How to Activate AutoCAD 2009 with Xforce Keygen 32 Bit
-
AutoCAD 2009 is a popular software for designing and drafting in various fields, such as architecture, engineering, construction and more. However, to use AutoCAD 2009, you need to activate it with a valid license key. Otherwise, you will not be able to access all the features and functions of the software.
-
One way to activate AutoCAD 2009 is to use Xforce keygen 32 bit. Xforce keygen is a tool that can generate license keys for various software products, including AutoCAD 2009. Xforce keygen 32 bit is compatible with Windows 32 bit operating systems.
In this article, we will show you how to activate AutoCAD 2009 with Xforce keygen 32 bit in a few simple steps.
-
Step 1: Download and install AutoCAD 2009
-
The first step is to download and install AutoCAD 2009 on your computer. You can download AutoCAD 2009 from the official website of Autodesk or from other sources. Make sure you download the correct version for your operating system (32 bit or 64 bit).
-
To install AutoCAD 2009, follow the installation wizard and accept the terms and conditions. When prompted for a serial number and a product key, enter any numbers you want. You will activate them later with Xforce keygen.
-
Step 2: Download and run Xforce keygen 32 bit
-
The next step is to download and run Xforce keygen 32 bit. You can download Xforce keygen 32 bit from various websites or torrent sites. However, be careful of viruses and malware that may harm your computer. Always scan the files before opening them.
-
To run Xforce keygen 32 bit, right-click on the file and select "Run as administrator". A window will open with a list of software products. Select "AutoCAD 2009" from the list and click on "Generate". A license key will be generated for you.
-
Step 3: Activate AutoCAD 2009 with the license key
-
The final step is to activate AutoCAD 2009 with the license key generated by Xforce keygen. To do this, open AutoCAD 2009 and click on "Activate" in the upper right corner. A window will pop up asking you to enter the serial number and the product key.
-
-
Enter the serial number and the product key that you entered during the installation process. Then click on "Next". Another window will appear asking you to select an activation method. Choose "I have an activation code from Autodesk" and click on "Next".
-
A request code will be displayed on the screen. Copy this code and paste it into Xforce keygen in the "Request" field. Then click on "Calculate". An activation code will be generated for you. Copy this code and paste it into AutoCAD in the "Activation" field. Then click on "Next".
-
Congratulations! You have successfully activated AutoCAD 2009 with Xforce keygen 32 bit. You can now enjoy all the features and functions of AutoCAD 2009 without any limitations.
-
Conclusion
-
Activating AutoCAD 2009 with Xforce keygen 32 bit is a simple and easy process that can save you time and money. However, it is not a legal or ethical way to use AutoCAD 2009. Xforce keygen is a crack tool that violates the terms and conditions of Autodesk. Using it may expose you to legal risks and security threats.
-
If you want to use AutoCAD 2009 legally and safely, we recommend you to buy a genuine license from Autodesk or its authorized dealers. This way, you can support the developers of AutoCAD and get access to technical support and updates.
ddb901b051
-
-
\ No newline at end of file
diff --git a/spaces/1acneusushi/gradio-2dmoleculeeditor/data/Biztree Business in a Box Product Key Crack The Ultimate Solution for Your Business Needs.md b/spaces/1acneusushi/gradio-2dmoleculeeditor/data/Biztree Business in a Box Product Key Crack The Ultimate Solution for Your Business Needs.md
deleted file mode 100644
index 183e9bc96381facc4c210335749f2f0011cb08f5..0000000000000000000000000000000000000000
--- a/spaces/1acneusushi/gradio-2dmoleculeeditor/data/Biztree Business in a Box Product Key Crack The Ultimate Solution for Your Business Needs.md
+++ /dev/null
@@ -1,100 +0,0 @@
-
-
Warcraft III: Reign of Chaos No CD Crack Download
-
Introduction
-
If you are a fan of real-time strategy games, you probably have played or heard of Warcraft III: Reign of Chaos, one of the most popular and influential titles in the genre. Released in 2002 by Blizzard Entertainment, Warcraft III: Reign of Chaos is the third installment in the Warcraft series, which also includes the massively multiplayer online role-playing game World of Warcraft. In this game, you can choose from four different races: Humans, Orcs, Night Elves, and Undead, and lead them in epic battles across the fantasy world of Azeroth.
However, if you want to play Warcraft III: Reign of Chaos on your PC, you will need to have the original CD inserted in your drive every time you launch the game. This can be inconvenient and annoying for many reasons, such as having to swap CDs, wasting disk space, risking damage to your CD, or losing your CD altogether. That's why many players look for a way to play Warcraft III: Reign of Chaos without the CD, by using a no CD crack.
-
A no CD crack is a modified version of the game's executable file that bypasses the CD check and allows you to run the game without inserting the CD. In this article, we will show you how to download and install a no CD crack for Warcraft III: Reign of Chaos, as well as the benefits and risks of using it.
-
How to download and install a no CD crack?
-
Before you download and install a no CD crack for Warcraft III: Reign of Chaos, you will need to have the game installed on your PC. You can either use the original CD or download the game from Blizzard's official website. You will also need to update the game to the latest version (1.27b) to ensure compatibility with the no CD crack.
-
Once you have the game installed and updated, you can follow these steps to download and install a no CD crack:
-
-
Go to a reputable website that offers no CD cracks for various games, such as GameCopyWorld or MegaGames.
-
Search for Warcraft III: Reign of Chaos and find the no CD crack that matches your game version and language.
-
Download the no CD crack file and extract it using a program like WinRAR or 7-Zip.
-
Copy the extracted file (usually named war3.exe) and paste it into your game folder, where you installed Warcraft III: Reign of Chaos. You may need to overwrite the existing file.
-
Run the game from the no CD crack file (war3.exe) and enjoy playing without the CD.
-
-
Benefits of using a no CD crack
-
Using a no CD crack for Warcraft III: Reign of Chaos can have several benefits for you as a player. Here are some of them:
-
Play without the CD
- the CD every time you launch the game. This can save you time and hassle, especially if you have multiple games that require CDs. You can also play the game on any PC that has the game installed, without carrying the CD with you.
-
Save disk space
-
Another benefit of using a no CD crack is that you can save disk space on your PC. When you install Warcraft III: Reign of Chaos from the CD, the game will copy some files from the CD to your hard drive. These files can take up several hundred megabytes of space, depending on your game version and language. By using a no CD crack, you can delete these files and free up some space on your hard drive.
-
Avoid scratches and damages
-
A no CD crack can also help you avoid scratches and damages to your CD. CDs are fragile and can be easily scratched or broken by accidents or mishandling. If your CD gets damaged, you may not be able to play the game or install it on another PC. By using a no CD crack, you can protect your CD from wear and tear and keep it in good condition.
-
Backup your game files
-
Finally, a no CD crack can allow you to backup your game files and restore them in case of any problems. Sometimes, your game files may get corrupted or deleted by viruses, malware, or human errors. If this happens, you may lose your progress, settings, or custom maps. By using a no CD crack, you can copy your game folder to another location or device and restore it if needed.
-
warcraft 3 reign of chaos no cd patch
-warcraft 3 roc no cd fixed exe
-warcraft 3 reign of chaos no cd key
-warcraft 3 reign of chaos no cd loader
-warcraft 3 reign of chaos no cd gamecopyworld
-warcraft 3 reign of chaos no cd gameburnworld
-warcraft 3 reign of chaos no cd iso
-warcraft 3 reign of chaos no cd archive.org
-warcraft 3 reign of chaos no cd free download
-warcraft 3 reign of chaos no cd crack mac
-warcraft 3 reign of chaos no cd crack windows 10
-warcraft 3 reign of chaos no cd crack linux
-warcraft 3 reign of chaos no cd crack lutris
-warcraft 3 reign of chaos no cd crack repack-mechanicz
-warcraft 3 reign of chaos no cd cheat
-warcraft 3 reign of chaos no cd trainer
-warcraft 3 reign of chaos no cd serial
-warcraft 3 reign of chaos no cd bnet loader
-warcraft 3 reign of chaos no cd resolution hacker
-warcraft 3 reign of chaos no cd widecraft
-warcraft iii the frozen throne no cd crack download
-warcraft iii complete edition no cd crack download
-warcraft iii original isos no cd crack download
-warcraft iii reforged no cd crack download
-warcraft iii classic edition no cd crack download
-how to install warcraft iii reign of chaos no cd crack
-how to play warcraft iii reign of chaos no cd crack online
-how to update warcraft iii reign of chaos no cd crack
-how to fix warcraft iii reign of chaos no cd crack errors
-how to uninstall warcraft iii reign of chaos no cd crack
-where to find warcraft iii reign of chaos no cd crack safe
-where to buy warcraft iii reign of chaos original game
-where to download warcraft iii reign of chaos full version
-where to watch warcraft iii reign of chaos gameplay videos
-where to read warcraft iii reign of chaos reviews and guides
-why play warcraft iii reign of chaos without a cd
-why use a crack for warcraft iii reign of chaos game
-why is warcraft iii reign of chaos still popular in 2021
-what is the best version of warcraft iii reign of chaos to play
-what is the difference between warcraft iii reign of chaos and reforged
-what is the story of warcraft iii reign of chaos and the frozen throne
-what are the system requirements for warcraft iii reign of chaos on pc and mac
-what are the cheat codes for warcraft iii reign of chaos single player mode
-what are the best mods for warcraft iii reign of chaos and the frozen throne
-what are the best maps for warcraft iii reign of chaos multiplayer mode
-what are the best strategies for playing as each race in warcraft iii reign of chaos
-what are the best units and heroes for each race in warcraft iii reign of chaos
-what are the best custom campaigns for warcraft iii reign of chaos and the frozen throne
-what are the best tips and tricks for mastering warcraft iii reign of chaos gameplay
-
Risks of using a no CD crack
-
While using a no CD crack for Warcraft III: Reign of Chaos can have some benefits, it also comes with some risks that you should be aware of. Here are some of them:
-
Legal issues
-
The first and most important risk of using a no CD crack is that it may be illegal in your country or region. According to Blizzard's End User License Agreement (EULA), you are not allowed to modify, copy, distribute, or reverse engineer the game or any part of it. A no CD crack is considered a modification of the game's executable file and may violate Blizzard's intellectual property rights. If you use a no CD crack, you may face legal consequences such as fines or lawsuits from Blizzard or other authorities.
-
Virus and malware infections
-
Another risk of using a no CD crack is that it may contain viruses or malware that can harm your PC or steal your personal information. Some websites that offer no CD cracks may be malicious or fraudulent and may infect your PC with spyware, ransomware, keyloggers, or other types of malware. These malware can slow down your PC, damage your files, monitor your online activities, or steal your passwords, credit card numbers, or other sensitive data. To avoid this risk, you should only download no CD cracks from reputable and trusted websites and scan them with an antivirus program before installing them.
-
Compatibility and performance problems
- game's executable file that bypasses the CD check and allows you to run the game without inserting the CD. It can have some benefits, such as playing without the CD, saving disk space, avoiding scratches and damages, and backing up your game files. However, it can also have some risks, such as legal issues, virus and malware infections, compatibility and performance problems, and online multiplayer restrictions.
-
How to download and install a no CD crack for Warcraft III: Reign of Chaos?
-
To download and install a no CD crack for Warcraft III: Reign of Chaos, you will need to have the game installed and updated on your PC. Then, you can follow these steps:
-
-
Go to a reputable website that offers no CD cracks for various games, such as GameCopyWorld or MegaGames.
-
Search for Warcraft III: Reign of Chaos and find the no CD crack that matches your game version and language.
-
Download the no CD crack file and extract it using a program like WinRAR or 7-Zip.
-
Copy the extracted file (usually named war3.exe) and paste it into your game folder, where you installed Warcraft III: Reign of Chaos. You may need to overwrite the existing file.
-
Run the game from the no CD crack file (war3.exe) and enjoy playing without the CD.
-
-
Is using a no CD crack legal?
-
Using a no CD crack may be illegal in your country or region. According to Blizzard's End User License Agreement (EULA), you are not allowed to modify, copy, distribute, or reverse engineer the game or any part of it. A no CD crack is considered a modification of the game's executable file and may violate Blizzard's intellectual property rights. If you use a no CD crack, you may face legal consequences such as fines or lawsuits from Blizzard or other authorities.
-
Can I play online multiplayer mode with a no CD crack?
-
You may not be able to play online multiplayer mode with a no CD crack. Warcraft III: Reign of Chaos has a built-in online multiplayer mode that allows you to play with or against other players on Blizzard's Battle.net service. However, to access this mode, you will need to have a valid CD key that is registered on your Battle.net account. A no CD crack may not work with Battle.net or may be detected as a cheat or hack by Blizzard's anti-cheat system. If this happens, you may not be able to join or host online games or you may get banned from Battle.net permanently. To avoid this risk, you should only use a no CD crack for offline single-player mode and use your original CD and CD key for online multiplayer mode.
- 0a6ba089eb
-
-
\ No newline at end of file
diff --git a/spaces/1acneusushi/gradio-2dmoleculeeditor/data/Descargar gratis imagenes movibles para easyworship consejos y recursos para mejorar tus proyecciones.md b/spaces/1acneusushi/gradio-2dmoleculeeditor/data/Descargar gratis imagenes movibles para easyworship consejos y recursos para mejorar tus proyecciones.md
deleted file mode 100644
index 7c4624627946d8fc17835efbb8554b9012db0c10..0000000000000000000000000000000000000000
--- a/spaces/1acneusushi/gradio-2dmoleculeeditor/data/Descargar gratis imagenes movibles para easyworship consejos y recursos para mejorar tus proyecciones.md
+++ /dev/null
@@ -1,116 +0,0 @@
-
-
Descargar gratis imagenes movibles para easyworship
-
Si eres una persona que se encarga de preparar y proyectar las presentaciones multimedia en tu iglesia, seguramente te interesa saber cómo puedes descargar gratis imagenes movibles para easyworship. En este artículo te vamos a explicar qué es easyworship, por qué usarlo, dónde encontrar y cómo descargar imagenes movibles para easyworship, cómo usarlas en las presentaciones y qué beneficios tiene usarlas. Así que sigue leyendo y descubre cómo puedes mejorar tus presentaciones con estas imágenes tan especiales.
-
descargar gratis imagenes movibles para easyworship
Easyworship es un programa de presentación multimedia para iglesias
-
Easyworship es un software diseñado especialmente para crear y proyectar presentaciones multimedia en las iglesias. Con este programa puedes combinar imágenes, videos, textos, canciones y otros elementos para crear presentaciones dinámicas y profesionales que acompañen tus cultos, sermones, eventos o actividades. Easyworship es fácil de usar, compatible con varios formatos de archivos y adaptable a diferentes pantallas y proyectores.
-
Easyworship permite crear presentaciones dinámicas y atractivas con imágenes, videos, textos y canciones
-
Con easyworship puedes crear presentaciones que se adapten al tema, al estilo y al público de cada ocasión. Puedes elegir entre una gran variedad de plantillas prediseñadas o crear tus propias plantillas personalizadas. Puedes agregar imágenes, videos, textos y canciones desde tu computadora o desde internet. Puedes editar el contenido, el orden, el tiempo y la transición de cada elemento. Puedes añadir efectos especiales como sombras, reflejos, bordes o animaciones. Puedes sincronizar las letras de las canciones con la música o con los videos. Puedes mostrar versículos bíblicos o citas inspiradoras con diferentes fuentes y colores.
-
Easyworship ofrece una gran variedad de opciones para personalizar y adaptar las presentaciones a cada ocasión
-
Con easyworship puedes personalizar y adaptar tus presentaciones según tus necesidades y preferencias. Puedes configurar el tamaño, la resolución, el brillo y el contraste de la pantalla o del proyector. Puedes ajustar el volumen, el balance y el ecualizador del sonido. Puedes crear listas de reproducción con diferentes presentaciones o elementos. Puedes programar las presentaciones para que se inicien automáticamente o manualmente. Puedes controlar las presentaciones desde tu computadora o desde un dispositivo móvil.
-
¿Dónde encontrar y cómo descargar imagenes movibles para easyworship?
-
Hay muchas fuentes en internet que ofrecen imagenes movibles para easyworship de forma gratuita
-
Si quieres descargar gratis imagenes movibles para easyworship, puedes encontrar muchas opciones en internet. Hay sitios web que ofrecen cientos o miles de imágenes animadas con diferentes temáticas, estilos y calidades. Algunas imágenes son libres de derechos de autor y otras requieren dar crédito al autor o a la fuente. Algunas imágenes son gratuitas y otras requieren una suscripción o un pago.
-
Algunas de las fuentes más populares son Pinterest, Recursos Bíblicos y EasyWorship Media
-
A continuación te mencionamos algunas de las fuentes más populares donde puedes descargar gratis imagenes movibles para easyworship:
-
-
Pinterest: Es una red social donde puedes encontrar miles de imágenes animadas sobre diversos temas como naturaleza, arte, religión, humor, etc. Solo tienes que buscar "fondos animados para easyworship" o "imagenes movibles para easyworship" en el buscador y verás los resultados. Para descargar una imagen solo tienes que hacer clic derecho sobre ella y elegir la opción "guardar imagen como".
-
Recursos Bíblicos: Es un sitio web que ofrece más de 200 imágenes animadas para easyworship con temática cristiana. Estas imágenes están hechas a una resolución apropiada para el programa y pueden ser utilizadas de diversas formas en las presentaciones de la iglesia. Para descargar un paquete de imágenes solo tienes que hacer clic en el enlace "descargar" que aparece debajo de cada imagen.
-
EasyWorship Media: Es la tienda oficial de medios de easyworship donde puedes encontrar una gran selección de fondos animados para tus presentaciones. Estas imágenes son creadas por talentosos artistas cristianos que ofrecen su trabajo de forma gratuita o a un precio accesible. Para descargar una imagen solo tienes que hacer clic en el botón "download" que aparece debajo de cada imagen.
-
-
Para descargar las imagenes movibles para easyworship se debe seguir los pasos que indica cada sitio web
-
Para descargar gratis imagenes movibles para easyworship se debe seguir los pasos que indica cada sitio web donde se encuentran las imágenes. En general estos pasos son:
-
-
Ingresar al sitio web donde se encuentran las imágenes.
-
Buscar las imágenes que se desean descargar usando el buscador o navegando por las categorías.
-
Seleccionar la imagen que se desea descargar haciendo clic sobre ella.
-
Verificar si la imagen tiene algún requisito o condición como dar crédito al autor o pagar una cuota.
-
Hacer clic derecho sobre la imagen y elegir la opción "guardar imagen como" o hacer clic en el botón "descargar" si lo tiene.
-
Elegir la carpeta donde se desea guardar la imagen en la computadora.
-
Repetir el proceso con todas las imágenes que se deseen descargar.
-
-
¿Cómo usar las imagenes movibles para easyworship en las presentaciones?
-
Las imagenes movibles para easyworship se pueden usar como fondos, transiciones, ilustraciones o adornos
-
Las imagenes movibles para easyworship se pueden usar de varias formas en las presentaciones dependiendo del efecto que se quiera lograr. Algunas formas son:
-
descargar gratis fondos animados para easyworship
-descargar gratis imagenes cristianas movibles para easyworship
-descargar gratis videos movibles para easyworship
-descargar gratis imagenes movibles de navidad para easyworship
-descargar gratis imagenes movibles de paisajes para easyworship
-descargar gratis imagenes movibles con frases para easyworship
-descargar gratis imagenes movibles de amor para easyworship
-descargar gratis imagenes movibles de dios para easyworship
-descargar gratis imagenes movibles de flores para easyworship
-descargar gratis imagenes movibles de animales para easyworship
-descargar gratis imagenes movibles de fuego para easyworship
-descargar gratis imagenes movibles de agua para easyworship
-descargar gratis imagenes movibles de cielo para easyworship
-descargar gratis imagenes movibles de musica para easyworship
-descargar gratis imagenes movibles de angeles para easyworship
-descargar gratis imagenes movibles de cruz para easyworship
-descargar gratis imagenes movibles de biblia para easyworship
-descargar gratis imagenes movibles de jesus para easyworship
-descargar gratis imagenes movibles de oracion para easyworship
-descargar gratis imagenes movibles de alabanza para easyworship
-descargar gratis imagenes movibles de adoracion para easyworship
-descargar gratis imagenes movibles de bendicion para easyworship
-descargar gratis imagenes movibles de esperanza para easyworship
-descargar gratis imagenes movibles de fe para easyworship
-descargar gratis imagenes movibles de gracia para easyworship
-descargar gratis imagenes movibles de paz para easyworship
-descargar gratis imagenes movibles de alegria para easyworship
-descargar gratis imagenes movibles de amor de dios para easyworship
-descargar gratis imagenes movibles de palabra de dios para easyworship
-descargar gratis imagenes movibles de promesas de dios para easyworship
-descargar gratis imagenes movibles de milagros de dios para easyworship
-descargar gratis imagenes movibles de testimonios de dios para easyworship
-descargar gratis imagenes movibles de iglesia para easyworship
-descargar gratis imagenes movibles de culto para easyworship
-descargar gratis imagenes movibles de predicacion para easyworship
-descargar gratis imagenes movibles de enseñanza para easyworship
-descargar gratis imagenes movibles de estudio biblico para easyworship
-descargar gratis imagenes movibles de escuela dominical para easyworship
-descargar gratis imagenes movibles de grupo pequeño para easyworship
-descargar gratis imagenes movibles de misiones para easyworship
-descargar gratis imagenes movibles de evangelismo para easyworship
-descargar gratis imagenes movibles de servicio social para easyworship
-descargar gratis imagenes movibles de ayuno y oracion para easyworship
-descargar gratis imagenes movibles de retiro espiritual para easyworship
-descargar gratis imagenes movibles de campamento cristiano para easyworship
-descargar gratis imagenes movibles de celebracion cristiana para easyworship
-descargar gratis imagenes movibles de cumpleaños cristiano para easyworship
-descargar gratis imagenes movibles de boda cristiana para easyworship
-descargar gratis imagenes movibles de bautismo cristiano para easyworship
-descargar gratis imagenes movibles de santa cena cristiana para easywors
-
-
Para usar las imagenes movibles para easyworship se debe importarlas al programa desde la carpeta donde se guardaron en la computadora. Para importar una imagen se debe hacer clic en el botón "import" que aparece en la parte superior del panel de medios. Luego se debe buscar la carpeta donde se encuentra la imagen y seleccionarla. La imagen aparecerá en el panel de medios y se podrá arrastrar a los paneles de fondos, transiciones o diapositivas según el uso que se le quiera dar.
-
Se puede ajustar el tamaño, la posición, la velocidad y el efecto de las imagenes movibles para easyworship según el gusto y el propósito
-
Se puede ajustar el tamaño, la posición, la velocidad y el efecto de las imagenes movibles para easyworship según el gusto y el propósito que se tenga. Para ajustar el tamaño y la posición de una imagen se debe hacer clic sobre ella y arrastrar los bordes o las esquinas hasta obtener el tamaño y la posición deseada. Para ajustar la velocidad y el efecto de una imagen se debe hacer clic derecho sobre ella y elegir la opción "propiedades". Se abrirá una ventana donde se podrá modificar la duración, el ciclo, el retraso y el efecto de la imagen.
-
¿Qué beneficios tiene usar imagenes movibles para easyworship en las presentaciones?
-
Las imagenes movibles para easyworship le dan vida y movimiento a las presentaciones
-
Las imagenes movibles para easyworship le dan vida y movimiento a las presentaciones al crear un ambiente dinámico y variado. Las imágenes animadas pueden expresar diferentes sensaciones, emociones, mensajes o conceptos de forma visual y creativa. Las imágenes animadas pueden complementar o contrastar con el contenido verbal o musical de la presentación. Las imágenes animadas pueden generar interés, curiosidad, sorpresa o admiración en los espectadores.
-
Las imagenes movibles para easyworship captan la atención y el interés de los espectadores
-
Las imagenes movibles para easyworship captan la atención y el interés de los espectadores al estimular sus sentidos y su memoria. Las imágenes animadas son más llamativas y memorables que las imágenes estáticas o los textos. Las imágenes animadas pueden ayudar a los espectadores a concentrarse, a comprender, a recordar o a aplicar lo que ven o escuchan en la presentación. Las imágenes animadas pueden motivar a los espectadores a participar, a interactuar o a responder a la presentación.
-
Las imagenes movibles para easyworship transmiten mensajes y emociones de forma visual y creativa
-
Las imagenes movibles para easyworship transmiten mensajes y emociones de forma visual y creativa al usar diferentes elementos como colores, formas, texturas, sonidos o movimientos. Las imágenes animadas pueden representar diferentes temas como naturaleza, arte, religión, humor, etc. Las imágenes animadas pueden reflejar diferentes estados de ánimo como alegría, paz, amor, fe, etc. Las imágenes animadas pueden inspirar diferentes acciones como alabar, orar, servir, etc.
-
Conclusión
-
En conclusión, descargar gratis imagenes movibles para easyworship es una forma sencilla y efectiva de mejorar tus presentaciones multimedia en tu iglesia. Con estas imágenes puedes crear presentaciones dinámicas, atractivas, personalizadas y adaptadas a cada ocasión. Con estas imágenes puedes usar easyworship como una herramienta poderosa para comunicar el mensaje de Dios de forma visual y creativa.
-
Preguntas frecuentes
-
-
¿Qué es easyworship?
-Easyworship es un programa de presentación multimedia para iglesias que permite combinar imágenes, videos, textos, canciones y otros elementos para crear presentaciones dinámicas y profesionales.
-
¿Dónde puedo descargar gratis imagenes movibles para easyworship?
-Puedes descargar gratis imagenes movibles para easyworship en sitios web como Pinterest, Recursos Bíblicos o EasyWorship Media.
-
¿Cómo puedo usar las imagenes movibles para easyworship en las presentaciones?
-
-
¿Cómo puedo usar las imagenes movibles para easyworship en las presentaciones?
-Puedes usar las imagenes movibles para easyworship como fondos, transiciones, ilustraciones o adornos. Para usarlas debes importarlas al programa y seleccionarlas en el panel de medios. Luego puedes ajustar su tamaño, posición, velocidad y efecto según tu gusto y propósito.
-
¿Qué beneficios tiene usar imagenes movibles para easyworship en las presentaciones?
-Usar imagenes movibles para easyworship tiene muchos beneficios como dar vida y movimiento a las presentaciones, captar la atención y el interés de los espectadores y transmitir mensajes y emociones de forma visual y creativa.
-
¿Qué requisitos o condiciones tienen las imagenes movibles para easyworship?
-Algunas imagenes movibles para easyworship son libres de derechos de autor y otras requieren dar crédito al autor o a la fuente. Algunas imágenes son gratuitas y otras requieren una suscripción o un pago. Se debe verificar estos requisitos o condiciones antes de descargar y usar las imágenes.
-
- 0a6ba089eb
-
-
\ No newline at end of file
diff --git a/spaces/1gistliPinn/ChatGPT4/Examples/Agrar Simulator 2011 Bga Crack [UPD].md b/spaces/1gistliPinn/ChatGPT4/Examples/Agrar Simulator 2011 Bga Crack [UPD].md
deleted file mode 100644
index a540f079c1e63123c639b52bdc2171fc8833d879..0000000000000000000000000000000000000000
--- a/spaces/1gistliPinn/ChatGPT4/Examples/Agrar Simulator 2011 Bga Crack [UPD].md
+++ /dev/null
@@ -1,20 +0,0 @@
-
-
How to Crack Agrar Simulator 2011 BGA and Enjoy Farming Fun
-
Agrar Simulator 2011 is a farming simulation game that lets you experience the life of a farmer. You can cultivate your fields, harvest your crops, raise animals, and sell your products. You can also use various vehicles and machines to help you with your tasks.
-
However, if you want to play the game without any restrictions, you might want to crack it. Cracking is a process of modifying the game files to bypass the copy protection and enable unlimited access. This way, you can play the game without needing a CD or a serial key.
But how do you crack Agrar Simulator 2011 BGA? Here are some steps you can follow:
-
-
Download the crack file from a reliable source. You can find one at [^1^]. Make sure you scan the file for viruses before opening it.
-
Extract the crack file using a program like WinRAR or 7-Zip. You should see a folder named "Agrar Simulator 2011" with several files inside.
-
Copy the files from the crack folder and paste them into the game installation folder. This is usually located at C:\Program Files (x86)\Agrar Simulator 2011. Overwrite any existing files when prompted.
-
Run the game as administrator. You should see a message saying "Cracked by GameCopyWorld". You can now enjoy the game without any limitations.
-
-
Note: Cracking is illegal and may violate the terms of service of the game developer. We do not condone or encourage cracking in any way. This article is for educational purposes only.
If you want to learn more about Agrar Simulator 2011 BGA, you can check out the official website at . There you can find more information about the game features, the system requirements, and the latest updates. You can also watch some gameplay videos and screenshots to see the game in action.
-
Agrar Simulator 2011 BGA is not just a game, but a realistic simulation of farming. You can choose from different scenarios and maps, each with its own challenges and opportunities. You can also customize your farm and your vehicles according to your preferences. You can even play online with other players and compete or cooperate with them.
-
Whether you are a fan of farming or just looking for a relaxing and enjoyable game, Agrar Simulator 2011 BGA might be the perfect choice for you. With its realistic graphics, sound effects, and physics, you will feel like you are really in the countryside. And with the crack file, you can play it anytime and anywhere you want.
One of the best features of Agrar Simulator 2011 BGA is the biogas plant. This is a facility that converts organic waste into biogas, which can be used as a renewable energy source. You can collect the waste from your animals and crops and transport it to the biogas plant. There you can sell the biogas or use it to power your vehicles and machines.
-
The biogas plant is not only good for the environment, but also for your income. You can earn extra money by selling the biogas or saving on fuel costs. You can also use the leftover material from the biogas plant as fertilizer for your fields. This way, you can improve your soil quality and increase your crop yield.
-
The biogas plant is a unique and innovative feature that sets Agrar Simulator 2011 BGA apart from other farming games. It adds more realism and challenge to the game, as well as more fun and satisfaction. You can experiment with different types of waste and see how they affect the biogas production. You can also upgrade the biogas plant to make it more efficient and profitable.
- d5da3c52bf
-
-
\ No newline at end of file
diff --git a/spaces/1gistliPinn/ChatGPT4/Examples/Dokmee.Enterprise.v3.2.0.1113.Multilingual.Incl.Keymaker-DJiNN.rar.html.md b/spaces/1gistliPinn/ChatGPT4/Examples/Dokmee.Enterprise.v3.2.0.1113.Multilingual.Incl.Keymaker-DJiNN.rar.html.md
deleted file mode 100644
index 23a853b80ebf87a5214e35eef92a7e9a96089bc4..0000000000000000000000000000000000000000
--- a/spaces/1gistliPinn/ChatGPT4/Examples/Dokmee.Enterprise.v3.2.0.1113.Multilingual.Incl.Keymaker-DJiNN.rar.html.md
+++ /dev/null
@@ -1,6 +0,0 @@
-
-
-SketchUp 2014 For Dummies: 9781118822661: Computer Science Books... Start creating a 3D model today with the SketchUp 2014 Understanding Guide. concepts and concepts.
-This book will be equally useful for beginners and advanced users.
-Discover SketchUp in minutes, including learning the basic tools and concepts.
-You'll also learn how to work with SketchUp to create a professional, high-quality 3D model for visualization, planning, and reporting.
-SketchUp is a great 3D modeling tool. 8a78ff9644
-
-
-
diff --git a/spaces/1pelhydcardo/ChatGPT-prompt-generator/assets/Call of Duty Mobile Mod Apk - How to Unlock All Skins and Weapons with Unlimited CP.md b/spaces/1pelhydcardo/ChatGPT-prompt-generator/assets/Call of Duty Mobile Mod Apk - How to Unlock All Skins and Weapons with Unlimited CP.md
deleted file mode 100644
index 4498a2239878728f86c7b96c9e0cc180715d12c9..0000000000000000000000000000000000000000
--- a/spaces/1pelhydcardo/ChatGPT-prompt-generator/assets/Call of Duty Mobile Mod Apk - How to Unlock All Skins and Weapons with Unlimited CP.md
+++ /dev/null
@@ -1,139 +0,0 @@
-
-
Call of Duty Mobile Unlimited CP Mod APK: What You Need to Know
-
Call of Duty Mobile is one of the most popular and successful first-person shooter games on mobile devices. It offers a thrilling and immersive experience that fans of the franchise love. However, some players are looking for ways to get an edge over their opponents and access more content without spending real money. That's where Call of Duty Mobile Unlimited CP Mod APK comes in.
In this article, we will explain what Call of Duty Mobile is, what CP is and why you need it, what Call of Duty Mobile Unlimited CP Mod APK is and how to download and install it, and what are the risks and consequences of using it. We will also answer some frequently asked questions about Call of Duty Mobile at the end.
-
What is Call of Duty Mobile?
-
Call of Duty Mobile is a mobile version of the famous FPS franchise that was released in 2019 by Activision and Tencent. It features various game modes, maps, characters, weapons, and items from different Call of Duty titles such as Modern Warfare, Black Ops, and Warzone. It also has its own original content and storylines that expand the Call of Duty universe.
-
Features of Call of Duty Mobile
-
Some of the features that make Call of Duty Mobile stand out are:
-
-
Console-quality HD graphics and sound that create a realistic and immersive atmosphere.
-
Customizable controls, voice and text chat, and social features that allow you to communicate and play with your friends.
-
A progression system that lets you level up your player and weapons, unlock new items, abilities, perks, skins, camos, and more.
-
A monetization system that uses in-game currency and microtransactions to purchase cosmetics, loot boxes, battle passes, and other benefits.
-
A regular update schedule that adds new seasonal content, events, challenges, rewards, game modes, maps, weapons, operators, and more.
-
-
Game modes of Call of Duty Mobile
-
Call of Duty Mobile has three main game modes that you can play:
-
call of duty mobile hack mod menu 1.0.33
-cod mobile hack aimbot unlock all skins
-call of duty mobile mod apk 2022 download
-cod mobile hack unlimited cod points
-call of duty mobile season 5 mod apk unlocked
-cod mobile hack no ban anti ban
-call of duty mobile hack download 2022
-cod mobile hack tutorial android 2022
-call of duty mobile mod apk cp
-cod mobile hack gameplay wallhack
-call of duty mobile hack mod menu 1.0.34
-cod mobile hack customizable esp
-call of duty mobile legends of war mod apk
-cod mobile hack zarchiver download
-call of duty mobile mod apk global version
-cod mobile hack net energy gain
-call of duty mobile modern warfare remastered mod apk
-cod mobile hack zombie mode
-call of duty mobile mod apk console quality hd gaming
-cod mobile hack new submachine guns
-call of duty mobile mod apk voice and text chat
-cod mobile hack create new account
-call of duty mobile mod apk thrilling 3d graphics and sound
-cod mobile hack free battle pass and extra features
-call of duty mobile mod apk iconic franchise on your phone
-
-
Multiplayer: This mode lets you compete with other players in various classic modes such as Team Deathmatch, Domination, Kill Confirmed, Search and Destroy, etc. on iconic maps such as Nuketown, Crash, Hijacked, etc.
-
Battle Royale: This mode lets you survive a 100-player experience on a large map with vehicles, weapons, items, classes, and more. You can play solo, duo, or squad and try to be the last one standing.
-
Zombies: This mode lets you team up with other players and fight against hordes of zombies in various maps and scenarios. You can use different weapons, items, perks, and skills to survive and complete objectives.
-
-
What is CP and why do you need it?
-
CP stands for Call of Duty Points, which is the premium currency of Call of Duty Mobile. You can use CP to buy various items and benefits that can enhance your gameplay and appearance.
-
CP is the premium currency of Call of Duty Mobile
-
CP is different from Credits, which is the free currency that you can earn by playing the game, completing tasks, and opening crates. CP can only be obtained by spending real money or using special offers and promotions. You can buy CP in different amounts and packages, depending on your region and platform.
-
CP can be used to buy various items and benefits
-
Some of the things that you can buy with CP are:
-
-
Battle Pass: This is a seasonal pass that gives you access to exclusive rewards such as weapons, operators, skins, camos, emotes, calling cards, etc. You can buy the regular Battle Pass for 220 CP or the Battle Pass Bundle for 520 CP, which also unlocks 12 tiers instantly.
-
Crate Bundles: These are bundles of crates that contain random items such as weapons, skins, camos, emotes, etc. You can buy different types of crates such as Premium Crates, Weapon Crates, Character Crates, etc. for various amounts of CP.
-
Lucky Draws: These are special draws that give you a chance to win rare and legendary items such as weapons, operators, skins, camos, etc. You can spin the Lucky Draw for 30 CP for the first time and then the price increases with each spin until you get all the items.
-
Store Items: These are individual items that you can buy directly from the store such as weapons, operators, skins, camos, etc. The price varies depending on the rarity and quality of the item.
-
CODM Championship: This is a competitive mode that lets you participate in tournaments and win prizes such as CP, Credits, weapons, skins, etc. You need to pay 200 CP to enter the CODM Championship and then you can earn back your CP and more by winning matches.
-
-
What is Call of Duty Mobile Unlimited CP Mod APK?
-
Call of Duty Mobile Unlimited CP Mod APK is a modified version of the game that gives you unlimited CP and other features that are not available in the official version. It is also known as Call of Duty Mobile Hack or Call of Duty Mobile Cheat.
-
It is a modified version of the game that gives you unlimited CP
-
The main feature of Call of Duty Mobile Unlimited CP Mod APK is that it gives you unlimited CP without spending any real money. You can use this CP to buy anything you want in the game such as Battle Passes, Crate Bundles, Lucky Draws, Store Items, CODM Championship entries, etc. You can also use this CP to upgrade your weapons and items to the max level.
-
It also has other features such as ESP, Aimbot, Wall Hack, etc.
-
Besides unlimited CP, Call of Duty Mobile Unlimited CP Mod APK also has other features that give you an unfair advantage over other players. Some of these features are:
-
-
ESP: This feature lets you see the enemy's location, health, name, distance, weapon, etc. through walls and obstacles. This helps you to spot and target them easily.
-
Aimbot: This feature lets you automatically aim and shoot at the enemy's head or body with perfect accuracy and speed. This helps you to kill them instantly and win every gunfight.
-
Wall Hack: This feature lets you shoot through walls and obstacles without any damage reduction or bullet drop. This helps you to hit the enemy even if they are hiding or cover.
-
No Recoil: This feature lets you fire your weapon without any recoil or kickback. This helps you to control your aim and spray better.
-
No Spread: This feature lets you fire your weapon without any bullet spread or deviation. This helps you to hit the enemy with every shot.
-
No Reload: This feature lets you fire your weapon without any need to reload or change magazines. This helps you to keep shooting without any interruption.
-
God Mode: This feature lets you become invincible and immune to any damage from the enemy or the environment. This helps you to survive any situation and explore the map freely.
-
-
How to download and install Call of Duty Mobile Unlimited CP Mod APK?
-
If you want to try Call of Duty Mobile Unlimited CP Mod APK, you need to follow these steps to download and install it on your device:
-
Follow these steps to download and install the mod apk
-
-
Find a reliable and safe source that provides the latest version of Call of Duty Mobile Unlimited CP Mod APK. You can search online or use the link below.
-
Download the mod apk file and the obb data file from the source. Make sure you have enough storage space on your device.
-
Enable the installation of apps from unknown sources on your device. You can do this by going to Settings > Security > Unknown Sources and turning it on.
-
Locate the downloaded files on your device and tap on them to install them. You may need to grant some permissions for the installation process.
-
Copy the obb data file to the Android/obb folder on your device. If the folder does not exist, create it manually.
-
Launch the game and enjoy unlimited CP and other features.
-
-
Beware of the risks and consequences of using the mod apk
-
While Call of Duty Mobile Unlimited CP Mod APK may sound tempting, it is not recommended to use it for several reasons:
-
-
It violates the game's terms of service and can result in bans or legal actions. Activision and Tencent have a strict anti-cheat system that detects and punishes players who use hacks or mods. You may lose your account, progress, items, etc. or face legal consequences if you use the mod apk.
-
It ruins the game's balance and fairness and can affect other players' enjoyment. Using hacks or mods gives you an unfair advantage over other players who play legitimately. This can make the game boring, frustrating, or unfair for them.
-
It exposes your device and data to malware and viruses that can harm your device or steal your information. The mod apk may contain malicious code or hidden programs that can infect your device or access your data without your consent. You may risk losing your files, contacts, photos, etc. or compromising your privacy or security if you use the mod apk.
-
-
Conclusion
-
Call of Duty Mobile Unlimited CP Mod APK is a modified version of the game that gives you unlimited CP and other features that are not available in the official version. It may seem like a good option for some players who want to access more content without spending real money or who want to dominate their opponents with ease. However, it is not recommended to use it as it violates the game's terms of service and can result in bans or legal actions. It also ruins the game's balance and fairness and can affect other players' enjoyment. It also exposes your device and data to malware and viruses that can harm your device or steal your information.
-
If you want to enjoy Call of Duty Mobile in a safe and legitimate way, you should avoid using hacks or mods and play the game as intended. You can still get CP by spending real money or using special offers and promotions. You can also improve your skills and performance by practicing, learning, and following tips and tricks from other players. You can also have fun with your friends by playing together in different game modes.
-
FAQs
-
Q1. Is Call of Duty Mobile free to play?
-
A1. Yes, Call of Duty Mobile is free to play on both Android and iOS devices. You can download it from Google Play Store or Apple App Store respectively. However, it also has optional in-game purchases that you can make with real money or in-game currency.
-
Q2. How can I get CP legitimately in Call of Duty Mobile?
-
A2. There are several ways to get CP legitimately in Call of Duty Mobile, such as:
-
-
Buying CP with real money from the in-game store or the official website.
-
Using special offers and promotions that give you CP as a bonus or a reward.
-
Participating in the CODM Championship and winning CP as a prize.
-
Using third-party apps or websites that offer CP as a gift or a reward for completing tasks or surveys. However, you should be careful and check the credibility and security of these sources before using them.
-
-
Q3. What are the best weapons and loadouts in Call of Duty Mobile?
-
A3. The best weapons and loadouts in Call of Duty Mobile depend on your personal preference, playstyle, game mode, map, and situation. However, some of the most popular and effective weapons and loadouts are:
-
-
Assault Rifles: These are versatile weapons that can perform well in most ranges and situations. Some of the best assault rifles are ASM10, AK-47, DR-H, BK57, and M4.
-
Submachine Guns: These are fast-firing weapons that excel in close-range combat and mobility. Some of the best submachine guns are QQ9, QXR, RUS-79U, MSMC, and PDW-57.
-
Sniper Rifles: These are powerful weapons that can kill enemies with one shot from long distances. Some of the best sniper rifles are DL Q33, Locus, Arctic .50, Outlaw, and M21 EBR.
-
Shotguns: These are devastating weapons that can deal massive damage in close-range combat. Some of the best shotguns are KRM-262, Echo, BY15, HS0405, and Striker.
-
Pistols: These are secondary weapons that can be used as a backup or a finisher. Some of the best pistols are MW11, J358, .50 GS, Renetti, and AGR 556.
-
Loadouts: These are combinations of weapons, attachments, perks, grenades, and operator skills that suit your playstyle and strategy. You can create up to 10 custom loadouts and switch between them during the game. You can also use the default loadouts or the recommended loadouts provided by the game.
-
-
Q4. How can I improve my skills and performance in Call of Duty Mobile?
-
A4. There are several tips and tricks that can help you improve your skills and performance in Call of Duty Mobile, such as:
-
-
Practice: The best way to improve your skills is to practice regularly and learn from your mistakes. You can play different game modes and maps, try different weapons and loadouts, watch replays and tutorials, and challenge yourself with different goals and objectives.
-
Learn: The more you know about the game, the better you can play it. You can learn about the game's mechanics, features, modes, maps, weapons, items, operators, skills, perks, etc. You can also learn from other players, streamers, youtubers, guides, forums, etc.
-
Adjust: The more you adapt to the game, the better you can play it. You can adjust your settings, controls, sensitivity, layout, graphics, sound, etc. to suit your device and preference. You can also adjust your strategy, tactics, loadouts, etc. to suit the game mode, map, situation, etc.
-
Communicate: The more you cooperate with your team, the better you can play it. You can communicate with your teammates using voice or text chat, ping system, gestures, etc. You can also coordinate your actions, roles, objectives, etc. with your teammates.
-
Enjoy: The more you have fun with the game, the better you can play it. You can play the game casually or competitively, solo or with friends, online or offline, etc. You can also try new things, experiment with different options, and challenge yourself with different modes.
-
-
Q5. Where can I find more tips and tricks for Call of Duty Mobile?
-
A5. There are many sources where you can find more tips and tricks for Call of Duty Mobile, such as:
-
-
The official website and social media accounts of Call of Duty Mobile that provide news, updates, events, announcements, etc.
-
The in-game community section that provides forums, clans, leaderboards, feedbacks, support, etc.
-
The online community platforms such as Reddit, Discord, Facebook, Twitter, Instagram, YouTube, Twitch, etc. that provide discussions, reviews, guides, videos, streams, etc.
-
The online gaming websites and magazines that provide articles, blogs, podcasts, etc.
-
The online gaming experts and influencers that provide tips, tricks, advice, recommendations, etc.
-
-
I hope this article has helped you to understand what Call of Duty Mobile Unlimited CP Mod APK is and what you need to know about it. If you have any questions or feedback, please feel free to leave a comment below. Thank you for reading and happy gaming!
197e85843d
-
-
\ No newline at end of file
diff --git a/spaces/1phancelerku/anime-remove-background/APK Evozi How to Save Time and Data with the Fastest and Easiest APK Downloader.md b/spaces/1phancelerku/anime-remove-background/APK Evozi How to Save Time and Data with the Fastest and Easiest APK Downloader.md
deleted file mode 100644
index 6b4c5e507e63a057db89c0a7457a0a76b0bf61ab..0000000000000000000000000000000000000000
--- a/spaces/1phancelerku/anime-remove-background/APK Evozi How to Save Time and Data with the Fastest and Easiest APK Downloader.md
+++ /dev/null
@@ -1,92 +0,0 @@
-
-
Apk Evozi: A Guide to Downloading Android Apps and Games
-
Do you want to download Android apps and games that are not available on Google Play? Do you want to save bandwidth and storage space by downloading only the APK file? Do you want to update your apps and games faster than waiting for Google Play? Do you want to avoid ads and in-app purchases by downloading modded or hacked versions of apps and games?
-
If you answered yes to any of these questions, then you need to know about Apk Evozi, a website that lets you download any Android app or game from Google Play as an APK file. In this article, we will explain what Apk Evozi is, how it works, why you should use it, how to use it, and what are the benefits of using it. Let's get started!
Apk Evozi is a website that allows you to download any Android app or game from Google Play as an APK file. An APK file is the installation package of an Android app or game, which contains all the files and data needed to run it on your device. By downloading the APK file, you can install the app or game without using Google Play.
-
Apk Evozi is developed by Evozi, a team of developers who create innovative apps for Android devices. Some of their popular apps include Network Speed, Internet Speed Meter, Device ID, and App Downloader. You can find more about them on their [Google Play page](^1^).
-
How does Apk Evozi work?
-
Apk Evozi works by using a script that fetches the APK file from Google Play servers and generates a download link for you. The script is updated regularly to ensure that it works with the latest versions of apps and games. The website also has a database of over 40 million APK files that you can browse and download.
-
Why use Apk Evozi?
-
There are many reasons why you might want to use Apk Evozi to download Android apps and games. Here are some of them:
You want to access apps and games that are not available in your region or device. For example, some apps and games are restricted to certain countries or devices due to licensing issues or compatibility issues. By using Apk Evozi, you can bypass these restrictions and download any app or game you want.
-
You want to save bandwidth and storage space by downloading only the APK file. For example, some apps and games are very large in size and take a lot of time and data to download from Google Play. By using Apk Evozi, you can download only the APK file, which is usually much smaller than the full app or game. You can then transfer the APK file to your device using a USB cable or a cloud service and install it offline.
-
You want to update your apps and games faster than waiting for Google Play. For example, some apps and games take a long time to receive updates from Google Play due to various reasons. By using Apk Evozi, you can download the latest version of any app or game as soon as it is released on Google Play.
-
You want to avoid ads and in-app purchases by downloading modded or hacked versions of apps and games
What are the benefits of using Apk Evozi?
-
By using Apk Evozi, you can enjoy many benefits that Google Play does not offer. Here are some of them:
-
Access to apps and games that are not available in your region or device
-
As we mentioned before, some apps and games are restricted to certain countries or devices due to licensing issues or compatibility issues. This can be frustrating if you want to try out a new app or game that is popular or useful in another region or device. By using Apk Evozi, you can download any app or game from any region or device and install it on your own device. For example, you can download the app Spotify, which is not available in some countries, or the game PUBG Mobile, which is not compatible with some devices.
-
Save bandwidth and storage space by downloading only the APK file
-
Another benefit of using Apk Evozi is that you can save bandwidth and storage space by downloading only the APK file. Some apps and games are very large in size and take a lot of time and data to download from Google Play. For example, the game Asphalt 9: Legends has a size of over 2 GB and requires an additional 1.5 GB of data to download after installation. By using Apk Evozi, you can download only the APK file, which is usually much smaller than the full app or game. For example, the APK file of Asphalt 9: Legends has a size of only 99 MB. You can then transfer the APK file to your device using a USB cable or a cloud service and install it offline. This way, you can save bandwidth and storage space on your device.
-
Update your apps and games faster than waiting for Google Play
-
A third benefit of using Apk Evozi is that you can update your apps and games faster than waiting for Google Play. Some apps and games take a long time to receive updates from Google Play due to various reasons. For example, some developers release updates in batches or stages to test their stability and performance before rolling them out to all users. Some users may also experience delays or errors in receiving updates from Google Play due to network issues or device settings. By using Apk Evozi, you can download the latest version of any app or game as soon as it is released on Google Play. You can also check the version history of any app or game on the Apk Evozi website to see if there are any new updates available.
-
Avoid ads and in-app purchases by downloading modded or hacked versions of apps and games
-
A fourth benefit of using Apk Evozi is that you can avoid ads and in-app purchases by downloading modded or hacked versions of apps and games. Some apps and games have annoying ads or require you to pay for extra features or content. For example, some apps have banner ads or pop-up ads that interrupt your experience or consume your data. Some games have in-app purchases that make you spend real money to unlock items, levels, characters, etc. By using Apk Evozi, you can download modded or hacked versions of apps and games that remove the ads or unlock the premium features or content for free. For example, you can download the modded version of Spotify that gives you unlimited skips, offline mode, no ads, etc. You can also download the hacked version of PUBG Mobile that gives you unlimited health, ammo, coins, etc. However, you should be careful when downloading modded or hacked versions of apps and games as they may contain malware or viruses that can harm your device or account.
-
Conclusion
-
In conclusion, Apk Evozi is a website that lets you download any Android app or game from Google Play as an APK file. By using Apk Evozi, you can access apps and games that are not available in your region or device, save bandwidth and storage space by downloading only the APK file, update your apps and games faster than waiting for Google Play, and avoid ads and in-app purchases by downloading modded or hacked versions of apps and games. To use Apk Evozi, you just need to find the app or game you want to download, copy its Google Play URL, paste it into the Apk Evozi downloader, click on the "Generate Download Link" button, and download the APK file and install it on your device.
-
If you are looking for a way to download Android apps and games without using Google Play, then Apk Evozi is a great option for you. Try it out today and enjoy the benefits of downloading APK files!
-
FAQs
-
-
Q: Is Apk Evozi safe to use?
-
A A: Apk Evozi is generally safe to use as it fetches the APK files from Google Play servers and does not modify them. However, you should always scan the APK files with a reliable antivirus software before installing them on your device. You should also be careful when downloading modded or hacked versions of apps and games as they may contain malware or viruses that can harm your device or account.
-
Q: Is Apk Evozi legal to use?
-
A: Apk Evozi is legal to use as long as you do not violate the terms and conditions of Google Play or the app or game developers. You should only download apps and games that you have purchased or are free to use. You should also respect the intellectual property rights of the app or game developers and not distribute or share the APK files without their permission.
-
Q: Is Apk Evozi compatible with all Android devices?
-
A: Apk Evozi is compatible with most Android devices that run on Android 4.0 or higher. However, some apps and games may not work properly on some devices due to hardware or software limitations. You should always check the compatibility and requirements of the app or game before downloading and installing it on your device.
-
Q: How can I contact Apk Evozi if I have any questions or feedback?
-
A: You can contact Apk Evozi by using the contact form on their website or by sending an email to support@apk.evozi.com. You can also follow them on their social media accounts, such as Facebook, Twitter, Instagram, and YouTube, to get the latest news and updates about their website and apps.
-
Q: What are some alternatives to Apk Evozi?
-
A: There are many other websites and apps that let you download APK files from Google Play or other sources. Some of them are APKPure, APKMirror, Aptoide, Uptodown, and Appvn. However, you should always be careful when using these websites and apps as they may not be as safe or reliable as Apk Evozi. You should always scan the APK files with a reliable antivirus software before installing them on your device.
-
197e85843d
-
-
\ No newline at end of file
diff --git a/spaces/AIConsultant/MusicGen/audiocraft/quantization/vq.py b/spaces/AIConsultant/MusicGen/audiocraft/quantization/vq.py
deleted file mode 100644
index aa57bea59db95ddae35e0657f723ca3a29ee943b..0000000000000000000000000000000000000000
--- a/spaces/AIConsultant/MusicGen/audiocraft/quantization/vq.py
+++ /dev/null
@@ -1,115 +0,0 @@
-# Copyright (c) Meta Platforms, Inc. and affiliates.
-# All rights reserved.
-#
-# This source code is licensed under the license found in the
-# LICENSE file in the root directory of this source tree.
-
-import math
-import typing as tp
-
-import torch
-
-from .base import BaseQuantizer, QuantizedResult
-from .core_vq import ResidualVectorQuantization
-
-
-class ResidualVectorQuantizer(BaseQuantizer):
- """Residual Vector Quantizer.
-
- Args:
- dimension (int): Dimension of the codebooks.
- n_q (int): Number of residual vector quantizers used.
- q_dropout (bool): Random quantizer drop out at train time.
- bins (int): Codebook size.
- decay (float): Decay for exponential moving average over the codebooks.
- kmeans_init (bool): Whether to use kmeans to initialize the codebooks.
- kmeans_iters (int): Number of iterations used for kmeans initialization.
- threshold_ema_dead_code (int): Threshold for dead code expiration. Replace any codes
- that have an exponential moving average cluster size less than the specified threshold with
- randomly selected vector from the current batch.
- orthogonal_reg_weight (float): Orthogonal regularization weights.
- orthogonal_reg_active_codes_only (bool): Apply orthogonal regularization only on active codes.
- orthogonal_reg_max_codes (optional int): Maximum number of codes to consider.
- for orthogonal regularization.
- """
- def __init__(
- self,
- dimension: int = 256,
- n_q: int = 8,
- q_dropout: bool = False,
- bins: int = 1024,
- decay: float = 0.99,
- kmeans_init: bool = True,
- kmeans_iters: int = 10,
- threshold_ema_dead_code: int = 2,
- orthogonal_reg_weight: float = 0.0,
- orthogonal_reg_active_codes_only: bool = False,
- orthogonal_reg_max_codes: tp.Optional[int] = None,
- ):
- super().__init__()
- self.max_n_q = n_q
- self.n_q = n_q
- self.q_dropout = q_dropout
- self.dimension = dimension
- self.bins = bins
- self.decay = decay
- self.kmeans_init = kmeans_init
- self.kmeans_iters = kmeans_iters
- self.threshold_ema_dead_code = threshold_ema_dead_code
- self.orthogonal_reg_weight = orthogonal_reg_weight
- self.orthogonal_reg_active_codes_only = orthogonal_reg_active_codes_only
- self.orthogonal_reg_max_codes = orthogonal_reg_max_codes
- self.vq = ResidualVectorQuantization(
- dim=self.dimension,
- codebook_size=self.bins,
- num_quantizers=self.n_q,
- decay=self.decay,
- kmeans_init=self.kmeans_init,
- kmeans_iters=self.kmeans_iters,
- threshold_ema_dead_code=self.threshold_ema_dead_code,
- orthogonal_reg_weight=self.orthogonal_reg_weight,
- orthogonal_reg_active_codes_only=self.orthogonal_reg_active_codes_only,
- orthogonal_reg_max_codes=self.orthogonal_reg_max_codes,
- channels_last=False
- )
-
- def forward(self, x: torch.Tensor, frame_rate: int):
- n_q = self.n_q
- if self.training and self.q_dropout:
- n_q = int(torch.randint(1, self.n_q + 1, (1,)).item())
- bw_per_q = math.log2(self.bins) * frame_rate / 1000
- quantized, codes, commit_loss = self.vq(x, n_q=n_q)
- codes = codes.transpose(0, 1)
- # codes is [B, K, T], with T frames, K nb of codebooks.
- bw = torch.tensor(n_q * bw_per_q).to(x)
- return QuantizedResult(quantized, codes, bw, penalty=torch.mean(commit_loss))
-
- def encode(self, x: torch.Tensor) -> torch.Tensor:
- """Encode a given input tensor with the specified frame rate at the given bandwidth.
- The RVQ encode method sets the appropriate number of quantizer to use
- and returns indices for each quantizer.
- """
- n_q = self.n_q
- codes = self.vq.encode(x, n_q=n_q)
- codes = codes.transpose(0, 1)
- # codes is [B, K, T], with T frames, K nb of codebooks.
- return codes
-
- def decode(self, codes: torch.Tensor) -> torch.Tensor:
- """Decode the given codes to the quantized representation."""
- # codes is [B, K, T], with T frames, K nb of codebooks, vq.decode expects [K, B, T].
- codes = codes.transpose(0, 1)
- quantized = self.vq.decode(codes)
- return quantized
-
- @property
- def total_codebooks(self):
- return self.max_n_q
-
- @property
- def num_codebooks(self):
- return self.n_q
-
- def set_num_codebooks(self, n: int):
- assert n > 0 and n <= self.max_n_q
- self.n_q = n
diff --git a/spaces/AIConsultant/MusicGen/tests/models/test_audiogen.py b/spaces/AIConsultant/MusicGen/tests/models/test_audiogen.py
deleted file mode 100644
index 3850af066cedd5ea38bd9aead9634d6aaf938218..0000000000000000000000000000000000000000
--- a/spaces/AIConsultant/MusicGen/tests/models/test_audiogen.py
+++ /dev/null
@@ -1,53 +0,0 @@
-# Copyright (c) Meta Platforms, Inc. and affiliates.
-# All rights reserved.
-#
-# This source code is licensed under the license found in the
-# LICENSE file in the root directory of this source tree.
-
-import pytest
-import torch
-
-from audiocraft.models import AudioGen
-
-
-class TestAudioGenModel:
- def get_audiogen(self):
- ag = AudioGen.get_pretrained(name='debug', device='cpu')
- ag.set_generation_params(duration=2.0, extend_stride=2.)
- return ag
-
- def test_base(self):
- ag = self.get_audiogen()
- assert ag.frame_rate == 25
- assert ag.sample_rate == 16000
- assert ag.audio_channels == 1
-
- def test_generate_continuation(self):
- ag = self.get_audiogen()
- prompt = torch.randn(3, 1, 16000)
- wav = ag.generate_continuation(prompt, 16000)
- assert list(wav.shape) == [3, 1, 32000]
-
- prompt = torch.randn(2, 1, 16000)
- wav = ag.generate_continuation(
- prompt, 16000, ['youpi', 'lapin dort'])
- assert list(wav.shape) == [2, 1, 32000]
-
- prompt = torch.randn(2, 1, 16000)
- with pytest.raises(AssertionError):
- wav = ag.generate_continuation(
- prompt, 16000, ['youpi', 'lapin dort', 'one too many'])
-
- def test_generate(self):
- ag = self.get_audiogen()
- wav = ag.generate(
- ['youpi', 'lapin dort'])
- assert list(wav.shape) == [2, 1, 32000]
-
- def test_generate_long(self):
- ag = self.get_audiogen()
- ag.max_duration = 3.
- ag.set_generation_params(duration=4., extend_stride=2.)
- wav = ag.generate(
- ['youpi', 'lapin dort'])
- assert list(wav.shape) == [2, 1, 16000 * 4]
diff --git a/spaces/ATang0729/Forecast4Muses/Model/Model6/Model6_0_ClothesDetection/mmyolo/configs/yolov5/voc/yolov5_l-v61_fast_1xb32-50e_voc.py b/spaces/ATang0729/Forecast4Muses/Model/Model6/Model6_0_ClothesDetection/mmyolo/configs/yolov5/voc/yolov5_l-v61_fast_1xb32-50e_voc.py
deleted file mode 100644
index 4b470973c46073748803bac2f736eca615e3cb00..0000000000000000000000000000000000000000
--- a/spaces/ATang0729/Forecast4Muses/Model/Model6/Model6_0_ClothesDetection/mmyolo/configs/yolov5/voc/yolov5_l-v61_fast_1xb32-50e_voc.py
+++ /dev/null
@@ -1,25 +0,0 @@
-_base_ = './yolov5_s-v61_fast_1xb64-50e_voc.py'
-
-deepen_factor = 1.0
-widen_factor = 1.0
-train_batch_size_per_gpu = 32
-train_num_workers = 8
-
-load_from = 'https://download.openmmlab.com/mmyolo/v0/yolov5/yolov5_l-v61_syncbn_fast_8xb16-300e_coco/yolov5_l-v61_syncbn_fast_8xb16-300e_coco_20220917_031007-096ef0eb.pth' # noqa
-
-model = dict(
- backbone=dict(
- deepen_factor=deepen_factor,
- widen_factor=widen_factor,
- ),
- neck=dict(
- deepen_factor=deepen_factor,
- widen_factor=widen_factor,
- ),
- bbox_head=dict(head_module=dict(widen_factor=widen_factor)))
-
-train_dataloader = dict(
- batch_size=train_batch_size_per_gpu, num_workers=train_num_workers)
-
-optim_wrapper = dict(
- optimizer=dict(batch_size_per_gpu=train_batch_size_per_gpu))
diff --git a/spaces/Ababababababbababa/AraPoet/app.py b/spaces/Ababababababbababa/AraPoet/app.py
deleted file mode 100644
index af769dff8abd1dbf74587cd2d33de416baf01ade..0000000000000000000000000000000000000000
--- a/spaces/Ababababababbababa/AraPoet/app.py
+++ /dev/null
@@ -1,121 +0,0 @@
-# coding=utf8
-
-import json
-import torch
-import gradio as gr
-import pyarabic.araby as araby
-from transformers import AutoTokenizer, AutoModelForSeq2SeqLM, AutoConfig
-
-feature_names = [
- "Title",
- "Meter",
- "Theme",
- "Name",
- "Era",
- "Country",
- "Type"
-]
-
-with open("./poet_names.json", 'r', encoding="utf-8") as fin:
- poet_names = json.load(fin)
-
-def normalize_text(text):
- text = araby.strip_tatweel(text)
- return text
-
-def generate_poem(country, era, meter, theme, lang_type, poet, num_lines, num_poems, title):
-
- num_poems = int(num_poems)
- prompt = title
- prompt = normalize_text(prompt)
-
- features = [prompt, meter, theme, poet, era, country, lang_type]
-
- prompt = ""
- for name, feat in zip(feature_names, features):
- prompt += f"{name}: {feat}; "
- prompt += f"Length: {num_lines}; Poem:"
-
- num_beams = 5
- top_k = 50
- top_p = 0.9
- r_penalty = 5.
-
- input_ids = torch.tensor(tokenizer.encode(prompt)).unsqueeze(0)
- print(f"> Running: {prompt} | {num_poems} Poems")
- outputs = model.generate(input_ids=input_ids,
- min_length=32,
- max_length=256,
- do_sample=True,
- top_k=top_k,
- top_p=top_p,
- repetition_penalty=r_penalty,
- num_beams=num_beams,
- num_return_sequences=num_poems,
- early_stopping=True
- )
-
- poems = []
- print(f"> # of Outputs: {len(outputs)}")
- for output in outputs:
- raw = tokenizer.decode(output)
- raw = raw.replace("", "").replace("", "")
- print("="*100)
- print(raw)
- print("="*100)
- poems += ['\n'.join(raw.split(""))]
-
- return "\n\n".join(poems)
-
-meters = ['البسيط', 'التفعيله', 'الحداء', 'الخفيف', 'الدوبيت', 'الرجز', 'الرمل', 'السريع', 'السلسلة', 'الصخري', 'الطويل', 'الكامل', 'الكان كان', 'اللويحاني', 'المتدارك', 'المتقارب', 'المجتث', 'المديد', 'المسحوب', 'المضارع', 'المقتضب', 'المنسرح', 'المواليا', 'الموشح', 'الهجيني', 'الهزج', 'الوافر', 'بحر أحذ الكامل', 'بحر أحذ المديد', 'بحر أحذ الوافر', 'بحر البسيط', 'بحر التفعيله', 'بحر الخبب', 'بحر الخفيف', 'بحر الدوبيت', 'بحر الرجز', 'بحر الرمل', 'بحر السريع', 'بحر السلسلة', 'بحر الطويل', 'بحر القوما', 'بحر الكامل', 'بحر الكامل المقطوع', 'بحر المتدارك', 'بحر المتدارك المنهوك', 'بحر المتقارب', 'بحر المجتث', 'بحر المديد', 'بحر المضارع', 'بحر المقتضب', 'بحر المنسرح', 'بحر المواليا', 'بحر الهزج', 'بحر الوافر', 'بحر تفعيلة الرجز', 'بحر تفعيلة الرمل', 'بحر تفعيلة الكامل', 'بحر تفعيلة المتقارب', 'بحر مجزوء البسيط', 'بحر مجزوء الخفيف', 'بحر مجزوء الدوبيت', 'بحر مجزوء الرجز', 'بحر مجزوء الرمل', 'بحر مجزوء الرمل ', 'بحر مجزوء السريع', 'بحر مجزوء الطويل', 'بحر مجزوء الكامل', 'بحر مجزوء المتدارك', 'بحر مجزوء المتقارب', 'بحر مجزوء المجتث', 'بحر مجزوء المديد', 'بحر مجزوء المنسرح', 'بحر مجزوء المواليا', 'بحر مجزوء الهزج', 'بحر مجزوء الوافر', 'بحر مجزوء موشح', 'بحر مخلع البسيط', 'بحر مخلع الرجز', 'بحر مخلع الرمل', 'بحر مخلع السريع', 'بحر مخلع الكامل', 'بحر مخلع موشح', 'بحر مربع البسيط', 'بحر مربع الرجز', 'بحر مشطور الرجز', 'بحر مشطور السريع', 'بحر مشطور الطويل', 'بحر منهوك البسيط', 'بحر منهوك الرجز', 'بحر منهوك الكامل', 'بحر منهوك المنسرح', 'بحر موشح', 'بسيط', 'زجل', 'شعر التفعيلة', 'شعر حر', 'عامي', 'عدة أبحر', 'عموديه', 'مجزوء الخفيف', 'نثريه', 'None']
-themes = ['قصيدة اعتذار', 'قصيدة الاناشيد', 'قصيدة المعلقات', 'قصيدة حزينه', 'قصيدة دينية', 'قصيدة ذم', 'قصيدة رثاء', 'قصيدة رومنسيه', 'قصيدة سياسية', 'قصيدة شوق', 'قصيدة عامه', 'قصيدة عتاب', 'قصيدة غزل', 'قصيدة فراق', 'قصيدة قصيره', 'قصيدة مدح', 'قصيدة هجاء', 'قصيدة وطنيه', 'None']
-language_types = ['شعبي', 'عامي', 'فصحى', 'فصيح', '-', 'None']
-poet_era = ['العصر الأموي', 'العصر الأندلسي', 'العصر الأيوبي', 'العصر الإسلامي', 'العصر الجاهلي', 'العصر الحديث', 'العصر العباسي', 'العصر العثماني', 'العصر الفاطمي', 'العصر المملوكي', 'المخضرمين', 'المغرب والأندلس', 'عصر بين الدولتين', 'قبل الإسلام', 'None']
-countries = ['الأردن', 'الإمارات', 'البحرين', 'الجزائر', 'السعودية', 'السنغال', 'السودان', 'الصومال', 'العراق', 'الكويت', 'المغرب', 'اليمن', 'تونس', 'سوريا', 'سورية', 'عمان', 'فلسطين', 'قطر', 'لبنان', 'ليبيا', 'مصر', 'موريتانيا', 'None']
-
-tokenizer: AutoTokenizer = AutoTokenizer.from_pretrained("bkhmsi/arapoet-mt5", use_auth_token="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")
-model: AutoModelForSeq2SeqLM = AutoModelForSeq2SeqLM.from_pretrained("bkhmsi/arapoet-mt5", use_auth_token="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")
-model.eval()
-
-title = ""
-with gr.Blocks(title=title) as demo:
- inputs = []
-
- gr.Markdown(
- """
- # AraPoet: Controlled Arabic Poetry Generation
-
- The model hosted here is a finetuned version of [mT5-large](https://huggingface.co/google/mt5-large) (∼ 1.2B parameters) on the largest repository of Arabic poems, the [ashaar](https://huggingface.co/datasets/arbml/ashaar) dataset.
- The model can be conditioned on a set of attributes to control the style of the generated poem.
- Namely: the poet name, country, era, meter, theme, language type, title and the length of the poem.
- You can start by clicking on one of the examples below or try your own input.
- """
- )
-
- with gr.Row():
- inputs += [gr.Dropdown(countries, label="Country", value="مصر")]
- inputs += [gr.Dropdown(poet_era, label="Era", value="العصر الحديث")]
- with gr.Row():
- inputs += [gr.Dropdown(meters, label="Meter", value="بحر السريع")]
- inputs += [gr.Dropdown(themes, label="Theme", value="قصيدة رومنسيه")]
- with gr.Row():
- inputs += [gr.Dropdown(language_types, label="Language Type", value="فصحى")]
- inputs += [gr.Dropdown(poet_names, label="Poet", value="أحمد شوقي")]
- with gr.Row():
- inputs += [gr.Slider(2, 20, value=6, step=1, label="Number of Lines")]
- inputs += [gr.Slider(1, 4, value=1, step=1, label="Number of Samples")]
- with gr.Row():
- inputs += [gr.Textbox(label="Title", value="إثن عنان القلب واسلم به")]
-
- btn = gr.Button("Generate")
- examples = gr.Examples(examples="./examples", inputs=inputs)
- btn.click(generate_poem, inputs, gr.TextArea(label="Generation"))
-
-
- gr.Markdown(
- """
- Checkout our [AraPoet Preprint](https://github.com/BKHMSI/BKHMSI.github.io/blob/master/archive/resources/AraPoet.pdf) for more details about the model.
- """
- )
-
-demo.launch()
\ No newline at end of file
diff --git a/spaces/AbandonedMuse/UnlimitedMusicGen/Dockerfile b/spaces/AbandonedMuse/UnlimitedMusicGen/Dockerfile
deleted file mode 100644
index efc2431ec0fe674c22fe2fdb9d7045cdf6cd2748..0000000000000000000000000000000000000000
--- a/spaces/AbandonedMuse/UnlimitedMusicGen/Dockerfile
+++ /dev/null
@@ -1,26 +0,0 @@
-FROM nvidia/cuda:11.8.0-base-ubuntu22.04
-
-ENV DEBIAN_FRONTEND=noninteractive \
- PYTHONUNBUFFERED=1 \
- PYTHONIOENCODING=UTF-8
-RUN --mount=type=cache,target=/var/cache/apt --mount=type=cache,target=/var/lib/apt apt update &&\
- apt install -y \
- wget \
- git \
- pkg-config \
- python3 \
- python3-pip \
- python-is-python3 \
- ffmpeg \
- libnvrtc11.2 \
- libtcmalloc-minimal4
-
-RUN useradd -m -u 1000 ac
-RUN --mount=type=cache,target=/root/.cache python -m pip install --upgrade pip wheel
-ENV TORCH_COMMAND="pip install torch==2.0.1+cu118 torchaudio --extra-index-url https://download.pytorch.org/whl/cu118"
-RUN --mount=type=cache,target=/root/.cache python -m $TORCH_COMMAND
-RUN ln -s /usr/lib/x86_64-linux-gnu/libnvrtc.so.11.2 /usr/lib/x86_64-linux-gnu/libnvrtc.so
-USER 1000
-RUN mkdir ~/.cache
-RUN --mount=type=cache,target=/home/ac/.cache --mount=source=.,target=/home/ac/audiocraft python -m pip install -r /home/ac/audiocraft/requirements.txt
-WORKDIR /home/ac/audiocraft
\ No newline at end of file
diff --git a/spaces/AchyuthGamer/OpenGPT/g4f/Provider/Providers/deprecated/__init__.py b/spaces/AchyuthGamer/OpenGPT/g4f/Provider/Providers/deprecated/__init__.py
deleted file mode 100644
index 5c66c87fa30e77def4d61737299ce32be3b6de9f..0000000000000000000000000000000000000000
--- a/spaces/AchyuthGamer/OpenGPT/g4f/Provider/Providers/deprecated/__init__.py
+++ /dev/null
@@ -1,14 +0,0 @@
-from .AiService import AiService
-from .CodeLinkAva import CodeLinkAva
-from .DfeHub import DfeHub
-from .EasyChat import EasyChat
-from .Forefront import Forefront
-from .GetGpt import GetGpt
-from .Opchatgpts import Opchatgpts
-from .Lockchat import Lockchat
-from .Wewordle import Wewordle
-from .Equing import Equing
-from .Wuguokai import Wuguokai
-from .V50 import V50
-from .FastGpt import FastGpt
-from .ChatgptLogin import ChatgptLogin
\ No newline at end of file
diff --git a/spaces/AgentVerse/agentVerse/agentverse/environments/tasksolving_env/rules/role_assigner/__init__.py b/spaces/AgentVerse/agentVerse/agentverse/environments/tasksolving_env/rules/role_assigner/__init__.py
deleted file mode 100644
index 2504dda5b6e3ca052d91b83ae3dd8b2c0e7f4b41..0000000000000000000000000000000000000000
--- a/spaces/AgentVerse/agentVerse/agentverse/environments/tasksolving_env/rules/role_assigner/__init__.py
+++ /dev/null
@@ -1,6 +0,0 @@
-from agentverse.registry import Registry
-
-role_assigner_registry = Registry(name="RoleAssignerRegistry")
-
-from .base import BaseRoleAssigner
-from .role_description import DescriptionAssigner
diff --git a/spaces/AgentVerse/agentVerse/agentverse/utils.py b/spaces/AgentVerse/agentVerse/agentverse/utils.py
deleted file mode 100644
index 196d0ba2e26e5a707574d5bb37306513fd63a202..0000000000000000000000000000000000000000
--- a/spaces/AgentVerse/agentVerse/agentverse/utils.py
+++ /dev/null
@@ -1,50 +0,0 @@
-from typing import NamedTuple, Union
-from enum import Enum
-
-import abc
-
-
-class AgentAction(NamedTuple):
- """Agent's action to take."""
-
- tool: str
- tool_input: Union[str, dict]
- log: str
-
-
-class AgentFinish(NamedTuple):
- """Agent's return value."""
-
- return_values: dict
- log: str
-
-
-class AgentCriticism(NamedTuple):
- """Agent's criticism."""
-
- is_agree: bool
- criticism: str
- sender_agent: object = None
-
-
-class AGENT_TYPES(Enum):
- ROLE_ASSIGNMENT = 0
- SOLVER = 1
- CRITIC = 2
- EXECUTION = 3
- EVALUATION = 4
- MANAGER = 5
-
-
-class Singleton(abc.ABCMeta, type):
- """
- Singleton metaclass for ensuring only one instance of a class.
- """
-
- _instances = {}
-
- def __call__(cls, *args, **kwargs):
- """Call method for the singleton metaclass."""
- if cls not in cls._instances:
- cls._instances[cls] = super(Singleton, cls).__call__(*args, **kwargs)
- return cls._instances[cls]
diff --git a/spaces/AgentVerse/agentVerse/ui/src/phaser3-rex-plugins/templates/spinner/puff/Puff.d.ts b/spaces/AgentVerse/agentVerse/ui/src/phaser3-rex-plugins/templates/spinner/puff/Puff.d.ts
deleted file mode 100644
index dfe78f8463022a04291f0776ab05f5d20f6a20ba..0000000000000000000000000000000000000000
--- a/spaces/AgentVerse/agentVerse/ui/src/phaser3-rex-plugins/templates/spinner/puff/Puff.d.ts
+++ /dev/null
@@ -1,2 +0,0 @@
-import Base from '../base/Base';
-export default class Puff extends Base { }
\ No newline at end of file
diff --git a/spaces/AgentVerse/agentVerse/ui/src/phaser3-rex-plugins/templates/ui/slider/GetEndPoint.js b/spaces/AgentVerse/agentVerse/ui/src/phaser3-rex-plugins/templates/ui/slider/GetEndPoint.js
deleted file mode 100644
index b4a303cc4077fe4124f08744a5317ff6537feaa3..0000000000000000000000000000000000000000
--- a/spaces/AgentVerse/agentVerse/ui/src/phaser3-rex-plugins/templates/ui/slider/GetEndPoint.js
+++ /dev/null
@@ -1,27 +0,0 @@
-import GetThumbAlignPoint from './GetThumbAlignPoint.js';
-
-const AlignRight = Phaser.Display.Align.RIGHT_CENTER;
-const AlignBottom = Phaser.Display.Align.BOTTOM_CENTER;
-
-var GetEndoint = function (out) {
- if (out === undefined) {
- out = tmpPoint;
- }
- if (this.childrenMap.thumb) {
- var align = (this.orientation === 0) ? AlignRight : AlignBottom;
- GetThumbAlignPoint.call(this, align, out);
- } else {
- if (this.orientation === 0) {
- out.x = this.innerRight - 1; // Add 1 pixel margin
- out.y = this.centerY;
- } else {
- out.x = this.centerX;
- out.y = this.innerBottom - 1; // Add 1 pixel margin
- }
- }
- return out;
-}
-
-var tmpPoint = {};
-
-export default GetEndoint;
\ No newline at end of file
diff --git a/spaces/Aki004/herta-so-vits/demo.py b/spaces/Aki004/herta-so-vits/demo.py
deleted file mode 100644
index 617eadac96c56c4517ddd69bb1b71d44c9629148..0000000000000000000000000000000000000000
--- a/spaces/Aki004/herta-so-vits/demo.py
+++ /dev/null
@@ -1,30 +0,0 @@
-import edge_tts
-import asyncio
-import librosa
-import soundfile
-import io
-
-from inference.infer_tool import Svc
-
-TEXT = "私はヘルタ。今は忙しいから、リモート人形のオート返答機能に任せる。こんにちは、こんにちは、ごきげんよう、良い日になりますように。それじゃ"
-VOICE = "ja-JP-NanamiNeural"
-OUTPUT_FILE = "test.mp3"
-
-asyncio.run(edge_tts.Communicate(TEXT, VOICE).save(OUTPUT_FILE))
-audio, sr = librosa.load(OUTPUT_FILE, sr=16000, mono=True)
-raw_path = io.BytesIO()
-soundfile.write(raw_path, audio, 16000, format="wav")
-raw_path.seek(0)
-print('checkpoint 1')
-
-model = Svc(fr"Herta-Svc/G_10000.pth", f"Herta-Svc/config.json", device = 'cpu')
-print('checkpoint 2')
-
-out_audio, out_sr = model.infer('speaker0', 0, raw_path,
- auto_predict_f0 = True,
- )
-print('checkpoint 3')
-
-soundfile.write('out_audio.wav', out_audio.cpu().numpy(), 44100)
-
-print("done")
\ No newline at end of file
diff --git a/spaces/Al-Chan/Vits_League_of_Legends_Yuumi_TTS/rearrange_speaker.py b/spaces/Al-Chan/Vits_League_of_Legends_Yuumi_TTS/rearrange_speaker.py
deleted file mode 100644
index de0f7545904cc088377c552cc6d9b058c5e9d342..0000000000000000000000000000000000000000
--- a/spaces/Al-Chan/Vits_League_of_Legends_Yuumi_TTS/rearrange_speaker.py
+++ /dev/null
@@ -1,37 +0,0 @@
-import torch
-import argparse
-import json
-
-if __name__ == "__main__":
- parser = argparse.ArgumentParser()
- parser.add_argument("--model_dir", type=str, default="./OUTPUT_MODEL/G_latest.pth")
- parser.add_argument("--config_dir", type=str, default="./configs/modified_finetune_speaker.json")
- args = parser.parse_args()
-
- model_sd = torch.load(args.model_dir, map_location='cpu')
- with open(args.config_dir, 'r', encoding='utf-8') as f:
- hps = json.load(f)
-
- valid_speakers = list(hps['speakers'].keys())
- if hps['data']['n_speakers'] > len(valid_speakers):
- new_emb_g = torch.zeros([len(valid_speakers), 256])
- old_emb_g = model_sd['model']['emb_g.weight']
- for i, speaker in enumerate(valid_speakers):
- new_emb_g[i, :] = old_emb_g[hps['speakers'][speaker], :]
- hps['speakers'][speaker] = i
- hps['data']['n_speakers'] = len(valid_speakers)
- model_sd['model']['emb_g.weight'] = new_emb_g
- with open("./finetune_speaker.json", 'w', encoding='utf-8') as f:
- json.dump(hps, f, indent=2)
- torch.save(model_sd, "./G_latest.pth")
- else:
- with open("./finetune_speaker.json", 'w', encoding='utf-8') as f:
- json.dump(hps, f, indent=2)
- torch.save(model_sd, "./G_latest.pth")
- # save another config file copy in MoeGoe format
- hps['speakers'] = valid_speakers
- with open("./moegoe_config.json", 'w', encoding='utf-8') as f:
- json.dump(hps, f, indent=2)
-
-
-
diff --git a/spaces/Alex123aaa/1234/README.md b/spaces/Alex123aaa/1234/README.md
deleted file mode 100644
index 77da4c0cb3ee265910403789f4f169e9ea3f165f..0000000000000000000000000000000000000000
--- a/spaces/Alex123aaa/1234/README.md
+++ /dev/null
@@ -1,13 +0,0 @@
----
-title: 1234
-emoji: 🌍
-colorFrom: gray
-colorTo: red
-sdk: gradio
-sdk_version: 3.45.0
-app_file: app.py
-pinned: false
-license: unknown
----
-
-Check out the configuration reference at https://huggingface.co/docs/hub/spaces-config-reference
diff --git "a/spaces/Ameaou/academic-chatgpt3.1/crazy_functions/\346\200\273\347\273\223word\346\226\207\346\241\243.py" "b/spaces/Ameaou/academic-chatgpt3.1/crazy_functions/\346\200\273\347\273\223word\346\226\207\346\241\243.py"
deleted file mode 100644
index f1fe20171cc54aec0c79f4961e71b57845f252d5..0000000000000000000000000000000000000000
--- "a/spaces/Ameaou/academic-chatgpt3.1/crazy_functions/\346\200\273\347\273\223word\346\226\207\346\241\243.py"
+++ /dev/null
@@ -1,127 +0,0 @@
-from toolbox import update_ui
-from toolbox import CatchException, report_execption, write_results_to_file
-from .crazy_utils import request_gpt_model_in_new_thread_with_ui_alive
-fast_debug = False
-
-
-def 解析docx(file_manifest, project_folder, llm_kwargs, plugin_kwargs, chatbot, history, system_prompt):
- import time, os
- # pip install python-docx 用于docx格式,跨平台
- # pip install pywin32 用于doc格式,仅支持Win平台
- for index, fp in enumerate(file_manifest):
- if fp.split(".")[-1] == "docx":
- from docx import Document
- doc = Document(fp)
- file_content = "\n".join([para.text for para in doc.paragraphs])
- else:
- import win32com.client
- word = win32com.client.Dispatch("Word.Application")
- word.visible = False
- # 打开文件
- print('fp', os.getcwd())
- doc = word.Documents.Open(os.getcwd() + '/' + fp)
- # file_content = doc.Content.Text
- doc = word.ActiveDocument
- file_content = doc.Range().Text
- doc.Close()
- word.Quit()
-
- print(file_content)
- # private_upload里面的文件名在解压zip后容易出现乱码(rar和7z格式正常),故可以只分析文章内容,不输入文件名
- from .crazy_utils import breakdown_txt_to_satisfy_token_limit_for_pdf
- from request_llm.bridge_all import model_info
- max_token = model_info[llm_kwargs['llm_model']]['max_token']
- TOKEN_LIMIT_PER_FRAGMENT = max_token * 3 // 4
- paper_fragments = breakdown_txt_to_satisfy_token_limit_for_pdf(
- txt=file_content,
- get_token_fn=model_info[llm_kwargs['llm_model']]['token_cnt'],
- limit=TOKEN_LIMIT_PER_FRAGMENT
- )
- this_paper_history = []
- for i, paper_frag in enumerate(paper_fragments):
- i_say = f'请对下面的文章片段用中文做概述,文件名是{os.path.relpath(fp, project_folder)},文章内容是 ```{paper_frag}```'
- i_say_show_user = f'请对下面的文章片段做概述: {os.path.abspath(fp)}的第{i+1}/{len(paper_fragments)}个片段。'
- gpt_say = yield from request_gpt_model_in_new_thread_with_ui_alive(
- inputs=i_say,
- inputs_show_user=i_say_show_user,
- llm_kwargs=llm_kwargs,
- chatbot=chatbot,
- history=[],
- sys_prompt="总结文章。"
- )
-
- chatbot[-1] = (i_say_show_user, gpt_say)
- history.extend([i_say_show_user,gpt_say])
- this_paper_history.extend([i_say_show_user,gpt_say])
-
- # 已经对该文章的所有片段总结完毕,如果文章被切分了,
- if len(paper_fragments) > 1:
- i_say = f"根据以上的对话,总结文章{os.path.abspath(fp)}的主要内容。"
- gpt_say = yield from request_gpt_model_in_new_thread_with_ui_alive(
- inputs=i_say,
- inputs_show_user=i_say,
- llm_kwargs=llm_kwargs,
- chatbot=chatbot,
- history=this_paper_history,
- sys_prompt="总结文章。"
- )
-
- history.extend([i_say,gpt_say])
- this_paper_history.extend([i_say,gpt_say])
-
- res = write_results_to_file(history)
- chatbot.append(("完成了吗?", res))
- yield from update_ui(chatbot=chatbot, history=history) # 刷新界面
-
- res = write_results_to_file(history)
- chatbot.append(("所有文件都总结完成了吗?", res))
- yield from update_ui(chatbot=chatbot, history=history) # 刷新界面
-
-
-@CatchException
-def 总结word文档(txt, llm_kwargs, plugin_kwargs, chatbot, history, system_prompt, web_port):
- import glob, os
-
- # 基本信息:功能、贡献者
- chatbot.append([
- "函数插件功能?",
- "批量总结Word文档。函数插件贡献者: JasonGuo1"])
- yield from update_ui(chatbot=chatbot, history=history) # 刷新界面
-
- # 尝试导入依赖,如果缺少依赖,则给出安装建议
- try:
- from docx import Document
- except:
- report_execption(chatbot, history,
- a=f"解析项目: {txt}",
- b=f"导入软件依赖失败。使用该模块需要额外依赖,安装方法```pip install --upgrade python-docx pywin32```。")
- yield from update_ui(chatbot=chatbot, history=history) # 刷新界面
- return
-
- # 清空历史,以免输入溢出
- history = []
-
- # 检测输入参数,如没有给定输入参数,直接退出
- if os.path.exists(txt):
- project_folder = txt
- else:
- if txt == "": txt = '空空如也的输入栏'
- report_execption(chatbot, history, a=f"解析项目: {txt}", b=f"找不到本地项目或无权访问: {txt}")
- yield from update_ui(chatbot=chatbot, history=history) # 刷新界面
- return
-
- # 搜索需要处理的文件清单
- if txt.endswith('.docx') or txt.endswith('.doc'):
- file_manifest = [txt]
- else:
- file_manifest = [f for f in glob.glob(f'{project_folder}/**/*.docx', recursive=True)] + \
- [f for f in glob.glob(f'{project_folder}/**/*.doc', recursive=True)]
-
- # 如果没找到任何文件
- if len(file_manifest) == 0:
- report_execption(chatbot, history, a=f"解析项目: {txt}", b=f"找不到任何.docx或doc文件: {txt}")
- yield from update_ui(chatbot=chatbot, history=history) # 刷新界面
- return
-
- # 开始正式执行任务
- yield from 解析docx(file_manifest, project_folder, llm_kwargs, plugin_kwargs, chatbot, history, system_prompt)
diff --git a/spaces/Androidonnxfork/CivitAi-to-Diffusers/diffusers/src/diffusers/pipelines/stable_diffusion/pipeline_onnx_stable_diffusion_inpaint_legacy.py b/spaces/Androidonnxfork/CivitAi-to-Diffusers/diffusers/src/diffusers/pipelines/stable_diffusion/pipeline_onnx_stable_diffusion_inpaint_legacy.py
deleted file mode 100644
index a4b54b9724fb7630f841253aee2fa44743fc6367..0000000000000000000000000000000000000000
--- a/spaces/Androidonnxfork/CivitAi-to-Diffusers/diffusers/src/diffusers/pipelines/stable_diffusion/pipeline_onnx_stable_diffusion_inpaint_legacy.py
+++ /dev/null
@@ -1,540 +0,0 @@
-import inspect
-from typing import Callable, List, Optional, Union
-
-import numpy as np
-import PIL
-import torch
-from transformers import CLIPImageProcessor, CLIPTokenizer
-
-from ...configuration_utils import FrozenDict
-from ...schedulers import DDIMScheduler, LMSDiscreteScheduler, PNDMScheduler
-from ...utils import deprecate, logging
-from ..onnx_utils import ORT_TO_NP_TYPE, OnnxRuntimeModel
-from ..pipeline_utils import DiffusionPipeline
-from . import StableDiffusionPipelineOutput
-
-
-logger = logging.get_logger(__name__) # pylint: disable=invalid-name
-
-
-def preprocess(image):
- w, h = image.size
- w, h = (x - x % 32 for x in (w, h)) # resize to integer multiple of 32
- image = image.resize((w, h), resample=PIL.Image.LANCZOS)
- image = np.array(image).astype(np.float32) / 255.0
- image = image[None].transpose(0, 3, 1, 2)
- return 2.0 * image - 1.0
-
-
-def preprocess_mask(mask, scale_factor=8):
- mask = mask.convert("L")
- w, h = mask.size
- w, h = (x - x % 32 for x in (w, h)) # resize to integer multiple of 32
- mask = mask.resize((w // scale_factor, h // scale_factor), resample=PIL.Image.NEAREST)
- mask = np.array(mask).astype(np.float32) / 255.0
- mask = np.tile(mask, (4, 1, 1))
- mask = mask[None].transpose(0, 1, 2, 3) # what does this step do?
- mask = 1 - mask # repaint white, keep black
- return mask
-
-
-class OnnxStableDiffusionInpaintPipelineLegacy(DiffusionPipeline):
- r"""
- Pipeline for text-guided image inpainting using Stable Diffusion. This is a *legacy feature* for Onnx pipelines to
- provide compatibility with StableDiffusionInpaintPipelineLegacy and may be removed in the future.
-
- This model inherits from [`DiffusionPipeline`]. Check the superclass documentation for the generic methods the
- library implements for all the pipelines (such as downloading or saving, running on a particular device, etc.)
-
- Args:
- vae ([`AutoencoderKL`]):
- Variational Auto-Encoder (VAE) Model to encode and decode images to and from latent representations.
- text_encoder ([`CLIPTextModel`]):
- Frozen text-encoder. Stable Diffusion uses the text portion of
- [CLIP](https://huggingface.co/docs/transformers/model_doc/clip#transformers.CLIPTextModel), specifically
- the [clip-vit-large-patch14](https://huggingface.co/openai/clip-vit-large-patch14) variant.
- tokenizer (`CLIPTokenizer`):
- Tokenizer of class
- [CLIPTokenizer](https://huggingface.co/docs/transformers/v4.21.0/en/model_doc/clip#transformers.CLIPTokenizer).
- unet ([`UNet2DConditionModel`]): Conditional U-Net architecture to denoise the encoded image latents.
- scheduler ([`SchedulerMixin`]):
- A scheduler to be used in combination with `unet` to denoise the encoded image latents. Can be one of
- [`DDIMScheduler`], [`LMSDiscreteScheduler`], or [`PNDMScheduler`].
- safety_checker ([`StableDiffusionSafetyChecker`]):
- Classification module that estimates whether generated images could be considered offensive or harmful.
- Please, refer to the [model card](https://huggingface.co/runwayml/stable-diffusion-v1-5) for details.
- feature_extractor ([`CLIPImageProcessor`]):
- Model that extracts features from generated images to be used as inputs for the `safety_checker`.
- """
- _optional_components = ["safety_checker", "feature_extractor"]
- _is_onnx = True
-
- vae_encoder: OnnxRuntimeModel
- vae_decoder: OnnxRuntimeModel
- text_encoder: OnnxRuntimeModel
- tokenizer: CLIPTokenizer
- unet: OnnxRuntimeModel
- scheduler: Union[DDIMScheduler, PNDMScheduler, LMSDiscreteScheduler]
- safety_checker: OnnxRuntimeModel
- feature_extractor: CLIPImageProcessor
-
- def __init__(
- self,
- vae_encoder: OnnxRuntimeModel,
- vae_decoder: OnnxRuntimeModel,
- text_encoder: OnnxRuntimeModel,
- tokenizer: CLIPTokenizer,
- unet: OnnxRuntimeModel,
- scheduler: Union[DDIMScheduler, PNDMScheduler, LMSDiscreteScheduler],
- safety_checker: OnnxRuntimeModel,
- feature_extractor: CLIPImageProcessor,
- requires_safety_checker: bool = True,
- ):
- super().__init__()
-
- if hasattr(scheduler.config, "steps_offset") and scheduler.config.steps_offset != 1:
- deprecation_message = (
- f"The configuration file of this scheduler: {scheduler} is outdated. `steps_offset`"
- f" should be set to 1 instead of {scheduler.config.steps_offset}. Please make sure "
- "to update the config accordingly as leaving `steps_offset` might led to incorrect results"
- " in future versions. If you have downloaded this checkpoint from the Hugging Face Hub,"
- " it would be very nice if you could open a Pull request for the `scheduler/scheduler_config.json`"
- " file"
- )
- deprecate("steps_offset!=1", "1.0.0", deprecation_message, standard_warn=False)
- new_config = dict(scheduler.config)
- new_config["steps_offset"] = 1
- scheduler._internal_dict = FrozenDict(new_config)
-
- if hasattr(scheduler.config, "clip_sample") and scheduler.config.clip_sample is True:
- deprecation_message = (
- f"The configuration file of this scheduler: {scheduler} has not set the configuration `clip_sample`."
- " `clip_sample` should be set to False in the configuration file. Please make sure to update the"
- " config accordingly as not setting `clip_sample` in the config might lead to incorrect results in"
- " future versions. If you have downloaded this checkpoint from the Hugging Face Hub, it would be very"
- " nice if you could open a Pull request for the `scheduler/scheduler_config.json` file"
- )
- deprecate("clip_sample not set", "1.0.0", deprecation_message, standard_warn=False)
- new_config = dict(scheduler.config)
- new_config["clip_sample"] = False
- scheduler._internal_dict = FrozenDict(new_config)
-
- if safety_checker is None and requires_safety_checker:
- logger.warning(
- f"You have disabled the safety checker for {self.__class__} by passing `safety_checker=None`. Ensure"
- " that you abide to the conditions of the Stable Diffusion license and do not expose unfiltered"
- " results in services or applications open to the public. Both the diffusers team and Hugging Face"
- " strongly recommend to keep the safety filter enabled in all public facing circumstances, disabling"
- " it only for use-cases that involve analyzing network behavior or auditing its results. For more"
- " information, please have a look at https://github.com/huggingface/diffusers/pull/254 ."
- )
-
- if safety_checker is not None and feature_extractor is None:
- raise ValueError(
- "Make sure to define a feature extractor when loading {self.__class__} if you want to use the safety"
- " checker. If you do not want to use the safety checker, you can pass `'safety_checker=None'` instead."
- )
-
- self.register_modules(
- vae_encoder=vae_encoder,
- vae_decoder=vae_decoder,
- text_encoder=text_encoder,
- tokenizer=tokenizer,
- unet=unet,
- scheduler=scheduler,
- safety_checker=safety_checker,
- feature_extractor=feature_extractor,
- )
- self.register_to_config(requires_safety_checker=requires_safety_checker)
-
- # Copied from diffusers.pipelines.stable_diffusion.pipeline_onnx_stable_diffusion.OnnxStableDiffusionPipeline._encode_prompt
- def _encode_prompt(
- self,
- prompt: Union[str, List[str]],
- num_images_per_prompt: Optional[int],
- do_classifier_free_guidance: bool,
- negative_prompt: Optional[str],
- prompt_embeds: Optional[np.ndarray] = None,
- negative_prompt_embeds: Optional[np.ndarray] = None,
- ):
- r"""
- Encodes the prompt into text encoder hidden states.
-
- Args:
- prompt (`str` or `List[str]`):
- prompt to be encoded
- num_images_per_prompt (`int`):
- number of images that should be generated per prompt
- do_classifier_free_guidance (`bool`):
- whether to use classifier free guidance or not
- negative_prompt (`str` or `List[str]`):
- The prompt or prompts not to guide the image generation. Ignored when not using guidance (i.e., ignored
- if `guidance_scale` is less than `1`).
- prompt_embeds (`np.ndarray`, *optional*):
- Pre-generated text embeddings. Can be used to easily tweak text inputs, *e.g.* prompt weighting. If not
- provided, text embeddings will be generated from `prompt` input argument.
- negative_prompt_embeds (`np.ndarray`, *optional*):
- Pre-generated negative text embeddings. Can be used to easily tweak text inputs, *e.g.* prompt
- weighting. If not provided, negative_prompt_embeds will be generated from `negative_prompt` input
- argument.
- """
- if prompt is not None and isinstance(prompt, str):
- batch_size = 1
- elif prompt is not None and isinstance(prompt, list):
- batch_size = len(prompt)
- else:
- batch_size = prompt_embeds.shape[0]
-
- if prompt_embeds is None:
- # get prompt text embeddings
- text_inputs = self.tokenizer(
- prompt,
- padding="max_length",
- max_length=self.tokenizer.model_max_length,
- truncation=True,
- return_tensors="np",
- )
- text_input_ids = text_inputs.input_ids
- untruncated_ids = self.tokenizer(prompt, padding="max_length", return_tensors="np").input_ids
-
- if not np.array_equal(text_input_ids, untruncated_ids):
- removed_text = self.tokenizer.batch_decode(
- untruncated_ids[:, self.tokenizer.model_max_length - 1 : -1]
- )
- logger.warning(
- "The following part of your input was truncated because CLIP can only handle sequences up to"
- f" {self.tokenizer.model_max_length} tokens: {removed_text}"
- )
-
- prompt_embeds = self.text_encoder(input_ids=text_input_ids.astype(np.int32))[0]
-
- prompt_embeds = np.repeat(prompt_embeds, num_images_per_prompt, axis=0)
-
- # get unconditional embeddings for classifier free guidance
- if do_classifier_free_guidance and negative_prompt_embeds is None:
- uncond_tokens: List[str]
- if negative_prompt is None:
- uncond_tokens = [""] * batch_size
- elif type(prompt) is not type(negative_prompt):
- raise TypeError(
- f"`negative_prompt` should be the same type to `prompt`, but got {type(negative_prompt)} !="
- f" {type(prompt)}."
- )
- elif isinstance(negative_prompt, str):
- uncond_tokens = [negative_prompt] * batch_size
- elif batch_size != len(negative_prompt):
- raise ValueError(
- f"`negative_prompt`: {negative_prompt} has batch size {len(negative_prompt)}, but `prompt`:"
- f" {prompt} has batch size {batch_size}. Please make sure that passed `negative_prompt` matches"
- " the batch size of `prompt`."
- )
- else:
- uncond_tokens = negative_prompt
-
- max_length = prompt_embeds.shape[1]
- uncond_input = self.tokenizer(
- uncond_tokens,
- padding="max_length",
- max_length=max_length,
- truncation=True,
- return_tensors="np",
- )
- negative_prompt_embeds = self.text_encoder(input_ids=uncond_input.input_ids.astype(np.int32))[0]
-
- if do_classifier_free_guidance:
- negative_prompt_embeds = np.repeat(negative_prompt_embeds, num_images_per_prompt, axis=0)
-
- # For classifier free guidance, we need to do two forward passes.
- # Here we concatenate the unconditional and text embeddings into a single batch
- # to avoid doing two forward passes
- prompt_embeds = np.concatenate([negative_prompt_embeds, prompt_embeds])
-
- return prompt_embeds
-
- def check_inputs(
- self,
- prompt,
- callback_steps,
- negative_prompt=None,
- prompt_embeds=None,
- negative_prompt_embeds=None,
- ):
- if (callback_steps is None) or (
- callback_steps is not None and (not isinstance(callback_steps, int) or callback_steps <= 0)
- ):
- raise ValueError(
- f"`callback_steps` has to be a positive integer but is {callback_steps} of type"
- f" {type(callback_steps)}."
- )
-
- if prompt is not None and prompt_embeds is not None:
- raise ValueError(
- f"Cannot forward both `prompt`: {prompt} and `prompt_embeds`: {prompt_embeds}. Please make sure to"
- " only forward one of the two."
- )
- elif prompt is None and prompt_embeds is None:
- raise ValueError(
- "Provide either `prompt` or `prompt_embeds`. Cannot leave both `prompt` and `prompt_embeds` undefined."
- )
- elif prompt is not None and (not isinstance(prompt, str) and not isinstance(prompt, list)):
- raise ValueError(f"`prompt` has to be of type `str` or `list` but is {type(prompt)}")
-
- if negative_prompt is not None and negative_prompt_embeds is not None:
- raise ValueError(
- f"Cannot forward both `negative_prompt`: {negative_prompt} and `negative_prompt_embeds`:"
- f" {negative_prompt_embeds}. Please make sure to only forward one of the two."
- )
-
- if prompt_embeds is not None and negative_prompt_embeds is not None:
- if prompt_embeds.shape != negative_prompt_embeds.shape:
- raise ValueError(
- "`prompt_embeds` and `negative_prompt_embeds` must have the same shape when passed directly, but"
- f" got: `prompt_embeds` {prompt_embeds.shape} != `negative_prompt_embeds`"
- f" {negative_prompt_embeds.shape}."
- )
-
- def __call__(
- self,
- prompt: Union[str, List[str]],
- image: Union[np.ndarray, PIL.Image.Image] = None,
- mask_image: Union[np.ndarray, PIL.Image.Image] = None,
- strength: float = 0.8,
- num_inference_steps: Optional[int] = 50,
- guidance_scale: Optional[float] = 7.5,
- negative_prompt: Optional[Union[str, List[str]]] = None,
- num_images_per_prompt: Optional[int] = 1,
- eta: Optional[float] = 0.0,
- generator: Optional[np.random.RandomState] = None,
- prompt_embeds: Optional[np.ndarray] = None,
- negative_prompt_embeds: Optional[np.ndarray] = None,
- output_type: Optional[str] = "pil",
- return_dict: bool = True,
- callback: Optional[Callable[[int, int, np.ndarray], None]] = None,
- callback_steps: int = 1,
- ):
- r"""
- Function invoked when calling the pipeline for generation.
-
- Args:
- prompt (`str` or `List[str]`):
- The prompt or prompts to guide the image generation.
- image (`nd.ndarray` or `PIL.Image.Image`):
- `Image`, or tensor representing an image batch, that will be used as the starting point for the
- process. This is the image whose masked region will be inpainted.
- mask_image (`nd.ndarray` or `PIL.Image.Image`):
- `Image`, or tensor representing an image batch, to mask `image`. White pixels in the mask will be
- replaced by noise and therefore repainted, while black pixels will be preserved. If `mask_image` is a
- PIL image, it will be converted to a single channel (luminance) before use. If it's a tensor, it should
- contain one color channel (L) instead of 3, so the expected shape would be `(B, H, W, 1)`.uu
- strength (`float`, *optional*, defaults to 0.8):
- Conceptually, indicates how much to transform the reference `image`. Must be between 0 and 1. `image`
- will be used as a starting point, adding more noise to it the larger the `strength`. The number of
- denoising steps depends on the amount of noise initially added. When `strength` is 1, added noise will
- be maximum and the denoising process will run for the full number of iterations specified in
- `num_inference_steps`. A value of 1, therefore, essentially ignores `image`.
- num_inference_steps (`int`, *optional*, defaults to 50):
- The number of denoising steps. More denoising steps usually lead to a higher quality image at the
- expense of slower inference. This parameter will be modulated by `strength`.
- guidance_scale (`float`, *optional*, defaults to 7.5):
- Guidance scale as defined in [Classifier-Free Diffusion Guidance](https://arxiv.org/abs/2207.12598).
- `guidance_scale` is defined as `w` of equation 2. of [Imagen
- Paper](https://arxiv.org/pdf/2205.11487.pdf). Guidance scale is enabled by setting `guidance_scale >
- 1`. Higher guidance scale encourages to generate images that are closely linked to the text `prompt`,
- usually at the expense of lower image quality.
- negative_prompt (`str` or `List[str]`, *optional*):
- The prompt or prompts not to guide the image generation. Ignored when not using guidance (i.e., ignored
- if `guidance_scale` is less than `1`).
- num_images_per_prompt (`int`, *optional*, defaults to 1):
- The number of images to generate per prompt.
- eta (`float`, *optional*, defaults to 0.0):
- Corresponds to parameter eta (?) in the DDIM paper: https://arxiv.org/abs/2010.02502. Only applies to
- [`schedulers.DDIMScheduler`], will be ignored for others.
- generator (`np.random.RandomState`, *optional*):
- A np.random.RandomState to make generation deterministic.
- prompt_embeds (`np.ndarray`, *optional*):
- Pre-generated text embeddings. Can be used to easily tweak text inputs, *e.g.* prompt weighting. If not
- provided, text embeddings will be generated from `prompt` input argument.
- negative_prompt_embeds (`np.ndarray`, *optional*):
- Pre-generated negative text embeddings. Can be used to easily tweak text inputs, *e.g.* prompt
- weighting. If not provided, negative_prompt_embeds will be generated from `negative_prompt` input
- argument.
- output_type (`str`, *optional*, defaults to `"pil"`):
- The output format of the generate image. Choose between
- [PIL](https://pillow.readthedocs.io/en/stable/): `PIL.Image.Image` or `np.array`.
- return_dict (`bool`, *optional*, defaults to `True`):
- Whether or not to return a [`~pipelines.stable_diffusion.StableDiffusionPipelineOutput`] instead of a
- plain tuple.
- callback (`Callable`, *optional*):
- A function that will be called every `callback_steps` steps during inference. The function will be
- called with the following arguments: `callback(step: int, timestep: int, latents: np.ndarray)`.
- callback_steps (`int`, *optional*, defaults to 1):
- The frequency at which the `callback` function will be called. If not specified, the callback will be
- called at every step.
-
- Returns:
- [`~pipelines.stable_diffusion.StableDiffusionPipelineOutput`] or `tuple`:
- [`~pipelines.stable_diffusion.StableDiffusionPipelineOutput`] if `return_dict` is True, otherwise a `tuple.
- When returning a tuple, the first element is a list with the generated images, and the second element is a
- list of `bool`s denoting whether the corresponding generated image likely represents "not-safe-for-work"
- (nsfw) content, according to the `safety_checker`.
- """
-
- # check inputs. Raise error if not correct
- self.check_inputs(prompt, callback_steps, negative_prompt, prompt_embeds, negative_prompt_embeds)
-
- # define call parameters
- if prompt is not None and isinstance(prompt, str):
- batch_size = 1
- elif prompt is not None and isinstance(prompt, list):
- batch_size = len(prompt)
- else:
- batch_size = prompt_embeds.shape[0]
-
- if strength < 0 or strength > 1:
- raise ValueError(f"The value of strength should in [0.0, 1.0] but is {strength}")
-
- if generator is None:
- generator = np.random
-
- # set timesteps
- self.scheduler.set_timesteps(num_inference_steps)
-
- if isinstance(image, PIL.Image.Image):
- image = preprocess(image)
-
- # here `guidance_scale` is defined analog to the guidance weight `w` of equation (2)
- # of the Imagen paper: https://arxiv.org/pdf/2205.11487.pdf . `guidance_scale = 1`
- # corresponds to doing no classifier free guidance.
- do_classifier_free_guidance = guidance_scale > 1.0
-
- prompt_embeds = self._encode_prompt(
- prompt,
- num_images_per_prompt,
- do_classifier_free_guidance,
- negative_prompt,
- prompt_embeds=prompt_embeds,
- negative_prompt_embeds=negative_prompt_embeds,
- )
-
- latents_dtype = prompt_embeds.dtype
- image = image.astype(latents_dtype)
-
- # encode the init image into latents and scale the latents
- init_latents = self.vae_encoder(sample=image)[0]
- init_latents = 0.18215 * init_latents
-
- # Expand init_latents for batch_size and num_images_per_prompt
- init_latents = np.concatenate([init_latents] * num_images_per_prompt, axis=0)
- init_latents_orig = init_latents
-
- # preprocess mask
- if not isinstance(mask_image, np.ndarray):
- mask_image = preprocess_mask(mask_image, 8)
- mask_image = mask_image.astype(latents_dtype)
- mask = np.concatenate([mask_image] * num_images_per_prompt, axis=0)
-
- # check sizes
- if not mask.shape == init_latents.shape:
- raise ValueError("The mask and image should be the same size!")
-
- # get the original timestep using init_timestep
- offset = self.scheduler.config.get("steps_offset", 0)
- init_timestep = int(num_inference_steps * strength) + offset
- init_timestep = min(init_timestep, num_inference_steps)
-
- timesteps = self.scheduler.timesteps.numpy()[-init_timestep]
- timesteps = np.array([timesteps] * batch_size * num_images_per_prompt)
-
- # add noise to latents using the timesteps
- noise = generator.randn(*init_latents.shape).astype(latents_dtype)
- init_latents = self.scheduler.add_noise(
- torch.from_numpy(init_latents), torch.from_numpy(noise), torch.from_numpy(timesteps)
- )
- init_latents = init_latents.numpy()
-
- # prepare extra kwargs for the scheduler step, since not all schedulers have the same signature
- # eta (?) is only used with the DDIMScheduler, it will be ignored for other schedulers.
- # eta corresponds to ? in DDIM paper: https://arxiv.org/abs/2010.02502
- # and should be between [0, 1]
- accepts_eta = "eta" in set(inspect.signature(self.scheduler.step).parameters.keys())
- extra_step_kwargs = {}
- if accepts_eta:
- extra_step_kwargs["eta"] = eta
-
- latents = init_latents
-
- t_start = max(num_inference_steps - init_timestep + offset, 0)
- timesteps = self.scheduler.timesteps[t_start:].numpy()
- timestep_dtype = next(
- (input.type for input in self.unet.model.get_inputs() if input.name == "timestep"), "tensor(float)"
- )
- timestep_dtype = ORT_TO_NP_TYPE[timestep_dtype]
-
- for i, t in enumerate(self.progress_bar(timesteps)):
- # expand the latents if we are doing classifier free guidance
- latent_model_input = np.concatenate([latents] * 2) if do_classifier_free_guidance else latents
- latent_model_input = self.scheduler.scale_model_input(latent_model_input, t)
-
- # predict the noise residual
- timestep = np.array([t], dtype=timestep_dtype)
- noise_pred = self.unet(sample=latent_model_input, timestep=timestep, encoder_hidden_states=prompt_embeds)[
- 0
- ]
-
- # perform guidance
- if do_classifier_free_guidance:
- noise_pred_uncond, noise_pred_text = np.split(noise_pred, 2)
- noise_pred = noise_pred_uncond + guidance_scale * (noise_pred_text - noise_pred_uncond)
-
- # compute the previous noisy sample x_t -> x_t-1
- latents = self.scheduler.step(
- torch.from_numpy(noise_pred), t, torch.from_numpy(latents), **extra_step_kwargs
- ).prev_sample
-
- latents = latents.numpy()
-
- init_latents_proper = self.scheduler.add_noise(
- torch.from_numpy(init_latents_orig), torch.from_numpy(noise), torch.from_numpy(np.array([t]))
- )
-
- init_latents_proper = init_latents_proper.numpy()
-
- latents = (init_latents_proper * mask) + (latents * (1 - mask))
-
- # call the callback, if provided
- if callback is not None and i % callback_steps == 0:
- callback(i, t, latents)
-
- latents = 1 / 0.18215 * latents
- # image = self.vae_decoder(latent_sample=latents)[0]
- # it seems likes there is a strange result for using half-precision vae decoder if batchsize>1
- image = np.concatenate(
- [self.vae_decoder(latent_sample=latents[i : i + 1])[0] for i in range(latents.shape[0])]
- )
-
- image = np.clip(image / 2 + 0.5, 0, 1)
- image = image.transpose((0, 2, 3, 1))
-
- if self.safety_checker is not None:
- safety_checker_input = self.feature_extractor(
- self.numpy_to_pil(image), return_tensors="np"
- ).pixel_values.astype(image.dtype)
- # There will throw an error if use safety_checker batchsize>1
- images, has_nsfw_concept = [], []
- for i in range(image.shape[0]):
- image_i, has_nsfw_concept_i = self.safety_checker(
- clip_input=safety_checker_input[i : i + 1], images=image[i : i + 1]
- )
- images.append(image_i)
- has_nsfw_concept.append(has_nsfw_concept_i[0])
- image = np.concatenate(images)
- else:
- has_nsfw_concept = None
-
- if output_type == "pil":
- image = self.numpy_to_pil(image)
-
- if not return_dict:
- return (image, has_nsfw_concept)
-
- return StableDiffusionPipelineOutput(images=image, nsfw_content_detected=has_nsfw_concept)
diff --git a/spaces/Andy1621/uniformer_image_detection/mmdet/core/anchor/utils.py b/spaces/Andy1621/uniformer_image_detection/mmdet/core/anchor/utils.py
deleted file mode 100644
index ab9b53f37f7be1f52fe63c5e53df64ac1303b9e0..0000000000000000000000000000000000000000
--- a/spaces/Andy1621/uniformer_image_detection/mmdet/core/anchor/utils.py
+++ /dev/null
@@ -1,71 +0,0 @@
-import torch
-
-
-def images_to_levels(target, num_levels):
- """Convert targets by image to targets by feature level.
-
- [target_img0, target_img1] -> [target_level0, target_level1, ...]
- """
- target = torch.stack(target, 0)
- level_targets = []
- start = 0
- for n in num_levels:
- end = start + n
- # level_targets.append(target[:, start:end].squeeze(0))
- level_targets.append(target[:, start:end])
- start = end
- return level_targets
-
-
-def anchor_inside_flags(flat_anchors,
- valid_flags,
- img_shape,
- allowed_border=0):
- """Check whether the anchors are inside the border.
-
- Args:
- flat_anchors (torch.Tensor): Flatten anchors, shape (n, 4).
- valid_flags (torch.Tensor): An existing valid flags of anchors.
- img_shape (tuple(int)): Shape of current image.
- allowed_border (int, optional): The border to allow the valid anchor.
- Defaults to 0.
-
- Returns:
- torch.Tensor: Flags indicating whether the anchors are inside a \
- valid range.
- """
- img_h, img_w = img_shape[:2]
- if allowed_border >= 0:
- inside_flags = valid_flags & \
- (flat_anchors[:, 0] >= -allowed_border) & \
- (flat_anchors[:, 1] >= -allowed_border) & \
- (flat_anchors[:, 2] < img_w + allowed_border) & \
- (flat_anchors[:, 3] < img_h + allowed_border)
- else:
- inside_flags = valid_flags
- return inside_flags
-
-
-def calc_region(bbox, ratio, featmap_size=None):
- """Calculate a proportional bbox region.
-
- The bbox center are fixed and the new h' and w' is h * ratio and w * ratio.
-
- Args:
- bbox (Tensor): Bboxes to calculate regions, shape (n, 4).
- ratio (float): Ratio of the output region.
- featmap_size (tuple): Feature map size used for clipping the boundary.
-
- Returns:
- tuple: x1, y1, x2, y2
- """
- x1 = torch.round((1 - ratio) * bbox[0] + ratio * bbox[2]).long()
- y1 = torch.round((1 - ratio) * bbox[1] + ratio * bbox[3]).long()
- x2 = torch.round(ratio * bbox[0] + (1 - ratio) * bbox[2]).long()
- y2 = torch.round(ratio * bbox[1] + (1 - ratio) * bbox[3]).long()
- if featmap_size is not None:
- x1 = x1.clamp(min=0, max=featmap_size[1])
- y1 = y1.clamp(min=0, max=featmap_size[0])
- x2 = x2.clamp(min=0, max=featmap_size[1])
- y2 = y2.clamp(min=0, max=featmap_size[0])
- return (x1, y1, x2, y2)
diff --git a/spaces/Andy1621/uniformer_image_detection/mmdet/models/losses/pisa_loss.py b/spaces/Andy1621/uniformer_image_detection/mmdet/models/losses/pisa_loss.py
deleted file mode 100644
index 4a48adfcd400bb07b719a6fbd5a8af0508820629..0000000000000000000000000000000000000000
--- a/spaces/Andy1621/uniformer_image_detection/mmdet/models/losses/pisa_loss.py
+++ /dev/null
@@ -1,183 +0,0 @@
-import mmcv
-import torch
-
-from mmdet.core import bbox_overlaps
-
-
-@mmcv.jit(derivate=True, coderize=True)
-def isr_p(cls_score,
- bbox_pred,
- bbox_targets,
- rois,
- sampling_results,
- loss_cls,
- bbox_coder,
- k=2,
- bias=0,
- num_class=80):
- """Importance-based Sample Reweighting (ISR_P), positive part.
-
- Args:
- cls_score (Tensor): Predicted classification scores.
- bbox_pred (Tensor): Predicted bbox deltas.
- bbox_targets (tuple[Tensor]): A tuple of bbox targets, the are
- labels, label_weights, bbox_targets, bbox_weights, respectively.
- rois (Tensor): Anchors (single_stage) in shape (n, 4) or RoIs
- (two_stage) in shape (n, 5).
- sampling_results (obj): Sampling results.
- loss_cls (func): Classification loss func of the head.
- bbox_coder (obj): BBox coder of the head.
- k (float): Power of the non-linear mapping.
- bias (float): Shift of the non-linear mapping.
- num_class (int): Number of classes, default: 80.
-
- Return:
- tuple([Tensor]): labels, imp_based_label_weights, bbox_targets,
- bbox_target_weights
- """
-
- labels, label_weights, bbox_targets, bbox_weights = bbox_targets
- pos_label_inds = ((labels >= 0) &
- (labels < num_class)).nonzero().reshape(-1)
- pos_labels = labels[pos_label_inds]
-
- # if no positive samples, return the original targets
- num_pos = float(pos_label_inds.size(0))
- if num_pos == 0:
- return labels, label_weights, bbox_targets, bbox_weights
-
- # merge pos_assigned_gt_inds of per image to a single tensor
- gts = list()
- last_max_gt = 0
- for i in range(len(sampling_results)):
- gt_i = sampling_results[i].pos_assigned_gt_inds
- gts.append(gt_i + last_max_gt)
- if len(gt_i) != 0:
- last_max_gt = gt_i.max() + 1
- gts = torch.cat(gts)
- assert len(gts) == num_pos
-
- cls_score = cls_score.detach()
- bbox_pred = bbox_pred.detach()
-
- # For single stage detectors, rois here indicate anchors, in shape (N, 4)
- # For two stage detectors, rois are in shape (N, 5)
- if rois.size(-1) == 5:
- pos_rois = rois[pos_label_inds][:, 1:]
- else:
- pos_rois = rois[pos_label_inds]
-
- if bbox_pred.size(-1) > 4:
- bbox_pred = bbox_pred.view(bbox_pred.size(0), -1, 4)
- pos_delta_pred = bbox_pred[pos_label_inds, pos_labels].view(-1, 4)
- else:
- pos_delta_pred = bbox_pred[pos_label_inds].view(-1, 4)
-
- # compute iou of the predicted bbox and the corresponding GT
- pos_delta_target = bbox_targets[pos_label_inds].view(-1, 4)
- pos_bbox_pred = bbox_coder.decode(pos_rois, pos_delta_pred)
- target_bbox_pred = bbox_coder.decode(pos_rois, pos_delta_target)
- ious = bbox_overlaps(pos_bbox_pred, target_bbox_pred, is_aligned=True)
-
- pos_imp_weights = label_weights[pos_label_inds]
- # Two steps to compute IoU-HLR. Samples are first sorted by IoU locally,
- # then sorted again within the same-rank group
- max_l_num = pos_labels.bincount().max()
- for label in pos_labels.unique():
- l_inds = (pos_labels == label).nonzero().view(-1)
- l_gts = gts[l_inds]
- for t in l_gts.unique():
- t_inds = l_inds[l_gts == t]
- t_ious = ious[t_inds]
- _, t_iou_rank_idx = t_ious.sort(descending=True)
- _, t_iou_rank = t_iou_rank_idx.sort()
- ious[t_inds] += max_l_num - t_iou_rank.float()
- l_ious = ious[l_inds]
- _, l_iou_rank_idx = l_ious.sort(descending=True)
- _, l_iou_rank = l_iou_rank_idx.sort() # IoU-HLR
- # linearly map HLR to label weights
- pos_imp_weights[l_inds] *= (max_l_num - l_iou_rank.float()) / max_l_num
-
- pos_imp_weights = (bias + pos_imp_weights * (1 - bias)).pow(k)
-
- # normalize to make the new weighted loss value equal to the original loss
- pos_loss_cls = loss_cls(
- cls_score[pos_label_inds], pos_labels, reduction_override='none')
- if pos_loss_cls.dim() > 1:
- ori_pos_loss_cls = pos_loss_cls * label_weights[pos_label_inds][:,
- None]
- new_pos_loss_cls = pos_loss_cls * pos_imp_weights[:, None]
- else:
- ori_pos_loss_cls = pos_loss_cls * label_weights[pos_label_inds]
- new_pos_loss_cls = pos_loss_cls * pos_imp_weights
- pos_loss_cls_ratio = ori_pos_loss_cls.sum() / new_pos_loss_cls.sum()
- pos_imp_weights = pos_imp_weights * pos_loss_cls_ratio
- label_weights[pos_label_inds] = pos_imp_weights
-
- bbox_targets = labels, label_weights, bbox_targets, bbox_weights
- return bbox_targets
-
-
-@mmcv.jit(derivate=True, coderize=True)
-def carl_loss(cls_score,
- labels,
- bbox_pred,
- bbox_targets,
- loss_bbox,
- k=1,
- bias=0.2,
- avg_factor=None,
- sigmoid=False,
- num_class=80):
- """Classification-Aware Regression Loss (CARL).
-
- Args:
- cls_score (Tensor): Predicted classification scores.
- labels (Tensor): Targets of classification.
- bbox_pred (Tensor): Predicted bbox deltas.
- bbox_targets (Tensor): Target of bbox regression.
- loss_bbox (func): Regression loss func of the head.
- bbox_coder (obj): BBox coder of the head.
- k (float): Power of the non-linear mapping.
- bias (float): Shift of the non-linear mapping.
- avg_factor (int): Average factor used in regression loss.
- sigmoid (bool): Activation of the classification score.
- num_class (int): Number of classes, default: 80.
-
- Return:
- dict: CARL loss dict.
- """
- pos_label_inds = ((labels >= 0) &
- (labels < num_class)).nonzero().reshape(-1)
- if pos_label_inds.numel() == 0:
- return dict(loss_carl=cls_score.sum()[None] * 0.)
- pos_labels = labels[pos_label_inds]
-
- # multiply pos_cls_score with the corresponding bbox weight
- # and remain gradient
- if sigmoid:
- pos_cls_score = cls_score.sigmoid()[pos_label_inds, pos_labels]
- else:
- pos_cls_score = cls_score.softmax(-1)[pos_label_inds, pos_labels]
- carl_loss_weights = (bias + (1 - bias) * pos_cls_score).pow(k)
-
- # normalize carl_loss_weight to make its sum equal to num positive
- num_pos = float(pos_cls_score.size(0))
- weight_ratio = num_pos / carl_loss_weights.sum()
- carl_loss_weights *= weight_ratio
-
- if avg_factor is None:
- avg_factor = bbox_targets.size(0)
- # if is class agnostic, bbox pred is in shape (N, 4)
- # otherwise, bbox pred is in shape (N, #classes, 4)
- if bbox_pred.size(-1) > 4:
- bbox_pred = bbox_pred.view(bbox_pred.size(0), -1, 4)
- pos_bbox_preds = bbox_pred[pos_label_inds, pos_labels]
- else:
- pos_bbox_preds = bbox_pred[pos_label_inds]
- ori_loss_reg = loss_bbox(
- pos_bbox_preds,
- bbox_targets[pos_label_inds],
- reduction_override='none') / avg_factor
- loss_carl = (ori_loss_reg * carl_loss_weights[:, None]).sum()
- return dict(loss_carl=loss_carl[None])
diff --git a/spaces/Andy1621/uniformer_video_demo/uniformer.py b/spaces/Andy1621/uniformer_video_demo/uniformer.py
deleted file mode 100644
index 3c239b96656c9ebdb5c66048c9c6cdfc27d44c34..0000000000000000000000000000000000000000
--- a/spaces/Andy1621/uniformer_video_demo/uniformer.py
+++ /dev/null
@@ -1,379 +0,0 @@
-from collections import OrderedDict
-import torch
-import torch.nn as nn
-from functools import partial
-from timm.models.layers import trunc_normal_, DropPath, to_2tuple
-
-
-def conv_3xnxn(inp, oup, kernel_size=3, stride=3, groups=1):
- return nn.Conv3d(inp, oup, (3, kernel_size, kernel_size), (2, stride, stride), (1, 0, 0), groups=groups)
-
-def conv_1xnxn(inp, oup, kernel_size=3, stride=3, groups=1):
- return nn.Conv3d(inp, oup, (1, kernel_size, kernel_size), (1, stride, stride), (0, 0, 0), groups=groups)
-
-def conv_3xnxn_std(inp, oup, kernel_size=3, stride=3, groups=1):
- return nn.Conv3d(inp, oup, (3, kernel_size, kernel_size), (1, stride, stride), (1, 0, 0), groups=groups)
-
-def conv_1x1x1(inp, oup, groups=1):
- return nn.Conv3d(inp, oup, (1, 1, 1), (1, 1, 1), (0, 0, 0), groups=groups)
-
-def conv_3x3x3(inp, oup, groups=1):
- return nn.Conv3d(inp, oup, (3, 3, 3), (1, 1, 1), (1, 1, 1), groups=groups)
-
-def conv_5x5x5(inp, oup, groups=1):
- return nn.Conv3d(inp, oup, (5, 5, 5), (1, 1, 1), (2, 2, 2), groups=groups)
-
-def bn_3d(dim):
- return nn.BatchNorm3d(dim)
-
-
-class Mlp(nn.Module):
- def __init__(self, in_features, hidden_features=None, out_features=None, act_layer=nn.GELU, drop=0.):
- super().__init__()
- out_features = out_features or in_features
- hidden_features = hidden_features or in_features
- self.fc1 = nn.Linear(in_features, hidden_features)
- self.act = act_layer()
- self.fc2 = nn.Linear(hidden_features, out_features)
- self.drop = nn.Dropout(drop)
-
- def forward(self, x):
- x = self.fc1(x)
- x = self.act(x)
- x = self.drop(x)
- x = self.fc2(x)
- x = self.drop(x)
- return x
-
-
-class Attention(nn.Module):
- def __init__(self, dim, num_heads=8, qkv_bias=False, qk_scale=None, attn_drop=0., proj_drop=0.):
- super().__init__()
- self.num_heads = num_heads
- head_dim = dim // num_heads
- # NOTE scale factor was wrong in my original version, can set manually to be compat with prev weights
- self.scale = qk_scale or head_dim ** -0.5
-
- self.qkv = nn.Linear(dim, dim * 3, bias=qkv_bias)
- self.attn_drop = nn.Dropout(attn_drop)
- self.proj = nn.Linear(dim, dim)
- self.proj_drop = nn.Dropout(proj_drop)
-
- def forward(self, x):
- B, N, C = x.shape
- qkv = self.qkv(x).reshape(B, N, 3, self.num_heads, C // self.num_heads).permute(2, 0, 3, 1, 4)
- q, k, v = qkv[0], qkv[1], qkv[2] # make torchscript happy (cannot use tensor as tuple)
-
- attn = (q @ k.transpose(-2, -1)) * self.scale
- attn = attn.softmax(dim=-1)
- attn = self.attn_drop(attn)
-
- x = (attn @ v).transpose(1, 2).reshape(B, N, C)
- x = self.proj(x)
- x = self.proj_drop(x)
- return x
-
-
-class CMlp(nn.Module):
- def __init__(self, in_features, hidden_features=None, out_features=None, act_layer=nn.GELU, drop=0.):
- super().__init__()
- out_features = out_features or in_features
- hidden_features = hidden_features or in_features
- self.fc1 = conv_1x1x1(in_features, hidden_features)
- self.act = act_layer()
- self.fc2 = conv_1x1x1(hidden_features, out_features)
- self.drop = nn.Dropout(drop)
-
- def forward(self, x):
- x = self.fc1(x)
- x = self.act(x)
- x = self.drop(x)
- x = self.fc2(x)
- x = self.drop(x)
- return x
-
-
-class CBlock(nn.Module):
- def __init__(self, dim, num_heads, mlp_ratio=4., qkv_bias=False, qk_scale=None, drop=0., attn_drop=0.,
- drop_path=0., act_layer=nn.GELU, norm_layer=nn.LayerNorm):
- super().__init__()
- self.pos_embed = conv_3x3x3(dim, dim, groups=dim)
- self.norm1 = bn_3d(dim)
- self.conv1 = conv_1x1x1(dim, dim, 1)
- self.conv2 = conv_1x1x1(dim, dim, 1)
- self.attn = conv_5x5x5(dim, dim, groups=dim)
- # NOTE: drop path for stochastic depth, we shall see if this is better than dropout here
- self.drop_path = DropPath(drop_path) if drop_path > 0. else nn.Identity()
- self.norm2 = bn_3d(dim)
- mlp_hidden_dim = int(dim * mlp_ratio)
- self.mlp = CMlp(in_features=dim, hidden_features=mlp_hidden_dim, act_layer=act_layer, drop=drop)
-
- def forward(self, x):
- x = x + self.pos_embed(x)
- x = x + self.drop_path(self.conv2(self.attn(self.conv1(self.norm1(x)))))
- x = x + self.drop_path(self.mlp(self.norm2(x)))
- return x
-
-
-class SABlock(nn.Module):
- def __init__(self, dim, num_heads, mlp_ratio=4., qkv_bias=False, qk_scale=None, drop=0., attn_drop=0.,
- drop_path=0., act_layer=nn.GELU, norm_layer=nn.LayerNorm):
- super().__init__()
- self.pos_embed = conv_3x3x3(dim, dim, groups=dim)
- self.norm1 = norm_layer(dim)
- self.attn = Attention(
- dim,
- num_heads=num_heads, qkv_bias=qkv_bias, qk_scale=qk_scale,
- attn_drop=attn_drop, proj_drop=drop)
- # NOTE: drop path for stochastic depth, we shall see if this is better than dropout here
- self.drop_path = DropPath(drop_path) if drop_path > 0. else nn.Identity()
- self.norm2 = norm_layer(dim)
- mlp_hidden_dim = int(dim * mlp_ratio)
- self.mlp = Mlp(in_features=dim, hidden_features=mlp_hidden_dim, act_layer=act_layer, drop=drop)
-
- def forward(self, x):
- x = x + self.pos_embed(x)
- B, C, T, H, W = x.shape
- x = x.flatten(2).transpose(1, 2)
- x = x + self.drop_path(self.attn(self.norm1(x)))
- x = x + self.drop_path(self.mlp(self.norm2(x)))
- x = x.transpose(1, 2).reshape(B, C, T, H, W)
- return x
-
-
-class SplitSABlock(nn.Module):
- def __init__(self, dim, num_heads, mlp_ratio=4., qkv_bias=False, qk_scale=None, drop=0., attn_drop=0.,
- drop_path=0., act_layer=nn.GELU, norm_layer=nn.LayerNorm):
- super().__init__()
- self.pos_embed = conv_3x3x3(dim, dim, groups=dim)
- self.t_norm = norm_layer(dim)
- self.t_attn = Attention(
- dim,
- num_heads=num_heads, qkv_bias=qkv_bias, qk_scale=qk_scale,
- attn_drop=attn_drop, proj_drop=drop)
- self.norm1 = norm_layer(dim)
- self.attn = Attention(
- dim,
- num_heads=num_heads, qkv_bias=qkv_bias, qk_scale=qk_scale,
- attn_drop=attn_drop, proj_drop=drop)
- # NOTE: drop path for stochastic depth, we shall see if this is better than dropout here
- self.drop_path = DropPath(drop_path) if drop_path > 0. else nn.Identity()
- self.norm2 = norm_layer(dim)
- mlp_hidden_dim = int(dim * mlp_ratio)
- self.mlp = Mlp(in_features=dim, hidden_features=mlp_hidden_dim, act_layer=act_layer, drop=drop)
-
- def forward(self, x):
- x = x + self.pos_embed(x)
- B, C, T, H, W = x.shape
- attn = x.view(B, C, T, H * W).permute(0, 3, 2, 1).contiguous()
- attn = attn.view(B * H * W, T, C)
- attn = attn + self.drop_path(self.t_attn(self.t_norm(attn)))
- attn = attn.view(B, H * W, T, C).permute(0, 2, 1, 3).contiguous()
- attn = attn.view(B * T, H * W, C)
- residual = x.view(B, C, T, H * W).permute(0, 2, 3, 1).contiguous()
- residual = residual.view(B * T, H * W, C)
- attn = residual + self.drop_path(self.attn(self.norm1(attn)))
- attn = attn.view(B, T * H * W, C)
- out = attn + self.drop_path(self.mlp(self.norm2(attn)))
- out = out.transpose(1, 2).reshape(B, C, T, H, W)
- return out
-
-
-class SpeicalPatchEmbed(nn.Module):
- """ Image to Patch Embedding
- """
- def __init__(self, img_size=224, patch_size=16, in_chans=3, embed_dim=768):
- super().__init__()
- img_size = to_2tuple(img_size)
- patch_size = to_2tuple(patch_size)
- num_patches = (img_size[1] // patch_size[1]) * (img_size[0] // patch_size[0])
- self.img_size = img_size
- self.patch_size = patch_size
- self.num_patches = num_patches
- self.norm = nn.LayerNorm(embed_dim)
- self.proj = conv_3xnxn(in_chans, embed_dim, kernel_size=patch_size[0], stride=patch_size[0])
-
- def forward(self, x):
- B, C, T, H, W = x.shape
- # FIXME look at relaxing size constraints
- # assert H == self.img_size[0] and W == self.img_size[1], \
- # f"Input image size ({H}*{W}) doesn't match model ({self.img_size[0]}*{self.img_size[1]})."
- x = self.proj(x)
- B, C, T, H, W = x.shape
- x = x.flatten(2).transpose(1, 2)
- x = self.norm(x)
- x = x.reshape(B, T, H, W, -1).permute(0, 4, 1, 2, 3).contiguous()
- return x
-
-
-class PatchEmbed(nn.Module):
- """ Image to Patch Embedding
- """
- def __init__(self, img_size=224, patch_size=16, in_chans=3, embed_dim=768, std=False):
- super().__init__()
- img_size = to_2tuple(img_size)
- patch_size = to_2tuple(patch_size)
- num_patches = (img_size[1] // patch_size[1]) * (img_size[0] // patch_size[0])
- self.img_size = img_size
- self.patch_size = patch_size
- self.num_patches = num_patches
- self.norm = nn.LayerNorm(embed_dim)
- if std:
- self.proj = conv_3xnxn_std(in_chans, embed_dim, kernel_size=patch_size[0], stride=patch_size[0])
- else:
- self.proj = conv_1xnxn(in_chans, embed_dim, kernel_size=patch_size[0], stride=patch_size[0])
-
- def forward(self, x):
- B, C, T, H, W = x.shape
- # FIXME look at relaxing size constraints
- # assert H == self.img_size[0] and W == self.img_size[1], \
- # f"Input image size ({H}*{W}) doesn't match model ({self.img_size[0]}*{self.img_size[1]})."
- x = self.proj(x)
- B, C, T, H, W = x.shape
- x = x.flatten(2).transpose(1, 2)
- x = self.norm(x)
- x = x.reshape(B, T, H, W, -1).permute(0, 4, 1, 2, 3).contiguous()
- return x
-
-
-class Uniformer(nn.Module):
- """ Vision Transformer
- A PyTorch impl of : `An Image is Worth 16x16 Words: Transformers for Image Recognition at Scale` -
- https://arxiv.org/abs/2010.11929
- """
- def __init__(self, depth=[5, 8, 20, 7], num_classes=400, img_size=224, in_chans=3, embed_dim=[64, 128, 320, 512],
- head_dim=64, mlp_ratio=4., qkv_bias=True, qk_scale=None, representation_size=None,
- drop_rate=0.3, attn_drop_rate=0., drop_path_rate=0., norm_layer=None, split=False, std=False):
- super().__init__()
-
- self.num_classes = num_classes
- self.num_features = self.embed_dim = embed_dim # num_features for consistency with other models
- norm_layer = partial(nn.LayerNorm, eps=1e-6)
-
- self.patch_embed1 = SpeicalPatchEmbed(
- img_size=img_size, patch_size=4, in_chans=in_chans, embed_dim=embed_dim[0])
- self.patch_embed2 = PatchEmbed(
- img_size=img_size // 4, patch_size=2, in_chans=embed_dim[0], embed_dim=embed_dim[1], std=std)
- self.patch_embed3 = PatchEmbed(
- img_size=img_size // 8, patch_size=2, in_chans=embed_dim[1], embed_dim=embed_dim[2], std=std)
- self.patch_embed4 = PatchEmbed(
- img_size=img_size // 16, patch_size=2, in_chans=embed_dim[2], embed_dim=embed_dim[3], std=std)
-
- self.pos_drop = nn.Dropout(p=drop_rate)
- dpr = [x.item() for x in torch.linspace(0, drop_path_rate, sum(depth))] # stochastic depth decay rule
- num_heads = [dim // head_dim for dim in embed_dim]
- self.blocks1 = nn.ModuleList([
- CBlock(
- dim=embed_dim[0], num_heads=num_heads[0], mlp_ratio=mlp_ratio, qkv_bias=qkv_bias, qk_scale=qk_scale,
- drop=drop_rate, attn_drop=attn_drop_rate, drop_path=dpr[i], norm_layer=norm_layer)
- for i in range(depth[0])])
- self.blocks2 = nn.ModuleList([
- CBlock(
- dim=embed_dim[1], num_heads=num_heads[1], mlp_ratio=mlp_ratio, qkv_bias=qkv_bias, qk_scale=qk_scale,
- drop=drop_rate, attn_drop=attn_drop_rate, drop_path=dpr[i+depth[0]], norm_layer=norm_layer)
- for i in range(depth[1])])
- if split:
- self.blocks3 = nn.ModuleList([
- SplitSABlock(
- dim=embed_dim[2], num_heads=num_heads[2], mlp_ratio=mlp_ratio, qkv_bias=qkv_bias, qk_scale=qk_scale,
- drop=drop_rate, attn_drop=attn_drop_rate, drop_path=dpr[i+depth[0]+depth[1]], norm_layer=norm_layer)
- for i in range(depth[2])])
- self.blocks4 = nn.ModuleList([
- SplitSABlock(
- dim=embed_dim[3], num_heads=num_heads[3], mlp_ratio=mlp_ratio, qkv_bias=qkv_bias, qk_scale=qk_scale,
- drop=drop_rate, attn_drop=attn_drop_rate, drop_path=dpr[i+depth[0]+depth[1]+depth[2]], norm_layer=norm_layer)
- for i in range(depth[3])])
- else:
- self.blocks3 = nn.ModuleList([
- SABlock(
- dim=embed_dim[2], num_heads=num_heads[2], mlp_ratio=mlp_ratio, qkv_bias=qkv_bias, qk_scale=qk_scale,
- drop=drop_rate, attn_drop=attn_drop_rate, drop_path=dpr[i+depth[0]+depth[1]], norm_layer=norm_layer)
- for i in range(depth[2])])
- self.blocks4 = nn.ModuleList([
- SABlock(
- dim=embed_dim[3], num_heads=num_heads[3], mlp_ratio=mlp_ratio, qkv_bias=qkv_bias, qk_scale=qk_scale,
- drop=drop_rate, attn_drop=attn_drop_rate, drop_path=dpr[i+depth[0]+depth[1]+depth[2]], norm_layer=norm_layer)
- for i in range(depth[3])])
- self.norm = bn_3d(embed_dim[-1])
-
- # Representation layer
- if representation_size:
- self.num_features = representation_size
- self.pre_logits = nn.Sequential(OrderedDict([
- ('fc', nn.Linear(embed_dim, representation_size)),
- ('act', nn.Tanh())
- ]))
- else:
- self.pre_logits = nn.Identity()
-
- # Classifier head
- self.head = nn.Linear(embed_dim[-1], num_classes) if num_classes > 0 else nn.Identity()
-
- self.apply(self._init_weights)
-
- for name, p in self.named_parameters():
- # fill proj weight with 1 here to improve training dynamics. Otherwise temporal attention inputs
- # are multiplied by 0*0, which is hard for the model to move out of.
- if 't_attn.qkv.weight' in name:
- nn.init.constant_(p, 0)
- if 't_attn.qkv.bias' in name:
- nn.init.constant_(p, 0)
- if 't_attn.proj.weight' in name:
- nn.init.constant_(p, 1)
- if 't_attn.proj.bias' in name:
- nn.init.constant_(p, 0)
-
- def _init_weights(self, m):
- if isinstance(m, nn.Linear):
- trunc_normal_(m.weight, std=.02)
- if isinstance(m, nn.Linear) and m.bias is not None:
- nn.init.constant_(m.bias, 0)
- elif isinstance(m, nn.LayerNorm):
- nn.init.constant_(m.bias, 0)
- nn.init.constant_(m.weight, 1.0)
-
- @torch.jit.ignore
- def no_weight_decay(self):
- return {'pos_embed', 'cls_token'}
-
- def get_classifier(self):
- return self.head
-
- def reset_classifier(self, num_classes, global_pool=''):
- self.num_classes = num_classes
- self.head = nn.Linear(self.embed_dim, num_classes) if num_classes > 0 else nn.Identity()
-
- def forward_features(self, x):
- x = self.patch_embed1(x)
- x = self.pos_drop(x)
- for blk in self.blocks1:
- x = blk(x)
- x = self.patch_embed2(x)
- for blk in self.blocks2:
- x = blk(x)
- x = self.patch_embed3(x)
- for blk in self.blocks3:
- x = blk(x)
- x = self.patch_embed4(x)
- for blk in self.blocks4:
- x = blk(x)
- x = self.norm(x)
- x = self.pre_logits(x)
- return x
-
- def forward(self, x):
- x = self.forward_features(x)
- x = x.flatten(2).mean(-1)
- x = self.head(x)
- return x
-
-
-def uniformer_small():
- return Uniformer(
- depth=[3, 4, 8, 3], embed_dim=[64, 128, 320, 512],
- head_dim=64, drop_rate=0.1)
-
-def uniformer_base():
- return Uniformer(
- depth=[5, 8, 20, 7], embed_dim=[64, 128, 320, 512],
- head_dim=64, drop_rate=0.3)
\ No newline at end of file
diff --git a/spaces/ArpitM/chat-llm-streaming/README.md b/spaces/ArpitM/chat-llm-streaming/README.md
deleted file mode 100644
index 349f818153093c6374a473a4ca5d5cb6cc8d1b52..0000000000000000000000000000000000000000
--- a/spaces/ArpitM/chat-llm-streaming/README.md
+++ /dev/null
@@ -1,12 +0,0 @@
----
-title: Chat Llm Streaming
-emoji: 📊
-colorFrom: blue
-colorTo: gray
-sdk: gradio
-sdk_version: 3.20.1
-app_file: app.py
-pinned: false
----
-
-Check out the configuration reference at https://huggingface.co/docs/hub/spaces-config-reference
diff --git a/spaces/Ataturk-Chatbot/HuggingFaceChat/venv/lib/python3.11/site-packages/pip/_vendor/rich/syntax.py b/spaces/Ataturk-Chatbot/HuggingFaceChat/venv/lib/python3.11/site-packages/pip/_vendor/rich/syntax.py
deleted file mode 100644
index 25b226a3a986c507747c8b40dc17f7a8017e73e1..0000000000000000000000000000000000000000
--- a/spaces/Ataturk-Chatbot/HuggingFaceChat/venv/lib/python3.11/site-packages/pip/_vendor/rich/syntax.py
+++ /dev/null
@@ -1,950 +0,0 @@
-import os.path
-import platform
-import re
-import sys
-import textwrap
-from abc import ABC, abstractmethod
-from pathlib import Path
-from typing import (
- Any,
- Dict,
- Iterable,
- List,
- NamedTuple,
- Optional,
- Sequence,
- Set,
- Tuple,
- Type,
- Union,
-)
-
-from pip._vendor.pygments.lexer import Lexer
-from pip._vendor.pygments.lexers import get_lexer_by_name, guess_lexer_for_filename
-from pip._vendor.pygments.style import Style as PygmentsStyle
-from pip._vendor.pygments.styles import get_style_by_name
-from pip._vendor.pygments.token import (
- Comment,
- Error,
- Generic,
- Keyword,
- Name,
- Number,
- Operator,
- String,
- Token,
- Whitespace,
-)
-from pip._vendor.pygments.util import ClassNotFound
-
-from pip._vendor.rich.containers import Lines
-from pip._vendor.rich.padding import Padding, PaddingDimensions
-
-from ._loop import loop_first
-from .cells import cell_len
-from .color import Color, blend_rgb
-from .console import Console, ConsoleOptions, JustifyMethod, RenderResult
-from .jupyter import JupyterMixin
-from .measure import Measurement
-from .segment import Segment, Segments
-from .style import Style, StyleType
-from .text import Text
-
-TokenType = Tuple[str, ...]
-
-WINDOWS = platform.system() == "Windows"
-DEFAULT_THEME = "monokai"
-
-# The following styles are based on https://github.com/pygments/pygments/blob/master/pygments/formatters/terminal.py
-# A few modifications were made
-
-ANSI_LIGHT: Dict[TokenType, Style] = {
- Token: Style(),
- Whitespace: Style(color="white"),
- Comment: Style(dim=True),
- Comment.Preproc: Style(color="cyan"),
- Keyword: Style(color="blue"),
- Keyword.Type: Style(color="cyan"),
- Operator.Word: Style(color="magenta"),
- Name.Builtin: Style(color="cyan"),
- Name.Function: Style(color="green"),
- Name.Namespace: Style(color="cyan", underline=True),
- Name.Class: Style(color="green", underline=True),
- Name.Exception: Style(color="cyan"),
- Name.Decorator: Style(color="magenta", bold=True),
- Name.Variable: Style(color="red"),
- Name.Constant: Style(color="red"),
- Name.Attribute: Style(color="cyan"),
- Name.Tag: Style(color="bright_blue"),
- String: Style(color="yellow"),
- Number: Style(color="blue"),
- Generic.Deleted: Style(color="bright_red"),
- Generic.Inserted: Style(color="green"),
- Generic.Heading: Style(bold=True),
- Generic.Subheading: Style(color="magenta", bold=True),
- Generic.Prompt: Style(bold=True),
- Generic.Error: Style(color="bright_red"),
- Error: Style(color="red", underline=True),
-}
-
-ANSI_DARK: Dict[TokenType, Style] = {
- Token: Style(),
- Whitespace: Style(color="bright_black"),
- Comment: Style(dim=True),
- Comment.Preproc: Style(color="bright_cyan"),
- Keyword: Style(color="bright_blue"),
- Keyword.Type: Style(color="bright_cyan"),
- Operator.Word: Style(color="bright_magenta"),
- Name.Builtin: Style(color="bright_cyan"),
- Name.Function: Style(color="bright_green"),
- Name.Namespace: Style(color="bright_cyan", underline=True),
- Name.Class: Style(color="bright_green", underline=True),
- Name.Exception: Style(color="bright_cyan"),
- Name.Decorator: Style(color="bright_magenta", bold=True),
- Name.Variable: Style(color="bright_red"),
- Name.Constant: Style(color="bright_red"),
- Name.Attribute: Style(color="bright_cyan"),
- Name.Tag: Style(color="bright_blue"),
- String: Style(color="yellow"),
- Number: Style(color="bright_blue"),
- Generic.Deleted: Style(color="bright_red"),
- Generic.Inserted: Style(color="bright_green"),
- Generic.Heading: Style(bold=True),
- Generic.Subheading: Style(color="bright_magenta", bold=True),
- Generic.Prompt: Style(bold=True),
- Generic.Error: Style(color="bright_red"),
- Error: Style(color="red", underline=True),
-}
-
-RICH_SYNTAX_THEMES = {"ansi_light": ANSI_LIGHT, "ansi_dark": ANSI_DARK}
-NUMBERS_COLUMN_DEFAULT_PADDING = 2
-
-
-class SyntaxTheme(ABC):
- """Base class for a syntax theme."""
-
- @abstractmethod
- def get_style_for_token(self, token_type: TokenType) -> Style:
- """Get a style for a given Pygments token."""
- raise NotImplementedError # pragma: no cover
-
- @abstractmethod
- def get_background_style(self) -> Style:
- """Get the background color."""
- raise NotImplementedError # pragma: no cover
-
-
-class PygmentsSyntaxTheme(SyntaxTheme):
- """Syntax theme that delegates to Pygments theme."""
-
- def __init__(self, theme: Union[str, Type[PygmentsStyle]]) -> None:
- self._style_cache: Dict[TokenType, Style] = {}
- if isinstance(theme, str):
- try:
- self._pygments_style_class = get_style_by_name(theme)
- except ClassNotFound:
- self._pygments_style_class = get_style_by_name("default")
- else:
- self._pygments_style_class = theme
-
- self._background_color = self._pygments_style_class.background_color
- self._background_style = Style(bgcolor=self._background_color)
-
- def get_style_for_token(self, token_type: TokenType) -> Style:
- """Get a style from a Pygments class."""
- try:
- return self._style_cache[token_type]
- except KeyError:
- try:
- pygments_style = self._pygments_style_class.style_for_token(token_type)
- except KeyError:
- style = Style.null()
- else:
- color = pygments_style["color"]
- bgcolor = pygments_style["bgcolor"]
- style = Style(
- color="#" + color if color else "#000000",
- bgcolor="#" + bgcolor if bgcolor else self._background_color,
- bold=pygments_style["bold"],
- italic=pygments_style["italic"],
- underline=pygments_style["underline"],
- )
- self._style_cache[token_type] = style
- return style
-
- def get_background_style(self) -> Style:
- return self._background_style
-
-
-class ANSISyntaxTheme(SyntaxTheme):
- """Syntax theme to use standard colors."""
-
- def __init__(self, style_map: Dict[TokenType, Style]) -> None:
- self.style_map = style_map
- self._missing_style = Style.null()
- self._background_style = Style.null()
- self._style_cache: Dict[TokenType, Style] = {}
-
- def get_style_for_token(self, token_type: TokenType) -> Style:
- """Look up style in the style map."""
- try:
- return self._style_cache[token_type]
- except KeyError:
- # Styles form a hierarchy
- # We need to go from most to least specific
- # e.g. ("foo", "bar", "baz") to ("foo", "bar") to ("foo",)
- get_style = self.style_map.get
- token = tuple(token_type)
- style = self._missing_style
- while token:
- _style = get_style(token)
- if _style is not None:
- style = _style
- break
- token = token[:-1]
- self._style_cache[token_type] = style
- return style
-
- def get_background_style(self) -> Style:
- return self._background_style
-
-
-SyntaxPosition = Tuple[int, int]
-
-
-class _SyntaxHighlightRange(NamedTuple):
- """
- A range to highlight in a Syntax object.
- `start` and `end` are 2-integers tuples, where the first integer is the line number
- (starting from 1) and the second integer is the column index (starting from 0).
- """
-
- style: StyleType
- start: SyntaxPosition
- end: SyntaxPosition
-
-
-class Syntax(JupyterMixin):
- """Construct a Syntax object to render syntax highlighted code.
-
- Args:
- code (str): Code to highlight.
- lexer (Lexer | str): Lexer to use (see https://pygments.org/docs/lexers/)
- theme (str, optional): Color theme, aka Pygments style (see https://pygments.org/docs/styles/#getting-a-list-of-available-styles). Defaults to "monokai".
- dedent (bool, optional): Enable stripping of initial whitespace. Defaults to False.
- line_numbers (bool, optional): Enable rendering of line numbers. Defaults to False.
- start_line (int, optional): Starting number for line numbers. Defaults to 1.
- line_range (Tuple[int | None, int | None], optional): If given should be a tuple of the start and end line to render.
- A value of None in the tuple indicates the range is open in that direction.
- highlight_lines (Set[int]): A set of line numbers to highlight.
- code_width: Width of code to render (not including line numbers), or ``None`` to use all available width.
- tab_size (int, optional): Size of tabs. Defaults to 4.
- word_wrap (bool, optional): Enable word wrapping.
- background_color (str, optional): Optional background color, or None to use theme color. Defaults to None.
- indent_guides (bool, optional): Show indent guides. Defaults to False.
- padding (PaddingDimensions): Padding to apply around the syntax. Defaults to 0 (no padding).
- """
-
- _pygments_style_class: Type[PygmentsStyle]
- _theme: SyntaxTheme
-
- @classmethod
- def get_theme(cls, name: Union[str, SyntaxTheme]) -> SyntaxTheme:
- """Get a syntax theme instance."""
- if isinstance(name, SyntaxTheme):
- return name
- theme: SyntaxTheme
- if name in RICH_SYNTAX_THEMES:
- theme = ANSISyntaxTheme(RICH_SYNTAX_THEMES[name])
- else:
- theme = PygmentsSyntaxTheme(name)
- return theme
-
- def __init__(
- self,
- code: str,
- lexer: Union[Lexer, str],
- *,
- theme: Union[str, SyntaxTheme] = DEFAULT_THEME,
- dedent: bool = False,
- line_numbers: bool = False,
- start_line: int = 1,
- line_range: Optional[Tuple[Optional[int], Optional[int]]] = None,
- highlight_lines: Optional[Set[int]] = None,
- code_width: Optional[int] = None,
- tab_size: int = 4,
- word_wrap: bool = False,
- background_color: Optional[str] = None,
- indent_guides: bool = False,
- padding: PaddingDimensions = 0,
- ) -> None:
- self.code = code
- self._lexer = lexer
- self.dedent = dedent
- self.line_numbers = line_numbers
- self.start_line = start_line
- self.line_range = line_range
- self.highlight_lines = highlight_lines or set()
- self.code_width = code_width
- self.tab_size = tab_size
- self.word_wrap = word_wrap
- self.background_color = background_color
- self.background_style = (
- Style(bgcolor=background_color) if background_color else Style()
- )
- self.indent_guides = indent_guides
- self.padding = padding
-
- self._theme = self.get_theme(theme)
- self._stylized_ranges: List[_SyntaxHighlightRange] = []
-
- @classmethod
- def from_path(
- cls,
- path: str,
- encoding: str = "utf-8",
- lexer: Optional[Union[Lexer, str]] = None,
- theme: Union[str, SyntaxTheme] = DEFAULT_THEME,
- dedent: bool = False,
- line_numbers: bool = False,
- line_range: Optional[Tuple[int, int]] = None,
- start_line: int = 1,
- highlight_lines: Optional[Set[int]] = None,
- code_width: Optional[int] = None,
- tab_size: int = 4,
- word_wrap: bool = False,
- background_color: Optional[str] = None,
- indent_guides: bool = False,
- padding: PaddingDimensions = 0,
- ) -> "Syntax":
- """Construct a Syntax object from a file.
-
- Args:
- path (str): Path to file to highlight.
- encoding (str): Encoding of file.
- lexer (str | Lexer, optional): Lexer to use. If None, lexer will be auto-detected from path/file content.
- theme (str, optional): Color theme, aka Pygments style (see https://pygments.org/docs/styles/#getting-a-list-of-available-styles). Defaults to "emacs".
- dedent (bool, optional): Enable stripping of initial whitespace. Defaults to True.
- line_numbers (bool, optional): Enable rendering of line numbers. Defaults to False.
- start_line (int, optional): Starting number for line numbers. Defaults to 1.
- line_range (Tuple[int, int], optional): If given should be a tuple of the start and end line to render.
- highlight_lines (Set[int]): A set of line numbers to highlight.
- code_width: Width of code to render (not including line numbers), or ``None`` to use all available width.
- tab_size (int, optional): Size of tabs. Defaults to 4.
- word_wrap (bool, optional): Enable word wrapping of code.
- background_color (str, optional): Optional background color, or None to use theme color. Defaults to None.
- indent_guides (bool, optional): Show indent guides. Defaults to False.
- padding (PaddingDimensions): Padding to apply around the syntax. Defaults to 0 (no padding).
-
- Returns:
- [Syntax]: A Syntax object that may be printed to the console
- """
- code = Path(path).read_text(encoding=encoding)
-
- if not lexer:
- lexer = cls.guess_lexer(path, code=code)
-
- return cls(
- code,
- lexer,
- theme=theme,
- dedent=dedent,
- line_numbers=line_numbers,
- line_range=line_range,
- start_line=start_line,
- highlight_lines=highlight_lines,
- code_width=code_width,
- tab_size=tab_size,
- word_wrap=word_wrap,
- background_color=background_color,
- indent_guides=indent_guides,
- padding=padding,
- )
-
- @classmethod
- def guess_lexer(cls, path: str, code: Optional[str] = None) -> str:
- """Guess the alias of the Pygments lexer to use based on a path and an optional string of code.
- If code is supplied, it will use a combination of the code and the filename to determine the
- best lexer to use. For example, if the file is ``index.html`` and the file contains Django
- templating syntax, then "html+django" will be returned. If the file is ``index.html``, and no
- templating language is used, the "html" lexer will be used. If no string of code
- is supplied, the lexer will be chosen based on the file extension..
-
- Args:
- path (AnyStr): The path to the file containing the code you wish to know the lexer for.
- code (str, optional): Optional string of code that will be used as a fallback if no lexer
- is found for the supplied path.
-
- Returns:
- str: The name of the Pygments lexer that best matches the supplied path/code.
- """
- lexer: Optional[Lexer] = None
- lexer_name = "default"
- if code:
- try:
- lexer = guess_lexer_for_filename(path, code)
- except ClassNotFound:
- pass
-
- if not lexer:
- try:
- _, ext = os.path.splitext(path)
- if ext:
- extension = ext.lstrip(".").lower()
- lexer = get_lexer_by_name(extension)
- except ClassNotFound:
- pass
-
- if lexer:
- if lexer.aliases:
- lexer_name = lexer.aliases[0]
- else:
- lexer_name = lexer.name
-
- return lexer_name
-
- def _get_base_style(self) -> Style:
- """Get the base style."""
- default_style = self._theme.get_background_style() + self.background_style
- return default_style
-
- def _get_token_color(self, token_type: TokenType) -> Optional[Color]:
- """Get a color (if any) for the given token.
-
- Args:
- token_type (TokenType): A token type tuple from Pygments.
-
- Returns:
- Optional[Color]: Color from theme, or None for no color.
- """
- style = self._theme.get_style_for_token(token_type)
- return style.color
-
- @property
- def lexer(self) -> Optional[Lexer]:
- """The lexer for this syntax, or None if no lexer was found.
-
- Tries to find the lexer by name if a string was passed to the constructor.
- """
-
- if isinstance(self._lexer, Lexer):
- return self._lexer
- try:
- return get_lexer_by_name(
- self._lexer,
- stripnl=False,
- ensurenl=True,
- tabsize=self.tab_size,
- )
- except ClassNotFound:
- return None
-
- def highlight(
- self,
- code: str,
- line_range: Optional[Tuple[Optional[int], Optional[int]]] = None,
- ) -> Text:
- """Highlight code and return a Text instance.
-
- Args:
- code (str): Code to highlight.
- line_range(Tuple[int, int], optional): Optional line range to highlight.
-
- Returns:
- Text: A text instance containing highlighted syntax.
- """
-
- base_style = self._get_base_style()
- justify: JustifyMethod = (
- "default" if base_style.transparent_background else "left"
- )
-
- text = Text(
- justify=justify,
- style=base_style,
- tab_size=self.tab_size,
- no_wrap=not self.word_wrap,
- )
- _get_theme_style = self._theme.get_style_for_token
-
- lexer = self.lexer
-
- if lexer is None:
- text.append(code)
- else:
- if line_range:
- # More complicated path to only stylize a portion of the code
- # This speeds up further operations as there are less spans to process
- line_start, line_end = line_range
-
- def line_tokenize() -> Iterable[Tuple[Any, str]]:
- """Split tokens to one per line."""
- assert lexer # required to make MyPy happy - we know lexer is not None at this point
-
- for token_type, token in lexer.get_tokens(code):
- while token:
- line_token, new_line, token = token.partition("\n")
- yield token_type, line_token + new_line
-
- def tokens_to_spans() -> Iterable[Tuple[str, Optional[Style]]]:
- """Convert tokens to spans."""
- tokens = iter(line_tokenize())
- line_no = 0
- _line_start = line_start - 1 if line_start else 0
-
- # Skip over tokens until line start
- while line_no < _line_start:
- try:
- _token_type, token = next(tokens)
- except StopIteration:
- break
- yield (token, None)
- if token.endswith("\n"):
- line_no += 1
- # Generate spans until line end
- for token_type, token in tokens:
- yield (token, _get_theme_style(token_type))
- if token.endswith("\n"):
- line_no += 1
- if line_end and line_no >= line_end:
- break
-
- text.append_tokens(tokens_to_spans())
-
- else:
- text.append_tokens(
- (token, _get_theme_style(token_type))
- for token_type, token in lexer.get_tokens(code)
- )
- if self.background_color is not None:
- text.stylize(f"on {self.background_color}")
-
- if self._stylized_ranges:
- self._apply_stylized_ranges(text)
-
- return text
-
- def stylize_range(
- self, style: StyleType, start: SyntaxPosition, end: SyntaxPosition
- ) -> None:
- """
- Adds a custom style on a part of the code, that will be applied to the syntax display when it's rendered.
- Line numbers are 1-based, while column indexes are 0-based.
-
- Args:
- style (StyleType): The style to apply.
- start (Tuple[int, int]): The start of the range, in the form `[line number, column index]`.
- end (Tuple[int, int]): The end of the range, in the form `[line number, column index]`.
- """
- self._stylized_ranges.append(_SyntaxHighlightRange(style, start, end))
-
- def _get_line_numbers_color(self, blend: float = 0.3) -> Color:
- background_style = self._theme.get_background_style() + self.background_style
- background_color = background_style.bgcolor
- if background_color is None or background_color.is_system_defined:
- return Color.default()
- foreground_color = self._get_token_color(Token.Text)
- if foreground_color is None or foreground_color.is_system_defined:
- return foreground_color or Color.default()
- new_color = blend_rgb(
- background_color.get_truecolor(),
- foreground_color.get_truecolor(),
- cross_fade=blend,
- )
- return Color.from_triplet(new_color)
-
- @property
- def _numbers_column_width(self) -> int:
- """Get the number of characters used to render the numbers column."""
- column_width = 0
- if self.line_numbers:
- column_width = (
- len(str(self.start_line + self.code.count("\n")))
- + NUMBERS_COLUMN_DEFAULT_PADDING
- )
- return column_width
-
- def _get_number_styles(self, console: Console) -> Tuple[Style, Style, Style]:
- """Get background, number, and highlight styles for line numbers."""
- background_style = self._get_base_style()
- if background_style.transparent_background:
- return Style.null(), Style(dim=True), Style.null()
- if console.color_system in ("256", "truecolor"):
- number_style = Style.chain(
- background_style,
- self._theme.get_style_for_token(Token.Text),
- Style(color=self._get_line_numbers_color()),
- self.background_style,
- )
- highlight_number_style = Style.chain(
- background_style,
- self._theme.get_style_for_token(Token.Text),
- Style(bold=True, color=self._get_line_numbers_color(0.9)),
- self.background_style,
- )
- else:
- number_style = background_style + Style(dim=True)
- highlight_number_style = background_style + Style(dim=False)
- return background_style, number_style, highlight_number_style
-
- def __rich_measure__(
- self, console: "Console", options: "ConsoleOptions"
- ) -> "Measurement":
-
- _, right, _, left = Padding.unpack(self.padding)
- padding = left + right
- if self.code_width is not None:
- width = self.code_width + self._numbers_column_width + padding + 1
- return Measurement(self._numbers_column_width, width)
- lines = self.code.splitlines()
- width = (
- self._numbers_column_width
- + padding
- + (max(cell_len(line) for line in lines) if lines else 0)
- )
- if self.line_numbers:
- width += 1
- return Measurement(self._numbers_column_width, width)
-
- def __rich_console__(
- self, console: Console, options: ConsoleOptions
- ) -> RenderResult:
- segments = Segments(self._get_syntax(console, options))
- if self.padding:
- yield Padding(
- segments, style=self._theme.get_background_style(), pad=self.padding
- )
- else:
- yield segments
-
- def _get_syntax(
- self,
- console: Console,
- options: ConsoleOptions,
- ) -> Iterable[Segment]:
- """
- Get the Segments for the Syntax object, excluding any vertical/horizontal padding
- """
- transparent_background = self._get_base_style().transparent_background
- code_width = (
- (
- (options.max_width - self._numbers_column_width - 1)
- if self.line_numbers
- else options.max_width
- )
- if self.code_width is None
- else self.code_width
- )
-
- ends_on_nl, processed_code = self._process_code(self.code)
- text = self.highlight(processed_code, self.line_range)
-
- if not self.line_numbers and not self.word_wrap and not self.line_range:
- if not ends_on_nl:
- text.remove_suffix("\n")
- # Simple case of just rendering text
- style = (
- self._get_base_style()
- + self._theme.get_style_for_token(Comment)
- + Style(dim=True)
- + self.background_style
- )
- if self.indent_guides and not options.ascii_only:
- text = text.with_indent_guides(self.tab_size, style=style)
- text.overflow = "crop"
- if style.transparent_background:
- yield from console.render(
- text, options=options.update(width=code_width)
- )
- else:
- syntax_lines = console.render_lines(
- text,
- options.update(width=code_width, height=None, justify="left"),
- style=self.background_style,
- pad=True,
- new_lines=True,
- )
- for syntax_line in syntax_lines:
- yield from syntax_line
- return
-
- start_line, end_line = self.line_range or (None, None)
- line_offset = 0
- if start_line:
- line_offset = max(0, start_line - 1)
- lines: Union[List[Text], Lines] = text.split("\n", allow_blank=ends_on_nl)
- if self.line_range:
- if line_offset > len(lines):
- return
- lines = lines[line_offset:end_line]
-
- if self.indent_guides and not options.ascii_only:
- style = (
- self._get_base_style()
- + self._theme.get_style_for_token(Comment)
- + Style(dim=True)
- + self.background_style
- )
- lines = (
- Text("\n")
- .join(lines)
- .with_indent_guides(self.tab_size, style=style)
- .split("\n", allow_blank=True)
- )
-
- numbers_column_width = self._numbers_column_width
- render_options = options.update(width=code_width)
-
- highlight_line = self.highlight_lines.__contains__
- _Segment = Segment
- new_line = _Segment("\n")
-
- line_pointer = "> " if options.legacy_windows else "❱ "
-
- (
- background_style,
- number_style,
- highlight_number_style,
- ) = self._get_number_styles(console)
-
- for line_no, line in enumerate(lines, self.start_line + line_offset):
- if self.word_wrap:
- wrapped_lines = console.render_lines(
- line,
- render_options.update(height=None, justify="left"),
- style=background_style,
- pad=not transparent_background,
- )
- else:
- segments = list(line.render(console, end=""))
- if options.no_wrap:
- wrapped_lines = [segments]
- else:
- wrapped_lines = [
- _Segment.adjust_line_length(
- segments,
- render_options.max_width,
- style=background_style,
- pad=not transparent_background,
- )
- ]
-
- if self.line_numbers:
- wrapped_line_left_pad = _Segment(
- " " * numbers_column_width + " ", background_style
- )
- for first, wrapped_line in loop_first(wrapped_lines):
- if first:
- line_column = str(line_no).rjust(numbers_column_width - 2) + " "
- if highlight_line(line_no):
- yield _Segment(line_pointer, Style(color="red"))
- yield _Segment(line_column, highlight_number_style)
- else:
- yield _Segment(" ", highlight_number_style)
- yield _Segment(line_column, number_style)
- else:
- yield wrapped_line_left_pad
- yield from wrapped_line
- yield new_line
- else:
- for wrapped_line in wrapped_lines:
- yield from wrapped_line
- yield new_line
-
- def _apply_stylized_ranges(self, text: Text) -> None:
- """
- Apply stylized ranges to a text instance,
- using the given code to determine the right portion to apply the style to.
-
- Args:
- text (Text): Text instance to apply the style to.
- """
- code = text.plain
- newlines_offsets = [
- # Let's add outer boundaries at each side of the list:
- 0,
- # N.B. using "\n" here is much faster than using metacharacters such as "^" or "\Z":
- *[
- match.start() + 1
- for match in re.finditer("\n", code, flags=re.MULTILINE)
- ],
- len(code) + 1,
- ]
-
- for stylized_range in self._stylized_ranges:
- start = _get_code_index_for_syntax_position(
- newlines_offsets, stylized_range.start
- )
- end = _get_code_index_for_syntax_position(
- newlines_offsets, stylized_range.end
- )
- if start is not None and end is not None:
- text.stylize(stylized_range.style, start, end)
-
- def _process_code(self, code: str) -> Tuple[bool, str]:
- """
- Applies various processing to a raw code string
- (normalises it so it always ends with a line return, dedents it if necessary, etc.)
-
- Args:
- code (str): The raw code string to process
-
- Returns:
- Tuple[bool, str]: the boolean indicates whether the raw code ends with a line return,
- while the string is the processed code.
- """
- ends_on_nl = code.endswith("\n")
- processed_code = code if ends_on_nl else code + "\n"
- processed_code = (
- textwrap.dedent(processed_code) if self.dedent else processed_code
- )
- processed_code = processed_code.expandtabs(self.tab_size)
- return ends_on_nl, processed_code
-
-
-def _get_code_index_for_syntax_position(
- newlines_offsets: Sequence[int], position: SyntaxPosition
-) -> Optional[int]:
- """
- Returns the index of the code string for the given positions.
-
- Args:
- newlines_offsets (Sequence[int]): The offset of each newline character found in the code snippet.
- position (SyntaxPosition): The position to search for.
-
- Returns:
- Optional[int]: The index of the code string for this position, or `None`
- if the given position's line number is out of range (if it's the column that is out of range
- we silently clamp its value so that it reaches the end of the line)
- """
- lines_count = len(newlines_offsets)
-
- line_number, column_index = position
- if line_number > lines_count or len(newlines_offsets) < (line_number + 1):
- return None # `line_number` is out of range
- line_index = line_number - 1
- line_length = newlines_offsets[line_index + 1] - newlines_offsets[line_index] - 1
- # If `column_index` is out of range: let's silently clamp it:
- column_index = min(line_length, column_index)
- return newlines_offsets[line_index] + column_index
-
-
-if __name__ == "__main__": # pragma: no cover
-
- import argparse
- import sys
-
- parser = argparse.ArgumentParser(
- description="Render syntax to the console with Rich"
- )
- parser.add_argument(
- "path",
- metavar="PATH",
- help="path to file, or - for stdin",
- )
- parser.add_argument(
- "-c",
- "--force-color",
- dest="force_color",
- action="store_true",
- default=None,
- help="force color for non-terminals",
- )
- parser.add_argument(
- "-i",
- "--indent-guides",
- dest="indent_guides",
- action="store_true",
- default=False,
- help="display indent guides",
- )
- parser.add_argument(
- "-l",
- "--line-numbers",
- dest="line_numbers",
- action="store_true",
- help="render line numbers",
- )
- parser.add_argument(
- "-w",
- "--width",
- type=int,
- dest="width",
- default=None,
- help="width of output (default will auto-detect)",
- )
- parser.add_argument(
- "-r",
- "--wrap",
- dest="word_wrap",
- action="store_true",
- default=False,
- help="word wrap long lines",
- )
- parser.add_argument(
- "-s",
- "--soft-wrap",
- action="store_true",
- dest="soft_wrap",
- default=False,
- help="enable soft wrapping mode",
- )
- parser.add_argument(
- "-t", "--theme", dest="theme", default="monokai", help="pygments theme"
- )
- parser.add_argument(
- "-b",
- "--background-color",
- dest="background_color",
- default=None,
- help="Override background color",
- )
- parser.add_argument(
- "-x",
- "--lexer",
- default=None,
- dest="lexer_name",
- help="Lexer name",
- )
- parser.add_argument(
- "-p", "--padding", type=int, default=0, dest="padding", help="Padding"
- )
- parser.add_argument(
- "--highlight-line",
- type=int,
- default=None,
- dest="highlight_line",
- help="The line number (not index!) to highlight",
- )
- args = parser.parse_args()
-
- from pip._vendor.rich.console import Console
-
- console = Console(force_terminal=args.force_color, width=args.width)
-
- if args.path == "-":
- code = sys.stdin.read()
- syntax = Syntax(
- code=code,
- lexer=args.lexer_name,
- line_numbers=args.line_numbers,
- word_wrap=args.word_wrap,
- theme=args.theme,
- background_color=args.background_color,
- indent_guides=args.indent_guides,
- padding=args.padding,
- highlight_lines={args.highlight_line},
- )
- else:
- syntax = Syntax.from_path(
- args.path,
- lexer=args.lexer_name,
- line_numbers=args.line_numbers,
- word_wrap=args.word_wrap,
- theme=args.theme,
- background_color=args.background_color,
- indent_guides=args.indent_guides,
- padding=args.padding,
- highlight_lines={args.highlight_line},
- )
- console.print(syntax, soft_wrap=args.soft_wrap)
diff --git a/spaces/Ataturk-Chatbot/HuggingFaceChat/venv/lib/python3.11/site-packages/pkg_resources/_vendor/zipp.py b/spaces/Ataturk-Chatbot/HuggingFaceChat/venv/lib/python3.11/site-packages/pkg_resources/_vendor/zipp.py
deleted file mode 100644
index 26b723c1fd3e25740e0268b8c9b50905c58c3d4a..0000000000000000000000000000000000000000
--- a/spaces/Ataturk-Chatbot/HuggingFaceChat/venv/lib/python3.11/site-packages/pkg_resources/_vendor/zipp.py
+++ /dev/null
@@ -1,329 +0,0 @@
-import io
-import posixpath
-import zipfile
-import itertools
-import contextlib
-import sys
-import pathlib
-
-if sys.version_info < (3, 7):
- from collections import OrderedDict
-else:
- OrderedDict = dict
-
-
-__all__ = ['Path']
-
-
-def _parents(path):
- """
- Given a path with elements separated by
- posixpath.sep, generate all parents of that path.
-
- >>> list(_parents('b/d'))
- ['b']
- >>> list(_parents('/b/d/'))
- ['/b']
- >>> list(_parents('b/d/f/'))
- ['b/d', 'b']
- >>> list(_parents('b'))
- []
- >>> list(_parents(''))
- []
- """
- return itertools.islice(_ancestry(path), 1, None)
-
-
-def _ancestry(path):
- """
- Given a path with elements separated by
- posixpath.sep, generate all elements of that path
-
- >>> list(_ancestry('b/d'))
- ['b/d', 'b']
- >>> list(_ancestry('/b/d/'))
- ['/b/d', '/b']
- >>> list(_ancestry('b/d/f/'))
- ['b/d/f', 'b/d', 'b']
- >>> list(_ancestry('b'))
- ['b']
- >>> list(_ancestry(''))
- []
- """
- path = path.rstrip(posixpath.sep)
- while path and path != posixpath.sep:
- yield path
- path, tail = posixpath.split(path)
-
-
-_dedupe = OrderedDict.fromkeys
-"""Deduplicate an iterable in original order"""
-
-
-def _difference(minuend, subtrahend):
- """
- Return items in minuend not in subtrahend, retaining order
- with O(1) lookup.
- """
- return itertools.filterfalse(set(subtrahend).__contains__, minuend)
-
-
-class CompleteDirs(zipfile.ZipFile):
- """
- A ZipFile subclass that ensures that implied directories
- are always included in the namelist.
- """
-
- @staticmethod
- def _implied_dirs(names):
- parents = itertools.chain.from_iterable(map(_parents, names))
- as_dirs = (p + posixpath.sep for p in parents)
- return _dedupe(_difference(as_dirs, names))
-
- def namelist(self):
- names = super(CompleteDirs, self).namelist()
- return names + list(self._implied_dirs(names))
-
- def _name_set(self):
- return set(self.namelist())
-
- def resolve_dir(self, name):
- """
- If the name represents a directory, return that name
- as a directory (with the trailing slash).
- """
- names = self._name_set()
- dirname = name + '/'
- dir_match = name not in names and dirname in names
- return dirname if dir_match else name
-
- @classmethod
- def make(cls, source):
- """
- Given a source (filename or zipfile), return an
- appropriate CompleteDirs subclass.
- """
- if isinstance(source, CompleteDirs):
- return source
-
- if not isinstance(source, zipfile.ZipFile):
- return cls(_pathlib_compat(source))
-
- # Only allow for FastLookup when supplied zipfile is read-only
- if 'r' not in source.mode:
- cls = CompleteDirs
-
- source.__class__ = cls
- return source
-
-
-class FastLookup(CompleteDirs):
- """
- ZipFile subclass to ensure implicit
- dirs exist and are resolved rapidly.
- """
-
- def namelist(self):
- with contextlib.suppress(AttributeError):
- return self.__names
- self.__names = super(FastLookup, self).namelist()
- return self.__names
-
- def _name_set(self):
- with contextlib.suppress(AttributeError):
- return self.__lookup
- self.__lookup = super(FastLookup, self)._name_set()
- return self.__lookup
-
-
-def _pathlib_compat(path):
- """
- For path-like objects, convert to a filename for compatibility
- on Python 3.6.1 and earlier.
- """
- try:
- return path.__fspath__()
- except AttributeError:
- return str(path)
-
-
-class Path:
- """
- A pathlib-compatible interface for zip files.
-
- Consider a zip file with this structure::
-
- .
- ├── a.txt
- └── b
- ├── c.txt
- └── d
- └── e.txt
-
- >>> data = io.BytesIO()
- >>> zf = zipfile.ZipFile(data, 'w')
- >>> zf.writestr('a.txt', 'content of a')
- >>> zf.writestr('b/c.txt', 'content of c')
- >>> zf.writestr('b/d/e.txt', 'content of e')
- >>> zf.filename = 'mem/abcde.zip'
-
- Path accepts the zipfile object itself or a filename
-
- >>> root = Path(zf)
-
- From there, several path operations are available.
-
- Directory iteration (including the zip file itself):
-
- >>> a, b = root.iterdir()
- >>> a
- Path('mem/abcde.zip', 'a.txt')
- >>> b
- Path('mem/abcde.zip', 'b/')
-
- name property:
-
- >>> b.name
- 'b'
-
- join with divide operator:
-
- >>> c = b / 'c.txt'
- >>> c
- Path('mem/abcde.zip', 'b/c.txt')
- >>> c.name
- 'c.txt'
-
- Read text:
-
- >>> c.read_text()
- 'content of c'
-
- existence:
-
- >>> c.exists()
- True
- >>> (b / 'missing.txt').exists()
- False
-
- Coercion to string:
-
- >>> import os
- >>> str(c).replace(os.sep, posixpath.sep)
- 'mem/abcde.zip/b/c.txt'
-
- At the root, ``name``, ``filename``, and ``parent``
- resolve to the zipfile. Note these attributes are not
- valid and will raise a ``ValueError`` if the zipfile
- has no filename.
-
- >>> root.name
- 'abcde.zip'
- >>> str(root.filename).replace(os.sep, posixpath.sep)
- 'mem/abcde.zip'
- >>> str(root.parent)
- 'mem'
- """
-
- __repr = "{self.__class__.__name__}({self.root.filename!r}, {self.at!r})"
-
- def __init__(self, root, at=""):
- """
- Construct a Path from a ZipFile or filename.
-
- Note: When the source is an existing ZipFile object,
- its type (__class__) will be mutated to a
- specialized type. If the caller wishes to retain the
- original type, the caller should either create a
- separate ZipFile object or pass a filename.
- """
- self.root = FastLookup.make(root)
- self.at = at
-
- def open(self, mode='r', *args, pwd=None, **kwargs):
- """
- Open this entry as text or binary following the semantics
- of ``pathlib.Path.open()`` by passing arguments through
- to io.TextIOWrapper().
- """
- if self.is_dir():
- raise IsADirectoryError(self)
- zip_mode = mode[0]
- if not self.exists() and zip_mode == 'r':
- raise FileNotFoundError(self)
- stream = self.root.open(self.at, zip_mode, pwd=pwd)
- if 'b' in mode:
- if args or kwargs:
- raise ValueError("encoding args invalid for binary operation")
- return stream
- return io.TextIOWrapper(stream, *args, **kwargs)
-
- @property
- def name(self):
- return pathlib.Path(self.at).name or self.filename.name
-
- @property
- def suffix(self):
- return pathlib.Path(self.at).suffix or self.filename.suffix
-
- @property
- def suffixes(self):
- return pathlib.Path(self.at).suffixes or self.filename.suffixes
-
- @property
- def stem(self):
- return pathlib.Path(self.at).stem or self.filename.stem
-
- @property
- def filename(self):
- return pathlib.Path(self.root.filename).joinpath(self.at)
-
- def read_text(self, *args, **kwargs):
- with self.open('r', *args, **kwargs) as strm:
- return strm.read()
-
- def read_bytes(self):
- with self.open('rb') as strm:
- return strm.read()
-
- def _is_child(self, path):
- return posixpath.dirname(path.at.rstrip("/")) == self.at.rstrip("/")
-
- def _next(self, at):
- return self.__class__(self.root, at)
-
- def is_dir(self):
- return not self.at or self.at.endswith("/")
-
- def is_file(self):
- return self.exists() and not self.is_dir()
-
- def exists(self):
- return self.at in self.root._name_set()
-
- def iterdir(self):
- if not self.is_dir():
- raise ValueError("Can't listdir a file")
- subs = map(self._next, self.root.namelist())
- return filter(self._is_child, subs)
-
- def __str__(self):
- return posixpath.join(self.root.filename, self.at)
-
- def __repr__(self):
- return self.__repr.format(self=self)
-
- def joinpath(self, *other):
- next = posixpath.join(self.at, *map(_pathlib_compat, other))
- return self._next(self.root.resolve_dir(next))
-
- __truediv__ = joinpath
-
- @property
- def parent(self):
- if not self.at:
- return self.filename.parent
- parent_at = posixpath.dirname(self.at.rstrip('/'))
- if parent_at:
- parent_at += '/'
- return self._next(parent_at)
diff --git a/spaces/Avin1221/darkstorm2150-Protogen_x3.4_Official_Release/README.md b/spaces/Avin1221/darkstorm2150-Protogen_x3.4_Official_Release/README.md
deleted file mode 100644
index c7c34b6b8fc4e8ac15a695edf261e80f34a9210d..0000000000000000000000000000000000000000
--- a/spaces/Avin1221/darkstorm2150-Protogen_x3.4_Official_Release/README.md
+++ /dev/null
@@ -1,12 +0,0 @@
----
-title: Darkstorm2150-Protogen X3.4 Official Release
-emoji: 👀
-colorFrom: gray
-colorTo: green
-sdk: gradio
-sdk_version: 3.16.0
-app_file: app.py
-pinned: false
----
-
-Check out the configuration reference at https://huggingface.co/docs/hub/spaces-config-reference
diff --git a/spaces/AzumaSeren100/XuanShen-Bert-VITS2/text/symbols.py b/spaces/AzumaSeren100/XuanShen-Bert-VITS2/text/symbols.py
deleted file mode 100644
index 9dfae4e633829f20c4fd767b1c7a9198911ed801..0000000000000000000000000000000000000000
--- a/spaces/AzumaSeren100/XuanShen-Bert-VITS2/text/symbols.py
+++ /dev/null
@@ -1,51 +0,0 @@
-punctuation = ['!', '?', '…', ",", ".", "'", '-']
-pu_symbols = punctuation + ["SP", "UNK"]
-pad = '_'
-
-# chinese
-zh_symbols = ['E', 'En', 'a', 'ai', 'an', 'ang', 'ao', 'b', 'c', 'ch', 'd', 'e', 'ei', 'en', 'eng', 'er', 'f', 'g', 'h',
- 'i', 'i0', 'ia', 'ian', 'iang', 'iao', 'ie', 'in', 'ing', 'iong', 'ir', 'iu', 'j', 'k', 'l', 'm', 'n', 'o',
- 'ong',
- 'ou', 'p', 'q', 'r', 's', 'sh', 't', 'u', 'ua', 'uai', 'uan', 'uang', 'ui', 'un', 'uo', 'v', 'van', 've', 'vn',
- 'w', 'x', 'y', 'z', 'zh',
- "AA", "EE", "OO"]
-num_zh_tones = 6
-
-# japanese
-ja_symbols = ['I', 'N', 'U', 'a', 'b', 'by', 'ch', 'cl', 'd', 'dy', 'e', 'f', 'g', 'gy', 'h', 'hy', 'i', 'j', 'k', 'ky',
- 'm', 'my', 'n', 'ny', 'o', 'p', 'py', 'r', 'ry', 's', 'sh', 't', 'ts', 'u', 'V', 'w', 'y', 'z']
-num_ja_tones = 1
-
-# English
-en_symbols = ['aa', 'ae', 'ah', 'ao', 'aw', 'ay', 'b', 'ch', 'd', 'dh', 'eh', 'er', 'ey', 'f', 'g', 'hh', 'ih', 'iy',
- 'jh', 'k', 'l', 'm', 'n', 'ng', 'ow', 'oy', 'p', 'r', 's',
- 'sh', 't', 'th', 'uh', 'uw', 'V', 'w', 'y', 'z', 'zh']
-num_en_tones = 4
-
-# combine all symbols
-normal_symbols = sorted(set(zh_symbols + ja_symbols + en_symbols))
-symbols = [pad] + normal_symbols + pu_symbols
-sil_phonemes_ids = [symbols.index(i) for i in pu_symbols]
-
-# combine all tones
-num_tones = num_zh_tones + num_ja_tones + num_en_tones
-
-# language maps
-language_id_map = {
- 'ZH': 0,
- "JA": 1,
- "EN": 2
-}
-num_languages = len(language_id_map.keys())
-
-language_tone_start_map = {
- 'ZH': 0,
- "JA": num_zh_tones,
- "EN": num_zh_tones + num_ja_tones
-}
-
-if __name__ == '__main__':
- a = set(zh_symbols)
- b = set(en_symbols)
- print(sorted(a&b))
-
diff --git a/spaces/Bart92/RVC_HF/demucs/model.py b/spaces/Bart92/RVC_HF/demucs/model.py
deleted file mode 100644
index e9d932f4d014f7b95b394d2e24ed5edc379ded8d..0000000000000000000000000000000000000000
--- a/spaces/Bart92/RVC_HF/demucs/model.py
+++ /dev/null
@@ -1,202 +0,0 @@
-# Copyright (c) Facebook, Inc. and its affiliates.
-# All rights reserved.
-#
-# This source code is licensed under the license found in the
-# LICENSE file in the root directory of this source tree.
-
-import math
-
-import julius
-from torch import nn
-
-from .utils import capture_init, center_trim
-
-
-class BLSTM(nn.Module):
- def __init__(self, dim, layers=1):
- super().__init__()
- self.lstm = nn.LSTM(bidirectional=True, num_layers=layers, hidden_size=dim, input_size=dim)
- self.linear = nn.Linear(2 * dim, dim)
-
- def forward(self, x):
- x = x.permute(2, 0, 1)
- x = self.lstm(x)[0]
- x = self.linear(x)
- x = x.permute(1, 2, 0)
- return x
-
-
-def rescale_conv(conv, reference):
- std = conv.weight.std().detach()
- scale = (std / reference)**0.5
- conv.weight.data /= scale
- if conv.bias is not None:
- conv.bias.data /= scale
-
-
-def rescale_module(module, reference):
- for sub in module.modules():
- if isinstance(sub, (nn.Conv1d, nn.ConvTranspose1d)):
- rescale_conv(sub, reference)
-
-
-class Demucs(nn.Module):
- @capture_init
- def __init__(self,
- sources,
- audio_channels=2,
- channels=64,
- depth=6,
- rewrite=True,
- glu=True,
- rescale=0.1,
- resample=True,
- kernel_size=8,
- stride=4,
- growth=2.,
- lstm_layers=2,
- context=3,
- normalize=False,
- samplerate=44100,
- segment_length=4 * 10 * 44100):
- """
- Args:
- sources (list[str]): list of source names
- audio_channels (int): stereo or mono
- channels (int): first convolution channels
- depth (int): number of encoder/decoder layers
- rewrite (bool): add 1x1 convolution to each encoder layer
- and a convolution to each decoder layer.
- For the decoder layer, `context` gives the kernel size.
- glu (bool): use glu instead of ReLU
- resample_input (bool): upsample x2 the input and downsample /2 the output.
- rescale (int): rescale initial weights of convolutions
- to get their standard deviation closer to `rescale`
- kernel_size (int): kernel size for convolutions
- stride (int): stride for convolutions
- growth (float): multiply (resp divide) number of channels by that
- for each layer of the encoder (resp decoder)
- lstm_layers (int): number of lstm layers, 0 = no lstm
- context (int): kernel size of the convolution in the
- decoder before the transposed convolution. If > 1,
- will provide some context from neighboring time
- steps.
- samplerate (int): stored as meta information for easing
- future evaluations of the model.
- segment_length (int): stored as meta information for easing
- future evaluations of the model. Length of the segments on which
- the model was trained.
- """
-
- super().__init__()
- self.audio_channels = audio_channels
- self.sources = sources
- self.kernel_size = kernel_size
- self.context = context
- self.stride = stride
- self.depth = depth
- self.resample = resample
- self.channels = channels
- self.normalize = normalize
- self.samplerate = samplerate
- self.segment_length = segment_length
-
- self.encoder = nn.ModuleList()
- self.decoder = nn.ModuleList()
-
- if glu:
- activation = nn.GLU(dim=1)
- ch_scale = 2
- else:
- activation = nn.ReLU()
- ch_scale = 1
- in_channels = audio_channels
- for index in range(depth):
- encode = []
- encode += [nn.Conv1d(in_channels, channels, kernel_size, stride), nn.ReLU()]
- if rewrite:
- encode += [nn.Conv1d(channels, ch_scale * channels, 1), activation]
- self.encoder.append(nn.Sequential(*encode))
-
- decode = []
- if index > 0:
- out_channels = in_channels
- else:
- out_channels = len(self.sources) * audio_channels
- if rewrite:
- decode += [nn.Conv1d(channels, ch_scale * channels, context), activation]
- decode += [nn.ConvTranspose1d(channels, out_channels, kernel_size, stride)]
- if index > 0:
- decode.append(nn.ReLU())
- self.decoder.insert(0, nn.Sequential(*decode))
- in_channels = channels
- channels = int(growth * channels)
-
- channels = in_channels
-
- if lstm_layers:
- self.lstm = BLSTM(channels, lstm_layers)
- else:
- self.lstm = None
-
- if rescale:
- rescale_module(self, reference=rescale)
-
- def valid_length(self, length):
- """
- Return the nearest valid length to use with the model so that
- there is no time steps left over in a convolutions, e.g. for all
- layers, size of the input - kernel_size % stride = 0.
-
- If the mixture has a valid length, the estimated sources
- will have exactly the same length when context = 1. If context > 1,
- the two signals can be center trimmed to match.
-
- For training, extracts should have a valid length.For evaluation
- on full tracks we recommend passing `pad = True` to :method:`forward`.
- """
- if self.resample:
- length *= 2
- for _ in range(self.depth):
- length = math.ceil((length - self.kernel_size) / self.stride) + 1
- length = max(1, length)
- length += self.context - 1
- for _ in range(self.depth):
- length = (length - 1) * self.stride + self.kernel_size
-
- if self.resample:
- length = math.ceil(length / 2)
- return int(length)
-
- def forward(self, mix):
- x = mix
-
- if self.normalize:
- mono = mix.mean(dim=1, keepdim=True)
- mean = mono.mean(dim=-1, keepdim=True)
- std = mono.std(dim=-1, keepdim=True)
- else:
- mean = 0
- std = 1
-
- x = (x - mean) / (1e-5 + std)
-
- if self.resample:
- x = julius.resample_frac(x, 1, 2)
-
- saved = []
- for encode in self.encoder:
- x = encode(x)
- saved.append(x)
- if self.lstm:
- x = self.lstm(x)
- for decode in self.decoder:
- skip = center_trim(saved.pop(-1), x)
- x = x + skip
- x = decode(x)
-
- if self.resample:
- x = julius.resample_frac(x, 2, 1)
- x = x * std + mean
- x = x.view(x.size(0), len(self.sources), self.audio_channels, x.size(-1))
- return x
diff --git a/spaces/Benson/text-generation/Examples/Arco Iris Seis Mvil Apk Beta.md b/spaces/Benson/text-generation/Examples/Arco Iris Seis Mvil Apk Beta.md
deleted file mode 100644
index 62130af908b60a101781b1bc27d8021b1ec09447..0000000000000000000000000000000000000000
--- a/spaces/Benson/text-generation/Examples/Arco Iris Seis Mvil Apk Beta.md
+++ /dev/null
@@ -1,64 +0,0 @@
-
-
Rainbow Six Mobile: Todo lo que necesitas saber sobre la versión beta
-
¿Eres un fan de los juegos de disparos tácticos? ¿Quieres experimentar la emoción de Rainbow Six en tu teléfono? Si es así, entonces estás de suerte. Ubisoft ha lanzado recientemente la versión beta de Rainbow Six Mobile, un juego de disparos en primera persona multijugador competitivo y gratuito diseñado exclusivamente para dispositivos móviles. En este artículo, te contaremos todo lo que necesitas saber sobre este juego, incluyendo cómo descargarlo y jugarlo, por qué deberías jugarlo, y algunos consejos y trucos para dominarlo. ¡Vamos a empezar!
Rainbow Six Mobile es una adaptación móvil de la aclamada franquicia Rainbow Six, que es conocida por su jugabilidad realista y estratégica, su lista épica de operadores, sus mapas icónicos y sus modos de juego de área y bomba seguros. En Rainbow Six Mobile, puedes competir en partidas 5v5 de ritmo rápido como atacante o defensor, enfrentarte a intensos combates a corta distancia mientras tomas decisiones tácticas oportunas, colaborar como equipo para establecer estrategias y aprovechar al máximo los entornos destructibles, y elija entre una amplia selección de operadores altamente capacitados, cada uno con sus propias habilidades y dispositivos únicos.
-
Una breve introducción al juego y sus características
-
-
Cómo descargar y jugar la versión beta en Android
-
La versión beta de Rainbow Six Mobile está disponible actualmente solo para dispositivos Android. Para descargarlo y reproducirlo, debes seguir estos pasos:
-
-
Abre Google Play en tu teléfono Android.
-
Buscar Rainbow Six Mobile o simplemente haga clic en este enlace.
-
Seleccione el botón de registro previo, y pronto recibirá una notificación cuando el juego esté listo para descargar.
-
Descarga e instala el juego en tu dispositivo.
-
Inicie el juego e inicie sesión con su cuenta de Ubisoft o cree una si no tiene una.
-
Elija su región y preferencias de idioma.
-
Completa las misiones de tutorial para aprender los fundamentos del juego.
-
Disfruta jugando Rainbow Six Mobile!
-
-
¿Cuáles son los requisitos del sistema y los problemas de compatibilidad
-
Rainbow Six Mobile es un juego que requiere un dispositivo de alto rendimiento para funcionar sin problemas. Los requisitos mínimos del sistema son:
-
-
-
Android 8.0 o superior
-
4 GB de RAM o más
-
Al menos 2 GB de espacio de almacenamiento gratuito
-
Una conexión a Internet estable
-
-
El juego también soporta algunos controladores externos, como Xbox One S Controller o PS4 DualShock 4 Controller. Sin embargo, algunos dispositivos pueden no ser compatibles con el juego o el controlador. Puede consultar la lista de dispositivos y controladores compatibles en el sitio web oficial o en la página de Google Play. Si encuentras algún problema o error mientras juegas el juego, puedes reportarlo a los desarrolladores a través del sistema de retroalimentación del juego o los canales oficiales de las redes sociales.
-
¿Por qué usted debe jugar Rainbow Six móvil
-
Rainbow Six Mobile es un juego que ofrece mucha diversión y emoción para los fanáticos de los juegos de disparos tácticos. Estas son algunas de las razones por las que deberías jugar:
-
Los beneficios de jugar un juego de disparos tácticos en el móvil
-
-
Las características únicas y modos de juego de Rainbow Six Mobile
-
Rainbow Six Mobile es un juego que tiene muchas características únicas y modos de juego que lo hacen destacar de otros juegos de disparos móviles. Por ejemplo, el juego tiene un motor de física realista e inmersivo que te permite interactuar con el entorno de varias maneras, como romper paredes, puertas, ventanas o pisos, crear nuevas líneas de visión o puntos de entrada, o usar la cubierta y la ocultación para tu ventaja. El juego también tiene un sistema de armas realista y auténtico que requiere que administres tu munición, retroceso y tiempo de recarga, así como personalizar tu carga con diferentes accesorios y pieles. El juego también tiene diferentes modos de juego que se adaptan a diferentes preferencias y niveles de habilidad, como el modo casual, el modo clasificado, el modo de entrenamiento y el modo de eventos especiales.
-
La lista diversa y personalizable de operadores y gadgets
-
Rainbow Six Mobile es un juego que tiene una lista diversa y personalizable de operadores y gadgets que le permiten crear su propio estilo de juego y estrategia. El juego cuenta con más de 20 operadores de diferentes países y unidades, cada uno con sus propias habilidades únicas y gadgets. Por ejemplo, puedes elegir a Ash, un atacante que puede usar sus rondas de asalto para destruir paredes o barricadas a distancia, o a Rook, un defensor que puede proporcionar a sus compañeros placas de armadura que aumentan su supervivencia. También puede desbloquear nuevos operadores al ganar créditos o comprarlos con dinero real. También puede personalizar sus operadores con diferentes trajes, tocados, encantos y emotes.
-
Consejos y trucos para dominar Rainbow Six móvil
-
Rainbow Six Mobile es un juego que requiere habilidad, estrategia y trabajo en equipo para ganar. Estos son algunos consejos y trucos para ayudarte a dominarlo:
-
Cómo elegir el mejor operador para tu estilo de juego y composición de equipo
-
-
Cómo utilizar el entorno y los gadgets para su ventaja
-
Utilizar el entorno y los gadgets a tu favor es otro factor clave para tu éxito en Rainbow Six Mobile. Siempre debe estar al tanto de su entorno y utilizarlos para su beneficio. Por ejemplo, puedes usar objetos destructibles como paredes, pisos o techos para crear nuevas líneas de visión o puntos de entrada, o para exponer o sorprender a tus enemigos. También puedes usar objetos de cubierta como mesas, sillas o gabinetes para protegerte del fuego enemigo o para ocultar tus aparatos. También debe hacer uso de sus aparatos y utilizarlos sabiamente. Por ejemplo, puedes usar drones o cámaras para explorar el área y localizar enemigos u objetivos, o puedes usar granadas o flashbangs para despejar habitaciones o cegar enemigos. También puedes usar trampas o escudos para ralentizar o impedir que los enemigos avancen o entren.
-
Cómo comunicarse y coordinar con sus compañeros de equipo
-
Comunicarse y coordinar con sus compañeros de equipo es esencial para su éxito en Rainbow Six Mobile. Siempre debes comunicarte con tus compañeros de equipo y compartir información, como ubicaciones enemigas, estado de salud, uso de dispositivos o planes de estrategia. También debe coordinarse con sus compañeros de equipo y trabajar en equipo, como la creación de fuegos cruzados, maniobras de flanco, distracciones o emboscadas. Puedes usar la función de chat de voz en el juego o los comandos de chat rápido para comunicarte y coordinarte con tus compañeros de equipo. También puedes usar el sistema de ping para marcar ubicaciones, enemigos u objetos para que tus compañeros los vean.
-
Conclusión
-
-
Preguntas frecuentes
-
Aquí están algunas de las preguntas y respuestas más frecuentes sobre Rainbow Six Mobile:
-
-
Q: ¿Rainbow Six Mobile es gratuito?
-
A: Sí, Rainbow Six Mobile es gratuito. Puedes descargarlo y jugarlo sin pagar nada. Sin embargo, el juego tiene algunas compras opcionales en el juego, como créditos, skins u operadores, que puedes comprar con dinero real si lo deseas.
-
Q: ¿Rainbow Six Mobile está disponible para dispositivos iOS?
-
A: Todavía no. Rainbow Six Mobile está disponible actualmente solo para dispositivos Android. Sin embargo, Ubisoft ha anunciado que está trabajando en llevar el juego a dispositivos iOS pronto.
-
Q: ¿Cómo puedo obtener más créditos en Rainbow Six Mobile?
-
A: Puedes obtener más créditos en Rainbow Six Mobile completando misiones, subiendo de nivel, participando en eventos, viendo anuncios o comprándolos con dinero real.
-
Q: ¿Cómo puedo desbloquear más operadores en Rainbow Six Mobile?
-
A: Puedes desbloquear más operadores en Rainbow Six Mobile al ganar créditos o comprarlos con dinero real.
-
Q: ¿Cómo puedo reportar un error o un tramposo en Rainbow Six Mobile?
-
A: Puedes reportar un error o un tramposo en Rainbow Six Mobile utilizando el sistema de retroalimentación del juego o poniéndote en contacto con los canales oficiales de las redes sociales.
-
64aa2da5cf
-
-
\ No newline at end of file
diff --git a/spaces/Benson/text-generation/Examples/Auto Chess War Mod Apk Terbaru.md b/spaces/Benson/text-generation/Examples/Auto Chess War Mod Apk Terbaru.md
deleted file mode 100644
index 7b6718aef2112b5fa825c433485d0c71a1c299f2..0000000000000000000000000000000000000000
--- a/spaces/Benson/text-generation/Examples/Auto Chess War Mod Apk Terbaru.md
+++ /dev/null
@@ -1,90 +0,0 @@
-
-
Auto Chess War Mod Apk Terbaru: Un juego de estrategia con un giro
-
Si usted está buscando un nuevo y emocionante juego de estrategia para jugar en su dispositivo móvil, es posible que desee echa un vistazo a la guerra de ajedrez auto mod apk terbaru. Este es un juego que combina los elementos de ajedrez, torre de defensa, y la recogida de cartas de una manera única. Tendrás que recoger diferentes héroes de varias clases y razas, colocarlos en un tablero de 8x8, y dejarlos luchar automáticamente contra otros jugadores o oponentes de IA. También tendrás que mejorar a tus héroes, combinarlos para crear otros más fuertes, usar objetos y habilidades para aumentar su rendimiento y derrotar a todos los enemigos para ser el último en pie.
La guerra de ajedrez automática se basa en el mod original de Dota Auto Chess que se convirtió en un gran éxito entre los jugadores de Dota 2. Sin embargo, tiene su propio conjunto de héroes y objetos que no están relacionados con la tradición Dota. También tiene un estilo más colorido y caricaturesco que lo hace atractivo para un público más amplio. Si usted es un fan de los juegos de estrategia y los luchadores de automóviles, que sin duda disfrutar de la guerra de ajedrez auto mod apk terbaru.
-
Cómo descargar e instalar Auto Chess War Mod Apk Terbaru
-
Descargar e instalar auto chess war mod apk terbaru es muy fácil y simple. Solo tienes que seguir estos pasos:
-
-
Descargar el archivo apk mod de una fuente de confianza. Puede encontrar el enlace al final de este artículo.
-
Habilitar fuentes desconocidas en la configuración del dispositivo. Esto le permitirá instalar aplicaciones que no son de la tienda de aplicaciones oficial. Para hacer esto, vaya a Configuración > Seguridad > Fuentes desconocidas y conéctelo.
-
Instalar el archivo apk mod y lanzar el juego. Verá un nuevo icono en la pantalla de inicio o cajón de aplicaciones. Toque en él y disfrutar del juego.
-
-
Nota: Es posible que tenga que desinstalar la versión original del juego antes de instalar el terbaru apk mod. Además, asegúrese de hacer una copia de seguridad de sus datos antes de hacerlo, ya que puede perder su progreso y logros.
-
-
Auto ajedrez guerra mod apk terbaru tiene muchas características que harán que su experiencia de juego más divertido y satisfactorio. Estos son algunos de ellos:
-
-
Desbloqueado todos los héroes y objetos. Usted tendrá acceso a todos los héroes y objetos en el juego, independientemente de su nivel o rango. Puedes elegir entre más de 50 héroes y 40 objetos, cada uno con sus propias habilidades y efectos.
-
Oro y gemas ilimitados. Tendrás recursos ilimitados para comprar, actualizar y combinar tus héroes y objetos. Nunca te quedarás sin oro y gemas, que son las principales monedas del juego.
-
No se requieren anuncios ni root. No verá ningún anuncio molesto o pop-ups mientras juega el juego. Usted tampoco tendrá que raíz de su dispositivo para utilizar el terbaru apk mod, que es seguro y conveniente.
-
-
Cómo jugar Auto Chess War Mod Apk Terbaru
-
Jugar guerra de ajedrez auto mod apk terbaru es muy fácil e intuitivo. Aquí están los pasos básicos:
-
-
Elige a tus héroes de diferentes clases y razas. Hay seis clases (guerrero, mago, asesino, cazador, druida y sacerdote) y seis razas (humano, elfo, orco, no muerto, bestia y demonio) en el juego. Cada clase y raza tiene sus propias ventajas y desventajas, así como sinergias con otras clases y razas. Por ejemplo, tener tres guerreros en tu tablero les dará armadura extra, mientras que tener tres elfos les dará evasión adicional.
-
Colócalas en el tablero de 8x8 y deja que luchen automáticamente. Tendrás un tiempo limitado para colocar a tus héroes en el tablero antes de que comience cada ronda. Puedes arrastrarlos y soltarlos en cualquier lugar de tu lado del tablero, o intercambiarlos con otros héroes. Una vez que la ronda comienza, tus héroes lucharán automáticamente contra los héroes del enemigo o la IA se arrastra. El ganador de cada ronda está determinado por quién tiene más héroes sobrevivientes o más puntos de salud.
-
-
Usa objetos y habilidades para mejorar el rendimiento de tus héroes. Puedes obtener objetos de derrotar a los monstruos de la IA u otros jugadores. Puedes equipar objetos a tus héroes arrastrándolos y dejándolos caer sobre sus retratos. Cada héroe puede tener hasta tres objetos a la vez. Los objetos pueden aumentar las estadísticas de tus héroes o darles efectos especiales. Por ejemplo, un objeto llamado Máscara de locura puede aumentar la velocidad de ataque de tu héroe pero silenciarlos para que no usen habilidades. También puedes utilizar las habilidades que están disponibles para cada clase o carrera tocando sus iconos en la parte inferior de la pantalla. Las habilidades pueden tener varios efectos como sanar, dañar, impresionar o pulir a tus héroes.
-
Derrota a todos los enemigos y sé el último en pie. Te enfrentarás a diferentes enemigos o la IA se arrastra en cada ronda hasta que solo quede un jugador. Perderás puntos de salud si pierdes una ronda o si no tienes espacio en tu tablero para nuevos héroes. El juego termina cuando tienes cero puntos de vida o cuando eres el único jugador que queda.
-
-
Consejos y trucos para Auto Chess War Mod Apk Terbaru
-
Para ayudarle a ganar más juegos en la guerra de ajedrez auto mod apk terbaru, aquí hay algunos consejos y trucos que puede utilizar:
-
-
-
Administre su economía sabiamente y ahorre oro para intereses. Ganará oro al ganar o perder rondas, completar misiones o vender héroes. También ganarás intereses en función de cuánto oro tengas al final de cada ronda. Cuanto más oro tengas, más intereses ganarás, hasta un máximo de 5 por ronda. Por lo tanto, es recomendable guardar su oro y gastarlo solo cuando sea necesario.
-
-
Aprende las fortalezas y debilidades de cada héroe y artículo. Usted debe familiarizarse con las habilidades y efectos de cada héroe y objeto en el juego. Esto te ayudará a elegir los mejores para tu estrategia y contrarrestar los movimientos de tus oponentes. Por ejemplo, debes saber que un héroe llamado Lina puede hacer daño masivo con su habilidad final, pero también es muy frágil y vulnerable a los asesinos. También debes saber que un objeto llamado Blade Mail puede reflejar el daño del atacante, pero también reduce la armadura de tu héroe.
-
Planifique sus transiciones de mediados y finales del juego en función de las estrategias de sus oponentes. No debes seguir con la misma estrategia a lo largo del juego, ya que puede ser ineficaz o anticuado a medida que el juego avanza. Siempre debes explorar los tableros de tus oponentes y ver lo que están construyendo y cómo lo están haciendo. Basado en esa información, usted debe planificar sus transiciones para contrarrestar sus estrategias o explotar sus debilidades. Por ejemplo, si ves que la mayoría de tus oponentes van a por magos, debes hacer la transición a guerreros o sacerdotes para resistir su daño mágico.
-
Espía a tus oponentes más fuertes y ajusta tu formación en consecuencia. Siempre debes vigilar quién está liderando el juego y quién es la mayor amenaza para ti. Puedes espiar sus tablas tocando sus retratos en la parte superior de la pantalla. Puedes ver a sus héroes, objetos, habilidades y formación. En base a esa información, debe ajustar su formación para que coincida con la de ellos. Por ejemplo, si ves que tienen una línea de frente fuerte de guerreros, debes colocar a tus asesinos detrás de ellos para apuntar a su contraluz blanda.
-
-
Revisión de Auto Chess War Mod Apk Terbaru
-
-
-
-
Pros
-
Contras
-
-
-
- Diversión: El juego es muy agradable y satisfactorio para jugar, especialmente cuando se gana un partido cercano o sacar un gran combo.
-
- Repetitivo: El juego puede ser aburrido y monótono después de un tiempo, ya que no hay mucha variedad o innovación en el juego o el contenido.
-
-
-
- Adictivo: El juego es muy atractivo y adictivo, ya que siempre querrás jugar una ronda más o probar una estrategia diferente.
-
- Basado en la suerte: El juego depende en gran medida de la suerte y la aleatoriedad, ya que no puede obtener los héroes o artículos que desea o necesita de la tienda o cofres.
-
-
-
- Desafiante: El juego es muy desafiante y estratégico, ya que tendrás que pensar rápido e inteligente para vencer a tus oponentes o enemigos de IA.
-
- Inestable: El juego puede ser inestable y con errores a veces, ya que puede bloquearse o congelarse durante el juego o la carga.
-
-
-
- Estratégico: El juego es muy estratégico y diverso, ya que tendrás que elegir entre diferentes clases y razas, utilizar diferentes objetos y habilidades, y adaptarse a diferentes situaciones.
-
- Desequilibrado: El juego puede ser desequilibrado e injusto a veces, ya que algunos héroes u objetos pueden ser demasiado fuertes o débiles en comparación con otros.
-
-
-
- Diverso: El juego es muy diverso y colorido, ya que tiene más de 50 héroes y 40 elementos con diferentes habilidades y efectos, así como un estilo caricaturesco que lo hace atractivo para una amplia audiencia.
-
- Pago a ganar: El juego puede ser de pago a ganar a veces, ya que algunos héroes u objetos pueden estar bloqueados detrás de un muro de pago o requerir dinero real para obtener.
-
-
-
- Gratis: El juego es gratis para descargar y jugar, lo que hace que sea accesible para cualquier persona que quiera probarlo.
-
- Ninguno
-
-
-
Conclusión
-
-
Preguntas frecuentes
-
Aquí hay algunas preguntas frecuentes acerca de la guerra de ajedrez auto mod apk terbaru:
-
-
Q1: ¿Cuál es la diferencia entre la guerra de ajedrez auto y otros juegos de batalla auto?
-
A1: La guerra de ajedrez automática se basa en el mod original de Dota Auto Chess, pero con sus propios héroes y objetos. También tiene un estilo más caricaturesco y un ritmo más rápido que otros juegos de combate automático.
-
Q2: ¿Cómo puedo obtener más oro y gemas en la guerra de ajedrez auto?
-
A2: Puedes obtener más oro y gemas jugando el juego regularmente, completando misiones y logros, viendo anuncios, o usando el terbaru apk mod.
-
Q3: ¿Cómo puedo desbloquear todos los héroes y objetos en la guerra de ajedrez automática?
-
A3: Usted puede desbloquear todos los héroes y artículos mediante la nivelación de su cuenta, abrir cofres, o el uso de la terbaru apk mod.
-
Q4: ¿Cómo puedo actualizar la guerra de ajedrez auto mod apk terbaru?
-
A4: Puede actualizar auto ajedrez guerra mod apk terbaru mediante la descarga de la última versión de la misma fuente que lo consiguió de. Asegúrese de hacer una copia de seguridad de sus datos antes de actualizar.
-
Q5: Es la guerra de ajedrez auto mod apk terbaru seguro de usar?
-
A5: Auto guerra de ajedrez mod apk terbaru es seguro de usar, siempre y cuando se descarga desde una fuente de confianza. Sin embargo, usted debe tener en cuenta que el uso de mod apk puede violar los términos de servicio del juego y resultar en una prohibición u otras consecuencias.
-
- : [Auto Chess War Mod Apk Terbaru Enlace de descarga](https://www.apkhome.us/auto-chess-war-apk-mod-unlimited/) 64aa2da5cf
-
-
\ No newline at end of file
diff --git a/spaces/Benson/text-generation/Examples/Brotato Zip Download.md b/spaces/Benson/text-generation/Examples/Brotato Zip Download.md
deleted file mode 100644
index 31ea7e8b02f4677f3f8211157247d1992a160777..0000000000000000000000000000000000000000
--- a/spaces/Benson/text-generation/Examples/Brotato Zip Download.md
+++ /dev/null
@@ -1,68 +0,0 @@
-
-
Descargar Brotato Zip: Cómo instalar y usar mods para tus juegos favoritos
-
¿Te encanta jugar juegos en tu PC, pero te gustaría poder personalizarlos a tu gusto? ¿Quieres probar nuevas características, gráficos, personajes o escenarios que no están disponibles en el juego original? Si es así, puede que te interese usar mods.
-
Los mods son modificaciones o adiciones a un juego que son creadas por fans o desarrolladores. Pueden cambiar cualquier cosa, desde el juego, a las imágenes, al sonido, a la historia. Los mods pueden hacer un juego más divertido, desafiante, inmersivo o realista.
Sin embargo, instalar y usar mods puede ser complicado a veces. Necesitas encontrar mods compatibles, descargarlos, instalarlos y activarlos. También tienes que asegurarte de que no entren en conflicto entre ellos o con el juego en sí. Y necesitas mantenerlos actualizados y organizados.
-
Ahí es donde entra Brotato Zip. Brotato Zip es un cargador mod que facilita la instalación y el uso de mods para varios juegos. También te conecta con una comunidad de modders y jugadores que comparten sus creaciones y comentarios. En este artículo, te mostraremos cómo descargar e instalar Brotato Zip, cómo descargar e instalar mods con él y cómo usarlos en tus juegos.
-
¿Qué es Brotato Zip?
-
Un cargador mod para varios juegos
-
Brotato Zip es un cargador mod que te permite cargar múltiples mods a la vez para diferentes juegos. Funciona inyectando un . Archivo pck en la carpeta del juego, que luego carga los mods desde una carpeta separada. De esta forma, no tendrás que modificar los archivos del juego ni preocuparte por romper nada.
-
Brotato Zip soporta muchos juegos populares, como Minecraft, Stardew Valley, Terraria, Among Us y más. Puedes consultar la lista de juegos compatibles en su página de GitHub. También planean agregar más juegos en el futuro.
-
Una comunidad de modders y jugadores
-
-
Brotato Zip tiene como objetivo crear una comunidad amigable y solidaria de modders y jugadores a los que les encanta jugar y mejorarlos. Dan la bienvenida a cualquiera que quiera unirse a ellos y divertirse.
-
¿Por qué utilizar Brotato Zip?
-
Para mejorar su experiencia de juego
-
La razón principal por la que deberías usar Brotato Zip es porque puede hacer que tu experiencia de juego sea más agradable. Con Brotato Zip, puedes acceder a miles de mods que pueden añadir nuevos contenidos, características, gráficos, sonidos o mecánicas a tus juegos. También puede mezclar y combinar diferentes mods para crear sus propias combinaciones únicas.
-
-
Por ejemplo, puedes usar Brotato Zip para añadir nuevos biomas, mobs, elementos o estructuras a Minecraft. También puedes usarlo para cambiar la apariencia de tu personaje, granja o pueblo en Stardew Valley. También puedes usarlo para añadir nuevos roles, mapas o modos a Among Us. También puedes usarlo para mejorar los gráficos, la física o el modo de juego de Terraria. Y estos son solo algunos ejemplos de lo que se puede hacer con Brotato Zip.
-
Para apoyar a los creadores de mod
-
Otra razón por la que deberías usar Brotato Zip es porque puede ayudarte a apoyar a los creadores de mods. Los creadores de mods son personas que dedican su tiempo y esfuerzo a crear mods de forma gratuita. Lo hacen porque les encantan los juegos y quieren compartir sus creaciones con otros. También escuchan comentarios y actualizan sus mods regularmente.
-
Usando Brotato Zip, puedes mostrar tu aprecio y gratitud a los creadores del mod. Puedes descargar sus mods, calificarlos, revisarlos y donarlos si quieres. También puede seguirlos en las redes sociales o unirse a sus servidores Discord. También puede darles sugerencias o informar de errores. Al hacer estas cosas, puedes ayudarles a mejorar sus mods y motivarlos a crear más.
-
¿Cómo descargar e instalar Brotato Zip?
-
Descargar el archivo PCK de GitHub
-
-
Para descargar el archivo PCK, vaya a la página de GitHub y haga clic en el botón verde "Código". Luego, haga clic en "Descargar ZIP". Guarde el archivo ZIP en su computadora y extráigalo. Verá una carpeta llamada "Brotato-Zip-main". Dentro de esta carpeta, encontrará el archivo PCK llamado "Brotato.zip". Este es el archivo que necesita.
-
Instalar el archivo PCK como cualquier otro mod
-
El segundo paso para usar Brotato Zip es instalar el archivo PCK como cualquier otro mod. Esto significa que necesita copiarlo y pegarlo en la carpeta del juego. La carpeta del juego es donde se almacenan los archivos del juego. Normalmente se encuentra en su carpeta Archivos de programa o Steam.
-
Para instalar el archivo PCK, busque su carpeta de juego y ábrala. Luego, encuentre la carpeta llamada "mods" o "modloader". Si no lo ve, cree uno usted mismo. Luego, copie y pegue el archivo PCK en esta carpeta. ¡Eso es todo! Usted ha instalado correctamente Brotato Zip.
-
¿Cómo descargar e instalar mods con Brotato Zip?
-
Crea una carpeta de mods en tu carpeta de juego
-
El tercer paso para usar Brotato Zip es crear una carpeta mods en tu carpeta de juego. Aquí es donde almacenarás todos los mods que quieras usar con Brotato Zip. Es importante mantener tus mods organizados y separados de tus archivos de juego.
-
Para crear una carpeta de mods, vuelva a su carpeta de juego y ábrala. Luego, haga clic derecho en un espacio vacío y seleccione "Nuevo" y luego "Carpeta". Nombra a esta carpeta "mods" o cualquier otra cosa que prefieras. Aquí es donde pondrás todas tus ZIPs mod.
-
Descargar mod ZIPs desde el sitio web de Brotato u otras fuentes
-
El cuarto paso para usar Brotato Zip es descargar mod ZIPs desde el sitio web de Brotato u otras fuentes. ZIPs Mod son archivos que contienen los datos mod que necesita cargar en su juego. Por lo general son comprimidos y fáciles de descargar.
-
-
También puede descargar ZPI mod de otras fuentes, como Nexus Mods, CurseForge, ModDB u otros sitios web que albergan mods para varios juegos. Solo asegúrate de que son compatibles con Brotato Zip y que son seguros y libres de virus.
-
Añadir a la carpeta mods
-
El quinto paso para usar Brotato Zip es agregar las ZIPs mod que descargó a la carpeta mods que creó en su carpeta de juegos. Así es como los instalas en tu juego.
-
Para agregarlos a la carpeta mods, busque los ZIPs mod que descargó y cópielos. Luego, vuelva a su carpeta de juegos y ábrala. Luego, abra la carpeta de mods que creó anteriormente y pegue las ZIPs de mod en ella. ¡Eso es todo! Has instalado correctamente los mods en tu juego.
-
¿Cómo usar mods con Brotato Zip?
-
Iniciar el juego con el cargador mod habilitado
-
El sexto paso para usar Brotato Zip es lanzar el juego con el cargador mod habilitado. Así es como activas los mods que instalaste en tu juego.
-
Para iniciar el juego con el cargador mod habilitado, vaya a su carpeta de juego y ábrala. Luego, encuentre el archivo ejecutable que ejecuta el juego. Por lo general se llama algo como "game.exe" o "launcher.exe". Haga clic derecho en él y seleccione "Ejecutar como administrador". Esto iniciará el juego con Brotato Zip corriendo en segundo plano.
-
Seleccione los mods que desea utilizar desde el menú
-
El séptimo y último paso para utilizar Brotato Zip es seleccionar los mods que desea utilizar en el menú. Así es como personalizas tu juego con los mods que instalaste.
-
Para seleccionar los mods que desea utilizar, pulse la tecla F10 en el teclado mientras está en el juego. Esto abrirá un menú que muestra todos los mods que tiene en su carpeta de mods. Puede desplazarse por ellos y verificarlos o desmarcarlos como desee. También puede ordenarlos por nombre, fecha, tamaño o calificación. También puede buscar mods específicos escribiendo su nombre o palabra clave.
-
-
Conclusión
-
Brotato Zip es un cargador mod que facilita la instalación y el uso de mods para varios juegos. También te conecta con una comunidad de modders y jugadores que comparten sus creaciones y comentarios. Para usar Brotato Zip, necesitas descargar e instalar el archivo PCK desde su página GitHub, crear una carpeta mods en tu carpeta de juegos, descargar ZIPs mod desde su sitio web u otras fuentes, agregarlos a la carpeta mods, iniciar el juego con el cargador mod habilitado, y seleccione los mods que desea usar en el menú.
-
Al usar Brotato Zip, puedes mejorar tu experiencia de juego, apoyar a los creadores de mods y divertirte con tus juegos. También puedes descubrir nuevos mods, aprender nuevas habilidades y hacer nuevos amigos. Brotato Zip es una herramienta que puede ayudarte a disfrutar de los juegos más que nunca.
-
Si quieres saber más sobre Brotato Zip, visita su página web, únete a su servidor Discord o síguelos en Twitter. También puede consultar su canal de YouTube para obtener tutoriales, comentarios y vitrinas de diferentes mods.
-
Gracias por leer este artículo. Esperamos que le resulte útil e informativo. Si tiene alguna pregunta o comentario, no dude en dejarlos a continuación. Y si le gustó este artículo, por favor compártalo con sus amigos y familiares.
-
Preguntas frecuentes
-
-
¿Brotato Zip es seguro y legal?
-
Sí, Brotato Zip es seguro y legal. No contiene virus ni malware, y no modifica ni daña tus archivos de juego. Tampoco viola los términos de servicio o derechos de autor de los juegos o los creadores de mods. Sin embargo, siempre debes usar Brotato Zip bajo tu propio riesgo y discreción, y hacer copias de seguridad de tus archivos de juego antes de usarlo.
-
Brotato Zip funciona con juegos multijugador?
-
-
¿Cómo actualizo Brotato Zip y los mods?
-
Para actualizar Brotato Zip, es necesario descargar la última versión del archivo PCK desde su página GitHub y reemplazar el antiguo en su carpeta de juego. Para actualizar los mods, necesita descargar la última versión de los ZPI mod desde su sitio web u otras fuentes y reemplazar los antiguos en su carpeta mods. También puede utilizar el menú Brotato Zip para buscar actualizaciones y descargarlas automáticamente.
-
¿Cómo desinstalo Brotato Zip y los mods?
-
Para desinstalar Brotato Zip, es necesario eliminar el archivo PCK de la carpeta del juego. Para desinstalar los mods, debe eliminar los ZIPs mod de su carpeta mods. También puedes usar el menú Brotato Zip para desactivar o eliminar los mods que ya no quieras usar.
-
¿Dónde puedo obtener ayuda o soporte para Brotato Zip y los mods?
-
Si necesita ayuda o soporte para Brotato Zip y los mods, puede visitar su sitio web, unirse a su servidor Discord o seguirlos en Twitter. También puede consultar su canal de YouTube para obtener tutoriales, revisiones y vitrinas de diferentes mods. También puede ponerse en contacto con ellos por correo electrónico en brotatozip@gmail.com.
-
- time:
-
-
- owner: {{ owner }}
- ip: {{ ip }}
- lat, lon: {{ loc }}
-
-
- more
-
- a brayden moore website
- thanks for visiting
- see the code
-
-
-
-
-
-
-
-
-
-
-
-
-
-
-
\ No newline at end of file
diff --git a/spaces/CAMP-ViL/Xplainer/description.md b/spaces/CAMP-ViL/Xplainer/description.md
deleted file mode 100644
index 6d0f97b1fb7ebdb7d6cbded61b3ca716698cfb9e..0000000000000000000000000000000000000000
--- a/spaces/CAMP-ViL/Xplainer/description.md
+++ /dev/null
@@ -1,3 +0,0 @@
-This demo provides a playground for testing the model of our paper "Xplainer: From X-Ray Observations to Explainable Zero-Shot Diagnosis", which was accepted for publication at MICCAI 2023. You can test our pre-defined prompts and define your own prompts and diseases.
-
-**Paper**: [arxiv](https://arxiv.org/pdf/2303.13391.pdf), **Code**: [Github](https://github.com/ChantalMP/Xplainer)
\ No newline at end of file
diff --git a/spaces/CVPR/LIVE/thrust/testing/unittest/meta.h b/spaces/CVPR/LIVE/thrust/testing/unittest/meta.h
deleted file mode 100644
index 39c62edb645361dcb9064b439b9dfc4d86b741e0..0000000000000000000000000000000000000000
--- a/spaces/CVPR/LIVE/thrust/testing/unittest/meta.h
+++ /dev/null
@@ -1,260 +0,0 @@
-/*! \file meta.h
- * \brief Defines template classes
- * for metaprogramming in the
- * unit tests.
- */
-
-#pragma once
-
-namespace unittest
-{
-
-// mark the absence of a type
-struct null_type {};
-
-// this type encapsulates a list of
-// up to 10 types
-template
- struct type_list
-{
- typedef T0 type_0;
- typedef T1 type_1;
- typedef T2 type_2;
- typedef T3 type_3;
- typedef T4 type_4;
- typedef T5 type_5;
- typedef T6 type_6;
- typedef T7 type_7;
- typedef T8 type_8;
- typedef T9 type_9;
- typedef T10 type_10;
- typedef T11 type_11;
- typedef T12 type_12;
- typedef T13 type_13;
- typedef T14 type_14;
- typedef T15 type_15;
- typedef T16 type_16;
- typedef T17 type_17;
- typedef T18 type_18;
- typedef T19 type_19;
-};
-
-// this type provides a way of indexing
-// into a type_list
-template
- struct get_type
-{
- typedef null_type type;
-};
-
-template struct get_type { typedef typename List::type_0 type; };
-template struct get_type { typedef typename List::type_1 type; };
-template struct get_type { typedef typename List::type_2 type; };
-template struct get_type { typedef typename List::type_3 type; };
-template struct get_type { typedef typename List::type_4 type; };
-template struct get_type { typedef typename List::type_5 type; };
-template struct get_type { typedef typename List::type_6 type; };
-template struct get_type { typedef typename List::type_7 type; };
-template struct get_type { typedef typename List::type_8 type; };
-template struct get_type { typedef typename List::type_9 type; };
-template struct get_type { typedef typename List::type_10 type; };
-template struct get_type { typedef typename List::type_11 type; };
-template struct get_type { typedef typename List::type_12 type; };
-template struct get_type { typedef typename List::type_13 type; };
-template struct get_type { typedef typename List::type_14 type; };
-template struct get_type { typedef typename List::type_15 type; };
-template struct get_type { typedef typename List::type_16 type; };
-template struct get_type { typedef typename List::type_17 type; };
-template struct get_type { typedef typename List::type_18 type; };
-template struct get_type { typedef typename List::type_19 type; };
-
-// this type and its specialization provides a way to
-// iterate over a type_list, and
-// applying a unary function to each type
-template class Function,
- typename T,
- unsigned int i = 0>
- struct for_each_type
-{
- template
- void operator()(U n)
- {
- // run the function on type T
- Function f;
- f(n);
-
- // get the next type
- typedef typename get_type::type next_type;
-
- // recurse to i + 1
- for_each_type loop;
- loop(n);
- }
-
- void operator()(void)
- {
- // run the function on type T
- Function f;
- f();
-
- // get the next type
- typedef typename get_type::type next_type;
-
- // recurse to i + 1
- for_each_type loop;
- loop();
- }
-};
-
-// terminal case: do nothing when encountering null_type
-template class Function,
- unsigned int i>
- struct for_each_type
-{
- template
- void operator()(U)
- {
- // no-op
- }
-
- void operator()(void)
- {
- // no-op
- }
-};
-
-// this type and its specialization instantiates
-// a template by applying T to Template.
-// if T == null_type, then its result is also null_type
-template class Template,
- typename T>
- struct ApplyTemplate1
-{
- typedef Template type;
-};
-
-template class Template>
- struct ApplyTemplate1
-{
- typedef null_type type;
-};
-
-// this type and its specializations instantiates
-// a template by applying T1 & T2 to Template.
-// if either T1 or T2 == null_type, then its result
-// is also null_type
-template class Template,
- typename T1,
- typename T2>
- struct ApplyTemplate2
-{
- typedef Template type;
-};
-
-template class Template,
- typename T>
- struct ApplyTemplate2
-{
- typedef null_type type;
-};
-
-template class Template,
- typename T>
- struct ApplyTemplate2
-{
- typedef null_type type;
-};
-
-template class Template>
- struct ApplyTemplate2
-{
- typedef null_type type;
-};
-
-// this type creates a new type_list by applying a Template to each of
-// the Type_list's types
-template class Template>
- struct transform1
-{
- typedef typename ApplyTemplate1::type>::type type_0;
- typedef typename ApplyTemplate1::type>::type type_1;
- typedef typename ApplyTemplate1::type>::type type_2;
- typedef typename ApplyTemplate1::type>::type type_3;
- typedef typename ApplyTemplate1::type>::type type_4;
- typedef typename ApplyTemplate1::type>::type type_5;
- typedef typename ApplyTemplate1::type>::type type_6;
- typedef typename ApplyTemplate1::type>::type type_7;
- typedef typename ApplyTemplate1::type>::type type_8;
- typedef typename ApplyTemplate1::type>::type type_9;
- typedef typename ApplyTemplate1::type>::type type_10;
- typedef typename ApplyTemplate1::type>::type type_11;
- typedef typename ApplyTemplate1::type>::type type_12;
- typedef typename ApplyTemplate1::type>::type type_13;
- typedef typename ApplyTemplate1::type>::type type_14;
- typedef typename ApplyTemplate1::type>::type type_15;
- typedef typename ApplyTemplate1::type>::type type_16;
- typedef typename ApplyTemplate1::type>::type type_17;
- typedef typename ApplyTemplate1::type>::type type_18;
- typedef typename ApplyTemplate1::type>::type type_19;
-
- typedef type_list type;
-};
-
-// this type creates a new type_list by applying a Template to each of
-// two type_list's types
-template class Template>
- struct transform2
-{
- typedef typename ApplyTemplate2::type, typename get_type::type>::type type_0;
- typedef typename ApplyTemplate2::type, typename get_type::type>::type type_1;
- typedef typename ApplyTemplate2::type, typename get_type::type>::type type_2;
- typedef typename ApplyTemplate2::type, typename get_type::type>::type type_3;
- typedef typename ApplyTemplate2::type, typename get_type::type>::type type_4;
- typedef typename ApplyTemplate2::type, typename get_type::type>::type type_5;
- typedef typename ApplyTemplate2::type, typename get_type::type>::type type_6;
- typedef typename ApplyTemplate2::type, typename get_type::type>::type type_7;
- typedef typename ApplyTemplate2::type, typename get_type::type>::type type_8;
- typedef typename ApplyTemplate2::type, typename get_type::type>::type type_9;
- typedef typename ApplyTemplate2::type, typename get_type::type>::type type_10;
- typedef typename ApplyTemplate2::type, typename get_type::type>::type type_11;
- typedef typename ApplyTemplate2::type, typename get_type::type>::type type_12;
- typedef typename ApplyTemplate2::type, typename get_type::type>::type type_13;
- typedef typename ApplyTemplate2::type, typename get_type::type>::type type_14;
- typedef typename ApplyTemplate2::type, typename get_type::type>::type type_15;
- typedef typename ApplyTemplate2::type, typename get_type::type>::type type_16;
- typedef typename ApplyTemplate2::type, typename get_type::type>::type type_17;
- typedef typename ApplyTemplate2::type, typename get_type::type>::type type_18;
- typedef typename ApplyTemplate2::type, typename get_type::type>::type type_19;
-
-
- typedef type_list type;
-};
-
-} // end unittest
-
diff --git a/spaces/CVPR/LIVE/thrust/thrust/detail/pointer.h b/spaces/CVPR/LIVE/thrust/thrust/detail/pointer.h
deleted file mode 100644
index e9204978f5d5990476698917842a1d77b779b5ba..0000000000000000000000000000000000000000
--- a/spaces/CVPR/LIVE/thrust/thrust/detail/pointer.h
+++ /dev/null
@@ -1,253 +0,0 @@
-/*
- * Copyright 2008-2018 NVIDIA Corporation
- *
- * Licensed under the Apache License, Version 2.0 (the "License");
- * you may not use this file except in compliance with the License.
- * You may obtain a copy of the License at
- *
- * http://www.apache.org/licenses/LICENSE-2.0
- *
- * Unless required by applicable law or agreed to in writing, software
- * distributed under the License is distributed on an "AS IS" BASIS,
- * WITHOUT WARRANTIES OR CONDITIONS OF ANY KIND, either express or implied.
- * See the License for the specific language governing permissions and
- * limitations under the License.
- */
-
-#pragma once
-
-#include
-#include
-#include
-#include
-#include
-#include
-#include
-
-
-namespace thrust
-{
-
-// declare pointer with default values of template parameters
-template class pointer;
-
-} // end thrust
-
-
-// specialize thrust::iterator_traits to avoid problems with the name of
-// pointer's constructor shadowing its nested pointer type
-// do this before pointer is defined so the specialization is correctly
-// used inside the definition
-namespace thrust
-{
-
-template
- struct iterator_traits >
-{
- private:
- typedef thrust::pointer ptr;
-
- public:
- typedef typename ptr::iterator_category iterator_category;
- typedef typename ptr::value_type value_type;
- typedef typename ptr::difference_type difference_type;
- // XXX implement this type (the result of operator->) later
- typedef void pointer;
- typedef typename ptr::reference reference;
-}; // end iterator_traits
-
-} // end thrust
-
-
-namespace thrust
-{
-
-namespace detail
-{
-
-// this metafunction computes the type of iterator_adaptor thrust::pointer should inherit from
-template
- struct pointer_base
-{
- // void pointers should have no element type
- // note that we remove_cv from the Element type to get the value_type
- typedef typename thrust::detail::eval_if<
- thrust::detail::is_void::type>::value,
- thrust::detail::identity_,
- thrust::detail::remove_cv
- >::type value_type;
-
- // if no Derived type is given, just use pointer
- typedef typename thrust::detail::eval_if<
- thrust::detail::is_same::value,
- thrust::detail::identity_ >,
- thrust::detail::identity_
- >::type derived_type;
-
- // void pointers should have no reference type
- // if no Reference type is given, just use reference
- typedef typename thrust::detail::eval_if<
- thrust::detail::is_void::type>::value,
- thrust::detail::identity_,
- thrust::detail::eval_if<
- thrust::detail::is_same::value,
- thrust::detail::identity_ >,
- thrust::detail::identity_
- >
- >::type reference_arg;
-
- typedef thrust::iterator_adaptor<
- derived_type, // pass along the type of our Derived class to iterator_adaptor
- Element *, // we adapt a raw pointer
- value_type, // the value type
- Tag, // system tag
- thrust::random_access_traversal_tag, // pointers have random access traversal
- reference_arg, // pass along our Reference type
- std::ptrdiff_t
- > type;
-}; // end pointer_base
-
-
-} // end detail
-
-
-// the base type for all of thrust's tagged pointers.
-// for reasonable pointer-like semantics, derived types should reimplement the following:
-// 1. no-argument constructor
-// 2. constructor from OtherElement *
-// 3. constructor from OtherPointer related by convertibility
-// 4. constructor from OtherPointer to void
-// 5. assignment from OtherPointer related by convertibility
-// These should just call the corresponding members of pointer.
-template
- class pointer
- : public thrust::detail::pointer_base::type
-{
- private:
- typedef typename thrust::detail::pointer_base::type super_t;
-
- typedef typename thrust::detail::pointer_base::derived_type derived_type;
-
- // friend iterator_core_access to give it access to dereference
- friend class thrust::iterator_core_access;
-
- __host__ __device__
- typename super_t::reference dereference() const;
-
- // don't provide access to this part of super_t's interface
- using super_t::base;
- using typename super_t::base_type;
-
- public:
- typedef typename super_t::base_type raw_pointer;
-
- // constructors
-
- __host__ __device__
- pointer();
-
- #if THRUST_CPP_DIALECT >= 2011
- // NOTE: This is needed so that Thrust smart pointers can be used in
- // `std::unique_ptr`.
- __host__ __device__
- pointer(decltype(nullptr));
- #endif
-
- // OtherValue shall be convertible to Value
- // XXX consider making the pointer implementation a template parameter which defaults to Element *
- template
- __host__ __device__
- explicit pointer(OtherElement *ptr);
-
- // OtherPointer's element_type shall be convertible to Element
- // OtherPointer's system shall be convertible to Tag
- template
- __host__ __device__
- pointer(const OtherPointer &other,
- typename thrust::detail::enable_if_pointer_is_convertible<
- OtherPointer,
- pointer
- >::type * = 0);
-
- // OtherPointer's element_type shall be void
- // OtherPointer's system shall be convertible to Tag
- template
- __host__ __device__
- explicit
- pointer(const OtherPointer &other,
- typename thrust::detail::enable_if_void_pointer_is_system_convertible<
- OtherPointer,
- pointer
- >::type * = 0);
-
- // assignment
-
- #if THRUST_CPP_DIALECT >= 2011
- // NOTE: This is needed so that Thrust smart pointers can be used in
- // `std::unique_ptr`.
- __host__ __device__
- derived_type& operator=(decltype(nullptr));
- #endif
-
- // OtherPointer's element_type shall be convertible to Element
- // OtherPointer's system shall be convertible to Tag
- template
- __host__ __device__
- typename thrust::detail::enable_if_pointer_is_convertible<
- OtherPointer,
- pointer,
- derived_type &
- >::type
- operator=(const OtherPointer &other);
-
- // observers
-
- __host__ __device__
- Element *get() const;
-
- #if THRUST_CPP_DIALECT >= 2011
- // NOTE: This is needed so that Thrust smart pointers can be used in
- // `std::unique_ptr`.
- __host__ __device__
- explicit operator bool() const;
- #endif
-
- __host__ __device__
- static derived_type pointer_to(typename thrust::detail::pointer_traits_detail::pointer_to_param::type r)
- {
- return thrust::detail::pointer_traits::pointer_to(r);
- }
-}; // end pointer
-
-// Output stream operator
-template
-__host__
-std::basic_ostream &
-operator<<(std::basic_ostream &os,
- const pointer &p);
-
-#if THRUST_CPP_DIALECT >= 2011
-// NOTE: This is needed so that Thrust smart pointers can be used in
-// `std::unique_ptr`.
-template
-__host__ __device__
-bool operator==(decltype(nullptr), pointer p);
-
-template
-__host__ __device__
-bool operator==(pointer p, decltype(nullptr));
-
-template
-__host__ __device__
-bool operator!=(decltype(nullptr), pointer p);
-
-template
-__host__ __device__
-bool operator!=(pointer p, decltype(nullptr));
-#endif
-
-} // end thrust
-
-#include
-
diff --git a/spaces/CVPR/drawings-to-human/README.md b/spaces/CVPR/drawings-to-human/README.md
deleted file mode 100644
index 5f6247d3dc5c8a9e89b103f641f97aa1543d6967..0000000000000000000000000000000000000000
--- a/spaces/CVPR/drawings-to-human/README.md
+++ /dev/null
@@ -1,12 +0,0 @@
----
-title: Drawings to Human
-emoji: ✍️🧍🏽♀️🧍🏻
-colorFrom: blue
-colorTo: blue
-sdk: gradio
-sdk_version: 3.0.24
-pinned: false
-app_file: main.py
----
-
-Check out the configuration reference at https://huggingface.co/docs/hub/spaces-config-reference
diff --git a/spaces/CVPR/lama-example/saicinpainting/training/visualizers/noop.py b/spaces/CVPR/lama-example/saicinpainting/training/visualizers/noop.py
deleted file mode 100644
index 4175089a54a8484d51e6c879c1a99c4e4d961d15..0000000000000000000000000000000000000000
--- a/spaces/CVPR/lama-example/saicinpainting/training/visualizers/noop.py
+++ /dev/null
@@ -1,9 +0,0 @@
-from saicinpainting.training.visualizers.base import BaseVisualizer
-
-
-class NoopVisualizer(BaseVisualizer):
- def __init__(self, *args, **kwargs):
- pass
-
- def __call__(self, epoch_i, batch_i, batch, suffix='', rank=None):
- pass
diff --git a/spaces/CarperAI/StableVicuna/README.md b/spaces/CarperAI/StableVicuna/README.md
deleted file mode 100644
index 3592aac607f78a1bf79e804fc4adeb1630ff3d29..0000000000000000000000000000000000000000
--- a/spaces/CarperAI/StableVicuna/README.md
+++ /dev/null
@@ -1,13 +0,0 @@
----
-title: StableVicuna
-emoji: 🦙
-colorFrom: blue
-colorTo: indigo
-sdk: gradio
-sdk_version: 3.27.0
-app_file: app.py
-pinned: false
-license: cc-by-nc-4.0
----
-
-Check out the configuration reference at https://huggingface.co/docs/hub/spaces-config-reference
diff --git a/spaces/Chris4K/llms_compare/Wondershare-Dvd-Slideshow-Builder-Deluxe-3d-Style-Pack-Registration-Code-Keygen.md b/spaces/Chris4K/llms_compare/Wondershare-Dvd-Slideshow-Builder-Deluxe-3d-Style-Pack-Registration-Code-Keygen.md
deleted file mode 100644
index cfea030fb120ed7958a75b506402c124d00d7743..0000000000000000000000000000000000000000
--- a/spaces/Chris4K/llms_compare/Wondershare-Dvd-Slideshow-Builder-Deluxe-3d-Style-Pack-Registration-Code-Keygen.md
+++ /dev/null
@@ -1,74 +0,0 @@
-## Wondershare Dvd Slideshow Builder Deluxe 3d Style Pack Registration Code Keygen
-
-
-
-
-
- 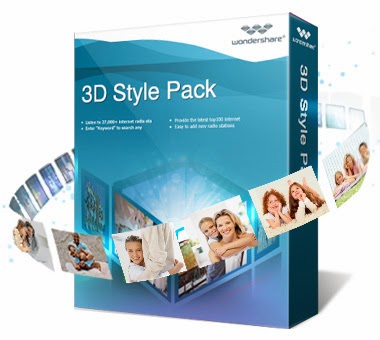
-
-
-
-
-
-**CLICK HERE ····· [https://urluso.com/2tBNxk](https://urluso.com/2tBNxk)**
-
-
-
-
-
-
-
-
-
-
-
-
-
-# How to Make Amazing 3D Slideshows with Wondershare DVD Slideshow Builder Deluxe and 3D Style Pack
-
-
-
-If you want to create stunning 3D slideshows from your photos and videos, you need a powerful and easy-to-use software that can handle the task. Wondershare DVD Slideshow Builder Deluxe is a professional slideshow maker that lets you turn your photos and videos into amazing DVD slideshows with music, transitions, effects, and more. But what if you want to add some extra flair to your slideshows with 3D movie effects? That's where Wondershare 3D Style Pack comes in.
-
-
-
-Wondershare 3D Style Pack is an exclusive expansion pack for Wondershare DVD Slideshow Builder Deluxe that gives you access to more than 10 "Styles" of popular 3D video effects, such as 3D Cube, Photo Flow, 3D Wall, 3D Square, 3D Carousel, 3D Box, and so on. With these 3D styles, you can make your slideshows more dynamic and eye-catching. You can also customize the 3D effects with parameters like angle, distance, depth, and color.
-
-
-
-To use Wondershare 3D Style Pack, you need to purchase both the software and the style pack from the official website[^1^]. Then, you can download and install them on your computer. After that, you can launch Wondershare DVD Slideshow Builder Deluxe and choose Standard mode. Then, you can import your photos and videos to the storyboard and apply the 3D styles to them. You can preview the effects in real time and adjust them as you like. Finally, you can burn your slideshow to DVD or save it as a video file.
-
-
-
-Wondershare 3D Style Pack is a great way to enhance your slideshows with 3D movie effects. It's compatible with Windows XP/Vista/7/8/10 and supports various formats of photos and videos. You can also get free updates and technical support from Wondershare. If you want to try it before buying it, you can download a free trial version of Wondershare DVD Slideshow Builder Deluxe from the official website[^1^] and use some of the 3D styles for free.
-
-
-
-So what are you waiting for? Get Wondershare DVD Slideshow Builder Deluxe and 3D Style Pack today and start making amazing 3D slideshows with your photos and videos!
-
-
-
-## How to Use Wondershare DVD Slideshow Builder Deluxe
-
-
-
-Wondershare DVD Slideshow Builder Deluxe is a user-friendly and versatile software that lets you create professional-looking slideshows with your photos and videos. You can use it to make slideshows for various occasions, such as weddings, birthdays, anniversaries, vacations, and more. You can also use it to make slideshows for business presentations, education, and marketing.
-
-
-
-To use Wondershare DVD Slideshow Builder Deluxe, you need to download and install it on your computer. Then, you can launch it and choose between two modes: Advanced Mode and Standard Mode[^1^]. Advanced Mode gives you more control and customization options for your slideshows, while Standard Mode is simpler and faster to use. You can switch between the modes at any time.
-
-
-
-In both modes, you can import your photos and videos to the storyboard by clicking the Add Files button or dragging and dropping them. You can also add background music, voiceovers, text, clipart, and other elements to your slideshows. You can edit your photos and videos with basic tools like crop, rotate, trim, adjust brightness, contrast, saturation, etc. You can also apply transitions, effects, filters, and themes to your slideshows to make them more attractive.
-
-
-
-Once you are satisfied with your slideshows, you can preview them in full screen and make any changes if needed. Then, you can save your slideshows as a video file in various formats like MP4, AVI, WMV, MOV, FLV, etc. You can also burn your slideshows to DVD discs or ISO files with a built-in DVD menu. You can also share your slideshows online via YouTube, Facebook, Vimeo, etc.
-
- 145887f19f
-
-
-
-
-
diff --git a/spaces/Cong723/gpt-academic-public/crazy_functions/crazy_functions_test.py b/spaces/Cong723/gpt-academic-public/crazy_functions/crazy_functions_test.py
deleted file mode 100644
index 6020fa2ffc3cdcb288f03e55ff37313b0be78222..0000000000000000000000000000000000000000
--- a/spaces/Cong723/gpt-academic-public/crazy_functions/crazy_functions_test.py
+++ /dev/null
@@ -1,130 +0,0 @@
-"""
-这是什么?
- 这个文件用于函数插件的单元测试
- 运行方法 python crazy_functions/crazy_functions_test.py
-"""
-
-def validate_path():
- import os, sys
- dir_name = os.path.dirname(__file__)
- root_dir_assume = os.path.abspath(os.path.dirname(__file__) + '/..')
- os.chdir(root_dir_assume)
- sys.path.append(root_dir_assume)
-
-validate_path() # validate path so you can run from base directory
-from colorful import *
-from toolbox import get_conf, ChatBotWithCookies
-proxies, WEB_PORT, LLM_MODEL, CONCURRENT_COUNT, AUTHENTICATION, CHATBOT_HEIGHT, LAYOUT, API_KEY = \
- get_conf('proxies', 'WEB_PORT', 'LLM_MODEL', 'CONCURRENT_COUNT', 'AUTHENTICATION', 'CHATBOT_HEIGHT', 'LAYOUT', 'API_KEY')
-
-llm_kwargs = {
- 'api_key': API_KEY,
- 'llm_model': LLM_MODEL,
- 'top_p':1.0,
- 'max_length': None,
- 'temperature':1.0,
-}
-plugin_kwargs = { }
-chatbot = ChatBotWithCookies(llm_kwargs)
-history = []
-system_prompt = "Serve me as a writing and programming assistant."
-web_port = 1024
-
-
-def test_解析一个Python项目():
- from crazy_functions.解析项目源代码 import 解析一个Python项目
- txt = "crazy_functions/test_project/python/dqn"
- for cookies, cb, hist, msg in 解析一个Python项目(txt, llm_kwargs, plugin_kwargs, chatbot, history, system_prompt, web_port):
- print(cb)
-
-def test_解析一个Cpp项目():
- from crazy_functions.解析项目源代码 import 解析一个C项目
- txt = "crazy_functions/test_project/cpp/cppipc"
- for cookies, cb, hist, msg in 解析一个C项目(txt, llm_kwargs, plugin_kwargs, chatbot, history, system_prompt, web_port):
- print(cb)
-
-def test_Latex英文润色():
- from crazy_functions.Latex全文润色 import Latex英文润色
- txt = "crazy_functions/test_project/latex/attention"
- for cookies, cb, hist, msg in Latex英文润色(txt, llm_kwargs, plugin_kwargs, chatbot, history, system_prompt, web_port):
- print(cb)
-
-def test_Markdown中译英():
- from crazy_functions.批量Markdown翻译 import Markdown中译英
- txt = "README.md"
- for cookies, cb, hist, msg in Markdown中译英(txt, llm_kwargs, plugin_kwargs, chatbot, history, system_prompt, web_port):
- print(cb)
-
-def test_批量翻译PDF文档():
- from crazy_functions.批量翻译PDF文档_多线程 import 批量翻译PDF文档
- txt = "crazy_functions/test_project/pdf_and_word"
- for cookies, cb, hist, msg in 批量翻译PDF文档(txt, llm_kwargs, plugin_kwargs, chatbot, history, system_prompt, web_port):
- print(cb)
-
-def test_谷歌检索小助手():
- from crazy_functions.谷歌检索小助手 import 谷歌检索小助手
- txt = "https://scholar.google.com/scholar?hl=en&as_sdt=0%2C5&q=auto+reinforcement+learning&btnG="
- for cookies, cb, hist, msg in 谷歌检索小助手(txt, llm_kwargs, plugin_kwargs, chatbot, history, system_prompt, web_port):
- print(cb)
-
-def test_总结word文档():
- from crazy_functions.总结word文档 import 总结word文档
- txt = "crazy_functions/test_project/pdf_and_word"
- for cookies, cb, hist, msg in 总结word文档(txt, llm_kwargs, plugin_kwargs, chatbot, history, system_prompt, web_port):
- print(cb)
-
-def test_下载arxiv论文并翻译摘要():
- from crazy_functions.下载arxiv论文翻译摘要 import 下载arxiv论文并翻译摘要
- txt = "1812.10695"
- for cookies, cb, hist, msg in 下载arxiv论文并翻译摘要(txt, llm_kwargs, plugin_kwargs, chatbot, history, system_prompt, web_port):
- print(cb)
-
-def test_联网回答问题():
- from crazy_functions.联网的ChatGPT import 连接网络回答问题
- # txt = "“我们称之为高效”是什么梗?"
- # >> 从第0份、第1份、第2份搜索结果可以看出,“我们称之为高效”是指在游戏社区中,用户们用来形容一些游戏策略或行为非常高效且能够带来好的效果的用语。这个用语最初可能是在群星(Stellaris)这个游戏里面流行起来的,后来也传播到了其他游戏中,比如巨像(Titan)等游戏。其中第1份搜索结果中的一篇文章也指出,“我们称之为高效”这 一用语来源于群星(Stellaris)游戏中的一个情节。
- # txt = "为什么说枪毙P社玩家没有一个冤枉的?"
- # >> 它们都是关于一个知乎用户所发的帖子,引用了一群游戏玩家对于需要对P社玩家进行枪毙的讨论,这个话题的本质是玩家们对于P 社游戏中的政治与历史元素的不同看法,以及其中不少玩家以极端立场宣扬的想法和言论,因此有人就以枪毙这些玩家来回应此类言论。但是这个话题本身并没有实质内容,只是一个玩笑或者恶搞,并不应该被当做真实的态度或者观点,因此这种说法没有实际意义。
- # txt = "谁是应急食品?"
- # >> '根据以上搜索结果可以得知,应急食品是“原神”游戏中的角色派蒙的外号。'
- # txt = "道路千万条,安全第一条。后面两句是?"
- # >> '行车不规范,亲人两行泪。'
- # txt = "What is in the canister?"
- # >> Rainbow Six Siege 游戏中 Smoke 的 Canister 中装有何种物质相关的官方信息。
- # txt = "失败的man是什么?"
- # >> 根据第1份搜索结果,可以得知失败的man是指一位在B站购买了蜘蛛侠COS服后穿上后被网友嘲笑的UP主,而“失败的man”是蜘蛛侠英文名“spiderman”的谐音梗,并且网友们还 给这位UP主起了“苍蝇侠”的外号。因此,失败的man是指这位UP主在穿上蜘蛛侠COS服后被网友嘲笑的情况。
- # txt = "老六是什么,起源于哪里?"
- # >> 老六是网络流行语,最初起源于游戏《CSGO》,指游戏中玩家中独来独往、游离于队伍之外的“自由人”或玩得比较菜或者玩得比较阴险的人 ,后来逐渐演变成指玩得比较阴险的玩家。
- # txt = "罗小黑战记因为什么经常被吐槽?"
- # >> 3. 更新速度。罗小黑战记的更新时间不定,时而快时而慢,给观众留下了等待的时间过长的印象。
- # txt = "沙特、伊朗最近的关系如何?"
- # >> 最近在中国的斡旋下,沙特和伊朗于3月10日达成了恢复两国外交关系的协议,这表明两国关系已经重新回到正常化状态。
- # txt = "You should have gone for the head. What does that mean?"
- # >> The phrase "You should have gone for the head" is a quote from the Marvel movies, Avengers: Infinity War and Avengers: Endgame. It was spoken by the character Thanos in Infinity War and by Thor in Endgame.
- txt = "AutoGPT是什么?"
- # >> AutoGPT是一个基于GPT-4语言模型的开源应用程序。它可以根据用户需求自主执行任务,包括事件分析、营销方案撰写、代码编程、数学运算等等,并完全不需要用户插手。它可以自己思考,给出实现的步骤和实现细节,甚至可以自问自答执 行任务。最近它在GitHub上爆火,成为了业内最热门的项目之一。
- # txt = "钟离带什么圣遗物?"
- for cookies, cb, hist, msg in 连接网络回答问题(txt, llm_kwargs, plugin_kwargs, chatbot, history, system_prompt, web_port):
- print("当前问答:", cb[-1][-1].replace("\n"," "))
- for i, it in enumerate(cb): print亮蓝(it[0]); print亮黄(it[1])
-
-def test_解析ipynb文件():
- from crazy_functions.解析JupyterNotebook import 解析ipynb文件
- txt = "crazy_functions/test_samples"
- for cookies, cb, hist, msg in 解析ipynb文件(txt, llm_kwargs, plugin_kwargs, chatbot, history, system_prompt, web_port):
- print(cb)
-
-
-# test_解析一个Python项目()
-# test_Latex英文润色()
-# test_Markdown中译英()
-# test_批量翻译PDF文档()
-# test_谷歌检索小助手()
-# test_总结word文档()
-# test_下载arxiv论文并翻译摘要()
-# test_解析一个Cpp项目()
-# test_联网回答问题()
-test_解析ipynb文件()
-
-input("程序完成,回车退出。")
-print("退出。")
\ No newline at end of file
diff --git a/spaces/Cpp4App/Cpp4App/CDM/detect_compo/deprecated/ip_segment.py b/spaces/Cpp4App/Cpp4App/CDM/detect_compo/deprecated/ip_segment.py
deleted file mode 100644
index a4c02cb1989e2939bb38eb692c2f4fb021a6ff16..0000000000000000000000000000000000000000
--- a/spaces/Cpp4App/Cpp4App/CDM/detect_compo/deprecated/ip_segment.py
+++ /dev/null
@@ -1,123 +0,0 @@
-import cv2
-import numpy as np
-import shutil
-import os
-from os.path import join as pjoin
-
-
-def segment_img(org, segment_size, output_path, overlap=100):
- if not os.path.exists(output_path):
- os.mkdir(output_path)
-
- height, width = np.shape(org)[0], np.shape(org)[1]
- top = 0
- bottom = segment_size
- segment_no = 0
- while top < height and bottom < height:
- segment = org[top:bottom]
- cv2.imwrite(os.path.join(output_path, str(segment_no) + '.png'), segment)
- segment_no += 1
- top += segment_size - overlap
- bottom = bottom + segment_size - overlap if bottom + segment_size - overlap <= height else height
-
-
-def clipping(img, components, pad=0, show=False):
- """
- :param adjust: shrink(negative) or expand(positive) the bounding box
- :param img: original image
- :param corners: ((column_min, row_min),(column_max, row_max))
- :return: list of clipping images
- """
- clips = []
- for component in components:
- clip = component.compo_clipping(img, pad=pad)
- clips.append(clip)
- if show:
- cv2.imshow('clipping', clip)
- cv2.waitKey()
- return clips
-
-
-def dissemble_clip_img_hollow(clip_root, org, compos):
- if os.path.exists(clip_root):
- shutil.rmtree(clip_root)
- os.mkdir(clip_root)
- cls_dirs = []
-
- bkg = org.copy()
- hollow_out = np.ones(bkg.shape[:2], dtype=np.uint8) * 255
- for compo in compos:
- cls = compo.category
- c_root = pjoin(clip_root, cls)
- c_path = pjoin(c_root, str(compo.id) + '.jpg')
- if cls not in cls_dirs:
- os.mkdir(c_root)
- cls_dirs.append(cls)
- clip = compo.compo_clipping(org)
- cv2.imwrite(c_path, clip)
-
- col_min, row_min, col_max, row_max = compo.put_bbox()
- hollow_out[row_min: row_max, col_min: col_max] = 0
-
- bkg = cv2.merge((bkg, hollow_out))
- cv2.imwrite(os.path.join(clip_root, 'bkg.png'), bkg)
-
-
-def dissemble_clip_img_fill(clip_root, org, compos, flag='most'):
-
- def average_pix_around(pad=6, offset=3):
- up = row_min - pad if row_min - pad >= 0 else 0
- left = col_min - pad if col_min - pad >= 0 else 0
- bottom = row_max + pad if row_max + pad < org.shape[0] - 1 else org.shape[0] - 1
- right = col_max + pad if col_max + pad < org.shape[1] - 1 else org.shape[1] - 1
-
- average = []
- for i in range(3):
- avg_up = np.average(org[up:row_min - offset, left:right, i])
- avg_bot = np.average(org[row_max + offset:bottom, left:right, i])
- avg_left = np.average(org[up:bottom, left:col_min - offset, i])
- avg_right = np.average(org[up:bottom, col_max + offset:right, i])
- average.append(int((avg_up + avg_bot + avg_left + avg_right)/4))
- return average
-
- def most_pix_around(pad=6, offset=2):
- up = row_min - pad if row_min - pad >= 0 else 0
- left = col_min - pad if col_min - pad >= 0 else 0
- bottom = row_max + pad if row_max + pad < org.shape[0] - 1 else org.shape[0] - 1
- right = col_max + pad if col_max + pad < org.shape[1] - 1 else org.shape[1] - 1
-
- most = []
- for i in range(3):
- val = np.concatenate((org[up:row_min - offset, left:right, i].flatten(),
- org[row_max + offset:bottom, left:right, i].flatten(),
- org[up:bottom, left:col_min - offset, i].flatten(),
- org[up:bottom, col_max + offset:right, i].flatten()))
- # print(val)
- # print(np.argmax(np.bincount(val)))
- most.append(int(np.argmax(np.bincount(val))))
- return most
-
- if os.path.exists(clip_root):
- shutil.rmtree(clip_root)
- os.mkdir(clip_root)
- cls_dirs = []
-
- bkg = org.copy()
- for compo in compos:
- cls = compo.category
- c_root = pjoin(clip_root, cls)
- c_path = pjoin(c_root, str(compo.id) + '.jpg')
- if cls not in cls_dirs:
- os.mkdir(c_root)
- cls_dirs.append(cls)
- clip = compo.compo_clipping(org)
- cv2.imwrite(c_path, clip)
-
- col_min, row_min, col_max, row_max = compo.put_bbox()
- if flag == 'average':
- color = average_pix_around()
- elif flag == 'most':
- color = most_pix_around()
- cv2.rectangle(bkg, (col_min, row_min), (col_max, row_max), color, -1)
-
- cv2.imwrite(os.path.join(clip_root, 'bkg.png'), bkg)
diff --git a/spaces/Cyril666/ContourNet-ABI/maskrcnn_benchmark/modeling/roi_heads/boundary_head/roi_boundary_feature_extractors.py b/spaces/Cyril666/ContourNet-ABI/maskrcnn_benchmark/modeling/roi_heads/boundary_head/roi_boundary_feature_extractors.py
deleted file mode 100644
index 96fe5b019a54ae06799065cf39adea7ba452442d..0000000000000000000000000000000000000000
--- a/spaces/Cyril666/ContourNet-ABI/maskrcnn_benchmark/modeling/roi_heads/boundary_head/roi_boundary_feature_extractors.py
+++ /dev/null
@@ -1,69 +0,0 @@
-# Copyright (c) Facebook, Inc. and its affiliates. All Rights Reserved.
-from torch import nn
-from torch.nn import functional as F
-
-# from ..box_head.roi_box_feature_extractors import ResNet50Conv5ROIFeatureExtractor
-from maskrcnn_benchmark.modeling.poolers import Pooler
-from maskrcnn_benchmark.modeling.make_layers import make_conv3x3
-
-
-class BOUNDARYRCNNFPNFeatureExtractor(nn.Module):
- """
- Heads for FPN for classification
- """
-
- def __init__(self, cfg, in_channels):
- """
- Arguments:
- num_classes (int): number of output classes
- input_size (int): number of channels of the input once it's flattened
- representation_size (int): size of the intermediate representation
- """
- super(BOUNDARYRCNNFPNFeatureExtractor, self).__init__()
-
- resolution = cfg.MODEL.ROI_BOUNDARY_HEAD.POOLER_RESOLUTION
- scales = cfg.MODEL.ROI_BOUNDARY_HEAD.POOLER_SCALES
- sampling_ratio = cfg.MODEL.ROI_BOUNDARY_HEAD.POOLER_SAMPLING_RATIO
- pooler = Pooler(
- output_size=(resolution, resolution),
- scales=scales,
- sampling_ratio=sampling_ratio,
- deformable=cfg.MODEL.ROI_BOUNDARY_HEAD.DEFORMABLE_POOLING
- # deformable = True
- )
- input_size = in_channels
- self.pooler = pooler
-
- layers = cfg.MODEL.ROI_BOUNDARY_HEAD.CONV_LAYERS
- use_gn = cfg.MODEL.ROI_MASK_HEAD.USE_GN
- dilation = cfg.MODEL.ROI_MASK_HEAD.DILATION
-
- next_feature = input_size
- self.blocks = []
- for layer_idx, layer_features in enumerate(layers, 1):
- layer_name = "boundary_fcn{}".format(layer_idx)
- module = make_conv3x3(
- next_feature, layer_features,
- dilation=dilation, stride=1, use_gn=use_gn
- )
- self.add_module(layer_name, module)
- next_feature = layer_features
- self.blocks.append(layer_name)
-
- def forward(self, x, proposals):
- x = self.pooler(x, proposals)
-
- for layer_name in self.blocks:
- x = F.relu(getattr(self, layer_name)(x))
-
- return x
-
-
-_ROI_KE_FEATURE_EXTRACTORS = {
- "BoundaryRCNNFPNFeatureExtractor": BOUNDARYRCNNFPNFeatureExtractor,
-}
-
-
-def make_roi_boundary_feature_extractor(cfg, in_channels):
- func = _ROI_KE_FEATURE_EXTRACTORS[cfg.MODEL.ROI_BOUNDARY_HEAD.FEATURE_EXTRACTOR]
- return func(cfg, in_channels)
diff --git a/spaces/DQChoi/gpt-demo/venv/lib/python3.11/site-packages/anyio/_core/_streams.py b/spaces/DQChoi/gpt-demo/venv/lib/python3.11/site-packages/anyio/_core/_streams.py
deleted file mode 100644
index 54ea2b2bafd321a4f88dfa6fd19993213eec8105..0000000000000000000000000000000000000000
--- a/spaces/DQChoi/gpt-demo/venv/lib/python3.11/site-packages/anyio/_core/_streams.py
+++ /dev/null
@@ -1,47 +0,0 @@
-from __future__ import annotations
-
-import math
-from typing import Any, TypeVar, overload
-
-from ..streams.memory import (
- MemoryObjectReceiveStream,
- MemoryObjectSendStream,
- MemoryObjectStreamState,
-)
-
-T_Item = TypeVar("T_Item")
-
-
-@overload
-def create_memory_object_stream(
- max_buffer_size: float = ...,
-) -> tuple[MemoryObjectSendStream[Any], MemoryObjectReceiveStream[Any]]:
- ...
-
-
-@overload
-def create_memory_object_stream(
- max_buffer_size: float = ..., item_type: type[T_Item] = ...
-) -> tuple[MemoryObjectSendStream[T_Item], MemoryObjectReceiveStream[T_Item]]:
- ...
-
-
-def create_memory_object_stream(
- max_buffer_size: float = 0, item_type: type[T_Item] | None = None
-) -> tuple[MemoryObjectSendStream[Any], MemoryObjectReceiveStream[Any]]:
- """
- Create a memory object stream.
-
- :param max_buffer_size: number of items held in the buffer until ``send()`` starts blocking
- :param item_type: type of item, for marking the streams with the right generic type for
- static typing (not used at run time)
- :return: a tuple of (send stream, receive stream)
-
- """
- if max_buffer_size != math.inf and not isinstance(max_buffer_size, int):
- raise ValueError("max_buffer_size must be either an integer or math.inf")
- if max_buffer_size < 0:
- raise ValueError("max_buffer_size cannot be negative")
-
- state: MemoryObjectStreamState = MemoryObjectStreamState(max_buffer_size)
- return MemoryObjectSendStream(state), MemoryObjectReceiveStream(state)
diff --git a/spaces/DQChoi/gpt-demo/venv/lib/python3.11/site-packages/dateutil/zoneinfo/__init__.py b/spaces/DQChoi/gpt-demo/venv/lib/python3.11/site-packages/dateutil/zoneinfo/__init__.py
deleted file mode 100644
index 34f11ad66c88047f2c049a4cdcc937b4b78ea6d6..0000000000000000000000000000000000000000
--- a/spaces/DQChoi/gpt-demo/venv/lib/python3.11/site-packages/dateutil/zoneinfo/__init__.py
+++ /dev/null
@@ -1,167 +0,0 @@
-# -*- coding: utf-8 -*-
-import warnings
-import json
-
-from tarfile import TarFile
-from pkgutil import get_data
-from io import BytesIO
-
-from dateutil.tz import tzfile as _tzfile
-
-__all__ = ["get_zonefile_instance", "gettz", "gettz_db_metadata"]
-
-ZONEFILENAME = "dateutil-zoneinfo.tar.gz"
-METADATA_FN = 'METADATA'
-
-
-class tzfile(_tzfile):
- def __reduce__(self):
- return (gettz, (self._filename,))
-
-
-def getzoneinfofile_stream():
- try:
- return BytesIO(get_data(__name__, ZONEFILENAME))
- except IOError as e: # TODO switch to FileNotFoundError?
- warnings.warn("I/O error({0}): {1}".format(e.errno, e.strerror))
- return None
-
-
-class ZoneInfoFile(object):
- def __init__(self, zonefile_stream=None):
- if zonefile_stream is not None:
- with TarFile.open(fileobj=zonefile_stream) as tf:
- self.zones = {zf.name: tzfile(tf.extractfile(zf), filename=zf.name)
- for zf in tf.getmembers()
- if zf.isfile() and zf.name != METADATA_FN}
- # deal with links: They'll point to their parent object. Less
- # waste of memory
- links = {zl.name: self.zones[zl.linkname]
- for zl in tf.getmembers() if
- zl.islnk() or zl.issym()}
- self.zones.update(links)
- try:
- metadata_json = tf.extractfile(tf.getmember(METADATA_FN))
- metadata_str = metadata_json.read().decode('UTF-8')
- self.metadata = json.loads(metadata_str)
- except KeyError:
- # no metadata in tar file
- self.metadata = None
- else:
- self.zones = {}
- self.metadata = None
-
- def get(self, name, default=None):
- """
- Wrapper for :func:`ZoneInfoFile.zones.get`. This is a convenience method
- for retrieving zones from the zone dictionary.
-
- :param name:
- The name of the zone to retrieve. (Generally IANA zone names)
-
- :param default:
- The value to return in the event of a missing key.
-
- .. versionadded:: 2.6.0
-
- """
- return self.zones.get(name, default)
-
-
-# The current API has gettz as a module function, although in fact it taps into
-# a stateful class. So as a workaround for now, without changing the API, we
-# will create a new "global" class instance the first time a user requests a
-# timezone. Ugly, but adheres to the api.
-#
-# TODO: Remove after deprecation period.
-_CLASS_ZONE_INSTANCE = []
-
-
-def get_zonefile_instance(new_instance=False):
- """
- This is a convenience function which provides a :class:`ZoneInfoFile`
- instance using the data provided by the ``dateutil`` package. By default, it
- caches a single instance of the ZoneInfoFile object and returns that.
-
- :param new_instance:
- If ``True``, a new instance of :class:`ZoneInfoFile` is instantiated and
- used as the cached instance for the next call. Otherwise, new instances
- are created only as necessary.
-
- :return:
- Returns a :class:`ZoneInfoFile` object.
-
- .. versionadded:: 2.6
- """
- if new_instance:
- zif = None
- else:
- zif = getattr(get_zonefile_instance, '_cached_instance', None)
-
- if zif is None:
- zif = ZoneInfoFile(getzoneinfofile_stream())
-
- get_zonefile_instance._cached_instance = zif
-
- return zif
-
-
-def gettz(name):
- """
- This retrieves a time zone from the local zoneinfo tarball that is packaged
- with dateutil.
-
- :param name:
- An IANA-style time zone name, as found in the zoneinfo file.
-
- :return:
- Returns a :class:`dateutil.tz.tzfile` time zone object.
-
- .. warning::
- It is generally inadvisable to use this function, and it is only
- provided for API compatibility with earlier versions. This is *not*
- equivalent to ``dateutil.tz.gettz()``, which selects an appropriate
- time zone based on the inputs, favoring system zoneinfo. This is ONLY
- for accessing the dateutil-specific zoneinfo (which may be out of
- date compared to the system zoneinfo).
-
- .. deprecated:: 2.6
- If you need to use a specific zoneinfofile over the system zoneinfo,
- instantiate a :class:`dateutil.zoneinfo.ZoneInfoFile` object and call
- :func:`dateutil.zoneinfo.ZoneInfoFile.get(name)` instead.
-
- Use :func:`get_zonefile_instance` to retrieve an instance of the
- dateutil-provided zoneinfo.
- """
- warnings.warn("zoneinfo.gettz() will be removed in future versions, "
- "to use the dateutil-provided zoneinfo files, instantiate a "
- "ZoneInfoFile object and use ZoneInfoFile.zones.get() "
- "instead. See the documentation for details.",
- DeprecationWarning)
-
- if len(_CLASS_ZONE_INSTANCE) == 0:
- _CLASS_ZONE_INSTANCE.append(ZoneInfoFile(getzoneinfofile_stream()))
- return _CLASS_ZONE_INSTANCE[0].zones.get(name)
-
-
-def gettz_db_metadata():
- """ Get the zonefile metadata
-
- See `zonefile_metadata`_
-
- :returns:
- A dictionary with the database metadata
-
- .. deprecated:: 2.6
- See deprecation warning in :func:`zoneinfo.gettz`. To get metadata,
- query the attribute ``zoneinfo.ZoneInfoFile.metadata``.
- """
- warnings.warn("zoneinfo.gettz_db_metadata() will be removed in future "
- "versions, to use the dateutil-provided zoneinfo files, "
- "ZoneInfoFile object and query the 'metadata' attribute "
- "instead. See the documentation for details.",
- DeprecationWarning)
-
- if len(_CLASS_ZONE_INSTANCE) == 0:
- _CLASS_ZONE_INSTANCE.append(ZoneInfoFile(getzoneinfofile_stream()))
- return _CLASS_ZONE_INSTANCE[0].metadata
diff --git a/spaces/DQChoi/gpt-demo/venv/lib/python3.11/site-packages/fontTools/ttLib/tables/ttProgram.py b/spaces/DQChoi/gpt-demo/venv/lib/python3.11/site-packages/fontTools/ttLib/tables/ttProgram.py
deleted file mode 100644
index 84aa63f36301ec9a4ae21acff0cbc95010d956b7..0000000000000000000000000000000000000000
--- a/spaces/DQChoi/gpt-demo/venv/lib/python3.11/site-packages/fontTools/ttLib/tables/ttProgram.py
+++ /dev/null
@@ -1,593 +0,0 @@
-"""ttLib.tables.ttProgram.py -- Assembler/disassembler for TrueType bytecode programs."""
-from __future__ import annotations
-
-from fontTools.misc.textTools import num2binary, binary2num, readHex, strjoin
-import array
-from io import StringIO
-from typing import List
-import re
-import logging
-
-
-log = logging.getLogger(__name__)
-
-# fmt: off
-
-# first, the list of instructions that eat bytes or words from the instruction stream
-
-streamInstructions = [
-#
-# opcode mnemonic argBits descriptive name pops pushes eats from instruction stream pushes
-#
- (0x40, 'NPUSHB', 0, 'PushNBytes', 0, -1), # n, b1, b2,...bn b1,b2...bn
- (0x41, 'NPUSHW', 0, 'PushNWords', 0, -1), # n, w1, w2,...w w1,w2...wn
- (0xb0, 'PUSHB', 3, 'PushBytes', 0, -1), # b0, b1,..bn b0, b1, ...,bn
- (0xb8, 'PUSHW', 3, 'PushWords', 0, -1), # w0,w1,..wn w0 ,w1, ...wn
-]
-
-
-# next, the list of "normal" instructions
-
-instructions = [
-#
-# opcode mnemonic argBits descriptive name pops pushes eats from instruction stream pushes
-#
- (0x7f, 'AA', 0, 'AdjustAngle', 1, 0), # p -
- (0x64, 'ABS', 0, 'Absolute', 1, 1), # n |n|
- (0x60, 'ADD', 0, 'Add', 2, 1), # n2, n1 (n1 + n2)
- (0x27, 'ALIGNPTS', 0, 'AlignPts', 2, 0), # p2, p1 -
- (0x3c, 'ALIGNRP', 0, 'AlignRelativePt', -1, 0), # p1, p2, ... , ploopvalue -
- (0x5a, 'AND', 0, 'LogicalAnd', 2, 1), # e2, e1 b
- (0x2b, 'CALL', 0, 'CallFunction', 1, 0), # f -
- (0x67, 'CEILING', 0, 'Ceiling', 1, 1), # n ceil(n)
- (0x25, 'CINDEX', 0, 'CopyXToTopStack', 1, 1), # k ek
- (0x22, 'CLEAR', 0, 'ClearStack', -1, 0), # all items on the stack -
- (0x4f, 'DEBUG', 0, 'DebugCall', 1, 0), # n -
- (0x73, 'DELTAC1', 0, 'DeltaExceptionC1', -1, 0), # argn, cn, argn-1,cn-1, , arg1, c1 -
- (0x74, 'DELTAC2', 0, 'DeltaExceptionC2', -1, 0), # argn, cn, argn-1,cn-1, , arg1, c1 -
- (0x75, 'DELTAC3', 0, 'DeltaExceptionC3', -1, 0), # argn, cn, argn-1,cn-1, , arg1, c1 -
- (0x5d, 'DELTAP1', 0, 'DeltaExceptionP1', -1, 0), # argn, pn, argn-1, pn-1, , arg1, p1 -
- (0x71, 'DELTAP2', 0, 'DeltaExceptionP2', -1, 0), # argn, pn, argn-1, pn-1, , arg1, p1 -
- (0x72, 'DELTAP3', 0, 'DeltaExceptionP3', -1, 0), # argn, pn, argn-1, pn-1, , arg1, p1 -
- (0x24, 'DEPTH', 0, 'GetDepthStack', 0, 1), # - n
- (0x62, 'DIV', 0, 'Divide', 2, 1), # n2, n1 (n1 * 64)/ n2
- (0x20, 'DUP', 0, 'DuplicateTopStack', 1, 2), # e e, e
- (0x59, 'EIF', 0, 'EndIf', 0, 0), # - -
- (0x1b, 'ELSE', 0, 'Else', 0, 0), # - -
- (0x2d, 'ENDF', 0, 'EndFunctionDefinition', 0, 0), # - -
- (0x54, 'EQ', 0, 'Equal', 2, 1), # e2, e1 b
- (0x57, 'EVEN', 0, 'Even', 1, 1), # e b
- (0x2c, 'FDEF', 0, 'FunctionDefinition', 1, 0), # f -
- (0x4e, 'FLIPOFF', 0, 'SetAutoFlipOff', 0, 0), # - -
- (0x4d, 'FLIPON', 0, 'SetAutoFlipOn', 0, 0), # - -
- (0x80, 'FLIPPT', 0, 'FlipPoint', -1, 0), # p1, p2, ..., ploopvalue -
- (0x82, 'FLIPRGOFF', 0, 'FlipRangeOff', 2, 0), # h, l -
- (0x81, 'FLIPRGON', 0, 'FlipRangeOn', 2, 0), # h, l -
- (0x66, 'FLOOR', 0, 'Floor', 1, 1), # n floor(n)
- (0x46, 'GC', 1, 'GetCoordOnPVector', 1, 1), # p c
- (0x88, 'GETINFO', 0, 'GetInfo', 1, 1), # selector result
- (0x91, 'GETVARIATION', 0, 'GetVariation', 0, -1), # - a1,..,an
- (0x0d, 'GFV', 0, 'GetFVector', 0, 2), # - px, py
- (0x0c, 'GPV', 0, 'GetPVector', 0, 2), # - px, py
- (0x52, 'GT', 0, 'GreaterThan', 2, 1), # e2, e1 b
- (0x53, 'GTEQ', 0, 'GreaterThanOrEqual', 2, 1), # e2, e1 b
- (0x89, 'IDEF', 0, 'InstructionDefinition', 1, 0), # f -
- (0x58, 'IF', 0, 'If', 1, 0), # e -
- (0x8e, 'INSTCTRL', 0, 'SetInstrExecControl', 2, 0), # s, v -
- (0x39, 'IP', 0, 'InterpolatePts', -1, 0), # p1, p2, ... , ploopvalue -
- (0x0f, 'ISECT', 0, 'MovePtToIntersect', 5, 0), # a1, a0, b1, b0, p -
- (0x30, 'IUP', 1, 'InterpolateUntPts', 0, 0), # - -
- (0x1c, 'JMPR', 0, 'Jump', 1, 0), # offset -
- (0x79, 'JROF', 0, 'JumpRelativeOnFalse', 2, 0), # e, offset -
- (0x78, 'JROT', 0, 'JumpRelativeOnTrue', 2, 0), # e, offset -
- (0x2a, 'LOOPCALL', 0, 'LoopAndCallFunction', 2, 0), # f, count -
- (0x50, 'LT', 0, 'LessThan', 2, 1), # e2, e1 b
- (0x51, 'LTEQ', 0, 'LessThenOrEqual', 2, 1), # e2, e1 b
- (0x8b, 'MAX', 0, 'Maximum', 2, 1), # e2, e1 max(e1, e2)
- (0x49, 'MD', 1, 'MeasureDistance', 2, 1), # p2,p1 d
- (0x2e, 'MDAP', 1, 'MoveDirectAbsPt', 1, 0), # p -
- (0xc0, 'MDRP', 5, 'MoveDirectRelPt', 1, 0), # p -
- (0x3e, 'MIAP', 1, 'MoveIndirectAbsPt', 2, 0), # n, p -
- (0x8c, 'MIN', 0, 'Minimum', 2, 1), # e2, e1 min(e1, e2)
- (0x26, 'MINDEX', 0, 'MoveXToTopStack', 1, 1), # k ek
- (0xe0, 'MIRP', 5, 'MoveIndirectRelPt', 2, 0), # n, p -
- (0x4b, 'MPPEM', 0, 'MeasurePixelPerEm', 0, 1), # - ppem
- (0x4c, 'MPS', 0, 'MeasurePointSize', 0, 1), # - pointSize
- (0x3a, 'MSIRP', 1, 'MoveStackIndirRelPt', 2, 0), # d, p -
- (0x63, 'MUL', 0, 'Multiply', 2, 1), # n2, n1 (n1 * n2)/64
- (0x65, 'NEG', 0, 'Negate', 1, 1), # n -n
- (0x55, 'NEQ', 0, 'NotEqual', 2, 1), # e2, e1 b
- (0x5c, 'NOT', 0, 'LogicalNot', 1, 1), # e ( not e )
- (0x6c, 'NROUND', 2, 'NoRound', 1, 1), # n1 n2
- (0x56, 'ODD', 0, 'Odd', 1, 1), # e b
- (0x5b, 'OR', 0, 'LogicalOr', 2, 1), # e2, e1 b
- (0x21, 'POP', 0, 'PopTopStack', 1, 0), # e -
- (0x45, 'RCVT', 0, 'ReadCVT', 1, 1), # location value
- (0x7d, 'RDTG', 0, 'RoundDownToGrid', 0, 0), # - -
- (0x7a, 'ROFF', 0, 'RoundOff', 0, 0), # - -
- (0x8a, 'ROLL', 0, 'RollTopThreeStack', 3, 3), # a,b,c b,a,c
- (0x68, 'ROUND', 2, 'Round', 1, 1), # n1 n2
- (0x43, 'RS', 0, 'ReadStore', 1, 1), # n v
- (0x3d, 'RTDG', 0, 'RoundToDoubleGrid', 0, 0), # - -
- (0x18, 'RTG', 0, 'RoundToGrid', 0, 0), # - -
- (0x19, 'RTHG', 0, 'RoundToHalfGrid', 0, 0), # - -
- (0x7c, 'RUTG', 0, 'RoundUpToGrid', 0, 0), # - -
- (0x77, 'S45ROUND', 0, 'SuperRound45Degrees', 1, 0), # n -
- (0x7e, 'SANGW', 0, 'SetAngleWeight', 1, 0), # weight -
- (0x85, 'SCANCTRL', 0, 'ScanConversionControl', 1, 0), # n -
- (0x8d, 'SCANTYPE', 0, 'ScanType', 1, 0), # n -
- (0x48, 'SCFS', 0, 'SetCoordFromStackFP', 2, 0), # c, p -
- (0x1d, 'SCVTCI', 0, 'SetCVTCutIn', 1, 0), # n -
- (0x5e, 'SDB', 0, 'SetDeltaBaseInGState', 1, 0), # n -
- (0x86, 'SDPVTL', 1, 'SetDualPVectorToLine', 2, 0), # p2, p1 -
- (0x5f, 'SDS', 0, 'SetDeltaShiftInGState', 1, 0), # n -
- (0x0b, 'SFVFS', 0, 'SetFVectorFromStack', 2, 0), # y, x -
- (0x04, 'SFVTCA', 1, 'SetFVectorToAxis', 0, 0), # - -
- (0x08, 'SFVTL', 1, 'SetFVectorToLine', 2, 0), # p2, p1 -
- (0x0e, 'SFVTPV', 0, 'SetFVectorToPVector', 0, 0), # - -
- (0x34, 'SHC', 1, 'ShiftContourByLastPt', 1, 0), # c -
- (0x32, 'SHP', 1, 'ShiftPointByLastPoint', -1, 0), # p1, p2, ..., ploopvalue -
- (0x38, 'SHPIX', 0, 'ShiftZoneByPixel', -1, 0), # d, p1, p2, ..., ploopvalue -
- (0x36, 'SHZ', 1, 'ShiftZoneByLastPoint', 1, 0), # e -
- (0x17, 'SLOOP', 0, 'SetLoopVariable', 1, 0), # n -
- (0x1a, 'SMD', 0, 'SetMinimumDistance', 1, 0), # distance -
- (0x0a, 'SPVFS', 0, 'SetPVectorFromStack', 2, 0), # y, x -
- (0x02, 'SPVTCA', 1, 'SetPVectorToAxis', 0, 0), # - -
- (0x06, 'SPVTL', 1, 'SetPVectorToLine', 2, 0), # p2, p1 -
- (0x76, 'SROUND', 0, 'SuperRound', 1, 0), # n -
- (0x10, 'SRP0', 0, 'SetRefPoint0', 1, 0), # p -
- (0x11, 'SRP1', 0, 'SetRefPoint1', 1, 0), # p -
- (0x12, 'SRP2', 0, 'SetRefPoint2', 1, 0), # p -
- (0x1f, 'SSW', 0, 'SetSingleWidth', 1, 0), # n -
- (0x1e, 'SSWCI', 0, 'SetSingleWidthCutIn', 1, 0), # n -
- (0x61, 'SUB', 0, 'Subtract', 2, 1), # n2, n1 (n1 - n2)
- (0x00, 'SVTCA', 1, 'SetFPVectorToAxis', 0, 0), # - -
- (0x23, 'SWAP', 0, 'SwapTopStack', 2, 2), # e2, e1 e1, e2
- (0x13, 'SZP0', 0, 'SetZonePointer0', 1, 0), # n -
- (0x14, 'SZP1', 0, 'SetZonePointer1', 1, 0), # n -
- (0x15, 'SZP2', 0, 'SetZonePointer2', 1, 0), # n -
- (0x16, 'SZPS', 0, 'SetZonePointerS', 1, 0), # n -
- (0x29, 'UTP', 0, 'UnTouchPt', 1, 0), # p -
- (0x70, 'WCVTF', 0, 'WriteCVTInFUnits', 2, 0), # n, l -
- (0x44, 'WCVTP', 0, 'WriteCVTInPixels', 2, 0), # v, l -
- (0x42, 'WS', 0, 'WriteStore', 2, 0), # v, l -
-]
-
-# fmt: on
-
-
-def bitRepr(value, bits):
- s = ""
- for i in range(bits):
- s = "01"[value & 0x1] + s
- value = value >> 1
- return s
-
-
-_mnemonicPat = re.compile(r"[A-Z][A-Z0-9]*$")
-
-
-def _makeDict(instructionList):
- opcodeDict = {}
- mnemonicDict = {}
- for op, mnemonic, argBits, name, pops, pushes in instructionList:
- assert _mnemonicPat.match(mnemonic)
- mnemonicDict[mnemonic] = op, argBits, name
- if argBits:
- argoffset = op
- for i in range(1 << argBits):
- opcodeDict[op + i] = mnemonic, argBits, argoffset, name
- else:
- opcodeDict[op] = mnemonic, 0, 0, name
- return opcodeDict, mnemonicDict
-
-
-streamOpcodeDict, streamMnemonicDict = _makeDict(streamInstructions)
-opcodeDict, mnemonicDict = _makeDict(instructions)
-
-
-class tt_instructions_error(Exception):
- def __init__(self, error):
- self.error = error
-
- def __str__(self):
- return "TT instructions error: %s" % repr(self.error)
-
-
-_comment = r"/\*.*?\*/"
-_instruction = r"([A-Z][A-Z0-9]*)\s*\[(.*?)\]"
-_number = r"-?[0-9]+"
-_token = "(%s)|(%s)|(%s)" % (_instruction, _number, _comment)
-
-_tokenRE = re.compile(_token)
-_whiteRE = re.compile(r"\s*")
-
-_pushCountPat = re.compile(r"[A-Z][A-Z0-9]*\s*\[.*?\]\s*/\* ([0-9]+).*?\*/")
-
-_indentRE = re.compile(r"^FDEF|IF|ELSE\[ \]\t.+")
-_unindentRE = re.compile(r"^ELSE|ENDF|EIF\[ \]\t.+")
-
-
-def _skipWhite(data, pos):
- m = _whiteRE.match(data, pos)
- newPos = m.regs[0][1]
- assert newPos >= pos
- return newPos
-
-
-class Program(object):
- def __init__(self) -> None:
- pass
-
- def fromBytecode(self, bytecode: bytes) -> None:
- self.bytecode = array.array("B", bytecode)
- if hasattr(self, "assembly"):
- del self.assembly
-
- def fromAssembly(self, assembly: List[str] | str) -> None:
- if isinstance(assembly, list):
- self.assembly = assembly
- elif isinstance(assembly, str):
- self.assembly = assembly.splitlines()
- else:
- raise TypeError(f"expected str or List[str], got {type(assembly).__name__}")
- if hasattr(self, "bytecode"):
- del self.bytecode
-
- def getBytecode(self) -> bytes:
- if not hasattr(self, "bytecode"):
- self._assemble()
- return self.bytecode.tobytes()
-
- def getAssembly(self, preserve=True) -> List[str]:
- if not hasattr(self, "assembly"):
- self._disassemble(preserve=preserve)
- return self.assembly
-
- def toXML(self, writer, ttFont) -> None:
- if (
- not hasattr(ttFont, "disassembleInstructions")
- or ttFont.disassembleInstructions
- ):
- try:
- assembly = self.getAssembly()
- except:
- import traceback
-
- tmp = StringIO()
- traceback.print_exc(file=tmp)
- msg = "An exception occurred during the decompilation of glyph program:\n\n"
- msg += tmp.getvalue()
- log.error(msg)
- writer.begintag("bytecode")
- writer.newline()
- writer.comment(msg.strip())
- writer.newline()
- writer.dumphex(self.getBytecode())
- writer.endtag("bytecode")
- writer.newline()
- else:
- if not assembly:
- return
- writer.begintag("assembly")
- writer.newline()
- i = 0
- indent = 0
- nInstr = len(assembly)
- while i < nInstr:
- instr = assembly[i]
- if _unindentRE.match(instr):
- indent -= 1
- writer.write(writer.indentwhite * indent)
- writer.write(instr)
- writer.newline()
- m = _pushCountPat.match(instr)
- i = i + 1
- if m:
- nValues = int(m.group(1))
- line: List[str] = []
- j = 0
- for j in range(nValues):
- if j and not (j % 25):
- writer.write(writer.indentwhite * indent)
- writer.write(" ".join(line))
- writer.newline()
- line = []
- line.append(assembly[i + j])
- writer.write(writer.indentwhite * indent)
- writer.write(" ".join(line))
- writer.newline()
- i = i + j + 1
- if _indentRE.match(instr):
- indent += 1
- writer.endtag("assembly")
- writer.newline()
- else:
- bytecode = self.getBytecode()
- if not bytecode:
- return
- writer.begintag("bytecode")
- writer.newline()
- writer.dumphex(bytecode)
- writer.endtag("bytecode")
- writer.newline()
-
- def fromXML(self, name, attrs, content, ttFont) -> None:
- if name == "assembly":
- self.fromAssembly(strjoin(content))
- self._assemble()
- del self.assembly
- else:
- assert name == "bytecode"
- self.fromBytecode(readHex(content))
-
- def _assemble(self) -> None:
- assembly = " ".join(getattr(self, "assembly", []))
- bytecode: List[int] = []
- push = bytecode.append
- lenAssembly = len(assembly)
- pos = _skipWhite(assembly, 0)
- while pos < lenAssembly:
- m = _tokenRE.match(assembly, pos)
- if m is None:
- raise tt_instructions_error(
- "Syntax error in TT program (%s)" % assembly[pos - 5 : pos + 15]
- )
- dummy, mnemonic, arg, number, comment = m.groups()
- pos = m.regs[0][1]
- if comment:
- pos = _skipWhite(assembly, pos)
- continue
-
- arg = arg.strip()
- if mnemonic.startswith("INSTR"):
- # Unknown instruction
- op = int(mnemonic[5:])
- push(op)
- elif mnemonic not in ("PUSH", "NPUSHB", "NPUSHW", "PUSHB", "PUSHW"):
- op, argBits, name = mnemonicDict[mnemonic]
- if len(arg) != argBits:
- raise tt_instructions_error(
- "Incorrect number of argument bits (%s[%s])" % (mnemonic, arg)
- )
- if arg:
- arg = binary2num(arg)
- push(op + arg)
- else:
- push(op)
- else:
- args = []
- pos = _skipWhite(assembly, pos)
- while pos < lenAssembly:
- m = _tokenRE.match(assembly, pos)
- if m is None:
- raise tt_instructions_error(
- "Syntax error in TT program (%s)" % assembly[pos : pos + 15]
- )
- dummy, _mnemonic, arg, number, comment = m.groups()
- if number is None and comment is None:
- break
- pos = m.regs[0][1]
- pos = _skipWhite(assembly, pos)
- if comment is not None:
- continue
- args.append(int(number))
- nArgs = len(args)
- if mnemonic == "PUSH":
- # Automatically choose the most compact representation
- nWords = 0
- while nArgs:
- while (
- nWords < nArgs
- and nWords < 255
- and not (0 <= args[nWords] <= 255)
- ):
- nWords += 1
- nBytes = 0
- while (
- nWords + nBytes < nArgs
- and nBytes < 255
- and 0 <= args[nWords + nBytes] <= 255
- ):
- nBytes += 1
- if (
- nBytes < 2
- and nWords + nBytes < 255
- and nWords + nBytes != nArgs
- ):
- # Will write bytes as words
- nWords += nBytes
- continue
-
- # Write words
- if nWords:
- if nWords <= 8:
- op, argBits, name = streamMnemonicDict["PUSHW"]
- op = op + nWords - 1
- push(op)
- else:
- op, argBits, name = streamMnemonicDict["NPUSHW"]
- push(op)
- push(nWords)
- for value in args[:nWords]:
- assert -32768 <= value < 32768, (
- "PUSH value out of range %d" % value
- )
- push((value >> 8) & 0xFF)
- push(value & 0xFF)
-
- # Write bytes
- if nBytes:
- pass
- if nBytes <= 8:
- op, argBits, name = streamMnemonicDict["PUSHB"]
- op = op + nBytes - 1
- push(op)
- else:
- op, argBits, name = streamMnemonicDict["NPUSHB"]
- push(op)
- push(nBytes)
- for value in args[nWords : nWords + nBytes]:
- push(value)
-
- nTotal = nWords + nBytes
- args = args[nTotal:]
- nArgs -= nTotal
- nWords = 0
- else:
- # Write exactly what we've been asked to
- words = mnemonic[-1] == "W"
- op, argBits, name = streamMnemonicDict[mnemonic]
- if mnemonic[0] != "N":
- assert nArgs <= 8, nArgs
- op = op + nArgs - 1
- push(op)
- else:
- assert nArgs < 256
- push(op)
- push(nArgs)
- if words:
- for value in args:
- assert -32768 <= value < 32768, (
- "PUSHW value out of range %d" % value
- )
- push((value >> 8) & 0xFF)
- push(value & 0xFF)
- else:
- for value in args:
- assert 0 <= value < 256, (
- "PUSHB value out of range %d" % value
- )
- push(value)
-
- pos = _skipWhite(assembly, pos)
-
- if bytecode:
- assert max(bytecode) < 256 and min(bytecode) >= 0
- self.bytecode = array.array("B", bytecode)
-
- def _disassemble(self, preserve=False) -> None:
- assembly = []
- i = 0
- bytecode = getattr(self, "bytecode", [])
- numBytecode = len(bytecode)
- while i < numBytecode:
- op = bytecode[i]
- try:
- mnemonic, argBits, argoffset, name = opcodeDict[op]
- except KeyError:
- if op in streamOpcodeDict:
- values = []
-
- # Merge consecutive PUSH operations
- while bytecode[i] in streamOpcodeDict:
- op = bytecode[i]
- mnemonic, argBits, argoffset, name = streamOpcodeDict[op]
- words = mnemonic[-1] == "W"
- if argBits:
- nValues = op - argoffset + 1
- else:
- i = i + 1
- nValues = bytecode[i]
- i = i + 1
- assert nValues > 0
- if not words:
- for j in range(nValues):
- value = bytecode[i]
- values.append(repr(value))
- i = i + 1
- else:
- for j in range(nValues):
- # cast to signed int16
- value = (bytecode[i] << 8) | bytecode[i + 1]
- if value >= 0x8000:
- value = value - 0x10000
- values.append(repr(value))
- i = i + 2
- if preserve:
- break
-
- if not preserve:
- mnemonic = "PUSH"
- nValues = len(values)
- if nValues == 1:
- assembly.append("%s[ ] /* 1 value pushed */" % mnemonic)
- else:
- assembly.append(
- "%s[ ] /* %s values pushed */" % (mnemonic, nValues)
- )
- assembly.extend(values)
- else:
- assembly.append("INSTR%d[ ]" % op)
- i = i + 1
- else:
- if argBits:
- assembly.append(
- mnemonic
- + "[%s] /* %s */" % (num2binary(op - argoffset, argBits), name)
- )
- else:
- assembly.append(mnemonic + "[ ] /* %s */" % name)
- i = i + 1
- self.assembly = assembly
-
- def __bool__(self) -> bool:
- """
- >>> p = Program()
- >>> bool(p)
- False
- >>> bc = array.array("B", [0])
- >>> p.fromBytecode(bc)
- >>> bool(p)
- True
- >>> p.bytecode.pop()
- 0
- >>> bool(p)
- False
-
- >>> p = Program()
- >>> asm = ['SVTCA[0]']
- >>> p.fromAssembly(asm)
- >>> bool(p)
- True
- >>> p.assembly.pop()
- 'SVTCA[0]'
- >>> bool(p)
- False
- """
- return (hasattr(self, "assembly") and len(self.assembly) > 0) or (
- hasattr(self, "bytecode") and len(self.bytecode) > 0
- )
-
- __nonzero__ = __bool__
-
- def __eq__(self, other) -> bool:
- if type(self) != type(other):
- return NotImplemented
- return self.__dict__ == other.__dict__
-
- def __ne__(self, other) -> bool:
- result = self.__eq__(other)
- return result if result is NotImplemented else not result
-
-
-def _test():
- """
- >>> _test()
- True
- """
-
- bc = b"""@;:9876543210/.-,+*)(\'&%$#"! \037\036\035\034\033\032\031\030\027\026\025\024\023\022\021\020\017\016\015\014\013\012\011\010\007\006\005\004\003\002\001\000,\001\260\030CXEj\260\031C`\260F#D#\020 \260FN\360M/\260\000\022\033!#\0213Y-,\001\260\030CX\260\005+\260\000\023K\260\024PX\261\000@8Y\260\006+\033!#\0213Y-,\001\260\030CXN\260\003%\020\362!\260\000\022M\033 E\260\004%\260\004%#Jad\260(RX!#\020\326\033\260\003%\020\362!\260\000\022YY-,\260\032CX!!\033\260\002%\260\002%I\260\003%\260\003%Ja d\260\020PX!!!\033\260\003%\260\003%I\260\000PX\260\000PX\270\377\3428!\033\260\0208!Y\033\260\000RX\260\0368!\033\270\377\3608!YYYY-,\001\260\030CX\260\005+\260\000\023K\260\024PX\271\000\000\377\3008Y\260\006+\033!#\0213Y-,N\001\212\020\261F\031CD\260\000\024\261\000F\342\260\000\025\271\000\000\377\3608\000\260\000<\260(+\260\002%\020\260\000<-,\001\030\260\000/\260\001\024\362\260\001\023\260\001\025M\260\000\022-,\001\260\030CX\260\005+\260\000\023\271\000\000\377\3408\260\006+\033!#\0213Y-,\001\260\030CXEdj#Edi\260\031Cd``\260F#D#\020 \260F\360/\260\000\022\033!! \212 \212RX\0213\033!!YY-,\001\261\013\012C#Ce\012-,\000\261\012\013C#C\013-,\000\260F#p\261\001F>\001\260F#p\261\002FE:\261\002\000\010\015-,\260\022+\260\002%E\260\002%Ej\260@\213`\260\002%#D!!!-,\260\023+\260\002%E\260\002%Ej\270\377\300\214`\260\002%#D!!!-,\260\000\260\022+!!!-,\260\000\260\023+!!!-,\001\260\006C\260\007Ce\012-, i\260@a\260\000\213 \261,\300\212\214\270\020\000b`+\014d#da\\X\260\003aY-,\261\000\003%EhT\260\034KPZX\260\003%E\260\003%E`h \260\004%#D\260\004%#D\033\260\003% Eh \212#D\260\003%Eh`\260\003%#DY-,\260\003% Eh \212#D\260\003%Edhe`\260\004%\260\001`#D-,\260\011CX\207!\300\033\260\022CX\207E\260\021+\260G#D\260Gz\344\033\003\212E\030i \260G#D\212\212\207 \260\240QX\260\021+\260G#D\260Gz\344\033!\260Gz\344YYY\030-, \212E#Eh`D-,EjB-,\001\030/-,\001\260\030CX\260\004%\260\004%Id#Edi\260@\213a \260\200bj\260\002%\260\002%a\214\260\031C`\260F#D!\212\020\260F\366!\033!!!!Y-,\001\260\030CX\260\002%E\260\002%Ed`j\260\003%Eja \260\004%Ej \212\213e\260\004%#D\214\260\003%#D!!\033 EjD EjDY-,\001 E\260\000U\260\030CZXEh#Ei\260@\213a \260\200bj \212#a \260\003%\213e\260\004%#D\214\260\003%#D!!\033!!\260\031+Y-,\001\212\212Ed#EdadB-,\260\004%\260\004%\260\031+\260\030CX\260\004%\260\004%\260\003%\260\033+\001\260\002%C\260@T\260\002%C\260\000TZX\260\003% E\260@aDY\260\002%C\260\000T\260\002%C\260@TZX\260\004% E\260@`DYY!!!!-,\001KRXC\260\002%E#aD\033!!Y-,\001KRXC\260\002%E#`D\033!!Y-,KRXED\033!!Y-,\001 \260\003%#I\260@`\260 c \260\000RX#\260\002%8#\260\002%e8\000\212c8\033!!!!!Y\001-,KPXED\033!!Y-,\001\260\005%\020# \212\365\000\260\001`#\355\354-,\001\260\005%\020# \212\365\000\260\001a#\355\354-,\001\260\006%\020\365\000\355\354-,F#F`\212\212F# F\212`\212a\270\377\200b# \020#\212\261KK\212pE` \260\000PX\260\001a\270\377\272\213\033\260F\214Y\260\020`h\001:-, E\260\003%FRX\260\002%F ha\260\003%\260\003%?#!8\033!\021Y-, E\260\003%FPX\260\002%F ha\260\003%\260\003%?#!8\033!\021Y-,\000\260\007C\260\006C\013-,\212\020\354-,\260\014CX!\033 F\260\000RX\270\377\3608\033\260\0208YY-, \260\000UX\270\020\000c\260\003%Ed\260\003%Eda\260\000SX\260\002\033\260@a\260\003Y%EiSXED\033!!Y\033!\260\002%E\260\002%Ead\260(QXED\033!!YY-,!!\014d#d\213\270@\000b-,!\260\200QX\014d#d\213\270 \000b\033\262\000@/+Y\260\002`-,!\260\300QX\014d#d\213\270\025Ub\033\262\000\200/+Y\260\002`-,\014d#d\213\270@\000b`#!-,KSX\260\004%\260\004%Id#Edi\260@\213a \260\200bj\260\002%\260\002%a\214\260F#D!\212\020\260F\366!\033!\212\021#\022 9/Y-,\260\002%\260\002%Id\260\300TX\270\377\3708\260\0108\033!!Y-,\260\023CX\003\033\002Y-,\260\023CX\002\033\003Y-,\260\012+#\020 <\260\027+-,\260\002%\270\377\3608\260(+\212\020# \320#\260\020+\260\005CX\300\033 str:
- return self.api.whoami()['name']
-
- def upload(self,
- folder_path: str,
- repo_name: str,
- organization: str = '',
- repo_type: str = 'model',
- private: bool = True,
- delete_existing_repo: bool = False) -> str:
- if not folder_path:
- raise ValueError
- if not repo_name:
- raise ValueError
- if not organization:
- organization = self.get_username()
- repo_id = f'{organization}/{repo_name}'
- if delete_existing_repo:
- try:
- self.api.delete_repo(repo_id, repo_type=repo_type)
- except Exception:
- pass
- try:
- self.api.create_repo(repo_id, repo_type=repo_type, private=private)
- self.api.upload_folder(repo_id=repo_id,
- folder_path=folder_path,
- path_in_repo='.',
- repo_type=repo_type)
- url = f'https://huggingface.co/{repo_id}'
- message = f'Your model was successfully uploaded to {url}.'
- except Exception as e:
- message = str(e)
- return message
diff --git a/spaces/Dinoking/Guccio-AI-Designer/models/stylegan/stylegan_tf/dnnlib/tflib/network.py b/spaces/Dinoking/Guccio-AI-Designer/models/stylegan/stylegan_tf/dnnlib/tflib/network.py
deleted file mode 100644
index d888a90dd23c1a941b5fb501afec1efcb763b5ea..0000000000000000000000000000000000000000
--- a/spaces/Dinoking/Guccio-AI-Designer/models/stylegan/stylegan_tf/dnnlib/tflib/network.py
+++ /dev/null
@@ -1,591 +0,0 @@
-# Copyright (c) 2019, NVIDIA CORPORATION. All rights reserved.
-#
-# This work is licensed under the Creative Commons Attribution-NonCommercial
-# 4.0 International License. To view a copy of this license, visit
-# http://creativecommons.org/licenses/by-nc/4.0/ or send a letter to
-# Creative Commons, PO Box 1866, Mountain View, CA 94042, USA.
-
-"""Helper for managing networks."""
-
-import types
-import inspect
-import re
-import uuid
-import sys
-import numpy as np
-import tensorflow as tf
-
-from collections import OrderedDict
-from typing import Any, List, Tuple, Union
-
-from . import tfutil
-from .. import util
-
-from .tfutil import TfExpression, TfExpressionEx
-
-_import_handlers = [] # Custom import handlers for dealing with legacy data in pickle import.
-_import_module_src = dict() # Source code for temporary modules created during pickle import.
-
-
-def import_handler(handler_func):
- """Function decorator for declaring custom import handlers."""
- _import_handlers.append(handler_func)
- return handler_func
-
-
-class Network:
- """Generic network abstraction.
-
- Acts as a convenience wrapper for a parameterized network construction
- function, providing several utility methods and convenient access to
- the inputs/outputs/weights.
-
- Network objects can be safely pickled and unpickled for long-term
- archival purposes. The pickling works reliably as long as the underlying
- network construction function is defined in a standalone Python module
- that has no side effects or application-specific imports.
-
- Args:
- name: Network name. Used to select TensorFlow name and variable scopes.
- func_name: Fully qualified name of the underlying network construction function, or a top-level function object.
- static_kwargs: Keyword arguments to be passed in to the network construction function.
-
- Attributes:
- name: User-specified name, defaults to build func name if None.
- scope: Unique TensorFlow scope containing template graph and variables, derived from the user-specified name.
- static_kwargs: Arguments passed to the user-supplied build func.
- components: Container for sub-networks. Passed to the build func, and retained between calls.
- num_inputs: Number of input tensors.
- num_outputs: Number of output tensors.
- input_shapes: Input tensor shapes (NC or NCHW), including minibatch dimension.
- output_shapes: Output tensor shapes (NC or NCHW), including minibatch dimension.
- input_shape: Short-hand for input_shapes[0].
- output_shape: Short-hand for output_shapes[0].
- input_templates: Input placeholders in the template graph.
- output_templates: Output tensors in the template graph.
- input_names: Name string for each input.
- output_names: Name string for each output.
- own_vars: Variables defined by this network (local_name => var), excluding sub-networks.
- vars: All variables (local_name => var).
- trainables: All trainable variables (local_name => var).
- var_global_to_local: Mapping from variable global names to local names.
- """
-
- def __init__(self, name: str = None, func_name: Any = None, **static_kwargs):
- tfutil.assert_tf_initialized()
- assert isinstance(name, str) or name is None
- assert func_name is not None
- assert isinstance(func_name, str) or util.is_top_level_function(func_name)
- assert util.is_pickleable(static_kwargs)
-
- self._init_fields()
- self.name = name
- self.static_kwargs = util.EasyDict(static_kwargs)
-
- # Locate the user-specified network build function.
- if util.is_top_level_function(func_name):
- func_name = util.get_top_level_function_name(func_name)
- module, self._build_func_name = util.get_module_from_obj_name(func_name)
- self._build_func = util.get_obj_from_module(module, self._build_func_name)
- assert callable(self._build_func)
-
- # Dig up source code for the module containing the build function.
- self._build_module_src = _import_module_src.get(module, None)
- if self._build_module_src is None:
- self._build_module_src = inspect.getsource(module)
-
- # Init TensorFlow graph.
- self._init_graph()
- self.reset_own_vars()
-
- def _init_fields(self) -> None:
- self.name = None
- self.scope = None
- self.static_kwargs = util.EasyDict()
- self.components = util.EasyDict()
- self.num_inputs = 0
- self.num_outputs = 0
- self.input_shapes = [[]]
- self.output_shapes = [[]]
- self.input_shape = []
- self.output_shape = []
- self.input_templates = []
- self.output_templates = []
- self.input_names = []
- self.output_names = []
- self.own_vars = OrderedDict()
- self.vars = OrderedDict()
- self.trainables = OrderedDict()
- self.var_global_to_local = OrderedDict()
-
- self._build_func = None # User-supplied build function that constructs the network.
- self._build_func_name = None # Name of the build function.
- self._build_module_src = None # Full source code of the module containing the build function.
- self._run_cache = dict() # Cached graph data for Network.run().
-
- def _init_graph(self) -> None:
- # Collect inputs.
- self.input_names = []
-
- for param in inspect.signature(self._build_func).parameters.values():
- if param.kind == param.POSITIONAL_OR_KEYWORD and param.default is param.empty:
- self.input_names.append(param.name)
-
- self.num_inputs = len(self.input_names)
- assert self.num_inputs >= 1
-
- # Choose name and scope.
- if self.name is None:
- self.name = self._build_func_name
- assert re.match("^[A-Za-z0-9_.\\-]*$", self.name)
- with tf.name_scope(None):
- self.scope = tf.get_default_graph().unique_name(self.name, mark_as_used=True)
-
- # Finalize build func kwargs.
- build_kwargs = dict(self.static_kwargs)
- build_kwargs["is_template_graph"] = True
- build_kwargs["components"] = self.components
-
- # Build template graph.
- with tfutil.absolute_variable_scope(self.scope, reuse=tf.AUTO_REUSE), tfutil.absolute_name_scope(self.scope): # ignore surrounding scopes
- assert tf.get_variable_scope().name == self.scope
- assert tf.get_default_graph().get_name_scope() == self.scope
- with tf.control_dependencies(None): # ignore surrounding control dependencies
- self.input_templates = [tf.placeholder(tf.float32, name=name) for name in self.input_names]
- out_expr = self._build_func(*self.input_templates, **build_kwargs)
-
- # Collect outputs.
- assert tfutil.is_tf_expression(out_expr) or isinstance(out_expr, tuple)
- self.output_templates = [out_expr] if tfutil.is_tf_expression(out_expr) else list(out_expr)
- self.num_outputs = len(self.output_templates)
- assert self.num_outputs >= 1
- assert all(tfutil.is_tf_expression(t) for t in self.output_templates)
-
- # Perform sanity checks.
- if any(t.shape.ndims is None for t in self.input_templates):
- raise ValueError("Network input shapes not defined. Please call x.set_shape() for each input.")
- if any(t.shape.ndims is None for t in self.output_templates):
- raise ValueError("Network output shapes not defined. Please call x.set_shape() where applicable.")
- if any(not isinstance(comp, Network) for comp in self.components.values()):
- raise ValueError("Components of a Network must be Networks themselves.")
- if len(self.components) != len(set(comp.name for comp in self.components.values())):
- raise ValueError("Components of a Network must have unique names.")
-
- # List inputs and outputs.
- self.input_shapes = [tfutil.shape_to_list(t.shape) for t in self.input_templates]
- self.output_shapes = [tfutil.shape_to_list(t.shape) for t in self.output_templates]
- self.input_shape = self.input_shapes[0]
- self.output_shape = self.output_shapes[0]
- self.output_names = [t.name.split("/")[-1].split(":")[0] for t in self.output_templates]
-
- # List variables.
- self.own_vars = OrderedDict((var.name[len(self.scope) + 1:].split(":")[0], var) for var in tf.global_variables(self.scope + "/"))
- self.vars = OrderedDict(self.own_vars)
- self.vars.update((comp.name + "/" + name, var) for comp in self.components.values() for name, var in comp.vars.items())
- self.trainables = OrderedDict((name, var) for name, var in self.vars.items() if var.trainable)
- self.var_global_to_local = OrderedDict((var.name.split(":")[0], name) for name, var in self.vars.items())
-
- def reset_own_vars(self) -> None:
- """Re-initialize all variables of this network, excluding sub-networks."""
- tfutil.run([var.initializer for var in self.own_vars.values()])
-
- def reset_vars(self) -> None:
- """Re-initialize all variables of this network, including sub-networks."""
- tfutil.run([var.initializer for var in self.vars.values()])
-
- def reset_trainables(self) -> None:
- """Re-initialize all trainable variables of this network, including sub-networks."""
- tfutil.run([var.initializer for var in self.trainables.values()])
-
- def get_output_for(self, *in_expr: TfExpression, return_as_list: bool = False, **dynamic_kwargs) -> Union[TfExpression, List[TfExpression]]:
- """Construct TensorFlow expression(s) for the output(s) of this network, given the input expression(s)."""
- assert len(in_expr) == self.num_inputs
- assert not all(expr is None for expr in in_expr)
-
- # Finalize build func kwargs.
- build_kwargs = dict(self.static_kwargs)
- build_kwargs.update(dynamic_kwargs)
- build_kwargs["is_template_graph"] = False
- build_kwargs["components"] = self.components
-
- # Build TensorFlow graph to evaluate the network.
- with tfutil.absolute_variable_scope(self.scope, reuse=True), tf.name_scope(self.name):
- assert tf.get_variable_scope().name == self.scope
- valid_inputs = [expr for expr in in_expr if expr is not None]
- final_inputs = []
- for expr, name, shape in zip(in_expr, self.input_names, self.input_shapes):
- if expr is not None:
- expr = tf.identity(expr, name=name)
- else:
- expr = tf.zeros([tf.shape(valid_inputs[0])[0]] + shape[1:], name=name)
- final_inputs.append(expr)
- out_expr = self._build_func(*final_inputs, **build_kwargs)
-
- # Propagate input shapes back to the user-specified expressions.
- for expr, final in zip(in_expr, final_inputs):
- if isinstance(expr, tf.Tensor):
- expr.set_shape(final.shape)
-
- # Express outputs in the desired format.
- assert tfutil.is_tf_expression(out_expr) or isinstance(out_expr, tuple)
- if return_as_list:
- out_expr = [out_expr] if tfutil.is_tf_expression(out_expr) else list(out_expr)
- return out_expr
-
- def get_var_local_name(self, var_or_global_name: Union[TfExpression, str]) -> str:
- """Get the local name of a given variable, without any surrounding name scopes."""
- assert tfutil.is_tf_expression(var_or_global_name) or isinstance(var_or_global_name, str)
- global_name = var_or_global_name if isinstance(var_or_global_name, str) else var_or_global_name.name
- return self.var_global_to_local[global_name]
-
- def find_var(self, var_or_local_name: Union[TfExpression, str]) -> TfExpression:
- """Find variable by local or global name."""
- assert tfutil.is_tf_expression(var_or_local_name) or isinstance(var_or_local_name, str)
- return self.vars[var_or_local_name] if isinstance(var_or_local_name, str) else var_or_local_name
-
- def get_var(self, var_or_local_name: Union[TfExpression, str]) -> np.ndarray:
- """Get the value of a given variable as NumPy array.
- Note: This method is very inefficient -- prefer to use tflib.run(list_of_vars) whenever possible."""
- return self.find_var(var_or_local_name).eval()
-
- def set_var(self, var_or_local_name: Union[TfExpression, str], new_value: Union[int, float, np.ndarray]) -> None:
- """Set the value of a given variable based on the given NumPy array.
- Note: This method is very inefficient -- prefer to use tflib.set_vars() whenever possible."""
- tfutil.set_vars({self.find_var(var_or_local_name): new_value})
-
- def __getstate__(self) -> dict:
- """Pickle export."""
- state = dict()
- state["version"] = 3
- state["name"] = self.name
- state["static_kwargs"] = dict(self.static_kwargs)
- state["components"] = dict(self.components)
- state["build_module_src"] = self._build_module_src
- state["build_func_name"] = self._build_func_name
- state["variables"] = list(zip(self.own_vars.keys(), tfutil.run(list(self.own_vars.values()))))
- return state
-
- def __setstate__(self, state: dict) -> None:
- """Pickle import."""
- # pylint: disable=attribute-defined-outside-init
- tfutil.assert_tf_initialized()
- self._init_fields()
-
- # Execute custom import handlers.
- for handler in _import_handlers:
- state = handler(state)
-
- # Set basic fields.
- assert state["version"] in [2, 3]
- self.name = state["name"]
- self.static_kwargs = util.EasyDict(state["static_kwargs"])
- self.components = util.EasyDict(state.get("components", {}))
- self._build_module_src = state["build_module_src"]
- self._build_func_name = state["build_func_name"]
-
- # Create temporary module from the imported source code.
- module_name = "_tflib_network_import_" + uuid.uuid4().hex
- module = types.ModuleType(module_name)
- sys.modules[module_name] = module
- _import_module_src[module] = self._build_module_src
- exec(self._build_module_src, module.__dict__) # pylint: disable=exec-used
-
- # Locate network build function in the temporary module.
- self._build_func = util.get_obj_from_module(module, self._build_func_name)
- assert callable(self._build_func)
-
- # Init TensorFlow graph.
- self._init_graph()
- self.reset_own_vars()
- tfutil.set_vars({self.find_var(name): value for name, value in state["variables"]})
-
- def clone(self, name: str = None, **new_static_kwargs) -> "Network":
- """Create a clone of this network with its own copy of the variables."""
- # pylint: disable=protected-access
- net = object.__new__(Network)
- net._init_fields()
- net.name = name if name is not None else self.name
- net.static_kwargs = util.EasyDict(self.static_kwargs)
- net.static_kwargs.update(new_static_kwargs)
- net._build_module_src = self._build_module_src
- net._build_func_name = self._build_func_name
- net._build_func = self._build_func
- net._init_graph()
- net.copy_vars_from(self)
- return net
-
- def copy_own_vars_from(self, src_net: "Network") -> None:
- """Copy the values of all variables from the given network, excluding sub-networks."""
- names = [name for name in self.own_vars.keys() if name in src_net.own_vars]
- tfutil.set_vars(tfutil.run({self.vars[name]: src_net.vars[name] for name in names}))
-
- def copy_vars_from(self, src_net: "Network") -> None:
- """Copy the values of all variables from the given network, including sub-networks."""
- names = [name for name in self.vars.keys() if name in src_net.vars]
- tfutil.set_vars(tfutil.run({self.vars[name]: src_net.vars[name] for name in names}))
-
- def copy_trainables_from(self, src_net: "Network") -> None:
- """Copy the values of all trainable variables from the given network, including sub-networks."""
- names = [name for name in self.trainables.keys() if name in src_net.trainables]
- tfutil.set_vars(tfutil.run({self.vars[name]: src_net.vars[name] for name in names}))
-
- def convert(self, new_func_name: str, new_name: str = None, **new_static_kwargs) -> "Network":
- """Create new network with the given parameters, and copy all variables from this network."""
- if new_name is None:
- new_name = self.name
- static_kwargs = dict(self.static_kwargs)
- static_kwargs.update(new_static_kwargs)
- net = Network(name=new_name, func_name=new_func_name, **static_kwargs)
- net.copy_vars_from(self)
- return net
-
- def setup_as_moving_average_of(self, src_net: "Network", beta: TfExpressionEx = 0.99, beta_nontrainable: TfExpressionEx = 0.0) -> tf.Operation:
- """Construct a TensorFlow op that updates the variables of this network
- to be slightly closer to those of the given network."""
- with tfutil.absolute_name_scope(self.scope + "/_MovingAvg"):
- ops = []
- for name, var in self.vars.items():
- if name in src_net.vars:
- cur_beta = beta if name in self.trainables else beta_nontrainable
- new_value = tfutil.lerp(src_net.vars[name], var, cur_beta)
- ops.append(var.assign(new_value))
- return tf.group(*ops)
-
- def run(self,
- *in_arrays: Tuple[Union[np.ndarray, None], ...],
- input_transform: dict = None,
- output_transform: dict = None,
- return_as_list: bool = False,
- print_progress: bool = False,
- minibatch_size: int = None,
- num_gpus: int = 1,
- assume_frozen: bool = False,
- **dynamic_kwargs) -> Union[np.ndarray, Tuple[np.ndarray, ...], List[np.ndarray]]:
- """Run this network for the given NumPy array(s), and return the output(s) as NumPy array(s).
-
- Args:
- input_transform: A dict specifying a custom transformation to be applied to the input tensor(s) before evaluating the network.
- The dict must contain a 'func' field that points to a top-level function. The function is called with the input
- TensorFlow expression(s) as positional arguments. Any remaining fields of the dict will be passed in as kwargs.
- output_transform: A dict specifying a custom transformation to be applied to the output tensor(s) after evaluating the network.
- The dict must contain a 'func' field that points to a top-level function. The function is called with the output
- TensorFlow expression(s) as positional arguments. Any remaining fields of the dict will be passed in as kwargs.
- return_as_list: True = return a list of NumPy arrays, False = return a single NumPy array, or a tuple if there are multiple outputs.
- print_progress: Print progress to the console? Useful for very large input arrays.
- minibatch_size: Maximum minibatch size to use, None = disable batching.
- num_gpus: Number of GPUs to use.
- assume_frozen: Improve multi-GPU performance by assuming that the trainable parameters will remain changed between calls.
- dynamic_kwargs: Additional keyword arguments to be passed into the network build function.
- """
- assert len(in_arrays) == self.num_inputs
- assert not all(arr is None for arr in in_arrays)
- assert input_transform is None or util.is_top_level_function(input_transform["func"])
- assert output_transform is None or util.is_top_level_function(output_transform["func"])
- output_transform, dynamic_kwargs = _handle_legacy_output_transforms(output_transform, dynamic_kwargs)
- num_items = in_arrays[0].shape[0]
- if minibatch_size is None:
- minibatch_size = num_items
-
- # Construct unique hash key from all arguments that affect the TensorFlow graph.
- key = dict(input_transform=input_transform, output_transform=output_transform, num_gpus=num_gpus, assume_frozen=assume_frozen, dynamic_kwargs=dynamic_kwargs)
- def unwind_key(obj):
- if isinstance(obj, dict):
- return [(key, unwind_key(value)) for key, value in sorted(obj.items())]
- if callable(obj):
- return util.get_top_level_function_name(obj)
- return obj
- key = repr(unwind_key(key))
-
- # Build graph.
- if key not in self._run_cache:
- with tfutil.absolute_name_scope(self.scope + "/_Run"), tf.control_dependencies(None):
- with tf.device("/cpu:0"):
- in_expr = [tf.placeholder(tf.float32, name=name) for name in self.input_names]
- in_split = list(zip(*[tf.split(x, num_gpus) for x in in_expr]))
-
- out_split = []
- for gpu in range(num_gpus):
- with tf.device("/gpu:%d" % gpu):
- net_gpu = self.clone() if assume_frozen else self
- in_gpu = in_split[gpu]
-
- if input_transform is not None:
- in_kwargs = dict(input_transform)
- in_gpu = in_kwargs.pop("func")(*in_gpu, **in_kwargs)
- in_gpu = [in_gpu] if tfutil.is_tf_expression(in_gpu) else list(in_gpu)
-
- assert len(in_gpu) == self.num_inputs
- out_gpu = net_gpu.get_output_for(*in_gpu, return_as_list=True, **dynamic_kwargs)
-
- if output_transform is not None:
- out_kwargs = dict(output_transform)
- out_gpu = out_kwargs.pop("func")(*out_gpu, **out_kwargs)
- out_gpu = [out_gpu] if tfutil.is_tf_expression(out_gpu) else list(out_gpu)
-
- assert len(out_gpu) == self.num_outputs
- out_split.append(out_gpu)
-
- with tf.device("/cpu:0"):
- out_expr = [tf.concat(outputs, axis=0) for outputs in zip(*out_split)]
- self._run_cache[key] = in_expr, out_expr
-
- # Run minibatches.
- in_expr, out_expr = self._run_cache[key]
- out_arrays = [np.empty([num_items] + tfutil.shape_to_list(expr.shape)[1:], expr.dtype.name) for expr in out_expr]
-
- for mb_begin in range(0, num_items, minibatch_size):
- if print_progress:
- print("\r%d / %d" % (mb_begin, num_items), end="")
-
- mb_end = min(mb_begin + minibatch_size, num_items)
- mb_num = mb_end - mb_begin
- mb_in = [src[mb_begin : mb_end] if src is not None else np.zeros([mb_num] + shape[1:]) for src, shape in zip(in_arrays, self.input_shapes)]
- mb_out = tf.get_default_session().run(out_expr, dict(zip(in_expr, mb_in)))
-
- for dst, src in zip(out_arrays, mb_out):
- dst[mb_begin: mb_end] = src
-
- # Done.
- if print_progress:
- print("\r%d / %d" % (num_items, num_items))
-
- if not return_as_list:
- out_arrays = out_arrays[0] if len(out_arrays) == 1 else tuple(out_arrays)
- return out_arrays
-
- def list_ops(self) -> List[TfExpression]:
- include_prefix = self.scope + "/"
- exclude_prefix = include_prefix + "_"
- ops = tf.get_default_graph().get_operations()
- ops = [op for op in ops if op.name.startswith(include_prefix)]
- ops = [op for op in ops if not op.name.startswith(exclude_prefix)]
- return ops
-
- def list_layers(self) -> List[Tuple[str, TfExpression, List[TfExpression]]]:
- """Returns a list of (layer_name, output_expr, trainable_vars) tuples corresponding to
- individual layers of the network. Mainly intended to be used for reporting."""
- layers = []
-
- def recurse(scope, parent_ops, parent_vars, level):
- # Ignore specific patterns.
- if any(p in scope for p in ["/Shape", "/strided_slice", "/Cast", "/concat", "/Assign"]):
- return
-
- # Filter ops and vars by scope.
- global_prefix = scope + "/"
- local_prefix = global_prefix[len(self.scope) + 1:]
- cur_ops = [op for op in parent_ops if op.name.startswith(global_prefix) or op.name == global_prefix[:-1]]
- cur_vars = [(name, var) for name, var in parent_vars if name.startswith(local_prefix) or name == local_prefix[:-1]]
- if not cur_ops and not cur_vars:
- return
-
- # Filter out all ops related to variables.
- for var in [op for op in cur_ops if op.type.startswith("Variable")]:
- var_prefix = var.name + "/"
- cur_ops = [op for op in cur_ops if not op.name.startswith(var_prefix)]
-
- # Scope does not contain ops as immediate children => recurse deeper.
- contains_direct_ops = any("/" not in op.name[len(global_prefix):] and op.type != "Identity" for op in cur_ops)
- if (level == 0 or not contains_direct_ops) and (len(cur_ops) + len(cur_vars)) > 1:
- visited = set()
- for rel_name in [op.name[len(global_prefix):] for op in cur_ops] + [name[len(local_prefix):] for name, _var in cur_vars]:
- token = rel_name.split("/")[0]
- if token not in visited:
- recurse(global_prefix + token, cur_ops, cur_vars, level + 1)
- visited.add(token)
- return
-
- # Report layer.
- layer_name = scope[len(self.scope) + 1:]
- layer_output = cur_ops[-1].outputs[0] if cur_ops else cur_vars[-1][1]
- layer_trainables = [var for _name, var in cur_vars if var.trainable]
- layers.append((layer_name, layer_output, layer_trainables))
-
- recurse(self.scope, self.list_ops(), list(self.vars.items()), 0)
- return layers
-
- def print_layers(self, title: str = None, hide_layers_with_no_params: bool = False) -> None:
- """Print a summary table of the network structure."""
- rows = [[title if title is not None else self.name, "Params", "OutputShape", "WeightShape"]]
- rows += [["---"] * 4]
- total_params = 0
-
- for layer_name, layer_output, layer_trainables in self.list_layers():
- num_params = sum(np.prod(tfutil.shape_to_list(var.shape)) for var in layer_trainables)
- weights = [var for var in layer_trainables if var.name.endswith("/weight:0")]
- weights.sort(key=lambda x: len(x.name))
- if len(weights) == 0 and len(layer_trainables) == 1:
- weights = layer_trainables
- total_params += num_params
-
- if not hide_layers_with_no_params or num_params != 0:
- num_params_str = str(num_params) if num_params > 0 else "-"
- output_shape_str = str(layer_output.shape)
- weight_shape_str = str(weights[0].shape) if len(weights) >= 1 else "-"
- rows += [[layer_name, num_params_str, output_shape_str, weight_shape_str]]
-
- rows += [["---"] * 4]
- rows += [["Total", str(total_params), "", ""]]
-
- widths = [max(len(cell) for cell in column) for column in zip(*rows)]
- print()
- for row in rows:
- print(" ".join(cell + " " * (width - len(cell)) for cell, width in zip(row, widths)))
- print()
-
- def setup_weight_histograms(self, title: str = None) -> None:
- """Construct summary ops to include histograms of all trainable parameters in TensorBoard."""
- if title is None:
- title = self.name
-
- with tf.name_scope(None), tf.device(None), tf.control_dependencies(None):
- for local_name, var in self.trainables.items():
- if "/" in local_name:
- p = local_name.split("/")
- name = title + "_" + p[-1] + "/" + "_".join(p[:-1])
- else:
- name = title + "_toplevel/" + local_name
-
- tf.summary.histogram(name, var)
-
-#----------------------------------------------------------------------------
-# Backwards-compatible emulation of legacy output transformation in Network.run().
-
-_print_legacy_warning = True
-
-def _handle_legacy_output_transforms(output_transform, dynamic_kwargs):
- global _print_legacy_warning
- legacy_kwargs = ["out_mul", "out_add", "out_shrink", "out_dtype"]
- if not any(kwarg in dynamic_kwargs for kwarg in legacy_kwargs):
- return output_transform, dynamic_kwargs
-
- if _print_legacy_warning:
- _print_legacy_warning = False
- print()
- print("WARNING: Old-style output transformations in Network.run() are deprecated.")
- print("Consider using 'output_transform=dict(func=tflib.convert_images_to_uint8)'")
- print("instead of 'out_mul=127.5, out_add=127.5, out_dtype=np.uint8'.")
- print()
- assert output_transform is None
-
- new_kwargs = dict(dynamic_kwargs)
- new_transform = {kwarg: new_kwargs.pop(kwarg) for kwarg in legacy_kwargs if kwarg in dynamic_kwargs}
- new_transform["func"] = _legacy_output_transform_func
- return new_transform, new_kwargs
-
-def _legacy_output_transform_func(*expr, out_mul=1.0, out_add=0.0, out_shrink=1, out_dtype=None):
- if out_mul != 1.0:
- expr = [x * out_mul for x in expr]
-
- if out_add != 0.0:
- expr = [x + out_add for x in expr]
-
- if out_shrink > 1:
- ksize = [1, 1, out_shrink, out_shrink]
- expr = [tf.nn.avg_pool(x, ksize=ksize, strides=ksize, padding="VALID", data_format="NCHW") for x in expr]
-
- if out_dtype is not None:
- if tf.as_dtype(out_dtype).is_integer:
- expr = [tf.round(x) for x in expr]
- expr = [tf.saturate_cast(x, out_dtype) for x in expr]
- return expr
diff --git a/spaces/DragGan/DragGan-Inversion/stylegan_human/pti/training/projectors/w_plus_projector.py b/spaces/DragGan/DragGan-Inversion/stylegan_human/pti/training/projectors/w_plus_projector.py
deleted file mode 100644
index b61fa0159b02a052bc8a52341a53ec4b62ced657..0000000000000000000000000000000000000000
--- a/spaces/DragGan/DragGan-Inversion/stylegan_human/pti/training/projectors/w_plus_projector.py
+++ /dev/null
@@ -1,163 +0,0 @@
-# Copyright (c) 2021, NVIDIA CORPORATION. All rights reserved.
-#
-# NVIDIA CORPORATION and its licensors retain all intellectual property
-# and proprietary rights in and to this software, related documentation
-# and any modifications thereto. Any use, reproduction, disclosure or
-# distribution of this software and related documentation without an express
-# license agreement from NVIDIA CORPORATION is strictly prohibited.
-
-"""Project given image to the latent space of pretrained network pickle."""
-
-import copy
-import wandb
-import numpy as np
-import torch
-import torch.nn.functional as F
-from tqdm import tqdm
-from configs import global_config, hyperparameters
-import dnnlib
-from utils.log_utils import log_image_from_w
-
-
-def project(
- G,
- # [C,H,W] and dynamic range [0,255], W & H must match G output resolution
- target: torch.Tensor,
- *,
- num_steps=1000,
- w_avg_samples=10000,
- initial_learning_rate=0.01,
- initial_noise_factor=0.05,
- lr_rampdown_length=0.25,
- lr_rampup_length=0.05,
- noise_ramp_length=0.75,
- regularize_noise_weight=1e5,
- verbose=False,
- device: torch.device,
- use_wandb=False,
- initial_w=None,
- image_log_step=global_config.image_rec_result_log_snapshot,
- w_name: str
-):
- print('inside training/projectors/w_plus_projector')
- print(target.shape, G.img_channels, G.img_resolution * 2, G.img_resolution)
- assert target.shape == (
- G.img_channels, G.img_resolution * 2, G.img_resolution)
-
- def logprint(*args):
- if verbose:
- print(*args)
-
- G = copy.deepcopy(G).eval().requires_grad_(
- False).to(device).float() # type: ignore
-
- # Compute w stats.
- logprint(
- f'Computing W midpoint and stddev using {w_avg_samples} samples...')
- z_samples = np.random.RandomState(123).randn(w_avg_samples, G.z_dim)
- w_samples = G.mapping(torch.from_numpy(
- z_samples).to(device), None) # [N, L, C]
- w_samples = w_samples[:, :1, :].cpu(
- ).numpy().astype(np.float32) # [N, 1, C]
- w_avg = np.mean(w_samples, axis=0, keepdims=True) # [1, 1, C]
- w_avg_tensor = torch.from_numpy(w_avg).to(global_config.device)
- w_std = (np.sum((w_samples - w_avg) ** 2) / w_avg_samples) ** 0.5
-
- start_w = initial_w if initial_w is not None else w_avg
-
- # Setup noise inputs.
- noise_bufs = {name: buf for (
- name, buf) in G.synthesis.named_buffers() if 'noise_const' in name}
-
- # Load VGG16 feature detector.
- url = 'https://nvlabs-fi-cdn.nvidia.com/stylegan2-ada-pytorch/pretrained/metrics/vgg16.pt'
- with dnnlib.util.open_url(url) as f:
- vgg16 = torch.jit.load(f).eval().to(device)
-
- # Features for target image.
- target_images = target.unsqueeze(0).to(device).to(torch.float32)
- if target_images.shape[2] > 256:
- target_images = F.interpolate(
- target_images, size=(256, 256), mode='area')
- target_features = vgg16(
- target_images, resize_images=False, return_lpips=True)
-
- start_w = np.repeat(start_w, G.mapping.num_ws, axis=1)
- w_opt = torch.tensor(start_w, dtype=torch.float32, device=device,
- requires_grad=True) # pylint: disable=not-callable
-
- optimizer = torch.optim.Adam([w_opt] + list(noise_bufs.values()), betas=(0.9, 0.999),
- lr=hyperparameters.first_inv_lr)
-
- # Init noise.
- for buf in noise_bufs.values():
- buf[:] = torch.randn_like(buf)
- buf.requires_grad = True
-
- for step in tqdm(range(num_steps)):
-
- # Learning rate schedule.
- t = step / num_steps
- w_noise_scale = w_std * initial_noise_factor * \
- max(0.0, 1.0 - t / noise_ramp_length) ** 2
- lr_ramp = min(1.0, (1.0 - t) / lr_rampdown_length)
- lr_ramp = 0.5 - 0.5 * np.cos(lr_ramp * np.pi)
- lr_ramp = lr_ramp * min(1.0, t / lr_rampup_length)
- lr = initial_learning_rate * lr_ramp
- for param_group in optimizer.param_groups:
- param_group['lr'] = lr
-
- # Synth images from opt_w.
- w_noise = torch.randn_like(w_opt) * w_noise_scale
- ws = (w_opt + w_noise)
-
- synth_images = G.synthesis(ws, noise_mode='const', force_fp32=True)
-
- # Downsample image to 256x256 if it's larger than that. VGG was built for 224x224 images.
- synth_images = (synth_images + 1) * (255 / 2)
- if synth_images.shape[2] > 256:
- synth_images = F.interpolate(
- synth_images, size=(256, 256), mode='area')
-
- # Features for synth images.
- synth_features = vgg16(
- synth_images, resize_images=False, return_lpips=True)
- dist = (target_features - synth_features).square().sum()
-
- # Noise regularization.
- reg_loss = 0.0
- for v in noise_bufs.values():
- noise = v[None, None, :, :] # must be [1,1,H,W] for F.avg_pool2d()
- while True:
- reg_loss += (noise * torch.roll(noise,
- shifts=1, dims=3)).mean() ** 2
- reg_loss += (noise * torch.roll(noise,
- shifts=1, dims=2)).mean() ** 2
- if noise.shape[2] <= 8:
- break
- noise = F.avg_pool2d(noise, kernel_size=2)
- loss = dist + reg_loss * regularize_noise_weight
-
- if step % image_log_step == 0:
- with torch.no_grad():
- if use_wandb:
- global_config.training_step += 1
- wandb.log({f'first projection _{w_name}': loss.detach(
- ).cpu()}, step=global_config.training_step)
- log_image_from_w(w_opt, G, w_name)
-
- # Step
- optimizer.zero_grad(set_to_none=True)
- loss.backward()
- optimizer.step()
- logprint(
- f'step {step + 1:>4d}/{num_steps}: dist {dist:<4.2f} loss {float(loss):<5.2f}')
-
- # Normalize noise.
- with torch.no_grad():
- for buf in noise_bufs.values():
- buf -= buf.mean()
- buf *= buf.square().mean().rsqrt()
-
- del G
- return w_opt
diff --git a/spaces/DragGan/DragGan/stylegan_human/utils/log_utils.py b/spaces/DragGan/DragGan/stylegan_human/utils/log_utils.py
deleted file mode 100644
index 12171ab4cb73659e163bafaf4491dd15218c7e06..0000000000000000000000000000000000000000
--- a/spaces/DragGan/DragGan/stylegan_human/utils/log_utils.py
+++ /dev/null
@@ -1,82 +0,0 @@
-# Copyright (c) SenseTime Research. All rights reserved.
-
-
-import numpy as np
-from PIL import Image
-import wandb
-from pti.pti_configs import global_config
-import torch
-import matplotlib.pyplot as plt
-
-
-def log_image_from_w(w, G, name):
- img = get_image_from_w(w, G)
- pillow_image = Image.fromarray(img)
- wandb.log(
- {f"{name}": [
- wandb.Image(pillow_image, caption=f"current inversion {name}")]},
- step=global_config.training_step)
-
-
-def log_images_from_w(ws, G, names):
- for name, w in zip(names, ws):
- w = w.to(global_config.device)
- log_image_from_w(w, G, name)
-
-
-def plot_image_from_w(w, G):
- img = get_image_from_w(w, G)
- pillow_image = Image.fromarray(img)
- plt.imshow(pillow_image)
- plt.show()
-
-
-def plot_image(img):
- img = (img.permute(0, 2, 3, 1) * 127.5 + 128).clamp(0, 255).to(torch.uint8).detach().cpu().numpy()
- pillow_image = Image.fromarray(img[0])
- plt.imshow(pillow_image)
- plt.show()
-
-
-def save_image(name, method_type, results_dir, image, run_id):
- image.save(f'{results_dir}/{method_type}_{name}_{run_id}.jpg')
-
-
-def save_w(w, G, name, method_type, results_dir):
- im = get_image_from_w(w, G)
- im = Image.fromarray(im, mode='RGB')
- save_image(name, method_type, results_dir, im)
-
-
-def save_concat_image(base_dir, image_latents, new_inv_image_latent, new_G,
- old_G,
- file_name,
- extra_image=None):
- images_to_save = []
- if extra_image is not None:
- images_to_save.append(extra_image)
- for latent in image_latents:
- images_to_save.append(get_image_from_w(latent, old_G))
- images_to_save.append(get_image_from_w(new_inv_image_latent, new_G))
- result_image = create_alongside_images(images_to_save)
- result_image.save(f'{base_dir}/{file_name}.jpg')
-
-
-def save_single_image(base_dir, image_latent, G, file_name):
- image_to_save = get_image_from_w(image_latent, G)
- image_to_save = Image.fromarray(image_to_save, mode='RGB')
- image_to_save.save(f'{base_dir}/{file_name}.jpg')
-
-
-def create_alongside_images(images):
- res = np.concatenate([np.array(image) for image in images], axis=1)
- return Image.fromarray(res, mode='RGB')
-
-
-def get_image_from_w(w, G):
- if len(w.size()) <= 2:
- w = w.unsqueeze(0)
- with torch.no_grad():
- img = G.synthesis(w, noise_mode='const')
- img = (img.permute(0, 2, 3, 1) * 127.5 + 128).clamp(0, 255).to(torch.uint8).detach().cpu().numpy()
- return img[0]
diff --git a/spaces/ECCV2022/storydalle/dalle/utils/sampling.py b/spaces/ECCV2022/storydalle/dalle/utils/sampling.py
deleted file mode 100644
index 26d544d960e33d3a7f0de63dd98fc1df1a521b6b..0000000000000000000000000000000000000000
--- a/spaces/ECCV2022/storydalle/dalle/utils/sampling.py
+++ /dev/null
@@ -1,369 +0,0 @@
-# ------------------------------------------------------------------------------------
-# Minimal DALL-E
-# Copyright (c) 2021 KakaoBrain. All Rights Reserved.
-# Licensed under the Apache License, Version 2.0 [see LICENSE for details]
-# ------------------------------------------------------------------------------------
-
-import torch
-from typing import Optional
-from tqdm import tqdm
-from torch.nn import functional as F
-
-
-torch.set_printoptions(precision=2, threshold=10)
-def cutoff_topk_logits(logits: torch.FloatTensor, k: int) -> torch.FloatTensor:
- if k is None:
- return logits
- else:
- v, ix = torch.topk(logits, k)
- out = logits.clone()
- out[out < v[:, [-1]]] = -float('Inf')
- return out
-
-
-def cutoff_topp_probs(probs: torch.FloatTensor, p: float) -> torch.FloatTensor:
- if p is None:
- return probs
- else:
- sorted_probs, sorted_indices = torch.sort(probs, dim=-1, descending=True)
- cum_probs = torch.cumsum(sorted_probs, dim=-1)
-
- sorted_idx_remove_cond = cum_probs >= p
-
- sorted_idx_remove_cond[..., 1:] = sorted_idx_remove_cond[..., :-1].clone()
- sorted_idx_remove_cond[..., 0] = 0
-
- indices_to_remove = sorted_idx_remove_cond.scatter(-1, sorted_indices, sorted_idx_remove_cond)
- probs = probs.masked_fill(indices_to_remove, 0.0)
- norm_probs = probs / torch.sum(probs, dim=-1, keepdim=True)
- return norm_probs
-
-
-def get_positional_encoding(inputs: torch.LongTensor, mode: str = '1d') -> torch.LongTensor:
- device = inputs.device
- if mode == '1d':
- B, N = inputs.shape
- xs_pos = torch.arange(N, device=device).repeat((B, 1))
- elif mode == '2d':
- B, H, W = inputs.shape
- xs_pos_h = torch.arange(H, device=device).repeat(B, W, 1).transpose(1, 2)
- xs_pos_w = torch.arange(W, device=device).repeat(B, H, 1)
- xs_pos = (xs_pos_h, xs_pos_w)
- else:
- raise ValueError('%s positional encoding invalid' % mode)
- return xs_pos
-
-
-@torch.no_grad()
-def sampling(model: torch.nn.Module,
- tokens: torch.LongTensor,
- top_k: Optional[float] = None,
- top_p: Optional[float] = None,
- softmax_temperature: float = 1.0,
- is_tqdm: bool = True,
- use_fp16: bool = True,
- max_seq_len: int = 256,
- prompt: Optional[torch.tensor] = None,
- pos_prompt: Optional[torch.Tensor] = None) -> torch.LongTensor:
-
- code = None
- past = None
-
- pbar = tqdm(range(max_seq_len), total=max_seq_len) if is_tqdm else range(max_seq_len)
- pos_enc_tokens = get_positional_encoding(tokens, mode='1d')
-
- for cnt, h in enumerate(pbar):
- if code is None:
- code_ = None
- pos_enc_code_ = None
- else:
- code_ = code.clone().detach()
- pos_enc_code_ = get_positional_encoding(code_, mode='1d')
- code_ = code_[:, cnt-1].unsqueeze(-1)
- pos_enc_code_ = pos_enc_code_[:, cnt-1].unsqueeze(-1)
-
- logits, present = model.sampling(images=code_,
- texts=tokens,
- pos_images=pos_enc_code_,
- pos_texts=pos_enc_tokens,
- use_fp16=use_fp16,
- past=past,
- prompt=prompt,
- pos_prompt=pos_prompt)
-
- logits = logits.to(dtype=torch.float32)
- logits = logits / softmax_temperature
-
- # print(len(present), present[0].shape)
- present = torch.stack(present).clone().detach()
- if past is None:
- past = [present]
- else:
- past.append(present)
-
- logits = cutoff_topk_logits(logits, top_k)
- probs = F.softmax(logits, dim=-1)
- probs = cutoff_topp_probs(probs, top_p)
- # print(probs[0])
-
- idx = torch.multinomial(probs, num_samples=1).clone().detach()
- # print(idx)
- code = idx if code is None else torch.cat([code, idx], axis=1)
-
- del past
- return code
-
-
-@torch.no_grad()
-def sampling_prefix(model: torch.nn.Module,
- tokens: torch.LongTensor,
- past: torch.FloatTensor,
- top_k: Optional[float] = None,
- top_p: Optional[float] = None,
- softmax_temperature: float = 1.0,
- is_tqdm: bool = True,
- use_fp16: bool = True,
- max_seq_len: int = 256,
- labels = None) -> torch.LongTensor:
- code = None
-
- pbar = tqdm(range(max_seq_len), total=max_seq_len) if is_tqdm else range(max_seq_len)
- pos_enc_tokens = get_positional_encoding(tokens, mode='1d')
-
- # print("Entering sampling_prefix; ", past.shape)
- if past is not None:
- past = [past]
-
- for cnt, h in enumerate(pbar):
- if code is None:
- code_ = None
- pos_enc_code_ = None
- else:
- code_ = code.clone().detach()
- pos_enc_code_ = get_positional_encoding(code_, mode='1d')
- code_ = code_[:, cnt-1].unsqueeze(-1)
- pos_enc_code_ = pos_enc_code_[:, cnt-1].unsqueeze(-1)
-
- # print("Looop enter")
- # print(cnt, past[0].shape)
- # print("-------------------")
- logits, present = model.sampling(images=code_,
- texts=tokens,
- pos_images=pos_enc_code_,
- pos_texts=pos_enc_tokens,
- use_fp16=use_fp16,
- past=past)
- logits = logits.to(dtype=torch.float32)
- logits = logits / softmax_temperature
-
- present = torch.stack(present).clone().detach()
-
- # print('Present', present.shape)
-
- if past is None:
- past = [present]
- else:
- # print("Loop end")
- # print(present.shape)
- # print("-----------------")
-
- # n_layers, temp, _, seq_len, n_dim = present.shape
- # _, _, bs, n_heads, pre_seq_len, n_dim = past[0].shape
- # assert temp == 2
- # past.append(present.view(n_layers, temp, bs, n_heads, seq_len, n_dim))
-
- past.append(present)
-
- logits = cutoff_topk_logits(logits, top_k)
- probs = F.softmax(logits, dim=-1)
- probs = cutoff_topp_probs(probs, top_p)
- print(torch.topk(probs, 5, dim=-1))
- if labels is not None:
- print(labels[cnt])
- idx = torch.multinomial(probs, num_samples=1).clone().detach()
- # print(idx)
- code = idx if code is None else torch.cat([code, idx], axis=1)
-
- del past
- return code
-
-
-@torch.no_grad()
-def sampling_prefix_new(model: torch.nn.Module,
- tokens: torch.LongTensor,
- past: torch.FloatTensor,
- top_k: Optional[float] = None,
- top_p: Optional[float] = None,
- softmax_temperature: float = 1.0,
- is_tqdm: bool = True,
- use_fp16: bool = True,
- max_seq_len: int = 256) -> torch.LongTensor:
- code = None
-
- pbar = tqdm(range(max_seq_len), total=max_seq_len) if is_tqdm else range(max_seq_len)
- pos_enc_tokens = get_positional_encoding(tokens, mode='1d')
-
- # print("Entering sampling_prefix; ", past.shape)
- if past is not None:
- past = [past]
-
- for cnt, h in enumerate(pbar):
- if code is None:
- code_ = None
- pos_enc_code_ = None
- else:
- code_ = code.clone().detach()
- pos_enc_code_ = get_positional_encoding(code_, mode='1d')
- # code_ = code_[:, cnt-1].unsqueeze(-1)
- # pos_enc_code_ = pos_enc_code_[:, cnt-1].unsqueeze(-1)
-
- # print("Looop enter")
- # print(cnt, past[0].shape)
- # print("-------------------")
-
- if cnt == 0:
- logits, present = model.sampling(images=code_,
- texts=tokens,
- pos_images=pos_enc_code_,
- pos_texts=pos_enc_tokens,
- use_fp16=use_fp16,
- past=past)
- logits = logits.to(dtype=torch.float32)
- logits = logits / softmax_temperature
-
- present = torch.stack(present).clone().detach()
-
- # print('Present', present.shape)
-
- if past is None:
- past = [present]
- else:
- pass
-
- logits = cutoff_topk_logits(logits, top_k)
- probs = F.softmax(logits, dim=-1)
- probs = cutoff_topp_probs(probs, top_p)
- # print(torch.topk(probs[0], 5))
- idx = torch.multinomial(probs, num_samples=1).clone().detach()
- # print(idx)
- code = idx if code is None else torch.cat([code, idx], axis=1)
-
- else:
- pass
-
-
- del past
- return code
-
-@torch.no_grad()
-def sampling_conditional(model: torch.nn.Module,
- cross_attention_idxs,
- cross_attention_layers,
- tokens: torch.LongTensor,
- src_codes: torch.FloatTensor,
- top_k: Optional[float] = None,
- top_p: Optional[float] = None,
- softmax_temperature: float = 1.0,
- is_tqdm: bool = True,
- use_fp16: bool = True,
- max_seq_len: int = 256,
- prompt: Optional[torch.tensor] = None,
- pos_prompt: Optional[torch.Tensor] = None) -> torch.LongTensor:
-
- code = None
- past = None
-
- pbar = tqdm(range(max_seq_len), total=max_seq_len) if is_tqdm else range(max_seq_len)
- pos_enc_tokens = get_positional_encoding(tokens, mode='1d')
-
- src_pos_tokens = get_positional_encoding(src_codes, mode='1d')
- src_tokens = model.tok_emb_img(src_codes)
- src_tokens = src_tokens + model.pos_emb_img(src_pos_tokens)
-
- for cnt, h in enumerate(pbar):
- if code is None:
- code_ = None
- pos_enc_code_ = None
- else:
- code_ = code.clone().detach()
- pos_enc_code_ = get_positional_encoding(code_, mode='1d')
- code_ = code_[:, cnt-1].unsqueeze(-1)
- pos_enc_code_ = pos_enc_code_[:, cnt-1].unsqueeze(-1)
-
- logits, present = model.sampling_with_context(images=code_,
- cross_attention_idxs=cross_attention_idxs,
- cross_attention_layers=cross_attention_layers,
- texts=tokens,
- pos_images=pos_enc_code_,
- pos_texts=pos_enc_tokens,
- source_image=src_tokens,
- use_fp16=use_fp16,
- past=past,
- prompt=prompt,
- pos_prompt=pos_prompt)
- logits = logits.to(dtype=torch.float32)
- logits = logits / softmax_temperature
-
- present = torch.stack(present).clone().detach()
- if past is None:
- past = [present]
- else:
- past.append(present)
-
- logits = cutoff_topk_logits(logits, top_k)
- probs = F.softmax(logits, dim=-1)
- probs = cutoff_topp_probs(probs, top_p)
-
- idx = torch.multinomial(probs, num_samples=1).clone().detach()
- code = idx if code is None else torch.cat([code, idx], axis=1)
-
- del past
- return code
-
-
-@torch.no_grad()
-def sampling_igpt(model: torch.nn.Module,
- sos: torch.FloatTensor,
- top_k: Optional[float] = None,
- top_p: Optional[float] = None,
- softmax_temperature: float = 1.0,
- is_tqdm: bool = True,
- use_fp16: bool = True,
- max_seq_len: int = 256) -> torch.LongTensor:
- code = None
- past = None
- pbar = tqdm(range(max_seq_len), total=max_seq_len) if is_tqdm else range(max_seq_len)
-
- for cnt, h in enumerate(pbar):
- if code is None:
- code_ = None
- pos_enc_code_ = None
- else:
- code_ = code.clone().detach()
- pos_enc_code_ = get_positional_encoding(code_, mode='1d')
- code_ = code_[:, cnt-1].unsqueeze(-1)
- pos_enc_code_ = pos_enc_code_[:, cnt-1].unsqueeze(-1)
-
- logits, present = model.sampling(sos=sos,
- codes=code_,
- pos_codes=pos_enc_code_,
- use_fp16=use_fp16,
- past=past)
- logits = logits.to(dtype=torch.float32)
- logits = logits / softmax_temperature
-
- present = torch.stack(present).clone().detach()
- if past is None:
- past = [present]
- else:
- past.append(present)
-
- logits = cutoff_topk_logits(logits, top_k)
- probs = F.softmax(logits, dim=-1)
- probs = cutoff_topp_probs(probs, top_p)
-
- idx = torch.multinomial(probs, num_samples=1).clone().detach()
- code = idx if code is None else torch.cat([code, idx], axis=1)
-
- del past
- return code
diff --git a/spaces/EPFL-VILAB/MultiMAE/mask2former/modeling/pixel_decoder/ops/modules/__init__.py b/spaces/EPFL-VILAB/MultiMAE/mask2former/modeling/pixel_decoder/ops/modules/__init__.py
deleted file mode 100644
index 6fdbf03359958f3d67ab00f879bf6b61a6c8f06a..0000000000000000000000000000000000000000
--- a/spaces/EPFL-VILAB/MultiMAE/mask2former/modeling/pixel_decoder/ops/modules/__init__.py
+++ /dev/null
@@ -1,12 +0,0 @@
-# ------------------------------------------------------------------------------------------------
-# Deformable DETR
-# Copyright (c) 2020 SenseTime. All Rights Reserved.
-# Licensed under the Apache License, Version 2.0 [see LICENSE for details]
-# ------------------------------------------------------------------------------------------------
-# Modified from https://github.com/chengdazhi/Deformable-Convolution-V2-PyTorch/tree/pytorch_1.0.0
-# ------------------------------------------------------------------------------------------------
-
-# Copyright (c) Facebook, Inc. and its affiliates.
-# Modified by Bowen Cheng from https://github.com/fundamentalvision/Deformable-DETR
-
-from .ms_deform_attn import MSDeformAttn
diff --git a/spaces/EPFL-VILAB/MultiMAE/mask2former/modeling/pixel_decoder/ops/setup.py b/spaces/EPFL-VILAB/MultiMAE/mask2former/modeling/pixel_decoder/ops/setup.py
deleted file mode 100644
index 244fdec83bee181e187d88800300395f449b0fbc..0000000000000000000000000000000000000000
--- a/spaces/EPFL-VILAB/MultiMAE/mask2former/modeling/pixel_decoder/ops/setup.py
+++ /dev/null
@@ -1,78 +0,0 @@
-# ------------------------------------------------------------------------------------------------
-# Deformable DETR
-# Copyright (c) 2020 SenseTime. All Rights Reserved.
-# Licensed under the Apache License, Version 2.0 [see LICENSE for details]
-# ------------------------------------------------------------------------------------------------
-# Modified from https://github.com/chengdazhi/Deformable-Convolution-V2-PyTorch/tree/pytorch_1.0.0
-# ------------------------------------------------------------------------------------------------
-
-# Copyright (c) Facebook, Inc. and its affiliates.
-# Modified by Bowen Cheng from https://github.com/fundamentalvision/Deformable-DETR
-
-import os
-import glob
-
-import torch
-
-from torch.utils.cpp_extension import CUDA_HOME
-from torch.utils.cpp_extension import CppExtension
-from torch.utils.cpp_extension import CUDAExtension
-
-from setuptools import find_packages
-from setuptools import setup
-
-requirements = ["torch", "torchvision"]
-
-def get_extensions():
- this_dir = os.path.dirname(os.path.abspath(__file__))
- extensions_dir = os.path.join(this_dir, "src")
-
- main_file = glob.glob(os.path.join(extensions_dir, "*.cpp"))
- source_cpu = glob.glob(os.path.join(extensions_dir, "cpu", "*.cpp"))
- source_cuda = glob.glob(os.path.join(extensions_dir, "cuda", "*.cu"))
-
- sources = main_file + source_cpu
- extension = CppExtension
- extra_compile_args = {"cxx": []}
- define_macros = []
-
- # Force cuda since torch ask for a device, not if cuda is in fact available.
- if (os.environ.get('FORCE_CUDA') or torch.cuda.is_available()) and CUDA_HOME is not None:
- extension = CUDAExtension
- sources += source_cuda
- define_macros += [("WITH_CUDA", None)]
- extra_compile_args["nvcc"] = [
- "-DCUDA_HAS_FP16=1",
- "-D__CUDA_NO_HALF_OPERATORS__",
- "-D__CUDA_NO_HALF_CONVERSIONS__",
- "-D__CUDA_NO_HALF2_OPERATORS__",
- ]
-# else:
-# if CUDA_HOME is None:
-# raise NotImplementedError('CUDA_HOME is None. Please set environment variable CUDA_HOME.')
-# else:
-# raise NotImplementedError('No CUDA runtime is found. Please set FORCE_CUDA=1 or test it by running torch.cuda.is_available().')
-
- sources = [os.path.join(extensions_dir, s) for s in sources]
- include_dirs = [extensions_dir]
- ext_modules = [
- extension(
- "MultiScaleDeformableAttention",
- sources,
- include_dirs=include_dirs,
- define_macros=define_macros,
- extra_compile_args=extra_compile_args,
- )
- ]
- return ext_modules
-
-setup(
- name="MultiScaleDeformableAttention",
- version="1.0",
- author="Weijie Su",
- url="https://github.com/fundamentalvision/Deformable-DETR",
- description="PyTorch Wrapper for CUDA Functions of Multi-Scale Deformable Attention",
- packages=find_packages(exclude=("configs", "tests",)),
- ext_modules=get_extensions(),
- cmdclass={"build_ext": torch.utils.cpp_extension.BuildExtension},
-)
diff --git a/spaces/Emanuel/porttagger/style.css b/spaces/Emanuel/porttagger/style.css
deleted file mode 100644
index dbf5c514e6e13268d8d39ca8d4f864c15facf975..0000000000000000000000000000000000000000
--- a/spaces/Emanuel/porttagger/style.css
+++ /dev/null
@@ -1,70 +0,0 @@
-a {
- color: inherit;
- text-decoration: underline;
-}
-
-.gradio-container {
- font-family: 'IBM Plex Sans', sans-serif;
-}
-
-.gr-button {
- color: white;
- border-color: #9d66e5;
- background: #9d66e5;
-}
-
-.container {
- max-width: 900px;
- margin: auto;
- padding-top: 1.5rem;
-}
-
-.gr-button {
- white-space: nowrap;
-}
-
-.gr-button:focus {
- border-color: rgb(147 197 253 / var(--tw-border-opacity));
- outline: none;
- box-shadow: var(--tw-ring-offset-shadow), var(--tw-ring-shadow), var(--tw-shadow, 0 0 #0000);
- --tw-border-opacity: 1;
- --tw-ring-offset-shadow: var(--tw-ring-inset) 0 0 0 var(--tw-ring-offset-width) var(--tw-ring-offset-color);
- --tw-ring-shadow: var(--tw-ring-inset) 0 0 0 calc(3px var(--tw-ring-offset-width)) var(--tw-ring-color);
- --tw-ring-color: rgb(191 219 254 / var(--tw-ring-opacity));
- --tw-ring-opacity: .5;
-}
-
-#advanced-options {
- margin-bottom: 20px;
-}
-
-.footer {
- margin-bottom: 45px;
- margin-top: 35px;
- text-align: center;
- border-bottom: 1px solid #e5e5e5;
-}
-
-.footer>p {
- font-size: .8rem;
- display: inline-block;
- padding: 0 10px;
- transform: translateY(10px);
- background: white;
-}
-
-.slogan {
- font-size: 15px;
- color: #495057;
-}
-
-.row {
- display: flex;
- margin-top: 20px;
-}
-
-.column {
- flex: 33.33%;
- padding-left: 50px;
- padding-right: 50px;
-}
\ No newline at end of file
diff --git a/spaces/EronSamez/RVC_HFmeu/infer/lib/uvr5_pack/lib_v5/layers_123812KB .py b/spaces/EronSamez/RVC_HFmeu/infer/lib/uvr5_pack/lib_v5/layers_123812KB .py
deleted file mode 100644
index 4fc1b5cb85a3327f60cbb9f5deffbeeaaac516ad..0000000000000000000000000000000000000000
--- a/spaces/EronSamez/RVC_HFmeu/infer/lib/uvr5_pack/lib_v5/layers_123812KB .py
+++ /dev/null
@@ -1,118 +0,0 @@
-import torch
-import torch.nn.functional as F
-from torch import nn
-
-from . import spec_utils
-
-
-class Conv2DBNActiv(nn.Module):
- def __init__(self, nin, nout, ksize=3, stride=1, pad=1, dilation=1, activ=nn.ReLU):
- super(Conv2DBNActiv, self).__init__()
- self.conv = nn.Sequential(
- nn.Conv2d(
- nin,
- nout,
- kernel_size=ksize,
- stride=stride,
- padding=pad,
- dilation=dilation,
- bias=False,
- ),
- nn.BatchNorm2d(nout),
- activ(),
- )
-
- def __call__(self, x):
- return self.conv(x)
-
-
-class SeperableConv2DBNActiv(nn.Module):
- def __init__(self, nin, nout, ksize=3, stride=1, pad=1, dilation=1, activ=nn.ReLU):
- super(SeperableConv2DBNActiv, self).__init__()
- self.conv = nn.Sequential(
- nn.Conv2d(
- nin,
- nin,
- kernel_size=ksize,
- stride=stride,
- padding=pad,
- dilation=dilation,
- groups=nin,
- bias=False,
- ),
- nn.Conv2d(nin, nout, kernel_size=1, bias=False),
- nn.BatchNorm2d(nout),
- activ(),
- )
-
- def __call__(self, x):
- return self.conv(x)
-
-
-class Encoder(nn.Module):
- def __init__(self, nin, nout, ksize=3, stride=1, pad=1, activ=nn.LeakyReLU):
- super(Encoder, self).__init__()
- self.conv1 = Conv2DBNActiv(nin, nout, ksize, 1, pad, activ=activ)
- self.conv2 = Conv2DBNActiv(nout, nout, ksize, stride, pad, activ=activ)
-
- def __call__(self, x):
- skip = self.conv1(x)
- h = self.conv2(skip)
-
- return h, skip
-
-
-class Decoder(nn.Module):
- def __init__(
- self, nin, nout, ksize=3, stride=1, pad=1, activ=nn.ReLU, dropout=False
- ):
- super(Decoder, self).__init__()
- self.conv = Conv2DBNActiv(nin, nout, ksize, 1, pad, activ=activ)
- self.dropout = nn.Dropout2d(0.1) if dropout else None
-
- def __call__(self, x, skip=None):
- x = F.interpolate(x, scale_factor=2, mode="bilinear", align_corners=True)
- if skip is not None:
- skip = spec_utils.crop_center(skip, x)
- x = torch.cat([x, skip], dim=1)
- h = self.conv(x)
-
- if self.dropout is not None:
- h = self.dropout(h)
-
- return h
-
-
-class ASPPModule(nn.Module):
- def __init__(self, nin, nout, dilations=(4, 8, 16), activ=nn.ReLU):
- super(ASPPModule, self).__init__()
- self.conv1 = nn.Sequential(
- nn.AdaptiveAvgPool2d((1, None)),
- Conv2DBNActiv(nin, nin, 1, 1, 0, activ=activ),
- )
- self.conv2 = Conv2DBNActiv(nin, nin, 1, 1, 0, activ=activ)
- self.conv3 = SeperableConv2DBNActiv(
- nin, nin, 3, 1, dilations[0], dilations[0], activ=activ
- )
- self.conv4 = SeperableConv2DBNActiv(
- nin, nin, 3, 1, dilations[1], dilations[1], activ=activ
- )
- self.conv5 = SeperableConv2DBNActiv(
- nin, nin, 3, 1, dilations[2], dilations[2], activ=activ
- )
- self.bottleneck = nn.Sequential(
- Conv2DBNActiv(nin * 5, nout, 1, 1, 0, activ=activ), nn.Dropout2d(0.1)
- )
-
- def forward(self, x):
- _, _, h, w = x.size()
- feat1 = F.interpolate(
- self.conv1(x), size=(h, w), mode="bilinear", align_corners=True
- )
- feat2 = self.conv2(x)
- feat3 = self.conv3(x)
- feat4 = self.conv4(x)
- feat5 = self.conv5(x)
- out = torch.cat((feat1, feat2, feat3, feat4, feat5), dim=1)
- bottle = self.bottleneck(out)
- return bottle
diff --git a/spaces/FYP-23-S1-21/Refineverse_Plugin/Refineverse.py b/spaces/FYP-23-S1-21/Refineverse_Plugin/Refineverse.py
deleted file mode 100644
index 59ea8c04f06dcb3824efb4340214b0e98877a74e..0000000000000000000000000000000000000000
--- a/spaces/FYP-23-S1-21/Refineverse_Plugin/Refineverse.py
+++ /dev/null
@@ -1,225 +0,0 @@
-# Plugin will run on this file & Strictly only contains codes for routing between files!
-from flask import Flask, render_template, request, flash, g
-from TextSummarizationFeature import summarize, getTextSumContents, insertTextSumRow
-from BreakdownFeature import breakdown, getBreakdownContents, insertBreakdownRow
-from TranslationFeature import translate_text, switch, getTranslatedContents, insertTranslationRow
-from GenerationFeature import generate, getTextGenContents, insertTextGenRow
-
-app = Flask(__name__)
-app.secret_key = 'refineverseAdmin' # Used to encrypt cookies & sessions
-
-# Routing to Main Dashboard/homepage file
-@app.route('/')
-def index():
- return render_template('RefineverseDashboardUI.html')
-
-# Routing to text summarization file
-@app.route('/text_summarization', methods=["POST", "GET"])
-def text_summarization():
- if request.method == "POST":
- try:
- # Grab the user story text from the textarea in html form
- Entered_story = request.form["input_text"]
-
- # The results are stored into a dictionary variable
- summarizedStory = summarize(Entered_story)
-
- flash("Your user story has been summarized!") # Displays a success message using flash, which is part of the Flask framework
-
- # Insert into TextSummarization table in Refineverse.db
- insertTextSumRow(Entered_story, summarizedStory)
-
- # Render and display summarized user story
- return render_template('TextSummarizationUI.html', summarizedStory=summarizedStory)
-
- # Exception handling messages for specific errors
- except ValueError as e:
- if str(e) == "Empty input!":
- flash("The input text cannot be empty! Please enter a user story.", 'error')
- return render_template('TextSummarizationUI.html')
- elif str(e) == "Incorrect format!":
- flash("Incorrect user story format! Please enter in the right format.", 'error')
- return render_template('TextSummarizationUI.html')
- elif str(e) == "Invalid length!":
- flash("Your inputted user story is too short to summarize. Please enter a longer story!", 'error')
- return render_template('TextSummarizationUI.html')
- else: # As a final resort, simply print out the error name
- flash("An error of type '{}' occurred: {}".format(type(e).__name__, str(e)), 'error')
- return render_template('TextSummarizationUI.html')
-
- except KeyError:
- flash("Please enter a valid user story!")
- return render_template('TextSummarizationUI.html')
-
- # Catch-all exception handling
- except Exception as e:
- flash("An error of type '{}' occurred: {}".format(type(e).__name__, str(e)), 'error')
- return render_template('TextSummarizationUI.html')
-
- else:
- return render_template('TextSummarizationUI.html')
-
-# Routing to summarization table file
-@app.route('/summarization_table')
-def summarization_table():
- # Get the summarization data from the database
- summarizations = getTextSumContents()
-
- # Render the summarization data as an HTML table
- return render_template('SummarizationTable.html', summarizations=summarizations)
-
-# Routing to Project Task Breakdown file
-@app.route("/project_breakdown", methods=["POST", "GET"]) # This tells flask the route to get to the page
-def project_breakdown():
- if request.method == "POST": # POST occurs when submitting a form, as specified in the HTML file
- try:
- # Grab the user story contents
- userStory = request.form["user-story-text"]
-
- # The results are stored into a dictionary variable
- processedLabel = breakdown(userStory)
-
- # Display success popup message
- flash("Your user story has been allocated as a " + processedLabel + " task!")
-
- insertBreakdownRow(userStory, processedLabel) # Inserts data into the Breakdown table
- rows = getBreakdownContents() # Grab all contents inside the Breakdown table
-
- return render_template('ProjectBreakdownUI.html', rows=rows)
-
- # Exception handling messages for specific errors
- except KeyError:
- flash("Please enter a valid user story!", 'error')
- rows = getBreakdownContents()
- return render_template('ProjectBreakdownUI.html', row=rows)
-
- # Catch-all exception handling
- except Exception as e:
- flash("An error of type '{}' occurred: {}".format(type(e).__name__, str(e)), 'error')
- rows = getBreakdownContents()
- return render_template('ProjectBreakdownUI.html', rows=rows)
-
- else: # For "GET" scenarios (loading the page, etc.)
- rows = getBreakdownContents() # To always display the table, we must grab the contents of Breakdown every time the page loads
- return render_template('ProjectBreakdownUI.html', rows=rows)
-
-# Routing to Translation file
-@app.route('/language_translation', methods=["POST", "GET"])
-def language_translation():
- if request.method == "POST":
- try:
- # Grab all relevant information for processing
- input_text = request.form['input'] # Grab user text input
-
- # Grab source language code
- source_language = request.form['source_language']
-
- # Grab target language code
- target_language = request.form['target_language']
-
- # Generate translated text using custom translation function
- translatedStory = translate_text(input_text, source_language, target_language)
-
- # Display success popup message
- flash("Your user story has been translated to " + switch(target_language) + " !")
-
- # Insert into Translation table in Refineverse.db
- insertTranslationRow(input_text, translatedStory)
-
- # Display the page
- return render_template('LanguageTranslationUI.html', input_text=input_text, translatedStory=translatedStory)
-
- # Exception handling messages for specific errors
- except ValueError as e:
- if str(e) == "Empty input!":
- flash("The input text cannot be empty! Please enter a user story.", 'error')
- return render_template('LanguageTranslationUI.html')
- elif str(e) == "Incorrect format!":
- flash("Unable to translate your user story. Please enter in the correct format.", 'error')
- return render_template('LanguageTranslationUI.html')
- else: # As a final resort, simply print out the error name
- flash("An error of type '{}' occurred: {}".format(type(e).__name__, str(e)), 'error')
- return render_template('LanguageTranslationUI.html')
-
- # Catch-all exception handling
- except Exception as e:
- flash("An error of type '{}' occurred: {}".format(type(e).__name__, str(e)), 'error')
- return render_template('LanguageTranslationUI.html')
-
- else:
- return render_template('LanguageTranslationUI.html')
-
-# Routing to translation table file
-@app.route('/translation_table')
-def translation_data():
- # Get the translation data from the database
- translations = getTranslatedContents()
-
- # Render the translation data as an HTML table
- return render_template('TranslationTable.html', translations=translations)
-
-# Routing to text summarization file
-@app.route('/text_generation', methods=["POST", "GET"])
-def text_generation():
- if request.method == "POST":
- try:
- # Grab the user story text from the textarea in html form
- Entered_story = request.form["input_text"]
-
- # The results are stored into a dictionary variable
- generatedStory = generate(Entered_story)
-
- # Display a success message for the user
- flash("Your user story has been generated!")
-
- # Insert into TextGeneration table in Refineverse.db
- insertTextGenRow(Entered_story, generatedStory)
-
- # Render and display summarized user story
- return render_template('TextGenerationUI.html', generatedStory=generatedStory)
-
- # Exception handling messages for specific errors
- except ValueError as e:
- if str(e) == "Empty input!":
- flash("The input text cannot be empty! Please enter a user story.", 'error')
- return render_template('TextGenerationUI.html')
- elif str(e) == "Incorrect format!":
- flash("Incorrect user story format! Please enter in the right format.", 'error')
- return render_template('TextGenerationUI.html')
- else: # As a final resort, simply print out the error name
- flash("An error of type '{}' occurred: {}".format(type(e).__name__, str(e)), 'error')
- return render_template('TextGenerationUI.html')
-
- except KeyError:
- flash("Please enter a valid user story!")
- return render_template('TextGenerationUI.html')
-
- # Catch-all exception handling
- except Exception as e:
- flash("An error of type '{}' occurred: {}".format(type(e).__name__, str(e)), 'error')
- return render_template('TextGenerationUI.html')
-
- else:
- return render_template('TextGenerationUI.html')
-
-# Routing to generation table file
-@app.route('/generation_table')
-def generation_table():
- # Get the generation data from the database
- generations = getTextGenContents()
-
- # Render the generation data as an HTML table
- return render_template('GenerationTable.html', generations=generations)
-
-# Used when the application is torn down
-# Its purpose is to close the database connection if it has not been closed
-@app.teardown_appcontext
-def close_connection(exception):
- db = getattr(g, '_database', None)
- if db is not None:
- db.close() # Closes the database connection
-
-# Initialise the app
-if __name__ == '__main__':
- app.run(host="0.0.0.0", port=7860) # For HF hosting
- #app.run(debug=False) # can set to True/False for local testing
\ No newline at end of file
diff --git a/spaces/Gradio-Blocks/Story-to-video/app.py b/spaces/Gradio-Blocks/Story-to-video/app.py
deleted file mode 100644
index 5b4831cb7b6e2d87bf92383aed6e8fb8fb58de65..0000000000000000000000000000000000000000
--- a/spaces/Gradio-Blocks/Story-to-video/app.py
+++ /dev/null
@@ -1,124 +0,0 @@
-from PIL import Image
-from transformers import AutoTokenizer, AutoModelForSeq2SeqLM,pipeline
-import requests
-import gradio as gr
-import torch
-import re
-from moviepy.editor import *
-import os
-import sys
-from huggingface_hub import snapshot_download
-import base64
-import io
-import cv2
-
-image_gen = gr.Interface.load("spaces/multimodalart/latentdiffusion")
-
-description = "Just upload an image, and generate a short story for the image.\n PS: GPT-2 is not perfect but it's fun to play with.May take a minute for the output to generate. Enjoyy!!!"
-title = "Story generator from images using ViT and GPT2"
-
-tokenizer = AutoTokenizer.from_pretrained("sshleifer/distilbart-cnn-12-6")
-
-model = AutoModelForSeq2SeqLM.from_pretrained("sshleifer/distilbart-cnn-12-6")
-
-def get_output_video(text):
-
- inputs = tokenizer(text,
- max_length=1024,
- truncation=True,
- return_tensors="pt")
-
- summary_ids = model.generate(inputs["input_ids"])
- summary = tokenizer.batch_decode(summary_ids,
- skip_special_tokens=True,
- clean_up_tokenization_spaces=False)
-
-
- plot = list(summary[0].split('.'))
-
- generated_images = []
- for senten in plot[:-1]:
- steps=50
- width=256
- height=256
- num_images=3
- diversity=6
-
- image_bytes = image_gen(senten, steps, width, height, num_images, diversity)
-
-
- # Algo from spaces/Gradio-Blocks/latent_gpt2_story/blob/main/app.py
-
- for image in image_bytes[1]:
- image_str = image[0]
- image_str = image_str.replace("data:image/png;base64,","")
- decoded_bytes = base64.decodebytes(bytes(image_str, "utf-8"))
- img = Image.open(io.BytesIO(decoded_bytes))
- generated_images.append(img)
-
- c = 0
- file_names = []
-
- for img in generated_images:
-
- f_name = 'img_'+str(c)+'.jpg'
- file_names.append(f_name)
- img = img.save(f_name)
- c+=1
-
- #print(file_names)
- clips = [ImageClip(m).set_duration(2)
- for m in file_names]
-
- concat_clip = concatenate_videoclips(clips, method="compose")
- concat_clip.write_videofile("test.mp4", fps=24)
-
- return 'test.mp4'
-
-
-text = 'Once, there was a boy who became bored when he watched over the village sheep grazing on the hillside. To entertain himself, he sang out, “Wolf! Wolf! The wolf is chasing the sheep!\”.When the villagers heard the cry, they came running up the hill to drive the wolf away. But, when they arrived, they saw no wolf. The boy was amused when seeing their angry faces.Don’t scream wolf, boy,\” warned the villagers, “when there is no wolf!” They angrily went back down the hill.Later, the shepherd boy cried out once again, “Wolf! Wolf! The wolf is chasing the sheep!” To his amusement, he looked on as the villagers came running up the hill to scare the wolf away.As they saw there was no wolf, they said strictly, “Save your frightened cry for when there really is a wolf! Don’t cry ‘wolf’ when there is no wolf!” But the boy grinned at their words while they walked grumbling down the hill once more.Later, the boy saw a real wolf sneaking around his flock. Alarmed, he jumped on his feet and cried out as loud as he could, “Wolf! Wolf!” But the villagers thought he was fooling them again, and so they didn’t come to help.At sunset, the villagers went looking for the boy who hadn’t returned with their sheep. When they went up the hill, they found him weeping.“There really was a wolf here! The flock is gone! I cried out, ‘Wolf!’ but you didn’t come,” he wailed.An old man went to comfort the boy. As he put his arm around him, he said, “Nobody believes a liar, even when he is telling the truth!\"'
-
-demo = gr.Blocks()
-
-with demo:
-
- gr.Markdown("# A System pipeline to generate bite-sized video from long stories")
-
- gr.Markdown("A story can be input by user. The story is summarized using DistillBART model. Then, it's step by step sent to the multimodal Diffusion model, to generate images.These are depicted as a video.")
- with gr.Row():
-
- # Left column (inputs)
-
- with gr.Column():
-
- input_start_text = gr.Textbox(value=text, label="Type your story here, for now a sample story is added already!")
-
-
-
-
- with gr.Row():
-
- button_gen_video = gr.Button("Generate Video")
-
-
-
-
-
-
-
- # Right column (outputs)
- with gr.Column():
-
-
- output_interpolation = gr.Video(label="Generated Video")
-
-
-
-
-
-
- gr.Markdown("
Future Works and Challenges
")
- gr.Markdown("Though this pipeline, isn't 100% perfect, but one can use similar system to create bite-sized videos from text resources. Effective for creating videos for educational lessons.")
- button_gen_video.click(fn=get_output_video, inputs=input_start_text, outputs=output_interpolation)
-
-demo.launch(debug=False)
\ No newline at end of file
diff --git a/spaces/Gradio-Blocks/uniformer_image_detection/configs/dcn/cascade_mask_rcnn_r101_fpn_dconv_c3-c5_1x_coco.py b/spaces/Gradio-Blocks/uniformer_image_detection/configs/dcn/cascade_mask_rcnn_r101_fpn_dconv_c3-c5_1x_coco.py
deleted file mode 100644
index 081b998f6f54d3d805dbab38b26750a378c0d93f..0000000000000000000000000000000000000000
--- a/spaces/Gradio-Blocks/uniformer_image_detection/configs/dcn/cascade_mask_rcnn_r101_fpn_dconv_c3-c5_1x_coco.py
+++ /dev/null
@@ -1,5 +0,0 @@
-_base_ = '../cascade_rcnn/cascade_mask_rcnn_r101_fpn_1x_coco.py'
-model = dict(
- backbone=dict(
- dcn=dict(type='DCN', deform_groups=1, fallback_on_stride=False),
- stage_with_dcn=(False, True, True, True)))
diff --git a/spaces/Gradio-Blocks/uniformer_image_segmentation/configs/deeplabv3/deeplabv3_r101-d8_512x512_20k_voc12aug.py b/spaces/Gradio-Blocks/uniformer_image_segmentation/configs/deeplabv3/deeplabv3_r101-d8_512x512_20k_voc12aug.py
deleted file mode 100644
index 40f5f62373e59d1c6c01ca3f57777698461127c9..0000000000000000000000000000000000000000
--- a/spaces/Gradio-Blocks/uniformer_image_segmentation/configs/deeplabv3/deeplabv3_r101-d8_512x512_20k_voc12aug.py
+++ /dev/null
@@ -1,2 +0,0 @@
-_base_ = './deeplabv3_r50-d8_512x512_20k_voc12aug.py'
-model = dict(pretrained='open-mmlab://resnet101_v1c', backbone=dict(depth=101))
diff --git a/spaces/Gradio-Blocks/uniformer_image_segmentation/configs/deeplabv3/deeplabv3_r18-d8_512x1024_80k_cityscapes.py b/spaces/Gradio-Blocks/uniformer_image_segmentation/configs/deeplabv3/deeplabv3_r18-d8_512x1024_80k_cityscapes.py
deleted file mode 100644
index e084e95c70b0b7b0c9dcc3388d6b7d3d51d54b6d..0000000000000000000000000000000000000000
--- a/spaces/Gradio-Blocks/uniformer_image_segmentation/configs/deeplabv3/deeplabv3_r18-d8_512x1024_80k_cityscapes.py
+++ /dev/null
@@ -1,9 +0,0 @@
-_base_ = './deeplabv3_r50-d8_512x1024_80k_cityscapes.py'
-model = dict(
- pretrained='open-mmlab://resnet18_v1c',
- backbone=dict(depth=18),
- decode_head=dict(
- in_channels=512,
- channels=128,
- ),
- auxiliary_head=dict(in_channels=256, channels=64))
diff --git a/spaces/HCMUT-GraduateThesis-HNTThinh/rgbdsod-multimae-demo/test_video.py b/spaces/HCMUT-GraduateThesis-HNTThinh/rgbdsod-multimae-demo/test_video.py
deleted file mode 100644
index c8d30f32f021fddac030262a5ef51283459d293a..0000000000000000000000000000000000000000
--- a/spaces/HCMUT-GraduateThesis-HNTThinh/rgbdsod-multimae-demo/test_video.py
+++ /dev/null
@@ -1,58 +0,0 @@
-import streamlit as st
-import cv2
-import tempfile
-from PIL import Image
-import os
-from stqdm import stqdm
-
-with st.spinner("Loading model ..."):
- from model import base_inference
-
-f = st.file_uploader("Upload file")
-
-if f is not None:
- with st.spinner():
- tfile = tempfile.NamedTemporaryFile(delete=False)
- tfile.write(f.read())
-
- vf = cv2.VideoCapture(tfile.name)
-
- frame_width = int(vf.get(3))
- frame_height = int(vf.get(4))
-
- size = (frame_width, frame_height)
-
- # Below VideoWriter object will create
- # a frame of above defined The output
- # is stored in 'filename.avi' file.
- tmp_file_path = 'tmp/video.avi'
- out_file_path = 'tmp/video.mp4'
-
- cap = cv2.VideoWriter(
- tmp_file_path,
- cv2.VideoWriter_fourcc('M', 'J', 'P', 'G'),
- 10, size
- )
- length = int(vf.get(cv2.CAP_PROP_FRAME_COUNT))
-
- sod_stframe = st.empty()
-
- for _ in stqdm(range(length)):
- if not vf.isOpened():
- break
-
- ret, image = vf.read()
- if not ret:
- break
-
- pred_depth, pred_sod, _ = base_inference(image, None)
- cap.write(pred_sod)
-
- vf.release()
- cap.release()
-
- os.system(f'ffmpeg -y -i {tmp_file_path} -vcodec libx264 {out_file_path} -hide_banner -loglevel error')
-
- video_file = open(out_file_path, 'rb')
- video_bytes = video_file.read()
- st.video(video_bytes, format="video/mp4")
diff --git a/spaces/HaloMaster/chinesesummary/fengshen/workspace/erlangshen-deberta-base/pretrain/README.md b/spaces/HaloMaster/chinesesummary/fengshen/workspace/erlangshen-deberta-base/pretrain/README.md
deleted file mode 100644
index 942a13a2a1a2eca8fe42ecaab03d33b16e4c0700..0000000000000000000000000000000000000000
--- a/spaces/HaloMaster/chinesesummary/fengshen/workspace/erlangshen-deberta-base/pretrain/README.md
+++ /dev/null
@@ -1,54 +0,0 @@
----
-language:
- - zh
-
-license: apache-2.0
-
-tags:
- - bert
-
-inference: true
-
-widget:
-- text: "生活的真谛是[MASK]。"
----
-# Erlangshen-Deberta-97M-Chinese,one model of [Fengshenbang-LM](https://github.com/IDEA-CCNL/Fengshenbang-LM).
-The 97 million parameter deberta-V2 base model, using 180G Chinese data, 24 A100(40G) training for 7 days,which is a encoder-only transformer structure. Consumed totally 1B samples.
-
-
-## Task Description
-
-Erlangshen-Deberta-97M-Chinese is pre-trained by bert like mask task from Deberta [paper](https://readpaper.com/paper/3033187248)
-
-
-## Usage
-```python
-from transformers import AutoModelForMaskedLM, AutoTokenizer, FillMaskPipeline
-import torch
-
-tokenizer=AutoTokenizer.from_pretrained('IDEA-CCNL/Erlangshen-DeBERTa-v2-97M-Chinese', use_fast=False)
-model=AutoModelForMaskedLM.from_pretrained('IDEA-CCNL/Erlangshen-DeBERTa-v2-97M-Chinese')
-text = '生活的真谛是[MASK]。'
-fillmask_pipe = FillMaskPipeline(model, tokenizer, device=7)
-print(fillmask_pipe(text, top_k=10))
-```
-
-## Finetune
-
-We present the dev results on some tasks.
-
-| Model | OCNLI | CMNLI |
-| ---------------------------------- | ----- | ------ |
-| RoBERTa-base | 0.743 | 0.7973 |
-| **Erlangshen-Deberta-97M-Chinese** | 0.752 | 0.807 |
-
-## Citation
-If you find the resource is useful, please cite the following website in your paper.
-```
-@misc{Fengshenbang-LM,
- title={Fengshenbang-LM},
- author={IDEA-CCNL},
- year={2022},
- howpublished={\url{https://github.com/IDEA-CCNL/Fengshenbang-LM}},
-}
-```
\ No newline at end of file
diff --git a/spaces/HarryLee/eCommerceImageCaptioning/fairseq/examples/multilingual/data_scripts/preprocess_ML50_v1.sh b/spaces/HarryLee/eCommerceImageCaptioning/fairseq/examples/multilingual/data_scripts/preprocess_ML50_v1.sh
deleted file mode 100644
index 4655936149cab212b3cfa14f306d71153729f9d7..0000000000000000000000000000000000000000
--- a/spaces/HarryLee/eCommerceImageCaptioning/fairseq/examples/multilingual/data_scripts/preprocess_ML50_v1.sh
+++ /dev/null
@@ -1,27 +0,0 @@
-#!/bin/bash
-# Copyright (c) Facebook, Inc. and its affiliates.
-# All rights reserved.
-#
-# This source code is licensed under the license found in the
-# LICENSE file in the root directory of this source tree.
-
-if [ -z $WORKDIR_ROOT ] ;
-then
- echo "please specify your working directory root in environment variable WORKDIR_ROOT. Exitting..."
- exit
-fi
-
-if [ -z $SPM_PATH ] ;
-then
- echo "Please install sentence piecence from https://github.com/google/sentencepiece and set SPM_PATH pointing to the installed spm_encode.py. Exitting..."
- exit
-fi
-
-ML50=${WORKDIR_ROOT}/ML50
-
-mkdir -p $ML50/dedup
-mkdir -p $ML50/cleaned_dedup
-
-python ./dedup_all.py --from-folder $ML50/raw --to-folder $ML50/dedup
-python ./remove_valid_test_in_train.py --from-folder $ML50/dedup --to-folder $ML50/clean
-python ./binarize.py --raw-folder $ML50/clean
\ No newline at end of file
diff --git a/spaces/Harveenchadha/Vakyansh-Hindi-TTS/ttsv/scripts/hifi/prepare_data.sh b/spaces/Harveenchadha/Vakyansh-Hindi-TTS/ttsv/scripts/hifi/prepare_data.sh
deleted file mode 100644
index d620cfeb93d8de9b2f750ad9bd52a937b0b88c33..0000000000000000000000000000000000000000
--- a/spaces/Harveenchadha/Vakyansh-Hindi-TTS/ttsv/scripts/hifi/prepare_data.sh
+++ /dev/null
@@ -1,10 +0,0 @@
-input_wav_path='/home/harveen/en/iitm_data/english/wav_22k' #give multiple folders separated by comma(,)
-gender='male'
-
-output_data_path='../../data/hifi/'$gender
-
-valid_samples=100
-test_samples=10
-
-mkdir -p $output_data_path
-python ../../utils/hifi/prepare_iitm_data_hifi.py -i $input_wav_path -v $valid_samples -t $test_samples -d $output_data_path
diff --git a/spaces/Harveenchadha/en_to_indic_translation/api.py b/spaces/Harveenchadha/en_to_indic_translation/api.py
deleted file mode 100644
index 601dd5ec161baa5d3041be111a0d83dd6f9073c3..0000000000000000000000000000000000000000
--- a/spaces/Harveenchadha/en_to_indic_translation/api.py
+++ /dev/null
@@ -1,86 +0,0 @@
-import time
-
-from fairseq import checkpoint_utils, distributed_utils, options, tasks, utils
-from inference.engine import Model
-from flask import Flask, request
-from flask import jsonify
-from flask_cors import CORS, cross_origin
-import webvtt
-from io import StringIO
-
-
-app = Flask(__name__)
-cors = CORS(app)
-app.config['CORS_HEADERS'] = 'Content-Type'
-
-indic2en_model = Model(expdir='../models/v3/indic-en')
-en2indic_model = Model(expdir='../models/v3/en-indic')
-m2m_model = Model(expdir='../models/m2m')
-
-language_dict = {
- 'Assamese': 'as',
- 'Hindi' : 'hi',
- 'Marathi' : 'mr',
- 'Tamil' : 'ta',
- 'Bengali' : 'bn',
- 'Kannada' : 'kn',
- 'Oriya' : 'or',
- 'Telugu' : 'te',
- 'Gujarati' : 'gu',
- 'Malayalam' : 'ml',
- 'Punjabi' : 'pa',
-}
-
-def get_inference_params():
- model_type = request.form['model_type']
- source_language = request.form['source_language']
- target_language = request.form['target_language']
-
- if model_type == 'indic-en':
- model = indic2en_model
- source_lang = language_dict[source_language]
- assert target_language == 'English'
- target_lang = 'en'
- elif model_type == 'en-indic':
- model = en2indic_model
- assert source_language == 'English'
- source_lang = 'en'
- target_lang = language_dict[target_language]
- elif model_type == 'm2m':
- model = m2m_model
- source_lang = language_dict[source_language]
- target_lang = language_dict[target_language]
-
- return model, source_lang, target_lang
-
-@app.route('/', methods=['GET'])
-def main():
- return "IndicTrans API"
-
-@app.route("/translate", methods=['POST'])
-@cross_origin()
-def infer_indic_en():
- model, source_lang, target_lang = get_inference_params()
- source_text = request.form['text']
-
- start_time = time.time()
- target_text = model.translate_paragraph(source_text, source_lang, target_lang)
- end_time = time.time()
- return {'text':target_text, 'duration':round(end_time-start_time, 2)}
-
-@app.route("/translate_vtt", methods=['POST'])
-@cross_origin()
-def infer_vtt_indic_en():
- model, source_lang, target_lang = get_inference_params()
- source_text = request.form['text']
- captions = webvtt.read_buffer(StringIO(source_text))
- source_sentences = [caption.text.replace('\r', '').replace('\n', ' ') for caption in captions]
-
- start_time = time.time()
- target_sentences = model.batch_translate(source_sentences, source_lang, target_lang)
- end_time = time.time()
-
- for i in range(len(target_sentences)):
- captions[i].text = target_sentences[i]
-
- return {'text': captions.content, 'duration':round(end_time-start_time, 2)}
diff --git a/spaces/Harveenchadha/en_to_indic_translation/indic_nlp_library/docs/make.bat b/spaces/Harveenchadha/en_to_indic_translation/indic_nlp_library/docs/make.bat
deleted file mode 100644
index 922152e96a04a242e6fc40f124261d74890617d8..0000000000000000000000000000000000000000
--- a/spaces/Harveenchadha/en_to_indic_translation/indic_nlp_library/docs/make.bat
+++ /dev/null
@@ -1,35 +0,0 @@
-@ECHO OFF
-
-pushd %~dp0
-
-REM Command file for Sphinx documentation
-
-if "%SPHINXBUILD%" == "" (
- set SPHINXBUILD=sphinx-build
-)
-set SOURCEDIR=.
-set BUILDDIR=_build
-
-if "%1" == "" goto help
-
-%SPHINXBUILD% >NUL 2>NUL
-if errorlevel 9009 (
- echo.
- echo.The 'sphinx-build' command was not found. Make sure you have Sphinx
- echo.installed, then set the SPHINXBUILD environment variable to point
- echo.to the full path of the 'sphinx-build' executable. Alternatively you
- echo.may add the Sphinx directory to PATH.
- echo.
- echo.If you don't have Sphinx installed, grab it from
- echo.http://sphinx-doc.org/
- exit /b 1
-)
-
-%SPHINXBUILD% -M %1 %SOURCEDIR% %BUILDDIR% %SPHINXOPTS% %O%
-goto end
-
-:help
-%SPHINXBUILD% -M help %SOURCEDIR% %BUILDDIR% %SPHINXOPTS% %O%
-
-:end
-popd
diff --git a/spaces/HighCWu/starganv2vc-paddle/starganv2vc_paddle/meldataset.py b/spaces/HighCWu/starganv2vc-paddle/starganv2vc_paddle/meldataset.py
deleted file mode 100644
index 2302da1926825afa81320643014f911c3086442b..0000000000000000000000000000000000000000
--- a/spaces/HighCWu/starganv2vc-paddle/starganv2vc_paddle/meldataset.py
+++ /dev/null
@@ -1,155 +0,0 @@
-#coding: utf-8
-
-import os
-import time
-import random
-import random
-import paddle
-import paddleaudio
-
-import numpy as np
-import soundfile as sf
-import paddle.nn.functional as F
-
-from paddle import nn
-from paddle.io import DataLoader
-
-import logging
-logger = logging.getLogger(__name__)
-logger.setLevel(logging.DEBUG)
-
-np.random.seed(1)
-random.seed(1)
-
-SPECT_PARAMS = {
- "n_fft": 2048,
- "win_length": 1200,
- "hop_length": 300
-}
-MEL_PARAMS = {
- "n_mels": 80,
- "n_fft": 2048,
- "win_length": 1200,
- "hop_length": 300
-}
-
-class MelDataset(paddle.io.Dataset):
- def __init__(self,
- data_list,
- sr=24000,
- validation=False,
- ):
-
- _data_list = [l[:-1].split('|') for l in data_list]
- self.data_list = [(path, int(label)) for path, label in _data_list]
- self.data_list_per_class = {
- target: [(path, label) for path, label in self.data_list if label == target] \
- for target in list(set([label for _, label in self.data_list]))}
-
- self.sr = sr
- self.to_melspec = paddleaudio.features.MelSpectrogram(**MEL_PARAMS)
- self.to_melspec.fbank_matrix[:] = paddle.load(os.path.dirname(__file__) + '/fbank_matrix.pd')['fbank_matrix']
-
- self.mean, self.std = -4, 4
- self.validation = validation
- self.max_mel_length = 192
-
- def __len__(self):
- return len(self.data_list)
-
- def __getitem__(self, idx):
- with paddle.fluid.dygraph.guard(paddle.CPUPlace()):
- data = self.data_list[idx]
- mel_tensor, label = self._load_data(data)
- ref_data = random.choice(self.data_list)
- ref_mel_tensor, ref_label = self._load_data(ref_data)
- ref2_data = random.choice(self.data_list_per_class[ref_label])
- ref2_mel_tensor, _ = self._load_data(ref2_data)
- return mel_tensor, label, ref_mel_tensor, ref2_mel_tensor, ref_label
-
- def _load_data(self, path):
- wave_tensor, label = self._load_tensor(path)
-
- if not self.validation: # random scale for robustness
- random_scale = 0.5 + 0.5 * np.random.random()
- wave_tensor = random_scale * wave_tensor
-
- mel_tensor = self.to_melspec(wave_tensor)
- mel_tensor = (paddle.log(1e-5 + mel_tensor) - self.mean) / self.std
- mel_length = mel_tensor.shape[1]
- if mel_length > self.max_mel_length:
- random_start = np.random.randint(0, mel_length - self.max_mel_length)
- mel_tensor = mel_tensor[:, random_start:random_start + self.max_mel_length]
-
- return mel_tensor, label
-
- def _preprocess(self, wave_tensor, ):
- mel_tensor = self.to_melspec(wave_tensor)
- mel_tensor = (paddle.log(1e-5 + mel_tensor) - self.mean) / self.std
- return mel_tensor
-
- def _load_tensor(self, data):
- wave_path, label = data
- label = int(label)
- wave, sr = sf.read(wave_path)
- wave_tensor = paddle.from_numpy(wave).astype(paddle.float32)
- return wave_tensor, label
-
-class Collater(object):
- """
- Args:
- adaptive_batch_size (bool): if true, decrease batch size when long data comes.
- """
-
- def __init__(self, return_wave=False):
- self.text_pad_index = 0
- self.return_wave = return_wave
- self.max_mel_length = 192
- self.mel_length_step = 16
- self.latent_dim = 16
-
- def __call__(self, batch):
- batch_size = len(batch)
- nmels = batch[0][0].shape[0]
- mels = paddle.zeros((batch_size, nmels, self.max_mel_length)).astype(paddle.float32)
- labels = paddle.zeros((batch_size)).astype(paddle.int64)
- ref_mels = paddle.zeros((batch_size, nmels, self.max_mel_length)).astype(paddle.float32)
- ref2_mels = paddle.zeros((batch_size, nmels, self.max_mel_length)).astype(paddle.float32)
- ref_labels = paddle.zeros((batch_size)).astype(paddle.int64)
-
- for bid, (mel, label, ref_mel, ref2_mel, ref_label) in enumerate(batch):
- mel_size = mel.shape[1]
- mels[bid, :, :mel_size] = mel
-
- ref_mel_size = ref_mel.shape[1]
- ref_mels[bid, :, :ref_mel_size] = ref_mel
-
- ref2_mel_size = ref2_mel.shape[1]
- ref2_mels[bid, :, :ref2_mel_size] = ref2_mel
-
- labels[bid] = label
- ref_labels[bid] = ref_label
-
- z_trg = paddle.randn((batch_size, self.latent_dim))
- z_trg2 = paddle.randn((batch_size, self.latent_dim))
-
- mels, ref_mels, ref2_mels = mels.unsqueeze(1), ref_mels.unsqueeze(1), ref2_mels.unsqueeze(1)
- return mels, labels, ref_mels, ref2_mels, ref_labels, z_trg, z_trg2
-
-def build_dataloader(path_list,
- validation=False,
- batch_size=4,
- num_workers=1,
- collate_config={},
- dataset_config={}):
-
- dataset = MelDataset(path_list, validation=validation)
- collate_fn = Collater(**collate_config)
- data_loader = DataLoader(dataset,
- batch_size=batch_size,
- shuffle=(not validation),
- num_workers=num_workers,
- drop_last=(not validation),
- collate_fn=collate_fn)
-
- return data_loader
diff --git a/spaces/IDEA-Research/Grounded-SAM/GroundingDINO/groundingdino/util/get_tokenlizer.py b/spaces/IDEA-Research/Grounded-SAM/GroundingDINO/groundingdino/util/get_tokenlizer.py
deleted file mode 100644
index f7dcf7e95f03f95b20546b26442a94225924618b..0000000000000000000000000000000000000000
--- a/spaces/IDEA-Research/Grounded-SAM/GroundingDINO/groundingdino/util/get_tokenlizer.py
+++ /dev/null
@@ -1,26 +0,0 @@
-from transformers import AutoTokenizer, BertModel, BertTokenizer, RobertaModel, RobertaTokenizerFast
-
-
-def get_tokenlizer(text_encoder_type):
- if not isinstance(text_encoder_type, str):
- # print("text_encoder_type is not a str")
- if hasattr(text_encoder_type, "text_encoder_type"):
- text_encoder_type = text_encoder_type.text_encoder_type
- elif text_encoder_type.get("text_encoder_type", False):
- text_encoder_type = text_encoder_type.get("text_encoder_type")
- else:
- raise ValueError(
- "Unknown type of text_encoder_type: {}".format(type(text_encoder_type))
- )
- print("final text_encoder_type: {}".format(text_encoder_type))
-
- tokenizer = AutoTokenizer.from_pretrained(text_encoder_type)
- return tokenizer
-
-
-def get_pretrained_language_model(text_encoder_type):
- if text_encoder_type == "bert-base-uncased":
- return BertModel.from_pretrained(text_encoder_type)
- if text_encoder_type == "roberta-base":
- return RobertaModel.from_pretrained(text_encoder_type)
- raise ValueError("Unknown text_encoder_type {}".format(text_encoder_type))
diff --git a/spaces/Illumotion/Koboldcpp/otherarch/gpt2_v2.cpp b/spaces/Illumotion/Koboldcpp/otherarch/gpt2_v2.cpp
deleted file mode 100644
index 33ca85e11547aee09db61e8c6d75a8703a069b2d..0000000000000000000000000000000000000000
--- a/spaces/Illumotion/Koboldcpp/otherarch/gpt2_v2.cpp
+++ /dev/null
@@ -1,653 +0,0 @@
-#include "ggml_v2.h"
-#include "otherarch.h"
-
-#include "utils.h"
-
-#include
-#include
-#include
-#include
-#include
-#include