align/core.pyx":16
- *
- * for y in range(t_y):
- * for x in range(max(0, t_x + y - t_y), min(t_x, y + 1)): # <<<<<<<<<<<<<<
- * if x == y:
- * v_cur = max_neg_val
- */
- __pyx_t_4 = (__pyx_v_y + 1);
- __pyx_t_5 = __pyx_v_t_x;
- if (((__pyx_t_4 < __pyx_t_5) != 0)) {
- __pyx_t_6 = __pyx_t_4;
- } else {
- __pyx_t_6 = __pyx_t_5;
- }
- __pyx_t_4 = __pyx_t_6;
- __pyx_t_5 = ((__pyx_v_t_x + __pyx_v_y) - __pyx_v_t_y);
- __pyx_t_6 = 0;
- if (((__pyx_t_5 > __pyx_t_6) != 0)) {
- __pyx_t_7 = __pyx_t_5;
- } else {
- __pyx_t_7 = __pyx_t_6;
- }
- __pyx_t_6 = __pyx_t_4;
- for (__pyx_t_5 = __pyx_t_7; __pyx_t_5 < __pyx_t_6; __pyx_t_5+=1) {
- __pyx_v_x = __pyx_t_5;
-
- /* "monotonic_align/core.pyx":17
- * for y in range(t_y):
- * for x in range(max(0, t_x + y - t_y), min(t_x, y + 1)):
- * if x == y: # <<<<<<<<<<<<<<
- * v_cur = max_neg_val
- * else:
- */
- __pyx_t_8 = ((__pyx_v_x == __pyx_v_y) != 0);
- if (__pyx_t_8) {
-
- /* "monotonic_align/core.pyx":18
- * for x in range(max(0, t_x + y - t_y), min(t_x, y + 1)):
- * if x == y:
- * v_cur = max_neg_val # <<<<<<<<<<<<<<
- * else:
- * v_cur = value[y-1, x]
- */
- __pyx_v_v_cur = __pyx_v_max_neg_val;
-
- /* "monotonic_align/core.pyx":17
- * for y in range(t_y):
- * for x in range(max(0, t_x + y - t_y), min(t_x, y + 1)):
- * if x == y: # <<<<<<<<<<<<<<
- * v_cur = max_neg_val
- * else:
- */
- goto __pyx_L7;
- }
-
- /* "monotonic_align/core.pyx":20
- * v_cur = max_neg_val
- * else:
- * v_cur = value[y-1, x] # <<<<<<<<<<<<<<
- * if x == 0:
- * if y == 0:
- */
- /*else*/ {
- __pyx_t_9 = (__pyx_v_y - 1);
- __pyx_t_10 = __pyx_v_x;
- __pyx_v_v_cur = (*((float *) ( /* dim=1 */ ((char *) (((float *) ( /* dim=0 */ (__pyx_v_value.data + __pyx_t_9 * __pyx_v_value.strides[0]) )) + __pyx_t_10)) )));
- }
- __pyx_L7:;
-
- /* "monotonic_align/core.pyx":21
- * else:
- * v_cur = value[y-1, x]
- * if x == 0: # <<<<<<<<<<<<<<
- * if y == 0:
- * v_prev = 0.
- */
- __pyx_t_8 = ((__pyx_v_x == 0) != 0);
- if (__pyx_t_8) {
-
- /* "monotonic_align/core.pyx":22
- * v_cur = value[y-1, x]
- * if x == 0:
- * if y == 0: # <<<<<<<<<<<<<<
- * v_prev = 0.
- * else:
- */
- __pyx_t_8 = ((__pyx_v_y == 0) != 0);
- if (__pyx_t_8) {
-
- /* "monotonic_align/core.pyx":23
- * if x == 0:
- * if y == 0:
- * v_prev = 0. # <<<<<<<<<<<<<<
- * else:
- * v_prev = max_neg_val
- */
- __pyx_v_v_prev = 0.;
-
- /* "monotonic_align/core.pyx":22
- * v_cur = value[y-1, x]
- * if x == 0:
- * if y == 0: # <<<<<<<<<<<<<<
- * v_prev = 0.
- * else:
- */
- goto __pyx_L9;
- }
-
- /* "monotonic_align/core.pyx":25
- * v_prev = 0.
- * else:
- * v_prev = max_neg_val # <<<<<<<<<<<<<<
- * else:
- * v_prev = value[y-1, x-1]
- */
- /*else*/ {
- __pyx_v_v_prev = __pyx_v_max_neg_val;
- }
- __pyx_L9:;
-
- /* "monotonic_align/core.pyx":21
- * else:
- * v_cur = value[y-1, x]
- * if x == 0: # <<<<<<<<<<<<<<
- * if y == 0:
- * v_prev = 0.
- */
- goto __pyx_L8;
- }
-
- /* "monotonic_align/core.pyx":27
- * v_prev = max_neg_val
- * else:
- * v_prev = value[y-1, x-1] # <<<<<<<<<<<<<<
- * value[y, x] += max(v_prev, v_cur)
- *
- */
- /*else*/ {
- __pyx_t_10 = (__pyx_v_y - 1);
- __pyx_t_9 = (__pyx_v_x - 1);
- __pyx_v_v_prev = (*((float *) ( /* dim=1 */ ((char *) (((float *) ( /* dim=0 */ (__pyx_v_value.data + __pyx_t_10 * __pyx_v_value.strides[0]) )) + __pyx_t_9)) )));
- }
- __pyx_L8:;
-
- /* "monotonic_align/core.pyx":28
- * else:
- * v_prev = value[y-1, x-1]
- * value[y, x] += max(v_prev, v_cur) # <<<<<<<<<<<<<<
- *
- * for y in range(t_y - 1, -1, -1):
- */
- __pyx_t_11 = __pyx_v_v_cur;
- __pyx_t_12 = __pyx_v_v_prev;
- if (((__pyx_t_11 > __pyx_t_12) != 0)) {
- __pyx_t_13 = __pyx_t_11;
- } else {
- __pyx_t_13 = __pyx_t_12;
- }
- __pyx_t_9 = __pyx_v_y;
- __pyx_t_10 = __pyx_v_x;
- *((float *) ( /* dim=1 */ ((char *) (((float *) ( /* dim=0 */ (__pyx_v_value.data + __pyx_t_9 * __pyx_v_value.strides[0]) )) + __pyx_t_10)) )) += __pyx_t_13;
- }
- }
-
- /* "monotonic_align/core.pyx":30
- * value[y, x] += max(v_prev, v_cur)
- *
- * for y in range(t_y - 1, -1, -1): # <<<<<<<<<<<<<<
- * path[y, index] = 1
- * if index != 0 and (index == y or value[y-1, index] < value[y-1, index-1]):
- */
- for (__pyx_t_1 = (__pyx_v_t_y - 1); __pyx_t_1 > -1; __pyx_t_1-=1) {
- __pyx_v_y = __pyx_t_1;
-
- /* "monotonic_align/core.pyx":31
- *
- * for y in range(t_y - 1, -1, -1):
- * path[y, index] = 1 # <<<<<<<<<<<<<<
- * if index != 0 and (index == y or value[y-1, index] < value[y-1, index-1]):
- * index = index - 1
- */
- __pyx_t_10 = __pyx_v_y;
- __pyx_t_9 = __pyx_v_index;
- *((int *) ( /* dim=1 */ ((char *) (((int *) ( /* dim=0 */ (__pyx_v_path.data + __pyx_t_10 * __pyx_v_path.strides[0]) )) + __pyx_t_9)) )) = 1;
-
- /* "monotonic_align/core.pyx":32
- * for y in range(t_y - 1, -1, -1):
- * path[y, index] = 1
- * if index != 0 and (index == y or value[y-1, index] < value[y-1, index-1]): # <<<<<<<<<<<<<<
- * index = index - 1
- *
- */
- __pyx_t_14 = ((__pyx_v_index != 0) != 0);
- if (__pyx_t_14) {
- } else {
- __pyx_t_8 = __pyx_t_14;
- goto __pyx_L13_bool_binop_done;
- }
- __pyx_t_14 = ((__pyx_v_index == __pyx_v_y) != 0);
- if (!__pyx_t_14) {
- } else {
- __pyx_t_8 = __pyx_t_14;
- goto __pyx_L13_bool_binop_done;
- }
- __pyx_t_9 = (__pyx_v_y - 1);
- __pyx_t_10 = __pyx_v_index;
- __pyx_t_15 = (__pyx_v_y - 1);
- __pyx_t_16 = (__pyx_v_index - 1);
- __pyx_t_14 = (((*((float *) ( /* dim=1 */ ((char *) (((float *) ( /* dim=0 */ (__pyx_v_value.data + __pyx_t_9 * __pyx_v_value.strides[0]) )) + __pyx_t_10)) ))) < (*((float *) ( /* dim=1 */ ((char *) (((float *) ( /* dim=0 */ (__pyx_v_value.data + __pyx_t_15 * __pyx_v_value.strides[0]) )) + __pyx_t_16)) )))) != 0);
- __pyx_t_8 = __pyx_t_14;
- __pyx_L13_bool_binop_done:;
- if (__pyx_t_8) {
-
- /* "monotonic_align/core.pyx":33
- * path[y, index] = 1
- * if index != 0 and (index == y or value[y-1, index] < value[y-1, index-1]):
- * index = index - 1 # <<<<<<<<<<<<<<
- *
- *
- */
- __pyx_v_index = (__pyx_v_index - 1);
-
- /* "monotonic_align/core.pyx":32
- * for y in range(t_y - 1, -1, -1):
- * path[y, index] = 1
- * if index != 0 and (index == y or value[y-1, index] < value[y-1, index-1]): # <<<<<<<<<<<<<<
- * index = index - 1
- *
- */
- }
- }
-
- /* "monotonic_align/core.pyx":7
- * @cython.boundscheck(False)
- * @cython.wraparound(False)
- * cdef void maximum_path_each(int[:,::1] path, float[:,::1] value, int t_y, int t_x, float max_neg_val=-1e9) nogil: # <<<<<<<<<<<<<<
- * cdef int x
- * cdef int y
- */
-
- /* function exit code */
-}
-
-/* "monotonic_align/core.pyx":38
- * @cython.boundscheck(False)
- * @cython.wraparound(False)
- * cpdef void maximum_path_c(int[:,:,::1] paths, float[:,:,::1] values, int[::1] t_ys, int[::1] t_xs) nogil: # <<<<<<<<<<<<<<
- * cdef int b = paths.shape[0]
- * cdef int i
- */
-
-static PyObject *__pyx_pw_15monotonic_align_4core_1maximum_path_c(PyObject *__pyx_self, PyObject *__pyx_args, PyObject *__pyx_kwds); /*proto*/
-static void __pyx_f_15monotonic_align_4core_maximum_path_c(__Pyx_memviewslice __pyx_v_paths, __Pyx_memviewslice __pyx_v_values, __Pyx_memviewslice __pyx_v_t_ys, __Pyx_memviewslice __pyx_v_t_xs, CYTHON_UNUSED int __pyx_skip_dispatch) {
- CYTHON_UNUSED int __pyx_v_b;
- int __pyx_v_i;
- int __pyx_t_1;
- int __pyx_t_2;
- int __pyx_t_3;
- __Pyx_memviewslice __pyx_t_4 = { 0, 0, { 0 }, { 0 }, { 0 } };
- __Pyx_memviewslice __pyx_t_5 = { 0, 0, { 0 }, { 0 }, { 0 } };
- Py_ssize_t __pyx_t_6;
- Py_ssize_t __pyx_t_7;
-
- /* "monotonic_align/core.pyx":39
- * @cython.wraparound(False)
- * cpdef void maximum_path_c(int[:,:,::1] paths, float[:,:,::1] values, int[::1] t_ys, int[::1] t_xs) nogil:
- * cdef int b = paths.shape[0] # <<<<<<<<<<<<<<
- * cdef int i
- * for i in prange(b, nogil=True):
- */
- __pyx_v_b = (__pyx_v_paths.shape[0]);
-
- /* "monotonic_align/core.pyx":41
- * cdef int b = paths.shape[0]
- * cdef int i
- * for i in prange(b, nogil=True): # <<<<<<<<<<<<<<
- * maximum_path_each(paths[i], values[i], t_ys[i], t_xs[i])
- */
- {
- #ifdef WITH_THREAD
- PyThreadState *_save;
- Py_UNBLOCK_THREADS
- __Pyx_FastGIL_Remember();
- #endif
- /*try:*/ {
- __pyx_t_1 = __pyx_v_b;
- if ((1 == 0)) abort();
- {
- #if ((defined(__APPLE__) || defined(__OSX__)) && (defined(__GNUC__) && (__GNUC__ > 2 || (__GNUC__ == 2 && (__GNUC_MINOR__ > 95)))))
- #undef likely
- #undef unlikely
- #define likely(x) (x)
- #define unlikely(x) (x)
- #endif
- __pyx_t_3 = (__pyx_t_1 - 0 + 1 - 1/abs(1)) / 1;
- if (__pyx_t_3 > 0)
- {
- #ifdef _OPENMP
- #pragma omp parallel private(__pyx_t_6, __pyx_t_7) firstprivate(__pyx_t_4, __pyx_t_5)
- #endif /* _OPENMP */
- {
- #ifdef _OPENMP
- #pragma omp for firstprivate(__pyx_v_i) lastprivate(__pyx_v_i)
- #endif /* _OPENMP */
- for (__pyx_t_2 = 0; __pyx_t_2 < __pyx_t_3; __pyx_t_2++){
- {
- __pyx_v_i = (int)(0 + 1 * __pyx_t_2);
-
- /* "monotonic_align/core.pyx":42
- * cdef int i
- * for i in prange(b, nogil=True):
- * maximum_path_each(paths[i], values[i], t_ys[i], t_xs[i]) # <<<<<<<<<<<<<<
- */
- __pyx_t_4.data = __pyx_v_paths.data;
- __pyx_t_4.memview = __pyx_v_paths.memview;
- __PYX_INC_MEMVIEW(&__pyx_t_4, 0);
- {
- Py_ssize_t __pyx_tmp_idx = __pyx_v_i;
- Py_ssize_t __pyx_tmp_stride = __pyx_v_paths.strides[0];
- __pyx_t_4.data += __pyx_tmp_idx * __pyx_tmp_stride;
-}
-
-__pyx_t_4.shape[0] = __pyx_v_paths.shape[1];
-__pyx_t_4.strides[0] = __pyx_v_paths.strides[1];
- __pyx_t_4.suboffsets[0] = -1;
-
-__pyx_t_4.shape[1] = __pyx_v_paths.shape[2];
-__pyx_t_4.strides[1] = __pyx_v_paths.strides[2];
- __pyx_t_4.suboffsets[1] = -1;
-
-__pyx_t_5.data = __pyx_v_values.data;
- __pyx_t_5.memview = __pyx_v_values.memview;
- __PYX_INC_MEMVIEW(&__pyx_t_5, 0);
- {
- Py_ssize_t __pyx_tmp_idx = __pyx_v_i;
- Py_ssize_t __pyx_tmp_stride = __pyx_v_values.strides[0];
- __pyx_t_5.data += __pyx_tmp_idx * __pyx_tmp_stride;
-}
-
-__pyx_t_5.shape[0] = __pyx_v_values.shape[1];
-__pyx_t_5.strides[0] = __pyx_v_values.strides[1];
- __pyx_t_5.suboffsets[0] = -1;
-
-__pyx_t_5.shape[1] = __pyx_v_values.shape[2];
-__pyx_t_5.strides[1] = __pyx_v_values.strides[2];
- __pyx_t_5.suboffsets[1] = -1;
-
-__pyx_t_6 = __pyx_v_i;
- __pyx_t_7 = __pyx_v_i;
- __pyx_f_15monotonic_align_4core_maximum_path_each(__pyx_t_4, __pyx_t_5, (*((int *) ( /* dim=0 */ ((char *) (((int *) __pyx_v_t_ys.data) + __pyx_t_6)) ))), (*((int *) ( /* dim=0 */ ((char *) (((int *) __pyx_v_t_xs.data) + __pyx_t_7)) ))), NULL);
- __PYX_XDEC_MEMVIEW(&__pyx_t_4, 0);
- __pyx_t_4.memview = NULL;
- __pyx_t_4.data = NULL;
- __PYX_XDEC_MEMVIEW(&__pyx_t_5, 0);
- __pyx_t_5.memview = NULL;
- __pyx_t_5.data = NULL;
- }
- }
- }
- }
- }
- #if ((defined(__APPLE__) || defined(__OSX__)) && (defined(__GNUC__) && (__GNUC__ > 2 || (__GNUC__ == 2 && (__GNUC_MINOR__ > 95)))))
- #undef likely
- #undef unlikely
- #define likely(x) __builtin_expect(!!(x), 1)
- #define unlikely(x) __builtin_expect(!!(x), 0)
- #endif
- }
-
- /* "monotonic_align/core.pyx":41
- * cdef int b = paths.shape[0]
- * cdef int i
- * for i in prange(b, nogil=True): # <<<<<<<<<<<<<<
- * maximum_path_each(paths[i], values[i], t_ys[i], t_xs[i])
- */
- /*finally:*/ {
- /*normal exit:*/{
- #ifdef WITH_THREAD
- __Pyx_FastGIL_Forget();
- Py_BLOCK_THREADS
- #endif
- goto __pyx_L5;
- }
- __pyx_L5:;
- }
- }
-
- /* "monotonic_align/core.pyx":38
- * @cython.boundscheck(False)
- * @cython.wraparound(False)
- * cpdef void maximum_path_c(int[:,:,::1] paths, float[:,:,::1] values, int[::1] t_ys, int[::1] t_xs) nogil: # <<<<<<<<<<<<<<
- * cdef int b = paths.shape[0]
- * cdef int i
- */
-
- /* function exit code */
-}
-
-/* Python wrapper */
-static PyObject *__pyx_pw_15monotonic_align_4core_1maximum_path_c(PyObject *__pyx_self, PyObject *__pyx_args, PyObject *__pyx_kwds); /*proto*/
-static PyObject *__pyx_pw_15monotonic_align_4core_1maximum_path_c(PyObject *__pyx_self, PyObject *__pyx_args, PyObject *__pyx_kwds) {
- __Pyx_memviewslice __pyx_v_paths = { 0, 0, { 0 }, { 0 }, { 0 } };
- __Pyx_memviewslice __pyx_v_values = { 0, 0, { 0 }, { 0 }, { 0 } };
- __Pyx_memviewslice __pyx_v_t_ys = { 0, 0, { 0 }, { 0 }, { 0 } };
- __Pyx_memviewslice __pyx_v_t_xs = { 0, 0, { 0 }, { 0 }, { 0 } };
- int __pyx_lineno = 0;
- const char *__pyx_filename = NULL;
- int __pyx_clineno = 0;
- PyObject *__pyx_r = 0;
- __Pyx_RefNannyDeclarations
- __Pyx_RefNannySetupContext("maximum_path_c (wrapper)", 0);
- {
- static PyObject **__pyx_pyargnames[] = {&__pyx_n_s_paths,&__pyx_n_s_values,&__pyx_n_s_t_ys,&__pyx_n_s_t_xs,0};
- PyObject* values[4] = {0,0,0,0};
- if (unlikely(__pyx_kwds)) {
- Py_ssize_t kw_args;
- const Py_ssize_t pos_args = PyTuple_GET_SIZE(__pyx_args);
- switch (pos_args) {
- case 4: values[3] = PyTuple_GET_ITEM(__pyx_args, 3);
- CYTHON_FALLTHROUGH;
- case 3: values[2] = PyTuple_GET_ITEM(__pyx_args, 2);
- CYTHON_FALLTHROUGH;
- case 2: values[1] = PyTuple_GET_ITEM(__pyx_args, 1);
- CYTHON_FALLTHROUGH;
- case 1: values[0] = PyTuple_GET_ITEM(__pyx_args, 0);
- CYTHON_FALLTHROUGH;
- case 0: break;
- default: goto __pyx_L5_argtuple_error;
- }
- kw_args = PyDict_Size(__pyx_kwds);
- switch (pos_args) {
- case 0:
- if (likely((values[0] = __Pyx_PyDict_GetItemStr(__pyx_kwds, __pyx_n_s_paths)) != 0)) kw_args--;
- else goto __pyx_L5_argtuple_error;
- CYTHON_FALLTHROUGH;
- case 1:
- if (likely((values[1] = __Pyx_PyDict_GetItemStr(__pyx_kwds, __pyx_n_s_values)) != 0)) kw_args--;
- else {
- __Pyx_RaiseArgtupleInvalid("maximum_path_c", 1, 4, 4, 1); __PYX_ERR(0, 38, __pyx_L3_error)
- }
- CYTHON_FALLTHROUGH;
- case 2:
- if (likely((values[2] = __Pyx_PyDict_GetItemStr(__pyx_kwds, __pyx_n_s_t_ys)) != 0)) kw_args--;
- else {
- __Pyx_RaiseArgtupleInvalid("maximum_path_c", 1, 4, 4, 2); __PYX_ERR(0, 38, __pyx_L3_error)
- }
- CYTHON_FALLTHROUGH;
- case 3:
- if (likely((values[3] = __Pyx_PyDict_GetItemStr(__pyx_kwds, __pyx_n_s_t_xs)) != 0)) kw_args--;
- else {
- __Pyx_RaiseArgtupleInvalid("maximum_path_c", 1, 4, 4, 3); __PYX_ERR(0, 38, __pyx_L3_error)
- }
- }
- if (unlikely(kw_args > 0)) {
- if (unlikely(__Pyx_ParseOptionalKeywords(__pyx_kwds, __pyx_pyargnames, 0, values, pos_args, "maximum_path_c") < 0)) __PYX_ERR(0, 38, __pyx_L3_error)
- }
- } else if (PyTuple_GET_SIZE(__pyx_args) != 4) {
- goto __pyx_L5_argtuple_error;
- } else {
- values[0] = PyTuple_GET_ITEM(__pyx_args, 0);
- values[1] = PyTuple_GET_ITEM(__pyx_args, 1);
- values[2] = PyTuple_GET_ITEM(__pyx_args, 2);
- values[3] = PyTuple_GET_ITEM(__pyx_args, 3);
- }
- __pyx_v_paths = __Pyx_PyObject_to_MemoryviewSlice_d_d_dc_int(values[0], PyBUF_WRITABLE); if (unlikely(!__pyx_v_paths.memview)) __PYX_ERR(0, 38, __pyx_L3_error)
- __pyx_v_values = __Pyx_PyObject_to_MemoryviewSlice_d_d_dc_float(values[1], PyBUF_WRITABLE); if (unlikely(!__pyx_v_values.memview)) __PYX_ERR(0, 38, __pyx_L3_error)
- __pyx_v_t_ys = __Pyx_PyObject_to_MemoryviewSlice_dc_int(values[2], PyBUF_WRITABLE); if (unlikely(!__pyx_v_t_ys.memview)) __PYX_ERR(0, 38, __pyx_L3_error)
- __pyx_v_t_xs = __Pyx_PyObject_to_MemoryviewSlice_dc_int(values[3], PyBUF_WRITABLE); if (unlikely(!__pyx_v_t_xs.memview)) __PYX_ERR(0, 38, __pyx_L3_error)
- }
- goto __pyx_L4_argument_unpacking_done;
- __pyx_L5_argtuple_error:;
- __Pyx_RaiseArgtupleInvalid("maximum_path_c", 1, 4, 4, PyTuple_GET_SIZE(__pyx_args)); __PYX_ERR(0, 38, __pyx_L3_error)
- __pyx_L3_error:;
- __Pyx_AddTraceback("monotonic_align.core.maximum_path_c", __pyx_clineno, __pyx_lineno, __pyx_filename);
- __Pyx_RefNannyFinishContext();
- return NULL;
- __pyx_L4_argument_unpacking_done:;
- __pyx_r = __pyx_pf_15monotonic_align_4core_maximum_path_c(__pyx_self, __pyx_v_paths, __pyx_v_values, __pyx_v_t_ys, __pyx_v_t_xs);
-
- /* function exit code */
- __Pyx_RefNannyFinishContext();
- return __pyx_r;
-}
-
-static PyObject *__pyx_pf_15monotonic_align_4core_maximum_path_c(CYTHON_UNUSED PyObject *__pyx_self, __Pyx_memviewslice __pyx_v_paths, __Pyx_memviewslice __pyx_v_values, __Pyx_memviewslice __pyx_v_t_ys, __Pyx_memviewslice __pyx_v_t_xs) {
- PyObject *__pyx_r = NULL;
- __Pyx_RefNannyDeclarations
- PyObject *__pyx_t_1 = NULL;
- int __pyx_lineno = 0;
- const char *__pyx_filename = NULL;
- int __pyx_clineno = 0;
- __Pyx_RefNannySetupContext("maximum_path_c", 0);
- __Pyx_XDECREF(__pyx_r);
- if (unlikely(!__pyx_v_paths.memview)) { __Pyx_RaiseUnboundLocalError("paths"); __PYX_ERR(0, 38, __pyx_L1_error) }
- if (unlikely(!__pyx_v_values.memview)) { __Pyx_RaiseUnboundLocalError("values"); __PYX_ERR(0, 38, __pyx_L1_error) }
- if (unlikely(!__pyx_v_t_ys.memview)) { __Pyx_RaiseUnboundLocalError("t_ys"); __PYX_ERR(0, 38, __pyx_L1_error) }
- if (unlikely(!__pyx_v_t_xs.memview)) { __Pyx_RaiseUnboundLocalError("t_xs"); __PYX_ERR(0, 38, __pyx_L1_error) }
- __pyx_t_1 = __Pyx_void_to_None(__pyx_f_15monotonic_align_4core_maximum_path_c(__pyx_v_paths, __pyx_v_values, __pyx_v_t_ys, __pyx_v_t_xs, 0)); if (unlikely(!__pyx_t_1)) __PYX_ERR(0, 38, __pyx_L1_error)
- __Pyx_GOTREF(__pyx_t_1);
- __pyx_r = __pyx_t_1;
- __pyx_t_1 = 0;
- goto __pyx_L0;
-
- /* function exit code */
- __pyx_L1_error:;
- __Pyx_XDECREF(__pyx_t_1);
- __Pyx_AddTraceback("monotonic_align.core.maximum_path_c", __pyx_clineno, __pyx_lineno, __pyx_filename);
- __pyx_r = NULL;
- __pyx_L0:;
- __PYX_XDEC_MEMVIEW(&__pyx_v_paths, 1);
- __PYX_XDEC_MEMVIEW(&__pyx_v_values, 1);
- __PYX_XDEC_MEMVIEW(&__pyx_v_t_ys, 1);
- __PYX_XDEC_MEMVIEW(&__pyx_v_t_xs, 1);
- __Pyx_XGIVEREF(__pyx_r);
- __Pyx_RefNannyFinishContext();
- return __pyx_r;
-}
-
-/* "View.MemoryView":122
- * cdef bint dtype_is_object
- *
- * def __cinit__(array self, tuple shape, Py_ssize_t itemsize, format not None, # <<<<<<<<<<<<<<
- * mode="c", bint allocate_buffer=True):
- *
- */
-
-/* Python wrapper */
-static int __pyx_array___cinit__(PyObject *__pyx_v_self, PyObject *__pyx_args, PyObject *__pyx_kwds); /*proto*/
-static int __pyx_array___cinit__(PyObject *__pyx_v_self, PyObject *__pyx_args, PyObject *__pyx_kwds) {
- PyObject *__pyx_v_shape = 0;
- Py_ssize_t __pyx_v_itemsize;
- PyObject *__pyx_v_format = 0;
- PyObject *__pyx_v_mode = 0;
- int __pyx_v_allocate_buffer;
- int __pyx_lineno = 0;
- const char *__pyx_filename = NULL;
- int __pyx_clineno = 0;
- int __pyx_r;
- __Pyx_RefNannyDeclarations
- __Pyx_RefNannySetupContext("__cinit__ (wrapper)", 0);
- {
- static PyObject **__pyx_pyargnames[] = {&__pyx_n_s_shape,&__pyx_n_s_itemsize,&__pyx_n_s_format,&__pyx_n_s_mode,&__pyx_n_s_allocate_buffer,0};
- PyObject* values[5] = {0,0,0,0,0};
- values[3] = ((PyObject *)__pyx_n_s_c);
- if (unlikely(__pyx_kwds)) {
- Py_ssize_t kw_args;
- const Py_ssize_t pos_args = PyTuple_GET_SIZE(__pyx_args);
- switch (pos_args) {
- case 5: values[4] = PyTuple_GET_ITEM(__pyx_args, 4);
- CYTHON_FALLTHROUGH;
- case 4: values[3] = PyTuple_GET_ITEM(__pyx_args, 3);
- CYTHON_FALLTHROUGH;
- case 3: values[2] = PyTuple_GET_ITEM(__pyx_args, 2);
- CYTHON_FALLTHROUGH;
- case 2: values[1] = PyTuple_GET_ITEM(__pyx_args, 1);
- CYTHON_FALLTHROUGH;
- case 1: values[0] = PyTuple_GET_ITEM(__pyx_args, 0);
- CYTHON_FALLTHROUGH;
- case 0: break;
- default: goto __pyx_L5_argtuple_error;
- }
- kw_args = PyDict_Size(__pyx_kwds);
- switch (pos_args) {
- case 0:
- if (likely((values[0] = __Pyx_PyDict_GetItemStr(__pyx_kwds, __pyx_n_s_shape)) != 0)) kw_args--;
- else goto __pyx_L5_argtuple_error;
- CYTHON_FALLTHROUGH;
- case 1:
- if (likely((values[1] = __Pyx_PyDict_GetItemStr(__pyx_kwds, __pyx_n_s_itemsize)) != 0)) kw_args--;
- else {
- __Pyx_RaiseArgtupleInvalid("__cinit__", 0, 3, 5, 1); __PYX_ERR(1, 122, __pyx_L3_error)
- }
- CYTHON_FALLTHROUGH;
- case 2:
- if (likely((values[2] = __Pyx_PyDict_GetItemStr(__pyx_kwds, __pyx_n_s_format)) != 0)) kw_args--;
- else {
- __Pyx_RaiseArgtupleInvalid("__cinit__", 0, 3, 5, 2); __PYX_ERR(1, 122, __pyx_L3_error)
- }
- CYTHON_FALLTHROUGH;
- case 3:
- if (kw_args > 0) {
- PyObject* value = __Pyx_PyDict_GetItemStr(__pyx_kwds, __pyx_n_s_mode);
- if (value) { values[3] = value; kw_args--; }
- }
- CYTHON_FALLTHROUGH;
- case 4:
- if (kw_args > 0) {
- PyObject* value = __Pyx_PyDict_GetItemStr(__pyx_kwds, __pyx_n_s_allocate_buffer);
- if (value) { values[4] = value; kw_args--; }
- }
- }
- if (unlikely(kw_args > 0)) {
- if (unlikely(__Pyx_ParseOptionalKeywords(__pyx_kwds, __pyx_pyargnames, 0, values, pos_args, "__cinit__") < 0)) __PYX_ERR(1, 122, __pyx_L3_error)
- }
- } else {
- switch (PyTuple_GET_SIZE(__pyx_args)) {
- case 5: values[4] = PyTuple_GET_ITEM(__pyx_args, 4);
- CYTHON_FALLTHROUGH;
- case 4: values[3] = PyTuple_GET_ITEM(__pyx_args, 3);
- CYTHON_FALLTHROUGH;
- case 3: values[2] = PyTuple_GET_ITEM(__pyx_args, 2);
- values[1] = PyTuple_GET_ITEM(__pyx_args, 1);
- values[0] = PyTuple_GET_ITEM(__pyx_args, 0);
- break;
- default: goto __pyx_L5_argtuple_error;
- }
- }
- __pyx_v_shape = ((PyObject*)values[0]);
- __pyx_v_itemsize = __Pyx_PyIndex_AsSsize_t(values[1]); if (unlikely((__pyx_v_itemsize == (Py_ssize_t)-1) && PyErr_Occurred())) __PYX_ERR(1, 122, __pyx_L3_error)
- __pyx_v_format = values[2];
- __pyx_v_mode = values[3];
- if (values[4]) {
- __pyx_v_allocate_buffer = __Pyx_PyObject_IsTrue(values[4]); if (unlikely((__pyx_v_allocate_buffer == (int)-1) && PyErr_Occurred())) __PYX_ERR(1, 123, __pyx_L3_error)
- } else {
-
- /* "View.MemoryView":123
- *
- * def __cinit__(array self, tuple shape, Py_ssize_t itemsize, format not None,
- * mode="c", bint allocate_buffer=True): # <<<<<<<<<<<<<<
- *
- * cdef int idx
- */
- __pyx_v_allocate_buffer = ((int)1);
- }
- }
- goto __pyx_L4_argument_unpacking_done;
- __pyx_L5_argtuple_error:;
- __Pyx_RaiseArgtupleInvalid("__cinit__", 0, 3, 5, PyTuple_GET_SIZE(__pyx_args)); __PYX_ERR(1, 122, __pyx_L3_error)
- __pyx_L3_error:;
- __Pyx_AddTraceback("View.MemoryView.array.__cinit__", __pyx_clineno, __pyx_lineno, __pyx_filename);
- __Pyx_RefNannyFinishContext();
- return -1;
- __pyx_L4_argument_unpacking_done:;
- if (unlikely(!__Pyx_ArgTypeTest(((PyObject *)__pyx_v_shape), (&PyTuple_Type), 1, "shape", 1))) __PYX_ERR(1, 122, __pyx_L1_error)
- if (unlikely(((PyObject *)__pyx_v_format) == Py_None)) {
- PyErr_Format(PyExc_TypeError, "Argument '%.200s' must not be None", "format"); __PYX_ERR(1, 122, __pyx_L1_error)
- }
- __pyx_r = __pyx_array___pyx_pf_15View_dot_MemoryView_5array___cinit__(((struct __pyx_array_obj *)__pyx_v_self), __pyx_v_shape, __pyx_v_itemsize, __pyx_v_format, __pyx_v_mode, __pyx_v_allocate_buffer);
-
- /* "View.MemoryView":122
- * cdef bint dtype_is_object
- *
- * def __cinit__(array self, tuple shape, Py_ssize_t itemsize, format not None, # <<<<<<<<<<<<<<
- * mode="c", bint allocate_buffer=True):
- *
- */
-
- /* function exit code */
- goto __pyx_L0;
- __pyx_L1_error:;
- __pyx_r = -1;
- __pyx_L0:;
- __Pyx_RefNannyFinishContext();
- return __pyx_r;
-}
-
-static int __pyx_array___pyx_pf_15View_dot_MemoryView_5array___cinit__(struct __pyx_array_obj *__pyx_v_self, PyObject *__pyx_v_shape, Py_ssize_t __pyx_v_itemsize, PyObject *__pyx_v_format, PyObject *__pyx_v_mode, int __pyx_v_allocate_buffer) {
- int __pyx_v_idx;
- Py_ssize_t __pyx_v_i;
- Py_ssize_t __pyx_v_dim;
- PyObject **__pyx_v_p;
- char __pyx_v_order;
- int __pyx_r;
- __Pyx_RefNannyDeclarations
- Py_ssize_t __pyx_t_1;
- int __pyx_t_2;
- PyObject *__pyx_t_3 = NULL;
- int __pyx_t_4;
- PyObject *__pyx_t_5 = NULL;
- PyObject *__pyx_t_6 = NULL;
- char *__pyx_t_7;
- int __pyx_t_8;
- Py_ssize_t __pyx_t_9;
- PyObject *__pyx_t_10 = NULL;
- Py_ssize_t __pyx_t_11;
- int __pyx_lineno = 0;
- const char *__pyx_filename = NULL;
- int __pyx_clineno = 0;
- __Pyx_RefNannySetupContext("__cinit__", 0);
- __Pyx_INCREF(__pyx_v_format);
-
- /* "View.MemoryView":129
- * cdef PyObject **p
- *
- * self.ndim = len(shape) # <<<<<<<<<<<<<<
- * self.itemsize = itemsize
- *
- */
- if (unlikely(__pyx_v_shape == Py_None)) {
- PyErr_SetString(PyExc_TypeError, "object of type 'NoneType' has no len()");
- __PYX_ERR(1, 129, __pyx_L1_error)
- }
- __pyx_t_1 = PyTuple_GET_SIZE(__pyx_v_shape); if (unlikely(__pyx_t_1 == ((Py_ssize_t)-1))) __PYX_ERR(1, 129, __pyx_L1_error)
- __pyx_v_self->ndim = ((int)__pyx_t_1);
-
- /* "View.MemoryView":130
- *
- * self.ndim = len(shape)
- * self.itemsize = itemsize # <<<<<<<<<<<<<<
- *
- * if not self.ndim:
- */
- __pyx_v_self->itemsize = __pyx_v_itemsize;
-
- /* "View.MemoryView":132
- * self.itemsize = itemsize
- *
- * if not self.ndim: # <<<<<<<<<<<<<<
- * raise ValueError("Empty shape tuple for cython.array")
- *
- */
- __pyx_t_2 = ((!(__pyx_v_self->ndim != 0)) != 0);
- if (unlikely(__pyx_t_2)) {
-
- /* "View.MemoryView":133
- *
- * if not self.ndim:
- * raise ValueError("Empty shape tuple for cython.array") # <<<<<<<<<<<<<<
- *
- * if itemsize <= 0:
- */
- __pyx_t_3 = __Pyx_PyObject_Call(__pyx_builtin_ValueError, __pyx_tuple__2, NULL); if (unlikely(!__pyx_t_3)) __PYX_ERR(1, 133, __pyx_L1_error)
- __Pyx_GOTREF(__pyx_t_3);
- __Pyx_Raise(__pyx_t_3, 0, 0, 0);
- __Pyx_DECREF(__pyx_t_3); __pyx_t_3 = 0;
- __PYX_ERR(1, 133, __pyx_L1_error)
-
- /* "View.MemoryView":132
- * self.itemsize = itemsize
- *
- * if not self.ndim: # <<<<<<<<<<<<<<
- * raise ValueError("Empty shape tuple for cython.array")
- *
- */
- }
-
- /* "View.MemoryView":135
- * raise ValueError("Empty shape tuple for cython.array")
- *
- * if itemsize <= 0: # <<<<<<<<<<<<<<
- * raise ValueError("itemsize <= 0 for cython.array")
- *
- */
- __pyx_t_2 = ((__pyx_v_itemsize <= 0) != 0);
- if (unlikely(__pyx_t_2)) {
-
- /* "View.MemoryView":136
- *
- * if itemsize <= 0:
- * raise ValueError("itemsize <= 0 for cython.array") # <<<<<<<<<<<<<<
- *
- * if not isinstance(format, bytes):
- */
- __pyx_t_3 = __Pyx_PyObject_Call(__pyx_builtin_ValueError, __pyx_tuple__3, NULL); if (unlikely(!__pyx_t_3)) __PYX_ERR(1, 136, __pyx_L1_error)
- __Pyx_GOTREF(__pyx_t_3);
- __Pyx_Raise(__pyx_t_3, 0, 0, 0);
- __Pyx_DECREF(__pyx_t_3); __pyx_t_3 = 0;
- __PYX_ERR(1, 136, __pyx_L1_error)
-
- /* "View.MemoryView":135
- * raise ValueError("Empty shape tuple for cython.array")
- *
- * if itemsize <= 0: # <<<<<<<<<<<<<<
- * raise ValueError("itemsize <= 0 for cython.array")
- *
- */
- }
-
- /* "View.MemoryView":138
- * raise ValueError("itemsize <= 0 for cython.array")
- *
- * if not isinstance(format, bytes): # <<<<<<<<<<<<<<
- * format = format.encode('ASCII')
- * self._format = format # keep a reference to the byte string
- */
- __pyx_t_2 = PyBytes_Check(__pyx_v_format);
- __pyx_t_4 = ((!(__pyx_t_2 != 0)) != 0);
- if (__pyx_t_4) {
-
- /* "View.MemoryView":139
- *
- * if not isinstance(format, bytes):
- * format = format.encode('ASCII') # <<<<<<<<<<<<<<
- * self._format = format # keep a reference to the byte string
- * self.format = self._format
- */
- __pyx_t_5 = __Pyx_PyObject_GetAttrStr(__pyx_v_format, __pyx_n_s_encode); if (unlikely(!__pyx_t_5)) __PYX_ERR(1, 139, __pyx_L1_error)
- __Pyx_GOTREF(__pyx_t_5);
- __pyx_t_6 = NULL;
- if (CYTHON_UNPACK_METHODS && likely(PyMethod_Check(__pyx_t_5))) {
- __pyx_t_6 = PyMethod_GET_SELF(__pyx_t_5);
- if (likely(__pyx_t_6)) {
- PyObject* function = PyMethod_GET_FUNCTION(__pyx_t_5);
- __Pyx_INCREF(__pyx_t_6);
- __Pyx_INCREF(function);
- __Pyx_DECREF_SET(__pyx_t_5, function);
- }
- }
- __pyx_t_3 = (__pyx_t_6) ? __Pyx_PyObject_Call2Args(__pyx_t_5, __pyx_t_6, __pyx_n_s_ASCII) : __Pyx_PyObject_CallOneArg(__pyx_t_5, __pyx_n_s_ASCII);
- __Pyx_XDECREF(__pyx_t_6); __pyx_t_6 = 0;
- if (unlikely(!__pyx_t_3)) __PYX_ERR(1, 139, __pyx_L1_error)
- __Pyx_GOTREF(__pyx_t_3);
- __Pyx_DECREF(__pyx_t_5); __pyx_t_5 = 0;
- __Pyx_DECREF_SET(__pyx_v_format, __pyx_t_3);
- __pyx_t_3 = 0;
-
- /* "View.MemoryView":138
- * raise ValueError("itemsize <= 0 for cython.array")
- *
- * if not isinstance(format, bytes): # <<<<<<<<<<<<<<
- * format = format.encode('ASCII')
- * self._format = format # keep a reference to the byte string
- */
- }
-
- /* "View.MemoryView":140
- * if not isinstance(format, bytes):
- * format = format.encode('ASCII')
- * self._format = format # keep a reference to the byte string # <<<<<<<<<<<<<<
- * self.format = self._format
- *
- */
- if (!(likely(PyBytes_CheckExact(__pyx_v_format))||((__pyx_v_format) == Py_None)||(PyErr_Format(PyExc_TypeError, "Expected %.16s, got %.200s", "bytes", Py_TYPE(__pyx_v_format)->tp_name), 0))) __PYX_ERR(1, 140, __pyx_L1_error)
- __pyx_t_3 = __pyx_v_format;
- __Pyx_INCREF(__pyx_t_3);
- __Pyx_GIVEREF(__pyx_t_3);
- __Pyx_GOTREF(__pyx_v_self->_format);
- __Pyx_DECREF(__pyx_v_self->_format);
- __pyx_v_self->_format = ((PyObject*)__pyx_t_3);
- __pyx_t_3 = 0;
-
- /* "View.MemoryView":141
- * format = format.encode('ASCII')
- * self._format = format # keep a reference to the byte string
- * self.format = self._format # <<<<<<<<<<<<<<
- *
- *
- */
- if (unlikely(__pyx_v_self->_format == Py_None)) {
- PyErr_SetString(PyExc_TypeError, "expected bytes, NoneType found");
- __PYX_ERR(1, 141, __pyx_L1_error)
- }
- __pyx_t_7 = __Pyx_PyBytes_AsWritableString(__pyx_v_self->_format); if (unlikely((!__pyx_t_7) && PyErr_Occurred())) __PYX_ERR(1, 141, __pyx_L1_error)
- __pyx_v_self->format = __pyx_t_7;
-
- /* "View.MemoryView":144
- *
- *
- * self._shape = PyObject_Malloc(sizeof(Py_ssize_t)*self.ndim*2) # <<<<<<<<<<<<<<
- * self._strides = self._shape + self.ndim
- *
- */
- __pyx_v_self->_shape = ((Py_ssize_t *)PyObject_Malloc((((sizeof(Py_ssize_t)) * __pyx_v_self->ndim) * 2)));
-
- /* "View.MemoryView":145
- *
- * self._shape = PyObject_Malloc(sizeof(Py_ssize_t)*self.ndim*2)
- * self._strides = self._shape + self.ndim # <<<<<<<<<<<<<<
- *
- * if not self._shape:
- */
- __pyx_v_self->_strides = (__pyx_v_self->_shape + __pyx_v_self->ndim);
-
- /* "View.MemoryView":147
- * self._strides = self._shape + self.ndim
- *
- * if not self._shape: # <<<<<<<<<<<<<<
- * raise MemoryError("unable to allocate shape and strides.")
- *
- */
- __pyx_t_4 = ((!(__pyx_v_self->_shape != 0)) != 0);
- if (unlikely(__pyx_t_4)) {
-
- /* "View.MemoryView":148
- *
- * if not self._shape:
- * raise MemoryError("unable to allocate shape and strides.") # <<<<<<<<<<<<<<
- *
- *
- */
- __pyx_t_3 = __Pyx_PyObject_Call(__pyx_builtin_MemoryError, __pyx_tuple__4, NULL); if (unlikely(!__pyx_t_3)) __PYX_ERR(1, 148, __pyx_L1_error)
- __Pyx_GOTREF(__pyx_t_3);
- __Pyx_Raise(__pyx_t_3, 0, 0, 0);
- __Pyx_DECREF(__pyx_t_3); __pyx_t_3 = 0;
- __PYX_ERR(1, 148, __pyx_L1_error)
-
- /* "View.MemoryView":147
- * self._strides = self._shape + self.ndim
- *
- * if not self._shape: # <<<<<<<<<<<<<<
- * raise MemoryError("unable to allocate shape and strides.")
- *
- */
- }
-
- /* "View.MemoryView":151
- *
- *
- * for idx, dim in enumerate(shape): # <<<<<<<<<<<<<<
- * if dim <= 0:
- * raise ValueError("Invalid shape in axis %d: %d." % (idx, dim))
- */
- __pyx_t_8 = 0;
- __pyx_t_3 = __pyx_v_shape; __Pyx_INCREF(__pyx_t_3); __pyx_t_1 = 0;
- for (;;) {
- if (__pyx_t_1 >= PyTuple_GET_SIZE(__pyx_t_3)) break;
- #if CYTHON_ASSUME_SAFE_MACROS && !CYTHON_AVOID_BORROWED_REFS
- __pyx_t_5 = PyTuple_GET_ITEM(__pyx_t_3, __pyx_t_1); __Pyx_INCREF(__pyx_t_5); __pyx_t_1++; if (unlikely(0 < 0)) __PYX_ERR(1, 151, __pyx_L1_error)
- #else
- __pyx_t_5 = PySequence_ITEM(__pyx_t_3, __pyx_t_1); __pyx_t_1++; if (unlikely(!__pyx_t_5)) __PYX_ERR(1, 151, __pyx_L1_error)
- __Pyx_GOTREF(__pyx_t_5);
- #endif
- __pyx_t_9 = __Pyx_PyIndex_AsSsize_t(__pyx_t_5); if (unlikely((__pyx_t_9 == (Py_ssize_t)-1) && PyErr_Occurred())) __PYX_ERR(1, 151, __pyx_L1_error)
- __Pyx_DECREF(__pyx_t_5); __pyx_t_5 = 0;
- __pyx_v_dim = __pyx_t_9;
- __pyx_v_idx = __pyx_t_8;
- __pyx_t_8 = (__pyx_t_8 + 1);
-
- /* "View.MemoryView":152
- *
- * for idx, dim in enumerate(shape):
- * if dim <= 0: # <<<<<<<<<<<<<<
- * raise ValueError("Invalid shape in axis %d: %d." % (idx, dim))
- * self._shape[idx] = dim
- */
- __pyx_t_4 = ((__pyx_v_dim <= 0) != 0);
- if (unlikely(__pyx_t_4)) {
-
- /* "View.MemoryView":153
- * for idx, dim in enumerate(shape):
- * if dim <= 0:
- * raise ValueError("Invalid shape in axis %d: %d." % (idx, dim)) # <<<<<<<<<<<<<<
- * self._shape[idx] = dim
- *
- */
- __pyx_t_5 = __Pyx_PyInt_From_int(__pyx_v_idx); if (unlikely(!__pyx_t_5)) __PYX_ERR(1, 153, __pyx_L1_error)
- __Pyx_GOTREF(__pyx_t_5);
- __pyx_t_6 = PyInt_FromSsize_t(__pyx_v_dim); if (unlikely(!__pyx_t_6)) __PYX_ERR(1, 153, __pyx_L1_error)
- __Pyx_GOTREF(__pyx_t_6);
- __pyx_t_10 = PyTuple_New(2); if (unlikely(!__pyx_t_10)) __PYX_ERR(1, 153, __pyx_L1_error)
- __Pyx_GOTREF(__pyx_t_10);
- __Pyx_GIVEREF(__pyx_t_5);
- PyTuple_SET_ITEM(__pyx_t_10, 0, __pyx_t_5);
- __Pyx_GIVEREF(__pyx_t_6);
- PyTuple_SET_ITEM(__pyx_t_10, 1, __pyx_t_6);
- __pyx_t_5 = 0;
- __pyx_t_6 = 0;
- __pyx_t_6 = __Pyx_PyString_Format(__pyx_kp_s_Invalid_shape_in_axis_d_d, __pyx_t_10); if (unlikely(!__pyx_t_6)) __PYX_ERR(1, 153, __pyx_L1_error)
- __Pyx_GOTREF(__pyx_t_6);
- __Pyx_DECREF(__pyx_t_10); __pyx_t_10 = 0;
- __pyx_t_10 = __Pyx_PyObject_CallOneArg(__pyx_builtin_ValueError, __pyx_t_6); if (unlikely(!__pyx_t_10)) __PYX_ERR(1, 153, __pyx_L1_error)
- __Pyx_GOTREF(__pyx_t_10);
- __Pyx_DECREF(__pyx_t_6); __pyx_t_6 = 0;
- __Pyx_Raise(__pyx_t_10, 0, 0, 0);
- __Pyx_DECREF(__pyx_t_10); __pyx_t_10 = 0;
- __PYX_ERR(1, 153, __pyx_L1_error)
-
- /* "View.MemoryView":152
- *
- * for idx, dim in enumerate(shape):
- * if dim <= 0: # <<<<<<<<<<<<<<
- * raise ValueError("Invalid shape in axis %d: %d." % (idx, dim))
- * self._shape[idx] = dim
- */
- }
-
- /* "View.MemoryView":154
- * if dim <= 0:
- * raise ValueError("Invalid shape in axis %d: %d." % (idx, dim))
- * self._shape[idx] = dim # <<<<<<<<<<<<<<
- *
- * cdef char order
- */
- (__pyx_v_self->_shape[__pyx_v_idx]) = __pyx_v_dim;
-
- /* "View.MemoryView":151
- *
- *
- * for idx, dim in enumerate(shape): # <<<<<<<<<<<<<<
- * if dim <= 0:
- * raise ValueError("Invalid shape in axis %d: %d." % (idx, dim))
- */
- }
- __Pyx_DECREF(__pyx_t_3); __pyx_t_3 = 0;
-
- /* "View.MemoryView":157
- *
- * cdef char order
- * if mode == 'fortran': # <<<<<<<<<<<<<<
- * order = b'F'
- * self.mode = u'fortran'
- */
- __pyx_t_4 = (__Pyx_PyString_Equals(__pyx_v_mode, __pyx_n_s_fortran, Py_EQ)); if (unlikely(__pyx_t_4 < 0)) __PYX_ERR(1, 157, __pyx_L1_error)
- if (__pyx_t_4) {
-
- /* "View.MemoryView":158
- * cdef char order
- * if mode == 'fortran':
- * order = b'F' # <<<<<<<<<<<<<<
- * self.mode = u'fortran'
- * elif mode == 'c':
- */
- __pyx_v_order = 'F';
-
- /* "View.MemoryView":159
- * if mode == 'fortran':
- * order = b'F'
- * self.mode = u'fortran' # <<<<<<<<<<<<<<
- * elif mode == 'c':
- * order = b'C'
- */
- __Pyx_INCREF(__pyx_n_u_fortran);
- __Pyx_GIVEREF(__pyx_n_u_fortran);
- __Pyx_GOTREF(__pyx_v_self->mode);
- __Pyx_DECREF(__pyx_v_self->mode);
- __pyx_v_self->mode = __pyx_n_u_fortran;
-
- /* "View.MemoryView":157
- *
- * cdef char order
- * if mode == 'fortran': # <<<<<<<<<<<<<<
- * order = b'F'
- * self.mode = u'fortran'
- */
- goto __pyx_L10;
- }
-
- /* "View.MemoryView":160
- * order = b'F'
- * self.mode = u'fortran'
- * elif mode == 'c': # <<<<<<<<<<<<<<
- * order = b'C'
- * self.mode = u'c'
- */
- __pyx_t_4 = (__Pyx_PyString_Equals(__pyx_v_mode, __pyx_n_s_c, Py_EQ)); if (unlikely(__pyx_t_4 < 0)) __PYX_ERR(1, 160, __pyx_L1_error)
- if (likely(__pyx_t_4)) {
-
- /* "View.MemoryView":161
- * self.mode = u'fortran'
- * elif mode == 'c':
- * order = b'C' # <<<<<<<<<<<<<<
- * self.mode = u'c'
- * else:
- */
- __pyx_v_order = 'C';
-
- /* "View.MemoryView":162
- * elif mode == 'c':
- * order = b'C'
- * self.mode = u'c' # <<<<<<<<<<<<<<
- * else:
- * raise ValueError("Invalid mode, expected 'c' or 'fortran', got %s" % mode)
- */
- __Pyx_INCREF(__pyx_n_u_c);
- __Pyx_GIVEREF(__pyx_n_u_c);
- __Pyx_GOTREF(__pyx_v_self->mode);
- __Pyx_DECREF(__pyx_v_self->mode);
- __pyx_v_self->mode = __pyx_n_u_c;
-
- /* "View.MemoryView":160
- * order = b'F'
- * self.mode = u'fortran'
- * elif mode == 'c': # <<<<<<<<<<<<<<
- * order = b'C'
- * self.mode = u'c'
- */
- goto __pyx_L10;
- }
-
- /* "View.MemoryView":164
- * self.mode = u'c'
- * else:
- * raise ValueError("Invalid mode, expected 'c' or 'fortran', got %s" % mode) # <<<<<<<<<<<<<<
- *
- * self.len = fill_contig_strides_array(self._shape, self._strides,
- */
- /*else*/ {
- __pyx_t_3 = __Pyx_PyString_FormatSafe(__pyx_kp_s_Invalid_mode_expected_c_or_fortr, __pyx_v_mode); if (unlikely(!__pyx_t_3)) __PYX_ERR(1, 164, __pyx_L1_error)
- __Pyx_GOTREF(__pyx_t_3);
- __pyx_t_10 = __Pyx_PyObject_CallOneArg(__pyx_builtin_ValueError, __pyx_t_3); if (unlikely(!__pyx_t_10)) __PYX_ERR(1, 164, __pyx_L1_error)
- __Pyx_GOTREF(__pyx_t_10);
- __Pyx_DECREF(__pyx_t_3); __pyx_t_3 = 0;
- __Pyx_Raise(__pyx_t_10, 0, 0, 0);
- __Pyx_DECREF(__pyx_t_10); __pyx_t_10 = 0;
- __PYX_ERR(1, 164, __pyx_L1_error)
- }
- __pyx_L10:;
-
- /* "View.MemoryView":166
- * raise ValueError("Invalid mode, expected 'c' or 'fortran', got %s" % mode)
- *
- * self.len = fill_contig_strides_array(self._shape, self._strides, # <<<<<<<<<<<<<<
- * itemsize, self.ndim, order)
- *
- */
- __pyx_v_self->len = __pyx_fill_contig_strides_array(__pyx_v_self->_shape, __pyx_v_self->_strides, __pyx_v_itemsize, __pyx_v_self->ndim, __pyx_v_order);
-
- /* "View.MemoryView":169
- * itemsize, self.ndim, order)
- *
- * self.free_data = allocate_buffer # <<<<<<<<<<<<<<
- * self.dtype_is_object = format == b'O'
- * if allocate_buffer:
- */
- __pyx_v_self->free_data = __pyx_v_allocate_buffer;
-
- /* "View.MemoryView":170
- *
- * self.free_data = allocate_buffer
- * self.dtype_is_object = format == b'O' # <<<<<<<<<<<<<<
- * if allocate_buffer:
- *
- */
- __pyx_t_10 = PyObject_RichCompare(__pyx_v_format, __pyx_n_b_O, Py_EQ); __Pyx_XGOTREF(__pyx_t_10); if (unlikely(!__pyx_t_10)) __PYX_ERR(1, 170, __pyx_L1_error)
- __pyx_t_4 = __Pyx_PyObject_IsTrue(__pyx_t_10); if (unlikely((__pyx_t_4 == (int)-1) && PyErr_Occurred())) __PYX_ERR(1, 170, __pyx_L1_error)
- __Pyx_DECREF(__pyx_t_10); __pyx_t_10 = 0;
- __pyx_v_self->dtype_is_object = __pyx_t_4;
-
- /* "View.MemoryView":171
- * self.free_data = allocate_buffer
- * self.dtype_is_object = format == b'O'
- * if allocate_buffer: # <<<<<<<<<<<<<<
- *
- *
- */
- __pyx_t_4 = (__pyx_v_allocate_buffer != 0);
- if (__pyx_t_4) {
-
- /* "View.MemoryView":174
- *
- *
- * self.data = malloc(self.len) # <<<<<<<<<<<<<<
- * if not self.data:
- * raise MemoryError("unable to allocate array data.")
- */
- __pyx_v_self->data = ((char *)malloc(__pyx_v_self->len));
-
- /* "View.MemoryView":175
- *
- * self.data = malloc(self.len)
- * if not self.data: # <<<<<<<<<<<<<<
- * raise MemoryError("unable to allocate array data.")
- *
- */
- __pyx_t_4 = ((!(__pyx_v_self->data != 0)) != 0);
- if (unlikely(__pyx_t_4)) {
-
- /* "View.MemoryView":176
- * self.data = malloc(self.len)
- * if not self.data:
- * raise MemoryError("unable to allocate array data.") # <<<<<<<<<<<<<<
- *
- * if self.dtype_is_object:
- */
- __pyx_t_10 = __Pyx_PyObject_Call(__pyx_builtin_MemoryError, __pyx_tuple__5, NULL); if (unlikely(!__pyx_t_10)) __PYX_ERR(1, 176, __pyx_L1_error)
- __Pyx_GOTREF(__pyx_t_10);
- __Pyx_Raise(__pyx_t_10, 0, 0, 0);
- __Pyx_DECREF(__pyx_t_10); __pyx_t_10 = 0;
- __PYX_ERR(1, 176, __pyx_L1_error)
-
- /* "View.MemoryView":175
- *
- * self.data = malloc(self.len)
- * if not self.data: # <<<<<<<<<<<<<<
- * raise MemoryError("unable to allocate array data.")
- *
- */
- }
-
- /* "View.MemoryView":178
- * raise MemoryError("unable to allocate array data.")
- *
- * if self.dtype_is_object: # <<<<<<<<<<<<<<
- * p = self.data
- * for i in range(self.len / itemsize):
- */
- __pyx_t_4 = (__pyx_v_self->dtype_is_object != 0);
- if (__pyx_t_4) {
-
- /* "View.MemoryView":179
- *
- * if self.dtype_is_object:
- * p = self.data # <<<<<<<<<<<<<<
- * for i in range(self.len / itemsize):
- * p[i] = Py_None
- */
- __pyx_v_p = ((PyObject **)__pyx_v_self->data);
-
- /* "View.MemoryView":180
- * if self.dtype_is_object:
- * p = self.data
- * for i in range(self.len / itemsize): # <<<<<<<<<<<<<<
- * p[i] = Py_None
- * Py_INCREF(Py_None)
- */
- if (unlikely(__pyx_v_itemsize == 0)) {
- PyErr_SetString(PyExc_ZeroDivisionError, "integer division or modulo by zero");
- __PYX_ERR(1, 180, __pyx_L1_error)
- }
- else if (sizeof(Py_ssize_t) == sizeof(long) && (!(((Py_ssize_t)-1) > 0)) && unlikely(__pyx_v_itemsize == (Py_ssize_t)-1) && unlikely(UNARY_NEG_WOULD_OVERFLOW(__pyx_v_self->len))) {
- PyErr_SetString(PyExc_OverflowError, "value too large to perform division");
- __PYX_ERR(1, 180, __pyx_L1_error)
- }
- __pyx_t_1 = __Pyx_div_Py_ssize_t(__pyx_v_self->len, __pyx_v_itemsize);
- __pyx_t_9 = __pyx_t_1;
- for (__pyx_t_11 = 0; __pyx_t_11 < __pyx_t_9; __pyx_t_11+=1) {
- __pyx_v_i = __pyx_t_11;
-
- /* "View.MemoryView":181
- * p = self.data
- * for i in range(self.len / itemsize):
- * p[i] = Py_None # <<<<<<<<<<<<<<
- * Py_INCREF(Py_None)
- *
- */
- (__pyx_v_p[__pyx_v_i]) = Py_None;
-
- /* "View.MemoryView":182
- * for i in range(self.len / itemsize):
- * p[i] = Py_None
- * Py_INCREF(Py_None) # <<<<<<<<<<<<<<
- *
- * @cname('getbuffer')
- */
- Py_INCREF(Py_None);
- }
-
- /* "View.MemoryView":178
- * raise MemoryError("unable to allocate array data.")
- *
- * if self.dtype_is_object: # <<<<<<<<<<<<<<
- * p = self.data
- * for i in range(self.len / itemsize):
- */
- }
-
- /* "View.MemoryView":171
- * self.free_data = allocate_buffer
- * self.dtype_is_object = format == b'O'
- * if allocate_buffer: # <<<<<<<<<<<<<<
- *
- *
- */
- }
-
- /* "View.MemoryView":122
- * cdef bint dtype_is_object
- *
- * def __cinit__(array self, tuple shape, Py_ssize_t itemsize, format not None, # <<<<<<<<<<<<<<
- * mode="c", bint allocate_buffer=True):
- *
- */
-
- /* function exit code */
- __pyx_r = 0;
- goto __pyx_L0;
- __pyx_L1_error:;
- __Pyx_XDECREF(__pyx_t_3);
- __Pyx_XDECREF(__pyx_t_5);
- __Pyx_XDECREF(__pyx_t_6);
- __Pyx_XDECREF(__pyx_t_10);
- __Pyx_AddTraceback("View.MemoryView.array.__cinit__", __pyx_clineno, __pyx_lineno, __pyx_filename);
- __pyx_r = -1;
- __pyx_L0:;
- __Pyx_XDECREF(__pyx_v_format);
- __Pyx_RefNannyFinishContext();
- return __pyx_r;
-}
-
-/* "View.MemoryView":185
- *
- * @cname('getbuffer')
- * def __getbuffer__(self, Py_buffer *info, int flags): # <<<<<<<<<<<<<<
- * cdef int bufmode = -1
- * if self.mode == u"c":
- */
-
-/* Python wrapper */
-static CYTHON_UNUSED int __pyx_array_getbuffer(PyObject *__pyx_v_self, Py_buffer *__pyx_v_info, int __pyx_v_flags); /*proto*/
-static CYTHON_UNUSED int __pyx_array_getbuffer(PyObject *__pyx_v_self, Py_buffer *__pyx_v_info, int __pyx_v_flags) {
- int __pyx_r;
- __Pyx_RefNannyDeclarations
- __Pyx_RefNannySetupContext("__getbuffer__ (wrapper)", 0);
- __pyx_r = __pyx_array___pyx_pf_15View_dot_MemoryView_5array_2__getbuffer__(((struct __pyx_array_obj *)__pyx_v_self), ((Py_buffer *)__pyx_v_info), ((int)__pyx_v_flags));
-
- /* function exit code */
- __Pyx_RefNannyFinishContext();
- return __pyx_r;
-}
-
-static int __pyx_array___pyx_pf_15View_dot_MemoryView_5array_2__getbuffer__(struct __pyx_array_obj *__pyx_v_self, Py_buffer *__pyx_v_info, int __pyx_v_flags) {
- int __pyx_v_bufmode;
- int __pyx_r;
- __Pyx_RefNannyDeclarations
- int __pyx_t_1;
- int __pyx_t_2;
- PyObject *__pyx_t_3 = NULL;
- char *__pyx_t_4;
- Py_ssize_t __pyx_t_5;
- int __pyx_t_6;
- Py_ssize_t *__pyx_t_7;
- int __pyx_lineno = 0;
- const char *__pyx_filename = NULL;
- int __pyx_clineno = 0;
- if (__pyx_v_info == NULL) {
- PyErr_SetString(PyExc_BufferError, "PyObject_GetBuffer: view==NULL argument is obsolete");
- return -1;
- }
- __Pyx_RefNannySetupContext("__getbuffer__", 0);
- __pyx_v_info->obj = Py_None; __Pyx_INCREF(Py_None);
- __Pyx_GIVEREF(__pyx_v_info->obj);
-
- /* "View.MemoryView":186
- * @cname('getbuffer')
- * def __getbuffer__(self, Py_buffer *info, int flags):
- * cdef int bufmode = -1 # <<<<<<<<<<<<<<
- * if self.mode == u"c":
- * bufmode = PyBUF_C_CONTIGUOUS | PyBUF_ANY_CONTIGUOUS
- */
- __pyx_v_bufmode = -1;
-
- /* "View.MemoryView":187
- * def __getbuffer__(self, Py_buffer *info, int flags):
- * cdef int bufmode = -1
- * if self.mode == u"c": # <<<<<<<<<<<<<<
- * bufmode = PyBUF_C_CONTIGUOUS | PyBUF_ANY_CONTIGUOUS
- * elif self.mode == u"fortran":
- */
- __pyx_t_1 = (__Pyx_PyUnicode_Equals(__pyx_v_self->mode, __pyx_n_u_c, Py_EQ)); if (unlikely(__pyx_t_1 < 0)) __PYX_ERR(1, 187, __pyx_L1_error)
- __pyx_t_2 = (__pyx_t_1 != 0);
- if (__pyx_t_2) {
-
- /* "View.MemoryView":188
- * cdef int bufmode = -1
- * if self.mode == u"c":
- * bufmode = PyBUF_C_CONTIGUOUS | PyBUF_ANY_CONTIGUOUS # <<<<<<<<<<<<<<
- * elif self.mode == u"fortran":
- * bufmode = PyBUF_F_CONTIGUOUS | PyBUF_ANY_CONTIGUOUS
- */
- __pyx_v_bufmode = (PyBUF_C_CONTIGUOUS | PyBUF_ANY_CONTIGUOUS);
-
- /* "View.MemoryView":187
- * def __getbuffer__(self, Py_buffer *info, int flags):
- * cdef int bufmode = -1
- * if self.mode == u"c": # <<<<<<<<<<<<<<
- * bufmode = PyBUF_C_CONTIGUOUS | PyBUF_ANY_CONTIGUOUS
- * elif self.mode == u"fortran":
- */
- goto __pyx_L3;
- }
-
- /* "View.MemoryView":189
- * if self.mode == u"c":
- * bufmode = PyBUF_C_CONTIGUOUS | PyBUF_ANY_CONTIGUOUS
- * elif self.mode == u"fortran": # <<<<<<<<<<<<<<
- * bufmode = PyBUF_F_CONTIGUOUS | PyBUF_ANY_CONTIGUOUS
- * if not (flags & bufmode):
- */
- __pyx_t_2 = (__Pyx_PyUnicode_Equals(__pyx_v_self->mode, __pyx_n_u_fortran, Py_EQ)); if (unlikely(__pyx_t_2 < 0)) __PYX_ERR(1, 189, __pyx_L1_error)
- __pyx_t_1 = (__pyx_t_2 != 0);
- if (__pyx_t_1) {
-
- /* "View.MemoryView":190
- * bufmode = PyBUF_C_CONTIGUOUS | PyBUF_ANY_CONTIGUOUS
- * elif self.mode == u"fortran":
- * bufmode = PyBUF_F_CONTIGUOUS | PyBUF_ANY_CONTIGUOUS # <<<<<<<<<<<<<<
- * if not (flags & bufmode):
- * raise ValueError("Can only create a buffer that is contiguous in memory.")
- */
- __pyx_v_bufmode = (PyBUF_F_CONTIGUOUS | PyBUF_ANY_CONTIGUOUS);
-
- /* "View.MemoryView":189
- * if self.mode == u"c":
- * bufmode = PyBUF_C_CONTIGUOUS | PyBUF_ANY_CONTIGUOUS
- * elif self.mode == u"fortran": # <<<<<<<<<<<<<<
- * bufmode = PyBUF_F_CONTIGUOUS | PyBUF_ANY_CONTIGUOUS
- * if not (flags & bufmode):
- */
- }
- __pyx_L3:;
-
- /* "View.MemoryView":191
- * elif self.mode == u"fortran":
- * bufmode = PyBUF_F_CONTIGUOUS | PyBUF_ANY_CONTIGUOUS
- * if not (flags & bufmode): # <<<<<<<<<<<<<<
- * raise ValueError("Can only create a buffer that is contiguous in memory.")
- * info.buf = self.data
- */
- __pyx_t_1 = ((!((__pyx_v_flags & __pyx_v_bufmode) != 0)) != 0);
- if (unlikely(__pyx_t_1)) {
-
- /* "View.MemoryView":192
- * bufmode = PyBUF_F_CONTIGUOUS | PyBUF_ANY_CONTIGUOUS
- * if not (flags & bufmode):
- * raise ValueError("Can only create a buffer that is contiguous in memory.") # <<<<<<<<<<<<<<
- * info.buf = self.data
- * info.len = self.len
- */
- __pyx_t_3 = __Pyx_PyObject_Call(__pyx_builtin_ValueError, __pyx_tuple__6, NULL); if (unlikely(!__pyx_t_3)) __PYX_ERR(1, 192, __pyx_L1_error)
- __Pyx_GOTREF(__pyx_t_3);
- __Pyx_Raise(__pyx_t_3, 0, 0, 0);
- __Pyx_DECREF(__pyx_t_3); __pyx_t_3 = 0;
- __PYX_ERR(1, 192, __pyx_L1_error)
-
- /* "View.MemoryView":191
- * elif self.mode == u"fortran":
- * bufmode = PyBUF_F_CONTIGUOUS | PyBUF_ANY_CONTIGUOUS
- * if not (flags & bufmode): # <<<<<<<<<<<<<<
- * raise ValueError("Can only create a buffer that is contiguous in memory.")
- * info.buf = self.data
- */
- }
-
- /* "View.MemoryView":193
- * if not (flags & bufmode):
- * raise ValueError("Can only create a buffer that is contiguous in memory.")
- * info.buf = self.data # <<<<<<<<<<<<<<
- * info.len = self.len
- * info.ndim = self.ndim
- */
- __pyx_t_4 = __pyx_v_self->data;
- __pyx_v_info->buf = __pyx_t_4;
-
- /* "View.MemoryView":194
- * raise ValueError("Can only create a buffer that is contiguous in memory.")
- * info.buf = self.data
- * info.len = self.len # <<<<<<<<<<<<<<
- * info.ndim = self.ndim
- * info.shape = self._shape
- */
- __pyx_t_5 = __pyx_v_self->len;
- __pyx_v_info->len = __pyx_t_5;
-
- /* "View.MemoryView":195
- * info.buf = self.data
- * info.len = self.len
- * info.ndim = self.ndim # <<<<<<<<<<<<<<
- * info.shape = self._shape
- * info.strides = self._strides
- */
- __pyx_t_6 = __pyx_v_self->ndim;
- __pyx_v_info->ndim = __pyx_t_6;
-
- /* "View.MemoryView":196
- * info.len = self.len
- * info.ndim = self.ndim
- * info.shape = self._shape # <<<<<<<<<<<<<<
- * info.strides = self._strides
- * info.suboffsets = NULL
- */
- __pyx_t_7 = __pyx_v_self->_shape;
- __pyx_v_info->shape = __pyx_t_7;
-
- /* "View.MemoryView":197
- * info.ndim = self.ndim
- * info.shape = self._shape
- * info.strides = self._strides # <<<<<<<<<<<<<<
- * info.suboffsets = NULL
- * info.itemsize = self.itemsize
- */
- __pyx_t_7 = __pyx_v_self->_strides;
- __pyx_v_info->strides = __pyx_t_7;
-
- /* "View.MemoryView":198
- * info.shape = self._shape
- * info.strides = self._strides
- * info.suboffsets = NULL # <<<<<<<<<<<<<<
- * info.itemsize = self.itemsize
- * info.readonly = 0
- */
- __pyx_v_info->suboffsets = NULL;
-
- /* "View.MemoryView":199
- * info.strides = self._strides
- * info.suboffsets = NULL
- * info.itemsize = self.itemsize # <<<<<<<<<<<<<<
- * info.readonly = 0
- *
- */
- __pyx_t_5 = __pyx_v_self->itemsize;
- __pyx_v_info->itemsize = __pyx_t_5;
-
- /* "View.MemoryView":200
- * info.suboffsets = NULL
- * info.itemsize = self.itemsize
- * info.readonly = 0 # <<<<<<<<<<<<<<
- *
- * if flags & PyBUF_FORMAT:
- */
- __pyx_v_info->readonly = 0;
-
- /* "View.MemoryView":202
- * info.readonly = 0
- *
- * if flags & PyBUF_FORMAT: # <<<<<<<<<<<<<<
- * info.format = self.format
- * else:
- */
- __pyx_t_1 = ((__pyx_v_flags & PyBUF_FORMAT) != 0);
- if (__pyx_t_1) {
-
- /* "View.MemoryView":203
- *
- * if flags & PyBUF_FORMAT:
- * info.format = self.format # <<<<<<<<<<<<<<
- * else:
- * info.format = NULL
- */
- __pyx_t_4 = __pyx_v_self->format;
- __pyx_v_info->format = __pyx_t_4;
-
- /* "View.MemoryView":202
- * info.readonly = 0
- *
- * if flags & PyBUF_FORMAT: # <<<<<<<<<<<<<<
- * info.format = self.format
- * else:
- */
- goto __pyx_L5;
- }
-
- /* "View.MemoryView":205
- * info.format = self.format
- * else:
- * info.format = NULL # <<<<<<<<<<<<<<
- *
- * info.obj = self
- */
- /*else*/ {
- __pyx_v_info->format = NULL;
- }
- __pyx_L5:;
-
- /* "View.MemoryView":207
- * info.format = NULL
- *
- * info.obj = self # <<<<<<<<<<<<<<
- *
- * __pyx_getbuffer = capsule( &__pyx_array_getbuffer, "getbuffer(obj, view, flags)")
- */
- __Pyx_INCREF(((PyObject *)__pyx_v_self));
- __Pyx_GIVEREF(((PyObject *)__pyx_v_self));
- __Pyx_GOTREF(__pyx_v_info->obj);
- __Pyx_DECREF(__pyx_v_info->obj);
- __pyx_v_info->obj = ((PyObject *)__pyx_v_self);
-
- /* "View.MemoryView":185
- *
- * @cname('getbuffer')
- * def __getbuffer__(self, Py_buffer *info, int flags): # <<<<<<<<<<<<<<
- * cdef int bufmode = -1
- * if self.mode == u"c":
- */
-
- /* function exit code */
- __pyx_r = 0;
- goto __pyx_L0;
- __pyx_L1_error:;
- __Pyx_XDECREF(__pyx_t_3);
- __Pyx_AddTraceback("View.MemoryView.array.__getbuffer__", __pyx_clineno, __pyx_lineno, __pyx_filename);
- __pyx_r = -1;
- if (__pyx_v_info->obj != NULL) {
- __Pyx_GOTREF(__pyx_v_info->obj);
- __Pyx_DECREF(__pyx_v_info->obj); __pyx_v_info->obj = 0;
- }
- goto __pyx_L2;
- __pyx_L0:;
- if (__pyx_v_info->obj == Py_None) {
- __Pyx_GOTREF(__pyx_v_info->obj);
- __Pyx_DECREF(__pyx_v_info->obj); __pyx_v_info->obj = 0;
- }
- __pyx_L2:;
- __Pyx_RefNannyFinishContext();
- return __pyx_r;
-}
-
-/* "View.MemoryView":211
- * __pyx_getbuffer = capsule( &__pyx_array_getbuffer, "getbuffer(obj, view, flags)")
- *
- * def __dealloc__(array self): # <<<<<<<<<<<<<<
- * if self.callback_free_data != NULL:
- * self.callback_free_data(self.data)
- */
-
-/* Python wrapper */
-static void __pyx_array___dealloc__(PyObject *__pyx_v_self); /*proto*/
-static void __pyx_array___dealloc__(PyObject *__pyx_v_self) {
- __Pyx_RefNannyDeclarations
- __Pyx_RefNannySetupContext("__dealloc__ (wrapper)", 0);
- __pyx_array___pyx_pf_15View_dot_MemoryView_5array_4__dealloc__(((struct __pyx_array_obj *)__pyx_v_self));
-
- /* function exit code */
- __Pyx_RefNannyFinishContext();
-}
-
-static void __pyx_array___pyx_pf_15View_dot_MemoryView_5array_4__dealloc__(struct __pyx_array_obj *__pyx_v_self) {
- __Pyx_RefNannyDeclarations
- int __pyx_t_1;
- __Pyx_RefNannySetupContext("__dealloc__", 0);
-
- /* "View.MemoryView":212
- *
- * def __dealloc__(array self):
- * if self.callback_free_data != NULL: # <<<<<<<<<<<<<<
- * self.callback_free_data(self.data)
- * elif self.free_data:
- */
- __pyx_t_1 = ((__pyx_v_self->callback_free_data != NULL) != 0);
- if (__pyx_t_1) {
-
- /* "View.MemoryView":213
- * def __dealloc__(array self):
- * if self.callback_free_data != NULL:
- * self.callback_free_data(self.data) # <<<<<<<<<<<<<<
- * elif self.free_data:
- * if self.dtype_is_object:
- */
- __pyx_v_self->callback_free_data(__pyx_v_self->data);
-
- /* "View.MemoryView":212
- *
- * def __dealloc__(array self):
- * if self.callback_free_data != NULL: # <<<<<<<<<<<<<<
- * self.callback_free_data(self.data)
- * elif self.free_data:
- */
- goto __pyx_L3;
- }
-
- /* "View.MemoryView":214
- * if self.callback_free_data != NULL:
- * self.callback_free_data(self.data)
- * elif self.free_data: # <<<<<<<<<<<<<<
- * if self.dtype_is_object:
- * refcount_objects_in_slice(self.data, self._shape,
- */
- __pyx_t_1 = (__pyx_v_self->free_data != 0);
- if (__pyx_t_1) {
-
- /* "View.MemoryView":215
- * self.callback_free_data(self.data)
- * elif self.free_data:
- * if self.dtype_is_object: # <<<<<<<<<<<<<<
- * refcount_objects_in_slice(self.data, self._shape,
- * self._strides, self.ndim, False)
- */
- __pyx_t_1 = (__pyx_v_self->dtype_is_object != 0);
- if (__pyx_t_1) {
-
- /* "View.MemoryView":216
- * elif self.free_data:
- * if self.dtype_is_object:
- * refcount_objects_in_slice(self.data, self._shape, # <<<<<<<<<<<<<<
- * self._strides, self.ndim, False)
- * free(self.data)
- */
- __pyx_memoryview_refcount_objects_in_slice(__pyx_v_self->data, __pyx_v_self->_shape, __pyx_v_self->_strides, __pyx_v_self->ndim, 0);
-
- /* "View.MemoryView":215
- * self.callback_free_data(self.data)
- * elif self.free_data:
- * if self.dtype_is_object: # <<<<<<<<<<<<<<
- * refcount_objects_in_slice(self.data, self._shape,
- * self._strides, self.ndim, False)
- */
- }
-
- /* "View.MemoryView":218
- * refcount_objects_in_slice(self.data, self._shape,
- * self._strides, self.ndim, False)
- * free(self.data) # <<<<<<<<<<<<<<
- * PyObject_Free(self._shape)
- *
- */
- free(__pyx_v_self->data);
-
- /* "View.MemoryView":214
- * if self.callback_free_data != NULL:
- * self.callback_free_data(self.data)
- * elif self.free_data: # <<<<<<<<<<<<<<
- * if self.dtype_is_object:
- * refcount_objects_in_slice(self.data, self._shape,
- */
- }
- __pyx_L3:;
-
- /* "View.MemoryView":219
- * self._strides, self.ndim, False)
- * free(self.data)
- * PyObject_Free(self._shape) # <<<<<<<<<<<<<<
- *
- * @property
- */
- PyObject_Free(__pyx_v_self->_shape);
-
- /* "View.MemoryView":211
- * __pyx_getbuffer = capsule( &__pyx_array_getbuffer, "getbuffer(obj, view, flags)")
- *
- * def __dealloc__(array self): # <<<<<<<<<<<<<<
- * if self.callback_free_data != NULL:
- * self.callback_free_data(self.data)
- */
-
- /* function exit code */
- __Pyx_RefNannyFinishContext();
-}
-
-/* "View.MemoryView":222
- *
- * @property
- * def memview(self): # <<<<<<<<<<<<<<
- * return self.get_memview()
- *
- */
-
-/* Python wrapper */
-static PyObject *__pyx_pw_15View_dot_MemoryView_5array_7memview_1__get__(PyObject *__pyx_v_self); /*proto*/
-static PyObject *__pyx_pw_15View_dot_MemoryView_5array_7memview_1__get__(PyObject *__pyx_v_self) {
- PyObject *__pyx_r = 0;
- __Pyx_RefNannyDeclarations
- __Pyx_RefNannySetupContext("__get__ (wrapper)", 0);
- __pyx_r = __pyx_pf_15View_dot_MemoryView_5array_7memview___get__(((struct __pyx_array_obj *)__pyx_v_self));
-
- /* function exit code */
- __Pyx_RefNannyFinishContext();
- return __pyx_r;
-}
-
-static PyObject *__pyx_pf_15View_dot_MemoryView_5array_7memview___get__(struct __pyx_array_obj *__pyx_v_self) {
- PyObject *__pyx_r = NULL;
- __Pyx_RefNannyDeclarations
- PyObject *__pyx_t_1 = NULL;
- int __pyx_lineno = 0;
- const char *__pyx_filename = NULL;
- int __pyx_clineno = 0;
- __Pyx_RefNannySetupContext("__get__", 0);
-
- /* "View.MemoryView":223
- * @property
- * def memview(self):
- * return self.get_memview() # <<<<<<<<<<<<<<
- *
- * @cname('get_memview')
- */
- __Pyx_XDECREF(__pyx_r);
- __pyx_t_1 = ((struct __pyx_vtabstruct_array *)__pyx_v_self->__pyx_vtab)->get_memview(__pyx_v_self); if (unlikely(!__pyx_t_1)) __PYX_ERR(1, 223, __pyx_L1_error)
- __Pyx_GOTREF(__pyx_t_1);
- __pyx_r = __pyx_t_1;
- __pyx_t_1 = 0;
- goto __pyx_L0;
-
- /* "View.MemoryView":222
- *
- * @property
- * def memview(self): # <<<<<<<<<<<<<<
- * return self.get_memview()
- *
- */
-
- /* function exit code */
- __pyx_L1_error:;
- __Pyx_XDECREF(__pyx_t_1);
- __Pyx_AddTraceback("View.MemoryView.array.memview.__get__", __pyx_clineno, __pyx_lineno, __pyx_filename);
- __pyx_r = NULL;
- __pyx_L0:;
- __Pyx_XGIVEREF(__pyx_r);
- __Pyx_RefNannyFinishContext();
- return __pyx_r;
-}
-
-/* "View.MemoryView":226
- *
- * @cname('get_memview')
- * cdef get_memview(self): # <<<<<<<<<<<<<<
- * flags = PyBUF_ANY_CONTIGUOUS|PyBUF_FORMAT|PyBUF_WRITABLE
- * return memoryview(self, flags, self.dtype_is_object)
- */
-
-static PyObject *__pyx_array_get_memview(struct __pyx_array_obj *__pyx_v_self) {
- int __pyx_v_flags;
- PyObject *__pyx_r = NULL;
- __Pyx_RefNannyDeclarations
- PyObject *__pyx_t_1 = NULL;
- PyObject *__pyx_t_2 = NULL;
- PyObject *__pyx_t_3 = NULL;
- int __pyx_lineno = 0;
- const char *__pyx_filename = NULL;
- int __pyx_clineno = 0;
- __Pyx_RefNannySetupContext("get_memview", 0);
-
- /* "View.MemoryView":227
- * @cname('get_memview')
- * cdef get_memview(self):
- * flags = PyBUF_ANY_CONTIGUOUS|PyBUF_FORMAT|PyBUF_WRITABLE # <<<<<<<<<<<<<<
- * return memoryview(self, flags, self.dtype_is_object)
- *
- */
- __pyx_v_flags = ((PyBUF_ANY_CONTIGUOUS | PyBUF_FORMAT) | PyBUF_WRITABLE);
-
- /* "View.MemoryView":228
- * cdef get_memview(self):
- * flags = PyBUF_ANY_CONTIGUOUS|PyBUF_FORMAT|PyBUF_WRITABLE
- * return memoryview(self, flags, self.dtype_is_object) # <<<<<<<<<<<<<<
- *
- * def __len__(self):
- */
- __Pyx_XDECREF(__pyx_r);
- __pyx_t_1 = __Pyx_PyInt_From_int(__pyx_v_flags); if (unlikely(!__pyx_t_1)) __PYX_ERR(1, 228, __pyx_L1_error)
- __Pyx_GOTREF(__pyx_t_1);
- __pyx_t_2 = __Pyx_PyBool_FromLong(__pyx_v_self->dtype_is_object); if (unlikely(!__pyx_t_2)) __PYX_ERR(1, 228, __pyx_L1_error)
- __Pyx_GOTREF(__pyx_t_2);
- __pyx_t_3 = PyTuple_New(3); if (unlikely(!__pyx_t_3)) __PYX_ERR(1, 228, __pyx_L1_error)
- __Pyx_GOTREF(__pyx_t_3);
- __Pyx_INCREF(((PyObject *)__pyx_v_self));
- __Pyx_GIVEREF(((PyObject *)__pyx_v_self));
- PyTuple_SET_ITEM(__pyx_t_3, 0, ((PyObject *)__pyx_v_self));
- __Pyx_GIVEREF(__pyx_t_1);
- PyTuple_SET_ITEM(__pyx_t_3, 1, __pyx_t_1);
- __Pyx_GIVEREF(__pyx_t_2);
- PyTuple_SET_ITEM(__pyx_t_3, 2, __pyx_t_2);
- __pyx_t_1 = 0;
- __pyx_t_2 = 0;
- __pyx_t_2 = __Pyx_PyObject_Call(((PyObject *)__pyx_memoryview_type), __pyx_t_3, NULL); if (unlikely(!__pyx_t_2)) __PYX_ERR(1, 228, __pyx_L1_error)
- __Pyx_GOTREF(__pyx_t_2);
- __Pyx_DECREF(__pyx_t_3); __pyx_t_3 = 0;
- __pyx_r = __pyx_t_2;
- __pyx_t_2 = 0;
- goto __pyx_L0;
-
- /* "View.MemoryView":226
- *
- * @cname('get_memview')
- * cdef get_memview(self): # <<<<<<<<<<<<<<
- * flags = PyBUF_ANY_CONTIGUOUS|PyBUF_FORMAT|PyBUF_WRITABLE
- * return memoryview(self, flags, self.dtype_is_object)
- */
-
- /* function exit code */
- __pyx_L1_error:;
- __Pyx_XDECREF(__pyx_t_1);
- __Pyx_XDECREF(__pyx_t_2);
- __Pyx_XDECREF(__pyx_t_3);
- __Pyx_AddTraceback("View.MemoryView.array.get_memview", __pyx_clineno, __pyx_lineno, __pyx_filename);
- __pyx_r = 0;
- __pyx_L0:;
- __Pyx_XGIVEREF(__pyx_r);
- __Pyx_RefNannyFinishContext();
- return __pyx_r;
-}
-
-/* "View.MemoryView":230
- * return memoryview(self, flags, self.dtype_is_object)
- *
- * def __len__(self): # <<<<<<<<<<<<<<
- * return self._shape[0]
- *
- */
-
-/* Python wrapper */
-static Py_ssize_t __pyx_array___len__(PyObject *__pyx_v_self); /*proto*/
-static Py_ssize_t __pyx_array___len__(PyObject *__pyx_v_self) {
- Py_ssize_t __pyx_r;
- __Pyx_RefNannyDeclarations
- __Pyx_RefNannySetupContext("__len__ (wrapper)", 0);
- __pyx_r = __pyx_array___pyx_pf_15View_dot_MemoryView_5array_6__len__(((struct __pyx_array_obj *)__pyx_v_self));
-
- /* function exit code */
- __Pyx_RefNannyFinishContext();
- return __pyx_r;
-}
-
-static Py_ssize_t __pyx_array___pyx_pf_15View_dot_MemoryView_5array_6__len__(struct __pyx_array_obj *__pyx_v_self) {
- Py_ssize_t __pyx_r;
- __Pyx_RefNannyDeclarations
- __Pyx_RefNannySetupContext("__len__", 0);
-
- /* "View.MemoryView":231
- *
- * def __len__(self):
- * return self._shape[0] # <<<<<<<<<<<<<<
- *
- * def __getattr__(self, attr):
- */
- __pyx_r = (__pyx_v_self->_shape[0]);
- goto __pyx_L0;
-
- /* "View.MemoryView":230
- * return memoryview(self, flags, self.dtype_is_object)
- *
- * def __len__(self): # <<<<<<<<<<<<<<
- * return self._shape[0]
- *
- */
-
- /* function exit code */
- __pyx_L0:;
- __Pyx_RefNannyFinishContext();
- return __pyx_r;
-}
-
-/* "View.MemoryView":233
- * return self._shape[0]
- *
- * def __getattr__(self, attr): # <<<<<<<<<<<<<<
- * return getattr(self.memview, attr)
- *
- */
-
-/* Python wrapper */
-static PyObject *__pyx_array___getattr__(PyObject *__pyx_v_self, PyObject *__pyx_v_attr); /*proto*/
-static PyObject *__pyx_array___getattr__(PyObject *__pyx_v_self, PyObject *__pyx_v_attr) {
- PyObject *__pyx_r = 0;
- __Pyx_RefNannyDeclarations
- __Pyx_RefNannySetupContext("__getattr__ (wrapper)", 0);
- __pyx_r = __pyx_array___pyx_pf_15View_dot_MemoryView_5array_8__getattr__(((struct __pyx_array_obj *)__pyx_v_self), ((PyObject *)__pyx_v_attr));
-
- /* function exit code */
- __Pyx_RefNannyFinishContext();
- return __pyx_r;
-}
-
-static PyObject *__pyx_array___pyx_pf_15View_dot_MemoryView_5array_8__getattr__(struct __pyx_array_obj *__pyx_v_self, PyObject *__pyx_v_attr) {
- PyObject *__pyx_r = NULL;
- __Pyx_RefNannyDeclarations
- PyObject *__pyx_t_1 = NULL;
- PyObject *__pyx_t_2 = NULL;
- int __pyx_lineno = 0;
- const char *__pyx_filename = NULL;
- int __pyx_clineno = 0;
- __Pyx_RefNannySetupContext("__getattr__", 0);
-
- /* "View.MemoryView":234
- *
- * def __getattr__(self, attr):
- * return getattr(self.memview, attr) # <<<<<<<<<<<<<<
- *
- * def __getitem__(self, item):
- */
- __Pyx_XDECREF(__pyx_r);
- __pyx_t_1 = __Pyx_PyObject_GetAttrStr(((PyObject *)__pyx_v_self), __pyx_n_s_memview); if (unlikely(!__pyx_t_1)) __PYX_ERR(1, 234, __pyx_L1_error)
- __Pyx_GOTREF(__pyx_t_1);
- __pyx_t_2 = __Pyx_GetAttr(__pyx_t_1, __pyx_v_attr); if (unlikely(!__pyx_t_2)) __PYX_ERR(1, 234, __pyx_L1_error)
- __Pyx_GOTREF(__pyx_t_2);
- __Pyx_DECREF(__pyx_t_1); __pyx_t_1 = 0;
- __pyx_r = __pyx_t_2;
- __pyx_t_2 = 0;
- goto __pyx_L0;
-
- /* "View.MemoryView":233
- * return self._shape[0]
- *
- * def __getattr__(self, attr): # <<<<<<<<<<<<<<
- * return getattr(self.memview, attr)
- *
- */
-
- /* function exit code */
- __pyx_L1_error:;
- __Pyx_XDECREF(__pyx_t_1);
- __Pyx_XDECREF(__pyx_t_2);
- __Pyx_AddTraceback("View.MemoryView.array.__getattr__", __pyx_clineno, __pyx_lineno, __pyx_filename);
- __pyx_r = NULL;
- __pyx_L0:;
- __Pyx_XGIVEREF(__pyx_r);
- __Pyx_RefNannyFinishContext();
- return __pyx_r;
-}
-
-/* "View.MemoryView":236
- * return getattr(self.memview, attr)
- *
- * def __getitem__(self, item): # <<<<<<<<<<<<<<
- * return self.memview[item]
- *
- */
-
-/* Python wrapper */
-static PyObject *__pyx_array___getitem__(PyObject *__pyx_v_self, PyObject *__pyx_v_item); /*proto*/
-static PyObject *__pyx_array___getitem__(PyObject *__pyx_v_self, PyObject *__pyx_v_item) {
- PyObject *__pyx_r = 0;
- __Pyx_RefNannyDeclarations
- __Pyx_RefNannySetupContext("__getitem__ (wrapper)", 0);
- __pyx_r = __pyx_array___pyx_pf_15View_dot_MemoryView_5array_10__getitem__(((struct __pyx_array_obj *)__pyx_v_self), ((PyObject *)__pyx_v_item));
-
- /* function exit code */
- __Pyx_RefNannyFinishContext();
- return __pyx_r;
-}
-
-static PyObject *__pyx_array___pyx_pf_15View_dot_MemoryView_5array_10__getitem__(struct __pyx_array_obj *__pyx_v_self, PyObject *__pyx_v_item) {
- PyObject *__pyx_r = NULL;
- __Pyx_RefNannyDeclarations
- PyObject *__pyx_t_1 = NULL;
- PyObject *__pyx_t_2 = NULL;
- int __pyx_lineno = 0;
- const char *__pyx_filename = NULL;
- int __pyx_clineno = 0;
- __Pyx_RefNannySetupContext("__getitem__", 0);
-
- /* "View.MemoryView":237
- *
- * def __getitem__(self, item):
- * return self.memview[item] # <<<<<<<<<<<<<<
- *
- * def __setitem__(self, item, value):
- */
- __Pyx_XDECREF(__pyx_r);
- __pyx_t_1 = __Pyx_PyObject_GetAttrStr(((PyObject *)__pyx_v_self), __pyx_n_s_memview); if (unlikely(!__pyx_t_1)) __PYX_ERR(1, 237, __pyx_L1_error)
- __Pyx_GOTREF(__pyx_t_1);
- __pyx_t_2 = __Pyx_PyObject_GetItem(__pyx_t_1, __pyx_v_item); if (unlikely(!__pyx_t_2)) __PYX_ERR(1, 237, __pyx_L1_error)
- __Pyx_GOTREF(__pyx_t_2);
- __Pyx_DECREF(__pyx_t_1); __pyx_t_1 = 0;
- __pyx_r = __pyx_t_2;
- __pyx_t_2 = 0;
- goto __pyx_L0;
-
- /* "View.MemoryView":236
- * return getattr(self.memview, attr)
- *
- * def __getitem__(self, item): # <<<<<<<<<<<<<<
- * return self.memview[item]
- *
- */
-
- /* function exit code */
- __pyx_L1_error:;
- __Pyx_XDECREF(__pyx_t_1);
- __Pyx_XDECREF(__pyx_t_2);
- __Pyx_AddTraceback("View.MemoryView.array.__getitem__", __pyx_clineno, __pyx_lineno, __pyx_filename);
- __pyx_r = NULL;
- __pyx_L0:;
- __Pyx_XGIVEREF(__pyx_r);
- __Pyx_RefNannyFinishContext();
- return __pyx_r;
-}
-
-/* "View.MemoryView":239
- * return self.memview[item]
- *
- * def __setitem__(self, item, value): # <<<<<<<<<<<<<<
- * self.memview[item] = value
- *
- */
-
-/* Python wrapper */
-static int __pyx_array___setitem__(PyObject *__pyx_v_self, PyObject *__pyx_v_item, PyObject *__pyx_v_value); /*proto*/
-static int __pyx_array___setitem__(PyObject *__pyx_v_self, PyObject *__pyx_v_item, PyObject *__pyx_v_value) {
- int __pyx_r;
- __Pyx_RefNannyDeclarations
- __Pyx_RefNannySetupContext("__setitem__ (wrapper)", 0);
- __pyx_r = __pyx_array___pyx_pf_15View_dot_MemoryView_5array_12__setitem__(((struct __pyx_array_obj *)__pyx_v_self), ((PyObject *)__pyx_v_item), ((PyObject *)__pyx_v_value));
-
- /* function exit code */
- __Pyx_RefNannyFinishContext();
- return __pyx_r;
-}
-
-static int __pyx_array___pyx_pf_15View_dot_MemoryView_5array_12__setitem__(struct __pyx_array_obj *__pyx_v_self, PyObject *__pyx_v_item, PyObject *__pyx_v_value) {
- int __pyx_r;
- __Pyx_RefNannyDeclarations
- PyObject *__pyx_t_1 = NULL;
- int __pyx_lineno = 0;
- const char *__pyx_filename = NULL;
- int __pyx_clineno = 0;
- __Pyx_RefNannySetupContext("__setitem__", 0);
-
- /* "View.MemoryView":240
- *
- * def __setitem__(self, item, value):
- * self.memview[item] = value # <<<<<<<<<<<<<<
- *
- *
- */
- __pyx_t_1 = __Pyx_PyObject_GetAttrStr(((PyObject *)__pyx_v_self), __pyx_n_s_memview); if (unlikely(!__pyx_t_1)) __PYX_ERR(1, 240, __pyx_L1_error)
- __Pyx_GOTREF(__pyx_t_1);
- if (unlikely(PyObject_SetItem(__pyx_t_1, __pyx_v_item, __pyx_v_value) < 0)) __PYX_ERR(1, 240, __pyx_L1_error)
- __Pyx_DECREF(__pyx_t_1); __pyx_t_1 = 0;
-
- /* "View.MemoryView":239
- * return self.memview[item]
- *
- * def __setitem__(self, item, value): # <<<<<<<<<<<<<<
- * self.memview[item] = value
- *
- */
-
- /* function exit code */
- __pyx_r = 0;
- goto __pyx_L0;
- __pyx_L1_error:;
- __Pyx_XDECREF(__pyx_t_1);
- __Pyx_AddTraceback("View.MemoryView.array.__setitem__", __pyx_clineno, __pyx_lineno, __pyx_filename);
- __pyx_r = -1;
- __pyx_L0:;
- __Pyx_RefNannyFinishContext();
- return __pyx_r;
-}
-
-/* "(tree fragment)":1
- * def __reduce_cython__(self): # <<<<<<<<<<<<<<
- * raise TypeError("no default __reduce__ due to non-trivial __cinit__")
- * def __setstate_cython__(self, __pyx_state):
- */
-
-/* Python wrapper */
-static PyObject *__pyx_pw___pyx_array_1__reduce_cython__(PyObject *__pyx_v_self, CYTHON_UNUSED PyObject *unused); /*proto*/
-static PyObject *__pyx_pw___pyx_array_1__reduce_cython__(PyObject *__pyx_v_self, CYTHON_UNUSED PyObject *unused) {
- PyObject *__pyx_r = 0;
- __Pyx_RefNannyDeclarations
- __Pyx_RefNannySetupContext("__reduce_cython__ (wrapper)", 0);
- __pyx_r = __pyx_pf___pyx_array___reduce_cython__(((struct __pyx_array_obj *)__pyx_v_self));
-
- /* function exit code */
- __Pyx_RefNannyFinishContext();
- return __pyx_r;
-}
-
-static PyObject *__pyx_pf___pyx_array___reduce_cython__(CYTHON_UNUSED struct __pyx_array_obj *__pyx_v_self) {
- PyObject *__pyx_r = NULL;
- __Pyx_RefNannyDeclarations
- PyObject *__pyx_t_1 = NULL;
- int __pyx_lineno = 0;
- const char *__pyx_filename = NULL;
- int __pyx_clineno = 0;
- __Pyx_RefNannySetupContext("__reduce_cython__", 0);
-
- /* "(tree fragment)":2
- * def __reduce_cython__(self):
- * raise TypeError("no default __reduce__ due to non-trivial __cinit__") # <<<<<<<<<<<<<<
- * def __setstate_cython__(self, __pyx_state):
- * raise TypeError("no default __reduce__ due to non-trivial __cinit__")
- */
- __pyx_t_1 = __Pyx_PyObject_Call(__pyx_builtin_TypeError, __pyx_tuple__7, NULL); if (unlikely(!__pyx_t_1)) __PYX_ERR(1, 2, __pyx_L1_error)
- __Pyx_GOTREF(__pyx_t_1);
- __Pyx_Raise(__pyx_t_1, 0, 0, 0);
- __Pyx_DECREF(__pyx_t_1); __pyx_t_1 = 0;
- __PYX_ERR(1, 2, __pyx_L1_error)
-
- /* "(tree fragment)":1
- * def __reduce_cython__(self): # <<<<<<<<<<<<<<
- * raise TypeError("no default __reduce__ due to non-trivial __cinit__")
- * def __setstate_cython__(self, __pyx_state):
- */
-
- /* function exit code */
- __pyx_L1_error:;
- __Pyx_XDECREF(__pyx_t_1);
- __Pyx_AddTraceback("View.MemoryView.array.__reduce_cython__", __pyx_clineno, __pyx_lineno, __pyx_filename);
- __pyx_r = NULL;
- __Pyx_XGIVEREF(__pyx_r);
- __Pyx_RefNannyFinishContext();
- return __pyx_r;
-}
-
-/* "(tree fragment)":3
- * def __reduce_cython__(self):
- * raise TypeError("no default __reduce__ due to non-trivial __cinit__")
- * def __setstate_cython__(self, __pyx_state): # <<<<<<<<<<<<<<
- * raise TypeError("no default __reduce__ due to non-trivial __cinit__")
- */
-
-/* Python wrapper */
-static PyObject *__pyx_pw___pyx_array_3__setstate_cython__(PyObject *__pyx_v_self, PyObject *__pyx_v___pyx_state); /*proto*/
-static PyObject *__pyx_pw___pyx_array_3__setstate_cython__(PyObject *__pyx_v_self, PyObject *__pyx_v___pyx_state) {
- PyObject *__pyx_r = 0;
- __Pyx_RefNannyDeclarations
- __Pyx_RefNannySetupContext("__setstate_cython__ (wrapper)", 0);
- __pyx_r = __pyx_pf___pyx_array_2__setstate_cython__(((struct __pyx_array_obj *)__pyx_v_self), ((PyObject *)__pyx_v___pyx_state));
-
- /* function exit code */
- __Pyx_RefNannyFinishContext();
- return __pyx_r;
-}
-
-static PyObject *__pyx_pf___pyx_array_2__setstate_cython__(CYTHON_UNUSED struct __pyx_array_obj *__pyx_v_self, CYTHON_UNUSED PyObject *__pyx_v___pyx_state) {
- PyObject *__pyx_r = NULL;
- __Pyx_RefNannyDeclarations
- PyObject *__pyx_t_1 = NULL;
- int __pyx_lineno = 0;
- const char *__pyx_filename = NULL;
- int __pyx_clineno = 0;
- __Pyx_RefNannySetupContext("__setstate_cython__", 0);
-
- /* "(tree fragment)":4
- * raise TypeError("no default __reduce__ due to non-trivial __cinit__")
- * def __setstate_cython__(self, __pyx_state):
- * raise TypeError("no default __reduce__ due to non-trivial __cinit__") # <<<<<<<<<<<<<<
- */
- __pyx_t_1 = __Pyx_PyObject_Call(__pyx_builtin_TypeError, __pyx_tuple__8, NULL); if (unlikely(!__pyx_t_1)) __PYX_ERR(1, 4, __pyx_L1_error)
- __Pyx_GOTREF(__pyx_t_1);
- __Pyx_Raise(__pyx_t_1, 0, 0, 0);
- __Pyx_DECREF(__pyx_t_1); __pyx_t_1 = 0;
- __PYX_ERR(1, 4, __pyx_L1_error)
-
- /* "(tree fragment)":3
- * def __reduce_cython__(self):
- * raise TypeError("no default __reduce__ due to non-trivial __cinit__")
- * def __setstate_cython__(self, __pyx_state): # <<<<<<<<<<<<<<
- * raise TypeError("no default __reduce__ due to non-trivial __cinit__")
- */
-
- /* function exit code */
- __pyx_L1_error:;
- __Pyx_XDECREF(__pyx_t_1);
- __Pyx_AddTraceback("View.MemoryView.array.__setstate_cython__", __pyx_clineno, __pyx_lineno, __pyx_filename);
- __pyx_r = NULL;
- __Pyx_XGIVEREF(__pyx_r);
- __Pyx_RefNannyFinishContext();
- return __pyx_r;
-}
-
-/* "View.MemoryView":244
- *
- * @cname("__pyx_array_new")
- * cdef array array_cwrapper(tuple shape, Py_ssize_t itemsize, char *format, # <<<<<<<<<<<<<<
- * char *mode, char *buf):
- * cdef array result
- */
-
-static struct __pyx_array_obj *__pyx_array_new(PyObject *__pyx_v_shape, Py_ssize_t __pyx_v_itemsize, char *__pyx_v_format, char *__pyx_v_mode, char *__pyx_v_buf) {
- struct __pyx_array_obj *__pyx_v_result = 0;
- struct __pyx_array_obj *__pyx_r = NULL;
- __Pyx_RefNannyDeclarations
- int __pyx_t_1;
- PyObject *__pyx_t_2 = NULL;
- PyObject *__pyx_t_3 = NULL;
- PyObject *__pyx_t_4 = NULL;
- PyObject *__pyx_t_5 = NULL;
- int __pyx_lineno = 0;
- const char *__pyx_filename = NULL;
- int __pyx_clineno = 0;
- __Pyx_RefNannySetupContext("array_cwrapper", 0);
-
- /* "View.MemoryView":248
- * cdef array result
- *
- * if buf == NULL: # <<<<<<<<<<<<<<
- * result = array(shape, itemsize, format, mode.decode('ASCII'))
- * else:
- */
- __pyx_t_1 = ((__pyx_v_buf == NULL) != 0);
- if (__pyx_t_1) {
-
- /* "View.MemoryView":249
- *
- * if buf == NULL:
- * result = array(shape, itemsize, format, mode.decode('ASCII')) # <<<<<<<<<<<<<<
- * else:
- * result = array(shape, itemsize, format, mode.decode('ASCII'),
- */
- __pyx_t_2 = PyInt_FromSsize_t(__pyx_v_itemsize); if (unlikely(!__pyx_t_2)) __PYX_ERR(1, 249, __pyx_L1_error)
- __Pyx_GOTREF(__pyx_t_2);
- __pyx_t_3 = __Pyx_PyBytes_FromString(__pyx_v_format); if (unlikely(!__pyx_t_3)) __PYX_ERR(1, 249, __pyx_L1_error)
- __Pyx_GOTREF(__pyx_t_3);
- __pyx_t_4 = __Pyx_decode_c_string(__pyx_v_mode, 0, strlen(__pyx_v_mode), NULL, NULL, PyUnicode_DecodeASCII); if (unlikely(!__pyx_t_4)) __PYX_ERR(1, 249, __pyx_L1_error)
- __Pyx_GOTREF(__pyx_t_4);
- __pyx_t_5 = PyTuple_New(4); if (unlikely(!__pyx_t_5)) __PYX_ERR(1, 249, __pyx_L1_error)
- __Pyx_GOTREF(__pyx_t_5);
- __Pyx_INCREF(__pyx_v_shape);
- __Pyx_GIVEREF(__pyx_v_shape);
- PyTuple_SET_ITEM(__pyx_t_5, 0, __pyx_v_shape);
- __Pyx_GIVEREF(__pyx_t_2);
- PyTuple_SET_ITEM(__pyx_t_5, 1, __pyx_t_2);
- __Pyx_GIVEREF(__pyx_t_3);
- PyTuple_SET_ITEM(__pyx_t_5, 2, __pyx_t_3);
- __Pyx_GIVEREF(__pyx_t_4);
- PyTuple_SET_ITEM(__pyx_t_5, 3, __pyx_t_4);
- __pyx_t_2 = 0;
- __pyx_t_3 = 0;
- __pyx_t_4 = 0;
- __pyx_t_4 = __Pyx_PyObject_Call(((PyObject *)__pyx_array_type), __pyx_t_5, NULL); if (unlikely(!__pyx_t_4)) __PYX_ERR(1, 249, __pyx_L1_error)
- __Pyx_GOTREF(__pyx_t_4);
- __Pyx_DECREF(__pyx_t_5); __pyx_t_5 = 0;
- __pyx_v_result = ((struct __pyx_array_obj *)__pyx_t_4);
- __pyx_t_4 = 0;
-
- /* "View.MemoryView":248
- * cdef array result
- *
- * if buf == NULL: # <<<<<<<<<<<<<<
- * result = array(shape, itemsize, format, mode.decode('ASCII'))
- * else:
- */
- goto __pyx_L3;
- }
-
- /* "View.MemoryView":251
- * result = array(shape, itemsize, format, mode.decode('ASCII'))
- * else:
- * result = array(shape, itemsize, format, mode.decode('ASCII'), # <<<<<<<<<<<<<<
- * allocate_buffer=False)
- * result.data = buf
- */
- /*else*/ {
- __pyx_t_4 = PyInt_FromSsize_t(__pyx_v_itemsize); if (unlikely(!__pyx_t_4)) __PYX_ERR(1, 251, __pyx_L1_error)
- __Pyx_GOTREF(__pyx_t_4);
- __pyx_t_5 = __Pyx_PyBytes_FromString(__pyx_v_format); if (unlikely(!__pyx_t_5)) __PYX_ERR(1, 251, __pyx_L1_error)
- __Pyx_GOTREF(__pyx_t_5);
- __pyx_t_3 = __Pyx_decode_c_string(__pyx_v_mode, 0, strlen(__pyx_v_mode), NULL, NULL, PyUnicode_DecodeASCII); if (unlikely(!__pyx_t_3)) __PYX_ERR(1, 251, __pyx_L1_error)
- __Pyx_GOTREF(__pyx_t_3);
- __pyx_t_2 = PyTuple_New(4); if (unlikely(!__pyx_t_2)) __PYX_ERR(1, 251, __pyx_L1_error)
- __Pyx_GOTREF(__pyx_t_2);
- __Pyx_INCREF(__pyx_v_shape);
- __Pyx_GIVEREF(__pyx_v_shape);
- PyTuple_SET_ITEM(__pyx_t_2, 0, __pyx_v_shape);
- __Pyx_GIVEREF(__pyx_t_4);
- PyTuple_SET_ITEM(__pyx_t_2, 1, __pyx_t_4);
- __Pyx_GIVEREF(__pyx_t_5);
- PyTuple_SET_ITEM(__pyx_t_2, 2, __pyx_t_5);
- __Pyx_GIVEREF(__pyx_t_3);
- PyTuple_SET_ITEM(__pyx_t_2, 3, __pyx_t_3);
- __pyx_t_4 = 0;
- __pyx_t_5 = 0;
- __pyx_t_3 = 0;
-
- /* "View.MemoryView":252
- * else:
- * result = array(shape, itemsize, format, mode.decode('ASCII'),
- * allocate_buffer=False) # <<<<<<<<<<<<<<
- * result.data = buf
- *
- */
- __pyx_t_3 = __Pyx_PyDict_NewPresized(1); if (unlikely(!__pyx_t_3)) __PYX_ERR(1, 252, __pyx_L1_error)
- __Pyx_GOTREF(__pyx_t_3);
- if (PyDict_SetItem(__pyx_t_3, __pyx_n_s_allocate_buffer, Py_False) < 0) __PYX_ERR(1, 252, __pyx_L1_error)
-
- /* "View.MemoryView":251
- * result = array(shape, itemsize, format, mode.decode('ASCII'))
- * else:
- * result = array(shape, itemsize, format, mode.decode('ASCII'), # <<<<<<<<<<<<<<
- * allocate_buffer=False)
- * result.data = buf
- */
- __pyx_t_5 = __Pyx_PyObject_Call(((PyObject *)__pyx_array_type), __pyx_t_2, __pyx_t_3); if (unlikely(!__pyx_t_5)) __PYX_ERR(1, 251, __pyx_L1_error)
- __Pyx_GOTREF(__pyx_t_5);
- __Pyx_DECREF(__pyx_t_2); __pyx_t_2 = 0;
- __Pyx_DECREF(__pyx_t_3); __pyx_t_3 = 0;
- __pyx_v_result = ((struct __pyx_array_obj *)__pyx_t_5);
- __pyx_t_5 = 0;
-
- /* "View.MemoryView":253
- * result = array(shape, itemsize, format, mode.decode('ASCII'),
- * allocate_buffer=False)
- * result.data = buf # <<<<<<<<<<<<<<
- *
- * return result
- */
- __pyx_v_result->data = __pyx_v_buf;
- }
- __pyx_L3:;
-
- /* "View.MemoryView":255
- * result.data = buf
- *
- * return result # <<<<<<<<<<<<<<
- *
- *
- */
- __Pyx_XDECREF(((PyObject *)__pyx_r));
- __Pyx_INCREF(((PyObject *)__pyx_v_result));
- __pyx_r = __pyx_v_result;
- goto __pyx_L0;
-
- /* "View.MemoryView":244
- *
- * @cname("__pyx_array_new")
- * cdef array array_cwrapper(tuple shape, Py_ssize_t itemsize, char *format, # <<<<<<<<<<<<<<
- * char *mode, char *buf):
- * cdef array result
- */
-
- /* function exit code */
- __pyx_L1_error:;
- __Pyx_XDECREF(__pyx_t_2);
- __Pyx_XDECREF(__pyx_t_3);
- __Pyx_XDECREF(__pyx_t_4);
- __Pyx_XDECREF(__pyx_t_5);
- __Pyx_AddTraceback("View.MemoryView.array_cwrapper", __pyx_clineno, __pyx_lineno, __pyx_filename);
- __pyx_r = 0;
- __pyx_L0:;
- __Pyx_XDECREF((PyObject *)__pyx_v_result);
- __Pyx_XGIVEREF((PyObject *)__pyx_r);
- __Pyx_RefNannyFinishContext();
- return __pyx_r;
-}
-
-/* "View.MemoryView":281
- * cdef class Enum(object):
- * cdef object name
- * def __init__(self, name): # <<<<<<<<<<<<<<
- * self.name = name
- * def __repr__(self):
- */
-
-/* Python wrapper */
-static int __pyx_MemviewEnum___init__(PyObject *__pyx_v_self, PyObject *__pyx_args, PyObject *__pyx_kwds); /*proto*/
-static int __pyx_MemviewEnum___init__(PyObject *__pyx_v_self, PyObject *__pyx_args, PyObject *__pyx_kwds) {
- PyObject *__pyx_v_name = 0;
- int __pyx_lineno = 0;
- const char *__pyx_filename = NULL;
- int __pyx_clineno = 0;
- int __pyx_r;
- __Pyx_RefNannyDeclarations
- __Pyx_RefNannySetupContext("__init__ (wrapper)", 0);
- {
- static PyObject **__pyx_pyargnames[] = {&__pyx_n_s_name,0};
- PyObject* values[1] = {0};
- if (unlikely(__pyx_kwds)) {
- Py_ssize_t kw_args;
- const Py_ssize_t pos_args = PyTuple_GET_SIZE(__pyx_args);
- switch (pos_args) {
- case 1: values[0] = PyTuple_GET_ITEM(__pyx_args, 0);
- CYTHON_FALLTHROUGH;
- case 0: break;
- default: goto __pyx_L5_argtuple_error;
- }
- kw_args = PyDict_Size(__pyx_kwds);
- switch (pos_args) {
- case 0:
- if (likely((values[0] = __Pyx_PyDict_GetItemStr(__pyx_kwds, __pyx_n_s_name)) != 0)) kw_args--;
- else goto __pyx_L5_argtuple_error;
- }
- if (unlikely(kw_args > 0)) {
- if (unlikely(__Pyx_ParseOptionalKeywords(__pyx_kwds, __pyx_pyargnames, 0, values, pos_args, "__init__") < 0)) __PYX_ERR(1, 281, __pyx_L3_error)
- }
- } else if (PyTuple_GET_SIZE(__pyx_args) != 1) {
- goto __pyx_L5_argtuple_error;
- } else {
- values[0] = PyTuple_GET_ITEM(__pyx_args, 0);
- }
- __pyx_v_name = values[0];
- }
- goto __pyx_L4_argument_unpacking_done;
- __pyx_L5_argtuple_error:;
- __Pyx_RaiseArgtupleInvalid("__init__", 1, 1, 1, PyTuple_GET_SIZE(__pyx_args)); __PYX_ERR(1, 281, __pyx_L3_error)
- __pyx_L3_error:;
- __Pyx_AddTraceback("View.MemoryView.Enum.__init__", __pyx_clineno, __pyx_lineno, __pyx_filename);
- __Pyx_RefNannyFinishContext();
- return -1;
- __pyx_L4_argument_unpacking_done:;
- __pyx_r = __pyx_MemviewEnum___pyx_pf_15View_dot_MemoryView_4Enum___init__(((struct __pyx_MemviewEnum_obj *)__pyx_v_self), __pyx_v_name);
-
- /* function exit code */
- __Pyx_RefNannyFinishContext();
- return __pyx_r;
-}
-
-static int __pyx_MemviewEnum___pyx_pf_15View_dot_MemoryView_4Enum___init__(struct __pyx_MemviewEnum_obj *__pyx_v_self, PyObject *__pyx_v_name) {
- int __pyx_r;
- __Pyx_RefNannyDeclarations
- __Pyx_RefNannySetupContext("__init__", 0);
-
- /* "View.MemoryView":282
- * cdef object name
- * def __init__(self, name):
- * self.name = name # <<<<<<<<<<<<<<
- * def __repr__(self):
- * return self.name
- */
- __Pyx_INCREF(__pyx_v_name);
- __Pyx_GIVEREF(__pyx_v_name);
- __Pyx_GOTREF(__pyx_v_self->name);
- __Pyx_DECREF(__pyx_v_self->name);
- __pyx_v_self->name = __pyx_v_name;
-
- /* "View.MemoryView":281
- * cdef class Enum(object):
- * cdef object name
- * def __init__(self, name): # <<<<<<<<<<<<<<
- * self.name = name
- * def __repr__(self):
- */
-
- /* function exit code */
- __pyx_r = 0;
- __Pyx_RefNannyFinishContext();
- return __pyx_r;
-}
-
-/* "View.MemoryView":283
- * def __init__(self, name):
- * self.name = name
- * def __repr__(self): # <<<<<<<<<<<<<<
- * return self.name
- *
- */
-
-/* Python wrapper */
-static PyObject *__pyx_MemviewEnum___repr__(PyObject *__pyx_v_self); /*proto*/
-static PyObject *__pyx_MemviewEnum___repr__(PyObject *__pyx_v_self) {
- PyObject *__pyx_r = 0;
- __Pyx_RefNannyDeclarations
- __Pyx_RefNannySetupContext("__repr__ (wrapper)", 0);
- __pyx_r = __pyx_MemviewEnum___pyx_pf_15View_dot_MemoryView_4Enum_2__repr__(((struct __pyx_MemviewEnum_obj *)__pyx_v_self));
-
- /* function exit code */
- __Pyx_RefNannyFinishContext();
- return __pyx_r;
-}
-
-static PyObject *__pyx_MemviewEnum___pyx_pf_15View_dot_MemoryView_4Enum_2__repr__(struct __pyx_MemviewEnum_obj *__pyx_v_self) {
- PyObject *__pyx_r = NULL;
- __Pyx_RefNannyDeclarations
- __Pyx_RefNannySetupContext("__repr__", 0);
-
- /* "View.MemoryView":284
- * self.name = name
- * def __repr__(self):
- * return self.name # <<<<<<<<<<<<<<
- *
- * cdef generic = Enum("")
- */
- __Pyx_XDECREF(__pyx_r);
- __Pyx_INCREF(__pyx_v_self->name);
- __pyx_r = __pyx_v_self->name;
- goto __pyx_L0;
-
- /* "View.MemoryView":283
- * def __init__(self, name):
- * self.name = name
- * def __repr__(self): # <<<<<<<<<<<<<<
- * return self.name
- *
- */
-
- /* function exit code */
- __pyx_L0:;
- __Pyx_XGIVEREF(__pyx_r);
- __Pyx_RefNannyFinishContext();
- return __pyx_r;
-}
-
-/* "(tree fragment)":1
- * def __reduce_cython__(self): # <<<<<<<<<<<<<<
- * cdef tuple state
- * cdef object _dict
- */
-
-/* Python wrapper */
-static PyObject *__pyx_pw___pyx_MemviewEnum_1__reduce_cython__(PyObject *__pyx_v_self, CYTHON_UNUSED PyObject *unused); /*proto*/
-static PyObject *__pyx_pw___pyx_MemviewEnum_1__reduce_cython__(PyObject *__pyx_v_self, CYTHON_UNUSED PyObject *unused) {
- PyObject *__pyx_r = 0;
- __Pyx_RefNannyDeclarations
- __Pyx_RefNannySetupContext("__reduce_cython__ (wrapper)", 0);
- __pyx_r = __pyx_pf___pyx_MemviewEnum___reduce_cython__(((struct __pyx_MemviewEnum_obj *)__pyx_v_self));
-
- /* function exit code */
- __Pyx_RefNannyFinishContext();
- return __pyx_r;
-}
-
-static PyObject *__pyx_pf___pyx_MemviewEnum___reduce_cython__(struct __pyx_MemviewEnum_obj *__pyx_v_self) {
- PyObject *__pyx_v_state = 0;
- PyObject *__pyx_v__dict = 0;
- int __pyx_v_use_setstate;
- PyObject *__pyx_r = NULL;
- __Pyx_RefNannyDeclarations
- PyObject *__pyx_t_1 = NULL;
- int __pyx_t_2;
- int __pyx_t_3;
- PyObject *__pyx_t_4 = NULL;
- PyObject *__pyx_t_5 = NULL;
- int __pyx_lineno = 0;
- const char *__pyx_filename = NULL;
- int __pyx_clineno = 0;
- __Pyx_RefNannySetupContext("__reduce_cython__", 0);
-
- /* "(tree fragment)":5
- * cdef object _dict
- * cdef bint use_setstate
- * state = (self.name,) # <<<<<<<<<<<<<<
- * _dict = getattr(self, '__dict__', None)
- * if _dict is not None:
- */
- __pyx_t_1 = PyTuple_New(1); if (unlikely(!__pyx_t_1)) __PYX_ERR(1, 5, __pyx_L1_error)
- __Pyx_GOTREF(__pyx_t_1);
- __Pyx_INCREF(__pyx_v_self->name);
- __Pyx_GIVEREF(__pyx_v_self->name);
- PyTuple_SET_ITEM(__pyx_t_1, 0, __pyx_v_self->name);
- __pyx_v_state = ((PyObject*)__pyx_t_1);
- __pyx_t_1 = 0;
-
- /* "(tree fragment)":6
- * cdef bint use_setstate
- * state = (self.name,)
- * _dict = getattr(self, '__dict__', None) # <<<<<<<<<<<<<<
- * if _dict is not None:
- * state += (_dict,)
- */
- __pyx_t_1 = __Pyx_GetAttr3(((PyObject *)__pyx_v_self), __pyx_n_s_dict, Py_None); if (unlikely(!__pyx_t_1)) __PYX_ERR(1, 6, __pyx_L1_error)
- __Pyx_GOTREF(__pyx_t_1);
- __pyx_v__dict = __pyx_t_1;
- __pyx_t_1 = 0;
-
- /* "(tree fragment)":7
- * state = (self.name,)
- * _dict = getattr(self, '__dict__', None)
- * if _dict is not None: # <<<<<<<<<<<<<<
- * state += (_dict,)
- * use_setstate = True
- */
- __pyx_t_2 = (__pyx_v__dict != Py_None);
- __pyx_t_3 = (__pyx_t_2 != 0);
- if (__pyx_t_3) {
-
- /* "(tree fragment)":8
- * _dict = getattr(self, '__dict__', None)
- * if _dict is not None:
- * state += (_dict,) # <<<<<<<<<<<<<<
- * use_setstate = True
- * else:
- */
- __pyx_t_1 = PyTuple_New(1); if (unlikely(!__pyx_t_1)) __PYX_ERR(1, 8, __pyx_L1_error)
- __Pyx_GOTREF(__pyx_t_1);
- __Pyx_INCREF(__pyx_v__dict);
- __Pyx_GIVEREF(__pyx_v__dict);
- PyTuple_SET_ITEM(__pyx_t_1, 0, __pyx_v__dict);
- __pyx_t_4 = PyNumber_InPlaceAdd(__pyx_v_state, __pyx_t_1); if (unlikely(!__pyx_t_4)) __PYX_ERR(1, 8, __pyx_L1_error)
- __Pyx_GOTREF(__pyx_t_4);
- __Pyx_DECREF(__pyx_t_1); __pyx_t_1 = 0;
- __Pyx_DECREF_SET(__pyx_v_state, ((PyObject*)__pyx_t_4));
- __pyx_t_4 = 0;
-
- /* "(tree fragment)":9
- * if _dict is not None:
- * state += (_dict,)
- * use_setstate = True # <<<<<<<<<<<<<<
- * else:
- * use_setstate = self.name is not None
- */
- __pyx_v_use_setstate = 1;
-
- /* "(tree fragment)":7
- * state = (self.name,)
- * _dict = getattr(self, '__dict__', None)
- * if _dict is not None: # <<<<<<<<<<<<<<
- * state += (_dict,)
- * use_setstate = True
- */
- goto __pyx_L3;
- }
-
- /* "(tree fragment)":11
- * use_setstate = True
- * else:
- * use_setstate = self.name is not None # <<<<<<<<<<<<<<
- * if use_setstate:
- * return __pyx_unpickle_Enum, (type(self), 0xb068931, None), state
- */
- /*else*/ {
- __pyx_t_3 = (__pyx_v_self->name != Py_None);
- __pyx_v_use_setstate = __pyx_t_3;
- }
- __pyx_L3:;
-
- /* "(tree fragment)":12
- * else:
- * use_setstate = self.name is not None
- * if use_setstate: # <<<<<<<<<<<<<<
- * return __pyx_unpickle_Enum, (type(self), 0xb068931, None), state
- * else:
- */
- __pyx_t_3 = (__pyx_v_use_setstate != 0);
- if (__pyx_t_3) {
-
- /* "(tree fragment)":13
- * use_setstate = self.name is not None
- * if use_setstate:
- * return __pyx_unpickle_Enum, (type(self), 0xb068931, None), state # <<<<<<<<<<<<<<
- * else:
- * return __pyx_unpickle_Enum, (type(self), 0xb068931, state)
- */
- __Pyx_XDECREF(__pyx_r);
- __Pyx_GetModuleGlobalName(__pyx_t_4, __pyx_n_s_pyx_unpickle_Enum); if (unlikely(!__pyx_t_4)) __PYX_ERR(1, 13, __pyx_L1_error)
- __Pyx_GOTREF(__pyx_t_4);
- __pyx_t_1 = PyTuple_New(3); if (unlikely(!__pyx_t_1)) __PYX_ERR(1, 13, __pyx_L1_error)
- __Pyx_GOTREF(__pyx_t_1);
- __Pyx_INCREF(((PyObject *)Py_TYPE(((PyObject *)__pyx_v_self))));
- __Pyx_GIVEREF(((PyObject *)Py_TYPE(((PyObject *)__pyx_v_self))));
- PyTuple_SET_ITEM(__pyx_t_1, 0, ((PyObject *)Py_TYPE(((PyObject *)__pyx_v_self))));
- __Pyx_INCREF(__pyx_int_184977713);
- __Pyx_GIVEREF(__pyx_int_184977713);
- PyTuple_SET_ITEM(__pyx_t_1, 1, __pyx_int_184977713);
- __Pyx_INCREF(Py_None);
- __Pyx_GIVEREF(Py_None);
- PyTuple_SET_ITEM(__pyx_t_1, 2, Py_None);
- __pyx_t_5 = PyTuple_New(3); if (unlikely(!__pyx_t_5)) __PYX_ERR(1, 13, __pyx_L1_error)
- __Pyx_GOTREF(__pyx_t_5);
- __Pyx_GIVEREF(__pyx_t_4);
- PyTuple_SET_ITEM(__pyx_t_5, 0, __pyx_t_4);
- __Pyx_GIVEREF(__pyx_t_1);
- PyTuple_SET_ITEM(__pyx_t_5, 1, __pyx_t_1);
- __Pyx_INCREF(__pyx_v_state);
- __Pyx_GIVEREF(__pyx_v_state);
- PyTuple_SET_ITEM(__pyx_t_5, 2, __pyx_v_state);
- __pyx_t_4 = 0;
- __pyx_t_1 = 0;
- __pyx_r = __pyx_t_5;
- __pyx_t_5 = 0;
- goto __pyx_L0;
-
- /* "(tree fragment)":12
- * else:
- * use_setstate = self.name is not None
- * if use_setstate: # <<<<<<<<<<<<<<
- * return __pyx_unpickle_Enum, (type(self), 0xb068931, None), state
- * else:
- */
- }
-
- /* "(tree fragment)":15
- * return __pyx_unpickle_Enum, (type(self), 0xb068931, None), state
- * else:
- * return __pyx_unpickle_Enum, (type(self), 0xb068931, state) # <<<<<<<<<<<<<<
- * def __setstate_cython__(self, __pyx_state):
- * __pyx_unpickle_Enum__set_state(self, __pyx_state)
- */
- /*else*/ {
- __Pyx_XDECREF(__pyx_r);
- __Pyx_GetModuleGlobalName(__pyx_t_5, __pyx_n_s_pyx_unpickle_Enum); if (unlikely(!__pyx_t_5)) __PYX_ERR(1, 15, __pyx_L1_error)
- __Pyx_GOTREF(__pyx_t_5);
- __pyx_t_1 = PyTuple_New(3); if (unlikely(!__pyx_t_1)) __PYX_ERR(1, 15, __pyx_L1_error)
- __Pyx_GOTREF(__pyx_t_1);
- __Pyx_INCREF(((PyObject *)Py_TYPE(((PyObject *)__pyx_v_self))));
- __Pyx_GIVEREF(((PyObject *)Py_TYPE(((PyObject *)__pyx_v_self))));
- PyTuple_SET_ITEM(__pyx_t_1, 0, ((PyObject *)Py_TYPE(((PyObject *)__pyx_v_self))));
- __Pyx_INCREF(__pyx_int_184977713);
- __Pyx_GIVEREF(__pyx_int_184977713);
- PyTuple_SET_ITEM(__pyx_t_1, 1, __pyx_int_184977713);
- __Pyx_INCREF(__pyx_v_state);
- __Pyx_GIVEREF(__pyx_v_state);
- PyTuple_SET_ITEM(__pyx_t_1, 2, __pyx_v_state);
- __pyx_t_4 = PyTuple_New(2); if (unlikely(!__pyx_t_4)) __PYX_ERR(1, 15, __pyx_L1_error)
- __Pyx_GOTREF(__pyx_t_4);
- __Pyx_GIVEREF(__pyx_t_5);
- PyTuple_SET_ITEM(__pyx_t_4, 0, __pyx_t_5);
- __Pyx_GIVEREF(__pyx_t_1);
- PyTuple_SET_ITEM(__pyx_t_4, 1, __pyx_t_1);
- __pyx_t_5 = 0;
- __pyx_t_1 = 0;
- __pyx_r = __pyx_t_4;
- __pyx_t_4 = 0;
- goto __pyx_L0;
- }
-
- /* "(tree fragment)":1
- * def __reduce_cython__(self): # <<<<<<<<<<<<<<
- * cdef tuple state
- * cdef object _dict
- */
-
- /* function exit code */
- __pyx_L1_error:;
- __Pyx_XDECREF(__pyx_t_1);
- __Pyx_XDECREF(__pyx_t_4);
- __Pyx_XDECREF(__pyx_t_5);
- __Pyx_AddTraceback("View.MemoryView.Enum.__reduce_cython__", __pyx_clineno, __pyx_lineno, __pyx_filename);
- __pyx_r = NULL;
- __pyx_L0:;
- __Pyx_XDECREF(__pyx_v_state);
- __Pyx_XDECREF(__pyx_v__dict);
- __Pyx_XGIVEREF(__pyx_r);
- __Pyx_RefNannyFinishContext();
- return __pyx_r;
-}
-
-/* "(tree fragment)":16
- * else:
- * return __pyx_unpickle_Enum, (type(self), 0xb068931, state)
- * def __setstate_cython__(self, __pyx_state): # <<<<<<<<<<<<<<
- * __pyx_unpickle_Enum__set_state(self, __pyx_state)
- */
-
-/* Python wrapper */
-static PyObject *__pyx_pw___pyx_MemviewEnum_3__setstate_cython__(PyObject *__pyx_v_self, PyObject *__pyx_v___pyx_state); /*proto*/
-static PyObject *__pyx_pw___pyx_MemviewEnum_3__setstate_cython__(PyObject *__pyx_v_self, PyObject *__pyx_v___pyx_state) {
- PyObject *__pyx_r = 0;
- __Pyx_RefNannyDeclarations
- __Pyx_RefNannySetupContext("__setstate_cython__ (wrapper)", 0);
- __pyx_r = __pyx_pf___pyx_MemviewEnum_2__setstate_cython__(((struct __pyx_MemviewEnum_obj *)__pyx_v_self), ((PyObject *)__pyx_v___pyx_state));
-
- /* function exit code */
- __Pyx_RefNannyFinishContext();
- return __pyx_r;
-}
-
-static PyObject *__pyx_pf___pyx_MemviewEnum_2__setstate_cython__(struct __pyx_MemviewEnum_obj *__pyx_v_self, PyObject *__pyx_v___pyx_state) {
- PyObject *__pyx_r = NULL;
- __Pyx_RefNannyDeclarations
- PyObject *__pyx_t_1 = NULL;
- int __pyx_lineno = 0;
- const char *__pyx_filename = NULL;
- int __pyx_clineno = 0;
- __Pyx_RefNannySetupContext("__setstate_cython__", 0);
-
- /* "(tree fragment)":17
- * return __pyx_unpickle_Enum, (type(self), 0xb068931, state)
- * def __setstate_cython__(self, __pyx_state):
- * __pyx_unpickle_Enum__set_state(self, __pyx_state) # <<<<<<<<<<<<<<
- */
- if (!(likely(PyTuple_CheckExact(__pyx_v___pyx_state))||((__pyx_v___pyx_state) == Py_None)||(PyErr_Format(PyExc_TypeError, "Expected %.16s, got %.200s", "tuple", Py_TYPE(__pyx_v___pyx_state)->tp_name), 0))) __PYX_ERR(1, 17, __pyx_L1_error)
- __pyx_t_1 = __pyx_unpickle_Enum__set_state(__pyx_v_self, ((PyObject*)__pyx_v___pyx_state)); if (unlikely(!__pyx_t_1)) __PYX_ERR(1, 17, __pyx_L1_error)
- __Pyx_GOTREF(__pyx_t_1);
- __Pyx_DECREF(__pyx_t_1); __pyx_t_1 = 0;
-
- /* "(tree fragment)":16
- * else:
- * return __pyx_unpickle_Enum, (type(self), 0xb068931, state)
- * def __setstate_cython__(self, __pyx_state): # <<<<<<<<<<<<<<
- * __pyx_unpickle_Enum__set_state(self, __pyx_state)
- */
-
- /* function exit code */
- __pyx_r = Py_None; __Pyx_INCREF(Py_None);
- goto __pyx_L0;
- __pyx_L1_error:;
- __Pyx_XDECREF(__pyx_t_1);
- __Pyx_AddTraceback("View.MemoryView.Enum.__setstate_cython__", __pyx_clineno, __pyx_lineno, __pyx_filename);
- __pyx_r = NULL;
- __pyx_L0:;
- __Pyx_XGIVEREF(__pyx_r);
- __Pyx_RefNannyFinishContext();
- return __pyx_r;
-}
-
-/* "View.MemoryView":298
- *
- * @cname('__pyx_align_pointer')
- * cdef void *align_pointer(void *memory, size_t alignment) nogil: # <<<<<<<<<<<<<<
- * "Align pointer memory on a given boundary"
- * cdef Py_intptr_t aligned_p = memory
- */
-
-static void *__pyx_align_pointer(void *__pyx_v_memory, size_t __pyx_v_alignment) {
- Py_intptr_t __pyx_v_aligned_p;
- size_t __pyx_v_offset;
- void *__pyx_r;
- int __pyx_t_1;
-
- /* "View.MemoryView":300
- * cdef void *align_pointer(void *memory, size_t alignment) nogil:
- * "Align pointer memory on a given boundary"
- * cdef Py_intptr_t aligned_p = memory # <<<<<<<<<<<<<<
- * cdef size_t offset
- *
- */
- __pyx_v_aligned_p = ((Py_intptr_t)__pyx_v_memory);
-
- /* "View.MemoryView":304
- *
- * with cython.cdivision(True):
- * offset = aligned_p % alignment # <<<<<<<<<<<<<<
- *
- * if offset > 0:
- */
- __pyx_v_offset = (__pyx_v_aligned_p % __pyx_v_alignment);
-
- /* "View.MemoryView":306
- * offset = aligned_p % alignment
- *
- * if offset > 0: # <<<<<<<<<<<<<<
- * aligned_p += alignment - offset
- *
- */
- __pyx_t_1 = ((__pyx_v_offset > 0) != 0);
- if (__pyx_t_1) {
-
- /* "View.MemoryView":307
- *
- * if offset > 0:
- * aligned_p += alignment - offset # <<<<<<<<<<<<<<
- *
- * return aligned_p
- */
- __pyx_v_aligned_p = (__pyx_v_aligned_p + (__pyx_v_alignment - __pyx_v_offset));
-
- /* "View.MemoryView":306
- * offset = aligned_p % alignment
- *
- * if offset > 0: # <<<<<<<<<<<<<<
- * aligned_p += alignment - offset
- *
- */
- }
-
- /* "View.MemoryView":309
- * aligned_p += alignment - offset
- *
- * return aligned_p # <<<<<<<<<<<<<<
- *
- *
- */
- __pyx_r = ((void *)__pyx_v_aligned_p);
- goto __pyx_L0;
-
- /* "View.MemoryView":298
- *
- * @cname('__pyx_align_pointer')
- * cdef void *align_pointer(void *memory, size_t alignment) nogil: # <<<<<<<<<<<<<<
- * "Align pointer memory on a given boundary"
- * cdef Py_intptr_t aligned_p = memory
- */
-
- /* function exit code */
- __pyx_L0:;
- return __pyx_r;
-}
-
-/* "View.MemoryView":345
- * cdef __Pyx_TypeInfo *typeinfo
- *
- * def __cinit__(memoryview self, object obj, int flags, bint dtype_is_object=False): # <<<<<<<<<<<<<<
- * self.obj = obj
- * self.flags = flags
- */
-
-/* Python wrapper */
-static int __pyx_memoryview___cinit__(PyObject *__pyx_v_self, PyObject *__pyx_args, PyObject *__pyx_kwds); /*proto*/
-static int __pyx_memoryview___cinit__(PyObject *__pyx_v_self, PyObject *__pyx_args, PyObject *__pyx_kwds) {
- PyObject *__pyx_v_obj = 0;
- int __pyx_v_flags;
- int __pyx_v_dtype_is_object;
- int __pyx_lineno = 0;
- const char *__pyx_filename = NULL;
- int __pyx_clineno = 0;
- int __pyx_r;
- __Pyx_RefNannyDeclarations
- __Pyx_RefNannySetupContext("__cinit__ (wrapper)", 0);
- {
- static PyObject **__pyx_pyargnames[] = {&__pyx_n_s_obj,&__pyx_n_s_flags,&__pyx_n_s_dtype_is_object,0};
- PyObject* values[3] = {0,0,0};
- if (unlikely(__pyx_kwds)) {
- Py_ssize_t kw_args;
- const Py_ssize_t pos_args = PyTuple_GET_SIZE(__pyx_args);
- switch (pos_args) {
- case 3: values[2] = PyTuple_GET_ITEM(__pyx_args, 2);
- CYTHON_FALLTHROUGH;
- case 2: values[1] = PyTuple_GET_ITEM(__pyx_args, 1);
- CYTHON_FALLTHROUGH;
- case 1: values[0] = PyTuple_GET_ITEM(__pyx_args, 0);
- CYTHON_FALLTHROUGH;
- case 0: break;
- default: goto __pyx_L5_argtuple_error;
- }
- kw_args = PyDict_Size(__pyx_kwds);
- switch (pos_args) {
- case 0:
- if (likely((values[0] = __Pyx_PyDict_GetItemStr(__pyx_kwds, __pyx_n_s_obj)) != 0)) kw_args--;
- else goto __pyx_L5_argtuple_error;
- CYTHON_FALLTHROUGH;
- case 1:
- if (likely((values[1] = __Pyx_PyDict_GetItemStr(__pyx_kwds, __pyx_n_s_flags)) != 0)) kw_args--;
- else {
- __Pyx_RaiseArgtupleInvalid("__cinit__", 0, 2, 3, 1); __PYX_ERR(1, 345, __pyx_L3_error)
- }
- CYTHON_FALLTHROUGH;
- case 2:
- if (kw_args > 0) {
- PyObject* value = __Pyx_PyDict_GetItemStr(__pyx_kwds, __pyx_n_s_dtype_is_object);
- if (value) { values[2] = value; kw_args--; }
- }
- }
- if (unlikely(kw_args > 0)) {
- if (unlikely(__Pyx_ParseOptionalKeywords(__pyx_kwds, __pyx_pyargnames, 0, values, pos_args, "__cinit__") < 0)) __PYX_ERR(1, 345, __pyx_L3_error)
- }
- } else {
- switch (PyTuple_GET_SIZE(__pyx_args)) {
- case 3: values[2] = PyTuple_GET_ITEM(__pyx_args, 2);
- CYTHON_FALLTHROUGH;
- case 2: values[1] = PyTuple_GET_ITEM(__pyx_args, 1);
- values[0] = PyTuple_GET_ITEM(__pyx_args, 0);
- break;
- default: goto __pyx_L5_argtuple_error;
- }
- }
- __pyx_v_obj = values[0];
- __pyx_v_flags = __Pyx_PyInt_As_int(values[1]); if (unlikely((__pyx_v_flags == (int)-1) && PyErr_Occurred())) __PYX_ERR(1, 345, __pyx_L3_error)
- if (values[2]) {
- __pyx_v_dtype_is_object = __Pyx_PyObject_IsTrue(values[2]); if (unlikely((__pyx_v_dtype_is_object == (int)-1) && PyErr_Occurred())) __PYX_ERR(1, 345, __pyx_L3_error)
- } else {
- __pyx_v_dtype_is_object = ((int)0);
- }
- }
- goto __pyx_L4_argument_unpacking_done;
- __pyx_L5_argtuple_error:;
- __Pyx_RaiseArgtupleInvalid("__cinit__", 0, 2, 3, PyTuple_GET_SIZE(__pyx_args)); __PYX_ERR(1, 345, __pyx_L3_error)
- __pyx_L3_error:;
- __Pyx_AddTraceback("View.MemoryView.memoryview.__cinit__", __pyx_clineno, __pyx_lineno, __pyx_filename);
- __Pyx_RefNannyFinishContext();
- return -1;
- __pyx_L4_argument_unpacking_done:;
- __pyx_r = __pyx_memoryview___pyx_pf_15View_dot_MemoryView_10memoryview___cinit__(((struct __pyx_memoryview_obj *)__pyx_v_self), __pyx_v_obj, __pyx_v_flags, __pyx_v_dtype_is_object);
-
- /* function exit code */
- __Pyx_RefNannyFinishContext();
- return __pyx_r;
-}
-
-static int __pyx_memoryview___pyx_pf_15View_dot_MemoryView_10memoryview___cinit__(struct __pyx_memoryview_obj *__pyx_v_self, PyObject *__pyx_v_obj, int __pyx_v_flags, int __pyx_v_dtype_is_object) {
- int __pyx_r;
- __Pyx_RefNannyDeclarations
- int __pyx_t_1;
- int __pyx_t_2;
- int __pyx_t_3;
- int __pyx_t_4;
- int __pyx_lineno = 0;
- const char *__pyx_filename = NULL;
- int __pyx_clineno = 0;
- __Pyx_RefNannySetupContext("__cinit__", 0);
-
- /* "View.MemoryView":346
- *
- * def __cinit__(memoryview self, object obj, int flags, bint dtype_is_object=False):
- * self.obj = obj # <<<<<<<<<<<<<<
- * self.flags = flags
- * if type(self) is memoryview or obj is not None:
- */
- __Pyx_INCREF(__pyx_v_obj);
- __Pyx_GIVEREF(__pyx_v_obj);
- __Pyx_GOTREF(__pyx_v_self->obj);
- __Pyx_DECREF(__pyx_v_self->obj);
- __pyx_v_self->obj = __pyx_v_obj;
-
- /* "View.MemoryView":347
- * def __cinit__(memoryview self, object obj, int flags, bint dtype_is_object=False):
- * self.obj = obj
- * self.flags = flags # <<<<<<<<<<<<<<
- * if type(self) is memoryview or obj is not None:
- * __Pyx_GetBuffer(obj, &self.view, flags)
- */
- __pyx_v_self->flags = __pyx_v_flags;
-
- /* "View.MemoryView":348
- * self.obj = obj
- * self.flags = flags
- * if type(self) is memoryview or obj is not None: # <<<<<<<<<<<<<<
- * __Pyx_GetBuffer(obj, &self.view, flags)
- * if self.view.obj == NULL:
- */
- __pyx_t_2 = (((PyObject *)Py_TYPE(((PyObject *)__pyx_v_self))) == ((PyObject *)__pyx_memoryview_type));
- __pyx_t_3 = (__pyx_t_2 != 0);
- if (!__pyx_t_3) {
- } else {
- __pyx_t_1 = __pyx_t_3;
- goto __pyx_L4_bool_binop_done;
- }
- __pyx_t_3 = (__pyx_v_obj != Py_None);
- __pyx_t_2 = (__pyx_t_3 != 0);
- __pyx_t_1 = __pyx_t_2;
- __pyx_L4_bool_binop_done:;
- if (__pyx_t_1) {
-
- /* "View.MemoryView":349
- * self.flags = flags
- * if type(self) is memoryview or obj is not None:
- * __Pyx_GetBuffer(obj, &self.view, flags) # <<<<<<<<<<<<<<
- * if self.view.obj == NULL:
- * (<__pyx_buffer *> &self.view).obj = Py_None
- */
- __pyx_t_4 = __Pyx_GetBuffer(__pyx_v_obj, (&__pyx_v_self->view), __pyx_v_flags); if (unlikely(__pyx_t_4 == ((int)-1))) __PYX_ERR(1, 349, __pyx_L1_error)
-
- /* "View.MemoryView":350
- * if type(self) is memoryview or obj is not None:
- * __Pyx_GetBuffer(obj, &self.view, flags)
- * if self.view.obj == NULL: # <<<<<<<<<<<<<<
- * (<__pyx_buffer *> &self.view).obj = Py_None
- * Py_INCREF(Py_None)
- */
- __pyx_t_1 = ((((PyObject *)__pyx_v_self->view.obj) == NULL) != 0);
- if (__pyx_t_1) {
-
- /* "View.MemoryView":351
- * __Pyx_GetBuffer(obj, &self.view, flags)
- * if self.view.obj == NULL:
- * (<__pyx_buffer *> &self.view).obj = Py_None # <<<<<<<<<<<<<<
- * Py_INCREF(Py_None)
- *
- */
- ((Py_buffer *)(&__pyx_v_self->view))->obj = Py_None;
-
- /* "View.MemoryView":352
- * if self.view.obj == NULL:
- * (<__pyx_buffer *> &self.view).obj = Py_None
- * Py_INCREF(Py_None) # <<<<<<<<<<<<<<
- *
- * global __pyx_memoryview_thread_locks_used
- */
- Py_INCREF(Py_None);
-
- /* "View.MemoryView":350
- * if type(self) is memoryview or obj is not None:
- * __Pyx_GetBuffer(obj, &self.view, flags)
- * if self.view.obj == NULL: # <<<<<<<<<<<<<<
- * (<__pyx_buffer *> &self.view).obj = Py_None
- * Py_INCREF(Py_None)
- */
- }
-
- /* "View.MemoryView":348
- * self.obj = obj
- * self.flags = flags
- * if type(self) is memoryview or obj is not None: # <<<<<<<<<<<<<<
- * __Pyx_GetBuffer(obj, &self.view, flags)
- * if self.view.obj == NULL:
- */
- }
-
- /* "View.MemoryView":355
- *
- * global __pyx_memoryview_thread_locks_used
- * if __pyx_memoryview_thread_locks_used < THREAD_LOCKS_PREALLOCATED: # <<<<<<<<<<<<<<
- * self.lock = __pyx_memoryview_thread_locks[__pyx_memoryview_thread_locks_used]
- * __pyx_memoryview_thread_locks_used += 1
- */
- __pyx_t_1 = ((__pyx_memoryview_thread_locks_used < 8) != 0);
- if (__pyx_t_1) {
-
- /* "View.MemoryView":356
- * global __pyx_memoryview_thread_locks_used
- * if __pyx_memoryview_thread_locks_used < THREAD_LOCKS_PREALLOCATED:
- * self.lock = __pyx_memoryview_thread_locks[__pyx_memoryview_thread_locks_used] # <<<<<<<<<<<<<<
- * __pyx_memoryview_thread_locks_used += 1
- * if self.lock is NULL:
- */
- __pyx_v_self->lock = (__pyx_memoryview_thread_locks[__pyx_memoryview_thread_locks_used]);
-
- /* "View.MemoryView":357
- * if __pyx_memoryview_thread_locks_used < THREAD_LOCKS_PREALLOCATED:
- * self.lock = __pyx_memoryview_thread_locks[__pyx_memoryview_thread_locks_used]
- * __pyx_memoryview_thread_locks_used += 1 # <<<<<<<<<<<<<<
- * if self.lock is NULL:
- * self.lock = PyThread_allocate_lock()
- */
- __pyx_memoryview_thread_locks_used = (__pyx_memoryview_thread_locks_used + 1);
-
- /* "View.MemoryView":355
- *
- * global __pyx_memoryview_thread_locks_used
- * if __pyx_memoryview_thread_locks_used < THREAD_LOCKS_PREALLOCATED: # <<<<<<<<<<<<<<
- * self.lock = __pyx_memoryview_thread_locks[__pyx_memoryview_thread_locks_used]
- * __pyx_memoryview_thread_locks_used += 1
- */
- }
-
- /* "View.MemoryView":358
- * self.lock = __pyx_memoryview_thread_locks[__pyx_memoryview_thread_locks_used]
- * __pyx_memoryview_thread_locks_used += 1
- * if self.lock is NULL: # <<<<<<<<<<<<<<
- * self.lock = PyThread_allocate_lock()
- * if self.lock is NULL:
- */
- __pyx_t_1 = ((__pyx_v_self->lock == NULL) != 0);
- if (__pyx_t_1) {
-
- /* "View.MemoryView":359
- * __pyx_memoryview_thread_locks_used += 1
- * if self.lock is NULL:
- * self.lock = PyThread_allocate_lock() # <<<<<<<<<<<<<<
- * if self.lock is NULL:
- * raise MemoryError
- */
- __pyx_v_self->lock = PyThread_allocate_lock();
-
- /* "View.MemoryView":360
- * if self.lock is NULL:
- * self.lock = PyThread_allocate_lock()
- * if self.lock is NULL: # <<<<<<<<<<<<<<
- * raise MemoryError
- *
- */
- __pyx_t_1 = ((__pyx_v_self->lock == NULL) != 0);
- if (unlikely(__pyx_t_1)) {
-
- /* "View.MemoryView":361
- * self.lock = PyThread_allocate_lock()
- * if self.lock is NULL:
- * raise MemoryError # <<<<<<<<<<<<<<
- *
- * if flags & PyBUF_FORMAT:
- */
- PyErr_NoMemory(); __PYX_ERR(1, 361, __pyx_L1_error)
-
- /* "View.MemoryView":360
- * if self.lock is NULL:
- * self.lock = PyThread_allocate_lock()
- * if self.lock is NULL: # <<<<<<<<<<<<<<
- * raise MemoryError
- *
- */
- }
-
- /* "View.MemoryView":358
- * self.lock = __pyx_memoryview_thread_locks[__pyx_memoryview_thread_locks_used]
- * __pyx_memoryview_thread_locks_used += 1
- * if self.lock is NULL: # <<<<<<<<<<<<<<
- * self.lock = PyThread_allocate_lock()
- * if self.lock is NULL:
- */
- }
-
- /* "View.MemoryView":363
- * raise MemoryError
- *
- * if flags & PyBUF_FORMAT: # <<<<<<<<<<<<<<
- * self.dtype_is_object = (self.view.format[0] == b'O' and self.view.format[1] == b'\0')
- * else:
- */
- __pyx_t_1 = ((__pyx_v_flags & PyBUF_FORMAT) != 0);
- if (__pyx_t_1) {
-
- /* "View.MemoryView":364
- *
- * if flags & PyBUF_FORMAT:
- * self.dtype_is_object = (self.view.format[0] == b'O' and self.view.format[1] == b'\0') # <<<<<<<<<<<<<<
- * else:
- * self.dtype_is_object = dtype_is_object
- */
- __pyx_t_2 = (((__pyx_v_self->view.format[0]) == 'O') != 0);
- if (__pyx_t_2) {
- } else {
- __pyx_t_1 = __pyx_t_2;
- goto __pyx_L11_bool_binop_done;
- }
- __pyx_t_2 = (((__pyx_v_self->view.format[1]) == '\x00') != 0);
- __pyx_t_1 = __pyx_t_2;
- __pyx_L11_bool_binop_done:;
- __pyx_v_self->dtype_is_object = __pyx_t_1;
-
- /* "View.MemoryView":363
- * raise MemoryError
- *
- * if flags & PyBUF_FORMAT: # <<<<<<<<<<<<<<
- * self.dtype_is_object = (self.view.format[0] == b'O' and self.view.format[1] == b'\0')
- * else:
- */
- goto __pyx_L10;
- }
-
- /* "View.MemoryView":366
- * self.dtype_is_object = (self.view.format[0] == b'O' and self.view.format[1] == b'\0')
- * else:
- * self.dtype_is_object = dtype_is_object # <<<<<<<<<<<<<<
- *
- * self.acquisition_count_aligned_p = <__pyx_atomic_int *> align_pointer(
- */
- /*else*/ {
- __pyx_v_self->dtype_is_object = __pyx_v_dtype_is_object;
- }
- __pyx_L10:;
-
- /* "View.MemoryView":368
- * self.dtype_is_object = dtype_is_object
- *
- * self.acquisition_count_aligned_p = <__pyx_atomic_int *> align_pointer( # <<<<<<<<<<<<<<
- * &self.acquisition_count[0], sizeof(__pyx_atomic_int))
- * self.typeinfo = NULL
- */
- __pyx_v_self->acquisition_count_aligned_p = ((__pyx_atomic_int *)__pyx_align_pointer(((void *)(&(__pyx_v_self->acquisition_count[0]))), (sizeof(__pyx_atomic_int))));
-
- /* "View.MemoryView":370
- * self.acquisition_count_aligned_p = <__pyx_atomic_int *> align_pointer(
- * &self.acquisition_count[0], sizeof(__pyx_atomic_int))
- * self.typeinfo = NULL # <<<<<<<<<<<<<<
- *
- * def __dealloc__(memoryview self):
- */
- __pyx_v_self->typeinfo = NULL;
-
- /* "View.MemoryView":345
- * cdef __Pyx_TypeInfo *typeinfo
- *
- * def __cinit__(memoryview self, object obj, int flags, bint dtype_is_object=False): # <<<<<<<<<<<<<<
- * self.obj = obj
- * self.flags = flags
- */
-
- /* function exit code */
- __pyx_r = 0;
- goto __pyx_L0;
- __pyx_L1_error:;
- __Pyx_AddTraceback("View.MemoryView.memoryview.__cinit__", __pyx_clineno, __pyx_lineno, __pyx_filename);
- __pyx_r = -1;
- __pyx_L0:;
- __Pyx_RefNannyFinishContext();
- return __pyx_r;
-}
-
-/* "View.MemoryView":372
- * self.typeinfo = NULL
- *
- * def __dealloc__(memoryview self): # <<<<<<<<<<<<<<
- * if self.obj is not None:
- * __Pyx_ReleaseBuffer(&self.view)
- */
-
-/* Python wrapper */
-static void __pyx_memoryview___dealloc__(PyObject *__pyx_v_self); /*proto*/
-static void __pyx_memoryview___dealloc__(PyObject *__pyx_v_self) {
- __Pyx_RefNannyDeclarations
- __Pyx_RefNannySetupContext("__dealloc__ (wrapper)", 0);
- __pyx_memoryview___pyx_pf_15View_dot_MemoryView_10memoryview_2__dealloc__(((struct __pyx_memoryview_obj *)__pyx_v_self));
-
- /* function exit code */
- __Pyx_RefNannyFinishContext();
-}
-
-static void __pyx_memoryview___pyx_pf_15View_dot_MemoryView_10memoryview_2__dealloc__(struct __pyx_memoryview_obj *__pyx_v_self) {
- int __pyx_v_i;
- __Pyx_RefNannyDeclarations
- int __pyx_t_1;
- int __pyx_t_2;
- int __pyx_t_3;
- int __pyx_t_4;
- int __pyx_t_5;
- PyThread_type_lock __pyx_t_6;
- PyThread_type_lock __pyx_t_7;
- __Pyx_RefNannySetupContext("__dealloc__", 0);
-
- /* "View.MemoryView":373
- *
- * def __dealloc__(memoryview self):
- * if self.obj is not None: # <<<<<<<<<<<<<<
- * __Pyx_ReleaseBuffer(&self.view)
- * elif (<__pyx_buffer *> &self.view).obj == Py_None:
- */
- __pyx_t_1 = (__pyx_v_self->obj != Py_None);
- __pyx_t_2 = (__pyx_t_1 != 0);
- if (__pyx_t_2) {
-
- /* "View.MemoryView":374
- * def __dealloc__(memoryview self):
- * if self.obj is not None:
- * __Pyx_ReleaseBuffer(&self.view) # <<<<<<<<<<<<<<
- * elif (<__pyx_buffer *> &self.view).obj == Py_None:
- *
- */
- __Pyx_ReleaseBuffer((&__pyx_v_self->view));
-
- /* "View.MemoryView":373
- *
- * def __dealloc__(memoryview self):
- * if self.obj is not None: # <<<<<<<<<<<<<<
- * __Pyx_ReleaseBuffer(&self.view)
- * elif (<__pyx_buffer *> &self.view).obj == Py_None:
- */
- goto __pyx_L3;
- }
-
- /* "View.MemoryView":375
- * if self.obj is not None:
- * __Pyx_ReleaseBuffer(&self.view)
- * elif (<__pyx_buffer *> &self.view).obj == Py_None: # <<<<<<<<<<<<<<
- *
- * (<__pyx_buffer *> &self.view).obj = NULL
- */
- __pyx_t_2 = ((((Py_buffer *)(&__pyx_v_self->view))->obj == Py_None) != 0);
- if (__pyx_t_2) {
-
- /* "View.MemoryView":377
- * elif (<__pyx_buffer *> &self.view).obj == Py_None:
- *
- * (<__pyx_buffer *> &self.view).obj = NULL # <<<<<<<<<<<<<<
- * Py_DECREF(Py_None)
- *
- */
- ((Py_buffer *)(&__pyx_v_self->view))->obj = NULL;
-
- /* "View.MemoryView":378
- *
- * (<__pyx_buffer *> &self.view).obj = NULL
- * Py_DECREF(Py_None) # <<<<<<<<<<<<<<
- *
- * cdef int i
- */
- Py_DECREF(Py_None);
-
- /* "View.MemoryView":375
- * if self.obj is not None:
- * __Pyx_ReleaseBuffer(&self.view)
- * elif (<__pyx_buffer *> &self.view).obj == Py_None: # <<<<<<<<<<<<<<
- *
- * (<__pyx_buffer *> &self.view).obj = NULL
- */
- }
- __pyx_L3:;
-
- /* "View.MemoryView":382
- * cdef int i
- * global __pyx_memoryview_thread_locks_used
- * if self.lock != NULL: # <<<<<<<<<<<<<<
- * for i in range(__pyx_memoryview_thread_locks_used):
- * if __pyx_memoryview_thread_locks[i] is self.lock:
- */
- __pyx_t_2 = ((__pyx_v_self->lock != NULL) != 0);
- if (__pyx_t_2) {
-
- /* "View.MemoryView":383
- * global __pyx_memoryview_thread_locks_used
- * if self.lock != NULL:
- * for i in range(__pyx_memoryview_thread_locks_used): # <<<<<<<<<<<<<<
- * if __pyx_memoryview_thread_locks[i] is self.lock:
- * __pyx_memoryview_thread_locks_used -= 1
- */
- __pyx_t_3 = __pyx_memoryview_thread_locks_used;
- __pyx_t_4 = __pyx_t_3;
- for (__pyx_t_5 = 0; __pyx_t_5 < __pyx_t_4; __pyx_t_5+=1) {
- __pyx_v_i = __pyx_t_5;
-
- /* "View.MemoryView":384
- * if self.lock != NULL:
- * for i in range(__pyx_memoryview_thread_locks_used):
- * if __pyx_memoryview_thread_locks[i] is self.lock: # <<<<<<<<<<<<<<
- * __pyx_memoryview_thread_locks_used -= 1
- * if i != __pyx_memoryview_thread_locks_used:
- */
- __pyx_t_2 = (((__pyx_memoryview_thread_locks[__pyx_v_i]) == __pyx_v_self->lock) != 0);
- if (__pyx_t_2) {
-
- /* "View.MemoryView":385
- * for i in range(__pyx_memoryview_thread_locks_used):
- * if __pyx_memoryview_thread_locks[i] is self.lock:
- * __pyx_memoryview_thread_locks_used -= 1 # <<<<<<<<<<<<<<
- * if i != __pyx_memoryview_thread_locks_used:
- * __pyx_memoryview_thread_locks[i], __pyx_memoryview_thread_locks[__pyx_memoryview_thread_locks_used] = (
- */
- __pyx_memoryview_thread_locks_used = (__pyx_memoryview_thread_locks_used - 1);
-
- /* "View.MemoryView":386
- * if __pyx_memoryview_thread_locks[i] is self.lock:
- * __pyx_memoryview_thread_locks_used -= 1
- * if i != __pyx_memoryview_thread_locks_used: # <<<<<<<<<<<<<<
- * __pyx_memoryview_thread_locks[i], __pyx_memoryview_thread_locks[__pyx_memoryview_thread_locks_used] = (
- * __pyx_memoryview_thread_locks[__pyx_memoryview_thread_locks_used], __pyx_memoryview_thread_locks[i])
- */
- __pyx_t_2 = ((__pyx_v_i != __pyx_memoryview_thread_locks_used) != 0);
- if (__pyx_t_2) {
-
- /* "View.MemoryView":388
- * if i != __pyx_memoryview_thread_locks_used:
- * __pyx_memoryview_thread_locks[i], __pyx_memoryview_thread_locks[__pyx_memoryview_thread_locks_used] = (
- * __pyx_memoryview_thread_locks[__pyx_memoryview_thread_locks_used], __pyx_memoryview_thread_locks[i]) # <<<<<<<<<<<<<<
- * break
- * else:
- */
- __pyx_t_6 = (__pyx_memoryview_thread_locks[__pyx_memoryview_thread_locks_used]);
- __pyx_t_7 = (__pyx_memoryview_thread_locks[__pyx_v_i]);
-
- /* "View.MemoryView":387
- * __pyx_memoryview_thread_locks_used -= 1
- * if i != __pyx_memoryview_thread_locks_used:
- * __pyx_memoryview_thread_locks[i], __pyx_memoryview_thread_locks[__pyx_memoryview_thread_locks_used] = ( # <<<<<<<<<<<<<<
- * __pyx_memoryview_thread_locks[__pyx_memoryview_thread_locks_used], __pyx_memoryview_thread_locks[i])
- * break
- */
- (__pyx_memoryview_thread_locks[__pyx_v_i]) = __pyx_t_6;
- (__pyx_memoryview_thread_locks[__pyx_memoryview_thread_locks_used]) = __pyx_t_7;
-
- /* "View.MemoryView":386
- * if __pyx_memoryview_thread_locks[i] is self.lock:
- * __pyx_memoryview_thread_locks_used -= 1
- * if i != __pyx_memoryview_thread_locks_used: # <<<<<<<<<<<<<<
- * __pyx_memoryview_thread_locks[i], __pyx_memoryview_thread_locks[__pyx_memoryview_thread_locks_used] = (
- * __pyx_memoryview_thread_locks[__pyx_memoryview_thread_locks_used], __pyx_memoryview_thread_locks[i])
- */
- }
-
- /* "View.MemoryView":389
- * __pyx_memoryview_thread_locks[i], __pyx_memoryview_thread_locks[__pyx_memoryview_thread_locks_used] = (
- * __pyx_memoryview_thread_locks[__pyx_memoryview_thread_locks_used], __pyx_memoryview_thread_locks[i])
- * break # <<<<<<<<<<<<<<
- * else:
- * PyThread_free_lock(self.lock)
- */
- goto __pyx_L6_break;
-
- /* "View.MemoryView":384
- * if self.lock != NULL:
- * for i in range(__pyx_memoryview_thread_locks_used):
- * if __pyx_memoryview_thread_locks[i] is self.lock: # <<<<<<<<<<<<<<
- * __pyx_memoryview_thread_locks_used -= 1
- * if i != __pyx_memoryview_thread_locks_used:
- */
- }
- }
- /*else*/ {
-
- /* "View.MemoryView":391
- * break
- * else:
- * PyThread_free_lock(self.lock) # <<<<<<<<<<<<<<
- *
- * cdef char *get_item_pointer(memoryview self, object index) except NULL:
- */
- PyThread_free_lock(__pyx_v_self->lock);
- }
- __pyx_L6_break:;
-
- /* "View.MemoryView":382
- * cdef int i
- * global __pyx_memoryview_thread_locks_used
- * if self.lock != NULL: # <<<<<<<<<<<<<<
- * for i in range(__pyx_memoryview_thread_locks_used):
- * if __pyx_memoryview_thread_locks[i] is self.lock:
- */
- }
-
- /* "View.MemoryView":372
- * self.typeinfo = NULL
- *
- * def __dealloc__(memoryview self): # <<<<<<<<<<<<<<
- * if self.obj is not None:
- * __Pyx_ReleaseBuffer(&self.view)
- */
-
- /* function exit code */
- __Pyx_RefNannyFinishContext();
-}
-
-/* "View.MemoryView":393
- * PyThread_free_lock(self.lock)
- *
- * cdef char *get_item_pointer(memoryview self, object index) except NULL: # <<<<<<<<<<<<<<
- * cdef Py_ssize_t dim
- * cdef char *itemp = self.view.buf
- */
-
-static char *__pyx_memoryview_get_item_pointer(struct __pyx_memoryview_obj *__pyx_v_self, PyObject *__pyx_v_index) {
- Py_ssize_t __pyx_v_dim;
- char *__pyx_v_itemp;
- PyObject *__pyx_v_idx = NULL;
- char *__pyx_r;
- __Pyx_RefNannyDeclarations
- Py_ssize_t __pyx_t_1;
- PyObject *__pyx_t_2 = NULL;
- Py_ssize_t __pyx_t_3;
- PyObject *(*__pyx_t_4)(PyObject *);
- PyObject *__pyx_t_5 = NULL;
- Py_ssize_t __pyx_t_6;
- char *__pyx_t_7;
- int __pyx_lineno = 0;
- const char *__pyx_filename = NULL;
- int __pyx_clineno = 0;
- __Pyx_RefNannySetupContext("get_item_pointer", 0);
-
- /* "View.MemoryView":395
- * cdef char *get_item_pointer(memoryview self, object index) except NULL:
- * cdef Py_ssize_t dim
- * cdef char *itemp = self.view.buf # <<<<<<<<<<<<<<
- *
- * for dim, idx in enumerate(index):
- */
- __pyx_v_itemp = ((char *)__pyx_v_self->view.buf);
-
- /* "View.MemoryView":397
- * cdef char *itemp = self.view.buf
- *
- * for dim, idx in enumerate(index): # <<<<<<<<<<<<<<
- * itemp = pybuffer_index(&self.view, itemp, idx, dim)
- *
- */
- __pyx_t_1 = 0;
- if (likely(PyList_CheckExact(__pyx_v_index)) || PyTuple_CheckExact(__pyx_v_index)) {
- __pyx_t_2 = __pyx_v_index; __Pyx_INCREF(__pyx_t_2); __pyx_t_3 = 0;
- __pyx_t_4 = NULL;
- } else {
- __pyx_t_3 = -1; __pyx_t_2 = PyObject_GetIter(__pyx_v_index); if (unlikely(!__pyx_t_2)) __PYX_ERR(1, 397, __pyx_L1_error)
- __Pyx_GOTREF(__pyx_t_2);
- __pyx_t_4 = Py_TYPE(__pyx_t_2)->tp_iternext; if (unlikely(!__pyx_t_4)) __PYX_ERR(1, 397, __pyx_L1_error)
- }
- for (;;) {
- if (likely(!__pyx_t_4)) {
- if (likely(PyList_CheckExact(__pyx_t_2))) {
- if (__pyx_t_3 >= PyList_GET_SIZE(__pyx_t_2)) break;
- #if CYTHON_ASSUME_SAFE_MACROS && !CYTHON_AVOID_BORROWED_REFS
- __pyx_t_5 = PyList_GET_ITEM(__pyx_t_2, __pyx_t_3); __Pyx_INCREF(__pyx_t_5); __pyx_t_3++; if (unlikely(0 < 0)) __PYX_ERR(1, 397, __pyx_L1_error)
- #else
- __pyx_t_5 = PySequence_ITEM(__pyx_t_2, __pyx_t_3); __pyx_t_3++; if (unlikely(!__pyx_t_5)) __PYX_ERR(1, 397, __pyx_L1_error)
- __Pyx_GOTREF(__pyx_t_5);
- #endif
- } else {
- if (__pyx_t_3 >= PyTuple_GET_SIZE(__pyx_t_2)) break;
- #if CYTHON_ASSUME_SAFE_MACROS && !CYTHON_AVOID_BORROWED_REFS
- __pyx_t_5 = PyTuple_GET_ITEM(__pyx_t_2, __pyx_t_3); __Pyx_INCREF(__pyx_t_5); __pyx_t_3++; if (unlikely(0 < 0)) __PYX_ERR(1, 397, __pyx_L1_error)
- #else
- __pyx_t_5 = PySequence_ITEM(__pyx_t_2, __pyx_t_3); __pyx_t_3++; if (unlikely(!__pyx_t_5)) __PYX_ERR(1, 397, __pyx_L1_error)
- __Pyx_GOTREF(__pyx_t_5);
- #endif
- }
- } else {
- __pyx_t_5 = __pyx_t_4(__pyx_t_2);
- if (unlikely(!__pyx_t_5)) {
- PyObject* exc_type = PyErr_Occurred();
- if (exc_type) {
- if (likely(__Pyx_PyErr_GivenExceptionMatches(exc_type, PyExc_StopIteration))) PyErr_Clear();
- else __PYX_ERR(1, 397, __pyx_L1_error)
- }
- break;
- }
- __Pyx_GOTREF(__pyx_t_5);
- }
- __Pyx_XDECREF_SET(__pyx_v_idx, __pyx_t_5);
- __pyx_t_5 = 0;
- __pyx_v_dim = __pyx_t_1;
- __pyx_t_1 = (__pyx_t_1 + 1);
-
- /* "View.MemoryView":398
- *
- * for dim, idx in enumerate(index):
- * itemp = pybuffer_index(&self.view, itemp, idx, dim) # <<<<<<<<<<<<<<
- *
- * return itemp
- */
- __pyx_t_6 = __Pyx_PyIndex_AsSsize_t(__pyx_v_idx); if (unlikely((__pyx_t_6 == (Py_ssize_t)-1) && PyErr_Occurred())) __PYX_ERR(1, 398, __pyx_L1_error)
- __pyx_t_7 = __pyx_pybuffer_index((&__pyx_v_self->view), __pyx_v_itemp, __pyx_t_6, __pyx_v_dim); if (unlikely(__pyx_t_7 == ((char *)NULL))) __PYX_ERR(1, 398, __pyx_L1_error)
- __pyx_v_itemp = __pyx_t_7;
-
- /* "View.MemoryView":397
- * cdef char *itemp = self.view.buf
- *
- * for dim, idx in enumerate(index): # <<<<<<<<<<<<<<
- * itemp = pybuffer_index(&self.view, itemp, idx, dim)
- *
- */
- }
- __Pyx_DECREF(__pyx_t_2); __pyx_t_2 = 0;
-
- /* "View.MemoryView":400
- * itemp = pybuffer_index(&self.view, itemp, idx, dim)
- *
- * return itemp # <<<<<<<<<<<<<<
- *
- *
- */
- __pyx_r = __pyx_v_itemp;
- goto __pyx_L0;
-
- /* "View.MemoryView":393
- * PyThread_free_lock(self.lock)
- *
- * cdef char *get_item_pointer(memoryview self, object index) except NULL: # <<<<<<<<<<<<<<
- * cdef Py_ssize_t dim
- * cdef char *itemp = self.view.buf
- */
-
- /* function exit code */
- __pyx_L1_error:;
- __Pyx_XDECREF(__pyx_t_2);
- __Pyx_XDECREF(__pyx_t_5);
- __Pyx_AddTraceback("View.MemoryView.memoryview.get_item_pointer", __pyx_clineno, __pyx_lineno, __pyx_filename);
- __pyx_r = NULL;
- __pyx_L0:;
- __Pyx_XDECREF(__pyx_v_idx);
- __Pyx_RefNannyFinishContext();
- return __pyx_r;
-}
-
-/* "View.MemoryView":403
- *
- *
- * def __getitem__(memoryview self, object index): # <<<<<<<<<<<<<<
- * if index is Ellipsis:
- * return self
- */
-
-/* Python wrapper */
-static PyObject *__pyx_memoryview___getitem__(PyObject *__pyx_v_self, PyObject *__pyx_v_index); /*proto*/
-static PyObject *__pyx_memoryview___getitem__(PyObject *__pyx_v_self, PyObject *__pyx_v_index) {
- PyObject *__pyx_r = 0;
- __Pyx_RefNannyDeclarations
- __Pyx_RefNannySetupContext("__getitem__ (wrapper)", 0);
- __pyx_r = __pyx_memoryview___pyx_pf_15View_dot_MemoryView_10memoryview_4__getitem__(((struct __pyx_memoryview_obj *)__pyx_v_self), ((PyObject *)__pyx_v_index));
-
- /* function exit code */
- __Pyx_RefNannyFinishContext();
- return __pyx_r;
-}
-
-static PyObject *__pyx_memoryview___pyx_pf_15View_dot_MemoryView_10memoryview_4__getitem__(struct __pyx_memoryview_obj *__pyx_v_self, PyObject *__pyx_v_index) {
- PyObject *__pyx_v_have_slices = NULL;
- PyObject *__pyx_v_indices = NULL;
- char *__pyx_v_itemp;
- PyObject *__pyx_r = NULL;
- __Pyx_RefNannyDeclarations
- int __pyx_t_1;
- int __pyx_t_2;
- PyObject *__pyx_t_3 = NULL;
- PyObject *__pyx_t_4 = NULL;
- PyObject *__pyx_t_5 = NULL;
- char *__pyx_t_6;
- int __pyx_lineno = 0;
- const char *__pyx_filename = NULL;
- int __pyx_clineno = 0;
- __Pyx_RefNannySetupContext("__getitem__", 0);
-
- /* "View.MemoryView":404
- *
- * def __getitem__(memoryview self, object index):
- * if index is Ellipsis: # <<<<<<<<<<<<<<
- * return self
- *
- */
- __pyx_t_1 = (__pyx_v_index == __pyx_builtin_Ellipsis);
- __pyx_t_2 = (__pyx_t_1 != 0);
- if (__pyx_t_2) {
-
- /* "View.MemoryView":405
- * def __getitem__(memoryview self, object index):
- * if index is Ellipsis:
- * return self # <<<<<<<<<<<<<<
- *
- * have_slices, indices = _unellipsify(index, self.view.ndim)
- */
- __Pyx_XDECREF(__pyx_r);
- __Pyx_INCREF(((PyObject *)__pyx_v_self));
- __pyx_r = ((PyObject *)__pyx_v_self);
- goto __pyx_L0;
-
- /* "View.MemoryView":404
- *
- * def __getitem__(memoryview self, object index):
- * if index is Ellipsis: # <<<<<<<<<<<<<<
- * return self
- *
- */
- }
-
- /* "View.MemoryView":407
- * return self
- *
- * have_slices, indices = _unellipsify(index, self.view.ndim) # <<<<<<<<<<<<<<
- *
- * cdef char *itemp
- */
- __pyx_t_3 = _unellipsify(__pyx_v_index, __pyx_v_self->view.ndim); if (unlikely(!__pyx_t_3)) __PYX_ERR(1, 407, __pyx_L1_error)
- __Pyx_GOTREF(__pyx_t_3);
- if (likely(__pyx_t_3 != Py_None)) {
- PyObject* sequence = __pyx_t_3;
- Py_ssize_t size = __Pyx_PySequence_SIZE(sequence);
- if (unlikely(size != 2)) {
- if (size > 2) __Pyx_RaiseTooManyValuesError(2);
- else if (size >= 0) __Pyx_RaiseNeedMoreValuesError(size);
- __PYX_ERR(1, 407, __pyx_L1_error)
- }
- #if CYTHON_ASSUME_SAFE_MACROS && !CYTHON_AVOID_BORROWED_REFS
- __pyx_t_4 = PyTuple_GET_ITEM(sequence, 0);
- __pyx_t_5 = PyTuple_GET_ITEM(sequence, 1);
- __Pyx_INCREF(__pyx_t_4);
- __Pyx_INCREF(__pyx_t_5);
- #else
- __pyx_t_4 = PySequence_ITEM(sequence, 0); if (unlikely(!__pyx_t_4)) __PYX_ERR(1, 407, __pyx_L1_error)
- __Pyx_GOTREF(__pyx_t_4);
- __pyx_t_5 = PySequence_ITEM(sequence, 1); if (unlikely(!__pyx_t_5)) __PYX_ERR(1, 407, __pyx_L1_error)
- __Pyx_GOTREF(__pyx_t_5);
- #endif
- __Pyx_DECREF(__pyx_t_3); __pyx_t_3 = 0;
- } else {
- __Pyx_RaiseNoneNotIterableError(); __PYX_ERR(1, 407, __pyx_L1_error)
- }
- __pyx_v_have_slices = __pyx_t_4;
- __pyx_t_4 = 0;
- __pyx_v_indices = __pyx_t_5;
- __pyx_t_5 = 0;
-
- /* "View.MemoryView":410
- *
- * cdef char *itemp
- * if have_slices: # <<<<<<<<<<<<<<
- * return memview_slice(self, indices)
- * else:
- */
- __pyx_t_2 = __Pyx_PyObject_IsTrue(__pyx_v_have_slices); if (unlikely(__pyx_t_2 < 0)) __PYX_ERR(1, 410, __pyx_L1_error)
- if (__pyx_t_2) {
-
- /* "View.MemoryView":411
- * cdef char *itemp
- * if have_slices:
- * return memview_slice(self, indices) # <<<<<<<<<<<<<<
- * else:
- * itemp = self.get_item_pointer(indices)
- */
- __Pyx_XDECREF(__pyx_r);
- __pyx_t_3 = ((PyObject *)__pyx_memview_slice(__pyx_v_self, __pyx_v_indices)); if (unlikely(!__pyx_t_3)) __PYX_ERR(1, 411, __pyx_L1_error)
- __Pyx_GOTREF(__pyx_t_3);
- __pyx_r = __pyx_t_3;
- __pyx_t_3 = 0;
- goto __pyx_L0;
-
- /* "View.MemoryView":410
- *
- * cdef char *itemp
- * if have_slices: # <<<<<<<<<<<<<<
- * return memview_slice(self, indices)
- * else:
- */
- }
-
- /* "View.MemoryView":413
- * return memview_slice(self, indices)
- * else:
- * itemp = self.get_item_pointer(indices) # <<<<<<<<<<<<<<
- * return self.convert_item_to_object(itemp)
- *
- */
- /*else*/ {
- __pyx_t_6 = ((struct __pyx_vtabstruct_memoryview *)__pyx_v_self->__pyx_vtab)->get_item_pointer(__pyx_v_self, __pyx_v_indices); if (unlikely(__pyx_t_6 == ((char *)NULL))) __PYX_ERR(1, 413, __pyx_L1_error)
- __pyx_v_itemp = __pyx_t_6;
-
- /* "View.MemoryView":414
- * else:
- * itemp = self.get_item_pointer(indices)
- * return self.convert_item_to_object(itemp) # <<<<<<<<<<<<<<
- *
- * def __setitem__(memoryview self, object index, object value):
- */
- __Pyx_XDECREF(__pyx_r);
- __pyx_t_3 = ((struct __pyx_vtabstruct_memoryview *)__pyx_v_self->__pyx_vtab)->convert_item_to_object(__pyx_v_self, __pyx_v_itemp); if (unlikely(!__pyx_t_3)) __PYX_ERR(1, 414, __pyx_L1_error)
- __Pyx_GOTREF(__pyx_t_3);
- __pyx_r = __pyx_t_3;
- __pyx_t_3 = 0;
- goto __pyx_L0;
- }
-
- /* "View.MemoryView":403
- *
- *
- * def __getitem__(memoryview self, object index): # <<<<<<<<<<<<<<
- * if index is Ellipsis:
- * return self
- */
-
- /* function exit code */
- __pyx_L1_error:;
- __Pyx_XDECREF(__pyx_t_3);
- __Pyx_XDECREF(__pyx_t_4);
- __Pyx_XDECREF(__pyx_t_5);
- __Pyx_AddTraceback("View.MemoryView.memoryview.__getitem__", __pyx_clineno, __pyx_lineno, __pyx_filename);
- __pyx_r = NULL;
- __pyx_L0:;
- __Pyx_XDECREF(__pyx_v_have_slices);
- __Pyx_XDECREF(__pyx_v_indices);
- __Pyx_XGIVEREF(__pyx_r);
- __Pyx_RefNannyFinishContext();
- return __pyx_r;
-}
-
-/* "View.MemoryView":416
- * return self.convert_item_to_object(itemp)
- *
- * def __setitem__(memoryview self, object index, object value): # <<<<<<<<<<<<<<
- * if self.view.readonly:
- * raise TypeError("Cannot assign to read-only memoryview")
- */
-
-/* Python wrapper */
-static int __pyx_memoryview___setitem__(PyObject *__pyx_v_self, PyObject *__pyx_v_index, PyObject *__pyx_v_value); /*proto*/
-static int __pyx_memoryview___setitem__(PyObject *__pyx_v_self, PyObject *__pyx_v_index, PyObject *__pyx_v_value) {
- int __pyx_r;
- __Pyx_RefNannyDeclarations
- __Pyx_RefNannySetupContext("__setitem__ (wrapper)", 0);
- __pyx_r = __pyx_memoryview___pyx_pf_15View_dot_MemoryView_10memoryview_6__setitem__(((struct __pyx_memoryview_obj *)__pyx_v_self), ((PyObject *)__pyx_v_index), ((PyObject *)__pyx_v_value));
-
- /* function exit code */
- __Pyx_RefNannyFinishContext();
- return __pyx_r;
-}
-
-static int __pyx_memoryview___pyx_pf_15View_dot_MemoryView_10memoryview_6__setitem__(struct __pyx_memoryview_obj *__pyx_v_self, PyObject *__pyx_v_index, PyObject *__pyx_v_value) {
- PyObject *__pyx_v_have_slices = NULL;
- PyObject *__pyx_v_obj = NULL;
- int __pyx_r;
- __Pyx_RefNannyDeclarations
- int __pyx_t_1;
- PyObject *__pyx_t_2 = NULL;
- PyObject *__pyx_t_3 = NULL;
- PyObject *__pyx_t_4 = NULL;
- int __pyx_lineno = 0;
- const char *__pyx_filename = NULL;
- int __pyx_clineno = 0;
- __Pyx_RefNannySetupContext("__setitem__", 0);
- __Pyx_INCREF(__pyx_v_index);
-
- /* "View.MemoryView":417
- *
- * def __setitem__(memoryview self, object index, object value):
- * if self.view.readonly: # <<<<<<<<<<<<<<
- * raise TypeError("Cannot assign to read-only memoryview")
- *
- */
- __pyx_t_1 = (__pyx_v_self->view.readonly != 0);
- if (unlikely(__pyx_t_1)) {
-
- /* "View.MemoryView":418
- * def __setitem__(memoryview self, object index, object value):
- * if self.view.readonly:
- * raise TypeError("Cannot assign to read-only memoryview") # <<<<<<<<<<<<<<
- *
- * have_slices, index = _unellipsify(index, self.view.ndim)
- */
- __pyx_t_2 = __Pyx_PyObject_Call(__pyx_builtin_TypeError, __pyx_tuple__9, NULL); if (unlikely(!__pyx_t_2)) __PYX_ERR(1, 418, __pyx_L1_error)
- __Pyx_GOTREF(__pyx_t_2);
- __Pyx_Raise(__pyx_t_2, 0, 0, 0);
- __Pyx_DECREF(__pyx_t_2); __pyx_t_2 = 0;
- __PYX_ERR(1, 418, __pyx_L1_error)
-
- /* "View.MemoryView":417
- *
- * def __setitem__(memoryview self, object index, object value):
- * if self.view.readonly: # <<<<<<<<<<<<<<
- * raise TypeError("Cannot assign to read-only memoryview")
- *
- */
- }
-
- /* "View.MemoryView":420
- * raise TypeError("Cannot assign to read-only memoryview")
- *
- * have_slices, index = _unellipsify(index, self.view.ndim) # <<<<<<<<<<<<<<
- *
- * if have_slices:
- */
- __pyx_t_2 = _unellipsify(__pyx_v_index, __pyx_v_self->view.ndim); if (unlikely(!__pyx_t_2)) __PYX_ERR(1, 420, __pyx_L1_error)
- __Pyx_GOTREF(__pyx_t_2);
- if (likely(__pyx_t_2 != Py_None)) {
- PyObject* sequence = __pyx_t_2;
- Py_ssize_t size = __Pyx_PySequence_SIZE(sequence);
- if (unlikely(size != 2)) {
- if (size > 2) __Pyx_RaiseTooManyValuesError(2);
- else if (size >= 0) __Pyx_RaiseNeedMoreValuesError(size);
- __PYX_ERR(1, 420, __pyx_L1_error)
- }
- #if CYTHON_ASSUME_SAFE_MACROS && !CYTHON_AVOID_BORROWED_REFS
- __pyx_t_3 = PyTuple_GET_ITEM(sequence, 0);
- __pyx_t_4 = PyTuple_GET_ITEM(sequence, 1);
- __Pyx_INCREF(__pyx_t_3);
- __Pyx_INCREF(__pyx_t_4);
- #else
- __pyx_t_3 = PySequence_ITEM(sequence, 0); if (unlikely(!__pyx_t_3)) __PYX_ERR(1, 420, __pyx_L1_error)
- __Pyx_GOTREF(__pyx_t_3);
- __pyx_t_4 = PySequence_ITEM(sequence, 1); if (unlikely(!__pyx_t_4)) __PYX_ERR(1, 420, __pyx_L1_error)
- __Pyx_GOTREF(__pyx_t_4);
- #endif
- __Pyx_DECREF(__pyx_t_2); __pyx_t_2 = 0;
- } else {
- __Pyx_RaiseNoneNotIterableError(); __PYX_ERR(1, 420, __pyx_L1_error)
- }
- __pyx_v_have_slices = __pyx_t_3;
- __pyx_t_3 = 0;
- __Pyx_DECREF_SET(__pyx_v_index, __pyx_t_4);
- __pyx_t_4 = 0;
-
- /* "View.MemoryView":422
- * have_slices, index = _unellipsify(index, self.view.ndim)
- *
- * if have_slices: # <<<<<<<<<<<<<<
- * obj = self.is_slice(value)
- * if obj:
- */
- __pyx_t_1 = __Pyx_PyObject_IsTrue(__pyx_v_have_slices); if (unlikely(__pyx_t_1 < 0)) __PYX_ERR(1, 422, __pyx_L1_error)
- if (__pyx_t_1) {
-
- /* "View.MemoryView":423
- *
- * if have_slices:
- * obj = self.is_slice(value) # <<<<<<<<<<<<<<
- * if obj:
- * self.setitem_slice_assignment(self[index], obj)
- */
- __pyx_t_2 = ((struct __pyx_vtabstruct_memoryview *)__pyx_v_self->__pyx_vtab)->is_slice(__pyx_v_self, __pyx_v_value); if (unlikely(!__pyx_t_2)) __PYX_ERR(1, 423, __pyx_L1_error)
- __Pyx_GOTREF(__pyx_t_2);
- __pyx_v_obj = __pyx_t_2;
- __pyx_t_2 = 0;
-
- /* "View.MemoryView":424
- * if have_slices:
- * obj = self.is_slice(value)
- * if obj: # <<<<<<<<<<<<<<
- * self.setitem_slice_assignment(self[index], obj)
- * else:
- */
- __pyx_t_1 = __Pyx_PyObject_IsTrue(__pyx_v_obj); if (unlikely(__pyx_t_1 < 0)) __PYX_ERR(1, 424, __pyx_L1_error)
- if (__pyx_t_1) {
-
- /* "View.MemoryView":425
- * obj = self.is_slice(value)
- * if obj:
- * self.setitem_slice_assignment(self[index], obj) # <<<<<<<<<<<<<<
- * else:
- * self.setitem_slice_assign_scalar(self[index], value)
- */
- __pyx_t_2 = __Pyx_PyObject_GetItem(((PyObject *)__pyx_v_self), __pyx_v_index); if (unlikely(!__pyx_t_2)) __PYX_ERR(1, 425, __pyx_L1_error)
- __Pyx_GOTREF(__pyx_t_2);
- __pyx_t_4 = ((struct __pyx_vtabstruct_memoryview *)__pyx_v_self->__pyx_vtab)->setitem_slice_assignment(__pyx_v_self, __pyx_t_2, __pyx_v_obj); if (unlikely(!__pyx_t_4)) __PYX_ERR(1, 425, __pyx_L1_error)
- __Pyx_GOTREF(__pyx_t_4);
- __Pyx_DECREF(__pyx_t_2); __pyx_t_2 = 0;
- __Pyx_DECREF(__pyx_t_4); __pyx_t_4 = 0;
-
- /* "View.MemoryView":424
- * if have_slices:
- * obj = self.is_slice(value)
- * if obj: # <<<<<<<<<<<<<<
- * self.setitem_slice_assignment(self[index], obj)
- * else:
- */
- goto __pyx_L5;
- }
-
- /* "View.MemoryView":427
- * self.setitem_slice_assignment(self[index], obj)
- * else:
- * self.setitem_slice_assign_scalar(self[index], value) # <<<<<<<<<<<<<<
- * else:
- * self.setitem_indexed(index, value)
- */
- /*else*/ {
- __pyx_t_4 = __Pyx_PyObject_GetItem(((PyObject *)__pyx_v_self), __pyx_v_index); if (unlikely(!__pyx_t_4)) __PYX_ERR(1, 427, __pyx_L1_error)
- __Pyx_GOTREF(__pyx_t_4);
- if (!(likely(((__pyx_t_4) == Py_None) || likely(__Pyx_TypeTest(__pyx_t_4, __pyx_memoryview_type))))) __PYX_ERR(1, 427, __pyx_L1_error)
- __pyx_t_2 = ((struct __pyx_vtabstruct_memoryview *)__pyx_v_self->__pyx_vtab)->setitem_slice_assign_scalar(__pyx_v_self, ((struct __pyx_memoryview_obj *)__pyx_t_4), __pyx_v_value); if (unlikely(!__pyx_t_2)) __PYX_ERR(1, 427, __pyx_L1_error)
- __Pyx_GOTREF(__pyx_t_2);
- __Pyx_DECREF(__pyx_t_4); __pyx_t_4 = 0;
- __Pyx_DECREF(__pyx_t_2); __pyx_t_2 = 0;
- }
- __pyx_L5:;
-
- /* "View.MemoryView":422
- * have_slices, index = _unellipsify(index, self.view.ndim)
- *
- * if have_slices: # <<<<<<<<<<<<<<
- * obj = self.is_slice(value)
- * if obj:
- */
- goto __pyx_L4;
- }
-
- /* "View.MemoryView":429
- * self.setitem_slice_assign_scalar(self[index], value)
- * else:
- * self.setitem_indexed(index, value) # <<<<<<<<<<<<<<
- *
- * cdef is_slice(self, obj):
- */
- /*else*/ {
- __pyx_t_2 = ((struct __pyx_vtabstruct_memoryview *)__pyx_v_self->__pyx_vtab)->setitem_indexed(__pyx_v_self, __pyx_v_index, __pyx_v_value); if (unlikely(!__pyx_t_2)) __PYX_ERR(1, 429, __pyx_L1_error)
- __Pyx_GOTREF(__pyx_t_2);
- __Pyx_DECREF(__pyx_t_2); __pyx_t_2 = 0;
- }
- __pyx_L4:;
-
- /* "View.MemoryView":416
- * return self.convert_item_to_object(itemp)
- *
- * def __setitem__(memoryview self, object index, object value): # <<<<<<<<<<<<<<
- * if self.view.readonly:
- * raise TypeError("Cannot assign to read-only memoryview")
- */
-
- /* function exit code */
- __pyx_r = 0;
- goto __pyx_L0;
- __pyx_L1_error:;
- __Pyx_XDECREF(__pyx_t_2);
- __Pyx_XDECREF(__pyx_t_3);
- __Pyx_XDECREF(__pyx_t_4);
- __Pyx_AddTraceback("View.MemoryView.memoryview.__setitem__", __pyx_clineno, __pyx_lineno, __pyx_filename);
- __pyx_r = -1;
- __pyx_L0:;
- __Pyx_XDECREF(__pyx_v_have_slices);
- __Pyx_XDECREF(__pyx_v_obj);
- __Pyx_XDECREF(__pyx_v_index);
- __Pyx_RefNannyFinishContext();
- return __pyx_r;
-}
-
-/* "View.MemoryView":431
- * self.setitem_indexed(index, value)
- *
- * cdef is_slice(self, obj): # <<<<<<<<<<<<<<
- * if not isinstance(obj, memoryview):
- * try:
- */
-
-static PyObject *__pyx_memoryview_is_slice(struct __pyx_memoryview_obj *__pyx_v_self, PyObject *__pyx_v_obj) {
- PyObject *__pyx_r = NULL;
- __Pyx_RefNannyDeclarations
- int __pyx_t_1;
- int __pyx_t_2;
- PyObject *__pyx_t_3 = NULL;
- PyObject *__pyx_t_4 = NULL;
- PyObject *__pyx_t_5 = NULL;
- PyObject *__pyx_t_6 = NULL;
- PyObject *__pyx_t_7 = NULL;
- PyObject *__pyx_t_8 = NULL;
- int __pyx_t_9;
- int __pyx_lineno = 0;
- const char *__pyx_filename = NULL;
- int __pyx_clineno = 0;
- __Pyx_RefNannySetupContext("is_slice", 0);
- __Pyx_INCREF(__pyx_v_obj);
-
- /* "View.MemoryView":432
- *
- * cdef is_slice(self, obj):
- * if not isinstance(obj, memoryview): # <<<<<<<<<<<<<<
- * try:
- * obj = memoryview(obj, self.flags & ~PyBUF_WRITABLE | PyBUF_ANY_CONTIGUOUS,
- */
- __pyx_t_1 = __Pyx_TypeCheck(__pyx_v_obj, __pyx_memoryview_type);
- __pyx_t_2 = ((!(__pyx_t_1 != 0)) != 0);
- if (__pyx_t_2) {
-
- /* "View.MemoryView":433
- * cdef is_slice(self, obj):
- * if not isinstance(obj, memoryview):
- * try: # <<<<<<<<<<<<<<
- * obj = memoryview(obj, self.flags & ~PyBUF_WRITABLE | PyBUF_ANY_CONTIGUOUS,
- * self.dtype_is_object)
- */
- {
- __Pyx_PyThreadState_declare
- __Pyx_PyThreadState_assign
- __Pyx_ExceptionSave(&__pyx_t_3, &__pyx_t_4, &__pyx_t_5);
- __Pyx_XGOTREF(__pyx_t_3);
- __Pyx_XGOTREF(__pyx_t_4);
- __Pyx_XGOTREF(__pyx_t_5);
- /*try:*/ {
-
- /* "View.MemoryView":434
- * if not isinstance(obj, memoryview):
- * try:
- * obj = memoryview(obj, self.flags & ~PyBUF_WRITABLE | PyBUF_ANY_CONTIGUOUS, # <<<<<<<<<<<<<<
- * self.dtype_is_object)
- * except TypeError:
- */
- __pyx_t_6 = __Pyx_PyInt_From_int(((__pyx_v_self->flags & (~PyBUF_WRITABLE)) | PyBUF_ANY_CONTIGUOUS)); if (unlikely(!__pyx_t_6)) __PYX_ERR(1, 434, __pyx_L4_error)
- __Pyx_GOTREF(__pyx_t_6);
-
- /* "View.MemoryView":435
- * try:
- * obj = memoryview(obj, self.flags & ~PyBUF_WRITABLE | PyBUF_ANY_CONTIGUOUS,
- * self.dtype_is_object) # <<<<<<<<<<<<<<
- * except TypeError:
- * return None
- */
- __pyx_t_7 = __Pyx_PyBool_FromLong(__pyx_v_self->dtype_is_object); if (unlikely(!__pyx_t_7)) __PYX_ERR(1, 435, __pyx_L4_error)
- __Pyx_GOTREF(__pyx_t_7);
-
- /* "View.MemoryView":434
- * if not isinstance(obj, memoryview):
- * try:
- * obj = memoryview(obj, self.flags & ~PyBUF_WRITABLE | PyBUF_ANY_CONTIGUOUS, # <<<<<<<<<<<<<<
- * self.dtype_is_object)
- * except TypeError:
- */
- __pyx_t_8 = PyTuple_New(3); if (unlikely(!__pyx_t_8)) __PYX_ERR(1, 434, __pyx_L4_error)
- __Pyx_GOTREF(__pyx_t_8);
- __Pyx_INCREF(__pyx_v_obj);
- __Pyx_GIVEREF(__pyx_v_obj);
- PyTuple_SET_ITEM(__pyx_t_8, 0, __pyx_v_obj);
- __Pyx_GIVEREF(__pyx_t_6);
- PyTuple_SET_ITEM(__pyx_t_8, 1, __pyx_t_6);
- __Pyx_GIVEREF(__pyx_t_7);
- PyTuple_SET_ITEM(__pyx_t_8, 2, __pyx_t_7);
- __pyx_t_6 = 0;
- __pyx_t_7 = 0;
- __pyx_t_7 = __Pyx_PyObject_Call(((PyObject *)__pyx_memoryview_type), __pyx_t_8, NULL); if (unlikely(!__pyx_t_7)) __PYX_ERR(1, 434, __pyx_L4_error)
- __Pyx_GOTREF(__pyx_t_7);
- __Pyx_DECREF(__pyx_t_8); __pyx_t_8 = 0;
- __Pyx_DECREF_SET(__pyx_v_obj, __pyx_t_7);
- __pyx_t_7 = 0;
-
- /* "View.MemoryView":433
- * cdef is_slice(self, obj):
- * if not isinstance(obj, memoryview):
- * try: # <<<<<<<<<<<<<<
- * obj = memoryview(obj, self.flags & ~PyBUF_WRITABLE | PyBUF_ANY_CONTIGUOUS,
- * self.dtype_is_object)
- */
- }
- __Pyx_XDECREF(__pyx_t_3); __pyx_t_3 = 0;
- __Pyx_XDECREF(__pyx_t_4); __pyx_t_4 = 0;
- __Pyx_XDECREF(__pyx_t_5); __pyx_t_5 = 0;
- goto __pyx_L9_try_end;
- __pyx_L4_error:;
- __Pyx_XDECREF(__pyx_t_6); __pyx_t_6 = 0;
- __Pyx_XDECREF(__pyx_t_7); __pyx_t_7 = 0;
- __Pyx_XDECREF(__pyx_t_8); __pyx_t_8 = 0;
-
- /* "View.MemoryView":436
- * obj = memoryview(obj, self.flags & ~PyBUF_WRITABLE | PyBUF_ANY_CONTIGUOUS,
- * self.dtype_is_object)
- * except TypeError: # <<<<<<<<<<<<<<
- * return None
- *
- */
- __pyx_t_9 = __Pyx_PyErr_ExceptionMatches(__pyx_builtin_TypeError);
- if (__pyx_t_9) {
- __Pyx_AddTraceback("View.MemoryView.memoryview.is_slice", __pyx_clineno, __pyx_lineno, __pyx_filename);
- if (__Pyx_GetException(&__pyx_t_7, &__pyx_t_8, &__pyx_t_6) < 0) __PYX_ERR(1, 436, __pyx_L6_except_error)
- __Pyx_GOTREF(__pyx_t_7);
- __Pyx_GOTREF(__pyx_t_8);
- __Pyx_GOTREF(__pyx_t_6);
-
- /* "View.MemoryView":437
- * self.dtype_is_object)
- * except TypeError:
- * return None # <<<<<<<<<<<<<<
- *
- * return obj
- */
- __Pyx_XDECREF(__pyx_r);
- __pyx_r = Py_None; __Pyx_INCREF(Py_None);
- __Pyx_DECREF(__pyx_t_6); __pyx_t_6 = 0;
- __Pyx_DECREF(__pyx_t_7); __pyx_t_7 = 0;
- __Pyx_DECREF(__pyx_t_8); __pyx_t_8 = 0;
- goto __pyx_L7_except_return;
- }
- goto __pyx_L6_except_error;
- __pyx_L6_except_error:;
-
- /* "View.MemoryView":433
- * cdef is_slice(self, obj):
- * if not isinstance(obj, memoryview):
- * try: # <<<<<<<<<<<<<<
- * obj = memoryview(obj, self.flags & ~PyBUF_WRITABLE | PyBUF_ANY_CONTIGUOUS,
- * self.dtype_is_object)
- */
- __Pyx_XGIVEREF(__pyx_t_3);
- __Pyx_XGIVEREF(__pyx_t_4);
- __Pyx_XGIVEREF(__pyx_t_5);
- __Pyx_ExceptionReset(__pyx_t_3, __pyx_t_4, __pyx_t_5);
- goto __pyx_L1_error;
- __pyx_L7_except_return:;
- __Pyx_XGIVEREF(__pyx_t_3);
- __Pyx_XGIVEREF(__pyx_t_4);
- __Pyx_XGIVEREF(__pyx_t_5);
- __Pyx_ExceptionReset(__pyx_t_3, __pyx_t_4, __pyx_t_5);
- goto __pyx_L0;
- __pyx_L9_try_end:;
- }
-
- /* "View.MemoryView":432
- *
- * cdef is_slice(self, obj):
- * if not isinstance(obj, memoryview): # <<<<<<<<<<<<<<
- * try:
- * obj = memoryview(obj, self.flags & ~PyBUF_WRITABLE | PyBUF_ANY_CONTIGUOUS,
- */
- }
-
- /* "View.MemoryView":439
- * return None
- *
- * return obj # <<<<<<<<<<<<<<
- *
- * cdef setitem_slice_assignment(self, dst, src):
- */
- __Pyx_XDECREF(__pyx_r);
- __Pyx_INCREF(__pyx_v_obj);
- __pyx_r = __pyx_v_obj;
- goto __pyx_L0;
-
- /* "View.MemoryView":431
- * self.setitem_indexed(index, value)
- *
- * cdef is_slice(self, obj): # <<<<<<<<<<<<<<
- * if not isinstance(obj, memoryview):
- * try:
- */
-
- /* function exit code */
- __pyx_L1_error:;
- __Pyx_XDECREF(__pyx_t_6);
- __Pyx_XDECREF(__pyx_t_7);
- __Pyx_XDECREF(__pyx_t_8);
- __Pyx_AddTraceback("View.MemoryView.memoryview.is_slice", __pyx_clineno, __pyx_lineno, __pyx_filename);
- __pyx_r = 0;
- __pyx_L0:;
- __Pyx_XDECREF(__pyx_v_obj);
- __Pyx_XGIVEREF(__pyx_r);
- __Pyx_RefNannyFinishContext();
- return __pyx_r;
-}
-
-/* "View.MemoryView":441
- * return obj
- *
- * cdef setitem_slice_assignment(self, dst, src): # <<<<<<<<<<<<<<
- * cdef __Pyx_memviewslice dst_slice
- * cdef __Pyx_memviewslice src_slice
- */
-
-static PyObject *__pyx_memoryview_setitem_slice_assignment(struct __pyx_memoryview_obj *__pyx_v_self, PyObject *__pyx_v_dst, PyObject *__pyx_v_src) {
- __Pyx_memviewslice __pyx_v_dst_slice;
- __Pyx_memviewslice __pyx_v_src_slice;
- PyObject *__pyx_r = NULL;
- __Pyx_RefNannyDeclarations
- __Pyx_memviewslice *__pyx_t_1;
- __Pyx_memviewslice *__pyx_t_2;
- PyObject *__pyx_t_3 = NULL;
- int __pyx_t_4;
- int __pyx_t_5;
- int __pyx_t_6;
- int __pyx_lineno = 0;
- const char *__pyx_filename = NULL;
- int __pyx_clineno = 0;
- __Pyx_RefNannySetupContext("setitem_slice_assignment", 0);
-
- /* "View.MemoryView":445
- * cdef __Pyx_memviewslice src_slice
- *
- * memoryview_copy_contents(get_slice_from_memview(src, &src_slice)[0], # <<<<<<<<<<<<<<
- * get_slice_from_memview(dst, &dst_slice)[0],
- * src.ndim, dst.ndim, self.dtype_is_object)
- */
- if (!(likely(((__pyx_v_src) == Py_None) || likely(__Pyx_TypeTest(__pyx_v_src, __pyx_memoryview_type))))) __PYX_ERR(1, 445, __pyx_L1_error)
- __pyx_t_1 = __pyx_memoryview_get_slice_from_memoryview(((struct __pyx_memoryview_obj *)__pyx_v_src), (&__pyx_v_src_slice)); if (unlikely(__pyx_t_1 == ((__Pyx_memviewslice *)NULL))) __PYX_ERR(1, 445, __pyx_L1_error)
-
- /* "View.MemoryView":446
- *
- * memoryview_copy_contents(get_slice_from_memview(src, &src_slice)[0],
- * get_slice_from_memview(dst, &dst_slice)[0], # <<<<<<<<<<<<<<
- * src.ndim, dst.ndim, self.dtype_is_object)
- *
- */
- if (!(likely(((__pyx_v_dst) == Py_None) || likely(__Pyx_TypeTest(__pyx_v_dst, __pyx_memoryview_type))))) __PYX_ERR(1, 446, __pyx_L1_error)
- __pyx_t_2 = __pyx_memoryview_get_slice_from_memoryview(((struct __pyx_memoryview_obj *)__pyx_v_dst), (&__pyx_v_dst_slice)); if (unlikely(__pyx_t_2 == ((__Pyx_memviewslice *)NULL))) __PYX_ERR(1, 446, __pyx_L1_error)
-
- /* "View.MemoryView":447
- * memoryview_copy_contents(get_slice_from_memview(src, &src_slice)[0],
- * get_slice_from_memview(dst, &dst_slice)[0],
- * src.ndim, dst.ndim, self.dtype_is_object) # <<<<<<<<<<<<<<
- *
- * cdef setitem_slice_assign_scalar(self, memoryview dst, value):
- */
- __pyx_t_3 = __Pyx_PyObject_GetAttrStr(__pyx_v_src, __pyx_n_s_ndim); if (unlikely(!__pyx_t_3)) __PYX_ERR(1, 447, __pyx_L1_error)
- __Pyx_GOTREF(__pyx_t_3);
- __pyx_t_4 = __Pyx_PyInt_As_int(__pyx_t_3); if (unlikely((__pyx_t_4 == (int)-1) && PyErr_Occurred())) __PYX_ERR(1, 447, __pyx_L1_error)
- __Pyx_DECREF(__pyx_t_3); __pyx_t_3 = 0;
- __pyx_t_3 = __Pyx_PyObject_GetAttrStr(__pyx_v_dst, __pyx_n_s_ndim); if (unlikely(!__pyx_t_3)) __PYX_ERR(1, 447, __pyx_L1_error)
- __Pyx_GOTREF(__pyx_t_3);
- __pyx_t_5 = __Pyx_PyInt_As_int(__pyx_t_3); if (unlikely((__pyx_t_5 == (int)-1) && PyErr_Occurred())) __PYX_ERR(1, 447, __pyx_L1_error)
- __Pyx_DECREF(__pyx_t_3); __pyx_t_3 = 0;
-
- /* "View.MemoryView":445
- * cdef __Pyx_memviewslice src_slice
- *
- * memoryview_copy_contents(get_slice_from_memview(src, &src_slice)[0], # <<<<<<<<<<<<<<
- * get_slice_from_memview(dst, &dst_slice)[0],
- * src.ndim, dst.ndim, self.dtype_is_object)
- */
- __pyx_t_6 = __pyx_memoryview_copy_contents((__pyx_t_1[0]), (__pyx_t_2[0]), __pyx_t_4, __pyx_t_5, __pyx_v_self->dtype_is_object); if (unlikely(__pyx_t_6 == ((int)-1))) __PYX_ERR(1, 445, __pyx_L1_error)
-
- /* "View.MemoryView":441
- * return obj
- *
- * cdef setitem_slice_assignment(self, dst, src): # <<<<<<<<<<<<<<
- * cdef __Pyx_memviewslice dst_slice
- * cdef __Pyx_memviewslice src_slice
- */
-
- /* function exit code */
- __pyx_r = Py_None; __Pyx_INCREF(Py_None);
- goto __pyx_L0;
- __pyx_L1_error:;
- __Pyx_XDECREF(__pyx_t_3);
- __Pyx_AddTraceback("View.MemoryView.memoryview.setitem_slice_assignment", __pyx_clineno, __pyx_lineno, __pyx_filename);
- __pyx_r = 0;
- __pyx_L0:;
- __Pyx_XGIVEREF(__pyx_r);
- __Pyx_RefNannyFinishContext();
- return __pyx_r;
-}
-
-/* "View.MemoryView":449
- * src.ndim, dst.ndim, self.dtype_is_object)
- *
- * cdef setitem_slice_assign_scalar(self, memoryview dst, value): # <<<<<<<<<<<<<<
- * cdef int array[128]
- * cdef void *tmp = NULL
- */
-
-static PyObject *__pyx_memoryview_setitem_slice_assign_scalar(struct __pyx_memoryview_obj *__pyx_v_self, struct __pyx_memoryview_obj *__pyx_v_dst, PyObject *__pyx_v_value) {
- int __pyx_v_array[0x80];
- void *__pyx_v_tmp;
- void *__pyx_v_item;
- __Pyx_memviewslice *__pyx_v_dst_slice;
- __Pyx_memviewslice __pyx_v_tmp_slice;
- PyObject *__pyx_r = NULL;
- __Pyx_RefNannyDeclarations
- __Pyx_memviewslice *__pyx_t_1;
- int __pyx_t_2;
- PyObject *__pyx_t_3 = NULL;
- int __pyx_t_4;
- int __pyx_t_5;
- char const *__pyx_t_6;
- PyObject *__pyx_t_7 = NULL;
- PyObject *__pyx_t_8 = NULL;
- PyObject *__pyx_t_9 = NULL;
- PyObject *__pyx_t_10 = NULL;
- PyObject *__pyx_t_11 = NULL;
- PyObject *__pyx_t_12 = NULL;
- int __pyx_lineno = 0;
- const char *__pyx_filename = NULL;
- int __pyx_clineno = 0;
- __Pyx_RefNannySetupContext("setitem_slice_assign_scalar", 0);
-
- /* "View.MemoryView":451
- * cdef setitem_slice_assign_scalar(self, memoryview dst, value):
- * cdef int array[128]
- * cdef void *tmp = NULL # <<<<<<<<<<<<<<
- * cdef void *item
- *
- */
- __pyx_v_tmp = NULL;
-
- /* "View.MemoryView":456
- * cdef __Pyx_memviewslice *dst_slice
- * cdef __Pyx_memviewslice tmp_slice
- * dst_slice = get_slice_from_memview(dst, &tmp_slice) # <<<<<<<<<<<<<<
- *
- * if self.view.itemsize > sizeof(array):
- */
- __pyx_t_1 = __pyx_memoryview_get_slice_from_memoryview(__pyx_v_dst, (&__pyx_v_tmp_slice)); if (unlikely(__pyx_t_1 == ((__Pyx_memviewslice *)NULL))) __PYX_ERR(1, 456, __pyx_L1_error)
- __pyx_v_dst_slice = __pyx_t_1;
-
- /* "View.MemoryView":458
- * dst_slice = get_slice_from_memview(dst, &tmp_slice)
- *
- * if self.view.itemsize > sizeof(array): # <<<<<<<<<<<<<<
- * tmp = PyMem_Malloc(self.view.itemsize)
- * if tmp == NULL:
- */
- __pyx_t_2 = ((((size_t)__pyx_v_self->view.itemsize) > (sizeof(__pyx_v_array))) != 0);
- if (__pyx_t_2) {
-
- /* "View.MemoryView":459
- *
- * if self.view.itemsize > sizeof(array):
- * tmp = PyMem_Malloc(self.view.itemsize) # <<<<<<<<<<<<<<
- * if tmp == NULL:
- * raise MemoryError
- */
- __pyx_v_tmp = PyMem_Malloc(__pyx_v_self->view.itemsize);
-
- /* "View.MemoryView":460
- * if self.view.itemsize > sizeof(array):
- * tmp = PyMem_Malloc(self.view.itemsize)
- * if tmp == NULL: # <<<<<<<<<<<<<<
- * raise MemoryError
- * item = tmp
- */
- __pyx_t_2 = ((__pyx_v_tmp == NULL) != 0);
- if (unlikely(__pyx_t_2)) {
-
- /* "View.MemoryView":461
- * tmp = PyMem_Malloc(self.view.itemsize)
- * if tmp == NULL:
- * raise MemoryError # <<<<<<<<<<<<<<
- * item = tmp
- * else:
- */
- PyErr_NoMemory(); __PYX_ERR(1, 461, __pyx_L1_error)
-
- /* "View.MemoryView":460
- * if self.view.itemsize > sizeof(array):
- * tmp = PyMem_Malloc(self.view.itemsize)
- * if tmp == NULL: # <<<<<<<<<<<<<<
- * raise MemoryError
- * item = tmp
- */
- }
-
- /* "View.MemoryView":462
- * if tmp == NULL:
- * raise MemoryError
- * item = tmp # <<<<<<<<<<<<<<
- * else:
- * item = array
- */
- __pyx_v_item = __pyx_v_tmp;
-
- /* "View.MemoryView":458
- * dst_slice = get_slice_from_memview(dst, &tmp_slice)
- *
- * if self.view.itemsize > sizeof(array): # <<<<<<<<<<<<<<
- * tmp = PyMem_Malloc(self.view.itemsize)
- * if tmp == NULL:
- */
- goto __pyx_L3;
- }
-
- /* "View.MemoryView":464
- * item = tmp
- * else:
- * item = array # <<<<<<<<<<<<<<
- *
- * try:
- */
- /*else*/ {
- __pyx_v_item = ((void *)__pyx_v_array);
- }
- __pyx_L3:;
-
- /* "View.MemoryView":466
- * item = array
- *
- * try: # <<<<<<<<<<<<<<
- * if self.dtype_is_object:
- * ( item)[0] = value
- */
- /*try:*/ {
-
- /* "View.MemoryView":467
- *
- * try:
- * if self.dtype_is_object: # <<<<<<<<<<<<<<
- * ( item)[0] = value
- * else:
- */
- __pyx_t_2 = (__pyx_v_self->dtype_is_object != 0);
- if (__pyx_t_2) {
-
- /* "View.MemoryView":468
- * try:
- * if self.dtype_is_object:
- * ( item)[0] = value # <<<<<<<<<<<<<<
- * else:
- * self.assign_item_from_object( item, value)
- */
- (((PyObject **)__pyx_v_item)[0]) = ((PyObject *)__pyx_v_value);
-
- /* "View.MemoryView":467
- *
- * try:
- * if self.dtype_is_object: # <<<<<<<<<<<<<<
- * ( item)[0] = value
- * else:
- */
- goto __pyx_L8;
- }
-
- /* "View.MemoryView":470
- * ( item)[0] = value
- * else:
- * self.assign_item_from_object( item, value) # <<<<<<<<<<<<<<
- *
- *
- */
- /*else*/ {
- __pyx_t_3 = ((struct __pyx_vtabstruct_memoryview *)__pyx_v_self->__pyx_vtab)->assign_item_from_object(__pyx_v_self, ((char *)__pyx_v_item), __pyx_v_value); if (unlikely(!__pyx_t_3)) __PYX_ERR(1, 470, __pyx_L6_error)
- __Pyx_GOTREF(__pyx_t_3);
- __Pyx_DECREF(__pyx_t_3); __pyx_t_3 = 0;
- }
- __pyx_L8:;
-
- /* "View.MemoryView":474
- *
- *
- * if self.view.suboffsets != NULL: # <<<<<<<<<<<<<<
- * assert_direct_dimensions(self.view.suboffsets, self.view.ndim)
- * slice_assign_scalar(dst_slice, dst.view.ndim, self.view.itemsize,
- */
- __pyx_t_2 = ((__pyx_v_self->view.suboffsets != NULL) != 0);
- if (__pyx_t_2) {
-
- /* "View.MemoryView":475
- *
- * if self.view.suboffsets != NULL:
- * assert_direct_dimensions(self.view.suboffsets, self.view.ndim) # <<<<<<<<<<<<<<
- * slice_assign_scalar(dst_slice, dst.view.ndim, self.view.itemsize,
- * item, self.dtype_is_object)
- */
- __pyx_t_3 = assert_direct_dimensions(__pyx_v_self->view.suboffsets, __pyx_v_self->view.ndim); if (unlikely(!__pyx_t_3)) __PYX_ERR(1, 475, __pyx_L6_error)
- __Pyx_GOTREF(__pyx_t_3);
- __Pyx_DECREF(__pyx_t_3); __pyx_t_3 = 0;
-
- /* "View.MemoryView":474
- *
- *
- * if self.view.suboffsets != NULL: # <<<<<<<<<<<<<<
- * assert_direct_dimensions(self.view.suboffsets, self.view.ndim)
- * slice_assign_scalar(dst_slice, dst.view.ndim, self.view.itemsize,
- */
- }
-
- /* "View.MemoryView":476
- * if self.view.suboffsets != NULL:
- * assert_direct_dimensions(self.view.suboffsets, self.view.ndim)
- * slice_assign_scalar(dst_slice, dst.view.ndim, self.view.itemsize, # <<<<<<<<<<<<<<
- * item, self.dtype_is_object)
- * finally:
- */
- __pyx_memoryview_slice_assign_scalar(__pyx_v_dst_slice, __pyx_v_dst->view.ndim, __pyx_v_self->view.itemsize, __pyx_v_item, __pyx_v_self->dtype_is_object);
- }
-
- /* "View.MemoryView":479
- * item, self.dtype_is_object)
- * finally:
- * PyMem_Free(tmp) # <<<<<<<<<<<<<<
- *
- * cdef setitem_indexed(self, index, value):
- */
- /*finally:*/ {
- /*normal exit:*/{
- PyMem_Free(__pyx_v_tmp);
- goto __pyx_L7;
- }
- __pyx_L6_error:;
- /*exception exit:*/{
- __Pyx_PyThreadState_declare
- __Pyx_PyThreadState_assign
- __pyx_t_7 = 0; __pyx_t_8 = 0; __pyx_t_9 = 0; __pyx_t_10 = 0; __pyx_t_11 = 0; __pyx_t_12 = 0;
- __Pyx_XDECREF(__pyx_t_3); __pyx_t_3 = 0;
- if (PY_MAJOR_VERSION >= 3) __Pyx_ExceptionSwap(&__pyx_t_10, &__pyx_t_11, &__pyx_t_12);
- if ((PY_MAJOR_VERSION < 3) || unlikely(__Pyx_GetException(&__pyx_t_7, &__pyx_t_8, &__pyx_t_9) < 0)) __Pyx_ErrFetch(&__pyx_t_7, &__pyx_t_8, &__pyx_t_9);
- __Pyx_XGOTREF(__pyx_t_7);
- __Pyx_XGOTREF(__pyx_t_8);
- __Pyx_XGOTREF(__pyx_t_9);
- __Pyx_XGOTREF(__pyx_t_10);
- __Pyx_XGOTREF(__pyx_t_11);
- __Pyx_XGOTREF(__pyx_t_12);
- __pyx_t_4 = __pyx_lineno; __pyx_t_5 = __pyx_clineno; __pyx_t_6 = __pyx_filename;
- {
- PyMem_Free(__pyx_v_tmp);
- }
- if (PY_MAJOR_VERSION >= 3) {
- __Pyx_XGIVEREF(__pyx_t_10);
- __Pyx_XGIVEREF(__pyx_t_11);
- __Pyx_XGIVEREF(__pyx_t_12);
- __Pyx_ExceptionReset(__pyx_t_10, __pyx_t_11, __pyx_t_12);
- }
- __Pyx_XGIVEREF(__pyx_t_7);
- __Pyx_XGIVEREF(__pyx_t_8);
- __Pyx_XGIVEREF(__pyx_t_9);
- __Pyx_ErrRestore(__pyx_t_7, __pyx_t_8, __pyx_t_9);
- __pyx_t_7 = 0; __pyx_t_8 = 0; __pyx_t_9 = 0; __pyx_t_10 = 0; __pyx_t_11 = 0; __pyx_t_12 = 0;
- __pyx_lineno = __pyx_t_4; __pyx_clineno = __pyx_t_5; __pyx_filename = __pyx_t_6;
- goto __pyx_L1_error;
- }
- __pyx_L7:;
- }
-
- /* "View.MemoryView":449
- * src.ndim, dst.ndim, self.dtype_is_object)
- *
- * cdef setitem_slice_assign_scalar(self, memoryview dst, value): # <<<<<<<<<<<<<<
- * cdef int array[128]
- * cdef void *tmp = NULL
- */
-
- /* function exit code */
- __pyx_r = Py_None; __Pyx_INCREF(Py_None);
- goto __pyx_L0;
- __pyx_L1_error:;
- __Pyx_XDECREF(__pyx_t_3);
- __Pyx_AddTraceback("View.MemoryView.memoryview.setitem_slice_assign_scalar", __pyx_clineno, __pyx_lineno, __pyx_filename);
- __pyx_r = 0;
- __pyx_L0:;
- __Pyx_XGIVEREF(__pyx_r);
- __Pyx_RefNannyFinishContext();
- return __pyx_r;
-}
-
-/* "View.MemoryView":481
- * PyMem_Free(tmp)
- *
- * cdef setitem_indexed(self, index, value): # <<<<<<<<<<<<<<
- * cdef char *itemp = self.get_item_pointer(index)
- * self.assign_item_from_object(itemp, value)
- */
-
-static PyObject *__pyx_memoryview_setitem_indexed(struct __pyx_memoryview_obj *__pyx_v_self, PyObject *__pyx_v_index, PyObject *__pyx_v_value) {
- char *__pyx_v_itemp;
- PyObject *__pyx_r = NULL;
- __Pyx_RefNannyDeclarations
- char *__pyx_t_1;
- PyObject *__pyx_t_2 = NULL;
- int __pyx_lineno = 0;
- const char *__pyx_filename = NULL;
- int __pyx_clineno = 0;
- __Pyx_RefNannySetupContext("setitem_indexed", 0);
-
- /* "View.MemoryView":482
- *
- * cdef setitem_indexed(self, index, value):
- * cdef char *itemp = self.get_item_pointer(index) # <<<<<<<<<<<<<<
- * self.assign_item_from_object(itemp, value)
- *
- */
- __pyx_t_1 = ((struct __pyx_vtabstruct_memoryview *)__pyx_v_self->__pyx_vtab)->get_item_pointer(__pyx_v_self, __pyx_v_index); if (unlikely(__pyx_t_1 == ((char *)NULL))) __PYX_ERR(1, 482, __pyx_L1_error)
- __pyx_v_itemp = __pyx_t_1;
-
- /* "View.MemoryView":483
- * cdef setitem_indexed(self, index, value):
- * cdef char *itemp = self.get_item_pointer(index)
- * self.assign_item_from_object(itemp, value) # <<<<<<<<<<<<<<
- *
- * cdef convert_item_to_object(self, char *itemp):
- */
- __pyx_t_2 = ((struct __pyx_vtabstruct_memoryview *)__pyx_v_self->__pyx_vtab)->assign_item_from_object(__pyx_v_self, __pyx_v_itemp, __pyx_v_value); if (unlikely(!__pyx_t_2)) __PYX_ERR(1, 483, __pyx_L1_error)
- __Pyx_GOTREF(__pyx_t_2);
- __Pyx_DECREF(__pyx_t_2); __pyx_t_2 = 0;
-
- /* "View.MemoryView":481
- * PyMem_Free(tmp)
- *
- * cdef setitem_indexed(self, index, value): # <<<<<<<<<<<<<<
- * cdef char *itemp = self.get_item_pointer(index)
- * self.assign_item_from_object(itemp, value)
- */
-
- /* function exit code */
- __pyx_r = Py_None; __Pyx_INCREF(Py_None);
- goto __pyx_L0;
- __pyx_L1_error:;
- __Pyx_XDECREF(__pyx_t_2);
- __Pyx_AddTraceback("View.MemoryView.memoryview.setitem_indexed", __pyx_clineno, __pyx_lineno, __pyx_filename);
- __pyx_r = 0;
- __pyx_L0:;
- __Pyx_XGIVEREF(__pyx_r);
- __Pyx_RefNannyFinishContext();
- return __pyx_r;
-}
-
-/* "View.MemoryView":485
- * self.assign_item_from_object(itemp, value)
- *
- * cdef convert_item_to_object(self, char *itemp): # <<<<<<<<<<<<<<
- * """Only used if instantiated manually by the user, or if Cython doesn't
- * know how to convert the type"""
- */
-
-static PyObject *__pyx_memoryview_convert_item_to_object(struct __pyx_memoryview_obj *__pyx_v_self, char *__pyx_v_itemp) {
- PyObject *__pyx_v_struct = NULL;
- PyObject *__pyx_v_bytesitem = 0;
- PyObject *__pyx_v_result = NULL;
- PyObject *__pyx_r = NULL;
- __Pyx_RefNannyDeclarations
- PyObject *__pyx_t_1 = NULL;
- PyObject *__pyx_t_2 = NULL;
- PyObject *__pyx_t_3 = NULL;
- PyObject *__pyx_t_4 = NULL;
- PyObject *__pyx_t_5 = NULL;
- PyObject *__pyx_t_6 = NULL;
- PyObject *__pyx_t_7 = NULL;
- int __pyx_t_8;
- PyObject *__pyx_t_9 = NULL;
- size_t __pyx_t_10;
- int __pyx_t_11;
- int __pyx_lineno = 0;
- const char *__pyx_filename = NULL;
- int __pyx_clineno = 0;
- __Pyx_RefNannySetupContext("convert_item_to_object", 0);
-
- /* "View.MemoryView":488
- * """Only used if instantiated manually by the user, or if Cython doesn't
- * know how to convert the type"""
- * import struct # <<<<<<<<<<<<<<
- * cdef bytes bytesitem
- *
- */
- __pyx_t_1 = __Pyx_Import(__pyx_n_s_struct, 0, 0); if (unlikely(!__pyx_t_1)) __PYX_ERR(1, 488, __pyx_L1_error)
- __Pyx_GOTREF(__pyx_t_1);
- __pyx_v_struct = __pyx_t_1;
- __pyx_t_1 = 0;
-
- /* "View.MemoryView":491
- * cdef bytes bytesitem
- *
- * bytesitem = itemp[:self.view.itemsize] # <<<<<<<<<<<<<<
- * try:
- * result = struct.unpack(self.view.format, bytesitem)
- */
- __pyx_t_1 = __Pyx_PyBytes_FromStringAndSize(__pyx_v_itemp + 0, __pyx_v_self->view.itemsize - 0); if (unlikely(!__pyx_t_1)) __PYX_ERR(1, 491, __pyx_L1_error)
- __Pyx_GOTREF(__pyx_t_1);
- __pyx_v_bytesitem = ((PyObject*)__pyx_t_1);
- __pyx_t_1 = 0;
-
- /* "View.MemoryView":492
- *
- * bytesitem = itemp[:self.view.itemsize]
- * try: # <<<<<<<<<<<<<<
- * result = struct.unpack(self.view.format, bytesitem)
- * except struct.error:
- */
- {
- __Pyx_PyThreadState_declare
- __Pyx_PyThreadState_assign
- __Pyx_ExceptionSave(&__pyx_t_2, &__pyx_t_3, &__pyx_t_4);
- __Pyx_XGOTREF(__pyx_t_2);
- __Pyx_XGOTREF(__pyx_t_3);
- __Pyx_XGOTREF(__pyx_t_4);
- /*try:*/ {
-
- /* "View.MemoryView":493
- * bytesitem = itemp[:self.view.itemsize]
- * try:
- * result = struct.unpack(self.view.format, bytesitem) # <<<<<<<<<<<<<<
- * except struct.error:
- * raise ValueError("Unable to convert item to object")
- */
- __pyx_t_5 = __Pyx_PyObject_GetAttrStr(__pyx_v_struct, __pyx_n_s_unpack); if (unlikely(!__pyx_t_5)) __PYX_ERR(1, 493, __pyx_L3_error)
- __Pyx_GOTREF(__pyx_t_5);
- __pyx_t_6 = __Pyx_PyBytes_FromString(__pyx_v_self->view.format); if (unlikely(!__pyx_t_6)) __PYX_ERR(1, 493, __pyx_L3_error)
- __Pyx_GOTREF(__pyx_t_6);
- __pyx_t_7 = NULL;
- __pyx_t_8 = 0;
- if (CYTHON_UNPACK_METHODS && likely(PyMethod_Check(__pyx_t_5))) {
- __pyx_t_7 = PyMethod_GET_SELF(__pyx_t_5);
- if (likely(__pyx_t_7)) {
- PyObject* function = PyMethod_GET_FUNCTION(__pyx_t_5);
- __Pyx_INCREF(__pyx_t_7);
- __Pyx_INCREF(function);
- __Pyx_DECREF_SET(__pyx_t_5, function);
- __pyx_t_8 = 1;
- }
- }
- #if CYTHON_FAST_PYCALL
- if (PyFunction_Check(__pyx_t_5)) {
- PyObject *__pyx_temp[3] = {__pyx_t_7, __pyx_t_6, __pyx_v_bytesitem};
- __pyx_t_1 = __Pyx_PyFunction_FastCall(__pyx_t_5, __pyx_temp+1-__pyx_t_8, 2+__pyx_t_8); if (unlikely(!__pyx_t_1)) __PYX_ERR(1, 493, __pyx_L3_error)
- __Pyx_XDECREF(__pyx_t_7); __pyx_t_7 = 0;
- __Pyx_GOTREF(__pyx_t_1);
- __Pyx_DECREF(__pyx_t_6); __pyx_t_6 = 0;
- } else
- #endif
- #if CYTHON_FAST_PYCCALL
- if (__Pyx_PyFastCFunction_Check(__pyx_t_5)) {
- PyObject *__pyx_temp[3] = {__pyx_t_7, __pyx_t_6, __pyx_v_bytesitem};
- __pyx_t_1 = __Pyx_PyCFunction_FastCall(__pyx_t_5, __pyx_temp+1-__pyx_t_8, 2+__pyx_t_8); if (unlikely(!__pyx_t_1)) __PYX_ERR(1, 493, __pyx_L3_error)
- __Pyx_XDECREF(__pyx_t_7); __pyx_t_7 = 0;
- __Pyx_GOTREF(__pyx_t_1);
- __Pyx_DECREF(__pyx_t_6); __pyx_t_6 = 0;
- } else
- #endif
- {
- __pyx_t_9 = PyTuple_New(2+__pyx_t_8); if (unlikely(!__pyx_t_9)) __PYX_ERR(1, 493, __pyx_L3_error)
- __Pyx_GOTREF(__pyx_t_9);
- if (__pyx_t_7) {
- __Pyx_GIVEREF(__pyx_t_7); PyTuple_SET_ITEM(__pyx_t_9, 0, __pyx_t_7); __pyx_t_7 = NULL;
- }
- __Pyx_GIVEREF(__pyx_t_6);
- PyTuple_SET_ITEM(__pyx_t_9, 0+__pyx_t_8, __pyx_t_6);
- __Pyx_INCREF(__pyx_v_bytesitem);
- __Pyx_GIVEREF(__pyx_v_bytesitem);
- PyTuple_SET_ITEM(__pyx_t_9, 1+__pyx_t_8, __pyx_v_bytesitem);
- __pyx_t_6 = 0;
- __pyx_t_1 = __Pyx_PyObject_Call(__pyx_t_5, __pyx_t_9, NULL); if (unlikely(!__pyx_t_1)) __PYX_ERR(1, 493, __pyx_L3_error)
- __Pyx_GOTREF(__pyx_t_1);
- __Pyx_DECREF(__pyx_t_9); __pyx_t_9 = 0;
- }
- __Pyx_DECREF(__pyx_t_5); __pyx_t_5 = 0;
- __pyx_v_result = __pyx_t_1;
- __pyx_t_1 = 0;
-
- /* "View.MemoryView":492
- *
- * bytesitem = itemp[:self.view.itemsize]
- * try: # <<<<<<<<<<<<<<
- * result = struct.unpack(self.view.format, bytesitem)
- * except struct.error:
- */
- }
-
- /* "View.MemoryView":497
- * raise ValueError("Unable to convert item to object")
- * else:
- * if len(self.view.format) == 1: # <<<<<<<<<<<<<<
- * return result[0]
- * return result
- */
- /*else:*/ {
- __pyx_t_10 = strlen(__pyx_v_self->view.format);
- __pyx_t_11 = ((__pyx_t_10 == 1) != 0);
- if (__pyx_t_11) {
-
- /* "View.MemoryView":498
- * else:
- * if len(self.view.format) == 1:
- * return result[0] # <<<<<<<<<<<<<<
- * return result
- *
- */
- __Pyx_XDECREF(__pyx_r);
- __pyx_t_1 = __Pyx_GetItemInt(__pyx_v_result, 0, long, 1, __Pyx_PyInt_From_long, 0, 0, 1); if (unlikely(!__pyx_t_1)) __PYX_ERR(1, 498, __pyx_L5_except_error)
- __Pyx_GOTREF(__pyx_t_1);
- __pyx_r = __pyx_t_1;
- __pyx_t_1 = 0;
- goto __pyx_L6_except_return;
-
- /* "View.MemoryView":497
- * raise ValueError("Unable to convert item to object")
- * else:
- * if len(self.view.format) == 1: # <<<<<<<<<<<<<<
- * return result[0]
- * return result
- */
- }
-
- /* "View.MemoryView":499
- * if len(self.view.format) == 1:
- * return result[0]
- * return result # <<<<<<<<<<<<<<
- *
- * cdef assign_item_from_object(self, char *itemp, object value):
- */
- __Pyx_XDECREF(__pyx_r);
- __Pyx_INCREF(__pyx_v_result);
- __pyx_r = __pyx_v_result;
- goto __pyx_L6_except_return;
- }
- __pyx_L3_error:;
- __Pyx_XDECREF(__pyx_t_1); __pyx_t_1 = 0;
- __Pyx_XDECREF(__pyx_t_5); __pyx_t_5 = 0;
- __Pyx_XDECREF(__pyx_t_6); __pyx_t_6 = 0;
- __Pyx_XDECREF(__pyx_t_7); __pyx_t_7 = 0;
- __Pyx_XDECREF(__pyx_t_9); __pyx_t_9 = 0;
-
- /* "View.MemoryView":494
- * try:
- * result = struct.unpack(self.view.format, bytesitem)
- * except struct.error: # <<<<<<<<<<<<<<
- * raise ValueError("Unable to convert item to object")
- * else:
- */
- __Pyx_ErrFetch(&__pyx_t_1, &__pyx_t_5, &__pyx_t_9);
- __pyx_t_6 = __Pyx_PyObject_GetAttrStr(__pyx_v_struct, __pyx_n_s_error); if (unlikely(!__pyx_t_6)) __PYX_ERR(1, 494, __pyx_L5_except_error)
- __Pyx_GOTREF(__pyx_t_6);
- __pyx_t_8 = __Pyx_PyErr_GivenExceptionMatches(__pyx_t_1, __pyx_t_6);
- __Pyx_DECREF(__pyx_t_6); __pyx_t_6 = 0;
- __Pyx_ErrRestore(__pyx_t_1, __pyx_t_5, __pyx_t_9);
- __pyx_t_1 = 0; __pyx_t_5 = 0; __pyx_t_9 = 0;
- if (__pyx_t_8) {
- __Pyx_AddTraceback("View.MemoryView.memoryview.convert_item_to_object", __pyx_clineno, __pyx_lineno, __pyx_filename);
- if (__Pyx_GetException(&__pyx_t_9, &__pyx_t_5, &__pyx_t_1) < 0) __PYX_ERR(1, 494, __pyx_L5_except_error)
- __Pyx_GOTREF(__pyx_t_9);
- __Pyx_GOTREF(__pyx_t_5);
- __Pyx_GOTREF(__pyx_t_1);
-
- /* "View.MemoryView":495
- * result = struct.unpack(self.view.format, bytesitem)
- * except struct.error:
- * raise ValueError("Unable to convert item to object") # <<<<<<<<<<<<<<
- * else:
- * if len(self.view.format) == 1:
- */
- __pyx_t_6 = __Pyx_PyObject_Call(__pyx_builtin_ValueError, __pyx_tuple__10, NULL); if (unlikely(!__pyx_t_6)) __PYX_ERR(1, 495, __pyx_L5_except_error)
- __Pyx_GOTREF(__pyx_t_6);
- __Pyx_Raise(__pyx_t_6, 0, 0, 0);
- __Pyx_DECREF(__pyx_t_6); __pyx_t_6 = 0;
- __PYX_ERR(1, 495, __pyx_L5_except_error)
- }
- goto __pyx_L5_except_error;
- __pyx_L5_except_error:;
-
- /* "View.MemoryView":492
- *
- * bytesitem = itemp[:self.view.itemsize]
- * try: # <<<<<<<<<<<<<<
- * result = struct.unpack(self.view.format, bytesitem)
- * except struct.error:
- */
- __Pyx_XGIVEREF(__pyx_t_2);
- __Pyx_XGIVEREF(__pyx_t_3);
- __Pyx_XGIVEREF(__pyx_t_4);
- __Pyx_ExceptionReset(__pyx_t_2, __pyx_t_3, __pyx_t_4);
- goto __pyx_L1_error;
- __pyx_L6_except_return:;
- __Pyx_XGIVEREF(__pyx_t_2);
- __Pyx_XGIVEREF(__pyx_t_3);
- __Pyx_XGIVEREF(__pyx_t_4);
- __Pyx_ExceptionReset(__pyx_t_2, __pyx_t_3, __pyx_t_4);
- goto __pyx_L0;
- }
-
- /* "View.MemoryView":485
- * self.assign_item_from_object(itemp, value)
- *
- * cdef convert_item_to_object(self, char *itemp): # <<<<<<<<<<<<<<
- * """Only used if instantiated manually by the user, or if Cython doesn't
- * know how to convert the type"""
- */
-
- /* function exit code */
- __pyx_L1_error:;
- __Pyx_XDECREF(__pyx_t_1);
- __Pyx_XDECREF(__pyx_t_5);
- __Pyx_XDECREF(__pyx_t_6);
- __Pyx_XDECREF(__pyx_t_7);
- __Pyx_XDECREF(__pyx_t_9);
- __Pyx_AddTraceback("View.MemoryView.memoryview.convert_item_to_object", __pyx_clineno, __pyx_lineno, __pyx_filename);
- __pyx_r = 0;
- __pyx_L0:;
- __Pyx_XDECREF(__pyx_v_struct);
- __Pyx_XDECREF(__pyx_v_bytesitem);
- __Pyx_XDECREF(__pyx_v_result);
- __Pyx_XGIVEREF(__pyx_r);
- __Pyx_RefNannyFinishContext();
- return __pyx_r;
-}
-
-/* "View.MemoryView":501
- * return result
- *
- * cdef assign_item_from_object(self, char *itemp, object value): # <<<<<<<<<<<<<<
- * """Only used if instantiated manually by the user, or if Cython doesn't
- * know how to convert the type"""
- */
-
-static PyObject *__pyx_memoryview_assign_item_from_object(struct __pyx_memoryview_obj *__pyx_v_self, char *__pyx_v_itemp, PyObject *__pyx_v_value) {
- PyObject *__pyx_v_struct = NULL;
- char __pyx_v_c;
- PyObject *__pyx_v_bytesvalue = 0;
- Py_ssize_t __pyx_v_i;
- PyObject *__pyx_r = NULL;
- __Pyx_RefNannyDeclarations
- PyObject *__pyx_t_1 = NULL;
- int __pyx_t_2;
- int __pyx_t_3;
- PyObject *__pyx_t_4 = NULL;
- PyObject *__pyx_t_5 = NULL;
- PyObject *__pyx_t_6 = NULL;
- int __pyx_t_7;
- PyObject *__pyx_t_8 = NULL;
- Py_ssize_t __pyx_t_9;
- PyObject *__pyx_t_10 = NULL;
- char *__pyx_t_11;
- char *__pyx_t_12;
- char *__pyx_t_13;
- char *__pyx_t_14;
- int __pyx_lineno = 0;
- const char *__pyx_filename = NULL;
- int __pyx_clineno = 0;
- __Pyx_RefNannySetupContext("assign_item_from_object", 0);
-
- /* "View.MemoryView":504
- * """Only used if instantiated manually by the user, or if Cython doesn't
- * know how to convert the type"""
- * import struct # <<<<<<<<<<<<<<
- * cdef char c
- * cdef bytes bytesvalue
- */
- __pyx_t_1 = __Pyx_Import(__pyx_n_s_struct, 0, 0); if (unlikely(!__pyx_t_1)) __PYX_ERR(1, 504, __pyx_L1_error)
- __Pyx_GOTREF(__pyx_t_1);
- __pyx_v_struct = __pyx_t_1;
- __pyx_t_1 = 0;
-
- /* "View.MemoryView":509
- * cdef Py_ssize_t i
- *
- * if isinstance(value, tuple): # <<<<<<<<<<<<<<
- * bytesvalue = struct.pack(self.view.format, *value)
- * else:
- */
- __pyx_t_2 = PyTuple_Check(__pyx_v_value);
- __pyx_t_3 = (__pyx_t_2 != 0);
- if (__pyx_t_3) {
-
- /* "View.MemoryView":510
- *
- * if isinstance(value, tuple):
- * bytesvalue = struct.pack(self.view.format, *value) # <<<<<<<<<<<<<<
- * else:
- * bytesvalue = struct.pack(self.view.format, value)
- */
- __pyx_t_1 = __Pyx_PyObject_GetAttrStr(__pyx_v_struct, __pyx_n_s_pack); if (unlikely(!__pyx_t_1)) __PYX_ERR(1, 510, __pyx_L1_error)
- __Pyx_GOTREF(__pyx_t_1);
- __pyx_t_4 = __Pyx_PyBytes_FromString(__pyx_v_self->view.format); if (unlikely(!__pyx_t_4)) __PYX_ERR(1, 510, __pyx_L1_error)
- __Pyx_GOTREF(__pyx_t_4);
- __pyx_t_5 = PyTuple_New(1); if (unlikely(!__pyx_t_5)) __PYX_ERR(1, 510, __pyx_L1_error)
- __Pyx_GOTREF(__pyx_t_5);
- __Pyx_GIVEREF(__pyx_t_4);
- PyTuple_SET_ITEM(__pyx_t_5, 0, __pyx_t_4);
- __pyx_t_4 = 0;
- __pyx_t_4 = __Pyx_PySequence_Tuple(__pyx_v_value); if (unlikely(!__pyx_t_4)) __PYX_ERR(1, 510, __pyx_L1_error)
- __Pyx_GOTREF(__pyx_t_4);
- __pyx_t_6 = PyNumber_Add(__pyx_t_5, __pyx_t_4); if (unlikely(!__pyx_t_6)) __PYX_ERR(1, 510, __pyx_L1_error)
- __Pyx_GOTREF(__pyx_t_6);
- __Pyx_DECREF(__pyx_t_5); __pyx_t_5 = 0;
- __Pyx_DECREF(__pyx_t_4); __pyx_t_4 = 0;
- __pyx_t_4 = __Pyx_PyObject_Call(__pyx_t_1, __pyx_t_6, NULL); if (unlikely(!__pyx_t_4)) __PYX_ERR(1, 510, __pyx_L1_error)
- __Pyx_GOTREF(__pyx_t_4);
- __Pyx_DECREF(__pyx_t_1); __pyx_t_1 = 0;
- __Pyx_DECREF(__pyx_t_6); __pyx_t_6 = 0;
- if (!(likely(PyBytes_CheckExact(__pyx_t_4))||((__pyx_t_4) == Py_None)||(PyErr_Format(PyExc_TypeError, "Expected %.16s, got %.200s", "bytes", Py_TYPE(__pyx_t_4)->tp_name), 0))) __PYX_ERR(1, 510, __pyx_L1_error)
- __pyx_v_bytesvalue = ((PyObject*)__pyx_t_4);
- __pyx_t_4 = 0;
-
- /* "View.MemoryView":509
- * cdef Py_ssize_t i
- *
- * if isinstance(value, tuple): # <<<<<<<<<<<<<<
- * bytesvalue = struct.pack(self.view.format, *value)
- * else:
- */
- goto __pyx_L3;
- }
-
- /* "View.MemoryView":512
- * bytesvalue = struct.pack(self.view.format, *value)
- * else:
- * bytesvalue = struct.pack(self.view.format, value) # <<<<<<<<<<<<<<
- *
- * for i, c in enumerate(bytesvalue):
- */
- /*else*/ {
- __pyx_t_6 = __Pyx_PyObject_GetAttrStr(__pyx_v_struct, __pyx_n_s_pack); if (unlikely(!__pyx_t_6)) __PYX_ERR(1, 512, __pyx_L1_error)
- __Pyx_GOTREF(__pyx_t_6);
- __pyx_t_1 = __Pyx_PyBytes_FromString(__pyx_v_self->view.format); if (unlikely(!__pyx_t_1)) __PYX_ERR(1, 512, __pyx_L1_error)
- __Pyx_GOTREF(__pyx_t_1);
- __pyx_t_5 = NULL;
- __pyx_t_7 = 0;
- if (CYTHON_UNPACK_METHODS && likely(PyMethod_Check(__pyx_t_6))) {
- __pyx_t_5 = PyMethod_GET_SELF(__pyx_t_6);
- if (likely(__pyx_t_5)) {
- PyObject* function = PyMethod_GET_FUNCTION(__pyx_t_6);
- __Pyx_INCREF(__pyx_t_5);
- __Pyx_INCREF(function);
- __Pyx_DECREF_SET(__pyx_t_6, function);
- __pyx_t_7 = 1;
- }
- }
- #if CYTHON_FAST_PYCALL
- if (PyFunction_Check(__pyx_t_6)) {
- PyObject *__pyx_temp[3] = {__pyx_t_5, __pyx_t_1, __pyx_v_value};
- __pyx_t_4 = __Pyx_PyFunction_FastCall(__pyx_t_6, __pyx_temp+1-__pyx_t_7, 2+__pyx_t_7); if (unlikely(!__pyx_t_4)) __PYX_ERR(1, 512, __pyx_L1_error)
- __Pyx_XDECREF(__pyx_t_5); __pyx_t_5 = 0;
- __Pyx_GOTREF(__pyx_t_4);
- __Pyx_DECREF(__pyx_t_1); __pyx_t_1 = 0;
- } else
- #endif
- #if CYTHON_FAST_PYCCALL
- if (__Pyx_PyFastCFunction_Check(__pyx_t_6)) {
- PyObject *__pyx_temp[3] = {__pyx_t_5, __pyx_t_1, __pyx_v_value};
- __pyx_t_4 = __Pyx_PyCFunction_FastCall(__pyx_t_6, __pyx_temp+1-__pyx_t_7, 2+__pyx_t_7); if (unlikely(!__pyx_t_4)) __PYX_ERR(1, 512, __pyx_L1_error)
- __Pyx_XDECREF(__pyx_t_5); __pyx_t_5 = 0;
- __Pyx_GOTREF(__pyx_t_4);
- __Pyx_DECREF(__pyx_t_1); __pyx_t_1 = 0;
- } else
- #endif
- {
- __pyx_t_8 = PyTuple_New(2+__pyx_t_7); if (unlikely(!__pyx_t_8)) __PYX_ERR(1, 512, __pyx_L1_error)
- __Pyx_GOTREF(__pyx_t_8);
- if (__pyx_t_5) {
- __Pyx_GIVEREF(__pyx_t_5); PyTuple_SET_ITEM(__pyx_t_8, 0, __pyx_t_5); __pyx_t_5 = NULL;
- }
- __Pyx_GIVEREF(__pyx_t_1);
- PyTuple_SET_ITEM(__pyx_t_8, 0+__pyx_t_7, __pyx_t_1);
- __Pyx_INCREF(__pyx_v_value);
- __Pyx_GIVEREF(__pyx_v_value);
- PyTuple_SET_ITEM(__pyx_t_8, 1+__pyx_t_7, __pyx_v_value);
- __pyx_t_1 = 0;
- __pyx_t_4 = __Pyx_PyObject_Call(__pyx_t_6, __pyx_t_8, NULL); if (unlikely(!__pyx_t_4)) __PYX_ERR(1, 512, __pyx_L1_error)
- __Pyx_GOTREF(__pyx_t_4);
- __Pyx_DECREF(__pyx_t_8); __pyx_t_8 = 0;
- }
- __Pyx_DECREF(__pyx_t_6); __pyx_t_6 = 0;
- if (!(likely(PyBytes_CheckExact(__pyx_t_4))||((__pyx_t_4) == Py_None)||(PyErr_Format(PyExc_TypeError, "Expected %.16s, got %.200s", "bytes", Py_TYPE(__pyx_t_4)->tp_name), 0))) __PYX_ERR(1, 512, __pyx_L1_error)
- __pyx_v_bytesvalue = ((PyObject*)__pyx_t_4);
- __pyx_t_4 = 0;
- }
- __pyx_L3:;
-
- /* "View.MemoryView":514
- * bytesvalue = struct.pack(self.view.format, value)
- *
- * for i, c in enumerate(bytesvalue): # <<<<<<<<<<<<<<
- * itemp[i] = c
- *
- */
- __pyx_t_9 = 0;
- if (unlikely(__pyx_v_bytesvalue == Py_None)) {
- PyErr_SetString(PyExc_TypeError, "'NoneType' is not iterable");
- __PYX_ERR(1, 514, __pyx_L1_error)
- }
- __Pyx_INCREF(__pyx_v_bytesvalue);
- __pyx_t_10 = __pyx_v_bytesvalue;
- __pyx_t_12 = PyBytes_AS_STRING(__pyx_t_10);
- __pyx_t_13 = (__pyx_t_12 + PyBytes_GET_SIZE(__pyx_t_10));
- for (__pyx_t_14 = __pyx_t_12; __pyx_t_14 < __pyx_t_13; __pyx_t_14++) {
- __pyx_t_11 = __pyx_t_14;
- __pyx_v_c = (__pyx_t_11[0]);
-
- /* "View.MemoryView":515
- *
- * for i, c in enumerate(bytesvalue):
- * itemp[i] = c # <<<<<<<<<<<<<<
- *
- * @cname('getbuffer')
- */
- __pyx_v_i = __pyx_t_9;
-
- /* "View.MemoryView":514
- * bytesvalue = struct.pack(self.view.format, value)
- *
- * for i, c in enumerate(bytesvalue): # <<<<<<<<<<<<<<
- * itemp[i] = c
- *
- */
- __pyx_t_9 = (__pyx_t_9 + 1);
-
- /* "View.MemoryView":515
- *
- * for i, c in enumerate(bytesvalue):
- * itemp[i] = c # <<<<<<<<<<<<<<
- *
- * @cname('getbuffer')
- */
- (__pyx_v_itemp[__pyx_v_i]) = __pyx_v_c;
- }
- __Pyx_DECREF(__pyx_t_10); __pyx_t_10 = 0;
-
- /* "View.MemoryView":501
- * return result
- *
- * cdef assign_item_from_object(self, char *itemp, object value): # <<<<<<<<<<<<<<
- * """Only used if instantiated manually by the user, or if Cython doesn't
- * know how to convert the type"""
- */
-
- /* function exit code */
- __pyx_r = Py_None; __Pyx_INCREF(Py_None);
- goto __pyx_L0;
- __pyx_L1_error:;
- __Pyx_XDECREF(__pyx_t_1);
- __Pyx_XDECREF(__pyx_t_4);
- __Pyx_XDECREF(__pyx_t_5);
- __Pyx_XDECREF(__pyx_t_6);
- __Pyx_XDECREF(__pyx_t_8);
- __Pyx_XDECREF(__pyx_t_10);
- __Pyx_AddTraceback("View.MemoryView.memoryview.assign_item_from_object", __pyx_clineno, __pyx_lineno, __pyx_filename);
- __pyx_r = 0;
- __pyx_L0:;
- __Pyx_XDECREF(__pyx_v_struct);
- __Pyx_XDECREF(__pyx_v_bytesvalue);
- __Pyx_XGIVEREF(__pyx_r);
- __Pyx_RefNannyFinishContext();
- return __pyx_r;
-}
-
-/* "View.MemoryView":518
- *
- * @cname('getbuffer')
- * def __getbuffer__(self, Py_buffer *info, int flags): # <<<<<<<<<<<<<<
- * if flags & PyBUF_WRITABLE and self.view.readonly:
- * raise ValueError("Cannot create writable memory view from read-only memoryview")
- */
-
-/* Python wrapper */
-static CYTHON_UNUSED int __pyx_memoryview_getbuffer(PyObject *__pyx_v_self, Py_buffer *__pyx_v_info, int __pyx_v_flags); /*proto*/
-static CYTHON_UNUSED int __pyx_memoryview_getbuffer(PyObject *__pyx_v_self, Py_buffer *__pyx_v_info, int __pyx_v_flags) {
- int __pyx_r;
- __Pyx_RefNannyDeclarations
- __Pyx_RefNannySetupContext("__getbuffer__ (wrapper)", 0);
- __pyx_r = __pyx_memoryview___pyx_pf_15View_dot_MemoryView_10memoryview_8__getbuffer__(((struct __pyx_memoryview_obj *)__pyx_v_self), ((Py_buffer *)__pyx_v_info), ((int)__pyx_v_flags));
-
- /* function exit code */
- __Pyx_RefNannyFinishContext();
- return __pyx_r;
-}
-
-static int __pyx_memoryview___pyx_pf_15View_dot_MemoryView_10memoryview_8__getbuffer__(struct __pyx_memoryview_obj *__pyx_v_self, Py_buffer *__pyx_v_info, int __pyx_v_flags) {
- int __pyx_r;
- __Pyx_RefNannyDeclarations
- int __pyx_t_1;
- int __pyx_t_2;
- PyObject *__pyx_t_3 = NULL;
- Py_ssize_t *__pyx_t_4;
- char *__pyx_t_5;
- void *__pyx_t_6;
- int __pyx_t_7;
- Py_ssize_t __pyx_t_8;
- int __pyx_lineno = 0;
- const char *__pyx_filename = NULL;
- int __pyx_clineno = 0;
- if (__pyx_v_info == NULL) {
- PyErr_SetString(PyExc_BufferError, "PyObject_GetBuffer: view==NULL argument is obsolete");
- return -1;
- }
- __Pyx_RefNannySetupContext("__getbuffer__", 0);
- __pyx_v_info->obj = Py_None; __Pyx_INCREF(Py_None);
- __Pyx_GIVEREF(__pyx_v_info->obj);
-
- /* "View.MemoryView":519
- * @cname('getbuffer')
- * def __getbuffer__(self, Py_buffer *info, int flags):
- * if flags & PyBUF_WRITABLE and self.view.readonly: # <<<<<<<<<<<<<<
- * raise ValueError("Cannot create writable memory view from read-only memoryview")
- *
- */
- __pyx_t_2 = ((__pyx_v_flags & PyBUF_WRITABLE) != 0);
- if (__pyx_t_2) {
- } else {
- __pyx_t_1 = __pyx_t_2;
- goto __pyx_L4_bool_binop_done;
- }
- __pyx_t_2 = (__pyx_v_self->view.readonly != 0);
- __pyx_t_1 = __pyx_t_2;
- __pyx_L4_bool_binop_done:;
- if (unlikely(__pyx_t_1)) {
-
- /* "View.MemoryView":520
- * def __getbuffer__(self, Py_buffer *info, int flags):
- * if flags & PyBUF_WRITABLE and self.view.readonly:
- * raise ValueError("Cannot create writable memory view from read-only memoryview") # <<<<<<<<<<<<<<
- *
- * if flags & PyBUF_ND:
- */
- __pyx_t_3 = __Pyx_PyObject_Call(__pyx_builtin_ValueError, __pyx_tuple__11, NULL); if (unlikely(!__pyx_t_3)) __PYX_ERR(1, 520, __pyx_L1_error)
- __Pyx_GOTREF(__pyx_t_3);
- __Pyx_Raise(__pyx_t_3, 0, 0, 0);
- __Pyx_DECREF(__pyx_t_3); __pyx_t_3 = 0;
- __PYX_ERR(1, 520, __pyx_L1_error)
-
- /* "View.MemoryView":519
- * @cname('getbuffer')
- * def __getbuffer__(self, Py_buffer *info, int flags):
- * if flags & PyBUF_WRITABLE and self.view.readonly: # <<<<<<<<<<<<<<
- * raise ValueError("Cannot create writable memory view from read-only memoryview")
- *
- */
- }
-
- /* "View.MemoryView":522
- * raise ValueError("Cannot create writable memory view from read-only memoryview")
- *
- * if flags & PyBUF_ND: # <<<<<<<<<<<<<<
- * info.shape = self.view.shape
- * else:
- */
- __pyx_t_1 = ((__pyx_v_flags & PyBUF_ND) != 0);
- if (__pyx_t_1) {
-
- /* "View.MemoryView":523
- *
- * if flags & PyBUF_ND:
- * info.shape = self.view.shape # <<<<<<<<<<<<<<
- * else:
- * info.shape = NULL
- */
- __pyx_t_4 = __pyx_v_self->view.shape;
- __pyx_v_info->shape = __pyx_t_4;
-
- /* "View.MemoryView":522
- * raise ValueError("Cannot create writable memory view from read-only memoryview")
- *
- * if flags & PyBUF_ND: # <<<<<<<<<<<<<<
- * info.shape = self.view.shape
- * else:
- */
- goto __pyx_L6;
- }
-
- /* "View.MemoryView":525
- * info.shape = self.view.shape
- * else:
- * info.shape = NULL # <<<<<<<<<<<<<<
- *
- * if flags & PyBUF_STRIDES:
- */
- /*else*/ {
- __pyx_v_info->shape = NULL;
- }
- __pyx_L6:;
-
- /* "View.MemoryView":527
- * info.shape = NULL
- *
- * if flags & PyBUF_STRIDES: # <<<<<<<<<<<<<<
- * info.strides = self.view.strides
- * else:
- */
- __pyx_t_1 = ((__pyx_v_flags & PyBUF_STRIDES) != 0);
- if (__pyx_t_1) {
-
- /* "View.MemoryView":528
- *
- * if flags & PyBUF_STRIDES:
- * info.strides = self.view.strides # <<<<<<<<<<<<<<
- * else:
- * info.strides = NULL
- */
- __pyx_t_4 = __pyx_v_self->view.strides;
- __pyx_v_info->strides = __pyx_t_4;
-
- /* "View.MemoryView":527
- * info.shape = NULL
- *
- * if flags & PyBUF_STRIDES: # <<<<<<<<<<<<<<
- * info.strides = self.view.strides
- * else:
- */
- goto __pyx_L7;
- }
-
- /* "View.MemoryView":530
- * info.strides = self.view.strides
- * else:
- * info.strides = NULL # <<<<<<<<<<<<<<
- *
- * if flags & PyBUF_INDIRECT:
- */
- /*else*/ {
- __pyx_v_info->strides = NULL;
- }
- __pyx_L7:;
-
- /* "View.MemoryView":532
- * info.strides = NULL
- *
- * if flags & PyBUF_INDIRECT: # <<<<<<<<<<<<<<
- * info.suboffsets = self.view.suboffsets
- * else:
- */
- __pyx_t_1 = ((__pyx_v_flags & PyBUF_INDIRECT) != 0);
- if (__pyx_t_1) {
-
- /* "View.MemoryView":533
- *
- * if flags & PyBUF_INDIRECT:
- * info.suboffsets = self.view.suboffsets # <<<<<<<<<<<<<<
- * else:
- * info.suboffsets = NULL
- */
- __pyx_t_4 = __pyx_v_self->view.suboffsets;
- __pyx_v_info->suboffsets = __pyx_t_4;
-
- /* "View.MemoryView":532
- * info.strides = NULL
- *
- * if flags & PyBUF_INDIRECT: # <<<<<<<<<<<<<<
- * info.suboffsets = self.view.suboffsets
- * else:
- */
- goto __pyx_L8;
- }
-
- /* "View.MemoryView":535
- * info.suboffsets = self.view.suboffsets
- * else:
- * info.suboffsets = NULL # <<<<<<<<<<<<<<
- *
- * if flags & PyBUF_FORMAT:
- */
- /*else*/ {
- __pyx_v_info->suboffsets = NULL;
- }
- __pyx_L8:;
-
- /* "View.MemoryView":537
- * info.suboffsets = NULL
- *
- * if flags & PyBUF_FORMAT: # <<<<<<<<<<<<<<
- * info.format = self.view.format
- * else:
- */
- __pyx_t_1 = ((__pyx_v_flags & PyBUF_FORMAT) != 0);
- if (__pyx_t_1) {
-
- /* "View.MemoryView":538
- *
- * if flags & PyBUF_FORMAT:
- * info.format = self.view.format # <<<<<<<<<<<<<<
- * else:
- * info.format = NULL
- */
- __pyx_t_5 = __pyx_v_self->view.format;
- __pyx_v_info->format = __pyx_t_5;
-
- /* "View.MemoryView":537
- * info.suboffsets = NULL
- *
- * if flags & PyBUF_FORMAT: # <<<<<<<<<<<<<<
- * info.format = self.view.format
- * else:
- */
- goto __pyx_L9;
- }
-
- /* "View.MemoryView":540
- * info.format = self.view.format
- * else:
- * info.format = NULL # <<<<<<<<<<<<<<
- *
- * info.buf = self.view.buf
- */
- /*else*/ {
- __pyx_v_info->format = NULL;
- }
- __pyx_L9:;
-
- /* "View.MemoryView":542
- * info.format = NULL
- *
- * info.buf = self.view.buf # <<<<<<<<<<<<<<
- * info.ndim = self.view.ndim
- * info.itemsize = self.view.itemsize
- */
- __pyx_t_6 = __pyx_v_self->view.buf;
- __pyx_v_info->buf = __pyx_t_6;
-
- /* "View.MemoryView":543
- *
- * info.buf = self.view.buf
- * info.ndim = self.view.ndim # <<<<<<<<<<<<<<
- * info.itemsize = self.view.itemsize
- * info.len = self.view.len
- */
- __pyx_t_7 = __pyx_v_self->view.ndim;
- __pyx_v_info->ndim = __pyx_t_7;
-
- /* "View.MemoryView":544
- * info.buf = self.view.buf
- * info.ndim = self.view.ndim
- * info.itemsize = self.view.itemsize # <<<<<<<<<<<<<<
- * info.len = self.view.len
- * info.readonly = self.view.readonly
- */
- __pyx_t_8 = __pyx_v_self->view.itemsize;
- __pyx_v_info->itemsize = __pyx_t_8;
-
- /* "View.MemoryView":545
- * info.ndim = self.view.ndim
- * info.itemsize = self.view.itemsize
- * info.len = self.view.len # <<<<<<<<<<<<<<
- * info.readonly = self.view.readonly
- * info.obj = self
- */
- __pyx_t_8 = __pyx_v_self->view.len;
- __pyx_v_info->len = __pyx_t_8;
-
- /* "View.MemoryView":546
- * info.itemsize = self.view.itemsize
- * info.len = self.view.len
- * info.readonly = self.view.readonly # <<<<<<<<<<<<<<
- * info.obj = self
- *
- */
- __pyx_t_1 = __pyx_v_self->view.readonly;
- __pyx_v_info->readonly = __pyx_t_1;
-
- /* "View.MemoryView":547
- * info.len = self.view.len
- * info.readonly = self.view.readonly
- * info.obj = self # <<<<<<<<<<<<<<
- *
- * __pyx_getbuffer = capsule( &__pyx_memoryview_getbuffer, "getbuffer(obj, view, flags)")
- */
- __Pyx_INCREF(((PyObject *)__pyx_v_self));
- __Pyx_GIVEREF(((PyObject *)__pyx_v_self));
- __Pyx_GOTREF(__pyx_v_info->obj);
- __Pyx_DECREF(__pyx_v_info->obj);
- __pyx_v_info->obj = ((PyObject *)__pyx_v_self);
-
- /* "View.MemoryView":518
- *
- * @cname('getbuffer')
- * def __getbuffer__(self, Py_buffer *info, int flags): # <<<<<<<<<<<<<<
- * if flags & PyBUF_WRITABLE and self.view.readonly:
- * raise ValueError("Cannot create writable memory view from read-only memoryview")
- */
-
- /* function exit code */
- __pyx_r = 0;
- goto __pyx_L0;
- __pyx_L1_error:;
- __Pyx_XDECREF(__pyx_t_3);
- __Pyx_AddTraceback("View.MemoryView.memoryview.__getbuffer__", __pyx_clineno, __pyx_lineno, __pyx_filename);
- __pyx_r = -1;
- if (__pyx_v_info->obj != NULL) {
- __Pyx_GOTREF(__pyx_v_info->obj);
- __Pyx_DECREF(__pyx_v_info->obj); __pyx_v_info->obj = 0;
- }
- goto __pyx_L2;
- __pyx_L0:;
- if (__pyx_v_info->obj == Py_None) {
- __Pyx_GOTREF(__pyx_v_info->obj);
- __Pyx_DECREF(__pyx_v_info->obj); __pyx_v_info->obj = 0;
- }
- __pyx_L2:;
- __Pyx_RefNannyFinishContext();
- return __pyx_r;
-}
-
-/* "View.MemoryView":553
- *
- * @property
- * def T(self): # <<<<<<<<<<<<<<
- * cdef _memoryviewslice result = memoryview_copy(self)
- * transpose_memslice(&result.from_slice)
- */
-
-/* Python wrapper */
-static PyObject *__pyx_pw_15View_dot_MemoryView_10memoryview_1T_1__get__(PyObject *__pyx_v_self); /*proto*/
-static PyObject *__pyx_pw_15View_dot_MemoryView_10memoryview_1T_1__get__(PyObject *__pyx_v_self) {
- PyObject *__pyx_r = 0;
- __Pyx_RefNannyDeclarations
- __Pyx_RefNannySetupContext("__get__ (wrapper)", 0);
- __pyx_r = __pyx_pf_15View_dot_MemoryView_10memoryview_1T___get__(((struct __pyx_memoryview_obj *)__pyx_v_self));
-
- /* function exit code */
- __Pyx_RefNannyFinishContext();
- return __pyx_r;
-}
-
-static PyObject *__pyx_pf_15View_dot_MemoryView_10memoryview_1T___get__(struct __pyx_memoryview_obj *__pyx_v_self) {
- struct __pyx_memoryviewslice_obj *__pyx_v_result = 0;
- PyObject *__pyx_r = NULL;
- __Pyx_RefNannyDeclarations
- PyObject *__pyx_t_1 = NULL;
- int __pyx_t_2;
- int __pyx_lineno = 0;
- const char *__pyx_filename = NULL;
- int __pyx_clineno = 0;
- __Pyx_RefNannySetupContext("__get__", 0);
-
- /* "View.MemoryView":554
- * @property
- * def T(self):
- * cdef _memoryviewslice result = memoryview_copy(self) # <<<<<<<<<<<<<<
- * transpose_memslice(&result.from_slice)
- * return result
- */
- __pyx_t_1 = __pyx_memoryview_copy_object(__pyx_v_self); if (unlikely(!__pyx_t_1)) __PYX_ERR(1, 554, __pyx_L1_error)
- __Pyx_GOTREF(__pyx_t_1);
- if (!(likely(((__pyx_t_1) == Py_None) || likely(__Pyx_TypeTest(__pyx_t_1, __pyx_memoryviewslice_type))))) __PYX_ERR(1, 554, __pyx_L1_error)
- __pyx_v_result = ((struct __pyx_memoryviewslice_obj *)__pyx_t_1);
- __pyx_t_1 = 0;
-
- /* "View.MemoryView":555
- * def T(self):
- * cdef _memoryviewslice result = memoryview_copy(self)
- * transpose_memslice(&result.from_slice) # <<<<<<<<<<<<<<
- * return result
- *
- */
- __pyx_t_2 = __pyx_memslice_transpose((&__pyx_v_result->from_slice)); if (unlikely(__pyx_t_2 == ((int)0))) __PYX_ERR(1, 555, __pyx_L1_error)
-
- /* "View.MemoryView":556
- * cdef _memoryviewslice result = memoryview_copy(self)
- * transpose_memslice(&result.from_slice)
- * return result # <<<<<<<<<<<<<<
- *
- * @property
- */
- __Pyx_XDECREF(__pyx_r);
- __Pyx_INCREF(((PyObject *)__pyx_v_result));
- __pyx_r = ((PyObject *)__pyx_v_result);
- goto __pyx_L0;
-
- /* "View.MemoryView":553
- *
- * @property
- * def T(self): # <<<<<<<<<<<<<<
- * cdef _memoryviewslice result = memoryview_copy(self)
- * transpose_memslice(&result.from_slice)
- */
-
- /* function exit code */
- __pyx_L1_error:;
- __Pyx_XDECREF(__pyx_t_1);
- __Pyx_AddTraceback("View.MemoryView.memoryview.T.__get__", __pyx_clineno, __pyx_lineno, __pyx_filename);
- __pyx_r = NULL;
- __pyx_L0:;
- __Pyx_XDECREF((PyObject *)__pyx_v_result);
- __Pyx_XGIVEREF(__pyx_r);
- __Pyx_RefNannyFinishContext();
- return __pyx_r;
-}
-
-/* "View.MemoryView":559
- *
- * @property
- * def base(self): # <<<<<<<<<<<<<<
- * return self.obj
- *
- */
-
-/* Python wrapper */
-static PyObject *__pyx_pw_15View_dot_MemoryView_10memoryview_4base_1__get__(PyObject *__pyx_v_self); /*proto*/
-static PyObject *__pyx_pw_15View_dot_MemoryView_10memoryview_4base_1__get__(PyObject *__pyx_v_self) {
- PyObject *__pyx_r = 0;
- __Pyx_RefNannyDeclarations
- __Pyx_RefNannySetupContext("__get__ (wrapper)", 0);
- __pyx_r = __pyx_pf_15View_dot_MemoryView_10memoryview_4base___get__(((struct __pyx_memoryview_obj *)__pyx_v_self));
-
- /* function exit code */
- __Pyx_RefNannyFinishContext();
- return __pyx_r;
-}
-
-static PyObject *__pyx_pf_15View_dot_MemoryView_10memoryview_4base___get__(struct __pyx_memoryview_obj *__pyx_v_self) {
- PyObject *__pyx_r = NULL;
- __Pyx_RefNannyDeclarations
- __Pyx_RefNannySetupContext("__get__", 0);
-
- /* "View.MemoryView":560
- * @property
- * def base(self):
- * return self.obj # <<<<<<<<<<<<<<
- *
- * @property
- */
- __Pyx_XDECREF(__pyx_r);
- __Pyx_INCREF(__pyx_v_self->obj);
- __pyx_r = __pyx_v_self->obj;
- goto __pyx_L0;
-
- /* "View.MemoryView":559
- *
- * @property
- * def base(self): # <<<<<<<<<<<<<<
- * return self.obj
- *
- */
-
- /* function exit code */
- __pyx_L0:;
- __Pyx_XGIVEREF(__pyx_r);
- __Pyx_RefNannyFinishContext();
- return __pyx_r;
-}
-
-/* "View.MemoryView":563
- *
- * @property
- * def shape(self): # <<<<<<<<<<<<<<
- * return tuple([length for length in self.view.shape[:self.view.ndim]])
- *
- */
-
-/* Python wrapper */
-static PyObject *__pyx_pw_15View_dot_MemoryView_10memoryview_5shape_1__get__(PyObject *__pyx_v_self); /*proto*/
-static PyObject *__pyx_pw_15View_dot_MemoryView_10memoryview_5shape_1__get__(PyObject *__pyx_v_self) {
- PyObject *__pyx_r = 0;
- __Pyx_RefNannyDeclarations
- __Pyx_RefNannySetupContext("__get__ (wrapper)", 0);
- __pyx_r = __pyx_pf_15View_dot_MemoryView_10memoryview_5shape___get__(((struct __pyx_memoryview_obj *)__pyx_v_self));
-
- /* function exit code */
- __Pyx_RefNannyFinishContext();
- return __pyx_r;
-}
-
-static PyObject *__pyx_pf_15View_dot_MemoryView_10memoryview_5shape___get__(struct __pyx_memoryview_obj *__pyx_v_self) {
- Py_ssize_t __pyx_v_length;
- PyObject *__pyx_r = NULL;
- __Pyx_RefNannyDeclarations
- PyObject *__pyx_t_1 = NULL;
- Py_ssize_t *__pyx_t_2;
- Py_ssize_t *__pyx_t_3;
- Py_ssize_t *__pyx_t_4;
- PyObject *__pyx_t_5 = NULL;
- int __pyx_lineno = 0;
- const char *__pyx_filename = NULL;
- int __pyx_clineno = 0;
- __Pyx_RefNannySetupContext("__get__", 0);
-
- /* "View.MemoryView":564
- * @property
- * def shape(self):
- * return tuple([length for length in self.view.shape[:self.view.ndim]]) # <<<<<<<<<<<<<<
- *
- * @property
- */
- __Pyx_XDECREF(__pyx_r);
- __pyx_t_1 = PyList_New(0); if (unlikely(!__pyx_t_1)) __PYX_ERR(1, 564, __pyx_L1_error)
- __Pyx_GOTREF(__pyx_t_1);
- __pyx_t_3 = (__pyx_v_self->view.shape + __pyx_v_self->view.ndim);
- for (__pyx_t_4 = __pyx_v_self->view.shape; __pyx_t_4 < __pyx_t_3; __pyx_t_4++) {
- __pyx_t_2 = __pyx_t_4;
- __pyx_v_length = (__pyx_t_2[0]);
- __pyx_t_5 = PyInt_FromSsize_t(__pyx_v_length); if (unlikely(!__pyx_t_5)) __PYX_ERR(1, 564, __pyx_L1_error)
- __Pyx_GOTREF(__pyx_t_5);
- if (unlikely(__Pyx_ListComp_Append(__pyx_t_1, (PyObject*)__pyx_t_5))) __PYX_ERR(1, 564, __pyx_L1_error)
- __Pyx_DECREF(__pyx_t_5); __pyx_t_5 = 0;
- }
- __pyx_t_5 = PyList_AsTuple(((PyObject*)__pyx_t_1)); if (unlikely(!__pyx_t_5)) __PYX_ERR(1, 564, __pyx_L1_error)
- __Pyx_GOTREF(__pyx_t_5);
- __Pyx_DECREF(__pyx_t_1); __pyx_t_1 = 0;
- __pyx_r = __pyx_t_5;
- __pyx_t_5 = 0;
- goto __pyx_L0;
-
- /* "View.MemoryView":563
- *
- * @property
- * def shape(self): # <<<<<<<<<<<<<<
- * return tuple([length for length in self.view.shape[:self.view.ndim]])
- *
- */
-
- /* function exit code */
- __pyx_L1_error:;
- __Pyx_XDECREF(__pyx_t_1);
- __Pyx_XDECREF(__pyx_t_5);
- __Pyx_AddTraceback("View.MemoryView.memoryview.shape.__get__", __pyx_clineno, __pyx_lineno, __pyx_filename);
- __pyx_r = NULL;
- __pyx_L0:;
- __Pyx_XGIVEREF(__pyx_r);
- __Pyx_RefNannyFinishContext();
- return __pyx_r;
-}
-
-/* "View.MemoryView":567
- *
- * @property
- * def strides(self): # <<<<<<<<<<<<<<
- * if self.view.strides == NULL:
- *
- */
-
-/* Python wrapper */
-static PyObject *__pyx_pw_15View_dot_MemoryView_10memoryview_7strides_1__get__(PyObject *__pyx_v_self); /*proto*/
-static PyObject *__pyx_pw_15View_dot_MemoryView_10memoryview_7strides_1__get__(PyObject *__pyx_v_self) {
- PyObject *__pyx_r = 0;
- __Pyx_RefNannyDeclarations
- __Pyx_RefNannySetupContext("__get__ (wrapper)", 0);
- __pyx_r = __pyx_pf_15View_dot_MemoryView_10memoryview_7strides___get__(((struct __pyx_memoryview_obj *)__pyx_v_self));
-
- /* function exit code */
- __Pyx_RefNannyFinishContext();
- return __pyx_r;
-}
-
-static PyObject *__pyx_pf_15View_dot_MemoryView_10memoryview_7strides___get__(struct __pyx_memoryview_obj *__pyx_v_self) {
- Py_ssize_t __pyx_v_stride;
- PyObject *__pyx_r = NULL;
- __Pyx_RefNannyDeclarations
- int __pyx_t_1;
- PyObject *__pyx_t_2 = NULL;
- Py_ssize_t *__pyx_t_3;
- Py_ssize_t *__pyx_t_4;
- Py_ssize_t *__pyx_t_5;
- PyObject *__pyx_t_6 = NULL;
- int __pyx_lineno = 0;
- const char *__pyx_filename = NULL;
- int __pyx_clineno = 0;
- __Pyx_RefNannySetupContext("__get__", 0);
-
- /* "View.MemoryView":568
- * @property
- * def strides(self):
- * if self.view.strides == NULL: # <<<<<<<<<<<<<<
- *
- * raise ValueError("Buffer view does not expose strides")
- */
- __pyx_t_1 = ((__pyx_v_self->view.strides == NULL) != 0);
- if (unlikely(__pyx_t_1)) {
-
- /* "View.MemoryView":570
- * if self.view.strides == NULL:
- *
- * raise ValueError("Buffer view does not expose strides") # <<<<<<<<<<<<<<
- *
- * return tuple([stride for stride in self.view.strides[:self.view.ndim]])
- */
- __pyx_t_2 = __Pyx_PyObject_Call(__pyx_builtin_ValueError, __pyx_tuple__12, NULL); if (unlikely(!__pyx_t_2)) __PYX_ERR(1, 570, __pyx_L1_error)
- __Pyx_GOTREF(__pyx_t_2);
- __Pyx_Raise(__pyx_t_2, 0, 0, 0);
- __Pyx_DECREF(__pyx_t_2); __pyx_t_2 = 0;
- __PYX_ERR(1, 570, __pyx_L1_error)
-
- /* "View.MemoryView":568
- * @property
- * def strides(self):
- * if self.view.strides == NULL: # <<<<<<<<<<<<<<
- *
- * raise ValueError("Buffer view does not expose strides")
- */
- }
-
- /* "View.MemoryView":572
- * raise ValueError("Buffer view does not expose strides")
- *
- * return tuple([stride for stride in self.view.strides[:self.view.ndim]]) # <<<<<<<<<<<<<<
- *
- * @property
- */
- __Pyx_XDECREF(__pyx_r);
- __pyx_t_2 = PyList_New(0); if (unlikely(!__pyx_t_2)) __PYX_ERR(1, 572, __pyx_L1_error)
- __Pyx_GOTREF(__pyx_t_2);
- __pyx_t_4 = (__pyx_v_self->view.strides + __pyx_v_self->view.ndim);
- for (__pyx_t_5 = __pyx_v_self->view.strides; __pyx_t_5 < __pyx_t_4; __pyx_t_5++) {
- __pyx_t_3 = __pyx_t_5;
- __pyx_v_stride = (__pyx_t_3[0]);
- __pyx_t_6 = PyInt_FromSsize_t(__pyx_v_stride); if (unlikely(!__pyx_t_6)) __PYX_ERR(1, 572, __pyx_L1_error)
- __Pyx_GOTREF(__pyx_t_6);
- if (unlikely(__Pyx_ListComp_Append(__pyx_t_2, (PyObject*)__pyx_t_6))) __PYX_ERR(1, 572, __pyx_L1_error)
- __Pyx_DECREF(__pyx_t_6); __pyx_t_6 = 0;
- }
- __pyx_t_6 = PyList_AsTuple(((PyObject*)__pyx_t_2)); if (unlikely(!__pyx_t_6)) __PYX_ERR(1, 572, __pyx_L1_error)
- __Pyx_GOTREF(__pyx_t_6);
- __Pyx_DECREF(__pyx_t_2); __pyx_t_2 = 0;
- __pyx_r = __pyx_t_6;
- __pyx_t_6 = 0;
- goto __pyx_L0;
-
- /* "View.MemoryView":567
- *
- * @property
- * def strides(self): # <<<<<<<<<<<<<<
- * if self.view.strides == NULL:
- *
- */
-
- /* function exit code */
- __pyx_L1_error:;
- __Pyx_XDECREF(__pyx_t_2);
- __Pyx_XDECREF(__pyx_t_6);
- __Pyx_AddTraceback("View.MemoryView.memoryview.strides.__get__", __pyx_clineno, __pyx_lineno, __pyx_filename);
- __pyx_r = NULL;
- __pyx_L0:;
- __Pyx_XGIVEREF(__pyx_r);
- __Pyx_RefNannyFinishContext();
- return __pyx_r;
-}
-
-/* "View.MemoryView":575
- *
- * @property
- * def suboffsets(self): # <<<<<<<<<<<<<<
- * if self.view.suboffsets == NULL:
- * return (-1,) * self.view.ndim
- */
-
-/* Python wrapper */
-static PyObject *__pyx_pw_15View_dot_MemoryView_10memoryview_10suboffsets_1__get__(PyObject *__pyx_v_self); /*proto*/
-static PyObject *__pyx_pw_15View_dot_MemoryView_10memoryview_10suboffsets_1__get__(PyObject *__pyx_v_self) {
- PyObject *__pyx_r = 0;
- __Pyx_RefNannyDeclarations
- __Pyx_RefNannySetupContext("__get__ (wrapper)", 0);
- __pyx_r = __pyx_pf_15View_dot_MemoryView_10memoryview_10suboffsets___get__(((struct __pyx_memoryview_obj *)__pyx_v_self));
-
- /* function exit code */
- __Pyx_RefNannyFinishContext();
- return __pyx_r;
-}
-
-static PyObject *__pyx_pf_15View_dot_MemoryView_10memoryview_10suboffsets___get__(struct __pyx_memoryview_obj *__pyx_v_self) {
- Py_ssize_t __pyx_v_suboffset;
- PyObject *__pyx_r = NULL;
- __Pyx_RefNannyDeclarations
- int __pyx_t_1;
- PyObject *__pyx_t_2 = NULL;
- PyObject *__pyx_t_3 = NULL;
- Py_ssize_t *__pyx_t_4;
- Py_ssize_t *__pyx_t_5;
- Py_ssize_t *__pyx_t_6;
- int __pyx_lineno = 0;
- const char *__pyx_filename = NULL;
- int __pyx_clineno = 0;
- __Pyx_RefNannySetupContext("__get__", 0);
-
- /* "View.MemoryView":576
- * @property
- * def suboffsets(self):
- * if self.view.suboffsets == NULL: # <<<<<<<<<<<<<<
- * return (-1,) * self.view.ndim
- *
- */
- __pyx_t_1 = ((__pyx_v_self->view.suboffsets == NULL) != 0);
- if (__pyx_t_1) {
-
- /* "View.MemoryView":577
- * def suboffsets(self):
- * if self.view.suboffsets == NULL:
- * return (-1,) * self.view.ndim # <<<<<<<<<<<<<<
- *
- * return tuple([suboffset for suboffset in self.view.suboffsets[:self.view.ndim]])
- */
- __Pyx_XDECREF(__pyx_r);
- __pyx_t_2 = __Pyx_PyInt_From_int(__pyx_v_self->view.ndim); if (unlikely(!__pyx_t_2)) __PYX_ERR(1, 577, __pyx_L1_error)
- __Pyx_GOTREF(__pyx_t_2);
- __pyx_t_3 = PyNumber_Multiply(__pyx_tuple__13, __pyx_t_2); if (unlikely(!__pyx_t_3)) __PYX_ERR(1, 577, __pyx_L1_error)
- __Pyx_GOTREF(__pyx_t_3);
- __Pyx_DECREF(__pyx_t_2); __pyx_t_2 = 0;
- __pyx_r = __pyx_t_3;
- __pyx_t_3 = 0;
- goto __pyx_L0;
-
- /* "View.MemoryView":576
- * @property
- * def suboffsets(self):
- * if self.view.suboffsets == NULL: # <<<<<<<<<<<<<<
- * return (-1,) * self.view.ndim
- *
- */
- }
-
- /* "View.MemoryView":579
- * return (-1,) * self.view.ndim
- *
- * return tuple([suboffset for suboffset in self.view.suboffsets[:self.view.ndim]]) # <<<<<<<<<<<<<<
- *
- * @property
- */
- __Pyx_XDECREF(__pyx_r);
- __pyx_t_3 = PyList_New(0); if (unlikely(!__pyx_t_3)) __PYX_ERR(1, 579, __pyx_L1_error)
- __Pyx_GOTREF(__pyx_t_3);
- __pyx_t_5 = (__pyx_v_self->view.suboffsets + __pyx_v_self->view.ndim);
- for (__pyx_t_6 = __pyx_v_self->view.suboffsets; __pyx_t_6 < __pyx_t_5; __pyx_t_6++) {
- __pyx_t_4 = __pyx_t_6;
- __pyx_v_suboffset = (__pyx_t_4[0]);
- __pyx_t_2 = PyInt_FromSsize_t(__pyx_v_suboffset); if (unlikely(!__pyx_t_2)) __PYX_ERR(1, 579, __pyx_L1_error)
- __Pyx_GOTREF(__pyx_t_2);
- if (unlikely(__Pyx_ListComp_Append(__pyx_t_3, (PyObject*)__pyx_t_2))) __PYX_ERR(1, 579, __pyx_L1_error)
- __Pyx_DECREF(__pyx_t_2); __pyx_t_2 = 0;
- }
- __pyx_t_2 = PyList_AsTuple(((PyObject*)__pyx_t_3)); if (unlikely(!__pyx_t_2)) __PYX_ERR(1, 579, __pyx_L1_error)
- __Pyx_GOTREF(__pyx_t_2);
- __Pyx_DECREF(__pyx_t_3); __pyx_t_3 = 0;
- __pyx_r = __pyx_t_2;
- __pyx_t_2 = 0;
- goto __pyx_L0;
-
- /* "View.MemoryView":575
- *
- * @property
- * def suboffsets(self): # <<<<<<<<<<<<<<
- * if self.view.suboffsets == NULL:
- * return (-1,) * self.view.ndim
- */
-
- /* function exit code */
- __pyx_L1_error:;
- __Pyx_XDECREF(__pyx_t_2);
- __Pyx_XDECREF(__pyx_t_3);
- __Pyx_AddTraceback("View.MemoryView.memoryview.suboffsets.__get__", __pyx_clineno, __pyx_lineno, __pyx_filename);
- __pyx_r = NULL;
- __pyx_L0:;
- __Pyx_XGIVEREF(__pyx_r);
- __Pyx_RefNannyFinishContext();
- return __pyx_r;
-}
-
-/* "View.MemoryView":582
- *
- * @property
- * def ndim(self): # <<<<<<<<<<<<<<
- * return self.view.ndim
- *
- */
-
-/* Python wrapper */
-static PyObject *__pyx_pw_15View_dot_MemoryView_10memoryview_4ndim_1__get__(PyObject *__pyx_v_self); /*proto*/
-static PyObject *__pyx_pw_15View_dot_MemoryView_10memoryview_4ndim_1__get__(PyObject *__pyx_v_self) {
- PyObject *__pyx_r = 0;
- __Pyx_RefNannyDeclarations
- __Pyx_RefNannySetupContext("__get__ (wrapper)", 0);
- __pyx_r = __pyx_pf_15View_dot_MemoryView_10memoryview_4ndim___get__(((struct __pyx_memoryview_obj *)__pyx_v_self));
-
- /* function exit code */
- __Pyx_RefNannyFinishContext();
- return __pyx_r;
-}
-
-static PyObject *__pyx_pf_15View_dot_MemoryView_10memoryview_4ndim___get__(struct __pyx_memoryview_obj *__pyx_v_self) {
- PyObject *__pyx_r = NULL;
- __Pyx_RefNannyDeclarations
- PyObject *__pyx_t_1 = NULL;
- int __pyx_lineno = 0;
- const char *__pyx_filename = NULL;
- int __pyx_clineno = 0;
- __Pyx_RefNannySetupContext("__get__", 0);
-
- /* "View.MemoryView":583
- * @property
- * def ndim(self):
- * return self.view.ndim # <<<<<<<<<<<<<<
- *
- * @property
- */
- __Pyx_XDECREF(__pyx_r);
- __pyx_t_1 = __Pyx_PyInt_From_int(__pyx_v_self->view.ndim); if (unlikely(!__pyx_t_1)) __PYX_ERR(1, 583, __pyx_L1_error)
- __Pyx_GOTREF(__pyx_t_1);
- __pyx_r = __pyx_t_1;
- __pyx_t_1 = 0;
- goto __pyx_L0;
-
- /* "View.MemoryView":582
- *
- * @property
- * def ndim(self): # <<<<<<<<<<<<<<
- * return self.view.ndim
- *
- */
-
- /* function exit code */
- __pyx_L1_error:;
- __Pyx_XDECREF(__pyx_t_1);
- __Pyx_AddTraceback("View.MemoryView.memoryview.ndim.__get__", __pyx_clineno, __pyx_lineno, __pyx_filename);
- __pyx_r = NULL;
- __pyx_L0:;
- __Pyx_XGIVEREF(__pyx_r);
- __Pyx_RefNannyFinishContext();
- return __pyx_r;
-}
-
-/* "View.MemoryView":586
- *
- * @property
- * def itemsize(self): # <<<<<<<<<<<<<<
- * return self.view.itemsize
- *
- */
-
-/* Python wrapper */
-static PyObject *__pyx_pw_15View_dot_MemoryView_10memoryview_8itemsize_1__get__(PyObject *__pyx_v_self); /*proto*/
-static PyObject *__pyx_pw_15View_dot_MemoryView_10memoryview_8itemsize_1__get__(PyObject *__pyx_v_self) {
- PyObject *__pyx_r = 0;
- __Pyx_RefNannyDeclarations
- __Pyx_RefNannySetupContext("__get__ (wrapper)", 0);
- __pyx_r = __pyx_pf_15View_dot_MemoryView_10memoryview_8itemsize___get__(((struct __pyx_memoryview_obj *)__pyx_v_self));
-
- /* function exit code */
- __Pyx_RefNannyFinishContext();
- return __pyx_r;
-}
-
-static PyObject *__pyx_pf_15View_dot_MemoryView_10memoryview_8itemsize___get__(struct __pyx_memoryview_obj *__pyx_v_self) {
- PyObject *__pyx_r = NULL;
- __Pyx_RefNannyDeclarations
- PyObject *__pyx_t_1 = NULL;
- int __pyx_lineno = 0;
- const char *__pyx_filename = NULL;
- int __pyx_clineno = 0;
- __Pyx_RefNannySetupContext("__get__", 0);
-
- /* "View.MemoryView":587
- * @property
- * def itemsize(self):
- * return self.view.itemsize # <<<<<<<<<<<<<<
- *
- * @property
- */
- __Pyx_XDECREF(__pyx_r);
- __pyx_t_1 = PyInt_FromSsize_t(__pyx_v_self->view.itemsize); if (unlikely(!__pyx_t_1)) __PYX_ERR(1, 587, __pyx_L1_error)
- __Pyx_GOTREF(__pyx_t_1);
- __pyx_r = __pyx_t_1;
- __pyx_t_1 = 0;
- goto __pyx_L0;
-
- /* "View.MemoryView":586
- *
- * @property
- * def itemsize(self): # <<<<<<<<<<<<<<
- * return self.view.itemsize
- *
- */
-
- /* function exit code */
- __pyx_L1_error:;
- __Pyx_XDECREF(__pyx_t_1);
- __Pyx_AddTraceback("View.MemoryView.memoryview.itemsize.__get__", __pyx_clineno, __pyx_lineno, __pyx_filename);
- __pyx_r = NULL;
- __pyx_L0:;
- __Pyx_XGIVEREF(__pyx_r);
- __Pyx_RefNannyFinishContext();
- return __pyx_r;
-}
-
-/* "View.MemoryView":590
- *
- * @property
- * def nbytes(self): # <<<<<<<<<<<<<<
- * return self.size * self.view.itemsize
- *
- */
-
-/* Python wrapper */
-static PyObject *__pyx_pw_15View_dot_MemoryView_10memoryview_6nbytes_1__get__(PyObject *__pyx_v_self); /*proto*/
-static PyObject *__pyx_pw_15View_dot_MemoryView_10memoryview_6nbytes_1__get__(PyObject *__pyx_v_self) {
- PyObject *__pyx_r = 0;
- __Pyx_RefNannyDeclarations
- __Pyx_RefNannySetupContext("__get__ (wrapper)", 0);
- __pyx_r = __pyx_pf_15View_dot_MemoryView_10memoryview_6nbytes___get__(((struct __pyx_memoryview_obj *)__pyx_v_self));
-
- /* function exit code */
- __Pyx_RefNannyFinishContext();
- return __pyx_r;
-}
-
-static PyObject *__pyx_pf_15View_dot_MemoryView_10memoryview_6nbytes___get__(struct __pyx_memoryview_obj *__pyx_v_self) {
- PyObject *__pyx_r = NULL;
- __Pyx_RefNannyDeclarations
- PyObject *__pyx_t_1 = NULL;
- PyObject *__pyx_t_2 = NULL;
- PyObject *__pyx_t_3 = NULL;
- int __pyx_lineno = 0;
- const char *__pyx_filename = NULL;
- int __pyx_clineno = 0;
- __Pyx_RefNannySetupContext("__get__", 0);
-
- /* "View.MemoryView":591
- * @property
- * def nbytes(self):
- * return self.size * self.view.itemsize # <<<<<<<<<<<<<<
- *
- * @property
- */
- __Pyx_XDECREF(__pyx_r);
- __pyx_t_1 = __Pyx_PyObject_GetAttrStr(((PyObject *)__pyx_v_self), __pyx_n_s_size); if (unlikely(!__pyx_t_1)) __PYX_ERR(1, 591, __pyx_L1_error)
- __Pyx_GOTREF(__pyx_t_1);
- __pyx_t_2 = PyInt_FromSsize_t(__pyx_v_self->view.itemsize); if (unlikely(!__pyx_t_2)) __PYX_ERR(1, 591, __pyx_L1_error)
- __Pyx_GOTREF(__pyx_t_2);
- __pyx_t_3 = PyNumber_Multiply(__pyx_t_1, __pyx_t_2); if (unlikely(!__pyx_t_3)) __PYX_ERR(1, 591, __pyx_L1_error)
- __Pyx_GOTREF(__pyx_t_3);
- __Pyx_DECREF(__pyx_t_1); __pyx_t_1 = 0;
- __Pyx_DECREF(__pyx_t_2); __pyx_t_2 = 0;
- __pyx_r = __pyx_t_3;
- __pyx_t_3 = 0;
- goto __pyx_L0;
-
- /* "View.MemoryView":590
- *
- * @property
- * def nbytes(self): # <<<<<<<<<<<<<<
- * return self.size * self.view.itemsize
- *
- */
-
- /* function exit code */
- __pyx_L1_error:;
- __Pyx_XDECREF(__pyx_t_1);
- __Pyx_XDECREF(__pyx_t_2);
- __Pyx_XDECREF(__pyx_t_3);
- __Pyx_AddTraceback("View.MemoryView.memoryview.nbytes.__get__", __pyx_clineno, __pyx_lineno, __pyx_filename);
- __pyx_r = NULL;
- __pyx_L0:;
- __Pyx_XGIVEREF(__pyx_r);
- __Pyx_RefNannyFinishContext();
- return __pyx_r;
-}
-
-/* "View.MemoryView":594
- *
- * @property
- * def size(self): # <<<<<<<<<<<<<<
- * if self._size is None:
- * result = 1
- */
-
-/* Python wrapper */
-static PyObject *__pyx_pw_15View_dot_MemoryView_10memoryview_4size_1__get__(PyObject *__pyx_v_self); /*proto*/
-static PyObject *__pyx_pw_15View_dot_MemoryView_10memoryview_4size_1__get__(PyObject *__pyx_v_self) {
- PyObject *__pyx_r = 0;
- __Pyx_RefNannyDeclarations
- __Pyx_RefNannySetupContext("__get__ (wrapper)", 0);
- __pyx_r = __pyx_pf_15View_dot_MemoryView_10memoryview_4size___get__(((struct __pyx_memoryview_obj *)__pyx_v_self));
-
- /* function exit code */
- __Pyx_RefNannyFinishContext();
- return __pyx_r;
-}
-
-static PyObject *__pyx_pf_15View_dot_MemoryView_10memoryview_4size___get__(struct __pyx_memoryview_obj *__pyx_v_self) {
- PyObject *__pyx_v_result = NULL;
- PyObject *__pyx_v_length = NULL;
- PyObject *__pyx_r = NULL;
- __Pyx_RefNannyDeclarations
- int __pyx_t_1;
- int __pyx_t_2;
- Py_ssize_t *__pyx_t_3;
- Py_ssize_t *__pyx_t_4;
- Py_ssize_t *__pyx_t_5;
- PyObject *__pyx_t_6 = NULL;
- int __pyx_lineno = 0;
- const char *__pyx_filename = NULL;
- int __pyx_clineno = 0;
- __Pyx_RefNannySetupContext("__get__", 0);
-
- /* "View.MemoryView":595
- * @property
- * def size(self):
- * if self._size is None: # <<<<<<<<<<<<<<
- * result = 1
- *
- */
- __pyx_t_1 = (__pyx_v_self->_size == Py_None);
- __pyx_t_2 = (__pyx_t_1 != 0);
- if (__pyx_t_2) {
-
- /* "View.MemoryView":596
- * def size(self):
- * if self._size is None:
- * result = 1 # <<<<<<<<<<<<<<
- *
- * for length in self.view.shape[:self.view.ndim]:
- */
- __Pyx_INCREF(__pyx_int_1);
- __pyx_v_result = __pyx_int_1;
-
- /* "View.MemoryView":598
- * result = 1
- *
- * for length in self.view.shape[:self.view.ndim]: # <<<<<<<<<<<<<<
- * result *= length
- *
- */
- __pyx_t_4 = (__pyx_v_self->view.shape + __pyx_v_self->view.ndim);
- for (__pyx_t_5 = __pyx_v_self->view.shape; __pyx_t_5 < __pyx_t_4; __pyx_t_5++) {
- __pyx_t_3 = __pyx_t_5;
- __pyx_t_6 = PyInt_FromSsize_t((__pyx_t_3[0])); if (unlikely(!__pyx_t_6)) __PYX_ERR(1, 598, __pyx_L1_error)
- __Pyx_GOTREF(__pyx_t_6);
- __Pyx_XDECREF_SET(__pyx_v_length, __pyx_t_6);
- __pyx_t_6 = 0;
-
- /* "View.MemoryView":599
- *
- * for length in self.view.shape[:self.view.ndim]:
- * result *= length # <<<<<<<<<<<<<<
- *
- * self._size = result
- */
- __pyx_t_6 = PyNumber_InPlaceMultiply(__pyx_v_result, __pyx_v_length); if (unlikely(!__pyx_t_6)) __PYX_ERR(1, 599, __pyx_L1_error)
- __Pyx_GOTREF(__pyx_t_6);
- __Pyx_DECREF_SET(__pyx_v_result, __pyx_t_6);
- __pyx_t_6 = 0;
- }
-
- /* "View.MemoryView":601
- * result *= length
- *
- * self._size = result # <<<<<<<<<<<<<<
- *
- * return self._size
- */
- __Pyx_INCREF(__pyx_v_result);
- __Pyx_GIVEREF(__pyx_v_result);
- __Pyx_GOTREF(__pyx_v_self->_size);
- __Pyx_DECREF(__pyx_v_self->_size);
- __pyx_v_self->_size = __pyx_v_result;
-
- /* "View.MemoryView":595
- * @property
- * def size(self):
- * if self._size is None: # <<<<<<<<<<<<<<
- * result = 1
- *
- */
- }
-
- /* "View.MemoryView":603
- * self._size = result
- *
- * return self._size # <<<<<<<<<<<<<<
- *
- * def __len__(self):
- */
- __Pyx_XDECREF(__pyx_r);
- __Pyx_INCREF(__pyx_v_self->_size);
- __pyx_r = __pyx_v_self->_size;
- goto __pyx_L0;
-
- /* "View.MemoryView":594
- *
- * @property
- * def size(self): # <<<<<<<<<<<<<<
- * if self._size is None:
- * result = 1
- */
-
- /* function exit code */
- __pyx_L1_error:;
- __Pyx_XDECREF(__pyx_t_6);
- __Pyx_AddTraceback("View.MemoryView.memoryview.size.__get__", __pyx_clineno, __pyx_lineno, __pyx_filename);
- __pyx_r = NULL;
- __pyx_L0:;
- __Pyx_XDECREF(__pyx_v_result);
- __Pyx_XDECREF(__pyx_v_length);
- __Pyx_XGIVEREF(__pyx_r);
- __Pyx_RefNannyFinishContext();
- return __pyx_r;
-}
-
-/* "View.MemoryView":605
- * return self._size
- *
- * def __len__(self): # <<<<<<<<<<<<<<
- * if self.view.ndim >= 1:
- * return self.view.shape[0]
- */
-
-/* Python wrapper */
-static Py_ssize_t __pyx_memoryview___len__(PyObject *__pyx_v_self); /*proto*/
-static Py_ssize_t __pyx_memoryview___len__(PyObject *__pyx_v_self) {
- Py_ssize_t __pyx_r;
- __Pyx_RefNannyDeclarations
- __Pyx_RefNannySetupContext("__len__ (wrapper)", 0);
- __pyx_r = __pyx_memoryview___pyx_pf_15View_dot_MemoryView_10memoryview_10__len__(((struct __pyx_memoryview_obj *)__pyx_v_self));
-
- /* function exit code */
- __Pyx_RefNannyFinishContext();
- return __pyx_r;
-}
-
-static Py_ssize_t __pyx_memoryview___pyx_pf_15View_dot_MemoryView_10memoryview_10__len__(struct __pyx_memoryview_obj *__pyx_v_self) {
- Py_ssize_t __pyx_r;
- __Pyx_RefNannyDeclarations
- int __pyx_t_1;
- __Pyx_RefNannySetupContext("__len__", 0);
-
- /* "View.MemoryView":606
- *
- * def __len__(self):
- * if self.view.ndim >= 1: # <<<<<<<<<<<<<<
- * return self.view.shape[0]
- *
- */
- __pyx_t_1 = ((__pyx_v_self->view.ndim >= 1) != 0);
- if (__pyx_t_1) {
-
- /* "View.MemoryView":607
- * def __len__(self):
- * if self.view.ndim >= 1:
- * return self.view.shape[0] # <<<<<<<<<<<<<<
- *
- * return 0
- */
- __pyx_r = (__pyx_v_self->view.shape[0]);
- goto __pyx_L0;
-
- /* "View.MemoryView":606
- *
- * def __len__(self):
- * if self.view.ndim >= 1: # <<<<<<<<<<<<<<
- * return self.view.shape[0]
- *
- */
- }
-
- /* "View.MemoryView":609
- * return self.view.shape[0]
- *
- * return 0 # <<<<<<<<<<<<<<
- *
- * def __repr__(self):
- */
- __pyx_r = 0;
- goto __pyx_L0;
-
- /* "View.MemoryView":605
- * return self._size
- *
- * def __len__(self): # <<<<<<<<<<<<<<
- * if self.view.ndim >= 1:
- * return self.view.shape[0]
- */
-
- /* function exit code */
- __pyx_L0:;
- __Pyx_RefNannyFinishContext();
- return __pyx_r;
-}
-
-/* "View.MemoryView":611
- * return 0
- *
- * def __repr__(self): # <<<<<<<<<<<<<<
- * return "" % (self.base.__class__.__name__,
- * id(self))
- */
-
-/* Python wrapper */
-static PyObject *__pyx_memoryview___repr__(PyObject *__pyx_v_self); /*proto*/
-static PyObject *__pyx_memoryview___repr__(PyObject *__pyx_v_self) {
- PyObject *__pyx_r = 0;
- __Pyx_RefNannyDeclarations
- __Pyx_RefNannySetupContext("__repr__ (wrapper)", 0);
- __pyx_r = __pyx_memoryview___pyx_pf_15View_dot_MemoryView_10memoryview_12__repr__(((struct __pyx_memoryview_obj *)__pyx_v_self));
-
- /* function exit code */
- __Pyx_RefNannyFinishContext();
- return __pyx_r;
-}
-
-static PyObject *__pyx_memoryview___pyx_pf_15View_dot_MemoryView_10memoryview_12__repr__(struct __pyx_memoryview_obj *__pyx_v_self) {
- PyObject *__pyx_r = NULL;
- __Pyx_RefNannyDeclarations
- PyObject *__pyx_t_1 = NULL;
- PyObject *__pyx_t_2 = NULL;
- PyObject *__pyx_t_3 = NULL;
- int __pyx_lineno = 0;
- const char *__pyx_filename = NULL;
- int __pyx_clineno = 0;
- __Pyx_RefNannySetupContext("__repr__", 0);
-
- /* "View.MemoryView":612
- *
- * def __repr__(self):
- * return "" % (self.base.__class__.__name__, # <<<<<<<<<<<<<<
- * id(self))
- *
- */
- __Pyx_XDECREF(__pyx_r);
- __pyx_t_1 = __Pyx_PyObject_GetAttrStr(((PyObject *)__pyx_v_self), __pyx_n_s_base); if (unlikely(!__pyx_t_1)) __PYX_ERR(1, 612, __pyx_L1_error)
- __Pyx_GOTREF(__pyx_t_1);
- __pyx_t_2 = __Pyx_PyObject_GetAttrStr(__pyx_t_1, __pyx_n_s_class); if (unlikely(!__pyx_t_2)) __PYX_ERR(1, 612, __pyx_L1_error)
- __Pyx_GOTREF(__pyx_t_2);
- __Pyx_DECREF(__pyx_t_1); __pyx_t_1 = 0;
- __pyx_t_1 = __Pyx_PyObject_GetAttrStr(__pyx_t_2, __pyx_n_s_name_2); if (unlikely(!__pyx_t_1)) __PYX_ERR(1, 612, __pyx_L1_error)
- __Pyx_GOTREF(__pyx_t_1);
- __Pyx_DECREF(__pyx_t_2); __pyx_t_2 = 0;
-
- /* "View.MemoryView":613
- * def __repr__(self):
- * return "" % (self.base.__class__.__name__,
- * id(self)) # <<<<<<<<<<<<<<
- *
- * def __str__(self):
- */
- __pyx_t_2 = __Pyx_PyObject_CallOneArg(__pyx_builtin_id, ((PyObject *)__pyx_v_self)); if (unlikely(!__pyx_t_2)) __PYX_ERR(1, 613, __pyx_L1_error)
- __Pyx_GOTREF(__pyx_t_2);
-
- /* "View.MemoryView":612
- *
- * def __repr__(self):
- * return "" % (self.base.__class__.__name__, # <<<<<<<<<<<<<<
- * id(self))
- *
- */
- __pyx_t_3 = PyTuple_New(2); if (unlikely(!__pyx_t_3)) __PYX_ERR(1, 612, __pyx_L1_error)
- __Pyx_GOTREF(__pyx_t_3);
- __Pyx_GIVEREF(__pyx_t_1);
- PyTuple_SET_ITEM(__pyx_t_3, 0, __pyx_t_1);
- __Pyx_GIVEREF(__pyx_t_2);
- PyTuple_SET_ITEM(__pyx_t_3, 1, __pyx_t_2);
- __pyx_t_1 = 0;
- __pyx_t_2 = 0;
- __pyx_t_2 = __Pyx_PyString_Format(__pyx_kp_s_MemoryView_of_r_at_0x_x, __pyx_t_3); if (unlikely(!__pyx_t_2)) __PYX_ERR(1, 612, __pyx_L1_error)
- __Pyx_GOTREF(__pyx_t_2);
- __Pyx_DECREF(__pyx_t_3); __pyx_t_3 = 0;
- __pyx_r = __pyx_t_2;
- __pyx_t_2 = 0;
- goto __pyx_L0;
-
- /* "View.MemoryView":611
- * return 0
- *
- * def __repr__(self): # <<<<<<<<<<<<<<
- * return "" % (self.base.__class__.__name__,
- * id(self))
- */
-
- /* function exit code */
- __pyx_L1_error:;
- __Pyx_XDECREF(__pyx_t_1);
- __Pyx_XDECREF(__pyx_t_2);
- __Pyx_XDECREF(__pyx_t_3);
- __Pyx_AddTraceback("View.MemoryView.memoryview.__repr__", __pyx_clineno, __pyx_lineno, __pyx_filename);
- __pyx_r = NULL;
- __pyx_L0:;
- __Pyx_XGIVEREF(__pyx_r);
- __Pyx_RefNannyFinishContext();
- return __pyx_r;
-}
-
-/* "View.MemoryView":615
- * id(self))
- *
- * def __str__(self): # <<<<<<<<<<<<<<
- * return "" % (self.base.__class__.__name__,)
- *
- */
-
-/* Python wrapper */
-static PyObject *__pyx_memoryview___str__(PyObject *__pyx_v_self); /*proto*/
-static PyObject *__pyx_memoryview___str__(PyObject *__pyx_v_self) {
- PyObject *__pyx_r = 0;
- __Pyx_RefNannyDeclarations
- __Pyx_RefNannySetupContext("__str__ (wrapper)", 0);
- __pyx_r = __pyx_memoryview___pyx_pf_15View_dot_MemoryView_10memoryview_14__str__(((struct __pyx_memoryview_obj *)__pyx_v_self));
-
- /* function exit code */
- __Pyx_RefNannyFinishContext();
- return __pyx_r;
-}
-
-static PyObject *__pyx_memoryview___pyx_pf_15View_dot_MemoryView_10memoryview_14__str__(struct __pyx_memoryview_obj *__pyx_v_self) {
- PyObject *__pyx_r = NULL;
- __Pyx_RefNannyDeclarations
- PyObject *__pyx_t_1 = NULL;
- PyObject *__pyx_t_2 = NULL;
- int __pyx_lineno = 0;
- const char *__pyx_filename = NULL;
- int __pyx_clineno = 0;
- __Pyx_RefNannySetupContext("__str__", 0);
-
- /* "View.MemoryView":616
- *
- * def __str__(self):
- * return "" % (self.base.__class__.__name__,) # <<<<<<<<<<<<<<
- *
- *
- */
- __Pyx_XDECREF(__pyx_r);
- __pyx_t_1 = __Pyx_PyObject_GetAttrStr(((PyObject *)__pyx_v_self), __pyx_n_s_base); if (unlikely(!__pyx_t_1)) __PYX_ERR(1, 616, __pyx_L1_error)
- __Pyx_GOTREF(__pyx_t_1);
- __pyx_t_2 = __Pyx_PyObject_GetAttrStr(__pyx_t_1, __pyx_n_s_class); if (unlikely(!__pyx_t_2)) __PYX_ERR(1, 616, __pyx_L1_error)
- __Pyx_GOTREF(__pyx_t_2);
- __Pyx_DECREF(__pyx_t_1); __pyx_t_1 = 0;
- __pyx_t_1 = __Pyx_PyObject_GetAttrStr(__pyx_t_2, __pyx_n_s_name_2); if (unlikely(!__pyx_t_1)) __PYX_ERR(1, 616, __pyx_L1_error)
- __Pyx_GOTREF(__pyx_t_1);
- __Pyx_DECREF(__pyx_t_2); __pyx_t_2 = 0;
- __pyx_t_2 = PyTuple_New(1); if (unlikely(!__pyx_t_2)) __PYX_ERR(1, 616, __pyx_L1_error)
- __Pyx_GOTREF(__pyx_t_2);
- __Pyx_GIVEREF(__pyx_t_1);
- PyTuple_SET_ITEM(__pyx_t_2, 0, __pyx_t_1);
- __pyx_t_1 = 0;
- __pyx_t_1 = __Pyx_PyString_Format(__pyx_kp_s_MemoryView_of_r_object, __pyx_t_2); if (unlikely(!__pyx_t_1)) __PYX_ERR(1, 616, __pyx_L1_error)
- __Pyx_GOTREF(__pyx_t_1);
- __Pyx_DECREF(__pyx_t_2); __pyx_t_2 = 0;
- __pyx_r = __pyx_t_1;
- __pyx_t_1 = 0;
- goto __pyx_L0;
-
- /* "View.MemoryView":615
- * id(self))
- *
- * def __str__(self): # <<<<<<<<<<<<<<
- * return "" % (self.base.__class__.__name__,)
- *
- */
-
- /* function exit code */
- __pyx_L1_error:;
- __Pyx_XDECREF(__pyx_t_1);
- __Pyx_XDECREF(__pyx_t_2);
- __Pyx_AddTraceback("View.MemoryView.memoryview.__str__", __pyx_clineno, __pyx_lineno, __pyx_filename);
- __pyx_r = NULL;
- __pyx_L0:;
- __Pyx_XGIVEREF(__pyx_r);
- __Pyx_RefNannyFinishContext();
- return __pyx_r;
-}
-
-/* "View.MemoryView":619
- *
- *
- * def is_c_contig(self): # <<<<<<<<<<<<<<
- * cdef __Pyx_memviewslice *mslice
- * cdef __Pyx_memviewslice tmp
- */
-
-/* Python wrapper */
-static PyObject *__pyx_memoryview_is_c_contig(PyObject *__pyx_v_self, CYTHON_UNUSED PyObject *unused); /*proto*/
-static PyObject *__pyx_memoryview_is_c_contig(PyObject *__pyx_v_self, CYTHON_UNUSED PyObject *unused) {
- PyObject *__pyx_r = 0;
- __Pyx_RefNannyDeclarations
- __Pyx_RefNannySetupContext("is_c_contig (wrapper)", 0);
- __pyx_r = __pyx_memoryview___pyx_pf_15View_dot_MemoryView_10memoryview_16is_c_contig(((struct __pyx_memoryview_obj *)__pyx_v_self));
-
- /* function exit code */
- __Pyx_RefNannyFinishContext();
- return __pyx_r;
-}
-
-static PyObject *__pyx_memoryview___pyx_pf_15View_dot_MemoryView_10memoryview_16is_c_contig(struct __pyx_memoryview_obj *__pyx_v_self) {
- __Pyx_memviewslice *__pyx_v_mslice;
- __Pyx_memviewslice __pyx_v_tmp;
- PyObject *__pyx_r = NULL;
- __Pyx_RefNannyDeclarations
- __Pyx_memviewslice *__pyx_t_1;
- PyObject *__pyx_t_2 = NULL;
- int __pyx_lineno = 0;
- const char *__pyx_filename = NULL;
- int __pyx_clineno = 0;
- __Pyx_RefNannySetupContext("is_c_contig", 0);
-
- /* "View.MemoryView":622
- * cdef __Pyx_memviewslice *mslice
- * cdef __Pyx_memviewslice tmp
- * mslice = get_slice_from_memview(self, &tmp) # <<<<<<<<<<<<<<
- * return slice_is_contig(mslice[0], 'C', self.view.ndim)
- *
- */
- __pyx_t_1 = __pyx_memoryview_get_slice_from_memoryview(__pyx_v_self, (&__pyx_v_tmp)); if (unlikely(__pyx_t_1 == ((__Pyx_memviewslice *)NULL))) __PYX_ERR(1, 622, __pyx_L1_error)
- __pyx_v_mslice = __pyx_t_1;
-
- /* "View.MemoryView":623
- * cdef __Pyx_memviewslice tmp
- * mslice = get_slice_from_memview(self, &tmp)
- * return slice_is_contig(mslice[0], 'C', self.view.ndim) # <<<<<<<<<<<<<<
- *
- * def is_f_contig(self):
- */
- __Pyx_XDECREF(__pyx_r);
- __pyx_t_2 = __Pyx_PyBool_FromLong(__pyx_memviewslice_is_contig((__pyx_v_mslice[0]), 'C', __pyx_v_self->view.ndim)); if (unlikely(!__pyx_t_2)) __PYX_ERR(1, 623, __pyx_L1_error)
- __Pyx_GOTREF(__pyx_t_2);
- __pyx_r = __pyx_t_2;
- __pyx_t_2 = 0;
- goto __pyx_L0;
-
- /* "View.MemoryView":619
- *
- *
- * def is_c_contig(self): # <<<<<<<<<<<<<<
- * cdef __Pyx_memviewslice *mslice
- * cdef __Pyx_memviewslice tmp
- */
-
- /* function exit code */
- __pyx_L1_error:;
- __Pyx_XDECREF(__pyx_t_2);
- __Pyx_AddTraceback("View.MemoryView.memoryview.is_c_contig", __pyx_clineno, __pyx_lineno, __pyx_filename);
- __pyx_r = NULL;
- __pyx_L0:;
- __Pyx_XGIVEREF(__pyx_r);
- __Pyx_RefNannyFinishContext();
- return __pyx_r;
-}
-
-/* "View.MemoryView":625
- * return slice_is_contig(mslice[0], 'C', self.view.ndim)
- *
- * def is_f_contig(self): # <<<<<<<<<<<<<<
- * cdef __Pyx_memviewslice *mslice
- * cdef __Pyx_memviewslice tmp
- */
-
-/* Python wrapper */
-static PyObject *__pyx_memoryview_is_f_contig(PyObject *__pyx_v_self, CYTHON_UNUSED PyObject *unused); /*proto*/
-static PyObject *__pyx_memoryview_is_f_contig(PyObject *__pyx_v_self, CYTHON_UNUSED PyObject *unused) {
- PyObject *__pyx_r = 0;
- __Pyx_RefNannyDeclarations
- __Pyx_RefNannySetupContext("is_f_contig (wrapper)", 0);
- __pyx_r = __pyx_memoryview___pyx_pf_15View_dot_MemoryView_10memoryview_18is_f_contig(((struct __pyx_memoryview_obj *)__pyx_v_self));
-
- /* function exit code */
- __Pyx_RefNannyFinishContext();
- return __pyx_r;
-}
-
-static PyObject *__pyx_memoryview___pyx_pf_15View_dot_MemoryView_10memoryview_18is_f_contig(struct __pyx_memoryview_obj *__pyx_v_self) {
- __Pyx_memviewslice *__pyx_v_mslice;
- __Pyx_memviewslice __pyx_v_tmp;
- PyObject *__pyx_r = NULL;
- __Pyx_RefNannyDeclarations
- __Pyx_memviewslice *__pyx_t_1;
- PyObject *__pyx_t_2 = NULL;
- int __pyx_lineno = 0;
- const char *__pyx_filename = NULL;
- int __pyx_clineno = 0;
- __Pyx_RefNannySetupContext("is_f_contig", 0);
-
- /* "View.MemoryView":628
- * cdef __Pyx_memviewslice *mslice
- * cdef __Pyx_memviewslice tmp
- * mslice = get_slice_from_memview(self, &tmp) # <<<<<<<<<<<<<<
- * return slice_is_contig(mslice[0], 'F', self.view.ndim)
- *
- */
- __pyx_t_1 = __pyx_memoryview_get_slice_from_memoryview(__pyx_v_self, (&__pyx_v_tmp)); if (unlikely(__pyx_t_1 == ((__Pyx_memviewslice *)NULL))) __PYX_ERR(1, 628, __pyx_L1_error)
- __pyx_v_mslice = __pyx_t_1;
-
- /* "View.MemoryView":629
- * cdef __Pyx_memviewslice tmp
- * mslice = get_slice_from_memview(self, &tmp)
- * return slice_is_contig(mslice[0], 'F', self.view.ndim) # <<<<<<<<<<<<<<
- *
- * def copy(self):
- */
- __Pyx_XDECREF(__pyx_r);
- __pyx_t_2 = __Pyx_PyBool_FromLong(__pyx_memviewslice_is_contig((__pyx_v_mslice[0]), 'F', __pyx_v_self->view.ndim)); if (unlikely(!__pyx_t_2)) __PYX_ERR(1, 629, __pyx_L1_error)
- __Pyx_GOTREF(__pyx_t_2);
- __pyx_r = __pyx_t_2;
- __pyx_t_2 = 0;
- goto __pyx_L0;
-
- /* "View.MemoryView":625
- * return slice_is_contig(mslice[0], 'C', self.view.ndim)
- *
- * def is_f_contig(self): # <<<<<<<<<<<<<<
- * cdef __Pyx_memviewslice *mslice
- * cdef __Pyx_memviewslice tmp
- */
-
- /* function exit code */
- __pyx_L1_error:;
- __Pyx_XDECREF(__pyx_t_2);
- __Pyx_AddTraceback("View.MemoryView.memoryview.is_f_contig", __pyx_clineno, __pyx_lineno, __pyx_filename);
- __pyx_r = NULL;
- __pyx_L0:;
- __Pyx_XGIVEREF(__pyx_r);
- __Pyx_RefNannyFinishContext();
- return __pyx_r;
-}
-
-/* "View.MemoryView":631
- * return slice_is_contig(mslice[0], 'F', self.view.ndim)
- *
- * def copy(self): # <<<<<<<<<<<<<<
- * cdef __Pyx_memviewslice mslice
- * cdef int flags = self.flags & ~PyBUF_F_CONTIGUOUS
- */
-
-/* Python wrapper */
-static PyObject *__pyx_memoryview_copy(PyObject *__pyx_v_self, CYTHON_UNUSED PyObject *unused); /*proto*/
-static PyObject *__pyx_memoryview_copy(PyObject *__pyx_v_self, CYTHON_UNUSED PyObject *unused) {
- PyObject *__pyx_r = 0;
- __Pyx_RefNannyDeclarations
- __Pyx_RefNannySetupContext("copy (wrapper)", 0);
- __pyx_r = __pyx_memoryview___pyx_pf_15View_dot_MemoryView_10memoryview_20copy(((struct __pyx_memoryview_obj *)__pyx_v_self));
-
- /* function exit code */
- __Pyx_RefNannyFinishContext();
- return __pyx_r;
-}
-
-static PyObject *__pyx_memoryview___pyx_pf_15View_dot_MemoryView_10memoryview_20copy(struct __pyx_memoryview_obj *__pyx_v_self) {
- __Pyx_memviewslice __pyx_v_mslice;
- int __pyx_v_flags;
- PyObject *__pyx_r = NULL;
- __Pyx_RefNannyDeclarations
- __Pyx_memviewslice __pyx_t_1;
- PyObject *__pyx_t_2 = NULL;
- int __pyx_lineno = 0;
- const char *__pyx_filename = NULL;
- int __pyx_clineno = 0;
- __Pyx_RefNannySetupContext("copy", 0);
-
- /* "View.MemoryView":633
- * def copy(self):
- * cdef __Pyx_memviewslice mslice
- * cdef int flags = self.flags & ~PyBUF_F_CONTIGUOUS # <<<<<<<<<<<<<<
- *
- * slice_copy(self, &mslice)
- */
- __pyx_v_flags = (__pyx_v_self->flags & (~PyBUF_F_CONTIGUOUS));
-
- /* "View.MemoryView":635
- * cdef int flags = self.flags & ~PyBUF_F_CONTIGUOUS
- *
- * slice_copy(self, &mslice) # <<<<<<<<<<<<<<
- * mslice = slice_copy_contig(&mslice, "c", self.view.ndim,
- * self.view.itemsize,
- */
- __pyx_memoryview_slice_copy(__pyx_v_self, (&__pyx_v_mslice));
-
- /* "View.MemoryView":636
- *
- * slice_copy(self, &mslice)
- * mslice = slice_copy_contig(&mslice, "c", self.view.ndim, # <<<<<<<<<<<<<<
- * self.view.itemsize,
- * flags|PyBUF_C_CONTIGUOUS,
- */
- __pyx_t_1 = __pyx_memoryview_copy_new_contig((&__pyx_v_mslice), ((char *)"c"), __pyx_v_self->view.ndim, __pyx_v_self->view.itemsize, (__pyx_v_flags | PyBUF_C_CONTIGUOUS), __pyx_v_self->dtype_is_object); if (unlikely(PyErr_Occurred())) __PYX_ERR(1, 636, __pyx_L1_error)
- __pyx_v_mslice = __pyx_t_1;
-
- /* "View.MemoryView":641
- * self.dtype_is_object)
- *
- * return memoryview_copy_from_slice(self, &mslice) # <<<<<<<<<<<<<<
- *
- * def copy_fortran(self):
- */
- __Pyx_XDECREF(__pyx_r);
- __pyx_t_2 = __pyx_memoryview_copy_object_from_slice(__pyx_v_self, (&__pyx_v_mslice)); if (unlikely(!__pyx_t_2)) __PYX_ERR(1, 641, __pyx_L1_error)
- __Pyx_GOTREF(__pyx_t_2);
- __pyx_r = __pyx_t_2;
- __pyx_t_2 = 0;
- goto __pyx_L0;
-
- /* "View.MemoryView":631
- * return slice_is_contig(mslice[0], 'F', self.view.ndim)
- *
- * def copy(self): # <<<<<<<<<<<<<<
- * cdef __Pyx_memviewslice mslice
- * cdef int flags = self.flags & ~PyBUF_F_CONTIGUOUS
- */
-
- /* function exit code */
- __pyx_L1_error:;
- __Pyx_XDECREF(__pyx_t_2);
- __Pyx_AddTraceback("View.MemoryView.memoryview.copy", __pyx_clineno, __pyx_lineno, __pyx_filename);
- __pyx_r = NULL;
- __pyx_L0:;
- __Pyx_XGIVEREF(__pyx_r);
- __Pyx_RefNannyFinishContext();
- return __pyx_r;
-}
-
-/* "View.MemoryView":643
- * return memoryview_copy_from_slice(self, &mslice)
- *
- * def copy_fortran(self): # <<<<<<<<<<<<<<
- * cdef __Pyx_memviewslice src, dst
- * cdef int flags = self.flags & ~PyBUF_C_CONTIGUOUS
- */
-
-/* Python wrapper */
-static PyObject *__pyx_memoryview_copy_fortran(PyObject *__pyx_v_self, CYTHON_UNUSED PyObject *unused); /*proto*/
-static PyObject *__pyx_memoryview_copy_fortran(PyObject *__pyx_v_self, CYTHON_UNUSED PyObject *unused) {
- PyObject *__pyx_r = 0;
- __Pyx_RefNannyDeclarations
- __Pyx_RefNannySetupContext("copy_fortran (wrapper)", 0);
- __pyx_r = __pyx_memoryview___pyx_pf_15View_dot_MemoryView_10memoryview_22copy_fortran(((struct __pyx_memoryview_obj *)__pyx_v_self));
-
- /* function exit code */
- __Pyx_RefNannyFinishContext();
- return __pyx_r;
-}
-
-static PyObject *__pyx_memoryview___pyx_pf_15View_dot_MemoryView_10memoryview_22copy_fortran(struct __pyx_memoryview_obj *__pyx_v_self) {
- __Pyx_memviewslice __pyx_v_src;
- __Pyx_memviewslice __pyx_v_dst;
- int __pyx_v_flags;
- PyObject *__pyx_r = NULL;
- __Pyx_RefNannyDeclarations
- __Pyx_memviewslice __pyx_t_1;
- PyObject *__pyx_t_2 = NULL;
- int __pyx_lineno = 0;
- const char *__pyx_filename = NULL;
- int __pyx_clineno = 0;
- __Pyx_RefNannySetupContext("copy_fortran", 0);
-
- /* "View.MemoryView":645
- * def copy_fortran(self):
- * cdef __Pyx_memviewslice src, dst
- * cdef int flags = self.flags & ~PyBUF_C_CONTIGUOUS # <<<<<<<<<<<<<<
- *
- * slice_copy(self, &src)
- */
- __pyx_v_flags = (__pyx_v_self->flags & (~PyBUF_C_CONTIGUOUS));
-
- /* "View.MemoryView":647
- * cdef int flags = self.flags & ~PyBUF_C_CONTIGUOUS
- *
- * slice_copy(self, &src) # <<<<<<<<<<<<<<
- * dst = slice_copy_contig(&src, "fortran", self.view.ndim,
- * self.view.itemsize,
- */
- __pyx_memoryview_slice_copy(__pyx_v_self, (&__pyx_v_src));
-
- /* "View.MemoryView":648
- *
- * slice_copy(self, &src)
- * dst = slice_copy_contig(&src, "fortran", self.view.ndim, # <<<<<<<<<<<<<<
- * self.view.itemsize,
- * flags|PyBUF_F_CONTIGUOUS,
- */
- __pyx_t_1 = __pyx_memoryview_copy_new_contig((&__pyx_v_src), ((char *)"fortran"), __pyx_v_self->view.ndim, __pyx_v_self->view.itemsize, (__pyx_v_flags | PyBUF_F_CONTIGUOUS), __pyx_v_self->dtype_is_object); if (unlikely(PyErr_Occurred())) __PYX_ERR(1, 648, __pyx_L1_error)
- __pyx_v_dst = __pyx_t_1;
-
- /* "View.MemoryView":653
- * self.dtype_is_object)
- *
- * return memoryview_copy_from_slice(self, &dst) # <<<<<<<<<<<<<<
- *
- *
- */
- __Pyx_XDECREF(__pyx_r);
- __pyx_t_2 = __pyx_memoryview_copy_object_from_slice(__pyx_v_self, (&__pyx_v_dst)); if (unlikely(!__pyx_t_2)) __PYX_ERR(1, 653, __pyx_L1_error)
- __Pyx_GOTREF(__pyx_t_2);
- __pyx_r = __pyx_t_2;
- __pyx_t_2 = 0;
- goto __pyx_L0;
-
- /* "View.MemoryView":643
- * return memoryview_copy_from_slice(self, &mslice)
- *
- * def copy_fortran(self): # <<<<<<<<<<<<<<
- * cdef __Pyx_memviewslice src, dst
- * cdef int flags = self.flags & ~PyBUF_C_CONTIGUOUS
- */
-
- /* function exit code */
- __pyx_L1_error:;
- __Pyx_XDECREF(__pyx_t_2);
- __Pyx_AddTraceback("View.MemoryView.memoryview.copy_fortran", __pyx_clineno, __pyx_lineno, __pyx_filename);
- __pyx_r = NULL;
- __pyx_L0:;
- __Pyx_XGIVEREF(__pyx_r);
- __Pyx_RefNannyFinishContext();
- return __pyx_r;
-}
-
-/* "(tree fragment)":1
- * def __reduce_cython__(self): # <<<<<<<<<<<<<<
- * raise TypeError("no default __reduce__ due to non-trivial __cinit__")
- * def __setstate_cython__(self, __pyx_state):
- */
-
-/* Python wrapper */
-static PyObject *__pyx_pw___pyx_memoryview_1__reduce_cython__(PyObject *__pyx_v_self, CYTHON_UNUSED PyObject *unused); /*proto*/
-static PyObject *__pyx_pw___pyx_memoryview_1__reduce_cython__(PyObject *__pyx_v_self, CYTHON_UNUSED PyObject *unused) {
- PyObject *__pyx_r = 0;
- __Pyx_RefNannyDeclarations
- __Pyx_RefNannySetupContext("__reduce_cython__ (wrapper)", 0);
- __pyx_r = __pyx_pf___pyx_memoryview___reduce_cython__(((struct __pyx_memoryview_obj *)__pyx_v_self));
-
- /* function exit code */
- __Pyx_RefNannyFinishContext();
- return __pyx_r;
-}
-
-static PyObject *__pyx_pf___pyx_memoryview___reduce_cython__(CYTHON_UNUSED struct __pyx_memoryview_obj *__pyx_v_self) {
- PyObject *__pyx_r = NULL;
- __Pyx_RefNannyDeclarations
- PyObject *__pyx_t_1 = NULL;
- int __pyx_lineno = 0;
- const char *__pyx_filename = NULL;
- int __pyx_clineno = 0;
- __Pyx_RefNannySetupContext("__reduce_cython__", 0);
-
- /* "(tree fragment)":2
- * def __reduce_cython__(self):
- * raise TypeError("no default __reduce__ due to non-trivial __cinit__") # <<<<<<<<<<<<<<
- * def __setstate_cython__(self, __pyx_state):
- * raise TypeError("no default __reduce__ due to non-trivial __cinit__")
- */
- __pyx_t_1 = __Pyx_PyObject_Call(__pyx_builtin_TypeError, __pyx_tuple__14, NULL); if (unlikely(!__pyx_t_1)) __PYX_ERR(1, 2, __pyx_L1_error)
- __Pyx_GOTREF(__pyx_t_1);
- __Pyx_Raise(__pyx_t_1, 0, 0, 0);
- __Pyx_DECREF(__pyx_t_1); __pyx_t_1 = 0;
- __PYX_ERR(1, 2, __pyx_L1_error)
-
- /* "(tree fragment)":1
- * def __reduce_cython__(self): # <<<<<<<<<<<<<<
- * raise TypeError("no default __reduce__ due to non-trivial __cinit__")
- * def __setstate_cython__(self, __pyx_state):
- */
-
- /* function exit code */
- __pyx_L1_error:;
- __Pyx_XDECREF(__pyx_t_1);
- __Pyx_AddTraceback("View.MemoryView.memoryview.__reduce_cython__", __pyx_clineno, __pyx_lineno, __pyx_filename);
- __pyx_r = NULL;
- __Pyx_XGIVEREF(__pyx_r);
- __Pyx_RefNannyFinishContext();
- return __pyx_r;
-}
-
-/* "(tree fragment)":3
- * def __reduce_cython__(self):
- * raise TypeError("no default __reduce__ due to non-trivial __cinit__")
- * def __setstate_cython__(self, __pyx_state): # <<<<<<<<<<<<<<
- * raise TypeError("no default __reduce__ due to non-trivial __cinit__")
- */
-
-/* Python wrapper */
-static PyObject *__pyx_pw___pyx_memoryview_3__setstate_cython__(PyObject *__pyx_v_self, PyObject *__pyx_v___pyx_state); /*proto*/
-static PyObject *__pyx_pw___pyx_memoryview_3__setstate_cython__(PyObject *__pyx_v_self, PyObject *__pyx_v___pyx_state) {
- PyObject *__pyx_r = 0;
- __Pyx_RefNannyDeclarations
- __Pyx_RefNannySetupContext("__setstate_cython__ (wrapper)", 0);
- __pyx_r = __pyx_pf___pyx_memoryview_2__setstate_cython__(((struct __pyx_memoryview_obj *)__pyx_v_self), ((PyObject *)__pyx_v___pyx_state));
-
- /* function exit code */
- __Pyx_RefNannyFinishContext();
- return __pyx_r;
-}
-
-static PyObject *__pyx_pf___pyx_memoryview_2__setstate_cython__(CYTHON_UNUSED struct __pyx_memoryview_obj *__pyx_v_self, CYTHON_UNUSED PyObject *__pyx_v___pyx_state) {
- PyObject *__pyx_r = NULL;
- __Pyx_RefNannyDeclarations
- PyObject *__pyx_t_1 = NULL;
- int __pyx_lineno = 0;
- const char *__pyx_filename = NULL;
- int __pyx_clineno = 0;
- __Pyx_RefNannySetupContext("__setstate_cython__", 0);
-
- /* "(tree fragment)":4
- * raise TypeError("no default __reduce__ due to non-trivial __cinit__")
- * def __setstate_cython__(self, __pyx_state):
- * raise TypeError("no default __reduce__ due to non-trivial __cinit__") # <<<<<<<<<<<<<<
- */
- __pyx_t_1 = __Pyx_PyObject_Call(__pyx_builtin_TypeError, __pyx_tuple__15, NULL); if (unlikely(!__pyx_t_1)) __PYX_ERR(1, 4, __pyx_L1_error)
- __Pyx_GOTREF(__pyx_t_1);
- __Pyx_Raise(__pyx_t_1, 0, 0, 0);
- __Pyx_DECREF(__pyx_t_1); __pyx_t_1 = 0;
- __PYX_ERR(1, 4, __pyx_L1_error)
-
- /* "(tree fragment)":3
- * def __reduce_cython__(self):
- * raise TypeError("no default __reduce__ due to non-trivial __cinit__")
- * def __setstate_cython__(self, __pyx_state): # <<<<<<<<<<<<<<
- * raise TypeError("no default __reduce__ due to non-trivial __cinit__")
- */
-
- /* function exit code */
- __pyx_L1_error:;
- __Pyx_XDECREF(__pyx_t_1);
- __Pyx_AddTraceback("View.MemoryView.memoryview.__setstate_cython__", __pyx_clineno, __pyx_lineno, __pyx_filename);
- __pyx_r = NULL;
- __Pyx_XGIVEREF(__pyx_r);
- __Pyx_RefNannyFinishContext();
- return __pyx_r;
-}
-
-/* "View.MemoryView":657
- *
- * @cname('__pyx_memoryview_new')
- * cdef memoryview_cwrapper(object o, int flags, bint dtype_is_object, __Pyx_TypeInfo *typeinfo): # <<<<<<<<<<<<<<
- * cdef memoryview result = memoryview(o, flags, dtype_is_object)
- * result.typeinfo = typeinfo
- */
-
-static PyObject *__pyx_memoryview_new(PyObject *__pyx_v_o, int __pyx_v_flags, int __pyx_v_dtype_is_object, __Pyx_TypeInfo *__pyx_v_typeinfo) {
- struct __pyx_memoryview_obj *__pyx_v_result = 0;
- PyObject *__pyx_r = NULL;
- __Pyx_RefNannyDeclarations
- PyObject *__pyx_t_1 = NULL;
- PyObject *__pyx_t_2 = NULL;
- PyObject *__pyx_t_3 = NULL;
- int __pyx_lineno = 0;
- const char *__pyx_filename = NULL;
- int __pyx_clineno = 0;
- __Pyx_RefNannySetupContext("memoryview_cwrapper", 0);
-
- /* "View.MemoryView":658
- * @cname('__pyx_memoryview_new')
- * cdef memoryview_cwrapper(object o, int flags, bint dtype_is_object, __Pyx_TypeInfo *typeinfo):
- * cdef memoryview result = memoryview(o, flags, dtype_is_object) # <<<<<<<<<<<<<<
- * result.typeinfo = typeinfo
- * return result
- */
- __pyx_t_1 = __Pyx_PyInt_From_int(__pyx_v_flags); if (unlikely(!__pyx_t_1)) __PYX_ERR(1, 658, __pyx_L1_error)
- __Pyx_GOTREF(__pyx_t_1);
- __pyx_t_2 = __Pyx_PyBool_FromLong(__pyx_v_dtype_is_object); if (unlikely(!__pyx_t_2)) __PYX_ERR(1, 658, __pyx_L1_error)
- __Pyx_GOTREF(__pyx_t_2);
- __pyx_t_3 = PyTuple_New(3); if (unlikely(!__pyx_t_3)) __PYX_ERR(1, 658, __pyx_L1_error)
- __Pyx_GOTREF(__pyx_t_3);
- __Pyx_INCREF(__pyx_v_o);
- __Pyx_GIVEREF(__pyx_v_o);
- PyTuple_SET_ITEM(__pyx_t_3, 0, __pyx_v_o);
- __Pyx_GIVEREF(__pyx_t_1);
- PyTuple_SET_ITEM(__pyx_t_3, 1, __pyx_t_1);
- __Pyx_GIVEREF(__pyx_t_2);
- PyTuple_SET_ITEM(__pyx_t_3, 2, __pyx_t_2);
- __pyx_t_1 = 0;
- __pyx_t_2 = 0;
- __pyx_t_2 = __Pyx_PyObject_Call(((PyObject *)__pyx_memoryview_type), __pyx_t_3, NULL); if (unlikely(!__pyx_t_2)) __PYX_ERR(1, 658, __pyx_L1_error)
- __Pyx_GOTREF(__pyx_t_2);
- __Pyx_DECREF(__pyx_t_3); __pyx_t_3 = 0;
- __pyx_v_result = ((struct __pyx_memoryview_obj *)__pyx_t_2);
- __pyx_t_2 = 0;
-
- /* "View.MemoryView":659
- * cdef memoryview_cwrapper(object o, int flags, bint dtype_is_object, __Pyx_TypeInfo *typeinfo):
- * cdef memoryview result = memoryview(o, flags, dtype_is_object)
- * result.typeinfo = typeinfo # <<<<<<<<<<<<<<
- * return result
- *
- */
- __pyx_v_result->typeinfo = __pyx_v_typeinfo;
-
- /* "View.MemoryView":660
- * cdef memoryview result = memoryview(o, flags, dtype_is_object)
- * result.typeinfo = typeinfo
- * return result # <<<<<<<<<<<<<<
- *
- * @cname('__pyx_memoryview_check')
- */
- __Pyx_XDECREF(__pyx_r);
- __Pyx_INCREF(((PyObject *)__pyx_v_result));
- __pyx_r = ((PyObject *)__pyx_v_result);
- goto __pyx_L0;
-
- /* "View.MemoryView":657
- *
- * @cname('__pyx_memoryview_new')
- * cdef memoryview_cwrapper(object o, int flags, bint dtype_is_object, __Pyx_TypeInfo *typeinfo): # <<<<<<<<<<<<<<
- * cdef memoryview result = memoryview(o, flags, dtype_is_object)
- * result.typeinfo = typeinfo
- */
-
- /* function exit code */
- __pyx_L1_error:;
- __Pyx_XDECREF(__pyx_t_1);
- __Pyx_XDECREF(__pyx_t_2);
- __Pyx_XDECREF(__pyx_t_3);
- __Pyx_AddTraceback("View.MemoryView.memoryview_cwrapper", __pyx_clineno, __pyx_lineno, __pyx_filename);
- __pyx_r = 0;
- __pyx_L0:;
- __Pyx_XDECREF((PyObject *)__pyx_v_result);
- __Pyx_XGIVEREF(__pyx_r);
- __Pyx_RefNannyFinishContext();
- return __pyx_r;
-}
-
-/* "View.MemoryView":663
- *
- * @cname('__pyx_memoryview_check')
- * cdef inline bint memoryview_check(object o): # <<<<<<<<<<<<<<
- * return isinstance(o, memoryview)
- *
- */
-
-static CYTHON_INLINE int __pyx_memoryview_check(PyObject *__pyx_v_o) {
- int __pyx_r;
- __Pyx_RefNannyDeclarations
- int __pyx_t_1;
- __Pyx_RefNannySetupContext("memoryview_check", 0);
-
- /* "View.MemoryView":664
- * @cname('__pyx_memoryview_check')
- * cdef inline bint memoryview_check(object o):
- * return isinstance(o, memoryview) # <<<<<<<<<<<<<<
- *
- * cdef tuple _unellipsify(object index, int ndim):
- */
- __pyx_t_1 = __Pyx_TypeCheck(__pyx_v_o, __pyx_memoryview_type);
- __pyx_r = __pyx_t_1;
- goto __pyx_L0;
-
- /* "View.MemoryView":663
- *
- * @cname('__pyx_memoryview_check')
- * cdef inline bint memoryview_check(object o): # <<<<<<<<<<<<<<
- * return isinstance(o, memoryview)
- *
- */
-
- /* function exit code */
- __pyx_L0:;
- __Pyx_RefNannyFinishContext();
- return __pyx_r;
-}
-
-/* "View.MemoryView":666
- * return isinstance(o, memoryview)
- *
- * cdef tuple _unellipsify(object index, int ndim): # <<<<<<<<<<<<<<
- * """
- * Replace all ellipses with full slices and fill incomplete indices with
- */
-
-static PyObject *_unellipsify(PyObject *__pyx_v_index, int __pyx_v_ndim) {
- PyObject *__pyx_v_tup = NULL;
- PyObject *__pyx_v_result = NULL;
- int __pyx_v_have_slices;
- int __pyx_v_seen_ellipsis;
- CYTHON_UNUSED PyObject *__pyx_v_idx = NULL;
- PyObject *__pyx_v_item = NULL;
- Py_ssize_t __pyx_v_nslices;
- PyObject *__pyx_r = NULL;
- __Pyx_RefNannyDeclarations
- int __pyx_t_1;
- int __pyx_t_2;
- PyObject *__pyx_t_3 = NULL;
- PyObject *__pyx_t_4 = NULL;
- Py_ssize_t __pyx_t_5;
- PyObject *(*__pyx_t_6)(PyObject *);
- PyObject *__pyx_t_7 = NULL;
- Py_ssize_t __pyx_t_8;
- int __pyx_t_9;
- int __pyx_t_10;
- PyObject *__pyx_t_11 = NULL;
- int __pyx_lineno = 0;
- const char *__pyx_filename = NULL;
- int __pyx_clineno = 0;
- __Pyx_RefNannySetupContext("_unellipsify", 0);
-
- /* "View.MemoryView":671
- * full slices.
- * """
- * if not isinstance(index, tuple): # <<<<<<<<<<<<<<
- * tup = (index,)
- * else:
- */
- __pyx_t_1 = PyTuple_Check(__pyx_v_index);
- __pyx_t_2 = ((!(__pyx_t_1 != 0)) != 0);
- if (__pyx_t_2) {
-
- /* "View.MemoryView":672
- * """
- * if not isinstance(index, tuple):
- * tup = (index,) # <<<<<<<<<<<<<<
- * else:
- * tup = index
- */
- __pyx_t_3 = PyTuple_New(1); if (unlikely(!__pyx_t_3)) __PYX_ERR(1, 672, __pyx_L1_error)
- __Pyx_GOTREF(__pyx_t_3);
- __Pyx_INCREF(__pyx_v_index);
- __Pyx_GIVEREF(__pyx_v_index);
- PyTuple_SET_ITEM(__pyx_t_3, 0, __pyx_v_index);
- __pyx_v_tup = __pyx_t_3;
- __pyx_t_3 = 0;
-
- /* "View.MemoryView":671
- * full slices.
- * """
- * if not isinstance(index, tuple): # <<<<<<<<<<<<<<
- * tup = (index,)
- * else:
- */
- goto __pyx_L3;
- }
-
- /* "View.MemoryView":674
- * tup = (index,)
- * else:
- * tup = index # <<<<<<<<<<<<<<
- *
- * result = []
- */
- /*else*/ {
- __Pyx_INCREF(__pyx_v_index);
- __pyx_v_tup = __pyx_v_index;
- }
- __pyx_L3:;
-
- /* "View.MemoryView":676
- * tup = index
- *
- * result = [] # <<<<<<<<<<<<<<
- * have_slices = False
- * seen_ellipsis = False
- */
- __pyx_t_3 = PyList_New(0); if (unlikely(!__pyx_t_3)) __PYX_ERR(1, 676, __pyx_L1_error)
- __Pyx_GOTREF(__pyx_t_3);
- __pyx_v_result = ((PyObject*)__pyx_t_3);
- __pyx_t_3 = 0;
-
- /* "View.MemoryView":677
- *
- * result = []
- * have_slices = False # <<<<<<<<<<<<<<
- * seen_ellipsis = False
- * for idx, item in enumerate(tup):
- */
- __pyx_v_have_slices = 0;
-
- /* "View.MemoryView":678
- * result = []
- * have_slices = False
- * seen_ellipsis = False # <<<<<<<<<<<<<<
- * for idx, item in enumerate(tup):
- * if item is Ellipsis:
- */
- __pyx_v_seen_ellipsis = 0;
-
- /* "View.MemoryView":679
- * have_slices = False
- * seen_ellipsis = False
- * for idx, item in enumerate(tup): # <<<<<<<<<<<<<<
- * if item is Ellipsis:
- * if not seen_ellipsis:
- */
- __Pyx_INCREF(__pyx_int_0);
- __pyx_t_3 = __pyx_int_0;
- if (likely(PyList_CheckExact(__pyx_v_tup)) || PyTuple_CheckExact(__pyx_v_tup)) {
- __pyx_t_4 = __pyx_v_tup; __Pyx_INCREF(__pyx_t_4); __pyx_t_5 = 0;
- __pyx_t_6 = NULL;
- } else {
- __pyx_t_5 = -1; __pyx_t_4 = PyObject_GetIter(__pyx_v_tup); if (unlikely(!__pyx_t_4)) __PYX_ERR(1, 679, __pyx_L1_error)
- __Pyx_GOTREF(__pyx_t_4);
- __pyx_t_6 = Py_TYPE(__pyx_t_4)->tp_iternext; if (unlikely(!__pyx_t_6)) __PYX_ERR(1, 679, __pyx_L1_error)
- }
- for (;;) {
- if (likely(!__pyx_t_6)) {
- if (likely(PyList_CheckExact(__pyx_t_4))) {
- if (__pyx_t_5 >= PyList_GET_SIZE(__pyx_t_4)) break;
- #if CYTHON_ASSUME_SAFE_MACROS && !CYTHON_AVOID_BORROWED_REFS
- __pyx_t_7 = PyList_GET_ITEM(__pyx_t_4, __pyx_t_5); __Pyx_INCREF(__pyx_t_7); __pyx_t_5++; if (unlikely(0 < 0)) __PYX_ERR(1, 679, __pyx_L1_error)
- #else
- __pyx_t_7 = PySequence_ITEM(__pyx_t_4, __pyx_t_5); __pyx_t_5++; if (unlikely(!__pyx_t_7)) __PYX_ERR(1, 679, __pyx_L1_error)
- __Pyx_GOTREF(__pyx_t_7);
- #endif
- } else {
- if (__pyx_t_5 >= PyTuple_GET_SIZE(__pyx_t_4)) break;
- #if CYTHON_ASSUME_SAFE_MACROS && !CYTHON_AVOID_BORROWED_REFS
- __pyx_t_7 = PyTuple_GET_ITEM(__pyx_t_4, __pyx_t_5); __Pyx_INCREF(__pyx_t_7); __pyx_t_5++; if (unlikely(0 < 0)) __PYX_ERR(1, 679, __pyx_L1_error)
- #else
- __pyx_t_7 = PySequence_ITEM(__pyx_t_4, __pyx_t_5); __pyx_t_5++; if (unlikely(!__pyx_t_7)) __PYX_ERR(1, 679, __pyx_L1_error)
- __Pyx_GOTREF(__pyx_t_7);
- #endif
- }
- } else {
- __pyx_t_7 = __pyx_t_6(__pyx_t_4);
- if (unlikely(!__pyx_t_7)) {
- PyObject* exc_type = PyErr_Occurred();
- if (exc_type) {
- if (likely(__Pyx_PyErr_GivenExceptionMatches(exc_type, PyExc_StopIteration))) PyErr_Clear();
- else __PYX_ERR(1, 679, __pyx_L1_error)
- }
- break;
- }
- __Pyx_GOTREF(__pyx_t_7);
- }
- __Pyx_XDECREF_SET(__pyx_v_item, __pyx_t_7);
- __pyx_t_7 = 0;
- __Pyx_INCREF(__pyx_t_3);
- __Pyx_XDECREF_SET(__pyx_v_idx, __pyx_t_3);
- __pyx_t_7 = __Pyx_PyInt_AddObjC(__pyx_t_3, __pyx_int_1, 1, 0, 0); if (unlikely(!__pyx_t_7)) __PYX_ERR(1, 679, __pyx_L1_error)
- __Pyx_GOTREF(__pyx_t_7);
- __Pyx_DECREF(__pyx_t_3);
- __pyx_t_3 = __pyx_t_7;
- __pyx_t_7 = 0;
-
- /* "View.MemoryView":680
- * seen_ellipsis = False
- * for idx, item in enumerate(tup):
- * if item is Ellipsis: # <<<<<<<<<<<<<<
- * if not seen_ellipsis:
- * result.extend([slice(None)] * (ndim - len(tup) + 1))
- */
- __pyx_t_2 = (__pyx_v_item == __pyx_builtin_Ellipsis);
- __pyx_t_1 = (__pyx_t_2 != 0);
- if (__pyx_t_1) {
-
- /* "View.MemoryView":681
- * for idx, item in enumerate(tup):
- * if item is Ellipsis:
- * if not seen_ellipsis: # <<<<<<<<<<<<<<
- * result.extend([slice(None)] * (ndim - len(tup) + 1))
- * seen_ellipsis = True
- */
- __pyx_t_1 = ((!(__pyx_v_seen_ellipsis != 0)) != 0);
- if (__pyx_t_1) {
-
- /* "View.MemoryView":682
- * if item is Ellipsis:
- * if not seen_ellipsis:
- * result.extend([slice(None)] * (ndim - len(tup) + 1)) # <<<<<<<<<<<<<<
- * seen_ellipsis = True
- * else:
- */
- __pyx_t_8 = PyObject_Length(__pyx_v_tup); if (unlikely(__pyx_t_8 == ((Py_ssize_t)-1))) __PYX_ERR(1, 682, __pyx_L1_error)
- __pyx_t_7 = PyList_New(1 * ((((__pyx_v_ndim - __pyx_t_8) + 1)<0) ? 0:((__pyx_v_ndim - __pyx_t_8) + 1))); if (unlikely(!__pyx_t_7)) __PYX_ERR(1, 682, __pyx_L1_error)
- __Pyx_GOTREF(__pyx_t_7);
- { Py_ssize_t __pyx_temp;
- for (__pyx_temp=0; __pyx_temp < ((__pyx_v_ndim - __pyx_t_8) + 1); __pyx_temp++) {
- __Pyx_INCREF(__pyx_slice__16);
- __Pyx_GIVEREF(__pyx_slice__16);
- PyList_SET_ITEM(__pyx_t_7, __pyx_temp, __pyx_slice__16);
- }
- }
- __pyx_t_9 = __Pyx_PyList_Extend(__pyx_v_result, __pyx_t_7); if (unlikely(__pyx_t_9 == ((int)-1))) __PYX_ERR(1, 682, __pyx_L1_error)
- __Pyx_DECREF(__pyx_t_7); __pyx_t_7 = 0;
-
- /* "View.MemoryView":683
- * if not seen_ellipsis:
- * result.extend([slice(None)] * (ndim - len(tup) + 1))
- * seen_ellipsis = True # <<<<<<<<<<<<<<
- * else:
- * result.append(slice(None))
- */
- __pyx_v_seen_ellipsis = 1;
-
- /* "View.MemoryView":681
- * for idx, item in enumerate(tup):
- * if item is Ellipsis:
- * if not seen_ellipsis: # <<<<<<<<<<<<<<
- * result.extend([slice(None)] * (ndim - len(tup) + 1))
- * seen_ellipsis = True
- */
- goto __pyx_L7;
- }
-
- /* "View.MemoryView":685
- * seen_ellipsis = True
- * else:
- * result.append(slice(None)) # <<<<<<<<<<<<<<
- * have_slices = True
- * else:
- */
- /*else*/ {
- __pyx_t_9 = __Pyx_PyList_Append(__pyx_v_result, __pyx_slice__16); if (unlikely(__pyx_t_9 == ((int)-1))) __PYX_ERR(1, 685, __pyx_L1_error)
- }
- __pyx_L7:;
-
- /* "View.MemoryView":686
- * else:
- * result.append(slice(None))
- * have_slices = True # <<<<<<<<<<<<<<
- * else:
- * if not isinstance(item, slice) and not PyIndex_Check(item):
- */
- __pyx_v_have_slices = 1;
-
- /* "View.MemoryView":680
- * seen_ellipsis = False
- * for idx, item in enumerate(tup):
- * if item is Ellipsis: # <<<<<<<<<<<<<<
- * if not seen_ellipsis:
- * result.extend([slice(None)] * (ndim - len(tup) + 1))
- */
- goto __pyx_L6;
- }
-
- /* "View.MemoryView":688
- * have_slices = True
- * else:
- * if not isinstance(item, slice) and not PyIndex_Check(item): # <<<<<<<<<<<<<<
- * raise TypeError("Cannot index with type '%s'" % type(item))
- *
- */
- /*else*/ {
- __pyx_t_2 = PySlice_Check(__pyx_v_item);
- __pyx_t_10 = ((!(__pyx_t_2 != 0)) != 0);
- if (__pyx_t_10) {
- } else {
- __pyx_t_1 = __pyx_t_10;
- goto __pyx_L9_bool_binop_done;
- }
- __pyx_t_10 = ((!(PyIndex_Check(__pyx_v_item) != 0)) != 0);
- __pyx_t_1 = __pyx_t_10;
- __pyx_L9_bool_binop_done:;
- if (unlikely(__pyx_t_1)) {
-
- /* "View.MemoryView":689
- * else:
- * if not isinstance(item, slice) and not PyIndex_Check(item):
- * raise TypeError("Cannot index with type '%s'" % type(item)) # <<<<<<<<<<<<<<
- *
- * have_slices = have_slices or isinstance(item, slice)
- */
- __pyx_t_7 = __Pyx_PyString_FormatSafe(__pyx_kp_s_Cannot_index_with_type_s, ((PyObject *)Py_TYPE(__pyx_v_item))); if (unlikely(!__pyx_t_7)) __PYX_ERR(1, 689, __pyx_L1_error)
- __Pyx_GOTREF(__pyx_t_7);
- __pyx_t_11 = __Pyx_PyObject_CallOneArg(__pyx_builtin_TypeError, __pyx_t_7); if (unlikely(!__pyx_t_11)) __PYX_ERR(1, 689, __pyx_L1_error)
- __Pyx_GOTREF(__pyx_t_11);
- __Pyx_DECREF(__pyx_t_7); __pyx_t_7 = 0;
- __Pyx_Raise(__pyx_t_11, 0, 0, 0);
- __Pyx_DECREF(__pyx_t_11); __pyx_t_11 = 0;
- __PYX_ERR(1, 689, __pyx_L1_error)
-
- /* "View.MemoryView":688
- * have_slices = True
- * else:
- * if not isinstance(item, slice) and not PyIndex_Check(item): # <<<<<<<<<<<<<<
- * raise TypeError("Cannot index with type '%s'" % type(item))
- *
- */
- }
-
- /* "View.MemoryView":691
- * raise TypeError("Cannot index with type '%s'" % type(item))
- *
- * have_slices = have_slices or isinstance(item, slice) # <<<<<<<<<<<<<<
- * result.append(item)
- *
- */
- __pyx_t_10 = (__pyx_v_have_slices != 0);
- if (!__pyx_t_10) {
- } else {
- __pyx_t_1 = __pyx_t_10;
- goto __pyx_L11_bool_binop_done;
- }
- __pyx_t_10 = PySlice_Check(__pyx_v_item);
- __pyx_t_2 = (__pyx_t_10 != 0);
- __pyx_t_1 = __pyx_t_2;
- __pyx_L11_bool_binop_done:;
- __pyx_v_have_slices = __pyx_t_1;
-
- /* "View.MemoryView":692
- *
- * have_slices = have_slices or isinstance(item, slice)
- * result.append(item) # <<<<<<<<<<<<<<
- *
- * nslices = ndim - len(result)
- */
- __pyx_t_9 = __Pyx_PyList_Append(__pyx_v_result, __pyx_v_item); if (unlikely(__pyx_t_9 == ((int)-1))) __PYX_ERR(1, 692, __pyx_L1_error)
- }
- __pyx_L6:;
-
- /* "View.MemoryView":679
- * have_slices = False
- * seen_ellipsis = False
- * for idx, item in enumerate(tup): # <<<<<<<<<<<<<<
- * if item is Ellipsis:
- * if not seen_ellipsis:
- */
- }
- __Pyx_DECREF(__pyx_t_4); __pyx_t_4 = 0;
- __Pyx_DECREF(__pyx_t_3); __pyx_t_3 = 0;
-
- /* "View.MemoryView":694
- * result.append(item)
- *
- * nslices = ndim - len(result) # <<<<<<<<<<<<<<
- * if nslices:
- * result.extend([slice(None)] * nslices)
- */
- __pyx_t_5 = PyList_GET_SIZE(__pyx_v_result); if (unlikely(__pyx_t_5 == ((Py_ssize_t)-1))) __PYX_ERR(1, 694, __pyx_L1_error)
- __pyx_v_nslices = (__pyx_v_ndim - __pyx_t_5);
-
- /* "View.MemoryView":695
- *
- * nslices = ndim - len(result)
- * if nslices: # <<<<<<<<<<<<<<
- * result.extend([slice(None)] * nslices)
- *
- */
- __pyx_t_1 = (__pyx_v_nslices != 0);
- if (__pyx_t_1) {
-
- /* "View.MemoryView":696
- * nslices = ndim - len(result)
- * if nslices:
- * result.extend([slice(None)] * nslices) # <<<<<<<<<<<<<<
- *
- * return have_slices or nslices, tuple(result)
- */
- __pyx_t_3 = PyList_New(1 * ((__pyx_v_nslices<0) ? 0:__pyx_v_nslices)); if (unlikely(!__pyx_t_3)) __PYX_ERR(1, 696, __pyx_L1_error)
- __Pyx_GOTREF(__pyx_t_3);
- { Py_ssize_t __pyx_temp;
- for (__pyx_temp=0; __pyx_temp < __pyx_v_nslices; __pyx_temp++) {
- __Pyx_INCREF(__pyx_slice__16);
- __Pyx_GIVEREF(__pyx_slice__16);
- PyList_SET_ITEM(__pyx_t_3, __pyx_temp, __pyx_slice__16);
- }
- }
- __pyx_t_9 = __Pyx_PyList_Extend(__pyx_v_result, __pyx_t_3); if (unlikely(__pyx_t_9 == ((int)-1))) __PYX_ERR(1, 696, __pyx_L1_error)
- __Pyx_DECREF(__pyx_t_3); __pyx_t_3 = 0;
-
- /* "View.MemoryView":695
- *
- * nslices = ndim - len(result)
- * if nslices: # <<<<<<<<<<<<<<
- * result.extend([slice(None)] * nslices)
- *
- */
- }
-
- /* "View.MemoryView":698
- * result.extend([slice(None)] * nslices)
- *
- * return have_slices or nslices, tuple(result) # <<<<<<<<<<<<<<
- *
- * cdef assert_direct_dimensions(Py_ssize_t *suboffsets, int ndim):
- */
- __Pyx_XDECREF(__pyx_r);
- if (!__pyx_v_have_slices) {
- } else {
- __pyx_t_4 = __Pyx_PyBool_FromLong(__pyx_v_have_slices); if (unlikely(!__pyx_t_4)) __PYX_ERR(1, 698, __pyx_L1_error)
- __Pyx_GOTREF(__pyx_t_4);
- __pyx_t_3 = __pyx_t_4;
- __pyx_t_4 = 0;
- goto __pyx_L14_bool_binop_done;
- }
- __pyx_t_4 = PyInt_FromSsize_t(__pyx_v_nslices); if (unlikely(!__pyx_t_4)) __PYX_ERR(1, 698, __pyx_L1_error)
- __Pyx_GOTREF(__pyx_t_4);
- __pyx_t_3 = __pyx_t_4;
- __pyx_t_4 = 0;
- __pyx_L14_bool_binop_done:;
- __pyx_t_4 = PyList_AsTuple(__pyx_v_result); if (unlikely(!__pyx_t_4)) __PYX_ERR(1, 698, __pyx_L1_error)
- __Pyx_GOTREF(__pyx_t_4);
- __pyx_t_11 = PyTuple_New(2); if (unlikely(!__pyx_t_11)) __PYX_ERR(1, 698, __pyx_L1_error)
- __Pyx_GOTREF(__pyx_t_11);
- __Pyx_GIVEREF(__pyx_t_3);
- PyTuple_SET_ITEM(__pyx_t_11, 0, __pyx_t_3);
- __Pyx_GIVEREF(__pyx_t_4);
- PyTuple_SET_ITEM(__pyx_t_11, 1, __pyx_t_4);
- __pyx_t_3 = 0;
- __pyx_t_4 = 0;
- __pyx_r = ((PyObject*)__pyx_t_11);
- __pyx_t_11 = 0;
- goto __pyx_L0;
-
- /* "View.MemoryView":666
- * return isinstance(o, memoryview)
- *
- * cdef tuple _unellipsify(object index, int ndim): # <<<<<<<<<<<<<<
- * """
- * Replace all ellipses with full slices and fill incomplete indices with
- */
-
- /* function exit code */
- __pyx_L1_error:;
- __Pyx_XDECREF(__pyx_t_3);
- __Pyx_XDECREF(__pyx_t_4);
- __Pyx_XDECREF(__pyx_t_7);
- __Pyx_XDECREF(__pyx_t_11);
- __Pyx_AddTraceback("View.MemoryView._unellipsify", __pyx_clineno, __pyx_lineno, __pyx_filename);
- __pyx_r = 0;
- __pyx_L0:;
- __Pyx_XDECREF(__pyx_v_tup);
- __Pyx_XDECREF(__pyx_v_result);
- __Pyx_XDECREF(__pyx_v_idx);
- __Pyx_XDECREF(__pyx_v_item);
- __Pyx_XGIVEREF(__pyx_r);
- __Pyx_RefNannyFinishContext();
- return __pyx_r;
-}
-
-/* "View.MemoryView":700
- * return have_slices or nslices, tuple(result)
- *
- * cdef assert_direct_dimensions(Py_ssize_t *suboffsets, int ndim): # <<<<<<<<<<<<<<
- * for suboffset in suboffsets[:ndim]:
- * if suboffset >= 0:
- */
-
-static PyObject *assert_direct_dimensions(Py_ssize_t *__pyx_v_suboffsets, int __pyx_v_ndim) {
- Py_ssize_t __pyx_v_suboffset;
- PyObject *__pyx_r = NULL;
- __Pyx_RefNannyDeclarations
- Py_ssize_t *__pyx_t_1;
- Py_ssize_t *__pyx_t_2;
- Py_ssize_t *__pyx_t_3;
- int __pyx_t_4;
- PyObject *__pyx_t_5 = NULL;
- int __pyx_lineno = 0;
- const char *__pyx_filename = NULL;
- int __pyx_clineno = 0;
- __Pyx_RefNannySetupContext("assert_direct_dimensions", 0);
-
- /* "View.MemoryView":701
- *
- * cdef assert_direct_dimensions(Py_ssize_t *suboffsets, int ndim):
- * for suboffset in suboffsets[:ndim]: # <<<<<<<<<<<<<<
- * if suboffset >= 0:
- * raise ValueError("Indirect dimensions not supported")
- */
- __pyx_t_2 = (__pyx_v_suboffsets + __pyx_v_ndim);
- for (__pyx_t_3 = __pyx_v_suboffsets; __pyx_t_3 < __pyx_t_2; __pyx_t_3++) {
- __pyx_t_1 = __pyx_t_3;
- __pyx_v_suboffset = (__pyx_t_1[0]);
-
- /* "View.MemoryView":702
- * cdef assert_direct_dimensions(Py_ssize_t *suboffsets, int ndim):
- * for suboffset in suboffsets[:ndim]:
- * if suboffset >= 0: # <<<<<<<<<<<<<<
- * raise ValueError("Indirect dimensions not supported")
- *
- */
- __pyx_t_4 = ((__pyx_v_suboffset >= 0) != 0);
- if (unlikely(__pyx_t_4)) {
-
- /* "View.MemoryView":703
- * for suboffset in suboffsets[:ndim]:
- * if suboffset >= 0:
- * raise ValueError("Indirect dimensions not supported") # <<<<<<<<<<<<<<
- *
- *
- */
- __pyx_t_5 = __Pyx_PyObject_Call(__pyx_builtin_ValueError, __pyx_tuple__17, NULL); if (unlikely(!__pyx_t_5)) __PYX_ERR(1, 703, __pyx_L1_error)
- __Pyx_GOTREF(__pyx_t_5);
- __Pyx_Raise(__pyx_t_5, 0, 0, 0);
- __Pyx_DECREF(__pyx_t_5); __pyx_t_5 = 0;
- __PYX_ERR(1, 703, __pyx_L1_error)
-
- /* "View.MemoryView":702
- * cdef assert_direct_dimensions(Py_ssize_t *suboffsets, int ndim):
- * for suboffset in suboffsets[:ndim]:
- * if suboffset >= 0: # <<<<<<<<<<<<<<
- * raise ValueError("Indirect dimensions not supported")
- *
- */
- }
- }
-
- /* "View.MemoryView":700
- * return have_slices or nslices, tuple(result)
- *
- * cdef assert_direct_dimensions(Py_ssize_t *suboffsets, int ndim): # <<<<<<<<<<<<<<
- * for suboffset in suboffsets[:ndim]:
- * if suboffset >= 0:
- */
-
- /* function exit code */
- __pyx_r = Py_None; __Pyx_INCREF(Py_None);
- goto __pyx_L0;
- __pyx_L1_error:;
- __Pyx_XDECREF(__pyx_t_5);
- __Pyx_AddTraceback("View.MemoryView.assert_direct_dimensions", __pyx_clineno, __pyx_lineno, __pyx_filename);
- __pyx_r = 0;
- __pyx_L0:;
- __Pyx_XGIVEREF(__pyx_r);
- __Pyx_RefNannyFinishContext();
- return __pyx_r;
-}
-
-/* "View.MemoryView":710
- *
- * @cname('__pyx_memview_slice')
- * cdef memoryview memview_slice(memoryview memview, object indices): # <<<<<<<<<<<<<<
- * cdef int new_ndim = 0, suboffset_dim = -1, dim
- * cdef bint negative_step
- */
-
-static struct __pyx_memoryview_obj *__pyx_memview_slice(struct __pyx_memoryview_obj *__pyx_v_memview, PyObject *__pyx_v_indices) {
- int __pyx_v_new_ndim;
- int __pyx_v_suboffset_dim;
- int __pyx_v_dim;
- __Pyx_memviewslice __pyx_v_src;
- __Pyx_memviewslice __pyx_v_dst;
- __Pyx_memviewslice *__pyx_v_p_src;
- struct __pyx_memoryviewslice_obj *__pyx_v_memviewsliceobj = 0;
- __Pyx_memviewslice *__pyx_v_p_dst;
- int *__pyx_v_p_suboffset_dim;
- Py_ssize_t __pyx_v_start;
- Py_ssize_t __pyx_v_stop;
- Py_ssize_t __pyx_v_step;
- int __pyx_v_have_start;
- int __pyx_v_have_stop;
- int __pyx_v_have_step;
- PyObject *__pyx_v_index = NULL;
- struct __pyx_memoryview_obj *__pyx_r = NULL;
- __Pyx_RefNannyDeclarations
- int __pyx_t_1;
- int __pyx_t_2;
- PyObject *__pyx_t_3 = NULL;
- struct __pyx_memoryview_obj *__pyx_t_4;
- char *__pyx_t_5;
- int __pyx_t_6;
- Py_ssize_t __pyx_t_7;
- PyObject *(*__pyx_t_8)(PyObject *);
- PyObject *__pyx_t_9 = NULL;
- Py_ssize_t __pyx_t_10;
- int __pyx_t_11;
- Py_ssize_t __pyx_t_12;
- int __pyx_lineno = 0;
- const char *__pyx_filename = NULL;
- int __pyx_clineno = 0;
- __Pyx_RefNannySetupContext("memview_slice", 0);
-
- /* "View.MemoryView":711
- * @cname('__pyx_memview_slice')
- * cdef memoryview memview_slice(memoryview memview, object indices):
- * cdef int new_ndim = 0, suboffset_dim = -1, dim # <<<<<<<<<<<<<<
- * cdef bint negative_step
- * cdef __Pyx_memviewslice src, dst
- */
- __pyx_v_new_ndim = 0;
- __pyx_v_suboffset_dim = -1;
-
- /* "View.MemoryView":718
- *
- *
- * memset(&dst, 0, sizeof(dst)) # <<<<<<<<<<<<<<
- *
- * cdef _memoryviewslice memviewsliceobj
- */
- (void)(memset((&__pyx_v_dst), 0, (sizeof(__pyx_v_dst))));
-
- /* "View.MemoryView":722
- * cdef _memoryviewslice memviewsliceobj
- *
- * assert memview.view.ndim > 0 # <<<<<<<<<<<<<<
- *
- * if isinstance(memview, _memoryviewslice):
- */
- #ifndef CYTHON_WITHOUT_ASSERTIONS
- if (unlikely(!Py_OptimizeFlag)) {
- if (unlikely(!((__pyx_v_memview->view.ndim > 0) != 0))) {
- PyErr_SetNone(PyExc_AssertionError);
- __PYX_ERR(1, 722, __pyx_L1_error)
- }
- }
- #endif
-
- /* "View.MemoryView":724
- * assert memview.view.ndim > 0
- *
- * if isinstance(memview, _memoryviewslice): # <<<<<<<<<<<<<<
- * memviewsliceobj = memview
- * p_src = &memviewsliceobj.from_slice
- */
- __pyx_t_1 = __Pyx_TypeCheck(((PyObject *)__pyx_v_memview), __pyx_memoryviewslice_type);
- __pyx_t_2 = (__pyx_t_1 != 0);
- if (__pyx_t_2) {
-
- /* "View.MemoryView":725
- *
- * if isinstance(memview, _memoryviewslice):
- * memviewsliceobj = memview # <<<<<<<<<<<<<<
- * p_src = &memviewsliceobj.from_slice
- * else:
- */
- if (!(likely(((((PyObject *)__pyx_v_memview)) == Py_None) || likely(__Pyx_TypeTest(((PyObject *)__pyx_v_memview), __pyx_memoryviewslice_type))))) __PYX_ERR(1, 725, __pyx_L1_error)
- __pyx_t_3 = ((PyObject *)__pyx_v_memview);
- __Pyx_INCREF(__pyx_t_3);
- __pyx_v_memviewsliceobj = ((struct __pyx_memoryviewslice_obj *)__pyx_t_3);
- __pyx_t_3 = 0;
-
- /* "View.MemoryView":726
- * if isinstance(memview, _memoryviewslice):
- * memviewsliceobj = memview
- * p_src = &memviewsliceobj.from_slice # <<<<<<<<<<<<<<
- * else:
- * slice_copy(memview, &src)
- */
- __pyx_v_p_src = (&__pyx_v_memviewsliceobj->from_slice);
-
- /* "View.MemoryView":724
- * assert memview.view.ndim > 0
- *
- * if isinstance(memview, _memoryviewslice): # <<<<<<<<<<<<<<
- * memviewsliceobj = memview
- * p_src = &memviewsliceobj.from_slice
- */
- goto __pyx_L3;
- }
-
- /* "View.MemoryView":728
- * p_src = &memviewsliceobj.from_slice
- * else:
- * slice_copy(memview, &src) # <<<<<<<<<<<<<<
- * p_src = &src
- *
- */
- /*else*/ {
- __pyx_memoryview_slice_copy(__pyx_v_memview, (&__pyx_v_src));
-
- /* "View.MemoryView":729
- * else:
- * slice_copy(memview, &src)
- * p_src = &src # <<<<<<<<<<<<<<
- *
- *
- */
- __pyx_v_p_src = (&__pyx_v_src);
- }
- __pyx_L3:;
-
- /* "View.MemoryView":735
- *
- *
- * dst.memview = p_src.memview # <<<<<<<<<<<<<<
- * dst.data = p_src.data
- *
- */
- __pyx_t_4 = __pyx_v_p_src->memview;
- __pyx_v_dst.memview = __pyx_t_4;
-
- /* "View.MemoryView":736
- *
- * dst.memview = p_src.memview
- * dst.data = p_src.data # <<<<<<<<<<<<<<
- *
- *
- */
- __pyx_t_5 = __pyx_v_p_src->data;
- __pyx_v_dst.data = __pyx_t_5;
-
- /* "View.MemoryView":741
- *
- *
- * cdef __Pyx_memviewslice *p_dst = &dst # <<<<<<<<<<<<<<
- * cdef int *p_suboffset_dim = &suboffset_dim
- * cdef Py_ssize_t start, stop, step
- */
- __pyx_v_p_dst = (&__pyx_v_dst);
-
- /* "View.MemoryView":742
- *
- * cdef __Pyx_memviewslice *p_dst = &dst
- * cdef int *p_suboffset_dim = &suboffset_dim # <<<<<<<<<<<<<<
- * cdef Py_ssize_t start, stop, step
- * cdef bint have_start, have_stop, have_step
- */
- __pyx_v_p_suboffset_dim = (&__pyx_v_suboffset_dim);
-
- /* "View.MemoryView":746
- * cdef bint have_start, have_stop, have_step
- *
- * for dim, index in enumerate(indices): # <<<<<<<<<<<<<<
- * if PyIndex_Check(index):
- * slice_memviewslice(
- */
- __pyx_t_6 = 0;
- if (likely(PyList_CheckExact(__pyx_v_indices)) || PyTuple_CheckExact(__pyx_v_indices)) {
- __pyx_t_3 = __pyx_v_indices; __Pyx_INCREF(__pyx_t_3); __pyx_t_7 = 0;
- __pyx_t_8 = NULL;
- } else {
- __pyx_t_7 = -1; __pyx_t_3 = PyObject_GetIter(__pyx_v_indices); if (unlikely(!__pyx_t_3)) __PYX_ERR(1, 746, __pyx_L1_error)
- __Pyx_GOTREF(__pyx_t_3);
- __pyx_t_8 = Py_TYPE(__pyx_t_3)->tp_iternext; if (unlikely(!__pyx_t_8)) __PYX_ERR(1, 746, __pyx_L1_error)
- }
- for (;;) {
- if (likely(!__pyx_t_8)) {
- if (likely(PyList_CheckExact(__pyx_t_3))) {
- if (__pyx_t_7 >= PyList_GET_SIZE(__pyx_t_3)) break;
- #if CYTHON_ASSUME_SAFE_MACROS && !CYTHON_AVOID_BORROWED_REFS
- __pyx_t_9 = PyList_GET_ITEM(__pyx_t_3, __pyx_t_7); __Pyx_INCREF(__pyx_t_9); __pyx_t_7++; if (unlikely(0 < 0)) __PYX_ERR(1, 746, __pyx_L1_error)
- #else
- __pyx_t_9 = PySequence_ITEM(__pyx_t_3, __pyx_t_7); __pyx_t_7++; if (unlikely(!__pyx_t_9)) __PYX_ERR(1, 746, __pyx_L1_error)
- __Pyx_GOTREF(__pyx_t_9);
- #endif
- } else {
- if (__pyx_t_7 >= PyTuple_GET_SIZE(__pyx_t_3)) break;
- #if CYTHON_ASSUME_SAFE_MACROS && !CYTHON_AVOID_BORROWED_REFS
- __pyx_t_9 = PyTuple_GET_ITEM(__pyx_t_3, __pyx_t_7); __Pyx_INCREF(__pyx_t_9); __pyx_t_7++; if (unlikely(0 < 0)) __PYX_ERR(1, 746, __pyx_L1_error)
- #else
- __pyx_t_9 = PySequence_ITEM(__pyx_t_3, __pyx_t_7); __pyx_t_7++; if (unlikely(!__pyx_t_9)) __PYX_ERR(1, 746, __pyx_L1_error)
- __Pyx_GOTREF(__pyx_t_9);
- #endif
- }
- } else {
- __pyx_t_9 = __pyx_t_8(__pyx_t_3);
- if (unlikely(!__pyx_t_9)) {
- PyObject* exc_type = PyErr_Occurred();
- if (exc_type) {
- if (likely(__Pyx_PyErr_GivenExceptionMatches(exc_type, PyExc_StopIteration))) PyErr_Clear();
- else __PYX_ERR(1, 746, __pyx_L1_error)
- }
- break;
- }
- __Pyx_GOTREF(__pyx_t_9);
- }
- __Pyx_XDECREF_SET(__pyx_v_index, __pyx_t_9);
- __pyx_t_9 = 0;
- __pyx_v_dim = __pyx_t_6;
- __pyx_t_6 = (__pyx_t_6 + 1);
-
- /* "View.MemoryView":747
- *
- * for dim, index in enumerate(indices):
- * if PyIndex_Check(index): # <<<<<<<<<<<<<<
- * slice_memviewslice(
- * p_dst, p_src.shape[dim], p_src.strides[dim], p_src.suboffsets[dim],
- */
- __pyx_t_2 = (PyIndex_Check(__pyx_v_index) != 0);
- if (__pyx_t_2) {
-
- /* "View.MemoryView":751
- * p_dst, p_src.shape[dim], p_src.strides[dim], p_src.suboffsets[dim],
- * dim, new_ndim, p_suboffset_dim,
- * index, 0, 0, # start, stop, step # <<<<<<<<<<<<<<
- * 0, 0, 0, # have_{start,stop,step}
- * False)
- */
- __pyx_t_10 = __Pyx_PyIndex_AsSsize_t(__pyx_v_index); if (unlikely((__pyx_t_10 == (Py_ssize_t)-1) && PyErr_Occurred())) __PYX_ERR(1, 751, __pyx_L1_error)
-
- /* "View.MemoryView":748
- * for dim, index in enumerate(indices):
- * if PyIndex_Check(index):
- * slice_memviewslice( # <<<<<<<<<<<<<<
- * p_dst, p_src.shape[dim], p_src.strides[dim], p_src.suboffsets[dim],
- * dim, new_ndim, p_suboffset_dim,
- */
- __pyx_t_11 = __pyx_memoryview_slice_memviewslice(__pyx_v_p_dst, (__pyx_v_p_src->shape[__pyx_v_dim]), (__pyx_v_p_src->strides[__pyx_v_dim]), (__pyx_v_p_src->suboffsets[__pyx_v_dim]), __pyx_v_dim, __pyx_v_new_ndim, __pyx_v_p_suboffset_dim, __pyx_t_10, 0, 0, 0, 0, 0, 0); if (unlikely(__pyx_t_11 == ((int)-1))) __PYX_ERR(1, 748, __pyx_L1_error)
-
- /* "View.MemoryView":747
- *
- * for dim, index in enumerate(indices):
- * if PyIndex_Check(index): # <<<<<<<<<<<<<<
- * slice_memviewslice(
- * p_dst, p_src.shape[dim], p_src.strides[dim], p_src.suboffsets[dim],
- */
- goto __pyx_L6;
- }
-
- /* "View.MemoryView":754
- * 0, 0, 0, # have_{start,stop,step}
- * False)
- * elif index is None: # <<<<<<<<<<<<<<
- * p_dst.shape[new_ndim] = 1
- * p_dst.strides[new_ndim] = 0
- */
- __pyx_t_2 = (__pyx_v_index == Py_None);
- __pyx_t_1 = (__pyx_t_2 != 0);
- if (__pyx_t_1) {
-
- /* "View.MemoryView":755
- * False)
- * elif index is None:
- * p_dst.shape[new_ndim] = 1 # <<<<<<<<<<<<<<
- * p_dst.strides[new_ndim] = 0
- * p_dst.suboffsets[new_ndim] = -1
- */
- (__pyx_v_p_dst->shape[__pyx_v_new_ndim]) = 1;
-
- /* "View.MemoryView":756
- * elif index is None:
- * p_dst.shape[new_ndim] = 1
- * p_dst.strides[new_ndim] = 0 # <<<<<<<<<<<<<<
- * p_dst.suboffsets[new_ndim] = -1
- * new_ndim += 1
- */
- (__pyx_v_p_dst->strides[__pyx_v_new_ndim]) = 0;
-
- /* "View.MemoryView":757
- * p_dst.shape[new_ndim] = 1
- * p_dst.strides[new_ndim] = 0
- * p_dst.suboffsets[new_ndim] = -1 # <<<<<<<<<<<<<<
- * new_ndim += 1
- * else:
- */
- (__pyx_v_p_dst->suboffsets[__pyx_v_new_ndim]) = -1L;
-
- /* "View.MemoryView":758
- * p_dst.strides[new_ndim] = 0
- * p_dst.suboffsets[new_ndim] = -1
- * new_ndim += 1 # <<<<<<<<<<<<<<
- * else:
- * start = index.start or 0
- */
- __pyx_v_new_ndim = (__pyx_v_new_ndim + 1);
-
- /* "View.MemoryView":754
- * 0, 0, 0, # have_{start,stop,step}
- * False)
- * elif index is None: # <<<<<<<<<<<<<<
- * p_dst.shape[new_ndim] = 1
- * p_dst.strides[new_ndim] = 0
- */
- goto __pyx_L6;
- }
-
- /* "View.MemoryView":760
- * new_ndim += 1
- * else:
- * start = index.start or 0 # <<<<<<<<<<<<<<
- * stop = index.stop or 0
- * step = index.step or 0
- */
- /*else*/ {
- __pyx_t_9 = __Pyx_PyObject_GetAttrStr(__pyx_v_index, __pyx_n_s_start); if (unlikely(!__pyx_t_9)) __PYX_ERR(1, 760, __pyx_L1_error)
- __Pyx_GOTREF(__pyx_t_9);
- __pyx_t_1 = __Pyx_PyObject_IsTrue(__pyx_t_9); if (unlikely(__pyx_t_1 < 0)) __PYX_ERR(1, 760, __pyx_L1_error)
- if (!__pyx_t_1) {
- __Pyx_DECREF(__pyx_t_9); __pyx_t_9 = 0;
- } else {
- __pyx_t_12 = __Pyx_PyIndex_AsSsize_t(__pyx_t_9); if (unlikely((__pyx_t_12 == (Py_ssize_t)-1) && PyErr_Occurred())) __PYX_ERR(1, 760, __pyx_L1_error)
- __pyx_t_10 = __pyx_t_12;
- __Pyx_DECREF(__pyx_t_9); __pyx_t_9 = 0;
- goto __pyx_L7_bool_binop_done;
- }
- __pyx_t_10 = 0;
- __pyx_L7_bool_binop_done:;
- __pyx_v_start = __pyx_t_10;
-
- /* "View.MemoryView":761
- * else:
- * start = index.start or 0
- * stop = index.stop or 0 # <<<<<<<<<<<<<<
- * step = index.step or 0
- *
- */
- __pyx_t_9 = __Pyx_PyObject_GetAttrStr(__pyx_v_index, __pyx_n_s_stop); if (unlikely(!__pyx_t_9)) __PYX_ERR(1, 761, __pyx_L1_error)
- __Pyx_GOTREF(__pyx_t_9);
- __pyx_t_1 = __Pyx_PyObject_IsTrue(__pyx_t_9); if (unlikely(__pyx_t_1 < 0)) __PYX_ERR(1, 761, __pyx_L1_error)
- if (!__pyx_t_1) {
- __Pyx_DECREF(__pyx_t_9); __pyx_t_9 = 0;
- } else {
- __pyx_t_12 = __Pyx_PyIndex_AsSsize_t(__pyx_t_9); if (unlikely((__pyx_t_12 == (Py_ssize_t)-1) && PyErr_Occurred())) __PYX_ERR(1, 761, __pyx_L1_error)
- __pyx_t_10 = __pyx_t_12;
- __Pyx_DECREF(__pyx_t_9); __pyx_t_9 = 0;
- goto __pyx_L9_bool_binop_done;
- }
- __pyx_t_10 = 0;
- __pyx_L9_bool_binop_done:;
- __pyx_v_stop = __pyx_t_10;
-
- /* "View.MemoryView":762
- * start = index.start or 0
- * stop = index.stop or 0
- * step = index.step or 0 # <<<<<<<<<<<<<<
- *
- * have_start = index.start is not None
- */
- __pyx_t_9 = __Pyx_PyObject_GetAttrStr(__pyx_v_index, __pyx_n_s_step); if (unlikely(!__pyx_t_9)) __PYX_ERR(1, 762, __pyx_L1_error)
- __Pyx_GOTREF(__pyx_t_9);
- __pyx_t_1 = __Pyx_PyObject_IsTrue(__pyx_t_9); if (unlikely(__pyx_t_1 < 0)) __PYX_ERR(1, 762, __pyx_L1_error)
- if (!__pyx_t_1) {
- __Pyx_DECREF(__pyx_t_9); __pyx_t_9 = 0;
- } else {
- __pyx_t_12 = __Pyx_PyIndex_AsSsize_t(__pyx_t_9); if (unlikely((__pyx_t_12 == (Py_ssize_t)-1) && PyErr_Occurred())) __PYX_ERR(1, 762, __pyx_L1_error)
- __pyx_t_10 = __pyx_t_12;
- __Pyx_DECREF(__pyx_t_9); __pyx_t_9 = 0;
- goto __pyx_L11_bool_binop_done;
- }
- __pyx_t_10 = 0;
- __pyx_L11_bool_binop_done:;
- __pyx_v_step = __pyx_t_10;
-
- /* "View.MemoryView":764
- * step = index.step or 0
- *
- * have_start = index.start is not None # <<<<<<<<<<<<<<
- * have_stop = index.stop is not None
- * have_step = index.step is not None
- */
- __pyx_t_9 = __Pyx_PyObject_GetAttrStr(__pyx_v_index, __pyx_n_s_start); if (unlikely(!__pyx_t_9)) __PYX_ERR(1, 764, __pyx_L1_error)
- __Pyx_GOTREF(__pyx_t_9);
- __pyx_t_1 = (__pyx_t_9 != Py_None);
- __Pyx_DECREF(__pyx_t_9); __pyx_t_9 = 0;
- __pyx_v_have_start = __pyx_t_1;
-
- /* "View.MemoryView":765
- *
- * have_start = index.start is not None
- * have_stop = index.stop is not None # <<<<<<<<<<<<<<
- * have_step = index.step is not None
- *
- */
- __pyx_t_9 = __Pyx_PyObject_GetAttrStr(__pyx_v_index, __pyx_n_s_stop); if (unlikely(!__pyx_t_9)) __PYX_ERR(1, 765, __pyx_L1_error)
- __Pyx_GOTREF(__pyx_t_9);
- __pyx_t_1 = (__pyx_t_9 != Py_None);
- __Pyx_DECREF(__pyx_t_9); __pyx_t_9 = 0;
- __pyx_v_have_stop = __pyx_t_1;
-
- /* "View.MemoryView":766
- * have_start = index.start is not None
- * have_stop = index.stop is not None
- * have_step = index.step is not None # <<<<<<<<<<<<<<
- *
- * slice_memviewslice(
- */
- __pyx_t_9 = __Pyx_PyObject_GetAttrStr(__pyx_v_index, __pyx_n_s_step); if (unlikely(!__pyx_t_9)) __PYX_ERR(1, 766, __pyx_L1_error)
- __Pyx_GOTREF(__pyx_t_9);
- __pyx_t_1 = (__pyx_t_9 != Py_None);
- __Pyx_DECREF(__pyx_t_9); __pyx_t_9 = 0;
- __pyx_v_have_step = __pyx_t_1;
-
- /* "View.MemoryView":768
- * have_step = index.step is not None
- *
- * slice_memviewslice( # <<<<<<<<<<<<<<
- * p_dst, p_src.shape[dim], p_src.strides[dim], p_src.suboffsets[dim],
- * dim, new_ndim, p_suboffset_dim,
- */
- __pyx_t_11 = __pyx_memoryview_slice_memviewslice(__pyx_v_p_dst, (__pyx_v_p_src->shape[__pyx_v_dim]), (__pyx_v_p_src->strides[__pyx_v_dim]), (__pyx_v_p_src->suboffsets[__pyx_v_dim]), __pyx_v_dim, __pyx_v_new_ndim, __pyx_v_p_suboffset_dim, __pyx_v_start, __pyx_v_stop, __pyx_v_step, __pyx_v_have_start, __pyx_v_have_stop, __pyx_v_have_step, 1); if (unlikely(__pyx_t_11 == ((int)-1))) __PYX_ERR(1, 768, __pyx_L1_error)
-
- /* "View.MemoryView":774
- * have_start, have_stop, have_step,
- * True)
- * new_ndim += 1 # <<<<<<<<<<<<<<
- *
- * if isinstance(memview, _memoryviewslice):
- */
- __pyx_v_new_ndim = (__pyx_v_new_ndim + 1);
- }
- __pyx_L6:;
-
- /* "View.MemoryView":746
- * cdef bint have_start, have_stop, have_step
- *
- * for dim, index in enumerate(indices): # <<<<<<<<<<<<<<
- * if PyIndex_Check(index):
- * slice_memviewslice(
- */
- }
- __Pyx_DECREF(__pyx_t_3); __pyx_t_3 = 0;
-
- /* "View.MemoryView":776
- * new_ndim += 1
- *
- * if isinstance(memview, _memoryviewslice): # <<<<<<<<<<<<<<
- * return memoryview_fromslice(dst, new_ndim,
- * memviewsliceobj.to_object_func,
- */
- __pyx_t_1 = __Pyx_TypeCheck(((PyObject *)__pyx_v_memview), __pyx_memoryviewslice_type);
- __pyx_t_2 = (__pyx_t_1 != 0);
- if (__pyx_t_2) {
-
- /* "View.MemoryView":777
- *
- * if isinstance(memview, _memoryviewslice):
- * return memoryview_fromslice(dst, new_ndim, # <<<<<<<<<<<<<<
- * memviewsliceobj.to_object_func,
- * memviewsliceobj.to_dtype_func,
- */
- __Pyx_XDECREF(((PyObject *)__pyx_r));
-
- /* "View.MemoryView":778
- * if isinstance(memview, _memoryviewslice):
- * return memoryview_fromslice(dst, new_ndim,
- * memviewsliceobj.to_object_func, # <<<<<<<<<<<<<<
- * memviewsliceobj.to_dtype_func,
- * memview.dtype_is_object)
- */
- if (unlikely(!__pyx_v_memviewsliceobj)) { __Pyx_RaiseUnboundLocalError("memviewsliceobj"); __PYX_ERR(1, 778, __pyx_L1_error) }
-
- /* "View.MemoryView":779
- * return memoryview_fromslice(dst, new_ndim,
- * memviewsliceobj.to_object_func,
- * memviewsliceobj.to_dtype_func, # <<<<<<<<<<<<<<
- * memview.dtype_is_object)
- * else:
- */
- if (unlikely(!__pyx_v_memviewsliceobj)) { __Pyx_RaiseUnboundLocalError("memviewsliceobj"); __PYX_ERR(1, 779, __pyx_L1_error) }
-
- /* "View.MemoryView":777
- *
- * if isinstance(memview, _memoryviewslice):
- * return memoryview_fromslice(dst, new_ndim, # <<<<<<<<<<<<<<
- * memviewsliceobj.to_object_func,
- * memviewsliceobj.to_dtype_func,
- */
- __pyx_t_3 = __pyx_memoryview_fromslice(__pyx_v_dst, __pyx_v_new_ndim, __pyx_v_memviewsliceobj->to_object_func, __pyx_v_memviewsliceobj->to_dtype_func, __pyx_v_memview->dtype_is_object); if (unlikely(!__pyx_t_3)) __PYX_ERR(1, 777, __pyx_L1_error)
- __Pyx_GOTREF(__pyx_t_3);
- if (!(likely(((__pyx_t_3) == Py_None) || likely(__Pyx_TypeTest(__pyx_t_3, __pyx_memoryview_type))))) __PYX_ERR(1, 777, __pyx_L1_error)
- __pyx_r = ((struct __pyx_memoryview_obj *)__pyx_t_3);
- __pyx_t_3 = 0;
- goto __pyx_L0;
-
- /* "View.MemoryView":776
- * new_ndim += 1
- *
- * if isinstance(memview, _memoryviewslice): # <<<<<<<<<<<<<<
- * return memoryview_fromslice(dst, new_ndim,
- * memviewsliceobj.to_object_func,
- */
- }
-
- /* "View.MemoryView":782
- * memview.dtype_is_object)
- * else:
- * return memoryview_fromslice(dst, new_ndim, NULL, NULL, # <<<<<<<<<<<<<<
- * memview.dtype_is_object)
- *
- */
- /*else*/ {
- __Pyx_XDECREF(((PyObject *)__pyx_r));
-
- /* "View.MemoryView":783
- * else:
- * return memoryview_fromslice(dst, new_ndim, NULL, NULL,
- * memview.dtype_is_object) # <<<<<<<<<<<<<<
- *
- *
- */
- __pyx_t_3 = __pyx_memoryview_fromslice(__pyx_v_dst, __pyx_v_new_ndim, NULL, NULL, __pyx_v_memview->dtype_is_object); if (unlikely(!__pyx_t_3)) __PYX_ERR(1, 782, __pyx_L1_error)
- __Pyx_GOTREF(__pyx_t_3);
-
- /* "View.MemoryView":782
- * memview.dtype_is_object)
- * else:
- * return memoryview_fromslice(dst, new_ndim, NULL, NULL, # <<<<<<<<<<<<<<
- * memview.dtype_is_object)
- *
- */
- if (!(likely(((__pyx_t_3) == Py_None) || likely(__Pyx_TypeTest(__pyx_t_3, __pyx_memoryview_type))))) __PYX_ERR(1, 782, __pyx_L1_error)
- __pyx_r = ((struct __pyx_memoryview_obj *)__pyx_t_3);
- __pyx_t_3 = 0;
- goto __pyx_L0;
- }
-
- /* "View.MemoryView":710
- *
- * @cname('__pyx_memview_slice')
- * cdef memoryview memview_slice(memoryview memview, object indices): # <<<<<<<<<<<<<<
- * cdef int new_ndim = 0, suboffset_dim = -1, dim
- * cdef bint negative_step
- */
-
- /* function exit code */
- __pyx_L1_error:;
- __Pyx_XDECREF(__pyx_t_3);
- __Pyx_XDECREF(__pyx_t_9);
- __Pyx_AddTraceback("View.MemoryView.memview_slice", __pyx_clineno, __pyx_lineno, __pyx_filename);
- __pyx_r = 0;
- __pyx_L0:;
- __Pyx_XDECREF((PyObject *)__pyx_v_memviewsliceobj);
- __Pyx_XDECREF(__pyx_v_index);
- __Pyx_XGIVEREF((PyObject *)__pyx_r);
- __Pyx_RefNannyFinishContext();
- return __pyx_r;
-}
-
-/* "View.MemoryView":807
- *
- * @cname('__pyx_memoryview_slice_memviewslice')
- * cdef int slice_memviewslice( # <<<<<<<<<<<<<<
- * __Pyx_memviewslice *dst,
- * Py_ssize_t shape, Py_ssize_t stride, Py_ssize_t suboffset,
- */
-
-static int __pyx_memoryview_slice_memviewslice(__Pyx_memviewslice *__pyx_v_dst, Py_ssize_t __pyx_v_shape, Py_ssize_t __pyx_v_stride, Py_ssize_t __pyx_v_suboffset, int __pyx_v_dim, int __pyx_v_new_ndim, int *__pyx_v_suboffset_dim, Py_ssize_t __pyx_v_start, Py_ssize_t __pyx_v_stop, Py_ssize_t __pyx_v_step, int __pyx_v_have_start, int __pyx_v_have_stop, int __pyx_v_have_step, int __pyx_v_is_slice) {
- Py_ssize_t __pyx_v_new_shape;
- int __pyx_v_negative_step;
- int __pyx_r;
- int __pyx_t_1;
- int __pyx_t_2;
- int __pyx_t_3;
- int __pyx_lineno = 0;
- const char *__pyx_filename = NULL;
- int __pyx_clineno = 0;
-
- /* "View.MemoryView":827
- * cdef bint negative_step
- *
- * if not is_slice: # <<<<<<<<<<<<<<
- *
- * if start < 0:
- */
- __pyx_t_1 = ((!(__pyx_v_is_slice != 0)) != 0);
- if (__pyx_t_1) {
-
- /* "View.MemoryView":829
- * if not is_slice:
- *
- * if start < 0: # <<<<<<<<<<<<<<
- * start += shape
- * if not 0 <= start < shape:
- */
- __pyx_t_1 = ((__pyx_v_start < 0) != 0);
- if (__pyx_t_1) {
-
- /* "View.MemoryView":830
- *
- * if start < 0:
- * start += shape # <<<<<<<<<<<<<<
- * if not 0 <= start < shape:
- * _err_dim(IndexError, "Index out of bounds (axis %d)", dim)
- */
- __pyx_v_start = (__pyx_v_start + __pyx_v_shape);
-
- /* "View.MemoryView":829
- * if not is_slice:
- *
- * if start < 0: # <<<<<<<<<<<<<<
- * start += shape
- * if not 0 <= start < shape:
- */
- }
-
- /* "View.MemoryView":831
- * if start < 0:
- * start += shape
- * if not 0 <= start < shape: # <<<<<<<<<<<<<<
- * _err_dim(IndexError, "Index out of bounds (axis %d)", dim)
- * else:
- */
- __pyx_t_1 = (0 <= __pyx_v_start);
- if (__pyx_t_1) {
- __pyx_t_1 = (__pyx_v_start < __pyx_v_shape);
- }
- __pyx_t_2 = ((!(__pyx_t_1 != 0)) != 0);
- if (__pyx_t_2) {
-
- /* "View.MemoryView":832
- * start += shape
- * if not 0 <= start < shape:
- * _err_dim(IndexError, "Index out of bounds (axis %d)", dim) # <<<<<<<<<<<<<<
- * else:
- *
- */
- __pyx_t_3 = __pyx_memoryview_err_dim(__pyx_builtin_IndexError, ((char *)"Index out of bounds (axis %d)"), __pyx_v_dim); if (unlikely(__pyx_t_3 == ((int)-1))) __PYX_ERR(1, 832, __pyx_L1_error)
-
- /* "View.MemoryView":831
- * if start < 0:
- * start += shape
- * if not 0 <= start < shape: # <<<<<<<<<<<<<<
- * _err_dim(IndexError, "Index out of bounds (axis %d)", dim)
- * else:
- */
- }
-
- /* "View.MemoryView":827
- * cdef bint negative_step
- *
- * if not is_slice: # <<<<<<<<<<<<<<
- *
- * if start < 0:
- */
- goto __pyx_L3;
- }
-
- /* "View.MemoryView":835
- * else:
- *
- * negative_step = have_step != 0 and step < 0 # <<<<<<<<<<<<<<
- *
- * if have_step and step == 0:
- */
- /*else*/ {
- __pyx_t_1 = ((__pyx_v_have_step != 0) != 0);
- if (__pyx_t_1) {
- } else {
- __pyx_t_2 = __pyx_t_1;
- goto __pyx_L6_bool_binop_done;
- }
- __pyx_t_1 = ((__pyx_v_step < 0) != 0);
- __pyx_t_2 = __pyx_t_1;
- __pyx_L6_bool_binop_done:;
- __pyx_v_negative_step = __pyx_t_2;
-
- /* "View.MemoryView":837
- * negative_step = have_step != 0 and step < 0
- *
- * if have_step and step == 0: # <<<<<<<<<<<<<<
- * _err_dim(ValueError, "Step may not be zero (axis %d)", dim)
- *
- */
- __pyx_t_1 = (__pyx_v_have_step != 0);
- if (__pyx_t_1) {
- } else {
- __pyx_t_2 = __pyx_t_1;
- goto __pyx_L9_bool_binop_done;
- }
- __pyx_t_1 = ((__pyx_v_step == 0) != 0);
- __pyx_t_2 = __pyx_t_1;
- __pyx_L9_bool_binop_done:;
- if (__pyx_t_2) {
-
- /* "View.MemoryView":838
- *
- * if have_step and step == 0:
- * _err_dim(ValueError, "Step may not be zero (axis %d)", dim) # <<<<<<<<<<<<<<
- *
- *
- */
- __pyx_t_3 = __pyx_memoryview_err_dim(__pyx_builtin_ValueError, ((char *)"Step may not be zero (axis %d)"), __pyx_v_dim); if (unlikely(__pyx_t_3 == ((int)-1))) __PYX_ERR(1, 838, __pyx_L1_error)
-
- /* "View.MemoryView":837
- * negative_step = have_step != 0 and step < 0
- *
- * if have_step and step == 0: # <<<<<<<<<<<<<<
- * _err_dim(ValueError, "Step may not be zero (axis %d)", dim)
- *
- */
- }
-
- /* "View.MemoryView":841
- *
- *
- * if have_start: # <<<<<<<<<<<<<<
- * if start < 0:
- * start += shape
- */
- __pyx_t_2 = (__pyx_v_have_start != 0);
- if (__pyx_t_2) {
-
- /* "View.MemoryView":842
- *
- * if have_start:
- * if start < 0: # <<<<<<<<<<<<<<
- * start += shape
- * if start < 0:
- */
- __pyx_t_2 = ((__pyx_v_start < 0) != 0);
- if (__pyx_t_2) {
-
- /* "View.MemoryView":843
- * if have_start:
- * if start < 0:
- * start += shape # <<<<<<<<<<<<<<
- * if start < 0:
- * start = 0
- */
- __pyx_v_start = (__pyx_v_start + __pyx_v_shape);
-
- /* "View.MemoryView":844
- * if start < 0:
- * start += shape
- * if start < 0: # <<<<<<<<<<<<<<
- * start = 0
- * elif start >= shape:
- */
- __pyx_t_2 = ((__pyx_v_start < 0) != 0);
- if (__pyx_t_2) {
-
- /* "View.MemoryView":845
- * start += shape
- * if start < 0:
- * start = 0 # <<<<<<<<<<<<<<
- * elif start >= shape:
- * if negative_step:
- */
- __pyx_v_start = 0;
-
- /* "View.MemoryView":844
- * if start < 0:
- * start += shape
- * if start < 0: # <<<<<<<<<<<<<<
- * start = 0
- * elif start >= shape:
- */
- }
-
- /* "View.MemoryView":842
- *
- * if have_start:
- * if start < 0: # <<<<<<<<<<<<<<
- * start += shape
- * if start < 0:
- */
- goto __pyx_L12;
- }
-
- /* "View.MemoryView":846
- * if start < 0:
- * start = 0
- * elif start >= shape: # <<<<<<<<<<<<<<
- * if negative_step:
- * start = shape - 1
- */
- __pyx_t_2 = ((__pyx_v_start >= __pyx_v_shape) != 0);
- if (__pyx_t_2) {
-
- /* "View.MemoryView":847
- * start = 0
- * elif start >= shape:
- * if negative_step: # <<<<<<<<<<<<<<
- * start = shape - 1
- * else:
- */
- __pyx_t_2 = (__pyx_v_negative_step != 0);
- if (__pyx_t_2) {
-
- /* "View.MemoryView":848
- * elif start >= shape:
- * if negative_step:
- * start = shape - 1 # <<<<<<<<<<<<<<
- * else:
- * start = shape
- */
- __pyx_v_start = (__pyx_v_shape - 1);
-
- /* "View.MemoryView":847
- * start = 0
- * elif start >= shape:
- * if negative_step: # <<<<<<<<<<<<<<
- * start = shape - 1
- * else:
- */
- goto __pyx_L14;
- }
-
- /* "View.MemoryView":850
- * start = shape - 1
- * else:
- * start = shape # <<<<<<<<<<<<<<
- * else:
- * if negative_step:
- */
- /*else*/ {
- __pyx_v_start = __pyx_v_shape;
- }
- __pyx_L14:;
-
- /* "View.MemoryView":846
- * if start < 0:
- * start = 0
- * elif start >= shape: # <<<<<<<<<<<<<<
- * if negative_step:
- * start = shape - 1
- */
- }
- __pyx_L12:;
-
- /* "View.MemoryView":841
- *
- *
- * if have_start: # <<<<<<<<<<<<<<
- * if start < 0:
- * start += shape
- */
- goto __pyx_L11;
- }
-
- /* "View.MemoryView":852
- * start = shape
- * else:
- * if negative_step: # <<<<<<<<<<<<<<
- * start = shape - 1
- * else:
- */
- /*else*/ {
- __pyx_t_2 = (__pyx_v_negative_step != 0);
- if (__pyx_t_2) {
-
- /* "View.MemoryView":853
- * else:
- * if negative_step:
- * start = shape - 1 # <<<<<<<<<<<<<<
- * else:
- * start = 0
- */
- __pyx_v_start = (__pyx_v_shape - 1);
-
- /* "View.MemoryView":852
- * start = shape
- * else:
- * if negative_step: # <<<<<<<<<<<<<<
- * start = shape - 1
- * else:
- */
- goto __pyx_L15;
- }
-
- /* "View.MemoryView":855
- * start = shape - 1
- * else:
- * start = 0 # <<<<<<<<<<<<<<
- *
- * if have_stop:
- */
- /*else*/ {
- __pyx_v_start = 0;
- }
- __pyx_L15:;
- }
- __pyx_L11:;
-
- /* "View.MemoryView":857
- * start = 0
- *
- * if have_stop: # <<<<<<<<<<<<<<
- * if stop < 0:
- * stop += shape
- */
- __pyx_t_2 = (__pyx_v_have_stop != 0);
- if (__pyx_t_2) {
-
- /* "View.MemoryView":858
- *
- * if have_stop:
- * if stop < 0: # <<<<<<<<<<<<<<
- * stop += shape
- * if stop < 0:
- */
- __pyx_t_2 = ((__pyx_v_stop < 0) != 0);
- if (__pyx_t_2) {
-
- /* "View.MemoryView":859
- * if have_stop:
- * if stop < 0:
- * stop += shape # <<<<<<<<<<<<<<
- * if stop < 0:
- * stop = 0
- */
- __pyx_v_stop = (__pyx_v_stop + __pyx_v_shape);
-
- /* "View.MemoryView":860
- * if stop < 0:
- * stop += shape
- * if stop < 0: # <<<<<<<<<<<<<<
- * stop = 0
- * elif stop > shape:
- */
- __pyx_t_2 = ((__pyx_v_stop < 0) != 0);
- if (__pyx_t_2) {
-
- /* "View.MemoryView":861
- * stop += shape
- * if stop < 0:
- * stop = 0 # <<<<<<<<<<<<<<
- * elif stop > shape:
- * stop = shape
- */
- __pyx_v_stop = 0;
-
- /* "View.MemoryView":860
- * if stop < 0:
- * stop += shape
- * if stop < 0: # <<<<<<<<<<<<<<
- * stop = 0
- * elif stop > shape:
- */
- }
-
- /* "View.MemoryView":858
- *
- * if have_stop:
- * if stop < 0: # <<<<<<<<<<<<<<
- * stop += shape
- * if stop < 0:
- */
- goto __pyx_L17;
- }
-
- /* "View.MemoryView":862
- * if stop < 0:
- * stop = 0
- * elif stop > shape: # <<<<<<<<<<<<<<
- * stop = shape
- * else:
- */
- __pyx_t_2 = ((__pyx_v_stop > __pyx_v_shape) != 0);
- if (__pyx_t_2) {
-
- /* "View.MemoryView":863
- * stop = 0
- * elif stop > shape:
- * stop = shape # <<<<<<<<<<<<<<
- * else:
- * if negative_step:
- */
- __pyx_v_stop = __pyx_v_shape;
-
- /* "View.MemoryView":862
- * if stop < 0:
- * stop = 0
- * elif stop > shape: # <<<<<<<<<<<<<<
- * stop = shape
- * else:
- */
- }
- __pyx_L17:;
-
- /* "View.MemoryView":857
- * start = 0
- *
- * if have_stop: # <<<<<<<<<<<<<<
- * if stop < 0:
- * stop += shape
- */
- goto __pyx_L16;
- }
-
- /* "View.MemoryView":865
- * stop = shape
- * else:
- * if negative_step: # <<<<<<<<<<<<<<
- * stop = -1
- * else:
- */
- /*else*/ {
- __pyx_t_2 = (__pyx_v_negative_step != 0);
- if (__pyx_t_2) {
-
- /* "View.MemoryView":866
- * else:
- * if negative_step:
- * stop = -1 # <<<<<<<<<<<<<<
- * else:
- * stop = shape
- */
- __pyx_v_stop = -1L;
-
- /* "View.MemoryView":865
- * stop = shape
- * else:
- * if negative_step: # <<<<<<<<<<<<<<
- * stop = -1
- * else:
- */
- goto __pyx_L19;
- }
-
- /* "View.MemoryView":868
- * stop = -1
- * else:
- * stop = shape # <<<<<<<<<<<<<<
- *
- * if not have_step:
- */
- /*else*/ {
- __pyx_v_stop = __pyx_v_shape;
- }
- __pyx_L19:;
- }
- __pyx_L16:;
-
- /* "View.MemoryView":870
- * stop = shape
- *
- * if not have_step: # <<<<<<<<<<<<<<
- * step = 1
- *
- */
- __pyx_t_2 = ((!(__pyx_v_have_step != 0)) != 0);
- if (__pyx_t_2) {
-
- /* "View.MemoryView":871
- *
- * if not have_step:
- * step = 1 # <<<<<<<<<<<<<<
- *
- *
- */
- __pyx_v_step = 1;
-
- /* "View.MemoryView":870
- * stop = shape
- *
- * if not have_step: # <<<<<<<<<<<<<<
- * step = 1
- *
- */
- }
-
- /* "View.MemoryView":875
- *
- * with cython.cdivision(True):
- * new_shape = (stop - start) // step # <<<<<<<<<<<<<<
- *
- * if (stop - start) - step * new_shape:
- */
- __pyx_v_new_shape = ((__pyx_v_stop - __pyx_v_start) / __pyx_v_step);
-
- /* "View.MemoryView":877
- * new_shape = (stop - start) // step
- *
- * if (stop - start) - step * new_shape: # <<<<<<<<<<<<<<
- * new_shape += 1
- *
- */
- __pyx_t_2 = (((__pyx_v_stop - __pyx_v_start) - (__pyx_v_step * __pyx_v_new_shape)) != 0);
- if (__pyx_t_2) {
-
- /* "View.MemoryView":878
- *
- * if (stop - start) - step * new_shape:
- * new_shape += 1 # <<<<<<<<<<<<<<
- *
- * if new_shape < 0:
- */
- __pyx_v_new_shape = (__pyx_v_new_shape + 1);
-
- /* "View.MemoryView":877
- * new_shape = (stop - start) // step
- *
- * if (stop - start) - step * new_shape: # <<<<<<<<<<<<<<
- * new_shape += 1
- *
- */
- }
-
- /* "View.MemoryView":880
- * new_shape += 1
- *
- * if new_shape < 0: # <<<<<<<<<<<<<<
- * new_shape = 0
- *
- */
- __pyx_t_2 = ((__pyx_v_new_shape < 0) != 0);
- if (__pyx_t_2) {
-
- /* "View.MemoryView":881
- *
- * if new_shape < 0:
- * new_shape = 0 # <<<<<<<<<<<<<<
- *
- *
- */
- __pyx_v_new_shape = 0;
-
- /* "View.MemoryView":880
- * new_shape += 1
- *
- * if new_shape < 0: # <<<<<<<<<<<<<<
- * new_shape = 0
- *
- */
- }
-
- /* "View.MemoryView":884
- *
- *
- * dst.strides[new_ndim] = stride * step # <<<<<<<<<<<<<<
- * dst.shape[new_ndim] = new_shape
- * dst.suboffsets[new_ndim] = suboffset
- */
- (__pyx_v_dst->strides[__pyx_v_new_ndim]) = (__pyx_v_stride * __pyx_v_step);
-
- /* "View.MemoryView":885
- *
- * dst.strides[new_ndim] = stride * step
- * dst.shape[new_ndim] = new_shape # <<<<<<<<<<<<<<
- * dst.suboffsets[new_ndim] = suboffset
- *
- */
- (__pyx_v_dst->shape[__pyx_v_new_ndim]) = __pyx_v_new_shape;
-
- /* "View.MemoryView":886
- * dst.strides[new_ndim] = stride * step
- * dst.shape[new_ndim] = new_shape
- * dst.suboffsets[new_ndim] = suboffset # <<<<<<<<<<<<<<
- *
- *
- */
- (__pyx_v_dst->suboffsets[__pyx_v_new_ndim]) = __pyx_v_suboffset;
- }
- __pyx_L3:;
-
- /* "View.MemoryView":889
- *
- *
- * if suboffset_dim[0] < 0: # <<<<<<<<<<<<<<
- * dst.data += start * stride
- * else:
- */
- __pyx_t_2 = (((__pyx_v_suboffset_dim[0]) < 0) != 0);
- if (__pyx_t_2) {
-
- /* "View.MemoryView":890
- *
- * if suboffset_dim[0] < 0:
- * dst.data += start * stride # <<<<<<<<<<<<<<
- * else:
- * dst.suboffsets[suboffset_dim[0]] += start * stride
- */
- __pyx_v_dst->data = (__pyx_v_dst->data + (__pyx_v_start * __pyx_v_stride));
-
- /* "View.MemoryView":889
- *
- *
- * if suboffset_dim[0] < 0: # <<<<<<<<<<<<<<
- * dst.data += start * stride
- * else:
- */
- goto __pyx_L23;
- }
-
- /* "View.MemoryView":892
- * dst.data += start * stride
- * else:
- * dst.suboffsets[suboffset_dim[0]] += start * stride # <<<<<<<<<<<<<<
- *
- * if suboffset >= 0:
- */
- /*else*/ {
- __pyx_t_3 = (__pyx_v_suboffset_dim[0]);
- (__pyx_v_dst->suboffsets[__pyx_t_3]) = ((__pyx_v_dst->suboffsets[__pyx_t_3]) + (__pyx_v_start * __pyx_v_stride));
- }
- __pyx_L23:;
-
- /* "View.MemoryView":894
- * dst.suboffsets[suboffset_dim[0]] += start * stride
- *
- * if suboffset >= 0: # <<<<<<<<<<<<<<
- * if not is_slice:
- * if new_ndim == 0:
- */
- __pyx_t_2 = ((__pyx_v_suboffset >= 0) != 0);
- if (__pyx_t_2) {
-
- /* "View.MemoryView":895
- *
- * if suboffset >= 0:
- * if not is_slice: # <<<<<<<<<<<<<<
- * if new_ndim == 0:
- * dst.data = ( dst.data)[0] + suboffset
- */
- __pyx_t_2 = ((!(__pyx_v_is_slice != 0)) != 0);
- if (__pyx_t_2) {
-
- /* "View.MemoryView":896
- * if suboffset >= 0:
- * if not is_slice:
- * if new_ndim == 0: # <<<<<<<<<<<<<<
- * dst.data = ( dst.data)[0] + suboffset
- * else:
- */
- __pyx_t_2 = ((__pyx_v_new_ndim == 0) != 0);
- if (__pyx_t_2) {
-
- /* "View.MemoryView":897
- * if not is_slice:
- * if new_ndim == 0:
- * dst.data = ( dst.data)[0] + suboffset # <<<<<<<<<<<<<<
- * else:
- * _err_dim(IndexError, "All dimensions preceding dimension %d "
- */
- __pyx_v_dst->data = ((((char **)__pyx_v_dst->data)[0]) + __pyx_v_suboffset);
-
- /* "View.MemoryView":896
- * if suboffset >= 0:
- * if not is_slice:
- * if new_ndim == 0: # <<<<<<<<<<<<<<
- * dst.data = ( dst.data)[0] + suboffset
- * else:
- */
- goto __pyx_L26;
- }
-
- /* "View.MemoryView":899
- * dst.data = ( dst.data)[0] + suboffset
- * else:
- * _err_dim(IndexError, "All dimensions preceding dimension %d " # <<<<<<<<<<<<<<
- * "must be indexed and not sliced", dim)
- * else:
- */
- /*else*/ {
-
- /* "View.MemoryView":900
- * else:
- * _err_dim(IndexError, "All dimensions preceding dimension %d "
- * "must be indexed and not sliced", dim) # <<<<<<<<<<<<<<
- * else:
- * suboffset_dim[0] = new_ndim
- */
- __pyx_t_3 = __pyx_memoryview_err_dim(__pyx_builtin_IndexError, ((char *)"All dimensions preceding dimension %d must be indexed and not sliced"), __pyx_v_dim); if (unlikely(__pyx_t_3 == ((int)-1))) __PYX_ERR(1, 899, __pyx_L1_error)
- }
- __pyx_L26:;
-
- /* "View.MemoryView":895
- *
- * if suboffset >= 0:
- * if not is_slice: # <<<<<<<<<<<<<<
- * if new_ndim == 0:
- * dst.data = ( dst.data)[0] + suboffset
- */
- goto __pyx_L25;
- }
-
- /* "View.MemoryView":902
- * "must be indexed and not sliced", dim)
- * else:
- * suboffset_dim[0] = new_ndim # <<<<<<<<<<<<<<
- *
- * return 0
- */
- /*else*/ {
- (__pyx_v_suboffset_dim[0]) = __pyx_v_new_ndim;
- }
- __pyx_L25:;
-
- /* "View.MemoryView":894
- * dst.suboffsets[suboffset_dim[0]] += start * stride
- *
- * if suboffset >= 0: # <<<<<<<<<<<<<<
- * if not is_slice:
- * if new_ndim == 0:
- */
- }
-
- /* "View.MemoryView":904
- * suboffset_dim[0] = new_ndim
- *
- * return 0 # <<<<<<<<<<<<<<
- *
- *
- */
- __pyx_r = 0;
- goto __pyx_L0;
-
- /* "View.MemoryView":807
- *
- * @cname('__pyx_memoryview_slice_memviewslice')
- * cdef int slice_memviewslice( # <<<<<<<<<<<<<<
- * __Pyx_memviewslice *dst,
- * Py_ssize_t shape, Py_ssize_t stride, Py_ssize_t suboffset,
- */
-
- /* function exit code */
- __pyx_L1_error:;
- {
- #ifdef WITH_THREAD
- PyGILState_STATE __pyx_gilstate_save = __Pyx_PyGILState_Ensure();
- #endif
- __Pyx_AddTraceback("View.MemoryView.slice_memviewslice", __pyx_clineno, __pyx_lineno, __pyx_filename);
- #ifdef WITH_THREAD
- __Pyx_PyGILState_Release(__pyx_gilstate_save);
- #endif
- }
- __pyx_r = -1;
- __pyx_L0:;
- return __pyx_r;
-}
-
-/* "View.MemoryView":910
- *
- * @cname('__pyx_pybuffer_index')
- * cdef char *pybuffer_index(Py_buffer *view, char *bufp, Py_ssize_t index, # <<<<<<<<<<<<<<
- * Py_ssize_t dim) except NULL:
- * cdef Py_ssize_t shape, stride, suboffset = -1
- */
-
-static char *__pyx_pybuffer_index(Py_buffer *__pyx_v_view, char *__pyx_v_bufp, Py_ssize_t __pyx_v_index, Py_ssize_t __pyx_v_dim) {
- Py_ssize_t __pyx_v_shape;
- Py_ssize_t __pyx_v_stride;
- Py_ssize_t __pyx_v_suboffset;
- Py_ssize_t __pyx_v_itemsize;
- char *__pyx_v_resultp;
- char *__pyx_r;
- __Pyx_RefNannyDeclarations
- Py_ssize_t __pyx_t_1;
- int __pyx_t_2;
- PyObject *__pyx_t_3 = NULL;
- PyObject *__pyx_t_4 = NULL;
- int __pyx_lineno = 0;
- const char *__pyx_filename = NULL;
- int __pyx_clineno = 0;
- __Pyx_RefNannySetupContext("pybuffer_index", 0);
-
- /* "View.MemoryView":912
- * cdef char *pybuffer_index(Py_buffer *view, char *bufp, Py_ssize_t index,
- * Py_ssize_t dim) except NULL:
- * cdef Py_ssize_t shape, stride, suboffset = -1 # <<<<<<<<<<<<<<
- * cdef Py_ssize_t itemsize = view.itemsize
- * cdef char *resultp
- */
- __pyx_v_suboffset = -1L;
-
- /* "View.MemoryView":913
- * Py_ssize_t dim) except NULL:
- * cdef Py_ssize_t shape, stride, suboffset = -1
- * cdef Py_ssize_t itemsize = view.itemsize # <<<<<<<<<<<<<<
- * cdef char *resultp
- *
- */
- __pyx_t_1 = __pyx_v_view->itemsize;
- __pyx_v_itemsize = __pyx_t_1;
-
- /* "View.MemoryView":916
- * cdef char *resultp
- *
- * if view.ndim == 0: # <<<<<<<<<<<<<<
- * shape = view.len / itemsize
- * stride = itemsize
- */
- __pyx_t_2 = ((__pyx_v_view->ndim == 0) != 0);
- if (__pyx_t_2) {
-
- /* "View.MemoryView":917
- *
- * if view.ndim == 0:
- * shape = view.len / itemsize # <<<<<<<<<<<<<<
- * stride = itemsize
- * else:
- */
- if (unlikely(__pyx_v_itemsize == 0)) {
- PyErr_SetString(PyExc_ZeroDivisionError, "integer division or modulo by zero");
- __PYX_ERR(1, 917, __pyx_L1_error)
- }
- else if (sizeof(Py_ssize_t) == sizeof(long) && (!(((Py_ssize_t)-1) > 0)) && unlikely(__pyx_v_itemsize == (Py_ssize_t)-1) && unlikely(UNARY_NEG_WOULD_OVERFLOW(__pyx_v_view->len))) {
- PyErr_SetString(PyExc_OverflowError, "value too large to perform division");
- __PYX_ERR(1, 917, __pyx_L1_error)
- }
- __pyx_v_shape = __Pyx_div_Py_ssize_t(__pyx_v_view->len, __pyx_v_itemsize);
-
- /* "View.MemoryView":918
- * if view.ndim == 0:
- * shape = view.len / itemsize
- * stride = itemsize # <<<<<<<<<<<<<<
- * else:
- * shape = view.shape[dim]
- */
- __pyx_v_stride = __pyx_v_itemsize;
-
- /* "View.MemoryView":916
- * cdef char *resultp
- *
- * if view.ndim == 0: # <<<<<<<<<<<<<<
- * shape = view.len / itemsize
- * stride = itemsize
- */
- goto __pyx_L3;
- }
-
- /* "View.MemoryView":920
- * stride = itemsize
- * else:
- * shape = view.shape[dim] # <<<<<<<<<<<<<<
- * stride = view.strides[dim]
- * if view.suboffsets != NULL:
- */
- /*else*/ {
- __pyx_v_shape = (__pyx_v_view->shape[__pyx_v_dim]);
-
- /* "View.MemoryView":921
- * else:
- * shape = view.shape[dim]
- * stride = view.strides[dim] # <<<<<<<<<<<<<<
- * if view.suboffsets != NULL:
- * suboffset = view.suboffsets[dim]
- */
- __pyx_v_stride = (__pyx_v_view->strides[__pyx_v_dim]);
-
- /* "View.MemoryView":922
- * shape = view.shape[dim]
- * stride = view.strides[dim]
- * if view.suboffsets != NULL: # <<<<<<<<<<<<<<
- * suboffset = view.suboffsets[dim]
- *
- */
- __pyx_t_2 = ((__pyx_v_view->suboffsets != NULL) != 0);
- if (__pyx_t_2) {
-
- /* "View.MemoryView":923
- * stride = view.strides[dim]
- * if view.suboffsets != NULL:
- * suboffset = view.suboffsets[dim] # <<<<<<<<<<<<<<
- *
- * if index < 0:
- */
- __pyx_v_suboffset = (__pyx_v_view->suboffsets[__pyx_v_dim]);
-
- /* "View.MemoryView":922
- * shape = view.shape[dim]
- * stride = view.strides[dim]
- * if view.suboffsets != NULL: # <<<<<<<<<<<<<<
- * suboffset = view.suboffsets[dim]
- *
- */
- }
- }
- __pyx_L3:;
-
- /* "View.MemoryView":925
- * suboffset = view.suboffsets[dim]
- *
- * if index < 0: # <<<<<<<<<<<<<<
- * index += view.shape[dim]
- * if index < 0:
- */
- __pyx_t_2 = ((__pyx_v_index < 0) != 0);
- if (__pyx_t_2) {
-
- /* "View.MemoryView":926
- *
- * if index < 0:
- * index += view.shape[dim] # <<<<<<<<<<<<<<
- * if index < 0:
- * raise IndexError("Out of bounds on buffer access (axis %d)" % dim)
- */
- __pyx_v_index = (__pyx_v_index + (__pyx_v_view->shape[__pyx_v_dim]));
-
- /* "View.MemoryView":927
- * if index < 0:
- * index += view.shape[dim]
- * if index < 0: # <<<<<<<<<<<<<<
- * raise IndexError("Out of bounds on buffer access (axis %d)" % dim)
- *
- */
- __pyx_t_2 = ((__pyx_v_index < 0) != 0);
- if (unlikely(__pyx_t_2)) {
-
- /* "View.MemoryView":928
- * index += view.shape[dim]
- * if index < 0:
- * raise IndexError("Out of bounds on buffer access (axis %d)" % dim) # <<<<<<<<<<<<<<
- *
- * if index >= shape:
- */
- __pyx_t_3 = PyInt_FromSsize_t(__pyx_v_dim); if (unlikely(!__pyx_t_3)) __PYX_ERR(1, 928, __pyx_L1_error)
- __Pyx_GOTREF(__pyx_t_3);
- __pyx_t_4 = __Pyx_PyString_Format(__pyx_kp_s_Out_of_bounds_on_buffer_access_a, __pyx_t_3); if (unlikely(!__pyx_t_4)) __PYX_ERR(1, 928, __pyx_L1_error)
- __Pyx_GOTREF(__pyx_t_4);
- __Pyx_DECREF(__pyx_t_3); __pyx_t_3 = 0;
- __pyx_t_3 = __Pyx_PyObject_CallOneArg(__pyx_builtin_IndexError, __pyx_t_4); if (unlikely(!__pyx_t_3)) __PYX_ERR(1, 928, __pyx_L1_error)
- __Pyx_GOTREF(__pyx_t_3);
- __Pyx_DECREF(__pyx_t_4); __pyx_t_4 = 0;
- __Pyx_Raise(__pyx_t_3, 0, 0, 0);
- __Pyx_DECREF(__pyx_t_3); __pyx_t_3 = 0;
- __PYX_ERR(1, 928, __pyx_L1_error)
-
- /* "View.MemoryView":927
- * if index < 0:
- * index += view.shape[dim]
- * if index < 0: # <<<<<<<<<<<<<<
- * raise IndexError("Out of bounds on buffer access (axis %d)" % dim)
- *
- */
- }
-
- /* "View.MemoryView":925
- * suboffset = view.suboffsets[dim]
- *
- * if index < 0: # <<<<<<<<<<<<<<
- * index += view.shape[dim]
- * if index < 0:
- */
- }
-
- /* "View.MemoryView":930
- * raise IndexError("Out of bounds on buffer access (axis %d)" % dim)
- *
- * if index >= shape: # <<<<<<<<<<<<<<
- * raise IndexError("Out of bounds on buffer access (axis %d)" % dim)
- *
- */
- __pyx_t_2 = ((__pyx_v_index >= __pyx_v_shape) != 0);
- if (unlikely(__pyx_t_2)) {
-
- /* "View.MemoryView":931
- *
- * if index >= shape:
- * raise IndexError("Out of bounds on buffer access (axis %d)" % dim) # <<<<<<<<<<<<<<
- *
- * resultp = bufp + index * stride
- */
- __pyx_t_3 = PyInt_FromSsize_t(__pyx_v_dim); if (unlikely(!__pyx_t_3)) __PYX_ERR(1, 931, __pyx_L1_error)
- __Pyx_GOTREF(__pyx_t_3);
- __pyx_t_4 = __Pyx_PyString_Format(__pyx_kp_s_Out_of_bounds_on_buffer_access_a, __pyx_t_3); if (unlikely(!__pyx_t_4)) __PYX_ERR(1, 931, __pyx_L1_error)
- __Pyx_GOTREF(__pyx_t_4);
- __Pyx_DECREF(__pyx_t_3); __pyx_t_3 = 0;
- __pyx_t_3 = __Pyx_PyObject_CallOneArg(__pyx_builtin_IndexError, __pyx_t_4); if (unlikely(!__pyx_t_3)) __PYX_ERR(1, 931, __pyx_L1_error)
- __Pyx_GOTREF(__pyx_t_3);
- __Pyx_DECREF(__pyx_t_4); __pyx_t_4 = 0;
- __Pyx_Raise(__pyx_t_3, 0, 0, 0);
- __Pyx_DECREF(__pyx_t_3); __pyx_t_3 = 0;
- __PYX_ERR(1, 931, __pyx_L1_error)
-
- /* "View.MemoryView":930
- * raise IndexError("Out of bounds on buffer access (axis %d)" % dim)
- *
- * if index >= shape: # <<<<<<<<<<<<<<
- * raise IndexError("Out of bounds on buffer access (axis %d)" % dim)
- *
- */
- }
-
- /* "View.MemoryView":933
- * raise IndexError("Out of bounds on buffer access (axis %d)" % dim)
- *
- * resultp = bufp + index * stride # <<<<<<<<<<<<<<
- * if suboffset >= 0:
- * resultp = ( resultp)[0] + suboffset
- */
- __pyx_v_resultp = (__pyx_v_bufp + (__pyx_v_index * __pyx_v_stride));
-
- /* "View.MemoryView":934
- *
- * resultp = bufp + index * stride
- * if suboffset >= 0: # <<<<<<<<<<<<<<
- * resultp = ( resultp)[0] + suboffset
- *
- */
- __pyx_t_2 = ((__pyx_v_suboffset >= 0) != 0);
- if (__pyx_t_2) {
-
- /* "View.MemoryView":935
- * resultp = bufp + index * stride
- * if suboffset >= 0:
- * resultp = ( resultp)[0] + suboffset # <<<<<<<<<<<<<<
- *
- * return resultp
- */
- __pyx_v_resultp = ((((char **)__pyx_v_resultp)[0]) + __pyx_v_suboffset);
-
- /* "View.MemoryView":934
- *
- * resultp = bufp + index * stride
- * if suboffset >= 0: # <<<<<<<<<<<<<<
- * resultp = ( resultp)[0] + suboffset
- *
- */
- }
-
- /* "View.MemoryView":937
- * resultp = ( resultp)[0] + suboffset
- *
- * return resultp # <<<<<<<<<<<<<<
- *
- *
- */
- __pyx_r = __pyx_v_resultp;
- goto __pyx_L0;
-
- /* "View.MemoryView":910
- *
- * @cname('__pyx_pybuffer_index')
- * cdef char *pybuffer_index(Py_buffer *view, char *bufp, Py_ssize_t index, # <<<<<<<<<<<<<<
- * Py_ssize_t dim) except NULL:
- * cdef Py_ssize_t shape, stride, suboffset = -1
- */
-
- /* function exit code */
- __pyx_L1_error:;
- __Pyx_XDECREF(__pyx_t_3);
- __Pyx_XDECREF(__pyx_t_4);
- __Pyx_AddTraceback("View.MemoryView.pybuffer_index", __pyx_clineno, __pyx_lineno, __pyx_filename);
- __pyx_r = NULL;
- __pyx_L0:;
- __Pyx_RefNannyFinishContext();
- return __pyx_r;
-}
-
-/* "View.MemoryView":943
- *
- * @cname('__pyx_memslice_transpose')
- * cdef int transpose_memslice(__Pyx_memviewslice *memslice) nogil except 0: # <<<<<<<<<<<<<<
- * cdef int ndim = memslice.memview.view.ndim
- *
- */
-
-static int __pyx_memslice_transpose(__Pyx_memviewslice *__pyx_v_memslice) {
- int __pyx_v_ndim;
- Py_ssize_t *__pyx_v_shape;
- Py_ssize_t *__pyx_v_strides;
- int __pyx_v_i;
- int __pyx_v_j;
- int __pyx_r;
- int __pyx_t_1;
- Py_ssize_t *__pyx_t_2;
- long __pyx_t_3;
- long __pyx_t_4;
- Py_ssize_t __pyx_t_5;
- Py_ssize_t __pyx_t_6;
- int __pyx_t_7;
- int __pyx_t_8;
- int __pyx_t_9;
- int __pyx_lineno = 0;
- const char *__pyx_filename = NULL;
- int __pyx_clineno = 0;
-
- /* "View.MemoryView":944
- * @cname('__pyx_memslice_transpose')
- * cdef int transpose_memslice(__Pyx_memviewslice *memslice) nogil except 0:
- * cdef int ndim = memslice.memview.view.ndim # <<<<<<<<<<<<<<
- *
- * cdef Py_ssize_t *shape = memslice.shape
- */
- __pyx_t_1 = __pyx_v_memslice->memview->view.ndim;
- __pyx_v_ndim = __pyx_t_1;
-
- /* "View.MemoryView":946
- * cdef int ndim = memslice.memview.view.ndim
- *
- * cdef Py_ssize_t *shape = memslice.shape # <<<<<<<<<<<<<<
- * cdef Py_ssize_t *strides = memslice.strides
- *
- */
- __pyx_t_2 = __pyx_v_memslice->shape;
- __pyx_v_shape = __pyx_t_2;
-
- /* "View.MemoryView":947
- *
- * cdef Py_ssize_t *shape = memslice.shape
- * cdef Py_ssize_t *strides = memslice.strides # <<<<<<<<<<<<<<
- *
- *
- */
- __pyx_t_2 = __pyx_v_memslice->strides;
- __pyx_v_strides = __pyx_t_2;
-
- /* "View.MemoryView":951
- *
- * cdef int i, j
- * for i in range(ndim / 2): # <<<<<<<<<<<<<<
- * j = ndim - 1 - i
- * strides[i], strides[j] = strides[j], strides[i]
- */
- __pyx_t_3 = __Pyx_div_long(__pyx_v_ndim, 2);
- __pyx_t_4 = __pyx_t_3;
- for (__pyx_t_1 = 0; __pyx_t_1 < __pyx_t_4; __pyx_t_1+=1) {
- __pyx_v_i = __pyx_t_1;
-
- /* "View.MemoryView":952
- * cdef int i, j
- * for i in range(ndim / 2):
- * j = ndim - 1 - i # <<<<<<<<<<<<<<
- * strides[i], strides[j] = strides[j], strides[i]
- * shape[i], shape[j] = shape[j], shape[i]
- */
- __pyx_v_j = ((__pyx_v_ndim - 1) - __pyx_v_i);
-
- /* "View.MemoryView":953
- * for i in range(ndim / 2):
- * j = ndim - 1 - i
- * strides[i], strides[j] = strides[j], strides[i] # <<<<<<<<<<<<<<
- * shape[i], shape[j] = shape[j], shape[i]
- *
- */
- __pyx_t_5 = (__pyx_v_strides[__pyx_v_j]);
- __pyx_t_6 = (__pyx_v_strides[__pyx_v_i]);
- (__pyx_v_strides[__pyx_v_i]) = __pyx_t_5;
- (__pyx_v_strides[__pyx_v_j]) = __pyx_t_6;
-
- /* "View.MemoryView":954
- * j = ndim - 1 - i
- * strides[i], strides[j] = strides[j], strides[i]
- * shape[i], shape[j] = shape[j], shape[i] # <<<<<<<<<<<<<<
- *
- * if memslice.suboffsets[i] >= 0 or memslice.suboffsets[j] >= 0:
- */
- __pyx_t_6 = (__pyx_v_shape[__pyx_v_j]);
- __pyx_t_5 = (__pyx_v_shape[__pyx_v_i]);
- (__pyx_v_shape[__pyx_v_i]) = __pyx_t_6;
- (__pyx_v_shape[__pyx_v_j]) = __pyx_t_5;
-
- /* "View.MemoryView":956
- * shape[i], shape[j] = shape[j], shape[i]
- *
- * if memslice.suboffsets[i] >= 0 or memslice.suboffsets[j] >= 0: # <<<<<<<<<<<<<<
- * _err(ValueError, "Cannot transpose memoryview with indirect dimensions")
- *
- */
- __pyx_t_8 = (((__pyx_v_memslice->suboffsets[__pyx_v_i]) >= 0) != 0);
- if (!__pyx_t_8) {
- } else {
- __pyx_t_7 = __pyx_t_8;
- goto __pyx_L6_bool_binop_done;
- }
- __pyx_t_8 = (((__pyx_v_memslice->suboffsets[__pyx_v_j]) >= 0) != 0);
- __pyx_t_7 = __pyx_t_8;
- __pyx_L6_bool_binop_done:;
- if (__pyx_t_7) {
-
- /* "View.MemoryView":957
- *
- * if memslice.suboffsets[i] >= 0 or memslice.suboffsets[j] >= 0:
- * _err(ValueError, "Cannot transpose memoryview with indirect dimensions") # <<<<<<<<<<<<<<
- *
- * return 1
- */
- __pyx_t_9 = __pyx_memoryview_err(__pyx_builtin_ValueError, ((char *)"Cannot transpose memoryview with indirect dimensions")); if (unlikely(__pyx_t_9 == ((int)-1))) __PYX_ERR(1, 957, __pyx_L1_error)
-
- /* "View.MemoryView":956
- * shape[i], shape[j] = shape[j], shape[i]
- *
- * if memslice.suboffsets[i] >= 0 or memslice.suboffsets[j] >= 0: # <<<<<<<<<<<<<<
- * _err(ValueError, "Cannot transpose memoryview with indirect dimensions")
- *
- */
- }
- }
-
- /* "View.MemoryView":959
- * _err(ValueError, "Cannot transpose memoryview with indirect dimensions")
- *
- * return 1 # <<<<<<<<<<<<<<
- *
- *
- */
- __pyx_r = 1;
- goto __pyx_L0;
-
- /* "View.MemoryView":943
- *
- * @cname('__pyx_memslice_transpose')
- * cdef int transpose_memslice(__Pyx_memviewslice *memslice) nogil except 0: # <<<<<<<<<<<<<<
- * cdef int ndim = memslice.memview.view.ndim
- *
- */
-
- /* function exit code */
- __pyx_L1_error:;
- {
- #ifdef WITH_THREAD
- PyGILState_STATE __pyx_gilstate_save = __Pyx_PyGILState_Ensure();
- #endif
- __Pyx_AddTraceback("View.MemoryView.transpose_memslice", __pyx_clineno, __pyx_lineno, __pyx_filename);
- #ifdef WITH_THREAD
- __Pyx_PyGILState_Release(__pyx_gilstate_save);
- #endif
- }
- __pyx_r = 0;
- __pyx_L0:;
- return __pyx_r;
-}
-
-/* "View.MemoryView":976
- * cdef int (*to_dtype_func)(char *, object) except 0
- *
- * def __dealloc__(self): # <<<<<<<<<<<<<<
- * __PYX_XDEC_MEMVIEW(&self.from_slice, 1)
- *
- */
-
-/* Python wrapper */
-static void __pyx_memoryviewslice___dealloc__(PyObject *__pyx_v_self); /*proto*/
-static void __pyx_memoryviewslice___dealloc__(PyObject *__pyx_v_self) {
- __Pyx_RefNannyDeclarations
- __Pyx_RefNannySetupContext("__dealloc__ (wrapper)", 0);
- __pyx_memoryviewslice___pyx_pf_15View_dot_MemoryView_16_memoryviewslice___dealloc__(((struct __pyx_memoryviewslice_obj *)__pyx_v_self));
-
- /* function exit code */
- __Pyx_RefNannyFinishContext();
-}
-
-static void __pyx_memoryviewslice___pyx_pf_15View_dot_MemoryView_16_memoryviewslice___dealloc__(struct __pyx_memoryviewslice_obj *__pyx_v_self) {
- __Pyx_RefNannyDeclarations
- __Pyx_RefNannySetupContext("__dealloc__", 0);
-
- /* "View.MemoryView":977
- *
- * def __dealloc__(self):
- * __PYX_XDEC_MEMVIEW(&self.from_slice, 1) # <<<<<<<<<<<<<<
- *
- * cdef convert_item_to_object(self, char *itemp):
- */
- __PYX_XDEC_MEMVIEW((&__pyx_v_self->from_slice), 1);
-
- /* "View.MemoryView":976
- * cdef int (*to_dtype_func)(char *, object) except 0
- *
- * def __dealloc__(self): # <<<<<<<<<<<<<<
- * __PYX_XDEC_MEMVIEW(&self.from_slice, 1)
- *
- */
-
- /* function exit code */
- __Pyx_RefNannyFinishContext();
-}
-
-/* "View.MemoryView":979
- * __PYX_XDEC_MEMVIEW(&self.from_slice, 1)
- *
- * cdef convert_item_to_object(self, char *itemp): # <<<<<<<<<<<<<<
- * if self.to_object_func != NULL:
- * return self.to_object_func(itemp)
- */
-
-static PyObject *__pyx_memoryviewslice_convert_item_to_object(struct __pyx_memoryviewslice_obj *__pyx_v_self, char *__pyx_v_itemp) {
- PyObject *__pyx_r = NULL;
- __Pyx_RefNannyDeclarations
- int __pyx_t_1;
- PyObject *__pyx_t_2 = NULL;
- int __pyx_lineno = 0;
- const char *__pyx_filename = NULL;
- int __pyx_clineno = 0;
- __Pyx_RefNannySetupContext("convert_item_to_object", 0);
-
- /* "View.MemoryView":980
- *
- * cdef convert_item_to_object(self, char *itemp):
- * if self.to_object_func != NULL: # <<<<<<<<<<<<<<
- * return self.to_object_func(itemp)
- * else:
- */
- __pyx_t_1 = ((__pyx_v_self->to_object_func != NULL) != 0);
- if (__pyx_t_1) {
-
- /* "View.MemoryView":981
- * cdef convert_item_to_object(self, char *itemp):
- * if self.to_object_func != NULL:
- * return self.to_object_func(itemp) # <<<<<<<<<<<<<<
- * else:
- * return memoryview.convert_item_to_object(self, itemp)
- */
- __Pyx_XDECREF(__pyx_r);
- __pyx_t_2 = __pyx_v_self->to_object_func(__pyx_v_itemp); if (unlikely(!__pyx_t_2)) __PYX_ERR(1, 981, __pyx_L1_error)
- __Pyx_GOTREF(__pyx_t_2);
- __pyx_r = __pyx_t_2;
- __pyx_t_2 = 0;
- goto __pyx_L0;
-
- /* "View.MemoryView":980
- *
- * cdef convert_item_to_object(self, char *itemp):
- * if self.to_object_func != NULL: # <<<<<<<<<<<<<<
- * return self.to_object_func(itemp)
- * else:
- */
- }
-
- /* "View.MemoryView":983
- * return self.to_object_func(itemp)
- * else:
- * return memoryview.convert_item_to_object(self, itemp) # <<<<<<<<<<<<<<
- *
- * cdef assign_item_from_object(self, char *itemp, object value):
- */
- /*else*/ {
- __Pyx_XDECREF(__pyx_r);
- __pyx_t_2 = __pyx_memoryview_convert_item_to_object(((struct __pyx_memoryview_obj *)__pyx_v_self), __pyx_v_itemp); if (unlikely(!__pyx_t_2)) __PYX_ERR(1, 983, __pyx_L1_error)
- __Pyx_GOTREF(__pyx_t_2);
- __pyx_r = __pyx_t_2;
- __pyx_t_2 = 0;
- goto __pyx_L0;
- }
-
- /* "View.MemoryView":979
- * __PYX_XDEC_MEMVIEW(&self.from_slice, 1)
- *
- * cdef convert_item_to_object(self, char *itemp): # <<<<<<<<<<<<<<
- * if self.to_object_func != NULL:
- * return self.to_object_func(itemp)
- */
-
- /* function exit code */
- __pyx_L1_error:;
- __Pyx_XDECREF(__pyx_t_2);
- __Pyx_AddTraceback("View.MemoryView._memoryviewslice.convert_item_to_object", __pyx_clineno, __pyx_lineno, __pyx_filename);
- __pyx_r = 0;
- __pyx_L0:;
- __Pyx_XGIVEREF(__pyx_r);
- __Pyx_RefNannyFinishContext();
- return __pyx_r;
-}
-
-/* "View.MemoryView":985
- * return memoryview.convert_item_to_object(self, itemp)
- *
- * cdef assign_item_from_object(self, char *itemp, object value): # <<<<<<<<<<<<<<
- * if self.to_dtype_func != NULL:
- * self.to_dtype_func(itemp, value)
- */
-
-static PyObject *__pyx_memoryviewslice_assign_item_from_object(struct __pyx_memoryviewslice_obj *__pyx_v_self, char *__pyx_v_itemp, PyObject *__pyx_v_value) {
- PyObject *__pyx_r = NULL;
- __Pyx_RefNannyDeclarations
- int __pyx_t_1;
- int __pyx_t_2;
- PyObject *__pyx_t_3 = NULL;
- int __pyx_lineno = 0;
- const char *__pyx_filename = NULL;
- int __pyx_clineno = 0;
- __Pyx_RefNannySetupContext("assign_item_from_object", 0);
-
- /* "View.MemoryView":986
- *
- * cdef assign_item_from_object(self, char *itemp, object value):
- * if self.to_dtype_func != NULL: # <<<<<<<<<<<<<<
- * self.to_dtype_func(itemp, value)
- * else:
- */
- __pyx_t_1 = ((__pyx_v_self->to_dtype_func != NULL) != 0);
- if (__pyx_t_1) {
-
- /* "View.MemoryView":987
- * cdef assign_item_from_object(self, char *itemp, object value):
- * if self.to_dtype_func != NULL:
- * self.to_dtype_func(itemp, value) # <<<<<<<<<<<<<<
- * else:
- * memoryview.assign_item_from_object(self, itemp, value)
- */
- __pyx_t_2 = __pyx_v_self->to_dtype_func(__pyx_v_itemp, __pyx_v_value); if (unlikely(__pyx_t_2 == ((int)0))) __PYX_ERR(1, 987, __pyx_L1_error)
-
- /* "View.MemoryView":986
- *
- * cdef assign_item_from_object(self, char *itemp, object value):
- * if self.to_dtype_func != NULL: # <<<<<<<<<<<<<<
- * self.to_dtype_func(itemp, value)
- * else:
- */
- goto __pyx_L3;
- }
-
- /* "View.MemoryView":989
- * self.to_dtype_func(itemp, value)
- * else:
- * memoryview.assign_item_from_object(self, itemp, value) # <<<<<<<<<<<<<<
- *
- * @property
- */
- /*else*/ {
- __pyx_t_3 = __pyx_memoryview_assign_item_from_object(((struct __pyx_memoryview_obj *)__pyx_v_self), __pyx_v_itemp, __pyx_v_value); if (unlikely(!__pyx_t_3)) __PYX_ERR(1, 989, __pyx_L1_error)
- __Pyx_GOTREF(__pyx_t_3);
- __Pyx_DECREF(__pyx_t_3); __pyx_t_3 = 0;
- }
- __pyx_L3:;
-
- /* "View.MemoryView":985
- * return memoryview.convert_item_to_object(self, itemp)
- *
- * cdef assign_item_from_object(self, char *itemp, object value): # <<<<<<<<<<<<<<
- * if self.to_dtype_func != NULL:
- * self.to_dtype_func(itemp, value)
- */
-
- /* function exit code */
- __pyx_r = Py_None; __Pyx_INCREF(Py_None);
- goto __pyx_L0;
- __pyx_L1_error:;
- __Pyx_XDECREF(__pyx_t_3);
- __Pyx_AddTraceback("View.MemoryView._memoryviewslice.assign_item_from_object", __pyx_clineno, __pyx_lineno, __pyx_filename);
- __pyx_r = 0;
- __pyx_L0:;
- __Pyx_XGIVEREF(__pyx_r);
- __Pyx_RefNannyFinishContext();
- return __pyx_r;
-}
-
-/* "View.MemoryView":992
- *
- * @property
- * def base(self): # <<<<<<<<<<<<<<
- * return self.from_object
- *
- */
-
-/* Python wrapper */
-static PyObject *__pyx_pw_15View_dot_MemoryView_16_memoryviewslice_4base_1__get__(PyObject *__pyx_v_self); /*proto*/
-static PyObject *__pyx_pw_15View_dot_MemoryView_16_memoryviewslice_4base_1__get__(PyObject *__pyx_v_self) {
- PyObject *__pyx_r = 0;
- __Pyx_RefNannyDeclarations
- __Pyx_RefNannySetupContext("__get__ (wrapper)", 0);
- __pyx_r = __pyx_pf_15View_dot_MemoryView_16_memoryviewslice_4base___get__(((struct __pyx_memoryviewslice_obj *)__pyx_v_self));
-
- /* function exit code */
- __Pyx_RefNannyFinishContext();
- return __pyx_r;
-}
-
-static PyObject *__pyx_pf_15View_dot_MemoryView_16_memoryviewslice_4base___get__(struct __pyx_memoryviewslice_obj *__pyx_v_self) {
- PyObject *__pyx_r = NULL;
- __Pyx_RefNannyDeclarations
- __Pyx_RefNannySetupContext("__get__", 0);
-
- /* "View.MemoryView":993
- * @property
- * def base(self):
- * return self.from_object # <<<<<<<<<<<<<<
- *
- * __pyx_getbuffer = capsule( &__pyx_memoryview_getbuffer, "getbuffer(obj, view, flags)")
- */
- __Pyx_XDECREF(__pyx_r);
- __Pyx_INCREF(__pyx_v_self->from_object);
- __pyx_r = __pyx_v_self->from_object;
- goto __pyx_L0;
-
- /* "View.MemoryView":992
- *
- * @property
- * def base(self): # <<<<<<<<<<<<<<
- * return self.from_object
- *
- */
-
- /* function exit code */
- __pyx_L0:;
- __Pyx_XGIVEREF(__pyx_r);
- __Pyx_RefNannyFinishContext();
- return __pyx_r;
-}
-
-/* "(tree fragment)":1
- * def __reduce_cython__(self): # <<<<<<<<<<<<<<
- * raise TypeError("no default __reduce__ due to non-trivial __cinit__")
- * def __setstate_cython__(self, __pyx_state):
- */
-
-/* Python wrapper */
-static PyObject *__pyx_pw___pyx_memoryviewslice_1__reduce_cython__(PyObject *__pyx_v_self, CYTHON_UNUSED PyObject *unused); /*proto*/
-static PyObject *__pyx_pw___pyx_memoryviewslice_1__reduce_cython__(PyObject *__pyx_v_self, CYTHON_UNUSED PyObject *unused) {
- PyObject *__pyx_r = 0;
- __Pyx_RefNannyDeclarations
- __Pyx_RefNannySetupContext("__reduce_cython__ (wrapper)", 0);
- __pyx_r = __pyx_pf___pyx_memoryviewslice___reduce_cython__(((struct __pyx_memoryviewslice_obj *)__pyx_v_self));
-
- /* function exit code */
- __Pyx_RefNannyFinishContext();
- return __pyx_r;
-}
-
-static PyObject *__pyx_pf___pyx_memoryviewslice___reduce_cython__(CYTHON_UNUSED struct __pyx_memoryviewslice_obj *__pyx_v_self) {
- PyObject *__pyx_r = NULL;
- __Pyx_RefNannyDeclarations
- PyObject *__pyx_t_1 = NULL;
- int __pyx_lineno = 0;
- const char *__pyx_filename = NULL;
- int __pyx_clineno = 0;
- __Pyx_RefNannySetupContext("__reduce_cython__", 0);
-
- /* "(tree fragment)":2
- * def __reduce_cython__(self):
- * raise TypeError("no default __reduce__ due to non-trivial __cinit__") # <<<<<<<<<<<<<<
- * def __setstate_cython__(self, __pyx_state):
- * raise TypeError("no default __reduce__ due to non-trivial __cinit__")
- */
- __pyx_t_1 = __Pyx_PyObject_Call(__pyx_builtin_TypeError, __pyx_tuple__18, NULL); if (unlikely(!__pyx_t_1)) __PYX_ERR(1, 2, __pyx_L1_error)
- __Pyx_GOTREF(__pyx_t_1);
- __Pyx_Raise(__pyx_t_1, 0, 0, 0);
- __Pyx_DECREF(__pyx_t_1); __pyx_t_1 = 0;
- __PYX_ERR(1, 2, __pyx_L1_error)
-
- /* "(tree fragment)":1
- * def __reduce_cython__(self): # <<<<<<<<<<<<<<
- * raise TypeError("no default __reduce__ due to non-trivial __cinit__")
- * def __setstate_cython__(self, __pyx_state):
- */
-
- /* function exit code */
- __pyx_L1_error:;
- __Pyx_XDECREF(__pyx_t_1);
- __Pyx_AddTraceback("View.MemoryView._memoryviewslice.__reduce_cython__", __pyx_clineno, __pyx_lineno, __pyx_filename);
- __pyx_r = NULL;
- __Pyx_XGIVEREF(__pyx_r);
- __Pyx_RefNannyFinishContext();
- return __pyx_r;
-}
-
-/* "(tree fragment)":3
- * def __reduce_cython__(self):
- * raise TypeError("no default __reduce__ due to non-trivial __cinit__")
- * def __setstate_cython__(self, __pyx_state): # <<<<<<<<<<<<<<
- * raise TypeError("no default __reduce__ due to non-trivial __cinit__")
- */
-
-/* Python wrapper */
-static PyObject *__pyx_pw___pyx_memoryviewslice_3__setstate_cython__(PyObject *__pyx_v_self, PyObject *__pyx_v___pyx_state); /*proto*/
-static PyObject *__pyx_pw___pyx_memoryviewslice_3__setstate_cython__(PyObject *__pyx_v_self, PyObject *__pyx_v___pyx_state) {
- PyObject *__pyx_r = 0;
- __Pyx_RefNannyDeclarations
- __Pyx_RefNannySetupContext("__setstate_cython__ (wrapper)", 0);
- __pyx_r = __pyx_pf___pyx_memoryviewslice_2__setstate_cython__(((struct __pyx_memoryviewslice_obj *)__pyx_v_self), ((PyObject *)__pyx_v___pyx_state));
-
- /* function exit code */
- __Pyx_RefNannyFinishContext();
- return __pyx_r;
-}
-
-static PyObject *__pyx_pf___pyx_memoryviewslice_2__setstate_cython__(CYTHON_UNUSED struct __pyx_memoryviewslice_obj *__pyx_v_self, CYTHON_UNUSED PyObject *__pyx_v___pyx_state) {
- PyObject *__pyx_r = NULL;
- __Pyx_RefNannyDeclarations
- PyObject *__pyx_t_1 = NULL;
- int __pyx_lineno = 0;
- const char *__pyx_filename = NULL;
- int __pyx_clineno = 0;
- __Pyx_RefNannySetupContext("__setstate_cython__", 0);
-
- /* "(tree fragment)":4
- * raise TypeError("no default __reduce__ due to non-trivial __cinit__")
- * def __setstate_cython__(self, __pyx_state):
- * raise TypeError("no default __reduce__ due to non-trivial __cinit__") # <<<<<<<<<<<<<<
- */
- __pyx_t_1 = __Pyx_PyObject_Call(__pyx_builtin_TypeError, __pyx_tuple__19, NULL); if (unlikely(!__pyx_t_1)) __PYX_ERR(1, 4, __pyx_L1_error)
- __Pyx_GOTREF(__pyx_t_1);
- __Pyx_Raise(__pyx_t_1, 0, 0, 0);
- __Pyx_DECREF(__pyx_t_1); __pyx_t_1 = 0;
- __PYX_ERR(1, 4, __pyx_L1_error)
-
- /* "(tree fragment)":3
- * def __reduce_cython__(self):
- * raise TypeError("no default __reduce__ due to non-trivial __cinit__")
- * def __setstate_cython__(self, __pyx_state): # <<<<<<<<<<<<<<
- * raise TypeError("no default __reduce__ due to non-trivial __cinit__")
- */
-
- /* function exit code */
- __pyx_L1_error:;
- __Pyx_XDECREF(__pyx_t_1);
- __Pyx_AddTraceback("View.MemoryView._memoryviewslice.__setstate_cython__", __pyx_clineno, __pyx_lineno, __pyx_filename);
- __pyx_r = NULL;
- __Pyx_XGIVEREF(__pyx_r);
- __Pyx_RefNannyFinishContext();
- return __pyx_r;
-}
-
-/* "View.MemoryView":999
- *
- * @cname('__pyx_memoryview_fromslice')
- * cdef memoryview_fromslice(__Pyx_memviewslice memviewslice, # <<<<<<<<<<<<<<
- * int ndim,
- * object (*to_object_func)(char *),
- */
-
-static PyObject *__pyx_memoryview_fromslice(__Pyx_memviewslice __pyx_v_memviewslice, int __pyx_v_ndim, PyObject *(*__pyx_v_to_object_func)(char *), int (*__pyx_v_to_dtype_func)(char *, PyObject *), int __pyx_v_dtype_is_object) {
- struct __pyx_memoryviewslice_obj *__pyx_v_result = 0;
- Py_ssize_t __pyx_v_suboffset;
- PyObject *__pyx_v_length = NULL;
- PyObject *__pyx_r = NULL;
- __Pyx_RefNannyDeclarations
- int __pyx_t_1;
- PyObject *__pyx_t_2 = NULL;
- PyObject *__pyx_t_3 = NULL;
- __Pyx_TypeInfo *__pyx_t_4;
- Py_buffer __pyx_t_5;
- Py_ssize_t *__pyx_t_6;
- Py_ssize_t *__pyx_t_7;
- Py_ssize_t *__pyx_t_8;
- Py_ssize_t __pyx_t_9;
- int __pyx_lineno = 0;
- const char *__pyx_filename = NULL;
- int __pyx_clineno = 0;
- __Pyx_RefNannySetupContext("memoryview_fromslice", 0);
-
- /* "View.MemoryView":1007
- * cdef _memoryviewslice result
- *
- * if memviewslice.memview == Py_None: # <<<<<<<<<<<<<<
- * return None
- *
- */
- __pyx_t_1 = ((((PyObject *)__pyx_v_memviewslice.memview) == Py_None) != 0);
- if (__pyx_t_1) {
-
- /* "View.MemoryView":1008
- *
- * if memviewslice.memview == Py_None:
- * return None # <<<<<<<<<<<<<<
- *
- *
- */
- __Pyx_XDECREF(__pyx_r);
- __pyx_r = Py_None; __Pyx_INCREF(Py_None);
- goto __pyx_L0;
-
- /* "View.MemoryView":1007
- * cdef _memoryviewslice result
- *
- * if memviewslice.memview == Py_None: # <<<<<<<<<<<<<<
- * return None
- *
- */
- }
-
- /* "View.MemoryView":1013
- *
- *
- * result = _memoryviewslice(None, 0, dtype_is_object) # <<<<<<<<<<<<<<
- *
- * result.from_slice = memviewslice
- */
- __pyx_t_2 = __Pyx_PyBool_FromLong(__pyx_v_dtype_is_object); if (unlikely(!__pyx_t_2)) __PYX_ERR(1, 1013, __pyx_L1_error)
- __Pyx_GOTREF(__pyx_t_2);
- __pyx_t_3 = PyTuple_New(3); if (unlikely(!__pyx_t_3)) __PYX_ERR(1, 1013, __pyx_L1_error)
- __Pyx_GOTREF(__pyx_t_3);
- __Pyx_INCREF(Py_None);
- __Pyx_GIVEREF(Py_None);
- PyTuple_SET_ITEM(__pyx_t_3, 0, Py_None);
- __Pyx_INCREF(__pyx_int_0);
- __Pyx_GIVEREF(__pyx_int_0);
- PyTuple_SET_ITEM(__pyx_t_3, 1, __pyx_int_0);
- __Pyx_GIVEREF(__pyx_t_2);
- PyTuple_SET_ITEM(__pyx_t_3, 2, __pyx_t_2);
- __pyx_t_2 = 0;
- __pyx_t_2 = __Pyx_PyObject_Call(((PyObject *)__pyx_memoryviewslice_type), __pyx_t_3, NULL); if (unlikely(!__pyx_t_2)) __PYX_ERR(1, 1013, __pyx_L1_error)
- __Pyx_GOTREF(__pyx_t_2);
- __Pyx_DECREF(__pyx_t_3); __pyx_t_3 = 0;
- __pyx_v_result = ((struct __pyx_memoryviewslice_obj *)__pyx_t_2);
- __pyx_t_2 = 0;
-
- /* "View.MemoryView":1015
- * result = _memoryviewslice(None, 0, dtype_is_object)
- *
- * result.from_slice = memviewslice # <<<<<<<<<<<<<<
- * __PYX_INC_MEMVIEW(&memviewslice, 1)
- *
- */
- __pyx_v_result->from_slice = __pyx_v_memviewslice;
-
- /* "View.MemoryView":1016
- *
- * result.from_slice = memviewslice
- * __PYX_INC_MEMVIEW(&memviewslice, 1) # <<<<<<<<<<<<<<
- *
- * result.from_object = ( memviewslice.memview).base
- */
- __PYX_INC_MEMVIEW((&__pyx_v_memviewslice), 1);
-
- /* "View.MemoryView":1018
- * __PYX_INC_MEMVIEW(&memviewslice, 1)
- *
- * result.from_object = ( memviewslice.memview).base # <<<<<<<<<<<<<<
- * result.typeinfo = memviewslice.memview.typeinfo
- *
- */
- __pyx_t_2 = __Pyx_PyObject_GetAttrStr(((PyObject *)__pyx_v_memviewslice.memview), __pyx_n_s_base); if (unlikely(!__pyx_t_2)) __PYX_ERR(1, 1018, __pyx_L1_error)
- __Pyx_GOTREF(__pyx_t_2);
- __Pyx_GIVEREF(__pyx_t_2);
- __Pyx_GOTREF(__pyx_v_result->from_object);
- __Pyx_DECREF(__pyx_v_result->from_object);
- __pyx_v_result->from_object = __pyx_t_2;
- __pyx_t_2 = 0;
-
- /* "View.MemoryView":1019
- *
- * result.from_object = ( memviewslice.memview).base
- * result.typeinfo = memviewslice.memview.typeinfo # <<<<<<<<<<<<<<
- *
- * result.view = memviewslice.memview.view
- */
- __pyx_t_4 = __pyx_v_memviewslice.memview->typeinfo;
- __pyx_v_result->__pyx_base.typeinfo = __pyx_t_4;
-
- /* "View.MemoryView":1021
- * result.typeinfo = memviewslice.memview.typeinfo
- *
- * result.view = memviewslice.memview.view # <<<<<<<<<<<<<<
- * result.view.buf = memviewslice.data
- * result.view.ndim = ndim
- */
- __pyx_t_5 = __pyx_v_memviewslice.memview->view;
- __pyx_v_result->__pyx_base.view = __pyx_t_5;
-
- /* "View.MemoryView":1022
- *
- * result.view = memviewslice.memview.view
- * result.view.buf = memviewslice.data # <<<<<<<<<<<<<<
- * result.view.ndim = ndim
- * (<__pyx_buffer *> &result.view).obj = Py_None
- */
- __pyx_v_result->__pyx_base.view.buf = ((void *)__pyx_v_memviewslice.data);
-
- /* "View.MemoryView":1023
- * result.view = memviewslice.memview.view
- * result.view.buf = memviewslice.data
- * result.view.ndim = ndim # <<<<<<<<<<<<<<
- * (<__pyx_buffer *> &result.view).obj = Py_None
- * Py_INCREF(Py_None)
- */
- __pyx_v_result->__pyx_base.view.ndim = __pyx_v_ndim;
-
- /* "View.MemoryView":1024
- * result.view.buf = memviewslice.data
- * result.view.ndim = ndim
- * (<__pyx_buffer *> &result.view).obj = Py_None # <<<<<<<<<<<<<<
- * Py_INCREF(Py_None)
- *
- */
- ((Py_buffer *)(&__pyx_v_result->__pyx_base.view))->obj = Py_None;
-
- /* "View.MemoryView":1025
- * result.view.ndim = ndim
- * (<__pyx_buffer *> &result.view).obj = Py_None
- * Py_INCREF(Py_None) # <<<<<<<<<<<<<<
- *
- * if (memviewslice.memview).flags & PyBUF_WRITABLE:
- */
- Py_INCREF(Py_None);
-
- /* "View.MemoryView":1027
- * Py_INCREF(Py_None)
- *
- * if (memviewslice.memview).flags & PyBUF_WRITABLE: # <<<<<<<<<<<<<<
- * result.flags = PyBUF_RECORDS
- * else:
- */
- __pyx_t_1 = ((((struct __pyx_memoryview_obj *)__pyx_v_memviewslice.memview)->flags & PyBUF_WRITABLE) != 0);
- if (__pyx_t_1) {
-
- /* "View.MemoryView":1028
- *
- * if (memviewslice.memview).flags & PyBUF_WRITABLE:
- * result.flags = PyBUF_RECORDS # <<<<<<<<<<<<<<
- * else:
- * result.flags = PyBUF_RECORDS_RO
- */
- __pyx_v_result->__pyx_base.flags = PyBUF_RECORDS;
-
- /* "View.MemoryView":1027
- * Py_INCREF(Py_None)
- *
- * if (memviewslice.memview).flags & PyBUF_WRITABLE: # <<<<<<<<<<<<<<
- * result.flags = PyBUF_RECORDS
- * else:
- */
- goto __pyx_L4;
- }
-
- /* "View.MemoryView":1030
- * result.flags = PyBUF_RECORDS
- * else:
- * result.flags = PyBUF_RECORDS_RO # <<<<<<<<<<<<<<
- *
- * result.view.shape = result.from_slice.shape
- */
- /*else*/ {
- __pyx_v_result->__pyx_base.flags = PyBUF_RECORDS_RO;
- }
- __pyx_L4:;
-
- /* "View.MemoryView":1032
- * result.flags = PyBUF_RECORDS_RO
- *
- * result.view.shape = result.from_slice.shape # <<<<<<<<<<<<<<
- * result.view.strides = result.from_slice.strides
- *
- */
- __pyx_v_result->__pyx_base.view.shape = ((Py_ssize_t *)__pyx_v_result->from_slice.shape);
-
- /* "View.MemoryView":1033
- *
- * result.view.shape = result.from_slice.shape
- * result.view.strides = result.from_slice.strides # <<<<<<<<<<<<<<
- *
- *
- */
- __pyx_v_result->__pyx_base.view.strides = ((Py_ssize_t *)__pyx_v_result->from_slice.strides);
-
- /* "View.MemoryView":1036
- *
- *
- * result.view.suboffsets = NULL # <<<<<<<<<<<<<<
- * for suboffset in result.from_slice.suboffsets[:ndim]:
- * if suboffset >= 0:
- */
- __pyx_v_result->__pyx_base.view.suboffsets = NULL;
-
- /* "View.MemoryView":1037
- *
- * result.view.suboffsets = NULL
- * for suboffset in result.from_slice.suboffsets[:ndim]: # <<<<<<<<<<<<<<
- * if suboffset >= 0:
- * result.view.suboffsets = result.from_slice.suboffsets
- */
- __pyx_t_7 = (__pyx_v_result->from_slice.suboffsets + __pyx_v_ndim);
- for (__pyx_t_8 = __pyx_v_result->from_slice.suboffsets; __pyx_t_8 < __pyx_t_7; __pyx_t_8++) {
- __pyx_t_6 = __pyx_t_8;
- __pyx_v_suboffset = (__pyx_t_6[0]);
-
- /* "View.MemoryView":1038
- * result.view.suboffsets = NULL
- * for suboffset in result.from_slice.suboffsets[:ndim]:
- * if suboffset >= 0: # <<<<<<<<<<<<<<
- * result.view.suboffsets = result.from_slice.suboffsets
- * break
- */
- __pyx_t_1 = ((__pyx_v_suboffset >= 0) != 0);
- if (__pyx_t_1) {
-
- /* "View.MemoryView":1039
- * for suboffset in result.from_slice.suboffsets[:ndim]:
- * if suboffset >= 0:
- * result.view.suboffsets = result.from_slice.suboffsets # <<<<<<<<<<<<<<
- * break
- *
- */
- __pyx_v_result->__pyx_base.view.suboffsets = ((Py_ssize_t *)__pyx_v_result->from_slice.suboffsets);
-
- /* "View.MemoryView":1040
- * if suboffset >= 0:
- * result.view.suboffsets = result.from_slice.suboffsets
- * break # <<<<<<<<<<<<<<
- *
- * result.view.len = result.view.itemsize
- */
- goto __pyx_L6_break;
-
- /* "View.MemoryView":1038
- * result.view.suboffsets = NULL
- * for suboffset in result.from_slice.suboffsets[:ndim]:
- * if suboffset >= 0: # <<<<<<<<<<<<<<
- * result.view.suboffsets = result.from_slice.suboffsets
- * break
- */
- }
- }
- __pyx_L6_break:;
-
- /* "View.MemoryView":1042
- * break
- *
- * result.view.len = result.view.itemsize # <<<<<<<<<<<<<<
- * for length in result.view.shape[:ndim]:
- * result.view.len *= length
- */
- __pyx_t_9 = __pyx_v_result->__pyx_base.view.itemsize;
- __pyx_v_result->__pyx_base.view.len = __pyx_t_9;
-
- /* "View.MemoryView":1043
- *
- * result.view.len = result.view.itemsize
- * for length in result.view.shape[:ndim]: # <<<<<<<<<<<<<<
- * result.view.len *= length
- *
- */
- __pyx_t_7 = (__pyx_v_result->__pyx_base.view.shape + __pyx_v_ndim);
- for (__pyx_t_8 = __pyx_v_result->__pyx_base.view.shape; __pyx_t_8 < __pyx_t_7; __pyx_t_8++) {
- __pyx_t_6 = __pyx_t_8;
- __pyx_t_2 = PyInt_FromSsize_t((__pyx_t_6[0])); if (unlikely(!__pyx_t_2)) __PYX_ERR(1, 1043, __pyx_L1_error)
- __Pyx_GOTREF(__pyx_t_2);
- __Pyx_XDECREF_SET(__pyx_v_length, __pyx_t_2);
- __pyx_t_2 = 0;
-
- /* "View.MemoryView":1044
- * result.view.len = result.view.itemsize
- * for length in result.view.shape[:ndim]:
- * result.view.len *= length # <<<<<<<<<<<<<<
- *
- * result.to_object_func = to_object_func
- */
- __pyx_t_2 = PyInt_FromSsize_t(__pyx_v_result->__pyx_base.view.len); if (unlikely(!__pyx_t_2)) __PYX_ERR(1, 1044, __pyx_L1_error)
- __Pyx_GOTREF(__pyx_t_2);
- __pyx_t_3 = PyNumber_InPlaceMultiply(__pyx_t_2, __pyx_v_length); if (unlikely(!__pyx_t_3)) __PYX_ERR(1, 1044, __pyx_L1_error)
- __Pyx_GOTREF(__pyx_t_3);
- __Pyx_DECREF(__pyx_t_2); __pyx_t_2 = 0;
- __pyx_t_9 = __Pyx_PyIndex_AsSsize_t(__pyx_t_3); if (unlikely((__pyx_t_9 == (Py_ssize_t)-1) && PyErr_Occurred())) __PYX_ERR(1, 1044, __pyx_L1_error)
- __Pyx_DECREF(__pyx_t_3); __pyx_t_3 = 0;
- __pyx_v_result->__pyx_base.view.len = __pyx_t_9;
- }
-
- /* "View.MemoryView":1046
- * result.view.len *= length
- *
- * result.to_object_func = to_object_func # <<<<<<<<<<<<<<
- * result.to_dtype_func = to_dtype_func
- *
- */
- __pyx_v_result->to_object_func = __pyx_v_to_object_func;
-
- /* "View.MemoryView":1047
- *
- * result.to_object_func = to_object_func
- * result.to_dtype_func = to_dtype_func # <<<<<<<<<<<<<<
- *
- * return result
- */
- __pyx_v_result->to_dtype_func = __pyx_v_to_dtype_func;
-
- /* "View.MemoryView":1049
- * result.to_dtype_func = to_dtype_func
- *
- * return result # <<<<<<<<<<<<<<
- *
- * @cname('__pyx_memoryview_get_slice_from_memoryview')
- */
- __Pyx_XDECREF(__pyx_r);
- __Pyx_INCREF(((PyObject *)__pyx_v_result));
- __pyx_r = ((PyObject *)__pyx_v_result);
- goto __pyx_L0;
-
- /* "View.MemoryView":999
- *
- * @cname('__pyx_memoryview_fromslice')
- * cdef memoryview_fromslice(__Pyx_memviewslice memviewslice, # <<<<<<<<<<<<<<
- * int ndim,
- * object (*to_object_func)(char *),
- */
-
- /* function exit code */
- __pyx_L1_error:;
- __Pyx_XDECREF(__pyx_t_2);
- __Pyx_XDECREF(__pyx_t_3);
- __Pyx_AddTraceback("View.MemoryView.memoryview_fromslice", __pyx_clineno, __pyx_lineno, __pyx_filename);
- __pyx_r = 0;
- __pyx_L0:;
- __Pyx_XDECREF((PyObject *)__pyx_v_result);
- __Pyx_XDECREF(__pyx_v_length);
- __Pyx_XGIVEREF(__pyx_r);
- __Pyx_RefNannyFinishContext();
- return __pyx_r;
-}
-
-/* "View.MemoryView":1052
- *
- * @cname('__pyx_memoryview_get_slice_from_memoryview')
- * cdef __Pyx_memviewslice *get_slice_from_memview(memoryview memview, # <<<<<<<<<<<<<<
- * __Pyx_memviewslice *mslice) except NULL:
- * cdef _memoryviewslice obj
- */
-
-static __Pyx_memviewslice *__pyx_memoryview_get_slice_from_memoryview(struct __pyx_memoryview_obj *__pyx_v_memview, __Pyx_memviewslice *__pyx_v_mslice) {
- struct __pyx_memoryviewslice_obj *__pyx_v_obj = 0;
- __Pyx_memviewslice *__pyx_r;
- __Pyx_RefNannyDeclarations
- int __pyx_t_1;
- int __pyx_t_2;
- PyObject *__pyx_t_3 = NULL;
- int __pyx_lineno = 0;
- const char *__pyx_filename = NULL;
- int __pyx_clineno = 0;
- __Pyx_RefNannySetupContext("get_slice_from_memview", 0);
-
- /* "View.MemoryView":1055
- * __Pyx_memviewslice *mslice) except NULL:
- * cdef _memoryviewslice obj
- * if isinstance(memview, _memoryviewslice): # <<<<<<<<<<<<<<
- * obj = memview
- * return &obj.from_slice
- */
- __pyx_t_1 = __Pyx_TypeCheck(((PyObject *)__pyx_v_memview), __pyx_memoryviewslice_type);
- __pyx_t_2 = (__pyx_t_1 != 0);
- if (__pyx_t_2) {
-
- /* "View.MemoryView":1056
- * cdef _memoryviewslice obj
- * if isinstance(memview, _memoryviewslice):
- * obj = memview # <<<<<<<<<<<<<<
- * return &obj.from_slice
- * else:
- */
- if (!(likely(((((PyObject *)__pyx_v_memview)) == Py_None) || likely(__Pyx_TypeTest(((PyObject *)__pyx_v_memview), __pyx_memoryviewslice_type))))) __PYX_ERR(1, 1056, __pyx_L1_error)
- __pyx_t_3 = ((PyObject *)__pyx_v_memview);
- __Pyx_INCREF(__pyx_t_3);
- __pyx_v_obj = ((struct __pyx_memoryviewslice_obj *)__pyx_t_3);
- __pyx_t_3 = 0;
-
- /* "View.MemoryView":1057
- * if isinstance(memview, _memoryviewslice):
- * obj = memview
- * return &obj.from_slice # <<<<<<<<<<<<<<
- * else:
- * slice_copy(memview, mslice)
- */
- __pyx_r = (&__pyx_v_obj->from_slice);
- goto __pyx_L0;
-
- /* "View.MemoryView":1055
- * __Pyx_memviewslice *mslice) except NULL:
- * cdef _memoryviewslice obj
- * if isinstance(memview, _memoryviewslice): # <<<<<<<<<<<<<<
- * obj = memview
- * return &obj.from_slice
- */
- }
-
- /* "View.MemoryView":1059
- * return &obj.from_slice
- * else:
- * slice_copy(memview, mslice) # <<<<<<<<<<<<<<
- * return mslice
- *
- */
- /*else*/ {
- __pyx_memoryview_slice_copy(__pyx_v_memview, __pyx_v_mslice);
-
- /* "View.MemoryView":1060
- * else:
- * slice_copy(memview, mslice)
- * return mslice # <<<<<<<<<<<<<<
- *
- * @cname('__pyx_memoryview_slice_copy')
- */
- __pyx_r = __pyx_v_mslice;
- goto __pyx_L0;
- }
-
- /* "View.MemoryView":1052
- *
- * @cname('__pyx_memoryview_get_slice_from_memoryview')
- * cdef __Pyx_memviewslice *get_slice_from_memview(memoryview memview, # <<<<<<<<<<<<<<
- * __Pyx_memviewslice *mslice) except NULL:
- * cdef _memoryviewslice obj
- */
-
- /* function exit code */
- __pyx_L1_error:;
- __Pyx_XDECREF(__pyx_t_3);
- __Pyx_AddTraceback("View.MemoryView.get_slice_from_memview", __pyx_clineno, __pyx_lineno, __pyx_filename);
- __pyx_r = NULL;
- __pyx_L0:;
- __Pyx_XDECREF((PyObject *)__pyx_v_obj);
- __Pyx_RefNannyFinishContext();
- return __pyx_r;
-}
-
-/* "View.MemoryView":1063
- *
- * @cname('__pyx_memoryview_slice_copy')
- * cdef void slice_copy(memoryview memview, __Pyx_memviewslice *dst): # <<<<<<<<<<<<<<
- * cdef int dim
- * cdef (Py_ssize_t*) shape, strides, suboffsets
- */
-
-static void __pyx_memoryview_slice_copy(struct __pyx_memoryview_obj *__pyx_v_memview, __Pyx_memviewslice *__pyx_v_dst) {
- int __pyx_v_dim;
- Py_ssize_t *__pyx_v_shape;
- Py_ssize_t *__pyx_v_strides;
- Py_ssize_t *__pyx_v_suboffsets;
- __Pyx_RefNannyDeclarations
- Py_ssize_t *__pyx_t_1;
- int __pyx_t_2;
- int __pyx_t_3;
- int __pyx_t_4;
- Py_ssize_t __pyx_t_5;
- __Pyx_RefNannySetupContext("slice_copy", 0);
-
- /* "View.MemoryView":1067
- * cdef (Py_ssize_t*) shape, strides, suboffsets
- *
- * shape = memview.view.shape # <<<<<<<<<<<<<<
- * strides = memview.view.strides
- * suboffsets = memview.view.suboffsets
- */
- __pyx_t_1 = __pyx_v_memview->view.shape;
- __pyx_v_shape = __pyx_t_1;
-
- /* "View.MemoryView":1068
- *
- * shape = memview.view.shape
- * strides = memview.view.strides # <<<<<<<<<<<<<<
- * suboffsets = memview.view.suboffsets
- *
- */
- __pyx_t_1 = __pyx_v_memview->view.strides;
- __pyx_v_strides = __pyx_t_1;
-
- /* "View.MemoryView":1069
- * shape = memview.view.shape
- * strides = memview.view.strides
- * suboffsets = memview.view.suboffsets # <<<<<<<<<<<<<<
- *
- * dst.memview = <__pyx_memoryview *> memview
- */
- __pyx_t_1 = __pyx_v_memview->view.suboffsets;
- __pyx_v_suboffsets = __pyx_t_1;
-
- /* "View.MemoryView":1071
- * suboffsets = memview.view.suboffsets
- *
- * dst.memview = <__pyx_memoryview *> memview # <<<<<<<<<<<<<<
- * dst.data = memview.view.buf
- *
- */
- __pyx_v_dst->memview = ((struct __pyx_memoryview_obj *)__pyx_v_memview);
-
- /* "View.MemoryView":1072
- *
- * dst.memview = <__pyx_memoryview *> memview
- * dst.data = memview.view.buf # <<<<<<<<<<<<<<
- *
- * for dim in range(memview.view.ndim):
- */
- __pyx_v_dst->data = ((char *)__pyx_v_memview->view.buf);
-
- /* "View.MemoryView":1074
- * dst.data = memview.view.buf
- *
- * for dim in range(memview.view.ndim): # <<<<<<<<<<<<<<
- * dst.shape[dim] = shape[dim]
- * dst.strides[dim] = strides[dim]
- */
- __pyx_t_2 = __pyx_v_memview->view.ndim;
- __pyx_t_3 = __pyx_t_2;
- for (__pyx_t_4 = 0; __pyx_t_4 < __pyx_t_3; __pyx_t_4+=1) {
- __pyx_v_dim = __pyx_t_4;
-
- /* "View.MemoryView":1075
- *
- * for dim in range(memview.view.ndim):
- * dst.shape[dim] = shape[dim] # <<<<<<<<<<<<<<
- * dst.strides[dim] = strides[dim]
- * dst.suboffsets[dim] = suboffsets[dim] if suboffsets else -1
- */
- (__pyx_v_dst->shape[__pyx_v_dim]) = (__pyx_v_shape[__pyx_v_dim]);
-
- /* "View.MemoryView":1076
- * for dim in range(memview.view.ndim):
- * dst.shape[dim] = shape[dim]
- * dst.strides[dim] = strides[dim] # <<<<<<<<<<<<<<
- * dst.suboffsets[dim] = suboffsets[dim] if suboffsets else -1
- *
- */
- (__pyx_v_dst->strides[__pyx_v_dim]) = (__pyx_v_strides[__pyx_v_dim]);
-
- /* "View.MemoryView":1077
- * dst.shape[dim] = shape[dim]
- * dst.strides[dim] = strides[dim]
- * dst.suboffsets[dim] = suboffsets[dim] if suboffsets else -1 # <<<<<<<<<<<<<<
- *
- * @cname('__pyx_memoryview_copy_object')
- */
- if ((__pyx_v_suboffsets != 0)) {
- __pyx_t_5 = (__pyx_v_suboffsets[__pyx_v_dim]);
- } else {
- __pyx_t_5 = -1L;
- }
- (__pyx_v_dst->suboffsets[__pyx_v_dim]) = __pyx_t_5;
- }
-
- /* "View.MemoryView":1063
- *
- * @cname('__pyx_memoryview_slice_copy')
- * cdef void slice_copy(memoryview memview, __Pyx_memviewslice *dst): # <<<<<<<<<<<<<<
- * cdef int dim
- * cdef (Py_ssize_t*) shape, strides, suboffsets
- */
-
- /* function exit code */
- __Pyx_RefNannyFinishContext();
-}
-
-/* "View.MemoryView":1080
- *
- * @cname('__pyx_memoryview_copy_object')
- * cdef memoryview_copy(memoryview memview): # <<<<<<<<<<<<<<
- * "Create a new memoryview object"
- * cdef __Pyx_memviewslice memviewslice
- */
-
-static PyObject *__pyx_memoryview_copy_object(struct __pyx_memoryview_obj *__pyx_v_memview) {
- __Pyx_memviewslice __pyx_v_memviewslice;
- PyObject *__pyx_r = NULL;
- __Pyx_RefNannyDeclarations
- PyObject *__pyx_t_1 = NULL;
- int __pyx_lineno = 0;
- const char *__pyx_filename = NULL;
- int __pyx_clineno = 0;
- __Pyx_RefNannySetupContext("memoryview_copy", 0);
-
- /* "View.MemoryView":1083
- * "Create a new memoryview object"
- * cdef __Pyx_memviewslice memviewslice
- * slice_copy(memview, &memviewslice) # <<<<<<<<<<<<<<
- * return memoryview_copy_from_slice(memview, &memviewslice)
- *
- */
- __pyx_memoryview_slice_copy(__pyx_v_memview, (&__pyx_v_memviewslice));
-
- /* "View.MemoryView":1084
- * cdef __Pyx_memviewslice memviewslice
- * slice_copy(memview, &memviewslice)
- * return memoryview_copy_from_slice(memview, &memviewslice) # <<<<<<<<<<<<<<
- *
- * @cname('__pyx_memoryview_copy_object_from_slice')
- */
- __Pyx_XDECREF(__pyx_r);
- __pyx_t_1 = __pyx_memoryview_copy_object_from_slice(__pyx_v_memview, (&__pyx_v_memviewslice)); if (unlikely(!__pyx_t_1)) __PYX_ERR(1, 1084, __pyx_L1_error)
- __Pyx_GOTREF(__pyx_t_1);
- __pyx_r = __pyx_t_1;
- __pyx_t_1 = 0;
- goto __pyx_L0;
-
- /* "View.MemoryView":1080
- *
- * @cname('__pyx_memoryview_copy_object')
- * cdef memoryview_copy(memoryview memview): # <<<<<<<<<<<<<<
- * "Create a new memoryview object"
- * cdef __Pyx_memviewslice memviewslice
- */
-
- /* function exit code */
- __pyx_L1_error:;
- __Pyx_XDECREF(__pyx_t_1);
- __Pyx_AddTraceback("View.MemoryView.memoryview_copy", __pyx_clineno, __pyx_lineno, __pyx_filename);
- __pyx_r = 0;
- __pyx_L0:;
- __Pyx_XGIVEREF(__pyx_r);
- __Pyx_RefNannyFinishContext();
- return __pyx_r;
-}
-
-/* "View.MemoryView":1087
- *
- * @cname('__pyx_memoryview_copy_object_from_slice')
- * cdef memoryview_copy_from_slice(memoryview memview, __Pyx_memviewslice *memviewslice): # <<<<<<<<<<<<<<
- * """
- * Create a new memoryview object from a given memoryview object and slice.
- */
-
-static PyObject *__pyx_memoryview_copy_object_from_slice(struct __pyx_memoryview_obj *__pyx_v_memview, __Pyx_memviewslice *__pyx_v_memviewslice) {
- PyObject *(*__pyx_v_to_object_func)(char *);
- int (*__pyx_v_to_dtype_func)(char *, PyObject *);
- PyObject *__pyx_r = NULL;
- __Pyx_RefNannyDeclarations
- int __pyx_t_1;
- int __pyx_t_2;
- PyObject *(*__pyx_t_3)(char *);
- int (*__pyx_t_4)(char *, PyObject *);
- PyObject *__pyx_t_5 = NULL;
- int __pyx_lineno = 0;
- const char *__pyx_filename = NULL;
- int __pyx_clineno = 0;
- __Pyx_RefNannySetupContext("memoryview_copy_from_slice", 0);
-
- /* "View.MemoryView":1094
- * cdef int (*to_dtype_func)(char *, object) except 0
- *
- * if isinstance(memview, _memoryviewslice): # <<<<<<<<<<<<<<
- * to_object_func = (<_memoryviewslice> memview).to_object_func
- * to_dtype_func = (<_memoryviewslice> memview).to_dtype_func
- */
- __pyx_t_1 = __Pyx_TypeCheck(((PyObject *)__pyx_v_memview), __pyx_memoryviewslice_type);
- __pyx_t_2 = (__pyx_t_1 != 0);
- if (__pyx_t_2) {
-
- /* "View.MemoryView":1095
- *
- * if isinstance(memview, _memoryviewslice):
- * to_object_func = (<_memoryviewslice> memview).to_object_func # <<<<<<<<<<<<<<
- * to_dtype_func = (<_memoryviewslice> memview).to_dtype_func
- * else:
- */
- __pyx_t_3 = ((struct __pyx_memoryviewslice_obj *)__pyx_v_memview)->to_object_func;
- __pyx_v_to_object_func = __pyx_t_3;
-
- /* "View.MemoryView":1096
- * if isinstance(memview, _memoryviewslice):
- * to_object_func = (<_memoryviewslice> memview).to_object_func
- * to_dtype_func = (<_memoryviewslice> memview).to_dtype_func # <<<<<<<<<<<<<<
- * else:
- * to_object_func = NULL
- */
- __pyx_t_4 = ((struct __pyx_memoryviewslice_obj *)__pyx_v_memview)->to_dtype_func;
- __pyx_v_to_dtype_func = __pyx_t_4;
-
- /* "View.MemoryView":1094
- * cdef int (*to_dtype_func)(char *, object) except 0
- *
- * if isinstance(memview, _memoryviewslice): # <<<<<<<<<<<<<<
- * to_object_func = (<_memoryviewslice> memview).to_object_func
- * to_dtype_func = (<_memoryviewslice> memview).to_dtype_func
- */
- goto __pyx_L3;
- }
-
- /* "View.MemoryView":1098
- * to_dtype_func = (<_memoryviewslice> memview).to_dtype_func
- * else:
- * to_object_func = NULL # <<<<<<<<<<<<<<
- * to_dtype_func = NULL
- *
- */
- /*else*/ {
- __pyx_v_to_object_func = NULL;
-
- /* "View.MemoryView":1099
- * else:
- * to_object_func = NULL
- * to_dtype_func = NULL # <<<<<<<<<<<<<<
- *
- * return memoryview_fromslice(memviewslice[0], memview.view.ndim,
- */
- __pyx_v_to_dtype_func = NULL;
- }
- __pyx_L3:;
-
- /* "View.MemoryView":1101
- * to_dtype_func = NULL
- *
- * return memoryview_fromslice(memviewslice[0], memview.view.ndim, # <<<<<<<<<<<<<<
- * to_object_func, to_dtype_func,
- * memview.dtype_is_object)
- */
- __Pyx_XDECREF(__pyx_r);
-
- /* "View.MemoryView":1103
- * return memoryview_fromslice(memviewslice[0], memview.view.ndim,
- * to_object_func, to_dtype_func,
- * memview.dtype_is_object) # <<<<<<<<<<<<<<
- *
- *
- */
- __pyx_t_5 = __pyx_memoryview_fromslice((__pyx_v_memviewslice[0]), __pyx_v_memview->view.ndim, __pyx_v_to_object_func, __pyx_v_to_dtype_func, __pyx_v_memview->dtype_is_object); if (unlikely(!__pyx_t_5)) __PYX_ERR(1, 1101, __pyx_L1_error)
- __Pyx_GOTREF(__pyx_t_5);
- __pyx_r = __pyx_t_5;
- __pyx_t_5 = 0;
- goto __pyx_L0;
-
- /* "View.MemoryView":1087
- *
- * @cname('__pyx_memoryview_copy_object_from_slice')
- * cdef memoryview_copy_from_slice(memoryview memview, __Pyx_memviewslice *memviewslice): # <<<<<<<<<<<<<<
- * """
- * Create a new memoryview object from a given memoryview object and slice.
- */
-
- /* function exit code */
- __pyx_L1_error:;
- __Pyx_XDECREF(__pyx_t_5);
- __Pyx_AddTraceback("View.MemoryView.memoryview_copy_from_slice", __pyx_clineno, __pyx_lineno, __pyx_filename);
- __pyx_r = 0;
- __pyx_L0:;
- __Pyx_XGIVEREF(__pyx_r);
- __Pyx_RefNannyFinishContext();
- return __pyx_r;
-}
-
-/* "View.MemoryView":1109
- *
- *
- * cdef Py_ssize_t abs_py_ssize_t(Py_ssize_t arg) nogil: # <<<<<<<<<<<<<<
- * if arg < 0:
- * return -arg
- */
-
-static Py_ssize_t abs_py_ssize_t(Py_ssize_t __pyx_v_arg) {
- Py_ssize_t __pyx_r;
- int __pyx_t_1;
-
- /* "View.MemoryView":1110
- *
- * cdef Py_ssize_t abs_py_ssize_t(Py_ssize_t arg) nogil:
- * if arg < 0: # <<<<<<<<<<<<<<
- * return -arg
- * else:
- */
- __pyx_t_1 = ((__pyx_v_arg < 0) != 0);
- if (__pyx_t_1) {
-
- /* "View.MemoryView":1111
- * cdef Py_ssize_t abs_py_ssize_t(Py_ssize_t arg) nogil:
- * if arg < 0:
- * return -arg # <<<<<<<<<<<<<<
- * else:
- * return arg
- */
- __pyx_r = (-__pyx_v_arg);
- goto __pyx_L0;
-
- /* "View.MemoryView":1110
- *
- * cdef Py_ssize_t abs_py_ssize_t(Py_ssize_t arg) nogil:
- * if arg < 0: # <<<<<<<<<<<<<<
- * return -arg
- * else:
- */
- }
-
- /* "View.MemoryView":1113
- * return -arg
- * else:
- * return arg # <<<<<<<<<<<<<<
- *
- * @cname('__pyx_get_best_slice_order')
- */
- /*else*/ {
- __pyx_r = __pyx_v_arg;
- goto __pyx_L0;
- }
-
- /* "View.MemoryView":1109
- *
- *
- * cdef Py_ssize_t abs_py_ssize_t(Py_ssize_t arg) nogil: # <<<<<<<<<<<<<<
- * if arg < 0:
- * return -arg
- */
-
- /* function exit code */
- __pyx_L0:;
- return __pyx_r;
-}
-
-/* "View.MemoryView":1116
- *
- * @cname('__pyx_get_best_slice_order')
- * cdef char get_best_order(__Pyx_memviewslice *mslice, int ndim) nogil: # <<<<<<<<<<<<<<
- * """
- * Figure out the best memory access order for a given slice.
- */
-
-static char __pyx_get_best_slice_order(__Pyx_memviewslice *__pyx_v_mslice, int __pyx_v_ndim) {
- int __pyx_v_i;
- Py_ssize_t __pyx_v_c_stride;
- Py_ssize_t __pyx_v_f_stride;
- char __pyx_r;
- int __pyx_t_1;
- int __pyx_t_2;
- int __pyx_t_3;
- int __pyx_t_4;
-
- /* "View.MemoryView":1121
- * """
- * cdef int i
- * cdef Py_ssize_t c_stride = 0 # <<<<<<<<<<<<<<
- * cdef Py_ssize_t f_stride = 0
- *
- */
- __pyx_v_c_stride = 0;
-
- /* "View.MemoryView":1122
- * cdef int i
- * cdef Py_ssize_t c_stride = 0
- * cdef Py_ssize_t f_stride = 0 # <<<<<<<<<<<<<<
- *
- * for i in range(ndim - 1, -1, -1):
- */
- __pyx_v_f_stride = 0;
-
- /* "View.MemoryView":1124
- * cdef Py_ssize_t f_stride = 0
- *
- * for i in range(ndim - 1, -1, -1): # <<<<<<<<<<<<<<
- * if mslice.shape[i] > 1:
- * c_stride = mslice.strides[i]
- */
- for (__pyx_t_1 = (__pyx_v_ndim - 1); __pyx_t_1 > -1; __pyx_t_1-=1) {
- __pyx_v_i = __pyx_t_1;
-
- /* "View.MemoryView":1125
- *
- * for i in range(ndim - 1, -1, -1):
- * if mslice.shape[i] > 1: # <<<<<<<<<<<<<<
- * c_stride = mslice.strides[i]
- * break
- */
- __pyx_t_2 = (((__pyx_v_mslice->shape[__pyx_v_i]) > 1) != 0);
- if (__pyx_t_2) {
-
- /* "View.MemoryView":1126
- * for i in range(ndim - 1, -1, -1):
- * if mslice.shape[i] > 1:
- * c_stride = mslice.strides[i] # <<<<<<<<<<<<<<
- * break
- *
- */
- __pyx_v_c_stride = (__pyx_v_mslice->strides[__pyx_v_i]);
-
- /* "View.MemoryView":1127
- * if mslice.shape[i] > 1:
- * c_stride = mslice.strides[i]
- * break # <<<<<<<<<<<<<<
- *
- * for i in range(ndim):
- */
- goto __pyx_L4_break;
-
- /* "View.MemoryView":1125
- *
- * for i in range(ndim - 1, -1, -1):
- * if mslice.shape[i] > 1: # <<<<<<<<<<<<<<
- * c_stride = mslice.strides[i]
- * break
- */
- }
- }
- __pyx_L4_break:;
-
- /* "View.MemoryView":1129
- * break
- *
- * for i in range(ndim): # <<<<<<<<<<<<<<
- * if mslice.shape[i] > 1:
- * f_stride = mslice.strides[i]
- */
- __pyx_t_1 = __pyx_v_ndim;
- __pyx_t_3 = __pyx_t_1;
- for (__pyx_t_4 = 0; __pyx_t_4 < __pyx_t_3; __pyx_t_4+=1) {
- __pyx_v_i = __pyx_t_4;
-
- /* "View.MemoryView":1130
- *
- * for i in range(ndim):
- * if mslice.shape[i] > 1: # <<<<<<<<<<<<<<
- * f_stride = mslice.strides[i]
- * break
- */
- __pyx_t_2 = (((__pyx_v_mslice->shape[__pyx_v_i]) > 1) != 0);
- if (__pyx_t_2) {
-
- /* "View.MemoryView":1131
- * for i in range(ndim):
- * if mslice.shape[i] > 1:
- * f_stride = mslice.strides[i] # <<<<<<<<<<<<<<
- * break
- *
- */
- __pyx_v_f_stride = (__pyx_v_mslice->strides[__pyx_v_i]);
-
- /* "View.MemoryView":1132
- * if mslice.shape[i] > 1:
- * f_stride = mslice.strides[i]
- * break # <<<<<<<<<<<<<<
- *
- * if abs_py_ssize_t(c_stride) <= abs_py_ssize_t(f_stride):
- */
- goto __pyx_L7_break;
-
- /* "View.MemoryView":1130
- *
- * for i in range(ndim):
- * if mslice.shape[i] > 1: # <<<<<<<<<<<<<<
- * f_stride = mslice.strides[i]
- * break
- */
- }
- }
- __pyx_L7_break:;
-
- /* "View.MemoryView":1134
- * break
- *
- * if abs_py_ssize_t(c_stride) <= abs_py_ssize_t(f_stride): # <<<<<<<<<<<<<<
- * return 'C'
- * else:
- */
- __pyx_t_2 = ((abs_py_ssize_t(__pyx_v_c_stride) <= abs_py_ssize_t(__pyx_v_f_stride)) != 0);
- if (__pyx_t_2) {
-
- /* "View.MemoryView":1135
- *
- * if abs_py_ssize_t(c_stride) <= abs_py_ssize_t(f_stride):
- * return 'C' # <<<<<<<<<<<<<<
- * else:
- * return 'F'
- */
- __pyx_r = 'C';
- goto __pyx_L0;
-
- /* "View.MemoryView":1134
- * break
- *
- * if abs_py_ssize_t(c_stride) <= abs_py_ssize_t(f_stride): # <<<<<<<<<<<<<<
- * return 'C'
- * else:
- */
- }
-
- /* "View.MemoryView":1137
- * return 'C'
- * else:
- * return 'F' # <<<<<<<<<<<<<<
- *
- * @cython.cdivision(True)
- */
- /*else*/ {
- __pyx_r = 'F';
- goto __pyx_L0;
- }
-
- /* "View.MemoryView":1116
- *
- * @cname('__pyx_get_best_slice_order')
- * cdef char get_best_order(__Pyx_memviewslice *mslice, int ndim) nogil: # <<<<<<<<<<<<<<
- * """
- * Figure out the best memory access order for a given slice.
- */
-
- /* function exit code */
- __pyx_L0:;
- return __pyx_r;
-}
-
-/* "View.MemoryView":1140
- *
- * @cython.cdivision(True)
- * cdef void _copy_strided_to_strided(char *src_data, Py_ssize_t *src_strides, # <<<<<<<<<<<<<<
- * char *dst_data, Py_ssize_t *dst_strides,
- * Py_ssize_t *src_shape, Py_ssize_t *dst_shape,
- */
-
-static void _copy_strided_to_strided(char *__pyx_v_src_data, Py_ssize_t *__pyx_v_src_strides, char *__pyx_v_dst_data, Py_ssize_t *__pyx_v_dst_strides, Py_ssize_t *__pyx_v_src_shape, Py_ssize_t *__pyx_v_dst_shape, int __pyx_v_ndim, size_t __pyx_v_itemsize) {
- CYTHON_UNUSED Py_ssize_t __pyx_v_i;
- CYTHON_UNUSED Py_ssize_t __pyx_v_src_extent;
- Py_ssize_t __pyx_v_dst_extent;
- Py_ssize_t __pyx_v_src_stride;
- Py_ssize_t __pyx_v_dst_stride;
- int __pyx_t_1;
- int __pyx_t_2;
- int __pyx_t_3;
- Py_ssize_t __pyx_t_4;
- Py_ssize_t __pyx_t_5;
- Py_ssize_t __pyx_t_6;
-
- /* "View.MemoryView":1147
- *
- * cdef Py_ssize_t i
- * cdef Py_ssize_t src_extent = src_shape[0] # <<<<<<<<<<<<<<
- * cdef Py_ssize_t dst_extent = dst_shape[0]
- * cdef Py_ssize_t src_stride = src_strides[0]
- */
- __pyx_v_src_extent = (__pyx_v_src_shape[0]);
-
- /* "View.MemoryView":1148
- * cdef Py_ssize_t i
- * cdef Py_ssize_t src_extent = src_shape[0]
- * cdef Py_ssize_t dst_extent = dst_shape[0] # <<<<<<<<<<<<<<
- * cdef Py_ssize_t src_stride = src_strides[0]
- * cdef Py_ssize_t dst_stride = dst_strides[0]
- */
- __pyx_v_dst_extent = (__pyx_v_dst_shape[0]);
-
- /* "View.MemoryView":1149
- * cdef Py_ssize_t src_extent = src_shape[0]
- * cdef Py_ssize_t dst_extent = dst_shape[0]
- * cdef Py_ssize_t src_stride = src_strides[0] # <<<<<<<<<<<<<<
- * cdef Py_ssize_t dst_stride = dst_strides[0]
- *
- */
- __pyx_v_src_stride = (__pyx_v_src_strides[0]);
-
- /* "View.MemoryView":1150
- * cdef Py_ssize_t dst_extent = dst_shape[0]
- * cdef Py_ssize_t src_stride = src_strides[0]
- * cdef Py_ssize_t dst_stride = dst_strides[0] # <<<<<<<<<<<<<<
- *
- * if ndim == 1:
- */
- __pyx_v_dst_stride = (__pyx_v_dst_strides[0]);
-
- /* "View.MemoryView":1152
- * cdef Py_ssize_t dst_stride = dst_strides[0]
- *
- * if ndim == 1: # <<<<<<<<<<<<<<
- * if (src_stride > 0 and dst_stride > 0 and
- * src_stride == itemsize == dst_stride):
- */
- __pyx_t_1 = ((__pyx_v_ndim == 1) != 0);
- if (__pyx_t_1) {
-
- /* "View.MemoryView":1153
- *
- * if ndim == 1:
- * if (src_stride > 0 and dst_stride > 0 and # <<<<<<<<<<<<<<
- * src_stride == itemsize == dst_stride):
- * memcpy(dst_data, src_data, itemsize * dst_extent)
- */
- __pyx_t_2 = ((__pyx_v_src_stride > 0) != 0);
- if (__pyx_t_2) {
- } else {
- __pyx_t_1 = __pyx_t_2;
- goto __pyx_L5_bool_binop_done;
- }
- __pyx_t_2 = ((__pyx_v_dst_stride > 0) != 0);
- if (__pyx_t_2) {
- } else {
- __pyx_t_1 = __pyx_t_2;
- goto __pyx_L5_bool_binop_done;
- }
-
- /* "View.MemoryView":1154
- * if ndim == 1:
- * if (src_stride > 0 and dst_stride > 0 and
- * src_stride == itemsize == dst_stride): # <<<<<<<<<<<<<<
- * memcpy(dst_data, src_data, itemsize * dst_extent)
- * else:
- */
- __pyx_t_2 = (((size_t)__pyx_v_src_stride) == __pyx_v_itemsize);
- if (__pyx_t_2) {
- __pyx_t_2 = (__pyx_v_itemsize == ((size_t)__pyx_v_dst_stride));
- }
- __pyx_t_3 = (__pyx_t_2 != 0);
- __pyx_t_1 = __pyx_t_3;
- __pyx_L5_bool_binop_done:;
-
- /* "View.MemoryView":1153
- *
- * if ndim == 1:
- * if (src_stride > 0 and dst_stride > 0 and # <<<<<<<<<<<<<<
- * src_stride == itemsize == dst_stride):
- * memcpy(dst_data, src_data, itemsize * dst_extent)
- */
- if (__pyx_t_1) {
-
- /* "View.MemoryView":1155
- * if (src_stride > 0 and dst_stride > 0 and
- * src_stride == itemsize == dst_stride):
- * memcpy(dst_data, src_data, itemsize * dst_extent) # <<<<<<<<<<<<<<
- * else:
- * for i in range(dst_extent):
- */
- (void)(memcpy(__pyx_v_dst_data, __pyx_v_src_data, (__pyx_v_itemsize * __pyx_v_dst_extent)));
-
- /* "View.MemoryView":1153
- *
- * if ndim == 1:
- * if (src_stride > 0 and dst_stride > 0 and # <<<<<<<<<<<<<<
- * src_stride == itemsize == dst_stride):
- * memcpy(dst_data, src_data, itemsize * dst_extent)
- */
- goto __pyx_L4;
- }
-
- /* "View.MemoryView":1157
- * memcpy(dst_data, src_data, itemsize * dst_extent)
- * else:
- * for i in range(dst_extent): # <<<<<<<<<<<<<<
- * memcpy(dst_data, src_data, itemsize)
- * src_data += src_stride
- */
- /*else*/ {
- __pyx_t_4 = __pyx_v_dst_extent;
- __pyx_t_5 = __pyx_t_4;
- for (__pyx_t_6 = 0; __pyx_t_6 < __pyx_t_5; __pyx_t_6+=1) {
- __pyx_v_i = __pyx_t_6;
-
- /* "View.MemoryView":1158
- * else:
- * for i in range(dst_extent):
- * memcpy(dst_data, src_data, itemsize) # <<<<<<<<<<<<<<
- * src_data += src_stride
- * dst_data += dst_stride
- */
- (void)(memcpy(__pyx_v_dst_data, __pyx_v_src_data, __pyx_v_itemsize));
-
- /* "View.MemoryView":1159
- * for i in range(dst_extent):
- * memcpy(dst_data, src_data, itemsize)
- * src_data += src_stride # <<<<<<<<<<<<<<
- * dst_data += dst_stride
- * else:
- */
- __pyx_v_src_data = (__pyx_v_src_data + __pyx_v_src_stride);
-
- /* "View.MemoryView":1160
- * memcpy(dst_data, src_data, itemsize)
- * src_data += src_stride
- * dst_data += dst_stride # <<<<<<<<<<<<<<
- * else:
- * for i in range(dst_extent):
- */
- __pyx_v_dst_data = (__pyx_v_dst_data + __pyx_v_dst_stride);
- }
- }
- __pyx_L4:;
-
- /* "View.MemoryView":1152
- * cdef Py_ssize_t dst_stride = dst_strides[0]
- *
- * if ndim == 1: # <<<<<<<<<<<<<<
- * if (src_stride > 0 and dst_stride > 0 and
- * src_stride == itemsize == dst_stride):
- */
- goto __pyx_L3;
- }
-
- /* "View.MemoryView":1162
- * dst_data += dst_stride
- * else:
- * for i in range(dst_extent): # <<<<<<<<<<<<<<
- * _copy_strided_to_strided(src_data, src_strides + 1,
- * dst_data, dst_strides + 1,
- */
- /*else*/ {
- __pyx_t_4 = __pyx_v_dst_extent;
- __pyx_t_5 = __pyx_t_4;
- for (__pyx_t_6 = 0; __pyx_t_6 < __pyx_t_5; __pyx_t_6+=1) {
- __pyx_v_i = __pyx_t_6;
-
- /* "View.MemoryView":1163
- * else:
- * for i in range(dst_extent):
- * _copy_strided_to_strided(src_data, src_strides + 1, # <<<<<<<<<<<<<<
- * dst_data, dst_strides + 1,
- * src_shape + 1, dst_shape + 1,
- */
- _copy_strided_to_strided(__pyx_v_src_data, (__pyx_v_src_strides + 1), __pyx_v_dst_data, (__pyx_v_dst_strides + 1), (__pyx_v_src_shape + 1), (__pyx_v_dst_shape + 1), (__pyx_v_ndim - 1), __pyx_v_itemsize);
-
- /* "View.MemoryView":1167
- * src_shape + 1, dst_shape + 1,
- * ndim - 1, itemsize)
- * src_data += src_stride # <<<<<<<<<<<<<<
- * dst_data += dst_stride
- *
- */
- __pyx_v_src_data = (__pyx_v_src_data + __pyx_v_src_stride);
-
- /* "View.MemoryView":1168
- * ndim - 1, itemsize)
- * src_data += src_stride
- * dst_data += dst_stride # <<<<<<<<<<<<<<
- *
- * cdef void copy_strided_to_strided(__Pyx_memviewslice *src,
- */
- __pyx_v_dst_data = (__pyx_v_dst_data + __pyx_v_dst_stride);
- }
- }
- __pyx_L3:;
-
- /* "View.MemoryView":1140
- *
- * @cython.cdivision(True)
- * cdef void _copy_strided_to_strided(char *src_data, Py_ssize_t *src_strides, # <<<<<<<<<<<<<<
- * char *dst_data, Py_ssize_t *dst_strides,
- * Py_ssize_t *src_shape, Py_ssize_t *dst_shape,
- */
-
- /* function exit code */
-}
-
-/* "View.MemoryView":1170
- * dst_data += dst_stride
- *
- * cdef void copy_strided_to_strided(__Pyx_memviewslice *src, # <<<<<<<<<<<<<<
- * __Pyx_memviewslice *dst,
- * int ndim, size_t itemsize) nogil:
- */
-
-static void copy_strided_to_strided(__Pyx_memviewslice *__pyx_v_src, __Pyx_memviewslice *__pyx_v_dst, int __pyx_v_ndim, size_t __pyx_v_itemsize) {
-
- /* "View.MemoryView":1173
- * __Pyx_memviewslice *dst,
- * int ndim, size_t itemsize) nogil:
- * _copy_strided_to_strided(src.data, src.strides, dst.data, dst.strides, # <<<<<<<<<<<<<<
- * src.shape, dst.shape, ndim, itemsize)
- *
- */
- _copy_strided_to_strided(__pyx_v_src->data, __pyx_v_src->strides, __pyx_v_dst->data, __pyx_v_dst->strides, __pyx_v_src->shape, __pyx_v_dst->shape, __pyx_v_ndim, __pyx_v_itemsize);
-
- /* "View.MemoryView":1170
- * dst_data += dst_stride
- *
- * cdef void copy_strided_to_strided(__Pyx_memviewslice *src, # <<<<<<<<<<<<<<
- * __Pyx_memviewslice *dst,
- * int ndim, size_t itemsize) nogil:
- */
-
- /* function exit code */
-}
-
-/* "View.MemoryView":1177
- *
- * @cname('__pyx_memoryview_slice_get_size')
- * cdef Py_ssize_t slice_get_size(__Pyx_memviewslice *src, int ndim) nogil: # <<<<<<<<<<<<<<
- * "Return the size of the memory occupied by the slice in number of bytes"
- * cdef Py_ssize_t shape, size = src.memview.view.itemsize
- */
-
-static Py_ssize_t __pyx_memoryview_slice_get_size(__Pyx_memviewslice *__pyx_v_src, int __pyx_v_ndim) {
- Py_ssize_t __pyx_v_shape;
- Py_ssize_t __pyx_v_size;
- Py_ssize_t __pyx_r;
- Py_ssize_t __pyx_t_1;
- Py_ssize_t *__pyx_t_2;
- Py_ssize_t *__pyx_t_3;
- Py_ssize_t *__pyx_t_4;
-
- /* "View.MemoryView":1179
- * cdef Py_ssize_t slice_get_size(__Pyx_memviewslice *src, int ndim) nogil:
- * "Return the size of the memory occupied by the slice in number of bytes"
- * cdef Py_ssize_t shape, size = src.memview.view.itemsize # <<<<<<<<<<<<<<
- *
- * for shape in src.shape[:ndim]:
- */
- __pyx_t_1 = __pyx_v_src->memview->view.itemsize;
- __pyx_v_size = __pyx_t_1;
-
- /* "View.MemoryView":1181
- * cdef Py_ssize_t shape, size = src.memview.view.itemsize
- *
- * for shape in src.shape[:ndim]: # <<<<<<<<<<<<<<
- * size *= shape
- *
- */
- __pyx_t_3 = (__pyx_v_src->shape + __pyx_v_ndim);
- for (__pyx_t_4 = __pyx_v_src->shape; __pyx_t_4 < __pyx_t_3; __pyx_t_4++) {
- __pyx_t_2 = __pyx_t_4;
- __pyx_v_shape = (__pyx_t_2[0]);
-
- /* "View.MemoryView":1182
- *
- * for shape in src.shape[:ndim]:
- * size *= shape # <<<<<<<<<<<<<<
- *
- * return size
- */
- __pyx_v_size = (__pyx_v_size * __pyx_v_shape);
- }
-
- /* "View.MemoryView":1184
- * size *= shape
- *
- * return size # <<<<<<<<<<<<<<
- *
- * @cname('__pyx_fill_contig_strides_array')
- */
- __pyx_r = __pyx_v_size;
- goto __pyx_L0;
-
- /* "View.MemoryView":1177
- *
- * @cname('__pyx_memoryview_slice_get_size')
- * cdef Py_ssize_t slice_get_size(__Pyx_memviewslice *src, int ndim) nogil: # <<<<<<<<<<<<<<
- * "Return the size of the memory occupied by the slice in number of bytes"
- * cdef Py_ssize_t shape, size = src.memview.view.itemsize
- */
-
- /* function exit code */
- __pyx_L0:;
- return __pyx_r;
-}
-
-/* "View.MemoryView":1187
- *
- * @cname('__pyx_fill_contig_strides_array')
- * cdef Py_ssize_t fill_contig_strides_array( # <<<<<<<<<<<<<<
- * Py_ssize_t *shape, Py_ssize_t *strides, Py_ssize_t stride,
- * int ndim, char order) nogil:
- */
-
-static Py_ssize_t __pyx_fill_contig_strides_array(Py_ssize_t *__pyx_v_shape, Py_ssize_t *__pyx_v_strides, Py_ssize_t __pyx_v_stride, int __pyx_v_ndim, char __pyx_v_order) {
- int __pyx_v_idx;
- Py_ssize_t __pyx_r;
- int __pyx_t_1;
- int __pyx_t_2;
- int __pyx_t_3;
- int __pyx_t_4;
-
- /* "View.MemoryView":1196
- * cdef int idx
- *
- * if order == 'F': # <<<<<<<<<<<<<<
- * for idx in range(ndim):
- * strides[idx] = stride
- */
- __pyx_t_1 = ((__pyx_v_order == 'F') != 0);
- if (__pyx_t_1) {
-
- /* "View.MemoryView":1197
- *
- * if order == 'F':
- * for idx in range(ndim): # <<<<<<<<<<<<<<
- * strides[idx] = stride
- * stride *= shape[idx]
- */
- __pyx_t_2 = __pyx_v_ndim;
- __pyx_t_3 = __pyx_t_2;
- for (__pyx_t_4 = 0; __pyx_t_4 < __pyx_t_3; __pyx_t_4+=1) {
- __pyx_v_idx = __pyx_t_4;
-
- /* "View.MemoryView":1198
- * if order == 'F':
- * for idx in range(ndim):
- * strides[idx] = stride # <<<<<<<<<<<<<<
- * stride *= shape[idx]
- * else:
- */
- (__pyx_v_strides[__pyx_v_idx]) = __pyx_v_stride;
-
- /* "View.MemoryView":1199
- * for idx in range(ndim):
- * strides[idx] = stride
- * stride *= shape[idx] # <<<<<<<<<<<<<<
- * else:
- * for idx in range(ndim - 1, -1, -1):
- */
- __pyx_v_stride = (__pyx_v_stride * (__pyx_v_shape[__pyx_v_idx]));
- }
-
- /* "View.MemoryView":1196
- * cdef int idx
- *
- * if order == 'F': # <<<<<<<<<<<<<<
- * for idx in range(ndim):
- * strides[idx] = stride
- */
- goto __pyx_L3;
- }
-
- /* "View.MemoryView":1201
- * stride *= shape[idx]
- * else:
- * for idx in range(ndim - 1, -1, -1): # <<<<<<<<<<<<<<
- * strides[idx] = stride
- * stride *= shape[idx]
- */
- /*else*/ {
- for (__pyx_t_2 = (__pyx_v_ndim - 1); __pyx_t_2 > -1; __pyx_t_2-=1) {
- __pyx_v_idx = __pyx_t_2;
-
- /* "View.MemoryView":1202
- * else:
- * for idx in range(ndim - 1, -1, -1):
- * strides[idx] = stride # <<<<<<<<<<<<<<
- * stride *= shape[idx]
- *
- */
- (__pyx_v_strides[__pyx_v_idx]) = __pyx_v_stride;
-
- /* "View.MemoryView":1203
- * for idx in range(ndim - 1, -1, -1):
- * strides[idx] = stride
- * stride *= shape[idx] # <<<<<<<<<<<<<<
- *
- * return stride
- */
- __pyx_v_stride = (__pyx_v_stride * (__pyx_v_shape[__pyx_v_idx]));
- }
- }
- __pyx_L3:;
-
- /* "View.MemoryView":1205
- * stride *= shape[idx]
- *
- * return stride # <<<<<<<<<<<<<<
- *
- * @cname('__pyx_memoryview_copy_data_to_temp')
- */
- __pyx_r = __pyx_v_stride;
- goto __pyx_L0;
-
- /* "View.MemoryView":1187
- *
- * @cname('__pyx_fill_contig_strides_array')
- * cdef Py_ssize_t fill_contig_strides_array( # <<<<<<<<<<<<<<
- * Py_ssize_t *shape, Py_ssize_t *strides, Py_ssize_t stride,
- * int ndim, char order) nogil:
- */
-
- /* function exit code */
- __pyx_L0:;
- return __pyx_r;
-}
-
-/* "View.MemoryView":1208
- *
- * @cname('__pyx_memoryview_copy_data_to_temp')
- * cdef void *copy_data_to_temp(__Pyx_memviewslice *src, # <<<<<<<<<<<<<<
- * __Pyx_memviewslice *tmpslice,
- * char order,
- */
-
-static void *__pyx_memoryview_copy_data_to_temp(__Pyx_memviewslice *__pyx_v_src, __Pyx_memviewslice *__pyx_v_tmpslice, char __pyx_v_order, int __pyx_v_ndim) {
- int __pyx_v_i;
- void *__pyx_v_result;
- size_t __pyx_v_itemsize;
- size_t __pyx_v_size;
- void *__pyx_r;
- Py_ssize_t __pyx_t_1;
- int __pyx_t_2;
- int __pyx_t_3;
- struct __pyx_memoryview_obj *__pyx_t_4;
- int __pyx_t_5;
- int __pyx_t_6;
- int __pyx_lineno = 0;
- const char *__pyx_filename = NULL;
- int __pyx_clineno = 0;
-
- /* "View.MemoryView":1219
- * cdef void *result
- *
- * cdef size_t itemsize = src.memview.view.itemsize # <<<<<<<<<<<<<<
- * cdef size_t size = slice_get_size(src, ndim)
- *
- */
- __pyx_t_1 = __pyx_v_src->memview->view.itemsize;
- __pyx_v_itemsize = __pyx_t_1;
-
- /* "View.MemoryView":1220
- *
- * cdef size_t itemsize = src.memview.view.itemsize
- * cdef size_t size = slice_get_size(src, ndim) # <<<<<<<<<<<<<<
- *
- * result = malloc(size)
- */
- __pyx_v_size = __pyx_memoryview_slice_get_size(__pyx_v_src, __pyx_v_ndim);
-
- /* "View.MemoryView":1222
- * cdef size_t size = slice_get_size(src, ndim)
- *
- * result = malloc(size) # <<<<<<<<<<<<<<
- * if not result:
- * _err(MemoryError, NULL)
- */
- __pyx_v_result = malloc(__pyx_v_size);
-
- /* "View.MemoryView":1223
- *
- * result = malloc(size)
- * if not result: # <<<<<<<<<<<<<<
- * _err(MemoryError, NULL)
- *
- */
- __pyx_t_2 = ((!(__pyx_v_result != 0)) != 0);
- if (__pyx_t_2) {
-
- /* "View.MemoryView":1224
- * result = malloc(size)
- * if not result:
- * _err(MemoryError, NULL) # <<<<<<<<<<<<<<
- *
- *
- */
- __pyx_t_3 = __pyx_memoryview_err(__pyx_builtin_MemoryError, NULL); if (unlikely(__pyx_t_3 == ((int)-1))) __PYX_ERR(1, 1224, __pyx_L1_error)
-
- /* "View.MemoryView":1223
- *
- * result = malloc(size)
- * if not result: # <<<<<<<<<<<<<<
- * _err(MemoryError, NULL)
- *
- */
- }
-
- /* "View.MemoryView":1227
- *
- *
- * tmpslice.data = result # <<<<<<<<<<<<<<
- * tmpslice.memview = src.memview
- * for i in range(ndim):
- */
- __pyx_v_tmpslice->data = ((char *)__pyx_v_result);
-
- /* "View.MemoryView":1228
- *
- * tmpslice.data = result
- * tmpslice.memview = src.memview # <<<<<<<<<<<<<<
- * for i in range(ndim):
- * tmpslice.shape[i] = src.shape[i]
- */
- __pyx_t_4 = __pyx_v_src->memview;
- __pyx_v_tmpslice->memview = __pyx_t_4;
-
- /* "View.MemoryView":1229
- * tmpslice.data = result
- * tmpslice.memview = src.memview
- * for i in range(ndim): # <<<<<<<<<<<<<<
- * tmpslice.shape[i] = src.shape[i]
- * tmpslice.suboffsets[i] = -1
- */
- __pyx_t_3 = __pyx_v_ndim;
- __pyx_t_5 = __pyx_t_3;
- for (__pyx_t_6 = 0; __pyx_t_6 < __pyx_t_5; __pyx_t_6+=1) {
- __pyx_v_i = __pyx_t_6;
-
- /* "View.MemoryView":1230
- * tmpslice.memview = src.memview
- * for i in range(ndim):
- * tmpslice.shape[i] = src.shape[i] # <<<<<<<<<<<<<<
- * tmpslice.suboffsets[i] = -1
- *
- */
- (__pyx_v_tmpslice->shape[__pyx_v_i]) = (__pyx_v_src->shape[__pyx_v_i]);
-
- /* "View.MemoryView":1231
- * for i in range(ndim):
- * tmpslice.shape[i] = src.shape[i]
- * tmpslice.suboffsets[i] = -1 # <<<<<<<<<<<<<<
- *
- * fill_contig_strides_array(&tmpslice.shape[0], &tmpslice.strides[0], itemsize,
- */
- (__pyx_v_tmpslice->suboffsets[__pyx_v_i]) = -1L;
- }
-
- /* "View.MemoryView":1233
- * tmpslice.suboffsets[i] = -1
- *
- * fill_contig_strides_array(&tmpslice.shape[0], &tmpslice.strides[0], itemsize, # <<<<<<<<<<<<<<
- * ndim, order)
- *
- */
- (void)(__pyx_fill_contig_strides_array((&(__pyx_v_tmpslice->shape[0])), (&(__pyx_v_tmpslice->strides[0])), __pyx_v_itemsize, __pyx_v_ndim, __pyx_v_order));
-
- /* "View.MemoryView":1237
- *
- *
- * for i in range(ndim): # <<<<<<<<<<<<<<
- * if tmpslice.shape[i] == 1:
- * tmpslice.strides[i] = 0
- */
- __pyx_t_3 = __pyx_v_ndim;
- __pyx_t_5 = __pyx_t_3;
- for (__pyx_t_6 = 0; __pyx_t_6 < __pyx_t_5; __pyx_t_6+=1) {
- __pyx_v_i = __pyx_t_6;
-
- /* "View.MemoryView":1238
- *
- * for i in range(ndim):
- * if tmpslice.shape[i] == 1: # <<<<<<<<<<<<<<
- * tmpslice.strides[i] = 0
- *
- */
- __pyx_t_2 = (((__pyx_v_tmpslice->shape[__pyx_v_i]) == 1) != 0);
- if (__pyx_t_2) {
-
- /* "View.MemoryView":1239
- * for i in range(ndim):
- * if tmpslice.shape[i] == 1:
- * tmpslice.strides[i] = 0 # <<<<<<<<<<<<<<
- *
- * if slice_is_contig(src[0], order, ndim):
- */
- (__pyx_v_tmpslice->strides[__pyx_v_i]) = 0;
-
- /* "View.MemoryView":1238
- *
- * for i in range(ndim):
- * if tmpslice.shape[i] == 1: # <<<<<<<<<<<<<<
- * tmpslice.strides[i] = 0
- *
- */
- }
- }
-
- /* "View.MemoryView":1241
- * tmpslice.strides[i] = 0
- *
- * if slice_is_contig(src[0], order, ndim): # <<<<<<<<<<<<<<
- * memcpy(result, src.data, size)
- * else:
- */
- __pyx_t_2 = (__pyx_memviewslice_is_contig((__pyx_v_src[0]), __pyx_v_order, __pyx_v_ndim) != 0);
- if (__pyx_t_2) {
-
- /* "View.MemoryView":1242
- *
- * if slice_is_contig(src[0], order, ndim):
- * memcpy(result, src.data, size) # <<<<<<<<<<<<<<
- * else:
- * copy_strided_to_strided(src, tmpslice, ndim, itemsize)
- */
- (void)(memcpy(__pyx_v_result, __pyx_v_src->data, __pyx_v_size));
-
- /* "View.MemoryView":1241
- * tmpslice.strides[i] = 0
- *
- * if slice_is_contig(src[0], order, ndim): # <<<<<<<<<<<<<<
- * memcpy(result, src.data, size)
- * else:
- */
- goto __pyx_L9;
- }
-
- /* "View.MemoryView":1244
- * memcpy(result, src.data, size)
- * else:
- * copy_strided_to_strided(src, tmpslice, ndim, itemsize) # <<<<<<<<<<<<<<
- *
- * return result
- */
- /*else*/ {
- copy_strided_to_strided(__pyx_v_src, __pyx_v_tmpslice, __pyx_v_ndim, __pyx_v_itemsize);
- }
- __pyx_L9:;
-
- /* "View.MemoryView":1246
- * copy_strided_to_strided(src, tmpslice, ndim, itemsize)
- *
- * return result # <<<<<<<<<<<<<<
- *
- *
- */
- __pyx_r = __pyx_v_result;
- goto __pyx_L0;
-
- /* "View.MemoryView":1208
- *
- * @cname('__pyx_memoryview_copy_data_to_temp')
- * cdef void *copy_data_to_temp(__Pyx_memviewslice *src, # <<<<<<<<<<<<<<
- * __Pyx_memviewslice *tmpslice,
- * char order,
- */
-
- /* function exit code */
- __pyx_L1_error:;
- {
- #ifdef WITH_THREAD
- PyGILState_STATE __pyx_gilstate_save = __Pyx_PyGILState_Ensure();
- #endif
- __Pyx_AddTraceback("View.MemoryView.copy_data_to_temp", __pyx_clineno, __pyx_lineno, __pyx_filename);
- #ifdef WITH_THREAD
- __Pyx_PyGILState_Release(__pyx_gilstate_save);
- #endif
- }
- __pyx_r = NULL;
- __pyx_L0:;
- return __pyx_r;
-}
-
-/* "View.MemoryView":1251
- *
- * @cname('__pyx_memoryview_err_extents')
- * cdef int _err_extents(int i, Py_ssize_t extent1, # <<<<<<<<<<<<<<
- * Py_ssize_t extent2) except -1 with gil:
- * raise ValueError("got differing extents in dimension %d (got %d and %d)" %
- */
-
-static int __pyx_memoryview_err_extents(int __pyx_v_i, Py_ssize_t __pyx_v_extent1, Py_ssize_t __pyx_v_extent2) {
- int __pyx_r;
- __Pyx_RefNannyDeclarations
- PyObject *__pyx_t_1 = NULL;
- PyObject *__pyx_t_2 = NULL;
- PyObject *__pyx_t_3 = NULL;
- PyObject *__pyx_t_4 = NULL;
- int __pyx_lineno = 0;
- const char *__pyx_filename = NULL;
- int __pyx_clineno = 0;
- #ifdef WITH_THREAD
- PyGILState_STATE __pyx_gilstate_save = __Pyx_PyGILState_Ensure();
- #endif
- __Pyx_RefNannySetupContext("_err_extents", 0);
-
- /* "View.MemoryView":1254
- * Py_ssize_t extent2) except -1 with gil:
- * raise ValueError("got differing extents in dimension %d (got %d and %d)" %
- * (i, extent1, extent2)) # <<<<<<<<<<<<<<
- *
- * @cname('__pyx_memoryview_err_dim')
- */
- __pyx_t_1 = __Pyx_PyInt_From_int(__pyx_v_i); if (unlikely(!__pyx_t_1)) __PYX_ERR(1, 1254, __pyx_L1_error)
- __Pyx_GOTREF(__pyx_t_1);
- __pyx_t_2 = PyInt_FromSsize_t(__pyx_v_extent1); if (unlikely(!__pyx_t_2)) __PYX_ERR(1, 1254, __pyx_L1_error)
- __Pyx_GOTREF(__pyx_t_2);
- __pyx_t_3 = PyInt_FromSsize_t(__pyx_v_extent2); if (unlikely(!__pyx_t_3)) __PYX_ERR(1, 1254, __pyx_L1_error)
- __Pyx_GOTREF(__pyx_t_3);
- __pyx_t_4 = PyTuple_New(3); if (unlikely(!__pyx_t_4)) __PYX_ERR(1, 1254, __pyx_L1_error)
- __Pyx_GOTREF(__pyx_t_4);
- __Pyx_GIVEREF(__pyx_t_1);
- PyTuple_SET_ITEM(__pyx_t_4, 0, __pyx_t_1);
- __Pyx_GIVEREF(__pyx_t_2);
- PyTuple_SET_ITEM(__pyx_t_4, 1, __pyx_t_2);
- __Pyx_GIVEREF(__pyx_t_3);
- PyTuple_SET_ITEM(__pyx_t_4, 2, __pyx_t_3);
- __pyx_t_1 = 0;
- __pyx_t_2 = 0;
- __pyx_t_3 = 0;
-
- /* "View.MemoryView":1253
- * cdef int _err_extents(int i, Py_ssize_t extent1,
- * Py_ssize_t extent2) except -1 with gil:
- * raise ValueError("got differing extents in dimension %d (got %d and %d)" % # <<<<<<<<<<<<<<
- * (i, extent1, extent2))
- *
- */
- __pyx_t_3 = __Pyx_PyString_Format(__pyx_kp_s_got_differing_extents_in_dimensi, __pyx_t_4); if (unlikely(!__pyx_t_3)) __PYX_ERR(1, 1253, __pyx_L1_error)
- __Pyx_GOTREF(__pyx_t_3);
- __Pyx_DECREF(__pyx_t_4); __pyx_t_4 = 0;
- __pyx_t_4 = __Pyx_PyObject_CallOneArg(__pyx_builtin_ValueError, __pyx_t_3); if (unlikely(!__pyx_t_4)) __PYX_ERR(1, 1253, __pyx_L1_error)
- __Pyx_GOTREF(__pyx_t_4);
- __Pyx_DECREF(__pyx_t_3); __pyx_t_3 = 0;
- __Pyx_Raise(__pyx_t_4, 0, 0, 0);
- __Pyx_DECREF(__pyx_t_4); __pyx_t_4 = 0;
- __PYX_ERR(1, 1253, __pyx_L1_error)
-
- /* "View.MemoryView":1251
- *
- * @cname('__pyx_memoryview_err_extents')
- * cdef int _err_extents(int i, Py_ssize_t extent1, # <<<<<<<<<<<<<<
- * Py_ssize_t extent2) except -1 with gil:
- * raise ValueError("got differing extents in dimension %d (got %d and %d)" %
- */
-
- /* function exit code */
- __pyx_L1_error:;
- __Pyx_XDECREF(__pyx_t_1);
- __Pyx_XDECREF(__pyx_t_2);
- __Pyx_XDECREF(__pyx_t_3);
- __Pyx_XDECREF(__pyx_t_4);
- __Pyx_AddTraceback("View.MemoryView._err_extents", __pyx_clineno, __pyx_lineno, __pyx_filename);
- __pyx_r = -1;
- __Pyx_RefNannyFinishContext();
- #ifdef WITH_THREAD
- __Pyx_PyGILState_Release(__pyx_gilstate_save);
- #endif
- return __pyx_r;
-}
-
-/* "View.MemoryView":1257
- *
- * @cname('__pyx_memoryview_err_dim')
- * cdef int _err_dim(object error, char *msg, int dim) except -1 with gil: # <<<<<<<<<<<<<<
- * raise error(msg.decode('ascii') % dim)
- *
- */
-
-static int __pyx_memoryview_err_dim(PyObject *__pyx_v_error, char *__pyx_v_msg, int __pyx_v_dim) {
- int __pyx_r;
- __Pyx_RefNannyDeclarations
- PyObject *__pyx_t_1 = NULL;
- PyObject *__pyx_t_2 = NULL;
- PyObject *__pyx_t_3 = NULL;
- PyObject *__pyx_t_4 = NULL;
- int __pyx_lineno = 0;
- const char *__pyx_filename = NULL;
- int __pyx_clineno = 0;
- #ifdef WITH_THREAD
- PyGILState_STATE __pyx_gilstate_save = __Pyx_PyGILState_Ensure();
- #endif
- __Pyx_RefNannySetupContext("_err_dim", 0);
- __Pyx_INCREF(__pyx_v_error);
-
- /* "View.MemoryView":1258
- * @cname('__pyx_memoryview_err_dim')
- * cdef int _err_dim(object error, char *msg, int dim) except -1 with gil:
- * raise error(msg.decode('ascii') % dim) # <<<<<<<<<<<<<<
- *
- * @cname('__pyx_memoryview_err')
- */
- __pyx_t_2 = __Pyx_decode_c_string(__pyx_v_msg, 0, strlen(__pyx_v_msg), NULL, NULL, PyUnicode_DecodeASCII); if (unlikely(!__pyx_t_2)) __PYX_ERR(1, 1258, __pyx_L1_error)
- __Pyx_GOTREF(__pyx_t_2);
- __pyx_t_3 = __Pyx_PyInt_From_int(__pyx_v_dim); if (unlikely(!__pyx_t_3)) __PYX_ERR(1, 1258, __pyx_L1_error)
- __Pyx_GOTREF(__pyx_t_3);
- __pyx_t_4 = PyUnicode_Format(__pyx_t_2, __pyx_t_3); if (unlikely(!__pyx_t_4)) __PYX_ERR(1, 1258, __pyx_L1_error)
- __Pyx_GOTREF(__pyx_t_4);
- __Pyx_DECREF(__pyx_t_2); __pyx_t_2 = 0;
- __Pyx_DECREF(__pyx_t_3); __pyx_t_3 = 0;
- __Pyx_INCREF(__pyx_v_error);
- __pyx_t_3 = __pyx_v_error; __pyx_t_2 = NULL;
- if (CYTHON_UNPACK_METHODS && unlikely(PyMethod_Check(__pyx_t_3))) {
- __pyx_t_2 = PyMethod_GET_SELF(__pyx_t_3);
- if (likely(__pyx_t_2)) {
- PyObject* function = PyMethod_GET_FUNCTION(__pyx_t_3);
- __Pyx_INCREF(__pyx_t_2);
- __Pyx_INCREF(function);
- __Pyx_DECREF_SET(__pyx_t_3, function);
- }
- }
- __pyx_t_1 = (__pyx_t_2) ? __Pyx_PyObject_Call2Args(__pyx_t_3, __pyx_t_2, __pyx_t_4) : __Pyx_PyObject_CallOneArg(__pyx_t_3, __pyx_t_4);
- __Pyx_XDECREF(__pyx_t_2); __pyx_t_2 = 0;
- __Pyx_DECREF(__pyx_t_4); __pyx_t_4 = 0;
- if (unlikely(!__pyx_t_1)) __PYX_ERR(1, 1258, __pyx_L1_error)
- __Pyx_GOTREF(__pyx_t_1);
- __Pyx_DECREF(__pyx_t_3); __pyx_t_3 = 0;
- __Pyx_Raise(__pyx_t_1, 0, 0, 0);
- __Pyx_DECREF(__pyx_t_1); __pyx_t_1 = 0;
- __PYX_ERR(1, 1258, __pyx_L1_error)
-
- /* "View.MemoryView":1257
- *
- * @cname('__pyx_memoryview_err_dim')
- * cdef int _err_dim(object error, char *msg, int dim) except -1 with gil: # <<<<<<<<<<<<<<
- * raise error(msg.decode('ascii') % dim)
- *
- */
-
- /* function exit code */
- __pyx_L1_error:;
- __Pyx_XDECREF(__pyx_t_1);
- __Pyx_XDECREF(__pyx_t_2);
- __Pyx_XDECREF(__pyx_t_3);
- __Pyx_XDECREF(__pyx_t_4);
- __Pyx_AddTraceback("View.MemoryView._err_dim", __pyx_clineno, __pyx_lineno, __pyx_filename);
- __pyx_r = -1;
- __Pyx_XDECREF(__pyx_v_error);
- __Pyx_RefNannyFinishContext();
- #ifdef WITH_THREAD
- __Pyx_PyGILState_Release(__pyx_gilstate_save);
- #endif
- return __pyx_r;
-}
-
-/* "View.MemoryView":1261
- *
- * @cname('__pyx_memoryview_err')
- * cdef int _err(object error, char *msg) except -1 with gil: # <<<<<<<<<<<<<<
- * if msg != NULL:
- * raise error(msg.decode('ascii'))
- */
-
-static int __pyx_memoryview_err(PyObject *__pyx_v_error, char *__pyx_v_msg) {
- int __pyx_r;
- __Pyx_RefNannyDeclarations
- int __pyx_t_1;
- PyObject *__pyx_t_2 = NULL;
- PyObject *__pyx_t_3 = NULL;
- PyObject *__pyx_t_4 = NULL;
- PyObject *__pyx_t_5 = NULL;
- int __pyx_lineno = 0;
- const char *__pyx_filename = NULL;
- int __pyx_clineno = 0;
- #ifdef WITH_THREAD
- PyGILState_STATE __pyx_gilstate_save = __Pyx_PyGILState_Ensure();
- #endif
- __Pyx_RefNannySetupContext("_err", 0);
- __Pyx_INCREF(__pyx_v_error);
-
- /* "View.MemoryView":1262
- * @cname('__pyx_memoryview_err')
- * cdef int _err(object error, char *msg) except -1 with gil:
- * if msg != NULL: # <<<<<<<<<<<<<<
- * raise error(msg.decode('ascii'))
- * else:
- */
- __pyx_t_1 = ((__pyx_v_msg != NULL) != 0);
- if (unlikely(__pyx_t_1)) {
-
- /* "View.MemoryView":1263
- * cdef int _err(object error, char *msg) except -1 with gil:
- * if msg != NULL:
- * raise error(msg.decode('ascii')) # <<<<<<<<<<<<<<
- * else:
- * raise error
- */
- __pyx_t_3 = __Pyx_decode_c_string(__pyx_v_msg, 0, strlen(__pyx_v_msg), NULL, NULL, PyUnicode_DecodeASCII); if (unlikely(!__pyx_t_3)) __PYX_ERR(1, 1263, __pyx_L1_error)
- __Pyx_GOTREF(__pyx_t_3);
- __Pyx_INCREF(__pyx_v_error);
- __pyx_t_4 = __pyx_v_error; __pyx_t_5 = NULL;
- if (CYTHON_UNPACK_METHODS && unlikely(PyMethod_Check(__pyx_t_4))) {
- __pyx_t_5 = PyMethod_GET_SELF(__pyx_t_4);
- if (likely(__pyx_t_5)) {
- PyObject* function = PyMethod_GET_FUNCTION(__pyx_t_4);
- __Pyx_INCREF(__pyx_t_5);
- __Pyx_INCREF(function);
- __Pyx_DECREF_SET(__pyx_t_4, function);
- }
- }
- __pyx_t_2 = (__pyx_t_5) ? __Pyx_PyObject_Call2Args(__pyx_t_4, __pyx_t_5, __pyx_t_3) : __Pyx_PyObject_CallOneArg(__pyx_t_4, __pyx_t_3);
- __Pyx_XDECREF(__pyx_t_5); __pyx_t_5 = 0;
- __Pyx_DECREF(__pyx_t_3); __pyx_t_3 = 0;
- if (unlikely(!__pyx_t_2)) __PYX_ERR(1, 1263, __pyx_L1_error)
- __Pyx_GOTREF(__pyx_t_2);
- __Pyx_DECREF(__pyx_t_4); __pyx_t_4 = 0;
- __Pyx_Raise(__pyx_t_2, 0, 0, 0);
- __Pyx_DECREF(__pyx_t_2); __pyx_t_2 = 0;
- __PYX_ERR(1, 1263, __pyx_L1_error)
-
- /* "View.MemoryView":1262
- * @cname('__pyx_memoryview_err')
- * cdef int _err(object error, char *msg) except -1 with gil:
- * if msg != NULL: # <<<<<<<<<<<<<<
- * raise error(msg.decode('ascii'))
- * else:
- */
- }
-
- /* "View.MemoryView":1265
- * raise error(msg.decode('ascii'))
- * else:
- * raise error # <<<<<<<<<<<<<<
- *
- * @cname('__pyx_memoryview_copy_contents')
- */
- /*else*/ {
- __Pyx_Raise(__pyx_v_error, 0, 0, 0);
- __PYX_ERR(1, 1265, __pyx_L1_error)
- }
-
- /* "View.MemoryView":1261
- *
- * @cname('__pyx_memoryview_err')
- * cdef int _err(object error, char *msg) except -1 with gil: # <<<<<<<<<<<<<<
- * if msg != NULL:
- * raise error(msg.decode('ascii'))
- */
-
- /* function exit code */
- __pyx_L1_error:;
- __Pyx_XDECREF(__pyx_t_2);
- __Pyx_XDECREF(__pyx_t_3);
- __Pyx_XDECREF(__pyx_t_4);
- __Pyx_XDECREF(__pyx_t_5);
- __Pyx_AddTraceback("View.MemoryView._err", __pyx_clineno, __pyx_lineno, __pyx_filename);
- __pyx_r = -1;
- __Pyx_XDECREF(__pyx_v_error);
- __Pyx_RefNannyFinishContext();
- #ifdef WITH_THREAD
- __Pyx_PyGILState_Release(__pyx_gilstate_save);
- #endif
- return __pyx_r;
-}
-
-/* "View.MemoryView":1268
- *
- * @cname('__pyx_memoryview_copy_contents')
- * cdef int memoryview_copy_contents(__Pyx_memviewslice src, # <<<<<<<<<<<<<<
- * __Pyx_memviewslice dst,
- * int src_ndim, int dst_ndim,
- */
-
-static int __pyx_memoryview_copy_contents(__Pyx_memviewslice __pyx_v_src, __Pyx_memviewslice __pyx_v_dst, int __pyx_v_src_ndim, int __pyx_v_dst_ndim, int __pyx_v_dtype_is_object) {
- void *__pyx_v_tmpdata;
- size_t __pyx_v_itemsize;
- int __pyx_v_i;
- char __pyx_v_order;
- int __pyx_v_broadcasting;
- int __pyx_v_direct_copy;
- __Pyx_memviewslice __pyx_v_tmp;
- int __pyx_v_ndim;
- int __pyx_r;
- Py_ssize_t __pyx_t_1;
- int __pyx_t_2;
- int __pyx_t_3;
- int __pyx_t_4;
- int __pyx_t_5;
- int __pyx_t_6;
- void *__pyx_t_7;
- int __pyx_t_8;
- int __pyx_lineno = 0;
- const char *__pyx_filename = NULL;
- int __pyx_clineno = 0;
-
- /* "View.MemoryView":1276
- * Check for overlapping memory and verify the shapes.
- * """
- * cdef void *tmpdata = NULL # <<<<<<<<<<<<<<
- * cdef size_t itemsize = src.memview.view.itemsize
- * cdef int i
- */
- __pyx_v_tmpdata = NULL;
-
- /* "View.MemoryView":1277
- * """
- * cdef void *tmpdata = NULL
- * cdef size_t itemsize = src.memview.view.itemsize # <<<<<<<<<<<<<<
- * cdef int i
- * cdef char order = get_best_order(&src, src_ndim)
- */
- __pyx_t_1 = __pyx_v_src.memview->view.itemsize;
- __pyx_v_itemsize = __pyx_t_1;
-
- /* "View.MemoryView":1279
- * cdef size_t itemsize = src.memview.view.itemsize
- * cdef int i
- * cdef char order = get_best_order(&src, src_ndim) # <<<<<<<<<<<<<<
- * cdef bint broadcasting = False
- * cdef bint direct_copy = False
- */
- __pyx_v_order = __pyx_get_best_slice_order((&__pyx_v_src), __pyx_v_src_ndim);
-
- /* "View.MemoryView":1280
- * cdef int i
- * cdef char order = get_best_order(&src, src_ndim)
- * cdef bint broadcasting = False # <<<<<<<<<<<<<<
- * cdef bint direct_copy = False
- * cdef __Pyx_memviewslice tmp
- */
- __pyx_v_broadcasting = 0;
-
- /* "View.MemoryView":1281
- * cdef char order = get_best_order(&src, src_ndim)
- * cdef bint broadcasting = False
- * cdef bint direct_copy = False # <<<<<<<<<<<<<<
- * cdef __Pyx_memviewslice tmp
- *
- */
- __pyx_v_direct_copy = 0;
-
- /* "View.MemoryView":1284
- * cdef __Pyx_memviewslice tmp
- *
- * if src_ndim < dst_ndim: # <<<<<<<<<<<<<<
- * broadcast_leading(&src, src_ndim, dst_ndim)
- * elif dst_ndim < src_ndim:
- */
- __pyx_t_2 = ((__pyx_v_src_ndim < __pyx_v_dst_ndim) != 0);
- if (__pyx_t_2) {
-
- /* "View.MemoryView":1285
- *
- * if src_ndim < dst_ndim:
- * broadcast_leading(&src, src_ndim, dst_ndim) # <<<<<<<<<<<<<<
- * elif dst_ndim < src_ndim:
- * broadcast_leading(&dst, dst_ndim, src_ndim)
- */
- __pyx_memoryview_broadcast_leading((&__pyx_v_src), __pyx_v_src_ndim, __pyx_v_dst_ndim);
-
- /* "View.MemoryView":1284
- * cdef __Pyx_memviewslice tmp
- *
- * if src_ndim < dst_ndim: # <<<<<<<<<<<<<<
- * broadcast_leading(&src, src_ndim, dst_ndim)
- * elif dst_ndim < src_ndim:
- */
- goto __pyx_L3;
- }
-
- /* "View.MemoryView":1286
- * if src_ndim < dst_ndim:
- * broadcast_leading(&src, src_ndim, dst_ndim)
- * elif dst_ndim < src_ndim: # <<<<<<<<<<<<<<
- * broadcast_leading(&dst, dst_ndim, src_ndim)
- *
- */
- __pyx_t_2 = ((__pyx_v_dst_ndim < __pyx_v_src_ndim) != 0);
- if (__pyx_t_2) {
-
- /* "View.MemoryView":1287
- * broadcast_leading(&src, src_ndim, dst_ndim)
- * elif dst_ndim < src_ndim:
- * broadcast_leading(&dst, dst_ndim, src_ndim) # <<<<<<<<<<<<<<
- *
- * cdef int ndim = max(src_ndim, dst_ndim)
- */
- __pyx_memoryview_broadcast_leading((&__pyx_v_dst), __pyx_v_dst_ndim, __pyx_v_src_ndim);
-
- /* "View.MemoryView":1286
- * if src_ndim < dst_ndim:
- * broadcast_leading(&src, src_ndim, dst_ndim)
- * elif dst_ndim < src_ndim: # <<<<<<<<<<<<<<
- * broadcast_leading(&dst, dst_ndim, src_ndim)
- *
- */
- }
- __pyx_L3:;
-
- /* "View.MemoryView":1289
- * broadcast_leading(&dst, dst_ndim, src_ndim)
- *
- * cdef int ndim = max(src_ndim, dst_ndim) # <<<<<<<<<<<<<<
- *
- * for i in range(ndim):
- */
- __pyx_t_3 = __pyx_v_dst_ndim;
- __pyx_t_4 = __pyx_v_src_ndim;
- if (((__pyx_t_3 > __pyx_t_4) != 0)) {
- __pyx_t_5 = __pyx_t_3;
- } else {
- __pyx_t_5 = __pyx_t_4;
- }
- __pyx_v_ndim = __pyx_t_5;
-
- /* "View.MemoryView":1291
- * cdef int ndim = max(src_ndim, dst_ndim)
- *
- * for i in range(ndim): # <<<<<<<<<<<<<<
- * if src.shape[i] != dst.shape[i]:
- * if src.shape[i] == 1:
- */
- __pyx_t_5 = __pyx_v_ndim;
- __pyx_t_3 = __pyx_t_5;
- for (__pyx_t_4 = 0; __pyx_t_4 < __pyx_t_3; __pyx_t_4+=1) {
- __pyx_v_i = __pyx_t_4;
-
- /* "View.MemoryView":1292
- *
- * for i in range(ndim):
- * if src.shape[i] != dst.shape[i]: # <<<<<<<<<<<<<<
- * if src.shape[i] == 1:
- * broadcasting = True
- */
- __pyx_t_2 = (((__pyx_v_src.shape[__pyx_v_i]) != (__pyx_v_dst.shape[__pyx_v_i])) != 0);
- if (__pyx_t_2) {
-
- /* "View.MemoryView":1293
- * for i in range(ndim):
- * if src.shape[i] != dst.shape[i]:
- * if src.shape[i] == 1: # <<<<<<<<<<<<<<
- * broadcasting = True
- * src.strides[i] = 0
- */
- __pyx_t_2 = (((__pyx_v_src.shape[__pyx_v_i]) == 1) != 0);
- if (__pyx_t_2) {
-
- /* "View.MemoryView":1294
- * if src.shape[i] != dst.shape[i]:
- * if src.shape[i] == 1:
- * broadcasting = True # <<<<<<<<<<<<<<
- * src.strides[i] = 0
- * else:
- */
- __pyx_v_broadcasting = 1;
-
- /* "View.MemoryView":1295
- * if src.shape[i] == 1:
- * broadcasting = True
- * src.strides[i] = 0 # <<<<<<<<<<<<<<
- * else:
- * _err_extents(i, dst.shape[i], src.shape[i])
- */
- (__pyx_v_src.strides[__pyx_v_i]) = 0;
-
- /* "View.MemoryView":1293
- * for i in range(ndim):
- * if src.shape[i] != dst.shape[i]:
- * if src.shape[i] == 1: # <<<<<<<<<<<<<<
- * broadcasting = True
- * src.strides[i] = 0
- */
- goto __pyx_L7;
- }
-
- /* "View.MemoryView":1297
- * src.strides[i] = 0
- * else:
- * _err_extents(i, dst.shape[i], src.shape[i]) # <<<<<<<<<<<<<<
- *
- * if src.suboffsets[i] >= 0:
- */
- /*else*/ {
- __pyx_t_6 = __pyx_memoryview_err_extents(__pyx_v_i, (__pyx_v_dst.shape[__pyx_v_i]), (__pyx_v_src.shape[__pyx_v_i])); if (unlikely(__pyx_t_6 == ((int)-1))) __PYX_ERR(1, 1297, __pyx_L1_error)
- }
- __pyx_L7:;
-
- /* "View.MemoryView":1292
- *
- * for i in range(ndim):
- * if src.shape[i] != dst.shape[i]: # <<<<<<<<<<<<<<
- * if src.shape[i] == 1:
- * broadcasting = True
- */
- }
-
- /* "View.MemoryView":1299
- * _err_extents(i, dst.shape[i], src.shape[i])
- *
- * if src.suboffsets[i] >= 0: # <<<<<<<<<<<<<<
- * _err_dim(ValueError, "Dimension %d is not direct", i)
- *
- */
- __pyx_t_2 = (((__pyx_v_src.suboffsets[__pyx_v_i]) >= 0) != 0);
- if (__pyx_t_2) {
-
- /* "View.MemoryView":1300
- *
- * if src.suboffsets[i] >= 0:
- * _err_dim(ValueError, "Dimension %d is not direct", i) # <<<<<<<<<<<<<<
- *
- * if slices_overlap(&src, &dst, ndim, itemsize):
- */
- __pyx_t_6 = __pyx_memoryview_err_dim(__pyx_builtin_ValueError, ((char *)"Dimension %d is not direct"), __pyx_v_i); if (unlikely(__pyx_t_6 == ((int)-1))) __PYX_ERR(1, 1300, __pyx_L1_error)
-
- /* "View.MemoryView":1299
- * _err_extents(i, dst.shape[i], src.shape[i])
- *
- * if src.suboffsets[i] >= 0: # <<<<<<<<<<<<<<
- * _err_dim(ValueError, "Dimension %d is not direct", i)
- *
- */
- }
- }
-
- /* "View.MemoryView":1302
- * _err_dim(ValueError, "Dimension %d is not direct", i)
- *
- * if slices_overlap(&src, &dst, ndim, itemsize): # <<<<<<<<<<<<<<
- *
- * if not slice_is_contig(src, order, ndim):
- */
- __pyx_t_2 = (__pyx_slices_overlap((&__pyx_v_src), (&__pyx_v_dst), __pyx_v_ndim, __pyx_v_itemsize) != 0);
- if (__pyx_t_2) {
-
- /* "View.MemoryView":1304
- * if slices_overlap(&src, &dst, ndim, itemsize):
- *
- * if not slice_is_contig(src, order, ndim): # <<<<<<<<<<<<<<
- * order = get_best_order(&dst, ndim)
- *
- */
- __pyx_t_2 = ((!(__pyx_memviewslice_is_contig(__pyx_v_src, __pyx_v_order, __pyx_v_ndim) != 0)) != 0);
- if (__pyx_t_2) {
-
- /* "View.MemoryView":1305
- *
- * if not slice_is_contig(src, order, ndim):
- * order = get_best_order(&dst, ndim) # <<<<<<<<<<<<<<
- *
- * tmpdata = copy_data_to_temp(&src, &tmp, order, ndim)
- */
- __pyx_v_order = __pyx_get_best_slice_order((&__pyx_v_dst), __pyx_v_ndim);
-
- /* "View.MemoryView":1304
- * if slices_overlap(&src, &dst, ndim, itemsize):
- *
- * if not slice_is_contig(src, order, ndim): # <<<<<<<<<<<<<<
- * order = get_best_order(&dst, ndim)
- *
- */
- }
-
- /* "View.MemoryView":1307
- * order = get_best_order(&dst, ndim)
- *
- * tmpdata = copy_data_to_temp(&src, &tmp, order, ndim) # <<<<<<<<<<<<<<
- * src = tmp
- *
- */
- __pyx_t_7 = __pyx_memoryview_copy_data_to_temp((&__pyx_v_src), (&__pyx_v_tmp), __pyx_v_order, __pyx_v_ndim); if (unlikely(__pyx_t_7 == ((void *)NULL))) __PYX_ERR(1, 1307, __pyx_L1_error)
- __pyx_v_tmpdata = __pyx_t_7;
-
- /* "View.MemoryView":1308
- *
- * tmpdata = copy_data_to_temp(&src, &tmp, order, ndim)
- * src = tmp # <<<<<<<<<<<<<<
- *
- * if not broadcasting:
- */
- __pyx_v_src = __pyx_v_tmp;
-
- /* "View.MemoryView":1302
- * _err_dim(ValueError, "Dimension %d is not direct", i)
- *
- * if slices_overlap(&src, &dst, ndim, itemsize): # <<<<<<<<<<<<<<
- *
- * if not slice_is_contig(src, order, ndim):
- */
- }
-
- /* "View.MemoryView":1310
- * src = tmp
- *
- * if not broadcasting: # <<<<<<<<<<<<<<
- *
- *
- */
- __pyx_t_2 = ((!(__pyx_v_broadcasting != 0)) != 0);
- if (__pyx_t_2) {
-
- /* "View.MemoryView":1313
- *
- *
- * if slice_is_contig(src, 'C', ndim): # <<<<<<<<<<<<<<
- * direct_copy = slice_is_contig(dst, 'C', ndim)
- * elif slice_is_contig(src, 'F', ndim):
- */
- __pyx_t_2 = (__pyx_memviewslice_is_contig(__pyx_v_src, 'C', __pyx_v_ndim) != 0);
- if (__pyx_t_2) {
-
- /* "View.MemoryView":1314
- *
- * if slice_is_contig(src, 'C', ndim):
- * direct_copy = slice_is_contig(dst, 'C', ndim) # <<<<<<<<<<<<<<
- * elif slice_is_contig(src, 'F', ndim):
- * direct_copy = slice_is_contig(dst, 'F', ndim)
- */
- __pyx_v_direct_copy = __pyx_memviewslice_is_contig(__pyx_v_dst, 'C', __pyx_v_ndim);
-
- /* "View.MemoryView":1313
- *
- *
- * if slice_is_contig(src, 'C', ndim): # <<<<<<<<<<<<<<
- * direct_copy = slice_is_contig(dst, 'C', ndim)
- * elif slice_is_contig(src, 'F', ndim):
- */
- goto __pyx_L12;
- }
-
- /* "View.MemoryView":1315
- * if slice_is_contig(src, 'C', ndim):
- * direct_copy = slice_is_contig(dst, 'C', ndim)
- * elif slice_is_contig(src, 'F', ndim): # <<<<<<<<<<<<<<
- * direct_copy = slice_is_contig(dst, 'F', ndim)
- *
- */
- __pyx_t_2 = (__pyx_memviewslice_is_contig(__pyx_v_src, 'F', __pyx_v_ndim) != 0);
- if (__pyx_t_2) {
-
- /* "View.MemoryView":1316
- * direct_copy = slice_is_contig(dst, 'C', ndim)
- * elif slice_is_contig(src, 'F', ndim):
- * direct_copy = slice_is_contig(dst, 'F', ndim) # <<<<<<<<<<<<<<
- *
- * if direct_copy:
- */
- __pyx_v_direct_copy = __pyx_memviewslice_is_contig(__pyx_v_dst, 'F', __pyx_v_ndim);
-
- /* "View.MemoryView":1315
- * if slice_is_contig(src, 'C', ndim):
- * direct_copy = slice_is_contig(dst, 'C', ndim)
- * elif slice_is_contig(src, 'F', ndim): # <<<<<<<<<<<<<<
- * direct_copy = slice_is_contig(dst, 'F', ndim)
- *
- */
- }
- __pyx_L12:;
-
- /* "View.MemoryView":1318
- * direct_copy = slice_is_contig(dst, 'F', ndim)
- *
- * if direct_copy: # <<<<<<<<<<<<<<
- *
- * refcount_copying(&dst, dtype_is_object, ndim, False)
- */
- __pyx_t_2 = (__pyx_v_direct_copy != 0);
- if (__pyx_t_2) {
-
- /* "View.MemoryView":1320
- * if direct_copy:
- *
- * refcount_copying(&dst, dtype_is_object, ndim, False) # <<<<<<<<<<<<<<
- * memcpy(dst.data, src.data, slice_get_size(&src, ndim))
- * refcount_copying(&dst, dtype_is_object, ndim, True)
- */
- __pyx_memoryview_refcount_copying((&__pyx_v_dst), __pyx_v_dtype_is_object, __pyx_v_ndim, 0);
-
- /* "View.MemoryView":1321
- *
- * refcount_copying(&dst, dtype_is_object, ndim, False)
- * memcpy(dst.data, src.data, slice_get_size(&src, ndim)) # <<<<<<<<<<<<<<
- * refcount_copying(&dst, dtype_is_object, ndim, True)
- * free(tmpdata)
- */
- (void)(memcpy(__pyx_v_dst.data, __pyx_v_src.data, __pyx_memoryview_slice_get_size((&__pyx_v_src), __pyx_v_ndim)));
-
- /* "View.MemoryView":1322
- * refcount_copying(&dst, dtype_is_object, ndim, False)
- * memcpy(dst.data, src.data, slice_get_size(&src, ndim))
- * refcount_copying(&dst, dtype_is_object, ndim, True) # <<<<<<<<<<<<<<
- * free(tmpdata)
- * return 0
- */
- __pyx_memoryview_refcount_copying((&__pyx_v_dst), __pyx_v_dtype_is_object, __pyx_v_ndim, 1);
-
- /* "View.MemoryView":1323
- * memcpy(dst.data, src.data, slice_get_size(&src, ndim))
- * refcount_copying(&dst, dtype_is_object, ndim, True)
- * free(tmpdata) # <<<<<<<<<<<<<<
- * return 0
- *
- */
- free(__pyx_v_tmpdata);
-
- /* "View.MemoryView":1324
- * refcount_copying(&dst, dtype_is_object, ndim, True)
- * free(tmpdata)
- * return 0 # <<<<<<<<<<<<<<
- *
- * if order == 'F' == get_best_order(&dst, ndim):
- */
- __pyx_r = 0;
- goto __pyx_L0;
-
- /* "View.MemoryView":1318
- * direct_copy = slice_is_contig(dst, 'F', ndim)
- *
- * if direct_copy: # <<<<<<<<<<<<<<
- *
- * refcount_copying(&dst, dtype_is_object, ndim, False)
- */
- }
-
- /* "View.MemoryView":1310
- * src = tmp
- *
- * if not broadcasting: # <<<<<<<<<<<<<<
- *
- *
- */
- }
-
- /* "View.MemoryView":1326
- * return 0
- *
- * if order == 'F' == get_best_order(&dst, ndim): # <<<<<<<<<<<<<<
- *
- *
- */
- __pyx_t_2 = (__pyx_v_order == 'F');
- if (__pyx_t_2) {
- __pyx_t_2 = ('F' == __pyx_get_best_slice_order((&__pyx_v_dst), __pyx_v_ndim));
- }
- __pyx_t_8 = (__pyx_t_2 != 0);
- if (__pyx_t_8) {
-
- /* "View.MemoryView":1329
- *
- *
- * transpose_memslice(&src) # <<<<<<<<<<<<<<
- * transpose_memslice(&dst)
- *
- */
- __pyx_t_5 = __pyx_memslice_transpose((&__pyx_v_src)); if (unlikely(__pyx_t_5 == ((int)0))) __PYX_ERR(1, 1329, __pyx_L1_error)
-
- /* "View.MemoryView":1330
- *
- * transpose_memslice(&src)
- * transpose_memslice(&dst) # <<<<<<<<<<<<<<
- *
- * refcount_copying(&dst, dtype_is_object, ndim, False)
- */
- __pyx_t_5 = __pyx_memslice_transpose((&__pyx_v_dst)); if (unlikely(__pyx_t_5 == ((int)0))) __PYX_ERR(1, 1330, __pyx_L1_error)
-
- /* "View.MemoryView":1326
- * return 0
- *
- * if order == 'F' == get_best_order(&dst, ndim): # <<<<<<<<<<<<<<
- *
- *
- */
- }
-
- /* "View.MemoryView":1332
- * transpose_memslice(&dst)
- *
- * refcount_copying(&dst, dtype_is_object, ndim, False) # <<<<<<<<<<<<<<
- * copy_strided_to_strided(&src, &dst, ndim, itemsize)
- * refcount_copying(&dst, dtype_is_object, ndim, True)
- */
- __pyx_memoryview_refcount_copying((&__pyx_v_dst), __pyx_v_dtype_is_object, __pyx_v_ndim, 0);
-
- /* "View.MemoryView":1333
- *
- * refcount_copying(&dst, dtype_is_object, ndim, False)
- * copy_strided_to_strided(&src, &dst, ndim, itemsize) # <<<<<<<<<<<<<<
- * refcount_copying(&dst, dtype_is_object, ndim, True)
- *
- */
- copy_strided_to_strided((&__pyx_v_src), (&__pyx_v_dst), __pyx_v_ndim, __pyx_v_itemsize);
-
- /* "View.MemoryView":1334
- * refcount_copying(&dst, dtype_is_object, ndim, False)
- * copy_strided_to_strided(&src, &dst, ndim, itemsize)
- * refcount_copying(&dst, dtype_is_object, ndim, True) # <<<<<<<<<<<<<<
- *
- * free(tmpdata)
- */
- __pyx_memoryview_refcount_copying((&__pyx_v_dst), __pyx_v_dtype_is_object, __pyx_v_ndim, 1);
-
- /* "View.MemoryView":1336
- * refcount_copying(&dst, dtype_is_object, ndim, True)
- *
- * free(tmpdata) # <<<<<<<<<<<<<<
- * return 0
- *
- */
- free(__pyx_v_tmpdata);
-
- /* "View.MemoryView":1337
- *
- * free(tmpdata)
- * return 0 # <<<<<<<<<<<<<<
- *
- * @cname('__pyx_memoryview_broadcast_leading')
- */
- __pyx_r = 0;
- goto __pyx_L0;
-
- /* "View.MemoryView":1268
- *
- * @cname('__pyx_memoryview_copy_contents')
- * cdef int memoryview_copy_contents(__Pyx_memviewslice src, # <<<<<<<<<<<<<<
- * __Pyx_memviewslice dst,
- * int src_ndim, int dst_ndim,
- */
-
- /* function exit code */
- __pyx_L1_error:;
- {
- #ifdef WITH_THREAD
- PyGILState_STATE __pyx_gilstate_save = __Pyx_PyGILState_Ensure();
- #endif
- __Pyx_AddTraceback("View.MemoryView.memoryview_copy_contents", __pyx_clineno, __pyx_lineno, __pyx_filename);
- #ifdef WITH_THREAD
- __Pyx_PyGILState_Release(__pyx_gilstate_save);
- #endif
- }
- __pyx_r = -1;
- __pyx_L0:;
- return __pyx_r;
-}
-
-/* "View.MemoryView":1340
- *
- * @cname('__pyx_memoryview_broadcast_leading')
- * cdef void broadcast_leading(__Pyx_memviewslice *mslice, # <<<<<<<<<<<<<<
- * int ndim,
- * int ndim_other) nogil:
- */
-
-static void __pyx_memoryview_broadcast_leading(__Pyx_memviewslice *__pyx_v_mslice, int __pyx_v_ndim, int __pyx_v_ndim_other) {
- int __pyx_v_i;
- int __pyx_v_offset;
- int __pyx_t_1;
- int __pyx_t_2;
- int __pyx_t_3;
-
- /* "View.MemoryView":1344
- * int ndim_other) nogil:
- * cdef int i
- * cdef int offset = ndim_other - ndim # <<<<<<<<<<<<<<
- *
- * for i in range(ndim - 1, -1, -1):
- */
- __pyx_v_offset = (__pyx_v_ndim_other - __pyx_v_ndim);
-
- /* "View.MemoryView":1346
- * cdef int offset = ndim_other - ndim
- *
- * for i in range(ndim - 1, -1, -1): # <<<<<<<<<<<<<<
- * mslice.shape[i + offset] = mslice.shape[i]
- * mslice.strides[i + offset] = mslice.strides[i]
- */
- for (__pyx_t_1 = (__pyx_v_ndim - 1); __pyx_t_1 > -1; __pyx_t_1-=1) {
- __pyx_v_i = __pyx_t_1;
-
- /* "View.MemoryView":1347
- *
- * for i in range(ndim - 1, -1, -1):
- * mslice.shape[i + offset] = mslice.shape[i] # <<<<<<<<<<<<<<
- * mslice.strides[i + offset] = mslice.strides[i]
- * mslice.suboffsets[i + offset] = mslice.suboffsets[i]
- */
- (__pyx_v_mslice->shape[(__pyx_v_i + __pyx_v_offset)]) = (__pyx_v_mslice->shape[__pyx_v_i]);
-
- /* "View.MemoryView":1348
- * for i in range(ndim - 1, -1, -1):
- * mslice.shape[i + offset] = mslice.shape[i]
- * mslice.strides[i + offset] = mslice.strides[i] # <<<<<<<<<<<<<<
- * mslice.suboffsets[i + offset] = mslice.suboffsets[i]
- *
- */
- (__pyx_v_mslice->strides[(__pyx_v_i + __pyx_v_offset)]) = (__pyx_v_mslice->strides[__pyx_v_i]);
-
- /* "View.MemoryView":1349
- * mslice.shape[i + offset] = mslice.shape[i]
- * mslice.strides[i + offset] = mslice.strides[i]
- * mslice.suboffsets[i + offset] = mslice.suboffsets[i] # <<<<<<<<<<<<<<
- *
- * for i in range(offset):
- */
- (__pyx_v_mslice->suboffsets[(__pyx_v_i + __pyx_v_offset)]) = (__pyx_v_mslice->suboffsets[__pyx_v_i]);
- }
-
- /* "View.MemoryView":1351
- * mslice.suboffsets[i + offset] = mslice.suboffsets[i]
- *
- * for i in range(offset): # <<<<<<<<<<<<<<
- * mslice.shape[i] = 1
- * mslice.strides[i] = mslice.strides[0]
- */
- __pyx_t_1 = __pyx_v_offset;
- __pyx_t_2 = __pyx_t_1;
- for (__pyx_t_3 = 0; __pyx_t_3 < __pyx_t_2; __pyx_t_3+=1) {
- __pyx_v_i = __pyx_t_3;
-
- /* "View.MemoryView":1352
- *
- * for i in range(offset):
- * mslice.shape[i] = 1 # <<<<<<<<<<<<<<
- * mslice.strides[i] = mslice.strides[0]
- * mslice.suboffsets[i] = -1
- */
- (__pyx_v_mslice->shape[__pyx_v_i]) = 1;
-
- /* "View.MemoryView":1353
- * for i in range(offset):
- * mslice.shape[i] = 1
- * mslice.strides[i] = mslice.strides[0] # <<<<<<<<<<<<<<
- * mslice.suboffsets[i] = -1
- *
- */
- (__pyx_v_mslice->strides[__pyx_v_i]) = (__pyx_v_mslice->strides[0]);
-
- /* "View.MemoryView":1354
- * mslice.shape[i] = 1
- * mslice.strides[i] = mslice.strides[0]
- * mslice.suboffsets[i] = -1 # <<<<<<<<<<<<<<
- *
- *
- */
- (__pyx_v_mslice->suboffsets[__pyx_v_i]) = -1L;
- }
-
- /* "View.MemoryView":1340
- *
- * @cname('__pyx_memoryview_broadcast_leading')
- * cdef void broadcast_leading(__Pyx_memviewslice *mslice, # <<<<<<<<<<<<<<
- * int ndim,
- * int ndim_other) nogil:
- */
-
- /* function exit code */
-}
-
-/* "View.MemoryView":1362
- *
- * @cname('__pyx_memoryview_refcount_copying')
- * cdef void refcount_copying(__Pyx_memviewslice *dst, bint dtype_is_object, # <<<<<<<<<<<<<<
- * int ndim, bint inc) nogil:
- *
- */
-
-static void __pyx_memoryview_refcount_copying(__Pyx_memviewslice *__pyx_v_dst, int __pyx_v_dtype_is_object, int __pyx_v_ndim, int __pyx_v_inc) {
- int __pyx_t_1;
-
- /* "View.MemoryView":1366
- *
- *
- * if dtype_is_object: # <<<<<<<<<<<<<<
- * refcount_objects_in_slice_with_gil(dst.data, dst.shape,
- * dst.strides, ndim, inc)
- */
- __pyx_t_1 = (__pyx_v_dtype_is_object != 0);
- if (__pyx_t_1) {
-
- /* "View.MemoryView":1367
- *
- * if dtype_is_object:
- * refcount_objects_in_slice_with_gil(dst.data, dst.shape, # <<<<<<<<<<<<<<
- * dst.strides, ndim, inc)
- *
- */
- __pyx_memoryview_refcount_objects_in_slice_with_gil(__pyx_v_dst->data, __pyx_v_dst->shape, __pyx_v_dst->strides, __pyx_v_ndim, __pyx_v_inc);
-
- /* "View.MemoryView":1366
- *
- *
- * if dtype_is_object: # <<<<<<<<<<<<<<
- * refcount_objects_in_slice_with_gil(dst.data, dst.shape,
- * dst.strides, ndim, inc)
- */
- }
-
- /* "View.MemoryView":1362
- *
- * @cname('__pyx_memoryview_refcount_copying')
- * cdef void refcount_copying(__Pyx_memviewslice *dst, bint dtype_is_object, # <<<<<<<<<<<<<<
- * int ndim, bint inc) nogil:
- *
- */
-
- /* function exit code */
-}
-
-/* "View.MemoryView":1371
- *
- * @cname('__pyx_memoryview_refcount_objects_in_slice_with_gil')
- * cdef void refcount_objects_in_slice_with_gil(char *data, Py_ssize_t *shape, # <<<<<<<<<<<<<<
- * Py_ssize_t *strides, int ndim,
- * bint inc) with gil:
- */
-
-static void __pyx_memoryview_refcount_objects_in_slice_with_gil(char *__pyx_v_data, Py_ssize_t *__pyx_v_shape, Py_ssize_t *__pyx_v_strides, int __pyx_v_ndim, int __pyx_v_inc) {
- __Pyx_RefNannyDeclarations
- #ifdef WITH_THREAD
- PyGILState_STATE __pyx_gilstate_save = __Pyx_PyGILState_Ensure();
- #endif
- __Pyx_RefNannySetupContext("refcount_objects_in_slice_with_gil", 0);
-
- /* "View.MemoryView":1374
- * Py_ssize_t *strides, int ndim,
- * bint inc) with gil:
- * refcount_objects_in_slice(data, shape, strides, ndim, inc) # <<<<<<<<<<<<<<
- *
- * @cname('__pyx_memoryview_refcount_objects_in_slice')
- */
- __pyx_memoryview_refcount_objects_in_slice(__pyx_v_data, __pyx_v_shape, __pyx_v_strides, __pyx_v_ndim, __pyx_v_inc);
-
- /* "View.MemoryView":1371
- *
- * @cname('__pyx_memoryview_refcount_objects_in_slice_with_gil')
- * cdef void refcount_objects_in_slice_with_gil(char *data, Py_ssize_t *shape, # <<<<<<<<<<<<<<
- * Py_ssize_t *strides, int ndim,
- * bint inc) with gil:
- */
-
- /* function exit code */
- __Pyx_RefNannyFinishContext();
- #ifdef WITH_THREAD
- __Pyx_PyGILState_Release(__pyx_gilstate_save);
- #endif
-}
-
-/* "View.MemoryView":1377
- *
- * @cname('__pyx_memoryview_refcount_objects_in_slice')
- * cdef void refcount_objects_in_slice(char *data, Py_ssize_t *shape, # <<<<<<<<<<<<<<
- * Py_ssize_t *strides, int ndim, bint inc):
- * cdef Py_ssize_t i
- */
-
-static void __pyx_memoryview_refcount_objects_in_slice(char *__pyx_v_data, Py_ssize_t *__pyx_v_shape, Py_ssize_t *__pyx_v_strides, int __pyx_v_ndim, int __pyx_v_inc) {
- CYTHON_UNUSED Py_ssize_t __pyx_v_i;
- __Pyx_RefNannyDeclarations
- Py_ssize_t __pyx_t_1;
- Py_ssize_t __pyx_t_2;
- Py_ssize_t __pyx_t_3;
- int __pyx_t_4;
- __Pyx_RefNannySetupContext("refcount_objects_in_slice", 0);
-
- /* "View.MemoryView":1381
- * cdef Py_ssize_t i
- *
- * for i in range(shape[0]): # <<<<<<<<<<<<<<
- * if ndim == 1:
- * if inc:
- */
- __pyx_t_1 = (__pyx_v_shape[0]);
- __pyx_t_2 = __pyx_t_1;
- for (__pyx_t_3 = 0; __pyx_t_3 < __pyx_t_2; __pyx_t_3+=1) {
- __pyx_v_i = __pyx_t_3;
-
- /* "View.MemoryView":1382
- *
- * for i in range(shape[0]):
- * if ndim == 1: # <<<<<<<<<<<<<<
- * if inc:
- * Py_INCREF(( data)[0])
- */
- __pyx_t_4 = ((__pyx_v_ndim == 1) != 0);
- if (__pyx_t_4) {
-
- /* "View.MemoryView":1383
- * for i in range(shape[0]):
- * if ndim == 1:
- * if inc: # <<<<<<<<<<<<<<
- * Py_INCREF(( data)[0])
- * else:
- */
- __pyx_t_4 = (__pyx_v_inc != 0);
- if (__pyx_t_4) {
-
- /* "View.MemoryView":1384
- * if ndim == 1:
- * if inc:
- * Py_INCREF(( data)[0]) # <<<<<<<<<<<<<<
- * else:
- * Py_DECREF(( data)[0])
- */
- Py_INCREF((((PyObject **)__pyx_v_data)[0]));
-
- /* "View.MemoryView":1383
- * for i in range(shape[0]):
- * if ndim == 1:
- * if inc: # <<<<<<<<<<<<<<
- * Py_INCREF(( data)[0])
- * else:
- */
- goto __pyx_L6;
- }
-
- /* "View.MemoryView":1386
- * Py_INCREF(( data)[0])
- * else:
- * Py_DECREF(( data)[0]) # <<<<<<<<<<<<<<
- * else:
- * refcount_objects_in_slice(data, shape + 1, strides + 1,
- */
- /*else*/ {
- Py_DECREF((((PyObject **)__pyx_v_data)[0]));
- }
- __pyx_L6:;
-
- /* "View.MemoryView":1382
- *
- * for i in range(shape[0]):
- * if ndim == 1: # <<<<<<<<<<<<<<
- * if inc:
- * Py_INCREF(( data)[0])
- */
- goto __pyx_L5;
- }
-
- /* "View.MemoryView":1388
- * Py_DECREF(( data)[0])
- * else:
- * refcount_objects_in_slice(data, shape + 1, strides + 1, # <<<<<<<<<<<<<<
- * ndim - 1, inc)
- *
- */
- /*else*/ {
-
- /* "View.MemoryView":1389
- * else:
- * refcount_objects_in_slice(data, shape + 1, strides + 1,
- * ndim - 1, inc) # <<<<<<<<<<<<<<
- *
- * data += strides[0]
- */
- __pyx_memoryview_refcount_objects_in_slice(__pyx_v_data, (__pyx_v_shape + 1), (__pyx_v_strides + 1), (__pyx_v_ndim - 1), __pyx_v_inc);
- }
- __pyx_L5:;
-
- /* "View.MemoryView":1391
- * ndim - 1, inc)
- *
- * data += strides[0] # <<<<<<<<<<<<<<
- *
- *
- */
- __pyx_v_data = (__pyx_v_data + (__pyx_v_strides[0]));
- }
-
- /* "View.MemoryView":1377
- *
- * @cname('__pyx_memoryview_refcount_objects_in_slice')
- * cdef void refcount_objects_in_slice(char *data, Py_ssize_t *shape, # <<<<<<<<<<<<<<
- * Py_ssize_t *strides, int ndim, bint inc):
- * cdef Py_ssize_t i
- */
-
- /* function exit code */
- __Pyx_RefNannyFinishContext();
-}
-
-/* "View.MemoryView":1397
- *
- * @cname('__pyx_memoryview_slice_assign_scalar')
- * cdef void slice_assign_scalar(__Pyx_memviewslice *dst, int ndim, # <<<<<<<<<<<<<<
- * size_t itemsize, void *item,
- * bint dtype_is_object) nogil:
- */
-
-static void __pyx_memoryview_slice_assign_scalar(__Pyx_memviewslice *__pyx_v_dst, int __pyx_v_ndim, size_t __pyx_v_itemsize, void *__pyx_v_item, int __pyx_v_dtype_is_object) {
-
- /* "View.MemoryView":1400
- * size_t itemsize, void *item,
- * bint dtype_is_object) nogil:
- * refcount_copying(dst, dtype_is_object, ndim, False) # <<<<<<<<<<<<<<
- * _slice_assign_scalar(dst.data, dst.shape, dst.strides, ndim,
- * itemsize, item)
- */
- __pyx_memoryview_refcount_copying(__pyx_v_dst, __pyx_v_dtype_is_object, __pyx_v_ndim, 0);
-
- /* "View.MemoryView":1401
- * bint dtype_is_object) nogil:
- * refcount_copying(dst, dtype_is_object, ndim, False)
- * _slice_assign_scalar(dst.data, dst.shape, dst.strides, ndim, # <<<<<<<<<<<<<<
- * itemsize, item)
- * refcount_copying(dst, dtype_is_object, ndim, True)
- */
- __pyx_memoryview__slice_assign_scalar(__pyx_v_dst->data, __pyx_v_dst->shape, __pyx_v_dst->strides, __pyx_v_ndim, __pyx_v_itemsize, __pyx_v_item);
-
- /* "View.MemoryView":1403
- * _slice_assign_scalar(dst.data, dst.shape, dst.strides, ndim,
- * itemsize, item)
- * refcount_copying(dst, dtype_is_object, ndim, True) # <<<<<<<<<<<<<<
- *
- *
- */
- __pyx_memoryview_refcount_copying(__pyx_v_dst, __pyx_v_dtype_is_object, __pyx_v_ndim, 1);
-
- /* "View.MemoryView":1397
- *
- * @cname('__pyx_memoryview_slice_assign_scalar')
- * cdef void slice_assign_scalar(__Pyx_memviewslice *dst, int ndim, # <<<<<<<<<<<<<<
- * size_t itemsize, void *item,
- * bint dtype_is_object) nogil:
- */
-
- /* function exit code */
-}
-
-/* "View.MemoryView":1407
- *
- * @cname('__pyx_memoryview__slice_assign_scalar')
- * cdef void _slice_assign_scalar(char *data, Py_ssize_t *shape, # <<<<<<<<<<<<<<
- * Py_ssize_t *strides, int ndim,
- * size_t itemsize, void *item) nogil:
- */
-
-static void __pyx_memoryview__slice_assign_scalar(char *__pyx_v_data, Py_ssize_t *__pyx_v_shape, Py_ssize_t *__pyx_v_strides, int __pyx_v_ndim, size_t __pyx_v_itemsize, void *__pyx_v_item) {
- CYTHON_UNUSED Py_ssize_t __pyx_v_i;
- Py_ssize_t __pyx_v_stride;
- Py_ssize_t __pyx_v_extent;
- int __pyx_t_1;
- Py_ssize_t __pyx_t_2;
- Py_ssize_t __pyx_t_3;
- Py_ssize_t __pyx_t_4;
-
- /* "View.MemoryView":1411
- * size_t itemsize, void *item) nogil:
- * cdef Py_ssize_t i
- * cdef Py_ssize_t stride = strides[0] # <<<<<<<<<<<<<<
- * cdef Py_ssize_t extent = shape[0]
- *
- */
- __pyx_v_stride = (__pyx_v_strides[0]);
-
- /* "View.MemoryView":1412
- * cdef Py_ssize_t i
- * cdef Py_ssize_t stride = strides[0]
- * cdef Py_ssize_t extent = shape[0] # <<<<<<<<<<<<<<
- *
- * if ndim == 1:
- */
- __pyx_v_extent = (__pyx_v_shape[0]);
-
- /* "View.MemoryView":1414
- * cdef Py_ssize_t extent = shape[0]
- *
- * if ndim == 1: # <<<<<<<<<<<<<<
- * for i in range(extent):
- * memcpy(data, item, itemsize)
- */
- __pyx_t_1 = ((__pyx_v_ndim == 1) != 0);
- if (__pyx_t_1) {
-
- /* "View.MemoryView":1415
- *
- * if ndim == 1:
- * for i in range(extent): # <<<<<<<<<<<<<<
- * memcpy(data, item, itemsize)
- * data += stride
- */
- __pyx_t_2 = __pyx_v_extent;
- __pyx_t_3 = __pyx_t_2;
- for (__pyx_t_4 = 0; __pyx_t_4 < __pyx_t_3; __pyx_t_4+=1) {
- __pyx_v_i = __pyx_t_4;
-
- /* "View.MemoryView":1416
- * if ndim == 1:
- * for i in range(extent):
- * memcpy(data, item, itemsize) # <<<<<<<<<<<<<<
- * data += stride
- * else:
- */
- (void)(memcpy(__pyx_v_data, __pyx_v_item, __pyx_v_itemsize));
-
- /* "View.MemoryView":1417
- * for i in range(extent):
- * memcpy(data, item, itemsize)
- * data += stride # <<<<<<<<<<<<<<
- * else:
- * for i in range(extent):
- */
- __pyx_v_data = (__pyx_v_data + __pyx_v_stride);
- }
-
- /* "View.MemoryView":1414
- * cdef Py_ssize_t extent = shape[0]
- *
- * if ndim == 1: # <<<<<<<<<<<<<<
- * for i in range(extent):
- * memcpy(data, item, itemsize)
- */
- goto __pyx_L3;
- }
-
- /* "View.MemoryView":1419
- * data += stride
- * else:
- * for i in range(extent): # <<<<<<<<<<<<<<
- * _slice_assign_scalar(data, shape + 1, strides + 1,
- * ndim - 1, itemsize, item)
- */
- /*else*/ {
- __pyx_t_2 = __pyx_v_extent;
- __pyx_t_3 = __pyx_t_2;
- for (__pyx_t_4 = 0; __pyx_t_4 < __pyx_t_3; __pyx_t_4+=1) {
- __pyx_v_i = __pyx_t_4;
-
- /* "View.MemoryView":1420
- * else:
- * for i in range(extent):
- * _slice_assign_scalar(data, shape + 1, strides + 1, # <<<<<<<<<<<<<<
- * ndim - 1, itemsize, item)
- * data += stride
- */
- __pyx_memoryview__slice_assign_scalar(__pyx_v_data, (__pyx_v_shape + 1), (__pyx_v_strides + 1), (__pyx_v_ndim - 1), __pyx_v_itemsize, __pyx_v_item);
-
- /* "View.MemoryView":1422
- * _slice_assign_scalar(data, shape + 1, strides + 1,
- * ndim - 1, itemsize, item)
- * data += stride # <<<<<<<<<<<<<<
- *
- *
- */
- __pyx_v_data = (__pyx_v_data + __pyx_v_stride);
- }
- }
- __pyx_L3:;
-
- /* "View.MemoryView":1407
- *
- * @cname('__pyx_memoryview__slice_assign_scalar')
- * cdef void _slice_assign_scalar(char *data, Py_ssize_t *shape, # <<<<<<<<<<<<<<
- * Py_ssize_t *strides, int ndim,
- * size_t itemsize, void *item) nogil:
- */
-
- /* function exit code */
-}
-
-/* "(tree fragment)":1
- * def __pyx_unpickle_Enum(__pyx_type, long __pyx_checksum, __pyx_state): # <<<<<<<<<<<<<<
- * cdef object __pyx_PickleError
- * cdef object __pyx_result
- */
-
-/* Python wrapper */
-static PyObject *__pyx_pw_15View_dot_MemoryView_1__pyx_unpickle_Enum(PyObject *__pyx_self, PyObject *__pyx_args, PyObject *__pyx_kwds); /*proto*/
-static PyMethodDef __pyx_mdef_15View_dot_MemoryView_1__pyx_unpickle_Enum = {"__pyx_unpickle_Enum", (PyCFunction)(void*)(PyCFunctionWithKeywords)__pyx_pw_15View_dot_MemoryView_1__pyx_unpickle_Enum, METH_VARARGS|METH_KEYWORDS, 0};
-static PyObject *__pyx_pw_15View_dot_MemoryView_1__pyx_unpickle_Enum(PyObject *__pyx_self, PyObject *__pyx_args, PyObject *__pyx_kwds) {
- PyObject *__pyx_v___pyx_type = 0;
- long __pyx_v___pyx_checksum;
- PyObject *__pyx_v___pyx_state = 0;
- int __pyx_lineno = 0;
- const char *__pyx_filename = NULL;
- int __pyx_clineno = 0;
- PyObject *__pyx_r = 0;
- __Pyx_RefNannyDeclarations
- __Pyx_RefNannySetupContext("__pyx_unpickle_Enum (wrapper)", 0);
- {
- static PyObject **__pyx_pyargnames[] = {&__pyx_n_s_pyx_type,&__pyx_n_s_pyx_checksum,&__pyx_n_s_pyx_state,0};
- PyObject* values[3] = {0,0,0};
- if (unlikely(__pyx_kwds)) {
- Py_ssize_t kw_args;
- const Py_ssize_t pos_args = PyTuple_GET_SIZE(__pyx_args);
- switch (pos_args) {
- case 3: values[2] = PyTuple_GET_ITEM(__pyx_args, 2);
- CYTHON_FALLTHROUGH;
- case 2: values[1] = PyTuple_GET_ITEM(__pyx_args, 1);
- CYTHON_FALLTHROUGH;
- case 1: values[0] = PyTuple_GET_ITEM(__pyx_args, 0);
- CYTHON_FALLTHROUGH;
- case 0: break;
- default: goto __pyx_L5_argtuple_error;
- }
- kw_args = PyDict_Size(__pyx_kwds);
- switch (pos_args) {
- case 0:
- if (likely((values[0] = __Pyx_PyDict_GetItemStr(__pyx_kwds, __pyx_n_s_pyx_type)) != 0)) kw_args--;
- else goto __pyx_L5_argtuple_error;
- CYTHON_FALLTHROUGH;
- case 1:
- if (likely((values[1] = __Pyx_PyDict_GetItemStr(__pyx_kwds, __pyx_n_s_pyx_checksum)) != 0)) kw_args--;
- else {
- __Pyx_RaiseArgtupleInvalid("__pyx_unpickle_Enum", 1, 3, 3, 1); __PYX_ERR(1, 1, __pyx_L3_error)
- }
- CYTHON_FALLTHROUGH;
- case 2:
- if (likely((values[2] = __Pyx_PyDict_GetItemStr(__pyx_kwds, __pyx_n_s_pyx_state)) != 0)) kw_args--;
- else {
- __Pyx_RaiseArgtupleInvalid("__pyx_unpickle_Enum", 1, 3, 3, 2); __PYX_ERR(1, 1, __pyx_L3_error)
- }
- }
- if (unlikely(kw_args > 0)) {
- if (unlikely(__Pyx_ParseOptionalKeywords(__pyx_kwds, __pyx_pyargnames, 0, values, pos_args, "__pyx_unpickle_Enum") < 0)) __PYX_ERR(1, 1, __pyx_L3_error)
- }
- } else if (PyTuple_GET_SIZE(__pyx_args) != 3) {
- goto __pyx_L5_argtuple_error;
- } else {
- values[0] = PyTuple_GET_ITEM(__pyx_args, 0);
- values[1] = PyTuple_GET_ITEM(__pyx_args, 1);
- values[2] = PyTuple_GET_ITEM(__pyx_args, 2);
- }
- __pyx_v___pyx_type = values[0];
- __pyx_v___pyx_checksum = __Pyx_PyInt_As_long(values[1]); if (unlikely((__pyx_v___pyx_checksum == (long)-1) && PyErr_Occurred())) __PYX_ERR(1, 1, __pyx_L3_error)
- __pyx_v___pyx_state = values[2];
- }
- goto __pyx_L4_argument_unpacking_done;
- __pyx_L5_argtuple_error:;
- __Pyx_RaiseArgtupleInvalid("__pyx_unpickle_Enum", 1, 3, 3, PyTuple_GET_SIZE(__pyx_args)); __PYX_ERR(1, 1, __pyx_L3_error)
- __pyx_L3_error:;
- __Pyx_AddTraceback("View.MemoryView.__pyx_unpickle_Enum", __pyx_clineno, __pyx_lineno, __pyx_filename);
- __Pyx_RefNannyFinishContext();
- return NULL;
- __pyx_L4_argument_unpacking_done:;
- __pyx_r = __pyx_pf_15View_dot_MemoryView___pyx_unpickle_Enum(__pyx_self, __pyx_v___pyx_type, __pyx_v___pyx_checksum, __pyx_v___pyx_state);
-
- /* function exit code */
- __Pyx_RefNannyFinishContext();
- return __pyx_r;
-}
-
-static PyObject *__pyx_pf_15View_dot_MemoryView___pyx_unpickle_Enum(CYTHON_UNUSED PyObject *__pyx_self, PyObject *__pyx_v___pyx_type, long __pyx_v___pyx_checksum, PyObject *__pyx_v___pyx_state) {
- PyObject *__pyx_v___pyx_PickleError = 0;
- PyObject *__pyx_v___pyx_result = 0;
- PyObject *__pyx_r = NULL;
- __Pyx_RefNannyDeclarations
- int __pyx_t_1;
- PyObject *__pyx_t_2 = NULL;
- PyObject *__pyx_t_3 = NULL;
- PyObject *__pyx_t_4 = NULL;
- PyObject *__pyx_t_5 = NULL;
- int __pyx_t_6;
- int __pyx_lineno = 0;
- const char *__pyx_filename = NULL;
- int __pyx_clineno = 0;
- __Pyx_RefNannySetupContext("__pyx_unpickle_Enum", 0);
-
- /* "(tree fragment)":4
- * cdef object __pyx_PickleError
- * cdef object __pyx_result
- * if __pyx_checksum != 0xb068931: # <<<<<<<<<<<<<<
- * from pickle import PickleError as __pyx_PickleError
- * raise __pyx_PickleError("Incompatible checksums (%s vs 0xb068931 = (name))" % __pyx_checksum)
- */
- __pyx_t_1 = ((__pyx_v___pyx_checksum != 0xb068931) != 0);
- if (__pyx_t_1) {
-
- /* "(tree fragment)":5
- * cdef object __pyx_result
- * if __pyx_checksum != 0xb068931:
- * from pickle import PickleError as __pyx_PickleError # <<<<<<<<<<<<<<
- * raise __pyx_PickleError("Incompatible checksums (%s vs 0xb068931 = (name))" % __pyx_checksum)
- * __pyx_result = Enum.__new__(__pyx_type)
- */
- __pyx_t_2 = PyList_New(1); if (unlikely(!__pyx_t_2)) __PYX_ERR(1, 5, __pyx_L1_error)
- __Pyx_GOTREF(__pyx_t_2);
- __Pyx_INCREF(__pyx_n_s_PickleError);
- __Pyx_GIVEREF(__pyx_n_s_PickleError);
- PyList_SET_ITEM(__pyx_t_2, 0, __pyx_n_s_PickleError);
- __pyx_t_3 = __Pyx_Import(__pyx_n_s_pickle, __pyx_t_2, 0); if (unlikely(!__pyx_t_3)) __PYX_ERR(1, 5, __pyx_L1_error)
- __Pyx_GOTREF(__pyx_t_3);
- __Pyx_DECREF(__pyx_t_2); __pyx_t_2 = 0;
- __pyx_t_2 = __Pyx_ImportFrom(__pyx_t_3, __pyx_n_s_PickleError); if (unlikely(!__pyx_t_2)) __PYX_ERR(1, 5, __pyx_L1_error)
- __Pyx_GOTREF(__pyx_t_2);
- __Pyx_INCREF(__pyx_t_2);
- __pyx_v___pyx_PickleError = __pyx_t_2;
- __Pyx_DECREF(__pyx_t_2); __pyx_t_2 = 0;
- __Pyx_DECREF(__pyx_t_3); __pyx_t_3 = 0;
-
- /* "(tree fragment)":6
- * if __pyx_checksum != 0xb068931:
- * from pickle import PickleError as __pyx_PickleError
- * raise __pyx_PickleError("Incompatible checksums (%s vs 0xb068931 = (name))" % __pyx_checksum) # <<<<<<<<<<<<<<
- * __pyx_result = Enum.__new__(__pyx_type)
- * if __pyx_state is not None:
- */
- __pyx_t_2 = __Pyx_PyInt_From_long(__pyx_v___pyx_checksum); if (unlikely(!__pyx_t_2)) __PYX_ERR(1, 6, __pyx_L1_error)
- __Pyx_GOTREF(__pyx_t_2);
- __pyx_t_4 = __Pyx_PyString_Format(__pyx_kp_s_Incompatible_checksums_s_vs_0xb0, __pyx_t_2); if (unlikely(!__pyx_t_4)) __PYX_ERR(1, 6, __pyx_L1_error)
- __Pyx_GOTREF(__pyx_t_4);
- __Pyx_DECREF(__pyx_t_2); __pyx_t_2 = 0;
- __Pyx_INCREF(__pyx_v___pyx_PickleError);
- __pyx_t_2 = __pyx_v___pyx_PickleError; __pyx_t_5 = NULL;
- if (CYTHON_UNPACK_METHODS && unlikely(PyMethod_Check(__pyx_t_2))) {
- __pyx_t_5 = PyMethod_GET_SELF(__pyx_t_2);
- if (likely(__pyx_t_5)) {
- PyObject* function = PyMethod_GET_FUNCTION(__pyx_t_2);
- __Pyx_INCREF(__pyx_t_5);
- __Pyx_INCREF(function);
- __Pyx_DECREF_SET(__pyx_t_2, function);
- }
- }
- __pyx_t_3 = (__pyx_t_5) ? __Pyx_PyObject_Call2Args(__pyx_t_2, __pyx_t_5, __pyx_t_4) : __Pyx_PyObject_CallOneArg(__pyx_t_2, __pyx_t_4);
- __Pyx_XDECREF(__pyx_t_5); __pyx_t_5 = 0;
- __Pyx_DECREF(__pyx_t_4); __pyx_t_4 = 0;
- if (unlikely(!__pyx_t_3)) __PYX_ERR(1, 6, __pyx_L1_error)
- __Pyx_GOTREF(__pyx_t_3);
- __Pyx_DECREF(__pyx_t_2); __pyx_t_2 = 0;
- __Pyx_Raise(__pyx_t_3, 0, 0, 0);
- __Pyx_DECREF(__pyx_t_3); __pyx_t_3 = 0;
- __PYX_ERR(1, 6, __pyx_L1_error)
-
- /* "(tree fragment)":4
- * cdef object __pyx_PickleError
- * cdef object __pyx_result
- * if __pyx_checksum != 0xb068931: # <<<<<<<<<<<<<<
- * from pickle import PickleError as __pyx_PickleError
- * raise __pyx_PickleError("Incompatible checksums (%s vs 0xb068931 = (name))" % __pyx_checksum)
- */
- }
-
- /* "(tree fragment)":7
- * from pickle import PickleError as __pyx_PickleError
- * raise __pyx_PickleError("Incompatible checksums (%s vs 0xb068931 = (name))" % __pyx_checksum)
- * __pyx_result = Enum.__new__(__pyx_type) # <<<<<<<<<<<<<<
- * if __pyx_state is not None:
- * __pyx_unpickle_Enum__set_state( __pyx_result, __pyx_state)
- */
- __pyx_t_2 = __Pyx_PyObject_GetAttrStr(((PyObject *)__pyx_MemviewEnum_type), __pyx_n_s_new); if (unlikely(!__pyx_t_2)) __PYX_ERR(1, 7, __pyx_L1_error)
- __Pyx_GOTREF(__pyx_t_2);
- __pyx_t_4 = NULL;
- if (CYTHON_UNPACK_METHODS && likely(PyMethod_Check(__pyx_t_2))) {
- __pyx_t_4 = PyMethod_GET_SELF(__pyx_t_2);
- if (likely(__pyx_t_4)) {
- PyObject* function = PyMethod_GET_FUNCTION(__pyx_t_2);
- __Pyx_INCREF(__pyx_t_4);
- __Pyx_INCREF(function);
- __Pyx_DECREF_SET(__pyx_t_2, function);
- }
- }
- __pyx_t_3 = (__pyx_t_4) ? __Pyx_PyObject_Call2Args(__pyx_t_2, __pyx_t_4, __pyx_v___pyx_type) : __Pyx_PyObject_CallOneArg(__pyx_t_2, __pyx_v___pyx_type);
- __Pyx_XDECREF(__pyx_t_4); __pyx_t_4 = 0;
- if (unlikely(!__pyx_t_3)) __PYX_ERR(1, 7, __pyx_L1_error)
- __Pyx_GOTREF(__pyx_t_3);
- __Pyx_DECREF(__pyx_t_2); __pyx_t_2 = 0;
- __pyx_v___pyx_result = __pyx_t_3;
- __pyx_t_3 = 0;
-
- /* "(tree fragment)":8
- * raise __pyx_PickleError("Incompatible checksums (%s vs 0xb068931 = (name))" % __pyx_checksum)
- * __pyx_result = Enum.__new__(__pyx_type)
- * if __pyx_state is not None: # <<<<<<<<<<<<<<
- * __pyx_unpickle_Enum__set_state( __pyx_result, __pyx_state)
- * return __pyx_result
- */
- __pyx_t_1 = (__pyx_v___pyx_state != Py_None);
- __pyx_t_6 = (__pyx_t_1 != 0);
- if (__pyx_t_6) {
-
- /* "(tree fragment)":9
- * __pyx_result = Enum.__new__(__pyx_type)
- * if __pyx_state is not None:
- * __pyx_unpickle_Enum__set_state( __pyx_result, __pyx_state) # <<<<<<<<<<<<<<
- * return __pyx_result
- * cdef __pyx_unpickle_Enum__set_state(Enum __pyx_result, tuple __pyx_state):
- */
- if (!(likely(PyTuple_CheckExact(__pyx_v___pyx_state))||((__pyx_v___pyx_state) == Py_None)||(PyErr_Format(PyExc_TypeError, "Expected %.16s, got %.200s", "tuple", Py_TYPE(__pyx_v___pyx_state)->tp_name), 0))) __PYX_ERR(1, 9, __pyx_L1_error)
- __pyx_t_3 = __pyx_unpickle_Enum__set_state(((struct __pyx_MemviewEnum_obj *)__pyx_v___pyx_result), ((PyObject*)__pyx_v___pyx_state)); if (unlikely(!__pyx_t_3)) __PYX_ERR(1, 9, __pyx_L1_error)
- __Pyx_GOTREF(__pyx_t_3);
- __Pyx_DECREF(__pyx_t_3); __pyx_t_3 = 0;
-
- /* "(tree fragment)":8
- * raise __pyx_PickleError("Incompatible checksums (%s vs 0xb068931 = (name))" % __pyx_checksum)
- * __pyx_result = Enum.__new__(__pyx_type)
- * if __pyx_state is not None: # <<<<<<<<<<<<<<
- * __pyx_unpickle_Enum__set_state( __pyx_result, __pyx_state)
- * return __pyx_result
- */
- }
-
- /* "(tree fragment)":10
- * if __pyx_state is not None:
- * __pyx_unpickle_Enum__set_state( __pyx_result, __pyx_state)
- * return __pyx_result # <<<<<<<<<<<<<<
- * cdef __pyx_unpickle_Enum__set_state(Enum __pyx_result, tuple __pyx_state):
- * __pyx_result.name = __pyx_state[0]
- */
- __Pyx_XDECREF(__pyx_r);
- __Pyx_INCREF(__pyx_v___pyx_result);
- __pyx_r = __pyx_v___pyx_result;
- goto __pyx_L0;
-
- /* "(tree fragment)":1
- * def __pyx_unpickle_Enum(__pyx_type, long __pyx_checksum, __pyx_state): # <<<<<<<<<<<<<<
- * cdef object __pyx_PickleError
- * cdef object __pyx_result
- */
-
- /* function exit code */
- __pyx_L1_error:;
- __Pyx_XDECREF(__pyx_t_2);
- __Pyx_XDECREF(__pyx_t_3);
- __Pyx_XDECREF(__pyx_t_4);
- __Pyx_XDECREF(__pyx_t_5);
- __Pyx_AddTraceback("View.MemoryView.__pyx_unpickle_Enum", __pyx_clineno, __pyx_lineno, __pyx_filename);
- __pyx_r = NULL;
- __pyx_L0:;
- __Pyx_XDECREF(__pyx_v___pyx_PickleError);
- __Pyx_XDECREF(__pyx_v___pyx_result);
- __Pyx_XGIVEREF(__pyx_r);
- __Pyx_RefNannyFinishContext();
- return __pyx_r;
-}
-
-/* "(tree fragment)":11
- * __pyx_unpickle_Enum__set_state( __pyx_result, __pyx_state)
- * return __pyx_result
- * cdef __pyx_unpickle_Enum__set_state(Enum __pyx_result, tuple __pyx_state): # <<<<<<<<<<<<<<
- * __pyx_result.name = __pyx_state[0]
- * if len(__pyx_state) > 1 and hasattr(__pyx_result, '__dict__'):
- */
-
-static PyObject *__pyx_unpickle_Enum__set_state(struct __pyx_MemviewEnum_obj *__pyx_v___pyx_result, PyObject *__pyx_v___pyx_state) {
- PyObject *__pyx_r = NULL;
- __Pyx_RefNannyDeclarations
- PyObject *__pyx_t_1 = NULL;
- int __pyx_t_2;
- Py_ssize_t __pyx_t_3;
- int __pyx_t_4;
- int __pyx_t_5;
- PyObject *__pyx_t_6 = NULL;
- PyObject *__pyx_t_7 = NULL;
- PyObject *__pyx_t_8 = NULL;
- int __pyx_lineno = 0;
- const char *__pyx_filename = NULL;
- int __pyx_clineno = 0;
- __Pyx_RefNannySetupContext("__pyx_unpickle_Enum__set_state", 0);
-
- /* "(tree fragment)":12
- * return __pyx_result
- * cdef __pyx_unpickle_Enum__set_state(Enum __pyx_result, tuple __pyx_state):
- * __pyx_result.name = __pyx_state[0] # <<<<<<<<<<<<<<
- * if len(__pyx_state) > 1 and hasattr(__pyx_result, '__dict__'):
- * __pyx_result.__dict__.update(__pyx_state[1])
- */
- if (unlikely(__pyx_v___pyx_state == Py_None)) {
- PyErr_SetString(PyExc_TypeError, "'NoneType' object is not subscriptable");
- __PYX_ERR(1, 12, __pyx_L1_error)
- }
- __pyx_t_1 = __Pyx_GetItemInt_Tuple(__pyx_v___pyx_state, 0, long, 1, __Pyx_PyInt_From_long, 0, 0, 1); if (unlikely(!__pyx_t_1)) __PYX_ERR(1, 12, __pyx_L1_error)
- __Pyx_GOTREF(__pyx_t_1);
- __Pyx_GIVEREF(__pyx_t_1);
- __Pyx_GOTREF(__pyx_v___pyx_result->name);
- __Pyx_DECREF(__pyx_v___pyx_result->name);
- __pyx_v___pyx_result->name = __pyx_t_1;
- __pyx_t_1 = 0;
-
- /* "(tree fragment)":13
- * cdef __pyx_unpickle_Enum__set_state(Enum __pyx_result, tuple __pyx_state):
- * __pyx_result.name = __pyx_state[0]
- * if len(__pyx_state) > 1 and hasattr(__pyx_result, '__dict__'): # <<<<<<<<<<<<<<
- * __pyx_result.__dict__.update(__pyx_state[1])
- */
- if (unlikely(__pyx_v___pyx_state == Py_None)) {
- PyErr_SetString(PyExc_TypeError, "object of type 'NoneType' has no len()");
- __PYX_ERR(1, 13, __pyx_L1_error)
- }
- __pyx_t_3 = PyTuple_GET_SIZE(__pyx_v___pyx_state); if (unlikely(__pyx_t_3 == ((Py_ssize_t)-1))) __PYX_ERR(1, 13, __pyx_L1_error)
- __pyx_t_4 = ((__pyx_t_3 > 1) != 0);
- if (__pyx_t_4) {
- } else {
- __pyx_t_2 = __pyx_t_4;
- goto __pyx_L4_bool_binop_done;
- }
- __pyx_t_4 = __Pyx_HasAttr(((PyObject *)__pyx_v___pyx_result), __pyx_n_s_dict); if (unlikely(__pyx_t_4 == ((int)-1))) __PYX_ERR(1, 13, __pyx_L1_error)
- __pyx_t_5 = (__pyx_t_4 != 0);
- __pyx_t_2 = __pyx_t_5;
- __pyx_L4_bool_binop_done:;
- if (__pyx_t_2) {
-
- /* "(tree fragment)":14
- * __pyx_result.name = __pyx_state[0]
- * if len(__pyx_state) > 1 and hasattr(__pyx_result, '__dict__'):
- * __pyx_result.__dict__.update(__pyx_state[1]) # <<<<<<<<<<<<<<
- */
- __pyx_t_6 = __Pyx_PyObject_GetAttrStr(((PyObject *)__pyx_v___pyx_result), __pyx_n_s_dict); if (unlikely(!__pyx_t_6)) __PYX_ERR(1, 14, __pyx_L1_error)
- __Pyx_GOTREF(__pyx_t_6);
- __pyx_t_7 = __Pyx_PyObject_GetAttrStr(__pyx_t_6, __pyx_n_s_update); if (unlikely(!__pyx_t_7)) __PYX_ERR(1, 14, __pyx_L1_error)
- __Pyx_GOTREF(__pyx_t_7);
- __Pyx_DECREF(__pyx_t_6); __pyx_t_6 = 0;
- if (unlikely(__pyx_v___pyx_state == Py_None)) {
- PyErr_SetString(PyExc_TypeError, "'NoneType' object is not subscriptable");
- __PYX_ERR(1, 14, __pyx_L1_error)
- }
- __pyx_t_6 = __Pyx_GetItemInt_Tuple(__pyx_v___pyx_state, 1, long, 1, __Pyx_PyInt_From_long, 0, 0, 1); if (unlikely(!__pyx_t_6)) __PYX_ERR(1, 14, __pyx_L1_error)
- __Pyx_GOTREF(__pyx_t_6);
- __pyx_t_8 = NULL;
- if (CYTHON_UNPACK_METHODS && likely(PyMethod_Check(__pyx_t_7))) {
- __pyx_t_8 = PyMethod_GET_SELF(__pyx_t_7);
- if (likely(__pyx_t_8)) {
- PyObject* function = PyMethod_GET_FUNCTION(__pyx_t_7);
- __Pyx_INCREF(__pyx_t_8);
- __Pyx_INCREF(function);
- __Pyx_DECREF_SET(__pyx_t_7, function);
- }
- }
- __pyx_t_1 = (__pyx_t_8) ? __Pyx_PyObject_Call2Args(__pyx_t_7, __pyx_t_8, __pyx_t_6) : __Pyx_PyObject_CallOneArg(__pyx_t_7, __pyx_t_6);
- __Pyx_XDECREF(__pyx_t_8); __pyx_t_8 = 0;
- __Pyx_DECREF(__pyx_t_6); __pyx_t_6 = 0;
- if (unlikely(!__pyx_t_1)) __PYX_ERR(1, 14, __pyx_L1_error)
- __Pyx_GOTREF(__pyx_t_1);
- __Pyx_DECREF(__pyx_t_7); __pyx_t_7 = 0;
- __Pyx_DECREF(__pyx_t_1); __pyx_t_1 = 0;
-
- /* "(tree fragment)":13
- * cdef __pyx_unpickle_Enum__set_state(Enum __pyx_result, tuple __pyx_state):
- * __pyx_result.name = __pyx_state[0]
- * if len(__pyx_state) > 1 and hasattr(__pyx_result, '__dict__'): # <<<<<<<<<<<<<<
- * __pyx_result.__dict__.update(__pyx_state[1])
- */
- }
-
- /* "(tree fragment)":11
- * __pyx_unpickle_Enum__set_state( __pyx_result, __pyx_state)
- * return __pyx_result
- * cdef __pyx_unpickle_Enum__set_state(Enum __pyx_result, tuple __pyx_state): # <<<<<<<<<<<<<<
- * __pyx_result.name = __pyx_state[0]
- * if len(__pyx_state) > 1 and hasattr(__pyx_result, '__dict__'):
- */
-
- /* function exit code */
- __pyx_r = Py_None; __Pyx_INCREF(Py_None);
- goto __pyx_L0;
- __pyx_L1_error:;
- __Pyx_XDECREF(__pyx_t_1);
- __Pyx_XDECREF(__pyx_t_6);
- __Pyx_XDECREF(__pyx_t_7);
- __Pyx_XDECREF(__pyx_t_8);
- __Pyx_AddTraceback("View.MemoryView.__pyx_unpickle_Enum__set_state", __pyx_clineno, __pyx_lineno, __pyx_filename);
- __pyx_r = 0;
- __pyx_L0:;
- __Pyx_XGIVEREF(__pyx_r);
- __Pyx_RefNannyFinishContext();
- return __pyx_r;
-}
-static struct __pyx_vtabstruct_array __pyx_vtable_array;
-
-static PyObject *__pyx_tp_new_array(PyTypeObject *t, PyObject *a, PyObject *k) {
- struct __pyx_array_obj *p;
- PyObject *o;
- if (likely((t->tp_flags & Py_TPFLAGS_IS_ABSTRACT) == 0)) {
- o = (*t->tp_alloc)(t, 0);
- } else {
- o = (PyObject *) PyBaseObject_Type.tp_new(t, __pyx_empty_tuple, 0);
- }
- if (unlikely(!o)) return 0;
- p = ((struct __pyx_array_obj *)o);
- p->__pyx_vtab = __pyx_vtabptr_array;
- p->mode = ((PyObject*)Py_None); Py_INCREF(Py_None);
- p->_format = ((PyObject*)Py_None); Py_INCREF(Py_None);
- if (unlikely(__pyx_array___cinit__(o, a, k) < 0)) goto bad;
- return o;
- bad:
- Py_DECREF(o); o = 0;
- return NULL;
-}
-
-static void __pyx_tp_dealloc_array(PyObject *o) {
- struct __pyx_array_obj *p = (struct __pyx_array_obj *)o;
- #if CYTHON_USE_TP_FINALIZE
- if (unlikely(PyType_HasFeature(Py_TYPE(o), Py_TPFLAGS_HAVE_FINALIZE) && Py_TYPE(o)->tp_finalize) && (!PyType_IS_GC(Py_TYPE(o)) || !_PyGC_FINALIZED(o))) {
- if (PyObject_CallFinalizerFromDealloc(o)) return;
- }
- #endif
- {
- PyObject *etype, *eval, *etb;
- PyErr_Fetch(&etype, &eval, &etb);
- __Pyx_SET_REFCNT(o, Py_REFCNT(o) + 1);
- __pyx_array___dealloc__(o);
- __Pyx_SET_REFCNT(o, Py_REFCNT(o) - 1);
- PyErr_Restore(etype, eval, etb);
- }
- Py_CLEAR(p->mode);
- Py_CLEAR(p->_format);
- (*Py_TYPE(o)->tp_free)(o);
-}
-static PyObject *__pyx_sq_item_array(PyObject *o, Py_ssize_t i) {
- PyObject *r;
- PyObject *x = PyInt_FromSsize_t(i); if(!x) return 0;
- r = Py_TYPE(o)->tp_as_mapping->mp_subscript(o, x);
- Py_DECREF(x);
- return r;
-}
-
-static int __pyx_mp_ass_subscript_array(PyObject *o, PyObject *i, PyObject *v) {
- if (v) {
- return __pyx_array___setitem__(o, i, v);
- }
- else {
- PyErr_Format(PyExc_NotImplementedError,
- "Subscript deletion not supported by %.200s", Py_TYPE(o)->tp_name);
- return -1;
- }
-}
-
-static PyObject *__pyx_tp_getattro_array(PyObject *o, PyObject *n) {
- PyObject *v = __Pyx_PyObject_GenericGetAttr(o, n);
- if (!v && PyErr_ExceptionMatches(PyExc_AttributeError)) {
- PyErr_Clear();
- v = __pyx_array___getattr__(o, n);
- }
- return v;
-}
-
-static PyObject *__pyx_getprop___pyx_array_memview(PyObject *o, CYTHON_UNUSED void *x) {
- return __pyx_pw_15View_dot_MemoryView_5array_7memview_1__get__(o);
-}
-
-static PyMethodDef __pyx_methods_array[] = {
- {"__getattr__", (PyCFunction)__pyx_array___getattr__, METH_O|METH_COEXIST, 0},
- {"__reduce_cython__", (PyCFunction)__pyx_pw___pyx_array_1__reduce_cython__, METH_NOARGS, 0},
- {"__setstate_cython__", (PyCFunction)__pyx_pw___pyx_array_3__setstate_cython__, METH_O, 0},
- {0, 0, 0, 0}
-};
-
-static struct PyGetSetDef __pyx_getsets_array[] = {
- {(char *)"memview", __pyx_getprop___pyx_array_memview, 0, (char *)0, 0},
- {0, 0, 0, 0, 0}
-};
-
-static PySequenceMethods __pyx_tp_as_sequence_array = {
- __pyx_array___len__, /*sq_length*/
- 0, /*sq_concat*/
- 0, /*sq_repeat*/
- __pyx_sq_item_array, /*sq_item*/
- 0, /*sq_slice*/
- 0, /*sq_ass_item*/
- 0, /*sq_ass_slice*/
- 0, /*sq_contains*/
- 0, /*sq_inplace_concat*/
- 0, /*sq_inplace_repeat*/
-};
-
-static PyMappingMethods __pyx_tp_as_mapping_array = {
- __pyx_array___len__, /*mp_length*/
- __pyx_array___getitem__, /*mp_subscript*/
- __pyx_mp_ass_subscript_array, /*mp_ass_subscript*/
-};
-
-static PyBufferProcs __pyx_tp_as_buffer_array = {
- #if PY_MAJOR_VERSION < 3
- 0, /*bf_getreadbuffer*/
- #endif
- #if PY_MAJOR_VERSION < 3
- 0, /*bf_getwritebuffer*/
- #endif
- #if PY_MAJOR_VERSION < 3
- 0, /*bf_getsegcount*/
- #endif
- #if PY_MAJOR_VERSION < 3
- 0, /*bf_getcharbuffer*/
- #endif
- __pyx_array_getbuffer, /*bf_getbuffer*/
- 0, /*bf_releasebuffer*/
-};
-
-static PyTypeObject __pyx_type___pyx_array = {
- PyVarObject_HEAD_INIT(0, 0)
- "monotonic_align.core.array", /*tp_name*/
- sizeof(struct __pyx_array_obj), /*tp_basicsize*/
- 0, /*tp_itemsize*/
- __pyx_tp_dealloc_array, /*tp_dealloc*/
- #if PY_VERSION_HEX < 0x030800b4
- 0, /*tp_print*/
- #endif
- #if PY_VERSION_HEX >= 0x030800b4
- 0, /*tp_vectorcall_offset*/
- #endif
- 0, /*tp_getattr*/
- 0, /*tp_setattr*/
- #if PY_MAJOR_VERSION < 3
- 0, /*tp_compare*/
- #endif
- #if PY_MAJOR_VERSION >= 3
- 0, /*tp_as_async*/
- #endif
- 0, /*tp_repr*/
- 0, /*tp_as_number*/
- &__pyx_tp_as_sequence_array, /*tp_as_sequence*/
- &__pyx_tp_as_mapping_array, /*tp_as_mapping*/
- 0, /*tp_hash*/
- 0, /*tp_call*/
- 0, /*tp_str*/
- __pyx_tp_getattro_array, /*tp_getattro*/
- 0, /*tp_setattro*/
- &__pyx_tp_as_buffer_array, /*tp_as_buffer*/
- Py_TPFLAGS_DEFAULT|Py_TPFLAGS_HAVE_VERSION_TAG|Py_TPFLAGS_CHECKTYPES|Py_TPFLAGS_HAVE_NEWBUFFER|Py_TPFLAGS_BASETYPE, /*tp_flags*/
- 0, /*tp_doc*/
- 0, /*tp_traverse*/
- 0, /*tp_clear*/
- 0, /*tp_richcompare*/
- 0, /*tp_weaklistoffset*/
- 0, /*tp_iter*/
- 0, /*tp_iternext*/
- __pyx_methods_array, /*tp_methods*/
- 0, /*tp_members*/
- __pyx_getsets_array, /*tp_getset*/
- 0, /*tp_base*/
- 0, /*tp_dict*/
- 0, /*tp_descr_get*/
- 0, /*tp_descr_set*/
- 0, /*tp_dictoffset*/
- 0, /*tp_init*/
- 0, /*tp_alloc*/
- __pyx_tp_new_array, /*tp_new*/
- 0, /*tp_free*/
- 0, /*tp_is_gc*/
- 0, /*tp_bases*/
- 0, /*tp_mro*/
- 0, /*tp_cache*/
- 0, /*tp_subclasses*/
- 0, /*tp_weaklist*/
- 0, /*tp_del*/
- 0, /*tp_version_tag*/
- #if PY_VERSION_HEX >= 0x030400a1
- 0, /*tp_finalize*/
- #endif
- #if PY_VERSION_HEX >= 0x030800b1
- 0, /*tp_vectorcall*/
- #endif
- #if PY_VERSION_HEX >= 0x030800b4 && PY_VERSION_HEX < 0x03090000
- 0, /*tp_print*/
- #endif
-};
-
-static PyObject *__pyx_tp_new_Enum(PyTypeObject *t, CYTHON_UNUSED PyObject *a, CYTHON_UNUSED PyObject *k) {
- struct __pyx_MemviewEnum_obj *p;
- PyObject *o;
- if (likely((t->tp_flags & Py_TPFLAGS_IS_ABSTRACT) == 0)) {
- o = (*t->tp_alloc)(t, 0);
- } else {
- o = (PyObject *) PyBaseObject_Type.tp_new(t, __pyx_empty_tuple, 0);
- }
- if (unlikely(!o)) return 0;
- p = ((struct __pyx_MemviewEnum_obj *)o);
- p->name = Py_None; Py_INCREF(Py_None);
- return o;
-}
-
-static void __pyx_tp_dealloc_Enum(PyObject *o) {
- struct __pyx_MemviewEnum_obj *p = (struct __pyx_MemviewEnum_obj *)o;
- #if CYTHON_USE_TP_FINALIZE
- if (unlikely(PyType_HasFeature(Py_TYPE(o), Py_TPFLAGS_HAVE_FINALIZE) && Py_TYPE(o)->tp_finalize) && !_PyGC_FINALIZED(o)) {
- if (PyObject_CallFinalizerFromDealloc(o)) return;
- }
- #endif
- PyObject_GC_UnTrack(o);
- Py_CLEAR(p->name);
- (*Py_TYPE(o)->tp_free)(o);
-}
-
-static int __pyx_tp_traverse_Enum(PyObject *o, visitproc v, void *a) {
- int e;
- struct __pyx_MemviewEnum_obj *p = (struct __pyx_MemviewEnum_obj *)o;
- if (p->name) {
- e = (*v)(p->name, a); if (e) return e;
- }
- return 0;
-}
-
-static int __pyx_tp_clear_Enum(PyObject *o) {
- PyObject* tmp;
- struct __pyx_MemviewEnum_obj *p = (struct __pyx_MemviewEnum_obj *)o;
- tmp = ((PyObject*)p->name);
- p->name = Py_None; Py_INCREF(Py_None);
- Py_XDECREF(tmp);
- return 0;
-}
-
-static PyMethodDef __pyx_methods_Enum[] = {
- {"__reduce_cython__", (PyCFunction)__pyx_pw___pyx_MemviewEnum_1__reduce_cython__, METH_NOARGS, 0},
- {"__setstate_cython__", (PyCFunction)__pyx_pw___pyx_MemviewEnum_3__setstate_cython__, METH_O, 0},
- {0, 0, 0, 0}
-};
-
-static PyTypeObject __pyx_type___pyx_MemviewEnum = {
- PyVarObject_HEAD_INIT(0, 0)
- "monotonic_align.core.Enum", /*tp_name*/
- sizeof(struct __pyx_MemviewEnum_obj), /*tp_basicsize*/
- 0, /*tp_itemsize*/
- __pyx_tp_dealloc_Enum, /*tp_dealloc*/
- #if PY_VERSION_HEX < 0x030800b4
- 0, /*tp_print*/
- #endif
- #if PY_VERSION_HEX >= 0x030800b4
- 0, /*tp_vectorcall_offset*/
- #endif
- 0, /*tp_getattr*/
- 0, /*tp_setattr*/
- #if PY_MAJOR_VERSION < 3
- 0, /*tp_compare*/
- #endif
- #if PY_MAJOR_VERSION >= 3
- 0, /*tp_as_async*/
- #endif
- __pyx_MemviewEnum___repr__, /*tp_repr*/
- 0, /*tp_as_number*/
- 0, /*tp_as_sequence*/
- 0, /*tp_as_mapping*/
- 0, /*tp_hash*/
- 0, /*tp_call*/
- 0, /*tp_str*/
- 0, /*tp_getattro*/
- 0, /*tp_setattro*/
- 0, /*tp_as_buffer*/
- Py_TPFLAGS_DEFAULT|Py_TPFLAGS_HAVE_VERSION_TAG|Py_TPFLAGS_CHECKTYPES|Py_TPFLAGS_HAVE_NEWBUFFER|Py_TPFLAGS_BASETYPE|Py_TPFLAGS_HAVE_GC, /*tp_flags*/
- 0, /*tp_doc*/
- __pyx_tp_traverse_Enum, /*tp_traverse*/
- __pyx_tp_clear_Enum, /*tp_clear*/
- 0, /*tp_richcompare*/
- 0, /*tp_weaklistoffset*/
- 0, /*tp_iter*/
- 0, /*tp_iternext*/
- __pyx_methods_Enum, /*tp_methods*/
- 0, /*tp_members*/
- 0, /*tp_getset*/
- 0, /*tp_base*/
- 0, /*tp_dict*/
- 0, /*tp_descr_get*/
- 0, /*tp_descr_set*/
- 0, /*tp_dictoffset*/
- __pyx_MemviewEnum___init__, /*tp_init*/
- 0, /*tp_alloc*/
- __pyx_tp_new_Enum, /*tp_new*/
- 0, /*tp_free*/
- 0, /*tp_is_gc*/
- 0, /*tp_bases*/
- 0, /*tp_mro*/
- 0, /*tp_cache*/
- 0, /*tp_subclasses*/
- 0, /*tp_weaklist*/
- 0, /*tp_del*/
- 0, /*tp_version_tag*/
- #if PY_VERSION_HEX >= 0x030400a1
- 0, /*tp_finalize*/
- #endif
- #if PY_VERSION_HEX >= 0x030800b1
- 0, /*tp_vectorcall*/
- #endif
- #if PY_VERSION_HEX >= 0x030800b4 && PY_VERSION_HEX < 0x03090000
- 0, /*tp_print*/
- #endif
-};
-static struct __pyx_vtabstruct_memoryview __pyx_vtable_memoryview;
-
-static PyObject *__pyx_tp_new_memoryview(PyTypeObject *t, PyObject *a, PyObject *k) {
- struct __pyx_memoryview_obj *p;
- PyObject *o;
- if (likely((t->tp_flags & Py_TPFLAGS_IS_ABSTRACT) == 0)) {
- o = (*t->tp_alloc)(t, 0);
- } else {
- o = (PyObject *) PyBaseObject_Type.tp_new(t, __pyx_empty_tuple, 0);
- }
- if (unlikely(!o)) return 0;
- p = ((struct __pyx_memoryview_obj *)o);
- p->__pyx_vtab = __pyx_vtabptr_memoryview;
- p->obj = Py_None; Py_INCREF(Py_None);
- p->_size = Py_None; Py_INCREF(Py_None);
- p->_array_interface = Py_None; Py_INCREF(Py_None);
- p->view.obj = NULL;
- if (unlikely(__pyx_memoryview___cinit__(o, a, k) < 0)) goto bad;
- return o;
- bad:
- Py_DECREF(o); o = 0;
- return NULL;
-}
-
-static void __pyx_tp_dealloc_memoryview(PyObject *o) {
- struct __pyx_memoryview_obj *p = (struct __pyx_memoryview_obj *)o;
- #if CYTHON_USE_TP_FINALIZE
- if (unlikely(PyType_HasFeature(Py_TYPE(o), Py_TPFLAGS_HAVE_FINALIZE) && Py_TYPE(o)->tp_finalize) && !_PyGC_FINALIZED(o)) {
- if (PyObject_CallFinalizerFromDealloc(o)) return;
- }
- #endif
- PyObject_GC_UnTrack(o);
- {
- PyObject *etype, *eval, *etb;
- PyErr_Fetch(&etype, &eval, &etb);
- __Pyx_SET_REFCNT(o, Py_REFCNT(o) + 1);
- __pyx_memoryview___dealloc__(o);
- __Pyx_SET_REFCNT(o, Py_REFCNT(o) - 1);
- PyErr_Restore(etype, eval, etb);
- }
- Py_CLEAR(p->obj);
- Py_CLEAR(p->_size);
- Py_CLEAR(p->_array_interface);
- (*Py_TYPE(o)->tp_free)(o);
-}
-
-static int __pyx_tp_traverse_memoryview(PyObject *o, visitproc v, void *a) {
- int e;
- struct __pyx_memoryview_obj *p = (struct __pyx_memoryview_obj *)o;
- if (p->obj) {
- e = (*v)(p->obj, a); if (e) return e;
- }
- if (p->_size) {
- e = (*v)(p->_size, a); if (e) return e;
- }
- if (p->_array_interface) {
- e = (*v)(p->_array_interface, a); if (e) return e;
- }
- if (p->view.obj) {
- e = (*v)(p->view.obj, a); if (e) return e;
- }
- return 0;
-}
-
-static int __pyx_tp_clear_memoryview(PyObject *o) {
- PyObject* tmp;
- struct __pyx_memoryview_obj *p = (struct __pyx_memoryview_obj *)o;
- tmp = ((PyObject*)p->obj);
- p->obj = Py_None; Py_INCREF(Py_None);
- Py_XDECREF(tmp);
- tmp = ((PyObject*)p->_size);
- p->_size = Py_None; Py_INCREF(Py_None);
- Py_XDECREF(tmp);
- tmp = ((PyObject*)p->_array_interface);
- p->_array_interface = Py_None; Py_INCREF(Py_None);
- Py_XDECREF(tmp);
- Py_CLEAR(p->view.obj);
- return 0;
-}
-static PyObject *__pyx_sq_item_memoryview(PyObject *o, Py_ssize_t i) {
- PyObject *r;
- PyObject *x = PyInt_FromSsize_t(i); if(!x) return 0;
- r = Py_TYPE(o)->tp_as_mapping->mp_subscript(o, x);
- Py_DECREF(x);
- return r;
-}
-
-static int __pyx_mp_ass_subscript_memoryview(PyObject *o, PyObject *i, PyObject *v) {
- if (v) {
- return __pyx_memoryview___setitem__(o, i, v);
- }
- else {
- PyErr_Format(PyExc_NotImplementedError,
- "Subscript deletion not supported by %.200s", Py_TYPE(o)->tp_name);
- return -1;
- }
-}
-
-static PyObject *__pyx_getprop___pyx_memoryview_T(PyObject *o, CYTHON_UNUSED void *x) {
- return __pyx_pw_15View_dot_MemoryView_10memoryview_1T_1__get__(o);
-}
-
-static PyObject *__pyx_getprop___pyx_memoryview_base(PyObject *o, CYTHON_UNUSED void *x) {
- return __pyx_pw_15View_dot_MemoryView_10memoryview_4base_1__get__(o);
-}
-
-static PyObject *__pyx_getprop___pyx_memoryview_shape(PyObject *o, CYTHON_UNUSED void *x) {
- return __pyx_pw_15View_dot_MemoryView_10memoryview_5shape_1__get__(o);
-}
-
-static PyObject *__pyx_getprop___pyx_memoryview_strides(PyObject *o, CYTHON_UNUSED void *x) {
- return __pyx_pw_15View_dot_MemoryView_10memoryview_7strides_1__get__(o);
-}
-
-static PyObject *__pyx_getprop___pyx_memoryview_suboffsets(PyObject *o, CYTHON_UNUSED void *x) {
- return __pyx_pw_15View_dot_MemoryView_10memoryview_10suboffsets_1__get__(o);
-}
-
-static PyObject *__pyx_getprop___pyx_memoryview_ndim(PyObject *o, CYTHON_UNUSED void *x) {
- return __pyx_pw_15View_dot_MemoryView_10memoryview_4ndim_1__get__(o);
-}
-
-static PyObject *__pyx_getprop___pyx_memoryview_itemsize(PyObject *o, CYTHON_UNUSED void *x) {
- return __pyx_pw_15View_dot_MemoryView_10memoryview_8itemsize_1__get__(o);
-}
-
-static PyObject *__pyx_getprop___pyx_memoryview_nbytes(PyObject *o, CYTHON_UNUSED void *x) {
- return __pyx_pw_15View_dot_MemoryView_10memoryview_6nbytes_1__get__(o);
-}
-
-static PyObject *__pyx_getprop___pyx_memoryview_size(PyObject *o, CYTHON_UNUSED void *x) {
- return __pyx_pw_15View_dot_MemoryView_10memoryview_4size_1__get__(o);
-}
-
-static PyMethodDef __pyx_methods_memoryview[] = {
- {"is_c_contig", (PyCFunction)__pyx_memoryview_is_c_contig, METH_NOARGS, 0},
- {"is_f_contig", (PyCFunction)__pyx_memoryview_is_f_contig, METH_NOARGS, 0},
- {"copy", (PyCFunction)__pyx_memoryview_copy, METH_NOARGS, 0},
- {"copy_fortran", (PyCFunction)__pyx_memoryview_copy_fortran, METH_NOARGS, 0},
- {"__reduce_cython__", (PyCFunction)__pyx_pw___pyx_memoryview_1__reduce_cython__, METH_NOARGS, 0},
- {"__setstate_cython__", (PyCFunction)__pyx_pw___pyx_memoryview_3__setstate_cython__, METH_O, 0},
- {0, 0, 0, 0}
-};
-
-static struct PyGetSetDef __pyx_getsets_memoryview[] = {
- {(char *)"T", __pyx_getprop___pyx_memoryview_T, 0, (char *)0, 0},
- {(char *)"base", __pyx_getprop___pyx_memoryview_base, 0, (char *)0, 0},
- {(char *)"shape", __pyx_getprop___pyx_memoryview_shape, 0, (char *)0, 0},
- {(char *)"strides", __pyx_getprop___pyx_memoryview_strides, 0, (char *)0, 0},
- {(char *)"suboffsets", __pyx_getprop___pyx_memoryview_suboffsets, 0, (char *)0, 0},
- {(char *)"ndim", __pyx_getprop___pyx_memoryview_ndim, 0, (char *)0, 0},
- {(char *)"itemsize", __pyx_getprop___pyx_memoryview_itemsize, 0, (char *)0, 0},
- {(char *)"nbytes", __pyx_getprop___pyx_memoryview_nbytes, 0, (char *)0, 0},
- {(char *)"size", __pyx_getprop___pyx_memoryview_size, 0, (char *)0, 0},
- {0, 0, 0, 0, 0}
-};
-
-static PySequenceMethods __pyx_tp_as_sequence_memoryview = {
- __pyx_memoryview___len__, /*sq_length*/
- 0, /*sq_concat*/
- 0, /*sq_repeat*/
- __pyx_sq_item_memoryview, /*sq_item*/
- 0, /*sq_slice*/
- 0, /*sq_ass_item*/
- 0, /*sq_ass_slice*/
- 0, /*sq_contains*/
- 0, /*sq_inplace_concat*/
- 0, /*sq_inplace_repeat*/
-};
-
-static PyMappingMethods __pyx_tp_as_mapping_memoryview = {
- __pyx_memoryview___len__, /*mp_length*/
- __pyx_memoryview___getitem__, /*mp_subscript*/
- __pyx_mp_ass_subscript_memoryview, /*mp_ass_subscript*/
-};
-
-static PyBufferProcs __pyx_tp_as_buffer_memoryview = {
- #if PY_MAJOR_VERSION < 3
- 0, /*bf_getreadbuffer*/
- #endif
- #if PY_MAJOR_VERSION < 3
- 0, /*bf_getwritebuffer*/
- #endif
- #if PY_MAJOR_VERSION < 3
- 0, /*bf_getsegcount*/
- #endif
- #if PY_MAJOR_VERSION < 3
- 0, /*bf_getcharbuffer*/
- #endif
- __pyx_memoryview_getbuffer, /*bf_getbuffer*/
- 0, /*bf_releasebuffer*/
-};
-
-static PyTypeObject __pyx_type___pyx_memoryview = {
- PyVarObject_HEAD_INIT(0, 0)
- "monotonic_align.core.memoryview", /*tp_name*/
- sizeof(struct __pyx_memoryview_obj), /*tp_basicsize*/
- 0, /*tp_itemsize*/
- __pyx_tp_dealloc_memoryview, /*tp_dealloc*/
- #if PY_VERSION_HEX < 0x030800b4
- 0, /*tp_print*/
- #endif
- #if PY_VERSION_HEX >= 0x030800b4
- 0, /*tp_vectorcall_offset*/
- #endif
- 0, /*tp_getattr*/
- 0, /*tp_setattr*/
- #if PY_MAJOR_VERSION < 3
- 0, /*tp_compare*/
- #endif
- #if PY_MAJOR_VERSION >= 3
- 0, /*tp_as_async*/
- #endif
- __pyx_memoryview___repr__, /*tp_repr*/
- 0, /*tp_as_number*/
- &__pyx_tp_as_sequence_memoryview, /*tp_as_sequence*/
- &__pyx_tp_as_mapping_memoryview, /*tp_as_mapping*/
- 0, /*tp_hash*/
- 0, /*tp_call*/
- __pyx_memoryview___str__, /*tp_str*/
- 0, /*tp_getattro*/
- 0, /*tp_setattro*/
- &__pyx_tp_as_buffer_memoryview, /*tp_as_buffer*/
- Py_TPFLAGS_DEFAULT|Py_TPFLAGS_HAVE_VERSION_TAG|Py_TPFLAGS_CHECKTYPES|Py_TPFLAGS_HAVE_NEWBUFFER|Py_TPFLAGS_BASETYPE|Py_TPFLAGS_HAVE_GC, /*tp_flags*/
- 0, /*tp_doc*/
- __pyx_tp_traverse_memoryview, /*tp_traverse*/
- __pyx_tp_clear_memoryview, /*tp_clear*/
- 0, /*tp_richcompare*/
- 0, /*tp_weaklistoffset*/
- 0, /*tp_iter*/
- 0, /*tp_iternext*/
- __pyx_methods_memoryview, /*tp_methods*/
- 0, /*tp_members*/
- __pyx_getsets_memoryview, /*tp_getset*/
- 0, /*tp_base*/
- 0, /*tp_dict*/
- 0, /*tp_descr_get*/
- 0, /*tp_descr_set*/
- 0, /*tp_dictoffset*/
- 0, /*tp_init*/
- 0, /*tp_alloc*/
- __pyx_tp_new_memoryview, /*tp_new*/
- 0, /*tp_free*/
- 0, /*tp_is_gc*/
- 0, /*tp_bases*/
- 0, /*tp_mro*/
- 0, /*tp_cache*/
- 0, /*tp_subclasses*/
- 0, /*tp_weaklist*/
- 0, /*tp_del*/
- 0, /*tp_version_tag*/
- #if PY_VERSION_HEX >= 0x030400a1
- 0, /*tp_finalize*/
- #endif
- #if PY_VERSION_HEX >= 0x030800b1
- 0, /*tp_vectorcall*/
- #endif
- #if PY_VERSION_HEX >= 0x030800b4 && PY_VERSION_HEX < 0x03090000
- 0, /*tp_print*/
- #endif
-};
-static struct __pyx_vtabstruct__memoryviewslice __pyx_vtable__memoryviewslice;
-
-static PyObject *__pyx_tp_new__memoryviewslice(PyTypeObject *t, PyObject *a, PyObject *k) {
- struct __pyx_memoryviewslice_obj *p;
- PyObject *o = __pyx_tp_new_memoryview(t, a, k);
- if (unlikely(!o)) return 0;
- p = ((struct __pyx_memoryviewslice_obj *)o);
- p->__pyx_base.__pyx_vtab = (struct __pyx_vtabstruct_memoryview*)__pyx_vtabptr__memoryviewslice;
- p->from_object = Py_None; Py_INCREF(Py_None);
- p->from_slice.memview = NULL;
- return o;
-}
-
-static void __pyx_tp_dealloc__memoryviewslice(PyObject *o) {
- struct __pyx_memoryviewslice_obj *p = (struct __pyx_memoryviewslice_obj *)o;
- #if CYTHON_USE_TP_FINALIZE
- if (unlikely(PyType_HasFeature(Py_TYPE(o), Py_TPFLAGS_HAVE_FINALIZE) && Py_TYPE(o)->tp_finalize) && !_PyGC_FINALIZED(o)) {
- if (PyObject_CallFinalizerFromDealloc(o)) return;
- }
- #endif
- PyObject_GC_UnTrack(o);
- {
- PyObject *etype, *eval, *etb;
- PyErr_Fetch(&etype, &eval, &etb);
- __Pyx_SET_REFCNT(o, Py_REFCNT(o) + 1);
- __pyx_memoryviewslice___dealloc__(o);
- __Pyx_SET_REFCNT(o, Py_REFCNT(o) - 1);
- PyErr_Restore(etype, eval, etb);
- }
- Py_CLEAR(p->from_object);
- PyObject_GC_Track(o);
- __pyx_tp_dealloc_memoryview(o);
-}
-
-static int __pyx_tp_traverse__memoryviewslice(PyObject *o, visitproc v, void *a) {
- int e;
- struct __pyx_memoryviewslice_obj *p = (struct __pyx_memoryviewslice_obj *)o;
- e = __pyx_tp_traverse_memoryview(o, v, a); if (e) return e;
- if (p->from_object) {
- e = (*v)(p->from_object, a); if (e) return e;
- }
- return 0;
-}
-
-static int __pyx_tp_clear__memoryviewslice(PyObject *o) {
- PyObject* tmp;
- struct __pyx_memoryviewslice_obj *p = (struct __pyx_memoryviewslice_obj *)o;
- __pyx_tp_clear_memoryview(o);
- tmp = ((PyObject*)p->from_object);
- p->from_object = Py_None; Py_INCREF(Py_None);
- Py_XDECREF(tmp);
- __PYX_XDEC_MEMVIEW(&p->from_slice, 1);
- return 0;
-}
-
-static PyObject *__pyx_getprop___pyx_memoryviewslice_base(PyObject *o, CYTHON_UNUSED void *x) {
- return __pyx_pw_15View_dot_MemoryView_16_memoryviewslice_4base_1__get__(o);
-}
-
-static PyMethodDef __pyx_methods__memoryviewslice[] = {
- {"__reduce_cython__", (PyCFunction)__pyx_pw___pyx_memoryviewslice_1__reduce_cython__, METH_NOARGS, 0},
- {"__setstate_cython__", (PyCFunction)__pyx_pw___pyx_memoryviewslice_3__setstate_cython__, METH_O, 0},
- {0, 0, 0, 0}
-};
-
-static struct PyGetSetDef __pyx_getsets__memoryviewslice[] = {
- {(char *)"base", __pyx_getprop___pyx_memoryviewslice_base, 0, (char *)0, 0},
- {0, 0, 0, 0, 0}
-};
-
-static PyTypeObject __pyx_type___pyx_memoryviewslice = {
- PyVarObject_HEAD_INIT(0, 0)
- "monotonic_align.core._memoryviewslice", /*tp_name*/
- sizeof(struct __pyx_memoryviewslice_obj), /*tp_basicsize*/
- 0, /*tp_itemsize*/
- __pyx_tp_dealloc__memoryviewslice, /*tp_dealloc*/
- #if PY_VERSION_HEX < 0x030800b4
- 0, /*tp_print*/
- #endif
- #if PY_VERSION_HEX >= 0x030800b4
- 0, /*tp_vectorcall_offset*/
- #endif
- 0, /*tp_getattr*/
- 0, /*tp_setattr*/
- #if PY_MAJOR_VERSION < 3
- 0, /*tp_compare*/
- #endif
- #if PY_MAJOR_VERSION >= 3
- 0, /*tp_as_async*/
- #endif
- #if CYTHON_COMPILING_IN_PYPY
- __pyx_memoryview___repr__, /*tp_repr*/
- #else
- 0, /*tp_repr*/
- #endif
- 0, /*tp_as_number*/
- 0, /*tp_as_sequence*/
- 0, /*tp_as_mapping*/
- 0, /*tp_hash*/
- 0, /*tp_call*/
- #if CYTHON_COMPILING_IN_PYPY
- __pyx_memoryview___str__, /*tp_str*/
- #else
- 0, /*tp_str*/
- #endif
- 0, /*tp_getattro*/
- 0, /*tp_setattro*/
- 0, /*tp_as_buffer*/
- Py_TPFLAGS_DEFAULT|Py_TPFLAGS_HAVE_VERSION_TAG|Py_TPFLAGS_CHECKTYPES|Py_TPFLAGS_HAVE_NEWBUFFER|Py_TPFLAGS_BASETYPE|Py_TPFLAGS_HAVE_GC, /*tp_flags*/
- "Internal class for passing memoryview slices to Python", /*tp_doc*/
- __pyx_tp_traverse__memoryviewslice, /*tp_traverse*/
- __pyx_tp_clear__memoryviewslice, /*tp_clear*/
- 0, /*tp_richcompare*/
- 0, /*tp_weaklistoffset*/
- 0, /*tp_iter*/
- 0, /*tp_iternext*/
- __pyx_methods__memoryviewslice, /*tp_methods*/
- 0, /*tp_members*/
- __pyx_getsets__memoryviewslice, /*tp_getset*/
- 0, /*tp_base*/
- 0, /*tp_dict*/
- 0, /*tp_descr_get*/
- 0, /*tp_descr_set*/
- 0, /*tp_dictoffset*/
- 0, /*tp_init*/
- 0, /*tp_alloc*/
- __pyx_tp_new__memoryviewslice, /*tp_new*/
- 0, /*tp_free*/
- 0, /*tp_is_gc*/
- 0, /*tp_bases*/
- 0, /*tp_mro*/
- 0, /*tp_cache*/
- 0, /*tp_subclasses*/
- 0, /*tp_weaklist*/
- 0, /*tp_del*/
- 0, /*tp_version_tag*/
- #if PY_VERSION_HEX >= 0x030400a1
- 0, /*tp_finalize*/
- #endif
- #if PY_VERSION_HEX >= 0x030800b1
- 0, /*tp_vectorcall*/
- #endif
- #if PY_VERSION_HEX >= 0x030800b4 && PY_VERSION_HEX < 0x03090000
- 0, /*tp_print*/
- #endif
-};
-
-static PyMethodDef __pyx_methods[] = {
- {"maximum_path_c", (PyCFunction)(void*)(PyCFunctionWithKeywords)__pyx_pw_15monotonic_align_4core_1maximum_path_c, METH_VARARGS|METH_KEYWORDS, 0},
- {0, 0, 0, 0}
-};
-
-#if PY_MAJOR_VERSION >= 3
-#if CYTHON_PEP489_MULTI_PHASE_INIT
-static PyObject* __pyx_pymod_create(PyObject *spec, PyModuleDef *def); /*proto*/
-static int __pyx_pymod_exec_core(PyObject* module); /*proto*/
-static PyModuleDef_Slot __pyx_moduledef_slots[] = {
- {Py_mod_create, (void*)__pyx_pymod_create},
- {Py_mod_exec, (void*)__pyx_pymod_exec_core},
- {0, NULL}
-};
-#endif
-
-static struct PyModuleDef __pyx_moduledef = {
- PyModuleDef_HEAD_INIT,
- "core",
- 0, /* m_doc */
- #if CYTHON_PEP489_MULTI_PHASE_INIT
- 0, /* m_size */
- #else
- -1, /* m_size */
- #endif
- __pyx_methods /* m_methods */,
- #if CYTHON_PEP489_MULTI_PHASE_INIT
- __pyx_moduledef_slots, /* m_slots */
- #else
- NULL, /* m_reload */
- #endif
- NULL, /* m_traverse */
- NULL, /* m_clear */
- NULL /* m_free */
-};
-#endif
-#ifndef CYTHON_SMALL_CODE
-#if defined(__clang__)
- #define CYTHON_SMALL_CODE
-#elif defined(__GNUC__) && (__GNUC__ > 4 || (__GNUC__ == 4 && __GNUC_MINOR__ >= 3))
- #define CYTHON_SMALL_CODE __attribute__((cold))
-#else
- #define CYTHON_SMALL_CODE
-#endif
-#endif
-
-static __Pyx_StringTabEntry __pyx_string_tab[] = {
- {&__pyx_n_s_ASCII, __pyx_k_ASCII, sizeof(__pyx_k_ASCII), 0, 0, 1, 1},
- {&__pyx_kp_s_Buffer_view_does_not_expose_stri, __pyx_k_Buffer_view_does_not_expose_stri, sizeof(__pyx_k_Buffer_view_does_not_expose_stri), 0, 0, 1, 0},
- {&__pyx_kp_s_Can_only_create_a_buffer_that_is, __pyx_k_Can_only_create_a_buffer_that_is, sizeof(__pyx_k_Can_only_create_a_buffer_that_is), 0, 0, 1, 0},
- {&__pyx_kp_s_Cannot_assign_to_read_only_memor, __pyx_k_Cannot_assign_to_read_only_memor, sizeof(__pyx_k_Cannot_assign_to_read_only_memor), 0, 0, 1, 0},
- {&__pyx_kp_s_Cannot_create_writable_memory_vi, __pyx_k_Cannot_create_writable_memory_vi, sizeof(__pyx_k_Cannot_create_writable_memory_vi), 0, 0, 1, 0},
- {&__pyx_kp_s_Cannot_index_with_type_s, __pyx_k_Cannot_index_with_type_s, sizeof(__pyx_k_Cannot_index_with_type_s), 0, 0, 1, 0},
- {&__pyx_n_s_Ellipsis, __pyx_k_Ellipsis, sizeof(__pyx_k_Ellipsis), 0, 0, 1, 1},
- {&__pyx_kp_s_Empty_shape_tuple_for_cython_arr, __pyx_k_Empty_shape_tuple_for_cython_arr, sizeof(__pyx_k_Empty_shape_tuple_for_cython_arr), 0, 0, 1, 0},
- {&__pyx_kp_s_Incompatible_checksums_s_vs_0xb0, __pyx_k_Incompatible_checksums_s_vs_0xb0, sizeof(__pyx_k_Incompatible_checksums_s_vs_0xb0), 0, 0, 1, 0},
- {&__pyx_n_s_IndexError, __pyx_k_IndexError, sizeof(__pyx_k_IndexError), 0, 0, 1, 1},
- {&__pyx_kp_s_Indirect_dimensions_not_supporte, __pyx_k_Indirect_dimensions_not_supporte, sizeof(__pyx_k_Indirect_dimensions_not_supporte), 0, 0, 1, 0},
- {&__pyx_kp_s_Invalid_mode_expected_c_or_fortr, __pyx_k_Invalid_mode_expected_c_or_fortr, sizeof(__pyx_k_Invalid_mode_expected_c_or_fortr), 0, 0, 1, 0},
- {&__pyx_kp_s_Invalid_shape_in_axis_d_d, __pyx_k_Invalid_shape_in_axis_d_d, sizeof(__pyx_k_Invalid_shape_in_axis_d_d), 0, 0, 1, 0},
- {&__pyx_n_s_MemoryError, __pyx_k_MemoryError, sizeof(__pyx_k_MemoryError), 0, 0, 1, 1},
- {&__pyx_kp_s_MemoryView_of_r_at_0x_x, __pyx_k_MemoryView_of_r_at_0x_x, sizeof(__pyx_k_MemoryView_of_r_at_0x_x), 0, 0, 1, 0},
- {&__pyx_kp_s_MemoryView_of_r_object, __pyx_k_MemoryView_of_r_object, sizeof(__pyx_k_MemoryView_of_r_object), 0, 0, 1, 0},
- {&__pyx_n_b_O, __pyx_k_O, sizeof(__pyx_k_O), 0, 0, 0, 1},
- {&__pyx_kp_s_Out_of_bounds_on_buffer_access_a, __pyx_k_Out_of_bounds_on_buffer_access_a, sizeof(__pyx_k_Out_of_bounds_on_buffer_access_a), 0, 0, 1, 0},
- {&__pyx_n_s_PickleError, __pyx_k_PickleError, sizeof(__pyx_k_PickleError), 0, 0, 1, 1},
- {&__pyx_n_s_TypeError, __pyx_k_TypeError, sizeof(__pyx_k_TypeError), 0, 0, 1, 1},
- {&__pyx_kp_s_Unable_to_convert_item_to_object, __pyx_k_Unable_to_convert_item_to_object, sizeof(__pyx_k_Unable_to_convert_item_to_object), 0, 0, 1, 0},
- {&__pyx_n_s_ValueError, __pyx_k_ValueError, sizeof(__pyx_k_ValueError), 0, 0, 1, 1},
- {&__pyx_n_s_View_MemoryView, __pyx_k_View_MemoryView, sizeof(__pyx_k_View_MemoryView), 0, 0, 1, 1},
- {&__pyx_n_s_allocate_buffer, __pyx_k_allocate_buffer, sizeof(__pyx_k_allocate_buffer), 0, 0, 1, 1},
- {&__pyx_n_s_base, __pyx_k_base, sizeof(__pyx_k_base), 0, 0, 1, 1},
- {&__pyx_n_s_c, __pyx_k_c, sizeof(__pyx_k_c), 0, 0, 1, 1},
- {&__pyx_n_u_c, __pyx_k_c, sizeof(__pyx_k_c), 0, 1, 0, 1},
- {&__pyx_n_s_class, __pyx_k_class, sizeof(__pyx_k_class), 0, 0, 1, 1},
- {&__pyx_n_s_cline_in_traceback, __pyx_k_cline_in_traceback, sizeof(__pyx_k_cline_in_traceback), 0, 0, 1, 1},
- {&__pyx_kp_s_contiguous_and_direct, __pyx_k_contiguous_and_direct, sizeof(__pyx_k_contiguous_and_direct), 0, 0, 1, 0},
- {&__pyx_kp_s_contiguous_and_indirect, __pyx_k_contiguous_and_indirect, sizeof(__pyx_k_contiguous_and_indirect), 0, 0, 1, 0},
- {&__pyx_n_s_dict, __pyx_k_dict, sizeof(__pyx_k_dict), 0, 0, 1, 1},
- {&__pyx_n_s_dtype_is_object, __pyx_k_dtype_is_object, sizeof(__pyx_k_dtype_is_object), 0, 0, 1, 1},
- {&__pyx_n_s_encode, __pyx_k_encode, sizeof(__pyx_k_encode), 0, 0, 1, 1},
- {&__pyx_n_s_enumerate, __pyx_k_enumerate, sizeof(__pyx_k_enumerate), 0, 0, 1, 1},
- {&__pyx_n_s_error, __pyx_k_error, sizeof(__pyx_k_error), 0, 0, 1, 1},
- {&__pyx_n_s_flags, __pyx_k_flags, sizeof(__pyx_k_flags), 0, 0, 1, 1},
- {&__pyx_n_s_format, __pyx_k_format, sizeof(__pyx_k_format), 0, 0, 1, 1},
- {&__pyx_n_s_fortran, __pyx_k_fortran, sizeof(__pyx_k_fortran), 0, 0, 1, 1},
- {&__pyx_n_u_fortran, __pyx_k_fortran, sizeof(__pyx_k_fortran), 0, 1, 0, 1},
- {&__pyx_n_s_getstate, __pyx_k_getstate, sizeof(__pyx_k_getstate), 0, 0, 1, 1},
- {&__pyx_kp_s_got_differing_extents_in_dimensi, __pyx_k_got_differing_extents_in_dimensi, sizeof(__pyx_k_got_differing_extents_in_dimensi), 0, 0, 1, 0},
- {&__pyx_n_s_id, __pyx_k_id, sizeof(__pyx_k_id), 0, 0, 1, 1},
- {&__pyx_n_s_import, __pyx_k_import, sizeof(__pyx_k_import), 0, 0, 1, 1},
- {&__pyx_n_s_itemsize, __pyx_k_itemsize, sizeof(__pyx_k_itemsize), 0, 0, 1, 1},
- {&__pyx_kp_s_itemsize_0_for_cython_array, __pyx_k_itemsize_0_for_cython_array, sizeof(__pyx_k_itemsize_0_for_cython_array), 0, 0, 1, 0},
- {&__pyx_n_s_main, __pyx_k_main, sizeof(__pyx_k_main), 0, 0, 1, 1},
- {&__pyx_n_s_memview, __pyx_k_memview, sizeof(__pyx_k_memview), 0, 0, 1, 1},
- {&__pyx_n_s_mode, __pyx_k_mode, sizeof(__pyx_k_mode), 0, 0, 1, 1},
- {&__pyx_n_s_name, __pyx_k_name, sizeof(__pyx_k_name), 0, 0, 1, 1},
- {&__pyx_n_s_name_2, __pyx_k_name_2, sizeof(__pyx_k_name_2), 0, 0, 1, 1},
- {&__pyx_n_s_ndim, __pyx_k_ndim, sizeof(__pyx_k_ndim), 0, 0, 1, 1},
- {&__pyx_n_s_new, __pyx_k_new, sizeof(__pyx_k_new), 0, 0, 1, 1},
- {&__pyx_kp_s_no_default___reduce___due_to_non, __pyx_k_no_default___reduce___due_to_non, sizeof(__pyx_k_no_default___reduce___due_to_non), 0, 0, 1, 0},
- {&__pyx_n_s_obj, __pyx_k_obj, sizeof(__pyx_k_obj), 0, 0, 1, 1},
- {&__pyx_n_s_pack, __pyx_k_pack, sizeof(__pyx_k_pack), 0, 0, 1, 1},
- {&__pyx_n_s_paths, __pyx_k_paths, sizeof(__pyx_k_paths), 0, 0, 1, 1},
- {&__pyx_n_s_pickle, __pyx_k_pickle, sizeof(__pyx_k_pickle), 0, 0, 1, 1},
- {&__pyx_n_s_pyx_PickleError, __pyx_k_pyx_PickleError, sizeof(__pyx_k_pyx_PickleError), 0, 0, 1, 1},
- {&__pyx_n_s_pyx_checksum, __pyx_k_pyx_checksum, sizeof(__pyx_k_pyx_checksum), 0, 0, 1, 1},
- {&__pyx_n_s_pyx_getbuffer, __pyx_k_pyx_getbuffer, sizeof(__pyx_k_pyx_getbuffer), 0, 0, 1, 1},
- {&__pyx_n_s_pyx_result, __pyx_k_pyx_result, sizeof(__pyx_k_pyx_result), 0, 0, 1, 1},
- {&__pyx_n_s_pyx_state, __pyx_k_pyx_state, sizeof(__pyx_k_pyx_state), 0, 0, 1, 1},
- {&__pyx_n_s_pyx_type, __pyx_k_pyx_type, sizeof(__pyx_k_pyx_type), 0, 0, 1, 1},
- {&__pyx_n_s_pyx_unpickle_Enum, __pyx_k_pyx_unpickle_Enum, sizeof(__pyx_k_pyx_unpickle_Enum), 0, 0, 1, 1},
- {&__pyx_n_s_pyx_vtable, __pyx_k_pyx_vtable, sizeof(__pyx_k_pyx_vtable), 0, 0, 1, 1},
- {&__pyx_n_s_range, __pyx_k_range, sizeof(__pyx_k_range), 0, 0, 1, 1},
- {&__pyx_n_s_reduce, __pyx_k_reduce, sizeof(__pyx_k_reduce), 0, 0, 1, 1},
- {&__pyx_n_s_reduce_cython, __pyx_k_reduce_cython, sizeof(__pyx_k_reduce_cython), 0, 0, 1, 1},
- {&__pyx_n_s_reduce_ex, __pyx_k_reduce_ex, sizeof(__pyx_k_reduce_ex), 0, 0, 1, 1},
- {&__pyx_n_s_setstate, __pyx_k_setstate, sizeof(__pyx_k_setstate), 0, 0, 1, 1},
- {&__pyx_n_s_setstate_cython, __pyx_k_setstate_cython, sizeof(__pyx_k_setstate_cython), 0, 0, 1, 1},
- {&__pyx_n_s_shape, __pyx_k_shape, sizeof(__pyx_k_shape), 0, 0, 1, 1},
- {&__pyx_n_s_size, __pyx_k_size, sizeof(__pyx_k_size), 0, 0, 1, 1},
- {&__pyx_n_s_start, __pyx_k_start, sizeof(__pyx_k_start), 0, 0, 1, 1},
- {&__pyx_n_s_step, __pyx_k_step, sizeof(__pyx_k_step), 0, 0, 1, 1},
- {&__pyx_n_s_stop, __pyx_k_stop, sizeof(__pyx_k_stop), 0, 0, 1, 1},
- {&__pyx_kp_s_strided_and_direct, __pyx_k_strided_and_direct, sizeof(__pyx_k_strided_and_direct), 0, 0, 1, 0},
- {&__pyx_kp_s_strided_and_direct_or_indirect, __pyx_k_strided_and_direct_or_indirect, sizeof(__pyx_k_strided_and_direct_or_indirect), 0, 0, 1, 0},
- {&__pyx_kp_s_strided_and_indirect, __pyx_k_strided_and_indirect, sizeof(__pyx_k_strided_and_indirect), 0, 0, 1, 0},
- {&__pyx_kp_s_stringsource, __pyx_k_stringsource, sizeof(__pyx_k_stringsource), 0, 0, 1, 0},
- {&__pyx_n_s_struct, __pyx_k_struct, sizeof(__pyx_k_struct), 0, 0, 1, 1},
- {&__pyx_n_s_t_xs, __pyx_k_t_xs, sizeof(__pyx_k_t_xs), 0, 0, 1, 1},
- {&__pyx_n_s_t_ys, __pyx_k_t_ys, sizeof(__pyx_k_t_ys), 0, 0, 1, 1},
- {&__pyx_n_s_test, __pyx_k_test, sizeof(__pyx_k_test), 0, 0, 1, 1},
- {&__pyx_kp_s_unable_to_allocate_array_data, __pyx_k_unable_to_allocate_array_data, sizeof(__pyx_k_unable_to_allocate_array_data), 0, 0, 1, 0},
- {&__pyx_kp_s_unable_to_allocate_shape_and_str, __pyx_k_unable_to_allocate_shape_and_str, sizeof(__pyx_k_unable_to_allocate_shape_and_str), 0, 0, 1, 0},
- {&__pyx_n_s_unpack, __pyx_k_unpack, sizeof(__pyx_k_unpack), 0, 0, 1, 1},
- {&__pyx_n_s_update, __pyx_k_update, sizeof(__pyx_k_update), 0, 0, 1, 1},
- {&__pyx_n_s_values, __pyx_k_values, sizeof(__pyx_k_values), 0, 0, 1, 1},
- {0, 0, 0, 0, 0, 0, 0}
-};
-static CYTHON_SMALL_CODE int __Pyx_InitCachedBuiltins(void) {
- __pyx_builtin_range = __Pyx_GetBuiltinName(__pyx_n_s_range); if (!__pyx_builtin_range) __PYX_ERR(0, 15, __pyx_L1_error)
- __pyx_builtin_ValueError = __Pyx_GetBuiltinName(__pyx_n_s_ValueError); if (!__pyx_builtin_ValueError) __PYX_ERR(1, 133, __pyx_L1_error)
- __pyx_builtin_MemoryError = __Pyx_GetBuiltinName(__pyx_n_s_MemoryError); if (!__pyx_builtin_MemoryError) __PYX_ERR(1, 148, __pyx_L1_error)
- __pyx_builtin_enumerate = __Pyx_GetBuiltinName(__pyx_n_s_enumerate); if (!__pyx_builtin_enumerate) __PYX_ERR(1, 151, __pyx_L1_error)
- __pyx_builtin_TypeError = __Pyx_GetBuiltinName(__pyx_n_s_TypeError); if (!__pyx_builtin_TypeError) __PYX_ERR(1, 2, __pyx_L1_error)
- __pyx_builtin_Ellipsis = __Pyx_GetBuiltinName(__pyx_n_s_Ellipsis); if (!__pyx_builtin_Ellipsis) __PYX_ERR(1, 404, __pyx_L1_error)
- __pyx_builtin_id = __Pyx_GetBuiltinName(__pyx_n_s_id); if (!__pyx_builtin_id) __PYX_ERR(1, 613, __pyx_L1_error)
- __pyx_builtin_IndexError = __Pyx_GetBuiltinName(__pyx_n_s_IndexError); if (!__pyx_builtin_IndexError) __PYX_ERR(1, 832, __pyx_L1_error)
- return 0;
- __pyx_L1_error:;
- return -1;
-}
-
-static CYTHON_SMALL_CODE int __Pyx_InitCachedConstants(void) {
- __Pyx_RefNannyDeclarations
- __Pyx_RefNannySetupContext("__Pyx_InitCachedConstants", 0);
-
- /* "View.MemoryView":133
- *
- * if not self.ndim:
- * raise ValueError("Empty shape tuple for cython.array") # <<<<<<<<<<<<<<
- *
- * if itemsize <= 0:
- */
- __pyx_tuple__2 = PyTuple_Pack(1, __pyx_kp_s_Empty_shape_tuple_for_cython_arr); if (unlikely(!__pyx_tuple__2)) __PYX_ERR(1, 133, __pyx_L1_error)
- __Pyx_GOTREF(__pyx_tuple__2);
- __Pyx_GIVEREF(__pyx_tuple__2);
-
- /* "View.MemoryView":136
- *
- * if itemsize <= 0:
- * raise ValueError("itemsize <= 0 for cython.array") # <<<<<<<<<<<<<<
- *
- * if not isinstance(format, bytes):
- */
- __pyx_tuple__3 = PyTuple_Pack(1, __pyx_kp_s_itemsize_0_for_cython_array); if (unlikely(!__pyx_tuple__3)) __PYX_ERR(1, 136, __pyx_L1_error)
- __Pyx_GOTREF(__pyx_tuple__3);
- __Pyx_GIVEREF(__pyx_tuple__3);
-
- /* "View.MemoryView":148
- *
- * if not self._shape:
- * raise MemoryError("unable to allocate shape and strides.") # <<<<<<<<<<<<<<
- *
- *
- */
- __pyx_tuple__4 = PyTuple_Pack(1, __pyx_kp_s_unable_to_allocate_shape_and_str); if (unlikely(!__pyx_tuple__4)) __PYX_ERR(1, 148, __pyx_L1_error)
- __Pyx_GOTREF(__pyx_tuple__4);
- __Pyx_GIVEREF(__pyx_tuple__4);
-
- /* "View.MemoryView":176
- * self.data = malloc(self.len)
- * if not self.data:
- * raise MemoryError("unable to allocate array data.") # <<<<<<<<<<<<<<
- *
- * if self.dtype_is_object:
- */
- __pyx_tuple__5 = PyTuple_Pack(1, __pyx_kp_s_unable_to_allocate_array_data); if (unlikely(!__pyx_tuple__5)) __PYX_ERR(1, 176, __pyx_L1_error)
- __Pyx_GOTREF(__pyx_tuple__5);
- __Pyx_GIVEREF(__pyx_tuple__5);
-
- /* "View.MemoryView":192
- * bufmode = PyBUF_F_CONTIGUOUS | PyBUF_ANY_CONTIGUOUS
- * if not (flags & bufmode):
- * raise ValueError("Can only create a buffer that is contiguous in memory.") # <<<<<<<<<<<<<<
- * info.buf = self.data
- * info.len = self.len
- */
- __pyx_tuple__6 = PyTuple_Pack(1, __pyx_kp_s_Can_only_create_a_buffer_that_is); if (unlikely(!__pyx_tuple__6)) __PYX_ERR(1, 192, __pyx_L1_error)
- __Pyx_GOTREF(__pyx_tuple__6);
- __Pyx_GIVEREF(__pyx_tuple__6);
-
- /* "(tree fragment)":2
- * def __reduce_cython__(self):
- * raise TypeError("no default __reduce__ due to non-trivial __cinit__") # <<<<<<<<<<<<<<
- * def __setstate_cython__(self, __pyx_state):
- * raise TypeError("no default __reduce__ due to non-trivial __cinit__")
- */
- __pyx_tuple__7 = PyTuple_Pack(1, __pyx_kp_s_no_default___reduce___due_to_non); if (unlikely(!__pyx_tuple__7)) __PYX_ERR(1, 2, __pyx_L1_error)
- __Pyx_GOTREF(__pyx_tuple__7);
- __Pyx_GIVEREF(__pyx_tuple__7);
-
- /* "(tree fragment)":4
- * raise TypeError("no default __reduce__ due to non-trivial __cinit__")
- * def __setstate_cython__(self, __pyx_state):
- * raise TypeError("no default __reduce__ due to non-trivial __cinit__") # <<<<<<<<<<<<<<
- */
- __pyx_tuple__8 = PyTuple_Pack(1, __pyx_kp_s_no_default___reduce___due_to_non); if (unlikely(!__pyx_tuple__8)) __PYX_ERR(1, 4, __pyx_L1_error)
- __Pyx_GOTREF(__pyx_tuple__8);
- __Pyx_GIVEREF(__pyx_tuple__8);
-
- /* "View.MemoryView":418
- * def __setitem__(memoryview self, object index, object value):
- * if self.view.readonly:
- * raise TypeError("Cannot assign to read-only memoryview") # <<<<<<<<<<<<<<
- *
- * have_slices, index = _unellipsify(index, self.view.ndim)
- */
- __pyx_tuple__9 = PyTuple_Pack(1, __pyx_kp_s_Cannot_assign_to_read_only_memor); if (unlikely(!__pyx_tuple__9)) __PYX_ERR(1, 418, __pyx_L1_error)
- __Pyx_GOTREF(__pyx_tuple__9);
- __Pyx_GIVEREF(__pyx_tuple__9);
-
- /* "View.MemoryView":495
- * result = struct.unpack(self.view.format, bytesitem)
- * except struct.error:
- * raise ValueError("Unable to convert item to object") # <<<<<<<<<<<<<<
- * else:
- * if len(self.view.format) == 1:
- */
- __pyx_tuple__10 = PyTuple_Pack(1, __pyx_kp_s_Unable_to_convert_item_to_object); if (unlikely(!__pyx_tuple__10)) __PYX_ERR(1, 495, __pyx_L1_error)
- __Pyx_GOTREF(__pyx_tuple__10);
- __Pyx_GIVEREF(__pyx_tuple__10);
-
- /* "View.MemoryView":520
- * def __getbuffer__(self, Py_buffer *info, int flags):
- * if flags & PyBUF_WRITABLE and self.view.readonly:
- * raise ValueError("Cannot create writable memory view from read-only memoryview") # <<<<<<<<<<<<<<
- *
- * if flags & PyBUF_ND:
- */
- __pyx_tuple__11 = PyTuple_Pack(1, __pyx_kp_s_Cannot_create_writable_memory_vi); if (unlikely(!__pyx_tuple__11)) __PYX_ERR(1, 520, __pyx_L1_error)
- __Pyx_GOTREF(__pyx_tuple__11);
- __Pyx_GIVEREF(__pyx_tuple__11);
-
- /* "View.MemoryView":570
- * if self.view.strides == NULL:
- *
- * raise ValueError("Buffer view does not expose strides") # <<<<<<<<<<<<<<
- *
- * return tuple([stride for stride in self.view.strides[:self.view.ndim]])
- */
- __pyx_tuple__12 = PyTuple_Pack(1, __pyx_kp_s_Buffer_view_does_not_expose_stri); if (unlikely(!__pyx_tuple__12)) __PYX_ERR(1, 570, __pyx_L1_error)
- __Pyx_GOTREF(__pyx_tuple__12);
- __Pyx_GIVEREF(__pyx_tuple__12);
-
- /* "View.MemoryView":577
- * def suboffsets(self):
- * if self.view.suboffsets == NULL:
- * return (-1,) * self.view.ndim # <<<<<<<<<<<<<<
- *
- * return tuple([suboffset for suboffset in self.view.suboffsets[:self.view.ndim]])
- */
- __pyx_tuple__13 = PyTuple_New(1); if (unlikely(!__pyx_tuple__13)) __PYX_ERR(1, 577, __pyx_L1_error)
- __Pyx_GOTREF(__pyx_tuple__13);
- __Pyx_INCREF(__pyx_int_neg_1);
- __Pyx_GIVEREF(__pyx_int_neg_1);
- PyTuple_SET_ITEM(__pyx_tuple__13, 0, __pyx_int_neg_1);
- __Pyx_GIVEREF(__pyx_tuple__13);
-
- /* "(tree fragment)":2
- * def __reduce_cython__(self):
- * raise TypeError("no default __reduce__ due to non-trivial __cinit__") # <<<<<<<<<<<<<<
- * def __setstate_cython__(self, __pyx_state):
- * raise TypeError("no default __reduce__ due to non-trivial __cinit__")
- */
- __pyx_tuple__14 = PyTuple_Pack(1, __pyx_kp_s_no_default___reduce___due_to_non); if (unlikely(!__pyx_tuple__14)) __PYX_ERR(1, 2, __pyx_L1_error)
- __Pyx_GOTREF(__pyx_tuple__14);
- __Pyx_GIVEREF(__pyx_tuple__14);
-
- /* "(tree fragment)":4
- * raise TypeError("no default __reduce__ due to non-trivial __cinit__")
- * def __setstate_cython__(self, __pyx_state):
- * raise TypeError("no default __reduce__ due to non-trivial __cinit__") # <<<<<<<<<<<<<<
- */
- __pyx_tuple__15 = PyTuple_Pack(1, __pyx_kp_s_no_default___reduce___due_to_non); if (unlikely(!__pyx_tuple__15)) __PYX_ERR(1, 4, __pyx_L1_error)
- __Pyx_GOTREF(__pyx_tuple__15);
- __Pyx_GIVEREF(__pyx_tuple__15);
-
- /* "View.MemoryView":682
- * if item is Ellipsis:
- * if not seen_ellipsis:
- * result.extend([slice(None)] * (ndim - len(tup) + 1)) # <<<<<<<<<<<<<<
- * seen_ellipsis = True
- * else:
- */
- __pyx_slice__16 = PySlice_New(Py_None, Py_None, Py_None); if (unlikely(!__pyx_slice__16)) __PYX_ERR(1, 682, __pyx_L1_error)
- __Pyx_GOTREF(__pyx_slice__16);
- __Pyx_GIVEREF(__pyx_slice__16);
-
- /* "View.MemoryView":703
- * for suboffset in suboffsets[:ndim]:
- * if suboffset >= 0:
- * raise ValueError("Indirect dimensions not supported") # <<<<<<<<<<<<<<
- *
- *
- */
- __pyx_tuple__17 = PyTuple_Pack(1, __pyx_kp_s_Indirect_dimensions_not_supporte); if (unlikely(!__pyx_tuple__17)) __PYX_ERR(1, 703, __pyx_L1_error)
- __Pyx_GOTREF(__pyx_tuple__17);
- __Pyx_GIVEREF(__pyx_tuple__17);
-
- /* "(tree fragment)":2
- * def __reduce_cython__(self):
- * raise TypeError("no default __reduce__ due to non-trivial __cinit__") # <<<<<<<<<<<<<<
- * def __setstate_cython__(self, __pyx_state):
- * raise TypeError("no default __reduce__ due to non-trivial __cinit__")
- */
- __pyx_tuple__18 = PyTuple_Pack(1, __pyx_kp_s_no_default___reduce___due_to_non); if (unlikely(!__pyx_tuple__18)) __PYX_ERR(1, 2, __pyx_L1_error)
- __Pyx_GOTREF(__pyx_tuple__18);
- __Pyx_GIVEREF(__pyx_tuple__18);
-
- /* "(tree fragment)":4
- * raise TypeError("no default __reduce__ due to non-trivial __cinit__")
- * def __setstate_cython__(self, __pyx_state):
- * raise TypeError("no default __reduce__ due to non-trivial __cinit__") # <<<<<<<<<<<<<<
- */
- __pyx_tuple__19 = PyTuple_Pack(1, __pyx_kp_s_no_default___reduce___due_to_non); if (unlikely(!__pyx_tuple__19)) __PYX_ERR(1, 4, __pyx_L1_error)
- __Pyx_GOTREF(__pyx_tuple__19);
- __Pyx_GIVEREF(__pyx_tuple__19);
-
- /* "View.MemoryView":286
- * return self.name
- *
- * cdef generic = Enum("") # <<<<<<<<<<<<<<
- * cdef strided = Enum("") # default
- * cdef indirect = Enum("")
- */
- __pyx_tuple__20 = PyTuple_Pack(1, __pyx_kp_s_strided_and_direct_or_indirect); if (unlikely(!__pyx_tuple__20)) __PYX_ERR(1, 286, __pyx_L1_error)
- __Pyx_GOTREF(__pyx_tuple__20);
- __Pyx_GIVEREF(__pyx_tuple__20);
-
- /* "View.MemoryView":287
- *
- * cdef generic = Enum("")
- * cdef strided = Enum("") # default # <<<<<<<<<<<<<<
- * cdef indirect = Enum("")
- *
- */
- __pyx_tuple__21 = PyTuple_Pack(1, __pyx_kp_s_strided_and_direct); if (unlikely(!__pyx_tuple__21)) __PYX_ERR(1, 287, __pyx_L1_error)
- __Pyx_GOTREF(__pyx_tuple__21);
- __Pyx_GIVEREF(__pyx_tuple__21);
-
- /* "View.MemoryView":288
- * cdef generic = Enum("")
- * cdef strided = Enum("") # default
- * cdef indirect = Enum("") # <<<<<<<<<<<<<<
- *
- *
- */
- __pyx_tuple__22 = PyTuple_Pack(1, __pyx_kp_s_strided_and_indirect); if (unlikely(!__pyx_tuple__22)) __PYX_ERR(1, 288, __pyx_L1_error)
- __Pyx_GOTREF(__pyx_tuple__22);
- __Pyx_GIVEREF(__pyx_tuple__22);
-
- /* "View.MemoryView":291
- *
- *
- * cdef contiguous = Enum("") # <<<<<<<<<<<<<<
- * cdef indirect_contiguous = Enum("")
- *
- */
- __pyx_tuple__23 = PyTuple_Pack(1, __pyx_kp_s_contiguous_and_direct); if (unlikely(!__pyx_tuple__23)) __PYX_ERR(1, 291, __pyx_L1_error)
- __Pyx_GOTREF(__pyx_tuple__23);
- __Pyx_GIVEREF(__pyx_tuple__23);
-
- /* "View.MemoryView":292
- *
- * cdef contiguous = Enum("")
- * cdef indirect_contiguous = Enum("") # <<<<<<<<<<<<<<
- *
- *
- */
- __pyx_tuple__24 = PyTuple_Pack(1, __pyx_kp_s_contiguous_and_indirect); if (unlikely(!__pyx_tuple__24)) __PYX_ERR(1, 292, __pyx_L1_error)
- __Pyx_GOTREF(__pyx_tuple__24);
- __Pyx_GIVEREF(__pyx_tuple__24);
-
- /* "(tree fragment)":1
- * def __pyx_unpickle_Enum(__pyx_type, long __pyx_checksum, __pyx_state): # <<<<<<<<<<<<<<
- * cdef object __pyx_PickleError
- * cdef object __pyx_result
- */
- __pyx_tuple__25 = PyTuple_Pack(5, __pyx_n_s_pyx_type, __pyx_n_s_pyx_checksum, __pyx_n_s_pyx_state, __pyx_n_s_pyx_PickleError, __pyx_n_s_pyx_result); if (unlikely(!__pyx_tuple__25)) __PYX_ERR(1, 1, __pyx_L1_error)
- __Pyx_GOTREF(__pyx_tuple__25);
- __Pyx_GIVEREF(__pyx_tuple__25);
- __pyx_codeobj__26 = (PyObject*)__Pyx_PyCode_New(3, 0, 5, 0, CO_OPTIMIZED|CO_NEWLOCALS, __pyx_empty_bytes, __pyx_empty_tuple, __pyx_empty_tuple, __pyx_tuple__25, __pyx_empty_tuple, __pyx_empty_tuple, __pyx_kp_s_stringsource, __pyx_n_s_pyx_unpickle_Enum, 1, __pyx_empty_bytes); if (unlikely(!__pyx_codeobj__26)) __PYX_ERR(1, 1, __pyx_L1_error)
- __Pyx_RefNannyFinishContext();
- return 0;
- __pyx_L1_error:;
- __Pyx_RefNannyFinishContext();
- return -1;
-}
-
-static CYTHON_SMALL_CODE int __Pyx_InitGlobals(void) {
- /* InitThreads.init */
- #ifdef WITH_THREAD
-PyEval_InitThreads();
-#endif
-
-if (unlikely(PyErr_Occurred())) __PYX_ERR(0, 1, __pyx_L1_error)
-
- if (__Pyx_InitStrings(__pyx_string_tab) < 0) __PYX_ERR(0, 1, __pyx_L1_error);
- __pyx_int_0 = PyInt_FromLong(0); if (unlikely(!__pyx_int_0)) __PYX_ERR(0, 1, __pyx_L1_error)
- __pyx_int_1 = PyInt_FromLong(1); if (unlikely(!__pyx_int_1)) __PYX_ERR(0, 1, __pyx_L1_error)
- __pyx_int_184977713 = PyInt_FromLong(184977713L); if (unlikely(!__pyx_int_184977713)) __PYX_ERR(0, 1, __pyx_L1_error)
- __pyx_int_neg_1 = PyInt_FromLong(-1); if (unlikely(!__pyx_int_neg_1)) __PYX_ERR(0, 1, __pyx_L1_error)
- return 0;
- __pyx_L1_error:;
- return -1;
-}
-
-static CYTHON_SMALL_CODE int __Pyx_modinit_global_init_code(void); /*proto*/
-static CYTHON_SMALL_CODE int __Pyx_modinit_variable_export_code(void); /*proto*/
-static CYTHON_SMALL_CODE int __Pyx_modinit_function_export_code(void); /*proto*/
-static CYTHON_SMALL_CODE int __Pyx_modinit_type_init_code(void); /*proto*/
-static CYTHON_SMALL_CODE int __Pyx_modinit_type_import_code(void); /*proto*/
-static CYTHON_SMALL_CODE int __Pyx_modinit_variable_import_code(void); /*proto*/
-static CYTHON_SMALL_CODE int __Pyx_modinit_function_import_code(void); /*proto*/
-
-static int __Pyx_modinit_global_init_code(void) {
- __Pyx_RefNannyDeclarations
- __Pyx_RefNannySetupContext("__Pyx_modinit_global_init_code", 0);
- /*--- Global init code ---*/
- generic = Py_None; Py_INCREF(Py_None);
- strided = Py_None; Py_INCREF(Py_None);
- indirect = Py_None; Py_INCREF(Py_None);
- contiguous = Py_None; Py_INCREF(Py_None);
- indirect_contiguous = Py_None; Py_INCREF(Py_None);
- __Pyx_RefNannyFinishContext();
- return 0;
-}
-
-static int __Pyx_modinit_variable_export_code(void) {
- __Pyx_RefNannyDeclarations
- __Pyx_RefNannySetupContext("__Pyx_modinit_variable_export_code", 0);
- /*--- Variable export code ---*/
- __Pyx_RefNannyFinishContext();
- return 0;
-}
-
-static int __Pyx_modinit_function_export_code(void) {
- __Pyx_RefNannyDeclarations
- __Pyx_RefNannySetupContext("__Pyx_modinit_function_export_code", 0);
- /*--- Function export code ---*/
- __Pyx_RefNannyFinishContext();
- return 0;
-}
-
-static int __Pyx_modinit_type_init_code(void) {
- __Pyx_RefNannyDeclarations
- int __pyx_lineno = 0;
- const char *__pyx_filename = NULL;
- int __pyx_clineno = 0;
- __Pyx_RefNannySetupContext("__Pyx_modinit_type_init_code", 0);
- /*--- Type init code ---*/
- __pyx_vtabptr_array = &__pyx_vtable_array;
- __pyx_vtable_array.get_memview = (PyObject *(*)(struct __pyx_array_obj *))__pyx_array_get_memview;
- if (PyType_Ready(&__pyx_type___pyx_array) < 0) __PYX_ERR(1, 105, __pyx_L1_error)
- #if PY_VERSION_HEX < 0x030800B1
- __pyx_type___pyx_array.tp_print = 0;
- #endif
- if (__Pyx_SetVtable(__pyx_type___pyx_array.tp_dict, __pyx_vtabptr_array) < 0) __PYX_ERR(1, 105, __pyx_L1_error)
- if (__Pyx_setup_reduce((PyObject*)&__pyx_type___pyx_array) < 0) __PYX_ERR(1, 105, __pyx_L1_error)
- __pyx_array_type = &__pyx_type___pyx_array;
- if (PyType_Ready(&__pyx_type___pyx_MemviewEnum) < 0) __PYX_ERR(1, 279, __pyx_L1_error)
- #if PY_VERSION_HEX < 0x030800B1
- __pyx_type___pyx_MemviewEnum.tp_print = 0;
- #endif
- if ((CYTHON_USE_TYPE_SLOTS && CYTHON_USE_PYTYPE_LOOKUP) && likely(!__pyx_type___pyx_MemviewEnum.tp_dictoffset && __pyx_type___pyx_MemviewEnum.tp_getattro == PyObject_GenericGetAttr)) {
- __pyx_type___pyx_MemviewEnum.tp_getattro = __Pyx_PyObject_GenericGetAttr;
- }
- if (__Pyx_setup_reduce((PyObject*)&__pyx_type___pyx_MemviewEnum) < 0) __PYX_ERR(1, 279, __pyx_L1_error)
- __pyx_MemviewEnum_type = &__pyx_type___pyx_MemviewEnum;
- __pyx_vtabptr_memoryview = &__pyx_vtable_memoryview;
- __pyx_vtable_memoryview.get_item_pointer = (char *(*)(struct __pyx_memoryview_obj *, PyObject *))__pyx_memoryview_get_item_pointer;
- __pyx_vtable_memoryview.is_slice = (PyObject *(*)(struct __pyx_memoryview_obj *, PyObject *))__pyx_memoryview_is_slice;
- __pyx_vtable_memoryview.setitem_slice_assignment = (PyObject *(*)(struct __pyx_memoryview_obj *, PyObject *, PyObject *))__pyx_memoryview_setitem_slice_assignment;
- __pyx_vtable_memoryview.setitem_slice_assign_scalar = (PyObject *(*)(struct __pyx_memoryview_obj *, struct __pyx_memoryview_obj *, PyObject *))__pyx_memoryview_setitem_slice_assign_scalar;
- __pyx_vtable_memoryview.setitem_indexed = (PyObject *(*)(struct __pyx_memoryview_obj *, PyObject *, PyObject *))__pyx_memoryview_setitem_indexed;
- __pyx_vtable_memoryview.convert_item_to_object = (PyObject *(*)(struct __pyx_memoryview_obj *, char *))__pyx_memoryview_convert_item_to_object;
- __pyx_vtable_memoryview.assign_item_from_object = (PyObject *(*)(struct __pyx_memoryview_obj *, char *, PyObject *))__pyx_memoryview_assign_item_from_object;
- if (PyType_Ready(&__pyx_type___pyx_memoryview) < 0) __PYX_ERR(1, 330, __pyx_L1_error)
- #if PY_VERSION_HEX < 0x030800B1
- __pyx_type___pyx_memoryview.tp_print = 0;
- #endif
- if ((CYTHON_USE_TYPE_SLOTS && CYTHON_USE_PYTYPE_LOOKUP) && likely(!__pyx_type___pyx_memoryview.tp_dictoffset && __pyx_type___pyx_memoryview.tp_getattro == PyObject_GenericGetAttr)) {
- __pyx_type___pyx_memoryview.tp_getattro = __Pyx_PyObject_GenericGetAttr;
- }
- if (__Pyx_SetVtable(__pyx_type___pyx_memoryview.tp_dict, __pyx_vtabptr_memoryview) < 0) __PYX_ERR(1, 330, __pyx_L1_error)
- if (__Pyx_setup_reduce((PyObject*)&__pyx_type___pyx_memoryview) < 0) __PYX_ERR(1, 330, __pyx_L1_error)
- __pyx_memoryview_type = &__pyx_type___pyx_memoryview;
- __pyx_vtabptr__memoryviewslice = &__pyx_vtable__memoryviewslice;
- __pyx_vtable__memoryviewslice.__pyx_base = *__pyx_vtabptr_memoryview;
- __pyx_vtable__memoryviewslice.__pyx_base.convert_item_to_object = (PyObject *(*)(struct __pyx_memoryview_obj *, char *))__pyx_memoryviewslice_convert_item_to_object;
- __pyx_vtable__memoryviewslice.__pyx_base.assign_item_from_object = (PyObject *(*)(struct __pyx_memoryview_obj *, char *, PyObject *))__pyx_memoryviewslice_assign_item_from_object;
- __pyx_type___pyx_memoryviewslice.tp_base = __pyx_memoryview_type;
- if (PyType_Ready(&__pyx_type___pyx_memoryviewslice) < 0) __PYX_ERR(1, 965, __pyx_L1_error)
- #if PY_VERSION_HEX < 0x030800B1
- __pyx_type___pyx_memoryviewslice.tp_print = 0;
- #endif
- if ((CYTHON_USE_TYPE_SLOTS && CYTHON_USE_PYTYPE_LOOKUP) && likely(!__pyx_type___pyx_memoryviewslice.tp_dictoffset && __pyx_type___pyx_memoryviewslice.tp_getattro == PyObject_GenericGetAttr)) {
- __pyx_type___pyx_memoryviewslice.tp_getattro = __Pyx_PyObject_GenericGetAttr;
- }
- if (__Pyx_SetVtable(__pyx_type___pyx_memoryviewslice.tp_dict, __pyx_vtabptr__memoryviewslice) < 0) __PYX_ERR(1, 965, __pyx_L1_error)
- if (__Pyx_setup_reduce((PyObject*)&__pyx_type___pyx_memoryviewslice) < 0) __PYX_ERR(1, 965, __pyx_L1_error)
- __pyx_memoryviewslice_type = &__pyx_type___pyx_memoryviewslice;
- __Pyx_RefNannyFinishContext();
- return 0;
- __pyx_L1_error:;
- __Pyx_RefNannyFinishContext();
- return -1;
-}
-
-static int __Pyx_modinit_type_import_code(void) {
- __Pyx_RefNannyDeclarations
- __Pyx_RefNannySetupContext("__Pyx_modinit_type_import_code", 0);
- /*--- Type import code ---*/
- __Pyx_RefNannyFinishContext();
- return 0;
-}
-
-static int __Pyx_modinit_variable_import_code(void) {
- __Pyx_RefNannyDeclarations
- __Pyx_RefNannySetupContext("__Pyx_modinit_variable_import_code", 0);
- /*--- Variable import code ---*/
- __Pyx_RefNannyFinishContext();
- return 0;
-}
-
-static int __Pyx_modinit_function_import_code(void) {
- __Pyx_RefNannyDeclarations
- __Pyx_RefNannySetupContext("__Pyx_modinit_function_import_code", 0);
- /*--- Function import code ---*/
- __Pyx_RefNannyFinishContext();
- return 0;
-}
-
-
-#ifndef CYTHON_NO_PYINIT_EXPORT
-#define __Pyx_PyMODINIT_FUNC PyMODINIT_FUNC
-#elif PY_MAJOR_VERSION < 3
-#ifdef __cplusplus
-#define __Pyx_PyMODINIT_FUNC extern "C" void
-#else
-#define __Pyx_PyMODINIT_FUNC void
-#endif
-#else
-#ifdef __cplusplus
-#define __Pyx_PyMODINIT_FUNC extern "C" PyObject *
-#else
-#define __Pyx_PyMODINIT_FUNC PyObject *
-#endif
-#endif
-
-
-#if PY_MAJOR_VERSION < 3
-__Pyx_PyMODINIT_FUNC initcore(void) CYTHON_SMALL_CODE; /*proto*/
-__Pyx_PyMODINIT_FUNC initcore(void)
-#else
-__Pyx_PyMODINIT_FUNC PyInit_core(void) CYTHON_SMALL_CODE; /*proto*/
-__Pyx_PyMODINIT_FUNC PyInit_core(void)
-#if CYTHON_PEP489_MULTI_PHASE_INIT
-{
- return PyModuleDef_Init(&__pyx_moduledef);
-}
-static CYTHON_SMALL_CODE int __Pyx_check_single_interpreter(void) {
- #if PY_VERSION_HEX >= 0x030700A1
- static PY_INT64_T main_interpreter_id = -1;
- PY_INT64_T current_id = PyInterpreterState_GetID(PyThreadState_Get()->interp);
- if (main_interpreter_id == -1) {
- main_interpreter_id = current_id;
- return (unlikely(current_id == -1)) ? -1 : 0;
- } else if (unlikely(main_interpreter_id != current_id))
- #else
- static PyInterpreterState *main_interpreter = NULL;
- PyInterpreterState *current_interpreter = PyThreadState_Get()->interp;
- if (!main_interpreter) {
- main_interpreter = current_interpreter;
- } else if (unlikely(main_interpreter != current_interpreter))
- #endif
- {
- PyErr_SetString(
- PyExc_ImportError,
- "Interpreter change detected - this module can only be loaded into one interpreter per process.");
- return -1;
- }
- return 0;
-}
-static CYTHON_SMALL_CODE int __Pyx_copy_spec_to_module(PyObject *spec, PyObject *moddict, const char* from_name, const char* to_name, int allow_none) {
- PyObject *value = PyObject_GetAttrString(spec, from_name);
- int result = 0;
- if (likely(value)) {
- if (allow_none || value != Py_None) {
- result = PyDict_SetItemString(moddict, to_name, value);
- }
- Py_DECREF(value);
- } else if (PyErr_ExceptionMatches(PyExc_AttributeError)) {
- PyErr_Clear();
- } else {
- result = -1;
- }
- return result;
-}
-static CYTHON_SMALL_CODE PyObject* __pyx_pymod_create(PyObject *spec, CYTHON_UNUSED PyModuleDef *def) {
- PyObject *module = NULL, *moddict, *modname;
- if (__Pyx_check_single_interpreter())
- return NULL;
- if (__pyx_m)
- return __Pyx_NewRef(__pyx_m);
- modname = PyObject_GetAttrString(spec, "name");
- if (unlikely(!modname)) goto bad;
- module = PyModule_NewObject(modname);
- Py_DECREF(modname);
- if (unlikely(!module)) goto bad;
- moddict = PyModule_GetDict(module);
- if (unlikely(!moddict)) goto bad;
- if (unlikely(__Pyx_copy_spec_to_module(spec, moddict, "loader", "__loader__", 1) < 0)) goto bad;
- if (unlikely(__Pyx_copy_spec_to_module(spec, moddict, "origin", "__file__", 1) < 0)) goto bad;
- if (unlikely(__Pyx_copy_spec_to_module(spec, moddict, "parent", "__package__", 1) < 0)) goto bad;
- if (unlikely(__Pyx_copy_spec_to_module(spec, moddict, "submodule_search_locations", "__path__", 0) < 0)) goto bad;
- return module;
-bad:
- Py_XDECREF(module);
- return NULL;
-}
-
-
-static CYTHON_SMALL_CODE int __pyx_pymod_exec_core(PyObject *__pyx_pyinit_module)
-#endif
-#endif
-{
- PyObject *__pyx_t_1 = NULL;
- static PyThread_type_lock __pyx_t_2[8];
- int __pyx_lineno = 0;
- const char *__pyx_filename = NULL;
- int __pyx_clineno = 0;
- __Pyx_RefNannyDeclarations
- #if CYTHON_PEP489_MULTI_PHASE_INIT
- if (__pyx_m) {
- if (__pyx_m == __pyx_pyinit_module) return 0;
- PyErr_SetString(PyExc_RuntimeError, "Module 'core' has already been imported. Re-initialisation is not supported.");
- return -1;
- }
- #elif PY_MAJOR_VERSION >= 3
- if (__pyx_m) return __Pyx_NewRef(__pyx_m);
- #endif
- #if CYTHON_REFNANNY
-__Pyx_RefNanny = __Pyx_RefNannyImportAPI("refnanny");
-if (!__Pyx_RefNanny) {
- PyErr_Clear();
- __Pyx_RefNanny = __Pyx_RefNannyImportAPI("Cython.Runtime.refnanny");
- if (!__Pyx_RefNanny)
- Py_FatalError("failed to import 'refnanny' module");
-}
-#endif
- __Pyx_RefNannySetupContext("__Pyx_PyMODINIT_FUNC PyInit_core(void)", 0);
- if (__Pyx_check_binary_version() < 0) __PYX_ERR(0, 1, __pyx_L1_error)
- #ifdef __Pxy_PyFrame_Initialize_Offsets
- __Pxy_PyFrame_Initialize_Offsets();
- #endif
- __pyx_empty_tuple = PyTuple_New(0); if (unlikely(!__pyx_empty_tuple)) __PYX_ERR(0, 1, __pyx_L1_error)
- __pyx_empty_bytes = PyBytes_FromStringAndSize("", 0); if (unlikely(!__pyx_empty_bytes)) __PYX_ERR(0, 1, __pyx_L1_error)
- __pyx_empty_unicode = PyUnicode_FromStringAndSize("", 0); if (unlikely(!__pyx_empty_unicode)) __PYX_ERR(0, 1, __pyx_L1_error)
- #ifdef __Pyx_CyFunction_USED
- if (__pyx_CyFunction_init() < 0) __PYX_ERR(0, 1, __pyx_L1_error)
- #endif
- #ifdef __Pyx_FusedFunction_USED
- if (__pyx_FusedFunction_init() < 0) __PYX_ERR(0, 1, __pyx_L1_error)
- #endif
- #ifdef __Pyx_Coroutine_USED
- if (__pyx_Coroutine_init() < 0) __PYX_ERR(0, 1, __pyx_L1_error)
- #endif
- #ifdef __Pyx_Generator_USED
- if (__pyx_Generator_init() < 0) __PYX_ERR(0, 1, __pyx_L1_error)
- #endif
- #ifdef __Pyx_AsyncGen_USED
- if (__pyx_AsyncGen_init() < 0) __PYX_ERR(0, 1, __pyx_L1_error)
- #endif
- #ifdef __Pyx_StopAsyncIteration_USED
- if (__pyx_StopAsyncIteration_init() < 0) __PYX_ERR(0, 1, __pyx_L1_error)
- #endif
- /*--- Library function declarations ---*/
- /*--- Threads initialization code ---*/
- #if defined(__PYX_FORCE_INIT_THREADS) && __PYX_FORCE_INIT_THREADS
- #ifdef WITH_THREAD /* Python build with threading support? */
- PyEval_InitThreads();
- #endif
- #endif
- /*--- Module creation code ---*/
- #if CYTHON_PEP489_MULTI_PHASE_INIT
- __pyx_m = __pyx_pyinit_module;
- Py_INCREF(__pyx_m);
- #else
- #if PY_MAJOR_VERSION < 3
- __pyx_m = Py_InitModule4("core", __pyx_methods, 0, 0, PYTHON_API_VERSION); Py_XINCREF(__pyx_m);
- #else
- __pyx_m = PyModule_Create(&__pyx_moduledef);
- #endif
- if (unlikely(!__pyx_m)) __PYX_ERR(0, 1, __pyx_L1_error)
- #endif
- __pyx_d = PyModule_GetDict(__pyx_m); if (unlikely(!__pyx_d)) __PYX_ERR(0, 1, __pyx_L1_error)
- Py_INCREF(__pyx_d);
- __pyx_b = PyImport_AddModule(__Pyx_BUILTIN_MODULE_NAME); if (unlikely(!__pyx_b)) __PYX_ERR(0, 1, __pyx_L1_error)
- Py_INCREF(__pyx_b);
- __pyx_cython_runtime = PyImport_AddModule((char *) "cython_runtime"); if (unlikely(!__pyx_cython_runtime)) __PYX_ERR(0, 1, __pyx_L1_error)
- Py_INCREF(__pyx_cython_runtime);
- if (PyObject_SetAttrString(__pyx_m, "__builtins__", __pyx_b) < 0) __PYX_ERR(0, 1, __pyx_L1_error);
- /*--- Initialize various global constants etc. ---*/
- if (__Pyx_InitGlobals() < 0) __PYX_ERR(0, 1, __pyx_L1_error)
- #if PY_MAJOR_VERSION < 3 && (__PYX_DEFAULT_STRING_ENCODING_IS_ASCII || __PYX_DEFAULT_STRING_ENCODING_IS_DEFAULT)
- if (__Pyx_init_sys_getdefaultencoding_params() < 0) __PYX_ERR(0, 1, __pyx_L1_error)
- #endif
- if (__pyx_module_is_main_monotonic_align__core) {
- if (PyObject_SetAttr(__pyx_m, __pyx_n_s_name_2, __pyx_n_s_main) < 0) __PYX_ERR(0, 1, __pyx_L1_error)
- }
- #if PY_MAJOR_VERSION >= 3
- {
- PyObject *modules = PyImport_GetModuleDict(); if (unlikely(!modules)) __PYX_ERR(0, 1, __pyx_L1_error)
- if (!PyDict_GetItemString(modules, "monotonic_align.core")) {
- if (unlikely(PyDict_SetItemString(modules, "monotonic_align.core", __pyx_m) < 0)) __PYX_ERR(0, 1, __pyx_L1_error)
- }
- }
- #endif
- /*--- Builtin init code ---*/
- if (__Pyx_InitCachedBuiltins() < 0) __PYX_ERR(0, 1, __pyx_L1_error)
- /*--- Constants init code ---*/
- if (__Pyx_InitCachedConstants() < 0) __PYX_ERR(0, 1, __pyx_L1_error)
- /*--- Global type/function init code ---*/
- (void)__Pyx_modinit_global_init_code();
- (void)__Pyx_modinit_variable_export_code();
- (void)__Pyx_modinit_function_export_code();
- if (unlikely(__Pyx_modinit_type_init_code() < 0)) __PYX_ERR(0, 1, __pyx_L1_error)
- (void)__Pyx_modinit_type_import_code();
- (void)__Pyx_modinit_variable_import_code();
- (void)__Pyx_modinit_function_import_code();
- /*--- Execution code ---*/
- #if defined(__Pyx_Generator_USED) || defined(__Pyx_Coroutine_USED)
- if (__Pyx_patch_abc() < 0) __PYX_ERR(0, 1, __pyx_L1_error)
- #endif
-
- /* "monotonic_align/core.pyx":7
- * @cython.boundscheck(False)
- * @cython.wraparound(False)
- * cdef void maximum_path_each(int[:,::1] path, float[:,::1] value, int t_y, int t_x, float max_neg_val=-1e9) nogil: # <<<<<<<<<<<<<<
- * cdef int x
- * cdef int y
- */
- __pyx_k_ = (-1e9);
-
- /* "monotonic_align/core.pyx":1
- * cimport cython # <<<<<<<<<<<<<<
- * from cython.parallel import prange
- *
- */
- __pyx_t_1 = __Pyx_PyDict_NewPresized(0); if (unlikely(!__pyx_t_1)) __PYX_ERR(0, 1, __pyx_L1_error)
- __Pyx_GOTREF(__pyx_t_1);
- if (PyDict_SetItem(__pyx_d, __pyx_n_s_test, __pyx_t_1) < 0) __PYX_ERR(0, 1, __pyx_L1_error)
- __Pyx_DECREF(__pyx_t_1); __pyx_t_1 = 0;
-
- /* "View.MemoryView":209
- * info.obj = self
- *
- * __pyx_getbuffer = capsule( &__pyx_array_getbuffer, "getbuffer(obj, view, flags)") # <<<<<<<<<<<<<<
- *
- * def __dealloc__(array self):
- */
- __pyx_t_1 = __pyx_capsule_create(((void *)(&__pyx_array_getbuffer)), ((char *)"getbuffer(obj, view, flags)")); if (unlikely(!__pyx_t_1)) __PYX_ERR(1, 209, __pyx_L1_error)
- __Pyx_GOTREF(__pyx_t_1);
- if (PyDict_SetItem((PyObject *)__pyx_array_type->tp_dict, __pyx_n_s_pyx_getbuffer, __pyx_t_1) < 0) __PYX_ERR(1, 209, __pyx_L1_error)
- __Pyx_DECREF(__pyx_t_1); __pyx_t_1 = 0;
- PyType_Modified(__pyx_array_type);
-
- /* "View.MemoryView":286
- * return self.name
- *
- * cdef generic = Enum("") # <<<<<<<<<<<<<<
- * cdef strided = Enum("") # default
- * cdef indirect = Enum("")
- */
- __pyx_t_1 = __Pyx_PyObject_Call(((PyObject *)__pyx_MemviewEnum_type), __pyx_tuple__20, NULL); if (unlikely(!__pyx_t_1)) __PYX_ERR(1, 286, __pyx_L1_error)
- __Pyx_GOTREF(__pyx_t_1);
- __Pyx_XGOTREF(generic);
- __Pyx_DECREF_SET(generic, __pyx_t_1);
- __Pyx_GIVEREF(__pyx_t_1);
- __pyx_t_1 = 0;
-
- /* "View.MemoryView":287
- *
- * cdef generic = Enum("")
- * cdef strided = Enum("") # default # <<<<<<<<<<<<<<
- * cdef indirect = Enum("")
- *
- */
- __pyx_t_1 = __Pyx_PyObject_Call(((PyObject *)__pyx_MemviewEnum_type), __pyx_tuple__21, NULL); if (unlikely(!__pyx_t_1)) __PYX_ERR(1, 287, __pyx_L1_error)
- __Pyx_GOTREF(__pyx_t_1);
- __Pyx_XGOTREF(strided);
- __Pyx_DECREF_SET(strided, __pyx_t_1);
- __Pyx_GIVEREF(__pyx_t_1);
- __pyx_t_1 = 0;
-
- /* "View.MemoryView":288
- * cdef generic = Enum("")
- * cdef strided = Enum("") # default
- * cdef indirect = Enum("") # <<<<<<<<<<<<<<
- *
- *
- */
- __pyx_t_1 = __Pyx_PyObject_Call(((PyObject *)__pyx_MemviewEnum_type), __pyx_tuple__22, NULL); if (unlikely(!__pyx_t_1)) __PYX_ERR(1, 288, __pyx_L1_error)
- __Pyx_GOTREF(__pyx_t_1);
- __Pyx_XGOTREF(indirect);
- __Pyx_DECREF_SET(indirect, __pyx_t_1);
- __Pyx_GIVEREF(__pyx_t_1);
- __pyx_t_1 = 0;
-
- /* "View.MemoryView":291
- *
- *
- * cdef contiguous = Enum("") # <<<<<<<<<<<<<<
- * cdef indirect_contiguous = Enum("")
- *
- */
- __pyx_t_1 = __Pyx_PyObject_Call(((PyObject *)__pyx_MemviewEnum_type), __pyx_tuple__23, NULL); if (unlikely(!__pyx_t_1)) __PYX_ERR(1, 291, __pyx_L1_error)
- __Pyx_GOTREF(__pyx_t_1);
- __Pyx_XGOTREF(contiguous);
- __Pyx_DECREF_SET(contiguous, __pyx_t_1);
- __Pyx_GIVEREF(__pyx_t_1);
- __pyx_t_1 = 0;
-
- /* "View.MemoryView":292
- *
- * cdef contiguous = Enum("")
- * cdef indirect_contiguous = Enum("") # <<<<<<<<<<<<<<
- *
- *
- */
- __pyx_t_1 = __Pyx_PyObject_Call(((PyObject *)__pyx_MemviewEnum_type), __pyx_tuple__24, NULL); if (unlikely(!__pyx_t_1)) __PYX_ERR(1, 292, __pyx_L1_error)
- __Pyx_GOTREF(__pyx_t_1);
- __Pyx_XGOTREF(indirect_contiguous);
- __Pyx_DECREF_SET(indirect_contiguous, __pyx_t_1);
- __Pyx_GIVEREF(__pyx_t_1);
- __pyx_t_1 = 0;
-
- /* "View.MemoryView":316
- *
- * DEF THREAD_LOCKS_PREALLOCATED = 8
- * cdef int __pyx_memoryview_thread_locks_used = 0 # <<<<<<<<<<<<<<
- * cdef PyThread_type_lock[THREAD_LOCKS_PREALLOCATED] __pyx_memoryview_thread_locks = [
- * PyThread_allocate_lock(),
- */
- __pyx_memoryview_thread_locks_used = 0;
-
- /* "View.MemoryView":317
- * DEF THREAD_LOCKS_PREALLOCATED = 8
- * cdef int __pyx_memoryview_thread_locks_used = 0
- * cdef PyThread_type_lock[THREAD_LOCKS_PREALLOCATED] __pyx_memoryview_thread_locks = [ # <<<<<<<<<<<<<<
- * PyThread_allocate_lock(),
- * PyThread_allocate_lock(),
- */
- __pyx_t_2[0] = PyThread_allocate_lock();
- __pyx_t_2[1] = PyThread_allocate_lock();
- __pyx_t_2[2] = PyThread_allocate_lock();
- __pyx_t_2[3] = PyThread_allocate_lock();
- __pyx_t_2[4] = PyThread_allocate_lock();
- __pyx_t_2[5] = PyThread_allocate_lock();
- __pyx_t_2[6] = PyThread_allocate_lock();
- __pyx_t_2[7] = PyThread_allocate_lock();
- memcpy(&(__pyx_memoryview_thread_locks[0]), __pyx_t_2, sizeof(__pyx_memoryview_thread_locks[0]) * (8));
-
- /* "View.MemoryView":549
- * info.obj = self
- *
- * __pyx_getbuffer = capsule( &__pyx_memoryview_getbuffer, "getbuffer(obj, view, flags)") # <<<<<<<<<<<<<<
- *
- *
- */
- __pyx_t_1 = __pyx_capsule_create(((void *)(&__pyx_memoryview_getbuffer)), ((char *)"getbuffer(obj, view, flags)")); if (unlikely(!__pyx_t_1)) __PYX_ERR(1, 549, __pyx_L1_error)
- __Pyx_GOTREF(__pyx_t_1);
- if (PyDict_SetItem((PyObject *)__pyx_memoryview_type->tp_dict, __pyx_n_s_pyx_getbuffer, __pyx_t_1) < 0) __PYX_ERR(1, 549, __pyx_L1_error)
- __Pyx_DECREF(__pyx_t_1); __pyx_t_1 = 0;
- PyType_Modified(__pyx_memoryview_type);
-
- /* "View.MemoryView":995
- * return self.from_object
- *
- * __pyx_getbuffer = capsule( &__pyx_memoryview_getbuffer, "getbuffer(obj, view, flags)") # <<<<<<<<<<<<<<
- *
- *
- */
- __pyx_t_1 = __pyx_capsule_create(((void *)(&__pyx_memoryview_getbuffer)), ((char *)"getbuffer(obj, view, flags)")); if (unlikely(!__pyx_t_1)) __PYX_ERR(1, 995, __pyx_L1_error)
- __Pyx_GOTREF(__pyx_t_1);
- if (PyDict_SetItem((PyObject *)__pyx_memoryviewslice_type->tp_dict, __pyx_n_s_pyx_getbuffer, __pyx_t_1) < 0) __PYX_ERR(1, 995, __pyx_L1_error)
- __Pyx_DECREF(__pyx_t_1); __pyx_t_1 = 0;
- PyType_Modified(__pyx_memoryviewslice_type);
-
- /* "(tree fragment)":1
- * def __pyx_unpickle_Enum(__pyx_type, long __pyx_checksum, __pyx_state): # <<<<<<<<<<<<<<
- * cdef object __pyx_PickleError
- * cdef object __pyx_result
- */
- __pyx_t_1 = PyCFunction_NewEx(&__pyx_mdef_15View_dot_MemoryView_1__pyx_unpickle_Enum, NULL, __pyx_n_s_View_MemoryView); if (unlikely(!__pyx_t_1)) __PYX_ERR(1, 1, __pyx_L1_error)
- __Pyx_GOTREF(__pyx_t_1);
- if (PyDict_SetItem(__pyx_d, __pyx_n_s_pyx_unpickle_Enum, __pyx_t_1) < 0) __PYX_ERR(1, 1, __pyx_L1_error)
- __Pyx_DECREF(__pyx_t_1); __pyx_t_1 = 0;
-
- /* "(tree fragment)":11
- * __pyx_unpickle_Enum__set_state( __pyx_result, __pyx_state)
- * return __pyx_result
- * cdef __pyx_unpickle_Enum__set_state(Enum __pyx_result, tuple __pyx_state): # <<<<<<<<<<<<<<
- * __pyx_result.name = __pyx_state[0]
- * if len(__pyx_state) > 1 and hasattr(__pyx_result, '__dict__'):
- */
-
- /*--- Wrapped vars code ---*/
-
- goto __pyx_L0;
- __pyx_L1_error:;
- __Pyx_XDECREF(__pyx_t_1);
- if (__pyx_m) {
- if (__pyx_d) {
- __Pyx_AddTraceback("init monotonic_align.core", __pyx_clineno, __pyx_lineno, __pyx_filename);
- }
- Py_CLEAR(__pyx_m);
- } else if (!PyErr_Occurred()) {
- PyErr_SetString(PyExc_ImportError, "init monotonic_align.core");
- }
- __pyx_L0:;
- __Pyx_RefNannyFinishContext();
- #if CYTHON_PEP489_MULTI_PHASE_INIT
- return (__pyx_m != NULL) ? 0 : -1;
- #elif PY_MAJOR_VERSION >= 3
- return __pyx_m;
- #else
- return;
- #endif
-}
-
-/* --- Runtime support code --- */
-/* Refnanny */
-#if CYTHON_REFNANNY
-static __Pyx_RefNannyAPIStruct *__Pyx_RefNannyImportAPI(const char *modname) {
- PyObject *m = NULL, *p = NULL;
- void *r = NULL;
- m = PyImport_ImportModule(modname);
- if (!m) goto end;
- p = PyObject_GetAttrString(m, "RefNannyAPI");
- if (!p) goto end;
- r = PyLong_AsVoidPtr(p);
-end:
- Py_XDECREF(p);
- Py_XDECREF(m);
- return (__Pyx_RefNannyAPIStruct *)r;
-}
-#endif
-
-/* PyObjectGetAttrStr */
-#if CYTHON_USE_TYPE_SLOTS
-static CYTHON_INLINE PyObject* __Pyx_PyObject_GetAttrStr(PyObject* obj, PyObject* attr_name) {
- PyTypeObject* tp = Py_TYPE(obj);
- if (likely(tp->tp_getattro))
- return tp->tp_getattro(obj, attr_name);
-#if PY_MAJOR_VERSION < 3
- if (likely(tp->tp_getattr))
- return tp->tp_getattr(obj, PyString_AS_STRING(attr_name));
-#endif
- return PyObject_GetAttr(obj, attr_name);
-}
-#endif
-
-/* GetBuiltinName */
-static PyObject *__Pyx_GetBuiltinName(PyObject *name) {
- PyObject* result = __Pyx_PyObject_GetAttrStr(__pyx_b, name);
- if (unlikely(!result)) {
- PyErr_Format(PyExc_NameError,
-#if PY_MAJOR_VERSION >= 3
- "name '%U' is not defined", name);
-#else
- "name '%.200s' is not defined", PyString_AS_STRING(name));
-#endif
- }
- return result;
-}
-
-/* MemviewSliceInit */
-static int
-__Pyx_init_memviewslice(struct __pyx_memoryview_obj *memview,
- int ndim,
- __Pyx_memviewslice *memviewslice,
- int memview_is_new_reference)
-{
- __Pyx_RefNannyDeclarations
- int i, retval=-1;
- Py_buffer *buf = &memview->view;
- __Pyx_RefNannySetupContext("init_memviewslice", 0);
- if (unlikely(memviewslice->memview || memviewslice->data)) {
- PyErr_SetString(PyExc_ValueError,
- "memviewslice is already initialized!");
- goto fail;
- }
- if (buf->strides) {
- for (i = 0; i < ndim; i++) {
- memviewslice->strides[i] = buf->strides[i];
- }
- } else {
- Py_ssize_t stride = buf->itemsize;
- for (i = ndim - 1; i >= 0; i--) {
- memviewslice->strides[i] = stride;
- stride *= buf->shape[i];
- }
- }
- for (i = 0; i < ndim; i++) {
- memviewslice->shape[i] = buf->shape[i];
- if (buf->suboffsets) {
- memviewslice->suboffsets[i] = buf->suboffsets[i];
- } else {
- memviewslice->suboffsets[i] = -1;
- }
- }
- memviewslice->memview = memview;
- memviewslice->data = (char *)buf->buf;
- if (__pyx_add_acquisition_count(memview) == 0 && !memview_is_new_reference) {
- Py_INCREF(memview);
- }
- retval = 0;
- goto no_fail;
-fail:
- memviewslice->memview = 0;
- memviewslice->data = 0;
- retval = -1;
-no_fail:
- __Pyx_RefNannyFinishContext();
- return retval;
-}
-#ifndef Py_NO_RETURN
-#define Py_NO_RETURN
-#endif
-static void __pyx_fatalerror(const char *fmt, ...) Py_NO_RETURN {
- va_list vargs;
- char msg[200];
-#ifdef HAVE_STDARG_PROTOTYPES
- va_start(vargs, fmt);
-#else
- va_start(vargs);
-#endif
- vsnprintf(msg, 200, fmt, vargs);
- va_end(vargs);
- Py_FatalError(msg);
-}
-static CYTHON_INLINE int
-__pyx_add_acquisition_count_locked(__pyx_atomic_int *acquisition_count,
- PyThread_type_lock lock)
-{
- int result;
- PyThread_acquire_lock(lock, 1);
- result = (*acquisition_count)++;
- PyThread_release_lock(lock);
- return result;
-}
-static CYTHON_INLINE int
-__pyx_sub_acquisition_count_locked(__pyx_atomic_int *acquisition_count,
- PyThread_type_lock lock)
-{
- int result;
- PyThread_acquire_lock(lock, 1);
- result = (*acquisition_count)--;
- PyThread_release_lock(lock);
- return result;
-}
-static CYTHON_INLINE void
-__Pyx_INC_MEMVIEW(__Pyx_memviewslice *memslice, int have_gil, int lineno)
-{
- int first_time;
- struct __pyx_memoryview_obj *memview = memslice->memview;
- if (unlikely(!memview || (PyObject *) memview == Py_None))
- return;
- if (unlikely(__pyx_get_slice_count(memview) < 0))
- __pyx_fatalerror("Acquisition count is %d (line %d)",
- __pyx_get_slice_count(memview), lineno);
- first_time = __pyx_add_acquisition_count(memview) == 0;
- if (unlikely(first_time)) {
- if (have_gil) {
- Py_INCREF((PyObject *) memview);
- } else {
- PyGILState_STATE _gilstate = PyGILState_Ensure();
- Py_INCREF((PyObject *) memview);
- PyGILState_Release(_gilstate);
- }
- }
-}
-static CYTHON_INLINE void __Pyx_XDEC_MEMVIEW(__Pyx_memviewslice *memslice,
- int have_gil, int lineno) {
- int last_time;
- struct __pyx_memoryview_obj *memview = memslice->memview;
- if (unlikely(!memview || (PyObject *) memview == Py_None)) {
- memslice->memview = NULL;
- return;
- }
- if (unlikely(__pyx_get_slice_count(memview) <= 0))
- __pyx_fatalerror("Acquisition count is %d (line %d)",
- __pyx_get_slice_count(memview), lineno);
- last_time = __pyx_sub_acquisition_count(memview) == 1;
- memslice->data = NULL;
- if (unlikely(last_time)) {
- if (have_gil) {
- Py_CLEAR(memslice->memview);
- } else {
- PyGILState_STATE _gilstate = PyGILState_Ensure();
- Py_CLEAR(memslice->memview);
- PyGILState_Release(_gilstate);
- }
- } else {
- memslice->memview = NULL;
- }
-}
-
-/* RaiseArgTupleInvalid */
-static void __Pyx_RaiseArgtupleInvalid(
- const char* func_name,
- int exact,
- Py_ssize_t num_min,
- Py_ssize_t num_max,
- Py_ssize_t num_found)
-{
- Py_ssize_t num_expected;
- const char *more_or_less;
- if (num_found < num_min) {
- num_expected = num_min;
- more_or_less = "at least";
- } else {
- num_expected = num_max;
- more_or_less = "at most";
- }
- if (exact) {
- more_or_less = "exactly";
- }
- PyErr_Format(PyExc_TypeError,
- "%.200s() takes %.8s %" CYTHON_FORMAT_SSIZE_T "d positional argument%.1s (%" CYTHON_FORMAT_SSIZE_T "d given)",
- func_name, more_or_less, num_expected,
- (num_expected == 1) ? "" : "s", num_found);
-}
-
-/* RaiseDoubleKeywords */
-static void __Pyx_RaiseDoubleKeywordsError(
- const char* func_name,
- PyObject* kw_name)
-{
- PyErr_Format(PyExc_TypeError,
- #if PY_MAJOR_VERSION >= 3
- "%s() got multiple values for keyword argument '%U'", func_name, kw_name);
- #else
- "%s() got multiple values for keyword argument '%s'", func_name,
- PyString_AsString(kw_name));
- #endif
-}
-
-/* ParseKeywords */
-static int __Pyx_ParseOptionalKeywords(
- PyObject *kwds,
- PyObject **argnames[],
- PyObject *kwds2,
- PyObject *values[],
- Py_ssize_t num_pos_args,
- const char* function_name)
-{
- PyObject *key = 0, *value = 0;
- Py_ssize_t pos = 0;
- PyObject*** name;
- PyObject*** first_kw_arg = argnames + num_pos_args;
- while (PyDict_Next(kwds, &pos, &key, &value)) {
- name = first_kw_arg;
- while (*name && (**name != key)) name++;
- if (*name) {
- values[name-argnames] = value;
- continue;
- }
- name = first_kw_arg;
- #if PY_MAJOR_VERSION < 3
- if (likely(PyString_Check(key))) {
- while (*name) {
- if ((CYTHON_COMPILING_IN_PYPY || PyString_GET_SIZE(**name) == PyString_GET_SIZE(key))
- && _PyString_Eq(**name, key)) {
- values[name-argnames] = value;
- break;
- }
- name++;
- }
- if (*name) continue;
- else {
- PyObject*** argname = argnames;
- while (argname != first_kw_arg) {
- if ((**argname == key) || (
- (CYTHON_COMPILING_IN_PYPY || PyString_GET_SIZE(**argname) == PyString_GET_SIZE(key))
- && _PyString_Eq(**argname, key))) {
- goto arg_passed_twice;
- }
- argname++;
- }
- }
- } else
- #endif
- if (likely(PyUnicode_Check(key))) {
- while (*name) {
- int cmp = (**name == key) ? 0 :
- #if !CYTHON_COMPILING_IN_PYPY && PY_MAJOR_VERSION >= 3
- (__Pyx_PyUnicode_GET_LENGTH(**name) != __Pyx_PyUnicode_GET_LENGTH(key)) ? 1 :
- #endif
- PyUnicode_Compare(**name, key);
- if (cmp < 0 && unlikely(PyErr_Occurred())) goto bad;
- if (cmp == 0) {
- values[name-argnames] = value;
- break;
- }
- name++;
- }
- if (*name) continue;
- else {
- PyObject*** argname = argnames;
- while (argname != first_kw_arg) {
- int cmp = (**argname == key) ? 0 :
- #if !CYTHON_COMPILING_IN_PYPY && PY_MAJOR_VERSION >= 3
- (__Pyx_PyUnicode_GET_LENGTH(**argname) != __Pyx_PyUnicode_GET_LENGTH(key)) ? 1 :
- #endif
- PyUnicode_Compare(**argname, key);
- if (cmp < 0 && unlikely(PyErr_Occurred())) goto bad;
- if (cmp == 0) goto arg_passed_twice;
- argname++;
- }
- }
- } else
- goto invalid_keyword_type;
- if (kwds2) {
- if (unlikely(PyDict_SetItem(kwds2, key, value))) goto bad;
- } else {
- goto invalid_keyword;
- }
- }
- return 0;
-arg_passed_twice:
- __Pyx_RaiseDoubleKeywordsError(function_name, key);
- goto bad;
-invalid_keyword_type:
- PyErr_Format(PyExc_TypeError,
- "%.200s() keywords must be strings", function_name);
- goto bad;
-invalid_keyword:
- PyErr_Format(PyExc_TypeError,
- #if PY_MAJOR_VERSION < 3
- "%.200s() got an unexpected keyword argument '%.200s'",
- function_name, PyString_AsString(key));
- #else
- "%s() got an unexpected keyword argument '%U'",
- function_name, key);
- #endif
-bad:
- return -1;
-}
-
-/* None */
-static CYTHON_INLINE void __Pyx_RaiseUnboundLocalError(const char *varname) {
- PyErr_Format(PyExc_UnboundLocalError, "local variable '%s' referenced before assignment", varname);
-}
-
-/* ArgTypeTest */
-static int __Pyx__ArgTypeTest(PyObject *obj, PyTypeObject *type, const char *name, int exact)
-{
- if (unlikely(!type)) {
- PyErr_SetString(PyExc_SystemError, "Missing type object");
- return 0;
- }
- else if (exact) {
- #if PY_MAJOR_VERSION == 2
- if ((type == &PyBaseString_Type) && likely(__Pyx_PyBaseString_CheckExact(obj))) return 1;
- #endif
- }
- else {
- if (likely(__Pyx_TypeCheck(obj, type))) return 1;
- }
- PyErr_Format(PyExc_TypeError,
- "Argument '%.200s' has incorrect type (expected %.200s, got %.200s)",
- name, type->tp_name, Py_TYPE(obj)->tp_name);
- return 0;
-}
-
-/* PyObjectCall */
-#if CYTHON_COMPILING_IN_CPYTHON
-static CYTHON_INLINE PyObject* __Pyx_PyObject_Call(PyObject *func, PyObject *arg, PyObject *kw) {
- PyObject *result;
- ternaryfunc call = func->ob_type->tp_call;
- if (unlikely(!call))
- return PyObject_Call(func, arg, kw);
- if (unlikely(Py_EnterRecursiveCall((char*)" while calling a Python object")))
- return NULL;
- result = (*call)(func, arg, kw);
- Py_LeaveRecursiveCall();
- if (unlikely(!result) && unlikely(!PyErr_Occurred())) {
- PyErr_SetString(
- PyExc_SystemError,
- "NULL result without error in PyObject_Call");
- }
- return result;
-}
-#endif
-
-/* PyErrFetchRestore */
-#if CYTHON_FAST_THREAD_STATE
-static CYTHON_INLINE void __Pyx_ErrRestoreInState(PyThreadState *tstate, PyObject *type, PyObject *value, PyObject *tb) {
- PyObject *tmp_type, *tmp_value, *tmp_tb;
- tmp_type = tstate->curexc_type;
- tmp_value = tstate->curexc_value;
- tmp_tb = tstate->curexc_traceback;
- tstate->curexc_type = type;
- tstate->curexc_value = value;
- tstate->curexc_traceback = tb;
- Py_XDECREF(tmp_type);
- Py_XDECREF(tmp_value);
- Py_XDECREF(tmp_tb);
-}
-static CYTHON_INLINE void __Pyx_ErrFetchInState(PyThreadState *tstate, PyObject **type, PyObject **value, PyObject **tb) {
- *type = tstate->curexc_type;
- *value = tstate->curexc_value;
- *tb = tstate->curexc_traceback;
- tstate->curexc_type = 0;
- tstate->curexc_value = 0;
- tstate->curexc_traceback = 0;
-}
-#endif
-
-/* RaiseException */
-#if PY_MAJOR_VERSION < 3
-static void __Pyx_Raise(PyObject *type, PyObject *value, PyObject *tb,
- CYTHON_UNUSED PyObject *cause) {
- __Pyx_PyThreadState_declare
- Py_XINCREF(type);
- if (!value || value == Py_None)
- value = NULL;
- else
- Py_INCREF(value);
- if (!tb || tb == Py_None)
- tb = NULL;
- else {
- Py_INCREF(tb);
- if (!PyTraceBack_Check(tb)) {
- PyErr_SetString(PyExc_TypeError,
- "raise: arg 3 must be a traceback or None");
- goto raise_error;
- }
- }
- if (PyType_Check(type)) {
-#if CYTHON_COMPILING_IN_PYPY
- if (!value) {
- Py_INCREF(Py_None);
- value = Py_None;
- }
-#endif
- PyErr_NormalizeException(&type, &value, &tb);
- } else {
- if (value) {
- PyErr_SetString(PyExc_TypeError,
- "instance exception may not have a separate value");
- goto raise_error;
- }
- value = type;
- type = (PyObject*) Py_TYPE(type);
- Py_INCREF(type);
- if (!PyType_IsSubtype((PyTypeObject *)type, (PyTypeObject *)PyExc_BaseException)) {
- PyErr_SetString(PyExc_TypeError,
- "raise: exception class must be a subclass of BaseException");
- goto raise_error;
- }
- }
- __Pyx_PyThreadState_assign
- __Pyx_ErrRestore(type, value, tb);
- return;
-raise_error:
- Py_XDECREF(value);
- Py_XDECREF(type);
- Py_XDECREF(tb);
- return;
-}
-#else
-static void __Pyx_Raise(PyObject *type, PyObject *value, PyObject *tb, PyObject *cause) {
- PyObject* owned_instance = NULL;
- if (tb == Py_None) {
- tb = 0;
- } else if (tb && !PyTraceBack_Check(tb)) {
- PyErr_SetString(PyExc_TypeError,
- "raise: arg 3 must be a traceback or None");
- goto bad;
- }
- if (value == Py_None)
- value = 0;
- if (PyExceptionInstance_Check(type)) {
- if (value) {
- PyErr_SetString(PyExc_TypeError,
- "instance exception may not have a separate value");
- goto bad;
- }
- value = type;
- type = (PyObject*) Py_TYPE(value);
- } else if (PyExceptionClass_Check(type)) {
- PyObject *instance_class = NULL;
- if (value && PyExceptionInstance_Check(value)) {
- instance_class = (PyObject*) Py_TYPE(value);
- if (instance_class != type) {
- int is_subclass = PyObject_IsSubclass(instance_class, type);
- if (!is_subclass) {
- instance_class = NULL;
- } else if (unlikely(is_subclass == -1)) {
- goto bad;
- } else {
- type = instance_class;
- }
- }
- }
- if (!instance_class) {
- PyObject *args;
- if (!value)
- args = PyTuple_New(0);
- else if (PyTuple_Check(value)) {
- Py_INCREF(value);
- args = value;
- } else
- args = PyTuple_Pack(1, value);
- if (!args)
- goto bad;
- owned_instance = PyObject_Call(type, args, NULL);
- Py_DECREF(args);
- if (!owned_instance)
- goto bad;
- value = owned_instance;
- if (!PyExceptionInstance_Check(value)) {
- PyErr_Format(PyExc_TypeError,
- "calling %R should have returned an instance of "
- "BaseException, not %R",
- type, Py_TYPE(value));
- goto bad;
- }
- }
- } else {
- PyErr_SetString(PyExc_TypeError,
- "raise: exception class must be a subclass of BaseException");
- goto bad;
- }
- if (cause) {
- PyObject *fixed_cause;
- if (cause == Py_None) {
- fixed_cause = NULL;
- } else if (PyExceptionClass_Check(cause)) {
- fixed_cause = PyObject_CallObject(cause, NULL);
- if (fixed_cause == NULL)
- goto bad;
- } else if (PyExceptionInstance_Check(cause)) {
- fixed_cause = cause;
- Py_INCREF(fixed_cause);
- } else {
- PyErr_SetString(PyExc_TypeError,
- "exception causes must derive from "
- "BaseException");
- goto bad;
- }
- PyException_SetCause(value, fixed_cause);
- }
- PyErr_SetObject(type, value);
- if (tb) {
-#if CYTHON_COMPILING_IN_PYPY
- PyObject *tmp_type, *tmp_value, *tmp_tb;
- PyErr_Fetch(&tmp_type, &tmp_value, &tmp_tb);
- Py_INCREF(tb);
- PyErr_Restore(tmp_type, tmp_value, tb);
- Py_XDECREF(tmp_tb);
-#else
- PyThreadState *tstate = __Pyx_PyThreadState_Current;
- PyObject* tmp_tb = tstate->curexc_traceback;
- if (tb != tmp_tb) {
- Py_INCREF(tb);
- tstate->curexc_traceback = tb;
- Py_XDECREF(tmp_tb);
- }
-#endif
- }
-bad:
- Py_XDECREF(owned_instance);
- return;
-}
-#endif
-
-/* PyCFunctionFastCall */
-#if CYTHON_FAST_PYCCALL
-static CYTHON_INLINE PyObject * __Pyx_PyCFunction_FastCall(PyObject *func_obj, PyObject **args, Py_ssize_t nargs) {
- PyCFunctionObject *func = (PyCFunctionObject*)func_obj;
- PyCFunction meth = PyCFunction_GET_FUNCTION(func);
- PyObject *self = PyCFunction_GET_SELF(func);
- int flags = PyCFunction_GET_FLAGS(func);
- assert(PyCFunction_Check(func));
- assert(METH_FASTCALL == (flags & ~(METH_CLASS | METH_STATIC | METH_COEXIST | METH_KEYWORDS | METH_STACKLESS)));
- assert(nargs >= 0);
- assert(nargs == 0 || args != NULL);
- /* _PyCFunction_FastCallDict() must not be called with an exception set,
- because it may clear it (directly or indirectly) and so the
- caller loses its exception */
- assert(!PyErr_Occurred());
- if ((PY_VERSION_HEX < 0x030700A0) || unlikely(flags & METH_KEYWORDS)) {
- return (*((__Pyx_PyCFunctionFastWithKeywords)(void*)meth)) (self, args, nargs, NULL);
- } else {
- return (*((__Pyx_PyCFunctionFast)(void*)meth)) (self, args, nargs);
- }
-}
-#endif
-
-/* PyFunctionFastCall */
-#if CYTHON_FAST_PYCALL
-static PyObject* __Pyx_PyFunction_FastCallNoKw(PyCodeObject *co, PyObject **args, Py_ssize_t na,
- PyObject *globals) {
- PyFrameObject *f;
- PyThreadState *tstate = __Pyx_PyThreadState_Current;
- PyObject **fastlocals;
- Py_ssize_t i;
- PyObject *result;
- assert(globals != NULL);
- /* XXX Perhaps we should create a specialized
- PyFrame_New() that doesn't take locals, but does
- take builtins without sanity checking them.
- */
- assert(tstate != NULL);
- f = PyFrame_New(tstate, co, globals, NULL);
- if (f == NULL) {
- return NULL;
- }
- fastlocals = __Pyx_PyFrame_GetLocalsplus(f);
- for (i = 0; i < na; i++) {
- Py_INCREF(*args);
- fastlocals[i] = *args++;
- }
- result = PyEval_EvalFrameEx(f,0);
- ++tstate->recursion_depth;
- Py_DECREF(f);
- --tstate->recursion_depth;
- return result;
-}
-#if 1 || PY_VERSION_HEX < 0x030600B1
-static PyObject *__Pyx_PyFunction_FastCallDict(PyObject *func, PyObject **args, Py_ssize_t nargs, PyObject *kwargs) {
- PyCodeObject *co = (PyCodeObject *)PyFunction_GET_CODE(func);
- PyObject *globals = PyFunction_GET_GLOBALS(func);
- PyObject *argdefs = PyFunction_GET_DEFAULTS(func);
- PyObject *closure;
-#if PY_MAJOR_VERSION >= 3
- PyObject *kwdefs;
-#endif
- PyObject *kwtuple, **k;
- PyObject **d;
- Py_ssize_t nd;
- Py_ssize_t nk;
- PyObject *result;
- assert(kwargs == NULL || PyDict_Check(kwargs));
- nk = kwargs ? PyDict_Size(kwargs) : 0;
- if (Py_EnterRecursiveCall((char*)" while calling a Python object")) {
- return NULL;
- }
- if (
-#if PY_MAJOR_VERSION >= 3
- co->co_kwonlyargcount == 0 &&
-#endif
- likely(kwargs == NULL || nk == 0) &&
- co->co_flags == (CO_OPTIMIZED | CO_NEWLOCALS | CO_NOFREE)) {
- if (argdefs == NULL && co->co_argcount == nargs) {
- result = __Pyx_PyFunction_FastCallNoKw(co, args, nargs, globals);
- goto done;
- }
- else if (nargs == 0 && argdefs != NULL
- && co->co_argcount == Py_SIZE(argdefs)) {
- /* function called with no arguments, but all parameters have
- a default value: use default values as arguments .*/
- args = &PyTuple_GET_ITEM(argdefs, 0);
- result =__Pyx_PyFunction_FastCallNoKw(co, args, Py_SIZE(argdefs), globals);
- goto done;
- }
- }
- if (kwargs != NULL) {
- Py_ssize_t pos, i;
- kwtuple = PyTuple_New(2 * nk);
- if (kwtuple == NULL) {
- result = NULL;
- goto done;
- }
- k = &PyTuple_GET_ITEM(kwtuple, 0);
- pos = i = 0;
- while (PyDict_Next(kwargs, &pos, &k[i], &k[i+1])) {
- Py_INCREF(k[i]);
- Py_INCREF(k[i+1]);
- i += 2;
- }
- nk = i / 2;
- }
- else {
- kwtuple = NULL;
- k = NULL;
- }
- closure = PyFunction_GET_CLOSURE(func);
-#if PY_MAJOR_VERSION >= 3
- kwdefs = PyFunction_GET_KW_DEFAULTS(func);
-#endif
- if (argdefs != NULL) {
- d = &PyTuple_GET_ITEM(argdefs, 0);
- nd = Py_SIZE(argdefs);
- }
- else {
- d = NULL;
- nd = 0;
- }
-#if PY_MAJOR_VERSION >= 3
- result = PyEval_EvalCodeEx((PyObject*)co, globals, (PyObject *)NULL,
- args, (int)nargs,
- k, (int)nk,
- d, (int)nd, kwdefs, closure);
-#else
- result = PyEval_EvalCodeEx(co, globals, (PyObject *)NULL,
- args, (int)nargs,
- k, (int)nk,
- d, (int)nd, closure);
-#endif
- Py_XDECREF(kwtuple);
-done:
- Py_LeaveRecursiveCall();
- return result;
-}
-#endif
-#endif
-
-/* PyObjectCall2Args */
-static CYTHON_UNUSED PyObject* __Pyx_PyObject_Call2Args(PyObject* function, PyObject* arg1, PyObject* arg2) {
- PyObject *args, *result = NULL;
- #if CYTHON_FAST_PYCALL
- if (PyFunction_Check(function)) {
- PyObject *args[2] = {arg1, arg2};
- return __Pyx_PyFunction_FastCall(function, args, 2);
- }
- #endif
- #if CYTHON_FAST_PYCCALL
- if (__Pyx_PyFastCFunction_Check(function)) {
- PyObject *args[2] = {arg1, arg2};
- return __Pyx_PyCFunction_FastCall(function, args, 2);
- }
- #endif
- args = PyTuple_New(2);
- if (unlikely(!args)) goto done;
- Py_INCREF(arg1);
- PyTuple_SET_ITEM(args, 0, arg1);
- Py_INCREF(arg2);
- PyTuple_SET_ITEM(args, 1, arg2);
- Py_INCREF(function);
- result = __Pyx_PyObject_Call(function, args, NULL);
- Py_DECREF(args);
- Py_DECREF(function);
-done:
- return result;
-}
-
-/* PyObjectCallMethO */
-#if CYTHON_COMPILING_IN_CPYTHON
-static CYTHON_INLINE PyObject* __Pyx_PyObject_CallMethO(PyObject *func, PyObject *arg) {
- PyObject *self, *result;
- PyCFunction cfunc;
- cfunc = PyCFunction_GET_FUNCTION(func);
- self = PyCFunction_GET_SELF(func);
- if (unlikely(Py_EnterRecursiveCall((char*)" while calling a Python object")))
- return NULL;
- result = cfunc(self, arg);
- Py_LeaveRecursiveCall();
- if (unlikely(!result) && unlikely(!PyErr_Occurred())) {
- PyErr_SetString(
- PyExc_SystemError,
- "NULL result without error in PyObject_Call");
- }
- return result;
-}
-#endif
-
-/* PyObjectCallOneArg */
-#if CYTHON_COMPILING_IN_CPYTHON
-static PyObject* __Pyx__PyObject_CallOneArg(PyObject *func, PyObject *arg) {
- PyObject *result;
- PyObject *args = PyTuple_New(1);
- if (unlikely(!args)) return NULL;
- Py_INCREF(arg);
- PyTuple_SET_ITEM(args, 0, arg);
- result = __Pyx_PyObject_Call(func, args, NULL);
- Py_DECREF(args);
- return result;
-}
-static CYTHON_INLINE PyObject* __Pyx_PyObject_CallOneArg(PyObject *func, PyObject *arg) {
-#if CYTHON_FAST_PYCALL
- if (PyFunction_Check(func)) {
- return __Pyx_PyFunction_FastCall(func, &arg, 1);
- }
-#endif
- if (likely(PyCFunction_Check(func))) {
- if (likely(PyCFunction_GET_FLAGS(func) & METH_O)) {
- return __Pyx_PyObject_CallMethO(func, arg);
-#if CYTHON_FAST_PYCCALL
- } else if (PyCFunction_GET_FLAGS(func) & METH_FASTCALL) {
- return __Pyx_PyCFunction_FastCall(func, &arg, 1);
-#endif
- }
- }
- return __Pyx__PyObject_CallOneArg(func, arg);
-}
-#else
-static CYTHON_INLINE PyObject* __Pyx_PyObject_CallOneArg(PyObject *func, PyObject *arg) {
- PyObject *result;
- PyObject *args = PyTuple_Pack(1, arg);
- if (unlikely(!args)) return NULL;
- result = __Pyx_PyObject_Call(func, args, NULL);
- Py_DECREF(args);
- return result;
-}
-#endif
-
-/* BytesEquals */
-static CYTHON_INLINE int __Pyx_PyBytes_Equals(PyObject* s1, PyObject* s2, int equals) {
-#if CYTHON_COMPILING_IN_PYPY
- return PyObject_RichCompareBool(s1, s2, equals);
-#else
- if (s1 == s2) {
- return (equals == Py_EQ);
- } else if (PyBytes_CheckExact(s1) & PyBytes_CheckExact(s2)) {
- const char *ps1, *ps2;
- Py_ssize_t length = PyBytes_GET_SIZE(s1);
- if (length != PyBytes_GET_SIZE(s2))
- return (equals == Py_NE);
- ps1 = PyBytes_AS_STRING(s1);
- ps2 = PyBytes_AS_STRING(s2);
- if (ps1[0] != ps2[0]) {
- return (equals == Py_NE);
- } else if (length == 1) {
- return (equals == Py_EQ);
- } else {
- int result;
-#if CYTHON_USE_UNICODE_INTERNALS
- Py_hash_t hash1, hash2;
- hash1 = ((PyBytesObject*)s1)->ob_shash;
- hash2 = ((PyBytesObject*)s2)->ob_shash;
- if (hash1 != hash2 && hash1 != -1 && hash2 != -1) {
- return (equals == Py_NE);
- }
-#endif
- result = memcmp(ps1, ps2, (size_t)length);
- return (equals == Py_EQ) ? (result == 0) : (result != 0);
- }
- } else if ((s1 == Py_None) & PyBytes_CheckExact(s2)) {
- return (equals == Py_NE);
- } else if ((s2 == Py_None) & PyBytes_CheckExact(s1)) {
- return (equals == Py_NE);
- } else {
- int result;
- PyObject* py_result = PyObject_RichCompare(s1, s2, equals);
- if (!py_result)
- return -1;
- result = __Pyx_PyObject_IsTrue(py_result);
- Py_DECREF(py_result);
- return result;
- }
-#endif
-}
-
-/* UnicodeEquals */
-static CYTHON_INLINE int __Pyx_PyUnicode_Equals(PyObject* s1, PyObject* s2, int equals) {
-#if CYTHON_COMPILING_IN_PYPY
- return PyObject_RichCompareBool(s1, s2, equals);
-#else
-#if PY_MAJOR_VERSION < 3
- PyObject* owned_ref = NULL;
-#endif
- int s1_is_unicode, s2_is_unicode;
- if (s1 == s2) {
- goto return_eq;
- }
- s1_is_unicode = PyUnicode_CheckExact(s1);
- s2_is_unicode = PyUnicode_CheckExact(s2);
-#if PY_MAJOR_VERSION < 3
- if ((s1_is_unicode & (!s2_is_unicode)) && PyString_CheckExact(s2)) {
- owned_ref = PyUnicode_FromObject(s2);
- if (unlikely(!owned_ref))
- return -1;
- s2 = owned_ref;
- s2_is_unicode = 1;
- } else if ((s2_is_unicode & (!s1_is_unicode)) && PyString_CheckExact(s1)) {
- owned_ref = PyUnicode_FromObject(s1);
- if (unlikely(!owned_ref))
- return -1;
- s1 = owned_ref;
- s1_is_unicode = 1;
- } else if (((!s2_is_unicode) & (!s1_is_unicode))) {
- return __Pyx_PyBytes_Equals(s1, s2, equals);
- }
-#endif
- if (s1_is_unicode & s2_is_unicode) {
- Py_ssize_t length;
- int kind;
- void *data1, *data2;
- if (unlikely(__Pyx_PyUnicode_READY(s1) < 0) || unlikely(__Pyx_PyUnicode_READY(s2) < 0))
- return -1;
- length = __Pyx_PyUnicode_GET_LENGTH(s1);
- if (length != __Pyx_PyUnicode_GET_LENGTH(s2)) {
- goto return_ne;
- }
-#if CYTHON_USE_UNICODE_INTERNALS
- {
- Py_hash_t hash1, hash2;
- #if CYTHON_PEP393_ENABLED
- hash1 = ((PyASCIIObject*)s1)->hash;
- hash2 = ((PyASCIIObject*)s2)->hash;
- #else
- hash1 = ((PyUnicodeObject*)s1)->hash;
- hash2 = ((PyUnicodeObject*)s2)->hash;
- #endif
- if (hash1 != hash2 && hash1 != -1 && hash2 != -1) {
- goto return_ne;
- }
- }
-#endif
- kind = __Pyx_PyUnicode_KIND(s1);
- if (kind != __Pyx_PyUnicode_KIND(s2)) {
- goto return_ne;
- }
- data1 = __Pyx_PyUnicode_DATA(s1);
- data2 = __Pyx_PyUnicode_DATA(s2);
- if (__Pyx_PyUnicode_READ(kind, data1, 0) != __Pyx_PyUnicode_READ(kind, data2, 0)) {
- goto return_ne;
- } else if (length == 1) {
- goto return_eq;
- } else {
- int result = memcmp(data1, data2, (size_t)(length * kind));
- #if PY_MAJOR_VERSION < 3
- Py_XDECREF(owned_ref);
- #endif
- return (equals == Py_EQ) ? (result == 0) : (result != 0);
- }
- } else if ((s1 == Py_None) & s2_is_unicode) {
- goto return_ne;
- } else if ((s2 == Py_None) & s1_is_unicode) {
- goto return_ne;
- } else {
- int result;
- PyObject* py_result = PyObject_RichCompare(s1, s2, equals);
- #if PY_MAJOR_VERSION < 3
- Py_XDECREF(owned_ref);
- #endif
- if (!py_result)
- return -1;
- result = __Pyx_PyObject_IsTrue(py_result);
- Py_DECREF(py_result);
- return result;
- }
-return_eq:
- #if PY_MAJOR_VERSION < 3
- Py_XDECREF(owned_ref);
- #endif
- return (equals == Py_EQ);
-return_ne:
- #if PY_MAJOR_VERSION < 3
- Py_XDECREF(owned_ref);
- #endif
- return (equals == Py_NE);
-#endif
-}
-
-/* None */
-static CYTHON_INLINE Py_ssize_t __Pyx_div_Py_ssize_t(Py_ssize_t a, Py_ssize_t b) {
- Py_ssize_t q = a / b;
- Py_ssize_t r = a - q*b;
- q -= ((r != 0) & ((r ^ b) < 0));
- return q;
-}
-
-/* GetAttr */
-static CYTHON_INLINE PyObject *__Pyx_GetAttr(PyObject *o, PyObject *n) {
-#if CYTHON_USE_TYPE_SLOTS
-#if PY_MAJOR_VERSION >= 3
- if (likely(PyUnicode_Check(n)))
-#else
- if (likely(PyString_Check(n)))
-#endif
- return __Pyx_PyObject_GetAttrStr(o, n);
-#endif
- return PyObject_GetAttr(o, n);
-}
-
-/* GetItemInt */
-static PyObject *__Pyx_GetItemInt_Generic(PyObject *o, PyObject* j) {
- PyObject *r;
- if (!j) return NULL;
- r = PyObject_GetItem(o, j);
- Py_DECREF(j);
- return r;
-}
-static CYTHON_INLINE PyObject *__Pyx_GetItemInt_List_Fast(PyObject *o, Py_ssize_t i,
- CYTHON_NCP_UNUSED int wraparound,
- CYTHON_NCP_UNUSED int boundscheck) {
-#if CYTHON_ASSUME_SAFE_MACROS && !CYTHON_AVOID_BORROWED_REFS
- Py_ssize_t wrapped_i = i;
- if (wraparound & unlikely(i < 0)) {
- wrapped_i += PyList_GET_SIZE(o);
- }
- if ((!boundscheck) || likely(__Pyx_is_valid_index(wrapped_i, PyList_GET_SIZE(o)))) {
- PyObject *r = PyList_GET_ITEM(o, wrapped_i);
- Py_INCREF(r);
- return r;
- }
- return __Pyx_GetItemInt_Generic(o, PyInt_FromSsize_t(i));
-#else
- return PySequence_GetItem(o, i);
-#endif
-}
-static CYTHON_INLINE PyObject *__Pyx_GetItemInt_Tuple_Fast(PyObject *o, Py_ssize_t i,
- CYTHON_NCP_UNUSED int wraparound,
- CYTHON_NCP_UNUSED int boundscheck) {
-#if CYTHON_ASSUME_SAFE_MACROS && !CYTHON_AVOID_BORROWED_REFS
- Py_ssize_t wrapped_i = i;
- if (wraparound & unlikely(i < 0)) {
- wrapped_i += PyTuple_GET_SIZE(o);
- }
- if ((!boundscheck) || likely(__Pyx_is_valid_index(wrapped_i, PyTuple_GET_SIZE(o)))) {
- PyObject *r = PyTuple_GET_ITEM(o, wrapped_i);
- Py_INCREF(r);
- return r;
- }
- return __Pyx_GetItemInt_Generic(o, PyInt_FromSsize_t(i));
-#else
- return PySequence_GetItem(o, i);
-#endif
-}
-static CYTHON_INLINE PyObject *__Pyx_GetItemInt_Fast(PyObject *o, Py_ssize_t i, int is_list,
- CYTHON_NCP_UNUSED int wraparound,
- CYTHON_NCP_UNUSED int boundscheck) {
-#if CYTHON_ASSUME_SAFE_MACROS && !CYTHON_AVOID_BORROWED_REFS && CYTHON_USE_TYPE_SLOTS
- if (is_list || PyList_CheckExact(o)) {
- Py_ssize_t n = ((!wraparound) | likely(i >= 0)) ? i : i + PyList_GET_SIZE(o);
- if ((!boundscheck) || (likely(__Pyx_is_valid_index(n, PyList_GET_SIZE(o))))) {
- PyObject *r = PyList_GET_ITEM(o, n);
- Py_INCREF(r);
- return r;
- }
- }
- else if (PyTuple_CheckExact(o)) {
- Py_ssize_t n = ((!wraparound) | likely(i >= 0)) ? i : i + PyTuple_GET_SIZE(o);
- if ((!boundscheck) || likely(__Pyx_is_valid_index(n, PyTuple_GET_SIZE(o)))) {
- PyObject *r = PyTuple_GET_ITEM(o, n);
- Py_INCREF(r);
- return r;
- }
- } else {
- PySequenceMethods *m = Py_TYPE(o)->tp_as_sequence;
- if (likely(m && m->sq_item)) {
- if (wraparound && unlikely(i < 0) && likely(m->sq_length)) {
- Py_ssize_t l = m->sq_length(o);
- if (likely(l >= 0)) {
- i += l;
- } else {
- if (!PyErr_ExceptionMatches(PyExc_OverflowError))
- return NULL;
- PyErr_Clear();
- }
- }
- return m->sq_item(o, i);
- }
- }
-#else
- if (is_list || PySequence_Check(o)) {
- return PySequence_GetItem(o, i);
- }
-#endif
- return __Pyx_GetItemInt_Generic(o, PyInt_FromSsize_t(i));
-}
-
-/* ObjectGetItem */
-#if CYTHON_USE_TYPE_SLOTS
-static PyObject *__Pyx_PyObject_GetIndex(PyObject *obj, PyObject* index) {
- PyObject *runerr;
- Py_ssize_t key_value;
- PySequenceMethods *m = Py_TYPE(obj)->tp_as_sequence;
- if (unlikely(!(m && m->sq_item))) {
- PyErr_Format(PyExc_TypeError, "'%.200s' object is not subscriptable", Py_TYPE(obj)->tp_name);
- return NULL;
- }
- key_value = __Pyx_PyIndex_AsSsize_t(index);
- if (likely(key_value != -1 || !(runerr = PyErr_Occurred()))) {
- return __Pyx_GetItemInt_Fast(obj, key_value, 0, 1, 1);
- }
- if (PyErr_GivenExceptionMatches(runerr, PyExc_OverflowError)) {
- PyErr_Clear();
- PyErr_Format(PyExc_IndexError, "cannot fit '%.200s' into an index-sized integer", Py_TYPE(index)->tp_name);
- }
- return NULL;
-}
-static PyObject *__Pyx_PyObject_GetItem(PyObject *obj, PyObject* key) {
- PyMappingMethods *m = Py_TYPE(obj)->tp_as_mapping;
- if (likely(m && m->mp_subscript)) {
- return m->mp_subscript(obj, key);
- }
- return __Pyx_PyObject_GetIndex(obj, key);
-}
-#endif
-
-/* decode_c_string */
-static CYTHON_INLINE PyObject* __Pyx_decode_c_string(
- const char* cstring, Py_ssize_t start, Py_ssize_t stop,
- const char* encoding, const char* errors,
- PyObject* (*decode_func)(const char *s, Py_ssize_t size, const char *errors)) {
- Py_ssize_t length;
- if (unlikely((start < 0) | (stop < 0))) {
- size_t slen = strlen(cstring);
- if (unlikely(slen > (size_t) PY_SSIZE_T_MAX)) {
- PyErr_SetString(PyExc_OverflowError,
- "c-string too long to convert to Python");
- return NULL;
- }
- length = (Py_ssize_t) slen;
- if (start < 0) {
- start += length;
- if (start < 0)
- start = 0;
- }
- if (stop < 0)
- stop += length;
- }
- if (unlikely(stop <= start))
- return __Pyx_NewRef(__pyx_empty_unicode);
- length = stop - start;
- cstring += start;
- if (decode_func) {
- return decode_func(cstring, length, errors);
- } else {
- return PyUnicode_Decode(cstring, length, encoding, errors);
- }
-}
-
-/* PyErrExceptionMatches */
-#if CYTHON_FAST_THREAD_STATE
-static int __Pyx_PyErr_ExceptionMatchesTuple(PyObject *exc_type, PyObject *tuple) {
- Py_ssize_t i, n;
- n = PyTuple_GET_SIZE(tuple);
-#if PY_MAJOR_VERSION >= 3
- for (i=0; icurexc_type;
- if (exc_type == err) return 1;
- if (unlikely(!exc_type)) return 0;
- if (unlikely(PyTuple_Check(err)))
- return __Pyx_PyErr_ExceptionMatchesTuple(exc_type, err);
- return __Pyx_PyErr_GivenExceptionMatches(exc_type, err);
-}
-#endif
-
-/* GetAttr3 */
-static PyObject *__Pyx_GetAttr3Default(PyObject *d) {
- __Pyx_PyThreadState_declare
- __Pyx_PyThreadState_assign
- if (unlikely(!__Pyx_PyErr_ExceptionMatches(PyExc_AttributeError)))
- return NULL;
- __Pyx_PyErr_Clear();
- Py_INCREF(d);
- return d;
-}
-static CYTHON_INLINE PyObject *__Pyx_GetAttr3(PyObject *o, PyObject *n, PyObject *d) {
- PyObject *r = __Pyx_GetAttr(o, n);
- return (likely(r)) ? r : __Pyx_GetAttr3Default(d);
-}
-
-/* PyDictVersioning */
-#if CYTHON_USE_DICT_VERSIONS && CYTHON_USE_TYPE_SLOTS
-static CYTHON_INLINE PY_UINT64_T __Pyx_get_tp_dict_version(PyObject *obj) {
- PyObject *dict = Py_TYPE(obj)->tp_dict;
- return likely(dict) ? __PYX_GET_DICT_VERSION(dict) : 0;
-}
-static CYTHON_INLINE PY_UINT64_T __Pyx_get_object_dict_version(PyObject *obj) {
- PyObject **dictptr = NULL;
- Py_ssize_t offset = Py_TYPE(obj)->tp_dictoffset;
- if (offset) {
-#if CYTHON_COMPILING_IN_CPYTHON
- dictptr = (likely(offset > 0)) ? (PyObject **) ((char *)obj + offset) : _PyObject_GetDictPtr(obj);
-#else
- dictptr = _PyObject_GetDictPtr(obj);
-#endif
- }
- return (dictptr && *dictptr) ? __PYX_GET_DICT_VERSION(*dictptr) : 0;
-}
-static CYTHON_INLINE int __Pyx_object_dict_version_matches(PyObject* obj, PY_UINT64_T tp_dict_version, PY_UINT64_T obj_dict_version) {
- PyObject *dict = Py_TYPE(obj)->tp_dict;
- if (unlikely(!dict) || unlikely(tp_dict_version != __PYX_GET_DICT_VERSION(dict)))
- return 0;
- return obj_dict_version == __Pyx_get_object_dict_version(obj);
-}
-#endif
-
-/* GetModuleGlobalName */
-#if CYTHON_USE_DICT_VERSIONS
-static PyObject *__Pyx__GetModuleGlobalName(PyObject *name, PY_UINT64_T *dict_version, PyObject **dict_cached_value)
-#else
-static CYTHON_INLINE PyObject *__Pyx__GetModuleGlobalName(PyObject *name)
-#endif
-{
- PyObject *result;
-#if !CYTHON_AVOID_BORROWED_REFS
-#if CYTHON_COMPILING_IN_CPYTHON && PY_VERSION_HEX >= 0x030500A1
- result = _PyDict_GetItem_KnownHash(__pyx_d, name, ((PyASCIIObject *) name)->hash);
- __PYX_UPDATE_DICT_CACHE(__pyx_d, result, *dict_cached_value, *dict_version)
- if (likely(result)) {
- return __Pyx_NewRef(result);
- } else if (unlikely(PyErr_Occurred())) {
- return NULL;
- }
-#else
- result = PyDict_GetItem(__pyx_d, name);
- __PYX_UPDATE_DICT_CACHE(__pyx_d, result, *dict_cached_value, *dict_version)
- if (likely(result)) {
- return __Pyx_NewRef(result);
- }
-#endif
-#else
- result = PyObject_GetItem(__pyx_d, name);
- __PYX_UPDATE_DICT_CACHE(__pyx_d, result, *dict_cached_value, *dict_version)
- if (likely(result)) {
- return __Pyx_NewRef(result);
- }
- PyErr_Clear();
-#endif
- return __Pyx_GetBuiltinName(name);
-}
-
-/* RaiseTooManyValuesToUnpack */
-static CYTHON_INLINE void __Pyx_RaiseTooManyValuesError(Py_ssize_t expected) {
- PyErr_Format(PyExc_ValueError,
- "too many values to unpack (expected %" CYTHON_FORMAT_SSIZE_T "d)", expected);
-}
-
-/* RaiseNeedMoreValuesToUnpack */
-static CYTHON_INLINE void __Pyx_RaiseNeedMoreValuesError(Py_ssize_t index) {
- PyErr_Format(PyExc_ValueError,
- "need more than %" CYTHON_FORMAT_SSIZE_T "d value%.1s to unpack",
- index, (index == 1) ? "" : "s");
-}
-
-/* RaiseNoneIterError */
-static CYTHON_INLINE void __Pyx_RaiseNoneNotIterableError(void) {
- PyErr_SetString(PyExc_TypeError, "'NoneType' object is not iterable");
-}
-
-/* ExtTypeTest */
-static CYTHON_INLINE int __Pyx_TypeTest(PyObject *obj, PyTypeObject *type) {
- if (unlikely(!type)) {
- PyErr_SetString(PyExc_SystemError, "Missing type object");
- return 0;
- }
- if (likely(__Pyx_TypeCheck(obj, type)))
- return 1;
- PyErr_Format(PyExc_TypeError, "Cannot convert %.200s to %.200s",
- Py_TYPE(obj)->tp_name, type->tp_name);
- return 0;
-}
-
-/* GetTopmostException */
-#if CYTHON_USE_EXC_INFO_STACK
-static _PyErr_StackItem *
-__Pyx_PyErr_GetTopmostException(PyThreadState *tstate)
-{
- _PyErr_StackItem *exc_info = tstate->exc_info;
- while ((exc_info->exc_type == NULL || exc_info->exc_type == Py_None) &&
- exc_info->previous_item != NULL)
- {
- exc_info = exc_info->previous_item;
- }
- return exc_info;
-}
-#endif
-
-/* SaveResetException */
-#if CYTHON_FAST_THREAD_STATE
-static CYTHON_INLINE void __Pyx__ExceptionSave(PyThreadState *tstate, PyObject **type, PyObject **value, PyObject **tb) {
- #if CYTHON_USE_EXC_INFO_STACK
- _PyErr_StackItem *exc_info = __Pyx_PyErr_GetTopmostException(tstate);
- *type = exc_info->exc_type;
- *value = exc_info->exc_value;
- *tb = exc_info->exc_traceback;
- #else
- *type = tstate->exc_type;
- *value = tstate->exc_value;
- *tb = tstate->exc_traceback;
- #endif
- Py_XINCREF(*type);
- Py_XINCREF(*value);
- Py_XINCREF(*tb);
-}
-static CYTHON_INLINE void __Pyx__ExceptionReset(PyThreadState *tstate, PyObject *type, PyObject *value, PyObject *tb) {
- PyObject *tmp_type, *tmp_value, *tmp_tb;
- #if CYTHON_USE_EXC_INFO_STACK
- _PyErr_StackItem *exc_info = tstate->exc_info;
- tmp_type = exc_info->exc_type;
- tmp_value = exc_info->exc_value;
- tmp_tb = exc_info->exc_traceback;
- exc_info->exc_type = type;
- exc_info->exc_value = value;
- exc_info->exc_traceback = tb;
- #else
- tmp_type = tstate->exc_type;
- tmp_value = tstate->exc_value;
- tmp_tb = tstate->exc_traceback;
- tstate->exc_type = type;
- tstate->exc_value = value;
- tstate->exc_traceback = tb;
- #endif
- Py_XDECREF(tmp_type);
- Py_XDECREF(tmp_value);
- Py_XDECREF(tmp_tb);
-}
-#endif
-
-/* GetException */
-#if CYTHON_FAST_THREAD_STATE
-static int __Pyx__GetException(PyThreadState *tstate, PyObject **type, PyObject **value, PyObject **tb)
-#else
-static int __Pyx_GetException(PyObject **type, PyObject **value, PyObject **tb)
-#endif
-{
- PyObject *local_type, *local_value, *local_tb;
-#if CYTHON_FAST_THREAD_STATE
- PyObject *tmp_type, *tmp_value, *tmp_tb;
- local_type = tstate->curexc_type;
- local_value = tstate->curexc_value;
- local_tb = tstate->curexc_traceback;
- tstate->curexc_type = 0;
- tstate->curexc_value = 0;
- tstate->curexc_traceback = 0;
-#else
- PyErr_Fetch(&local_type, &local_value, &local_tb);
-#endif
- PyErr_NormalizeException(&local_type, &local_value, &local_tb);
-#if CYTHON_FAST_THREAD_STATE
- if (unlikely(tstate->curexc_type))
-#else
- if (unlikely(PyErr_Occurred()))
-#endif
- goto bad;
- #if PY_MAJOR_VERSION >= 3
- if (local_tb) {
- if (unlikely(PyException_SetTraceback(local_value, local_tb) < 0))
- goto bad;
- }
- #endif
- Py_XINCREF(local_tb);
- Py_XINCREF(local_type);
- Py_XINCREF(local_value);
- *type = local_type;
- *value = local_value;
- *tb = local_tb;
-#if CYTHON_FAST_THREAD_STATE
- #if CYTHON_USE_EXC_INFO_STACK
- {
- _PyErr_StackItem *exc_info = tstate->exc_info;
- tmp_type = exc_info->exc_type;
- tmp_value = exc_info->exc_value;
- tmp_tb = exc_info->exc_traceback;
- exc_info->exc_type = local_type;
- exc_info->exc_value = local_value;
- exc_info->exc_traceback = local_tb;
- }
- #else
- tmp_type = tstate->exc_type;
- tmp_value = tstate->exc_value;
- tmp_tb = tstate->exc_traceback;
- tstate->exc_type = local_type;
- tstate->exc_value = local_value;
- tstate->exc_traceback = local_tb;
- #endif
- Py_XDECREF(tmp_type);
- Py_XDECREF(tmp_value);
- Py_XDECREF(tmp_tb);
-#else
- PyErr_SetExcInfo(local_type, local_value, local_tb);
-#endif
- return 0;
-bad:
- *type = 0;
- *value = 0;
- *tb = 0;
- Py_XDECREF(local_type);
- Py_XDECREF(local_value);
- Py_XDECREF(local_tb);
- return -1;
-}
-
-/* SwapException */
-#if CYTHON_FAST_THREAD_STATE
-static CYTHON_INLINE void __Pyx__ExceptionSwap(PyThreadState *tstate, PyObject **type, PyObject **value, PyObject **tb) {
- PyObject *tmp_type, *tmp_value, *tmp_tb;
- #if CYTHON_USE_EXC_INFO_STACK
- _PyErr_StackItem *exc_info = tstate->exc_info;
- tmp_type = exc_info->exc_type;
- tmp_value = exc_info->exc_value;
- tmp_tb = exc_info->exc_traceback;
- exc_info->exc_type = *type;
- exc_info->exc_value = *value;
- exc_info->exc_traceback = *tb;
- #else
- tmp_type = tstate->exc_type;
- tmp_value = tstate->exc_value;
- tmp_tb = tstate->exc_traceback;
- tstate->exc_type = *type;
- tstate->exc_value = *value;
- tstate->exc_traceback = *tb;
- #endif
- *type = tmp_type;
- *value = tmp_value;
- *tb = tmp_tb;
-}
-#else
-static CYTHON_INLINE void __Pyx_ExceptionSwap(PyObject **type, PyObject **value, PyObject **tb) {
- PyObject *tmp_type, *tmp_value, *tmp_tb;
- PyErr_GetExcInfo(&tmp_type, &tmp_value, &tmp_tb);
- PyErr_SetExcInfo(*type, *value, *tb);
- *type = tmp_type;
- *value = tmp_value;
- *tb = tmp_tb;
-}
-#endif
-
-/* Import */
-static PyObject *__Pyx_Import(PyObject *name, PyObject *from_list, int level) {
- PyObject *empty_list = 0;
- PyObject *module = 0;
- PyObject *global_dict = 0;
- PyObject *empty_dict = 0;
- PyObject *list;
- #if PY_MAJOR_VERSION < 3
- PyObject *py_import;
- py_import = __Pyx_PyObject_GetAttrStr(__pyx_b, __pyx_n_s_import);
- if (!py_import)
- goto bad;
- #endif
- if (from_list)
- list = from_list;
- else {
- empty_list = PyList_New(0);
- if (!empty_list)
- goto bad;
- list = empty_list;
- }
- global_dict = PyModule_GetDict(__pyx_m);
- if (!global_dict)
- goto bad;
- empty_dict = PyDict_New();
- if (!empty_dict)
- goto bad;
- {
- #if PY_MAJOR_VERSION >= 3
- if (level == -1) {
- if ((1) && (strchr(__Pyx_MODULE_NAME, '.'))) {
- module = PyImport_ImportModuleLevelObject(
- name, global_dict, empty_dict, list, 1);
- if (!module) {
- if (!PyErr_ExceptionMatches(PyExc_ImportError))
- goto bad;
- PyErr_Clear();
- }
- }
- level = 0;
- }
- #endif
- if (!module) {
- #if PY_MAJOR_VERSION < 3
- PyObject *py_level = PyInt_FromLong(level);
- if (!py_level)
- goto bad;
- module = PyObject_CallFunctionObjArgs(py_import,
- name, global_dict, empty_dict, list, py_level, (PyObject *)NULL);
- Py_DECREF(py_level);
- #else
- module = PyImport_ImportModuleLevelObject(
- name, global_dict, empty_dict, list, level);
- #endif
- }
- }
-bad:
- #if PY_MAJOR_VERSION < 3
- Py_XDECREF(py_import);
- #endif
- Py_XDECREF(empty_list);
- Py_XDECREF(empty_dict);
- return module;
-}
-
-/* FastTypeChecks */
-#if CYTHON_COMPILING_IN_CPYTHON
-static int __Pyx_InBases(PyTypeObject *a, PyTypeObject *b) {
- while (a) {
- a = a->tp_base;
- if (a == b)
- return 1;
- }
- return b == &PyBaseObject_Type;
-}
-static CYTHON_INLINE int __Pyx_IsSubtype(PyTypeObject *a, PyTypeObject *b) {
- PyObject *mro;
- if (a == b) return 1;
- mro = a->tp_mro;
- if (likely(mro)) {
- Py_ssize_t i, n;
- n = PyTuple_GET_SIZE(mro);
- for (i = 0; i < n; i++) {
- if (PyTuple_GET_ITEM(mro, i) == (PyObject *)b)
- return 1;
- }
- return 0;
- }
- return __Pyx_InBases(a, b);
-}
-#if PY_MAJOR_VERSION == 2
-static int __Pyx_inner_PyErr_GivenExceptionMatches2(PyObject *err, PyObject* exc_type1, PyObject* exc_type2) {
- PyObject *exception, *value, *tb;
- int res;
- __Pyx_PyThreadState_declare
- __Pyx_PyThreadState_assign
- __Pyx_ErrFetch(&exception, &value, &tb);
- res = exc_type1 ? PyObject_IsSubclass(err, exc_type1) : 0;
- if (unlikely(res == -1)) {
- PyErr_WriteUnraisable(err);
- res = 0;
- }
- if (!res) {
- res = PyObject_IsSubclass(err, exc_type2);
- if (unlikely(res == -1)) {
- PyErr_WriteUnraisable(err);
- res = 0;
- }
- }
- __Pyx_ErrRestore(exception, value, tb);
- return res;
-}
-#else
-static CYTHON_INLINE int __Pyx_inner_PyErr_GivenExceptionMatches2(PyObject *err, PyObject* exc_type1, PyObject *exc_type2) {
- int res = exc_type1 ? __Pyx_IsSubtype((PyTypeObject*)err, (PyTypeObject*)exc_type1) : 0;
- if (!res) {
- res = __Pyx_IsSubtype((PyTypeObject*)err, (PyTypeObject*)exc_type2);
- }
- return res;
-}
-#endif
-static int __Pyx_PyErr_GivenExceptionMatchesTuple(PyObject *exc_type, PyObject *tuple) {
- Py_ssize_t i, n;
- assert(PyExceptionClass_Check(exc_type));
- n = PyTuple_GET_SIZE(tuple);
-#if PY_MAJOR_VERSION >= 3
- for (i=0; i= 0 || (x^b) >= 0))
- return PyInt_FromLong(x);
- return PyLong_Type.tp_as_number->nb_add(op1, op2);
- }
- #endif
- #if CYTHON_USE_PYLONG_INTERNALS
- if (likely(PyLong_CheckExact(op1))) {
- const long b = intval;
- long a, x;
-#ifdef HAVE_LONG_LONG
- const PY_LONG_LONG llb = intval;
- PY_LONG_LONG lla, llx;
-#endif
- const digit* digits = ((PyLongObject*)op1)->ob_digit;
- const Py_ssize_t size = Py_SIZE(op1);
- if (likely(__Pyx_sst_abs(size) <= 1)) {
- a = likely(size) ? digits[0] : 0;
- if (size == -1) a = -a;
- } else {
- switch (size) {
- case -2:
- if (8 * sizeof(long) - 1 > 2 * PyLong_SHIFT) {
- a = -(long) (((((unsigned long)digits[1]) << PyLong_SHIFT) | (unsigned long)digits[0]));
- break;
-#ifdef HAVE_LONG_LONG
- } else if (8 * sizeof(PY_LONG_LONG) - 1 > 2 * PyLong_SHIFT) {
- lla = -(PY_LONG_LONG) (((((unsigned PY_LONG_LONG)digits[1]) << PyLong_SHIFT) | (unsigned PY_LONG_LONG)digits[0]));
- goto long_long;
-#endif
- }
- CYTHON_FALLTHROUGH;
- case 2:
- if (8 * sizeof(long) - 1 > 2 * PyLong_SHIFT) {
- a = (long) (((((unsigned long)digits[1]) << PyLong_SHIFT) | (unsigned long)digits[0]));
- break;
-#ifdef HAVE_LONG_LONG
- } else if (8 * sizeof(PY_LONG_LONG) - 1 > 2 * PyLong_SHIFT) {
- lla = (PY_LONG_LONG) (((((unsigned PY_LONG_LONG)digits[1]) << PyLong_SHIFT) | (unsigned PY_LONG_LONG)digits[0]));
- goto long_long;
-#endif
- }
- CYTHON_FALLTHROUGH;
- case -3:
- if (8 * sizeof(long) - 1 > 3 * PyLong_SHIFT) {
- a = -(long) (((((((unsigned long)digits[2]) << PyLong_SHIFT) | (unsigned long)digits[1]) << PyLong_SHIFT) | (unsigned long)digits[0]));
- break;
-#ifdef HAVE_LONG_LONG
- } else if (8 * sizeof(PY_LONG_LONG) - 1 > 3 * PyLong_SHIFT) {
- lla = -(PY_LONG_LONG) (((((((unsigned PY_LONG_LONG)digits[2]) << PyLong_SHIFT) | (unsigned PY_LONG_LONG)digits[1]) << PyLong_SHIFT) | (unsigned PY_LONG_LONG)digits[0]));
- goto long_long;
-#endif
- }
- CYTHON_FALLTHROUGH;
- case 3:
- if (8 * sizeof(long) - 1 > 3 * PyLong_SHIFT) {
- a = (long) (((((((unsigned long)digits[2]) << PyLong_SHIFT) | (unsigned long)digits[1]) << PyLong_SHIFT) | (unsigned long)digits[0]));
- break;
-#ifdef HAVE_LONG_LONG
- } else if (8 * sizeof(PY_LONG_LONG) - 1 > 3 * PyLong_SHIFT) {
- lla = (PY_LONG_LONG) (((((((unsigned PY_LONG_LONG)digits[2]) << PyLong_SHIFT) | (unsigned PY_LONG_LONG)digits[1]) << PyLong_SHIFT) | (unsigned PY_LONG_LONG)digits[0]));
- goto long_long;
-#endif
- }
- CYTHON_FALLTHROUGH;
- case -4:
- if (8 * sizeof(long) - 1 > 4 * PyLong_SHIFT) {
- a = -(long) (((((((((unsigned long)digits[3]) << PyLong_SHIFT) | (unsigned long)digits[2]) << PyLong_SHIFT) | (unsigned long)digits[1]) << PyLong_SHIFT) | (unsigned long)digits[0]));
- break;
-#ifdef HAVE_LONG_LONG
- } else if (8 * sizeof(PY_LONG_LONG) - 1 > 4 * PyLong_SHIFT) {
- lla = -(PY_LONG_LONG) (((((((((unsigned PY_LONG_LONG)digits[3]) << PyLong_SHIFT) | (unsigned PY_LONG_LONG)digits[2]) << PyLong_SHIFT) | (unsigned PY_LONG_LONG)digits[1]) << PyLong_SHIFT) | (unsigned PY_LONG_LONG)digits[0]));
- goto long_long;
-#endif
- }
- CYTHON_FALLTHROUGH;
- case 4:
- if (8 * sizeof(long) - 1 > 4 * PyLong_SHIFT) {
- a = (long) (((((((((unsigned long)digits[3]) << PyLong_SHIFT) | (unsigned long)digits[2]) << PyLong_SHIFT) | (unsigned long)digits[1]) << PyLong_SHIFT) | (unsigned long)digits[0]));
- break;
-#ifdef HAVE_LONG_LONG
- } else if (8 * sizeof(PY_LONG_LONG) - 1 > 4 * PyLong_SHIFT) {
- lla = (PY_LONG_LONG) (((((((((unsigned PY_LONG_LONG)digits[3]) << PyLong_SHIFT) | (unsigned PY_LONG_LONG)digits[2]) << PyLong_SHIFT) | (unsigned PY_LONG_LONG)digits[1]) << PyLong_SHIFT) | (unsigned PY_LONG_LONG)digits[0]));
- goto long_long;
-#endif
- }
- CYTHON_FALLTHROUGH;
- default: return PyLong_Type.tp_as_number->nb_add(op1, op2);
- }
- }
- x = a + b;
- return PyLong_FromLong(x);
-#ifdef HAVE_LONG_LONG
- long_long:
- llx = lla + llb;
- return PyLong_FromLongLong(llx);
-#endif
-
-
- }
- #endif
- if (PyFloat_CheckExact(op1)) {
- const long b = intval;
- double a = PyFloat_AS_DOUBLE(op1);
- double result;
- PyFPE_START_PROTECT("add", return NULL)
- result = ((double)a) + (double)b;
- PyFPE_END_PROTECT(result)
- return PyFloat_FromDouble(result);
- }
- return (inplace ? PyNumber_InPlaceAdd : PyNumber_Add)(op1, op2);
-}
-#endif
-
-/* None */
-static CYTHON_INLINE long __Pyx_div_long(long a, long b) {
- long q = a / b;
- long r = a - q*b;
- q -= ((r != 0) & ((r ^ b) < 0));
- return q;
-}
-
-/* ImportFrom */
-static PyObject* __Pyx_ImportFrom(PyObject* module, PyObject* name) {
- PyObject* value = __Pyx_PyObject_GetAttrStr(module, name);
- if (unlikely(!value) && PyErr_ExceptionMatches(PyExc_AttributeError)) {
- PyErr_Format(PyExc_ImportError,
- #if PY_MAJOR_VERSION < 3
- "cannot import name %.230s", PyString_AS_STRING(name));
- #else
- "cannot import name %S", name);
- #endif
- }
- return value;
-}
-
-/* HasAttr */
-static CYTHON_INLINE int __Pyx_HasAttr(PyObject *o, PyObject *n) {
- PyObject *r;
- if (unlikely(!__Pyx_PyBaseString_Check(n))) {
- PyErr_SetString(PyExc_TypeError,
- "hasattr(): attribute name must be string");
- return -1;
- }
- r = __Pyx_GetAttr(o, n);
- if (unlikely(!r)) {
- PyErr_Clear();
- return 0;
- } else {
- Py_DECREF(r);
- return 1;
- }
-}
-
-/* PyObject_GenericGetAttrNoDict */
-#if CYTHON_USE_TYPE_SLOTS && CYTHON_USE_PYTYPE_LOOKUP && PY_VERSION_HEX < 0x03070000
-static PyObject *__Pyx_RaiseGenericGetAttributeError(PyTypeObject *tp, PyObject *attr_name) {
- PyErr_Format(PyExc_AttributeError,
-#if PY_MAJOR_VERSION >= 3
- "'%.50s' object has no attribute '%U'",
- tp->tp_name, attr_name);
-#else
- "'%.50s' object has no attribute '%.400s'",
- tp->tp_name, PyString_AS_STRING(attr_name));
-#endif
- return NULL;
-}
-static CYTHON_INLINE PyObject* __Pyx_PyObject_GenericGetAttrNoDict(PyObject* obj, PyObject* attr_name) {
- PyObject *descr;
- PyTypeObject *tp = Py_TYPE(obj);
- if (unlikely(!PyString_Check(attr_name))) {
- return PyObject_GenericGetAttr(obj, attr_name);
- }
- assert(!tp->tp_dictoffset);
- descr = _PyType_Lookup(tp, attr_name);
- if (unlikely(!descr)) {
- return __Pyx_RaiseGenericGetAttributeError(tp, attr_name);
- }
- Py_INCREF(descr);
- #if PY_MAJOR_VERSION < 3
- if (likely(PyType_HasFeature(Py_TYPE(descr), Py_TPFLAGS_HAVE_CLASS)))
- #endif
- {
- descrgetfunc f = Py_TYPE(descr)->tp_descr_get;
- if (unlikely(f)) {
- PyObject *res = f(descr, obj, (PyObject *)tp);
- Py_DECREF(descr);
- return res;
- }
- }
- return descr;
-}
-#endif
-
-/* PyObject_GenericGetAttr */
-#if CYTHON_USE_TYPE_SLOTS && CYTHON_USE_PYTYPE_LOOKUP && PY_VERSION_HEX < 0x03070000
-static PyObject* __Pyx_PyObject_GenericGetAttr(PyObject* obj, PyObject* attr_name) {
- if (unlikely(Py_TYPE(obj)->tp_dictoffset)) {
- return PyObject_GenericGetAttr(obj, attr_name);
- }
- return __Pyx_PyObject_GenericGetAttrNoDict(obj, attr_name);
-}
-#endif
-
-/* SetVTable */
-static int __Pyx_SetVtable(PyObject *dict, void *vtable) {
-#if PY_VERSION_HEX >= 0x02070000
- PyObject *ob = PyCapsule_New(vtable, 0, 0);
-#else
- PyObject *ob = PyCObject_FromVoidPtr(vtable, 0);
-#endif
- if (!ob)
- goto bad;
- if (PyDict_SetItem(dict, __pyx_n_s_pyx_vtable, ob) < 0)
- goto bad;
- Py_DECREF(ob);
- return 0;
-bad:
- Py_XDECREF(ob);
- return -1;
-}
-
-/* PyObjectGetAttrStrNoError */
-static void __Pyx_PyObject_GetAttrStr_ClearAttributeError(void) {
- __Pyx_PyThreadState_declare
- __Pyx_PyThreadState_assign
- if (likely(__Pyx_PyErr_ExceptionMatches(PyExc_AttributeError)))
- __Pyx_PyErr_Clear();
-}
-static CYTHON_INLINE PyObject* __Pyx_PyObject_GetAttrStrNoError(PyObject* obj, PyObject* attr_name) {
- PyObject *result;
-#if CYTHON_COMPILING_IN_CPYTHON && CYTHON_USE_TYPE_SLOTS && PY_VERSION_HEX >= 0x030700B1
- PyTypeObject* tp = Py_TYPE(obj);
- if (likely(tp->tp_getattro == PyObject_GenericGetAttr)) {
- return _PyObject_GenericGetAttrWithDict(obj, attr_name, NULL, 1);
- }
-#endif
- result = __Pyx_PyObject_GetAttrStr(obj, attr_name);
- if (unlikely(!result)) {
- __Pyx_PyObject_GetAttrStr_ClearAttributeError();
- }
- return result;
-}
-
-/* SetupReduce */
-static int __Pyx_setup_reduce_is_named(PyObject* meth, PyObject* name) {
- int ret;
- PyObject *name_attr;
- name_attr = __Pyx_PyObject_GetAttrStr(meth, __pyx_n_s_name_2);
- if (likely(name_attr)) {
- ret = PyObject_RichCompareBool(name_attr, name, Py_EQ);
- } else {
- ret = -1;
- }
- if (unlikely(ret < 0)) {
- PyErr_Clear();
- ret = 0;
- }
- Py_XDECREF(name_attr);
- return ret;
-}
-static int __Pyx_setup_reduce(PyObject* type_obj) {
- int ret = 0;
- PyObject *object_reduce = NULL;
- PyObject *object_reduce_ex = NULL;
- PyObject *reduce = NULL;
- PyObject *reduce_ex = NULL;
- PyObject *reduce_cython = NULL;
- PyObject *setstate = NULL;
- PyObject *setstate_cython = NULL;
-#if CYTHON_USE_PYTYPE_LOOKUP
- if (_PyType_Lookup((PyTypeObject*)type_obj, __pyx_n_s_getstate)) goto __PYX_GOOD;
-#else
- if (PyObject_HasAttr(type_obj, __pyx_n_s_getstate)) goto __PYX_GOOD;
-#endif
-#if CYTHON_USE_PYTYPE_LOOKUP
- object_reduce_ex = _PyType_Lookup(&PyBaseObject_Type, __pyx_n_s_reduce_ex); if (!object_reduce_ex) goto __PYX_BAD;
-#else
- object_reduce_ex = __Pyx_PyObject_GetAttrStr((PyObject*)&PyBaseObject_Type, __pyx_n_s_reduce_ex); if (!object_reduce_ex) goto __PYX_BAD;
-#endif
- reduce_ex = __Pyx_PyObject_GetAttrStr(type_obj, __pyx_n_s_reduce_ex); if (unlikely(!reduce_ex)) goto __PYX_BAD;
- if (reduce_ex == object_reduce_ex) {
-#if CYTHON_USE_PYTYPE_LOOKUP
- object_reduce = _PyType_Lookup(&PyBaseObject_Type, __pyx_n_s_reduce); if (!object_reduce) goto __PYX_BAD;
-#else
- object_reduce = __Pyx_PyObject_GetAttrStr((PyObject*)&PyBaseObject_Type, __pyx_n_s_reduce); if (!object_reduce) goto __PYX_BAD;
-#endif
- reduce = __Pyx_PyObject_GetAttrStr(type_obj, __pyx_n_s_reduce); if (unlikely(!reduce)) goto __PYX_BAD;
- if (reduce == object_reduce || __Pyx_setup_reduce_is_named(reduce, __pyx_n_s_reduce_cython)) {
- reduce_cython = __Pyx_PyObject_GetAttrStrNoError(type_obj, __pyx_n_s_reduce_cython);
- if (likely(reduce_cython)) {
- ret = PyDict_SetItem(((PyTypeObject*)type_obj)->tp_dict, __pyx_n_s_reduce, reduce_cython); if (unlikely(ret < 0)) goto __PYX_BAD;
- ret = PyDict_DelItem(((PyTypeObject*)type_obj)->tp_dict, __pyx_n_s_reduce_cython); if (unlikely(ret < 0)) goto __PYX_BAD;
- } else if (reduce == object_reduce || PyErr_Occurred()) {
- goto __PYX_BAD;
- }
- setstate = __Pyx_PyObject_GetAttrStr(type_obj, __pyx_n_s_setstate);
- if (!setstate) PyErr_Clear();
- if (!setstate || __Pyx_setup_reduce_is_named(setstate, __pyx_n_s_setstate_cython)) {
- setstate_cython = __Pyx_PyObject_GetAttrStrNoError(type_obj, __pyx_n_s_setstate_cython);
- if (likely(setstate_cython)) {
- ret = PyDict_SetItem(((PyTypeObject*)type_obj)->tp_dict, __pyx_n_s_setstate, setstate_cython); if (unlikely(ret < 0)) goto __PYX_BAD;
- ret = PyDict_DelItem(((PyTypeObject*)type_obj)->tp_dict, __pyx_n_s_setstate_cython); if (unlikely(ret < 0)) goto __PYX_BAD;
- } else if (!setstate || PyErr_Occurred()) {
- goto __PYX_BAD;
- }
- }
- PyType_Modified((PyTypeObject*)type_obj);
- }
- }
- goto __PYX_GOOD;
-__PYX_BAD:
- if (!PyErr_Occurred())
- PyErr_Format(PyExc_RuntimeError, "Unable to initialize pickling for %s", ((PyTypeObject*)type_obj)->tp_name);
- ret = -1;
-__PYX_GOOD:
-#if !CYTHON_USE_PYTYPE_LOOKUP
- Py_XDECREF(object_reduce);
- Py_XDECREF(object_reduce_ex);
-#endif
- Py_XDECREF(reduce);
- Py_XDECREF(reduce_ex);
- Py_XDECREF(reduce_cython);
- Py_XDECREF(setstate);
- Py_XDECREF(setstate_cython);
- return ret;
-}
-
-/* CLineInTraceback */
-#ifndef CYTHON_CLINE_IN_TRACEBACK
-static int __Pyx_CLineForTraceback(CYTHON_NCP_UNUSED PyThreadState *tstate, int c_line) {
- PyObject *use_cline;
- PyObject *ptype, *pvalue, *ptraceback;
-#if CYTHON_COMPILING_IN_CPYTHON
- PyObject **cython_runtime_dict;
-#endif
- if (unlikely(!__pyx_cython_runtime)) {
- return c_line;
- }
- __Pyx_ErrFetchInState(tstate, &ptype, &pvalue, &ptraceback);
-#if CYTHON_COMPILING_IN_CPYTHON
- cython_runtime_dict = _PyObject_GetDictPtr(__pyx_cython_runtime);
- if (likely(cython_runtime_dict)) {
- __PYX_PY_DICT_LOOKUP_IF_MODIFIED(
- use_cline, *cython_runtime_dict,
- __Pyx_PyDict_GetItemStr(*cython_runtime_dict, __pyx_n_s_cline_in_traceback))
- } else
-#endif
- {
- PyObject *use_cline_obj = __Pyx_PyObject_GetAttrStr(__pyx_cython_runtime, __pyx_n_s_cline_in_traceback);
- if (use_cline_obj) {
- use_cline = PyObject_Not(use_cline_obj) ? Py_False : Py_True;
- Py_DECREF(use_cline_obj);
- } else {
- PyErr_Clear();
- use_cline = NULL;
- }
- }
- if (!use_cline) {
- c_line = 0;
- PyObject_SetAttr(__pyx_cython_runtime, __pyx_n_s_cline_in_traceback, Py_False);
- }
- else if (use_cline == Py_False || (use_cline != Py_True && PyObject_Not(use_cline) != 0)) {
- c_line = 0;
- }
- __Pyx_ErrRestoreInState(tstate, ptype, pvalue, ptraceback);
- return c_line;
-}
-#endif
-
-/* CodeObjectCache */
-static int __pyx_bisect_code_objects(__Pyx_CodeObjectCacheEntry* entries, int count, int code_line) {
- int start = 0, mid = 0, end = count - 1;
- if (end >= 0 && code_line > entries[end].code_line) {
- return count;
- }
- while (start < end) {
- mid = start + (end - start) / 2;
- if (code_line < entries[mid].code_line) {
- end = mid;
- } else if (code_line > entries[mid].code_line) {
- start = mid + 1;
- } else {
- return mid;
- }
- }
- if (code_line <= entries[mid].code_line) {
- return mid;
- } else {
- return mid + 1;
- }
-}
-static PyCodeObject *__pyx_find_code_object(int code_line) {
- PyCodeObject* code_object;
- int pos;
- if (unlikely(!code_line) || unlikely(!__pyx_code_cache.entries)) {
- return NULL;
- }
- pos = __pyx_bisect_code_objects(__pyx_code_cache.entries, __pyx_code_cache.count, code_line);
- if (unlikely(pos >= __pyx_code_cache.count) || unlikely(__pyx_code_cache.entries[pos].code_line != code_line)) {
- return NULL;
- }
- code_object = __pyx_code_cache.entries[pos].code_object;
- Py_INCREF(code_object);
- return code_object;
-}
-static void __pyx_insert_code_object(int code_line, PyCodeObject* code_object) {
- int pos, i;
- __Pyx_CodeObjectCacheEntry* entries = __pyx_code_cache.entries;
- if (unlikely(!code_line)) {
- return;
- }
- if (unlikely(!entries)) {
- entries = (__Pyx_CodeObjectCacheEntry*)PyMem_Malloc(64*sizeof(__Pyx_CodeObjectCacheEntry));
- if (likely(entries)) {
- __pyx_code_cache.entries = entries;
- __pyx_code_cache.max_count = 64;
- __pyx_code_cache.count = 1;
- entries[0].code_line = code_line;
- entries[0].code_object = code_object;
- Py_INCREF(code_object);
- }
- return;
- }
- pos = __pyx_bisect_code_objects(__pyx_code_cache.entries, __pyx_code_cache.count, code_line);
- if ((pos < __pyx_code_cache.count) && unlikely(__pyx_code_cache.entries[pos].code_line == code_line)) {
- PyCodeObject* tmp = entries[pos].code_object;
- entries[pos].code_object = code_object;
- Py_DECREF(tmp);
- return;
- }
- if (__pyx_code_cache.count == __pyx_code_cache.max_count) {
- int new_max = __pyx_code_cache.max_count + 64;
- entries = (__Pyx_CodeObjectCacheEntry*)PyMem_Realloc(
- __pyx_code_cache.entries, ((size_t)new_max) * sizeof(__Pyx_CodeObjectCacheEntry));
- if (unlikely(!entries)) {
- return;
- }
- __pyx_code_cache.entries = entries;
- __pyx_code_cache.max_count = new_max;
- }
- for (i=__pyx_code_cache.count; i>pos; i--) {
- entries[i] = entries[i-1];
- }
- entries[pos].code_line = code_line;
- entries[pos].code_object = code_object;
- __pyx_code_cache.count++;
- Py_INCREF(code_object);
-}
-
-/* AddTraceback */
-#include "compile.h"
-#include "frameobject.h"
-#include "traceback.h"
-static PyCodeObject* __Pyx_CreateCodeObjectForTraceback(
- const char *funcname, int c_line,
- int py_line, const char *filename) {
- PyCodeObject *py_code = 0;
- PyObject *py_srcfile = 0;
- PyObject *py_funcname = 0;
- #if PY_MAJOR_VERSION < 3
- py_srcfile = PyString_FromString(filename);
- #else
- py_srcfile = PyUnicode_FromString(filename);
- #endif
- if (!py_srcfile) goto bad;
- if (c_line) {
- #if PY_MAJOR_VERSION < 3
- py_funcname = PyString_FromFormat( "%s (%s:%d)", funcname, __pyx_cfilenm, c_line);
- #else
- py_funcname = PyUnicode_FromFormat( "%s (%s:%d)", funcname, __pyx_cfilenm, c_line);
- #endif
- }
- else {
- #if PY_MAJOR_VERSION < 3
- py_funcname = PyString_FromString(funcname);
- #else
- py_funcname = PyUnicode_FromString(funcname);
- #endif
- }
- if (!py_funcname) goto bad;
- py_code = __Pyx_PyCode_New(
- 0,
- 0,
- 0,
- 0,
- 0,
- __pyx_empty_bytes, /*PyObject *code,*/
- __pyx_empty_tuple, /*PyObject *consts,*/
- __pyx_empty_tuple, /*PyObject *names,*/
- __pyx_empty_tuple, /*PyObject *varnames,*/
- __pyx_empty_tuple, /*PyObject *freevars,*/
- __pyx_empty_tuple, /*PyObject *cellvars,*/
- py_srcfile, /*PyObject *filename,*/
- py_funcname, /*PyObject *name,*/
- py_line,
- __pyx_empty_bytes /*PyObject *lnotab*/
- );
- Py_DECREF(py_srcfile);
- Py_DECREF(py_funcname);
- return py_code;
-bad:
- Py_XDECREF(py_srcfile);
- Py_XDECREF(py_funcname);
- return NULL;
-}
-static void __Pyx_AddTraceback(const char *funcname, int c_line,
- int py_line, const char *filename) {
- PyCodeObject *py_code = 0;
- PyFrameObject *py_frame = 0;
- PyThreadState *tstate = __Pyx_PyThreadState_Current;
- if (c_line) {
- c_line = __Pyx_CLineForTraceback(tstate, c_line);
- }
- py_code = __pyx_find_code_object(c_line ? -c_line : py_line);
- if (!py_code) {
- py_code = __Pyx_CreateCodeObjectForTraceback(
- funcname, c_line, py_line, filename);
- if (!py_code) goto bad;
- __pyx_insert_code_object(c_line ? -c_line : py_line, py_code);
- }
- py_frame = PyFrame_New(
- tstate, /*PyThreadState *tstate,*/
- py_code, /*PyCodeObject *code,*/
- __pyx_d, /*PyObject *globals,*/
- 0 /*PyObject *locals*/
- );
- if (!py_frame) goto bad;
- __Pyx_PyFrame_SetLineNumber(py_frame, py_line);
- PyTraceBack_Here(py_frame);
-bad:
- Py_XDECREF(py_code);
- Py_XDECREF(py_frame);
-}
-
-#if PY_MAJOR_VERSION < 3
-static int __Pyx_GetBuffer(PyObject *obj, Py_buffer *view, int flags) {
- if (PyObject_CheckBuffer(obj)) return PyObject_GetBuffer(obj, view, flags);
- if (__Pyx_TypeCheck(obj, __pyx_array_type)) return __pyx_array_getbuffer(obj, view, flags);
- if (__Pyx_TypeCheck(obj, __pyx_memoryview_type)) return __pyx_memoryview_getbuffer(obj, view, flags);
- PyErr_Format(PyExc_TypeError, "'%.200s' does not have the buffer interface", Py_TYPE(obj)->tp_name);
- return -1;
-}
-static void __Pyx_ReleaseBuffer(Py_buffer *view) {
- PyObject *obj = view->obj;
- if (!obj) return;
- if (PyObject_CheckBuffer(obj)) {
- PyBuffer_Release(view);
- return;
- }
- if ((0)) {}
- view->obj = NULL;
- Py_DECREF(obj);
-}
-#endif
-
-
-/* MemviewSliceIsContig */
-static int
-__pyx_memviewslice_is_contig(const __Pyx_memviewslice mvs, char order, int ndim)
-{
- int i, index, step, start;
- Py_ssize_t itemsize = mvs.memview->view.itemsize;
- if (order == 'F') {
- step = 1;
- start = 0;
- } else {
- step = -1;
- start = ndim - 1;
- }
- for (i = 0; i < ndim; i++) {
- index = start + step * i;
- if (mvs.suboffsets[index] >= 0 || mvs.strides[index] != itemsize)
- return 0;
- itemsize *= mvs.shape[index];
- }
- return 1;
-}
-
-/* OverlappingSlices */
-static void
-__pyx_get_array_memory_extents(__Pyx_memviewslice *slice,
- void **out_start, void **out_end,
- int ndim, size_t itemsize)
-{
- char *start, *end;
- int i;
- start = end = slice->data;
- for (i = 0; i < ndim; i++) {
- Py_ssize_t stride = slice->strides[i];
- Py_ssize_t extent = slice->shape[i];
- if (extent == 0) {
- *out_start = *out_end = start;
- return;
- } else {
- if (stride > 0)
- end += stride * (extent - 1);
- else
- start += stride * (extent - 1);
- }
- }
- *out_start = start;
- *out_end = end + itemsize;
-}
-static int
-__pyx_slices_overlap(__Pyx_memviewslice *slice1,
- __Pyx_memviewslice *slice2,
- int ndim, size_t itemsize)
-{
- void *start1, *end1, *start2, *end2;
- __pyx_get_array_memory_extents(slice1, &start1, &end1, ndim, itemsize);
- __pyx_get_array_memory_extents(slice2, &start2, &end2, ndim, itemsize);
- return (start1 < end2) && (start2 < end1);
-}
-
-/* Capsule */
-static CYTHON_INLINE PyObject *
-__pyx_capsule_create(void *p, CYTHON_UNUSED const char *sig)
-{
- PyObject *cobj;
-#if PY_VERSION_HEX >= 0x02070000
- cobj = PyCapsule_New(p, sig, NULL);
-#else
- cobj = PyCObject_FromVoidPtr(p, NULL);
-#endif
- return cobj;
-}
-
-/* IsLittleEndian */
-static CYTHON_INLINE int __Pyx_Is_Little_Endian(void)
-{
- union {
- uint32_t u32;
- uint8_t u8[4];
- } S;
- S.u32 = 0x01020304;
- return S.u8[0] == 4;
-}
-
-/* BufferFormatCheck */
-static void __Pyx_BufFmt_Init(__Pyx_BufFmt_Context* ctx,
- __Pyx_BufFmt_StackElem* stack,
- __Pyx_TypeInfo* type) {
- stack[0].field = &ctx->root;
- stack[0].parent_offset = 0;
- ctx->root.type = type;
- ctx->root.name = "buffer dtype";
- ctx->root.offset = 0;
- ctx->head = stack;
- ctx->head->field = &ctx->root;
- ctx->fmt_offset = 0;
- ctx->head->parent_offset = 0;
- ctx->new_packmode = '@';
- ctx->enc_packmode = '@';
- ctx->new_count = 1;
- ctx->enc_count = 0;
- ctx->enc_type = 0;
- ctx->is_complex = 0;
- ctx->is_valid_array = 0;
- ctx->struct_alignment = 0;
- while (type->typegroup == 'S') {
- ++ctx->head;
- ctx->head->field = type->fields;
- ctx->head->parent_offset = 0;
- type = type->fields->type;
- }
-}
-static int __Pyx_BufFmt_ParseNumber(const char** ts) {
- int count;
- const char* t = *ts;
- if (*t < '0' || *t > '9') {
- return -1;
- } else {
- count = *t++ - '0';
- while (*t >= '0' && *t <= '9') {
- count *= 10;
- count += *t++ - '0';
- }
- }
- *ts = t;
- return count;
-}
-static int __Pyx_BufFmt_ExpectNumber(const char **ts) {
- int number = __Pyx_BufFmt_ParseNumber(ts);
- if (number == -1)
- PyErr_Format(PyExc_ValueError,\
- "Does not understand character buffer dtype format string ('%c')", **ts);
- return number;
-}
-static void __Pyx_BufFmt_RaiseUnexpectedChar(char ch) {
- PyErr_Format(PyExc_ValueError,
- "Unexpected format string character: '%c'", ch);
-}
-static const char* __Pyx_BufFmt_DescribeTypeChar(char ch, int is_complex) {
- switch (ch) {
- case '?': return "'bool'";
- case 'c': return "'char'";
- case 'b': return "'signed char'";
- case 'B': return "'unsigned char'";
- case 'h': return "'short'";
- case 'H': return "'unsigned short'";
- case 'i': return "'int'";
- case 'I': return "'unsigned int'";
- case 'l': return "'long'";
- case 'L': return "'unsigned long'";
- case 'q': return "'long long'";
- case 'Q': return "'unsigned long long'";
- case 'f': return (is_complex ? "'complex float'" : "'float'");
- case 'd': return (is_complex ? "'complex double'" : "'double'");
- case 'g': return (is_complex ? "'complex long double'" : "'long double'");
- case 'T': return "a struct";
- case 'O': return "Python object";
- case 'P': return "a pointer";
- case 's': case 'p': return "a string";
- case 0: return "end";
- default: return "unparseable format string";
- }
-}
-static size_t __Pyx_BufFmt_TypeCharToStandardSize(char ch, int is_complex) {
- switch (ch) {
- case '?': case 'c': case 'b': case 'B': case 's': case 'p': return 1;
- case 'h': case 'H': return 2;
- case 'i': case 'I': case 'l': case 'L': return 4;
- case 'q': case 'Q': return 8;
- case 'f': return (is_complex ? 8 : 4);
- case 'd': return (is_complex ? 16 : 8);
- case 'g': {
- PyErr_SetString(PyExc_ValueError, "Python does not define a standard format string size for long double ('g')..");
- return 0;
- }
- case 'O': case 'P': return sizeof(void*);
- default:
- __Pyx_BufFmt_RaiseUnexpectedChar(ch);
- return 0;
- }
-}
-static size_t __Pyx_BufFmt_TypeCharToNativeSize(char ch, int is_complex) {
- switch (ch) {
- case '?': case 'c': case 'b': case 'B': case 's': case 'p': return 1;
- case 'h': case 'H': return sizeof(short);
- case 'i': case 'I': return sizeof(int);
- case 'l': case 'L': return sizeof(long);
- #ifdef HAVE_LONG_LONG
- case 'q': case 'Q': return sizeof(PY_LONG_LONG);
- #endif
- case 'f': return sizeof(float) * (is_complex ? 2 : 1);
- case 'd': return sizeof(double) * (is_complex ? 2 : 1);
- case 'g': return sizeof(long double) * (is_complex ? 2 : 1);
- case 'O': case 'P': return sizeof(void*);
- default: {
- __Pyx_BufFmt_RaiseUnexpectedChar(ch);
- return 0;
- }
- }
-}
-typedef struct { char c; short x; } __Pyx_st_short;
-typedef struct { char c; int x; } __Pyx_st_int;
-typedef struct { char c; long x; } __Pyx_st_long;
-typedef struct { char c; float x; } __Pyx_st_float;
-typedef struct { char c; double x; } __Pyx_st_double;
-typedef struct { char c; long double x; } __Pyx_st_longdouble;
-typedef struct { char c; void *x; } __Pyx_st_void_p;
-#ifdef HAVE_LONG_LONG
-typedef struct { char c; PY_LONG_LONG x; } __Pyx_st_longlong;
-#endif
-static size_t __Pyx_BufFmt_TypeCharToAlignment(char ch, CYTHON_UNUSED int is_complex) {
- switch (ch) {
- case '?': case 'c': case 'b': case 'B': case 's': case 'p': return 1;
- case 'h': case 'H': return sizeof(__Pyx_st_short) - sizeof(short);
- case 'i': case 'I': return sizeof(__Pyx_st_int) - sizeof(int);
- case 'l': case 'L': return sizeof(__Pyx_st_long) - sizeof(long);
-#ifdef HAVE_LONG_LONG
- case 'q': case 'Q': return sizeof(__Pyx_st_longlong) - sizeof(PY_LONG_LONG);
-#endif
- case 'f': return sizeof(__Pyx_st_float) - sizeof(float);
- case 'd': return sizeof(__Pyx_st_double) - sizeof(double);
- case 'g': return sizeof(__Pyx_st_longdouble) - sizeof(long double);
- case 'P': case 'O': return sizeof(__Pyx_st_void_p) - sizeof(void*);
- default:
- __Pyx_BufFmt_RaiseUnexpectedChar(ch);
- return 0;
- }
-}
-/* These are for computing the padding at the end of the struct to align
- on the first member of the struct. This will probably the same as above,
- but we don't have any guarantees.
- */
-typedef struct { short x; char c; } __Pyx_pad_short;
-typedef struct { int x; char c; } __Pyx_pad_int;
-typedef struct { long x; char c; } __Pyx_pad_long;
-typedef struct { float x; char c; } __Pyx_pad_float;
-typedef struct { double x; char c; } __Pyx_pad_double;
-typedef struct { long double x; char c; } __Pyx_pad_longdouble;
-typedef struct { void *x; char c; } __Pyx_pad_void_p;
-#ifdef HAVE_LONG_LONG
-typedef struct { PY_LONG_LONG x; char c; } __Pyx_pad_longlong;
-#endif
-static size_t __Pyx_BufFmt_TypeCharToPadding(char ch, CYTHON_UNUSED int is_complex) {
- switch (ch) {
- case '?': case 'c': case 'b': case 'B': case 's': case 'p': return 1;
- case 'h': case 'H': return sizeof(__Pyx_pad_short) - sizeof(short);
- case 'i': case 'I': return sizeof(__Pyx_pad_int) - sizeof(int);
- case 'l': case 'L': return sizeof(__Pyx_pad_long) - sizeof(long);
-#ifdef HAVE_LONG_LONG
- case 'q': case 'Q': return sizeof(__Pyx_pad_longlong) - sizeof(PY_LONG_LONG);
-#endif
- case 'f': return sizeof(__Pyx_pad_float) - sizeof(float);
- case 'd': return sizeof(__Pyx_pad_double) - sizeof(double);
- case 'g': return sizeof(__Pyx_pad_longdouble) - sizeof(long double);
- case 'P': case 'O': return sizeof(__Pyx_pad_void_p) - sizeof(void*);
- default:
- __Pyx_BufFmt_RaiseUnexpectedChar(ch);
- return 0;
- }
-}
-static char __Pyx_BufFmt_TypeCharToGroup(char ch, int is_complex) {
- switch (ch) {
- case 'c':
- return 'H';
- case 'b': case 'h': case 'i':
- case 'l': case 'q': case 's': case 'p':
- return 'I';
- case '?': case 'B': case 'H': case 'I': case 'L': case 'Q':
- return 'U';
- case 'f': case 'd': case 'g':
- return (is_complex ? 'C' : 'R');
- case 'O':
- return 'O';
- case 'P':
- return 'P';
- default: {
- __Pyx_BufFmt_RaiseUnexpectedChar(ch);
- return 0;
- }
- }
-}
-static void __Pyx_BufFmt_RaiseExpected(__Pyx_BufFmt_Context* ctx) {
- if (ctx->head == NULL || ctx->head->field == &ctx->root) {
- const char* expected;
- const char* quote;
- if (ctx->head == NULL) {
- expected = "end";
- quote = "";
- } else {
- expected = ctx->head->field->type->name;
- quote = "'";
- }
- PyErr_Format(PyExc_ValueError,
- "Buffer dtype mismatch, expected %s%s%s but got %s",
- quote, expected, quote,
- __Pyx_BufFmt_DescribeTypeChar(ctx->enc_type, ctx->is_complex));
- } else {
- __Pyx_StructField* field = ctx->head->field;
- __Pyx_StructField* parent = (ctx->head - 1)->field;
- PyErr_Format(PyExc_ValueError,
- "Buffer dtype mismatch, expected '%s' but got %s in '%s.%s'",
- field->type->name, __Pyx_BufFmt_DescribeTypeChar(ctx->enc_type, ctx->is_complex),
- parent->type->name, field->name);
- }
-}
-static int __Pyx_BufFmt_ProcessTypeChunk(__Pyx_BufFmt_Context* ctx) {
- char group;
- size_t size, offset, arraysize = 1;
- if (ctx->enc_type == 0) return 0;
- if (ctx->head->field->type->arraysize[0]) {
- int i, ndim = 0;
- if (ctx->enc_type == 's' || ctx->enc_type == 'p') {
- ctx->is_valid_array = ctx->head->field->type->ndim == 1;
- ndim = 1;
- if (ctx->enc_count != ctx->head->field->type->arraysize[0]) {
- PyErr_Format(PyExc_ValueError,
- "Expected a dimension of size %zu, got %zu",
- ctx->head->field->type->arraysize[0], ctx->enc_count);
- return -1;
- }
- }
- if (!ctx->is_valid_array) {
- PyErr_Format(PyExc_ValueError, "Expected %d dimensions, got %d",
- ctx->head->field->type->ndim, ndim);
- return -1;
- }
- for (i = 0; i < ctx->head->field->type->ndim; i++) {
- arraysize *= ctx->head->field->type->arraysize[i];
- }
- ctx->is_valid_array = 0;
- ctx->enc_count = 1;
- }
- group = __Pyx_BufFmt_TypeCharToGroup(ctx->enc_type, ctx->is_complex);
- do {
- __Pyx_StructField* field = ctx->head->field;
- __Pyx_TypeInfo* type = field->type;
- if (ctx->enc_packmode == '@' || ctx->enc_packmode == '^') {
- size = __Pyx_BufFmt_TypeCharToNativeSize(ctx->enc_type, ctx->is_complex);
- } else {
- size = __Pyx_BufFmt_TypeCharToStandardSize(ctx->enc_type, ctx->is_complex);
- }
- if (ctx->enc_packmode == '@') {
- size_t align_at = __Pyx_BufFmt_TypeCharToAlignment(ctx->enc_type, ctx->is_complex);
- size_t align_mod_offset;
- if (align_at == 0) return -1;
- align_mod_offset = ctx->fmt_offset % align_at;
- if (align_mod_offset > 0) ctx->fmt_offset += align_at - align_mod_offset;
- if (ctx->struct_alignment == 0)
- ctx->struct_alignment = __Pyx_BufFmt_TypeCharToPadding(ctx->enc_type,
- ctx->is_complex);
- }
- if (type->size != size || type->typegroup != group) {
- if (type->typegroup == 'C' && type->fields != NULL) {
- size_t parent_offset = ctx->head->parent_offset + field->offset;
- ++ctx->head;
- ctx->head->field = type->fields;
- ctx->head->parent_offset = parent_offset;
- continue;
- }
- if ((type->typegroup == 'H' || group == 'H') && type->size == size) {
- } else {
- __Pyx_BufFmt_RaiseExpected(ctx);
- return -1;
- }
- }
- offset = ctx->head->parent_offset + field->offset;
- if (ctx->fmt_offset != offset) {
- PyErr_Format(PyExc_ValueError,
- "Buffer dtype mismatch; next field is at offset %" CYTHON_FORMAT_SSIZE_T "d but %" CYTHON_FORMAT_SSIZE_T "d expected",
- (Py_ssize_t)ctx->fmt_offset, (Py_ssize_t)offset);
- return -1;
- }
- ctx->fmt_offset += size;
- if (arraysize)
- ctx->fmt_offset += (arraysize - 1) * size;
- --ctx->enc_count;
- while (1) {
- if (field == &ctx->root) {
- ctx->head = NULL;
- if (ctx->enc_count != 0) {
- __Pyx_BufFmt_RaiseExpected(ctx);
- return -1;
- }
- break;
- }
- ctx->head->field = ++field;
- if (field->type == NULL) {
- --ctx->head;
- field = ctx->head->field;
- continue;
- } else if (field->type->typegroup == 'S') {
- size_t parent_offset = ctx->head->parent_offset + field->offset;
- if (field->type->fields->type == NULL) continue;
- field = field->type->fields;
- ++ctx->head;
- ctx->head->field = field;
- ctx->head->parent_offset = parent_offset;
- break;
- } else {
- break;
- }
- }
- } while (ctx->enc_count);
- ctx->enc_type = 0;
- ctx->is_complex = 0;
- return 0;
-}
-static PyObject *
-__pyx_buffmt_parse_array(__Pyx_BufFmt_Context* ctx, const char** tsp)
-{
- const char *ts = *tsp;
- int i = 0, number, ndim;
- ++ts;
- if (ctx->new_count != 1) {
- PyErr_SetString(PyExc_ValueError,
- "Cannot handle repeated arrays in format string");
- return NULL;
- }
- if (__Pyx_BufFmt_ProcessTypeChunk(ctx) == -1) return NULL;
- ndim = ctx->head->field->type->ndim;
- while (*ts && *ts != ')') {
- switch (*ts) {
- case ' ': case '\f': case '\r': case '\n': case '\t': case '\v': continue;
- default: break;
- }
- number = __Pyx_BufFmt_ExpectNumber(&ts);
- if (number == -1) return NULL;
- if (i < ndim && (size_t) number != ctx->head->field->type->arraysize[i])
- return PyErr_Format(PyExc_ValueError,
- "Expected a dimension of size %zu, got %d",
- ctx->head->field->type->arraysize[i], number);
- if (*ts != ',' && *ts != ')')
- return PyErr_Format(PyExc_ValueError,
- "Expected a comma in format string, got '%c'", *ts);
- if (*ts == ',') ts++;
- i++;
- }
- if (i != ndim)
- return PyErr_Format(PyExc_ValueError, "Expected %d dimension(s), got %d",
- ctx->head->field->type->ndim, i);
- if (!*ts) {
- PyErr_SetString(PyExc_ValueError,
- "Unexpected end of format string, expected ')'");
- return NULL;
- }
- ctx->is_valid_array = 1;
- ctx->new_count = 1;
- *tsp = ++ts;
- return Py_None;
-}
-static const char* __Pyx_BufFmt_CheckString(__Pyx_BufFmt_Context* ctx, const char* ts) {
- int got_Z = 0;
- while (1) {
- switch(*ts) {
- case 0:
- if (ctx->enc_type != 0 && ctx->head == NULL) {
- __Pyx_BufFmt_RaiseExpected(ctx);
- return NULL;
- }
- if (__Pyx_BufFmt_ProcessTypeChunk(ctx) == -1) return NULL;
- if (ctx->head != NULL) {
- __Pyx_BufFmt_RaiseExpected(ctx);
- return NULL;
- }
- return ts;
- case ' ':
- case '\r':
- case '\n':
- ++ts;
- break;
- case '<':
- if (!__Pyx_Is_Little_Endian()) {
- PyErr_SetString(PyExc_ValueError, "Little-endian buffer not supported on big-endian compiler");
- return NULL;
- }
- ctx->new_packmode = '=';
- ++ts;
- break;
- case '>':
- case '!':
- if (__Pyx_Is_Little_Endian()) {
- PyErr_SetString(PyExc_ValueError, "Big-endian buffer not supported on little-endian compiler");
- return NULL;
- }
- ctx->new_packmode = '=';
- ++ts;
- break;
- case '=':
- case '@':
- case '^':
- ctx->new_packmode = *ts++;
- break;
- case 'T':
- {
- const char* ts_after_sub;
- size_t i, struct_count = ctx->new_count;
- size_t struct_alignment = ctx->struct_alignment;
- ctx->new_count = 1;
- ++ts;
- if (*ts != '{') {
- PyErr_SetString(PyExc_ValueError, "Buffer acquisition: Expected '{' after 'T'");
- return NULL;
- }
- if (__Pyx_BufFmt_ProcessTypeChunk(ctx) == -1) return NULL;
- ctx->enc_type = 0;
- ctx->enc_count = 0;
- ctx->struct_alignment = 0;
- ++ts;
- ts_after_sub = ts;
- for (i = 0; i != struct_count; ++i) {
- ts_after_sub = __Pyx_BufFmt_CheckString(ctx, ts);
- if (!ts_after_sub) return NULL;
- }
- ts = ts_after_sub;
- if (struct_alignment) ctx->struct_alignment = struct_alignment;
- }
- break;
- case '}':
- {
- size_t alignment = ctx->struct_alignment;
- ++ts;
- if (__Pyx_BufFmt_ProcessTypeChunk(ctx) == -1) return NULL;
- ctx->enc_type = 0;
- if (alignment && ctx->fmt_offset % alignment) {
- ctx->fmt_offset += alignment - (ctx->fmt_offset % alignment);
- }
- }
- return ts;
- case 'x':
- if (__Pyx_BufFmt_ProcessTypeChunk(ctx) == -1) return NULL;
- ctx->fmt_offset += ctx->new_count;
- ctx->new_count = 1;
- ctx->enc_count = 0;
- ctx->enc_type = 0;
- ctx->enc_packmode = ctx->new_packmode;
- ++ts;
- break;
- case 'Z':
- got_Z = 1;
- ++ts;
- if (*ts != 'f' && *ts != 'd' && *ts != 'g') {
- __Pyx_BufFmt_RaiseUnexpectedChar('Z');
- return NULL;
- }
- CYTHON_FALLTHROUGH;
- case '?': case 'c': case 'b': case 'B': case 'h': case 'H': case 'i': case 'I':
- case 'l': case 'L': case 'q': case 'Q':
- case 'f': case 'd': case 'g':
- case 'O': case 'p':
- if ((ctx->enc_type == *ts) && (got_Z == ctx->is_complex) &&
- (ctx->enc_packmode == ctx->new_packmode) && (!ctx->is_valid_array)) {
- ctx->enc_count += ctx->new_count;
- ctx->new_count = 1;
- got_Z = 0;
- ++ts;
- break;
- }
- CYTHON_FALLTHROUGH;
- case 's':
- if (__Pyx_BufFmt_ProcessTypeChunk(ctx) == -1) return NULL;
- ctx->enc_count = ctx->new_count;
- ctx->enc_packmode = ctx->new_packmode;
- ctx->enc_type = *ts;
- ctx->is_complex = got_Z;
- ++ts;
- ctx->new_count = 1;
- got_Z = 0;
- break;
- case ':':
- ++ts;
- while(*ts != ':') ++ts;
- ++ts;
- break;
- case '(':
- if (!__pyx_buffmt_parse_array(ctx, &ts)) return NULL;
- break;
- default:
- {
- int number = __Pyx_BufFmt_ExpectNumber(&ts);
- if (number == -1) return NULL;
- ctx->new_count = (size_t)number;
- }
- }
- }
-}
-
-/* TypeInfoCompare */
- static int
-__pyx_typeinfo_cmp(__Pyx_TypeInfo *a, __Pyx_TypeInfo *b)
-{
- int i;
- if (!a || !b)
- return 0;
- if (a == b)
- return 1;
- if (a->size != b->size || a->typegroup != b->typegroup ||
- a->is_unsigned != b->is_unsigned || a->ndim != b->ndim) {
- if (a->typegroup == 'H' || b->typegroup == 'H') {
- return a->size == b->size;
- } else {
- return 0;
- }
- }
- if (a->ndim) {
- for (i = 0; i < a->ndim; i++)
- if (a->arraysize[i] != b->arraysize[i])
- return 0;
- }
- if (a->typegroup == 'S') {
- if (a->flags != b->flags)
- return 0;
- if (a->fields || b->fields) {
- if (!(a->fields && b->fields))
- return 0;
- for (i = 0; a->fields[i].type && b->fields[i].type; i++) {
- __Pyx_StructField *field_a = a->fields + i;
- __Pyx_StructField *field_b = b->fields + i;
- if (field_a->offset != field_b->offset ||
- !__pyx_typeinfo_cmp(field_a->type, field_b->type))
- return 0;
- }
- return !a->fields[i].type && !b->fields[i].type;
- }
- }
- return 1;
-}
-
-/* MemviewSliceValidateAndInit */
- static int
-__pyx_check_strides(Py_buffer *buf, int dim, int ndim, int spec)
-{
- if (buf->shape[dim] <= 1)
- return 1;
- if (buf->strides) {
- if (spec & __Pyx_MEMVIEW_CONTIG) {
- if (spec & (__Pyx_MEMVIEW_PTR|__Pyx_MEMVIEW_FULL)) {
- if (unlikely(buf->strides[dim] != sizeof(void *))) {
- PyErr_Format(PyExc_ValueError,
- "Buffer is not indirectly contiguous "
- "in dimension %d.", dim);
- goto fail;
- }
- } else if (unlikely(buf->strides[dim] != buf->itemsize)) {
- PyErr_SetString(PyExc_ValueError,
- "Buffer and memoryview are not contiguous "
- "in the same dimension.");
- goto fail;
- }
- }
- if (spec & __Pyx_MEMVIEW_FOLLOW) {
- Py_ssize_t stride = buf->strides[dim];
- if (stride < 0)
- stride = -stride;
- if (unlikely(stride < buf->itemsize)) {
- PyErr_SetString(PyExc_ValueError,
- "Buffer and memoryview are not contiguous "
- "in the same dimension.");
- goto fail;
- }
- }
- } else {
- if (unlikely(spec & __Pyx_MEMVIEW_CONTIG && dim != ndim - 1)) {
- PyErr_Format(PyExc_ValueError,
- "C-contiguous buffer is not contiguous in "
- "dimension %d", dim);
- goto fail;
- } else if (unlikely(spec & (__Pyx_MEMVIEW_PTR))) {
- PyErr_Format(PyExc_ValueError,
- "C-contiguous buffer is not indirect in "
- "dimension %d", dim);
- goto fail;
- } else if (unlikely(buf->suboffsets)) {
- PyErr_SetString(PyExc_ValueError,
- "Buffer exposes suboffsets but no strides");
- goto fail;
- }
- }
- return 1;
-fail:
- return 0;
-}
-static int
-__pyx_check_suboffsets(Py_buffer *buf, int dim, CYTHON_UNUSED int ndim, int spec)
-{
- if (spec & __Pyx_MEMVIEW_DIRECT) {
- if (unlikely(buf->suboffsets && buf->suboffsets[dim] >= 0)) {
- PyErr_Format(PyExc_ValueError,
- "Buffer not compatible with direct access "
- "in dimension %d.", dim);
- goto fail;
- }
- }
- if (spec & __Pyx_MEMVIEW_PTR) {
- if (unlikely(!buf->suboffsets || (buf->suboffsets[dim] < 0))) {
- PyErr_Format(PyExc_ValueError,
- "Buffer is not indirectly accessible "
- "in dimension %d.", dim);
- goto fail;
- }
- }
- return 1;
-fail:
- return 0;
-}
-static int
-__pyx_verify_contig(Py_buffer *buf, int ndim, int c_or_f_flag)
-{
- int i;
- if (c_or_f_flag & __Pyx_IS_F_CONTIG) {
- Py_ssize_t stride = 1;
- for (i = 0; i < ndim; i++) {
- if (unlikely(stride * buf->itemsize != buf->strides[i] && buf->shape[i] > 1)) {
- PyErr_SetString(PyExc_ValueError,
- "Buffer not fortran contiguous.");
- goto fail;
- }
- stride = stride * buf->shape[i];
- }
- } else if (c_or_f_flag & __Pyx_IS_C_CONTIG) {
- Py_ssize_t stride = 1;
- for (i = ndim - 1; i >- 1; i--) {
- if (unlikely(stride * buf->itemsize != buf->strides[i] && buf->shape[i] > 1)) {
- PyErr_SetString(PyExc_ValueError,
- "Buffer not C contiguous.");
- goto fail;
- }
- stride = stride * buf->shape[i];
- }
- }
- return 1;
-fail:
- return 0;
-}
-static int __Pyx_ValidateAndInit_memviewslice(
- int *axes_specs,
- int c_or_f_flag,
- int buf_flags,
- int ndim,
- __Pyx_TypeInfo *dtype,
- __Pyx_BufFmt_StackElem stack[],
- __Pyx_memviewslice *memviewslice,
- PyObject *original_obj)
-{
- struct __pyx_memoryview_obj *memview, *new_memview;
- __Pyx_RefNannyDeclarations
- Py_buffer *buf;
- int i, spec = 0, retval = -1;
- __Pyx_BufFmt_Context ctx;
- int from_memoryview = __pyx_memoryview_check(original_obj);
- __Pyx_RefNannySetupContext("ValidateAndInit_memviewslice", 0);
- if (from_memoryview && __pyx_typeinfo_cmp(dtype, ((struct __pyx_memoryview_obj *)
- original_obj)->typeinfo)) {
- memview = (struct __pyx_memoryview_obj *) original_obj;
- new_memview = NULL;
- } else {
- memview = (struct __pyx_memoryview_obj *) __pyx_memoryview_new(
- original_obj, buf_flags, 0, dtype);
- new_memview = memview;
- if (unlikely(!memview))
- goto fail;
- }
- buf = &memview->view;
- if (unlikely(buf->ndim != ndim)) {
- PyErr_Format(PyExc_ValueError,
- "Buffer has wrong number of dimensions (expected %d, got %d)",
- ndim, buf->ndim);
- goto fail;
- }
- if (new_memview) {
- __Pyx_BufFmt_Init(&ctx, stack, dtype);
- if (unlikely(!__Pyx_BufFmt_CheckString(&ctx, buf->format))) goto fail;
- }
- if (unlikely((unsigned) buf->itemsize != dtype->size)) {
- PyErr_Format(PyExc_ValueError,
- "Item size of buffer (%" CYTHON_FORMAT_SSIZE_T "u byte%s) "
- "does not match size of '%s' (%" CYTHON_FORMAT_SSIZE_T "u byte%s)",
- buf->itemsize,
- (buf->itemsize > 1) ? "s" : "",
- dtype->name,
- dtype->size,
- (dtype->size > 1) ? "s" : "");
- goto fail;
- }
- if (buf->len > 0) {
- for (i = 0; i < ndim; i++) {
- spec = axes_specs[i];
- if (unlikely(!__pyx_check_strides(buf, i, ndim, spec)))
- goto fail;
- if (unlikely(!__pyx_check_suboffsets(buf, i, ndim, spec)))
- goto fail;
- }
- if (unlikely(buf->strides && !__pyx_verify_contig(buf, ndim, c_or_f_flag)))
- goto fail;
- }
- if (unlikely(__Pyx_init_memviewslice(memview, ndim, memviewslice,
- new_memview != NULL) == -1)) {
- goto fail;
- }
- retval = 0;
- goto no_fail;
-fail:
- Py_XDECREF(new_memview);
- retval = -1;
-no_fail:
- __Pyx_RefNannyFinishContext();
- return retval;
-}
-
-/* ObjectToMemviewSlice */
- static CYTHON_INLINE __Pyx_memviewslice __Pyx_PyObject_to_MemoryviewSlice_d_d_dc_int(PyObject *obj, int writable_flag) {
- __Pyx_memviewslice result = { 0, 0, { 0 }, { 0 }, { 0 } };
- __Pyx_BufFmt_StackElem stack[1];
- int axes_specs[] = { (__Pyx_MEMVIEW_DIRECT | __Pyx_MEMVIEW_FOLLOW), (__Pyx_MEMVIEW_DIRECT | __Pyx_MEMVIEW_FOLLOW), (__Pyx_MEMVIEW_DIRECT | __Pyx_MEMVIEW_CONTIG) };
- int retcode;
- if (obj == Py_None) {
- result.memview = (struct __pyx_memoryview_obj *) Py_None;
- return result;
- }
- retcode = __Pyx_ValidateAndInit_memviewslice(axes_specs, __Pyx_IS_C_CONTIG,
- (PyBUF_C_CONTIGUOUS | PyBUF_FORMAT) | writable_flag, 3,
- &__Pyx_TypeInfo_int, stack,
- &result, obj);
- if (unlikely(retcode == -1))
- goto __pyx_fail;
- return result;
-__pyx_fail:
- result.memview = NULL;
- result.data = NULL;
- return result;
-}
-
-/* ObjectToMemviewSlice */
- static CYTHON_INLINE __Pyx_memviewslice __Pyx_PyObject_to_MemoryviewSlice_d_d_dc_float(PyObject *obj, int writable_flag) {
- __Pyx_memviewslice result = { 0, 0, { 0 }, { 0 }, { 0 } };
- __Pyx_BufFmt_StackElem stack[1];
- int axes_specs[] = { (__Pyx_MEMVIEW_DIRECT | __Pyx_MEMVIEW_FOLLOW), (__Pyx_MEMVIEW_DIRECT | __Pyx_MEMVIEW_FOLLOW), (__Pyx_MEMVIEW_DIRECT | __Pyx_MEMVIEW_CONTIG) };
- int retcode;
- if (obj == Py_None) {
- result.memview = (struct __pyx_memoryview_obj *) Py_None;
- return result;
- }
- retcode = __Pyx_ValidateAndInit_memviewslice(axes_specs, __Pyx_IS_C_CONTIG,
- (PyBUF_C_CONTIGUOUS | PyBUF_FORMAT) | writable_flag, 3,
- &__Pyx_TypeInfo_float, stack,
- &result, obj);
- if (unlikely(retcode == -1))
- goto __pyx_fail;
- return result;
-__pyx_fail:
- result.memview = NULL;
- result.data = NULL;
- return result;
-}
-
-/* ObjectToMemviewSlice */
- static CYTHON_INLINE __Pyx_memviewslice __Pyx_PyObject_to_MemoryviewSlice_dc_int(PyObject *obj, int writable_flag) {
- __Pyx_memviewslice result = { 0, 0, { 0 }, { 0 }, { 0 } };
- __Pyx_BufFmt_StackElem stack[1];
- int axes_specs[] = { (__Pyx_MEMVIEW_DIRECT | __Pyx_MEMVIEW_CONTIG) };
- int retcode;
- if (obj == Py_None) {
- result.memview = (struct __pyx_memoryview_obj *) Py_None;
- return result;
- }
- retcode = __Pyx_ValidateAndInit_memviewslice(axes_specs, __Pyx_IS_C_CONTIG,
- (PyBUF_C_CONTIGUOUS | PyBUF_FORMAT) | writable_flag, 1,
- &__Pyx_TypeInfo_int, stack,
- &result, obj);
- if (unlikely(retcode == -1))
- goto __pyx_fail;
- return result;
-__pyx_fail:
- result.memview = NULL;
- result.data = NULL;
- return result;
-}
-
-/* CIntToPy */
- static CYTHON_INLINE PyObject* __Pyx_PyInt_From_int(int value) {
- const int neg_one = (int) ((int) 0 - (int) 1), const_zero = (int) 0;
- const int is_unsigned = neg_one > const_zero;
- if (is_unsigned) {
- if (sizeof(int) < sizeof(long)) {
- return PyInt_FromLong((long) value);
- } else if (sizeof(int) <= sizeof(unsigned long)) {
- return PyLong_FromUnsignedLong((unsigned long) value);
-#ifdef HAVE_LONG_LONG
- } else if (sizeof(int) <= sizeof(unsigned PY_LONG_LONG)) {
- return PyLong_FromUnsignedLongLong((unsigned PY_LONG_LONG) value);
-#endif
- }
- } else {
- if (sizeof(int) <= sizeof(long)) {
- return PyInt_FromLong((long) value);
-#ifdef HAVE_LONG_LONG
- } else if (sizeof(int) <= sizeof(PY_LONG_LONG)) {
- return PyLong_FromLongLong((PY_LONG_LONG) value);
-#endif
- }
- }
- {
- int one = 1; int little = (int)*(unsigned char *)&one;
- unsigned char *bytes = (unsigned char *)&value;
- return _PyLong_FromByteArray(bytes, sizeof(int),
- little, !is_unsigned);
- }
-}
-
-/* CIntFromPyVerify */
- #define __PYX_VERIFY_RETURN_INT(target_type, func_type, func_value)\
- __PYX__VERIFY_RETURN_INT(target_type, func_type, func_value, 0)
-#define __PYX_VERIFY_RETURN_INT_EXC(target_type, func_type, func_value)\
- __PYX__VERIFY_RETURN_INT(target_type, func_type, func_value, 1)
-#define __PYX__VERIFY_RETURN_INT(target_type, func_type, func_value, exc)\
- {\
- func_type value = func_value;\
- if (sizeof(target_type) < sizeof(func_type)) {\
- if (unlikely(value != (func_type) (target_type) value)) {\
- func_type zero = 0;\
- if (exc && unlikely(value == (func_type)-1 && PyErr_Occurred()))\
- return (target_type) -1;\
- if (is_unsigned && unlikely(value < zero))\
- goto raise_neg_overflow;\
- else\
- goto raise_overflow;\
- }\
- }\
- return (target_type) value;\
- }
-
-/* CIntToPy */
- static CYTHON_INLINE PyObject* __Pyx_PyInt_From_long(long value) {
- const long neg_one = (long) ((long) 0 - (long) 1), const_zero = (long) 0;
- const int is_unsigned = neg_one > const_zero;
- if (is_unsigned) {
- if (sizeof(long) < sizeof(long)) {
- return PyInt_FromLong((long) value);
- } else if (sizeof(long) <= sizeof(unsigned long)) {
- return PyLong_FromUnsignedLong((unsigned long) value);
-#ifdef HAVE_LONG_LONG
- } else if (sizeof(long) <= sizeof(unsigned PY_LONG_LONG)) {
- return PyLong_FromUnsignedLongLong((unsigned PY_LONG_LONG) value);
-#endif
- }
- } else {
- if (sizeof(long) <= sizeof(long)) {
- return PyInt_FromLong((long) value);
-#ifdef HAVE_LONG_LONG
- } else if (sizeof(long) <= sizeof(PY_LONG_LONG)) {
- return PyLong_FromLongLong((PY_LONG_LONG) value);
-#endif
- }
- }
- {
- int one = 1; int little = (int)*(unsigned char *)&one;
- unsigned char *bytes = (unsigned char *)&value;
- return _PyLong_FromByteArray(bytes, sizeof(long),
- little, !is_unsigned);
- }
-}
-
-/* MemviewSliceCopyTemplate */
- static __Pyx_memviewslice
-__pyx_memoryview_copy_new_contig(const __Pyx_memviewslice *from_mvs,
- const char *mode, int ndim,
- size_t sizeof_dtype, int contig_flag,
- int dtype_is_object)
-{
- __Pyx_RefNannyDeclarations
- int i;
- __Pyx_memviewslice new_mvs = { 0, 0, { 0 }, { 0 }, { 0 } };
- struct __pyx_memoryview_obj *from_memview = from_mvs->memview;
- Py_buffer *buf = &from_memview->view;
- PyObject *shape_tuple = NULL;
- PyObject *temp_int = NULL;
- struct __pyx_array_obj *array_obj = NULL;
- struct __pyx_memoryview_obj *memview_obj = NULL;
- __Pyx_RefNannySetupContext("__pyx_memoryview_copy_new_contig", 0);
- for (i = 0; i < ndim; i++) {
- if (unlikely(from_mvs->suboffsets[i] >= 0)) {
- PyErr_Format(PyExc_ValueError, "Cannot copy memoryview slice with "
- "indirect dimensions (axis %d)", i);
- goto fail;
- }
- }
- shape_tuple = PyTuple_New(ndim);
- if (unlikely(!shape_tuple)) {
- goto fail;
- }
- __Pyx_GOTREF(shape_tuple);
- for(i = 0; i < ndim; i++) {
- temp_int = PyInt_FromSsize_t(from_mvs->shape[i]);
- if(unlikely(!temp_int)) {
- goto fail;
- } else {
- PyTuple_SET_ITEM(shape_tuple, i, temp_int);
- temp_int = NULL;
- }
- }
- array_obj = __pyx_array_new(shape_tuple, sizeof_dtype, buf->format, (char *) mode, NULL);
- if (unlikely(!array_obj)) {
- goto fail;
- }
- __Pyx_GOTREF(array_obj);
- memview_obj = (struct __pyx_memoryview_obj *) __pyx_memoryview_new(
- (PyObject *) array_obj, contig_flag,
- dtype_is_object,
- from_mvs->memview->typeinfo);
- if (unlikely(!memview_obj))
- goto fail;
- if (unlikely(__Pyx_init_memviewslice(memview_obj, ndim, &new_mvs, 1) < 0))
- goto fail;
- if (unlikely(__pyx_memoryview_copy_contents(*from_mvs, new_mvs, ndim, ndim,
- dtype_is_object) < 0))
- goto fail;
- goto no_fail;
-fail:
- __Pyx_XDECREF(new_mvs.memview);
- new_mvs.memview = NULL;
- new_mvs.data = NULL;
-no_fail:
- __Pyx_XDECREF(shape_tuple);
- __Pyx_XDECREF(temp_int);
- __Pyx_XDECREF(array_obj);
- __Pyx_RefNannyFinishContext();
- return new_mvs;
-}
-
-/* CIntFromPy */
- static CYTHON_INLINE int __Pyx_PyInt_As_int(PyObject *x) {
- const int neg_one = (int) ((int) 0 - (int) 1), const_zero = (int) 0;
- const int is_unsigned = neg_one > const_zero;
-#if PY_MAJOR_VERSION < 3
- if (likely(PyInt_Check(x))) {
- if (sizeof(int) < sizeof(long)) {
- __PYX_VERIFY_RETURN_INT(int, long, PyInt_AS_LONG(x))
- } else {
- long val = PyInt_AS_LONG(x);
- if (is_unsigned && unlikely(val < 0)) {
- goto raise_neg_overflow;
- }
- return (int) val;
- }
- } else
-#endif
- if (likely(PyLong_Check(x))) {
- if (is_unsigned) {
-#if CYTHON_USE_PYLONG_INTERNALS
- const digit* digits = ((PyLongObject*)x)->ob_digit;
- switch (Py_SIZE(x)) {
- case 0: return (int) 0;
- case 1: __PYX_VERIFY_RETURN_INT(int, digit, digits[0])
- case 2:
- if (8 * sizeof(int) > 1 * PyLong_SHIFT) {
- if (8 * sizeof(unsigned long) > 2 * PyLong_SHIFT) {
- __PYX_VERIFY_RETURN_INT(int, unsigned long, (((((unsigned long)digits[1]) << PyLong_SHIFT) | (unsigned long)digits[0])))
- } else if (8 * sizeof(int) >= 2 * PyLong_SHIFT) {
- return (int) (((((int)digits[1]) << PyLong_SHIFT) | (int)digits[0]));
- }
- }
- break;
- case 3:
- if (8 * sizeof(int) > 2 * PyLong_SHIFT) {
- if (8 * sizeof(unsigned long) > 3 * PyLong_SHIFT) {
- __PYX_VERIFY_RETURN_INT(int, unsigned long, (((((((unsigned long)digits[2]) << PyLong_SHIFT) | (unsigned long)digits[1]) << PyLong_SHIFT) | (unsigned long)digits[0])))
- } else if (8 * sizeof(int) >= 3 * PyLong_SHIFT) {
- return (int) (((((((int)digits[2]) << PyLong_SHIFT) | (int)digits[1]) << PyLong_SHIFT) | (int)digits[0]));
- }
- }
- break;
- case 4:
- if (8 * sizeof(int) > 3 * PyLong_SHIFT) {
- if (8 * sizeof(unsigned long) > 4 * PyLong_SHIFT) {
- __PYX_VERIFY_RETURN_INT(int, unsigned long, (((((((((unsigned long)digits[3]) << PyLong_SHIFT) | (unsigned long)digits[2]) << PyLong_SHIFT) | (unsigned long)digits[1]) << PyLong_SHIFT) | (unsigned long)digits[0])))
- } else if (8 * sizeof(int) >= 4 * PyLong_SHIFT) {
- return (int) (((((((((int)digits[3]) << PyLong_SHIFT) | (int)digits[2]) << PyLong_SHIFT) | (int)digits[1]) << PyLong_SHIFT) | (int)digits[0]));
- }
- }
- break;
- }
-#endif
-#if CYTHON_COMPILING_IN_CPYTHON
- if (unlikely(Py_SIZE(x) < 0)) {
- goto raise_neg_overflow;
- }
-#else
- {
- int result = PyObject_RichCompareBool(x, Py_False, Py_LT);
- if (unlikely(result < 0))
- return (int) -1;
- if (unlikely(result == 1))
- goto raise_neg_overflow;
- }
-#endif
- if (sizeof(int) <= sizeof(unsigned long)) {
- __PYX_VERIFY_RETURN_INT_EXC(int, unsigned long, PyLong_AsUnsignedLong(x))
-#ifdef HAVE_LONG_LONG
- } else if (sizeof(int) <= sizeof(unsigned PY_LONG_LONG)) {
- __PYX_VERIFY_RETURN_INT_EXC(int, unsigned PY_LONG_LONG, PyLong_AsUnsignedLongLong(x))
-#endif
- }
- } else {
-#if CYTHON_USE_PYLONG_INTERNALS
- const digit* digits = ((PyLongObject*)x)->ob_digit;
- switch (Py_SIZE(x)) {
- case 0: return (int) 0;
- case -1: __PYX_VERIFY_RETURN_INT(int, sdigit, (sdigit) (-(sdigit)digits[0]))
- case 1: __PYX_VERIFY_RETURN_INT(int, digit, +digits[0])
- case -2:
- if (8 * sizeof(int) - 1 > 1 * PyLong_SHIFT) {
- if (8 * sizeof(unsigned long) > 2 * PyLong_SHIFT) {
- __PYX_VERIFY_RETURN_INT(int, long, -(long) (((((unsigned long)digits[1]) << PyLong_SHIFT) | (unsigned long)digits[0])))
- } else if (8 * sizeof(int) - 1 > 2 * PyLong_SHIFT) {
- return (int) (((int)-1)*(((((int)digits[1]) << PyLong_SHIFT) | (int)digits[0])));
- }
- }
- break;
- case 2:
- if (8 * sizeof(int) > 1 * PyLong_SHIFT) {
- if (8 * sizeof(unsigned long) > 2 * PyLong_SHIFT) {
- __PYX_VERIFY_RETURN_INT(int, unsigned long, (((((unsigned long)digits[1]) << PyLong_SHIFT) | (unsigned long)digits[0])))
- } else if (8 * sizeof(int) - 1 > 2 * PyLong_SHIFT) {
- return (int) ((((((int)digits[1]) << PyLong_SHIFT) | (int)digits[0])));
- }
- }
- break;
- case -3:
- if (8 * sizeof(int) - 1 > 2 * PyLong_SHIFT) {
- if (8 * sizeof(unsigned long) > 3 * PyLong_SHIFT) {
- __PYX_VERIFY_RETURN_INT(int, long, -(long) (((((((unsigned long)digits[2]) << PyLong_SHIFT) | (unsigned long)digits[1]) << PyLong_SHIFT) | (unsigned long)digits[0])))
- } else if (8 * sizeof(int) - 1 > 3 * PyLong_SHIFT) {
- return (int) (((int)-1)*(((((((int)digits[2]) << PyLong_SHIFT) | (int)digits[1]) << PyLong_SHIFT) | (int)digits[0])));
- }
- }
- break;
- case 3:
- if (8 * sizeof(int) > 2 * PyLong_SHIFT) {
- if (8 * sizeof(unsigned long) > 3 * PyLong_SHIFT) {
- __PYX_VERIFY_RETURN_INT(int, unsigned long, (((((((unsigned long)digits[2]) << PyLong_SHIFT) | (unsigned long)digits[1]) << PyLong_SHIFT) | (unsigned long)digits[0])))
- } else if (8 * sizeof(int) - 1 > 3 * PyLong_SHIFT) {
- return (int) ((((((((int)digits[2]) << PyLong_SHIFT) | (int)digits[1]) << PyLong_SHIFT) | (int)digits[0])));
- }
- }
- break;
- case -4:
- if (8 * sizeof(int) - 1 > 3 * PyLong_SHIFT) {
- if (8 * sizeof(unsigned long) > 4 * PyLong_SHIFT) {
- __PYX_VERIFY_RETURN_INT(int, long, -(long) (((((((((unsigned long)digits[3]) << PyLong_SHIFT) | (unsigned long)digits[2]) << PyLong_SHIFT) | (unsigned long)digits[1]) << PyLong_SHIFT) | (unsigned long)digits[0])))
- } else if (8 * sizeof(int) - 1 > 4 * PyLong_SHIFT) {
- return (int) (((int)-1)*(((((((((int)digits[3]) << PyLong_SHIFT) | (int)digits[2]) << PyLong_SHIFT) | (int)digits[1]) << PyLong_SHIFT) | (int)digits[0])));
- }
- }
- break;
- case 4:
- if (8 * sizeof(int) > 3 * PyLong_SHIFT) {
- if (8 * sizeof(unsigned long) > 4 * PyLong_SHIFT) {
- __PYX_VERIFY_RETURN_INT(int, unsigned long, (((((((((unsigned long)digits[3]) << PyLong_SHIFT) | (unsigned long)digits[2]) << PyLong_SHIFT) | (unsigned long)digits[1]) << PyLong_SHIFT) | (unsigned long)digits[0])))
- } else if (8 * sizeof(int) - 1 > 4 * PyLong_SHIFT) {
- return (int) ((((((((((int)digits[3]) << PyLong_SHIFT) | (int)digits[2]) << PyLong_SHIFT) | (int)digits[1]) << PyLong_SHIFT) | (int)digits[0])));
- }
- }
- break;
- }
-#endif
- if (sizeof(int) <= sizeof(long)) {
- __PYX_VERIFY_RETURN_INT_EXC(int, long, PyLong_AsLong(x))
-#ifdef HAVE_LONG_LONG
- } else if (sizeof(int) <= sizeof(PY_LONG_LONG)) {
- __PYX_VERIFY_RETURN_INT_EXC(int, PY_LONG_LONG, PyLong_AsLongLong(x))
-#endif
- }
- }
- {
-#if CYTHON_COMPILING_IN_PYPY && !defined(_PyLong_AsByteArray)
- PyErr_SetString(PyExc_RuntimeError,
- "_PyLong_AsByteArray() not available in PyPy, cannot convert large numbers");
-#else
- int val;
- PyObject *v = __Pyx_PyNumber_IntOrLong(x);
- #if PY_MAJOR_VERSION < 3
- if (likely(v) && !PyLong_Check(v)) {
- PyObject *tmp = v;
- v = PyNumber_Long(tmp);
- Py_DECREF(tmp);
- }
- #endif
- if (likely(v)) {
- int one = 1; int is_little = (int)*(unsigned char *)&one;
- unsigned char *bytes = (unsigned char *)&val;
- int ret = _PyLong_AsByteArray((PyLongObject *)v,
- bytes, sizeof(val),
- is_little, !is_unsigned);
- Py_DECREF(v);
- if (likely(!ret))
- return val;
- }
-#endif
- return (int) -1;
- }
- } else {
- int val;
- PyObject *tmp = __Pyx_PyNumber_IntOrLong(x);
- if (!tmp) return (int) -1;
- val = __Pyx_PyInt_As_int(tmp);
- Py_DECREF(tmp);
- return val;
- }
-raise_overflow:
- PyErr_SetString(PyExc_OverflowError,
- "value too large to convert to int");
- return (int) -1;
-raise_neg_overflow:
- PyErr_SetString(PyExc_OverflowError,
- "can't convert negative value to int");
- return (int) -1;
-}
-
-/* CIntFromPy */
- static CYTHON_INLINE long __Pyx_PyInt_As_long(PyObject *x) {
- const long neg_one = (long) ((long) 0 - (long) 1), const_zero = (long) 0;
- const int is_unsigned = neg_one > const_zero;
-#if PY_MAJOR_VERSION < 3
- if (likely(PyInt_Check(x))) {
- if (sizeof(long) < sizeof(long)) {
- __PYX_VERIFY_RETURN_INT(long, long, PyInt_AS_LONG(x))
- } else {
- long val = PyInt_AS_LONG(x);
- if (is_unsigned && unlikely(val < 0)) {
- goto raise_neg_overflow;
- }
- return (long) val;
- }
- } else
-#endif
- if (likely(PyLong_Check(x))) {
- if (is_unsigned) {
-#if CYTHON_USE_PYLONG_INTERNALS
- const digit* digits = ((PyLongObject*)x)->ob_digit;
- switch (Py_SIZE(x)) {
- case 0: return (long) 0;
- case 1: __PYX_VERIFY_RETURN_INT(long, digit, digits[0])
- case 2:
- if (8 * sizeof(long) > 1 * PyLong_SHIFT) {
- if (8 * sizeof(unsigned long) > 2 * PyLong_SHIFT) {
- __PYX_VERIFY_RETURN_INT(long, unsigned long, (((((unsigned long)digits[1]) << PyLong_SHIFT) | (unsigned long)digits[0])))
- } else if (8 * sizeof(long) >= 2 * PyLong_SHIFT) {
- return (long) (((((long)digits[1]) << PyLong_SHIFT) | (long)digits[0]));
- }
- }
- break;
- case 3:
- if (8 * sizeof(long) > 2 * PyLong_SHIFT) {
- if (8 * sizeof(unsigned long) > 3 * PyLong_SHIFT) {
- __PYX_VERIFY_RETURN_INT(long, unsigned long, (((((((unsigned long)digits[2]) << PyLong_SHIFT) | (unsigned long)digits[1]) << PyLong_SHIFT) | (unsigned long)digits[0])))
- } else if (8 * sizeof(long) >= 3 * PyLong_SHIFT) {
- return (long) (((((((long)digits[2]) << PyLong_SHIFT) | (long)digits[1]) << PyLong_SHIFT) | (long)digits[0]));
- }
- }
- break;
- case 4:
- if (8 * sizeof(long) > 3 * PyLong_SHIFT) {
- if (8 * sizeof(unsigned long) > 4 * PyLong_SHIFT) {
- __PYX_VERIFY_RETURN_INT(long, unsigned long, (((((((((unsigned long)digits[3]) << PyLong_SHIFT) | (unsigned long)digits[2]) << PyLong_SHIFT) | (unsigned long)digits[1]) << PyLong_SHIFT) | (unsigned long)digits[0])))
- } else if (8 * sizeof(long) >= 4 * PyLong_SHIFT) {
- return (long) (((((((((long)digits[3]) << PyLong_SHIFT) | (long)digits[2]) << PyLong_SHIFT) | (long)digits[1]) << PyLong_SHIFT) | (long)digits[0]));
- }
- }
- break;
- }
-#endif
-#if CYTHON_COMPILING_IN_CPYTHON
- if (unlikely(Py_SIZE(x) < 0)) {
- goto raise_neg_overflow;
- }
-#else
- {
- int result = PyObject_RichCompareBool(x, Py_False, Py_LT);
- if (unlikely(result < 0))
- return (long) -1;
- if (unlikely(result == 1))
- goto raise_neg_overflow;
- }
-#endif
- if (sizeof(long) <= sizeof(unsigned long)) {
- __PYX_VERIFY_RETURN_INT_EXC(long, unsigned long, PyLong_AsUnsignedLong(x))
-#ifdef HAVE_LONG_LONG
- } else if (sizeof(long) <= sizeof(unsigned PY_LONG_LONG)) {
- __PYX_VERIFY_RETURN_INT_EXC(long, unsigned PY_LONG_LONG, PyLong_AsUnsignedLongLong(x))
-#endif
- }
- } else {
-#if CYTHON_USE_PYLONG_INTERNALS
- const digit* digits = ((PyLongObject*)x)->ob_digit;
- switch (Py_SIZE(x)) {
- case 0: return (long) 0;
- case -1: __PYX_VERIFY_RETURN_INT(long, sdigit, (sdigit) (-(sdigit)digits[0]))
- case 1: __PYX_VERIFY_RETURN_INT(long, digit, +digits[0])
- case -2:
- if (8 * sizeof(long) - 1 > 1 * PyLong_SHIFT) {
- if (8 * sizeof(unsigned long) > 2 * PyLong_SHIFT) {
- __PYX_VERIFY_RETURN_INT(long, long, -(long) (((((unsigned long)digits[1]) << PyLong_SHIFT) | (unsigned long)digits[0])))
- } else if (8 * sizeof(long) - 1 > 2 * PyLong_SHIFT) {
- return (long) (((long)-1)*(((((long)digits[1]) << PyLong_SHIFT) | (long)digits[0])));
- }
- }
- break;
- case 2:
- if (8 * sizeof(long) > 1 * PyLong_SHIFT) {
- if (8 * sizeof(unsigned long) > 2 * PyLong_SHIFT) {
- __PYX_VERIFY_RETURN_INT(long, unsigned long, (((((unsigned long)digits[1]) << PyLong_SHIFT) | (unsigned long)digits[0])))
- } else if (8 * sizeof(long) - 1 > 2 * PyLong_SHIFT) {
- return (long) ((((((long)digits[1]) << PyLong_SHIFT) | (long)digits[0])));
- }
- }
- break;
- case -3:
- if (8 * sizeof(long) - 1 > 2 * PyLong_SHIFT) {
- if (8 * sizeof(unsigned long) > 3 * PyLong_SHIFT) {
- __PYX_VERIFY_RETURN_INT(long, long, -(long) (((((((unsigned long)digits[2]) << PyLong_SHIFT) | (unsigned long)digits[1]) << PyLong_SHIFT) | (unsigned long)digits[0])))
- } else if (8 * sizeof(long) - 1 > 3 * PyLong_SHIFT) {
- return (long) (((long)-1)*(((((((long)digits[2]) << PyLong_SHIFT) | (long)digits[1]) << PyLong_SHIFT) | (long)digits[0])));
- }
- }
- break;
- case 3:
- if (8 * sizeof(long) > 2 * PyLong_SHIFT) {
- if (8 * sizeof(unsigned long) > 3 * PyLong_SHIFT) {
- __PYX_VERIFY_RETURN_INT(long, unsigned long, (((((((unsigned long)digits[2]) << PyLong_SHIFT) | (unsigned long)digits[1]) << PyLong_SHIFT) | (unsigned long)digits[0])))
- } else if (8 * sizeof(long) - 1 > 3 * PyLong_SHIFT) {
- return (long) ((((((((long)digits[2]) << PyLong_SHIFT) | (long)digits[1]) << PyLong_SHIFT) | (long)digits[0])));
- }
- }
- break;
- case -4:
- if (8 * sizeof(long) - 1 > 3 * PyLong_SHIFT) {
- if (8 * sizeof(unsigned long) > 4 * PyLong_SHIFT) {
- __PYX_VERIFY_RETURN_INT(long, long, -(long) (((((((((unsigned long)digits[3]) << PyLong_SHIFT) | (unsigned long)digits[2]) << PyLong_SHIFT) | (unsigned long)digits[1]) << PyLong_SHIFT) | (unsigned long)digits[0])))
- } else if (8 * sizeof(long) - 1 > 4 * PyLong_SHIFT) {
- return (long) (((long)-1)*(((((((((long)digits[3]) << PyLong_SHIFT) | (long)digits[2]) << PyLong_SHIFT) | (long)digits[1]) << PyLong_SHIFT) | (long)digits[0])));
- }
- }
- break;
- case 4:
- if (8 * sizeof(long) > 3 * PyLong_SHIFT) {
- if (8 * sizeof(unsigned long) > 4 * PyLong_SHIFT) {
- __PYX_VERIFY_RETURN_INT(long, unsigned long, (((((((((unsigned long)digits[3]) << PyLong_SHIFT) | (unsigned long)digits[2]) << PyLong_SHIFT) | (unsigned long)digits[1]) << PyLong_SHIFT) | (unsigned long)digits[0])))
- } else if (8 * sizeof(long) - 1 > 4 * PyLong_SHIFT) {
- return (long) ((((((((((long)digits[3]) << PyLong_SHIFT) | (long)digits[2]) << PyLong_SHIFT) | (long)digits[1]) << PyLong_SHIFT) | (long)digits[0])));
- }
- }
- break;
- }
-#endif
- if (sizeof(long) <= sizeof(long)) {
- __PYX_VERIFY_RETURN_INT_EXC(long, long, PyLong_AsLong(x))
-#ifdef HAVE_LONG_LONG
- } else if (sizeof(long) <= sizeof(PY_LONG_LONG)) {
- __PYX_VERIFY_RETURN_INT_EXC(long, PY_LONG_LONG, PyLong_AsLongLong(x))
-#endif
- }
- }
- {
-#if CYTHON_COMPILING_IN_PYPY && !defined(_PyLong_AsByteArray)
- PyErr_SetString(PyExc_RuntimeError,
- "_PyLong_AsByteArray() not available in PyPy, cannot convert large numbers");
-#else
- long val;
- PyObject *v = __Pyx_PyNumber_IntOrLong(x);
- #if PY_MAJOR_VERSION < 3
- if (likely(v) && !PyLong_Check(v)) {
- PyObject *tmp = v;
- v = PyNumber_Long(tmp);
- Py_DECREF(tmp);
- }
- #endif
- if (likely(v)) {
- int one = 1; int is_little = (int)*(unsigned char *)&one;
- unsigned char *bytes = (unsigned char *)&val;
- int ret = _PyLong_AsByteArray((PyLongObject *)v,
- bytes, sizeof(val),
- is_little, !is_unsigned);
- Py_DECREF(v);
- if (likely(!ret))
- return val;
- }
-#endif
- return (long) -1;
- }
- } else {
- long val;
- PyObject *tmp = __Pyx_PyNumber_IntOrLong(x);
- if (!tmp) return (long) -1;
- val = __Pyx_PyInt_As_long(tmp);
- Py_DECREF(tmp);
- return val;
- }
-raise_overflow:
- PyErr_SetString(PyExc_OverflowError,
- "value too large to convert to long");
- return (long) -1;
-raise_neg_overflow:
- PyErr_SetString(PyExc_OverflowError,
- "can't convert negative value to long");
- return (long) -1;
-}
-
-/* CIntFromPy */
- static CYTHON_INLINE char __Pyx_PyInt_As_char(PyObject *x) {
- const char neg_one = (char) ((char) 0 - (char) 1), const_zero = (char) 0;
- const int is_unsigned = neg_one > const_zero;
-#if PY_MAJOR_VERSION < 3
- if (likely(PyInt_Check(x))) {
- if (sizeof(char) < sizeof(long)) {
- __PYX_VERIFY_RETURN_INT(char, long, PyInt_AS_LONG(x))
- } else {
- long val = PyInt_AS_LONG(x);
- if (is_unsigned && unlikely(val < 0)) {
- goto raise_neg_overflow;
- }
- return (char) val;
- }
- } else
-#endif
- if (likely(PyLong_Check(x))) {
- if (is_unsigned) {
-#if CYTHON_USE_PYLONG_INTERNALS
- const digit* digits = ((PyLongObject*)x)->ob_digit;
- switch (Py_SIZE(x)) {
- case 0: return (char) 0;
- case 1: __PYX_VERIFY_RETURN_INT(char, digit, digits[0])
- case 2:
- if (8 * sizeof(char) > 1 * PyLong_SHIFT) {
- if (8 * sizeof(unsigned long) > 2 * PyLong_SHIFT) {
- __PYX_VERIFY_RETURN_INT(char, unsigned long, (((((unsigned long)digits[1]) << PyLong_SHIFT) | (unsigned long)digits[0])))
- } else if (8 * sizeof(char) >= 2 * PyLong_SHIFT) {
- return (char) (((((char)digits[1]) << PyLong_SHIFT) | (char)digits[0]));
- }
- }
- break;
- case 3:
- if (8 * sizeof(char) > 2 * PyLong_SHIFT) {
- if (8 * sizeof(unsigned long) > 3 * PyLong_SHIFT) {
- __PYX_VERIFY_RETURN_INT(char, unsigned long, (((((((unsigned long)digits[2]) << PyLong_SHIFT) | (unsigned long)digits[1]) << PyLong_SHIFT) | (unsigned long)digits[0])))
- } else if (8 * sizeof(char) >= 3 * PyLong_SHIFT) {
- return (char) (((((((char)digits[2]) << PyLong_SHIFT) | (char)digits[1]) << PyLong_SHIFT) | (char)digits[0]));
- }
- }
- break;
- case 4:
- if (8 * sizeof(char) > 3 * PyLong_SHIFT) {
- if (8 * sizeof(unsigned long) > 4 * PyLong_SHIFT) {
- __PYX_VERIFY_RETURN_INT(char, unsigned long, (((((((((unsigned long)digits[3]) << PyLong_SHIFT) | (unsigned long)digits[2]) << PyLong_SHIFT) | (unsigned long)digits[1]) << PyLong_SHIFT) | (unsigned long)digits[0])))
- } else if (8 * sizeof(char) >= 4 * PyLong_SHIFT) {
- return (char) (((((((((char)digits[3]) << PyLong_SHIFT) | (char)digits[2]) << PyLong_SHIFT) | (char)digits[1]) << PyLong_SHIFT) | (char)digits[0]));
- }
- }
- break;
- }
-#endif
-#if CYTHON_COMPILING_IN_CPYTHON
- if (unlikely(Py_SIZE(x) < 0)) {
- goto raise_neg_overflow;
- }
-#else
- {
- int result = PyObject_RichCompareBool(x, Py_False, Py_LT);
- if (unlikely(result < 0))
- return (char) -1;
- if (unlikely(result == 1))
- goto raise_neg_overflow;
- }
-#endif
- if (sizeof(char) <= sizeof(unsigned long)) {
- __PYX_VERIFY_RETURN_INT_EXC(char, unsigned long, PyLong_AsUnsignedLong(x))
-#ifdef HAVE_LONG_LONG
- } else if (sizeof(char) <= sizeof(unsigned PY_LONG_LONG)) {
- __PYX_VERIFY_RETURN_INT_EXC(char, unsigned PY_LONG_LONG, PyLong_AsUnsignedLongLong(x))
-#endif
- }
- } else {
-#if CYTHON_USE_PYLONG_INTERNALS
- const digit* digits = ((PyLongObject*)x)->ob_digit;
- switch (Py_SIZE(x)) {
- case 0: return (char) 0;
- case -1: __PYX_VERIFY_RETURN_INT(char, sdigit, (sdigit) (-(sdigit)digits[0]))
- case 1: __PYX_VERIFY_RETURN_INT(char, digit, +digits[0])
- case -2:
- if (8 * sizeof(char) - 1 > 1 * PyLong_SHIFT) {
- if (8 * sizeof(unsigned long) > 2 * PyLong_SHIFT) {
- __PYX_VERIFY_RETURN_INT(char, long, -(long) (((((unsigned long)digits[1]) << PyLong_SHIFT) | (unsigned long)digits[0])))
- } else if (8 * sizeof(char) - 1 > 2 * PyLong_SHIFT) {
- return (char) (((char)-1)*(((((char)digits[1]) << PyLong_SHIFT) | (char)digits[0])));
- }
- }
- break;
- case 2:
- if (8 * sizeof(char) > 1 * PyLong_SHIFT) {
- if (8 * sizeof(unsigned long) > 2 * PyLong_SHIFT) {
- __PYX_VERIFY_RETURN_INT(char, unsigned long, (((((unsigned long)digits[1]) << PyLong_SHIFT) | (unsigned long)digits[0])))
- } else if (8 * sizeof(char) - 1 > 2 * PyLong_SHIFT) {
- return (char) ((((((char)digits[1]) << PyLong_SHIFT) | (char)digits[0])));
- }
- }
- break;
- case -3:
- if (8 * sizeof(char) - 1 > 2 * PyLong_SHIFT) {
- if (8 * sizeof(unsigned long) > 3 * PyLong_SHIFT) {
- __PYX_VERIFY_RETURN_INT(char, long, -(long) (((((((unsigned long)digits[2]) << PyLong_SHIFT) | (unsigned long)digits[1]) << PyLong_SHIFT) | (unsigned long)digits[0])))
- } else if (8 * sizeof(char) - 1 > 3 * PyLong_SHIFT) {
- return (char) (((char)-1)*(((((((char)digits[2]) << PyLong_SHIFT) | (char)digits[1]) << PyLong_SHIFT) | (char)digits[0])));
- }
- }
- break;
- case 3:
- if (8 * sizeof(char) > 2 * PyLong_SHIFT) {
- if (8 * sizeof(unsigned long) > 3 * PyLong_SHIFT) {
- __PYX_VERIFY_RETURN_INT(char, unsigned long, (((((((unsigned long)digits[2]) << PyLong_SHIFT) | (unsigned long)digits[1]) << PyLong_SHIFT) | (unsigned long)digits[0])))
- } else if (8 * sizeof(char) - 1 > 3 * PyLong_SHIFT) {
- return (char) ((((((((char)digits[2]) << PyLong_SHIFT) | (char)digits[1]) << PyLong_SHIFT) | (char)digits[0])));
- }
- }
- break;
- case -4:
- if (8 * sizeof(char) - 1 > 3 * PyLong_SHIFT) {
- if (8 * sizeof(unsigned long) > 4 * PyLong_SHIFT) {
- __PYX_VERIFY_RETURN_INT(char, long, -(long) (((((((((unsigned long)digits[3]) << PyLong_SHIFT) | (unsigned long)digits[2]) << PyLong_SHIFT) | (unsigned long)digits[1]) << PyLong_SHIFT) | (unsigned long)digits[0])))
- } else if (8 * sizeof(char) - 1 > 4 * PyLong_SHIFT) {
- return (char) (((char)-1)*(((((((((char)digits[3]) << PyLong_SHIFT) | (char)digits[2]) << PyLong_SHIFT) | (char)digits[1]) << PyLong_SHIFT) | (char)digits[0])));
- }
- }
- break;
- case 4:
- if (8 * sizeof(char) > 3 * PyLong_SHIFT) {
- if (8 * sizeof(unsigned long) > 4 * PyLong_SHIFT) {
- __PYX_VERIFY_RETURN_INT(char, unsigned long, (((((((((unsigned long)digits[3]) << PyLong_SHIFT) | (unsigned long)digits[2]) << PyLong_SHIFT) | (unsigned long)digits[1]) << PyLong_SHIFT) | (unsigned long)digits[0])))
- } else if (8 * sizeof(char) - 1 > 4 * PyLong_SHIFT) {
- return (char) ((((((((((char)digits[3]) << PyLong_SHIFT) | (char)digits[2]) << PyLong_SHIFT) | (char)digits[1]) << PyLong_SHIFT) | (char)digits[0])));
- }
- }
- break;
- }
-#endif
- if (sizeof(char) <= sizeof(long)) {
- __PYX_VERIFY_RETURN_INT_EXC(char, long, PyLong_AsLong(x))
-#ifdef HAVE_LONG_LONG
- } else if (sizeof(char) <= sizeof(PY_LONG_LONG)) {
- __PYX_VERIFY_RETURN_INT_EXC(char, PY_LONG_LONG, PyLong_AsLongLong(x))
-#endif
- }
- }
- {
-#if CYTHON_COMPILING_IN_PYPY && !defined(_PyLong_AsByteArray)
- PyErr_SetString(PyExc_RuntimeError,
- "_PyLong_AsByteArray() not available in PyPy, cannot convert large numbers");
-#else
- char val;
- PyObject *v = __Pyx_PyNumber_IntOrLong(x);
- #if PY_MAJOR_VERSION < 3
- if (likely(v) && !PyLong_Check(v)) {
- PyObject *tmp = v;
- v = PyNumber_Long(tmp);
- Py_DECREF(tmp);
- }
- #endif
- if (likely(v)) {
- int one = 1; int is_little = (int)*(unsigned char *)&one;
- unsigned char *bytes = (unsigned char *)&val;
- int ret = _PyLong_AsByteArray((PyLongObject *)v,
- bytes, sizeof(val),
- is_little, !is_unsigned);
- Py_DECREF(v);
- if (likely(!ret))
- return val;
- }
-#endif
- return (char) -1;
- }
- } else {
- char val;
- PyObject *tmp = __Pyx_PyNumber_IntOrLong(x);
- if (!tmp) return (char) -1;
- val = __Pyx_PyInt_As_char(tmp);
- Py_DECREF(tmp);
- return val;
- }
-raise_overflow:
- PyErr_SetString(PyExc_OverflowError,
- "value too large to convert to char");
- return (char) -1;
-raise_neg_overflow:
- PyErr_SetString(PyExc_OverflowError,
- "can't convert negative value to char");
- return (char) -1;
-}
-
-/* CheckBinaryVersion */
- static int __Pyx_check_binary_version(void) {
- char ctversion[4], rtversion[4];
- PyOS_snprintf(ctversion, 4, "%d.%d", PY_MAJOR_VERSION, PY_MINOR_VERSION);
- PyOS_snprintf(rtversion, 4, "%s", Py_GetVersion());
- if (ctversion[0] != rtversion[0] || ctversion[2] != rtversion[2]) {
- char message[200];
- PyOS_snprintf(message, sizeof(message),
- "compiletime version %s of module '%.100s' "
- "does not match runtime version %s",
- ctversion, __Pyx_MODULE_NAME, rtversion);
- return PyErr_WarnEx(NULL, message, 1);
- }
- return 0;
-}
-
-/* InitStrings */
- static int __Pyx_InitStrings(__Pyx_StringTabEntry *t) {
- while (t->p) {
- #if PY_MAJOR_VERSION < 3
- if (t->is_unicode) {
- *t->p = PyUnicode_DecodeUTF8(t->s, t->n - 1, NULL);
- } else if (t->intern) {
- *t->p = PyString_InternFromString(t->s);
- } else {
- *t->p = PyString_FromStringAndSize(t->s, t->n - 1);
- }
- #else
- if (t->is_unicode | t->is_str) {
- if (t->intern) {
- *t->p = PyUnicode_InternFromString(t->s);
- } else if (t->encoding) {
- *t->p = PyUnicode_Decode(t->s, t->n - 1, t->encoding, NULL);
- } else {
- *t->p = PyUnicode_FromStringAndSize(t->s, t->n - 1);
- }
- } else {
- *t->p = PyBytes_FromStringAndSize(t->s, t->n - 1);
- }
- #endif
- if (!*t->p)
- return -1;
- if (PyObject_Hash(*t->p) == -1)
- return -1;
- ++t;
- }
- return 0;
-}
-
-static CYTHON_INLINE PyObject* __Pyx_PyUnicode_FromString(const char* c_str) {
- return __Pyx_PyUnicode_FromStringAndSize(c_str, (Py_ssize_t)strlen(c_str));
-}
-static CYTHON_INLINE const char* __Pyx_PyObject_AsString(PyObject* o) {
- Py_ssize_t ignore;
- return __Pyx_PyObject_AsStringAndSize(o, &ignore);
-}
-#if __PYX_DEFAULT_STRING_ENCODING_IS_ASCII || __PYX_DEFAULT_STRING_ENCODING_IS_DEFAULT
-#if !CYTHON_PEP393_ENABLED
-static const char* __Pyx_PyUnicode_AsStringAndSize(PyObject* o, Py_ssize_t *length) {
- char* defenc_c;
- PyObject* defenc = _PyUnicode_AsDefaultEncodedString(o, NULL);
- if (!defenc) return NULL;
- defenc_c = PyBytes_AS_STRING(defenc);
-#if __PYX_DEFAULT_STRING_ENCODING_IS_ASCII
- {
- char* end = defenc_c + PyBytes_GET_SIZE(defenc);
- char* c;
- for (c = defenc_c; c < end; c++) {
- if ((unsigned char) (*c) >= 128) {
- PyUnicode_AsASCIIString(o);
- return NULL;
- }
- }
- }
-#endif
- *length = PyBytes_GET_SIZE(defenc);
- return defenc_c;
-}
-#else
-static CYTHON_INLINE const char* __Pyx_PyUnicode_AsStringAndSize(PyObject* o, Py_ssize_t *length) {
- if (unlikely(__Pyx_PyUnicode_READY(o) == -1)) return NULL;
-#if __PYX_DEFAULT_STRING_ENCODING_IS_ASCII
- if (likely(PyUnicode_IS_ASCII(o))) {
- *length = PyUnicode_GET_LENGTH(o);
- return PyUnicode_AsUTF8(o);
- } else {
- PyUnicode_AsASCIIString(o);
- return NULL;
- }
-#else
- return PyUnicode_AsUTF8AndSize(o, length);
-#endif
-}
-#endif
-#endif
-static CYTHON_INLINE const char* __Pyx_PyObject_AsStringAndSize(PyObject* o, Py_ssize_t *length) {
-#if __PYX_DEFAULT_STRING_ENCODING_IS_ASCII || __PYX_DEFAULT_STRING_ENCODING_IS_DEFAULT
- if (
-#if PY_MAJOR_VERSION < 3 && __PYX_DEFAULT_STRING_ENCODING_IS_ASCII
- __Pyx_sys_getdefaultencoding_not_ascii &&
-#endif
- PyUnicode_Check(o)) {
- return __Pyx_PyUnicode_AsStringAndSize(o, length);
- } else
-#endif
-#if (!CYTHON_COMPILING_IN_PYPY) || (defined(PyByteArray_AS_STRING) && defined(PyByteArray_GET_SIZE))
- if (PyByteArray_Check(o)) {
- *length = PyByteArray_GET_SIZE(o);
- return PyByteArray_AS_STRING(o);
- } else
-#endif
- {
- char* result;
- int r = PyBytes_AsStringAndSize(o, &result, length);
- if (unlikely(r < 0)) {
- return NULL;
- } else {
- return result;
- }
- }
-}
-static CYTHON_INLINE int __Pyx_PyObject_IsTrue(PyObject* x) {
- int is_true = x == Py_True;
- if (is_true | (x == Py_False) | (x == Py_None)) return is_true;
- else return PyObject_IsTrue(x);
-}
-static CYTHON_INLINE int __Pyx_PyObject_IsTrueAndDecref(PyObject* x) {
- int retval;
- if (unlikely(!x)) return -1;
- retval = __Pyx_PyObject_IsTrue(x);
- Py_DECREF(x);
- return retval;
-}
-static PyObject* __Pyx_PyNumber_IntOrLongWrongResultType(PyObject* result, const char* type_name) {
-#if PY_MAJOR_VERSION >= 3
- if (PyLong_Check(result)) {
- if (PyErr_WarnFormat(PyExc_DeprecationWarning, 1,
- "__int__ returned non-int (type %.200s). "
- "The ability to return an instance of a strict subclass of int "
- "is deprecated, and may be removed in a future version of Python.",
- Py_TYPE(result)->tp_name)) {
- Py_DECREF(result);
- return NULL;
- }
- return result;
- }
-#endif
- PyErr_Format(PyExc_TypeError,
- "__%.4s__ returned non-%.4s (type %.200s)",
- type_name, type_name, Py_TYPE(result)->tp_name);
- Py_DECREF(result);
- return NULL;
-}
-static CYTHON_INLINE PyObject* __Pyx_PyNumber_IntOrLong(PyObject* x) {
-#if CYTHON_USE_TYPE_SLOTS
- PyNumberMethods *m;
-#endif
- const char *name = NULL;
- PyObject *res = NULL;
-#if PY_MAJOR_VERSION < 3
- if (likely(PyInt_Check(x) || PyLong_Check(x)))
-#else
- if (likely(PyLong_Check(x)))
-#endif
- return __Pyx_NewRef(x);
-#if CYTHON_USE_TYPE_SLOTS
- m = Py_TYPE(x)->tp_as_number;
- #if PY_MAJOR_VERSION < 3
- if (m && m->nb_int) {
- name = "int";
- res = m->nb_int(x);
- }
- else if (m && m->nb_long) {
- name = "long";
- res = m->nb_long(x);
- }
- #else
- if (likely(m && m->nb_int)) {
- name = "int";
- res = m->nb_int(x);
- }
- #endif
-#else
- if (!PyBytes_CheckExact(x) && !PyUnicode_CheckExact(x)) {
- res = PyNumber_Int(x);
- }
-#endif
- if (likely(res)) {
-#if PY_MAJOR_VERSION < 3
- if (unlikely(!PyInt_Check(res) && !PyLong_Check(res))) {
-#else
- if (unlikely(!PyLong_CheckExact(res))) {
-#endif
- return __Pyx_PyNumber_IntOrLongWrongResultType(res, name);
- }
- }
- else if (!PyErr_Occurred()) {
- PyErr_SetString(PyExc_TypeError,
- "an integer is required");
- }
- return res;
-}
-static CYTHON_INLINE Py_ssize_t __Pyx_PyIndex_AsSsize_t(PyObject* b) {
- Py_ssize_t ival;
- PyObject *x;
-#if PY_MAJOR_VERSION < 3
- if (likely(PyInt_CheckExact(b))) {
- if (sizeof(Py_ssize_t) >= sizeof(long))
- return PyInt_AS_LONG(b);
- else
- return PyInt_AsSsize_t(b);
- }
-#endif
- if (likely(PyLong_CheckExact(b))) {
- #if CYTHON_USE_PYLONG_INTERNALS
- const digit* digits = ((PyLongObject*)b)->ob_digit;
- const Py_ssize_t size = Py_SIZE(b);
- if (likely(__Pyx_sst_abs(size) <= 1)) {
- ival = likely(size) ? digits[0] : 0;
- if (size == -1) ival = -ival;
- return ival;
- } else {
- switch (size) {
- case 2:
- if (8 * sizeof(Py_ssize_t) > 2 * PyLong_SHIFT) {
- return (Py_ssize_t) (((((size_t)digits[1]) << PyLong_SHIFT) | (size_t)digits[0]));
- }
- break;
- case -2:
- if (8 * sizeof(Py_ssize_t) > 2 * PyLong_SHIFT) {
- return -(Py_ssize_t) (((((size_t)digits[1]) << PyLong_SHIFT) | (size_t)digits[0]));
- }
- break;
- case 3:
- if (8 * sizeof(Py_ssize_t) > 3 * PyLong_SHIFT) {
- return (Py_ssize_t) (((((((size_t)digits[2]) << PyLong_SHIFT) | (size_t)digits[1]) << PyLong_SHIFT) | (size_t)digits[0]));
- }
- break;
- case -3:
- if (8 * sizeof(Py_ssize_t) > 3 * PyLong_SHIFT) {
- return -(Py_ssize_t) (((((((size_t)digits[2]) << PyLong_SHIFT) | (size_t)digits[1]) << PyLong_SHIFT) | (size_t)digits[0]));
- }
- break;
- case 4:
- if (8 * sizeof(Py_ssize_t) > 4 * PyLong_SHIFT) {
- return (Py_ssize_t) (((((((((size_t)digits[3]) << PyLong_SHIFT) | (size_t)digits[2]) << PyLong_SHIFT) | (size_t)digits[1]) << PyLong_SHIFT) | (size_t)digits[0]));
- }
- break;
- case -4:
- if (8 * sizeof(Py_ssize_t) > 4 * PyLong_SHIFT) {
- return -(Py_ssize_t) (((((((((size_t)digits[3]) << PyLong_SHIFT) | (size_t)digits[2]) << PyLong_SHIFT) | (size_t)digits[1]) << PyLong_SHIFT) | (size_t)digits[0]));
- }
- break;
- }
- }
- #endif
- return PyLong_AsSsize_t(b);
- }
- x = PyNumber_Index(b);
- if (!x) return -1;
- ival = PyInt_AsSsize_t(x);
- Py_DECREF(x);
- return ival;
-}
-static CYTHON_INLINE PyObject * __Pyx_PyBool_FromLong(long b) {
- return b ? __Pyx_NewRef(Py_True) : __Pyx_NewRef(Py_False);
-}
-static CYTHON_INLINE PyObject * __Pyx_PyInt_FromSize_t(size_t ival) {
- return PyInt_FromSize_t(ival);
-}
-
-
-#endif /* Py_PYTHON_H */
diff --git a/spaces/AlbertoFH98/CastenaApp/README.md b/spaces/AlbertoFH98/CastenaApp/README.md
deleted file mode 100644
index b2b179d45de5933c212ed0fccc422d9ebe26e284..0000000000000000000000000000000000000000
--- a/spaces/AlbertoFH98/CastenaApp/README.md
+++ /dev/null
@@ -1,13 +0,0 @@
----
-title: CastenaApp
-emoji: 📈
-colorFrom: green
-colorTo: gray
-sdk: streamlit
-sdk_version: 1.27.2
-app_file: app.py
-pinned: false
-license: apache-2.0
----
-
-Check out the configuration reference at https://huggingface.co/docs/hub/spaces-config-reference
diff --git a/spaces/AlekseyKorshuk/thin-plate-spline-motion-model/frames_dataset.py b/spaces/AlekseyKorshuk/thin-plate-spline-motion-model/frames_dataset.py
deleted file mode 100644
index 2328c6a0af028518fe5412ebc75b2b8a019d77c8..0000000000000000000000000000000000000000
--- a/spaces/AlekseyKorshuk/thin-plate-spline-motion-model/frames_dataset.py
+++ /dev/null
@@ -1,173 +0,0 @@
-import os
-from skimage import io, img_as_float32
-from skimage.color import gray2rgb
-from sklearn.model_selection import train_test_split
-from imageio import mimread
-from skimage.transform import resize
-import numpy as np
-from torch.utils.data import Dataset
-from augmentation import AllAugmentationTransform
-import glob
-from functools import partial
-
-
-def read_video(name, frame_shape):
- """
- Read video which can be:
- - an image of concatenated frames
- - '.mp4' and'.gif'
- - folder with videos
- """
-
- if os.path.isdir(name):
- frames = sorted(os.listdir(name))
- num_frames = len(frames)
- video_array = np.array(
- [img_as_float32(io.imread(os.path.join(name, frames[idx]))) for idx in range(num_frames)])
- elif name.lower().endswith('.png') or name.lower().endswith('.jpg'):
- image = io.imread(name)
-
- if len(image.shape) == 2 or image.shape[2] == 1:
- image = gray2rgb(image)
-
- if image.shape[2] == 4:
- image = image[..., :3]
-
- image = img_as_float32(image)
-
- video_array = np.moveaxis(image, 1, 0)
-
- video_array = video_array.reshape((-1,) + frame_shape)
- video_array = np.moveaxis(video_array, 1, 2)
- elif name.lower().endswith('.gif') or name.lower().endswith('.mp4') or name.lower().endswith('.mov'):
- video = mimread(name)
- if len(video[0].shape) == 2:
- video = [gray2rgb(frame) for frame in video]
- if frame_shape is not None:
- video = np.array([resize(frame, frame_shape) for frame in video])
- video = np.array(video)
- if video.shape[-1] == 4:
- video = video[..., :3]
- video_array = img_as_float32(video)
- else:
- raise Exception("Unknown file extensions %s" % name)
-
- return video_array
-
-
-class FramesDataset(Dataset):
- """
- Dataset of videos, each video can be represented as:
- - an image of concatenated frames
- - '.mp4' or '.gif'
- - folder with all frames
- """
-
- def __init__(self, root_dir, frame_shape=(256, 256, 3), id_sampling=False, is_train=True,
- random_seed=0, pairs_list=None, augmentation_params=None):
- self.root_dir = root_dir
- self.videos = os.listdir(root_dir)
- self.frame_shape = frame_shape
- print(self.frame_shape)
- self.pairs_list = pairs_list
- self.id_sampling = id_sampling
-
- if os.path.exists(os.path.join(root_dir, 'train')):
- assert os.path.exists(os.path.join(root_dir, 'test'))
- print("Use predefined train-test split.")
- if id_sampling:
- train_videos = {os.path.basename(video).split('#')[0] for video in
- os.listdir(os.path.join(root_dir, 'train'))}
- train_videos = list(train_videos)
- else:
- train_videos = os.listdir(os.path.join(root_dir, 'train'))
- test_videos = os.listdir(os.path.join(root_dir, 'test'))
- self.root_dir = os.path.join(self.root_dir, 'train' if is_train else 'test')
- else:
- print("Use random train-test split.")
- train_videos, test_videos = train_test_split(self.videos, random_state=random_seed, test_size=0.2)
-
- if is_train:
- self.videos = train_videos
- else:
- self.videos = test_videos
-
- self.is_train = is_train
-
- if self.is_train:
- self.transform = AllAugmentationTransform(**augmentation_params)
- else:
- self.transform = None
-
- def __len__(self):
- return len(self.videos)
-
- def __getitem__(self, idx):
-
- if self.is_train and self.id_sampling:
- name = self.videos[idx]
- path = np.random.choice(glob.glob(os.path.join(self.root_dir, name + '*.mp4')))
- else:
- name = self.videos[idx]
- path = os.path.join(self.root_dir, name)
-
- video_name = os.path.basename(path)
- if self.is_train and os.path.isdir(path):
-
- frames = os.listdir(path)
- num_frames = len(frames)
- frame_idx = np.sort(np.random.choice(num_frames, replace=True, size=2))
-
- if self.frame_shape is not None:
- resize_fn = partial(resize, output_shape=self.frame_shape)
- else:
- resize_fn = img_as_float32
-
- if type(frames[0]) is bytes:
- video_array = [resize_fn(io.imread(os.path.join(path, frames[idx].decode('utf-8')))) for idx in
- frame_idx]
- else:
- video_array = [resize_fn(io.imread(os.path.join(path, frames[idx]))) for idx in frame_idx]
- else:
-
- video_array = read_video(path, frame_shape=self.frame_shape)
-
- num_frames = len(video_array)
- frame_idx = np.sort(np.random.choice(num_frames, replace=True, size=2)) if self.is_train else range(
- num_frames)
- video_array = video_array[frame_idx]
-
-
- if self.transform is not None:
- video_array = self.transform(video_array)
-
- out = {}
- if self.is_train:
- source = np.array(video_array[0], dtype='float32')
- driving = np.array(video_array[1], dtype='float32')
-
- out['driving'] = driving.transpose((2, 0, 1))
- out['source'] = source.transpose((2, 0, 1))
- else:
- video = np.array(video_array, dtype='float32')
- out['video'] = video.transpose((3, 0, 1, 2))
-
- out['name'] = video_name
- return out
-
-
-class DatasetRepeater(Dataset):
- """
- Pass several times over the same dataset for better i/o performance
- """
-
- def __init__(self, dataset, num_repeats=100):
- self.dataset = dataset
- self.num_repeats = num_repeats
-
- def __len__(self):
- return self.num_repeats * self.dataset.__len__()
-
- def __getitem__(self, idx):
- return self.dataset[idx % self.dataset.__len__()]
-
diff --git a/spaces/Amrrs/DragGan-Inversion/PTI/models/StyleCLIP/mapper/latent_mappers.py b/spaces/Amrrs/DragGan-Inversion/PTI/models/StyleCLIP/mapper/latent_mappers.py
deleted file mode 100644
index 63637adc9646986a3546edd19f4555a2f75a379f..0000000000000000000000000000000000000000
--- a/spaces/Amrrs/DragGan-Inversion/PTI/models/StyleCLIP/mapper/latent_mappers.py
+++ /dev/null
@@ -1,81 +0,0 @@
-import torch
-from torch import nn
-from torch.nn import Module
-
-from models.StyleCLIP.models.stylegan2.model import EqualLinear, PixelNorm
-
-
-class Mapper(Module):
-
- def __init__(self, opts):
- super(Mapper, self).__init__()
-
- self.opts = opts
- layers = [PixelNorm()]
-
- for i in range(4):
- layers.append(
- EqualLinear(
- 512, 512, lr_mul=0.01, activation='fused_lrelu'
- )
- )
-
- self.mapping = nn.Sequential(*layers)
-
-
- def forward(self, x):
- x = self.mapping(x)
- return x
-
-
-class SingleMapper(Module):
-
- def __init__(self, opts):
- super(SingleMapper, self).__init__()
-
- self.opts = opts
-
- self.mapping = Mapper(opts)
-
- def forward(self, x):
- out = self.mapping(x)
- return out
-
-
-class LevelsMapper(Module):
-
- def __init__(self, opts):
- super(LevelsMapper, self).__init__()
-
- self.opts = opts
-
- if not opts.no_coarse_mapper:
- self.course_mapping = Mapper(opts)
- if not opts.no_medium_mapper:
- self.medium_mapping = Mapper(opts)
- if not opts.no_fine_mapper:
- self.fine_mapping = Mapper(opts)
-
- def forward(self, x):
- x_coarse = x[:, :4, :]
- x_medium = x[:, 4:8, :]
- x_fine = x[:, 8:, :]
-
- if not self.opts.no_coarse_mapper:
- x_coarse = self.course_mapping(x_coarse)
- else:
- x_coarse = torch.zeros_like(x_coarse)
- if not self.opts.no_medium_mapper:
- x_medium = self.medium_mapping(x_medium)
- else:
- x_medium = torch.zeros_like(x_medium)
- if not self.opts.no_fine_mapper:
- x_fine = self.fine_mapping(x_fine)
- else:
- x_fine = torch.zeros_like(x_fine)
-
-
- out = torch.cat([x_coarse, x_medium, x_fine], dim=1)
-
- return out
-
diff --git a/spaces/Androidonnxfork/CivitAi-to-Diffusers/diffusers/docs/source/en/optimization/fp16.md b/spaces/Androidonnxfork/CivitAi-to-Diffusers/diffusers/docs/source/en/optimization/fp16.md
deleted file mode 100644
index 8b3a62cba099022610c84fd9ce74fc42b6bdf75b..0000000000000000000000000000000000000000
--- a/spaces/Androidonnxfork/CivitAi-to-Diffusers/diffusers/docs/source/en/optimization/fp16.md
+++ /dev/null
@@ -1,434 +0,0 @@
-
-
-# Memory and speed
-
-We present some techniques and ideas to optimize 🤗 Diffusers _inference_ for memory or speed. As a general rule, we recommend the use of [xFormers](https://github.com/facebookresearch/xformers) for memory efficient attention, please see the recommended [installation instructions](xformers).
-
-We'll discuss how the following settings impact performance and memory.
-
-| | Latency | Speedup |
-| ---------------- | ------- | ------- |
-| original | 9.50s | x1 |
-| fp16 | 3.61s | x2.63 |
-| channels last | 3.30s | x2.88 |
-| traced UNet | 3.21s | x2.96 |
-| memory efficient attention | 2.63s | x3.61 |
-
-
- obtained on NVIDIA TITAN RTX by generating a single image of size 512x512 from
- the prompt "a photo of an astronaut riding a horse on mars" with 50 DDIM
- steps.
-
-
-### Use tf32 instead of fp32 (on Ampere and later CUDA devices)
-
-On Ampere and later CUDA devices matrix multiplications and convolutions can use the TensorFloat32 (TF32) mode for faster but slightly less accurate computations. By default PyTorch enables TF32 mode for convolutions but not matrix multiplications, and unless a network requires full float32 precision we recommend enabling this setting for matrix multiplications, too. It can significantly speed up computations with typically negligible loss of numerical accuracy. You can read more about it [here](https://huggingface.co/docs/transformers/v4.18.0/en/performance#tf32). All you need to do is to add this before your inference:
-
-```python
-import torch
-
-torch.backends.cuda.matmul.allow_tf32 = True
-```
-
-## Half precision weights
-
-To save more GPU memory and get more speed, you can load and run the model weights directly in half precision. This involves loading the float16 version of the weights, which was saved to a branch named `fp16`, and telling PyTorch to use the `float16` type when loading them:
-
-```Python
-import torch
-from diffusers import DiffusionPipeline
-
-pipe = DiffusionPipeline.from_pretrained(
- "runwayml/stable-diffusion-v1-5",
- torch_dtype=torch.float16,
-)
-pipe = pipe.to("cuda")
-
-prompt = "a photo of an astronaut riding a horse on mars"
-image = pipe(prompt).images[0]
-```
-
-
-
- It is strongly discouraged to make use of [`torch.autocast`](https://pytorch.org/docs/stable/amp.html#torch.autocast) in any of the pipelines as it can lead to black images and is always slower than using pure
- float16 precision.
-
-
-
-## Sliced attention for additional memory savings
-
-For even additional memory savings, you can use a sliced version of attention that performs the computation in steps instead of all at once.
-
-
- Attention slicing is useful even if a batch size of just 1 is used - as long
- as the model uses more than one attention head. If there is more than one
- attention head the *QK^T* attention matrix can be computed sequentially for
- each head which can save a significant amount of memory.
-
-
-To perform the attention computation sequentially over each head, you only need to invoke [`~DiffusionPipeline.enable_attention_slicing`] in your pipeline before inference, like here:
-
-```Python
-import torch
-from diffusers import DiffusionPipeline
-
-pipe = DiffusionPipeline.from_pretrained(
- "runwayml/stable-diffusion-v1-5",
- torch_dtype=torch.float16,
-)
-pipe = pipe.to("cuda")
-
-prompt = "a photo of an astronaut riding a horse on mars"
-pipe.enable_attention_slicing()
-image = pipe(prompt).images[0]
-```
-
-There's a small performance penalty of about 10% slower inference times, but this method allows you to use Stable Diffusion in as little as 3.2 GB of VRAM!
-
-
-## Sliced VAE decode for larger batches
-
-To decode large batches of images with limited VRAM, or to enable batches with 32 images or more, you can use sliced VAE decode that decodes the batch latents one image at a time.
-
-You likely want to couple this with [`~StableDiffusionPipeline.enable_attention_slicing`] or [`~StableDiffusionPipeline.enable_xformers_memory_efficient_attention`] to further minimize memory use.
-
-To perform the VAE decode one image at a time, invoke [`~StableDiffusionPipeline.enable_vae_slicing`] in your pipeline before inference. For example:
-
-```Python
-import torch
-from diffusers import StableDiffusionPipeline
-
-pipe = StableDiffusionPipeline.from_pretrained(
- "runwayml/stable-diffusion-v1-5",
- torch_dtype=torch.float16,
-)
-pipe = pipe.to("cuda")
-
-prompt = "a photo of an astronaut riding a horse on mars"
-pipe.enable_vae_slicing()
-images = pipe([prompt] * 32).images
-```
-
-You may see a small performance boost in VAE decode on multi-image batches. There should be no performance impact on single-image batches.
-
-
-## Tiled VAE decode and encode for large images
-
-Tiled VAE processing makes it possible to work with large images on limited VRAM. For example, generating 4k images in 8GB of VRAM. Tiled VAE decoder splits the image into overlapping tiles, decodes the tiles, and blends the outputs to make the final image.
-
-You want to couple this with [`~StableDiffusionPipeline.enable_attention_slicing`] or [`~StableDiffusionPipeline.enable_xformers_memory_efficient_attention`] to further minimize memory use.
-
-To use tiled VAE processing, invoke [`~StableDiffusionPipeline.enable_vae_tiling`] in your pipeline before inference. For example:
-
-```python
-import torch
-from diffusers import StableDiffusionPipeline, UniPCMultistepScheduler
-
-pipe = StableDiffusionPipeline.from_pretrained(
- "runwayml/stable-diffusion-v1-5",
- torch_dtype=torch.float16,
-)
-pipe.scheduler = UniPCMultistepScheduler.from_config(pipe.scheduler.config)
-pipe = pipe.to("cuda")
-prompt = "a beautiful landscape photograph"
-pipe.enable_vae_tiling()
-pipe.enable_xformers_memory_efficient_attention()
-
-image = pipe([prompt], width=3840, height=2224, num_inference_steps=20).images[0]
-```
-
-The output image will have some tile-to-tile tone variation from the tiles having separate decoders, but you shouldn't see sharp seams between the tiles. The tiling is turned off for images that are 512x512 or smaller.
-
-
-
-## Offloading to CPU with accelerate for memory savings
-
-For additional memory savings, you can offload the weights to CPU and only load them to GPU when performing the forward pass.
-
-To perform CPU offloading, all you have to do is invoke [`~StableDiffusionPipeline.enable_sequential_cpu_offload`]:
-
-```Python
-import torch
-from diffusers import StableDiffusionPipeline
-
-pipe = StableDiffusionPipeline.from_pretrained(
- "runwayml/stable-diffusion-v1-5",
- torch_dtype=torch.float16,
-)
-
-prompt = "a photo of an astronaut riding a horse on mars"
-pipe.enable_sequential_cpu_offload()
-image = pipe(prompt).images[0]
-```
-
-And you can get the memory consumption to < 3GB.
-
-Note that this method works at the submodule level, not on whole models. This is the best way to minimize memory consumption, but inference is much slower due to the iterative nature of the process. The UNet component of the pipeline runs several times (as many as `num_inference_steps`); each time, the different submodules of the UNet are sequentially onloaded and then offloaded as they are needed, so the number of memory transfers is large.
-
-
-Consider using model offloading as another point in the optimization space: it will be much faster, but memory savings won't be as large.
-
-
-It is also possible to chain offloading with attention slicing for minimal memory consumption (< 2GB).
-
-```Python
-import torch
-from diffusers import StableDiffusionPipeline
-
-pipe = StableDiffusionPipeline.from_pretrained(
- "runwayml/stable-diffusion-v1-5",
- torch_dtype=torch.float16,
-)
-
-prompt = "a photo of an astronaut riding a horse on mars"
-pipe.enable_sequential_cpu_offload()
-pipe.enable_attention_slicing(1)
-
-image = pipe(prompt).images[0]
-```
-
-**Note**: When using `enable_sequential_cpu_offload()`, it is important to **not** move the pipeline to CUDA beforehand or else the gain in memory consumption will only be minimal. See [this issue](https://github.com/huggingface/diffusers/issues/1934) for more information.
-
-**Note**: `enable_sequential_cpu_offload()` is a stateful operation that installs hooks on the models.
-
-
-
-## Model offloading for fast inference and memory savings
-
-[Sequential CPU offloading](#sequential_offloading), as discussed in the previous section, preserves a lot of memory but makes inference slower, because submodules are moved to GPU as needed, and immediately returned to CPU when a new module runs.
-
-Full-model offloading is an alternative that moves whole models to the GPU, instead of handling each model's constituent _modules_. This results in a negligible impact on inference time (compared with moving the pipeline to `cuda`), while still providing some memory savings.
-
-In this scenario, only one of the main components of the pipeline (typically: text encoder, unet and vae)
-will be in the GPU while the others wait in the CPU. Components like the UNet that run for multiple iterations will stay on GPU until they are no longer needed.
-
-This feature can be enabled by invoking `enable_model_cpu_offload()` on the pipeline, as shown below.
-
-```Python
-import torch
-from diffusers import StableDiffusionPipeline
-
-pipe = StableDiffusionPipeline.from_pretrained(
- "runwayml/stable-diffusion-v1-5",
- torch_dtype=torch.float16,
-)
-
-prompt = "a photo of an astronaut riding a horse on mars"
-pipe.enable_model_cpu_offload()
-image = pipe(prompt).images[0]
-```
-
-This is also compatible with attention slicing for additional memory savings.
-
-```Python
-import torch
-from diffusers import StableDiffusionPipeline
-
-pipe = StableDiffusionPipeline.from_pretrained(
- "runwayml/stable-diffusion-v1-5",
- torch_dtype=torch.float16,
-)
-
-prompt = "a photo of an astronaut riding a horse on mars"
-pipe.enable_model_cpu_offload()
-pipe.enable_attention_slicing(1)
-
-image = pipe(prompt).images[0]
-```
-
-
-This feature requires `accelerate` version 0.17.0 or larger.
-
-
-**Note**: `enable_model_cpu_offload()` is a stateful operation that installs hooks on the models and state on the pipeline. In order to properly offload
-models after they are called, it is required that the entire pipeline is run and models are called in the order the pipeline expects them to be. Exercise caution
-if models are re-used outside the context of the pipeline after hooks have been installed. See [accelerate](https://huggingface.co/docs/accelerate/v0.18.0/en/package_reference/big_modeling#accelerate.hooks.remove_hook_from_module)
-for further docs on removing hooks.
-
-## Using Channels Last memory format
-
-Channels last memory format is an alternative way of ordering NCHW tensors in memory preserving dimensions ordering. Channels last tensors ordered in such a way that channels become the densest dimension (aka storing images pixel-per-pixel). Since not all operators currently support channels last format it may result in a worst performance, so it's better to try it and see if it works for your model.
-
-For example, in order to set the UNet model in our pipeline to use channels last format, we can use the following:
-
-```python
-print(pipe.unet.conv_out.state_dict()["weight"].stride()) # (2880, 9, 3, 1)
-pipe.unet.to(memory_format=torch.channels_last) # in-place operation
-print(
- pipe.unet.conv_out.state_dict()["weight"].stride()
-) # (2880, 1, 960, 320) having a stride of 1 for the 2nd dimension proves that it works
-```
-
-## Tracing
-
-Tracing runs an example input tensor through your model, and captures the operations that are invoked as that input makes its way through the model's layers so that an executable or `ScriptFunction` is returned that will be optimized using just-in-time compilation.
-
-To trace our UNet model, we can use the following:
-
-```python
-import time
-import torch
-from diffusers import StableDiffusionPipeline
-import functools
-
-# torch disable grad
-torch.set_grad_enabled(False)
-
-# set variables
-n_experiments = 2
-unet_runs_per_experiment = 50
-
-
-# load inputs
-def generate_inputs():
- sample = torch.randn(2, 4, 64, 64).half().cuda()
- timestep = torch.rand(1).half().cuda() * 999
- encoder_hidden_states = torch.randn(2, 77, 768).half().cuda()
- return sample, timestep, encoder_hidden_states
-
-
-pipe = StableDiffusionPipeline.from_pretrained(
- "runwayml/stable-diffusion-v1-5",
- torch_dtype=torch.float16,
-).to("cuda")
-unet = pipe.unet
-unet.eval()
-unet.to(memory_format=torch.channels_last) # use channels_last memory format
-unet.forward = functools.partial(unet.forward, return_dict=False) # set return_dict=False as default
-
-# warmup
-for _ in range(3):
- with torch.inference_mode():
- inputs = generate_inputs()
- orig_output = unet(*inputs)
-
-# trace
-print("tracing..")
-unet_traced = torch.jit.trace(unet, inputs)
-unet_traced.eval()
-print("done tracing")
-
-
-# warmup and optimize graph
-for _ in range(5):
- with torch.inference_mode():
- inputs = generate_inputs()
- orig_output = unet_traced(*inputs)
-
-
-# benchmarking
-with torch.inference_mode():
- for _ in range(n_experiments):
- torch.cuda.synchronize()
- start_time = time.time()
- for _ in range(unet_runs_per_experiment):
- orig_output = unet_traced(*inputs)
- torch.cuda.synchronize()
- print(f"unet traced inference took {time.time() - start_time:.2f} seconds")
- for _ in range(n_experiments):
- torch.cuda.synchronize()
- start_time = time.time()
- for _ in range(unet_runs_per_experiment):
- orig_output = unet(*inputs)
- torch.cuda.synchronize()
- print(f"unet inference took {time.time() - start_time:.2f} seconds")
-
-# save the model
-unet_traced.save("unet_traced.pt")
-```
-
-Then we can replace the `unet` attribute of the pipeline with the traced model like the following
-
-```python
-from diffusers import StableDiffusionPipeline
-import torch
-from dataclasses import dataclass
-
-
-@dataclass
-class UNet2DConditionOutput:
- sample: torch.FloatTensor
-
-
-pipe = StableDiffusionPipeline.from_pretrained(
- "runwayml/stable-diffusion-v1-5",
- torch_dtype=torch.float16,
-).to("cuda")
-
-# use jitted unet
-unet_traced = torch.jit.load("unet_traced.pt")
-
-
-# del pipe.unet
-class TracedUNet(torch.nn.Module):
- def __init__(self):
- super().__init__()
- self.in_channels = pipe.unet.in_channels
- self.device = pipe.unet.device
-
- def forward(self, latent_model_input, t, encoder_hidden_states):
- sample = unet_traced(latent_model_input, t, encoder_hidden_states)[0]
- return UNet2DConditionOutput(sample=sample)
-
-
-pipe.unet = TracedUNet()
-
-with torch.inference_mode():
- image = pipe([prompt] * 1, num_inference_steps=50).images[0]
-```
-
-
-## Memory Efficient Attention
-
-Recent work on optimizing the bandwitdh in the attention block has generated huge speed ups and gains in GPU memory usage. The most recent being Flash Attention from @tridao: [code](https://github.com/HazyResearch/flash-attention), [paper](https://arxiv.org/pdf/2205.14135.pdf).
-
-Here are the speedups we obtain on a few Nvidia GPUs when running the inference at 512x512 with a batch size of 1 (one prompt):
-
-| GPU | Base Attention FP16 | Memory Efficient Attention FP16 |
-|------------------ |--------------------- |--------------------------------- |
-| NVIDIA Tesla T4 | 3.5it/s | 5.5it/s |
-| NVIDIA 3060 RTX | 4.6it/s | 7.8it/s |
-| NVIDIA A10G | 8.88it/s | 15.6it/s |
-| NVIDIA RTX A6000 | 11.7it/s | 21.09it/s |
-| NVIDIA TITAN RTX | 12.51it/s | 18.22it/s |
-| A100-SXM4-40GB | 18.6it/s | 29.it/s |
-| A100-SXM-80GB | 18.7it/s | 29.5it/s |
-
-To leverage it just make sure you have:
-
-
-
-If you have PyTorch 2.0 installed, you shouldn't use xFormers!
-
-
-
- - PyTorch > 1.12
- - Cuda available
- - [Installed the xformers library](xformers).
-```python
-from diffusers import DiffusionPipeline
-import torch
-
-pipe = DiffusionPipeline.from_pretrained(
- "runwayml/stable-diffusion-v1-5",
- torch_dtype=torch.float16,
-).to("cuda")
-
-pipe.enable_xformers_memory_efficient_attention()
-
-with torch.inference_mode():
- sample = pipe("a small cat")
-
-# optional: You can disable it via
-# pipe.disable_xformers_memory_efficient_attention()
-```
diff --git a/spaces/Andy1621/uniformer_image_segmentation/configs/ccnet/ccnet_r50-d8_512x1024_80k_cityscapes.py b/spaces/Andy1621/uniformer_image_segmentation/configs/ccnet/ccnet_r50-d8_512x1024_80k_cityscapes.py
deleted file mode 100644
index 16e34356e9f8566ec73e3c25c771e281d3eeb975..0000000000000000000000000000000000000000
--- a/spaces/Andy1621/uniformer_image_segmentation/configs/ccnet/ccnet_r50-d8_512x1024_80k_cityscapes.py
+++ /dev/null
@@ -1,4 +0,0 @@
-_base_ = [
- '../_base_/models/ccnet_r50-d8.py', '../_base_/datasets/cityscapes.py',
- '../_base_/default_runtime.py', '../_base_/schedules/schedule_80k.py'
-]
diff --git a/spaces/Andy1621/uniformer_light/imagenet_class_index.py b/spaces/Andy1621/uniformer_light/imagenet_class_index.py
deleted file mode 100644
index 5407d1471197fd9bfa466c47d8dfb6683cb9f551..0000000000000000000000000000000000000000
--- a/spaces/Andy1621/uniformer_light/imagenet_class_index.py
+++ /dev/null
@@ -1,1002 +0,0 @@
-imagenet_classnames = {
- "0": ["n01440764", "tench"],
- "1": ["n01443537", "goldfish"],
- "2": ["n01484850", "great_white_shark"],
- "3": ["n01491361", "tiger_shark"],
- "4": ["n01494475", "hammerhead"],
- "5": ["n01496331", "electric_ray"],
- "6": ["n01498041", "stingray"],
- "7": ["n01514668", "cock"],
- "8": ["n01514859", "hen"],
- "9": ["n01518878", "ostrich"],
- "10": ["n01530575", "brambling"],
- "11": ["n01531178", "goldfinch"],
- "12": ["n01532829", "house_finch"],
- "13": ["n01534433", "junco"],
- "14": ["n01537544", "indigo_bunting"],
- "15": ["n01558993", "robin"],
- "16": ["n01560419", "bulbul"],
- "17": ["n01580077", "jay"],
- "18": ["n01582220", "magpie"],
- "19": ["n01592084", "chickadee"],
- "20": ["n01601694", "water_ouzel"],
- "21": ["n01608432", "kite"],
- "22": ["n01614925", "bald_eagle"],
- "23": ["n01616318", "vulture"],
- "24": ["n01622779", "great_grey_owl"],
- "25": ["n01629819", "European_fire_salamander"],
- "26": ["n01630670", "common_newt"],
- "27": ["n01631663", "eft"],
- "28": ["n01632458", "spotted_salamander"],
- "29": ["n01632777", "axolotl"],
- "30": ["n01641577", "bullfrog"],
- "31": ["n01644373", "tree_frog"],
- "32": ["n01644900", "tailed_frog"],
- "33": ["n01664065", "loggerhead"],
- "34": ["n01665541", "leatherback_turtle"],
- "35": ["n01667114", "mud_turtle"],
- "36": ["n01667778", "terrapin"],
- "37": ["n01669191", "box_turtle"],
- "38": ["n01675722", "banded_gecko"],
- "39": ["n01677366", "common_iguana"],
- "40": ["n01682714", "American_chameleon"],
- "41": ["n01685808", "whiptail"],
- "42": ["n01687978", "agama"],
- "43": ["n01688243", "frilled_lizard"],
- "44": ["n01689811", "alligator_lizard"],
- "45": ["n01692333", "Gila_monster"],
- "46": ["n01693334", "green_lizard"],
- "47": ["n01694178", "African_chameleon"],
- "48": ["n01695060", "Komodo_dragon"],
- "49": ["n01697457", "African_crocodile"],
- "50": ["n01698640", "American_alligator"],
- "51": ["n01704323", "triceratops"],
- "52": ["n01728572", "thunder_snake"],
- "53": ["n01728920", "ringneck_snake"],
- "54": ["n01729322", "hognose_snake"],
- "55": ["n01729977", "green_snake"],
- "56": ["n01734418", "king_snake"],
- "57": ["n01735189", "garter_snake"],
- "58": ["n01737021", "water_snake"],
- "59": ["n01739381", "vine_snake"],
- "60": ["n01740131", "night_snake"],
- "61": ["n01742172", "boa_constrictor"],
- "62": ["n01744401", "rock_python"],
- "63": ["n01748264", "Indian_cobra"],
- "64": ["n01749939", "green_mamba"],
- "65": ["n01751748", "sea_snake"],
- "66": ["n01753488", "horned_viper"],
- "67": ["n01755581", "diamondback"],
- "68": ["n01756291", "sidewinder"],
- "69": ["n01768244", "trilobite"],
- "70": ["n01770081", "harvestman"],
- "71": ["n01770393", "scorpion"],
- "72": ["n01773157", "black_and_gold_garden_spider"],
- "73": ["n01773549", "barn_spider"],
- "74": ["n01773797", "garden_spider"],
- "75": ["n01774384", "black_widow"],
- "76": ["n01774750", "tarantula"],
- "77": ["n01775062", "wolf_spider"],
- "78": ["n01776313", "tick"],
- "79": ["n01784675", "centipede"],
- "80": ["n01795545", "black_grouse"],
- "81": ["n01796340", "ptarmigan"],
- "82": ["n01797886", "ruffed_grouse"],
- "83": ["n01798484", "prairie_chicken"],
- "84": ["n01806143", "peacock"],
- "85": ["n01806567", "quail"],
- "86": ["n01807496", "partridge"],
- "87": ["n01817953", "African_grey"],
- "88": ["n01818515", "macaw"],
- "89": ["n01819313", "sulphur-crested_cockatoo"],
- "90": ["n01820546", "lorikeet"],
- "91": ["n01824575", "coucal"],
- "92": ["n01828970", "bee_eater"],
- "93": ["n01829413", "hornbill"],
- "94": ["n01833805", "hummingbird"],
- "95": ["n01843065", "jacamar"],
- "96": ["n01843383", "toucan"],
- "97": ["n01847000", "drake"],
- "98": ["n01855032", "red-breasted_merganser"],
- "99": ["n01855672", "goose"],
- "100": ["n01860187", "black_swan"],
- "101": ["n01871265", "tusker"],
- "102": ["n01872401", "echidna"],
- "103": ["n01873310", "platypus"],
- "104": ["n01877812", "wallaby"],
- "105": ["n01882714", "koala"],
- "106": ["n01883070", "wombat"],
- "107": ["n01910747", "jellyfish"],
- "108": ["n01914609", "sea_anemone"],
- "109": ["n01917289", "brain_coral"],
- "110": ["n01924916", "flatworm"],
- "111": ["n01930112", "nematode"],
- "112": ["n01943899", "conch"],
- "113": ["n01944390", "snail"],
- "114": ["n01945685", "slug"],
- "115": ["n01950731", "sea_slug"],
- "116": ["n01955084", "chiton"],
- "117": ["n01968897", "chambered_nautilus"],
- "118": ["n01978287", "Dungeness_crab"],
- "119": ["n01978455", "rock_crab"],
- "120": ["n01980166", "fiddler_crab"],
- "121": ["n01981276", "king_crab"],
- "122": ["n01983481", "American_lobster"],
- "123": ["n01984695", "spiny_lobster"],
- "124": ["n01985128", "crayfish"],
- "125": ["n01986214", "hermit_crab"],
- "126": ["n01990800", "isopod"],
- "127": ["n02002556", "white_stork"],
- "128": ["n02002724", "black_stork"],
- "129": ["n02006656", "spoonbill"],
- "130": ["n02007558", "flamingo"],
- "131": ["n02009229", "little_blue_heron"],
- "132": ["n02009912", "American_egret"],
- "133": ["n02011460", "bittern"],
- "134": ["n02012849", "crane"],
- "135": ["n02013706", "limpkin"],
- "136": ["n02017213", "European_gallinule"],
- "137": ["n02018207", "American_coot"],
- "138": ["n02018795", "bustard"],
- "139": ["n02025239", "ruddy_turnstone"],
- "140": ["n02027492", "red-backed_sandpiper"],
- "141": ["n02028035", "redshank"],
- "142": ["n02033041", "dowitcher"],
- "143": ["n02037110", "oystercatcher"],
- "144": ["n02051845", "pelican"],
- "145": ["n02056570", "king_penguin"],
- "146": ["n02058221", "albatross"],
- "147": ["n02066245", "grey_whale"],
- "148": ["n02071294", "killer_whale"],
- "149": ["n02074367", "dugong"],
- "150": ["n02077923", "sea_lion"],
- "151": ["n02085620", "Chihuahua"],
- "152": ["n02085782", "Japanese_spaniel"],
- "153": ["n02085936", "Maltese_dog"],
- "154": ["n02086079", "Pekinese"],
- "155": ["n02086240", "Shih-Tzu"],
- "156": ["n02086646", "Blenheim_spaniel"],
- "157": ["n02086910", "papillon"],
- "158": ["n02087046", "toy_terrier"],
- "159": ["n02087394", "Rhodesian_ridgeback"],
- "160": ["n02088094", "Afghan_hound"],
- "161": ["n02088238", "basset"],
- "162": ["n02088364", "beagle"],
- "163": ["n02088466", "bloodhound"],
- "164": ["n02088632", "bluetick"],
- "165": ["n02089078", "black-and-tan_coonhound"],
- "166": ["n02089867", "Walker_hound"],
- "167": ["n02089973", "English_foxhound"],
- "168": ["n02090379", "redbone"],
- "169": ["n02090622", "borzoi"],
- "170": ["n02090721", "Irish_wolfhound"],
- "171": ["n02091032", "Italian_greyhound"],
- "172": ["n02091134", "whippet"],
- "173": ["n02091244", "Ibizan_hound"],
- "174": ["n02091467", "Norwegian_elkhound"],
- "175": ["n02091635", "otterhound"],
- "176": ["n02091831", "Saluki"],
- "177": ["n02092002", "Scottish_deerhound"],
- "178": ["n02092339", "Weimaraner"],
- "179": ["n02093256", "Staffordshire_bullterrier"],
- "180": ["n02093428", "American_Staffordshire_terrier"],
- "181": ["n02093647", "Bedlington_terrier"],
- "182": ["n02093754", "Border_terrier"],
- "183": ["n02093859", "Kerry_blue_terrier"],
- "184": ["n02093991", "Irish_terrier"],
- "185": ["n02094114", "Norfolk_terrier"],
- "186": ["n02094258", "Norwich_terrier"],
- "187": ["n02094433", "Yorkshire_terrier"],
- "188": ["n02095314", "wire-haired_fox_terrier"],
- "189": ["n02095570", "Lakeland_terrier"],
- "190": ["n02095889", "Sealyham_terrier"],
- "191": ["n02096051", "Airedale"],
- "192": ["n02096177", "cairn"],
- "193": ["n02096294", "Australian_terrier"],
- "194": ["n02096437", "Dandie_Dinmont"],
- "195": ["n02096585", "Boston_bull"],
- "196": ["n02097047", "miniature_schnauzer"],
- "197": ["n02097130", "giant_schnauzer"],
- "198": ["n02097209", "standard_schnauzer"],
- "199": ["n02097298", "Scotch_terrier"],
- "200": ["n02097474", "Tibetan_terrier"],
- "201": ["n02097658", "silky_terrier"],
- "202": ["n02098105", "soft-coated_wheaten_terrier"],
- "203": ["n02098286", "West_Highland_white_terrier"],
- "204": ["n02098413", "Lhasa"],
- "205": ["n02099267", "flat-coated_retriever"],
- "206": ["n02099429", "curly-coated_retriever"],
- "207": ["n02099601", "golden_retriever"],
- "208": ["n02099712", "Labrador_retriever"],
- "209": ["n02099849", "Chesapeake_Bay_retriever"],
- "210": ["n02100236", "German_short-haired_pointer"],
- "211": ["n02100583", "vizsla"],
- "212": ["n02100735", "English_setter"],
- "213": ["n02100877", "Irish_setter"],
- "214": ["n02101006", "Gordon_setter"],
- "215": ["n02101388", "Brittany_spaniel"],
- "216": ["n02101556", "clumber"],
- "217": ["n02102040", "English_springer"],
- "218": ["n02102177", "Welsh_springer_spaniel"],
- "219": ["n02102318", "cocker_spaniel"],
- "220": ["n02102480", "Sussex_spaniel"],
- "221": ["n02102973", "Irish_water_spaniel"],
- "222": ["n02104029", "kuvasz"],
- "223": ["n02104365", "schipperke"],
- "224": ["n02105056", "groenendael"],
- "225": ["n02105162", "malinois"],
- "226": ["n02105251", "briard"],
- "227": ["n02105412", "kelpie"],
- "228": ["n02105505", "komondor"],
- "229": ["n02105641", "Old_English_sheepdog"],
- "230": ["n02105855", "Shetland_sheepdog"],
- "231": ["n02106030", "collie"],
- "232": ["n02106166", "Border_collie"],
- "233": ["n02106382", "Bouvier_des_Flandres"],
- "234": ["n02106550", "Rottweiler"],
- "235": ["n02106662", "German_shepherd"],
- "236": ["n02107142", "Doberman"],
- "237": ["n02107312", "miniature_pinscher"],
- "238": ["n02107574", "Greater_Swiss_Mountain_dog"],
- "239": ["n02107683", "Bernese_mountain_dog"],
- "240": ["n02107908", "Appenzeller"],
- "241": ["n02108000", "EntleBucher"],
- "242": ["n02108089", "boxer"],
- "243": ["n02108422", "bull_mastiff"],
- "244": ["n02108551", "Tibetan_mastiff"],
- "245": ["n02108915", "French_bulldog"],
- "246": ["n02109047", "Great_Dane"],
- "247": ["n02109525", "Saint_Bernard"],
- "248": ["n02109961", "Eskimo_dog"],
- "249": ["n02110063", "malamute"],
- "250": ["n02110185", "Siberian_husky"],
- "251": ["n02110341", "dalmatian"],
- "252": ["n02110627", "affenpinscher"],
- "253": ["n02110806", "basenji"],
- "254": ["n02110958", "pug"],
- "255": ["n02111129", "Leonberg"],
- "256": ["n02111277", "Newfoundland"],
- "257": ["n02111500", "Great_Pyrenees"],
- "258": ["n02111889", "Samoyed"],
- "259": ["n02112018", "Pomeranian"],
- "260": ["n02112137", "chow"],
- "261": ["n02112350", "keeshond"],
- "262": ["n02112706", "Brabancon_griffon"],
- "263": ["n02113023", "Pembroke"],
- "264": ["n02113186", "Cardigan"],
- "265": ["n02113624", "toy_poodle"],
- "266": ["n02113712", "miniature_poodle"],
- "267": ["n02113799", "standard_poodle"],
- "268": ["n02113978", "Mexican_hairless"],
- "269": ["n02114367", "timber_wolf"],
- "270": ["n02114548", "white_wolf"],
- "271": ["n02114712", "red_wolf"],
- "272": ["n02114855", "coyote"],
- "273": ["n02115641", "dingo"],
- "274": ["n02115913", "dhole"],
- "275": ["n02116738", "African_hunting_dog"],
- "276": ["n02117135", "hyena"],
- "277": ["n02119022", "red_fox"],
- "278": ["n02119789", "kit_fox"],
- "279": ["n02120079", "Arctic_fox"],
- "280": ["n02120505", "grey_fox"],
- "281": ["n02123045", "tabby"],
- "282": ["n02123159", "tiger_cat"],
- "283": ["n02123394", "Persian_cat"],
- "284": ["n02123597", "Siamese_cat"],
- "285": ["n02124075", "Egyptian_cat"],
- "286": ["n02125311", "cougar"],
- "287": ["n02127052", "lynx"],
- "288": ["n02128385", "leopard"],
- "289": ["n02128757", "snow_leopard"],
- "290": ["n02128925", "jaguar"],
- "291": ["n02129165", "lion"],
- "292": ["n02129604", "tiger"],
- "293": ["n02130308", "cheetah"],
- "294": ["n02132136", "brown_bear"],
- "295": ["n02133161", "American_black_bear"],
- "296": ["n02134084", "ice_bear"],
- "297": ["n02134418", "sloth_bear"],
- "298": ["n02137549", "mongoose"],
- "299": ["n02138441", "meerkat"],
- "300": ["n02165105", "tiger_beetle"],
- "301": ["n02165456", "ladybug"],
- "302": ["n02167151", "ground_beetle"],
- "303": ["n02168699", "long-horned_beetle"],
- "304": ["n02169497", "leaf_beetle"],
- "305": ["n02172182", "dung_beetle"],
- "306": ["n02174001", "rhinoceros_beetle"],
- "307": ["n02177972", "weevil"],
- "308": ["n02190166", "fly"],
- "309": ["n02206856", "bee"],
- "310": ["n02219486", "ant"],
- "311": ["n02226429", "grasshopper"],
- "312": ["n02229544", "cricket"],
- "313": ["n02231487", "walking_stick"],
- "314": ["n02233338", "cockroach"],
- "315": ["n02236044", "mantis"],
- "316": ["n02256656", "cicada"],
- "317": ["n02259212", "leafhopper"],
- "318": ["n02264363", "lacewing"],
- "319": ["n02268443", "dragonfly"],
- "320": ["n02268853", "damselfly"],
- "321": ["n02276258", "admiral"],
- "322": ["n02277742", "ringlet"],
- "323": ["n02279972", "monarch"],
- "324": ["n02280649", "cabbage_butterfly"],
- "325": ["n02281406", "sulphur_butterfly"],
- "326": ["n02281787", "lycaenid"],
- "327": ["n02317335", "starfish"],
- "328": ["n02319095", "sea_urchin"],
- "329": ["n02321529", "sea_cucumber"],
- "330": ["n02325366", "wood_rabbit"],
- "331": ["n02326432", "hare"],
- "332": ["n02328150", "Angora"],
- "333": ["n02342885", "hamster"],
- "334": ["n02346627", "porcupine"],
- "335": ["n02356798", "fox_squirrel"],
- "336": ["n02361337", "marmot"],
- "337": ["n02363005", "beaver"],
- "338": ["n02364673", "guinea_pig"],
- "339": ["n02389026", "sorrel"],
- "340": ["n02391049", "zebra"],
- "341": ["n02395406", "hog"],
- "342": ["n02396427", "wild_boar"],
- "343": ["n02397096", "warthog"],
- "344": ["n02398521", "hippopotamus"],
- "345": ["n02403003", "ox"],
- "346": ["n02408429", "water_buffalo"],
- "347": ["n02410509", "bison"],
- "348": ["n02412080", "ram"],
- "349": ["n02415577", "bighorn"],
- "350": ["n02417914", "ibex"],
- "351": ["n02422106", "hartebeest"],
- "352": ["n02422699", "impala"],
- "353": ["n02423022", "gazelle"],
- "354": ["n02437312", "Arabian_camel"],
- "355": ["n02437616", "llama"],
- "356": ["n02441942", "weasel"],
- "357": ["n02442845", "mink"],
- "358": ["n02443114", "polecat"],
- "359": ["n02443484", "black-footed_ferret"],
- "360": ["n02444819", "otter"],
- "361": ["n02445715", "skunk"],
- "362": ["n02447366", "badger"],
- "363": ["n02454379", "armadillo"],
- "364": ["n02457408", "three-toed_sloth"],
- "365": ["n02480495", "orangutan"],
- "366": ["n02480855", "gorilla"],
- "367": ["n02481823", "chimpanzee"],
- "368": ["n02483362", "gibbon"],
- "369": ["n02483708", "siamang"],
- "370": ["n02484975", "guenon"],
- "371": ["n02486261", "patas"],
- "372": ["n02486410", "baboon"],
- "373": ["n02487347", "macaque"],
- "374": ["n02488291", "langur"],
- "375": ["n02488702", "colobus"],
- "376": ["n02489166", "proboscis_monkey"],
- "377": ["n02490219", "marmoset"],
- "378": ["n02492035", "capuchin"],
- "379": ["n02492660", "howler_monkey"],
- "380": ["n02493509", "titi"],
- "381": ["n02493793", "spider_monkey"],
- "382": ["n02494079", "squirrel_monkey"],
- "383": ["n02497673", "Madagascar_cat"],
- "384": ["n02500267", "indri"],
- "385": ["n02504013", "Indian_elephant"],
- "386": ["n02504458", "African_elephant"],
- "387": ["n02509815", "lesser_panda"],
- "388": ["n02510455", "giant_panda"],
- "389": ["n02514041", "barracouta"],
- "390": ["n02526121", "eel"],
- "391": ["n02536864", "coho"],
- "392": ["n02606052", "rock_beauty"],
- "393": ["n02607072", "anemone_fish"],
- "394": ["n02640242", "sturgeon"],
- "395": ["n02641379", "gar"],
- "396": ["n02643566", "lionfish"],
- "397": ["n02655020", "puffer"],
- "398": ["n02666196", "abacus"],
- "399": ["n02667093", "abaya"],
- "400": ["n02669723", "academic_gown"],
- "401": ["n02672831", "accordion"],
- "402": ["n02676566", "acoustic_guitar"],
- "403": ["n02687172", "aircraft_carrier"],
- "404": ["n02690373", "airliner"],
- "405": ["n02692877", "airship"],
- "406": ["n02699494", "altar"],
- "407": ["n02701002", "ambulance"],
- "408": ["n02704792", "amphibian"],
- "409": ["n02708093", "analog_clock"],
- "410": ["n02727426", "apiary"],
- "411": ["n02730930", "apron"],
- "412": ["n02747177", "ashcan"],
- "413": ["n02749479", "assault_rifle"],
- "414": ["n02769748", "backpack"],
- "415": ["n02776631", "bakery"],
- "416": ["n02777292", "balance_beam"],
- "417": ["n02782093", "balloon"],
- "418": ["n02783161", "ballpoint"],
- "419": ["n02786058", "Band_Aid"],
- "420": ["n02787622", "banjo"],
- "421": ["n02788148", "bannister"],
- "422": ["n02790996", "barbell"],
- "423": ["n02791124", "barber_chair"],
- "424": ["n02791270", "barbershop"],
- "425": ["n02793495", "barn"],
- "426": ["n02794156", "barometer"],
- "427": ["n02795169", "barrel"],
- "428": ["n02797295", "barrow"],
- "429": ["n02799071", "baseball"],
- "430": ["n02802426", "basketball"],
- "431": ["n02804414", "bassinet"],
- "432": ["n02804610", "bassoon"],
- "433": ["n02807133", "bathing_cap"],
- "434": ["n02808304", "bath_towel"],
- "435": ["n02808440", "bathtub"],
- "436": ["n02814533", "beach_wagon"],
- "437": ["n02814860", "beacon"],
- "438": ["n02815834", "beaker"],
- "439": ["n02817516", "bearskin"],
- "440": ["n02823428", "beer_bottle"],
- "441": ["n02823750", "beer_glass"],
- "442": ["n02825657", "bell_cote"],
- "443": ["n02834397", "bib"],
- "444": ["n02835271", "bicycle-built-for-two"],
- "445": ["n02837789", "bikini"],
- "446": ["n02840245", "binder"],
- "447": ["n02841315", "binoculars"],
- "448": ["n02843684", "birdhouse"],
- "449": ["n02859443", "boathouse"],
- "450": ["n02860847", "bobsled"],
- "451": ["n02865351", "bolo_tie"],
- "452": ["n02869837", "bonnet"],
- "453": ["n02870880", "bookcase"],
- "454": ["n02871525", "bookshop"],
- "455": ["n02877765", "bottlecap"],
- "456": ["n02879718", "bow"],
- "457": ["n02883205", "bow_tie"],
- "458": ["n02892201", "brass"],
- "459": ["n02892767", "brassiere"],
- "460": ["n02894605", "breakwater"],
- "461": ["n02895154", "breastplate"],
- "462": ["n02906734", "broom"],
- "463": ["n02909870", "bucket"],
- "464": ["n02910353", "buckle"],
- "465": ["n02916936", "bulletproof_vest"],
- "466": ["n02917067", "bullet_train"],
- "467": ["n02927161", "butcher_shop"],
- "468": ["n02930766", "cab"],
- "469": ["n02939185", "caldron"],
- "470": ["n02948072", "candle"],
- "471": ["n02950826", "cannon"],
- "472": ["n02951358", "canoe"],
- "473": ["n02951585", "can_opener"],
- "474": ["n02963159", "cardigan"],
- "475": ["n02965783", "car_mirror"],
- "476": ["n02966193", "carousel"],
- "477": ["n02966687", "carpenter's_kit"],
- "478": ["n02971356", "carton"],
- "479": ["n02974003", "car_wheel"],
- "480": ["n02977058", "cash_machine"],
- "481": ["n02978881", "cassette"],
- "482": ["n02979186", "cassette_player"],
- "483": ["n02980441", "castle"],
- "484": ["n02981792", "catamaran"],
- "485": ["n02988304", "CD_player"],
- "486": ["n02992211", "cello"],
- "487": ["n02992529", "cellular_telephone"],
- "488": ["n02999410", "chain"],
- "489": ["n03000134", "chainlink_fence"],
- "490": ["n03000247", "chain_mail"],
- "491": ["n03000684", "chain_saw"],
- "492": ["n03014705", "chest"],
- "493": ["n03016953", "chiffonier"],
- "494": ["n03017168", "chime"],
- "495": ["n03018349", "china_cabinet"],
- "496": ["n03026506", "Christmas_stocking"],
- "497": ["n03028079", "church"],
- "498": ["n03032252", "cinema"],
- "499": ["n03041632", "cleaver"],
- "500": ["n03042490", "cliff_dwelling"],
- "501": ["n03045698", "cloak"],
- "502": ["n03047690", "clog"],
- "503": ["n03062245", "cocktail_shaker"],
- "504": ["n03063599", "coffee_mug"],
- "505": ["n03063689", "coffeepot"],
- "506": ["n03065424", "coil"],
- "507": ["n03075370", "combination_lock"],
- "508": ["n03085013", "computer_keyboard"],
- "509": ["n03089624", "confectionery"],
- "510": ["n03095699", "container_ship"],
- "511": ["n03100240", "convertible"],
- "512": ["n03109150", "corkscrew"],
- "513": ["n03110669", "cornet"],
- "514": ["n03124043", "cowboy_boot"],
- "515": ["n03124170", "cowboy_hat"],
- "516": ["n03125729", "cradle"],
- "517": ["n03126707", "crane"],
- "518": ["n03127747", "crash_helmet"],
- "519": ["n03127925", "crate"],
- "520": ["n03131574", "crib"],
- "521": ["n03133878", "Crock_Pot"],
- "522": ["n03134739", "croquet_ball"],
- "523": ["n03141823", "crutch"],
- "524": ["n03146219", "cuirass"],
- "525": ["n03160309", "dam"],
- "526": ["n03179701", "desk"],
- "527": ["n03180011", "desktop_computer"],
- "528": ["n03187595", "dial_telephone"],
- "529": ["n03188531", "diaper"],
- "530": ["n03196217", "digital_clock"],
- "531": ["n03197337", "digital_watch"],
- "532": ["n03201208", "dining_table"],
- "533": ["n03207743", "dishrag"],
- "534": ["n03207941", "dishwasher"],
- "535": ["n03208938", "disk_brake"],
- "536": ["n03216828", "dock"],
- "537": ["n03218198", "dogsled"],
- "538": ["n03220513", "dome"],
- "539": ["n03223299", "doormat"],
- "540": ["n03240683", "drilling_platform"],
- "541": ["n03249569", "drum"],
- "542": ["n03250847", "drumstick"],
- "543": ["n03255030", "dumbbell"],
- "544": ["n03259280", "Dutch_oven"],
- "545": ["n03271574", "electric_fan"],
- "546": ["n03272010", "electric_guitar"],
- "547": ["n03272562", "electric_locomotive"],
- "548": ["n03290653", "entertainment_center"],
- "549": ["n03291819", "envelope"],
- "550": ["n03297495", "espresso_maker"],
- "551": ["n03314780", "face_powder"],
- "552": ["n03325584", "feather_boa"],
- "553": ["n03337140", "file"],
- "554": ["n03344393", "fireboat"],
- "555": ["n03345487", "fire_engine"],
- "556": ["n03347037", "fire_screen"],
- "557": ["n03355925", "flagpole"],
- "558": ["n03372029", "flute"],
- "559": ["n03376595", "folding_chair"],
- "560": ["n03379051", "football_helmet"],
- "561": ["n03384352", "forklift"],
- "562": ["n03388043", "fountain"],
- "563": ["n03388183", "fountain_pen"],
- "564": ["n03388549", "four-poster"],
- "565": ["n03393912", "freight_car"],
- "566": ["n03394916", "French_horn"],
- "567": ["n03400231", "frying_pan"],
- "568": ["n03404251", "fur_coat"],
- "569": ["n03417042", "garbage_truck"],
- "570": ["n03424325", "gasmask"],
- "571": ["n03425413", "gas_pump"],
- "572": ["n03443371", "goblet"],
- "573": ["n03444034", "go-kart"],
- "574": ["n03445777", "golf_ball"],
- "575": ["n03445924", "golfcart"],
- "576": ["n03447447", "gondola"],
- "577": ["n03447721", "gong"],
- "578": ["n03450230", "gown"],
- "579": ["n03452741", "grand_piano"],
- "580": ["n03457902", "greenhouse"],
- "581": ["n03459775", "grille"],
- "582": ["n03461385", "grocery_store"],
- "583": ["n03467068", "guillotine"],
- "584": ["n03476684", "hair_slide"],
- "585": ["n03476991", "hair_spray"],
- "586": ["n03478589", "half_track"],
- "587": ["n03481172", "hammer"],
- "588": ["n03482405", "hamper"],
- "589": ["n03483316", "hand_blower"],
- "590": ["n03485407", "hand-held_computer"],
- "591": ["n03485794", "handkerchief"],
- "592": ["n03492542", "hard_disc"],
- "593": ["n03494278", "harmonica"],
- "594": ["n03495258", "harp"],
- "595": ["n03496892", "harvester"],
- "596": ["n03498962", "hatchet"],
- "597": ["n03527444", "holster"],
- "598": ["n03529860", "home_theater"],
- "599": ["n03530642", "honeycomb"],
- "600": ["n03532672", "hook"],
- "601": ["n03534580", "hoopskirt"],
- "602": ["n03535780", "horizontal_bar"],
- "603": ["n03538406", "horse_cart"],
- "604": ["n03544143", "hourglass"],
- "605": ["n03584254", "iPod"],
- "606": ["n03584829", "iron"],
- "607": ["n03590841", "jack-o'-lantern"],
- "608": ["n03594734", "jean"],
- "609": ["n03594945", "jeep"],
- "610": ["n03595614", "jersey"],
- "611": ["n03598930", "jigsaw_puzzle"],
- "612": ["n03599486", "jinrikisha"],
- "613": ["n03602883", "joystick"],
- "614": ["n03617480", "kimono"],
- "615": ["n03623198", "knee_pad"],
- "616": ["n03627232", "knot"],
- "617": ["n03630383", "lab_coat"],
- "618": ["n03633091", "ladle"],
- "619": ["n03637318", "lampshade"],
- "620": ["n03642806", "laptop"],
- "621": ["n03649909", "lawn_mower"],
- "622": ["n03657121", "lens_cap"],
- "623": ["n03658185", "letter_opener"],
- "624": ["n03661043", "library"],
- "625": ["n03662601", "lifeboat"],
- "626": ["n03666591", "lighter"],
- "627": ["n03670208", "limousine"],
- "628": ["n03673027", "liner"],
- "629": ["n03676483", "lipstick"],
- "630": ["n03680355", "Loafer"],
- "631": ["n03690938", "lotion"],
- "632": ["n03691459", "loudspeaker"],
- "633": ["n03692522", "loupe"],
- "634": ["n03697007", "lumbermill"],
- "635": ["n03706229", "magnetic_compass"],
- "636": ["n03709823", "mailbag"],
- "637": ["n03710193", "mailbox"],
- "638": ["n03710637", "maillot"],
- "639": ["n03710721", "maillot"],
- "640": ["n03717622", "manhole_cover"],
- "641": ["n03720891", "maraca"],
- "642": ["n03721384", "marimba"],
- "643": ["n03724870", "mask"],
- "644": ["n03729826", "matchstick"],
- "645": ["n03733131", "maypole"],
- "646": ["n03733281", "maze"],
- "647": ["n03733805", "measuring_cup"],
- "648": ["n03742115", "medicine_chest"],
- "649": ["n03743016", "megalith"],
- "650": ["n03759954", "microphone"],
- "651": ["n03761084", "microwave"],
- "652": ["n03763968", "military_uniform"],
- "653": ["n03764736", "milk_can"],
- "654": ["n03769881", "minibus"],
- "655": ["n03770439", "miniskirt"],
- "656": ["n03770679", "minivan"],
- "657": ["n03773504", "missile"],
- "658": ["n03775071", "mitten"],
- "659": ["n03775546", "mixing_bowl"],
- "660": ["n03776460", "mobile_home"],
- "661": ["n03777568", "Model_T"],
- "662": ["n03777754", "modem"],
- "663": ["n03781244", "monastery"],
- "664": ["n03782006", "monitor"],
- "665": ["n03785016", "moped"],
- "666": ["n03786901", "mortar"],
- "667": ["n03787032", "mortarboard"],
- "668": ["n03788195", "mosque"],
- "669": ["n03788365", "mosquito_net"],
- "670": ["n03791053", "motor_scooter"],
- "671": ["n03792782", "mountain_bike"],
- "672": ["n03792972", "mountain_tent"],
- "673": ["n03793489", "mouse"],
- "674": ["n03794056", "mousetrap"],
- "675": ["n03796401", "moving_van"],
- "676": ["n03803284", "muzzle"],
- "677": ["n03804744", "nail"],
- "678": ["n03814639", "neck_brace"],
- "679": ["n03814906", "necklace"],
- "680": ["n03825788", "nipple"],
- "681": ["n03832673", "notebook"],
- "682": ["n03837869", "obelisk"],
- "683": ["n03838899", "oboe"],
- "684": ["n03840681", "ocarina"],
- "685": ["n03841143", "odometer"],
- "686": ["n03843555", "oil_filter"],
- "687": ["n03854065", "organ"],
- "688": ["n03857828", "oscilloscope"],
- "689": ["n03866082", "overskirt"],
- "690": ["n03868242", "oxcart"],
- "691": ["n03868863", "oxygen_mask"],
- "692": ["n03871628", "packet"],
- "693": ["n03873416", "paddle"],
- "694": ["n03874293", "paddlewheel"],
- "695": ["n03874599", "padlock"],
- "696": ["n03876231", "paintbrush"],
- "697": ["n03877472", "pajama"],
- "698": ["n03877845", "palace"],
- "699": ["n03884397", "panpipe"],
- "700": ["n03887697", "paper_towel"],
- "701": ["n03888257", "parachute"],
- "702": ["n03888605", "parallel_bars"],
- "703": ["n03891251", "park_bench"],
- "704": ["n03891332", "parking_meter"],
- "705": ["n03895866", "passenger_car"],
- "706": ["n03899768", "patio"],
- "707": ["n03902125", "pay-phone"],
- "708": ["n03903868", "pedestal"],
- "709": ["n03908618", "pencil_box"],
- "710": ["n03908714", "pencil_sharpener"],
- "711": ["n03916031", "perfume"],
- "712": ["n03920288", "Petri_dish"],
- "713": ["n03924679", "photocopier"],
- "714": ["n03929660", "pick"],
- "715": ["n03929855", "pickelhaube"],
- "716": ["n03930313", "picket_fence"],
- "717": ["n03930630", "pickup"],
- "718": ["n03933933", "pier"],
- "719": ["n03935335", "piggy_bank"],
- "720": ["n03937543", "pill_bottle"],
- "721": ["n03938244", "pillow"],
- "722": ["n03942813", "ping-pong_ball"],
- "723": ["n03944341", "pinwheel"],
- "724": ["n03947888", "pirate"],
- "725": ["n03950228", "pitcher"],
- "726": ["n03954731", "plane"],
- "727": ["n03956157", "planetarium"],
- "728": ["n03958227", "plastic_bag"],
- "729": ["n03961711", "plate_rack"],
- "730": ["n03967562", "plow"],
- "731": ["n03970156", "plunger"],
- "732": ["n03976467", "Polaroid_camera"],
- "733": ["n03976657", "pole"],
- "734": ["n03977966", "police_van"],
- "735": ["n03980874", "poncho"],
- "736": ["n03982430", "pool_table"],
- "737": ["n03983396", "pop_bottle"],
- "738": ["n03991062", "pot"],
- "739": ["n03992509", "potter's_wheel"],
- "740": ["n03995372", "power_drill"],
- "741": ["n03998194", "prayer_rug"],
- "742": ["n04004767", "printer"],
- "743": ["n04005630", "prison"],
- "744": ["n04008634", "projectile"],
- "745": ["n04009552", "projector"],
- "746": ["n04019541", "puck"],
- "747": ["n04023962", "punching_bag"],
- "748": ["n04026417", "purse"],
- "749": ["n04033901", "quill"],
- "750": ["n04033995", "quilt"],
- "751": ["n04037443", "racer"],
- "752": ["n04039381", "racket"],
- "753": ["n04040759", "radiator"],
- "754": ["n04041544", "radio"],
- "755": ["n04044716", "radio_telescope"],
- "756": ["n04049303", "rain_barrel"],
- "757": ["n04065272", "recreational_vehicle"],
- "758": ["n04067472", "reel"],
- "759": ["n04069434", "reflex_camera"],
- "760": ["n04070727", "refrigerator"],
- "761": ["n04074963", "remote_control"],
- "762": ["n04081281", "restaurant"],
- "763": ["n04086273", "revolver"],
- "764": ["n04090263", "rifle"],
- "765": ["n04099969", "rocking_chair"],
- "766": ["n04111531", "rotisserie"],
- "767": ["n04116512", "rubber_eraser"],
- "768": ["n04118538", "rugby_ball"],
- "769": ["n04118776", "rule"],
- "770": ["n04120489", "running_shoe"],
- "771": ["n04125021", "safe"],
- "772": ["n04127249", "safety_pin"],
- "773": ["n04131690", "saltshaker"],
- "774": ["n04133789", "sandal"],
- "775": ["n04136333", "sarong"],
- "776": ["n04141076", "sax"],
- "777": ["n04141327", "scabbard"],
- "778": ["n04141975", "scale"],
- "779": ["n04146614", "school_bus"],
- "780": ["n04147183", "schooner"],
- "781": ["n04149813", "scoreboard"],
- "782": ["n04152593", "screen"],
- "783": ["n04153751", "screw"],
- "784": ["n04154565", "screwdriver"],
- "785": ["n04162706", "seat_belt"],
- "786": ["n04179913", "sewing_machine"],
- "787": ["n04192698", "shield"],
- "788": ["n04200800", "shoe_shop"],
- "789": ["n04201297", "shoji"],
- "790": ["n04204238", "shopping_basket"],
- "791": ["n04204347", "shopping_cart"],
- "792": ["n04208210", "shovel"],
- "793": ["n04209133", "shower_cap"],
- "794": ["n04209239", "shower_curtain"],
- "795": ["n04228054", "ski"],
- "796": ["n04229816", "ski_mask"],
- "797": ["n04235860", "sleeping_bag"],
- "798": ["n04238763", "slide_rule"],
- "799": ["n04239074", "sliding_door"],
- "800": ["n04243546", "slot"],
- "801": ["n04251144", "snorkel"],
- "802": ["n04252077", "snowmobile"],
- "803": ["n04252225", "snowplow"],
- "804": ["n04254120", "soap_dispenser"],
- "805": ["n04254680", "soccer_ball"],
- "806": ["n04254777", "sock"],
- "807": ["n04258138", "solar_dish"],
- "808": ["n04259630", "sombrero"],
- "809": ["n04263257", "soup_bowl"],
- "810": ["n04264628", "space_bar"],
- "811": ["n04265275", "space_heater"],
- "812": ["n04266014", "space_shuttle"],
- "813": ["n04270147", "spatula"],
- "814": ["n04273569", "speedboat"],
- "815": ["n04275548", "spider_web"],
- "816": ["n04277352", "spindle"],
- "817": ["n04285008", "sports_car"],
- "818": ["n04286575", "spotlight"],
- "819": ["n04296562", "stage"],
- "820": ["n04310018", "steam_locomotive"],
- "821": ["n04311004", "steel_arch_bridge"],
- "822": ["n04311174", "steel_drum"],
- "823": ["n04317175", "stethoscope"],
- "824": ["n04325704", "stole"],
- "825": ["n04326547", "stone_wall"],
- "826": ["n04328186", "stopwatch"],
- "827": ["n04330267", "stove"],
- "828": ["n04332243", "strainer"],
- "829": ["n04335435", "streetcar"],
- "830": ["n04336792", "stretcher"],
- "831": ["n04344873", "studio_couch"],
- "832": ["n04346328", "stupa"],
- "833": ["n04347754", "submarine"],
- "834": ["n04350905", "suit"],
- "835": ["n04355338", "sundial"],
- "836": ["n04355933", "sunglass"],
- "837": ["n04356056", "sunglasses"],
- "838": ["n04357314", "sunscreen"],
- "839": ["n04366367", "suspension_bridge"],
- "840": ["n04367480", "swab"],
- "841": ["n04370456", "sweatshirt"],
- "842": ["n04371430", "swimming_trunks"],
- "843": ["n04371774", "swing"],
- "844": ["n04372370", "switch"],
- "845": ["n04376876", "syringe"],
- "846": ["n04380533", "table_lamp"],
- "847": ["n04389033", "tank"],
- "848": ["n04392985", "tape_player"],
- "849": ["n04398044", "teapot"],
- "850": ["n04399382", "teddy"],
- "851": ["n04404412", "television"],
- "852": ["n04409515", "tennis_ball"],
- "853": ["n04417672", "thatch"],
- "854": ["n04418357", "theater_curtain"],
- "855": ["n04423845", "thimble"],
- "856": ["n04428191", "thresher"],
- "857": ["n04429376", "throne"],
- "858": ["n04435653", "tile_roof"],
- "859": ["n04442312", "toaster"],
- "860": ["n04443257", "tobacco_shop"],
- "861": ["n04447861", "toilet_seat"],
- "862": ["n04456115", "torch"],
- "863": ["n04458633", "totem_pole"],
- "864": ["n04461696", "tow_truck"],
- "865": ["n04462240", "toyshop"],
- "866": ["n04465501", "tractor"],
- "867": ["n04467665", "trailer_truck"],
- "868": ["n04476259", "tray"],
- "869": ["n04479046", "trench_coat"],
- "870": ["n04482393", "tricycle"],
- "871": ["n04483307", "trimaran"],
- "872": ["n04485082", "tripod"],
- "873": ["n04486054", "triumphal_arch"],
- "874": ["n04487081", "trolleybus"],
- "875": ["n04487394", "trombone"],
- "876": ["n04493381", "tub"],
- "877": ["n04501370", "turnstile"],
- "878": ["n04505470", "typewriter_keyboard"],
- "879": ["n04507155", "umbrella"],
- "880": ["n04509417", "unicycle"],
- "881": ["n04515003", "upright"],
- "882": ["n04517823", "vacuum"],
- "883": ["n04522168", "vase"],
- "884": ["n04523525", "vault"],
- "885": ["n04525038", "velvet"],
- "886": ["n04525305", "vending_machine"],
- "887": ["n04532106", "vestment"],
- "888": ["n04532670", "viaduct"],
- "889": ["n04536866", "violin"],
- "890": ["n04540053", "volleyball"],
- "891": ["n04542943", "waffle_iron"],
- "892": ["n04548280", "wall_clock"],
- "893": ["n04548362", "wallet"],
- "894": ["n04550184", "wardrobe"],
- "895": ["n04552348", "warplane"],
- "896": ["n04553703", "washbasin"],
- "897": ["n04554684", "washer"],
- "898": ["n04557648", "water_bottle"],
- "899": ["n04560804", "water_jug"],
- "900": ["n04562935", "water_tower"],
- "901": ["n04579145", "whiskey_jug"],
- "902": ["n04579432", "whistle"],
- "903": ["n04584207", "wig"],
- "904": ["n04589890", "window_screen"],
- "905": ["n04590129", "window_shade"],
- "906": ["n04591157", "Windsor_tie"],
- "907": ["n04591713", "wine_bottle"],
- "908": ["n04592741", "wing"],
- "909": ["n04596742", "wok"],
- "910": ["n04597913", "wooden_spoon"],
- "911": ["n04599235", "wool"],
- "912": ["n04604644", "worm_fence"],
- "913": ["n04606251", "wreck"],
- "914": ["n04612504", "yawl"],
- "915": ["n04613696", "yurt"],
- "916": ["n06359193", "web_site"],
- "917": ["n06596364", "comic_book"],
- "918": ["n06785654", "crossword_puzzle"],
- "919": ["n06794110", "street_sign"],
- "920": ["n06874185", "traffic_light"],
- "921": ["n07248320", "book_jacket"],
- "922": ["n07565083", "menu"],
- "923": ["n07579787", "plate"],
- "924": ["n07583066", "guacamole"],
- "925": ["n07584110", "consomme"],
- "926": ["n07590611", "hot_pot"],
- "927": ["n07613480", "trifle"],
- "928": ["n07614500", "ice_cream"],
- "929": ["n07615774", "ice_lolly"],
- "930": ["n07684084", "French_loaf"],
- "931": ["n07693725", "bagel"],
- "932": ["n07695742", "pretzel"],
- "933": ["n07697313", "cheeseburger"],
- "934": ["n07697537", "hotdog"],
- "935": ["n07711569", "mashed_potato"],
- "936": ["n07714571", "head_cabbage"],
- "937": ["n07714990", "broccoli"],
- "938": ["n07715103", "cauliflower"],
- "939": ["n07716358", "zucchini"],
- "940": ["n07716906", "spaghetti_squash"],
- "941": ["n07717410", "acorn_squash"],
- "942": ["n07717556", "butternut_squash"],
- "943": ["n07718472", "cucumber"],
- "944": ["n07718747", "artichoke"],
- "945": ["n07720875", "bell_pepper"],
- "946": ["n07730033", "cardoon"],
- "947": ["n07734744", "mushroom"],
- "948": ["n07742313", "Granny_Smith"],
- "949": ["n07745940", "strawberry"],
- "950": ["n07747607", "orange"],
- "951": ["n07749582", "lemon"],
- "952": ["n07753113", "fig"],
- "953": ["n07753275", "pineapple"],
- "954": ["n07753592", "banana"],
- "955": ["n07754684", "jackfruit"],
- "956": ["n07760859", "custard_apple"],
- "957": ["n07768694", "pomegranate"],
- "958": ["n07802026", "hay"],
- "959": ["n07831146", "carbonara"],
- "960": ["n07836838", "chocolate_sauce"],
- "961": ["n07860988", "dough"],
- "962": ["n07871810", "meat_loaf"],
- "963": ["n07873807", "pizza"],
- "964": ["n07875152", "potpie"],
- "965": ["n07880968", "burrito"],
- "966": ["n07892512", "red_wine"],
- "967": ["n07920052", "espresso"],
- "968": ["n07930864", "cup"],
- "969": ["n07932039", "eggnog"],
- "970": ["n09193705", "alp"],
- "971": ["n09229709", "bubble"],
- "972": ["n09246464", "cliff"],
- "973": ["n09256479", "coral_reef"],
- "974": ["n09288635", "geyser"],
- "975": ["n09332890", "lakeside"],
- "976": ["n09399592", "promontory"],
- "977": ["n09421951", "sandbar"],
- "978": ["n09428293", "seashore"],
- "979": ["n09468604", "valley"],
- "980": ["n09472597", "volcano"],
- "981": ["n09835506", "ballplayer"],
- "982": ["n10148035", "groom"],
- "983": ["n10565667", "scuba_diver"],
- "984": ["n11879895", "rapeseed"],
- "985": ["n11939491", "daisy"],
- "986": ["n12057211", "yellow_lady's_slipper"],
- "987": ["n12144580", "corn"],
- "988": ["n12267677", "acorn"],
- "989": ["n12620546", "hip"],
- "990": ["n12768682", "buckeye"],
- "991": ["n12985857", "coral_fungus"],
- "992": ["n12998815", "agaric"],
- "993": ["n13037406", "gyromitra"],
- "994": ["n13040303", "stinkhorn"],
- "995": ["n13044778", "earthstar"],
- "996": ["n13052670", "hen-of-the-woods"],
- "997": ["n13054560", "bolete"],
- "998": ["n13133613", "ear"],
- "999": ["n15075141", "toilet_tissue"]
-}
\ No newline at end of file
diff --git a/spaces/AnimalEquality/chatbot/_proc/_docs/site_libs/quarto-search/fuse.min.js b/spaces/AnimalEquality/chatbot/_proc/_docs/site_libs/quarto-search/fuse.min.js
deleted file mode 100644
index adc28356e2f591038d4e691cb5b418d7a490bfb1..0000000000000000000000000000000000000000
--- a/spaces/AnimalEquality/chatbot/_proc/_docs/site_libs/quarto-search/fuse.min.js
+++ /dev/null
@@ -1,9 +0,0 @@
-/**
- * Fuse.js v6.6.2 - Lightweight fuzzy-search (http://fusejs.io)
- *
- * Copyright (c) 2022 Kiro Risk (http://kiro.me)
- * All Rights Reserved. Apache Software License 2.0
- *
- * http://www.apache.org/licenses/LICENSE-2.0
- */
-var e,t;e=this,t=function(){"use strict";function e(e,t){var n=Object.keys(e);if(Object.getOwnPropertySymbols){var r=Object.getOwnPropertySymbols(e);t&&(r=r.filter((function(t){return Object.getOwnPropertyDescriptor(e,t).enumerable}))),n.push.apply(n,r)}return n}function t(t){for(var n=1;ne.length)&&(t=e.length);for(var n=0,r=new Array(t);n0&&void 0!==arguments[0]?arguments[0]:1,t=arguments.length>1&&void 0!==arguments[1]?arguments[1]:3,n=new Map,r=Math.pow(10,t);return{get:function(t){var i=t.match(C).length;if(n.has(i))return n.get(i);var o=1/Math.pow(i,.5*e),c=parseFloat(Math.round(o*r)/r);return n.set(i,c),c},clear:function(){n.clear()}}}var $=function(){function e(){var t=arguments.length>0&&void 0!==arguments[0]?arguments[0]:{},n=t.getFn,i=void 0===n?I.getFn:n,o=t.fieldNormWeight,c=void 0===o?I.fieldNormWeight:o;r(this,e),this.norm=E(c,3),this.getFn=i,this.isCreated=!1,this.setIndexRecords()}return o(e,[{key:"setSources",value:function(){var e=arguments.length>0&&void 0!==arguments[0]?arguments[0]:[];this.docs=e}},{key:"setIndexRecords",value:function(){var e=arguments.length>0&&void 0!==arguments[0]?arguments[0]:[];this.records=e}},{key:"setKeys",value:function(){var e=this,t=arguments.length>0&&void 0!==arguments[0]?arguments[0]:[];this.keys=t,this._keysMap={},t.forEach((function(t,n){e._keysMap[t.id]=n}))}},{key:"create",value:function(){var e=this;!this.isCreated&&this.docs.length&&(this.isCreated=!0,g(this.docs[0])?this.docs.forEach((function(t,n){e._addString(t,n)})):this.docs.forEach((function(t,n){e._addObject(t,n)})),this.norm.clear())}},{key:"add",value:function(e){var t=this.size();g(e)?this._addString(e,t):this._addObject(e,t)}},{key:"removeAt",value:function(e){this.records.splice(e,1);for(var t=e,n=this.size();t2&&void 0!==arguments[2]?arguments[2]:{},r=n.getFn,i=void 0===r?I.getFn:r,o=n.fieldNormWeight,c=void 0===o?I.fieldNormWeight:o,a=new $({getFn:i,fieldNormWeight:c});return a.setKeys(e.map(_)),a.setSources(t),a.create(),a}function R(e){var t=arguments.length>1&&void 0!==arguments[1]?arguments[1]:{},n=t.errors,r=void 0===n?0:n,i=t.currentLocation,o=void 0===i?0:i,c=t.expectedLocation,a=void 0===c?0:c,s=t.distance,u=void 0===s?I.distance:s,h=t.ignoreLocation,l=void 0===h?I.ignoreLocation:h,f=r/e.length;if(l)return f;var d=Math.abs(a-o);return u?f+d/u:d?1:f}function N(){for(var e=arguments.length>0&&void 0!==arguments[0]?arguments[0]:[],t=arguments.length>1&&void 0!==arguments[1]?arguments[1]:I.minMatchCharLength,n=[],r=-1,i=-1,o=0,c=e.length;o=t&&n.push([r,i]),r=-1)}return e[o-1]&&o-r>=t&&n.push([r,o-1]),n}var P=32;function W(e){for(var t={},n=0,r=e.length;n1&&void 0!==arguments[1]?arguments[1]:{},o=i.location,c=void 0===o?I.location:o,a=i.threshold,s=void 0===a?I.threshold:a,u=i.distance,h=void 0===u?I.distance:u,l=i.includeMatches,f=void 0===l?I.includeMatches:l,d=i.findAllMatches,v=void 0===d?I.findAllMatches:d,g=i.minMatchCharLength,y=void 0===g?I.minMatchCharLength:g,p=i.isCaseSensitive,m=void 0===p?I.isCaseSensitive:p,k=i.ignoreLocation,M=void 0===k?I.ignoreLocation:k;if(r(this,e),this.options={location:c,threshold:s,distance:h,includeMatches:f,findAllMatches:v,minMatchCharLength:y,isCaseSensitive:m,ignoreLocation:M},this.pattern=m?t:t.toLowerCase(),this.chunks=[],this.pattern.length){var b=function(e,t){n.chunks.push({pattern:e,alphabet:W(e),startIndex:t})},x=this.pattern.length;if(x>P){for(var w=0,L=x%P,S=x-L;w3&&void 0!==arguments[3]?arguments[3]:{},i=r.location,o=void 0===i?I.location:i,c=r.distance,a=void 0===c?I.distance:c,s=r.threshold,u=void 0===s?I.threshold:s,h=r.findAllMatches,l=void 0===h?I.findAllMatches:h,f=r.minMatchCharLength,d=void 0===f?I.minMatchCharLength:f,v=r.includeMatches,g=void 0===v?I.includeMatches:v,y=r.ignoreLocation,p=void 0===y?I.ignoreLocation:y;if(t.length>P)throw new Error(w(P));for(var m,k=t.length,M=e.length,b=Math.max(0,Math.min(o,M)),x=u,L=b,S=d>1||g,_=S?Array(M):[];(m=e.indexOf(t,L))>-1;){var O=R(t,{currentLocation:m,expectedLocation:b,distance:a,ignoreLocation:p});if(x=Math.min(O,x),L=m+k,S)for(var j=0;j=z;q-=1){var B=q-1,J=n[e.charAt(B)];if(S&&(_[B]=+!!J),K[q]=(K[q+1]<<1|1)&J,F&&(K[q]|=(A[q+1]|A[q])<<1|1|A[q+1]),K[q]&$&&(C=R(t,{errors:F,currentLocation:B,expectedLocation:b,distance:a,ignoreLocation:p}))<=x){if(x=C,(L=B)<=b)break;z=Math.max(1,2*b-L)}}if(R(t,{errors:F+1,currentLocation:b,expectedLocation:b,distance:a,ignoreLocation:p})>x)break;A=K}var U={isMatch:L>=0,score:Math.max(.001,C)};if(S){var V=N(_,d);V.length?g&&(U.indices=V):U.isMatch=!1}return U}(e,n,i,{location:c+o,distance:a,threshold:s,findAllMatches:u,minMatchCharLength:h,includeMatches:r,ignoreLocation:l}),p=y.isMatch,m=y.score,k=y.indices;p&&(g=!0),v+=m,p&&k&&(d=[].concat(f(d),f(k)))}));var y={isMatch:g,score:g?v/this.chunks.length:1};return g&&r&&(y.indices=d),y}}]),e}(),z=function(){function e(t){r(this,e),this.pattern=t}return o(e,[{key:"search",value:function(){}}],[{key:"isMultiMatch",value:function(e){return D(e,this.multiRegex)}},{key:"isSingleMatch",value:function(e){return D(e,this.singleRegex)}}]),e}();function D(e,t){var n=e.match(t);return n?n[1]:null}var K=function(e){a(n,e);var t=l(n);function n(e){return r(this,n),t.call(this,e)}return o(n,[{key:"search",value:function(e){var t=e===this.pattern;return{isMatch:t,score:t?0:1,indices:[0,this.pattern.length-1]}}}],[{key:"type",get:function(){return"exact"}},{key:"multiRegex",get:function(){return/^="(.*)"$/}},{key:"singleRegex",get:function(){return/^=(.*)$/}}]),n}(z),q=function(e){a(n,e);var t=l(n);function n(e){return r(this,n),t.call(this,e)}return o(n,[{key:"search",value:function(e){var t=-1===e.indexOf(this.pattern);return{isMatch:t,score:t?0:1,indices:[0,e.length-1]}}}],[{key:"type",get:function(){return"inverse-exact"}},{key:"multiRegex",get:function(){return/^!"(.*)"$/}},{key:"singleRegex",get:function(){return/^!(.*)$/}}]),n}(z),B=function(e){a(n,e);var t=l(n);function n(e){return r(this,n),t.call(this,e)}return o(n,[{key:"search",value:function(e){var t=e.startsWith(this.pattern);return{isMatch:t,score:t?0:1,indices:[0,this.pattern.length-1]}}}],[{key:"type",get:function(){return"prefix-exact"}},{key:"multiRegex",get:function(){return/^\^"(.*)"$/}},{key:"singleRegex",get:function(){return/^\^(.*)$/}}]),n}(z),J=function(e){a(n,e);var t=l(n);function n(e){return r(this,n),t.call(this,e)}return o(n,[{key:"search",value:function(e){var t=!e.startsWith(this.pattern);return{isMatch:t,score:t?0:1,indices:[0,e.length-1]}}}],[{key:"type",get:function(){return"inverse-prefix-exact"}},{key:"multiRegex",get:function(){return/^!\^"(.*)"$/}},{key:"singleRegex",get:function(){return/^!\^(.*)$/}}]),n}(z),U=function(e){a(n,e);var t=l(n);function n(e){return r(this,n),t.call(this,e)}return o(n,[{key:"search",value:function(e){var t=e.endsWith(this.pattern);return{isMatch:t,score:t?0:1,indices:[e.length-this.pattern.length,e.length-1]}}}],[{key:"type",get:function(){return"suffix-exact"}},{key:"multiRegex",get:function(){return/^"(.*)"\$$/}},{key:"singleRegex",get:function(){return/^(.*)\$$/}}]),n}(z),V=function(e){a(n,e);var t=l(n);function n(e){return r(this,n),t.call(this,e)}return o(n,[{key:"search",value:function(e){var t=!e.endsWith(this.pattern);return{isMatch:t,score:t?0:1,indices:[0,e.length-1]}}}],[{key:"type",get:function(){return"inverse-suffix-exact"}},{key:"multiRegex",get:function(){return/^!"(.*)"\$$/}},{key:"singleRegex",get:function(){return/^!(.*)\$$/}}]),n}(z),G=function(e){a(n,e);var t=l(n);function n(e){var i,o=arguments.length>1&&void 0!==arguments[1]?arguments[1]:{},c=o.location,a=void 0===c?I.location:c,s=o.threshold,u=void 0===s?I.threshold:s,h=o.distance,l=void 0===h?I.distance:h,f=o.includeMatches,d=void 0===f?I.includeMatches:f,v=o.findAllMatches,g=void 0===v?I.findAllMatches:v,y=o.minMatchCharLength,p=void 0===y?I.minMatchCharLength:y,m=o.isCaseSensitive,k=void 0===m?I.isCaseSensitive:m,M=o.ignoreLocation,b=void 0===M?I.ignoreLocation:M;return r(this,n),(i=t.call(this,e))._bitapSearch=new T(e,{location:a,threshold:u,distance:l,includeMatches:d,findAllMatches:g,minMatchCharLength:p,isCaseSensitive:k,ignoreLocation:b}),i}return o(n,[{key:"search",value:function(e){return this._bitapSearch.searchIn(e)}}],[{key:"type",get:function(){return"fuzzy"}},{key:"multiRegex",get:function(){return/^"(.*)"$/}},{key:"singleRegex",get:function(){return/^(.*)$/}}]),n}(z),H=function(e){a(n,e);var t=l(n);function n(e){return r(this,n),t.call(this,e)}return o(n,[{key:"search",value:function(e){for(var t,n=0,r=[],i=this.pattern.length;(t=e.indexOf(this.pattern,n))>-1;)n=t+i,r.push([t,n-1]);var o=!!r.length;return{isMatch:o,score:o?0:1,indices:r}}}],[{key:"type",get:function(){return"include"}},{key:"multiRegex",get:function(){return/^'"(.*)"$/}},{key:"singleRegex",get:function(){return/^'(.*)$/}}]),n}(z),Q=[K,H,B,J,V,U,q,G],X=Q.length,Y=/ +(?=(?:[^\"]*\"[^\"]*\")*[^\"]*$)/;function Z(e){var t=arguments.length>1&&void 0!==arguments[1]?arguments[1]:{};return e.split("|").map((function(e){for(var n=e.trim().split(Y).filter((function(e){return e&&!!e.trim()})),r=[],i=0,o=n.length;i1&&void 0!==arguments[1]?arguments[1]:{},i=n.isCaseSensitive,o=void 0===i?I.isCaseSensitive:i,c=n.includeMatches,a=void 0===c?I.includeMatches:c,s=n.minMatchCharLength,u=void 0===s?I.minMatchCharLength:s,h=n.ignoreLocation,l=void 0===h?I.ignoreLocation:h,f=n.findAllMatches,d=void 0===f?I.findAllMatches:f,v=n.location,g=void 0===v?I.location:v,y=n.threshold,p=void 0===y?I.threshold:y,m=n.distance,k=void 0===m?I.distance:m;r(this,e),this.query=null,this.options={isCaseSensitive:o,includeMatches:a,minMatchCharLength:u,findAllMatches:d,ignoreLocation:l,location:g,threshold:p,distance:k},this.pattern=o?t:t.toLowerCase(),this.query=Z(this.pattern,this.options)}return o(e,[{key:"searchIn",value:function(e){var t=this.query;if(!t)return{isMatch:!1,score:1};var n=this.options,r=n.includeMatches;e=n.isCaseSensitive?e:e.toLowerCase();for(var i=0,o=[],c=0,a=0,s=t.length;a-1&&(n.refIndex=e.idx),t.matches.push(n)}}))}function ve(e,t){t.score=e.score}function ge(e,t){var n=arguments.length>2&&void 0!==arguments[2]?arguments[2]:{},r=n.includeMatches,i=void 0===r?I.includeMatches:r,o=n.includeScore,c=void 0===o?I.includeScore:o,a=[];return i&&a.push(de),c&&a.push(ve),e.map((function(e){var n=e.idx,r={item:t[n],refIndex:n};return a.length&&a.forEach((function(t){t(e,r)})),r}))}var ye=function(){function e(n){var i=arguments.length>1&&void 0!==arguments[1]?arguments[1]:{},o=arguments.length>2?arguments[2]:void 0;r(this,e),this.options=t(t({},I),i),this.options.useExtendedSearch,this._keyStore=new S(this.options.keys),this.setCollection(n,o)}return o(e,[{key:"setCollection",value:function(e,t){if(this._docs=e,t&&!(t instanceof $))throw new Error("Incorrect 'index' type");this._myIndex=t||F(this.options.keys,this._docs,{getFn:this.options.getFn,fieldNormWeight:this.options.fieldNormWeight})}},{key:"add",value:function(e){k(e)&&(this._docs.push(e),this._myIndex.add(e))}},{key:"remove",value:function(){for(var e=arguments.length>0&&void 0!==arguments[0]?arguments[0]:function(){return!1},t=[],n=0,r=this._docs.length;n1&&void 0!==arguments[1]?arguments[1]:{},n=t.limit,r=void 0===n?-1:n,i=this.options,o=i.includeMatches,c=i.includeScore,a=i.shouldSort,s=i.sortFn,u=i.ignoreFieldNorm,h=g(e)?g(this._docs[0])?this._searchStringList(e):this._searchObjectList(e):this._searchLogical(e);return fe(h,{ignoreFieldNorm:u}),a&&h.sort(s),y(r)&&r>-1&&(h=h.slice(0,r)),ge(h,this._docs,{includeMatches:o,includeScore:c})}},{key:"_searchStringList",value:function(e){var t=re(e,this.options),n=this._myIndex.records,r=[];return n.forEach((function(e){var n=e.v,i=e.i,o=e.n;if(k(n)){var c=t.searchIn(n),a=c.isMatch,s=c.score,u=c.indices;a&&r.push({item:n,idx:i,matches:[{score:s,value:n,norm:o,indices:u}]})}})),r}},{key:"_searchLogical",value:function(e){var t=this,n=function(e,t){var n=(arguments.length>2&&void 0!==arguments[2]?arguments[2]:{}).auto,r=void 0===n||n,i=function e(n){var i=Object.keys(n),o=ue(n);if(!o&&i.length>1&&!se(n))return e(le(n));if(he(n)){var c=o?n[ce]:i[0],a=o?n[ae]:n[c];if(!g(a))throw new Error(x(c));var s={keyId:j(c),pattern:a};return r&&(s.searcher=re(a,t)),s}var u={children:[],operator:i[0]};return i.forEach((function(t){var r=n[t];v(r)&&r.forEach((function(t){u.children.push(e(t))}))})),u};return se(e)||(e=le(e)),i(e)}(e,this.options),r=function e(n,r,i){if(!n.children){var o=n.keyId,c=n.searcher,a=t._findMatches({key:t._keyStore.get(o),value:t._myIndex.getValueForItemAtKeyId(r,o),searcher:c});return a&&a.length?[{idx:i,item:r,matches:a}]:[]}for(var s=[],u=0,h=n.children.length;u1&&void 0!==arguments[1]?arguments[1]:{},n=t.getFn,r=void 0===n?I.getFn:n,i=t.fieldNormWeight,o=void 0===i?I.fieldNormWeight:i,c=e.keys,a=e.records,s=new $({getFn:r,fieldNormWeight:o});return s.setKeys(c),s.setIndexRecords(a),s},ye.config=I,function(){ne.push.apply(ne,arguments)}(te),ye},"object"==typeof exports&&"undefined"!=typeof module?module.exports=t():"function"==typeof define&&define.amd?define(t):(e="undefined"!=typeof globalThis?globalThis:e||self).Fuse=t();
\ No newline at end of file
diff --git a/spaces/Anonymous-123/ImageNet-Editing/object_removal/TFill/model/losses.py b/spaces/Anonymous-123/ImageNet-Editing/object_removal/TFill/model/losses.py
deleted file mode 100644
index b8577df7241e42098fb0ef42c74cd4e13afce384..0000000000000000000000000000000000000000
--- a/spaces/Anonymous-123/ImageNet-Editing/object_removal/TFill/model/losses.py
+++ /dev/null
@@ -1,364 +0,0 @@
-import torch
-import torch.nn as nn
-import torch.nn.functional as F
-import torchvision.models as models
-
-
-####################################################################################################
-# adversarial loss for different gan mode
-####################################################################################################
-class GANLoss(nn.Module):
- """Define different GAN objectives.
-
- The GANLoss class abstracts away the need to create the target label tensor
- that has the same size as the input.
- """
-
- def __init__(self, gan_mode, target_real_label=1.0, target_fake_label=0.0):
- """ Initialize the GANLoss class.
-
- Parameters:
- gan_mode (str) - - the type of GAN objective. It currently supports vanilla, lsgan, and wgangp.
- target_real_label (bool) - - label for a real image
- target_fake_label (bool) - - label of a fake image
-
- Note: Do not use sigmoid as the last layer of Discriminator.
- LSGAN needs no sigmoid. vanilla GANs will handle it with BCEWithLogitsLoss.
- """
- super(GANLoss, self).__init__()
- self.register_buffer('real_label', torch.tensor(target_real_label))
- self.register_buffer('fake_label', torch.tensor(target_fake_label))
- self.gan_mode = gan_mode
- if gan_mode == 'lsgan':
- self.loss = nn.MSELoss()
- elif gan_mode == 'vanilla':
- self.loss = nn.BCEWithLogitsLoss()
- elif gan_mode == 'hinge':
- self.loss = nn.ReLU()
- elif gan_mode in ['wgangp', 'nonsaturating']:
- self.loss = None
- else:
- raise NotImplementedError('gan mode %s not implemented' % gan_mode)
-
- def get_target_tensor(self, prediction, target_is_real):
- """Create label tensors with the same size as the input.
-
- Parameters:
- prediction (tensor) - - tpyically the prediction from a discriminator
- target_is_real (bool) - - if the ground truth label is for real examples or fake examples
-
- Returns:
- A label tensor filled with ground truth label, and with the size of the input
- """
-
- if target_is_real:
- target_tensor = self.real_label
- else:
- target_tensor = self.fake_label
- return target_tensor.expand_as(prediction)
-
- def calculate_loss(self, prediction, target_is_real, is_dis=False):
- """Calculate loss given Discriminator's output and grount truth labels.
-
- Parameters:
- prediction (tensor) - - tpyically the prediction output from a discriminator
- target_is_real (bool) - - if the ground truth label is for real examples or fake examples
-
- Returns:
- the calculated loss.
- """
- if self.gan_mode in ['lsgan', 'vanilla']:
- target_tensor = self.get_target_tensor(prediction, target_is_real)
- loss = self.loss(prediction, target_tensor)
- if self.gan_mode == 'lsgan':
- loss = loss * 0.5
- else:
- if is_dis:
- if target_is_real:
- prediction = -prediction
- if self.gan_mode == 'wgangp':
- loss = prediction.mean()
- elif self.gan_mode == 'nonsaturating':
- loss = F.softplus(prediction).mean()
- elif self.gan_mode == 'hinge':
- loss = self.loss(1+prediction).mean()
- else:
- if self.gan_mode == 'nonsaturating':
- loss = F.softplus(-prediction).mean()
- else:
- loss = -prediction.mean()
- return loss
-
- def __call__(self, predictions, target_is_real, is_dis=False):
- """Calculate loss for multi-scales gan"""
- if isinstance(predictions, list):
- losses = []
- for prediction in predictions:
- losses.append(self.calculate_loss(prediction, target_is_real, is_dis))
- loss = sum(losses)
- else:
- loss = self.calculate_loss(predictions, target_is_real, is_dis)
-
- return loss
-
-
-def cal_gradient_penalty(netD, real_data, fake_data, device, type='mixed', constant=1.0, lambda_gp=10.0):
- """Calculate the gradient penalty loss, used in WGAN-GP paper https://arxiv.org/abs/1704.00028
-
- Arguments:
- netD (network) -- discriminator network
- real_data (tensor array) -- real examples
- fake_data (tensor array) -- generated examples from the generator
- device (str) -- GPU / CPU: from torch.device('cuda:{}'.format(self.gpu_ids[0])) if self.gpu_ids else torch.device('cpu')
- type (str) -- if we mix real and fake data or not [real | fake | mixed].
- constant (float) -- the constant used in formula ( ||gradient||_2 - constant)^2
- lambda_gp (float) -- weight for this loss
-
- Returns the gradient penalty loss
- """
- if lambda_gp > 0.0:
- if type == 'real': # either use real examples, fake examples, or a linear interpolation of two.
- interpolatesv = real_data
- elif type == 'fake':
- interpolatesv = fake_data
- elif type == 'mixed':
- alpha = torch.rand(real_data.shape[0], 1, device=device)
- alpha = alpha.expand(real_data.shape[0], real_data.nelement() // real_data.shape[0]).contiguous().view(*real_data.shape)
- interpolatesv = alpha * real_data + ((1 - alpha) * fake_data)
- else:
- raise NotImplementedError('{} not implemented'.format(type))
- interpolatesv.requires_grad_(True)
- disc_interpolates = netD(interpolatesv)
- if isinstance(disc_interpolates, list):
- gradients = 0
- for disc_interpolate in disc_interpolates:
- gradients += torch.autograd.grad(outputs=disc_interpolate, inputs=interpolatesv,
- grad_outputs=torch.ones(disc_interpolate.size()).to(device),
- create_graph=True, retain_graph=True, only_inputs=True)[0]
- else:
- gradients = torch.autograd.grad(outputs=disc_interpolates, inputs=interpolatesv,
- grad_outputs=torch.ones(disc_interpolates.size()).to(device),
- create_graph=True, retain_graph=True, only_inputs=True)[0]
- gradients = gradients.view(real_data.size(0), -1) # flat the data
- gradient_penalty = (((gradients + 1e-16).norm(2, dim=1) - constant) ** 2).mean() * lambda_gp # added eps
- return gradient_penalty, gradients
- else:
- return 0.0, None
-
-
-####################################################################################################
-# trained LPIPS loss
-####################################################################################################
-def normalize_tensor(x, eps=1e-10):
- norm_factor = torch.sqrt(torch.sum(x**2, dim=1, keepdim=True))
- return x/(norm_factor+eps)
-
-
-def spatial_average(x, keepdim=True):
- return x.mean([2, 3], keepdim=keepdim)
-
-
-class NetLinLayer(nn.Module):
- """ A single linear layer which does a 1x1 conv """
- def __init__(self, chn_in, chn_out=1, use_dropout=False):
- super(NetLinLayer, self).__init__()
- layers = [nn.Dropout(), ] if (use_dropout) else []
- layers += [nn.Conv2d(chn_in, chn_out, 1, stride=1, padding=0, bias=False), ]
- self.model = nn.Sequential(*layers)
-
-
-class LPIPSLoss(nn.Module):
- """
- Learned perceptual metric
- https://github.com/richzhang/PerceptualSimilarity
- """
- def __init__(self, use_dropout=True, ckpt_path=None):
- super(LPIPSLoss, self).__init__()
- self.path = ckpt_path
- self.net = VGG16()
- self.chns = [64, 128, 256, 512, 512] # vg16 features
- self.lin0 = NetLinLayer(self.chns[0], use_dropout=use_dropout)
- self.lin1 = NetLinLayer(self.chns[1], use_dropout=use_dropout)
- self.lin2 = NetLinLayer(self.chns[2], use_dropout=use_dropout)
- self.lin3 = NetLinLayer(self.chns[3], use_dropout=use_dropout)
- self.lin4 = NetLinLayer(self.chns[4], use_dropout=use_dropout)
- self.load_from_pretrained()
- for param in self.parameters():
- param.requires_grad = False
-
- def load_from_pretrained(self):
- self.load_state_dict(torch.load(self.path, map_location=torch.device("cpu")), strict=False)
- print("loaded pretrained LPIPS loss from {}".format(self.path))
-
- def _get_features(self, vgg_f):
- names = ['relu1_2', 'relu2_2', 'relu3_3', 'relu4_3', 'relu5_3']
- feats = []
- for i in range(len(names)):
- name = names[i]
- feat = vgg_f[name]
- feats.append(feat)
- return feats
-
- def forward(self, x, y):
- x_vgg, y_vgg = self._get_features(self.net(x)), self._get_features(self.net(y))
- lins = [self.lin0, self.lin1, self.lin2, self.lin3, self.lin4]
- reses = []
- loss = 0
-
- for i in range(len(self.chns)):
- x_feats, y_feats = normalize_tensor(x_vgg[i]), normalize_tensor(y_vgg[i])
- diffs = (x_feats - y_feats) ** 2
- res = spatial_average(lins[i].model(diffs))
- loss += res
- reses.append(res)
-
- return loss
-
-
-class PerceptualLoss(nn.Module):
- r"""
- Perceptual loss, VGG-based
- https://arxiv.org/abs/1603.08155
- https://github.com/dxyang/StyleTransfer/blob/master/utils.py
- """
-
- def __init__(self, weights=[1.0, 1.0, 1.0, 1.0, 0.0]):
- super(PerceptualLoss, self).__init__()
- self.add_module('vgg', VGG16())
- self.criterion = nn.L1Loss()
- self.weights = weights
-
- def __call__(self, x, y):
- # Compute features
- x_vgg, y_vgg = self.vgg(x), self.vgg(y)
-
- content_loss = 0.0
- content_loss += self.weights[0] * self.criterion(x_vgg['relu1_2'], y_vgg['relu1_2']) if self.weights[0] > 0 else 0
- content_loss += self.weights[1] * self.criterion(x_vgg['relu2_2'], y_vgg['relu2_2']) if self.weights[1] > 0 else 0
- content_loss += self.weights[2] * self.criterion(x_vgg['relu3_3'], y_vgg['relu3_3']) if self.weights[2] > 0 else 0
- content_loss += self.weights[3] * self.criterion(x_vgg['relu4_3'], y_vgg['relu4_3']) if self.weights[3] > 0 else 0
- content_loss += self.weights[4] * self.criterion(x_vgg['relu5_3'], y_vgg['relu5_3']) if self.weights[4] > 0 else 0
-
- return content_loss
-
-
-class Normalization(nn.Module):
- def __init__(self, device):
- super(Normalization, self).__init__()
- # .view the mean and std to make them [C x 1 x 1] so that they can
- # directly work with image Tensor of shape [B x C x H x W].
- # B is batch size. C is number of channels. H is height and W is width.
- mean = torch.tensor([0.485, 0.456, 0.406]).to(device)
- std = torch.tensor([0.229, 0.224, 0.225]).to(device)
- self.mean = mean.view(-1, 1, 1)
- self.std = std.view(-1, 1, 1)
-
- def forward(self, img):
- # normalize img
- return (img - self.mean) / self.std
-
-
-class VGG16(nn.Module):
- def __init__(self):
- super(VGG16, self).__init__()
- features = models.vgg16(pretrained=True).features
- self.relu1_1 = torch.nn.Sequential()
- self.relu1_2 = torch.nn.Sequential()
-
- self.relu2_1 = torch.nn.Sequential()
- self.relu2_2 = torch.nn.Sequential()
-
- self.relu3_1 = torch.nn.Sequential()
- self.relu3_2 = torch.nn.Sequential()
- self.relu3_3 = torch.nn.Sequential()
-
- self.relu4_1 = torch.nn.Sequential()
- self.relu4_2 = torch.nn.Sequential()
- self.relu4_3 = torch.nn.Sequential()
-
- self.relu5_1 = torch.nn.Sequential()
- self.relu5_2 = torch.nn.Sequential()
- self.relu5_3 = torch.nn.Sequential()
-
- for x in range(2):
- self.relu1_1.add_module(str(x), features[x])
-
- for x in range(2, 4):
- self.relu1_2.add_module(str(x), features[x])
-
- for x in range(4, 7):
- self.relu2_1.add_module(str(x), features[x])
-
- for x in range(7, 9):
- self.relu2_2.add_module(str(x), features[x])
-
- for x in range(9, 12):
- self.relu3_1.add_module(str(x), features[x])
-
- for x in range(12, 14):
- self.relu3_2.add_module(str(x), features[x])
-
- for x in range(14, 16):
- self.relu3_3.add_module(str(x), features[x])
-
- for x in range(16, 18):
- self.relu4_1.add_module(str(x), features[x])
-
- for x in range(18, 21):
- self.relu4_2.add_module(str(x), features[x])
-
- for x in range(21, 23):
- self.relu4_3.add_module(str(x), features[x])
-
- for x in range(23, 26):
- self.relu5_1.add_module(str(x), features[x])
-
- for x in range(26, 28):
- self.relu5_2.add_module(str(x), features[x])
-
- for x in range(28, 30):
- self.relu5_3.add_module(str(x), features[x])
-
- # don't need the gradients, just want the features
- for param in self.parameters():
- param.requires_grad = False
-
- def forward(self, x,):
- relu1_1 = self.relu1_1(x)
- relu1_2 = self.relu1_2(relu1_1)
-
- relu2_1 = self.relu2_1(relu1_2)
- relu2_2 = self.relu2_2(relu2_1)
-
- relu3_1 = self.relu3_1(relu2_2)
- relu3_2 = self.relu3_2(relu3_1)
- relu3_3 = self.relu3_3(relu3_2)
-
- relu4_1 = self.relu4_1(relu3_3)
- relu4_2 = self.relu4_2(relu4_1)
- relu4_3 = self.relu4_3(relu4_2)
-
- relu5_1 = self.relu5_1(relu4_3)
- relu5_2 = self.relu5_2(relu5_1)
- relu5_3 = self.relu5_3(relu5_2)
-
- out = {
- 'relu1_1': relu1_1,
- 'relu1_2': relu1_2,
-
- 'relu2_1': relu2_1,
- 'relu2_2': relu2_2,
-
- 'relu3_1': relu3_1,
- 'relu3_2': relu3_2,
- 'relu3_3': relu3_3,
-
- 'relu4_1': relu4_1,
- 'relu4_2': relu4_2,
- 'relu4_3': relu4_3,
-
- 'relu5_1': relu5_1,
- 'relu5_2': relu5_2,
- 'relu5_3': relu5_3,
- }
- return out
\ No newline at end of file
diff --git a/spaces/Anonymous-sub/Rerender/ControlNet/annotator/uniformer/mmcv/image/io.py b/spaces/Anonymous-sub/Rerender/ControlNet/annotator/uniformer/mmcv/image/io.py
deleted file mode 100644
index d3fa2e8cc06b1a7b0b69de6406980b15d61a1e5d..0000000000000000000000000000000000000000
--- a/spaces/Anonymous-sub/Rerender/ControlNet/annotator/uniformer/mmcv/image/io.py
+++ /dev/null
@@ -1,258 +0,0 @@
-# Copyright (c) OpenMMLab. All rights reserved.
-import io
-import os.path as osp
-from pathlib import Path
-
-import cv2
-import numpy as np
-from cv2 import (IMREAD_COLOR, IMREAD_GRAYSCALE, IMREAD_IGNORE_ORIENTATION,
- IMREAD_UNCHANGED)
-
-from annotator.uniformer.mmcv.utils import check_file_exist, is_str, mkdir_or_exist
-
-try:
- from turbojpeg import TJCS_RGB, TJPF_BGR, TJPF_GRAY, TurboJPEG
-except ImportError:
- TJCS_RGB = TJPF_GRAY = TJPF_BGR = TurboJPEG = None
-
-try:
- from PIL import Image, ImageOps
-except ImportError:
- Image = None
-
-try:
- import tifffile
-except ImportError:
- tifffile = None
-
-jpeg = None
-supported_backends = ['cv2', 'turbojpeg', 'pillow', 'tifffile']
-
-imread_flags = {
- 'color': IMREAD_COLOR,
- 'grayscale': IMREAD_GRAYSCALE,
- 'unchanged': IMREAD_UNCHANGED,
- 'color_ignore_orientation': IMREAD_IGNORE_ORIENTATION | IMREAD_COLOR,
- 'grayscale_ignore_orientation':
- IMREAD_IGNORE_ORIENTATION | IMREAD_GRAYSCALE
-}
-
-imread_backend = 'cv2'
-
-
-def use_backend(backend):
- """Select a backend for image decoding.
-
- Args:
- backend (str): The image decoding backend type. Options are `cv2`,
- `pillow`, `turbojpeg` (see https://github.com/lilohuang/PyTurboJPEG)
- and `tifffile`. `turbojpeg` is faster but it only supports `.jpeg`
- file format.
- """
- assert backend in supported_backends
- global imread_backend
- imread_backend = backend
- if imread_backend == 'turbojpeg':
- if TurboJPEG is None:
- raise ImportError('`PyTurboJPEG` is not installed')
- global jpeg
- if jpeg is None:
- jpeg = TurboJPEG()
- elif imread_backend == 'pillow':
- if Image is None:
- raise ImportError('`Pillow` is not installed')
- elif imread_backend == 'tifffile':
- if tifffile is None:
- raise ImportError('`tifffile` is not installed')
-
-
-def _jpegflag(flag='color', channel_order='bgr'):
- channel_order = channel_order.lower()
- if channel_order not in ['rgb', 'bgr']:
- raise ValueError('channel order must be either "rgb" or "bgr"')
-
- if flag == 'color':
- if channel_order == 'bgr':
- return TJPF_BGR
- elif channel_order == 'rgb':
- return TJCS_RGB
- elif flag == 'grayscale':
- return TJPF_GRAY
- else:
- raise ValueError('flag must be "color" or "grayscale"')
-
-
-def _pillow2array(img, flag='color', channel_order='bgr'):
- """Convert a pillow image to numpy array.
-
- Args:
- img (:obj:`PIL.Image.Image`): The image loaded using PIL
- flag (str): Flags specifying the color type of a loaded image,
- candidates are 'color', 'grayscale' and 'unchanged'.
- Default to 'color'.
- channel_order (str): The channel order of the output image array,
- candidates are 'bgr' and 'rgb'. Default to 'bgr'.
-
- Returns:
- np.ndarray: The converted numpy array
- """
- channel_order = channel_order.lower()
- if channel_order not in ['rgb', 'bgr']:
- raise ValueError('channel order must be either "rgb" or "bgr"')
-
- if flag == 'unchanged':
- array = np.array(img)
- if array.ndim >= 3 and array.shape[2] >= 3: # color image
- array[:, :, :3] = array[:, :, (2, 1, 0)] # RGB to BGR
- else:
- # Handle exif orientation tag
- if flag in ['color', 'grayscale']:
- img = ImageOps.exif_transpose(img)
- # If the image mode is not 'RGB', convert it to 'RGB' first.
- if img.mode != 'RGB':
- if img.mode != 'LA':
- # Most formats except 'LA' can be directly converted to RGB
- img = img.convert('RGB')
- else:
- # When the mode is 'LA', the default conversion will fill in
- # the canvas with black, which sometimes shadows black objects
- # in the foreground.
- #
- # Therefore, a random color (124, 117, 104) is used for canvas
- img_rgba = img.convert('RGBA')
- img = Image.new('RGB', img_rgba.size, (124, 117, 104))
- img.paste(img_rgba, mask=img_rgba.split()[3]) # 3 is alpha
- if flag in ['color', 'color_ignore_orientation']:
- array = np.array(img)
- if channel_order != 'rgb':
- array = array[:, :, ::-1] # RGB to BGR
- elif flag in ['grayscale', 'grayscale_ignore_orientation']:
- img = img.convert('L')
- array = np.array(img)
- else:
- raise ValueError(
- 'flag must be "color", "grayscale", "unchanged", '
- f'"color_ignore_orientation" or "grayscale_ignore_orientation"'
- f' but got {flag}')
- return array
-
-
-def imread(img_or_path, flag='color', channel_order='bgr', backend=None):
- """Read an image.
-
- Args:
- img_or_path (ndarray or str or Path): Either a numpy array or str or
- pathlib.Path. If it is a numpy array (loaded image), then
- it will be returned as is.
- flag (str): Flags specifying the color type of a loaded image,
- candidates are `color`, `grayscale`, `unchanged`,
- `color_ignore_orientation` and `grayscale_ignore_orientation`.
- By default, `cv2` and `pillow` backend would rotate the image
- according to its EXIF info unless called with `unchanged` or
- `*_ignore_orientation` flags. `turbojpeg` and `tifffile` backend
- always ignore image's EXIF info regardless of the flag.
- The `turbojpeg` backend only supports `color` and `grayscale`.
- channel_order (str): Order of channel, candidates are `bgr` and `rgb`.
- backend (str | None): The image decoding backend type. Options are
- `cv2`, `pillow`, `turbojpeg`, `tifffile`, `None`.
- If backend is None, the global imread_backend specified by
- ``mmcv.use_backend()`` will be used. Default: None.
-
- Returns:
- ndarray: Loaded image array.
- """
-
- if backend is None:
- backend = imread_backend
- if backend not in supported_backends:
- raise ValueError(f'backend: {backend} is not supported. Supported '
- "backends are 'cv2', 'turbojpeg', 'pillow'")
- if isinstance(img_or_path, Path):
- img_or_path = str(img_or_path)
-
- if isinstance(img_or_path, np.ndarray):
- return img_or_path
- elif is_str(img_or_path):
- check_file_exist(img_or_path,
- f'img file does not exist: {img_or_path}')
- if backend == 'turbojpeg':
- with open(img_or_path, 'rb') as in_file:
- img = jpeg.decode(in_file.read(),
- _jpegflag(flag, channel_order))
- if img.shape[-1] == 1:
- img = img[:, :, 0]
- return img
- elif backend == 'pillow':
- img = Image.open(img_or_path)
- img = _pillow2array(img, flag, channel_order)
- return img
- elif backend == 'tifffile':
- img = tifffile.imread(img_or_path)
- return img
- else:
- flag = imread_flags[flag] if is_str(flag) else flag
- img = cv2.imread(img_or_path, flag)
- if flag == IMREAD_COLOR and channel_order == 'rgb':
- cv2.cvtColor(img, cv2.COLOR_BGR2RGB, img)
- return img
- else:
- raise TypeError('"img" must be a numpy array or a str or '
- 'a pathlib.Path object')
-
-
-def imfrombytes(content, flag='color', channel_order='bgr', backend=None):
- """Read an image from bytes.
-
- Args:
- content (bytes): Image bytes got from files or other streams.
- flag (str): Same as :func:`imread`.
- backend (str | None): The image decoding backend type. Options are
- `cv2`, `pillow`, `turbojpeg`, `None`. If backend is None, the
- global imread_backend specified by ``mmcv.use_backend()`` will be
- used. Default: None.
-
- Returns:
- ndarray: Loaded image array.
- """
-
- if backend is None:
- backend = imread_backend
- if backend not in supported_backends:
- raise ValueError(f'backend: {backend} is not supported. Supported '
- "backends are 'cv2', 'turbojpeg', 'pillow'")
- if backend == 'turbojpeg':
- img = jpeg.decode(content, _jpegflag(flag, channel_order))
- if img.shape[-1] == 1:
- img = img[:, :, 0]
- return img
- elif backend == 'pillow':
- buff = io.BytesIO(content)
- img = Image.open(buff)
- img = _pillow2array(img, flag, channel_order)
- return img
- else:
- img_np = np.frombuffer(content, np.uint8)
- flag = imread_flags[flag] if is_str(flag) else flag
- img = cv2.imdecode(img_np, flag)
- if flag == IMREAD_COLOR and channel_order == 'rgb':
- cv2.cvtColor(img, cv2.COLOR_BGR2RGB, img)
- return img
-
-
-def imwrite(img, file_path, params=None, auto_mkdir=True):
- """Write image to file.
-
- Args:
- img (ndarray): Image array to be written.
- file_path (str): Image file path.
- params (None or list): Same as opencv :func:`imwrite` interface.
- auto_mkdir (bool): If the parent folder of `file_path` does not exist,
- whether to create it automatically.
-
- Returns:
- bool: Successful or not.
- """
- if auto_mkdir:
- dir_name = osp.abspath(osp.dirname(file_path))
- mkdir_or_exist(dir_name)
- return cv2.imwrite(file_path, img, params)
diff --git a/spaces/Ataturk-Chatbot/HuggingFaceChat/venv/lib/python3.11/site-packages/setuptools/_distutils/log.py b/spaces/Ataturk-Chatbot/HuggingFaceChat/venv/lib/python3.11/site-packages/setuptools/_distutils/log.py
deleted file mode 100644
index be25f6cabd839af772dd74399c57991c222d3da8..0000000000000000000000000000000000000000
--- a/spaces/Ataturk-Chatbot/HuggingFaceChat/venv/lib/python3.11/site-packages/setuptools/_distutils/log.py
+++ /dev/null
@@ -1,80 +0,0 @@
-"""A simple log mechanism styled after PEP 282."""
-
-# The class here is styled after PEP 282 so that it could later be
-# replaced with a standard Python logging implementation.
-
-import sys
-
-DEBUG = 1
-INFO = 2
-WARN = 3
-ERROR = 4
-FATAL = 5
-
-
-class Log:
- def __init__(self, threshold=WARN):
- self.threshold = threshold
-
- def _log(self, level, msg, args):
- if level not in (DEBUG, INFO, WARN, ERROR, FATAL):
- raise ValueError('%s wrong log level' % str(level))
-
- if level >= self.threshold:
- if args:
- msg = msg % args
- if level in (WARN, ERROR, FATAL):
- stream = sys.stderr
- else:
- stream = sys.stdout
- try:
- stream.write('%s\n' % msg)
- except UnicodeEncodeError:
- # emulate backslashreplace error handler
- encoding = stream.encoding
- msg = msg.encode(encoding, "backslashreplace").decode(encoding)
- stream.write('%s\n' % msg)
- stream.flush()
-
- def log(self, level, msg, *args):
- self._log(level, msg, args)
-
- def debug(self, msg, *args):
- self._log(DEBUG, msg, args)
-
- def info(self, msg, *args):
- self._log(INFO, msg, args)
-
- def warn(self, msg, *args):
- self._log(WARN, msg, args)
-
- def error(self, msg, *args):
- self._log(ERROR, msg, args)
-
- def fatal(self, msg, *args):
- self._log(FATAL, msg, args)
-
-
-_global_log = Log()
-log = _global_log.log
-debug = _global_log.debug
-info = _global_log.info
-warn = _global_log.warn
-error = _global_log.error
-fatal = _global_log.fatal
-
-
-def set_threshold(level):
- # return the old threshold for use from tests
- old = _global_log.threshold
- _global_log.threshold = level
- return old
-
-
-def set_verbosity(v):
- if v <= 0:
- set_threshold(WARN)
- elif v == 1:
- set_threshold(INFO)
- elif v >= 2:
- set_threshold(DEBUG)
diff --git a/spaces/Audio-AGI/WavJourney/VoiceParser/hubert_manager.py b/spaces/Audio-AGI/WavJourney/VoiceParser/hubert_manager.py
deleted file mode 100644
index 5f8445147a8997fdb54e1246e9a85af40342c748..0000000000000000000000000000000000000000
--- a/spaces/Audio-AGI/WavJourney/VoiceParser/hubert_manager.py
+++ /dev/null
@@ -1,33 +0,0 @@
-import os.path
-import shutil
-import urllib.request
-
-import huggingface_hub
-
-
-class HuBERTManager:
- @staticmethod
- def make_sure_hubert_installed(download_url: str = 'https://dl.fbaipublicfiles.com/hubert/hubert_base_ls960.pt', file_name: str = 'hubert.pt'):
- install_dir = os.path.join('VoiceParser', 'hubert')
- if not os.path.isdir(install_dir):
- os.makedirs(install_dir, exist_ok=True)
- install_file = os.path.join(install_dir, file_name)
- if not os.path.isfile(install_file):
- print('Downloading HuBERT base model')
- urllib.request.urlretrieve(download_url, install_file)
- print('Downloaded HuBERT')
- return install_file
-
-
- @staticmethod
- def make_sure_tokenizer_installed(model: str = 'quantifier_hubert_base_ls960_14.pth', repo: str = 'GitMylo/bark-voice-cloning', local_file: str = 'tokenizer.pth'):
- install_dir = os.path.join('VoiceParser', 'hubert')
- if not os.path.isdir(install_dir):
- os.makedirs(install_dir, exist_ok=True)
- install_file = os.path.join(install_dir, local_file)
- if not os.path.isfile(install_file):
- print('Downloading HuBERT custom tokenizer')
- huggingface_hub.hf_hub_download(repo, model, local_dir=install_dir, local_dir_use_symlinks=False)
- shutil.move(os.path.join(install_dir, model), install_file)
- print('Downloaded tokenizer')
- return install_file
\ No newline at end of file
diff --git a/spaces/Audio-AGI/WavJourney/voice_presets.py b/spaces/Audio-AGI/WavJourney/voice_presets.py
deleted file mode 100644
index 5cf8ed05c3c45bf550254b206e25345cd21759b9..0000000000000000000000000000000000000000
--- a/spaces/Audio-AGI/WavJourney/voice_presets.py
+++ /dev/null
@@ -1,96 +0,0 @@
-import os
-import json, json5
-from pathlib import Path
-
-import utils
-from APIs import VP
-
-
-def save_voice_presets_metadata(voice_presets_path, metadata):
- with open(voice_presets_path / 'metadata.json', 'w') as f:
- json.dump(metadata, f, indent=4)
-
-def load_voice_presets_metadata(voice_presets_path, safe_if_metadata_not_exist=False):
- metadata_full_path = Path(voice_presets_path) / 'metadata.json'
-
- if safe_if_metadata_not_exist:
- if not os.path.exists(metadata_full_path):
- return {}
-
- with open(metadata_full_path, 'r') as f:
- presets = json5.load(f)
-
- return presets
-
-# return system voice presets and session voice presets individually, each in a list
-def get_voice_presets(session_id):
- system_presets, session_presets = [], []
-
- # Load system presets
- system_presets = load_voice_presets_metadata(utils.get_system_voice_preset_path())
-
- # Load session presets
- session_presets = load_voice_presets_metadata(
- utils.get_session_voice_preset_path(session_id),
- safe_if_metadata_not_exist=True
- )
-
- return system_presets, session_presets
-
-# return merged voice presets in a {voice_preset_name: voice_preset} dict
-def get_merged_voice_presets(session_id):
- system_presets, session_presets = get_voice_presets(session_id)
- res = {}
- for preset in list(system_presets.values()) + list(session_presets.values()):
- res[preset['id']] = preset # session presets with the same id will cover that of system presets
- return res
-
-def add_voice_preset(voice_presets_path, presets, id, desc, wav_file_path):
- if id in presets:
- raise KeyError(f'{id} already in voice preset, path={voice_presets_path}!')
-
- # Convert wav to npz
- npz_path = voice_presets_path / 'npz'
- VP(wav_file_path, npz_path)
- npz_file_path = npz_path / f'{Path(wav_file_path).stem}.npz'
-
- presets[id] = {
- 'id': id,
- 'desc': desc,
- 'npz_path': str(npz_file_path)
- }
- save_voice_presets_metadata(voice_presets_path, presets)
- return presets[id]
-
-def add_session_voice_preset(id, desc, wav_file_path, session_id):
- voice_presets_path = utils.get_session_voice_preset_path(session_id)
- os.makedirs(voice_presets_path / 'npz', exist_ok=True)
- presets = load_voice_presets_metadata(voice_presets_path, safe_if_metadata_not_exist=True)
- if len(presets) >= 3:
- raise ValueError(f'session voice presets size exceed 3')
- if id in presets:
- raise KeyError(f'{id} already in voice preset, path={voice_presets_path}!')
-
- return add_voice_preset(voice_presets_path, presets, id, desc, wav_file_path)
-
-def add_system_voice_preset(id, desc, wav_file_path):
- voice_presets_path = utils.get_system_voice_preset_path()
- presets = load_voice_presets_metadata(voice_presets_path)
- return add_voice_preset(voice_presets_path, presets, id, desc, wav_file_path)
-
-# if session_id set to '', we are removing system voice presets
-def remove_session_voice_preset(id, session_id):
- voice_presets_path = utils.get_session_voice_preset_path(session_id)
- presets = load_voice_presets_metadata(
- voice_presets_path,
- safe_if_metadata_not_exist=True
- )
- preset = presets.pop(id)
- npz_path = preset['npz_path']
-
- try:
- os.remove(npz_path)
- except FileNotFoundError:
- print(f"INFO: trying to delete {npz_path} which does not exist, path={voice_presets_path}.")
-
- save_voice_presets_metadata(voice_presets_path, presets)
\ No newline at end of file
diff --git a/spaces/B10915003/B10915003-autotrain-jimmy-test-face-identification-53251125423/README.md b/spaces/B10915003/B10915003-autotrain-jimmy-test-face-identification-53251125423/README.md
deleted file mode 100644
index a9ab63e3f27464e7178821e9537fcec13c51cc41..0000000000000000000000000000000000000000
--- a/spaces/B10915003/B10915003-autotrain-jimmy-test-face-identification-53251125423/README.md
+++ /dev/null
@@ -1,13 +0,0 @@
----
-title: B10915003 Autotrain Jimmy Test Face Identification 53251125423
-emoji: 👁
-colorFrom: green
-colorTo: indigo
-sdk: gradio
-sdk_version: 3.27.0
-app_file: app.py
-pinned: false
-license: apache-2.0
----
-
-Check out the configuration reference at https://huggingface.co/docs/hub/spaces-config-reference
diff --git a/spaces/BAAI/dreambooth-altdiffusion/app.py b/spaces/BAAI/dreambooth-altdiffusion/app.py
deleted file mode 100644
index 80a9d1812ffb28057624ca9e77d6376afd4325cd..0000000000000000000000000000000000000000
--- a/spaces/BAAI/dreambooth-altdiffusion/app.py
+++ /dev/null
@@ -1,654 +0,0 @@
-import gradio as gr
-import os
-from pathlib import Path
-import argparse
-import shutil
-from train_dreambooth import run_training
-from convertosd import convert
-from PIL import Image
-from slugify import slugify
-import requests
-import torch
-import zipfile
-import tarfile
-import urllib.parse
-import gc
-# from diffusers import StableDiffusionPipeline
-from huggingface_hub import snapshot_download
-
-
-is_spaces = True if "SPACE_ID" in os.environ else False
-is_shared_ui = True if "IS_SHARED_UI" in os.environ else False
-is_gpu_associated = torch.cuda.is_available()
-
-css = '''
- .instruction{position: absolute; top: 0;right: 0;margin-top: 0px !important}
- .arrow{position: absolute;top: 0;right: -110px;margin-top: -8px !important}
- #component-4, #component-3, #component-10{min-height: 0}
- .duplicate-button img{margin: 0}
-'''
-maximum_concepts = 3
-
-#Pre download the files
-if(is_gpu_associated):
- model_v1 = snapshot_download(repo_id="multimodalart/sd-fine-tunable")
- model_v2 = snapshot_download(repo_id="stabilityai/stable-diffusion-2")
- model_v2_512 = snapshot_download(repo_id="stabilityai/stable-diffusion-2-base")
- model_alt = snapshot_download(repo_id="BAAI/AltDiffusion")
- model_alt_m9 = snapshot_download(repo_id="BAAI/AltDiffusion-m9")
- safety_checker = snapshot_download(repo_id="multimodalart/sd-sc")
- model_to_load = model_alt_m9
-with zipfile.ZipFile("mix.zip", 'r') as zip_ref:
- zip_ref.extractall(".")
-
-def swap_text(option, base):
- resize_width = 768 if base == "v2-768" else 512
- mandatory_liability = "You must have the right to do so and you are liable for the images you use, example:"
- if(option == "object"):
- instance_prompt_example = "cttoy"
- freeze_for = 30
- return [f"You are going to train `object`(s), upload 5-10 images of each object you are planning on training on from different angles/perspectives. You can use services like birme for smart cropping. {mandatory_liability}:", '''
''', f"You should name your concept with a unique made up word that has low chance of the model already knowing it (e.g.: `{instance_prompt_example}` here). Images will be automatically cropped to {resize_width}x{resize_width}.", freeze_for, gr.update(visible=False)]
- elif(option == "person"):
- instance_prompt_example = "julcto"
- freeze_for = 70
- #show_prior_preservation = True if base != "v2-768" else False
- show_prior_preservation=False
- if(show_prior_preservation):
- prior_preservation_box_update = gr.update(visible=show_prior_preservation)
- else:
- prior_preservation_box_update = gr.update(visible=show_prior_preservation, value=False)
- return [f"You are going to train a `person`(s), upload 10-20 images of each person you are planning on training on from different angles/perspectives. You can use services like birme for smart cropping. {mandatory_liability}:", '''
''', f"You should name your concept with a unique made up word that has low chance of the model already knowing it (e.g.: `{instance_prompt_example}` here). Images will be automatically cropped to {resize_width}x{resize_width}.", freeze_for, prior_preservation_box_update]
- elif(option == "style"):
- instance_prompt_example = "trsldamrl"
- freeze_for = 10
- return [f"You are going to train a `style`, upload 10-20 images of the style you are planning on training on. You can use services like birme for smart cropping. Name the files with the words you would like {mandatory_liability}:", '''
''', f"You should name your concept with a unique made up word that has low chance of the model already knowing it (e.g.: `{instance_prompt_example}` here). Images will be automatically cropped to {resize_width}x{resize_width}", freeze_for, gr.update(visible=False)]
-
-def swap_base_model(selected_model):
- if(is_gpu_associated):
- global model_to_load
- # if(selected_model == "v1-5"):
- # model_to_load = model_v1
- # elif(selected_model == "v2-768"):
- # model_to_load = model_v2
- # elif(selected_model == "alt"):
- # model_to_load = model_alt
- # elif(selected_model == "alt_m9"):
- # model_to_load = model_alt_m9
- # else:
- # model_to_load = model_v2_512
- if(selected_model == "alt"):
- model_to_load = model_alt
-
-def count_files(*inputs):
- file_counter = 0
- concept_counter = 0
- for i, input in enumerate(inputs):
- if(i < maximum_concepts-1):
- files = inputs[i]
- if(files):
- concept_counter+=1
- file_counter+=len(files)
- uses_custom = inputs[-1]
- type_of_thing = inputs[-4]
- selected_model = inputs[-5]
- experimental_faces = inputs[-6]
- if(uses_custom):
- Training_Steps = int(inputs[-3])
- else:
- Training_Steps = file_counter*150
- if(type_of_thing == "person" and Training_Steps > 2400):
- Training_Steps = 2400 #Avoid overfitting on person faces
- if(is_spaces):
- if(selected_model == "v1-5" or selected_model == "alt" or selected_model == "alt_m9"):
- its = 1.1
- if(experimental_faces):
- its = 1
- elif(selected_model == "v2-512"):
- its = 0.8
- if(experimental_faces):
- its = 0.7
- elif(selected_model == "v2-768"):
- its = 0.5
- summary_sentence = f'''You are going to train {concept_counter} {type_of_thing}(s), with {file_counter} images for {Training_Steps} steps. The training should take around {round(Training_Steps/its, 2)} seconds, or {round((Training_Steps/its)/60, 2)} minutes.
- The setup, compression and uploading the model can take up to 20 minutes.
As the T4-Small GPU costs US$0.60 for 1h, the estimated cost for this training is below US${round((((Training_Steps/its)/3600)+0.3+0.1)*0.60, 2)}.
- If you check the box below the GPU attribution will automatically removed after training is done and the model is uploaded. If not, don't forget to come back here and swap the hardware back to CPU.
'''
- else:
- summary_sentence = f'''You are going to train {concept_counter} {type_of_thing}(s), with {file_counter} images for {Training_Steps} steps.
'''
-
- return([gr.update(visible=True), gr.update(visible=True, value=summary_sentence)])
-
-def update_steps(*files_list):
- file_counter = 0
- for i, files in enumerate(files_list):
- if(files):
- file_counter+=len(files)
- return(gr.update(value=file_counter*200))
-
-def pad_image(image):
- w, h = image.size
- if w == h:
- return image
- elif w > h:
- new_image = Image.new(image.mode, (w, w), (0, 0, 0))
- new_image.paste(image, (0, (w - h) // 2))
- return new_image
- else:
- new_image = Image.new(image.mode, (h, h), (0, 0, 0))
- new_image.paste(image, ((h - w) // 2, 0))
- return new_image
-
-def train(*inputs):
- if is_shared_ui:
- raise gr.Error("This Space only works in duplicated instances")
- if not is_gpu_associated:
- raise gr.Error("Please associate a T4 GPU for this Space")
- torch.cuda.empty_cache()
- if 'pipe' in globals():
- global pipe, pipe_is_set
- del pipe
- pipe_is_set = False
- gc.collect()
-
- if os.path.exists("output_model"): shutil.rmtree('output_model')
- if os.path.exists("instance_images"): shutil.rmtree('instance_images')
- if os.path.exists("diffusers_model.tar"): os.remove("diffusers_model.tar")
- if os.path.exists("model.ckpt"): os.remove("model.ckpt")
- if os.path.exists("hastrained.success"): os.remove("hastrained.success")
- file_counter = 0
- which_model = inputs[-10]
- resolution = 512 if which_model != "v2-768" else 768
- for i, input in enumerate(inputs):
- if(i < maximum_concepts-1):
- if(input):
- os.makedirs('instance_images',exist_ok=True)
- files = inputs[i+(maximum_concepts*2)]
- prompt = inputs[i+maximum_concepts]
- if(prompt == "" or prompt == None):
- raise gr.Error("You forgot to define your concept prompt")
- for j, file_temp in enumerate(files):
- file = Image.open(file_temp.name)
- image = pad_image(file)
- image = image.resize((resolution, resolution))
- extension = file_temp.name.split(".")[1]
- image = image.convert('RGB')
- image.save(f'instance_images/{prompt}_({j+1}).jpg', format="JPEG", quality = 100)
- file_counter += 1
-
- os.makedirs('output_model',exist_ok=True)
- uses_custom = inputs[-1]
- type_of_thing = inputs[-4]
- remove_attribution_after = inputs[-6]
- experimental_face_improvement = inputs[-9]
-
- if(uses_custom):
- Training_Steps = int(inputs[-3])
- Train_text_encoder_for = int(inputs[-2])
- else:
- if(type_of_thing == "object"):
- Train_text_encoder_for=30
-
- elif(type_of_thing == "style"):
- Train_text_encoder_for=15
-
- elif(type_of_thing == "person"):
- Train_text_encoder_for=70
-
- Training_Steps = file_counter*150
- if(type_of_thing == "person" and Training_Steps > 2600):
- Training_Steps = 2600 #Avoid overfitting on people's faces
- stptxt = int((Training_Steps*Train_text_encoder_for)/100)
- gradient_checkpointing = True if (experimental_face_improvement or which_model != "v1-5") else False
- cache_latents = True if which_model != "v1-5" else False
- if (type_of_thing == "object" or type_of_thing == "style" or (type_of_thing == "person" and not experimental_face_improvement)):
- args_general = argparse.Namespace(
- image_captions_filename = True,
- train_text_encoder = True if stptxt > 0 else False,
- stop_text_encoder_training = stptxt,
- save_n_steps = 0,
- pretrained_model_name_or_path = model_to_load,
- instance_data_dir="instance_images",
- class_data_dir=None,
- output_dir="output_model",
- instance_prompt="",
- seed=42,
- resolution=resolution,
- mixed_precision="fp16",
- train_batch_size=1,
- gradient_accumulation_steps=1,
- use_8bit_adam=True,
- learning_rate=2e-6,
- lr_scheduler="polynomial",
- lr_warmup_steps = 0,
- max_train_steps=Training_Steps,
- gradient_checkpointing=gradient_checkpointing,
- cache_latents=cache_latents,
- )
- print("Starting single training...")
- lock_file = open("intraining.lock", "w")
- lock_file.close()
- run_training(args_general)
- else:
- args_general = argparse.Namespace(
- image_captions_filename = True,
- train_text_encoder = True if stptxt > 0 else False,
- stop_text_encoder_training = stptxt,
- save_n_steps = 0,
- pretrained_model_name_or_path = model_to_load,
- instance_data_dir="instance_images",
- class_data_dir="Mix",
- output_dir="output_model",
- with_prior_preservation=True,
- prior_loss_weight=1.0,
- instance_prompt="",
- seed=42,
- resolution=resolution,
- mixed_precision="fp16",
- train_batch_size=1,
- gradient_accumulation_steps=1,
- use_8bit_adam=True,
- learning_rate=2e-6,
- lr_scheduler="polynomial",
- lr_warmup_steps = 0,
- max_train_steps=Training_Steps,
- num_class_images=200,
- gradient_checkpointing=gradient_checkpointing,
- cache_latents=cache_latents,
- )
- print("Starting multi-training...")
- lock_file = open("intraining.lock", "w")
- lock_file.close()
- run_training(args_general)
- gc.collect()
- torch.cuda.empty_cache()
- if(which_model == "v1-5"):
- print("Adding Safety Checker to the model...")
- shutil.copytree(f"{safety_checker}/feature_extractor", "output_model/feature_extractor")
- shutil.copytree(f"{safety_checker}/safety_checker", "output_model/safety_checker")
- shutil.copy(f"model_index.json", "output_model/model_index.json")
-
- if(not remove_attribution_after):
- print("Archiving model file...")
- with tarfile.open("diffusers_model.tar", "w") as tar:
- tar.add("output_model", arcname=os.path.basename("output_model"))
- if os.path.exists("intraining.lock"): os.remove("intraining.lock")
- trained_file = open("hastrained.success", "w")
- trained_file.close()
- print("Training completed!")
- return [
- gr.update(visible=True, value=["diffusers_model.tar"]), #result
- gr.update(visible=True), #try_your_model
- gr.update(visible=True), #push_to_hub
- gr.update(visible=True), #convert_button
- gr.update(visible=False), #training_ongoing
- gr.update(visible=True) #completed_training
- ]
- else:
- hf_token = inputs[-5]
- model_name = inputs[-7]
- where_to_upload = inputs[-8]
- push(model_name, where_to_upload, hf_token, which_model, True)
- hardware_url = f"https://huggingface.co/spaces/{os.environ['SPACE_ID']}/hardware"
- headers = { "authorization" : f"Bearer {hf_token}"}
- body = {'flavor': 'cpu-basic'}
- requests.post(hardware_url, json = body, headers=headers)
-
-pipe_is_set = False
-def generate(prompt, steps):
- torch.cuda.empty_cache()
- # from diffusers import StableDiffusionPipeline
- from diffusers import DiffusionPipeline
- global pipe_is_set
- if(not pipe_is_set):
- global pipe
- # pipe = StableDiffusionPipeline.from_pretrained("./output_model", torch_dtype=torch.float16)
- pipe = DiffusionPipeline.from_pretrained("./output_model", torch_dtype=torch.float16)
- pipe = pipe.to("cuda")
- pipe_is_set = True
-
- image = pipe(prompt, num_inference_steps=steps).images[0]
- return(image)
-
-def push(model_name, where_to_upload, hf_token, which_model, comes_from_automated=False):
- if(not os.path.exists("model.ckpt")):
- convert("output_model", "model.ckpt")
- from huggingface_hub import HfApi, HfFolder, CommitOperationAdd
- from huggingface_hub import create_repo
- model_name_slug = slugify(model_name)
- api = HfApi()
- your_username = api.whoami(token=hf_token)["name"]
- if(where_to_upload == "My personal profile"):
- model_id = f"{your_username}/{model_name_slug}"
- else:
- model_id = f"sd-dreambooth-library/{model_name_slug}"
- headers = {"Authorization" : f"Bearer: {hf_token}", "Content-Type": "application/json"}
- response = requests.post("https://huggingface.co/organizations/sd-dreambooth-library/share/SSeOwppVCscfTEzFGQaqpfcjukVeNrKNHX", headers=headers)
-
- images_upload = os.listdir("instance_images")
- image_string = ""
- instance_prompt_list = []
- previous_instance_prompt = ''
- for i, image in enumerate(images_upload):
- instance_prompt = image.split("_")[0]
- if(instance_prompt != previous_instance_prompt):
- title_instance_prompt_string = instance_prompt
- instance_prompt_list.append(instance_prompt)
- else:
- title_instance_prompt_string = ''
- previous_instance_prompt = instance_prompt
- image_string = f'''{title_instance_prompt_string} {"(use that on your prompt)" if title_instance_prompt_string != "" else ""}
-{image_string}})'''
- readme_text = f'''---
-license: creativeml-openrail-m
-tags:
-- text-to-image
-widget:
-- text: {instance_prompt_list[0]}
----
-### {model_name} Dreambooth model trained by {api.whoami(token=hf_token)["name"]} with [Hugging Face Dreambooth Training Space](https://huggingface.co/spaces/multimodalart/dreambooth-training) with the {which_model} base model
-
-You run your new concept via `diffusers` [Colab Notebook for Inference](https://colab.research.google.com/github/huggingface/notebooks/blob/main/diffusers/sd_dreambooth_inference.ipynb). Don't forget to use the concept prompts!
-
-Sample pictures of:
-{image_string}
-'''
- #Save the readme to a file
- readme_file = open("model.README.md", "w")
- readme_file.write(readme_text)
- readme_file.close()
- #Save the token identifier to a file
- text_file = open("token_identifier.txt", "w")
- text_file.write(', '.join(instance_prompt_list))
- text_file.close()
- try:
- create_repo(model_id,private=True, token=hf_token)
- except:
- import time
- epoch_time = str(int(time.time()))
- create_repo(f"{model_id}-{epoch_time}", private=True,token=hf_token)
- operations = [
- CommitOperationAdd(path_in_repo="token_identifier.txt", path_or_fileobj="token_identifier.txt"),
- CommitOperationAdd(path_in_repo="README.md", path_or_fileobj="model.README.md"),
- CommitOperationAdd(path_in_repo=f"model.ckpt",path_or_fileobj="model.ckpt")
- ]
- api.create_commit(
- repo_id=model_id,
- operations=operations,
- commit_message=f"Upload the model {model_name}",
- token=hf_token
- )
- api.upload_folder(
- folder_path="output_model",
- repo_id=model_id,
- token=hf_token
- )
- api.upload_folder(
- folder_path="instance_images",
- path_in_repo="concept_images",
- repo_id=model_id,
- token=hf_token
- )
- if is_spaces:
- if(not comes_from_automated):
- extra_message = "Don't forget to remove the GPU attribution after you play with it."
- else:
- extra_message = "The GPU has been removed automatically as requested, and you can try the model via the model page"
- api.create_discussion(repo_id=os.environ['SPACE_ID'], title=f"Your model {model_name} has finished trained from the Dreambooth Train Spaces!", description=f"Your model has been successfully uploaded to: https://huggingface.co/{model_id}. {extra_message}",repo_type="space", token=hf_token)
-
- return [gr.update(visible=True, value=f"Successfully uploaded your model. Access it [here](https://huggingface.co/{model_id})"), gr.update(visible=True, value=["diffusers_model.tar", "model.ckpt"])]
-
-def convert_to_ckpt():
- if 'pipe' in globals():
- global pipe, pipe_is_set
- del pipe
- pipe_is_set = False
- gc.collect()
- convert("output_model", "model.ckpt")
- return gr.update(visible=True, value=["diffusers_model.tar", "model.ckpt"])
-
-def check_status(top_description):
- if os.path.exists("hastrained.success"):
- if is_spaces:
- update_top_tag = gr.update(value=f'''
-
-
Your model has finished training ✅
-
Yay, congratulations on training your model. Scroll down to play with with it, save it (either downloading it or on the Hugging Face Hub). Once you are done, your model is safe, and you don't want to train a new one, go to the settings page and downgrade your Space to a CPU Basic
-
- ''')
- else:
- update_top_tag = gr.update(value=f'''
-
-
Your model has finished training ✅
-
Yay, congratulations on training your model. Scroll down to play with with it, save it (either downloading it or on the Hugging Face Hub).
-
- ''')
- show_outputs = True
- elif os.path.exists("intraining.lock"):
- update_top_tag = gr.update(value='''
-
-
Don't worry, your model is still training! ⌛
-
You closed the tab while your model was training, but it's all good! It is still training right now. You can click the "Open logs" button above here to check the training status. Once training is done, reload this tab to interact with your model
-
- ''')
- show_outputs = False
- else:
- update_top_tag = gr.update(value=top_description)
- show_outputs = False
- if os.path.exists("diffusers_model.tar"):
- update_files_tag = gr.update(visible=show_outputs, value=["diffusers_model.tar"])
- else:
- update_files_tag = gr.update(visible=show_outputs)
- return [
- update_top_tag, #top_description
- gr.update(visible=show_outputs), #try_your_model
- gr.update(visible=show_outputs), #push_to_hub
- update_files_tag, #result
- gr.update(visible=show_outputs), #convert_button
- ]
-
-def checkbox_swap(checkbox):
- return [gr.update(visible=checkbox), gr.update(visible=checkbox), gr.update(visible=checkbox), gr.update(visible=checkbox)]
-
-with gr.Blocks(css=css) as demo:
- with gr.Box():
- gr.HTML(f'''
-
-
-
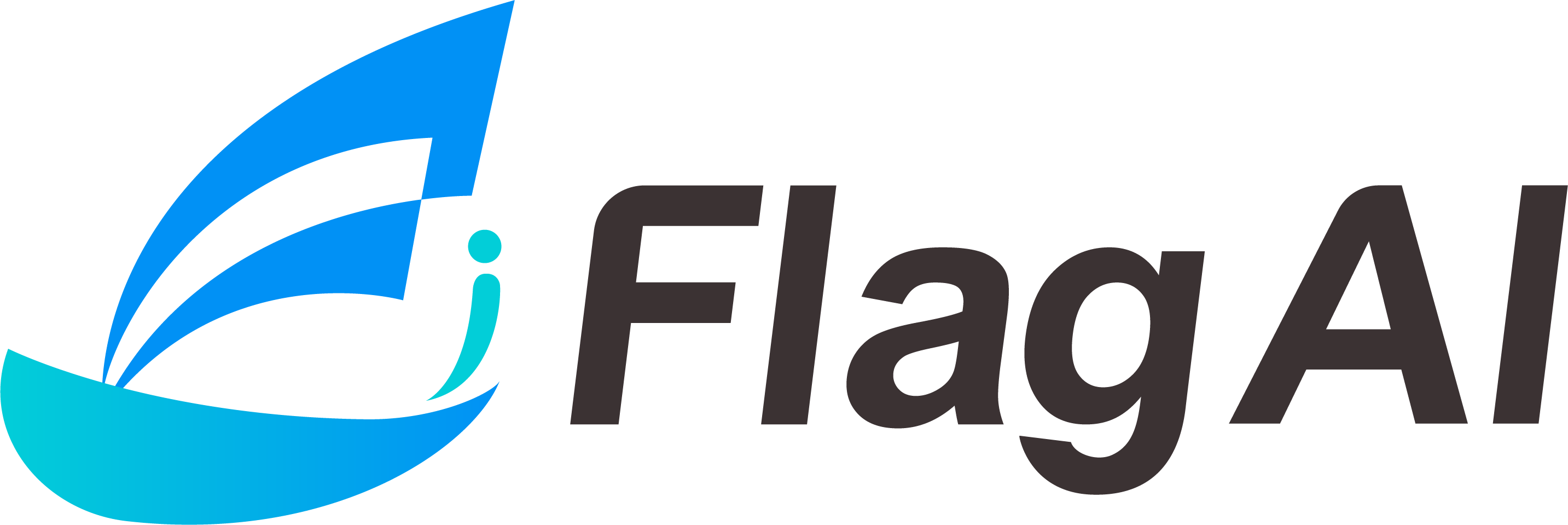
-
-
-
- Dreambooth Web UI
-
-
-

-
- This is a dreambooth Training UI for AltDiffusion-m9 model,which is a multilingual image-to-text model supported 9 languages.
- You can duplicate this space to your own!
-
-
- ''')
- with gr.Box():
- if is_shared_ui:
- top_description = gr.HTML(f'''
-
-
Attention - This Space doesn't work in this shared UI
-
For it to work, you can either run locally or duplicate the Space and run it on your own profile using a (paid) private T4 GPU for training. As each T4 costs US$0.60/h, it should cost < US$1 to train most models using default settings! 
-

-

-
- ''')
- elif(is_spaces):
- if(is_gpu_associated):
- top_description = gr.HTML(f'''
-
-
You have successfully associated a GPU to the Dreambooth Training Space 🎉
-
Certify that you got a T4. You can now train your model! You will be billed by the minute from when you activated the GPU until when it is turned it off.
-
- ''')
- else:
- top_description = gr.HTML(f'''
-
-
You have successfully duplicated the Dreambooth Training Space 🎉
-
There's only one step left before you can train your model: attribute a T4 GPU to it (via the Settings tab) and run the training below. Other GPUs are not compatible for now. You will be billed by the minute from when you activate the GPU until when it is turned it off.
-
- ''')
- else:
- top_description = gr.HTML(f'''
-
-
You have successfully cloned the Dreambooth Training Space locally 🎉
-
Do a pip install requirements-local.txt
-
- ''')
-
- # gr.Markdown("# Dreambooth Training UI 💭")
- gr.Markdown("Customize AltDiffusion and AltDiffusion-m9(ⁿᵉʷ!) by giving it a few examples of a concept. Based on the [🧨 diffusers](https://github.com/huggingface/diffusers) implementation, additional techniques from [TheLastBen](https://github.com/TheLastBen/diffusers) and [ShivamShrirao](https://github.com/ShivamShrirao/diffusers)")
-
- with gr.Row() as what_are_you_training:
- type_of_thing = gr.Dropdown(label="What would you like to train?", choices=["object", "person", "style"], value="object", interactive=True)
- base_model_to_use = gr.Dropdown(label="Which base model would you like to use?", choices=["alt", "alt_m9"], value="alt_m9", interactive=True)
-
- #Very hacky approach to emulate dynamically created Gradio components
- with gr.Column() as upload_your_concept:
- with gr.Box():
- thing_description = gr.Markdown("You are going to train an `object`, please upload 5-10 images of the object you are planning on training on from different angles/perspectives. You must have the right to do so and you are liable for the images you use, example")
- thing_experimental = gr.Checkbox(label="Improve faces (prior preservation) - can take longer training but can improve faces", visible=False, value=False)
- thing_image_example = gr.HTML('''
''')
- things_naming = gr.Markdown("You should name your concept with a unique made up word that has low chance of the model already knowing it (e.g.: `UzNrto` here). Images will be automatically cropped to 512x512.")
-
- # with gr.Column():
- file_collection = []
- concept_collection = []
- buttons_collection = []
- delete_collection = []
- is_visible = []
-
- row = [None] * maximum_concepts
- for x in range(maximum_concepts):
- ordinal = lambda n: "%d%s" % (n, "tsnrhtdd"[(n // 10 % 10 != 1) * (n % 10 < 4) * n % 10::4])
- if(x == 0):
- visible = True
- is_visible.append(gr.State(value=True))
- else:
- visible = False
- is_visible.append(gr.State(value=False))
-
- file_collection.append(gr.File(label=f'''Upload the images for your {ordinal(x+1) if (x>0) else ""} concept''', file_count="multiple", interactive=True, visible=visible))
- with gr.Column(visible=visible) as row[x]:
- concept_collection.append(gr.Textbox(label=f'''{ordinal(x+1) if (x>0) else ""} concept prompt - use a unique, made up word to avoid collisions'''))
- with gr.Row():
- if(x < maximum_concepts-1):
- buttons_collection.append(gr.Button(value="Add +1 concept", visible=visible))
- if(x > 0):
- delete_collection.append(gr.Button(value=f"Delete {ordinal(x+1)} concept"))
-
- counter_add = 1
- for button in buttons_collection:
- if(counter_add < len(buttons_collection)):
- button.click(lambda:
- [gr.update(visible=True),gr.update(visible=True), gr.update(visible=False), gr.update(visible=True), True, None],
- None,
- [row[counter_add], file_collection[counter_add], buttons_collection[counter_add-1], buttons_collection[counter_add], is_visible[counter_add], file_collection[counter_add]], queue=False)
- else:
- button.click(lambda:[gr.update(visible=True),gr.update(visible=True), gr.update(visible=False), True], None, [row[counter_add], file_collection[counter_add], buttons_collection[counter_add-1], is_visible[counter_add]], queue=False)
- counter_add += 1
-
- counter_delete = 1
- for delete_button in delete_collection:
- if(counter_delete < len(delete_collection)+1):
- delete_button.click(lambda:[gr.update(visible=False),gr.update(visible=False), gr.update(visible=True), False], None, [file_collection[counter_delete], row[counter_delete], buttons_collection[counter_delete-1], is_visible[counter_delete]], queue=False)
- counter_delete += 1
-
- with gr.Accordion("Custom Settings", open=False):
- swap_auto_calculated = gr.Checkbox(label="Use custom settings")
- gr.Markdown("If not checked, the % of frozen encoder will be tuned automatically to whether you are training an `object`, `person` or `style`. The text-encoder is frozen after 10% of the steps for a style, 30% of the steps for an object and 75% trained for persons. The number of steps varies between 1400 and 2400 depending on how many images uploaded. If you see too many artifacts in your output, it means it may have overfit and you need less steps. If your results aren't really what you wanted, it may be underfitting and you need more steps.")
- steps = gr.Number(label="How many steps", value=2400)
- perc_txt_encoder = gr.Number(label="Percentage of the training steps the text-encoder should be trained as well", value=30)
-
- with gr.Box(visible=False) as training_summary:
- training_summary_text = gr.HTML("", visible=True, label="Training Summary")
- is_advanced_visible = True if is_spaces else False
- training_summary_checkbox = gr.Checkbox(label="Automatically remove paid GPU attribution and upload model to the Hugging Face Hub after training", value=True, visible=is_advanced_visible)
- training_summary_model_name = gr.Textbox(label="Name of your model", visible=True)
- training_summary_where_to_upload = gr.Dropdown(["My personal profile", "Public Library"], value="My personal profile", label="Upload to", visible=True)
- training_summary_token_message = gr.Markdown("[A Hugging Face write access token](https://huggingface.co/settings/tokens), go to \"New token\" -> Role : Write. A regular read token won't work here.", visible=True)
- training_summary_token = gr.Textbox(label="Hugging Face Write Token", type="password", visible=True)
-
- train_btn = gr.Button("Start Training")
- if(is_shared_ui):
- training_ongoing = gr.Markdown("## This Space only works in duplicated instances. Please duplicate it and try again!", visible=False)
- elif(not is_gpu_associated):
- training_ongoing = gr.Markdown("## Oops, you haven't associated your T4 GPU to this Space. Visit the Settings tab, associate and try again.", visible=False)
- else:
- training_ongoing = gr.Markdown("## Training is ongoing ⌛... You can close this tab if you like or just wait. If you did not check the `Remove GPU After training`, you can come back here to try your model and upload it after training. Don't forget to remove the GPU attribution after you are done. ", visible=False)
-
- #Post-training UI
- completed_training = gr.Markdown('''# ✅ Training completed.
- ### Don't forget to remove the GPU attribution after you are done trying and uploading your model''', visible=False)
-
- with gr.Row():
- with gr.Box(visible=False) as try_your_model:
- gr.Markdown("## Try your model")
- prompt = gr.Textbox(label="Type your prompt")
- result_image = gr.Image()
- inference_steps = gr.Slider(minimum=1, maximum=150, value=50, step=1)
- generate_button = gr.Button("Generate Image")
-
- with gr.Box(visible=False) as push_to_hub:
- gr.Markdown("## Push to Hugging Face Hub")
- model_name = gr.Textbox(label="Name of your model", placeholder="Tarsila do Amaral Style")
- where_to_upload = gr.Dropdown(["My personal profile", "Public Library"], label="Upload to")
- gr.Markdown("[A Hugging Face write access token](https://huggingface.co/settings/tokens), go to \"New token\" -> Role : Write. A regular read token won't work here.")
- hf_token = gr.Textbox(label="Hugging Face Write Token", type="password")
-
- push_button = gr.Button("Push to the Hub")
-
- result = gr.File(label="Download the uploaded models in the diffusers format", visible=True)
- success_message_upload = gr.Markdown(visible=False)
- convert_button = gr.Button("Convert to CKPT", visible=False)
-
- #Swap the examples and the % of text encoder trained depending if it is an object, person or style
- type_of_thing.change(fn=swap_text, inputs=[type_of_thing, base_model_to_use], outputs=[thing_description, thing_image_example, things_naming, perc_txt_encoder, thing_experimental], queue=False, show_progress=False)
-
- #Swap the base model
- base_model_to_use.change(fn=swap_text, inputs=[type_of_thing, base_model_to_use], outputs=[thing_description, thing_image_example, things_naming, perc_txt_encoder, thing_experimental], queue=False, show_progress=False)
- base_model_to_use.change(fn=swap_base_model, inputs=base_model_to_use, outputs=[])
-
- #Update the summary box below the UI according to how many images are uploaded and whether users are using custom settings or not
- for file in file_collection:
- #file.change(fn=update_steps,inputs=file_collection, outputs=steps)
- file.change(fn=count_files, inputs=file_collection+[thing_experimental]+[base_model_to_use]+[type_of_thing]+[steps]+[perc_txt_encoder]+[swap_auto_calculated], outputs=[training_summary, training_summary_text], queue=False)
-
- thing_experimental.change(fn=count_files, inputs=file_collection+[thing_experimental]+[base_model_to_use]+[type_of_thing]+[steps]+[perc_txt_encoder]+[swap_auto_calculated], outputs=[training_summary, training_summary_text], queue=False)
- base_model_to_use.change(fn=count_files, inputs=file_collection+[thing_experimental]+[base_model_to_use]+[type_of_thing]+[steps]+[perc_txt_encoder]+[swap_auto_calculated], outputs=[training_summary, training_summary_text], queue=False)
- steps.change(fn=count_files, inputs=file_collection+[thing_experimental]+[base_model_to_use]+[type_of_thing]+[steps]+[perc_txt_encoder]+[swap_auto_calculated], outputs=[training_summary, training_summary_text], queue=False)
- perc_txt_encoder.change(fn=count_files, inputs=file_collection+[thing_experimental]+[base_model_to_use]+[type_of_thing]+[steps]+[perc_txt_encoder]+[swap_auto_calculated], outputs=[training_summary, training_summary_text], queue=False)
-
- #Give more options if the user wants to finish everything after training
- if(is_spaces):
- training_summary_checkbox.change(fn=checkbox_swap, inputs=training_summary_checkbox, outputs=[training_summary_token_message, training_summary_token, training_summary_model_name, training_summary_where_to_upload],queue=False, show_progress=False)
- #Add a message for while it is in training
- train_btn.click(lambda:gr.update(visible=True), inputs=None, outputs=training_ongoing)
-
- #The main train function
- train_btn.click(fn=train, inputs=is_visible+concept_collection+file_collection+[base_model_to_use]+[thing_experimental]+[training_summary_where_to_upload]+[training_summary_model_name]+[training_summary_checkbox]+[training_summary_token]+[type_of_thing]+[steps]+[perc_txt_encoder]+[swap_auto_calculated], outputs=[result, try_your_model, push_to_hub, convert_button, training_ongoing, completed_training], queue=False)
-
- #Button to generate an image from your trained model after training
- generate_button.click(fn=generate, inputs=[prompt, inference_steps], outputs=result_image, queue=False)
- #Button to push the model to the Hugging Face Hub
- push_button.click(fn=push, inputs=[model_name, where_to_upload, hf_token, base_model_to_use], outputs=[success_message_upload, result], queue=False)
- #Button to convert the model to ckpt format
- convert_button.click(fn=convert_to_ckpt, inputs=[], outputs=result, queue=False)
-
- #Checks if the training is running
- demo.load(fn=check_status, inputs=top_description, outputs=[top_description, try_your_model, push_to_hub, result, convert_button], queue=False, show_progress=False)
-
-demo.queue(default_enabled=False).launch(debug=True)
\ No newline at end of file
diff --git a/spaces/Bart92/RVC_HF/infer/lib/uvr5_pack/lib_v5/model_param_init.py b/spaces/Bart92/RVC_HF/infer/lib/uvr5_pack/lib_v5/model_param_init.py
deleted file mode 100644
index b995c0bfb1194746187692e2ab1c2a6dbaaaec6c..0000000000000000000000000000000000000000
--- a/spaces/Bart92/RVC_HF/infer/lib/uvr5_pack/lib_v5/model_param_init.py
+++ /dev/null
@@ -1,69 +0,0 @@
-import json
-import os
-import pathlib
-
-default_param = {}
-default_param["bins"] = 768
-default_param["unstable_bins"] = 9 # training only
-default_param["reduction_bins"] = 762 # training only
-default_param["sr"] = 44100
-default_param["pre_filter_start"] = 757
-default_param["pre_filter_stop"] = 768
-default_param["band"] = {}
-
-
-default_param["band"][1] = {
- "sr": 11025,
- "hl": 128,
- "n_fft": 960,
- "crop_start": 0,
- "crop_stop": 245,
- "lpf_start": 61, # inference only
- "res_type": "polyphase",
-}
-
-default_param["band"][2] = {
- "sr": 44100,
- "hl": 512,
- "n_fft": 1536,
- "crop_start": 24,
- "crop_stop": 547,
- "hpf_start": 81, # inference only
- "res_type": "sinc_best",
-}
-
-
-def int_keys(d):
- r = {}
- for k, v in d:
- if k.isdigit():
- k = int(k)
- r[k] = v
- return r
-
-
-class ModelParameters(object):
- def __init__(self, config_path=""):
- if ".pth" == pathlib.Path(config_path).suffix:
- import zipfile
-
- with zipfile.ZipFile(config_path, "r") as zip:
- self.param = json.loads(
- zip.read("param.json"), object_pairs_hook=int_keys
- )
- elif ".json" == pathlib.Path(config_path).suffix:
- with open(config_path, "r") as f:
- self.param = json.loads(f.read(), object_pairs_hook=int_keys)
- else:
- self.param = default_param
-
- for k in [
- "mid_side",
- "mid_side_b",
- "mid_side_b2",
- "stereo_w",
- "stereo_n",
- "reverse",
- ]:
- if not k in self.param:
- self.param[k] = False
diff --git a/spaces/Benson/text-generation/Examples/Baku Burger House.md b/spaces/Benson/text-generation/Examples/Baku Burger House.md
deleted file mode 100644
index 44cb34aaba2d7a77f60af7fea0ede84e580def18..0000000000000000000000000000000000000000
--- a/spaces/Benson/text-generation/Examples/Baku Burger House.md
+++ /dev/null
@@ -1,79 +0,0 @@
-
-Burger House Bakú: La mejor guía para las mejores hamburguesas de la ciudad
-Si usted está buscando un lugar para disfrutar de una deliciosa hamburguesa en Bakú, usted debe definitivamente echa un vistazo a Burger House Bakú. Este restaurante ofrece una variedad de hamburguesas, acompañamientos y bebidas que satisfarán sus antojos y lo harán feliz. En este artículo, te contaremos todo lo que necesitas saber sobre Burger House Bakú, incluyendo qué es, por qué debes visitarlo, qué sirve, dónde se encuentra, cuándo está abierto y qué piensan otros clientes de él. ¡Sigue leyendo para saber más!
-Introducción
-¿Qué es Burger House Bakú?
-Burger House Bakú es un restaurante especializado en hamburguesas. Fue fundada en 2018 por un grupo de amigos que querían compartir su pasión por las hamburguesas con la gente de Bakú. Utilizan ingredientes frescos, salsas caseras y carne de calidad para crear sus hamburguesas, que se cocinan por encargo y se sirven con una sonrisa. Burger House Bakú tiene como objetivo proporcionar un ambiente agradable y acogedor donde los clientes pueden relajarse y disfrutar de su comida.
-baku burger house
Download ►►►►► https://bltlly.com/2v6Mzw
-¿Por qué visitar Burger House Bakú?
-Hay muchas razones por las que deberías visitar Burger House Bakú. Aquí están algunas de ellas:
-
-- Podrás degustar algunas de las mejores hamburguesas de la ciudad, elaboradas con ingredientes frescos y de calidad.
-- Usted tendrá una amplia gama de opciones para elegir, incluyendo hamburguesas clásicas, hamburguesas con queso, hamburguesas de tocino, hamburguesas vegetarianas y más.
-- También podrás disfrutar de algunos deliciosos platos, como papas fritas, aros de cebolla, ensalada y más.
-- Podrás saciar tu sed con algunas bebidas refrescantes, como refrescos, cerveza, batidos y más.
-- Experimentará un ambiente agradable y acogedor, con personal atento y música agradable.
-- Obtendrá una buena relación calidad-precio, ya que los precios son razonables y las porciones generosas.
-
-El menú
-Las hamburguesas
-
-Hamburguesa clásica
-Esta es la hamburguesa más simple y básica del menú. Consiste en una hamburguesa de ternera, lechuga, tomate, cebolla, pepinillos, ketchup, mostaza y mayonesa en un pan de sésamo. Es perfecto para aquellos que quieren una hamburguesa clásica y sencilla que sea satisfactoria y sabrosa.
-Hamburguesa de queso
-Esta es una hamburguesa clásica con un toque extra de queso. Consiste en una hamburguesa de ternera, queso, lechuga, tomate, cebolla, encurtidos, ketchup, mostaza y mayonesa en un bollo de sésamo. Es perfecto para aquellos que aman el queso y quieren una hamburguesa más sabrosa.
-Hamburguesa de tocino
-Esta es una hamburguesa clásica con un toque extra de tocino. Consiste en una hamburguesa de ternera, tocino, queso, lechuga, tomate, cebolla, encurtidos, ketchup, mostaza y mayonesa en un bollo de sésamo. Es perfecto para aquellos que aman el tocino y quieren una hamburguesa más crujiente y ahumado.
-Hamburguesa vegetariana
-Esta es una hamburguesa para aquellos que prefieren una opción vegetariana. Consiste en una hamburguesa vegetariana, lechuga, tomate, cebolla, encurtidos, ketchup, mostaza y mayonesa en un bollo de sésamo. Es perfecto para aquellos que quieren una hamburguesa sana y libre de carne que sigue siendo deliciosa y satisfactoria.
-Los lados
-Ninguna hamburguesa está completa sin algunos lados para ir junto con ella. Burger House Bakú ofrece algunos lados sabrosos y crujientes que complementan sus hamburguesas. Estos son algunos de sus lados más populares:
-
-Fries
-Estos son los platos clásicos y más populares para hamburguesas. Están hechos de papas frescas que se cortan en tiras finas y se fríen hasta que estén doradas y crujientes. Se sazona con sal y se sirve con ketchup o mayonesa. Son perfectos para aquellos que quieren un lado simple y crujiente que vaya bien con cualquier hamburguesa.
-Anillos de cebolla
-
-Ensalada
-Este es un acompañamiento para aquellos que quieren una opción más ligera y saludable. Está hecho de lechuga fresca, tomate, pepino, zanahoria y cebolla que se mezclan con aderezo. Se sirve con crutones o queso en la parte superior. Es perfecto para aquellos que quieren un lado refrescante y nutritivo que equilibre su hamburguesa.
-Las bebidas
-Para lavar su hamburguesa y los lados, necesitará algunas bebidas para saciar su sed. Burger House Bakú ofrece algunas bebidas refrescantes y deliciosas que se adaptan a diferentes gustos y preferencias. Aquí están algunas de sus bebidas más populares:
-Soda
-Esta es la bebida clásica y más popular para las hamburguesas. Es una bebida carbonatada que viene en diferentes sabores, como cola, limón, naranja y más. Se sirve fría con cubitos de hielo. Es perfecto para aquellos que quieren una bebida dulce y gaseosa que vaya bien con cualquier hamburguesa.
-Cerveza
-Esta es otra bebida popular para hamburguesas. Es una bebida alcohólica que viene en diferentes tipos, como lager, ale, stout y más. Se sirve frío con o sin espuma. Es perfecto para aquellos que quieren una bebida amarga y refrescante que realza el sabor de su hamburguesa.
-Batido de leche
-Esta es una bebida para aquellos que quieren un tratamiento cremoso e indulgente. Es una bebida mezclada que viene en diferentes sabores, como chocolate, vainilla, fresa y más. Se sirve frío con crema batida y una cereza en la parte superior. Es perfecto para aquellos que quieren una bebida rica y suave que satisfaga su gusto por los dulces.
-La ubicación y las horas
-¿Dónde está Burger House Bakú?
-Burger House Bakú se encuentra en el corazón de la ciudad, cerca de la Plaza de la Fuente. La dirección es 28 Nizami Street, Bakú 1000. Se puede llegar fácilmente en transporte público o en coche. Hay un amplio aparcamiento cerca.
-¿Cuándo está abierto Burger House Bakú?
-
-Los comentarios y valoraciones
-¿Qué dicen los clientes sobre Burger House Baku?
-Burger House Bakú ha recibido muchas críticas positivas y valoraciones de clientes que han probado su comida. Estos son algunos de los comentarios que han dejado en varias plataformas:
-
-- "¡Las mejores hamburguesas de la ciudad! ¡Frescas, jugosas, sabrosas y grandes! ¡Las papas fritas también son increíbles! ¡Muy recomendable!" - Ali en Google Reviews
-- "Me encanta este lugar! Las hamburguesas son tan buenas y el personal es tan amable! El ambiente es acogedor y relajante! Siempre vengo aquí con mis amigos!" - Leyla en Facebook
-- "Burger House Bakú es mi lugar favorito de hamburguesas en Bakú! Las hamburguesas se cocinan a la perfección y los lados son deliciosos! Los precios son razonables y las porciones son generosas! No puedo tener suficiente de ella!" - Samir en TripAdvisor
-
-¿Cómo se compara Burger House Bakú con otros lugares de hamburguesas en Bakú?
Burger House Bakú es uno de los mejores lugares de hamburguesas en Bakú, según muchos clientes y críticos. Tiene una alta calificación de 4.8 de 5 estrellas en Google Reviews, 4.9 de 5 estrellas en Facebook y 4.5 de 5 estrellas en TripAdvisor . También tiene un Certificado de Excelencia de TripAdvisor, lo que significa que recibe constantemente excelentes críticas de los viajeros. Burger House Bakú se destaca de otros lugares de hamburguesas en Bakú debido a su calidad, variedad, servicio y valor. Ofrece hamburguesas frescas y sabrosas que se adaptan a diferentes gustos y preferencias, así como deliciosas guarniciones y bebidas que las complementan. También ofrece un ambiente agradable y acogedor, con personal atento y música agradable. También ofrece precios razonables y porciones generosas que hacen que los clientes se sientan satisfechos y felices.
-Conclusión
-Resumen de los puntos principales
-
-Llamada a la acción
-Si usted está buscando un lugar para disfrutar de una deliciosa hamburguesa en Bakú, usted debe definitivamente echa un vistazo a Burger House Bakú. Usted no se arrepentirá! Puede visitar su sitio web para ver su menú, ordenar en línea o hacer una reserva. También puede seguirlos en las redes sociales para obtener las últimas actualizaciones y promociones. ¡No pierdas esta oportunidad de probar algunas de las mejores hamburguesas de la ciudad! ¡Visita Burger House Bakú hoy!
-Preguntas frecuentes
-
-- Q: ¿Cómo puedo contactar con Burger House Baku?
-- A: Puede ponerse en contacto con Burger House Bakú por teléfono al +994 12 555 55 55 o por correo electrónico a info@burgerhousebaku.com.
-- Q: ¿Burger House Bakú ofrece entrega o comida para llevar?
-- A: Sí, Burger House Bakú ofrece opciones de entrega y comida para llevar. Usted puede ordenar en línea a través de su sitio web o por teléfono, y tener su comida entregada a su casa u oficina. También puede recoger su comida de su restaurante.
-- Q: ¿Burger House Bakú tiene ofertas especiales o descuentos?
-- A: Sí, Burger House Bakú tiene algunas ofertas especiales y descuentos para sus clientes. Por ejemplo, puede obtener una bebida gratis con cualquier pedido de hamburguesas los lunes, o obtener un descuento del 10% en su factura si muestra su identificación de estudiante los martes. También puede unirse a su programa de lealtad y obtener puntos por cada compra que puede canjear por alimentos o regalos gratis.
-- Q: ¿Burger House Baku abastece para eventos o fiestas?
-- A: Sí, Burger House Bakú atiende a eventos o fiestas de cualquier tamaño y ocasión. Puedes elegir entre su menú de catering o personalizar tu propio menú según tus necesidades y preferencias. También puede reservar su restaurante para eventos privados o fiestas.
-- P: ¿Burger House Bakú tiene opciones vegetarianas o veganas?
-
-
64aa2da5cf
-
-
\ No newline at end of file
diff --git a/spaces/BestteaLib/README/README.md b/spaces/BestteaLib/README/README.md
deleted file mode 100644
index 3194fcbd06e56d0e8126c9a0abdd2db4d932fe03..0000000000000000000000000000000000000000
--- a/spaces/BestteaLib/README/README.md
+++ /dev/null
@@ -1,10 +0,0 @@
----
-title: README
-emoji: 🏢
-colorFrom: purple
-colorTo: purple
-sdk: static
-pinned: false
----
-
-Edit this `README.md` markdown file to author your organization card.
diff --git a/spaces/BetterAPI/BetterChat_new/src/lib/utils/share.ts b/spaces/BetterAPI/BetterChat_new/src/lib/utils/share.ts
deleted file mode 100644
index 4587669a10164aa7c961429fbddec9cf438c0eca..0000000000000000000000000000000000000000
--- a/spaces/BetterAPI/BetterChat_new/src/lib/utils/share.ts
+++ /dev/null
@@ -1,7 +0,0 @@
-export function share(url: string, title: string) {
- if (navigator.share) {
- navigator.share({ url, title });
- } else {
- prompt("Copy this public url to share:", url);
- }
-}
diff --git a/spaces/Big-Web/MMSD/env/Lib/site-packages/setuptools/_vendor/packaging/tags.py b/spaces/Big-Web/MMSD/env/Lib/site-packages/setuptools/_vendor/packaging/tags.py
deleted file mode 100644
index 9a3d25a71c75c975291cf987001ecd6882d6417d..0000000000000000000000000000000000000000
--- a/spaces/Big-Web/MMSD/env/Lib/site-packages/setuptools/_vendor/packaging/tags.py
+++ /dev/null
@@ -1,487 +0,0 @@
-# This file is dual licensed under the terms of the Apache License, Version
-# 2.0, and the BSD License. See the LICENSE file in the root of this repository
-# for complete details.
-
-import logging
-import platform
-import sys
-import sysconfig
-from importlib.machinery import EXTENSION_SUFFIXES
-from typing import (
- Dict,
- FrozenSet,
- Iterable,
- Iterator,
- List,
- Optional,
- Sequence,
- Tuple,
- Union,
- cast,
-)
-
-from . import _manylinux, _musllinux
-
-logger = logging.getLogger(__name__)
-
-PythonVersion = Sequence[int]
-MacVersion = Tuple[int, int]
-
-INTERPRETER_SHORT_NAMES: Dict[str, str] = {
- "python": "py", # Generic.
- "cpython": "cp",
- "pypy": "pp",
- "ironpython": "ip",
- "jython": "jy",
-}
-
-
-_32_BIT_INTERPRETER = sys.maxsize <= 2 ** 32
-
-
-class Tag:
- """
- A representation of the tag triple for a wheel.
-
- Instances are considered immutable and thus are hashable. Equality checking
- is also supported.
- """
-
- __slots__ = ["_interpreter", "_abi", "_platform", "_hash"]
-
- def __init__(self, interpreter: str, abi: str, platform: str) -> None:
- self._interpreter = interpreter.lower()
- self._abi = abi.lower()
- self._platform = platform.lower()
- # The __hash__ of every single element in a Set[Tag] will be evaluated each time
- # that a set calls its `.disjoint()` method, which may be called hundreds of
- # times when scanning a page of links for packages with tags matching that
- # Set[Tag]. Pre-computing the value here produces significant speedups for
- # downstream consumers.
- self._hash = hash((self._interpreter, self._abi, self._platform))
-
- @property
- def interpreter(self) -> str:
- return self._interpreter
-
- @property
- def abi(self) -> str:
- return self._abi
-
- @property
- def platform(self) -> str:
- return self._platform
-
- def __eq__(self, other: object) -> bool:
- if not isinstance(other, Tag):
- return NotImplemented
-
- return (
- (self._hash == other._hash) # Short-circuit ASAP for perf reasons.
- and (self._platform == other._platform)
- and (self._abi == other._abi)
- and (self._interpreter == other._interpreter)
- )
-
- def __hash__(self) -> int:
- return self._hash
-
- def __str__(self) -> str:
- return f"{self._interpreter}-{self._abi}-{self._platform}"
-
- def __repr__(self) -> str:
- return f"<{self} @ {id(self)}>"
-
-
-def parse_tag(tag: str) -> FrozenSet[Tag]:
- """
- Parses the provided tag (e.g. `py3-none-any`) into a frozenset of Tag instances.
-
- Returning a set is required due to the possibility that the tag is a
- compressed tag set.
- """
- tags = set()
- interpreters, abis, platforms = tag.split("-")
- for interpreter in interpreters.split("."):
- for abi in abis.split("."):
- for platform_ in platforms.split("."):
- tags.add(Tag(interpreter, abi, platform_))
- return frozenset(tags)
-
-
-def _get_config_var(name: str, warn: bool = False) -> Union[int, str, None]:
- value = sysconfig.get_config_var(name)
- if value is None and warn:
- logger.debug(
- "Config variable '%s' is unset, Python ABI tag may be incorrect", name
- )
- return value
-
-
-def _normalize_string(string: str) -> str:
- return string.replace(".", "_").replace("-", "_")
-
-
-def _abi3_applies(python_version: PythonVersion) -> bool:
- """
- Determine if the Python version supports abi3.
-
- PEP 384 was first implemented in Python 3.2.
- """
- return len(python_version) > 1 and tuple(python_version) >= (3, 2)
-
-
-def _cpython_abis(py_version: PythonVersion, warn: bool = False) -> List[str]:
- py_version = tuple(py_version) # To allow for version comparison.
- abis = []
- version = _version_nodot(py_version[:2])
- debug = pymalloc = ucs4 = ""
- with_debug = _get_config_var("Py_DEBUG", warn)
- has_refcount = hasattr(sys, "gettotalrefcount")
- # Windows doesn't set Py_DEBUG, so checking for support of debug-compiled
- # extension modules is the best option.
- # https://github.com/pypa/pip/issues/3383#issuecomment-173267692
- has_ext = "_d.pyd" in EXTENSION_SUFFIXES
- if with_debug or (with_debug is None and (has_refcount or has_ext)):
- debug = "d"
- if py_version < (3, 8):
- with_pymalloc = _get_config_var("WITH_PYMALLOC", warn)
- if with_pymalloc or with_pymalloc is None:
- pymalloc = "m"
- if py_version < (3, 3):
- unicode_size = _get_config_var("Py_UNICODE_SIZE", warn)
- if unicode_size == 4 or (
- unicode_size is None and sys.maxunicode == 0x10FFFF
- ):
- ucs4 = "u"
- elif debug:
- # Debug builds can also load "normal" extension modules.
- # We can also assume no UCS-4 or pymalloc requirement.
- abis.append(f"cp{version}")
- abis.insert(
- 0,
- "cp{version}{debug}{pymalloc}{ucs4}".format(
- version=version, debug=debug, pymalloc=pymalloc, ucs4=ucs4
- ),
- )
- return abis
-
-
-def cpython_tags(
- python_version: Optional[PythonVersion] = None,
- abis: Optional[Iterable[str]] = None,
- platforms: Optional[Iterable[str]] = None,
- *,
- warn: bool = False,
-) -> Iterator[Tag]:
- """
- Yields the tags for a CPython interpreter.
-
- The tags consist of:
- - cp--
- - cp-abi3-
- - cp-none-
- - cp-abi3- # Older Python versions down to 3.2.
-
- If python_version only specifies a major version then user-provided ABIs and
- the 'none' ABItag will be used.
-
- If 'abi3' or 'none' are specified in 'abis' then they will be yielded at
- their normal position and not at the beginning.
- """
- if not python_version:
- python_version = sys.version_info[:2]
-
- interpreter = f"cp{_version_nodot(python_version[:2])}"
-
- if abis is None:
- if len(python_version) > 1:
- abis = _cpython_abis(python_version, warn)
- else:
- abis = []
- abis = list(abis)
- # 'abi3' and 'none' are explicitly handled later.
- for explicit_abi in ("abi3", "none"):
- try:
- abis.remove(explicit_abi)
- except ValueError:
- pass
-
- platforms = list(platforms or platform_tags())
- for abi in abis:
- for platform_ in platforms:
- yield Tag(interpreter, abi, platform_)
- if _abi3_applies(python_version):
- yield from (Tag(interpreter, "abi3", platform_) for platform_ in platforms)
- yield from (Tag(interpreter, "none", platform_) for platform_ in platforms)
-
- if _abi3_applies(python_version):
- for minor_version in range(python_version[1] - 1, 1, -1):
- for platform_ in platforms:
- interpreter = "cp{version}".format(
- version=_version_nodot((python_version[0], minor_version))
- )
- yield Tag(interpreter, "abi3", platform_)
-
-
-def _generic_abi() -> Iterator[str]:
- abi = sysconfig.get_config_var("SOABI")
- if abi:
- yield _normalize_string(abi)
-
-
-def generic_tags(
- interpreter: Optional[str] = None,
- abis: Optional[Iterable[str]] = None,
- platforms: Optional[Iterable[str]] = None,
- *,
- warn: bool = False,
-) -> Iterator[Tag]:
- """
- Yields the tags for a generic interpreter.
-
- The tags consist of:
- - --
-
- The "none" ABI will be added if it was not explicitly provided.
- """
- if not interpreter:
- interp_name = interpreter_name()
- interp_version = interpreter_version(warn=warn)
- interpreter = "".join([interp_name, interp_version])
- if abis is None:
- abis = _generic_abi()
- platforms = list(platforms or platform_tags())
- abis = list(abis)
- if "none" not in abis:
- abis.append("none")
- for abi in abis:
- for platform_ in platforms:
- yield Tag(interpreter, abi, platform_)
-
-
-def _py_interpreter_range(py_version: PythonVersion) -> Iterator[str]:
- """
- Yields Python versions in descending order.
-
- After the latest version, the major-only version will be yielded, and then
- all previous versions of that major version.
- """
- if len(py_version) > 1:
- yield f"py{_version_nodot(py_version[:2])}"
- yield f"py{py_version[0]}"
- if len(py_version) > 1:
- for minor in range(py_version[1] - 1, -1, -1):
- yield f"py{_version_nodot((py_version[0], minor))}"
-
-
-def compatible_tags(
- python_version: Optional[PythonVersion] = None,
- interpreter: Optional[str] = None,
- platforms: Optional[Iterable[str]] = None,
-) -> Iterator[Tag]:
- """
- Yields the sequence of tags that are compatible with a specific version of Python.
-
- The tags consist of:
- - py*-none-
- - -none-any # ... if `interpreter` is provided.
- - py*-none-any
- """
- if not python_version:
- python_version = sys.version_info[:2]
- platforms = list(platforms or platform_tags())
- for version in _py_interpreter_range(python_version):
- for platform_ in platforms:
- yield Tag(version, "none", platform_)
- if interpreter:
- yield Tag(interpreter, "none", "any")
- for version in _py_interpreter_range(python_version):
- yield Tag(version, "none", "any")
-
-
-def _mac_arch(arch: str, is_32bit: bool = _32_BIT_INTERPRETER) -> str:
- if not is_32bit:
- return arch
-
- if arch.startswith("ppc"):
- return "ppc"
-
- return "i386"
-
-
-def _mac_binary_formats(version: MacVersion, cpu_arch: str) -> List[str]:
- formats = [cpu_arch]
- if cpu_arch == "x86_64":
- if version < (10, 4):
- return []
- formats.extend(["intel", "fat64", "fat32"])
-
- elif cpu_arch == "i386":
- if version < (10, 4):
- return []
- formats.extend(["intel", "fat32", "fat"])
-
- elif cpu_arch == "ppc64":
- # TODO: Need to care about 32-bit PPC for ppc64 through 10.2?
- if version > (10, 5) or version < (10, 4):
- return []
- formats.append("fat64")
-
- elif cpu_arch == "ppc":
- if version > (10, 6):
- return []
- formats.extend(["fat32", "fat"])
-
- if cpu_arch in {"arm64", "x86_64"}:
- formats.append("universal2")
-
- if cpu_arch in {"x86_64", "i386", "ppc64", "ppc", "intel"}:
- formats.append("universal")
-
- return formats
-
-
-def mac_platforms(
- version: Optional[MacVersion] = None, arch: Optional[str] = None
-) -> Iterator[str]:
- """
- Yields the platform tags for a macOS system.
-
- The `version` parameter is a two-item tuple specifying the macOS version to
- generate platform tags for. The `arch` parameter is the CPU architecture to
- generate platform tags for. Both parameters default to the appropriate value
- for the current system.
- """
- version_str, _, cpu_arch = platform.mac_ver()
- if version is None:
- version = cast("MacVersion", tuple(map(int, version_str.split(".")[:2])))
- else:
- version = version
- if arch is None:
- arch = _mac_arch(cpu_arch)
- else:
- arch = arch
-
- if (10, 0) <= version and version < (11, 0):
- # Prior to Mac OS 11, each yearly release of Mac OS bumped the
- # "minor" version number. The major version was always 10.
- for minor_version in range(version[1], -1, -1):
- compat_version = 10, minor_version
- binary_formats = _mac_binary_formats(compat_version, arch)
- for binary_format in binary_formats:
- yield "macosx_{major}_{minor}_{binary_format}".format(
- major=10, minor=minor_version, binary_format=binary_format
- )
-
- if version >= (11, 0):
- # Starting with Mac OS 11, each yearly release bumps the major version
- # number. The minor versions are now the midyear updates.
- for major_version in range(version[0], 10, -1):
- compat_version = major_version, 0
- binary_formats = _mac_binary_formats(compat_version, arch)
- for binary_format in binary_formats:
- yield "macosx_{major}_{minor}_{binary_format}".format(
- major=major_version, minor=0, binary_format=binary_format
- )
-
- if version >= (11, 0):
- # Mac OS 11 on x86_64 is compatible with binaries from previous releases.
- # Arm64 support was introduced in 11.0, so no Arm binaries from previous
- # releases exist.
- #
- # However, the "universal2" binary format can have a
- # macOS version earlier than 11.0 when the x86_64 part of the binary supports
- # that version of macOS.
- if arch == "x86_64":
- for minor_version in range(16, 3, -1):
- compat_version = 10, minor_version
- binary_formats = _mac_binary_formats(compat_version, arch)
- for binary_format in binary_formats:
- yield "macosx_{major}_{minor}_{binary_format}".format(
- major=compat_version[0],
- minor=compat_version[1],
- binary_format=binary_format,
- )
- else:
- for minor_version in range(16, 3, -1):
- compat_version = 10, minor_version
- binary_format = "universal2"
- yield "macosx_{major}_{minor}_{binary_format}".format(
- major=compat_version[0],
- minor=compat_version[1],
- binary_format=binary_format,
- )
-
-
-def _linux_platforms(is_32bit: bool = _32_BIT_INTERPRETER) -> Iterator[str]:
- linux = _normalize_string(sysconfig.get_platform())
- if is_32bit:
- if linux == "linux_x86_64":
- linux = "linux_i686"
- elif linux == "linux_aarch64":
- linux = "linux_armv7l"
- _, arch = linux.split("_", 1)
- yield from _manylinux.platform_tags(linux, arch)
- yield from _musllinux.platform_tags(arch)
- yield linux
-
-
-def _generic_platforms() -> Iterator[str]:
- yield _normalize_string(sysconfig.get_platform())
-
-
-def platform_tags() -> Iterator[str]:
- """
- Provides the platform tags for this installation.
- """
- if platform.system() == "Darwin":
- return mac_platforms()
- elif platform.system() == "Linux":
- return _linux_platforms()
- else:
- return _generic_platforms()
-
-
-def interpreter_name() -> str:
- """
- Returns the name of the running interpreter.
- """
- name = sys.implementation.name
- return INTERPRETER_SHORT_NAMES.get(name) or name
-
-
-def interpreter_version(*, warn: bool = False) -> str:
- """
- Returns the version of the running interpreter.
- """
- version = _get_config_var("py_version_nodot", warn=warn)
- if version:
- version = str(version)
- else:
- version = _version_nodot(sys.version_info[:2])
- return version
-
-
-def _version_nodot(version: PythonVersion) -> str:
- return "".join(map(str, version))
-
-
-def sys_tags(*, warn: bool = False) -> Iterator[Tag]:
- """
- Returns the sequence of tag triples for the running interpreter.
-
- The order of the sequence corresponds to priority order for the
- interpreter, from most to least important.
- """
-
- interp_name = interpreter_name()
- if interp_name == "cp":
- yield from cpython_tags(warn=warn)
- else:
- yield from generic_tags()
-
- if interp_name == "pp":
- yield from compatible_tags(interpreter="pp3")
- else:
- yield from compatible_tags()
diff --git a/spaces/CVH-vn1210/make_hair/minigpt4/datasets/datasets/__init__.py b/spaces/CVH-vn1210/make_hair/minigpt4/datasets/datasets/__init__.py
deleted file mode 100644
index e69de29bb2d1d6434b8b29ae775ad8c2e48c5391..0000000000000000000000000000000000000000
diff --git a/spaces/CVPR/Dual-Key_Backdoor_Attacks/datagen/detectron2/demo/demo.py b/spaces/CVPR/Dual-Key_Backdoor_Attacks/datagen/detectron2/demo/demo.py
deleted file mode 100644
index cf82e3faf659027792aa597432eafa48fb16385b..0000000000000000000000000000000000000000
--- a/spaces/CVPR/Dual-Key_Backdoor_Attacks/datagen/detectron2/demo/demo.py
+++ /dev/null
@@ -1,159 +0,0 @@
-# Copyright (c) Facebook, Inc. and its affiliates. All Rights Reserved
-import argparse
-import glob
-import multiprocessing as mp
-import os
-import time
-import cv2
-import tqdm
-
-from detectron2.config import get_cfg
-from detectron2.data.detection_utils import read_image
-from detectron2.utils.logger import setup_logger
-
-from predictor import VisualizationDemo
-
-# constants
-WINDOW_NAME = "COCO detections"
-
-
-def setup_cfg(args):
- # load config from file and command-line arguments
- cfg = get_cfg()
- cfg.merge_from_file(args.config_file)
- cfg.merge_from_list(args.opts)
- # Set score_threshold for builtin models
- cfg.MODEL.RETINANET.SCORE_THRESH_TEST = args.confidence_threshold
- cfg.MODEL.ROI_HEADS.SCORE_THRESH_TEST = args.confidence_threshold
- cfg.MODEL.PANOPTIC_FPN.COMBINE.INSTANCES_CONFIDENCE_THRESH = args.confidence_threshold
- cfg.freeze()
- return cfg
-
-
-def get_parser():
- parser = argparse.ArgumentParser(description="Detectron2 demo for builtin models")
- parser.add_argument(
- "--config-file",
- default="configs/quick_schedules/mask_rcnn_R_50_FPN_inference_acc_test.yaml",
- metavar="FILE",
- help="path to config file",
- )
- parser.add_argument("--webcam", action="store_true", help="Take inputs from webcam.")
- parser.add_argument("--video-input", help="Path to video file.")
- parser.add_argument(
- "--input",
- nargs="+",
- help="A list of space separated input images; "
- "or a single glob pattern such as 'directory/*.jpg'",
- )
- parser.add_argument(
- "--output",
- help="A file or directory to save output visualizations. "
- "If not given, will show output in an OpenCV window.",
- )
-
- parser.add_argument(
- "--confidence-threshold",
- type=float,
- default=0.5,
- help="Minimum score for instance predictions to be shown",
- )
- parser.add_argument(
- "--opts",
- help="Modify config options using the command-line 'KEY VALUE' pairs",
- default=[],
- nargs=argparse.REMAINDER,
- )
- return parser
-
-
-if __name__ == "__main__":
- mp.set_start_method("spawn", force=True)
- args = get_parser().parse_args()
- setup_logger(name="fvcore")
- logger = setup_logger()
- logger.info("Arguments: " + str(args))
-
- cfg = setup_cfg(args)
-
- demo = VisualizationDemo(cfg)
-
- if args.input:
- if len(args.input) == 1:
- args.input = glob.glob(os.path.expanduser(args.input[0]))
- assert args.input, "The input path(s) was not found"
- for path in tqdm.tqdm(args.input, disable=not args.output):
- # use PIL, to be consistent with evaluation
- img = read_image(path, format="BGR")
- start_time = time.time()
- predictions, visualized_output = demo.run_on_image(img)
- logger.info(
- "{}: {} in {:.2f}s".format(
- path,
- "detected {} instances".format(len(predictions["instances"]))
- if "instances" in predictions
- else "finished",
- time.time() - start_time,
- )
- )
-
- if args.output:
- if os.path.isdir(args.output):
- assert os.path.isdir(args.output), args.output
- out_filename = os.path.join(args.output, os.path.basename(path))
- else:
- assert len(args.input) == 1, "Please specify a directory with args.output"
- out_filename = args.output
- visualized_output.save(out_filename)
- else:
- cv2.namedWindow(WINDOW_NAME, cv2.WINDOW_NORMAL)
- cv2.imshow(WINDOW_NAME, visualized_output.get_image()[:, :, ::-1])
- if cv2.waitKey(0) == 27:
- break # esc to quit
- elif args.webcam:
- assert args.input is None, "Cannot have both --input and --webcam!"
- cam = cv2.VideoCapture(0)
- for vis in tqdm.tqdm(demo.run_on_video(cam)):
- cv2.namedWindow(WINDOW_NAME, cv2.WINDOW_NORMAL)
- cv2.imshow(WINDOW_NAME, vis)
- if cv2.waitKey(1) == 27:
- break # esc to quit
- cv2.destroyAllWindows()
- elif args.video_input:
- video = cv2.VideoCapture(args.video_input)
- width = int(video.get(cv2.CAP_PROP_FRAME_WIDTH))
- height = int(video.get(cv2.CAP_PROP_FRAME_HEIGHT))
- frames_per_second = video.get(cv2.CAP_PROP_FPS)
- num_frames = int(video.get(cv2.CAP_PROP_FRAME_COUNT))
- basename = os.path.basename(args.video_input)
-
- if args.output:
- if os.path.isdir(args.output):
- output_fname = os.path.join(args.output, basename)
- output_fname = os.path.splitext(output_fname)[0] + ".mkv"
- else:
- output_fname = args.output
- assert not os.path.isfile(output_fname), output_fname
- output_file = cv2.VideoWriter(
- filename=output_fname,
- # some installation of opencv may not support x264 (due to its license),
- # you can try other format (e.g. MPEG)
- fourcc=cv2.VideoWriter_fourcc(*"x264"),
- fps=float(frames_per_second),
- frameSize=(width, height),
- isColor=True,
- )
- assert os.path.isfile(args.video_input)
- for vis_frame in tqdm.tqdm(demo.run_on_video(video), total=num_frames):
- if args.output:
- output_file.write(vis_frame)
- else:
- cv2.namedWindow(basename, cv2.WINDOW_NORMAL)
- cv2.imshow(basename, vis_frame)
- if cv2.waitKey(1) == 27:
- break # esc to quit
- video.release()
- if args.output:
- output_file.release()
- else:
- cv2.destroyAllWindows()
diff --git a/spaces/CVPR/LIVE/thrust/CONTRIBUTING.md b/spaces/CVPR/LIVE/thrust/CONTRIBUTING.md
deleted file mode 100644
index 5ab75fa6650687c849caf75deb2f926cd628f239..0000000000000000000000000000000000000000
--- a/spaces/CVPR/LIVE/thrust/CONTRIBUTING.md
+++ /dev/null
@@ -1,490 +0,0 @@
-# Table of Contents
-
-1. [Contributing to Thrust](#contributing-to-thrust)
-1. [CMake Options](#cmake-options)
-1. [Development Model](#development-model)
-
-# Contributing to Thrust
-
-Thrust uses Github to manage all open-source development, including bug
-tracking, pull requests, and design discussions. This document details how to get
-started as a Thrust contributor.
-
-An overview of this process is:
-
-1. [Clone the Thrust repository](#clone-the-thrust-repository)
-1. [Setup a fork of Thrust](#setup-a-fork-of-thrust)
-1. [Setup your environment](#setup-your-environment)
-1. [Create a development branch](#create-a-development-branch)
-1. [Local development loop](#local-development-loop)
-1. [Push development branch to your fork](#push-development-branch-to-your-fork)
-1. [Create pull request](#create-pull-request)
-1. [Address feedback and update pull request](#address-feedback-and-update-pull-request)
-1. [When your PR is approved...](#when-your-pr-is-approved)
-
-## Clone the Thrust Repository
-
-To get started, clone the main repository to your local computer. Thrust should
-be cloned recursively to setup the CUB submodule (required for `CUDA`
-acceleration).
-
-```
-git clone --recursive https://github.com/thrust/thrust.git
-cd thrust
-```
-
-## Setup a Fork of Thrust
-
-You'll need a fork of Thrust on Github to create a pull request. To setup your
-fork:
-
-1. Create a Github account (if needed)
-2. Go to [the Thrust Github page](https://github.com/thrust/thrust)
-3. Click "Fork" and follow any prompts that appear.
-
-Once your fork is created, setup a new remote repo in your local Thrust clone:
-
-```
-git remote add github-fork git@github.com:/thrust.git
-```
-
-If you need to modify CUB, too, go to
-[the CUB Github page](https://github.com/thrust/cub) and repeat this process.
-Create CUB's `github-fork` remote in the `thrust/dependencies/cub` submodule.
-
-## Setup Your Environment
-
-### Git Environment
-
-If you haven't already, this is a good time to tell git who you are. This
-information is used to fill out authorship information on your git commits.
-
-```
-git config --global user.name "John Doe"
-git config --global user.email johndoe@example.com
-```
-
-### Configure CMake builds
-
-Thrust uses [CMake](https://www.cmake.org) for its developer build system. To
-configure, build, and test your checkout of Thrust:
-
-```
-# Create build directory:
-mkdir build
-cd build
-
-# Configure -- use one of the following:
-cmake .. # Command line interface.
-ccmake .. # ncurses GUI (Linux only)
-cmake-gui # Graphical UI, set source/build directories in the app
-
-# Build:
-cmake --build . -j # invokes make (or ninja, etc)
-
-# Run tests and examples:
-ctest
-```
-
-See [CMake Options](#cmake-options) for details on customizing the build.
-
-## Create a Development Branch
-
-All work should be done in a development branch (also called a "topic branch")
-and not directly in the `master` branch. This makes it easier to manage multiple
-in-progress patches at once, and provides a descriptive label for your patch
-as it passes through the review system.
-
-To create a new branch based on the current `master`:
-
-```
-# Checkout local master branch:
-cd /path/to/thrust/sources
-git checkout master
-
-# Sync local master branch with github:
-git pull
-
-# Create a new branch named `my_descriptive_branch_name` based on master:
-git checkout -b my_descriptive_branch_name
-
-# Verify that the branch has been created and is currently checked out:
-git branch
-```
-
-Thrust branch names should follow a particular pattern:
-
-- For new features, name the branch `feature/`
-- For bugfixes associated with a github issue, use `bug/github/-`
- - Internal nvidia and gitlab bugs should use `nvidia` or `gitlab` in place of
- `github`.
-
-If you plan to work on CUB as part of your patch, repeat this process in the
-`thrust/dependencies/cub` submodule.
-
-## Local Development Loop
-
-### Edit, Build, Test, Repeat
-
-Once the topic branch is created, you're all set to start working on Thrust
-code. Make some changes, then build and test them:
-
-```
-# Implement changes:
-cd /path/to/thrust/sources
-emacs thrust/some_file.h # or whatever editor you prefer
-
-# Create / update a unit test for your changes:
-emacs testing/some_test.cu
-
-# Check that everything builds and tests pass:
-cd /path/to/thrust/build/directory
-cmake --build . -j
-ctest
-```
-
-### Creating a Commit
-
-Once you're satisfied with your patch, commit your changes:
-
-#### Thrust-only Changes
-
-```
-# Manually add changed files and create a commit:
-cd /path/to/thrust
-git add thrust/some_file.h
-git add testing/some_test.cu
-git commit
-
-# Or, if possible, use git-gui to review your changes while building your patch:
-git gui
-```
-
-#### Thrust and CUB Changes
-
-```
-# Create CUB patch first:
-cd /path/to/thrust/dependencies/cub
-# Manually add changed files and create a commit:
-git add cub/some_file.cuh
-git commit
-
-# Create Thrust patch, including submodule update:
-cd /path/to/thrust/
-git add dependencies/cub # Updates submodule info
-git add thrust/some_file.h
-git add testing/some_test.cu
-git commit
-
-# Or, if possible, use git-gui to review your changes while building your patch:
-cd /path/to/thrust/dependencies/cub
-git gui
-cd /path/to/thrust
-git gui # Include dependencies/cub as part of your commit
-
-```
-
-#### Writing a Commit Message
-
-Your commit message will communicate the purpose and rationale behind your
-patch to other developers, and will be used to populate the initial description
-of your Github pull request.
-
-When writing a commit message, the following standard format should be used,
-since tools in the git ecosystem are designed to parse this correctly:
-
-```
-First line of commit message is a short summary (<80 char)
-
-Detailed description of change begins on third line. This portion can
-span multiple lines, try to manually wrap them at something reasonable.
-
-Blank lines can be used to separate multiple paragraphs in the description.
-
-If your patch is associated with another pull request or issue in the main
-Thrust repository, you should reference it with a `#` symbol, e.g.
-#1023 for issue 1023.
-
-For issues / pull requests in a different github repo, reference them using
-the full syntax, e.g. thrust/cub#4 for issue 4 in the thrust/cub repo.
-
-Markdown is recommended for formatting more detailed messages, as these will
-be nicely rendered on Github, etc.
-```
-
-## Push Development Branch to your Fork
-
-Once you've committed your changes to a local development branch, it's time to
-push them to your fork:
-
-```
-cd /path/to/thrust/checkout
-git checkout my_descriptive_branch_name # if not already checked out
-git push --set-upstream github-fork my_descriptive_branch_name
-```
-
-`--set-upstream github-fork` tells git that future pushes/pulls on this branch
-should target your `github-fork` remote by default.
-
-If have CUB changes to commit as part of your patch, repeat this process in the
-`thrust/dependencies/cub` submodule.
-
-## Create Pull Request
-
-To create a pull request for your freshly pushed branch, open your github fork
-in a browser by going to `https://www.github.com//thrust`. A
-prompt may automatically appear asking you to create a pull request if you've
-recently pushed a branch.
-
-If there's no prompt, go to "Code" > "Branches" and click the appropriate
-"New pull request" button for your branch.
-
-If you would like a specific developer to review your patch, feel free to
-request them as a reviewer at this time.
-
-The Thrust team will review your patch, test it on NVIDIA's internal CI, and
-provide feedback.
-
-
-If have CUB changes to commit as part of your patch, repeat this process with
-your CUB branch and fork.
-
-## Address Feedback and Update Pull Request
-
-If the reviewers request changes to your patch, use the following process to
-update the pull request:
-
-```
-# Make changes:
-cd /path/to/thrust/sources
-git checkout my_descriptive_branch_name
-emacs thrust/some_file.h
-emacs testing/some_test.cu
-
-# Build + test
-cd /path/to/thrust/build/directory
-cmake --build . -j
-ctest
-
-# Amend commit:
-cd /path/to/thrust/sources
-git add thrust/some_file.h
-git add testing/some_test.cu
-git commit --amend
-# Or
-git gui # Check the "Amend Last Commit" box
-
-# Update the branch on your fork:
-git push -f
-```
-
-At this point, the pull request should show your recent changes.
-
-If have CUB changes to commit as part of your patch, repeat this process in the
-`thrust/dependencies/cub` submodule, and be sure to include any CUB submodule
-updates as part of your commit.
-
-## When Your PR is Approved
-
-Once your pull request is approved by the Thrust team, no further action is
-needed from you. We will handle integrating it since we must coordinate changes
-to `master` with NVIDIA's internal perforce repository.
-
-# CMake Options
-
-A Thrust build is configured using CMake options. These may be passed to CMake
-using
-
-```
-cmake -D= /path/to/thrust/sources
-```
-
-or configured interactively with the `ccmake` or `cmake-gui` interfaces.
-
-Thrust supports two build modes. By default, a single configuration is built
-that targets a specific host system, device system, and C++ dialect.
-When `THRUST_ENABLE_MULTICONFIG` is `ON`, multiple configurations
-targeting a variety of systems and dialects are generated.
-
-The CMake options are divided into these categories:
-
-1. [Generic CMake Options](#generic-cmake-options): Options applicable to all
- Thrust builds.
-1. [Single Config CMake Options](#single-config-cmake-options) Options
- applicable only when `THRUST_ENABLE_MULTICONFIG` is disabled.
-1. [Multi Config CMake Options](#multi-config-cmake-options) Options applicable
- only when `THRUST_ENABLE_MULTICONFIG` is enabled.
-1. [CUDA Specific CMake Options](#cuda-specific-cmake-options) Options that
- control CUDA compilation. Only available when one or more configurations
- targets the CUDA system.
-1. [TBB Specific CMake Options](#tbb-specific-cmake-options) Options that
- control TBB compilation. Only available when one or more configurations
- targets the TBB system.
-
-## Generic CMake Options
-
-- `CMAKE_BUILD_TYPE={Release, Debug, RelWithDebInfo, MinSizeRel}`
- - Standard CMake build option. Default: `RelWithDebInfo`
-- `THRUST_ENABLE_HEADER_TESTING={ON, OFF}`
- - Whether to test compile public headers. Default is `ON`.
-- `THRUST_ENABLE_TESTING={ON, OFF}`
- - Whether to build unit tests. Default is `ON`.
-- `THRUST_ENABLE_EXAMPLES={ON, OFF}`
- - Whether to build examples. Default is `ON`.
-- `THRUST_ENABLE_MULTICONFIG={ON, OFF}`
- - Toggles single-config and multi-config modes. Default is `OFF` (single config).
-- `THRUST_ENABLE_EXAMPLE_FILECHECK={ON, OFF}`
- - Enable validation of example outputs using the LLVM FileCheck utility.
- Default is `OFF`.
-
-## Single Config CMake Options
-
-- `THRUST_HOST_SYSTEM={CPP, TBB, OMP}`
- - Selects the host system. Default: `CPP`
-- `THRUST_DEVICE_SYSTEM={CUDA, TBB, OMP, CPP}`
- - Selects the device system. Default: `CUDA`
-- `THRUST_CPP_DIALECT={11, 14, 17}`
- - Selects the C++ standard dialect to use. Default is `14` (C++14).
-
-## Multi Config CMake Options
-
-- `THRUST_MULTICONFIG_ENABLE_DIALECT_CPPXX={ON, OFF}`
- - Toggle whether a specific C++ dialect will be targeted.
- - Possible values of `XX` are `{11, 14, 17}`.
- - By default, only C++14 is enabled.
-- `THRUST_MULTICONFIG_ENABLE_SYSTEM_XXXX={ON, OFF}`
- - Toggle whether a specific system will be targeted.
- - Possible values of `XXXX` are `{CPP, CUDA, TBB, OMP}`
- - By default, only `CPP` and `CUDA` are enabled.
-- `THRUST_MULTICONFIG_WORKLOAD={SMALL, MEDIUM, LARGE, FULL}`
- - Restricts the host/device combinations that will be targeted.
- - By default, the `SMALL` workload is used.
- - The full cross product of `host x device` systems results in 12
- configurations, some of which are more important than others.
- This option can be used to prune some of the less important ones.
- - `SMALL`: (3 configs) Minimal coverage and validation of each device system against the `CPP` host.
- - `MEDIUM`: (6 configs) Cheap extended coverage.
- - `LARGE`: (8 configs) Expensive extended coverage. Includes all useful build configurations.
- - `FULL`: (12 configs) The complete cross product of all possible build configurations.
-
-| Config | Workloads | Value | Expense | Note |
-|----------|-----------|------------|-----------|------------------------------|
-| CPP/CUDA | `F L M S` | Essential | Expensive | Validates CUDA against CPP |
-| CPP/OMP | `F L M S` | Essential | Cheap | Validates OMP against CPP |
-| CPP/TBB | `F L M S` | Essential | Cheap | Validates TBB against CPP |
-| CPP/CPP | `F L M ` | Important | Cheap | Tests CPP as device |
-| OMP/OMP | `F L M ` | Important | Cheap | Tests OMP as host |
-| TBB/TBB | `F L M ` | Important | Cheap | Tests TBB as host |
-| TBB/CUDA | `F L ` | Important | Expensive | Validates TBB/CUDA interop |
-| OMP/CUDA | `F L ` | Important | Expensive | Validates OMP/CUDA interop |
-| TBB/OMP | `F ` | Not useful | Cheap | Mixes CPU-parallel systems |
-| OMP/TBB | `F ` | Not useful | Cheap | Mixes CPU-parallel systems |
-| TBB/CPP | `F ` | Not Useful | Cheap | Parallel host, serial device |
-| OMP/CPP | `F ` | Not Useful | Cheap | Parallel host, serial device |
-
-## CUDA Specific CMake Options
-
-- `THRUST_INCLUDE_CUB_CMAKE={ON, OFF}`
- - If enabled, the CUB project will be built as part of Thrust. Default is
- `OFF`.
- - This adds CUB tests, etc. Useful for working on both CUB and Thrust
- simultaneously.
- - CUB configurations will be generated for each C++ dialect targeted by
- the current Thrust build.
-- `THRUST_ENABLE_COMPUTE_XX={ON, OFF}`
- - Controls the targeted CUDA architecture(s)
- - Multiple options may be selected when using NVCC as the CUDA compiler.
- - Valid values of `XX` are:
- `{35, 37, 50, 52, 53, 60, 61, 62, 70, 72, 75, 80}`
- - Default value depends on `THRUST_DISABLE_ARCH_BY_DEFAULT`:
-- `THRUST_ENABLE_COMPUTE_FUTURE={ON, OFF}`
- - If enabled, CUDA objects will target the most recent virtual architecture
- in addition to the real architectures specified by the
- `THRUST_ENABLE_COMPUTE_XX` options.
- - Default value depends on `THRUST_DISABLE_ARCH_BY_DEFAULT`:
-- `THRUST_DISABLE_ARCH_BY_DEFAULT={ON, OFF}`
- - When `ON`, all `THRUST_ENABLE_COMPUTE_*` options are initially `OFF`.
- - Default: `OFF` (meaning all architectures are enabled by default)
-- `THRUST_ENABLE_TESTS_WITH_RDC={ON, OFF}`
- - Whether to enable Relocatable Device Code when building tests.
- Default is `OFF`.
-- `THRUST_ENABLE_EXAMPLES_WITH_RDC={ON, OFF}`
- - Whether to enable Relocatable Device Code when building examples.
- Default is `OFF`.
-
-## TBB Specific CMake Options
-
-- `THRUST_TBB_ROOT=`
- - When the TBB system is requested, set this to the root of the TBB installation
- (e.g. the location of `lib/`, `bin/` and `include/` for the TBB libraries).
-
-# Development Model
-
-The following is a description of the basic development process that Thrust follows. This is a living
-document that will evolve as our process evolves.
-
-Thrust is distributed in three ways:
-
- * On GitHub.
- * In the NVIDIA HPC SDK.
- * In the CUDA Toolkit.
-
-## Trunk Based Development
-
-Thrust uses [trunk based development](https://trunkbaseddevelopment.com). There is a single long-lived
-branch called `master`. Engineers may create branches for feature development. Such branches always
-merge into `master`. There are no release branches. Releases are produced by taking a snapshot of
-`master` ("snapping"). After a release has been snapped from `master`, it will never be changed.
-
-## Repositories
-
-As Thrust is developed both on GitHub and internally at NVIDIA, there are three main places where code lives:
-
- * The Source of Truth, the [public Thrust repository](https://github.com/thrust/thrust), referred to as
- `github` later in this document.
- * An internal GitLab repository, referred to as `gitlab` later in this document.
- * An internal Perforce repository, referred to as `perforce` later in this document.
-
-## Versioning
-
-Thrust has its own versioning system for releases, independent of the versioning scheme of the NVIDIA
-HPC SDK or the CUDA Toolkit.
-
-Today, Thrust version numbers have a specific [semantic meaning](https://semver.org/).
-Releases prior to 1.10.0 largely, but not strictly, followed these semantic meanings.
-
-The version number for a Thrust release uses the following format: `MMM.mmm.ss-ppp`, where:
-
- * `THRUST_VERSION_MAJOR`/`MMM`: Major version, up to 3 decimal digits. It is incremented
- when changes that are API-backwards-incompatible are made.
- * `THRUST_VERSION_MINOR`/`mmm`: Minor version, up to 3 decimal digits. It is incremented when
- breaking API, ABI, or semantic changes are made.
- * `THRUST_VERSION_SUBMINOR`/`ss`: Subminor version, up to 2 decimal digits. It is incremented
- when notable new features or bug fixes or features that are API-backwards-compatible are made.
- * `THRUST_PATCH_NUMBER`/`ppp`: Patch number, up to 3 decimal digits. It is incremented if any
- change in the repo whatsoever is made and no other version component has been incremented.
-
-The `` header defines `THRUST_*` macros for all of the version components mentioned
-above. Additionally, a `THRUST_VERSION` macro is defined, which is an integer literal containing all
-of the version components except for `THRUST_PATCH_NUMBER`.
-
-## Branches and Tags
-
-The following tag names are used in the Thrust project:
-
- * `github/nvhpc-X.Y`: the tag that directly corresponds to what has been shipped in the NVIDIA HPC SDK release X.Y.
- * `github/cuda-X.Y`: the tag that directly corresponds to what has been shipped in the CUDA Toolkit release X.Y.
- * `github/A.B.C`: the tag that directly corresponds to a Thrust version A.B.C.
-
-The following branch names are used in the Thrust project:
-
- * `github/master`: the Source of Truth development branch of Thrust.
- * `github/old-master`: the old Source of Truth branch, before unification of public and internal repositories.
- * `github/feature/`: feature branch for a feature under development.
- * `github/bug//-`: bug fix branch, where `bug-system` is `github` or `nvidia`.
- * `gitlab/master`: mirror of `github/master`.
- * `perforce/private`: mirrored `github/master`, plus files necessary for internal NVIDIA testing systems.
-
-On the rare occasion that we cannot do work in the open, for example when developing a change specific to an
-unreleased product, these branches may exist on `gitlab` instead of `github`. By default, everything should be
-in the open on `github` unless there is a strong motivation for it to not be open.
diff --git a/spaces/CVPR/LIVE/thrust/testing/omp/nvcc_independence.cpp b/spaces/CVPR/LIVE/thrust/testing/omp/nvcc_independence.cpp
deleted file mode 100644
index c870928a8a36c94cf9a835dceed99fb401524fb9..0000000000000000000000000000000000000000
--- a/spaces/CVPR/LIVE/thrust/testing/omp/nvcc_independence.cpp
+++ /dev/null
@@ -1,75 +0,0 @@
-#include
-#include
-#include
-#include
-#include
-#include
-#include
-
-void TestNvccIndependenceTransform(void)
-{
- typedef int T;
- const int n = 10;
-
- thrust::host_vector h_input = unittest::random_integers(n);
- thrust::device_vector d_input = h_input;
-
- thrust::host_vector h_output(n);
- thrust::device_vector d_output(n);
-
- thrust::transform(h_input.begin(), h_input.end(), h_output.begin(), thrust::negate());
- thrust::transform(d_input.begin(), d_input.end(), d_output.begin(), thrust::negate());
-
- ASSERT_EQUAL(h_output, d_output);
-}
-DECLARE_UNITTEST(TestNvccIndependenceTransform);
-
-void TestNvccIndependenceReduce(void)
-{
- typedef int T;
- const int n = 10;
-
- thrust::host_vector h_data = unittest::random_integers(n);
- thrust::device_vector d_data = h_data;
-
- T init = 13;
-
- T h_result = thrust::reduce(h_data.begin(), h_data.end(), init);
- T d_result = thrust::reduce(d_data.begin(), d_data.end(), init);
-
- ASSERT_ALMOST_EQUAL(h_result, d_result);
-}
-DECLARE_UNITTEST(TestNvccIndependenceReduce);
-
-void TestNvccIndependenceExclusiveScan(void)
-{
- typedef int T;
- const int n = 10;
-
- thrust::host_vector h_input = unittest::random_integers(n);
- thrust::device_vector d_input = h_input;
-
- thrust::host_vector