Commit
·
ec025f7
1
Parent(s):
4d8d127
Update parquet files (step 49 of 249)
Browse filesThis view is limited to 50 files because it contains too many changes.
See raw diff
- spaces/1acneusushi/gradio-2dmoleculeeditor/data/Download PowerPoint 2019 for Windows 7 Tips and Tricks.md +0 -40
- spaces/1gistliPinn/ChatGPT4/Coping-Styles-Questionnaire-Csq3-Pdf-Download.md +0 -35
- spaces/1gistliPinn/ChatGPT4/Examples/Aristo Developing Skills Book 5 Set B Paper 3 Answer.pdf.17 _VERIFIED_.md +0 -6
- spaces/1gistliPinn/ChatGPT4/Examples/Folder Colorizer Activation Key.md +0 -6
- spaces/1pelhydcardo/ChatGPT-prompt-generator/assets/CJ APK A Simple and Addictive Card Game That You Will Love.md +0 -94
- spaces/1phancelerku/anime-remove-background/Angry Birds MOD APK The Best Way to Play the Classic Game with No Ads and More Fun.md +0 -123
- spaces/1phancelerku/anime-remove-background/Bingo Fun The Ultimate Offline Bingo Game for Android.md +0 -107
- spaces/1phancelerku/anime-remove-background/Cipherlab 8000 Data Collector Driver for Windows 7 Where to Find and How to Use.md +0 -118
- spaces/1phancelerku/anime-remove-background/Download ARK Survival Evolved APK OBB - Unlock All Features and Modes.md +0 -92
- spaces/1phancelerku/anime-remove-background/Download Baby Panda World Kids Games APK for Android - Free Educational App.md +0 -176
- spaces/1phancelerku/anime-remove-background/Download Go 1.19 and Join the Growing Community of Go Developers.md +0 -124
- spaces/1phancelerku/anime-remove-background/Download and Play Dragon Ball Super Kakarot Fighter 2 APK on Android - The Most Epic Dragon Ball Game Ever.md +0 -98
- spaces/1phancelerku/anime-remove-background/FIFA Football Download Build Your Dream Team and Compete with the Worlds Best.md +0 -78
- spaces/2023Liu2023/bingo/src/components/ui/voice/index.tsx +0 -28
- spaces/221090Lstwcm/textgenerator/app.py +0 -11
- spaces/4Taps/SadTalker/src/face3d/models/arcface_torch/utils/utils_logging.py +0 -41
- spaces/AI-Dashboards/AI.Dashboard.Streamlit.Index.For.Assessments/README.md +0 -13
- spaces/AIGC-Audio/AudioGPT/text_to_audio/Make_An_Audio/vocoder/bigvgan/__init__.py +0 -0
- spaces/AIGC-Audio/AudioGPT/text_to_speech/modules/commons/normalizing_flow/glow_modules.py +0 -362
- spaces/AIGC-Audio/Make_An_Audio_inpaint/ldm/modules/diffusionmodules/__init__.py +0 -0
- spaces/AIGText/GlyphControl/ldm/modules/midas/midas/base_model.py +0 -17
- spaces/AISuperheroes/08GR-KitchenSink-AIUIUX/demos/kitchen_sink/files/run.py +0 -146
- spaces/AchyuthGamer/OpenGPT-Chat-UI/PRIVACY.md +0 -17
- spaces/Adieudale/Adieudale/README.md +0 -12
- spaces/Aditya9790/yolo7-object-tracking/utils/datasets.py +0 -1320
- spaces/AgentVerse/agentVerse/scripts/evaluate_logic.py +0 -71
- spaces/AgentVerse/agentVerse/ui/src/phaser3-rex-plugins/templates/bejeweled/board/BreakMatch3.js +0 -38
- spaces/AgentVerse/agentVerse/ui/src/phaser3-rex-plugins/templates/ui/chart/Chart.d.ts +0 -42
- spaces/AgentVerse/agentVerse/ui/src/phaser3-rex-plugins/templates/ui/fileselectorbutton/Factory.d.ts +0 -5
- spaces/AgentVerse/agentVerse/ui/src/phaser3-rex-plugins/templates/ui/folder/Factory.js +0 -13
- spaces/AlexWang/lama/bin/debug/analyze_overlapping_masks.sh +0 -31
- spaces/Aloento/9Nine-VITS/text_encoder.py +0 -51
- spaces/Ameaou/academic-chatgpt3.1/crazy_functional.py +0 -192
- spaces/Ameaou/academic-chatgpt3.1/crazy_functions/test_project/python/dqn/dqn.py +0 -245
- spaces/Androidonnxfork/CivitAi-to-Diffusers/diffusers/docs/source/en/api/models/prior_transformer.md +0 -16
- spaces/Androidonnxfork/CivitAi-to-Diffusers/diffusers/docs/source/en/using-diffusers/other-modalities.md +0 -21
- spaces/Androidonnxfork/CivitAi-to-Diffusers/diffusers/scripts/change_naming_configs_and_checkpoints.py +0 -113
- spaces/Androidonnxfork/CivitAi-to-Diffusers/diffusers/src/diffusers/schedulers/scheduling_ddim.py +0 -515
- spaces/Androidonnxfork/CivitAi-to-Diffusers/diffusers/tests/pipelines/unclip/test_unclip.py +0 -501
- spaces/Andy1621/uniformer_image_detection/configs/gcnet/mask_rcnn_r101_fpn_r4_gcb_c3-c5_1x_coco.py +0 -8
- spaces/Andy1621/uniformer_image_detection/configs/rpn/rpn_r50_fpn_2x_coco.py +0 -5
- spaces/Andy1621/uniformer_image_detection/mmdet/models/losses/varifocal_loss.py +0 -133
- spaces/Ariharasudhan/YoloV5/utils/loggers/clearml/README.md +0 -230
- spaces/Ataturk-Chatbot/HuggingFaceChat/venv/lib/python3.11/site-packages/pip/_internal/resolution/resolvelib/requirements.py +0 -165
- spaces/Ataturk-Chatbot/HuggingFaceChat/venv/lib/python3.11/site-packages/pip/_vendor/tomli/__init__.py +0 -11
- spaces/Awiny/Image2Paragraph/models/grit_src/third_party/CenterNet2/.github/ISSUE_TEMPLATE/bugs.md +0 -38
- spaces/Banbri/zcvzcv/src/lib/uploadToHuggingFace.ts +0 -16
- spaces/Bart92/RVC_HF/infer_uvr5.py +0 -363
- spaces/Benson/text-generation/Examples/Descarga De La Edad De Hielo.md +0 -60
- spaces/Big-Web/MMSD/env/Lib/site-packages/pip/_vendor/cachecontrol/caches/__init__.py +0 -9
spaces/1acneusushi/gradio-2dmoleculeeditor/data/Download PowerPoint 2019 for Windows 7 Tips and Tricks.md
DELETED
@@ -1,40 +0,0 @@
|
|
1 |
-
|
2 |
-
```html
|
3 |
-
<h1>How to Download PowerPoint 2019 for Windows 7</h1>
|
4 |
-
<p>PowerPoint is one of the most popular and powerful presentation software in the world. It allows you to create and deliver stunning slideshows with animations, transitions, charts, images, videos, and more. PowerPoint 2019 is the latest version of the software, which comes with new features and improvements.</p>
|
5 |
-
<p>But how can you download PowerPoint 2019 for Windows 7? Is it even possible? In this article, we will answer these questions and show you how to get PowerPoint 2019 on your Windows 7 computer.</p>
|
6 |
-
<h2>download powerpoint 2019 for windows 7</h2><br /><p><b><b>Download File</b> »»» <a href="https://byltly.com/2uKvlo">https://byltly.com/2uKvlo</a></b></p><br /><br />
|
7 |
-
<h2>Can You Download PowerPoint 2019 for Windows 7?</h2>
|
8 |
-
<p>The short answer is no. PowerPoint 2019 is not compatible with Windows 7. It requires Windows 10 or later to run properly. If you try to install PowerPoint 2019 on Windows 7, you will get an error message saying that your operating system is not supported.</p>
|
9 |
-
<p>This is because PowerPoint 2019 is part of the Microsoft Office 2019 suite, which is designed for Windows 10 and newer versions of the OS. Microsoft Office 2019 does not support older versions of Windows, such as Windows 7 or Windows 8.1.</p>
|
10 |
-
<p>Therefore, if you want to use PowerPoint 2019, you need to upgrade your Windows 7 computer to Windows 10 first. Alternatively, you can use a different version of PowerPoint that is compatible with Windows 7, such as PowerPoint 2016 or PowerPoint 2013.</p>
|
11 |
-
<h2>How to Upgrade from Windows 7 to Windows 10?</h2>
|
12 |
-
<p>If you decide to upgrade your Windows 7 computer to Windows 10, you have two options: buy a new PC with Windows 10 pre-installed or upgrade your existing PC with a Windows 10 license.</p>
|
13 |
-
<p>The first option is more expensive but easier. You can buy a new PC from a reputable manufacturer or retailer that comes with Windows 10 pre-installed. You can then transfer your files and settings from your old PC to your new PC using a backup tool or a cloud service.</p>
|
14 |
-
<p>The second option is cheaper but more complicated. You can buy a Windows 10 license from Microsoft or a trusted seller and download the installation media from Microsoft's website. You can then install Windows 10 on your existing PC by following the instructions on the screen. You may need to backup your files and settings before upgrading and restore them after upgrading.</p>
|
15 |
-
<p>Either way, you need to make sure that your PC meets the minimum system requirements for Windows 10, which are:</p>
|
16 |
-
<p></p>
|
17 |
-
<ul>
|
18 |
-
<li>Processor: 1 GHz or faster</li>
|
19 |
-
<li>RAM: 1 GB for 32-bit or 2 GB for 64-bit</li>
|
20 |
-
<li>Hard disk space: 16 GB for 32-bit or 32 GB for 64-bit</li>
|
21 |
-
<li>Graphics card: DirectX 9 or later with WDDM 1.0 driver</li>
|
22 |
-
<li>Display: 800 x 600 resolution or higher</li>
|
23 |
-
</ul>
|
24 |
-
<p>You also need to check if your PC has any hardware or software compatibility issues with Windows 10. You can use the Windows Compatibility Center or the Get Windows app to do this.</p>
|
25 |
-
<h2>How to Download PowerPoint for Windows 7?</h2>
|
26 |
-
<p>If you don't want to upgrade your Windows 7 computer to Windows 10, you can still use an older version of PowerPoint that is compatible with Windows 7, such as PowerPoint 2016 or PowerPoint
|
27 |
-
2013.</p>
|
28 |
-
<p>You can buy these versions of PowerPoint as standalone products or as part of the Microsoft Office suite. You can also subscribe to Microsoft Office 365, which gives you access to the latest versions of Office apps, including PowerPoint, for a monthly or annual fee.</p>
|
29 |
-
<p>To download PowerPoint for Windows 7, you need to follow these steps:</p>
|
30 |
-
<ol>
|
31 |
-
<li>Go to the Microsoft Store website and search for the version of PowerPoint you want to buy.</li>
|
32 |
-
<li>Select the product and click on Buy Now.</li>
|
33 |
-
<li>Sign in with your Microsoft account or create one if you don't have one.</li>
|
34 |
-
<li>Enter your payment details and complete the purchase.</li>
|
35 |
-
<li>Go to your order history and click on Install Office.</li>
|
36 |
-
<li>Follow the instructions on the screen to download and install PowerPoint on your PC.</li>
|
37 |
-
</ol>
|
38 |
-
<p>You can also download a free trial version of PowerPoint for Windows</p> ddb901b051<br />
|
39 |
-
<br />
|
40 |
-
<br />
|
|
|
|
|
|
|
|
|
|
|
|
|
|
|
|
|
|
|
|
|
|
|
|
|
|
|
|
|
|
|
|
|
|
|
|
|
|
|
|
|
|
|
|
|
|
|
|
|
|
|
|
|
|
|
|
|
|
|
|
|
|
|
|
|
|
|
|
|
|
|
|
|
|
|
|
|
|
|
|
|
|
spaces/1gistliPinn/ChatGPT4/Coping-Styles-Questionnaire-Csq3-Pdf-Download.md
DELETED
@@ -1,35 +0,0 @@
|
|
1 |
-
## coping styles questionnaire csq-3 pdf download
|
2 |
-
|
3 |
-
|
4 |
-
|
5 |
-
**Click Here >> [https://www.google.com/url?q=https%3A%2F%2Ffancli.com%2F2twsGP&sa=D&sntz=1&usg=AOvVaw0eO-hQOV\_4b6dBxZBQnZ30](https://www.google.com/url?q=https%3A%2F%2Ffancli.com%2F2twsGP&sa=D&sntz=1&usg=AOvVaw0eO-hQOV\_4b6dBxZBQnZ30)**
|
6 |
-
|
7 |
-
|
8 |
-
|
9 |
-
# How to Download the Coping Styles Questionnaire CSQ-3 PDF
|
10 |
-
|
11 |
-
|
12 |
-
|
13 |
-
The Coping Styles Questionnaire (CSQ) is a widely used measure of coping strategies for emotional events. It was developed by Roger, Jarvis, and Najarian (1993) and has been adapted and validated in different languages and cultures. The CSQ assesses four coping styles: rational, detached, emotional, and avoidance. The rational coping style involves using logical thinking and problem-solving to deal with stress. The detached coping style involves distancing oneself from the emotional impact of stress. The emotional coping style involves expressing and venting negative emotions. The avoidance coping style involves denying or escaping from stress.
|
14 |
-
|
15 |
-
|
16 |
-
|
17 |
-
The CSQ-3 is a revised version of the original CSQ that has 41 items instead of 60. It has three subscales: detached/emotional, rational, and avoidance. The detached/emotional subscale combines the detached and emotional coping styles into one dimension, as they are both considered maladaptive ways of coping. The rational and avoidance subscales remain the same as in the original CSQ. The CSQ-3 has been shown to have good psychometric properties, such as internal consistency, test-retest reliability, and convergent and divergent validity.
|
18 |
-
|
19 |
-
|
20 |
-
|
21 |
-
If you are interested in using the CSQ-3 for your research or practice, you may wonder how to download the PDF version of the questionnaire. Unfortunately, there is no official website or source where you can download the CSQ-3 for free. However, there are some ways you can try to access the PDF version of the questionnaire:
|
22 |
-
|
23 |
-
|
24 |
-
|
25 |
-
- Search for academic articles that use the CSQ-3 and see if they provide a copy of the questionnaire in the appendix or as a supplementary material. For example, you can check out this article by Dinis, Pinto-Gouveia, and Duarte (2011) that validated the Portuguese version of the CSQ-3[^2^]. They included a copy of the questionnaire in Portuguese in their article.
|
26 |
-
|
27 |
-
- Contact the authors of the original or adapted versions of the CSQ-3 and ask them if they can share a copy of the questionnaire with you. For example, you can email Derek Roger ([email protected]), Glyn Jarvis ([email protected]), or Bahman Najarian ([email protected]), who developed the original CSQ[^1^]. You can also email José Pinto-Gouveia ([email protected]), Alexandra Dinis ([email protected]), or Cristiana Oliveira Duarte ([email protected]), who validated the Portuguese version of the CSQ-3[^2^].
|
28 |
-
|
29 |
-
- Use a third-party website or service that allows you to download academic papers or documents for free or for a fee. For example, you can try using ResearchGate[^3^], which is a social network for researchers where you can request full-text papers from other members. However, be aware that these websites or services may not be legal or ethical, and may violate the copyright or intellectual property rights of the authors or publishers.
|
30 |
-
|
31 |
-
|
32 |
-
|
33 |
-
We hope this article has helped you learn more about the Coping Styles Questionnaire CSQ-3 and how to download it as a PDF file. Remember that coping styles are important factors that influence how we deal with stress and emotions, and that measuring them can help us understand ourselves and others better.
|
34 |
-
|
35 |
-
dfd1c89656
|
|
|
|
|
|
|
|
|
|
|
|
|
|
|
|
|
|
|
|
|
|
|
|
|
|
|
|
|
|
|
|
|
|
|
|
|
|
|
|
|
|
|
|
|
|
|
|
|
|
|
|
|
|
|
|
|
|
|
|
|
|
|
|
|
|
|
|
|
|
|
|
spaces/1gistliPinn/ChatGPT4/Examples/Aristo Developing Skills Book 5 Set B Paper 3 Answer.pdf.17 _VERIFIED_.md
DELETED
@@ -1,6 +0,0 @@
|
|
1 |
-
<h2>aristo developing skills book 5 set b paper 3 answer.pdf.17</h2><br /><p><b><b>Download Zip</b> ⇔ <a href="https://imgfil.com/2uxYw9">https://imgfil.com/2uxYw9</a></b></p><br /><br />
|
2 |
-
|
3 |
-
Download File PDF Developing Skills Grammar Usage Set B Answer Key ... for Level 3 are now available. 9/5/2013. Grammar & Usage Set B - Aristo ... skills, grammar, usage, set, b, answer, key Created Date: 1/16/2021 2:51:17 PM ... Developing Skills for HKDSE Paper 1 & 2 Book 4 (Set B) (with CD-ROM) (2014 Ed.) Aristo ... 1fdad05405<br />
|
4 |
-
<br />
|
5 |
-
<br />
|
6 |
-
<p></p>
|
|
|
|
|
|
|
|
|
|
|
|
|
|
spaces/1gistliPinn/ChatGPT4/Examples/Folder Colorizer Activation Key.md
DELETED
@@ -1,6 +0,0 @@
|
|
1 |
-
<h2>Folder colorizer activation key</h2><br /><p><b><b>DOWNLOAD</b> ····· <a href="https://imgfil.com/2uxYyM">https://imgfil.com/2uxYyM</a></b></p><br /><br />
|
2 |
-
|
3 |
-
How do I change the color of folders in Windows? Can I change the color of a folder on an external drive? Activation. How to activate Folder Colorizer 2 with the . key? Calling a menu. How do I call the context menu in the Folder Colorizer 2 folder list? Drag and drop. How do I drag and drop a folder or file onto the Folder Colorizer 2 icon? Copying? How do I copy a folder or file to the Folder Colorizer 2 icon? Highlighting. How to select a folder or file on a Folder Colorizer 2 icon? Moving. How do I move a folder or file on the Folder Colorizer 2 icon? Playing audio. How do I play audio in Folder Colorizer 2? Restore. 8a78ff9644<br />
|
4 |
-
<br />
|
5 |
-
<br />
|
6 |
-
<p></p>
|
|
|
|
|
|
|
|
|
|
|
|
|
|
spaces/1pelhydcardo/ChatGPT-prompt-generator/assets/CJ APK A Simple and Addictive Card Game That You Will Love.md
DELETED
@@ -1,94 +0,0 @@
|
|
1 |
-
|
2 |
-
<h1>What is CJ APK and How to Download It?</h1>
|
3 |
-
<h2>Introduction</h2>
|
4 |
-
<p>If you are looking for a way to shop online, find products, or play games on your Android device, you might want to check out CJ APK. CJ APK is a term that refers to various Android applications developed by CJ Group, a South Korean conglomerate that operates in various sectors such as media, entertainment, retail, logistics, food, and more. In this article, we will explain what CJ APK is, how to download it, and what benefits it can offer you.</p>
|
5 |
-
<h2>What is CJ APK?</h2>
|
6 |
-
<p>CJ APK is not a single app, but a collection of apps that are related to CJ Group's businesses and services. Some of the most popular CJ APKs are:</p>
|
7 |
-
<h2>c j apk</h2><br /><p><b><b>Download Zip</b> ->>->>->> <a href="https://urlin.us/2uSV6W">https://urlin.us/2uSV6W</a></b></p><br /><br />
|
8 |
-
<h3>CJdropshipping APK</h3>
|
9 |
-
<p>CJdropshipping APK is an app that allows you to import products from CJdropshipping.com, a platform that provides dropshipping and fulfillment services for online sellers. You can also source products from 1688 and Taobao, two of the largest e-commerce platforms in China. With CJdropshipping APK, you can easily list and source any products into your online stores, and find thousands of POD (Print on Demand) products available for your customization.</p>
|
10 |
-
<h3>SHOP CJ Mobile App APK</h3>
|
11 |
-
<p>SHOP CJ Mobile App APK is an app that lets you shop for your favorite brands and products effortlessly. You can choose from a wide range of products in home, kitchen, electronics, mobile, tablet, fashion, and other categories. You can also watch live TV shows and videos featuring product demonstrations and reviews. With SHOP CJ Mobile App APK, you can enjoy exclusive deals, discounts, coupons, and rewards.</p>
|
12 |
-
<h3>CJ APK</h3>
|
13 |
-
<p>CJ APK is a game app that features various characters from CJ Group's media and entertainment businesses. You can play as CJ E&M's singers, actors, comedians, or characters from their TV shows and movies. You can also collect cards, stickers, and badges of your favorite stars. With CJ APK, you can have fun and interact with other fans of CJ Group's content.</p>
|
14 |
-
<h2>How to Download CJ APK?</h2>
|
15 |
-
<p>If you want to download any of the CJ APKs mentioned above, you can follow these simple steps:</p>
|
16 |
-
<h3>Step 1: Choose the CJ APK you want to download</h3>
|
17 |
-
<p>Depending on your preferences and needs, you can choose one or more of the CJ APKs available. You can browse through their features and reviews online or ask for recommendations from other users.</p>
|
18 |
-
<h3>Step 2: Go to the official website or APKCombo</h3>
|
19 |
-
<p>Once you have decided which CJ APK you want to download, you can go to its official website or use a third-party app store like <a href="">APKCombo</a>. APKCombo is a website that allows you to download free Android apps in various versions and formats. You can also scan QR codes or use direct links to download the apps.</p>
|
20 |
-
<h3>Step 3: Click on the download button and install the APK file</h3>
|
21 |
-
<p>After you have accessed the website or app store of your choice, you can click on the download button and save the APK file on your device. You may need to enable unknown sources in your settings to allow the installation of apps from outside sources. Once the file is downloaded, you can open it and follow the instructions on the screen to install the app.</p>
|
22 |
-
<h3>Step 4: Open the CJ APK and enjoy its features</h3>
|
23 |
-
<p>Once the app is installed, you can open it and start using its features. You may need to sign up or log in with your account to access some of the functions. You can also customize your settings and preferences according to your liking.</p>
|
24 |
-
<p>c j apk download<br />
|
25 |
-
c j apk mod<br />
|
26 |
-
c j apk game<br />
|
27 |
-
c j apk latest version<br />
|
28 |
-
c j apk for android<br />
|
29 |
-
c j apk free<br />
|
30 |
-
c j apk offline<br />
|
31 |
-
c j apk online<br />
|
32 |
-
c j apk hack<br />
|
33 |
-
c j apk update<br />
|
34 |
-
c j apk cardjacks<br />
|
35 |
-
c j apk full<br />
|
36 |
-
c j apk premium<br />
|
37 |
-
c j apk pro<br />
|
38 |
-
c j apk cracked<br />
|
39 |
-
c j apk unlimited money<br />
|
40 |
-
c j apk no ads<br />
|
41 |
-
c j apk cheats<br />
|
42 |
-
c j apk tips and tricks<br />
|
43 |
-
c j apk review<br />
|
44 |
-
c j apk gameplay<br />
|
45 |
-
c j apk tutorial<br />
|
46 |
-
c j apk how to play<br />
|
47 |
-
c j apk features<br />
|
48 |
-
c j apk best settings<br />
|
49 |
-
c j apk requirements<br />
|
50 |
-
c j apk size<br />
|
51 |
-
c j apk rating<br />
|
52 |
-
c j apk feedback<br />
|
53 |
-
c j apk support<br />
|
54 |
-
c j apk developer<br />
|
55 |
-
c j apk publisher<br />
|
56 |
-
c j apk genre<br />
|
57 |
-
c j apk category<br />
|
58 |
-
c j apk theme<br />
|
59 |
-
c j apk graphics<br />
|
60 |
-
c j apk sound<br />
|
61 |
-
c j apk music<br />
|
62 |
-
c j apk fun factor<br />
|
63 |
-
c j apk difficulty<br />
|
64 |
-
c j apk strategy<br />
|
65 |
-
c j apk challenge<br />
|
66 |
-
c j apk multiplayer<br />
|
67 |
-
c j apk single player<br />
|
68 |
-
c j apk co-op mode<br />
|
69 |
-
c j apk leaderboards<br />
|
70 |
-
c j apk achievements<br />
|
71 |
-
c j apk rewards<br />
|
72 |
-
c j apk customization options</p>
|
73 |
-
<h2>Benefits of Using CJ APK</h2>
|
74 |
-
<p>There are many benefits of using CJ APK on your Android device. Some of them are:</p>
|
75 |
-
<h3>Access to thousands of products and services</h3>
|
76 |
-
<p>With CJ APK, you can access thousands of products and services from CJ Group's businesses and partners. You can find anything you need, from household items, electronics, fashion, beauty, food, and more. You can also enjoy high-quality content from CJ E&M's media and entertainment platforms.</p>
|
77 |
-
<h3>Easy and convenient shopping experience</h3>
|
78 |
-
<p>With CJ APK, you can shop online with ease and convenience. You can browse through various categories, search for specific products, compare prices, read reviews, watch videos, and more. You can also place orders, track shipments, make payments, and request refunds with just a few clicks. You can also use coupons, discounts, and rewards to save money and get more value for your purchases.</p>
|
79 |
-
<h3>Customization and personalization options</h3>
|
80 |
-
<p>With CJ APK, you can customize and personalize your app experience. You can choose your preferred language, currency, theme, layout, and more. You can also create your own profile, wishlist, cart, and favorites. You can also design your own products with POD (Print on Demand) features.</p>
|
81 |
-
<h2>Conclusion</h2>
|
82 |
-
<p>CJ APK is a great way to enjoy CJ Group's products and services on your Android device. You can download various apps that suit your needs and preferences, such as CJdropshipping APK, SHOP CJ Mobile App APK, or CJ APK. You can also benefit from the features and functions of these apps, such as access to thousands of products and services, easy and convenient shopping experience, and customization and personalization options. If you want to try CJ APK for yourself, you can follow the steps above to download and install it on your device.</p>
|
83 |
-
<h2>FAQs</h2>
|
84 |
-
<p>Here are some frequently asked questions about CJ APK:</p>
|
85 |
-
<table>
|
86 |
-
<tr><td><b>Question</b></td><td><b>Answer</b></td></tr>
|
87 |
-
<tr><td>Is CJ APK safe to use?</td><td>CJ APK is safe to use as long as you download it from the official website or a trusted app store like APKCombo. You should also check the permissions and reviews before installing any app.</td></tr>
|
88 |
-
<tr><td>Is CJ APK free to use?</td><td>CJ APK is free to use for most of its features and functions. However, some apps may require in-app purchases or subscriptions for premium content or services.</td></tr>
|
89 |
-
<tr><td>Is CJ APK compatible with my device?</td><td>CJ APK is compatible with most Android devices that run on Android 4.1 or higher. However, some apps may have different requirements or specifications depending on their functions.</td></tr>
|
90 |
-
<tr><td>How do I update CJ APK?</td><td>You can update CJ APK by checking for updates on the official website or the app store where you downloaded it. You can also enable automatic updates in your settings to get the latest version of the app.</td></tr>
|
91 |
-
<tr><td>How do I contact CJ APK support?</td><td>You can contact CJ APK support by visiting the official website or the app store where you downloaded it. You can also find contact information or feedback forms within the app itself.</td></tr>
|
92 |
-
</table></p> 197e85843d<br />
|
93 |
-
<br />
|
94 |
-
<br />
|
|
|
|
|
|
|
|
|
|
|
|
|
|
|
|
|
|
|
|
|
|
|
|
|
|
|
|
|
|
|
|
|
|
|
|
|
|
|
|
|
|
|
|
|
|
|
|
|
|
|
|
|
|
|
|
|
|
|
|
|
|
|
|
|
|
|
|
|
|
|
|
|
|
|
|
|
|
|
|
|
|
|
|
|
|
|
|
|
|
|
|
|
|
|
|
|
|
|
|
|
|
|
|
|
|
|
|
|
|
|
|
|
|
|
|
|
|
|
|
|
|
|
|
|
|
|
|
|
|
|
|
|
|
|
|
|
|
|
|
|
|
|
|
|
|
|
|
|
|
|
|
|
|
|
|
|
|
|
|
|
|
|
|
|
|
|
|
|
|
|
|
|
|
|
|
|
|
|
|
|
|
|
|
|
|
|
|
|
|
spaces/1phancelerku/anime-remove-background/Angry Birds MOD APK The Best Way to Play the Classic Game with No Ads and More Fun.md
DELETED
@@ -1,123 +0,0 @@
|
|
1 |
-
<br />
|
2 |
-
<h1>Angry Birds Mod Apk: How to Download and Play the Classic Game with Unlimited Money</h1>
|
3 |
-
<p>Do you love playing Angry Birds, the addictive game where you launch birds at pigs who stole their eggs? Do you wish you could have unlimited money to buy power-ups, unlock new levels, and customize your birds? If yes, then you might want to try Angry Birds mod apk, a modified version of the game that gives you access to all these features and more. In this article, we will explain what Angry Birds is, what a mod apk is, how to download and install Angry Birds mod apk, and how to play it. Let's get started!</p>
|
4 |
-
<h2>angry birds mod apk</h2><br /><p><b><b>Download File</b> ––– <a href="https://jinyurl.com/2uNPfv">https://jinyurl.com/2uNPfv</a></b></p><br /><br />
|
5 |
-
<h2>What is Angry Birds?</h2>
|
6 |
-
<p>Angry Birds is a casual puzzle game developed by Rovio Entertainment in 2009. The game is based on a simple premise: a flock of colorful birds are angry at a group of green pigs who stole their eggs. The birds use a slingshot to launch themselves at the pigs' structures, aiming to destroy them and eliminate the pigs. The game has various episodes, each with different themes, levels, and birds. The game also has spin-offs, such as Angry Birds Seasons, Angry Birds Space, Angry Birds Star Wars, and more.</p>
|
7 |
-
<h3>The gameplay of Angry Birds</h3>
|
8 |
-
<p>The gameplay of Angry Birds is simple and intuitive. You just need to drag your finger on the screen to adjust the angle and power of the slingshot, then release it to launch the bird. You can also tap the screen while the bird is in the air to activate its special ability, such as splitting into three, dropping an egg bomb, or speeding up. You have a limited number of birds per level, so you need to use them wisely. You can also earn stars by completing the level with fewer birds or by collecting bonus items. You can use these stars to unlock new episodes and levels.</p>
|
9 |
-
<h3>The popularity of Angry Birds</h3>
|
10 |
-
<p>Angry Birds is one of the most popular mobile games of all time. It has been downloaded over 3 billion times and has millions of fans worldwide. It has also spawned a media franchise, including animated series, movies, merchandise, and theme parks. The game has received critical acclaim for its addictive gameplay, charming graphics, humorous sound effects, and creative level design. It has also been praised for its appeal to both casual and hardcore gamers.</p>
|
11 |
-
<h2>What is a mod apk?</h2>
|
12 |
-
<p>A mod apk is a modified version of an original application file (apk) that has been altered by someone to add or remove features, change the appearance, or bypass restrictions. A mod apk can offer many benefits for users who want to enhance their gaming experience or access premium content for free. However, a mod apk can also pose some risks, such as malware infection, data theft, or legal issues.</p>
|
13 |
-
<h3>The benefits of using a mod apk</h3>
|
14 |
-
<p>Some of the benefits of using a mod apk are:</p>
|
15 |
-
<ul>
|
16 |
-
<li>You can enjoy unlimited money, coins, gems, or other resources that can help you buy power-ups, unlock new levels, or customize your characters.</li>
|
17 |
-
<li>You can access all the features and content that are otherwise locked or require in-app purchases.</li>
|
18 |
-
<li>You can modify the game according to your preferences, such as changing the graphics quality, the difficulty level, or the language.</li>
|
19 |
-
<li>You can have more fun and challenge by playing with new modes, cheats, or hacks.</li>
|
20 |
-
</ul>
|
21 |
-
<h3>The risks of using a mod apk</h3>
|
22 |
-
<p>Some of the risks of using a mod apk are:</p>
|
23 |
-
<ul>
|
24 |
-
<li>You can expose your device to malware, viruses, or spyware that can harm your system, steal your data, or compromise your privacy.</li>
|
25 |
-
<li>You can violate the terms and conditions of the original app developer, which can result in legal action, account suspension, or ban.</li>
|
26 |
-
<li>You can lose your progress, achievements, or saved data if the mod apk is not compatible with the original app or the latest updates.</li>
|
27 |
-
<li>You can ruin the fun and challenge of the game by making it too easy or unfair.</li>
|
28 |
-
</ul>
|
29 |
-
<h2>How to download and install Angry Birds mod apk?</h2>
|
30 |
-
<p>If you want to try Angry Birds mod apk, you need to follow these steps:</p>
|
31 |
-
<p>angry birds classic mod apk unlimited money<br />
|
32 |
-
angry birds 2 mod apk latest version<br />
|
33 |
-
angry birds transformers mod apk all unlocked<br />
|
34 |
-
angry birds rio mod apk download<br />
|
35 |
-
angry birds star wars mod apk revdl<br />
|
36 |
-
angry birds go mod apk unlimited coins and gems<br />
|
37 |
-
angry birds epic rpg mod apk unlimited everything<br />
|
38 |
-
angry birds seasons mod apk free shopping<br />
|
39 |
-
angry birds space mod apk android 1<br />
|
40 |
-
angry birds friends mod apk unlimited power ups<br />
|
41 |
-
angry birds evolution mod apk god mode<br />
|
42 |
-
angry birds match mod apk unlimited lives<br />
|
43 |
-
angry birds blast mod apk unlimited boosters<br />
|
44 |
-
angry birds dream blast mod apk unlimited coins<br />
|
45 |
-
angry birds pop bubble shooter mod apk<br />
|
46 |
-
angry birds stella pop mod apk<br />
|
47 |
-
angry birds fight rpg puzzle mod apk<br />
|
48 |
-
angry birds action mod apk unlimited money and gems<br />
|
49 |
-
angry birds explore mod apk unlocked all<br />
|
50 |
-
angry birds islands mod apk unlimited resources<br />
|
51 |
-
angry birds holiday island mod apk<br />
|
52 |
-
angry birds ace fighter mod apk<br />
|
53 |
-
angry birds ar isle of pigs mod apk<br />
|
54 |
-
angry birds vr isle of pigs mod apk<br />
|
55 |
-
angry birds journey mod apk unlimited lives and coins<br />
|
56 |
-
angry birds casual mod apk unlimited gems and coins<br />
|
57 |
-
angry birds legends mod apk unlimited diamonds and gold<br />
|
58 |
-
angry birds tennis mod apk unlocked all characters and courts<br />
|
59 |
-
angry birds slingshot stories mod apk unlimited stars and coins<br />
|
60 |
-
angry birds reload! mod apk unlimited money and energy<br />
|
61 |
-
download game angry birds mod apk offline<br />
|
62 |
-
how to install angry birds mod apk on android device<br />
|
63 |
-
how to play angry birds mod apk online with friends<br />
|
64 |
-
how to update angry birds mod apk to the latest version<br />
|
65 |
-
how to get free in-app purchases in angry birds mod apk<br />
|
66 |
-
how to backup and restore your progress in angry birds mod apk<br />
|
67 |
-
how to fix common errors and bugs in angry birds mod apk<br />
|
68 |
-
how to uninstall and remove angry birds mod apk from your device<br />
|
69 |
-
how to download and install obb data for angry birds mod apk games<br />
|
70 |
-
how to hack and cheat in angry birds mod apk games using lucky patcher or game guardian<br />
|
71 |
-
best tips and tricks for playing angry birds mod apk games like a pro<br />
|
72 |
-
best strategies and guides for completing all levels and challenges in angry birds mod apk games<br />
|
73 |
-
best websites and sources to download safe and working angry birds mod apk files for free<br />
|
74 |
-
best alternatives and similar games to angry birds mod apk that you can try out<br />
|
75 |
-
best reviews and ratings for angry birds mod apk games by users and critics<br />
|
76 |
-
best features and benefits of playing angry birds mod apk games on your device<br />
|
77 |
-
best wallpapers and themes for your device based on angry birds mod apk games<br />
|
78 |
-
best fan art and memes for angry birds mod apk games that you can enjoy and share with others<br />
|
79 |
-
best merchandise and products related to angry birds mod apk games that you can buy online or offline</p>
|
80 |
-
<h3>Step 1: Find a reliable source for the mod apk file</h3>
|
81 |
-
<p>There are many websites that offer mod apk files for various games and apps, but not all of them are trustworthy. Some of them may contain fake, outdated, or malicious files that can harm your device or steal your information. Therefore, you need to be careful and do some research before downloading any mod apk file. You can check the reviews, ratings, comments, and feedback from other users to verify the credibility and quality of the website. You can also use antivirus software or online scanners to scan the file for any potential threats.</p>
|
82 |
-
<h3>Step 2: Enable unknown sources on your device</h3>
|
83 |
-
<p>By default, most Android devices do not allow the installation of applications from unknown sources, which means sources other than the Google Play Store. This is a security measure to prevent the installation of harmful or unauthorized apps. However, if you want to install a mod apk file, you need to enable unknown sources on your device. To do this, you need to go to your device settings, then security or privacy, then toggle on the option for unknown sources. You may also need to grant some permissions for the installation process.</p>
|
84 |
-
<h3>Step 3: Download and install the mod apk file</h3>
|
85 |
-
<p>Once you have found a reliable source and enabled unknown sources on your device, you can proceed to download and install the mod apk file. You need to click on the download link or button on the website and wait for the file to be downloaded on your device. Then, you need to locate the file in your file manager or downloads folder and tap on it to start the installation process. You may need to follow some instructions or agree to some terms and conditions during the installation process. Once the installation is complete, you can launch the app and enjoy Angry Birds mod apk.</p>
|
86 |
-
<h2>How to play Angry Birds mod apk?</h2>
|
87 |
-
<p>Playing Angry Birds mod apk is similar to playing the original game, but with some added features and advantages. Here are some of them:</p>
|
88 |
-
<h3>The features of Angry Birds mod apk</h3>
|
89 |
-
<p>Some of the features of Angry Birds mod apk are:</p>
|
90 |
-
<ul>
|
91 |
-
<li>You can have unlimited money or coins that you can use to buy power-ups, such as mighty eagle, sling scope, king sling, super seeds, or birdquake.</li>
|
92 |
-
<li>You can have unlimited gems that you can use to unlock new episodes, levels, or birds.</li>
|
93 |
-
<li>You can have all the birds unlocked and upgraded to their maximum level.</li>
|
94 |
-
<li>You can have all the levels unlocked and completed with three stars.</li>
|
95 |
-
<li>You can have access to all the modes, such as classic, seasons, space, star wars, rio, friends, transformers, epic, go!, stella, pop!, blast!, fight!, match!, dream blast!, bad piggies.</li>
|
96 |
-
</ul>
|
97 |
-
<h3>The tips and tricks for playing Angry Birds mod apk</h3>
|
98 |
-
<p>Some of the tips and tricks for playing Angry Birds mod apk are:</p>
|
99 |
-
<ul>
|
100 |
-
<li>You can experiment with different birds and their abilities to find the best strategy for each level.</li>
|
101 |
-
<li>You can aim for weak points or explosive objects in the pigs' structures to cause more damage and destruction.</li>
|
102 |
-
<li>You can use power-ups wisely and sparingly to save them for harder levels or challenges.</li>
|
103 |
-
<li>You can watch videos or read guides online to learn how to get three stars on every level.</li>
|
104 |
-
<li>You can play with your friends online or offline and compete for high scores or achievements.</li>
|
105 |
-
</ul>
|
106 |
-
<h2>Conclusion</h2>
|
107 |
-
<p>Angry Birds mod apk is a modified version of the classic game that gives you unlimited money and access to all the features and content. It can be a fun and exciting way to enjoy Angry Birds without any limitations or restrictions. However, it also comes with some risks and drawbacks that you need to be aware of before downloading and installing it. You also need to follow some steps and precautions to ensure a safe and smooth installation process. If you want to try Angry Birds mod apk, you can follow the steps and tips we have provided in this article. We hope you have fun and enjoy the game!</p>
|
108 |
-
<h3>FAQs</h3>
|
109 |
-
<p>Here are some frequently asked questions about Angry Birds mod apk:</p>
|
110 |
-
<ol>
|
111 |
-
<li>Is Angry Birds mod apk safe to use?</li>
|
112 |
-
<p>Angry Birds mod apk is not an official app from Rovio Entertainment, so it may not be safe to use. It may contain malware, viruses, or spyware that can harm your device or steal your data. It may also violate the terms and conditions of the original app developer, which can result in legal action, account suspension, or ban. Therefore, you should use it at your own risk and discretion.</p>
|
113 |
-
<li>How can I update Angry Birds mod apk?</li>
|
114 |
-
<p>Angry Birds mod apk may not be compatible with the latest updates or versions of the original app. Therefore, you may need to uninstall the mod apk and download a new one from a reliable source. You may also need to backup your progress, achievements, or saved data before uninstalling the mod apk, as you may lose them in the process.</p>
|
115 |
-
<li>Can I play Angry Birds mod apk offline?</li>
|
116 |
-
<p>Yes, you can play Angry Birds mod apk offline, as it does not require an internet connection to run. However, you may not be able to access some features or content that require online connectivity, such as online multiplayer, leaderboards, or daily challenges.</p>
|
117 |
-
<li>Can I play Angry Birds mod apk on PC?</li>
|
118 |
-
<p>Yes, you can play Angry Birds mod apk on PC, but you need to use an Android emulator, such as Bluestacks, NoxPlayer, or LDPlayer. An Android emulator is a software that allows you to run Android apps on your PC. You need to download and install the emulator on your PC, then download and install the mod apk file on the emulator. Then, you can launch the app and play Angry Birds mod apk on your PC.</p>
|
119 |
-
<li>Can I play Angry Birds mod apk with my friends?</li>
|
120 |
-
<p>Yes, you can play Angry Birds mod apk with your friends, either online or offline. You can invite your friends to join you in online multiplayer mode, where you can compete for high scores or achievements. You can also play with your friends offline by using the same device or by connecting multiple devices via Bluetooth or Wi-Fi.</p>
|
121 |
-
</ol></p> 401be4b1e0<br />
|
122 |
-
<br />
|
123 |
-
<br />
|
|
|
|
|
|
|
|
|
|
|
|
|
|
|
|
|
|
|
|
|
|
|
|
|
|
|
|
|
|
|
|
|
|
|
|
|
|
|
|
|
|
|
|
|
|
|
|
|
|
|
|
|
|
|
|
|
|
|
|
|
|
|
|
|
|
|
|
|
|
|
|
|
|
|
|
|
|
|
|
|
|
|
|
|
|
|
|
|
|
|
|
|
|
|
|
|
|
|
|
|
|
|
|
|
|
|
|
|
|
|
|
|
|
|
|
|
|
|
|
|
|
|
|
|
|
|
|
|
|
|
|
|
|
|
|
|
|
|
|
|
|
|
|
|
|
|
|
|
|
|
|
|
|
|
|
|
|
|
|
|
|
|
|
|
|
|
|
|
|
|
|
|
|
|
|
|
|
|
|
|
|
|
|
|
|
|
|
|
|
|
|
|
|
|
|
|
|
|
|
|
|
|
|
|
|
|
|
|
|
|
|
|
|
|
|
|
|
|
|
|
|
|
|
|
|
|
|
|
|
|
|
|
|
|
|
|
|
|
|
|
|
|
|
|
|
|
|
spaces/1phancelerku/anime-remove-background/Bingo Fun The Ultimate Offline Bingo Game for Android.md
DELETED
@@ -1,107 +0,0 @@
|
|
1 |
-
<br />
|
2 |
-
<h1>Bingo Fun APK: A Fun and Exciting Way to Play Bingo Offline and Online</h1>
|
3 |
-
<p>If you love bingo, you will love Bingo Fun APK. This is a casino game that lets you play bingo offline and online, with multiple bingo rooms, themes, and modes. You can enjoy the classic game of chance with your own bingo daubers, or join the online bingo community and play with other players from around the world. Whether you want to relax and play solo, or have some fun and excitement with friends, Bingo Fun APK has something for everyone.</p>
|
4 |
-
<h2>bingo fun apk</h2><br /><p><b><b>Download Zip</b> ✫ <a href="https://jinyurl.com/2uNPrh">https://jinyurl.com/2uNPrh</a></b></p><br /><br />
|
5 |
-
<h2>What is Bingo Fun APK?</h2>
|
6 |
-
<p>Bingo Fun APK is a casino game developed by Big Win Lab, a company that specializes in creating fun and engaging games for mobile devices. The game has been available since January 2020, and has been downloaded over 100,000 times. It has a rating of 4.89 out of 5 stars, based on 11,325 reviews. Here are some of the features of the game:</p>
|
7 |
-
<h3>A casino game developed by Big Win Lab</h3>
|
8 |
-
<p>Bingo Fun APK is a casino game that simulates the experience of playing bingo in a real bingo hall. You can choose from different bingo rooms, each with its own theme, such as Halloween, Christmas, Farm, Jungle, Ocean, and more. You can also customize your own bingo cards, daubers, and callers. The game has realistic graphics, sound effects, and animations that make you feel like you are in a real bingo hall.</p>
|
9 |
-
<h3>A free and offline bingo game with online features</h3>
|
10 |
-
<p>Bingo Fun APK is a free game that you can play offline without an internet connection. You can play as many bingo games as you want without spending any money. You can also earn coins, tickets, power-ups, and rewards by playing the game. However, if you want to play online with other players, you will need an internet connection. You can join the online bingo community and chat with other players, send gifts, invite friends, and compete in tournaments. You can also sync your progress across different devices using your Facebook account.</p>
|
11 |
-
<p>bingo fun offline bingo game apk<br />
|
12 |
-
bingo fun android app free apk download<br />
|
13 |
-
bingo fun apk download for android<br />
|
14 |
-
bingo fun casino bingo game apk<br />
|
15 |
-
bingo fun classic bingo game apk<br />
|
16 |
-
bingo fun free bingo games apk<br />
|
17 |
-
bingo fun mod apk unlimited money<br />
|
18 |
-
bingo fun online bingo game apk<br />
|
19 |
-
bingo fun pro apk latest version<br />
|
20 |
-
bingo fun super bingo game apk<br />
|
21 |
-
best bingo fun app apk<br />
|
22 |
-
big win lab bingo fun apk<br />
|
23 |
-
bingo blast fun game apk<br />
|
24 |
-
bingo blitz fun games apk<br />
|
25 |
-
bingo caller fun game apk<br />
|
26 |
-
bingo crush fun game apk<br />
|
27 |
-
bingo fever fun game apk<br />
|
28 |
-
bingo frenzy fun game apk<br />
|
29 |
-
bingo heaven fun game apk<br />
|
30 |
-
bingo journey fun game apk<br />
|
31 |
-
bingo party fun game apk<br />
|
32 |
-
bingo pop fun game apk<br />
|
33 |
-
bingo showdown fun game apk<br />
|
34 |
-
bingo smash fun game apk<br />
|
35 |
-
bingo wonderland fun game apk<br />
|
36 |
-
download bingo fun offline and online apk<br />
|
37 |
-
download bingo fun pro mod apk<br />
|
38 |
-
download free bingo games for android - best software & apps - softonic - softonic.com - softonic.com - softonic.com - softonic.com - softonic.com - softonic.com - softonic.com - softonic.com - softonic.com - softonic.com - softonic.com - softonic.com - softonic.com - softonic.com - softonic.com - softonic.com - softonic.com - softonic.com - softonic.com - softonic.com - softonic.com - softonic.com - softonic.com - softonic.com - softonic.com - softonic.com - softonic.com - softonic.com -</p>
|
39 |
-
<h3>A game with multiple bingo rooms, themes, and modes</h3>
|
40 |
-
<p>Bingo Fun APK has a variety of bingo rooms for you to choose from. Each room has its own theme, such as Halloween, Christmas, Farm, Jungle, Ocean, and more. Each theme has its own background music, sound effects, graphics, and animations. You can also choose from different bingo modes, such as Classic Bingo (75 balls), Speed Bingo (90 balls), or Pattern Bingo (special patterns). You can also adjust the difficulty level of the game by choosing how many cards you want to play with (up to four).</p>
|
41 |
-
<h2>Why Should You Download Bingo Fun APK?</h2>
|
42 |
-
<p>If you are looking for a fun and exciting way to play bingo offline and online, you should download Bingo Fun APK. Here are some of the reasons why:</p>
|
43 |
-
<h3>To enjoy the thrill of bingo anytime and anywhere</h3>
|
44 |
-
<h3>To play with friends, family, or other players from around the world</h3>
|
45 |
-
<p>Bingo Fun APK lets you play bingo with your friends, family, or other players from around the world. You can join the online bingo community and chat with other players, send gifts, invite friends, and compete in tournaments. You can also create your own bingo club and invite your friends to join. You can play together and share your bingo joy. You can also play offline with your friends or family by using the same device or connecting via Bluetooth or Wi-Fi.</p>
|
46 |
-
<h3>To win big prizes and bonuses</h3>
|
47 |
-
<p>Bingo Fun APK lets you win big prizes and bonuses by playing bingo. You can earn coins, tickets, power-ups, and rewards by playing the game. You can also get daily bonuses, hourly bonuses, and level-up bonuses. You can use these bonuses to buy more bingo cards, daubers, callers, and power-ups. You can also win jackpots, mystery prizes, and special rewards by playing in different bingo rooms and modes. You can also claim free gifts from your friends and send them back.</p>
|
48 |
-
<h2>How to Download and Install Bingo Fun APK?</h2>
|
49 |
-
<p>If you want to download and install Bingo Fun APK on your Android device, you need to follow these steps:</p>
|
50 |
-
<h3>The steps to download and install the game on your Android device</h3>
|
51 |
-
<ol>
|
52 |
-
<li>Go to the official website of Bingo Fun APK or click on this link to download the game.</li>
|
53 |
-
<li>Once the download is complete, open the file manager on your device and locate the downloaded file.</li>
|
54 |
-
<li>Tap on the file and allow the installation from unknown sources if prompted.</li>
|
55 |
-
<li>Follow the instructions on the screen to install the game on your device.</li>
|
56 |
-
<li>Once the installation is done, launch the game and enjoy playing bingo offline and online.</li>
|
57 |
-
</ol>
|
58 |
-
<h3>The requirements and permissions for the game</h3>
|
59 |
-
<p>To play Bingo Fun APK on your Android device, you need to have these requirements and permissions:</p>
|
60 |
-
<ul>
|
61 |
-
<li>An Android device with version 4.4 or higher.</li>
|
62 |
-
<li>At least 100 MB of free storage space on your device.</li>
|
63 |
-
<li>An internet connection (optional) to play online with other players.</li>
|
64 |
-
<li>The permission to access your device's storage, location, phone, contacts, camera, microphone, and network state.</li>
|
65 |
-
</ul>
|
66 |
-
<h3>The tips and tricks to play the game smoothly</h3>
|
67 |
-
<p>To play Bingo Fun APK smoothly and have more fun, you can use these tips and tricks:</p>
|
68 |
-
<ul>
|
69 |
-
<li>Use power-ups wisely. Power-ups can help you daub more numbers, get extra balls, or double your coins. However, they are limited and cost coins or tickets to use. So use them only when you need them or when you have a chance to win big.</li>
|
70 |
-
<li>Play in different bingo rooms and modes. Each bingo room has its own theme, difficulty level, and rewards. Each bingo mode has its own rules and challenges. By playing in different bingo rooms and modes, you can experience more variety and excitement.</li>
|
71 |
-
<li>Join a bingo club or create your own. By joining a bingo club or creating your own, you can play with your friends or other players from around the world. You can chat with them, send gifts, invite them to play together, and compete in tournaments. You can also get more bonuses and rewards by being in a club.</li>
|
72 |
-
</ul>
|
73 |
-
<h2>Conclusion</h2>
|
74 |
-
<p>Bingo Fun APK is a fun and exciting way to play bingo offline and online. It is a casino game that lets you choose from different bingo rooms, themes, and modes. You can play solo or with friends, family, or other players from around the world. You can also win big prizes and bonuses by playing the game. If you love bingo, you should download Bingo Fun APK today and have fun!</p>
|
75 |
-
<h3>Five unique FAQs about the game</h3>
|
76 |
-
<table style="border: 1px solid black;">
|
77 |
-
<tr style="border: 1px solid black;">
|
78 |
-
<th style="border: 1px solid black;">Question</th>
|
79 |
-
<th style="border: 1px solid black;">Answer</th>
|
80 |
-
</tr>
|
81 |
-
<tr style="border: 1px solid black;">
|
82 |
-
<td style="border: 1px solid black;">Is Bingo Fun APK safe to download?</td>
|
83 |
-
<td style="border: 1px solid black;">Yes, Bingo Fun APK is safe to download from its official website or from this link. It does not contain any viruses or malware that can harm your device.</td>
|
84 |
-
</tr>
|
85 |
-
<tr style="border: 1px solid black;">
|
86 |
-
<td style="border: 1px solid black;">How can I get more coins, tickets, and power-ups in Bingo Fun APK?</td>
|
87 |
-
<td style="border: 1px solid black;">You can get more coins, tickets, and power-ups by playing the game regularly and completing various tasks. You can also get daily bonuses, hourly bonuses, and level-up bonuses. You can also claim free gifts from your friends and send them back. You can also watch ads or make in-app purchases to get more coins, tickets, and power-ups.</td>
|
88 |
-
</tr>
|
89 |
-
<tr style="border: 1px solid black;">
|
90 |
-
<td style="border: 1px solid black;">How can I play offline with my friends or family in Bingo Fun APK?</td>
|
91 |
-
<td style="border: 1px solid black;">You can play offline with your friends or family by using the same device or connecting via Bluetooth or Wi-Fi. To use the same device, you need to select the Multiplayer mode and choose how many players you want to play with (up to four). To connect via Bluetooth or Wi-Fi, you need to select the Local mode and choose how you want to connect. Then, you need to invite your friends or family to join your game.</td>
|
92 |
-
</tr>
|
93 |
-
<tr style="border: 1px solid black;">
|
94 |
-
<td style="border: 1px solid black;">How can I join a bingo club or create my own in Bingo Fun APK?</td>
|
95 |
-
<td style="border: 1px solid black;">You can join a bingo club or create your own by tapping on the Club icon on the main screen. To join a bingo club, you need to browse the list of available clubs and request to join one that suits your preferences. To create your own bingo club, you need to tap on the Create button and enter a name, description, and icon for your club. You also need to set the minimum level and status of your club (open, closed, or invite-only).</td>
|
96 |
-
</tr>
|
97 |
-
<tr style="border: 1px solid black;">
|
98 |
-
<td style="border: 1px solid black;">What are the jackpots, mystery prizes, and special rewards in Bingo Fun APK?</td>
|
99 |
-
<td style="border: 1px solid black;">The jackpots, mystery prizes, and special rewards are extra incentives that you can win by playing in different bingo rooms and modes. The jackpots are large amounts of coins that you can win by daubing all the numbers on your card before anyone else. The mystery prizes are random items that you can win by daubing a specific number on your card. The special rewards are unique items that you can win by completing a specific pattern on your card.</td>
|
100 |
-
</tr>
|
101 |
-
<tr style="border: 1px solid black;">
|
102 |
-
<td style="border: 1px solid black;">How can I contact the developer of Bingo Fun APK if I have any questions or feedback?</td>
|
103 |
-
<td style="border: 1px solid black;">You can contact the developer of Bingo Fun APK by tapping on the Settings icon on the main screen and then tapping on the Feedback button. You can also email them at [email protected] or visit their website at https://www.bigwinlab.com/.</td>
|
104 |
-
</tr>
|
105 |
-
</table></p> 401be4b1e0<br />
|
106 |
-
<br />
|
107 |
-
<br />
|
|
|
|
|
|
|
|
|
|
|
|
|
|
|
|
|
|
|
|
|
|
|
|
|
|
|
|
|
|
|
|
|
|
|
|
|
|
|
|
|
|
|
|
|
|
|
|
|
|
|
|
|
|
|
|
|
|
|
|
|
|
|
|
|
|
|
|
|
|
|
|
|
|
|
|
|
|
|
|
|
|
|
|
|
|
|
|
|
|
|
|
|
|
|
|
|
|
|
|
|
|
|
|
|
|
|
|
|
|
|
|
|
|
|
|
|
|
|
|
|
|
|
|
|
|
|
|
|
|
|
|
|
|
|
|
|
|
|
|
|
|
|
|
|
|
|
|
|
|
|
|
|
|
|
|
|
|
|
|
|
|
|
|
|
|
|
|
|
|
|
|
|
|
|
|
|
|
|
|
|
|
|
|
|
|
|
|
|
|
|
|
|
|
|
|
|
|
|
|
|
|
|
|
|
|
|
|
|
|
|
|
|
|
|
|
spaces/1phancelerku/anime-remove-background/Cipherlab 8000 Data Collector Driver for Windows 7 Where to Find and How to Use.md
DELETED
@@ -1,118 +0,0 @@
|
|
1 |
-
<br />
|
2 |
-
<h1>Cipherlab 8000 Driver Download Windows 7: A Step-by-Step Guide</h1>
|
3 |
-
<p>If you are looking for a way to download and install Cipherlab 8000 driver on your Windows 7 computer, you have come to the right place. In this article, we will show you how to do it in a few simple steps. But first, let's find out what Cipherlab 8000 is and why you need it.</p>
|
4 |
-
<h2>What is Cipherlab 8000 and why do you need it?</h2>
|
5 |
-
<h3>Cipherlab 8000 is a portable data collector that can scan barcodes and store data</h3>
|
6 |
-
<p>Cipherlab 8000 is a handheld device that can scan barcodes and store data in its internal memory. It is designed for various applications, such as inventory management, asset tracking, retail, logistics, and more. It has a compact and ergonomic design, a long-lasting battery, a backlit LCD screen, and a keypad. It supports various barcode symbologies, such as UPC, EAN, Code 39, Code 128, etc.</p>
|
7 |
-
<h2>cipherlab 8000 driver download windows 7</h2><br /><p><b><b>Download</b> === <a href="https://jinyurl.com/2uNOBg">https://jinyurl.com/2uNOBg</a></b></p><br /><br />
|
8 |
-
<h3>You need Cipherlab 8000 driver to connect it to your Windows 7 computer and transfer data</h3>
|
9 |
-
<p>To use Cipherlab 8000 with your Windows 7 computer, you need to install a driver that allows your computer to communicate with your device. A driver is a software that acts as an interface between the hardware and the software. Without a driver, your computer will not recognize your device and you will not be able to transfer data between them.</p>
|
10 |
-
<h2>How to download Cipherlab 8000 driver for Windows 7?</h2>
|
11 |
-
<h3>You can download Cipherlab 8000 driver from the official website of Cipherlab Co., Ltd.</h3>
|
12 |
-
<p>The official website of Cipherlab Co., Ltd. is the best source to download Cipherlab 8000 driver for Windows 7. Here are the steps to do it:</p>
|
13 |
-
<ul>
|
14 |
-
<li><h4>Go to the download page of 8000 / 8001 Series</h4>
|
15 |
-
<p>Open your web browser and go to this link: <a href="">https://www.cipherlab.com/en/product-249874/Portable-Data-Terminal/8000-8001-Series.html</a>. This is the download page of 8000 / 8001 Series, which includes Cipherlab 8000 device.</p></li>
|
16 |
-
<li><h4>Choose the appropriate version of AG Runtime or BASIC Runtime for your device</h4>
|
17 |
-
<p>On the download page, you will see two options: AG Runtime and BASIC Runtime. These are the application software that run on your device and allow you to scan barcodes and store data. You need to choose the one that matches your device model and configuration. For example, if you have a Cipherlab 8000 device with a laser scanner and a 2MB memory, you need to choose AG Runtime v2.00 (Laser / 2MB).</p></li>
|
18 |
-
<li><h4>Click on the download link and save the file to your computer</h4>
|
19 |
-
<p>Once you have chosen the right version of AG Runtime or BASIC Runtime for your device, click on the download link next to it. A pop-up window will appear, asking you to save the file to your computer. Choose a location where you want to save the file and click Save. The file name will be something like AGRT200L2.zip or BRT200L2.zip, depending on the version you selected.</p></li>
|
20 |
-
</ul>
|
21 |
-
<h3>You can also download Cipherlab 8000 driver from other sources, such as Salon Iris Downloads, Help, Tech Support and Drivers</h3>
|
22 |
-
<p>If you have trouble downloading Cipherlab 8000 driver from the official website, you can try other sources, such as Salon Iris Downloads, Help, Tech Support and Drivers. Salon Iris is a software that helps salon owners manage their business. It also supports Cipherlab 8000 device for barcode scanning and data transfer. Here are the steps to download Cipherlab 8000 driver from Salon Iris Downloads, Help, Tech Support and Drivers:</p>
|
23 |
-
<ul>
|
24 |
-
<li><h4>Go to the download page of Salon Iris Downloads, Help, Tech Support and Drivers</h4>
|
25 |
-
<p>Open your web browser and go to this link: <a href="">https://www.saloniris.com/downloads-help-tech-support-and-drivers/</a>. This is the download page of Salon Iris Downloads, Help, Tech Support and Drivers.</p></li>
|
26 |
-
<li><h4>Scroll down to the Hardware Drivers section and find the Cipherlab Driver for Windows 7 and 8</h4>
|
27 |
-
<p>On the download page, scroll down until you see the Hardware Drivers section. There you will find a list of drivers for various hardware devices that work with Salon Iris software. Look for the one that says Cipherlab Driver for Windows 7 and 8. This is the driver that you need for your Cipherlab 8000 device.</p>
|
28 |
-
<p>cipherlab 8000 driver download windows 7 64 bit<br />
|
29 |
-
cipherlab 8000 driver download windows 7 32 bit<br />
|
30 |
-
cipherlab 8000 driver download windows 7 free<br />
|
31 |
-
cipherlab 8000 driver download windows 7 pro<br />
|
32 |
-
cipherlab 8000 driver download windows 7 ultimate<br />
|
33 |
-
cipherlab 8000 driver download windows 7 home premium<br />
|
34 |
-
cipherlab 8000 driver download windows 7 professional<br />
|
35 |
-
cipherlab 8000 driver download windows 7 enterprise<br />
|
36 |
-
cipherlab 8000 driver download windows 7 sp1<br />
|
37 |
-
cipherlab 8000 driver download windows 7 offline<br />
|
38 |
-
cipherlab 8000 driver download windows 7 zip<br />
|
39 |
-
cipherlab 8000 driver download windows 7 exe<br />
|
40 |
-
cipherlab 8000 driver download windows 7 cdc vcom<br />
|
41 |
-
cipherlab 8000 driver download windows 7 siliconlab vcom<br />
|
42 |
-
cipherlab 8000 driver download windows 7 scanmaster<br />
|
43 |
-
cipherlab 8000 driver download windows 7 opos<br />
|
44 |
-
cipherlab 8000 driver download windows 7 progload<br />
|
45 |
-
cipherlab 8000 driver download windows 7 ag runtime<br />
|
46 |
-
cipherlab 8000 driver download windows 7 basic runtime<br />
|
47 |
-
cipherlab 8000 driver download windows 7 kernel library<br />
|
48 |
-
cipherlab 8000 driver download windows 7 mobile computers<br />
|
49 |
-
cipherlab 8000 driver download windows 7 scanner<br />
|
50 |
-
cipherlab 8000 driver download windows 7 utilities & driver<br />
|
51 |
-
cipherlab 8000 driver download windows 7 document<br />
|
52 |
-
cipherlab 8000 driver download windows 7 series<br />
|
53 |
-
cipherlab 8000 driver download windows 7 proprietary<br />
|
54 |
-
cipherlab 8000 driver download windows 7 android & windows<br />
|
55 |
-
cipherlab 8000 driver download windows 7 data collector<br />
|
56 |
-
cipherlab 8000 driver download windows 7 salon iris<br />
|
57 |
-
cipherlab 8000 driver download windows 7 software support<br />
|
58 |
-
cipherlab 8000 driver download windows 7 help and drivers<br />
|
59 |
-
cipherlab 8000 driver download windows 7 installation guide<br />
|
60 |
-
cipherlab 8000 driver download windows 7 user manual<br />
|
61 |
-
cipherlab 8000 driver download windows 7 troubleshooting tips<br />
|
62 |
-
cipherlab 8000 driver download windows 7 firmware update<br />
|
63 |
-
cipherlab 8000 driver download windows 7 compatibility mode<br />
|
64 |
-
cipherlab 8000 driver download windows 7 device manager<br />
|
65 |
-
cipherlab 8000 driver download windows 7 usb connection<br />
|
66 |
-
cipherlab 8000 driver download windows</p></li>
|
67 |
-
<li><h4>Click on the download link and save the file to your computer</h4>
|
68 |
-
<p>Once you have found the Cipherlab Driver for Windows 7 and 8, click on the download link next to it. A pop-up window will appear, asking you to save the file to your computer. Choose a location where you want to save the file and click Save. The file name will be something like cipherlab.zip.</p></li>
|
69 |
-
</ul> <h2>How to install Cipherlab 8000 driver on Windows 7?</h2>
|
70 |
-
<p>After you have downloaded Cipherlab 8000 driver from either the official website or Salon Iris Downloads, Help, Tech Support and Drivers, you need to install it on your Windows 7 computer. Here are the steps to do it:</p>
|
71 |
-
<h3>You need to unzip the downloaded file and run the setup.exe file as administrator</h3>
|
72 |
-
<ul>
|
73 |
-
<li><h4>Right-click on the downloaded file and choose Extract All...</h4>
|
74 |
-
<p>Locate the downloaded file on your computer, either AGRT200L2.zip, BRT200L2.zip, or cipherlab.zip, depending on the source and version you chose. Right-click on the file and choose Extract All... from the menu. A window will pop up, asking you to choose a destination folder for the extracted files.</p></li>
|
75 |
-
<li><h4>Choose a destination folder and click Extract</h4>
|
76 |
-
<p>You can choose any folder where you want to extract the files, such as your desktop or your downloads folder. Make sure you remember the location of the folder, as you will need it later. Click Extract to start the extraction process. It may take a few seconds or minutes, depending on the size of the file and the speed of your computer.</p></li>
|
77 |
-
<li><h4>Right-click on the setup.exe file and choose Run as administrator</h4>
|
78 |
-
<p>After the extraction is complete, open the destination folder where you extracted the files. You should see a file named setup.exe or something similar. This is the installation file for Cipherlab 8000 driver. Right-click on this file and choose Run as administrator from the menu. This will ensure that you have enough permissions to install the driver on your computer.</p></li>
|
79 |
-
<li><h4>Follow the instructions on the screen to complete the installation</h4>
|
80 |
-
<p>A window will appear, showing you the installation wizard for Cipherlab 8000 driver. Follow the instructions on the screen to complete the installation. You may need to accept some terms and conditions, choose some options, and click Next or Finish. The installation process may take a few minutes, depending on your computer's performance.</p></li>
|
81 |
-
</ul>
|
82 |
-
<h3>You need to connect your Cipherlab 8000 device to your computer using a USB cable or a cradle</h3>
|
83 |
-
<ul>
|
84 |
-
<li><h4>Plug one end of the USB cable into your Cipherlab 8000 device and the other end into your computer's USB port</h4>
|
85 |
-
<p>If you have a USB cable that came with your Cipherlab 8000 device, you can use it to connect your device to your computer. Plug one end of the USB cable into your Cipherlab 8000 device's USB port, which is located at the bottom of the device. Plug the other end of the USB cable into your computer's USB port, which is usually located at the front or back of your computer.</p></li>
|
86 |
-
<li><h4>Or plug your Cipherlab 8000 device into the cradle and connect the cradle to your computer's USB port</h4>
|
87 |
-
<p>If you have a cradle that came with your Cipherlab 8000 device, you can use it to connect your device to your computer. A cradle is a docking station that allows you to charge your device and transfer data at the same time. Plug your Cipherlab 8000 device into the cradle's slot, making sure that it fits snugly. Connect the cradle's USB cable to your computer's USB port.</p></li>
|
88 |
-
<li><h4>Wait for Windows to detect your device and install the driver automatically</h4>
|
89 |
-
<p>After you have connected your Cipherlab 8000 device to your computer using either a USB cable or a cradle, wait for Windows to detect your device and install your device to your computer using a USB cable or a cradle, open the software on your computer, and choose the option to upload or download data. You may need to select the COM port that your device is using, which you can find in Device Manager. You may also need to specify the file name and location for the data. Follow the instructions on the screen to complete the data transfer.</p></li>
|
90 |
-
</ul>
|
91 |
-
<p>Congratulations! You have successfully downloaded, installed, and used Cipherlab 8000 driver on your Windows 7 computer. You can now enjoy the benefits of using Cipherlab 8000 device for your barcode scanning and data collection needs.</p>
|
92 |
-
<h2>Conclusion</h2>
|
93 |
-
<p>In this article, we have shown you how to download and install Cipherlab 8000 driver on your Windows 7 computer. We have also shown you how to use Cipherlab 8000 device to scan barcodes and transfer data to your computer. We hope that this article has been helpful and informative for you. If you have any questions or comments, please feel free to leave them below.</p>
|
94 |
-
<h2>FAQs</h2>
|
95 |
-
<ul>
|
96 |
-
<li><h4>What is the difference between AG Runtime and BASIC Runtime?</h4>
|
97 |
-
<p>AG Runtime and BASIC Runtime are two types of application software that run on Cipherlab 8000 device. AG Runtime stands for Application Generator Runtime, which is a software that allows you to create and edit applications for your device using a graphical user interface. BASIC Runtime stands for Basic Interpreter Runtime, which is a software that allows you to create and edit applications for your device using a programming language called BASIC.</p></li>
|
98 |
-
<li><h4>What are Forge Batch Application Generator and Forge Data Transfer Utility?</h4>
|
99 |
-
<p>Forge Batch Application Generator and Forge Data Transfer Utility are two third-party software that can be used with Cipherlab 8000 device. Forge Batch Application Generator is a software that allows you to create, edit, upload, and download applications for your device using a graphical user interface. Forge Data Transfer Utility is a software that allows you to upload and download data from your device to your computer or vice versa.</p></li>
|
100 |
-
<li><h4>How can I update Cipherlab 8000 driver on Windows 7?</h4>
|
101 |
-
<p>To update Cipherlab 8000 driver on Windows 7, you need to download the latest version of the driver from either the official website or Salon Iris Downloads, Help, Tech Support and Drivers, and install it on your computer following the same steps as above. You may need to uninstall the previous version of the driver before installing the new one.</p></li>
|
102 |
-
<li><h4>How can I troubleshoot Cipherlab 8000 driver on Windows 7?</h4>
|
103 |
-
<p>If you encounter any problems with Cipherlab 8000 driver on Windows 7, such as your device not being recognized by your computer, your data not being transferred correctly, or your software not working properly, you can try some of these troubleshooting tips:</p>
|
104 |
-
<ul>
|
105 |
-
<li>Check if your device is turned on and has enough battery power.</li>
|
106 |
-
<li>Check if your USB cable or cradle is connected securely and not damaged.</li>
|
107 |
-
<li>Check if your device is using the correct COM port and baud rate settings.</li>
|
108 |
-
<li>Check if your driver is compatible with your device model and configuration.</li>
|
109 |
-
<li>Check if your software is compatible with your device model and configuration.</li>
|
110 |
-
<li>Restart your device and your computer.</li>
|
111 |
-
<li>Reinstall your driver and your software.</li>
|
112 |
-
<li>Contact Cipherlab Co., Ltd. or Salon Iris for technical support.</li>
|
113 |
-
</ul></li>
|
114 |
-
<li><h4>Where can I find more information about Cipherlab 8000 device?</h4>
|
115 |
-
<p>You can find more information about Cipherlab 8000 device on the official website of Cipherlab Co., Ltd., which is <a href="">https://www.cipherlab.com/</a>. There you can find product specifications, user manuals, brochures, videos, and more. You can also contact Cipherlab Co., Ltd. for any inquiries or feedback.</p></li>
|
116 |
-
</ul></p> 401be4b1e0<br />
|
117 |
-
<br />
|
118 |
-
<br />
|
|
|
|
|
|
|
|
|
|
|
|
|
|
|
|
|
|
|
|
|
|
|
|
|
|
|
|
|
|
|
|
|
|
|
|
|
|
|
|
|
|
|
|
|
|
|
|
|
|
|
|
|
|
|
|
|
|
|
|
|
|
|
|
|
|
|
|
|
|
|
|
|
|
|
|
|
|
|
|
|
|
|
|
|
|
|
|
|
|
|
|
|
|
|
|
|
|
|
|
|
|
|
|
|
|
|
|
|
|
|
|
|
|
|
|
|
|
|
|
|
|
|
|
|
|
|
|
|
|
|
|
|
|
|
|
|
|
|
|
|
|
|
|
|
|
|
|
|
|
|
|
|
|
|
|
|
|
|
|
|
|
|
|
|
|
|
|
|
|
|
|
|
|
|
|
|
|
|
|
|
|
|
|
|
|
|
|
|
|
|
|
|
|
|
|
|
|
|
|
|
|
|
|
|
|
|
|
|
|
|
|
|
|
|
|
|
|
|
|
|
|
|
|
|
|
|
|
|
|
|
|
|
|
|
|
|
|
spaces/1phancelerku/anime-remove-background/Download ARK Survival Evolved APK OBB - Unlock All Features and Modes.md
DELETED
@@ -1,92 +0,0 @@
|
|
1 |
-
<br />
|
2 |
-
<h1>How to Download OBB ARK Survival Evolved</h1>
|
3 |
-
<p>ARK Survival Evolved is one of the most popular and immersive action-adventure survival games on the market. It allows you to explore, craft, tame, and fight in a vast open world full of dinosaurs and other creatures. However, if you want to enjoy the full experience of this game on your Android device, you will need to download and install the OBB files along with the APK file. In this article, we will explain what OBB files are, why you need them, and how to install them on your Android device.</p>
|
4 |
-
<h2>What is ARK Survival Evolved?</h2>
|
5 |
-
<h3>Features and gameplay</h3>
|
6 |
-
<p>ARK Survival Evolved is a game that combines prehistoric and modern concepts for players to survive and explore an endless land. You can choose to play solo or with other players online, and use your cunning to kill or tame the primeval creatures roaming the land. You can also build shelters, craft items, grow crops, research technologies, and customize your character. The game features a dynamic day-night cycle, weather system, and realistic physics. You can also experience different maps and modes, such as Ragnarok, Valguero, Genesis, and more.</p>
|
7 |
-
<h2>download obb ark survival evolved</h2><br /><p><b><b>Download Zip</b> === <a href="https://jinyurl.com/2uNPC8">https://jinyurl.com/2uNPC8</a></b></p><br /><br />
|
8 |
-
<h3>Download options and requirements</h3>
|
9 |
-
<p>ARK Survival Evolved is available for download on various platforms, such as PC, Xbox One, PlayStation 4, Nintendo Switch, iOS, and Android. However, the game is not free to play, and you will need to purchase it from the official store or website of your platform. For Android devices, you can buy the game from the Google Play Store for $19.99. The game requires Android 7.0 or higher, and at least 2 GB of RAM and 2 GB of storage space. You will also need an additional 2 GB of storage space for the OBB files.</p>
|
10 |
-
<h2>What are OBB files and why do you need them?</h2>
|
11 |
-
<h3>The difference between APK and OBB files</h3>
|
12 |
-
<p>An APK file is the main file format for installing applications on Android devices. It contains all the necessary code, resources, and metadata for the app to function properly. However, some apps may require additional data that is not stored in the APK file, such as graphics, sound, or video. These data are stored in OBB files, which are expansion files that complement the APK file. OBB files usually have a larger size than APK files, and they are stored in a separate folder on your device.</p>
|
13 |
-
<h3>The benefits of OBB files for ARK Survival Evolved</h3>
|
14 |
-
<p>As you can imagine, ARK Survival Evolved is a game that has a lot of data that cannot be compressed into a single APK file. The game has high-quality graphics, sound effects, music, animations, and more that enhance the gameplay experience. Therefore, you will need to download the OBB files along with the APK file to enjoy the full features of the game. The OBB files will also allow you to update the game without having to download the entire APK file again.</p>
|
15 |
-
<h2>How to install OBB files on Android devices</h2>
|
16 |
-
<h3>Step 1: Allow unknown sources</h3>
|
17 |
-
<p>Before you can install any APK or OBB file on your Android device, you need to enable the option to allow unknown sources. This option allows you to install apps from sources other than the Google Play Store. To do this, go to Settings > Security > Unknown Sources and toggle it on. You may also need to grant permission to specific apps that you use to download or install APK or OBB files.</p>
|
18 |
-
<h3>Step <h3>Step 2: Download the OBB files from a reliable source</h3>
|
19 |
-
<p>Once you have enabled the unknown sources option, you can proceed to download the OBB files for ARK Survival Evolved. You can find many websites that offer the OBB files for free, but you need to be careful and choose a reliable and safe source. Some websites may contain malware, viruses, or fake files that can harm your device or compromise your data. Therefore, we recommend you to use a trusted and verified website, such as [APKPure] or [APKMody]. These websites provide the latest and original OBB files for ARK Survival Evolved, as well as other popular games and apps.</p>
|
20 |
-
<h3>Step 3: Extract and copy the OBB folder to the destination path</h3>
|
21 |
-
<p>After you have downloaded the OBB files, you will need to extract and copy them to the correct location on your device. The OBB files are usually compressed in a ZIP or RAR format, so you will need a file manager app that can extract them, such as [ZArchiver] or [RAR]. To extract and copy the OBB files, follow these steps:</p>
|
22 |
-
<ul>
|
23 |
-
<li>Open the file manager app and locate the downloaded OBB files.</li>
|
24 |
-
<li>Select the OBB files and tap on Extract.</li>
|
25 |
-
<li>Choose a destination folder where you want to extract the files. You can create a new folder or use an existing one.</li>
|
26 |
-
<li>Wait for the extraction process to finish.</li>
|
27 |
-
<li>After the extraction is done, you will see a folder named com.studiowildcard.wardrumstudios.ark. This is the OBB folder for ARK Survival Evolved.</li>
|
28 |
-
<li>Copy this folder and paste it to the following path: Internal Storage > Android > obb. If you don't see the obb folder, you can create one.</li>
|
29 |
-
</ul>
|
30 |
-
<h3>Step 4: Launch the game and enjoy</h3>
|
31 |
-
<p>Now that you have installed the OBB files, you can launch the game and enjoy it on your Android device. To do this, simply tap on the ARK Survival Evolved icon on your home screen or app drawer. The game will verify the OBB files and load the data. You may need to grant some permissions to the game, such as storage access, location access, etc. Once the game is loaded, you can start playing and exploring the world of ARK Survival Evolved.</p>
|
32 |
-
<h2>Conclusion</h2>
|
33 |
-
<p>In this article, we have shown you how to download and install the OBB files for ARK Survival Evolved on your Android device. By following these simple steps, you can enjoy the full features and graphics of this amazing game. We hope you found this article helpful and informative. If you have any questions or feedback, please feel free to leave a comment below.</p>
|
34 |
-
<p>download obb ark survival evolved apk<br />
|
35 |
-
download obb ark survival evolved mod<br />
|
36 |
-
download obb ark survival evolved android<br />
|
37 |
-
download obb ark survival evolved latest version<br />
|
38 |
-
download obb ark survival evolved free<br />
|
39 |
-
download obb ark survival evolved unlimited amber<br />
|
40 |
-
download obb ark survival evolved offline<br />
|
41 |
-
download obb ark survival evolved xapk<br />
|
42 |
-
download obb ark survival evolved apkcombo<br />
|
43 |
-
download obb ark survival evolved apk pure<br />
|
44 |
-
download obb ark survival evolved apk mod<br />
|
45 |
-
download obb ark survival evolved apk data<br />
|
46 |
-
download obb ark survival evolved apk + data<br />
|
47 |
-
download obb ark survival evolved apk + obb<br />
|
48 |
-
download obb ark survival evolved apk + mod<br />
|
49 |
-
download obb ark survival evolved apk + unlimited amber<br />
|
50 |
-
download obb ark survival evolved apk + offline<br />
|
51 |
-
download obb ark survival evolved apk + xapk<br />
|
52 |
-
download obb ark survival evolved apk + apkcombo<br />
|
53 |
-
download obb ark survival evolved apk + apk pure<br />
|
54 |
-
download obb ark survival evolved mod apk<br />
|
55 |
-
download obb ark survival evolved mod apk + data<br />
|
56 |
-
download obb ark survival evolved mod apk + obb<br />
|
57 |
-
download obb ark survival evolved mod apk + unlimited amber<br />
|
58 |
-
download obb ark survival evolved mod apk + offline<br />
|
59 |
-
download obb ark survival evolved mod apk + xapk<br />
|
60 |
-
download obb ark survival evolved mod apk + apkcombo<br />
|
61 |
-
download obb ark survival evolved mod apk + apk pure<br />
|
62 |
-
download obb ark survival evolved android apk<br />
|
63 |
-
download obb ark survival evolved android mod<br />
|
64 |
-
download obb ark survival evolved android data<br />
|
65 |
-
download obb ark survival evolved android xapk<br />
|
66 |
-
download obb ark survival evolved android offline<br />
|
67 |
-
download obb ark survival evolved android unlimited amber<br />
|
68 |
-
download obb ark survival evolved latest version apk<br />
|
69 |
-
download obb ark survival evolved latest version mod<br />
|
70 |
-
download obb ark survival evolved latest version data<br />
|
71 |
-
download obb ark survival evolved latest version xapk<br />
|
72 |
-
download obb ark survival evolved latest version offline<br />
|
73 |
-
download obb ark survival evolved latest version unlimited amber<br />
|
74 |
-
download obb ark survival evolved free apk<br />
|
75 |
-
download obb ark survival evolved free mod<br />
|
76 |
-
download obb ark survival evolved free data<br />
|
77 |
-
download obb ark survival evolved free xapk<br />
|
78 |
-
download obb ark survival evolved free offline<br />
|
79 |
-
download obb ark survival evolved free unlimited amber</p>
|
80 |
-
<h2>FAQs</h2>
|
81 |
-
<h4>What is the size of the OBB files for ARK Survival Evolved?</h4>
|
82 |
-
<p>The size of the OBB files for ARK Survival Evolved may vary depending on the version and update of the game. However, as of June 2023, the latest version of the game (v2.0.25) has an OBB file size of about 2 GB.</p>
|
83 |
-
<h4>Can I play ARK Survival Evolved offline?</h4>
|
84 |
-
<p>No, you cannot play ARK Survival Evolved offline. The game requires an internet connection to run and access its online features, such as multiplayer mode, cloud save, etc.</p>
|
85 |
-
<h4>Can I transfer my progress from one device to another?</h4>
|
86 |
-
<p>Yes, you can transfer your progress from one device to another by using your Google Play account. To do this, you need to sign in with your Google Play account on both devices and enable cloud save in the game settings. Then, you can sync your progress across devices.</p>
|
87 |
-
<h4>How can I update ARK Survival Evolved?</h4>
|
88 |
-
<p>To update ARK Survival Evolved, you need to download and install the latest APK and OBB files from a reliable source. You can also check for updates from within the game settings or from the Google Play Store.</p>
|
89 |
-
<h4>How can I get more resources and items in ARK Survival Evolved?</h4>
|
90 |
-
<p>You can get more resources and items in ARK Survival Evolved by exploring, harvesting, crafting, trading, or buying them with real money. You can also use cheats or mods to get unlimited resources and items, but this may affect your gameplay experience or cause errors.</p> 401be4b1e0<br />
|
91 |
-
<br />
|
92 |
-
<br />
|
|
|
|
|
|
|
|
|
|
|
|
|
|
|
|
|
|
|
|
|
|
|
|
|
|
|
|
|
|
|
|
|
|
|
|
|
|
|
|
|
|
|
|
|
|
|
|
|
|
|
|
|
|
|
|
|
|
|
|
|
|
|
|
|
|
|
|
|
|
|
|
|
|
|
|
|
|
|
|
|
|
|
|
|
|
|
|
|
|
|
|
|
|
|
|
|
|
|
|
|
|
|
|
|
|
|
|
|
|
|
|
|
|
|
|
|
|
|
|
|
|
|
|
|
|
|
|
|
|
|
|
|
|
|
|
|
|
|
|
|
|
|
|
|
|
|
|
|
|
|
|
|
|
|
|
|
|
|
|
|
|
|
|
|
|
|
|
|
|
|
|
|
|
|
|
|
|
|
|
|
|
|
|
|
|
spaces/1phancelerku/anime-remove-background/Download Baby Panda World Kids Games APK for Android - Free Educational App.md
DELETED
@@ -1,176 +0,0 @@
|
|
1 |
-
<br />
|
2 |
-
<h1>Baby Panda World: A Fun and Educational Game for Kids</h1>
|
3 |
-
<p>Do you want to give your kids a fun and educational game that they can play on their Android devices? If yes, then you should check out Baby Panda World, a game developed by BabyBus that offers 130+ popular activities for kids in one app. In this article, we will tell you everything you need to know about Baby Panda World, including what it is, how to download and install it, how to play it, and why you should play it. We will also share some reviews and ratings from other users and parents who have tried the game. So, let's get started!</p>
|
4 |
-
<h2>baby panda world download apk</h2><br /><p><b><b>Download</b> ⚙⚙⚙ <a href="https://jinyurl.com/2uNPRD">https://jinyurl.com/2uNPRD</a></b></p><br /><br />
|
5 |
-
<h2>What is Baby Panda World?</h2>
|
6 |
-
<p>Baby Panda World is an educational game that allows kids to explore the world and create their own story. They can choose from different scenes, such as the supermarket, the hospital, the farm, the airport, the amusement park, and more. They can also play with popular BabyBus characters, such as Kiki, Miumiu, Whiskers, Hank, Rudolph, and more. They can interact with various objects and characters in each scene, such as buying groceries, taking care of animals, flying a plane, riding a roller coaster, and more. They can also learn about 8 major fields of knowledge: science, painting, music, math, language, emotional intelligence, health, and society.</p>
|
7 |
-
<h3>Features of Baby Panda World</h3>
|
8 |
-
<h4>Explore the world and create your own story</h4>
|
9 |
-
<p>Baby Panda World gives kids the freedom to explore the world and create their own story. They can choose from different scenes that represent different aspects of life. They can also switch between scenes anytime they want. They can use their imagination and creativity to make their own story in each scene. For example, they can pretend to be a doctor in the hospital, a farmer in the farm, a pilot in the airport, or a customer in the supermarket. They can also make up their own dialogues and scenarios with the characters they meet.</p>
|
10 |
-
<h4>Learn about 8 major fields of knowledge</h4>
|
11 |
-
<p>Baby Panda World also helps kids learn about 8 major fields of knowledge: science, painting, music, math, language, emotional intelligence, health, and society. Each scene has different activities that teach kids something new and interesting. For example, they can learn about gravity in the science museum, colors in the painting studio, instruments in the music room, numbers in the math classroom, words in the library, emotions in the kindergarten, hygiene in the bathroom, and manners in the restaurant. They can also take quizzes and challenges to test their knowledge.</p>
|
12 |
-
<p>baby panda world apk free download<br />
|
13 |
-
download baby panda world kids games<br />
|
14 |
-
baby panda world android game download<br />
|
15 |
-
baby panda world app download for pc<br />
|
16 |
-
baby panda world mod apk download<br />
|
17 |
-
how to download baby panda world on tablet<br />
|
18 |
-
baby panda world latest version apk download<br />
|
19 |
-
baby panda world offline game download<br />
|
20 |
-
baby panda world full apk download<br />
|
21 |
-
baby panda world hack apk download<br />
|
22 |
-
baby panda world game download for laptop<br />
|
23 |
-
baby panda world unlimited coins apk download<br />
|
24 |
-
baby panda world online game download<br />
|
25 |
-
baby panda world app store download<br />
|
26 |
-
baby panda world premium apk download<br />
|
27 |
-
baby panda world game download for windows 10<br />
|
28 |
-
baby panda world no ads apk download<br />
|
29 |
-
baby panda world play store download<br />
|
30 |
-
baby panda world pro apk download<br />
|
31 |
-
baby panda world game download for mac<br />
|
32 |
-
baby panda world unlocked apk download<br />
|
33 |
-
baby panda world game download for android tv<br />
|
34 |
-
baby panda world paid apk download<br />
|
35 |
-
baby panda world game free download for pc<br />
|
36 |
-
baby panda world cracked apk download<br />
|
37 |
-
baby panda world game download for ios<br />
|
38 |
-
baby panda world vip apk download<br />
|
39 |
-
baby panda world game free download for mobile<br />
|
40 |
-
baby panda world patched apk download<br />
|
41 |
-
baby panda world game install and download<br />
|
42 |
-
baby panda world ad free apk download<br />
|
43 |
-
baby panda world game free download for tablet<br />
|
44 |
-
baby panda world modded apk download<br />
|
45 |
-
baby panda world game free online no download<br />
|
46 |
-
baby panda world all games unlocked apk download<br />
|
47 |
-
baby panda world game free download for laptop<br />
|
48 |
-
baby panda world cheat apk download<br />
|
49 |
-
baby panda world game free play without downloading<br />
|
50 |
-
baby panda world all access apk download<br />
|
51 |
-
baby panda world game free to play and download</p>
|
52 |
-
<h4>Play with popular BabyBus characters</h4>
|
53 |
-
<p>Baby Panda World also features popular BabyBus characters that kids love. They can play with Kiki, Miumiu, Whiskers, Hank, Rudolph, and more. They can also dress them up with different outfits and accessories. They can also interact with them in different ways. For example, they can hug them, tickle them, feed them, play games with them, or take photos with them. They can also hear them talk and sing in different languages, such as English, Chinese, Japanese, Korean, and more.</p>
|
54 |
-
<h3>How to download Baby Panda World APK?</h3>
|
55 |
-
<p>Baby Panda World is available for free on Google Play Store, but if you want to download the APK file for some reason, you can do so from other sources. Here are some of the websites where you can download Baby Panda World APK:</p>
|
56 |
-
<h4>Download from Google Play Store</h4>
|
57 |
-
<p>The easiest and safest way to download Baby Panda World APK is from Google Play Store. You just need to follow these steps:</p>
|
58 |
-
<ol>
|
59 |
-
<li>Open Google Play Store on your Android device.</li>
|
60 |
-
<li>Search for Baby Panda World or click <a href="">here</a>.</li>
|
61 |
-
<li>Tap on the Install button and wait for the download to finish.</li>
|
62 |
-
<li>Enjoy playing Baby Panda World!</li>
|
63 |
-
</ol>
|
64 |
-
<h4>Download from AppBrain</h4>
|
65 |
-
<p>Another option to download Baby Panda World APK is from AppBrain, a website that offers free and paid apps for Android. You just need to follow these steps:</p>
|
66 |
-
<ol>
|
67 |
-
<li>Open AppBrain on your browser or click <a href="">here</a>.</li>
|
68 |
-
<li>Search for Baby Panda World or click <a href="">here</a>.</li>
|
69 |
-
<li>Tap on the Download button and wait for the download to start.</li>
|
70 |
-
<li>Save the APK file on your device.</li>
|
71 |
-
</ol>
|
72 |
-
<h4>Download from APKCombo</h4>
|
73 |
-
<p>A third option to download Baby Panda World APK is from APKCombo, a website that offers APK files for various apps and games. You just need to follow these steps:</p>
|
74 |
-
<ol>
|
75 |
-
<li>Open APKCombo on your browser or click <a href="">here</a>.</li>
|
76 |
-
<li>Search for Baby Panda World or click <a href="">here</a>.</li>
|
77 |
-
<li>Select the version and variant of the app that you want to download.</li>
|
78 |
-
<li>Tap on the Download button and wait for the download to start.</li>
|
79 |
-
<li>Save the APK file on your device.</li>
|
80 |
-
</ol>
|
81 |
-
<h3>How to install Baby Panda World APK?</h3>
|
82 |
-
<p>After downloading the APK file of Baby Panda World, you need to install it on your device. You just need to follow these steps:</p>
|
83 |
-
<h4>Enable unknown sources</h4>
|
84 |
-
<p>Before installing any APK file, you need to enable unknown sources on your device. This will allow you to install apps from sources other than Google Play Store. To do this, you need to:</p>
|
85 |
-
<ol>
|
86 |
-
<li>Go to Settings on your device.</li>
|
87 |
-
<li>Select Security or Privacy or Applications (depending on your device).</li>
|
88 |
-
<li>Find and toggle on Unknown Sources or Allow Installation of Apps from Unknown Sources (depending on your device).</li>
|
89 |
-
</ol>
|
90 |
-
<h4>Locate the downloaded file</h4>
|
91 |
-
<p>After enabling unknown sources, you need to locate the downloaded file of Baby Panda World APK. To do this, you need to:</p>
|
92 |
-
<ol>
|
93 |
-
<li>Go to File Manager or Downloads or My Files (depending on your device).</li>
|
94 |
-
<li>Find and tap on the APK file of Baby Panda World.</li>
|
95 |
-
</ol>
|
96 |
-
<h4>Tap to install and launch the app</h4>
|
97 |
-
<p>After locating the file, you need to tap on it to install and launch the app. To do this, you need to:</p>
|
98 |
-
<ol>
|
99 |
-
<li>Tap on the Install button and wait for the installation to finish.</li>
|
100 |
-
<li>Tap on the Open button or find and tap on the app icon on your home screen or app drawer.</li>
|
101 |
-
<li>Enjoy playing Baby Panda World!</li>
|
102 |
-
</ol>
|
103 |
-
<h3>How to play Baby Panda World?</h3>
|
104 |
-
<p>Baby Panda World is very easy and fun to play. You just need to follow these steps:</p>
|
105 |
-
<h4>Choose a scene and a character</h4>
|
106 |
-
<p>The first thing you need to do is choose a scene and a character that you want to play with. To do this, you need to:</p>
|
107 |
-
<ol>
|
108 |
-
<li>Swipe left or right on the screen to see different scenes.</li>
|
109 |
-
<li>Tap on the scene that you want to enter.</li>
|
110 |
-
<li>Select a character that you want to play with from the bottom of the screen.</li>
|
111 |
-
</ol>
|
112 |
-
<h4>Interact with objects and characters</h4>
|
113 |
-
<p>The next thing you need to do is interact with objects and characters in each scene. To do this, you need to:</p>
|
114 |
-
<ol>
|
115 |
-
<li>Tap or drag on any object or character that you see on the screen.</li>
|
116 |
-
<li>See what happens when you interact with them. For example, you can buy food, cook meals, play instruments, solve puzzles, and more.</li>
|
117 |
-
<li>Listen to the sounds and voices that accompany each interaction. For example, you can hear the cashier say the price, the animals make noises, the music play, and more.</li>
|
118 |
-
</ol>
|
119 |
-
<h4>Collect coins and unlock more content</h4>
|
120 |
-
<p>The last thing you need to do is collect coins and unlock more content in the game. To do this, you need to:</p>
|
121 |
-
<ol>
|
122 |
-
<li>Complete tasks and challenges in each scene. For example, you can help the doctor treat the patients, help the farmer harvest the crops, help the pilot land the plane, and more.</li>
|
123 |
-
<li>Earn coins for each task and challenge that you complete.</li>
|
124 |
-
<li>Use the coins to unlock more scenes, characters, outfits, and accessories in the game.</li>
|
125 |
-
</ol>
|
126 |
-
<h2>Why should you play Baby Panda World?</h2>
|
127 |
-
<p>Baby Panda World is not only a fun and entertaining game, but also a beneficial and educational one. Here are some of the reasons why you should play Baby Panda World:</p>
|
128 |
-
<h3>Benefits of playing Baby Panda World</h3>
|
129 |
-
<h4>Stimulate creativity and imagination</h4>
|
130 |
-
<p>Baby Panda World stimulates kids' creativity and imagination by allowing them to create their own story in each scene. They can use their imagination to make up their own dialogues and scenarios with the characters they meet. They can also use their creativity to dress up their characters with different outfits and accessories. They can also express their artistic skills by painting, drawing, or coloring in the game.</p>
|
131 |
-
<h4>Develop cognitive and social skills</h4>
|
132 |
-
<p>Baby Panda World develops kids' cognitive and social skills by teaching them about 8 major fields of knowledge. They can learn about science, painting, music, math, language, emotional intelligence, health, and society in a fun and interactive way. They can also develop their memory, logic, problem-solving, and critical thinking skills by taking quizzes and challenges in the game. They can also develop their communication, cooperation, and empathy skills by interacting with different characters in the game.</p>
|
133 |
-
<h4>Enhance curiosity and interest in learning</h4>
|
134 |
-
<p>Baby Panda World enhances kids' curiosity and interest in learning by exposing them to different aspects of life. They can explore different scenes that represent different environments and situations. They can also learn new facts and information about various topics and subjects. They can also discover new things and experiences by trying out different activities and games in the game.</p>
|
135 |
-
<h3>Reviews and ratings of Baby Panda World</h3>
|
136 |
-
<h4>Positive feedback from users and parents</h4>
|
137 |
-
<p>Baby Panda World has received positive feedback from users and parents who have tried the game. Here are some of the comments that they have left on Google Play Store:</p>
|
138 |
-
<ul>
|
139 |
-
<li>"My kids love this game so much. They play it every day. They learn a lot from it. They have fun with it. It's a great game for kids."</li>
|
140 |
-
<li>"This is the best game ever. It has so many scenes and activities. It's like a mini world for kids. It's very educational and entertaining."</li>
|
141 |
-
<li>"I highly recommend this game for kids. It's very creative and interactive. It's very easy to use and understand. It's very cute and colorful."</li>
|
142 |
-
</ul>
|
143 |
-
<h4>High ratings and rankings on app stores</h4>
|
144 |
-
<p>Baby Panda World has also received high ratings and rankings on app stores. Here are some of the statistics that show its popularity:</p>
|
145 |
-
<table>
|
146 |
-
<tr><th>App Store</th><th>Rating</th><th>Ranking</th></tr>
|
147 |
-
<tr><td>Google Play Store</td><td>4.5 out of 5 stars</td><td>#1 in Educational Games for Kids</td></tr>
|
148 |
-
<tr><td>AppBrain</td><td>4 out of 5 stars</td><td>#2 in Educational Games for Kids</td></tr>
|
149 |
-
<tr><td>APKCombo</td><td>4 out of 5 stars</td><td>#3 in Educational Games for Kids</td></tr>
|
150 |
-
</table>
|
151 |
-
<h4>Suggestions for improvement and updates</h4>
|
152 |
-
<p>Baby Panda World is not perfect, however, and there are some suggestions for improvement and updates from users and parents who have tried the game. Here are some of the issues that they have raised on Google Play Store:</p>
|
153 |
-
<ul>
|
154 |
-
<li>"The game is good but it needs more scenes and characters. It gets boring after playing for a while."</li>
|
155 |
-
<li>"The game is nice but it has too many ads. It's annoying when they pop up every few minutes."</li>
|
156 |
-
<li>"The game is fun but it has some bugs and glitches. It sometimes crashes or freezes."</li>
|
157 |
-
</ul>
|
158 |
-
<p>We hope that the developers of Baby Panda World will address these issues and provide more updates and improvements to the game in the future.</p>
|
159 |
-
<h2>Conclusion</h2>
|
160 |
-
<p>Baby Panda World is a fun and educational game for kids that allows them to explore the world and create their own story. They can play with popular BabyBus characters, interact with various objects and characters, learn about 8 major fields of knowledge, and collect coins and unlock more content. The game has received positive feedback from users and parents, as well as high ratings and rankings on app stores. However, the game also has some room for improvement and updates, such as adding more scenes and characters, reducing ads, and fixing bugs and glitches. Overall, Baby Panda World is a great game for kids that we recommend you to try.</p>
|
161 |
-
<h3>FAQs</h3>
|
162 |
-
<p>Here are some of the frequently asked questions about Baby Panda World:</p>
|
163 |
-
<ul>
|
164 |
-
<li><b>Q: Is Baby Panda World free?</b></li>
|
165 |
-
<li>A: Yes, Baby Panda World is free to download and play. However, it contains ads and in-app purchases that you can disable or buy if you want.</li>
|
166 |
-
<li><b>Q: Is Baby Panda World safe for kids?</b></li>
|
167 |
-
<li>A: Yes, Baby Panda World is safe for kids. It does not contain any violence, gore, or inappropriate content. It also does not collect any personal information from the users.</li>
|
168 |
-
<li><b>Q: What are the age requirements for Baby Panda World?</b></li>
|
169 |
-
<li>A: Baby Panda World is suitable for kids of all ages, but it is especially designed for kids aged 3 to 8 years old.</li>
|
170 |
-
<li><b>Q: What are the device requirements for Baby Panda World?</b></li>
|
171 |
-
<li>A: Baby Panda World requires Android 4.4 or higher to run smoothly. It also requires at least 300 MB of free storage space on your device.</li>
|
172 |
-
<li><b>Q: How can I contact the developers of Baby Panda World?</b></li>
|
173 |
-
<li>A: You can contact the developers of Baby Panda World by sending an email to <a href="mailto:[email protected]">[email protected]</a> or by visiting their website at <a href="http://www.babybus.com">www.babybus.com</a>.</li>
|
174 |
-
</ul></p> 401be4b1e0<br />
|
175 |
-
<br />
|
176 |
-
<br />
|
|
|
|
|
|
|
|
|
|
|
|
|
|
|
|
|
|
|
|
|
|
|
|
|
|
|
|
|
|
|
|
|
|
|
|
|
|
|
|
|
|
|
|
|
|
|
|
|
|
|
|
|
|
|
|
|
|
|
|
|
|
|
|
|
|
|
|
|
|
|
|
|
|
|
|
|
|
|
|
|
|
|
|
|
|
|
|
|
|
|
|
|
|
|
|
|
|
|
|
|
|
|
|
|
|
|
|
|
|
|
|
|
|
|
|
|
|
|
|
|
|
|
|
|
|
|
|
|
|
|
|
|
|
|
|
|
|
|
|
|
|
|
|
|
|
|
|
|
|
|
|
|
|
|
|
|
|
|
|
|
|
|
|
|
|
|
|
|
|
|
|
|
|
|
|
|
|
|
|
|
|
|
|
|
|
|
|
|
|
|
|
|
|
|
|
|
|
|
|
|
|
|
|
|
|
|
|
|
|
|
|
|
|
|
|
|
|
|
|
|
|
|
|
|
|
|
|
|
|
|
|
|
|
|
|
|
|
|
|
|
|
|
|
|
|
|
|
|
|
|
|
|
|
|
|
|
|
|
|
|
|
|
|
|
|
|
|
|
|
|
|
|
|
|
|
|
|
|
|
|
|
|
|
|
|
|
|
|
|
|
|
|
|
|
|
|
|
|
|
|
|
|
|
|
|
|
|
|
|
|
|
|
|
|
|
|
|
|
|
|
|
|
|
|
|
|
|
|
|
|
|
|
|
|
|
|
|
|
|
|
|
|
|
|
|
|
|
|
|
|
|
|
|
spaces/1phancelerku/anime-remove-background/Download Go 1.19 and Join the Growing Community of Go Developers.md
DELETED
@@ -1,124 +0,0 @@
|
|
1 |
-
<br />
|
2 |
-
<h1>How to Download Go 1.19</h1>
|
3 |
-
<p>Go is a popular programming language that is designed for simplicity, concurrency, and performance. It is widely used for developing web applications, microservices, command-line tools, and more.</p>
|
4 |
-
<p>In this article, you will learn how to download and install Go 1.19, the latest version of Go as of August 2022. You will also learn about some of the new features, benefits, and improvements that Go 1.19 offers compared to previous versions.</p>
|
5 |
-
<h2>download go 1.19</h2><br /><p><b><b>Download</b> » <a href="https://jinyurl.com/2uNLFA">https://jinyurl.com/2uNLFA</a></b></p><br /><br />
|
6 |
-
<h2>Prerequisites for Installing Go 1.19</h2>
|
7 |
-
<p>Before you download and install Go 1.19, you need to make sure that your system meets the following requirements:</p>
|
8 |
-
<ul>
|
9 |
-
<li>You have an active internet connection to download the Go binary or source file.</li>
|
10 |
-
<li>You have enough disk space to store the Go installation files (about 100 MB).</li>
|
11 |
-
<li>You have a supported operating system and processor architecture. Go 1.19 supports Windows, macOS, Linux, FreeBSD, and other Unix-like systems on x86-64, ARM64, ARMv6, PPC64LE, S390X, LoongArch64, and RISC-V architectures.</li>
|
12 |
-
</ul>
|
13 |
-
<h2>Downloading Go 1.19 from the Official Website</h2>
|
14 |
-
<p>The easiest way to download Go 1.19 is from the official website at <a href="(^4^)">https://go.dev/doc/install</a>. Here you can find the download links for different operating systems and architectures.</p>
|
15 |
-
<h3>Choosing the Right File for Your System</h3>
|
16 |
-
<p>The file name and kind of the Go installation file depend on your operating system and processor architecture. For example, if you are using Windows on a x86-64 machine, you can choose either go1.20.4.windows-amd64.zip (archive file) or go1.20.4.windows-amd64.msi (installer file). If you are using Linux on a ARM64 machine, you can choose go1.20.4.linux-arm64.tar.gz (archive file).</p>
|
17 |
-
<p>You can find the full list of file names and kinds for different systems in <a href="(^5^)">https://go.dev/dl/</a>. You can also use the featured downloads section to quickly select the most common options.</p>
|
18 |
-
<h3>Extracting and Installing Go 1.19</h3>
|
19 |
-
<p>The steps for extracting and installing Go 1.19 vary depending on your operating system and file type.</p>
|
20 |
-
<p>How to download and install go 1.19 on Linux<br />
|
21 |
-
Go 1.19 release notes and new features<br />
|
22 |
-
Download go 1.19 for Windows 7 or later<br />
|
23 |
-
Go 1.19 generics and doc comments examples<br />
|
24 |
-
Download go 1.19 for macOS ARM64<br />
|
25 |
-
Go 1.19 memory model and sync/atomic package<br />
|
26 |
-
Download go 1.19 source code and build from scratch<br />
|
27 |
-
Go 1.19 performance and implementation improvements<br />
|
28 |
-
Download go 1.19 for RISC-V architecture<br />
|
29 |
-
Go 1.19 garbage collector and soft memory limit<br />
|
30 |
-
Download go 1.19 for Loongson LoongArch architecture<br />
|
31 |
-
Go 1.19 feedback and bug reports<br />
|
32 |
-
Download go 1.19 beta and release candidates<br />
|
33 |
-
Go 1.19 tutorial and getting started guide<br />
|
34 |
-
Download go 1.19 for Android and iOS devices<br />
|
35 |
-
Go 1.19 modules and checksum database<br />
|
36 |
-
Download go 1.19 for Docker and Kubernetes<br />
|
37 |
-
Go 1.19 testing and benchmarking tools<br />
|
38 |
-
Download go 1.19 for web development and frameworks<br />
|
39 |
-
Go 1.19 debugging and profiling tools<br />
|
40 |
-
Download go 1.19 for data science and machine learning<br />
|
41 |
-
Go 1.19 concurrency and parallelism features<br />
|
42 |
-
Download go 1.19 for blockchain and cryptocurrency development<br />
|
43 |
-
Go 1.19 standard library and packages<br />
|
44 |
-
Download go 1.19 for game development and graphics<br />
|
45 |
-
Go 1.19 best practices and style guide<br />
|
46 |
-
Download go 1.19 for cloud computing and serverless functions<br />
|
47 |
-
Go 1.19 security and cryptography features<br />
|
48 |
-
Download go 1.19 for embedded systems and IoT devices<br />
|
49 |
-
Go 1.19 error handling and logging features<br />
|
50 |
-
Download go 1.19 for desktop applications and GUI frameworks<br />
|
51 |
-
Go 1.19 networking and HTTP features<br />
|
52 |
-
Download go 1.19 for microservices and RESTful APIs<br />
|
53 |
-
Go 1.19 database and SQL features<br />
|
54 |
-
Download go 1.19 for command-line tools and scripts<br />
|
55 |
-
Go 1.19 text processing and regular expressions features<br />
|
56 |
-
Download go 1.19 for artificial intelligence and natural language processing<br />
|
57 |
-
Go 1.19 file system and OS features<br />
|
58 |
-
Download go 1.19 for image processing and computer vision<br />
|
59 |
-
Go 1.19 reflection and code generation features</p>
|
60 |
-
<p>If you are using Windows and downloaded an installer file (.msi), you can simply double-click on it and follow the instructions on the screen.</p>
|
61 |
-
<p>If you are using Windows and downloaded an archive file (.zip), you can extract it to any folder you want (for example, C:\Go) using a tool like WinZip or 7-Zip. Then you need to add C:\Go\bin (or whatever folder you chose) to your PATH environment variable.</p>
|
62 |
-
<p>If you are using macOS and downloaded an installer file (.pkg), you can double-click on it and follow the instructions on the screen.</p>
|
63 |
-
<p>If you are If you are using macOS and downloaded an archive file (.tar.gz), you can extract it to /usr/local/go using the following command in a terminal: sudo tar -C /usr/local -xzf go1.20.4.darwin-amd64.tar.gz Then you need to add /usr/local/go/bin to your PATH environment variable. If you are using Linux or another Unix-like system and downloaded an archive file (.tar.gz), you can extract it to /usr/local/go using the following command in a terminal: sudo tar -C /usr/local -xzf go1.20.4.linux-amd64.tar.gz Then you need to add /usr/local/go/bin to your PATH environment variable. <h2>Verifying the Installation of Go 1.19</h2>
|
64 |
-
<p>After you have extracted and installed Go 1.19, you can verify that it is working properly by checking the version and path of Go using the go command.</p>
|
65 |
-
<p>Open a terminal or command prompt and type: go version You should see something like: go version go1.20.4 linux/amd64 This means that you have successfully installed Go 1.19 on your system.</p>
|
66 |
-
<p>You can also check the path of Go by typing: go env GOROOT You should see something like: /usr/local/go This means that Go is installed in /usr/local/go on your system.</p>
|
67 |
-
<h2>Writing Your First Go Program with Go 1.19</h2>
|
68 |
-
<p>Now that you have downloaded and installed Go 1.19, you can start writing your first Go program with it. In this section, you will learn how to create a simple Hello World program that prints "Hello, world!" to the standard output.</p>
|
69 |
-
<h3>Creating a Hello World Program</h3>
|
70 |
-
<p>To create a Hello World program in Go, you need to do the following steps:</p>
|
71 |
-
<ol>
|
72 |
-
<li>Create a folder for your project (for example, hello) and change into it.</li>
|
73 |
-
<li>Create a file named main.go with the following content: <pre><code>package main import "fmt" func main() fmt.Println("Hello, world!") </code></pre>
|
74 |
-
This is the simplest Go program that consists of a main package, an import statement, and a main function. The fmt package provides formatting and printing functions, and the Println function prints a line of text to the standard output.</li>
|
75 |
-
<li>Save the file and close it.</li>
|
76 |
-
</ol>
|
77 |
-
<h3>Running and Building Your Program</h3>
|
78 |
-
<p>To run your Hello World program, you can use the go run command in a terminal or command prompt:</p>
|
79 |
-
go run main.go You should see something like: Hello, world! This means that your program has executed successfully. To build your Hello World program, you can use the go build command in a terminal or command prompt: go build main.go This will create an executable file named main (or main.exe on Windows) in the same folder as your source file. You can run this file by typing: ./main or main.exe You should see the same output as before. <h2>Conclusion</h2>
|
80 |
-
<p>In this article, you have learned how to download and install Go 1.19, the latest version of Go as of August 2022. You have also learned about some of the new features, benefits, and improvements that Go 1.19 offers compared to previous versions. Finally, you have written your first Go program with Go 1.19 and learned how to run and build it.</p>
|
81 |
-
<p>If you want to learn more about Go and how to use it for various projects, you can check out some of these resources:</p>
|
82 |
-
<ul>
|
83 |
-
<li><a href="">https://go.dev/</a>: The official website of Go that provides documentation, tutorials, blog posts, community links, and more.</li>
|
84 |
-
<li><a href="">https://gobyexample.com/</a>: A website that shows how to use various features of Go by example.</li>
|
85 |
-
<li><a href="">https://tour.golang.org/</a>: An interactive online tour that introduces you to the basics of Go.</li>
|
86 |
-
<li><a href="">https://golang.org/doc/effective_go.html</a>: A guide that shows how to write clear and idiomatic Go code.</li>
|
87 |
-
<li><a href="">https://golang.org/ref/spec</a>: The official specification of the Go language.</li>
|
88 |
-
</ul>
|
89 |
-
<h2>FAQs</h2>
|
90 |
-
<p>Here are some frequently asked questions about Go 1.19:</p>
|
91 |
-
<h4>What are some of the new features in Go 1.19?</h4>
|
92 |
-
<p>Some of the new features in Go 1.19 href="">https://go.dev/doc/install</a>.</li>
|
93 |
-
<li>Extract and install Go 1.19 following the instructions for your system as explained in the previous section.</li>
|
94 |
-
<li>Update your PATH environment variable to point to the new Go installation folder.</li>
|
95 |
-
<li>Rebuild any Go packages or programs that you have installed or written using the go install or go build commands.</li>
|
96 |
-
</ol>
|
97 |
-
<p>You can also use the go get command to download and install the latest version of any Go package or module from a remote repository.</p>
|
98 |
-
<h4>How can I use generics in Go 1.19?</h4>
|
99 |
-
<p>To use generics in Go 1.19, you need to do the following steps:</p>
|
100 |
-
<ol>
|
101 |
-
<li>Define a generic parameter using square brackets after the function or type name. For example, to define a generic function that adds two values of any type, you can write: <pre><code>func add[T any](a, b T) T return a + b </code></pre>
|
102 |
-
The [T any] syntax means that T is a generic parameter that can be any type.</li>
|
103 |
-
<li>Optionally, specify a constraint for the generic parameter using the type keyword. A constraint is a type or interface that restricts the possible values of the generic parameter. For example, to define a generic function that compares two values of any comparable type, you can write: <pre><code>func max[T comparable](a, b T) T if a > b return a return b </code></pre>
|
104 |
-
The [T comparable] syntax means that T is a generic parameter that can be any type that supports the comparison operators (such as ==, <, >, etc.). The comparable keyword is a predeclared constraint that represents this set of types.</li>
|
105 |
-
<li>Use type inference to call or instantiate the generic function or type without specifying the generic argument explicitly. For example, to call the add function with two integers, you can write: <pre><code>x := add(1, 2) // x is an int </code></pre>
|
106 |
-
The compiler will infer that T is int based on the arguments passed to the function.</li>
|
107 |
-
<li>Alternatively, use type arguments to call or instantiate the generic function or type with an explicit generic argument. For example, to call the add function with two strings, you can write: <pre><code>y := add[string]("Hello, ", "world!") // y is a string </code></pre>
|
108 |
-
The [string] syntax means that T is string for this call.</li>
|
109 |
-
</ol>
|
110 |
-
<h4>How can I embed interfaces with overlapping method sets in Go 1.19?</h4>
|
111 |
-
<p>To embed interfaces with overlapping method sets in Go 1.19, you need to do the following steps:</p>
|
112 |
-
<ol>
|
113 |
-
<li>Define two or more interfaces that have methods with the same name but different signatures. The signatures must be distinguishable by the number or types of parameters or results. For example, you can define two interfaces that have a Read method with different signatures: <pre><code>type Reader interface Read(p []byte) (int, error) type StringReader interface Read() string </code></pre>
|
114 |
-
The Read methods have different signatures because they have different numbers and types of parameters and results.</li>
|
115 |
-
<li>Define another interface that embeds the interfaces with overlapping methods. For example, you can define an interface that embeds both Reader and StringReader: <pre><code>type ReaderStringer interface Reader StringReader </code></pre>
|
116 |
-
This interface inherits both Read methods from the embedded interfaces.</li>
|
117 |
-
<li>Implement the interface with overlapping methods by providing concrete methods for each signature. For example, you can implement the ReaderStringer interface by defining a struct that has both Read methods: <pre><code>type MyReader struct data string func (r *MyReader) Read(p []byte) (int, error) // copy data to p and return number of bytes and error func (r *MyReader) Read() string // return data as a string </code></pre>
|
118 |
-
This struct satisfies the ReaderStringer interface because it has both Read methods with different signatures.</li>
|
119 |
-
<li>Use type assertions or reflections to access the specific method of the interface with overlapping methods. For example, you can use a type assertion to call the Read method of StringReader on a value of ReaderStringer: <pre><code>var rs ReaderStringer = &MyReader"Hello" s := rs.(StringReader).Read() // s is "Hello" </code></pre>
|
120 |
-
The rs.(StringReader) syntax means that rs is converted to StringReader and then its Read method is called.</li>
|
121 |
-
</ol>
|
122 |
-
<h2></h2></p> 197e85843d<br />
|
123 |
-
<br />
|
124 |
-
<br />
|
|
|
|
|
|
|
|
|
|
|
|
|
|
|
|
|
|
|
|
|
|
|
|
|
|
|
|
|
|
|
|
|
|
|
|
|
|
|
|
|
|
|
|
|
|
|
|
|
|
|
|
|
|
|
|
|
|
|
|
|
|
|
|
|
|
|
|
|
|
|
|
|
|
|
|
|
|
|
|
|
|
|
|
|
|
|
|
|
|
|
|
|
|
|
|
|
|
|
|
|
|
|
|
|
|
|
|
|
|
|
|
|
|
|
|
|
|
|
|
|
|
|
|
|
|
|
|
|
|
|
|
|
|
|
|
|
|
|
|
|
|
|
|
|
|
|
|
|
|
|
|
|
|
|
|
|
|
|
|
|
|
|
|
|
|
|
|
|
|
|
|
|
|
|
|
|
|
|
|
|
|
|
|
|
|
|
|
|
|
|
|
|
|
|
|
|
|
|
|
|
|
|
|
|
|
|
|
|
|
|
|
|
|
|
|
|
|
|
|
|
|
|
|
|
|
|
|
|
|
|
|
|
|
|
|
|
|
|
|
|
|
|
|
|
|
|
|
|
|
spaces/1phancelerku/anime-remove-background/Download and Play Dragon Ball Super Kakarot Fighter 2 APK on Android - The Most Epic Dragon Ball Game Ever.md
DELETED
@@ -1,98 +0,0 @@
|
|
1 |
-
|
2 |
-
<h1>Dragon Ball Super Kakarot Fighter 2 APK Download: A Guide for Android Users</h1>
|
3 |
-
<p>If you are a fan of Dragon Ball, you might have heard of Dragon Ball Super Kakarot Fighter 2, a modded version of a popular game called Dragon Ball Tap Battle. This game is not available on the official app stores, but you can download it as an APK file and install it on your Android device. In this article, we will tell you everything you need to know about this game, including its features, requirements, and installation steps.</p>
|
4 |
-
<h2>What is Dragon Ball Super Kakarot Fighter 2?</h2>
|
5 |
-
<p>Dragon Ball Super Kakarot Fighter 2 is a fan-made game that is based on the original Dragon Ball Tap Battle, which was released by Bandai Namco Entertainment in 2013. The game is a 2D fighting game that allows you to control your favorite characters from the Dragon Ball series and fight against other players or the computer. The game has a simple tap-based control system that makes it easy to play even for beginners.</p>
|
6 |
-
<h2>dragon ball super kakarot fighter 2 apk download</h2><br /><p><b><b>DOWNLOAD</b> 🗹 <a href="https://jinyurl.com/2uNNjg">https://jinyurl.com/2uNNjg</a></b></p><br /><br />
|
7 |
-
<h3>A modded version of Dragon Ball Tap Battle</h3>
|
8 |
-
<p>Dragon Ball Super Kakarot Fighter 2 is not an official game, but a modded version that was created by AnthonyBrayanVE, a fan of the series. The modder added a lot of new content and features to the original game, such as new characters, stages, skills, transformations, graphics, and sound effects. The modder also improved the gameplay and the balance of the game, making it more fun and challenging.</p>
|
9 |
-
<h3>Features of the game</h3>
|
10 |
-
<p>Dragon Ball Super Kakarot Fighter 2 has a lot of features that make it stand out from other Dragon Ball games. Here are some of them:</p>
|
11 |
-
<h4>New characters and stages</h4>
|
12 |
-
<p>The game has over 100 characters from different sagas and movies of the Dragon Ball series, including Goku, Vegeta, Gohan, Piccolo, Frieza, Cell, Buu, Beerus, Whis, Broly, Jiren, Gogeta, Vegito, and many more. You can also unlock some hidden characters by completing certain missions or challenges. The game also has over 50 stages from various locations in the Dragon Ball universe, such as Planet Namek, Earth, Hell, Tournament of Power, Future Trunks' timeline, and more.</p>
|
13 |
-
<h4>New skills and transformations</h4>
|
14 |
-
<p>The game allows you to customize your characters with different skills and transformations that can enhance their abilities and change their appearance. You can choose from various skills such as Kamehameha, Final Flash, Spirit Bomb, Big Bang Attack, Death Beam, Special Beam Cannon, Solar Flare, Ki Blast Cannon, Instant Transmission, Kaioken, Fusion Dance, Potara Earrings, and more. You can also transform your characters into different forms such as Super Saiyan, Super Saiyan God, Super Saiyan Blue, Ultra Instinct, Golden Frieza, Perfect Cell, Majin Buu, Legendary Super Saiyan Broly,</p> <p>and more.</p>
|
15 |
-
<h4>Improved graphics and sound effects</h4 <p>The game has improved graphics and sound effects that make it more realistic and immersive. The characters and the stages are more detailed and colorful, and the animations are smoother and faster. The game also has new sound effects and voice clips that match the characters and the situations. You can hear the characters shouting their attacks, taunting their opponents, or expressing their emotions. You can also hear the sound of the punches, kicks, blasts, explosions, and transformations.</p>
|
16 |
-
<h2>How to download and install Dragon Ball Super Kakarot Fighter 2 APK?</h2>
|
17 |
-
<p>If you want to play Dragon Ball Super Kakarot Fighter 2 on your Android device, you need to download and install the APK file and the OBB data file of the game. These files are not available on the official app stores, so you need to find a trusted source that provides them. You also need to make sure that your device meets the requirements for the game.</p>
|
18 |
-
<p>dragon ball z kakarot fighter 2 apk free download<br />
|
19 |
-
dragon ball super kakarot 2 tap battle mod apk<br />
|
20 |
-
dragon ball z kakarot mobile game apk download<br />
|
21 |
-
dragon ball super kakarot fighter 2 android game<br />
|
22 |
-
dragon ball z kakarot fighter 2 apk offline<br />
|
23 |
-
dragon ball super kakarot 2 tap battle download<br />
|
24 |
-
dragon ball z kakarot mobile apk mod<br />
|
25 |
-
dragon ball super kakarot fighter 2 gameplay<br />
|
26 |
-
dragon ball z kakarot fighter 2 apk online<br />
|
27 |
-
dragon ball super kakarot 2 tap battle apk mod<br />
|
28 |
-
dragon ball z kakarot mobile game download<br />
|
29 |
-
dragon ball super kakarot fighter 2 android download<br />
|
30 |
-
dragon ball z kakarot fighter 2 apk latest version<br />
|
31 |
-
dragon ball super kakarot 2 tap battle mod by anthonybrayanve<br />
|
32 |
-
dragon ball z kakarot mobile apk free download<br />
|
33 |
-
dragon ball super kakarot fighter 2 android apk<br />
|
34 |
-
dragon ball z kakarot fighter 2 apk update<br />
|
35 |
-
dragon ball super kakarot 2 tap battle mod download<br />
|
36 |
-
dragon ball z kakarot mobile game mod apk<br />
|
37 |
-
dragon ball super kakarot fighter 2 android game download<br />
|
38 |
-
dragon ball z kakarot fighter 2 apk full version<br />
|
39 |
-
dragon ball super kakarot 2 tap battle apk free download<br />
|
40 |
-
dragon ball z kakarot mobile game online<br />
|
41 |
-
dragon ball super kakarot fighter 2 android gameplay<br />
|
42 |
-
dragon ball z kakarot fighter 2 apk no verification<br />
|
43 |
-
dragon ball super kakarot 2 tap battle mod apk download<br />
|
44 |
-
dragon ball z kakarot mobile game offline<br />
|
45 |
-
dragon ball super kakarot fighter 2 android mod apk<br />
|
46 |
-
dragon ball z kakarot fighter 2 apk obb download<br />
|
47 |
-
dragon ball super kakarot 2 tap battle apk latest version<br />
|
48 |
-
dragon ball z kakarot mobile game review<br />
|
49 |
-
dragon ball super kakarot fighter 2 android offline<br />
|
50 |
-
dragon ball z kakarot fighter 2 apk unlimited money<br />
|
51 |
-
dragon ball super kakarot 2 tap battle mod by anthonybrayanve apk<br />
|
52 |
-
dragon ball z kakarot mobile game for android<br />
|
53 |
-
dragon ball super kakarot fighter 2 android online<br />
|
54 |
-
dragon ball z kakarot fighter 2 apk hack download<br />
|
55 |
-
dragon ball super kakarot 2 tap battle mod features<br />
|
56 |
-
dragon ball z kakarot mobile game for ios<br />
|
57 |
-
dragon ball super kakarot fighter 2 android update<br />
|
58 |
-
dragon ball z kakarot fighter 2 apk cheats<br />
|
59 |
-
dragon ball super kakarot 2 tap battle mod characters<br />
|
60 |
-
dragon ball z kakarot mobile game trailer<br />
|
61 |
-
dragon ball super kakarot fighter 2 android requirements<br />
|
62 |
-
dragon ball z kakarot fighter 2 apk data download<br />
|
63 |
-
dragon ball super kakarot 2 tap battle mod gameplay<br />
|
64 |
-
dragon ball z kakarot mobile game release date<br />
|
65 |
-
dragon ball super kakarot fighter 2 android emulator<br />
|
66 |
-
dragon ball z kakarot fighter 2 apk revdl</p>
|
67 |
-
<h3>Requirements for the game</h3>
|
68 |
-
<p>Before you download and install the game, you need to check if your device meets the following requirements:</p>
|
69 |
-
<h4>Android device with at least 2 GB of RAM and 1 GB of storage space</h4>
|
70 |
-
<p>The game is a large and heavy game that requires a lot of memory and storage space to run smoothly. You need to have at least 2 GB of RAM and 1 GB of free storage space on your device. If your device has less than that, you might experience lagging, crashing, or loading issues.</p>
|
71 |
-
<h4>Internet connection for downloading the APK file and the OBB data file</h4>
|
72 |
-
<p>The game is not an online game, but you need an internet connection to download the APK file and the OBB data file of the game. These files are usually large in size, so you need a fast and stable internet connection to download them without any interruption or corruption.</p>
|
73 |
-
<h3>Steps to follow</h3>
|
74 |
-
<p>Once you have checked the requirements and found a trusted source for the files, you can follow these steps to download and install the game:</p>
|
75 |
-
<h4>Download the APK file and the OBB data file from a trusted source</h4>
|
76 |
-
<p>The first step is to download the APK file and the OBB data file of the game from a trusted source. You can search for these files on Google or use a link provided by a reliable website or YouTube channel. Make sure that the files are compatible with your device and have no viruses or malware. You can use an antivirus app to scan the files before downloading them.</p>
|
77 |
-
<h4>Enable unknown sources on your device settings</h4>
|
78 |
-
<p>The second step is to enable unknown sources on your device settings. This will allow you to install apps that are not from the official app stores. To do this, go to your device settings, then security, then unknown sources, and turn it on. You might see a warning message that says installing apps from unknown sources can harm your device, but don't worry, as long as you trust the source of the files, there is no risk.</p>
|
79 |
-
<h4>Install the APK file and extract the OBB data file to the Android/OBB folder</h4>
|
80 |
-
<p>The third step is to install the APK file and extract the OBB data file to the Android/OBB folder on your device. To do this, locate the downloaded files on your device storage, then tap on the APK file to start the installation process. Follow the instructions on the screen until the installation is complete. Then, use a file manager app to extract or unzip the OBB data file to the Android/OBB folder on your device. Make sure that you create a folder with the name of the game inside the OBB folder and place the extracted data file inside it.</p>
|
81 |
-
<h4>Launch the game and enjoy</h4>
|
82 |
-
<p>The final step is to launch the game and enjoy playing it. To do this, go to your app drawer or home screen and tap on the icon of the game. The game will start loading and ask for some permissions. Grant them and wait for a few seconds until the game is ready. Then, you can choose your mode, character, stage, skill, transformation, and start fighting.</p>
|
83 |
-
<h2>Conclusion</h2>
|
84 |
-
<p>Dragon Ball Super Kakarot Fighter 2 APK is a fun and exciting game for Dragon Ball fans who want to experience a new and improved version of Dragon Ball Tap Battle. The game offers a lot of content and customization options for the players who want to create their own battles with their favorite characters. The game is easy to download and install on Android devices as long as you follow our guide carefully.</p>
|
85 |
-
<p>If you have any questions or problems with downloading or installing Dragon Ball Super Kakarot Fighter 2 APK, feel free to leave a comment below or contact us through our website. We will try our best to help you out.</p <p>Here are some FAQs that you might have about Dragon Ball Super Kakarot Fighter 2 APK:</p>
|
86 |
-
<h3>FAQs</h3>
|
87 |
-
<p><b>Q: Is Dragon Ball Super Kakarot Fighter 2 APK safe to download and install?</b></p>
|
88 |
-
<p>A: Yes, as long as you download the APK file and the OBB data file from a trusted source and scan them with an antivirus app before installing them. You should also enable unknown sources on your device settings only for this game and disable it after the installation is done.</p>
|
89 |
-
<p><b>Q: Is Dragon Ball Super Kakarot Fighter 2 APK free to play?</b></p>
|
90 |
-
<p>A: Yes, the game is free to play and does not require any in-app purchases or subscriptions. However, you might see some ads or pop-ups while playing the game, which you can skip or close.</p>
|
91 |
-
<p><b>Q: Can I play Dragon Ball Super Kakarot Fighter 2 APK offline?</b></p>
|
92 |
-
<p>A: Yes, you can play the game offline once you have downloaded and installed the APK file and the OBB data file. You only need an internet connection to download the files and update the game if there are any new versions available.</p>
|
93 |
-
<p><b>Q: Can I play Dragon Ball Super Kakarot Fighter 2 APK with my friends?</b></p>
|
94 |
-
<p>A: Yes, you can play the game with your friends by using the multiplayer mode. You can either join an online room or create your own room and invite your friends to join. You can also chat with your friends and other players in the game.</p>
|
95 |
-
<p><b>Q: How can I update Dragon Ball Super Kakarot Fighter 2 APK?</b></p>
|
96 |
-
<p>A: You can update the game by downloading and installing the latest version of the APK file and the OBB data file from the same source that you used before. You should also delete the old version of the game before installing the new one.</p> 197e85843d<br />
|
97 |
-
<br />
|
98 |
-
<br />
|
|
|
|
|
|
|
|
|
|
|
|
|
|
|
|
|
|
|
|
|
|
|
|
|
|
|
|
|
|
|
|
|
|
|
|
|
|
|
|
|
|
|
|
|
|
|
|
|
|
|
|
|
|
|
|
|
|
|
|
|
|
|
|
|
|
|
|
|
|
|
|
|
|
|
|
|
|
|
|
|
|
|
|
|
|
|
|
|
|
|
|
|
|
|
|
|
|
|
|
|
|
|
|
|
|
|
|
|
|
|
|
|
|
|
|
|
|
|
|
|
|
|
|
|
|
|
|
|
|
|
|
|
|
|
|
|
|
|
|
|
|
|
|
|
|
|
|
|
|
|
|
|
|
|
|
|
|
|
|
|
|
|
|
|
|
|
|
|
|
|
|
|
|
|
|
|
|
|
|
|
|
|
|
|
|
|
|
|
|
|
|
|
|
|
|
|
|
spaces/1phancelerku/anime-remove-background/FIFA Football Download Build Your Dream Team and Compete with the Worlds Best.md
DELETED
@@ -1,78 +0,0 @@
|
|
1 |
-
<br />
|
2 |
-
<h1>FIFA Football Download: How to Play the Best Soccer Game on Your Mobile Device</h1>
|
3 |
-
<h2>Introduction</h2>
|
4 |
-
<p>If you are a soccer fan, you probably know about FIFA, the most popular and realistic soccer video game series from EA Sports. But did you know that you can also play FIFA on your mobile device? That's right, FIFA Football is a free-to-play mobile game that lets you build your own ultimate team, compete in various modes, and enjoy the thrill of soccer anytime, anywhere. In this article, we will show you how to download FIFA Football on your Android or iOS device, and how to play it and enjoy its features.</p>
|
5 |
-
<h2>fifa football download</h2><br /><p><b><b>Download File</b> »»» <a href="https://jinyurl.com/2uNTwN">https://jinyurl.com/2uNTwN</a></b></p><br /><br />
|
6 |
-
<h2>How to download FIFA Football on Android and iOS devices</h2>
|
7 |
-
<h3>Step 1: Go to the official website or app store</h3>
|
8 |
-
<p>The first step to download FIFA Football is to go to the official website of EA Sports FIFA or the app store of your device. You can find FIFA Football on Google Play Store for Android devices, or on Apple App Store for iOS devices. Alternatively, you can scan the QR code on the website to get the direct link to the app.</p>
|
9 |
-
<h3>Step 2: Choose your device and download the app</h3>
|
10 |
-
<p>The next step is to choose your device and download the app. The app size is about 100 MB, so make sure you have enough space on your device and a stable internet connection. The app is compatible with Android devices running Android 6.0 or higher, and iOS devices running iOS 12.0 or higher. The app is also rated for everyone, so you can enjoy it with your family and friends.</p>
|
11 |
-
<h3>Step 3: Launch the app and sign in with your EA account</h3>
|
12 |
-
<p>The final step is to launch the app and sign in with your EA account. If you don't have an EA account, you can create one for free by following the instructions on the screen. You will need an EA account to access all the features of FIFA Football, such as online multiplayer, leaderboards, rewards, and more. You can also link your Facebook account to sync your progress across devices.</p>
|
13 |
-
<h2>How to play FIFA Football and enjoy its features</h2>
|
14 |
-
<h3>Build your ultimate team with star players from the biggest leagues and top teams</h3>
|
15 |
-
<p>One of the main features of FIFA Football is that you can build your own ultimate team with star players from over 30 leagues and 600 teams, including Ligue 1 Uber Eats, Premier League, LaLiga Santander, Bundesliga, Serie A TIM, and more. You can collect player items and put your favorite soccer stars to the test, such as Kylian Mbappé, Virgil van Dijk, Son Heung-min, Kai Havertz, Christian Pulisic, Vinicius Jr, Pedri, João Félix, Jude Bellingham, Alphonso Davies, Dušan Vlahović, and more. You can also score big with world soccer icons like Paolo Maldini, Ronaldinho, and more.</p>
|
16 |
-
<h3>Relive the world's greatest soccer tournament with FIFA World Cup mode</h3>
|
17 |
-
<p>Another feature of FIFA Football is that you can relive the world's greatest soccer tournament with FIFA World Cup mode. This is the only licensed FIFA World Cup 2022 mobile game where you can replay the official tournament brackets with any of the 32 qualified nations. You can <p>experience the excitement and drama of the world's biggest soccer stage, and compete for glory and honor. You can also customize your team with exclusive World Cup kits, badges, and player items.</p>
|
18 |
-
<h3>Experience immersive next-level soccer simulation with realistic graphics and sound effects</h3>
|
19 |
-
<p>FIFA Football is not just a game, it's a simulation of soccer that will make you feel like you are on the pitch. The game features realistic graphics and sound effects that will immerse you in the action. You can see the players' faces, expressions, movements, and skills in high definition, and hear the crowd cheering, chanting, and reacting to every goal, foul, and save. You can also enjoy the commentary from famous soccer personalities like Martin Tyler, Alan Smith, Derek Rae, Lee Dixon, and more.</p>
|
20 |
-
<h3>Manage your own dream team with manager mode and strategic gameplay</h3>
|
21 |
-
<p>If you want to take your soccer experience to the next level, you can try the manager mode and strategic gameplay of FIFA Football. In manager mode, you can take charge of your own club and make all the decisions, from transfers, formations, tactics, training, to finances. You can also compete in various leagues and tournaments, and climb the ranks from amateur to legendary. In strategic gameplay, you can use your skills and knowledge to outsmart your opponents and win matches. You can choose from different play styles, such as attack, defense, balanced, or custom. You can also use advanced controls, such as gestures, buttons, or virtual sticks.</p>
|
22 |
-
<p>fifa football download for pc<br />
|
23 |
-
fifa football download for android<br />
|
24 |
-
fifa football download apk<br />
|
25 |
-
fifa football download free<br />
|
26 |
-
fifa football download offline<br />
|
27 |
-
fifa football download 2023<br />
|
28 |
-
fifa football download for windows 10<br />
|
29 |
-
fifa football download for ios<br />
|
30 |
-
fifa football download mod apk<br />
|
31 |
-
fifa football download game<br />
|
32 |
-
fifa football download for laptop<br />
|
33 |
-
fifa football download full version<br />
|
34 |
-
fifa football download for mac<br />
|
35 |
-
fifa football download size<br />
|
36 |
-
fifa football download play store<br />
|
37 |
-
fifa football download link<br />
|
38 |
-
fifa football download highly compressed<br />
|
39 |
-
fifa football download without internet<br />
|
40 |
-
fifa football download latest version<br />
|
41 |
-
fifa football download hack<br />
|
42 |
-
fifa football download obb<br />
|
43 |
-
fifa football download uptodown<br />
|
44 |
-
fifa football download for pc windows 7<br />
|
45 |
-
fifa football download online<br />
|
46 |
-
fifa football download 2022<br />
|
47 |
-
fifa football download for mobile<br />
|
48 |
-
fifa football download softonic<br />
|
49 |
-
fifa football download with commentary<br />
|
50 |
-
fifa football download data<br />
|
51 |
-
fifa football download app store<br />
|
52 |
-
fifa football download unlimited money<br />
|
53 |
-
fifa football download for chromebook<br />
|
54 |
-
fifa football download pc game free full version<br />
|
55 |
-
fifa football download in jio phone<br />
|
56 |
-
fifa football download 2021<br />
|
57 |
-
fifa football download for pc windows 10 64 bit<br />
|
58 |
-
fifa football download website<br />
|
59 |
-
fifa football download rexdl<br />
|
60 |
-
fifa football download revdl<br />
|
61 |
-
fifa football download update<br />
|
62 |
-
fifa football download apk + data + obb offline<br />
|
63 |
-
fifa football download for pc windows 8.1 64 bit free full version</p>
|
64 |
-
<h2>Conclusion</h2>
|
65 |
-
<p>FIFA Football is the best soccer game for your mobile device. It lets you download it for free and play it anytime, anywhere. It lets you build your ultimate team with star players from over 30 leagues and 600 teams. It lets you relive the world's greatest soccer tournament with FIFA World Cup mode. It lets you experience immersive next-level soccer simulation with realistic graphics and sound effects. And it lets you manage your own dream team with manager mode and strategic gameplay. What are you waiting for? Download FIFA Football today and enjoy the beautiful game!</p>
|
66 |
-
<h2>FAQs</h2>
|
67 |
-
<h4>Q: How much space do I need to download FIFA Football?</h4>
|
68 |
-
<p>A: The app size is about 100 MB, but you may need additional space for updates and data files.</p>
|
69 |
-
<h4>Q: Do I need an internet connection to play FIFA Football?</h4>
|
70 |
-
<p>A: Yes, you need an internet connection to access all the features of FIFA Football, such as online multiplayer, leaderboards, rewards, and more.</p>
|
71 |
-
<h4>Q: Can I play FIFA Football offline?</h4>
|
72 |
-
<p>A: Yes, you can play some modes offline, such as FIFA World Cup mode and manager mode.</p>
|
73 |
-
<h4>Q: How can I get more coins and gems in FIFA Football?</h4>
|
74 |
-
<p>A: You can get more coins and gems by completing daily objectives, participating in events, winning matches, or purchasing them with real money.</p>
|
75 |
-
<h4>Q: How can I contact EA Sports for support or feedback?</h4>
|
76 |
-
<p>A: You can contact EA Sports by visiting their help center, sending them an email, or following them on social media.</p> 197e85843d<br />
|
77 |
-
<br />
|
78 |
-
<br />
|
|
|
|
|
|
|
|
|
|
|
|
|
|
|
|
|
|
|
|
|
|
|
|
|
|
|
|
|
|
|
|
|
|
|
|
|
|
|
|
|
|
|
|
|
|
|
|
|
|
|
|
|
|
|
|
|
|
|
|
|
|
|
|
|
|
|
|
|
|
|
|
|
|
|
|
|
|
|
|
|
|
|
|
|
|
|
|
|
|
|
|
|
|
|
|
|
|
|
|
|
|
|
|
|
|
|
|
|
|
|
|
|
|
|
|
|
|
|
|
|
|
|
|
|
|
|
|
|
|
|
|
|
|
|
|
|
|
|
|
|
|
|
|
|
|
|
|
|
|
|
|
|
|
|
|
|
|
spaces/2023Liu2023/bingo/src/components/ui/voice/index.tsx
DELETED
@@ -1,28 +0,0 @@
|
|
1 |
-
import './index.scss'
|
2 |
-
|
3 |
-
export interface VoiceProps extends CSSPropertyRule {
|
4 |
-
num?: number;
|
5 |
-
duration?: number;
|
6 |
-
}
|
7 |
-
export default function Voice({ duration = 400, num = 7, ...others }) {
|
8 |
-
return (
|
9 |
-
<div className="voice-button" { ...others }>
|
10 |
-
{Array.from({ length: num }).map((_, index) => {
|
11 |
-
const randomDuration = Math.random() * 100 + duration
|
12 |
-
const initialDelay = Math.random() * 2 * duration
|
13 |
-
const initialScale = Math.sin((index + 1) * Math.PI / num)
|
14 |
-
return (
|
15 |
-
<div
|
16 |
-
className="voice-button-item"
|
17 |
-
key={index}
|
18 |
-
style={{
|
19 |
-
animationDelay: initialDelay + 'ms',
|
20 |
-
animationDuration: randomDuration + 'ms',
|
21 |
-
transform: `scale(${initialScale})`
|
22 |
-
}}
|
23 |
-
/>
|
24 |
-
)
|
25 |
-
})}
|
26 |
-
</div>
|
27 |
-
)
|
28 |
-
}
|
|
|
|
|
|
|
|
|
|
|
|
|
|
|
|
|
|
|
|
|
|
|
|
|
|
|
|
|
|
|
|
|
|
|
|
|
|
|
|
|
|
|
|
|
|
|
|
|
|
|
|
|
|
|
|
|
|
spaces/221090Lstwcm/textgenerator/app.py
DELETED
@@ -1,11 +0,0 @@
|
|
1 |
-
#libraries
|
2 |
-
import gradio as gr
|
3 |
-
from gradio.mix import Parallel
|
4 |
-
|
5 |
-
#variables, functions and parameters
|
6 |
-
model1=gr.Interface.load("huggingface/gpt2")
|
7 |
-
model2=gr.Interface.load("huggingface/EleutherAI/gpt-j-6B")
|
8 |
-
model3=gr.Interface.load("huggingface/distilgpt2")
|
9 |
-
|
10 |
-
#funcations, parameters and variables
|
11 |
-
gr.Parallel(model1,model2,model3).launch()
|
|
|
|
|
|
|
|
|
|
|
|
|
|
|
|
|
|
|
|
|
|
|
|
spaces/4Taps/SadTalker/src/face3d/models/arcface_torch/utils/utils_logging.py
DELETED
@@ -1,41 +0,0 @@
|
|
1 |
-
import logging
|
2 |
-
import os
|
3 |
-
import sys
|
4 |
-
|
5 |
-
|
6 |
-
class AverageMeter(object):
|
7 |
-
"""Computes and stores the average and current value
|
8 |
-
"""
|
9 |
-
|
10 |
-
def __init__(self):
|
11 |
-
self.val = None
|
12 |
-
self.avg = None
|
13 |
-
self.sum = None
|
14 |
-
self.count = None
|
15 |
-
self.reset()
|
16 |
-
|
17 |
-
def reset(self):
|
18 |
-
self.val = 0
|
19 |
-
self.avg = 0
|
20 |
-
self.sum = 0
|
21 |
-
self.count = 0
|
22 |
-
|
23 |
-
def update(self, val, n=1):
|
24 |
-
self.val = val
|
25 |
-
self.sum += val * n
|
26 |
-
self.count += n
|
27 |
-
self.avg = self.sum / self.count
|
28 |
-
|
29 |
-
|
30 |
-
def init_logging(rank, models_root):
|
31 |
-
if rank == 0:
|
32 |
-
log_root = logging.getLogger()
|
33 |
-
log_root.setLevel(logging.INFO)
|
34 |
-
formatter = logging.Formatter("Training: %(asctime)s-%(message)s")
|
35 |
-
handler_file = logging.FileHandler(os.path.join(models_root, "training.log"))
|
36 |
-
handler_stream = logging.StreamHandler(sys.stdout)
|
37 |
-
handler_file.setFormatter(formatter)
|
38 |
-
handler_stream.setFormatter(formatter)
|
39 |
-
log_root.addHandler(handler_file)
|
40 |
-
log_root.addHandler(handler_stream)
|
41 |
-
log_root.info('rank_id: %d' % rank)
|
|
|
|
|
|
|
|
|
|
|
|
|
|
|
|
|
|
|
|
|
|
|
|
|
|
|
|
|
|
|
|
|
|
|
|
|
|
|
|
|
|
|
|
|
|
|
|
|
|
|
|
|
|
|
|
|
|
|
|
|
|
|
|
|
|
|
|
|
|
|
|
|
|
|
|
|
|
|
|
|
|
|
|
spaces/AI-Dashboards/AI.Dashboard.Streamlit.Index.For.Assessments/README.md
DELETED
@@ -1,13 +0,0 @@
|
|
1 |
-
---
|
2 |
-
title: AI.Dashboard.Streamlit.Index.For.Assessments
|
3 |
-
emoji: 👁
|
4 |
-
colorFrom: yellow
|
5 |
-
colorTo: red
|
6 |
-
sdk: streamlit
|
7 |
-
sdk_version: 1.17.0
|
8 |
-
app_file: app.py
|
9 |
-
pinned: false
|
10 |
-
license: mit
|
11 |
-
---
|
12 |
-
|
13 |
-
Check out the configuration reference at https://huggingface.co/docs/hub/spaces-config-reference
|
|
|
|
|
|
|
|
|
|
|
|
|
|
|
|
|
|
|
|
|
|
|
|
|
|
|
|
spaces/AIGC-Audio/AudioGPT/text_to_audio/Make_An_Audio/vocoder/bigvgan/__init__.py
DELETED
File without changes
|
spaces/AIGC-Audio/AudioGPT/text_to_speech/modules/commons/normalizing_flow/glow_modules.py
DELETED
@@ -1,362 +0,0 @@
|
|
1 |
-
import scipy
|
2 |
-
from torch.nn import functional as F
|
3 |
-
import torch
|
4 |
-
from torch import nn
|
5 |
-
import numpy as np
|
6 |
-
from text_to_speech.modules.commons.wavenet import WN
|
7 |
-
from text_to_speech.modules.tts.glow import utils
|
8 |
-
|
9 |
-
|
10 |
-
class ActNorm(nn.Module):
|
11 |
-
def __init__(self, channels, ddi=False, **kwargs):
|
12 |
-
super().__init__()
|
13 |
-
self.channels = channels
|
14 |
-
self.initialized = not ddi
|
15 |
-
|
16 |
-
self.logs = nn.Parameter(torch.zeros(1, channels, 1))
|
17 |
-
self.bias = nn.Parameter(torch.zeros(1, channels, 1))
|
18 |
-
|
19 |
-
def forward(self, x, x_mask=None, reverse=False, **kwargs):
|
20 |
-
if x_mask is None:
|
21 |
-
x_mask = torch.ones(x.size(0), 1, x.size(2)).to(device=x.device, dtype=x.dtype)
|
22 |
-
x_len = torch.sum(x_mask, [1, 2])
|
23 |
-
if not self.initialized:
|
24 |
-
self.initialize(x, x_mask)
|
25 |
-
self.initialized = True
|
26 |
-
|
27 |
-
if reverse:
|
28 |
-
z = (x - self.bias) * torch.exp(-self.logs) * x_mask
|
29 |
-
logdet = torch.sum(-self.logs) * x_len
|
30 |
-
else:
|
31 |
-
z = (self.bias + torch.exp(self.logs) * x) * x_mask
|
32 |
-
logdet = torch.sum(self.logs) * x_len # [b]
|
33 |
-
return z, logdet
|
34 |
-
|
35 |
-
def store_inverse(self):
|
36 |
-
pass
|
37 |
-
|
38 |
-
def set_ddi(self, ddi):
|
39 |
-
self.initialized = not ddi
|
40 |
-
|
41 |
-
def initialize(self, x, x_mask):
|
42 |
-
with torch.no_grad():
|
43 |
-
denom = torch.sum(x_mask, [0, 2])
|
44 |
-
m = torch.sum(x * x_mask, [0, 2]) / denom
|
45 |
-
m_sq = torch.sum(x * x * x_mask, [0, 2]) / denom
|
46 |
-
v = m_sq - (m ** 2)
|
47 |
-
logs = 0.5 * torch.log(torch.clamp_min(v, 1e-6))
|
48 |
-
|
49 |
-
bias_init = (-m * torch.exp(-logs)).view(*self.bias.shape).to(dtype=self.bias.dtype)
|
50 |
-
logs_init = (-logs).view(*self.logs.shape).to(dtype=self.logs.dtype)
|
51 |
-
|
52 |
-
self.bias.data.copy_(bias_init)
|
53 |
-
self.logs.data.copy_(logs_init)
|
54 |
-
|
55 |
-
|
56 |
-
class InvConvNear(nn.Module):
|
57 |
-
def __init__(self, channels, n_split=4, no_jacobian=False, lu=True, n_sqz=2, **kwargs):
|
58 |
-
super().__init__()
|
59 |
-
assert (n_split % 2 == 0)
|
60 |
-
self.channels = channels
|
61 |
-
self.n_split = n_split
|
62 |
-
self.n_sqz = n_sqz
|
63 |
-
self.no_jacobian = no_jacobian
|
64 |
-
|
65 |
-
w_init = torch.qr(torch.FloatTensor(self.n_split, self.n_split).normal_())[0]
|
66 |
-
if torch.det(w_init) < 0:
|
67 |
-
w_init[:, 0] = -1 * w_init[:, 0]
|
68 |
-
self.lu = lu
|
69 |
-
if lu:
|
70 |
-
# LU decomposition can slightly speed up the inverse
|
71 |
-
np_p, np_l, np_u = scipy.linalg.lu(w_init)
|
72 |
-
np_s = np.diag(np_u)
|
73 |
-
np_sign_s = np.sign(np_s)
|
74 |
-
np_log_s = np.log(np.abs(np_s))
|
75 |
-
np_u = np.triu(np_u, k=1)
|
76 |
-
l_mask = np.tril(np.ones(w_init.shape, dtype=float), -1)
|
77 |
-
eye = np.eye(*w_init.shape, dtype=float)
|
78 |
-
|
79 |
-
self.register_buffer('p', torch.Tensor(np_p.astype(float)))
|
80 |
-
self.register_buffer('sign_s', torch.Tensor(np_sign_s.astype(float)))
|
81 |
-
self.l = nn.Parameter(torch.Tensor(np_l.astype(float)), requires_grad=True)
|
82 |
-
self.log_s = nn.Parameter(torch.Tensor(np_log_s.astype(float)), requires_grad=True)
|
83 |
-
self.u = nn.Parameter(torch.Tensor(np_u.astype(float)), requires_grad=True)
|
84 |
-
self.register_buffer('l_mask', torch.Tensor(l_mask))
|
85 |
-
self.register_buffer('eye', torch.Tensor(eye))
|
86 |
-
else:
|
87 |
-
self.weight = nn.Parameter(w_init)
|
88 |
-
|
89 |
-
def forward(self, x, x_mask=None, reverse=False, **kwargs):
|
90 |
-
b, c, t = x.size()
|
91 |
-
assert (c % self.n_split == 0)
|
92 |
-
if x_mask is None:
|
93 |
-
x_mask = 1
|
94 |
-
x_len = torch.ones((b,), dtype=x.dtype, device=x.device) * t
|
95 |
-
else:
|
96 |
-
x_len = torch.sum(x_mask, [1, 2])
|
97 |
-
|
98 |
-
x = x.view(b, self.n_sqz, c // self.n_split, self.n_split // self.n_sqz, t)
|
99 |
-
x = x.permute(0, 1, 3, 2, 4).contiguous().view(b, self.n_split, c // self.n_split, t)
|
100 |
-
|
101 |
-
if self.lu:
|
102 |
-
self.weight, log_s = self._get_weight()
|
103 |
-
logdet = log_s.sum()
|
104 |
-
logdet = logdet * (c / self.n_split) * x_len
|
105 |
-
else:
|
106 |
-
logdet = torch.logdet(self.weight) * (c / self.n_split) * x_len # [b]
|
107 |
-
|
108 |
-
if reverse:
|
109 |
-
if hasattr(self, "weight_inv"):
|
110 |
-
weight = self.weight_inv
|
111 |
-
else:
|
112 |
-
weight = torch.inverse(self.weight.float()).to(dtype=self.weight.dtype)
|
113 |
-
logdet = -logdet
|
114 |
-
else:
|
115 |
-
weight = self.weight
|
116 |
-
if self.no_jacobian:
|
117 |
-
logdet = 0
|
118 |
-
|
119 |
-
weight = weight.view(self.n_split, self.n_split, 1, 1)
|
120 |
-
z = F.conv2d(x, weight)
|
121 |
-
|
122 |
-
z = z.view(b, self.n_sqz, self.n_split // self.n_sqz, c // self.n_split, t)
|
123 |
-
z = z.permute(0, 1, 3, 2, 4).contiguous().view(b, c, t) * x_mask
|
124 |
-
return z, logdet
|
125 |
-
|
126 |
-
def _get_weight(self):
|
127 |
-
l, log_s, u = self.l, self.log_s, self.u
|
128 |
-
l = l * self.l_mask + self.eye
|
129 |
-
u = u * self.l_mask.transpose(0, 1).contiguous() + torch.diag(self.sign_s * torch.exp(log_s))
|
130 |
-
weight = torch.matmul(self.p, torch.matmul(l, u))
|
131 |
-
return weight, log_s
|
132 |
-
|
133 |
-
def store_inverse(self):
|
134 |
-
weight, _ = self._get_weight()
|
135 |
-
self.weight_inv = torch.inverse(weight.float()).to(next(self.parameters()).device)
|
136 |
-
|
137 |
-
|
138 |
-
class InvConv(nn.Module):
|
139 |
-
def __init__(self, channels, no_jacobian=False, lu=True, **kwargs):
|
140 |
-
super().__init__()
|
141 |
-
w_shape = [channels, channels]
|
142 |
-
w_init = np.linalg.qr(np.random.randn(*w_shape))[0].astype(float)
|
143 |
-
LU_decomposed = lu
|
144 |
-
if not LU_decomposed:
|
145 |
-
# Sample a random orthogonal matrix:
|
146 |
-
self.register_parameter("weight", nn.Parameter(torch.Tensor(w_init)))
|
147 |
-
else:
|
148 |
-
np_p, np_l, np_u = scipy.linalg.lu(w_init)
|
149 |
-
np_s = np.diag(np_u)
|
150 |
-
np_sign_s = np.sign(np_s)
|
151 |
-
np_log_s = np.log(np.abs(np_s))
|
152 |
-
np_u = np.triu(np_u, k=1)
|
153 |
-
l_mask = np.tril(np.ones(w_shape, dtype=float), -1)
|
154 |
-
eye = np.eye(*w_shape, dtype=float)
|
155 |
-
|
156 |
-
self.register_buffer('p', torch.Tensor(np_p.astype(float)))
|
157 |
-
self.register_buffer('sign_s', torch.Tensor(np_sign_s.astype(float)))
|
158 |
-
self.l = nn.Parameter(torch.Tensor(np_l.astype(float)))
|
159 |
-
self.log_s = nn.Parameter(torch.Tensor(np_log_s.astype(float)))
|
160 |
-
self.u = nn.Parameter(torch.Tensor(np_u.astype(float)))
|
161 |
-
self.l_mask = torch.Tensor(l_mask)
|
162 |
-
self.eye = torch.Tensor(eye)
|
163 |
-
self.w_shape = w_shape
|
164 |
-
self.LU = LU_decomposed
|
165 |
-
self.weight = None
|
166 |
-
|
167 |
-
def get_weight(self, device, reverse):
|
168 |
-
w_shape = self.w_shape
|
169 |
-
self.p = self.p.to(device)
|
170 |
-
self.sign_s = self.sign_s.to(device)
|
171 |
-
self.l_mask = self.l_mask.to(device)
|
172 |
-
self.eye = self.eye.to(device)
|
173 |
-
l = self.l * self.l_mask + self.eye
|
174 |
-
u = self.u * self.l_mask.transpose(0, 1).contiguous() + torch.diag(self.sign_s * torch.exp(self.log_s))
|
175 |
-
dlogdet = self.log_s.sum()
|
176 |
-
if not reverse:
|
177 |
-
w = torch.matmul(self.p, torch.matmul(l, u))
|
178 |
-
else:
|
179 |
-
l = torch.inverse(l.double()).float()
|
180 |
-
u = torch.inverse(u.double()).float()
|
181 |
-
w = torch.matmul(u, torch.matmul(l, self.p.inverse()))
|
182 |
-
return w.view(w_shape[0], w_shape[1], 1), dlogdet
|
183 |
-
|
184 |
-
def forward(self, x, x_mask=None, reverse=False, **kwargs):
|
185 |
-
"""
|
186 |
-
log-det = log|abs(|W|)| * pixels
|
187 |
-
"""
|
188 |
-
b, c, t = x.size()
|
189 |
-
if x_mask is None:
|
190 |
-
x_len = torch.ones((b,), dtype=x.dtype, device=x.device) * t
|
191 |
-
else:
|
192 |
-
x_len = torch.sum(x_mask, [1, 2])
|
193 |
-
logdet = 0
|
194 |
-
if not reverse:
|
195 |
-
weight, dlogdet = self.get_weight(x.device, reverse)
|
196 |
-
z = F.conv1d(x, weight)
|
197 |
-
if logdet is not None:
|
198 |
-
logdet = logdet + dlogdet * x_len
|
199 |
-
return z, logdet
|
200 |
-
else:
|
201 |
-
if self.weight is None:
|
202 |
-
weight, dlogdet = self.get_weight(x.device, reverse)
|
203 |
-
else:
|
204 |
-
weight, dlogdet = self.weight, self.dlogdet
|
205 |
-
z = F.conv1d(x, weight)
|
206 |
-
if logdet is not None:
|
207 |
-
logdet = logdet - dlogdet * x_len
|
208 |
-
return z, logdet
|
209 |
-
|
210 |
-
def store_inverse(self):
|
211 |
-
self.weight, self.dlogdet = self.get_weight('cuda', reverse=True)
|
212 |
-
|
213 |
-
|
214 |
-
class CouplingBlock(nn.Module):
|
215 |
-
def __init__(self, in_channels, hidden_channels, kernel_size, dilation_rate, n_layers,
|
216 |
-
gin_channels=0, p_dropout=0, sigmoid_scale=False, wn=None):
|
217 |
-
super().__init__()
|
218 |
-
self.in_channels = in_channels
|
219 |
-
self.hidden_channels = hidden_channels
|
220 |
-
self.kernel_size = kernel_size
|
221 |
-
self.dilation_rate = dilation_rate
|
222 |
-
self.n_layers = n_layers
|
223 |
-
self.gin_channels = gin_channels
|
224 |
-
self.p_dropout = p_dropout
|
225 |
-
self.sigmoid_scale = sigmoid_scale
|
226 |
-
|
227 |
-
start = torch.nn.Conv1d(in_channels // 2, hidden_channels, 1)
|
228 |
-
start = torch.nn.utils.weight_norm(start)
|
229 |
-
self.start = start
|
230 |
-
# Initializing last layer to 0 makes the affine coupling layers
|
231 |
-
# do nothing at first. This helps with training stability
|
232 |
-
end = torch.nn.Conv1d(hidden_channels, in_channels, 1)
|
233 |
-
end.weight.data.zero_()
|
234 |
-
end.bias.data.zero_()
|
235 |
-
self.end = end
|
236 |
-
self.wn = WN(hidden_channels, kernel_size, dilation_rate, n_layers, gin_channels, p_dropout)
|
237 |
-
if wn is not None:
|
238 |
-
self.wn.in_layers = wn.in_layers
|
239 |
-
self.wn.res_skip_layers = wn.res_skip_layers
|
240 |
-
|
241 |
-
def forward(self, x, x_mask=None, reverse=False, g=None, **kwargs):
|
242 |
-
if x_mask is None:
|
243 |
-
x_mask = 1
|
244 |
-
x_0, x_1 = x[:, :self.in_channels // 2], x[:, self.in_channels // 2:]
|
245 |
-
|
246 |
-
x = self.start(x_0) * x_mask
|
247 |
-
x = self.wn(x, x_mask, g)
|
248 |
-
out = self.end(x)
|
249 |
-
|
250 |
-
z_0 = x_0
|
251 |
-
m = out[:, :self.in_channels // 2, :]
|
252 |
-
logs = out[:, self.in_channels // 2:, :]
|
253 |
-
if self.sigmoid_scale:
|
254 |
-
logs = torch.log(1e-6 + torch.sigmoid(logs + 2))
|
255 |
-
if reverse:
|
256 |
-
z_1 = (x_1 - m) * torch.exp(-logs) * x_mask
|
257 |
-
logdet = torch.sum(-logs * x_mask, [1, 2])
|
258 |
-
else:
|
259 |
-
z_1 = (m + torch.exp(logs) * x_1) * x_mask
|
260 |
-
logdet = torch.sum(logs * x_mask, [1, 2])
|
261 |
-
z = torch.cat([z_0, z_1], 1)
|
262 |
-
return z, logdet
|
263 |
-
|
264 |
-
def store_inverse(self):
|
265 |
-
self.wn.remove_weight_norm()
|
266 |
-
|
267 |
-
|
268 |
-
class Glow(nn.Module):
|
269 |
-
def __init__(self,
|
270 |
-
in_channels,
|
271 |
-
hidden_channels,
|
272 |
-
kernel_size,
|
273 |
-
dilation_rate,
|
274 |
-
n_blocks,
|
275 |
-
n_layers,
|
276 |
-
p_dropout=0.,
|
277 |
-
n_split=4,
|
278 |
-
n_sqz=2,
|
279 |
-
sigmoid_scale=False,
|
280 |
-
gin_channels=0,
|
281 |
-
inv_conv_type='near',
|
282 |
-
share_cond_layers=False,
|
283 |
-
share_wn_layers=0,
|
284 |
-
):
|
285 |
-
super().__init__()
|
286 |
-
|
287 |
-
self.in_channels = in_channels
|
288 |
-
self.hidden_channels = hidden_channels
|
289 |
-
self.kernel_size = kernel_size
|
290 |
-
self.dilation_rate = dilation_rate
|
291 |
-
self.n_blocks = n_blocks
|
292 |
-
self.n_layers = n_layers
|
293 |
-
self.p_dropout = p_dropout
|
294 |
-
self.n_split = n_split
|
295 |
-
self.n_sqz = n_sqz
|
296 |
-
self.sigmoid_scale = sigmoid_scale
|
297 |
-
self.gin_channels = gin_channels
|
298 |
-
self.share_cond_layers = share_cond_layers
|
299 |
-
if gin_channels != 0 and share_cond_layers:
|
300 |
-
cond_layer = torch.nn.Conv1d(gin_channels * n_sqz, 2 * hidden_channels * n_layers, 1)
|
301 |
-
self.cond_layer = torch.nn.utils.weight_norm(cond_layer, name='weight')
|
302 |
-
wn = None
|
303 |
-
self.flows = nn.ModuleList()
|
304 |
-
for b in range(n_blocks):
|
305 |
-
self.flows.append(ActNorm(channels=in_channels * n_sqz))
|
306 |
-
if inv_conv_type == 'near':
|
307 |
-
self.flows.append(InvConvNear(channels=in_channels * n_sqz, n_split=n_split, n_sqz=n_sqz))
|
308 |
-
if inv_conv_type == 'invconv':
|
309 |
-
self.flows.append(InvConv(channels=in_channels * n_sqz))
|
310 |
-
if share_wn_layers > 0:
|
311 |
-
if b % share_wn_layers == 0:
|
312 |
-
wn = WN(hidden_channels, kernel_size, dilation_rate, n_layers, gin_channels * n_sqz,
|
313 |
-
p_dropout, share_cond_layers)
|
314 |
-
self.flows.append(
|
315 |
-
CouplingBlock(
|
316 |
-
in_channels * n_sqz,
|
317 |
-
hidden_channels,
|
318 |
-
kernel_size=kernel_size,
|
319 |
-
dilation_rate=dilation_rate,
|
320 |
-
n_layers=n_layers,
|
321 |
-
gin_channels=gin_channels * n_sqz,
|
322 |
-
p_dropout=p_dropout,
|
323 |
-
sigmoid_scale=sigmoid_scale,
|
324 |
-
wn=wn
|
325 |
-
))
|
326 |
-
|
327 |
-
def forward(self, x, x_mask=None, g=None, reverse=False, return_hiddens=False):
|
328 |
-
logdet_tot = 0
|
329 |
-
if not reverse:
|
330 |
-
flows = self.flows
|
331 |
-
else:
|
332 |
-
flows = reversed(self.flows)
|
333 |
-
if return_hiddens:
|
334 |
-
hs = []
|
335 |
-
if self.n_sqz > 1:
|
336 |
-
x, x_mask_ = utils.squeeze(x, x_mask, self.n_sqz)
|
337 |
-
if g is not None:
|
338 |
-
g, _ = utils.squeeze(g, x_mask, self.n_sqz)
|
339 |
-
x_mask = x_mask_
|
340 |
-
if self.share_cond_layers and g is not None:
|
341 |
-
g = self.cond_layer(g)
|
342 |
-
for f in flows:
|
343 |
-
x, logdet = f(x, x_mask, g=g, reverse=reverse)
|
344 |
-
if return_hiddens:
|
345 |
-
hs.append(x)
|
346 |
-
logdet_tot += logdet
|
347 |
-
if self.n_sqz > 1:
|
348 |
-
x, x_mask = utils.unsqueeze(x, x_mask, self.n_sqz)
|
349 |
-
if return_hiddens:
|
350 |
-
return x, logdet_tot, hs
|
351 |
-
return x, logdet_tot
|
352 |
-
|
353 |
-
def store_inverse(self):
|
354 |
-
def remove_weight_norm(m):
|
355 |
-
try:
|
356 |
-
nn.utils.remove_weight_norm(m)
|
357 |
-
except ValueError: # this module didn't have weight norm
|
358 |
-
return
|
359 |
-
|
360 |
-
self.apply(remove_weight_norm)
|
361 |
-
for f in self.flows:
|
362 |
-
f.store_inverse()
|
|
|
|
|
|
|
|
|
|
|
|
|
|
|
|
|
|
|
|
|
|
|
|
|
|
|
|
|
|
|
|
|
|
|
|
|
|
|
|
|
|
|
|
|
|
|
|
|
|
|
|
|
|
|
|
|
|
|
|
|
|
|
|
|
|
|
|
|
|
|
|
|
|
|
|
|
|
|
|
|
|
|
|
|
|
|
|
|
|
|
|
|
|
|
|
|
|
|
|
|
|
|
|
|
|
|
|
|
|
|
|
|
|
|
|
|
|
|
|
|
|
|
|
|
|
|
|
|
|
|
|
|
|
|
|
|
|
|
|
|
|
|
|
|
|
|
|
|
|
|
|
|
|
|
|
|
|
|
|
|
|
|
|
|
|
|
|
|
|
|
|
|
|
|
|
|
|
|
|
|
|
|
|
|
|
|
|
|
|
|
|
|
|
|
|
|
|
|
|
|
|
|
|
|
|
|
|
|
|
|
|
|
|
|
|
|
|
|
|
|
|
|
|
|
|
|
|
|
|
|
|
|
|
|
|
|
|
|
|
|
|
|
|
|
|
|
|
|
|
|
|
|
|
|
|
|
|
|
|
|
|
|
|
|
|
|
|
|
|
|
|
|
|
|
|
|
|
|
|
|
|
|
|
|
|
|
|
|
|
|
|
|
|
|
|
|
|
|
|
|
|
|
|
|
|
|
|
|
|
|
|
|
|
|
|
|
|
|
|
|
|
|
|
|
|
|
|
|
|
|
|
|
|
|
|
|
|
|
|
|
|
|
|
|
|
|
|
|
|
|
|
|
|
|
|
|
|
|
|
|
|
|
|
|
|
|
|
|
|
|
|
|
|
|
|
|
|
|
|
|
|
|
|
|
|
|
|
|
|
|
|
|
|
|
|
|
|
|
|
|
|
|
|
|
|
|
|
|
|
|
|
|
|
|
|
|
|
|
|
|
|
|
|
|
|
|
|
|
|
|
|
|
|
|
|
|
|
|
|
|
|
|
|
|
|
|
|
|
|
|
|
|
|
|
|
|
|
|
|
|
|
|
|
|
|
|
|
|
|
|
|
|
|
|
|
|
|
|
|
|
|
|
|
|
|
|
|
|
|
|
|
|
|
|
|
|
|
|
|
|
|
|
|
|
|
|
|
|
|
|
|
|
|
|
|
|
|
|
|
|
|
|
|
|
|
|
|
|
|
|
|
|
|
|
|
|
|
|
|
|
|
|
|
|
|
|
|
|
|
|
|
|
|
|
|
|
|
|
|
|
|
|
|
|
|
|
|
|
|
|
|
|
|
|
|
|
|
|
|
|
|
|
|
|
|
|
|
|
|
|
|
|
|
|
|
|
|
|
|
|
|
|
|
|
|
|
|
|
|
|
|
|
|
|
|
|
|
|
|
|
|
|
|
|
|
|
|
|
|
|
|
|
|
|
|
|
|
|
|
|
|
|
|
|
|
|
|
|
|
|
|
|
|
|
|
|
|
|
|
|
|
|
|
|
|
|
|
|
|
|
|
|
|
|
|
|
|
|
|
|
|
|
|
|
|
|
|
|
|
|
|
|
|
|
|
|
|
|
|
|
|
|
|
|
|
|
|
|
|
|
|
|
|
|
|
|
|
|
|
|
|
|
|
|
|
spaces/AIGC-Audio/Make_An_Audio_inpaint/ldm/modules/diffusionmodules/__init__.py
DELETED
File without changes
|
spaces/AIGText/GlyphControl/ldm/modules/midas/midas/base_model.py
DELETED
@@ -1,17 +0,0 @@
|
|
1 |
-
import torch
|
2 |
-
import os
|
3 |
-
|
4 |
-
|
5 |
-
class BaseModel(torch.nn.Module):
|
6 |
-
def load(self, path):
|
7 |
-
"""Load model from file.
|
8 |
-
|
9 |
-
Args:
|
10 |
-
path (str): file path
|
11 |
-
"""
|
12 |
-
parameters = torch.load(path, map_location=torch.device('cpu'))
|
13 |
-
|
14 |
-
if "optimizer" in parameters:
|
15 |
-
parameters = parameters["model"]
|
16 |
-
|
17 |
-
self.load_state_dict(parameters)
|
|
|
|
|
|
|
|
|
|
|
|
|
|
|
|
|
|
|
|
|
|
|
|
|
|
|
|
|
|
|
|
|
|
|
|
spaces/AISuperheroes/08GR-KitchenSink-AIUIUX/demos/kitchen_sink/files/run.py
DELETED
@@ -1,146 +0,0 @@
|
|
1 |
-
import os
|
2 |
-
import json
|
3 |
-
import numpy as np
|
4 |
-
import gradio as gr
|
5 |
-
|
6 |
-
CHOICES = ["foo", "bar", "baz"]
|
7 |
-
JSONOBJ = """{"items":{"item":[{"id": "0001","type": null,"is_good": false,"ppu": 0.55,"batters":{"batter":[{ "id": "1001", "type": "Regular" },{ "id": "1002", "type": "Chocolate" },{ "id": "1003", "type": "Blueberry" },{ "id": "1004", "type": "Devil's Food" }]},"topping":[{ "id": "5001", "type": "None" },{ "id": "5002", "type": "Glazed" },{ "id": "5005", "type": "Sugar" },{ "id": "5007", "type": "Powdered Sugar" },{ "id": "5006", "type": "Chocolate with Sprinkles" },{ "id": "5003", "type": "Chocolate" },{ "id": "5004", "type": "Maple" }]}]}}"""
|
8 |
-
|
9 |
-
def fn(
|
10 |
-
text1,
|
11 |
-
text2,
|
12 |
-
num,
|
13 |
-
slider1,
|
14 |
-
slider2,
|
15 |
-
single_checkbox,
|
16 |
-
checkboxes,
|
17 |
-
radio,
|
18 |
-
dropdown,
|
19 |
-
im1,
|
20 |
-
im2,
|
21 |
-
im3,
|
22 |
-
im4,
|
23 |
-
video,
|
24 |
-
audio1,
|
25 |
-
audio2,
|
26 |
-
file,
|
27 |
-
df1,
|
28 |
-
df2,
|
29 |
-
):
|
30 |
-
return (
|
31 |
-
(text1 if single_checkbox else text2)
|
32 |
-
+ ", selected:"
|
33 |
-
+ ", ".join(checkboxes), # Text
|
34 |
-
{
|
35 |
-
"positive": num / (num + slider1 + slider2),
|
36 |
-
"negative": slider1 / (num + slider1 + slider2),
|
37 |
-
"neutral": slider2 / (num + slider1 + slider2),
|
38 |
-
}, # Label
|
39 |
-
(audio1[0], np.flipud(audio1[1]))
|
40 |
-
if audio1 is not None else os.path.join(os.path.dirname(__file__), "files/cantina.wav"), # Audio
|
41 |
-
np.flipud(im1)
|
42 |
-
if im1 is not None else os.path.join(os.path.dirname(__file__), "files/cheetah1.jpg"), # Image
|
43 |
-
video
|
44 |
-
if video is not None else os.path.join(os.path.dirname(__file__), "files/world.mp4"), # Video
|
45 |
-
[
|
46 |
-
("The", "art"),
|
47 |
-
("quick brown", "adj"),
|
48 |
-
("fox", "nn"),
|
49 |
-
("jumped", "vrb"),
|
50 |
-
("testing testing testing", None),
|
51 |
-
("over", "prp"),
|
52 |
-
("the", "art"),
|
53 |
-
("testing", None),
|
54 |
-
("lazy", "adj"),
|
55 |
-
("dogs", "nn"),
|
56 |
-
(".", "punc"),
|
57 |
-
] + [(f"test {x}", f"test {x}") for x in range(10)], # HighlightedText
|
58 |
-
[
|
59 |
-
("The testing testing testing", None),
|
60 |
-
("over", 0.6),
|
61 |
-
("the", 0.2),
|
62 |
-
("testing", None),
|
63 |
-
("lazy", -0.1),
|
64 |
-
("dogs", 0.4),
|
65 |
-
(".", 0),
|
66 |
-
] + [(f"test", x / 10) for x in range(-10, 10)], # HighlightedText
|
67 |
-
json.loads(JSONOBJ), # JSON
|
68 |
-
"<button style='background-color: red'>Click Me: " + radio + "</button>", # HTML
|
69 |
-
os.path.join(os.path.dirname(__file__), "files/titanic.csv"),
|
70 |
-
df1, # Dataframe
|
71 |
-
np.random.randint(0, 10, (4, 4)), # Dataframe
|
72 |
-
df2, # Timeseries
|
73 |
-
)
|
74 |
-
|
75 |
-
|
76 |
-
demo = gr.Interface(
|
77 |
-
fn,
|
78 |
-
inputs=[
|
79 |
-
gr.Textbox(value="Lorem ipsum", label="Textbox"),
|
80 |
-
gr.Textbox(lines=3, placeholder="Type here..", label="Textbox 2"),
|
81 |
-
gr.Number(label="Number", value=42),
|
82 |
-
gr.Slider(10, 20, value=15, label="Slider: 10 - 20"),
|
83 |
-
gr.Slider(maximum=20, step=0.04, label="Slider: step @ 0.04"),
|
84 |
-
gr.Checkbox(label="Checkbox"),
|
85 |
-
gr.CheckboxGroup(label="CheckboxGroup", choices=CHOICES, value=CHOICES[0:2]),
|
86 |
-
gr.Radio(label="Radio", choices=CHOICES, value=CHOICES[2]),
|
87 |
-
gr.Dropdown(label="Dropdown", choices=CHOICES),
|
88 |
-
gr.Image(label="Image"),
|
89 |
-
gr.Image(label="Image w/ Cropper", tool="select"),
|
90 |
-
gr.Image(label="Sketchpad", source="canvas"),
|
91 |
-
gr.Image(label="Webcam", source="webcam"),
|
92 |
-
gr.Video(label="Video"),
|
93 |
-
gr.Audio(label="Audio"),
|
94 |
-
gr.Audio(label="Microphone", source="microphone"),
|
95 |
-
gr.File(label="File"),
|
96 |
-
gr.Dataframe(label="Dataframe", headers=["Name", "Age", "Gender"]),
|
97 |
-
gr.Timeseries(x="time", y=["price", "value"], colors=["pink", "purple"]),
|
98 |
-
],
|
99 |
-
outputs=[
|
100 |
-
gr.Textbox(label="Textbox"),
|
101 |
-
gr.Label(label="Label"),
|
102 |
-
gr.Audio(label="Audio"),
|
103 |
-
gr.Image(label="Image"),
|
104 |
-
gr.Video(label="Video"),
|
105 |
-
gr.HighlightedText(label="HighlightedText", color_map={"punc": "pink", "test 0": "blue"}),
|
106 |
-
gr.HighlightedText(label="HighlightedText", show_legend=True),
|
107 |
-
gr.JSON(label="JSON"),
|
108 |
-
gr.HTML(label="HTML"),
|
109 |
-
gr.File(label="File"),
|
110 |
-
gr.Dataframe(label="Dataframe"),
|
111 |
-
gr.Dataframe(label="Numpy"),
|
112 |
-
gr.Timeseries(x="time", y=["price", "value"], label="Timeseries"),
|
113 |
-
],
|
114 |
-
examples=[
|
115 |
-
[
|
116 |
-
"the quick brown fox",
|
117 |
-
"jumps over the lazy dog",
|
118 |
-
10,
|
119 |
-
12,
|
120 |
-
4,
|
121 |
-
True,
|
122 |
-
["foo", "baz"],
|
123 |
-
"baz",
|
124 |
-
"bar",
|
125 |
-
os.path.join(os.path.dirname(__file__), "files/cheetah1.jpg"),
|
126 |
-
os.path.join(os.path.dirname(__file__), "files/cheetah1.jpg"),
|
127 |
-
os.path.join(os.path.dirname(__file__), "files/cheetah1.jpg"),
|
128 |
-
os.path.join(os.path.dirname(__file__), "files/cheetah1.jpg"),
|
129 |
-
os.path.join(os.path.dirname(__file__), "files/world.mp4"),
|
130 |
-
os.path.join(os.path.dirname(__file__), "files/cantina.wav"),
|
131 |
-
os.path.join(os.path.dirname(__file__), "files/cantina.wav"),
|
132 |
-
os.path.join(os.path.dirname(__file__), "files/titanic.csv"),
|
133 |
-
[[1, 2, 3], [3, 4, 5]],
|
134 |
-
os.path.join(os.path.dirname(__file__), "files/time.csv"),
|
135 |
-
]
|
136 |
-
]
|
137 |
-
* 3,
|
138 |
-
theme="default",
|
139 |
-
title="Gradio AI UI UX",
|
140 |
-
cache_examples=False,
|
141 |
-
description="Try out all the components!",
|
142 |
-
article="Learn more about [Gradio](http://gradio.app)",
|
143 |
-
)
|
144 |
-
|
145 |
-
if __name__ == "__main__":
|
146 |
-
demo.launch()
|
|
|
|
|
|
|
|
|
|
|
|
|
|
|
|
|
|
|
|
|
|
|
|
|
|
|
|
|
|
|
|
|
|
|
|
|
|
|
|
|
|
|
|
|
|
|
|
|
|
|
|
|
|
|
|
|
|
|
|
|
|
|
|
|
|
|
|
|
|
|
|
|
|
|
|
|
|
|
|
|
|
|
|
|
|
|
|
|
|
|
|
|
|
|
|
|
|
|
|
|
|
|
|
|
|
|
|
|
|
|
|
|
|
|
|
|
|
|
|
|
|
|
|
|
|
|
|
|
|
|
|
|
|
|
|
|
|
|
|
|
|
|
|
|
|
|
|
|
|
|
|
|
|
|
|
|
|
|
|
|
|
|
|
|
|
|
|
|
|
|
|
|
|
|
|
|
|
|
|
|
|
|
|
|
|
|
|
|
|
|
|
|
|
|
|
|
|
|
|
|
|
|
|
|
|
|
|
|
|
|
|
|
|
|
|
|
|
|
|
|
|
|
|
|
|
|
|
|
|
|
|
|
|
|
|
|
|
|
|
|
|
|
|
|
|
|
|
|
|
|
|
|
|
|
|
|
|
|
|
|
|
|
|
|
|
|
|
|
|
|
|
|
|
|
|
|
|
|
|
|
|
|
|
|
|
|
|
|
|
|
|
|
|
spaces/AchyuthGamer/OpenGPT-Chat-UI/PRIVACY.md
DELETED
@@ -1,17 +0,0 @@
|
|
1 |
-
# About & Privacy - BlindChat
|
2 |
-
|
3 |
-
## Privacy
|
4 |
-
|
5 |
-
<em>Last updated: September 15, 2023</em>
|
6 |
-
|
7 |
-
No conversations are recorded. All computation happens on your device, and conversations are stored locally in the browser’s cache.
|
8 |
-
|
9 |
-
We don’t and never will see your data, so we cannot train on your data. Your data remains yours.
|
10 |
-
|
11 |
-
## About
|
12 |
-
|
13 |
-
BlindChat is an open-source project to provide fully in-browser and private Conversational AI.
|
14 |
-
|
15 |
-
It is currently developed and maintained by [Mithril Security](https://www.mithrilsecurity.io/), a startup aiming to make AI more private.
|
16 |
-
|
17 |
-
You can find more information on our [Github](https://github.com/mithril-security/blind_chat/), join us on our [Discord](https://discord.com/invite/TxEHagpWd4), or directly [contact us](mailto:[email protected]).
|
|
|
|
|
|
|
|
|
|
|
|
|
|
|
|
|
|
|
|
|
|
|
|
|
|
|
|
|
|
|
|
|
|
|
|
spaces/Adieudale/Adieudale/README.md
DELETED
@@ -1,12 +0,0 @@
|
|
1 |
-
---
|
2 |
-
title: Adieudale
|
3 |
-
emoji: 😻
|
4 |
-
colorFrom: gray
|
5 |
-
colorTo: pink
|
6 |
-
sdk: docker
|
7 |
-
pinned: false
|
8 |
-
license: mit
|
9 |
-
app_port: 8080
|
10 |
-
---
|
11 |
-
|
12 |
-
Check out the configuration reference at https://huggingface.co/docs/hub/spaces-config-reference
|
|
|
|
|
|
|
|
|
|
|
|
|
|
|
|
|
|
|
|
|
|
|
|
|
|
spaces/Aditya9790/yolo7-object-tracking/utils/datasets.py
DELETED
@@ -1,1320 +0,0 @@
|
|
1 |
-
# Dataset utils and dataloaders
|
2 |
-
|
3 |
-
import glob
|
4 |
-
import logging
|
5 |
-
import math
|
6 |
-
import os
|
7 |
-
import random
|
8 |
-
import shutil
|
9 |
-
import time
|
10 |
-
from itertools import repeat
|
11 |
-
from multiprocessing.pool import ThreadPool
|
12 |
-
from pathlib import Path
|
13 |
-
from threading import Thread
|
14 |
-
|
15 |
-
import cv2
|
16 |
-
import numpy as np
|
17 |
-
import torch
|
18 |
-
import torch.nn.functional as F
|
19 |
-
from PIL import Image, ExifTags
|
20 |
-
from torch.utils.data import Dataset
|
21 |
-
from tqdm import tqdm
|
22 |
-
|
23 |
-
import pickle
|
24 |
-
from copy import deepcopy
|
25 |
-
#from pycocotools import mask as maskUtils
|
26 |
-
from torchvision.utils import save_image
|
27 |
-
from torchvision.ops import roi_pool, roi_align, ps_roi_pool, ps_roi_align
|
28 |
-
|
29 |
-
from utils.general import check_requirements, xyxy2xywh, xywh2xyxy, xywhn2xyxy, xyn2xy, segment2box, segments2boxes, \
|
30 |
-
resample_segments, clean_str
|
31 |
-
from utils.torch_utils import torch_distributed_zero_first
|
32 |
-
|
33 |
-
# Parameters
|
34 |
-
help_url = 'https://github.com/ultralytics/yolov5/wiki/Train-Custom-Data'
|
35 |
-
img_formats = ['bmp', 'jpg', 'jpeg', 'png', 'tif', 'tiff', 'dng', 'webp', 'mpo'] # acceptable image suffixes
|
36 |
-
vid_formats = ['mov', 'avi', 'mp4', 'mpg', 'mpeg', 'm4v', 'wmv', 'mkv'] # acceptable video suffixes
|
37 |
-
logger = logging.getLogger(__name__)
|
38 |
-
|
39 |
-
# Get orientation exif tag
|
40 |
-
for orientation in ExifTags.TAGS.keys():
|
41 |
-
if ExifTags.TAGS[orientation] == 'Orientation':
|
42 |
-
break
|
43 |
-
|
44 |
-
|
45 |
-
def get_hash(files):
|
46 |
-
# Returns a single hash value of a list of files
|
47 |
-
return sum(os.path.getsize(f) for f in files if os.path.isfile(f))
|
48 |
-
|
49 |
-
|
50 |
-
def exif_size(img):
|
51 |
-
# Returns exif-corrected PIL size
|
52 |
-
s = img.size # (width, height)
|
53 |
-
try:
|
54 |
-
rotation = dict(img._getexif().items())[orientation]
|
55 |
-
if rotation == 6: # rotation 270
|
56 |
-
s = (s[1], s[0])
|
57 |
-
elif rotation == 8: # rotation 90
|
58 |
-
s = (s[1], s[0])
|
59 |
-
except:
|
60 |
-
pass
|
61 |
-
|
62 |
-
return s
|
63 |
-
|
64 |
-
|
65 |
-
def create_dataloader(path, imgsz, batch_size, stride, opt, hyp=None, augment=False, cache=False, pad=0.0, rect=False,
|
66 |
-
rank=-1, world_size=1, workers=8, image_weights=False, quad=False, prefix=''):
|
67 |
-
# Make sure only the first process in DDP process the dataset first, and the following others can use the cache
|
68 |
-
with torch_distributed_zero_first(rank):
|
69 |
-
dataset = LoadImagesAndLabels(path, imgsz, batch_size,
|
70 |
-
augment=augment, # augment images
|
71 |
-
hyp=hyp, # augmentation hyperparameters
|
72 |
-
rect=rect, # rectangular training
|
73 |
-
cache_images=cache,
|
74 |
-
single_cls=opt.single_cls,
|
75 |
-
stride=int(stride),
|
76 |
-
pad=pad,
|
77 |
-
image_weights=image_weights,
|
78 |
-
prefix=prefix)
|
79 |
-
|
80 |
-
batch_size = min(batch_size, len(dataset))
|
81 |
-
nw = min([os.cpu_count() // world_size, batch_size if batch_size > 1 else 0, workers]) # number of workers
|
82 |
-
sampler = torch.utils.data.distributed.DistributedSampler(dataset) if rank != -1 else None
|
83 |
-
loader = torch.utils.data.DataLoader if image_weights else InfiniteDataLoader
|
84 |
-
# Use torch.utils.data.DataLoader() if dataset.properties will update during training else InfiniteDataLoader()
|
85 |
-
dataloader = loader(dataset,
|
86 |
-
batch_size=batch_size,
|
87 |
-
num_workers=nw,
|
88 |
-
sampler=sampler,
|
89 |
-
pin_memory=True,
|
90 |
-
collate_fn=LoadImagesAndLabels.collate_fn4 if quad else LoadImagesAndLabels.collate_fn)
|
91 |
-
return dataloader, dataset
|
92 |
-
|
93 |
-
|
94 |
-
class InfiniteDataLoader(torch.utils.data.dataloader.DataLoader):
|
95 |
-
""" Dataloader that reuses workers
|
96 |
-
|
97 |
-
Uses same syntax as vanilla DataLoader
|
98 |
-
"""
|
99 |
-
|
100 |
-
def __init__(self, *args, **kwargs):
|
101 |
-
super().__init__(*args, **kwargs)
|
102 |
-
object.__setattr__(self, 'batch_sampler', _RepeatSampler(self.batch_sampler))
|
103 |
-
self.iterator = super().__iter__()
|
104 |
-
|
105 |
-
def __len__(self):
|
106 |
-
return len(self.batch_sampler.sampler)
|
107 |
-
|
108 |
-
def __iter__(self):
|
109 |
-
for i in range(len(self)):
|
110 |
-
yield next(self.iterator)
|
111 |
-
|
112 |
-
|
113 |
-
class _RepeatSampler(object):
|
114 |
-
""" Sampler that repeats forever
|
115 |
-
|
116 |
-
Args:
|
117 |
-
sampler (Sampler)
|
118 |
-
"""
|
119 |
-
|
120 |
-
def __init__(self, sampler):
|
121 |
-
self.sampler = sampler
|
122 |
-
|
123 |
-
def __iter__(self):
|
124 |
-
while True:
|
125 |
-
yield from iter(self.sampler)
|
126 |
-
|
127 |
-
|
128 |
-
class LoadImages: # for inference
|
129 |
-
def __init__(self, path, img_size=640, stride=32):
|
130 |
-
p = str(Path(path).absolute()) # os-agnostic absolute path
|
131 |
-
if '*' in p:
|
132 |
-
files = sorted(glob.glob(p, recursive=True)) # glob
|
133 |
-
elif os.path.isdir(p):
|
134 |
-
files = sorted(glob.glob(os.path.join(p, '*.*'))) # dir
|
135 |
-
elif os.path.isfile(p):
|
136 |
-
files = [p] # files
|
137 |
-
else:
|
138 |
-
raise Exception(f'ERROR: {p} does not exist')
|
139 |
-
|
140 |
-
images = [x for x in files if x.split('.')[-1].lower() in img_formats]
|
141 |
-
videos = [x for x in files if x.split('.')[-1].lower() in vid_formats]
|
142 |
-
ni, nv = len(images), len(videos)
|
143 |
-
|
144 |
-
self.img_size = img_size
|
145 |
-
self.stride = stride
|
146 |
-
self.files = images + videos
|
147 |
-
self.nf = ni + nv # number of files
|
148 |
-
self.video_flag = [False] * ni + [True] * nv
|
149 |
-
self.mode = 'image'
|
150 |
-
if any(videos):
|
151 |
-
self.new_video(videos[0]) # new video
|
152 |
-
else:
|
153 |
-
self.cap = None
|
154 |
-
assert self.nf > 0, f'No images or videos found in {p}. ' \
|
155 |
-
f'Supported formats are:\nimages: {img_formats}\nvideos: {vid_formats}'
|
156 |
-
|
157 |
-
def __iter__(self):
|
158 |
-
self.count = 0
|
159 |
-
return self
|
160 |
-
|
161 |
-
def __next__(self):
|
162 |
-
if self.count == self.nf:
|
163 |
-
raise StopIteration
|
164 |
-
path = self.files[self.count]
|
165 |
-
|
166 |
-
if self.video_flag[self.count]:
|
167 |
-
# Read video
|
168 |
-
self.mode = 'video'
|
169 |
-
ret_val, img0 = self.cap.read()
|
170 |
-
if not ret_val:
|
171 |
-
self.count += 1
|
172 |
-
self.cap.release()
|
173 |
-
if self.count == self.nf: # last video
|
174 |
-
raise StopIteration
|
175 |
-
else:
|
176 |
-
path = self.files[self.count]
|
177 |
-
self.new_video(path)
|
178 |
-
ret_val, img0 = self.cap.read()
|
179 |
-
|
180 |
-
self.frame += 1
|
181 |
-
print(f'video {self.count + 1}/{self.nf} ({self.frame}/{self.nframes}) {path}: ', end='')
|
182 |
-
|
183 |
-
else:
|
184 |
-
# Read image
|
185 |
-
self.count += 1
|
186 |
-
img0 = cv2.imread(path) # BGR
|
187 |
-
assert img0 is not None, 'Image Not Found ' + path
|
188 |
-
#print(f'image {self.count}/{self.nf} {path}: ', end='')
|
189 |
-
|
190 |
-
# Padded resize
|
191 |
-
img = letterbox(img0, self.img_size, stride=self.stride)[0]
|
192 |
-
|
193 |
-
# Convert
|
194 |
-
img = img[:, :, ::-1].transpose(2, 0, 1) # BGR to RGB, to 3x416x416
|
195 |
-
img = np.ascontiguousarray(img)
|
196 |
-
|
197 |
-
return path, img, img0, self.cap
|
198 |
-
|
199 |
-
def new_video(self, path):
|
200 |
-
self.frame = 0
|
201 |
-
self.cap = cv2.VideoCapture(path)
|
202 |
-
self.nframes = int(self.cap.get(cv2.CAP_PROP_FRAME_COUNT))
|
203 |
-
|
204 |
-
def __len__(self):
|
205 |
-
return self.nf # number of files
|
206 |
-
|
207 |
-
|
208 |
-
class LoadWebcam: # for inference
|
209 |
-
def __init__(self, pipe='0', img_size=640, stride=32):
|
210 |
-
self.img_size = img_size
|
211 |
-
self.stride = stride
|
212 |
-
|
213 |
-
if pipe.isnumeric():
|
214 |
-
pipe = eval(pipe) # local camera
|
215 |
-
# pipe = 'rtsp://192.168.1.64/1' # IP camera
|
216 |
-
# pipe = 'rtsp://username:[email protected]/1' # IP camera with login
|
217 |
-
# pipe = 'http://wmccpinetop.axiscam.net/mjpg/video.mjpg' # IP golf camera
|
218 |
-
|
219 |
-
self.pipe = pipe
|
220 |
-
self.cap = cv2.VideoCapture(pipe) # video capture object
|
221 |
-
self.cap.set(cv2.CAP_PROP_BUFFERSIZE, 3) # set buffer size
|
222 |
-
|
223 |
-
def __iter__(self):
|
224 |
-
self.count = -1
|
225 |
-
return self
|
226 |
-
|
227 |
-
def __next__(self):
|
228 |
-
self.count += 1
|
229 |
-
if cv2.waitKey(1) == ord('q'): # q to quit
|
230 |
-
self.cap.release()
|
231 |
-
cv2.destroyAllWindows()
|
232 |
-
raise StopIteration
|
233 |
-
|
234 |
-
# Read frame
|
235 |
-
if self.pipe == 0: # local camera
|
236 |
-
ret_val, img0 = self.cap.read()
|
237 |
-
img0 = cv2.flip(img0, 1) # flip left-right
|
238 |
-
else: # IP camera
|
239 |
-
n = 0
|
240 |
-
while True:
|
241 |
-
n += 1
|
242 |
-
self.cap.grab()
|
243 |
-
if n % 30 == 0: # skip frames
|
244 |
-
ret_val, img0 = self.cap.retrieve()
|
245 |
-
if ret_val:
|
246 |
-
break
|
247 |
-
|
248 |
-
# Print
|
249 |
-
assert ret_val, f'Camera Error {self.pipe}'
|
250 |
-
img_path = 'webcam.jpg'
|
251 |
-
print(f'webcam {self.count}: ', end='')
|
252 |
-
|
253 |
-
# Padded resize
|
254 |
-
img = letterbox(img0, self.img_size, stride=self.stride)[0]
|
255 |
-
|
256 |
-
# Convert
|
257 |
-
img = img[:, :, ::-1].transpose(2, 0, 1) # BGR to RGB, to 3x416x416
|
258 |
-
img = np.ascontiguousarray(img)
|
259 |
-
|
260 |
-
return img_path, img, img0, None
|
261 |
-
|
262 |
-
def __len__(self):
|
263 |
-
return 0
|
264 |
-
|
265 |
-
|
266 |
-
class LoadStreams: # multiple IP or RTSP cameras
|
267 |
-
def __init__(self, sources='streams.txt', img_size=640, stride=32):
|
268 |
-
self.mode = 'stream'
|
269 |
-
self.img_size = img_size
|
270 |
-
self.stride = stride
|
271 |
-
|
272 |
-
if os.path.isfile(sources):
|
273 |
-
with open(sources, 'r') as f:
|
274 |
-
sources = [x.strip() for x in f.read().strip().splitlines() if len(x.strip())]
|
275 |
-
else:
|
276 |
-
sources = [sources]
|
277 |
-
|
278 |
-
n = len(sources)
|
279 |
-
self.imgs = [None] * n
|
280 |
-
self.sources = [clean_str(x) for x in sources] # clean source names for later
|
281 |
-
for i, s in enumerate(sources):
|
282 |
-
# Start the thread to read frames from the video stream
|
283 |
-
print(f'{i + 1}/{n}: {s}... ', end='')
|
284 |
-
url = eval(s) if s.isnumeric() else s
|
285 |
-
if 'youtube.com/' in str(url) or 'youtu.be/' in str(url): # if source is YouTube video
|
286 |
-
check_requirements(('pafy', 'youtube_dl'))
|
287 |
-
import pafy
|
288 |
-
url = pafy.new(url).getbest(preftype="mp4").url
|
289 |
-
cap = cv2.VideoCapture(url)
|
290 |
-
assert cap.isOpened(), f'Failed to open {s}'
|
291 |
-
w = int(cap.get(cv2.CAP_PROP_FRAME_WIDTH))
|
292 |
-
h = int(cap.get(cv2.CAP_PROP_FRAME_HEIGHT))
|
293 |
-
self.fps = cap.get(cv2.CAP_PROP_FPS) % 100
|
294 |
-
|
295 |
-
_, self.imgs[i] = cap.read() # guarantee first frame
|
296 |
-
thread = Thread(target=self.update, args=([i, cap]), daemon=True)
|
297 |
-
print(f' success ({w}x{h} at {self.fps:.2f} FPS).')
|
298 |
-
thread.start()
|
299 |
-
print('') # newline
|
300 |
-
|
301 |
-
# check for common shapes
|
302 |
-
s = np.stack([letterbox(x, self.img_size, stride=self.stride)[0].shape for x in self.imgs], 0) # shapes
|
303 |
-
self.rect = np.unique(s, axis=0).shape[0] == 1 # rect inference if all shapes equal
|
304 |
-
if not self.rect:
|
305 |
-
print('WARNING: Different stream shapes detected. For optimal performance supply similarly-shaped streams.')
|
306 |
-
|
307 |
-
def update(self, index, cap):
|
308 |
-
# Read next stream frame in a daemon thread
|
309 |
-
n = 0
|
310 |
-
while cap.isOpened():
|
311 |
-
n += 1
|
312 |
-
# _, self.imgs[index] = cap.read()
|
313 |
-
cap.grab()
|
314 |
-
if n == 4: # read every 4th frame
|
315 |
-
success, im = cap.retrieve()
|
316 |
-
self.imgs[index] = im if success else self.imgs[index] * 0
|
317 |
-
n = 0
|
318 |
-
time.sleep(1 / self.fps) # wait time
|
319 |
-
|
320 |
-
def __iter__(self):
|
321 |
-
self.count = -1
|
322 |
-
return self
|
323 |
-
|
324 |
-
def __next__(self):
|
325 |
-
self.count += 1
|
326 |
-
img0 = self.imgs.copy()
|
327 |
-
if cv2.waitKey(1) == ord('q'): # q to quit
|
328 |
-
cv2.destroyAllWindows()
|
329 |
-
raise StopIteration
|
330 |
-
|
331 |
-
# Letterbox
|
332 |
-
img = [letterbox(x, self.img_size, auto=self.rect, stride=self.stride)[0] for x in img0]
|
333 |
-
|
334 |
-
# Stack
|
335 |
-
img = np.stack(img, 0)
|
336 |
-
|
337 |
-
# Convert
|
338 |
-
img = img[:, :, :, ::-1].transpose(0, 3, 1, 2) # BGR to RGB, to bsx3x416x416
|
339 |
-
img = np.ascontiguousarray(img)
|
340 |
-
|
341 |
-
return self.sources, img, img0, None
|
342 |
-
|
343 |
-
def __len__(self):
|
344 |
-
return 0 # 1E12 frames = 32 streams at 30 FPS for 30 years
|
345 |
-
|
346 |
-
|
347 |
-
def img2label_paths(img_paths):
|
348 |
-
# Define label paths as a function of image paths
|
349 |
-
sa, sb = os.sep + 'images' + os.sep, os.sep + 'labels' + os.sep # /images/, /labels/ substrings
|
350 |
-
return ['txt'.join(x.replace(sa, sb, 1).rsplit(x.split('.')[-1], 1)) for x in img_paths]
|
351 |
-
|
352 |
-
|
353 |
-
class LoadImagesAndLabels(Dataset): # for training/testing
|
354 |
-
def __init__(self, path, img_size=640, batch_size=16, augment=False, hyp=None, rect=False, image_weights=False,
|
355 |
-
cache_images=False, single_cls=False, stride=32, pad=0.0, prefix=''):
|
356 |
-
self.img_size = img_size
|
357 |
-
self.augment = augment
|
358 |
-
self.hyp = hyp
|
359 |
-
self.image_weights = image_weights
|
360 |
-
self.rect = False if image_weights else rect
|
361 |
-
self.mosaic = self.augment and not self.rect # load 4 images at a time into a mosaic (only during training)
|
362 |
-
self.mosaic_border = [-img_size // 2, -img_size // 2]
|
363 |
-
self.stride = stride
|
364 |
-
self.path = path
|
365 |
-
#self.albumentations = Albumentations() if augment else None
|
366 |
-
|
367 |
-
try:
|
368 |
-
f = [] # image files
|
369 |
-
for p in path if isinstance(path, list) else [path]:
|
370 |
-
p = Path(p) # os-agnostic
|
371 |
-
if p.is_dir(): # dir
|
372 |
-
f += glob.glob(str(p / '**' / '*.*'), recursive=True)
|
373 |
-
# f = list(p.rglob('**/*.*')) # pathlib
|
374 |
-
elif p.is_file(): # file
|
375 |
-
with open(p, 'r') as t:
|
376 |
-
t = t.read().strip().splitlines()
|
377 |
-
parent = str(p.parent) + os.sep
|
378 |
-
f += [x.replace('./', parent) if x.startswith('./') else x for x in t] # local to global path
|
379 |
-
# f += [p.parent / x.lstrip(os.sep) for x in t] # local to global path (pathlib)
|
380 |
-
else:
|
381 |
-
raise Exception(f'{prefix}{p} does not exist')
|
382 |
-
self.img_files = sorted([x.replace('/', os.sep) for x in f if x.split('.')[-1].lower() in img_formats])
|
383 |
-
# self.img_files = sorted([x for x in f if x.suffix[1:].lower() in img_formats]) # pathlib
|
384 |
-
assert self.img_files, f'{prefix}No images found'
|
385 |
-
except Exception as e:
|
386 |
-
raise Exception(f'{prefix}Error loading data from {path}: {e}\nSee {help_url}')
|
387 |
-
|
388 |
-
# Check cache
|
389 |
-
self.label_files = img2label_paths(self.img_files) # labels
|
390 |
-
cache_path = (p if p.is_file() else Path(self.label_files[0]).parent).with_suffix('.cache') # cached labels
|
391 |
-
if cache_path.is_file():
|
392 |
-
cache, exists = torch.load(cache_path), True # load
|
393 |
-
#if cache['hash'] != get_hash(self.label_files + self.img_files) or 'version' not in cache: # changed
|
394 |
-
# cache, exists = self.cache_labels(cache_path, prefix), False # re-cache
|
395 |
-
else:
|
396 |
-
cache, exists = self.cache_labels(cache_path, prefix), False # cache
|
397 |
-
|
398 |
-
# Display cache
|
399 |
-
nf, nm, ne, nc, n = cache.pop('results') # found, missing, empty, corrupted, total
|
400 |
-
if exists:
|
401 |
-
d = f"Scanning '{cache_path}' images and labels... {nf} found, {nm} missing, {ne} empty, {nc} corrupted"
|
402 |
-
tqdm(None, desc=prefix + d, total=n, initial=n) # display cache results
|
403 |
-
assert nf > 0 or not augment, f'{prefix}No labels in {cache_path}. Can not train without labels. See {help_url}'
|
404 |
-
|
405 |
-
# Read cache
|
406 |
-
cache.pop('hash') # remove hash
|
407 |
-
cache.pop('version') # remove version
|
408 |
-
labels, shapes, self.segments = zip(*cache.values())
|
409 |
-
self.labels = list(labels)
|
410 |
-
self.shapes = np.array(shapes, dtype=np.float64)
|
411 |
-
self.img_files = list(cache.keys()) # update
|
412 |
-
self.label_files = img2label_paths(cache.keys()) # update
|
413 |
-
if single_cls:
|
414 |
-
for x in self.labels:
|
415 |
-
x[:, 0] = 0
|
416 |
-
|
417 |
-
n = len(shapes) # number of images
|
418 |
-
bi = np.floor(np.arange(n) / batch_size).astype(int) # batch index
|
419 |
-
nb = bi[-1] + 1 # number of batches
|
420 |
-
self.batch = bi # batch index of image
|
421 |
-
self.n = n
|
422 |
-
self.indices = range(n)
|
423 |
-
|
424 |
-
# Rectangular Training
|
425 |
-
if self.rect:
|
426 |
-
# Sort by aspect ratio
|
427 |
-
s = self.shapes # wh
|
428 |
-
ar = s[:, 1] / s[:, 0] # aspect ratio
|
429 |
-
irect = ar.argsort()
|
430 |
-
self.img_files = [self.img_files[i] for i in irect]
|
431 |
-
self.label_files = [self.label_files[i] for i in irect]
|
432 |
-
self.labels = [self.labels[i] for i in irect]
|
433 |
-
self.shapes = s[irect] # wh
|
434 |
-
ar = ar[irect]
|
435 |
-
|
436 |
-
# Set training image shapes
|
437 |
-
shapes = [[1, 1]] * nb
|
438 |
-
for i in range(nb):
|
439 |
-
ari = ar[bi == i]
|
440 |
-
mini, maxi = ari.min(), ari.max()
|
441 |
-
if maxi < 1:
|
442 |
-
shapes[i] = [maxi, 1]
|
443 |
-
elif mini > 1:
|
444 |
-
shapes[i] = [1, 1 / mini]
|
445 |
-
|
446 |
-
self.batch_shapes = np.ceil(np.array(shapes) * img_size / stride + pad).astype(int) * stride
|
447 |
-
|
448 |
-
# Cache images into memory for faster training (WARNING: large datasets may exceed system RAM)
|
449 |
-
self.imgs = [None] * n
|
450 |
-
if cache_images:
|
451 |
-
if cache_images == 'disk':
|
452 |
-
self.im_cache_dir = Path(Path(self.img_files[0]).parent.as_posix() + '_npy')
|
453 |
-
self.img_npy = [self.im_cache_dir / Path(f).with_suffix('.npy').name for f in self.img_files]
|
454 |
-
self.im_cache_dir.mkdir(parents=True, exist_ok=True)
|
455 |
-
gb = 0 # Gigabytes of cached images
|
456 |
-
self.img_hw0, self.img_hw = [None] * n, [None] * n
|
457 |
-
results = ThreadPool(8).imap(lambda x: load_image(*x), zip(repeat(self), range(n)))
|
458 |
-
pbar = tqdm(enumerate(results), total=n)
|
459 |
-
for i, x in pbar:
|
460 |
-
if cache_images == 'disk':
|
461 |
-
if not self.img_npy[i].exists():
|
462 |
-
np.save(self.img_npy[i].as_posix(), x[0])
|
463 |
-
gb += self.img_npy[i].stat().st_size
|
464 |
-
else:
|
465 |
-
self.imgs[i], self.img_hw0[i], self.img_hw[i] = x
|
466 |
-
gb += self.imgs[i].nbytes
|
467 |
-
pbar.desc = f'{prefix}Caching images ({gb / 1E9:.1f}GB)'
|
468 |
-
pbar.close()
|
469 |
-
|
470 |
-
def cache_labels(self, path=Path('./labels.cache'), prefix=''):
|
471 |
-
# Cache dataset labels, check images and read shapes
|
472 |
-
x = {} # dict
|
473 |
-
nm, nf, ne, nc = 0, 0, 0, 0 # number missing, found, empty, duplicate
|
474 |
-
pbar = tqdm(zip(self.img_files, self.label_files), desc='Scanning images', total=len(self.img_files))
|
475 |
-
for i, (im_file, lb_file) in enumerate(pbar):
|
476 |
-
try:
|
477 |
-
# verify images
|
478 |
-
im = Image.open(im_file)
|
479 |
-
im.verify() # PIL verify
|
480 |
-
shape = exif_size(im) # image size
|
481 |
-
segments = [] # instance segments
|
482 |
-
assert (shape[0] > 9) & (shape[1] > 9), f'image size {shape} <10 pixels'
|
483 |
-
assert im.format.lower() in img_formats, f'invalid image format {im.format}'
|
484 |
-
|
485 |
-
# verify labels
|
486 |
-
if os.path.isfile(lb_file):
|
487 |
-
nf += 1 # label found
|
488 |
-
with open(lb_file, 'r') as f:
|
489 |
-
l = [x.split() for x in f.read().strip().splitlines()]
|
490 |
-
if any([len(x) > 8 for x in l]): # is segment
|
491 |
-
classes = np.array([x[0] for x in l], dtype=np.float32)
|
492 |
-
segments = [np.array(x[1:], dtype=np.float32).reshape(-1, 2) for x in l] # (cls, xy1...)
|
493 |
-
l = np.concatenate((classes.reshape(-1, 1), segments2boxes(segments)), 1) # (cls, xywh)
|
494 |
-
l = np.array(l, dtype=np.float32)
|
495 |
-
if len(l):
|
496 |
-
assert l.shape[1] == 5, 'labels require 5 columns each'
|
497 |
-
assert (l >= 0).all(), 'negative labels'
|
498 |
-
assert (l[:, 1:] <= 1).all(), 'non-normalized or out of bounds coordinate labels'
|
499 |
-
assert np.unique(l, axis=0).shape[0] == l.shape[0], 'duplicate labels'
|
500 |
-
else:
|
501 |
-
ne += 1 # label empty
|
502 |
-
l = np.zeros((0, 5), dtype=np.float32)
|
503 |
-
else:
|
504 |
-
nm += 1 # label missing
|
505 |
-
l = np.zeros((0, 5), dtype=np.float32)
|
506 |
-
x[im_file] = [l, shape, segments]
|
507 |
-
except Exception as e:
|
508 |
-
nc += 1
|
509 |
-
print(f'{prefix}WARNING: Ignoring corrupted image and/or label {im_file}: {e}')
|
510 |
-
|
511 |
-
pbar.desc = f"{prefix}Scanning '{path.parent / path.stem}' images and labels... " \
|
512 |
-
f"{nf} found, {nm} missing, {ne} empty, {nc} corrupted"
|
513 |
-
pbar.close()
|
514 |
-
|
515 |
-
if nf == 0:
|
516 |
-
print(f'{prefix}WARNING: No labels found in {path}. See {help_url}')
|
517 |
-
|
518 |
-
x['hash'] = get_hash(self.label_files + self.img_files)
|
519 |
-
x['results'] = nf, nm, ne, nc, i + 1
|
520 |
-
x['version'] = 0.1 # cache version
|
521 |
-
torch.save(x, path) # save for next time
|
522 |
-
logging.info(f'{prefix}New cache created: {path}')
|
523 |
-
return x
|
524 |
-
|
525 |
-
def __len__(self):
|
526 |
-
return len(self.img_files)
|
527 |
-
|
528 |
-
# def __iter__(self):
|
529 |
-
# self.count = -1
|
530 |
-
# print('ran dataset iter')
|
531 |
-
# #self.shuffled_vector = np.random.permutation(self.nF) if self.augment else np.arange(self.nF)
|
532 |
-
# return self
|
533 |
-
|
534 |
-
def __getitem__(self, index):
|
535 |
-
index = self.indices[index] # linear, shuffled, or image_weights
|
536 |
-
|
537 |
-
hyp = self.hyp
|
538 |
-
mosaic = self.mosaic and random.random() < hyp['mosaic']
|
539 |
-
if mosaic:
|
540 |
-
# Load mosaic
|
541 |
-
if random.random() < 0.8:
|
542 |
-
img, labels = load_mosaic(self, index)
|
543 |
-
else:
|
544 |
-
img, labels = load_mosaic9(self, index)
|
545 |
-
shapes = None
|
546 |
-
|
547 |
-
# MixUp https://arxiv.org/pdf/1710.09412.pdf
|
548 |
-
if random.random() < hyp['mixup']:
|
549 |
-
if random.random() < 0.8:
|
550 |
-
img2, labels2 = load_mosaic(self, random.randint(0, len(self.labels) - 1))
|
551 |
-
else:
|
552 |
-
img2, labels2 = load_mosaic9(self, random.randint(0, len(self.labels) - 1))
|
553 |
-
r = np.random.beta(8.0, 8.0) # mixup ratio, alpha=beta=8.0
|
554 |
-
img = (img * r + img2 * (1 - r)).astype(np.uint8)
|
555 |
-
labels = np.concatenate((labels, labels2), 0)
|
556 |
-
|
557 |
-
else:
|
558 |
-
# Load image
|
559 |
-
img, (h0, w0), (h, w) = load_image(self, index)
|
560 |
-
|
561 |
-
# Letterbox
|
562 |
-
shape = self.batch_shapes[self.batch[index]] if self.rect else self.img_size # final letterboxed shape
|
563 |
-
img, ratio, pad = letterbox(img, shape, auto=False, scaleup=self.augment)
|
564 |
-
shapes = (h0, w0), ((h / h0, w / w0), pad) # for COCO mAP rescaling
|
565 |
-
|
566 |
-
labels = self.labels[index].copy()
|
567 |
-
if labels.size: # normalized xywh to pixel xyxy format
|
568 |
-
labels[:, 1:] = xywhn2xyxy(labels[:, 1:], ratio[0] * w, ratio[1] * h, padw=pad[0], padh=pad[1])
|
569 |
-
|
570 |
-
if self.augment:
|
571 |
-
# Augment imagespace
|
572 |
-
if not mosaic:
|
573 |
-
img, labels = random_perspective(img, labels,
|
574 |
-
degrees=hyp['degrees'],
|
575 |
-
translate=hyp['translate'],
|
576 |
-
scale=hyp['scale'],
|
577 |
-
shear=hyp['shear'],
|
578 |
-
perspective=hyp['perspective'])
|
579 |
-
|
580 |
-
|
581 |
-
#img, labels = self.albumentations(img, labels)
|
582 |
-
|
583 |
-
# Augment colorspace
|
584 |
-
augment_hsv(img, hgain=hyp['hsv_h'], sgain=hyp['hsv_s'], vgain=hyp['hsv_v'])
|
585 |
-
|
586 |
-
# Apply cutouts
|
587 |
-
# if random.random() < 0.9:
|
588 |
-
# labels = cutout(img, labels)
|
589 |
-
|
590 |
-
if random.random() < hyp['paste_in']:
|
591 |
-
sample_labels, sample_images, sample_masks = [], [], []
|
592 |
-
while len(sample_labels) < 30:
|
593 |
-
sample_labels_, sample_images_, sample_masks_ = load_samples(self, random.randint(0, len(self.labels) - 1))
|
594 |
-
sample_labels += sample_labels_
|
595 |
-
sample_images += sample_images_
|
596 |
-
sample_masks += sample_masks_
|
597 |
-
#print(len(sample_labels))
|
598 |
-
if len(sample_labels) == 0:
|
599 |
-
break
|
600 |
-
labels = pastein(img, labels, sample_labels, sample_images, sample_masks)
|
601 |
-
|
602 |
-
nL = len(labels) # number of labels
|
603 |
-
if nL:
|
604 |
-
labels[:, 1:5] = xyxy2xywh(labels[:, 1:5]) # convert xyxy to xywh
|
605 |
-
labels[:, [2, 4]] /= img.shape[0] # normalized height 0-1
|
606 |
-
labels[:, [1, 3]] /= img.shape[1] # normalized width 0-1
|
607 |
-
|
608 |
-
if self.augment:
|
609 |
-
# flip up-down
|
610 |
-
if random.random() < hyp['flipud']:
|
611 |
-
img = np.flipud(img)
|
612 |
-
if nL:
|
613 |
-
labels[:, 2] = 1 - labels[:, 2]
|
614 |
-
|
615 |
-
# flip left-right
|
616 |
-
if random.random() < hyp['fliplr']:
|
617 |
-
img = np.fliplr(img)
|
618 |
-
if nL:
|
619 |
-
labels[:, 1] = 1 - labels[:, 1]
|
620 |
-
|
621 |
-
labels_out = torch.zeros((nL, 6))
|
622 |
-
if nL:
|
623 |
-
labels_out[:, 1:] = torch.from_numpy(labels)
|
624 |
-
|
625 |
-
# Convert
|
626 |
-
img = img[:, :, ::-1].transpose(2, 0, 1) # BGR to RGB, to 3x416x416
|
627 |
-
img = np.ascontiguousarray(img)
|
628 |
-
|
629 |
-
return torch.from_numpy(img), labels_out, self.img_files[index], shapes
|
630 |
-
|
631 |
-
@staticmethod
|
632 |
-
def collate_fn(batch):
|
633 |
-
img, label, path, shapes = zip(*batch) # transposed
|
634 |
-
for i, l in enumerate(label):
|
635 |
-
l[:, 0] = i # add target image index for build_targets()
|
636 |
-
return torch.stack(img, 0), torch.cat(label, 0), path, shapes
|
637 |
-
|
638 |
-
@staticmethod
|
639 |
-
def collate_fn4(batch):
|
640 |
-
img, label, path, shapes = zip(*batch) # transposed
|
641 |
-
n = len(shapes) // 4
|
642 |
-
img4, label4, path4, shapes4 = [], [], path[:n], shapes[:n]
|
643 |
-
|
644 |
-
ho = torch.tensor([[0., 0, 0, 1, 0, 0]])
|
645 |
-
wo = torch.tensor([[0., 0, 1, 0, 0, 0]])
|
646 |
-
s = torch.tensor([[1, 1, .5, .5, .5, .5]]) # scale
|
647 |
-
for i in range(n): # zidane torch.zeros(16,3,720,1280) # BCHW
|
648 |
-
i *= 4
|
649 |
-
if random.random() < 0.5:
|
650 |
-
im = F.interpolate(img[i].unsqueeze(0).float(), scale_factor=2., mode='bilinear', align_corners=False)[
|
651 |
-
0].type(img[i].type())
|
652 |
-
l = label[i]
|
653 |
-
else:
|
654 |
-
im = torch.cat((torch.cat((img[i], img[i + 1]), 1), torch.cat((img[i + 2], img[i + 3]), 1)), 2)
|
655 |
-
l = torch.cat((label[i], label[i + 1] + ho, label[i + 2] + wo, label[i + 3] + ho + wo), 0) * s
|
656 |
-
img4.append(im)
|
657 |
-
label4.append(l)
|
658 |
-
|
659 |
-
for i, l in enumerate(label4):
|
660 |
-
l[:, 0] = i # add target image index for build_targets()
|
661 |
-
|
662 |
-
return torch.stack(img4, 0), torch.cat(label4, 0), path4, shapes4
|
663 |
-
|
664 |
-
|
665 |
-
# Ancillary functions --------------------------------------------------------------------------------------------------
|
666 |
-
def load_image(self, index):
|
667 |
-
# loads 1 image from dataset, returns img, original hw, resized hw
|
668 |
-
img = self.imgs[index]
|
669 |
-
if img is None: # not cached
|
670 |
-
path = self.img_files[index]
|
671 |
-
img = cv2.imread(path) # BGR
|
672 |
-
assert img is not None, 'Image Not Found ' + path
|
673 |
-
h0, w0 = img.shape[:2] # orig hw
|
674 |
-
r = self.img_size / max(h0, w0) # resize image to img_size
|
675 |
-
if r != 1: # always resize down, only resize up if training with augmentation
|
676 |
-
interp = cv2.INTER_AREA if r < 1 and not self.augment else cv2.INTER_LINEAR
|
677 |
-
img = cv2.resize(img, (int(w0 * r), int(h0 * r)), interpolation=interp)
|
678 |
-
return img, (h0, w0), img.shape[:2] # img, hw_original, hw_resized
|
679 |
-
else:
|
680 |
-
return self.imgs[index], self.img_hw0[index], self.img_hw[index] # img, hw_original, hw_resized
|
681 |
-
|
682 |
-
|
683 |
-
def augment_hsv(img, hgain=0.5, sgain=0.5, vgain=0.5):
|
684 |
-
r = np.random.uniform(-1, 1, 3) * [hgain, sgain, vgain] + 1 # random gains
|
685 |
-
hue, sat, val = cv2.split(cv2.cvtColor(img, cv2.COLOR_BGR2HSV))
|
686 |
-
dtype = img.dtype # uint8
|
687 |
-
|
688 |
-
x = np.arange(0, 256, dtype=np.int16)
|
689 |
-
lut_hue = ((x * r[0]) % 180).astype(dtype)
|
690 |
-
lut_sat = np.clip(x * r[1], 0, 255).astype(dtype)
|
691 |
-
lut_val = np.clip(x * r[2], 0, 255).astype(dtype)
|
692 |
-
|
693 |
-
img_hsv = cv2.merge((cv2.LUT(hue, lut_hue), cv2.LUT(sat, lut_sat), cv2.LUT(val, lut_val))).astype(dtype)
|
694 |
-
cv2.cvtColor(img_hsv, cv2.COLOR_HSV2BGR, dst=img) # no return needed
|
695 |
-
|
696 |
-
|
697 |
-
def hist_equalize(img, clahe=True, bgr=False):
|
698 |
-
# Equalize histogram on BGR image 'img' with img.shape(n,m,3) and range 0-255
|
699 |
-
yuv = cv2.cvtColor(img, cv2.COLOR_BGR2YUV if bgr else cv2.COLOR_RGB2YUV)
|
700 |
-
if clahe:
|
701 |
-
c = cv2.createCLAHE(clipLimit=2.0, tileGridSize=(8, 8))
|
702 |
-
yuv[:, :, 0] = c.apply(yuv[:, :, 0])
|
703 |
-
else:
|
704 |
-
yuv[:, :, 0] = cv2.equalizeHist(yuv[:, :, 0]) # equalize Y channel histogram
|
705 |
-
return cv2.cvtColor(yuv, cv2.COLOR_YUV2BGR if bgr else cv2.COLOR_YUV2RGB) # convert YUV image to RGB
|
706 |
-
|
707 |
-
|
708 |
-
def load_mosaic(self, index):
|
709 |
-
# loads images in a 4-mosaic
|
710 |
-
|
711 |
-
labels4, segments4 = [], []
|
712 |
-
s = self.img_size
|
713 |
-
yc, xc = [int(random.uniform(-x, 2 * s + x)) for x in self.mosaic_border] # mosaic center x, y
|
714 |
-
indices = [index] + random.choices(self.indices, k=3) # 3 additional image indices
|
715 |
-
for i, index in enumerate(indices):
|
716 |
-
# Load image
|
717 |
-
img, _, (h, w) = load_image(self, index)
|
718 |
-
|
719 |
-
# place img in img4
|
720 |
-
if i == 0: # top left
|
721 |
-
img4 = np.full((s * 2, s * 2, img.shape[2]), 114, dtype=np.uint8) # base image with 4 tiles
|
722 |
-
x1a, y1a, x2a, y2a = max(xc - w, 0), max(yc - h, 0), xc, yc # xmin, ymin, xmax, ymax (large image)
|
723 |
-
x1b, y1b, x2b, y2b = w - (x2a - x1a), h - (y2a - y1a), w, h # xmin, ymin, xmax, ymax (small image)
|
724 |
-
elif i == 1: # top right
|
725 |
-
x1a, y1a, x2a, y2a = xc, max(yc - h, 0), min(xc + w, s * 2), yc
|
726 |
-
x1b, y1b, x2b, y2b = 0, h - (y2a - y1a), min(w, x2a - x1a), h
|
727 |
-
elif i == 2: # bottom left
|
728 |
-
x1a, y1a, x2a, y2a = max(xc - w, 0), yc, xc, min(s * 2, yc + h)
|
729 |
-
x1b, y1b, x2b, y2b = w - (x2a - x1a), 0, w, min(y2a - y1a, h)
|
730 |
-
elif i == 3: # bottom right
|
731 |
-
x1a, y1a, x2a, y2a = xc, yc, min(xc + w, s * 2), min(s * 2, yc + h)
|
732 |
-
x1b, y1b, x2b, y2b = 0, 0, min(w, x2a - x1a), min(y2a - y1a, h)
|
733 |
-
|
734 |
-
img4[y1a:y2a, x1a:x2a] = img[y1b:y2b, x1b:x2b] # img4[ymin:ymax, xmin:xmax]
|
735 |
-
padw = x1a - x1b
|
736 |
-
padh = y1a - y1b
|
737 |
-
|
738 |
-
# Labels
|
739 |
-
labels, segments = self.labels[index].copy(), self.segments[index].copy()
|
740 |
-
if labels.size:
|
741 |
-
labels[:, 1:] = xywhn2xyxy(labels[:, 1:], w, h, padw, padh) # normalized xywh to pixel xyxy format
|
742 |
-
segments = [xyn2xy(x, w, h, padw, padh) for x in segments]
|
743 |
-
labels4.append(labels)
|
744 |
-
segments4.extend(segments)
|
745 |
-
|
746 |
-
# Concat/clip labels
|
747 |
-
labels4 = np.concatenate(labels4, 0)
|
748 |
-
for x in (labels4[:, 1:], *segments4):
|
749 |
-
np.clip(x, 0, 2 * s, out=x) # clip when using random_perspective()
|
750 |
-
# img4, labels4 = replicate(img4, labels4) # replicate
|
751 |
-
|
752 |
-
# Augment
|
753 |
-
#img4, labels4, segments4 = remove_background(img4, labels4, segments4)
|
754 |
-
#sample_segments(img4, labels4, segments4, probability=self.hyp['copy_paste'])
|
755 |
-
img4, labels4, segments4 = copy_paste(img4, labels4, segments4, probability=self.hyp['copy_paste'])
|
756 |
-
img4, labels4 = random_perspective(img4, labels4, segments4,
|
757 |
-
degrees=self.hyp['degrees'],
|
758 |
-
translate=self.hyp['translate'],
|
759 |
-
scale=self.hyp['scale'],
|
760 |
-
shear=self.hyp['shear'],
|
761 |
-
perspective=self.hyp['perspective'],
|
762 |
-
border=self.mosaic_border) # border to remove
|
763 |
-
|
764 |
-
return img4, labels4
|
765 |
-
|
766 |
-
|
767 |
-
def load_mosaic9(self, index):
|
768 |
-
# loads images in a 9-mosaic
|
769 |
-
|
770 |
-
labels9, segments9 = [], []
|
771 |
-
s = self.img_size
|
772 |
-
indices = [index] + random.choices(self.indices, k=8) # 8 additional image indices
|
773 |
-
for i, index in enumerate(indices):
|
774 |
-
# Load image
|
775 |
-
img, _, (h, w) = load_image(self, index)
|
776 |
-
|
777 |
-
# place img in img9
|
778 |
-
if i == 0: # center
|
779 |
-
img9 = np.full((s * 3, s * 3, img.shape[2]), 114, dtype=np.uint8) # base image with 4 tiles
|
780 |
-
h0, w0 = h, w
|
781 |
-
c = s, s, s + w, s + h # xmin, ymin, xmax, ymax (base) coordinates
|
782 |
-
elif i == 1: # top
|
783 |
-
c = s, s - h, s + w, s
|
784 |
-
elif i == 2: # top right
|
785 |
-
c = s + wp, s - h, s + wp + w, s
|
786 |
-
elif i == 3: # right
|
787 |
-
c = s + w0, s, s + w0 + w, s + h
|
788 |
-
elif i == 4: # bottom right
|
789 |
-
c = s + w0, s + hp, s + w0 + w, s + hp + h
|
790 |
-
elif i == 5: # bottom
|
791 |
-
c = s + w0 - w, s + h0, s + w0, s + h0 + h
|
792 |
-
elif i == 6: # bottom left
|
793 |
-
c = s + w0 - wp - w, s + h0, s + w0 - wp, s + h0 + h
|
794 |
-
elif i == 7: # left
|
795 |
-
c = s - w, s + h0 - h, s, s + h0
|
796 |
-
elif i == 8: # top left
|
797 |
-
c = s - w, s + h0 - hp - h, s, s + h0 - hp
|
798 |
-
|
799 |
-
padx, pady = c[:2]
|
800 |
-
x1, y1, x2, y2 = [max(x, 0) for x in c] # allocate coords
|
801 |
-
|
802 |
-
# Labels
|
803 |
-
labels, segments = self.labels[index].copy(), self.segments[index].copy()
|
804 |
-
if labels.size:
|
805 |
-
labels[:, 1:] = xywhn2xyxy(labels[:, 1:], w, h, padx, pady) # normalized xywh to pixel xyxy format
|
806 |
-
segments = [xyn2xy(x, w, h, padx, pady) for x in segments]
|
807 |
-
labels9.append(labels)
|
808 |
-
segments9.extend(segments)
|
809 |
-
|
810 |
-
# Image
|
811 |
-
img9[y1:y2, x1:x2] = img[y1 - pady:, x1 - padx:] # img9[ymin:ymax, xmin:xmax]
|
812 |
-
hp, wp = h, w # height, width previous
|
813 |
-
|
814 |
-
# Offset
|
815 |
-
yc, xc = [int(random.uniform(0, s)) for _ in self.mosaic_border] # mosaic center x, y
|
816 |
-
img9 = img9[yc:yc + 2 * s, xc:xc + 2 * s]
|
817 |
-
|
818 |
-
# Concat/clip labels
|
819 |
-
labels9 = np.concatenate(labels9, 0)
|
820 |
-
labels9[:, [1, 3]] -= xc
|
821 |
-
labels9[:, [2, 4]] -= yc
|
822 |
-
c = np.array([xc, yc]) # centers
|
823 |
-
segments9 = [x - c for x in segments9]
|
824 |
-
|
825 |
-
for x in (labels9[:, 1:], *segments9):
|
826 |
-
np.clip(x, 0, 2 * s, out=x) # clip when using random_perspective()
|
827 |
-
# img9, labels9 = replicate(img9, labels9) # replicate
|
828 |
-
|
829 |
-
# Augment
|
830 |
-
#img9, labels9, segments9 = remove_background(img9, labels9, segments9)
|
831 |
-
img9, labels9, segments9 = copy_paste(img9, labels9, segments9, probability=self.hyp['copy_paste'])
|
832 |
-
img9, labels9 = random_perspective(img9, labels9, segments9,
|
833 |
-
degrees=self.hyp['degrees'],
|
834 |
-
translate=self.hyp['translate'],
|
835 |
-
scale=self.hyp['scale'],
|
836 |
-
shear=self.hyp['shear'],
|
837 |
-
perspective=self.hyp['perspective'],
|
838 |
-
border=self.mosaic_border) # border to remove
|
839 |
-
|
840 |
-
return img9, labels9
|
841 |
-
|
842 |
-
|
843 |
-
def load_samples(self, index):
|
844 |
-
# loads images in a 4-mosaic
|
845 |
-
|
846 |
-
labels4, segments4 = [], []
|
847 |
-
s = self.img_size
|
848 |
-
yc, xc = [int(random.uniform(-x, 2 * s + x)) for x in self.mosaic_border] # mosaic center x, y
|
849 |
-
indices = [index] + random.choices(self.indices, k=3) # 3 additional image indices
|
850 |
-
for i, index in enumerate(indices):
|
851 |
-
# Load image
|
852 |
-
img, _, (h, w) = load_image(self, index)
|
853 |
-
|
854 |
-
# place img in img4
|
855 |
-
if i == 0: # top left
|
856 |
-
img4 = np.full((s * 2, s * 2, img.shape[2]), 114, dtype=np.uint8) # base image with 4 tiles
|
857 |
-
x1a, y1a, x2a, y2a = max(xc - w, 0), max(yc - h, 0), xc, yc # xmin, ymin, xmax, ymax (large image)
|
858 |
-
x1b, y1b, x2b, y2b = w - (x2a - x1a), h - (y2a - y1a), w, h # xmin, ymin, xmax, ymax (small image)
|
859 |
-
elif i == 1: # top right
|
860 |
-
x1a, y1a, x2a, y2a = xc, max(yc - h, 0), min(xc + w, s * 2), yc
|
861 |
-
x1b, y1b, x2b, y2b = 0, h - (y2a - y1a), min(w, x2a - x1a), h
|
862 |
-
elif i == 2: # bottom left
|
863 |
-
x1a, y1a, x2a, y2a = max(xc - w, 0), yc, xc, min(s * 2, yc + h)
|
864 |
-
x1b, y1b, x2b, y2b = w - (x2a - x1a), 0, w, min(y2a - y1a, h)
|
865 |
-
elif i == 3: # bottom right
|
866 |
-
x1a, y1a, x2a, y2a = xc, yc, min(xc + w, s * 2), min(s * 2, yc + h)
|
867 |
-
x1b, y1b, x2b, y2b = 0, 0, min(w, x2a - x1a), min(y2a - y1a, h)
|
868 |
-
|
869 |
-
img4[y1a:y2a, x1a:x2a] = img[y1b:y2b, x1b:x2b] # img4[ymin:ymax, xmin:xmax]
|
870 |
-
padw = x1a - x1b
|
871 |
-
padh = y1a - y1b
|
872 |
-
|
873 |
-
# Labels
|
874 |
-
labels, segments = self.labels[index].copy(), self.segments[index].copy()
|
875 |
-
if labels.size:
|
876 |
-
labels[:, 1:] = xywhn2xyxy(labels[:, 1:], w, h, padw, padh) # normalized xywh to pixel xyxy format
|
877 |
-
segments = [xyn2xy(x, w, h, padw, padh) for x in segments]
|
878 |
-
labels4.append(labels)
|
879 |
-
segments4.extend(segments)
|
880 |
-
|
881 |
-
# Concat/clip labels
|
882 |
-
labels4 = np.concatenate(labels4, 0)
|
883 |
-
for x in (labels4[:, 1:], *segments4):
|
884 |
-
np.clip(x, 0, 2 * s, out=x) # clip when using random_perspective()
|
885 |
-
# img4, labels4 = replicate(img4, labels4) # replicate
|
886 |
-
|
887 |
-
# Augment
|
888 |
-
#img4, labels4, segments4 = remove_background(img4, labels4, segments4)
|
889 |
-
sample_labels, sample_images, sample_masks = sample_segments(img4, labels4, segments4, probability=0.5)
|
890 |
-
|
891 |
-
return sample_labels, sample_images, sample_masks
|
892 |
-
|
893 |
-
|
894 |
-
def copy_paste(img, labels, segments, probability=0.5):
|
895 |
-
# Implement Copy-Paste augmentation https://arxiv.org/abs/2012.07177, labels as nx5 np.array(cls, xyxy)
|
896 |
-
n = len(segments)
|
897 |
-
if probability and n:
|
898 |
-
h, w, c = img.shape # height, width, channels
|
899 |
-
im_new = np.zeros(img.shape, np.uint8)
|
900 |
-
for j in random.sample(range(n), k=round(probability * n)):
|
901 |
-
l, s = labels[j], segments[j]
|
902 |
-
box = w - l[3], l[2], w - l[1], l[4]
|
903 |
-
ioa = bbox_ioa(box, labels[:, 1:5]) # intersection over area
|
904 |
-
if (ioa < 0.30).all(): # allow 30% obscuration of existing labels
|
905 |
-
labels = np.concatenate((labels, [[l[0], *box]]), 0)
|
906 |
-
segments.append(np.concatenate((w - s[:, 0:1], s[:, 1:2]), 1))
|
907 |
-
cv2.drawContours(im_new, [segments[j].astype(np.int32)], -1, (255, 255, 255), cv2.FILLED)
|
908 |
-
|
909 |
-
result = cv2.bitwise_and(src1=img, src2=im_new)
|
910 |
-
result = cv2.flip(result, 1) # augment segments (flip left-right)
|
911 |
-
i = result > 0 # pixels to replace
|
912 |
-
# i[:, :] = result.max(2).reshape(h, w, 1) # act over ch
|
913 |
-
img[i] = result[i] # cv2.imwrite('debug.jpg', img) # debug
|
914 |
-
|
915 |
-
return img, labels, segments
|
916 |
-
|
917 |
-
|
918 |
-
def remove_background(img, labels, segments):
|
919 |
-
# Implement Copy-Paste augmentation https://arxiv.org/abs/2012.07177, labels as nx5 np.array(cls, xyxy)
|
920 |
-
n = len(segments)
|
921 |
-
h, w, c = img.shape # height, width, channels
|
922 |
-
im_new = np.zeros(img.shape, np.uint8)
|
923 |
-
img_new = np.ones(img.shape, np.uint8) * 114
|
924 |
-
for j in range(n):
|
925 |
-
cv2.drawContours(im_new, [segments[j].astype(np.int32)], -1, (255, 255, 255), cv2.FILLED)
|
926 |
-
|
927 |
-
result = cv2.bitwise_and(src1=img, src2=im_new)
|
928 |
-
|
929 |
-
i = result > 0 # pixels to replace
|
930 |
-
img_new[i] = result[i] # cv2.imwrite('debug.jpg', img) # debug
|
931 |
-
|
932 |
-
return img_new, labels, segments
|
933 |
-
|
934 |
-
|
935 |
-
def sample_segments(img, labels, segments, probability=0.5):
|
936 |
-
# Implement Copy-Paste augmentation https://arxiv.org/abs/2012.07177, labels as nx5 np.array(cls, xyxy)
|
937 |
-
n = len(segments)
|
938 |
-
sample_labels = []
|
939 |
-
sample_images = []
|
940 |
-
sample_masks = []
|
941 |
-
if probability and n:
|
942 |
-
h, w, c = img.shape # height, width, channels
|
943 |
-
for j in random.sample(range(n), k=round(probability * n)):
|
944 |
-
l, s = labels[j], segments[j]
|
945 |
-
box = l[1].astype(int).clip(0,w-1), l[2].astype(int).clip(0,h-1), l[3].astype(int).clip(0,w-1), l[4].astype(int).clip(0,h-1)
|
946 |
-
|
947 |
-
#print(box)
|
948 |
-
if (box[2] <= box[0]) or (box[3] <= box[1]):
|
949 |
-
continue
|
950 |
-
|
951 |
-
sample_labels.append(l[0])
|
952 |
-
|
953 |
-
mask = np.zeros(img.shape, np.uint8)
|
954 |
-
|
955 |
-
cv2.drawContours(mask, [segments[j].astype(np.int32)], -1, (255, 255, 255), cv2.FILLED)
|
956 |
-
sample_masks.append(mask[box[1]:box[3],box[0]:box[2],:])
|
957 |
-
|
958 |
-
result = cv2.bitwise_and(src1=img, src2=mask)
|
959 |
-
i = result > 0 # pixels to replace
|
960 |
-
mask[i] = result[i] # cv2.imwrite('debug.jpg', img) # debug
|
961 |
-
#print(box)
|
962 |
-
sample_images.append(mask[box[1]:box[3],box[0]:box[2],:])
|
963 |
-
|
964 |
-
return sample_labels, sample_images, sample_masks
|
965 |
-
|
966 |
-
|
967 |
-
def replicate(img, labels):
|
968 |
-
# Replicate labels
|
969 |
-
h, w = img.shape[:2]
|
970 |
-
boxes = labels[:, 1:].astype(int)
|
971 |
-
x1, y1, x2, y2 = boxes.T
|
972 |
-
s = ((x2 - x1) + (y2 - y1)) / 2 # side length (pixels)
|
973 |
-
for i in s.argsort()[:round(s.size * 0.5)]: # smallest indices
|
974 |
-
x1b, y1b, x2b, y2b = boxes[i]
|
975 |
-
bh, bw = y2b - y1b, x2b - x1b
|
976 |
-
yc, xc = int(random.uniform(0, h - bh)), int(random.uniform(0, w - bw)) # offset x, y
|
977 |
-
x1a, y1a, x2a, y2a = [xc, yc, xc + bw, yc + bh]
|
978 |
-
img[y1a:y2a, x1a:x2a] = img[y1b:y2b, x1b:x2b] # img4[ymin:ymax, xmin:xmax]
|
979 |
-
labels = np.append(labels, [[labels[i, 0], x1a, y1a, x2a, y2a]], axis=0)
|
980 |
-
|
981 |
-
return img, labels
|
982 |
-
|
983 |
-
|
984 |
-
def letterbox(img, new_shape=(640, 640), color=(114, 114, 114), auto=True, scaleFill=False, scaleup=True, stride=32):
|
985 |
-
# Resize and pad image while meeting stride-multiple constraints
|
986 |
-
shape = img.shape[:2] # current shape [height, width]
|
987 |
-
if isinstance(new_shape, int):
|
988 |
-
new_shape = (new_shape, new_shape)
|
989 |
-
|
990 |
-
# Scale ratio (new / old)
|
991 |
-
r = min(new_shape[0] / shape[0], new_shape[1] / shape[1])
|
992 |
-
if not scaleup: # only scale down, do not scale up (for better test mAP)
|
993 |
-
r = min(r, 1.0)
|
994 |
-
|
995 |
-
# Compute padding
|
996 |
-
ratio = r, r # width, height ratios
|
997 |
-
new_unpad = int(round(shape[1] * r)), int(round(shape[0] * r))
|
998 |
-
dw, dh = new_shape[1] - new_unpad[0], new_shape[0] - new_unpad[1] # wh padding
|
999 |
-
if auto: # minimum rectangle
|
1000 |
-
dw, dh = np.mod(dw, stride), np.mod(dh, stride) # wh padding
|
1001 |
-
elif scaleFill: # stretch
|
1002 |
-
dw, dh = 0.0, 0.0
|
1003 |
-
new_unpad = (new_shape[1], new_shape[0])
|
1004 |
-
ratio = new_shape[1] / shape[1], new_shape[0] / shape[0] # width, height ratios
|
1005 |
-
|
1006 |
-
dw /= 2 # divide padding into 2 sides
|
1007 |
-
dh /= 2
|
1008 |
-
|
1009 |
-
if shape[::-1] != new_unpad: # resize
|
1010 |
-
img = cv2.resize(img, new_unpad, interpolation=cv2.INTER_LINEAR)
|
1011 |
-
top, bottom = int(round(dh - 0.1)), int(round(dh + 0.1))
|
1012 |
-
left, right = int(round(dw - 0.1)), int(round(dw + 0.1))
|
1013 |
-
img = cv2.copyMakeBorder(img, top, bottom, left, right, cv2.BORDER_CONSTANT, value=color) # add border
|
1014 |
-
return img, ratio, (dw, dh)
|
1015 |
-
|
1016 |
-
|
1017 |
-
def random_perspective(img, targets=(), segments=(), degrees=10, translate=.1, scale=.1, shear=10, perspective=0.0,
|
1018 |
-
border=(0, 0)):
|
1019 |
-
# torchvision.transforms.RandomAffine(degrees=(-10, 10), translate=(.1, .1), scale=(.9, 1.1), shear=(-10, 10))
|
1020 |
-
# targets = [cls, xyxy]
|
1021 |
-
|
1022 |
-
height = img.shape[0] + border[0] * 2 # shape(h,w,c)
|
1023 |
-
width = img.shape[1] + border[1] * 2
|
1024 |
-
|
1025 |
-
# Center
|
1026 |
-
C = np.eye(3)
|
1027 |
-
C[0, 2] = -img.shape[1] / 2 # x translation (pixels)
|
1028 |
-
C[1, 2] = -img.shape[0] / 2 # y translation (pixels)
|
1029 |
-
|
1030 |
-
# Perspective
|
1031 |
-
P = np.eye(3)
|
1032 |
-
P[2, 0] = random.uniform(-perspective, perspective) # x perspective (about y)
|
1033 |
-
P[2, 1] = random.uniform(-perspective, perspective) # y perspective (about x)
|
1034 |
-
|
1035 |
-
# Rotation and Scale
|
1036 |
-
R = np.eye(3)
|
1037 |
-
a = random.uniform(-degrees, degrees)
|
1038 |
-
# a += random.choice([-180, -90, 0, 90]) # add 90deg rotations to small rotations
|
1039 |
-
s = random.uniform(1 - scale, 1.1 + scale)
|
1040 |
-
# s = 2 ** random.uniform(-scale, scale)
|
1041 |
-
R[:2] = cv2.getRotationMatrix2D(angle=a, center=(0, 0), scale=s)
|
1042 |
-
|
1043 |
-
# Shear
|
1044 |
-
S = np.eye(3)
|
1045 |
-
S[0, 1] = math.tan(random.uniform(-shear, shear) * math.pi / 180) # x shear (deg)
|
1046 |
-
S[1, 0] = math.tan(random.uniform(-shear, shear) * math.pi / 180) # y shear (deg)
|
1047 |
-
|
1048 |
-
# Translation
|
1049 |
-
T = np.eye(3)
|
1050 |
-
T[0, 2] = random.uniform(0.5 - translate, 0.5 + translate) * width # x translation (pixels)
|
1051 |
-
T[1, 2] = random.uniform(0.5 - translate, 0.5 + translate) * height # y translation (pixels)
|
1052 |
-
|
1053 |
-
# Combined rotation matrix
|
1054 |
-
M = T @ S @ R @ P @ C # order of operations (right to left) is IMPORTANT
|
1055 |
-
if (border[0] != 0) or (border[1] != 0) or (M != np.eye(3)).any(): # image changed
|
1056 |
-
if perspective:
|
1057 |
-
img = cv2.warpPerspective(img, M, dsize=(width, height), borderValue=(114, 114, 114))
|
1058 |
-
else: # affine
|
1059 |
-
img = cv2.warpAffine(img, M[:2], dsize=(width, height), borderValue=(114, 114, 114))
|
1060 |
-
|
1061 |
-
# Visualize
|
1062 |
-
# import matplotlib.pyplot as plt
|
1063 |
-
# ax = plt.subplots(1, 2, figsize=(12, 6))[1].ravel()
|
1064 |
-
# ax[0].imshow(img[:, :, ::-1]) # base
|
1065 |
-
# ax[1].imshow(img2[:, :, ::-1]) # warped
|
1066 |
-
|
1067 |
-
# Transform label coordinates
|
1068 |
-
n = len(targets)
|
1069 |
-
if n:
|
1070 |
-
use_segments = any(x.any() for x in segments)
|
1071 |
-
new = np.zeros((n, 4))
|
1072 |
-
if use_segments: # warp segments
|
1073 |
-
segments = resample_segments(segments) # upsample
|
1074 |
-
for i, segment in enumerate(segments):
|
1075 |
-
xy = np.ones((len(segment), 3))
|
1076 |
-
xy[:, :2] = segment
|
1077 |
-
xy = xy @ M.T # transform
|
1078 |
-
xy = xy[:, :2] / xy[:, 2:3] if perspective else xy[:, :2] # perspective rescale or affine
|
1079 |
-
|
1080 |
-
# clip
|
1081 |
-
new[i] = segment2box(xy, width, height)
|
1082 |
-
|
1083 |
-
else: # warp boxes
|
1084 |
-
xy = np.ones((n * 4, 3))
|
1085 |
-
xy[:, :2] = targets[:, [1, 2, 3, 4, 1, 4, 3, 2]].reshape(n * 4, 2) # x1y1, x2y2, x1y2, x2y1
|
1086 |
-
xy = xy @ M.T # transform
|
1087 |
-
xy = (xy[:, :2] / xy[:, 2:3] if perspective else xy[:, :2]).reshape(n, 8) # perspective rescale or affine
|
1088 |
-
|
1089 |
-
# create new boxes
|
1090 |
-
x = xy[:, [0, 2, 4, 6]]
|
1091 |
-
y = xy[:, [1, 3, 5, 7]]
|
1092 |
-
new = np.concatenate((x.min(1), y.min(1), x.max(1), y.max(1))).reshape(4, n).T
|
1093 |
-
|
1094 |
-
# clip
|
1095 |
-
new[:, [0, 2]] = new[:, [0, 2]].clip(0, width)
|
1096 |
-
new[:, [1, 3]] = new[:, [1, 3]].clip(0, height)
|
1097 |
-
|
1098 |
-
# filter candidates
|
1099 |
-
i = box_candidates(box1=targets[:, 1:5].T * s, box2=new.T, area_thr=0.01 if use_segments else 0.10)
|
1100 |
-
targets = targets[i]
|
1101 |
-
targets[:, 1:5] = new[i]
|
1102 |
-
|
1103 |
-
return img, targets
|
1104 |
-
|
1105 |
-
|
1106 |
-
def box_candidates(box1, box2, wh_thr=2, ar_thr=20, area_thr=0.1, eps=1e-16): # box1(4,n), box2(4,n)
|
1107 |
-
# Compute candidate boxes: box1 before augment, box2 after augment, wh_thr (pixels), aspect_ratio_thr, area_ratio
|
1108 |
-
w1, h1 = box1[2] - box1[0], box1[3] - box1[1]
|
1109 |
-
w2, h2 = box2[2] - box2[0], box2[3] - box2[1]
|
1110 |
-
ar = np.maximum(w2 / (h2 + eps), h2 / (w2 + eps)) # aspect ratio
|
1111 |
-
return (w2 > wh_thr) & (h2 > wh_thr) & (w2 * h2 / (w1 * h1 + eps) > area_thr) & (ar < ar_thr) # candidates
|
1112 |
-
|
1113 |
-
|
1114 |
-
def bbox_ioa(box1, box2):
|
1115 |
-
# Returns the intersection over box2 area given box1, box2. box1 is 4, box2 is nx4. boxes are x1y1x2y2
|
1116 |
-
box2 = box2.transpose()
|
1117 |
-
|
1118 |
-
# Get the coordinates of bounding boxes
|
1119 |
-
b1_x1, b1_y1, b1_x2, b1_y2 = box1[0], box1[1], box1[2], box1[3]
|
1120 |
-
b2_x1, b2_y1, b2_x2, b2_y2 = box2[0], box2[1], box2[2], box2[3]
|
1121 |
-
|
1122 |
-
# Intersection area
|
1123 |
-
inter_area = (np.minimum(b1_x2, b2_x2) - np.maximum(b1_x1, b2_x1)).clip(0) * \
|
1124 |
-
(np.minimum(b1_y2, b2_y2) - np.maximum(b1_y1, b2_y1)).clip(0)
|
1125 |
-
|
1126 |
-
# box2 area
|
1127 |
-
box2_area = (b2_x2 - b2_x1) * (b2_y2 - b2_y1) + 1e-16
|
1128 |
-
|
1129 |
-
# Intersection over box2 area
|
1130 |
-
return inter_area / box2_area
|
1131 |
-
|
1132 |
-
|
1133 |
-
def cutout(image, labels):
|
1134 |
-
# Applies image cutout augmentation https://arxiv.org/abs/1708.04552
|
1135 |
-
h, w = image.shape[:2]
|
1136 |
-
|
1137 |
-
# create random masks
|
1138 |
-
scales = [0.5] * 1 + [0.25] * 2 + [0.125] * 4 + [0.0625] * 8 + [0.03125] * 16 # image size fraction
|
1139 |
-
for s in scales:
|
1140 |
-
mask_h = random.randint(1, int(h * s))
|
1141 |
-
mask_w = random.randint(1, int(w * s))
|
1142 |
-
|
1143 |
-
# box
|
1144 |
-
xmin = max(0, random.randint(0, w) - mask_w // 2)
|
1145 |
-
ymin = max(0, random.randint(0, h) - mask_h // 2)
|
1146 |
-
xmax = min(w, xmin + mask_w)
|
1147 |
-
ymax = min(h, ymin + mask_h)
|
1148 |
-
|
1149 |
-
# apply random color mask
|
1150 |
-
image[ymin:ymax, xmin:xmax] = [random.randint(64, 191) for _ in range(3)]
|
1151 |
-
|
1152 |
-
# return unobscured labels
|
1153 |
-
if len(labels) and s > 0.03:
|
1154 |
-
box = np.array([xmin, ymin, xmax, ymax], dtype=np.float32)
|
1155 |
-
ioa = bbox_ioa(box, labels[:, 1:5]) # intersection over area
|
1156 |
-
labels = labels[ioa < 0.60] # remove >60% obscured labels
|
1157 |
-
|
1158 |
-
return labels
|
1159 |
-
|
1160 |
-
|
1161 |
-
def pastein(image, labels, sample_labels, sample_images, sample_masks):
|
1162 |
-
# Applies image cutout augmentation https://arxiv.org/abs/1708.04552
|
1163 |
-
h, w = image.shape[:2]
|
1164 |
-
|
1165 |
-
# create random masks
|
1166 |
-
scales = [0.75] * 2 + [0.5] * 4 + [0.25] * 4 + [0.125] * 4 + [0.0625] * 6 # image size fraction
|
1167 |
-
for s in scales:
|
1168 |
-
if random.random() < 0.2:
|
1169 |
-
continue
|
1170 |
-
mask_h = random.randint(1, int(h * s))
|
1171 |
-
mask_w = random.randint(1, int(w * s))
|
1172 |
-
|
1173 |
-
# box
|
1174 |
-
xmin = max(0, random.randint(0, w) - mask_w // 2)
|
1175 |
-
ymin = max(0, random.randint(0, h) - mask_h // 2)
|
1176 |
-
xmax = min(w, xmin + mask_w)
|
1177 |
-
ymax = min(h, ymin + mask_h)
|
1178 |
-
|
1179 |
-
box = np.array([xmin, ymin, xmax, ymax], dtype=np.float32)
|
1180 |
-
if len(labels):
|
1181 |
-
ioa = bbox_ioa(box, labels[:, 1:5]) # intersection over area
|
1182 |
-
else:
|
1183 |
-
ioa = np.zeros(1)
|
1184 |
-
|
1185 |
-
if (ioa < 0.30).all() and len(sample_labels) and (xmax > xmin+20) and (ymax > ymin+20): # allow 30% obscuration of existing labels
|
1186 |
-
sel_ind = random.randint(0, len(sample_labels)-1)
|
1187 |
-
#print(len(sample_labels))
|
1188 |
-
#print(sel_ind)
|
1189 |
-
#print((xmax-xmin, ymax-ymin))
|
1190 |
-
#print(image[ymin:ymax, xmin:xmax].shape)
|
1191 |
-
#print([[sample_labels[sel_ind], *box]])
|
1192 |
-
#print(labels.shape)
|
1193 |
-
hs, ws, cs = sample_images[sel_ind].shape
|
1194 |
-
r_scale = min((ymax-ymin)/hs, (xmax-xmin)/ws)
|
1195 |
-
r_w = int(ws*r_scale)
|
1196 |
-
r_h = int(hs*r_scale)
|
1197 |
-
|
1198 |
-
if (r_w > 10) and (r_h > 10):
|
1199 |
-
r_mask = cv2.resize(sample_masks[sel_ind], (r_w, r_h))
|
1200 |
-
r_image = cv2.resize(sample_images[sel_ind], (r_w, r_h))
|
1201 |
-
temp_crop = image[ymin:ymin+r_h, xmin:xmin+r_w]
|
1202 |
-
m_ind = r_mask > 0
|
1203 |
-
if m_ind.astype(np.int32).sum() > 60:
|
1204 |
-
temp_crop[m_ind] = r_image[m_ind]
|
1205 |
-
#print(sample_labels[sel_ind])
|
1206 |
-
#print(sample_images[sel_ind].shape)
|
1207 |
-
#print(temp_crop.shape)
|
1208 |
-
box = np.array([xmin, ymin, xmin+r_w, ymin+r_h], dtype=np.float32)
|
1209 |
-
if len(labels):
|
1210 |
-
labels = np.concatenate((labels, [[sample_labels[sel_ind], *box]]), 0)
|
1211 |
-
else:
|
1212 |
-
labels = np.array([[sample_labels[sel_ind], *box]])
|
1213 |
-
|
1214 |
-
image[ymin:ymin+r_h, xmin:xmin+r_w] = temp_crop
|
1215 |
-
|
1216 |
-
return labels
|
1217 |
-
|
1218 |
-
class Albumentations:
|
1219 |
-
# YOLOv5 Albumentations class (optional, only used if package is installed)
|
1220 |
-
def __init__(self):
|
1221 |
-
self.transform = None
|
1222 |
-
import albumentations as A
|
1223 |
-
|
1224 |
-
self.transform = A.Compose([
|
1225 |
-
A.CLAHE(p=0.01),
|
1226 |
-
A.RandomBrightnessContrast(brightness_limit=0.2, contrast_limit=0.2, p=0.01),
|
1227 |
-
A.RandomGamma(gamma_limit=[80, 120], p=0.01),
|
1228 |
-
A.Blur(p=0.01),
|
1229 |
-
A.MedianBlur(p=0.01),
|
1230 |
-
A.ToGray(p=0.01),
|
1231 |
-
A.ImageCompression(quality_lower=75, p=0.01),],
|
1232 |
-
bbox_params=A.BboxParams(format='pascal_voc', label_fields=['class_labels']))
|
1233 |
-
|
1234 |
-
#logging.info(colorstr('albumentations: ') + ', '.join(f'{x}' for x in self.transform.transforms if x.p))
|
1235 |
-
|
1236 |
-
def __call__(self, im, labels, p=1.0):
|
1237 |
-
if self.transform and random.random() < p:
|
1238 |
-
new = self.transform(image=im, bboxes=labels[:, 1:], class_labels=labels[:, 0]) # transformed
|
1239 |
-
im, labels = new['image'], np.array([[c, *b] for c, b in zip(new['class_labels'], new['bboxes'])])
|
1240 |
-
return im, labels
|
1241 |
-
|
1242 |
-
|
1243 |
-
def create_folder(path='./new'):
|
1244 |
-
# Create folder
|
1245 |
-
if os.path.exists(path):
|
1246 |
-
shutil.rmtree(path) # delete output folder
|
1247 |
-
os.makedirs(path) # make new output folder
|
1248 |
-
|
1249 |
-
|
1250 |
-
def flatten_recursive(path='../coco'):
|
1251 |
-
# Flatten a recursive directory by bringing all files to top level
|
1252 |
-
new_path = Path(path + '_flat')
|
1253 |
-
create_folder(new_path)
|
1254 |
-
for file in tqdm(glob.glob(str(Path(path)) + '/**/*.*', recursive=True)):
|
1255 |
-
shutil.copyfile(file, new_path / Path(file).name)
|
1256 |
-
|
1257 |
-
|
1258 |
-
def extract_boxes(path='../coco/'): # from utils.datasets import *; extract_boxes('../coco128')
|
1259 |
-
# Convert detection dataset into classification dataset, with one directory per class
|
1260 |
-
|
1261 |
-
path = Path(path) # images dir
|
1262 |
-
shutil.rmtree(path / 'classifier') if (path / 'classifier').is_dir() else None # remove existing
|
1263 |
-
files = list(path.rglob('*.*'))
|
1264 |
-
n = len(files) # number of files
|
1265 |
-
for im_file in tqdm(files, total=n):
|
1266 |
-
if im_file.suffix[1:] in img_formats:
|
1267 |
-
# image
|
1268 |
-
im = cv2.imread(str(im_file))[..., ::-1] # BGR to RGB
|
1269 |
-
h, w = im.shape[:2]
|
1270 |
-
|
1271 |
-
# labels
|
1272 |
-
lb_file = Path(img2label_paths([str(im_file)])[0])
|
1273 |
-
if Path(lb_file).exists():
|
1274 |
-
with open(lb_file, 'r') as f:
|
1275 |
-
lb = np.array([x.split() for x in f.read().strip().splitlines()], dtype=np.float32) # labels
|
1276 |
-
|
1277 |
-
for j, x in enumerate(lb):
|
1278 |
-
c = int(x[0]) # class
|
1279 |
-
f = (path / 'classifier') / f'{c}' / f'{path.stem}_{im_file.stem}_{j}.jpg' # new filename
|
1280 |
-
if not f.parent.is_dir():
|
1281 |
-
f.parent.mkdir(parents=True)
|
1282 |
-
|
1283 |
-
b = x[1:] * [w, h, w, h] # box
|
1284 |
-
# b[2:] = b[2:].max() # rectangle to square
|
1285 |
-
b[2:] = b[2:] * 1.2 + 3 # pad
|
1286 |
-
b = xywh2xyxy(b.reshape(-1, 4)).ravel().astype(np.int)
|
1287 |
-
|
1288 |
-
b[[0, 2]] = np.clip(b[[0, 2]], 0, w) # clip boxes outside of image
|
1289 |
-
b[[1, 3]] = np.clip(b[[1, 3]], 0, h)
|
1290 |
-
assert cv2.imwrite(str(f), im[b[1]:b[3], b[0]:b[2]]), f'box failure in {f}'
|
1291 |
-
|
1292 |
-
|
1293 |
-
def autosplit(path='../coco', weights=(0.9, 0.1, 0.0), annotated_only=False):
|
1294 |
-
""" Autosplit a dataset into train/val/test splits and save path/autosplit_*.txt files
|
1295 |
-
Usage: from utils.datasets import *; autosplit('../coco')
|
1296 |
-
Arguments
|
1297 |
-
path: Path to images directory
|
1298 |
-
weights: Train, val, test weights (list)
|
1299 |
-
annotated_only: Only use images with an annotated txt file
|
1300 |
-
"""
|
1301 |
-
path = Path(path) # images dir
|
1302 |
-
files = sum([list(path.rglob(f"*.{img_ext}")) for img_ext in img_formats], []) # image files only
|
1303 |
-
n = len(files) # number of files
|
1304 |
-
indices = random.choices([0, 1, 2], weights=weights, k=n) # assign each image to a split
|
1305 |
-
|
1306 |
-
txt = ['autosplit_train.txt', 'autosplit_val.txt', 'autosplit_test.txt'] # 3 txt files
|
1307 |
-
[(path / x).unlink() for x in txt if (path / x).exists()] # remove existing
|
1308 |
-
|
1309 |
-
print(f'Autosplitting images from {path}' + ', using *.txt labeled images only' * annotated_only)
|
1310 |
-
for i, img in tqdm(zip(indices, files), total=n):
|
1311 |
-
if not annotated_only or Path(img2label_paths([str(img)])[0]).exists(): # check label
|
1312 |
-
with open(path / txt[i], 'a') as f:
|
1313 |
-
f.write(str(img) + '\n') # add image to txt file
|
1314 |
-
|
1315 |
-
|
1316 |
-
def load_segmentations(self, index):
|
1317 |
-
key = '/work/handsomejw66/coco17/' + self.img_files[index]
|
1318 |
-
#print(key)
|
1319 |
-
# /work/handsomejw66/coco17/
|
1320 |
-
return self.segs[key]
|
|
|
|
|
|
|
|
|
|
|
|
|
|
|
|
|
|
|
|
|
|
|
|
|
|
|
|
|
|
|
|
|
|
|
|
|
|
|
|
|
|
|
|
|
|
|
|
|
|
|
|
|
|
|
|
|
|
|
|
|
|
|
|
|
|
|
|
|
|
|
|
|
|
|
|
|
|
|
|
|
|
|
|
|
|
|
|
|
|
|
|
|
|
|
|
|
|
|
|
|
|
|
|
|
|
|
|
|
|
|
|
|
|
|
|
|
|
|
|
|
|
|
|
|
|
|
|
|
|
|
|
|
|
|
|
|
|
|
|
|
|
|
|
|
|
|
|
|
|
|
|
|
|
|
|
|
|
|
|
|
|
|
|
|
|
|
|
|
|
|
|
|
|
|
|
|
|
|
|
|
|
|
|
|
|
|
|
|
|
|
|
|
|
|
|
|
|
|
|
|
|
|
|
|
|
|
|
|
|
|
|
|
|
|
|
|
|
|
|
|
|
|
|
|
|
|
|
|
|
|
|
|
|
|
|
|
|
|
|
|
|
|
|
|
|
|
|
|
|
|
|
|
|
|
|
|
|
|
|
|
|
|
|
|
|
|
|
|
|
|
|
|
|
|
|
|
|
|
|
|
|
|
|
|
|
|
|
|
|
|
|
|
|
|
|
|
|
|
|
|
|
|
|
|
|
|
|
|
|
|
|
|
|
|
|
|
|
|
|
|
|
|
|
|
|
|
|
|
|
|
|
|
|
|
|
|
|
|
|
|
|
|
|
|
|
|
|
|
|
|
|
|
|
|
|
|
|
|
|
|
|
|
|
|
|
|
|
|
|
|
|
|
|
|
|
|
|
|
|
|
|
|
|
|
|
|
|
|
|
|
|
|
|
|
|
|
|
|
|
|
|
|
|
|
|
|
|
|
|
|
|
|
|
|
|
|
|
|
|
|
|
|
|
|
|
|
|
|
|
|
|
|
|
|
|
|
|
|
|
|
|
|
|
|
|
|
|
|
|
|
|
|
|
|
|
|
|
|
|
|
|
|
|
|
|
|
|
|
|
|
|
|
|
|
|
|
|
|
|
|
|
|
|
|
|
|
|
|
|
|
|
|
|
|
|
|
|
|
|
|
|
|
|
|
|
|
|
|
|
|
|
|
|
|
|
|
|
|
|
|
|
|
|
|
|
|
|
|
|
|
|
|
|
|
|
|
|
|
|
|
|
|
|
|
|
|
|
|
|
|
|
|
|
|
|
|
|
|
|
|
|
|
|
|
|
|
|
|
|
|
|
|
|
|
|
|
|
|
|
|
|
|
|
|
|
|
|
|
|
|
|
|
|
|
|
|
|
|
|
|
|
|
|
|
|
|
|
|
|
|
|
|
|
|
|
|
|
|
|
|
|
|
|
|
|
|
|
|
|
|
|
|
|
|
|
|
|
|
|
|
|
|
|
|
|
|
|
|
|
|
|
|
|
|
|
|
|
|
|
|
|
|
|
|
|
|
|
|
|
|
|
|
|
|
|
|
|
|
|
|
|
|
|
|
|
|
|
|
|
|
|
|
|
|
|
|
|
|
|
|
|
|
|
|
|
|
|
|
|
|
|
|
|
|
|
|
|
|
|
|
|
|
|
|
|
|
|
|
|
|
|
|
|
|
|
|
|
|
|
|
|
|
|
|
|
|
|
|
|
|
|
|
|
|
|
|
|
|
|
|
|
|
|
|
|
|
|
|
|
|
|
|
|
|
|
|
|
|
|
|
|
|
|
|
|
|
|
|
|
|
|
|
|
|
|
|
|
|
|
|
|
|
|
|
|
|
|
|
|
|
|
|
|
|
|
|
|
|
|
|
|
|
|
|
|
|
|
|
|
|
|
|
|
|
|
|
|
|
|
|
|
|
|
|
|
|
|
|
|
|
|
|
|
|
|
|
|
|
|
|
|
|
|
|
|
|
|
|
|
|
|
|
|
|
|
|
|
|
|
|
|
|
|
|
|
|
|
|
|
|
|
|
|
|
|
|
|
|
|
|
|
|
|
|
|
|
|
|
|
|
|
|
|
|
|
|
|
|
|
|
|
|
|
|
|
|
|
|
|
|
|
|
|
|
|
|
|
|
|
|
|
|
|
|
|
|
|
|
|
|
|
|
|
|
|
|
|
|
|
|
|
|
|
|
|
|
|
|
|
|
|
|
|
|
|
|
|
|
|
|
|
|
|
|
|
|
|
|
|
|
|
|
|
|
|
|
|
|
|
|
|
|
|
|
|
|
|
|
|
|
|
|
|
|
|
|
|
|
|
|
|
|
|
|
|
|
|
|
|
|
|
|
|
|
|
|
|
|
|
|
|
|
|
|
|
|
|
|
|
|
|
|
|
|
|
|
|
|
|
|
|
|
|
|
|
|
|
|
|
|
|
|
|
|
|
|
|
|
|
|
|
|
|
|
|
|
|
|
|
|
|
|
|
|
|
|
|
|
|
|
|
|
|
|
|
|
|
|
|
|
|
|
|
|
|
|
|
|
|
|
|
|
|
|
|
|
|
|
|
|
|
|
|
|
|
|
|
|
|
|
|
|
|
|
|
|
|
|
|
|
|
|
|
|
|
|
|
|
|
|
|
|
|
|
|
|
|
|
|
|
|
|
|
|
|
|
|
|
|
|
|
|
|
|
|
|
|
|
|
|
|
|
|
|
|
|
|
|
|
|
|
|
|
|
|
|
|
|
|
|
|
|
|
|
|
|
|
|
|
|
|
|
|
|
|
|
|
|
|
|
|
|
|
|
|
|
|
|
|
|
|
|
|
|
|
|
|
|
|
|
|
|
|
|
|
|
|
|
|
|
|
|
|
|
|
|
|
|
|
|
|
|
|
|
|
|
|
|
|
|
|
|
|
|
|
|
|
|
|
|
|
|
|
|
|
|
|
|
|
|
|
|
|
|
|
|
|
|
|
|
|
|
|
|
|
|
|
|
|
|
|
|
|
|
|
|
|
|
|
|
|
|
|
|
|
|
|
|
|
|
|
|
|
|
|
|
|
|
|
|
|
|
|
|
|
|
|
|
|
|
|
|
|
|
|
|
|
|
|
|
|
|
|
|
|
|
|
|
|
|
|
|
|
|
|
|
|
|
|
|
|
|
|
|
|
|
|
|
|
|
|
|
|
|
|
|
|
|
|
|
|
|
|
|
|
|
|
|
|
|
|
|
|
|
|
|
|
|
|
|
|
|
|
|
|
|
|
|
|
|
|
|
|
|
|
|
|
|
|
|
|
|
|
|
|
|
|
|
|
|
|
|
|
|
|
|
|
|
|
|
|
|
|
|
|
|
|
|
|
|
|
|
|
|
|
|
|
|
|
|
|
|
|
|
|
|
|
|
|
|
|
|
|
|
|
|
|
|
|
|
|
|
|
|
|
|
|
|
|
|
|
|
|
|
|
|
|
|
|
|
|
|
|
|
|
|
|
|
|
|
|
|
|
|
|
|
|
|
|
|
|
|
|
|
|
|
|
|
|
|
|
|
|
|
|
|
|
|
|
|
|
|
|
|
|
|
|
|
|
|
|
|
|
|
|
|
|
|
|
|
|
|
|
|
|
|
|
|
|
|
|
|
|
|
|
|
|
|
|
|
|
|
|
|
|
|
|
|
|
|
|
|
|
|
|
|
|
|
|
|
|
|
|
|
|
|
|
|
|
|
|
|
|
|
|
|
|
|
|
|
|
|
|
|
|
|
|
|
|
|
|
|
|
|
|
|
|
|
|
|
|
|
|
|
|
|
|
|
|
|
|
|
|
|
|
|
|
|
|
|
|
|
|
|
|
|
|
|
|
|
|
|
|
|
|
|
|
|
|
|
|
|
|
|
|
|
|
|
|
|
|
|
|
|
|
|
|
|
|
|
|
|
|
|
|
|
|
|
|
|
|
|
|
|
|
|
|
|
|
|
|
|
|
|
|
|
|
|
|
|
|
|
|
|
|
|
|
|
|
|
|
|
|
|
|
|
|
|
|
|
|
|
|
|
|
|
|
|
|
|
|
|
|
|
|
|
|
|
|
|
|
|
|
|
|
|
|
|
|
|
|
|
|
|
|
|
|
|
|
|
|
|
|
|
|
|
|
|
|
|
|
|
|
|
|
|
|
|
|
|
|
|
|
|
|
|
|
|
|
|
|
|
|
|
|
|
|
|
|
|
|
|
|
|
|
|
|
|
|
|
|
|
|
|
|
|
|
|
|
|
|
|
|
|
|
|
|
|
|
|
|
|
|
|
|
|
|
|
|
|
|
|
|
|
|
|
|
|
|
|
|
|
|
|
|
|
|
|
|
|
|
|
|
|
|
|
|
|
|
|
|
|
|
|
|
|
|
|
|
|
|
|
|
|
|
|
|
|
|
|
|
|
|
|
|
|
|
|
|
|
|
|
|
|
|
|
|
|
|
|
|
|
|
|
|
|
|
|
|
|
|
|
|
|
|
|
|
|
|
|
|
|
|
|
|
|
|
|
|
|
|
|
|
|
|
|
|
|
|
|
|
|
|
|
|
|
|
|
|
|
|
|
|
|
|
|
|
|
|
|
|
|
|
|
|
|
|
|
|
|
|
|
|
|
|
|
|
|
|
|
|
|
|
|
|
|
|
|
|
|
|
|
|
|
|
|
|
|
|
|
|
|
|
|
|
|
|
|
|
|
|
|
|
|
|
|
|
|
|
|
|
|
|
|
|
|
|
|
|
|
|
|
|
|
|
|
|
|
|
|
|
|
|
|
|
|
|
|
|
|
|
|
|
|
|
|
|
|
|
|
|
|
|
|
|
|
|
|
|
|
|
|
|
|
|
|
|
|
|
|
|
|
|
|
|
|
|
|
|
|
|
|
|
|
|
|
|
|
|
|
|
|
|
|
|
|
|
|
|
|
|
|
|
|
|
|
|
|
|
|
|
|
|
|
|
|
|
|
|
|
|
|
|
|
|
|
|
|
|
|
|
|
|
|
|
|
|
|
|
|
|
|
|
|
|
|
|
|
|
|
|
|
|
|
|
|
|
|
|
|
|
|
|
|
|
|
|
|
|
|
|
|
|
|
|
|
|
|
|
|
|
|
|
|
|
|
|
|
|
|
|
|
|
|
|
|
|
|
|
|
|
|
|
|
|
|
|
|
|
|
|
|
|
|
|
|
|
|
|
|
|
|
|
|
|
|
|
|
|
|
|
|
|
|
|
|
|
|
|
|
|
|
|
|
|
|
|
|
|
|
|
|
|
|
|
|
|
|
|
|
|
|
|
|
|
|
|
|
|
|
|
|
|
|
|
|
|
|
|
|
|
|
|
|
|
|
|
|
|
|
|
|
|
|
|
|
|
|
|
|
|
|
|
|
|
|
|
|
|
|
|
|
|
|
|
|
|
|
|
|
|
|
|
|
|
|
|
|
|
|
|
|
|
|
|
|
|
|
|
|
|
|
|
|
|
|
|
|
|
|
|
|
|
|
|
|
|
|
|
|
|
|
|
|
|
|
|
|
|
|
|
|
|
|
|
|
|
|
|
|
|
|
|
|
|
|
|
|
|
|
|
|
|
|
|
|
|
|
|
|
|
|
|
|
|
|
|
|
|
|
|
|
|
|
|
|
|
|
|
|
|
|
|
|
|
|
|
|
|
|
|
|
|
|
|
|
|
|
|
|
|
|
|
|
|
|
|
|
|
|
|
|
|
|
|
|
|
|
|
|
|
|
|
|
|
|
|
|
|
|
|
|
|
|
|
|
|
|
|
|
|
|
|
|
|
|
|
|
|
|
|
|
|
|
|
|
|
|
|
|
|
|
|
|
|
|
|
|
|
|
|
|
|
|
|
|
|
|
|
|
|
|
|
|
|
|
|
|
|
|
|
|
|
|
|
|
|
|
|
|
|
|
|
|
|
|
|
|
|
|
|
|
|
|
|
|
|
|
|
|
|
spaces/AgentVerse/agentVerse/scripts/evaluate_logic.py
DELETED
@@ -1,71 +0,0 @@
|
|
1 |
-
import re
|
2 |
-
import json
|
3 |
-
import subprocess
|
4 |
-
from importlib import reload
|
5 |
-
from argparse import ArgumentParser
|
6 |
-
|
7 |
-
parser = ArgumentParser()
|
8 |
-
parser.add_argument("--path", type=str, required=True)
|
9 |
-
parser.add_argument("--max_line", type=int, default=1000000000000)
|
10 |
-
args = parser.parse_args()
|
11 |
-
|
12 |
-
|
13 |
-
def check_corr(result: str, correct_solution: str, tol: float = 1e-3):
|
14 |
-
result = result.replace(",", "")
|
15 |
-
if result.strip() == correct_solution.strip():
|
16 |
-
return 1
|
17 |
-
try:
|
18 |
-
result = float(result.strip())
|
19 |
-
correct_solution = float(correct_solution.strip())
|
20 |
-
return abs(result - correct_solution) < tol
|
21 |
-
except:
|
22 |
-
return 0
|
23 |
-
|
24 |
-
|
25 |
-
final_accs = []
|
26 |
-
err_cnts = []
|
27 |
-
for i in range(2):
|
28 |
-
acc = 0
|
29 |
-
total = 0
|
30 |
-
err_cnt = 0
|
31 |
-
with open(args.path) as f:
|
32 |
-
for idx, line in enumerate(f):
|
33 |
-
if idx == args.max_line:
|
34 |
-
break
|
35 |
-
line = json.loads(line)
|
36 |
-
label = str(line["label"])
|
37 |
-
if i == 0:
|
38 |
-
response = line["response"]
|
39 |
-
else:
|
40 |
-
if line["logs"][0]["module"] == "Role Assigner":
|
41 |
-
response = line["logs"][1]["content"]
|
42 |
-
else:
|
43 |
-
response = line["logs"][0]["content"]
|
44 |
-
total += 1
|
45 |
-
result = re.findall(r"\\boxed\{(.+?)\}", response)
|
46 |
-
if len(result) == 0:
|
47 |
-
err_cnt += 1
|
48 |
-
# print(response)
|
49 |
-
continue
|
50 |
-
result = result[0]
|
51 |
-
result = re.sub(r"\\text\{.+\}?", "", result)
|
52 |
-
result = (
|
53 |
-
result.replace("rd", "")
|
54 |
-
.replace("nd", "")
|
55 |
-
.replace("st", "")
|
56 |
-
.replace("th", "")
|
57 |
-
.replace("House", "")
|
58 |
-
.replace("house", "")
|
59 |
-
.replace("\\", "")
|
60 |
-
)
|
61 |
-
|
62 |
-
# acc += check_corr(result, label)
|
63 |
-
try:
|
64 |
-
acc += int(result) == int(label)
|
65 |
-
except:
|
66 |
-
print(result)
|
67 |
-
|
68 |
-
final_accs.append(acc / total)
|
69 |
-
err_cnts.append(err_cnt)
|
70 |
-
print(final_accs)
|
71 |
-
print(err_cnts)
|
|
|
|
|
|
|
|
|
|
|
|
|
|
|
|
|
|
|
|
|
|
|
|
|
|
|
|
|
|
|
|
|
|
|
|
|
|
|
|
|
|
|
|
|
|
|
|
|
|
|
|
|
|
|
|
|
|
|
|
|
|
|
|
|
|
|
|
|
|
|
|
|
|
|
|
|
|
|
|
|
|
|
|
|
|
|
|
|
|
|
|
|
|
|
|
|
|
|
|
|
|
|
|
|
|
|
|
|
|
|
|
|
|
|
|
|
|
|
|
|
|
|
|
|
|
|
|
|
|
|
|
|
|
|
|
|
|
|
|
|
|
|
|
spaces/AgentVerse/agentVerse/ui/src/phaser3-rex-plugins/templates/bejeweled/board/BreakMatch3.js
DELETED
@@ -1,38 +0,0 @@
|
|
1 |
-
/*
|
2 |
-
1. Pick each match3 line
|
3 |
-
2. Pick a random chess in this match3 line
|
4 |
-
3. Change symbol to a different value of all neighbors
|
5 |
-
*/
|
6 |
-
|
7 |
-
import RefreshSymbolCache from './match/RefreshSymbolCache.js';
|
8 |
-
import GetMatchN from './match/GetMatchN.js';
|
9 |
-
import RandomSymbol from './chess/RandomSymobl.js';
|
10 |
-
|
11 |
-
const GetRandom = Phaser.Utils.Array.GetRandom;
|
12 |
-
|
13 |
-
var BreakMatch3 = function () {
|
14 |
-
var tileZ = this.chessTileZ,
|
15 |
-
scope = this.chessCallbackScope,
|
16 |
-
symbols = this.candidateSymbols;
|
17 |
-
|
18 |
-
RefreshSymbolCache.call(this); // only refresh symbol cache once
|
19 |
-
GetMatchN.call(this, 3, function (result, board) {
|
20 |
-
// Pick a random chess in this match3 line
|
21 |
-
var tileXY = GetRandom(result.tileXY);
|
22 |
-
var chess = board.tileXYZToChess(tileXY.x, tileXY.y, tileZ);
|
23 |
-
var neighborChess = board.getNeighborChess(chess, null);
|
24 |
-
// collect symbols of all neighbors
|
25 |
-
var excluded = [];
|
26 |
-
for (var i = 0, cnt = neighborChess.length; i < cnt; i++) {
|
27 |
-
excluded.push(neighborChess[i].getData('symbol'));
|
28 |
-
}
|
29 |
-
var newSymbol = RandomSymbol(board, tileXY.x, tileXY.y, symbols, scope, excluded);
|
30 |
-
if (newSymbol != null) {
|
31 |
-
// Change symbol to a different value of all neighbors.
|
32 |
-
// It also fires 'changedata_symbol' event.
|
33 |
-
chess.setData('symbol', newSymbol);
|
34 |
-
}
|
35 |
-
});
|
36 |
-
}
|
37 |
-
|
38 |
-
export default BreakMatch3;
|
|
|
|
|
|
|
|
|
|
|
|
|
|
|
|
|
|
|
|
|
|
|
|
|
|
|
|
|
|
|
|
|
|
|
|
|
|
|
|
|
|
|
|
|
|
|
|
|
|
|
|
|
|
|
|
|
|
|
|
|
|
|
|
|
|
|
|
|
|
|
|
|
|
|
|
|
|
spaces/AgentVerse/agentVerse/ui/src/phaser3-rex-plugins/templates/ui/chart/Chart.d.ts
DELETED
@@ -1,42 +0,0 @@
|
|
1 |
-
// import * as Phaser from 'phaser';
|
2 |
-
import Canvas from '../canvas/Canvas';
|
3 |
-
|
4 |
-
export default Chart;
|
5 |
-
|
6 |
-
declare namespace Chart {
|
7 |
-
type IndexType = number | string;
|
8 |
-
|
9 |
-
interface IConfig {
|
10 |
-
|
11 |
-
}
|
12 |
-
}
|
13 |
-
|
14 |
-
declare class Chart extends Canvas {
|
15 |
-
constructor(
|
16 |
-
scene: Phaser.Scene,
|
17 |
-
x: number, y: number,
|
18 |
-
width: number, height: number,
|
19 |
-
config?: Chart.IConfig
|
20 |
-
);
|
21 |
-
|
22 |
-
setChart(config: Chart.IConfig): this;
|
23 |
-
|
24 |
-
getChartDataset(
|
25 |
-
datasetIndex: Chart.IndexType
|
26 |
-
): { [index: Chart.IndexType]: number };
|
27 |
-
|
28 |
-
getChartData(
|
29 |
-
datasetIndex: Chart.IndexType,
|
30 |
-
dataIndex: Chart.IndexType
|
31 |
-
): number;
|
32 |
-
|
33 |
-
setChartData(
|
34 |
-
datasetIndex: Chart.IndexType,
|
35 |
-
dataIndex: Chart.IndexType,
|
36 |
-
value: number
|
37 |
-
): this;
|
38 |
-
|
39 |
-
updateChart(): this;
|
40 |
-
|
41 |
-
chart: any;
|
42 |
-
}
|
|
|
|
|
|
|
|
|
|
|
|
|
|
|
|
|
|
|
|
|
|
|
|
|
|
|
|
|
|
|
|
|
|
|
|
|
|
|
|
|
|
|
|
|
|
|
|
|
|
|
|
|
|
|
|
|
|
|
|
|
|
|
|
|
|
|
|
|
|
|
|
|
|
|
|
|
|
|
|
|
|
|
|
|
|
spaces/AgentVerse/agentVerse/ui/src/phaser3-rex-plugins/templates/ui/fileselectorbutton/Factory.d.ts
DELETED
@@ -1,5 +0,0 @@
|
|
1 |
-
import FileSelectorButton from './FileSelectorButton.js';
|
2 |
-
|
3 |
-
export default function (
|
4 |
-
config?: FileSelectorButton.IConfig
|
5 |
-
): FileSelectorButton;
|
|
|
|
|
|
|
|
|
|
|
|
spaces/AgentVerse/agentVerse/ui/src/phaser3-rex-plugins/templates/ui/folder/Factory.js
DELETED
@@ -1,13 +0,0 @@
|
|
1 |
-
import Folder from './Folder.js';
|
2 |
-
import ObjectFactory from '../ObjectFactory.js';
|
3 |
-
import SetValue from '../../../plugins/utils/object/SetValue.js';
|
4 |
-
|
5 |
-
ObjectFactory.register('folder', function (config) {
|
6 |
-
var gameObject = new Folder(this.scene, config);
|
7 |
-
this.scene.add.existing(gameObject);
|
8 |
-
return gameObject;
|
9 |
-
});
|
10 |
-
|
11 |
-
SetValue(window, 'RexPlugins.UI.Folder', Folder);
|
12 |
-
|
13 |
-
export default Folder;
|
|
|
|
|
|
|
|
|
|
|
|
|
|
|
|
|
|
|
|
|
|
|
|
|
|
|
|
spaces/AlexWang/lama/bin/debug/analyze_overlapping_masks.sh
DELETED
@@ -1,31 +0,0 @@
|
|
1 |
-
#!/bin/bash
|
2 |
-
|
3 |
-
BASEDIR="$(dirname $0)"
|
4 |
-
|
5 |
-
# paths are valid for mml7
|
6 |
-
|
7 |
-
# select images
|
8 |
-
#ls /data/inpainting/work/data/train | shuf | head -2000 | xargs -n1 -I{} cp {} /data/inpainting/mask_analysis/src
|
9 |
-
|
10 |
-
# generate masks
|
11 |
-
#"$BASEDIR/../gen_debug_mask_dataset.py" \
|
12 |
-
# "$BASEDIR/../../configs/debug_mask_gen.yaml" \
|
13 |
-
# "/data/inpainting/mask_analysis/src" \
|
14 |
-
# "/data/inpainting/mask_analysis/generated"
|
15 |
-
|
16 |
-
# predict
|
17 |
-
#"$BASEDIR/../predict.py" \
|
18 |
-
# model.path="simple_pix2pix2_gap_sdpl_novgg_large_b18_ffc075_batch8x15/saved_checkpoint/r.suvorov_2021-04-30_14-41-12_train_simple_pix2pix2_gap_sdpl_novgg_large_b18_ffc075_batch8x15_epoch22-step-574999" \
|
19 |
-
# indir="/data/inpainting/mask_analysis/generated" \
|
20 |
-
# outdir="/data/inpainting/mask_analysis/predicted" \
|
21 |
-
# dataset.img_suffix=.jpg \
|
22 |
-
# +out_ext=.jpg
|
23 |
-
|
24 |
-
# analyze good and bad samples
|
25 |
-
"$BASEDIR/../analyze_errors.py" \
|
26 |
-
--only-report \
|
27 |
-
--n-jobs 8 \
|
28 |
-
"$BASEDIR/../../configs/analyze_mask_errors.yaml" \
|
29 |
-
"/data/inpainting/mask_analysis/small/generated" \
|
30 |
-
"/data/inpainting/mask_analysis/small/predicted" \
|
31 |
-
"/data/inpainting/mask_analysis/small/report"
|
|
|
|
|
|
|
|
|
|
|
|
|
|
|
|
|
|
|
|
|
|
|
|
|
|
|
|
|
|
|
|
|
|
|
|
|
|
|
|
|
|
|
|
|
|
|
|
|
|
|
|
|
|
|
|
|
|
|
|
|
|
|
|
spaces/Aloento/9Nine-VITS/text_encoder.py
DELETED
@@ -1,51 +0,0 @@
|
|
1 |
-
import math
|
2 |
-
|
3 |
-
import torch
|
4 |
-
from torch import nn
|
5 |
-
|
6 |
-
import attentions
|
7 |
-
import commons
|
8 |
-
|
9 |
-
|
10 |
-
class TextEncoder(nn.Module):
|
11 |
-
def __init__(self,
|
12 |
-
n_vocab,
|
13 |
-
out_channels,
|
14 |
-
hidden_channels,
|
15 |
-
filter_channels,
|
16 |
-
n_heads,
|
17 |
-
n_layers,
|
18 |
-
kernel_size,
|
19 |
-
p_dropout):
|
20 |
-
super().__init__()
|
21 |
-
self.n_vocab = n_vocab
|
22 |
-
self.out_channels = out_channels
|
23 |
-
self.hidden_channels = hidden_channels
|
24 |
-
self.filter_channels = filter_channels
|
25 |
-
self.n_heads = n_heads
|
26 |
-
self.n_layers = n_layers
|
27 |
-
self.kernel_size = kernel_size
|
28 |
-
self.p_dropout = p_dropout
|
29 |
-
|
30 |
-
self.emb = nn.Embedding(n_vocab, hidden_channels)
|
31 |
-
nn.init.normal_(self.emb.weight, 0.0, hidden_channels ** -0.5)
|
32 |
-
|
33 |
-
self.encoder = attentions.Encoder(
|
34 |
-
hidden_channels,
|
35 |
-
filter_channels,
|
36 |
-
n_heads,
|
37 |
-
n_layers,
|
38 |
-
kernel_size,
|
39 |
-
p_dropout)
|
40 |
-
self.proj = nn.Conv1d(hidden_channels, out_channels * 2, 1)
|
41 |
-
|
42 |
-
def forward(self, x, x_lengths):
|
43 |
-
x = self.emb(x) * math.sqrt(self.hidden_channels) # [b, t, h]
|
44 |
-
x = torch.transpose(x, 1, -1) # [b, h, t]
|
45 |
-
x_mask = torch.unsqueeze(commons.sequence_mask(x_lengths, x.size(2)), 1).to(x.dtype)
|
46 |
-
|
47 |
-
x = self.encoder(x * x_mask, x_mask)
|
48 |
-
stats = self.proj(x) * x_mask
|
49 |
-
|
50 |
-
m, logs = torch.split(stats, self.out_channels, dim=1)
|
51 |
-
return x, m, logs, x_mask
|
|
|
|
|
|
|
|
|
|
|
|
|
|
|
|
|
|
|
|
|
|
|
|
|
|
|
|
|
|
|
|
|
|
|
|
|
|
|
|
|
|
|
|
|
|
|
|
|
|
|
|
|
|
|
|
|
|
|
|
|
|
|
|
|
|
|
|
|
|
|
|
|
|
|
|
|
|
|
|
|
|
|
|
|
|
|
|
|
|
|
|
|
|
|
|
|
|
|
|
|
|
|
|
spaces/Ameaou/academic-chatgpt3.1/crazy_functional.py
DELETED
@@ -1,192 +0,0 @@
|
|
1 |
-
from toolbox import HotReload # HotReload 的意思是热更新,修改函数插件后,不需要重启程序,代码直接生效
|
2 |
-
|
3 |
-
|
4 |
-
def get_crazy_functions():
|
5 |
-
###################### 第一组插件 ###########################
|
6 |
-
from crazy_functions.读文章写摘要 import 读文章写摘要
|
7 |
-
from crazy_functions.生成函数注释 import 批量生成函数注释
|
8 |
-
from crazy_functions.解析项目源代码 import 解析项目本身
|
9 |
-
from crazy_functions.解析项目源代码 import 解析一个Python项目
|
10 |
-
from crazy_functions.解析项目源代码 import 解析一个C项目的头文件
|
11 |
-
from crazy_functions.解析项目源代码 import 解析一个C项目
|
12 |
-
from crazy_functions.解析项目源代码 import 解析一个Golang项目
|
13 |
-
from crazy_functions.解析项目源代码 import 解析一个Java项目
|
14 |
-
from crazy_functions.解析项目源代码 import 解析一个Rect项目
|
15 |
-
from crazy_functions.高级功能函数模板 import 高阶功能模板函数
|
16 |
-
from crazy_functions.代码重写为全英文_多线程 import 全项目切换英文
|
17 |
-
from crazy_functions.Latex全文润色 import Latex英文润色
|
18 |
-
from crazy_functions.询问多个大语言模型 import 同时问询
|
19 |
-
from crazy_functions.解析项目源代码 import 解析一个Lua项目
|
20 |
-
from crazy_functions.解析项目源代码 import 解析一个CSharp项目
|
21 |
-
from crazy_functions.总结word文档 import 总结word文档
|
22 |
-
function_plugins = {
|
23 |
-
|
24 |
-
"解析整个Python项目": {
|
25 |
-
"Color": "stop", # 按钮颜色
|
26 |
-
"Function": HotReload(解析一个Python项目)
|
27 |
-
},
|
28 |
-
"批量总结Word文档": {
|
29 |
-
"Color": "stop",
|
30 |
-
"Function": HotReload(总结word文档)
|
31 |
-
},
|
32 |
-
"解析整个C++项目头文件": {
|
33 |
-
"Color": "stop", # 按钮颜色
|
34 |
-
"AsButton": False, # 加入下拉菜单中
|
35 |
-
"Function": HotReload(解析一个C项目的头文件)
|
36 |
-
},
|
37 |
-
"解析整个C++项目(.cpp/.hpp/.c/.h)": {
|
38 |
-
"Color": "stop", # 按钮颜色
|
39 |
-
"AsButton": False, # 加入下拉菜单中
|
40 |
-
"Function": HotReload(解析一个C项目)
|
41 |
-
},
|
42 |
-
"解析整个Go项目": {
|
43 |
-
"Color": "stop", # 按钮颜色
|
44 |
-
"AsButton": False, # 加入下拉菜单中
|
45 |
-
"Function": HotReload(解析一个Golang项目)
|
46 |
-
},
|
47 |
-
"解析整个Java项目": {
|
48 |
-
"Color": "stop", # 按钮颜色
|
49 |
-
"AsButton": False, # 加入下拉菜单中
|
50 |
-
"Function": HotReload(解析一个Java项目)
|
51 |
-
},
|
52 |
-
"解析整个React项目": {
|
53 |
-
"Color": "stop", # 按钮颜色
|
54 |
-
"AsButton": False, # 加入下拉菜单中
|
55 |
-
"Function": HotReload(解析一个Rect项目)
|
56 |
-
},
|
57 |
-
"解析整个Lua项目": {
|
58 |
-
"Color": "stop", # 按钮颜色
|
59 |
-
"AsButton": False, # 加入下拉菜单中
|
60 |
-
"Function": HotReload(解析一个Lua项目)
|
61 |
-
},
|
62 |
-
"解析整个CSharp项目": {
|
63 |
-
"Color": "stop", # 按钮颜色
|
64 |
-
"AsButton": False, # 加入下拉菜单中
|
65 |
-
"Function": HotReload(解析一个CSharp项目)
|
66 |
-
},
|
67 |
-
"读Tex论文写摘要": {
|
68 |
-
"Color": "stop", # 按钮颜色
|
69 |
-
"Function": HotReload(读文章写摘要)
|
70 |
-
},
|
71 |
-
"批量生成函数注释": {
|
72 |
-
"Color": "stop", # 按钮颜色
|
73 |
-
"Function": HotReload(批量生成函数注释)
|
74 |
-
},
|
75 |
-
"[多线程Demo] 解析此项目本身(源码自译解)": {
|
76 |
-
"Function": HotReload(解析项目本身)
|
77 |
-
},
|
78 |
-
"[多线程demo] 把本项目源代码切换成全英文": {
|
79 |
-
# HotReload 的意思是热更新,修改函数插件代码后,不需要重启程序,代码直接生效
|
80 |
-
"AsButton": False, # 加入下拉菜单中
|
81 |
-
"Function": HotReload(全项目切换英文)
|
82 |
-
},
|
83 |
-
"[函数插件模板Demo] 历史上的今天": {
|
84 |
-
# HotReload 的意思是热更新,修改函数插件代码后,不需要重启程序,代码直接生效
|
85 |
-
"Function": HotReload(高阶功能模板函数)
|
86 |
-
},
|
87 |
-
|
88 |
-
}
|
89 |
-
###################### 第二组插件 ###########################
|
90 |
-
# [第二组插件]: 经过充分测试
|
91 |
-
from crazy_functions.批量总结PDF文档 import 批量总结PDF文档
|
92 |
-
from crazy_functions.批量总结PDF文档pdfminer import 批量总结PDF文档pdfminer
|
93 |
-
from crazy_functions.批量翻译PDF文档_多线程 import 批量翻译PDF文档
|
94 |
-
from crazy_functions.谷歌检索小助手 import 谷歌检索小助手
|
95 |
-
from crazy_functions.理解PDF文档内容 import 理解PDF文档内容标准文件输入
|
96 |
-
from crazy_functions.Latex全文润色 import Latex中文润色
|
97 |
-
from crazy_functions.Latex全文翻译 import Latex中译英
|
98 |
-
from crazy_functions.Latex全文翻译 import Latex英译中
|
99 |
-
from crazy_functions.批量Markdown翻译 import Markdown中译英
|
100 |
-
from crazy_functions.批量Markdown翻译 import Markdown英译中
|
101 |
-
|
102 |
-
function_plugins.update({
|
103 |
-
"批量翻译PDF文档(多线程)": {
|
104 |
-
"Color": "stop",
|
105 |
-
"AsButton": True, # 加入下拉菜单中
|
106 |
-
"Function": HotReload(批量翻译PDF文档)
|
107 |
-
},
|
108 |
-
"询问多个GPT模型": {
|
109 |
-
"Color": "stop", # 按钮颜色
|
110 |
-
"Function": HotReload(同时问询)
|
111 |
-
},
|
112 |
-
"[测试功能] 批量总结PDF文档": {
|
113 |
-
"Color": "stop",
|
114 |
-
"AsButton": False, # 加入下拉菜单中
|
115 |
-
# HotReload 的意思是热更新,修改函数插件代码后,不需要重启程序,代码直接生效
|
116 |
-
"Function": HotReload(批量总结PDF文档)
|
117 |
-
},
|
118 |
-
"[测试功能] 批量总结PDF文档pdfminer": {
|
119 |
-
"Color": "stop",
|
120 |
-
"AsButton": False, # 加入下拉菜单中
|
121 |
-
"Function": HotReload(批量总结PDF文档pdfminer)
|
122 |
-
},
|
123 |
-
"谷歌学术检索助手(输入谷歌学术搜索页url)": {
|
124 |
-
"Color": "stop",
|
125 |
-
"AsButton": False, # 加入下拉菜单中
|
126 |
-
"Function": HotReload(谷歌检索小助手)
|
127 |
-
},
|
128 |
-
|
129 |
-
"理解PDF文档内容 (模仿ChatPDF)": {
|
130 |
-
# HotReload 的意思是热更新,修改函数插件代码后,不需要重启程序,代码直接生效
|
131 |
-
"Color": "stop",
|
132 |
-
"AsButton": False, # 加入下拉菜单中
|
133 |
-
"Function": HotReload(理解PDF文档内容标准文件输入)
|
134 |
-
},
|
135 |
-
"[测试功能] 英文Latex项目全文润色(输入路径或上传压缩包)": {
|
136 |
-
# HotReload 的意思是热更新,修改函数插件代码后,不需要重启程序,代码直接生效
|
137 |
-
"Color": "stop",
|
138 |
-
"AsButton": False, # 加入下拉菜单中
|
139 |
-
"Function": HotReload(Latex英文润色)
|
140 |
-
},
|
141 |
-
"[测试功能] 中文Latex项目全文润色(输入路径或上传压缩包)": {
|
142 |
-
# HotReload 的意思是热更新,修改函数插件代码后,不需要重启程序,代码直接生效
|
143 |
-
"Color": "stop",
|
144 |
-
"AsButton": False, # 加入下拉菜单中
|
145 |
-
"Function": HotReload(Latex中文润色)
|
146 |
-
},
|
147 |
-
"[测试功能] Latex项目全文中译英(输入路径或上传压缩包)": {
|
148 |
-
# HotReload 的意思是热更新,修改函数插件代码后,不需要重启程序,代码直接生效
|
149 |
-
"Color": "stop",
|
150 |
-
"AsButton": False, # 加入下拉菜单中
|
151 |
-
"Function": HotReload(Latex中译英)
|
152 |
-
},
|
153 |
-
"[测试功能] Latex项目全文英译中(输入路径或上传压缩包)": {
|
154 |
-
# HotReload 的意思是热更新,修改函数插件代码后,不需要重启程序,代码直接生效
|
155 |
-
"Color": "stop",
|
156 |
-
"AsButton": False, # 加入下拉菜单中
|
157 |
-
"Function": HotReload(Latex英译中)
|
158 |
-
},
|
159 |
-
"[测试功能] 批量Markdown中译英(输入路径或上传压缩包)": {
|
160 |
-
# HotReload 的意思是热更新,修改函数插件代码后,不需要重启程序,代码直接生效
|
161 |
-
"Color": "stop",
|
162 |
-
"AsButton": False, # 加入下拉菜单中
|
163 |
-
"Function": HotReload(Markdown中译英)
|
164 |
-
},
|
165 |
-
"[测试功能] 批量Markdown英译中(输入路径或上传压缩包)": {
|
166 |
-
# HotReload 的意思是热更新,修改函数插件代码后,不需要重启程序,代码直接生效
|
167 |
-
"Color": "stop",
|
168 |
-
"AsButton": False, # 加入下拉菜单中
|
169 |
-
"Function": HotReload(Markdown英译中)
|
170 |
-
},
|
171 |
-
|
172 |
-
})
|
173 |
-
|
174 |
-
###################### 第三组插件 ###########################
|
175 |
-
# [第三组插件]: 尚未充分测试的函数插件,放在这里
|
176 |
-
try:
|
177 |
-
from crazy_functions.下载arxiv论文翻译摘要 import 下载arxiv论文并翻译摘要
|
178 |
-
function_plugins.update({
|
179 |
-
"一键下载arxiv论文并翻译摘要(先在input输入编号,如1812.10695)": {
|
180 |
-
"Color": "stop",
|
181 |
-
"AsButton": False, # 加入下拉菜单中
|
182 |
-
"Function": HotReload(下载arxiv论文并翻译摘要)
|
183 |
-
}
|
184 |
-
})
|
185 |
-
|
186 |
-
except Exception as err:
|
187 |
-
print(f'[下载arxiv论文并翻译摘要] 插件导入失败 {str(err)}')
|
188 |
-
|
189 |
-
|
190 |
-
|
191 |
-
###################### 第n组插件 ###########################
|
192 |
-
return function_plugins
|
|
|
|
|
|
|
|
|
|
|
|
|
|
|
|
|
|
|
|
|
|
|
|
|
|
|
|
|
|
|
|
|
|
|
|
|
|
|
|
|
|
|
|
|
|
|
|
|
|
|
|
|
|
|
|
|
|
|
|
|
|
|
|
|
|
|
|
|
|
|
|
|
|
|
|
|
|
|
|
|
|
|
|
|
|
|
|
|
|
|
|
|
|
|
|
|
|
|
|
|
|
|
|
|
|
|
|
|
|
|
|
|
|
|
|
|
|
|
|
|
|
|
|
|
|
|
|
|
|
|
|
|
|
|
|
|
|
|
|
|
|
|
|
|
|
|
|
|
|
|
|
|
|
|
|
|
|
|
|
|
|
|
|
|
|
|
|
|
|
|
|
|
|
|
|
|
|
|
|
|
|
|
|
|
|
|
|
|
|
|
|
|
|
|
|
|
|
|
|
|
|
|
|
|
|
|
|
|
|
|
|
|
|
|
|
|
|
|
|
|
|
|
|
|
|
|
|
|
|
|
|
|
|
|
|
|
|
|
|
|
|
|
|
|
|
|
|
|
|
|
|
|
|
|
|
|
|
|
|
|
|
|
|
|
|
|
|
|
|
|
|
|
|
|
|
|
|
|
|
|
|
|
|
|
|
|
|
|
|
|
|
|
|
|
|
|
|
|
|
|
|
|
|
|
|
|
|
|
|
|
|
|
|
|
|
|
|
|
|
|
|
|
|
|
|
|
|
|
|
|
|
|
|
|
|
|
|
|
|
|
|
|
|
|
|
|
|
|
|
|
|
|
|
|
|
|
|
|
|
|
|
|
|
|
|
|
|
|
|
|
|
|
|
|
|
|
|
|
|
|
|
|
|
|
|
spaces/Ameaou/academic-chatgpt3.1/crazy_functions/test_project/python/dqn/dqn.py
DELETED
@@ -1,245 +0,0 @@
|
|
1 |
-
from typing import Any, Dict, List, Optional, Tuple, Type, Union
|
2 |
-
|
3 |
-
import gym
|
4 |
-
import numpy as np
|
5 |
-
import torch as th
|
6 |
-
from torch.nn import functional as F
|
7 |
-
|
8 |
-
from stable_baselines3.common import logger
|
9 |
-
from stable_baselines3.common.off_policy_algorithm import OffPolicyAlgorithm
|
10 |
-
from stable_baselines3.common.preprocessing import maybe_transpose
|
11 |
-
from stable_baselines3.common.type_aliases import GymEnv, MaybeCallback, Schedule
|
12 |
-
from stable_baselines3.common.utils import get_linear_fn, is_vectorized_observation, polyak_update
|
13 |
-
from stable_baselines3.dqn.policies import DQNPolicy
|
14 |
-
|
15 |
-
|
16 |
-
class DQN(OffPolicyAlgorithm):
|
17 |
-
"""
|
18 |
-
Deep Q-Network (DQN)
|
19 |
-
|
20 |
-
Paper: https://arxiv.org/abs/1312.5602, https://www.nature.com/articles/nature14236
|
21 |
-
Default hyperparameters are taken from the nature paper,
|
22 |
-
except for the optimizer and learning rate that were taken from Stable Baselines defaults.
|
23 |
-
|
24 |
-
:param policy: The policy model to use (MlpPolicy, CnnPolicy, ...)
|
25 |
-
:param env: The environment to learn from (if registered in Gym, can be str)
|
26 |
-
:param learning_rate: The learning rate, it can be a function
|
27 |
-
of the current progress remaining (from 1 to 0)
|
28 |
-
:param buffer_size: size of the replay buffer
|
29 |
-
:param learning_starts: how many steps of the model to collect transitions for before learning starts
|
30 |
-
:param batch_size: Minibatch size for each gradient update
|
31 |
-
:param tau: the soft update coefficient ("Polyak update", between 0 and 1) default 1 for hard update
|
32 |
-
:param gamma: the discount factor
|
33 |
-
:param train_freq: Update the model every ``train_freq`` steps. Alternatively pass a tuple of frequency and unit
|
34 |
-
like ``(5, "step")`` or ``(2, "episode")``.
|
35 |
-
:param gradient_steps: How many gradient steps to do after each rollout (see ``train_freq``)
|
36 |
-
Set to ``-1`` means to do as many gradient steps as steps done in the environment
|
37 |
-
during the rollout.
|
38 |
-
:param optimize_memory_usage: Enable a memory efficient variant of the replay buffer
|
39 |
-
at a cost of more complexity.
|
40 |
-
See https://github.com/DLR-RM/stable-baselines3/issues/37#issuecomment-637501195
|
41 |
-
:param target_update_interval: update the target network every ``target_update_interval``
|
42 |
-
environment steps.
|
43 |
-
:param exploration_fraction: fraction of entire training period over which the exploration rate is reduced
|
44 |
-
:param exploration_initial_eps: initial value of random action probability
|
45 |
-
:param exploration_final_eps: final value of random action probability
|
46 |
-
:param max_grad_norm: The maximum value for the gradient clipping
|
47 |
-
:param tensorboard_log: the log location for tensorboard (if None, no logging)
|
48 |
-
:param create_eval_env: Whether to create a second environment that will be
|
49 |
-
used for evaluating the agent periodically. (Only available when passing string for the environment)
|
50 |
-
:param policy_kwargs: additional arguments to be passed to the policy on creation
|
51 |
-
:param verbose: the verbosity level: 0 no output, 1 info, 2 debug
|
52 |
-
:param seed: Seed for the pseudo random generators
|
53 |
-
:param device: Device (cpu, cuda, ...) on which the code should be run.
|
54 |
-
Setting it to auto, the code will be run on the GPU if possible.
|
55 |
-
:param _init_setup_model: Whether or not to build the network at the creation of the instance
|
56 |
-
"""
|
57 |
-
|
58 |
-
def __init__(
|
59 |
-
self,
|
60 |
-
policy: Union[str, Type[DQNPolicy]],
|
61 |
-
env: Union[GymEnv, str],
|
62 |
-
learning_rate: Union[float, Schedule] = 1e-4,
|
63 |
-
buffer_size: int = 1000000,
|
64 |
-
learning_starts: int = 50000,
|
65 |
-
batch_size: Optional[int] = 32,
|
66 |
-
tau: float = 1.0,
|
67 |
-
gamma: float = 0.99,
|
68 |
-
train_freq: Union[int, Tuple[int, str]] = 4,
|
69 |
-
gradient_steps: int = 1,
|
70 |
-
optimize_memory_usage: bool = False,
|
71 |
-
target_update_interval: int = 10000,
|
72 |
-
exploration_fraction: float = 0.1,
|
73 |
-
exploration_initial_eps: float = 1.0,
|
74 |
-
exploration_final_eps: float = 0.05,
|
75 |
-
max_grad_norm: float = 10,
|
76 |
-
tensorboard_log: Optional[str] = None,
|
77 |
-
create_eval_env: bool = False,
|
78 |
-
policy_kwargs: Optional[Dict[str, Any]] = None,
|
79 |
-
verbose: int = 0,
|
80 |
-
seed: Optional[int] = None,
|
81 |
-
device: Union[th.device, str] = "auto",
|
82 |
-
_init_setup_model: bool = True,
|
83 |
-
):
|
84 |
-
|
85 |
-
super(DQN, self).__init__(
|
86 |
-
policy,
|
87 |
-
env,
|
88 |
-
DQNPolicy,
|
89 |
-
learning_rate,
|
90 |
-
buffer_size,
|
91 |
-
learning_starts,
|
92 |
-
batch_size,
|
93 |
-
tau,
|
94 |
-
gamma,
|
95 |
-
train_freq,
|
96 |
-
gradient_steps,
|
97 |
-
action_noise=None, # No action noise
|
98 |
-
policy_kwargs=policy_kwargs,
|
99 |
-
tensorboard_log=tensorboard_log,
|
100 |
-
verbose=verbose,
|
101 |
-
device=device,
|
102 |
-
create_eval_env=create_eval_env,
|
103 |
-
seed=seed,
|
104 |
-
sde_support=False,
|
105 |
-
optimize_memory_usage=optimize_memory_usage,
|
106 |
-
supported_action_spaces=(gym.spaces.Discrete,),
|
107 |
-
)
|
108 |
-
|
109 |
-
self.exploration_initial_eps = exploration_initial_eps
|
110 |
-
self.exploration_final_eps = exploration_final_eps
|
111 |
-
self.exploration_fraction = exploration_fraction
|
112 |
-
self.target_update_interval = target_update_interval
|
113 |
-
self.max_grad_norm = max_grad_norm
|
114 |
-
# "epsilon" for the epsilon-greedy exploration
|
115 |
-
self.exploration_rate = 0.0
|
116 |
-
# Linear schedule will be defined in `_setup_model()`
|
117 |
-
self.exploration_schedule = None
|
118 |
-
self.q_net, self.q_net_target = None, None
|
119 |
-
|
120 |
-
if _init_setup_model:
|
121 |
-
self._setup_model()
|
122 |
-
|
123 |
-
def _setup_model(self) -> None:
|
124 |
-
super(DQN, self)._setup_model()
|
125 |
-
self._create_aliases()
|
126 |
-
self.exploration_schedule = get_linear_fn(
|
127 |
-
self.exploration_initial_eps, self.exploration_final_eps, self.exploration_fraction
|
128 |
-
)
|
129 |
-
|
130 |
-
def _create_aliases(self) -> None:
|
131 |
-
self.q_net = self.policy.q_net
|
132 |
-
self.q_net_target = self.policy.q_net_target
|
133 |
-
|
134 |
-
def _on_step(self) -> None:
|
135 |
-
"""
|
136 |
-
Update the exploration rate and target network if needed.
|
137 |
-
This method is called in ``collect_rollouts()`` after each step in the environment.
|
138 |
-
"""
|
139 |
-
if self.num_timesteps % self.target_update_interval == 0:
|
140 |
-
polyak_update(self.q_net.parameters(), self.q_net_target.parameters(), self.tau)
|
141 |
-
|
142 |
-
self.exploration_rate = self.exploration_schedule(self._current_progress_remaining)
|
143 |
-
logger.record("rollout/exploration rate", self.exploration_rate)
|
144 |
-
|
145 |
-
def train(self, gradient_steps: int, batch_size: int = 100) -> None:
|
146 |
-
# Update learning rate according to schedule
|
147 |
-
self._update_learning_rate(self.policy.optimizer)
|
148 |
-
|
149 |
-
losses = []
|
150 |
-
for _ in range(gradient_steps):
|
151 |
-
# Sample replay buffer
|
152 |
-
replay_data = self.replay_buffer.sample(batch_size, env=self._vec_normalize_env)
|
153 |
-
|
154 |
-
with th.no_grad():
|
155 |
-
# Compute the next Q-values using the target network
|
156 |
-
next_q_values = self.q_net_target(replay_data.next_observations)
|
157 |
-
# Follow greedy policy: use the one with the highest value
|
158 |
-
next_q_values, _ = next_q_values.max(dim=1)
|
159 |
-
# Avoid potential broadcast issue
|
160 |
-
next_q_values = next_q_values.reshape(-1, 1)
|
161 |
-
# 1-step TD target
|
162 |
-
target_q_values = replay_data.rewards + (1 - replay_data.dones) * self.gamma * next_q_values
|
163 |
-
|
164 |
-
# Get current Q-values estimates
|
165 |
-
current_q_values = self.q_net(replay_data.observations)
|
166 |
-
|
167 |
-
# Retrieve the q-values for the actions from the replay buffer
|
168 |
-
current_q_values = th.gather(current_q_values, dim=1, index=replay_data.actions.long())
|
169 |
-
|
170 |
-
# Compute Huber loss (less sensitive to outliers)
|
171 |
-
loss = F.smooth_l1_loss(current_q_values, target_q_values)
|
172 |
-
losses.append(loss.item())
|
173 |
-
|
174 |
-
# Optimize the policy
|
175 |
-
self.policy.optimizer.zero_grad()
|
176 |
-
loss.backward()
|
177 |
-
# Clip gradient norm
|
178 |
-
th.nn.utils.clip_grad_norm_(self.policy.parameters(), self.max_grad_norm)
|
179 |
-
self.policy.optimizer.step()
|
180 |
-
|
181 |
-
# Increase update counter
|
182 |
-
self._n_updates += gradient_steps
|
183 |
-
|
184 |
-
logger.record("train/n_updates", self._n_updates, exclude="tensorboard")
|
185 |
-
logger.record("train/loss", np.mean(losses))
|
186 |
-
|
187 |
-
def predict(
|
188 |
-
self,
|
189 |
-
observation: np.ndarray,
|
190 |
-
state: Optional[np.ndarray] = None,
|
191 |
-
mask: Optional[np.ndarray] = None,
|
192 |
-
deterministic: bool = False,
|
193 |
-
) -> Tuple[np.ndarray, Optional[np.ndarray]]:
|
194 |
-
"""
|
195 |
-
Overrides the base_class predict function to include epsilon-greedy exploration.
|
196 |
-
|
197 |
-
:param observation: the input observation
|
198 |
-
:param state: The last states (can be None, used in recurrent policies)
|
199 |
-
:param mask: The last masks (can be None, used in recurrent policies)
|
200 |
-
:param deterministic: Whether or not to return deterministic actions.
|
201 |
-
:return: the model's action and the next state
|
202 |
-
(used in recurrent policies)
|
203 |
-
"""
|
204 |
-
if not deterministic and np.random.rand() < self.exploration_rate:
|
205 |
-
if is_vectorized_observation(maybe_transpose(observation, self.observation_space), self.observation_space):
|
206 |
-
n_batch = observation.shape[0]
|
207 |
-
action = np.array([self.action_space.sample() for _ in range(n_batch)])
|
208 |
-
else:
|
209 |
-
action = np.array(self.action_space.sample())
|
210 |
-
else:
|
211 |
-
action, state = self.policy.predict(observation, state, mask, deterministic)
|
212 |
-
return action, state
|
213 |
-
|
214 |
-
def learn(
|
215 |
-
self,
|
216 |
-
total_timesteps: int,
|
217 |
-
callback: MaybeCallback = None,
|
218 |
-
log_interval: int = 4,
|
219 |
-
eval_env: Optional[GymEnv] = None,
|
220 |
-
eval_freq: int = -1,
|
221 |
-
n_eval_episodes: int = 5,
|
222 |
-
tb_log_name: str = "DQN",
|
223 |
-
eval_log_path: Optional[str] = None,
|
224 |
-
reset_num_timesteps: bool = True,
|
225 |
-
) -> OffPolicyAlgorithm:
|
226 |
-
|
227 |
-
return super(DQN, self).learn(
|
228 |
-
total_timesteps=total_timesteps,
|
229 |
-
callback=callback,
|
230 |
-
log_interval=log_interval,
|
231 |
-
eval_env=eval_env,
|
232 |
-
eval_freq=eval_freq,
|
233 |
-
n_eval_episodes=n_eval_episodes,
|
234 |
-
tb_log_name=tb_log_name,
|
235 |
-
eval_log_path=eval_log_path,
|
236 |
-
reset_num_timesteps=reset_num_timesteps,
|
237 |
-
)
|
238 |
-
|
239 |
-
def _excluded_save_params(self) -> List[str]:
|
240 |
-
return super(DQN, self)._excluded_save_params() + ["q_net", "q_net_target"]
|
241 |
-
|
242 |
-
def _get_torch_save_params(self) -> Tuple[List[str], List[str]]:
|
243 |
-
state_dicts = ["policy", "policy.optimizer"]
|
244 |
-
|
245 |
-
return state_dicts, []
|
|
|
|
|
|
|
|
|
|
|
|
|
|
|
|
|
|
|
|
|
|
|
|
|
|
|
|
|
|
|
|
|
|
|
|
|
|
|
|
|
|
|
|
|
|
|
|
|
|
|
|
|
|
|
|
|
|
|
|
|
|
|
|
|
|
|
|
|
|
|
|
|
|
|
|
|
|
|
|
|
|
|
|
|
|
|
|
|
|
|
|
|
|
|
|
|
|
|
|
|
|
|
|
|
|
|
|
|
|
|
|
|
|
|
|
|
|
|
|
|
|
|
|
|
|
|
|
|
|
|
|
|
|
|
|
|
|
|
|
|
|
|
|
|
|
|
|
|
|
|
|
|
|
|
|
|
|
|
|
|
|
|
|
|
|
|
|
|
|
|
|
|
|
|
|
|
|
|
|
|
|
|
|
|
|
|
|
|
|
|
|
|
|
|
|
|
|
|
|
|
|
|
|
|
|
|
|
|
|
|
|
|
|
|
|
|
|
|
|
|
|
|
|
|
|
|
|
|
|
|
|
|
|
|
|
|
|
|
|
|
|
|
|
|
|
|
|
|
|
|
|
|
|
|
|
|
|
|
|
|
|
|
|
|
|
|
|
|
|
|
|
|
|
|
|
|
|
|
|
|
|
|
|
|
|
|
|
|
|
|
|
|
|
|
|
|
|
|
|
|
|
|
|
|
|
|
|
|
|
|
|
|
|
|
|
|
|
|
|
|
|
|
|
|
|
|
|
|
|
|
|
|
|
|
|
|
|
|
|
|
|
|
|
|
|
|
|
|
|
|
|
|
|
|
|
|
|
|
|
|
|
|
|
|
|
|
|
|
|
|
|
|
|
|
|
|
|
|
|
|
|
|
|
|
|
|
|
|
|
|
|
|
|
|
|
|
|
|
|
|
|
|
|
|
|
|
|
|
|
|
|
|
|
|
|
|
|
|
|
|
|
|
|
|
|
|
|
|
|
|
|
|
|
|
|
|
|
|
|
|
|
|
|
|
|
|
|
|
|
|
|
|
|
|
|
|
|
|
|
|
|
|
|
|
|
|
|
|
|
|
|
|
|
|
|
|
|
|
|
|
|
|
|
|
|
|
|
|
|
|
|
spaces/Androidonnxfork/CivitAi-to-Diffusers/diffusers/docs/source/en/api/models/prior_transformer.md
DELETED
@@ -1,16 +0,0 @@
|
|
1 |
-
# Prior Transformer
|
2 |
-
|
3 |
-
The Prior Transformer was originally introduced in [Hierarchical Text-Conditional Image Generation with CLIP Latents
|
4 |
-
](https://huggingface.co/papers/2204.06125) by Ramesh et al. It is used to predict CLIP image embeddings from CLIP text embeddings; image embeddings are predicted through a denoising diffusion process.
|
5 |
-
|
6 |
-
The abstract from the paper is:
|
7 |
-
|
8 |
-
*Contrastive models like CLIP have been shown to learn robust representations of images that capture both semantics and style. To leverage these representations for image generation, we propose a two-stage model: a prior that generates a CLIP image embedding given a text caption, and a decoder that generates an image conditioned on the image embedding. We show that explicitly generating image representations improves image diversity with minimal loss in photorealism and caption similarity. Our decoders conditioned on image representations can also produce variations of an image that preserve both its semantics and style, while varying the non-essential details absent from the image representation. Moreover, the joint embedding space of CLIP enables language-guided image manipulations in a zero-shot fashion. We use diffusion models for the decoder and experiment with both autoregressive and diffusion models for the prior, finding that the latter are computationally more efficient and produce higher-quality samples.*
|
9 |
-
|
10 |
-
## PriorTransformer
|
11 |
-
|
12 |
-
[[autodoc]] PriorTransformer
|
13 |
-
|
14 |
-
## PriorTransformerOutput
|
15 |
-
|
16 |
-
[[autodoc]] models.prior_transformer.PriorTransformerOutput
|
|
|
|
|
|
|
|
|
|
|
|
|
|
|
|
|
|
|
|
|
|
|
|
|
|
|
|
|
|
|
|
|
|
spaces/Androidonnxfork/CivitAi-to-Diffusers/diffusers/docs/source/en/using-diffusers/other-modalities.md
DELETED
@@ -1,21 +0,0 @@
|
|
1 |
-
<!--Copyright 2023 The HuggingFace Team. All rights reserved.
|
2 |
-
|
3 |
-
Licensed under the Apache License, Version 2.0 (the "License"); you may not use this file except in compliance with
|
4 |
-
the License. You may obtain a copy of the License at
|
5 |
-
|
6 |
-
http://www.apache.org/licenses/LICENSE-2.0
|
7 |
-
|
8 |
-
Unless required by applicable law or agreed to in writing, software distributed under the License is distributed on
|
9 |
-
an "AS IS" BASIS, WITHOUT WARRANTIES OR CONDITIONS OF ANY KIND, either express or implied. See the License for the
|
10 |
-
specific language governing permissions and limitations under the License.
|
11 |
-
-->
|
12 |
-
|
13 |
-
# Using Diffusers with other modalities
|
14 |
-
|
15 |
-
Diffusers is in the process of expanding to modalities other than images.
|
16 |
-
|
17 |
-
Example type | Colab | Pipeline |
|
18 |
-
:-------------------------:|:-------------------------:|:-------------------------:|
|
19 |
-
[Molecule conformation](https://www.nature.com/subjects/molecular-conformation#:~:text=Definition,to%20changes%20in%20their%20environment.) generation | [](https://colab.research.google.com/github/huggingface/notebooks/blob/main/diffusers/geodiff_molecule_conformation.ipynb) | ❌
|
20 |
-
|
21 |
-
More coming soon!
|
|
|
|
|
|
|
|
|
|
|
|
|
|
|
|
|
|
|
|
|
|
|
|
|
|
|
|
|
|
|
|
|
|
|
|
|
|
|
|
|
|
|
|
spaces/Androidonnxfork/CivitAi-to-Diffusers/diffusers/scripts/change_naming_configs_and_checkpoints.py
DELETED
@@ -1,113 +0,0 @@
|
|
1 |
-
# coding=utf-8
|
2 |
-
# Copyright 2023 The HuggingFace Inc. team.
|
3 |
-
#
|
4 |
-
# Licensed under the Apache License, Version 2.0 (the "License");
|
5 |
-
# you may not use this file except in compliance with the License.
|
6 |
-
# You may obtain a copy of the License at
|
7 |
-
#
|
8 |
-
# http://www.apache.org/licenses/LICENSE-2.0
|
9 |
-
#
|
10 |
-
# Unless required by applicable law or agreed to in writing, software
|
11 |
-
# distributed under the License is distributed on an "AS IS" BASIS,
|
12 |
-
# WITHOUT WARRANTIES OR CONDITIONS OF ANY KIND, either express or implied.
|
13 |
-
# See the License for the specific language governing permissions and
|
14 |
-
# limitations under the License.
|
15 |
-
""" Conversion script for the LDM checkpoints. """
|
16 |
-
|
17 |
-
import argparse
|
18 |
-
import json
|
19 |
-
import os
|
20 |
-
|
21 |
-
import torch
|
22 |
-
from transformers.file_utils import has_file
|
23 |
-
|
24 |
-
from diffusers import UNet2DConditionModel, UNet2DModel
|
25 |
-
|
26 |
-
|
27 |
-
do_only_config = False
|
28 |
-
do_only_weights = True
|
29 |
-
do_only_renaming = False
|
30 |
-
|
31 |
-
|
32 |
-
if __name__ == "__main__":
|
33 |
-
parser = argparse.ArgumentParser()
|
34 |
-
|
35 |
-
parser.add_argument(
|
36 |
-
"--repo_path",
|
37 |
-
default=None,
|
38 |
-
type=str,
|
39 |
-
required=True,
|
40 |
-
help="The config json file corresponding to the architecture.",
|
41 |
-
)
|
42 |
-
|
43 |
-
parser.add_argument("--dump_path", default=None, type=str, required=True, help="Path to the output model.")
|
44 |
-
|
45 |
-
args = parser.parse_args()
|
46 |
-
|
47 |
-
config_parameters_to_change = {
|
48 |
-
"image_size": "sample_size",
|
49 |
-
"num_res_blocks": "layers_per_block",
|
50 |
-
"block_channels": "block_out_channels",
|
51 |
-
"down_blocks": "down_block_types",
|
52 |
-
"up_blocks": "up_block_types",
|
53 |
-
"downscale_freq_shift": "freq_shift",
|
54 |
-
"resnet_num_groups": "norm_num_groups",
|
55 |
-
"resnet_act_fn": "act_fn",
|
56 |
-
"resnet_eps": "norm_eps",
|
57 |
-
"num_head_channels": "attention_head_dim",
|
58 |
-
}
|
59 |
-
|
60 |
-
key_parameters_to_change = {
|
61 |
-
"time_steps": "time_proj",
|
62 |
-
"mid": "mid_block",
|
63 |
-
"downsample_blocks": "down_blocks",
|
64 |
-
"upsample_blocks": "up_blocks",
|
65 |
-
}
|
66 |
-
|
67 |
-
subfolder = "" if has_file(args.repo_path, "config.json") else "unet"
|
68 |
-
|
69 |
-
with open(os.path.join(args.repo_path, subfolder, "config.json"), "r", encoding="utf-8") as reader:
|
70 |
-
text = reader.read()
|
71 |
-
config = json.loads(text)
|
72 |
-
|
73 |
-
if do_only_config:
|
74 |
-
for key in config_parameters_to_change.keys():
|
75 |
-
config.pop(key, None)
|
76 |
-
|
77 |
-
if has_file(args.repo_path, "config.json"):
|
78 |
-
model = UNet2DModel(**config)
|
79 |
-
else:
|
80 |
-
class_name = UNet2DConditionModel if "ldm-text2im-large-256" in args.repo_path else UNet2DModel
|
81 |
-
model = class_name(**config)
|
82 |
-
|
83 |
-
if do_only_config:
|
84 |
-
model.save_config(os.path.join(args.repo_path, subfolder))
|
85 |
-
|
86 |
-
config = dict(model.config)
|
87 |
-
|
88 |
-
if do_only_renaming:
|
89 |
-
for key, value in config_parameters_to_change.items():
|
90 |
-
if key in config:
|
91 |
-
config[value] = config[key]
|
92 |
-
del config[key]
|
93 |
-
|
94 |
-
config["down_block_types"] = [k.replace("UNetRes", "") for k in config["down_block_types"]]
|
95 |
-
config["up_block_types"] = [k.replace("UNetRes", "") for k in config["up_block_types"]]
|
96 |
-
|
97 |
-
if do_only_weights:
|
98 |
-
state_dict = torch.load(os.path.join(args.repo_path, subfolder, "diffusion_pytorch_model.bin"))
|
99 |
-
|
100 |
-
new_state_dict = {}
|
101 |
-
for param_key, param_value in state_dict.items():
|
102 |
-
if param_key.endswith(".op.bias") or param_key.endswith(".op.weight"):
|
103 |
-
continue
|
104 |
-
has_changed = False
|
105 |
-
for key, new_key in key_parameters_to_change.items():
|
106 |
-
if not has_changed and param_key.split(".")[0] == key:
|
107 |
-
new_state_dict[".".join([new_key] + param_key.split(".")[1:])] = param_value
|
108 |
-
has_changed = True
|
109 |
-
if not has_changed:
|
110 |
-
new_state_dict[param_key] = param_value
|
111 |
-
|
112 |
-
model.load_state_dict(new_state_dict)
|
113 |
-
model.save_pretrained(os.path.join(args.repo_path, subfolder))
|
|
|
|
|
|
|
|
|
|
|
|
|
|
|
|
|
|
|
|
|
|
|
|
|
|
|
|
|
|
|
|
|
|
|
|
|
|
|
|
|
|
|
|
|
|
|
|
|
|
|
|
|
|
|
|
|
|
|
|
|
|
|
|
|
|
|
|
|
|
|
|
|
|
|
|
|
|
|
|
|
|
|
|
|
|
|
|
|
|
|
|
|
|
|
|
|
|
|
|
|
|
|
|
|
|
|
|
|
|
|
|
|
|
|
|
|
|
|
|
|
|
|
|
|
|
|
|
|
|
|
|
|
|
|
|
|
|
|
|
|
|
|
|
|
|
|
|
|
|
|
|
|
|
|
|
|
|
|
|
|
|
|
|
|
|
|
|
|
|
|
|
|
|
|
|
|
|
|
|
|
|
|
|
|
|
|
|
|
|
|
|
|
|
|
|
|
|
|
|
|
|
|
|
|
|
|
|
|
|
|
|
|
|
|
|
|
|
|
|
|
|
|
|
|
|
|
|
spaces/Androidonnxfork/CivitAi-to-Diffusers/diffusers/src/diffusers/schedulers/scheduling_ddim.py
DELETED
@@ -1,515 +0,0 @@
|
|
1 |
-
# Copyright 2023 Stanford University Team and The HuggingFace Team. All rights reserved.
|
2 |
-
#
|
3 |
-
# Licensed under the Apache License, Version 2.0 (the "License");
|
4 |
-
# you may not use this file except in compliance with the License.
|
5 |
-
# You may obtain a copy of the License at
|
6 |
-
#
|
7 |
-
# http://www.apache.org/licenses/LICENSE-2.0
|
8 |
-
#
|
9 |
-
# Unless required by applicable law or agreed to in writing, software
|
10 |
-
# distributed under the License is distributed on an "AS IS" BASIS,
|
11 |
-
# WITHOUT WARRANTIES OR CONDITIONS OF ANY KIND, either express or implied.
|
12 |
-
# See the License for the specific language governing permissions and
|
13 |
-
# limitations under the License.
|
14 |
-
|
15 |
-
# DISCLAIMER: This code is strongly influenced by https://github.com/pesser/pytorch_diffusion
|
16 |
-
# and https://github.com/hojonathanho/diffusion
|
17 |
-
|
18 |
-
import math
|
19 |
-
from dataclasses import dataclass
|
20 |
-
from typing import List, Optional, Tuple, Union
|
21 |
-
|
22 |
-
import numpy as np
|
23 |
-
import torch
|
24 |
-
|
25 |
-
from ..configuration_utils import ConfigMixin, register_to_config
|
26 |
-
from ..utils import BaseOutput, randn_tensor
|
27 |
-
from .scheduling_utils import KarrasDiffusionSchedulers, SchedulerMixin
|
28 |
-
|
29 |
-
|
30 |
-
@dataclass
|
31 |
-
# Copied from diffusers.schedulers.scheduling_ddpm.DDPMSchedulerOutput with DDPM->DDIM
|
32 |
-
class DDIMSchedulerOutput(BaseOutput):
|
33 |
-
"""
|
34 |
-
Output class for the scheduler's step function output.
|
35 |
-
|
36 |
-
Args:
|
37 |
-
prev_sample (`torch.FloatTensor` of shape `(batch_size, num_channels, height, width)` for images):
|
38 |
-
Computed sample (x_{t-1}) of previous timestep. `prev_sample` should be used as next model input in the
|
39 |
-
denoising loop.
|
40 |
-
pred_original_sample (`torch.FloatTensor` of shape `(batch_size, num_channels, height, width)` for images):
|
41 |
-
The predicted denoised sample (x_{0}) based on the model output from the current timestep.
|
42 |
-
`pred_original_sample` can be used to preview progress or for guidance.
|
43 |
-
"""
|
44 |
-
|
45 |
-
prev_sample: torch.FloatTensor
|
46 |
-
pred_original_sample: Optional[torch.FloatTensor] = None
|
47 |
-
|
48 |
-
|
49 |
-
# Copied from diffusers.schedulers.scheduling_ddpm.betas_for_alpha_bar
|
50 |
-
def betas_for_alpha_bar(
|
51 |
-
num_diffusion_timesteps,
|
52 |
-
max_beta=0.999,
|
53 |
-
alpha_transform_type="cosine",
|
54 |
-
):
|
55 |
-
"""
|
56 |
-
Create a beta schedule that discretizes the given alpha_t_bar function, which defines the cumulative product of
|
57 |
-
(1-beta) over time from t = [0,1].
|
58 |
-
|
59 |
-
Contains a function alpha_bar that takes an argument t and transforms it to the cumulative product of (1-beta) up
|
60 |
-
to that part of the diffusion process.
|
61 |
-
|
62 |
-
|
63 |
-
Args:
|
64 |
-
num_diffusion_timesteps (`int`): the number of betas to produce.
|
65 |
-
max_beta (`float`): the maximum beta to use; use values lower than 1 to
|
66 |
-
prevent singularities.
|
67 |
-
alpha_transform_type (`str`, *optional*, default to `cosine`): the type of noise schedule for alpha_bar.
|
68 |
-
Choose from `cosine` or `exp`
|
69 |
-
|
70 |
-
Returns:
|
71 |
-
betas (`np.ndarray`): the betas used by the scheduler to step the model outputs
|
72 |
-
"""
|
73 |
-
if alpha_transform_type == "cosine":
|
74 |
-
|
75 |
-
def alpha_bar_fn(t):
|
76 |
-
return math.cos((t + 0.008) / 1.008 * math.pi / 2) ** 2
|
77 |
-
|
78 |
-
elif alpha_transform_type == "exp":
|
79 |
-
|
80 |
-
def alpha_bar_fn(t):
|
81 |
-
return math.exp(t * -12.0)
|
82 |
-
|
83 |
-
else:
|
84 |
-
raise ValueError(f"Unsupported alpha_tranform_type: {alpha_transform_type}")
|
85 |
-
|
86 |
-
betas = []
|
87 |
-
for i in range(num_diffusion_timesteps):
|
88 |
-
t1 = i / num_diffusion_timesteps
|
89 |
-
t2 = (i + 1) / num_diffusion_timesteps
|
90 |
-
betas.append(min(1 - alpha_bar_fn(t2) / alpha_bar_fn(t1), max_beta))
|
91 |
-
return torch.tensor(betas, dtype=torch.float32)
|
92 |
-
|
93 |
-
|
94 |
-
def rescale_zero_terminal_snr(betas):
|
95 |
-
"""
|
96 |
-
Rescales betas to have zero terminal SNR Based on https://arxiv.org/pdf/2305.08891.pdf (Algorithm 1)
|
97 |
-
|
98 |
-
|
99 |
-
Args:
|
100 |
-
betas (`torch.FloatTensor`):
|
101 |
-
the betas that the scheduler is being initialized with.
|
102 |
-
|
103 |
-
Returns:
|
104 |
-
`torch.FloatTensor`: rescaled betas with zero terminal SNR
|
105 |
-
"""
|
106 |
-
# Convert betas to alphas_bar_sqrt
|
107 |
-
alphas = 1.0 - betas
|
108 |
-
alphas_cumprod = torch.cumprod(alphas, dim=0)
|
109 |
-
alphas_bar_sqrt = alphas_cumprod.sqrt()
|
110 |
-
|
111 |
-
# Store old values.
|
112 |
-
alphas_bar_sqrt_0 = alphas_bar_sqrt[0].clone()
|
113 |
-
alphas_bar_sqrt_T = alphas_bar_sqrt[-1].clone()
|
114 |
-
|
115 |
-
# Shift so the last timestep is zero.
|
116 |
-
alphas_bar_sqrt -= alphas_bar_sqrt_T
|
117 |
-
|
118 |
-
# Scale so the first timestep is back to the old value.
|
119 |
-
alphas_bar_sqrt *= alphas_bar_sqrt_0 / (alphas_bar_sqrt_0 - alphas_bar_sqrt_T)
|
120 |
-
|
121 |
-
# Convert alphas_bar_sqrt to betas
|
122 |
-
alphas_bar = alphas_bar_sqrt**2 # Revert sqrt
|
123 |
-
alphas = alphas_bar[1:] / alphas_bar[:-1] # Revert cumprod
|
124 |
-
alphas = torch.cat([alphas_bar[0:1], alphas])
|
125 |
-
betas = 1 - alphas
|
126 |
-
|
127 |
-
return betas
|
128 |
-
|
129 |
-
|
130 |
-
class DDIMScheduler(SchedulerMixin, ConfigMixin):
|
131 |
-
"""
|
132 |
-
Denoising diffusion implicit models is a scheduler that extends the denoising procedure introduced in denoising
|
133 |
-
diffusion probabilistic models (DDPMs) with non-Markovian guidance.
|
134 |
-
|
135 |
-
[`~ConfigMixin`] takes care of storing all config attributes that are passed in the scheduler's `__init__`
|
136 |
-
function, such as `num_train_timesteps`. They can be accessed via `scheduler.config.num_train_timesteps`.
|
137 |
-
[`SchedulerMixin`] provides general loading and saving functionality via the [`SchedulerMixin.save_pretrained`] and
|
138 |
-
[`~SchedulerMixin.from_pretrained`] functions.
|
139 |
-
|
140 |
-
For more details, see the original paper: https://arxiv.org/abs/2010.02502
|
141 |
-
|
142 |
-
Args:
|
143 |
-
num_train_timesteps (`int`): number of diffusion steps used to train the model.
|
144 |
-
beta_start (`float`): the starting `beta` value of inference.
|
145 |
-
beta_end (`float`): the final `beta` value.
|
146 |
-
beta_schedule (`str`):
|
147 |
-
the beta schedule, a mapping from a beta range to a sequence of betas for stepping the model. Choose from
|
148 |
-
`linear`, `scaled_linear`, or `squaredcos_cap_v2`.
|
149 |
-
trained_betas (`np.ndarray`, optional):
|
150 |
-
option to pass an array of betas directly to the constructor to bypass `beta_start`, `beta_end` etc.
|
151 |
-
clip_sample (`bool`, default `True`):
|
152 |
-
option to clip predicted sample for numerical stability.
|
153 |
-
clip_sample_range (`float`, default `1.0`):
|
154 |
-
the maximum magnitude for sample clipping. Valid only when `clip_sample=True`.
|
155 |
-
set_alpha_to_one (`bool`, default `True`):
|
156 |
-
each diffusion step uses the value of alphas product at that step and at the previous one. For the final
|
157 |
-
step there is no previous alpha. When this option is `True` the previous alpha product is fixed to `1`,
|
158 |
-
otherwise it uses the value of alpha at step 0.
|
159 |
-
steps_offset (`int`, default `0`):
|
160 |
-
an offset added to the inference steps. You can use a combination of `offset=1` and
|
161 |
-
`set_alpha_to_one=False`, to make the last step use step 0 for the previous alpha product, as done in
|
162 |
-
stable diffusion.
|
163 |
-
prediction_type (`str`, default `epsilon`, optional):
|
164 |
-
prediction type of the scheduler function, one of `epsilon` (predicting the noise of the diffusion
|
165 |
-
process), `sample` (directly predicting the noisy sample`) or `v_prediction` (see section 2.4
|
166 |
-
https://imagen.research.google/video/paper.pdf)
|
167 |
-
thresholding (`bool`, default `False`):
|
168 |
-
whether to use the "dynamic thresholding" method (introduced by Imagen, https://arxiv.org/abs/2205.11487).
|
169 |
-
Note that the thresholding method is unsuitable for latent-space diffusion models (such as
|
170 |
-
stable-diffusion).
|
171 |
-
dynamic_thresholding_ratio (`float`, default `0.995`):
|
172 |
-
the ratio for the dynamic thresholding method. Default is `0.995`, the same as Imagen
|
173 |
-
(https://arxiv.org/abs/2205.11487). Valid only when `thresholding=True`.
|
174 |
-
sample_max_value (`float`, default `1.0`):
|
175 |
-
the threshold value for dynamic thresholding. Valid only when `thresholding=True`.
|
176 |
-
timestep_spacing (`str`, default `"leading"`):
|
177 |
-
The way the timesteps should be scaled. Refer to Table 2. of [Common Diffusion Noise Schedules and Sample
|
178 |
-
Steps are Flawed](https://arxiv.org/abs/2305.08891) for more information.
|
179 |
-
rescale_betas_zero_snr (`bool`, default `False`):
|
180 |
-
whether to rescale the betas to have zero terminal SNR (proposed by https://arxiv.org/pdf/2305.08891.pdf).
|
181 |
-
This can enable the model to generate very bright and dark samples instead of limiting it to samples with
|
182 |
-
medium brightness. Loosely related to
|
183 |
-
[`--offset_noise`](https://github.com/huggingface/diffusers/blob/74fd735eb073eb1d774b1ab4154a0876eb82f055/examples/dreambooth/train_dreambooth.py#L506).
|
184 |
-
"""
|
185 |
-
|
186 |
-
_compatibles = [e.name for e in KarrasDiffusionSchedulers]
|
187 |
-
order = 1
|
188 |
-
|
189 |
-
@register_to_config
|
190 |
-
def __init__(
|
191 |
-
self,
|
192 |
-
num_train_timesteps: int = 1000,
|
193 |
-
beta_start: float = 0.0001,
|
194 |
-
beta_end: float = 0.02,
|
195 |
-
beta_schedule: str = "linear",
|
196 |
-
trained_betas: Optional[Union[np.ndarray, List[float]]] = None,
|
197 |
-
clip_sample: bool = True,
|
198 |
-
set_alpha_to_one: bool = True,
|
199 |
-
steps_offset: int = 0,
|
200 |
-
prediction_type: str = "epsilon",
|
201 |
-
thresholding: bool = False,
|
202 |
-
dynamic_thresholding_ratio: float = 0.995,
|
203 |
-
clip_sample_range: float = 1.0,
|
204 |
-
sample_max_value: float = 1.0,
|
205 |
-
timestep_spacing: str = "leading",
|
206 |
-
rescale_betas_zero_snr: bool = False,
|
207 |
-
):
|
208 |
-
if trained_betas is not None:
|
209 |
-
self.betas = torch.tensor(trained_betas, dtype=torch.float32)
|
210 |
-
elif beta_schedule == "linear":
|
211 |
-
self.betas = torch.linspace(beta_start, beta_end, num_train_timesteps, dtype=torch.float32)
|
212 |
-
elif beta_schedule == "scaled_linear":
|
213 |
-
# this schedule is very specific to the latent diffusion model.
|
214 |
-
self.betas = (
|
215 |
-
torch.linspace(beta_start**0.5, beta_end**0.5, num_train_timesteps, dtype=torch.float32) ** 2
|
216 |
-
)
|
217 |
-
elif beta_schedule == "squaredcos_cap_v2":
|
218 |
-
# Glide cosine schedule
|
219 |
-
self.betas = betas_for_alpha_bar(num_train_timesteps)
|
220 |
-
else:
|
221 |
-
raise NotImplementedError(f"{beta_schedule} does is not implemented for {self.__class__}")
|
222 |
-
|
223 |
-
# Rescale for zero SNR
|
224 |
-
if rescale_betas_zero_snr:
|
225 |
-
self.betas = rescale_zero_terminal_snr(self.betas)
|
226 |
-
|
227 |
-
self.alphas = 1.0 - self.betas
|
228 |
-
self.alphas_cumprod = torch.cumprod(self.alphas, dim=0)
|
229 |
-
|
230 |
-
# At every step in ddim, we are looking into the previous alphas_cumprod
|
231 |
-
# For the final step, there is no previous alphas_cumprod because we are already at 0
|
232 |
-
# `set_alpha_to_one` decides whether we set this parameter simply to one or
|
233 |
-
# whether we use the final alpha of the "non-previous" one.
|
234 |
-
self.final_alpha_cumprod = torch.tensor(1.0) if set_alpha_to_one else self.alphas_cumprod[0]
|
235 |
-
|
236 |
-
# standard deviation of the initial noise distribution
|
237 |
-
self.init_noise_sigma = 1.0
|
238 |
-
|
239 |
-
# setable values
|
240 |
-
self.num_inference_steps = None
|
241 |
-
self.timesteps = torch.from_numpy(np.arange(0, num_train_timesteps)[::-1].copy().astype(np.int64))
|
242 |
-
|
243 |
-
def scale_model_input(self, sample: torch.FloatTensor, timestep: Optional[int] = None) -> torch.FloatTensor:
|
244 |
-
"""
|
245 |
-
Ensures interchangeability with schedulers that need to scale the denoising model input depending on the
|
246 |
-
current timestep.
|
247 |
-
|
248 |
-
Args:
|
249 |
-
sample (`torch.FloatTensor`): input sample
|
250 |
-
timestep (`int`, optional): current timestep
|
251 |
-
|
252 |
-
Returns:
|
253 |
-
`torch.FloatTensor`: scaled input sample
|
254 |
-
"""
|
255 |
-
return sample
|
256 |
-
|
257 |
-
def _get_variance(self, timestep, prev_timestep):
|
258 |
-
alpha_prod_t = self.alphas_cumprod[timestep]
|
259 |
-
alpha_prod_t_prev = self.alphas_cumprod[prev_timestep] if prev_timestep >= 0 else self.final_alpha_cumprod
|
260 |
-
beta_prod_t = 1 - alpha_prod_t
|
261 |
-
beta_prod_t_prev = 1 - alpha_prod_t_prev
|
262 |
-
|
263 |
-
variance = (beta_prod_t_prev / beta_prod_t) * (1 - alpha_prod_t / alpha_prod_t_prev)
|
264 |
-
|
265 |
-
return variance
|
266 |
-
|
267 |
-
# Copied from diffusers.schedulers.scheduling_ddpm.DDPMScheduler._threshold_sample
|
268 |
-
def _threshold_sample(self, sample: torch.FloatTensor) -> torch.FloatTensor:
|
269 |
-
"""
|
270 |
-
"Dynamic thresholding: At each sampling step we set s to a certain percentile absolute pixel value in xt0 (the
|
271 |
-
prediction of x_0 at timestep t), and if s > 1, then we threshold xt0 to the range [-s, s] and then divide by
|
272 |
-
s. Dynamic thresholding pushes saturated pixels (those near -1 and 1) inwards, thereby actively preventing
|
273 |
-
pixels from saturation at each step. We find that dynamic thresholding results in significantly better
|
274 |
-
photorealism as well as better image-text alignment, especially when using very large guidance weights."
|
275 |
-
|
276 |
-
https://arxiv.org/abs/2205.11487
|
277 |
-
"""
|
278 |
-
dtype = sample.dtype
|
279 |
-
batch_size, channels, height, width = sample.shape
|
280 |
-
|
281 |
-
if dtype not in (torch.float32, torch.float64):
|
282 |
-
sample = sample.float() # upcast for quantile calculation, and clamp not implemented for cpu half
|
283 |
-
|
284 |
-
# Flatten sample for doing quantile calculation along each image
|
285 |
-
sample = sample.reshape(batch_size, channels * height * width)
|
286 |
-
|
287 |
-
abs_sample = sample.abs() # "a certain percentile absolute pixel value"
|
288 |
-
|
289 |
-
s = torch.quantile(abs_sample, self.config.dynamic_thresholding_ratio, dim=1)
|
290 |
-
s = torch.clamp(
|
291 |
-
s, min=1, max=self.config.sample_max_value
|
292 |
-
) # When clamped to min=1, equivalent to standard clipping to [-1, 1]
|
293 |
-
|
294 |
-
s = s.unsqueeze(1) # (batch_size, 1) because clamp will broadcast along dim=0
|
295 |
-
sample = torch.clamp(sample, -s, s) / s # "we threshold xt0 to the range [-s, s] and then divide by s"
|
296 |
-
|
297 |
-
sample = sample.reshape(batch_size, channels, height, width)
|
298 |
-
sample = sample.to(dtype)
|
299 |
-
|
300 |
-
return sample
|
301 |
-
|
302 |
-
def set_timesteps(self, num_inference_steps: int, device: Union[str, torch.device] = None):
|
303 |
-
"""
|
304 |
-
Sets the discrete timesteps used for the diffusion chain. Supporting function to be run before inference.
|
305 |
-
|
306 |
-
Args:
|
307 |
-
num_inference_steps (`int`):
|
308 |
-
the number of diffusion steps used when generating samples with a pre-trained model.
|
309 |
-
"""
|
310 |
-
|
311 |
-
if num_inference_steps > self.config.num_train_timesteps:
|
312 |
-
raise ValueError(
|
313 |
-
f"`num_inference_steps`: {num_inference_steps} cannot be larger than `self.config.train_timesteps`:"
|
314 |
-
f" {self.config.num_train_timesteps} as the unet model trained with this scheduler can only handle"
|
315 |
-
f" maximal {self.config.num_train_timesteps} timesteps."
|
316 |
-
)
|
317 |
-
|
318 |
-
self.num_inference_steps = num_inference_steps
|
319 |
-
|
320 |
-
# "linspace", "leading", "trailing" corresponds to annotation of Table 2. of https://arxiv.org/abs/2305.08891
|
321 |
-
if self.config.timestep_spacing == "linspace":
|
322 |
-
timesteps = (
|
323 |
-
np.linspace(0, self.config.num_train_timesteps - 1, num_inference_steps)
|
324 |
-
.round()[::-1]
|
325 |
-
.copy()
|
326 |
-
.astype(np.int64)
|
327 |
-
)
|
328 |
-
elif self.config.timestep_spacing == "leading":
|
329 |
-
step_ratio = self.config.num_train_timesteps // self.num_inference_steps
|
330 |
-
# creates integer timesteps by multiplying by ratio
|
331 |
-
# casting to int to avoid issues when num_inference_step is power of 3
|
332 |
-
timesteps = (np.arange(0, num_inference_steps) * step_ratio).round()[::-1].copy().astype(np.int64)
|
333 |
-
timesteps += self.config.steps_offset
|
334 |
-
elif self.config.timestep_spacing == "trailing":
|
335 |
-
step_ratio = self.config.num_train_timesteps / self.num_inference_steps
|
336 |
-
# creates integer timesteps by multiplying by ratio
|
337 |
-
# casting to int to avoid issues when num_inference_step is power of 3
|
338 |
-
timesteps = np.round(np.arange(self.config.num_train_timesteps, 0, -step_ratio)).astype(np.int64)
|
339 |
-
timesteps -= 1
|
340 |
-
else:
|
341 |
-
raise ValueError(
|
342 |
-
f"{self.config.timestep_spacing} is not supported. Please make sure to choose one of 'leading' or 'trailing'."
|
343 |
-
)
|
344 |
-
|
345 |
-
self.timesteps = torch.from_numpy(timesteps).to(device)
|
346 |
-
|
347 |
-
def step(
|
348 |
-
self,
|
349 |
-
model_output: torch.FloatTensor,
|
350 |
-
timestep: int,
|
351 |
-
sample: torch.FloatTensor,
|
352 |
-
eta: float = 0.0,
|
353 |
-
use_clipped_model_output: bool = False,
|
354 |
-
generator=None,
|
355 |
-
variance_noise: Optional[torch.FloatTensor] = None,
|
356 |
-
return_dict: bool = True,
|
357 |
-
) -> Union[DDIMSchedulerOutput, Tuple]:
|
358 |
-
"""
|
359 |
-
Predict the sample at the previous timestep by reversing the SDE. Core function to propagate the diffusion
|
360 |
-
process from the learned model outputs (most often the predicted noise).
|
361 |
-
|
362 |
-
Args:
|
363 |
-
model_output (`torch.FloatTensor`): direct output from learned diffusion model.
|
364 |
-
timestep (`int`): current discrete timestep in the diffusion chain.
|
365 |
-
sample (`torch.FloatTensor`):
|
366 |
-
current instance of sample being created by diffusion process.
|
367 |
-
eta (`float`): weight of noise for added noise in diffusion step.
|
368 |
-
use_clipped_model_output (`bool`): if `True`, compute "corrected" `model_output` from the clipped
|
369 |
-
predicted original sample. Necessary because predicted original sample is clipped to [-1, 1] when
|
370 |
-
`self.config.clip_sample` is `True`. If no clipping has happened, "corrected" `model_output` would
|
371 |
-
coincide with the one provided as input and `use_clipped_model_output` will have not effect.
|
372 |
-
generator: random number generator.
|
373 |
-
variance_noise (`torch.FloatTensor`): instead of generating noise for the variance using `generator`, we
|
374 |
-
can directly provide the noise for the variance itself. This is useful for methods such as
|
375 |
-
CycleDiffusion. (https://arxiv.org/abs/2210.05559)
|
376 |
-
return_dict (`bool`): option for returning tuple rather than DDIMSchedulerOutput class
|
377 |
-
|
378 |
-
Returns:
|
379 |
-
[`~schedulers.scheduling_utils.DDIMSchedulerOutput`] or `tuple`:
|
380 |
-
[`~schedulers.scheduling_utils.DDIMSchedulerOutput`] if `return_dict` is True, otherwise a `tuple`. When
|
381 |
-
returning a tuple, the first element is the sample tensor.
|
382 |
-
|
383 |
-
"""
|
384 |
-
if self.num_inference_steps is None:
|
385 |
-
raise ValueError(
|
386 |
-
"Number of inference steps is 'None', you need to run 'set_timesteps' after creating the scheduler"
|
387 |
-
)
|
388 |
-
|
389 |
-
# See formulas (12) and (16) of DDIM paper https://arxiv.org/pdf/2010.02502.pdf
|
390 |
-
# Ideally, read DDIM paper in-detail understanding
|
391 |
-
|
392 |
-
# Notation (<variable name> -> <name in paper>
|
393 |
-
# - pred_noise_t -> e_theta(x_t, t)
|
394 |
-
# - pred_original_sample -> f_theta(x_t, t) or x_0
|
395 |
-
# - std_dev_t -> sigma_t
|
396 |
-
# - eta -> η
|
397 |
-
# - pred_sample_direction -> "direction pointing to x_t"
|
398 |
-
# - pred_prev_sample -> "x_t-1"
|
399 |
-
|
400 |
-
# 1. get previous step value (=t-1)
|
401 |
-
prev_timestep = timestep - self.config.num_train_timesteps // self.num_inference_steps
|
402 |
-
|
403 |
-
# 2. compute alphas, betas
|
404 |
-
alpha_prod_t = self.alphas_cumprod[timestep]
|
405 |
-
alpha_prod_t_prev = self.alphas_cumprod[prev_timestep] if prev_timestep >= 0 else self.final_alpha_cumprod
|
406 |
-
|
407 |
-
beta_prod_t = 1 - alpha_prod_t
|
408 |
-
|
409 |
-
# 3. compute predicted original sample from predicted noise also called
|
410 |
-
# "predicted x_0" of formula (12) from https://arxiv.org/pdf/2010.02502.pdf
|
411 |
-
if self.config.prediction_type == "epsilon":
|
412 |
-
pred_original_sample = (sample - beta_prod_t ** (0.5) * model_output) / alpha_prod_t ** (0.5)
|
413 |
-
pred_epsilon = model_output
|
414 |
-
elif self.config.prediction_type == "sample":
|
415 |
-
pred_original_sample = model_output
|
416 |
-
pred_epsilon = (sample - alpha_prod_t ** (0.5) * pred_original_sample) / beta_prod_t ** (0.5)
|
417 |
-
elif self.config.prediction_type == "v_prediction":
|
418 |
-
pred_original_sample = (alpha_prod_t**0.5) * sample - (beta_prod_t**0.5) * model_output
|
419 |
-
pred_epsilon = (alpha_prod_t**0.5) * model_output + (beta_prod_t**0.5) * sample
|
420 |
-
else:
|
421 |
-
raise ValueError(
|
422 |
-
f"prediction_type given as {self.config.prediction_type} must be one of `epsilon`, `sample`, or"
|
423 |
-
" `v_prediction`"
|
424 |
-
)
|
425 |
-
|
426 |
-
# 4. Clip or threshold "predicted x_0"
|
427 |
-
if self.config.thresholding:
|
428 |
-
pred_original_sample = self._threshold_sample(pred_original_sample)
|
429 |
-
elif self.config.clip_sample:
|
430 |
-
pred_original_sample = pred_original_sample.clamp(
|
431 |
-
-self.config.clip_sample_range, self.config.clip_sample_range
|
432 |
-
)
|
433 |
-
|
434 |
-
# 5. compute variance: "sigma_t(η)" -> see formula (16)
|
435 |
-
# σ_t = sqrt((1 − α_t−1)/(1 − α_t)) * sqrt(1 − α_t/α_t−1)
|
436 |
-
variance = self._get_variance(timestep, prev_timestep)
|
437 |
-
std_dev_t = eta * variance ** (0.5)
|
438 |
-
|
439 |
-
if use_clipped_model_output:
|
440 |
-
# the pred_epsilon is always re-derived from the clipped x_0 in Glide
|
441 |
-
pred_epsilon = (sample - alpha_prod_t ** (0.5) * pred_original_sample) / beta_prod_t ** (0.5)
|
442 |
-
|
443 |
-
# 6. compute "direction pointing to x_t" of formula (12) from https://arxiv.org/pdf/2010.02502.pdf
|
444 |
-
pred_sample_direction = (1 - alpha_prod_t_prev - std_dev_t**2) ** (0.5) * pred_epsilon
|
445 |
-
|
446 |
-
# 7. compute x_t without "random noise" of formula (12) from https://arxiv.org/pdf/2010.02502.pdf
|
447 |
-
prev_sample = alpha_prod_t_prev ** (0.5) * pred_original_sample + pred_sample_direction
|
448 |
-
|
449 |
-
if eta > 0:
|
450 |
-
if variance_noise is not None and generator is not None:
|
451 |
-
raise ValueError(
|
452 |
-
"Cannot pass both generator and variance_noise. Please make sure that either `generator` or"
|
453 |
-
" `variance_noise` stays `None`."
|
454 |
-
)
|
455 |
-
|
456 |
-
if variance_noise is None:
|
457 |
-
variance_noise = randn_tensor(
|
458 |
-
model_output.shape, generator=generator, device=model_output.device, dtype=model_output.dtype
|
459 |
-
)
|
460 |
-
variance = std_dev_t * variance_noise
|
461 |
-
|
462 |
-
prev_sample = prev_sample + variance
|
463 |
-
|
464 |
-
if not return_dict:
|
465 |
-
return (prev_sample,)
|
466 |
-
|
467 |
-
return DDIMSchedulerOutput(prev_sample=prev_sample, pred_original_sample=pred_original_sample)
|
468 |
-
|
469 |
-
# Copied from diffusers.schedulers.scheduling_ddpm.DDPMScheduler.add_noise
|
470 |
-
def add_noise(
|
471 |
-
self,
|
472 |
-
original_samples: torch.FloatTensor,
|
473 |
-
noise: torch.FloatTensor,
|
474 |
-
timesteps: torch.IntTensor,
|
475 |
-
) -> torch.FloatTensor:
|
476 |
-
# Make sure alphas_cumprod and timestep have same device and dtype as original_samples
|
477 |
-
alphas_cumprod = self.alphas_cumprod.to(device=original_samples.device, dtype=original_samples.dtype)
|
478 |
-
timesteps = timesteps.to(original_samples.device)
|
479 |
-
|
480 |
-
sqrt_alpha_prod = alphas_cumprod[timesteps] ** 0.5
|
481 |
-
sqrt_alpha_prod = sqrt_alpha_prod.flatten()
|
482 |
-
while len(sqrt_alpha_prod.shape) < len(original_samples.shape):
|
483 |
-
sqrt_alpha_prod = sqrt_alpha_prod.unsqueeze(-1)
|
484 |
-
|
485 |
-
sqrt_one_minus_alpha_prod = (1 - alphas_cumprod[timesteps]) ** 0.5
|
486 |
-
sqrt_one_minus_alpha_prod = sqrt_one_minus_alpha_prod.flatten()
|
487 |
-
while len(sqrt_one_minus_alpha_prod.shape) < len(original_samples.shape):
|
488 |
-
sqrt_one_minus_alpha_prod = sqrt_one_minus_alpha_prod.unsqueeze(-1)
|
489 |
-
|
490 |
-
noisy_samples = sqrt_alpha_prod * original_samples + sqrt_one_minus_alpha_prod * noise
|
491 |
-
return noisy_samples
|
492 |
-
|
493 |
-
# Copied from diffusers.schedulers.scheduling_ddpm.DDPMScheduler.get_velocity
|
494 |
-
def get_velocity(
|
495 |
-
self, sample: torch.FloatTensor, noise: torch.FloatTensor, timesteps: torch.IntTensor
|
496 |
-
) -> torch.FloatTensor:
|
497 |
-
# Make sure alphas_cumprod and timestep have same device and dtype as sample
|
498 |
-
alphas_cumprod = self.alphas_cumprod.to(device=sample.device, dtype=sample.dtype)
|
499 |
-
timesteps = timesteps.to(sample.device)
|
500 |
-
|
501 |
-
sqrt_alpha_prod = alphas_cumprod[timesteps] ** 0.5
|
502 |
-
sqrt_alpha_prod = sqrt_alpha_prod.flatten()
|
503 |
-
while len(sqrt_alpha_prod.shape) < len(sample.shape):
|
504 |
-
sqrt_alpha_prod = sqrt_alpha_prod.unsqueeze(-1)
|
505 |
-
|
506 |
-
sqrt_one_minus_alpha_prod = (1 - alphas_cumprod[timesteps]) ** 0.5
|
507 |
-
sqrt_one_minus_alpha_prod = sqrt_one_minus_alpha_prod.flatten()
|
508 |
-
while len(sqrt_one_minus_alpha_prod.shape) < len(sample.shape):
|
509 |
-
sqrt_one_minus_alpha_prod = sqrt_one_minus_alpha_prod.unsqueeze(-1)
|
510 |
-
|
511 |
-
velocity = sqrt_alpha_prod * noise - sqrt_one_minus_alpha_prod * sample
|
512 |
-
return velocity
|
513 |
-
|
514 |
-
def __len__(self):
|
515 |
-
return self.config.num_train_timesteps
|
|
|
|
|
|
|
|
|
|
|
|
|
|
|
|
|
|
|
|
|
|
|
|
|
|
|
|
|
|
|
|
|
|
|
|
|
|
|
|
|
|
|
|
|
|
|
|
|
|
|
|
|
|
|
|
|
|
|
|
|
|
|
|
|
|
|
|
|
|
|
|
|
|
|
|
|
|
|
|
|
|
|
|
|
|
|
|
|
|
|
|
|
|
|
|
|
|
|
|
|
|
|
|
|
|
|
|
|
|
|
|
|
|
|
|
|
|
|
|
|
|
|
|
|
|
|
|
|
|
|
|
|
|
|
|
|
|
|
|
|
|
|
|
|
|
|
|
|
|
|
|
|
|
|
|
|
|
|
|
|
|
|
|
|
|
|
|
|
|
|
|
|
|
|
|
|
|
|
|
|
|
|
|
|
|
|
|
|
|
|
|
|
|
|
|
|
|
|
|
|
|
|
|
|
|
|
|
|
|
|
|
|
|
|
|
|
|
|
|
|
|
|
|
|
|
|
|
|
|
|
|
|
|
|
|
|
|
|
|
|
|
|
|
|
|
|
|
|
|
|
|
|
|
|
|
|
|
|
|
|
|
|
|
|
|
|
|
|
|
|
|
|
|
|
|
|
|
|
|
|
|
|
|
|
|
|
|
|
|
|
|
|
|
|
|
|
|
|
|
|
|
|
|
|
|
|
|
|
|
|
|
|
|
|
|
|
|
|
|
|
|
|
|
|
|
|
|
|
|
|
|
|
|
|
|
|
|
|
|
|
|
|
|
|
|
|
|
|
|
|
|
|
|
|
|
|
|
|
|
|
|
|
|
|
|
|
|
|
|
|
|
|
|
|
|
|
|
|
|
|
|
|
|
|
|
|
|
|
|
|
|
|
|
|
|
|
|
|
|
|
|
|
|
|
|
|
|
|
|
|
|
|
|
|
|
|
|
|
|
|
|
|
|
|
|
|
|
|
|
|
|
|
|
|
|
|
|
|
|
|
|
|
|
|
|
|
|
|
|
|
|
|
|
|
|
|
|
|
|
|
|
|
|
|
|
|
|
|
|
|
|
|
|
|
|
|
|
|
|
|
|
|
|
|
|
|
|
|
|
|
|
|
|
|
|
|
|
|
|
|
|
|
|
|
|
|
|
|
|
|
|
|
|
|
|
|
|
|
|
|
|
|
|
|
|
|
|
|
|
|
|
|
|
|
|
|
|
|
|
|
|
|
|
|
|
|
|
|
|
|
|
|
|
|
|
|
|
|
|
|
|
|
|
|
|
|
|
|
|
|
|
|
|
|
|
|
|
|
|
|
|
|
|
|
|
|
|
|
|
|
|
|
|
|
|
|
|
|
|
|
|
|
|
|
|
|
|
|
|
|
|
|
|
|
|
|
|
|
|
|
|
|
|
|
|
|
|
|
|
|
|
|
|
|
|
|
|
|
|
|
|
|
|
|
|
|
|
|
|
|
|
|
|
|
|
|
|
|
|
|
|
|
|
|
|
|
|
|
|
|
|
|
|
|
|
|
|
|
|
|
|
|
|
|
|
|
|
|
|
|
|
|
|
|
|
|
|
|
|
|
|
|
|
|
|
|
|
|
|
|
|
|
|
|
|
|
|
|
|
|
|
|
|
|
|
|
|
|
|
|
|
|
|
|
|
|
|
|
|
|
|
|
|
|
|
|
|
|
|
|
|
|
|
|
|
|
|
|
|
|
|
|
|
|
|
|
|
|
|
|
|
|
|
|
|
|
|
|
|
|
|
|
|
|
|
|
|
|
|
|
|
|
|
|
|
|
|
|
|
|
|
|
|
|
|
|
|
|
|
|
|
|
|
|
|
|
|
|
|
|
|
|
|
|
|
|
|
|
|
|
|
|
|
|
|
|
|
|
|
|
|
|
|
|
|
|
|
|
|
|
|
|
|
|
|
|
|
|
|
|
|
|
|
|
|
|
|
|
|
|
|
|
|
|
|
|
|
|
|
|
|
|
|
|
|
|
|
|
|
|
|
|
|
|
|
|
|
|
|
|
|
|
|
|
|
|
|
|
|
|
|
|
|
|
|
|
|
|
|
|
|
|
|
|
|
|
|
|
|
|
|
|
|
|
|
|
|
|
|
|
|
|
|
|
|
|
|
|
|
|
|
|
|
|
|
|
|
|
|
|
|
|
|
|
|
|
|
|
|
|
|
|
|
|
|
|
|
|
|
|
|
|
|
|
|
|
|
|
|
|
|
|
|
|
|
|
|
|
|
|
|
|
|
|
|
|
|
|
|
|
|
|
|
|
|
|
|
|
|
|
|
|
|
|
|
|
|
|
|
|
|
spaces/Androidonnxfork/CivitAi-to-Diffusers/diffusers/tests/pipelines/unclip/test_unclip.py
DELETED
@@ -1,501 +0,0 @@
|
|
1 |
-
# coding=utf-8
|
2 |
-
# Copyright 2023 HuggingFace Inc.
|
3 |
-
#
|
4 |
-
# Licensed under the Apache License, Version 2.0 (the "License");
|
5 |
-
# you may not use this file except in compliance with the License.
|
6 |
-
# You may obtain a copy of the License at
|
7 |
-
#
|
8 |
-
# http://www.apache.org/licenses/LICENSE-2.0
|
9 |
-
#
|
10 |
-
# Unless required by applicable law or agreed to in writing, software
|
11 |
-
# distributed under the License is distributed on an "AS IS" BASIS,
|
12 |
-
# WITHOUT WARRANTIES OR CONDITIONS OF ANY KIND, either express or implied.
|
13 |
-
# See the License for the specific language governing permissions and
|
14 |
-
# limitations under the License.
|
15 |
-
|
16 |
-
import gc
|
17 |
-
import unittest
|
18 |
-
|
19 |
-
import numpy as np
|
20 |
-
import torch
|
21 |
-
from transformers import CLIPTextConfig, CLIPTextModelWithProjection, CLIPTokenizer
|
22 |
-
|
23 |
-
from diffusers import PriorTransformer, UnCLIPPipeline, UnCLIPScheduler, UNet2DConditionModel, UNet2DModel
|
24 |
-
from diffusers.pipelines.unclip.text_proj import UnCLIPTextProjModel
|
25 |
-
from diffusers.utils import load_numpy, nightly, slow, torch_device
|
26 |
-
from diffusers.utils.testing_utils import enable_full_determinism, require_torch_gpu, skip_mps
|
27 |
-
|
28 |
-
from ..pipeline_params import TEXT_TO_IMAGE_BATCH_PARAMS, TEXT_TO_IMAGE_PARAMS
|
29 |
-
from ..test_pipelines_common import PipelineTesterMixin, assert_mean_pixel_difference
|
30 |
-
|
31 |
-
|
32 |
-
enable_full_determinism()
|
33 |
-
|
34 |
-
|
35 |
-
class UnCLIPPipelineFastTests(PipelineTesterMixin, unittest.TestCase):
|
36 |
-
pipeline_class = UnCLIPPipeline
|
37 |
-
params = TEXT_TO_IMAGE_PARAMS - {
|
38 |
-
"negative_prompt",
|
39 |
-
"height",
|
40 |
-
"width",
|
41 |
-
"negative_prompt_embeds",
|
42 |
-
"guidance_scale",
|
43 |
-
"prompt_embeds",
|
44 |
-
"cross_attention_kwargs",
|
45 |
-
}
|
46 |
-
batch_params = TEXT_TO_IMAGE_BATCH_PARAMS
|
47 |
-
required_optional_params = [
|
48 |
-
"generator",
|
49 |
-
"return_dict",
|
50 |
-
"prior_num_inference_steps",
|
51 |
-
"decoder_num_inference_steps",
|
52 |
-
"super_res_num_inference_steps",
|
53 |
-
]
|
54 |
-
test_xformers_attention = False
|
55 |
-
|
56 |
-
@property
|
57 |
-
def text_embedder_hidden_size(self):
|
58 |
-
return 32
|
59 |
-
|
60 |
-
@property
|
61 |
-
def time_input_dim(self):
|
62 |
-
return 32
|
63 |
-
|
64 |
-
@property
|
65 |
-
def block_out_channels_0(self):
|
66 |
-
return self.time_input_dim
|
67 |
-
|
68 |
-
@property
|
69 |
-
def time_embed_dim(self):
|
70 |
-
return self.time_input_dim * 4
|
71 |
-
|
72 |
-
@property
|
73 |
-
def cross_attention_dim(self):
|
74 |
-
return 100
|
75 |
-
|
76 |
-
@property
|
77 |
-
def dummy_tokenizer(self):
|
78 |
-
tokenizer = CLIPTokenizer.from_pretrained("hf-internal-testing/tiny-random-clip")
|
79 |
-
return tokenizer
|
80 |
-
|
81 |
-
@property
|
82 |
-
def dummy_text_encoder(self):
|
83 |
-
torch.manual_seed(0)
|
84 |
-
config = CLIPTextConfig(
|
85 |
-
bos_token_id=0,
|
86 |
-
eos_token_id=2,
|
87 |
-
hidden_size=self.text_embedder_hidden_size,
|
88 |
-
projection_dim=self.text_embedder_hidden_size,
|
89 |
-
intermediate_size=37,
|
90 |
-
layer_norm_eps=1e-05,
|
91 |
-
num_attention_heads=4,
|
92 |
-
num_hidden_layers=5,
|
93 |
-
pad_token_id=1,
|
94 |
-
vocab_size=1000,
|
95 |
-
)
|
96 |
-
return CLIPTextModelWithProjection(config)
|
97 |
-
|
98 |
-
@property
|
99 |
-
def dummy_prior(self):
|
100 |
-
torch.manual_seed(0)
|
101 |
-
|
102 |
-
model_kwargs = {
|
103 |
-
"num_attention_heads": 2,
|
104 |
-
"attention_head_dim": 12,
|
105 |
-
"embedding_dim": self.text_embedder_hidden_size,
|
106 |
-
"num_layers": 1,
|
107 |
-
}
|
108 |
-
|
109 |
-
model = PriorTransformer(**model_kwargs)
|
110 |
-
return model
|
111 |
-
|
112 |
-
@property
|
113 |
-
def dummy_text_proj(self):
|
114 |
-
torch.manual_seed(0)
|
115 |
-
|
116 |
-
model_kwargs = {
|
117 |
-
"clip_embeddings_dim": self.text_embedder_hidden_size,
|
118 |
-
"time_embed_dim": self.time_embed_dim,
|
119 |
-
"cross_attention_dim": self.cross_attention_dim,
|
120 |
-
}
|
121 |
-
|
122 |
-
model = UnCLIPTextProjModel(**model_kwargs)
|
123 |
-
return model
|
124 |
-
|
125 |
-
@property
|
126 |
-
def dummy_decoder(self):
|
127 |
-
torch.manual_seed(0)
|
128 |
-
|
129 |
-
model_kwargs = {
|
130 |
-
"sample_size": 32,
|
131 |
-
# RGB in channels
|
132 |
-
"in_channels": 3,
|
133 |
-
# Out channels is double in channels because predicts mean and variance
|
134 |
-
"out_channels": 6,
|
135 |
-
"down_block_types": ("ResnetDownsampleBlock2D", "SimpleCrossAttnDownBlock2D"),
|
136 |
-
"up_block_types": ("SimpleCrossAttnUpBlock2D", "ResnetUpsampleBlock2D"),
|
137 |
-
"mid_block_type": "UNetMidBlock2DSimpleCrossAttn",
|
138 |
-
"block_out_channels": (self.block_out_channels_0, self.block_out_channels_0 * 2),
|
139 |
-
"layers_per_block": 1,
|
140 |
-
"cross_attention_dim": self.cross_attention_dim,
|
141 |
-
"attention_head_dim": 4,
|
142 |
-
"resnet_time_scale_shift": "scale_shift",
|
143 |
-
"class_embed_type": "identity",
|
144 |
-
}
|
145 |
-
|
146 |
-
model = UNet2DConditionModel(**model_kwargs)
|
147 |
-
return model
|
148 |
-
|
149 |
-
@property
|
150 |
-
def dummy_super_res_kwargs(self):
|
151 |
-
return {
|
152 |
-
"sample_size": 64,
|
153 |
-
"layers_per_block": 1,
|
154 |
-
"down_block_types": ("ResnetDownsampleBlock2D", "ResnetDownsampleBlock2D"),
|
155 |
-
"up_block_types": ("ResnetUpsampleBlock2D", "ResnetUpsampleBlock2D"),
|
156 |
-
"block_out_channels": (self.block_out_channels_0, self.block_out_channels_0 * 2),
|
157 |
-
"in_channels": 6,
|
158 |
-
"out_channels": 3,
|
159 |
-
}
|
160 |
-
|
161 |
-
@property
|
162 |
-
def dummy_super_res_first(self):
|
163 |
-
torch.manual_seed(0)
|
164 |
-
|
165 |
-
model = UNet2DModel(**self.dummy_super_res_kwargs)
|
166 |
-
return model
|
167 |
-
|
168 |
-
@property
|
169 |
-
def dummy_super_res_last(self):
|
170 |
-
# seeded differently to get different unet than `self.dummy_super_res_first`
|
171 |
-
torch.manual_seed(1)
|
172 |
-
|
173 |
-
model = UNet2DModel(**self.dummy_super_res_kwargs)
|
174 |
-
return model
|
175 |
-
|
176 |
-
def get_dummy_components(self):
|
177 |
-
prior = self.dummy_prior
|
178 |
-
decoder = self.dummy_decoder
|
179 |
-
text_proj = self.dummy_text_proj
|
180 |
-
text_encoder = self.dummy_text_encoder
|
181 |
-
tokenizer = self.dummy_tokenizer
|
182 |
-
super_res_first = self.dummy_super_res_first
|
183 |
-
super_res_last = self.dummy_super_res_last
|
184 |
-
|
185 |
-
prior_scheduler = UnCLIPScheduler(
|
186 |
-
variance_type="fixed_small_log",
|
187 |
-
prediction_type="sample",
|
188 |
-
num_train_timesteps=1000,
|
189 |
-
clip_sample_range=5.0,
|
190 |
-
)
|
191 |
-
|
192 |
-
decoder_scheduler = UnCLIPScheduler(
|
193 |
-
variance_type="learned_range",
|
194 |
-
prediction_type="epsilon",
|
195 |
-
num_train_timesteps=1000,
|
196 |
-
)
|
197 |
-
|
198 |
-
super_res_scheduler = UnCLIPScheduler(
|
199 |
-
variance_type="fixed_small_log",
|
200 |
-
prediction_type="epsilon",
|
201 |
-
num_train_timesteps=1000,
|
202 |
-
)
|
203 |
-
|
204 |
-
components = {
|
205 |
-
"prior": prior,
|
206 |
-
"decoder": decoder,
|
207 |
-
"text_proj": text_proj,
|
208 |
-
"text_encoder": text_encoder,
|
209 |
-
"tokenizer": tokenizer,
|
210 |
-
"super_res_first": super_res_first,
|
211 |
-
"super_res_last": super_res_last,
|
212 |
-
"prior_scheduler": prior_scheduler,
|
213 |
-
"decoder_scheduler": decoder_scheduler,
|
214 |
-
"super_res_scheduler": super_res_scheduler,
|
215 |
-
}
|
216 |
-
|
217 |
-
return components
|
218 |
-
|
219 |
-
def get_dummy_inputs(self, device, seed=0):
|
220 |
-
if str(device).startswith("mps"):
|
221 |
-
generator = torch.manual_seed(seed)
|
222 |
-
else:
|
223 |
-
generator = torch.Generator(device=device).manual_seed(seed)
|
224 |
-
inputs = {
|
225 |
-
"prompt": "horse",
|
226 |
-
"generator": generator,
|
227 |
-
"prior_num_inference_steps": 2,
|
228 |
-
"decoder_num_inference_steps": 2,
|
229 |
-
"super_res_num_inference_steps": 2,
|
230 |
-
"output_type": "numpy",
|
231 |
-
}
|
232 |
-
return inputs
|
233 |
-
|
234 |
-
def test_unclip(self):
|
235 |
-
device = "cpu"
|
236 |
-
|
237 |
-
components = self.get_dummy_components()
|
238 |
-
|
239 |
-
pipe = self.pipeline_class(**components)
|
240 |
-
pipe = pipe.to(device)
|
241 |
-
|
242 |
-
pipe.set_progress_bar_config(disable=None)
|
243 |
-
|
244 |
-
output = pipe(**self.get_dummy_inputs(device))
|
245 |
-
image = output.images
|
246 |
-
|
247 |
-
image_from_tuple = pipe(
|
248 |
-
**self.get_dummy_inputs(device),
|
249 |
-
return_dict=False,
|
250 |
-
)[0]
|
251 |
-
|
252 |
-
image_slice = image[0, -3:, -3:, -1]
|
253 |
-
image_from_tuple_slice = image_from_tuple[0, -3:, -3:, -1]
|
254 |
-
|
255 |
-
assert image.shape == (1, 64, 64, 3)
|
256 |
-
|
257 |
-
expected_slice = np.array(
|
258 |
-
[
|
259 |
-
0.9997,
|
260 |
-
0.9988,
|
261 |
-
0.0028,
|
262 |
-
0.9997,
|
263 |
-
0.9984,
|
264 |
-
0.9965,
|
265 |
-
0.0029,
|
266 |
-
0.9986,
|
267 |
-
0.0025,
|
268 |
-
]
|
269 |
-
)
|
270 |
-
|
271 |
-
assert np.abs(image_slice.flatten() - expected_slice).max() < 1e-2
|
272 |
-
assert np.abs(image_from_tuple_slice.flatten() - expected_slice).max() < 1e-2
|
273 |
-
|
274 |
-
def test_unclip_passed_text_embed(self):
|
275 |
-
device = torch.device("cpu")
|
276 |
-
|
277 |
-
class DummyScheduler:
|
278 |
-
init_noise_sigma = 1
|
279 |
-
|
280 |
-
components = self.get_dummy_components()
|
281 |
-
|
282 |
-
pipe = self.pipeline_class(**components)
|
283 |
-
pipe = pipe.to(device)
|
284 |
-
|
285 |
-
prior = components["prior"]
|
286 |
-
decoder = components["decoder"]
|
287 |
-
super_res_first = components["super_res_first"]
|
288 |
-
tokenizer = components["tokenizer"]
|
289 |
-
text_encoder = components["text_encoder"]
|
290 |
-
|
291 |
-
generator = torch.Generator(device=device).manual_seed(0)
|
292 |
-
dtype = prior.dtype
|
293 |
-
batch_size = 1
|
294 |
-
|
295 |
-
shape = (batch_size, prior.config.embedding_dim)
|
296 |
-
prior_latents = pipe.prepare_latents(
|
297 |
-
shape, dtype=dtype, device=device, generator=generator, latents=None, scheduler=DummyScheduler()
|
298 |
-
)
|
299 |
-
shape = (batch_size, decoder.config.in_channels, decoder.config.sample_size, decoder.config.sample_size)
|
300 |
-
decoder_latents = pipe.prepare_latents(
|
301 |
-
shape, dtype=dtype, device=device, generator=generator, latents=None, scheduler=DummyScheduler()
|
302 |
-
)
|
303 |
-
|
304 |
-
shape = (
|
305 |
-
batch_size,
|
306 |
-
super_res_first.config.in_channels // 2,
|
307 |
-
super_res_first.config.sample_size,
|
308 |
-
super_res_first.config.sample_size,
|
309 |
-
)
|
310 |
-
super_res_latents = pipe.prepare_latents(
|
311 |
-
shape, dtype=dtype, device=device, generator=generator, latents=None, scheduler=DummyScheduler()
|
312 |
-
)
|
313 |
-
|
314 |
-
pipe.set_progress_bar_config(disable=None)
|
315 |
-
|
316 |
-
prompt = "this is a prompt example"
|
317 |
-
|
318 |
-
generator = torch.Generator(device=device).manual_seed(0)
|
319 |
-
output = pipe(
|
320 |
-
[prompt],
|
321 |
-
generator=generator,
|
322 |
-
prior_num_inference_steps=2,
|
323 |
-
decoder_num_inference_steps=2,
|
324 |
-
super_res_num_inference_steps=2,
|
325 |
-
prior_latents=prior_latents,
|
326 |
-
decoder_latents=decoder_latents,
|
327 |
-
super_res_latents=super_res_latents,
|
328 |
-
output_type="np",
|
329 |
-
)
|
330 |
-
image = output.images
|
331 |
-
|
332 |
-
text_inputs = tokenizer(
|
333 |
-
prompt,
|
334 |
-
padding="max_length",
|
335 |
-
max_length=tokenizer.model_max_length,
|
336 |
-
return_tensors="pt",
|
337 |
-
)
|
338 |
-
text_model_output = text_encoder(text_inputs.input_ids)
|
339 |
-
text_attention_mask = text_inputs.attention_mask
|
340 |
-
|
341 |
-
generator = torch.Generator(device=device).manual_seed(0)
|
342 |
-
image_from_text = pipe(
|
343 |
-
generator=generator,
|
344 |
-
prior_num_inference_steps=2,
|
345 |
-
decoder_num_inference_steps=2,
|
346 |
-
super_res_num_inference_steps=2,
|
347 |
-
prior_latents=prior_latents,
|
348 |
-
decoder_latents=decoder_latents,
|
349 |
-
super_res_latents=super_res_latents,
|
350 |
-
text_model_output=text_model_output,
|
351 |
-
text_attention_mask=text_attention_mask,
|
352 |
-
output_type="np",
|
353 |
-
)[0]
|
354 |
-
|
355 |
-
# make sure passing text embeddings manually is identical
|
356 |
-
assert np.abs(image - image_from_text).max() < 1e-4
|
357 |
-
|
358 |
-
# Overriding PipelineTesterMixin::test_attention_slicing_forward_pass
|
359 |
-
# because UnCLIP GPU undeterminism requires a looser check.
|
360 |
-
@skip_mps
|
361 |
-
def test_attention_slicing_forward_pass(self):
|
362 |
-
test_max_difference = torch_device == "cpu"
|
363 |
-
|
364 |
-
self._test_attention_slicing_forward_pass(test_max_difference=test_max_difference, expected_max_diff=0.01)
|
365 |
-
|
366 |
-
# Overriding PipelineTesterMixin::test_inference_batch_single_identical
|
367 |
-
# because UnCLIP undeterminism requires a looser check.
|
368 |
-
@skip_mps
|
369 |
-
def test_inference_batch_single_identical(self):
|
370 |
-
test_max_difference = torch_device == "cpu"
|
371 |
-
relax_max_difference = True
|
372 |
-
additional_params_copy_to_batched_inputs = [
|
373 |
-
"prior_num_inference_steps",
|
374 |
-
"decoder_num_inference_steps",
|
375 |
-
"super_res_num_inference_steps",
|
376 |
-
]
|
377 |
-
|
378 |
-
self._test_inference_batch_single_identical(
|
379 |
-
test_max_difference=test_max_difference,
|
380 |
-
relax_max_difference=relax_max_difference,
|
381 |
-
additional_params_copy_to_batched_inputs=additional_params_copy_to_batched_inputs,
|
382 |
-
)
|
383 |
-
|
384 |
-
def test_inference_batch_consistent(self):
|
385 |
-
additional_params_copy_to_batched_inputs = [
|
386 |
-
"prior_num_inference_steps",
|
387 |
-
"decoder_num_inference_steps",
|
388 |
-
"super_res_num_inference_steps",
|
389 |
-
]
|
390 |
-
|
391 |
-
if torch_device == "mps":
|
392 |
-
# TODO: MPS errors with larger batch sizes
|
393 |
-
batch_sizes = [2, 3]
|
394 |
-
self._test_inference_batch_consistent(
|
395 |
-
batch_sizes=batch_sizes,
|
396 |
-
additional_params_copy_to_batched_inputs=additional_params_copy_to_batched_inputs,
|
397 |
-
)
|
398 |
-
else:
|
399 |
-
self._test_inference_batch_consistent(
|
400 |
-
additional_params_copy_to_batched_inputs=additional_params_copy_to_batched_inputs
|
401 |
-
)
|
402 |
-
|
403 |
-
@skip_mps
|
404 |
-
def test_dict_tuple_outputs_equivalent(self):
|
405 |
-
return super().test_dict_tuple_outputs_equivalent()
|
406 |
-
|
407 |
-
@skip_mps
|
408 |
-
def test_save_load_local(self):
|
409 |
-
return super().test_save_load_local()
|
410 |
-
|
411 |
-
@skip_mps
|
412 |
-
def test_save_load_optional_components(self):
|
413 |
-
return super().test_save_load_optional_components()
|
414 |
-
|
415 |
-
|
416 |
-
@nightly
|
417 |
-
class UnCLIPPipelineCPUIntegrationTests(unittest.TestCase):
|
418 |
-
def tearDown(self):
|
419 |
-
# clean up the VRAM after each test
|
420 |
-
super().tearDown()
|
421 |
-
gc.collect()
|
422 |
-
torch.cuda.empty_cache()
|
423 |
-
|
424 |
-
def test_unclip_karlo_cpu_fp32(self):
|
425 |
-
expected_image = load_numpy(
|
426 |
-
"https://huggingface.co/datasets/hf-internal-testing/diffusers-images/resolve/main"
|
427 |
-
"/unclip/karlo_v1_alpha_horse_cpu.npy"
|
428 |
-
)
|
429 |
-
|
430 |
-
pipeline = UnCLIPPipeline.from_pretrained("kakaobrain/karlo-v1-alpha")
|
431 |
-
pipeline.set_progress_bar_config(disable=None)
|
432 |
-
|
433 |
-
generator = torch.manual_seed(0)
|
434 |
-
output = pipeline(
|
435 |
-
"horse",
|
436 |
-
num_images_per_prompt=1,
|
437 |
-
generator=generator,
|
438 |
-
output_type="np",
|
439 |
-
)
|
440 |
-
|
441 |
-
image = output.images[0]
|
442 |
-
|
443 |
-
assert image.shape == (256, 256, 3)
|
444 |
-
assert np.abs(expected_image - image).max() < 1e-1
|
445 |
-
|
446 |
-
|
447 |
-
@slow
|
448 |
-
@require_torch_gpu
|
449 |
-
class UnCLIPPipelineIntegrationTests(unittest.TestCase):
|
450 |
-
def tearDown(self):
|
451 |
-
# clean up the VRAM after each test
|
452 |
-
super().tearDown()
|
453 |
-
gc.collect()
|
454 |
-
torch.cuda.empty_cache()
|
455 |
-
|
456 |
-
def test_unclip_karlo(self):
|
457 |
-
expected_image = load_numpy(
|
458 |
-
"https://huggingface.co/datasets/hf-internal-testing/diffusers-images/resolve/main"
|
459 |
-
"/unclip/karlo_v1_alpha_horse_fp16.npy"
|
460 |
-
)
|
461 |
-
|
462 |
-
pipeline = UnCLIPPipeline.from_pretrained("kakaobrain/karlo-v1-alpha", torch_dtype=torch.float16)
|
463 |
-
pipeline = pipeline.to(torch_device)
|
464 |
-
pipeline.set_progress_bar_config(disable=None)
|
465 |
-
|
466 |
-
generator = torch.Generator(device="cpu").manual_seed(0)
|
467 |
-
output = pipeline(
|
468 |
-
"horse",
|
469 |
-
generator=generator,
|
470 |
-
output_type="np",
|
471 |
-
)
|
472 |
-
|
473 |
-
image = output.images[0]
|
474 |
-
|
475 |
-
assert image.shape == (256, 256, 3)
|
476 |
-
|
477 |
-
assert_mean_pixel_difference(image, expected_image)
|
478 |
-
|
479 |
-
def test_unclip_pipeline_with_sequential_cpu_offloading(self):
|
480 |
-
torch.cuda.empty_cache()
|
481 |
-
torch.cuda.reset_max_memory_allocated()
|
482 |
-
torch.cuda.reset_peak_memory_stats()
|
483 |
-
|
484 |
-
pipe = UnCLIPPipeline.from_pretrained("kakaobrain/karlo-v1-alpha", torch_dtype=torch.float16)
|
485 |
-
pipe = pipe.to(torch_device)
|
486 |
-
pipe.set_progress_bar_config(disable=None)
|
487 |
-
pipe.enable_attention_slicing()
|
488 |
-
pipe.enable_sequential_cpu_offload()
|
489 |
-
|
490 |
-
_ = pipe(
|
491 |
-
"horse",
|
492 |
-
num_images_per_prompt=1,
|
493 |
-
prior_num_inference_steps=2,
|
494 |
-
decoder_num_inference_steps=2,
|
495 |
-
super_res_num_inference_steps=2,
|
496 |
-
output_type="np",
|
497 |
-
)
|
498 |
-
|
499 |
-
mem_bytes = torch.cuda.max_memory_allocated()
|
500 |
-
# make sure that less than 7 GB is allocated
|
501 |
-
assert mem_bytes < 7 * 10**9
|
|
|
|
|
|
|
|
|
|
|
|
|
|
|
|
|
|
|
|
|
|
|
|
|
|
|
|
|
|
|
|
|
|
|
|
|
|
|
|
|
|
|
|
|
|
|
|
|
|
|
|
|
|
|
|
|
|
|
|
|
|
|
|
|
|
|
|
|
|
|
|
|
|
|
|
|
|
|
|
|
|
|
|
|
|
|
|
|
|
|
|
|
|
|
|
|
|
|
|
|
|
|
|
|
|
|
|
|
|
|
|
|
|
|
|
|
|
|
|
|
|
|
|
|
|
|
|
|
|
|
|
|
|
|
|
|
|
|
|
|
|
|
|
|
|
|
|
|
|
|
|
|
|
|
|
|
|
|
|
|
|
|
|
|
|
|
|
|
|
|
|
|
|
|
|
|
|
|
|
|
|
|
|
|
|
|
|
|
|
|
|
|
|
|
|
|
|
|
|
|
|
|
|
|
|
|
|
|
|
|
|
|
|
|
|
|
|
|
|
|
|
|
|
|
|
|
|
|
|
|
|
|
|
|
|
|
|
|
|
|
|
|
|
|
|
|
|
|
|
|
|
|
|
|
|
|
|
|
|
|
|
|
|
|
|
|
|
|
|
|
|
|
|
|
|
|
|
|
|
|
|
|
|
|
|
|
|
|
|
|
|
|
|
|
|
|
|
|
|
|
|
|
|
|
|
|
|
|
|
|
|
|
|
|
|
|
|
|
|
|
|
|
|
|
|
|
|
|
|
|
|
|
|
|
|
|
|
|
|
|
|
|
|
|
|
|
|
|
|
|
|
|
|
|
|
|
|
|
|
|
|
|
|
|
|
|
|
|
|
|
|
|
|
|
|
|
|
|
|
|
|
|
|
|
|
|
|
|
|
|
|
|
|
|
|
|
|
|
|
|
|
|
|
|
|
|
|
|
|
|
|
|
|
|
|
|
|
|
|
|
|
|
|
|
|
|
|
|
|
|
|
|
|
|
|
|
|
|
|
|
|
|
|
|
|
|
|
|
|
|
|
|
|
|
|
|
|
|
|
|
|
|
|
|
|
|
|
|
|
|
|
|
|
|
|
|
|
|
|
|
|
|
|
|
|
|
|
|
|
|
|
|
|
|
|
|
|
|
|
|
|
|
|
|
|
|
|
|
|
|
|
|
|
|
|
|
|
|
|
|
|
|
|
|
|
|
|
|
|
|
|
|
|
|
|
|
|
|
|
|
|
|
|
|
|
|
|
|
|
|
|
|
|
|
|
|
|
|
|
|
|
|
|
|
|
|
|
|
|
|
|
|
|
|
|
|
|
|
|
|
|
|
|
|
|
|
|
|
|
|
|
|
|
|
|
|
|
|
|
|
|
|
|
|
|
|
|
|
|
|
|
|
|
|
|
|
|
|
|
|
|
|
|
|
|
|
|
|
|
|
|
|
|
|
|
|
|
|
|
|
|
|
|
|
|
|
|
|
|
|
|
|
|
|
|
|
|
|
|
|
|
|
|
|
|
|
|
|
|
|
|
|
|
|
|
|
|
|
|
|
|
|
|
|
|
|
|
|
|
|
|
|
|
|
|
|
|
|
|
|
|
|
|
|
|
|
|
|
|
|
|
|
|
|
|
|
|
|
|
|
|
|
|
|
|
|
|
|
|
|
|
|
|
|
|
|
|
|
|
|
|
|
|
|
|
|
|
|
|
|
|
|
|
|
|
|
|
|
|
|
|
|
|
|
|
|
|
|
|
|
|
|
|
|
|
|
|
|
|
|
|
|
|
|
|
|
|
|
|
|
|
|
|
|
|
|
|
|
|
|
|
|
|
|
|
|
|
|
|
|
|
|
|
|
|
|
|
|
|
|
|
|
|
|
|
|
|
|
|
|
|
|
|
|
|
|
|
|
|
|
|
|
|
|
|
|
|
|
|
|
|
|
|
|
|
|
|
|
|
|
|
|
|
|
|
|
|
|
|
|
|
|
|
|
|
|
|
|
|
|
|
|
|
|
|
|
|
|
|
|
|
|
|
|
|
|
|
|
|
|
|
|
|
|
|
|
|
|
|
|
|
|
|
|
|
|
|
|
|
|
|
|
|
|
|
|
|
|
|
|
|
|
|
|
|
|
|
|
|
|
|
|
|
|
|
|
|
|
|
|
|
|
|
|
|
|
|
|
|
|
|
|
|
|
|
|
|
|
|
|
|
|
|
|
|
|
|
|
|
|
|
|
|
|
|
|
|
|
|
|
|
|
|
|
|
|
|
|
|
|
|
|
|
spaces/Andy1621/uniformer_image_detection/configs/gcnet/mask_rcnn_r101_fpn_r4_gcb_c3-c5_1x_coco.py
DELETED
@@ -1,8 +0,0 @@
|
|
1 |
-
_base_ = '../mask_rcnn/mask_rcnn_r101_fpn_1x_coco.py'
|
2 |
-
model = dict(
|
3 |
-
backbone=dict(plugins=[
|
4 |
-
dict(
|
5 |
-
cfg=dict(type='ContextBlock', ratio=1. / 4),
|
6 |
-
stages=(False, True, True, True),
|
7 |
-
position='after_conv3')
|
8 |
-
]))
|
|
|
|
|
|
|
|
|
|
|
|
|
|
|
|
|
|
spaces/Andy1621/uniformer_image_detection/configs/rpn/rpn_r50_fpn_2x_coco.py
DELETED
@@ -1,5 +0,0 @@
|
|
1 |
-
_base_ = './rpn_r50_fpn_1x_coco.py'
|
2 |
-
|
3 |
-
# learning policy
|
4 |
-
lr_config = dict(step=[16, 22])
|
5 |
-
runner = dict(type='EpochBasedRunner', max_epochs=24)
|
|
|
|
|
|
|
|
|
|
|
|
spaces/Andy1621/uniformer_image_detection/mmdet/models/losses/varifocal_loss.py
DELETED
@@ -1,133 +0,0 @@
|
|
1 |
-
import mmcv
|
2 |
-
import torch.nn as nn
|
3 |
-
import torch.nn.functional as F
|
4 |
-
|
5 |
-
from ..builder import LOSSES
|
6 |
-
from .utils import weight_reduce_loss
|
7 |
-
|
8 |
-
|
9 |
-
@mmcv.jit(derivate=True, coderize=True)
|
10 |
-
def varifocal_loss(pred,
|
11 |
-
target,
|
12 |
-
weight=None,
|
13 |
-
alpha=0.75,
|
14 |
-
gamma=2.0,
|
15 |
-
iou_weighted=True,
|
16 |
-
reduction='mean',
|
17 |
-
avg_factor=None):
|
18 |
-
"""`Varifocal Loss <https://arxiv.org/abs/2008.13367>`_
|
19 |
-
|
20 |
-
Args:
|
21 |
-
pred (torch.Tensor): The prediction with shape (N, C), C is the
|
22 |
-
number of classes
|
23 |
-
target (torch.Tensor): The learning target of the iou-aware
|
24 |
-
classification score with shape (N, C), C is the number of classes.
|
25 |
-
weight (torch.Tensor, optional): The weight of loss for each
|
26 |
-
prediction. Defaults to None.
|
27 |
-
alpha (float, optional): A balance factor for the negative part of
|
28 |
-
Varifocal Loss, which is different from the alpha of Focal Loss.
|
29 |
-
Defaults to 0.75.
|
30 |
-
gamma (float, optional): The gamma for calculating the modulating
|
31 |
-
factor. Defaults to 2.0.
|
32 |
-
iou_weighted (bool, optional): Whether to weight the loss of the
|
33 |
-
positive example with the iou target. Defaults to True.
|
34 |
-
reduction (str, optional): The method used to reduce the loss into
|
35 |
-
a scalar. Defaults to 'mean'. Options are "none", "mean" and
|
36 |
-
"sum".
|
37 |
-
avg_factor (int, optional): Average factor that is used to average
|
38 |
-
the loss. Defaults to None.
|
39 |
-
"""
|
40 |
-
# pred and target should be of the same size
|
41 |
-
assert pred.size() == target.size()
|
42 |
-
pred_sigmoid = pred.sigmoid()
|
43 |
-
target = target.type_as(pred)
|
44 |
-
if iou_weighted:
|
45 |
-
focal_weight = target * (target > 0.0).float() + \
|
46 |
-
alpha * (pred_sigmoid - target).abs().pow(gamma) * \
|
47 |
-
(target <= 0.0).float()
|
48 |
-
else:
|
49 |
-
focal_weight = (target > 0.0).float() + \
|
50 |
-
alpha * (pred_sigmoid - target).abs().pow(gamma) * \
|
51 |
-
(target <= 0.0).float()
|
52 |
-
loss = F.binary_cross_entropy_with_logits(
|
53 |
-
pred, target, reduction='none') * focal_weight
|
54 |
-
loss = weight_reduce_loss(loss, weight, reduction, avg_factor)
|
55 |
-
return loss
|
56 |
-
|
57 |
-
|
58 |
-
@LOSSES.register_module()
|
59 |
-
class VarifocalLoss(nn.Module):
|
60 |
-
|
61 |
-
def __init__(self,
|
62 |
-
use_sigmoid=True,
|
63 |
-
alpha=0.75,
|
64 |
-
gamma=2.0,
|
65 |
-
iou_weighted=True,
|
66 |
-
reduction='mean',
|
67 |
-
loss_weight=1.0):
|
68 |
-
"""`Varifocal Loss <https://arxiv.org/abs/2008.13367>`_
|
69 |
-
|
70 |
-
Args:
|
71 |
-
use_sigmoid (bool, optional): Whether the prediction is
|
72 |
-
used for sigmoid or softmax. Defaults to True.
|
73 |
-
alpha (float, optional): A balance factor for the negative part of
|
74 |
-
Varifocal Loss, which is different from the alpha of Focal
|
75 |
-
Loss. Defaults to 0.75.
|
76 |
-
gamma (float, optional): The gamma for calculating the modulating
|
77 |
-
factor. Defaults to 2.0.
|
78 |
-
iou_weighted (bool, optional): Whether to weight the loss of the
|
79 |
-
positive examples with the iou target. Defaults to True.
|
80 |
-
reduction (str, optional): The method used to reduce the loss into
|
81 |
-
a scalar. Defaults to 'mean'. Options are "none", "mean" and
|
82 |
-
"sum".
|
83 |
-
loss_weight (float, optional): Weight of loss. Defaults to 1.0.
|
84 |
-
"""
|
85 |
-
super(VarifocalLoss, self).__init__()
|
86 |
-
assert use_sigmoid is True, \
|
87 |
-
'Only sigmoid varifocal loss supported now.'
|
88 |
-
assert alpha >= 0.0
|
89 |
-
self.use_sigmoid = use_sigmoid
|
90 |
-
self.alpha = alpha
|
91 |
-
self.gamma = gamma
|
92 |
-
self.iou_weighted = iou_weighted
|
93 |
-
self.reduction = reduction
|
94 |
-
self.loss_weight = loss_weight
|
95 |
-
|
96 |
-
def forward(self,
|
97 |
-
pred,
|
98 |
-
target,
|
99 |
-
weight=None,
|
100 |
-
avg_factor=None,
|
101 |
-
reduction_override=None):
|
102 |
-
"""Forward function.
|
103 |
-
|
104 |
-
Args:
|
105 |
-
pred (torch.Tensor): The prediction.
|
106 |
-
target (torch.Tensor): The learning target of the prediction.
|
107 |
-
weight (torch.Tensor, optional): The weight of loss for each
|
108 |
-
prediction. Defaults to None.
|
109 |
-
avg_factor (int, optional): Average factor that is used to average
|
110 |
-
the loss. Defaults to None.
|
111 |
-
reduction_override (str, optional): The reduction method used to
|
112 |
-
override the original reduction method of the loss.
|
113 |
-
Options are "none", "mean" and "sum".
|
114 |
-
|
115 |
-
Returns:
|
116 |
-
torch.Tensor: The calculated loss
|
117 |
-
"""
|
118 |
-
assert reduction_override in (None, 'none', 'mean', 'sum')
|
119 |
-
reduction = (
|
120 |
-
reduction_override if reduction_override else self.reduction)
|
121 |
-
if self.use_sigmoid:
|
122 |
-
loss_cls = self.loss_weight * varifocal_loss(
|
123 |
-
pred,
|
124 |
-
target,
|
125 |
-
weight,
|
126 |
-
alpha=self.alpha,
|
127 |
-
gamma=self.gamma,
|
128 |
-
iou_weighted=self.iou_weighted,
|
129 |
-
reduction=reduction,
|
130 |
-
avg_factor=avg_factor)
|
131 |
-
else:
|
132 |
-
raise NotImplementedError
|
133 |
-
return loss_cls
|
|
|
|
|
|
|
|
|
|
|
|
|
|
|
|
|
|
|
|
|
|
|
|
|
|
|
|
|
|
|
|
|
|
|
|
|
|
|
|
|
|
|
|
|
|
|
|
|
|
|
|
|
|
|
|
|
|
|
|
|
|
|
|
|
|
|
|
|
|
|
|
|
|
|
|
|
|
|
|
|
|
|
|
|
|
|
|
|
|
|
|
|
|
|
|
|
|
|
|
|
|
|
|
|
|
|
|
|
|
|
|
|
|
|
|
|
|
|
|
|
|
|
|
|
|
|
|
|
|
|
|
|
|
|
|
|
|
|
|
|
|
|
|
|
|
|
|
|
|
|
|
|
|
|
|
|
|
|
|
|
|
|
|
|
|
|
|
|
|
|
|
|
|
|
|
|
|
|
|
|
|
|
|
|
|
|
|
|
|
|
|
|
|
|
|
|
|
|
|
|
|
|
|
|
|
|
|
|
|
|
|
|
|
|
|
|
|
|
|
|
|
|
|
|
|
|
|
|
|
|
|
|
|
|
|
|
|
|
|
|
|
|
|
|
|
|
|
|
|
|
|
|
|
|
|
|
|
|
|
|
|
|
|
|
|
|
|
spaces/Ariharasudhan/YoloV5/utils/loggers/clearml/README.md
DELETED
@@ -1,230 +0,0 @@
|
|
1 |
-
# ClearML Integration
|
2 |
-
|
3 |
-
<img align="center" src="https://github.com/thepycoder/clearml_screenshots/raw/main/logos_dark.png#gh-light-mode-only" alt="Clear|ML"><img align="center" src="https://github.com/thepycoder/clearml_screenshots/raw/main/logos_light.png#gh-dark-mode-only" alt="Clear|ML">
|
4 |
-
|
5 |
-
## About ClearML
|
6 |
-
|
7 |
-
[ClearML](https://cutt.ly/yolov5-tutorial-clearml) is an [open-source](https://github.com/allegroai/clearml) toolbox designed to save you time ⏱️.
|
8 |
-
|
9 |
-
🔨 Track every YOLOv5 training run in the <b>experiment manager</b>
|
10 |
-
|
11 |
-
🔧 Version and easily access your custom training data with the integrated ClearML <b>Data Versioning Tool</b>
|
12 |
-
|
13 |
-
🔦 <b>Remotely train and monitor</b> your YOLOv5 training runs using ClearML Agent
|
14 |
-
|
15 |
-
🔬 Get the very best mAP using ClearML <b>Hyperparameter Optimization</b>
|
16 |
-
|
17 |
-
🔭 Turn your newly trained <b>YOLOv5 model into an API</b> with just a few commands using ClearML Serving
|
18 |
-
|
19 |
-
<br />
|
20 |
-
And so much more. It's up to you how many of these tools you want to use, you can stick to the experiment manager, or chain them all together into an impressive pipeline!
|
21 |
-
<br />
|
22 |
-
<br />
|
23 |
-
|
24 |
-

|
25 |
-
|
26 |
-
|
27 |
-
<br />
|
28 |
-
<br />
|
29 |
-
|
30 |
-
## 🦾 Setting Things Up
|
31 |
-
|
32 |
-
To keep track of your experiments and/or data, ClearML needs to communicate to a server. You have 2 options to get one:
|
33 |
-
|
34 |
-
Either sign up for free to the [ClearML Hosted Service](https://cutt.ly/yolov5-tutorial-clearml) or you can set up your own server, see [here](https://clear.ml/docs/latest/docs/deploying_clearml/clearml_server). Even the server is open-source, so even if you're dealing with sensitive data, you should be good to go!
|
35 |
-
|
36 |
-
1. Install the `clearml` python package:
|
37 |
-
|
38 |
-
```bash
|
39 |
-
pip install clearml
|
40 |
-
```
|
41 |
-
|
42 |
-
1. Connect the ClearML SDK to the server by [creating credentials](https://app.clear.ml/settings/workspace-configuration) (go right top to Settings -> Workspace -> Create new credentials), then execute the command below and follow the instructions:
|
43 |
-
|
44 |
-
```bash
|
45 |
-
clearml-init
|
46 |
-
```
|
47 |
-
|
48 |
-
That's it! You're done 😎
|
49 |
-
|
50 |
-
<br />
|
51 |
-
|
52 |
-
## 🚀 Training YOLOv5 With ClearML
|
53 |
-
|
54 |
-
To enable ClearML experiment tracking, simply install the ClearML pip package.
|
55 |
-
|
56 |
-
```bash
|
57 |
-
pip install clearml>=1.2.0
|
58 |
-
```
|
59 |
-
|
60 |
-
This will enable integration with the YOLOv5 training script. Every training run from now on, will be captured and stored by the ClearML experiment manager.
|
61 |
-
|
62 |
-
If you want to change the `project_name` or `task_name`, use the `--project` and `--name` arguments of the `train.py` script, by default the project will be called `YOLOv5` and the task `Training`.
|
63 |
-
PLEASE NOTE: ClearML uses `/` as a delimter for subprojects, so be careful when using `/` in your project name!
|
64 |
-
|
65 |
-
```bash
|
66 |
-
python train.py --img 640 --batch 16 --epochs 3 --data coco128.yaml --weights yolov5s.pt --cache
|
67 |
-
```
|
68 |
-
|
69 |
-
or with custom project and task name:
|
70 |
-
```bash
|
71 |
-
python train.py --project my_project --name my_training --img 640 --batch 16 --epochs 3 --data coco128.yaml --weights yolov5s.pt --cache
|
72 |
-
```
|
73 |
-
|
74 |
-
This will capture:
|
75 |
-
- Source code + uncommitted changes
|
76 |
-
- Installed packages
|
77 |
-
- (Hyper)parameters
|
78 |
-
- Model files (use `--save-period n` to save a checkpoint every n epochs)
|
79 |
-
- Console output
|
80 |
-
- Scalars (mAP_0.5, mAP_0.5:0.95, precision, recall, losses, learning rates, ...)
|
81 |
-
- General info such as machine details, runtime, creation date etc.
|
82 |
-
- All produced plots such as label correlogram and confusion matrix
|
83 |
-
- Images with bounding boxes per epoch
|
84 |
-
- Mosaic per epoch
|
85 |
-
- Validation images per epoch
|
86 |
-
- ...
|
87 |
-
|
88 |
-
That's a lot right? 🤯
|
89 |
-
Now, we can visualize all of this information in the ClearML UI to get an overview of our training progress. Add custom columns to the table view (such as e.g. mAP_0.5) so you can easily sort on the best performing model. Or select multiple experiments and directly compare them!
|
90 |
-
|
91 |
-
There even more we can do with all of this information, like hyperparameter optimization and remote execution, so keep reading if you want to see how that works!
|
92 |
-
|
93 |
-
<br />
|
94 |
-
|
95 |
-
## 🔗 Dataset Version Management
|
96 |
-
|
97 |
-
Versioning your data separately from your code is generally a good idea and makes it easy to aqcuire the latest version too. This repository supports supplying a dataset version ID and it will make sure to get the data if it's not there yet. Next to that, this workflow also saves the used dataset ID as part of the task parameters, so you will always know for sure which data was used in which experiment!
|
98 |
-
|
99 |
-

|
100 |
-
|
101 |
-
### Prepare Your Dataset
|
102 |
-
|
103 |
-
The YOLOv5 repository supports a number of different datasets by using yaml files containing their information. By default datasets are downloaded to the `../datasets` folder in relation to the repository root folder. So if you downloaded the `coco128` dataset using the link in the yaml or with the scripts provided by yolov5, you get this folder structure:
|
104 |
-
|
105 |
-
```
|
106 |
-
..
|
107 |
-
|_ yolov5
|
108 |
-
|_ datasets
|
109 |
-
|_ coco128
|
110 |
-
|_ images
|
111 |
-
|_ labels
|
112 |
-
|_ LICENSE
|
113 |
-
|_ README.txt
|
114 |
-
```
|
115 |
-
But this can be any dataset you wish. Feel free to use your own, as long as you keep to this folder structure.
|
116 |
-
|
117 |
-
Next, ⚠️**copy the corresponding yaml file to the root of the dataset folder**⚠️. This yaml files contains the information ClearML will need to properly use the dataset. You can make this yourself too, of course, just follow the structure of the example yamls.
|
118 |
-
|
119 |
-
Basically we need the following keys: `path`, `train`, `test`, `val`, `nc`, `names`.
|
120 |
-
|
121 |
-
```
|
122 |
-
..
|
123 |
-
|_ yolov5
|
124 |
-
|_ datasets
|
125 |
-
|_ coco128
|
126 |
-
|_ images
|
127 |
-
|_ labels
|
128 |
-
|_ coco128.yaml # <---- HERE!
|
129 |
-
|_ LICENSE
|
130 |
-
|_ README.txt
|
131 |
-
```
|
132 |
-
|
133 |
-
### Upload Your Dataset
|
134 |
-
|
135 |
-
To get this dataset into ClearML as a versionned dataset, go to the dataset root folder and run the following command:
|
136 |
-
```bash
|
137 |
-
cd coco128
|
138 |
-
clearml-data sync --project YOLOv5 --name coco128 --folder .
|
139 |
-
```
|
140 |
-
|
141 |
-
The command `clearml-data sync` is actually a shorthand command. You could also run these commands one after the other:
|
142 |
-
```bash
|
143 |
-
# Optionally add --parent <parent_dataset_id> if you want to base
|
144 |
-
# this version on another dataset version, so no duplicate files are uploaded!
|
145 |
-
clearml-data create --name coco128 --project YOLOv5
|
146 |
-
clearml-data add --files .
|
147 |
-
clearml-data close
|
148 |
-
```
|
149 |
-
|
150 |
-
### Run Training Using A ClearML Dataset
|
151 |
-
|
152 |
-
Now that you have a ClearML dataset, you can very simply use it to train custom YOLOv5 🚀 models!
|
153 |
-
|
154 |
-
```bash
|
155 |
-
python train.py --img 640 --batch 16 --epochs 3 --data clearml://<your_dataset_id> --weights yolov5s.pt --cache
|
156 |
-
```
|
157 |
-
|
158 |
-
<br />
|
159 |
-
|
160 |
-
## 👀 Hyperparameter Optimization
|
161 |
-
|
162 |
-
Now that we have our experiments and data versioned, it's time to take a look at what we can build on top!
|
163 |
-
|
164 |
-
Using the code information, installed packages and environment details, the experiment itself is now **completely reproducible**. In fact, ClearML allows you to clone an experiment and even change its parameters. We can then just rerun it with these new parameters automatically, this is basically what HPO does!
|
165 |
-
|
166 |
-
To **run hyperparameter optimization locally**, we've included a pre-made script for you. Just make sure a training task has been run at least once, so it is in the ClearML experiment manager, we will essentially clone it and change its hyperparameters.
|
167 |
-
|
168 |
-
You'll need to fill in the ID of this `template task` in the script found at `utils/loggers/clearml/hpo.py` and then just run it :) You can change `task.execute_locally()` to `task.execute()` to put it in a ClearML queue and have a remote agent work on it instead.
|
169 |
-
|
170 |
-
```bash
|
171 |
-
# To use optuna, install it first, otherwise you can change the optimizer to just be RandomSearch
|
172 |
-
pip install optuna
|
173 |
-
python utils/loggers/clearml/hpo.py
|
174 |
-
```
|
175 |
-
|
176 |
-

|
177 |
-
|
178 |
-
## 🤯 Remote Execution (advanced)
|
179 |
-
|
180 |
-
Running HPO locally is really handy, but what if we want to run our experiments on a remote machine instead? Maybe you have access to a very powerful GPU machine on-site or you have some budget to use cloud GPUs.
|
181 |
-
This is where the ClearML Agent comes into play. Check out what the agent can do here:
|
182 |
-
|
183 |
-
- [YouTube video](https://youtu.be/MX3BrXnaULs)
|
184 |
-
- [Documentation](https://clear.ml/docs/latest/docs/clearml_agent)
|
185 |
-
|
186 |
-
In short: every experiment tracked by the experiment manager contains enough information to reproduce it on a different machine (installed packages, uncommitted changes etc.). So a ClearML agent does just that: it listens to a queue for incoming tasks and when it finds one, it recreates the environment and runs it while still reporting scalars, plots etc. to the experiment manager.
|
187 |
-
|
188 |
-
You can turn any machine (a cloud VM, a local GPU machine, your own laptop ... ) into a ClearML agent by simply running:
|
189 |
-
```bash
|
190 |
-
clearml-agent daemon --queue <queues_to_listen_to> [--docker]
|
191 |
-
```
|
192 |
-
|
193 |
-
### Cloning, Editing And Enqueuing
|
194 |
-
|
195 |
-
With our agent running, we can give it some work. Remember from the HPO section that we can clone a task and edit the hyperparameters? We can do that from the interface too!
|
196 |
-
|
197 |
-
🪄 Clone the experiment by right clicking it
|
198 |
-
|
199 |
-
🎯 Edit the hyperparameters to what you wish them to be
|
200 |
-
|
201 |
-
⏳ Enqueue the task to any of the queues by right clicking it
|
202 |
-
|
203 |
-

|
204 |
-
|
205 |
-
### Executing A Task Remotely
|
206 |
-
|
207 |
-
Now you can clone a task like we explained above, or simply mark your current script by adding `task.execute_remotely()` and on execution it will be put into a queue, for the agent to start working on!
|
208 |
-
|
209 |
-
To run the YOLOv5 training script remotely, all you have to do is add this line to the training.py script after the clearml logger has been instatiated:
|
210 |
-
```python
|
211 |
-
# ...
|
212 |
-
# Loggers
|
213 |
-
data_dict = None
|
214 |
-
if RANK in {-1, 0}:
|
215 |
-
loggers = Loggers(save_dir, weights, opt, hyp, LOGGER) # loggers instance
|
216 |
-
if loggers.clearml:
|
217 |
-
loggers.clearml.task.execute_remotely(queue='my_queue') # <------ ADD THIS LINE
|
218 |
-
# Data_dict is either None is user did not choose for ClearML dataset or is filled in by ClearML
|
219 |
-
data_dict = loggers.clearml.data_dict
|
220 |
-
# ...
|
221 |
-
```
|
222 |
-
When running the training script after this change, python will run the script up until that line, after which it will package the code and send it to the queue instead!
|
223 |
-
|
224 |
-
### Autoscaling workers
|
225 |
-
|
226 |
-
ClearML comes with autoscalers too! This tool will automatically spin up new remote machines in the cloud of your choice (AWS, GCP, Azure) and turn them into ClearML agents for you whenever there are experiments detected in the queue. Once the tasks are processed, the autoscaler will automatically shut down the remote machines and you stop paying!
|
227 |
-
|
228 |
-
Check out the autoscalers getting started video below.
|
229 |
-
|
230 |
-
[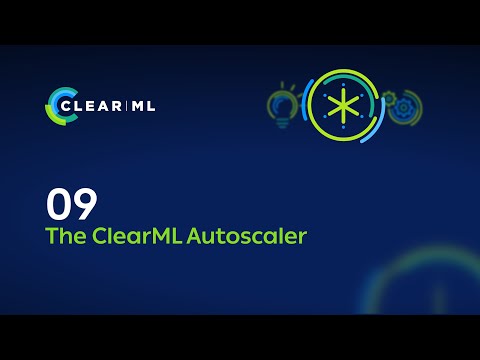](https://youtu.be/j4XVMAaUt3E)
|
|
|
|
|
|
|
|
|
|
|
|
|
|
|
|
|
|
|
|
|
|
|
|
|
|
|
|
|
|
|
|
|
|
|
|
|
|
|
|
|
|
|
|
|
|
|
|
|
|
|
|
|
|
|
|
|
|
|
|
|
|
|
|
|
|
|
|
|
|
|
|
|
|
|
|
|
|
|
|
|
|
|
|
|
|
|
|
|
|
|
|
|
|
|
|
|
|
|
|
|
|
|
|
|
|
|
|
|
|
|
|
|
|
|
|
|
|
|
|
|
|
|
|
|
|
|
|
|
|
|
|
|
|
|
|
|
|
|
|
|
|
|
|
|
|
|
|
|
|
|
|
|
|
|
|
|
|
|
|
|
|
|
|
|
|
|
|
|
|
|
|
|
|
|
|
|
|
|
|
|
|
|
|
|
|
|
|
|
|
|
|
|
|
|
|
|
|
|
|
|
|
|
|
|
|
|
|
|
|
|
|
|
|
|
|
|
|
|
|
|
|
|
|
|
|
|
|
|
|
|
|
|
|
|
|
|
|
|
|
|
|
|
|
|
|
|
|
|
|
|
|
|
|
|
|
|
|
|
|
|
|
|
|
|
|
|
|
|
|
|
|
|
|
|
|
|
|
|
|
|
|
|
|
|
|
|
|
|
|
|
|
|
|
|
|
|
|
|
|
|
|
|
|
|
|
|
|
|
|
|
|
|
|
|
|
|
|
|
|
|
|
|
|
|
|
|
|
|
|
|
|
|
|
|
|
|
|
|
|
|
|
|
|
|
|
|
|
|
|
|
|
|
|
|
|
|
|
|
|
|
|
|
|
|
|
|
|
|
|
|
|
|
|
|
|
|
|
|
|
|
|
|
|
|
|
|
|
|
|
|
|
|
|
|
|
|
|
|
|
|
|
|
|
|
|
|
|
|
|
|
|
|
|
|
|
|
|
|
|
|
|
|
|
|
|
|
|
|
|
|
|
|
|
|
|
|
|
|
|
|
|
|
|
|
|
|
|
|
|
|
|
|
|
|
|
|
|
|
|
|
|
spaces/Ataturk-Chatbot/HuggingFaceChat/venv/lib/python3.11/site-packages/pip/_internal/resolution/resolvelib/requirements.py
DELETED
@@ -1,165 +0,0 @@
|
|
1 |
-
from pip._vendor.packaging.specifiers import SpecifierSet
|
2 |
-
from pip._vendor.packaging.utils import NormalizedName, canonicalize_name
|
3 |
-
|
4 |
-
from pip._internal.req.req_install import InstallRequirement
|
5 |
-
|
6 |
-
from .base import Candidate, CandidateLookup, Requirement, format_name
|
7 |
-
|
8 |
-
|
9 |
-
class ExplicitRequirement(Requirement):
|
10 |
-
def __init__(self, candidate: Candidate) -> None:
|
11 |
-
self.candidate = candidate
|
12 |
-
|
13 |
-
def __str__(self) -> str:
|
14 |
-
return str(self.candidate)
|
15 |
-
|
16 |
-
def __repr__(self) -> str:
|
17 |
-
return "{class_name}({candidate!r})".format(
|
18 |
-
class_name=self.__class__.__name__,
|
19 |
-
candidate=self.candidate,
|
20 |
-
)
|
21 |
-
|
22 |
-
@property
|
23 |
-
def project_name(self) -> NormalizedName:
|
24 |
-
# No need to canonicalize - the candidate did this
|
25 |
-
return self.candidate.project_name
|
26 |
-
|
27 |
-
@property
|
28 |
-
def name(self) -> str:
|
29 |
-
# No need to canonicalize - the candidate did this
|
30 |
-
return self.candidate.name
|
31 |
-
|
32 |
-
def format_for_error(self) -> str:
|
33 |
-
return self.candidate.format_for_error()
|
34 |
-
|
35 |
-
def get_candidate_lookup(self) -> CandidateLookup:
|
36 |
-
return self.candidate, None
|
37 |
-
|
38 |
-
def is_satisfied_by(self, candidate: Candidate) -> bool:
|
39 |
-
return candidate == self.candidate
|
40 |
-
|
41 |
-
|
42 |
-
class SpecifierRequirement(Requirement):
|
43 |
-
def __init__(self, ireq: InstallRequirement) -> None:
|
44 |
-
assert ireq.link is None, "This is a link, not a specifier"
|
45 |
-
self._ireq = ireq
|
46 |
-
self._extras = frozenset(ireq.extras)
|
47 |
-
|
48 |
-
def __str__(self) -> str:
|
49 |
-
return str(self._ireq.req)
|
50 |
-
|
51 |
-
def __repr__(self) -> str:
|
52 |
-
return "{class_name}({requirement!r})".format(
|
53 |
-
class_name=self.__class__.__name__,
|
54 |
-
requirement=str(self._ireq.req),
|
55 |
-
)
|
56 |
-
|
57 |
-
@property
|
58 |
-
def project_name(self) -> NormalizedName:
|
59 |
-
assert self._ireq.req, "Specifier-backed ireq is always PEP 508"
|
60 |
-
return canonicalize_name(self._ireq.req.name)
|
61 |
-
|
62 |
-
@property
|
63 |
-
def name(self) -> str:
|
64 |
-
return format_name(self.project_name, self._extras)
|
65 |
-
|
66 |
-
def format_for_error(self) -> str:
|
67 |
-
# Convert comma-separated specifiers into "A, B, ..., F and G"
|
68 |
-
# This makes the specifier a bit more "human readable", without
|
69 |
-
# risking a change in meaning. (Hopefully! Not all edge cases have
|
70 |
-
# been checked)
|
71 |
-
parts = [s.strip() for s in str(self).split(",")]
|
72 |
-
if len(parts) == 0:
|
73 |
-
return ""
|
74 |
-
elif len(parts) == 1:
|
75 |
-
return parts[0]
|
76 |
-
|
77 |
-
return ", ".join(parts[:-1]) + " and " + parts[-1]
|
78 |
-
|
79 |
-
def get_candidate_lookup(self) -> CandidateLookup:
|
80 |
-
return None, self._ireq
|
81 |
-
|
82 |
-
def is_satisfied_by(self, candidate: Candidate) -> bool:
|
83 |
-
assert candidate.name == self.name, (
|
84 |
-
f"Internal issue: Candidate is not for this requirement "
|
85 |
-
f"{candidate.name} vs {self.name}"
|
86 |
-
)
|
87 |
-
# We can safely always allow prereleases here since PackageFinder
|
88 |
-
# already implements the prerelease logic, and would have filtered out
|
89 |
-
# prerelease candidates if the user does not expect them.
|
90 |
-
assert self._ireq.req, "Specifier-backed ireq is always PEP 508"
|
91 |
-
spec = self._ireq.req.specifier
|
92 |
-
return spec.contains(candidate.version, prereleases=True)
|
93 |
-
|
94 |
-
|
95 |
-
class RequiresPythonRequirement(Requirement):
|
96 |
-
"""A requirement representing Requires-Python metadata."""
|
97 |
-
|
98 |
-
def __init__(self, specifier: SpecifierSet, match: Candidate) -> None:
|
99 |
-
self.specifier = specifier
|
100 |
-
self._candidate = match
|
101 |
-
|
102 |
-
def __str__(self) -> str:
|
103 |
-
return f"Python {self.specifier}"
|
104 |
-
|
105 |
-
def __repr__(self) -> str:
|
106 |
-
return "{class_name}({specifier!r})".format(
|
107 |
-
class_name=self.__class__.__name__,
|
108 |
-
specifier=str(self.specifier),
|
109 |
-
)
|
110 |
-
|
111 |
-
@property
|
112 |
-
def project_name(self) -> NormalizedName:
|
113 |
-
return self._candidate.project_name
|
114 |
-
|
115 |
-
@property
|
116 |
-
def name(self) -> str:
|
117 |
-
return self._candidate.name
|
118 |
-
|
119 |
-
def format_for_error(self) -> str:
|
120 |
-
return str(self)
|
121 |
-
|
122 |
-
def get_candidate_lookup(self) -> CandidateLookup:
|
123 |
-
if self.specifier.contains(self._candidate.version, prereleases=True):
|
124 |
-
return self._candidate, None
|
125 |
-
return None, None
|
126 |
-
|
127 |
-
def is_satisfied_by(self, candidate: Candidate) -> bool:
|
128 |
-
assert candidate.name == self._candidate.name, "Not Python candidate"
|
129 |
-
# We can safely always allow prereleases here since PackageFinder
|
130 |
-
# already implements the prerelease logic, and would have filtered out
|
131 |
-
# prerelease candidates if the user does not expect them.
|
132 |
-
return self.specifier.contains(candidate.version, prereleases=True)
|
133 |
-
|
134 |
-
|
135 |
-
class UnsatisfiableRequirement(Requirement):
|
136 |
-
"""A requirement that cannot be satisfied."""
|
137 |
-
|
138 |
-
def __init__(self, name: NormalizedName) -> None:
|
139 |
-
self._name = name
|
140 |
-
|
141 |
-
def __str__(self) -> str:
|
142 |
-
return f"{self._name} (unavailable)"
|
143 |
-
|
144 |
-
def __repr__(self) -> str:
|
145 |
-
return "{class_name}({name!r})".format(
|
146 |
-
class_name=self.__class__.__name__,
|
147 |
-
name=str(self._name),
|
148 |
-
)
|
149 |
-
|
150 |
-
@property
|
151 |
-
def project_name(self) -> NormalizedName:
|
152 |
-
return self._name
|
153 |
-
|
154 |
-
@property
|
155 |
-
def name(self) -> str:
|
156 |
-
return self._name
|
157 |
-
|
158 |
-
def format_for_error(self) -> str:
|
159 |
-
return str(self)
|
160 |
-
|
161 |
-
def get_candidate_lookup(self) -> CandidateLookup:
|
162 |
-
return None, None
|
163 |
-
|
164 |
-
def is_satisfied_by(self, candidate: Candidate) -> bool:
|
165 |
-
return False
|
|
|
|
|
|
|
|
|
|
|
|
|
|
|
|
|
|
|
|
|
|
|
|
|
|
|
|
|
|
|
|
|
|
|
|
|
|
|
|
|
|
|
|
|
|
|
|
|
|
|
|
|
|
|
|
|
|
|
|
|
|
|
|
|
|
|
|
|
|
|
|
|
|
|
|
|
|
|
|
|
|
|
|
|
|
|
|
|
|
|
|
|
|
|
|
|
|
|
|
|
|
|
|
|
|
|
|
|
|
|
|
|
|
|
|
|
|
|
|
|
|
|
|
|
|
|
|
|
|
|
|
|
|
|
|
|
|
|
|
|
|
|
|
|
|
|
|
|
|
|
|
|
|
|
|
|
|
|
|
|
|
|
|
|
|
|
|
|
|
|
|
|
|
|
|
|
|
|
|
|
|
|
|
|
|
|
|
|
|
|
|
|
|
|
|
|
|
|
|
|
|
|
|
|
|
|
|
|
|
|
|
|
|
|
|
|
|
|
|
|
|
|
|
|
|
|
|
|
|
|
|
|
|
|
|
|
|
|
|
|
|
|
|
|
|
|
|
|
|
|
|
|
|
|
|
|
|
|
|
|
|
|
|
|
|
|
|
|
|
|
|
|
|
|
|
|
|
|
|
|
|
|
|
|
|
|
|
|
|
|
|
|
|
|
|
|
|
|
|
|
|
|
|
|
|
|
|
|
|
|
|
|
|
|
|
|
|
|
|
|
|
|
|
|
|
|
|
|
|
|
|
spaces/Ataturk-Chatbot/HuggingFaceChat/venv/lib/python3.11/site-packages/pip/_vendor/tomli/__init__.py
DELETED
@@ -1,11 +0,0 @@
|
|
1 |
-
# SPDX-License-Identifier: MIT
|
2 |
-
# SPDX-FileCopyrightText: 2021 Taneli Hukkinen
|
3 |
-
# Licensed to PSF under a Contributor Agreement.
|
4 |
-
|
5 |
-
__all__ = ("loads", "load", "TOMLDecodeError")
|
6 |
-
__version__ = "2.0.1" # DO NOT EDIT THIS LINE MANUALLY. LET bump2version UTILITY DO IT
|
7 |
-
|
8 |
-
from ._parser import TOMLDecodeError, load, loads
|
9 |
-
|
10 |
-
# Pretend this exception was created here.
|
11 |
-
TOMLDecodeError.__module__ = __name__
|
|
|
|
|
|
|
|
|
|
|
|
|
|
|
|
|
|
|
|
|
|
|
|
spaces/Awiny/Image2Paragraph/models/grit_src/third_party/CenterNet2/.github/ISSUE_TEMPLATE/bugs.md
DELETED
@@ -1,38 +0,0 @@
|
|
1 |
-
---
|
2 |
-
name: "🐛 Bugs"
|
3 |
-
about: Report bugs in detectron2
|
4 |
-
title: Please read & provide the following
|
5 |
-
|
6 |
-
---
|
7 |
-
|
8 |
-
## Instructions To Reproduce the 🐛 Bug:
|
9 |
-
1. Full runnable code or full changes you made:
|
10 |
-
```
|
11 |
-
If making changes to the project itself, please use output of the following command:
|
12 |
-
git rev-parse HEAD; git diff
|
13 |
-
|
14 |
-
<put code or diff here>
|
15 |
-
```
|
16 |
-
2. What exact command you run:
|
17 |
-
3. __Full logs__ or other relevant observations:
|
18 |
-
```
|
19 |
-
<put logs here>
|
20 |
-
```
|
21 |
-
4. please simplify the steps as much as possible so they do not require additional resources to
|
22 |
-
run, such as a private dataset.
|
23 |
-
|
24 |
-
## Expected behavior:
|
25 |
-
|
26 |
-
If there are no obvious error in "full logs" provided above,
|
27 |
-
please tell us the expected behavior.
|
28 |
-
|
29 |
-
## Environment:
|
30 |
-
|
31 |
-
Provide your environment information using the following command:
|
32 |
-
```
|
33 |
-
wget -nc -q https://github.com/facebookresearch/detectron2/raw/main/detectron2/utils/collect_env.py && python collect_env.py
|
34 |
-
```
|
35 |
-
|
36 |
-
If your issue looks like an installation issue / environment issue,
|
37 |
-
please first try to solve it yourself with the instructions in
|
38 |
-
https://detectron2.readthedocs.io/tutorials/install.html#common-installation-issues
|
|
|
|
|
|
|
|
|
|
|
|
|
|
|
|
|
|
|
|
|
|
|
|
|
|
|
|
|
|
|
|
|
|
|
|
|
|
|
|
|
|
|
|
|
|
|
|
|
|
|
|
|
|
|
|
|
|
|
|
|
|
|
|
|
|
|
|
|
|
|
|
|
|
|
|
|
|
spaces/Banbri/zcvzcv/src/lib/uploadToHuggingFace.ts
DELETED
@@ -1,16 +0,0 @@
|
|
1 |
-
export async function uploadToHuggingFace(file: File) {
|
2 |
-
const UPLOAD_URL = 'https://huggingface.co/uploads'
|
3 |
-
|
4 |
-
const response = await fetch(UPLOAD_URL, {
|
5 |
-
method: 'POST',
|
6 |
-
headers: {
|
7 |
-
'Content-Type': file.type,
|
8 |
-
'X-Requested-With': 'XMLHttpRequest',
|
9 |
-
},
|
10 |
-
body: file, /// <- File inherits from Blob
|
11 |
-
})
|
12 |
-
|
13 |
-
const url = await response.text()
|
14 |
-
|
15 |
-
return url
|
16 |
-
}
|
|
|
|
|
|
|
|
|
|
|
|
|
|
|
|
|
|
|
|
|
|
|
|
|
|
|
|
|
|
|
|
|
|
spaces/Bart92/RVC_HF/infer_uvr5.py
DELETED
@@ -1,363 +0,0 @@
|
|
1 |
-
import os, sys, torch, warnings, pdb
|
2 |
-
|
3 |
-
now_dir = os.getcwd()
|
4 |
-
sys.path.append(now_dir)
|
5 |
-
from json import load as ll
|
6 |
-
|
7 |
-
warnings.filterwarnings("ignore")
|
8 |
-
import librosa
|
9 |
-
import importlib
|
10 |
-
import numpy as np
|
11 |
-
import hashlib, math
|
12 |
-
from tqdm import tqdm
|
13 |
-
from lib.uvr5_pack.lib_v5 import spec_utils
|
14 |
-
from lib.uvr5_pack.utils import _get_name_params, inference
|
15 |
-
from lib.uvr5_pack.lib_v5.model_param_init import ModelParameters
|
16 |
-
import soundfile as sf
|
17 |
-
from lib.uvr5_pack.lib_v5.nets_new import CascadedNet
|
18 |
-
from lib.uvr5_pack.lib_v5 import nets_61968KB as nets
|
19 |
-
|
20 |
-
|
21 |
-
class _audio_pre_:
|
22 |
-
def __init__(self, agg, model_path, device, is_half):
|
23 |
-
self.model_path = model_path
|
24 |
-
self.device = device
|
25 |
-
self.data = {
|
26 |
-
# Processing Options
|
27 |
-
"postprocess": False,
|
28 |
-
"tta": False,
|
29 |
-
# Constants
|
30 |
-
"window_size": 512,
|
31 |
-
"agg": agg,
|
32 |
-
"high_end_process": "mirroring",
|
33 |
-
}
|
34 |
-
mp = ModelParameters("lib/uvr5_pack/lib_v5/modelparams/4band_v2.json")
|
35 |
-
model = nets.CascadedASPPNet(mp.param["bins"] * 2)
|
36 |
-
cpk = torch.load(model_path, map_location="cpu")
|
37 |
-
model.load_state_dict(cpk)
|
38 |
-
model.eval()
|
39 |
-
if is_half:
|
40 |
-
model = model.half().to(device)
|
41 |
-
else:
|
42 |
-
model = model.to(device)
|
43 |
-
|
44 |
-
self.mp = mp
|
45 |
-
self.model = model
|
46 |
-
|
47 |
-
def _path_audio_(self, music_file, ins_root=None, vocal_root=None, format="flac"):
|
48 |
-
if ins_root is None and vocal_root is None:
|
49 |
-
return "No save root."
|
50 |
-
name = os.path.basename(music_file)
|
51 |
-
if ins_root is not None:
|
52 |
-
os.makedirs(ins_root, exist_ok=True)
|
53 |
-
if vocal_root is not None:
|
54 |
-
os.makedirs(vocal_root, exist_ok=True)
|
55 |
-
X_wave, y_wave, X_spec_s, y_spec_s = {}, {}, {}, {}
|
56 |
-
bands_n = len(self.mp.param["band"])
|
57 |
-
# print(bands_n)
|
58 |
-
for d in range(bands_n, 0, -1):
|
59 |
-
bp = self.mp.param["band"][d]
|
60 |
-
if d == bands_n: # high-end band
|
61 |
-
(
|
62 |
-
X_wave[d],
|
63 |
-
_,
|
64 |
-
) = librosa.core.load(
|
65 |
-
music_file,
|
66 |
-
bp["sr"],
|
67 |
-
False,
|
68 |
-
dtype=np.float32,
|
69 |
-
res_type=bp["res_type"],
|
70 |
-
)
|
71 |
-
if X_wave[d].ndim == 1:
|
72 |
-
X_wave[d] = np.asfortranarray([X_wave[d], X_wave[d]])
|
73 |
-
else: # lower bands
|
74 |
-
X_wave[d] = librosa.core.resample(
|
75 |
-
X_wave[d + 1],
|
76 |
-
self.mp.param["band"][d + 1]["sr"],
|
77 |
-
bp["sr"],
|
78 |
-
res_type=bp["res_type"],
|
79 |
-
)
|
80 |
-
# Stft of wave source
|
81 |
-
X_spec_s[d] = spec_utils.wave_to_spectrogram_mt(
|
82 |
-
X_wave[d],
|
83 |
-
bp["hl"],
|
84 |
-
bp["n_fft"],
|
85 |
-
self.mp.param["mid_side"],
|
86 |
-
self.mp.param["mid_side_b2"],
|
87 |
-
self.mp.param["reverse"],
|
88 |
-
)
|
89 |
-
# pdb.set_trace()
|
90 |
-
if d == bands_n and self.data["high_end_process"] != "none":
|
91 |
-
input_high_end_h = (bp["n_fft"] // 2 - bp["crop_stop"]) + (
|
92 |
-
self.mp.param["pre_filter_stop"] - self.mp.param["pre_filter_start"]
|
93 |
-
)
|
94 |
-
input_high_end = X_spec_s[d][
|
95 |
-
:, bp["n_fft"] // 2 - input_high_end_h : bp["n_fft"] // 2, :
|
96 |
-
]
|
97 |
-
|
98 |
-
X_spec_m = spec_utils.combine_spectrograms(X_spec_s, self.mp)
|
99 |
-
aggresive_set = float(self.data["agg"] / 100)
|
100 |
-
aggressiveness = {
|
101 |
-
"value": aggresive_set,
|
102 |
-
"split_bin": self.mp.param["band"][1]["crop_stop"],
|
103 |
-
}
|
104 |
-
with torch.no_grad():
|
105 |
-
pred, X_mag, X_phase = inference(
|
106 |
-
X_spec_m, self.device, self.model, aggressiveness, self.data
|
107 |
-
)
|
108 |
-
# Postprocess
|
109 |
-
if self.data["postprocess"]:
|
110 |
-
pred_inv = np.clip(X_mag - pred, 0, np.inf)
|
111 |
-
pred = spec_utils.mask_silence(pred, pred_inv)
|
112 |
-
y_spec_m = pred * X_phase
|
113 |
-
v_spec_m = X_spec_m - y_spec_m
|
114 |
-
|
115 |
-
if ins_root is not None:
|
116 |
-
if self.data["high_end_process"].startswith("mirroring"):
|
117 |
-
input_high_end_ = spec_utils.mirroring(
|
118 |
-
self.data["high_end_process"], y_spec_m, input_high_end, self.mp
|
119 |
-
)
|
120 |
-
wav_instrument = spec_utils.cmb_spectrogram_to_wave(
|
121 |
-
y_spec_m, self.mp, input_high_end_h, input_high_end_
|
122 |
-
)
|
123 |
-
else:
|
124 |
-
wav_instrument = spec_utils.cmb_spectrogram_to_wave(y_spec_m, self.mp)
|
125 |
-
print("%s instruments done" % name)
|
126 |
-
if format in ["wav", "flac"]:
|
127 |
-
sf.write(
|
128 |
-
os.path.join(
|
129 |
-
ins_root,
|
130 |
-
"instrument_{}_{}.{}".format(name, self.data["agg"], format),
|
131 |
-
),
|
132 |
-
(np.array(wav_instrument) * 32768).astype("int16"),
|
133 |
-
self.mp.param["sr"],
|
134 |
-
) #
|
135 |
-
else:
|
136 |
-
path = os.path.join(
|
137 |
-
ins_root, "instrument_{}_{}.wav".format(name, self.data["agg"])
|
138 |
-
)
|
139 |
-
sf.write(
|
140 |
-
path,
|
141 |
-
(np.array(wav_instrument) * 32768).astype("int16"),
|
142 |
-
self.mp.param["sr"],
|
143 |
-
)
|
144 |
-
if os.path.exists(path):
|
145 |
-
os.system(
|
146 |
-
"ffmpeg -i %s -vn %s -q:a 2 -y"
|
147 |
-
% (path, path[:-4] + ".%s" % format)
|
148 |
-
)
|
149 |
-
if vocal_root is not None:
|
150 |
-
if self.data["high_end_process"].startswith("mirroring"):
|
151 |
-
input_high_end_ = spec_utils.mirroring(
|
152 |
-
self.data["high_end_process"], v_spec_m, input_high_end, self.mp
|
153 |
-
)
|
154 |
-
wav_vocals = spec_utils.cmb_spectrogram_to_wave(
|
155 |
-
v_spec_m, self.mp, input_high_end_h, input_high_end_
|
156 |
-
)
|
157 |
-
else:
|
158 |
-
wav_vocals = spec_utils.cmb_spectrogram_to_wave(v_spec_m, self.mp)
|
159 |
-
print("%s vocals done" % name)
|
160 |
-
if format in ["wav", "flac"]:
|
161 |
-
sf.write(
|
162 |
-
os.path.join(
|
163 |
-
vocal_root,
|
164 |
-
"vocal_{}_{}.{}".format(name, self.data["agg"], format),
|
165 |
-
),
|
166 |
-
(np.array(wav_vocals) * 32768).astype("int16"),
|
167 |
-
self.mp.param["sr"],
|
168 |
-
)
|
169 |
-
else:
|
170 |
-
path = os.path.join(
|
171 |
-
vocal_root, "vocal_{}_{}.wav".format(name, self.data["agg"])
|
172 |
-
)
|
173 |
-
sf.write(
|
174 |
-
path,
|
175 |
-
(np.array(wav_vocals) * 32768).astype("int16"),
|
176 |
-
self.mp.param["sr"],
|
177 |
-
)
|
178 |
-
if os.path.exists(path):
|
179 |
-
os.system(
|
180 |
-
"ffmpeg -i %s -vn %s -q:a 2 -y"
|
181 |
-
% (path, path[:-4] + ".%s" % format)
|
182 |
-
)
|
183 |
-
|
184 |
-
|
185 |
-
class _audio_pre_new:
|
186 |
-
def __init__(self, agg, model_path, device, is_half):
|
187 |
-
self.model_path = model_path
|
188 |
-
self.device = device
|
189 |
-
self.data = {
|
190 |
-
# Processing Options
|
191 |
-
"postprocess": False,
|
192 |
-
"tta": False,
|
193 |
-
# Constants
|
194 |
-
"window_size": 512,
|
195 |
-
"agg": agg,
|
196 |
-
"high_end_process": "mirroring",
|
197 |
-
}
|
198 |
-
mp = ModelParameters("lib/uvr5_pack/lib_v5/modelparams/4band_v3.json")
|
199 |
-
nout = 64 if "DeReverb" in model_path else 48
|
200 |
-
model = CascadedNet(mp.param["bins"] * 2, nout)
|
201 |
-
cpk = torch.load(model_path, map_location="cpu")
|
202 |
-
model.load_state_dict(cpk)
|
203 |
-
model.eval()
|
204 |
-
if is_half:
|
205 |
-
model = model.half().to(device)
|
206 |
-
else:
|
207 |
-
model = model.to(device)
|
208 |
-
|
209 |
-
self.mp = mp
|
210 |
-
self.model = model
|
211 |
-
|
212 |
-
def _path_audio_(
|
213 |
-
self, music_file, vocal_root=None, ins_root=None, format="flac"
|
214 |
-
): # 3个VR模型vocal和ins是反的
|
215 |
-
if ins_root is None and vocal_root is None:
|
216 |
-
return "No save root."
|
217 |
-
name = os.path.basename(music_file)
|
218 |
-
if ins_root is not None:
|
219 |
-
os.makedirs(ins_root, exist_ok=True)
|
220 |
-
if vocal_root is not None:
|
221 |
-
os.makedirs(vocal_root, exist_ok=True)
|
222 |
-
X_wave, y_wave, X_spec_s, y_spec_s = {}, {}, {}, {}
|
223 |
-
bands_n = len(self.mp.param["band"])
|
224 |
-
# print(bands_n)
|
225 |
-
for d in range(bands_n, 0, -1):
|
226 |
-
bp = self.mp.param["band"][d]
|
227 |
-
if d == bands_n: # high-end band
|
228 |
-
(
|
229 |
-
X_wave[d],
|
230 |
-
_,
|
231 |
-
) = librosa.core.load(
|
232 |
-
music_file,
|
233 |
-
bp["sr"],
|
234 |
-
False,
|
235 |
-
dtype=np.float32,
|
236 |
-
res_type=bp["res_type"],
|
237 |
-
)
|
238 |
-
if X_wave[d].ndim == 1:
|
239 |
-
X_wave[d] = np.asfortranarray([X_wave[d], X_wave[d]])
|
240 |
-
else: # lower bands
|
241 |
-
X_wave[d] = librosa.core.resample(
|
242 |
-
X_wave[d + 1],
|
243 |
-
self.mp.param["band"][d + 1]["sr"],
|
244 |
-
bp["sr"],
|
245 |
-
res_type=bp["res_type"],
|
246 |
-
)
|
247 |
-
# Stft of wave source
|
248 |
-
X_spec_s[d] = spec_utils.wave_to_spectrogram_mt(
|
249 |
-
X_wave[d],
|
250 |
-
bp["hl"],
|
251 |
-
bp["n_fft"],
|
252 |
-
self.mp.param["mid_side"],
|
253 |
-
self.mp.param["mid_side_b2"],
|
254 |
-
self.mp.param["reverse"],
|
255 |
-
)
|
256 |
-
# pdb.set_trace()
|
257 |
-
if d == bands_n and self.data["high_end_process"] != "none":
|
258 |
-
input_high_end_h = (bp["n_fft"] // 2 - bp["crop_stop"]) + (
|
259 |
-
self.mp.param["pre_filter_stop"] - self.mp.param["pre_filter_start"]
|
260 |
-
)
|
261 |
-
input_high_end = X_spec_s[d][
|
262 |
-
:, bp["n_fft"] // 2 - input_high_end_h : bp["n_fft"] // 2, :
|
263 |
-
]
|
264 |
-
|
265 |
-
X_spec_m = spec_utils.combine_spectrograms(X_spec_s, self.mp)
|
266 |
-
aggresive_set = float(self.data["agg"] / 100)
|
267 |
-
aggressiveness = {
|
268 |
-
"value": aggresive_set,
|
269 |
-
"split_bin": self.mp.param["band"][1]["crop_stop"],
|
270 |
-
}
|
271 |
-
with torch.no_grad():
|
272 |
-
pred, X_mag, X_phase = inference(
|
273 |
-
X_spec_m, self.device, self.model, aggressiveness, self.data
|
274 |
-
)
|
275 |
-
# Postprocess
|
276 |
-
if self.data["postprocess"]:
|
277 |
-
pred_inv = np.clip(X_mag - pred, 0, np.inf)
|
278 |
-
pred = spec_utils.mask_silence(pred, pred_inv)
|
279 |
-
y_spec_m = pred * X_phase
|
280 |
-
v_spec_m = X_spec_m - y_spec_m
|
281 |
-
|
282 |
-
if ins_root is not None:
|
283 |
-
if self.data["high_end_process"].startswith("mirroring"):
|
284 |
-
input_high_end_ = spec_utils.mirroring(
|
285 |
-
self.data["high_end_process"], y_spec_m, input_high_end, self.mp
|
286 |
-
)
|
287 |
-
wav_instrument = spec_utils.cmb_spectrogram_to_wave(
|
288 |
-
y_spec_m, self.mp, input_high_end_h, input_high_end_
|
289 |
-
)
|
290 |
-
else:
|
291 |
-
wav_instrument = spec_utils.cmb_spectrogram_to_wave(y_spec_m, self.mp)
|
292 |
-
print("%s instruments done" % name)
|
293 |
-
if format in ["wav", "flac"]:
|
294 |
-
sf.write(
|
295 |
-
os.path.join(
|
296 |
-
ins_root,
|
297 |
-
"instrument_{}_{}.{}".format(name, self.data["agg"], format),
|
298 |
-
),
|
299 |
-
(np.array(wav_instrument) * 32768).astype("int16"),
|
300 |
-
self.mp.param["sr"],
|
301 |
-
) #
|
302 |
-
else:
|
303 |
-
path = os.path.join(
|
304 |
-
ins_root, "instrument_{}_{}.wav".format(name, self.data["agg"])
|
305 |
-
)
|
306 |
-
sf.write(
|
307 |
-
path,
|
308 |
-
(np.array(wav_instrument) * 32768).astype("int16"),
|
309 |
-
self.mp.param["sr"],
|
310 |
-
)
|
311 |
-
if os.path.exists(path):
|
312 |
-
os.system(
|
313 |
-
"ffmpeg -i %s -vn %s -q:a 2 -y"
|
314 |
-
% (path, path[:-4] + ".%s" % format)
|
315 |
-
)
|
316 |
-
if vocal_root is not None:
|
317 |
-
if self.data["high_end_process"].startswith("mirroring"):
|
318 |
-
input_high_end_ = spec_utils.mirroring(
|
319 |
-
self.data["high_end_process"], v_spec_m, input_high_end, self.mp
|
320 |
-
)
|
321 |
-
wav_vocals = spec_utils.cmb_spectrogram_to_wave(
|
322 |
-
v_spec_m, self.mp, input_high_end_h, input_high_end_
|
323 |
-
)
|
324 |
-
else:
|
325 |
-
wav_vocals = spec_utils.cmb_spectrogram_to_wave(v_spec_m, self.mp)
|
326 |
-
print("%s vocals done" % name)
|
327 |
-
if format in ["wav", "flac"]:
|
328 |
-
sf.write(
|
329 |
-
os.path.join(
|
330 |
-
vocal_root,
|
331 |
-
"vocal_{}_{}.{}".format(name, self.data["agg"], format),
|
332 |
-
),
|
333 |
-
(np.array(wav_vocals) * 32768).astype("int16"),
|
334 |
-
self.mp.param["sr"],
|
335 |
-
)
|
336 |
-
else:
|
337 |
-
path = os.path.join(
|
338 |
-
vocal_root, "vocal_{}_{}.wav".format(name, self.data["agg"])
|
339 |
-
)
|
340 |
-
sf.write(
|
341 |
-
path,
|
342 |
-
(np.array(wav_vocals) * 32768).astype("int16"),
|
343 |
-
self.mp.param["sr"],
|
344 |
-
)
|
345 |
-
if os.path.exists(path):
|
346 |
-
os.system(
|
347 |
-
"ffmpeg -i %s -vn %s -q:a 2 -y"
|
348 |
-
% (path, path[:-4] + ".%s" % format)
|
349 |
-
)
|
350 |
-
|
351 |
-
|
352 |
-
if __name__ == "__main__":
|
353 |
-
device = "cuda"
|
354 |
-
is_half = True
|
355 |
-
# model_path = "uvr5_weights/2_HP-UVR.pth"
|
356 |
-
# model_path = "uvr5_weights/VR-DeEchoDeReverb.pth"
|
357 |
-
# model_path = "uvr5_weights/VR-DeEchoNormal.pth"
|
358 |
-
model_path = "uvr5_weights/DeEchoNormal.pth"
|
359 |
-
# pre_fun = _audio_pre_(model_path=model_path, device=device, is_half=True,agg=10)
|
360 |
-
pre_fun = _audio_pre_new(model_path=model_path, device=device, is_half=True, agg=10)
|
361 |
-
audio_path = "雪雪伴奏对消HP5.wav"
|
362 |
-
save_path = "opt"
|
363 |
-
pre_fun._path_audio_(audio_path, save_path, save_path)
|
|
|
|
|
|
|
|
|
|
|
|
|
|
|
|
|
|
|
|
|
|
|
|
|
|
|
|
|
|
|
|
|
|
|
|
|
|
|
|
|
|
|
|
|
|
|
|
|
|
|
|
|
|
|
|
|
|
|
|
|
|
|
|
|
|
|
|
|
|
|
|
|
|
|
|
|
|
|
|
|
|
|
|
|
|
|
|
|
|
|
|
|
|
|
|
|
|
|
|
|
|
|
|
|
|
|
|
|
|
|
|
|
|
|
|
|
|
|
|
|
|
|
|
|
|
|
|
|
|
|
|
|
|
|
|
|
|
|
|
|
|
|
|
|
|
|
|
|
|
|
|
|
|
|
|
|
|
|
|
|
|
|
|
|
|
|
|
|
|
|
|
|
|
|
|
|
|
|
|
|
|
|
|
|
|
|
|
|
|
|
|
|
|
|
|
|
|
|
|
|
|
|
|
|
|
|
|
|
|
|
|
|
|
|
|
|
|
|
|
|
|
|
|
|
|
|
|
|
|
|
|
|
|
|
|
|
|
|
|
|
|
|
|
|
|
|
|
|
|
|
|
|
|
|
|
|
|
|
|
|
|
|
|
|
|
|
|
|
|
|
|
|
|
|
|
|
|
|
|
|
|
|
|
|
|
|
|
|
|
|
|
|
|
|
|
|
|
|
|
|
|
|
|
|
|
|
|
|
|
|
|
|
|
|
|
|
|
|
|
|
|
|
|
|
|
|
|
|
|
|
|
|
|
|
|
|
|
|
|
|
|
|
|
|
|
|
|
|
|
|
|
|
|
|
|
|
|
|
|
|
|
|
|
|
|
|
|
|
|
|
|
|
|
|
|
|
|
|
|
|
|
|
|
|
|
|
|
|
|
|
|
|
|
|
|
|
|
|
|
|
|
|
|
|
|
|
|
|
|
|
|
|
|
|
|
|
|
|
|
|
|
|
|
|
|
|
|
|
|
|
|
|
|
|
|
|
|
|
|
|
|
|
|
|
|
|
|
|
|
|
|
|
|
|
|
|
|
|
|
|
|
|
|
|
|
|
|
|
|
|
|
|
|
|
|
|
|
|
|
|
|
|
|
|
|
|
|
|
|
|
|
|
|
|
|
|
|
|
|
|
|
|
|
|
|
|
|
|
|
|
|
|
|
|
|
|
|
|
|
|
|
|
|
|
|
|
|
|
|
|
|
|
|
|
|
|
|
|
|
|
|
|
|
|
|
|
|
|
|
|
|
|
|
|
|
|
|
|
|
|
|
|
|
|
|
|
|
|
|
|
|
|
|
|
|
|
|
|
|
|
|
|
|
|
|
|
|
|
|
|
|
|
|
|
|
|
|
|
|
|
|
|
|
|
|
|
|
|
|
|
|
|
|
|
|
|
|
|
|
|
|
|
|
|
|
|
|
|
|
|
|
|
|
|
|
|
|
|
|
|
|
|
|
|
|
|
|
|
|
|
|
|
|
|
|
|
|
|
|
|
|
|
|
|
|
|
|
|
|
|
|
|
|
|
|
|
|
|
|
|
|
|
|
|
|
|
|
|
|
|
|
|
|
|
|
|
|
|
|
|
|
|
|
|
|
|
|
|
|
|
|
|
|
|
|
|
|
|
|
|
|
|
|
|
|
|
|
spaces/Benson/text-generation/Examples/Descarga De La Edad De Hielo.md
DELETED
@@ -1,60 +0,0 @@
|
|
1 |
-
<br />
|
2 |
-
<h1>Edad de Hielo AR: Una aplicación genial para dar vida al mundo prehistórico</h1>
|
3 |
-
<p>¿Alguna vez te has preguntado cómo sería ver un mamut lanudo, un tigre de dientes de sable, o un oso de cara corta en la vida real? Bueno, ahora puedes, gracias a Ice Age AR, una aplicación gratuita de realidad aumentada que te permite dar vida a tus personajes favoritos de Ice Age: Collision Course. En este artículo, te contaremos todo lo que necesitas saber sobre esta increíble aplicación, cómo usarla y por qué deberías probarla. </p>
|
4 |
-
<h2>descarga de la edad de hielo</h2><br /><p><b><b>Download</b> ->>->>->> <a href="https://bltlly.com/2v6Ma0">https://bltlly.com/2v6Ma0</a></b></p><br /><br />
|
5 |
-
<h2>¿Qué es la edad de hielo AR? </h2>
|
6 |
-
<p>Ice Age AR es una aplicación que utiliza la tecnología de realidad aumentada para crear modelos 3D realistas de los animales de la Edad de Hielo en la pantalla de tu smartphone o tablet. La realidad aumentada (RA) es una tecnología que superpone imágenes digitales sobre el mundo real, creando una experiencia inmersiva e interactiva. Puede utilizar la cámara de su dispositivo para escanear marcadores especiales que desencadenan las secuencias de AR, y luego ver como los animales aparecen delante de usted. También puedes interactuar con ellos, pasearlos, tomar fotos y compartirlas con tus amigos. </p>
|
7 |
-
<h3>Las características de la aplicación</h3>
|
8 |
-
<p>Ice Age AR ofrece nueve experiencias diferentes de realidad aumentada, incluyendo:</p>
|
9 |
-
<ul>
|
10 |
-
<li>Conoce a Manny, Sid y Diego en AMAZING LIFE SIZE MODE. Puedes ver lo grandes que eran estos animales y compararlos contigo mismo. </li>
|
11 |
-
<li>Tome uno de sus héroes sub-cero para un paseo! Hacer rugir Diego o ver torpe Sid pose. Puedes controlar sus movimientos y expresiones con gestos simples. </li>
|
12 |
-
<li>Libera dos personajes a la vez en el modo interactivo DOS JUGADORES. Juega con un amigo y cada uno puede controlar un personaje en una escena interactiva. </li>
|
13 |
-
<li>Toma el control de Scrat en el ESPACIO. Ayúdalo a encontrar su bellota y volar su nave espacial evitando asteroides. </li>
|
14 |
-
</ul>
|
15 |
-
|
16 |
-
<h3>Los requisitos de la aplicación</h3>
|
17 |
-
<p>Esta aplicación está disponible para dispositivos Android e iOS, pero solo funciona en combinación con el libro "Ice Age: Collision Course - Bring the herd to life". El libro contiene los marcadores de realidad aumentada que activan la aplicación. Puede comprar el libro en línea o en su librería local. Alternativamente, puede descargar una página de prueba desde la propia aplicación. </p>
|
18 |
-
<p></p>
|
19 |
-
<p>Para usar la aplicación, necesita un teléfono inteligente o tableta compatible con una cámara orientada hacia atrás y una conexión a Internet. La aplicación es gratuita para descargar y usar, pero puede contener anuncios y compras en la aplicación. </p>
|
20 |
-
<h3>Los comentarios de la aplicación</h3>
|
21 |
-
<p>Ice Age AR ha recibido críticas positivas de usuarios que lo han probado. Estos son algunos de sus comentarios:</p>
|
22 |
-
<blockquote>"Esta aplicación es genial!!!!! Me encanta cómo se puede tomar una foto, pero no se puede ver el rebaño en la vida real, pero yo estaba un poco esperando que, pero es una buena aplicación lo recomiendo" - Thomas Y amigos Historias</blockquote>
|
23 |
-
<blockquote>"Me encantó, funcionó perfectamente, lástima que no poseo el libro." - Jake MF</blockquote>
|
24 |
-
<blockquote>"¡Prepárate para una increíble experiencia de Realidad Aumentada de la Era de Hielo! Descarga esta aplicación de realidad aumentada GRATIS para dar vida a tus personajes favoritos de Ice Age: Collision Course." - App Store descripción</blockquote>
|
25 |
-
<h2>¿Cómo usar la Edad de Hielo AR? </h2>
|
26 |
-
<p>Usar Ice Age AR es muy fácil y divertido. Solo sigue estos sencillos pasos:</p>
|
27 |
-
<h3>Descargar e instalar la aplicación <h3>Descargar e instalar la aplicación</h3>
|
28 |
-
<p>Lo primero que tienes que hacer es descargar e instalar la aplicación Ice Age AR en tu dispositivo. Puedes encontrarlo en Google Play Store o en App Store, dependiendo de tu dispositivo. Solo busca "Ice Age AR" y busca el icono de la aplicación que muestra Scrat sosteniendo una bellota. Toca el botón de instalación y espera a que la aplicación se descargue e instale. </p>
|
29 |
-
<h3>Encuentra los marcadores AR</h3>
|
30 |
-
|
31 |
-
<h3>Iniciar la aplicación y escanear los marcadores</h3>
|
32 |
-
<p>Una vez que tenga la aplicación y los marcadores de RA listos, puede iniciar la aplicación y comenzar a escanear los marcadores. Para ello, es necesario abrir la aplicación y toque en el "Escanear" botón. Luego, debes apuntar la cámara de tu dispositivo a uno de los marcadores de RA y esperar unos segundos. La aplicación detectará automáticamente el marcador y lanzará la secuencia AR correspondiente. Verá una pantalla de carga con algunas instrucciones y consejos, y luego verá que el animal aparece en su pantalla. </p>
|
33 |
-
<h3>Interactuar con los caracteres</h3>
|
34 |
-
<p>Después de ver el animal en tu pantalla, puedes interactuar con él de diferentes maneras. Puedes usar tus dedos para tocar, deslizar, pellizcar o girar la pantalla para cambiar el ángulo, el tamaño o la posición del animal. También puede usar comandos de voz o gestos para hacer que el animal se mueva o haga sonidos. Por ejemplo, puedes decir "Rugir" para hacer rugir a Diego, o "Saltar" para hacer saltar a Sid. También puedes tocar diferentes partes del cuerpo del animal para ver lo que sucede. </p>
|
35 |
-
<h3>Toma fotos y compártelas</h3>
|
36 |
-
<p>Una de las mejores características de Ice Age AR es que puedes tomar fotos de ti mismo con los animales y compartirlas con tus amigos. Para hacer esto, debe tocar el icono de la cámara en la esquina inferior derecha de la pantalla. Esto abrirá un modo selfie donde puedes verte a ti mismo y al animal en la misma pantalla. Puede ajustar su posición y posar como desee, y luego toque en el botón de obturación para tomar una foto. La foto se guardará en la galería de tu dispositivo, y también puedes compartirla directamente desde la aplicación por correo electrónico, redes sociales o aplicaciones de mensajería. </p>
|
37 |
-
<h2>¿Por qué usar Ice Age AR? </h2>
|
38 |
-
<p>Ice Age AR no es solo una aplicación divertida y entretenida, sino también educativa y creativa. Estas son algunas de las razones por las que deberías usarla:</p>
|
39 |
-
<h3>Aprende sobre los animales de la Edad de Hielo</h3>
|
40 |
-
|
41 |
-
<h3>Diviértete con tus amigos y familiares</h3>
|
42 |
-
<p>Ice Age AR también es una gran manera de divertirse con sus amigos y familiares. Pueden jugar juntos en el modo de dos jugadores, donde cada uno puede controlar un personaje en una escena interactiva. También puedes tomar fotos junto con tus personajes favoritos y compartirlas en línea. Incluso puedes crear tus propias historias o escenarios usando Ice Age AR como una herramienta para la imaginación y la creatividad. </p>
|
43 |
-
<h3>Experimenta la realidad aumentada</h3>
|
44 |
-
<p>Ice Age AR también es una gran manera de experimentar la tecnología de realidad aumentada, que se está volviendo más popular y accesible en los últimos años. La realidad aumentada es una tecnología que mejora su percepción de la realidad mediante la adición de elementos digitales a la misma. Puede crear efectos increíbles que te hacen sentir como si estuvieras en otro mundo o tiempo. También puede ofrecer nuevas posibilidades de aprendizaje, entretenimiento, comunicación y arte. </p>
|
45 |
-
<h2>Conclusión</h2>
|
46 |
-
<p>Ice Age AR es una aplicación genial que da vida al mundo prehistórico usando tecnología de realidad aumentada. Te permite interactuar con modelos 3D realistas de animales de la Edad de Hielo en la pantalla de tu smartphone o tablet. También puedes aprender sobre ellos, divertirte con ellos, tomar fotos con ellos y compartirlas con tus amigos. Todo lo que necesitas es un dispositivo compatible, un dispositivo compatible, la aplicación y el libro con los marcadores AR. También puede descargar una página de prueba de la propia aplicación para probarlo. Si eres un fanático de la Edad de Hielo o simplemente tienes curiosidad por el mundo prehistórico, definitivamente deberías probar Ice Age AR. Es una app genial que te hará sentir como si estuvieras en otra época. </p>
|
47 |
-
<h2>Preguntas frecuentes</h2>
|
48 |
-
<p>Aquí están algunas de las preguntas más frecuentes sobre la Edad de Hielo AR:</p>
|
49 |
-
<h3>Q: ¿Es segura la RA de la edad de hielo para los niños? </h3>
|
50 |
-
|
51 |
-
<h3>Q: ¿Cómo puedo obtener más marcadores AR? </h3>
|
52 |
-
<p>A: La única manera de conseguir más marcadores de AR es comprar el libro "Ice Age: Collision Course - Bring the herd to life". El libro tiene 32 páginas, cada una con un marcador RA diferente. Puede comprar el libro en línea o en su librería local. Alternativamente, puede descargar una página de prueba de la propia aplicación, que cuenta con Manny, Sid y Diego.</p>
|
53 |
-
<h3>Q: ¿Cómo puedo cambiar entre diferentes idiomas? </h3>
|
54 |
-
<p>A: Ice Age AR admite dos idiomas: inglés y francés. Puede cambiar entre ellos pulsando en el icono de configuración en la esquina superior izquierda de la pantalla. A continuación, puede seleccionar su idioma preferido en el menú desplegable. </p>
|
55 |
-
<h3>Q: ¿Cómo puedo quitar anuncios o desbloquear funciones premium? </h3>
|
56 |
-
<p>A: Ice Age AR es gratis de descargar y usar, pero puede contener anuncios y compras en la aplicación. Puede eliminar anuncios o desbloquear funciones premium tocando el icono de la tienda en la esquina superior derecha de la pantalla. Luego, puede elegir entre diferentes opciones, como eliminar anuncios por $0.99 o desbloquear todos los caracteres por $4.99. </p>
|
57 |
-
<h3>Q: ¿Cómo puedo contactar a los desarrolladores o reportar un problema? </h3>
|
58 |
-
<p>A: Si tienes alguna pregunta, comentario, o problemas con Ice Age AR, puedes contactar a los desarrolladores tocando el icono de información en la esquina superior izquierda de la pantalla. Luego, puede seleccionar "Contáctenos" o "Reportar un problema" en el menú. También puede visitar su sitio web en www.iceagear.com o seguirlos en Facebook o Twitter.</p> 64aa2da5cf<br />
|
59 |
-
<br />
|
60 |
-
<br />
|
|
|
|
|
|
|
|
|
|
|
|
|
|
|
|
|
|
|
|
|
|
|
|
|
|
|
|
|
|
|
|
|
|
|
|
|
|
|
|
|
|
|
|
|
|
|
|
|
|
|
|
|
|
|
|
|
|
|
|
|
|
|
|
|
|
|
|
|
|
|
|
|
|
|
|
|
|
|
|
|
|
|
|
|
|
|
|
|
|
|
|
|
|
|
|
|
|
|
|
|
|
|
|
|
|
|
|
|
|
|
|
|
|
|
|
|
|
|
|
|
|
spaces/Big-Web/MMSD/env/Lib/site-packages/pip/_vendor/cachecontrol/caches/__init__.py
DELETED
@@ -1,9 +0,0 @@
|
|
1 |
-
# SPDX-FileCopyrightText: 2015 Eric Larson
|
2 |
-
#
|
3 |
-
# SPDX-License-Identifier: Apache-2.0
|
4 |
-
|
5 |
-
from .file_cache import FileCache, SeparateBodyFileCache
|
6 |
-
from .redis_cache import RedisCache
|
7 |
-
|
8 |
-
|
9 |
-
__all__ = ["FileCache", "SeparateBodyFileCache", "RedisCache"]
|
|
|
|
|
|
|
|
|
|
|
|
|
|
|
|
|
|
|
|