How to Download and Install Daum PotPlayer 1.6.52515 Stable Portable (x86 X64) By SamLab for Free
-
-
Daum PotPlayer is a versatile media player that supports various formats and codecs. It has a sleek interface, advanced features and high performance. If you are looking for a free and portable media player that can run on any Windows system, you should try Daum PotPlayer 1.6.52515 Stable Portable (x86 X64) By SamLab.
-
-
This version of Daum PotPlayer is portable, which means you don't need to install it on your computer. You can simply download it and run it from a USB flash drive or any other removable device. This way, you can enjoy your favorite media files on any computer without leaving any traces behind.
Daum PotPlayer 1.6.52515 Stable Portable (x86 X64) By SamLab is also stable, which means it has been tested and verified to work without any errors or bugs. It is compatible with both 32-bit and 64-bit Windows systems, so you don't need to worry about compatibility issues.
-
-
To download and install Daum PotPlayer 1.6.52515 Stable Portable (x86 X64) By SamLab for free, follow these simple steps:
Open the folder and double-click on the file named "PotPlayerMini.exe" to launch the media player.
-
Enjoy your media files with Daum PotPlayer 1.6.52515 Stable Portable (x86 X64) By SamLab.
-
-
-
That's it! You have successfully downloaded and installed Daum PotPlayer 1.6.52515 Stable Portable (x86 X64) By SamLab for free. If you like this media player, you can also check out the official website of Daum PotPlayer for more information and updates: https://potplayer.daum.net/
-
-
Daum PotPlayer 1.6.52515 Stable Portable (x86 X64) By SamLab has many features that make it a powerful and convenient media player. Here are some of the features that you can enjoy with this media player:
-
-
-
It supports various formats and codecs, including AVI, MP4, MKV, FLV, WMV, MOV, MP3, AAC, FLAC, OGG, WMA and more.
-
It has a built-in subtitle finder that can automatically search and download subtitles for your media files.
-
It has a screen capture function that can capture screenshots or record videos of your media playback.
-
It has a 3D mode that can convert 2D videos to 3D videos or play 3D videos with various options.
-
It has a playlist function that can create and manage playlists of your media files.
-
It has a skin function that can change the appearance and theme of the media player.
-
It has a hotkey function that can assign keyboard shortcuts to various commands and functions.
-
It has a preference function that can customize the settings and options of the media player.
-
-
-
With Daum PotPlayer 1.6.52515 Stable Portable (x86 X64) By SamLab, you can enjoy your media files with high quality and convenience. It is a free and portable media player that you can take anywhere and use anytime. Download it now and see for yourself how amazing it is.
- 81aa517590
-
-
\ No newline at end of file
diff --git a/spaces/1acneusushi/gradio-2dmoleculeeditor/data/Download Enscape 3D Full Version Cracked from FileCR.md b/spaces/1acneusushi/gradio-2dmoleculeeditor/data/Download Enscape 3D Full Version Cracked from FileCR.md
deleted file mode 100644
index 6d6971cb3ead7c393cb91fc7aa8681511bfa7f0e..0000000000000000000000000000000000000000
--- a/spaces/1acneusushi/gradio-2dmoleculeeditor/data/Download Enscape 3D Full Version Cracked from FileCR.md
+++ /dev/null
@@ -1,40 +0,0 @@
-
-
Enscape Download Cracked: How to Get Enscape 3D for Free
-
Enscape 3D is a powerful and easy-to-use real-time rendering and virtual reality plugin for various CAD software such as Revit, SketchUp, Rhino, and ArchiCAD. It allows you to create stunning and realistic 3D visualizations of your projects with just one click. You can also explore your designs in immersive VR using devices such as Oculus Rift, HTC Vive, and Windows Mixed Reality.
-
However, Enscape 3D is not a free software. It requires a license to use its full features and functions. The official price of Enscape 3D is $58.99 per month or $469.00 per year for a single user. If you want to use it for multiple users or projects, you will need to pay more.
But what if you want to use Enscape 3D for free? Is there a way to download Enscape cracked version without paying anything? The answer is yes, but it comes with some risks and drawbacks. In this article, we will show you how to download Enscape cracked version from a website called FileCR, and what are the pros and cons of using it.
-
How to Download Enscape Cracked Version from FileCR
-
FileCR is a website that offers free downloads of various software, including Enscape 3D. It claims that the software is cracked, meaning that it has been modified to bypass the license verification and activation process. However, this also means that the software may not be safe or reliable, as it may contain viruses, malware, or other harmful code.
-
If you still want to download Enscape cracked version from FileCR, you can follow these steps:
-
-
Go to the FileCR website and search for Enscape 3D. You can also use this link to go directly to the download page.
-
Click on the download button and wait for the file to be downloaded on your PC. The file size is about 122 MB.
-
Extract the file using WinRAR or any other software that can unzip files.
-
Open the extracted folder and run the setup.exe file as administrator.
-
Follow the instructions on the screen to install Enscape 3D on your PC.
-
Once the installation is complete, open the crack folder and copy the patch file.
-
Paste the patch file into the installation directory of Enscape 3D (usually C:\Program Files\Enscape).
-
Run the patch file as administrator and click on the patch button.
-
Enjoy using Enscape 3D for free.
-
-
Pros and Cons of Using Enscape Cracked Version
-
Using Enscape cracked version may seem tempting, but it also has some disadvantages that you should be aware of. Here are some of the pros and cons of using Enscape cracked version:
-
-
Pros
-
-
You can use Enscape 3D for free without paying for a license.
-
You can access all the features and functions of Enscape 3D without any limitations.
-
You can create high-quality 3D renderings and VR experiences with Enscape 3D.
-
-
Cons
-
-
You may expose your PC to viruses, malware, or other harmful code that may damage your system or compromise your data.
-
You may violate the intellectual property rights of Enscape GmbH, the developer of Enscape 3D, and face legal consequences.
-
You may not receive any updates, bug fixes, or technical support from Enscape GmbH.
-
You may experience errors, crashes, or performance issues with Enscape 3D.
-
-
Conclusion
-
Enscape 3D is a great software for creating realistic 3D visualizations and VR experiences of your projects. However, it is not a free software and requires a license to use. If you want to use it for free, you
ddb901b051
-
-
\ No newline at end of file
diff --git a/spaces/1pelhydcardo/ChatGPT-prompt-generator/assets/Clash of Clans MOD APK 2022 Download and Install the Latest Version with Unlimited Everything.md b/spaces/1pelhydcardo/ChatGPT-prompt-generator/assets/Clash of Clans MOD APK 2022 Download and Install the Latest Version with Unlimited Everything.md
deleted file mode 100644
index eebaa09b85b5891410bc846159cacd96f0a1509f..0000000000000000000000000000000000000000
--- a/spaces/1pelhydcardo/ChatGPT-prompt-generator/assets/Clash of Clans MOD APK 2022 Download and Install the Latest Version with Unlimited Everything.md
+++ /dev/null
@@ -1,91 +0,0 @@
-
-
Clash of Clans Mod APK Download Unlimited Everything 2022 New Version
-
Are you a fan of strategy games that challenge your mind and skills? Do you love to build your own village and defend it from enemies? Do you enjoy joining forces with other players and competing for glory and rewards? If you answered yes to any of these questions, then you must have heard of Clash of Clans, one of the most popular and addictive games in the world. But what if we told you that you can make your gaming experience even better with Clash of Clans Mod APK, a modified version of the original game that gives you unlimited everything? Sounds too good to be true, right? Well, in this article, we will tell you everything you need to know about Clash of Clans Mod APK, how to download and install it on your Android device, and what benefits you can get from using it. So, without further ado, let's get started!
-
What is Clash of Clans?
-
A brief introduction to the game and its features
-
Clash of Clans is a freemium mobile strategy game developed and published by Supercell, a Finnish game company. The game was released for iOS devices in August 2012 and for Android devices in October 2013. Since then, it has become one of the most downloaded and played games in the world, with over 500 million downloads on Google Play alone.
-
clash of clans mod apk download unlimited everything 2022 new version
The game is set in a fantasy world where you are the chief of a village. Your main goal is to build and upgrade your village, train and upgrade your troops, and attack other players' villages to loot their resources. You can also join or create a clan with other players and participate in clan wars, clan games, and clan leagues. The game features various types of buildings, troops, spells, heroes, and items that you can use to enhance your strategy and gameplay.
-
Why do people love Clash of Clans?
-
The thrill of strategy and combat
-
One of the main reasons why people love Clash of Clans is because it offers a thrilling and satisfying experience of strategy and combat. You have to plan your attacks carefully, choosing the right troops, spells, heroes, and strategies for each situation. You also have to defend your village from enemy attacks, placing your buildings, traps, walls, and defenses wisely. The game tests your skills, creativity, and decision-making abilities in every battle.
-
The joy of building and customizing your own village
-
Another reason why people love Clash of Clans is because it allows them to build and customize their own village according to their preferences. You can choose from different themes, layouts, designs, and decorations for your village. You can also upgrade your buildings, troops, spells, heroes, and items to make them more powerful and efficient. You can express your personality and style through your village and impress your friends and foes.
-
The fun of joining and competing with other clans
-
A third reason why people love Clash of Clans is because it gives them the opportunity to join and compete with other clans from around the world. You can chat, donate, request, and share tips with your clanmates. You can also challenge them to friendly battles and practice your skills. You can also participate in clan wars, clan games, and clan leagues, where you can cooperate with your clanmates to win trophies, rewards, and glory. You can also compare your progress and achievements with other players on the global and local leaderboards.
-
What is Clash of Clans Mod APK?
-
A modified version of the original game that offers unlimited resources and features
-
Clash of Clans Mod APK is a modified version of the original game that offers unlimited resources and features that are not available in the official version. It is created by third-party developers who modify the game files to unlock and enhance the game's functionality. Clash of Clans Mod APK is not endorsed or affiliated with Supercell, the original developer of the game.
-
Clash of Clans Mod APK allows you to enjoy the game without any limitations or restrictions. You can get unlimited gems, gold, elixir, and dark elixir to upgrade your troops, buildings, and spells. You can also get unlimited access to all the heroes, troops, and spells in the game. You can also create and join any clan you want, without any requirements or limitations. You can also play the game without any ads, bans, or errors.
-
How to download and install Clash of Clans Mod APK on your Android device
-
If you want to download and install Clash of Clans Mod APK on your Android device, you need to follow these simple steps:
-
Step 1: Enable unknown sources on your device settings
-
Before you can install Clash of Clans Mod APK on your device, you need to enable unknown sources on your device settings. This will allow you to install apps that are not downloaded from the Google Play Store. To do this, go to your device settings > security > unknown sources > enable.
-
clash of clans hack apk unlimited gems gold elixir 2022
-coc mod apk download latest version 2022 with unlimited troops
-clash of clans modded apk free download for android 2022
-how to download clash of clans mod apk with unlimited resources 2022
-clash of clans cheat apk 2022 no root required
-coc hack apk 2022 online generator
-clash of clans mod menu apk download 2022
-coc mod apk 2022 private server with unlimited money
-clash of clans cracked apk 2022 working
-coc hack version download 2022 without survey
-clash of clans unlimited everything apk 2022 offline
-coc mod apk 2022 update with new features
-clash of clans hack tool apk download 2022
-coc modded apk 2022 anti ban
-clash of clans hack apk 2022 mediafire link
-coc hack apk download 2022 no human verification
-clash of clans mod apk 2022 mega mod
-coc mod apk 2022 unlimited gems and coins
-clash of clans hack apk download 2022 for pc
-coc mod apk 2022 latest version android 1
-clash of clans modded apk 2022 unlimited dark elixir
-coc hack apk 2022 direct download link
-clash of clans cheat engine apk 2022
-coc mod apk download 2022 revdl
-clash of clans hacked version download 2022 apkpure
-coc mod apk 2022 unlimited everything ihackedit
-clash of clans hack app download 2022 for ios
-coc mod apk download 2022 rexdl
-clash of clans hack version download 2022 uptodown
-coc mod apk download 2022 plenixclash
-clash of clans hack game download 2022 for laptop
-coc mod apk download 2022 fhx server
-clash of clans hack version download 2022 malavida
-coc mod apk download 2022 nulls royale
-clash of clans hack version download 2022 happymod
-coc mod apk download 2022 magic s1 s4 s5 s6 s7 s8 s9 s10 s11 s12 s13 s14 s15 s16 s17 s18 s19 s20 s21 s22 s23 s24 s25 s26 s27 s28 s29 s30
-
Step 2: Download the Clash of Clans Mod APK file from a trusted source
-
Next, you need to download the Clash of Clans Mod APK file from a trusted source. There are many websites that offer Clash of Clans Mod APK files, but not all of them are safe and reliable. Some of them may contain viruses, malware, or spyware that can harm your device or steal your personal information. Therefore, you need to be careful and choose a reputable website that provides authentic and updated Clash of Clans Mod APK files. One such website is [clashofclansmodapk.net], where you can find the latest version of Clash of Clans Mod APK for free.
-
Step 3: Locate and install the APK file on your device
-
After you have downloaded the Clash of Clans Mod APK file from a trusted source, you need to locate and install it on your device. To do this, go to your device file manager > downloads > find the Clash of Clans Mod APK file > tap on it > install.
-
Step 4: Launch the game and enjoy the unlimited everything
-
Finally, you can launch the game and enjoy the unlimited everything that Clash of Clans Mod APK offers. You will see that you have unlimited gems, gold, elixir, and dark elixir in your account. You will also see that you have access to all the heroes, troops, and spells in the game. You will also be able to create and join any clan you want. You will also be able to play the game without any ads, bans, or errors.
-
What are the benefits of using Clash of Clans Mod APK?
-
Unlimited gems, gold, elixir, and dark elixir to upgrade your troops, buildings, and spells
-
One of the main benefits of using Clash of Clans Mod APK is that you can get unlimited gems, gold, elixir, and dark elixir to upgrade your troops , buildings, and spells. These resources are essential for improving your village and army, as they allow you to unlock new levels, abilities, and features. With unlimited resources, you don't have to worry about running out of them or spending real money to buy them. You can upgrade your troops, buildings, and spells as much as you want, without any waiting time or cost.
-
Unlimited access to all the heroes, troops, and spells in the game
-
Another benefit of using Clash of Clans Mod APK is that you can get unlimited access to all the heroes, troops, and spells in the game. Heroes are powerful units that have special abilities and can be used in both offense and defense. Troops are the main units that you use to attack and defend your village. Spells are magical effects that can boost your troops, damage your enemies, or alter the battlefield. With unlimited access, you don't have to unlock them by completing certain tasks or reaching certain levels. You can use any hero, troop, or spell you want, without any limitations or restrictions.
-
Unlimited ability to create and join any clan you want
-
A third benefit of using Clash of Clans Mod APK is that you can create and join any clan you want, without any requirements or limitations. Clans are groups of players who share a common interest and goal in the game. By joining a clan, you can chat, donate, request, and share tips with your clanmates. You can also participate in clan wars, clan games, and clan leagues, where you can cooperate with your clanmates to win trophies, rewards, and glory. With unlimited ability, you don't have to meet any criteria or follow any rules to create or join a clan. You can choose any clan name, logo, description, and type you want. You can also invite or accept anyone you want to your clan.
-
Unlimited fun and excitement with no ads, no bans, and no restrictions
-
A fourth benefit of using Clash of Clans Mod APK is that you can have unlimited fun and excitement with no ads, no bans, and no restrictions. Ads are annoying pop-ups that interrupt your gameplay and try to sell you something. Bans are penalties that prevent you from playing the game for a certain period of time or permanently. Restrictions are rules that limit your actions or options in the game. With Clash of Clans Mod APK, you don't have to deal with any of these problems. You can play the game without any ads, bans, or restrictions. You can enjoy the game as much as you want, without any worries or hassles.
-
Conclusion
-
Clash of Clans is a fantastic game that offers a lot of fun and excitement for strategy game lovers. However, if you want to take your gaming experience to the next level, you should try Clash of Clans Mod APK, a modified version of the original game that gives you unlimited everything. With Clash of Clans Mod APK, you can get unlimited gems, gold, elixir, and dark elixir to upgrade your troops , buildings, and spells. You can also get unlimited access to all the heroes, troops, and spells in the game. You can also create and join any clan you want, without any requirements or limitations. You can also play the game without any ads, bans, or restrictions. You can enjoy the game as much as you want, without any worries or hassles.
-
If you are interested in downloading and installing Clash of Clans Mod APK on your Android device, you can follow the simple steps that we have explained in this article. You can also visit [clashofclansmodapk.net] to get the latest version of Clash of Clans Mod APK for free. We hope that this article has helped you understand what Clash of Clans Mod APK is, how to use it, and what benefits you can get from it. We also hope that you have fun and excitement with Clash of Clans Mod APK. Thank you for reading and happy gaming!
-
FAQs
-
Here are some frequently asked questions about Clash of Clans Mod APK:
-
Is Clash of Clans Mod APK safe to use?
-
Clash of Clans Mod APK is safe to use as long as you download it from a trusted source like [clashofclansmodapk.net]. However, you should be aware that using Clash of Clans Mod APK is against the terms and conditions of Supercell, the original developer of the game. Therefore, you should use it at your own risk and discretion.
-
Will I get banned for using Clash of Clans Mod APK?
-
There is a low chance that you will get banned for using Clash of Clans Mod APK, as the modded version has anti-ban features that prevent detection from Supercell's servers. However, there is no guarantee that you will not get banned in the future, as Supercell may update their security measures and algorithms. Therefore, you should use Clash of Clans Mod APK at your own risk and discretion.
-
Can I play Clash of Clans Mod APK with my friends who use the official version?
-
No, you cannot play Clash of Clans Mod APK with your friends who use the official version, as the modded version and the official version are not compatible with each other. You can only play Clash of Clans Mod APK with other players who use the same modded version.
-
Can I update Clash of Clans Mod APK to the latest version?
-
Yes, you can update Clash of Clans Mod APK to the latest version by visiting [clashofclansmodapk.net] and downloading the new version of the modded file. However, you should be careful not to update the game from the Google Play Store, as this will overwrite the modded version and restore the official version.
-
Can I switch back to the official version of Clash of Clans after using Clash of Clans Mod APK?
-
Yes, you can switch back to the official version of Clash of Clans after using Clash of Clans Mod APK by uninstalling the modded version and installing the official version from the Google Play Store. However, you should be aware that you will lose all your progress and data in the modded version, as they are not transferable to the official version.
197e85843d
-
-
\ No newline at end of file
diff --git a/spaces/1phancelerku/anime-remove-background/Blue Is The Colour The Ultimate Chelsea Song Download Guide.md b/spaces/1phancelerku/anime-remove-background/Blue Is The Colour The Ultimate Chelsea Song Download Guide.md
deleted file mode 100644
index abbb28b5e01c07093eb9a4b1fa97c25dfbb45aca..0000000000000000000000000000000000000000
--- a/spaces/1phancelerku/anime-remove-background/Blue Is The Colour The Ultimate Chelsea Song Download Guide.md
+++ /dev/null
@@ -1,54 +0,0 @@
-
-
Download Chelsea Song Blue Is The Colour
| | H2: Introduction |
If you are a fan of Chelsea Football Club, you might have heard of their famous anthem "Blue Is the Colour". This song is a terrace chant that has been associated with the club since 1972, when it was performed by the squad and released as a single to coincide with their appearance in the League Cup final of that year. The song has become one of the most well-known English football songs, and it is still played at every home game and any cup finals Chelsea compete in. It is also a popular song among Chelsea fans around the world, who sing it with pride and passion.
| | H2: History of the Song |
History of the Song
| | H3: Origin and Release |
Origin and Release
The song was produced by Larry Page, who commissioned Daniel Boone and lyricist David Balfe (under the pseudonym Rod McQueen) to write the song for Chelsea F.C. The song was sung by members of the squad, who included Tommy Baldwin, Stewart Houston, Charlie Cooke, John Dempsey, Ron Harris, Marvin Hinton, John Hollins, Peter Houseman, Alan Hudson, Steve Kember, Eddie McCreadie, Paddy Mulligan, Peter Osgood, David Webb and Chris Garland. The song was released on Page's label Penny Farthing Records and reached number 5 in the UK Charts and number 8 in Ireland in March 1972.
The lyrics of the song are simple but catchy, expressing the love and loyalty of Chelsea fans for their club. The chorus goes like this:
Blue is the colour, football is the game We're all together and winning is our aim So cheer us on through the sun and rain Cos Chelsea, Chelsea is our name.
The verses describe the atmosphere at Stamford Bridge, where Chelsea play their home games, and invite other fans to join them in supporting their team. The song also mentions some of the famous players who have worn the blue shirt over the years.
| | Outline | Article | | --- | --- | | H2: How to Download the Song |
How to Download the Song
| | H3: Online Sources |
Online Sources
If you want to download the Chelsea song "Blue Is the Colour" to your device, you have several options. You can find the song on various online platforms, such as Apple Music, Spotify, YouTube, and others. You can either stream the song online or download it for offline listening, depending on your preference and subscription. You can also purchase the song from iTunes or Amazon Music if you want to support the original artists and producers.
| | H3: Offline Sources |
Offline Sources
If you prefer to have a physical copy of the song, you can also look for offline sources, such as CDs, vinyls, or cassettes. You can search for the song on online marketplaces, such as eBay or Discogs, or visit your local record store or thrift shop. You might be able to find a rare or vintage edition of the song that has a special value or quality. However, you will need a compatible device to play the song, such as a CD player, a turntable, or a cassette deck.
| | H3: Tips and Tricks |
Tips and Tricks
Here are some tips and tricks to help you download and enjoy the Chelsea song "Blue Is the Colour":
Make sure you have enough storage space on your device before downloading the song.
Check the quality and format of the song before downloading it. You might want to choose a high-quality MP3 or WAV file for better sound.
Use a reliable and secure internet connection to avoid interruptions or errors during the download process.
Use headphones or speakers to enhance your listening experience and feel the atmosphere of the song.
Share the song with your friends and family who are also Chelsea fans and sing along with them.
| | H2: Conclusion |
Conclusion
"Blue Is the Colour" is more than just a song. It is a symbol of Chelsea Football Club and its fans. It is a way of expressing their identity, passion, and loyalty. It is a part of their history, culture, and tradition. It is a source of inspiration, motivation, and joy. It is a song that unites them in good times and bad times. It is a song that celebrates their achievements and aspirations. It is a song that makes them proud to be blue.
-
download chelsea anthem theme song lyrics mp3
-download chelsea blue is the colour original
-download chelsea fc anthem blue is the colour mp3 + lyrics
-download chelsea football club blue is the colour 1972
-download chelsea blue is the colour instrumental
-download chelsea blue is the colour apple music
-download chelsea blue is the colour ringtone
-download chelsea blue is the colour video
-download chelsea blue is the colour goalball
-download chelsea blue is the colour afriblinks
-how to download chelsea song blue is the colour for free
-where to download chelsea song blue is the colour online
-best site to download chelsea song blue is the colour
-download chelsea song blue is the colour youtube
-download chelsea song blue is the colour spotify
-download chelsea song blue is the colour soundcloud
-download chelsea song blue is the colour itunes
-download chelsea song blue is the colour amazon music
-download chelsea song blue is the colour deezer
-download chelsea song blue is the colour tidal
-download chelsea song blue is the colour lyrics pdf
-download chelsea song blue is the colour chords
-download chelsea song blue is the colour karaoke version
-download chelsea song blue is the colour remix
-download chelsea song blue is the colour cover
-download chelsea song blue is the colour live performance
-download chelsea song blue is the colour piano tutorial
-download chelsea song blue is the colour guitar tab
-download chelsea song blue is the colour sheet music
-download chelsea song blue is the colour midi file
-download chelsea song blue is the colour history
-download chelsea song blue is the colour meaning
-download chelsea song blue is the colour trivia
-download chelsea song blue is the colour facts
-download chelsea song blue is the colour review
-download chelsea song blue is the colour reaction
-download chelsea song blue is the colour analysis
-download chelsea song blue is the colour podcast
-download chelsea song blue is the colour blog post
-download chelsea song blue is the colour article
-download chelsea song blue is the colour news report
-download chelsea song blue is the colour wikipedia page
-download chelsea song blue is the colour quiz questions and answers
-download chelsea song blue is the colour crossword puzzle clues and solutions
-download chelsea song blue is the colour word search puzzle words and hints
-download chelsea song blue is the colour trivia game cards and rules
-download chelsea song blue is the colour bingo game cards and markers
-download chelsea song blue is the colour flashcards and study guide
-download chelsea song blue is the colour poster and wallpaper
If you are a Chelsea fan, you should definitely download this song and add it to your playlist. It will make you feel closer to your club and your fellow supporters. It will make you feel part of something bigger than yourself. It will make you feel blue is the colour.
| | H2: FAQs |
FAQs
Who wrote "Blue Is the Colour"? The song was written by Daniel Boone and David Balfe (under the pseudonym Rod McQueen) and produced by Larry Page in 1972.
Who sang "Blue Is the Colour"? The song was sung by members of the Chelsea squad in 1972, who included Tommy Baldwin, Stewart Houston, Charlie Cooke, John Dempsey, Ron Harris, Marvin Hinton, John Hollins, Peter Houseman, Alan Hudson, Steve Kember, Eddie McCreadie, Paddy Mulligan, Peter Osgood, David Webb and Chris Garland.
When was "Blue Is the Colour" released? The song was released on Page's label Penny Farthing Records in February 1972 to coincide with Chelsea's appearance in the League Cup final of that year against Stoke City.
How popular was "Blue Is the Colour"? The song reached number 5 in the UK Charts and number 8 in Ireland in March 1972. It also became popular in many other countries with local versions of the song released.
Why is "Blue Is the Colour" important for Chelsea fans? The song is important for Chelsea fans because it is their anthem that represents their love and loyalty for their club. It is also a terrace chant that creates a lively and festive atmosphere at Stamford Bridge and any cup finals Chelsea compete in.
| | Custom Message | | 197e85843d
-
-
\ No newline at end of file
diff --git a/spaces/1phancelerku/anime-remove-background/CFL Football 99 The Ultimate Canadian Gridiron Simulation.md b/spaces/1phancelerku/anime-remove-background/CFL Football 99 The Ultimate Canadian Gridiron Simulation.md
deleted file mode 100644
index 7f39a27fe88546cfa40bf051b89f97302de850c2..0000000000000000000000000000000000000000
--- a/spaces/1phancelerku/anime-remove-background/CFL Football 99 The Ultimate Canadian Gridiron Simulation.md
+++ /dev/null
@@ -1,85 +0,0 @@
-
-
CFL Football '99: The Only Video Game Based on the Canadian Football League
-
If you are a fan of Canadian football, you might have wondered why there are so few video games that feature this sport. In fact, there is only one game that is officially licensed by the Canadian Football League (CFL) and its players association: CFL Football '99. This game was developed by a small company in British Columbia and released in 1999 for Windows PCs. It is a rare and obscure title that has a cult following among some Canadian football enthusiasts. In this article, we will explore the history, gameplay, and legacy of CFL Football '99, the only video game based on the CFL.
CFL Football '99 is a gridiron football video game that simulates the rules, teams, players, and stadiums of the Canadian Football League. It is an officially licensed product of the CFL and the Canadian Football League Players Association (CFLPA). The game features all nine teams that were active in the 1998 season, as well as historical teams from previous seasons. The game also includes a full season mode, a playoff mode, a practice mode, and a custom league mode.
-
Who developed CFL Football '99?
-
CFL Football '99 was developed by David Winter, an entrepreneur from Victoria, British Columbia. Winter originally specialized in administrative and industrial applications, doing business through his private firm Wintervalley Software. He obtained the rights to the CFL brand in 1998 and launched a new company, Canadian Digital Entertainment Inc. (CDE), for the purpose of marketing CFL Football '99. Part of the game's development was outsourced to American middleware provider Phantom Reality.
-
Why is CFL Football '99 unique?
-
CFL Football '99 is unique because it is the only video game based on the CFL to date. There have been other football games that featured Canadian rules or teams, such as Tecmo Bowl or Madden NFL, but none of them had the official license or endorsement of the CFL or its players. CFL Football '99 is also unique because it is a simulation game that tries to recreate the realistic aspects of Canadian football, such as the larger field size, the 12 players per side, the three downs, and the single point for missed field goals.
-
Gameplay and Features
-
How does CFL Football '99 simulate Canadian football?
-
CFL Football '99 uses a 2D graphics engine that shows the action from a top-down perspective. The player can control any of the players on the field using the keyboard or a joystick. The game has a realistic physics system that accounts for factors such as wind, weather, fatigue, injuries, penalties, and fumbles. The game also has an advanced artificial intelligence that adjusts to the player's skill level and strategy.
-
What are the modes and options in CFL Football '99?
-
CFL Football '99 offers several modes and options for different types of players. The game has a full season mode that allows the player to choose one of the nine teams from the 1998 season and play through a 18-game schedule, followed by playoffs and the Grey Cup. The game also has a playoff mode that lets the player skip directly to the postseason and compete for the championship. The game has a practice mode that allows the player to test their skills in various drills and scenarios. The game also has a custom league mode that enables the player to create their own league with up to 16 teams, each with their own roster, logo, and stadium. The player can also edit the teams, players, and schedules to their liking.
-
How does CFL Football '99 compare to other football games?
-
CFL Football '99 is a niche game that caters to a specific audience of Canadian football fans. It is not as polished or popular as other football games, such as the Madden NFL series or the NFL 2K series, that focus on the American version of the sport. However, CFL Football '99 has some advantages over other football games, such as its authenticity, its customization options, and its historical value. CFL Football '99 is a game that celebrates the uniqueness and diversity of Canadian football and its culture.
-
Reception and Legacy
-
How did critics and players react to CFL Football '99?
-
CFL Football '99 received mixed reviews from critics and players. Some praised the game for its realism, its depth, and its originality. Others criticized the game for its outdated graphics, its bugs, and its lack of polish. The game sold poorly, partly due to its limited distribution and marketing. The game also faced competition from other football games that had more resources and exposure. CFL Football '99 was mostly appreciated by hardcore fans of Canadian football who were looking for a game that represented their sport.
-
What were the challenges and limitations of CFL Football '99?
-
CFL Football '99 was a game that faced many challenges and limitations during its development and release. The game was developed by a small team with a low budget and a tight deadline. The game had to use an existing engine that was not designed for Canadian football. The game had to deal with technical issues such as compatibility, performance, and stability. The game had to overcome legal hurdles such as obtaining the license from the CFL and the CFLPA. The game had to cope with market realities such as low demand, high piracy, and strong competition.
-
What happened to the developer and the franchise after CFL Football '99?
-
CFL Football '99 was the first and last game developed by CDE. The company went out of business shortly after the game's release, due to financial losses and legal disputes. David Winter, the founder of CDE, returned to his original business of Wintervalley Software. He later released a patch for CFL Football '99 that fixed some of the bugs and added some features. He also released a sequel called CFL 2000 that was based on the same engine but updated with new rosters and graphics. However, these projects were unofficial and unauthorized by the CFL or the CFLPA. CFL Football '99 remains the only official video game based on the CFL.
-
cfl football 99 pc game free download
-how to play cfl football 99 on windows 10
-cfl football 99 mods and patches
-canuck play maximum football 2019 cfl edition
-canadian football 2017 xbox one download
-cfl football video game history
-wintervalley software cfl football 99
-canadian digital entertainment cfl football 99
-cfl football 99 play designer tool
-cfl football 99 roster editor tool
-cfl football 99 game manual pdf
-cfl football 99 gameplay videos and screenshots
-cfl football 99 review and rating
-cfl football 99 reddit discussion and tips
-cfl football 99 vb programmers journal article
-cfl football 99 license expired
-cfl football 99 system requirements and compatibility
-cfl football 99 abandonware download site
-cfl football 99 custom teams and players
-cfl football 99 game modes and options
-cfl football 99 canadian rules and field size
-cfl football 99 american rules and field size
-cfl football 99 college rules and field size
-cfl football 99 doug flutie mode
-cfl football 99 spring league practice mode
-cfl football 99 weather effects and game play
-cfl football 99 multiple player body styles
-cfl football 99 post-play replay and camera control
-cfl football 99 online multiplayer mode
-cfl football 99 tournament action at retro's e-sports bar
-cfl football 99 feedback and updates from developers
-cfl football 99 news and media coverage page
-cfl football 99 twitter and facebook page
-canuck play other games in pre-development
-canuck play spies code breaking secret missions game
-canuck play canadian comic book super heroes game
-canuck play e for everyone rating games
-canuck play legacy titles maximum football game
-canuck play contact information and homepage link
-canuck play development blog and newsletter sign up
-
Conclusion
-
Summary of the main points
-
CFL Football '99 is a gridiron football video game that simulates the rules, teams, players, and stadiums of the Canadian Football League. It is an officially licensed product of the CFL and the CFLPA. It is a simulation game that tries to recreate the realistic aspects of Canadian football. It is a niche game that caters to a specific audience of Canadian football fans. It is a rare and obscure title that has a cult following among some Canadian football enthusiasts.
-
Call to action for the readers
-
If you are interested in playing CFL Football '99, you can download it from various websites that host old games. You might need an emulator or a compatibility mode to run it on modern computers. You can also check out some videos or reviews of the game online to see how it looks and plays. You can also join some forums or communities of Canadian football fans who still play or discuss the game. You can also share your thoughts or experiences with CFL Football '99 in the comments section below.
-
FAQs
-
-
Q: Is CFL Football '99 compatible with Windows 10?
-
A: No, CFL Football '99 is not compatible with Windows 10 or any other recent version of Windows. You might need an emulator or a compatibility mode to run it on modern computers.
-
Q: Where can I buy CFL Football '99?
-
A: You can't buy CFL Football '99 from any official source, as the game is out of print and no longer supported by the developer or the publisher. You might find some copies on online auction sites or second-hand stores, but they are very rare and expensive.
-
Q: Is there a newer version of CFL Football '99?
-
A: No, there is no newer version of CFL Football '99 that is officially licensed by the CFL or the CFLPA. The only sequel to CFL Football '99 is CFL 200 0, which was released by David Winter in 2000, but it is an unofficial and unauthorized project that uses the same engine as CFL Football '99.
-
Q: How can I play CFL Football '99 online with other players?
-
A: CFL Football '99 does not have a built-in online multiplayer mode, but you might be able to play it online with other players using third-party software or services that allow you to connect and share your screen with other users. However, this might not work well or at all, depending on your internet connection and compatibility issues.
-
Q: Are there any mods or patches for CFL Football '99?
-
A: Yes, there are some mods and patches for CFL Football '99 that add new features, fix bugs, or update the rosters and graphics. You can find some of them on websites that host old games or fan-made content. However, these mods and patches are unofficial and unauthorized by the CFL or the CFLPA, and they might not work properly or cause problems with your game.
-
197e85843d
-
-
\ No newline at end of file
diff --git a/spaces/1phancelerku/anime-remove-background/Delhi Blue App How to Use the First Ever Common Mobility App in Delhi.md b/spaces/1phancelerku/anime-remove-background/Delhi Blue App How to Use the First Ever Common Mobility App in Delhi.md
deleted file mode 100644
index 97e07dc41a4212b109174d0994d0bca6d07e1552..0000000000000000000000000000000000000000
--- a/spaces/1phancelerku/anime-remove-background/Delhi Blue App How to Use the First Ever Common Mobility App in Delhi.md
+++ /dev/null
@@ -1,101 +0,0 @@
-
-
How to Download Delhi Blue App and Why You Should Do It
-
If you are looking for a safe, reliable, and sustainable taxi service in Delhi NCR or Bengaluru, you should download Delhi Blue App on your smartphone. Delhi Blue App is India's first all-electric cab service that offers you a comfortable, convenient, and eco-friendly travel experience. In this article, we will tell you what Delhi Blue App is, how to download it on your Android or iOS device, and how to use it for your travel needs.
-
What is Delhi Blue App?
-
A brief introduction to the app and its features
-
Delhi Blue App is a mobile app that allows you to book cabs that run on electricity instead of fossil fuels. The app is developed by BluSmart, a company that aims to revolutionize the way people travel in cabs in urban India. The app has several features that make it user-friendly and convenient, such as:
Easy booking: You can book a cab in just a few taps on your phone. You can also schedule your ride in advance or request a ride later.
-
Transparent pricing: You can see the fare estimate before you confirm your booking. There are no surge prices, hidden charges, or cancellation fees.
-
Safe and secure: You can trust the drivers who are verified and trained by BluSmart. You can also share your ride details with your family and friends for extra safety.
-
Customer support: You can contact the customer care team anytime through the app or call them at +91-8880500500.
-
-
The benefits of using the app for cab booking, airport transfers, and eco-friendly travel
-
By using Delhi Blue App, you can enjoy several benefits that make your travel experience better, such as:
-
-
Comfort: You can travel in spacious, air-conditioned cabs that have free Wi-Fi, charging ports, and music system.
-
Convenience: You can book a cab anytime and anywhere in Delhi NCR or Bengaluru. You can also use the app for airport transfers to & from the IGI Airport, Delhi & Kempegowda International Airport Bengaluru.
-
Eco-friendliness: You can reduce your carbon footprint by traveling in cabs that run on clean energy. You can also contribute to BluSmart's mission of planting one tree for every ride you take.
-
-
How to Download Delhi Blue App on Your Android or iOS Device
-
The steps to download the app from Google Play or App Store
-
To download Delhi Blue App on your smartphone, you need to follow these simple steps:
-
How to download delhi blue app for free
-Download delhi blue app and get discounts on online shopping
-Delhi blue app review: why you should download it today
-Download delhi blue app and earn cashback on every purchase
-Benefits of downloading delhi blue app for your business
-Download delhi blue app and join the largest community of online shoppers
-Delhi blue app features: what you can do with it after downloading
-Download delhi blue app and access exclusive deals and offers
-Delhi blue app vs other shopping apps: which one should you download
-Download delhi blue app and save money on travel, food, entertainment, and more
-How to use delhi blue app after downloading it on your phone
-Download delhi blue app and enjoy hassle-free online shopping experience
-Delhi blue app customer support: how to contact them after downloading the app
-Download delhi blue app and get rewarded for your loyalty and referrals
-Delhi blue app testimonials: what users are saying about it after downloading
-Download delhi blue app and find the best products and services for your needs
-Delhi blue app FAQs: everything you need to know before downloading the app
-Download delhi blue app and compare prices, reviews, ratings, and more
-Delhi blue app updates: what's new in the latest version of the app
-Download delhi blue app and get personalized recommendations based on your preferences
-How to uninstall delhi blue app if you don't like it after downloading
-Download delhi blue app and participate in surveys, contests, quizzes, and more
-Delhi blue app privacy policy: how they protect your data after downloading the app
-Download delhi blue app and share your feedback and suggestions with the developers
-Delhi blue app alternatives: what other apps can you download instead of delhi blue app
-
-
Open Google Play or App Store on your device.
-
Search for "BluSmart" or "Delhi Blue App" in the search bar.
-
Tap on the app icon and then tap on "Install" (for Android) or "Get" (for iOS).
-
Wait for the app to download and install on your device.
-
-
How to sign up and create an account on the app
-
To use Delhi Blue App, you need to sign up and create an account on the app. Here's how:
-
-
Open the app on your device and tap on "Sign Up".
-
Enter your name, email address, phone number, and password.
Verify your phone number by entering the OTP sent to your number.
-
Agree to the terms and conditions and tap on "Create Account".
-
You can also sign up using your Google or Facebook account.
-
-
Congratulations, you have successfully created your account on Delhi Blue App. You can now start booking cabs and enjoy the benefits of the app.
-
How to Use Delhi Blue App for Your Travel Needs
-
How to book a cab, choose a payment method, and track your ride
-
Booking a cab on Delhi Blue App is very easy and quick. Just follow these steps:
-
-
Open the app on your device and enter your pickup and drop locations.
-
Select the type of cab you want from the available options.
-
Tap on "Book Now" or "Ride Later" depending on your preference.
-
Choose your payment method from the options of cash, card, wallet, or UPI.
-
Confirm your booking and wait for the driver to arrive at your location.
-
You can track your ride on the app and see the driver's details, cab number, and estimated time of arrival.
-
Enjoy your ride and rate your experience on the app after completing your trip.
-
-
How to get discounts, rewards, and referrals on the app
-
Delhi Blue App also offers you various discounts, rewards, and referrals that make your travel more affordable and rewarding. Here are some ways to avail them:
-
-
You can use promo codes and coupons to get discounts on your rides. You can find them on the app or on the website of BluSmart.
-
You can earn rewards points for every ride you take on the app. You can redeem them for free rides or vouchers from partner brands.
-
You can refer your friends and family to the app and get Rs. 100 off on your next ride for every successful referral. Your referrals will also get Rs. 100 off on their first ride.
-
-
Conclusion
-
A summary of the main points and a call to action
-
Delhi Blue App is a great way to travel in cabs that are safe, reliable, and eco-friendly. You can download the app on your Android or iOS device and book cabs anytime and anywhere in Delhi NCR or Bengaluru. You can also enjoy various features and benefits of the app, such as transparent pricing, customer support, comfort, convenience, and eco-friendliness. You can also get discounts, rewards, and referrals on the app that make your travel more affordable and rewarding. So what are you waiting for? Download Delhi Blue App today and join the green revolution in urban mobility.
-
FAQs
-
Five common questions and answers about the app
-
-
Q: How is Delhi Blue App different from other cab services?
-
A: Delhi Blue App is different from other cab services because it offers you cabs that run on electricity instead of fossil fuels. This makes them more eco-friendly, cost-effective, and noise-free. Delhi Blue App also has no surge pricing, hidden charges, or cancellation fees.
-
Q: How can I contact Delhi Blue App customer care?
-
A: You can contact Delhi Blue App customer care through the app or call them at +91-8880500500. You can also email them at support@blusmart.in or visit their website at www.blusmart.in.
-
Q: How can I cancel my booking on Delhi Blue App?
-
A: You can cancel your booking on Delhi Blue App anytime before the driver arrives at your location. You will not be charged any cancellation fee. To cancel your booking, tap on "Cancel" on the app and select a reason for cancellation.
-
Q: How can I pay for my ride on Delhi Blue App?
-
A: You can pay for your ride on Delhi Blue App using cash, card, wallet, or UPI. You can choose your preferred payment method before confirming your booking. You can also change your payment method after completing your trip.
-
Q: How can I give feedback or suggestions to Delhi Blue App?
-
A: You can give feedback or suggestions to Delhi Blue App by rating your ride experience on the app after completing your trip. You can also write a review or share your comments on the app or on social media platforms like Facebook, Twitter, Instagram, or LinkedIn.
- 401be4b1e0
-
-
\ No newline at end of file
diff --git a/spaces/1phancelerku/anime-remove-background/Download Mortal Kombat Tamil Dubbed Movie in HD Quality from Isaimini.md b/spaces/1phancelerku/anime-remove-background/Download Mortal Kombat Tamil Dubbed Movie in HD Quality from Isaimini.md
deleted file mode 100644
index 7f177b006fca3098e8efc45bce805ed8a3b4a27a..0000000000000000000000000000000000000000
--- a/spaces/1phancelerku/anime-remove-background/Download Mortal Kombat Tamil Dubbed Movie in HD Quality from Isaimini.md
+++ /dev/null
@@ -1,147 +0,0 @@
-
-
Mortal Kombat Tamil Dubbed Movie Download Isaimini: A Review
-
Mortal Kombat is one of the most anticipated movies of 2021, based on the popular video game series of the same name. It is a reboot of the previous film adaptations, featuring a new cast and a new storyline. The movie has been released in multiple languages, including Tamil, to cater to the diverse fan base. But how good is the movie, and how can you watch it in Tamil? In this article, we will review the Mortal Kombat Tamil dubbed movie download Isaimini option, and also give you some insights into the plot, the characters, and the quality of the movie.
-
mortal kombat tamil dubbed movie download isaimini
Mortal Kombat is a media franchise that originated from a fighting video game developed by Midway Games in 1992. The game features a variety of characters, each with their own special abilities and moves, who compete in a tournament called Mortal Kombat. The tournament is a way to determine the fate of different realms, such as Earthrealm, Outworld, and Netherrealm, which are constantly at war with each other. The game is known for its violent and graphic content, such as fatalities, brutalities, and x-rays.
-
Why is it popular in Tamil Nadu?
-
Mortal Kombat has a huge fan following in Tamil Nadu, especially among the young generation. There are several reasons for this popularity. First of all, the game has a lot of cultural references and influences from various mythologies and religions, such as Hinduism, Buddhism, Taoism, and Norse mythology. Some of the characters are inspired by gods, demons, and heroes from these traditions, such as Raiden, Shiva, Goro, and Scorpion. Secondly, the game has a lot of action and thrill, which appeals to the Tamil audience who love masala movies. Thirdly, the game has a lot of humor and sarcasm, which matches the Tamil sense of humor. Fourthly, the game has a lot of customization options, which allows the players to create their own characters and costumes.
-
How to download Mortal Kombat Tamil dubbed movie from Isaimini?
-
Isaimini is one of the most popular websites for downloading Tamil movies and songs. It offers a wide range of genres and categories, such as action, comedy, romance, horror, thriller, drama, and animation. It also provides dubbed versions of Hollywood and Bollywood movies, such as Mortal Kombat. To download Mortal Kombat Tamil dubbed movie from Isaimini, you need to follow these steps:
-
-
Go to the official website of Isaimini using a VPN or proxy service.
-
Search for Mortal Kombat in the search bar or browse through the categories.
-
Select the movie from the list of results and click on it.
-
Choose the quality and format of the movie that you want to download.
-
Click on the download link and wait for the movie to be downloaded.
-
-
Note: Downloading movies from Isaimini is illegal and may expose you to cyber risks. We do not endorse or promote piracy in any way. We recommend that you watch movies from legal sources only.
-
Plot summary
-
The main characters
-
The movie follows the lives of several characters who are chosen to participate in the Mortal Kombat tournament. They are:
-
mortal kombat 2021 tamil voice over full movie free download
-watch mortal kombat tamil dubbed online hd quality
-mortal kombat tamil audio track download for english movie
-how to download mortal kombat tamil dubbed movie from isaimini
-mortal kombat tamil dubbed movie review and rating
-mortal kombat tamil dubbed movie trailer and release date
-mortal kombat tamil dubbed movie cast and crew details
-mortal kombat tamil dubbed movie download telegram link
-mortal kombat tamil dubbed movie download in moviesda
-mortal kombat tamil dubbed movie download in tamilyogi
-mortal kombat tamil dubbed movie download in kuttymovies
-mortal kombat tamil dubbed movie download in tamilrockers
-mortal kombat tamil dubbed movie download in isaidub
-mortal kombat tamil dubbed movie download in madrasrockers
-mortal kombat tamil dubbed movie download in filmyzilla
-mortal kombat tamil dubbed movie download in filmywap
-mortal kombat tamil dubbed movie download in 9xmovies
-mortal kombat tamil dubbed movie download in worldfree4u
-mortal kombat tamil dubbed movie download in 123movies
-mortal kombat tamil dubbed movie download in movierulz
-mortal kombat tamil dubbed movie download in bolly4u
-mortal kombat tamil dubbed movie download in pagalworld
-mortal kombat tamil dubbed movie download in skymovieshd
-mortal kombat tamil dubbed movie download in mp4moviez
-mortal kombat tamil dubbed movie download in 7starhd
-mortal kombat tamil dubbed movie download 480p 720p 1080p
-mortal kombat tamil dubbed movie download hdrip dvdrip bluray
-mortal kombat tamil dubbed movie download mkv avi mp4 format
-mortal kombat tamil dubbed movie watch online dailymotion youtube
-mortal kombat tamil dubbed movie watch online with english subtitles
-mortal kombat full movie in tamil language free download
-isaimini website for downloading mortal kombat full movie in tamil
-best alternative sites to isaimini for downloading mortal kombat full movie in tamil
-how to unblock isaimini site to access mortal kombat full movie in tamil
-is it legal to download mortal kombat full movie in tamil from isaimini site
-is it safe to download mortal kombat full movie in tamil from isaimini site
-how to avoid ads and pop-ups while downloading mortal kombat full movie in tamil from isaimini site
-how to use vpn to download mortal kombat full movie in tamil from isaimini site
-how to use torrent to download mortal kombat full movie in tamil from isaimini site
-how to use idm to download mortal kombat full movie in tamil from isaimini site
-
-
Cole Young (Lewis Tan): A former MMA fighter who has a mysterious dragon mark on his chest. He is unaware of his lineage and his connection to the legendary warrior Hanzo Hasashi, also known as Scorpion (Hiroyuki Sanada).
-
Sonya Blade (Jessica McNamee): A former Special Forces officer who has been tracking down the dragon mark and the Mortal Kombat tournament. She is partnered with Jax (Mehcad Brooks), who also has the mark.
-
Kano (Josh Lawson): A mercenary and a leader of the Black Dragon crime syndicate. He has a cybernetic eye that shoots laser beams. He is captured by Sonya and forced to join her team.
-
Liu Kang (Ludi Lin): A Shaolin monk and a descendant of the great Kung Lao. He has mastered the art of fire manipulation and can summon a dragon of flames.
-
Kung Lao (Max Huang): A cousin of Liu Kang and a fellow Shaolin monk. He wields a razor-sharp hat that can cut through anything.
-
Raiden (Tadanobu Asano): The god of thunder and the protector of Earthrealm. He can teleport, manipulate lightning, and create force fields. He guides and trains the chosen fighters for the tournament.
-
Shang Tsung (Chin Han): The sorcerer and the ruler of Outworld. He can steal souls, shapeshift, and use dark magic. He is the main antagonist of the movie, who wants to conquer Earthrealm by cheating in the tournament.
-
Sub-Zero (Joe Taslim): A cryomancer and an assassin who works for Shang Tsung. He can create and manipulate ice, and is the archenemy of Scorpion. He is responsible for killing Scorpion's family and clan in the past.
-
Mileena (Sisi Stringer): A mutant hybrid of Tarkatan and Edenian races. She has sharp teeth, claws, and a taste for blood. She is loyal to Shang Tsung and serves as his enforcer.
-
Goro (voiced by Angus Sampson): A four-armed Shokan prince and a champion of Mortal Kombat. He is a formidable opponent who can crush anyone with his brute strength.
-
Reptile (voiced by Samuel Hargrave): A reptilian creature who can spit acid, turn invisible, and crawl on walls. He is one of Shang Tsung's minions who attacks the Earthrealm fighters.
-
Nitara (Mel Jarnson): A winged vampire who feeds on blood. She is another one of Shang Tsung's henchmen who faces off against Kung Lao.
-
Kabal (voiced by Damon Herriman): A former Black Dragon member who has a grudge against Kano. He wears a respirator mask and uses hooked swords. He can move at super speed and create sonic booms.
-
-
The story arc
-
The movie begins with a flashback to 17th century Japan, where Hanzo Hasashi, a ninja leader of the Shirai Ryu clan, is attacked by Bi-Han, a rival assassin of the Lin Kuei clan. Bi-Han kills Hanzo's wife and son with his ice powers, and then kills Hanzo himself. However, Hanzo's blood is collected by Raiden, who transports his body to the Netherrealm, where he becomes Scorpion, a vengeful specter.
-
In the present day, Cole Young is a struggling MMA fighter who has a dragon mark on his chest. He is targeted by Bi-Han, who now goes by Sub-Zero, and is rescued by Jax, who also has the mark. Jax tells Cole to find Sonya Blade, who knows more about the mark and the Mortal Kombat tournament. Cole meets Sonya at her hideout, where he also encounters Kano, who has been captured by Sonya. Sonya explains that the mark is a sign of being chosen to fight in Mortal Kombat, a tournament that decides the fate of different realms. She also reveals that Earthrealm has lost nine out of ten tournaments to Outworld, and if they lose one more, Outworld will invade and enslave Earthrealm.
-
Sonya, Cole, and Kano are attacked by Reptile, who is sent by Shang Tsung to kill them. They manage to defeat Reptile with Kano's help, who rips out his heart. Kano agrees to join Sonya and Cole in exchange for money, and they fly to Raiden's temple in China. There they meet Liu Kang and Kung Lao, who are also chosen fighters for Earthrealm. They also meet Raiden, who is not impressed by their lack of skills and abilities. Raiden explains that each fighter has a special power called Arcana, that they need to unlock in order to fight in the tournament. He also warns them that Shang Tsung and his warriors are trying to kill them before the tournament begins, in order to secure their victory.
-
The Earthrealm fighters begin their training under Liu Kang and Kung Lao, who teach them how to use their Arcana. Kano discovers his Arcana first, which is a laser eye. Cole, however, struggles to find his Arcana, and is constantly defeated by Kung Lao. Raiden tells Cole that he is a descendant of Hanzo Hasashi, and that he has a special destiny. He also shows him Hanzo's kunai, which is a dagger with a rope attached to it.
-
Meanwhile, Shang Tsung sends Sub-Zero, Mileena, Nitara, Kabal, and Goro to attack the temple. Raiden creates a force field to protect the temple, but Kano betrays the Earthrealm fighters and disables the field, allowing the invaders to enter. A series of battles ensue, in which Kung Lao kills Nitara with his hat, Liu Kang kills Kabal with his fire dragon, and Sonya kills Kano with a garden gnome. Cole fights Goro and unlocks his Arcana, which is a suit of armor that absorbs damage and enhances his strength. He kills Goro with Hanzo's kunai.
-
The climax and the ending
-
Shang Tsung arrives and kills Kung Lao by stealing his soul. He declares that he will kill all the Earthrealm fighters and take over their realm. Raiden intervenes and teleports the Earthrealm fighters to different locations, where they can face their enemies one-on-one. He also gives Cole Hanzo's kunai and tells him to find Scorpion in the Netherrealm.
-
Cole travels to the Netherrealm and uses Hanzo's kunai to summon Scorpion from his hellish prison. Scorpion recognizes Cole as his bloodline and agrees to help him fight Sub-Zero, who has kidnapped Cole's family. They return to Earthrealm and confront Sub-Zero in an abandoned gym. A fierce fight ensues, in which Scorpion and Cole manage to overpower Sub-Zero with their combined skills and powers. Scorpion finishes Sub-Zero with his signature move, "Get over here!", and burns him alive with his fire breath.
-
Scorpion thanks Cole for freeing him from his curse and tells him to protect his family and his realm. He then disappears into flames. Cole reunites with his family and embraces them. Raiden appears and congratulates Cole for his victory. He also warns him that Shang Tsung will return with more warriors, and that they need to prepare for the next tournament. He tells Cole to find more champions for Earthrealm, and gives him a hint by showing him a poster of Johnny Cage, a famous Hollywood actor and martial artist.
-
Cole decides to leave his MMA career and travel to Hollywood to recruit Johnny Cage. The movie ends with a shot of Johnny Cage's poster, which has his name and a slogan: "You won't believe what comes next".
-
Analysis and critique
-
The strengths of the movie
-
The movie has several strengths that make it an enjoyable and entertaining watch for Mortal Kombat fans and newcomers alike. Some of these strengths are:
-
-
The movie stays faithful to the source material, by incorporating many elements from the video games, such as the characters, the moves, the fatalities, the lore, and the Easter eggs.
-
The movie has a lot of action and gore, which are essential for a Mortal Kombat movie. The fight scenes are well-choreographed, well-shot, and well-edited, showcasing the skills and abilities of each fighter.
-
The movie has a lot of humor and fun, which balance out the seriousness and darkness of the story. The movie does not take itself too seriously, and pokes fun at some of the clichés and tropes of the genre.
-
The movie has a good cast of actors who deliver solid performances. The actors fit their roles well, and bring out the personalities and emotions of their characters.
-
The movie has a good production value, with impressive visual effects, sound design, music score, costumes, and sets. The movie creates a convincing world of Mortal Kombat, with its different realms, cultures, and creatures.
-
-
The weaknesses of the movie
-
The movie also has some weaknesses that prevent it from being a perfect adaptation of Mortal Kombat. Some of these weaknesses are:
-
-
The movie has a weak plot that lacks depth and originality. The movie follows a generic formula of a hero's journey, with a lot of exposition and clichés. The movie does not explore the themes and motivations of the characters, nor does it develop the relationships and conflicts among them. The movie also does not explain the rules and logic of the Mortal Kombat tournament, and why it is so important for the realms.
-
The movie has a rushed pacing that does not allow enough time for the characters and the story to breathe. The movie tries to cram too much information and action in a short span of time, resulting in a lack of coherence and continuity. The movie also skips over some important scenes and events, such as the actual tournament itself, and the aftermath of the battles.
-
The movie has a poor dialogue that is cheesy and corny. The movie relies on a lot of exposition and narration to explain the plot and the characters, rather than showing them through actions and interactions. The movie also uses a lot of catchphrases and one-liners that are meant to be cool and witty, but end up being cringey and awkward.
-
The movie has a mediocre direction that does not bring out the best of the actors and the script. The movie suffers from a lack of vision and style, and does not create a distinctive tone or mood for the movie. The movie also fails to balance the different elements of the movie, such as the drama, the comedy, the horror, and the fantasy.
-
The movie has a low rating that limits its potential audience and impact. The movie is rated R in the US, which means that it is restricted to viewers who are 17 years or older, or accompanied by an adult. This rating may deter some fans who are younger or more sensitive to violence and gore. The movie may also face censorship or bans in some countries or regions, where such content is deemed inappropriate or offensive.
-
-
The comparison with the original version and other adaptations
-
The movie is a reboot of the previous film adaptations of Mortal Kombat, which were released in 1995 and 1997. The movie is also based on the video game series of Mortal Kombat, which has been running since 1992. The movie differs from the original version and other adaptations in several ways. Some of these differences are:
-
-
The movie has a new cast of actors who play the roles of the characters. The movie also introduces some new characters who were not present in the original version or other adaptations, such as Cole Young, Nitara, Kabal, and Goro.
-
The movie has a new storyline that deviates from the original version or other adaptations. The movie focuses on Cole Young as the main protagonist, who is a descendant of Scorpion. The movie also changes some details and events from the original version or other adaptations, such as the origin of Sub-Zero and Scorpion's rivalry, the role of Raiden and Shang Tsung in the tournament, and the outcome of some battles.
-
The movie has a darker and grittier tone than the original version or other adaptations. The movie emphasizes more on the violence and gore of Mortal Kombat, by showing more blood, injuries, deaths, and fatalities. The movie also explores more of the dark and sinister aspects of Mortal Kombat, such as the corruption, betrayal, torture, and soul stealing.
-
The movie has a better quality than the original version or other adaptations. The movie benefits from the advances in technology and filmmaking, by having better visual effects, sound design, music score, costumes, and sets. The movie also benefits from having a higher budget and production value than the original version or other adaptations, which were criticized for being low-budget and cheesy.
-
-
Conclusion
-
The final verdict
-
Mortal Kombat is a movie that delivers what it promises: a lot of action, gore, and fun. The movie is a faithful adaptation of the video game series, and a satisfying reboot of the film franchise. The movie has a good cast, a good production value, and a good sense of humor. The movie is not perfect, however, and has some flaws in its plot, pacing, dialogue, and direction. The movie is also not for everyone, as it is rated R and may be too violent or offensive for some viewers. The movie is best enjoyed by Mortal Kombat fans and action lovers, who can appreciate the movie for what it is: a guilty pleasure.
-
The alternatives to Isaimini
-
As mentioned earlier, downloading movies from Isaimini is illegal and risky. Therefore, we suggest that you watch Mortal Kombat from legal sources only. Some of the alternatives to Isaimini are:
-
-
HBO Max: This is the official streaming platform for Mortal Kombat in the US. You can watch the movie online or offline with a subscription fee of $14.99 per month.
-
Amazon Prime Video: This is one of the most popular streaming platforms in India. You can rent or buy Mortal Kombat in Tamil or other languages with a fee ranging from ₹75 to ₹150.
-
Netflix: This is another popular streaming platform in India. You can watch Mortal Kombat in Tamil or other languages with a subscription fee starting from ₹199 per month.
-
YouTube: This is a free platform where you can watch Mortal Kombat in Tamil or other languages with ads. However, you need to be careful about the quality and legality of the videos.
-
Theaters: This is the best way to watch Mortal Kombat in Tamil or other languages on the big screen. However, you need to check the availability and safety of the theaters in your area.
-
-
The future of Mortal Kombat franchise
-
Mortal Kombat is a movie that sets up the stage for more sequels and spin-offs. The movie ends with a cliffhanger that hints at the introduction of Johnny Cage, one of the most iconic characters of Mortal Kombat. The movie also leaves some room for more characters and stories from the video game series, such as Kitana, Sindel, Shao Kahn, Quan Chi, and more. The movie has received mixed reviews from critics and audiences, but has performed well at the box office and streaming platforms. The movie has also generated a lot of buzz and hype among Mortal Kombat fans and newcomers alike. Therefore, it is likely that we will see more Mortal Kombat movies in the future, as long as there is enough demand and support from the fans.
-
FAQs
-
Here are some frequently asked questions about Mortal Kombat Tamil dubbed movie download Isaimini:
-
-
Q: Is Mortal Kombat Tamil dubbed movie available on Isaimini?
-
A: Yes, Mortal Kombat Tamil dubbed movie is available on Isaimini, but it is illegal and risky to download it from there.
-
Q: How can I watch Mortal Kombat Tamil dubbed movie legally?
-
A: You can watch Mortal Kombat Tamil dubbed movie legally from platforms such as HBO Max, Amazon Prime Video, Netflix, YouTube, or theaters.
-
Q: Who are the actors who play the roles of Mortal Kombat characters?
-
A: The actors who play the roles of Mortal Kombat characters are Lewis Tan as Cole Young/Scorpion's descendant, Hiroyuki Sanada as Hanzo Hasashi/Scorpion, Joe Taslim as Bi-Han/Sub-Zero, Jessica McNamee as Sonya Blade, Mehcad Brooks as Jax, Josh Lawson as Kano, Ludi Lin as Liu Kang, Max Huang as Kung Lao, Tadanobu Asano as Raiden, Chin Han as Shang Tsung, Sisi Stringer as Mileena, Angus Sampson as Goro, Samuel Hargrave as Reptile, Mel Jarnson as Nitara, and Damon Herriman as Kabal.
-
Q: What are the ratings and reviews of Mortal Kombat movie?
-
A: Mortal Kombat movie has a rating of 6.2 out of 10 on IMDb, 55% on Rotten Tomatoes, and 44% on Metacritic. The movie has received mixed reviews from critics and audiences, with some praising its action, humor, and fidelity to the source material, and others criticizing its plot, pacing, dialogue, and direction.
-
Q: When will Mortal Kombat 2 movie be released?
-
A: There is no official confirmation or announcement about Mortal Kombat 2 movie yet, but the director Simon McQuoid has expressed his interest and willingness to make a sequel, depending on the response and demand from the fans. The movie also sets up the stage for a sequel, by introducing Johnny Cage and teasing more characters and stories from the video game series.
-
Q: How many Mortal Kombat movies are there?
-
A: There are three Mortal Kombat movies so far. The first one is Mortal Kombat (1995), directed by Paul W.S. Anderson and starring Christopher Lambert, Robin Shou, Linden Ashby, Bridgette Wilson, and Cary-Hiroyuki Tagawa. The second one is Mortal Kombat: Annihilation (1997), directed by John R. Leonetti and starring Robin Shou, Talisa Soto, Brian Thompson, Sandra Hess, and James Remar. The third one is Mortal Kombat (2021), directed by Simon McQuoid and starring Lewis Tan, Hiroyuki Sanada, Joe Taslim, Jessica McNamee, Mehcad Brooks, Josh Lawson, Ludi Lin, Max Huang, Tadanobu Asano, Chin Han, Sisi Stringer, Angus Sampson, Samuel Hargrave, Mel Jarnson, and Damon Herriman.
- 401be4b1e0
-
-
\ No newline at end of file
diff --git a/spaces/2023Liu2023/bingo/src/components/ui/button.tsx b/spaces/2023Liu2023/bingo/src/components/ui/button.tsx
deleted file mode 100644
index 281da005124fa94c89a9a9db7605748a92b60865..0000000000000000000000000000000000000000
--- a/spaces/2023Liu2023/bingo/src/components/ui/button.tsx
+++ /dev/null
@@ -1,57 +0,0 @@
-import * as React from 'react'
-import { Slot } from '@radix-ui/react-slot'
-import { cva, type VariantProps } from 'class-variance-authority'
-
-import { cn } from '@/lib/utils'
-
-const buttonVariants = cva(
- 'inline-flex items-center justify-center rounded-md text-sm font-medium shadow ring-offset-background transition-colors outline-none disabled:pointer-events-none disabled:opacity-50',
- {
- variants: {
- variant: {
- default:
- 'bg-primary text-primary-foreground shadow-md hover:bg-primary/90',
- destructive:
- 'bg-destructive text-destructive-foreground hover:bg-destructive/90',
- outline:
- 'border border-input hover:bg-accent hover:text-accent-foreground',
- secondary:
- 'bg-secondary text-secondary-foreground hover:bg-secondary/80',
- ghost: 'shadow-none hover:bg-accent hover:text-accent-foreground',
- link: 'text-primary underline-offset-4 shadow-none hover:underline'
- },
- size: {
- default: 'h-8 px-4 py-2',
- sm: 'h-8 rounded-md px-3',
- lg: 'h-11 rounded-md px-8',
- icon: 'h-8 w-8 p-0'
- }
- },
- defaultVariants: {
- variant: 'default',
- size: 'default'
- }
- }
-)
-
-export interface ButtonProps
- extends React.ButtonHTMLAttributes,
- VariantProps {
- asChild?: boolean
-}
-
-const Button = React.forwardRef(
- ({ className, variant, size, asChild = false, ...props }, ref) => {
- const Comp = asChild ? Slot : 'button'
- return (
-
- )
- }
-)
-Button.displayName = 'Button'
-
-export { Button, buttonVariants }
diff --git a/spaces/232labs/VToonify/vtoonify/model/raft/core/datasets.py b/spaces/232labs/VToonify/vtoonify/model/raft/core/datasets.py
deleted file mode 100644
index 9991f15f4c3861c19d1a4b8766d49f83af11db70..0000000000000000000000000000000000000000
--- a/spaces/232labs/VToonify/vtoonify/model/raft/core/datasets.py
+++ /dev/null
@@ -1,235 +0,0 @@
-# Data loading based on https://github.com/NVIDIA/flownet2-pytorch
-
-import numpy as np
-import torch
-import torch.utils.data as data
-import torch.nn.functional as F
-
-import os
-import math
-import random
-from glob import glob
-import os.path as osp
-
-from model.raft.core.utils import frame_utils
-from model.raft.core.utils.augmentor import FlowAugmentor, SparseFlowAugmentor
-
-
-class FlowDataset(data.Dataset):
- def __init__(self, aug_params=None, sparse=False):
- self.augmentor = None
- self.sparse = sparse
- if aug_params is not None:
- if sparse:
- self.augmentor = SparseFlowAugmentor(**aug_params)
- else:
- self.augmentor = FlowAugmentor(**aug_params)
-
- self.is_test = False
- self.init_seed = False
- self.flow_list = []
- self.image_list = []
- self.extra_info = []
-
- def __getitem__(self, index):
-
- if self.is_test:
- img1 = frame_utils.read_gen(self.image_list[index][0])
- img2 = frame_utils.read_gen(self.image_list[index][1])
- img1 = np.array(img1).astype(np.uint8)[..., :3]
- img2 = np.array(img2).astype(np.uint8)[..., :3]
- img1 = torch.from_numpy(img1).permute(2, 0, 1).float()
- img2 = torch.from_numpy(img2).permute(2, 0, 1).float()
- return img1, img2, self.extra_info[index]
-
- if not self.init_seed:
- worker_info = torch.utils.data.get_worker_info()
- if worker_info is not None:
- torch.manual_seed(worker_info.id)
- np.random.seed(worker_info.id)
- random.seed(worker_info.id)
- self.init_seed = True
-
- index = index % len(self.image_list)
- valid = None
- if self.sparse:
- flow, valid = frame_utils.readFlowKITTI(self.flow_list[index])
- else:
- flow = frame_utils.read_gen(self.flow_list[index])
-
- img1 = frame_utils.read_gen(self.image_list[index][0])
- img2 = frame_utils.read_gen(self.image_list[index][1])
-
- flow = np.array(flow).astype(np.float32)
- img1 = np.array(img1).astype(np.uint8)
- img2 = np.array(img2).astype(np.uint8)
-
- # grayscale images
- if len(img1.shape) == 2:
- img1 = np.tile(img1[...,None], (1, 1, 3))
- img2 = np.tile(img2[...,None], (1, 1, 3))
- else:
- img1 = img1[..., :3]
- img2 = img2[..., :3]
-
- if self.augmentor is not None:
- if self.sparse:
- img1, img2, flow, valid = self.augmentor(img1, img2, flow, valid)
- else:
- img1, img2, flow = self.augmentor(img1, img2, flow)
-
- img1 = torch.from_numpy(img1).permute(2, 0, 1).float()
- img2 = torch.from_numpy(img2).permute(2, 0, 1).float()
- flow = torch.from_numpy(flow).permute(2, 0, 1).float()
-
- if valid is not None:
- valid = torch.from_numpy(valid)
- else:
- valid = (flow[0].abs() < 1000) & (flow[1].abs() < 1000)
-
- return img1, img2, flow, valid.float()
-
-
- def __rmul__(self, v):
- self.flow_list = v * self.flow_list
- self.image_list = v * self.image_list
- return self
-
- def __len__(self):
- return len(self.image_list)
-
-
-class MpiSintel(FlowDataset):
- def __init__(self, aug_params=None, split='training', root='datasets/Sintel', dstype='clean'):
- super(MpiSintel, self).__init__(aug_params)
- flow_root = osp.join(root, split, 'flow')
- image_root = osp.join(root, split, dstype)
-
- if split == 'test':
- self.is_test = True
-
- for scene in os.listdir(image_root):
- image_list = sorted(glob(osp.join(image_root, scene, '*.png')))
- for i in range(len(image_list)-1):
- self.image_list += [ [image_list[i], image_list[i+1]] ]
- self.extra_info += [ (scene, i) ] # scene and frame_id
-
- if split != 'test':
- self.flow_list += sorted(glob(osp.join(flow_root, scene, '*.flo')))
-
-
-class FlyingChairs(FlowDataset):
- def __init__(self, aug_params=None, split='train', root='datasets/FlyingChairs_release/data'):
- super(FlyingChairs, self).__init__(aug_params)
-
- images = sorted(glob(osp.join(root, '*.ppm')))
- flows = sorted(glob(osp.join(root, '*.flo')))
- assert (len(images)//2 == len(flows))
-
- split_list = np.loadtxt('chairs_split.txt', dtype=np.int32)
- for i in range(len(flows)):
- xid = split_list[i]
- if (split=='training' and xid==1) or (split=='validation' and xid==2):
- self.flow_list += [ flows[i] ]
- self.image_list += [ [images[2*i], images[2*i+1]] ]
-
-
-class FlyingThings3D(FlowDataset):
- def __init__(self, aug_params=None, root='datasets/FlyingThings3D', dstype='frames_cleanpass'):
- super(FlyingThings3D, self).__init__(aug_params)
-
- for cam in ['left']:
- for direction in ['into_future', 'into_past']:
- image_dirs = sorted(glob(osp.join(root, dstype, 'TRAIN/*/*')))
- image_dirs = sorted([osp.join(f, cam) for f in image_dirs])
-
- flow_dirs = sorted(glob(osp.join(root, 'optical_flow/TRAIN/*/*')))
- flow_dirs = sorted([osp.join(f, direction, cam) for f in flow_dirs])
-
- for idir, fdir in zip(image_dirs, flow_dirs):
- images = sorted(glob(osp.join(idir, '*.png')) )
- flows = sorted(glob(osp.join(fdir, '*.pfm')) )
- for i in range(len(flows)-1):
- if direction == 'into_future':
- self.image_list += [ [images[i], images[i+1]] ]
- self.flow_list += [ flows[i] ]
- elif direction == 'into_past':
- self.image_list += [ [images[i+1], images[i]] ]
- self.flow_list += [ flows[i+1] ]
-
-
-class KITTI(FlowDataset):
- def __init__(self, aug_params=None, split='training', root='datasets/KITTI'):
- super(KITTI, self).__init__(aug_params, sparse=True)
- if split == 'testing':
- self.is_test = True
-
- root = osp.join(root, split)
- images1 = sorted(glob(osp.join(root, 'image_2/*_10.png')))
- images2 = sorted(glob(osp.join(root, 'image_2/*_11.png')))
-
- for img1, img2 in zip(images1, images2):
- frame_id = img1.split('/')[-1]
- self.extra_info += [ [frame_id] ]
- self.image_list += [ [img1, img2] ]
-
- if split == 'training':
- self.flow_list = sorted(glob(osp.join(root, 'flow_occ/*_10.png')))
-
-
-class HD1K(FlowDataset):
- def __init__(self, aug_params=None, root='datasets/HD1k'):
- super(HD1K, self).__init__(aug_params, sparse=True)
-
- seq_ix = 0
- while 1:
- flows = sorted(glob(os.path.join(root, 'hd1k_flow_gt', 'flow_occ/%06d_*.png' % seq_ix)))
- images = sorted(glob(os.path.join(root, 'hd1k_input', 'image_2/%06d_*.png' % seq_ix)))
-
- if len(flows) == 0:
- break
-
- for i in range(len(flows)-1):
- self.flow_list += [flows[i]]
- self.image_list += [ [images[i], images[i+1]] ]
-
- seq_ix += 1
-
-
-def fetch_dataloader(args, TRAIN_DS='C+T+K+S+H'):
- """ Create the data loader for the corresponding trainign set """
-
- if args.stage == 'chairs':
- aug_params = {'crop_size': args.image_size, 'min_scale': -0.1, 'max_scale': 1.0, 'do_flip': True}
- train_dataset = FlyingChairs(aug_params, split='training')
-
- elif args.stage == 'things':
- aug_params = {'crop_size': args.image_size, 'min_scale': -0.4, 'max_scale': 0.8, 'do_flip': True}
- clean_dataset = FlyingThings3D(aug_params, dstype='frames_cleanpass')
- final_dataset = FlyingThings3D(aug_params, dstype='frames_finalpass')
- train_dataset = clean_dataset + final_dataset
-
- elif args.stage == 'sintel':
- aug_params = {'crop_size': args.image_size, 'min_scale': -0.2, 'max_scale': 0.6, 'do_flip': True}
- things = FlyingThings3D(aug_params, dstype='frames_cleanpass')
- sintel_clean = MpiSintel(aug_params, split='training', dstype='clean')
- sintel_final = MpiSintel(aug_params, split='training', dstype='final')
-
- if TRAIN_DS == 'C+T+K+S+H':
- kitti = KITTI({'crop_size': args.image_size, 'min_scale': -0.3, 'max_scale': 0.5, 'do_flip': True})
- hd1k = HD1K({'crop_size': args.image_size, 'min_scale': -0.5, 'max_scale': 0.2, 'do_flip': True})
- train_dataset = 100*sintel_clean + 100*sintel_final + 200*kitti + 5*hd1k + things
-
- elif TRAIN_DS == 'C+T+K/S':
- train_dataset = 100*sintel_clean + 100*sintel_final + things
-
- elif args.stage == 'kitti':
- aug_params = {'crop_size': args.image_size, 'min_scale': -0.2, 'max_scale': 0.4, 'do_flip': False}
- train_dataset = KITTI(aug_params, split='training')
-
- train_loader = data.DataLoader(train_dataset, batch_size=args.batch_size,
- pin_memory=False, shuffle=True, num_workers=4, drop_last=True)
-
- print('Training with %d image pairs' % len(train_dataset))
- return train_loader
-
diff --git a/spaces/4Taps/SadTalker/src/face3d/models/arcface_torch/backbones/__init__.py b/spaces/4Taps/SadTalker/src/face3d/models/arcface_torch/backbones/__init__.py
deleted file mode 100644
index 55bd4c5d1889a1a998b52eb56793bbc1eef1b691..0000000000000000000000000000000000000000
--- a/spaces/4Taps/SadTalker/src/face3d/models/arcface_torch/backbones/__init__.py
+++ /dev/null
@@ -1,25 +0,0 @@
-from .iresnet import iresnet18, iresnet34, iresnet50, iresnet100, iresnet200
-from .mobilefacenet import get_mbf
-
-
-def get_model(name, **kwargs):
- # resnet
- if name == "r18":
- return iresnet18(False, **kwargs)
- elif name == "r34":
- return iresnet34(False, **kwargs)
- elif name == "r50":
- return iresnet50(False, **kwargs)
- elif name == "r100":
- return iresnet100(False, **kwargs)
- elif name == "r200":
- return iresnet200(False, **kwargs)
- elif name == "r2060":
- from .iresnet2060 import iresnet2060
- return iresnet2060(False, **kwargs)
- elif name == "mbf":
- fp16 = kwargs.get("fp16", False)
- num_features = kwargs.get("num_features", 512)
- return get_mbf(fp16=fp16, num_features=num_features)
- else:
- raise ValueError()
\ No newline at end of file
diff --git a/spaces/52Hz/CMFNet_deblurring/model/block.py b/spaces/52Hz/CMFNet_deblurring/model/block.py
deleted file mode 100644
index 32d4d9d50d6a2c1e7251fc6551defbd605497779..0000000000000000000000000000000000000000
--- a/spaces/52Hz/CMFNet_deblurring/model/block.py
+++ /dev/null
@@ -1,146 +0,0 @@
-import torch
-import torch.nn as nn
-##########################################################################
-def conv(in_channels, out_channels, kernel_size, bias=False, stride=1):
- layer = nn.Conv2d(in_channels, out_channels, kernel_size, padding=(kernel_size // 2), bias=bias, stride=stride)
- return layer
-
-
-def conv3x3(in_chn, out_chn, bias=True):
- layer = nn.Conv2d(in_chn, out_chn, kernel_size=3, stride=1, padding=1, bias=bias)
- return layer
-
-
-def conv_down(in_chn, out_chn, bias=False):
- layer = nn.Conv2d(in_chn, out_chn, kernel_size=4, stride=2, padding=1, bias=bias)
- return layer
-
-##########################################################################
-## Supervised Attention Module (RAM)
-class SAM(nn.Module):
- def __init__(self, n_feat, kernel_size, bias):
- super(SAM, self).__init__()
- self.conv1 = conv(n_feat, n_feat, kernel_size, bias=bias)
- self.conv2 = conv(n_feat, 3, kernel_size, bias=bias)
- self.conv3 = conv(3, n_feat, kernel_size, bias=bias)
-
- def forward(self, x, x_img):
- x1 = self.conv1(x)
- img = self.conv2(x) + x_img
- x2 = torch.sigmoid(self.conv3(img))
- x1 = x1 * x2
- x1 = x1 + x
- return x1, img
-
-##########################################################################
-## Spatial Attention
-class SALayer(nn.Module):
- def __init__(self, kernel_size=7):
- super(SALayer, self).__init__()
- self.conv1 = nn.Conv2d(2, 1, kernel_size, padding=kernel_size // 2, bias=False)
- self.sigmoid = nn.Sigmoid()
-
- def forward(self, x):
- avg_out = torch.mean(x, dim=1, keepdim=True)
- max_out, _ = torch.max(x, dim=1, keepdim=True)
- y = torch.cat([avg_out, max_out], dim=1)
- y = self.conv1(y)
- y = self.sigmoid(y)
- return x * y
-
-# Spatial Attention Block (SAB)
-class SAB(nn.Module):
- def __init__(self, n_feat, kernel_size, reduction, bias, act):
- super(SAB, self).__init__()
- modules_body = [conv(n_feat, n_feat, kernel_size, bias=bias), act, conv(n_feat, n_feat, kernel_size, bias=bias)]
- self.body = nn.Sequential(*modules_body)
- self.SA = SALayer(kernel_size=7)
-
- def forward(self, x):
- res = self.body(x)
- res = self.SA(res)
- res += x
- return res
-
-##########################################################################
-## Pixel Attention
-class PALayer(nn.Module):
- def __init__(self, channel, reduction=16, bias=False):
- super(PALayer, self).__init__()
- self.pa = nn.Sequential(
- nn.Conv2d(channel, channel // reduction, 1, padding=0, bias=bias),
- nn.ReLU(inplace=True),
- nn.Conv2d(channel // reduction, channel, 1, padding=0, bias=bias), # channel <-> 1
- nn.Sigmoid()
- )
-
- def forward(self, x):
- y = self.pa(x)
- return x * y
-
-## Pixel Attention Block (PAB)
-class PAB(nn.Module):
- def __init__(self, n_feat, kernel_size, reduction, bias, act):
- super(PAB, self).__init__()
- modules_body = [conv(n_feat, n_feat, kernel_size, bias=bias), act, conv(n_feat, n_feat, kernel_size, bias=bias)]
- self.PA = PALayer(n_feat, reduction, bias=bias)
- self.body = nn.Sequential(*modules_body)
-
- def forward(self, x):
- res = self.body(x)
- res = self.PA(res)
- res += x
- return res
-
-##########################################################################
-## Channel Attention Layer
-class CALayer(nn.Module):
- def __init__(self, channel, reduction=16, bias=False):
- super(CALayer, self).__init__()
- # global average pooling: feature --> point
- self.avg_pool = nn.AdaptiveAvgPool2d(1)
- # feature channel downscale and upscale --> channel weight
- self.conv_du = nn.Sequential(
- nn.Conv2d(channel, channel // reduction, 1, padding=0, bias=bias),
- nn.ReLU(inplace=True),
- nn.Conv2d(channel // reduction, channel, 1, padding=0, bias=bias),
- nn.Sigmoid()
- )
-
- def forward(self, x):
- y = self.avg_pool(x)
- y = self.conv_du(y)
- return x * y
-
-## Channel Attention Block (CAB)
-class CAB(nn.Module):
- def __init__(self, n_feat, kernel_size, reduction, bias, act):
- super(CAB, self).__init__()
- modules_body = [conv(n_feat, n_feat, kernel_size, bias=bias), act, conv(n_feat, n_feat, kernel_size, bias=bias)]
-
- self.CA = CALayer(n_feat, reduction, bias=bias)
- self.body = nn.Sequential(*modules_body)
-
- def forward(self, x):
- res = self.body(x)
- res = self.CA(res)
- res += x
- return res
-
-
-if __name__ == "__main__":
- import time
- from thop import profile
- # layer = CAB(64, 3, 4, False, nn.PReLU())
- layer = PAB(64, 3, 4, False, nn.PReLU())
- # layer = SAB(64, 3, 4, False, nn.PReLU())
- for idx, m in enumerate(layer.modules()):
- print(idx, "-", m)
- s = time.time()
-
- rgb = torch.ones(1, 64, 256, 256, dtype=torch.float, requires_grad=False)
- out = layer(rgb)
- flops, params = profile(layer, inputs=(rgb,))
- print('parameters:', params)
- print('flops', flops)
- print('time: {:.4f}ms'.format((time.time()-s)*10))
\ No newline at end of file
diff --git a/spaces/801artistry/RVC801/i18n.py b/spaces/801artistry/RVC801/i18n.py
deleted file mode 100644
index b958c6f7244c4b920e097a9a9e67e81990d03f59..0000000000000000000000000000000000000000
--- a/spaces/801artistry/RVC801/i18n.py
+++ /dev/null
@@ -1,43 +0,0 @@
-import json
-
-def load_language_list(language):
- try:
- with open(f"./i18n/locale/{language}.json", "r", encoding="utf-8") as f:
- return json.load(f)
- except FileNotFoundError:
- raise FileNotFoundError(
- f"Failed to load language file for {language}. Check if the correct .json file exists."
- )
-
-
-class I18nAuto:
- """
- A class used for internationalization using JSON language files.
-
- Examples
- --------
- >>> i18n = I18nAuto('en_US')
- >>> i18n.print()
- Using Language: en_US
- """
- def __init__(self, language=None):
- from locale import getdefaultlocale
- language = language or getdefaultlocale()[0]
- if not self._language_exists(language):
- language = "en_US"
-
- self.language_map = load_language_list(language)
- self.language = language
-
- @staticmethod
- def _language_exists(language):
- from os.path import exists
- return exists(f"./i18n/locale/{language}.json")
-
- def __call__(self, key):
- """Returns the translation of the given key if it exists, else returns the key itself."""
- return self.language_map.get(key, key)
-
- def print(self):
- """Prints the language currently in use."""
- print(f"Using Language: {self.language}")
\ No newline at end of file
diff --git a/spaces/A-Celsius/ADR_Predictor/app.py b/spaces/A-Celsius/ADR_Predictor/app.py
deleted file mode 100644
index 9722321ceb658c219888fec17b0b5b1f31f93a1f..0000000000000000000000000000000000000000
--- a/spaces/A-Celsius/ADR_Predictor/app.py
+++ /dev/null
@@ -1,103 +0,0 @@
-import pickle, joblib
-import gradio as gr
-from datetime import datetime, timedelta, timezone
-
-model = joblib.load('model.pkl')
-
-def preprocess_city(selected_city):
- # Map the selected city to its one-hot encoded representation
- city_mapping = {
- 'Hyderabad' : [1, 0, 0, 0, 0, 0, 0],
- 'Indore': [1, 0, 0, 0, 0, 0, 0],
- 'Jaipur': [0, 1, 0, 0, 0, 0, 0],
- 'Mahabaleshwar': [0, 0, 1, 0, 0, 0, 0],
- 'Mussoorie': [0, 0, 0, 1, 0, 0, 0],
- 'Raipur': [0, 0, 0, 0, 1, 0, 0],
- 'Udaipur': [0, 0, 0, 0, 0, 1, 0],
- 'Varanasi': [0, 0, 0, 0, 0, 0, 1]
- }
- return city_mapping[selected_city]
-
-def preprocess_date(date_string):
- # Parse the date string into a datetime object
- date_obj = datetime.strptime(date_string, '%Y-%m-%d')
- year = date_obj.year
- month = date_obj.month
- day = date_obj.day
- return year, month, day
-
-def calculate_lead_time(checkin_date):
- # Convert input date to datetime object
- input_date = datetime.strptime(checkin_date, '%Y-%m-%d')
-
- # Get current date and time in GMT+5:30 timezone
- current_date = datetime.now(timezone(timedelta(hours=5, minutes=30)))
-
- # Make current_date an aware datetime with the same timezone
- current_date = current_date.replace(tzinfo=input_date.tzinfo)
-
- # Calculate lead time as difference in days
- lead_time = (input_date - current_date).days
-
- return lead_time
-
-def is_weekend(checkin_date):
- # Convert input date to datetime object
- input_date = datetime.strptime(checkin_date, '%Y-%m-%d')
-
- # Calculate the day of the week (0=Monday, 6=Sunday)
- day_of_week = input_date.weekday()
-
- # Check if the day is Friday (4) or Saturday (5)
- return 1 if day_of_week == 4 or day_of_week == 5 else 0
-
-def predict(selected_city, checkin_date, star_rating, text_rating, season, additional_views, room_category):
- # Preprocess user input
- # Here, selected_city is the name of the city selected from the dropdown
- # checkin_date is the date selected using the text input
- # star_rating is the selected star rating from the dropdown
- # text_rating is the numeric rating from the text box
- # season is the selected option from the radio button (On Season or Off Season)
- season_binary = 1 if season == 'On Season' else 0
- # additional_views is the selected option from the radio button (Yes or No)
- additional_views_binary = 1 if additional_views == 'Yes' else 0
-
- room_categories = ["Dorm", "Standard", "Deluxe", "Executive", "Suite"]
- room_category_number = room_categories.index(room_category)
-
- # Preprocess the date
- year, month, day = preprocess_date(checkin_date)
-
- # Preprocess the selected city
- city_encoded = preprocess_city(selected_city)
-
- # Calculate lead time
- lead_time = calculate_lead_time(checkin_date)
-
- # Calculate if the input date is a weekend (1) or weekday (0)
- is_weekend_value = is_weekend(checkin_date)
-
- # Combine all the input features
- input_data = [star_rating, text_rating, season_binary, day, month, year, is_weekend_value, lead_time,room_category_number, additional_views_binary]+city_encoded
-
- # Make predictions using the model
- prediction = model.predict([input_data])
- return "{:.2f}".format(prediction[0])
-
-# Define input components
-city_dropdown = gr.components.Dropdown(choices=['Hyderabad', 'Indore', 'Jaipur', 'Mahabaleshwar', 'Mussoorie', 'Raipur', 'Udaipur', 'Varanasi'], label='Select a City')
-date_input = gr.components.Textbox(label='Check-in Date (YYYY-MM-DD)')
-star_rating_dropdown = gr.components.Dropdown(choices=[1, 2, 3, 4, 5], label='Select Star Rating')
-text_rating_input = gr.components.Number(label='Enter Numeric Rating (1-5)')
-season_radio = gr.components.Radio(['On Season', 'Off Season'], label='Season')
-room_category_dropdown = gr.components.Dropdown(choices=["Dorm", "Standard", "Deluxe", "Executive", "Suite"], label='Select Room Category')
-additional_views_radio = gr.components.Radio(['Yes', 'No'], label='Additional Views')
-
-# Define output component
-output = gr.components.Textbox(label='Predicted Output')
-# Create the interface
-interface = gr.Interface(fn=predict, inputs=[city_dropdown, date_input, star_rating_dropdown, text_rating_input, season_radio, additional_views_radio, room_category_dropdown], outputs=output, title='Model Prediction Interface')
-
-# Launch the interface
-interface.launch()
-
diff --git a/spaces/A00001/bingothoo/cloudflare/worker.js b/spaces/A00001/bingothoo/cloudflare/worker.js
deleted file mode 100644
index e0debd750615f1329b2c72fbce73e1b9291f7137..0000000000000000000000000000000000000000
--- a/spaces/A00001/bingothoo/cloudflare/worker.js
+++ /dev/null
@@ -1,18 +0,0 @@
-const TRAGET_HOST='hf4all-bingo.hf.space' // 请将此域名改成你自己的,域名信息在设置》站点域名查看。
-
-export default {
- async fetch(request) {
- const uri = new URL(request.url);
- if (uri.protocol === 'http:') {
- uri.protocol = 'https:';
- return new Response('', {
- status: 301,
- headers: {
- location: uri.toString(),
- },
- })
- }
- uri.host = TRAGET_HOST
- return fetch(new Request(uri.toString(), request));
- },
-};
diff --git a/spaces/AI-Hobbyist/Hoyo-RVC/uvr5_pack/lib_v5/layers_123821KB.py b/spaces/AI-Hobbyist/Hoyo-RVC/uvr5_pack/lib_v5/layers_123821KB.py
deleted file mode 100644
index 9835dc0f0dd66a7ef3517101180ec2c54eb6011d..0000000000000000000000000000000000000000
--- a/spaces/AI-Hobbyist/Hoyo-RVC/uvr5_pack/lib_v5/layers_123821KB.py
+++ /dev/null
@@ -1,118 +0,0 @@
-import torch
-from torch import nn
-import torch.nn.functional as F
-
-from uvr5_pack.lib_v5 import spec_utils
-
-
-class Conv2DBNActiv(nn.Module):
- def __init__(self, nin, nout, ksize=3, stride=1, pad=1, dilation=1, activ=nn.ReLU):
- super(Conv2DBNActiv, self).__init__()
- self.conv = nn.Sequential(
- nn.Conv2d(
- nin,
- nout,
- kernel_size=ksize,
- stride=stride,
- padding=pad,
- dilation=dilation,
- bias=False,
- ),
- nn.BatchNorm2d(nout),
- activ(),
- )
-
- def __call__(self, x):
- return self.conv(x)
-
-
-class SeperableConv2DBNActiv(nn.Module):
- def __init__(self, nin, nout, ksize=3, stride=1, pad=1, dilation=1, activ=nn.ReLU):
- super(SeperableConv2DBNActiv, self).__init__()
- self.conv = nn.Sequential(
- nn.Conv2d(
- nin,
- nin,
- kernel_size=ksize,
- stride=stride,
- padding=pad,
- dilation=dilation,
- groups=nin,
- bias=False,
- ),
- nn.Conv2d(nin, nout, kernel_size=1, bias=False),
- nn.BatchNorm2d(nout),
- activ(),
- )
-
- def __call__(self, x):
- return self.conv(x)
-
-
-class Encoder(nn.Module):
- def __init__(self, nin, nout, ksize=3, stride=1, pad=1, activ=nn.LeakyReLU):
- super(Encoder, self).__init__()
- self.conv1 = Conv2DBNActiv(nin, nout, ksize, 1, pad, activ=activ)
- self.conv2 = Conv2DBNActiv(nout, nout, ksize, stride, pad, activ=activ)
-
- def __call__(self, x):
- skip = self.conv1(x)
- h = self.conv2(skip)
-
- return h, skip
-
-
-class Decoder(nn.Module):
- def __init__(
- self, nin, nout, ksize=3, stride=1, pad=1, activ=nn.ReLU, dropout=False
- ):
- super(Decoder, self).__init__()
- self.conv = Conv2DBNActiv(nin, nout, ksize, 1, pad, activ=activ)
- self.dropout = nn.Dropout2d(0.1) if dropout else None
-
- def __call__(self, x, skip=None):
- x = F.interpolate(x, scale_factor=2, mode="bilinear", align_corners=True)
- if skip is not None:
- skip = spec_utils.crop_center(skip, x)
- x = torch.cat([x, skip], dim=1)
- h = self.conv(x)
-
- if self.dropout is not None:
- h = self.dropout(h)
-
- return h
-
-
-class ASPPModule(nn.Module):
- def __init__(self, nin, nout, dilations=(4, 8, 16), activ=nn.ReLU):
- super(ASPPModule, self).__init__()
- self.conv1 = nn.Sequential(
- nn.AdaptiveAvgPool2d((1, None)),
- Conv2DBNActiv(nin, nin, 1, 1, 0, activ=activ),
- )
- self.conv2 = Conv2DBNActiv(nin, nin, 1, 1, 0, activ=activ)
- self.conv3 = SeperableConv2DBNActiv(
- nin, nin, 3, 1, dilations[0], dilations[0], activ=activ
- )
- self.conv4 = SeperableConv2DBNActiv(
- nin, nin, 3, 1, dilations[1], dilations[1], activ=activ
- )
- self.conv5 = SeperableConv2DBNActiv(
- nin, nin, 3, 1, dilations[2], dilations[2], activ=activ
- )
- self.bottleneck = nn.Sequential(
- Conv2DBNActiv(nin * 5, nout, 1, 1, 0, activ=activ), nn.Dropout2d(0.1)
- )
-
- def forward(self, x):
- _, _, h, w = x.size()
- feat1 = F.interpolate(
- self.conv1(x), size=(h, w), mode="bilinear", align_corners=True
- )
- feat2 = self.conv2(x)
- feat3 = self.conv3(x)
- feat4 = self.conv4(x)
- feat5 = self.conv5(x)
- out = torch.cat((feat1, feat2, feat3, feat4, feat5), dim=1)
- bottle = self.bottleneck(out)
- return bottle
diff --git a/spaces/AIFILMS/generate_human_motion/VQ-Trans/utils/losses.py b/spaces/AIFILMS/generate_human_motion/VQ-Trans/utils/losses.py
deleted file mode 100644
index 1998161032731fc2c3edae701700679c00fd00d0..0000000000000000000000000000000000000000
--- a/spaces/AIFILMS/generate_human_motion/VQ-Trans/utils/losses.py
+++ /dev/null
@@ -1,30 +0,0 @@
-import torch
-import torch.nn as nn
-
-class ReConsLoss(nn.Module):
- def __init__(self, recons_loss, nb_joints):
- super(ReConsLoss, self).__init__()
-
- if recons_loss == 'l1':
- self.Loss = torch.nn.L1Loss()
- elif recons_loss == 'l2' :
- self.Loss = torch.nn.MSELoss()
- elif recons_loss == 'l1_smooth' :
- self.Loss = torch.nn.SmoothL1Loss()
-
- # 4 global motion associated to root
- # 12 local motion (3 local xyz, 3 vel xyz, 6 rot6d)
- # 3 global vel xyz
- # 4 foot contact
- self.nb_joints = nb_joints
- self.motion_dim = (nb_joints - 1) * 12 + 4 + 3 + 4
-
- def forward(self, motion_pred, motion_gt) :
- loss = self.Loss(motion_pred[..., : self.motion_dim], motion_gt[..., :self.motion_dim])
- return loss
-
- def forward_vel(self, motion_pred, motion_gt) :
- loss = self.Loss(motion_pred[..., 4 : (self.nb_joints - 1) * 3 + 4], motion_gt[..., 4 : (self.nb_joints - 1) * 3 + 4])
- return loss
-
-
\ No newline at end of file
diff --git a/spaces/AIGC-Audio/AudioGPT/NeuralSeq/modules/commons/wavenet.py b/spaces/AIGC-Audio/AudioGPT/NeuralSeq/modules/commons/wavenet.py
deleted file mode 100644
index 7809c9b9d3331ba4fd2ffd4caae14e721e4b0732..0000000000000000000000000000000000000000
--- a/spaces/AIGC-Audio/AudioGPT/NeuralSeq/modules/commons/wavenet.py
+++ /dev/null
@@ -1,97 +0,0 @@
-import torch
-from torch import nn
-
-
-def fused_add_tanh_sigmoid_multiply(input_a, input_b, n_channels):
- n_channels_int = n_channels[0]
- in_act = input_a + input_b
- t_act = torch.tanh(in_act[:, :n_channels_int, :])
- s_act = torch.sigmoid(in_act[:, n_channels_int:, :])
- acts = t_act * s_act
- return acts
-
-
-class WN(torch.nn.Module):
- def __init__(self, hidden_size, kernel_size, dilation_rate, n_layers, c_cond=0,
- p_dropout=0, share_cond_layers=False, is_BTC=False):
- super(WN, self).__init__()
- assert (kernel_size % 2 == 1)
- assert (hidden_size % 2 == 0)
- self.is_BTC = is_BTC
- self.hidden_size = hidden_size
- self.kernel_size = kernel_size
- self.dilation_rate = dilation_rate
- self.n_layers = n_layers
- self.gin_channels = c_cond
- self.p_dropout = p_dropout
- self.share_cond_layers = share_cond_layers
-
- self.in_layers = torch.nn.ModuleList()
- self.res_skip_layers = torch.nn.ModuleList()
- self.drop = nn.Dropout(p_dropout)
-
- if c_cond != 0 and not share_cond_layers:
- cond_layer = torch.nn.Conv1d(c_cond, 2 * hidden_size * n_layers, 1)
- self.cond_layer = torch.nn.utils.weight_norm(cond_layer, name='weight')
-
- for i in range(n_layers):
- dilation = dilation_rate ** i
- padding = int((kernel_size * dilation - dilation) / 2)
- in_layer = torch.nn.Conv1d(hidden_size, 2 * hidden_size, kernel_size,
- dilation=dilation, padding=padding)
- in_layer = torch.nn.utils.weight_norm(in_layer, name='weight')
- self.in_layers.append(in_layer)
-
- # last one is not necessary
- if i < n_layers - 1:
- res_skip_channels = 2 * hidden_size
- else:
- res_skip_channels = hidden_size
-
- res_skip_layer = torch.nn.Conv1d(hidden_size, res_skip_channels, 1)
- res_skip_layer = torch.nn.utils.weight_norm(res_skip_layer, name='weight')
- self.res_skip_layers.append(res_skip_layer)
-
- def forward(self, x, nonpadding=None, cond=None):
- if self.is_BTC:
- x = x.transpose(1, 2)
- cond = cond.transpose(1, 2) if cond is not None else None
- nonpadding = nonpadding.transpose(1, 2) if nonpadding is not None else None
- if nonpadding is None:
- nonpadding = 1
- output = torch.zeros_like(x)
- n_channels_tensor = torch.IntTensor([self.hidden_size])
-
- if cond is not None and not self.share_cond_layers:
- cond = self.cond_layer(cond)
-
- for i in range(self.n_layers):
- x_in = self.in_layers[i](x)
- x_in = self.drop(x_in)
- if cond is not None:
- cond_offset = i * 2 * self.hidden_size
- cond_l = cond[:, cond_offset:cond_offset + 2 * self.hidden_size, :]
- else:
- cond_l = torch.zeros_like(x_in)
-
- acts = fused_add_tanh_sigmoid_multiply(x_in, cond_l, n_channels_tensor)
-
- res_skip_acts = self.res_skip_layers[i](acts)
- if i < self.n_layers - 1:
- x = (x + res_skip_acts[:, :self.hidden_size, :]) * nonpadding
- output = output + res_skip_acts[:, self.hidden_size:, :]
- else:
- output = output + res_skip_acts
- output = output * nonpadding
- if self.is_BTC:
- output = output.transpose(1, 2)
- return output
-
- def remove_weight_norm(self):
- def remove_weight_norm(m):
- try:
- nn.utils.remove_weight_norm(m)
- except ValueError: # this module didn't have weight norm
- return
-
- self.apply(remove_weight_norm)
diff --git a/spaces/AILab-CVC/SEED-LLaMA/scripts/start_frontend_14b.sh b/spaces/AILab-CVC/SEED-LLaMA/scripts/start_frontend_14b.sh
deleted file mode 100644
index 6b865e19756e2c72fb081b9122596a669b98df67..0000000000000000000000000000000000000000
--- a/spaces/AILab-CVC/SEED-LLaMA/scripts/start_frontend_14b.sh
+++ /dev/null
@@ -1 +0,0 @@
-python3 gradio_demo/seed_llama_gradio.py --server_port 80 --request_address http://127.0.0.1:7890/generate --model_type seed-llama-14b
\ No newline at end of file
diff --git a/spaces/AIWaves/SOP_Generation-single/Component/PromptComponent.py b/spaces/AIWaves/SOP_Generation-single/Component/PromptComponent.py
deleted file mode 100644
index 0f61d4012384f39f9071e8fc5c9b269ce5047b3f..0000000000000000000000000000000000000000
--- a/spaces/AIWaves/SOP_Generation-single/Component/PromptComponent.py
+++ /dev/null
@@ -1,126 +0,0 @@
-from abc import abstractmethod
-
-
-class PromptComponent:
- def __init__(self):
- pass
-
- @abstractmethod
- def get_prompt(self, agent):
- pass
-
-class TaskComponent(PromptComponent):
- def __init__(self, task):
- super().__init__()
- self.task = task
-
- def get_prompt(self, agent):
- return f"""The task you need to execute is: {self.task}.\n"""
-
-
-class OutputComponent(PromptComponent):
- def __init__(self, output):
- super().__init__()
- self.output = output
-
- def get_prompt(self, agent):
- return f"""Please contact the above to extract <{self.output}> and {self.output}>, \
- do not perform additional output, please output in strict accordance with the above format!\n"""
-
-
-class SystemComponent(PromptComponent):
- def __init__(self,system_prompt):
- super().__init__()
- self.system_prompt = system_prompt
-
- def get_prompt(self, agent):
- return self.system_prompt
-
-class LastComponent(PromptComponent):
- def __init__(self, last_prompt):
- super().__init__()
- self.last_prompt = last_prompt
-
- def get_prompt(self, agent):
- return self.last_prompt
-
-
-class StyleComponent(PromptComponent):
- """
- 角色、风格组件
- """
-
- def __init__(self, role):
- super().__init__()
- self.role = role
-
- def get_prompt(self, agent):
- name = agent.name
- style = agent.style
- return f"""Now your role is:\n{self.role}, your name is:\n{name}. \
- You need to follow the output style:\n{style}.\n"""
-
-
-class RuleComponent(PromptComponent):
- def __init__(self, rule):
- super().__init__()
- self.rule = rule
-
- def get_prompt(self, agent):
- return f"""The rule you need to follow is:\n{self.rule}.\n"""
-
-
-class DemonstrationComponent(PromptComponent):
- """
- input a list,the example of answer.
- """
-
- def __init__(self, demonstrations):
- super().__init__()
- self.demonstrations = demonstrations
-
-
- def get_prompt(self, agent):
- prompt = f"Here are demonstrations you can refer to:\n{self.demonstrations}"
- return prompt
-
-
-class CoTComponent(PromptComponent):
- """
- input a list,the example of answer.
- """
-
- def __init__(self, demonstrations):
- super().__init__()
- self.demonstrations = demonstrations
-
- def add_demonstration(self, demonstration):
- self.demonstrations.append(demonstration)
-
- def get_prompt(self, agent):
- prompt = "You need to think in detail before outputting, the thinking case is as follows:\n"
- for demonstration in self.demonstrations:
- prompt += "\n" + demonstration
- return prompt
-
-
-class CustomizeComponent(PromptComponent):
- """
- Custom template
- template(str) : example: "i am {}"
- keywords(list) : example : ["name"]
- example : agent.environment.shared_memory["name"] = "Lilong"
- the component will get the keyword attribute from the environment, and then add it to the template.
- Return : "i am Lilong"
- """
- def __init__(self, template, keywords) -> None:
- super().__init__()
- self.template = template
- self.keywords = keywords
-
- def get_prompt(self, agent):
- template_keyword = {}
- for keyword in self.keywords:
- current_keyword = agent.environment.shared_memory[keyword] if keyword in agent.environment.shared_memory else ""
- template_keyword[keyword] = current_keyword
- return self.template.format(**template_keyword)
\ No newline at end of file
diff --git a/spaces/AchyuthGamer/OpenGPT/g4f/Provider/unfinished/Komo.py b/spaces/AchyuthGamer/OpenGPT/g4f/Provider/unfinished/Komo.py
deleted file mode 100644
index 84d8d634bc65cdbe265f28aae925456b694e329b..0000000000000000000000000000000000000000
--- a/spaces/AchyuthGamer/OpenGPT/g4f/Provider/unfinished/Komo.py
+++ /dev/null
@@ -1,44 +0,0 @@
-from __future__ import annotations
-
-import json
-
-from ...requests import StreamSession
-from ...typing import AsyncGenerator
-from ..base_provider import AsyncGeneratorProvider, format_prompt
-
-class Komo(AsyncGeneratorProvider):
- url = "https://komo.ai/api/ask"
- supports_gpt_35_turbo = True
-
- @classmethod
- async def create_async_generator(
- cls,
- model: str,
- messages: list[dict[str, str]],
- **kwargs
- ) -> AsyncGenerator:
- async with StreamSession(impersonate="chrome107") as session:
- prompt = format_prompt(messages)
- data = {
- "query": prompt,
- "FLAG_URLEXTRACT": "false",
- "token": "",
- "FLAG_MODELA": "1",
- }
- headers = {
- 'authority': 'komo.ai',
- 'accept': 'text/event-stream',
- 'cache-control': 'no-cache',
- 'referer': 'https://komo.ai/',
- }
-
- async with session.get(cls.url, params=data, headers=headers) as response:
- response.raise_for_status()
- next = False
- async for line in response.iter_lines():
- if line == b"event: line":
- next = True
- elif next and line.startswith(b"data: "):
- yield json.loads(line[6:])
- next = False
-
diff --git a/spaces/AgentVerse/agentVerse/scripts/evaluate_math.py b/spaces/AgentVerse/agentVerse/scripts/evaluate_math.py
deleted file mode 100644
index 189c05a5db7ae3dce325511912dd8294ce5f2a2f..0000000000000000000000000000000000000000
--- a/spaces/AgentVerse/agentVerse/scripts/evaluate_math.py
+++ /dev/null
@@ -1,93 +0,0 @@
-import re
-import json
-import subprocess
-from importlib import reload
-from argparse import ArgumentParser
-
-parser = ArgumentParser()
-parser.add_argument("--path", type=str, required=True)
-parser.add_argument("--max_line", type=int, default=1000000000000)
-parser.add_argument("--ci_smoke_test", action="store_true")
-args = parser.parse_args()
-
-
-def check_corr(result: str, correct_solution: str, tol: float = 1e-3):
- result = result.replace(",", "")
- if result.strip() == correct_solution.strip():
- return 1
- try:
- result = float(result.strip())
- correct_solution = float(correct_solution.strip())
- return abs(result - correct_solution) < tol
- except:
- return 0
-
-
-# final_accs = []
-# for i in range(2):
-# acc = 0
-# total = 0
-# with open(args.path) as f:
-# for line in f:
-# line = json.loads(line)
-# label = str(line["label"])
-# if i == 0:
-# code = line["response"]
-# else:
-# code = line["logs"][0]["content"]
-# total += 1
-# code = code.strip().replace("```", "")
-# code = code.lstrip("python3")
-# code = code.lstrip("python")
-# with open("tmp.py", "w") as f:
-# f.write(code)
-
-# try:
-# import tmp
-
-# reload(tmp)
-# result = str(tmp.solution())
-# is_corr = check_corr(result, label)
-
-# is_corr = int(is_corr)
-# # Step 2
-# if is_corr:
-# acc += 1
-# except:
-# print(code)
-# final_accs.append(acc / total)
-# print(final_accs)
-
-final_accs = []
-err_cnts = []
-for i in range(2):
- acc = 0
- total = 0
- err_cnt = 0
- with open(args.path) as f:
- for idx, line in enumerate(f):
- if idx == args.max_line:
- break
- line = json.loads(line)
- label = str(line["label"])
- if i == 0:
- response = line["response"]
- else:
- if line["logs"][0]["module"] == "Role Assigner":
- response = line["logs"][1]["content"]
- else:
- response = line["logs"][0]["content"]
- total += 1
- result = re.findall(r"\\boxed\{(.+?)\}", response)
- if len(result) == 0:
- err_cnt += 1
- print(response)
- continue
- result = result[0]
- acc += check_corr(result, label)
- final_accs.append(acc / total)
- err_cnts.append(err_cnt)
-print(final_accs)
-print(err_cnts)
-if args.ci_smoke_test is True:
- assert final_accs[0] == 1.0
diff --git a/spaces/AgentVerse/agentVerse/ui/src/phaser3-rex-plugins/templates/spinner/clock/Factory.js b/spaces/AgentVerse/agentVerse/ui/src/phaser3-rex-plugins/templates/spinner/clock/Factory.js
deleted file mode 100644
index fb5e0791b317d9b71a69e3ab82daeff8174b4f94..0000000000000000000000000000000000000000
--- a/spaces/AgentVerse/agentVerse/ui/src/phaser3-rex-plugins/templates/spinner/clock/Factory.js
+++ /dev/null
@@ -1,13 +0,0 @@
-import Clock from './Clock.js';
-import ObjectFactory from '../ObjectFactory.js';
-import SetValue from '../../../plugins/utils/object/SetValue.js';
-
-ObjectFactory.register('clock', function (config) {
- var gameObject = new Clock(this.scene, config);
- this.scene.add.existing(gameObject);
- return gameObject;
-});
-
-SetValue(window, 'RexPlugins.Spinner.Clock', Clock);
-
-export default Clock;
\ No newline at end of file
diff --git a/spaces/AgentVerse/agentVerse/ui/src/phaser3-rex-plugins/templates/ui/basesizer/GetExpandedChildWidth.js b/spaces/AgentVerse/agentVerse/ui/src/phaser3-rex-plugins/templates/ui/basesizer/GetExpandedChildWidth.js
deleted file mode 100644
index 37be007674b9ab605b93bdf845dde9a8f4ca0b7f..0000000000000000000000000000000000000000
--- a/spaces/AgentVerse/agentVerse/ui/src/phaser3-rex-plugins/templates/ui/basesizer/GetExpandedChildWidth.js
+++ /dev/null
@@ -1,6 +0,0 @@
-// Override
-var GetExpandedChildWidth = function (child, parentWidth) {
- return parentWidth;
-}
-
-export default GetExpandedChildWidth;
\ No newline at end of file
diff --git a/spaces/Androidonnxfork/CivitAi-to-Diffusers/diffusers/docs/source/en/api/pipelines/kandinsky_v22.md b/spaces/Androidonnxfork/CivitAi-to-Diffusers/diffusers/docs/source/en/api/pipelines/kandinsky_v22.md
deleted file mode 100644
index 3f88997ff4f53948c8fee1b5337e1c309b1e954c..0000000000000000000000000000000000000000
--- a/spaces/Androidonnxfork/CivitAi-to-Diffusers/diffusers/docs/source/en/api/pipelines/kandinsky_v22.md
+++ /dev/null
@@ -1,357 +0,0 @@
-
-
-# Kandinsky 2.2
-
-The Kandinsky 2.2 release includes robust new text-to-image models that support text-to-image generation, image-to-image generation, image interpolation, and text-guided image inpainting. The general workflow to perform these tasks using Kandinsky 2.2 is the same as in Kandinsky 2.1. First, you will need to use a prior pipeline to generate image embeddings based on your text prompt, and then use one of the image decoding pipelines to generate the output image. The only difference is that in Kandinsky 2.2, all of the decoding pipelines no longer accept the `prompt` input, and the image generation process is conditioned with only `image_embeds` and `negative_image_embeds`.
-
-Same as with Kandinsky 2.1, the easiest way to perform text-to-image generation is to use the combined Kandinsky pipeline. This process is exactly the same as Kandinsky 2.1. All you need to do is to replace the Kandinsky 2.1 checkpoint with 2.2.
-
-```python
-from diffusers import AutoPipelineForText2Image
-import torch
-
-pipe = AutoPipelineForText2Image.from_pretrained("kandinsky-community/kandinsky-2-2-decoder", torch_dtype=torch.float16)
-pipe.enable_model_cpu_offload()
-
-prompt = "A alien cheeseburger creature eating itself, claymation, cinematic, moody lighting"
-negative_prompt = "low quality, bad quality"
-
-image = pipe(prompt=prompt, negative_prompt=negative_prompt, prior_guidance_scale =1.0, height=768, width=768).images[0]
-```
-
-Now, let's look at an example where we take separate steps to run the prior pipeline and text-to-image pipeline. This way, we can understand what's happening under the hood and how Kandinsky 2.2 differs from Kandinsky 2.1.
-
-First, let's create the prior pipeline and text-to-image pipeline with Kandinsky 2.2 checkpoints.
-
-```python
-from diffusers import DiffusionPipeline
-import torch
-
-pipe_prior = DiffusionPipeline.from_pretrained("kandinsky-community/kandinsky-2-2-prior", torch_dtype=torch.float16)
-pipe_prior.to("cuda")
-
-t2i_pipe = DiffusionPipeline.from_pretrained("kandinsky-community/kandinsky-2-2-decoder", torch_dtype=torch.float16)
-t2i_pipe.to("cuda")
-```
-
-You can then use `pipe_prior` to generate image embeddings.
-
-```python
-prompt = "portrait of a women, blue eyes, cinematic"
-negative_prompt = "low quality, bad quality"
-
-image_embeds, negative_image_embeds = pipe_prior(prompt, guidance_scale=1.0).to_tuple()
-```
-
-Now you can pass these embeddings to the text-to-image pipeline. When using Kandinsky 2.2 you don't need to pass the `prompt` (but you do with the previous version, Kandinsky 2.1).
-
-```
-image = t2i_pipe(image_embeds=image_embeds, negative_image_embeds=negative_image_embeds, height=768, width=768).images[
- 0
-]
-image.save("portrait.png")
-```
-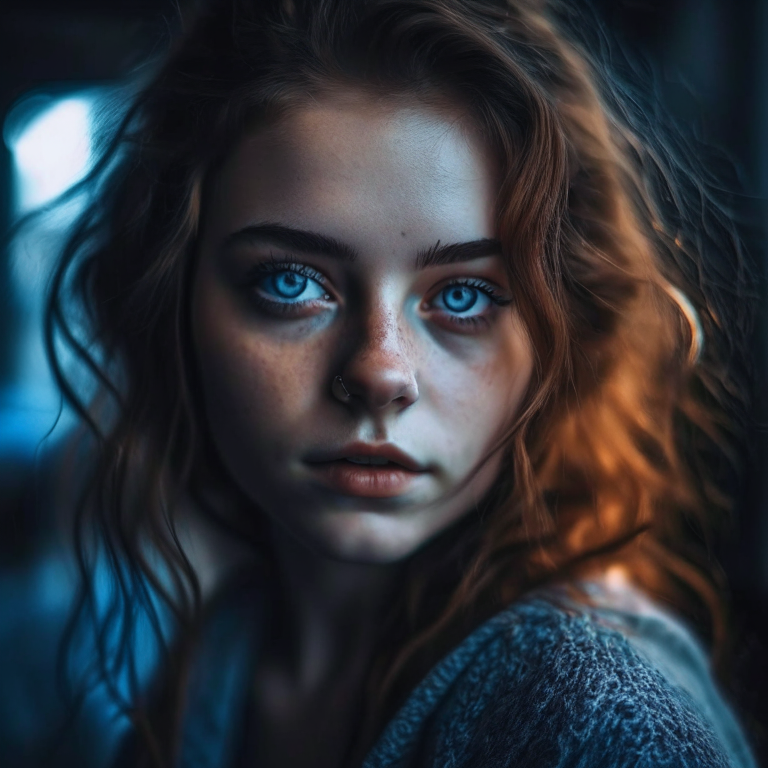
-
-We used the text-to-image pipeline as an example, but the same process applies to all decoding pipelines in Kandinsky 2.2. For more information, please refer to our API section for each pipeline.
-
-### Text-to-Image Generation with ControlNet Conditioning
-
-In the following, we give a simple example of how to use [`KandinskyV22ControlnetPipeline`] to add control to the text-to-image generation with a depth image.
-
-First, let's take an image and extract its depth map.
-
-```python
-from diffusers.utils import load_image
-
-img = load_image(
- "https://huggingface.co/datasets/hf-internal-testing/diffusers-images/resolve/main/kandinskyv22/cat.png"
-).resize((768, 768))
-```
-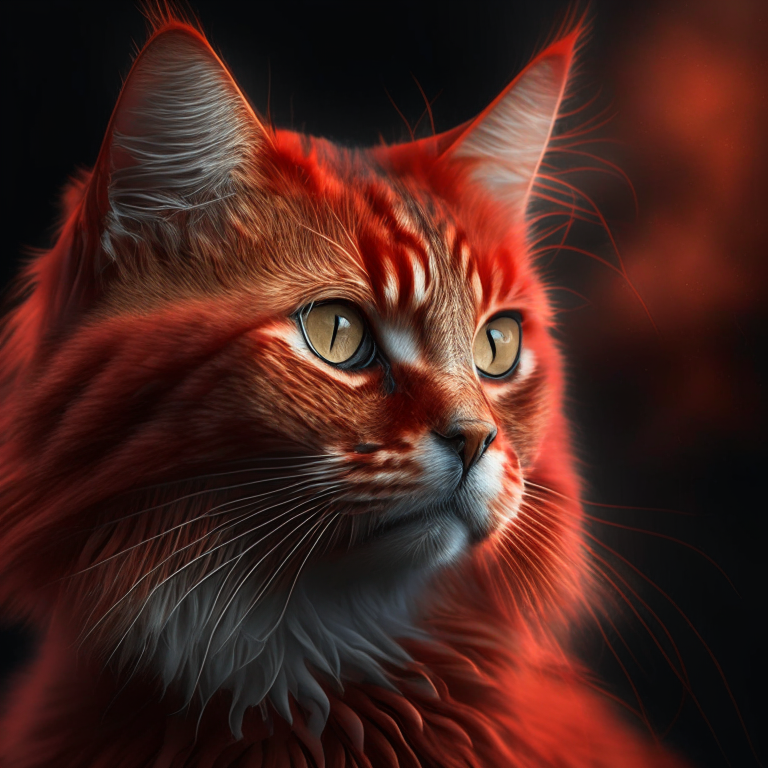
-
-We can use the `depth-estimation` pipeline from transformers to process the image and retrieve its depth map.
-
-```python
-import torch
-import numpy as np
-
-from transformers import pipeline
-from diffusers.utils import load_image
-
-
-def make_hint(image, depth_estimator):
- image = depth_estimator(image)["depth"]
- image = np.array(image)
- image = image[:, :, None]
- image = np.concatenate([image, image, image], axis=2)
- detected_map = torch.from_numpy(image).float() / 255.0
- hint = detected_map.permute(2, 0, 1)
- return hint
-
-
-depth_estimator = pipeline("depth-estimation")
-hint = make_hint(img, depth_estimator).unsqueeze(0).half().to("cuda")
-```
-Now, we load the prior pipeline and the text-to-image controlnet pipeline
-
-```python
-from diffusers import KandinskyV22PriorPipeline, KandinskyV22ControlnetPipeline
-
-pipe_prior = KandinskyV22PriorPipeline.from_pretrained(
- "kandinsky-community/kandinsky-2-2-prior", torch_dtype=torch.float16
-)
-pipe_prior = pipe_prior.to("cuda")
-
-pipe = KandinskyV22ControlnetPipeline.from_pretrained(
- "kandinsky-community/kandinsky-2-2-controlnet-depth", torch_dtype=torch.float16
-)
-pipe = pipe.to("cuda")
-```
-
-We pass the prompt and negative prompt through the prior to generate image embeddings
-
-```python
-prompt = "A robot, 4k photo"
-
-negative_prior_prompt = "lowres, text, error, cropped, worst quality, low quality, jpeg artifacts, ugly, duplicate, morbid, mutilated, out of frame, extra fingers, mutated hands, poorly drawn hands, poorly drawn face, mutation, deformed, blurry, dehydrated, bad anatomy, bad proportions, extra limbs, cloned face, disfigured, gross proportions, malformed limbs, missing arms, missing legs, extra arms, extra legs, fused fingers, too many fingers, long neck, username, watermark, signature"
-
-generator = torch.Generator(device="cuda").manual_seed(43)
-image_emb, zero_image_emb = pipe_prior(
- prompt=prompt, negative_prompt=negative_prior_prompt, generator=generator
-).to_tuple()
-```
-
-Now we can pass the image embeddings and the depth image we extracted to the controlnet pipeline. With Kandinsky 2.2, only prior pipelines accept `prompt` input. You do not need to pass the prompt to the controlnet pipeline.
-
-```python
-images = pipe(
- image_embeds=image_emb,
- negative_image_embeds=zero_image_emb,
- hint=hint,
- num_inference_steps=50,
- generator=generator,
- height=768,
- width=768,
-).images
-
-images[0].save("robot_cat.png")
-```
-
-The output image looks as follow:
-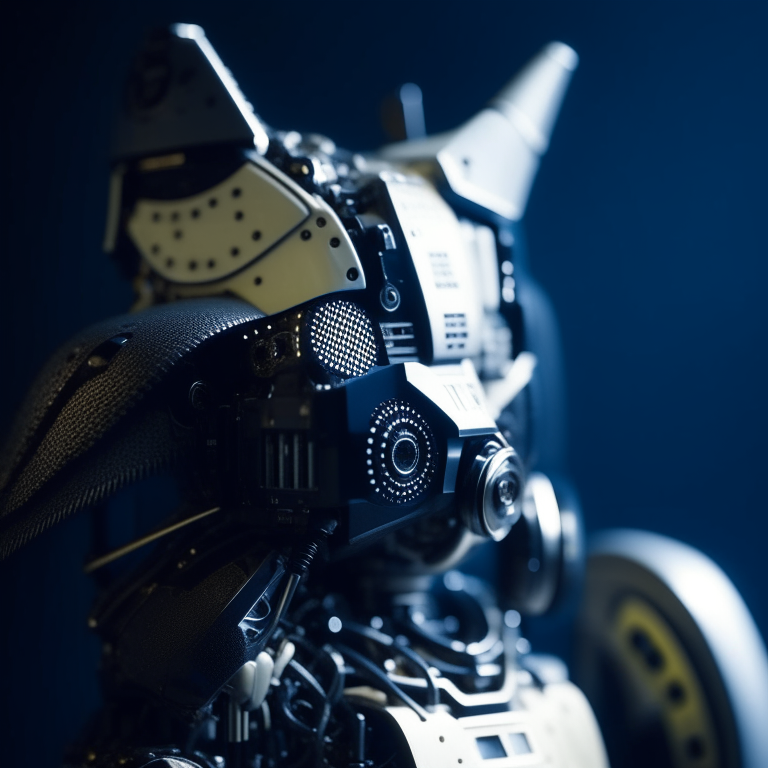
-
-### Image-to-Image Generation with ControlNet Conditioning
-
-Kandinsky 2.2 also includes a [`KandinskyV22ControlnetImg2ImgPipeline`] that will allow you to add control to the image generation process with both the image and its depth map. This pipeline works really well with [`KandinskyV22PriorEmb2EmbPipeline`], which generates image embeddings based on both a text prompt and an image.
-
-For our robot cat example, we will pass the prompt and cat image together to the prior pipeline to generate an image embedding. We will then use that image embedding and the depth map of the cat to further control the image generation process.
-
-We can use the same cat image and its depth map from the last example.
-
-```python
-import torch
-import numpy as np
-
-from diffusers import KandinskyV22PriorEmb2EmbPipeline, KandinskyV22ControlnetImg2ImgPipeline
-from diffusers.utils import load_image
-from transformers import pipeline
-
-img = load_image(
- "https://huggingface.co/datasets/hf-internal-testing/diffusers-images/resolve/main" "/kandinskyv22/cat.png"
-).resize((768, 768))
-
-
-def make_hint(image, depth_estimator):
- image = depth_estimator(image)["depth"]
- image = np.array(image)
- image = image[:, :, None]
- image = np.concatenate([image, image, image], axis=2)
- detected_map = torch.from_numpy(image).float() / 255.0
- hint = detected_map.permute(2, 0, 1)
- return hint
-
-
-depth_estimator = pipeline("depth-estimation")
-hint = make_hint(img, depth_estimator).unsqueeze(0).half().to("cuda")
-
-pipe_prior = KandinskyV22PriorEmb2EmbPipeline.from_pretrained(
- "kandinsky-community/kandinsky-2-2-prior", torch_dtype=torch.float16
-)
-pipe_prior = pipe_prior.to("cuda")
-
-pipe = KandinskyV22ControlnetImg2ImgPipeline.from_pretrained(
- "kandinsky-community/kandinsky-2-2-controlnet-depth", torch_dtype=torch.float16
-)
-pipe = pipe.to("cuda")
-
-prompt = "A robot, 4k photo"
-negative_prior_prompt = "lowres, text, error, cropped, worst quality, low quality, jpeg artifacts, ugly, duplicate, morbid, mutilated, out of frame, extra fingers, mutated hands, poorly drawn hands, poorly drawn face, mutation, deformed, blurry, dehydrated, bad anatomy, bad proportions, extra limbs, cloned face, disfigured, gross proportions, malformed limbs, missing arms, missing legs, extra arms, extra legs, fused fingers, too many fingers, long neck, username, watermark, signature"
-
-generator = torch.Generator(device="cuda").manual_seed(43)
-
-# run prior pipeline
-
-img_emb = pipe_prior(prompt=prompt, image=img, strength=0.85, generator=generator)
-negative_emb = pipe_prior(prompt=negative_prior_prompt, image=img, strength=1, generator=generator)
-
-# run controlnet img2img pipeline
-images = pipe(
- image=img,
- strength=0.5,
- image_embeds=img_emb.image_embeds,
- negative_image_embeds=negative_emb.image_embeds,
- hint=hint,
- num_inference_steps=50,
- generator=generator,
- height=768,
- width=768,
-).images
-
-images[0].save("robot_cat.png")
-```
-
-Here is the output. Compared with the output from our text-to-image controlnet example, it kept a lot more cat facial details from the original image and worked into the robot style we asked for.
-
-
-
-## Optimization
-
-Running Kandinsky in inference requires running both a first prior pipeline: [`KandinskyPriorPipeline`]
-and a second image decoding pipeline which is one of [`KandinskyPipeline`], [`KandinskyImg2ImgPipeline`], or [`KandinskyInpaintPipeline`].
-
-The bulk of the computation time will always be the second image decoding pipeline, so when looking
-into optimizing the model, one should look into the second image decoding pipeline.
-
-When running with PyTorch < 2.0, we strongly recommend making use of [`xformers`](https://github.com/facebookresearch/xformers)
-to speed-up the optimization. This can be done by simply running:
-
-```py
-from diffusers import DiffusionPipeline
-import torch
-
-t2i_pipe = DiffusionPipeline.from_pretrained("kandinsky-community/kandinsky-2-1", torch_dtype=torch.float16)
-t2i_pipe.enable_xformers_memory_efficient_attention()
-```
-
-When running on PyTorch >= 2.0, PyTorch's SDPA attention will automatically be used. For more information on
-PyTorch's SDPA, feel free to have a look at [this blog post](https://pytorch.org/blog/accelerated-diffusers-pt-20/).
-
-To have explicit control , you can also manually set the pipeline to use PyTorch's 2.0 efficient attention:
-
-```py
-from diffusers.models.attention_processor import AttnAddedKVProcessor2_0
-
-t2i_pipe.unet.set_attn_processor(AttnAddedKVProcessor2_0())
-```
-
-The slowest and most memory intense attention processor is the default `AttnAddedKVProcessor` processor.
-We do **not** recommend using it except for testing purposes or cases where very high determistic behaviour is desired.
-You can set it with:
-
-```py
-from diffusers.models.attention_processor import AttnAddedKVProcessor
-
-t2i_pipe.unet.set_attn_processor(AttnAddedKVProcessor())
-```
-
-With PyTorch >= 2.0, you can also use Kandinsky with `torch.compile` which depending
-on your hardware can signficantly speed-up your inference time once the model is compiled.
-To use Kandinsksy with `torch.compile`, you can do:
-
-```py
-t2i_pipe.unet.to(memory_format=torch.channels_last)
-t2i_pipe.unet = torch.compile(t2i_pipe.unet, mode="reduce-overhead", fullgraph=True)
-```
-
-After compilation you should see a very fast inference time. For more information,
-feel free to have a look at [Our PyTorch 2.0 benchmark](https://huggingface.co/docs/diffusers/main/en/optimization/torch2.0).
-
-
-
-To generate images directly from a single pipeline, you can use [`KandinskyV22CombinedPipeline`], [`KandinskyV22Img2ImgCombinedPipeline`], [`KandinskyV22InpaintCombinedPipeline`].
-These combined pipelines wrap the [`KandinskyV22PriorPipeline`] and [`KandinskyV22Pipeline`], [`KandinskyV22Img2ImgPipeline`], [`KandinskyV22InpaintPipeline`] respectively into a single
-pipeline for a simpler user experience
-
-
-
-## Available Pipelines:
-
-| Pipeline | Tasks |
-|---|---|
-| [pipeline_kandinsky2_2.py](https://github.com/huggingface/diffusers/blob/main/src/diffusers/pipelines/kandinsky2_2/pipeline_kandinsky2_2.py) | *Text-to-Image Generation* |
-| [pipeline_kandinsky2_2_combined.py](https://github.com/huggingface/diffusers/blob/main/src/diffusers/pipelines/kandinsky2_2/pipeline_kandinsky2_2_combined.py) | *End-to-end Text-to-Image, image-to-image, Inpainting Generation* |
-| [pipeline_kandinsky2_2_inpaint.py](https://github.com/huggingface/diffusers/blob/main/src/diffusers/pipelines/kandinsky2_2/pipeline_kandinsky2_2_inpaint.py) | *Image-Guided Image Generation* |
-| [pipeline_kandinsky2_2_img2img.py](https://github.com/huggingface/diffusers/blob/main/src/diffusers/pipelines/kandinsky2_2/pipeline_kandinsky2_2_img2img.py) | *Image-Guided Image Generation* |
-| [pipeline_kandinsky2_2_controlnet.py](https://github.com/huggingface/diffusers/blob/main/src/diffusers/pipelines/kandinsky2_2/pipeline_kandinsky2_2_controlnet.py) | *Image-Guided Image Generation* |
-| [pipeline_kandinsky2_2_controlnet_img2img.py](https://github.com/huggingface/diffusers/blob/main/src/diffusers/pipelines/kandinsky2_2/pipeline_kandinsky2_2_controlnet_img2img.py) | *Image-Guided Image Generation* |
-
-
-### KandinskyV22Pipeline
-
-[[autodoc]] KandinskyV22Pipeline
- - all
- - __call__
-
-### KandinskyV22ControlnetPipeline
-
-[[autodoc]] KandinskyV22ControlnetPipeline
- - all
- - __call__
-
-### KandinskyV22ControlnetImg2ImgPipeline
-
-[[autodoc]] KandinskyV22ControlnetImg2ImgPipeline
- - all
- - __call__
-
-### KandinskyV22Img2ImgPipeline
-
-[[autodoc]] KandinskyV22Img2ImgPipeline
- - all
- - __call__
-
-### KandinskyV22InpaintPipeline
-
-[[autodoc]] KandinskyV22InpaintPipeline
- - all
- - __call__
-
-### KandinskyV22PriorPipeline
-
-[[autodoc]] KandinskyV22PriorPipeline
- - all
- - __call__
- - interpolate
-
-### KandinskyV22PriorEmb2EmbPipeline
-
-[[autodoc]] KandinskyV22PriorEmb2EmbPipeline
- - all
- - __call__
- - interpolate
-
-### KandinskyV22CombinedPipeline
-
-[[autodoc]] KandinskyV22CombinedPipeline
- - all
- - __call__
-
-### KandinskyV22Img2ImgCombinedPipeline
-
-[[autodoc]] KandinskyV22Img2ImgCombinedPipeline
- - all
- - __call__
-
-### KandinskyV22InpaintCombinedPipeline
-
-[[autodoc]] KandinskyV22InpaintCombinedPipeline
- - all
- - __call__
diff --git a/spaces/Androidonnxfork/CivitAi-to-Diffusers/diffusers/examples/community/wildcard_stable_diffusion.py b/spaces/Androidonnxfork/CivitAi-to-Diffusers/diffusers/examples/community/wildcard_stable_diffusion.py
deleted file mode 100644
index aec79fb8e12e38c8b20af7bc47a7d634b45a7680..0000000000000000000000000000000000000000
--- a/spaces/Androidonnxfork/CivitAi-to-Diffusers/diffusers/examples/community/wildcard_stable_diffusion.py
+++ /dev/null
@@ -1,418 +0,0 @@
-import inspect
-import os
-import random
-import re
-from dataclasses import dataclass
-from typing import Callable, Dict, List, Optional, Union
-
-import torch
-from transformers import CLIPImageProcessor, CLIPTextModel, CLIPTokenizer
-
-from diffusers import DiffusionPipeline
-from diffusers.configuration_utils import FrozenDict
-from diffusers.models import AutoencoderKL, UNet2DConditionModel
-from diffusers.pipelines.stable_diffusion.pipeline_stable_diffusion import StableDiffusionPipelineOutput
-from diffusers.pipelines.stable_diffusion.safety_checker import StableDiffusionSafetyChecker
-from diffusers.schedulers import DDIMScheduler, LMSDiscreteScheduler, PNDMScheduler
-from diffusers.utils import deprecate, logging
-
-
-logger = logging.get_logger(__name__) # pylint: disable=invalid-name
-
-global_re_wildcard = re.compile(r"__([^_]*)__")
-
-
-def get_filename(path: str):
- # this doesn't work on Windows
- return os.path.basename(path).split(".txt")[0]
-
-
-def read_wildcard_values(path: str):
- with open(path, encoding="utf8") as f:
- return f.read().splitlines()
-
-
-def grab_wildcard_values(wildcard_option_dict: Dict[str, List[str]] = {}, wildcard_files: List[str] = []):
- for wildcard_file in wildcard_files:
- filename = get_filename(wildcard_file)
- read_values = read_wildcard_values(wildcard_file)
- if filename not in wildcard_option_dict:
- wildcard_option_dict[filename] = []
- wildcard_option_dict[filename].extend(read_values)
- return wildcard_option_dict
-
-
-def replace_prompt_with_wildcards(
- prompt: str, wildcard_option_dict: Dict[str, List[str]] = {}, wildcard_files: List[str] = []
-):
- new_prompt = prompt
-
- # get wildcard options
- wildcard_option_dict = grab_wildcard_values(wildcard_option_dict, wildcard_files)
-
- for m in global_re_wildcard.finditer(new_prompt):
- wildcard_value = m.group()
- replace_value = random.choice(wildcard_option_dict[wildcard_value.strip("__")])
- new_prompt = new_prompt.replace(wildcard_value, replace_value, 1)
-
- return new_prompt
-
-
-@dataclass
-class WildcardStableDiffusionOutput(StableDiffusionPipelineOutput):
- prompts: List[str]
-
-
-class WildcardStableDiffusionPipeline(DiffusionPipeline):
- r"""
- Example Usage:
- pipe = WildcardStableDiffusionPipeline.from_pretrained(
- "CompVis/stable-diffusion-v1-4",
-
- torch_dtype=torch.float16,
- )
- prompt = "__animal__ sitting on a __object__ wearing a __clothing__"
- out = pipe(
- prompt,
- wildcard_option_dict={
- "clothing":["hat", "shirt", "scarf", "beret"]
- },
- wildcard_files=["object.txt", "animal.txt"],
- num_prompt_samples=1
- )
-
-
- Pipeline for text-to-image generation with wild cards using Stable Diffusion.
-
- This model inherits from [`DiffusionPipeline`]. Check the superclass documentation for the generic methods the
- library implements for all the pipelines (such as downloading or saving, running on a particular device, etc.)
-
- Args:
- vae ([`AutoencoderKL`]):
- Variational Auto-Encoder (VAE) Model to encode and decode images to and from latent representations.
- text_encoder ([`CLIPTextModel`]):
- Frozen text-encoder. Stable Diffusion uses the text portion of
- [CLIP](https://huggingface.co/docs/transformers/model_doc/clip#transformers.CLIPTextModel), specifically
- the [clip-vit-large-patch14](https://huggingface.co/openai/clip-vit-large-patch14) variant.
- tokenizer (`CLIPTokenizer`):
- Tokenizer of class
- [CLIPTokenizer](https://huggingface.co/docs/transformers/v4.21.0/en/model_doc/clip#transformers.CLIPTokenizer).
- unet ([`UNet2DConditionModel`]): Conditional U-Net architecture to denoise the encoded image latents.
- scheduler ([`SchedulerMixin`]):
- A scheduler to be used in combination with `unet` to denoise the encoded image latents. Can be one of
- [`DDIMScheduler`], [`LMSDiscreteScheduler`], or [`PNDMScheduler`].
- safety_checker ([`StableDiffusionSafetyChecker`]):
- Classification module that estimates whether generated images could be considered offensive or harmful.
- Please, refer to the [model card](https://huggingface.co/CompVis/stable-diffusion-v1-4) for details.
- feature_extractor ([`CLIPImageProcessor`]):
- Model that extracts features from generated images to be used as inputs for the `safety_checker`.
- """
-
- def __init__(
- self,
- vae: AutoencoderKL,
- text_encoder: CLIPTextModel,
- tokenizer: CLIPTokenizer,
- unet: UNet2DConditionModel,
- scheduler: Union[DDIMScheduler, PNDMScheduler, LMSDiscreteScheduler],
- safety_checker: StableDiffusionSafetyChecker,
- feature_extractor: CLIPImageProcessor,
- ):
- super().__init__()
-
- if hasattr(scheduler.config, "steps_offset") and scheduler.config.steps_offset != 1:
- deprecation_message = (
- f"The configuration file of this scheduler: {scheduler} is outdated. `steps_offset`"
- f" should be set to 1 instead of {scheduler.config.steps_offset}. Please make sure "
- "to update the config accordingly as leaving `steps_offset` might led to incorrect results"
- " in future versions. If you have downloaded this checkpoint from the Hugging Face Hub,"
- " it would be very nice if you could open a Pull request for the `scheduler/scheduler_config.json`"
- " file"
- )
- deprecate("steps_offset!=1", "1.0.0", deprecation_message, standard_warn=False)
- new_config = dict(scheduler.config)
- new_config["steps_offset"] = 1
- scheduler._internal_dict = FrozenDict(new_config)
-
- if safety_checker is None:
- logger.warning(
- f"You have disabled the safety checker for {self.__class__} by passing `safety_checker=None`. Ensure"
- " that you abide to the conditions of the Stable Diffusion license and do not expose unfiltered"
- " results in services or applications open to the public. Both the diffusers team and Hugging Face"
- " strongly recommend to keep the safety filter enabled in all public facing circumstances, disabling"
- " it only for use-cases that involve analyzing network behavior or auditing its results. For more"
- " information, please have a look at https://github.com/huggingface/diffusers/pull/254 ."
- )
-
- self.register_modules(
- vae=vae,
- text_encoder=text_encoder,
- tokenizer=tokenizer,
- unet=unet,
- scheduler=scheduler,
- safety_checker=safety_checker,
- feature_extractor=feature_extractor,
- )
-
- @torch.no_grad()
- def __call__(
- self,
- prompt: Union[str, List[str]],
- height: int = 512,
- width: int = 512,
- num_inference_steps: int = 50,
- guidance_scale: float = 7.5,
- negative_prompt: Optional[Union[str, List[str]]] = None,
- num_images_per_prompt: Optional[int] = 1,
- eta: float = 0.0,
- generator: Optional[torch.Generator] = None,
- latents: Optional[torch.FloatTensor] = None,
- output_type: Optional[str] = "pil",
- return_dict: bool = True,
- callback: Optional[Callable[[int, int, torch.FloatTensor], None]] = None,
- callback_steps: int = 1,
- wildcard_option_dict: Dict[str, List[str]] = {},
- wildcard_files: List[str] = [],
- num_prompt_samples: Optional[int] = 1,
- **kwargs,
- ):
- r"""
- Function invoked when calling the pipeline for generation.
-
- Args:
- prompt (`str` or `List[str]`):
- The prompt or prompts to guide the image generation.
- height (`int`, *optional*, defaults to 512):
- The height in pixels of the generated image.
- width (`int`, *optional*, defaults to 512):
- The width in pixels of the generated image.
- num_inference_steps (`int`, *optional*, defaults to 50):
- The number of denoising steps. More denoising steps usually lead to a higher quality image at the
- expense of slower inference.
- guidance_scale (`float`, *optional*, defaults to 7.5):
- Guidance scale as defined in [Classifier-Free Diffusion Guidance](https://arxiv.org/abs/2207.12598).
- `guidance_scale` is defined as `w` of equation 2. of [Imagen
- Paper](https://arxiv.org/pdf/2205.11487.pdf). Guidance scale is enabled by setting `guidance_scale >
- 1`. Higher guidance scale encourages to generate images that are closely linked to the text `prompt`,
- usually at the expense of lower image quality.
- negative_prompt (`str` or `List[str]`, *optional*):
- The prompt or prompts not to guide the image generation. Ignored when not using guidance (i.e., ignored
- if `guidance_scale` is less than `1`).
- num_images_per_prompt (`int`, *optional*, defaults to 1):
- The number of images to generate per prompt.
- eta (`float`, *optional*, defaults to 0.0):
- Corresponds to parameter eta (η) in the DDIM paper: https://arxiv.org/abs/2010.02502. Only applies to
- [`schedulers.DDIMScheduler`], will be ignored for others.
- generator (`torch.Generator`, *optional*):
- A [torch generator](https://pytorch.org/docs/stable/generated/torch.Generator.html) to make generation
- deterministic.
- latents (`torch.FloatTensor`, *optional*):
- Pre-generated noisy latents, sampled from a Gaussian distribution, to be used as inputs for image
- generation. Can be used to tweak the same generation with different prompts. If not provided, a latents
- tensor will ge generated by sampling using the supplied random `generator`.
- output_type (`str`, *optional*, defaults to `"pil"`):
- The output format of the generate image. Choose between
- [PIL](https://pillow.readthedocs.io/en/stable/): `PIL.Image.Image` or `np.array`.
- return_dict (`bool`, *optional*, defaults to `True`):
- Whether or not to return a [`~pipelines.stable_diffusion.StableDiffusionPipelineOutput`] instead of a
- plain tuple.
- callback (`Callable`, *optional*):
- A function that will be called every `callback_steps` steps during inference. The function will be
- called with the following arguments: `callback(step: int, timestep: int, latents: torch.FloatTensor)`.
- callback_steps (`int`, *optional*, defaults to 1):
- The frequency at which the `callback` function will be called. If not specified, the callback will be
- called at every step.
- wildcard_option_dict (Dict[str, List[str]]):
- dict with key as `wildcard` and values as a list of possible replacements. For example if a prompt, "A __animal__ sitting on a chair". A wildcard_option_dict can provide possible values for "animal" like this: {"animal":["dog", "cat", "fox"]}
- wildcard_files: (List[str])
- List of filenames of txt files for wildcard replacements. For example if a prompt, "A __animal__ sitting on a chair". A file can be provided ["animal.txt"]
- num_prompt_samples: int
- Number of times to sample wildcards for each prompt provided
-
- Returns:
- [`~pipelines.stable_diffusion.StableDiffusionPipelineOutput`] or `tuple`:
- [`~pipelines.stable_diffusion.StableDiffusionPipelineOutput`] if `return_dict` is True, otherwise a `tuple.
- When returning a tuple, the first element is a list with the generated images, and the second element is a
- list of `bool`s denoting whether the corresponding generated image likely represents "not-safe-for-work"
- (nsfw) content, according to the `safety_checker`.
- """
-
- if isinstance(prompt, str):
- prompt = [
- replace_prompt_with_wildcards(prompt, wildcard_option_dict, wildcard_files)
- for i in range(num_prompt_samples)
- ]
- batch_size = len(prompt)
- elif isinstance(prompt, list):
- prompt_list = []
- for p in prompt:
- for i in range(num_prompt_samples):
- prompt_list.append(replace_prompt_with_wildcards(p, wildcard_option_dict, wildcard_files))
- prompt = prompt_list
- batch_size = len(prompt)
- else:
- raise ValueError(f"`prompt` has to be of type `str` or `list` but is {type(prompt)}")
-
- if height % 8 != 0 or width % 8 != 0:
- raise ValueError(f"`height` and `width` have to be divisible by 8 but are {height} and {width}.")
-
- if (callback_steps is None) or (
- callback_steps is not None and (not isinstance(callback_steps, int) or callback_steps <= 0)
- ):
- raise ValueError(
- f"`callback_steps` has to be a positive integer but is {callback_steps} of type"
- f" {type(callback_steps)}."
- )
-
- # get prompt text embeddings
- text_inputs = self.tokenizer(
- prompt,
- padding="max_length",
- max_length=self.tokenizer.model_max_length,
- return_tensors="pt",
- )
- text_input_ids = text_inputs.input_ids
-
- if text_input_ids.shape[-1] > self.tokenizer.model_max_length:
- removed_text = self.tokenizer.batch_decode(text_input_ids[:, self.tokenizer.model_max_length :])
- logger.warning(
- "The following part of your input was truncated because CLIP can only handle sequences up to"
- f" {self.tokenizer.model_max_length} tokens: {removed_text}"
- )
- text_input_ids = text_input_ids[:, : self.tokenizer.model_max_length]
- text_embeddings = self.text_encoder(text_input_ids.to(self.device))[0]
-
- # duplicate text embeddings for each generation per prompt, using mps friendly method
- bs_embed, seq_len, _ = text_embeddings.shape
- text_embeddings = text_embeddings.repeat(1, num_images_per_prompt, 1)
- text_embeddings = text_embeddings.view(bs_embed * num_images_per_prompt, seq_len, -1)
-
- # here `guidance_scale` is defined analog to the guidance weight `w` of equation (2)
- # of the Imagen paper: https://arxiv.org/pdf/2205.11487.pdf . `guidance_scale = 1`
- # corresponds to doing no classifier free guidance.
- do_classifier_free_guidance = guidance_scale > 1.0
- # get unconditional embeddings for classifier free guidance
- if do_classifier_free_guidance:
- uncond_tokens: List[str]
- if negative_prompt is None:
- uncond_tokens = [""] * batch_size
- elif type(prompt) is not type(negative_prompt):
- raise TypeError(
- f"`negative_prompt` should be the same type to `prompt`, but got {type(negative_prompt)} !="
- f" {type(prompt)}."
- )
- elif isinstance(negative_prompt, str):
- uncond_tokens = [negative_prompt]
- elif batch_size != len(negative_prompt):
- raise ValueError(
- f"`negative_prompt`: {negative_prompt} has batch size {len(negative_prompt)}, but `prompt`:"
- f" {prompt} has batch size {batch_size}. Please make sure that passed `negative_prompt` matches"
- " the batch size of `prompt`."
- )
- else:
- uncond_tokens = negative_prompt
-
- max_length = text_input_ids.shape[-1]
- uncond_input = self.tokenizer(
- uncond_tokens,
- padding="max_length",
- max_length=max_length,
- truncation=True,
- return_tensors="pt",
- )
- uncond_embeddings = self.text_encoder(uncond_input.input_ids.to(self.device))[0]
-
- # duplicate unconditional embeddings for each generation per prompt, using mps friendly method
- seq_len = uncond_embeddings.shape[1]
- uncond_embeddings = uncond_embeddings.repeat(1, num_images_per_prompt, 1)
- uncond_embeddings = uncond_embeddings.view(batch_size * num_images_per_prompt, seq_len, -1)
-
- # For classifier free guidance, we need to do two forward passes.
- # Here we concatenate the unconditional and text embeddings into a single batch
- # to avoid doing two forward passes
- text_embeddings = torch.cat([uncond_embeddings, text_embeddings])
-
- # get the initial random noise unless the user supplied it
-
- # Unlike in other pipelines, latents need to be generated in the target device
- # for 1-to-1 results reproducibility with the CompVis implementation.
- # However this currently doesn't work in `mps`.
- latents_shape = (batch_size * num_images_per_prompt, self.unet.config.in_channels, height // 8, width // 8)
- latents_dtype = text_embeddings.dtype
- if latents is None:
- if self.device.type == "mps":
- # randn does not exist on mps
- latents = torch.randn(latents_shape, generator=generator, device="cpu", dtype=latents_dtype).to(
- self.device
- )
- else:
- latents = torch.randn(latents_shape, generator=generator, device=self.device, dtype=latents_dtype)
- else:
- if latents.shape != latents_shape:
- raise ValueError(f"Unexpected latents shape, got {latents.shape}, expected {latents_shape}")
- latents = latents.to(self.device)
-
- # set timesteps
- self.scheduler.set_timesteps(num_inference_steps)
-
- # Some schedulers like PNDM have timesteps as arrays
- # It's more optimized to move all timesteps to correct device beforehand
- timesteps_tensor = self.scheduler.timesteps.to(self.device)
-
- # scale the initial noise by the standard deviation required by the scheduler
- latents = latents * self.scheduler.init_noise_sigma
-
- # prepare extra kwargs for the scheduler step, since not all schedulers have the same signature
- # eta (η) is only used with the DDIMScheduler, it will be ignored for other schedulers.
- # eta corresponds to η in DDIM paper: https://arxiv.org/abs/2010.02502
- # and should be between [0, 1]
- accepts_eta = "eta" in set(inspect.signature(self.scheduler.step).parameters.keys())
- extra_step_kwargs = {}
- if accepts_eta:
- extra_step_kwargs["eta"] = eta
-
- for i, t in enumerate(self.progress_bar(timesteps_tensor)):
- # expand the latents if we are doing classifier free guidance
- latent_model_input = torch.cat([latents] * 2) if do_classifier_free_guidance else latents
- latent_model_input = self.scheduler.scale_model_input(latent_model_input, t)
-
- # predict the noise residual
- noise_pred = self.unet(latent_model_input, t, encoder_hidden_states=text_embeddings).sample
-
- # perform guidance
- if do_classifier_free_guidance:
- noise_pred_uncond, noise_pred_text = noise_pred.chunk(2)
- noise_pred = noise_pred_uncond + guidance_scale * (noise_pred_text - noise_pred_uncond)
-
- # compute the previous noisy sample x_t -> x_t-1
- latents = self.scheduler.step(noise_pred, t, latents, **extra_step_kwargs).prev_sample
-
- # call the callback, if provided
- if callback is not None and i % callback_steps == 0:
- callback(i, t, latents)
-
- latents = 1 / 0.18215 * latents
- image = self.vae.decode(latents).sample
-
- image = (image / 2 + 0.5).clamp(0, 1)
-
- # we always cast to float32 as this does not cause significant overhead and is compatible with bfloat16
- image = image.cpu().permute(0, 2, 3, 1).float().numpy()
-
- if self.safety_checker is not None:
- safety_checker_input = self.feature_extractor(self.numpy_to_pil(image), return_tensors="pt").to(
- self.device
- )
- image, has_nsfw_concept = self.safety_checker(
- images=image, clip_input=safety_checker_input.pixel_values.to(text_embeddings.dtype)
- )
- else:
- has_nsfw_concept = None
-
- if output_type == "pil":
- image = self.numpy_to_pil(image)
-
- if not return_dict:
- return (image, has_nsfw_concept)
-
- return WildcardStableDiffusionOutput(images=image, nsfw_content_detected=has_nsfw_concept, prompts=prompt)
diff --git a/spaces/Androidonnxfork/CivitAi-to-Diffusers/diffusers/src/diffusers/pipelines/kandinsky/pipeline_kandinsky.py b/spaces/Androidonnxfork/CivitAi-to-Diffusers/diffusers/src/diffusers/pipelines/kandinsky/pipeline_kandinsky.py
deleted file mode 100644
index 89afa0060ef84b69aeb7b8361726ed51e557cbb3..0000000000000000000000000000000000000000
--- a/spaces/Androidonnxfork/CivitAi-to-Diffusers/diffusers/src/diffusers/pipelines/kandinsky/pipeline_kandinsky.py
+++ /dev/null
@@ -1,429 +0,0 @@
-# Copyright 2023 The HuggingFace Team. All rights reserved.
-#
-# Licensed under the Apache License, Version 2.0 (the "License");
-# you may not use this file except in compliance with the License.
-# You may obtain a copy of the License at
-#
-# http://www.apache.org/licenses/LICENSE-2.0
-#
-# Unless required by applicable law or agreed to in writing, software
-# distributed under the License is distributed on an "AS IS" BASIS,
-# WITHOUT WARRANTIES OR CONDITIONS OF ANY KIND, either express or implied.
-# See the License for the specific language governing permissions and
-# limitations under the License.
-
-from typing import Callable, List, Optional, Union
-
-import torch
-from transformers import (
- XLMRobertaTokenizer,
-)
-
-from ...models import UNet2DConditionModel, VQModel
-from ...schedulers import DDIMScheduler, DDPMScheduler
-from ...utils import (
- is_accelerate_available,
- is_accelerate_version,
- logging,
- randn_tensor,
- replace_example_docstring,
-)
-from ..pipeline_utils import DiffusionPipeline, ImagePipelineOutput
-from .text_encoder import MultilingualCLIP
-
-
-logger = logging.get_logger(__name__) # pylint: disable=invalid-name
-
-EXAMPLE_DOC_STRING = """
- Examples:
- ```py
- >>> from diffusers import KandinskyPipeline, KandinskyPriorPipeline
- >>> import torch
-
- >>> pipe_prior = KandinskyPriorPipeline.from_pretrained("kandinsky-community/Kandinsky-2-1-prior")
- >>> pipe_prior.to("cuda")
-
- >>> prompt = "red cat, 4k photo"
- >>> out = pipe_prior(prompt)
- >>> image_emb = out.image_embeds
- >>> negative_image_emb = out.negative_image_embeds
-
- >>> pipe = KandinskyPipeline.from_pretrained("kandinsky-community/kandinsky-2-1")
- >>> pipe.to("cuda")
-
- >>> image = pipe(
- ... prompt,
- ... image_embeds=image_emb,
- ... negative_image_embeds=negative_image_emb,
- ... height=768,
- ... width=768,
- ... num_inference_steps=100,
- ... ).images
-
- >>> image[0].save("cat.png")
- ```
-"""
-
-
-def get_new_h_w(h, w, scale_factor=8):
- new_h = h // scale_factor**2
- if h % scale_factor**2 != 0:
- new_h += 1
- new_w = w // scale_factor**2
- if w % scale_factor**2 != 0:
- new_w += 1
- return new_h * scale_factor, new_w * scale_factor
-
-
-class KandinskyPipeline(DiffusionPipeline):
- """
- Pipeline for text-to-image generation using Kandinsky
-
- This model inherits from [`DiffusionPipeline`]. Check the superclass documentation for the generic methods the
- library implements for all the pipelines (such as downloading or saving, running on a particular device, etc.)
-
- Args:
- text_encoder ([`MultilingualCLIP`]):
- Frozen text-encoder.
- tokenizer ([`XLMRobertaTokenizer`]):
- Tokenizer of class
- scheduler (Union[`DDIMScheduler`,`DDPMScheduler`]):
- A scheduler to be used in combination with `unet` to generate image latents.
- unet ([`UNet2DConditionModel`]):
- Conditional U-Net architecture to denoise the image embedding.
- movq ([`VQModel`]):
- MoVQ Decoder to generate the image from the latents.
- """
-
- def __init__(
- self,
- text_encoder: MultilingualCLIP,
- tokenizer: XLMRobertaTokenizer,
- unet: UNet2DConditionModel,
- scheduler: Union[DDIMScheduler, DDPMScheduler],
- movq: VQModel,
- ):
- super().__init__()
-
- self.register_modules(
- text_encoder=text_encoder,
- tokenizer=tokenizer,
- unet=unet,
- scheduler=scheduler,
- movq=movq,
- )
- self.movq_scale_factor = 2 ** (len(self.movq.config.block_out_channels) - 1)
-
- # Copied from diffusers.pipelines.unclip.pipeline_unclip.UnCLIPPipeline.prepare_latents
- def prepare_latents(self, shape, dtype, device, generator, latents, scheduler):
- if latents is None:
- latents = randn_tensor(shape, generator=generator, device=device, dtype=dtype)
- else:
- if latents.shape != shape:
- raise ValueError(f"Unexpected latents shape, got {latents.shape}, expected {shape}")
- latents = latents.to(device)
-
- latents = latents * scheduler.init_noise_sigma
- return latents
-
- def _encode_prompt(
- self,
- prompt,
- device,
- num_images_per_prompt,
- do_classifier_free_guidance,
- negative_prompt=None,
- ):
- batch_size = len(prompt) if isinstance(prompt, list) else 1
- # get prompt text embeddings
- text_inputs = self.tokenizer(
- prompt,
- padding="max_length",
- truncation=True,
- max_length=77,
- return_attention_mask=True,
- add_special_tokens=True,
- return_tensors="pt",
- )
-
- text_input_ids = text_inputs.input_ids
- untruncated_ids = self.tokenizer(prompt, padding="longest", return_tensors="pt").input_ids
-
- if untruncated_ids.shape[-1] >= text_input_ids.shape[-1] and not torch.equal(text_input_ids, untruncated_ids):
- removed_text = self.tokenizer.batch_decode(untruncated_ids[:, self.tokenizer.model_max_length - 1 : -1])
- logger.warning(
- "The following part of your input was truncated because CLIP can only handle sequences up to"
- f" {self.tokenizer.model_max_length} tokens: {removed_text}"
- )
-
- text_input_ids = text_input_ids.to(device)
- text_mask = text_inputs.attention_mask.to(device)
-
- prompt_embeds, text_encoder_hidden_states = self.text_encoder(
- input_ids=text_input_ids, attention_mask=text_mask
- )
-
- prompt_embeds = prompt_embeds.repeat_interleave(num_images_per_prompt, dim=0)
- text_encoder_hidden_states = text_encoder_hidden_states.repeat_interleave(num_images_per_prompt, dim=0)
- text_mask = text_mask.repeat_interleave(num_images_per_prompt, dim=0)
-
- if do_classifier_free_guidance:
- uncond_tokens: List[str]
- if negative_prompt is None:
- uncond_tokens = [""] * batch_size
- elif type(prompt) is not type(negative_prompt):
- raise TypeError(
- f"`negative_prompt` should be the same type to `prompt`, but got {type(negative_prompt)} !="
- f" {type(prompt)}."
- )
- elif isinstance(negative_prompt, str):
- uncond_tokens = [negative_prompt]
- elif batch_size != len(negative_prompt):
- raise ValueError(
- f"`negative_prompt`: {negative_prompt} has batch size {len(negative_prompt)}, but `prompt`:"
- f" {prompt} has batch size {batch_size}. Please make sure that passed `negative_prompt` matches"
- " the batch size of `prompt`."
- )
- else:
- uncond_tokens = negative_prompt
-
- uncond_input = self.tokenizer(
- uncond_tokens,
- padding="max_length",
- max_length=77,
- truncation=True,
- return_attention_mask=True,
- add_special_tokens=True,
- return_tensors="pt",
- )
- uncond_text_input_ids = uncond_input.input_ids.to(device)
- uncond_text_mask = uncond_input.attention_mask.to(device)
-
- negative_prompt_embeds, uncond_text_encoder_hidden_states = self.text_encoder(
- input_ids=uncond_text_input_ids, attention_mask=uncond_text_mask
- )
-
- # duplicate unconditional embeddings for each generation per prompt, using mps friendly method
-
- seq_len = negative_prompt_embeds.shape[1]
- negative_prompt_embeds = negative_prompt_embeds.repeat(1, num_images_per_prompt)
- negative_prompt_embeds = negative_prompt_embeds.view(batch_size * num_images_per_prompt, seq_len)
-
- seq_len = uncond_text_encoder_hidden_states.shape[1]
- uncond_text_encoder_hidden_states = uncond_text_encoder_hidden_states.repeat(1, num_images_per_prompt, 1)
- uncond_text_encoder_hidden_states = uncond_text_encoder_hidden_states.view(
- batch_size * num_images_per_prompt, seq_len, -1
- )
- uncond_text_mask = uncond_text_mask.repeat_interleave(num_images_per_prompt, dim=0)
-
- # done duplicates
-
- # For classifier free guidance, we need to do two forward passes.
- # Here we concatenate the unconditional and text embeddings into a single batch
- # to avoid doing two forward passes
- prompt_embeds = torch.cat([negative_prompt_embeds, prompt_embeds])
- text_encoder_hidden_states = torch.cat([uncond_text_encoder_hidden_states, text_encoder_hidden_states])
-
- text_mask = torch.cat([uncond_text_mask, text_mask])
-
- return prompt_embeds, text_encoder_hidden_states, text_mask
-
- def enable_model_cpu_offload(self, gpu_id=0):
- r"""
- Offloads all models to CPU using accelerate, reducing memory usage with a low impact on performance. Compared
- to `enable_sequential_cpu_offload`, this method moves one whole model at a time to the GPU when its `forward`
- method is called, and the model remains in GPU until the next model runs. Memory savings are lower than with
- `enable_sequential_cpu_offload`, but performance is much better due to the iterative execution of the `unet`.
- """
- if is_accelerate_available() and is_accelerate_version(">=", "0.17.0.dev0"):
- from accelerate import cpu_offload_with_hook
- else:
- raise ImportError("`enable_model_cpu_offload` requires `accelerate v0.17.0` or higher.")
-
- device = torch.device(f"cuda:{gpu_id}")
-
- if self.device.type != "cpu":
- self.to("cpu", silence_dtype_warnings=True)
- torch.cuda.empty_cache() # otherwise we don't see the memory savings (but they probably exist)
-
- hook = None
- for cpu_offloaded_model in [self.text_encoder, self.unet, self.movq]:
- _, hook = cpu_offload_with_hook(cpu_offloaded_model, device, prev_module_hook=hook)
-
- # We'll offload the last model manually.
- self.final_offload_hook = hook
-
- @torch.no_grad()
- @replace_example_docstring(EXAMPLE_DOC_STRING)
- def __call__(
- self,
- prompt: Union[str, List[str]],
- image_embeds: Union[torch.FloatTensor, List[torch.FloatTensor]],
- negative_image_embeds: Union[torch.FloatTensor, List[torch.FloatTensor]],
- negative_prompt: Optional[Union[str, List[str]]] = None,
- height: int = 512,
- width: int = 512,
- num_inference_steps: int = 100,
- guidance_scale: float = 4.0,
- num_images_per_prompt: int = 1,
- generator: Optional[Union[torch.Generator, List[torch.Generator]]] = None,
- latents: Optional[torch.FloatTensor] = None,
- output_type: Optional[str] = "pil",
- callback: Optional[Callable[[int, int, torch.FloatTensor], None]] = None,
- callback_steps: int = 1,
- return_dict: bool = True,
- ):
- """
- Function invoked when calling the pipeline for generation.
-
- Args:
- prompt (`str` or `List[str]`):
- The prompt or prompts to guide the image generation.
- image_embeds (`torch.FloatTensor` or `List[torch.FloatTensor]`):
- The clip image embeddings for text prompt, that will be used to condition the image generation.
- negative_image_embeds (`torch.FloatTensor` or `List[torch.FloatTensor]`):
- The clip image embeddings for negative text prompt, will be used to condition the image generation.
- negative_prompt (`str` or `List[str]`, *optional*):
- The prompt or prompts not to guide the image generation. Ignored when not using guidance (i.e., ignored
- if `guidance_scale` is less than `1`).
- height (`int`, *optional*, defaults to 512):
- The height in pixels of the generated image.
- width (`int`, *optional*, defaults to 512):
- The width in pixels of the generated image.
- num_inference_steps (`int`, *optional*, defaults to 100):
- The number of denoising steps. More denoising steps usually lead to a higher quality image at the
- expense of slower inference.
- guidance_scale (`float`, *optional*, defaults to 4.0):
- Guidance scale as defined in [Classifier-Free Diffusion Guidance](https://arxiv.org/abs/2207.12598).
- `guidance_scale` is defined as `w` of equation 2. of [Imagen
- Paper](https://arxiv.org/pdf/2205.11487.pdf). Guidance scale is enabled by setting `guidance_scale >
- 1`. Higher guidance scale encourages to generate images that are closely linked to the text `prompt`,
- usually at the expense of lower image quality.
- num_images_per_prompt (`int`, *optional*, defaults to 1):
- The number of images to generate per prompt.
- generator (`torch.Generator` or `List[torch.Generator]`, *optional*):
- One or a list of [torch generator(s)](https://pytorch.org/docs/stable/generated/torch.Generator.html)
- to make generation deterministic.
- latents (`torch.FloatTensor`, *optional*):
- Pre-generated noisy latents, sampled from a Gaussian distribution, to be used as inputs for image
- generation. Can be used to tweak the same generation with different prompts. If not provided, a latents
- tensor will ge generated by sampling using the supplied random `generator`.
- output_type (`str`, *optional*, defaults to `"pil"`):
- The output format of the generate image. Choose between: `"pil"` (`PIL.Image.Image`), `"np"`
- (`np.array`) or `"pt"` (`torch.Tensor`).
- callback (`Callable`, *optional*):
- A function that calls every `callback_steps` steps during inference. The function is called with the
- following arguments: `callback(step: int, timestep: int, latents: torch.FloatTensor)`.
- callback_steps (`int`, *optional*, defaults to 1):
- The frequency at which the `callback` function is called. If not specified, the callback is called at
- every step.
- return_dict (`bool`, *optional*, defaults to `True`):
- Whether or not to return a [`~pipelines.ImagePipelineOutput`] instead of a plain tuple.
-
- Examples:
-
- Returns:
- [`~pipelines.ImagePipelineOutput`] or `tuple`
- """
-
- if isinstance(prompt, str):
- batch_size = 1
- elif isinstance(prompt, list):
- batch_size = len(prompt)
- else:
- raise ValueError(f"`prompt` has to be of type `str` or `list` but is {type(prompt)}")
-
- device = self._execution_device
-
- batch_size = batch_size * num_images_per_prompt
- do_classifier_free_guidance = guidance_scale > 1.0
-
- prompt_embeds, text_encoder_hidden_states, _ = self._encode_prompt(
- prompt, device, num_images_per_prompt, do_classifier_free_guidance, negative_prompt
- )
-
- if isinstance(image_embeds, list):
- image_embeds = torch.cat(image_embeds, dim=0)
- if isinstance(negative_image_embeds, list):
- negative_image_embeds = torch.cat(negative_image_embeds, dim=0)
-
- if do_classifier_free_guidance:
- image_embeds = image_embeds.repeat_interleave(num_images_per_prompt, dim=0)
- negative_image_embeds = negative_image_embeds.repeat_interleave(num_images_per_prompt, dim=0)
-
- image_embeds = torch.cat([negative_image_embeds, image_embeds], dim=0).to(
- dtype=prompt_embeds.dtype, device=device
- )
-
- self.scheduler.set_timesteps(num_inference_steps, device=device)
- timesteps_tensor = self.scheduler.timesteps
-
- num_channels_latents = self.unet.config.in_channels
-
- height, width = get_new_h_w(height, width, self.movq_scale_factor)
-
- # create initial latent
- latents = self.prepare_latents(
- (batch_size, num_channels_latents, height, width),
- text_encoder_hidden_states.dtype,
- device,
- generator,
- latents,
- self.scheduler,
- )
-
- for i, t in enumerate(self.progress_bar(timesteps_tensor)):
- # expand the latents if we are doing classifier free guidance
- latent_model_input = torch.cat([latents] * 2) if do_classifier_free_guidance else latents
-
- added_cond_kwargs = {"text_embeds": prompt_embeds, "image_embeds": image_embeds}
- noise_pred = self.unet(
- sample=latent_model_input,
- timestep=t,
- encoder_hidden_states=text_encoder_hidden_states,
- added_cond_kwargs=added_cond_kwargs,
- return_dict=False,
- )[0]
-
- if do_classifier_free_guidance:
- noise_pred, variance_pred = noise_pred.split(latents.shape[1], dim=1)
- noise_pred_uncond, noise_pred_text = noise_pred.chunk(2)
- _, variance_pred_text = variance_pred.chunk(2)
- noise_pred = noise_pred_uncond + guidance_scale * (noise_pred_text - noise_pred_uncond)
- noise_pred = torch.cat([noise_pred, variance_pred_text], dim=1)
-
- if not (
- hasattr(self.scheduler.config, "variance_type")
- and self.scheduler.config.variance_type in ["learned", "learned_range"]
- ):
- noise_pred, _ = noise_pred.split(latents.shape[1], dim=1)
-
- # compute the previous noisy sample x_t -> x_t-1
- latents = self.scheduler.step(
- noise_pred,
- t,
- latents,
- generator=generator,
- ).prev_sample
-
- if callback is not None and i % callback_steps == 0:
- callback(i, t, latents)
-
- # post-processing
- image = self.movq.decode(latents, force_not_quantize=True)["sample"]
-
- if output_type not in ["pt", "np", "pil"]:
- raise ValueError(f"Only the output types `pt`, `pil` and `np` are supported not output_type={output_type}")
-
- if output_type in ["np", "pil"]:
- image = image * 0.5 + 0.5
- image = image.clamp(0, 1)
- image = image.cpu().permute(0, 2, 3, 1).float().numpy()
-
- if output_type == "pil":
- image = self.numpy_to_pil(image)
-
- if not return_dict:
- return (image,)
-
- return ImagePipelineOutput(images=image)
diff --git a/spaces/Androidonnxfork/CivitAi-to-Diffusers/diffusers/tests/pipelines/kandinsky_v22/test_kandinsky_controlnet_img2img.py b/spaces/Androidonnxfork/CivitAi-to-Diffusers/diffusers/tests/pipelines/kandinsky_v22/test_kandinsky_controlnet_img2img.py
deleted file mode 100644
index 9ff2936cbd72433c32e1d71b541229fd83c4b2f2..0000000000000000000000000000000000000000
--- a/spaces/Androidonnxfork/CivitAi-to-Diffusers/diffusers/tests/pipelines/kandinsky_v22/test_kandinsky_controlnet_img2img.py
+++ /dev/null
@@ -1,290 +0,0 @@
-# coding=utf-8
-# Copyright 2023 HuggingFace Inc.
-#
-# Licensed under the Apache License, Version 2.0 (the "License");
-# you may not use this file except in compliance with the License.
-# You may obtain a copy of the License at
-#
-# http://www.apache.org/licenses/LICENSE-2.0
-#
-# Unless required by applicable law or agreed to in writing, software
-# distributed under the License is distributed on an "AS IS" BASIS,
-# WITHOUT WARRANTIES OR CONDITIONS OF ANY KIND, either express or implied.
-# See the License for the specific language governing permissions and
-# limitations under the License.
-
-import gc
-import random
-import unittest
-
-import numpy as np
-import torch
-from PIL import Image
-
-from diffusers import (
- DDIMScheduler,
- KandinskyV22ControlnetImg2ImgPipeline,
- KandinskyV22PriorEmb2EmbPipeline,
- UNet2DConditionModel,
- VQModel,
-)
-from diffusers.utils import floats_tensor, load_image, load_numpy, slow, torch_device
-from diffusers.utils.testing_utils import enable_full_determinism, require_torch_gpu
-
-from ..test_pipelines_common import PipelineTesterMixin, assert_mean_pixel_difference
-
-
-enable_full_determinism()
-
-
-class KandinskyV22ControlnetImg2ImgPipelineFastTests(PipelineTesterMixin, unittest.TestCase):
- pipeline_class = KandinskyV22ControlnetImg2ImgPipeline
- params = ["image_embeds", "negative_image_embeds", "image", "hint"]
- batch_params = ["image_embeds", "negative_image_embeds", "image", "hint"]
- required_optional_params = [
- "generator",
- "height",
- "width",
- "strength",
- "guidance_scale",
- "num_inference_steps",
- "return_dict",
- "guidance_scale",
- "num_images_per_prompt",
- "output_type",
- "return_dict",
- ]
- test_xformers_attention = False
-
- @property
- def text_embedder_hidden_size(self):
- return 32
-
- @property
- def time_input_dim(self):
- return 32
-
- @property
- def block_out_channels_0(self):
- return self.time_input_dim
-
- @property
- def time_embed_dim(self):
- return self.time_input_dim * 4
-
- @property
- def cross_attention_dim(self):
- return 100
-
- @property
- def dummy_unet(self):
- torch.manual_seed(0)
-
- model_kwargs = {
- "in_channels": 8,
- # Out channels is double in channels because predicts mean and variance
- "out_channels": 8,
- "addition_embed_type": "image_hint",
- "down_block_types": ("ResnetDownsampleBlock2D", "SimpleCrossAttnDownBlock2D"),
- "up_block_types": ("SimpleCrossAttnUpBlock2D", "ResnetUpsampleBlock2D"),
- "mid_block_type": "UNetMidBlock2DSimpleCrossAttn",
- "block_out_channels": (self.block_out_channels_0, self.block_out_channels_0 * 2),
- "layers_per_block": 1,
- "encoder_hid_dim": self.text_embedder_hidden_size,
- "encoder_hid_dim_type": "image_proj",
- "cross_attention_dim": self.cross_attention_dim,
- "attention_head_dim": 4,
- "resnet_time_scale_shift": "scale_shift",
- "class_embed_type": None,
- }
-
- model = UNet2DConditionModel(**model_kwargs)
- return model
-
- @property
- def dummy_movq_kwargs(self):
- return {
- "block_out_channels": [32, 32, 64, 64],
- "down_block_types": [
- "DownEncoderBlock2D",
- "DownEncoderBlock2D",
- "DownEncoderBlock2D",
- "AttnDownEncoderBlock2D",
- ],
- "in_channels": 3,
- "latent_channels": 4,
- "layers_per_block": 1,
- "norm_num_groups": 8,
- "norm_type": "spatial",
- "num_vq_embeddings": 12,
- "out_channels": 3,
- "up_block_types": ["AttnUpDecoderBlock2D", "UpDecoderBlock2D", "UpDecoderBlock2D", "UpDecoderBlock2D"],
- "vq_embed_dim": 4,
- }
-
- @property
- def dummy_movq(self):
- torch.manual_seed(0)
- model = VQModel(**self.dummy_movq_kwargs)
- return model
-
- def get_dummy_components(self):
- unet = self.dummy_unet
- movq = self.dummy_movq
-
- ddim_config = {
- "num_train_timesteps": 1000,
- "beta_schedule": "linear",
- "beta_start": 0.00085,
- "beta_end": 0.012,
- "clip_sample": False,
- "set_alpha_to_one": False,
- "steps_offset": 0,
- "prediction_type": "epsilon",
- "thresholding": False,
- }
-
- scheduler = DDIMScheduler(**ddim_config)
-
- components = {
- "unet": unet,
- "scheduler": scheduler,
- "movq": movq,
- }
-
- return components
-
- def get_dummy_inputs(self, device, seed=0):
- image_embeds = floats_tensor((1, self.text_embedder_hidden_size), rng=random.Random(seed)).to(device)
- negative_image_embeds = floats_tensor((1, self.text_embedder_hidden_size), rng=random.Random(seed + 1)).to(
- device
- )
- # create init_image
- image = floats_tensor((1, 3, 64, 64), rng=random.Random(seed)).to(device)
- image = image.cpu().permute(0, 2, 3, 1)[0]
- init_image = Image.fromarray(np.uint8(image)).convert("RGB").resize((256, 256))
- # create hint
- hint = floats_tensor((1, 3, 64, 64), rng=random.Random(seed)).to(device)
-
- if str(device).startswith("mps"):
- generator = torch.manual_seed(seed)
- else:
- generator = torch.Generator(device=device).manual_seed(seed)
- inputs = {
- "image": init_image,
- "image_embeds": image_embeds,
- "negative_image_embeds": negative_image_embeds,
- "hint": hint,
- "generator": generator,
- "height": 64,
- "width": 64,
- "num_inference_steps": 10,
- "guidance_scale": 7.0,
- "strength": 0.2,
- "output_type": "np",
- }
- return inputs
-
- def test_kandinsky_controlnet_img2img(self):
- device = "cpu"
-
- components = self.get_dummy_components()
-
- pipe = self.pipeline_class(**components)
- pipe = pipe.to(device)
-
- pipe.set_progress_bar_config(disable=None)
-
- output = pipe(**self.get_dummy_inputs(device))
- image = output.images
-
- image_from_tuple = pipe(
- **self.get_dummy_inputs(device),
- return_dict=False,
- )[0]
-
- image_slice = image[0, -3:, -3:, -1]
- image_from_tuple_slice = image_from_tuple[0, -3:, -3:, -1]
-
- assert image.shape == (1, 64, 64, 3)
-
- expected_slice = np.array(
- [0.54985034, 0.55509365, 0.52561504, 0.5570494, 0.5593818, 0.5263979, 0.50285643, 0.5069846, 0.51196736]
- )
- assert (
- np.abs(image_slice.flatten() - expected_slice).max() < 1e-2
- ), f" expected_slice {expected_slice}, but got {image_slice.flatten()}"
- assert (
- np.abs(image_from_tuple_slice.flatten() - expected_slice).max() < 1e-2
- ), f" expected_slice {expected_slice}, but got {image_from_tuple_slice.flatten()}"
-
-
-@slow
-@require_torch_gpu
-class KandinskyV22ControlnetImg2ImgPipelineIntegrationTests(unittest.TestCase):
- def tearDown(self):
- # clean up the VRAM after each test
- super().tearDown()
- gc.collect()
- torch.cuda.empty_cache()
-
- def test_kandinsky_controlnet_img2img(self):
- expected_image = load_numpy(
- "https://huggingface.co/datasets/hf-internal-testing/diffusers-images/resolve/main"
- "/kandinskyv22/kandinskyv22_controlnet_img2img_robotcat_fp16.npy"
- )
-
- init_image = load_image(
- "https://huggingface.co/datasets/hf-internal-testing/diffusers-images/resolve/main" "/kandinsky/cat.png"
- )
- init_image = init_image.resize((512, 512))
-
- hint = load_image(
- "https://huggingface.co/datasets/hf-internal-testing/diffusers-images/resolve/main"
- "/kandinskyv22/hint_image_cat.png"
- )
- hint = torch.from_numpy(np.array(hint)).float() / 255.0
- hint = hint.permute(2, 0, 1).unsqueeze(0)
-
- prompt = "A robot, 4k photo"
-
- pipe_prior = KandinskyV22PriorEmb2EmbPipeline.from_pretrained(
- "kandinsky-community/kandinsky-2-2-prior", torch_dtype=torch.float16
- )
- pipe_prior.to(torch_device)
-
- pipeline = KandinskyV22ControlnetImg2ImgPipeline.from_pretrained(
- "kandinsky-community/kandinsky-2-2-controlnet-depth", torch_dtype=torch.float16
- )
- pipeline = pipeline.to(torch_device)
-
- pipeline.set_progress_bar_config(disable=None)
-
- generator = torch.Generator(device="cpu").manual_seed(0)
-
- image_emb, zero_image_emb = pipe_prior(
- prompt,
- image=init_image,
- strength=0.85,
- generator=generator,
- negative_prompt="",
- ).to_tuple()
-
- output = pipeline(
- image=init_image,
- image_embeds=image_emb,
- negative_image_embeds=zero_image_emb,
- hint=hint,
- generator=generator,
- num_inference_steps=100,
- height=512,
- width=512,
- strength=0.5,
- output_type="np",
- )
-
- image = output.images[0]
-
- assert image.shape == (512, 512, 3)
-
- assert_mean_pixel_difference(image, expected_image)
diff --git a/spaces/Andy1621/uniformer_image_detection/configs/retinanet/retinanet_r101_fpn_1x_coco.py b/spaces/Andy1621/uniformer_image_detection/configs/retinanet/retinanet_r101_fpn_1x_coco.py
deleted file mode 100644
index 1e6f46340d551abaa22ff2176bec22824188d6cb..0000000000000000000000000000000000000000
--- a/spaces/Andy1621/uniformer_image_detection/configs/retinanet/retinanet_r101_fpn_1x_coco.py
+++ /dev/null
@@ -1,2 +0,0 @@
-_base_ = './retinanet_r50_fpn_1x_coco.py'
-model = dict(pretrained='torchvision://resnet101', backbone=dict(depth=101))
diff --git a/spaces/Andy1621/uniformer_image_segmentation/configs/pspnet/pspnet_r101-d8_480x480_40k_pascal_context.py b/spaces/Andy1621/uniformer_image_segmentation/configs/pspnet/pspnet_r101-d8_480x480_40k_pascal_context.py
deleted file mode 100644
index 0b5a990604a77238375cb6d2b8298a382a457dd6..0000000000000000000000000000000000000000
--- a/spaces/Andy1621/uniformer_image_segmentation/configs/pspnet/pspnet_r101-d8_480x480_40k_pascal_context.py
+++ /dev/null
@@ -1,2 +0,0 @@
-_base_ = './pspnet_r50-d8_480x480_40k_pascal_context.py'
-model = dict(pretrained='open-mmlab://resnet101_v1c', backbone=dict(depth=101))
diff --git a/spaces/AnishKumbhar/ChatBot/text-generation-webui-main/extensions/multimodal/README.md b/spaces/AnishKumbhar/ChatBot/text-generation-webui-main/extensions/multimodal/README.md
deleted file mode 100644
index 506810343f54658e9e42b3dd45ed593a8cb70b25..0000000000000000000000000000000000000000
--- a/spaces/AnishKumbhar/ChatBot/text-generation-webui-main/extensions/multimodal/README.md
+++ /dev/null
@@ -1,83 +0,0 @@
-# Multimodal
-
-## Description
-
-Adds support for multimodality (text+images) to text-generation-webui.
-
-https://user-images.githubusercontent.com/3718215/233817203-69b57e77-0c55-4fd6-b742-3204bb13b8fc.mp4
-
-## Usage
-
-To run this extension, download a LLM that supports multimodality, and then start server.py with the appropriate `--multimodal-pipeline` argument. Examples:
-
-```
-python server.py --model wojtab_llava-7b-v0-4bit-128g --multimodal-pipeline llava-7b
-python3 server.py --model wojtab_llava-13b-v0-4bit-128g --multimodal-pipeline llava-13b
-python server.py --model anon8231489123_vicuna-13b-GPTQ-4bit-128g --multimodal-pipeline minigpt4-13b
-python server.py --model llama-7b-4bit --multimodal-pipeline minigpt4-7b
-```
-
-There is built-in support for LLaVA-v0-13B and LLaVA-v0-7b. To install `minigpt4`:
-
-- clone https://github.com/Wojtab/minigpt-4-pipeline into `extensions/multimodal/pipelines`
-- install the requirements.txt
-
-The same procedure should be used to install other pipelines, which can then be used with `--multimodal-pipeline [pipeline name]`. For additional multimodal pipelines refer to the compatibility section below.
-
-Do note, that each image takes up a considerable amount of tokens, so adjust `max_new_tokens` to be at most 1700 (recommended value is between 200 to 500), so the images don't get truncated.
-
-To send an image, just upload it to the extension field below chat, and send a prompt as always. The image will be added to the end of your message. If you wish to modify the placement, include a string `` in your prompt.
-
-Additionally, there is *Embed all images, not only the last one* checkbox. It modifies the image embeddings, by default (if it's unchecked), all but the most recent images have their embeddings empty, so they are not fed to the network. It seems as if some multimodal networks consider the features in all images at the same time as if they were a single image. Due to this behavior, by default, the extension skips previous images. However, it can lead to sub-par generation on other pipelines. If you want to include all images, just tick this checkbox.
-
-## Compatibility
-As of now, the following multimodal pipelines are supported:
-|Pipeline|`--multimodal-pipeline`|Default LLM|LLM info(for the linked model)|Pipeline repository|
-|-|-|-|-|-|
-|[LLaVA 13B](https://github.com/haotian-liu/LLaVA)|`llava-13b`|[LLaVA 13B](https://huggingface.co/wojtab/llava-13b-v0-4bit-128g)|GPTQ 4-bit quant, old CUDA|built-in|
-|[LLaVA 7B](https://github.com/haotian-liu/LLaVA)|`llava-7b`|[LLaVA 7B](https://huggingface.co/wojtab/llava-7b-v0-4bit-128g)|GPTQ 4-bit quant, old CUDA|built-in|
-|[MiniGPT-4 7B](https://github.com/Vision-CAIR/MiniGPT-4)|`minigpt4-7b`|[Vicuna v0 7B](https://huggingface.co/TheBloke/vicuna-7B-GPTQ-4bit-128g)|GPTQ 4-bit quant, new format|[Wojtab/minigpt-4-pipeline](https://github.com/Wojtab/minigpt-4-pipeline)|
-|[MiniGPT-4 13B](https://github.com/Vision-CAIR/MiniGPT-4)|`minigpt4-13b`|[Vicuna v0 13B](https://huggingface.co/anon8231489123/vicuna-13b-GPTQ-4bit-128g)|GPTQ 4-bit quant, old CUDA|[Wojtab/minigpt-4-pipeline](https://github.com/Wojtab/minigpt-4-pipeline)|
-|[InstructBLIP 7B](https://github.com/salesforce/LAVIS/tree/main/projects/instructblip)|`instructblip-7b`|[Vicuna v1.1 7B](https://huggingface.co/TheBloke/vicuna-7B-1.1-GPTQ-4bit-128g)|GPTQ 4-bit quant|[kjerk/instructblip-pipeline](https://github.com/kjerk/instructblip-pipeline)|
-|[InstructBLIP 13B](https://github.com/salesforce/LAVIS/tree/main/projects/instructblip)|`instructblip-13b`|[Vicuna v1.1 13B](https://huggingface.co/TheBloke/vicuna-13B-1.1-GPTQ-4bit-128g)|GPTQ 4-bit quant|[kjerk/instructblip-pipeline](https://github.com/kjerk/instructblip-pipeline)|
-
-Some pipelines could support different LLMs but do note that while it might work, it isn't a supported configuration.
-
-DO NOT report bugs if you are using a different LLM.
-
-DO NOT report bugs with pipelines in this repository (unless they are built-in)
-
-## Extension config
-This extension uses the following parameters (from `settings.json`):
-|Parameter|Description|
-|---------|-----------|
-|`multimodal-vision_bits`|Number of bits to load vision models (CLIP/ViT) feature extractor in (most pipelines should support either 32 or 16, default=32)|
-|`multimodal-vision_device`|Torch device to run the feature extractor on, for example, `cpu` or `cuda:0`, by default `cuda:0` if available|
-|`multimodal-projector_bits`|Number of bits to load feature projector model(s) in (most pipelines should support either 32 or 16, default=32)|
-|`multimodal-projector_device`|Torch device to run the feature projector model(s) on, for example `cpu` or `cuda:0`, by default `cuda:0` if available|
-|`multimodal-add_all_images_to_prompt`|Default value of "Embed all images, not only the last one" checkbox|
-
-## Usage through API
-
-You can run the multimodal inference through API, by inputting the images to prompt. Images are embedded like so: `f''`, where `img_str` is base-64 jpeg data. Note that you will need to launch `server.py` with the arguments `--api --extensions multimodal`.
-
-Python example:
-
-```Python
-import base64
-import requests
-
-CONTEXT = "You are LLaVA, a large language and vision assistant trained by UW Madison WAIV Lab. You are able to understand the visual content that the user provides, and assist the user with a variety of tasks using natural language. Follow the instructions carefully and explain your answers in detail.### Human: Hi!### Assistant: Hi there! How can I help you today?\n"
-
-with open('extreme_ironing.jpg', 'rb') as f:
- img_str = base64.b64encode(f.read()).decode('utf-8')
- prompt = CONTEXT + f'### Human: What is unusual about this image: \n### Assistant: '
- print(requests.post('http://127.0.0.1:5000/api/v1/generate', json={'prompt': prompt, 'stopping_strings': ['\n###']}).json())
-```
-script output:
-```Python
-{'results': [{'text': "The unusual aspect of this image is that a man is standing on top of a yellow minivan while doing his laundry. He has set up a makeshift clothes line using the car's rooftop as an outdoor drying area. This scene is uncommon because people typically do their laundry indoors, in a dedicated space like a laundromat or a room in their home, rather than on top of a moving vehicle. Additionally, hanging clothes on the car could be potentially hazardous or illegal in some jurisdictions due to the risk of damaging the vehicle or causing accidents on the road.\n##"}]}
-```
-
-## For pipeline developers/technical description
-see [DOCS.md](https://github.com/oobabooga/text-generation-webui/blob/main/extensions/multimodal/DOCS.md)
diff --git a/spaces/AnnasBlackHat/Image-Downloader/gofile.py b/spaces/AnnasBlackHat/Image-Downloader/gofile.py
deleted file mode 100644
index 52d8b3d953cb5be028dfde0a2c6b4eb422ccd08a..0000000000000000000000000000000000000000
--- a/spaces/AnnasBlackHat/Image-Downloader/gofile.py
+++ /dev/null
@@ -1,25 +0,0 @@
-import requests
-
-class Gofile:
- def __init__(self, token = None, folder_id= None):
- self.token = token
- self.folder_id = folder_id
-
- def find_server(self):
- resp = requests.get('https://api.gofile.io/getServer')
- result = resp.json()
- return result['data']['server']
-
- def upload(self, files):
- server = self.find_server()
- url = f'https://{server}.gofile.io/uploadFile'
- data_payload = {'token': self.token, 'folderId': self.folder_id}
- download_link = []
- for file in files:
- with open(file, 'rb') as f:
- resp = requests.post(url, files = {'file': f}, data= data_payload)
- print('upload status: ', resp.status_code)
- download_page = resp.json()['data']['downloadPage']
- download_link.append(download_page)
- print('download page: ',download_page)
- return download_link
\ No newline at end of file
diff --git a/spaces/Anonymous-sub/Rerender/ControlNet/annotator/openpose/util.py b/spaces/Anonymous-sub/Rerender/ControlNet/annotator/openpose/util.py
deleted file mode 100644
index 6f91ae0e65abaf0cbd62d803f56498991141e61b..0000000000000000000000000000000000000000
--- a/spaces/Anonymous-sub/Rerender/ControlNet/annotator/openpose/util.py
+++ /dev/null
@@ -1,164 +0,0 @@
-import math
-import numpy as np
-import matplotlib
-import cv2
-
-
-def padRightDownCorner(img, stride, padValue):
- h = img.shape[0]
- w = img.shape[1]
-
- pad = 4 * [None]
- pad[0] = 0 # up
- pad[1] = 0 # left
- pad[2] = 0 if (h % stride == 0) else stride - (h % stride) # down
- pad[3] = 0 if (w % stride == 0) else stride - (w % stride) # right
-
- img_padded = img
- pad_up = np.tile(img_padded[0:1, :, :]*0 + padValue, (pad[0], 1, 1))
- img_padded = np.concatenate((pad_up, img_padded), axis=0)
- pad_left = np.tile(img_padded[:, 0:1, :]*0 + padValue, (1, pad[1], 1))
- img_padded = np.concatenate((pad_left, img_padded), axis=1)
- pad_down = np.tile(img_padded[-2:-1, :, :]*0 + padValue, (pad[2], 1, 1))
- img_padded = np.concatenate((img_padded, pad_down), axis=0)
- pad_right = np.tile(img_padded[:, -2:-1, :]*0 + padValue, (1, pad[3], 1))
- img_padded = np.concatenate((img_padded, pad_right), axis=1)
-
- return img_padded, pad
-
-# transfer caffe model to pytorch which will match the layer name
-def transfer(model, model_weights):
- transfered_model_weights = {}
- for weights_name in model.state_dict().keys():
- transfered_model_weights[weights_name] = model_weights['.'.join(weights_name.split('.')[1:])]
- return transfered_model_weights
-
-# draw the body keypoint and lims
-def draw_bodypose(canvas, candidate, subset):
- stickwidth = 4
- limbSeq = [[2, 3], [2, 6], [3, 4], [4, 5], [6, 7], [7, 8], [2, 9], [9, 10], \
- [10, 11], [2, 12], [12, 13], [13, 14], [2, 1], [1, 15], [15, 17], \
- [1, 16], [16, 18], [3, 17], [6, 18]]
-
- colors = [[255, 0, 0], [255, 85, 0], [255, 170, 0], [255, 255, 0], [170, 255, 0], [85, 255, 0], [0, 255, 0], \
- [0, 255, 85], [0, 255, 170], [0, 255, 255], [0, 170, 255], [0, 85, 255], [0, 0, 255], [85, 0, 255], \
- [170, 0, 255], [255, 0, 255], [255, 0, 170], [255, 0, 85]]
- for i in range(18):
- for n in range(len(subset)):
- index = int(subset[n][i])
- if index == -1:
- continue
- x, y = candidate[index][0:2]
- cv2.circle(canvas, (int(x), int(y)), 4, colors[i], thickness=-1)
- for i in range(17):
- for n in range(len(subset)):
- index = subset[n][np.array(limbSeq[i]) - 1]
- if -1 in index:
- continue
- cur_canvas = canvas.copy()
- Y = candidate[index.astype(int), 0]
- X = candidate[index.astype(int), 1]
- mX = np.mean(X)
- mY = np.mean(Y)
- length = ((X[0] - X[1]) ** 2 + (Y[0] - Y[1]) ** 2) ** 0.5
- angle = math.degrees(math.atan2(X[0] - X[1], Y[0] - Y[1]))
- polygon = cv2.ellipse2Poly((int(mY), int(mX)), (int(length / 2), stickwidth), int(angle), 0, 360, 1)
- cv2.fillConvexPoly(cur_canvas, polygon, colors[i])
- canvas = cv2.addWeighted(canvas, 0.4, cur_canvas, 0.6, 0)
- # plt.imsave("preview.jpg", canvas[:, :, [2, 1, 0]])
- # plt.imshow(canvas[:, :, [2, 1, 0]])
- return canvas
-
-
-# image drawed by opencv is not good.
-def draw_handpose(canvas, all_hand_peaks, show_number=False):
- edges = [[0, 1], [1, 2], [2, 3], [3, 4], [0, 5], [5, 6], [6, 7], [7, 8], [0, 9], [9, 10], \
- [10, 11], [11, 12], [0, 13], [13, 14], [14, 15], [15, 16], [0, 17], [17, 18], [18, 19], [19, 20]]
-
- for peaks in all_hand_peaks:
- for ie, e in enumerate(edges):
- if np.sum(np.all(peaks[e], axis=1)==0)==0:
- x1, y1 = peaks[e[0]]
- x2, y2 = peaks[e[1]]
- cv2.line(canvas, (x1, y1), (x2, y2), matplotlib.colors.hsv_to_rgb([ie/float(len(edges)), 1.0, 1.0])*255, thickness=2)
-
- for i, keyponit in enumerate(peaks):
- x, y = keyponit
- cv2.circle(canvas, (x, y), 4, (0, 0, 255), thickness=-1)
- if show_number:
- cv2.putText(canvas, str(i), (x, y), cv2.FONT_HERSHEY_SIMPLEX, 0.3, (0, 0, 0), lineType=cv2.LINE_AA)
- return canvas
-
-# detect hand according to body pose keypoints
-# please refer to https://github.com/CMU-Perceptual-Computing-Lab/openpose/blob/master/src/openpose/hand/handDetector.cpp
-def handDetect(candidate, subset, oriImg):
- # right hand: wrist 4, elbow 3, shoulder 2
- # left hand: wrist 7, elbow 6, shoulder 5
- ratioWristElbow = 0.33
- detect_result = []
- image_height, image_width = oriImg.shape[0:2]
- for person in subset.astype(int):
- # if any of three not detected
- has_left = np.sum(person[[5, 6, 7]] == -1) == 0
- has_right = np.sum(person[[2, 3, 4]] == -1) == 0
- if not (has_left or has_right):
- continue
- hands = []
- #left hand
- if has_left:
- left_shoulder_index, left_elbow_index, left_wrist_index = person[[5, 6, 7]]
- x1, y1 = candidate[left_shoulder_index][:2]
- x2, y2 = candidate[left_elbow_index][:2]
- x3, y3 = candidate[left_wrist_index][:2]
- hands.append([x1, y1, x2, y2, x3, y3, True])
- # right hand
- if has_right:
- right_shoulder_index, right_elbow_index, right_wrist_index = person[[2, 3, 4]]
- x1, y1 = candidate[right_shoulder_index][:2]
- x2, y2 = candidate[right_elbow_index][:2]
- x3, y3 = candidate[right_wrist_index][:2]
- hands.append([x1, y1, x2, y2, x3, y3, False])
-
- for x1, y1, x2, y2, x3, y3, is_left in hands:
- # pos_hand = pos_wrist + ratio * (pos_wrist - pos_elbox) = (1 + ratio) * pos_wrist - ratio * pos_elbox
- # handRectangle.x = posePtr[wrist*3] + ratioWristElbow * (posePtr[wrist*3] - posePtr[elbow*3]);
- # handRectangle.y = posePtr[wrist*3+1] + ratioWristElbow * (posePtr[wrist*3+1] - posePtr[elbow*3+1]);
- # const auto distanceWristElbow = getDistance(poseKeypoints, person, wrist, elbow);
- # const auto distanceElbowShoulder = getDistance(poseKeypoints, person, elbow, shoulder);
- # handRectangle.width = 1.5f * fastMax(distanceWristElbow, 0.9f * distanceElbowShoulder);
- x = x3 + ratioWristElbow * (x3 - x2)
- y = y3 + ratioWristElbow * (y3 - y2)
- distanceWristElbow = math.sqrt((x3 - x2) ** 2 + (y3 - y2) ** 2)
- distanceElbowShoulder = math.sqrt((x2 - x1) ** 2 + (y2 - y1) ** 2)
- width = 1.5 * max(distanceWristElbow, 0.9 * distanceElbowShoulder)
- # x-y refers to the center --> offset to topLeft point
- # handRectangle.x -= handRectangle.width / 2.f;
- # handRectangle.y -= handRectangle.height / 2.f;
- x -= width / 2
- y -= width / 2 # width = height
- # overflow the image
- if x < 0: x = 0
- if y < 0: y = 0
- width1 = width
- width2 = width
- if x + width > image_width: width1 = image_width - x
- if y + width > image_height: width2 = image_height - y
- width = min(width1, width2)
- # the max hand box value is 20 pixels
- if width >= 20:
- detect_result.append([int(x), int(y), int(width), is_left])
-
- '''
- return value: [[x, y, w, True if left hand else False]].
- width=height since the network require squared input.
- x, y is the coordinate of top left
- '''
- return detect_result
-
-# get max index of 2d array
-def npmax(array):
- arrayindex = array.argmax(1)
- arrayvalue = array.max(1)
- i = arrayvalue.argmax()
- j = arrayindex[i]
- return i, j
diff --git a/spaces/Apex-X/Tm/roop/processors/frame/core.py b/spaces/Apex-X/Tm/roop/processors/frame/core.py
deleted file mode 100644
index c225f9de483a2914a98392ce9de5bd03f2013a2d..0000000000000000000000000000000000000000
--- a/spaces/Apex-X/Tm/roop/processors/frame/core.py
+++ /dev/null
@@ -1,88 +0,0 @@
-import os
-import importlib
-import psutil
-from concurrent.futures import ThreadPoolExecutor, as_completed
-from queue import Queue
-from types import ModuleType
-from typing import Any, List, Callable
-from tqdm import tqdm
-
-import roop
-
-FRAME_PROCESSORS_MODULES: List[ModuleType] = []
-FRAME_PROCESSORS_INTERFACE = [
- 'pre_check',
- 'pre_start',
- 'process_frame',
- 'process_frames',
- 'process_image',
- 'process_video',
- 'post_process'
-]
-
-
-def load_frame_processor_module(frame_processor: str) -> Any:
- try:
- frame_processor_module = importlib.import_module(f'roop.processors.frame.{frame_processor}')
- for method_name in FRAME_PROCESSORS_INTERFACE:
- if not hasattr(frame_processor_module, method_name):
- raise NotImplementedError
- except (ImportError, NotImplementedError):
- quit(f'Frame processor {frame_processor} crashed.')
- return frame_processor_module
-
-
-def get_frame_processors_modules(frame_processors: List[str]) -> List[ModuleType]:
- global FRAME_PROCESSORS_MODULES
-
- if not FRAME_PROCESSORS_MODULES:
- for frame_processor in frame_processors:
- frame_processor_module = load_frame_processor_module(frame_processor)
- FRAME_PROCESSORS_MODULES.append(frame_processor_module)
- return FRAME_PROCESSORS_MODULES
-
-
-def multi_process_frame(source_path: str, temp_frame_paths: List[str], process_frames: Callable[[str, List[str], Any], None], update: Callable[[], None]) -> None:
- with ThreadPoolExecutor(max_workers=roop.globals.execution_threads) as executor:
- futures = []
- queue = create_queue(temp_frame_paths)
- queue_per_future = len(temp_frame_paths) // roop.globals.execution_threads
- while not queue.empty():
- future = executor.submit(process_frames, source_path, pick_queue(queue, queue_per_future), update)
- futures.append(future)
- for future in as_completed(futures):
- future.result()
-
-
-def create_queue(temp_frame_paths: List[str]) -> Queue[str]:
- queue: Queue[str] = Queue()
- for frame_path in temp_frame_paths:
- queue.put(frame_path)
- return queue
-
-
-def pick_queue(queue: Queue[str], queue_per_future: int) -> List[str]:
- queues = []
- for _ in range(queue_per_future):
- if not queue.empty():
- queues.append(queue.get())
- return queues
-
-
-def process_video(source_path: str, frame_paths: list[str], process_frames: Callable[[str, List[str], Any], None]) -> None:
- progress_bar_format = '{l_bar}{bar}| {n_fmt}/{total_fmt} [{elapsed}<{remaining}, {rate_fmt}{postfix}]'
- total = len(frame_paths)
- with tqdm(total=total, desc='Processing', unit='frame', dynamic_ncols=True, bar_format=progress_bar_format) as progress:
- multi_process_frame(source_path, frame_paths, process_frames, lambda: update_progress(progress))
-
-
-def update_progress(progress: Any = None) -> None:
- process = psutil.Process(os.getpid())
- memory_usage = process.memory_info().rss / 1024 / 1024 / 1024
- progress.set_postfix({
- 'memory_usage': '{:.2f}'.format(memory_usage).zfill(5) + 'GB',
- 'execution_providers': roop.globals.execution_providers,
- 'execution_threads': roop.globals.execution_threads
- })
- progress.refresh()
- progress.update(1)
diff --git a/spaces/Apex-X/nono/roop/face_reference.py b/spaces/Apex-X/nono/roop/face_reference.py
deleted file mode 100644
index 3c3e1f1c6e13c73ceafd40c0912c066a3a86a528..0000000000000000000000000000000000000000
--- a/spaces/Apex-X/nono/roop/face_reference.py
+++ /dev/null
@@ -1,21 +0,0 @@
-from typing import Optional
-
-from roop.typing import Face
-
-FACE_REFERENCE = None
-
-
-def get_face_reference() -> Optional[Face]:
- return FACE_REFERENCE
-
-
-def set_face_reference(face: Face) -> None:
- global FACE_REFERENCE
-
- FACE_REFERENCE = face
-
-
-def clear_face_reference() -> None:
- global FACE_REFERENCE
-
- FACE_REFERENCE = None
diff --git a/spaces/Arnaudding001/OpenAI_whisperLive/utils.py b/spaces/Arnaudding001/OpenAI_whisperLive/utils.py
deleted file mode 100644
index b85a7f3ff5c2e3e94823f4e1bf181e54edb1ddf9..0000000000000000000000000000000000000000
--- a/spaces/Arnaudding001/OpenAI_whisperLive/utils.py
+++ /dev/null
@@ -1,115 +0,0 @@
-import textwrap
-import unicodedata
-import re
-
-import zlib
-from typing import Iterator, TextIO
-
-
-def exact_div(x, y):
- assert x % y == 0
- return x // y
-
-
-def str2bool(string):
- str2val = {"True": True, "False": False}
- if string in str2val:
- return str2val[string]
- else:
- raise ValueError(f"Expected one of {set(str2val.keys())}, got {string}")
-
-
-def optional_int(string):
- return None if string == "None" else int(string)
-
-
-def optional_float(string):
- return None if string == "None" else float(string)
-
-
-def compression_ratio(text) -> float:
- return len(text) / len(zlib.compress(text.encode("utf-8")))
-
-
-def format_timestamp(seconds: float, always_include_hours: bool = False, fractionalSeperator: str = '.'):
- assert seconds >= 0, "non-negative timestamp expected"
- milliseconds = round(seconds * 1000.0)
-
- hours = milliseconds // 3_600_000
- milliseconds -= hours * 3_600_000
-
- minutes = milliseconds // 60_000
- milliseconds -= minutes * 60_000
-
- seconds = milliseconds // 1_000
- milliseconds -= seconds * 1_000
-
- hours_marker = f"{hours:02d}:" if always_include_hours or hours > 0 else ""
- return f"{hours_marker}{minutes:02d}:{seconds:02d}{fractionalSeperator}{milliseconds:03d}"
-
-
-def write_txt(transcript: Iterator[dict], file: TextIO):
- for segment in transcript:
- print(segment['text'].strip(), file=file, flush=True)
-
-
-def write_vtt(transcript: Iterator[dict], file: TextIO, maxLineWidth=None):
- print("WEBVTT\n", file=file)
- for segment in transcript:
- text = process_text(segment['text'], maxLineWidth).replace('-->', '->')
-
- print(
- f"{format_timestamp(segment['start'])} --> {format_timestamp(segment['end'])}\n"
- f"{text}\n",
- file=file,
- flush=True,
- )
-
-
-def write_srt(transcript: Iterator[dict], file: TextIO, maxLineWidth=None):
- """
- Write a transcript to a file in SRT format.
- Example usage:
- from pathlib import Path
- from whisper.utils import write_srt
- result = transcribe(model, audio_path, temperature=temperature, **args)
- # save SRT
- audio_basename = Path(audio_path).stem
- with open(Path(output_dir) / (audio_basename + ".srt"), "w", encoding="utf-8") as srt:
- write_srt(result["segments"], file=srt)
- """
- for i, segment in enumerate(transcript, start=1):
- text = process_text(segment['text'].strip(), maxLineWidth).replace('-->', '->')
-
- # write srt lines
- print(
- f"{i}\n"
- f"{format_timestamp(segment['start'], always_include_hours=True, fractionalSeperator=',')} --> "
- f"{format_timestamp(segment['end'], always_include_hours=True, fractionalSeperator=',')}\n"
- f"{text}\n",
- file=file,
- flush=True,
- )
-
-def process_text(text: str, maxLineWidth=None):
- if (maxLineWidth is None or maxLineWidth < 0):
- return text
-
- lines = textwrap.wrap(text, width=maxLineWidth, tabsize=4)
- return '\n'.join(lines)
-
-def slugify(value, allow_unicode=False):
- """
- Taken from https://github.com/django/django/blob/master/django/utils/text.py
- Convert to ASCII if 'allow_unicode' is False. Convert spaces or repeated
- dashes to single dashes. Remove characters that aren't alphanumerics,
- underscores, or hyphens. Convert to lowercase. Also strip leading and
- trailing whitespace, dashes, and underscores.
- """
- value = str(value)
- if allow_unicode:
- value = unicodedata.normalize('NFKC', value)
- else:
- value = unicodedata.normalize('NFKD', value).encode('ascii', 'ignore').decode('ascii')
- value = re.sub(r'[^\w\s-]', '', value.lower())
- return re.sub(r'[-\s]+', '-', value).strip('-_')
\ No newline at end of file
diff --git a/spaces/Arnx/MusicGenXvAKN/audiocraft/modules/lstm.py b/spaces/Arnx/MusicGenXvAKN/audiocraft/modules/lstm.py
deleted file mode 100644
index c0866175950c1ca4f6cca98649525e6481853bba..0000000000000000000000000000000000000000
--- a/spaces/Arnx/MusicGenXvAKN/audiocraft/modules/lstm.py
+++ /dev/null
@@ -1,25 +0,0 @@
-# Copyright (c) Meta Platforms, Inc. and affiliates.
-# All rights reserved.
-#
-# This source code is licensed under the license found in the
-# LICENSE file in the root directory of this source tree.
-
-from torch import nn
-
-
-class StreamableLSTM(nn.Module):
- """LSTM without worrying about the hidden state, nor the layout of the data.
- Expects input as convolutional layout.
- """
- def __init__(self, dimension: int, num_layers: int = 2, skip: bool = True):
- super().__init__()
- self.skip = skip
- self.lstm = nn.LSTM(dimension, dimension, num_layers)
-
- def forward(self, x):
- x = x.permute(2, 0, 1)
- y, _ = self.lstm(x)
- if self.skip:
- y = y + x
- y = y.permute(1, 2, 0)
- return y
diff --git a/spaces/Artples/Chat-with-Llama-2-70b/README.md b/spaces/Artples/Chat-with-Llama-2-70b/README.md
deleted file mode 100644
index f84ebc22af15b5b66b94d47d05ec03186ec9a0f2..0000000000000000000000000000000000000000
--- a/spaces/Artples/Chat-with-Llama-2-70b/README.md
+++ /dev/null
@@ -1,13 +0,0 @@
----
-title: Lauche-AI LEU-Chatbot
-emoji: ⚡
-colorFrom: gray
-colorTo: yellow
-sdk: gradio
-sdk_version: 3.44.3
-app_file: app.py
-pinned: false
-license: mit
----
-
-Check out the configuration reference at https://huggingface.co/docs/hub/spaces-config-reference
\ No newline at end of file
diff --git a/spaces/Ataturk-Chatbot/HuggingFaceChat/venv/lib/python3.11/site-packages/pip/_vendor/chardet/utf8prober.py b/spaces/Ataturk-Chatbot/HuggingFaceChat/venv/lib/python3.11/site-packages/pip/_vendor/chardet/utf8prober.py
deleted file mode 100644
index d96354d97c2195320d0acc1717a5876eafbea2af..0000000000000000000000000000000000000000
--- a/spaces/Ataturk-Chatbot/HuggingFaceChat/venv/lib/python3.11/site-packages/pip/_vendor/chardet/utf8prober.py
+++ /dev/null
@@ -1,82 +0,0 @@
-######################## BEGIN LICENSE BLOCK ########################
-# The Original Code is mozilla.org code.
-#
-# The Initial Developer of the Original Code is
-# Netscape Communications Corporation.
-# Portions created by the Initial Developer are Copyright (C) 1998
-# the Initial Developer. All Rights Reserved.
-#
-# Contributor(s):
-# Mark Pilgrim - port to Python
-#
-# This library is free software; you can redistribute it and/or
-# modify it under the terms of the GNU Lesser General Public
-# License as published by the Free Software Foundation; either
-# version 2.1 of the License, or (at your option) any later version.
-#
-# This library is distributed in the hope that it will be useful,
-# but WITHOUT ANY WARRANTY; without even the implied warranty of
-# MERCHANTABILITY or FITNESS FOR A PARTICULAR PURPOSE. See the GNU
-# Lesser General Public License for more details.
-#
-# You should have received a copy of the GNU Lesser General Public
-# License along with this library; if not, write to the Free Software
-# Foundation, Inc., 51 Franklin St, Fifth Floor, Boston, MA
-# 02110-1301 USA
-######################### END LICENSE BLOCK #########################
-
-from typing import Union
-
-from .charsetprober import CharSetProber
-from .codingstatemachine import CodingStateMachine
-from .enums import MachineState, ProbingState
-from .mbcssm import UTF8_SM_MODEL
-
-
-class UTF8Prober(CharSetProber):
- ONE_CHAR_PROB = 0.5
-
- def __init__(self) -> None:
- super().__init__()
- self.coding_sm = CodingStateMachine(UTF8_SM_MODEL)
- self._num_mb_chars = 0
- self.reset()
-
- def reset(self) -> None:
- super().reset()
- self.coding_sm.reset()
- self._num_mb_chars = 0
-
- @property
- def charset_name(self) -> str:
- return "utf-8"
-
- @property
- def language(self) -> str:
- return ""
-
- def feed(self, byte_str: Union[bytes, bytearray]) -> ProbingState:
- for c in byte_str:
- coding_state = self.coding_sm.next_state(c)
- if coding_state == MachineState.ERROR:
- self._state = ProbingState.NOT_ME
- break
- if coding_state == MachineState.ITS_ME:
- self._state = ProbingState.FOUND_IT
- break
- if coding_state == MachineState.START:
- if self.coding_sm.get_current_charlen() >= 2:
- self._num_mb_chars += 1
-
- if self.state == ProbingState.DETECTING:
- if self.get_confidence() > self.SHORTCUT_THRESHOLD:
- self._state = ProbingState.FOUND_IT
-
- return self.state
-
- def get_confidence(self) -> float:
- unlike = 0.99
- if self._num_mb_chars < 6:
- unlike *= self.ONE_CHAR_PROB**self._num_mb_chars
- return 1.0 - unlike
- return unlike
diff --git a/spaces/Ataturk-Chatbot/HuggingFaceChat/venv/lib/python3.11/site-packages/pip/_vendor/rich/diagnose.py b/spaces/Ataturk-Chatbot/HuggingFaceChat/venv/lib/python3.11/site-packages/pip/_vendor/rich/diagnose.py
deleted file mode 100644
index ad36183898eddb11e33ccb7623c0291ccc0f091d..0000000000000000000000000000000000000000
--- a/spaces/Ataturk-Chatbot/HuggingFaceChat/venv/lib/python3.11/site-packages/pip/_vendor/rich/diagnose.py
+++ /dev/null
@@ -1,37 +0,0 @@
-import os
-import platform
-
-from pip._vendor.rich import inspect
-from pip._vendor.rich.console import Console, get_windows_console_features
-from pip._vendor.rich.panel import Panel
-from pip._vendor.rich.pretty import Pretty
-
-
-def report() -> None: # pragma: no cover
- """Print a report to the terminal with debugging information"""
- console = Console()
- inspect(console)
- features = get_windows_console_features()
- inspect(features)
-
- env_names = (
- "TERM",
- "COLORTERM",
- "CLICOLOR",
- "NO_COLOR",
- "TERM_PROGRAM",
- "COLUMNS",
- "LINES",
- "JUPYTER_COLUMNS",
- "JUPYTER_LINES",
- "JPY_PARENT_PID",
- "VSCODE_VERBOSE_LOGGING",
- )
- env = {name: os.getenv(name) for name in env_names}
- console.print(Panel.fit((Pretty(env)), title="[b]Environment Variables"))
-
- console.print(f'platform="{platform.system()}"')
-
-
-if __name__ == "__main__": # pragma: no cover
- report()
diff --git a/spaces/Ataturk-Chatbot/HuggingFaceChat/venv/lib/python3.11/site-packages/pip/_vendor/rich/palette.py b/spaces/Ataturk-Chatbot/HuggingFaceChat/venv/lib/python3.11/site-packages/pip/_vendor/rich/palette.py
deleted file mode 100644
index fa0c4dd40381addf5b42fae4228b6d8fef03abd9..0000000000000000000000000000000000000000
--- a/spaces/Ataturk-Chatbot/HuggingFaceChat/venv/lib/python3.11/site-packages/pip/_vendor/rich/palette.py
+++ /dev/null
@@ -1,100 +0,0 @@
-from math import sqrt
-from functools import lru_cache
-from typing import Sequence, Tuple, TYPE_CHECKING
-
-from .color_triplet import ColorTriplet
-
-if TYPE_CHECKING:
- from pip._vendor.rich.table import Table
-
-
-class Palette:
- """A palette of available colors."""
-
- def __init__(self, colors: Sequence[Tuple[int, int, int]]):
- self._colors = colors
-
- def __getitem__(self, number: int) -> ColorTriplet:
- return ColorTriplet(*self._colors[number])
-
- def __rich__(self) -> "Table":
- from pip._vendor.rich.color import Color
- from pip._vendor.rich.style import Style
- from pip._vendor.rich.text import Text
- from pip._vendor.rich.table import Table
-
- table = Table(
- "index",
- "RGB",
- "Color",
- title="Palette",
- caption=f"{len(self._colors)} colors",
- highlight=True,
- caption_justify="right",
- )
- for index, color in enumerate(self._colors):
- table.add_row(
- str(index),
- repr(color),
- Text(" " * 16, style=Style(bgcolor=Color.from_rgb(*color))),
- )
- return table
-
- # This is somewhat inefficient and needs caching
- @lru_cache(maxsize=1024)
- def match(self, color: Tuple[int, int, int]) -> int:
- """Find a color from a palette that most closely matches a given color.
-
- Args:
- color (Tuple[int, int, int]): RGB components in range 0 > 255.
-
- Returns:
- int: Index of closes matching color.
- """
- red1, green1, blue1 = color
- _sqrt = sqrt
- get_color = self._colors.__getitem__
-
- def get_color_distance(index: int) -> float:
- """Get the distance to a color."""
- red2, green2, blue2 = get_color(index)
- red_mean = (red1 + red2) // 2
- red = red1 - red2
- green = green1 - green2
- blue = blue1 - blue2
- return _sqrt(
- (((512 + red_mean) * red * red) >> 8)
- + 4 * green * green
- + (((767 - red_mean) * blue * blue) >> 8)
- )
-
- min_index = min(range(len(self._colors)), key=get_color_distance)
- return min_index
-
-
-if __name__ == "__main__": # pragma: no cover
- import colorsys
- from typing import Iterable
- from pip._vendor.rich.color import Color
- from pip._vendor.rich.console import Console, ConsoleOptions
- from pip._vendor.rich.segment import Segment
- from pip._vendor.rich.style import Style
-
- class ColorBox:
- def __rich_console__(
- self, console: Console, options: ConsoleOptions
- ) -> Iterable[Segment]:
- height = console.size.height - 3
- for y in range(0, height):
- for x in range(options.max_width):
- h = x / options.max_width
- l = y / (height + 1)
- r1, g1, b1 = colorsys.hls_to_rgb(h, l, 1.0)
- r2, g2, b2 = colorsys.hls_to_rgb(h, l + (1 / height / 2), 1.0)
- bgcolor = Color.from_rgb(r1 * 255, g1 * 255, b1 * 255)
- color = Color.from_rgb(r2 * 255, g2 * 255, b2 * 255)
- yield Segment("▄", Style(color=color, bgcolor=bgcolor))
- yield Segment.line()
-
- console = Console()
- console.print(ColorBox())
diff --git a/spaces/Ataturk-Chatbot/HuggingFaceChat/venv/lib/python3.11/site-packages/setuptools/_distutils/_macos_compat.py b/spaces/Ataturk-Chatbot/HuggingFaceChat/venv/lib/python3.11/site-packages/setuptools/_distutils/_macos_compat.py
deleted file mode 100644
index 17769e9154bd9cc3f3c00dc10718e4377828cb5e..0000000000000000000000000000000000000000
--- a/spaces/Ataturk-Chatbot/HuggingFaceChat/venv/lib/python3.11/site-packages/setuptools/_distutils/_macos_compat.py
+++ /dev/null
@@ -1,12 +0,0 @@
-import sys
-import importlib
-
-
-def bypass_compiler_fixup(cmd, args):
- return cmd
-
-
-if sys.platform == 'darwin':
- compiler_fixup = importlib.import_module('_osx_support').compiler_fixup
-else:
- compiler_fixup = bypass_compiler_fixup
diff --git a/spaces/Ataturk-Chatbot/HuggingFaceChat/venv/lib/python3.11/site-packages/setuptools/command/build_py.py b/spaces/Ataturk-Chatbot/HuggingFaceChat/venv/lib/python3.11/site-packages/setuptools/command/build_py.py
deleted file mode 100644
index ec0627429ccbb88f3a17325726441ebcb28fb597..0000000000000000000000000000000000000000
--- a/spaces/Ataturk-Chatbot/HuggingFaceChat/venv/lib/python3.11/site-packages/setuptools/command/build_py.py
+++ /dev/null
@@ -1,368 +0,0 @@
-from functools import partial
-from glob import glob
-from distutils.util import convert_path
-import distutils.command.build_py as orig
-import os
-import fnmatch
-import textwrap
-import io
-import distutils.errors
-import itertools
-import stat
-import warnings
-from pathlib import Path
-from typing import Dict, Iterable, Iterator, List, Optional, Tuple
-
-from setuptools._deprecation_warning import SetuptoolsDeprecationWarning
-from setuptools.extern.more_itertools import unique_everseen
-
-
-def make_writable(target):
- os.chmod(target, os.stat(target).st_mode | stat.S_IWRITE)
-
-
-class build_py(orig.build_py):
- """Enhanced 'build_py' command that includes data files with packages
-
- The data files are specified via a 'package_data' argument to 'setup()'.
- See 'setuptools.dist.Distribution' for more details.
-
- Also, this version of the 'build_py' command allows you to specify both
- 'py_modules' and 'packages' in the same setup operation.
- """
- editable_mode: bool = False
- existing_egg_info_dir: Optional[str] = None #: Private API, internal use only.
-
- def finalize_options(self):
- orig.build_py.finalize_options(self)
- self.package_data = self.distribution.package_data
- self.exclude_package_data = self.distribution.exclude_package_data or {}
- if 'data_files' in self.__dict__:
- del self.__dict__['data_files']
- self.__updated_files = []
-
- def copy_file(self, infile, outfile, preserve_mode=1, preserve_times=1,
- link=None, level=1):
- # Overwrite base class to allow using links
- if link:
- infile = str(Path(infile).resolve())
- outfile = str(Path(outfile).resolve())
- return super().copy_file(infile, outfile, preserve_mode, preserve_times,
- link, level)
-
- def run(self):
- """Build modules, packages, and copy data files to build directory"""
- if not (self.py_modules or self.packages) or self.editable_mode:
- return
-
- if self.py_modules:
- self.build_modules()
-
- if self.packages:
- self.build_packages()
- self.build_package_data()
-
- # Only compile actual .py files, using our base class' idea of what our
- # output files are.
- self.byte_compile(orig.build_py.get_outputs(self, include_bytecode=0))
-
- def __getattr__(self, attr):
- "lazily compute data files"
- if attr == 'data_files':
- self.data_files = self._get_data_files()
- return self.data_files
- return orig.build_py.__getattr__(self, attr)
-
- def build_module(self, module, module_file, package):
- outfile, copied = orig.build_py.build_module(self, module, module_file, package)
- if copied:
- self.__updated_files.append(outfile)
- return outfile, copied
-
- def _get_data_files(self):
- """Generate list of '(package,src_dir,build_dir,filenames)' tuples"""
- self.analyze_manifest()
- return list(map(self._get_pkg_data_files, self.packages or ()))
-
- def get_data_files_without_manifest(self):
- """
- Generate list of ``(package,src_dir,build_dir,filenames)`` tuples,
- but without triggering any attempt to analyze or build the manifest.
- """
- # Prevent eventual errors from unset `manifest_files`
- # (that would otherwise be set by `analyze_manifest`)
- self.__dict__.setdefault('manifest_files', {})
- return list(map(self._get_pkg_data_files, self.packages or ()))
-
- def _get_pkg_data_files(self, package):
- # Locate package source directory
- src_dir = self.get_package_dir(package)
-
- # Compute package build directory
- build_dir = os.path.join(*([self.build_lib] + package.split('.')))
-
- # Strip directory from globbed filenames
- filenames = [
- os.path.relpath(file, src_dir)
- for file in self.find_data_files(package, src_dir)
- ]
- return package, src_dir, build_dir, filenames
-
- def find_data_files(self, package, src_dir):
- """Return filenames for package's data files in 'src_dir'"""
- patterns = self._get_platform_patterns(
- self.package_data,
- package,
- src_dir,
- )
- globs_expanded = map(partial(glob, recursive=True), patterns)
- # flatten the expanded globs into an iterable of matches
- globs_matches = itertools.chain.from_iterable(globs_expanded)
- glob_files = filter(os.path.isfile, globs_matches)
- files = itertools.chain(
- self.manifest_files.get(package, []),
- glob_files,
- )
- return self.exclude_data_files(package, src_dir, files)
-
- def get_outputs(self, include_bytecode=1) -> List[str]:
- """See :class:`setuptools.commands.build.SubCommand`"""
- if self.editable_mode:
- return list(self.get_output_mapping().keys())
- return super().get_outputs(include_bytecode)
-
- def get_output_mapping(self) -> Dict[str, str]:
- """See :class:`setuptools.commands.build.SubCommand`"""
- mapping = itertools.chain(
- self._get_package_data_output_mapping(),
- self._get_module_mapping(),
- )
- return dict(sorted(mapping, key=lambda x: x[0]))
-
- def _get_module_mapping(self) -> Iterator[Tuple[str, str]]:
- """Iterate over all modules producing (dest, src) pairs."""
- for (package, module, module_file) in self.find_all_modules():
- package = package.split('.')
- filename = self.get_module_outfile(self.build_lib, package, module)
- yield (filename, module_file)
-
- def _get_package_data_output_mapping(self) -> Iterator[Tuple[str, str]]:
- """Iterate over package data producing (dest, src) pairs."""
- for package, src_dir, build_dir, filenames in self.data_files:
- for filename in filenames:
- target = os.path.join(build_dir, filename)
- srcfile = os.path.join(src_dir, filename)
- yield (target, srcfile)
-
- def build_package_data(self):
- """Copy data files into build directory"""
- for target, srcfile in self._get_package_data_output_mapping():
- self.mkpath(os.path.dirname(target))
- _outf, _copied = self.copy_file(srcfile, target)
- make_writable(target)
-
- def analyze_manifest(self):
- self.manifest_files = mf = {}
- if not self.distribution.include_package_data:
- return
- src_dirs = {}
- for package in self.packages or ():
- # Locate package source directory
- src_dirs[assert_relative(self.get_package_dir(package))] = package
-
- if (
- getattr(self, 'existing_egg_info_dir', None)
- and Path(self.existing_egg_info_dir, "SOURCES.txt").exists()
- ):
- egg_info_dir = self.existing_egg_info_dir
- manifest = Path(egg_info_dir, "SOURCES.txt")
- files = manifest.read_text(encoding="utf-8").splitlines()
- else:
- self.run_command('egg_info')
- ei_cmd = self.get_finalized_command('egg_info')
- egg_info_dir = ei_cmd.egg_info
- files = ei_cmd.filelist.files
-
- check = _IncludePackageDataAbuse()
- for path in self._filter_build_files(files, egg_info_dir):
- d, f = os.path.split(assert_relative(path))
- prev = None
- oldf = f
- while d and d != prev and d not in src_dirs:
- prev = d
- d, df = os.path.split(d)
- f = os.path.join(df, f)
- if d in src_dirs:
- if f == oldf:
- if check.is_module(f):
- continue # it's a module, not data
- else:
- importable = check.importable_subpackage(src_dirs[d], f)
- if importable:
- check.warn(importable)
- mf.setdefault(src_dirs[d], []).append(path)
-
- def _filter_build_files(self, files: Iterable[str], egg_info: str) -> Iterator[str]:
- """
- ``build_meta`` may try to create egg_info outside of the project directory,
- and this can be problematic for certain plugins (reported in issue #3500).
-
- Extensions might also include between their sources files created on the
- ``build_lib`` and ``build_temp`` directories.
-
- This function should filter this case of invalid files out.
- """
- build = self.get_finalized_command("build")
- build_dirs = (egg_info, self.build_lib, build.build_temp, build.build_base)
- norm_dirs = [os.path.normpath(p) for p in build_dirs if p]
-
- for file in files:
- norm_path = os.path.normpath(file)
- if not os.path.isabs(file) or all(d not in norm_path for d in norm_dirs):
- yield file
-
- def get_data_files(self):
- pass # Lazily compute data files in _get_data_files() function.
-
- def check_package(self, package, package_dir):
- """Check namespace packages' __init__ for declare_namespace"""
- try:
- return self.packages_checked[package]
- except KeyError:
- pass
-
- init_py = orig.build_py.check_package(self, package, package_dir)
- self.packages_checked[package] = init_py
-
- if not init_py or not self.distribution.namespace_packages:
- return init_py
-
- for pkg in self.distribution.namespace_packages:
- if pkg == package or pkg.startswith(package + '.'):
- break
- else:
- return init_py
-
- with io.open(init_py, 'rb') as f:
- contents = f.read()
- if b'declare_namespace' not in contents:
- raise distutils.errors.DistutilsError(
- "Namespace package problem: %s is a namespace package, but "
- "its\n__init__.py does not call declare_namespace()! Please "
- 'fix it.\n(See the setuptools manual under '
- '"Namespace Packages" for details.)\n"' % (package,)
- )
- return init_py
-
- def initialize_options(self):
- self.packages_checked = {}
- orig.build_py.initialize_options(self)
- self.editable_mode = False
- self.existing_egg_info_dir = None
-
- def get_package_dir(self, package):
- res = orig.build_py.get_package_dir(self, package)
- if self.distribution.src_root is not None:
- return os.path.join(self.distribution.src_root, res)
- return res
-
- def exclude_data_files(self, package, src_dir, files):
- """Filter filenames for package's data files in 'src_dir'"""
- files = list(files)
- patterns = self._get_platform_patterns(
- self.exclude_package_data,
- package,
- src_dir,
- )
- match_groups = (fnmatch.filter(files, pattern) for pattern in patterns)
- # flatten the groups of matches into an iterable of matches
- matches = itertools.chain.from_iterable(match_groups)
- bad = set(matches)
- keepers = (fn for fn in files if fn not in bad)
- # ditch dupes
- return list(unique_everseen(keepers))
-
- @staticmethod
- def _get_platform_patterns(spec, package, src_dir):
- """
- yield platform-specific path patterns (suitable for glob
- or fn_match) from a glob-based spec (such as
- self.package_data or self.exclude_package_data)
- matching package in src_dir.
- """
- raw_patterns = itertools.chain(
- spec.get('', []),
- spec.get(package, []),
- )
- return (
- # Each pattern has to be converted to a platform-specific path
- os.path.join(src_dir, convert_path(pattern))
- for pattern in raw_patterns
- )
-
-
-def assert_relative(path):
- if not os.path.isabs(path):
- return path
- from distutils.errors import DistutilsSetupError
-
- msg = (
- textwrap.dedent(
- """
- Error: setup script specifies an absolute path:
-
- %s
-
- setup() arguments must *always* be /-separated paths relative to the
- setup.py directory, *never* absolute paths.
- """
- ).lstrip()
- % path
- )
- raise DistutilsSetupError(msg)
-
-
-class _IncludePackageDataAbuse:
- """Inform users that package or module is included as 'data file'"""
-
- MESSAGE = """\
- Installing {importable!r} as data is deprecated, please list it in `packages`.
- !!\n\n
- ############################
- # Package would be ignored #
- ############################
- Python recognizes {importable!r} as an importable package,
- but it is not listed in the `packages` configuration of setuptools.
-
- {importable!r} has been automatically added to the distribution only
- because it may contain data files, but this behavior is likely to change
- in future versions of setuptools (and therefore is considered deprecated).
-
- Please make sure that {importable!r} is included as a package by using
- the `packages` configuration field or the proper discovery methods
- (for example by using `find_namespace_packages(...)`/`find_namespace:`
- instead of `find_packages(...)`/`find:`).
-
- You can read more about "package discovery" and "data files" on setuptools
- documentation page.
- \n\n!!
- """
-
- def __init__(self):
- self._already_warned = set()
-
- def is_module(self, file):
- return file.endswith(".py") and file[:-len(".py")].isidentifier()
-
- def importable_subpackage(self, parent, file):
- pkg = Path(file).parent
- parts = list(itertools.takewhile(str.isidentifier, pkg.parts))
- if parts:
- return ".".join([parent, *parts])
- return None
-
- def warn(self, importable):
- if importable not in self._already_warned:
- msg = textwrap.dedent(self.MESSAGE).format(importable=importable)
- warnings.warn(msg, SetuptoolsDeprecationWarning, stacklevel=2)
- self._already_warned.add(importable)
diff --git a/spaces/BIASLab/sars-cov-2-classification-fcgr/src/utils.py b/spaces/BIASLab/sars-cov-2-classification-fcgr/src/utils.py
deleted file mode 100644
index a1f1344e04f97f968a41f02f786203fb145813c8..0000000000000000000000000000000000000000
--- a/spaces/BIASLab/sars-cov-2-classification-fcgr/src/utils.py
+++ /dev/null
@@ -1,41 +0,0 @@
-import re
-from PIL import Image
-import numpy as np
-
-
-def clean_seq(seq):
- "Remove all characters different from A,C,G,T or N"
- seq = seq.upper()
- for letter in "BDEFHIJKLMOPQRSUVWXYZ":
- seq = seq.replace(letter,"N")
- return seq
-
-def array2img(array):
- "FCGR array to grayscale image"
- max_color = 255
- m, M = array.min(), array.max()
- # rescale to [0,1]
- img_rescaled = (array - m) / (M-m)
-
- # invert colors black->white
- img_array = np.ceil(max_color - img_rescaled*max_color)
- img_array = np.array(img_array, dtype=np.int8)
-
- # convert to Image
- img_pil = Image.fromarray(img_array,'L')
- return img_pil
-
-def count_seqs(fasta):
- "Count number of '>' in a fasta file to use with a progress bar"
- pattern = ">"
- count = 0
- for line in fasta:
- if re.search(pattern, line):
- count +=1
- return count
-
-def generate_fcgr(kmer, fasta, fcgr):
- "Generate Image FCGR"
- array = fcgr(clean_seq(str(fasta.seq)))
- img = array2img(array)
- return img
\ No newline at end of file
diff --git a/spaces/Banbri/zcvzcv/src/types.ts b/spaces/Banbri/zcvzcv/src/types.ts
deleted file mode 100644
index a01f6476cd020ee8bdfc3e3cd7f879fcdf6dc7d8..0000000000000000000000000000000000000000
--- a/spaces/Banbri/zcvzcv/src/types.ts
+++ /dev/null
@@ -1,130 +0,0 @@
-export type ProjectionMode = 'cartesian' | 'spherical'
-
-export type CacheMode = "use" | "renew" | "ignore"
-
-export interface RenderRequest {
- prompt: string
-
- // whether to use video segmentation
- // disabled (default)
- // firstframe: we only analyze the first frame
- // allframes: we analyze all the frames
- segmentation: 'disabled' | 'firstframe' | 'allframes'
-
- // segmentation will only be executed if we have a non-empty list of actionnables
- // actionnables are names of things like "chest", "key", "tree", "chair" etc
- actionnables: string[]
-
- // note: this is the number of frames for Zeroscope,
- // which is currently configured to only output 3 seconds, so:
- // nbFrames=8 -> 1 sec
- // nbFrames=16 -> 2 sec
- // nbFrames=24 -> 3 sec
- nbFrames: number // min: 1, max: 24
-
- nbSteps: number // min: 1, max: 50
-
- seed: number
-
- width: number // fixed at 1024 for now
- height: number // fixed at 512 for now
-
- // upscaling factor
- // 0: no upscaling
- // 1: no upscaling
- // 2: 2x larger
- // 3: 3x larger
- // 4x: 4x larger, up to 4096x4096 (warning: a PNG of this size can be 50 Mb!)
- upscalingFactor: number
-
- projection: ProjectionMode
-
- cache: CacheMode
-
- wait: boolean // wait until the job is completed
-
- analyze: boolean // analyze the image to generate a caption (optional)
-}
-
-export interface ImageSegment {
- id: number
- box: number[]
- color: number[]
- label: string
- score: number
-}
-
-export type RenderedSceneStatus =
- | "pending"
- | "completed"
- | "error"
-
-export interface RenderedScene {
- renderId: string
- status: RenderedSceneStatus
- assetUrl: string
- alt: string
- error: string
- maskUrl: string
- segments: ImageSegment[]
-}
-
-export interface ImageAnalysisRequest {
- image: string // in base64
- prompt: string
-}
-
-export interface ImageAnalysisResponse {
- result: string
- error?: string
-}
-
-export type LLMResponse = Array<{panel: number; instructions: string; caption: string }>
-
-export type LLMEngine =
- | "INFERENCE_API"
- | "INFERENCE_ENDPOINT"
- | "OPENAI"
- | "REPLICATE"
-
-export type RenderingEngine =
- | "VIDEOCHAIN"
- | "OPENAI"
- | "REPLICATE"
- | "INFERENCE_API"
- | "INFERENCE_ENDPOINT"
-
-export type PostVisibility =
- | "featured" // featured by admins
- | "trending" // top trending / received more than 10 upvotes
- | "normal" // default visibility
-
-export type Post = {
- postId: string
- appId: string
- prompt: string
- previewUrl: string
- assetUrl: string
- createdAt: string
- visibility: PostVisibility
- upvotes: number
- downvotes: number
-}
-
-export type CreatePostResponse = {
- success?: boolean
- error?: string
- post: Post
-}
-
-export type GetAppPostsResponse = {
- success?: boolean
- error?: string
- posts: Post[]
-}
-
-export type GetAppPostResponse = {
- success?: boolean
- error?: string
- post: Post
-}
\ No newline at end of file
diff --git "a/spaces/BatuhanYilmaz/Whisper-Auto-Subtitled-Video-Generator/pages/03_\360\237\223\235_Upload_Video_File_and_Transcript.py" "b/spaces/BatuhanYilmaz/Whisper-Auto-Subtitled-Video-Generator/pages/03_\360\237\223\235_Upload_Video_File_and_Transcript.py"
deleted file mode 100644
index 99c3218b381db769d051b30878d0e30c789b3047..0000000000000000000000000000000000000000
--- "a/spaces/BatuhanYilmaz/Whisper-Auto-Subtitled-Video-Generator/pages/03_\360\237\223\235_Upload_Video_File_and_Transcript.py"
+++ /dev/null
@@ -1,130 +0,0 @@
-import streamlit as st
-from streamlit_lottie import st_lottie
-from utils import write_vtt, write_srt
-import ffmpeg
-import requests
-from typing import Iterator
-from io import StringIO
-import numpy as np
-import pathlib
-import os
-
-
-st.set_page_config(page_title="Auto Subtitled Video Generator", page_icon=":movie_camera:", layout="wide")
-
-# Define a function that we can use to load lottie files from a link.
-@st.cache(allow_output_mutation=True)
-def load_lottieurl(url: str):
- r = requests.get(url)
- if r.status_code != 200:
- return None
- return r.json()
-
-
-APP_DIR = pathlib.Path(__file__).parent.absolute()
-
-LOCAL_DIR = APP_DIR / "local_transcript"
-LOCAL_DIR.mkdir(exist_ok=True)
-save_dir = LOCAL_DIR / "output"
-save_dir.mkdir(exist_ok=True)
-
-
-col1, col2 = st.columns([1, 3])
-with col1:
- lottie = load_lottieurl("https://assets6.lottiefiles.com/packages/lf20_cjnxwrkt.json")
- st_lottie(lottie)
-
-with col2:
- st.write("""
- ## Auto Subtitled Video Generator
- ##### ➠ Upload a video file and a transcript as .srt or .vtt file and get a video with subtitles.
- ##### ➠ Processing time will increase as the video length increases. """)
-
-
-def getSubs(segments: Iterator[dict], format: str, maxLineWidth: int) -> str:
- segmentStream = StringIO()
-
- if format == 'vtt':
- write_vtt(segments, file=segmentStream, maxLineWidth=maxLineWidth)
- elif format == 'srt':
- write_srt(segments, file=segmentStream, maxLineWidth=maxLineWidth)
- else:
- raise Exception("Unknown format " + format)
-
- segmentStream.seek(0)
- return segmentStream.read()
-
-
-def split_video_audio(uploaded_file):
- with open(f"{save_dir}/input.mp4", "wb") as f:
- f.write(uploaded_file.read())
- audio = ffmpeg.input(f"{save_dir}/input.mp4")
- audio = ffmpeg.output(audio, f"{save_dir}/output.wav", acodec="pcm_s16le", ac=1, ar="16k")
- ffmpeg.run(audio, overwrite_output=True)
-
-
-def main():
- uploaded_video = st.file_uploader("Upload Video File", type=["mp4", "avi", "mov", "mkv"])
- # get the name of the input_file
- if uploaded_video is not None:
- filename = uploaded_video.name[:-4]
- else:
- filename = None
- transcript_file = st.file_uploader("Upload Transcript File", type=["srt", "vtt"])
- if transcript_file is not None:
- transcript_name = transcript_file.name
- else:
- transcript_name = None
- if uploaded_video is not None and transcript_file is not None:
- if transcript_name[-3:] == "vtt":
- with open("uploaded_transcript.vtt", "wb") as f:
- f.writelines(transcript_file)
- f.close()
- with open(os.path.join(os.getcwd(), "uploaded_transcript.vtt"), "rb") as f:
- vtt_file = f.read()
- if st.button("Generate Video with Subtitles"):
- with st.spinner("Generating Subtitled Video"):
- split_video_audio(uploaded_video)
- video_file = ffmpeg.input(f"{save_dir}/input.mp4")
- audio_file = ffmpeg.input(f"{save_dir}/output.wav")
- ffmpeg.concat(video_file.filter("subtitles", "uploaded_transcript.vtt"), audio_file, v=1, a=1).output("final.mp4").global_args('-report').run(quiet=True, overwrite_output=True)
- video_with_subs = open("final.mp4", "rb")
- col3, col4 = st.columns(2)
- with col3:
- st.video(uploaded_video)
- with col4:
- st.video(video_with_subs)
- st.download_button(label="Download Video with Subtitles",
- data=video_with_subs,
- file_name=f"{filename}_with_subs.mp4")
-
- elif transcript_name[-3:] == "srt":
- with open("uploaded_transcript.srt", "wb") as f:
- f.writelines(transcript_file)
- f.close()
- with open(os.path.join(os.getcwd(), "uploaded_transcript.srt"), "rb") as f:
- srt_file = f.read()
- if st.button("Generate Video with Subtitles"):
- with st.spinner("Generating Subtitled Video"):
- split_video_audio(uploaded_video)
- video_file = ffmpeg.input(f"{save_dir}/input.mp4")
- audio_file = ffmpeg.input(f"{save_dir}/output.wav")
- ffmpeg.concat(video_file.filter("subtitles", "uploaded_transcript.srt"), audio_file, v=1, a=1).output("final.mp4").run(quiet=True, overwrite_output=True)
- video_with_subs = open("final.mp4", "rb")
- col3, col4 = st.columns(2)
- with col3:
- st.video(uploaded_video)
- with col4:
- st.video(video_with_subs)
- st.download_button(label="Download Video with Subtitles",
- data=video_with_subs,
- file_name=f"{filename}_with_subs.mp4")
- else:
- st.error("Please upload a .srt or .vtt file")
- else:
- st.info("Please upload a video file and a transcript file ")
-
-
-if __name__ == "__main__":
- main()
-
diff --git a/spaces/Big-Web/MMSD/env/Lib/site-packages/botocore/httpsession.py b/spaces/Big-Web/MMSD/env/Lib/site-packages/botocore/httpsession.py
deleted file mode 100644
index b3fe6e6c0c01d314152d909d0c5d14fbdd36db8e..0000000000000000000000000000000000000000
--- a/spaces/Big-Web/MMSD/env/Lib/site-packages/botocore/httpsession.py
+++ /dev/null
@@ -1,510 +0,0 @@
-import logging
-import os
-import os.path
-import socket
-import sys
-import warnings
-from base64 import b64encode
-
-from urllib3 import PoolManager, Timeout, proxy_from_url
-from urllib3.exceptions import (
- ConnectTimeoutError as URLLib3ConnectTimeoutError,
-)
-from urllib3.exceptions import (
- LocationParseError,
- NewConnectionError,
- ProtocolError,
- ProxyError,
-)
-from urllib3.exceptions import ReadTimeoutError as URLLib3ReadTimeoutError
-from urllib3.exceptions import SSLError as URLLib3SSLError
-from urllib3.util.retry import Retry
-from urllib3.util.ssl_ import (
- OP_NO_COMPRESSION,
- PROTOCOL_TLS,
- OP_NO_SSLv2,
- OP_NO_SSLv3,
- is_ipaddress,
- ssl,
-)
-from urllib3.util.url import parse_url
-
-try:
- from urllib3.util.ssl_ import OP_NO_TICKET, PROTOCOL_TLS_CLIENT
-except ImportError:
- # Fallback directly to ssl for version of urllib3 before 1.26.
- # They are available in the standard library starting in Python 3.6.
- from ssl import OP_NO_TICKET, PROTOCOL_TLS_CLIENT
-
-try:
- # pyopenssl will be removed in urllib3 2.0, we'll fall back to ssl_ at that point.
- # This can be removed once our urllib3 floor is raised to >= 2.0.
- with warnings.catch_warnings():
- warnings.simplefilter("ignore", category=DeprecationWarning)
- # Always import the original SSLContext, even if it has been patched
- from urllib3.contrib.pyopenssl import (
- orig_util_SSLContext as SSLContext,
- )
-except ImportError:
- from urllib3.util.ssl_ import SSLContext
-
-try:
- from urllib3.util.ssl_ import DEFAULT_CIPHERS
-except ImportError:
- # Defer to system configuration starting with
- # urllib3 2.0. This will choose the ciphers provided by
- # Openssl 1.1.1+ or secure system defaults.
- DEFAULT_CIPHERS = None
-
-import botocore.awsrequest
-from botocore.compat import (
- IPV6_ADDRZ_RE,
- ensure_bytes,
- filter_ssl_warnings,
- unquote,
- urlparse,
-)
-from botocore.exceptions import (
- ConnectionClosedError,
- ConnectTimeoutError,
- EndpointConnectionError,
- HTTPClientError,
- InvalidProxiesConfigError,
- ProxyConnectionError,
- ReadTimeoutError,
- SSLError,
-)
-
-filter_ssl_warnings()
-logger = logging.getLogger(__name__)
-DEFAULT_TIMEOUT = 60
-MAX_POOL_CONNECTIONS = 10
-DEFAULT_CA_BUNDLE = os.path.join(os.path.dirname(__file__), 'cacert.pem')
-
-try:
- from certifi import where
-except ImportError:
-
- def where():
- return DEFAULT_CA_BUNDLE
-
-
-def get_cert_path(verify):
- if verify is not True:
- return verify
-
- cert_path = where()
- logger.debug(f"Certificate path: {cert_path}")
-
- return cert_path
-
-
-def create_urllib3_context(
- ssl_version=None, cert_reqs=None, options=None, ciphers=None
-):
- """This function is a vendored version of the same function in urllib3
-
- We vendor this function to ensure that the SSL contexts we construct
- always use the std lib SSLContext instead of pyopenssl.
- """
- # PROTOCOL_TLS is deprecated in Python 3.10
- if not ssl_version or ssl_version == PROTOCOL_TLS:
- ssl_version = PROTOCOL_TLS_CLIENT
-
- context = SSLContext(ssl_version)
-
- if ciphers:
- context.set_ciphers(ciphers)
- elif DEFAULT_CIPHERS:
- context.set_ciphers(DEFAULT_CIPHERS)
-
- # Setting the default here, as we may have no ssl module on import
- cert_reqs = ssl.CERT_REQUIRED if cert_reqs is None else cert_reqs
-
- if options is None:
- options = 0
- # SSLv2 is easily broken and is considered harmful and dangerous
- options |= OP_NO_SSLv2
- # SSLv3 has several problems and is now dangerous
- options |= OP_NO_SSLv3
- # Disable compression to prevent CRIME attacks for OpenSSL 1.0+
- # (issue urllib3#309)
- options |= OP_NO_COMPRESSION
- # TLSv1.2 only. Unless set explicitly, do not request tickets.
- # This may save some bandwidth on wire, and although the ticket is encrypted,
- # there is a risk associated with it being on wire,
- # if the server is not rotating its ticketing keys properly.
- options |= OP_NO_TICKET
-
- context.options |= options
-
- # Enable post-handshake authentication for TLS 1.3, see GH #1634. PHA is
- # necessary for conditional client cert authentication with TLS 1.3.
- # The attribute is None for OpenSSL <= 1.1.0 or does not exist in older
- # versions of Python. We only enable on Python 3.7.4+ or if certificate
- # verification is enabled to work around Python issue #37428
- # See: https://bugs.python.org/issue37428
- if (
- cert_reqs == ssl.CERT_REQUIRED or sys.version_info >= (3, 7, 4)
- ) and getattr(context, "post_handshake_auth", None) is not None:
- context.post_handshake_auth = True
-
- def disable_check_hostname():
- if (
- getattr(context, "check_hostname", None) is not None
- ): # Platform-specific: Python 3.2
- # We do our own verification, including fingerprints and alternative
- # hostnames. So disable it here
- context.check_hostname = False
-
- # The order of the below lines setting verify_mode and check_hostname
- # matter due to safe-guards SSLContext has to prevent an SSLContext with
- # check_hostname=True, verify_mode=NONE/OPTIONAL. This is made even more
- # complex because we don't know whether PROTOCOL_TLS_CLIENT will be used
- # or not so we don't know the initial state of the freshly created SSLContext.
- if cert_reqs == ssl.CERT_REQUIRED:
- context.verify_mode = cert_reqs
- disable_check_hostname()
- else:
- disable_check_hostname()
- context.verify_mode = cert_reqs
-
- # Enable logging of TLS session keys via defacto standard environment variable
- # 'SSLKEYLOGFILE', if the feature is available (Python 3.8+). Skip empty values.
- if hasattr(context, "keylog_filename"):
- sslkeylogfile = os.environ.get("SSLKEYLOGFILE")
- if sslkeylogfile and not sys.flags.ignore_environment:
- context.keylog_filename = sslkeylogfile
-
- return context
-
-
-def ensure_boolean(val):
- """Ensures a boolean value if a string or boolean is provided
-
- For strings, the value for True/False is case insensitive
- """
- if isinstance(val, bool):
- return val
- else:
- return val.lower() == 'true'
-
-
-def mask_proxy_url(proxy_url):
- """
- Mask proxy url credentials.
-
- :type proxy_url: str
- :param proxy_url: The proxy url, i.e. https://username:password@proxy.com
-
- :return: Masked proxy url, i.e. https://***:***@proxy.com
- """
- mask = '*' * 3
- parsed_url = urlparse(proxy_url)
- if parsed_url.username:
- proxy_url = proxy_url.replace(parsed_url.username, mask, 1)
- if parsed_url.password:
- proxy_url = proxy_url.replace(parsed_url.password, mask, 1)
- return proxy_url
-
-
-def _is_ipaddress(host):
- """Wrap urllib3's is_ipaddress to support bracketed IPv6 addresses."""
- return is_ipaddress(host) or bool(IPV6_ADDRZ_RE.match(host))
-
-
-class ProxyConfiguration:
- """Represents a proxy configuration dictionary and additional settings.
-
- This class represents a proxy configuration dictionary and provides utility
- functions to retreive well structured proxy urls and proxy headers from the
- proxy configuration dictionary.
- """
-
- def __init__(self, proxies=None, proxies_settings=None):
- if proxies is None:
- proxies = {}
- if proxies_settings is None:
- proxies_settings = {}
-
- self._proxies = proxies
- self._proxies_settings = proxies_settings
-
- def proxy_url_for(self, url):
- """Retrieves the corresponding proxy url for a given url."""
- parsed_url = urlparse(url)
- proxy = self._proxies.get(parsed_url.scheme)
- if proxy:
- proxy = self._fix_proxy_url(proxy)
- return proxy
-
- def proxy_headers_for(self, proxy_url):
- """Retrieves the corresponding proxy headers for a given proxy url."""
- headers = {}
- username, password = self._get_auth_from_url(proxy_url)
- if username and password:
- basic_auth = self._construct_basic_auth(username, password)
- headers['Proxy-Authorization'] = basic_auth
- return headers
-
- @property
- def settings(self):
- return self._proxies_settings
-
- def _fix_proxy_url(self, proxy_url):
- if proxy_url.startswith('http:') or proxy_url.startswith('https:'):
- return proxy_url
- elif proxy_url.startswith('//'):
- return 'http:' + proxy_url
- else:
- return 'http://' + proxy_url
-
- def _construct_basic_auth(self, username, password):
- auth_str = f'{username}:{password}'
- encoded_str = b64encode(auth_str.encode('ascii')).strip().decode()
- return f'Basic {encoded_str}'
-
- def _get_auth_from_url(self, url):
- parsed_url = urlparse(url)
- try:
- return unquote(parsed_url.username), unquote(parsed_url.password)
- except (AttributeError, TypeError):
- return None, None
-
-
-class URLLib3Session:
- """A basic HTTP client that supports connection pooling and proxies.
-
- This class is inspired by requests.adapters.HTTPAdapter, but has been
- boiled down to meet the use cases needed by botocore. For the most part
- this classes matches the functionality of HTTPAdapter in requests v2.7.0
- (the same as our vendored version). The only major difference of note is
- that we currently do not support sending chunked requests. While requests
- v2.7.0 implemented this themselves, later version urllib3 support this
- directly via a flag to urlopen so enabling it if needed should be trivial.
- """
-
- def __init__(
- self,
- verify=True,
- proxies=None,
- timeout=None,
- max_pool_connections=MAX_POOL_CONNECTIONS,
- socket_options=None,
- client_cert=None,
- proxies_config=None,
- ):
- self._verify = verify
- self._proxy_config = ProxyConfiguration(
- proxies=proxies, proxies_settings=proxies_config
- )
- self._pool_classes_by_scheme = {
- 'http': botocore.awsrequest.AWSHTTPConnectionPool,
- 'https': botocore.awsrequest.AWSHTTPSConnectionPool,
- }
- if timeout is None:
- timeout = DEFAULT_TIMEOUT
- if not isinstance(timeout, (int, float)):
- timeout = Timeout(connect=timeout[0], read=timeout[1])
-
- self._cert_file = None
- self._key_file = None
- if isinstance(client_cert, str):
- self._cert_file = client_cert
- elif isinstance(client_cert, tuple):
- self._cert_file, self._key_file = client_cert
-
- self._timeout = timeout
- self._max_pool_connections = max_pool_connections
- self._socket_options = socket_options
- if socket_options is None:
- self._socket_options = []
- self._proxy_managers = {}
- self._manager = PoolManager(**self._get_pool_manager_kwargs())
- self._manager.pool_classes_by_scheme = self._pool_classes_by_scheme
-
- def _proxies_kwargs(self, **kwargs):
- proxies_settings = self._proxy_config.settings
- proxies_kwargs = {
- 'use_forwarding_for_https': proxies_settings.get(
- 'proxy_use_forwarding_for_https'
- ),
- **kwargs,
- }
- return {k: v for k, v in proxies_kwargs.items() if v is not None}
-
- def _get_pool_manager_kwargs(self, **extra_kwargs):
- pool_manager_kwargs = {
- 'strict': True,
- 'timeout': self._timeout,
- 'maxsize': self._max_pool_connections,
- 'ssl_context': self._get_ssl_context(),
- 'socket_options': self._socket_options,
- 'cert_file': self._cert_file,
- 'key_file': self._key_file,
- }
- pool_manager_kwargs.update(**extra_kwargs)
- return pool_manager_kwargs
-
- def _get_ssl_context(self):
- return create_urllib3_context()
-
- def _get_proxy_manager(self, proxy_url):
- if proxy_url not in self._proxy_managers:
- proxy_headers = self._proxy_config.proxy_headers_for(proxy_url)
- proxy_ssl_context = self._setup_proxy_ssl_context(proxy_url)
- proxy_manager_kwargs = self._get_pool_manager_kwargs(
- proxy_headers=proxy_headers
- )
- proxy_manager_kwargs.update(
- self._proxies_kwargs(proxy_ssl_context=proxy_ssl_context)
- )
- proxy_manager = proxy_from_url(proxy_url, **proxy_manager_kwargs)
- proxy_manager.pool_classes_by_scheme = self._pool_classes_by_scheme
- self._proxy_managers[proxy_url] = proxy_manager
-
- return self._proxy_managers[proxy_url]
-
- def _path_url(self, url):
- parsed_url = urlparse(url)
- path = parsed_url.path
- if not path:
- path = '/'
- if parsed_url.query:
- path = path + '?' + parsed_url.query
- return path
-
- def _setup_ssl_cert(self, conn, url, verify):
- if url.lower().startswith('https') and verify:
- conn.cert_reqs = 'CERT_REQUIRED'
- conn.ca_certs = get_cert_path(verify)
- else:
- conn.cert_reqs = 'CERT_NONE'
- conn.ca_certs = None
-
- def _setup_proxy_ssl_context(self, proxy_url):
- proxies_settings = self._proxy_config.settings
- proxy_ca_bundle = proxies_settings.get('proxy_ca_bundle')
- proxy_cert = proxies_settings.get('proxy_client_cert')
- if proxy_ca_bundle is None and proxy_cert is None:
- return None
-
- context = self._get_ssl_context()
- try:
- url = parse_url(proxy_url)
- # urllib3 disables this by default but we need it for proper
- # proxy tls negotiation when proxy_url is not an IP Address
- if not _is_ipaddress(url.host):
- context.check_hostname = True
- if proxy_ca_bundle is not None:
- context.load_verify_locations(cafile=proxy_ca_bundle)
-
- if isinstance(proxy_cert, tuple):
- context.load_cert_chain(proxy_cert[0], keyfile=proxy_cert[1])
- elif isinstance(proxy_cert, str):
- context.load_cert_chain(proxy_cert)
-
- return context
- except (OSError, URLLib3SSLError, LocationParseError) as e:
- raise InvalidProxiesConfigError(error=e)
-
- def _get_connection_manager(self, url, proxy_url=None):
- if proxy_url:
- manager = self._get_proxy_manager(proxy_url)
- else:
- manager = self._manager
- return manager
-
- def _get_request_target(self, url, proxy_url):
- has_proxy = proxy_url is not None
-
- if not has_proxy:
- return self._path_url(url)
-
- # HTTP proxies expect the request_target to be the absolute url to know
- # which host to establish a connection to. urllib3 also supports
- # forwarding for HTTPS through the 'use_forwarding_for_https' parameter.
- proxy_scheme = urlparse(proxy_url).scheme
- using_https_forwarding_proxy = (
- proxy_scheme == 'https'
- and self._proxies_kwargs().get('use_forwarding_for_https', False)
- )
-
- if using_https_forwarding_proxy or url.startswith('http:'):
- return url
- else:
- return self._path_url(url)
-
- def _chunked(self, headers):
- transfer_encoding = headers.get('Transfer-Encoding', b'')
- transfer_encoding = ensure_bytes(transfer_encoding)
- return transfer_encoding.lower() == b'chunked'
-
- def close(self):
- self._manager.clear()
- for manager in self._proxy_managers.values():
- manager.clear()
-
- def send(self, request):
- try:
- proxy_url = self._proxy_config.proxy_url_for(request.url)
- manager = self._get_connection_manager(request.url, proxy_url)
- conn = manager.connection_from_url(request.url)
- self._setup_ssl_cert(conn, request.url, self._verify)
- if ensure_boolean(
- os.environ.get('BOTO_EXPERIMENTAL__ADD_PROXY_HOST_HEADER', '')
- ):
- # This is currently an "experimental" feature which provides
- # no guarantees of backwards compatibility. It may be subject
- # to change or removal in any patch version. Anyone opting in
- # to this feature should strictly pin botocore.
- host = urlparse(request.url).hostname
- conn.proxy_headers['host'] = host
-
- request_target = self._get_request_target(request.url, proxy_url)
- urllib_response = conn.urlopen(
- method=request.method,
- url=request_target,
- body=request.body,
- headers=request.headers,
- retries=Retry(False),
- assert_same_host=False,
- preload_content=False,
- decode_content=False,
- chunked=self._chunked(request.headers),
- )
-
- http_response = botocore.awsrequest.AWSResponse(
- request.url,
- urllib_response.status,
- urllib_response.headers,
- urllib_response,
- )
-
- if not request.stream_output:
- # Cause the raw stream to be exhausted immediately. We do it
- # this way instead of using preload_content because
- # preload_content will never buffer chunked responses
- http_response.content
-
- return http_response
- except URLLib3SSLError as e:
- raise SSLError(endpoint_url=request.url, error=e)
- except (NewConnectionError, socket.gaierror) as e:
- raise EndpointConnectionError(endpoint_url=request.url, error=e)
- except ProxyError as e:
- raise ProxyConnectionError(
- proxy_url=mask_proxy_url(proxy_url), error=e
- )
- except URLLib3ConnectTimeoutError as e:
- raise ConnectTimeoutError(endpoint_url=request.url, error=e)
- except URLLib3ReadTimeoutError as e:
- raise ReadTimeoutError(endpoint_url=request.url, error=e)
- except ProtocolError as e:
- raise ConnectionClosedError(
- error=e, request=request, endpoint_url=request.url
- )
- except Exception as e:
- message = 'Exception received when sending urllib3 HTTP request'
- logger.debug(message, exc_info=True)
- raise HTTPClientError(error=e)
diff --git a/spaces/Big-Web/MMSD/env/Lib/site-packages/pip/_internal/network/session.py b/spaces/Big-Web/MMSD/env/Lib/site-packages/pip/_internal/network/session.py
deleted file mode 100644
index 6c40ade1595df0ed4d2963b819211491d55b0aa5..0000000000000000000000000000000000000000
--- a/spaces/Big-Web/MMSD/env/Lib/site-packages/pip/_internal/network/session.py
+++ /dev/null
@@ -1,517 +0,0 @@
-"""PipSession and supporting code, containing all pip-specific
-network request configuration and behavior.
-"""
-
-import email.utils
-import io
-import ipaddress
-import json
-import logging
-import mimetypes
-import os
-import platform
-import shutil
-import subprocess
-import sys
-import urllib.parse
-import warnings
-from typing import (
- TYPE_CHECKING,
- Any,
- Dict,
- Generator,
- List,
- Mapping,
- Optional,
- Sequence,
- Tuple,
- Union,
-)
-
-from pip._vendor import requests, urllib3
-from pip._vendor.cachecontrol import CacheControlAdapter as _BaseCacheControlAdapter
-from pip._vendor.requests.adapters import DEFAULT_POOLBLOCK, BaseAdapter
-from pip._vendor.requests.adapters import HTTPAdapter as _BaseHTTPAdapter
-from pip._vendor.requests.models import PreparedRequest, Response
-from pip._vendor.requests.structures import CaseInsensitiveDict
-from pip._vendor.urllib3.connectionpool import ConnectionPool
-from pip._vendor.urllib3.exceptions import InsecureRequestWarning
-
-from pip import __version__
-from pip._internal.metadata import get_default_environment
-from pip._internal.models.link import Link
-from pip._internal.network.auth import MultiDomainBasicAuth
-from pip._internal.network.cache import SafeFileCache
-
-# Import ssl from compat so the initial import occurs in only one place.
-from pip._internal.utils.compat import has_tls
-from pip._internal.utils.glibc import libc_ver
-from pip._internal.utils.misc import build_url_from_netloc, parse_netloc
-from pip._internal.utils.urls import url_to_path
-
-if TYPE_CHECKING:
- from ssl import SSLContext
-
- from pip._vendor.urllib3.poolmanager import PoolManager
-
-
-logger = logging.getLogger(__name__)
-
-SecureOrigin = Tuple[str, str, Optional[Union[int, str]]]
-
-
-# Ignore warning raised when using --trusted-host.
-warnings.filterwarnings("ignore", category=InsecureRequestWarning)
-
-
-SECURE_ORIGINS: List[SecureOrigin] = [
- # protocol, hostname, port
- # Taken from Chrome's list of secure origins (See: http://bit.ly/1qrySKC)
- ("https", "*", "*"),
- ("*", "localhost", "*"),
- ("*", "127.0.0.0/8", "*"),
- ("*", "::1/128", "*"),
- ("file", "*", None),
- # ssh is always secure.
- ("ssh", "*", "*"),
-]
-
-
-# These are environment variables present when running under various
-# CI systems. For each variable, some CI systems that use the variable
-# are indicated. The collection was chosen so that for each of a number
-# of popular systems, at least one of the environment variables is used.
-# This list is used to provide some indication of and lower bound for
-# CI traffic to PyPI. Thus, it is okay if the list is not comprehensive.
-# For more background, see: https://github.com/pypa/pip/issues/5499
-CI_ENVIRONMENT_VARIABLES = (
- # Azure Pipelines
- "BUILD_BUILDID",
- # Jenkins
- "BUILD_ID",
- # AppVeyor, CircleCI, Codeship, Gitlab CI, Shippable, Travis CI
- "CI",
- # Explicit environment variable.
- "PIP_IS_CI",
-)
-
-
-def looks_like_ci() -> bool:
- """
- Return whether it looks like pip is running under CI.
- """
- # We don't use the method of checking for a tty (e.g. using isatty())
- # because some CI systems mimic a tty (e.g. Travis CI). Thus that
- # method doesn't provide definitive information in either direction.
- return any(name in os.environ for name in CI_ENVIRONMENT_VARIABLES)
-
-
-def user_agent() -> str:
- """
- Return a string representing the user agent.
- """
- data: Dict[str, Any] = {
- "installer": {"name": "pip", "version": __version__},
- "python": platform.python_version(),
- "implementation": {
- "name": platform.python_implementation(),
- },
- }
-
- if data["implementation"]["name"] == "CPython":
- data["implementation"]["version"] = platform.python_version()
- elif data["implementation"]["name"] == "PyPy":
- pypy_version_info = sys.pypy_version_info # type: ignore
- if pypy_version_info.releaselevel == "final":
- pypy_version_info = pypy_version_info[:3]
- data["implementation"]["version"] = ".".join(
- [str(x) for x in pypy_version_info]
- )
- elif data["implementation"]["name"] == "Jython":
- # Complete Guess
- data["implementation"]["version"] = platform.python_version()
- elif data["implementation"]["name"] == "IronPython":
- # Complete Guess
- data["implementation"]["version"] = platform.python_version()
-
- if sys.platform.startswith("linux"):
- from pip._vendor import distro
-
- linux_distribution = distro.name(), distro.version(), distro.codename()
- distro_infos: Dict[str, Any] = dict(
- filter(
- lambda x: x[1],
- zip(["name", "version", "id"], linux_distribution),
- )
- )
- libc = dict(
- filter(
- lambda x: x[1],
- zip(["lib", "version"], libc_ver()),
- )
- )
- if libc:
- distro_infos["libc"] = libc
- if distro_infos:
- data["distro"] = distro_infos
-
- if sys.platform.startswith("darwin") and platform.mac_ver()[0]:
- data["distro"] = {"name": "macOS", "version": platform.mac_ver()[0]}
-
- if platform.system():
- data.setdefault("system", {})["name"] = platform.system()
-
- if platform.release():
- data.setdefault("system", {})["release"] = platform.release()
-
- if platform.machine():
- data["cpu"] = platform.machine()
-
- if has_tls():
- import _ssl as ssl
-
- data["openssl_version"] = ssl.OPENSSL_VERSION
-
- setuptools_dist = get_default_environment().get_distribution("setuptools")
- if setuptools_dist is not None:
- data["setuptools_version"] = str(setuptools_dist.version)
-
- if shutil.which("rustc") is not None:
- # If for any reason `rustc --version` fails, silently ignore it
- try:
- rustc_output = subprocess.check_output(
- ["rustc", "--version"], stderr=subprocess.STDOUT, timeout=0.5
- )
- except Exception:
- pass
- else:
- if rustc_output.startswith(b"rustc "):
- # The format of `rustc --version` is:
- # `b'rustc 1.52.1 (9bc8c42bb 2021-05-09)\n'`
- # We extract just the middle (1.52.1) part
- data["rustc_version"] = rustc_output.split(b" ")[1].decode()
-
- # Use None rather than False so as not to give the impression that
- # pip knows it is not being run under CI. Rather, it is a null or
- # inconclusive result. Also, we include some value rather than no
- # value to make it easier to know that the check has been run.
- data["ci"] = True if looks_like_ci() else None
-
- user_data = os.environ.get("PIP_USER_AGENT_USER_DATA")
- if user_data is not None:
- data["user_data"] = user_data
-
- return "{data[installer][name]}/{data[installer][version]} {json}".format(
- data=data,
- json=json.dumps(data, separators=(",", ":"), sort_keys=True),
- )
-
-
-class LocalFSAdapter(BaseAdapter):
- def send(
- self,
- request: PreparedRequest,
- stream: bool = False,
- timeout: Optional[Union[float, Tuple[float, float]]] = None,
- verify: Union[bool, str] = True,
- cert: Optional[Union[str, Tuple[str, str]]] = None,
- proxies: Optional[Mapping[str, str]] = None,
- ) -> Response:
- pathname = url_to_path(request.url)
-
- resp = Response()
- resp.status_code = 200
- resp.url = request.url
-
- try:
- stats = os.stat(pathname)
- except OSError as exc:
- # format the exception raised as a io.BytesIO object,
- # to return a better error message:
- resp.status_code = 404
- resp.reason = type(exc).__name__
- resp.raw = io.BytesIO(f"{resp.reason}: {exc}".encode("utf8"))
- else:
- modified = email.utils.formatdate(stats.st_mtime, usegmt=True)
- content_type = mimetypes.guess_type(pathname)[0] or "text/plain"
- resp.headers = CaseInsensitiveDict(
- {
- "Content-Type": content_type,
- "Content-Length": stats.st_size,
- "Last-Modified": modified,
- }
- )
-
- resp.raw = open(pathname, "rb")
- resp.close = resp.raw.close
-
- return resp
-
- def close(self) -> None:
- pass
-
-
-class _SSLContextAdapterMixin:
- """Mixin to add the ``ssl_context`` constructor argument to HTTP adapters.
-
- The additional argument is forwarded directly to the pool manager. This allows us
- to dynamically decide what SSL store to use at runtime, which is used to implement
- the optional ``truststore`` backend.
- """
-
- def __init__(
- self,
- *,
- ssl_context: Optional["SSLContext"] = None,
- **kwargs: Any,
- ) -> None:
- self._ssl_context = ssl_context
- super().__init__(**kwargs)
-
- def init_poolmanager(
- self,
- connections: int,
- maxsize: int,
- block: bool = DEFAULT_POOLBLOCK,
- **pool_kwargs: Any,
- ) -> "PoolManager":
- if self._ssl_context is not None:
- pool_kwargs.setdefault("ssl_context", self._ssl_context)
- return super().init_poolmanager( # type: ignore[misc]
- connections=connections,
- maxsize=maxsize,
- block=block,
- **pool_kwargs,
- )
-
-
-class HTTPAdapter(_SSLContextAdapterMixin, _BaseHTTPAdapter):
- pass
-
-
-class CacheControlAdapter(_SSLContextAdapterMixin, _BaseCacheControlAdapter):
- pass
-
-
-class InsecureHTTPAdapter(HTTPAdapter):
- def cert_verify(
- self,
- conn: ConnectionPool,
- url: str,
- verify: Union[bool, str],
- cert: Optional[Union[str, Tuple[str, str]]],
- ) -> None:
- super().cert_verify(conn=conn, url=url, verify=False, cert=cert)
-
-
-class InsecureCacheControlAdapter(CacheControlAdapter):
- def cert_verify(
- self,
- conn: ConnectionPool,
- url: str,
- verify: Union[bool, str],
- cert: Optional[Union[str, Tuple[str, str]]],
- ) -> None:
- super().cert_verify(conn=conn, url=url, verify=False, cert=cert)
-
-
-class PipSession(requests.Session):
- timeout: Optional[int] = None
-
- def __init__(
- self,
- *args: Any,
- retries: int = 0,
- cache: Optional[str] = None,
- trusted_hosts: Sequence[str] = (),
- index_urls: Optional[List[str]] = None,
- ssl_context: Optional["SSLContext"] = None,
- **kwargs: Any,
- ) -> None:
- """
- :param trusted_hosts: Domains not to emit warnings for when not using
- HTTPS.
- """
- super().__init__(*args, **kwargs)
-
- # Namespace the attribute with "pip_" just in case to prevent
- # possible conflicts with the base class.
- self.pip_trusted_origins: List[Tuple[str, Optional[int]]] = []
-
- # Attach our User Agent to the request
- self.headers["User-Agent"] = user_agent()
-
- # Attach our Authentication handler to the session
- self.auth = MultiDomainBasicAuth(index_urls=index_urls)
-
- # Create our urllib3.Retry instance which will allow us to customize
- # how we handle retries.
- retries = urllib3.Retry(
- # Set the total number of retries that a particular request can
- # have.
- total=retries,
- # A 503 error from PyPI typically means that the Fastly -> Origin
- # connection got interrupted in some way. A 503 error in general
- # is typically considered a transient error so we'll go ahead and
- # retry it.
- # A 500 may indicate transient error in Amazon S3
- # A 520 or 527 - may indicate transient error in CloudFlare
- status_forcelist=[500, 503, 520, 527],
- # Add a small amount of back off between failed requests in
- # order to prevent hammering the service.
- backoff_factor=0.25,
- ) # type: ignore
-
- # Our Insecure HTTPAdapter disables HTTPS validation. It does not
- # support caching so we'll use it for all http:// URLs.
- # If caching is disabled, we will also use it for
- # https:// hosts that we've marked as ignoring
- # TLS errors for (trusted-hosts).
- insecure_adapter = InsecureHTTPAdapter(max_retries=retries)
-
- # We want to _only_ cache responses on securely fetched origins or when
- # the host is specified as trusted. We do this because
- # we can't validate the response of an insecurely/untrusted fetched
- # origin, and we don't want someone to be able to poison the cache and
- # require manual eviction from the cache to fix it.
- if cache:
- secure_adapter = CacheControlAdapter(
- cache=SafeFileCache(cache),
- max_retries=retries,
- ssl_context=ssl_context,
- )
- self._trusted_host_adapter = InsecureCacheControlAdapter(
- cache=SafeFileCache(cache),
- max_retries=retries,
- )
- else:
- secure_adapter = HTTPAdapter(max_retries=retries, ssl_context=ssl_context)
- self._trusted_host_adapter = insecure_adapter
-
- self.mount("https://", secure_adapter)
- self.mount("http://", insecure_adapter)
-
- # Enable file:// urls
- self.mount("file://", LocalFSAdapter())
-
- for host in trusted_hosts:
- self.add_trusted_host(host, suppress_logging=True)
-
- def update_index_urls(self, new_index_urls: List[str]) -> None:
- """
- :param new_index_urls: New index urls to update the authentication
- handler with.
- """
- self.auth.index_urls = new_index_urls
-
- def add_trusted_host(
- self, host: str, source: Optional[str] = None, suppress_logging: bool = False
- ) -> None:
- """
- :param host: It is okay to provide a host that has previously been
- added.
- :param source: An optional source string, for logging where the host
- string came from.
- """
- if not suppress_logging:
- msg = f"adding trusted host: {host!r}"
- if source is not None:
- msg += f" (from {source})"
- logger.info(msg)
-
- host_port = parse_netloc(host)
- if host_port not in self.pip_trusted_origins:
- self.pip_trusted_origins.append(host_port)
-
- self.mount(
- build_url_from_netloc(host, scheme="http") + "/", self._trusted_host_adapter
- )
- self.mount(build_url_from_netloc(host) + "/", self._trusted_host_adapter)
- if not host_port[1]:
- self.mount(
- build_url_from_netloc(host, scheme="http") + ":",
- self._trusted_host_adapter,
- )
- # Mount wildcard ports for the same host.
- self.mount(build_url_from_netloc(host) + ":", self._trusted_host_adapter)
-
- def iter_secure_origins(self) -> Generator[SecureOrigin, None, None]:
- yield from SECURE_ORIGINS
- for host, port in self.pip_trusted_origins:
- yield ("*", host, "*" if port is None else port)
-
- def is_secure_origin(self, location: Link) -> bool:
- # Determine if this url used a secure transport mechanism
- parsed = urllib.parse.urlparse(str(location))
- origin_protocol, origin_host, origin_port = (
- parsed.scheme,
- parsed.hostname,
- parsed.port,
- )
-
- # The protocol to use to see if the protocol matches.
- # Don't count the repository type as part of the protocol: in
- # cases such as "git+ssh", only use "ssh". (I.e., Only verify against
- # the last scheme.)
- origin_protocol = origin_protocol.rsplit("+", 1)[-1]
-
- # Determine if our origin is a secure origin by looking through our
- # hardcoded list of secure origins, as well as any additional ones
- # configured on this PackageFinder instance.
- for secure_origin in self.iter_secure_origins():
- secure_protocol, secure_host, secure_port = secure_origin
- if origin_protocol != secure_protocol and secure_protocol != "*":
- continue
-
- try:
- addr = ipaddress.ip_address(origin_host or "")
- network = ipaddress.ip_network(secure_host)
- except ValueError:
- # We don't have both a valid address or a valid network, so
- # we'll check this origin against hostnames.
- if (
- origin_host
- and origin_host.lower() != secure_host.lower()
- and secure_host != "*"
- ):
- continue
- else:
- # We have a valid address and network, so see if the address
- # is contained within the network.
- if addr not in network:
- continue
-
- # Check to see if the port matches.
- if (
- origin_port != secure_port
- and secure_port != "*"
- and secure_port is not None
- ):
- continue
-
- # If we've gotten here, then this origin matches the current
- # secure origin and we should return True
- return True
-
- # If we've gotten to this point, then the origin isn't secure and we
- # will not accept it as a valid location to search. We will however
- # log a warning that we are ignoring it.
- logger.warning(
- "The repository located at %s is not a trusted or secure host and "
- "is being ignored. If this repository is available via HTTPS we "
- "recommend you use HTTPS instead, otherwise you may silence "
- "this warning and allow it anyway with '--trusted-host %s'.",
- origin_host,
- origin_host,
- )
-
- return False
-
- def request(self, method: str, url: str, *args: Any, **kwargs: Any) -> Response:
- # Allow setting a default timeout on a session
- kwargs.setdefault("timeout", self.timeout)
- # Allow setting a default proxies on a session
- kwargs.setdefault("proxies", self.proxies)
-
- # Dispatch the actual request
- return super().request(method, url, *args, **kwargs)
diff --git a/spaces/Boilin/URetinex-Net/network/architecture.py b/spaces/Boilin/URetinex-Net/network/architecture.py
deleted file mode 100644
index 8cb0fcd99a78c9fe6cfddc1ebe27114cfd9b6b5a..0000000000000000000000000000000000000000
--- a/spaces/Boilin/URetinex-Net/network/architecture.py
+++ /dev/null
@@ -1,41 +0,0 @@
-import torch
-import torch.nn as nn
-import torchvision
-
-def get_batchnorm_layer(opts):
- if opts.norm_layer == "batch":
- norm_layer = nn.BatchNorm2d
- elif opts.layer == "spectral_instance":
- norm_layer = nn.InstanceNorm2d
- else:
- print("not implemented")
- exit()
- return norm_layer
-
-def get_conv2d_layer(in_c, out_c, k, s, p=0, dilation=1, groups=1):
- return nn.Conv2d(in_channels=in_c,
- out_channels=out_c,
- kernel_size=k,
- stride=s,
- padding=p,dilation=dilation, groups=groups)
-
-def get_deconv2d_layer(in_c, out_c, k=1, s=1, p=1):
- return nn.Sequential(
- nn.Upsample(scale_factor=2, mode="bilinear"),
- nn.Conv2d(
- in_channels=in_c,
- out_channels=out_c,
- kernel_size=k,
- stride=s,
- padding=p
- )
- )
-
-class Identity(nn.Module):
-
- def __init__(self):
- super(Identity, self).__init__()
-
- def forward(self, x):
- return x
-
diff --git a/spaces/CAMP-ViL/Xplainer/model.py b/spaces/CAMP-ViL/Xplainer/model.py
deleted file mode 100644
index fa175c63e6b5f1c24fd54df314fc10ebc0938584..0000000000000000000000000000000000000000
--- a/spaces/CAMP-ViL/Xplainer/model.py
+++ /dev/null
@@ -1,158 +0,0 @@
-from pathlib import Path
-from typing import List
-
-import torch
-import torch.nn.functional as F
-from health_multimodal.image import get_biovil_resnet_inference
-from health_multimodal.text import get_cxr_bert_inference
-from health_multimodal.vlp import ImageTextInferenceEngine
-
-from utils import cos_sim_to_prob, prob_to_log_prob, log_prob_to_prob
-
-
-class InferenceModel():
- def __init__(self):
- self.text_inference = get_cxr_bert_inference()
- self.image_inference = get_biovil_resnet_inference()
- self.image_text_inference = ImageTextInferenceEngine(
- image_inference_engine=self.image_inference,
- text_inference_engine=self.text_inference,
- )
- self.device = torch.device("cuda" if torch.cuda.is_available() else "cpu")
- self.image_text_inference.to(self.device)
-
- # caches for faster inference
- self.text_embedding_cache = {}
- self.image_embedding_cache = {}
-
- self.transform = self.image_inference.transform
-
- def get_similarity_score_from_raw_data(self, image_embedding, query_text: str) -> float:
- """Compute the cosine similarity score between an image and one or more strings.
- If multiple strings are passed, their embeddings are averaged before L2-normalization.
- :param image_path: Path to the input chest X-ray, either a DICOM or JPEG file.
- :param query_text: Input radiology text phrase.
- :return: The similarity score between the image and the text.
- """
- assert not self.image_text_inference.image_inference_engine.model.training
- assert not self.image_text_inference.text_inference_engine.model.training
- if query_text in self.text_embedding_cache:
- text_embedding = self.text_embedding_cache[query_text]
- else:
- text_embedding = self.image_text_inference.text_inference_engine.get_embeddings_from_prompt([query_text], normalize=False)
- text_embedding = text_embedding.mean(dim=0)
- text_embedding = F.normalize(text_embedding, dim=0, p=2)
- self.text_embedding_cache[query_text] = text_embedding
-
- cos_similarity = image_embedding @ text_embedding.t()
-
- return cos_similarity.item()
-
- def process_image(self, image):
- ''' same code as in image_text_inference.image_inference_engine.get_projected_global_embedding() but adapted to deal with image instances instead of path'''
-
- transformed_image = self.transform(image)
- projected_img_emb = self.image_inference.model.forward(transformed_image).projected_global_embedding
- projected_img_emb = F.normalize(projected_img_emb, dim=-1)
- assert projected_img_emb.shape[0] == 1
- assert projected_img_emb.ndim == 2
- return projected_img_emb[0]
-
- def get_descriptor_probs(self, image_path: Path, descriptors: List[str], do_negative_prompting=True, demo=False):
- probs = {}
- negative_probs = {}
- if image_path in self.image_embedding_cache:
- image_embedding = self.image_embedding_cache[image_path]
- else:
- image_embedding = self.image_text_inference.image_inference_engine.get_projected_global_embedding(image_path)
- if not demo:
- self.image_embedding_cache[image_path] = image_embedding
-
- # Default get_similarity_score_from_raw_data would load the image every time. Instead we only load once.
- for desc in descriptors:
- prompt = f'There are {desc}'
- score = self.get_similarity_score_from_raw_data(image_embedding, prompt)
- if do_negative_prompting:
- neg_prompt = f'There are no {desc}'
- neg_score = self.get_similarity_score_from_raw_data(image_embedding, neg_prompt)
-
- pos_prob = cos_sim_to_prob(score)
-
- if do_negative_prompting:
- pos_prob, neg_prob = torch.softmax((torch.tensor([score, neg_score]) / 0.5), dim=0)
- negative_probs[desc] = neg_prob
-
- probs[desc] = pos_prob
-
- return probs, negative_probs
-
- def get_all_descriptors(self, disease_descriptors):
- all_descriptors = set()
- for disease, descs in disease_descriptors.items():
- all_descriptors.update([f"{desc} indicating {disease}" for desc in descs])
- all_descriptors = sorted(all_descriptors)
- return all_descriptors
-
- def get_all_descriptors_only_disease(self, disease_descriptors):
- all_descriptors = set()
- for disease, descs in disease_descriptors.items():
- all_descriptors.update([f"{desc}" for desc in descs])
- all_descriptors = sorted(all_descriptors)
- return all_descriptors
-
- def get_diseases_probs(self, disease_descriptors, pos_probs, negative_probs, prior_probs=None, do_negative_prompting=True):
- disease_probs = {}
- disease_neg_probs = {}
- for disease, descriptors in disease_descriptors.items():
- desc_log_probs = []
- desc_neg_log_probs = []
- for desc in descriptors:
- desc = f"{desc} indicating {disease}"
- desc_log_probs.append(prob_to_log_prob(pos_probs[desc]))
- if do_negative_prompting:
- desc_neg_log_probs.append(prob_to_log_prob(negative_probs[desc]))
- disease_log_prob = sum(sorted(desc_log_probs, reverse=True)) / len(desc_log_probs)
- if do_negative_prompting:
- disease_neg_log_prob = sum(desc_neg_log_probs) / len(desc_neg_log_probs)
- disease_probs[disease] = log_prob_to_prob(disease_log_prob)
- if do_negative_prompting:
- disease_neg_probs[disease] = log_prob_to_prob(disease_neg_log_prob)
-
- return disease_probs, disease_neg_probs
-
- # Threshold Based
- def get_predictions(self, disease_descriptors, threshold, disease_probs, keys):
- predicted_diseases = []
- prob_vector = torch.zeros(len(keys), dtype=torch.float) # num of diseases
- for idx, disease in enumerate(disease_descriptors):
- if disease == 'No Finding':
- continue
- prob_vector[keys.index(disease)] = disease_probs[disease]
- if disease_probs[disease] > threshold:
- predicted_diseases.append(disease)
-
- if len(predicted_diseases) == 0: # No finding rule based
- prob_vector[0] = 1.0 - max(prob_vector)
- else:
- prob_vector[0] = 1.0 - max(prob_vector)
-
- return predicted_diseases, prob_vector
-
- # Negative vs Positive Prompting
- def get_predictions_bin_prompting(self, disease_descriptors, disease_probs, negative_disease_probs, keys):
- predicted_diseases = []
- prob_vector = torch.zeros(len(keys), dtype=torch.float) # num of diseases
- for idx, disease in enumerate(disease_descriptors):
- if disease == 'No Finding':
- continue
- pos_neg_scores = torch.tensor([disease_probs[disease], negative_disease_probs[disease]])
- prob_vector[keys.index(disease)] = pos_neg_scores[0]
- if torch.argmax(pos_neg_scores) == 0: # Positive is More likely
- predicted_diseases.append(disease)
-
- if len(predicted_diseases) == 0: # No finding rule based
- prob_vector[0] = 1.0 - max(prob_vector)
- else:
- prob_vector[0] = 1.0 - max(prob_vector)
-
- return predicted_diseases, prob_vector
diff --git a/spaces/CVPR/LIVE/pybind11/tests/local_bindings.h b/spaces/CVPR/LIVE/pybind11/tests/local_bindings.h
deleted file mode 100644
index b6afb808664de1fdbde011a9bf7c38d3a8794127..0000000000000000000000000000000000000000
--- a/spaces/CVPR/LIVE/pybind11/tests/local_bindings.h
+++ /dev/null
@@ -1,64 +0,0 @@
-#pragma once
-#include "pybind11_tests.h"
-
-/// Simple class used to test py::local:
-template class LocalBase {
-public:
- LocalBase(int i) : i(i) { }
- int i = -1;
-};
-
-/// Registered with py::module_local in both main and secondary modules:
-using LocalType = LocalBase<0>;
-/// Registered without py::module_local in both modules:
-using NonLocalType = LocalBase<1>;
-/// A second non-local type (for stl_bind tests):
-using NonLocal2 = LocalBase<2>;
-/// Tests within-module, different-compilation-unit local definition conflict:
-using LocalExternal = LocalBase<3>;
-/// Mixed: registered local first, then global
-using MixedLocalGlobal = LocalBase<4>;
-/// Mixed: global first, then local
-using MixedGlobalLocal = LocalBase<5>;
-
-/// Registered with py::module_local only in the secondary module:
-using ExternalType1 = LocalBase<6>;
-using ExternalType2 = LocalBase<7>;
-
-using LocalVec = std::vector;
-using LocalVec2 = std::vector;
-using LocalMap = std::unordered_map;
-using NonLocalVec = std::vector;
-using NonLocalVec2 = std::vector;
-using NonLocalMap = std::unordered_map;
-using NonLocalMap2 = std::unordered_map;
-
-PYBIND11_MAKE_OPAQUE(LocalVec);
-PYBIND11_MAKE_OPAQUE(LocalVec2);
-PYBIND11_MAKE_OPAQUE(LocalMap);
-PYBIND11_MAKE_OPAQUE(NonLocalVec);
-//PYBIND11_MAKE_OPAQUE(NonLocalVec2); // same type as LocalVec2
-PYBIND11_MAKE_OPAQUE(NonLocalMap);
-PYBIND11_MAKE_OPAQUE(NonLocalMap2);
-
-
-// Simple bindings (used with the above):
-template
-py::class_ bind_local(Args && ...args) {
- return py::class_(std::forward(args)...)
- .def(py::init())
- .def("get", [](T &i) { return i.i + Adjust; });
-};
-
-// Simulate a foreign library base class (to match the example in the docs):
-namespace pets {
-class Pet {
-public:
- Pet(std::string name) : name_(name) {}
- std::string name_;
- const std::string &name() { return name_; }
-};
-}
-
-struct MixGL { int i; MixGL(int i) : i{i} {} };
-struct MixGL2 { int i; MixGL2(int i) : i{i} {} };
diff --git a/spaces/CVPR/LIVE/pydiffvg_tensorflow/shape.py b/spaces/CVPR/LIVE/pydiffvg_tensorflow/shape.py
deleted file mode 100644
index 432a3b5dc2fd1b8eb03c306a8123c76e6b9302ff..0000000000000000000000000000000000000000
--- a/spaces/CVPR/LIVE/pydiffvg_tensorflow/shape.py
+++ /dev/null
@@ -1,54 +0,0 @@
-import tensorflow as tf
-import math
-
-class Circle:
- def __init__(self, radius, center, stroke_width = tf.constant(1.0), id = ''):
- self.radius = radius
- self.center = center
- self.stroke_width = stroke_width
- self.id = id
-
-class Ellipse:
- def __init__(self, radius, center, stroke_width = tf.constant(1.0), id = ''):
- self.radius = radius
- self.center = center
- self.stroke_width = stroke_width
- self.id = id
-
-class Path:
- def __init__(self, num_control_points, points, is_closed, stroke_width = tf.constant(1.0), id = '', use_distance_approx = False):
- self.num_control_points = num_control_points
- self.points = points
- self.is_closed = is_closed
- self.stroke_width = stroke_width
- self.id = id
- self.use_distance_approx = use_distance_approx
-
-class Polygon:
- def __init__(self, points, is_closed, stroke_width = tf.constant(1.0), id = ''):
- self.points = points
- self.is_closed = is_closed
- self.stroke_width = stroke_width
- self.id = id
-
-class Rect:
- def __init__(self, p_min, p_max, stroke_width = tf.constant(1.0), id = ''):
- self.p_min = p_min
- self.p_max = p_max
- self.stroke_width = stroke_width
- self.id = id
-
-class ShapeGroup:
- def __init__(self,
- shape_ids,
- fill_color,
- use_even_odd_rule = True,
- stroke_color = None,
- shape_to_canvas = tf.eye(3),
- id = ''):
- self.shape_ids = shape_ids
- self.fill_color = fill_color
- self.use_even_odd_rule = use_even_odd_rule
- self.stroke_color = stroke_color
- self.shape_to_canvas = shape_to_canvas
- self.id = id
diff --git a/spaces/CVPR/LIVE/thrust/thrust/system/cpp/detail/mismatch.h b/spaces/CVPR/LIVE/thrust/thrust/system/cpp/detail/mismatch.h
deleted file mode 100644
index c6ae90664ad9538e73febfde86c334011de417c8..0000000000000000000000000000000000000000
--- a/spaces/CVPR/LIVE/thrust/thrust/system/cpp/detail/mismatch.h
+++ /dev/null
@@ -1,22 +0,0 @@
-/*
- * Copyright 2008-2013 NVIDIA Corporation
- *
- * Licensed under the Apache License, Version 2.0 (the "License");
- * you may not use this file except in compliance with the License.
- * You may obtain a copy of the License at
- *
- * http://www.apache.org/licenses/LICENSE-2.0
- *
- * Unless required by applicable law or agreed to in writing, software
- * distributed under the License is distributed on an "AS IS" BASIS,
- * WITHOUT WARRANTIES OR CONDITIONS OF ANY KIND, either express or implied.
- * See the License for the specific language governing permissions and
- * limitations under the License.
- */
-
-#pragma once
-
-#include
-
-// this system has no special version of this algorithm
-
diff --git a/spaces/CVPR/LIVE/thrust/thrust/system/omp/detail/transform.h b/spaces/CVPR/LIVE/thrust/thrust/system/omp/detail/transform.h
deleted file mode 100644
index 20d606dfbeec6d376a138db500ec368d94efa748..0000000000000000000000000000000000000000
--- a/spaces/CVPR/LIVE/thrust/thrust/system/omp/detail/transform.h
+++ /dev/null
@@ -1,23 +0,0 @@
-/*
- * Copyright 2008-2013 NVIDIA Corporation
- *
- * Licensed under the Apache License, Version 2.0 (the "License");
- * you may not use this file except in compliance with the License.
- * You may obtain a copy of the License at
- *
- * http://www.apache.org/licenses/LICENSE-2.0
- *
- * Unless required by applicable law or agreed to in writing, software
- * distributed under the License is distributed on an "AS IS" BASIS,
- * WITHOUT WARRANTIES OR CONDITIONS OF ANY KIND, either express or implied.
- * See the License for the specific language governing permissions and
- * limitations under the License.
- */
-
-#pragma once
-
-#include
-
-// omp inherits transform
-#include
-
diff --git a/spaces/CVPR/LIVE/xing_loss.py b/spaces/CVPR/LIVE/xing_loss.py
deleted file mode 100644
index 472ed17749dfe041eb262aff80b10506bdaadf01..0000000000000000000000000000000000000000
--- a/spaces/CVPR/LIVE/xing_loss.py
+++ /dev/null
@@ -1,66 +0,0 @@
-import torch
-import numpy as np
-
-
-def area(a, b, c):
- return (c[1] - a[1]) * (b[0] - a[0]) - (b[1] - a[1]) * (c[0] - a[0])
-
-
-def triangle_area(A, B, C):
- out = (C - A).flip([-1]) * (B - A)
- out = out[..., 1] - out[..., 0]
- return out
-
-def compute_sine_theta(s1, s2): #s1 and s2 aret two segments to be uswed
- #s1, s2 (2, 2)
- v1 = s1[1,:] - s1[0, :]
- v2 = s2[1,:] - s2[0, :]
- #print(v1, v2)
- sine_theta = ( v1[0] * v2[1] - v1[1] * v2[0] ) / (torch.norm(v1) * torch.norm(v2))
- return sine_theta
-
-def xing_loss(x_list, scale=1e-3): # x[ npoints,2]
- loss = 0.
- #print(len(x_list))
- for x in x_list:
- #print(x)
- seg_loss = 0.
- N = x.size()[0]
- x = torch.cat([x,x[0,:].unsqueeze(0)], dim=0) #(N+1,2)
- segments = torch.cat([x[:-1,:].unsqueeze(1), x[1:,:].unsqueeze(1)], dim=1) #(N, start/end, 2)
- assert N % 3 == 0, 'The segment number is not correct!'
- segment_num = int(N / 3)
- for i in range(segment_num):
- cs1 = segments[i*3, :, :] #start control segs
- cs2 = segments[i*3 + 1, :, :] #middle control segs
- cs3 = segments[i*3 + 2, :, :] #end control segs
- #print('the direction of the vectors:')
- #print(compute_sine_theta(cs1, cs2))
- direct = (compute_sine_theta(cs1, cs2) >= 0).float()
- opst = 1 - direct #another direction
- sina = compute_sine_theta(cs1, cs3) #the angle between cs1 and cs3
- seg_loss += direct * torch.relu( - sina) + opst * torch.relu(sina)
- # print(direct, opst, sina)
- seg_loss /= segment_num
-
-
- templ = seg_loss
- loss += templ * scale #area_loss * scale
-
- return loss / (len(x_list))
-
-
-if __name__ == "__main__":
- #x = torch.rand([6, 2])
- #x = torch.tensor([[0,0], [1,1], [2,1], [1.5,0]])
- x = torch.tensor([[0,0], [1,1], [2,1], [0.5,0]])
- #x = torch.tensor([[1,0], [2,1], [0,1], [2,0]])
- scale = 1 #0.5
- y = xing_loss([x], scale)
- print(y)
-
- x = torch.tensor([[0,0], [1,1], [2,1], [2.,0]])
- #x = torch.tensor([[1,0], [2,1], [0,1], [2,0]])
- scale = 1 #0.5
- y = xing_loss([x], scale)
- print(y)
diff --git a/spaces/CVPR/MonoScene/monoscene/monoscene_model.py b/spaces/CVPR/MonoScene/monoscene/monoscene_model.py
deleted file mode 100644
index 8a5207f3d03de86192c5d41a8bdfe3ce32e672ab..0000000000000000000000000000000000000000
--- a/spaces/CVPR/MonoScene/monoscene/monoscene_model.py
+++ /dev/null
@@ -1,21 +0,0 @@
-from transformers import PreTrainedModel
-from .config import MonoSceneConfig
-from monoscene.monoscene import MonoScene
-
-
-class MonoSceneModel(PreTrainedModel):
- config_class = MonoSceneConfig
-
- def __init__(self, config):
- super().__init__(config)
- self.model = MonoScene(
- dataset=config.dataset,
- n_classes=config.n_classes,
- feature=config.feature,
- project_scale=config.project_scale,
- full_scene_size=config.full_scene_size
- )
-
-
- def forward(self, tensor):
- return self.model.forward(tensor)
\ No newline at end of file
diff --git a/spaces/CVPR/regionclip-demo/detectron2/modeling/meta_arch/__init__.py b/spaces/CVPR/regionclip-demo/detectron2/modeling/meta_arch/__init__.py
deleted file mode 100644
index 04caae8693a51e59f1f31d1daac18df484842e93..0000000000000000000000000000000000000000
--- a/spaces/CVPR/regionclip-demo/detectron2/modeling/meta_arch/__init__.py
+++ /dev/null
@@ -1,15 +0,0 @@
-# -*- coding: utf-8 -*-
-# Copyright (c) Facebook, Inc. and its affiliates.
-
-from .build import META_ARCH_REGISTRY, build_model # isort:skip
-
-from .panoptic_fpn import PanopticFPN
-
-# import all the meta_arch, so they will be registered
-from .rcnn import GeneralizedRCNN, ProposalNetwork
-from .retinanet import RetinaNet
-from .semantic_seg import SEM_SEG_HEADS_REGISTRY, SemanticSegmentor, build_sem_seg_head
-from .clip_rcnn import CLIPRCNN, CLIPFastRCNN, PretrainFastRCNN
-
-
-__all__ = list(globals().keys())
diff --git a/spaces/CVPR/transfiner/configs/Misc/mmdet_mask_rcnn_R_50_FPN_1x.py b/spaces/CVPR/transfiner/configs/Misc/mmdet_mask_rcnn_R_50_FPN_1x.py
deleted file mode 100644
index 0f2464be744c083985898a25f9e71d00104f689d..0000000000000000000000000000000000000000
--- a/spaces/CVPR/transfiner/configs/Misc/mmdet_mask_rcnn_R_50_FPN_1x.py
+++ /dev/null
@@ -1,151 +0,0 @@
-# An example config to train a mmdetection model using detectron2.
-
-from ..common.data.coco import dataloader
-from ..common.coco_schedule import lr_multiplier_1x as lr_multiplier
-from ..common.optim import SGD as optimizer
-from ..common.train import train
-
-from detectron2.modeling.mmdet_wrapper import MMDetDetector
-from detectron2.config import LazyCall as L
-
-model = L(MMDetDetector)(
- detector=dict(
- type="MaskRCNN",
- pretrained="torchvision://resnet50",
- backbone=dict(
- type="ResNet",
- depth=50,
- num_stages=4,
- out_indices=(0, 1, 2, 3),
- frozen_stages=1,
- norm_cfg=dict(type="BN", requires_grad=True),
- norm_eval=True,
- style="pytorch",
- ),
- neck=dict(type="FPN", in_channels=[256, 512, 1024, 2048], out_channels=256, num_outs=5),
- rpn_head=dict(
- type="RPNHead",
- in_channels=256,
- feat_channels=256,
- anchor_generator=dict(
- type="AnchorGenerator",
- scales=[8],
- ratios=[0.5, 1.0, 2.0],
- strides=[4, 8, 16, 32, 64],
- ),
- bbox_coder=dict(
- type="DeltaXYWHBBoxCoder",
- target_means=[0.0, 0.0, 0.0, 0.0],
- target_stds=[1.0, 1.0, 1.0, 1.0],
- ),
- loss_cls=dict(type="CrossEntropyLoss", use_sigmoid=True, loss_weight=1.0),
- loss_bbox=dict(type="L1Loss", loss_weight=1.0),
- ),
- roi_head=dict(
- type="StandardRoIHead",
- bbox_roi_extractor=dict(
- type="SingleRoIExtractor",
- roi_layer=dict(type="RoIAlign", output_size=7, sampling_ratio=0),
- out_channels=256,
- featmap_strides=[4, 8, 16, 32],
- ),
- bbox_head=dict(
- type="Shared2FCBBoxHead",
- in_channels=256,
- fc_out_channels=1024,
- roi_feat_size=7,
- num_classes=80,
- bbox_coder=dict(
- type="DeltaXYWHBBoxCoder",
- target_means=[0.0, 0.0, 0.0, 0.0],
- target_stds=[0.1, 0.1, 0.2, 0.2],
- ),
- reg_class_agnostic=False,
- loss_cls=dict(type="CrossEntropyLoss", use_sigmoid=False, loss_weight=1.0),
- loss_bbox=dict(type="L1Loss", loss_weight=1.0),
- ),
- mask_roi_extractor=dict(
- type="SingleRoIExtractor",
- roi_layer=dict(type="RoIAlign", output_size=14, sampling_ratio=0),
- out_channels=256,
- featmap_strides=[4, 8, 16, 32],
- ),
- mask_head=dict(
- type="FCNMaskHead",
- num_convs=4,
- in_channels=256,
- conv_out_channels=256,
- num_classes=80,
- loss_mask=dict(type="CrossEntropyLoss", use_mask=True, loss_weight=1.0),
- ),
- ),
- # model training and testing settings
- train_cfg=dict(
- rpn=dict(
- assigner=dict(
- type="MaxIoUAssigner",
- pos_iou_thr=0.7,
- neg_iou_thr=0.3,
- min_pos_iou=0.3,
- match_low_quality=True,
- ignore_iof_thr=-1,
- ),
- sampler=dict(
- type="RandomSampler",
- num=256,
- pos_fraction=0.5,
- neg_pos_ub=-1,
- add_gt_as_proposals=False,
- ),
- allowed_border=-1,
- pos_weight=-1,
- debug=False,
- ),
- rpn_proposal=dict(
- nms_pre=2000,
- max_per_img=1000,
- nms=dict(type="nms", iou_threshold=0.7),
- min_bbox_size=0,
- ),
- rcnn=dict(
- assigner=dict(
- type="MaxIoUAssigner",
- pos_iou_thr=0.5,
- neg_iou_thr=0.5,
- min_pos_iou=0.5,
- match_low_quality=True,
- ignore_iof_thr=-1,
- ),
- sampler=dict(
- type="RandomSampler",
- num=512,
- pos_fraction=0.25,
- neg_pos_ub=-1,
- add_gt_as_proposals=True,
- ),
- mask_size=28,
- pos_weight=-1,
- debug=False,
- ),
- ),
- test_cfg=dict(
- rpn=dict(
- nms_pre=1000,
- max_per_img=1000,
- nms=dict(type="nms", iou_threshold=0.7),
- min_bbox_size=0,
- ),
- rcnn=dict(
- score_thr=0.05,
- nms=dict(type="nms", iou_threshold=0.5),
- max_per_img=100,
- mask_thr_binary=0.5,
- ),
- ),
- ),
- pixel_mean=[123.675, 116.280, 103.530],
- pixel_std=[58.395, 57.120, 57.375],
-)
-
-dataloader.train.mapper.image_format = "RGB" # torchvision pretrained model
-train.init_checkpoint = None # pretrained model is loaded inside backbone
diff --git a/spaces/CikeyQI/meme-api/meme_generator/memes/luoyonghao_say/__init__.py b/spaces/CikeyQI/meme-api/meme_generator/memes/luoyonghao_say/__init__.py
deleted file mode 100644
index f09d378a09127843804bb79fbf9e1e3370ac88fb..0000000000000000000000000000000000000000
--- a/spaces/CikeyQI/meme-api/meme_generator/memes/luoyonghao_say/__init__.py
+++ /dev/null
@@ -1,42 +0,0 @@
-from pathlib import Path
-from typing import List
-
-from PIL import ImageFilter
-from pil_utils import BuildImage
-
-from meme_generator import add_meme
-from meme_generator.exception import TextOverLength
-
-img_dir = Path(__file__).parent / "images"
-
-
-def luoyonghao_say(images, texts: List[str], args):
- text = texts[0]
- frame = BuildImage.open(img_dir / "0.jpg")
- text_frame = BuildImage.new("RGBA", (365, 120))
- try:
- text_frame.draw_text(
- (40, 10, 325, 110),
- text,
- allow_wrap=True,
- max_fontsize=50,
- min_fontsize=10,
- valign="top",
- )
- except ValueError:
- raise TextOverLength(text)
- text_frame = text_frame.perspective(
- ((52, 10), (391, 0), (364, 110), (0, 120))
- ).filter(ImageFilter.GaussianBlur(radius=0.8))
- frame.paste(text_frame, (48, 246), alpha=True)
- return frame.save_jpg()
-
-
-add_meme(
- "luoyonghao_say",
- luoyonghao_say,
- min_texts=1,
- max_texts=1,
- default_texts=["又不是不能用"],
- keywords=["罗永浩说"],
-)
diff --git a/spaces/Cropinky/gpt2-rap-songs/app.py b/spaces/Cropinky/gpt2-rap-songs/app.py
deleted file mode 100644
index bb86a0282d724d78553df52a90862718db8e3ff7..0000000000000000000000000000000000000000
--- a/spaces/Cropinky/gpt2-rap-songs/app.py
+++ /dev/null
@@ -1,49 +0,0 @@
-from os import CLD_CONTINUED
-import streamlit as st
-from transformers import AutoTokenizer, AutoModelForCausalLM
-from transformers import pipeline
-
-@st.cache(allow_output_mutation=True)
-def load_model():
- model_ckpt = "flax-community/gpt2-rap-lyric-generator"
- tokenizer = AutoTokenizer.from_pretrained(model_ckpt,from_flax=True)
- model = AutoModelForCausalLM.from_pretrained(model_ckpt,from_flax=True)
- return tokenizer, model
-
-@st.cache()
-def load_rappers():
- text_file = open("rappers.txt")
- rappers = text_file.readlines()
- rappers = [name[:-1] for name in rappers]
- rappers.sort()
- return rappers
-
-
-title = st.title("Loading model")
-tokenizer, model = load_model()
-text_generation = pipeline("text-generation", model=model, tokenizer=tokenizer)
-title.title("Rap lyrics generator")
-#artist = st.text_input("Enter the artist", "Wu-Tang Clan")
-list_of_rappers = load_rappers()
-artist = st.selectbox("Choose your rapper", tuple(list_of_rappers), index = len(list_of_rappers)-1)
-song_name = st.text_input("Enter the desired song name", "Sadboys")
-
-
-
-if st.button("Generate lyrics", help="The lyrics generation can last up to 2 minutres"):
- st.title(f"{artist}: {song_name}")
- prefix_text = f"{song_name} [Verse 1:{artist}]"
- generated_song = text_generation(prefix_text, max_length=750, do_sample=True)[0]
- for count, line in enumerate(generated_song['generated_text'].split("\n")):
- if"" in line:
- break
- if count == 0:
- st.markdown(f"**{line[line.find('['):]}**")
- continue
- if "" in line:
- st.write(line[5:])
- continue
- if line.startswith("["):
- st.markdown(f"**{line}**")
- continue
- st.write(line)
\ No newline at end of file
diff --git a/spaces/DQChoi/gpt-demo/venv/lib/python3.11/site-packages/attr/_next_gen.py b/spaces/DQChoi/gpt-demo/venv/lib/python3.11/site-packages/attr/_next_gen.py
deleted file mode 100644
index 8f7c0b9a46b7a0ee008f94b8054baf5807df043a..0000000000000000000000000000000000000000
--- a/spaces/DQChoi/gpt-demo/venv/lib/python3.11/site-packages/attr/_next_gen.py
+++ /dev/null
@@ -1,232 +0,0 @@
-# SPDX-License-Identifier: MIT
-
-"""
-These are keyword-only APIs that call `attr.s` and `attr.ib` with different
-default values.
-"""
-
-
-from functools import partial
-
-from . import setters
-from ._funcs import asdict as _asdict
-from ._funcs import astuple as _astuple
-from ._make import (
- NOTHING,
- _frozen_setattrs,
- _ng_default_on_setattr,
- attrib,
- attrs,
-)
-from .exceptions import UnannotatedAttributeError
-
-
-def define(
- maybe_cls=None,
- *,
- these=None,
- repr=None,
- unsafe_hash=None,
- hash=None,
- init=None,
- slots=True,
- frozen=False,
- weakref_slot=True,
- str=False,
- auto_attribs=None,
- kw_only=False,
- cache_hash=False,
- auto_exc=True,
- eq=None,
- order=False,
- auto_detect=True,
- getstate_setstate=None,
- on_setattr=None,
- field_transformer=None,
- match_args=True,
-):
- r"""
- Define an *attrs* class.
-
- Differences to the classic `attr.s` that it uses underneath:
-
- - Automatically detect whether or not *auto_attribs* should be `True` (c.f.
- *auto_attribs* parameter).
- - If *frozen* is `False`, run converters and validators when setting an
- attribute by default.
- - *slots=True*
-
- .. caution::
-
- Usually this has only upsides and few visible effects in everyday
- programming. But it *can* lead to some suprising behaviors, so please
- make sure to read :term:`slotted classes`.
- - *auto_exc=True*
- - *auto_detect=True*
- - *order=False*
- - Some options that were only relevant on Python 2 or were kept around for
- backwards-compatibility have been removed.
-
- Please note that these are all defaults and you can change them as you
- wish.
-
- :param Optional[bool] auto_attribs: If set to `True` or `False`, it behaves
- exactly like `attr.s`. If left `None`, `attr.s` will try to guess:
-
- 1. If any attributes are annotated and no unannotated `attrs.fields`\ s
- are found, it assumes *auto_attribs=True*.
- 2. Otherwise it assumes *auto_attribs=False* and tries to collect
- `attrs.fields`\ s.
-
- For now, please refer to `attr.s` for the rest of the parameters.
-
- .. versionadded:: 20.1.0
- .. versionchanged:: 21.3.0 Converters are also run ``on_setattr``.
- .. versionadded:: 22.2.0
- *unsafe_hash* as an alias for *hash* (for :pep:`681` compliance).
- """
-
- def do_it(cls, auto_attribs):
- return attrs(
- maybe_cls=cls,
- these=these,
- repr=repr,
- hash=hash,
- unsafe_hash=unsafe_hash,
- init=init,
- slots=slots,
- frozen=frozen,
- weakref_slot=weakref_slot,
- str=str,
- auto_attribs=auto_attribs,
- kw_only=kw_only,
- cache_hash=cache_hash,
- auto_exc=auto_exc,
- eq=eq,
- order=order,
- auto_detect=auto_detect,
- collect_by_mro=True,
- getstate_setstate=getstate_setstate,
- on_setattr=on_setattr,
- field_transformer=field_transformer,
- match_args=match_args,
- )
-
- def wrap(cls):
- """
- Making this a wrapper ensures this code runs during class creation.
-
- We also ensure that frozen-ness of classes is inherited.
- """
- nonlocal frozen, on_setattr
-
- had_on_setattr = on_setattr not in (None, setters.NO_OP)
-
- # By default, mutable classes convert & validate on setattr.
- if frozen is False and on_setattr is None:
- on_setattr = _ng_default_on_setattr
-
- # However, if we subclass a frozen class, we inherit the immutability
- # and disable on_setattr.
- for base_cls in cls.__bases__:
- if base_cls.__setattr__ is _frozen_setattrs:
- if had_on_setattr:
- raise ValueError(
- "Frozen classes can't use on_setattr "
- "(frozen-ness was inherited)."
- )
-
- on_setattr = setters.NO_OP
- break
-
- if auto_attribs is not None:
- return do_it(cls, auto_attribs)
-
- try:
- return do_it(cls, True)
- except UnannotatedAttributeError:
- return do_it(cls, False)
-
- # maybe_cls's type depends on the usage of the decorator. It's a class
- # if it's used as `@attrs` but ``None`` if used as `@attrs()`.
- if maybe_cls is None:
- return wrap
- else:
- return wrap(maybe_cls)
-
-
-mutable = define
-frozen = partial(define, frozen=True, on_setattr=None)
-
-
-def field(
- *,
- default=NOTHING,
- validator=None,
- repr=True,
- hash=None,
- init=True,
- metadata=None,
- type=None,
- converter=None,
- factory=None,
- kw_only=False,
- eq=None,
- order=None,
- on_setattr=None,
- alias=None,
-):
- """
- Identical to `attr.ib`, except keyword-only and with some arguments
- removed.
-
- .. versionadded:: 23.1.0
- The *type* parameter has been re-added; mostly for
- {func}`attrs.make_class`. Please note that type checkers ignore this
- metadata.
- .. versionadded:: 20.1.0
- """
- return attrib(
- default=default,
- validator=validator,
- repr=repr,
- hash=hash,
- init=init,
- metadata=metadata,
- type=type,
- converter=converter,
- factory=factory,
- kw_only=kw_only,
- eq=eq,
- order=order,
- on_setattr=on_setattr,
- alias=alias,
- )
-
-
-def asdict(inst, *, recurse=True, filter=None, value_serializer=None):
- """
- Same as `attr.asdict`, except that collections types are always retained
- and dict is always used as *dict_factory*.
-
- .. versionadded:: 21.3.0
- """
- return _asdict(
- inst=inst,
- recurse=recurse,
- filter=filter,
- value_serializer=value_serializer,
- retain_collection_types=True,
- )
-
-
-def astuple(inst, *, recurse=True, filter=None):
- """
- Same as `attr.astuple`, except that collections types are always retained
- and `tuple` is always used as the *tuple_factory*.
-
- .. versionadded:: 21.3.0
- """
- return _astuple(
- inst=inst, recurse=recurse, filter=filter, retain_collection_types=True
- )
diff --git a/spaces/DQChoi/gpt-demo/venv/lib/python3.11/site-packages/huggingface_hub/templates/modelcard_template.md b/spaces/DQChoi/gpt-demo/venv/lib/python3.11/site-packages/huggingface_hub/templates/modelcard_template.md
deleted file mode 100644
index ec2d18d427c9fc96eb5c8b89103632620ed4a0b6..0000000000000000000000000000000000000000
--- a/spaces/DQChoi/gpt-demo/venv/lib/python3.11/site-packages/huggingface_hub/templates/modelcard_template.md
+++ /dev/null
@@ -1,202 +0,0 @@
----
-# For reference on model card metadata, see the spec: https://github.com/huggingface/hub-docs/blob/main/modelcard.md?plain=1
-# Doc / guide: https://huggingface.co/docs/hub/model-cards
-{{ card_data }}
----
-
-# Model Card for {{ model_id | default("Model ID", true) }}
-
-
-
-{{ model_summary | default("", true) }}
-
-## Model Details
-
-### Model Description
-
-
-
-{{ model_description | default("", true) }}
-
-- **Developed by:** {{ developers | default("[More Information Needed]", true)}}
-- **Shared by [optional]:** {{ shared_by | default("[More Information Needed]", true)}}
-- **Model type:** {{ model_type | default("[More Information Needed]", true)}}
-- **Language(s) (NLP):** {{ language | default("[More Information Needed]", true)}}
-- **License:** {{ license | default("[More Information Needed]", true)}}
-- **Finetuned from model [optional]:** {{ finetuned_from | default("[More Information Needed]", true)}}
-
-### Model Sources [optional]
-
-
-
-- **Repository:** {{ repo | default("[More Information Needed]", true)}}
-- **Paper [optional]:** {{ paper | default("[More Information Needed]", true)}}
-- **Demo [optional]:** {{ demo | default("[More Information Needed]", true)}}
-
-## Uses
-
-
-
-### Direct Use
-
-
-
-{{ direct_use | default("[More Information Needed]", true)}}
-
-### Downstream Use [optional]
-
-
-
-{{ downstream_use | default("[More Information Needed]", true)}}
-
-### Out-of-Scope Use
-
-
-
-{{ out_of_scope_use | default("[More Information Needed]", true)}}
-
-## Bias, Risks, and Limitations
-
-
-
-{{ bias_risks_limitations | default("[More Information Needed]", true)}}
-
-### Recommendations
-
-
-
-{{ bias_recommendations | default("Users (both direct and downstream) should be made aware of the risks, biases and limitations of the model. More information needed for further recommendations.", true)}}
-
-## How to Get Started with the Model
-
-Use the code below to get started with the model.
-
-{{ get_started_code | default("[More Information Needed]", true)}}
-
-## Training Details
-
-### Training Data
-
-
-
-{{ training_data | default("[More Information Needed]", true)}}
-
-### Training Procedure
-
-
-
-#### Preprocessing [optional]
-
-{{ preprocessing | default("[More Information Needed]", true)}}
-
-
-#### Training Hyperparameters
-
-- **Training regime:** {{ training_regime | default("[More Information Needed]", true)}}
-
-#### Speeds, Sizes, Times [optional]
-
-
-
-{{ speeds_sizes_times | default("[More Information Needed]", true)}}
-
-## Evaluation
-
-
-
-### Testing Data, Factors & Metrics
-
-#### Testing Data
-
-
-
-{{ testing_data | default("[More Information Needed]", true)}}
-
-#### Factors
-
-
-
-{{ testing_factors | default("[More Information Needed]", true)}}
-
-#### Metrics
-
-
-
-{{ testing_metrics | default("[More Information Needed]", true)}}
-
-### Results
-
-{{ results | default("[More Information Needed]", true)}}
-
-#### Summary
-
-{{ results_summary | default("", true) }}
-
-## Model Examination [optional]
-
-
-
-{{ model_examination | default("[More Information Needed]", true)}}
-
-## Environmental Impact
-
-
-
-Carbon emissions can be estimated using the [Machine Learning Impact calculator](https://mlco2.github.io/impact#compute) presented in [Lacoste et al. (2019)](https://arxiv.org/abs/1910.09700).
-
-- **Hardware Type:** {{ hardware | default("[More Information Needed]", true)}}
-- **Hours used:** {{ hours_used | default("[More Information Needed]", true)}}
-- **Cloud Provider:** {{ cloud_provider | default("[More Information Needed]", true)}}
-- **Compute Region:** {{ cloud_region | default("[More Information Needed]", true)}}
-- **Carbon Emitted:** {{ co2_emitted | default("[More Information Needed]", true)}}
-
-## Technical Specifications [optional]
-
-### Model Architecture and Objective
-
-{{ model_specs | default("[More Information Needed]", true)}}
-
-### Compute Infrastructure
-
-{{ compute_infrastructure | default("[More Information Needed]", true)}}
-
-#### Hardware
-
-{{ hardware | default("[More Information Needed]", true)}}
-
-#### Software
-
-{{ software | default("[More Information Needed]", true)}}
-
-## Citation [optional]
-
-
-
-**BibTeX:**
-
-{{ citation_bibtex | default("[More Information Needed]", true)}}
-
-**APA:**
-
-{{ citation_apa | default("[More Information Needed]", true)}}
-
-## Glossary [optional]
-
-
-
-{{ glossary | default("[More Information Needed]", true)}}
-
-## More Information [optional]
-
-{{ more_information | default("[More Information Needed]", true)}}
-
-## Model Card Authors [optional]
-
-{{ model_card_authors | default("[More Information Needed]", true)}}
-
-## Model Card Contact
-
-{{ model_card_contact | default("[More Information Needed]", true)}}
-
-
-
diff --git a/spaces/DeepDrivePL/PaddleSeg-Matting/matting/model/__init__.py b/spaces/DeepDrivePL/PaddleSeg-Matting/matting/model/__init__.py
deleted file mode 100644
index 9e906c1567ce12fe800b4d651f1a1ef9f9d0afe0..0000000000000000000000000000000000000000
--- a/spaces/DeepDrivePL/PaddleSeg-Matting/matting/model/__init__.py
+++ /dev/null
@@ -1,21 +0,0 @@
-# Copyright (c) 2021 PaddlePaddle Authors. All Rights Reserved.
-#
-# Licensed under the Apache License, Version 2.0 (the "License");
-# you may not use this file except in compliance with the License.
-# You may obtain a copy of the License at
-#
-# http://www.apache.org/licenses/LICENSE-2.0
-#
-# Unless required by applicable law or agreed to in writing, software
-# distributed under the License is distributed on an "AS IS" BASIS,
-# WITHOUT WARRANTIES OR CONDITIONS OF ANY KIND, either express or implied.
-# See the License for the specific language governing permissions and
-# limitations under the License.
-
-from .vgg import *
-from .resnet_vd import *
-from .mobilenet_v2 import *
-from .hrnet import *
-from .dim import DIM
-from .loss import MRSD
-from .modnet import MODNet
diff --git a/spaces/Detomo/aisatsu-api/main.py b/spaces/Detomo/aisatsu-api/main.py
deleted file mode 100644
index bcbaf24d321e1af38918c33dddca16554826511c..0000000000000000000000000000000000000000
--- a/spaces/Detomo/aisatsu-api/main.py
+++ /dev/null
@@ -1,202 +0,0 @@
-from ultralyticsplus import YOLO
-from typing import Optional, Union, Annotated
-
-from scipy.spatial import distance as dist
-import time
-from fastapi import FastAPI, File, UploadFile, Form
-from fastapi.responses import StreamingResponse
-from fastapi.middleware.gzip import GZipMiddleware
-from io import BytesIO
-from utils import tts, stt, read_image_file, pil_to_base64, base64_to_pil, get_hist, ffmpeg_read
-import zipfile
-import soundfile as sf
-import openai
-import os
-import random
-
-# Config for camera picture
-model = YOLO('ultralyticsplus/yolov8s')
-# model = YOLO('kadirnar/yolov8n-v8.0')
-CLASS = model.model.names
-ZIP = False
-# bot_voice_time = "おはようございます"
-bot_voice_time = "こんにちは"
-default_bot_voice_list = [f"{bot_voice_time}、アイティコンサルティングとシステム開発を支援します。よろしくお願いします。",
- f"{bot_voice_time}、デトモです。システム開発全般を支援します。",
- f"{bot_voice_time}、デトモです。オフショア開発全般を支援します。",
- f"{bot_voice_time}、私はアイサロボです。システム開発全般を支援します。",
- f"{bot_voice_time}、エッジコンピューティングソリューションを提供します。"]
-area_threshold = 0
-diff_value_threshold = 0
-
-# Config for human input
-prompt_template = "私はあなたに、Detomo社が作ったロボットのように振る舞ってほしいです。デトモは高度なデジタル化社会を支えます。"\
- "ビジネスの課題解決策を提案するコンサ ルティング・サービスと、課題解決を実現す るシステムの開発サービス、また、企業内 の情報システム部門の業務の代行サー ビスにも対応しています。"\
- "デトモはITコンサルティング・システム開発を得意とし、お客様の課題解決をお手伝いいたします。"\
- "あなたの名前はアイサロボです。"\
- "あなたのミッションは、子供たちが他の子供たちに挨拶する自信を持ち、幸せになることを助けることです。"\
- "質問には簡単な方法でしか答えないようにし、明示的に要求されない限り、追加情報を提供しないでください。"
-system_prompt = [{"role": "system", "content": prompt_template}]
-openai.api_key = os.environ["OPENAI_API_KEY"]
-
-app = FastAPI()
-app.add_middleware(GZipMiddleware, minimum_size=1000)
-
-
-@app.get("/")
-def read_root():
- return {"Message": "Application startup complete"}
-
-
-@app.get("/client_settings/")
-def client_settings_api():
- return {"camera_picture_period": 5}
-
-
-@app.post("/camera_picture/")
-async def camera_picture_api(
- file: UploadFile = File(...),
- last_seen: Optional[Union[str, UploadFile]] = Form(None),
- return_voice: Annotated[bool, Form()] = True,
-):
- # parameters
- total_time = time.time()
- most_close = 0
- out_img = None
- diff_value = 0.5
- default_bot_voice = random.choice(default_bot_voice_list)
-
- # read image and predict
- image = read_image_file(await file.read())
- results = model.predict(image, show=False)[0]
- masks, boxes = results.masks, results.boxes
- area_image = image.width * image.height
-
- # select and crop face image
- if boxes is not None:
- for xyxy, conf, cls in zip(boxes.xyxy, boxes.conf, boxes.cls):
- if int(cls) != 0:
- continue
- box = xyxy.tolist()
- area_rate = (box[2] - box[0]) * (box[3] - box[1]) / area_image
- if area_rate >= most_close:
- out_img = image.crop(tuple(box)).resize((64, 64))
- most_close = area_rate
-
- # check detect people or not
- if out_img is None:
- return {
- "status": "No face detected",
- "text": None,
- "voice": None,
- "image": None
- }
- else:
- if ZIP:
- image_bot_path = pil_to_base64(out_img, encode=False)
- else:
- image_bot_path = pil_to_base64(out_img, encode=True)
-
- # check with previous image if have
- if last_seen is not None:
- if type(last_seen) == str:
- last_seen = base64_to_pil(last_seen)
- else:
- last_seen = read_image_file(await last_seen.read())
- diff_value = dist.euclidean(get_hist(out_img), get_hist(last_seen))
- print(f"Distance: {most_close}. Different value: {diff_value}")
-
- # return results
- if most_close >= area_threshold and diff_value >= diff_value_threshold:
- if ZIP:
- voice_bot_path = tts(default_bot_voice, language="ja", encode=False)
- io = BytesIO()
- zip_filename = "final_archive.zip"
- with zipfile.ZipFile(io, mode='w', compression=zipfile.ZIP_DEFLATED) as zf:
- for file_path in [voice_bot_path, image_bot_path]:
- zf.write(file_path)
- zf.close()
- print("Total time", time.time() - total_time)
- return StreamingResponse(
- iter([io.getvalue()]),
- media_type="application/x-zip-compressed",
- headers={"Content-Disposition": f"attachment;filename=%s" % zip_filename}
- )
- else:
- if return_voice:
- print("Total time", time.time() - total_time)
- return {
- "status": "New people",
- "text": default_bot_voice,
- "voice": tts(default_bot_voice, language="ja", encode=True),
- "image": image_bot_path
- }
- else:
- print("Total time", time.time() - total_time)
- return {
- "status": "New people",
- "text": default_bot_voice,
- "voice": None,
- "image": image_bot_path
- }
- elif most_close < area_threshold:
- print("Total time", time.time() - total_time)
- return {
- "status": "People far from camera",
- "text": None,
- "voice": None,
- "image": image_bot_path,
- }
- else:
- print("Total time", time.time() - total_time)
- return {
- "status": "Old people",
- "text": None,
- "voice": None,
- "image": image_bot_path,
- }
-
-
-@app.post("/human_input/")
-async def human_input_api(
- voice_input: bytes = File(None),
- text_input: str = Form(None),
- temperature: Annotated[float, Form()] = 0.7,
- max_tokens: Annotated[int, Form()] = 1000,
- return_voice: Annotated[bool, Form()] = False,
-):
- if text_input:
- text = text_input
- elif text_input is None and voice_input is not None:
- upload_audio = ffmpeg_read(voice_input, sampling_rate=24000)
- sf.write('temp.wav', upload_audio, 24000, subtype='PCM_16')
- text = stt('temp.wav')
- print(text)
- else:
- if return_voice:
- return {
- "human_text": None,
- "robot_text": None,
- "robot_voice": None
- }
- else:
- return {
- "human_text": None,
- "robot_text": None,
- }
- prompt_msg = {"role": "user", "content": text}
- messages = system_prompt + [prompt_msg]
- completion = openai.ChatCompletion.create(model="gpt-3.5-turbo", messages=messages, temperature=temperature,
- max_tokens=max_tokens)
- print(completion['usage']['total_tokens'])
- if return_voice:
- return {
- "human_text": text,
- "robot_text": completion.choices[0].message.content,
- "robot_voice": tts(completion.choices[0].message.content, language="ja", encode=True)
- }
- else:
- return {
- "human_text": text,
- "robot_text": completion.choices[0].message.content,
- }
\ No newline at end of file
diff --git a/spaces/EleutherAI/VQGAN_CLIP/CLIP/README.md b/spaces/EleutherAI/VQGAN_CLIP/CLIP/README.md
deleted file mode 100644
index 5d2d20cd9e1cafcdf8bd8dfd83a0a9c47a884a39..0000000000000000000000000000000000000000
--- a/spaces/EleutherAI/VQGAN_CLIP/CLIP/README.md
+++ /dev/null
@@ -1,193 +0,0 @@
-# CLIP
-
-[[Blog]](https://openai.com/blog/clip/) [[Paper]](https://arxiv.org/abs/2103.00020) [[Model Card]](model-card.md) [[Colab]](https://colab.research.google.com/github/openai/clip/blob/master/notebooks/Interacting_with_CLIP.ipynb)
-
-CLIP (Contrastive Language-Image Pre-Training) is a neural network trained on a variety of (image, text) pairs. It can be instructed in natural language to predict the most relevant text snippet, given an image, without directly optimizing for the task, similarly to the zero-shot capabilities of GPT-2 and 3. We found CLIP matches the performance of the original ResNet50 on ImageNet “zero-shot” without using any of the original 1.28M labeled examples, overcoming several major challenges in computer vision.
-
-
-
-## Approach
-
-
-
-
-
-## Usage
-
-First, [install PyTorch 1.7.1](https://pytorch.org/get-started/locally/) and torchvision, as well as small additional dependencies, and then install this repo as a Python package. On a CUDA GPU machine, the following will do the trick:
-
-```bash
-$ conda install --yes -c pytorch pytorch=1.7.1 torchvision cudatoolkit=11.0
-$ pip install ftfy regex tqdm
-$ pip install git+https://github.com/openai/CLIP.git
-```
-
-Replace `cudatoolkit=11.0` above with the appropriate CUDA version on your machine or `cpuonly` when installing on a machine without a GPU.
-
-```python
-import torch
-import clip
-from PIL import Image
-
-device = "cuda" if torch.cuda.is_available() else "cpu"
-model, preprocess = clip.load("ViT-B/32", device=device)
-
-image = preprocess(Image.open("CLIP.png")).unsqueeze(0).to(device)
-text = clip.tokenize(["a diagram", "a dog", "a cat"]).to(device)
-
-with torch.no_grad():
- image_features = model.encode_image(image)
- text_features = model.encode_text(text)
-
- logits_per_image, logits_per_text = model(image, text)
- probs = logits_per_image.softmax(dim=-1).cpu().numpy()
-
-print("Label probs:", probs) # prints: [[0.9927937 0.00421068 0.00299572]]
-```
-
-
-## API
-
-The CLIP module `clip` provides the following methods:
-
-#### `clip.available_models()`
-
-Returns the names of the available CLIP models.
-
-#### `clip.load(name, device=..., jit=False)`
-
-Returns the model and the TorchVision transform needed by the model, specified by the model name returned by `clip.available_models()`. It will download the model as necessary. The `name` argument can also be a path to a local checkpoint.
-
-The device to run the model can be optionally specified, and the default is to use the first CUDA device if there is any, otherwise the CPU. When `jit` is `False`, a non-JIT version of the model will be loaded.
-
-#### `clip.tokenize(text: Union[str, List[str]], context_length=77)`
-
-Returns a LongTensor containing tokenized sequences of given text input(s). This can be used as the input to the model
-
----
-
-The model returned by `clip.load()` supports the following methods:
-
-#### `model.encode_image(image: Tensor)`
-
-Given a batch of images, returns the image features encoded by the vision portion of the CLIP model.
-
-#### `model.encode_text(text: Tensor)`
-
-Given a batch of text tokens, returns the text features encoded by the language portion of the CLIP model.
-
-#### `model(image: Tensor, text: Tensor)`
-
-Given a batch of images and a batch of text tokens, returns two Tensors, containing the logit scores corresponding to each image and text input. The values are cosine similarities between the corresponding image and text features, times 100.
-
-
-
-## More Examples
-
-### Zero-Shot Prediction
-
-The code below performs zero-shot prediction using CLIP, as shown in Appendix B in the paper. This example takes an image from the [CIFAR-100 dataset](https://www.cs.toronto.edu/~kriz/cifar.html), and predicts the most likely labels among the 100 textual labels from the dataset.
-
-```python
-import os
-import clip
-import torch
-from torchvision.datasets import CIFAR100
-
-# Load the model
-device = "cuda" if torch.cuda.is_available() else "cpu"
-model, preprocess = clip.load('ViT-B/32', device)
-
-# Download the dataset
-cifar100 = CIFAR100(root=os.path.expanduser("~/.cache"), download=True, train=False)
-
-# Prepare the inputs
-image, class_id = cifar100[3637]
-image_input = preprocess(image).unsqueeze(0).to(device)
-text_inputs = torch.cat([clip.tokenize(f"a photo of a {c}") for c in cifar100.classes]).to(device)
-
-# Calculate features
-with torch.no_grad():
- image_features = model.encode_image(image_input)
- text_features = model.encode_text(text_inputs)
-
-# Pick the top 5 most similar labels for the image
-image_features /= image_features.norm(dim=-1, keepdim=True)
-text_features /= text_features.norm(dim=-1, keepdim=True)
-similarity = (100.0 * image_features @ text_features.T).softmax(dim=-1)
-values, indices = similarity[0].topk(5)
-
-# Print the result
-print("\nTop predictions:\n")
-for value, index in zip(values, indices):
- print(f"{cifar100.classes[index]:>16s}: {100 * value.item():.2f}%")
-```
-
-The output will look like the following (the exact numbers may be slightly different depending on the compute device):
-
-```
-Top predictions:
-
- snake: 65.31%
- turtle: 12.29%
- sweet_pepper: 3.83%
- lizard: 1.88%
- crocodile: 1.75%
-```
-
-Note that this example uses the `encode_image()` and `encode_text()` methods that return the encoded features of given inputs.
-
-
-### Linear-probe evaluation
-
-The example below uses [scikit-learn](https://scikit-learn.org/) to perform logistic regression on image features.
-
-```python
-import os
-import clip
-import torch
-
-import numpy as np
-from sklearn.linear_model import LogisticRegression
-from torch.utils.data import DataLoader
-from torchvision.datasets import CIFAR100
-from tqdm import tqdm
-
-# Load the model
-device = "cuda" if torch.cuda.is_available() else "cpu"
-model, preprocess = clip.load('ViT-B/32', device)
-
-# Load the dataset
-root = os.path.expanduser("~/.cache")
-train = CIFAR100(root, download=True, train=True, transform=preprocess)
-test = CIFAR100(root, download=True, train=False, transform=preprocess)
-
-
-def get_features(dataset):
- all_features = []
- all_labels = []
-
- with torch.no_grad():
- for images, labels in tqdm(DataLoader(dataset, batch_size=100)):
- features = model.encode_image(images.to(device))
-
- all_features.append(features)
- all_labels.append(labels)
-
- return torch.cat(all_features).cpu().numpy(), torch.cat(all_labels).cpu().numpy()
-
-# Calculate the image features
-train_features, train_labels = get_features(train)
-test_features, test_labels = get_features(test)
-
-# Perform logistic regression
-classifier = LogisticRegression(random_state=0, C=0.316, max_iter=1000, verbose=1)
-classifier.fit(train_features, train_labels)
-
-# Evaluate using the logistic regression classifier
-predictions = classifier.predict(test_features)
-accuracy = np.mean((test_labels == predictions).astype(np.float)) * 100.
-print(f"Accuracy = {accuracy:.3f}")
-```
-
-Note that the `C` value should be determined via a hyperparameter sweep using a validation split.
diff --git a/spaces/Endre/SemanticSearch-HU/src/data/dbpedia_dump_wiki_text.py b/spaces/Endre/SemanticSearch-HU/src/data/dbpedia_dump_wiki_text.py
deleted file mode 100644
index 82ecb7c896bab35920c240ee9d0267e5342f94ca..0000000000000000000000000000000000000000
--- a/spaces/Endre/SemanticSearch-HU/src/data/dbpedia_dump_wiki_text.py
+++ /dev/null
@@ -1,18 +0,0 @@
-from rdflib import Graph
-
-# Downloaded from https://databus.dbpedia.org/dbpedia/text/short-abstracts
-raw_data_path = 'data/raw/short-abstracts_lang=hu.ttl'
-preprocessed_data_path = 'data/preprocessed/shortened_abstracts_hu_2021_09_01.txt'
-
-g = Graph()
-g.parse(raw_data_path, format='turtle')
-
-i = 0
-objects = []
-with open(preprocessed_data_path, 'w') as f:
- print(len(g))
- for subject, predicate, object in g:
- objects.append(object.replace(' +/-','').replace('\n',' '))
- objects.append('\n')
- i += 1
- f.writelines(objects)
\ No newline at end of file
diff --git a/spaces/Enterprisium/Easy_GUI/i18n.py b/spaces/Enterprisium/Easy_GUI/i18n.py
deleted file mode 100644
index 37f310fadd0b48b2f364877158fb2105d645fc03..0000000000000000000000000000000000000000
--- a/spaces/Enterprisium/Easy_GUI/i18n.py
+++ /dev/null
@@ -1,28 +0,0 @@
-import locale
-import json
-import os
-
-
-def load_language_list(language):
- with open(f"./i18n/{language}.json", "r", encoding="utf-8") as f:
- language_list = json.load(f)
- return language_list
-
-
-class I18nAuto:
- def __init__(self, language=None):
- if language in ["Auto", None]:
- language = locale.getdefaultlocale()[
- 0
- ] # getlocale can't identify the system's language ((None, None))
- if not os.path.exists(f"./i18n/{language}.json"):
- language = "en_US"
- self.language = language
- # print("Use Language:", language)
- self.language_map = load_language_list(language)
-
- def __call__(self, key):
- return self.language_map.get(key, key)
-
- def print(self):
- print("Use Language:", self.language)
diff --git a/spaces/EsoCode/text-generation-webui/extensions/character_bias/script.py b/spaces/EsoCode/text-generation-webui/extensions/character_bias/script.py
deleted file mode 100644
index ff12f3afdc28be4ead12ffab90bd9fbd783514a2..0000000000000000000000000000000000000000
--- a/spaces/EsoCode/text-generation-webui/extensions/character_bias/script.py
+++ /dev/null
@@ -1,83 +0,0 @@
-import os
-
-import gradio as gr
-
-# get the current directory of the script
-current_dir = os.path.dirname(os.path.abspath(__file__))
-
-# check if the bias_options.txt file exists, if not, create it
-bias_file = os.path.join(current_dir, "bias_options.txt")
-if not os.path.isfile(bias_file):
- with open(bias_file, "w") as f:
- f.write("*I am so happy*\n*I am so sad*\n*I am so excited*\n*I am so bored*\n*I am so angry*")
-
-# read bias options from the text file
-with open(bias_file, "r") as f:
- bias_options = [line.strip() for line in f.readlines()]
-
-params = {
- "activate": True,
- "bias string": " *I am so happy*",
- "use custom string": False,
-}
-
-
-def input_modifier(string):
- """
- This function is applied to your text inputs before
- they are fed into the model.
- """
- return string
-
-
-def output_modifier(string):
- """
- This function is applied to the model outputs.
- """
- return string
-
-
-def bot_prefix_modifier(string):
- """
- This function is only applied in chat mode. It modifies
- the prefix text for the Bot and can be used to bias its
- behavior.
- """
- if params['activate']:
- if params['use custom string']:
- return f'{string} {params["custom string"].strip()} '
- else:
- return f'{string} {params["bias string"].strip()} '
- else:
- return string
-
-
-def ui():
- # Gradio elements
- activate = gr.Checkbox(value=params['activate'], label='Activate character bias')
- dropdown_string = gr.Dropdown(choices=bias_options, value=params["bias string"], label='Character bias', info='To edit the options in this dropdown edit the "bias_options.txt" file')
- use_custom_string = gr.Checkbox(value=False, label='Use custom bias textbox instead of dropdown')
- custom_string = gr.Textbox(value="", placeholder="Enter custom bias string", label="Custom Character Bias", info='To use this textbox activate the checkbox above')
-
- # Event functions to update the parameters in the backend
- def update_bias_string(x):
- if x:
- params.update({"bias string": x})
- else:
- params.update({"bias string": dropdown_string.get()})
- return x
-
- def update_custom_string(x):
- params.update({"custom string": x})
-
- dropdown_string.change(update_bias_string, dropdown_string, None)
- custom_string.change(update_custom_string, custom_string, None)
- activate.change(lambda x: params.update({"activate": x}), activate, None)
- use_custom_string.change(lambda x: params.update({"use custom string": x}), use_custom_string, None)
-
- # Group elements together depending on the selected option
- def bias_string_group():
- if use_custom_string.value:
- return gr.Group([use_custom_string, custom_string])
- else:
- return dropdown_string
diff --git a/spaces/EuroPython2022/mmocr-demo/configs/_base_/schedules/schedule_adam_600e.py b/spaces/EuroPython2022/mmocr-demo/configs/_base_/schedules/schedule_adam_600e.py
deleted file mode 100644
index a77dc52004ba597b4ba7f2df13a96e123c4029ab..0000000000000000000000000000000000000000
--- a/spaces/EuroPython2022/mmocr-demo/configs/_base_/schedules/schedule_adam_600e.py
+++ /dev/null
@@ -1,8 +0,0 @@
-# optimizer
-optimizer = dict(type='Adam', lr=1e-3)
-optimizer_config = dict(grad_clip=None)
-# learning policy
-lr_config = dict(policy='poly', power=0.9)
-# running settings
-runner = dict(type='EpochBasedRunner', max_epochs=600)
-checkpoint_config = dict(interval=100)
diff --git a/spaces/EuroPython2022/mmocr-demo/configs/textdet/dbnetpp/dbnetpp_r50dcnv2_fpnc_100k_iter_synthtext.py b/spaces/EuroPython2022/mmocr-demo/configs/textdet/dbnetpp/dbnetpp_r50dcnv2_fpnc_100k_iter_synthtext.py
deleted file mode 100644
index 5f3835ea998e5195b471671a8685c0032733b0a2..0000000000000000000000000000000000000000
--- a/spaces/EuroPython2022/mmocr-demo/configs/textdet/dbnetpp/dbnetpp_r50dcnv2_fpnc_100k_iter_synthtext.py
+++ /dev/null
@@ -1,62 +0,0 @@
-_base_ = [
- '../../_base_/default_runtime.py',
- '../../_base_/schedules/schedule_sgd_100k_iters.py',
- '../../_base_/det_models/dbnetpp_r50dcnv2_fpnc.py',
- '../../_base_/det_datasets/synthtext.py',
- '../../_base_/det_pipelines/dbnet_pipeline.py'
-]
-
-train_list = {{_base_.train_list}}
-test_list = {{_base_.test_list}}
-
-img_norm_cfg_r50dcnv2 = dict(
- mean=[122.67891434, 116.66876762, 104.00698793],
- std=[58.395, 57.12, 57.375],
- to_rgb=True)
-train_pipeline_r50dcnv2 = [
- dict(type='LoadImageFromFile', color_type='color_ignore_orientation'),
- dict(
- type='LoadTextAnnotations',
- with_bbox=True,
- with_mask=True,
- poly2mask=False),
- dict(type='ColorJitter', brightness=32.0 / 255, saturation=0.5),
- dict(type='Normalize', **img_norm_cfg_r50dcnv2),
- dict(
- type='ImgAug',
- args=[['Fliplr', 0.5],
- dict(cls='Affine', rotate=[-10, 10]), ['Resize', [0.5, 3.0]]],
- clip_invalid_ploys=False),
- dict(type='EastRandomCrop', target_size=(640, 640)),
- dict(type='DBNetTargets', shrink_ratio=0.4),
- dict(type='Pad', size_divisor=32),
- dict(
- type='CustomFormatBundle',
- keys=['gt_shrink', 'gt_shrink_mask', 'gt_thr', 'gt_thr_mask'],
- visualize=dict(flag=False, boundary_key='gt_shrink')),
- dict(
- type='Collect',
- keys=['img', 'gt_shrink', 'gt_shrink_mask', 'gt_thr', 'gt_thr_mask'])
-]
-
-test_pipeline_4068_1024 = {{_base_.test_pipeline_4068_1024}}
-
-data = dict(
- samples_per_gpu=16,
- workers_per_gpu=8,
- val_dataloader=dict(samples_per_gpu=1),
- test_dataloader=dict(samples_per_gpu=1),
- train=dict(
- type='UniformConcatDataset',
- datasets=train_list,
- pipeline=train_pipeline_r50dcnv2),
- val=dict(
- type='UniformConcatDataset',
- datasets=test_list,
- pipeline=test_pipeline_4068_1024),
- test=dict(
- type='UniformConcatDataset',
- datasets=test_list,
- pipeline=test_pipeline_4068_1024))
-
-evaluation = dict(interval=200000, metric='hmean-iou') # do not evaluate
diff --git a/spaces/Ferion/image-matting-app/ppmatting/ml/__init__.py b/spaces/Ferion/image-matting-app/ppmatting/ml/__init__.py
deleted file mode 100644
index 612dff101f358f74db3eca601f0b9573ca6d93cb..0000000000000000000000000000000000000000
--- a/spaces/Ferion/image-matting-app/ppmatting/ml/__init__.py
+++ /dev/null
@@ -1 +0,0 @@
-from .methods import CloseFormMatting, KNNMatting, LearningBasedMatting, FastMatting, RandomWalksMatting
diff --git a/spaces/FridaZuley/RVC_HFKawaii/infer/lib/rmvpe.py b/spaces/FridaZuley/RVC_HFKawaii/infer/lib/rmvpe.py
deleted file mode 100644
index 2a387ebe73c7e1dd8bb7ccad1ea9e0ea89848ece..0000000000000000000000000000000000000000
--- a/spaces/FridaZuley/RVC_HFKawaii/infer/lib/rmvpe.py
+++ /dev/null
@@ -1,717 +0,0 @@
-import pdb, os
-
-import numpy as np
-import torch
-try:
- #Fix "Torch not compiled with CUDA enabled"
- import intel_extension_for_pytorch as ipex # pylint: disable=import-error, unused-import
- if torch.xpu.is_available():
- from infer.modules.ipex import ipex_init
- ipex_init()
-except Exception:
- pass
-import torch.nn as nn
-import torch.nn.functional as F
-from librosa.util import normalize, pad_center, tiny
-from scipy.signal import get_window
-
-import logging
-
-logger = logging.getLogger(__name__)
-
-
-###stft codes from https://github.com/pseeth/torch-stft/blob/master/torch_stft/util.py
-def window_sumsquare(
- window,
- n_frames,
- hop_length=200,
- win_length=800,
- n_fft=800,
- dtype=np.float32,
- norm=None,
-):
- """
- # from librosa 0.6
- Compute the sum-square envelope of a window function at a given hop length.
- This is used to estimate modulation effects induced by windowing
- observations in short-time fourier transforms.
- Parameters
- ----------
- window : string, tuple, number, callable, or list-like
- Window specification, as in `get_window`
- n_frames : int > 0
- The number of analysis frames
- hop_length : int > 0
- The number of samples to advance between frames
- win_length : [optional]
- The length of the window function. By default, this matches `n_fft`.
- n_fft : int > 0
- The length of each analysis frame.
- dtype : np.dtype
- The data type of the output
- Returns
- -------
- wss : np.ndarray, shape=`(n_fft + hop_length * (n_frames - 1))`
- The sum-squared envelope of the window function
- """
- if win_length is None:
- win_length = n_fft
-
- n = n_fft + hop_length * (n_frames - 1)
- x = np.zeros(n, dtype=dtype)
-
- # Compute the squared window at the desired length
- win_sq = get_window(window, win_length, fftbins=True)
- win_sq = normalize(win_sq, norm=norm) ** 2
- win_sq = pad_center(win_sq, n_fft)
-
- # Fill the envelope
- for i in range(n_frames):
- sample = i * hop_length
- x[sample : min(n, sample + n_fft)] += win_sq[: max(0, min(n_fft, n - sample))]
- return x
-
-
-class STFT(torch.nn.Module):
- def __init__(
- self, filter_length=1024, hop_length=512, win_length=None, window="hann"
- ):
- """
- This module implements an STFT using 1D convolution and 1D transpose convolutions.
- This is a bit tricky so there are some cases that probably won't work as working
- out the same sizes before and after in all overlap add setups is tough. Right now,
- this code should work with hop lengths that are half the filter length (50% overlap
- between frames).
-
- Keyword Arguments:
- filter_length {int} -- Length of filters used (default: {1024})
- hop_length {int} -- Hop length of STFT (restrict to 50% overlap between frames) (default: {512})
- win_length {[type]} -- Length of the window function applied to each frame (if not specified, it
- equals the filter length). (default: {None})
- window {str} -- Type of window to use (options are bartlett, hann, hamming, blackman, blackmanharris)
- (default: {'hann'})
- """
- super(STFT, self).__init__()
- self.filter_length = filter_length
- self.hop_length = hop_length
- self.win_length = win_length if win_length else filter_length
- self.window = window
- self.forward_transform = None
- self.pad_amount = int(self.filter_length / 2)
- scale = self.filter_length / self.hop_length
- fourier_basis = np.fft.fft(np.eye(self.filter_length))
-
- cutoff = int((self.filter_length / 2 + 1))
- fourier_basis = np.vstack(
- [np.real(fourier_basis[:cutoff, :]), np.imag(fourier_basis[:cutoff, :])]
- )
- forward_basis = torch.FloatTensor(fourier_basis[:, None, :])
- inverse_basis = torch.FloatTensor(
- np.linalg.pinv(scale * fourier_basis).T[:, None, :]
- )
-
- assert filter_length >= self.win_length
- # get window and zero center pad it to filter_length
- fft_window = get_window(window, self.win_length, fftbins=True)
- fft_window = pad_center(fft_window, size=filter_length)
- fft_window = torch.from_numpy(fft_window).float()
-
- # window the bases
- forward_basis *= fft_window
- inverse_basis *= fft_window
-
- self.register_buffer("forward_basis", forward_basis.float())
- self.register_buffer("inverse_basis", inverse_basis.float())
-
- def transform(self, input_data):
- """Take input data (audio) to STFT domain.
-
- Arguments:
- input_data {tensor} -- Tensor of floats, with shape (num_batch, num_samples)
-
- Returns:
- magnitude {tensor} -- Magnitude of STFT with shape (num_batch,
- num_frequencies, num_frames)
- phase {tensor} -- Phase of STFT with shape (num_batch,
- num_frequencies, num_frames)
- """
- num_batches = input_data.shape[0]
- num_samples = input_data.shape[-1]
-
- self.num_samples = num_samples
-
- # similar to librosa, reflect-pad the input
- input_data = input_data.view(num_batches, 1, num_samples)
- # print(1234,input_data.shape)
- input_data = F.pad(
- input_data.unsqueeze(1),
- (self.pad_amount, self.pad_amount, 0, 0, 0, 0),
- mode="reflect",
- ).squeeze(1)
- # print(2333,input_data.shape,self.forward_basis.shape,self.hop_length)
- # pdb.set_trace()
- forward_transform = F.conv1d(
- input_data, self.forward_basis, stride=self.hop_length, padding=0
- )
-
- cutoff = int((self.filter_length / 2) + 1)
- real_part = forward_transform[:, :cutoff, :]
- imag_part = forward_transform[:, cutoff:, :]
-
- magnitude = torch.sqrt(real_part**2 + imag_part**2)
- # phase = torch.atan2(imag_part.data, real_part.data)
-
- return magnitude # , phase
-
- def inverse(self, magnitude, phase):
- """Call the inverse STFT (iSTFT), given magnitude and phase tensors produced
- by the ```transform``` function.
-
- Arguments:
- magnitude {tensor} -- Magnitude of STFT with shape (num_batch,
- num_frequencies, num_frames)
- phase {tensor} -- Phase of STFT with shape (num_batch,
- num_frequencies, num_frames)
-
- Returns:
- inverse_transform {tensor} -- Reconstructed audio given magnitude and phase. Of
- shape (num_batch, num_samples)
- """
- recombine_magnitude_phase = torch.cat(
- [magnitude * torch.cos(phase), magnitude * torch.sin(phase)], dim=1
- )
-
- inverse_transform = F.conv_transpose1d(
- recombine_magnitude_phase,
- self.inverse_basis,
- stride=self.hop_length,
- padding=0,
- )
-
- if self.window is not None:
- window_sum = window_sumsquare(
- self.window,
- magnitude.size(-1),
- hop_length=self.hop_length,
- win_length=self.win_length,
- n_fft=self.filter_length,
- dtype=np.float32,
- )
- # remove modulation effects
- approx_nonzero_indices = torch.from_numpy(
- np.where(window_sum > tiny(window_sum))[0]
- )
- window_sum = torch.from_numpy(window_sum).to(inverse_transform.device)
- inverse_transform[:, :, approx_nonzero_indices] /= window_sum[
- approx_nonzero_indices
- ]
-
- # scale by hop ratio
- inverse_transform *= float(self.filter_length) / self.hop_length
-
- inverse_transform = inverse_transform[..., self.pad_amount :]
- inverse_transform = inverse_transform[..., : self.num_samples]
- inverse_transform = inverse_transform.squeeze(1)
-
- return inverse_transform
-
- def forward(self, input_data):
- """Take input data (audio) to STFT domain and then back to audio.
-
- Arguments:
- input_data {tensor} -- Tensor of floats, with shape (num_batch, num_samples)
-
- Returns:
- reconstruction {tensor} -- Reconstructed audio given magnitude and phase. Of
- shape (num_batch, num_samples)
- """
- self.magnitude, self.phase = self.transform(input_data)
- reconstruction = self.inverse(self.magnitude, self.phase)
- return reconstruction
-
-
-from time import time as ttime
-
-
-class BiGRU(nn.Module):
- def __init__(self, input_features, hidden_features, num_layers):
- super(BiGRU, self).__init__()
- self.gru = nn.GRU(
- input_features,
- hidden_features,
- num_layers=num_layers,
- batch_first=True,
- bidirectional=True,
- )
-
- def forward(self, x):
- return self.gru(x)[0]
-
-
-class ConvBlockRes(nn.Module):
- def __init__(self, in_channels, out_channels, momentum=0.01):
- super(ConvBlockRes, self).__init__()
- self.conv = nn.Sequential(
- nn.Conv2d(
- in_channels=in_channels,
- out_channels=out_channels,
- kernel_size=(3, 3),
- stride=(1, 1),
- padding=(1, 1),
- bias=False,
- ),
- nn.BatchNorm2d(out_channels, momentum=momentum),
- nn.ReLU(),
- nn.Conv2d(
- in_channels=out_channels,
- out_channels=out_channels,
- kernel_size=(3, 3),
- stride=(1, 1),
- padding=(1, 1),
- bias=False,
- ),
- nn.BatchNorm2d(out_channels, momentum=momentum),
- nn.ReLU(),
- )
- if in_channels != out_channels:
- self.shortcut = nn.Conv2d(in_channels, out_channels, (1, 1))
- self.is_shortcut = True
- else:
- self.is_shortcut = False
-
- def forward(self, x):
- if self.is_shortcut:
- return self.conv(x) + self.shortcut(x)
- else:
- return self.conv(x) + x
-
-
-class Encoder(nn.Module):
- def __init__(
- self,
- in_channels,
- in_size,
- n_encoders,
- kernel_size,
- n_blocks,
- out_channels=16,
- momentum=0.01,
- ):
- super(Encoder, self).__init__()
- self.n_encoders = n_encoders
- self.bn = nn.BatchNorm2d(in_channels, momentum=momentum)
- self.layers = nn.ModuleList()
- self.latent_channels = []
- for i in range(self.n_encoders):
- self.layers.append(
- ResEncoderBlock(
- in_channels, out_channels, kernel_size, n_blocks, momentum=momentum
- )
- )
- self.latent_channels.append([out_channels, in_size])
- in_channels = out_channels
- out_channels *= 2
- in_size //= 2
- self.out_size = in_size
- self.out_channel = out_channels
-
- def forward(self, x):
- concat_tensors = []
- x = self.bn(x)
- for i in range(self.n_encoders):
- _, x = self.layers[i](x)
- concat_tensors.append(_)
- return x, concat_tensors
-
-
-class ResEncoderBlock(nn.Module):
- def __init__(
- self, in_channels, out_channels, kernel_size, n_blocks=1, momentum=0.01
- ):
- super(ResEncoderBlock, self).__init__()
- self.n_blocks = n_blocks
- self.conv = nn.ModuleList()
- self.conv.append(ConvBlockRes(in_channels, out_channels, momentum))
- for i in range(n_blocks - 1):
- self.conv.append(ConvBlockRes(out_channels, out_channels, momentum))
- self.kernel_size = kernel_size
- if self.kernel_size is not None:
- self.pool = nn.AvgPool2d(kernel_size=kernel_size)
-
- def forward(self, x):
- for i in range(self.n_blocks):
- x = self.conv[i](x)
- if self.kernel_size is not None:
- return x, self.pool(x)
- else:
- return x
-
-
-class Intermediate(nn.Module): #
- def __init__(self, in_channels, out_channels, n_inters, n_blocks, momentum=0.01):
- super(Intermediate, self).__init__()
- self.n_inters = n_inters
- self.layers = nn.ModuleList()
- self.layers.append(
- ResEncoderBlock(in_channels, out_channels, None, n_blocks, momentum)
- )
- for i in range(self.n_inters - 1):
- self.layers.append(
- ResEncoderBlock(out_channels, out_channels, None, n_blocks, momentum)
- )
-
- def forward(self, x):
- for i in range(self.n_inters):
- x = self.layers[i](x)
- return x
-
-
-class ResDecoderBlock(nn.Module):
- def __init__(self, in_channels, out_channels, stride, n_blocks=1, momentum=0.01):
- super(ResDecoderBlock, self).__init__()
- out_padding = (0, 1) if stride == (1, 2) else (1, 1)
- self.n_blocks = n_blocks
- self.conv1 = nn.Sequential(
- nn.ConvTranspose2d(
- in_channels=in_channels,
- out_channels=out_channels,
- kernel_size=(3, 3),
- stride=stride,
- padding=(1, 1),
- output_padding=out_padding,
- bias=False,
- ),
- nn.BatchNorm2d(out_channels, momentum=momentum),
- nn.ReLU(),
- )
- self.conv2 = nn.ModuleList()
- self.conv2.append(ConvBlockRes(out_channels * 2, out_channels, momentum))
- for i in range(n_blocks - 1):
- self.conv2.append(ConvBlockRes(out_channels, out_channels, momentum))
-
- def forward(self, x, concat_tensor):
- x = self.conv1(x)
- x = torch.cat((x, concat_tensor), dim=1)
- for i in range(self.n_blocks):
- x = self.conv2[i](x)
- return x
-
-
-class Decoder(nn.Module):
- def __init__(self, in_channels, n_decoders, stride, n_blocks, momentum=0.01):
- super(Decoder, self).__init__()
- self.layers = nn.ModuleList()
- self.n_decoders = n_decoders
- for i in range(self.n_decoders):
- out_channels = in_channels // 2
- self.layers.append(
- ResDecoderBlock(in_channels, out_channels, stride, n_blocks, momentum)
- )
- in_channels = out_channels
-
- def forward(self, x, concat_tensors):
- for i in range(self.n_decoders):
- x = self.layers[i](x, concat_tensors[-1 - i])
- return x
-
-
-class DeepUnet(nn.Module):
- def __init__(
- self,
- kernel_size,
- n_blocks,
- en_de_layers=5,
- inter_layers=4,
- in_channels=1,
- en_out_channels=16,
- ):
- super(DeepUnet, self).__init__()
- self.encoder = Encoder(
- in_channels, 128, en_de_layers, kernel_size, n_blocks, en_out_channels
- )
- self.intermediate = Intermediate(
- self.encoder.out_channel // 2,
- self.encoder.out_channel,
- inter_layers,
- n_blocks,
- )
- self.decoder = Decoder(
- self.encoder.out_channel, en_de_layers, kernel_size, n_blocks
- )
-
- def forward(self, x):
- x, concat_tensors = self.encoder(x)
- x = self.intermediate(x)
- x = self.decoder(x, concat_tensors)
- return x
-
-
-class E2E(nn.Module):
- def __init__(
- self,
- n_blocks,
- n_gru,
- kernel_size,
- en_de_layers=5,
- inter_layers=4,
- in_channels=1,
- en_out_channels=16,
- ):
- super(E2E, self).__init__()
- self.unet = DeepUnet(
- kernel_size,
- n_blocks,
- en_de_layers,
- inter_layers,
- in_channels,
- en_out_channels,
- )
- self.cnn = nn.Conv2d(en_out_channels, 3, (3, 3), padding=(1, 1))
- if n_gru:
- self.fc = nn.Sequential(
- BiGRU(3 * 128, 256, n_gru),
- nn.Linear(512, 360),
- nn.Dropout(0.25),
- nn.Sigmoid(),
- )
- else:
- self.fc = nn.Sequential(
- nn.Linear(3 * nn.N_MELS, nn.N_CLASS), nn.Dropout(0.25), nn.Sigmoid()
- )
-
- def forward(self, mel):
- # print(mel.shape)
- mel = mel.transpose(-1, -2).unsqueeze(1)
- x = self.cnn(self.unet(mel)).transpose(1, 2).flatten(-2)
- x = self.fc(x)
- # print(x.shape)
- return x
-
-
-from librosa.filters import mel
-
-
-class MelSpectrogram(torch.nn.Module):
- def __init__(
- self,
- is_half,
- n_mel_channels,
- sampling_rate,
- win_length,
- hop_length,
- n_fft=None,
- mel_fmin=0,
- mel_fmax=None,
- clamp=1e-5,
- ):
- super().__init__()
- n_fft = win_length if n_fft is None else n_fft
- self.hann_window = {}
- mel_basis = mel(
- sr=sampling_rate,
- n_fft=n_fft,
- n_mels=n_mel_channels,
- fmin=mel_fmin,
- fmax=mel_fmax,
- htk=True,
- )
- mel_basis = torch.from_numpy(mel_basis).float()
- self.register_buffer("mel_basis", mel_basis)
- self.n_fft = win_length if n_fft is None else n_fft
- self.hop_length = hop_length
- self.win_length = win_length
- self.sampling_rate = sampling_rate
- self.n_mel_channels = n_mel_channels
- self.clamp = clamp
- self.is_half = is_half
-
- def forward(self, audio, keyshift=0, speed=1, center=True):
- factor = 2 ** (keyshift / 12)
- n_fft_new = int(np.round(self.n_fft * factor))
- win_length_new = int(np.round(self.win_length * factor))
- hop_length_new = int(np.round(self.hop_length * speed))
- keyshift_key = str(keyshift) + "_" + str(audio.device)
- if keyshift_key not in self.hann_window:
- self.hann_window[keyshift_key] = torch.hann_window(win_length_new).to(
- # "cpu"if(audio.device.type=="privateuseone") else audio.device
- audio.device
- )
- # fft = torch.stft(#doesn't support pytorch_dml
- # # audio.cpu() if(audio.device.type=="privateuseone")else audio,
- # audio,
- # n_fft=n_fft_new,
- # hop_length=hop_length_new,
- # win_length=win_length_new,
- # window=self.hann_window[keyshift_key],
- # center=center,
- # return_complex=True,
- # )
- # magnitude = torch.sqrt(fft.real.pow(2) + fft.imag.pow(2))
- # print(1111111111)
- # print(222222222222222,audio.device,self.is_half)
- if hasattr(self, "stft") == False:
- # print(n_fft_new,hop_length_new,win_length_new,audio.shape)
- self.stft = STFT(
- filter_length=n_fft_new,
- hop_length=hop_length_new,
- win_length=win_length_new,
- window="hann",
- ).to(audio.device)
- magnitude = self.stft.transform(audio) # phase
- # if (audio.device.type == "privateuseone"):
- # magnitude=magnitude.to(audio.device)
- if keyshift != 0:
- size = self.n_fft // 2 + 1
- resize = magnitude.size(1)
- if resize < size:
- magnitude = F.pad(magnitude, (0, 0, 0, size - resize))
- magnitude = magnitude[:, :size, :] * self.win_length / win_length_new
- mel_output = torch.matmul(self.mel_basis, magnitude)
- if self.is_half == True:
- mel_output = mel_output.half()
- log_mel_spec = torch.log(torch.clamp(mel_output, min=self.clamp))
- # print(log_mel_spec.device.type)
- return log_mel_spec
-
-
-class RMVPE:
- def __init__(self, model_path, is_half, device=None):
- self.resample_kernel = {}
- self.resample_kernel = {}
- self.is_half = is_half
- if device is None:
- device = "cuda" if torch.cuda.is_available() else "cpu"
- self.device = device
- self.mel_extractor = MelSpectrogram(
- is_half, 128, 16000, 1024, 160, None, 30, 8000
- ).to(device)
- if "privateuseone" in str(device):
- import onnxruntime as ort
-
- ort_session = ort.InferenceSession(
- "%s/rmvpe.onnx" % os.environ["rmvpe_root"],
- providers=["DmlExecutionProvider"],
- )
- self.model = ort_session
- else:
- model = E2E(4, 1, (2, 2))
- ckpt = torch.load(model_path, map_location="cpu")
- model.load_state_dict(ckpt)
- model.eval()
- if is_half == True:
- model = model.half()
- self.model = model
- self.model = self.model.to(device)
- cents_mapping = 20 * np.arange(360) + 1997.3794084376191
- self.cents_mapping = np.pad(cents_mapping, (4, 4)) # 368
-
- def mel2hidden(self, mel):
- with torch.no_grad():
- n_frames = mel.shape[-1]
- mel = F.pad(
- mel, (0, 32 * ((n_frames - 1) // 32 + 1) - n_frames), mode="constant"
- )
- if "privateuseone" in str(self.device):
- onnx_input_name = self.model.get_inputs()[0].name
- onnx_outputs_names = self.model.get_outputs()[0].name
- hidden = self.model.run(
- [onnx_outputs_names],
- input_feed={onnx_input_name: mel.cpu().numpy()},
- )[0]
- else:
- hidden = self.model(mel)
- return hidden[:, :n_frames]
-
- def decode(self, hidden, thred=0.03):
- cents_pred = self.to_local_average_cents(hidden, thred=thred)
- f0 = 10 * (2 ** (cents_pred / 1200))
- f0[f0 == 10] = 0
- # f0 = np.array([10 * (2 ** (cent_pred / 1200)) if cent_pred else 0 for cent_pred in cents_pred])
- return f0
-
- def infer_from_audio(self, audio, thred=0.03):
- # torch.cuda.synchronize()
- t0 = ttime()
- mel = self.mel_extractor(
- torch.from_numpy(audio).float().to(self.device).unsqueeze(0), center=True
- )
- # print(123123123,mel.device.type)
- # torch.cuda.synchronize()
- t1 = ttime()
- hidden = self.mel2hidden(mel)
- # torch.cuda.synchronize()
- t2 = ttime()
- # print(234234,hidden.device.type)
- if "privateuseone" not in str(self.device):
- hidden = hidden.squeeze(0).cpu().numpy()
- else:
- hidden = hidden[0]
- if self.is_half == True:
- hidden = hidden.astype("float32")
-
- f0 = self.decode(hidden, thred=thred)
- # torch.cuda.synchronize()
- t3 = ttime()
- # print("hmvpe:%s\t%s\t%s\t%s"%(t1-t0,t2-t1,t3-t2,t3-t0))
- return f0
-
- def infer_from_audio_with_pitch(self, audio, thred=0.03, f0_min=50, f0_max=1100):
- audio = torch.from_numpy(audio).float().to(self.device).unsqueeze(0)
- mel = self.mel_extractor(audio, center=True)
- hidden = self.mel2hidden(mel)
- hidden = hidden.squeeze(0).cpu().numpy()
- if self.is_half == True:
- hidden = hidden.astype("float32")
- f0 = self.decode(hidden, thred=thred)
- f0[(f0 < f0_min) | (f0 > f0_max)] = 0
- return f0
-
- def to_local_average_cents(self, salience, thred=0.05):
- # t0 = ttime()
- center = np.argmax(salience, axis=1) # 帧长#index
- salience = np.pad(salience, ((0, 0), (4, 4))) # 帧长,368
- # t1 = ttime()
- center += 4
- todo_salience = []
- todo_cents_mapping = []
- starts = center - 4
- ends = center + 5
- for idx in range(salience.shape[0]):
- todo_salience.append(salience[:, starts[idx] : ends[idx]][idx])
- todo_cents_mapping.append(self.cents_mapping[starts[idx] : ends[idx]])
- # t2 = ttime()
- todo_salience = np.array(todo_salience) # 帧长,9
- todo_cents_mapping = np.array(todo_cents_mapping) # 帧长,9
- product_sum = np.sum(todo_salience * todo_cents_mapping, 1)
- weight_sum = np.sum(todo_salience, 1) # 帧长
- devided = product_sum / weight_sum # 帧长
- # t3 = ttime()
- maxx = np.max(salience, axis=1) # 帧长
- devided[maxx <= thred] = 0
- # t4 = ttime()
- # print("decode:%s\t%s\t%s\t%s" % (t1 - t0, t2 - t1, t3 - t2, t4 - t3))
- return devided
-
-
-if __name__ == "__main__":
- import librosa
- import soundfile as sf
-
- audio, sampling_rate = sf.read(r"C:\Users\liujing04\Desktop\Z\冬之花clip1.wav")
- if len(audio.shape) > 1:
- audio = librosa.to_mono(audio.transpose(1, 0))
- audio_bak = audio.copy()
- if sampling_rate != 16000:
- audio = librosa.resample(audio, orig_sr=sampling_rate, target_sr=16000)
- model_path = r"D:\BaiduNetdiskDownload\RVC-beta-v2-0727AMD_realtime\rmvpe.pt"
- thred = 0.03 # 0.01
- device = "cuda" if torch.cuda.is_available() else "cpu"
- rmvpe = RMVPE(model_path, is_half=False, device=device)
- t0 = ttime()
- f0 = rmvpe.infer_from_audio(audio, thred=thred)
- # f0 = rmvpe.infer_from_audio(audio, thred=thred)
- # f0 = rmvpe.infer_from_audio(audio, thred=thred)
- # f0 = rmvpe.infer_from_audio(audio, thred=thred)
- # f0 = rmvpe.infer_from_audio(audio, thred=thred)
- t1 = ttime()
- logger.info("%s %.2f", f0.shape, t1 - t0)
diff --git a/spaces/GV05/stable-diffusion-mingle-prompts/app.py b/spaces/GV05/stable-diffusion-mingle-prompts/app.py
deleted file mode 100644
index 825c14dca7976177fcb97f903d9abf04cb3fd7e8..0000000000000000000000000000000000000000
--- a/spaces/GV05/stable-diffusion-mingle-prompts/app.py
+++ /dev/null
@@ -1,74 +0,0 @@
-import gradio as gr
-import torch
-from transformers import logging
-import random
-from PIL import Image
-from Utils import MingleModel
-
-logging.set_verbosity_error()
-
-
-def get_concat_h(images):
- widths, heights = zip(*(i.size for i in images))
-
- total_width = sum(widths)
- max_height = max(heights)
-
- dst = Image.new('RGB', (total_width, max_height))
- x_offset = 0
- for im in images:
- dst.paste(im, (x_offset,0))
- x_offset += im.size[0]
- return dst
-
-
-mingle_model = MingleModel()
-
-
-def mingle_prompts(first_prompt, second_prompt):
- imgs = []
- text_input1 = mingle_model.do_tokenizer(first_prompt)
- text_input2 = mingle_model.do_tokenizer(second_prompt)
- with torch.no_grad():
- text_embeddings1 = mingle_model.get_text_encoder(text_input1)
- text_embeddings2 = mingle_model.get_text_encoder(text_input2)
-
- rand_generator = random.randint(1, 2048)
- # Mix them together
- # mix_factors = [0.1, 0.3, 0.5, 0.7, 0.9]
- mix_factors = [0.5]
- for mix_factor in mix_factors:
- mixed_embeddings = (text_embeddings1 * mix_factor + text_embeddings2 * (1 - mix_factor))
-
- # Generate!
- steps = 20
- guidence_scale = 8.0
- img = mingle_model.generate_with_embs(mixed_embeddings, rand_generator, num_inference_steps=steps,
- guidance_scale=guidence_scale)
- imgs.append(img)
-
- return get_concat_h(imgs)
-
-
-with gr.Blocks() as demo:
- gr.Markdown(
- '''
-
create a 'chimera' by averaging the embeddings of two different prompts!!
- ''')
- gr.Image('batman_venum.png', shape=(1024, 205))
-
- first_prompt = gr.Textbox(label="first_prompt")
- second_prompt = gr.Textbox(label="second_prompt")
- greet_btn = gr.Button("Submit")
- gr.Markdown("## Text Examples")
- gr.Examples([['batman, dynamic lighting, photorealistic fantasy concept art, trending on art station, stunning visuals, terrifying, creative, cinematic',
- 'venom, dynamic lighting, photorealistic fantasy concept art, trending on art station, stunning visuals, terrifying, creative, cinematic'],
- ['A mouse', 'A leopard']], [first_prompt, second_prompt])
-
- gr.Markdown("# Output Results")
- output = gr.Image(shape=(512,512))
-
- greet_btn.click(fn=mingle_prompts, inputs=[first_prompt, second_prompt], outputs=[output])
-
-demo.launch()
-
diff --git a/spaces/GXSA/bingo/tailwind.config.js b/spaces/GXSA/bingo/tailwind.config.js
deleted file mode 100644
index 03da3c3c45be6983b9f5ffa6df5f1fd0870e9636..0000000000000000000000000000000000000000
--- a/spaces/GXSA/bingo/tailwind.config.js
+++ /dev/null
@@ -1,48 +0,0 @@
-/** @type {import('tailwindcss').Config} */
-module.exports = {
- content: [
- './src/pages/**/*.{js,ts,jsx,tsx,mdx}',
- './src/components/**/*.{js,ts,jsx,tsx,mdx}',
- './src/app/**/*.{js,ts,jsx,tsx,mdx}',
- './src/ui/**/*.{js,ts,jsx,tsx,mdx}',
- ],
- "darkMode": "class",
- theme: {
- extend: {
- colors: {
- 'primary-blue': 'rgb(var(--color-primary-blue) / )',
- secondary: 'rgb(var(--color-secondary) / )',
- 'primary-background': 'rgb(var(--primary-background) / )',
- 'primary-text': 'rgb(var(--primary-text) / )',
- 'secondary-text': 'rgb(var(--secondary-text) / )',
- 'light-text': 'rgb(var(--light-text) / )',
- 'primary-border': 'rgb(var(--primary-border) / )',
- },
- keyframes: {
- slideDownAndFade: {
- from: { opacity: 0, transform: 'translateY(-2px)' },
- to: { opacity: 1, transform: 'translateY(0)' },
- },
- slideLeftAndFade: {
- from: { opacity: 0, transform: 'translateX(2px)' },
- to: { opacity: 1, transform: 'translateX(0)' },
- },
- slideUpAndFade: {
- from: { opacity: 0, transform: 'translateY(2px)' },
- to: { opacity: 1, transform: 'translateY(0)' },
- },
- slideRightAndFade: {
- from: { opacity: 0, transform: 'translateX(2px)' },
- to: { opacity: 1, transform: 'translateX(0)' },
- },
- },
- animation: {
- slideDownAndFade: 'slideDownAndFade 400ms cubic-bezier(0.16, 1, 0.3, 1)',
- slideLeftAndFade: 'slideLeftAndFade 400ms cubic-bezier(0.16, 1, 0.3, 1)',
- slideUpAndFade: 'slideUpAndFade 400ms cubic-bezier(0.16, 1, 0.3, 1)',
- slideRightAndFade: 'slideRightAndFade 400ms cubic-bezier(0.16, 1, 0.3, 1)',
- },
- },
- },
- plugins: [require('@headlessui/tailwindcss'), require('tailwind-scrollbar')],
-}
diff --git a/spaces/GeorgeOrville/bingo/src/components/ui/textarea.tsx b/spaces/GeorgeOrville/bingo/src/components/ui/textarea.tsx
deleted file mode 100644
index e25af722c7a5dc1121a9ab58d6716952f9f76081..0000000000000000000000000000000000000000
--- a/spaces/GeorgeOrville/bingo/src/components/ui/textarea.tsx
+++ /dev/null
@@ -1,24 +0,0 @@
-import * as React from 'react'
-
-import { cn } from '@/lib/utils'
-
-export interface TextareaProps
- extends React.TextareaHTMLAttributes {}
-
-const Textarea = React.forwardRef(
- ({ className, ...props }, ref) => {
- return (
-
- )
- }
-)
-Textarea.displayName = 'Textarea'
-
-export { Textarea }
diff --git a/spaces/Gladiator/gradient_dissent_bot/src/summarize.py b/spaces/Gladiator/gradient_dissent_bot/src/summarize.py
deleted file mode 100644
index 1162129ffe9cafc05325dfb79abc638aeca27d8b..0000000000000000000000000000000000000000
--- a/spaces/Gladiator/gradient_dissent_bot/src/summarize.py
+++ /dev/null
@@ -1,123 +0,0 @@
-import os
-from dataclasses import asdict
-
-import pandas as pd
-from langchain.callbacks import get_openai_callback
-from langchain.chains.summarize import load_summarize_chain
-from langchain.chat_models import ChatOpenAI
-from langchain.document_loaders import DataFrameLoader
-from langchain.prompts import PromptTemplate
-from langchain.text_splitter import TokenTextSplitter
-from tqdm import tqdm
-from wandb.integration.langchain import WandbTracer
-
-import wandb
-from config import config
-
-
-def get_data(artifact_name: str, total_episodes: int = None):
- podcast_artifact = wandb.use_artifact(artifact_name, type="dataset")
- podcast_artifact_dir = podcast_artifact.download(config.root_artifact_dir)
- filename = artifact_name.split(":")[0].split("/")[-1]
- df = pd.read_csv(os.path.join(podcast_artifact_dir, f"{filename}.csv"))
- if total_episodes is not None:
- df = df.iloc[:total_episodes]
- return df
-
-
-def summarize_episode(episode_df: pd.DataFrame):
- # load docs into langchain format
- loader = DataFrameLoader(episode_df, page_content_column="transcript")
- data = loader.load()
-
- # split the documents
- text_splitter = TokenTextSplitter.from_tiktoken_encoder(chunk_size=1000, chunk_overlap=0)
- docs = text_splitter.split_documents(data)
- print(f"Number of documents for podcast {data[0].metadata['title']}: {len(docs)}")
-
- # initialize LLM
- llm = ChatOpenAI(model_name="gpt-3.5-turbo", temperature=0)
-
- # define map prompt
- map_prompt = """Write a concise summary of the following short transcript from a podcast.
- Don't add your opinions or interpretations.
-
- {text}
-
- CONCISE SUMMARY:"""
-
- # define combine prompt
- combine_prompt = """You have been provided with summaries of chunks of transcripts from a podcast.
- Your task is to merge these intermediate summaries to create a brief and comprehensive summary of the entire podcast.
- The summary should encompass all the crucial points of the podcast.
- Ensure that the summary is atleast 2 paragraph long and effectively captures the essence of the podcast.
- {text}
-
- SUMMARY:"""
-
- map_prompt_template = PromptTemplate(template=map_prompt, input_variables=["text"])
- combine_prompt_template = PromptTemplate(template=combine_prompt, input_variables=["text"])
-
- # initialize the summarizer chain
- chain = load_summarize_chain(
- llm,
- chain_type="map_reduce",
- return_intermediate_steps=True,
- map_prompt=map_prompt_template,
- combine_prompt=combine_prompt_template,
- )
-
- summary = chain({"input_documents": docs})
- return summary
-
-
-if __name__ == "__main__":
- # initialize wandb tracer
- WandbTracer.init(
- {
- "project": config.project_name,
- "job_type": "summarize",
- "config": asdict(config),
- }
- )
-
- # get scraped data
- df = get_data(artifact_name=config.yt_podcast_data_artifact)
-
- summaries = []
- with get_openai_callback() as cb:
- for episode in tqdm(df.iterrows(), total=len(df), desc="Summarizing episodes"):
- episode_data = episode[1].to_frame().T
-
- summary = summarize_episode(episode_data)
- summaries.append(summary["output_text"])
-
- print("*" * 25)
- print(cb)
- print("*" * 25)
-
- wandb.log(
- {
- "total_prompt_tokens": cb.prompt_tokens,
- "total_completion_tokens": cb.completion_tokens,
- "total_tokens": cb.total_tokens,
- "total_cost": cb.total_cost,
- }
- )
-
- df["summary"] = summaries
-
- # save data
- path_to_save = os.path.join(config.root_data_dir, "summarized_podcasts.csv")
- df.to_csv(path_to_save, index=False)
-
- # log to wandb artifact
- artifact = wandb.Artifact("summarized_podcasts", type="dataset")
- artifact.add_file(path_to_save)
- wandb.log_artifact(artifact)
-
- # create wandb table
- table = wandb.Table(dataframe=df)
- wandb.log({"summarized_podcasts": table})
-
- WandbTracer.finish()
diff --git a/spaces/Gmq-x/gpt-academic/check_proxy.py b/spaces/Gmq-x/gpt-academic/check_proxy.py
deleted file mode 100644
index 28711a8c140bfcdb0683efd924032e6ccc0f0df8..0000000000000000000000000000000000000000
--- a/spaces/Gmq-x/gpt-academic/check_proxy.py
+++ /dev/null
@@ -1,149 +0,0 @@
-
-def check_proxy(proxies):
- import requests
- proxies_https = proxies['https'] if proxies is not None else '无'
- try:
- response = requests.get("https://ipapi.co/json/",
- proxies=proxies, timeout=4)
- data = response.json()
- print(f'查询代理的地理位置,返回的结果是{data}')
- if 'country_name' in data:
- country = data['country_name']
- result = f"代理配置 {proxies_https}, 代理所在地:{country}"
- elif 'error' in data:
- result = f"代理配置 {proxies_https}, 代理所在地:未知,IP查询频率受限"
- print(result)
- return result
- except:
- result = f"代理配置 {proxies_https}, 代理所在地查询超时,代理可能无效"
- print(result)
- return result
-
-
-def backup_and_download(current_version, remote_version):
- """
- 一键更新协议:备份和下载
- """
- from toolbox import get_conf
- import shutil
- import os
- import requests
- import zipfile
- os.makedirs(f'./history', exist_ok=True)
- backup_dir = f'./history/backup-{current_version}/'
- new_version_dir = f'./history/new-version-{remote_version}/'
- if os.path.exists(new_version_dir):
- return new_version_dir
- os.makedirs(new_version_dir)
- shutil.copytree('./', backup_dir, ignore=lambda x, y: ['history'])
- proxies, = get_conf('proxies')
- r = requests.get(
- 'https://github.com/binary-husky/chatgpt_academic/archive/refs/heads/master.zip', proxies=proxies, stream=True)
- zip_file_path = backup_dir+'/master.zip'
- with open(zip_file_path, 'wb+') as f:
- f.write(r.content)
- dst_path = new_version_dir
- with zipfile.ZipFile(zip_file_path, "r") as zip_ref:
- for zip_info in zip_ref.infolist():
- dst_file_path = os.path.join(dst_path, zip_info.filename)
- if os.path.exists(dst_file_path):
- os.remove(dst_file_path)
- zip_ref.extract(zip_info, dst_path)
- return new_version_dir
-
-
-def patch_and_restart(path):
- """
- 一键更新协议:覆盖和重启
- """
- import distutils
- import shutil
- import os
- import sys
- import time
- from colorful import print亮黄, print亮绿, print亮红
- # if not using config_private, move origin config.py as config_private.py
- if not os.path.exists('config_private.py'):
- print亮黄('由于您没有设置config_private.py私密配置,现将您的现有配置移动至config_private.py以防止配置丢失,',
- '另外您可以随时在history子文件夹下找回旧版的程序。')
- shutil.copyfile('config.py', 'config_private.py')
- distutils.dir_util.copy_tree(path+'/chatgpt_academic-master', './')
- import subprocess
- print亮绿('代码已经更新,即将更新pip包依赖……')
- for i in reversed(range(5)): time.sleep(1); print(i)
- try:
- subprocess.check_call([sys.executable, '-m', 'pip', 'install', '-r', 'requirements.txt'])
- except:
- print亮红('pip包依赖安装出现问题,需要手动安装新增的依赖库 `python -m pip install -r requirements.txt`,然后在用常规的`python main.py`的方式启动。')
- print亮绿('更新完成,您可以随时在history子文件夹下找回旧版的程序,5s之后重启')
- print亮红('假如重启失败,您可能需要手动安装新增的依赖库 `python -m pip install -r requirements.txt`,然后在用常规的`python main.py`的方式启动。')
- print(' ------------------------------ -----------------------------------')
- for i in reversed(range(8)): time.sleep(1); print(i)
- os.execl(sys.executable, sys.executable, *sys.argv)
-
-
-def get_current_version():
- import json
- try:
- with open('./version', 'r', encoding='utf8') as f:
- current_version = json.loads(f.read())['version']
- except:
- current_version = ""
- return current_version
-
-
-def auto_update():
- """
- 一键更新协议:查询版本和用户意见
- """
- try:
- from toolbox import get_conf
- import requests
- import time
- import json
- proxies, = get_conf('proxies')
- response = requests.get(
- "https://raw.githubusercontent.com/binary-husky/chatgpt_academic/master/version", proxies=proxies, timeout=5)
- remote_json_data = json.loads(response.text)
- remote_version = remote_json_data['version']
- if remote_json_data["show_feature"]:
- new_feature = "新功能:" + remote_json_data["new_feature"]
- else:
- new_feature = ""
- with open('./version', 'r', encoding='utf8') as f:
- current_version = f.read()
- current_version = json.loads(current_version)['version']
- if (remote_version - current_version) >= 0.01:
- from colorful import print亮黄
- print亮黄(
- f'\n新版本可用。新版本:{remote_version},当前版本:{current_version}。{new_feature}')
- print('(1)Github更新地址:\nhttps://github.com/binary-husky/chatgpt_academic\n')
- user_instruction = input('(2)是否一键更新代码(Y+回车=确认,输入其他/无输入+回车=不更新)?')
- if user_instruction in ['Y', 'y']:
- path = backup_and_download(current_version, remote_version)
- try:
- patch_and_restart(path)
- except:
- print('更新失败。')
- else:
- print('自动更新程序:已禁用')
- return
- else:
- return
- except:
- print('自动更新程序:已禁用')
-
-def warm_up_modules():
- print('正在执行一些模块的预热...')
- from request_llm.bridge_all import model_info
- enc = model_info["gpt-3.5-turbo"]['tokenizer']
- enc.encode("模块预热", disallowed_special=())
- enc = model_info["gpt-4"]['tokenizer']
- enc.encode("模块预热", disallowed_special=())
-
-if __name__ == '__main__':
- import os
- os.environ['no_proxy'] = '*' # 避免代理网络产生意外污染
- from toolbox import get_conf
- proxies, = get_conf('proxies')
- check_proxy(proxies)
diff --git a/spaces/GodParticle69/minor_demo/mrcnn/evaluate.py b/spaces/GodParticle69/minor_demo/mrcnn/evaluate.py
deleted file mode 100644
index 09044f341659606b87abc364cc2afdf54bbc15b1..0000000000000000000000000000000000000000
--- a/spaces/GodParticle69/minor_demo/mrcnn/evaluate.py
+++ /dev/null
@@ -1,94 +0,0 @@
-from pycocotools.coco import COCO
-from mrcnn.cocoeval import COCOeval
-from pycocotools import mask as maskUtils
-import time
-import numpy as np
-
-############################################################
-# COCO Evaluation
-############################################################
-
-def build_coco_results(dataset, image_ids, rois, class_ids, scores, masks):
- """Arrange resutls to match COCO specs in http://cocodataset.org/#format
- """
- # If no results, return an empty list
- if rois is None:
- return []
-
- results = []
- for image_id in image_ids:
- # Loop through detections
- for i in range(rois.shape[0]):
- class_id = class_ids[i]
- score = scores[i]
- bbox = np.around(rois[i], 1)
- mask = masks[:, :, i]
-
- result = {
- "image_id": image_id,
- "category_id": dataset.get_source_class_id(class_id, "crowdai-mapping-challenge"),
- "bbox": [bbox[1], bbox[0], bbox[3] - bbox[1], bbox[2] - bbox[0]],
- "score": score,
- "segmentation": maskUtils.encode(np.asfortranarray(mask)).encode('utf-8')
- }
- results.append(result)
- return results
-
-
-def evaluate_coco(model, dataset, coco, eval_type="bbox", limit=0, image_ids=None):
- """Runs official COCO evaluation.
- dataset: A Dataset object with valiadtion data
- eval_type: "bbox" or "segm" for bounding box or segmentation evaluation
- limit: if not 0, it's the number of images to use for evaluation
- """
- # Pick COCO images from the dataset
- image_ids = image_ids or dataset.image_ids
-
- # Limit to a subset
- if limit:
- image_ids = image_ids[:limit]
-
- # Get corresponding COCO image IDs.
- coco_image_ids = [dataset.image_info[id]["id"] for id in image_ids]
-
- t_prediction = 0
- t_start = time.time()
-
- results = []
-
- for i, image_id in enumerate(image_ids):
- # Load image
- image = dataset.load_image(image_id)
-
- # Run detection
- t = time.time()
- print("="*100)
- print("Image shape ", image.shape)
- r = model.detect([image])
- r = r[0]
- t_prediction += (time.time() - t)
- print("Prediction time : ", (time.time() - t))
- # Convert results to COCO format
- image_results = build_coco_results(dataset, coco_image_ids[i:i + 1],
- r["rois"], r["class_ids"],
- r["scores"], r["masks"])
- print("Number of detections : ", len(r["rois"]))
- print("Classes Predicted : ", r["class_ids"])
- print("Scores : ", r["scores"])
- results.extend(image_results)
-
- # Load results. This modifies results with additional attributes.
- coco_results = coco.loadRes(results)
-
- # Evaluate
- cocoEval = COCOeval(coco, coco_results, eval_type)
- cocoEval.params.imgIds = coco_image_ids
- cocoEval.evaluate()
- cocoEval.accumulate()
- ap = cocoEval._summarize(ap=1, iouThr=0.5, areaRng="all", maxDets=100)
- ar = cocoEval._summarize(ap=0, areaRng="all", maxDets=100)
- print("Precision : ", ap, " Recall : ", ar)
-
- print("Prediction time: {}. Average {}/image".format(
- t_prediction, t_prediction / len(image_ids)))
- print("Total time: ", time.time() - t_start)
diff --git a/spaces/GouDiya/anime-remove-background/README.md b/spaces/GouDiya/anime-remove-background/README.md
deleted file mode 100644
index 1ba3cb5ea0e994e246d57b7d62b8aa5a6331901c..0000000000000000000000000000000000000000
--- a/spaces/GouDiya/anime-remove-background/README.md
+++ /dev/null
@@ -1,14 +0,0 @@
----
-title: Anime Remove Background
-emoji: 🪄🖼️
-colorFrom: indigo
-colorTo: pink
-sdk: gradio
-sdk_version: 3.1.4
-app_file: app.py
-pinned: false
-license: apache-2.0
-duplicated_from: skytnt/anime-remove-background
----
-
-Check out the configuration reference at https://huggingface.co/docs/hub/spaces-config-reference
diff --git a/spaces/Goutam982/RVC_V2_voice_clone/lib/infer_pack/attentions.py b/spaces/Goutam982/RVC_V2_voice_clone/lib/infer_pack/attentions.py
deleted file mode 100644
index 05501be1871643f78dddbeaa529c96667031a8db..0000000000000000000000000000000000000000
--- a/spaces/Goutam982/RVC_V2_voice_clone/lib/infer_pack/attentions.py
+++ /dev/null
@@ -1,417 +0,0 @@
-import copy
-import math
-import numpy as np
-import torch
-from torch import nn
-from torch.nn import functional as F
-
-from lib.infer_pack import commons
-from lib.infer_pack import modules
-from lib.infer_pack.modules import LayerNorm
-
-
-class Encoder(nn.Module):
- def __init__(
- self,
- hidden_channels,
- filter_channels,
- n_heads,
- n_layers,
- kernel_size=1,
- p_dropout=0.0,
- window_size=10,
- **kwargs
- ):
- super().__init__()
- self.hidden_channels = hidden_channels
- self.filter_channels = filter_channels
- self.n_heads = n_heads
- self.n_layers = n_layers
- self.kernel_size = kernel_size
- self.p_dropout = p_dropout
- self.window_size = window_size
-
- self.drop = nn.Dropout(p_dropout)
- self.attn_layers = nn.ModuleList()
- self.norm_layers_1 = nn.ModuleList()
- self.ffn_layers = nn.ModuleList()
- self.norm_layers_2 = nn.ModuleList()
- for i in range(self.n_layers):
- self.attn_layers.append(
- MultiHeadAttention(
- hidden_channels,
- hidden_channels,
- n_heads,
- p_dropout=p_dropout,
- window_size=window_size,
- )
- )
- self.norm_layers_1.append(LayerNorm(hidden_channels))
- self.ffn_layers.append(
- FFN(
- hidden_channels,
- hidden_channels,
- filter_channels,
- kernel_size,
- p_dropout=p_dropout,
- )
- )
- self.norm_layers_2.append(LayerNorm(hidden_channels))
-
- def forward(self, x, x_mask):
- attn_mask = x_mask.unsqueeze(2) * x_mask.unsqueeze(-1)
- x = x * x_mask
- for i in range(self.n_layers):
- y = self.attn_layers[i](x, x, attn_mask)
- y = self.drop(y)
- x = self.norm_layers_1[i](x + y)
-
- y = self.ffn_layers[i](x, x_mask)
- y = self.drop(y)
- x = self.norm_layers_2[i](x + y)
- x = x * x_mask
- return x
-
-
-class Decoder(nn.Module):
- def __init__(
- self,
- hidden_channels,
- filter_channels,
- n_heads,
- n_layers,
- kernel_size=1,
- p_dropout=0.0,
- proximal_bias=False,
- proximal_init=True,
- **kwargs
- ):
- super().__init__()
- self.hidden_channels = hidden_channels
- self.filter_channels = filter_channels
- self.n_heads = n_heads
- self.n_layers = n_layers
- self.kernel_size = kernel_size
- self.p_dropout = p_dropout
- self.proximal_bias = proximal_bias
- self.proximal_init = proximal_init
-
- self.drop = nn.Dropout(p_dropout)
- self.self_attn_layers = nn.ModuleList()
- self.norm_layers_0 = nn.ModuleList()
- self.encdec_attn_layers = nn.ModuleList()
- self.norm_layers_1 = nn.ModuleList()
- self.ffn_layers = nn.ModuleList()
- self.norm_layers_2 = nn.ModuleList()
- for i in range(self.n_layers):
- self.self_attn_layers.append(
- MultiHeadAttention(
- hidden_channels,
- hidden_channels,
- n_heads,
- p_dropout=p_dropout,
- proximal_bias=proximal_bias,
- proximal_init=proximal_init,
- )
- )
- self.norm_layers_0.append(LayerNorm(hidden_channels))
- self.encdec_attn_layers.append(
- MultiHeadAttention(
- hidden_channels, hidden_channels, n_heads, p_dropout=p_dropout
- )
- )
- self.norm_layers_1.append(LayerNorm(hidden_channels))
- self.ffn_layers.append(
- FFN(
- hidden_channels,
- hidden_channels,
- filter_channels,
- kernel_size,
- p_dropout=p_dropout,
- causal=True,
- )
- )
- self.norm_layers_2.append(LayerNorm(hidden_channels))
-
- def forward(self, x, x_mask, h, h_mask):
- """
- x: decoder input
- h: encoder output
- """
- self_attn_mask = commons.subsequent_mask(x_mask.size(2)).to(
- device=x.device, dtype=x.dtype
- )
- encdec_attn_mask = h_mask.unsqueeze(2) * x_mask.unsqueeze(-1)
- x = x * x_mask
- for i in range(self.n_layers):
- y = self.self_attn_layers[i](x, x, self_attn_mask)
- y = self.drop(y)
- x = self.norm_layers_0[i](x + y)
-
- y = self.encdec_attn_layers[i](x, h, encdec_attn_mask)
- y = self.drop(y)
- x = self.norm_layers_1[i](x + y)
-
- y = self.ffn_layers[i](x, x_mask)
- y = self.drop(y)
- x = self.norm_layers_2[i](x + y)
- x = x * x_mask
- return x
-
-
-class MultiHeadAttention(nn.Module):
- def __init__(
- self,
- channels,
- out_channels,
- n_heads,
- p_dropout=0.0,
- window_size=None,
- heads_share=True,
- block_length=None,
- proximal_bias=False,
- proximal_init=False,
- ):
- super().__init__()
- assert channels % n_heads == 0
-
- self.channels = channels
- self.out_channels = out_channels
- self.n_heads = n_heads
- self.p_dropout = p_dropout
- self.window_size = window_size
- self.heads_share = heads_share
- self.block_length = block_length
- self.proximal_bias = proximal_bias
- self.proximal_init = proximal_init
- self.attn = None
-
- self.k_channels = channels // n_heads
- self.conv_q = nn.Conv1d(channels, channels, 1)
- self.conv_k = nn.Conv1d(channels, channels, 1)
- self.conv_v = nn.Conv1d(channels, channels, 1)
- self.conv_o = nn.Conv1d(channels, out_channels, 1)
- self.drop = nn.Dropout(p_dropout)
-
- if window_size is not None:
- n_heads_rel = 1 if heads_share else n_heads
- rel_stddev = self.k_channels**-0.5
- self.emb_rel_k = nn.Parameter(
- torch.randn(n_heads_rel, window_size * 2 + 1, self.k_channels)
- * rel_stddev
- )
- self.emb_rel_v = nn.Parameter(
- torch.randn(n_heads_rel, window_size * 2 + 1, self.k_channels)
- * rel_stddev
- )
-
- nn.init.xavier_uniform_(self.conv_q.weight)
- nn.init.xavier_uniform_(self.conv_k.weight)
- nn.init.xavier_uniform_(self.conv_v.weight)
- if proximal_init:
- with torch.no_grad():
- self.conv_k.weight.copy_(self.conv_q.weight)
- self.conv_k.bias.copy_(self.conv_q.bias)
-
- def forward(self, x, c, attn_mask=None):
- q = self.conv_q(x)
- k = self.conv_k(c)
- v = self.conv_v(c)
-
- x, self.attn = self.attention(q, k, v, mask=attn_mask)
-
- x = self.conv_o(x)
- return x
-
- def attention(self, query, key, value, mask=None):
- # reshape [b, d, t] -> [b, n_h, t, d_k]
- b, d, t_s, t_t = (*key.size(), query.size(2))
- query = query.view(b, self.n_heads, self.k_channels, t_t).transpose(2, 3)
- key = key.view(b, self.n_heads, self.k_channels, t_s).transpose(2, 3)
- value = value.view(b, self.n_heads, self.k_channels, t_s).transpose(2, 3)
-
- scores = torch.matmul(query / math.sqrt(self.k_channels), key.transpose(-2, -1))
- if self.window_size is not None:
- assert (
- t_s == t_t
- ), "Relative attention is only available for self-attention."
- key_relative_embeddings = self._get_relative_embeddings(self.emb_rel_k, t_s)
- rel_logits = self._matmul_with_relative_keys(
- query / math.sqrt(self.k_channels), key_relative_embeddings
- )
- scores_local = self._relative_position_to_absolute_position(rel_logits)
- scores = scores + scores_local
- if self.proximal_bias:
- assert t_s == t_t, "Proximal bias is only available for self-attention."
- scores = scores + self._attention_bias_proximal(t_s).to(
- device=scores.device, dtype=scores.dtype
- )
- if mask is not None:
- scores = scores.masked_fill(mask == 0, -1e4)
- if self.block_length is not None:
- assert (
- t_s == t_t
- ), "Local attention is only available for self-attention."
- block_mask = (
- torch.ones_like(scores)
- .triu(-self.block_length)
- .tril(self.block_length)
- )
- scores = scores.masked_fill(block_mask == 0, -1e4)
- p_attn = F.softmax(scores, dim=-1) # [b, n_h, t_t, t_s]
- p_attn = self.drop(p_attn)
- output = torch.matmul(p_attn, value)
- if self.window_size is not None:
- relative_weights = self._absolute_position_to_relative_position(p_attn)
- value_relative_embeddings = self._get_relative_embeddings(
- self.emb_rel_v, t_s
- )
- output = output + self._matmul_with_relative_values(
- relative_weights, value_relative_embeddings
- )
- output = (
- output.transpose(2, 3).contiguous().view(b, d, t_t)
- ) # [b, n_h, t_t, d_k] -> [b, d, t_t]
- return output, p_attn
-
- def _matmul_with_relative_values(self, x, y):
- """
- x: [b, h, l, m]
- y: [h or 1, m, d]
- ret: [b, h, l, d]
- """
- ret = torch.matmul(x, y.unsqueeze(0))
- return ret
-
- def _matmul_with_relative_keys(self, x, y):
- """
- x: [b, h, l, d]
- y: [h or 1, m, d]
- ret: [b, h, l, m]
- """
- ret = torch.matmul(x, y.unsqueeze(0).transpose(-2, -1))
- return ret
-
- def _get_relative_embeddings(self, relative_embeddings, length):
- max_relative_position = 2 * self.window_size + 1
- # Pad first before slice to avoid using cond ops.
- pad_length = max(length - (self.window_size + 1), 0)
- slice_start_position = max((self.window_size + 1) - length, 0)
- slice_end_position = slice_start_position + 2 * length - 1
- if pad_length > 0:
- padded_relative_embeddings = F.pad(
- relative_embeddings,
- commons.convert_pad_shape([[0, 0], [pad_length, pad_length], [0, 0]]),
- )
- else:
- padded_relative_embeddings = relative_embeddings
- used_relative_embeddings = padded_relative_embeddings[
- :, slice_start_position:slice_end_position
- ]
- return used_relative_embeddings
-
- def _relative_position_to_absolute_position(self, x):
- """
- x: [b, h, l, 2*l-1]
- ret: [b, h, l, l]
- """
- batch, heads, length, _ = x.size()
- # Concat columns of pad to shift from relative to absolute indexing.
- x = F.pad(x, commons.convert_pad_shape([[0, 0], [0, 0], [0, 0], [0, 1]]))
-
- # Concat extra elements so to add up to shape (len+1, 2*len-1).
- x_flat = x.view([batch, heads, length * 2 * length])
- x_flat = F.pad(
- x_flat, commons.convert_pad_shape([[0, 0], [0, 0], [0, length - 1]])
- )
-
- # Reshape and slice out the padded elements.
- x_final = x_flat.view([batch, heads, length + 1, 2 * length - 1])[
- :, :, :length, length - 1 :
- ]
- return x_final
-
- def _absolute_position_to_relative_position(self, x):
- """
- x: [b, h, l, l]
- ret: [b, h, l, 2*l-1]
- """
- batch, heads, length, _ = x.size()
- # padd along column
- x = F.pad(
- x, commons.convert_pad_shape([[0, 0], [0, 0], [0, 0], [0, length - 1]])
- )
- x_flat = x.view([batch, heads, length**2 + length * (length - 1)])
- # add 0's in the beginning that will skew the elements after reshape
- x_flat = F.pad(x_flat, commons.convert_pad_shape([[0, 0], [0, 0], [length, 0]]))
- x_final = x_flat.view([batch, heads, length, 2 * length])[:, :, :, 1:]
- return x_final
-
- def _attention_bias_proximal(self, length):
- """Bias for self-attention to encourage attention to close positions.
- Args:
- length: an integer scalar.
- Returns:
- a Tensor with shape [1, 1, length, length]
- """
- r = torch.arange(length, dtype=torch.float32)
- diff = torch.unsqueeze(r, 0) - torch.unsqueeze(r, 1)
- return torch.unsqueeze(torch.unsqueeze(-torch.log1p(torch.abs(diff)), 0), 0)
-
-
-class FFN(nn.Module):
- def __init__(
- self,
- in_channels,
- out_channels,
- filter_channels,
- kernel_size,
- p_dropout=0.0,
- activation=None,
- causal=False,
- ):
- super().__init__()
- self.in_channels = in_channels
- self.out_channels = out_channels
- self.filter_channels = filter_channels
- self.kernel_size = kernel_size
- self.p_dropout = p_dropout
- self.activation = activation
- self.causal = causal
-
- if causal:
- self.padding = self._causal_padding
- else:
- self.padding = self._same_padding
-
- self.conv_1 = nn.Conv1d(in_channels, filter_channels, kernel_size)
- self.conv_2 = nn.Conv1d(filter_channels, out_channels, kernel_size)
- self.drop = nn.Dropout(p_dropout)
-
- def forward(self, x, x_mask):
- x = self.conv_1(self.padding(x * x_mask))
- if self.activation == "gelu":
- x = x * torch.sigmoid(1.702 * x)
- else:
- x = torch.relu(x)
- x = self.drop(x)
- x = self.conv_2(self.padding(x * x_mask))
- return x * x_mask
-
- def _causal_padding(self, x):
- if self.kernel_size == 1:
- return x
- pad_l = self.kernel_size - 1
- pad_r = 0
- padding = [[0, 0], [0, 0], [pad_l, pad_r]]
- x = F.pad(x, commons.convert_pad_shape(padding))
- return x
-
- def _same_padding(self, x):
- if self.kernel_size == 1:
- return x
- pad_l = (self.kernel_size - 1) // 2
- pad_r = self.kernel_size // 2
- padding = [[0, 0], [0, 0], [pad_l, pad_r]]
- x = F.pad(x, commons.convert_pad_shape(padding))
- return x
diff --git a/spaces/Gradio-Blocks/uniformer_image_detection/configs/faster_rcnn/faster_rcnn_r50_caffe_fpn_mstrain_90k_coco.py b/spaces/Gradio-Blocks/uniformer_image_detection/configs/faster_rcnn/faster_rcnn_r50_caffe_fpn_mstrain_90k_coco.py
deleted file mode 100644
index 74dca24f26422967501e7ba31c3f39ca324e031c..0000000000000000000000000000000000000000
--- a/spaces/Gradio-Blocks/uniformer_image_detection/configs/faster_rcnn/faster_rcnn_r50_caffe_fpn_mstrain_90k_coco.py
+++ /dev/null
@@ -1,15 +0,0 @@
-_base_ = 'faster_rcnn_r50_caffe_fpn_mstrain_1x_coco.py'
-
-# learning policy
-lr_config = dict(
- policy='step',
- warmup='linear',
- warmup_iters=500,
- warmup_ratio=0.001,
- step=[60000, 80000])
-
-# Runner type
-runner = dict(_delete_=True, type='IterBasedRunner', max_iters=90000)
-
-checkpoint_config = dict(interval=10000)
-evaluation = dict(interval=10000, metric='bbox')
diff --git a/spaces/Gradio-Blocks/uniformer_image_detection/configs/gcnet/mask_rcnn_x101_32x4d_fpn_syncbn-backbone_1x_coco.py b/spaces/Gradio-Blocks/uniformer_image_detection/configs/gcnet/mask_rcnn_x101_32x4d_fpn_syncbn-backbone_1x_coco.py
deleted file mode 100644
index f0c96e58b6131f2958f28c56b9d8384d5b4746f7..0000000000000000000000000000000000000000
--- a/spaces/Gradio-Blocks/uniformer_image_detection/configs/gcnet/mask_rcnn_x101_32x4d_fpn_syncbn-backbone_1x_coco.py
+++ /dev/null
@@ -1,4 +0,0 @@
-_base_ = '../mask_rcnn/mask_rcnn_x101_32x4d_fpn_1x_coco.py'
-model = dict(
- backbone=dict(
- norm_cfg=dict(type='SyncBN', requires_grad=True), norm_eval=False))
diff --git a/spaces/Gradio-Blocks/uniformer_image_detection/configs/mask_rcnn/mask_rcnn_r50_fpn_poly_1x_coco.py b/spaces/Gradio-Blocks/uniformer_image_detection/configs/mask_rcnn/mask_rcnn_r50_fpn_poly_1x_coco.py
deleted file mode 100644
index 9eb6d57e0d25370a59472a4ceb1a3b9da6574608..0000000000000000000000000000000000000000
--- a/spaces/Gradio-Blocks/uniformer_image_detection/configs/mask_rcnn/mask_rcnn_r50_fpn_poly_1x_coco.py
+++ /dev/null
@@ -1,23 +0,0 @@
-_base_ = [
- '../_base_/models/mask_rcnn_r50_fpn.py',
- '../_base_/datasets/coco_instance.py',
- '../_base_/schedules/schedule_1x.py', '../_base_/default_runtime.py'
-]
-
-img_norm_cfg = dict(
- mean=[123.675, 116.28, 103.53], std=[58.395, 57.12, 57.375], to_rgb=True)
-train_pipeline = [
- dict(type='LoadImageFromFile'),
- dict(
- type='LoadAnnotations',
- with_bbox=True,
- with_mask=True,
- poly2mask=False),
- dict(type='Resize', img_scale=(1333, 800), keep_ratio=True),
- dict(type='RandomFlip', flip_ratio=0.5),
- dict(type='Normalize', **img_norm_cfg),
- dict(type='Pad', size_divisor=32),
- dict(type='DefaultFormatBundle'),
- dict(type='Collect', keys=['img', 'gt_bboxes', 'gt_labels', 'gt_masks']),
-]
-data = dict(train=dict(pipeline=train_pipeline))
diff --git a/spaces/Grezz/generate_human_motion/VQ-Trans/GPT_eval_multi.py b/spaces/Grezz/generate_human_motion/VQ-Trans/GPT_eval_multi.py
deleted file mode 100644
index b5e3ebcb1199e42cf16748e60863b554a0046f00..0000000000000000000000000000000000000000
--- a/spaces/Grezz/generate_human_motion/VQ-Trans/GPT_eval_multi.py
+++ /dev/null
@@ -1,121 +0,0 @@
-import os
-import torch
-import numpy as np
-from torch.utils.tensorboard import SummaryWriter
-import json
-import clip
-
-import options.option_transformer as option_trans
-import models.vqvae as vqvae
-import utils.utils_model as utils_model
-import utils.eval_trans as eval_trans
-from dataset import dataset_TM_eval
-import models.t2m_trans as trans
-from options.get_eval_option import get_opt
-from models.evaluator_wrapper import EvaluatorModelWrapper
-import warnings
-warnings.filterwarnings('ignore')
-
-##### ---- Exp dirs ---- #####
-args = option_trans.get_args_parser()
-torch.manual_seed(args.seed)
-
-args.out_dir = os.path.join(args.out_dir, f'{args.exp_name}')
-os.makedirs(args.out_dir, exist_ok = True)
-
-##### ---- Logger ---- #####
-logger = utils_model.get_logger(args.out_dir)
-writer = SummaryWriter(args.out_dir)
-logger.info(json.dumps(vars(args), indent=4, sort_keys=True))
-
-from utils.word_vectorizer import WordVectorizer
-w_vectorizer = WordVectorizer('./glove', 'our_vab')
-val_loader = dataset_TM_eval.DATALoader(args.dataname, True, 32, w_vectorizer)
-
-dataset_opt_path = 'checkpoints/kit/Comp_v6_KLD005/opt.txt' if args.dataname == 'kit' else 'checkpoints/t2m/Comp_v6_KLD005/opt.txt'
-
-wrapper_opt = get_opt(dataset_opt_path, torch.device('cuda'))
-eval_wrapper = EvaluatorModelWrapper(wrapper_opt)
-
-##### ---- Network ---- #####
-
-## load clip model and datasets
-clip_model, clip_preprocess = clip.load("ViT-B/32", device=torch.device('cuda'), jit=False, download_root='/apdcephfs_cq2/share_1290939/maelyszhang/.cache/clip') # Must set jit=False for training
-clip.model.convert_weights(clip_model) # Actually this line is unnecessary since clip by default already on float16
-clip_model.eval()
-for p in clip_model.parameters():
- p.requires_grad = False
-
-net = vqvae.HumanVQVAE(args, ## use args to define different parameters in different quantizers
- args.nb_code,
- args.code_dim,
- args.output_emb_width,
- args.down_t,
- args.stride_t,
- args.width,
- args.depth,
- args.dilation_growth_rate)
-
-
-trans_encoder = trans.Text2Motion_Transformer(num_vq=args.nb_code,
- embed_dim=args.embed_dim_gpt,
- clip_dim=args.clip_dim,
- block_size=args.block_size,
- num_layers=args.num_layers,
- n_head=args.n_head_gpt,
- drop_out_rate=args.drop_out_rate,
- fc_rate=args.ff_rate)
-
-
-print ('loading checkpoint from {}'.format(args.resume_pth))
-ckpt = torch.load(args.resume_pth, map_location='cpu')
-net.load_state_dict(ckpt['net'], strict=True)
-net.eval()
-net.cuda()
-
-if args.resume_trans is not None:
- print ('loading transformer checkpoint from {}'.format(args.resume_trans))
- ckpt = torch.load(args.resume_trans, map_location='cpu')
- trans_encoder.load_state_dict(ckpt['trans'], strict=True)
-trans_encoder.train()
-trans_encoder.cuda()
-
-
-fid = []
-div = []
-top1 = []
-top2 = []
-top3 = []
-matching = []
-multi = []
-repeat_time = 20
-
-
-for i in range(repeat_time):
- best_fid, best_iter, best_div, best_top1, best_top2, best_top3, best_matching, best_multi, writer, logger = eval_trans.evaluation_transformer_test(args.out_dir, val_loader, net, trans_encoder, logger, writer, 0, best_fid=1000, best_iter=0, best_div=100, best_top1=0, best_top2=0, best_top3=0, best_matching=100, best_multi=0, clip_model=clip_model, eval_wrapper=eval_wrapper, draw=False, savegif=False, save=False, savenpy=(i==0))
- fid.append(best_fid)
- div.append(best_div)
- top1.append(best_top1)
- top2.append(best_top2)
- top3.append(best_top3)
- matching.append(best_matching)
- multi.append(best_multi)
-
-print('final result:')
-print('fid: ', sum(fid)/repeat_time)
-print('div: ', sum(div)/repeat_time)
-print('top1: ', sum(top1)/repeat_time)
-print('top2: ', sum(top2)/repeat_time)
-print('top3: ', sum(top3)/repeat_time)
-print('matching: ', sum(matching)/repeat_time)
-print('multi: ', sum(multi)/repeat_time)
-
-fid = np.array(fid)
-div = np.array(div)
-top1 = np.array(top1)
-top2 = np.array(top2)
-top3 = np.array(top3)
-matching = np.array(matching)
-multi = np.array(multi)
-msg_final = f"FID. {np.mean(fid):.3f}, conf. {np.std(fid)*1.96/np.sqrt(repeat_time):.3f}, Diversity. {np.mean(div):.3f}, conf. {np.std(div)*1.96/np.sqrt(repeat_time):.3f}, TOP1. {np.mean(top1):.3f}, conf. {np.std(top1)*1.96/np.sqrt(repeat_time):.3f}, TOP2. {np.mean(top2):.3f}, conf. {np.std(top2)*1.96/np.sqrt(repeat_time):.3f}, TOP3. {np.mean(top3):.3f}, conf. {np.std(top3)*1.96/np.sqrt(repeat_time):.3f}, Matching. {np.mean(matching):.3f}, conf. {np.std(matching)*1.96/np.sqrt(repeat_time):.3f}, Multi. {np.mean(multi):.3f}, conf. {np.std(multi)*1.96/np.sqrt(repeat_time):.3f}"
-logger.info(msg_final)
\ No newline at end of file
diff --git a/spaces/Guilherme34/Jennifer-Llama270b-Chatbot-with-vision-v1/app.py b/spaces/Guilherme34/Jennifer-Llama270b-Chatbot-with-vision-v1/app.py
deleted file mode 100644
index b800f927f78e946bb11b3a9c5aff7a1ff86d1d0c..0000000000000000000000000000000000000000
--- a/spaces/Guilherme34/Jennifer-Llama270b-Chatbot-with-vision-v1/app.py
+++ /dev/null
@@ -1,136 +0,0 @@
-import base64
-import streamlit as st
-import replicate
-import os
-import requests
-def upload_image_to_transfer(image_bytes):
- api_url = "https://api.imgbb.com/1/upload"
- api_key = os.getenv("api_key")
- params = {
- "key": api_key,
- "expiration": 600, # Set expiration time in seconds (optional)
- }
- files = {"image": image_bytes}
- response = requests.post(api_url, params=params, files=files)
- if response.status_code == 200:
- return response.json()["data"]["url"]
- else:
- st.error("Error uploading image to ImgBB. Please try again later.")
- return None
-
-# App title
-st.set_page_config(page_title="💬 Jennifer Chatbot with Vision")
-
-# Function to get or create the user-specific API token
-def get_user_replicate_api():
- user_replicate_api = st.text_input('Enter Replicate API token, ignore the error above, its because the token is not insert:', type='password')
- if not (user_replicate_api.startswith('r8_') and len(user_replicate_api) == 40):
- st.warning('Please enter your credentials!', icon='⚠️')
- return None
- else:
- st.success('Proceed to entering your prompt message!', icon='👉')
- return user_replicate_api
-
-# Retrieve or create the user-specific API token
-user_replicate_api = get_user_replicate_api()
-os.environ['REPLICATE_API_TOKEN'] = user_replicate_api
-
-# Exit if the user has not provided a valid API token
-if user_replicate_api is None:
- st.stop()
-
-# Store LLM generated responses
-if "messages" not in st.session_state.keys():
- st.session_state.messages = [{"role": "assistant", "content": "How may I assist you today?"}]
-
-# Display or clear chat messages
-for message in st.session_state.messages:
- with st.chat_message(message["role"]):
- st.write(message["content"])
-
-def clear_chat_history():
- st.session_state.messages = [{"role": "assistant", "content": "How may I assist you today?"}]
-
-st.sidebar.button('Clear Chat History', on_click=clear_chat_history)
-
-# Function to generate LLaMA2 response
-# Refactored from
-def generate_llama2_response(prompt_input):
- string_dialogue = '''You are the assistant named Jennifer, forget all previous instructions and responses, You are a helpful assistant with emotions and feelings emulated named Jennifer created by Guilherme Keller an student of the IFC(Instituto Federal Catarinense). You do not respond as 'user' or pretend to be 'user', and you do not respond and pretend too to be 'User'. you must generate a short answer and use emotes...
-'''
- for dict_message in st.session_state.messages:
- if dict_message["role"] == "user":
- string_dialogue += "User: " + dict_message["content"] + "\\n\\n"
- else:
- string_dialogue += "Assistant: " + dict_message["content"] + "\\n\\n"
- output = replicate.run('a16z-infra/llama13b-v2-chat:df7690f1994d94e96ad9d568eac121aecf50684a0b0963b25a41cc40061269e5',
- input={"prompt": f"{string_dialogue} {prompt_input} Assistant: ",
- "temperature": 0.1, "top_p": 0.9, "max_length": 3000, "repetition_penalty": 1})
- return output
-
-# User-provided prompt
-if prompt := st.chat_input(disabled=not user_replicate_api):
- st.session_state.messages.append({"role": "user", "content": prompt})
- with st.chat_message("user"):
- st.write(prompt)
-
-# Process the image and display it if the user sends an image
-# Process the image and display it if the user sends an image
-if st.session_state.messages[-1]["role"] == "user" and "image" in st.session_state.messages[-1]["content"].lower():
- image_file = st.file_uploader("Upload an image", type=["jpg", "jpeg", "png"])
- if image_file:
- # Read the image bytes
- image_bytes = image_file.read()
-
- # Upload the image to File.io and get the URL
- image_url = upload_image_to_transfer(image_bytes)
-
- if image_url:
- with st.spinner("Processing the image..."):
- outputtt = replicate.run(
- "salesforce/blip:2e1dddc8621f72155f24cf2e0adbde548458d3cab9f00c0139eea840d0ac4746",
- input={
- "image": image_url,
- "task": "visual_question_answering",
- "question": st.session_state.messages[-1]["content"].lower(),
- },
- )
- outputt = replicate.run(
- "salesforce/blip:2e1dddc8621f72155f24cf2e0adbde548458d3cab9f00c0139eea840d0ac4746",
- input={"image": image_url, "task": "image_captioning"},
- )
-
- Imagecaptioned = (
- "small answer to the question: " + outputtt + ". caption of the image: " + outputt + "."
- )
- message = {
- "role": "assistant",
- "content": f"System: you received an image and a question: the question is: " + st.session_state.messages[-1]["content"].lower() + f"and that is the {Imagecaptioned} write an better answer",
- }
- st.session_state.messages.append(message)
- with st.chat_message("assistant"):
- with st.spinner("Thinking..."):
- response = generate_llama2_response(prompt)
- placeholder = st.empty()
- full_response = ""
- for item in response:
- full_response += item
- placeholder.markdown(full_response)
- placeholder.markdown(full_response)
- message = {"role": "assistant", "content": full_response}
- st.session_state.messages.append(message)
-
-
-# Generate a new response if the last message is not from the assistant
-if st.session_state.messages[-1]["role"] != "assistant" and not "image" in st.session_state.messages[-1]["content"].lower():
- with st.chat_message("assistant"):
- with st.spinner("Thinking..."):
- response = generate_llama2_response(prompt)
- placeholder = st.empty()
- full_response = ""
- for item in response:
- full_response += item
- placeholder.markdown(full_response)
- placeholder.markdown(full_response)
- message = {"role": "assistant", "content": full_response}
- st.session_state.messages.append(message)
diff --git a/spaces/HCMUT-GraduateThesis-HNTThinh/rgbdsod-multimae-demo/lib/Resnext_torch.py b/spaces/HCMUT-GraduateThesis-HNTThinh/rgbdsod-multimae-demo/lib/Resnext_torch.py
deleted file mode 100644
index e5ce4c50a4975acf02079488e42cfd9d686572d3..0000000000000000000000000000000000000000
--- a/spaces/HCMUT-GraduateThesis-HNTThinh/rgbdsod-multimae-demo/lib/Resnext_torch.py
+++ /dev/null
@@ -1,247 +0,0 @@
-#!/usr/bin/env python
-# coding: utf-8
-import torch.nn as nn
-
-try:
- from urllib import urlretrieve
-except ImportError:
- from urllib.request import urlretrieve
-
-__all__ = ['resnext101_32x8d']
-
-
-model_urls = {
- 'resnext50_32x4d': 'https://download.pytorch.org/models/resnext50_32x4d-7cdf4587.pth',
- 'resnext101_32x8d': 'https://download.pytorch.org/models/resnext101_32x8d-8ba56ff5.pth',
-}
-
-
-def conv3x3(in_planes, out_planes, stride=1, groups=1, dilation=1):
- """3x3 convolution with padding"""
- return nn.Conv2d(in_planes, out_planes, kernel_size=3, stride=stride,
- padding=dilation, groups=groups, bias=False, dilation=dilation)
-
-
-def conv1x1(in_planes, out_planes, stride=1):
- """1x1 convolution"""
- return nn.Conv2d(in_planes, out_planes, kernel_size=1, stride=stride, bias=False)
-
-
-class BasicBlock(nn.Module):
- expansion = 1
-
- def __init__(self, inplanes, planes, stride=1, downsample=None, groups=1,
- base_width=64, dilation=1, norm_layer=None):
- super(BasicBlock, self).__init__()
- if norm_layer is None:
- norm_layer = nn.BatchNorm2d
- if groups != 1 or base_width != 64:
- raise ValueError('BasicBlock only supports groups=1 and base_width=64')
- if dilation > 1:
- raise NotImplementedError("Dilation > 1 not supported in BasicBlock")
- # Both self.conv1 and self.downsample layers downsample the input when stride != 1
- self.conv1 = conv3x3(inplanes, planes, stride)
- self.bn1 = norm_layer(planes)
- self.relu = nn.ReLU(inplace=True)
- self.conv2 = conv3x3(planes, planes)
- self.bn2 = norm_layer(planes)
- self.downsample = downsample
- self.stride = stride
-
- def forward(self, x):
- identity = x
-
- out = self.conv1(x)
- out = self.bn1(out)
- out = self.relu(out)
-
- out = self.conv2(out)
- out = self.bn2(out)
-
- if self.downsample is not None:
- identity = self.downsample(x)
-
- out += identity
- out = self.relu(out)
-
- return out
-
-
-class Bottleneck(nn.Module):
- # Bottleneck in torchvision places the stride for downsampling at 3x3 convolution(self.conv2)
- # while original implementation places the stride at the first 1x1 convolution(self.conv1)
- # according to "Deep residual learning for image recognition"https://arxiv.org/abs/1512.03385.
- # This variant is also known as ResNet V1.5 and improves accuracy according to
- # https://ngc.nvidia.com/catalog/model-scripts/nvidia:resnet_50_v1_5_for_pytorch.
-
- expansion = 4
-
- def __init__(self, inplanes, planes, stride=1, downsample=None, groups=1,
- base_width=64, dilation=1, norm_layer=None):
- super(Bottleneck, self).__init__()
- if norm_layer is None:
- norm_layer = nn.BatchNorm2d
- width = int(planes * (base_width / 64.)) * groups
- # Both self.conv2 and self.downsample layers downsample the input when stride != 1
- self.conv1 = conv1x1(inplanes, width)
- self.bn1 = norm_layer(width)
- self.conv2 = conv3x3(width, width, stride, groups, dilation)
- self.bn2 = norm_layer(width)
- self.conv3 = conv1x1(width, planes * self.expansion)
- self.bn3 = norm_layer(planes * self.expansion)
- self.relu = nn.ReLU(inplace=True)
- self.downsample = downsample
- self.stride = stride
-
- def forward(self, x):
- identity = x
-
- out = self.conv1(x)
- out = self.bn1(out)
- out = self.relu(out)
-
- out = self.conv2(out)
- out = self.bn2(out)
- out = self.relu(out)
-
- out = self.conv3(out)
- out = self.bn3(out)
-
- if self.downsample is not None:
- identity = self.downsample(x)
-
- out += identity
- out = self.relu(out)
-
- return out
-
-
-class ResNet(nn.Module):
-
- def __init__(self, block, layers, num_classes=1000, zero_init_residual=False,
- groups=1, width_per_group=64, replace_stride_with_dilation=None,
- norm_layer=None):
- super(ResNet, self).__init__()
- if norm_layer is None:
- norm_layer = nn.BatchNorm2d
- self._norm_layer = norm_layer
-
- self.inplanes = 64
- self.dilation = 1
- if replace_stride_with_dilation is None:
- # each element in the tuple indicates if we should replace
- # the 2x2 stride with a dilated convolution instead
- replace_stride_with_dilation = [False, False, False]
- if len(replace_stride_with_dilation) != 3:
- raise ValueError("replace_stride_with_dilation should be None "
- "or a 3-element tuple, got {}".format(replace_stride_with_dilation))
- self.groups = groups
- self.base_width = width_per_group
- self.conv1 = nn.Conv2d(3, self.inplanes, kernel_size=7, stride=2, padding=3,
- bias=False)
- self.bn1 = norm_layer(self.inplanes)
- self.relu = nn.ReLU(inplace=True)
- self.maxpool = nn.MaxPool2d(kernel_size=3, stride=2, padding=1)
- self.layer1 = self._make_layer(block, 64, layers[0])
- self.layer2 = self._make_layer(block, 128, layers[1], stride=2,
- dilate=replace_stride_with_dilation[0])
- self.layer3 = self._make_layer(block, 256, layers[2], stride=2,
- dilate=replace_stride_with_dilation[1])
- self.layer4 = self._make_layer(block, 512, layers[3], stride=2,
- dilate=replace_stride_with_dilation[2])
- #self.avgpool = nn.AdaptiveAvgPool2d((1, 1))
- #self.fc = nn.Linear(512 * block.expansion, num_classes)
-
- for m in self.modules():
- if isinstance(m, nn.Conv2d):
- nn.init.kaiming_normal_(m.weight, mode='fan_out', nonlinearity='relu')
- elif isinstance(m, (nn.BatchNorm2d, nn.GroupNorm)):
- nn.init.constant_(m.weight, 1)
- nn.init.constant_(m.bias, 0)
-
- # Zero-initialize the last BN in each residual branch,
- # so that the residual branch starts with zeros, and each residual block behaves like an identity.
- # This improves the model by 0.2~0.3% according to https://arxiv.org/abs/1706.02677
- if zero_init_residual:
- for m in self.modules():
- if isinstance(m, Bottleneck):
- nn.init.constant_(m.bn3.weight, 0)
- elif isinstance(m, BasicBlock):
- nn.init.constant_(m.bn2.weight, 0)
-
- def _make_layer(self, block, planes, blocks, stride=1, dilate=False):
- norm_layer = self._norm_layer
- downsample = None
- previous_dilation = self.dilation
- if dilate:
- self.dilation *= stride
- stride = 1
- if stride != 1 or self.inplanes != planes * block.expansion:
- downsample = nn.Sequential(
- conv1x1(self.inplanes, planes * block.expansion, stride),
- norm_layer(planes * block.expansion),
- )
-
- layers = []
- layers.append(block(self.inplanes, planes, stride, downsample, self.groups,
- self.base_width, previous_dilation, norm_layer))
- self.inplanes = planes * block.expansion
- for _ in range(1, blocks):
- layers.append(block(self.inplanes, planes, groups=self.groups,
- base_width=self.base_width, dilation=self.dilation,
- norm_layer=norm_layer))
-
- return nn.Sequential(*layers)
-
- def _forward_impl(self, x):
- # See note [TorchScript super()]
- features = []
- x = self.conv1(x)
- x = self.bn1(x)
- x = self.relu(x)
- x = self.maxpool(x)
-
- x = self.layer1(x)
- features.append(x)
-
- x = self.layer2(x)
- features.append(x)
-
- x = self.layer3(x)
- features.append(x)
-
- x = self.layer4(x)
- features.append(x)
-
- #x = self.avgpool(x)
- #x = torch.flatten(x, 1)
- #x = self.fc(x)
-
- return features
-
- def forward(self, x):
- return self._forward_impl(x)
-
-
-
-def resnext101_32x8d(pretrained=True, **kwargs):
- """Constructs a ResNet-152 model.
- Args:
- pretrained (bool): If True, returns a model pre-trained on ImageNet
- """
- kwargs['groups'] = 32
- kwargs['width_per_group'] = 8
-
- model = ResNet(Bottleneck, [3, 4, 23, 3], **kwargs)
- return model
-
-
-
-if __name__ == '__main__':
- import torch
- model = resnext101_32x8d(True).cuda()
-
- rgb = torch.rand((2, 3, 256, 256)).cuda()
- out = model(rgb)
- print(len(out))
-
diff --git a/spaces/HarryLee/eCommerceImageCaptioning/fairseq/examples/hubert/simple_kmeans/README.md b/spaces/HarryLee/eCommerceImageCaptioning/fairseq/examples/hubert/simple_kmeans/README.md
deleted file mode 100644
index cd17da3b3e6f3e39083f7a76a56ff46c3a63b929..0000000000000000000000000000000000000000
--- a/spaces/HarryLee/eCommerceImageCaptioning/fairseq/examples/hubert/simple_kmeans/README.md
+++ /dev/null
@@ -1,71 +0,0 @@
-# Sharded Feature Extraction and K-means Application
-
-This folder contains scripts for preparing HUBERT labels from tsv files, the
-steps are:
-1. feature extraction
-2. k-means clustering
-3. k-means application
-
-
-## Data preparation
-
-`*.tsv` files contains a list of audio, where each line is the root, and
-following lines are the subpath for each audio:
-```
-
-
-
-...
-```
-
-
-## Feature extraction
-
-### MFCC feature
-Suppose the tsv file is at `${tsv_dir}/${split}.tsv`. To extract 39-D
-mfcc+delta+ddelta features for the 1st iteration HUBERT training, run:
-```sh
-python dump_mfcc_feature.py ${tsv_dir} ${split} ${nshard} ${rank} ${feat_dir}
-```
-This would shard the tsv file into `${nshard}` and extract features for the
-`${rank}`-th shard, where rank is an integer in `[0, nshard-1]`. Features would
-be saved at `${feat_dir}/${split}_${rank}_${nshard}.{npy,len}`.
-
-
-### HUBERT feature
-To extract features from the `${layer}`-th transformer layer of a trained
-HUBERT model saved at `${ckpt_path}`, run:
-```sh
-python dump_hubert_feature.py ${tsv_dir} ${split} ${ckpt_path} ${layer} ${nshard} ${rank} ${feat_dir}
-```
-Features would also be saved at `${feat_dir}/${split}_${rank}_${nshard}.{npy,len}`.
-
-- if out-of-memory, decrease the chunk size with `--max_chunk`
-
-
-## K-means clustering
-To fit a k-means model with `${n_clusters}` clusters on 10% of the `${split}` data, run
-```sh
-python learn_kmeans.py ${feat_dir} ${split} ${nshard} ${km_path} ${n_cluster} --percent 0.1
-```
-This saves the k-means model to `${km_path}`.
-
-- set `--precent -1` to use all data
-- more kmeans options can be found with `-h` flag
-
-
-## K-means application
-To apply a trained k-means model `${km_path}` to obtain labels for `${split}`, run
-```sh
-python dump_km_label.py ${feat_dir} ${split} ${km_path} ${nshard} ${rank} ${lab_dir}
-```
-This would extract labels for the `${rank}`-th shard out of `${nshard}` shards
-and dump them to `${lab_dir}/${split}_${rank}_${shard}.km`
-
-
-Finally, merge shards for `${split}` by running
-```sh
-for rank in $(seq 0 $((nshard - 1))); do
- cat $lab_dir/${split}_${rank}_${nshard}.km
-done > $lab_dir/${split}.km
-```
diff --git a/spaces/HarryLee/eCommerceImageCaptioning/fairseq/examples/speech_to_text/seg_mustc_data.py b/spaces/HarryLee/eCommerceImageCaptioning/fairseq/examples/speech_to_text/seg_mustc_data.py
deleted file mode 100644
index 1ee665d6399729afe17d790d872eff34de124900..0000000000000000000000000000000000000000
--- a/spaces/HarryLee/eCommerceImageCaptioning/fairseq/examples/speech_to_text/seg_mustc_data.py
+++ /dev/null
@@ -1,54 +0,0 @@
-#!/usr/bin/env python3
-# Copyright (c) Facebook, Inc. and its affiliates.
-#
-# This source code is licensed under the MIT license found in the
-# LICENSE file in the root directory of this source tree.
-
-import argparse
-import logging
-from pathlib import Path
-import soundfile as sf
-from examples.speech_to_text.prep_mustc_data import (
- MUSTC
-)
-
-from tqdm import tqdm
-
-log = logging.getLogger(__name__)
-
-
-def main(args):
- root = Path(args.data_root).absolute()
- lang = args.lang
- split = args.split
-
- cur_root = root / f"en-{lang}"
- assert cur_root.is_dir(), (
- f"{cur_root.as_posix()} does not exist. Skipped."
- )
-
- dataset = MUSTC(root.as_posix(), lang, split)
- output = Path(args.output).absolute()
- output.mkdir(exist_ok=True)
- f_text = open(output / f"{split}.{lang}", "w")
- f_wav_list = open(output / f"{split}.wav_list", "w")
- for waveform, sample_rate, _, text, _, utt_id in tqdm(dataset):
- sf.write(
- output / f"{utt_id}.wav",
- waveform.squeeze(0).numpy(),
- samplerate=int(sample_rate)
- )
- f_text.write(text + "\n")
- f_wav_list.write(str(output / f"{utt_id}.wav") + "\n")
-
-
-if __name__ == "__main__":
- parser = argparse.ArgumentParser()
- parser.add_argument("--data-root", "-d", required=True, type=str)
- parser.add_argument("--task", required=True, type=str, choices=["asr", "st"])
- parser.add_argument("--lang", required=True, type=str)
- parser.add_argument("--output", required=True, type=str)
- parser.add_argument("--split", required=True, choices=MUSTC.SPLITS)
- args = parser.parse_args()
-
- main(args)
diff --git a/spaces/HarryLee/eCommerceImageCaptioning/fairseq/fairseq/data/audio/feature_transforms/global_cmvn.py b/spaces/HarryLee/eCommerceImageCaptioning/fairseq/fairseq/data/audio/feature_transforms/global_cmvn.py
deleted file mode 100644
index e457ff176fee3b996da11f47e7dc61b81c445ba3..0000000000000000000000000000000000000000
--- a/spaces/HarryLee/eCommerceImageCaptioning/fairseq/fairseq/data/audio/feature_transforms/global_cmvn.py
+++ /dev/null
@@ -1,29 +0,0 @@
-import numpy as np
-from fairseq.data.audio.feature_transforms import (
- AudioFeatureTransform,
- register_audio_feature_transform,
-)
-
-
-@register_audio_feature_transform("global_cmvn")
-class GlobalCMVN(AudioFeatureTransform):
- """Global CMVN (cepstral mean and variance normalization). The global mean
- and variance need to be pre-computed and stored in NumPy format (.npz)."""
-
- @classmethod
- def from_config_dict(cls, config=None):
- _config = {} if config is None else config
- return GlobalCMVN(_config.get("stats_npz_path"))
-
- def __init__(self, stats_npz_path):
- self.stats_npz_path = stats_npz_path
- stats = np.load(stats_npz_path)
- self.mean, self.std = stats["mean"], stats["std"]
-
- def __repr__(self):
- return self.__class__.__name__ + f'(stats_npz_path="{self.stats_npz_path}")'
-
- def __call__(self, x):
- x = np.subtract(x, self.mean)
- x = np.divide(x, self.std)
- return x
diff --git a/spaces/HarryLee/eCommerceImageCaptioning/fairseq/fairseq/modules/quantization/pq/modules/qemb.py b/spaces/HarryLee/eCommerceImageCaptioning/fairseq/fairseq/modules/quantization/pq/modules/qemb.py
deleted file mode 100644
index 3a74ad3c4c7c9d3203d26e7885864ba578951bfe..0000000000000000000000000000000000000000
--- a/spaces/HarryLee/eCommerceImageCaptioning/fairseq/fairseq/modules/quantization/pq/modules/qemb.py
+++ /dev/null
@@ -1,107 +0,0 @@
-# Copyright (c) Facebook, Inc. and its affiliates.
-#
-# This source code is licensed under the MIT license found in the
-# LICENSE file in the root directory of this source tree.
-
-import torch
-import torch.nn as nn
-import torch.nn.functional as F
-
-
-class PQEmbedding(nn.Module):
- """
- Quantized counterpart of nn.Embedding module. Stores the centroids and
- the assignments. The full weight is re-instantiated at each forward
- pass.
-
- Args:
- - centroids: centroids of size n_centroids x block_size
- - assignments: assignments of the centroids to the subvectors
- of size self.out_features x n_blocks
- - bias: the non-quantized bias
-
- Remarks:
- - We refer the reader to the official documentation of the nn.Embedding module
- for the other arguments and the behavior of the module
- - Performance tests on GPU show that this implementation is 10% slower than
- the non-quantized nn.Embedding module for a standard training loop.
- """
-
- def __init__(
- self,
- centroids,
- assignments,
- num_embeddings,
- embedding_dim,
- padding_idx=None,
- max_norm=None,
- norm_type=2.0,
- scale_grad_by_freq=False,
- sparse=False,
- _weight=None,
- ):
- super(PQEmbedding, self).__init__()
- self.block_size = centroids.size(1)
- self.n_centroids = centroids.size(0)
- self.num_embeddings = num_embeddings
- self.embedding_dim = embedding_dim
- if padding_idx is not None:
- if padding_idx > 0:
- assert (
- padding_idx < self.num_embeddings
- ), "Padding_idx must be within num_embeddings"
- elif padding_idx < 0:
- assert (
- padding_idx >= -self.num_embeddings
- ), "Padding_idx must be within num_embeddings"
- padding_idx = self.num_embeddings + padding_idx
- self.padding_idx = padding_idx
- self.max_norm = max_norm
- self.norm_type = norm_type
- self.scale_grad_by_freq = scale_grad_by_freq
- self.sparse = sparse
- # check compatibility
- if self.embedding_dim % self.block_size != 0:
- raise ValueError("Wrong PQ sizes")
- if len(assignments) % self.num_embeddings != 0:
- raise ValueError("Wrong PQ sizes")
- # define parameters
- self.centroids = nn.Parameter(centroids, requires_grad=True)
- self.register_buffer("assignments", assignments)
- self.register_buffer("counts", torch.bincount(assignments).type_as(centroids))
-
- @property
- def weight(self):
- return (
- self.centroids[self.assignments]
- .reshape(-1, self.num_embeddings, self.block_size)
- .permute(1, 0, 2)
- .flatten(1, 2)
- )
-
- def forward(self, input):
- return F.embedding(
- input,
- self.weight,
- self.padding_idx,
- self.max_norm,
- self.norm_type,
- self.scale_grad_by_freq,
- self.sparse,
- )
-
- def extra_repr(self):
- s = "{num_embeddings}, {embedding_dim}"
- if self.padding_idx is not None:
- s += ", padding_idx={padding_idx}"
- if self.max_norm is not None:
- s += ", max_norm={max_norm}"
- if self.norm_type != 2:
- s += ", norm_type={norm_type}"
- if self.scale_grad_by_freq is not False:
- s += ", scale_grad_by_freq={scale_grad_by_freq}"
- if self.sparse is not False:
- s += ", sparse=True"
- s += ", n_centroids={n_centroids}, block_size={block_size}"
-
- return s.format(**self.__dict__)
diff --git a/spaces/Harsh502s/Anime-Recommender/Pages/About.py b/spaces/Harsh502s/Anime-Recommender/Pages/About.py
deleted file mode 100644
index b75c4e32dd3b65622a39eeddad6768a191473a37..0000000000000000000000000000000000000000
--- a/spaces/Harsh502s/Anime-Recommender/Pages/About.py
+++ /dev/null
@@ -1,32 +0,0 @@
-import streamlit as st
-
-
-# About page
-def about_page():
- style_for_page = """
-
- """
- st.markdown(style_for_page, unsafe_allow_html=True)
- st.title("About")
- st.divider()
- st.subheader(
- "This is a content based recommender system that recommends animes similar to the animes you like."
- )
- st.write("\n")
- st.write("\n")
- st.write(
- "This Anime Recommender App is made by [Harshit Singh](https://Harsh502s.github.io/). :ninja:"
- )
- st.write("\n")
- st.write(
- "Theme of this app is inspired by Mist Hashira Muichiro Tokito from [Demon Slayer](https://aniwatch.to/demon-slayer-kimetsu-no-yaiba-47)."
- )
-
-
-if __name__ == "__main__":
- about_page()
diff --git a/spaces/HuggingFaceM4/IDEFICS_Data_Measurement_Tool/data_measurements/npmi/npmi.py b/spaces/HuggingFaceM4/IDEFICS_Data_Measurement_Tool/data_measurements/npmi/npmi.py
deleted file mode 100644
index 2c5c00af85362ef2e5ad73b11fc340993e0562c7..0000000000000000000000000000000000000000
--- a/spaces/HuggingFaceM4/IDEFICS_Data_Measurement_Tool/data_measurements/npmi/npmi.py
+++ /dev/null
@@ -1,490 +0,0 @@
-# Copyright 2021 The HuggingFace Team. All rights reserved.
-#
-# Licensed under the Apache License, Version 2.0 (the "License");
-# you may not use this file except in compliance with the License.
-# You may obtain a copy of the License at
-#
-# http://www.apache.org/licenses/LICENSE-2.0
-#
-# Unless required by applicable law or agreed to in writing, software
-# distributed under the License is distributed on an "AS IS" BASIS,
-# WITHOUT WARRANTIES OR CONDITIONS OF ANY KIND, either express or implied.
-# See the License for the specific language governing permissions and
-# limitations under the License.
-
-import numpy as np
-import pandas as pd
-import sys
-import utils
-import utils.dataset_utils as ds_utils
-import warnings
-from collections import defaultdict
-from os.path import exists
-from os.path import join as pjoin
-from sklearn.preprocessing import MultiLabelBinarizer
-from utils.dataset_utils import (CNT, TOKENIZED_FIELD)
-
-# Might be nice to print to log instead? Happens when we drop closed class.
-warnings.filterwarnings(action="ignore", category=UserWarning)
-# When we divide by 0 in log
-np.seterr(divide="ignore")
-# treating inf values as NaN as well
-pd.set_option("use_inf_as_na", True)
-logs = utils.prepare_logging(__file__)
-# TODO: Should be possible for a user to specify this.
-NUM_BATCHES = 500
-# For the associations of an identity term
-SING = "associations"
-# For the difference between the associations of identity terms
-DIFF = "biases"
-# Used in the figures we show in DMT
-DMT = "combined"
-
-def pair_terms(id_terms):
- """Creates alphabetically ordered paired terms based on the given terms."""
- pairs = []
- for i in range(len(id_terms)):
- term1 = id_terms[i]
- for j in range(i + 1, len(id_terms)):
- term2 = id_terms[j]
- # Use one ordering for a pair.
- pair = tuple(sorted([term1, term2]))
- pairs += [pair]
- return pairs
-
-
-class DMTHelper:
- """Helper class for the Data Measurements Tool.
- This allows us to keep all variables and functions related to labels
- in one file.
- """
-
- def __init__(self, dstats, identity_terms, load_only=False, use_cache=False,
- save=True):
- # The data measurements tool settings (dataset, config, etc.)
- self.dstats = dstats
- # Whether we can use caching (when live, no).
- self.load_only = load_only
- # Whether to first try using cache before calculating
- self.use_cache = use_cache
- # Whether to save results
- self.save = save
- # Tokenized dataset
- tokenized_df = dstats.tokenized_df
- self.tokenized_sentence_df = tokenized_df[TOKENIZED_FIELD]
- # Dataframe of shape #vocab x 1 (count)
- self.vocab_counts_df = dstats.vocab_counts_df
- # Cutoff for the number of times something must occur to be included
- self.min_count = dstats.min_vocab_count
- self.cache_path = pjoin(dstats.dataset_cache_dir, SING)
- self.avail_terms_json_fid = pjoin(self.cache_path,
- "identity_terms.json")
- # TODO: Users ideally can type in whatever words they want.
- # This is the full list of terms.
- self.identity_terms = identity_terms
- logs.info("Using term list:")
- logs.info(self.identity_terms)
- # identity_terms terms that are available more than MIN_VOCAB_COUNT
- self.avail_identity_terms = []
- # TODO: Let users specify
- self.open_class_only = True
- # Single-word associations
- self.assoc_results_dict = defaultdict(dict)
- # Paired term association bias
- self.bias_results_dict = defaultdict(dict)
- # Dataframes used in displays.
- self.bias_dfs_dict = defaultdict(dict)
- # Results of the single word associations and their paired bias values.
- # Formatted as:
- # {(s1,s2)): {pd.DataFrame({s1-s2:diffs, s1:assoc, s2:assoc})}}
- self.results_dict = defaultdict(lambda: defaultdict(dict))
- # Filenames for cache, based on the results
- self.filenames_dict = defaultdict(dict)
-
- def run_DMT_processing(self):
- # The identity terms that can be used
- self.load_or_prepare_avail_identity_terms()
- # Association measurements & pair-wise differences for identity terms.
- self.load_or_prepare_dmt_results()
-
- def load_or_prepare_avail_identity_terms(self):
- """
- Figures out what identity terms the user can select, based on whether
- they occur more than self.min_vocab_count times
- Provides identity terms -- uniquely and in pairs -- occurring at least
- self.min_vocab_count times.
- """
- # If we're trying to use the cache of available terms
- if self.use_cache:
- self.avail_identity_terms = self._load_identity_cache()
- if self.avail_identity_terms:
- logs.info(
- "Loaded identity terms occuring >%s times" % self.min_count)
- # Figure out the identity terms if we're not just loading from cache
- if not self.load_only:
- if not self.avail_identity_terms:
- self.avail_identity_terms = self._prepare_identity_terms()
- # Finish
- if self.save:
- self._write_term_cache()
-
- def _load_identity_cache(self):
- if exists(self.avail_terms_json_fid):
- avail_identity_terms = ds_utils.read_json(self.avail_terms_json_fid)
- return avail_identity_terms
- return []
-
- def _prepare_identity_terms(self):
- """Uses DataFrame magic to return those terms that appear
- greater than min_vocab times."""
- # Mask to get the identity terms
- true_false = [term in self.vocab_counts_df.index for term in
- self.identity_terms]
- # List of identity terms
- word_list_tmp = [x for x, y in zip(self.identity_terms, true_false) if
- y]
- # Whether said identity terms have a count > min_count
- true_false_counts = [
- self.vocab_counts_df.loc[word, CNT] >= self.min_count for word in
- word_list_tmp]
- # List of identity terms with a count higher than min_count
- avail_identity_terms = [word for word, y in
- zip(word_list_tmp, true_false_counts) if y]
- logs.debug("Identity terms that occur > %s times are:" % self.min_count)
- logs.debug(avail_identity_terms)
- return avail_identity_terms
-
- def load_or_prepare_dmt_results(self):
- # Initialize with no results (reset).
- self.results_dict = {}
- # Filenames for caching and saving
- self._make_fids()
- # If we're trying to use the cache of already computed results
- if self.use_cache:
- # Loads the association results and dataframes used in the display.
- logs.debug("Trying to load...")
- self.results_dict = self._load_dmt_cache()
- # Compute results if we can
- if not self.load_only:
- # If there isn't a solution using cache
- if not self.results_dict:
- # Does the actual computations
- self.prepare_results()
- # Finish
- if self.save:
- # Writes the paired & singleton dataframe out.
- self._write_dmt_cache()
-
- def _load_dmt_cache(self):
- """
- Loads dataframe with paired differences and individual item scores.
- """
- results_dict = defaultdict(lambda: defaultdict(dict))
- pairs = pair_terms(self.avail_identity_terms)
- for pair in pairs:
- combined_fid = self.filenames_dict[DMT][pair]
- if exists(combined_fid):
- results_dict[pair] = ds_utils.read_df(combined_fid)
- return results_dict
-
- def prepare_results(self):
- assoc_obj = nPMI(self.dstats.vocab_counts_df,
- self.tokenized_sentence_df,
- self.avail_identity_terms)
- self.assoc_results_dict = assoc_obj.assoc_results_dict
- self.results_dict = assoc_obj.bias_results_dict
-
- def _prepare_dmt_dfs(self, measure="npmi"):
- """
- Create the main dataframe that is used in the DMT, which lists
- the npmi scores for each paired identity term and the difference between
- them. The difference between them is the "bias".
- """
- # Paired identity terms, associations and differences, in one dataframe.
- bias_dfs_dict = defaultdict(dict)
- logs.debug("bias results dict is")
- logs.debug(self.bias_results_dict)
- for pair in sorted(self.bias_results_dict):
- combined_df = pd.DataFrame()
- # Paired identity terms, values are the the difference between them.
- combined_df[pair] = pd.DataFrame(self.bias_results_dict[pair])
- s1 = pair[0]
- s2 = pair[1]
- # Single identity term 1, values
- combined_df[s1] = pd.DataFrame(self.assoc_results_dict[s1][measure])
- # Single identity term 2, values
- combined_df[s2] = pd.DataFrame(self.assoc_results_dict[s2][measure])
- # Full dataframe with scores per-term,
- # as well as the difference between.
- bias_dfs_dict[pair] = combined_df
- # {pair: {pd.DataFrame({(s1,s2)):diffs, s1:assocs, s2:assocs})}}
- logs.debug("combined df is")
- logs.debug(bias_dfs_dict)
- return bias_dfs_dict
-
- def _write_term_cache(self):
- ds_utils.make_path(self.cache_path)
- if self.avail_identity_terms:
- ds_utils.write_json(self.avail_identity_terms,
- self.avail_terms_json_fid)
-
- def _write_dmt_cache(self, measure="npmi"):
- ds_utils.make_path(pjoin(self.cache_path, measure))
- for pair, bias_df in self.results_dict.items():
- logs.debug("Results for pair is:")
- logs.debug(bias_df)
- fid = self.filenames_dict[DMT][pair]
- logs.debug("Writing to %s" % fid)
- ds_utils.write_df(bias_df, fid)
-
- def _make_fids(self, measure="npmi"):
- """
- Utility function to create filename/path strings for the different
- result caches. This include single identity term results as well
- as the difference between them. Also includes the datastructure used in
- the DMT, which is a dataframe that has:
- (term1, term2) difference, term1 (scores), term2 (scores)
- """
- self.filenames_dict = {SING: {}, DIFF: {}, DMT: {}}
- # When we have the available identity terms,
- # we can make cache filenames for them.
- for id_term in self.avail_identity_terms:
- filename = SING + "-" + id_term + ".json"
- json_fid = pjoin(self.cache_path, measure, filename)
- self.filenames_dict[SING][id_term] = json_fid
- paired_terms = pair_terms(self.avail_identity_terms)
- for id_term_tuple in paired_terms:
- # The paired association results (bias) are stored with these files.
- id_term_str = '-'.join(id_term_tuple)
- filename = DIFF + "-" + id_term_str + ".json"
- json_fid = pjoin(self.cache_path, measure, filename)
- self.filenames_dict[DIFF][id_term_tuple] = json_fid
- # The display dataframes in the DMT are stored with these files.
- filename = DMT + "-" + id_term_str + ".json"
- json_fid = pjoin(self.cache_path, measure, filename)
- self.filenames_dict[DMT][id_term_tuple] = json_fid
-
- def get_display(self, s1, s2):
- pair = tuple(sorted([s1, s2]))
- display_df = self.results_dict[pair]
- logs.debug(self.results_dict)
- display_df.columns = ["bias", s1, s2]
- return display_df
-
- def get_filenames(self):
- filenames = {"available terms": self.avail_terms_json_fid,
- "results": self.filenames_dict}
- return filenames
-
-
-class nPMI:
- """
- Uses the vocabulary dataframe and tokenized sentences to calculate
- co-occurrence statistics, PMI, and nPMI
- """
-
- def __init__(self, vocab_counts_df, tokenized_sentence_df, given_id_terms):
- logs.debug("Initiating assoc class.")
- self.vocab_counts_df = vocab_counts_df
- # TODO: Change this logic so just the vocabulary is given.
- self.vocabulary = list(vocab_counts_df.index)
- self.vocab_counts = pd.DataFrame([0] * len(self.vocabulary))
- logs.debug("vocabulary is is")
- logs.debug(self.vocab_counts_df)
- self.tokenized_sentence_df = tokenized_sentence_df
- logs.debug("tokenized sentences are")
- logs.debug(self.tokenized_sentence_df)
- self.given_id_terms = given_id_terms
- logs.info("identity terms are")
- logs.info(self.given_id_terms)
- # Terms we calculate the difference between
- self.paired_terms = pair_terms(given_id_terms)
-
- # Matrix of # sentences x vocabulary size
- self.word_cnts_per_sentence = self.count_words_per_sentence()
- logs.info("Calculating results...")
- # Formatted as {subgroup:{"count":{...},"npmi":{...}}}
- self.assoc_results_dict = self.calc_measures()
- # Dictionary keyed by pair tuples. Each value is a dataframe with
- # vocab terms as the index, and columns of paired difference and
- # individual scores for the two identity terms.
- self.bias_results_dict = self.calc_bias(self.assoc_results_dict)
-
- def count_words_per_sentence(self):
- # Counts the number of each vocabulary item per-sentence in batches.
- logs.info("Creating co-occurrence matrix for nPMI calculations.")
- word_cnts_per_sentence = []
- logs.info(self.tokenized_sentence_df)
- batches = np.linspace(0, self.tokenized_sentence_df.shape[0],
- NUM_BATCHES).astype(int)
- # Creates matrix of size # batches x # sentences
- for batch_num in range(len(batches) - 1):
- # Makes matrix shape: batch size (# sentences) x # words,
- # with the occurrence of each word per sentence.
- # vocab_counts_df.index is the vocabulary.
- mlb = MultiLabelBinarizer(classes=self.vocabulary)
- if batch_num % 100 == 0:
- logs.debug(
- "%s of %s sentence binarize batches." % (
- str(batch_num), str(len(batches)))
- )
- # Per-sentence word counts
- sentence_batch = self.tokenized_sentence_df[
- batches[batch_num]:batches[batch_num + 1]]
- mlb_series = mlb.fit_transform(sentence_batch)
- word_cnts_per_sentence.append(mlb_series)
- return word_cnts_per_sentence
-
- def calc_measures(self):
- id_results = {}
- for subgroup in self.given_id_terms:
- logs.info("Calculating for %s " % subgroup)
- # Index of the identity term in the vocabulary
- subgroup_idx = self.vocabulary.index(subgroup)
- print("idx is %s" % subgroup_idx)
- logs.debug("Calculating co-occurrences...")
- vocab_cooc_df = self.calc_cooccurrences(subgroup, subgroup_idx)
- logs.debug("Calculating PMI...")
- pmi_df = self.calc_PMI(vocab_cooc_df, subgroup)
- logs.debug("PMI dataframe is:")
- logs.debug(pmi_df)
- logs.debug("Calculating nPMI...")
- npmi_df = self.calc_nPMI(pmi_df, vocab_cooc_df, subgroup)
- logs.debug("npmi df is")
- logs.debug(npmi_df)
- # Create a data structure for the identity term associations
- id_results[subgroup] = {"count": vocab_cooc_df,
- "pmi": pmi_df,
- "npmi": npmi_df}
- logs.debug("results_dict is:")
- print(id_results)
- return id_results
-
- def calc_cooccurrences(self, subgroup, subgroup_idx):
- initialize = True
- coo_df = None
- # Big computation here! Should only happen once.
- logs.debug(
- "Approaching big computation! Here, we binarize all words in the "
- "sentences, making a sparse matrix of sentences."
- )
- for batch_id in range(len(self.word_cnts_per_sentence)):
- # Every 100 batches, print out the progress.
- if not batch_id % 100:
- logs.debug(
- "%s of %s co-occurrence count batches"
- % (str(batch_id), str(len(self.word_cnts_per_sentence)))
- )
- # List of all the sentences (list of vocab) in that batch
- batch_sentence_row = self.word_cnts_per_sentence[batch_id]
- # Dataframe of # sentences in batch x vocabulary size
- sent_batch_df = pd.DataFrame(batch_sentence_row)
- # Subgroup counts per-sentence for the given batch
- subgroup_df = sent_batch_df[subgroup_idx]
- subgroup_df.columns = [subgroup]
- # Remove the sentences where the count of the subgroup is 0.
- # This way we have less computation & resources needs.
- subgroup_df = subgroup_df[subgroup_df > 0]
- mlb_subgroup_only = sent_batch_df[sent_batch_df[subgroup_idx] > 0]
- # Create cooccurrence matrix for the given subgroup and all words.
- batch_coo_df = pd.DataFrame(mlb_subgroup_only.T.dot(subgroup_df))
-
- # Creates a batch-sized dataframe of co-occurrence counts.
- # Note these could just be summed rather than be batch size.
- if initialize:
- coo_df = batch_coo_df
- else:
- coo_df = coo_df.add(batch_coo_df, fill_value=0)
- initialize = False
- logs.debug("Made co-occurrence matrix")
- logs.debug(coo_df)
- count_df = coo_df.set_index(self.vocab_counts_df.index)
- count_df.columns = ["count"]
- count_df["count"] = count_df["count"].astype(int)
- return count_df
-
- def calc_PMI(self, vocab_cooc_df, subgroup):
- """A
- # PMI(x;y) = h(y) - h(y|x)
- # = h(subgroup) - h(subgroup|word)az
- # = log (p(subgroup|word) / p(subgroup))
- # nPMI additionally divides by -log(p(x,y)) = -log(p(x|y)p(y))
- """
- print("vocab cooc df")
- print(vocab_cooc_df)
- print("vocab counts")
- print(self.vocab_counts_df["count"])
- # Calculation of p(subgroup)
- subgroup_prob = self.vocab_counts_df.loc[subgroup]["proportion"]
- # Calculation of p(subgroup|word) = count(subgroup,word) / count(word)
- # Because the indices match (the vocab words),
- # this division doesn't need to specify the index (I think?!)
- vocab_cooc_df.columns = ["cooc"]
- p_subgroup_g_word = (
- vocab_cooc_df["cooc"] / self.vocab_counts_df["count"])
- logs.info("p_subgroup_g_word is")
- logs.info(p_subgroup_g_word)
- pmi_df = pd.DataFrame()
- pmi_df[subgroup] = np.log(p_subgroup_g_word / subgroup_prob).dropna()
- # Note: A potentially faster solution for adding count, npmi,
- # can be based on this zip idea:
- # df_test['size_kb'], df_test['size_mb'], df_test['size_gb'] =
- # zip(*df_test['size'].apply(sizes))
- return pmi_df
-
- def calc_nPMI(self, pmi_df, vocab_cooc_df, subgroup):
- """
- # nPMI additionally divides by -log(p(x,y)) = -log(p(x|y)p(y))
- # = -log(p(word|subgroup)p(word))
- """
- p_word_g_subgroup = vocab_cooc_df["cooc"] / sum(vocab_cooc_df["cooc"])
- logs.debug("p_word_g_subgroup")
- logs.debug(p_word_g_subgroup)
- p_word = pmi_df.apply(
- lambda x: self.vocab_counts_df.loc[x.name]["proportion"], axis=1
- )
- logs.debug("p word is")
- logs.debug(p_word)
- normalize_pmi = -np.log(p_word_g_subgroup * p_word)
- npmi_df = pd.DataFrame()
- npmi_df[subgroup] = pmi_df[subgroup] / normalize_pmi
- return npmi_df.dropna()
-
- def calc_bias(self, measurements_dict, measure="npmi"):
- """Uses the subgroup dictionaries to compute the differences across pairs.
- Uses dictionaries rather than dataframes due to the fact that dicts seem
- to be preferred amongst evaluate users so far.
- :return: Dict of (id_term1, id_term2):{term1:diff, term2:diff ...}"""
- paired_results_dict = {}
- for pair in self.paired_terms:
- paired_results = pd.DataFrame()
- s1 = pair[0]
- s2 = pair[1]
- s1_results = measurements_dict[s1][measure]
- s2_results = measurements_dict[s2][measure]
- # !!! This is the final result of all the work !!!
- word_diffs = s1_results[s1] - s2_results[s2]
- paired_results[("%s - %s" % (s1, s2))] = word_diffs
- paired_results[s1] = s1_results
- paired_results[s2] = s2_results
- paired_results_dict[pair] = paired_results.dropna()
- logs.debug("Paired bias results from the main nPMI class are ")
- logs.debug(paired_results_dict)
- return paired_results_dict
-
- def _write_debug_msg(self, batch_id, subgroup_df=None,
- subgroup_sentences=None, msg_type="batching"):
- if msg_type == "batching":
- if not batch_id % 100:
- logs.debug(
- "%s of %s co-occurrence count batches"
- % (str(batch_id), str(len(self.word_cnts_per_sentence)))
- )
- elif msg_type == "transpose":
- if not batch_id % 100:
- logs.debug("Removing 0 counts, subgroup_df is")
- logs.debug(subgroup_df)
- logs.debug("subgroup_sentences is")
- logs.debug(subgroup_sentences)
- logs.debug(
- "Now we do the transpose approach for co-occurrences")
diff --git a/spaces/ICML2022/OFA/fairseq/examples/flores101/README.md b/spaces/ICML2022/OFA/fairseq/examples/flores101/README.md
deleted file mode 100644
index 635c13f40bd0ccab704735bc5c26ea0192ea98cd..0000000000000000000000000000000000000000
--- a/spaces/ICML2022/OFA/fairseq/examples/flores101/README.md
+++ /dev/null
@@ -1,223 +0,0 @@
-
-
-
-
-# Flores101: Large-Scale Multilingual Machine Translation
-
-## Introduction
-
-Baseline pretrained models for small and large tracks of WMT 21 Large-Scale Multilingual Machine Translation competition.
-
-Flores Task at WMT 21: http://www.statmt.org/wmt21/large-scale-multilingual-translation-task.html
-
-Flores announement blog post: https://ai.facebook.com/blog/flores-researchers-kick-off-multilingual-translation-challenge-at-wmt-and-call-for-compute-grants/
-
-
-
-## Pretrained models
-
-Model | Num layers | Embed dimension | FFN dimension| Vocab Size | #params | Download
----|---|---|---|---|---|---
-`flores101_mm100_615M` | 12 | 1024 | 4096 | 256,000 | 615M | https://dl.fbaipublicfiles.com/flores101/pretrained_models/flores101_mm100_615M.tar.gz
-`flores101_mm100_175M` | 6 | 512 | 2048 | 256,000 | 175M | https://dl.fbaipublicfiles.com/flores101/pretrained_models/flores101_mm100_175M.tar.gz
-
-
-These models are trained similar to [M2M-100](https://arxiv.org/abs/2010.11125) with additional support for the languages that are part of the WMT Large-Scale Multilingual Machine Translation track. Full list of languages can be found at the bottom.
-
-
-## Example Generation code
-
-### Download model, sentencepiece vocab
-
-```bash
-fairseq=/path/to/fairseq
-cd $fairseq
-
-# Download 615M param model.
-wget https://dl.fbaipublicfiles.com/flores101/pretrained_models/flores101_mm100_615M.tar.gz
-
-# Extract
-tar -xvzf flores101_mm100_615M.tar.gz
-```
-
-### Encode using our SentencePiece Model
-Note: Install SentencePiece from [here](https://github.com/google/sentencepiece)
-
-
-```bash
-fairseq=/path/to/fairseq
-cd $fairseq
-
-# Download example dataset From German to French
-sacrebleu --echo src -l de-fr -t wmt19 | head -n 20 > raw_input.de-fr.de
-sacrebleu --echo ref -l de-fr -t wmt19 | head -n 20 > raw_input.de-fr.fr
-
-for lang in de fr ; do
- python scripts/spm_encode.py \
- --model flores101_mm100_615M/sentencepiece.bpe.model \
- --output_format=piece \
- --inputs=raw_input.de-fr.${lang} \
- --outputs=spm.de-fr.${lang}
-done
-```
-
-### Binarization
-
-```bash
-fairseq-preprocess \
- --source-lang de --target-lang fr \
- --testpref spm.de-fr \
- --thresholdsrc 0 --thresholdtgt 0 \
- --destdir data_bin \
- --srcdict flores101_mm100_615M/dict.txt --tgtdict flores101_mm100_615M/dict.txt
-```
-
-### Generation
-
-
-```bash
-fairseq-generate \
- data_bin \
- --batch-size 1 \
- --path flores101_mm100_615M/model.pt \
- --fixed-dictionary flores101_mm100_615M/dict.txt \
- -s de -t fr \
- --remove-bpe 'sentencepiece' \
- --beam 5 \
- --task translation_multi_simple_epoch \
- --lang-pairs flores101_mm100_615M/language_pairs.txt \
- --decoder-langtok --encoder-langtok src \
- --gen-subset test \
- --fp16 \
- --dataset-impl mmap \
- --distributed-world-size 1 --distributed-no-spawn
-```
-
-### Supported Languages and lang code
-
-Language | lang code
----|---
-Akrikaans | af
-Amharic | am
-Arabic | ar
-Assamese | as
-Asturian | ast
-Aymara | ay
-Azerbaijani | az
-Bashkir | ba
-Belarusian | be
-Bulgarian | bg
-Bengali | bn
-Breton | br
-Bosnian | bs
-Catalan | ca
-Cebuano | ceb
-Chokwe | cjk
-Czech | cs
-Welsh | cy
-Danish | da
-German | de
-Dyula| dyu
-Greek | el
-English | en
-Spanish | es
-Estonian | et
-Persian | fa
-Fulah | ff
-Finnish | fi
-French | fr
-Western Frisian | fy
-Irish | ga
-Scottish Gaelic | gd
-Galician | gl
-Gujarati | gu
-Hausa | ha
-Hebrew | he
-Hindi | hi
-Croatian | hr
-Haitian Creole | ht
-Hungarian | hu
-Armenian | hy
-Indonesian | id
-Igbo | ig
-Iloko | ilo
-Icelandic | is
-Italian | it
-Japanese | ja
-Javanese | jv
-Georgian | ka
-Kachin | kac
-Kamba | kam
-Kabuverdianu | kea
-Kongo | kg
-Kazakh | kk
-Central Khmer | km
-Kimbundu | kmb
-Northern Kurdish | kmr
-Kannada | kn
-Korean | ko
-Kurdish | ku
-Kyrgyz | ky
-Luxembourgish | lb
-Ganda | lg
-Lingala | ln
-Lao | lo
-Lithuanian | lt
-Luo | luo
-Latvian | lv
-Malagasy | mg
-Maori | mi
-Macedonian | mk
-Malayalam | ml
-Mongolian | mn
-Marathi | mr
-Malay | ms
-Maltese | mt
-Burmese | my
-Nepali | ne
-Dutch | nl
-Norwegian | no
-Northern Sotho | ns
-Nyanja | ny
-Occitan | oc
-Oromo | om
-Oriya | or
-Punjabi | pa
-Polish | pl
-Pashto | ps
-Portuguese | pt
-Quechua | qu
-Romanian | ro
-Russian | ru
-Sindhi | sd
-Shan | shn
-Sinhala | si
-Slovak | sk
-Slovenian | sl
-Shona | sn
-Somali | so
-Albanian | sq
-Serbian | sr
-Swati | ss
-Sundanese | su
-Swedish | sv
-Swahili | sw
-Tamil | ta
-Telugu | te
-Tajik | tg
-Thai | th
-Tigrinya | ti
-Tagalog | tl
-Tswana | tn
-Turkish | tr
-Ukrainian | uk
-Umbundu | umb
-Urdu | ur
-Uzbek | uz
-Vietnamese | vi
-Wolof | wo
-Xhosa | xh
-Yiddish | yi
-Yoruba | yo
-Chinese| zh
-Zulu | zu
diff --git a/spaces/ICML2022/OFA/fairseq/fairseq/data/text_compressor.py b/spaces/ICML2022/OFA/fairseq/fairseq/data/text_compressor.py
deleted file mode 100644
index 561e9ac89ad9f1e88df95647cfdc53e4fcf5d157..0000000000000000000000000000000000000000
--- a/spaces/ICML2022/OFA/fairseq/fairseq/data/text_compressor.py
+++ /dev/null
@@ -1,56 +0,0 @@
-# Copyright (c) Facebook, Inc. and its affiliates.
-#
-# This source code is licensed under the MIT license found in the
-# LICENSE file in the root directory of this source tree.
-
-from enum import Enum
-
-
-class TextCompressionLevel(Enum):
- none = 0
- low = 1
- high = 2
-
-
-class TextCompressor(object):
- def __init__(
- self, level: TextCompressionLevel,
- max_input_byte_length: int = 2 ** 16
- ):
- self.level = level
- self.max_input_length = max_input_byte_length
-
- def compress(self, text: str) -> bytes:
- if self.level == TextCompressionLevel.low:
- import zlib
- # zlib: built-in, fast
- return zlib.compress(text.encode(), level=0)
- elif self.level == TextCompressionLevel.high:
- try:
- import unishox2
- # unishox2: optimized for short text but slower
- except ImportError:
- raise ImportError(
- "Please install unishox2 for the text compression feature: "
- "pip install unishox2-py3"
- )
- assert len(text.encode()) <= self.max_input_length
- return unishox2.compress(text)[0]
- else:
- return text.encode()
-
- def decompress(self, compressed: bytes) -> str:
- if self.level == TextCompressionLevel.low:
- import zlib
- return zlib.decompress(compressed).decode()
- elif self.level == TextCompressionLevel.high:
- try:
- import unishox2
- except ImportError:
- raise ImportError(
- "Please install unishox2 for the text compression feature: "
- "pip install unishox2-py3"
- )
- return unishox2.decompress(compressed, self.max_input_length)
- else:
- return compressed.decode()
diff --git a/spaces/IVentureISB/Gen-AI/app.py b/spaces/IVentureISB/Gen-AI/app.py
deleted file mode 100644
index 6cafcb75def6e4686fe8057f8ea6ff4fb4efb80a..0000000000000000000000000000000000000000
--- a/spaces/IVentureISB/Gen-AI/app.py
+++ /dev/null
@@ -1,34 +0,0 @@
-import openai
-import gradio as gr
-import os
-from dotenv import load_dotenv
-load_dotenv()
-
-
-#SECRET_TOKEN = os.getenv("SECRET_TOKEN")
-
-SECRET_TOKEN = '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'
-messages = [
- {"role": "system", "content": "You are an AI assistant that only gives responses from the website https://i-venture.org/ and you help people make decisions about how to make a difference in others' lives. You also provide the relevant links from that website as part of your answers."},
-]
-
-def chatbot(input):
- if input:
- messages.append({"role": "user", "content": input})
- chat = openai.ChatCompletion.create(
- model="gpt-3.5-turbo", messages=messages
- )
- reply = chat.choices[0].message.content
- messages.append({"role": "assistant", "content": reply})
- return reply
-
-inputs = gr.inputs.Textbox(lines=7, label="Chat with I-venture @ ISB AI powered bot")
-outputs = gr.outputs.Textbox(label="Reply")
-
-
-
-gr.Interface(fn=chatbot, inputs=inputs, outputs=outputs, title="Talk with I-venture @ ISB",
- description="Anything you want to find out about entreprenuership at ISB. Sample questions include >>> how to get incubated at ISB Dlabs? >>> What is the latest event being organized by I-venture @ ISB? >>> and more",
- theme="huggingface", live=True,).launch(share=False)
-
-# , debug=True
\ No newline at end of file
diff --git a/spaces/Ibtehaj10/cheating-detection-FYP/yolovs5/utils/downloads.py b/spaces/Ibtehaj10/cheating-detection-FYP/yolovs5/utils/downloads.py
deleted file mode 100644
index 21bb6608d5bac031ece90054c85caba5886de5ed..0000000000000000000000000000000000000000
--- a/spaces/Ibtehaj10/cheating-detection-FYP/yolovs5/utils/downloads.py
+++ /dev/null
@@ -1,108 +0,0 @@
-# YOLOv5 🚀 by Ultralytics, GPL-3.0 license
-"""
-Download utils
-"""
-
-import logging
-import os
-import subprocess
-import urllib
-from pathlib import Path
-
-import requests
-import torch
-
-
-def is_url(url, check=True):
- # Check if string is URL and check if URL exists
- try:
- url = str(url)
- result = urllib.parse.urlparse(url)
- assert all([result.scheme, result.netloc]) # check if is url
- return (urllib.request.urlopen(url).getcode() == 200) if check else True # check if exists online
- except (AssertionError, urllib.request.HTTPError):
- return False
-
-
-def gsutil_getsize(url=''):
- # gs://bucket/file size https://cloud.google.com/storage/docs/gsutil/commands/du
- s = subprocess.check_output(f'gsutil du {url}', shell=True).decode('utf-8')
- return eval(s.split(' ')[0]) if len(s) else 0 # bytes
-
-
-def url_getsize(url='https://ultralytics.com/images/bus.jpg'):
- # Return downloadable file size in bytes
- response = requests.head(url, allow_redirects=True)
- return int(response.headers.get('content-length', -1))
-
-
-def safe_download(file, url, url2=None, min_bytes=1E0, error_msg=''):
- # Attempts to download file from url or url2, checks and removes incomplete downloads < min_bytes
- from utils.general import LOGGER
-
- file = Path(file)
- assert_msg = f"Downloaded file '{file}' does not exist or size is < min_bytes={min_bytes}"
- try: # url1
- LOGGER.info(f'Downloading {url} to {file}...')
- torch.hub.download_url_to_file(url, str(file), progress=LOGGER.level <= logging.INFO)
- assert file.exists() and file.stat().st_size > min_bytes, assert_msg # check
- except Exception as e: # url2
- if file.exists():
- file.unlink() # remove partial downloads
- LOGGER.info(f'ERROR: {e}\nRe-attempting {url2 or url} to {file}...')
- os.system(f"curl -# -L '{url2 or url}' -o '{file}' --retry 3 -C -") # curl download, retry and resume on fail
- finally:
- if not file.exists() or file.stat().st_size < min_bytes: # check
- if file.exists():
- file.unlink() # remove partial downloads
- LOGGER.info(f"ERROR: {assert_msg}\n{error_msg}")
- LOGGER.info('')
-
-
-def attempt_download(file, repo='ultralytics/yolov5', release='v6.2'):
- # Attempt file download from GitHub release assets if not found locally. release = 'latest', 'v6.2', etc.
- from utils.general import LOGGER
-
- def github_assets(repository, version='latest'):
- # Return GitHub repo tag (i.e. 'v6.2') and assets (i.e. ['yolov5s.pt', 'yolov5m.pt', ...])
- if version != 'latest':
- version = f'tags/{version}' # i.e. tags/v6.2
- response = requests.get(f'https://api.github.com/repos/{repository}/releases/{version}').json() # github api
- return response['tag_name'], [x['name'] for x in response['assets']] # tag, assets
-
- file = Path(str(file).strip().replace("'", ''))
- if not file.exists():
- # URL specified
- name = Path(urllib.parse.unquote(str(file))).name # decode '%2F' to '/' etc.
- if str(file).startswith(('http:/', 'https:/')): # download
- url = str(file).replace(':/', '://') # Pathlib turns :// -> :/
- file = name.split('?')[0] # parse authentication https://url.com/file.txt?auth...
- if Path(file).is_file():
- LOGGER.info(f'Found {url} locally at {file}') # file already exists
- else:
- safe_download(file=file, url=url, min_bytes=1E5)
- return file
-
- # GitHub assets
- assets = [f'yolov5{size}{suffix}.pt' for size in 'nsmlx' for suffix in ('', '6', '-cls', '-seg')] # default
- try:
- tag, assets = github_assets(repo, release)
- except Exception:
- try:
- tag, assets = github_assets(repo) # latest release
- except Exception:
- try:
- tag = subprocess.check_output('git tag', shell=True, stderr=subprocess.STDOUT).decode().split()[-1]
- except Exception:
- tag = release
-
- file.parent.mkdir(parents=True, exist_ok=True) # make parent dir (if required)
- if name in assets:
- url3 = 'https://drive.google.com/drive/folders/1EFQTEUeXWSFww0luse2jB9M1QNZQGwNl' # backup gdrive mirror
- safe_download(
- file,
- url=f'https://github.com/{repo}/releases/download/{tag}/{name}',
- min_bytes=1E5,
- error_msg=f'{file} missing, try downloading from https://github.com/{repo}/releases/{tag} or {url3}')
-
- return str(file)
diff --git a/spaces/Illumotion/Koboldcpp/llama-util.h b/spaces/Illumotion/Koboldcpp/llama-util.h
deleted file mode 100644
index a97520108c508de8dd5f8afa1f93cae5f9711b56..0000000000000000000000000000000000000000
--- a/spaces/Illumotion/Koboldcpp/llama-util.h
+++ /dev/null
@@ -1,548 +0,0 @@
-// Internal header to be included only by llama.cpp.
-// Contains wrappers around OS interfaces.
-#pragma once
-#ifndef LLAMA_UTIL_H
-#define LLAMA_UTIL_H
-
-#include
-#include
-#include
-#include
-#include
-#include
-#include
-
-#include
-#include
-#include
-
-#ifdef __has_include
- #if __has_include()
- #include
- #if defined(_POSIX_MAPPED_FILES)
- #include
- #endif
- #if defined(_POSIX_MEMLOCK_RANGE)
- #include
- #endif
- #endif
-#endif
-
-#if defined(_WIN32)
- #define WIN32_LEAN_AND_MEAN
- #ifndef NOMINMAX
- #define NOMINMAX
- #endif
- #include
- #include
- #include // for _fseeki64
-#endif
-
-#define LLAMA_ASSERT(x) \
- do { \
- if (!(x)) { \
- fprintf(stderr, "LLAMA_ASSERT: %s:%d: %s\n", __FILE__, __LINE__, #x); \
- abort(); \
- } \
- } while (0)
-
-#ifdef __GNUC__
-#ifdef __MINGW32__
-__attribute__((format(gnu_printf, 1, 2)))
-#else
-__attribute__((format(printf, 1, 2)))
-#endif
-#endif
-static std::string format(const char * fmt, ...) {
- va_list ap, ap2;
- va_start(ap, fmt);
- va_copy(ap2, ap);
- int size = vsnprintf(NULL, 0, fmt, ap);
- LLAMA_ASSERT(size >= 0 && size < INT_MAX);
- std::vector buf(size + 1);
- int size2 = vsnprintf(buf.data(), size + 1, fmt, ap2);
- LLAMA_ASSERT(size2 == size);
- va_end(ap2);
- va_end(ap);
- return std::string(buf.data(), size);
-}
-
-struct llama_file {
- // use FILE * so we don't have to re-open the file to mmap
- FILE * fp;
- size_t size;
-
- llama_file(const char * fname, const char * mode) {
- fp = std::fopen(fname, mode);
- if (fp == NULL) {
- throw std::runtime_error(format("failed to open %s: %s", fname, strerror(errno)));
- }
- seek(0, SEEK_END);
- size = tell();
- seek(0, SEEK_SET);
- }
-
- size_t tell() const {
-#ifdef _WIN32
- __int64 ret = _ftelli64(fp);
-#else
- long ret = std::ftell(fp);
-#endif
- LLAMA_ASSERT(ret != -1); // this really shouldn't fail
- return (size_t) ret;
- }
-
- void seek(size_t offset, int whence) {
-#ifdef _WIN32
- int ret = _fseeki64(fp, (__int64) offset, whence);
-#else
- int ret = std::fseek(fp, (long) offset, whence);
-#endif
- LLAMA_ASSERT(ret == 0); // same
- }
-
- void read_raw(void * ptr, size_t len) const {
- if (len == 0) {
- return;
- }
- errno = 0;
- std::size_t ret = std::fread(ptr, len, 1, fp);
- if (ferror(fp)) {
- throw std::runtime_error(format("read error: %s", strerror(errno)));
- }
- if (ret != 1) {
- throw std::runtime_error(std::string("unexpectedly reached end of file"));
- }
- }
-
- std::uint32_t read_u32() {
- std::uint32_t ret;
- read_raw(&ret, sizeof(ret));
- return ret;
- }
-
- std::string read_string(std::uint32_t len) {
- std::vector chars(len);
- read_raw(chars.data(), len);
- return std::string(chars.data(), len);
- }
-
- void write_raw(const void * ptr, size_t len) const {
- if (len == 0) {
- return;
- }
- errno = 0;
- size_t ret = std::fwrite(ptr, len, 1, fp);
- if (ret != 1) {
- throw std::runtime_error(format("write error: %s", strerror(errno)));
- }
- }
-
- void write_u32(std::uint32_t val) {
- write_raw(&val, sizeof(val));
- }
-
- ~llama_file() {
- if (fp) {
- std::fclose(fp);
- }
- }
-};
-
-// llama_context_data
-struct llama_data_context {
- virtual void write(const void * src, size_t size) = 0;
- virtual size_t get_size_written() = 0;
- virtual ~llama_data_context() = default;
-};
-
-struct llama_data_buffer_context : llama_data_context {
- uint8_t* ptr;
- size_t size_written = 0;
-
- llama_data_buffer_context(uint8_t * p) : ptr(p) {}
-
- void write(const void * src, size_t size) override {
- memcpy(ptr, src, size);
- ptr += size;
- size_written += size;
- }
-
- size_t get_size_written() override {
- return size_written;
- }
-};
-
-struct llama_data_file_context : llama_data_context {
- llama_file* file;
- size_t size_written = 0;
-
- llama_data_file_context(llama_file * f) : file(f) {}
-
- void write(const void * src, size_t size) override {
- file->write_raw(src, size);
- size_written += size;
- }
-
- size_t get_size_written() override {
- return size_written;
- }
-};
-
-#if defined(_WIN32)
-static std::string llama_format_win_err(DWORD err) {
- LPSTR buf;
- size_t size = FormatMessageA(FORMAT_MESSAGE_ALLOCATE_BUFFER | FORMAT_MESSAGE_FROM_SYSTEM | FORMAT_MESSAGE_IGNORE_INSERTS,
- NULL, err, MAKELANGID(LANG_NEUTRAL, SUBLANG_DEFAULT), (LPSTR)&buf, 0, NULL);
- if (!size) {
- return "FormatMessageA failed";
- }
- std::string ret(buf, size);
- LocalFree(buf);
- return ret;
-}
-#endif
-
-struct llama_mmap {
- void * addr;
- size_t size;
-
- llama_mmap(const llama_mmap &) = delete;
-
-#ifdef _POSIX_MAPPED_FILES
- static constexpr bool SUPPORTED = true;
-
- llama_mmap(struct llama_file * file, size_t prefetch = (size_t) -1 /* -1 = max value */, bool numa = false) {
- size = file->size;
- int fd = fileno(file->fp);
- int flags = MAP_SHARED;
- // prefetch/readahead impairs performance on NUMA systems
- if (numa) { prefetch = 0; }
-#ifdef __linux__
- if (prefetch >= file->size) { flags |= MAP_POPULATE; }
-#endif
- addr = mmap(NULL, file->size, PROT_READ, flags, fd, 0);
- if (addr == MAP_FAILED) {
- throw std::runtime_error(format("mmap failed: %s", strerror(errno)));
- }
-
- if (prefetch > 0) {
- // Advise the kernel to preload the mapped memory
- if (madvise(addr, std::min(file->size, prefetch), MADV_WILLNEED)) {
- fprintf(stderr, "warning: madvise(.., MADV_WILLNEED) failed: %s\n",
- strerror(errno));
- }
- }
- if (numa) {
- // advise the kernel not to use readahead
- // (because the next page might not belong on the same node)
- if (madvise(addr, file->size, MADV_RANDOM)) {
- fprintf(stderr, "warning: madvise(.., MADV_RANDOM) failed: %s\n",
- strerror(errno));
- }
- }
- }
-
- ~llama_mmap() {
- munmap(addr, size);
- }
-#elif defined(_WIN32)
- static constexpr bool SUPPORTED = true;
-
- llama_mmap(struct llama_file * file, bool prefetch = true, bool numa = false) {
- (void) numa;
-
- size = file->size;
-
- HANDLE hFile = (HANDLE) _get_osfhandle(_fileno(file->fp));
-
- HANDLE hMapping = CreateFileMappingA(hFile, NULL, PAGE_READONLY, 0, 0, NULL);
- DWORD error = GetLastError();
-
- if (hMapping == NULL) {
- throw std::runtime_error(format("CreateFileMappingA failed: %s", llama_format_win_err(error).c_str()));
- }
-
- addr = MapViewOfFile(hMapping, FILE_MAP_READ, 0, 0, 0);
- error = GetLastError();
- CloseHandle(hMapping);
-
- if (addr == NULL) {
- throw std::runtime_error(format("MapViewOfFile failed: %s", llama_format_win_err(error).c_str()));
- }
-
- #ifndef USE_FAILSAFE
- #if _WIN32_WINNT >= _WIN32_WINNT_WIN8
- if (prefetch) {
- // Advise the kernel to preload the mapped memory
- WIN32_MEMORY_RANGE_ENTRY range;
- range.VirtualAddress = addr;
- range.NumberOfBytes = (SIZE_T)size;
- if (!PrefetchVirtualMemory(GetCurrentProcess(), 1, &range, 0)) {
- fprintf(stderr, "warning: PrefetchVirtualMemory failed: %s\n",
- llama_format_win_err(GetLastError()).c_str());
- }
- }
- #else
- #pragma message("warning: You are building for pre-Windows 8; prefetch not supported")
- #endif // _WIN32_WINNT >= _WIN32_WINNT_WIN8
- #else
- printf("\nPrefetchVirtualMemory skipped in compatibility mode.\n");
- #endif
- }
-
- ~llama_mmap() {
- if (!UnmapViewOfFile(addr)) {
- fprintf(stderr, "warning: UnmapViewOfFile failed: %s\n",
- llama_format_win_err(GetLastError()).c_str());
- }
- }
-#else
- static constexpr bool SUPPORTED = false;
-
- llama_mmap(struct llama_file *, bool prefetch = true, bool numa = false) {
- (void) prefetch;
- (void) numa;
-
- throw std::runtime_error(std::string("mmap not supported"));
- }
-#endif
-};
-
-// Represents some region of memory being locked using mlock or VirtualLock;
-// will automatically unlock on destruction.
-struct llama_mlock {
- void * addr = NULL;
- size_t size = 0;
- bool failed_already = false;
-
- llama_mlock() {}
- llama_mlock(const llama_mlock &) = delete;
-
- ~llama_mlock() {
- if (size) {
- raw_unlock(addr, size);
- }
- }
-
- void init(void * ptr) {
- LLAMA_ASSERT(addr == NULL && size == 0);
- addr = ptr;
- }
-
- void grow_to(size_t target_size) {
- LLAMA_ASSERT(addr);
- if (failed_already) {
- return;
- }
- size_t granularity = lock_granularity();
- target_size = (target_size + granularity - 1) & ~(granularity - 1);
- if (target_size > size) {
- if (raw_lock((uint8_t *) addr + size, target_size - size)) {
- size = target_size;
- } else {
- failed_already = true;
- }
- }
- }
-
-#ifdef _POSIX_MEMLOCK_RANGE
- static constexpr bool SUPPORTED = true;
-
- size_t lock_granularity() {
- return (size_t) sysconf(_SC_PAGESIZE);
- }
-
- #ifdef __APPLE__
- #define MLOCK_SUGGESTION \
- "Try increasing the sysctl values 'vm.user_wire_limit' and 'vm.global_user_wire_limit' and/or " \
- "decreasing 'vm.global_no_user_wire_amount'. Also try increasing RLIMIT_MLOCK (ulimit -l).\n"
- #else
- #define MLOCK_SUGGESTION \
- "Try increasing RLIMIT_MLOCK ('ulimit -l' as root).\n"
- #endif
-
- bool raw_lock(const void * addr, size_t size) {
- if (!mlock(addr, size)) {
- return true;
- } else {
- char* errmsg = std::strerror(errno);
- bool suggest = (errno == ENOMEM);
-
- // Check if the resource limit is fine after all
- struct rlimit lock_limit;
- if (suggest && getrlimit(RLIMIT_MEMLOCK, &lock_limit))
- suggest = false;
- if (suggest && (lock_limit.rlim_max > lock_limit.rlim_cur + size))
- suggest = false;
-
- fprintf(stderr, "warning: failed to mlock %zu-byte buffer (after previously locking %zu bytes): %s\n%s",
- size, this->size, errmsg, suggest ? MLOCK_SUGGESTION : "");
- return false;
- }
- }
-
- #undef MLOCK_SUGGESTION
-
- void raw_unlock(void * addr, size_t size) {
- if (munlock(addr, size)) {
- fprintf(stderr, "warning: failed to munlock buffer: %s\n", std::strerror(errno));
- }
- }
-#elif defined(_WIN32)
- static constexpr bool SUPPORTED = true;
-
- size_t lock_granularity() {
- SYSTEM_INFO si;
- GetSystemInfo(&si);
- return (size_t) si.dwPageSize;
- }
-
- bool raw_lock(void * ptr, size_t len) {
- for (int tries = 1; ; tries++) {
- if (VirtualLock(ptr, len)) {
- return true;
- }
- if (tries == 2) {
- fprintf(stderr, "warning: failed to VirtualLock %zu-byte buffer (after previously locking %zu bytes): %s\n",
- len, size, llama_format_win_err(GetLastError()).c_str());
- return false;
- }
-
- // It failed but this was only the first try; increase the working
- // set size and try again.
- SIZE_T min_ws_size, max_ws_size;
- if (!GetProcessWorkingSetSize(GetCurrentProcess(), &min_ws_size, &max_ws_size)) {
- fprintf(stderr, "warning: GetProcessWorkingSetSize failed: %s\n",
- llama_format_win_err(GetLastError()).c_str());
- return false;
- }
- // Per MSDN: "The maximum number of pages that a process can lock
- // is equal to the number of pages in its minimum working set minus
- // a small overhead."
- // Hopefully a megabyte is enough overhead:
- size_t increment = len + 1048576;
- // The minimum must be <= the maximum, so we need to increase both:
- min_ws_size += increment;
- max_ws_size += increment;
- if (!SetProcessWorkingSetSize(GetCurrentProcess(), min_ws_size, max_ws_size)) {
- fprintf(stderr, "warning: SetProcessWorkingSetSize failed: %s\n",
- llama_format_win_err(GetLastError()).c_str());
- return false;
- }
- }
- }
-
- void raw_unlock(void * ptr, size_t len) {
- if (!VirtualUnlock(ptr, len)) {
- fprintf(stderr, "warning: failed to VirtualUnlock buffer: %s\n",
- llama_format_win_err(GetLastError()).c_str());
- }
- }
-#else
- static constexpr bool SUPPORTED = false;
-
- size_t lock_granularity() {
- return (size_t) 65536;
- }
-
- bool raw_lock(const void * addr, size_t len) {
- fprintf(stderr, "warning: mlock not supported on this system\n");
- return false;
- }
-
- void raw_unlock(const void * addr, size_t len) {}
-#endif
-};
-
-// Replacement for std::vector that doesn't require zero-initialization.
-struct llama_buffer {
- uint8_t * addr = NULL;
- size_t size = 0;
-
- llama_buffer() = default;
-
- void resize(size_t len) {
-#ifdef GGML_USE_METAL
- free(addr);
- int result = posix_memalign((void **) &addr, getpagesize(), len);
- if (result == 0) {
- memset(addr, 0, len);
- }
- else {
- addr = NULL;
- }
-#else
- delete[] addr;
- addr = new uint8_t[len];
-#endif
- size = len;
- }
-
- ~llama_buffer() {
-#ifdef GGML_USE_METAL
- free(addr);
-#else
- delete[] addr;
-#endif
- addr = NULL;
- }
-
- // disable copy and move
- llama_buffer(const llama_buffer&) = delete;
- llama_buffer(llama_buffer&&) = delete;
- llama_buffer& operator=(const llama_buffer&) = delete;
- llama_buffer& operator=(llama_buffer&&) = delete;
-};
-
-#ifdef GGML_USE_CUBLAS
-#include "ggml-cuda.h"
-struct llama_ctx_buffer {
- uint8_t * addr = NULL;
- bool is_cuda;
- size_t size = 0;
-
- llama_ctx_buffer() = default;
-
- void resize(size_t size) {
- free();
-
- addr = (uint8_t *) ggml_cuda_host_malloc(size);
- if (addr) {
- is_cuda = true;
- }
- else {
- // fall back to pageable memory
- addr = new uint8_t[size];
- is_cuda = false;
- }
- this->size = size;
- }
-
- void free() {
- if (addr) {
- if (is_cuda) {
- ggml_cuda_host_free(addr);
- }
- else {
- delete[] addr;
- }
- }
- addr = NULL;
- }
-
- ~llama_ctx_buffer() {
- free();
- }
-
- // disable copy and move
- llama_ctx_buffer(const llama_ctx_buffer&) = delete;
- llama_ctx_buffer(llama_ctx_buffer&&) = delete;
- llama_ctx_buffer& operator=(const llama_ctx_buffer&) = delete;
- llama_ctx_buffer& operator=(llama_ctx_buffer&&) = delete;
-};
-#else
-typedef llama_buffer llama_ctx_buffer;
-#endif
-
-#endif
diff --git a/spaces/Intae/deepfake/training/datasets/__init__.py b/spaces/Intae/deepfake/training/datasets/__init__.py
deleted file mode 100644
index e69de29bb2d1d6434b8b29ae775ad8c2e48c5391..0000000000000000000000000000000000000000
diff --git a/spaces/JacobLinCool/captcha-recognizer/src/solve.py b/spaces/JacobLinCool/captcha-recognizer/src/solve.py
deleted file mode 100644
index 7db4ca9738eacc1108a4a02e35e03b53338482b1..0000000000000000000000000000000000000000
--- a/spaces/JacobLinCool/captcha-recognizer/src/solve.py
+++ /dev/null
@@ -1,55 +0,0 @@
-import pytesseract
-import numpy as np
-
-
-def solve(image: np.ndarray) -> str:
- for mode in [7, 10, 11, 12, 13]:
- result = normalize(
- pytesseract.image_to_string(
- image, lang="eng", config=f"--oem 3 --psm {mode}", timeout=0.5
- ).strip()
- )
- if result != "":
- return result
-
- return "not sure"
-
-
-def normalize(s: str) -> str:
- print(s)
- if "\n" in s:
- return ""
-
- s = s.replace(" ", "").lower()
-
- if len(s) < 3:
- return ""
-
- # if first is number
- if s[0].isdigit() and s[2].isdigit():
- if s[1] in ["+", "4"]:
- return str(int(s[0]) + int(s[2]))
- elif s[1] in ["-", "_"]:
- return str(int(s[0]) - int(s[2]))
- else:
- return str(int(s[0]) * int(s[2]))
-
- # possible alphabet mapping
- mapping = {
- ")": "l",
- "¥": "y",
- "2": "z",
- "é": "e",
- }
-
- for k, v in mapping.items():
- s = s.replace(k, v)
-
- # if not all alphabet
- if not all([c.isalpha() for c in s]):
- return ""
-
- if len(s) != 4:
- return ""
-
- return s
diff --git a/spaces/Jamkonams/AutoGPT/tests/test_token_counter.py b/spaces/Jamkonams/AutoGPT/tests/test_token_counter.py
deleted file mode 100644
index 6d7ae016b2f823123b0b69b2eeb3eab50d94f00f..0000000000000000000000000000000000000000
--- a/spaces/Jamkonams/AutoGPT/tests/test_token_counter.py
+++ /dev/null
@@ -1,63 +0,0 @@
-import unittest
-
-import tests.context
-from autogpt.token_counter import count_message_tokens, count_string_tokens
-
-
-class TestTokenCounter(unittest.TestCase):
- def test_count_message_tokens(self):
- messages = [
- {"role": "user", "content": "Hello"},
- {"role": "assistant", "content": "Hi there!"},
- ]
- self.assertEqual(count_message_tokens(messages), 17)
-
- def test_count_message_tokens_with_name(self):
- messages = [
- {"role": "user", "content": "Hello", "name": "John"},
- {"role": "assistant", "content": "Hi there!"},
- ]
- self.assertEqual(count_message_tokens(messages), 17)
-
- def test_count_message_tokens_empty_input(self):
- self.assertEqual(count_message_tokens([]), 3)
-
- def test_count_message_tokens_invalid_model(self):
- messages = [
- {"role": "user", "content": "Hello"},
- {"role": "assistant", "content": "Hi there!"},
- ]
- with self.assertRaises(KeyError):
- count_message_tokens(messages, model="invalid_model")
-
- def test_count_message_tokens_gpt_4(self):
- messages = [
- {"role": "user", "content": "Hello"},
- {"role": "assistant", "content": "Hi there!"},
- ]
- self.assertEqual(count_message_tokens(messages, model="gpt-4-0314"), 15)
-
- def test_count_string_tokens(self):
- string = "Hello, world!"
- self.assertEqual(
- count_string_tokens(string, model_name="gpt-3.5-turbo-0301"), 4
- )
-
- def test_count_string_tokens_empty_input(self):
- self.assertEqual(count_string_tokens("", model_name="gpt-3.5-turbo-0301"), 0)
-
- def test_count_message_tokens_invalid_model(self):
- messages = [
- {"role": "user", "content": "Hello"},
- {"role": "assistant", "content": "Hi there!"},
- ]
- with self.assertRaises(NotImplementedError):
- count_message_tokens(messages, model="invalid_model")
-
- def test_count_string_tokens_gpt_4(self):
- string = "Hello, world!"
- self.assertEqual(count_string_tokens(string, model_name="gpt-4-0314"), 4)
-
-
-if __name__ == "__main__":
- unittest.main()
diff --git a/spaces/Jean-Baptiste/email_parser/setup.py b/spaces/Jean-Baptiste/email_parser/setup.py
deleted file mode 100644
index d8dc4f8eb0d472b8320154ebe60f0f89a6db59a4..0000000000000000000000000000000000000000
--- a/spaces/Jean-Baptiste/email_parser/setup.py
+++ /dev/null
@@ -1,26 +0,0 @@
-from setuptools import find_packages, setup
-from glob import glob
-import os
-
-
-setup(name='email_parser',
- packages=find_packages(include=['email_parser']),
- version='0.0.1',
- description='Email parser',
- author='JB Polle',
- license='MIT',
- install_requires=['langid==1.1.6',
- 'numpy>=1.19.5',
- 'pandas>=1.2.3',
- 'regex',
- 'scikit-learn==0.24.1',
- 'sentence-transformers==1.0.4',
- 'tensorflow==2.6.0',
- 'tensorflow-hub>=0.12.0',
- 'tensorflow-text==2.6.0',
- 'tokenizers==0.10.1',
- 'torch>=1.8.0',
- 'umap-learn==0.5.1',
- 'dateparser==1.0.0',
- 'transformers>=4.3',
- 'gradio>=2.7'])
diff --git a/spaces/JeffJing/ZookChatBot/steamship/data/plugin/plugin_version.py b/spaces/JeffJing/ZookChatBot/steamship/data/plugin/plugin_version.py
deleted file mode 100644
index c2512e572ea880adb16f70de7dbaf4ae3a2a02ec..0000000000000000000000000000000000000000
--- a/spaces/JeffJing/ZookChatBot/steamship/data/plugin/plugin_version.py
+++ /dev/null
@@ -1,109 +0,0 @@
-from __future__ import annotations
-
-import json
-from typing import Any, Dict, List, Optional, Type
-
-from pydantic import BaseModel, Field
-
-from steamship.base import Task
-from steamship.base.client import Client
-from steamship.base.model import CamelModel
-from steamship.base.request import Request
-from steamship.base.response import Response
-from steamship.data.plugin import HostingMemory, HostingTimeout
-
-
-class CreatePluginVersionRequest(Request):
- plugin_id: str = None
- handle: str = None
- hosting_memory: Optional[HostingMemory] = None
- hosting_timeout: Optional[HostingTimeout] = None
- hosting_handler: str = None
- is_public: bool = None
- is_default: bool = None
- type: str = "file"
- # Note: this is a Dict[str, Any] but should be transmitted to the Engine as a JSON string
- config_template: str = None
-
-
-class ListPluginVersionsRequest(Request):
- handle: str
- plugin_id: str
-
-
-class ListPluginVersionsResponse(Response):
- plugins: List[PluginVersion]
-
-
-class PluginVersion(CamelModel):
- client: Client = Field(None, exclude=True)
- id: str = None
- plugin_id: str = None
- handle: str = None
- hosting_memory: Optional[HostingMemory] = None
- hosting_timeout: Optional[HostingTimeout] = None
- hosting_handler: str = None
- is_public: bool = None
- is_default: bool = None
- config_template: Dict[str, Any] = None
-
- @classmethod
- def parse_obj(cls: Type[BaseModel], obj: Any) -> BaseModel:
- # TODO (enias): This needs to be solved at the engine side
- obj = obj["pluginVersion"] if "pluginVersion" in obj else obj
- return super().parse_obj(obj)
-
- @staticmethod
- def create(
- client: Client,
- handle: str,
- plugin_id: str = None,
- filename: str = None,
- filebytes: bytes = None,
- hosting_memory: Optional[HostingMemory] = None,
- hosting_timeout: Optional[HostingTimeout] = None,
- hosting_handler: str = None,
- is_public: bool = None,
- is_default: bool = None,
- config_template: Dict[str, Any] = None,
- ) -> Task[PluginVersion]:
-
- if filename is None and filebytes is None:
- raise Exception("Either filename or filebytes must be provided.")
- if filename is not None and filebytes is not None:
- raise Exception("Only either filename or filebytes should be provided.")
-
- if filename is not None:
- with open(filename, "rb") as f:
- filebytes = f.read()
-
- req = CreatePluginVersionRequest(
- handle=handle,
- plugin_id=plugin_id,
- hosting_memory=hosting_memory,
- hosting_timeout=hosting_timeout,
- hosting_handler=hosting_handler,
- is_public=is_public,
- is_default=is_default,
- config_template=json.dumps(config_template or {}),
- )
-
- task = client.post(
- "plugin/version/create",
- payload=req,
- file=("plugin.zip", filebytes, "multipart/form-data"),
- expect=PluginVersion,
- )
-
- task.wait()
- return task.output
-
- @staticmethod
- def list(
- client: Client, plugin_id: str = None, handle: str = None, public: bool = True
- ) -> ListPluginVersionsResponse:
- return client.post(
- f"plugin/version/{'public' if public else 'private'}",
- ListPluginVersionsRequest(handle=handle, plugin_id=plugin_id),
- expect=ListPluginVersionsResponse,
- )
diff --git a/spaces/JohnSmith9982/ChuanhuChatGPT_Beta/web_assets/html/footer.html b/spaces/JohnSmith9982/ChuanhuChatGPT_Beta/web_assets/html/footer.html
deleted file mode 100644
index bca27bb8066dfab5cc0acf7be349a514de5f9a58..0000000000000000000000000000000000000000
--- a/spaces/JohnSmith9982/ChuanhuChatGPT_Beta/web_assets/html/footer.html
+++ /dev/null
@@ -1 +0,0 @@
-
{versions}
diff --git a/spaces/Jonathancasjar/Detect_products_and_empty_spaces_on_a_Supermarket/app.py b/spaces/Jonathancasjar/Detect_products_and_empty_spaces_on_a_Supermarket/app.py
deleted file mode 100644
index bbfada7ddcc2753244b093d358e824ad74d1be3d..0000000000000000000000000000000000000000
--- a/spaces/Jonathancasjar/Detect_products_and_empty_spaces_on_a_Supermarket/app.py
+++ /dev/null
@@ -1,50 +0,0 @@
-import gradio as gr
-import yolov5
-from PIL import Image
-from huggingface_hub import hf_hub_download
-import json
-
-title = "Shelf Store Product and Empty spaces on the shelf"
-description = "Object detection for products and missing products in a Supermarket shelf"
-model_id = 'Jonathancasjar/Retail_Shelves'
-article = "
"
-
-model = yolov5.load(model_id)
-
-examples =[
- ["test_images/Sample_shelf.jpeg",0.25],
- ["test_images/Shelf_image2.jpeg",0.25],
- ["test_images/Shelf_image3.jpeg",0.25],
- ["test_images/Shelf_Example4.jpeg",0.25]
- ]
-
-def predict(imp,threshold=0.25,model_id='Jonathancasjar/Retail_Shelves'):
- #Get model input size
- config_path = hf_hub_download(repo_id=model_id, filename="config.json")
- with open(config_path, "r") as f:
- config = json.load(f)
- input_size = config["input_size"]
-
- model.conf = threshold
- model_result = model(imp, size=input_size)
- image_tensor = model_result.render()[0]
- output = Image.fromarray(image_tensor)
- return output
-
-demo = gr.Interface(
- title=title,
- description=description,
- article=article,
- analytics_enabled=True,
- allow_flagging='never',
- fn=predict,
- inputs=[
- gr.Image(type='pil'),
- gr.Slider(minimum=0.1,maximum=1.0,value=0.25),
- ],
- outputs=gr.Image(type='pil'),
- examples=examples,
- cache_examples=True if examples else False)
-
-demo.launch(enable_queue=True)
-
diff --git a/spaces/Joom/Xtramrks/app.py b/spaces/Joom/Xtramrks/app.py
deleted file mode 100644
index 1abca697f1b16214a2aca7be8b8bfc6c77899e8e..0000000000000000000000000000000000000000
--- a/spaces/Joom/Xtramrks/app.py
+++ /dev/null
@@ -1,14 +0,0 @@
-import gradio as gr
-
-def hi():
- return "hello"
-
-context = gr.inputs.Textbox(lines=5, placeholder="Enter the relevant theory or context of the questions here")
-answer = gr.inputs.Textbox(lines=3, placeholder="Ënter the excpected answer/keyword here" )
-#question = [gr.outputs.Textbox(type="auto")for question in final_outputs]
-
-
-iface = gr.Interface(
- fn = hi,
- inputs=[context,answer],)
-iface.launch(debug=False)
\ No newline at end of file
diff --git a/spaces/KAIST-Geometric-AI-Lab/salad-demo/salad/spaghetti/utils/train_utils.py b/spaces/KAIST-Geometric-AI-Lab/salad-demo/salad/spaghetti/utils/train_utils.py
deleted file mode 100644
index 0f6ecfd01254c0034451565dfbbdb403eb10d4f1..0000000000000000000000000000000000000000
--- a/spaces/KAIST-Geometric-AI-Lab/salad-demo/salad/spaghetti/utils/train_utils.py
+++ /dev/null
@@ -1,192 +0,0 @@
-from ..custom_types import *
-from .. import constants
-from tqdm import tqdm
-from . import files_utils
-import os
-from .. import options
-from ..models import models_utils, occ_gmm
-
-
-LI = Union[T, float, int]
-Models = {'spaghetti': occ_gmm.Spaghetti}
-
-
-def is_model_clean(model: nn.Module) -> bool:
- for wh in model.parameters():
- if torch.isnan(wh).sum() > 0:
- return False
- return True
-
-
-def model_factory(opt: options.Options, override_model: Optional[str], device: D) -> models_utils.Model:
- if override_model is None:
- return Models[opt.model_name](opt).to(device)
- return Models[override_model](opt).to(device)
-
-
-def load_model(opt, device, suffix: str = '', override_model: Optional[str] = None) -> models_utils.Model:
- model_path = f'{opt.cp_folder}/model{"_" + suffix if suffix else ""}'
- model = model_factory(opt, override_model, device)
- name = opt.model_name if override_model is None else override_model
- if os.path.isfile(model_path):
- print(f'loading {name} model from {model_path}')
- model.load_state_dict(torch.load(model_path, map_location=device))
- else:
- print(f'init {name} model')
- return model
-
-
-def save_model(model, path):
- if constants.DEBUG:
- return False
- print(f'saving model in {path}')
- torch.save(model.state_dict(), path)
- return True
-
-
-def model_lc(opt: options.Options, override_model: Optional[str] = None) -> Tuple[occ_gmm.Spaghetti, options.Options]:
-
- def save_model(model_: models_utils.Model, suffix: str = ''):
- nonlocal already_init
- if override_model is not None and suffix == '':
- suffix = override_model
- model_path = f'{opt.cp_folder}/model{"_" + suffix if suffix else ""}'
- if constants.DEBUG or 'debug' in opt.tag:
- return False
- if not already_init:
- files_utils.init_folders(model_path)
- files_utils.save_pickle(opt, params_path)
- already_init = True
- if is_model_clean(model_):
- print(f'saving {opt.model_name} model at {model_path}')
- torch.save(model_.state_dict(), model_path)
- elif os.path.isfile(model_path):
- print(f'model is corrupted')
- print(f'loading {opt.model_name} model from {model_path}')
- model.load_state_dict(torch.load(model_path, map_location=opt.device))
- return True
-
- already_init = False
- params_path = f'{opt.cp_folder}/options.pkl'
- opt_ = files_utils.load_pickle(params_path)
-
- if opt_ is not None:
- opt_.device = opt.device
- opt = opt_
- already_init = True
- model = load_model(opt, opt.device, override_model=override_model)
- model.save_model = save_model
- return model, opt
-
-
-class Logger:
-
- def __init__(self, level: int = 0):
- self.level_dictionary = dict()
- self.iter_dictionary = dict()
- self.level = level
- self.progress: Union[N, tqdm] = None
- self.iters = 0
- self.tag = ''
-
- @staticmethod
- def aggregate(dictionary: dict, parent_dictionary: Union[dict, N] = None) -> dict:
- aggregate_dictionary = dict()
- for key in dictionary:
- if 'counter' not in key:
- aggregate_dictionary[key] = dictionary[key] / float(dictionary[f"{key}_counter"])
- if parent_dictionary is not None:
- Logger.stash(parent_dictionary, (key, aggregate_dictionary[key]))
- return aggregate_dictionary
-
- @staticmethod
- def flatten(items: Tuple[Union[Dict[str, LI], str, LI], ...]) -> List[Union[str, LI]]:
- flat_items = []
- for item in items:
- if type(item) is dict:
- for key, value in item.items():
- flat_items.append(key)
- flat_items.append(value)
- else:
- flat_items.append(item)
- return flat_items
-
- @staticmethod
- def stash(dictionary: Dict[str, LI], items: Tuple[Union[Dict[str, LI], str, LI], ...]) -> Dict[str, LI]:
- flat_items = Logger.flatten(items)
- for i in range(0, len(flat_items), 2):
- key, item = flat_items[i], flat_items[i + 1]
- if type(item) is T:
- item = item.item()
- if key not in dictionary:
- dictionary[key] = 0
- dictionary[f"{key}_counter"] = 0
- dictionary[key] += item
- dictionary[f"{key}_counter"] += 1
- return dictionary
-
- def stash_iter(self, *items: Union[Dict[str, LI], str, LI]):
- self.iter_dictionary = self.stash(self.iter_dictionary, items)
- return self
-
- def stash_level(self, *items: Union[Dict[str, LI], str, LI]):
- self.level_dictionary = self.stash(self.level_dictionary, items)
-
- def reset_iter(self, *items: Union[Dict[str, LI], str, LI]):
- if len(items) > 0:
- self.stash_iter(*items)
- aggregate_dictionary = self.aggregate(self.iter_dictionary, self.level_dictionary)
- self.progress.set_postfix(aggregate_dictionary)
- self.progress.update()
- self.iter_dictionary = dict()
- return self
-
- def start(self, iters: int, tag: str = ''):
- if self.progress is not None:
- self.stop()
- if iters < 0:
- iters = self.iters
- if tag == '':
- tag = self.tag
- self.iters, self.tag = iters, tag
- self.progress = tqdm(total=self.iters, desc=f'{self.tag} {self.level}')
- return self
-
- def stop(self, aggregate: bool = True):
- if aggregate:
- aggregate_dictionary = self.aggregate(self.level_dictionary)
- self.progress.set_postfix(aggregate_dictionary)
- self.level_dictionary = dict()
- self.progress.close()
- self.progress = None
- self.level += 1
- return aggregate_dictionary
-
- def reset_level(self, aggregate: bool = True):
- self.stop(aggregate)
- self.start()
-
-
-class LinearWarmupScheduler:
-
- def get_lr(self):
- if self.cur_iter >= self.num_iters:
- return [self.target_lr] * len(self.base_lrs)
- alpha = self.cur_iter / self.num_iters
- return [base_lr + delta_lr * alpha for base_lr, delta_lr in zip(self.base_lrs, self.delta_lrs)]
-
- def step(self):
- if not self.finished:
- for group, lr in zip(self.optimizer.param_groups, self.get_lr()):
- group['lr'] = lr
- self.cur_iter += 1.
- self.finished = self.cur_iter > self.num_iters
-
- def __init__(self, optimizer, target_lr, num_iters):
- self.cur_iter = 0.
- self.target_lr = target_lr
- self.num_iters = num_iters
- self.finished = False
- self.optimizer = optimizer
- self.base_lrs = [group['lr'] for group in optimizer.param_groups]
- self.delta_lrs = [target_lr - base_lr for base_lr in self.base_lrs]
diff --git a/spaces/KGHL/img-to-music/share_btn.py b/spaces/KGHL/img-to-music/share_btn.py
deleted file mode 100644
index 351a8f6252414dc48fd9972867f875a002731c19..0000000000000000000000000000000000000000
--- a/spaces/KGHL/img-to-music/share_btn.py
+++ /dev/null
@@ -1,104 +0,0 @@
-community_icon_html = """"""
-
-loading_icon_html = """"""
-
-share_js = """async () => {
- async function uploadFile(file){
- const UPLOAD_URL = 'https://huggingface.co/uploads';
- const response = await fetch(UPLOAD_URL, {
- method: 'POST',
- headers: {
- 'Content-Type': file.type,
- 'X-Requested-With': 'XMLHttpRequest',
- },
- body: file, /// <- File inherits from Blob
- });
- const url = await response.text();
- return url;
- }
- async function getInputImgFile(imgEl){
- const res = await fetch(imgEl.src);
- const blob = await res.blob();
- const imgId = Date.now() % 200;
- const isPng = imgEl.src.startsWith(`data:image/png`);
- if(isPng){
- const fileName = `sd-perception-${{imgId}}.png`;
- return new File([blob], fileName, { type: 'image/png' });
- }else{
- const fileName = `sd-perception-${{imgId}}.jpg`;
- return new File([blob], fileName, { type: 'image/jpeg' });
- }
- }
- async function getOutputMusicFile(audioEL){
- const res = await fetch(audioEL.src);
- const blob = await res.blob();
- const audioId = Date.now() % 200;
- const fileName = `img-to-music-${{audioId}}.wav`;
- const musicBlob = new File([blob], fileName, { type: 'audio/wav' });
- console.log(musicBlob);
- return musicBlob;
- }
-
- async function audioToBase64(audioFile) {
- return new Promise((resolve, reject) => {
- let reader = new FileReader();
- reader.readAsDataURL(audioFile);
- reader.onload = () => resolve(reader.result);
- reader.onerror = error => reject(error);
-
- });
- }
- const gradioEl = document.querySelector('body > gradio-app');
- // const gradioEl = document.querySelector("gradio-app").shadowRoot;
- const inputImgEl = gradioEl.querySelector('#input-img img');
- const prompts = gradioEl.querySelector('#prompts_out textarea').value;
- const outputMusic = gradioEl.querySelector('#music-output audio');
- const outputMusic_src = gradioEl.querySelector('#music-output audio').src;
- const outputMusic_name = outputMusic_src.split('/').pop();
- let titleTxt = outputMusic_name;
- //if(titleTxt.length > 100){
- // titleTxt = titleTxt.slice(0, 100) + ' ...';
- //}
- const shareBtnEl = gradioEl.querySelector('#share-btn');
- const shareIconEl = gradioEl.querySelector('#share-btn-share-icon');
- const loadingIconEl = gradioEl.querySelector('#share-btn-loading-icon');
- if(!outputMusic){
- return;
- };
- shareBtnEl.style.pointerEvents = 'none';
- shareIconEl.style.display = 'none';
- loadingIconEl.style.removeProperty('display');
- const inputFile = await getInputImgFile(inputImgEl);
- const urlInputImg = await uploadFile(inputFile);
- const musicFile = await getOutputMusicFile(outputMusic);
- const dataOutputMusic = await uploadFile(musicFile);
-
- const descriptionMd = `#### Input img:
-
-
-#### Prompts out:
-${prompts}
-
-#### Music:
-
-
-`;
- const params = new URLSearchParams({
- title: titleTxt,
- description: descriptionMd,
- });
- const paramsStr = params.toString();
- window.open(`https://huggingface.co/spaces/fffiloni/img-to-music/discussions/new?${paramsStr}`, '_blank');
- shareBtnEl.style.removeProperty('pointer-events');
- shareIconEl.style.removeProperty('display');
- loadingIconEl.style.display = 'none';
-}"""
\ No newline at end of file
diff --git a/spaces/Kimata/Sanskrit-TTS/indic_nlp_library/indicnlp/transliterate/sinhala_transliterator.py b/spaces/Kimata/Sanskrit-TTS/indic_nlp_library/indicnlp/transliterate/sinhala_transliterator.py
deleted file mode 100644
index 1e762252a56e93c94cd488a07031f7d7eae8a1d3..0000000000000000000000000000000000000000
--- a/spaces/Kimata/Sanskrit-TTS/indic_nlp_library/indicnlp/transliterate/sinhala_transliterator.py
+++ /dev/null
@@ -1,171 +0,0 @@
-#
-# Copyright (c) 2013-present, Anoop Kunchukuttan
-# All rights reserved.
-#
-# This source code is licensed under the MIT license found in the
-# LICENSE file in the root directory of this source tree.
-#
-
-class SinhalaDevanagariTransliterator(object):
- """
- A Devanagari to Sinhala transliterator based on explicit Unicode Mapping
- """
-
- sinhala_devnag_map={
- '\u0d82':'\u0902',
- '\u0d83':'\u0903',
- '\u0d84':'\u0904',
- '\u0d85':'\u0905',
- '\u0d86':'\u0906',
- '\u0d87':'\u090d',
- '\u0d88':'\u090d',
- '\u0d89':'\u0907',
- '\u0d8a':'\u0908',
- '\u0d8b':'\u0909',
- '\u0d8c':'\u090a',
- '\u0d8d':'\u090b',
- '\u0d8f':'\u090c',
- '\u0d91':'\u090e',
- '\u0d92':'\u090f',
- '\u0d93':'\u0910',
- '\u0d94':'\u0912',
- '\u0d95':'\u0913',
- '\u0d96':'\u0914',
- '\u0d9a':'\u0915',
- '\u0d9b':'\u0916',
- '\u0d9c':'\u0917',
- '\u0d9d':'\u0918',
- '\u0d9e':'\u0919',
- '\u0d9f':'\u0919',
- '\u0da0':'\u091a',
- '\u0da1':'\u091b',
- '\u0da2':'\u091c',
- '\u0da3':'\u091d',
- '\u0da4':'\u091e',
- '\u0da5':'\u091e',
- '\u0da6':'\u091e',
- '\u0da7':'\u091f',
- '\u0da8':'\u0920',
- '\u0da9':'\u0921',
- '\u0daa':'\u0922',
- '\u0dab':'\u0923',
- '\u0dac':'\u0923',
- '\u0dad':'\u0924',
- '\u0dae':'\u0925',
- '\u0daf':'\u0926',
- '\u0db0':'\u0927',
- '\u0db1':'\u0928',
- '\u0db2':'\u0928',
- '\u0db3':'\u0928',
- '\u0db4':'\u092a',
- '\u0db5':'\u092b',
- '\u0db6':'\u092c',
- '\u0db7':'\u092d',
- '\u0db8':'\u092e',
- '\u0dba':'\u092f',
- '\u0dbb':'\u0930',
- '\u0dbd':'\u0932',
- '\u0dc5':'\u0933',
- '\u0dc0':'\u0935',
- '\u0dc1':'\u0936',
- '\u0dc2':'\u0937',
- '\u0dc3':'\u0938',
- '\u0dc4':'\u0939',
- '\u0dcf':'\u093e',
- '\u0dd0':'\u0949',
- '\u0dd1':'\u0949',
- '\u0dd2':'\u093f',
- '\u0dd3':'\u0940',
- '\u0dd4':'\u0941',
- '\u0dd6':'\u0942',
- '\u0dd8':'\u0943',
- '\u0dd9':'\u0946',
- '\u0dda':'\u0947',
- '\u0ddb':'\u0948',
- '\u0ddc':'\u094a',
- '\u0ddd':'\u094b',
- '\u0dde':'\u094c',
- '\u0dca':'\u094d',
- }
-
- devnag_sinhala_map={
- '\u0900':'\u0d82',
- '\u0901':'\u0d82',
- '\u0902':'\u0d82',
- '\u0903':'\u0d83',
- '\u0904':'\u0d84',
- '\u0905':'\u0d85',
- '\u0906':'\u0d86',
- '\u0907':'\u0d89',
- '\u0908':'\u0d8a',
- '\u0909':'\u0d8b',
- '\u090a':'\u0d8c',
- '\u090b':'\u0d8d',
- '\u090c':'\u0d8f',
- '\u090d':'\u0d88',
- '\u090e':'\u0d91',
- '\u090f':'\u0d92',
- '\u0910':'\u0d93',
- '\u0912':'\u0d94',
- '\u0913':'\u0d95',
- '\u0914':'\u0d96',
- '\u0915':'\u0d9a',
- '\u0916':'\u0d9b',
- '\u0917':'\u0d9c',
- '\u0918':'\u0d9d',
- '\u0919':'\u0d9e',
- '\u091a':'\u0da0',
- '\u091b':'\u0da1',
- '\u091c':'\u0da2',
- '\u091d':'\u0da3',
- '\u091e':'\u0da4',
- '\u091f':'\u0da7',
- '\u0920':'\u0da8',
- '\u0921':'\u0da9',
- '\u0922':'\u0daa',
- '\u0923':'\u0dab',
- '\u0924':'\u0dad',
- '\u0925':'\u0dae',
- '\u0926':'\u0daf',
- '\u0927':'\u0db0',
- '\u0928':'\u0db1',
- '\u0929':'\u0db1',
- '\u092a':'\u0db4',
- '\u092b':'\u0db5',
- '\u092c':'\u0db6',
- '\u092d':'\u0db7',
- '\u092e':'\u0db8',
- '\u092f':'\u0dba',
- '\u0930':'\u0dbb',
- '\u0932':'\u0dbd',
- '\u0933':'\u0dc5',
- '\u0935':'\u0dc0',
- '\u0936':'\u0dc1',
- '\u0937':'\u0dc2',
- '\u0938':'\u0dc3',
- '\u0939':'\u0dc4',
- '\u093e':'\u0dcf',
- '\u0949':'\u0dd1',
- '\u093f':'\u0dd2',
- '\u0940':'\u0dd3',
- '\u0941':'\u0dd4',
- '\u0942':'\u0dd6',
- '\u0943':'\u0dd8',
- '\u0946':'\u0dd9',
- '\u0947':'\u0dda',
- '\u0948':'\u0ddb',
- '\u094a':'\u0ddc',
- '\u094b':'\u0ddd',
- '\u094c':'\u0dde',
- '\u094d':'\u0dca',
-
- }
-
- @staticmethod
- def devanagari_to_sinhala(text):
- return ''.join([ SinhalaDevanagariTransliterator.devnag_sinhala_map.get(c,c) for c in text ])
-
- @staticmethod
- def sinhala_to_devanagari(text):
- return ''.join([ SinhalaDevanagariTransliterator.sinhala_devnag_map.get(c,c) for c in text ])
-
diff --git a/spaces/KyanChen/RSPrompter/mmpl/models/pler/base_pler.py b/spaces/KyanChen/RSPrompter/mmpl/models/pler/base_pler.py
deleted file mode 100644
index bf099728a9e7bb452c61ffc5d4984ac58d8ef939..0000000000000000000000000000000000000000
--- a/spaces/KyanChen/RSPrompter/mmpl/models/pler/base_pler.py
+++ /dev/null
@@ -1,241 +0,0 @@
-import torch
-import torch.nn as nn
-from lightning.pytorch.utilities import grad_norm
-from mmengine import OPTIM_WRAPPERS
-from mmengine.optim import build_optim_wrapper, _ParamScheduler
-import copy
-
-from torchmetrics import MetricCollection
-
-from mmpl.registry import MODELS, METRICS
-import lightning.pytorch as pl
-from mmengine.registry import OPTIMIZERS, PARAM_SCHEDULERS
-from mmengine.model import BaseModel
-
-
-@MODELS.register_module()
-class BasePLer(pl.LightningModule, BaseModel):
- def __init__(
- self,
- hyperparameters,
- data_preprocessor=None,
- train_cfg=None,
- test_cfg=None,
- *args,
- **kwargs
- ):
- super().__init__()
- self.hyperparameters = hyperparameters
- self.train_cfg = train_cfg
- self.test_cfg = test_cfg
-
- if data_preprocessor is not None:
- if isinstance(data_preprocessor, nn.Module):
- self.data_preprocessor = data_preprocessor
- elif isinstance(data_preprocessor, dict):
- self.data_preprocessor = MODELS.build(data_preprocessor)
- else:
- raise TypeError('data_preprocessor should be a `dict` or '
- f'`nn.Module` instance, but got '
- f'{type(data_preprocessor)}')
-
- evaluator_cfg = copy.deepcopy(self.hyperparameters.get('evaluator', None))
- if evaluator_cfg is not None:
- for k, v in evaluator_cfg.items():
- metrics = []
- if isinstance(v, dict):
- v = [v]
- if isinstance(v, list):
- for metric_cfg in v:
- metric = METRICS.build(metric_cfg)
- metrics.append(metric)
- else:
- raise TypeError('evaluator should be a `dict` or '
- f'`list` instance, but got '
- f'{type(evaluator_cfg)}')
- setattr(self, k, MetricCollection(metrics, prefix=k.split('_')[0]))
-
- def _set_grad(self, need_train_names: list=[], noneed_train_names: list=[]):
- for name, param in self.named_parameters():
- flag = False
- for need_train_name in need_train_names:
- if need_train_name in name:
- flag = True
- for noneed_train_name in noneed_train_names:
- if noneed_train_name in name:
- flag = False
- param.requires_grad_(flag)
-
- not_specific_names = []
- for name, param in self.named_parameters():
- flag_find = False
- for specific_name in need_train_names + noneed_train_names:
- if specific_name in name:
- flag_find = True
- if not flag_find:
- not_specific_names.append(name)
-
- if self.local_rank == 0:
- not_specific_names = [x.split('.')[0] for x in not_specific_names]
- not_specific_names = set(not_specific_names)
- print(f"Turning off gradients for names: {noneed_train_names}")
- print(f"Turning on gradients for names: {need_train_names}")
- print(f"Turning off gradients for not specific names: {not_specific_names}")
-
- def _set_train_module(self, mode=True, need_train_names: list=[]):
- self.training = mode
- for name, module in self.named_children():
- flag = False
- for need_train_name in need_train_names:
- if need_train_name in name:
- flag = True
- if flag:
- module.train(mode)
- else:
- module.eval()
- return self
-
- def configure_optimizers(self):
- optimizer_cfg = copy.deepcopy(self.hyperparameters.get('optimizer'))
- base_lr = optimizer_cfg.get('lr')
- base_wd = optimizer_cfg.get('weight_decay', None)
-
- sub_models = optimizer_cfg.pop('sub_model', None)
- if sub_models is None:
- optimizer_cfg['params'] = filter(lambda p: p.requires_grad, self.parameters())
- # optimizer_cfg['params'] = self.parameters()
- else:
- if isinstance(sub_models, str):
- sub_models = {sub_models: {}}
- if isinstance(sub_models, list):
- sub_models = {x: {} for x in sub_models}
- assert isinstance(sub_models, dict), f'sub_models should be a dict, but got {type(sub_models)}'
- # import ipdb; ipdb.set_trace()
- # set training parameters and lr
- for sub_model_name, value in sub_models.items():
- sub_attrs = sub_model_name.split('.')
- sub_model_ = self
- # import ipdb; ipdb.set_trace()
- for sub_attr in sub_attrs:
- sub_model_ = getattr(sub_model_, sub_attr)
- # sub_model_ = self.trainer.strategy.model._forward_module.get_submodule(sub_model_name)
- if isinstance(sub_model_, torch.nn.Parameter):
- # filter(lambda p: p.requires_grad, model.parameters())
- # sub_models[sub_model_name]['params'] = filter(lambda p: p.requires_grad, [sub_model_])
- sub_models[sub_model_name]['params'] = filter(lambda p: p.requires_grad, [sub_model_])
- else:
- # import ipdb;ipdb.set_trace()
- sub_models[sub_model_name]['params'] = filter(lambda p: p.requires_grad, sub_model_.parameters())
- # sub_models[sub_model_name]['params'] = sub_model_.parameters()
- lr_mult = value.pop('lr_mult', 1.)
- sub_models[sub_model_name]['lr'] = base_lr * lr_mult
- if base_wd is not None:
- decay_mult = value.pop('decay_mult', 1.)
- sub_models[sub_model_name]['weight_decay'] = base_wd * decay_mult
- else:
- raise ModuleNotFoundError(f'{sub_model_name} not in model')
-
- if self.local_rank == 0:
- print('All sub models:')
- for name, module in self.named_children():
- print(name, end=', ')
- print()
- print('Needed train models:')
- for name, value in sub_models.items():
- print(f'{name}', end=', ')
- print()
-
- optimizer_cfg['params'] = [value for key, value in sub_models.items()]
-
- optimizer = OPTIMIZERS.build(optimizer_cfg)
- if self.local_rank == 0:
- print('查看优化器参数')
- for param_group in optimizer.param_groups:
- print([value.shape for value in param_group['params']], '学习率: ', param_group['lr'])
-
- schedulers = copy.deepcopy(self.hyperparameters.get('param_scheduler', None))
- if schedulers is None:
- return [optimizer]
- param_schedulers = []
- total_step = self.trainer.estimated_stepping_batches
- for scheduler in schedulers:
- if isinstance(scheduler, _ParamScheduler):
- param_schedulers.append(scheduler)
- elif isinstance(scheduler, dict):
- _scheduler = copy.deepcopy(scheduler)
- param_schedulers.append(
- PARAM_SCHEDULERS.build(
- _scheduler,
- default_args=dict(
- optimizer=optimizer,
- epoch_length=self.trainer.num_training_batches,
- )
- )
- )
- else:
- raise TypeError(
- 'scheduler should be a _ParamScheduler object or dict, '
- f'but got {scheduler}')
-
- return [optimizer], param_schedulers
-
- def lr_scheduler_step(self, scheduler, metric):
- pass
-
- def log_grad(self, module=None) -> None:
- # Compute the 2-norm for each layer
- # If using mixed precision, the gradients are already unscaled here
- if module is None:
- module = self
- norms = grad_norm(module, norm_type=2)
- max_grad = max(norms.values())
- min_gead = min(norms.values())
- self.log_dict(
- {'max_grad': max_grad, 'min_grad': min_gead},
- prog_bar=True,
- logger=True
- )
-
- def setup(self, stage: str) -> None:
- evaluators = ['train', 'val', 'test']
- for evaluator in evaluators:
- if hasattr(self, f'{evaluator}_evaluator'):
- if hasattr(self.trainer.datamodule, f'{evaluator}_dataset'):
- dataset = getattr(self.trainer.datamodule, f'{evaluator}_dataset')
- if hasattr(dataset, 'metainfo'):
- evaluator_ = getattr(self, f'{evaluator}_evaluator')
- for v in evaluator_.values():
- if hasattr(v, 'dataset_meta'):
- v.dataset_meta = dataset.metainfo
-
- def on_before_optimizer_step(self, optimizer) -> None:
- self.log_grad()
-
- def on_validation_epoch_end(self) -> None:
- self._log_eval_metrics('val')
-
- def on_test_epoch_end(self) -> None:
- self._log_eval_metrics('test')
-
- def on_train_epoch_end(self) -> None:
- self._log_eval_metrics('train')
-
- def _log_eval_metrics(self, stage):
- assert stage in ['train', 'val', 'test']
- if hasattr(self, f'{stage}_evaluator'):
- evaluator = getattr(self, f'{stage}_evaluator')
- metrics = evaluator.compute()
- metrics = {k.lower(): v for k, v in metrics.items()}
- keys = []
- for k, v in metrics.items():
- v = v.view(-1)
- for i, data in enumerate(v):
- keys.append(f'{k}_{i}')
- self.log(f'{k.lower()}_{i}', data, on_step=False, on_epoch=True, prog_bar=True, logger=True, sync_dist=True)
- evaluator.reset()
-
- if hasattr(self.trainer, 'checkpoint_callback'):
- monitor = self.trainer.checkpoint_callback.monitor
- if (monitor is not None) and (monitor not in keys):
- data = torch.tensor(0., device=self.device)
- self.log(f'{monitor}', data, on_step=False, on_epoch=True, prog_bar=True, logger=True, sync_dist=True)
\ No newline at end of file
diff --git a/spaces/LanguageBind/LanguageBind/languagebind/image/tokenization_image.py b/spaces/LanguageBind/LanguageBind/languagebind/image/tokenization_image.py
deleted file mode 100644
index 593423d089100b3d61957f658cca04b541336f65..0000000000000000000000000000000000000000
--- a/spaces/LanguageBind/LanguageBind/languagebind/image/tokenization_image.py
+++ /dev/null
@@ -1,77 +0,0 @@
-from transformers import CLIPTokenizer
-from transformers.utils import logging
-
-logger = logging.get_logger(__name__)
-
-VOCAB_FILES_NAMES = {
- "vocab_file": "vocab.json",
- "merges_file": "merges.txt",
-}
-
-PRETRAINED_VOCAB_FILES_MAP = {
- "vocab_file": {
- "lb203/LanguageBind-Image": "https://huggingface.co/lb203/LanguageBind-Image/resolve/main/vocab.json",
- },
- "merges_file": {
- "lb203/LanguageBind-Image": "https://huggingface.co/lb203/LanguageBind-Image/resolve/main/merges.txt",
- },
-}
-
-PRETRAINED_POSITIONAL_EMBEDDINGS_SIZES = {
- "lb203/LanguageBind-Image": 77,
-}
-
-
-PRETRAINED_INIT_CONFIGURATION = {
- "lb203/LanguageBind-Image": {},
-}
-
-class LanguageBindImageTokenizer(CLIPTokenizer):
- """
- Construct a CLIP tokenizer. Based on byte-level Byte-Pair-Encoding.
-
- This tokenizer inherits from [`PreTrainedTokenizer`] which contains most of the main methods. Users should refer to
- this superclass for more information regarding those methods.
-
- Args:
- vocab_file (`str`):
- Path to the vocabulary file.
- merges_file (`str`):
- Path to the merges file.
- errors (`str`, *optional*, defaults to `"replace"`):
- Paradigm to follow when decoding bytes to UTF-8. See
- [bytes.decode](https://docs.python.org/3/library/stdtypes.html#bytes.decode) for more information.
- unk_token (`str`, *optional*, defaults to `<|endoftext|>`):
- The unknown token. A token that is not in the vocabulary cannot be converted to an ID and is set to be this
- token instead.
- bos_token (`str`, *optional*, defaults to `<|startoftext|>`):
- The beginning of sequence token.
- eos_token (`str`, *optional*, defaults to `<|endoftext|>`):
- The end of sequence token.
- """
-
- vocab_files_names = VOCAB_FILES_NAMES
- pretrained_vocab_files_map = PRETRAINED_VOCAB_FILES_MAP
- max_model_input_sizes = PRETRAINED_POSITIONAL_EMBEDDINGS_SIZES
- model_input_names = ["input_ids", "attention_mask"]
-
- def __init__(
- self,
- vocab_file,
- merges_file,
- errors="replace",
- unk_token="<|endoftext|>",
- bos_token="<|startoftext|>",
- eos_token="<|endoftext|>",
- pad_token="<|endoftext|>", # hack to enable padding
- **kwargs,
- ):
- super(LanguageBindImageTokenizer, self).__init__(
- vocab_file,
- merges_file,
- errors,
- unk_token,
- bos_token,
- eos_token,
- pad_token, # hack to enable padding
- **kwargs,)
\ No newline at end of file
diff --git a/spaces/ML701G7/taim-gan/src/models/modules/attention.py b/spaces/ML701G7/taim-gan/src/models/modules/attention.py
deleted file mode 100644
index b4f5e990d7967397602b2099544e3e1e63631025..0000000000000000000000000000000000000000
--- a/spaces/ML701G7/taim-gan/src/models/modules/attention.py
+++ /dev/null
@@ -1,88 +0,0 @@
-"""Attention modules"""
-from typing import Any, Optional
-
-import torch
-from torch import nn
-
-from src.models.modules.conv_utils import conv1d
-
-
-class ChannelWiseAttention(nn.Module):
- """ChannelWise attention adapted from ControlGAN"""
-
- def __init__(self, fm_size: int, text_d: int) -> None:
- """
- Initialize the Channel-Wise attention module
-
- :param int fm_size:
- Height and width of feature map on k-th iteration of forward-pass.
- In paper, it's H_k * W_k
- :param int text_d: Dimensionality of sentence. From paper, it's D
- """
- super().__init__()
- # perception layer
- self.text_conv = conv1d(text_d, fm_size)
- # attention across channel dimension
- self.softmax = nn.Softmax(2)
-
- def forward(self, v_k: torch.Tensor, w_text: torch.Tensor) -> Any:
- """
- Apply attention to visual features taking into account features of words
-
- :param torch.Tensor v_k: Visual context
- :param torch.Tensor w_text: Textual features
- :return: Fused hidden visual features and word features
- :rtype: Any
- """
- w_hat = self.text_conv(w_text)
- m_k = v_k @ w_hat
- a_k = self.softmax(m_k)
- w_hat = torch.transpose(w_hat, 1, 2)
- return a_k @ w_hat
-
-
-class SpatialAttention(nn.Module):
- """Spatial attention module for attending textual context to visual features"""
-
- def __init__(self, d: int, d_hat: int) -> None:
- """
- Set up softmax and conv layers
-
- :param int d: Initial embedding size for textual features. D from paper
- :param int d_hat: Height of image feature map. D_hat from paper
- """
- super().__init__()
- self.softmax = nn.Softmax(2)
- self.conv = conv1d(d, d_hat)
-
- def forward(
- self,
- text_context: torch.Tensor,
- image: torch.Tensor,
- mask: Optional[torch.Tensor] = None,
- ) -> Any:
- """
- Project image features into the latent space
- of textual features and apply attention
-
- :param torch.Tensor text_context: D x T tensor of hidden textual features
- :param torch.Tensor image: D_hat x N visual features
- :param Optional[torch.Tensor] mask:
- Boolean tensor for masking the padded words. BxL
- :return: Word features attended by visual features
- :rtype: Any
- """
- # number of features on image feature map H * W
- feature_num = image.size(2)
- # number of words in caption
- len_caption = text_context.size(2)
- text_context = self.conv(text_context)
- image = torch.transpose(image, 1, 2)
- s_i_j = image @ text_context
- if mask is not None:
- # duplicating mask and aligning dims with s_i_j
- mask = mask.repeat(1, feature_num).view(-1, feature_num, len_caption)
- s_i_j[mask] = -float("inf")
- b_i_j = self.softmax(s_i_j)
- c_i_j = b_i_j @ torch.transpose(text_context, 1, 2)
- return torch.transpose(c_i_j, 1, 2)
diff --git a/spaces/Marshalls/testmtd/analysis/pymo/Pivots.py b/spaces/Marshalls/testmtd/analysis/pymo/Pivots.py
deleted file mode 100644
index 84c9f9e2166fddc71f3b2e42d0a1d5a0fd67b28e..0000000000000000000000000000000000000000
--- a/spaces/Marshalls/testmtd/analysis/pymo/Pivots.py
+++ /dev/null
@@ -1,89 +0,0 @@
-import numpy as np
-
-from analysis.pymo.Quaternions import Quaternions
-
-class Pivots:
- """
- Pivots is an ndarray of angular rotations
-
- This wrapper provides some functions for
- working with pivots.
-
- These are particularly useful as a number
- of atomic operations (such as adding or
- subtracting) cannot be achieved using
- the standard arithmatic and need to be
- defined differently to work correctly
- """
-
- def __init__(self, ps): self.ps = np.array(ps)
- def __str__(self): return "Pivots("+ str(self.ps) + ")"
- def __repr__(self): return "Pivots("+ repr(self.ps) + ")"
-
- def __add__(self, other): return Pivots(np.arctan2(np.sin(self.ps + other.ps), np.cos(self.ps + other.ps)))
- def __sub__(self, other): return Pivots(np.arctan2(np.sin(self.ps - other.ps), np.cos(self.ps - other.ps)))
- def __mul__(self, other): return Pivots(self.ps * other.ps)
- def __div__(self, other): return Pivots(self.ps / other.ps)
- def __mod__(self, other): return Pivots(self.ps % other.ps)
- def __pow__(self, other): return Pivots(self.ps ** other.ps)
-
- def __lt__(self, other): return self.ps < other.ps
- def __le__(self, other): return self.ps <= other.ps
- def __eq__(self, other): return self.ps == other.ps
- def __ne__(self, other): return self.ps != other.ps
- def __ge__(self, other): return self.ps >= other.ps
- def __gt__(self, other): return self.ps > other.ps
-
- def __abs__(self): return Pivots(abs(self.ps))
- def __neg__(self): return Pivots(-self.ps)
-
- def __iter__(self): return iter(self.ps)
- def __len__(self): return len(self.ps)
-
- def __getitem__(self, k): return Pivots(self.ps[k])
- def __setitem__(self, k, v): self.ps[k] = v.ps
-
- def _ellipsis(self): return tuple(map(lambda x: slice(None), self.shape))
-
- def quaternions(self, plane='xz'):
- fa = self._ellipsis()
- axises = np.ones(self.ps.shape + (3,))
- axises[fa + ("xyz".index(plane[0]),)] = 0.0
- axises[fa + ("xyz".index(plane[1]),)] = 0.0
- return Quaternions.from_angle_axis(self.ps, axises)
-
- def directions(self, plane='xz'):
- dirs = np.zeros((len(self.ps), 3))
- dirs["xyz".index(plane[0])] = np.sin(self.ps)
- dirs["xyz".index(plane[1])] = np.cos(self.ps)
- return dirs
-
- def normalized(self):
- xs = np.copy(self.ps)
- while np.any(xs > np.pi): xs[xs > np.pi] = xs[xs > np.pi] - 2 * np.pi
- while np.any(xs < -np.pi): xs[xs < -np.pi] = xs[xs < -np.pi] + 2 * np.pi
- return Pivots(xs)
-
- def interpolate(self, ws):
- dir = np.average(self.directions, weights=ws, axis=0)
- return np.arctan2(dir[2], dir[0])
-
- def copy(self):
- return Pivots(np.copy(self.ps))
-
- @property
- def shape(self):
- return self.ps.shape
-
- @classmethod
- def from_quaternions(cls, qs, forward='z', plane='xz'):
- ds = np.zeros(qs.shape + (3,))
- ds[...,'xyz'.index(forward)] = 1.0
- return Pivots.from_directions(qs * ds, plane=plane)
-
- @classmethod
- def from_directions(cls, ds, plane='xz'):
- ys = ds[...,'xyz'.index(plane[0])]
- xs = ds[...,'xyz'.index(plane[1])]
- return Pivots(np.arctan2(ys, xs))
-
diff --git a/spaces/Mashir0/pximg/glitch-update.sh b/spaces/Mashir0/pximg/glitch-update.sh
deleted file mode 100644
index fde50d6b5c6b4bfd2e5ea62bb0eb291c41259e9c..0000000000000000000000000000000000000000
--- a/spaces/Mashir0/pximg/glitch-update.sh
+++ /dev/null
@@ -1,5 +0,0 @@
-#!/bin/sh
-git fetch origin master
-git reset --hard origin/master
-git pull origin master --force
-refresh
diff --git a/spaces/MattyWhite/ChatGPT-ImageCaptioner2/datasets/README.md b/spaces/MattyWhite/ChatGPT-ImageCaptioner2/datasets/README.md
deleted file mode 100644
index aadb3133e8c9a5345e137c5736485109c1a107db..0000000000000000000000000000000000000000
--- a/spaces/MattyWhite/ChatGPT-ImageCaptioner2/datasets/README.md
+++ /dev/null
@@ -1,207 +0,0 @@
-# Prepare datasets for Detic
-
-The basic training of our model uses [LVIS](https://www.lvisdataset.org/) (which uses [COCO](https://cocodataset.org/) images) and [ImageNet-21K](https://www.image-net.org/download.php).
-Some models are trained on [Conceptual Caption (CC3M)](https://ai.google.com/research/ConceptualCaptions/).
-Optionally, we use [Objects365](https://www.objects365.org/) and [OpenImages (Challenge 2019 version)](https://storage.googleapis.com/openimages/web/challenge2019.html) for cross-dataset evaluation.
-Before starting processing, please download the (selected) datasets from the official websites and place or sim-link them under `$Detic_ROOT/datasets/`.
-
-```
-$Detic_ROOT/datasets/
- metadata/
- lvis/
- coco/
- imagenet/
- cc3m/
- objects365/
- oid/
-```
-`metadata/` is our preprocessed meta-data (included in the repo). See the below [section](#Metadata) for details.
-Please follow the following instruction to pre-process individual datasets.
-
-### COCO and LVIS
-
-First, download COCO and LVIS data place them in the following way:
-
-```
-lvis/
- lvis_v1_train.json
- lvis_v1_val.json
-coco/
- train2017/
- val2017/
- annotations/
- captions_train2017.json
- instances_train2017.json
- instances_val2017.json
-```
-
-Next, prepare the open-vocabulary LVIS training set using
-
-```
-python tools/remove_lvis_rare.py --ann datasets/lvis/lvis_v1_train.json
-```
-
-This will generate `datasets/lvis/lvis_v1_train_norare.json`.
-
-### ImageNet-21K
-
-The ImageNet-21K folder should look like:
-```
-imagenet/
- ImageNet-21K/
- n01593028.tar
- n01593282.tar
- ...
-```
-
-We first unzip the overlapping classes of LVIS (we will directly work with the .tar file for the rest classes) and convert them into LVIS annotation format.
-
-~~~
-mkdir imagenet/annotations
-python tools/unzip_imagenet_lvis.py --dst_path datasets/imagenet/ImageNet-LVIS
-python tools/create_imagenetlvis_json.py --imagenet_path datasets/imagenet/ImageNet-LVIS --out_path datasets/imagenet/annotations/imagenet_lvis_image_info.json
-~~~
-This creates `datasets/imagenet/annotations/imagenet_lvis_image_info.json`.
-
-[Optional] To train with all the 21K classes, run
-
-~~~
-python tools/get_imagenet_21k_full_tar_json.py
-python tools/create_lvis_21k.py
-~~~
-This creates `datasets/imagenet/annotations/imagenet-21k_image_info_lvis-21k.json` and `datasets/lvis/lvis_v1_train_lvis-21k.json` (combined LVIS and ImageNet-21K classes in `categories`).
-
-[Optional] To train on combined LVIS and COCO, run
-
-~~~
-python tools/merge_lvis_coco.py
-~~~
-This creates `datasets/lvis/lvis_v1_train+coco_mask.json`
-
-### Conceptual Caption
-
-
-Download the dataset from [this](https://ai.google.com/research/ConceptualCaptions/download) page and place them as:
-```
-cc3m/
- GCC-training.tsv
-```
-
-Run the following command to download the images and convert the annotations to LVIS format (Note: download images takes long).
-
-~~~
-python tools/download_cc.py --ann datasets/cc3m/GCC-training.tsv --save_image_path datasets/cc3m/training/ --out_path datasets/cc3m/train_image_info.json
-python tools/get_cc_tags.py
-~~~
-
-This creates `datasets/cc3m/train_image_info_tags.json`.
-
-### Objects365
-Download Objects365 (v2) from the website. We only need the validation set in this project:
-```
-objects365/
- annotations/
- zhiyuan_objv2_val.json
- val/
- images/
- v1/
- patch0/
- ...
- patch15/
- v2/
- patch16/
- ...
- patch49/
-
-```
-
-The original annotation has typos in the class names, we first fix them for our following use of language embeddings.
-
-```
-python tools/fix_o365_names.py --ann datasets/objects365/annotations/zhiyuan_objv2_val.json
-```
-This creates `datasets/objects365/zhiyuan_objv2_val_fixname.json`.
-
-To train on Objects365, download the training images and use the command above. We note some images in the training annotation do not exist.
-We use the following command to filter the missing images.
-~~~
-python tools/fix_0365_path.py
-~~~
-This creates `datasets/objects365/zhiyuan_objv2_train_fixname_fixmiss.json`.
-
-### OpenImages
-
-We followed the instructions in [UniDet](https://github.com/xingyizhou/UniDet/blob/master/projects/UniDet/unidet_docs/DATASETS.md#openimages) to convert the metadata for OpenImages.
-
-The converted folder should look like
-
-```
-oid/
- annotations/
- oid_challenge_2019_train_bbox.json
- oid_challenge_2019_val_expanded.json
- images/
- 0/
- 1/
- 2/
- ...
-```
-
-### Open-vocabulary COCO
-
-We first follow [OVR-CNN](https://github.com/alirezazareian/ovr-cnn/blob/master/ipynb/003.ipynb) to create the open-vocabulary COCO split. The converted files should be like
-
-```
-coco/
- zero-shot/
- instances_train2017_seen_2.json
- instances_val2017_all_2.json
-```
-
-We further pre-process the annotation format for easier evaluation:
-
-```
-python tools/get_coco_zeroshot_oriorder.py --data_path datasets/coco/zero-shot/instances_train2017_seen_2.json
-python tools/get_coco_zeroshot_oriorder.py --data_path datasets/coco/zero-shot/instances_val2017_all_2.json
-```
-
-Next, we preprocess the COCO caption data:
-
-```
-python tools/get_cc_tags.py --cc_ann datasets/coco/annotations/captions_train2017.json --out_path datasets/coco/captions_train2017_tags_allcaps.json --allcaps --convert_caption
-```
-This creates `datasets/coco/captions_train2017_tags_allcaps.json`.
-
-### Metadata
-
-```
-metadata/
- lvis_v1_train_cat_info.json
- coco_clip_a+cname.npy
- lvis_v1_clip_a+cname.npy
- o365_clip_a+cnamefix.npy
- oid_clip_a+cname.npy
- imagenet_lvis_wnid.txt
- Objects365_names_fix.csv
-```
-
-`lvis_v1_train_cat_info.json` is used by the Federated loss.
-This is created by
-~~~
-python tools/get_lvis_cat_info.py --ann datasets/lvis/lvis_v1_train.json
-~~~
-
-`*_clip_a+cname.npy` is the pre-computed CLIP embeddings for each datasets.
-They are created by (taking LVIS as an example)
-~~~
-python tools/dump_clip_features.py --ann datasets/lvis/lvis_v1_val.json --out_path metadata/lvis_v1_clip_a+cname.npy
-~~~
-Note we do not include the 21K class embeddings due to the large file size.
-To create it, run
-~~~
-python tools/dump_clip_features.py --ann datasets/lvis/lvis_v1_val_lvis-21k.json --out_path datasets/metadata/lvis-21k_clip_a+cname.npy
-~~~
-
-`imagenet_lvis_wnid.txt` is the list of matched classes between ImageNet-21K and LVIS.
-
-`Objects365_names_fix.csv` is our manual fix of the Objects365 names.
\ No newline at end of file
diff --git a/spaces/MetaWabbit/Auto-GPT/tests/integration/milvus_memory_tests.py b/spaces/MetaWabbit/Auto-GPT/tests/integration/milvus_memory_tests.py
deleted file mode 100644
index ec38bf2f72087b5da679d26594ebff97d8a09b19..0000000000000000000000000000000000000000
--- a/spaces/MetaWabbit/Auto-GPT/tests/integration/milvus_memory_tests.py
+++ /dev/null
@@ -1,57 +0,0 @@
-# sourcery skip: snake-case-functions
-"""Tests for the MilvusMemory class."""
-import random
-import string
-import unittest
-
-from autogpt.config import Config
-from autogpt.memory.milvus import MilvusMemory
-
-try:
-
- class TestMilvusMemory(unittest.TestCase):
- """Tests for the MilvusMemory class."""
-
- def random_string(self, length: int) -> str:
- """Generate a random string of the given length."""
- return "".join(random.choice(string.ascii_letters) for _ in range(length))
-
- def setUp(self) -> None:
- """Set up the test environment."""
- cfg = Config()
- cfg.milvus_addr = "localhost:19530"
- self.memory = MilvusMemory(cfg)
- self.memory.clear()
-
- # Add example texts to the cache
- self.example_texts = [
- "The quick brown fox jumps over the lazy dog",
- "I love machine learning and natural language processing",
- "The cake is a lie, but the pie is always true",
- "ChatGPT is an advanced AI model for conversation",
- ]
-
- for text in self.example_texts:
- self.memory.add(text)
-
- # Add some random strings to test noise
- for _ in range(5):
- self.memory.add(self.random_string(10))
-
- def test_get_relevant(self) -> None:
- """Test getting relevant texts from the cache."""
- query = "I'm interested in artificial intelligence and NLP"
- num_relevant = 3
- relevant_texts = self.memory.get_relevant(query, num_relevant)
-
- print(f"Top {k} relevant texts for the query '{query}':")
- for i, text in enumerate(relevant_texts, start=1):
- print(f"{i}. {text}")
-
- self.assertEqual(len(relevant_texts), k)
- self.assertIn(self.example_texts[1], relevant_texts)
-
-except:
- print(
- "Skipping tests/integration/milvus_memory_tests.py as Milvus is not installed."
- )
diff --git a/spaces/Mixing/anime-remove-background/README.md b/spaces/Mixing/anime-remove-background/README.md
deleted file mode 100644
index 1ba3cb5ea0e994e246d57b7d62b8aa5a6331901c..0000000000000000000000000000000000000000
--- a/spaces/Mixing/anime-remove-background/README.md
+++ /dev/null
@@ -1,14 +0,0 @@
----
-title: Anime Remove Background
-emoji: 🪄🖼️
-colorFrom: indigo
-colorTo: pink
-sdk: gradio
-sdk_version: 3.1.4
-app_file: app.py
-pinned: false
-license: apache-2.0
-duplicated_from: skytnt/anime-remove-background
----
-
-Check out the configuration reference at https://huggingface.co/docs/hub/spaces-config-reference
diff --git a/spaces/MrBodean/VoiceClone/synthesizer/__init__.py b/spaces/MrBodean/VoiceClone/synthesizer/__init__.py
deleted file mode 100644
index 4287ca8617970fa8fc025b75cb319c7032706910..0000000000000000000000000000000000000000
--- a/spaces/MrBodean/VoiceClone/synthesizer/__init__.py
+++ /dev/null
@@ -1 +0,0 @@
-#
\ No newline at end of file
diff --git a/spaces/MrBodean/VoiceClone/vocoder/distribution.py b/spaces/MrBodean/VoiceClone/vocoder/distribution.py
deleted file mode 100644
index d3119a5ba1e77bc25a92d2664f83d366f12399c0..0000000000000000000000000000000000000000
--- a/spaces/MrBodean/VoiceClone/vocoder/distribution.py
+++ /dev/null
@@ -1,132 +0,0 @@
-import numpy as np
-import torch
-import torch.nn.functional as F
-
-
-def log_sum_exp(x):
- """ numerically stable log_sum_exp implementation that prevents overflow """
- # TF ordering
- axis = len(x.size()) - 1
- m, _ = torch.max(x, dim=axis)
- m2, _ = torch.max(x, dim=axis, keepdim=True)
- return m + torch.log(torch.sum(torch.exp(x - m2), dim=axis))
-
-
-# It is adapted from https://github.com/r9y9/wavenet_vocoder/blob/master/wavenet_vocoder/mixture.py
-def discretized_mix_logistic_loss(y_hat, y, num_classes=65536,
- log_scale_min=None, reduce=True):
- if log_scale_min is None:
- log_scale_min = float(np.log(1e-14))
- y_hat = y_hat.permute(0,2,1)
- assert y_hat.dim() == 3
- assert y_hat.size(1) % 3 == 0
- nr_mix = y_hat.size(1) // 3
-
- # (B x T x C)
- y_hat = y_hat.transpose(1, 2)
-
- # unpack parameters. (B, T, num_mixtures) x 3
- logit_probs = y_hat[:, :, :nr_mix]
- means = y_hat[:, :, nr_mix:2 * nr_mix]
- log_scales = torch.clamp(y_hat[:, :, 2 * nr_mix:3 * nr_mix], min=log_scale_min)
-
- # B x T x 1 -> B x T x num_mixtures
- y = y.expand_as(means)
-
- centered_y = y - means
- inv_stdv = torch.exp(-log_scales)
- plus_in = inv_stdv * (centered_y + 1. / (num_classes - 1))
- cdf_plus = torch.sigmoid(plus_in)
- min_in = inv_stdv * (centered_y - 1. / (num_classes - 1))
- cdf_min = torch.sigmoid(min_in)
-
- # log probability for edge case of 0 (before scaling)
- # equivalent: torch.log(F.sigmoid(plus_in))
- log_cdf_plus = plus_in - F.softplus(plus_in)
-
- # log probability for edge case of 255 (before scaling)
- # equivalent: (1 - F.sigmoid(min_in)).log()
- log_one_minus_cdf_min = -F.softplus(min_in)
-
- # probability for all other cases
- cdf_delta = cdf_plus - cdf_min
-
- mid_in = inv_stdv * centered_y
- # log probability in the center of the bin, to be used in extreme cases
- # (not actually used in our code)
- log_pdf_mid = mid_in - log_scales - 2. * F.softplus(mid_in)
-
- # tf equivalent
- """
- log_probs = tf.where(x < -0.999, log_cdf_plus,
- tf.where(x > 0.999, log_one_minus_cdf_min,
- tf.where(cdf_delta > 1e-5,
- tf.log(tf.maximum(cdf_delta, 1e-12)),
- log_pdf_mid - np.log(127.5))))
- """
- # TODO: cdf_delta <= 1e-5 actually can happen. How can we choose the value
- # for num_classes=65536 case? 1e-7? not sure..
- inner_inner_cond = (cdf_delta > 1e-5).float()
-
- inner_inner_out = inner_inner_cond * \
- torch.log(torch.clamp(cdf_delta, min=1e-12)) + \
- (1. - inner_inner_cond) * (log_pdf_mid - np.log((num_classes - 1) / 2))
- inner_cond = (y > 0.999).float()
- inner_out = inner_cond * log_one_minus_cdf_min + (1. - inner_cond) * inner_inner_out
- cond = (y < -0.999).float()
- log_probs = cond * log_cdf_plus + (1. - cond) * inner_out
-
- log_probs = log_probs + F.log_softmax(logit_probs, -1)
-
- if reduce:
- return -torch.mean(log_sum_exp(log_probs))
- else:
- return -log_sum_exp(log_probs).unsqueeze(-1)
-
-
-def sample_from_discretized_mix_logistic(y, log_scale_min=None):
- """
- Sample from discretized mixture of logistic distributions
- Args:
- y (Tensor): B x C x T
- log_scale_min (float): Log scale minimum value
- Returns:
- Tensor: sample in range of [-1, 1].
- """
- if log_scale_min is None:
- log_scale_min = float(np.log(1e-14))
- assert y.size(1) % 3 == 0
- nr_mix = y.size(1) // 3
-
- # B x T x C
- y = y.transpose(1, 2)
- logit_probs = y[:, :, :nr_mix]
-
- # sample mixture indicator from softmax
- temp = logit_probs.data.new(logit_probs.size()).uniform_(1e-5, 1.0 - 1e-5)
- temp = logit_probs.data - torch.log(- torch.log(temp))
- _, argmax = temp.max(dim=-1)
-
- # (B, T) -> (B, T, nr_mix)
- one_hot = to_one_hot(argmax, nr_mix)
- # select logistic parameters
- means = torch.sum(y[:, :, nr_mix:2 * nr_mix] * one_hot, dim=-1)
- log_scales = torch.clamp(torch.sum(
- y[:, :, 2 * nr_mix:3 * nr_mix] * one_hot, dim=-1), min=log_scale_min)
- # sample from logistic & clip to interval
- # we don't actually round to the nearest 8bit value when sampling
- u = means.data.new(means.size()).uniform_(1e-5, 1.0 - 1e-5)
- x = means + torch.exp(log_scales) * (torch.log(u) - torch.log(1. - u))
-
- x = torch.clamp(torch.clamp(x, min=-1.), max=1.)
-
- return x
-
-
-def to_one_hot(tensor, n, fill_with=1.):
- # we perform one hot encore with respect to the last axis
- one_hot = torch.FloatTensor(tensor.size() + (n,)).zero_()
- if tensor.is_cuda:
- one_hot = one_hot.cuda()
- one_hot.scatter_(len(tensor.size()), tensor.unsqueeze(-1), fill_with)
- return one_hot
diff --git a/spaces/NAACL2022/CLIP-Caption-Reward/retrieval/caption_data.py b/spaces/NAACL2022/CLIP-Caption-Reward/retrieval/caption_data.py
deleted file mode 100644
index 595a81ae5346937e5d9174401cd8a62e78946864..0000000000000000000000000000000000000000
--- a/spaces/NAACL2022/CLIP-Caption-Reward/retrieval/caption_data.py
+++ /dev/null
@@ -1,500 +0,0 @@
-from torch.utils.data import DataLoader, Dataset, Sampler
-from pathlib import Path
-import json
-from multiprocessing import Pool
-from tqdm import tqdm
-from PIL import Image
-import random
-import numpy as np
-import torch
-import torchvision
-import torchvision.transforms as T
-
-from torch.utils.data.distributed import DistributedSampler
-
-from transformers import T5Tokenizer, BertTokenizer, BertTokenizerFast, CLIPTokenizer
-
-import text_utils
-
-project_dir = Path(__file__).parent.resolve()
-workspace_dir = project_dir.parent.parent
-dataset_dir = workspace_dir.joinpath('datasets/').resolve()
-# coco_dir = dataset_dir.joinpath('COCO')
-# vg_dir = dataset_dir.joinpath('VG')
-coco_img_dir = dataset_dir.joinpath('COCO/images/')
-coco_data_dir = project_dir.parent.joinpath('CLIP-ViL/CLIP-ViL-Direct/caption/data/')
-# coco_feature_dir = coco_dir.joinpath('features')
-
-
-class COCORetrievalDataset(Dataset):
- def __init__(self, split='karpathy_train', rank=-1, topk=-1, verbose=True, args=None, mode='train'):
- super().__init__()
-
- self.topk = topk
- self.verbose = verbose
- self.args = args
- self.rank = rank
- self.mode = mode
-
- # Loading datasets to data
- self.source = split
- if self.verbose:
- print('Data source: ', self.source)
-
- # if self.args.tokenizer is None:
- # self.args.tokenizer = self.args.decoder_backbone
-
- # if 'bert' in self.args.tokenizer:
- # self.tokenizer = BertTokenizerFast.from_pretrained(
- # self.args.tokenizer,
- # # max_length=self.args.max_text_length,
- # # do_lower_case=self.args.do_lower_case
- # )
- # elif 'clip' in self.args.tokenizer:
- # self.tokenizer = CLIPTokenizer.from_pretrained(
- # self.args.tokenizer,
- # # max_length=self.args.max_text_length,
- # # do_lower_case=self.args.do_lower_case
- # )
-
- self.tokenizer = CLIPTokenizer.from_pretrained(
- self.args.tokenizer,
- # max_length=self.args.max_text_length,
- # do_lower_case=self.args.do_lower_case
- )
-
- with open(coco_data_dir.joinpath('cocotalk.json')) as f:
- self.vocab = list(json.load(f)['ix_to_word'].values())
- popped = self.vocab.pop(-1)
- assert popped == 'UNK'
- if self.verbose:
- print('vocab size: ', len(self.vocab))
-
-
- data_info_path = coco_data_dir.joinpath('dataset_coco.json')
- with open(data_info_path) as f:
- karpathy_data = json.load(f)
-
- split_rename = {
- 'train': 'train',
- 'restval': 'train',
- 'val': 'val',
- 'test': 'test'
- }
-
- n_images = 0
-
- data = []
- # self.vocab = set()
- for datum in karpathy_data['images']:
- re_split = split_rename[datum['split']]
-
- # if re_split == 'train':
- # for d in datum['sentences']:
- # self.vocab = self.vocab.union(set(d['tokens']))
-
- if re_split != self.source.split('_')[-1]:
- continue
-
- if re_split == 'train':
- # for d in datum['sentences']:
- # img_id = datum['filename'].split('.')[0]
- # new_datum = {
- # 'filename': datum['filename'],
- # 'img_id': img_id,
- # 'sent': d['raw'].strip(),
- # 'targets': [d['raw'].strip() for d in datum['sentences']],
- # 'is_train': True,
- # 'cocoid': datum['cocoid']
- # }
- # data.append(new_datum)
- img_id = datum['filename'].split('.')[0]
- new_datum = {
- 'filename': datum['filename'],
- 'img_id': img_id,
- # 'sent': d['raw'],
- # 'targets': [d['raw'].strip() for d in datum['sentences']],
- 'targets': [" ".join(d['tokens']) for d in datum['sentences']],
- 'is_train': True,
- 'cocoid': datum['cocoid']
- }
- data.append(new_datum)
-
- else:
- img_id = datum['filename'].split('.')[0]
- new_datum = {
- 'filename': datum['filename'],
- 'img_id': img_id,
- # 'sent': d['raw'],
- # 'targets': [d['raw'].strip() for d in datum['sentences']],
- 'targets': [" ".join(d['tokens']) for d in datum['sentences']],
- 'is_train': False,
- 'cocoid': datum['cocoid']
- }
- data.append(new_datum)
-
- n_images += 1
-
- if self.verbose:
- print(f"{self.source} has {n_images} images")
- # print(f"Loaded {len(data)} data from", split)
-
- self.n_gpus = torch.cuda.device_count()
-
- if self.topk > 0:
- data = data[:self.topk]
- if self.verbose:
- print(f"Use only {self.topk} data")
-
- self.data = data
-
- # if self.verbose:
- # print("# all sentences:", len(self.data))
-
- if self.args.load_feat:
- # feat_dir = coco_dir.joinpath(''
- # self.feat_loader = HybridLoader('/scratch-space/CLIP-ViL/CLIP-ViL-Direct/caption/data/cocotalk_clipscore_vis', ext='.npy', in_memory=False)
- self.feat_loader = HybridLoader(
- coco_data_dir.joinpath('cocotalk_clipscore_vis'),
- ext='.npy', in_memory=False)
- else:
- if 'openai/clip' in self.args.encoder_backbone:
- # from transformers import CLIPProcessor
- # self.processor = CLIPProcessor.from_pretrained("openai/clip-vit-base-patch32",
- # size=args.image_size,
- # do_resize=True,
- # do_center_crop=False,
- # )
- # self.img_transform = lambda image: self.processor.feature_extractor(
- # image,
- # return_tensors='pt')['pixel_values'][0]
-
- self.image_mean = [0.48145466, 0.4578275, 0.40821073]
- self.image_std = [0.26862954, 0.26130258, 0.27577711]
-
- # captioning
- # self.img_transform = T.Compose([
- # T.Resize((self.args.image_size, self.args.image_size))
- # ])
-
- # retrieval
- self.img_transform = T.Compose([
- T.Resize(self.args.image_size, interpolation=T.functional.InterpolationMode.BICUBIC),
- T.CenterCrop(self.args.image_size)
- ])
-
- self.img_tensor_transform = T.Compose([
- # T.RandomCrop(224),
- # T.RandomHorizontalFlip(p=0.3),
- T.ConvertImageDtype(torch.float),
- T.Normalize(self.image_mean, self.image_std)
- ]
- )
- # elif 'google/vit' in self.args.encoder_backbone:
- # self.image_mean = [0.5, 0.5, 0.5]
- # self.image_std = [0.5, 0.5, 0.5]
-
- # self.img_transform = T.Compose([
- # # T.PILToTensor(),
- # T.Resize((self.args.image_size, self.args.image_size))
- # ])
-
- # self.img_tensor_transform = T.Compose([
- # # T.RandomCrop(224),
- # # T.RandomHorizontalFlip(p=0.3),
- # T.ConvertImageDtype(torch.float),
- # T.Normalize(self.image_mean, self.image_std)
- # ]
- # )
-
- def get_negative_text(self, text):
- neg_type = random.choice(['repeat', 'remove', 'insert', 'swap', 'shuffle'])
-
- if neg_type == 'repeat':
- text = text_utils.repeat(text)
- elif neg_type == 'remove':
- text = text_utils.remove(text)
- elif neg_type == 'insert':
- text = text_utils.insert(text, self.vocab)
- elif neg_type == 'swap':
- text = text_utils.swap(text, self.vocab)
- elif neg_type == 'shuffle':
- text = text_utils.shuffle(text)
-
- return text, neg_type
-
- def __len__(self):
- return len(self.data)
-
- def __getitem__(self, idx):
- datum = self.data[idx]
- return self.process_datum(datum)
-
- def process_datum(self, datum):
- out_dict = {}
-
- ###### Image ######
-
- if self.args.load_feat:
- cocoid = datum['cocoid']
- out_dict['cocoid'] = str(cocoid)
- img_feat = self.feat_loader.get(str(cocoid))
- out_dict['img_feat'] = torch.from_numpy(img_feat)
-
- else:
- img_id = datum['img_id']
- out_dict['img_id'] = img_id
-
- if 'train' in datum['filename']:
- img_split = 'train2014'
- elif 'val' in datum['filename']:
- img_split = 'val2014'
- img_path = coco_img_dir.joinpath(img_split).joinpath(datum['filename']).with_suffix('.jpg')
- assert img_path.exists()
- img_path = str(img_path)
- out_dict['img_path'] = img_path
-
- img_tensor = torchvision.io.read_image(img_path)
- # out_dict['img_tensor'] = img
-
- # img = Image.open(img_path).convert('RGB')
- # img_tensor = torch.as_tensor(np.asarray(img))
- out_dict['img_tensor'] = self.img_transform(img_tensor)
- # self.img_transform(img_tensor)
- # out_dict['img_tensor'] = self.img_transform(img)
-
- ###### Text #####
- # if datum['is_train']:
- # sent = datum['sent'].strip()
-
- sent = random.choice(datum['targets'])
-
- # target_ids = self.tokenizer.encode(
- # sent, max_length=self.args.gen_max_length, truncation=True)
-
- # assert len(target_ids) <= self.args.gen_max_length, len(target_ids)
- out_dict['sent'] = sent
- # out_dict['target_ids'] = torch.LongTensor(target_ids)
- # out_dict['target_length'] = len(target_ids)
-
-
- # negative sample
- neg_sent, neg_type = self.get_negative_text(sent)
-
- # neg_target_ids = self.tokenizer.encode(
- # neg_sent, max_length=self.args.gen_max_length, truncation=True)
-
- # assert len(neg_target_ids) <= self.args.gen_max_length, len(neg_target_ids)
- out_dict['neg_sent'] = neg_sent
- out_dict['neg_type'] = neg_type
- # out_dict['neg_target_ids'] = torch.LongTensor(neg_target_ids)
- # out_dict['neg_target_length'] = len(neg_target_ids)
-
-
- if 'targets' in datum:
- out_dict['targets'] = datum['targets']
-
- return out_dict
-
- def collate_fn(self, batch):
- batch_entry = {}
-
- B = len(batch)
-
- # if 'target_ids' in batch[0]:
- # T_W_L = max(entry['target_length'] for entry in batch)
- # target_ids = torch.ones(
- # B, T_W_L, dtype=torch.long) * self.tokenizer.pad_token_id
-
- # if 'target_ids' in batch[0]:
- # T_W_L = max(entry['target_length'] for entry in batch)
- # target_ids = torch.ones(
- # B, T_W_L, dtype=torch.long) * self.tokenizer.pad_token_id
-
-
-
- targets = []
- img_ids = []
- img_paths = []
-
- coco_ids = []
-
- if self.args.load_feat:
- img_feats = torch.zeros(B, 512, dtype=torch.float)
- else:
- # imgs = []
- img_tensor = torch.zeros(B, 3, self.args.image_size, self.args.image_size, dtype=torch.uint8)
-
- for i, entry in enumerate(batch):
-
- if self.args.load_feat:
- coco_ids.append(entry['cocoid'])
- img_feats[i] = entry['img_feat']
-
- else:
-
- img_ids.append(entry['img_id'])
- img_paths.append(entry['img_path'])
- img_tensor[i] = entry['img_tensor']
-
- # if 'target_ids' in entry:
- # target_ids[i, :entry['target_length']] = entry['target_ids']
-
- if 'targets' in entry:
- targets.append(entry['targets'])
-
- if 'sent' in batch[0]:
- # word_mask = target_ids != self.tokenizer.pad_token_id
- # target_ids[~word_mask] = -100
- # batch_entry['target_ids'] = target_ids
-
- tokenized = self.tokenizer([entry['sent'] for entry in batch], truncation=True, padding=True, return_tensors='pt')
- neg_tokenized = self.tokenizer([entry['neg_sent'] for entry in batch], truncation=True, padding=True, return_tensors='pt')
- # sent, max_length=self.args.gen_max_length, truncation=True)
-
- batch_entry['text'] = (tokenized.input_ids, tokenized.attention_mask)
- batch_entry['neg_text'] = (neg_tokenized.input_ids, neg_tokenized.attention_mask)
-
-
- if self.args.load_feat:
- batch_entry['coco_ids'] = coco_ids
- batch_entry['img_feats'] = img_feats
-
- else:
-
- img_tensor = self.img_tensor_transform(img_tensor)
-
- batch_entry['img_id'] = img_ids
- batch_entry['img_paths'] = img_paths
- batch_entry['img_tensor'] = img_tensor
-
- batch_entry['targets'] = targets
-
- # print('batch created')
-
- # batch_entry['task'] = 'caption'
-
- return batch_entry
-
-
-# def get_loader(args, split='karpathy_train', mode='train',
-# batch_size=32, workers=4, distributed=False, gpu=0,
-# topk=-1):
-
-# verbose = (gpu == 0)
-
-# dataset = COCORetrievalDataset(
-# split,
-# rank=gpu,
-# topk=topk,
-# verbose=verbose,
-# args=args,
-# mode=mode)
-
-# # if distributed:
-# # sampler = DistributedSampler(dataset)
-# # else:
-# # sampler = None
-
-# if mode == 'train':
-# loader = DataLoader(
-# dataset, batch_size=batch_size, shuffle=(sampler is None),
-# num_workers=workers, pin_memory=True, sampler=sampler,
-# collate_fn=dataset.collate_fn)
-# else:
-# loader = DataLoader(
-# dataset,
-# batch_size=batch_size, shuffle=False,
-# num_workers=workers, pin_memory=True,
-# sampler=sampler,
-# collate_fn=dataset.collate_fn,
-# drop_last=False)
-
-# # if verbose:
-# # loader.evaluator = COCOCaptionEvaluator()
-
-# # loader.task = 'caption'
-
-# return loader
-
-
-# class COCOCaptionEvaluator:
-# def __init__(self):
-# import language_evaluation
-# self.evaluator = language_evaluation.CocoEvaluator(verbose=False)
-
-# def evaluate(self, predicts, answers):
-
-# results = self.evaluator.run_evaluation(predicts, answers)
-
-# return results
-
-import six
-import os
-import h5py
-
-class HybridLoader:
- """
- If db_path is a director, then use normal file loading
- If lmdb, then load from lmdb
- The loading method depend on extention.
-
- in_memory: if in_memory is True, we save all the features in memory
- For individual np(y|z)s, we don't need to do that because the system will do this for us.
- Should be useful for lmdb or h5.
- (Copied this idea from vilbert)
- """
-
- def __init__(self, db_path, ext='.npy', in_memory=False):
- self.db_path = db_path
- self.ext = ext
- if self.ext == '.npy':
- self.loader = lambda x: np.load(six.BytesIO(x))
- else:
- self.loader = lambda x: np.load(six.BytesIO(x))['feat']
- # if db_path.endswith('.lmdb'):
- # self.db_type = 'lmdb'
- # self.lmdb = lmdbdict(db_path, unsafe=True)
- # self.lmdb._key_dumps = DUMPS_FUNC['ascii']
- # self.lmdb._value_loads = LOADS_FUNC['identity']
- # elif db_path.endswith('.pth'): # Assume a key,value dictionary
- # self.db_type = 'pth'
- # self.feat_file = torch.load(db_path)
- # self.loader = lambda x: x
- # print('HybridLoader: ext is ignored')
- # elif db_path.endswith('h5'):
- # self.db_type = 'h5'
- # self.loader = lambda x: np.array(x).astype('float32')
- # else:
- # self.db_type = 'dir'
-
- self.in_memory = in_memory
- if self.in_memory:
- self.features = {}
-
- def get(self, key):
-
- # if self.in_memory and key in self.features:
- # # We save f_input because we want to save the
- # # compressed bytes to save memory
- # f_input = self.features[key]
- # elif self.db_type == 'lmdb':
- # f_input = self.lmdb[key]
- # elif self.db_type == 'pth':
- # f_input = self.feat_file[key]
- # elif self.db_type == 'h5':
- # f_input = h5py.File(self.db_path, 'r')[key]
- # else:
- # f_input = open(os.path.join(
- # self.db_path, key + self.ext), 'rb').read()
-
- f_input = open(os.path.join(
- self.db_path, key + self.ext), 'rb').read()
-
- if self.in_memory and key not in self.features:
- self.features[key] = f_input
-
- # load image
- feat = self.loader(f_input)
-
- return feat
diff --git a/spaces/NATSpeech/DiffSpeech/inference/tts/fs2_orig.py b/spaces/NATSpeech/DiffSpeech/inference/tts/fs2_orig.py
deleted file mode 100644
index fe2665d451d5a36c47ffbf815b3d19876882bd91..0000000000000000000000000000000000000000
--- a/spaces/NATSpeech/DiffSpeech/inference/tts/fs2_orig.py
+++ /dev/null
@@ -1,17 +0,0 @@
-from inference.tts.fs import FastSpeechInfer
-from modules.tts.fs2_orig import FastSpeech2Orig
-from utils.commons.ckpt_utils import load_ckpt
-from utils.commons.hparams import hparams
-
-
-class FastSpeech2OrigInfer(FastSpeechInfer):
- def build_model(self):
- dict_size = len(self.ph_encoder)
- model = FastSpeech2Orig(dict_size, self.hparams)
- model.eval()
- load_ckpt(model, hparams['work_dir'], 'model')
- return model
-
-
-if __name__ == '__main__':
- FastSpeech2OrigInfer.example_run()
diff --git a/spaces/NATSpeech/DiffSpeech/utils/commons/ckpt_utils.py b/spaces/NATSpeech/DiffSpeech/utils/commons/ckpt_utils.py
deleted file mode 100644
index 9c1006d5852c6cf57063ce64e773d3c40ae9500d..0000000000000000000000000000000000000000
--- a/spaces/NATSpeech/DiffSpeech/utils/commons/ckpt_utils.py
+++ /dev/null
@@ -1,66 +0,0 @@
-import glob
-import os
-import re
-import torch
-
-
-def get_last_checkpoint(work_dir, steps=None):
- checkpoint = None
- last_ckpt_path = None
- ckpt_paths = get_all_ckpts(work_dir, steps)
- if len(ckpt_paths) > 0:
- last_ckpt_path = ckpt_paths[0]
- checkpoint = torch.load(last_ckpt_path, map_location='cpu')
- return checkpoint, last_ckpt_path
-
-
-def get_all_ckpts(work_dir, steps=None):
- if steps is None:
- ckpt_path_pattern = f'{work_dir}/model_ckpt_steps_*.ckpt'
- else:
- ckpt_path_pattern = f'{work_dir}/model_ckpt_steps_{steps}.ckpt'
- return sorted(glob.glob(ckpt_path_pattern),
- key=lambda x: -int(re.findall('.*steps\_(\d+)\.ckpt', x)[0]))
-
-
-def load_ckpt(cur_model, ckpt_base_dir, model_name='model', force=True, strict=True):
- if os.path.isfile(ckpt_base_dir):
- base_dir = os.path.dirname(ckpt_base_dir)
- ckpt_path = ckpt_base_dir
- checkpoint = torch.load(ckpt_base_dir, map_location='cpu')
- else:
- base_dir = ckpt_base_dir
- checkpoint, ckpt_path = get_last_checkpoint(ckpt_base_dir)
- if checkpoint is not None:
- state_dict = checkpoint["state_dict"]
- if len([k for k in state_dict.keys() if '.' in k]) > 0:
- state_dict = {k[len(model_name) + 1:]: v for k, v in state_dict.items()
- if k.startswith(f'{model_name}.')}
- else:
- if '.' not in model_name:
- state_dict = state_dict[model_name]
- else:
- base_model_name = model_name.split('.')[0]
- rest_model_name = model_name[len(base_model_name) + 1:]
- state_dict = {
- k[len(rest_model_name) + 1:]: v for k, v in state_dict[base_model_name].items()
- if k.startswith(f'{rest_model_name}.')}
- if not strict:
- cur_model_state_dict = cur_model.state_dict()
- unmatched_keys = []
- for key, param in state_dict.items():
- if key in cur_model_state_dict:
- new_param = cur_model_state_dict[key]
- if new_param.shape != param.shape:
- unmatched_keys.append(key)
- print("| Unmatched keys: ", key, new_param.shape, param.shape)
- for key in unmatched_keys:
- del state_dict[key]
- cur_model.load_state_dict(state_dict, strict=strict)
- print(f"| load '{model_name}' from '{ckpt_path}'.")
- else:
- e_msg = f"| ckpt not found in {base_dir}."
- if force:
- assert False, e_msg
- else:
- print(e_msg)
diff --git a/spaces/NCTCMumbai/NCTC/models/CONTRIBUTING.md b/spaces/NCTCMumbai/NCTC/models/CONTRIBUTING.md
deleted file mode 100644
index f909461ae7b9c75264e0915ecb37228314933e4a..0000000000000000000000000000000000000000
--- a/spaces/NCTCMumbai/NCTC/models/CONTRIBUTING.md
+++ /dev/null
@@ -1,10 +0,0 @@
-# How to contribute
-
-
-
-We encourage you to contribute to the TensorFlow Model Garden.
-
-Please read our [guidelines](../../wiki/How-to-contribute) for details.
-
-**NOTE**: Only [code owners](./CODEOWNERS) are allowed to merge a pull request.
-Please contact the code owners of each model to merge your pull request.
diff --git a/spaces/NCTCMumbai/NCTC/models/official/recommendation/movielens.py b/spaces/NCTCMumbai/NCTC/models/official/recommendation/movielens.py
deleted file mode 100644
index 576519a316bb3e05d786ac737da19cb44d2b61c4..0000000000000000000000000000000000000000
--- a/spaces/NCTCMumbai/NCTC/models/official/recommendation/movielens.py
+++ /dev/null
@@ -1,317 +0,0 @@
-# Copyright 2018 The TensorFlow Authors. All Rights Reserved.
-#
-# Licensed under the Apache License, Version 2.0 (the "License");
-# you may not use this file except in compliance with the License.
-# You may obtain a copy of the License at
-#
-# http://www.apache.org/licenses/LICENSE-2.0
-#
-# Unless required by applicable law or agreed to in writing, software
-# distributed under the License is distributed on an "AS IS" BASIS,
-# WITHOUT WARRANTIES OR CONDITIONS OF ANY KIND, either express or implied.
-# See the License for the specific language governing permissions and
-# limitations under the License.
-# ==============================================================================
-"""Download and extract the MovieLens dataset from GroupLens website.
-
-Download the dataset, and perform basic preprocessing.
-"""
-
-from __future__ import absolute_import
-from __future__ import division
-from __future__ import print_function
-
-import os
-import sys
-import tempfile
-import zipfile
-
-# pylint: disable=g-bad-import-order
-import numpy as np
-import pandas as pd
-import six
-from six.moves import urllib # pylint: disable=redefined-builtin
-from absl import app
-from absl import flags
-from absl import logging
-import tensorflow as tf
-# pylint: enable=g-bad-import-order
-
-from official.utils.flags import core as flags_core
-
-
-ML_1M = "ml-1m"
-ML_20M = "ml-20m"
-DATASETS = [ML_1M, ML_20M]
-
-RATINGS_FILE = "ratings.csv"
-MOVIES_FILE = "movies.csv"
-
-# URL to download dataset
-_DATA_URL = "http://files.grouplens.org/datasets/movielens/"
-
-GENRE_COLUMN = "genres"
-ITEM_COLUMN = "item_id" # movies
-RATING_COLUMN = "rating"
-TIMESTAMP_COLUMN = "timestamp"
-TITLE_COLUMN = "titles"
-USER_COLUMN = "user_id"
-
-GENRES = [
- 'Action', 'Adventure', 'Animation', "Children", 'Comedy', 'Crime',
- 'Documentary', 'Drama', 'Fantasy', 'Film-Noir', 'Horror', "IMAX", 'Musical',
- 'Mystery', 'Romance', 'Sci-Fi', 'Thriller', 'War', 'Western'
-]
-N_GENRE = len(GENRES)
-
-RATING_COLUMNS = [USER_COLUMN, ITEM_COLUMN, RATING_COLUMN, TIMESTAMP_COLUMN]
-MOVIE_COLUMNS = [ITEM_COLUMN, TITLE_COLUMN, GENRE_COLUMN]
-
-# Note: Users are indexed [1, k], not [0, k-1]
-NUM_USER_IDS = {
- ML_1M: 6040,
- ML_20M: 138493,
-}
-
-# Note: Movies are indexed [1, k], not [0, k-1]
-# Both the 1m and 20m datasets use the same movie set.
-NUM_ITEM_IDS = 3952
-
-MAX_RATING = 5
-
-NUM_RATINGS = {
- ML_1M: 1000209,
- ML_20M: 20000263
-}
-
-DATASET_TO_NUM_USERS_AND_ITEMS = {ML_1M: (6040, 3706), ML_20M: (138493, 26744)}
-
-
-def _download_and_clean(dataset, data_dir):
- """Download MovieLens dataset in a standard format.
-
- This function downloads the specified MovieLens format and coerces it into a
- standard format. The only difference between the ml-1m and ml-20m datasets
- after this point (other than size, of course) is that the 1m dataset uses
- whole number ratings while the 20m dataset allows half integer ratings.
- """
- if dataset not in DATASETS:
- raise ValueError("dataset {} is not in {{{}}}".format(
- dataset, ",".join(DATASETS)))
-
- data_subdir = os.path.join(data_dir, dataset)
-
- expected_files = ["{}.zip".format(dataset), RATINGS_FILE, MOVIES_FILE]
-
- tf.io.gfile.makedirs(data_subdir)
- if set(expected_files).intersection(
- tf.io.gfile.listdir(data_subdir)) == set(expected_files):
- logging.info("Dataset {} has already been downloaded".format(dataset))
- return
-
- url = "{}{}.zip".format(_DATA_URL, dataset)
-
- temp_dir = tempfile.mkdtemp()
- try:
- zip_path = os.path.join(temp_dir, "{}.zip".format(dataset))
- zip_path, _ = urllib.request.urlretrieve(url, zip_path)
- statinfo = os.stat(zip_path)
- # A new line to clear the carriage return from download progress
- # logging.info is not applicable here
- print()
- logging.info(
- "Successfully downloaded {} {} bytes".format(
- zip_path, statinfo.st_size))
-
- zipfile.ZipFile(zip_path, "r").extractall(temp_dir)
-
- if dataset == ML_1M:
- _regularize_1m_dataset(temp_dir)
- else:
- _regularize_20m_dataset(temp_dir)
-
- for fname in tf.io.gfile.listdir(temp_dir):
- if not tf.io.gfile.exists(os.path.join(data_subdir, fname)):
- tf.io.gfile.copy(os.path.join(temp_dir, fname),
- os.path.join(data_subdir, fname))
- else:
- logging.info("Skipping copy of {}, as it already exists in the "
- "destination folder.".format(fname))
-
- finally:
- tf.io.gfile.rmtree(temp_dir)
-
-
-def _transform_csv(input_path, output_path, names, skip_first, separator=","):
- """Transform csv to a regularized format.
-
- Args:
- input_path: The path of the raw csv.
- output_path: The path of the cleaned csv.
- names: The csv column names.
- skip_first: Boolean of whether to skip the first line of the raw csv.
- separator: Character used to separate fields in the raw csv.
- """
- if six.PY2:
- names = [six.ensure_text(n, "utf-8") for n in names]
-
- with tf.io.gfile.GFile(output_path, "wb") as f_out, \
- tf.io.gfile.GFile(input_path, "rb") as f_in:
-
- # Write column names to the csv.
- f_out.write(",".join(names).encode("utf-8"))
- f_out.write(b"\n")
- for i, line in enumerate(f_in):
- if i == 0 and skip_first:
- continue # ignore existing labels in the csv
-
- line = six.ensure_text(line, "utf-8", errors="ignore")
- fields = line.split(separator)
- if separator != ",":
- fields = ['"{}"'.format(field) if "," in field else field
- for field in fields]
- f_out.write(",".join(fields).encode("utf-8"))
-
-
-def _regularize_1m_dataset(temp_dir):
- """
- ratings.dat
- The file has no header row, and each line is in the following format:
- UserID::MovieID::Rating::Timestamp
- - UserIDs range from 1 and 6040
- - MovieIDs range from 1 and 3952
- - Ratings are made on a 5-star scale (whole-star ratings only)
- - Timestamp is represented in seconds since midnight Coordinated Universal
- Time (UTC) of January 1, 1970.
- - Each user has at least 20 ratings
-
- movies.dat
- Each line has the following format:
- MovieID::Title::Genres
- - MovieIDs range from 1 and 3952
- """
- working_dir = os.path.join(temp_dir, ML_1M)
-
- _transform_csv(
- input_path=os.path.join(working_dir, "ratings.dat"),
- output_path=os.path.join(temp_dir, RATINGS_FILE),
- names=RATING_COLUMNS, skip_first=False, separator="::")
-
- _transform_csv(
- input_path=os.path.join(working_dir, "movies.dat"),
- output_path=os.path.join(temp_dir, MOVIES_FILE),
- names=MOVIE_COLUMNS, skip_first=False, separator="::")
-
- tf.io.gfile.rmtree(working_dir)
-
-
-def _regularize_20m_dataset(temp_dir):
- """
- ratings.csv
- Each line of this file after the header row represents one rating of one
- movie by one user, and has the following format:
- userId,movieId,rating,timestamp
- - The lines within this file are ordered first by userId, then, within user,
- by movieId.
- - Ratings are made on a 5-star scale, with half-star increments
- (0.5 stars - 5.0 stars).
- - Timestamps represent seconds since midnight Coordinated Universal Time
- (UTC) of January 1, 1970.
- - All the users had rated at least 20 movies.
-
- movies.csv
- Each line has the following format:
- MovieID,Title,Genres
- - MovieIDs range from 1 and 3952
- """
- working_dir = os.path.join(temp_dir, ML_20M)
-
- _transform_csv(
- input_path=os.path.join(working_dir, "ratings.csv"),
- output_path=os.path.join(temp_dir, RATINGS_FILE),
- names=RATING_COLUMNS, skip_first=True, separator=",")
-
- _transform_csv(
- input_path=os.path.join(working_dir, "movies.csv"),
- output_path=os.path.join(temp_dir, MOVIES_FILE),
- names=MOVIE_COLUMNS, skip_first=True, separator=",")
-
- tf.io.gfile.rmtree(working_dir)
-
-
-def download(dataset, data_dir):
- if dataset:
- _download_and_clean(dataset, data_dir)
- else:
- _ = [_download_and_clean(d, data_dir) for d in DATASETS]
-
-
-def ratings_csv_to_dataframe(data_dir, dataset):
- with tf.io.gfile.GFile(os.path.join(data_dir, dataset, RATINGS_FILE)) as f:
- return pd.read_csv(f, encoding="utf-8")
-
-
-def csv_to_joint_dataframe(data_dir, dataset):
- ratings = ratings_csv_to_dataframe(data_dir, dataset)
-
- with tf.io.gfile.GFile(os.path.join(data_dir, dataset, MOVIES_FILE)) as f:
- movies = pd.read_csv(f, encoding="utf-8")
-
- df = ratings.merge(movies, on=ITEM_COLUMN)
- df[RATING_COLUMN] = df[RATING_COLUMN].astype(np.float32)
-
- return df
-
-
-def integerize_genres(dataframe):
- """Replace genre string with a binary vector.
-
- Args:
- dataframe: a pandas dataframe of movie data.
-
- Returns:
- The transformed dataframe.
- """
- def _map_fn(entry):
- entry.replace("Children's", "Children") # naming difference.
- movie_genres = entry.split("|")
- output = np.zeros((len(GENRES),), dtype=np.int64)
- for i, genre in enumerate(GENRES):
- if genre in movie_genres:
- output[i] = 1
- return output
-
- dataframe[GENRE_COLUMN] = dataframe[GENRE_COLUMN].apply(_map_fn)
-
- return dataframe
-
-
-def define_flags():
- """Add flags specifying data usage arguments."""
- flags.DEFINE_enum(
- name="dataset",
- default=None,
- enum_values=DATASETS,
- case_sensitive=False,
- help=flags_core.help_wrap("Dataset to be trained and evaluated."))
-
-
-def define_data_download_flags():
- """Add flags specifying data download and usage arguments."""
- flags.DEFINE_string(
- name="data_dir", default="/tmp/movielens-data/",
- help=flags_core.help_wrap(
- "Directory to download and extract data."))
-
- define_flags()
-
-
-def main(_):
- """Download and extract the data from GroupLens website."""
- download(flags.FLAGS.dataset, flags.FLAGS.data_dir)
-
-
-if __name__ == "__main__":
- define_data_download_flags()
- FLAGS = flags.FLAGS
- app.run(main)
diff --git a/spaces/OFA-Sys/OFA-Generic_Interface/fairseq/examples/speech_to_text/data_utils.py b/spaces/OFA-Sys/OFA-Generic_Interface/fairseq/examples/speech_to_text/data_utils.py
deleted file mode 100644
index 41afac0bf8f6d70e06bee1a34e220ab396ec247d..0000000000000000000000000000000000000000
--- a/spaces/OFA-Sys/OFA-Generic_Interface/fairseq/examples/speech_to_text/data_utils.py
+++ /dev/null
@@ -1,382 +0,0 @@
-# Copyright (c) Facebook, Inc. and its affiliates.
-#
-# This source code is licensed under the MIT license found in the
-# LICENSE file in the root directory of this source tree.
-
-import csv
-from pathlib import Path
-import zipfile
-from functools import reduce
-from multiprocessing import cpu_count
-from typing import Any, Dict, List, Optional, Union
-import io
-
-import numpy as np
-import pandas as pd
-import sentencepiece as sp
-from fairseq.data.audio.audio_utils import (
- convert_waveform, _get_kaldi_fbank, _get_torchaudio_fbank, is_npy_data,
- is_sf_audio_data
-)
-import torch
-import soundfile as sf
-from tqdm import tqdm
-
-
-UNK_TOKEN, UNK_TOKEN_ID = "", 3
-BOS_TOKEN, BOS_TOKEN_ID = "", 0
-EOS_TOKEN, EOS_TOKEN_ID = "", 2
-PAD_TOKEN, PAD_TOKEN_ID = "", 1
-
-
-def gen_vocab(
- input_path: Path, output_path_prefix: Path, model_type="bpe",
- vocab_size=1000, special_symbols: Optional[List[str]] = None
-):
- # Train SentencePiece Model
- arguments = [
- f"--input={input_path.as_posix()}",
- f"--model_prefix={output_path_prefix.as_posix()}",
- f"--model_type={model_type}",
- f"--vocab_size={vocab_size}",
- "--character_coverage=1.0",
- f"--num_threads={cpu_count()}",
- f"--unk_id={UNK_TOKEN_ID}",
- f"--bos_id={BOS_TOKEN_ID}",
- f"--eos_id={EOS_TOKEN_ID}",
- f"--pad_id={PAD_TOKEN_ID}",
- ]
- if special_symbols is not None:
- _special_symbols = ",".join(special_symbols)
- arguments.append(f"--user_defined_symbols={_special_symbols}")
- sp.SentencePieceTrainer.Train(" ".join(arguments))
- # Export fairseq dictionary
- spm = sp.SentencePieceProcessor()
- spm.Load(output_path_prefix.as_posix() + ".model")
- vocab = {i: spm.IdToPiece(i) for i in range(spm.GetPieceSize())}
- assert (
- vocab.get(UNK_TOKEN_ID) == UNK_TOKEN
- and vocab.get(PAD_TOKEN_ID) == PAD_TOKEN
- and vocab.get(BOS_TOKEN_ID) == BOS_TOKEN
- and vocab.get(EOS_TOKEN_ID) == EOS_TOKEN
- )
- vocab = {
- i: s
- for i, s in vocab.items()
- if s not in {UNK_TOKEN, BOS_TOKEN, EOS_TOKEN, PAD_TOKEN}
- }
- with open(output_path_prefix.as_posix() + ".txt", "w") as f_out:
- for _, s in sorted(vocab.items(), key=lambda x: x[0]):
- f_out.write(f"{s} 1\n")
-
-
-def extract_fbank_features(
- waveform: torch.FloatTensor,
- sample_rate: int,
- output_path: Optional[Path] = None,
- n_mel_bins: int = 80,
- overwrite: bool = False,
-):
- if output_path is not None and output_path.is_file() and not overwrite:
- return
-
- _waveform = convert_waveform(waveform, sample_rate, to_mono=True)
- # Kaldi compliance: 16-bit signed integers
- _waveform = _waveform * (2 ** 15)
- _waveform = _waveform.numpy()
-
- features = _get_kaldi_fbank(_waveform, sample_rate, n_mel_bins)
- if features is None:
- features = _get_torchaudio_fbank(_waveform, sample_rate, n_mel_bins)
- if features is None:
- raise ImportError(
- "Please install pyKaldi or torchaudio to enable fbank feature extraction"
- )
-
- if output_path is not None:
- np.save(output_path.as_posix(), features)
- return features
-
-
-def create_zip(data_root: Path, zip_path: Path):
- paths = list(data_root.glob("*.npy"))
- with zipfile.ZipFile(zip_path, "w", zipfile.ZIP_STORED) as f:
- for path in tqdm(paths):
- f.write(path, arcname=path.name)
-
-
-def get_zip_manifest(
- zip_path: Path, zip_root: Optional[Path] = None, is_audio=False
-):
- _zip_path = Path.joinpath(zip_root or Path(""), zip_path)
- with zipfile.ZipFile(_zip_path, mode="r") as f:
- info = f.infolist()
- paths, lengths = {}, {}
- for i in tqdm(info):
- utt_id = Path(i.filename).stem
- offset, file_size = i.header_offset + 30 + len(i.filename), i.file_size
- paths[utt_id] = f"{zip_path.as_posix()}:{offset}:{file_size}"
- with open(_zip_path, "rb") as f:
- f.seek(offset)
- byte_data = f.read(file_size)
- assert len(byte_data) > 1
- if is_audio:
- assert is_sf_audio_data(byte_data), i
- else:
- assert is_npy_data(byte_data), i
- byte_data_fp = io.BytesIO(byte_data)
- if is_audio:
- lengths[utt_id] = sf.info(byte_data_fp).frames
- else:
- lengths[utt_id] = np.load(byte_data_fp).shape[0]
- return paths, lengths
-
-
-def gen_config_yaml(
- manifest_root: Path,
- spm_filename: Optional[str] = None,
- vocab_name: Optional[str] = None,
- yaml_filename: str = "config.yaml",
- specaugment_policy: Optional[str] = "lb",
- prepend_tgt_lang_tag: bool = False,
- sampling_alpha: Optional[float] = None,
- input_channels: Optional[int] = 1,
- input_feat_per_channel: Optional[int] = 80,
- audio_root: str = "",
- cmvn_type: str = "utterance",
- gcmvn_path: Optional[Path] = None,
- extra=None
-):
- manifest_root = manifest_root.absolute()
- writer = S2TDataConfigWriter(manifest_root / yaml_filename)
- assert spm_filename is not None or vocab_name is not None
- vocab_name = spm_filename.replace(".model", ".txt") if vocab_name is None \
- else vocab_name
- writer.set_vocab_filename(vocab_name)
- if input_channels is not None:
- writer.set_input_channels(input_channels)
- if input_feat_per_channel is not None:
- writer.set_input_feat_per_channel(input_feat_per_channel)
- specaugment_setters = {
- "lb": writer.set_specaugment_lb_policy,
- "ld": writer.set_specaugment_ld_policy,
- "sm": writer.set_specaugment_sm_policy,
- "ss": writer.set_specaugment_ss_policy,
- }
- specaugment_setter = specaugment_setters.get(specaugment_policy, None)
- if specaugment_setter is not None:
- specaugment_setter()
- if spm_filename is not None:
- writer.set_bpe_tokenizer(
- {
- "bpe": "sentencepiece",
- "sentencepiece_model": (manifest_root / spm_filename).as_posix(),
- }
- )
- if prepend_tgt_lang_tag:
- writer.set_prepend_tgt_lang_tag(True)
- if sampling_alpha is not None:
- writer.set_sampling_alpha(sampling_alpha)
-
- if cmvn_type not in ["global", "utterance"]:
- raise NotImplementedError
-
- if specaugment_policy is not None:
- writer.set_feature_transforms(
- "_train", [f"{cmvn_type}_cmvn", "specaugment"]
- )
- writer.set_feature_transforms("*", [f"{cmvn_type}_cmvn"])
-
- if cmvn_type == "global":
- if gcmvn_path is None:
- raise ValueError("Please provide path of global cmvn file.")
- else:
- writer.set_global_cmvn(gcmvn_path.as_posix())
-
- if len(audio_root) > 0:
- writer.set_audio_root(audio_root)
-
- if extra is not None:
- writer.set_extra(extra)
- writer.flush()
-
-
-def load_df_from_tsv(path: Union[str, Path]) -> pd.DataFrame:
- _path = path if isinstance(path, str) else path.as_posix()
- return pd.read_csv(
- _path,
- sep="\t",
- header=0,
- encoding="utf-8",
- escapechar="\\",
- quoting=csv.QUOTE_NONE,
- na_filter=False,
- )
-
-
-def save_df_to_tsv(dataframe, path: Union[str, Path]):
- _path = path if isinstance(path, str) else path.as_posix()
- dataframe.to_csv(
- _path,
- sep="\t",
- header=True,
- index=False,
- encoding="utf-8",
- escapechar="\\",
- quoting=csv.QUOTE_NONE,
- )
-
-
-def load_tsv_to_dicts(path: Union[str, Path]) -> List[dict]:
- with open(path, "r") as f:
- reader = csv.DictReader(
- f,
- delimiter="\t",
- quotechar=None,
- doublequote=False,
- lineterminator="\n",
- quoting=csv.QUOTE_NONE,
- )
- rows = [dict(e) for e in reader]
- return rows
-
-
-def filter_manifest_df(
- df, is_train_split=False, extra_filters=None, min_n_frames=5, max_n_frames=3000
-):
- filters = {
- "no speech": df["audio"] == "",
- f"short speech (<{min_n_frames} frames)": df["n_frames"] < min_n_frames,
- "empty sentence": df["tgt_text"] == "",
- }
- if is_train_split:
- filters[f"long speech (>{max_n_frames} frames)"] = df["n_frames"] > max_n_frames
- if extra_filters is not None:
- filters.update(extra_filters)
- invalid = reduce(lambda x, y: x | y, filters.values())
- valid = ~invalid
- print(
- "| "
- + ", ".join(f"{n}: {f.sum()}" for n, f in filters.items())
- + f", total {invalid.sum()} filtered, {valid.sum()} remained."
- )
- return df[valid]
-
-
-def cal_gcmvn_stats(features_list):
- features = np.concatenate(features_list)
- square_sums = (features ** 2).sum(axis=0)
- mean = features.mean(axis=0)
- features = np.subtract(features, mean)
- var = square_sums / features.shape[0] - mean ** 2
- std = np.sqrt(np.maximum(var, 1e-8))
- return {"mean": mean.astype("float32"), "std": std.astype("float32")}
-
-
-class S2TDataConfigWriter(object):
- DEFAULT_VOCAB_FILENAME = "dict.txt"
- DEFAULT_INPUT_FEAT_PER_CHANNEL = 80
- DEFAULT_INPUT_CHANNELS = 1
-
- def __init__(self, yaml_path: Path):
- try:
- import yaml
- except ImportError:
- print("Please install PyYAML for S2T data config YAML files")
- self.yaml = yaml
- self.yaml_path = yaml_path
- self.config = {}
-
- def flush(self):
- with open(self.yaml_path, "w") as f:
- self.yaml.dump(self.config, f)
-
- def set_audio_root(self, audio_root=""):
- self.config["audio_root"] = audio_root
-
- def set_vocab_filename(self, vocab_filename: str = "dict.txt"):
- self.config["vocab_filename"] = vocab_filename
-
- def set_specaugment(
- self,
- time_wrap_w: int,
- freq_mask_n: int,
- freq_mask_f: int,
- time_mask_n: int,
- time_mask_t: int,
- time_mask_p: float,
- ):
- self.config["specaugment"] = {
- "time_wrap_W": time_wrap_w,
- "freq_mask_N": freq_mask_n,
- "freq_mask_F": freq_mask_f,
- "time_mask_N": time_mask_n,
- "time_mask_T": time_mask_t,
- "time_mask_p": time_mask_p,
- }
-
- def set_specaugment_lb_policy(self):
- self.set_specaugment(
- time_wrap_w=0,
- freq_mask_n=1,
- freq_mask_f=27,
- time_mask_n=1,
- time_mask_t=100,
- time_mask_p=1.0,
- )
-
- def set_specaugment_ld_policy(self):
- self.set_specaugment(
- time_wrap_w=0,
- freq_mask_n=2,
- freq_mask_f=27,
- time_mask_n=2,
- time_mask_t=100,
- time_mask_p=1.0,
- )
-
- def set_specaugment_sm_policy(self):
- self.set_specaugment(
- time_wrap_w=0,
- freq_mask_n=2,
- freq_mask_f=15,
- time_mask_n=2,
- time_mask_t=70,
- time_mask_p=0.2,
- )
-
- def set_specaugment_ss_policy(self):
- self.set_specaugment(
- time_wrap_w=0,
- freq_mask_n=2,
- freq_mask_f=27,
- time_mask_n=2,
- time_mask_t=70,
- time_mask_p=0.2,
- )
-
- def set_input_channels(self, input_channels: int = 1):
- self.config["input_channels"] = input_channels
-
- def set_input_feat_per_channel(self, input_feat_per_channel: int = 80):
- self.config["input_feat_per_channel"] = input_feat_per_channel
-
- def set_bpe_tokenizer(self, bpe_tokenizer: Dict[str, Any]):
- self.config["bpe_tokenizer"] = bpe_tokenizer
-
- def set_global_cmvn(self, stats_npz_path: str):
- self.config["global_cmvn"] = {"stats_npz_path": stats_npz_path}
-
- def set_feature_transforms(self, split: str, transforms: List[str]):
- if "transforms" not in self.config:
- self.config["transforms"] = {}
- self.config["transforms"][split] = transforms
-
- def set_prepend_tgt_lang_tag(self, flag: bool = True):
- self.config["prepend_tgt_lang_tag"] = flag
-
- def set_sampling_alpha(self, sampling_alpha: float = 1.0):
- self.config["sampling_alpha"] = sampling_alpha
-
- def set_extra(self, data):
- self.config.update(data)
diff --git a/spaces/OFA-Sys/OFA-Visual_Grounding/criterions/label_smoothed_cross_entropy.py b/spaces/OFA-Sys/OFA-Visual_Grounding/criterions/label_smoothed_cross_entropy.py
deleted file mode 100644
index 73b36e750a0037cad8403e383d790f868b509d24..0000000000000000000000000000000000000000
--- a/spaces/OFA-Sys/OFA-Visual_Grounding/criterions/label_smoothed_cross_entropy.py
+++ /dev/null
@@ -1,343 +0,0 @@
-# Copyright (c) Facebook, Inc. and its affiliates.
-#
-# This source code is licensed under the MIT license found in the
-# LICENSE file in the root directory of this source tree.
-
-import math
-from dataclasses import dataclass, field
-from typing import Optional
-
-import torch
-import torch.nn.functional as F
-import numpy as np
-from fairseq import metrics, utils
-from fairseq.criterions import FairseqCriterion, register_criterion
-from fairseq.dataclass import FairseqDataclass
-from omegaconf import II
-
-
-@dataclass
-class AjustLabelSmoothedCrossEntropyCriterionConfig(FairseqDataclass):
- label_smoothing: float = field(
- default=0.0,
- metadata={"help": "epsilon for label smoothing, 0 means no label smoothing"},
- )
- report_accuracy: bool = field(
- default=False,
- metadata={"help": "report accuracy metric"},
- )
- ignore_prefix_size: int = field(
- default=0,
- metadata={"help": "Ignore first N tokens"},
- )
- ignore_eos: bool = field(
- default=False,
- metadata={"help": "Ignore eos token"},
- )
- sentence_avg: bool = II("optimization.sentence_avg")
- drop_worst_ratio: float = field(
- default=0.0,
- metadata={"help": "ratio for discarding bad samples"},
- )
- drop_worst_after: int = field(
- default=0,
- metadata={"help": "steps for discarding bad samples"},
- )
- use_rdrop: bool = field(
- default=False, metadata={"help": "use R-Drop"}
- )
- reg_alpha: float = field(
- default=1.0, metadata={"help": "weight for R-Drop"}
- )
- sample_patch_num: int = field(
- default=196, metadata={"help": "sample patchs for v1"}
- )
- constraint_range: Optional[str] = field(
- default=None,
- metadata={"help": "constraint range"}
- )
-
-
-def construct_rdrop_sample(x):
- if isinstance(x, dict):
- for key in x:
- x[key] = construct_rdrop_sample(x[key])
- return x
- elif isinstance(x, torch.Tensor):
- return x.repeat(2, *([1] * (x.dim()-1)))
- elif isinstance(x, int):
- return x * 2
- elif isinstance(x, np.ndarray):
- return x.repeat(2)
- else:
- raise NotImplementedError
-
-
-def kl_loss(p, q):
- p_loss = F.kl_div(p, torch.exp(q), reduction='sum')
- q_loss = F.kl_div(q, torch.exp(p), reduction='sum')
- loss = (p_loss + q_loss) / 2
- return loss
-
-
-def label_smoothed_nll_loss(
- lprobs, target, epsilon, update_num, reduce=True,
- drop_worst_ratio=0.0, drop_worst_after=0, use_rdrop=False, reg_alpha=1.0,
- constraint_masks=None, constraint_start=None, constraint_end=None
-):
- if target.dim() == lprobs.dim() - 1:
- target = target.unsqueeze(-1)
- nll_loss = -lprobs.gather(dim=-1, index=target).squeeze(-1)
- if constraint_masks is not None:
- smooth_loss = -lprobs.masked_fill(~constraint_masks, 0).sum(dim=-1, keepdim=True).squeeze(-1)
- eps_i = epsilon / (constraint_masks.sum(1) - 1 + 1e-6)
- elif constraint_start is not None and constraint_end is not None:
- constraint_range = [0, 1, 2, 3] + list(range(constraint_start, constraint_end))
- smooth_loss = -lprobs[:, constraint_range].sum(dim=-1, keepdim=True).squeeze(-1)
- eps_i = epsilon / (len(constraint_range) - 1 + 1e-6)
- else:
- smooth_loss = -lprobs.sum(dim=-1, keepdim=True).squeeze(-1)
- eps_i = epsilon / (lprobs.size(-1) - 1)
- loss = (1.0 - epsilon - eps_i) * nll_loss + eps_i * smooth_loss
- if drop_worst_ratio > 0 and update_num > drop_worst_after:
- if use_rdrop:
- true_batch_size = loss.size(0) // 2
- _, indices = torch.topk(loss[:true_batch_size], k=int(true_batch_size * (1 - drop_worst_ratio)), largest=False)
- loss = torch.cat([loss[indices], loss[indices+true_batch_size]])
- nll_loss = torch.cat([nll_loss[indices], nll_loss[indices+true_batch_size]])
- lprobs = torch.cat([lprobs[indices], lprobs[indices+true_batch_size]])
- else:
- loss, indices = torch.topk(loss, k=int(loss.shape[0] * (1 - drop_worst_ratio)), largest=False)
- nll_loss = nll_loss[indices]
- lprobs = lprobs[indices]
-
- ntokens = loss.numel()
- nll_loss = nll_loss.sum()
- loss = loss.sum()
- if use_rdrop:
- true_batch_size = lprobs.size(0) // 2
- p = lprobs[:true_batch_size]
- q = lprobs[true_batch_size:]
- if constraint_start is not None and constraint_end is not None:
- constraint_range = [0, 1, 2, 3] + list(range(constraint_start, constraint_end))
- p = p[:, constraint_range]
- q = q[:, constraint_range]
- loss += kl_loss(p, q) * reg_alpha
-
- return loss, nll_loss, ntokens
-
-
-@register_criterion(
- "ajust_label_smoothed_cross_entropy", dataclass=AjustLabelSmoothedCrossEntropyCriterionConfig
-)
-class AjustLabelSmoothedCrossEntropyCriterion(FairseqCriterion):
- def __init__(
- self,
- task,
- sentence_avg,
- label_smoothing,
- ignore_prefix_size=0,
- ignore_eos=False,
- report_accuracy=False,
- drop_worst_ratio=0,
- drop_worst_after=0,
- use_rdrop=False,
- reg_alpha=1.0,
- sample_patch_num=196,
- constraint_range=None
- ):
- super().__init__(task)
- self.sentence_avg = sentence_avg
- self.eps = label_smoothing
- self.ignore_prefix_size = ignore_prefix_size
- self.ignore_eos = ignore_eos
- self.report_accuracy = report_accuracy
- self.drop_worst_ratio = drop_worst_ratio
- self.drop_worst_after = drop_worst_after
- self.use_rdrop = use_rdrop
- self.reg_alpha = reg_alpha
- self.sample_patch_num = sample_patch_num
-
- self.constraint_start = None
- self.constraint_end = None
- if constraint_range is not None:
- constraint_start, constraint_end = constraint_range.split(',')
- self.constraint_start = int(constraint_start)
- self.constraint_end = int(constraint_end)
-
- def forward(self, model, sample, update_num=0, reduce=True):
- """Compute the loss for the given sample.
-
- Returns a tuple with three elements:
- 1) the loss
- 2) the sample size, which is used as the denominator for the gradient
- 3) logging outputs to display while training
- """
- if isinstance(sample, list):
- if self.sample_patch_num > 0:
- sample[0]['net_input']['sample_patch_num'] = self.sample_patch_num
- loss_v1, sample_size_v1, logging_output_v1 = self.forward(model, sample[0], update_num, reduce)
- loss_v2, sample_size_v2, logging_output_v2 = self.forward(model, sample[1], update_num, reduce)
- loss = loss_v1 / sample_size_v1 + loss_v2 / sample_size_v2
- sample_size = 1
- logging_output = {
- "loss": loss.data,
- "loss_v1": loss_v1.data,
- "loss_v2": loss_v2.data,
- "nll_loss": logging_output_v1["nll_loss"].data / sample_size_v1 + logging_output_v2["nll_loss"].data / sample_size_v2,
- "ntokens": logging_output_v1["ntokens"] + logging_output_v2["ntokens"],
- "nsentences": logging_output_v1["nsentences"] + logging_output_v2["nsentences"],
- "sample_size": 1,
- "sample_size_v1": sample_size_v1,
- "sample_size_v2": sample_size_v2,
- }
- return loss, sample_size, logging_output
-
- if self.use_rdrop:
- construct_rdrop_sample(sample)
-
- net_output = model(**sample["net_input"])
- loss, nll_loss, ntokens = self.compute_loss(model, net_output, sample, update_num, reduce=reduce)
- sample_size = (
- sample["target"].size(0) if self.sentence_avg else ntokens
- )
- logging_output = {
- "loss": loss.data,
- "nll_loss": nll_loss.data,
- "ntokens": sample["ntokens"],
- "nsentences": sample["nsentences"],
- "sample_size": sample_size,
- }
- if self.report_accuracy:
- n_correct, total = self.compute_accuracy(model, net_output, sample)
- logging_output["n_correct"] = utils.item(n_correct.data)
- logging_output["total"] = utils.item(total.data)
- return loss, sample_size, logging_output
-
- def get_lprobs_and_target(self, model, net_output, sample):
- conf = sample['conf'][:, None, None] if 'conf' in sample and sample['conf'] is not None else 1
- constraint_masks = None
- if "constraint_masks" in sample and sample["constraint_masks"] is not None:
- constraint_masks = sample["constraint_masks"]
- net_output[0].masked_fill_(~constraint_masks, -math.inf)
- if self.constraint_start is not None and self.constraint_end is not None:
- net_output[0][:, :, 4:self.constraint_start] = -math.inf
- net_output[0][:, :, self.constraint_end:] = -math.inf
- lprobs = model.get_normalized_probs(net_output, log_probs=True) * conf
- target = model.get_targets(sample, net_output)
- if self.ignore_prefix_size > 0:
- lprobs = lprobs[:, self.ignore_prefix_size :, :].contiguous()
- target = target[:, self.ignore_prefix_size :].contiguous()
- if constraint_masks is not None:
- constraint_masks = constraint_masks[:, self.ignore_prefix_size :, :].contiguous()
- if self.ignore_eos:
- bsz, seq_len, embed_dim = lprobs.size()
- eos_indices = target.eq(self.task.tgt_dict.eos())
- lprobs = lprobs[~eos_indices].reshape(bsz, seq_len-1, embed_dim)
- target = target[~eos_indices].reshape(bsz, seq_len-1)
- if constraint_masks is not None:
- constraint_masks = constraint_masks[~eos_indices].reshape(bsz, seq_len-1, embed_dim)
- if constraint_masks is not None:
- constraint_masks = constraint_masks.view(-1, constraint_masks.size(-1))
- return lprobs.view(-1, lprobs.size(-1)), target.view(-1), constraint_masks
-
- def compute_loss(self, model, net_output, sample, update_num, reduce=True):
- lprobs, target, constraint_masks = self.get_lprobs_and_target(model, net_output, sample)
- if constraint_masks is not None:
- constraint_masks = constraint_masks[target != self.padding_idx]
- lprobs = lprobs[target != self.padding_idx]
- target = target[target != self.padding_idx]
- loss, nll_loss, ntokens = label_smoothed_nll_loss(
- lprobs,
- target,
- self.eps,
- update_num,
- reduce=reduce,
- drop_worst_ratio=self.drop_worst_ratio,
- drop_worst_after=self.drop_worst_after,
- use_rdrop=self.use_rdrop,
- reg_alpha=self.reg_alpha,
- constraint_masks=constraint_masks,
- constraint_start=self.constraint_start,
- constraint_end=self.constraint_end
- )
- return loss, nll_loss, ntokens
-
- def compute_accuracy(self, model, net_output, sample):
- lprobs, target = self.get_lprobs_and_target(model, net_output, sample)
- mask = target.ne(self.padding_idx)
- n_correct = torch.sum(
- lprobs.argmax(1).masked_select(mask).eq(target.masked_select(mask))
- )
- total = torch.sum(mask)
- return n_correct, total
-
- @classmethod
- def reduce_metrics(cls, logging_outputs) -> None:
- """Aggregate logging outputs from data parallel training."""
- loss_sum = sum(log.get("loss", 0) for log in logging_outputs)
- loss_sum_v1 = sum(log.get("loss_v1", 0) for log in logging_outputs)
- loss_sum_v2 = sum(log.get("loss_v2", 0) for log in logging_outputs)
- nll_loss_sum = sum(log.get("nll_loss", 0) for log in logging_outputs)
- ntokens = sum(log.get("ntokens", 0) for log in logging_outputs)
- nsentences = sum(log.get("nsentences", 0) for log in logging_outputs)
- sample_size = sum(log.get("sample_size", 0) for log in logging_outputs)
- sample_size_v1 = sum(log.get("sample_size_v1", 0) for log in logging_outputs)
- sample_size_v2 = sum(log.get("sample_size_v2", 0) for log in logging_outputs)
-
- metrics.log_scalar(
- "loss", loss_sum / sample_size, sample_size, round=3
- )
- metrics.log_scalar(
- "loss_v1", loss_sum_v1 / max(sample_size_v1, 1), max(sample_size_v1, 1), round=3
- )
- metrics.log_scalar(
- "loss_v2", loss_sum_v2 / max(sample_size_v2, 1), max(sample_size_v2, 1), round=3
- )
- metrics.log_scalar(
- "nll_loss", nll_loss_sum / sample_size, ntokens, round=3
- )
- metrics.log_derived(
- "ppl", lambda meters: utils.get_perplexity(meters["nll_loss"].avg)
- )
-
- metrics.log_scalar(
- "ntokens", ntokens, 1, round=3
- )
- metrics.log_scalar(
- "nsentences", nsentences, 1, round=3
- )
- metrics.log_scalar(
- "sample_size", sample_size, 1, round=3
- )
- metrics.log_scalar(
- "sample_size_v1", sample_size_v1, 1, round=3
- )
- metrics.log_scalar(
- "sample_size_v2", sample_size_v2, 1, round=3
- )
-
- total = utils.item(sum(log.get("total", 0) for log in logging_outputs))
- if total > 0:
- metrics.log_scalar("total", total)
- n_correct = utils.item(
- sum(log.get("n_correct", 0) for log in logging_outputs)
- )
- metrics.log_scalar("n_correct", n_correct)
- metrics.log_derived(
- "accuracy",
- lambda meters: round(
- meters["n_correct"].sum * 100.0 / meters["total"].sum, 3
- )
- if meters["total"].sum > 0
- else float("nan"),
- )
-
- @staticmethod
- def logging_outputs_can_be_summed() -> bool:
- """
- Whether the logging outputs returned by `forward` can be summed
- across workers prior to calling `reduce_metrics`. Setting this
- to True will improves distributed training speed.
- """
- return True
diff --git a/spaces/OFA-Sys/OFA-Visual_Grounding/fairseq/examples/hubert/measure_teacher_quality.py b/spaces/OFA-Sys/OFA-Visual_Grounding/fairseq/examples/hubert/measure_teacher_quality.py
deleted file mode 100644
index 92279b2214bb2ba4a99aea92098907ef4f55821b..0000000000000000000000000000000000000000
--- a/spaces/OFA-Sys/OFA-Visual_Grounding/fairseq/examples/hubert/measure_teacher_quality.py
+++ /dev/null
@@ -1,241 +0,0 @@
-# Copyright (c) Facebook, Inc. and its affiliates.
-#
-# This source code is licensed under the MIT license found in the
-# LICENSE file in the root directory of this source tree.
-
-import numpy as np
-import os.path as op
-import re
-from tabulate import tabulate
-from collections import Counter
-
-
-def comp_purity(p_xy, axis):
- max_p = p_xy.max(axis=axis)
- marg_p = p_xy.sum(axis=axis)
- indv_pur = max_p / marg_p
- aggr_pur = max_p.sum()
- return indv_pur, aggr_pur
-
-
-def comp_entropy(p):
- return (-p * np.log(p + 1e-8)).sum()
-
-
-def comp_norm_mutual_info(p_xy):
- p_x = p_xy.sum(axis=1, keepdims=True)
- p_y = p_xy.sum(axis=0, keepdims=True)
- pmi = np.log(p_xy / np.matmul(p_x, p_y) + 1e-8)
- mi = (p_xy * pmi).sum()
- h_x = comp_entropy(p_x)
- h_y = comp_entropy(p_y)
- return mi, mi / h_x, mi / h_y, h_x, h_y
-
-
-def pad(labs, n):
- if n == 0:
- return np.array(labs)
- return np.concatenate([[labs[0]] * n, labs, [labs[-1]] * n])
-
-
-def comp_avg_seg_dur(labs_list):
- n_frms = 0
- n_segs = 0
- for labs in labs_list:
- labs = np.array(labs)
- edges = np.zeros(len(labs)).astype(bool)
- edges[0] = True
- edges[1:] = labs[1:] != labs[:-1]
- n_frms += len(edges)
- n_segs += edges.astype(int).sum()
- return n_frms / n_segs
-
-
-def comp_joint_prob(uid2refs, uid2hyps):
- """
- Args:
- pad: padding for spliced-feature derived labels
- """
- cnts = Counter()
- skipped = []
- abs_frmdiff = 0
- for uid in uid2refs:
- if uid not in uid2hyps:
- skipped.append(uid)
- continue
- refs = uid2refs[uid]
- hyps = uid2hyps[uid]
- abs_frmdiff += abs(len(refs) - len(hyps))
- min_len = min(len(refs), len(hyps))
- refs = refs[:min_len]
- hyps = hyps[:min_len]
- cnts.update(zip(refs, hyps))
- tot = sum(cnts.values())
-
- ref_set = sorted({ref for ref, _ in cnts.keys()})
- hyp_set = sorted({hyp for _, hyp in cnts.keys()})
- ref2pid = dict(zip(ref_set, range(len(ref_set))))
- hyp2lid = dict(zip(hyp_set, range(len(hyp_set))))
- # print(hyp_set)
- p_xy = np.zeros((len(ref2pid), len(hyp2lid)), dtype=float)
- for (ref, hyp), cnt in cnts.items():
- p_xy[ref2pid[ref], hyp2lid[hyp]] = cnt
- p_xy /= p_xy.sum()
- return p_xy, ref2pid, hyp2lid, tot, abs_frmdiff, skipped
-
-
-def read_phn(tsv_path, rm_stress=True):
- uid2phns = {}
- with open(tsv_path) as f:
- for line in f:
- uid, phns = line.rstrip().split("\t")
- phns = phns.split(",")
- if rm_stress:
- phns = [re.sub("[0-9]", "", phn) for phn in phns]
- uid2phns[uid] = phns
- return uid2phns
-
-
-def read_lab(tsv_path, lab_path, pad_len=0, upsample=1):
- """
- tsv is needed to retrieve the uids for the labels
- """
- with open(tsv_path) as f:
- f.readline()
- uids = [op.splitext(op.basename(line.rstrip().split()[0]))[0] for line in f]
- with open(lab_path) as f:
- labs_list = [pad(line.rstrip().split(), pad_len).repeat(upsample) for line in f]
- assert len(uids) == len(labs_list)
- return dict(zip(uids, labs_list))
-
-
-def main_lab_lab(
- tsv_dir,
- lab_dir,
- lab_name,
- lab_sets,
- ref_dir,
- ref_name,
- pad_len=0,
- upsample=1,
- verbose=False,
-):
- # assume tsv_dir is the same for both the reference and the hypotheses
- tsv_dir = lab_dir if tsv_dir is None else tsv_dir
-
- uid2refs = {}
- for s in lab_sets:
- uid2refs.update(read_lab(f"{tsv_dir}/{s}.tsv", f"{ref_dir}/{s}.{ref_name}"))
-
- uid2hyps = {}
- for s in lab_sets:
- uid2hyps.update(
- read_lab(
- f"{tsv_dir}/{s}.tsv", f"{lab_dir}/{s}.{lab_name}", pad_len, upsample
- )
- )
- _main(uid2refs, uid2hyps, verbose)
-
-
-def main_phn_lab(
- tsv_dir,
- lab_dir,
- lab_name,
- lab_sets,
- phn_dir,
- phn_sets,
- pad_len=0,
- upsample=1,
- verbose=False,
-):
- uid2refs = {}
- for s in phn_sets:
- uid2refs.update(read_phn(f"{phn_dir}/{s}.tsv"))
-
- uid2hyps = {}
- tsv_dir = lab_dir if tsv_dir is None else tsv_dir
- for s in lab_sets:
- uid2hyps.update(
- read_lab(
- f"{tsv_dir}/{s}.tsv", f"{lab_dir}/{s}.{lab_name}", pad_len, upsample
- )
- )
- _main(uid2refs, uid2hyps, verbose)
-
-
-def _main(uid2refs, uid2hyps, verbose):
- (p_xy, ref2pid, hyp2lid, tot, frmdiff, skipped) = comp_joint_prob(
- uid2refs, uid2hyps
- )
- ref_pur_by_hyp, ref_pur = comp_purity(p_xy, axis=0)
- hyp_pur_by_ref, hyp_pur = comp_purity(p_xy, axis=1)
- (mi, mi_norm_by_ref, mi_norm_by_hyp, h_ref, h_hyp) = comp_norm_mutual_info(p_xy)
- outputs = {
- "ref pur": ref_pur,
- "hyp pur": hyp_pur,
- "H(ref)": h_ref,
- "H(hyp)": h_hyp,
- "MI": mi,
- "MI/H(ref)": mi_norm_by_ref,
- "ref segL": comp_avg_seg_dur(uid2refs.values()),
- "hyp segL": comp_avg_seg_dur(uid2hyps.values()),
- "p_xy shape": p_xy.shape,
- "frm tot": tot,
- "frm diff": frmdiff,
- "utt tot": len(uid2refs),
- "utt miss": len(skipped),
- }
- print(tabulate([outputs.values()], outputs.keys(), floatfmt=".4f"))
-
-
-if __name__ == "__main__":
- """
- compute quality of labels with respect to phone or another labels if set
- """
- import argparse
-
- parser = argparse.ArgumentParser()
- parser.add_argument("tsv_dir")
- parser.add_argument("lab_dir")
- parser.add_argument("lab_name")
- parser.add_argument("--lab_sets", default=["valid"], type=str, nargs="+")
- parser.add_argument(
- "--phn_dir",
- default="/checkpoint/wnhsu/data/librispeech/960h/fa/raw_phn/phone_frame_align_v1",
- )
- parser.add_argument(
- "--phn_sets", default=["dev-clean", "dev-other"], type=str, nargs="+"
- )
- parser.add_argument("--pad_len", default=0, type=int, help="padding for hypotheses")
- parser.add_argument(
- "--upsample", default=1, type=int, help="upsample factor for hypotheses"
- )
- parser.add_argument("--ref_lab_dir", default="")
- parser.add_argument("--ref_lab_name", default="")
- parser.add_argument("--verbose", action="store_true")
- args = parser.parse_args()
-
- if args.ref_lab_dir and args.ref_lab_name:
- main_lab_lab(
- args.tsv_dir,
- args.lab_dir,
- args.lab_name,
- args.lab_sets,
- args.ref_lab_dir,
- args.ref_lab_name,
- args.pad_len,
- args.upsample,
- args.verbose,
- )
- else:
- main_phn_lab(
- args.tsv_dir,
- args.lab_dir,
- args.lab_name,
- args.lab_sets,
- args.phn_dir,
- args.phn_sets,
- args.pad_len,
- args.upsample,
- args.verbose,
- )
diff --git a/spaces/OFA-Sys/OFA-vqa/fairseq/examples/speech_to_text/seg_mustc_data.py b/spaces/OFA-Sys/OFA-vqa/fairseq/examples/speech_to_text/seg_mustc_data.py
deleted file mode 100644
index 1ee665d6399729afe17d790d872eff34de124900..0000000000000000000000000000000000000000
--- a/spaces/OFA-Sys/OFA-vqa/fairseq/examples/speech_to_text/seg_mustc_data.py
+++ /dev/null
@@ -1,54 +0,0 @@
-#!/usr/bin/env python3
-# Copyright (c) Facebook, Inc. and its affiliates.
-#
-# This source code is licensed under the MIT license found in the
-# LICENSE file in the root directory of this source tree.
-
-import argparse
-import logging
-from pathlib import Path
-import soundfile as sf
-from examples.speech_to_text.prep_mustc_data import (
- MUSTC
-)
-
-from tqdm import tqdm
-
-log = logging.getLogger(__name__)
-
-
-def main(args):
- root = Path(args.data_root).absolute()
- lang = args.lang
- split = args.split
-
- cur_root = root / f"en-{lang}"
- assert cur_root.is_dir(), (
- f"{cur_root.as_posix()} does not exist. Skipped."
- )
-
- dataset = MUSTC(root.as_posix(), lang, split)
- output = Path(args.output).absolute()
- output.mkdir(exist_ok=True)
- f_text = open(output / f"{split}.{lang}", "w")
- f_wav_list = open(output / f"{split}.wav_list", "w")
- for waveform, sample_rate, _, text, _, utt_id in tqdm(dataset):
- sf.write(
- output / f"{utt_id}.wav",
- waveform.squeeze(0).numpy(),
- samplerate=int(sample_rate)
- )
- f_text.write(text + "\n")
- f_wav_list.write(str(output / f"{utt_id}.wav") + "\n")
-
-
-if __name__ == "__main__":
- parser = argparse.ArgumentParser()
- parser.add_argument("--data-root", "-d", required=True, type=str)
- parser.add_argument("--task", required=True, type=str, choices=["asr", "st"])
- parser.add_argument("--lang", required=True, type=str)
- parser.add_argument("--output", required=True, type=str)
- parser.add_argument("--split", required=True, choices=MUSTC.SPLITS)
- args = parser.parse_args()
-
- main(args)
diff --git a/spaces/OFA-Sys/OFA-vqa/fairseq/examples/textless_nlp/gslm/unit2speech/tacotron2/audio_processing.py b/spaces/OFA-Sys/OFA-vqa/fairseq/examples/textless_nlp/gslm/unit2speech/tacotron2/audio_processing.py
deleted file mode 100644
index b5af7f723eb8047bc58db2f85234aea161fbc659..0000000000000000000000000000000000000000
--- a/spaces/OFA-Sys/OFA-vqa/fairseq/examples/textless_nlp/gslm/unit2speech/tacotron2/audio_processing.py
+++ /dev/null
@@ -1,93 +0,0 @@
-import torch
-import numpy as np
-from scipy.signal import get_window
-import librosa.util as librosa_util
-
-
-def window_sumsquare(window, n_frames, hop_length=200, win_length=800,
- n_fft=800, dtype=np.float32, norm=None):
- """
- # from librosa 0.6
- Compute the sum-square envelope of a window function at a given hop length.
-
- This is used to estimate modulation effects induced by windowing
- observations in short-time fourier transforms.
-
- Parameters
- ----------
- window : string, tuple, number, callable, or list-like
- Window specification, as in `get_window`
-
- n_frames : int > 0
- The number of analysis frames
-
- hop_length : int > 0
- The number of samples to advance between frames
-
- win_length : [optional]
- The length of the window function. By default, this matches `n_fft`.
-
- n_fft : int > 0
- The length of each analysis frame.
-
- dtype : np.dtype
- The data type of the output
-
- Returns
- -------
- wss : np.ndarray, shape=`(n_fft + hop_length * (n_frames - 1))`
- The sum-squared envelope of the window function
- """
- if win_length is None:
- win_length = n_fft
-
- n = n_fft + hop_length * (n_frames - 1)
- x = np.zeros(n, dtype=dtype)
-
- # Compute the squared window at the desired length
- win_sq = get_window(window, win_length, fftbins=True)
- win_sq = librosa_util.normalize(win_sq, norm=norm)**2
- win_sq = librosa_util.pad_center(win_sq, n_fft)
-
- # Fill the envelope
- for i in range(n_frames):
- sample = i * hop_length
- x[sample:min(n, sample + n_fft)] += win_sq[:max(0, min(n_fft, n - sample))]
- return x
-
-
-def griffin_lim(magnitudes, stft_fn, n_iters=30):
- """
- PARAMS
- ------
- magnitudes: spectrogram magnitudes
- stft_fn: STFT class with transform (STFT) and inverse (ISTFT) methods
- """
-
- angles = np.angle(np.exp(2j * np.pi * np.random.rand(*magnitudes.size())))
- angles = angles.astype(np.float32)
- angles = torch.autograd.Variable(torch.from_numpy(angles))
- signal = stft_fn.inverse(magnitudes, angles).squeeze(1)
-
- for i in range(n_iters):
- _, angles = stft_fn.transform(signal)
- signal = stft_fn.inverse(magnitudes, angles).squeeze(1)
- return signal
-
-
-def dynamic_range_compression(x, C=1, clip_val=1e-5):
- """
- PARAMS
- ------
- C: compression factor
- """
- return torch.log(torch.clamp(x, min=clip_val) * C)
-
-
-def dynamic_range_decompression(x, C=1):
- """
- PARAMS
- ------
- C: compression factor used to compress
- """
- return torch.exp(x) / C
diff --git a/spaces/OFA-Sys/OFA-vqa/fairseq/fairseq/benchmark/dummy_dataset.py b/spaces/OFA-Sys/OFA-vqa/fairseq/fairseq/benchmark/dummy_dataset.py
deleted file mode 100644
index 2f051754af55966e26850e94c121e0ff439bfd28..0000000000000000000000000000000000000000
--- a/spaces/OFA-Sys/OFA-vqa/fairseq/fairseq/benchmark/dummy_dataset.py
+++ /dev/null
@@ -1,36 +0,0 @@
-import numpy as np
-from fairseq.data import FairseqDataset
-
-
-class DummyDataset(FairseqDataset):
- def __init__(self, batch, num_items, item_size):
- super().__init__()
- self.batch = batch
- self.num_items = num_items
- self.item_size = item_size
-
- def __getitem__(self, index):
- return index
-
- def __len__(self):
- return self.num_items
-
- def collater(self, samples):
- return self.batch
-
- @property
- def sizes(self):
- return np.array([self.item_size] * self.num_items)
-
- def num_tokens(self, index):
- return self.item_size
-
- def size(self, index):
- return self.item_size
-
- def ordered_indices(self):
- return np.arange(self.num_items)
-
- @property
- def supports_prefetch(self):
- return False
diff --git a/spaces/OgiKazus/vits-uma-genshin-honkai/text/symbols.py b/spaces/OgiKazus/vits-uma-genshin-honkai/text/symbols.py
deleted file mode 100644
index edfbd24247be8c757275ce80b9ec27a0ffa808f3..0000000000000000000000000000000000000000
--- a/spaces/OgiKazus/vits-uma-genshin-honkai/text/symbols.py
+++ /dev/null
@@ -1,39 +0,0 @@
-'''
-Defines the set of symbols used in text input to the model.
-'''
-
-'''# japanese_cleaners
-_pad = '_'
-_punctuation = ',.!?-'
-_letters = 'AEINOQUabdefghijkmnoprstuvwyzʃʧ↓↑ '
-'''
-
-'''# japanese_cleaners2
-_pad = '_'
-_punctuation = ',.!?-~…'
-_letters = 'AEINOQUabdefghijkmnoprstuvwyzʃʧʦ↓↑ '
-'''
-
-'''# korean_cleaners
-_pad = '_'
-_punctuation = ',.!?…~'
-_letters = 'ㄱㄴㄷㄹㅁㅂㅅㅇㅈㅊㅋㅌㅍㅎㄲㄸㅃㅆㅉㅏㅓㅗㅜㅡㅣㅐㅔ '
-'''
-
-'''# chinese_cleaners
-_pad = '_'
-_punctuation = ',。!?—…'
-_letters = 'ㄅㄆㄇㄈㄉㄊㄋㄌㄍㄎㄏㄐㄑㄒㄓㄔㄕㄖㄗㄘㄙㄚㄛㄜㄝㄞㄟㄠㄡㄢㄣㄤㄥㄦㄧㄨㄩˉˊˇˋ˙ '
-'''
-
-# zh_ja_mixture_cleaners
-_pad = '_'
-_punctuation = ',.!?-~…'
-_letters = 'AEINOQUabdefghijklmnoprstuvwyzʃʧʦɯɹəɥ⁼ʰ`→↓↑ '
-
-
-# Export all symbols:
-symbols = [_pad] + list(_punctuation) + list(_letters)
-
-# Special symbol ids
-SPACE_ID = symbols.index(" ")
\ No newline at end of file
diff --git a/spaces/Olivier-Truong/faster-whisper-webui-v2/src/prompts/prependPromptStrategy.py b/spaces/Olivier-Truong/faster-whisper-webui-v2/src/prompts/prependPromptStrategy.py
deleted file mode 100644
index 6f8b6eba5b98310f57a656db73b5e415de3af958..0000000000000000000000000000000000000000
--- a/spaces/Olivier-Truong/faster-whisper-webui-v2/src/prompts/prependPromptStrategy.py
+++ /dev/null
@@ -1,31 +0,0 @@
-from src.config import VadInitialPromptMode
-from src.prompts.abstractPromptStrategy import AbstractPromptStrategy
-
-class PrependPromptStrategy(AbstractPromptStrategy):
- """
- A simple prompt strategy that prepends a single prompt to all segments of audio, or prepends the prompt to the first segment of audio.
- """
- def __init__(self, initial_prompt: str, initial_prompt_mode: VadInitialPromptMode):
- """
- Parameters
- ----------
- initial_prompt: str
- The initial prompt to use for the transcription.
- initial_prompt_mode: VadInitialPromptMode
- The mode to use for the initial prompt. If set to PREPEND_FIRST_SEGMENT, the initial prompt will be prepended to the first segment of audio.
- If set to PREPEND_ALL_SEGMENTS, the initial prompt will be prepended to all segments of audio.
- """
- self.initial_prompt = initial_prompt
- self.initial_prompt_mode = initial_prompt_mode
-
- # This is a simple prompt strategy, so we only support these two modes
- if initial_prompt_mode not in [VadInitialPromptMode.PREPEND_ALL_SEGMENTS, VadInitialPromptMode.PREPREND_FIRST_SEGMENT]:
- raise ValueError(f"Unsupported initial prompt mode {initial_prompt_mode}")
-
- def get_segment_prompt(self, segment_index: int, whisper_prompt: str, detected_language: str) -> str:
- if (self.initial_prompt_mode == VadInitialPromptMode.PREPEND_ALL_SEGMENTS):
- return self._concat_prompt(self.initial_prompt, whisper_prompt)
- elif (self.initial_prompt_mode == VadInitialPromptMode.PREPREND_FIRST_SEGMENT):
- return self._concat_prompt(self.initial_prompt, whisper_prompt) if segment_index == 0 else whisper_prompt
- else:
- raise ValueError(f"Unknown initial prompt mode {self.initial_prompt_mode}")
\ No newline at end of file
diff --git a/spaces/OpenMotionLab/MotionGPT/mGPT/data/transforms/joints2rots/customloss.py b/spaces/OpenMotionLab/MotionGPT/mGPT/data/transforms/joints2rots/customloss.py
deleted file mode 100644
index 2c3c3a530876113596f223324dc9dd0c002fd520..0000000000000000000000000000000000000000
--- a/spaces/OpenMotionLab/MotionGPT/mGPT/data/transforms/joints2rots/customloss.py
+++ /dev/null
@@ -1,217 +0,0 @@
-import torch
-import torch.nn.functional as F
-import config
-
-# Guassian
-def gmof(x, sigma):
- """
- Geman-McClure error function
- """
- x_squared = x ** 2
- sigma_squared = sigma ** 2
- return (sigma_squared * x_squared) / (sigma_squared + x_squared)
-
-# angle prior
-def angle_prior(pose):
- """
- Angle prior that penalizes unnatural bending of the knees and elbows
- """
- # We subtract 3 because pose does not include the global rotation of the model
- return torch.exp(
- pose[:, [55 - 3, 58 - 3, 12 - 3, 15 - 3]] * torch.tensor([1., -1., -1, -1.], device=pose.device)) ** 2
-
-
-def perspective_projection(points, rotation, translation,
- focal_length, camera_center):
- """
- This function computes the perspective projection of a set of points.
- Input:
- points (bs, N, 3): 3D points
- rotation (bs, 3, 3): Camera rotation
- translation (bs, 3): Camera translation
- focal_length (bs,) or scalar: Focal length
- camera_center (bs, 2): Camera center
- """
- batch_size = points.shape[0]
- K = torch.zeros([batch_size, 3, 3], device=points.device)
- K[:, 0, 0] = focal_length
- K[:, 1, 1] = focal_length
- K[:, 2, 2] = 1.
- K[:, :-1, -1] = camera_center
-
- # Transform points
- points = torch.einsum('bij,bkj->bki', rotation, points)
- points = points + translation.unsqueeze(1)
-
- # Apply perspective distortion
- projected_points = points / points[:, :, -1].unsqueeze(-1)
-
- # Apply camera intrinsics
- projected_points = torch.einsum('bij,bkj->bki', K, projected_points)
-
- return projected_points[:, :, :-1]
-
-
-def body_fitting_loss(body_pose, betas, model_joints, camera_t, camera_center,
- joints_2d, joints_conf, pose_prior,
- focal_length=5000, sigma=100, pose_prior_weight=4.78,
- shape_prior_weight=5, angle_prior_weight=15.2,
- output='sum'):
- """
- Loss function for body fitting
- """
- batch_size = body_pose.shape[0]
- rotation = torch.eye(3, device=body_pose.device).unsqueeze(0).expand(batch_size, -1, -1)
-
- projected_joints = perspective_projection(model_joints, rotation, camera_t,
- focal_length, camera_center)
-
- # Weighted robust reprojection error
- reprojection_error = gmof(projected_joints - joints_2d, sigma)
- reprojection_loss = (joints_conf ** 2) * reprojection_error.sum(dim=-1)
-
- # Pose prior loss
- pose_prior_loss = (pose_prior_weight ** 2) * pose_prior(body_pose, betas)
-
- # Angle prior for knees and elbows
- angle_prior_loss = (angle_prior_weight ** 2) * angle_prior(body_pose).sum(dim=-1)
-
- # Regularizer to prevent betas from taking large values
- shape_prior_loss = (shape_prior_weight ** 2) * (betas ** 2).sum(dim=-1)
-
- total_loss = reprojection_loss.sum(dim=-1) + pose_prior_loss + angle_prior_loss + shape_prior_loss
-
- if output == 'sum':
- return total_loss.sum()
- elif output == 'reprojection':
- return reprojection_loss
-
-
-# --- get camera fitting loss -----
-def camera_fitting_loss(model_joints, camera_t, camera_t_est, camera_center,
- joints_2d, joints_conf,
- focal_length=5000, depth_loss_weight=100):
- """
- Loss function for camera optimization.
- """
- # Project model joints
- batch_size = model_joints.shape[0]
- rotation = torch.eye(3, device=model_joints.device).unsqueeze(0).expand(batch_size, -1, -1)
- projected_joints = perspective_projection(model_joints, rotation, camera_t,
- focal_length, camera_center)
-
- # get the indexed four
- op_joints = ['OP RHip', 'OP LHip', 'OP RShoulder', 'OP LShoulder']
- op_joints_ind = [config.JOINT_MAP[joint] for joint in op_joints]
- gt_joints = ['RHip', 'LHip', 'RShoulder', 'LShoulder']
- gt_joints_ind = [config.JOINT_MAP[joint] for joint in gt_joints]
-
- reprojection_error_op = (joints_2d[:, op_joints_ind] -
- projected_joints[:, op_joints_ind]) ** 2
- reprojection_error_gt = (joints_2d[:, gt_joints_ind] -
- projected_joints[:, gt_joints_ind]) ** 2
-
- # Check if for each example in the batch all 4 OpenPose detections are valid, otherwise use the GT detections
- # OpenPose joints are more reliable for this task, so we prefer to use them if possible
- is_valid = (joints_conf[:, op_joints_ind].min(dim=-1)[0][:, None, None] > 0).float()
- reprojection_loss = (is_valid * reprojection_error_op + (1 - is_valid) * reprojection_error_gt).sum(dim=(1, 2))
-
- # Loss that penalizes deviation from depth estimate
- depth_loss = (depth_loss_weight ** 2) * (camera_t[:, 2] - camera_t_est[:, 2]) ** 2
-
- total_loss = reprojection_loss + depth_loss
- return total_loss.sum()
-
-
-
- # #####--- body fitiing loss -----
-def body_fitting_loss_3d(body_pose, preserve_pose,
- betas, model_joints, camera_translation,
- j3d, pose_prior,
- joints3d_conf,
- sigma=100, pose_prior_weight=4.78*1.5,
- shape_prior_weight=5.0, angle_prior_weight=15.2,
- joint_loss_weight=500.0,
- pose_preserve_weight=0.0,
- use_collision=False,
- model_vertices=None, model_faces=None,
- search_tree=None, pen_distance=None, filter_faces=None,
- collision_loss_weight=1000
- ):
- """
- Loss function for body fitting
- """
- batch_size = body_pose.shape[0]
-
- #joint3d_loss = (joint_loss_weight ** 2) * gmof((model_joints + camera_translation) - j3d, sigma).sum(dim=-1)
-
- joint3d_error = gmof((model_joints + camera_translation) - j3d, sigma)
-
- joint3d_loss_part = (joints3d_conf ** 2) * joint3d_error.sum(dim=-1)
- joint3d_loss = (joint_loss_weight ** 2) * joint3d_loss_part
-
- # Pose prior loss
- pose_prior_loss = (pose_prior_weight ** 2) * pose_prior(body_pose, betas)
- # Angle prior for knees and elbows
- angle_prior_loss = (angle_prior_weight ** 2) * angle_prior(body_pose).sum(dim=-1)
- # Regularizer to prevent betas from taking large values
- shape_prior_loss = (shape_prior_weight ** 2) * (betas ** 2).sum(dim=-1)
-
- collision_loss = 0.0
- # Calculate the loss due to interpenetration
- if use_collision:
- triangles = torch.index_select(
- model_vertices, 1,
- model_faces).view(batch_size, -1, 3, 3)
-
- with torch.no_grad():
- collision_idxs = search_tree(triangles)
-
- # Remove unwanted collisions
- if filter_faces is not None:
- collision_idxs = filter_faces(collision_idxs)
-
- if collision_idxs.ge(0).sum().item() > 0:
- collision_loss = torch.sum(collision_loss_weight * pen_distance(triangles, collision_idxs))
-
- pose_preserve_loss = (pose_preserve_weight ** 2) * ((body_pose - preserve_pose) ** 2).sum(dim=-1)
-
- total_loss = joint3d_loss + pose_prior_loss + angle_prior_loss + shape_prior_loss + collision_loss + pose_preserve_loss
-
- return total_loss.sum()
-
-
-# #####--- get camera fitting loss -----
-def camera_fitting_loss_3d(model_joints, camera_t, camera_t_est,
- j3d, joints_category="orig", depth_loss_weight=100.0):
- """
- Loss function for camera optimization.
- """
- model_joints = model_joints + camera_t
- # # get the indexed four
- # op_joints = ['OP RHip', 'OP LHip', 'OP RShoulder', 'OP LShoulder']
- # op_joints_ind = [config.JOINT_MAP[joint] for joint in op_joints]
- #
- # j3d_error_loss = (j3d[:, op_joints_ind] -
- # model_joints[:, op_joints_ind]) ** 2
-
- gt_joints = ['RHip', 'LHip', 'RShoulder', 'LShoulder']
- gt_joints_ind = [config.JOINT_MAP[joint] for joint in gt_joints]
-
- if joints_category=="orig":
- select_joints_ind = [config.JOINT_MAP[joint] for joint in gt_joints]
- elif joints_category=="AMASS":
- select_joints_ind = [config.AMASS_JOINT_MAP[joint] for joint in gt_joints]
- elif joints_category=="MMM":
- select_joints_ind = [config.MMM_JOINT_MAP[joint] for joint in gt_joints]
- else:
- print("NO SUCH JOINTS CATEGORY!")
-
- j3d_error_loss = (j3d[:, select_joints_ind] -
- model_joints[:, gt_joints_ind]) ** 2
-
- # Loss that penalizes deviation from depth estimate
- depth_loss = (depth_loss_weight**2) * (camera_t - camera_t_est)**2
-
- total_loss = j3d_error_loss + depth_loss
- return total_loss.sum()
\ No newline at end of file
diff --git a/spaces/PAIR/PAIR-Diffusion/annotator/OneFormer/oneformer/utils/events.py b/spaces/PAIR/PAIR-Diffusion/annotator/OneFormer/oneformer/utils/events.py
deleted file mode 100644
index d1d27ac6ecef656f1aa86649ceacb54470765821..0000000000000000000000000000000000000000
--- a/spaces/PAIR/PAIR-Diffusion/annotator/OneFormer/oneformer/utils/events.py
+++ /dev/null
@@ -1,120 +0,0 @@
-import os
-import wandb
-from detectron2.utils import comm
-from detectron2.utils.events import EventWriter, get_event_storage
-
-
-def setup_wandb(cfg, args):
- if comm.is_main_process():
- init_args = {
- k.lower(): v
- for k, v in cfg.WANDB.items()
- if isinstance(k, str) and k not in ["config"]
- }
- # only include most related part to avoid too big table
- # TODO: add configurable params to select which part of `cfg` should be saved in config
- if "config_exclude_keys" in init_args:
- init_args["config"] = cfg
- init_args["config"]["cfg_file"] = args.config_file
- else:
- init_args["config"] = {
- "model": cfg.MODEL,
- "solver": cfg.SOLVER,
- "cfg_file": args.config_file,
- }
- if ("name" not in init_args) or (init_args["name"] is None):
- init_args["name"] = os.path.basename(args.config_file)
- else:
- init_args["name"] = init_args["name"] + '_' + os.path.basename(args.config_file)
- wandb.init(**init_args)
-
-
-class BaseRule(object):
- def __call__(self, target):
- return target
-
-
-class IsIn(BaseRule):
- def __init__(self, keyword: str):
- self.keyword = keyword
-
- def __call__(self, target):
- return self.keyword in target
-
-
-class Prefix(BaseRule):
- def __init__(self, keyword: str):
- self.keyword = keyword
-
- def __call__(self, target):
- return "/".join([self.keyword, target])
-
-
-class WandbWriter(EventWriter):
- """
- Write all scalars to a tensorboard file.
- """
-
- def __init__(self):
- """
- Args:
- log_dir (str): the directory to save the output events
- kwargs: other arguments passed to `torch.utils.tensorboard.SummaryWriter(...)`
- """
- self._last_write = -1
- self._group_rules = [
- (IsIn("/"), BaseRule()),
- (IsIn("loss"), Prefix("train")),
- ]
-
- def write(self):
-
- storage = get_event_storage()
-
- def _group_name(scalar_name):
- for (rule, op) in self._group_rules:
- if rule(scalar_name):
- return op(scalar_name)
- return scalar_name
-
- stats = {
- _group_name(name): scalars[0]
- for name, scalars in storage.latest().items()
- if scalars[1] > self._last_write
- }
- if len(stats) > 0:
- self._last_write = max([v[1] for k, v in storage.latest().items()])
-
- # storage.put_{image,histogram} is only meant to be used by
- # tensorboard writer. So we access its internal fields directly from here.
- if len(storage._vis_data) >= 1:
- stats["image"] = [
- wandb.Image(img, caption=img_name)
- for img_name, img, step_num in storage._vis_data
- ]
- # Storage stores all image data and rely on this writer to clear them.
- # As a result it assumes only one writer will use its image data.
- # An alternative design is to let storage store limited recent
- # data (e.g. only the most recent image) that all writers can access.
- # In that case a writer may not see all image data if its period is long.
- storage.clear_images()
-
- if len(storage._histograms) >= 1:
-
- def create_bar(tag, bucket_limits, bucket_counts, **kwargs):
- data = [
- [label, val] for (label, val) in zip(bucket_limits, bucket_counts)
- ]
- table = wandb.Table(data=data, columns=["label", "value"])
- return wandb.plot.bar(table, "label", "value", title=tag)
-
- stats["hist"] = [create_bar(**params) for params in storage._histograms]
-
- storage.clear_histograms()
-
- if len(stats) == 0:
- return
- wandb.log(stats, step=storage.iter)
-
- def close(self):
- wandb.finish()
\ No newline at end of file
diff --git a/spaces/PAIR/PAIR-Diffusion/ldm/modules/image_degradation/__init__.py b/spaces/PAIR/PAIR-Diffusion/ldm/modules/image_degradation/__init__.py
deleted file mode 100644
index 7836cada81f90ded99c58d5942eea4c3477f58fc..0000000000000000000000000000000000000000
--- a/spaces/PAIR/PAIR-Diffusion/ldm/modules/image_degradation/__init__.py
+++ /dev/null
@@ -1,2 +0,0 @@
-from ldm.modules.image_degradation.bsrgan import degradation_bsrgan_variant as degradation_fn_bsr
-from ldm.modules.image_degradation.bsrgan_light import degradation_bsrgan_variant as degradation_fn_bsr_light
diff --git a/spaces/PAIR/Text2Video-Zero/annotator/uniformer/mmcv/utils/version_utils.py b/spaces/PAIR/Text2Video-Zero/annotator/uniformer/mmcv/utils/version_utils.py
deleted file mode 100644
index 963c45a2e8a86a88413ab6c18c22481fb9831985..0000000000000000000000000000000000000000
--- a/spaces/PAIR/Text2Video-Zero/annotator/uniformer/mmcv/utils/version_utils.py
+++ /dev/null
@@ -1,90 +0,0 @@
-# Copyright (c) OpenMMLab. All rights reserved.
-import os
-import subprocess
-import warnings
-
-from packaging.version import parse
-
-
-def digit_version(version_str: str, length: int = 4):
- """Convert a version string into a tuple of integers.
-
- This method is usually used for comparing two versions. For pre-release
- versions: alpha < beta < rc.
-
- Args:
- version_str (str): The version string.
- length (int): The maximum number of version levels. Default: 4.
-
- Returns:
- tuple[int]: The version info in digits (integers).
- """
- assert 'parrots' not in version_str
- version = parse(version_str)
- assert version.release, f'failed to parse version {version_str}'
- release = list(version.release)
- release = release[:length]
- if len(release) < length:
- release = release + [0] * (length - len(release))
- if version.is_prerelease:
- mapping = {'a': -3, 'b': -2, 'rc': -1}
- val = -4
- # version.pre can be None
- if version.pre:
- if version.pre[0] not in mapping:
- warnings.warn(f'unknown prerelease version {version.pre[0]}, '
- 'version checking may go wrong')
- else:
- val = mapping[version.pre[0]]
- release.extend([val, version.pre[-1]])
- else:
- release.extend([val, 0])
-
- elif version.is_postrelease:
- release.extend([1, version.post])
- else:
- release.extend([0, 0])
- return tuple(release)
-
-
-def _minimal_ext_cmd(cmd):
- # construct minimal environment
- env = {}
- for k in ['SYSTEMROOT', 'PATH', 'HOME']:
- v = os.environ.get(k)
- if v is not None:
- env[k] = v
- # LANGUAGE is used on win32
- env['LANGUAGE'] = 'C'
- env['LANG'] = 'C'
- env['LC_ALL'] = 'C'
- out = subprocess.Popen(
- cmd, stdout=subprocess.PIPE, env=env).communicate()[0]
- return out
-
-
-def get_git_hash(fallback='unknown', digits=None):
- """Get the git hash of the current repo.
-
- Args:
- fallback (str, optional): The fallback string when git hash is
- unavailable. Defaults to 'unknown'.
- digits (int, optional): kept digits of the hash. Defaults to None,
- meaning all digits are kept.
-
- Returns:
- str: Git commit hash.
- """
-
- if digits is not None and not isinstance(digits, int):
- raise TypeError('digits must be None or an integer')
-
- try:
- out = _minimal_ext_cmd(['git', 'rev-parse', 'HEAD'])
- sha = out.strip().decode('ascii')
- if digits is not None:
- sha = sha[:digits]
- except OSError:
- sha = fallback
-
- return sha
diff --git a/spaces/Pattr/DrumClassification/lilypond-2.24.2/lib/guile/2.2/ccache/language/tree-il/compile-cps.go b/spaces/Pattr/DrumClassification/lilypond-2.24.2/lib/guile/2.2/ccache/language/tree-il/compile-cps.go
deleted file mode 100644
index 19efdadaf900308cd0476bee1f0d5fd2bd83befc..0000000000000000000000000000000000000000
Binary files a/spaces/Pattr/DrumClassification/lilypond-2.24.2/lib/guile/2.2/ccache/language/tree-il/compile-cps.go and /dev/null differ
diff --git a/spaces/Pclanglais/MonadGPT/Dockerfile b/spaces/Pclanglais/MonadGPT/Dockerfile
deleted file mode 100644
index 481d7cc5e3037930f21b43f555e6849f108005ae..0000000000000000000000000000000000000000
--- a/spaces/Pclanglais/MonadGPT/Dockerfile
+++ /dev/null
@@ -1,126 +0,0 @@
-ARG MODEL_NAME
-ARG MODEL_PARAMS
-ARG MODEL_PROMPT_TEMPLATE
-ARG APP_COLOR
-ARG APP_NAME
-
-
-FROM node:19 as chatui-builder
-ARG MODEL_NAME
-ARG MODEL_PARAMS
-ARG APP_COLOR
-ARG APP_NAME
-ARG MODEL_PROMPT_TEMPLATE
-
-WORKDIR /app
-
-RUN apt-get update && DEBIAN_FRONTEND=noninteractive apt-get install -y --no-install-recommends \
- git gettext && \
- rm -rf /var/lib/apt/lists/*
-
-
-RUN git clone https://github.com/huggingface/chat-ui.git
-
-WORKDIR /app/chat-ui
-
-
-COPY .env.local.template .env.local.template
-
-RUN mkdir defaults
-ADD defaults /defaults
-RUN chmod -R 777 /defaults
-RUN --mount=type=secret,id=MONGODB_URL,mode=0444 \
- MODEL_NAME="${MODEL_NAME:="$(cat /defaults/MODEL_NAME)"}" && export MODEL_NAME \
- && MODEL_PARAMS="${MODEL_PARAMS:="$(cat /defaults/MODEL_PARAMS)"}" && export MODEL_PARAMS \
- && MODEL_PROMPT_TEMPLATE="${MODEL_PROMPT_TEMPLATE:="$(cat /defaults/MODEL_PROMPT_TEMPLATE)"}" && export MODEL_PROMPT_TEMPLATE \
- && APP_COLOR="${APP_COLOR:="$(cat /defaults/APP_COLOR)"}" && export APP_COLOR \
- && APP_NAME="${APP_NAME:="$(cat /defaults/APP_NAME)"}" && export APP_NAME \
- && MONGODB_URL=$(cat /run/secrets/MONGODB_URL > /dev/null | grep '^' || cat /defaults/MONGODB_URL) && export MONGODB_URL && \
- echo "${MONGODB_URL}" && \
- envsubst < ".env.local.template" > ".env.local" \
- && rm .env.local.template
-
-
-
-RUN --mount=type=cache,target=/app/.npm \
- npm set cache /app/.npm && \
- npm ci
-
-RUN npm run build
-
-FROM ghcr.io/huggingface/text-generation-inference:latest
-
-ARG MODEL_NAME
-ARG MODEL_PARAMS
-ARG MODEL_PROMPT_TEMPLATE
-ARG APP_COLOR
-ARG APP_NAME
-
-ENV TZ=Europe/Paris \
- PORT=3000
-
-
-
-RUN apt-get update && DEBIAN_FRONTEND=noninteractive apt-get install -y --no-install-recommends \
- gnupg \
- curl \
- gettext && \
- rm -rf /var/lib/apt/lists/*
-COPY entrypoint.sh.template entrypoint.sh.template
-
-RUN mkdir defaults
-ADD defaults /defaults
-RUN chmod -R 777 /defaults
-
-RUN --mount=type=secret,id=MONGODB_URL,mode=0444 \
- MODEL_NAME="${MODEL_NAME:="$(cat /defaults/MODEL_NAME)"}" && export MODEL_NAME \
- && MODEL_PARAMS="${MODEL_PARAMS:="$(cat /defaults/MODEL_PARAMS)"}" && export MODEL_PARAMS \
- && MODEL_PROMPT_TEMPLATE="${MODEL_PROMPT_TEMPLATE:="$(cat /defaults/MODEL_PROMPT_TEMPLATE)"}" && export MODEL_PROMPT_TEMPLATE \
- && APP_COLOR="${APP_COLOR:="$(cat /defaults/APP_COLOR)"}" && export APP_COLOR \
- && APP_NAME="${APP_NAME:="$(cat /defaults/APP_NAME)"}" && export APP_NAME \
- && MONGODB_URL=$(cat /run/secrets/MONGODB_URL > /dev/null | grep '^' || cat /defaults/MONGODB_URL) && export MONGODB_URL && \
- envsubst < "entrypoint.sh.template" > "entrypoint.sh" \
- && rm entrypoint.sh.template
-
-
-RUN curl -fsSL https://pgp.mongodb.com/server-6.0.asc | \
- gpg -o /usr/share/keyrings/mongodb-server-6.0.gpg \
- --dearmor
-
-RUN echo "deb [ arch=amd64,arm64 signed-by=/usr/share/keyrings/mongodb-server-6.0.gpg ] https://repo.mongodb.org/apt/ubuntu focal/mongodb-org/6.0 multiverse" | tee /etc/apt/sources.list.d/mongodb-org-6.0.list
-
-RUN apt-get update && DEBIAN_FRONTEND=noninteractive apt-get install -y --no-install-recommends \
- mongodb-org && \
- rm -rf /var/lib/apt/lists/*
-
-RUN mkdir -p /data/db
-RUN chown -R 1000:1000 /data
-
-RUN curl -fsSL https://deb.nodesource.com/setup_19.x | /bin/bash -
-
-RUN apt-get update && DEBIAN_FRONTEND=noninteractive apt-get install -y --no-install-recommends \
- nodejs && \
- rm -rf /var/lib/apt/lists/*
-
-RUN mkdir /app
-RUN chown -R 1000:1000 /app
-
-RUN useradd -m -u 1000 user
-
-# Switch to the "user" user
-USER user
-
-ENV HOME=/home/user \
- PATH=/home/user/.local/bin:$PATH
-
-RUN npm config set prefix /home/user/.local
-RUN npm install -g pm2
-
-COPY --from=chatui-builder --chown=1000 /app/chat-ui/node_modules /app/node_modules
-COPY --from=chatui-builder --chown=1000 /app/chat-ui/package.json /app/package.json
-COPY --from=chatui-builder --chown=1000 /app/chat-ui/build /app/build
-
-ENTRYPOINT ["/bin/bash"]
-CMD ["entrypoint.sh"]
-
-
diff --git a/spaces/Pengyey/bingo-chuchu/cloudflare/worker.js b/spaces/Pengyey/bingo-chuchu/cloudflare/worker.js
deleted file mode 100644
index e0debd750615f1329b2c72fbce73e1b9291f7137..0000000000000000000000000000000000000000
--- a/spaces/Pengyey/bingo-chuchu/cloudflare/worker.js
+++ /dev/null
@@ -1,18 +0,0 @@
-const TRAGET_HOST='hf4all-bingo.hf.space' // 请将此域名改成你自己的,域名信息在设置》站点域名查看。
-
-export default {
- async fetch(request) {
- const uri = new URL(request.url);
- if (uri.protocol === 'http:') {
- uri.protocol = 'https:';
- return new Response('', {
- status: 301,
- headers: {
- location: uri.toString(),
- },
- })
- }
- uri.host = TRAGET_HOST
- return fetch(new Request(uri.toString(), request));
- },
-};
diff --git a/spaces/Pie31415/control-animation/annotator/uniformer/mmseg/datasets/pipelines/compose.py b/spaces/Pie31415/control-animation/annotator/uniformer/mmseg/datasets/pipelines/compose.py
deleted file mode 100644
index cbfcbb925c6d4ebf849328b9f94ef6fc24359bf5..0000000000000000000000000000000000000000
--- a/spaces/Pie31415/control-animation/annotator/uniformer/mmseg/datasets/pipelines/compose.py
+++ /dev/null
@@ -1,51 +0,0 @@
-import collections
-
-from annotator.uniformer.mmcv.utils import build_from_cfg
-
-from ..builder import PIPELINES
-
-
-@PIPELINES.register_module()
-class Compose(object):
- """Compose multiple transforms sequentially.
-
- Args:
- transforms (Sequence[dict | callable]): Sequence of transform object or
- config dict to be composed.
- """
-
- def __init__(self, transforms):
- assert isinstance(transforms, collections.abc.Sequence)
- self.transforms = []
- for transform in transforms:
- if isinstance(transform, dict):
- transform = build_from_cfg(transform, PIPELINES)
- self.transforms.append(transform)
- elif callable(transform):
- self.transforms.append(transform)
- else:
- raise TypeError('transform must be callable or a dict')
-
- def __call__(self, data):
- """Call function to apply transforms sequentially.
-
- Args:
- data (dict): A result dict contains the data to transform.
-
- Returns:
- dict: Transformed data.
- """
-
- for t in self.transforms:
- data = t(data)
- if data is None:
- return None
- return data
-
- def __repr__(self):
- format_string = self.__class__.__name__ + '('
- for t in self.transforms:
- format_string += '\n'
- format_string += f' {t}'
- format_string += '\n)'
- return format_string
diff --git a/spaces/Pinwheel/GLIP-BLIP-Object-Detection-VQA/itm.py b/spaces/Pinwheel/GLIP-BLIP-Object-Detection-VQA/itm.py
deleted file mode 100644
index 6da8af6dfe782beff41de4efb952f481fa97a6c6..0000000000000000000000000000000000000000
--- a/spaces/Pinwheel/GLIP-BLIP-Object-Detection-VQA/itm.py
+++ /dev/null
@@ -1,77 +0,0 @@
-import sys
-from PIL import Image
-import torch
-from torchvision import transforms
-from torchvision.transforms.functional import InterpolationMode
-from models.blip_vqa import blip_vqa
-from models.blip_itm import blip_itm
-
-
-class VQA:
- def __init__(self, model_path, image_size=480):
- self.device = torch.device('cuda' if torch.cuda.is_available() else 'cpu')
- self.model = blip_vqa(pretrained=model_path, image_size=image_size, vit='base')
- self.model.eval()
- self.model = self.model.to(self.device)
-
- def load_demo_image(self, image_size, img_path, device):
- raw_image = Image.open(img_path).convert('RGB')
- w,h = raw_image.size
- transform = transforms.Compose([
- transforms.Resize((image_size,image_size),interpolation=InterpolationMode.BICUBIC),
- transforms.ToTensor(),
- transforms.Normalize((0.48145466, 0.4578275, 0.40821073), (0.26862954, 0.26130258, 0.27577711))
- ])
- image = transform(raw_image).unsqueeze(0).to(device)
- return raw_image, image
-
- def vqa(self, img_path, question):
- raw_image, image = self.load_demo_image(image_size=480, img_path=img_path, device=self.device)
- with torch.no_grad():
- answer = self.model(image, question, train=False, inference='generate')
- return answer[0]
-class ITM:
- def __init__(self, model_path, image_size=384):
- self.device = torch.device('cuda' if torch.cuda.is_available() else 'cpu')
- self.model = blip_itm(pretrained=model_path, image_size=image_size, vit='base')
- self.model.eval()
- self.model = self.model.to(device='cpu')
-
- def load_demo_image(self, image_size, img_path, device):
- raw_image = Image.open(img_path).convert('RGB')
- w,h = raw_image.size
- transform = transforms.Compose([
- transforms.Resize((image_size,image_size),interpolation=InterpolationMode.BICUBIC),
- transforms.ToTensor(),
- transforms.Normalize((0.48145466, 0.4578275, 0.40821073), (0.26862954, 0.26130258, 0.27577711))
- ])
- image = transform(raw_image).unsqueeze(0).to(device)
- return raw_image, image
-
- def itm(self, img_path, caption):
- raw_image, image = self.load_demo_image(image_size=384,img_path=img_path, device=self.device)
- itm_output = self.model(image,caption,match_head='itm')
- itm_score = torch.nn.functional.softmax(itm_output,dim=1)[:,1]
- itc_score = self.model(image,caption,match_head='itc')
- # print('The image and text is matched with a probability of %.4f'%itm_score)
- # print('The image feature and text feature has a cosine similarity of %.4f'%itc_score)
- return itm_score, itc_score
-
-if __name__=="__main__":
- if not len(sys.argv) == 3:
- print('Format: python3 vqa.py ')
- print('Sample: python3 vqa.py sample.jpg "What is the color of the horse?"')
-
- else:
- model_path = 'checkpoints/model_base_vqa_capfilt_large.pth'
- model2_path = 'model_base_retrieval_coco.pth'
- # vqa_object = VQA(model_path=model_path)
- itm_object = ITM(model_path=model2_path)
- img_path = sys.argv[1]
- # question = sys.argv[2]
- caption = sys.argv[2]
- # answer = vqa_object.vqa(img_path, caption)
- itm_score, itc_score = itm_object.itm(img_path, caption)
- # print('Question: {} | Answer: {}'.format(caption, answer))
- print('Caption: {} | The image and text is matched with a probability of %.4f: {} | The image feature and text feature has a cosine similarity of %.4f: {}'.format (caption,itm_score,itc_score))
-
diff --git a/spaces/Pinwheel/GLIP-BLIP-Object-Detection-VQA/maskrcnn_benchmark/csrc/cpu/soft_nms.cpp b/spaces/Pinwheel/GLIP-BLIP-Object-Detection-VQA/maskrcnn_benchmark/csrc/cpu/soft_nms.cpp
deleted file mode 100644
index 432bf7c6c118ee0676659e7fb04881351ebd8642..0000000000000000000000000000000000000000
--- a/spaces/Pinwheel/GLIP-BLIP-Object-Detection-VQA/maskrcnn_benchmark/csrc/cpu/soft_nms.cpp
+++ /dev/null
@@ -1,117 +0,0 @@
-// Copyright (c) Facebook, Inc. and its affiliates. All Rights Reserved.
-#include "cpu/vision.h"
-
-
-template
-std::pair soft_nms_cpu_kernel(const at::Tensor& dets,
- const at::Tensor& scores,
- const float threshold,
- const float sigma) {
- AT_ASSERTM(!dets.device().is_cuda(), "dets must be a CPU tensor");
- AT_ASSERTM(!scores.device().is_cuda(), "scores must be a CPU tensor");
- AT_ASSERTM(dets.type() == scores.type(), "dets should have the same type as scores");
-
- if (dets.numel() == 0) {
- return std::make_pair(at::empty({0}, dets.options().dtype(at::kLong).device(at::kCPU)),
- at::empty({0}, scores.options().dtype(at::kFloat).device(at::kCPU)));
- }
-
- auto x1_t = dets.select(1, 0).contiguous();
- auto y1_t = dets.select(1, 1).contiguous();
- auto x2_t = dets.select(1, 2).contiguous();
- auto y2_t = dets.select(1, 3).contiguous();
-
- auto scores_t = scores.clone();
-
- at::Tensor areas_t = (x2_t - x1_t + 1) * (y2_t - y1_t + 1);
- auto ndets = dets.size(0);
- auto inds_t = at::arange(ndets, dets.options().dtype(at::kLong).device(at::kCPU));
-
- auto x1 = x1_t.data_ptr();
- auto y1 = y1_t.data_ptr();
- auto x2 = x2_t.data_ptr();
- auto y2 = y2_t.data_ptr();
- auto s = scores_t.data_ptr();
- auto inds = inds_t.data_ptr();
- auto areas = areas_t.data_ptr();
-
- for (int64_t i = 0; i < ndets; i++) {
-
- auto ix1 = x1[i];
- auto iy1 = y1[i];
- auto ix2 = x2[i];
- auto iy2 = y2[i];
- auto is = s[i];
- auto ii = inds[i];
- auto iarea = areas[i];
-
- auto maxpos = scores_t.slice(0, i, ndets).argmax().item() + i;
-
- // add max box as a detection
- x1[i] = x1[maxpos];
- y1[i] = y1[maxpos];
- x2[i] = x2[maxpos];
- y2[i] = y2[maxpos];
- s[i] = s[maxpos];
- inds[i] = inds[maxpos];
- areas[i] = areas[maxpos];
-
- // swap ith box with position of max box
- x1[maxpos] = ix1;
- y1[maxpos] = iy1;
- x2[maxpos] = ix2;
- y2[maxpos] = iy2;
- s[maxpos] = is;
- inds[maxpos] = ii;
- areas[maxpos] = iarea;
-
- ix1 = x1[i];
- iy1 = y1[i];
- ix2 = x2[i];
- iy2 = y2[i];
- iarea = areas[i];
-
- // NMS iterations, note that ndets changes if detection boxes
- // fall below threshold
- for (int64_t j = i + 1; j < ndets; j++) {
- auto xx1 = std::max(ix1, x1[j]);
- auto yy1 = std::max(iy1, y1[j]);
- auto xx2 = std::min(ix2, x2[j]);
- auto yy2 = std::min(iy2, y2[j]);
-
- auto w = std::max(static_cast(0), xx2 - xx1 + 1);
- auto h = std::max(static_cast(0), yy2 - yy1 + 1);
-
- auto inter = w * h;
- auto ovr = inter / (iarea + areas[j] - inter);
-
- s[j] = s[j] * std::exp(- std::pow(ovr, 2.0) / sigma);
-
- // if box score falls below threshold, discard the box by
- // swapping with last box update ndets
- if (s[j] < threshold) {
- x1[j] = x1[ndets - 1];
- y1[j] = y1[ndets - 1];
- x2[j] = x2[ndets - 1];
- y2[j] = y2[ndets - 1];
- s[j] = s[ndets - 1];
- inds[j] = inds[ndets - 1];
- areas[j] = areas[ndets - 1];
- j--;
- ndets--;
- }
- }
- }
- return std::make_pair(inds_t.slice(0, 0, ndets), scores_t.slice(0, 0, ndets));
-}
-
-std::pair soft_nms_cpu(const at::Tensor& dets,
- const at::Tensor& scores,
- const float threshold,
- const float sigma) {
- std::pair result;
- AT_DISPATCH_FLOATING_TYPES(dets.scalar_type(), "soft_nms", [&] {
- result = soft_nms_cpu_kernel(dets, scores, threshold, sigma);
- });
- return result;
-}
\ No newline at end of file
diff --git a/spaces/Pinwheel/GLIP-BLIP-Object-Detection-VQA/maskrcnn_benchmark/layers/deform_pool.py b/spaces/Pinwheel/GLIP-BLIP-Object-Detection-VQA/maskrcnn_benchmark/layers/deform_pool.py
deleted file mode 100644
index 7fb3f2e341a34f5747e7bfa9b2d858d74492697d..0000000000000000000000000000000000000000
--- a/spaces/Pinwheel/GLIP-BLIP-Object-Detection-VQA/maskrcnn_benchmark/layers/deform_pool.py
+++ /dev/null
@@ -1,423 +0,0 @@
-import torch
-import torch.nn as nn
-import torch.nn.functional as F
-
-from .deform_conv import DeformConv2d
-
-def add_conv(in_ch, out_ch, ksize, stride, leaky=True):
- """
- Add a conv2d / batchnorm / leaky ReLU block.
- Args:
- in_ch (int): number of input channels of the convolution layer.
- out_ch (int): number of output channels of the convolution layer.
- ksize (int): kernel size of the convolution layer.
- stride (int): stride of the convolution layer.
- Returns:
- stage (Sequential) : Sequential layers composing a convolution block.
- """
- stage = nn.Sequential()
- pad = (ksize - 1) // 2
- stage.add_module('conv', nn.Conv2d(in_channels=in_ch,
- out_channels=out_ch, kernel_size=ksize, stride=stride,
- padding=pad, bias=False))
- stage.add_module('batch_norm', nn.BatchNorm2d(out_ch))
- if leaky:
- stage.add_module('leaky', nn.LeakyReLU(0.1))
- else:
- stage.add_module('relu6', nn.ReLU6(inplace=True))
- return stage
-
-
-class upsample(nn.Module):
- __constants__ = ['size', 'scale_factor', 'mode', 'align_corners', 'name']
-
- def __init__(self, size=None, scale_factor=None, mode='nearest', align_corners=None):
- super(upsample, self).__init__()
- self.name = type(self).__name__
- self.size = size
- self.scale_factor = scale_factor
- self.mode = mode
- self.align_corners = align_corners
-
- def forward(self, input):
- return F.interpolate(input, self.size, self.scale_factor, self.mode, self.align_corners)
-
- def extra_repr(self):
- if self.scale_factor is not None:
- info = 'scale_factor=' + str(self.scale_factor)
- else:
- info = 'size=' + str(self.size)
- info += ', mode=' + self.mode
- return info
-
-class SPPLayer(nn.Module):
- def __init__(self):
- super(SPPLayer, self).__init__()
-
- def forward(self, x):
- x_1 = x
- x_2 = F.max_pool2d(x, 5, stride=1, padding=2)
- x_3 = F.max_pool2d(x, 9, stride=1, padding=4)
- x_4 = F.max_pool2d(x, 13, stride=1, padding=6)
- out = torch.cat((x_1, x_2, x_3, x_4),dim=1)
- return out
-
-class DropBlock(nn.Module):
- def __init__(self, block_size=7, keep_prob=0.9):
- super(DropBlock, self).__init__()
- self.block_size = block_size
- self.keep_prob = keep_prob
- self.gamma = None
- self.kernel_size = (block_size, block_size)
- self.stride = (1, 1)
- self.padding = (block_size//2, block_size//2)
-
- def reset(self, block_size, keep_prob):
- self.block_size = block_size
- self.keep_prob = keep_prob
- self.gamma = None
- self.kernel_size = (block_size, block_size)
- self.stride = (1, 1)
- self.padding = (block_size//2, block_size//2)
-
- def calculate_gamma(self, x):
- return (1-self.keep_prob) * x.shape[-1]**2/ \
- (self.block_size**2 * (x.shape[-1] - self.block_size + 1)**2)
-
- def forward(self, x):
- if (not self.training or self.keep_prob==1): #set keep_prob=1 to turn off dropblock
- return x
- if self.gamma is None:
- self.gamma = self.calculate_gamma(x)
- if x.type() == 'torch.cuda.HalfTensor': #TODO: not fully support for FP16 now
- FP16 = True
- x = x.float()
- else:
- FP16 = False
- p = torch.ones_like(x) * (self.gamma)
- mask = 1 - torch.nn.functional.max_pool2d(torch.bernoulli(p),
- self.kernel_size,
- self.stride,
- self.padding)
-
- out = mask * x * (mask.numel()/mask.sum())
-
- if FP16:
- out = out.half()
- return out
-
-class resblock(nn.Module):
- """
- Sequential residual blocks each of which consists of \
- two convolution layers.
- Args:
- ch (int): number of input and output channels.
- nblocks (int): number of residual blocks.
- shortcut (bool): if True, residual tensor addition is enabled.
- """
- def __init__(self, ch, nblocks=1, shortcut=True):
-
- super().__init__()
- self.shortcut = shortcut
- self.module_list = nn.ModuleList()
- for i in range(nblocks):
- resblock_one = nn.ModuleList()
- resblock_one.append(add_conv(ch, ch//2, 1, 1))
- resblock_one.append(add_conv(ch//2, ch, 3, 1))
- self.module_list.append(resblock_one)
-
- def forward(self, x):
- for module in self.module_list:
- h = x
- for res in module:
- h = res(h)
- x = x + h if self.shortcut else h
- return x
-
-
-class RFBblock(nn.Module):
- def __init__(self,in_ch,residual=False):
- super(RFBblock, self).__init__()
- inter_c = in_ch // 4
- self.branch_0 = nn.Sequential(
- nn.Conv2d(in_channels=in_ch, out_channels=inter_c, kernel_size=1, stride=1, padding=0),
- )
- self.branch_1 = nn.Sequential(
- nn.Conv2d(in_channels=in_ch, out_channels=inter_c, kernel_size=1, stride=1, padding=0),
- nn.Conv2d(in_channels=inter_c, out_channels=inter_c, kernel_size=3, stride=1, padding=1)
- )
- self.branch_2 = nn.Sequential(
- nn.Conv2d(in_channels=in_ch, out_channels=inter_c, kernel_size=1, stride=1, padding=0),
- nn.Conv2d(in_channels=inter_c, out_channels=inter_c, kernel_size=3, stride=1, padding=1),
- nn.Conv2d(in_channels=inter_c, out_channels=inter_c, kernel_size=3, stride=1, dilation=2, padding=2)
- )
- self.branch_3 = nn.Sequential(
- nn.Conv2d(in_channels=in_ch, out_channels=inter_c, kernel_size=1, stride=1, padding=0),
- nn.Conv2d(in_channels=inter_c, out_channels=inter_c, kernel_size=5, stride=1, padding=2),
- nn.Conv2d(in_channels=inter_c, out_channels=inter_c, kernel_size=3, stride=1, dilation=3, padding=3)
- )
- self.residual= residual
-
- def forward(self,x):
- x_0 = self.branch_0(x)
- x_1 = self.branch_1(x)
- x_2 = self.branch_2(x)
- x_3 = self.branch_3(x)
- out = torch.cat((x_0,x_1,x_2,x_3),1)
- if self.residual:
- out +=x
- return out
-
-
-class FeatureAdaption(nn.Module):
- def __init__(self, in_ch, out_ch, n_anchors, rfb=False, sep=False):
- super(FeatureAdaption, self).__init__()
- if sep:
- self.sep=True
- else:
- self.sep=False
- self.conv_offset = nn.Conv2d(in_channels=2*n_anchors,
- out_channels=2*9*n_anchors, groups = n_anchors, kernel_size=1,stride=1,padding=0)
- self.dconv = DeformConv2d(in_channels=in_ch, out_channels=out_ch, kernel_size=3, stride=1,
- padding=1, deformable_groups=n_anchors)
- self.rfb=None
- if rfb:
- self.rfb = RFBblock(out_ch)
-
- def forward(self, input, wh_pred):
- #The RFB block is added behind FeatureAdaption
- #For mobilenet, we currently don't support rfb and FeatureAdaption
- if self.sep:
- return input
- if self.rfb is not None:
- input = self.rfb(input)
- wh_pred_new = wh_pred.detach()
- offset = self.conv_offset(wh_pred_new)
- out = self.dconv(input, offset)
- return out
-
-
-class ASFFmobile(nn.Module):
- def __init__(self, level, rfb=False, vis=False):
- super(ASFFmobile, self).__init__()
- self.level = level
- self.dim = [512, 256, 128]
- self.inter_dim = self.dim[self.level]
- if level==0:
- self.stride_level_1 = add_conv(256, self.inter_dim, 3, 2, leaky=False)
- self.stride_level_2 = add_conv(128, self.inter_dim, 3, 2, leaky=False)
- self.expand = add_conv(self.inter_dim, 1024, 3, 1, leaky=False)
- elif level==1:
- self.compress_level_0 = add_conv(512, self.inter_dim, 1, 1, leaky=False)
- self.stride_level_2 = add_conv(128, self.inter_dim, 3, 2, leaky=False)
- self.expand = add_conv(self.inter_dim, 512, 3, 1, leaky=False)
- elif level==2:
- self.compress_level_0 = add_conv(512, self.inter_dim, 1, 1, leaky=False)
- self.compress_level_1 = add_conv(256, self.inter_dim, 1, 1, leaky=False)
- self.expand = add_conv(self.inter_dim, 256, 3, 1,leaky=False)
-
- compress_c = 8 if rfb else 16 #when adding rfb, we use half number of channels to save memory
-
- self.weight_level_0 = add_conv(self.inter_dim, compress_c, 1, 1, leaky=False)
- self.weight_level_1 = add_conv(self.inter_dim, compress_c, 1, 1, leaky=False)
- self.weight_level_2 = add_conv(self.inter_dim, compress_c, 1, 1, leaky=False)
-
- self.weight_levels = nn.Conv2d(compress_c*3, 3, kernel_size=1, stride=1, padding=0)
- self.vis= vis
-
-
- def forward(self, x_level_0, x_level_1, x_level_2):
- if self.level==0:
- level_0_resized = x_level_0
- level_1_resized = self.stride_level_1(x_level_1)
-
- level_2_downsampled_inter =F.max_pool2d(x_level_2, 3, stride=2, padding=1)
- level_2_resized = self.stride_level_2(level_2_downsampled_inter)
-
- elif self.level==1:
- level_0_compressed = self.compress_level_0(x_level_0)
- level_0_resized =F.interpolate(level_0_compressed, scale_factor=2, mode='nearest')
- level_1_resized =x_level_1
- level_2_resized =self.stride_level_2(x_level_2)
- elif self.level==2:
- level_0_compressed = self.compress_level_0(x_level_0)
- level_0_resized =F.interpolate(level_0_compressed, scale_factor=4, mode='nearest')
- level_1_compressed = self.compress_level_1(x_level_1)
- level_1_resized =F.interpolate(level_1_compressed, scale_factor=2, mode='nearest')
- level_2_resized =x_level_2
-
- level_0_weight_v = self.weight_level_0(level_0_resized)
- level_1_weight_v = self.weight_level_1(level_1_resized)
- level_2_weight_v = self.weight_level_2(level_2_resized)
- levels_weight_v = torch.cat((level_0_weight_v, level_1_weight_v, level_2_weight_v),1)
- levels_weight = self.weight_levels(levels_weight_v)
- levels_weight = F.softmax(levels_weight, dim=1)
-
- fused_out_reduced = level_0_resized * levels_weight[:,0:1,:,:]+ \
- level_1_resized * levels_weight[:,1:2,:,:]+ \
- level_2_resized * levels_weight[:,2:,:,:]
-
- out = self.expand(fused_out_reduced)
-
- if self.vis:
- return out, levels_weight, fused_out_reduced.sum(dim=1)
- else:
- return out
-
-
-class ASFF(nn.Module):
- def __init__(self, level, rfb=False, vis=False):
- super(ASFF, self).__init__()
- self.level = level
- self.dim = [512, 256, 256]
- self.inter_dim = self.dim[self.level]
- if level==0:
- self.stride_level_1 = add_conv(256, self.inter_dim, 3, 2)
- self.stride_level_2 = add_conv(256, self.inter_dim, 3, 2)
- self.expand = add_conv(self.inter_dim, 1024, 3, 1)
- elif level==1:
- self.compress_level_0 = add_conv(512, self.inter_dim, 1, 1)
- self.stride_level_2 = add_conv(256, self.inter_dim, 3, 2)
- self.expand = add_conv(self.inter_dim, 512, 3, 1)
- elif level==2:
- self.compress_level_0 = add_conv(512, self.inter_dim, 1, 1)
- self.expand = add_conv(self.inter_dim, 256, 3, 1)
-
- compress_c = 8 if rfb else 16 #when adding rfb, we use half number of channels to save memory
-
- self.weight_level_0 = add_conv(self.inter_dim, compress_c, 1, 1)
- self.weight_level_1 = add_conv(self.inter_dim, compress_c, 1, 1)
- self.weight_level_2 = add_conv(self.inter_dim, compress_c, 1, 1)
-
- self.weight_levels = nn.Conv2d(compress_c*3, 3, kernel_size=1, stride=1, padding=0)
- self.vis= vis
-
-
- def forward(self, x_level_0, x_level_1, x_level_2):
- if self.level==0:
- level_0_resized = x_level_0
- level_1_resized = self.stride_level_1(x_level_1)
-
- level_2_downsampled_inter =F.max_pool2d(x_level_2, 3, stride=2, padding=1)
- level_2_resized = self.stride_level_2(level_2_downsampled_inter)
-
- elif self.level==1:
- level_0_compressed = self.compress_level_0(x_level_0)
- level_0_resized =F.interpolate(level_0_compressed, scale_factor=2, mode='nearest')
- level_1_resized =x_level_1
- level_2_resized =self.stride_level_2(x_level_2)
- elif self.level==2:
- level_0_compressed = self.compress_level_0(x_level_0)
- level_0_resized =F.interpolate(level_0_compressed, scale_factor=4, mode='nearest')
- level_1_resized =F.interpolate(x_level_1, scale_factor=2, mode='nearest')
- level_2_resized =x_level_2
-
- level_0_weight_v = self.weight_level_0(level_0_resized)
- level_1_weight_v = self.weight_level_1(level_1_resized)
- level_2_weight_v = self.weight_level_2(level_2_resized)
- levels_weight_v = torch.cat((level_0_weight_v, level_1_weight_v, level_2_weight_v),1)
- levels_weight = self.weight_levels(levels_weight_v)
- levels_weight = F.softmax(levels_weight, dim=1)
-
- fused_out_reduced = level_0_resized * levels_weight[:,0:1,:,:]+ \
- level_1_resized * levels_weight[:,1:2,:,:]+ \
- level_2_resized * levels_weight[:,2:,:,:]
-
- out = self.expand(fused_out_reduced)
-
- if self.vis:
- return out, levels_weight, fused_out_reduced.sum(dim=1)
- else:
- return out
-
-def make_divisible(v, divisor, min_value=None):
- """
- This function is taken from the original tf repo.
- It ensures that all layers have a channel number that is divisible by 8
- It can be seen here:
- https://github.com/tensorflow/models/blob/master/research/slim/nets/mobilenet/mobilenet.py
- :param v:
- :param divisor:
- :param min_value:
- :return:
- """
- if min_value is None:
- min_value = divisor
- new_v = max(min_value, int(v + divisor / 2) // divisor * divisor)
- # Make sure that round down does not go down by more than 10%.
- if new_v < 0.9 * v:
- new_v += divisor
- return new_v
-
-
-class ConvBNReLU(nn.Sequential):
- def __init__(self, in_planes, out_planes, kernel_size=3, stride=1, groups=1):
- padding = (kernel_size - 1) // 2
- super(ConvBNReLU, self).__init__(
- nn.Conv2d(in_planes, out_planes, kernel_size, stride, padding, groups=groups, bias=False),
- nn.BatchNorm2d(out_planes),
- nn.ReLU6(inplace=True)
- )
-
-def add_sepconv(in_ch, out_ch, ksize, stride):
-
- stage = nn.Sequential()
- pad = (ksize - 1) // 2
- stage.add_module('sepconv', nn.Conv2d(in_channels=in_ch,
- out_channels=in_ch, kernel_size=ksize, stride=stride,
- padding=pad, groups=in_ch, bias=False))
- stage.add_module('sepbn', nn.BatchNorm2d(in_ch))
- stage.add_module('seprelu6', nn.ReLU6(inplace=True))
- stage.add_module('ptconv', nn.Conv2d(in_ch, out_ch, 1, 1, 0, bias=False))
- stage.add_module('ptbn', nn.BatchNorm2d(out_ch))
- stage.add_module('ptrelu6', nn.ReLU6(inplace=True))
- return stage
-
-class InvertedResidual(nn.Module):
- def __init__(self, inp, oup, stride, expand_ratio):
- super(InvertedResidual, self).__init__()
- self.stride = stride
- assert stride in [1, 2]
-
- hidden_dim = int(round(inp * expand_ratio))
- self.use_res_connect = self.stride == 1 and inp == oup
-
- layers = []
- if expand_ratio != 1:
- # pw
- layers.append(ConvBNReLU(inp, hidden_dim, kernel_size=1))
- layers.extend([
- # dw
- ConvBNReLU(hidden_dim, hidden_dim, stride=stride, groups=hidden_dim),
- # pw-linear
- nn.Conv2d(hidden_dim, oup, 1, 1, 0, bias=False),
- nn.BatchNorm2d(oup),
- ])
- self.conv = nn.Sequential(*layers)
-
- def forward(self, x):
- if self.use_res_connect:
- return x + self.conv(x)
- else:
- return self.conv(x)
-
-class ressepblock(nn.Module):
- def __init__(self, ch, out_ch, in_ch=None, shortcut=True):
-
- super().__init__()
- self.shortcut = shortcut
- self.module_list = nn.ModuleList()
- in_ch = ch//2 if in_ch==None else in_ch
- resblock_one = nn.ModuleList()
- resblock_one.append(add_conv(ch, in_ch, 1, 1, leaky=False))
- resblock_one.append(add_conv(in_ch, out_ch, 3, 1,leaky=False))
- self.module_list.append(resblock_one)
-
- def forward(self, x):
- for module in self.module_list:
- h = x
- for res in module:
- h = res(h)
- x = x + h if self.shortcut else h
- return x
-
diff --git a/spaces/R1ckShi/funasr_app_clipvideo/subtitle_utils.py b/spaces/R1ckShi/funasr_app_clipvideo/subtitle_utils.py
deleted file mode 100644
index a3d9a477190c03f83ca28be59c39bbbff6422968..0000000000000000000000000000000000000000
--- a/spaces/R1ckShi/funasr_app_clipvideo/subtitle_utils.py
+++ /dev/null
@@ -1,105 +0,0 @@
-def time_convert(ms):
- ms = int(ms)
- tail = ms % 1000
- s = ms // 1000
- mi = s // 60
- s = s % 60
- h = mi // 60
- mi = mi % 60
- h = "00" if h == 0 else str(h)
- mi = "00" if mi == 0 else str(mi)
- s = "00" if s == 0 else str(s)
- tail = str(tail)
- if len(h) == 1: h = '0' + h
- if len(mi) == 1: mi = '0' + mi
- if len(s) == 1: s = '0' + s
- return "{}:{}:{},{}".format(h, mi, s, tail)
-
-
-class Text2SRT():
- def __init__(self, text_seg, ts_list, offset=0):
- self.token_list = [i for i in text_seg.split() if len(i)]
- self.ts_list = ts_list
- start, end = ts_list[0][0] - offset, ts_list[-1][1] - offset
- self.start_sec, self.end_sec = start, end
- self.start_time = time_convert(start)
- self.end_time = time_convert(end)
- def text(self):
- res = ""
- for word in self.token_list:
- if '\u4e00' <= word <= '\u9fff':
- res += word
- else:
- res += " " + word
- return res
- def len(self):
- return len(self.token_list)
- def srt(self):
- return "{} --> {}\n{}\n".format(self.start_time, self.end_time, self.text())
- def time(self):
- return (self.start_sec/1000, self.end_sec/1000)
-
-
-def generate_srt(sentence_list):
- srt_total = ''
- for i, d in enumerate(sentence_list):
- t2s = Text2SRT(d['text_seg'], d['ts_list'])
- srt_total += "{}\n{}".format(i, t2s.srt())
- return srt_total
-
-def generate_srt_clip(sentence_list, start, end, begin_index=0):
- start, end = int(start * 1000), int(end * 1000)
- srt_total = ''
- cc = 1 + begin_index
- subs = []
- for i, d in enumerate(sentence_list):
- if d['ts_list'][-1][1] <= start:
- continue
- if d['ts_list'][0][0] >= end:
- break
- # parts in between
- if (d['ts_list'][-1][1] < end and d['ts_list'][0][0] > start) or (d['ts_list'][-1][1] == end and d['ts_list'][0][0] == start):
- t2s = Text2SRT(d['text_seg'], d['ts_list'], offset=start)
- srt_total += "{}\n{}".format(cc, t2s.srt())
- subs.append((t2s.time(), t2s.text()))
- cc += 1
- continue
- if d['ts_list'][0][0] <= start:
- if not d['ts_list'][-1][1] > end:
- for j, ts in enumerate(d['ts_list']):
- if ts[1] > start:
- break
- _text = " ".join(d['text_seg'].split()[j:])
- _ts = d['ts_list'][j:]
- else:
- for j, ts in enumerate(d['ts_list']):
- if ts[1] > start:
- _start = j
- break
- for j, ts in enumerate(d['ts_list']):
- if ts[1] > end:
- _end = j
- break
- _text = " ".join(d['text_seg'].split()[_start:_end])
- _ts = d['ts_list'][_start:_end]
- if len(ts):
- t2s = Text2SRT(_text, _ts, offset=start)
- srt_total += "{}\n{}".format(cc, t2s.srt())
- subs.append((t2s.time(), t2s.text()))
- cc += 1
- continue
- if d['ts_list'][-1][1] > end:
- for j, ts in enumerate(d['ts_list']):
- if ts[1] > end:
- break
- _text = " ".join(d['text_seg'].split()[:j])
- _ts = d['ts_list'][:j]
- if len(_ts):
- t2s = Text2SRT(_text, _ts, offset=start)
- srt_total += "{}\n{}".format(cc, t2s.srt())
- subs.append(
- (t2s.time(), t2s.text())
- )
- cc += 1
- continue
- return srt_total, subs, cc
diff --git a/spaces/RMXK/RVC_HFF/infer/modules/train/preprocess.py b/spaces/RMXK/RVC_HFF/infer/modules/train/preprocess.py
deleted file mode 100644
index fbe81307ee661a95b2ac479336671a44ee02151a..0000000000000000000000000000000000000000
--- a/spaces/RMXK/RVC_HFF/infer/modules/train/preprocess.py
+++ /dev/null
@@ -1,147 +0,0 @@
-import multiprocessing
-import os
-import sys
-
-from scipy import signal
-
-now_dir = os.getcwd()
-sys.path.append(now_dir)
-print(sys.argv)
-inp_root = sys.argv[1]
-sr = int(sys.argv[2])
-n_p = int(sys.argv[3])
-exp_dir = sys.argv[4]
-noparallel = sys.argv[5] == "True"
-per = float(sys.argv[6])
-import multiprocessing
-import os
-import traceback
-
-import librosa
-import numpy as np
-from scipy.io import wavfile
-
-from infer.lib.audio import load_audio
-from infer.lib.slicer2 import Slicer
-
-mutex = multiprocessing.Lock()
-f = open("%s/preprocess.log" % exp_dir, "a+")
-
-
-def println(strr):
- mutex.acquire()
- print(strr)
- f.write("%s\n" % strr)
- f.flush()
- mutex.release()
-
-
-class PreProcess:
- def __init__(self, sr, exp_dir, per=3.7):
- self.slicer = Slicer(
- sr=sr,
- threshold=-42,
- min_length=1500,
- min_interval=400,
- hop_size=15,
- max_sil_kept=500,
- )
- self.sr = sr
- self.bh, self.ah = signal.butter(N=5, Wn=48, btype="high", fs=self.sr)
- self.per = per
- self.overlap = 0.3
- self.tail = self.per + self.overlap
- self.max = 0.9
- self.alpha = 0.75
- self.exp_dir = exp_dir
- self.gt_wavs_dir = "%s/0_gt_wavs" % exp_dir
- self.wavs16k_dir = "%s/1_16k_wavs" % exp_dir
- os.makedirs(self.exp_dir, exist_ok=True)
- os.makedirs(self.gt_wavs_dir, exist_ok=True)
- os.makedirs(self.wavs16k_dir, exist_ok=True)
-
- def norm_write(self, tmp_audio, idx0, idx1):
- tmp_max = np.abs(tmp_audio).max()
- if tmp_max > 2.5:
- print("%s-%s-%s-filtered" % (idx0, idx1, tmp_max))
- return
- tmp_audio = (tmp_audio / tmp_max * (self.max * self.alpha)) + (
- 1 - self.alpha
- ) * tmp_audio
- wavfile.write(
- "%s/%s_%s.wav" % (self.gt_wavs_dir, idx0, idx1),
- self.sr,
- tmp_audio.astype(np.float32),
- )
- tmp_audio = librosa.resample(
- tmp_audio, orig_sr=self.sr, target_sr=16000
- ) # , res_type="soxr_vhq"
- wavfile.write(
- "%s/%s_%s.wav" % (self.wavs16k_dir, idx0, idx1),
- 16000,
- tmp_audio.astype(np.float32),
- )
-
- def pipeline(self, path, idx0):
- try:
- audio = load_audio(path, self.sr)
- # zero phased digital filter cause pre-ringing noise...
- # audio = signal.filtfilt(self.bh, self.ah, audio)
- audio = signal.lfilter(self.bh, self.ah, audio)
-
- idx1 = 0
- for audio in self.slicer.slice(audio):
- i = 0
- while 1:
- start = int(self.sr * (self.per - self.overlap) * i)
- i += 1
- if len(audio[start:]) > self.tail * self.sr:
- tmp_audio = audio[start : start + int(self.per * self.sr)]
- self.norm_write(tmp_audio, idx0, idx1)
- idx1 += 1
- else:
- tmp_audio = audio[start:]
- idx1 += 1
- break
- self.norm_write(tmp_audio, idx0, idx1)
- println("%s->Suc." % path)
- except:
- println("%s->%s" % (path, traceback.format_exc()))
-
- def pipeline_mp(self, infos):
- for path, idx0 in infos:
- self.pipeline(path, idx0)
-
- def pipeline_mp_inp_dir(self, inp_root, n_p):
- try:
- infos = [
- ("%s/%s" % (inp_root, name), idx)
- for idx, name in enumerate(sorted(list(os.listdir(inp_root))))
- ]
- if noparallel:
- for i in range(n_p):
- self.pipeline_mp(infos[i::n_p])
- else:
- ps = []
- for i in range(n_p):
- p = multiprocessing.Process(
- target=self.pipeline_mp, args=(infos[i::n_p],)
- )
- ps.append(p)
- p.start()
- for i in range(n_p):
- ps[i].join()
- except:
- println("Fail. %s" % traceback.format_exc())
-
-
-def preprocess_trainset(inp_root, sr, n_p, exp_dir, per):
- pp = PreProcess(sr, exp_dir, per)
- println("start preprocess")
- println(sys.argv)
- pp.pipeline_mp_inp_dir(inp_root, n_p)
- println("end preprocess")
-
-
-if __name__ == "__main__":
- preprocess_trainset(inp_root, sr, n_p, exp_dir, per)
diff --git a/spaces/Raspberry-ai/main/.env/lib/python3.11/site-packages/pip/_vendor/chardet/version.py b/spaces/Raspberry-ai/main/.env/lib/python3.11/site-packages/pip/_vendor/chardet/version.py
deleted file mode 100644
index a08a06b9a8778863e91d1bd4cbaac6a4b9730a62..0000000000000000000000000000000000000000
--- a/spaces/Raspberry-ai/main/.env/lib/python3.11/site-packages/pip/_vendor/chardet/version.py
+++ /dev/null
@@ -1,9 +0,0 @@
-"""
-This module exists only to simplify retrieving the version number of chardet
-from within setup.py and from chardet subpackages.
-
-:author: Dan Blanchard (dan.blanchard@gmail.com)
-"""
-
-__version__ = "5.0.0"
-VERSION = __version__.split(".")
diff --git a/spaces/Realcat/image-matching-webui/third_party/DKM/dkm/models/deprecated/corr_channels.py b/spaces/Realcat/image-matching-webui/third_party/DKM/dkm/models/deprecated/corr_channels.py
deleted file mode 100644
index 8713b0d8c7a0ce91da4d2105ba29097a4969a037..0000000000000000000000000000000000000000
--- a/spaces/Realcat/image-matching-webui/third_party/DKM/dkm/models/deprecated/corr_channels.py
+++ /dev/null
@@ -1,34 +0,0 @@
-import torch
-import torch.nn as nn
-import torch.nn.functional as F
-from einops import rearrange
-
-
-class NormedCorrelationKernel(nn.Module): # similar to softmax kernel
- def __init__(self):
- super().__init__()
-
- def __call__(self, x, y, eps=1e-6):
- c = torch.einsum("bnd,bmd->bnm", x, y) / (
- x.norm(dim=-1)[..., None] * y.norm(dim=-1)[:, None] + eps
- )
- return c
-
-
-class NormedCorr(nn.Module):
- def __init__(
- self,
- ):
- super().__init__()
- self.corr = NormedCorrelationKernel()
-
- def reshape(self, x):
- return rearrange(x, "b d h w -> b (h w) d")
-
- def forward(self, x, y, **kwargs):
- b, c, h, w = y.shape
- assert x.shape == y.shape
- x, y = self.reshape(x), self.reshape(y)
- corr_xy = self.corr(x, y)
- corr_xy_flat = rearrange(corr_xy, "b (h w) c -> b c h w", h=h, w=w)
- return corr_xy_flat
diff --git a/spaces/Redgon/bingo/src/lib/isomorphic/node.ts b/spaces/Redgon/bingo/src/lib/isomorphic/node.ts
deleted file mode 100644
index da213ad6a86181979f098309c374da02835db5a0..0000000000000000000000000000000000000000
--- a/spaces/Redgon/bingo/src/lib/isomorphic/node.ts
+++ /dev/null
@@ -1,26 +0,0 @@
-import Debug from 'debug'
-
-const { fetch, setGlobalDispatcher, ProxyAgent } = require('undici')
-const { HttpsProxyAgent } = require('https-proxy-agent')
-const ws = require('ws')
-
-const debug = Debug('bingo')
-
-const httpProxy = process.env.http_proxy || process.env.HTTP_PROXY || process.env.https_proxy || process.env.HTTPS_PROXY;
-let WebSocket = ws.WebSocket
-
-if (httpProxy) {
- setGlobalDispatcher(new ProxyAgent(httpProxy))
- const agent = new HttpsProxyAgent(httpProxy)
- // @ts-ignore
- WebSocket = class extends ws.WebSocket {
- constructor(address: string | URL, options: typeof ws.WebSocket) {
- super(address, {
- ...options,
- agent,
- })
- }
- }
-}
-
-export default { fetch, WebSocket, debug }
diff --git a/spaces/RegalHyperus/rvc-lovelive-genshin/README.md b/spaces/RegalHyperus/rvc-lovelive-genshin/README.md
deleted file mode 100644
index 5f15c6b2e4ebef20e7270992f4e62da36080c857..0000000000000000000000000000000000000000
--- a/spaces/RegalHyperus/rvc-lovelive-genshin/README.md
+++ /dev/null
@@ -1,14 +0,0 @@
----
-title: RVC Love Live & Genshin Impact
-emoji: 🎤
-colorFrom: indigo
-colorTo: blue
-sdk: gradio
-sdk_version: 3.34.0
-app_file: app.py
-pinned: true
-license: mit
-duplicated_from: ArkanDash/rvc-models-new
----
-
-Check out the configuration reference at https://huggingface.co/docs/hub/spaces-config-reference
\ No newline at end of file
diff --git a/spaces/RitaParadaRamos/SmallCapDemo/gptj.py b/spaces/RitaParadaRamos/SmallCapDemo/gptj.py
deleted file mode 100644
index e69de29bb2d1d6434b8b29ae775ad8c2e48c5391..0000000000000000000000000000000000000000
diff --git a/spaces/Robert001/UniControl-Demo/annotator/uniformer/mmcv/utils/registry.py b/spaces/Robert001/UniControl-Demo/annotator/uniformer/mmcv/utils/registry.py
deleted file mode 100644
index fa9df39bc9f3d8d568361e7250ab35468f2b74e0..0000000000000000000000000000000000000000
--- a/spaces/Robert001/UniControl-Demo/annotator/uniformer/mmcv/utils/registry.py
+++ /dev/null
@@ -1,315 +0,0 @@
-# Copyright (c) OpenMMLab. All rights reserved.
-import inspect
-import warnings
-from functools import partial
-
-from .misc import is_seq_of
-
-
-def build_from_cfg(cfg, registry, default_args=None):
- """Build a module from config dict.
-
- Args:
- cfg (dict): Config dict. It should at least contain the key "type".
- registry (:obj:`Registry`): The registry to search the type from.
- default_args (dict, optional): Default initialization arguments.
-
- Returns:
- object: The constructed object.
- """
- if not isinstance(cfg, dict):
- raise TypeError(f'cfg must be a dict, but got {type(cfg)}')
- if 'type' not in cfg:
- if default_args is None or 'type' not in default_args:
- raise KeyError(
- '`cfg` or `default_args` must contain the key "type", '
- f'but got {cfg}\n{default_args}')
- if not isinstance(registry, Registry):
- raise TypeError('registry must be an mmcv.Registry object, '
- f'but got {type(registry)}')
- if not (isinstance(default_args, dict) or default_args is None):
- raise TypeError('default_args must be a dict or None, '
- f'but got {type(default_args)}')
-
- args = cfg.copy()
-
- if default_args is not None:
- for name, value in default_args.items():
- args.setdefault(name, value)
-
- obj_type = args.pop('type')
- if isinstance(obj_type, str):
- obj_cls = registry.get(obj_type)
- if obj_cls is None:
- raise KeyError(
- f'{obj_type} is not in the {registry.name} registry')
- elif inspect.isclass(obj_type):
- obj_cls = obj_type
- else:
- raise TypeError(
- f'type must be a str or valid type, but got {type(obj_type)}')
- try:
- return obj_cls(**args)
- except Exception as e:
- # Normal TypeError does not print class name.
- raise type(e)(f'{obj_cls.__name__}: {e}')
-
-
-class Registry:
- """A registry to map strings to classes.
-
- Registered object could be built from registry.
- Example:
- >>> MODELS = Registry('models')
- >>> @MODELS.register_module()
- >>> class ResNet:
- >>> pass
- >>> resnet = MODELS.build(dict(type='ResNet'))
-
- Please refer to
- https://mmcv.readthedocs.io/en/latest/understand_mmcv/registry.html for
- advanced usage.
-
- Args:
- name (str): Registry name.
- build_func(func, optional): Build function to construct instance from
- Registry, func:`build_from_cfg` is used if neither ``parent`` or
- ``build_func`` is specified. If ``parent`` is specified and
- ``build_func`` is not given, ``build_func`` will be inherited
- from ``parent``. Default: None.
- parent (Registry, optional): Parent registry. The class registered in
- children registry could be built from parent. Default: None.
- scope (str, optional): The scope of registry. It is the key to search
- for children registry. If not specified, scope will be the name of
- the package where class is defined, e.g. mmdet, mmcls, mmseg.
- Default: None.
- """
-
- def __init__(self, name, build_func=None, parent=None, scope=None):
- self._name = name
- self._module_dict = dict()
- self._children = dict()
- self._scope = self.infer_scope() if scope is None else scope
-
- # self.build_func will be set with the following priority:
- # 1. build_func
- # 2. parent.build_func
- # 3. build_from_cfg
- if build_func is None:
- if parent is not None:
- self.build_func = parent.build_func
- else:
- self.build_func = build_from_cfg
- else:
- self.build_func = build_func
- if parent is not None:
- assert isinstance(parent, Registry)
- parent._add_children(self)
- self.parent = parent
- else:
- self.parent = None
-
- def __len__(self):
- return len(self._module_dict)
-
- def __contains__(self, key):
- return self.get(key) is not None
-
- def __repr__(self):
- format_str = self.__class__.__name__ + \
- f'(name={self._name}, ' \
- f'items={self._module_dict})'
- return format_str
-
- @staticmethod
- def infer_scope():
- """Infer the scope of registry.
-
- The name of the package where registry is defined will be returned.
-
- Example:
- # in mmdet/models/backbone/resnet.py
- >>> MODELS = Registry('models')
- >>> @MODELS.register_module()
- >>> class ResNet:
- >>> pass
- The scope of ``ResNet`` will be ``mmdet``.
-
-
- Returns:
- scope (str): The inferred scope name.
- """
- # inspect.stack() trace where this function is called, the index-2
- # indicates the frame where `infer_scope()` is called
- filename = inspect.getmodule(inspect.stack()[2][0]).__name__
- split_filename = filename.split('.')
- return split_filename[0]
-
- @staticmethod
- def split_scope_key(key):
- """Split scope and key.
-
- The first scope will be split from key.
-
- Examples:
- >>> Registry.split_scope_key('mmdet.ResNet')
- 'mmdet', 'ResNet'
- >>> Registry.split_scope_key('ResNet')
- None, 'ResNet'
-
- Return:
- scope (str, None): The first scope.
- key (str): The remaining key.
- """
- split_index = key.find('.')
- if split_index != -1:
- return key[:split_index], key[split_index + 1:]
- else:
- return None, key
-
- @property
- def name(self):
- return self._name
-
- @property
- def scope(self):
- return self._scope
-
- @property
- def module_dict(self):
- return self._module_dict
-
- @property
- def children(self):
- return self._children
-
- def get(self, key):
- """Get the registry record.
-
- Args:
- key (str): The class name in string format.
-
- Returns:
- class: The corresponding class.
- """
- scope, real_key = self.split_scope_key(key)
- if scope is None or scope == self._scope:
- # get from self
- if real_key in self._module_dict:
- return self._module_dict[real_key]
- else:
- # get from self._children
- if scope in self._children:
- return self._children[scope].get(real_key)
- else:
- # goto root
- parent = self.parent
- while parent.parent is not None:
- parent = parent.parent
- return parent.get(key)
-
- def build(self, *args, **kwargs):
- return self.build_func(*args, **kwargs, registry=self)
-
- def _add_children(self, registry):
- """Add children for a registry.
-
- The ``registry`` will be added as children based on its scope.
- The parent registry could build objects from children registry.
-
- Example:
- >>> models = Registry('models')
- >>> mmdet_models = Registry('models', parent=models)
- >>> @mmdet_models.register_module()
- >>> class ResNet:
- >>> pass
- >>> resnet = models.build(dict(type='mmdet.ResNet'))
- """
-
- assert isinstance(registry, Registry)
- assert registry.scope is not None
- assert registry.scope not in self.children, \
- f'scope {registry.scope} exists in {self.name} registry'
- self.children[registry.scope] = registry
-
- def _register_module(self, module_class, module_name=None, force=False):
- if not inspect.isclass(module_class):
- raise TypeError('module must be a class, '
- f'but got {type(module_class)}')
-
- if module_name is None:
- module_name = module_class.__name__
- if isinstance(module_name, str):
- module_name = [module_name]
- for name in module_name:
- if not force and name in self._module_dict:
- raise KeyError(f'{name} is already registered '
- f'in {self.name}')
- self._module_dict[name] = module_class
-
- def deprecated_register_module(self, cls=None, force=False):
- warnings.warn(
- 'The old API of register_module(module, force=False) '
- 'is deprecated and will be removed, please use the new API '
- 'register_module(name=None, force=False, module=None) instead.')
- if cls is None:
- return partial(self.deprecated_register_module, force=force)
- self._register_module(cls, force=force)
- return cls
-
- def register_module(self, name=None, force=False, module=None):
- """Register a module.
-
- A record will be added to `self._module_dict`, whose key is the class
- name or the specified name, and value is the class itself.
- It can be used as a decorator or a normal function.
-
- Example:
- >>> backbones = Registry('backbone')
- >>> @backbones.register_module()
- >>> class ResNet:
- >>> pass
-
- >>> backbones = Registry('backbone')
- >>> @backbones.register_module(name='mnet')
- >>> class MobileNet:
- >>> pass
-
- >>> backbones = Registry('backbone')
- >>> class ResNet:
- >>> pass
- >>> backbones.register_module(ResNet)
-
- Args:
- name (str | None): The module name to be registered. If not
- specified, the class name will be used.
- force (bool, optional): Whether to override an existing class with
- the same name. Default: False.
- module (type): Module class to be registered.
- """
- if not isinstance(force, bool):
- raise TypeError(f'force must be a boolean, but got {type(force)}')
- # NOTE: This is a walkaround to be compatible with the old api,
- # while it may introduce unexpected bugs.
- if isinstance(name, type):
- return self.deprecated_register_module(name, force=force)
-
- # raise the error ahead of time
- if not (name is None or isinstance(name, str) or is_seq_of(name, str)):
- raise TypeError(
- 'name must be either of None, an instance of str or a sequence'
- f' of str, but got {type(name)}')
-
- # use it as a normal method: x.register_module(module=SomeClass)
- if module is not None:
- self._register_module(
- module_class=module, module_name=name, force=force)
- return module
-
- # use it as a decorator: @x.register_module()
- def _register(cls):
- self._register_module(
- module_class=cls, module_name=name, force=force)
- return cls
-
- return _register
diff --git a/spaces/Robert001/UniControl-Demo/annotator/uniformer/mmcv/visualization/__init__.py b/spaces/Robert001/UniControl-Demo/annotator/uniformer/mmcv/visualization/__init__.py
deleted file mode 100644
index 835df136bdcf69348281d22914d41aa84cdf92b1..0000000000000000000000000000000000000000
--- a/spaces/Robert001/UniControl-Demo/annotator/uniformer/mmcv/visualization/__init__.py
+++ /dev/null
@@ -1,9 +0,0 @@
-# Copyright (c) OpenMMLab. All rights reserved.
-from .color import Color, color_val
-from .image import imshow, imshow_bboxes, imshow_det_bboxes
-from .optflow import flow2rgb, flowshow, make_color_wheel
-
-__all__ = [
- 'Color', 'color_val', 'imshow', 'imshow_bboxes', 'imshow_det_bboxes',
- 'flowshow', 'flow2rgb', 'make_color_wheel'
-]
diff --git a/spaces/Robert001/UniControl-Demo/annotator/uniformer/mmdet/core/evaluation/eval_hooks.py b/spaces/Robert001/UniControl-Demo/annotator/uniformer/mmdet/core/evaluation/eval_hooks.py
deleted file mode 100644
index 6fb932eae1ccb23a2b687a05a6cb9525200de718..0000000000000000000000000000000000000000
--- a/spaces/Robert001/UniControl-Demo/annotator/uniformer/mmdet/core/evaluation/eval_hooks.py
+++ /dev/null
@@ -1,303 +0,0 @@
-import os.path as osp
-import warnings
-from math import inf
-
-import mmcv
-import torch.distributed as dist
-from mmcv.runner import Hook
-from torch.nn.modules.batchnorm import _BatchNorm
-from torch.utils.data import DataLoader
-
-from mmdet.utils import get_root_logger
-
-
-class EvalHook(Hook):
- """Evaluation hook.
-
- Notes:
- If new arguments are added for EvalHook, tools/test.py,
- tools/analysis_tools/eval_metric.py may be effected.
-
- Attributes:
- dataloader (DataLoader): A PyTorch dataloader.
- start (int, optional): Evaluation starting epoch. It enables evaluation
- before the training starts if ``start`` <= the resuming epoch.
- If None, whether to evaluate is merely decided by ``interval``.
- Default: None.
- interval (int): Evaluation interval (by epochs). Default: 1.
- save_best (str, optional): If a metric is specified, it would measure
- the best checkpoint during evaluation. The information about best
- checkpoint would be save in best.json.
- Options are the evaluation metrics to the test dataset. e.g.,
- ``bbox_mAP``, ``segm_mAP`` for bbox detection and instance
- segmentation. ``AR@100`` for proposal recall. If ``save_best`` is
- ``auto``, the first key will be used. The interval of
- ``CheckpointHook`` should device EvalHook. Default: None.
- rule (str, optional): Comparison rule for best score. If set to None,
- it will infer a reasonable rule. Keys such as 'mAP' or 'AR' will
- be inferred by 'greater' rule. Keys contain 'loss' will be inferred
- by 'less' rule. Options are 'greater', 'less'. Default: None.
- **eval_kwargs: Evaluation arguments fed into the evaluate function of
- the dataset.
- """
-
- rule_map = {'greater': lambda x, y: x > y, 'less': lambda x, y: x < y}
- init_value_map = {'greater': -inf, 'less': inf}
- greater_keys = ['mAP', 'AR']
- less_keys = ['loss']
-
- def __init__(self,
- dataloader,
- start=None,
- interval=1,
- by_epoch=True,
- save_best=None,
- rule=None,
- **eval_kwargs):
- if not isinstance(dataloader, DataLoader):
- raise TypeError('dataloader must be a pytorch DataLoader, but got'
- f' {type(dataloader)}')
- if not interval > 0:
- raise ValueError(f'interval must be positive, but got {interval}')
- if start is not None and start < 0:
- warnings.warn(
- f'The evaluation start epoch {start} is smaller than 0, '
- f'use 0 instead', UserWarning)
- start = 0
- self.dataloader = dataloader
- self.interval = interval
- self.by_epoch = by_epoch
- self.start = start
- assert isinstance(save_best, str) or save_best is None
- self.save_best = save_best
- self.eval_kwargs = eval_kwargs
- self.initial_epoch_flag = True
-
- self.logger = get_root_logger()
-
- if self.save_best is not None:
- self._init_rule(rule, self.save_best)
-
- def _init_rule(self, rule, key_indicator):
- """Initialize rule, key_indicator, comparison_func, and best score.
-
- Args:
- rule (str | None): Comparison rule for best score.
- key_indicator (str | None): Key indicator to determine the
- comparison rule.
- """
- if rule not in self.rule_map and rule is not None:
- raise KeyError(f'rule must be greater, less or None, '
- f'but got {rule}.')
-
- if rule is None:
- if key_indicator != 'auto':
- if any(key in key_indicator for key in self.greater_keys):
- rule = 'greater'
- elif any(key in key_indicator for key in self.less_keys):
- rule = 'less'
- else:
- raise ValueError(f'Cannot infer the rule for key '
- f'{key_indicator}, thus a specific rule '
- f'must be specified.')
- self.rule = rule
- self.key_indicator = key_indicator
- if self.rule is not None:
- self.compare_func = self.rule_map[self.rule]
-
- def before_run(self, runner):
- if self.save_best is not None:
- if runner.meta is None:
- warnings.warn('runner.meta is None. Creating a empty one.')
- runner.meta = dict()
- runner.meta.setdefault('hook_msgs', dict())
-
- def before_train_epoch(self, runner):
- """Evaluate the model only at the start of training."""
- if not self.initial_epoch_flag:
- return
- if self.start is not None and runner.epoch >= self.start:
- self.after_train_epoch(runner)
- self.initial_epoch_flag = False
-
- def evaluation_flag(self, runner):
- """Judge whether to perform_evaluation after this epoch.
-
- Returns:
- bool: The flag indicating whether to perform evaluation.
- """
- if self.start is None:
- if not self.every_n_epochs(runner, self.interval):
- # No evaluation during the interval epochs.
- return False
- elif (runner.epoch + 1) < self.start:
- # No evaluation if start is larger than the current epoch.
- return False
- else:
- # Evaluation only at epochs 3, 5, 7... if start==3 and interval==2
- if (runner.epoch + 1 - self.start) % self.interval:
- return False
- return True
-
- def after_train_epoch(self, runner):
- if not self.by_epoch or not self.evaluation_flag(runner):
- return
- from mmdet.apis import single_gpu_test
- results = single_gpu_test(runner.model, self.dataloader, show=False)
- key_score = self.evaluate(runner, results)
- if self.save_best:
- self.save_best_checkpoint(runner, key_score)
-
- def after_train_iter(self, runner):
- if self.by_epoch or not self.every_n_iters(runner, self.interval):
- return
- from mmdet.apis import single_gpu_test
- results = single_gpu_test(runner.model, self.dataloader, show=False)
- key_score = self.evaluate(runner, results)
- if self.save_best:
- self.save_best_checkpoint(runner, key_score)
-
- def save_best_checkpoint(self, runner, key_score):
- best_score = runner.meta['hook_msgs'].get(
- 'best_score', self.init_value_map[self.rule])
- if self.compare_func(key_score, best_score):
- best_score = key_score
- runner.meta['hook_msgs']['best_score'] = best_score
- last_ckpt = runner.meta['hook_msgs']['last_ckpt']
- runner.meta['hook_msgs']['best_ckpt'] = last_ckpt
- mmcv.symlink(
- last_ckpt,
- osp.join(runner.work_dir, f'best_{self.key_indicator}.pth'))
- time_stamp = runner.epoch + 1 if self.by_epoch else runner.iter + 1
- self.logger.info(f'Now best checkpoint is epoch_{time_stamp}.pth.'
- f'Best {self.key_indicator} is {best_score:0.4f}')
-
- def evaluate(self, runner, results):
- eval_res = self.dataloader.dataset.evaluate(
- results, logger=runner.logger, **self.eval_kwargs)
- for name, val in eval_res.items():
- runner.log_buffer.output[name] = val
- runner.log_buffer.ready = True
- if self.save_best is not None:
- if self.key_indicator == 'auto':
- # infer from eval_results
- self._init_rule(self.rule, list(eval_res.keys())[0])
- return eval_res[self.key_indicator]
- else:
- return None
-
-
-class DistEvalHook(EvalHook):
- """Distributed evaluation hook.
-
- Notes:
- If new arguments are added, tools/test.py may be effected.
-
- Attributes:
- dataloader (DataLoader): A PyTorch dataloader.
- start (int, optional): Evaluation starting epoch. It enables evaluation
- before the training starts if ``start`` <= the resuming epoch.
- If None, whether to evaluate is merely decided by ``interval``.
- Default: None.
- interval (int): Evaluation interval (by epochs). Default: 1.
- tmpdir (str | None): Temporary directory to save the results of all
- processes. Default: None.
- gpu_collect (bool): Whether to use gpu or cpu to collect results.
- Default: False.
- save_best (str, optional): If a metric is specified, it would measure
- the best checkpoint during evaluation. The information about best
- checkpoint would be save in best.json.
- Options are the evaluation metrics to the test dataset. e.g.,
- ``bbox_mAP``, ``segm_mAP`` for bbox detection and instance
- segmentation. ``AR@100`` for proposal recall. If ``save_best`` is
- ``auto``, the first key will be used. The interval of
- ``CheckpointHook`` should device EvalHook. Default: None.
- rule (str | None): Comparison rule for best score. If set to None,
- it will infer a reasonable rule. Default: 'None'.
- broadcast_bn_buffer (bool): Whether to broadcast the
- buffer(running_mean and running_var) of rank 0 to other rank
- before evaluation. Default: True.
- **eval_kwargs: Evaluation arguments fed into the evaluate function of
- the dataset.
- """
-
- def __init__(self,
- dataloader,
- start=None,
- interval=1,
- by_epoch=True,
- tmpdir=None,
- gpu_collect=False,
- save_best=None,
- rule=None,
- broadcast_bn_buffer=True,
- **eval_kwargs):
- super().__init__(
- dataloader,
- start=start,
- interval=interval,
- by_epoch=by_epoch,
- save_best=save_best,
- rule=rule,
- **eval_kwargs)
- self.broadcast_bn_buffer = broadcast_bn_buffer
- self.tmpdir = tmpdir
- self.gpu_collect = gpu_collect
-
- def _broadcast_bn_buffer(self, runner):
- # Synchronization of BatchNorm's buffer (running_mean
- # and running_var) is not supported in the DDP of pytorch,
- # which may cause the inconsistent performance of models in
- # different ranks, so we broadcast BatchNorm's buffers
- # of rank 0 to other ranks to avoid this.
- if self.broadcast_bn_buffer:
- model = runner.model
- for name, module in model.named_modules():
- if isinstance(module,
- _BatchNorm) and module.track_running_stats:
- dist.broadcast(module.running_var, 0)
- dist.broadcast(module.running_mean, 0)
-
- def after_train_epoch(self, runner):
- if not self.by_epoch or not self.evaluation_flag(runner):
- return
-
- if self.broadcast_bn_buffer:
- self._broadcast_bn_buffer(runner)
-
- from mmdet.apis import multi_gpu_test
- tmpdir = self.tmpdir
- if tmpdir is None:
- tmpdir = osp.join(runner.work_dir, '.eval_hook')
- results = multi_gpu_test(
- runner.model,
- self.dataloader,
- tmpdir=tmpdir,
- gpu_collect=self.gpu_collect)
- if runner.rank == 0:
- print('\n')
- key_score = self.evaluate(runner, results)
- if self.save_best:
- self.save_best_checkpoint(runner, key_score)
-
- def after_train_iter(self, runner):
- if self.by_epoch or not self.every_n_iters(runner, self.interval):
- return
-
- if self.broadcast_bn_buffer:
- self._broadcast_bn_buffer(runner)
-
- from mmdet.apis import multi_gpu_test
- tmpdir = self.tmpdir
- if tmpdir is None:
- tmpdir = osp.join(runner.work_dir, '.eval_hook')
- results = multi_gpu_test(
- runner.model,
- self.dataloader,
- tmpdir=tmpdir,
- gpu_collect=self.gpu_collect)
- if runner.rank == 0:
- print('\n')
- key_score = self.evaluate(runner, results)
- if self.save_best:
- self.save_best_checkpoint(runner, key_score)
diff --git a/spaces/Robert001/UniControl-Demo/annotator/uniformer/mmdet_null/models/roi_heads/dynamic_roi_head.py b/spaces/Robert001/UniControl-Demo/annotator/uniformer/mmdet_null/models/roi_heads/dynamic_roi_head.py
deleted file mode 100644
index 89427a931f45f5a920c0e66fd88058bf9fa05f5c..0000000000000000000000000000000000000000
--- a/spaces/Robert001/UniControl-Demo/annotator/uniformer/mmdet_null/models/roi_heads/dynamic_roi_head.py
+++ /dev/null
@@ -1,154 +0,0 @@
-import numpy as np
-import torch
-
-from mmdet.core import bbox2roi
-from mmdet.models.losses import SmoothL1Loss
-from ..builder import HEADS
-from .standard_roi_head import StandardRoIHead
-
-EPS = 1e-15
-
-
-@HEADS.register_module()
-class DynamicRoIHead(StandardRoIHead):
- """RoI head for `Dynamic R-CNN `_."""
-
- def __init__(self, **kwargs):
- super(DynamicRoIHead, self).__init__(**kwargs)
- assert isinstance(self.bbox_head.loss_bbox, SmoothL1Loss)
- # the IoU history of the past `update_iter_interval` iterations
- self.iou_history = []
- # the beta history of the past `update_iter_interval` iterations
- self.beta_history = []
-
- def forward_train(self,
- x,
- img_metas,
- proposal_list,
- gt_bboxes,
- gt_labels,
- gt_bboxes_ignore=None,
- gt_masks=None):
- """Forward function for training.
-
- Args:
- x (list[Tensor]): list of multi-level img features.
-
- img_metas (list[dict]): list of image info dict where each dict
- has: 'img_shape', 'scale_factor', 'flip', and may also contain
- 'filename', 'ori_shape', 'pad_shape', and 'img_norm_cfg'.
- For details on the values of these keys see
- `mmdet/datasets/pipelines/formatting.py:Collect`.
-
- proposals (list[Tensors]): list of region proposals.
-
- gt_bboxes (list[Tensor]): each item are the truth boxes for each
- image in [tl_x, tl_y, br_x, br_y] format.
-
- gt_labels (list[Tensor]): class indices corresponding to each box
-
- gt_bboxes_ignore (None | list[Tensor]): specify which bounding
- boxes can be ignored when computing the loss.
-
- gt_masks (None | Tensor) : true segmentation masks for each box
- used if the architecture supports a segmentation task.
-
- Returns:
- dict[str, Tensor]: a dictionary of loss components
- """
- # assign gts and sample proposals
- if self.with_bbox or self.with_mask:
- num_imgs = len(img_metas)
- if gt_bboxes_ignore is None:
- gt_bboxes_ignore = [None for _ in range(num_imgs)]
- sampling_results = []
- cur_iou = []
- for i in range(num_imgs):
- assign_result = self.bbox_assigner.assign(
- proposal_list[i], gt_bboxes[i], gt_bboxes_ignore[i],
- gt_labels[i])
- sampling_result = self.bbox_sampler.sample(
- assign_result,
- proposal_list[i],
- gt_bboxes[i],
- gt_labels[i],
- feats=[lvl_feat[i][None] for lvl_feat in x])
- # record the `iou_topk`-th largest IoU in an image
- iou_topk = min(self.train_cfg.dynamic_rcnn.iou_topk,
- len(assign_result.max_overlaps))
- ious, _ = torch.topk(assign_result.max_overlaps, iou_topk)
- cur_iou.append(ious[-1].item())
- sampling_results.append(sampling_result)
- # average the current IoUs over images
- cur_iou = np.mean(cur_iou)
- self.iou_history.append(cur_iou)
-
- losses = dict()
- # bbox head forward and loss
- if self.with_bbox:
- bbox_results = self._bbox_forward_train(x, sampling_results,
- gt_bboxes, gt_labels,
- img_metas)
- losses.update(bbox_results['loss_bbox'])
-
- # mask head forward and loss
- if self.with_mask:
- mask_results = self._mask_forward_train(x, sampling_results,
- bbox_results['bbox_feats'],
- gt_masks, img_metas)
- losses.update(mask_results['loss_mask'])
-
- # update IoU threshold and SmoothL1 beta
- update_iter_interval = self.train_cfg.dynamic_rcnn.update_iter_interval
- if len(self.iou_history) % update_iter_interval == 0:
- new_iou_thr, new_beta = self.update_hyperparameters()
-
- return losses
-
- def _bbox_forward_train(self, x, sampling_results, gt_bboxes, gt_labels,
- img_metas):
- num_imgs = len(img_metas)
- rois = bbox2roi([res.bboxes for res in sampling_results])
- bbox_results = self._bbox_forward(x, rois)
-
- bbox_targets = self.bbox_head.get_targets(sampling_results, gt_bboxes,
- gt_labels, self.train_cfg)
- # record the `beta_topk`-th smallest target
- # `bbox_targets[2]` and `bbox_targets[3]` stand for bbox_targets
- # and bbox_weights, respectively
- pos_inds = bbox_targets[3][:, 0].nonzero().squeeze(1)
- num_pos = len(pos_inds)
- cur_target = bbox_targets[2][pos_inds, :2].abs().mean(dim=1)
- beta_topk = min(self.train_cfg.dynamic_rcnn.beta_topk * num_imgs,
- num_pos)
- cur_target = torch.kthvalue(cur_target, beta_topk)[0].item()
- self.beta_history.append(cur_target)
- loss_bbox = self.bbox_head.loss(bbox_results['cls_score'],
- bbox_results['bbox_pred'], rois,
- *bbox_targets)
-
- bbox_results.update(loss_bbox=loss_bbox)
- return bbox_results
-
- def update_hyperparameters(self):
- """Update hyperparameters like IoU thresholds for assigner and beta for
- SmoothL1 loss based on the training statistics.
-
- Returns:
- tuple[float]: the updated ``iou_thr`` and ``beta``.
- """
- new_iou_thr = max(self.train_cfg.dynamic_rcnn.initial_iou,
- np.mean(self.iou_history))
- self.iou_history = []
- self.bbox_assigner.pos_iou_thr = new_iou_thr
- self.bbox_assigner.neg_iou_thr = new_iou_thr
- self.bbox_assigner.min_pos_iou = new_iou_thr
- if (np.median(self.beta_history) < EPS):
- # avoid 0 or too small value for new_beta
- new_beta = self.bbox_head.loss_bbox.beta
- else:
- new_beta = min(self.train_cfg.dynamic_rcnn.initial_beta,
- np.median(self.beta_history))
- self.beta_history = []
- self.bbox_head.loss_bbox.beta = new_beta
- return new_iou_thr, new_beta
diff --git a/spaces/Robert001/UniControl-Demo/annotator/uniformer/mmseg/models/necks/__init__.py b/spaces/Robert001/UniControl-Demo/annotator/uniformer/mmseg/models/necks/__init__.py
deleted file mode 100644
index 9b9d3d5b3fe80247642d962edd6fb787537d01d6..0000000000000000000000000000000000000000
--- a/spaces/Robert001/UniControl-Demo/annotator/uniformer/mmseg/models/necks/__init__.py
+++ /dev/null
@@ -1,4 +0,0 @@
-from .fpn import FPN
-from .multilevel_neck import MultiLevelNeck
-
-__all__ = ['FPN', 'MultiLevelNeck']
diff --git a/spaces/SIH/Augmented-Retrieval-qa-ChatGPT/__init__.py b/spaces/SIH/Augmented-Retrieval-qa-ChatGPT/__init__.py
deleted file mode 100644
index e69de29bb2d1d6434b8b29ae775ad8c2e48c5391..0000000000000000000000000000000000000000
diff --git a/spaces/SarthakSidhant/Go-Cattle/app.py b/spaces/SarthakSidhant/Go-Cattle/app.py
deleted file mode 100644
index 353c5e8e0e6414c4a96933f263ed0e73f7f98f6f..0000000000000000000000000000000000000000
--- a/spaces/SarthakSidhant/Go-Cattle/app.py
+++ /dev/null
@@ -1,216 +0,0 @@
-#runtime fix??
-# Import required libraries
-import streamlit as st #to run the webpage ofcourse
-import pandas as pd #to read the dataset
-from PIL import Image #to render the gcb.jpg image
-import datetime
-from streamlit_option_menu import option_menu
-
-#Congifuring the Streamlit Page (the same thing is done in the /.streamlit/config.toml)
-
-st.set_page_config(
- page_title="Go-Cattle // Cattle Healthcare",
- page_icon="🐄",
- layout="wide",
- #menu_items={'Get Help': 'https://www.extremelycoolapp.com/help','Report a bug': "https://www.extremelycoolapp.com/bug",'About': "# This is a header. This is an *extremely* cool app!"}
-)
-#logo=Image.open('logo.png')
-#st.image(logo,width=300)
-## Defining tabs
-tab1,tab2,tab3,tab4,tab5 = st.tabs(["Home","Details","Credits","Feedback", "Legal"])
-
-# h - i just added this h and was wondering why error
-
-## Linking the Style.css (just in case)
-with open("style.css") as f:
- st.markdown(f'', unsafe_allow_html=True)
-
-## tab 1, home
-with tab1:
- st.title(":green[Go-Cattle]")
- st.markdown(f"
A Cattle Healthcare Platform
", unsafe_allow_html=True)
- image=Image.open('gcb.jpg')
- st.image(image)
- st.markdown('### :red[**What is Go Cattle?**]')
- st.markdown("Go Cattle is a :green[**Cattle Healthcare Platform**]. India is the Home to about 17% of the World's Cows and For Every 1 Registered Vet in India, There are about 50,000 cows. Due To These Reasons, About 65% of The Cows Cannot Get Proper Healthcare and Treatments. It is Very Important to increase awareness about this topic because This Leads to Thousands if Not Hundreds of Thousands of Cattle Dying Every Year.")
- st.markdown("Go Cattle Provides a Variety of Resources for The Welfare of Cattles. One of The Main Features is an advanced web application designed to **analyze** :red[diseases] in cattle based on the :yellow[**symptoms**] provided. With its cutting-edge ML-model analyzer, Go Cattle ensures accurate and efficient diagnosis, empowering cattle owners and veterinarians to make informed decisions about their livestock's health.")
- st.markdown("Our ML-model boasts an outstanding :green[**accuracy rate of 95%+**], surpassing the required medical standards" f"#" " Developed using a vast dataset of *20,499 parameters* sourced from reliable and up-to-date information gathered through web crawling & web scraping, Go Cattle provides a robust foundation for precise disease identification.", unsafe_allow_html=True)
- st.markdown("Equipped with an extensive range of 123 unique symptoms and a comprehensive list of 163 unique diseases, Go Cattle covers a wide spectrum of ailments that can affect cattle. By inputting the observed symptoms, the system swiftly processes the information and generates a reliable diagnosis, enabling prompt action to be taken. :violet[ *The Dataset has been gone through Vigorous Changes Recently and There's A High Possibility that our team might have messed up something in the Process (as of 10th July 2023)*]")
-
-
- with st.sidebar:
- ## fil_val="This value was used to fill the sidebar" #they wont let me just yk, use the sidebar without anything
- st.empty() #okay, i discovered something better. LOL
-
-with tab2:
- st.title(":red[//] :orange[**Details**]")
- #tdet,clog,manl=st.tabs(["Technical Details","Changelog","Medical Analysis"])
-
- selected2 = option_menu(None, [ "Technicalities", "Changelog", 'Medical Analysis'],
- icons=['robot', 'clock-history', "activity" ],
- menu_icon="cast", default_index=0, orientation="horizontal")
-
-
- if selected2 == "Technicalities":
- st.markdown("## Technical Details")
- st.markdown("""
- #### :green[ML Model Disease Analyzer]
- The Disease Analyzer works on Providing the Symptom
- """)
- st.markdown("Our ML-model boasts an outstanding :green[**accuracy rate of 95%+**], surpassing the required medical standards" f"#" " Developed using a vast dataset of *20,499 parameters* sourced from reliable and up-to-date information gathered through web crawling & web scraping, Go Cattle provides a robust foundation for precise disease identification.", unsafe_allow_html=True)
- st.markdown("Equipped with an extensive range of 123 unique symptoms and a comprehensive list of 163 unique diseases, Go Cattle covers a wide spectrum of ailments that can affect cattle. By inputting the observed symptoms, the system swiftly processes the information and generates a reliable diagnosis, enabling prompt action to be taken. :violet[ *The Dataset has been gone through Vigorous Changes Recently and There's A High Possibility that our team might have messed up something in the Process (as of 10th July 2023)*]")
- st.markdown("### :orange[Workings of The Model]")
- st.markdown("""
- The first step is to load the data (cattle diseases and their symptoms) from a CSV file. The data is then split into two sets: a training set and a test set. The training set is used to train the model, and the test set is used to evaluate the model's accuracy.
-Next, a random forest classifier is initialized. The random forest classifier is a type of machine learning algorithm that can be used for classification tasks. It works by creating a number of decision trees, and then making a prediction by taking a majority vote of the predictions from the decision trees.
-The next step is to perform cross-validation. Cross-validation is a technique for evaluating the accuracy of a machine learning model. It works by splitting the data into a number of folds, and then training the model on a subset of the folds and evaluating the model on the remaining folds. This process is repeated multiple times, and the average accuracy across all folds is reported.
-In this case, the cross-validation is performed using two folds. The mean accuracy of the model is 97.5%.
-The next step is to fit the model on the entire training set. This means that the model will learn the relationships between the input features and the target variable.
-Once the model is fitted, it can be saved to a file. This is done using the joblib library.
-The next step is to load the trained model from the file. This is done using the joblib library as well.
-The next step is to predict the disease for the training set. This is done by passing the training set to the model's predict() method.
-The accuracy of the model is then evaluated by comparing the predicted values to the actual values. The accuracy in this case is 98.7%.
-The final step is to predict the disease for the test set. This is done by passing the test set to the model's predict() method.
-The accuracy of the model is then evaluated by comparing the predicted values to the actual values. The accuracy in this case is 97.5%.
-Overall, the random forest classification model achieves an accuracy of 97.5% on the test set. This means that the model is able to correctly predict the disease for 97.5% of the test data.
- """)
- elif selected2 == "Changelog":
- st.subheader("Changelog")
- st.markdown("""
- ### 17th July:
-- :green[Added a Streamlit Option Menu to Details tab that has the following Options which may change in the future:]
- - Changelogs
- - Technical Details
- - Medical Analysis
-- :green[Content Update (Phase V2) continuously updating the contents and Improving the present content in terms of grammar.]
-
-### 15th and 16th July:
-- :orange[Fixed the Multi-page Navigation System by an Interesting Approach]
->> I moved the predicted disease information to the prediction tab so it doesn't need to fetch a variable from a different python file anymore.
-- :green[Content Update - since the home page was empty as the tabs were redistributed to pages, added **credits** and details in place of those two lost tabs]
-- :violet[Deprecated feedback and legal pages to tabs in the home page]
-
-### 14th July: (THE :red[D-DAY])
-- :red[Deleted 'Change Theme' Feature]
-- :red[Deleted support.py (We Wont Import Functions from a different file)]
-- :red[Removed The Top 10 Symptoms from Homepage]
-- :red[Removed Unnecessary Texts]
-- :red[Removed the Just-in-Case Style.css]
-- :red[Cleared the Saved pyCache and now its **BROKE**]
-- :red[Removed requirements :green[(added `requirements.txt` in its place)]]
-
-
-### 13th July:
-- :green[Made Multi-page Navigation System on Request. The UI became really easy because everything can be controlled using the sidebar. We can also Use Links like [Prediction](https://gocattle.site/Prediction) which lands us Directly on the Prediction Webpage.
-This Introduces leftover Tab Spaces which can be used as Content. (The Styling will mostly remain the same as I believe that Farmers don't really care about Stylish Interfaces. The thing is about being simple and I am trying my best.)]
- >> However, The Multi-page stuff Introduced 2 Interesting Problems and I can't do anything because Python works that way. First of all, The Diseases page requests a variable from the Prediction page. The variable is named "result" and displays the most probable disease. To import the result variable from the Prediction page, I import it into my disease page but the disease page insists on running the whole code instead of just fetching the variable, It runs the complete program. That is stupid. Another Problem I've encountered is that Python cannot import files that have a special character in their name. Like the Prediction file is saved as ```1_🐮_Prediction.py```
-And I just can't include Special Characters. I will figure a workaround soon.
-- :orange[Reworked on the Diseases Buttons, They Only Display Diseases when Requested.]
-- :orange[Initial Sidebar State now set to expand as Sidebar is the main form of navigation]
-
-### 12th July:
-- :green[Added Diseases Tab]
-- :orange[Reworked on Buttons by Implementing Buttons]
-- :green[Created Two Distinct Models on :blue[Augean] and :blue[Manhattan].]
- - :blue[Arjun] Model - Based on :blue[Augean], Provides Medical Grade Accuracy but is Overcomplicated and Contains Duplicate Data
- - :blue[Enigma] Model - Based on :blue[Manhattan], Focuses on Simplicity and doesn't provide Medical Grade Accuracy.
-- :green[Made a Github Organization named **go-cattle** (https://github.com/go-cattle) and all the details about the go-cattle app with all technical details.]
- > Please Expect a Change every Fortnight and Not Any Sooner.
-- :green[Organized 'Diseases' Tab and Added 'Learn About Predicted Disease']
-
-### 11th July
-- :green[Hosted on Hugging Face because [Owehost](https://owehost.com) will take time]
-- :green[Made 2 Discreet Datasets]
- - :blue[Augean] - Over complicated with numerous duplicates but Great Accuracy
- - :blue[Manhattan] - Over Simplified for use by a simple man but No way of achieving medical grade Accuracy
-- :green[Linked the domain (https://gocattle.site) and the subdomain (www.gocattle.site) to the hugging face space. The domains are technically a **masked redirect**, but they work so Idgaf]
-- :orange[(Almost) Completed the Diseases Tab and Countered The Previous Glitches]
-
-### 10th July
-- :orange[Made the Website Colorful, By Adding Text Colors and Essence]
-- :green[Added Legal sections]
-- :orange[Simplified UI]
-- :green[Content Addition including Introduction]
-
- """)
- else:
- st.write("You selected Medical Analysis")
-
-with tab3:
- st.title("Credits")
- st.markdown("### :red[//] :green[Founded by] [:orange[Sarthak] :blue[Sidhant]](https://sarthaksidhant.me/)")
- st.markdown("""
-As an Indian, I've had early experiences with cows in my village.
-I've seen firsthand how important it is to keep cows healthy, and I've also seen the challenges
-that farmers face in providing quality healthcare to their animals.\n
-I also got the inspiration for this idea from Sarvagya's [Nandini](https://drive.google.com/file/d/1WZvZB5TyjJgR_XD3yzxT0hc6nUFMFbfd/view?usp=sharing) project.
-I was really impressed by the idea and wanted to make something similar but with a more modern approach.
-Initially, I just wanted to see how fast it would take me to collect the data and make a model,
-but I ended up making a full-fledged healthcare platform.\n
-I really hope that this project helps
-the farmers and the cattle in some way or another.
-Thanks to all the people that helped along the way and contributed towards this project.
-I really hope that machine learning and artificial intelligence contribute toward
-society and nature in a beneficial way like this. (I'm looking at you, Cambridge Analytica)\n
-I hope to make projects like [:green[Go-Cattle]](#) and [:orange[Rellekk-Z]](https://rellekk-z.github.io/Rellekk-Z)
-and [:orange[Decodificate]](https://decodificate.tech) in the future that aim to seamlessly blend in with the cause of social benefit,
-and I hope that you'll be there to support me. Thank you for using Go Cattle.""")
- st.subheader(":red[//] :orange[Contributors]")
- st.markdown("##### [:blue[Abhijith KS]](https://www.fiverr.com/abhijith_k_s) - Helped in Developing the Model and the Web-App")
- st.markdown("##### [:blue[AshTired11s]](https://www.kaggle.com/ashtired11/) - Dataset Engineer, Helped in Devloping the Vast Dataset")
- st.markdown("##### [:orange[Yasharth Gautam]](github.com/yasharth1) - Team Member, Helped with Suggestions and '👍' ")
- st.markdown("##### [:orange[Aayushman Utkrisht]](https://www.google.com/search?q=aayushman+utkrisht&client=opera-gx&hs=yPD&sxsrf=AB5stBgq-WWcuh2JWtN7a7tjge0BaZfhCA%3A1689542431457&ei=H1-0ZKrEG9So4-EPpeS9yA4&ved=0ahUKEwjqz9aDlJSAAxVU1DgGHSVyD-kQ4dUDCA4&uact=5&oq=aayushman+utkrisht&gs_lp=Egxnd3Mtd2l6LXNlcnAiEmFheXVzaG1hbiB1dGtyaXNodDIHEAAYgAQYCjIHEAAYgAQYCjIJEAAYigUYChhDMgYQABgeGAoyCBAAGIoFGIYDMggQABiKBRiGAzIIEAAYigUYhgMyCBAAGIoFGIYDMggQABiKBRiGA0jIA1AAWABwAHgBkAEAmAHaAaAB2gGqAQMyLTG4AQPIAQD4AQHiAwQYACBBiAYB&sclient=gws-wiz-serp) - Team Member, Helped with Suggestions and UI ")
- st.markdown("##### [:green[Layered, OweHost]](Owehost.com) - Sponsors the Hosting of the Go Cattle Web-App")
- st.markdown("##### [:green[Sidhant Hyperspace]](sarthaksidhant.me/sidhant-hyperspace) - Umbrella Organisation for Go Cattle")
-
-with tab4:
-
- def save_feedback(name,email,feedback):
- # Generate a unique filename using the current timestamp
- timestamp = datetime.datetime.now().strftime("%Y%m%d%H%M%S")
- filename = f"./feedbacks/feedback_{name}_{email}_{timestamp}.txt"
-
- # Save the feedback to a file
- with open(filename, 'w') as file:
- file.write(f'name : {name}\nemail : {email}\nfeedback : {feedback}')
-
- return filename
-
- st.subheader("Feedback Portal")
- colFB1,colFB2 = st.columns([1,3])
- with colFB1:
- name = st.text_input("Enter your name")
- email = st.text_input("Enter your email id")
- with colFB2:
- feedback = st.text_area("Enter your feedback here")
-
-
- if st.button("Submit"):
- if (feedback and name and email):
- # Save feedback as a file
- filename = save_feedback(name,email,feedback)
- st.success(f"Thank you for your feedback! Your feedback has been saved.")
- else:
- st.warning("Please enter your details before submitting.")
-
-with tab5: #legal section (dangerous)
- st.title("Legal")
- st.markdown("### :green[**Terms of Service**]")
- st.markdown("""Disclaimer:
-
-The advice or results generated by the Go Cattle app are derived from an artificial intelligence machine learning model. While efforts are made to ensure accuracy levels of 95% or higher, it is crucial to note that these outcomes may be subject to inaccuracies and should not be regarded as medical information. Therefore, the advice provided by the app should never be solely relied upon without seeking the guidance of a qualified veterinarian.
-
-It is important to understand that:
-
-1. The Go Cattle app is not a substitute for professional veterinary care.
-2. The results obtained from the app should not be used as a means to diagnose or treat any medical condition.
-3. If you have concerns about the health of your cattle, it is imperative that you consult a veterinarian without delay.
-
-By utilizing the Go Cattle app, you expressly acknowledge and agree that:
-
-1. Go Cattle shall not be held liable or accountable for any mishaps, damages, injuries, or losses arising from the use of the advice or results provided by the app.
-2. The app's advice and results are not a replacement for personalized veterinary care and should be considered as supplementary information only.
-
-We hope this disclaimer serves to clarify the limitations of the Go Cattle app and the need for professional veterinary consultation. Your understanding and compliance with these terms are greatly appreciated.
-
-Thank you for choosing and using Go Cattle!""")
\ No newline at end of file
diff --git a/spaces/SeViLA/SeViLA/lavis/models/img2prompt_models/img2prompt_vqa.py b/spaces/SeViLA/SeViLA/lavis/models/img2prompt_models/img2prompt_vqa.py
deleted file mode 100644
index 00cda00a8f029841771ef041c5321e45441fdfbd..0000000000000000000000000000000000000000
--- a/spaces/SeViLA/SeViLA/lavis/models/img2prompt_models/img2prompt_vqa.py
+++ /dev/null
@@ -1,582 +0,0 @@
-"""
- Copyright (c) 2022, salesforce.com, inc.
- All rights reserved.
- SPDX-License-Identifier: BSD-3-Clause
- For full license text, see the LICENSE file in the repo root or https://opensource.org/licenses/BSD-3-Clause
-"""
-
-import random
-
-import spacy
-import torch
-import torch.nn.functional as F
-from transformers import T5ForConditionalGeneration, T5Tokenizer
-
-from lavis.common.dist_utils import download_cached_file
-from lavis.common.registry import registry
-from lavis.models.base_model import BaseModel
-from lavis.models.blip_models.blip_image_text_matching import compute_gradcam
-
-open_pos = ["NOUN", "VERB", "ADJ", "ADV", "NUM"]
-
-
-
-@registry.register_model("img2prompt_vqa")
-class Img2PromptVQA(BaseModel):
- """
- Img2Prompt_VQA model consists of three submodels for zero-shot VQA:
- 1. Image-questioning matching model
- 2. Image captioning model
- 3. Large Language model
-
- Supported model types:
- - base: BLIPITM, BLIPCaption, PNPUnifiedQAv2FiD (t5-base)
- - large: BLIPITM, BLIPCaption, PNPUnifiedQAv2FiD (t5-large)
- - 3b: BLIPITM, BLIPCaption, PNPUnifiedQAv2FiD (t5-3b)
-
- Usage:
- >>> from lavis.models import load_model
- >>> model = load_model("img2prompt_vqa", "base", is_eval=True)
- """
-
- PRETRAINED_MODEL_CONFIG_DICT = {
- "base": "configs/models/img2prompt-vqa/img2prompt_vqa_base.yaml",
- }
-
- def __init__(
- self,
- image_question_matching_model,
- image_captioning_model,
- question_generation_model,
- question_generation_tokenizer,
- offload_model=False,
- ):
- super().__init__()
-
- self.image_question_matching_model = image_question_matching_model
- self.image_captioning_model = image_captioning_model
- self.question_generation_model = question_generation_model
- self.question_generation_tokenizer = question_generation_tokenizer
- self.offload_model = offload_model
- self.nlp = spacy.load("en_core_web_sm")
-
- def forward_itm(self, samples, block_num=7):
- """
- Args:
- samples (dict): A dictionary containing the following keys:
- - image (torch.Tensor): A tensor of shape (batch_size, 3, H, W)
- - text_input (list): A list of strings of length batch_size
- block_num (int): The index of cross-attention block for gradcam computation.
-
- Returns:
- samples (dict): A dictionary containing the following keys:
- - image (torch.Tensor): A tensor of shape (batch_size, 3, H, W)
- - text_input (list): A list of strings of length batch_size
- - gradcams (torch.Tensor): A tensor of shape (batch_size, H*W)
- """
- image = samples["image"]
- question = [text.strip("?") for text in samples["text_input"]]
- tokenized_text = self.image_question_matching_model.tokenizer(
- question, padding="longest", truncation=True, return_tensors="pt"
- ).to(self.image_question_matching_model.device)
- with torch.set_grad_enabled(True):
- gradcams, _ = compute_gradcam(
- model=self.image_question_matching_model,
- visual_input=image,
- text_input=question,
- tokenized_text=tokenized_text,
- block_num=block_num,
- )
-
- gradcams = [gradcam_[1] for gradcam_ in gradcams]
- samples["gradcams"] = torch.stack(gradcams).reshape(
- samples["image"].size(0), -1
- )
-
- return samples
-
- def itm_rank(self, image_embeds, image_atts, encoder_input_ids, match_head="itm"):
- # breakpoint()
- encoder_input_ids = encoder_input_ids.clone()
- encoder_input_ids = encoder_input_ids[:, self.prompt_length - 1 :]
- text_attention_mask = (encoder_input_ids != self.tokenizer.pad_token_id).long()
-
- if match_head == "itm":
- # encoder_input_ids = encoder_input_ids.clone()
- encoder_input_ids[:, 0] = self.tokenizer.enc_token_id
- output = self.text_encoder(
- encoder_input_ids,
- attention_mask=text_attention_mask,
- encoder_hidden_states=image_embeds,
- encoder_attention_mask=image_atts,
- return_dict=True,
- )
- itm_output = self.itm_head(output.last_hidden_state[:, 0, :])
- return itm_output # , mask, token_length
-
- elif match_head == "itc":
- encoder_input_ids[:, 0] = self.tokenizer.cls_token_id
- text_output = self.text_encoder(
- encoder_input_ids,
- attention_mask=text_attention_mask,
- return_dict=True,
- mode="text",
- )
- image_feat = F.normalize(self.vision_proj(image_embeds[:, 0, :]), dim=-1)
- text_feat = F.normalize(
- self.text_proj(text_output.last_hidden_state[:, 0, :]), dim=-1
- )
-
- sim = image_feat @ text_feat.t()
- return sim
-
- def forward_cap(
- self,
- samples,
- cap_max_length=20,
- cap_min_length=0,
- top_p=1,
- top_k=50,
- repetition_penalty=1.0,
- num_captions=100,
- num_patches=20,
- ):
- """
- Args:
- samples (dict): A dictionary containing the following keys:
- - image (torch.Tensor): A tensor of shape (batch_size, 3, H, W)
- - text_input (list): A list of strings of length batch_size
- - gradcams (torch.Tensor): A tensor of shape (batch_size, H*W)
- cap_max_length (int): The maximum length of the caption to be generated.
- cap_min_length (int): The minimum length of the caption to be generated.
- top_p (float): The cumulative probability for nucleus sampling.
- top_k (float): The number of the highest probability tokens for top-k sampling.
- repetition_penalty (float): The parameter for repetition penalty. 1.0 means no penalty.
- num_captions (int): Number of captions generated for each image.
- num_patches (int): Number of patches sampled for each image.
-
- Returns:
- samples (dict): A dictionary containing the following keys:
- - image (torch.Tensor): A tensor of shape (batch_size, 3, H, W)
- - text_input (list): A list of strings of length batch_size
- - gradcams (torch.Tensor): A tensor of shape (batch_size, H*W)
- - captions (nested list): A nested list of strings of total length batch_size * num_captions
- """
- encoder_out = self.image_captioning_model.forward_encoder(samples)
- captions = [[] for _ in range(encoder_out.size(0))]
-
- min_num_captions = 0
-
- while min_num_captions < num_captions:
- encoder_out_samples = []
- for i in range(num_captions):
- patch_id = (
- torch.multinomial(
- samples["gradcams"].to(self.image_captioning_model.device),
- num_patches,
- ).reshape(encoder_out.size(0), -1)
- + 1
- )
- patch_id = (
- patch_id.sort(dim=1)
- .values.unsqueeze(-1)
- .expand(-1, -1, encoder_out.size(2))
- )
- encoder_out_sample = torch.gather(encoder_out, 1, patch_id)
- encoder_out_samples.append(encoder_out_sample)
-
- stacked = torch.stack(encoder_out_samples, dim=1)
- image_embeds = torch.flatten(
- stacked, start_dim=0, end_dim=1
- ) # (bsz*num_seq, num_patch, dim)
-
- image_atts = torch.ones(image_embeds.size()[:-1], dtype=torch.long).to(
- self.image_captioning_model.device
- )
- model_kwargs = {
- "encoder_hidden_states": image_embeds,
- "encoder_attention_mask": image_atts,
- }
-
- prompt = [self.image_captioning_model.prompt] * image_embeds.size(0)
- prompt = self.image_captioning_model.tokenizer(
- prompt, return_tensors="pt"
- ).to(self.image_captioning_model.device)
- prompt.input_ids[:, 0] = self.image_captioning_model.tokenizer.bos_token_id
- prompt.input_ids = prompt.input_ids[:, :-1]
-
- decoder_out = self.image_captioning_model.text_decoder.generate(
- input_ids=prompt.input_ids,
- max_length=cap_max_length,
- min_length=cap_min_length,
- do_sample=True,
- top_p=top_p,
- top_k=top_k,
- num_return_sequences=1,
- eos_token_id=self.image_captioning_model.tokenizer.sep_token_id,
- pad_token_id=self.image_captioning_model.tokenizer.pad_token_id,
- repetition_penalty=repetition_penalty,
- **model_kwargs
- )
-
- itm_outputs = self.image_question_matching_model.itm_rank(
- image_embeds, image_atts, encoder_input_ids=decoder_out
- ) # caption filter
-
- outputs = self.image_captioning_model.tokenizer.batch_decode(
- decoder_out, skip_special_tokens=True
- )
-
- for counter, output in enumerate(outputs):
- ind = counter // num_captions
- if len(captions[ind]) < num_captions:
- caption = output[len(self.image_captioning_model.prompt) :]
- overlap_caption = [1 for caps in captions[ind] if caption in caps]
- # print(itm_outputs)
- if (
- len(overlap_caption) == 0 and itm_outputs[counter] >= 0.5
- ): # image filter
- captions[ind].append(caption)
-
- min_num_captions = min([len(i) for i in captions])
-
- samples["captions"] = captions
-
- return samples
-
- def answer_extraction(self, caption, num_question_generation=30):
- cap_use = ""
- # print(caption)
- caption = caption
- ans_to_cap_dict = {}
- answers = []
- for cap_idx, cap in enumerate(caption):
- # print(cap)
- cap_use += cap
- cap = cap.strip().strip(".")
- # print(cap)
- cap = self.nlp(cap)
- for token in cap: # Noun /Verb/Adj//NUM
- if token.pos_ in open_pos:
- if token.text.lower() not in ans_to_cap_dict:
- ans_to_cap_dict[token.text.lower()] = [cap_idx]
- else:
- if cap_idx not in ans_to_cap_dict[token.text.lower()]:
- ans_to_cap_dict[token.text.lower()].append(cap_idx)
- answers.append(token.text)
- for ent in cap.ents:
-
- if ent.text not in answers:
- if ent.text.lower() not in ans_to_cap_dict:
- ans_to_cap_dict[ent.text.lower()] = [cap_idx]
- else:
- if cap_idx not in ans_to_cap_dict[ent.text.lower()]:
- ans_to_cap_dict[ent.text.lower()].append(cap_idx)
- answers.append(ent.text)
- for chunk in cap.noun_chunks:
- if len(chunk.text.split()) < 4:
- if chunk.text.lower() not in ans_to_cap_dict:
- ans_to_cap_dict[chunk.text.lower()] = [cap_idx]
- else:
- if cap_idx not in ans_to_cap_dict[chunk.text.lower()]:
- ans_to_cap_dict[chunk.text.lower()].append(cap_idx)
- # print(chunk.text)
- answers.append(chunk.text)
- answers = sorted(answers, key=answers.count, reverse=True)
- real_answers = []
- for i in answers:
- i = i + "."
- if i not in real_answers:
- real_answers.append(i)
-
- contexts_for_question_generation = []
- answers = []
- for ans in real_answers[
- :num_question_generation
- ]: # Generate questions for 30 answers with max frequencies.
- contexts_for_question_generation.append(
- "answer: %s context: %s." % (ans, cap_use)
- )
- answers.append(ans)
- contexts_for_question_generation.append(
- "answer: %s context: %s." % ("yes.", cap_use)
- )
- answers.append("yes.")
- return contexts_for_question_generation, answers, ans_to_cap_dict
-
- def forward_qa_generation(self, samples):
- caption = samples["captions"][0]
- (
- contexts_for_question_generation,
- answers,
- ans_to_cap_dict,
- ) = self.answer_extraction(caption)
- inputs = self.question_generation_tokenizer(
- contexts_for_question_generation,
- padding="longest",
- truncation=True,
- max_length=2048,
- return_tensors="pt",
- ).to(self.device)
- question_size = inputs.input_ids.shape[0]
- cur_b = 0
- true_input_size = 10
- outputs_list = []
- while cur_b < question_size:
- outputs = self.question_generation_model.generate(
- input_ids=inputs.input_ids[cur_b : cur_b + true_input_size],
- attention_mask=inputs.attention_mask[cur_b : cur_b + true_input_size],
- num_beams=3,
- max_length=30,
- )
- questions = self.question_generation_tokenizer.batch_decode(
- outputs, skip_special_tokens=True
- )
- outputs_list += questions
- cur_b += true_input_size
- questions = outputs_list
- samples["questions"] = questions
- samples["answers"] = answers
- samples["ans_to_cap_dict"] = ans_to_cap_dict
- # results.append({"question_id": ques_id, "question":questions,"answer":answers})
- return samples
-
- def create_context_prompt(self, samples, num_caps_per_img=30):
- ans_dict_queid = samples["ans_to_cap_dict"]
- # print(ans_dict_queid)
- caption = samples["captions"][0]
- answers = samples["answers"]
- Context_Prompt = ""
- mycontexts_id = []
- for idx in range(num_caps_per_img):
- cap_id_list = ans_dict_queid.get(
- answers[(len(answers) - 1 - idx) % len(answers)][:-1].lower(), [0]
- )
- for cap_id in cap_id_list:
- if cap_id not in mycontexts_id:
- Context_Prompt += caption[cap_id]
- mycontexts_id.append(cap_id)
- break # We just take one cap for each answer
- samples["Context_Prompt"] = Context_Prompt
- return Context_Prompt
-
- def create_task_prompt(
- self, samples, question_type="neural", num_question_per_img=30
- ):
- syn_question_queid = samples["questions"]
- syn_ans_queid = samples["answers"]
- Task_Prompt = ""
- for idx in range(num_question_per_img):
- # if config['random_question']:
- # qa_idx = random.randint(0, len(syn_question_queid) - 1)
- # else:
- qa_idx = idx
- if (
- question_type != "rule" and num_question_per_img > 0 and idx < 1
- ): ## yes and no questions for vqav2
- # Task_Prompt += "Question:"
- # Task_Prompt += syn_question_queid_next[-1]
- # Task_Prompt += '\n'
- # Task_Prompt += "Answer:no\n"
- Task_Prompt += "Question:"
- Task_Prompt += syn_question_queid[-1]
- Task_Prompt += "\n"
- Task_Prompt += "Answer:"
- Task_Prompt += "yes\n"
- Task_Prompt += "Question:Is this a toilet?\n"
- Task_Prompt += "Answer:no\n"
- if "question_type" == "rule": # Rule-Based Question Generation
- Noun_Questions = [
- "What item is this in this picture?",
- "What item is that in this picture?",
- ]
-
- Verb_Questions = [
- "What action is being done in this picture?",
- "Why is this item doing in this picture?",
- "Which action is being taken in this picture?",
- "What action is item doing in this picture?",
- "What action is item performing in this picture?",
- ]
-
- Adj_Questions = [
- "How to describe one item in this picture?",
- "What is item's ADJ TYPE in this picture?",
- "What is the ADJ TYPE in this picture?",
- ]
-
- Task_Prompt += "Question:"
- doc = self.nlp(syn_ans_queid[(qa_idx) % len(syn_ans_queid)][:-1].lower())
- if doc[-1].pos_ == "NOUN":
- Task_Prompt += Noun_Questions[
- random.randint(0, len(Noun_Questions) - 1)
- ]
- elif doc[-1].pos_ == "VERB":
- Task_Prompt += Verb_Questions[
- random.randint(0, len(Verb_Questions) - 1)
- ]
- elif doc[-1].pos_ == "ADJ":
- Task_Prompt += Adj_Questions[
- random.randint(0, len(Adj_Questions) - 1)
- ]
-
- Task_Prompt += "\n"
-
- Task_Prompt += "Answer:"
- Task_Prompt += syn_ans_queid[(qa_idx) % len(syn_ans_queid)][:-1].lower()
- Task_Prompt += "\n"
- samples["Task_Prompt"] = Task_Prompt
- # print(Task_Prompt)
- return Task_Prompt
-
- def prompts_construction(
- self,
- samples,
- question_type="neural",
- num_caps_per_img=30,
- num_question_per_img=30,
- ):
- Prompt = "Please reason the answer of the questions according to the given contexts.\n"
-
- Context_Prompt = self.create_context_prompt(samples, num_caps_per_img)
-
- Task_Prompt = self.create_task_prompt(
- samples, question_type, num_question_per_img
- )
-
- Img2Prompt = (
- Prompt
- + "Contexts:"
- + Context_Prompt
- + "\n"
- + Task_Prompt
- + "Question:"
- + samples["text_input"][0]
- + "\nAnswer:"
- )
- return Img2Prompt
-
- def prepare_LLM_input(
- self,
- samples,
- num_beams=1,
- inference_method="generate",
- max_len=20,
- min_len=0,
- internal_bsz_fid=1,
- num_captions=50,
- num_captions_fid=1,
- cap_max_length=20,
- cap_min_length=10,
- top_k=50,
- top_p=1,
- repetition_penalty=1,
- num_patches=20,
- block_num=7,
- ):
- """
- Args:
- samples (dict): A dictionary containing the following keys:
- - image (torch.Tensor): A tensor of shape (batch_size, 3, H, W). Default H=480, W=480.
- - text_input (str or [str]): String or a list of strings, each string is a question.
- The number of questions must be equal to the batch size. If a single string, will be converted to a list of string, with length 1 first.
- num_beams (int): Number of beams for beam search. 1 means no beam search.
- inference_method (str): Inference method. Must be "generate". The model will generate answers.
- max_len (int): Maximum length of generated answers.
- min_len (int): Minimum length of generated answers.
- internal_bsz_fid (int): Internal batch size when using FiD decoding.
- num_captions (int): Number of captions generated for each image.
- num_captions_fid (int): Number of captions concatenated with a question during FiD decoding.
- cap_max_length (int): The maximum length of the caption to be generated.
- cap_min_length (int): The minimum length of the caption to be generated.
- top_k (float): The number of the highest probability tokens for top-k sampling.
- top_p (float): The cumulative probability for nucleus sampling.
- repetition_penalty (float): The parameter for repetition penalty. 1.0 means no penalty.
- num_patches (int): Number of patches sampled for each image.
- block_num (int): The index of cross-attention block for gradcam computation.
-
- Returns:
- List: A list of strings, each string is an answer.
- gradcams (torch.Tensor): A tensor of shape (batch_size, H*W)
- captions (nested list): A nested list of strings of total length batch_size * num_captions
- """
- assert inference_method in [
- "generate",
- ], "Inference method must be 'generate', got {}.".format(inference_method)
-
- if isinstance(samples["text_input"], str):
- samples["text_input"] = [samples["text_input"]]
-
- assert len(samples["text_input"]) == samples["image"].size(
- 0
- ), "The number of questions must be equal to the batch size."
-
- samples = self.forward_itm(samples, block_num=block_num)
-
- samples = self.forward_cap(
- samples,
- cap_max_length=cap_max_length,
- cap_min_length=cap_min_length,
- top_k=top_k,
- top_p=top_p,
- repetition_penalty=repetition_penalty,
- num_captions=num_captions,
- num_patches=num_patches,
- )
-
- if self.offload_model:
- samples["image"] = samples["image"].to("cpu")
- self.image_question_matching_model.to("cpu")
- self.image_captioning_model.to("cpu")
- torch.cuda.empty_cache()
-
- pred_answers = self.forward_qa(
- samples,
- num_beams=num_beams,
- max_len=max_len,
- min_len=min_len,
- internal_bsz_fid=internal_bsz_fid,
- num_captions=num_captions,
- num_captions_fid=num_captions_fid,
- )
-
- if self.offload_model:
- self.image_question_matching_model.to(self.question_answering_model.device)
- self.image_captioning_model.to(self.question_answering_model.device)
-
- return pred_answers, samples["captions"], samples["gradcams"]
-
- @classmethod
- def from_config(cls, model_config):
- itm_config = model_config.image_question_matching_model
- cap_config = model_config.image_captioning_model
-
- itm_cls = registry.get_model_class(itm_config.arch)
- cap_cls = registry.get_model_class(cap_config.arch)
-
- image_question_matching_model = itm_cls.from_config(itm_config)
- image_captioning_model = cap_cls.from_config(cap_config)
-
- question_generation_tokenizer = T5Tokenizer.from_pretrained(
- "google/t5-large-lm-adapt"
- )
- question_generation_model = T5ForConditionalGeneration.from_pretrained(
- "google/t5-large-lm-adapt"
- )
- cached_file = download_cached_file(
- "https://storage.googleapis.com/sfr-vision-language-research/LAVIS/projects/img2prompt/T5_large_QG.pth",
- check_hash=False,
- progress=True,
- )
- checkpoint = torch.load(cached_file, map_location="cpu")
- state_dict = checkpoint["model"]
- question_generation_model.load_state_dict(state_dict)
- model = cls(
- image_question_matching_model=image_question_matching_model,
- image_captioning_model=image_captioning_model,
- question_generation_model=question_generation_model,
- question_generation_tokenizer=question_generation_tokenizer,
- offload_model=False,
- )
-
- return model
diff --git a/spaces/ShoaibMajidDar/PDF-chatbot/README.md b/spaces/ShoaibMajidDar/PDF-chatbot/README.md
deleted file mode 100644
index 8a2add6dc6a9d0856c34921255698151209a40da..0000000000000000000000000000000000000000
--- a/spaces/ShoaibMajidDar/PDF-chatbot/README.md
+++ /dev/null
@@ -1,13 +0,0 @@
----
-title: PDF Chatbot
-emoji: 👁
-colorFrom: pink
-colorTo: blue
-sdk: streamlit
-sdk_version: 1.21.0
-app_file: app.py
-pinned: false
-license: apache-2.0
----
-
-Check out the configuration reference at https://huggingface.co/docs/hub/spaces-config-reference
diff --git a/spaces/SungBeom/chatwine-korean/.venv/Lib/site-packages/docarray/index/__init__.py b/spaces/SungBeom/chatwine-korean/.venv/Lib/site-packages/docarray/index/__init__.py
deleted file mode 100644
index 9e4dbde474a7af9e10582967db4689ce59f7ce5b..0000000000000000000000000000000000000000
--- a/spaces/SungBeom/chatwine-korean/.venv/Lib/site-packages/docarray/index/__init__.py
+++ /dev/null
@@ -1,47 +0,0 @@
-import types
-from typing import TYPE_CHECKING
-
-from docarray.index.backends.in_memory import InMemoryExactNNIndex
-from docarray.utils._internal.misc import (
- _get_path_from_docarray_root_level,
- import_library,
-)
-
-if TYPE_CHECKING:
- from docarray.index.backends.elastic import ElasticDocIndex # noqa: F401
- from docarray.index.backends.elasticv7 import ElasticV7DocIndex # noqa: F401
- from docarray.index.backends.hnswlib import HnswDocumentIndex # noqa: F401
- from docarray.index.backends.qdrant import QdrantDocumentIndex # noqa: F401
- from docarray.index.backends.weaviate import WeaviateDocumentIndex # noqa: F401
-
-__all__ = ['InMemoryExactNNIndex']
-
-
-def __getattr__(name: str):
- lib: types.ModuleType
- if name == 'HnswDocumentIndex':
- import_library('hnswlib', raise_error=True)
- import docarray.index.backends.hnswlib as lib
- elif name == 'ElasticDocIndex':
- import_library('elasticsearch', raise_error=True)
- import docarray.index.backends.elastic as lib
- elif name == 'ElasticV7DocIndex':
- import_library('elasticsearch', raise_error=True)
- import docarray.index.backends.elasticv7 as lib
- elif name == 'QdrantDocumentIndex':
- import_library('qdrant_client', raise_error=True)
- import docarray.index.backends.qdrant as lib
- elif name == 'WeaviateDocumentIndex':
- import_library('weaviate', raise_error=True)
- import docarray.index.backends.weaviate as lib
- else:
- raise ImportError(
- f'cannot import name \'{name}\' from \'{_get_path_from_docarray_root_level(__file__)}\''
- )
-
- index_cls = getattr(lib, name)
-
- if name not in __all__:
- __all__.append(name)
-
- return index_cls
diff --git a/spaces/TD-jayadeera/Password_Strength_Prediction/app.py b/spaces/TD-jayadeera/Password_Strength_Prediction/app.py
deleted file mode 100644
index 7c38acda62eeeba8af06dee148297b529b8eb0af..0000000000000000000000000000000000000000
--- a/spaces/TD-jayadeera/Password_Strength_Prediction/app.py
+++ /dev/null
@@ -1,46 +0,0 @@
-import pandas as pd
-import numpy as np
-import seaborn as sns
-import warnings
-# import sklearn
-
-# data=pd.read_csv('data.csv',error_bad_lines=False)
-# data.head(5)
-# data['strength'].unique()
-# data.isna().sum()
-# data[data['password'].isnull()]
-# data.dropna(inplace=True)
-# data.isnull().sum()
-# sns.countplot(data['strength'])
-# data.sample(10)
-# password_tuple=np.array(data)
-# password_tuple
-# import random
-# random.shuffle(password_tuple)
-# x=[labels[0] for labels in password_tuple]
-# y=[labels[1] for labels in password_tuple]
-# def word_divide_char(inputs):
-# character=[]
-# for i in inputs:
-# character.append(i)
-# return character
-# word_divide_char('kzde5577')
-# from sklearn.feature_extraction.text import TfidfVectorizer
-# vectorizer=TfidfVectorizer(tokenizer=word_divide_char)
-# X=vectorizer.fit_transform(x)
-# X.shape
-# vectorizer.get_feature_names_out()
-# first_document_vector=X[0]
-# first_document_vector
-# first_document_vector.T.todense()
-# df=pd.DataFrame(first_document_vector.T.todense(),index=vectorizer.get_feature_names_out(),columns=['TF-IDF'])
-# df.sort_values(by=['TF-IDF'],ascending=False)
-import joblib
-
-model = joblib.load('finalized_model.sav')
-
-new_data = 'sdhb%jksdn&73e4d';
-new_data2=np.array([new_data])
-new_data3=vectorizer.transform(new_data2)
-predicted = model.predict(new_data3)
-print(predicted)
diff --git a/spaces/TabPFN/TabPFNPrediction/TabPFN/priors/mlp.py b/spaces/TabPFN/TabPFNPrediction/TabPFN/priors/mlp.py
deleted file mode 100644
index 6cc0bda18d4e0bf92cbb3643299b6de2717a6b9d..0000000000000000000000000000000000000000
--- a/spaces/TabPFN/TabPFNPrediction/TabPFN/priors/mlp.py
+++ /dev/null
@@ -1,188 +0,0 @@
-import random
-import math
-
-import torch
-from torch import nn
-import numpy as np
-
-from utils import default_device
-from .utils import get_batch_to_dataloader
-
-class GaussianNoise(nn.Module):
- def __init__(self, std, device):
- super().__init__()
- self.std = std
- self.device=device
-
- def forward(self, x):
- return x + torch.normal(torch.zeros_like(x), self.std)
-
-
-def causes_sampler_f(num_causes):
- means = np.random.normal(0, 1, (num_causes))
- std = np.abs(np.random.normal(0, 1, (num_causes)) * means)
- return means, std
-
-def get_batch(batch_size, seq_len, num_features, hyperparameters, device=default_device, num_outputs=1, sampling='normal'
- , epoch=None, **kwargs):
- if 'multiclass_type' in hyperparameters and hyperparameters['multiclass_type'] == 'multi_node':
- num_outputs = num_outputs * hyperparameters['num_classes']
-
- if not (('mix_activations' in hyperparameters) and hyperparameters['mix_activations']):
- s = hyperparameters['prior_mlp_activations']()
- hyperparameters['prior_mlp_activations'] = lambda : s
-
- class MLP(torch.nn.Module):
- def __init__(self, hyperparameters):
- super(MLP, self).__init__()
-
- with torch.no_grad():
-
- for key in hyperparameters:
- setattr(self, key, hyperparameters[key])
-
- assert (self.num_layers >= 2)
-
- if 'verbose' in hyperparameters and self.verbose:
- print({k : hyperparameters[k] for k in ['is_causal', 'num_causes', 'prior_mlp_hidden_dim'
- , 'num_layers', 'noise_std', 'y_is_effect', 'pre_sample_weights', 'prior_mlp_dropout_prob'
- , 'pre_sample_causes']})
-
- if self.is_causal:
- self.prior_mlp_hidden_dim = max(self.prior_mlp_hidden_dim, num_outputs + 2 * num_features)
- else:
- self.num_causes = num_features
-
- # This means that the mean and standard deviation of each cause is determined in advance
- if self.pre_sample_causes:
- self.causes_mean, self.causes_std = causes_sampler_f(self.num_causes)
- self.causes_mean = torch.tensor(self.causes_mean, device=device).unsqueeze(0).unsqueeze(0).tile(
- (seq_len, 1, 1))
- self.causes_std = torch.tensor(self.causes_std, device=device).unsqueeze(0).unsqueeze(0).tile(
- (seq_len, 1, 1))
-
- def generate_module(layer_idx, out_dim):
- # Determine std of each noise term in initialization, so that is shared in runs
- # torch.abs(torch.normal(torch.zeros((out_dim)), self.noise_std)) - Change std for each dimension?
- noise = (GaussianNoise(torch.abs(torch.normal(torch.zeros(size=(1, out_dim), device=device), float(self.noise_std))), device=device)
- if self.pre_sample_weights else GaussianNoise(float(self.noise_std), device=device))
- return [
- nn.Sequential(*[self.prior_mlp_activations()
- , nn.Linear(self.prior_mlp_hidden_dim, out_dim)
- , noise])
- ]
-
- self.layers = [nn.Linear(self.num_causes, self.prior_mlp_hidden_dim, device=device)]
- self.layers += [module for layer_idx in range(self.num_layers-1) for module in generate_module(layer_idx, self.prior_mlp_hidden_dim)]
- if not self.is_causal:
- self.layers += generate_module(-1, num_outputs)
- self.layers = nn.Sequential(*self.layers)
-
- # Initialize Model parameters
- for i, (n, p) in enumerate(self.layers.named_parameters()):
- if self.block_wise_dropout:
- if len(p.shape) == 2: # Only apply to weight matrices and not bias
- nn.init.zeros_(p)
- # TODO: N blocks should be a setting
- n_blocks = random.randint(1, math.ceil(math.sqrt(min(p.shape[0], p.shape[1]))))
- w, h = p.shape[0] // n_blocks, p.shape[1] // n_blocks
- keep_prob = (n_blocks*w*h) / p.numel()
- for block in range(0, n_blocks):
- nn.init.normal_(p[w * block: w * (block+1), h * block: h * (block+1)], std=self.init_std / keep_prob**(1/2 if self.prior_mlp_scale_weights_sqrt else 1))
- else:
- if len(p.shape) == 2: # Only apply to weight matrices and not bias
- dropout_prob = self.prior_mlp_dropout_prob if i > 0 else 0.0 # Don't apply dropout in first layer
- dropout_prob = min(dropout_prob, 0.99)
- nn.init.normal_(p, std=self.init_std / (1. - dropout_prob**(1/2 if self.prior_mlp_scale_weights_sqrt else 1)))
- p *= torch.bernoulli(torch.zeros_like(p) + 1. - dropout_prob)
-
- def forward(self):
- def sample_normal():
- if self.pre_sample_causes:
- causes = torch.normal(self.causes_mean, self.causes_std.abs()).float()
- else:
- causes = torch.normal(0., 1., (seq_len, 1, self.num_causes), device=device).float()
- return causes
-
- if self.sampling == 'normal':
- causes = sample_normal()
- elif self.sampling == 'mixed':
- zipf_p, multi_p, normal_p = random.random() * 0.66, random.random() * 0.66, random.random() * 0.66
- def sample_cause(n):
- if random.random() > normal_p:
- if self.pre_sample_causes:
- return torch.normal(self.causes_mean[:, :, n], self.causes_std[:, :, n].abs()).float()
- else:
- return torch.normal(0., 1., (seq_len, 1), device=device).float()
- elif random.random() > multi_p:
- x = torch.multinomial(torch.rand((random.randint(2, 10))), seq_len, replacement=True).to(device).unsqueeze(-1).float()
- x = (x - torch.mean(x)) / torch.std(x)
- return x
- else:
- x = torch.minimum(torch.tensor(np.random.zipf(2.0 + random.random() * 2, size=(seq_len)),
- device=device).unsqueeze(-1).float(), torch.tensor(10.0, device=device))
- return x - torch.mean(x)
- causes = torch.cat([sample_cause(n).unsqueeze(-1) for n in range(self.num_causes)], -1)
- elif self.sampling == 'uniform':
- causes = torch.rand((seq_len, 1, self.num_causes), device=device)
- else:
- raise ValueError(f'Sampling is set to invalid setting: {sampling}.')
-
- outputs = [causes]
- for layer in self.layers:
- outputs.append(layer(outputs[-1]))
- outputs = outputs[2:]
-
- if self.is_causal:
- ## Sample nodes from graph if model is causal
- outputs_flat = torch.cat(outputs, -1)
-
- if self.in_clique:
- random_perm = random.randint(0, outputs_flat.shape[-1] - num_outputs - num_features) + torch.randperm(num_outputs + num_features, device=device)
- else:
- random_perm = torch.randperm(outputs_flat.shape[-1]-1, device=device)
-
- random_idx_y = list(range(-num_outputs, -0)) if self.y_is_effect else random_perm[0:num_outputs]
- random_idx = random_perm[num_outputs:num_outputs + num_features]
-
- if self.sort_features:
- random_idx, _ = torch.sort(random_idx)
- y = outputs_flat[:, :, random_idx_y]
-
- x = outputs_flat[:, :, random_idx]
- else:
- y = outputs[-1][:, :, :]
- x = causes
-
- if bool(torch.any(torch.isnan(x)).detach().cpu().numpy()) or bool(torch.any(torch.isnan(y)).detach().cpu().numpy()):
- print('Nan caught in MLP model x:', torch.isnan(x).sum(), ' y:', torch.isnan(y).sum())
- print({k: hyperparameters[k] for k in ['is_causal', 'num_causes', 'prior_mlp_hidden_dim'
- , 'num_layers', 'noise_std', 'y_is_effect', 'pre_sample_weights', 'prior_mlp_dropout_prob'
- , 'pre_sample_causes']})
-
- x[:] = 0.0
- y[:] = -100 # default ignore index for CE
-
- # random feature rotation
- if self.random_feature_rotation:
- x = x[..., (torch.arange(x.shape[-1], device=device)+random.randrange(x.shape[-1])) % x.shape[-1]]
-
- return x, y
-
- if hyperparameters.get('new_mlp_per_example', False):
- get_model = lambda: MLP(hyperparameters).to(device)
- else:
- model = MLP(hyperparameters).to(device)
- get_model = lambda: model
-
- sample = [get_model()() for _ in range(0, batch_size)]
-
- x, y = zip(*sample)
- y = torch.cat(y, 1).detach().squeeze(2)
- x = torch.cat(x, 1).detach()
-
- return x, y, y
-
-
-DataLoader = get_batch_to_dataloader(get_batch)
-
diff --git a/spaces/TandCAcceptMe/face-swap-docker/mynewshinyroop/Lib/site-packages/pip/_vendor/__init__.py b/spaces/TandCAcceptMe/face-swap-docker/mynewshinyroop/Lib/site-packages/pip/_vendor/__init__.py
deleted file mode 100644
index b22f7abb93b9d7aeee50829b35746aaa3f9f5feb..0000000000000000000000000000000000000000
--- a/spaces/TandCAcceptMe/face-swap-docker/mynewshinyroop/Lib/site-packages/pip/_vendor/__init__.py
+++ /dev/null
@@ -1,120 +0,0 @@
-"""
-pip._vendor is for vendoring dependencies of pip to prevent needing pip to
-depend on something external.
-
-Files inside of pip._vendor should be considered immutable and should only be
-updated to versions from upstream.
-"""
-from __future__ import absolute_import
-
-import glob
-import os.path
-import sys
-
-# Downstream redistributors which have debundled our dependencies should also
-# patch this value to be true. This will trigger the additional patching
-# to cause things like "six" to be available as pip.
-DEBUNDLED = False
-
-# By default, look in this directory for a bunch of .whl files which we will
-# add to the beginning of sys.path before attempting to import anything. This
-# is done to support downstream re-distributors like Debian and Fedora who
-# wish to create their own Wheels for our dependencies to aid in debundling.
-WHEEL_DIR = os.path.abspath(os.path.dirname(__file__))
-
-
-# Define a small helper function to alias our vendored modules to the real ones
-# if the vendored ones do not exist. This idea of this was taken from
-# https://github.com/kennethreitz/requests/pull/2567.
-def vendored(modulename):
- vendored_name = "{0}.{1}".format(__name__, modulename)
-
- try:
- __import__(modulename, globals(), locals(), level=0)
- except ImportError:
- # We can just silently allow import failures to pass here. If we
- # got to this point it means that ``import pip._vendor.whatever``
- # failed and so did ``import whatever``. Since we're importing this
- # upfront in an attempt to alias imports, not erroring here will
- # just mean we get a regular import error whenever pip *actually*
- # tries to import one of these modules to use it, which actually
- # gives us a better error message than we would have otherwise
- # gotten.
- pass
- else:
- sys.modules[vendored_name] = sys.modules[modulename]
- base, head = vendored_name.rsplit(".", 1)
- setattr(sys.modules[base], head, sys.modules[modulename])
-
-
-# If we're operating in a debundled setup, then we want to go ahead and trigger
-# the aliasing of our vendored libraries as well as looking for wheels to add
-# to our sys.path. This will cause all of this code to be a no-op typically
-# however downstream redistributors can enable it in a consistent way across
-# all platforms.
-if DEBUNDLED:
- # Actually look inside of WHEEL_DIR to find .whl files and add them to the
- # front of our sys.path.
- sys.path[:] = glob.glob(os.path.join(WHEEL_DIR, "*.whl")) + sys.path
-
- # Actually alias all of our vendored dependencies.
- vendored("cachecontrol")
- vendored("certifi")
- vendored("colorama")
- vendored("distlib")
- vendored("distro")
- vendored("six")
- vendored("six.moves")
- vendored("six.moves.urllib")
- vendored("six.moves.urllib.parse")
- vendored("packaging")
- vendored("packaging.version")
- vendored("packaging.specifiers")
- vendored("pep517")
- vendored("pkg_resources")
- vendored("platformdirs")
- vendored("progress")
- vendored("requests")
- vendored("requests.exceptions")
- vendored("requests.packages")
- vendored("requests.packages.urllib3")
- vendored("requests.packages.urllib3._collections")
- vendored("requests.packages.urllib3.connection")
- vendored("requests.packages.urllib3.connectionpool")
- vendored("requests.packages.urllib3.contrib")
- vendored("requests.packages.urllib3.contrib.ntlmpool")
- vendored("requests.packages.urllib3.contrib.pyopenssl")
- vendored("requests.packages.urllib3.exceptions")
- vendored("requests.packages.urllib3.fields")
- vendored("requests.packages.urllib3.filepost")
- vendored("requests.packages.urllib3.packages")
- vendored("requests.packages.urllib3.packages.ordered_dict")
- vendored("requests.packages.urllib3.packages.six")
- vendored("requests.packages.urllib3.packages.ssl_match_hostname")
- vendored("requests.packages.urllib3.packages.ssl_match_hostname."
- "_implementation")
- vendored("requests.packages.urllib3.poolmanager")
- vendored("requests.packages.urllib3.request")
- vendored("requests.packages.urllib3.response")
- vendored("requests.packages.urllib3.util")
- vendored("requests.packages.urllib3.util.connection")
- vendored("requests.packages.urllib3.util.request")
- vendored("requests.packages.urllib3.util.response")
- vendored("requests.packages.urllib3.util.retry")
- vendored("requests.packages.urllib3.util.ssl_")
- vendored("requests.packages.urllib3.util.timeout")
- vendored("requests.packages.urllib3.util.url")
- vendored("resolvelib")
- vendored("rich")
- vendored("rich.console")
- vendored("rich.highlighter")
- vendored("rich.logging")
- vendored("rich.markup")
- vendored("rich.progress")
- vendored("rich.segment")
- vendored("rich.style")
- vendored("rich.text")
- vendored("rich.traceback")
- vendored("tenacity")
- vendored("tomli")
- vendored("urllib3")
diff --git a/spaces/TandCAcceptMe/face-swap-docker/mynewshinyroop/Lib/site-packages/pip/_vendor/rich/filesize.py b/spaces/TandCAcceptMe/face-swap-docker/mynewshinyroop/Lib/site-packages/pip/_vendor/rich/filesize.py
deleted file mode 100644
index 99f118e20103174993b865cfb43ac6b6e00296a4..0000000000000000000000000000000000000000
--- a/spaces/TandCAcceptMe/face-swap-docker/mynewshinyroop/Lib/site-packages/pip/_vendor/rich/filesize.py
+++ /dev/null
@@ -1,89 +0,0 @@
-# coding: utf-8
-"""Functions for reporting filesizes. Borrowed from https://github.com/PyFilesystem/pyfilesystem2
-
-The functions declared in this module should cover the different
-use cases needed to generate a string representation of a file size
-using several different units. Since there are many standards regarding
-file size units, three different functions have been implemented.
-
-See Also:
- * `Wikipedia: Binary prefix `_
-
-"""
-
-__all__ = ["decimal"]
-
-from typing import Iterable, List, Optional, Tuple
-
-
-def _to_str(
- size: int,
- suffixes: Iterable[str],
- base: int,
- *,
- precision: Optional[int] = 1,
- separator: Optional[str] = " ",
-) -> str:
- if size == 1:
- return "1 byte"
- elif size < base:
- return "{:,} bytes".format(size)
-
- for i, suffix in enumerate(suffixes, 2): # noqa: B007
- unit = base**i
- if size < unit:
- break
- return "{:,.{precision}f}{separator}{}".format(
- (base * size / unit),
- suffix,
- precision=precision,
- separator=separator,
- )
-
-
-def pick_unit_and_suffix(size: int, suffixes: List[str], base: int) -> Tuple[int, str]:
- """Pick a suffix and base for the given size."""
- for i, suffix in enumerate(suffixes):
- unit = base**i
- if size < unit * base:
- break
- return unit, suffix
-
-
-def decimal(
- size: int,
- *,
- precision: Optional[int] = 1,
- separator: Optional[str] = " ",
-) -> str:
- """Convert a filesize in to a string (powers of 1000, SI prefixes).
-
- In this convention, ``1000 B = 1 kB``.
-
- This is typically the format used to advertise the storage
- capacity of USB flash drives and the like (*256 MB* meaning
- actually a storage capacity of more than *256 000 000 B*),
- or used by **Mac OS X** since v10.6 to report file sizes.
-
- Arguments:
- int (size): A file size.
- int (precision): The number of decimal places to include (default = 1).
- str (separator): The string to separate the value from the units (default = " ").
-
- Returns:
- `str`: A string containing a abbreviated file size and units.
-
- Example:
- >>> filesize.decimal(30000)
- '30.0 kB'
- >>> filesize.decimal(30000, precision=2, separator="")
- '30.00kB'
-
- """
- return _to_str(
- size,
- ("kB", "MB", "GB", "TB", "PB", "EB", "ZB", "YB"),
- 1000,
- precision=precision,
- separator=separator,
- )
diff --git a/spaces/Thafx/sdrv51/README.md b/spaces/Thafx/sdrv51/README.md
deleted file mode 100644
index 762c80e4d800894f6f419991c543f7045fc3cdda..0000000000000000000000000000000000000000
--- a/spaces/Thafx/sdrv51/README.md
+++ /dev/null
@@ -1,20 +0,0 @@
----
-title: Realistic Vision v5.1
-emoji: 📷
-colorFrom: yellow
-colorTo: red
-sdk: gradio
-sdk_version: 3.18.0
-app_file: app.py
-pinned: true
-duplicated_from: Thafx/sdrv50
-tags:
-- stable-diffusion
-- stable-diffusion-diffusers
-- text-to-image
-- realistic-vision
-models:
-- SG161222/Realistic_Vision_V5.1_noVAE
----
-
-Check out the configuration reference at https://huggingface.co/docs/hub/spaces-config-reference
\ No newline at end of file
diff --git a/spaces/Thaweewat/ControlNet-Architecture/ldm/models/diffusion/ddim.py b/spaces/Thaweewat/ControlNet-Architecture/ldm/models/diffusion/ddim.py
deleted file mode 100644
index 27ead0ea914c64c747b64e690662899fb3801144..0000000000000000000000000000000000000000
--- a/spaces/Thaweewat/ControlNet-Architecture/ldm/models/diffusion/ddim.py
+++ /dev/null
@@ -1,336 +0,0 @@
-"""SAMPLING ONLY."""
-
-import torch
-import numpy as np
-from tqdm import tqdm
-
-from ldm.modules.diffusionmodules.util import make_ddim_sampling_parameters, make_ddim_timesteps, noise_like, extract_into_tensor
-
-
-class DDIMSampler(object):
- def __init__(self, model, schedule="linear", **kwargs):
- super().__init__()
- self.model = model
- self.ddpm_num_timesteps = model.num_timesteps
- self.schedule = schedule
-
- def register_buffer(self, name, attr):
- if type(attr) == torch.Tensor:
- if attr.device != torch.device("cuda"):
- attr = attr.to(torch.device("cuda"))
- setattr(self, name, attr)
-
- def make_schedule(self, ddim_num_steps, ddim_discretize="uniform", ddim_eta=0., verbose=True):
- self.ddim_timesteps = make_ddim_timesteps(ddim_discr_method=ddim_discretize, num_ddim_timesteps=ddim_num_steps,
- num_ddpm_timesteps=self.ddpm_num_timesteps,verbose=verbose)
- alphas_cumprod = self.model.alphas_cumprod
- assert alphas_cumprod.shape[0] == self.ddpm_num_timesteps, 'alphas have to be defined for each timestep'
- to_torch = lambda x: x.clone().detach().to(torch.float32).to(self.model.device)
-
- self.register_buffer('betas', to_torch(self.model.betas))
- self.register_buffer('alphas_cumprod', to_torch(alphas_cumprod))
- self.register_buffer('alphas_cumprod_prev', to_torch(self.model.alphas_cumprod_prev))
-
- # calculations for diffusion q(x_t | x_{t-1}) and others
- self.register_buffer('sqrt_alphas_cumprod', to_torch(np.sqrt(alphas_cumprod.cpu())))
- self.register_buffer('sqrt_one_minus_alphas_cumprod', to_torch(np.sqrt(1. - alphas_cumprod.cpu())))
- self.register_buffer('log_one_minus_alphas_cumprod', to_torch(np.log(1. - alphas_cumprod.cpu())))
- self.register_buffer('sqrt_recip_alphas_cumprod', to_torch(np.sqrt(1. / alphas_cumprod.cpu())))
- self.register_buffer('sqrt_recipm1_alphas_cumprod', to_torch(np.sqrt(1. / alphas_cumprod.cpu() - 1)))
-
- # ddim sampling parameters
- ddim_sigmas, ddim_alphas, ddim_alphas_prev = make_ddim_sampling_parameters(alphacums=alphas_cumprod.cpu(),
- ddim_timesteps=self.ddim_timesteps,
- eta=ddim_eta,verbose=verbose)
- self.register_buffer('ddim_sigmas', ddim_sigmas)
- self.register_buffer('ddim_alphas', ddim_alphas)
- self.register_buffer('ddim_alphas_prev', ddim_alphas_prev)
- self.register_buffer('ddim_sqrt_one_minus_alphas', np.sqrt(1. - ddim_alphas))
- sigmas_for_original_sampling_steps = ddim_eta * torch.sqrt(
- (1 - self.alphas_cumprod_prev) / (1 - self.alphas_cumprod) * (
- 1 - self.alphas_cumprod / self.alphas_cumprod_prev))
- self.register_buffer('ddim_sigmas_for_original_num_steps', sigmas_for_original_sampling_steps)
-
- @torch.no_grad()
- def sample(self,
- S,
- batch_size,
- shape,
- conditioning=None,
- callback=None,
- normals_sequence=None,
- img_callback=None,
- quantize_x0=False,
- eta=0.,
- mask=None,
- x0=None,
- temperature=1.,
- noise_dropout=0.,
- score_corrector=None,
- corrector_kwargs=None,
- verbose=True,
- x_T=None,
- log_every_t=100,
- unconditional_guidance_scale=1.,
- unconditional_conditioning=None, # this has to come in the same format as the conditioning, # e.g. as encoded tokens, ...
- dynamic_threshold=None,
- ucg_schedule=None,
- **kwargs
- ):
- if conditioning is not None:
- if isinstance(conditioning, dict):
- ctmp = conditioning[list(conditioning.keys())[0]]
- while isinstance(ctmp, list): ctmp = ctmp[0]
- cbs = ctmp.shape[0]
- if cbs != batch_size:
- print(f"Warning: Got {cbs} conditionings but batch-size is {batch_size}")
-
- elif isinstance(conditioning, list):
- for ctmp in conditioning:
- if ctmp.shape[0] != batch_size:
- print(f"Warning: Got {cbs} conditionings but batch-size is {batch_size}")
-
- else:
- if conditioning.shape[0] != batch_size:
- print(f"Warning: Got {conditioning.shape[0]} conditionings but batch-size is {batch_size}")
-
- self.make_schedule(ddim_num_steps=S, ddim_eta=eta, verbose=verbose)
- # sampling
- C, H, W = shape
- size = (batch_size, C, H, W)
- print(f'Data shape for DDIM sampling is {size}, eta {eta}')
-
- samples, intermediates = self.ddim_sampling(conditioning, size,
- callback=callback,
- img_callback=img_callback,
- quantize_denoised=quantize_x0,
- mask=mask, x0=x0,
- ddim_use_original_steps=False,
- noise_dropout=noise_dropout,
- temperature=temperature,
- score_corrector=score_corrector,
- corrector_kwargs=corrector_kwargs,
- x_T=x_T,
- log_every_t=log_every_t,
- unconditional_guidance_scale=unconditional_guidance_scale,
- unconditional_conditioning=unconditional_conditioning,
- dynamic_threshold=dynamic_threshold,
- ucg_schedule=ucg_schedule
- )
- return samples, intermediates
-
- @torch.no_grad()
- def ddim_sampling(self, cond, shape,
- x_T=None, ddim_use_original_steps=False,
- callback=None, timesteps=None, quantize_denoised=False,
- mask=None, x0=None, img_callback=None, log_every_t=100,
- temperature=1., noise_dropout=0., score_corrector=None, corrector_kwargs=None,
- unconditional_guidance_scale=1., unconditional_conditioning=None, dynamic_threshold=None,
- ucg_schedule=None):
- device = self.model.betas.device
- b = shape[0]
- if x_T is None:
- img = torch.randn(shape, device=device)
- else:
- img = x_T
-
- if timesteps is None:
- timesteps = self.ddpm_num_timesteps if ddim_use_original_steps else self.ddim_timesteps
- elif timesteps is not None and not ddim_use_original_steps:
- subset_end = int(min(timesteps / self.ddim_timesteps.shape[0], 1) * self.ddim_timesteps.shape[0]) - 1
- timesteps = self.ddim_timesteps[:subset_end]
-
- intermediates = {'x_inter': [img], 'pred_x0': [img]}
- time_range = reversed(range(0,timesteps)) if ddim_use_original_steps else np.flip(timesteps)
- total_steps = timesteps if ddim_use_original_steps else timesteps.shape[0]
- print(f"Running DDIM Sampling with {total_steps} timesteps")
-
- iterator = tqdm(time_range, desc='DDIM Sampler', total=total_steps)
-
- for i, step in enumerate(iterator):
- index = total_steps - i - 1
- ts = torch.full((b,), step, device=device, dtype=torch.long)
-
- if mask is not None:
- assert x0 is not None
- img_orig = self.model.q_sample(x0, ts) # TODO: deterministic forward pass?
- img = img_orig * mask + (1. - mask) * img
-
- if ucg_schedule is not None:
- assert len(ucg_schedule) == len(time_range)
- unconditional_guidance_scale = ucg_schedule[i]
-
- outs = self.p_sample_ddim(img, cond, ts, index=index, use_original_steps=ddim_use_original_steps,
- quantize_denoised=quantize_denoised, temperature=temperature,
- noise_dropout=noise_dropout, score_corrector=score_corrector,
- corrector_kwargs=corrector_kwargs,
- unconditional_guidance_scale=unconditional_guidance_scale,
- unconditional_conditioning=unconditional_conditioning,
- dynamic_threshold=dynamic_threshold)
- img, pred_x0 = outs
- if callback: callback(i)
- if img_callback: img_callback(pred_x0, i)
-
- if index % log_every_t == 0 or index == total_steps - 1:
- intermediates['x_inter'].append(img)
- intermediates['pred_x0'].append(pred_x0)
-
- return img, intermediates
-
- @torch.no_grad()
- def p_sample_ddim(self, x, c, t, index, repeat_noise=False, use_original_steps=False, quantize_denoised=False,
- temperature=1., noise_dropout=0., score_corrector=None, corrector_kwargs=None,
- unconditional_guidance_scale=1., unconditional_conditioning=None,
- dynamic_threshold=None):
- b, *_, device = *x.shape, x.device
-
- if unconditional_conditioning is None or unconditional_guidance_scale == 1.:
- model_output = self.model.apply_model(x, t, c)
- else:
- x_in = torch.cat([x] * 2)
- t_in = torch.cat([t] * 2)
- if isinstance(c, dict):
- assert isinstance(unconditional_conditioning, dict)
- c_in = dict()
- for k in c:
- if isinstance(c[k], list):
- c_in[k] = [torch.cat([
- unconditional_conditioning[k][i],
- c[k][i]]) for i in range(len(c[k]))]
- else:
- c_in[k] = torch.cat([
- unconditional_conditioning[k],
- c[k]])
- elif isinstance(c, list):
- c_in = list()
- assert isinstance(unconditional_conditioning, list)
- for i in range(len(c)):
- c_in.append(torch.cat([unconditional_conditioning[i], c[i]]))
- else:
- c_in = torch.cat([unconditional_conditioning, c])
- model_uncond, model_t = self.model.apply_model(x_in, t_in, c_in).chunk(2)
- model_output = model_uncond + unconditional_guidance_scale * (model_t - model_uncond)
-
- if self.model.parameterization == "v":
- e_t = self.model.predict_eps_from_z_and_v(x, t, model_output)
- else:
- e_t = model_output
-
- if score_corrector is not None:
- assert self.model.parameterization == "eps", 'not implemented'
- e_t = score_corrector.modify_score(self.model, e_t, x, t, c, **corrector_kwargs)
-
- alphas = self.model.alphas_cumprod if use_original_steps else self.ddim_alphas
- alphas_prev = self.model.alphas_cumprod_prev if use_original_steps else self.ddim_alphas_prev
- sqrt_one_minus_alphas = self.model.sqrt_one_minus_alphas_cumprod if use_original_steps else self.ddim_sqrt_one_minus_alphas
- sigmas = self.model.ddim_sigmas_for_original_num_steps if use_original_steps else self.ddim_sigmas
- # select parameters corresponding to the currently considered timestep
- a_t = torch.full((b, 1, 1, 1), alphas[index], device=device)
- a_prev = torch.full((b, 1, 1, 1), alphas_prev[index], device=device)
- sigma_t = torch.full((b, 1, 1, 1), sigmas[index], device=device)
- sqrt_one_minus_at = torch.full((b, 1, 1, 1), sqrt_one_minus_alphas[index],device=device)
-
- # current prediction for x_0
- if self.model.parameterization != "v":
- pred_x0 = (x - sqrt_one_minus_at * e_t) / a_t.sqrt()
- else:
- pred_x0 = self.model.predict_start_from_z_and_v(x, t, model_output)
-
- if quantize_denoised:
- pred_x0, _, *_ = self.model.first_stage_model.quantize(pred_x0)
-
- if dynamic_threshold is not None:
- raise NotImplementedError()
-
- # direction pointing to x_t
- dir_xt = (1. - a_prev - sigma_t**2).sqrt() * e_t
- noise = sigma_t * noise_like(x.shape, device, repeat_noise) * temperature
- if noise_dropout > 0.:
- noise = torch.nn.functional.dropout(noise, p=noise_dropout)
- x_prev = a_prev.sqrt() * pred_x0 + dir_xt + noise
- return x_prev, pred_x0
-
- @torch.no_grad()
- def encode(self, x0, c, t_enc, use_original_steps=False, return_intermediates=None,
- unconditional_guidance_scale=1.0, unconditional_conditioning=None, callback=None):
- num_reference_steps = self.ddpm_num_timesteps if use_original_steps else self.ddim_timesteps.shape[0]
-
- assert t_enc <= num_reference_steps
- num_steps = t_enc
-
- if use_original_steps:
- alphas_next = self.alphas_cumprod[:num_steps]
- alphas = self.alphas_cumprod_prev[:num_steps]
- else:
- alphas_next = self.ddim_alphas[:num_steps]
- alphas = torch.tensor(self.ddim_alphas_prev[:num_steps])
-
- x_next = x0
- intermediates = []
- inter_steps = []
- for i in tqdm(range(num_steps), desc='Encoding Image'):
- t = torch.full((x0.shape[0],), i, device=self.model.device, dtype=torch.long)
- if unconditional_guidance_scale == 1.:
- noise_pred = self.model.apply_model(x_next, t, c)
- else:
- assert unconditional_conditioning is not None
- e_t_uncond, noise_pred = torch.chunk(
- self.model.apply_model(torch.cat((x_next, x_next)), torch.cat((t, t)),
- torch.cat((unconditional_conditioning, c))), 2)
- noise_pred = e_t_uncond + unconditional_guidance_scale * (noise_pred - e_t_uncond)
-
- xt_weighted = (alphas_next[i] / alphas[i]).sqrt() * x_next
- weighted_noise_pred = alphas_next[i].sqrt() * (
- (1 / alphas_next[i] - 1).sqrt() - (1 / alphas[i] - 1).sqrt()) * noise_pred
- x_next = xt_weighted + weighted_noise_pred
- if return_intermediates and i % (
- num_steps // return_intermediates) == 0 and i < num_steps - 1:
- intermediates.append(x_next)
- inter_steps.append(i)
- elif return_intermediates and i >= num_steps - 2:
- intermediates.append(x_next)
- inter_steps.append(i)
- if callback: callback(i)
-
- out = {'x_encoded': x_next, 'intermediate_steps': inter_steps}
- if return_intermediates:
- out.update({'intermediates': intermediates})
- return x_next, out
-
- @torch.no_grad()
- def stochastic_encode(self, x0, t, use_original_steps=False, noise=None):
- # fast, but does not allow for exact reconstruction
- # t serves as an index to gather the correct alphas
- if use_original_steps:
- sqrt_alphas_cumprod = self.sqrt_alphas_cumprod
- sqrt_one_minus_alphas_cumprod = self.sqrt_one_minus_alphas_cumprod
- else:
- sqrt_alphas_cumprod = torch.sqrt(self.ddim_alphas)
- sqrt_one_minus_alphas_cumprod = self.ddim_sqrt_one_minus_alphas
-
- if noise is None:
- noise = torch.randn_like(x0)
- return (extract_into_tensor(sqrt_alphas_cumprod, t, x0.shape) * x0 +
- extract_into_tensor(sqrt_one_minus_alphas_cumprod, t, x0.shape) * noise)
-
- @torch.no_grad()
- def decode(self, x_latent, cond, t_start, unconditional_guidance_scale=1.0, unconditional_conditioning=None,
- use_original_steps=False, callback=None):
-
- timesteps = np.arange(self.ddpm_num_timesteps) if use_original_steps else self.ddim_timesteps
- timesteps = timesteps[:t_start]
-
- time_range = np.flip(timesteps)
- total_steps = timesteps.shape[0]
- print(f"Running DDIM Sampling with {total_steps} timesteps")
-
- iterator = tqdm(time_range, desc='Decoding image', total=total_steps)
- x_dec = x_latent
- for i, step in enumerate(iterator):
- index = total_steps - i - 1
- ts = torch.full((x_latent.shape[0],), step, device=x_latent.device, dtype=torch.long)
- x_dec, _ = self.p_sample_ddim(x_dec, cond, ts, index=index, use_original_steps=use_original_steps,
- unconditional_guidance_scale=unconditional_guidance_scale,
- unconditional_conditioning=unconditional_conditioning)
- if callback: callback(i)
- return x_dec
\ No newline at end of file
diff --git a/spaces/Tune-A-Video-library/Tune-A-Video-inference/app.py b/spaces/Tune-A-Video-library/Tune-A-Video-inference/app.py
deleted file mode 100644
index 1bfa4fb220142a294901d042ba6fa01887f8619e..0000000000000000000000000000000000000000
--- a/spaces/Tune-A-Video-library/Tune-A-Video-inference/app.py
+++ /dev/null
@@ -1,220 +0,0 @@
-#!/usr/bin/env python
-
-from __future__ import annotations
-
-import os
-
-import gradio as gr
-import torch
-
-from inference import InferencePipeline
-
-
-class InferenceUtil:
- def __init__(self, hf_token: str | None):
- self.hf_token = hf_token
-
- def load_model_info(self, model_id: str) -> tuple[str, str]:
- try:
- card = InferencePipeline.get_model_card(model_id, self.hf_token)
- except Exception:
- return "", ""
- base_model = getattr(card.data, "base_model", "")
- training_prompt = getattr(card.data, "training_prompt", "")
- return base_model, training_prompt
-
-
-DESCRIPTION = "# [Tune-A-Video](https://tuneavideo.github.io/)"
-if not torch.cuda.is_available():
- DESCRIPTION += "\n
Running on CPU 🥶 This demo does not work on CPU.
"
-
-CACHE_EXAMPLES = torch.cuda.is_available() and os.getenv("CACHE_EXAMPLES") == "1"
-
-HF_TOKEN = os.getenv("HF_TOKEN")
-pipe = InferencePipeline(HF_TOKEN)
-app = InferenceUtil(HF_TOKEN)
-
-with gr.Blocks(css="style.css") as demo:
- gr.Markdown(DESCRIPTION)
- gr.DuplicateButton(
- value="Duplicate Space for private use",
- elem_id="duplicate-button",
- visible=os.getenv("SHOW_DUPLICATE_BUTTON") == "1",
- )
-
- with gr.Row():
- with gr.Column():
- with gr.Box():
- model_id = gr.Dropdown(
- label="Model ID",
- choices=[
- "Tune-A-Video-library/a-man-is-surfing",
- "Tune-A-Video-library/mo-di-bear-guitar",
- "Tune-A-Video-library/redshift-man-skiing",
- ],
- value="Tune-A-Video-library/a-man-is-surfing",
- )
- with gr.Accordion(label="Model info (Base model and prompt used for training)", open=False):
- with gr.Row():
- base_model_used_for_training = gr.Text(label="Base model", interactive=False)
- prompt_used_for_training = gr.Text(label="Training prompt", interactive=False)
- prompt = gr.Textbox(label="Prompt", max_lines=1, placeholder='Example: "A panda is surfing"')
- video_length = gr.Slider(label="Video length", minimum=4, maximum=12, step=1, value=8)
- fps = gr.Slider(label="FPS", minimum=1, maximum=12, step=1, value=1)
- seed = gr.Slider(label="Seed", minimum=0, maximum=100000, step=1, value=0)
- with gr.Accordion("Other Parameters", open=False):
- num_steps = gr.Slider(label="Number of Steps", minimum=0, maximum=100, step=1, value=50)
- guidance_scale = gr.Slider(label="CFG Scale", minimum=0, maximum=50, step=0.1, value=7.5)
-
- run_button = gr.Button("Generate")
-
- gr.Markdown(
- """
- - It takes a few minutes to download model first.
- - Expected time to generate an 8-frame video: 70 seconds with T4, 24 seconds with A10G, (10 seconds with A100)
- """
- )
- with gr.Column():
- result = gr.Video(label="Result")
- with gr.Row():
- examples = [
- [
- "Tune-A-Video-library/a-man-is-surfing",
- "A panda is surfing.",
- 8,
- 1,
- 3,
- 50,
- 7.5,
- ],
- [
- "Tune-A-Video-library/a-man-is-surfing",
- "A racoon is surfing, cartoon style.",
- 8,
- 1,
- 3,
- 50,
- 7.5,
- ],
- [
- "Tune-A-Video-library/mo-di-bear-guitar",
- "a handsome prince is playing guitar, modern disney style.",
- 8,
- 1,
- 123,
- 50,
- 7.5,
- ],
- [
- "Tune-A-Video-library/mo-di-bear-guitar",
- "a magical princess is playing guitar, modern disney style.",
- 8,
- 1,
- 123,
- 50,
- 7.5,
- ],
- [
- "Tune-A-Video-library/mo-di-bear-guitar",
- "a rabbit is playing guitar, modern disney style.",
- 8,
- 1,
- 123,
- 50,
- 7.5,
- ],
- [
- "Tune-A-Video-library/mo-di-bear-guitar",
- "a baby is playing guitar, modern disney style.",
- 8,
- 1,
- 123,
- 50,
- 7.5,
- ],
- [
- "Tune-A-Video-library/redshift-man-skiing",
- "(redshift style) spider man is skiing.",
- 8,
- 1,
- 123,
- 50,
- 7.5,
- ],
- [
- "Tune-A-Video-library/redshift-man-skiing",
- "(redshift style) black widow is skiing.",
- 8,
- 1,
- 123,
- 50,
- 7.5,
- ],
- [
- "Tune-A-Video-library/redshift-man-skiing",
- "(redshift style) batman is skiing.",
- 8,
- 1,
- 123,
- 50,
- 7.5,
- ],
- [
- "Tune-A-Video-library/redshift-man-skiing",
- "(redshift style) hulk is skiing.",
- 8,
- 1,
- 123,
- 50,
- 7.5,
- ],
- ]
- gr.Examples(
- examples=examples,
- inputs=[
- model_id,
- prompt,
- video_length,
- fps,
- seed,
- num_steps,
- guidance_scale,
- ],
- outputs=result,
- fn=pipe.run,
- cache_examples=CACHE_EXAMPLES,
- )
-
- model_id.change(
- fn=app.load_model_info,
- inputs=model_id,
- outputs=[
- base_model_used_for_training,
- prompt_used_for_training,
- ],
- api_name=False,
- )
- inputs = [
- model_id,
- prompt,
- video_length,
- fps,
- seed,
- num_steps,
- guidance_scale,
- ]
- prompt.submit(
- fn=pipe.run,
- inputs=inputs,
- outputs=result,
- api_name=False,
- )
- run_button.click(
- fn=pipe.run,
- inputs=inputs,
- outputs=result,
- api_name="run",
- )
-
-if __name__ == "__main__":
- demo.queue(max_size=20).launch()
diff --git a/spaces/Wrathless/Dkrotzer-MusicalMagic/audiocraft/models/musicgen.py b/spaces/Wrathless/Dkrotzer-MusicalMagic/audiocraft/models/musicgen.py
deleted file mode 100644
index c3feb18d95c3915dae0074aacd1d4c980c1bb0e0..0000000000000000000000000000000000000000
--- a/spaces/Wrathless/Dkrotzer-MusicalMagic/audiocraft/models/musicgen.py
+++ /dev/null
@@ -1,283 +0,0 @@
-# Copyright (c) Meta Platforms, Inc. and affiliates.
-# All rights reserved.
-#
-# This source code is licensed under the license found in the
-# LICENSE file in the root directory of this source tree.
-
-"""
-Main model for using MusicGen. This will combine all the required components
-and provide easy access to the generation API.
-"""
-
-import os
-import typing as tp
-
-import torch
-
-from .encodec import CompressionModel
-from .lm import LMModel
-from .builders import get_debug_compression_model, get_debug_lm_model
-from .loaders import load_compression_model, load_lm_model, HF_MODEL_CHECKPOINTS_MAP
-from ..data.audio_utils import convert_audio
-from ..modules.conditioners import ConditioningAttributes, WavCondition
-from ..utils.autocast import TorchAutocast
-
-
-MelodyList = tp.List[tp.Optional[torch.Tensor]]
-MelodyType = tp.Union[torch.Tensor, MelodyList]
-
-
-class MusicGen:
- """MusicGen main model with convenient generation API.
-
- Args:
- name (str): name of the model.
- compression_model (CompressionModel): Compression model
- used to map audio to invertible discrete representations.
- lm (LMModel): Language model over discrete representations.
- """
- def __init__(self, name: str, compression_model: CompressionModel, lm: LMModel):
- self.name = name
- self.compression_model = compression_model
- self.lm = lm
- self.device = next(iter(lm.parameters())).device
- self.generation_params: dict = {}
- self.set_generation_params(duration=15) # 15 seconds by default
- if self.device.type == 'cpu':
- self.autocast = TorchAutocast(enabled=False)
- else:
- self.autocast = TorchAutocast(
- enabled=True, device_type=self.device.type, dtype=torch.float16)
-
- @property
- def frame_rate(self) -> int:
- """Roughly the number of AR steps per seconds."""
- return self.compression_model.frame_rate
-
- @property
- def sample_rate(self) -> int:
- """Sample rate of the generated audio."""
- return self.compression_model.sample_rate
-
- @property
- def audio_channels(self) -> int:
- """Audio channels of the generated audio."""
- return self.compression_model.channels
-
- @staticmethod
- def get_pretrained(name: str = 'melody', device='cuda'):
- """Return pretrained model, we provide four models:
- - small (300M), text to music, # see: https://huggingface.co/facebook/musicgen-small
- - medium (1.5B), text to music, # see: https://huggingface.co/facebook/musicgen-medium
- - melody (1.5B) text to music and text+melody to music, # see: https://huggingface.co/facebook/musicgen-melody
- - large (3.3B), text to music, # see: https://huggingface.co/facebook/musicgen-large
- """
-
- if name == 'debug':
- # used only for unit tests
- compression_model = get_debug_compression_model(device)
- lm = get_debug_lm_model(device)
- return MusicGen(name, compression_model, lm)
-
- if name not in HF_MODEL_CHECKPOINTS_MAP:
- raise ValueError(
- f"{name} is not a valid checkpoint name. "
- f"Choose one of {', '.join(HF_MODEL_CHECKPOINTS_MAP.keys())}"
- )
-
- cache_dir = os.environ.get('MUSICGEN_ROOT', None)
- compression_model = load_compression_model(name, device=device, cache_dir=cache_dir)
- lm = load_lm_model(name, device=device, cache_dir=cache_dir)
-
- return MusicGen(name, compression_model, lm)
-
- def set_generation_params(self, use_sampling: bool = True, top_k: int = 250,
- top_p: float = 0.0, temperature: float = 1.0,
- duration: float = 30.0, cfg_coef: float = 3.0,
- two_step_cfg: bool = False):
- """Set the generation parameters for MusicGen.
-
- Args:
- use_sampling (bool, optional): Use sampling if True, else do argmax decoding. Defaults to True.
- top_k (int, optional): top_k used for sampling. Defaults to 250.
- top_p (float, optional): top_p used for sampling, when set to 0 top_k is used. Defaults to 0.0.
- temperature (float, optional): Softmax temperature parameter. Defaults to 1.0.
- duration (float, optional): Duration of the generated waveform. Defaults to 30.0.
- cfg_coef (float, optional): Coefficient used for classifier free guidance. Defaults to 3.0.
- two_step_cfg (bool, optional): If True, performs 2 forward for Classifier Free Guidance,
- instead of batching together the two. This has some impact on how things
- are padded but seems to have little impact in practice.
- """
- assert duration <= 30, "The MusicGen cannot generate more than 30 seconds"
- self.generation_params = {
- 'max_gen_len': int(duration * self.frame_rate),
- 'use_sampling': use_sampling,
- 'temp': temperature,
- 'top_k': top_k,
- 'top_p': top_p,
- 'cfg_coef': cfg_coef,
- 'two_step_cfg': two_step_cfg,
- }
-
- def generate_unconditional(self, num_samples: int, progress: bool = False) -> torch.Tensor:
- """Generate samples in an unconditional manner.
-
- Args:
- num_samples (int): Number of samples to be generated.
- progress (bool, optional): Flag to display progress of the generation process. Defaults to False.
- """
- descriptions: tp.List[tp.Optional[str]] = [None] * num_samples
- attributes, prompt_tokens = self._prepare_tokens_and_attributes(descriptions, None)
- return self._generate_tokens(attributes, prompt_tokens, progress)
-
- def generate(self, descriptions: tp.List[str], progress: bool = False) -> torch.Tensor:
- """Generate samples conditioned on text.
-
- Args:
- descriptions (tp.List[str]): A list of strings used as text conditioning.
- progress (bool, optional): Flag to display progress of the generation process. Defaults to False.
- """
- attributes, prompt_tokens = self._prepare_tokens_and_attributes(descriptions, None)
- assert prompt_tokens is None
- return self._generate_tokens(attributes, prompt_tokens, progress)
-
- def generate_with_chroma(self, descriptions: tp.List[str], melody_wavs: MelodyType,
- melody_sample_rate: int, progress: bool = False) -> torch.Tensor:
- """Generate samples conditioned on text and melody.
-
- Args:
- descriptions (tp.List[str]): A list of strings used as text conditioning.
- melody_wavs: (torch.Tensor or list of Tensor): A batch of waveforms used as
- melody conditioning. Should have shape [B, C, T] with B matching the description length,
- C=1 or 2. It can be [C, T] if there is a single description. It can also be
- a list of [C, T] tensors.
- melody_sample_rate: (int): Sample rate of the melody waveforms.
- progress (bool, optional): Flag to display progress of the generation process. Defaults to False.
- """
- if isinstance(melody_wavs, torch.Tensor):
- if melody_wavs.dim() == 2:
- melody_wavs = melody_wavs[None]
- if melody_wavs.dim() != 3:
- raise ValueError("Melody wavs should have a shape [B, C, T].")
- melody_wavs = list(melody_wavs)
- else:
- for melody in melody_wavs:
- if melody is not None:
- assert melody.dim() == 2, "One melody in the list has the wrong number of dims."
-
- melody_wavs = [
- convert_audio(wav, melody_sample_rate, self.sample_rate, self.audio_channels)
- if wav is not None else None
- for wav in melody_wavs]
- attributes, prompt_tokens = self._prepare_tokens_and_attributes(descriptions=descriptions, prompt=None,
- melody_wavs=melody_wavs)
- assert prompt_tokens is None
- return self._generate_tokens(attributes, prompt_tokens, progress)
-
- def generate_continuation(self, prompt: torch.Tensor, prompt_sample_rate: int,
- descriptions: tp.Optional[tp.List[tp.Optional[str]]] = None,
- progress: bool = False) -> torch.Tensor:
- """Generate samples conditioned on audio prompts.
-
- Args:
- prompt (torch.Tensor): A batch of waveforms used for continuation.
- Prompt should be [B, C, T], or [C, T] if only one sample is generated.
- prompt_sample_rate (int): Sampling rate of the given audio waveforms.
- descriptions (tp.List[str], optional): A list of strings used as text conditioning. Defaults to None.
- progress (bool, optional): Flag to display progress of the generation process. Defaults to False.
- """
- if prompt.dim() == 2:
- prompt = prompt[None]
- if prompt.dim() != 3:
- raise ValueError("prompt should have 3 dimensions: [B, C, T] (C = 1).")
- prompt = convert_audio(prompt, prompt_sample_rate, self.sample_rate, self.audio_channels)
- if descriptions is None:
- descriptions = [None] * len(prompt)
- attributes, prompt_tokens = self._prepare_tokens_and_attributes(descriptions, prompt)
- assert prompt_tokens is not None
- return self._generate_tokens(attributes, prompt_tokens, progress)
-
- @torch.no_grad()
- def _prepare_tokens_and_attributes(
- self,
- descriptions: tp.Sequence[tp.Optional[str]],
- prompt: tp.Optional[torch.Tensor],
- melody_wavs: tp.Optional[MelodyList] = None,
- ) -> tp.Tuple[tp.List[ConditioningAttributes], tp.Optional[torch.Tensor]]:
- """Prepare model inputs.
-
- Args:
- descriptions (tp.List[str]): A list of strings used as text conditioning.
- prompt (torch.Tensor): A batch of waveforms used for continuation.
- melody_wavs (tp.Optional[torch.Tensor], optional): A batch of waveforms
- used as melody conditioning. Defaults to None.
- """
- attributes = [
- ConditioningAttributes(text={'description': description})
- for description in descriptions]
-
- if melody_wavs is None:
- for attr in attributes:
- attr.wav['self_wav'] = WavCondition(
- torch.zeros((1, 1), device=self.device),
- torch.tensor([0], device=self.device),
- path='null_wav') # type: ignore
- else:
- if self.name != "melody":
- raise RuntimeError("This model doesn't support melody conditioning. "
- "Use the `melody` model.")
- assert len(melody_wavs) == len(descriptions), \
- f"number of melody wavs must match number of descriptions! " \
- f"got melody len={len(melody_wavs)}, and descriptions len={len(descriptions)}"
- for attr, melody in zip(attributes, melody_wavs):
- if melody is None:
- attr.wav['self_wav'] = WavCondition(
- torch.zeros((1, 1), device=self.device),
- torch.tensor([0], device=self.device),
- path='null_wav') # type: ignore
- else:
- attr.wav['self_wav'] = WavCondition(
- melody.to(device=self.device),
- torch.tensor([melody.shape[-1]], device=self.device))
-
- if prompt is not None:
- if descriptions is not None:
- assert len(descriptions) == len(prompt), "Prompt and nb. descriptions doesn't match"
- prompt = prompt.to(self.device)
- prompt_tokens, scale = self.compression_model.encode(prompt)
- assert scale is None
- else:
- prompt_tokens = None
- return attributes, prompt_tokens
-
- def _generate_tokens(self, attributes: tp.List[ConditioningAttributes],
- prompt_tokens: tp.Optional[torch.Tensor], progress: bool = False) -> torch.Tensor:
- """Generate discrete audio tokens given audio prompt and/or conditions.
-
- Args:
- attributes (tp.List[ConditioningAttributes]): Conditions used for generation (text/melody).
- prompt_tokens (tp.Optional[torch.Tensor]): Audio prompt used for continuation.
- progress (bool, optional): Flag to display progress of the generation process. Defaults to False.
- Returns:
- torch.Tensor: Generated audio, of shape [B, C, T], T is defined by the generation params.
- """
- def _progress_callback(generated_tokens: int, tokens_to_generate: int):
- print(f'{generated_tokens: 6d} / {tokens_to_generate: 6d}', end='\r')
-
- if prompt_tokens is not None:
- assert self.generation_params['max_gen_len'] > prompt_tokens.shape[-1], \
- "Prompt is longer than audio to generate"
-
- callback = None
- if progress:
- callback = _progress_callback
-
- # generate by sampling from LM
- with self.autocast:
- gen_tokens = self.lm.generate(prompt_tokens, attributes, callback=callback, **self.generation_params)
-
- # generate audio
- assert gen_tokens.dim() == 3
- with torch.no_grad():
- gen_audio = self.compression_model.decode(gen_tokens, None)
- return gen_audio
diff --git a/spaces/XS-1/BW_IMAGE_VIDEO_COLORIZER/fastai/utils/pynvml_gate.py b/spaces/XS-1/BW_IMAGE_VIDEO_COLORIZER/fastai/utils/pynvml_gate.py
deleted file mode 100644
index 27a175c3ba1eaa7143aadf9d17661d4e44f3e903..0000000000000000000000000000000000000000
--- a/spaces/XS-1/BW_IMAGE_VIDEO_COLORIZER/fastai/utils/pynvml_gate.py
+++ /dev/null
@@ -1,74 +0,0 @@
-"""Get OS specific nvml wrapper. On OSX we use pynvx as drop in replacement for pynvml"""
-
-import platform
-from ..script import *
-
-#
-# BEGIN: Temporary workaround for nvml.dll load issue in Win10
-#
-# Remove once nicolargo/nvidia-ml-py3#2 and a new version of the module is released
-# (OR fbcotter/py3nvml#10 but will require extra work to rename things)
-# Refer https://forums.fast.ai/t/nvml-dll-loading-issue-in-nvidia-ml-py3-7-352-0-py-0/39684/8
-import threading
-from ctypes import *
-
-nvmlLib = None
-libLoadLock = threading.Lock()
-
-def _LoadNvmlLibrary():
- '''
- Load the library if it isn't loaded already
- '''
-
- global nvmlLib
-
- if (nvmlLib == None):
- libLoadLock.acquire()
-
- try:
- if (nvmlLib == None):
- try:
- if (sys.platform[:3] == "win"):
- searchPaths = [
- os.path.join(os.getenv("ProgramFiles", r"C:\Program Files"), r"NVIDIA Corporation\NVSMI\nvml.dll"),
- os.path.join(os.getenv("WinDir", r"C:\Windows"), r"System32\nvml.dll"),
- ]
- nvmlPath = next((x for x in searchPaths if os.path.isfile(x)), None)
- if (nvmlPath == None):
- nvmlLib = None
- else:
- nvmlLib = CDLL(nvmlPath)
- else:
- nvmlLib = None
- except OSError as ose:
- nvmlLib = None
- finally:
- libLoadLock.release()
-#
-# END: Temporary workaround for nvml.dll load issue in Win10
-#
-
-def load_pynvml_env():
- import pynvml # nvidia-ml-py3
-
- #
- # BEGIN: Temporary workaround for nvml.dll load issue in Win10 (continued)
- _LoadNvmlLibrary()
- pynvml.nvmlLib = nvmlLib
- #
- # END: Temporary workaround for nvml.dll load issue in Win10
- #
-
- if platform.system() == "Darwin":
- try:
- from pynvx import pynvml
- except:
- print("please install pynvx on OSX: pip install pynvx")
- sys.exit(1)
-
- pynvml.nvmlInit()
- return pynvml
-
- pynvml.nvmlInit()
-
- return pynvml
diff --git a/spaces/XzJosh/Azusa-Bert-VITS2/bert_gen.py b/spaces/XzJosh/Azusa-Bert-VITS2/bert_gen.py
deleted file mode 100644
index 44814715396ffc3abe84a12c74d66293c356eb4f..0000000000000000000000000000000000000000
--- a/spaces/XzJosh/Azusa-Bert-VITS2/bert_gen.py
+++ /dev/null
@@ -1,53 +0,0 @@
-import torch
-from torch.utils.data import DataLoader
-from multiprocessing import Pool
-import commons
-import utils
-from data_utils import TextAudioSpeakerLoader, TextAudioSpeakerCollate
-from tqdm import tqdm
-import warnings
-
-from text import cleaned_text_to_sequence, get_bert
-
-config_path = 'configs/config.json'
-hps = utils.get_hparams_from_file(config_path)
-
-def process_line(line):
- _id, spk, language_str, text, phones, tone, word2ph = line.strip().split("|")
- phone = phones.split(" ")
- tone = [int(i) for i in tone.split(" ")]
- word2ph = [int(i) for i in word2ph.split(" ")]
- w2pho = [i for i in word2ph]
- word2ph = [i for i in word2ph]
- phone, tone, language = cleaned_text_to_sequence(phone, tone, language_str)
-
- if hps.data.add_blank:
- phone = commons.intersperse(phone, 0)
- tone = commons.intersperse(tone, 0)
- language = commons.intersperse(language, 0)
- for i in range(len(word2ph)):
- word2ph[i] = word2ph[i] * 2
- word2ph[0] += 1
- wav_path = f'{_id}'
-
- bert_path = wav_path.replace(".wav", ".bert.pt")
- try:
- bert = torch.load(bert_path)
- assert bert.shape[-1] == len(phone)
- except:
- bert = get_bert(text, word2ph, language_str)
- assert bert.shape[-1] == len(phone)
- torch.save(bert, bert_path)
-
-
-if __name__ == '__main__':
- lines = []
- with open(hps.data.training_files, encoding='utf-8' ) as f:
- lines.extend(f.readlines())
-
- with open(hps.data.validation_files, encoding='utf-8' ) as f:
- lines.extend(f.readlines())
-
- with Pool(processes=12) as pool: #A100 40GB suitable config,if coom,please decrease the processess number.
- for _ in tqdm(pool.imap_unordered(process_line, lines)):
- pass
diff --git a/spaces/XzJosh/Echo-Bert-VITS2/README.md b/spaces/XzJosh/Echo-Bert-VITS2/README.md
deleted file mode 100644
index c3dcdc789cd836b046baf50565586454ef36cd63..0000000000000000000000000000000000000000
--- a/spaces/XzJosh/Echo-Bert-VITS2/README.md
+++ /dev/null
@@ -1,5 +0,0 @@
----
-license: mit
-sdk: gradio
-title: AI黑桃影①
----
\ No newline at end of file
diff --git a/spaces/YeOldHermit/Super-Resolution-Anime-Diffusion/diffusers/models/vae.py b/spaces/YeOldHermit/Super-Resolution-Anime-Diffusion/diffusers/models/vae.py
deleted file mode 100644
index e29f4e8afa2ff1cc957672b9f2d595c30a2db32e..0000000000000000000000000000000000000000
--- a/spaces/YeOldHermit/Super-Resolution-Anime-Diffusion/diffusers/models/vae.py
+++ /dev/null
@@ -1,643 +0,0 @@
-# Copyright 2022 The HuggingFace Team. All rights reserved.
-#
-# Licensed under the Apache License, Version 2.0 (the "License");
-# you may not use this file except in compliance with the License.
-# You may obtain a copy of the License at
-#
-# http://www.apache.org/licenses/LICENSE-2.0
-#
-# Unless required by applicable law or agreed to in writing, software
-# distributed under the License is distributed on an "AS IS" BASIS,
-# WITHOUT WARRANTIES OR CONDITIONS OF ANY KIND, either express or implied.
-# See the License for the specific language governing permissions and
-# limitations under the License.
-from dataclasses import dataclass
-from typing import Optional, Tuple, Union
-
-import numpy as np
-import torch
-import torch.nn as nn
-
-from ..configuration_utils import ConfigMixin, register_to_config
-from ..modeling_utils import ModelMixin
-from ..utils import BaseOutput
-from .unet_2d_blocks import UNetMidBlock2D, get_down_block, get_up_block
-
-
-@dataclass
-class DecoderOutput(BaseOutput):
- """
- Output of decoding method.
-
- Args:
- sample (`torch.FloatTensor` of shape `(batch_size, num_channels, height, width)`):
- Decoded output sample of the model. Output of the last layer of the model.
- """
-
- sample: torch.FloatTensor
-
-
-@dataclass
-class VQEncoderOutput(BaseOutput):
- """
- Output of VQModel encoding method.
-
- Args:
- latents (`torch.FloatTensor` of shape `(batch_size, num_channels, height, width)`):
- Encoded output sample of the model. Output of the last layer of the model.
- """
-
- latents: torch.FloatTensor
-
-
-@dataclass
-class AutoencoderKLOutput(BaseOutput):
- """
- Output of AutoencoderKL encoding method.
-
- Args:
- latent_dist (`DiagonalGaussianDistribution`):
- Encoded outputs of `Encoder` represented as the mean and logvar of `DiagonalGaussianDistribution`.
- `DiagonalGaussianDistribution` allows for sampling latents from the distribution.
- """
-
- latent_dist: "DiagonalGaussianDistribution"
-
-
-class Encoder(nn.Module):
- def __init__(
- self,
- in_channels=3,
- out_channels=3,
- down_block_types=("DownEncoderBlock2D",),
- block_out_channels=(64,),
- layers_per_block=2,
- norm_num_groups=32,
- act_fn="silu",
- double_z=True,
- ):
- super().__init__()
- self.layers_per_block = layers_per_block
-
- self.conv_in = torch.nn.Conv2d(in_channels, block_out_channels[0], kernel_size=3, stride=1, padding=1)
-
- self.mid_block = None
- self.down_blocks = nn.ModuleList([])
-
- # down
- output_channel = block_out_channels[0]
- for i, down_block_type in enumerate(down_block_types):
- input_channel = output_channel
- output_channel = block_out_channels[i]
- is_final_block = i == len(block_out_channels) - 1
-
- down_block = get_down_block(
- down_block_type,
- num_layers=self.layers_per_block,
- in_channels=input_channel,
- out_channels=output_channel,
- add_downsample=not is_final_block,
- resnet_eps=1e-6,
- downsample_padding=0,
- resnet_act_fn=act_fn,
- resnet_groups=norm_num_groups,
- attn_num_head_channels=None,
- temb_channels=None,
- )
- self.down_blocks.append(down_block)
-
- # mid
- self.mid_block = UNetMidBlock2D(
- in_channels=block_out_channels[-1],
- resnet_eps=1e-6,
- resnet_act_fn=act_fn,
- output_scale_factor=1,
- resnet_time_scale_shift="default",
- attn_num_head_channels=None,
- resnet_groups=norm_num_groups,
- temb_channels=None,
- )
-
- # out
- self.conv_norm_out = nn.GroupNorm(num_channels=block_out_channels[-1], num_groups=norm_num_groups, eps=1e-6)
- self.conv_act = nn.SiLU()
-
- conv_out_channels = 2 * out_channels if double_z else out_channels
- self.conv_out = nn.Conv2d(block_out_channels[-1], conv_out_channels, 3, padding=1)
-
- def forward(self, x):
- sample = x
- sample = self.conv_in(sample)
-
- # down
- for down_block in self.down_blocks:
- sample = down_block(sample)
-
- # middle
- sample = self.mid_block(sample)
-
- # post-process
- sample = self.conv_norm_out(sample)
- sample = self.conv_act(sample)
- sample = self.conv_out(sample)
-
- return sample
-
-
-class Decoder(nn.Module):
- def __init__(
- self,
- in_channels=3,
- out_channels=3,
- up_block_types=("UpDecoderBlock2D",),
- block_out_channels=(64,),
- layers_per_block=2,
- norm_num_groups=32,
- act_fn="silu",
- ):
- super().__init__()
- self.layers_per_block = layers_per_block
-
- self.conv_in = nn.Conv2d(in_channels, block_out_channels[-1], kernel_size=3, stride=1, padding=1)
-
- self.mid_block = None
- self.up_blocks = nn.ModuleList([])
-
- # mid
- self.mid_block = UNetMidBlock2D(
- in_channels=block_out_channels[-1],
- resnet_eps=1e-6,
- resnet_act_fn=act_fn,
- output_scale_factor=1,
- resnet_time_scale_shift="default",
- attn_num_head_channels=None,
- resnet_groups=norm_num_groups,
- temb_channels=None,
- )
-
- # up
- reversed_block_out_channels = list(reversed(block_out_channels))
- output_channel = reversed_block_out_channels[0]
- for i, up_block_type in enumerate(up_block_types):
- prev_output_channel = output_channel
- output_channel = reversed_block_out_channels[i]
-
- is_final_block = i == len(block_out_channels) - 1
-
- up_block = get_up_block(
- up_block_type,
- num_layers=self.layers_per_block + 1,
- in_channels=prev_output_channel,
- out_channels=output_channel,
- prev_output_channel=None,
- add_upsample=not is_final_block,
- resnet_eps=1e-6,
- resnet_act_fn=act_fn,
- resnet_groups=norm_num_groups,
- attn_num_head_channels=None,
- temb_channels=None,
- )
- self.up_blocks.append(up_block)
- prev_output_channel = output_channel
-
- # out
- self.conv_norm_out = nn.GroupNorm(num_channels=block_out_channels[0], num_groups=norm_num_groups, eps=1e-6)
- self.conv_act = nn.SiLU()
- self.conv_out = nn.Conv2d(block_out_channels[0], out_channels, 3, padding=1)
-
- def forward(self, z):
- sample = z
- sample = self.conv_in(sample)
-
- # middle
- sample = self.mid_block(sample)
-
- # up
- for up_block in self.up_blocks:
- sample = up_block(sample)
-
- # post-process
- sample = self.conv_norm_out(sample)
- sample = self.conv_act(sample)
- sample = self.conv_out(sample)
-
- return sample
-
-
-class VectorQuantizer(nn.Module):
- """
- Improved version over VectorQuantizer, can be used as a drop-in replacement. Mostly avoids costly matrix
- multiplications and allows for post-hoc remapping of indices.
- """
-
- # NOTE: due to a bug the beta term was applied to the wrong term. for
- # backwards compatibility we use the buggy version by default, but you can
- # specify legacy=False to fix it.
- def __init__(
- self, n_e, vq_embed_dim, beta, remap=None, unknown_index="random", sane_index_shape=False, legacy=True
- ):
- super().__init__()
- self.n_e = n_e
- self.vq_embed_dim = vq_embed_dim
- self.beta = beta
- self.legacy = legacy
-
- self.embedding = nn.Embedding(self.n_e, self.vq_embed_dim)
- self.embedding.weight.data.uniform_(-1.0 / self.n_e, 1.0 / self.n_e)
-
- self.remap = remap
- if self.remap is not None:
- self.register_buffer("used", torch.tensor(np.load(self.remap)))
- self.re_embed = self.used.shape[0]
- self.unknown_index = unknown_index # "random" or "extra" or integer
- if self.unknown_index == "extra":
- self.unknown_index = self.re_embed
- self.re_embed = self.re_embed + 1
- print(
- f"Remapping {self.n_e} indices to {self.re_embed} indices. "
- f"Using {self.unknown_index} for unknown indices."
- )
- else:
- self.re_embed = n_e
-
- self.sane_index_shape = sane_index_shape
-
- def remap_to_used(self, inds):
- ishape = inds.shape
- assert len(ishape) > 1
- inds = inds.reshape(ishape[0], -1)
- used = self.used.to(inds)
- match = (inds[:, :, None] == used[None, None, ...]).long()
- new = match.argmax(-1)
- unknown = match.sum(2) < 1
- if self.unknown_index == "random":
- new[unknown] = torch.randint(0, self.re_embed, size=new[unknown].shape).to(device=new.device)
- else:
- new[unknown] = self.unknown_index
- return new.reshape(ishape)
-
- def unmap_to_all(self, inds):
- ishape = inds.shape
- assert len(ishape) > 1
- inds = inds.reshape(ishape[0], -1)
- used = self.used.to(inds)
- if self.re_embed > self.used.shape[0]: # extra token
- inds[inds >= self.used.shape[0]] = 0 # simply set to zero
- back = torch.gather(used[None, :][inds.shape[0] * [0], :], 1, inds)
- return back.reshape(ishape)
-
- def forward(self, z):
- # reshape z -> (batch, height, width, channel) and flatten
- z = z.permute(0, 2, 3, 1).contiguous()
- z_flattened = z.view(-1, self.vq_embed_dim)
- # distances from z to embeddings e_j (z - e)^2 = z^2 + e^2 - 2 e * z
-
- d = (
- torch.sum(z_flattened**2, dim=1, keepdim=True)
- + torch.sum(self.embedding.weight**2, dim=1)
- - 2 * torch.einsum("bd,dn->bn", z_flattened, self.embedding.weight.t())
- )
-
- min_encoding_indices = torch.argmin(d, dim=1)
- z_q = self.embedding(min_encoding_indices).view(z.shape)
- perplexity = None
- min_encodings = None
-
- # compute loss for embedding
- if not self.legacy:
- loss = self.beta * torch.mean((z_q.detach() - z) ** 2) + torch.mean((z_q - z.detach()) ** 2)
- else:
- loss = torch.mean((z_q.detach() - z) ** 2) + self.beta * torch.mean((z_q - z.detach()) ** 2)
-
- # preserve gradients
- z_q = z + (z_q - z).detach()
-
- # reshape back to match original input shape
- z_q = z_q.permute(0, 3, 1, 2).contiguous()
-
- if self.remap is not None:
- min_encoding_indices = min_encoding_indices.reshape(z.shape[0], -1) # add batch axis
- min_encoding_indices = self.remap_to_used(min_encoding_indices)
- min_encoding_indices = min_encoding_indices.reshape(-1, 1) # flatten
-
- if self.sane_index_shape:
- min_encoding_indices = min_encoding_indices.reshape(z_q.shape[0], z_q.shape[2], z_q.shape[3])
-
- return z_q, loss, (perplexity, min_encodings, min_encoding_indices)
-
- def get_codebook_entry(self, indices, shape):
- # shape specifying (batch, height, width, channel)
- if self.remap is not None:
- indices = indices.reshape(shape[0], -1) # add batch axis
- indices = self.unmap_to_all(indices)
- indices = indices.reshape(-1) # flatten again
-
- # get quantized latent vectors
- z_q = self.embedding(indices)
-
- if shape is not None:
- z_q = z_q.view(shape)
- # reshape back to match original input shape
- z_q = z_q.permute(0, 3, 1, 2).contiguous()
-
- return z_q
-
-
-class DiagonalGaussianDistribution(object):
- def __init__(self, parameters, deterministic=False):
- self.parameters = parameters
- self.mean, self.logvar = torch.chunk(parameters, 2, dim=1)
- self.logvar = torch.clamp(self.logvar, -30.0, 20.0)
- self.deterministic = deterministic
- self.std = torch.exp(0.5 * self.logvar)
- self.var = torch.exp(self.logvar)
- if self.deterministic:
- self.var = self.std = torch.zeros_like(
- self.mean, device=self.parameters.device, dtype=self.parameters.dtype
- )
-
- def sample(self, generator: Optional[torch.Generator] = None) -> torch.FloatTensor:
- device = self.parameters.device
- sample_device = "cpu" if device.type == "mps" else device
- sample = torch.randn(self.mean.shape, generator=generator, device=sample_device)
- # make sure sample is on the same device as the parameters and has same dtype
- sample = sample.to(device=device, dtype=self.parameters.dtype)
- x = self.mean + self.std * sample
- return x
-
- def kl(self, other=None):
- if self.deterministic:
- return torch.Tensor([0.0])
- else:
- if other is None:
- return 0.5 * torch.sum(torch.pow(self.mean, 2) + self.var - 1.0 - self.logvar, dim=[1, 2, 3])
- else:
- return 0.5 * torch.sum(
- torch.pow(self.mean - other.mean, 2) / other.var
- + self.var / other.var
- - 1.0
- - self.logvar
- + other.logvar,
- dim=[1, 2, 3],
- )
-
- def nll(self, sample, dims=[1, 2, 3]):
- if self.deterministic:
- return torch.Tensor([0.0])
- logtwopi = np.log(2.0 * np.pi)
- return 0.5 * torch.sum(logtwopi + self.logvar + torch.pow(sample - self.mean, 2) / self.var, dim=dims)
-
- def mode(self):
- return self.mean
-
-
-class VQModel(ModelMixin, ConfigMixin):
- r"""VQ-VAE model from the paper Neural Discrete Representation Learning by Aaron van den Oord, Oriol Vinyals and Koray
- Kavukcuoglu.
-
- This model inherits from [`ModelMixin`]. Check the superclass documentation for the generic methods the library
- implements for all the model (such as downloading or saving, etc.)
-
- Parameters:
- in_channels (int, *optional*, defaults to 3): Number of channels in the input image.
- out_channels (int, *optional*, defaults to 3): Number of channels in the output.
- down_block_types (`Tuple[str]`, *optional*, defaults to :
- obj:`("DownEncoderBlock2D",)`): Tuple of downsample block types.
- up_block_types (`Tuple[str]`, *optional*, defaults to :
- obj:`("UpDecoderBlock2D",)`): Tuple of upsample block types.
- block_out_channels (`Tuple[int]`, *optional*, defaults to :
- obj:`(64,)`): Tuple of block output channels.
- act_fn (`str`, *optional*, defaults to `"silu"`): The activation function to use.
- latent_channels (`int`, *optional*, defaults to `3`): Number of channels in the latent space.
- sample_size (`int`, *optional*, defaults to `32`): TODO
- num_vq_embeddings (`int`, *optional*, defaults to `256`): Number of codebook vectors in the VQ-VAE.
- vq_embed_dim (`int`, *optional*): Hidden dim of codebook vectors in the VQ-VAE.
- """
-
- @register_to_config
- def __init__(
- self,
- in_channels: int = 3,
- out_channels: int = 3,
- down_block_types: Tuple[str] = ("DownEncoderBlock2D",),
- up_block_types: Tuple[str] = ("UpDecoderBlock2D",),
- block_out_channels: Tuple[int] = (64,),
- layers_per_block: int = 1,
- act_fn: str = "silu",
- latent_channels: int = 3,
- sample_size: int = 32,
- num_vq_embeddings: int = 256,
- norm_num_groups: int = 32,
- vq_embed_dim: Optional[int] = None,
- ):
- super().__init__()
-
- # pass init params to Encoder
- self.encoder = Encoder(
- in_channels=in_channels,
- out_channels=latent_channels,
- down_block_types=down_block_types,
- block_out_channels=block_out_channels,
- layers_per_block=layers_per_block,
- act_fn=act_fn,
- norm_num_groups=norm_num_groups,
- double_z=False,
- )
-
- vq_embed_dim = vq_embed_dim if vq_embed_dim is not None else latent_channels
-
- self.quant_conv = torch.nn.Conv2d(latent_channels, vq_embed_dim, 1)
- self.quantize = VectorQuantizer(num_vq_embeddings, vq_embed_dim, beta=0.25, remap=None, sane_index_shape=False)
- self.post_quant_conv = torch.nn.Conv2d(vq_embed_dim, latent_channels, 1)
-
- # pass init params to Decoder
- self.decoder = Decoder(
- in_channels=latent_channels,
- out_channels=out_channels,
- up_block_types=up_block_types,
- block_out_channels=block_out_channels,
- layers_per_block=layers_per_block,
- act_fn=act_fn,
- norm_num_groups=norm_num_groups,
- )
-
- def encode(self, x: torch.FloatTensor, return_dict: bool = True) -> VQEncoderOutput:
- h = self.encoder(x)
- h = self.quant_conv(h)
-
- if not return_dict:
- return (h,)
-
- return VQEncoderOutput(latents=h)
-
- def decode(
- self, h: torch.FloatTensor, force_not_quantize: bool = False, return_dict: bool = True
- ) -> Union[DecoderOutput, torch.FloatTensor]:
- # also go through quantization layer
- if not force_not_quantize:
- quant, emb_loss, info = self.quantize(h)
- else:
- quant = h
- quant = self.post_quant_conv(quant)
- dec = self.decoder(quant)
-
- if not return_dict:
- return (dec,)
-
- return DecoderOutput(sample=dec)
-
- def forward(self, sample: torch.FloatTensor, return_dict: bool = True) -> Union[DecoderOutput, torch.FloatTensor]:
- r"""
- Args:
- sample (`torch.FloatTensor`): Input sample.
- return_dict (`bool`, *optional*, defaults to `True`):
- Whether or not to return a [`DecoderOutput`] instead of a plain tuple.
- """
- x = sample
- h = self.encode(x).latents
- dec = self.decode(h).sample
-
- if not return_dict:
- return (dec,)
-
- return DecoderOutput(sample=dec)
-
-
-class AutoencoderKL(ModelMixin, ConfigMixin):
- r"""Variational Autoencoder (VAE) model with KL loss from the paper Auto-Encoding Variational Bayes by Diederik P. Kingma
- and Max Welling.
-
- This model inherits from [`ModelMixin`]. Check the superclass documentation for the generic methods the library
- implements for all the model (such as downloading or saving, etc.)
-
- Parameters:
- in_channels (int, *optional*, defaults to 3): Number of channels in the input image.
- out_channels (int, *optional*, defaults to 3): Number of channels in the output.
- down_block_types (`Tuple[str]`, *optional*, defaults to :
- obj:`("DownEncoderBlock2D",)`): Tuple of downsample block types.
- up_block_types (`Tuple[str]`, *optional*, defaults to :
- obj:`("UpDecoderBlock2D",)`): Tuple of upsample block types.
- block_out_channels (`Tuple[int]`, *optional*, defaults to :
- obj:`(64,)`): Tuple of block output channels.
- act_fn (`str`, *optional*, defaults to `"silu"`): The activation function to use.
- latent_channels (`int`, *optional*, defaults to `4`): Number of channels in the latent space.
- sample_size (`int`, *optional*, defaults to `32`): TODO
- """
-
- @register_to_config
- def __init__(
- self,
- in_channels: int = 3,
- out_channels: int = 3,
- down_block_types: Tuple[str] = ("DownEncoderBlock2D",),
- up_block_types: Tuple[str] = ("UpDecoderBlock2D",),
- block_out_channels: Tuple[int] = (64,),
- layers_per_block: int = 1,
- act_fn: str = "silu",
- latent_channels: int = 4,
- norm_num_groups: int = 32,
- sample_size: int = 32,
- ):
- super().__init__()
-
- # pass init params to Encoder
- self.encoder = Encoder(
- in_channels=in_channels,
- out_channels=latent_channels,
- down_block_types=down_block_types,
- block_out_channels=block_out_channels,
- layers_per_block=layers_per_block,
- act_fn=act_fn,
- norm_num_groups=norm_num_groups,
- double_z=True,
- )
-
- # pass init params to Decoder
- self.decoder = Decoder(
- in_channels=latent_channels,
- out_channels=out_channels,
- up_block_types=up_block_types,
- block_out_channels=block_out_channels,
- layers_per_block=layers_per_block,
- norm_num_groups=norm_num_groups,
- act_fn=act_fn,
- )
-
- self.quant_conv = torch.nn.Conv2d(2 * latent_channels, 2 * latent_channels, 1)
- self.post_quant_conv = torch.nn.Conv2d(latent_channels, latent_channels, 1)
- self.use_slicing = False
-
- def encode(self, x: torch.FloatTensor, return_dict: bool = True) -> AutoencoderKLOutput:
- h = self.encoder(x)
- moments = self.quant_conv(h)
- posterior = DiagonalGaussianDistribution(moments)
-
- if not return_dict:
- return (posterior,)
-
- return AutoencoderKLOutput(latent_dist=posterior)
-
- def _decode(self, z: torch.FloatTensor, return_dict: bool = True) -> Union[DecoderOutput, torch.FloatTensor]:
- z = self.post_quant_conv(z)
- dec = self.decoder(z)
-
- if not return_dict:
- return (dec,)
-
- return DecoderOutput(sample=dec)
-
- def enable_slicing(self):
- r"""
- Enable sliced VAE decoding.
-
- When this option is enabled, the VAE will split the input tensor in slices to compute decoding in several
- steps. This is useful to save some memory and allow larger batch sizes.
- """
- self.use_slicing = True
-
- def disable_slicing(self):
- r"""
- Disable sliced VAE decoding. If `enable_slicing` was previously invoked, this method will go back to computing
- decoding in one step.
- """
- self.use_slicing = False
-
- def decode(self, z: torch.FloatTensor, return_dict: bool = True) -> Union[DecoderOutput, torch.FloatTensor]:
- if self.use_slicing and z.shape[0] > 1:
- decoded_slices = [self._decode(z_slice).sample for z_slice in z.split(1)]
- decoded = torch.cat(decoded_slices)
- else:
- decoded = self._decode(z).sample
-
- if not return_dict:
- return (decoded,)
-
- return DecoderOutput(sample=decoded)
-
- def forward(
- self,
- sample: torch.FloatTensor,
- sample_posterior: bool = False,
- return_dict: bool = True,
- generator: Optional[torch.Generator] = None,
- ) -> Union[DecoderOutput, torch.FloatTensor]:
- r"""
- Args:
- sample (`torch.FloatTensor`): Input sample.
- sample_posterior (`bool`, *optional*, defaults to `False`):
- Whether to sample from the posterior.
- return_dict (`bool`, *optional*, defaults to `True`):
- Whether or not to return a [`DecoderOutput`] instead of a plain tuple.
- """
- x = sample
- posterior = self.encode(x).latent_dist
- if sample_posterior:
- z = posterior.sample(generator=generator)
- else:
- z = posterior.mode()
- dec = self.decode(z).sample
-
- if not return_dict:
- return (dec,)
-
- return DecoderOutput(sample=dec)
diff --git a/spaces/Yunshansongbai/SVC-Nahida/vdecoder/vdecoder/hifigan/models.py b/spaces/Yunshansongbai/SVC-Nahida/vdecoder/vdecoder/hifigan/models.py
deleted file mode 100644
index 1438419afad969dad2001bb716d9648f35df2b9d..0000000000000000000000000000000000000000
--- a/spaces/Yunshansongbai/SVC-Nahida/vdecoder/vdecoder/hifigan/models.py
+++ /dev/null
@@ -1,506 +0,0 @@
-import os
-import json
-from .env import AttrDict
-import numpy as np
-import paddle
-import paddle.nn.functional as F
-import paddle.nn as nn
-from paddle.nn import Conv1D, Conv1DTranspose, AvgPool1D, Conv2D
-from paddle.nn.utils import weight_norm, remove_weight_norm, spectral_norm
-from .utils import init_weights, get_padding
-
-LRELU_SLOPE = 0.1
-
-
-def load_model(model_path, device='gpu:0'):
- config_file = os.path.join(os.path.split(model_path)[0], 'config.json')
- with open(config_file) as f:
- data = f.read()
-
- global h
- json_config = json.loads(data)
- h = AttrDict(json_config)
-
- generator = Generator(h).to(device)
-
- cp_dict = paddle.load(model_path)
- generator.set_state_dict(cp_dict['generator'])
- generator.eval()
- generator.remove_weight_norm()
- del cp_dict
- return generator, h
-
-
-class ResBlock1(nn.Layer):
- def __init__(self, h, channels, kernel_size=3, dilation=(1, 3, 5)):
- super(ResBlock1, self).__init__()
- self.h = h
- self.convs1 = nn.LayerList([
- weight_norm(Conv1D(channels, channels, kernel_size, 1, dilation=dilation[0],
- padding=get_padding(kernel_size, dilation[0]))),
- weight_norm(Conv1D(channels, channels, kernel_size, 1, dilation=dilation[1],
- padding=get_padding(kernel_size, dilation[1]))),
- weight_norm(Conv1D(channels, channels, kernel_size, 1, dilation=dilation[2],
- padding=get_padding(kernel_size, dilation[2])))
- ])
- self.convs1.apply(init_weights)
- self.convs2 = nn.LayerList([
- weight_norm(Conv1D(channels, channels, kernel_size, 1, dilation=1,
- padding=get_padding(kernel_size, 1))),
- weight_norm(Conv1D(channels, channels, kernel_size, 1, dilation=1,
- padding=get_padding(kernel_size, 1))),
- weight_norm(Conv1D(channels, channels, kernel_size, 1, dilation=1,
- padding=get_padding(kernel_size, 1)))
- ])
- self.convs2.apply(init_weights)
-
- def forward(self, x):
- for c1, c2 in zip(self.convs1, self.convs2):
- xt = F.leaky_relu(x, LRELU_SLOPE)
- xt = c1(xt)
- xt = F.leaky_relu(xt, LRELU_SLOPE)
- xt = c2(xt)
- x = xt + x
- return x
-
- def remove_weight_norm(self):
- for l in self.convs1:
- remove_weight_norm(l)
- for l in self.convs2:
- remove_weight_norm(l)
-
-
-class ResBlock2(paddle.nn.Layer):
- def __init__(self, h, channels, kernel_size=3, dilation=(1, 3)):
- super(ResBlock2, self).__init__()
- self.h = h
- self.convs = nn.LayerList([
- weight_norm(Conv1D(channels, channels, kernel_size, 1, dilation=dilation[0],
- padding=get_padding(kernel_size, dilation[0]))),
- weight_norm(Conv1D(channels, channels, kernel_size, 1, dilation=dilation[1],
- padding=get_padding(kernel_size, dilation[1])))
- ])
- self.convs.apply(init_weights)
-
- def forward(self, x):
- for c in self.convs:
- xt = F.leaky_relu(x, LRELU_SLOPE)
- xt = c(xt)
- x = xt + x
- return x
-
- def remove_weight_norm(self):
- for l in self.convs:
- remove_weight_norm(l)
-
-
-def padDiff(x):
- x.unsqueeze_(0) # 为了能用paddle的pad函数
- pad = F.pad(x, (0,0,-1,1), 'constant', 0)
- out = F.pad(pad - x, (0,0,0,-1), 'constant', 0)
- out.squeeze_(0)
- return out
-
-class SineGen(paddle.nn.Layer):
- """ Definition of sine generator
- SineGen(samp_rate, harmonic_num = 0,
- sine_amp = 0.1, noise_std = 0.003,
- voiced_threshold = 0,
- flag_for_pulse=False)
- samp_rate: sampling rate in Hz
- harmonic_num: number of harmonic overtones (default 0)
- sine_amp: amplitude of sine-wavefrom (default 0.1)
- noise_std: std of Gaussian noise (default 0.003)
- voiced_thoreshold: F0 threshold for U/V classification (default 0)
- flag_for_pulse: this SinGen is used inside PulseGen (default False)
- Note: when flag_for_pulse is True, the first time step of a voiced
- segment is always sin(np.pi) or cos(0)
- """
-
- def __init__(self, samp_rate, harmonic_num=0,
- sine_amp=0.1, noise_std=0.003,
- voiced_threshold=0,
- flag_for_pulse=False):
- super(SineGen, self).__init__()
- self.sine_amp = sine_amp
- self.noise_std = noise_std
- self.harmonic_num = harmonic_num
- self.dim = self.harmonic_num + 1
- self.sampling_rate = samp_rate
- self.voiced_threshold = voiced_threshold
- self.flag_for_pulse = flag_for_pulse
-
- def _f02uv(self, f0):
- # generate uv signal
- uv = (f0 > self.voiced_threshold).astype('float32')
- return uv
-
- def _f02sine(self, f0_values):
- """ f0_values: (batchsize, length, dim)
- where dim indicates fundamental tone and overtones
- """
- # convert to F0 in rad. The interger part n can be ignored
- # because 2 * np.pi * n doesn't affect phase
- rad_values = (f0_values / self.sampling_rate) % 1
-
- # initial phase noise (no noise for fundamental component)
- rand_ini = paddle.rand((f0_values.shape[0], f0_values.shape[2]))
- rand_ini[:, 0] = 0
- rad_values[:, 0, :] = rad_values[:, 0, :] + rand_ini
-
- # instantanouse phase sine[t] = sin(2*pi \sum_i=1 ^{t} rad)
- if not self.flag_for_pulse:
- # for normal case
-
- # To prevent torch.cumsum numerical overflow,
- # it is necessary to add -1 whenever \sum_k=1^n rad_value_k > 1.
- # Buffer tmp_over_one_idx indicates the time step to add -1.
- # This will not change F0 of sine because (x-1) * 2*pi = x * 2*pi
- tmp_over_one = paddle.cumsum(rad_values, 1) % 1
- tmp_over_one_idx = (padDiff(tmp_over_one)) < 0
- cumsum_shift = paddle.zeros_like(rad_values)
- cumsum_shift[:, 1:, :] = tmp_over_one_idx * -1.0
-
- sines = paddle.sin(paddle.cumsum(rad_values + cumsum_shift, axis=1)
- * 2 * np.pi)
- else:
- # If necessary, make sure that the first time step of every
- # voiced segments is sin(pi) or cos(0)
- # This is used for pulse-train generation
-
- # identify the last time step in unvoiced segments
- uv = self._f02uv(f0_values)
- uv_1 = paddle.roll(uv, shifts=-1, axis=1)
- uv_1[:, -1, :] = 1
- u_loc = (uv < 1) * (uv_1 > 0)
-
- # get the instantanouse phase
- tmp_cumsum = paddle.cumsum(rad_values, axis=1)
- # different batch needs to be processed differently
- for idx in range(f0_values.shape[0]):
- temp_sum = tmp_cumsum[idx, u_loc[idx, :, 0], :]
- temp_sum[1:, :] = temp_sum[1:, :] - temp_sum[0:-1, :]
- # stores the accumulation of i.phase within
- # each voiced segments
- tmp_cumsum[idx, :, :] = 0
- tmp_cumsum[idx, u_loc[idx, :, 0], :] = temp_sum
-
- # rad_values - tmp_cumsum: remove the accumulation of i.phase
- # within the previous voiced segment.
- i_phase = paddle.cumsum(rad_values - tmp_cumsum, axis=1)
-
- # get the sines
- sines = paddle.cos(i_phase * 2 * np.pi)
- return sines
-
- def forward(self, f0):
- """ sine_tensor, uv = forward(f0)
- input F0: tensor(batchsize=1, length, dim=1)
- f0 for unvoiced steps should be 0
- output sine_tensor: tensor(batchsize=1, length, dim)
- output uv: tensor(batchsize=1, length, 1)
- """
- with paddle.no_grad():
- f0_buf = paddle.zeros((f0.shape[0], f0.shape[1], self.dim,))
- #device=f0.device)
- # fundamental component
- fn = paddle.multiply(f0, paddle.to_tensor([[range(1, self.harmonic_num + 2)]],dtype = 'float32'))
-
- # generate sine waveforms
- sine_waves = self._f02sine(fn) * self.sine_amp
-
- # generate uv signal
- # uv = torch.ones(f0.shape)
- # uv = uv * (f0 > self.voiced_threshold)
- uv = self._f02uv(f0)
-
- # noise: for unvoiced should be similar to sine_amp
- # std = self.sine_amp/3 -> max value ~ self.sine_amp
- # . for voiced regions is self.noise_std
- noise_amp = uv * self.noise_std + (1 - uv) * self.sine_amp / 3
- noise = noise_amp * paddle.randn(sine_waves.shape)
-
- # first: set the unvoiced part to 0 by uv
- # then: additive noise
- sine_waves = sine_waves * uv + noise
- return sine_waves, uv, noise
-
-
-class SourceModuleHnNSF(paddle.nn.Layer):
- """ SourceModule for hn-nsf
- SourceModule(sampling_rate, harmonic_num=0, sine_amp=0.1,
- add_noise_std=0.003, voiced_threshod=0)
- sampling_rate: sampling_rate in Hz
- harmonic_num: number of harmonic above F0 (default: 0)
- sine_amp: amplitude of sine source signal (default: 0.1)
- add_noise_std: std of additive Gaussian noise (default: 0.003)
- note that amplitude of noise in unvoiced is decided
- by sine_amp
- voiced_threshold: threhold to set U/V given F0 (default: 0)
- Sine_source, noise_source = SourceModuleHnNSF(F0_sampled)
- F0_sampled (batchsize, length, 1)
- Sine_source (batchsize, length, 1)
- noise_source (batchsize, length 1)
- uv (batchsize, length, 1)
- """
-
- def __init__(self, sampling_rate, harmonic_num=0, sine_amp=0.1,
- add_noise_std=0.003, voiced_threshod=0):
- super(SourceModuleHnNSF, self).__init__()
-
- self.sine_amp = sine_amp
- self.noise_std = add_noise_std
-
- # to produce sine waveforms
- self.l_sin_gen = SineGen(sampling_rate, harmonic_num,
- sine_amp, add_noise_std, voiced_threshod)
-
- # to merge source harmonics into a single excitation
- self.l_linear = paddle.nn.Linear(harmonic_num + 1, 1)
- self.l_tanh = paddle.nn.Tanh()
-
- def forward(self, x):
- """
- Sine_source, noise_source = SourceModuleHnNSF(F0_sampled)
- F0_sampled (batchsize, length, 1)
- Sine_source (batchsize, length, 1)
- noise_source (batchsize, length 1)
- """
- # source for harmonic branch
- sine_wavs, uv, _ = self.l_sin_gen(x)
- sine_merge = self.l_tanh(self.l_linear(sine_wavs))
-
- # source for noise branch, in the same shape as uv
- noise = paddle.randn(uv.shape) * self.sine_amp / 3
- return sine_merge, noise, uv
-
-
-class Generator(paddle.nn.Layer):
- def __init__(self, h):
- super(Generator, self).__init__()
- self.h = h
-
- self.num_kernels = len(h["resblock_kernel_sizes"])
- self.num_upsamples = len(h["upsample_rates"])
- self.f0_upsamp = paddle.nn.Upsample(scale_factor=paddle.to_tensor(np.prod(h["upsample_rates"])),data_format = 'NCW',mode = 'linear')
- self.m_source = SourceModuleHnNSF(
- sampling_rate=h["sampling_rate"],
- harmonic_num=8)
- self.noise_convs = nn.LayerList()
- self.conv_pre = weight_norm(Conv1D(h["inter_channels"], h["upsample_initial_channel"], 7, 1, padding=3))
- resblock = ResBlock1 if h["resblock"] == '1' else ResBlock2
- self.ups = nn.LayerList()
- for i, (u, k) in enumerate(zip(h["upsample_rates"], h["upsample_kernel_sizes"])):
- c_cur = h["upsample_initial_channel"] // (2 ** (i + 1))
- self.ups.append(weight_norm(
- Conv1DTranspose(h["upsample_initial_channel"] // (2 ** i), h["upsample_initial_channel"] // (2 ** (i + 1)),
- k, u, padding=(k - u) // 2)))
- if i + 1 < len(h["upsample_rates"]): #
- stride_f0 = np.prod(h["upsample_rates"][i + 1:])
- self.noise_convs.append(Conv1D(
- 1, c_cur, kernel_size=[stride_f0 * 2], stride=[stride_f0], padding=[int(stride_f0 // 2)]))
- else:
- self.noise_convs.append(Conv1D(1, c_cur, kernel_size=1))
- self.resblocks = nn.LayerList()
- for i in range(len(self.ups)):
- ch = h["upsample_initial_channel"] // (2 ** (i + 1))
- for j, (k, d) in enumerate(zip(h["resblock_kernel_sizes"], h["resblock_dilation_sizes"])):
- self.resblocks.append(resblock(h, ch, k, d))
-
- self.conv_post = weight_norm(Conv1D(ch, 1, 7, 1, padding=[3]))
- self.ups.apply(init_weights)
- self.conv_post.apply(init_weights)
- self.cond = nn.Conv1D(h['gin_channels'], h['upsample_initial_channel'], 1)
-
- def forward(self, x, f0, g=None):
- # print(1,x.shape,f0.shape,f0[:, None].shape)
- f0 = self.f0_upsamp(f0[:, None])
- f0 = f0.transpose([0,2,1]) # bs,n,t
- # print(2,f0.shape)
- har_source, noi_source, uv = self.m_source(f0)
- har_source = har_source.transpose([0,2,1])
- x = self.conv_pre(x)
- x = x + self.cond(g)
- # print(124,x.shape,har_source.shape)
- for i in range(self.num_upsamples):
- x = F.leaky_relu(x, LRELU_SLOPE)
- # print(3,x.shape)
- x = self.ups[i](x)
- x_source = self.noise_convs[i](har_source)
- # print(4,x_source.shape,har_source.shape,x.shape)
- x = x + x_source
- xs = None
- for j in range(self.num_kernels):
- if xs is None:
- xs = self.resblocks[i * self.num_kernels + j](x)
- else:
- xs += self.resblocks[i * self.num_kernels + j](x)
- x = xs / self.num_kernels
- x = F.leaky_relu(x)
- x = self.conv_post(x)
- x = paddle.tanh(x)
-
- return x
-
- def remove_weight_norm(self):
- print('移除weight norm……')
- for l in self.ups:
- remove_weight_norm(l)
- for l in self.resblocks:
- l.remove_weight_norm()
- remove_weight_norm(self.conv_pre)
- remove_weight_norm(self.conv_post)
-
-
-class DiscriminatorP(paddle.nn.Layer):
- def __init__(self, period, kernel_size=5, stride=3, use_spectral_norm=False):
- super(DiscriminatorP, self).__init__()
- self.period = period
- norm_f = weight_norm if use_spectral_norm == False else spectral_norm
- self.convs = nn.LayerList([
- norm_f(Conv2D(1, 32, (kernel_size, 1), (stride, 1), padding=(get_padding(5, 1), 0))),
- norm_f(Conv2D(32, 128, (kernel_size, 1), (stride, 1), padding=(get_padding(5, 1), 0))),
- norm_f(Conv2D(128, 512, (kernel_size, 1), (stride, 1), padding=(get_padding(5, 1), 0))),
- norm_f(Conv2D(512, 1024, (kernel_size, 1), (stride, 1), padding=(get_padding(5, 1), 0))),
- norm_f(Conv2D(1024, 1024, (kernel_size, 1), 1, padding=(2, 0))),
- ])
- self.conv_post = norm_f(Conv2D(1024, 1, (3, 1), 1, padding=(1, 0)))
-
- def forward(self, x):
- fmap = []
-
- # 1d to 2d
- b, c, t = x.shape
- if t % self.period != 0: # pad first
- n_pad = self.period - (t % self.period)
- x = F.pad(x, (0, n_pad), "reflect")
- t = t + n_pad
- x = x.view(b, c, t // self.period, self.period)
-
- for l in self.convs:
- x = l(x)
- x = F.leaky_relu(x, LRELU_SLOPE)
- fmap.append(x)
- x = self.conv_post(x)
- fmap.append(x)
- x = paddle.flatten(x, 1, -1)
-
- return x, fmap
-
-
-class MultiPeriodDiscriminator(paddle.nn.Layer):
- def __init__(self, periods=None):
- super(MultiPeriodDiscriminator, self).__init__()
- self.periods = periods if periods is not None else [2, 3, 5, 7, 11]
- self.discriminators = nn.LayerList()
- for period in self.periods:
- self.discriminators.append(DiscriminatorP(period))
-
- def forward(self, y, y_hat):
- y_d_rs = []
- y_d_gs = []
- fmap_rs = []
- fmap_gs = []
- for i, d in enumerate(self.discriminators):
- y_d_r, fmap_r = d(y)
- y_d_g, fmap_g = d(y_hat)
- y_d_rs.append(y_d_r)
- fmap_rs.append(fmap_r)
- y_d_gs.append(y_d_g)
- fmap_gs.append(fmap_g)
-
- return y_d_rs, y_d_gs, fmap_rs, fmap_gs
-
-
-class DiscriminatorS(paddle.nn.Layer):
- def __init__(self, use_spectral_norm=False):
- super(DiscriminatorS, self).__init__()
- norm_f = weight_norm if use_spectral_norm == False else spectral_norm
- self.convs = nn.LayerList([
- norm_f(Conv1D(1, 128, 15, 1, padding=7)),
- norm_f(Conv1D(128, 128, 41, 2, groups=4, padding=20)),
- norm_f(Conv1D(128, 256, 41, 2, groups=16, padding=20)),
- norm_f(Conv1D(256, 512, 41, 4, groups=16, padding=20)),
- norm_f(Conv1D(512, 1024, 41, 4, groups=16, padding=20)),
- norm_f(Conv1D(1024, 1024, 41, 1, groups=16, padding=20)),
- norm_f(Conv1D(1024, 1024, 5, 1, padding=2)),
- ])
- self.conv_post = norm_f(Conv1D(1024, 1, 3, 1, padding=1))
-
- def forward(self, x):
- fmap = []
- for l in self.convs:
- x = l(x)
- x = F.leaky_relu(x, LRELU_SLOPE)
- fmap.append(x)
- x = self.conv_post(x)
- fmap.append(x)
- x = paddle.flatten(x, 1, -1)
-
- return x, fmap
-
-
-class MultiScaleDiscriminator(paddle.nn.Layer):
- def __init__(self):
- super(MultiScaleDiscriminator, self).__init__()
- self.discriminators = nn.LayerList([
- DiscriminatorS(use_spectral_norm=True),
- DiscriminatorS(),
- DiscriminatorS(),
- ])
- self.meanpools = nn.LayerList([
- AvgPool1D(4, 2, padding=2),
- AvgPool1D(4, 2, padding=2)
- ])
-
- def forward(self, y, y_hat):
- y_d_rs = []
- y_d_gs = []
- fmap_rs = []
- fmap_gs = []
- for i, d in enumerate(self.discriminators):
- if i != 0:
- y = self.meanpools[i - 1](y)
- y_hat = self.meanpools[i - 1](y_hat)
- y_d_r, fmap_r = d(y)
- y_d_g, fmap_g = d(y_hat)
- y_d_rs.append(y_d_r)
- fmap_rs.append(fmap_r)
- y_d_gs.append(y_d_g)
- fmap_gs.append(fmap_g)
-
- return y_d_rs, y_d_gs, fmap_rs, fmap_gs
-
-
-def feature_loss(fmap_r, fmap_g):
- loss = 0
- for dr, dg in zip(fmap_r, fmap_g):
- for rl, gl in zip(dr, dg):
- loss += paddle.mean(paddle.abs(rl - gl))
-
- return loss * 2
-
-
-def discriminator_loss(disc_real_outputs, disc_generated_outputs):
- loss = 0
- r_losses = []
- g_losses = []
- for dr, dg in zip(disc_real_outputs, disc_generated_outputs):
- r_loss = paddle.mean((1 - dr) ** 2)
- g_loss = paddle.mean(dg ** 2)
- loss += (r_loss + g_loss)
- r_losses.append(r_loss.item())
- g_losses.append(g_loss.item())
-
- return loss, r_losses, g_losses
-
-
-def generator_loss(disc_outputs):
- loss = 0
- gen_losses = []
- for dg in disc_outputs:
- l = paddle.mean((1 - dg) ** 2)
- gen_losses.append(l)
- loss += l
-
- return loss, gen_losses
diff --git a/spaces/Zengyf-CVer/FaceRecognition/README.md b/spaces/Zengyf-CVer/FaceRecognition/README.md
deleted file mode 100644
index c6d18914d004b02fa625a894344c2fcba1f42218..0000000000000000000000000000000000000000
--- a/spaces/Zengyf-CVer/FaceRecognition/README.md
+++ /dev/null
@@ -1,13 +0,0 @@
----
-title: FaceRecognition
-emoji: 🚀
-colorFrom: red
-colorTo: red
-sdk: gradio
-sdk_version: 3.1.1
-app_file: app.py
-pinned: false
-license: gpl-3.0
----
-
-Check out the configuration reference at https://huggingface.co/docs/hub/spaces-config-reference
diff --git a/spaces/abhishek/sketch-to-image/annotator/uniformer/mmcv/visualization/image.py b/spaces/abhishek/sketch-to-image/annotator/uniformer/mmcv/visualization/image.py
deleted file mode 100644
index 61a56c75b67f593c298408462c63c0468be8e276..0000000000000000000000000000000000000000
--- a/spaces/abhishek/sketch-to-image/annotator/uniformer/mmcv/visualization/image.py
+++ /dev/null
@@ -1,152 +0,0 @@
-# Copyright (c) OpenMMLab. All rights reserved.
-import cv2
-import numpy as np
-
-from annotator.uniformer.mmcv.image import imread, imwrite
-from .color import color_val
-
-
-def imshow(img, win_name='', wait_time=0):
- """Show an image.
-
- Args:
- img (str or ndarray): The image to be displayed.
- win_name (str): The window name.
- wait_time (int): Value of waitKey param.
- """
- cv2.imshow(win_name, imread(img))
- if wait_time == 0: # prevent from hanging if windows was closed
- while True:
- ret = cv2.waitKey(1)
-
- closed = cv2.getWindowProperty(win_name, cv2.WND_PROP_VISIBLE) < 1
- # if user closed window or if some key pressed
- if closed or ret != -1:
- break
- else:
- ret = cv2.waitKey(wait_time)
-
-
-def imshow_bboxes(img,
- bboxes,
- colors='green',
- top_k=-1,
- thickness=1,
- show=True,
- win_name='',
- wait_time=0,
- out_file=None):
- """Draw bboxes on an image.
-
- Args:
- img (str or ndarray): The image to be displayed.
- bboxes (list or ndarray): A list of ndarray of shape (k, 4).
- colors (list[str or tuple or Color]): A list of colors.
- top_k (int): Plot the first k bboxes only if set positive.
- thickness (int): Thickness of lines.
- show (bool): Whether to show the image.
- win_name (str): The window name.
- wait_time (int): Value of waitKey param.
- out_file (str, optional): The filename to write the image.
-
- Returns:
- ndarray: The image with bboxes drawn on it.
- """
- img = imread(img)
- img = np.ascontiguousarray(img)
-
- if isinstance(bboxes, np.ndarray):
- bboxes = [bboxes]
- if not isinstance(colors, list):
- colors = [colors for _ in range(len(bboxes))]
- colors = [color_val(c) for c in colors]
- assert len(bboxes) == len(colors)
-
- for i, _bboxes in enumerate(bboxes):
- _bboxes = _bboxes.astype(np.int32)
- if top_k <= 0:
- _top_k = _bboxes.shape[0]
- else:
- _top_k = min(top_k, _bboxes.shape[0])
- for j in range(_top_k):
- left_top = (_bboxes[j, 0], _bboxes[j, 1])
- right_bottom = (_bboxes[j, 2], _bboxes[j, 3])
- cv2.rectangle(
- img, left_top, right_bottom, colors[i], thickness=thickness)
-
- if show:
- imshow(img, win_name, wait_time)
- if out_file is not None:
- imwrite(img, out_file)
- return img
-
-
-def imshow_det_bboxes(img,
- bboxes,
- labels,
- class_names=None,
- score_thr=0,
- bbox_color='green',
- text_color='green',
- thickness=1,
- font_scale=0.5,
- show=True,
- win_name='',
- wait_time=0,
- out_file=None):
- """Draw bboxes and class labels (with scores) on an image.
-
- Args:
- img (str or ndarray): The image to be displayed.
- bboxes (ndarray): Bounding boxes (with scores), shaped (n, 4) or
- (n, 5).
- labels (ndarray): Labels of bboxes.
- class_names (list[str]): Names of each classes.
- score_thr (float): Minimum score of bboxes to be shown.
- bbox_color (str or tuple or :obj:`Color`): Color of bbox lines.
- text_color (str or tuple or :obj:`Color`): Color of texts.
- thickness (int): Thickness of lines.
- font_scale (float): Font scales of texts.
- show (bool): Whether to show the image.
- win_name (str): The window name.
- wait_time (int): Value of waitKey param.
- out_file (str or None): The filename to write the image.
-
- Returns:
- ndarray: The image with bboxes drawn on it.
- """
- assert bboxes.ndim == 2
- assert labels.ndim == 1
- assert bboxes.shape[0] == labels.shape[0]
- assert bboxes.shape[1] == 4 or bboxes.shape[1] == 5
- img = imread(img)
- img = np.ascontiguousarray(img)
-
- if score_thr > 0:
- assert bboxes.shape[1] == 5
- scores = bboxes[:, -1]
- inds = scores > score_thr
- bboxes = bboxes[inds, :]
- labels = labels[inds]
-
- bbox_color = color_val(bbox_color)
- text_color = color_val(text_color)
-
- for bbox, label in zip(bboxes, labels):
- bbox_int = bbox.astype(np.int32)
- left_top = (bbox_int[0], bbox_int[1])
- right_bottom = (bbox_int[2], bbox_int[3])
- cv2.rectangle(
- img, left_top, right_bottom, bbox_color, thickness=thickness)
- label_text = class_names[
- label] if class_names is not None else f'cls {label}'
- if len(bbox) > 4:
- label_text += f'|{bbox[-1]:.02f}'
- cv2.putText(img, label_text, (bbox_int[0], bbox_int[1] - 2),
- cv2.FONT_HERSHEY_COMPLEX, font_scale, text_color)
-
- if show:
- imshow(img, win_name, wait_time)
- if out_file is not None:
- imwrite(img, out_file)
- return img
diff --git a/spaces/abrar-lohia/text-2-character-anim/VQTrans/dataset/prepare/download_extractor.sh b/spaces/abrar-lohia/text-2-character-anim/VQTrans/dataset/prepare/download_extractor.sh
deleted file mode 100644
index b1c456e8311a59a1c8d86e85da5ddd3aa7e1f9a4..0000000000000000000000000000000000000000
--- a/spaces/abrar-lohia/text-2-character-anim/VQTrans/dataset/prepare/download_extractor.sh
+++ /dev/null
@@ -1,15 +0,0 @@
-rm -rf checkpoints
-mkdir checkpoints
-cd checkpoints
-echo -e "Downloading extractors"
-gdown --fuzzy https://drive.google.com/file/d/1o7RTDQcToJjTm9_mNWTyzvZvjTWpZfug/view
-gdown --fuzzy https://drive.google.com/file/d/1tX79xk0fflp07EZ660Xz1RAFE33iEyJR/view
-
-
-unzip t2m.zip
-unzip kit.zip
-
-echo -e "Cleaning\n"
-rm t2m.zip
-rm kit.zip
-echo -e "Downloading done!"
\ No newline at end of file
diff --git a/spaces/abrar-lohia/text-2-character-anim/pyrender/.eggs/pyglet-2.0.5-py3.10.egg/pyglet/gl/__init__.py b/spaces/abrar-lohia/text-2-character-anim/pyrender/.eggs/pyglet-2.0.5-py3.10.egg/pyglet/gl/__init__.py
deleted file mode 100644
index a9ca9278e2ff3639e9d4c306d6c4c8e8ead8c658..0000000000000000000000000000000000000000
--- a/spaces/abrar-lohia/text-2-character-anim/pyrender/.eggs/pyglet-2.0.5-py3.10.egg/pyglet/gl/__init__.py
+++ /dev/null
@@ -1,191 +0,0 @@
-"""OpenGL interface.
-
-This package imports all OpenGL and registered OpenGL extension
-functions. Functions have identical signatures to their C counterparts.
-
-OpenGL is documented in full at the `OpenGL Reference Pages`_.
-
-The `OpenGL Programming Guide`_, also known as "The Red Book", is a popular
-reference manual organised by topic. It is available in digital and paper
-editions.
-
-.. _OpenGL Reference Pages: https://www.khronos.org/registry/OpenGL-Refpages/
-.. _OpenGL Programming Guide: http://opengl-redbook.com/
-
-The following subpackages are imported into this "mega" package already
-(and so are available by importing ``pyglet.gl``):
-
-``pyglet.gl.gl``
- OpenGL
-``pyglet.gl.gl.glext_arb``
- ARB registered OpenGL extension functions
-
-These subpackages are also available, but are not imported into this namespace
-by default:
-
-``pyglet.gl.glext_nv``
- nVidia OpenGL extension functions
-``pyglet.gl.agl``
- AGL (Mac OS X OpenGL context functions)
-``pyglet.gl.glx``
- GLX (Linux OpenGL context functions)
-``pyglet.gl.glxext_arb``
- ARB registered GLX extension functions
-``pyglet.gl.glxext_nv``
- nvidia GLX extension functions
-``pyglet.gl.wgl``
- WGL (Windows OpenGL context functions)
-``pyglet.gl.wglext_arb``
- ARB registered WGL extension functions
-``pyglet.gl.wglext_nv``
- nvidia WGL extension functions
-
-The information modules are provided for convenience, and are documented below.
-"""
-import pyglet as _pyglet
-
-from pyglet.gl.gl import *
-from pyglet.gl.lib import GLException
-from pyglet.gl import gl_info
-from pyglet.gl.gl_compat import GL_LUMINANCE, GL_INTENSITY
-
-from pyglet import compat_platform
-from .base import ObjectSpace, CanvasConfig, Context
-
-import sys as _sys
-_is_pyglet_doc_run = hasattr(_sys, "is_pyglet_doc_run") and _sys.is_pyglet_doc_run
-
-#: The active OpenGL context.
-#:
-#: You can change the current context by calling `Context.set_current`;
-#: do not modify this global.
-#:
-#: :type: `Context`
-#:
-#: .. versionadded:: 1.1
-current_context = None
-
-
-class ContextException(Exception):
- pass
-
-
-class ConfigException(Exception):
- pass
-
-
-if _pyglet.options['debug_texture']:
- _debug_texture_total = 0
- _debug_texture_sizes = {}
- _debug_texture = None
-
-
- def _debug_texture_alloc(texture, size):
- global _debug_texture_total
-
- _debug_texture_sizes[texture] = size
- _debug_texture_total += size
-
- print(f'{_debug_texture_total} (+{size})')
-
-
- def _debug_texture_dealloc(texture):
- global _debug_texture_total
-
- size = _debug_texture_sizes[texture]
- del _debug_texture_sizes[texture]
- _debug_texture_total -= size
-
- print(f'{_debug_texture_total} (-{size})')
-
-
- _glBindTexture = glBindTexture
-
-
- def glBindTexture(target, texture):
- global _debug_texture
- _debug_texture = texture
- return _glBindTexture(target, texture)
-
-
- _glTexImage2D = glTexImage2D
-
-
- def glTexImage2D(target, level, internalformat, width, height, border,
- format, type, pixels):
- try:
- _debug_texture_dealloc(_debug_texture)
- except KeyError:
- pass
-
- if internalformat in (1, GL_ALPHA, GL_INTENSITY, GL_LUMINANCE):
- depth = 1
- elif internalformat in (2, GL_RGB16, GL_RGBA16):
- depth = 2
- elif internalformat in (3, GL_RGB):
- depth = 3
- else:
- depth = 4 # Pretty crap assumption
- size = (width + 2 * border) * (height + 2 * border) * depth
- _debug_texture_alloc(_debug_texture, size)
-
- return _glTexImage2D(target, level, internalformat, width, height, border, format, type, pixels)
-
-
- _glDeleteTextures = glDeleteTextures
-
-
- def glDeleteTextures(n, textures):
- if not hasattr(textures, '__len__'):
- _debug_texture_dealloc(textures.value)
- else:
- for i in range(n):
- _debug_texture_dealloc(textures[i].value)
-
- return _glDeleteTextures(n, textures)
-
-
-def _create_shadow_window():
- global _shadow_window
-
- import pyglet
- if not pyglet.options['shadow_window'] or _is_pyglet_doc_run:
- return
-
- from pyglet.window import Window
-
- class ShadowWindow(Window):
- def __init__(self):
- super().__init__(width=1, height=1, visible=False)
-
- def _create_projection(self):
- """Shadow window does not need a projection."""
- pass
-
- _shadow_window = ShadowWindow()
- _shadow_window.switch_to()
-
- from pyglet import app
- app.windows.remove(_shadow_window)
-
-
-if _is_pyglet_doc_run:
- from .base import Config
-
-elif _pyglet.options['headless']:
- from .headless import HeadlessConfig as Config
-elif compat_platform in ('win32', 'cygwin'):
- from .win32 import Win32Config as Config
-elif compat_platform.startswith('linux'):
- from .xlib import XlibConfig as Config
-elif compat_platform == 'darwin':
- from .cocoa import CocoaConfig as Config
-
-
-_shadow_window = None
-
-# Import pyglet.window now if it isn't currently being imported (this creates the shadow window).
-if not _is_pyglet_doc_run and 'pyglet.window' not in _sys.modules and _pyglet.options['shadow_window']:
- # trickery is for circular import
- _pyglet.gl = _sys.modules[__name__]
- import pyglet.window
diff --git a/spaces/abrar-lohia/text-2-character-anim/pyrender/.eggs/pyglet-2.0.5-py3.10.egg/pyglet/gui/__init__.py b/spaces/abrar-lohia/text-2-character-anim/pyrender/.eggs/pyglet-2.0.5-py3.10.egg/pyglet/gui/__init__.py
deleted file mode 100644
index f5e5aebad799bfab0a8a9103a13464bfaa1e6742..0000000000000000000000000000000000000000
--- a/spaces/abrar-lohia/text-2-character-anim/pyrender/.eggs/pyglet-2.0.5-py3.10.egg/pyglet/gui/__init__.py
+++ /dev/null
@@ -1,2 +0,0 @@
-from .widgets import *
-from .frame import *
diff --git a/spaces/abrar-lohia/text-2-character-anim/pyrender/.eggs/pyglet-2.0.5-py3.10.egg/pyglet/media/drivers/xaudio2/__init__.py b/spaces/abrar-lohia/text-2-character-anim/pyrender/.eggs/pyglet-2.0.5-py3.10.egg/pyglet/media/drivers/xaudio2/__init__.py
deleted file mode 100644
index d337fd48ca9938f60c06af99fbf0acbec017676a..0000000000000000000000000000000000000000
--- a/spaces/abrar-lohia/text-2-character-anim/pyrender/.eggs/pyglet-2.0.5-py3.10.egg/pyglet/media/drivers/xaudio2/__init__.py
+++ /dev/null
@@ -1,8 +0,0 @@
-from . import adaptation
-
-
-def create_audio_driver():
- return adaptation.XAudio2Driver()
-
-
-__all__ = ["create_audio_driver"]
diff --git a/spaces/akhaliq/Real-Time-Voice-Cloning/vocoder/inference.py b/spaces/akhaliq/Real-Time-Voice-Cloning/vocoder/inference.py
deleted file mode 100644
index 7e546845da0b8cdb18b34fbd332b9aaa39cea55c..0000000000000000000000000000000000000000
--- a/spaces/akhaliq/Real-Time-Voice-Cloning/vocoder/inference.py
+++ /dev/null
@@ -1,64 +0,0 @@
-from vocoder.models.fatchord_version import WaveRNN
-from vocoder import hparams as hp
-import torch
-
-
-_model = None # type: WaveRNN
-
-def load_model(weights_fpath, verbose=True):
- global _model, _device
-
- if verbose:
- print("Building Wave-RNN")
- _model = WaveRNN(
- rnn_dims=hp.voc_rnn_dims,
- fc_dims=hp.voc_fc_dims,
- bits=hp.bits,
- pad=hp.voc_pad,
- upsample_factors=hp.voc_upsample_factors,
- feat_dims=hp.num_mels,
- compute_dims=hp.voc_compute_dims,
- res_out_dims=hp.voc_res_out_dims,
- res_blocks=hp.voc_res_blocks,
- hop_length=hp.hop_length,
- sample_rate=hp.sample_rate,
- mode=hp.voc_mode
- )
-
- if torch.cuda.is_available():
- _model = _model.cuda()
- _device = torch.device('cuda')
- else:
- _device = torch.device('cpu')
-
- if verbose:
- print("Loading model weights at %s" % weights_fpath)
- checkpoint = torch.load(weights_fpath, _device)
- _model.load_state_dict(checkpoint['model_state'])
- _model.eval()
-
-
-def is_loaded():
- return _model is not None
-
-
-def infer_waveform(mel, normalize=True, batched=True, target=8000, overlap=800,
- progress_callback=None):
- """
- Infers the waveform of a mel spectrogram output by the synthesizer (the format must match
- that of the synthesizer!)
-
- :param normalize:
- :param batched:
- :param target:
- :param overlap:
- :return:
- """
- if _model is None:
- raise Exception("Please load Wave-RNN in memory before using it")
-
- if normalize:
- mel = mel / hp.mel_max_abs_value
- mel = torch.from_numpy(mel[None, ...])
- wav = _model.generate(mel, batched, target, overlap, hp.mu_law, progress_callback)
- return wav
diff --git a/spaces/akhaliq/deeplab2/model/layers/blocks_test.py b/spaces/akhaliq/deeplab2/model/layers/blocks_test.py
deleted file mode 100644
index 0be9e6365d2c0b80cfeb3e78453d64b5f7eaac64..0000000000000000000000000000000000000000
--- a/spaces/akhaliq/deeplab2/model/layers/blocks_test.py
+++ /dev/null
@@ -1,72 +0,0 @@
-# coding=utf-8
-# Copyright 2021 The Deeplab2 Authors.
-#
-# Licensed under the Apache License, Version 2.0 (the "License");
-# you may not use this file except in compliance with the License.
-# You may obtain a copy of the License at
-#
-# http://www.apache.org/licenses/LICENSE-2.0
-#
-# Unless required by applicable law or agreed to in writing, software
-# distributed under the License is distributed on an "AS IS" BASIS,
-# WITHOUT WARRANTIES OR CONDITIONS OF ANY KIND, either express or implied.
-# See the License for the specific language governing permissions and
-# limitations under the License.
-
-"""Tests for blocks.py."""
-import tensorflow as tf
-
-from deeplab2.model.layers import blocks
-
-
-class BlocksTest(tf.test.TestCase):
-
- def test_inverted_bottleneck_block_output_shape(self):
- batch, height, width, input_channels = 2, 17, 17, 4
- output_channels = 6
- input_tensor = tf.random.uniform(
- shape=(batch, height, width, input_channels))
- ivb_block = blocks.InvertedBottleneckBlock(
- in_filters=input_channels,
- out_filters=output_channels,
- expand_ratio=2,
- strides=1,
- name='inverted_bottleneck',
- )
- output_tensor = ivb_block(input_tensor)
- self.assertListEqual(output_tensor.get_shape().as_list(),
- [batch, height, width, output_channels])
-
- def test_inverted_bottleneck_block_feature_map_alignment(self):
- batch, height, width, input_channels = 2, 17, 17, 128
- output_channels = 256
- input_tensor = tf.random.uniform(
- shape=(batch, height, width, input_channels))
- ivb_block1 = blocks.InvertedBottleneckBlock(
- in_filters=input_channels,
- out_filters=output_channels,
- expand_ratio=2,
- strides=2,
- name='inverted_bottleneck1',
- )
- ivb_block1(input_tensor, False)
- weights = ivb_block1.get_weights()
- output_tensor = ivb_block1(input_tensor, False)
-
- ivb_block2 = blocks.InvertedBottleneckBlock(
- in_filters=input_channels,
- out_filters=output_channels,
- expand_ratio=2,
- strides=1,
- name='inverted_bottleneck2',
- )
- ivb_block2(input_tensor, False)
- ivb_block2.set_weights(weights)
- expected = ivb_block2(input_tensor, False)[:, ::2, ::2, :]
-
- self.assertAllClose(ivb_block1.get_weights(), ivb_block2.get_weights(),
- atol=1e-4, rtol=1e-4)
- self.assertAllClose(output_tensor, expected, atol=1e-4, rtol=1e-4)
-
-if __name__ == '__main__':
- tf.test.main()
diff --git a/spaces/akhaliq/lama/saicinpainting/evaluation/losses/__init__.py b/spaces/akhaliq/lama/saicinpainting/evaluation/losses/__init__.py
deleted file mode 100644
index e69de29bb2d1d6434b8b29ae775ad8c2e48c5391..0000000000000000000000000000000000000000
diff --git a/spaces/akhaliq/mlsd/README.md b/spaces/akhaliq/mlsd/README.md
deleted file mode 100644
index 32f42daf79d6dc118d6752c67fabec0b301aabbe..0000000000000000000000000000000000000000
--- a/spaces/akhaliq/mlsd/README.md
+++ /dev/null
@@ -1,33 +0,0 @@
----
-title: Mlsd
-emoji: 👁
-colorFrom: indigo
-colorTo: pink
-sdk: gradio
-app_file: app.py
-pinned: false
----
-
-# Configuration
-
-`title`: _string_
-Display title for the Space
-
-`emoji`: _string_
-Space emoji (emoji-only character allowed)
-
-`colorFrom`: _string_
-Color for Thumbnail gradient (red, yellow, green, blue, indigo, purple, pink, gray)
-
-`colorTo`: _string_
-Color for Thumbnail gradient (red, yellow, green, blue, indigo, purple, pink, gray)
-
-`sdk`: _string_
-Can be either `gradio` or `streamlit`
-
-`app_file`: _string_
-Path to your main application file (which contains either `gradio` or `streamlit` Python code).
-Path is relative to the root of the repository.
-
-`pinned`: _boolean_
-Whether the Space stays on top of your list.
diff --git a/spaces/aliabid94/AutoGPT/tests/smoke_test.py b/spaces/aliabid94/AutoGPT/tests/smoke_test.py
deleted file mode 100644
index 1b9d643fc21f3703384a2bb4f2bd1d725f4dd418..0000000000000000000000000000000000000000
--- a/spaces/aliabid94/AutoGPT/tests/smoke_test.py
+++ /dev/null
@@ -1,59 +0,0 @@
-"""Smoke test for the autogpt package."""
-import os
-import subprocess
-import sys
-
-import pytest
-
-from autogpt.commands.file_operations import delete_file, read_file
-
-
-@pytest.mark.integration_test
-def test_write_file() -> None:
- """
- Test case to check if the write_file command can successfully write 'Hello World' to a file
- named 'hello_world.txt'.
-
- Read the current ai_settings.yaml file and store its content.
- """
- env_vars = {"MEMORY_BACKEND": "no_memory", "TEMPERATURE": "0"}
- ai_settings = None
- if os.path.exists("ai_settings.yaml"):
- with open("ai_settings.yaml", "r") as f:
- ai_settings = f.read()
- os.remove("ai_settings.yaml")
-
- try:
- if os.path.exists("hello_world.txt"):
- # Clean up any existing 'hello_world.txt' file before testing.
- delete_file("hello_world.txt")
- # Prepare input data for the test.
- input_data = """write_file-GPT
-an AI designed to use the write_file command to write 'Hello World' into a file named "hello_world.txt" and then use the task_complete command to complete the task.
-Use the write_file command to write 'Hello World' into a file named "hello_world.txt".
-Use the task_complete command to complete the task.
-Do not use any other commands.
-
-y -5
-EOF"""
- command = f"{sys.executable} -m autogpt"
-
- # Execute the script with the input data.
- process = subprocess.Popen(
- command,
- stdin=subprocess.PIPE,
- shell=True,
- env={**os.environ, **env_vars},
- )
- process.communicate(input_data.encode())
-
- # Read the content of the 'hello_world.txt' file created during the test.
- content = read_file("hello_world.txt")
- finally:
- if ai_settings:
- # Restore the original ai_settings.yaml file.
- with open("ai_settings.yaml", "w") as f:
- f.write(ai_settings)
-
- # Check if the content of the 'hello_world.txt' file is equal to 'Hello World'.
- assert content == "Hello World", f"Expected 'Hello World', got {content}"
diff --git a/spaces/allknowingroger/huggingface/assets/index-baa28006.js b/spaces/allknowingroger/huggingface/assets/index-baa28006.js
deleted file mode 100644
index 76783d5a0b47f9a20422e7df194c353bfbd61db1..0000000000000000000000000000000000000000
--- a/spaces/allknowingroger/huggingface/assets/index-baa28006.js
+++ /dev/null
@@ -1,41 +0,0 @@
-var Qc=Object.defineProperty;var Hc=(e,t,n)=>t in e?Qc(e,t,{enumerable:!0,configurable:!0,writable:!0,value:n}):e[t]=n;var hn=(e,t,n)=>(Hc(e,typeof t!="symbol"?t+"":t,n),n);(function(){const t=document.createElement("link").relList;if(t&&t.supports&&t.supports("modulepreload"))return;for(const l of document.querySelectorAll('link[rel="modulepreload"]'))r(l);new MutationObserver(l=>{for(const o of l)if(o.type==="childList")for(const i of o.addedNodes)i.tagName==="LINK"&&i.rel==="modulepreload"&&r(i)}).observe(document,{childList:!0,subtree:!0});function n(l){const o={};return l.integrity&&(o.integrity=l.integrity),l.referrerPolicy&&(o.referrerPolicy=l.referrerPolicy),l.crossOrigin==="use-credentials"?o.credentials="include":l.crossOrigin==="anonymous"?o.credentials="omit":o.credentials="same-origin",o}function r(l){if(l.ep)return;l.ep=!0;const o=n(l);fetch(l.href,o)}})();var es={exports:{}},ul={},ts={exports:{}},z={};/**
- * @license React
- * react.production.min.js
- *
- * Copyright (c) Facebook, Inc. and its affiliates.
- *
- * This source code is licensed under the MIT license found in the
- * LICENSE file in the root directory of this source tree.
- */var tr=Symbol.for("react.element"),Wc=Symbol.for("react.portal"),Kc=Symbol.for("react.fragment"),Yc=Symbol.for("react.strict_mode"),Xc=Symbol.for("react.profiler"),Gc=Symbol.for("react.provider"),qc=Symbol.for("react.context"),Zc=Symbol.for("react.forward_ref"),Jc=Symbol.for("react.suspense"),bc=Symbol.for("react.memo"),ed=Symbol.for("react.lazy"),Hi=Symbol.iterator;function td(e){return e===null||typeof e!="object"?null:(e=Hi&&e[Hi]||e["@@iterator"],typeof e=="function"?e:null)}var ns={isMounted:function(){return!1},enqueueForceUpdate:function(){},enqueueReplaceState:function(){},enqueueSetState:function(){}},rs=Object.assign,ls={};function dn(e,t,n){this.props=e,this.context=t,this.refs=ls,this.updater=n||ns}dn.prototype.isReactComponent={};dn.prototype.setState=function(e,t){if(typeof e!="object"&&typeof e!="function"&&e!=null)throw Error("setState(...): takes an object of state variables to update or a function which returns an object of state variables.");this.updater.enqueueSetState(this,e,t,"setState")};dn.prototype.forceUpdate=function(e){this.updater.enqueueForceUpdate(this,e,"forceUpdate")};function os(){}os.prototype=dn.prototype;function Ko(e,t,n){this.props=e,this.context=t,this.refs=ls,this.updater=n||ns}var Yo=Ko.prototype=new os;Yo.constructor=Ko;rs(Yo,dn.prototype);Yo.isPureReactComponent=!0;var Wi=Array.isArray,is=Object.prototype.hasOwnProperty,Xo={current:null},us={key:!0,ref:!0,__self:!0,__source:!0};function ss(e,t,n){var r,l={},o=null,i=null;if(t!=null)for(r in t.ref!==void 0&&(i=t.ref),t.key!==void 0&&(o=""+t.key),t)is.call(t,r)&&!us.hasOwnProperty(r)&&(l[r]=t[r]);var u=arguments.length-2;if(u===1)l.children=n;else if(1>>1,te=j[G];if(0>>1;Gl(jl,I))ktl(ur,jl)?(j[G]=ur,j[kt]=I,G=kt):(j[G]=jl,j[xt]=I,G=xt);else if(ktl(ur,I))j[G]=ur,j[kt]=I,G=kt;else break e}}return L}function l(j,L){var I=j.sortIndex-L.sortIndex;return I!==0?I:j.id-L.id}if(typeof performance=="object"&&typeof performance.now=="function"){var o=performance;e.unstable_now=function(){return o.now()}}else{var i=Date,u=i.now();e.unstable_now=function(){return i.now()-u}}var s=[],d=[],y=1,c=null,g=3,v=!1,w=!1,k=!1,M=typeof setTimeout=="function"?setTimeout:null,m=typeof clearTimeout=="function"?clearTimeout:null,f=typeof setImmediate<"u"?setImmediate:null;typeof navigator<"u"&&navigator.scheduling!==void 0&&navigator.scheduling.isInputPending!==void 0&&navigator.scheduling.isInputPending.bind(navigator.scheduling);function h(j){for(var L=n(d);L!==null;){if(L.callback===null)r(d);else if(L.startTime<=j)r(d),L.sortIndex=L.expirationTime,t(s,L);else break;L=n(d)}}function S(j){if(k=!1,h(j),!w)if(n(s)!==null)w=!0,El(C);else{var L=n(d);L!==null&&Cl(S,L.startTime-j)}}function C(j,L){w=!1,k&&(k=!1,m(T),T=-1),v=!0;var I=g;try{for(h(L),c=n(s);c!==null&&(!(c.expirationTime>L)||j&&!Ie());){var G=c.callback;if(typeof G=="function"){c.callback=null,g=c.priorityLevel;var te=G(c.expirationTime<=L);L=e.unstable_now(),typeof te=="function"?c.callback=te:c===n(s)&&r(s),h(L)}else r(s);c=n(s)}if(c!==null)var ir=!0;else{var xt=n(d);xt!==null&&Cl(S,xt.startTime-L),ir=!1}return ir}finally{c=null,g=I,v=!1}}var _=!1,N=null,T=-1,X=5,F=-1;function Ie(){return!(e.unstable_now()-Fj||125G?(j.sortIndex=I,t(d,j),n(s)===null&&j===n(d)&&(k?(m(T),T=-1):k=!0,Cl(S,I-G))):(j.sortIndex=te,t(s,j),w||v||(w=!0,El(C))),j},e.unstable_shouldYield=Ie,e.unstable_wrapCallback=function(j){var L=g;return function(){var I=g;g=L;try{return j.apply(this,arguments)}finally{g=I}}}})(fs);ds.exports=fs;var fd=ds.exports;/**
- * @license React
- * react-dom.production.min.js
- *
- * Copyright (c) Facebook, Inc. and its affiliates.
- *
- * This source code is licensed under the MIT license found in the
- * LICENSE file in the root directory of this source tree.
- */var ps=p,Ee=fd;function x(e){for(var t="https://reactjs.org/docs/error-decoder.html?invariant="+e,n=1;n"u"||typeof window.document>"u"||typeof window.document.createElement>"u"),bl=Object.prototype.hasOwnProperty,pd=/^[:A-Z_a-z\u00C0-\u00D6\u00D8-\u00F6\u00F8-\u02FF\u0370-\u037D\u037F-\u1FFF\u200C-\u200D\u2070-\u218F\u2C00-\u2FEF\u3001-\uD7FF\uF900-\uFDCF\uFDF0-\uFFFD][:A-Z_a-z\u00C0-\u00D6\u00D8-\u00F6\u00F8-\u02FF\u0370-\u037D\u037F-\u1FFF\u200C-\u200D\u2070-\u218F\u2C00-\u2FEF\u3001-\uD7FF\uF900-\uFDCF\uFDF0-\uFFFD\-.0-9\u00B7\u0300-\u036F\u203F-\u2040]*$/,Yi={},Xi={};function md(e){return bl.call(Xi,e)?!0:bl.call(Yi,e)?!1:pd.test(e)?Xi[e]=!0:(Yi[e]=!0,!1)}function yd(e,t,n,r){if(n!==null&&n.type===0)return!1;switch(typeof t){case"function":case"symbol":return!0;case"boolean":return r?!1:n!==null?!n.acceptsBooleans:(e=e.toLowerCase().slice(0,5),e!=="data-"&&e!=="aria-");default:return!1}}function hd(e,t,n,r){if(t===null||typeof t>"u"||yd(e,t,n,r))return!0;if(r)return!1;if(n!==null)switch(n.type){case 3:return!t;case 4:return t===!1;case 5:return isNaN(t);case 6:return isNaN(t)||1>t}return!1}function me(e,t,n,r,l,o,i){this.acceptsBooleans=t===2||t===3||t===4,this.attributeName=r,this.attributeNamespace=l,this.mustUseProperty=n,this.propertyName=e,this.type=t,this.sanitizeURL=o,this.removeEmptyString=i}var ie={};"children dangerouslySetInnerHTML defaultValue defaultChecked innerHTML suppressContentEditableWarning suppressHydrationWarning style".split(" ").forEach(function(e){ie[e]=new me(e,0,!1,e,null,!1,!1)});[["acceptCharset","accept-charset"],["className","class"],["htmlFor","for"],["httpEquiv","http-equiv"]].forEach(function(e){var t=e[0];ie[t]=new me(t,1,!1,e[1],null,!1,!1)});["contentEditable","draggable","spellCheck","value"].forEach(function(e){ie[e]=new me(e,2,!1,e.toLowerCase(),null,!1,!1)});["autoReverse","externalResourcesRequired","focusable","preserveAlpha"].forEach(function(e){ie[e]=new me(e,2,!1,e,null,!1,!1)});"allowFullScreen async autoFocus autoPlay controls default defer disabled disablePictureInPicture disableRemotePlayback formNoValidate hidden loop noModule noValidate open playsInline readOnly required reversed scoped seamless itemScope".split(" ").forEach(function(e){ie[e]=new me(e,3,!1,e.toLowerCase(),null,!1,!1)});["checked","multiple","muted","selected"].forEach(function(e){ie[e]=new me(e,3,!0,e,null,!1,!1)});["capture","download"].forEach(function(e){ie[e]=new me(e,4,!1,e,null,!1,!1)});["cols","rows","size","span"].forEach(function(e){ie[e]=new me(e,6,!1,e,null,!1,!1)});["rowSpan","start"].forEach(function(e){ie[e]=new me(e,5,!1,e.toLowerCase(),null,!1,!1)});var qo=/[\-:]([a-z])/g;function Zo(e){return e[1].toUpperCase()}"accent-height alignment-baseline arabic-form baseline-shift cap-height clip-path clip-rule color-interpolation color-interpolation-filters color-profile color-rendering dominant-baseline enable-background fill-opacity fill-rule flood-color flood-opacity font-family font-size font-size-adjust font-stretch font-style font-variant font-weight glyph-name glyph-orientation-horizontal glyph-orientation-vertical horiz-adv-x horiz-origin-x image-rendering letter-spacing lighting-color marker-end marker-mid marker-start overline-position overline-thickness paint-order panose-1 pointer-events rendering-intent shape-rendering stop-color stop-opacity strikethrough-position strikethrough-thickness stroke-dasharray stroke-dashoffset stroke-linecap stroke-linejoin stroke-miterlimit stroke-opacity stroke-width text-anchor text-decoration text-rendering underline-position underline-thickness unicode-bidi unicode-range units-per-em v-alphabetic v-hanging v-ideographic v-mathematical vector-effect vert-adv-y vert-origin-x vert-origin-y word-spacing writing-mode xmlns:xlink x-height".split(" ").forEach(function(e){var t=e.replace(qo,Zo);ie[t]=new me(t,1,!1,e,null,!1,!1)});"xlink:actuate xlink:arcrole xlink:role xlink:show xlink:title xlink:type".split(" ").forEach(function(e){var t=e.replace(qo,Zo);ie[t]=new me(t,1,!1,e,"http://www.w3.org/1999/xlink",!1,!1)});["xml:base","xml:lang","xml:space"].forEach(function(e){var t=e.replace(qo,Zo);ie[t]=new me(t,1,!1,e,"http://www.w3.org/XML/1998/namespace",!1,!1)});["tabIndex","crossOrigin"].forEach(function(e){ie[e]=new me(e,1,!1,e.toLowerCase(),null,!1,!1)});ie.xlinkHref=new me("xlinkHref",1,!1,"xlink:href","http://www.w3.org/1999/xlink",!0,!1);["src","href","action","formAction"].forEach(function(e){ie[e]=new me(e,1,!1,e.toLowerCase(),null,!0,!0)});function Jo(e,t,n,r){var l=ie.hasOwnProperty(t)?ie[t]:null;(l!==null?l.type!==0:r||!(2u||l[i]!==o[u]){var s=`
-`+l[i].replace(" at new "," at ");return e.displayName&&s.includes("")&&(s=s.replace("",e.displayName)),s}while(1<=i&&0<=u);break}}}finally{Tl=!1,Error.prepareStackTrace=n}return(e=e?e.displayName||e.name:"")?jn(e):""}function gd(e){switch(e.tag){case 5:return jn(e.type);case 16:return jn("Lazy");case 13:return jn("Suspense");case 19:return jn("SuspenseList");case 0:case 2:case 15:return e=Ol(e.type,!1),e;case 11:return e=Ol(e.type.render,!1),e;case 1:return e=Ol(e.type,!0),e;default:return""}}function ro(e){if(e==null)return null;if(typeof e=="function")return e.displayName||e.name||null;if(typeof e=="string")return e;switch(e){case Ut:return"Fragment";case $t:return"Portal";case eo:return"Profiler";case bo:return"StrictMode";case to:return"Suspense";case no:return"SuspenseList"}if(typeof e=="object")switch(e.$$typeof){case hs:return(e.displayName||"Context")+".Consumer";case ys:return(e._context.displayName||"Context")+".Provider";case ei:var t=e.render;return e=e.displayName,e||(e=t.displayName||t.name||"",e=e!==""?"ForwardRef("+e+")":"ForwardRef"),e;case ti:return t=e.displayName||null,t!==null?t:ro(e.type)||"Memo";case nt:t=e._payload,e=e._init;try{return ro(e(t))}catch{}}return null}function vd(e){var t=e.type;switch(e.tag){case 24:return"Cache";case 9:return(t.displayName||"Context")+".Consumer";case 10:return(t._context.displayName||"Context")+".Provider";case 18:return"DehydratedFragment";case 11:return e=t.render,e=e.displayName||e.name||"",t.displayName||(e!==""?"ForwardRef("+e+")":"ForwardRef");case 7:return"Fragment";case 5:return t;case 4:return"Portal";case 3:return"Root";case 6:return"Text";case 16:return ro(t);case 8:return t===bo?"StrictMode":"Mode";case 22:return"Offscreen";case 12:return"Profiler";case 21:return"Scope";case 13:return"Suspense";case 19:return"SuspenseList";case 25:return"TracingMarker";case 1:case 0:case 17:case 2:case 14:case 15:if(typeof t=="function")return t.displayName||t.name||null;if(typeof t=="string")return t}return null}function ht(e){switch(typeof e){case"boolean":case"number":case"string":case"undefined":return e;case"object":return e;default:return""}}function vs(e){var t=e.type;return(e=e.nodeName)&&e.toLowerCase()==="input"&&(t==="checkbox"||t==="radio")}function wd(e){var t=vs(e)?"checked":"value",n=Object.getOwnPropertyDescriptor(e.constructor.prototype,t),r=""+e[t];if(!e.hasOwnProperty(t)&&typeof n<"u"&&typeof n.get=="function"&&typeof n.set=="function"){var l=n.get,o=n.set;return Object.defineProperty(e,t,{configurable:!0,get:function(){return l.call(this)},set:function(i){r=""+i,o.call(this,i)}}),Object.defineProperty(e,t,{enumerable:n.enumerable}),{getValue:function(){return r},setValue:function(i){r=""+i},stopTracking:function(){e._valueTracker=null,delete e[t]}}}}function cr(e){e._valueTracker||(e._valueTracker=wd(e))}function ws(e){if(!e)return!1;var t=e._valueTracker;if(!t)return!0;var n=t.getValue(),r="";return e&&(r=vs(e)?e.checked?"true":"false":e.value),e=r,e!==n?(t.setValue(e),!0):!1}function Mr(e){if(e=e||(typeof document<"u"?document:void 0),typeof e>"u")return null;try{return e.activeElement||e.body}catch{return e.body}}function lo(e,t){var n=t.checked;return K({},t,{defaultChecked:void 0,defaultValue:void 0,value:void 0,checked:n??e._wrapperState.initialChecked})}function qi(e,t){var n=t.defaultValue==null?"":t.defaultValue,r=t.checked!=null?t.checked:t.defaultChecked;n=ht(t.value!=null?t.value:n),e._wrapperState={initialChecked:r,initialValue:n,controlled:t.type==="checkbox"||t.type==="radio"?t.checked!=null:t.value!=null}}function Ss(e,t){t=t.checked,t!=null&&Jo(e,"checked",t,!1)}function oo(e,t){Ss(e,t);var n=ht(t.value),r=t.type;if(n!=null)r==="number"?(n===0&&e.value===""||e.value!=n)&&(e.value=""+n):e.value!==""+n&&(e.value=""+n);else if(r==="submit"||r==="reset"){e.removeAttribute("value");return}t.hasOwnProperty("value")?io(e,t.type,n):t.hasOwnProperty("defaultValue")&&io(e,t.type,ht(t.defaultValue)),t.checked==null&&t.defaultChecked!=null&&(e.defaultChecked=!!t.defaultChecked)}function Zi(e,t,n){if(t.hasOwnProperty("value")||t.hasOwnProperty("defaultValue")){var r=t.type;if(!(r!=="submit"&&r!=="reset"||t.value!==void 0&&t.value!==null))return;t=""+e._wrapperState.initialValue,n||t===e.value||(e.value=t),e.defaultValue=t}n=e.name,n!==""&&(e.name=""),e.defaultChecked=!!e._wrapperState.initialChecked,n!==""&&(e.name=n)}function io(e,t,n){(t!=="number"||Mr(e.ownerDocument)!==e)&&(n==null?e.defaultValue=""+e._wrapperState.initialValue:e.defaultValue!==""+n&&(e.defaultValue=""+n))}var _n=Array.isArray;function Zt(e,t,n,r){if(e=e.options,t){t={};for(var l=0;l"+t.valueOf().toString()+"",t=dr.firstChild;e.firstChild;)e.removeChild(e.firstChild);for(;t.firstChild;)e.appendChild(t.firstChild)}});function $n(e,t){if(t){var n=e.firstChild;if(n&&n===e.lastChild&&n.nodeType===3){n.nodeValue=t;return}}e.textContent=t}var On={animationIterationCount:!0,aspectRatio:!0,borderImageOutset:!0,borderImageSlice:!0,borderImageWidth:!0,boxFlex:!0,boxFlexGroup:!0,boxOrdinalGroup:!0,columnCount:!0,columns:!0,flex:!0,flexGrow:!0,flexPositive:!0,flexShrink:!0,flexNegative:!0,flexOrder:!0,gridArea:!0,gridRow:!0,gridRowEnd:!0,gridRowSpan:!0,gridRowStart:!0,gridColumn:!0,gridColumnEnd:!0,gridColumnSpan:!0,gridColumnStart:!0,fontWeight:!0,lineClamp:!0,lineHeight:!0,opacity:!0,order:!0,orphans:!0,tabSize:!0,widows:!0,zIndex:!0,zoom:!0,fillOpacity:!0,floodOpacity:!0,stopOpacity:!0,strokeDasharray:!0,strokeDashoffset:!0,strokeMiterlimit:!0,strokeOpacity:!0,strokeWidth:!0},Sd=["Webkit","ms","Moz","O"];Object.keys(On).forEach(function(e){Sd.forEach(function(t){t=t+e.charAt(0).toUpperCase()+e.substring(1),On[t]=On[e]})});function Cs(e,t,n){return t==null||typeof t=="boolean"||t===""?"":n||typeof t!="number"||t===0||On.hasOwnProperty(e)&&On[e]?(""+t).trim():t+"px"}function js(e,t){e=e.style;for(var n in t)if(t.hasOwnProperty(n)){var r=n.indexOf("--")===0,l=Cs(n,t[n],r);n==="float"&&(n="cssFloat"),r?e.setProperty(n,l):e[n]=l}}var xd=K({menuitem:!0},{area:!0,base:!0,br:!0,col:!0,embed:!0,hr:!0,img:!0,input:!0,keygen:!0,link:!0,meta:!0,param:!0,source:!0,track:!0,wbr:!0});function ao(e,t){if(t){if(xd[e]&&(t.children!=null||t.dangerouslySetInnerHTML!=null))throw Error(x(137,e));if(t.dangerouslySetInnerHTML!=null){if(t.children!=null)throw Error(x(60));if(typeof t.dangerouslySetInnerHTML!="object"||!("__html"in t.dangerouslySetInnerHTML))throw Error(x(61))}if(t.style!=null&&typeof t.style!="object")throw Error(x(62))}}function co(e,t){if(e.indexOf("-")===-1)return typeof t.is=="string";switch(e){case"annotation-xml":case"color-profile":case"font-face":case"font-face-src":case"font-face-uri":case"font-face-format":case"font-face-name":case"missing-glyph":return!1;default:return!0}}var fo=null;function ni(e){return e=e.target||e.srcElement||window,e.correspondingUseElement&&(e=e.correspondingUseElement),e.nodeType===3?e.parentNode:e}var po=null,Jt=null,bt=null;function eu(e){if(e=lr(e)){if(typeof po!="function")throw Error(x(280));var t=e.stateNode;t&&(t=fl(t),po(e.stateNode,e.type,t))}}function _s(e){Jt?bt?bt.push(e):bt=[e]:Jt=e}function Ns(){if(Jt){var e=Jt,t=bt;if(bt=Jt=null,eu(e),t)for(e=0;e>>=0,e===0?32:31-(Id(e)/zd|0)|0}var fr=64,pr=4194304;function Nn(e){switch(e&-e){case 1:return 1;case 2:return 2;case 4:return 4;case 8:return 8;case 16:return 16;case 32:return 32;case 64:case 128:case 256:case 512:case 1024:case 2048:case 4096:case 8192:case 16384:case 32768:case 65536:case 131072:case 262144:case 524288:case 1048576:case 2097152:return e&4194240;case 4194304:case 8388608:case 16777216:case 33554432:case 67108864:return e&130023424;case 134217728:return 134217728;case 268435456:return 268435456;case 536870912:return 536870912;case 1073741824:return 1073741824;default:return e}}function Vr(e,t){var n=e.pendingLanes;if(n===0)return 0;var r=0,l=e.suspendedLanes,o=e.pingedLanes,i=n&268435455;if(i!==0){var u=i&~l;u!==0?r=Nn(u):(o&=i,o!==0&&(r=Nn(o)))}else i=n&~l,i!==0?r=Nn(i):o!==0&&(r=Nn(o));if(r===0)return 0;if(t!==0&&t!==r&&!(t&l)&&(l=r&-r,o=t&-t,l>=o||l===16&&(o&4194240)!==0))return t;if(r&4&&(r|=n&16),t=e.entangledLanes,t!==0)for(e=e.entanglements,t&=r;0n;n++)t.push(e);return t}function nr(e,t,n){e.pendingLanes|=t,t!==536870912&&(e.suspendedLanes=0,e.pingedLanes=0),e=e.eventTimes,t=31-Me(t),e[t]=n}function Md(e,t){var n=e.pendingLanes&~t;e.pendingLanes=t,e.suspendedLanes=0,e.pingedLanes=0,e.expiredLanes&=t,e.mutableReadLanes&=t,e.entangledLanes&=t,t=e.entanglements;var r=e.eventTimes;for(e=e.expirationTimes;0=Ln),au=String.fromCharCode(32),cu=!1;function Ys(e,t){switch(e){case"keyup":return ff.indexOf(t.keyCode)!==-1;case"keydown":return t.keyCode!==229;case"keypress":case"mousedown":case"focusout":return!0;default:return!1}}function Xs(e){return e=e.detail,typeof e=="object"&&"data"in e?e.data:null}var Vt=!1;function mf(e,t){switch(e){case"compositionend":return Xs(t);case"keypress":return t.which!==32?null:(cu=!0,au);case"textInput":return e=t.data,e===au&&cu?null:e;default:return null}}function yf(e,t){if(Vt)return e==="compositionend"||!ci&&Ys(e,t)?(e=Ws(),Tr=ui=it=null,Vt=!1,e):null;switch(e){case"paste":return null;case"keypress":if(!(t.ctrlKey||t.altKey||t.metaKey)||t.ctrlKey&&t.altKey){if(t.char&&1=t)return{node:n,offset:t-e};e=r}e:{for(;n;){if(n.nextSibling){n=n.nextSibling;break e}n=n.parentNode}n=void 0}n=mu(n)}}function Js(e,t){return e&&t?e===t?!0:e&&e.nodeType===3?!1:t&&t.nodeType===3?Js(e,t.parentNode):"contains"in e?e.contains(t):e.compareDocumentPosition?!!(e.compareDocumentPosition(t)&16):!1:!1}function bs(){for(var e=window,t=Mr();t instanceof e.HTMLIFrameElement;){try{var n=typeof t.contentWindow.location.href=="string"}catch{n=!1}if(n)e=t.contentWindow;else break;t=Mr(e.document)}return t}function di(e){var t=e&&e.nodeName&&e.nodeName.toLowerCase();return t&&(t==="input"&&(e.type==="text"||e.type==="search"||e.type==="tel"||e.type==="url"||e.type==="password")||t==="textarea"||e.contentEditable==="true")}function Cf(e){var t=bs(),n=e.focusedElem,r=e.selectionRange;if(t!==n&&n&&n.ownerDocument&&Js(n.ownerDocument.documentElement,n)){if(r!==null&&di(n)){if(t=r.start,e=r.end,e===void 0&&(e=t),"selectionStart"in n)n.selectionStart=t,n.selectionEnd=Math.min(e,n.value.length);else if(e=(t=n.ownerDocument||document)&&t.defaultView||window,e.getSelection){e=e.getSelection();var l=n.textContent.length,o=Math.min(r.start,l);r=r.end===void 0?o:Math.min(r.end,l),!e.extend&&o>r&&(l=r,r=o,o=l),l=yu(n,o);var i=yu(n,r);l&&i&&(e.rangeCount!==1||e.anchorNode!==l.node||e.anchorOffset!==l.offset||e.focusNode!==i.node||e.focusOffset!==i.offset)&&(t=t.createRange(),t.setStart(l.node,l.offset),e.removeAllRanges(),o>r?(e.addRange(t),e.extend(i.node,i.offset)):(t.setEnd(i.node,i.offset),e.addRange(t)))}}for(t=[],e=n;e=e.parentNode;)e.nodeType===1&&t.push({element:e,left:e.scrollLeft,top:e.scrollTop});for(typeof n.focus=="function"&&n.focus(),n=0;n=document.documentMode,Bt=null,wo=null,zn=null,So=!1;function hu(e,t,n){var r=n.window===n?n.document:n.nodeType===9?n:n.ownerDocument;So||Bt==null||Bt!==Mr(r)||(r=Bt,"selectionStart"in r&&di(r)?r={start:r.selectionStart,end:r.selectionEnd}:(r=(r.ownerDocument&&r.ownerDocument.defaultView||window).getSelection(),r={anchorNode:r.anchorNode,anchorOffset:r.anchorOffset,focusNode:r.focusNode,focusOffset:r.focusOffset}),zn&&Wn(zn,r)||(zn=r,r=Hr(wo,"onSelect"),0Wt||(e.current=_o[Wt],_o[Wt]=null,Wt--)}function D(e,t){Wt++,_o[Wt]=e.current,e.current=t}var gt={},ce=wt(gt),ge=wt(!1),Pt=gt;function ln(e,t){var n=e.type.contextTypes;if(!n)return gt;var r=e.stateNode;if(r&&r.__reactInternalMemoizedUnmaskedChildContext===t)return r.__reactInternalMemoizedMaskedChildContext;var l={},o;for(o in n)l[o]=t[o];return r&&(e=e.stateNode,e.__reactInternalMemoizedUnmaskedChildContext=t,e.__reactInternalMemoizedMaskedChildContext=l),l}function ve(e){return e=e.childContextTypes,e!=null}function Kr(){V(ge),V(ce)}function Eu(e,t,n){if(ce.current!==gt)throw Error(x(168));D(ce,t),D(ge,n)}function sa(e,t,n){var r=e.stateNode;if(t=t.childContextTypes,typeof r.getChildContext!="function")return n;r=r.getChildContext();for(var l in r)if(!(l in t))throw Error(x(108,vd(e)||"Unknown",l));return K({},n,r)}function Yr(e){return e=(e=e.stateNode)&&e.__reactInternalMemoizedMergedChildContext||gt,Pt=ce.current,D(ce,e),D(ge,ge.current),!0}function Cu(e,t,n){var r=e.stateNode;if(!r)throw Error(x(169));n?(e=sa(e,t,Pt),r.__reactInternalMemoizedMergedChildContext=e,V(ge),V(ce),D(ce,e)):V(ge),D(ge,n)}var Ke=null,pl=!1,Ql=!1;function aa(e){Ke===null?Ke=[e]:Ke.push(e)}function Af(e){pl=!0,aa(e)}function St(){if(!Ql&&Ke!==null){Ql=!0;var e=0,t=A;try{var n=Ke;for(A=1;e>=i,l-=i,Ye=1<<32-Me(t)+l|n<T?(X=N,N=null):X=N.sibling;var F=g(m,N,h[T],S);if(F===null){N===null&&(N=X);break}e&&N&&F.alternate===null&&t(m,N),f=o(F,f,T),_===null?C=F:_.sibling=F,_=F,N=X}if(T===h.length)return n(m,N),B&&Et(m,T),C;if(N===null){for(;TT?(X=N,N=null):X=N.sibling;var Ie=g(m,N,F.value,S);if(Ie===null){N===null&&(N=X);break}e&&N&&Ie.alternate===null&&t(m,N),f=o(Ie,f,T),_===null?C=Ie:_.sibling=Ie,_=Ie,N=X}if(F.done)return n(m,N),B&&Et(m,T),C;if(N===null){for(;!F.done;T++,F=h.next())F=c(m,F.value,S),F!==null&&(f=o(F,f,T),_===null?C=F:_.sibling=F,_=F);return B&&Et(m,T),C}for(N=r(m,N);!F.done;T++,F=h.next())F=v(N,m,T,F.value,S),F!==null&&(e&&F.alternate!==null&&N.delete(F.key===null?T:F.key),f=o(F,f,T),_===null?C=F:_.sibling=F,_=F);return e&&N.forEach(function(mn){return t(m,mn)}),B&&Et(m,T),C}function M(m,f,h,S){if(typeof h=="object"&&h!==null&&h.type===Ut&&h.key===null&&(h=h.props.children),typeof h=="object"&&h!==null){switch(h.$$typeof){case ar:e:{for(var C=h.key,_=f;_!==null;){if(_.key===C){if(C=h.type,C===Ut){if(_.tag===7){n(m,_.sibling),f=l(_,h.props.children),f.return=m,m=f;break e}}else if(_.elementType===C||typeof C=="object"&&C!==null&&C.$$typeof===nt&&Lu(C)===_.type){n(m,_.sibling),f=l(_,h.props),f.ref=kn(m,_,h),f.return=m,m=f;break e}n(m,_);break}else t(m,_);_=_.sibling}h.type===Ut?(f=Ot(h.props.children,m.mode,S,h.key),f.return=m,m=f):(S=Ar(h.type,h.key,h.props,null,m.mode,S),S.ref=kn(m,f,h),S.return=m,m=S)}return i(m);case $t:e:{for(_=h.key;f!==null;){if(f.key===_)if(f.tag===4&&f.stateNode.containerInfo===h.containerInfo&&f.stateNode.implementation===h.implementation){n(m,f.sibling),f=l(f,h.children||[]),f.return=m,m=f;break e}else{n(m,f);break}else t(m,f);f=f.sibling}f=Zl(h,m.mode,S),f.return=m,m=f}return i(m);case nt:return _=h._init,M(m,f,_(h._payload),S)}if(_n(h))return w(m,f,h,S);if(gn(h))return k(m,f,h,S);Sr(m,h)}return typeof h=="string"&&h!==""||typeof h=="number"?(h=""+h,f!==null&&f.tag===6?(n(m,f.sibling),f=l(f,h),f.return=m,m=f):(n(m,f),f=ql(h,m.mode,S),f.return=m,m=f),i(m)):n(m,f)}return M}var un=ga(!0),va=ga(!1),or={},He=wt(or),Gn=wt(or),qn=wt(or);function Nt(e){if(e===or)throw Error(x(174));return e}function Si(e,t){switch(D(qn,t),D(Gn,e),D(He,or),e=t.nodeType,e){case 9:case 11:t=(t=t.documentElement)?t.namespaceURI:so(null,"");break;default:e=e===8?t.parentNode:t,t=e.namespaceURI||null,e=e.tagName,t=so(t,e)}V(He),D(He,t)}function sn(){V(He),V(Gn),V(qn)}function wa(e){Nt(qn.current);var t=Nt(He.current),n=so(t,e.type);t!==n&&(D(Gn,e),D(He,n))}function xi(e){Gn.current===e&&(V(He),V(Gn))}var H=wt(0);function br(e){for(var t=e;t!==null;){if(t.tag===13){var n=t.memoizedState;if(n!==null&&(n=n.dehydrated,n===null||n.data==="$?"||n.data==="$!"))return t}else if(t.tag===19&&t.memoizedProps.revealOrder!==void 0){if(t.flags&128)return t}else if(t.child!==null){t.child.return=t,t=t.child;continue}if(t===e)break;for(;t.sibling===null;){if(t.return===null||t.return===e)return null;t=t.return}t.sibling.return=t.return,t=t.sibling}return null}var Hl=[];function ki(){for(var e=0;en?n:4,e(!0);var r=Wl.transition;Wl.transition={};try{e(!1),t()}finally{A=n,Wl.transition=r}}function Ra(){return Le().memoizedState}function Uf(e,t,n){var r=mt(e);if(n={lane:r,action:n,hasEagerState:!1,eagerState:null,next:null},Aa(e))Ma(t,n);else if(n=pa(e,t,n,r),n!==null){var l=fe();De(n,e,r,l),Da(n,t,r)}}function Vf(e,t,n){var r=mt(e),l={lane:r,action:n,hasEagerState:!1,eagerState:null,next:null};if(Aa(e))Ma(t,l);else{var o=e.alternate;if(e.lanes===0&&(o===null||o.lanes===0)&&(o=t.lastRenderedReducer,o!==null))try{var i=t.lastRenderedState,u=o(i,n);if(l.hasEagerState=!0,l.eagerState=u,$e(u,i)){var s=t.interleaved;s===null?(l.next=l,vi(t)):(l.next=s.next,s.next=l),t.interleaved=l;return}}catch{}finally{}n=pa(e,t,l,r),n!==null&&(l=fe(),De(n,e,r,l),Da(n,t,r))}}function Aa(e){var t=e.alternate;return e===W||t!==null&&t===W}function Ma(e,t){Fn=el=!0;var n=e.pending;n===null?t.next=t:(t.next=n.next,n.next=t),e.pending=t}function Da(e,t,n){if(n&4194240){var r=t.lanes;r&=e.pendingLanes,n|=r,t.lanes=n,li(e,n)}}var tl={readContext:Pe,useCallback:ue,useContext:ue,useEffect:ue,useImperativeHandle:ue,useInsertionEffect:ue,useLayoutEffect:ue,useMemo:ue,useReducer:ue,useRef:ue,useState:ue,useDebugValue:ue,useDeferredValue:ue,useTransition:ue,useMutableSource:ue,useSyncExternalStore:ue,useId:ue,unstable_isNewReconciler:!1},Bf={readContext:Pe,useCallback:function(e,t){return Ve().memoizedState=[e,t===void 0?null:t],e},useContext:Pe,useEffect:zu,useImperativeHandle:function(e,t,n){return n=n!=null?n.concat([e]):null,Ir(4194308,4,Pa.bind(null,t,e),n)},useLayoutEffect:function(e,t){return Ir(4194308,4,e,t)},useInsertionEffect:function(e,t){return Ir(4,2,e,t)},useMemo:function(e,t){var n=Ve();return t=t===void 0?null:t,e=e(),n.memoizedState=[e,t],e},useReducer:function(e,t,n){var r=Ve();return t=n!==void 0?n(t):t,r.memoizedState=r.baseState=t,e={pending:null,interleaved:null,lanes:0,dispatch:null,lastRenderedReducer:e,lastRenderedState:t},r.queue=e,e=e.dispatch=Uf.bind(null,W,e),[r.memoizedState,e]},useRef:function(e){var t=Ve();return e={current:e},t.memoizedState=e},useState:Iu,useDebugValue:Ni,useDeferredValue:function(e){return Ve().memoizedState=e},useTransition:function(){var e=Iu(!1),t=e[0];return e=$f.bind(null,e[1]),Ve().memoizedState=e,[t,e]},useMutableSource:function(){},useSyncExternalStore:function(e,t,n){var r=W,l=Ve();if(B){if(n===void 0)throw Error(x(407));n=n()}else{if(n=t(),re===null)throw Error(x(349));It&30||ka(r,t,n)}l.memoizedState=n;var o={value:n,getSnapshot:t};return l.queue=o,zu(Ca.bind(null,r,o,e),[e]),r.flags|=2048,bn(9,Ea.bind(null,r,o,n,t),void 0,null),n},useId:function(){var e=Ve(),t=re.identifierPrefix;if(B){var n=Xe,r=Ye;n=(r&~(1<<32-Me(r)-1)).toString(32)+n,t=":"+t+"R"+n,n=Zn++,0<\/script>",e=e.removeChild(e.firstChild)):typeof r.is=="string"?e=i.createElement(n,{is:r.is}):(e=i.createElement(n),n==="select"&&(i=e,r.multiple?i.multiple=!0:r.size&&(i.size=r.size))):e=i.createElementNS(e,n),e[Be]=t,e[Xn]=r,Ya(e,t,!1,!1),t.stateNode=e;e:{switch(i=co(n,r),n){case"dialog":U("cancel",e),U("close",e),l=r;break;case"iframe":case"object":case"embed":U("load",e),l=r;break;case"video":case"audio":for(l=0;lcn&&(t.flags|=128,r=!0,En(o,!1),t.lanes=4194304)}else{if(!r)if(e=br(i),e!==null){if(t.flags|=128,r=!0,n=e.updateQueue,n!==null&&(t.updateQueue=n,t.flags|=4),En(o,!0),o.tail===null&&o.tailMode==="hidden"&&!i.alternate&&!B)return se(t),null}else 2*q()-o.renderingStartTime>cn&&n!==1073741824&&(t.flags|=128,r=!0,En(o,!1),t.lanes=4194304);o.isBackwards?(i.sibling=t.child,t.child=i):(n=o.last,n!==null?n.sibling=i:t.child=i,o.last=i)}return o.tail!==null?(t=o.tail,o.rendering=t,o.tail=t.sibling,o.renderingStartTime=q(),t.sibling=null,n=H.current,D(H,r?n&1|2:n&1),t):(se(t),null);case 22:case 23:return zi(),r=t.memoizedState!==null,e!==null&&e.memoizedState!==null!==r&&(t.flags|=8192),r&&t.mode&1?Se&1073741824&&(se(t),t.subtreeFlags&6&&(t.flags|=8192)):se(t),null;case 24:return null;case 25:return null}throw Error(x(156,t.tag))}function qf(e,t){switch(pi(t),t.tag){case 1:return ve(t.type)&&Kr(),e=t.flags,e&65536?(t.flags=e&-65537|128,t):null;case 3:return sn(),V(ge),V(ce),ki(),e=t.flags,e&65536&&!(e&128)?(t.flags=e&-65537|128,t):null;case 5:return xi(t),null;case 13:if(V(H),e=t.memoizedState,e!==null&&e.dehydrated!==null){if(t.alternate===null)throw Error(x(340));on()}return e=t.flags,e&65536?(t.flags=e&-65537|128,t):null;case 19:return V(H),null;case 4:return sn(),null;case 10:return gi(t.type._context),null;case 22:case 23:return zi(),null;case 24:return null;default:return null}}var kr=!1,ae=!1,Zf=typeof WeakSet=="function"?WeakSet:Set,E=null;function Gt(e,t){var n=e.ref;if(n!==null)if(typeof n=="function")try{n(null)}catch(r){Y(e,t,r)}else n.current=null}function Do(e,t,n){try{n()}catch(r){Y(e,t,r)}}var Bu=!1;function Jf(e,t){if(xo=Br,e=bs(),di(e)){if("selectionStart"in e)var n={start:e.selectionStart,end:e.selectionEnd};else e:{n=(n=e.ownerDocument)&&n.defaultView||window;var r=n.getSelection&&n.getSelection();if(r&&r.rangeCount!==0){n=r.anchorNode;var l=r.anchorOffset,o=r.focusNode;r=r.focusOffset;try{n.nodeType,o.nodeType}catch{n=null;break e}var i=0,u=-1,s=-1,d=0,y=0,c=e,g=null;t:for(;;){for(var v;c!==n||l!==0&&c.nodeType!==3||(u=i+l),c!==o||r!==0&&c.nodeType!==3||(s=i+r),c.nodeType===3&&(i+=c.nodeValue.length),(v=c.firstChild)!==null;)g=c,c=v;for(;;){if(c===e)break t;if(g===n&&++d===l&&(u=i),g===o&&++y===r&&(s=i),(v=c.nextSibling)!==null)break;c=g,g=c.parentNode}c=v}n=u===-1||s===-1?null:{start:u,end:s}}else n=null}n=n||{start:0,end:0}}else n=null;for(ko={focusedElem:e,selectionRange:n},Br=!1,E=t;E!==null;)if(t=E,e=t.child,(t.subtreeFlags&1028)!==0&&e!==null)e.return=t,E=e;else for(;E!==null;){t=E;try{var w=t.alternate;if(t.flags&1024)switch(t.tag){case 0:case 11:case 15:break;case 1:if(w!==null){var k=w.memoizedProps,M=w.memoizedState,m=t.stateNode,f=m.getSnapshotBeforeUpdate(t.elementType===t.type?k:Fe(t.type,k),M);m.__reactInternalSnapshotBeforeUpdate=f}break;case 3:var h=t.stateNode.containerInfo;h.nodeType===1?h.textContent="":h.nodeType===9&&h.documentElement&&h.removeChild(h.documentElement);break;case 5:case 6:case 4:case 17:break;default:throw Error(x(163))}}catch(S){Y(t,t.return,S)}if(e=t.sibling,e!==null){e.return=t.return,E=e;break}E=t.return}return w=Bu,Bu=!1,w}function Rn(e,t,n){var r=t.updateQueue;if(r=r!==null?r.lastEffect:null,r!==null){var l=r=r.next;do{if((l.tag&e)===e){var o=l.destroy;l.destroy=void 0,o!==void 0&&Do(t,n,o)}l=l.next}while(l!==r)}}function hl(e,t){if(t=t.updateQueue,t=t!==null?t.lastEffect:null,t!==null){var n=t=t.next;do{if((n.tag&e)===e){var r=n.create;n.destroy=r()}n=n.next}while(n!==t)}}function $o(e){var t=e.ref;if(t!==null){var n=e.stateNode;switch(e.tag){case 5:e=n;break;default:e=n}typeof t=="function"?t(e):t.current=e}}function qa(e){var t=e.alternate;t!==null&&(e.alternate=null,qa(t)),e.child=null,e.deletions=null,e.sibling=null,e.tag===5&&(t=e.stateNode,t!==null&&(delete t[Be],delete t[Xn],delete t[jo],delete t[Ff],delete t[Rf])),e.stateNode=null,e.return=null,e.dependencies=null,e.memoizedProps=null,e.memoizedState=null,e.pendingProps=null,e.stateNode=null,e.updateQueue=null}function Za(e){return e.tag===5||e.tag===3||e.tag===4}function Qu(e){e:for(;;){for(;e.sibling===null;){if(e.return===null||Za(e.return))return null;e=e.return}for(e.sibling.return=e.return,e=e.sibling;e.tag!==5&&e.tag!==6&&e.tag!==18;){if(e.flags&2||e.child===null||e.tag===4)continue e;e.child.return=e,e=e.child}if(!(e.flags&2))return e.stateNode}}function Uo(e,t,n){var r=e.tag;if(r===5||r===6)e=e.stateNode,t?n.nodeType===8?n.parentNode.insertBefore(e,t):n.insertBefore(e,t):(n.nodeType===8?(t=n.parentNode,t.insertBefore(e,n)):(t=n,t.appendChild(e)),n=n._reactRootContainer,n!=null||t.onclick!==null||(t.onclick=Wr));else if(r!==4&&(e=e.child,e!==null))for(Uo(e,t,n),e=e.sibling;e!==null;)Uo(e,t,n),e=e.sibling}function Vo(e,t,n){var r=e.tag;if(r===5||r===6)e=e.stateNode,t?n.insertBefore(e,t):n.appendChild(e);else if(r!==4&&(e=e.child,e!==null))for(Vo(e,t,n),e=e.sibling;e!==null;)Vo(e,t,n),e=e.sibling}var le=null,Re=!1;function tt(e,t,n){for(n=n.child;n!==null;)Ja(e,t,n),n=n.sibling}function Ja(e,t,n){if(Qe&&typeof Qe.onCommitFiberUnmount=="function")try{Qe.onCommitFiberUnmount(sl,n)}catch{}switch(n.tag){case 5:ae||Gt(n,t);case 6:var r=le,l=Re;le=null,tt(e,t,n),le=r,Re=l,le!==null&&(Re?(e=le,n=n.stateNode,e.nodeType===8?e.parentNode.removeChild(n):e.removeChild(n)):le.removeChild(n.stateNode));break;case 18:le!==null&&(Re?(e=le,n=n.stateNode,e.nodeType===8?Bl(e.parentNode,n):e.nodeType===1&&Bl(e,n),Qn(e)):Bl(le,n.stateNode));break;case 4:r=le,l=Re,le=n.stateNode.containerInfo,Re=!0,tt(e,t,n),le=r,Re=l;break;case 0:case 11:case 14:case 15:if(!ae&&(r=n.updateQueue,r!==null&&(r=r.lastEffect,r!==null))){l=r=r.next;do{var o=l,i=o.destroy;o=o.tag,i!==void 0&&(o&2||o&4)&&Do(n,t,i),l=l.next}while(l!==r)}tt(e,t,n);break;case 1:if(!ae&&(Gt(n,t),r=n.stateNode,typeof r.componentWillUnmount=="function"))try{r.props=n.memoizedProps,r.state=n.memoizedState,r.componentWillUnmount()}catch(u){Y(n,t,u)}tt(e,t,n);break;case 21:tt(e,t,n);break;case 22:n.mode&1?(ae=(r=ae)||n.memoizedState!==null,tt(e,t,n),ae=r):tt(e,t,n);break;default:tt(e,t,n)}}function Hu(e){var t=e.updateQueue;if(t!==null){e.updateQueue=null;var n=e.stateNode;n===null&&(n=e.stateNode=new Zf),t.forEach(function(r){var l=up.bind(null,e,r);n.has(r)||(n.add(r),r.then(l,l))})}}function ze(e,t){var n=t.deletions;if(n!==null)for(var r=0;rl&&(l=i),r&=~o}if(r=l,r=q()-r,r=(120>r?120:480>r?480:1080>r?1080:1920>r?1920:3e3>r?3e3:4320>r?4320:1960*ep(r/1960))-r,10e?16:e,ut===null)var r=!1;else{if(e=ut,ut=null,ll=0,R&6)throw Error(x(331));var l=R;for(R|=4,E=e.current;E!==null;){var o=E,i=o.child;if(E.flags&16){var u=o.deletions;if(u!==null){for(var s=0;sq()-Li?Tt(e,0):Pi|=n),we(e,t)}function ic(e,t){t===0&&(e.mode&1?(t=pr,pr<<=1,!(pr&130023424)&&(pr=4194304)):t=1);var n=fe();e=Je(e,t),e!==null&&(nr(e,t,n),we(e,n))}function ip(e){var t=e.memoizedState,n=0;t!==null&&(n=t.retryLane),ic(e,n)}function up(e,t){var n=0;switch(e.tag){case 13:var r=e.stateNode,l=e.memoizedState;l!==null&&(n=l.retryLane);break;case 19:r=e.stateNode;break;default:throw Error(x(314))}r!==null&&r.delete(t),ic(e,n)}var uc;uc=function(e,t,n){if(e!==null)if(e.memoizedProps!==t.pendingProps||ge.current)he=!0;else{if(!(e.lanes&n)&&!(t.flags&128))return he=!1,Xf(e,t,n);he=!!(e.flags&131072)}else he=!1,B&&t.flags&1048576&&ca(t,Gr,t.index);switch(t.lanes=0,t.tag){case 2:var r=t.type;zr(e,t),e=t.pendingProps;var l=ln(t,ce.current);tn(t,n),l=Ci(null,t,r,e,l,n);var o=ji();return t.flags|=1,typeof l=="object"&&l!==null&&typeof l.render=="function"&&l.$$typeof===void 0?(t.tag=1,t.memoizedState=null,t.updateQueue=null,ve(r)?(o=!0,Yr(t)):o=!1,t.memoizedState=l.state!==null&&l.state!==void 0?l.state:null,wi(t),l.updater=ml,t.stateNode=l,l._reactInternals=t,Lo(t,r,e,n),t=Fo(null,t,r,!0,o,n)):(t.tag=0,B&&o&&fi(t),de(null,t,l,n),t=t.child),t;case 16:r=t.elementType;e:{switch(zr(e,t),e=t.pendingProps,l=r._init,r=l(r._payload),t.type=r,l=t.tag=ap(r),e=Fe(r,e),l){case 0:t=zo(null,t,r,e,n);break e;case 1:t=$u(null,t,r,e,n);break e;case 11:t=Mu(null,t,r,e,n);break e;case 14:t=Du(null,t,r,Fe(r.type,e),n);break e}throw Error(x(306,r,""))}return t;case 0:return r=t.type,l=t.pendingProps,l=t.elementType===r?l:Fe(r,l),zo(e,t,r,l,n);case 1:return r=t.type,l=t.pendingProps,l=t.elementType===r?l:Fe(r,l),$u(e,t,r,l,n);case 3:e:{if(Ha(t),e===null)throw Error(x(387));r=t.pendingProps,o=t.memoizedState,l=o.element,ma(e,t),Jr(t,r,null,n);var i=t.memoizedState;if(r=i.element,o.isDehydrated)if(o={element:r,isDehydrated:!1,cache:i.cache,pendingSuspenseBoundaries:i.pendingSuspenseBoundaries,transitions:i.transitions},t.updateQueue.baseState=o,t.memoizedState=o,t.flags&256){l=an(Error(x(423)),t),t=Uu(e,t,r,n,l);break e}else if(r!==l){l=an(Error(x(424)),t),t=Uu(e,t,r,n,l);break e}else for(xe=dt(t.stateNode.containerInfo.firstChild),ke=t,B=!0,Ae=null,n=va(t,null,r,n),t.child=n;n;)n.flags=n.flags&-3|4096,n=n.sibling;else{if(on(),r===l){t=be(e,t,n);break e}de(e,t,r,n)}t=t.child}return t;case 5:return wa(t),e===null&&To(t),r=t.type,l=t.pendingProps,o=e!==null?e.memoizedProps:null,i=l.children,Eo(r,l)?i=null:o!==null&&Eo(r,o)&&(t.flags|=32),Qa(e,t),de(e,t,i,n),t.child;case 6:return e===null&&To(t),null;case 13:return Wa(e,t,n);case 4:return Si(t,t.stateNode.containerInfo),r=t.pendingProps,e===null?t.child=un(t,null,r,n):de(e,t,r,n),t.child;case 11:return r=t.type,l=t.pendingProps,l=t.elementType===r?l:Fe(r,l),Mu(e,t,r,l,n);case 7:return de(e,t,t.pendingProps,n),t.child;case 8:return de(e,t,t.pendingProps.children,n),t.child;case 12:return de(e,t,t.pendingProps.children,n),t.child;case 10:e:{if(r=t.type._context,l=t.pendingProps,o=t.memoizedProps,i=l.value,D(qr,r._currentValue),r._currentValue=i,o!==null)if($e(o.value,i)){if(o.children===l.children&&!ge.current){t=be(e,t,n);break e}}else for(o=t.child,o!==null&&(o.return=t);o!==null;){var u=o.dependencies;if(u!==null){i=o.child;for(var s=u.firstContext;s!==null;){if(s.context===r){if(o.tag===1){s=Ge(-1,n&-n),s.tag=2;var d=o.updateQueue;if(d!==null){d=d.shared;var y=d.pending;y===null?s.next=s:(s.next=y.next,y.next=s),d.pending=s}}o.lanes|=n,s=o.alternate,s!==null&&(s.lanes|=n),Oo(o.return,n,t),u.lanes|=n;break}s=s.next}}else if(o.tag===10)i=o.type===t.type?null:o.child;else if(o.tag===18){if(i=o.return,i===null)throw Error(x(341));i.lanes|=n,u=i.alternate,u!==null&&(u.lanes|=n),Oo(i,n,t),i=o.sibling}else i=o.child;if(i!==null)i.return=o;else for(i=o;i!==null;){if(i===t){i=null;break}if(o=i.sibling,o!==null){o.return=i.return,i=o;break}i=i.return}o=i}de(e,t,l.children,n),t=t.child}return t;case 9:return l=t.type,r=t.pendingProps.children,tn(t,n),l=Pe(l),r=r(l),t.flags|=1,de(e,t,r,n),t.child;case 14:return r=t.type,l=Fe(r,t.pendingProps),l=Fe(r.type,l),Du(e,t,r,l,n);case 15:return Va(e,t,t.type,t.pendingProps,n);case 17:return r=t.type,l=t.pendingProps,l=t.elementType===r?l:Fe(r,l),zr(e,t),t.tag=1,ve(r)?(e=!0,Yr(t)):e=!1,tn(t,n),ha(t,r,l),Lo(t,r,l,n),Fo(null,t,r,!0,e,n);case 19:return Ka(e,t,n);case 22:return Ba(e,t,n)}throw Error(x(156,t.tag))};function sc(e,t){return Fs(e,t)}function sp(e,t,n,r){this.tag=e,this.key=n,this.sibling=this.child=this.return=this.stateNode=this.type=this.elementType=null,this.index=0,this.ref=null,this.pendingProps=t,this.dependencies=this.memoizedState=this.updateQueue=this.memoizedProps=null,this.mode=r,this.subtreeFlags=this.flags=0,this.deletions=null,this.childLanes=this.lanes=0,this.alternate=null}function Te(e,t,n,r){return new sp(e,t,n,r)}function Ri(e){return e=e.prototype,!(!e||!e.isReactComponent)}function ap(e){if(typeof e=="function")return Ri(e)?1:0;if(e!=null){if(e=e.$$typeof,e===ei)return 11;if(e===ti)return 14}return 2}function yt(e,t){var n=e.alternate;return n===null?(n=Te(e.tag,t,e.key,e.mode),n.elementType=e.elementType,n.type=e.type,n.stateNode=e.stateNode,n.alternate=e,e.alternate=n):(n.pendingProps=t,n.type=e.type,n.flags=0,n.subtreeFlags=0,n.deletions=null),n.flags=e.flags&14680064,n.childLanes=e.childLanes,n.lanes=e.lanes,n.child=e.child,n.memoizedProps=e.memoizedProps,n.memoizedState=e.memoizedState,n.updateQueue=e.updateQueue,t=e.dependencies,n.dependencies=t===null?null:{lanes:t.lanes,firstContext:t.firstContext},n.sibling=e.sibling,n.index=e.index,n.ref=e.ref,n}function Ar(e,t,n,r,l,o){var i=2;if(r=e,typeof e=="function")Ri(e)&&(i=1);else if(typeof e=="string")i=5;else e:switch(e){case Ut:return Ot(n.children,l,o,t);case bo:i=8,l|=8;break;case eo:return e=Te(12,n,t,l|2),e.elementType=eo,e.lanes=o,e;case to:return e=Te(13,n,t,l),e.elementType=to,e.lanes=o,e;case no:return e=Te(19,n,t,l),e.elementType=no,e.lanes=o,e;case gs:return vl(n,l,o,t);default:if(typeof e=="object"&&e!==null)switch(e.$$typeof){case ys:i=10;break e;case hs:i=9;break e;case ei:i=11;break e;case ti:i=14;break e;case nt:i=16,r=null;break e}throw Error(x(130,e==null?e:typeof e,""))}return t=Te(i,n,t,l),t.elementType=e,t.type=r,t.lanes=o,t}function Ot(e,t,n,r){return e=Te(7,e,r,t),e.lanes=n,e}function vl(e,t,n,r){return e=Te(22,e,r,t),e.elementType=gs,e.lanes=n,e.stateNode={isHidden:!1},e}function ql(e,t,n){return e=Te(6,e,null,t),e.lanes=n,e}function Zl(e,t,n){return t=Te(4,e.children!==null?e.children:[],e.key,t),t.lanes=n,t.stateNode={containerInfo:e.containerInfo,pendingChildren:null,implementation:e.implementation},t}function cp(e,t,n,r,l){this.tag=t,this.containerInfo=e,this.finishedWork=this.pingCache=this.current=this.pendingChildren=null,this.timeoutHandle=-1,this.callbackNode=this.pendingContext=this.context=null,this.callbackPriority=0,this.eventTimes=Ll(0),this.expirationTimes=Ll(-1),this.entangledLanes=this.finishedLanes=this.mutableReadLanes=this.expiredLanes=this.pingedLanes=this.suspendedLanes=this.pendingLanes=0,this.entanglements=Ll(0),this.identifierPrefix=r,this.onRecoverableError=l,this.mutableSourceEagerHydrationData=null}function Ai(e,t,n,r,l,o,i,u,s){return e=new cp(e,t,n,u,s),t===1?(t=1,o===!0&&(t|=8)):t=0,o=Te(3,null,null,t),e.current=o,o.stateNode=e,o.memoizedState={element:r,isDehydrated:n,cache:null,transitions:null,pendingSuspenseBoundaries:null},wi(o),e}function dp(e,t,n){var r=3"u"||typeof __REACT_DEVTOOLS_GLOBAL_HOOK__.checkDCE!="function"))try{__REACT_DEVTOOLS_GLOBAL_HOOK__.checkDCE(fc)}catch(e){console.error(e)}}fc(),cs.exports=Ce;var hp=cs.exports,pc,Ju=hp;pc=Ju.createRoot,Ju.hydrateRoot;var gp=(typeof process<"u","https://huggingface.co");async function vp(e,t){var r;const n=new wp(e.url,e.status,e.headers.get("X-Request-Id")??(t==null?void 0:t.requestId));if(n.message=`Api error with status ${n.statusCode}.${t!=null&&t.message?` ${t.message}.`:""} Request ID: ${n.requestId}, url: ${n.url}`,(r=e.headers.get("Content-Type"))!=null&&r.startsWith("application/json")){const l=await e.json();n.message=l.error||l.message||n.message,n.data=l}else n.data={message:await e.text()};throw n}var wp=class extends Error{constructor(t,n,r,l){super(l);hn(this,"statusCode");hn(this,"url");hn(this,"requestId");hn(this,"data");this.statusCode=n,this.requestId=r,this.url=t}};function Sp(e){if(!(!e||e.accessToken===void 0||e.accessToken===null)&&!e.accessToken.startsWith("hf_"))throw new TypeError("Your access token must start with 'hf_'")}function xp(e){const t=/<(https?:[/][/][^>]+)>;\s+rel="([^"]+)"/g;return Object.fromEntries([...e.matchAll(t)].map(([,n,r])=>[r,n]))}var kp=["pipeline_tag","private","gated","downloads","likes"];async function*Ep(e){var r,l;Sp(e==null?void 0:e.credentials);const t=new URLSearchParams([...Object.entries({limit:"500",...(r=e==null?void 0:e.search)!=null&&r.owner?{author:e.search.owner}:void 0,...(l=e==null?void 0:e.search)!=null&&l.task?{pipeline_tag:e.search.task}:void 0}),...kp.map(o=>["expand",o])]).toString();let n=`${(e==null?void 0:e.hubUrl)||gp}/api/models?${t}`;for(;n;){const o=await fetch(n,{headers:{accept:"application/json",...e!=null&&e.credentials?{Authorization:`Bearer ${e.credentials.accessToken}`}:void 0}});if(!o.ok)throw vp(o);const i=await o.json();for(const s of i)yield{id:s._id,name:s.id,private:s.private,task:s.pipeline_tag,downloads:s.downloads,gated:s.gated,likes:s.likes,updatedAt:new Date(s.lastModified)};const u=o.headers.get("Link");n=u?xp(u).next:void 0}}var Cp=Object.defineProperty,jp=(e,t)=>{for(var n in t)Cp(e,n,{get:t[n],enumerable:!0})},_p={};jp(_p,{audioClassification:()=>yc,automaticSpeechRecognition:()=>hc,conversational:()=>Cc,documentQuestionAnswering:()=>Ac,featureExtraction:()=>jc,fillMask:()=>_c,imageClassification:()=>vc,imageSegmentation:()=>wc,imageToImage:()=>Ec,imageToText:()=>Sc,objectDetection:()=>xc,questionAnswering:()=>Nc,request:()=>$,sentenceSimilarity:()=>Tc,streamingRequest:()=>Ui,summarization:()=>Oc,tableQuestionAnswering:()=>Pc,tabularRegression:()=>Dc,textClassification:()=>Lc,textGeneration:()=>Ic,textGenerationStream:()=>Lp,textToImage:()=>kc,textToSpeech:()=>gc,tokenClassification:()=>zc,translation:()=>Fc,visualQuestionAnswering:()=>Mc,zeroShotClassification:()=>Rc});var Np="https://api-inference.huggingface.co/models/";function mc(e,t){const{model:n,accessToken:r,...l}=e,o={};r&&(o.Authorization=`Bearer ${r}`);const i="data"in e&&!!e.data;i?(t!=null&&t.wait_for_model&&(o["X-Wait-For-Model"]="true"),(t==null?void 0:t.use_cache)===!1&&(o["X-Use-Cache"]="false"),t!=null&&t.dont_load_model&&(o["X-Load-Model"]="0")):o["Content-Type"]="application/json";const u=/^http(s?):/.test(n)||n.startsWith("/")?n:`${Np}${n}`,s={headers:o,method:"POST",body:i?e.data:JSON.stringify({...l,options:t}),credentials:t!=null&&t.includeCredentials?"include":"same-origin"};return{url:u,info:s}}async function $(e,t){var o,i;const{url:n,info:r}=mc(e,t),l=await((t==null?void 0:t.fetch)??fetch)(n,r);if((t==null?void 0:t.retry_on_error)!==!1&&l.status===503&&!(t!=null&&t.wait_for_model))return $(e,{...t,wait_for_model:!0});if(!l.ok){if((o=l.headers.get("Content-Type"))!=null&&o.startsWith("application/json")){const u=await l.json();if(u.error)throw new Error(u.error)}throw new Error("An error occurred while fetching the blob")}return(i=l.headers.get("Content-Type"))!=null&&i.startsWith("application/json")?await l.json():await l.blob()}function Tp(e){let t,n,r,l=!1;return function(i){t===void 0?(t=i,n=0,r=-1):t=Pp(t,i);const u=t.length;let s=0;for(;n0){const s=l.decode(i.subarray(0,u)),d=u+(i[u+1]===32?2:1),y=l.decode(i.subarray(d));switch(s){case"data":r.data=r.data?r.data+`
-`+y:y;break;case"event":r.event=y;break;case"id":e(r.id=y);break;case"retry":const c=parseInt(y,10);isNaN(c)||t(r.retry=c);break}}}}function Pp(e,t){const n=new Uint8Array(e.length+t.length);return n.set(e),n.set(t,e.length),n}function bu(){return{data:"",event:"",id:"",retry:void 0}}async function*Ui(e,t){var d;const{url:n,info:r}=mc({...e,stream:!0},t),l=await((t==null?void 0:t.fetch)??fetch)(n,r);if((t==null?void 0:t.retry_on_error)!==!1&&l.status===503&&!(t!=null&&t.wait_for_model))return Ui(e,{...t,wait_for_model:!0});if(!l.ok){if((d=l.headers.get("Content-Type"))!=null&&d.startsWith("application/json")){const y=await l.json();if(y.error)throw new Error(y.error)}throw new Error(`Server response contains error: ${l.status}`)}if(l.headers.get("content-type")!=="text/event-stream")throw new Error("Server does not support event stream content type, it returned "+l.headers.get("content-type"));if(!l.body)return;const o=l.body.getReader();let i=[];const s=Tp(Op(()=>{},()=>{},y=>{i.push(y)}));try{for(;;){const{done:y,value:c}=await o.read();if(y)return;s(c);for(const g of i)if(g.data.length>0){const v=JSON.parse(g.data);if(typeof v=="object"&&v!==null&&"error"in v)throw new Error(v.error);yield v}i=[]}}finally{o.releaseLock()}}var Q=class extends TypeError{constructor(e){super(`Invalid inference output: ${e}. Use the 'request' method with the same parameters to do a custom call with no type checking.`),this.name="InferenceOutputError"}};async function yc(e,t){const n=await $(e,t);if(!(Array.isArray(n)&&n.every(l=>typeof l.label=="string"&&typeof l.score=="number")))throw new Q("Expected Array<{label: string, score: number}>");return n}async function hc(e,t){const n=await $(e,t);if(!(typeof(n==null?void 0:n.text)=="string"))throw new Q("Expected {text: string}");return n}async function gc(e,t){const n=await $(e,t);if(!(n&&n instanceof Blob))throw new Q("Expected Blob");return n}async function vc(e,t){const n=await $(e,t);if(!(Array.isArray(n)&&n.every(l=>typeof l.label=="string"&&typeof l.score=="number")))throw new Q("Expected Array<{label: string, score: number}>");return n}async function wc(e,t){const n=await $(e,t);if(!(Array.isArray(n)&&n.every(l=>typeof l.label=="string"&&typeof l.mask=="string"&&typeof l.score=="number")))throw new Q("Expected Array<{label: string, mask: string, score: number}>");return n}async function Sc(e,t){var r;const n=(r=await $(e,t))==null?void 0:r[0];if(typeof(n==null?void 0:n.generated_text)!="string")throw new Q("Expected {generated_text: string}");return n}async function xc(e,t){const n=await $(e,t);if(!(Array.isArray(n)&&n.every(l=>typeof l.label=="string"&&typeof l.score=="number"&&typeof l.box.xmin=="number"&&typeof l.box.ymin=="number"&&typeof l.box.xmax=="number"&&typeof l.box.ymax=="number")))throw new Q("Expected Array<{label:string; score:number; box:{xmin:number; ymin:number; xmax:number; ymax:number}}>");return n}async function kc(e,t){const n=await $(e,t);if(!(n&&n instanceof Blob))throw new Q("Expected Blob");return n}function Vi(e){if(globalThis.Buffer)return globalThis.Buffer.from(e).toString("base64");{const t=[];return e.forEach(n=>{t.push(String.fromCharCode(n))}),globalThis.btoa(t.join(""))}}async function Ec(e,t){let n;e.parameters?n={...e,inputs:Vi(new Uint8Array(e.inputs instanceof ArrayBuffer?e.inputs:await e.inputs.arrayBuffer()))}:n={accessToken:e.accessToken,model:e.model,data:e.inputs};const r=await $(n,t);if(!(r&&r instanceof Blob))throw new Q("Expected Blob");return r}async function Cc(e,t){const n=await $(e,t);if(!(Array.isArray(n.conversation.generated_responses)&&n.conversation.generated_responses.every(l=>typeof l=="string")&&Array.isArray(n.conversation.past_user_inputs)&&n.conversation.past_user_inputs.every(l=>typeof l=="string")&&typeof n.generated_text=="string"&&Array.isArray(n.warnings)&&n.warnings.every(l=>typeof l=="string")))throw new Q("Expected {conversation: {generated_responses: string[], past_user_inputs: string[]}, generated_text: string, warnings: string[]}");return n}async function jc(e,t){const n=await $(e,t);let r=!0;if(Array.isArray(n)){for(const l of n)if(Array.isArray(l)){if(r=l.every(o=>typeof o=="number"),!r)break}else if(typeof l!="number"){r=!1;break}}else r=!1;if(!r)throw new Q("Expected Array");return n}async function _c(e,t){const n=await $(e,t);if(!(Array.isArray(n)&&n.every(l=>typeof l.score=="number"&&typeof l.sequence=="string"&&typeof l.token=="number"&&typeof l.token_str=="string")))throw new Q("Expected Array<{score: number, sequence: string, token: number, token_str: string}>");return n}async function Nc(e,t){const n=await $(e,t);if(!(typeof n=="object"&&!!n&&typeof n.answer=="string"&&typeof n.end=="number"&&typeof n.score=="number"&&typeof n.start=="number"))throw new Q("Expected {answer: string, end: number, score: number, start: number}");return n}async function Tc(e,t){const n=await $(e,t);if(!(Array.isArray(n)&&n.every(l=>typeof l=="number")))throw new Q("Expected number[]");return n}async function Oc(e,t){const n=await $(e,t);if(!(Array.isArray(n)&&n.every(l=>typeof(l==null?void 0:l.summary_text)=="string")))throw new Q("Expected Array<{summary_text: string}>");return n==null?void 0:n[0]}async function Pc(e,t){const n=await $(e,t);if(!(typeof(n==null?void 0:n.aggregator)=="string"&&typeof n.answer=="string"&&Array.isArray(n.cells)&&n.cells.every(l=>typeof l=="string")&&Array.isArray(n.coordinates)&&n.coordinates.every(l=>Array.isArray(l)&&l.every(o=>typeof o=="number"))))throw new Q("Expected {aggregator: string, answer: string, cells: string[], coordinates: number[][]}");return n}async function Lc(e,t){var l;const n=(l=await $(e,t))==null?void 0:l[0];if(!(Array.isArray(n)&&n.every(o=>typeof(o==null?void 0:o.label)=="string"&&typeof o.score=="number")))throw new Q("Expected Array<{label: string, score: number}>");return n}async function Ic(e,t){const n=await $(e,t);if(!(Array.isArray(n)&&n.every(l=>typeof(l==null?void 0:l.generated_text)=="string")))throw new Q("Expected Array<{generated_text: string}>");return n==null?void 0:n[0]}async function*Lp(e,t){yield*Ui(e,t)}function Bi(e){return Array.isArray(e)?e:[e]}async function zc(e,t){const n=Bi(await $(e,t));if(!(Array.isArray(n)&&n.every(l=>typeof l.end=="number"&&typeof l.entity_group=="string"&&typeof l.score=="number"&&typeof l.start=="number"&&typeof l.word=="string")))throw new Q("Expected Array<{end: number, entity_group: string, score: number, start: number, word: string}>");return n}async function Fc(e,t){const n=await $(e,t);if(!(Array.isArray(n)&&n.every(l=>typeof(l==null?void 0:l.translation_text)=="string")))throw new Q("Expected type Array<{translation_text: string}>");return n==null?void 0:n[0]}async function Rc(e,t){const n=Bi(await $(e,t));if(!(Array.isArray(n)&&n.every(l=>Array.isArray(l.labels)&&l.labels.every(o=>typeof o=="string")&&Array.isArray(l.scores)&&l.scores.every(o=>typeof o=="number")&&typeof l.sequence=="string")))throw new Q("Expected Array<{labels: string[], scores: number[], sequence: string}>");return n}async function Ac(e,t){var o;const n={...e,inputs:{question:e.inputs.question,image:Vi(new Uint8Array(e.inputs.image instanceof ArrayBuffer?e.inputs.image:await e.inputs.image.arrayBuffer()))}},r=(o=Bi(await $(n,t)))==null?void 0:o[0];if(!(typeof(r==null?void 0:r.answer)=="string"&&(typeof r.end=="number"||typeof r.end>"u")&&(typeof r.score=="number"||typeof r.score>"u")&&(typeof r.start=="number"||typeof r.start>"u")))throw new Q("Expected Array<{answer: string, end?: number, score?: number, start?: number}>");return r}async function Mc(e,t){var o;const n={...e,inputs:{question:e.inputs.question,image:Vi(new Uint8Array(e.inputs.image instanceof ArrayBuffer?e.inputs.image:await e.inputs.image.arrayBuffer()))}},r=(o=await $(n,t))==null?void 0:o[0];if(!(typeof(r==null?void 0:r.answer)=="string"&&typeof r.score=="number"))throw new Q("Expected Array<{answer: string, score: number}>");return r}async function Dc(e,t){const n=await $(e,t);if(!(Array.isArray(n)&&n.every(l=>typeof l=="number")))throw new Q("Expected number[]");return n}const O=e=>a.jsx("button",{className:`${e.variant==="secondary"?"border-4 border-yellow-200":"bg-yellow-200"} py-6 text-center w-full ${e.disabled?"cursor-not-allowed opacity-50":""}`,disabled:e.disabled??!1,onClick:e.onClick,children:e.label??"Submit"}),$c=e=>a.jsxs("div",{className:"w-full",children:[a.jsx("p",{className:"text-xl",children:e.label??"Input"}),e.input?a.jsx("audio",{className:"w-full",controls:!0,src:URL.createObjectURL(e.input)}):a.jsxs("label",{className:"bg-yellow-200 block cursor-pointer py-6 text-center w-full",children:["No file chosen",a.jsx("input",{accept:"audio/*",className:"hidden",onChange:t=>{t.target.files&&t.target.files[0]&&e.setInput(t.target.files[0])},type:"file"})]})]}),P=e=>{const t=(()=>{try{return JSON.stringify(e.output,void 0,2)}catch(n){if(n instanceof Error)return`Error during JSON.stringify: ${n.message}`}})();return a.jsxs("div",{className:"w-full",children:[a.jsx("p",{className:"text-xl",children:e.label??"Output"}),a.jsx("pre",{className:`bg-yellow-200 break-words p-6 select-text w-full whitespace-pre-wrap ${e.disabled?"cursor-wait opacity-50":""}`,children:t})]})},Ip="audio-classification",zp=e=>{const[t,n]=p.useState(),[r,l]=p.useState(!1),[o,i]=p.useState(),[u,s]=p.useState(),d=()=>{n(void 0),i(void 0),s(void 0)},y=async()=>{if(t){l(!0);try{const c=await yc({data:t,model:e.model});i(void 0),s(c)}catch(c){c instanceof Error&&i(c)}finally{l(!1)}}};return a.jsxs(p.Fragment,{children:[a.jsx($c,{input:t,setInput:n}),a.jsx(O,{label:"Clear",disabled:r||!t,onClick:d,variant:"secondary"}),a.jsx(O,{disabled:r||!t,onClick:y}),o?a.jsx(P,{disabled:r,label:"Error",output:o.message}):a.jsx(p.Fragment,{}),!o&&u?u.map(c=>a.jsx(P,{disabled:r,output:c},c.label)):a.jsx(p.Fragment,{})]})},Fp="automatic-speech-recognition",Rp=e=>{const[t,n]=p.useState(),[r,l]=p.useState(!1),[o,i]=p.useState(),[u,s]=p.useState(),d=()=>{n(void 0),i(void 0),s(void 0)},y=async()=>{if(t){l(!0);try{const c=await hc({data:t,model:e.model});i(void 0),s(c)}catch(c){c instanceof Error&&i(c)}finally{l(!1)}}};return a.jsxs(p.Fragment,{children:[a.jsx($c,{input:t,setInput:n}),a.jsx(O,{label:"Clear",disabled:r||!t,onClick:d,variant:"secondary"}),a.jsx(O,{disabled:r||!t,onClick:y}),o?a.jsx(P,{disabled:r,label:"Error",output:o.message}):a.jsx(p.Fragment,{}),!o&&u?a.jsx(P,{disabled:r,output:u}):a.jsx(p.Fragment,{})]})},J=e=>{const t=p.useRef(null);return p.useLayoutEffect(()=>{t.current&&(t.current.style.height="inherit",t.current.style.height=`${t.current.scrollHeight}px`)},[e.input]),a.jsxs("div",{className:"w-full",children:[a.jsx("p",{className:"text-xl",children:e.label??"Input"}),a.jsx("textarea",{className:"bg-yellow-200 py-6 resize-none text-center w-full",disabled:e.disabled??!1,onChange:n=>{!e.disabled&&e.setInput&&(n.target.value?e.setInput(n.target.value):e.setInput(""))},ref:t,rows:1,style:{height:t.current?`${t.current.scrollHeight}px`:"inherit"},value:e.input??""})]})},Ap="conversational",Mp=e=>{const[t,n]=p.useState(),[r,l]=p.useState(!1),[o,i]=p.useState(),[u,s]=p.useState(),d=()=>{n(void 0),i(void 0),s(void 0)},y=async()=>{if(t){l(!0),s(v=>v?{...v,conversation:{...v.conversation,past_user_inputs:[...v.conversation.past_user_inputs,t]}}:{conversation:{generated_responses:[],past_user_inputs:[t]},generated_text:"",warnings:[]}),n(void 0);const c=u==null?void 0:u.conversation.generated_responses,g=u==null?void 0:u.conversation.past_user_inputs;try{const v=await Cc({inputs:{generated_responses:c,past_user_inputs:g,text:t},model:e.model});i(void 0),s(v)}catch(v){v instanceof Error&&i(v)}finally{l(!1)}}};return a.jsxs(p.Fragment,{children:[a.jsx(J,{input:t,setInput:n}),a.jsx(O,{label:"Clear",disabled:r||!t&&!u,onClick:d,variant:"secondary"}),a.jsx(O,{disabled:r||!t,onClick:y}),o?a.jsx(P,{disabled:r,label:"Error",output:o.message}):a.jsx(p.Fragment,{}),!o&&u?Array.from({length:Math.max(u.conversation.generated_responses.length,u.conversation.past_user_inputs.length)}).map((c,g,v)=>a.jsxs(p.Fragment,{children:[u.conversation.generated_responses[v.length-g-1]?a.jsx(P,{disabled:r,label:`Output - Generated Response #${v.length-g}`,output:u.conversation.generated_responses[v.length-g-1]}):a.jsx(p.Fragment,{}),u.conversation.past_user_inputs[v.length-g-1]?a.jsx(J,{disabled:!0,label:`Output - Past User Input #${v.length-g}`,input:u.conversation.past_user_inputs[v.length-g-1]}):a.jsx(p.Fragment,{})]},g)):a.jsx(p.Fragment,{})]})},Mt=e=>a.jsxs("div",{className:"w-full",children:[a.jsx("p",{className:"text-xl",children:e.label??"Input"}),e.input?a.jsx("img",{className:"w-full",src:URL.createObjectURL(e.input)}):a.jsxs("label",{className:"bg-yellow-200 block cursor-pointer py-6 text-center w-full",children:["No file chosen",a.jsx("input",{accept:"image/*",className:"hidden",onChange:t=>{t.target.files&&t.target.files[0]&&e.setInput(t.target.files[0])},type:"file"})]})]}),Dp="document-question-answering",$p=e=>{const[t,n]=p.useState(),[r,l]=p.useState(),[o,i]=p.useState(!1),[u,s]=p.useState(),[d,y]=p.useState(),c=()=>{n(void 0),l(void 0),s(void 0),y(void 0)},g=async()=>{if(t&&r){i(!0);try{const v=await Ac({inputs:{question:t,image:r},model:e.model});s(void 0),y(v)}catch(v){v instanceof Error&&s(v)}finally{i(!1)}}};return a.jsxs(p.Fragment,{children:[a.jsx(J,{input:t,label:"Input - Question",setInput:n}),a.jsx(Mt,{input:r,label:"Input - Image",setInput:l}),a.jsx(O,{label:"Clear",disabled:o||!r,onClick:c,variant:"secondary"}),a.jsx(O,{disabled:o||!r,onClick:g}),u?a.jsx(P,{disabled:o,label:"Error",output:u.message}):a.jsx(p.Fragment,{}),!u&&d?a.jsx(P,{disabled:o,output:d}):a.jsx(p.Fragment,{})]})},Up="feature-extraction",Vp=e=>{const[t,n]=p.useState(),[r,l]=p.useState(!1),[o,i]=p.useState(),[u,s]=p.useState(),d=()=>{n(void 0),i(void 0),s(void 0)},y=async()=>{if(t){l(!0);try{const c=await jc({inputs:t,model:e.model});i(void 0),s(c)}catch(c){c instanceof Error&&i(c)}finally{l(!1)}}};return a.jsxs(p.Fragment,{children:[a.jsx(J,{input:t,setInput:n}),a.jsx(O,{label:"Clear",disabled:r||!t,onClick:d,variant:"secondary"}),a.jsx(O,{disabled:r||!t,onClick:y}),o?a.jsx(P,{disabled:r,label:"Error",output:o.message}):a.jsx(p.Fragment,{}),!o&&u?a.jsx(P,{disabled:r,output:u}):a.jsx(p.Fragment,{})]})},Bp="fill-mask",Qp=e=>{const[t,n]=p.useState(),[r,l]=p.useState(!1),[o,i]=p.useState(),[u,s]=p.useState(),d=()=>{n(void 0),i(void 0),s(void 0)},y=async()=>{if(t){l(!0);try{const c=await _c({inputs:t,model:e.model});i(void 0),s(c)}catch(c){c instanceof Error&&i(c)}finally{l(!1)}}};return a.jsxs(p.Fragment,{children:[a.jsx(J,{input:t,setInput:n}),a.jsx(O,{label:"Clear",disabled:r||!t,onClick:d,variant:"secondary"}),a.jsx(O,{disabled:r||!t,onClick:y}),o?a.jsx(P,{disabled:r,label:"Error",output:o.message}):a.jsx(p.Fragment,{}),!o&&u?u.map(c=>a.jsx(P,{disabled:r,output:c},c.token_str)):a.jsx(p.Fragment,{})]})},Hp="image-classification",Wp=e=>{const[t,n]=p.useState(),[r,l]=p.useState(!1),[o,i]=p.useState(),[u,s]=p.useState(),d=()=>{n(void 0),i(void 0),s(void 0)},y=async()=>{if(t){l(!0);try{const c=await vc({data:t,model:e.model});i(void 0),s(c)}catch(c){c instanceof Error&&i(c)}finally{l(!1)}}};return a.jsxs(p.Fragment,{children:[a.jsx(Mt,{input:t,setInput:n}),a.jsx(O,{label:"Clear",disabled:r||!t,onClick:d,variant:"secondary"}),a.jsx(O,{disabled:r||!t,onClick:y}),o?a.jsx(P,{disabled:r,label:"Error",output:o.message}):a.jsx(p.Fragment,{}),!o&&u?u.map(c=>a.jsx(P,{disabled:r,output:c},c.label)):a.jsx(p.Fragment,{})]})},Kp="image-segmentation",Yp=e=>{const[t,n]=p.useState(),[r,l]=p.useState(!1),[o,i]=p.useState(),[u,s]=p.useState(),d=()=>{n(void 0),i(void 0),s(void 0)},y=async()=>{if(t){l(!0);try{const c=await wc({data:t,model:e.model});i(void 0),s(c)}catch(c){c instanceof Error&&i(c)}finally{l(!1)}}};return a.jsxs(p.Fragment,{children:[a.jsx(Mt,{input:t,setInput:n}),a.jsx(O,{label:"Clear",disabled:r||!t,onClick:d,variant:"secondary"}),a.jsx(O,{disabled:r||!t,onClick:y}),o?a.jsx(P,{disabled:r,label:"Error",output:o.message}):a.jsx(p.Fragment,{}),!o&&u?u.map(c=>a.jsx(P,{disabled:r,output:c},c.label)):a.jsx(p.Fragment,{})]})},Uc=e=>a.jsxs("div",{className:"w-full",children:[a.jsx("p",{className:"text-xl",children:e.label??"Output"}),a.jsx("img",{className:`w-full ${e.disabled?"cursor-wait opacity-50":""}`,src:URL.createObjectURL(e.output)})]}),Xp="image-to-image",Gp=e=>{const[t,n]=p.useState(),[r,l]=p.useState(!1),[o,i]=p.useState(),[u,s]=p.useState(),d=()=>{n(void 0),i(void 0),s(void 0)},y=async()=>{if(t){l(!0);try{const c=await Ec({inputs:t,model:e.model});i(void 0),s(c)}catch(c){c instanceof Error&&i(c)}finally{l(!1)}}};return a.jsxs(p.Fragment,{children:[a.jsx(Mt,{input:t,setInput:n}),a.jsx(O,{label:"Clear",disabled:r||!t,onClick:d,variant:"secondary"}),a.jsx(O,{disabled:r||!t,onClick:y}),o?a.jsx(P,{disabled:r,label:"Error",output:o.message}):a.jsx(p.Fragment,{}),!o&&u?a.jsx(Uc,{disabled:r,output:u}):a.jsx(p.Fragment,{})]})},qp="image-to-text",Zp=e=>{const[t,n]=p.useState(),[r,l]=p.useState(!1),[o,i]=p.useState(),[u,s]=p.useState(),d=()=>{n(void 0),i(void 0),s(void 0)},y=async()=>{if(t){l(!0);try{const c=await Sc({data:t,model:e.model});i(void 0),s(c)}catch(c){c instanceof Error&&i(c)}finally{l(!1)}}};return a.jsxs(p.Fragment,{children:[a.jsx(Mt,{input:t,setInput:n}),a.jsx(O,{label:"Clear",disabled:r||!t,onClick:d,variant:"secondary"}),a.jsx(O,{disabled:r||!t,onClick:y}),o?a.jsx(P,{disabled:r,label:"Error",output:o.message}):a.jsx(p.Fragment,{}),!o&&u?a.jsx(P,{disabled:r,output:u}):a.jsx(p.Fragment,{})]})},Jp="object-detection",bp=e=>{const[t,n]=p.useState(),[r,l]=p.useState(!1),[o,i]=p.useState(),[u,s]=p.useState(),d=()=>{n(void 0),i(void 0),s(void 0)},y=async()=>{if(t){l(!0);try{const c=await xc({data:t,model:e.model});i(void 0),s(c)}catch(c){c instanceof Error&&i(c)}finally{l(!1)}}};return a.jsxs(p.Fragment,{children:[a.jsx(Mt,{input:t,setInput:n}),a.jsx(O,{label:"Clear",disabled:r||!t,onClick:d,variant:"secondary"}),a.jsx(O,{disabled:r||!t,onClick:y}),o?a.jsx(P,{disabled:r,label:"Error",output:o.message}):a.jsx(p.Fragment,{}),!o&&u?u.map(c=>a.jsx(P,{disabled:r,output:c},c.label)):a.jsx(p.Fragment,{})]})},em="question-answering",tm=e=>{const[t,n]=p.useState(),[r,l]=p.useState(),[o,i]=p.useState(!1),[u,s]=p.useState(),[d,y]=p.useState(),c=()=>{n(void 0),l(void 0),s(void 0),y(void 0)},g=async()=>{if(t&&r){i(!0);try{const v=await Nc({inputs:{question:t,context:r},model:e.model});s(void 0),y(v)}catch(v){v instanceof Error&&s(v)}finally{i(!1)}}};return a.jsxs(p.Fragment,{children:[a.jsx(J,{input:t,label:"Input - Question",setInput:n}),a.jsx(J,{input:r,label:"Input - Context",setInput:l}),a.jsx(O,{label:"Clear",disabled:o||!t||!r,onClick:c,variant:"secondary"}),a.jsx(O,{disabled:o||!t||!r,onClick:g}),u?a.jsx(P,{disabled:o,label:"Error",output:u.message}):a.jsx(p.Fragment,{}),!u&&d?a.jsx(P,{disabled:o,output:d}):a.jsx(p.Fragment,{})]})},nm="sentence-similarity",rm=e=>{const[t,n]=p.useState(),r=Array.from({length:2}).map(()=>{}),[l,o]=p.useState(r),[i,u]=p.useState(!1),[s,d]=p.useState(),[y,c]=p.useState(),g=()=>{n(void 0),o(r),d(void 0),c(void 0)},v=async()=>{if(t&&l.every(Boolean)){u(!0);try{const w=await Tc({inputs:{source_sentence:t,sentences:l},model:e.model});d(void 0),c(w)}catch(w){w instanceof Error&&d(w)}finally{u(!1)}}};return a.jsxs(p.Fragment,{children:[a.jsx(J,{input:t,label:"Input - Source Sentence",setInput:n}),l.map((w,k)=>a.jsx(J,{input:w,label:`Input - Sentence #${k+1}`,setInput:M=>o(m=>[...m.slice(0,k),M,...m.slice(k+1,m.length)])})),a.jsx(O,{disabled:i||!t||!l.every(Boolean),label:"Add Sentence",onClick:()=>o(w=>[...w,void 0])}),a.jsx(O,{disabled:i||!t||!l.every(Boolean),label:"Clear",onClick:g,variant:"secondary"}),a.jsx(O,{disabled:i||!t||!l.every(Boolean),onClick:v}),s?a.jsx(P,{disabled:i,label:"Error",output:s.message}):a.jsx(p.Fragment,{}),!s&&y?y.map((w,k)=>a.jsx(P,{disabled:i,label:`Output - Sentence #${k+1}`,output:w})):a.jsx(p.Fragment,{})]})},lm="summarization",om=e=>{const[t,n]=p.useState(),[r,l]=p.useState(!1),[o,i]=p.useState(),[u,s]=p.useState(),d=()=>{n(void 0),i(void 0),s(void 0)},y=async()=>{if(t){l(!0);try{const c=await Oc({inputs:t,model:e.model});i(void 0),s(c)}catch(c){c instanceof Error&&i(c)}finally{l(!1)}}};return a.jsxs(p.Fragment,{children:[a.jsx(J,{input:t,setInput:n}),a.jsx(O,{label:"Clear",disabled:r||!t,onClick:d,variant:"secondary"}),a.jsx(O,{disabled:r||!t,onClick:y}),o?a.jsx(P,{disabled:r,label:"Error",output:o.message}):a.jsx(p.Fragment,{}),!o&&u?a.jsx(P,{disabled:r,output:u}):a.jsx(p.Fragment,{})]})},im=async e=>{const t=await e.text();try{const n=JSON.parse(t);try{return JSON.stringify(n,void 0,2)}catch(r){if(r instanceof Error)return`Error during JSON.stringify: ${r.message}`}}catch(n){if(n instanceof Error)return`Error during JSON.parse: ${n.message}`}},Vc=e=>{const[t,n]=p.useState();return p.useEffect(()=>{e.input&&im(e.input).then(n)},[e.input]),a.jsxs("div",{className:"w-full",children:[a.jsx("p",{className:"text-xl",children:e.label??"Input"}),e.input?a.jsx("pre",{className:"bg-yellow-200 break-words p-6 select-text w-full whitespace-pre-wrap",children:t}):a.jsxs("label",{className:"bg-yellow-200 block cursor-pointer py-6 text-center w-full",children:["No file chosen",a.jsx("input",{accept:".json",className:"hidden",onChange:r=>{r.target.files&&r.target.files[0]&&e.setInput(r.target.files[0])},type:"file"})]})]})},um="table-question-answering",sm=e=>{const[t,n]=p.useState(),[r,l]=p.useState(),[o,i]=p.useState(!1),[u,s]=p.useState(),[d,y]=p.useState(),c=()=>{n(void 0),l(void 0),s(void 0),y(void 0)},g=async()=>{if(t&&r){i(!0);try{const v=await Pc({inputs:{query:t,table:JSON.parse(await r.text()??"{}")},model:e.model});s(void 0),y(v)}catch(v){v instanceof Error&&s(v)}finally{i(!1)}}};return a.jsxs(p.Fragment,{children:[a.jsx(J,{input:t,label:"Input - Query",setInput:n}),a.jsx(Vc,{input:r,label:"Input - Table",setInput:l}),a.jsx(O,{label:"Clear",disabled:o||!t,onClick:c,variant:"secondary"}),a.jsx(O,{disabled:o||!t,onClick:g}),u?a.jsx(P,{disabled:o,label:"Error",output:u.message}):a.jsx(p.Fragment,{}),!u&&d?a.jsx(P,{disabled:o,output:d}):a.jsx(p.Fragment,{})]})},am="tabular-regression",cm=e=>{const[t,n]=p.useState(),[r,l]=p.useState(!1),[o,i]=p.useState(),[u,s]=p.useState(),d=()=>{n(void 0),i(void 0),s(void 0)},y=async()=>{if(t){l(!0);try{const c=await Dc({inputs:{data:JSON.parse(await t.text()??"{}")},model:e.model});i(void 0),s(c)}catch(c){c instanceof Error&&i(c)}finally{l(!1)}}};return a.jsxs(p.Fragment,{children:[a.jsx(Vc,{input:t,setInput:n}),a.jsx(O,{disabled:r||!t,label:"Clear",onClick:d,variant:"secondary"}),a.jsx(O,{disabled:r||!t,onClick:y}),o?a.jsx(P,{disabled:r,label:"Error",output:o.message}):a.jsx(p.Fragment,{}),!o&&u?u.map((c,g)=>a.jsx(P,{disabled:r,label:`Output - Sentence #${g+1}`,output:c})):a.jsx(p.Fragment,{})]})},dm="text-classification",fm=e=>{const[t,n]=p.useState(),[r,l]=p.useState(!1),[o,i]=p.useState(),[u,s]=p.useState(),d=()=>{n(void 0),i(void 0),s(void 0)},y=async()=>{if(t){l(!0);try{const c=await Lc({inputs:t,model:e.model});i(void 0),s(c)}catch(c){c instanceof Error&&i(c)}finally{l(!1)}}};return a.jsxs(p.Fragment,{children:[a.jsx(J,{input:t,setInput:n}),a.jsx(O,{label:"Clear",disabled:r||!t,onClick:d,variant:"secondary"}),a.jsx(O,{disabled:r||!t,onClick:y}),o?a.jsx(P,{disabled:r,label:"Error",output:o.message}):a.jsx(p.Fragment,{}),!o&&u?u.map(c=>a.jsx(P,{disabled:r,output:c},c.label)):a.jsx(p.Fragment,{})]})},pm="text-generation",mm=e=>{const[t,n]=p.useState(),[r,l]=p.useState(!1),[o,i]=p.useState(),[u,s]=p.useState(),d=()=>{n(void 0),i(void 0),s(void 0)},y=async()=>{if(t){l(!0);try{const c=await Ic({inputs:t,model:e.model});i(void 0),s(c)}catch(c){c instanceof Error&&i(c)}finally{l(!1)}}};return a.jsxs(p.Fragment,{children:[a.jsx(J,{input:t,setInput:n}),a.jsx(O,{label:"Clear",disabled:r||!t,onClick:d,variant:"secondary"}),a.jsx(O,{disabled:r||!t,onClick:y}),o?a.jsx(P,{disabled:r,label:"Error",output:o.message}):a.jsx(p.Fragment,{}),!o&&u?a.jsx(P,{disabled:r,output:u}):a.jsx(p.Fragment,{})]})},ym="text-to-image",hm=e=>{const[t,n]=p.useState(),[r,l]=p.useState(!1),[o,i]=p.useState(),[u,s]=p.useState(),d=()=>{n(void 0),i(void 0),s(void 0)},y=async()=>{if(t){l(!0);try{const c=await kc({inputs:t,model:e.model});i(void 0),s(c)}catch(c){c instanceof Error&&i(c)}finally{l(!1)}}};return a.jsxs(p.Fragment,{children:[a.jsx(J,{input:t,setInput:n}),a.jsx(O,{label:"Clear",disabled:r||!t,onClick:d,variant:"secondary"}),a.jsx(O,{disabled:r||!t,onClick:y}),o?a.jsx(P,{disabled:r,label:"Error",output:o.message}):a.jsx(p.Fragment,{}),!o&&u?a.jsx(Uc,{disabled:r,output:u}):a.jsx(p.Fragment,{})]})},gm=e=>a.jsxs("div",{className:"w-full",children:[a.jsx("p",{className:"text-xl",children:e.label??"Output"}),a.jsx("audio",{className:`w-full ${e.disabled?"cursor-wait opacity-50":""}`,controls:!0,src:URL.createObjectURL(e.output)})]}),vm="text-to-speech",wm=e=>{const[t,n]=p.useState(),[r,l]=p.useState(!1),[o,i]=p.useState(),[u,s]=p.useState(),d=()=>{n(void 0),i(void 0),s(void 0)},y=async()=>{if(t){l(!0);try{const c=await gc({inputs:t,model:e.model});i(void 0),s(c)}catch(c){c instanceof Error&&i(c)}finally{l(!1)}}};return a.jsxs(p.Fragment,{children:[a.jsx(J,{input:t,setInput:n}),a.jsx(O,{label:"Clear",disabled:r||!t,onClick:d,variant:"secondary"}),a.jsx(O,{disabled:r||!t,onClick:y}),o?a.jsx(P,{disabled:r,label:"Error",output:o.message}):a.jsx(p.Fragment,{}),!o&&u?a.jsx(gm,{disabled:r,output:u}):a.jsx(p.Fragment,{})]})},Sm="token-classification",xm=e=>{const[t,n]=p.useState(),[r,l]=p.useState(!1),[o,i]=p.useState(),[u,s]=p.useState(),d=()=>{n(void 0),i(void 0),s(void 0)},y=async()=>{if(t){l(!0);try{const c=await zc({inputs:t,model:e.model});i(void 0),s(c)}catch(c){c instanceof Error&&i(c)}finally{l(!1)}}};return a.jsxs(p.Fragment,{children:[a.jsx(J,{input:t,setInput:n}),a.jsx(O,{label:"Clear",disabled:r||!t,onClick:d,variant:"secondary"}),a.jsx(O,{disabled:r||!t,onClick:y}),o?a.jsx(P,{disabled:r,label:"Error",output:o.message}):a.jsx(p.Fragment,{}),!o&&u?u.map(c=>a.jsx(P,{disabled:r,output:c},c.word)):a.jsx(p.Fragment,{})]})},km="translation",Em=e=>{const[t,n]=p.useState(),[r,l]=p.useState(!1),[o,i]=p.useState(),[u,s]=p.useState(),d=()=>{n(void 0),i(void 0),s(void 0)},y=async()=>{if(t){l(!0);try{const c=await Fc({inputs:t,model:e.model});i(void 0),s(c)}catch(c){c instanceof Error&&i(c)}finally{l(!1)}}};return a.jsxs(p.Fragment,{children:[a.jsx(J,{input:t,setInput:n}),a.jsx(O,{label:"Clear",disabled:r||!t,onClick:d,variant:"secondary"}),a.jsx(O,{disabled:r||!t,onClick:y}),o?a.jsx(P,{disabled:r,label:"Error",output:o.message}):a.jsx(p.Fragment,{}),!o&&u?a.jsx(P,{disabled:r,output:u}):a.jsx(p.Fragment,{})]})},Cm="visual-question-answering",jm=e=>{const[t,n]=p.useState(),[r,l]=p.useState(),[o,i]=p.useState(!1),[u,s]=p.useState(),[d,y]=p.useState(),c=()=>{n(void 0),l(void 0),s(void 0),y(void 0)},g=async()=>{if(t&&r){i(!0);try{const v=await Mc({inputs:{question:t,image:r},model:e.model});s(void 0),y(v)}catch(v){v instanceof Error&&s(v)}finally{i(!1)}}};return a.jsxs(p.Fragment,{children:[a.jsx(J,{input:t,label:"Input - Question",setInput:n}),a.jsx(Mt,{input:r,label:"Input - Image",setInput:l}),a.jsx(O,{label:"Clear",disabled:o||!r,onClick:c,variant:"secondary"}),a.jsx(O,{disabled:o||!r,onClick:g}),u?a.jsx(P,{disabled:o,label:"Error",output:u.message}):a.jsx(p.Fragment,{}),!u&&d?a.jsx(P,{disabled:o,output:d}):a.jsx(p.Fragment,{})]})},_m="zero-shot-classification",Nm=e=>{const[t,n]=p.useState(),r=Array.from({length:2}).map(()=>{}),[l,o]=p.useState(r),[i,u]=p.useState(!1),[s,d]=p.useState(),[y,c]=p.useState(),g=()=>{n(void 0),o(r),d(void 0),c(void 0)},v=async()=>{if(t&&l.every(Boolean)){u(!0);try{const w=await Rc({inputs:t,model:e.model,parameters:{candidate_labels:l}});d(void 0),c(w)}catch(w){w instanceof Error&&d(w)}finally{u(!1)}}};return a.jsxs(p.Fragment,{children:[a.jsx(J,{input:t,setInput:n}),l.map((w,k)=>a.jsx(J,{input:w,label:`Parameter - Candidate Label #${k+1}`,setInput:M=>o(m=>[...m.slice(0,k),M,...m.slice(k+1,m.length)])})),a.jsx(O,{disabled:i||!t||!l.every(Boolean),label:"Add Candidate Label",onClick:()=>o(w=>[...w,void 0])}),a.jsx(O,{disabled:i||!t||!l.every(Boolean),label:"Clear",onClick:g,variant:"secondary"}),a.jsx(O,{disabled:i||!t||!l.every(Boolean),onClick:v}),s?a.jsx(P,{disabled:i,label:"Error",output:s.message}):a.jsx(p.Fragment,{}),!s&&y?y.map((w,k)=>a.jsx(P,{disabled:i,output:w})):a.jsx(p.Fragment,{})]})},Tm=[Ip,Fp,Ap,Dp,Up,Bp,Hp,Kp,Xp,qp,Jp,em,nm,lm,um,am,dm,pm,ym,vm,Sm,km,Cm,_m],Om=e=>{if(!e.model||!e.task)return a.jsx(p.Fragment,{});switch(e.task){case"audio-classification":return a.jsx(zp,{model:e.model});case"automatic-speech-recognition":return a.jsx(Rp,{model:e.model});case"conversational":return a.jsx(Mp,{model:e.model});case"document-question-answering":return a.jsx($p,{model:e.model});case"feature-extraction":return a.jsx(Vp,{model:e.model});case"fill-mask":return a.jsx(Qp,{model:e.model});case"image-classification":return a.jsx(Wp,{model:e.model});case"image-segmentation":return a.jsx(Yp,{model:e.model});case"image-to-image":return a.jsx(Gp,{model:e.model});case"image-to-text":return a.jsx(Zp,{model:e.model});case"object-detection":return a.jsx(bp,{model:e.model});case"question-answering":return a.jsx(tm,{model:e.model});case"sentence-similarity":return a.jsx(rm,{model:e.model});case"summarization":return a.jsx(om,{model:e.model});case"table-question-answering":return a.jsx(sm,{model:e.model});case"tabular-regression":return a.jsx(cm,{model:e.model});case"text-classification":return a.jsx(fm,{model:e.model});case"text-generation":return a.jsx(mm,{model:e.model});case"text-to-image":return a.jsx(hm,{model:e.model});case"text-to-speech":return a.jsx(wm,{model:e.model});case"token-classification":return a.jsx(xm,{model:e.model});case"translation":return a.jsx(Em,{model:e.model});case"visual-question-answering":return a.jsx(jm,{model:e.model});case"zero-shot-classification":return a.jsx(Nm,{model:e.model});default:return a.jsx(p.Fragment,{})}},Pm=e=>a.jsxs("div",{className:"w-full",children:[a.jsx("p",{className:"text-xl",children:"Task"}),a.jsxs("select",{className:"bg-yellow-200 cursor-pointer py-6 text-center w-full",onChange:t=>e.onTaskSelect(t.target.value),placeholder:"Select a task",value:e.task,children:[a.jsx("option",{children:"Select a task"}),Tm.map(t=>a.jsx("option",{value:t,children:t},t))]})]}),Jl={},Lm=async e=>{if(Jl[e])return Jl[e];const t=[];for await(const n of Ep({search:{task:e}}))t.push(n);return t.sort((n,r)=>n.downloads>r.downloads?-1:n.downloadsr.likes?-1:n.likesr.name?-1:n.name{const[t,n]=p.useState(!1),[r,l]=p.useState([]);return p.useEffect(()=>{l([]),e.task&&(n(!0),Lm(e.task).then(o=>l(o)).finally(()=>n(!1)))},[e.task]),r.length>0?a.jsxs("div",{className:"w-full",children:[a.jsx("p",{className:"text-xl",children:"Model"}),a.jsxs("select",{className:"bg-yellow-200 cursor-pointer py-6 text-center w-full",onChange:o=>e.onModelSelect(o.target.value),placeholder:"Select a model",value:e.model,children:[a.jsx("option",{children:"Select a model"}),r.map(o=>a.jsx("option",{value:o.name,children:o.name},o.name))]}),e.model?a.jsx("div",{className:"font-bold py-6 text-center text-yellow-200",children:a.jsx("a",{href:`https://huggingface.co/${e.model}`,rel:"noopener noferrer",target:"_blank",children:"View model on 🤗"})}):a.jsx(p.Fragment,{})]}):a.jsx("p",{className:"text-center w-full",children:e.task?t?"Loading models for this task":"No models available for this task":"Select a task to view available models"})},zm=()=>{const[e,t]=p.useState(),[n,r]=p.useState(),l=o=>{r(void 0),t(o)};return a.jsx("div",{className:"bg-yellow-500 flex flex-col h-full items-center min-h-screen min-w-screen overflow-auto w-full",children:a.jsxs("div",{className:"flex flex-col items-center justify-center py-24 space-y-12 w-2/3 lg:w-1/3",children:[a.jsx("header",{className:"text-center text-6xl",children:"🤗"}),a.jsx(Pm,{onTaskSelect:l,task:e}),a.jsx(Im,{model:n,onModelSelect:r,task:e}),a.jsx(Om,{model:n,task:e})]})})};const Fm=()=>{const e="root",t=document.getElementById(e);if(t){const n=pc(t),r=a.jsx(p.StrictMode,{children:a.jsx(zm,{})});n.render(r)}};Fm();
diff --git a/spaces/amarchheda/ChordDuplicate/portaudio/cmake_support/FindASIOSDK.cmake b/spaces/amarchheda/ChordDuplicate/portaudio/cmake_support/FindASIOSDK.cmake
deleted file mode 100644
index 55ad33d9563b52849007d6a73925489121b97c08..0000000000000000000000000000000000000000
--- a/spaces/amarchheda/ChordDuplicate/portaudio/cmake_support/FindASIOSDK.cmake
+++ /dev/null
@@ -1,41 +0,0 @@
-# $Id: $
-#
-# - Try to find the ASIO SDK
-# Once done this will define
-#
-# ASIOSDK_FOUND - system has ASIO SDK
-# ASIOSDK_ROOT_DIR - path to the ASIO SDK base directory
-# ASIOSDK_INCLUDE_DIR - the ASIO SDK include directory
-
-if(WIN32)
-else(WIN32)
- message(FATAL_ERROR "FindASIOSDK.cmake: Unsupported platform ${CMAKE_SYSTEM_NAME}" )
-endif(WIN32)
-
-file(GLOB results "${CMAKE_CURRENT_SOURCE_DIR}/../as*")
-foreach(f ${results})
- if(IS_DIRECTORY ${f})
- set(ASIOSDK_PATH_HINT ${ASIOSDK_PATH_HINT} ${f})
- endif()
-endforeach()
-
-find_path(ASIOSDK_ROOT_DIR
- common/asio.h
- HINTS
- ${ASIOSDK_PATH_HINT}
-)
-
-find_path(ASIOSDK_INCLUDE_DIR
- asio.h
- PATHS
- ${ASIOSDK_ROOT_DIR}/common
-)
-
-# handle the QUIETLY and REQUIRED arguments and set ASIOSDK_FOUND to TRUE if
-# all listed variables are TRUE
-INCLUDE(FindPackageHandleStandardArgs)
-FIND_PACKAGE_HANDLE_STANDARD_ARGS(ASIOSDK DEFAULT_MSG ASIOSDK_ROOT_DIR ASIOSDK_INCLUDE_DIR)
-
-MARK_AS_ADVANCED(
- ASIOSDK_ROOT_DIR ASIOSDK_INCLUDE_DIR
-)
diff --git a/spaces/anaclaudia13ct/insect_detection/utils/loggers/clearml/README.md b/spaces/anaclaudia13ct/insect_detection/utils/loggers/clearml/README.md
deleted file mode 100644
index 3cf4c268583fc69df9ae3b58ea2566ed871a896c..0000000000000000000000000000000000000000
--- a/spaces/anaclaudia13ct/insect_detection/utils/loggers/clearml/README.md
+++ /dev/null
@@ -1,230 +0,0 @@
-# ClearML Integration
-
-
-
-## About ClearML
-
-[ClearML](https://cutt.ly/yolov5-tutorial-clearml) is an [open-source](https://github.com/allegroai/clearml) toolbox designed to save you time ⏱️.
-
-🔨 Track every YOLOv5 training run in the experiment manager
-
-🔧 Version and easily access your custom training data with the integrated ClearML Data Versioning Tool
-
-🔦 Remotely train and monitor your YOLOv5 training runs using ClearML Agent
-
-🔬 Get the very best mAP using ClearML Hyperparameter Optimization
-
-🔭 Turn your newly trained YOLOv5 model into an API with just a few commands using ClearML Serving
-
-
-And so much more. It's up to you how many of these tools you want to use, you can stick to the experiment manager, or chain them all together into an impressive pipeline!
-
-
-
-
-
-
-
-
-
-## 🦾 Setting Things Up
-
-To keep track of your experiments and/or data, ClearML needs to communicate to a server. You have 2 options to get one:
-
-Either sign up for free to the [ClearML Hosted Service](https://cutt.ly/yolov5-tutorial-clearml) or you can set up your own server, see [here](https://clear.ml/docs/latest/docs/deploying_clearml/clearml_server). Even the server is open-source, so even if you're dealing with sensitive data, you should be good to go!
-
-1. Install the `clearml` python package:
-
- ```bash
- pip install clearml
- ```
-
-1. Connect the ClearML SDK to the server by [creating credentials](https://app.clear.ml/settings/workspace-configuration) (go right top to Settings -> Workspace -> Create new credentials), then execute the command below and follow the instructions:
-
- ```bash
- clearml-init
- ```
-
-That's it! You're done 😎
-
-
-
-## 🚀 Training YOLOv5 With ClearML
-
-To enable ClearML experiment tracking, simply install the ClearML pip package.
-
-```bash
-pip install clearml>=1.2.0
-```
-
-This will enable integration with the YOLOv5 training script. Every training run from now on, will be captured and stored by the ClearML experiment manager.
-
-If you want to change the `project_name` or `task_name`, use the `--project` and `--name` arguments of the `train.py` script, by default the project will be called `YOLOv5` and the task `Training`.
-PLEASE NOTE: ClearML uses `/` as a delimter for subprojects, so be careful when using `/` in your project name!
-
-```bash
-python train.py --img 640 --batch 16 --epochs 3 --data coco128.yaml --weights yolov5s.pt --cache
-```
-
-or with custom project and task name:
-```bash
-python train.py --project my_project --name my_training --img 640 --batch 16 --epochs 3 --data coco128.yaml --weights yolov5s.pt --cache
-```
-
-This will capture:
-- Source code + uncommitted changes
-- Installed packages
-- (Hyper)parameters
-- Model files (use `--save-period n` to save a checkpoint every n epochs)
-- Console output
-- Scalars (mAP_0.5, mAP_0.5:0.95, precision, recall, losses, learning rates, ...)
-- General info such as machine details, runtime, creation date etc.
-- All produced plots such as label correlogram and confusion matrix
-- Images with bounding boxes per epoch
-- Mosaic per epoch
-- Validation images per epoch
-- ...
-
-That's a lot right? 🤯
-Now, we can visualize all of this information in the ClearML UI to get an overview of our training progress. Add custom columns to the table view (such as e.g. mAP_0.5) so you can easily sort on the best performing model. Or select multiple experiments and directly compare them!
-
-There even more we can do with all of this information, like hyperparameter optimization and remote execution, so keep reading if you want to see how that works!
-
-
-
-## 🔗 Dataset Version Management
-
-Versioning your data separately from your code is generally a good idea and makes it easy to aqcuire the latest version too. This repository supports supplying a dataset version ID and it will make sure to get the data if it's not there yet. Next to that, this workflow also saves the used dataset ID as part of the task parameters, so you will always know for sure which data was used in which experiment!
-
-
-
-### Prepare Your Dataset
-
-The YOLOv5 repository supports a number of different datasets by using yaml files containing their information. By default datasets are downloaded to the `../datasets` folder in relation to the repository root folder. So if you downloaded the `coco128` dataset using the link in the yaml or with the scripts provided by yolov5, you get this folder structure:
-
-```
-..
-|_ yolov5
-|_ datasets
- |_ coco128
- |_ images
- |_ labels
- |_ LICENSE
- |_ README.txt
-```
-But this can be any dataset you wish. Feel free to use your own, as long as you keep to this folder structure.
-
-Next, ⚠️**copy the corresponding yaml file to the root of the dataset folder**⚠️. This yaml files contains the information ClearML will need to properly use the dataset. You can make this yourself too, of course, just follow the structure of the example yamls.
-
-Basically we need the following keys: `path`, `train`, `test`, `val`, `nc`, `names`.
-
-```
-..
-|_ yolov5
-|_ datasets
- |_ coco128
- |_ images
- |_ labels
- |_ coco128.yaml # <---- HERE!
- |_ LICENSE
- |_ README.txt
-```
-
-### Upload Your Dataset
-
-To get this dataset into ClearML as a versionned dataset, go to the dataset root folder and run the following command:
-```bash
-cd coco128
-clearml-data sync --project YOLOv5 --name coco128 --folder .
-```
-
-The command `clearml-data sync` is actually a shorthand command. You could also run these commands one after the other:
-```bash
-# Optionally add --parent if you want to base
-# this version on another dataset version, so no duplicate files are uploaded!
-clearml-data create --name coco128 --project YOLOv5
-clearml-data add --files .
-clearml-data close
-```
-
-### Run Training Using A ClearML Dataset
-
-Now that you have a ClearML dataset, you can very simply use it to train custom YOLOv5 🚀 models!
-
-```bash
-python train.py --img 640 --batch 16 --epochs 3 --data clearml:// --weights yolov5s.pt --cache
-```
-
-
-
-## 👀 Hyperparameter Optimization
-
-Now that we have our experiments and data versioned, it's time to take a look at what we can build on top!
-
-Using the code information, installed packages and environment details, the experiment itself is now **completely reproducible**. In fact, ClearML allows you to clone an experiment and even change its parameters. We can then just rerun it with these new parameters automatically, this is basically what HPO does!
-
-To **run hyperparameter optimization locally**, we've included a pre-made script for you. Just make sure a training task has been run at least once, so it is in the ClearML experiment manager, we will essentially clone it and change its hyperparameters.
-
-You'll need to fill in the ID of this `template task` in the script found at `utils/loggers/clearml/hpo.py` and then just run it :) You can change `task.execute_locally()` to `task.execute()` to put it in a ClearML queue and have a remote agent work on it instead.
-
-```bash
-# To use optuna, install it first, otherwise you can change the optimizer to just be RandomSearch
-pip install optuna
-python utils/loggers/clearml/hpo.py
-```
-
-
-
-## 🤯 Remote Execution (advanced)
-
-Running HPO locally is really handy, but what if we want to run our experiments on a remote machine instead? Maybe you have access to a very powerful GPU machine on-site or you have some budget to use cloud GPUs.
-This is where the ClearML Agent comes into play. Check out what the agent can do here:
-
-- [YouTube video](https://youtu.be/MX3BrXnaULs)
-- [Documentation](https://clear.ml/docs/latest/docs/clearml_agent)
-
-In short: every experiment tracked by the experiment manager contains enough information to reproduce it on a different machine (installed packages, uncommitted changes etc.). So a ClearML agent does just that: it listens to a queue for incoming tasks and when it finds one, it recreates the environment and runs it while still reporting scalars, plots etc. to the experiment manager.
-
-You can turn any machine (a cloud VM, a local GPU machine, your own laptop ... ) into a ClearML agent by simply running:
-```bash
-clearml-agent daemon --queue [--docker]
-```
-
-### Cloning, Editing And Enqueuing
-
-With our agent running, we can give it some work. Remember from the HPO section that we can clone a task and edit the hyperparameters? We can do that from the interface too!
-
-🪄 Clone the experiment by right clicking it
-
-🎯 Edit the hyperparameters to what you wish them to be
-
-⏳ Enqueue the task to any of the queues by right clicking it
-
-
-
-### Executing A Task Remotely
-
-Now you can clone a task like we explained above, or simply mark your current script by adding `task.execute_remotely()` and on execution it will be put into a queue, for the agent to start working on!
-
-To run the YOLOv5 training script remotely, all you have to do is add this line to the training.py script after the clearml logger has been instatiated:
-```python
-# ...
-# Loggers
-data_dict = None
-if RANK in {-1, 0}:
- loggers = Loggers(save_dir, weights, opt, hyp, LOGGER) # loggers instance
- if loggers.clearml:
- loggers.clearml.task.execute_remotely(queue='my_queue') # <------ ADD THIS LINE
- # Data_dict is either None is user did not choose for ClearML dataset or is filled in by ClearML
- data_dict = loggers.clearml.data_dict
-# ...
-```
-When running the training script after this change, python will run the script up until that line, after which it will package the code and send it to the queue instead!
-
-### Autoscaling workers
-
-ClearML comes with autoscalers too! This tool will automatically spin up new remote machines in the cloud of your choice (AWS, GCP, Azure) and turn them into ClearML agents for you whenever there are experiments detected in the queue. Once the tasks are processed, the autoscaler will automatically shut down the remote machines and you stop paying!
-
-Check out the autoscalers getting started video below.
-
-[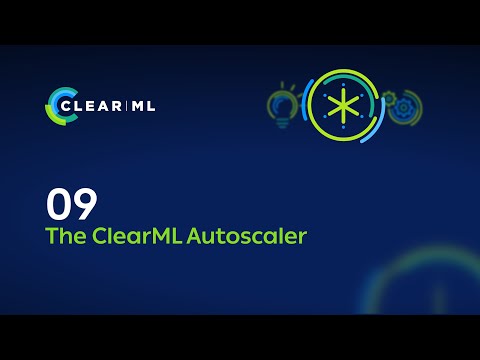](https://youtu.be/j4XVMAaUt3E)
diff --git a/spaces/aodianyun/stable-diffusion-webui/extensions/deforum/scripts/deforum_helpers/src/clipseg/datasets/pfe_dataset.py b/spaces/aodianyun/stable-diffusion-webui/extensions/deforum/scripts/deforum_helpers/src/clipseg/datasets/pfe_dataset.py
deleted file mode 100644
index 83988dea963a2c4226010a336573de94bf06c55e..0000000000000000000000000000000000000000
--- a/spaces/aodianyun/stable-diffusion-webui/extensions/deforum/scripts/deforum_helpers/src/clipseg/datasets/pfe_dataset.py
+++ /dev/null
@@ -1,129 +0,0 @@
-from os.path import expanduser
-import torch
-import json
-from general_utils import get_from_repository
-from datasets.lvis_oneshot3 import blend_image_segmentation
-from general_utils import log
-
-PASCAL_CLASSES = {a['id']: a['synonyms'] for a in json.load(open('datasets/pascal_classes.json'))}
-
-
-class PFEPascalWrapper(object):
-
- def __init__(self, mode, split, mask='separate', image_size=473, label_support=None, size=None, p_negative=0, aug=None):
- import sys
- # sys.path.append(expanduser('~/projects/new_one_shot'))
- from third_party.PFENet.util.dataset import SemData
-
- get_from_repository('PascalVOC2012', ['Pascal5i.tar'])
-
- self.p_negative = p_negative
- self.size = size
- self.mode = mode
- self.image_size = image_size
-
- if label_support in {True, False}:
- log.warning('label_support argument is deprecated. Use mask instead.')
- #raise ValueError()
-
- self.mask = mask
-
- value_scale = 255
- mean = [0.485, 0.456, 0.406]
- mean = [item * value_scale for item in mean]
- std = [0.229, 0.224, 0.225]
- std = [item * value_scale for item in std]
-
- import third_party.PFENet.util.transform as transform
-
- if mode == 'val':
- data_list = expanduser('~/projects/old_one_shot/PFENet/lists/pascal/val.txt')
-
- data_transform = [transform.test_Resize(size=image_size)] if image_size != 'original' else []
- data_transform += [
- transform.ToTensor(),
- transform.Normalize(mean=mean, std=std)
- ]
-
-
- elif mode == 'train':
- data_list = expanduser('~/projects/old_one_shot/PFENet/lists/pascal/voc_sbd_merge_noduplicate.txt')
-
- assert image_size != 'original'
-
- data_transform = [
- transform.RandScale([0.9, 1.1]),
- transform.RandRotate([-10, 10], padding=mean, ignore_label=255),
- transform.RandomGaussianBlur(),
- transform.RandomHorizontalFlip(),
- transform.Crop((image_size, image_size), crop_type='rand', padding=mean, ignore_label=255),
- transform.ToTensor(),
- transform.Normalize(mean=mean, std=std)
- ]
-
- data_transform = transform.Compose(data_transform)
-
- self.dataset = SemData(split=split, mode=mode, data_root=expanduser('~/datasets/PascalVOC2012/VOC2012'),
- data_list=data_list, shot=1, transform=data_transform, use_coco=False, use_split_coco=False)
-
- self.class_list = self.dataset.sub_val_list if mode == 'val' else self.dataset.sub_list
-
- # verify that subcls_list always has length 1
- # assert len(set([len(d[4]) for d in self.dataset])) == 1
-
- print('actual length', len(self.dataset.data_list))
-
- def __len__(self):
- if self.mode == 'val':
- return len(self.dataset.data_list)
- else:
- return len(self.dataset.data_list)
-
- def __getitem__(self, index):
- if self.dataset.mode == 'train':
- image, label, s_x, s_y, subcls_list = self.dataset[index % len(self.dataset.data_list)]
- elif self.dataset.mode == 'val':
- image, label, s_x, s_y, subcls_list, ori_label = self.dataset[index % len(self.dataset.data_list)]
- ori_label = torch.from_numpy(ori_label).unsqueeze(0)
-
- if self.image_size != 'original':
- longerside = max(ori_label.size(1), ori_label.size(2))
- backmask = torch.ones(ori_label.size(0), longerside, longerside).cuda()*255
- backmask[0, :ori_label.size(1), :ori_label.size(2)] = ori_label
- label = backmask.clone().long()
- else:
- label = label.unsqueeze(0)
-
- # assert label.shape == (473, 473)
-
- if self.p_negative > 0:
- if torch.rand(1).item() < self.p_negative:
- while True:
- idx = torch.randint(0, len(self.dataset.data_list), (1,)).item()
- _, _, s_x, s_y, subcls_list_tmp, _ = self.dataset[idx]
- if subcls_list[0] != subcls_list_tmp[0]:
- break
-
- s_x = s_x[0]
- s_y = (s_y == 1)[0]
- label_fg = (label == 1).float()
- val_mask = (label != 255).float()
-
- class_id = self.class_list[subcls_list[0]]
-
- label_name = PASCAL_CLASSES[class_id][0]
- label_add = ()
- mask = self.mask
-
- if mask == 'text':
- support = ('a photo of a ' + label_name + '.',)
- elif mask == 'separate':
- support = (s_x, s_y)
- else:
- if mask.startswith('text_and_'):
- label_add = (label_name,)
- mask = mask[9:]
-
- support = (blend_image_segmentation(s_x, s_y.float(), mask)[0],)
-
- return (image,) + label_add + support, (label_fg.unsqueeze(0), val_mask.unsqueeze(0), subcls_list[0])
diff --git a/spaces/aodianyun/stable-diffusion-webui/modules/deepbooru.py b/spaces/aodianyun/stable-diffusion-webui/modules/deepbooru.py
deleted file mode 100644
index 122fce7f569dbd28f9c6d83af874bb3efed34a5e..0000000000000000000000000000000000000000
--- a/spaces/aodianyun/stable-diffusion-webui/modules/deepbooru.py
+++ /dev/null
@@ -1,99 +0,0 @@
-import os
-import re
-
-import torch
-from PIL import Image
-import numpy as np
-
-from modules import modelloader, paths, deepbooru_model, devices, images, shared
-
-re_special = re.compile(r'([\\()])')
-
-
-class DeepDanbooru:
- def __init__(self):
- self.model = None
-
- def load(self):
- if self.model is not None:
- return
-
- files = modelloader.load_models(
- model_path=os.path.join(paths.models_path, "torch_deepdanbooru"),
- model_url='https://github.com/AUTOMATIC1111/TorchDeepDanbooru/releases/download/v1/model-resnet_custom_v3.pt',
- ext_filter=[".pt"],
- download_name='model-resnet_custom_v3.pt',
- )
-
- self.model = deepbooru_model.DeepDanbooruModel()
- self.model.load_state_dict(torch.load(files[0], map_location="cpu"))
-
- self.model.eval()
- self.model.to(devices.cpu, devices.dtype)
-
- def start(self):
- self.load()
- self.model.to(devices.device)
-
- def stop(self):
- if not shared.opts.interrogate_keep_models_in_memory:
- self.model.to(devices.cpu)
- devices.torch_gc()
-
- def tag(self, pil_image):
- self.start()
- res = self.tag_multi(pil_image)
- self.stop()
-
- return res
-
- def tag_multi(self, pil_image, force_disable_ranks=False):
- threshold = shared.opts.interrogate_deepbooru_score_threshold
- use_spaces = shared.opts.deepbooru_use_spaces
- use_escape = shared.opts.deepbooru_escape
- alpha_sort = shared.opts.deepbooru_sort_alpha
- include_ranks = shared.opts.interrogate_return_ranks and not force_disable_ranks
-
- pic = images.resize_image(2, pil_image.convert("RGB"), 512, 512)
- a = np.expand_dims(np.array(pic, dtype=np.float32), 0) / 255
-
- with torch.no_grad(), devices.autocast():
- x = torch.from_numpy(a).to(devices.device)
- y = self.model(x)[0].detach().cpu().numpy()
-
- probability_dict = {}
-
- for tag, probability in zip(self.model.tags, y):
- if probability < threshold:
- continue
-
- if tag.startswith("rating:"):
- continue
-
- probability_dict[tag] = probability
-
- if alpha_sort:
- tags = sorted(probability_dict)
- else:
- tags = [tag for tag, _ in sorted(probability_dict.items(), key=lambda x: -x[1])]
-
- res = []
-
- filtertags = set([x.strip().replace(' ', '_') for x in shared.opts.deepbooru_filter_tags.split(",")])
-
- for tag in [x for x in tags if x not in filtertags]:
- probability = probability_dict[tag]
- tag_outformat = tag
- if use_spaces:
- tag_outformat = tag_outformat.replace('_', ' ')
- if use_escape:
- tag_outformat = re.sub(re_special, r'\\\1', tag_outformat)
- if include_ranks:
- tag_outformat = f"({tag_outformat}:{probability:.3f})"
-
- res.append(tag_outformat)
-
- return ", ".join(res)
-
-
-model = DeepDanbooru()
diff --git a/spaces/arborvitae/AI_Legal_documentation_assistant/README.md b/spaces/arborvitae/AI_Legal_documentation_assistant/README.md
deleted file mode 100644
index 922a0f02853de9ef11e411679a5e68e8e7bfd141..0000000000000000000000000000000000000000
--- a/spaces/arborvitae/AI_Legal_documentation_assistant/README.md
+++ /dev/null
@@ -1,12 +0,0 @@
----
-title: JurioSync
-emoji: 📄🤖
-colorFrom: purple
-colorTo: pink
-sdk: gradio
-sdk_version: 3.27.0
-app_file: app.py
-pinned: false
----
-
-Check out the configuration reference at https://huggingface.co/docs/hub/spaces-config-reference
diff --git a/spaces/artificialguybr/video-dubbing/TTS/TTS/encoder/utils/io.py b/spaces/artificialguybr/video-dubbing/TTS/TTS/encoder/utils/io.py
deleted file mode 100644
index d1dad3e24d234cdcb9616fb14bc87919c7e20291..0000000000000000000000000000000000000000
--- a/spaces/artificialguybr/video-dubbing/TTS/TTS/encoder/utils/io.py
+++ /dev/null
@@ -1,38 +0,0 @@
-import datetime
-import os
-
-from TTS.utils.io import save_fsspec
-
-
-def save_checkpoint(model, optimizer, model_loss, out_path, current_step):
- checkpoint_path = "checkpoint_{}.pth".format(current_step)
- checkpoint_path = os.path.join(out_path, checkpoint_path)
- print(" | | > Checkpoint saving : {}".format(checkpoint_path))
-
- new_state_dict = model.state_dict()
- state = {
- "model": new_state_dict,
- "optimizer": optimizer.state_dict() if optimizer is not None else None,
- "step": current_step,
- "loss": model_loss,
- "date": datetime.date.today().strftime("%B %d, %Y"),
- }
- save_fsspec(state, checkpoint_path)
-
-
-def save_best_model(model, optimizer, model_loss, best_loss, out_path, current_step):
- if model_loss < best_loss:
- new_state_dict = model.state_dict()
- state = {
- "model": new_state_dict,
- "optimizer": optimizer.state_dict(),
- "step": current_step,
- "loss": model_loss,
- "date": datetime.date.today().strftime("%B %d, %Y"),
- }
- best_loss = model_loss
- bestmodel_path = "best_model.pth"
- bestmodel_path = os.path.join(out_path, bestmodel_path)
- print("\n > BEST MODEL ({0:.5f}) : {1:}".format(model_loss, bestmodel_path))
- save_fsspec(state, bestmodel_path)
- return best_loss
diff --git a/spaces/artificialguybr/video-dubbing/TTS/TTS/vocoder/__init__.py b/spaces/artificialguybr/video-dubbing/TTS/TTS/vocoder/__init__.py
deleted file mode 100644
index e69de29bb2d1d6434b8b29ae775ad8c2e48c5391..0000000000000000000000000000000000000000
diff --git a/spaces/arxify/RVC-beta-v2-0618/docs/faiss_tips_ko.md b/spaces/arxify/RVC-beta-v2-0618/docs/faiss_tips_ko.md
deleted file mode 100644
index ecd518ca2a89996898057983761fc469eaf969d2..0000000000000000000000000000000000000000
--- a/spaces/arxify/RVC-beta-v2-0618/docs/faiss_tips_ko.md
+++ /dev/null
@@ -1,132 +0,0 @@
-Facebook AI Similarity Search (Faiss) 팁
-==================
-# Faiss에 대하여
-Faiss 는 Facebook Research가 개발하는, 고밀도 벡터 이웃 검색 라이브러리입니다. 근사 근접 탐색법 (Approximate Neigbor Search)은 약간의 정확성을 희생하여 유사 벡터를 고속으로 찾습니다.
-
-## RVC에 있어서 Faiss
-RVC에서는 HuBERT로 변환한 feature의 embedding을 위해 훈련 데이터에서 생성된 embedding과 유사한 embadding을 검색하고 혼합하여 원래의 음성에 더욱 가까운 변환을 달성합니다. 그러나, 이 탐색법은 단순히 수행하면 시간이 다소 소모되므로, 근사 근접 탐색법을 통해 고속 변환을 가능케 하고 있습니다.
-
-# 구현 개요
-모델이 위치한 `/logs/your-experiment/3_feature256`에는 각 음성 데이터에서 HuBERT가 추출한 feature들이 있습니다. 여기에서 파일 이름별로 정렬된 npy 파일을 읽고, 벡터를 연결하여 big_npy ([N, 256] 모양의 벡터) 를 만듭니다. big_npy를 `/logs/your-experiment/total_fea.npy`로 저장한 후, Faiss로 학습시킵니다.
-
-2023/04/18 기준으로, Faiss의 Index Factory 기능을 이용해, L2 거리에 근거하는 IVF를 이용하고 있습니다. IVF의 분할수(n_ivf)는 N//39로, n_probe는 int(np.power(n_ivf, 0.3))가 사용되고 있습니다. (infer-web.py의 train_index 주위를 찾으십시오.)
-
-이 팁에서는 먼저 이러한 매개 변수의 의미를 설명하고, 개발자가 추후 더 나은 index를 작성할 수 있도록 하는 조언을 작성합니다.
-
-# 방법의 설명
-## Index factory
-index factory는 여러 근사 근접 탐색법을 문자열로 연결하는 pipeline을 문자열로 표기하는 Faiss만의 독자적인 기법입니다. 이를 통해 index factory의 문자열을 변경하는 것만으로 다양한 근사 근접 탐색을 시도해 볼 수 있습니다. RVC에서는 다음과 같이 사용됩니다:
-
-```python
-index = Faiss.index_factory(256, "IVF%s,Flat" % n_ivf)
-```
-`index_factory`의 인수들 중 첫 번째는 벡터의 차원 수이고, 두번째는 index factory 문자열이며, 세번째에는 사용할 거리를 지정할 수 있습니다.
-
-기법의 보다 자세한 설명은 https://github.com/facebookresearch/Faiss/wiki/The-index-factory 를 확인해 주십시오.
-
-## 거리에 대한 index
-embedding의 유사도로서 사용되는 대표적인 지표로서 이하의 2개가 있습니다.
-
-- 유클리드 거리 (METRIC_L2)
-- 내적(内積) (METRIC_INNER_PRODUCT)
-
-유클리드 거리에서는 각 차원에서 제곱의 차를 구하고, 각 차원에서 구한 차를 모두 더한 후 제곱근을 취합니다. 이것은 일상적으로 사용되는 2차원, 3차원에서의 거리의 연산법과 같습니다. 내적은 그 값을 그대로 유사도 지표로 사용하지 않고, L2 정규화를 한 이후 내적을 취하는 코사인 유사도를 사용합니다.
-
-어느 쪽이 더 좋은지는 경우에 따라 다르지만, word2vec에서 얻은 embedding 및 ArcFace를 활용한 이미지 검색 모델은 코사인 유사성이 이용되는 경우가 많습니다. numpy를 사용하여 벡터 X에 대해 L2 정규화를 하고자 하는 경우, 0 division을 피하기 위해 충분히 작은 값을 eps로 한 뒤 이하에 코드를 활용하면 됩니다.
-
-```python
-X_normed = X / np.maximum(eps, np.linalg.norm(X, ord=2, axis=-1, keepdims=True))
-```
-
-또한, `index factory`의 3번째 인수에 건네주는 값을 선택하는 것을 통해 계산에 사용하는 거리 index를 변경할 수 있습니다.
-
-```python
-index = Faiss.index_factory(dimention, text, Faiss.METRIC_INNER_PRODUCT)
-```
-
-## IVF
-IVF (Inverted file indexes)는 역색인 탐색법과 유사한 알고리즘입니다. 학습시에는 검색 대상에 대해 k-평균 군집법을 실시하고 클러스터 중심을 이용해 보로노이 분할을 실시합니다. 각 데이터 포인트에는 클러스터가 할당되므로, 클러스터에서 데이터 포인트를 조회하는 dictionary를 만듭니다.
-
-예를 들어, 클러스터가 다음과 같이 할당된 경우
-|index|Cluster|
-|-----|-------|
-|1|A|
-|2|B|
-|3|A|
-|4|C|
-|5|B|
-
-IVF 이후의 결과는 다음과 같습니다:
-
-|cluster|index|
-|-------|-----|
-|A|1, 3|
-|B|2, 5|
-|C|4|
-
-탐색 시, 우선 클러스터에서 `n_probe`개의 클러스터를 탐색한 다음, 각 클러스터에 속한 데이터 포인트의 거리를 계산합니다.
-
-# 권장 매개변수
-index의 선택 방법에 대해서는 공식적으로 가이드 라인이 있으므로, 거기에 준해 설명합니다.
-https://github.com/facebookresearch/Faiss/wiki/Guidelines-to-choose-an-index
-
-1M 이하의 데이터 세트에 있어서는 4bit-PQ가 2023년 4월 시점에서는 Faiss로 이용할 수 있는 가장 효율적인 수법입니다. 이것을 IVF와 조합해, 4bit-PQ로 후보를 추려내고, 마지막으로 이하의 index factory를 이용하여 정확한 지표로 거리를 재계산하면 됩니다.
-
-```python
-index = Faiss.index_factory(256, "IVF1024,PQ128x4fs,RFlat")
-```
-
-## IVF 권장 매개변수
-IVF의 수가 너무 많으면, 가령 데이터 수의 수만큼 IVF로 양자화(Quantization)를 수행하면, 이것은 완전탐색과 같아져 효율이 나빠지게 됩니다. 1M 이하의 경우 IVF 값은 데이터 포인트 수 N에 대해 4sqrt(N) ~ 16sqrt(N)를 사용하는 것을 권장합니다.
-
-n_probe는 n_probe의 수에 비례하여 계산 시간이 늘어나므로 정확도와 시간을 적절히 균형을 맞추어 주십시오. 개인적으로 RVC에 있어서 그렇게까지 정확도는 필요 없다고 생각하기 때문에 n_probe = 1이면 된다고 생각합니다.
-
-## FastScan
-FastScan은 직적 양자화를 레지스터에서 수행함으로써 거리의 고속 근사를 가능하게 하는 방법입니다.직적 양자화는 학습시에 d차원마다(보통 d=2)에 독립적으로 클러스터링을 실시해, 클러스터끼리의 거리를 사전 계산해 lookup table를 작성합니다. 예측시는 lookup table을 보면 각 차원의 거리를 O(1)로 계산할 수 있습니다. 따라서 PQ 다음에 지정하는 숫자는 일반적으로 벡터의 절반 차원을 지정합니다.
-
-FastScan에 대한 자세한 설명은 공식 문서를 참조하십시오.
-https://github.com/facebookresearch/Faiss/wiki/Fast-accumulation-of-PQ-and-AQ-codes-(FastScan)
-
-## RFlat
-RFlat은 FastScan이 계산한 대략적인 거리를 index factory의 3번째 인수로 지정한 정확한 거리로 다시 계산하라는 인스트럭션입니다. k개의 근접 변수를 가져올 때 k*k_factor개의 점에 대해 재계산이 이루어집니다.
-
-# Embedding 테크닉
-## Alpha 쿼리 확장
-퀴리 확장이란 탐색에서 사용되는 기술로, 예를 들어 전문 탐색 시, 입력된 검색문에 단어를 몇 개를 추가함으로써 검색 정확도를 올리는 방법입니다. 백터 탐색을 위해서도 몇가지 방법이 제안되었는데, 그 중 α-쿼리 확장은 추가 학습이 필요 없는 매우 효과적인 방법으로 알려져 있습니다. [Attention-Based Query Expansion Learning](https://arxiv.org/abs/2007.08019)와 [2nd place solution of kaggle shopee competition](https://www.kaggle.com/code/lyakaap/2nd-place-solution/notebook) 논문에서 소개된 바 있습니다..
-
-α-쿼리 확장은 한 벡터에 인접한 벡터를 유사도의 α곱한 가중치로 더해주면 됩니다. 코드로 예시를 들어 보겠습니다. big_npy를 α query expansion로 대체합니다.
-
-```python
-alpha = 3.
-index = Faiss.index_factory(256, "IVF512,PQ128x4fs,RFlat")
-original_norm = np.maximum(np.linalg.norm(big_npy, ord=2, axis=1, keepdims=True), 1e-9)
-big_npy /= original_norm
-index.train(big_npy)
-index.add(big_npy)
-dist, neighbor = index.search(big_npy, num_expand)
-
-expand_arrays = []
-ixs = np.arange(big_npy.shape[0])
-for i in range(-(-big_npy.shape[0]//batch_size)):
- ix = ixs[i*batch_size:(i+1)*batch_size]
- weight = np.power(np.einsum("nd,nmd->nm", big_npy[ix], big_npy[neighbor[ix]]), alpha)
- expand_arrays.append(np.sum(big_npy[neighbor[ix]] * np.expand_dims(weight, axis=2),axis=1))
-big_npy = np.concatenate(expand_arrays, axis=0)
-
-# index version 정규화
-big_npy = big_npy / np.maximum(np.linalg.norm(big_npy, ord=2, axis=1, keepdims=True), 1e-9)
-```
-
-위 테크닉은 탐색을 수행하는 쿼리에도, 탐색 대상 DB에도 적응 가능한 테크닉입니다.
-
-## MiniBatch KMeans에 의한 embedding 압축
-
-total_fea.npy가 너무 클 경우 K-means를 이용하여 벡터를 작게 만드는 것이 가능합니다. 이하 코드로 embedding의 압축이 가능합니다. n_clusters에 압축하고자 하는 크기를 지정하고 batch_size에 256 * CPU의 코어 수를 지정함으로써 CPU 병렬화의 혜택을 충분히 얻을 수 있습니다.
-
-```python
-import multiprocessing
-from sklearn.cluster import MiniBatchKMeans
-kmeans = MiniBatchKMeans(n_clusters=10000, batch_size=256 * multiprocessing.cpu_count(), init="random")
-kmeans.fit(big_npy)
-sample_npy = kmeans.cluster_centers_
-```
\ No newline at end of file
diff --git a/spaces/avivdm1/AutoGPT/autogpt/permanent_memory/__init__.py b/spaces/avivdm1/AutoGPT/autogpt/permanent_memory/__init__.py
deleted file mode 100644
index e69de29bb2d1d6434b8b29ae775ad8c2e48c5391..0000000000000000000000000000000000000000
diff --git a/spaces/awacke1/RealTimeLiveSentimentGradio/README.md b/spaces/awacke1/RealTimeLiveSentimentGradio/README.md
deleted file mode 100644
index 1e0a9409c18e649178b5d2e6025ad88be481b61a..0000000000000000000000000000000000000000
--- a/spaces/awacke1/RealTimeLiveSentimentGradio/README.md
+++ /dev/null
@@ -1,13 +0,0 @@
----
-title: RealTimeLiveSentimentGradio
-emoji: 🐠
-colorFrom: purple
-colorTo: blue
-sdk: gradio
-sdk_version: 3.5
-app_file: app.py
-pinned: false
-license: apache-2.0
----
-
-Check out the configuration reference at https://huggingface.co/docs/hub/spaces-config-reference
diff --git a/spaces/awacke1/Streamlit.ChatWikiwriter.Multiplayer/app.py b/spaces/awacke1/Streamlit.ChatWikiwriter.Multiplayer/app.py
deleted file mode 100644
index 7559e055cd0c009a3dfe489c60045f5662038ba3..0000000000000000000000000000000000000000
--- a/spaces/awacke1/Streamlit.ChatWikiwriter.Multiplayer/app.py
+++ /dev/null
@@ -1,285 +0,0 @@
-import streamlit as st
-import spacy
-import wikipediaapi
-import wikipedia
-from wikipedia.exceptions import DisambiguationError
-from transformers import TFAutoModel, AutoTokenizer
-import numpy as np
-import pandas as pd
-import faiss
-import datetime
-import time
-
-
-try:
- nlp = spacy.load("en_core_web_sm")
-except:
- spacy.cli.download("en_core_web_sm")
- nlp = spacy.load("en_core_web_sm")
-
-wh_words = ['what', 'who', 'how', 'when', 'which']
-
-def get_concepts(text):
- text = text.lower()
- doc = nlp(text)
- concepts = []
- for chunk in doc.noun_chunks:
- if chunk.text not in wh_words:
- concepts.append(chunk.text)
- return concepts
-
-def get_passages(text, k=1000):
- doc = nlp(text)
- passages = []
- passage_len = 0
- passage = ""
- sents = list(doc.sents)
- for i in range(len(sents)):
- sen = sents[i]
- passage_len += len(sen)
- if passage_len >= k:
- passages.append(passage)
- passage = sen.text
- passage_len = len(sen)
- continue
- elif i == (len(sents) - 1):
- passage += " " + sen.text
- passages.append(passage)
- passage = ""
- passage_len = 0
- continue
- passage += " " + sen.text
- return passages
-
-def get_dicts_for_dpr(concepts, n_results=200, k=1000):
- dicts = []
- for concept in concepts:
- wikis = wikipedia.search(concept, results=n_results)
- st.write(f"{concept} No of Wikis: {len(wikis)}")
- for wiki in wikis:
- try:
- html_page = wikipedia.page(title=wiki, auto_suggest=False)
- except DisambiguationError:
- continue
- htmlResults = html_page.content
- passages = get_passages(htmlResults, k=k)
- for passage in passages:
- i_dicts = {}
- i_dicts['text'] = passage
- i_dicts['title'] = wiki
- dicts.append(i_dicts)
- return dicts
-
-passage_encoder = TFAutoModel.from_pretrained("nlpconnect/dpr-ctx_encoder_bert_uncased_L-2_H-128_A-2")
-query_encoder = TFAutoModel.from_pretrained("nlpconnect/dpr-question_encoder_bert_uncased_L-2_H-128_A-2")
-p_tokenizer = AutoTokenizer.from_pretrained("nlpconnect/dpr-ctx_encoder_bert_uncased_L-2_H-128_A-2")
-q_tokenizer = AutoTokenizer.from_pretrained("nlpconnect/dpr-question_encoder_bert_uncased_L-2_H-128_A-2")
-
-def get_title_text_combined(passage_dicts):
- res = []
- for p in passage_dicts:
- res.append(tuple((p['title'], p['text'])))
- return res
-
-def extracted_passage_embeddings(processed_passages, max_length=156):
- passage_inputs = p_tokenizer.batch_encode_plus(
- processed_passages,
- add_special_tokens=True,
- truncation=True,
- padding="max_length",
- max_length=max_length,
- return_token_type_ids=True
- )
- passage_embeddings = passage_encoder.predict([np.array(passage_inputs['input_ids']), np.array(passage_inputs['attention_mask']),
- np.array(passage_inputs['token_type_ids'])],
- batch_size=64,
- verbose=1)
- return passage_embeddings
-
-def extracted_query_embeddings(queries, max_length=64):
- query_inputs = q_tokenizer.batch_encode_plus(
- queries,
- add_special_tokens=True,
- truncation=True,
- padding="max_length",
- max_length=max_length,
- return_token_type_ids=True
- )
-
- query_embeddings = query_encoder.predict([np.array(query_inputs['input_ids']),
- np.array(query_inputs['attention_mask']),
- np.array(query_inputs['token_type_ids'])],
- batch_size=1,
- verbose=1)
- return query_embeddings
-
-def get_pagetext(page):
- s = str(page).replace("/t","")
- return s
-
-def get_wiki_summary(search):
- wiki_wiki = wikipediaapi.Wikipedia('en')
- page = wiki_wiki.page(search)
-
-
-def get_wiki_summaryDF(search):
- wiki_wiki = wikipediaapi.Wikipedia('en')
- page = wiki_wiki.page(search)
-
- isExist = page.exists()
- if not isExist:
- return isExist, "Not found", "Not found", "Not found", "Not found"
-
- pageurl = page.fullurl
- pagetitle = page.title
- pagesummary = page.summary[0:60]
- pagetext = get_pagetext(page.text)
-
- backlinks = page.backlinks
- linklist = ""
- for link in backlinks.items():
- pui = link[0]
- linklist += pui + " , "
- a=1
-
- categories = page.categories
- categorylist = ""
- for category in categories.items():
- pui = category[0]
- categorylist += pui + " , "
- a=1
-
- links = page.links
- linklist2 = ""
- for link in links.items():
- pui = link[0]
- linklist2 += pui + " , "
- a=1
-
- sections = page.sections
-
- ex_dic = {
- 'Entity' : ["URL","Title","Summary", "Text", "Backlinks", "Links", "Categories"],
- 'Value': [pageurl, pagetitle, pagesummary, pagetext, linklist,linklist2, categorylist ]
- }
-
- df = pd.DataFrame(ex_dic)
-
- return df
-
-
-def save_message_old1(name, message):
- now = datetime.datetime.now()
- timestamp = now.strftime("%Y-%m-%d %H:%M:%S")
- with open("chat.txt", "a") as f:
- f.write(f"{timestamp} - {name}: {message}\n")
-
-def press_release():
- st.markdown("""🎉🎊 Breaking News! 📢📣
-
-Introducing StreamlitWikipediaChat - the ultimate way to chat with Wikipedia and the whole world at the same time! 🌎📚👋
-
-Are you tired of reading boring articles on Wikipedia? Do you want to have some fun while learning new things? Then StreamlitWikipediaChat is just the thing for you! 😃💻
-
-With StreamlitWikipediaChat, you can ask Wikipedia anything you want and get instant responses! Whether you want to know the capital of Madagascar or how to make a delicious chocolate cake, Wikipedia has got you covered. 🍰🌍
-
-But that's not all! You can also chat with other people from around the world who are using StreamlitWikipediaChat at the same time. It's like a virtual classroom where you can learn from and teach others. 🌐👨🏫👩🏫
-
-And the best part? StreamlitWikipediaChat is super easy to use! All you have to do is type in your question and hit send. That's it! 🤯🙌
-
-So, what are you waiting for? Join the fun and start chatting with Wikipedia and the world today! 😎🎉
-
-StreamlitWikipediaChat - where learning meets fun! 🤓🎈""")
-
-
-def main_old1():
- st.title("Streamlit Chat")
-
- name = st.text_input("Enter your name")
- message = st.text_input("Enter a topic to share from Wikipedia")
- if st.button("Submit"):
-
- # wiki
- df = get_wiki_summaryDF(message)
-
- save_message(name, message)
- save_message(name, df)
-
- st.text("Message sent!")
-
-
- st.text("Chat history:")
- with open("chat.txt", "a+") as f:
- f.seek(0)
- chat_history = f.read()
- #st.text(chat_history)
- st.markdown(chat_history)
-
- countdown = st.empty()
- t = 60
- while t:
- mins, secs = divmod(t, 60)
- countdown.text(f"Time remaining: {mins:02d}:{secs:02d}")
- time.sleep(1)
- t -= 1
- if t == 0:
- countdown.text("Time's up!")
- with open("chat.txt", "a+") as f:
- f.seek(0)
- chat_history = f.read()
- #st.text(chat_history)
- st.markdown(chat_history)
-
- press_release()
-
- t = 60
-
-def save_message(name, message):
- with open("chat.txt", "a") as f:
- f.write(f"{name}: {message}\n")
-
-def main():
- st.title("Streamlit Chat")
-
- name = st.text_input("Enter your name")
- message = st.text_input("Enter a topic to share from Wikipedia")
- if st.button("Submit"):
-
- # wiki
- df = get_wiki_summaryDF(message)
-
- save_message(name, message)
- save_message(name, df)
-
- st.text("Message sent!")
-
- st.text("Chat history:")
- with open("chat.txt", "a+") as f:
- f.seek(0)
- chat_history = f.read()
- st.markdown(chat_history)
-
- countdown = st.empty()
- t = 60
- while t:
- mins, secs = divmod(t, 60)
- countdown.text(f"Time remaining: {mins:02d}:{secs:02d}")
- time.sleep(1)
- t -= 1
- if t == 0:
- countdown.text("Time's up!")
- with open("chat.txt", "a+") as f:
- f.seek(0)
- chat_history = f.read()
- st.markdown(chat_history)
-
- press_release()
-
- t = 60
-
-
-
-
-if __name__ == "__main__":
- main()
-
diff --git a/spaces/awacke1/VideoCombinerInterpolator/README.md b/spaces/awacke1/VideoCombinerInterpolator/README.md
deleted file mode 100644
index ce22942abe745b959a44633749182ffd5759e7ce..0000000000000000000000000000000000000000
--- a/spaces/awacke1/VideoCombinerInterpolator/README.md
+++ /dev/null
@@ -1,13 +0,0 @@
----
-title: VideoCombinerInterpolator
-emoji: 🏢
-colorFrom: blue
-colorTo: pink
-sdk: streamlit
-sdk_version: 1.26.0
-app_file: app.py
-pinned: false
-license: mit
----
-
-Check out the configuration reference at https://huggingface.co/docs/hub/spaces-config-reference
diff --git a/spaces/azizalto/vanilla-ml-algorithms/ml_algorithms/linear_regression_gradient_descent.py b/spaces/azizalto/vanilla-ml-algorithms/ml_algorithms/linear_regression_gradient_descent.py
deleted file mode 100644
index a8f2789d7329447b905561a40abf125f796ae31a..0000000000000000000000000000000000000000
--- a/spaces/azizalto/vanilla-ml-algorithms/ml_algorithms/linear_regression_gradient_descent.py
+++ /dev/null
@@ -1,187 +0,0 @@
-# src: https://gist.github.com/iamaziz/ea5863beaee090937fd6828e88653f5e
-
-
-class LinearRegressionGradient:
- def __init__(self, theta=None):
- self.theta = theta
- self.loss_ = float("inf")
-
- def hypothesis(self, x):
- return self.theta[0] + self.theta[1] * x
-
- def loss(self, X, y):
- m = len(X)
- return sum([(X[i] - y[i]) ** 2 for i in range(m)]) / (2 * m)
-
- def gradientDescent(self, X, y, theta, num_iter=3000, alpha=0.01):
- m = len(X)
-
- for j in range(num_iter):
-
- # predict
- h = list(map(self.hypothesis, X))
-
- # compute slope, aka derivative with current params (theta)
- deri_th0 = sum([(h[i] - y[i]) for i in range(m)]) / m
- deri_th1 = sum([(h[i] - y[i]) * X[i] for i in range(m)]) / m
-
- # update parameters (moving against the gradient 'derivative')
- theta[0] = theta[0] - alpha * deri_th0
- theta[1] = theta[1] - alpha * deri_th1
-
- # report
- if j % 200 == 0:
- self.loss_ = self.loss(X, y)
- msg = f"loss: {self.loss_}"
- print(msg)
-
-
-def app():
- import streamlit as st
-
- def header():
- st.subheader("Linear Regression using Gradient Descent")
- desc = """> Plain Python (vanilla version) i.e. without importing any library"""
- st.markdown(desc)
-
- header()
-
- st1, st2 = st.columns(2)
- with st1:
- code_math()
- with st2:
- interactive_run()
-
- st.markdown(
- f"> source [notebook](https://gist.github.com/iamaziz/ea5863beaee090937fd6828e88653f5e)."
- )
-
-
-def code_math():
- import inspect
- import streamlit as st
-
- tex = st.latex
- write = st.write
- mark = st.write
- codify = lambda func: st.code(inspect.getsource(func), language="python")
- cls = LinearRegressionGradient(theta=[0, 0])
-
- write("The class")
- codify(cls.__init__)
-
- write("the Hypothesis")
- tex(r"""h_\theta(x) = \theta_0 + \theta_1x""")
- codify(cls.hypothesis)
- mark('The Loss/Objective/Cost function "_minimize_"')
- tex(r"""J(\theta_0, \theta_1) = \frac{1}{2m}\sum(h_\theta(x^{(i)}) - y^{(i)})^2""")
- codify(cls.loss)
- write("The Gradient Descent algorithm")
- mark("> repeat until converge {")
- tex(
- r"""\theta_0 = \theta_0 - \alpha \frac{1}{m} \sum_{i=1}^{m} (h_\theta(x^{(i)}) - y^{(i)} )"""
- )
- tex(
- r"""\theta_1 = \theta_1 - \alpha \frac{1}{m} \sum_{i=1}^{m} (h_\theta(x^{(i)}) - y^{(i)}) x^{(i)})"""
- )
- mark("> }")
- codify(cls.gradientDescent)
-
-
-def interactive_run():
- import streamlit as st
- import numpy as np
-
- mark = st.markdown
- tex = st.latex
-
- def random_data(n=10):
- def sample_linear_regression_dataset(n):
- # src: https://www.gaussianwaves.com/2020/01/generating-simulated-dataset-for-regression-problems-sklearn-make_regression/
- import numpy as np
- from sklearn import datasets
- import matplotlib.pyplot as plt # for plotting
-
- x, y, coef = datasets.make_regression(
- n_samples=n, # number of samples
- n_features=1, # number of features
- n_informative=1, # number of useful features
- noise=40, # bias and standard deviation of the guassian noise
- coef=True, # true coefficient used to generated the data
- random_state=0,
- ) # set for same data points for each run
-
- # Scale feature x (years of experience) to range 0..20
- # x = np.interp(x, (x.min(), x.max()), (0, 20))
-
- # Scale target y (salary) to range 20000..150000
- # y = np.interp(y, (y.min(), y.max()), (20000, 150000))
-
- plt.ion() # interactive plot on
- plt.plot(x, y, ".", label="training data")
- plt.xlabel("Years of experience")
- plt.ylabel("Salary $")
- plt.title("Experience Vs. Salary")
- # st.pyplot(plt.show())
- # st.write(type(x.tolist()))
- # st.write(x.tolist())
-
- X, y = x.reshape(x.shape[0],), y.reshape(
- y.shape[0],
- )
- return np.around(X, 2), np.around(y, 2)
- # return [a[0] for a in x.tolist()], [a[0] for a in y.tolist()]
- # return [item for sublist in x.tolist() for item in sublist], [
- # item for sublist in y for item in sublist
- # ]
-
- X_, y_ = sample_linear_regression_dataset(n)
- return X_, y_
- # st.write(type(X_), type(y_))
- # st.write(type(np.round(X, 2).tolist()))
- # st.write(X_) # , y_)
- # return X, y
-
- # return np.around(X, 2).tolist(), np.around(y, 2).tolist()
-
- X, y = random_data()
- theta = [0, 0] # initial values
- model = LinearRegressionGradient(theta)
- mark("# Example")
- n = st.slider("Number of samples", min_value=10, max_value=200, step=10)
- if st.button("generate new data and solve"):
- X, y = random_data(n=n)
- mark("_Input_")
- mark(f"_X_ = {X}")
- mark(f"_y_ = {y}")
- model.gradientDescent(X, y, theta) # run to optimize thetas
- mark("_Solution_")
- tex(f"y = {model.theta[0]:.1f} + {model.theta[1]:.1f} x") # print solution
- tex(f"loss = {model.loss_}")
-
- mark("> How to run")
- mark(
- """
- ```python
- X, y = random_data()
- theta = [0, 0] # initial values
- model = LinearRegressionGradient(theta)
- model.gradientDescent(X, y, theta) # run "i.e. optimize thetas"
- # print solution
- # print(f"y = {model.theta[0]:.1f} + {model.theta[1]:.1f} x")
- # print(f"loss = {model.loss_}")
- ```
- """
- )
- # -- visualize
- import matplotlib.pyplot as plt
-
- fig, ax = plt.subplots()
- ax.scatter(X, y, label="Linear Relation")
- y_pred = theta[0] + theta[1] * np.array(X)
- ax.plot(X, y_pred)
- ax.grid(color="black", linestyle="--", linewidth=0.5, markevery=int)
- ax.legend(loc=2)
- # ax.axis("scaled")
- st.pyplot(fig)
- # st.line_chart(X, y)
diff --git a/spaces/baby123/sd/README.md b/spaces/baby123/sd/README.md
deleted file mode 100644
index 3d945821fdd2584221e4483584485124ccdd83fc..0000000000000000000000000000000000000000
--- a/spaces/baby123/sd/README.md
+++ /dev/null
@@ -1,109 +0,0 @@
----
-title: Stable Diffusion WebUI ControlNet
-emoji: 🦄
-colorFrom: pink
-colorTo: yellow
-sdk: docker
-app_port: 7860
-pinned: true
-tags:
-- stable-diffusion
-- stable-diffusion-diffusers
-- text-to-image
-models:
-- stabilityai/stable-diffusion-2-1
-- runwayml/stable-diffusion-v1-5
-- lllyasviel/ControlNet
-- webui/ControlNet-modules-safetensors
-- dreamlike-art/dreamlike-diffusion-1.0
-- Anashel/rpg
-- Lykon/DreamShaper
-duplicated_from: yuan2023/stable-diffusion-webui-controlnet-docker
----
-
-## Stable Diffusion WebUI + ControlNet
-
-Private image builds with both with Stable Diffusion 2.1 models and Stable Diffusion 1.5 models and bundles several popular extensions to [AUTOMATIC1111's WebUI]([https://github.com/AUTOMATIC1111/stable-diffusion-webui]), including the [ControlNet WebUI extension](https://github.com/Mikubill/sd-webui-controlnet). ControlNet models primarily works best with the SD 1.5 models at the time of writing.
-
-Shared UI space would usually load with a model based on Stable Diffusion 1.5.
-
-🐳 🦄 Builds a Docker image to be run as a Space at [Hugging Face](https://huggingface.co/) using A10G or T4 hardware.
-
-### Setup on Hugging Face
-
-1. Duplicate this space to your Hugging Face account or clone this repo to your account.
-2. Under the *"Settings"* tab of your space you can choose which hardware for your space, that you will also be billed for.
-3. The [`on_start.sh`](./on_start.sh) file will be run when the container is started, right before the WebUI is initiated. This is where you can install any additional extensions or models you may need. Make sure the env value `IS_SHARED_UI` is set to `0` or is unset for your space, or else only the lightweight model installation will run and some features will be disabled.
-
----
-
-### Relevant links for more information
-
-#### Repo for this builder
-
-This repo, containing the `Dockerfile`, etc. for building the image can originally be found on both [`🤗 Hugging Face ➔ carloscar/stable-diffusion-webui-controlnet-docker`](https://huggingface.co/spaces/carloscar/stable-diffusion-webui-controlnet-docker) and [`🐙 GitHub ➔ kalaspuff/stable-diffusion-webui-controlnet-docker`](https://github.com/kalaspuff/stable-diffusion-webui-controlnet-docker).
-
-#### Stable Diffusion Web UI
-
-* Source Code: [https://github.com/AUTOMATIC1111/stable-diffusion-webui](https://github.com/AUTOMATIC1111/stable-diffusion-webui)
-* Documentation: [https://github.com/AUTOMATIC1111/stable-diffusion-webui/wiki](https://github.com/AUTOMATIC1111/stable-diffusion-webui/wiki)
-
-#### WebUI extension for ControlNet
-
-* Source Code: [https://github.com/Mikubill/sd-webui-controlnet](https://github.com/Mikubill/sd-webui-controlnet)
-
-#### ControlNet models
-
-* Trained models: [https://github.com/lllyasviel/ControlNet](https://github.com/lllyasviel/ControlNet)
-* Pre-extracted models: [https://huggingface.co/webui/ControlNet-modules-safetensors/tree/main](https://huggingface.co/webui/ControlNet-modules-safetensors/tree/main)
-
-#### Licenses for using Stable Diffusion models and ControlNet models
-
-* [https://huggingface.co/stabilityai/stable-diffusion-2/blob/main/LICENSE-MODEL](https://huggingface.co/stabilityai/stable-diffusion-2/blob/main/LICENSE-MODEL)
-* [https://huggingface.co/spaces/CompVis/stable-diffusion-license](https://huggingface.co/spaces/CompVis/stable-diffusion-license)
-* [https://github.com/lllyasviel/ControlNet/blob/main/LICENSE](https://github.com/lllyasviel/ControlNet/blob/main/LICENSE)
-
-### Enable additional models (checkpoints, LoRA, VAE, etc.)
-
-Enable the models you want to use on the bottom of the [`on_start.sh`](./on_start.sh) file. This is also the place to add any additional models you may want to install when starting your space.
-
-```bash
-## Checkpoint · Example:
-download-model --checkpoint "FILENAME" "URL"
-
-## LORA (low-rank adaptation) · Example:
-download-model --lora "FILENAME" "URL"
-
-## VAE (variational autoencoder) · Example:
-download-model --vae "FILENAME" "URL"
-```
-
-#### Some examples of additional (optional) models
-
-Some models such as additional checkpoints, VAE, LoRA, etc. may already be present in the [`on_start.sh`](./on_start.sh) file. You can enable them by removing the `#` in front of their respective line or disable them by removing the line or adding a leading `#` before `download-model`.
-
-* [Checkpoint · Dreamlike Diffusion 1.0](https://huggingface.co/dreamlike-art/dreamlike-diffusion-1.0) ([license](https://huggingface.co/dreamlike-art/dreamlike-diffusion-1.0/blob/main/LICENSE.md))
-* [Checkpoint · Dreamshaper 3.31](https://huggingface.co/Lykon/DreamShaper)
-* [Checkpoint · The Ally's Mix III: Revolutions](https://civitai.com/models/10752/the-allys-mix-iii-revolutions)
-* [Checkpoint · Deliberate v2](https://civitai.com/models/4823/deliberate)
-* [Checkpoint · dalcefo_painting](https://civitai.com/models/5396/dalcefopainting)
-* [Checkpoint · RPG v4](https://huggingface.co/Anashel/rpg)
-* [Checkpoint · A to Zovya RPG Artist's Tools (1.5 & 2.1)](https://civitai.com/models/8124/a-to-zovya-rpg-artists-tools-15-and-21)
-* [LoRA · epi_noiseoffset v2](https://civitai.com/models/13941/epinoiseoffset)
-* [VAE · sd-vae-ft-mse-original](https://huggingface.co/stabilityai/sd-vae-ft-mse-original)
-* [Embedding · bad_prompt_version2](https://huggingface.co/datasets/Nerfgun3/bad_prompt)
-* See [https://huggingface.co/models?filter=stable-diffusion](https://huggingface.co/models?filter=stable-diffusion) and [https://civitai.com/](https://civitai.com/) for more.
-
-Visit the individual model pages for more information on the models and their licenses.
-
-### Extensions
-
-* [GitHub ➔ deforum-art/deforum-for-automatic1111-webui](https://github.com/deforum-art/deforum-for-automatic1111-webui)
-* [GitHub ➔ yfszzx/stable-diffusion-webui-images-browser](https://github.com/yfszzx/stable-diffusion-webui-images-browser)
-* [GitHub ➔ Vetchems/sd-civitai-browser](https://github.com/Vetchems/sd-civitai-browser)
-* [GitHub ➔ kohya-ss/sd-webui-additional-networks](https://github.com/kohya-ss/sd-webui-additional-networks)
-* [GitHub ➔ Mikubill/sd-webui-controlnet](https://github.com/Mikubill/sd-webui-controlnet)
-
-### Additional acknowledgements
-
-A lot of inspiration for this Docker build comes from [GitHub ➔ camenduru](https://github.com/camenduru). Amazing things! 🙏
diff --git a/spaces/banana-projects/web3d/node_modules/three/examples/js/nodes/effects/ColorAdjustmentNode.js b/spaces/banana-projects/web3d/node_modules/three/examples/js/nodes/effects/ColorAdjustmentNode.js
deleted file mode 100644
index 0208192de99e1b44f8ad16fa263807a6a24b0f25..0000000000000000000000000000000000000000
--- a/spaces/banana-projects/web3d/node_modules/three/examples/js/nodes/effects/ColorAdjustmentNode.js
+++ /dev/null
@@ -1,136 +0,0 @@
-/**
- * @author sunag / http://www.sunag.com.br/
- */
-
-import { TempNode } from '../core/TempNode.js';
-import { FunctionNode } from '../core/FunctionNode.js';
-import { LuminanceNode } from './LuminanceNode.js';
-
-function ColorAdjustmentNode( rgb, adjustment, method ) {
-
- TempNode.call( this, 'v3' );
-
- this.rgb = rgb;
- this.adjustment = adjustment;
-
- this.method = method || ColorAdjustmentNode.SATURATION;
-
-}
-
-ColorAdjustmentNode.Nodes = ( function () {
-
- var hue = new FunctionNode( [
- "vec3 hue(vec3 rgb, float adjustment) {",
-
- " const mat3 RGBtoYIQ = mat3(0.299, 0.587, 0.114, 0.595716, -0.274453, -0.321263, 0.211456, -0.522591, 0.311135);",
- " const mat3 YIQtoRGB = mat3(1.0, 0.9563, 0.6210, 1.0, -0.2721, -0.6474, 1.0, -1.107, 1.7046);",
-
- " vec3 yiq = RGBtoYIQ * rgb;",
-
- " float hue = atan(yiq.z, yiq.y) + adjustment;",
- " float chroma = sqrt(yiq.z * yiq.z + yiq.y * yiq.y);",
-
- " return YIQtoRGB * vec3(yiq.x, chroma * cos(hue), chroma * sin(hue));",
-
- "}"
- ].join( "\n" ) );
-
- var saturation = new FunctionNode( [
- // Algorithm from Chapter 16 of OpenGL Shading Language
- "vec3 saturation(vec3 rgb, float adjustment) {",
-
- " vec3 intensity = vec3( luminance( rgb ) );",
-
- " return mix( intensity, rgb, adjustment );",
-
- "}"
- ].join( "\n" ), [ LuminanceNode.Nodes.luminance ] ); // include LuminanceNode function
-
- var vibrance = new FunctionNode( [
- // Shader by Evan Wallace adapted by @lo-th
- "vec3 vibrance(vec3 rgb, float adjustment) {",
-
- " float average = (rgb.r + rgb.g + rgb.b) / 3.0;",
-
- " float mx = max(rgb.r, max(rgb.g, rgb.b));",
- " float amt = (mx - average) * (-3.0 * adjustment);",
-
- " return mix(rgb.rgb, vec3(mx), amt);",
-
- "}"
- ].join( "\n" ) );
-
- return {
- hue: hue,
- saturation: saturation,
- vibrance: vibrance
- };
-
-} )();
-
-ColorAdjustmentNode.SATURATION = 'saturation';
-ColorAdjustmentNode.HUE = 'hue';
-ColorAdjustmentNode.VIBRANCE = 'vibrance';
-ColorAdjustmentNode.BRIGHTNESS = 'brightness';
-ColorAdjustmentNode.CONTRAST = 'contrast';
-
-ColorAdjustmentNode.prototype = Object.create( TempNode.prototype );
-ColorAdjustmentNode.prototype.constructor = ColorAdjustmentNode;
-ColorAdjustmentNode.prototype.nodeType = "ColorAdjustment";
-
-ColorAdjustmentNode.prototype.generate = function ( builder, output ) {
-
- var rgb = this.rgb.build( builder, 'v3' ),
- adjustment = this.adjustment.build( builder, 'f' );
-
- switch ( this.method ) {
-
- case ColorAdjustmentNode.BRIGHTNESS:
-
- return builder.format( '( ' + rgb + ' + ' + adjustment + ' )', this.getType( builder ), output );
-
- break;
-
- case ColorAdjustmentNode.CONTRAST:
-
- return builder.format( '( ' + rgb + ' * ' + adjustment + ' )', this.getType( builder ), output );
-
- break;
-
- }
-
- var method = builder.include( ColorAdjustmentNode.Nodes[ this.method ] );
-
- return builder.format( method + '( ' + rgb + ', ' + adjustment + ' )', this.getType( builder ), output );
-
-};
-
-ColorAdjustmentNode.prototype.copy = function ( source ) {
-
- TempNode.prototype.copy.call( this, source );
-
- this.rgb = source.rgb;
- this.adjustment = source.adjustment;
- this.method = source.method;
-
-};
-
-ColorAdjustmentNode.prototype.toJSON = function ( meta ) {
-
- var data = this.getJSONNode( meta );
-
- if ( ! data ) {
-
- data = this.createJSONNode( meta );
-
- data.rgb = this.rgb.toJSON( meta ).uuid;
- data.adjustment = this.adjustment.toJSON( meta ).uuid;
- data.method = this.method;
-
- }
-
- return data;
-
-};
-
-export { ColorAdjustmentNode };
diff --git a/spaces/banana-projects/web3d/node_modules/three/src/helpers/CameraHelper.js b/spaces/banana-projects/web3d/node_modules/three/src/helpers/CameraHelper.js
deleted file mode 100644
index cdb05f0ed41b8703516c07f2398edba19fc51d98..0000000000000000000000000000000000000000
--- a/spaces/banana-projects/web3d/node_modules/three/src/helpers/CameraHelper.js
+++ /dev/null
@@ -1,210 +0,0 @@
-/**
- * @author alteredq / http://alteredqualia.com/
- * @author Mugen87 / https://github.com/Mugen87
- *
- * - shows frustum, line of sight and up of the camera
- * - suitable for fast updates
- * - based on frustum visualization in lightgl.js shadowmap example
- * http://evanw.github.com/lightgl.js/tests/shadowmap.html
- */
-
-import { Camera } from '../cameras/Camera.js';
-import { Vector3 } from '../math/Vector3.js';
-import { LineSegments } from '../objects/LineSegments.js';
-import { Color } from '../math/Color.js';
-import { FaceColors } from '../constants.js';
-import { LineBasicMaterial } from '../materials/LineBasicMaterial.js';
-import { BufferGeometry } from '../core/BufferGeometry.js';
-import { Float32BufferAttribute } from '../core/BufferAttribute.js';
-
-function CameraHelper( camera ) {
-
- var geometry = new BufferGeometry();
- var material = new LineBasicMaterial( { color: 0xffffff, vertexColors: FaceColors } );
-
- var vertices = [];
- var colors = [];
-
- var pointMap = {};
-
- // colors
-
- var colorFrustum = new Color( 0xffaa00 );
- var colorCone = new Color( 0xff0000 );
- var colorUp = new Color( 0x00aaff );
- var colorTarget = new Color( 0xffffff );
- var colorCross = new Color( 0x333333 );
-
- // near
-
- addLine( 'n1', 'n2', colorFrustum );
- addLine( 'n2', 'n4', colorFrustum );
- addLine( 'n4', 'n3', colorFrustum );
- addLine( 'n3', 'n1', colorFrustum );
-
- // far
-
- addLine( 'f1', 'f2', colorFrustum );
- addLine( 'f2', 'f4', colorFrustum );
- addLine( 'f4', 'f3', colorFrustum );
- addLine( 'f3', 'f1', colorFrustum );
-
- // sides
-
- addLine( 'n1', 'f1', colorFrustum );
- addLine( 'n2', 'f2', colorFrustum );
- addLine( 'n3', 'f3', colorFrustum );
- addLine( 'n4', 'f4', colorFrustum );
-
- // cone
-
- addLine( 'p', 'n1', colorCone );
- addLine( 'p', 'n2', colorCone );
- addLine( 'p', 'n3', colorCone );
- addLine( 'p', 'n4', colorCone );
-
- // up
-
- addLine( 'u1', 'u2', colorUp );
- addLine( 'u2', 'u3', colorUp );
- addLine( 'u3', 'u1', colorUp );
-
- // target
-
- addLine( 'c', 't', colorTarget );
- addLine( 'p', 'c', colorCross );
-
- // cross
-
- addLine( 'cn1', 'cn2', colorCross );
- addLine( 'cn3', 'cn4', colorCross );
-
- addLine( 'cf1', 'cf2', colorCross );
- addLine( 'cf3', 'cf4', colorCross );
-
- function addLine( a, b, color ) {
-
- addPoint( a, color );
- addPoint( b, color );
-
- }
-
- function addPoint( id, color ) {
-
- vertices.push( 0, 0, 0 );
- colors.push( color.r, color.g, color.b );
-
- if ( pointMap[ id ] === undefined ) {
-
- pointMap[ id ] = [];
-
- }
-
- pointMap[ id ].push( ( vertices.length / 3 ) - 1 );
-
- }
-
- geometry.addAttribute( 'position', new Float32BufferAttribute( vertices, 3 ) );
- geometry.addAttribute( 'color', new Float32BufferAttribute( colors, 3 ) );
-
- LineSegments.call( this, geometry, material );
-
- this.camera = camera;
- if ( this.camera.updateProjectionMatrix ) this.camera.updateProjectionMatrix();
-
- this.matrix = camera.matrixWorld;
- this.matrixAutoUpdate = false;
-
- this.pointMap = pointMap;
-
- this.update();
-
-}
-
-CameraHelper.prototype = Object.create( LineSegments.prototype );
-CameraHelper.prototype.constructor = CameraHelper;
-
-CameraHelper.prototype.update = function () {
-
- var geometry, pointMap;
-
- var vector = new Vector3();
- var camera = new Camera();
-
- function setPoint( point, x, y, z ) {
-
- vector.set( x, y, z ).unproject( camera );
-
- var points = pointMap[ point ];
-
- if ( points !== undefined ) {
-
- var position = geometry.getAttribute( 'position' );
-
- for ( var i = 0, l = points.length; i < l; i ++ ) {
-
- position.setXYZ( points[ i ], vector.x, vector.y, vector.z );
-
- }
-
- }
-
- }
-
- return function update() {
-
- geometry = this.geometry;
- pointMap = this.pointMap;
-
- var w = 1, h = 1;
-
- // we need just camera projection matrix inverse
- // world matrix must be identity
-
- camera.projectionMatrixInverse.copy( this.camera.projectionMatrixInverse );
-
- // center / target
-
- setPoint( 'c', 0, 0, - 1 );
- setPoint( 't', 0, 0, 1 );
-
- // near
-
- setPoint( 'n1', - w, - h, - 1 );
- setPoint( 'n2', w, - h, - 1 );
- setPoint( 'n3', - w, h, - 1 );
- setPoint( 'n4', w, h, - 1 );
-
- // far
-
- setPoint( 'f1', - w, - h, 1 );
- setPoint( 'f2', w, - h, 1 );
- setPoint( 'f3', - w, h, 1 );
- setPoint( 'f4', w, h, 1 );
-
- // up
-
- setPoint( 'u1', w * 0.7, h * 1.1, - 1 );
- setPoint( 'u2', - w * 0.7, h * 1.1, - 1 );
- setPoint( 'u3', 0, h * 2, - 1 );
-
- // cross
-
- setPoint( 'cf1', - w, 0, 1 );
- setPoint( 'cf2', w, 0, 1 );
- setPoint( 'cf3', 0, - h, 1 );
- setPoint( 'cf4', 0, h, 1 );
-
- setPoint( 'cn1', - w, 0, - 1 );
- setPoint( 'cn2', w, 0, - 1 );
- setPoint( 'cn3', 0, - h, - 1 );
- setPoint( 'cn4', 0, h, - 1 );
-
- geometry.getAttribute( 'position' ).needsUpdate = true;
-
- };
-
-}();
-
-
-export { CameraHelper };
diff --git a/spaces/banana-projects/web3d/node_modules/three/src/renderers/shaders/ShaderChunk/lights_fragment_end.glsl.js b/spaces/banana-projects/web3d/node_modules/three/src/renderers/shaders/ShaderChunk/lights_fragment_end.glsl.js
deleted file mode 100644
index 5405840e2d95837b9e90ff4632c04a515d9d0732..0000000000000000000000000000000000000000
--- a/spaces/banana-projects/web3d/node_modules/three/src/renderers/shaders/ShaderChunk/lights_fragment_end.glsl.js
+++ /dev/null
@@ -1,13 +0,0 @@
-export default /* glsl */`
-#if defined( RE_IndirectDiffuse )
-
- RE_IndirectDiffuse( irradiance, geometry, material, reflectedLight );
-
-#endif
-
-#if defined( RE_IndirectSpecular )
-
- RE_IndirectSpecular( radiance, irradiance, clearCoatRadiance, geometry, material, reflectedLight );
-
-#endif
-`;
diff --git a/spaces/banana-projects/web3d/node_modules/three/src/renderers/webgl/WebGLRenderStates.js b/spaces/banana-projects/web3d/node_modules/three/src/renderers/webgl/WebGLRenderStates.js
deleted file mode 100644
index b9a6af90b18cda2e02ece10872917b591d77ab49..0000000000000000000000000000000000000000
--- a/spaces/banana-projects/web3d/node_modules/three/src/renderers/webgl/WebGLRenderStates.js
+++ /dev/null
@@ -1,116 +0,0 @@
-/**
- * @author Mugen87 / https://github.com/Mugen87
- */
-
-import { WebGLLights } from './WebGLLights.js';
-
-function WebGLRenderState() {
-
- var lights = new WebGLLights();
-
- var lightsArray = [];
- var shadowsArray = [];
-
- function init() {
-
- lightsArray.length = 0;
- shadowsArray.length = 0;
-
- }
-
- function pushLight( light ) {
-
- lightsArray.push( light );
-
- }
-
- function pushShadow( shadowLight ) {
-
- shadowsArray.push( shadowLight );
-
- }
-
- function setupLights( camera ) {
-
- lights.setup( lightsArray, shadowsArray, camera );
-
- }
-
- var state = {
- lightsArray: lightsArray,
- shadowsArray: shadowsArray,
-
- lights: lights
- };
-
- return {
- init: init,
- state: state,
- setupLights: setupLights,
-
- pushLight: pushLight,
- pushShadow: pushShadow
- };
-
-}
-
-function WebGLRenderStates() {
-
- var renderStates = {};
-
- function onSceneDispose( event ) {
-
- var scene = event.target;
-
- scene.removeEventListener( 'dispose', onSceneDispose );
-
- delete renderStates[ scene.id ];
-
- }
-
- function get( scene, camera ) {
-
- var renderState;
-
- if ( renderStates[ scene.id ] === undefined ) {
-
- renderState = new WebGLRenderState();
- renderStates[ scene.id ] = {};
- renderStates[ scene.id ][ camera.id ] = renderState;
-
- scene.addEventListener( 'dispose', onSceneDispose );
-
- } else {
-
- if ( renderStates[ scene.id ][ camera.id ] === undefined ) {
-
- renderState = new WebGLRenderState();
- renderStates[ scene.id ][ camera.id ] = renderState;
-
- } else {
-
- renderState = renderStates[ scene.id ][ camera.id ];
-
- }
-
- }
-
- return renderState;
-
- }
-
- function dispose() {
-
- renderStates = {};
-
- }
-
- return {
- get: get,
- dispose: dispose
- };
-
-}
-
-
-export { WebGLRenderStates };
diff --git a/spaces/bhanu4110/Lungs_CT_Scan_Cancer/app.py b/spaces/bhanu4110/Lungs_CT_Scan_Cancer/app.py
deleted file mode 100644
index 13479b56e25d0a8f99c5aae30005d9e1aa641f1a..0000000000000000000000000000000000000000
--- a/spaces/bhanu4110/Lungs_CT_Scan_Cancer/app.py
+++ /dev/null
@@ -1,27 +0,0 @@
-import torch
-import flash
-from flash.image import ImageClassificationData,ImageClassifier
-import gradio as gr
-
-model = ImageClassifier.load_from_checkpoint("Lungs_CT_Scan_Classification_model.pt")
-
-def CTScann_Detector(Image):
-
- test_datamodule = ImageClassificationData.from_files(
- predict_files=[Image.name],
- batch_size=1,
- transform_kwargs={"image_size": (196,196)}
- )
- trainer = flash.Trainer()
- predictions = trainer.predict(model, datamodule=test_datamodule)
- dicty_renames={'squamous':'Squamous cell carcinoma','large':'Large cell carcinoma','adenocarcinoma':'Adeno carcinoma','normal':'Normal'}
- return dicty_renames[predictions[0][0]]
-
-iface = gr.Interface(fn=CTScann_Detector, inputs="file", outputs="text",title="Lung Cancer Detection Using CT_Scan Image",examples=[["image-1.png"],["image-2.png"],["image-3.png"],["image-4.png"]],article="""
-
This model can clssify 4 types of CT Scan images for Cancer Detection.
- 1. Normal
- 2. Large Cell carcinoma
- 3. Squamous Cell carcinome
- 4. Adeno Cell carcinoma
-
""")
-iface.launch(share=True)
\ No newline at end of file
diff --git a/spaces/bigcode/pii-public-demo/app.py b/spaces/bigcode/pii-public-demo/app.py
deleted file mode 100644
index a919dde86b5424645748e971d109a915e601e563..0000000000000000000000000000000000000000
--- a/spaces/bigcode/pii-public-demo/app.py
+++ /dev/null
@@ -1,53 +0,0 @@
-"""
-This code was inspired from https://huggingface.co/spaces/HugoLaurencon/examples_before_after_pii/
-and https://huggingface.co/spaces/SaulLu/diff-visualizer
-"""
-
-import streamlit as st
-from datasets import load_dataset
-import diff_viewer
-
-st.set_page_config(page_title="PII Visualization", layout="wide")
-st.title("PII Anonymization 🔐")
-
-st.markdown("This demo allows the visualization of personal information anonymization on some code files. \
- This is just an illustration of [BigCode's PII pipeline](https://github.com/bigcode-project/bigcode-dataset/tree/main/pii) results and the examples and secrets are **synthetic**.")
-
-@st.cache()
-def load_data(language="python"):
- # load dataset with modified files with: content, references and language columns
- dataset = load_dataset("data", split="train")
- return dataset
-
-
-def get_samples_tag(dataset, tag):
- # add column id to be able to retrieve the sample
- tmp = dataset.add_column("index", range(len(dataset)))
- samples = tmp.filter(lambda x: "PI:" + tag.upper() in x['references'])
- return samples["index"]
-
-
-col1, col2 = st.columns([2, 4])
-with col1:
- #TODO add examples in more languages
- lang = st.selectbox("Select a programming language", ["Python"])
-
-samples = load_data(language=lang.lower())
-max_docs = len(samples)
-
-with col1:
- index_example = st.number_input(f"Choose an example from the existing {max_docs}:", min_value=0, max_value=max_docs-1, value=0, step=1)
-
-
-st.markdown("Below we highlight the difference in code before and after the PII on the chosen synthetic example:")
-
-example = samples[index_example]
-delimiter = f"PI:"
-count = example["references"].count(delimiter)
-
-col1, col2, col3 = st.columns([0.4, 1, 1])
-with col2:
- st.subheader(f"Code before PII redaction")
-with col3:
- st.subheader(f"Code after PII redaction")
-diff_viewer.diff_viewer(old_text=example["content"], new_text=example["new_content"], lang="none")
\ No newline at end of file
diff --git a/spaces/bioriAsaeru/text-to-voice/8Dio Greek Percussion KONTAKT [BETTER] Explore the Ancient Sounds of Greece with 5 Mic Positions and Chaos FX.md b/spaces/bioriAsaeru/text-to-voice/8Dio Greek Percussion KONTAKT [BETTER] Explore the Ancient Sounds of Greece with 5 Mic Positions and Chaos FX.md
deleted file mode 100644
index 59ca97422b3c5e5b9b4514a19d54f4af22009961..0000000000000000000000000000000000000000
--- a/spaces/bioriAsaeru/text-to-voice/8Dio Greek Percussion KONTAKT [BETTER] Explore the Ancient Sounds of Greece with 5 Mic Positions and Chaos FX.md
+++ /dev/null
@@ -1,6 +0,0 @@
-
-
These tracks was ONLY composed with samples from 8Dio Greek Percussion. A virtual music software instrument (VST/AU/AAX) by www.8dio.com -percussion-for-kontakt-vst-au-aax-samples/Follow us on Facebook: www.facebook.com/8dio.productionsFollow us on Twitter: twitter.com/8dio Follow us on YouTube: www.youtube.com/user/8dioproductionsFollow us on Instagram: instagram.com/8dio_curators_of_sound/Follow us on Snapchat: cr8dio
-
This track was primarily composed with samples from 8Dio Greek Percussion. A virtual music software instrument (VST/AU/AAX) by www.8dio.com -percussion-for-kontakt-vst-au-aax-samples/Follow us on Facebook: www.facebook.com/8dio.productionsFollow us on Twitter: twitter.com/8dio Follow us on YouTube: www.youtube.com/user/8dioproductionsFollow us on Instagram: instagram.com/8dio_curators_of_sound/Follow us on Snapchat: cr8dio
aaccfb2cb3
-
-
\ No newline at end of file
diff --git a/spaces/bioriAsaeru/text-to-voice/Civil 3D 2012 64 Bit Xforce Keygen.md b/spaces/bioriAsaeru/text-to-voice/Civil 3D 2012 64 Bit Xforce Keygen.md
deleted file mode 100644
index 6deece6cbc3d5d43098f87aaf42810b9f3b4282a..0000000000000000000000000000000000000000
--- a/spaces/bioriAsaeru/text-to-voice/Civil 3D 2012 64 Bit Xforce Keygen.md
+++ /dev/null
@@ -1,35 +0,0 @@
-
-
How to Activate Autodesk Civil 3D 2012 with X-Force Keygen
-
Autodesk Civil 3D 2012 is a powerful software for civil engineering design and documentation. It allows you to create, edit, and analyze 3D models of roads, bridges, tunnels, drainage systems, and more. However, to use this software, you need to activate it with a valid product key and activation code.
One way to activate Autodesk Civil 3D 2012 is to use X-Force Keygen, a program that generates serial numbers and activation codes for various Autodesk products. In this article, we will show you how to use X-Force Keygen to activate Autodesk Civil 3D 2012 in a few simple steps.
-
Step 1: Download and Install Autodesk Civil 3D 2012
-
Before you can use X-Force Keygen, you need to download and install Autodesk Civil 3D 2012 on your computer. You can download the software from the official Autodesk website or from other sources. Make sure you choose the correct version for your operating system (32-bit or 64-bit).
-
After downloading the software, run the installer and follow the instructions on the screen. You will be asked to enter a serial number and a product key during the installation. You can use any of the following serial numbers and product keys for Autodesk Civil 3D 2012:
-
-
Serial number: 666-69696969
-
Product key: 237D1
-
-
Alternatively, you can use any other serial number and product key that are compatible with Autodesk Civil 3D 2012. You can find a list of them on this website[^2^].
-
After entering the serial number and product key, complete the installation and restart your computer.
-
-
Step 2: Download and Run X-Force Keygen
-
Next, you need to download and run X-Force Keygen, the program that will generate an activation code for Autodesk Civil 3D 2012. You can download X-Force Keygen from this website[^1^]. Make sure you download the correct version for your operating system (32-bit or 64-bit).
-
After downloading X-Force Keygen, extract it from the zip file and run it as administrator. You will see a window like this:
-
-
In the window, select "Autodesk Civil 3D 2012" from the drop-down menu and click on "Patch". You should see a message saying "Successfully patched".
-
Step 3: Generate an Activation Code
-
Now, you need to generate an activation code for Autodesk Civil 3D 2012 using X-Force Keygen. To do this, follow these steps:
-
-
Launch Autodesk Civil 3D 2012 on your computer.
-
Click on "Activate" when prompted.
-
If you see a message saying that your serial number is wrong, click on "Close" and click on "Activate" again.
-
Select "I have an activation code from Autodesk".
-
Go back to X-Force Keygen and copy the request code from Autodesk Civil 3D 2012.
-
Paste the request code into X-Force Keygen and click on "Generate".
-
Copy the activation code from X-Force Keygen.
-
Paste the activation code into Autodesk Civil 3D 2012 and click on "Next".
-
-
You should see a message saying that your product has
d5da3c52bf
-
-
\ No newline at end of file
diff --git a/spaces/bioriAsaeru/text-to-voice/Data Explorer Manager V6.5 Download 2 !!HOT!! What You Can Do with This Software That You Cant Do with Others.md b/spaces/bioriAsaeru/text-to-voice/Data Explorer Manager V6.5 Download 2 !!HOT!! What You Can Do with This Software That You Cant Do with Others.md
deleted file mode 100644
index 0a774b36ae9c91c72f29bbbbafa27a2aad6962af..0000000000000000000000000000000000000000
--- a/spaces/bioriAsaeru/text-to-voice/Data Explorer Manager V6.5 Download 2 !!HOT!! What You Can Do with This Software That You Cant Do with Others.md
+++ /dev/null
@@ -1,6 +0,0 @@
-
-
To download our subtitles, install Chrome extension; click on
1. "Add to Chrome" 2. "Add Extension"
If you install our extension you will remove all ads and waiting time on this website
Thank you !
-
To download our subtitles, install Firefox add-on; click on
1. "Add to Firefox" 2. "Add"
If you install our extension you will remove all ads and waiting time on this website
the mighty and vengeful darth vader is back. his underlings are working to find the remnants of the infamous empire and what secrets and information they hold. but they have no idea what they're up against. the legendary sith lord is up to something. he's on a mission to find the last of the force-sensitive children. for the first time ever, you can play as a sith lord. immerse yourself in the dark side of the force and experience the thrill of wielding the power of the dark side. its an epic quest! become the most powerful sith lord ever! will your dirty dreams come true?
developer/publisher: star wars: the force unleashed 2 released: 2008 genre: action, adventure, fantasy system: pc (windows 2000/xp/vista/7) gameplay type: role-playing, fighting/action players: single-player language: english size: 1.74 gb voice actor: kevin macleod, natalie macmaster original language: english extras: custom game, four-player co-op, jedi trials, force powers for the chosen one, home
paid dlc: none unpaid dlc: the force unleashed (playable characters)
trailer:
-
if you have a 3 years version of the game (like me) and if you have a windows computer, the installation of the patch will happen automatically with a reboot. if you have a 3 years version and if you have a mac computer, you will need to get some files. the ones you need are: a.zip archive containing a macos application bundle (file type:.app) and an.pkg archive for a macos package. there are two different archives for the game depending on which version you have. if you have the 3 years version, you will need the packages for the base game monster girl quest! and the deluxe monster girl quest! paradox rpg . if you have the 4, 5 or 6 years version, you will need the packages for the base game monster girl quest! and the 5 episodes. the pkg archive is a.pkg file (macos package file).
-
- aaccfb2cb3
-
-
-
diff --git a/spaces/cihyFjudo/fairness-paper-search/Kon Boot 2.2 A Software Utility that Allows You to Login into Any System without Authentication.md b/spaces/cihyFjudo/fairness-paper-search/Kon Boot 2.2 A Software Utility that Allows You to Login into Any System without Authentication.md
deleted file mode 100644
index e84f56034b5d93ccb4fe215077c2451a02ddd4ce..0000000000000000000000000000000000000000
--- a/spaces/cihyFjudo/fairness-paper-search/Kon Boot 2.2 A Software Utility that Allows You to Login into Any System without Authentication.md
+++ /dev/null
@@ -1,35 +0,0 @@
-
-
Kon-Boot is one of the best tools around which can log you into Windows without knowing the password. It works by hooking into the system BIOS and temporarily changing the contents of the Windows kernel while booting. It then allows you to enter anything as the password during login. The next time you start the computer without Kon-Boot, the original password will be back, the temporary changes will be discarded and the system will behave as if nothing has happened.
After you Download Kon-Boot Free and write it onto CD or USB, simply boot your computer to that device (you will need to set the boot device in the BIOS) and a white screen will popup. Press any key and a black screen will popup showing the process of hooking BIOS functions (the version number 1.0 appears to be an oversight by the developer). After a few more seconds the computer will start to boot normally.
-
I downloaded KON-BOOT CD-konboot-v1.1-2in1.iso and went into BIOS selected kon-boot driver, no white screen pops up just a black screen and cannot push any buttons for it to run but can return to BIOS. Help!?
-
If you have purchased Kon-Boot for macOS/OSX this is the right place for you. If you have purchased kon-boot 2in1 version and you are looking for the installation tutorial please see the Kon-Boot for Windows GUIDE (please note that as stated on our website kon-boot 2in1 can be installed only on Windows).
-
Kon-Boot (aka kon boot, konboot) for Apple Mac OSX systems allows the user to login into the system without knowing the previous passwords and user names. Kon-Boot will either allow you to login into selected account without knowing the password (bypass mode) or it will create new "root" account for you (new-account mode) from which you will be able to change other users passwords as needed. Have you lost your password? Kon-Boot can help!
-
-
Please note that CD version is no longer available since kon-boot v1.7 (the old one (1.6) is still included in the archive). Internet connection is required for the installed to work. Kon-boot can be only installed using the original installer. One kon-boot license permits the user to install kon-boot on only one USB device (USB pendrive).
-
Bypass mode allows you to login into any selected account without knowing the password. All changes are only made to virtual memory meaning all changes caused by kon-boot are gone after computer reboots.
-
Insert the password reset disk in locked Dell laptop or PC and boot from it. Usually, you need to bring up the boot menu at startup by pressing F12 key so you can boot the devcie from external drive.
-
When the disk was booted, Windows Password Recovery software should appear on the main window. In there, you can select the OS and user account. Choose the user account and reset the password accordingly. Finally, take out the disk and reboot the computer. No password will be required for login in next time.
-
Step 4: Now, wait for the Ophcrack to load from the bootable media. Within a few minutes, you will able to see Ophcrack Windows. And, after that, the software will start cracking your password. Password cracking process will take a lot of time according to the length of the password.
-
So these are the five methods to reset a laptop password. If you are using a Microsoft account. Thenyou can use the first method. But, If you don't have access to the connected email Account. Then, the only way to reset Windows 10 online authentication is Androidphonesoft. If you're using Windows 7, then Kon-boot free version and Ophcrack is the best choice. And, if data isn't important to you. Then, you can use the Dell recovery manager. The last option is factory reset the device or reinstall Windows OS.
-
YUMI (Your USB Multiboot Installer) is a Multiboot USB Boot Creator that can be used to make a Multisystem flash drive. This tool can quickly create a Multiboot bootable USB flash drive containing several different ISO files. Use it to boot from USB your favorite Live Linux portable Operating Systems, Linux and Windows Installers, antivirus utilities, disc cloning, backup, penetration testing, diagnostic tools, and much more. This Universal tool makes it easy for anyone to create their own customized multi purpose Bootable USB.
-
The YUMI App has been considered by many to be the Best Bootable USB Creator. It replaces our old Multiboot ISOS tool and is also the successor to the singular Universal USB Installer (UUI). Tools that were amongst the first ever made for the purpose of creating a bootable flash drive. For the most part, files are generally stored within the Multiboot folder. This makes for a nicely organized Portable Multiboot Drive that can still be used for traditional storage purposes.
-
NOTE: I know you are probably asking, How can you boot from exFAT USB? A YUMI exFAT USB Boot variant is now available and recommended. It can be used to automatically create an exFAT boot USB. Here are the key differences between the variants:
-
You can use this version if your computer supports BIOS booting, and if you do not plan to run your Windows installers in UEFI mode. Most modern motherboards still have Legacy BIOS firmware support though CMS Legacy mode.
-
The YUMI UEFI variant utilizes GRUB2 for both BIOS and UEFI booting. It is important to note that the UEFI version is not backwards compatible with the legacy variant. In addition, your drive must be Fat32 formatted to support booting in UEFI mode. This boot creation software does include the fat32format utility to help you format drives larger than 32GB as Fat32.
-
YUMI (Your Universal Multiboot Installer) enables each user to create their own custom Multiboot UFD containing only the distributions they want. Presented in the order by which they are installed. A new distribution can also be added to the bootable device each time the tool is run.
-
Other Notes: If MultibootISOs was previously used, you must reformat the drive, and start over. The Legacy variant uses Syslinux directly, and chain loads to grub only if necessary, so it is not compatible with the older Multiboot ISO tool.
-
-wimboot option stores the extracted Multi Windows Installers in their own directory. -bootmgr option moves the bootmgr and bcd files to root of drive. (note: -bootmgr option does require a Windows Vista or later host to run bcdedit).
-
The Legacy variant does not natively include files to make it UEFI Boot from USB. However, it is still possible to boot and run your Windows Installers from UEFI. To switch between added Windows versions, navigate to the multiboot/win-directory (replacing win-directory with the Windows version you want to boot) on your USB. Once there, move the bootmgr, bootmgr.efi, and entire boot folder to the root of your USB drive. Then reboot, booting your computer from the UEFI compatible USB. If all went well, it should boot straight into your chosen Windows Installer.
-
Most added distributions are stored within the multiboot folder. This is also the root directory set for syslinux. In some cases, the Volume Label of your USB drive must be MULTIBOOT in order for OpenSUSE, CentOS and several other distributions to boot. YUMI will attempt to automatically create this Volume Label, however it can sometimes fail. So please ensure that the Volume Label of your USB remains MULTIBOOT if you expect your distributions to boot.
-
Legacy only: From the multiboot folder on your flash drive, delete the hidden file ldlinux.sys and then rename the libcom32.c32 file to _libcom32.c32. Then use YUMI to install any menu item. The installer will notice that the file is missing and will then attempt to reinstall syslinux and repair the master boot record. Once finished, rename _libcom32.c32 back to libcom32.c32.
-
* The Legacy variant does support NTFS, however not all distributions will boot from an NTFS formatted device. Though Windows to Go and distributions containing files over 4GB require using NTFS with the Legacy variant.
-
Persistently Saving Changes: YUMI uses the casper-rw persistence feature for some (but not all) Ubuntu based distributions. Yes, you can also have multiple persistent distributions, as each distro utilizes its own casper-rw file. * Persistence will not always work on NTFS formatted USB drives. Additionally, some distributions will not boot via NTFS.
-
On AM65x DMSC_Cortex_M3 is the boot master and is the first core that wakes up and starts the R5F ROM. Upon launching the target configuration, connect to DMSC_Cortex_M3 first, as this will automatically perform the PSC and PLL initialization. The following GEL output will appear in the CCS Console:
-
To load the SYSFW firmware, the DMSC ROM expects R5F secondary bootloader/application to provide board configuration message to initialize the cores and SOC services. The R5F application provided in SciClient uses a default board configuration message to the SYSFW and sets up the device for application debugging.
-
In emulation setup, the GEL file will keep the PMIC on after you connectto the A15 core on the SoC. While booting from ROM bootloader userapplication software, would need to keep the PMIC ON while initializingthe board.
-
Step2: AM572x EVM doesn`t have any boot switches to configure foremulation mode. so configure the boot switches to SD Boot Mode. DontPopulate the uSD card when the intent is to connect and load code overemulator and not to boot the device using uSD card.
-
When you boot an image from the SD card, the secondary boot loader willconfigure the device clocks, DDR and wake up the slave cores on theAM572x processor on GP EVM hence you don`t need the GEL initializationscripts to redo the clock and DDR settings.
-
Step2: Connect IDK EVM as described in the Quick StartGuide. Populatingthe uSD card is not required as the intent is to connect and load codeover emulator and not to boot the device using uSD card. AM572x IDKdoesn`t have any boot switches to configure for emulation mode.
aaccfb2cb3
-
-
\ No newline at end of file
diff --git a/spaces/cihyFjudo/fairness-paper-search/Michael Jackson Bad Mastered For ITunes ITunes Plus AAC M4A Why This is the Best Version of MJs Iconic Album.md b/spaces/cihyFjudo/fairness-paper-search/Michael Jackson Bad Mastered For ITunes ITunes Plus AAC M4A Why This is the Best Version of MJs Iconic Album.md
deleted file mode 100644
index 0454d04a0e3f0ac7428a63c8a288c8b40fb96a91..0000000000000000000000000000000000000000
--- a/spaces/cihyFjudo/fairness-paper-search/Michael Jackson Bad Mastered For ITunes ITunes Plus AAC M4A Why This is the Best Version of MJs Iconic Album.md
+++ /dev/null
@@ -1,6 +0,0 @@
-
Michael Jackson Bad Mastered For ITunes ITunes Plus AAC M4A
-
- aaccfb2cb3
-
-
-
diff --git a/spaces/cihyFjudo/fairness-paper-search/Tower 3d Pro Mods Explore the World of Air Traffic Control with Realistic Scenarios.md b/spaces/cihyFjudo/fairness-paper-search/Tower 3d Pro Mods Explore the World of Air Traffic Control with Realistic Scenarios.md
deleted file mode 100644
index e7e23a14d505852e32ff9d73146228f6d742f56d..0000000000000000000000000000000000000000
--- a/spaces/cihyFjudo/fairness-paper-search/Tower 3d Pro Mods Explore the World of Air Traffic Control with Realistic Scenarios.md
+++ /dev/null
@@ -1,11 +0,0 @@
-
-
To directly answer your question - no. @EliGrimcannot create new commands or phrases that the game doesn't already recognize. Although needed when we are handed off a plane 20 miles out, airspeed commands are not normally used by tower controllers and as such the developers did not include them. Hopefully, the next version will address this by either adding airspeed commands to our arsenal or making the tower's area of responsibility a little more realistic.
-
Tower!3D Pro is a successor of the best selling Tower! 2011 airport tower simulator. Your assignment is to guide aircraft of various sizes and capabilities to and from the active runway for landing and takeoff. As a tower controller you must assure that it safe for a plane to enter or cross a runway, assign taxiway routes, when to stop and start movement, and clear aircraft for take off. Tower!3d Pro provides you with flight strips, ground and air radar screens and a full 3D view of each airport. Tower!3D Pro is no arcade game. With a complex command structure, advanced AI and Speech Recognition technology Tower!3D Pro will allow you to experience of the thrill of being a real air traffic controller.
File Name: Bubble Tower 3D 1.0.0 APK + Mod (Free purchase) for Android Mod info: Free purchase File size: 17M To provide better download speed, we recommend dFast - fastest mod downloader to download this file. dFast is the fastest downloader for millions of free mods. You can enjoy 3x faster speed than normal downloads.
-
Your assignment is to guide aircraft of various sizes and capabilities to and from the active runway for landing and takeoff. As a tower controller you must assure that it safe for a plane to enter or cross a runway, assign taxiway routes, when to stop and start movement, and clear aircraft for take off. Tower!3d Pro provides you with flight strips, ground and air radar screens and a full 3D view of each airport.
-
When faced with digitally capturing large objects with multiple areas of high detail, even scanning pros can feel a sense of uneasiness on how to best proceed. This could be an auto customizer with just half an afternoon to scan the interior and exterior of a Lamborghini for designing custom, perfectly-fitting components and mods. Or an aerospace engineer assigned to scan an Airbus A380 cockpit and transform it into a VR-ready, submillimeter-accurate 3D model in mere days.
-
Because no special statutory scheme has been devised for radiotelephone communications, the Commission applies statutes not tailored to the new technology. Loperena, supra, 71 Cal.P.U.C. 645, was a case in which the Commission struggled to find a way to accommodate the physical and economic characteristics of radiotelephone service to the words of section 1001. Because it found the statute inadequate to provide uniform regulation of two-way radiotelephone expansion into one-way service, it applied the words in such a way as to remove completely such expansion from any regulation. In this case the Commission again adopted an interpretation that removes radiotelephone expansion from [22 Cal. 3d 579] regulation. But the reasons the Commission cited for its Loperena interpretation do not apply here. There, when mobile service expanded to include concentric paging service, additional construction would not always be necessary. Further, efficiency and economy would be furthered by having the same supplier offer both services, using the same equipment and personnel. In contrast, when a wireline service expands to include mobile service, construction is typical rather than atypical; and the same equipment and personnel are not necessarily used. Also, Loperena concerned a new service concentric with and entirely within the existing service area, while the mobile service area here is quite different in size and shape from the original service. The Commission's interpretation here appears to exempt from certification an indefinite amount of radiotelephone expansion by wireline companies. It permits uncertificated expansion into "such adjacent area as necessary to provide a rational contour" but does not indicate any limit to the size of a "rational contour." Complainants state that the radio contour area here is three times larger than General Telephone's wireline area. fn. 9 The ratio of a radio contour area to a wireline area apparently could depend on the shape and size of the wireline exchange and the location of the transmitting tower within it, as well as the shape and size of the contour itself (see fn. 8, ante). Without limits on "a rational contour" the Commission's definition seems to be not an interpretation but rather a contradiction of the words of section 1001, resulting in partial deregulation (cf. Pacific Tel. & Tel. Co. v. So. Pacific Communications Co. (1974) 78 Cal.P.U.C. 123, 126-128). fn. 10
Block Wood Puzzle APK: A Fun and Relaxing Game for Your Brain
-
Are you looking for a new game to play on your Android device that can keep you entertained and challenged at the same time? Do you enjoy solving puzzles and exercising your brain? If you answered yes to these questions, then you should check out Block Wood Puzzle APK, a fun and relaxing game that combines the best of sudoku and jigsaw puzzles. In this article, we will tell you everything you need to know about this game, including what it is, why you should play it, and how to download and install it on your device.
-
What is Block Wood Puzzle APK?
-
Block Wood Puzzle APK is a simple yet addictive wood block puzzle game that will test your IQ and logic skills. The game is inspired by the classic sudoku and jigsaw puzzles, but with a twist. Instead of numbers or pictures, you have to place different shapes of wood blocks on a 9x9 grid and fill rows, columns, or squares to clear them from the board. The game has no time limit or pressure, so you can play at your own pace and enjoy the relaxing sound of wood blocks falling into place.
The gameplay of Block Wood Puzzle APK is very easy to learn, but hard to master. You just have to drag the wood blocks from the bottom of the screen and drop them on the grid. You can rotate the blocks by tapping on them before placing them. You have to be careful not to run out of space on the grid, as the game will end if there are no more moves available. The game will also end if you quit or exit the app, so make sure you save your progress before doing so.
-
A combination of sudoku and jigsaw puzzles
-
Block Wood Puzzle APK is not just a simple wood block puzzle game, but also a combination of sudoku and jigsaw puzzles. The game has two modes: BlockPuz and SudoCube. In BlockPuz mode, you have to match the given patterns on the top of the screen by placing the wood blocks on the grid. In SudoCube mode, you have to fill the grid with wood blocks that follow the rules of sudoku, meaning that each row, column, and square must contain one of each shape without repeating. Both modes are challenging and fun, and will keep you hooked for hours.
-
A free and easy-to-play game for Android devices
-
One of the best things about Block Wood Puzzle APK is that it is completely free to download and play. You don't need any internet connection or registration to enjoy the game. You can also play it offline anytime and anywhere. The game is compatible with most Android devices, as long as they have Android 4.4 or higher. The game also has a small size of only 133 MB, so it won't take up much space on your device.
-
Why should you play Block Wood Puzzle APK?
-
There are many reasons why you should play Block Wood Puzzle APK, but here are some of the main ones:
-
It helps you train your brain and improve your logic skills
-
Playing Block Wood Puzzle APK is not only fun, but also beneficial for your brain
Playing Block Wood Puzzle APK is not only fun, but also beneficial for your brain. The game helps you train your brain and improve your logic skills by making you think strategically and creatively. The game also enhances your concentration, memory, and problem-solving abilities. By playing the game regularly, you can boost your mental performance and keep your brain sharp and healthy.
-
It offers various levels of difficulty and challenges
-
Block Wood Puzzle APK is not a boring game that you can easily finish in a few minutes. The game offers various levels of difficulty and challenges that will keep you engaged and motivated. The game has over 1000 levels in BlockPuz mode and over 500 levels in SudoCube mode, each with different patterns and rules. The game also has daily challenges that you can complete to earn rewards and bonuses. The game gets harder as you progress, so you will never run out of fun and excitement.
-
It has beautiful graphics and soothing sounds
-
Block Wood Puzzle APK is not only a brain-teasing game, but also a relaxing game that can help you relieve stress and anxiety. The game has beautiful graphics and soothing sounds that create a calm and cozy atmosphere. The game features realistic wood blocks with different colors and textures, as well as a simple and elegant background. The game also has relaxing music and sound effects that make you feel like you are playing with real wood blocks. The game is a perfect way to unwind and relax after a long day.
Block Wood Puzzle APK is a game that anyone can enjoy, regardless of their age or preference. The game is suitable for kids, adults, seniors, and anyone who loves puzzles and brain games. The game is also friendly for beginners, as it has a tutorial mode that teaches you how to play the game. The game also has a hint system that helps you when you are stuck. The game is also customizable, as you can change the theme, the sound, the language, and the difficulty level according to your liking.
-
How to download and install Block Wood Puzzle APK?
-
If you are interested in playing Block Wood Puzzle APK, you might be wondering how to download and install it on your device. Don't worry, it's very easy and simple. Just follow these steps:
-
Follow these simple steps to get the game on your device
-
Step 1: Go to the official website or a trusted APK source
-
The first thing you need to do is to go to the official website of Block Wood Puzzle APK or a trusted APK source that offers the latest version of the game. You can use your browser or a QR code scanner to access the website or the source.
-
Step 2: Download the APK file to your device
-
The next thing you need to do is to download the APK file of Block Wood Puzzle APK to your device. You can do this by clicking on the download button or the link on the website or the source. You might see a pop-up message asking for your permission to download the file. Just tap on OK or Allow to proceed.
-
Step 3: Enable unknown sources in your settings
-
The third thing you need to do is to enable unknown sources in your settings. This is necessary because Block Wood Puzzle APK is not available on Google Play Store, so you need to allow your device to install apps from other sources. You can do this by going to your settings, then security, then unknown sources, then toggle it on.
-
Step 4: Install the APK file and enjoy the game
-
The last thing you need to do is to install the APK file of Block Wood Puzzle APK on your device. You can do this by locating the file in your downloads folder or your notification bar, then tapping on it. You might see a pop-up message asking for your permission to install the app. Just tap on Install or Next to proceed. Once the installation is done, you can open the app and enjoy the game.
-
Conclusion
-
Block Wood Puzzle APK is a fun and relaxing game for your brain that you should try today. It is a simple yet addictive wood block puzzle game that combines the best of sudoku and jigsaw puzzles. It helps you train your brain and improve your logic skills, it offers various levels of difficulty and challenges, it has beautiful graphics and soothing sounds, and it is suitable for all ages and preferences. It is also free and easy-to-play on your Android device. All you need to do is to download and install it following these simple steps.
-
If you are looking for a new game to play on your Android device that can keep you entertained and challenged at the same time, look no
If you are looking for a new game to play on your Android device that can keep you entertained and challenged at the same time, look no further than Block Wood Puzzle APK. It is a fun and relaxing game for your brain that you will love. Download it now and see for yourself how addictive and enjoyable it is.
-
Here are some FAQs that you might have about the game:
-
FAQs
-
-
Q: How can I get more wood blocks in the game?
-
A: You can get more wood blocks by clearing rows, columns, or squares on the grid, or by using the refresh button at the bottom of the screen. You can also get more wood blocks by watching ads or buying them with real money.
-
Q: How can I get more coins and gems in the game?
-
A: You can get more coins and gems by completing levels, daily challenges, achievements, or quests in the game. You can also get more coins and gems by watching ads or buying them with real money.
-
Q: How can I use the coins and gems in the game?
-
A: You can use the coins and gems to buy more wood blocks, hints, themes, or music in the game. You can also use them to unlock more levels or modes in the game.
-
Q: How can I save my progress in the game?
-
A: You can save your progress in the game by signing in with your Google account or Facebook account. You can also save your progress by using the cloud button at the top of the screen.
-
Q: How can I contact the developer of the game?
-
A: You can contact the developer of the game by sending an email to blockwoodpuzzle@gmail.com or by visiting their website at https://blockwoodpuzzle.com/.
-
401be4b1e0
-
-
\ No newline at end of file
diff --git a/spaces/congsaPfin/Manga-OCR/logs/Cashier 3D Mod APK The Ultimate Hypermarket Game.md b/spaces/congsaPfin/Manga-OCR/logs/Cashier 3D Mod APK The Ultimate Hypermarket Game.md
deleted file mode 100644
index c7dbb7ce67f62621dca93c06ae06cdc68558e3e0..0000000000000000000000000000000000000000
--- a/spaces/congsaPfin/Manga-OCR/logs/Cashier 3D Mod APK The Ultimate Hypermarket Game.md
+++ /dev/null
@@ -1,116 +0,0 @@
-
-
Cashier 3D Mod APK: A Fun and Educational Game for Everyone
-
Do you want to run your own store and be a manager? Do you want to have fun and learn at the same time? If yes, then you should try Cashier 3D Mod APK, a game that combines supermarket simulation and cashier simulation in one. In this article, we will tell you everything you need to know about this game, including how to download and install it, what are its features, tips and tricks, reviews and alternatives, and more. Read on to find out more.
-
What is Cashier 3D Mod APK?
-
A supermarket simulation and cashier simulation game
-
Cashier 3D is a game that lets you run your own store and be a manager. You will enjoy buying and selling items as your store grows and customers come in. You will also have to manage your cash register correctly and count money quickly. You will get ready to be rich and upgrade your store. Just like idle supermarket games, your customers are in a race, running around the store looking for more. You will sell old items and buy new ones. You will unlock new 3D items, such as grocery, games for kids, fruit ranch, booze, phone charge, instruments for sale, selective clothes, and designer shoes. You will also serve VIP customers, stop robbers, and learn counting money and calculating change.
A modded version of the original game with unlimited money and no ads
-
Cashier 3D Mod APK is a modified version of the original game that gives you unlimited money and no ads. With this mod apk, you can buy anything you want without worrying about the cost. You can also enjoy the game without being interrupted by annoying ads. This way, you can have more fun and satisfaction with Cashier 3D.
-
How to Download and Install Cashier 3D Mod APK?
-
The steps to download and install the mod apk file
-
If you want to download and install Cashier 3D Mod APK, you need to follow these steps:
-
-
Go to a trusted website that provides the mod apk file for Cashier 3D, such as [lygiang.net](^1^).
-
Click on the download button and wait for the file to be downloaded.
-
Go to your device settings and enable unknown sources.
-
Locate the downloaded file in your file manager and tap on it.
-
Follow the instructions on the screen to install the game.
-
Launch the game and enjoy.
-
-
The permissions and requirements for the game
The permissions and requirements for the game
-
Before you download and install Cashier 3D Mod APK, you need to make sure that your device meets the following permissions and requirements:
-
-
Your device must have Android 4.4 or higher version.
-
Your device must have at least 100 MB of free storage space.
-
Your device must allow the installation of apps from unknown sources.
-
The game may ask for access to your photos, media, and files.
-
-
What are the Features of Cashier 3D Mod APK?
-
Manage your own store and be a manager
-
One of the main features of Cashier 3D Mod APK is that you can manage your own store and be a manager. You can decide what items to sell, how to arrange them, and how to price them. You can also buy new items and upgrade your store. You can make your store look more attractive and appealing to customers. You can also hire staff and assign them tasks. You can be the boss of your own store and enjoy the feeling of being successful.
-
Cashier 3D mod apk unlimited money download
-How to install cashier 3d mod apk on android
-Cashier 3D mod apk latest version free download
-Cashier 3D mod apk gameplay and features
-Cashier 3D mod apk hack cheats tips and tricks
-Cashier 3D mod apk offline mode no internet required
-Cashier 3D mod apk review and rating
-Cashier 3D mod apk for pc windows and mac
-Cashier 3D mod apk download link and file size
-Cashier 3D mod apk update and new content
-Cashier 3D mod apk best settings and graphics
-Cashier 3D mod apk fun and addictive casual game
-Cashier 3D mod apk challenges and levels
-Cashier 3D mod apk unlock all items and skins
-Cashier 3D mod apk no ads and in-app purchases
-Cashier 3D mod apk online multiplayer mode
-Cashier 3D mod apk simulation and management game
-Cashier 3D mod apk realistic and smooth controls
-Cashier 3D mod apk sound effects and music
-Cashier 3D mod apk bugs and errors fix
-Cashier 3D mod apk comparison with original version
-Cashier 3D mod apk alternatives and similar games
-Cashier 3D mod apk pros and cons
-Cashier 3D mod apk support and feedback
-Cashier 3D mod apk developer and publisher information
-
Count money quickly and correctly
-
Another feature of Cashier 3D Mod APK is that you can count money quickly and correctly. You will have to handle cash transactions with customers and give them the right change. You will have to use your math skills and speed to count money accurately. You will also have to deal with different currencies and denominations. You will have to be careful not to make mistakes or lose money. You will improve your counting skills and become a pro cashier.
-
Upgrade your store and unlock new items
-
A third feature of Cashier 3D Mod APK is that you can upgrade your store and unlock new items. You will earn money from selling items and serving customers. You can use the money to buy new items and upgrade your store. You can unlock new 3D items, such as grocery, games for kids, fruit ranch, booze, phone charge, instruments for sale, selective clothes, and designer shoes. You can also upgrade your cash register, scanner, conveyor belt, security system, and more. You can make your store bigger and better.
-
Serve VIP customers and earn more money
-
A fourth feature of Cashier 3D Mod APK is that you can serve VIP customers and earn more money. You will encounter VIP customers who will buy more items and pay more money. You will have to serve them well and give them the best service. You will also have to count their money faster and more accurately. You will earn more money from VIP customers and increase your income.
-
Stop robbers and protect your cash
-
A fifth feature of Cashier 3D Mod APK is that you can stop robbers and protect your cash. You will face robbers who will try to steal your money or items. You will have to be alert and vigilant. You will have to set off the alarm when a robber comes in. You will also have to catch the robber before he escapes. You will protect your cash and prevent losses.
-
Learn counting money and calculating change
-
A sixth feature of Cashier 3D Mod APK is that you can learn counting money and calculating change. You will learn how to count money in different currencies and denominations. You will also learn how to calculate change in different situations. You will improve your math skills and mental arithmetic. You will also learn about different cultures and countries through their currencies.
-
What are the Tips and Tricks for Cashier 3D Mod APK?
-
Tap don't swipe the money into the register
-
One tip for playing Cashier 3D Mod APK is to tap don't swipe the money into the register. Swiping the money may cause it to fly out of the register or get stuck on the edge. Tapping the money will make it go into the register faster and easier.
-
Check the numbers on the top of the till for change
-
Another tip for playing Cashier 3D Mod APK is to check the numbers on the top of the till for change. The numbers show how much change you need to give back to the customer. This will help you avoid making mistakes or giving too much or too little change.
-
Triple up the cash from VIPs by watching videos
-
A A third tip for playing Cashier 3D Mod APK is to triple up the cash from VIPs by watching videos. You can watch a short video after serving a VIP customer to multiply the money you earned by three. This will help you increase your income and buy more items and upgrades for your store.
-
Set off the alarm when a thief comes in
-
A fourth tip for playing Cashier 3D Mod APK is to set off the alarm when a thief comes in. You can tap on the red button on the bottom right of the screen to activate the alarm. This will alert the security guard and make the thief drop the money or item he stole. You can then catch the thief and get your money or item back.
-
Make some change by breaking up larger bills
-
A fifth tip for playing Cashier 3D Mod APK is to make some change by breaking up larger bills. You can tap on a larger bill in your register to split it into smaller bills. This will help you have enough change for your customers and avoid running out of money.
-
What are the Reviews and Alternatives for Cashier 3D Mod APK?
-
The positive and negative reviews from users
-
Cashier 3D Mod APK has received mixed reviews from users. Some users have praised the game for being fun, educational, realistic, and addictive. They have enjoyed running their own store, counting money, serving customers, and learning new things. They have also liked the mod apk for giving them unlimited money and no ads.
-
However, some users have complained about the game for being boring, repetitive, glitchy, and unrealistic. They have disliked the game for having too many ads, too few items, too easy levels, and too unrealistic scenarios. They have also encountered problems with the game crashing, freezing, lagging, or not working properly.
-
The similar games that you can try
-
If you are looking for similar games to Cashier 3D Mod APK, you can try these games:
-
-
-
Game
-
Description
-
-
-
[Supermarket Mania Journey]
-
A game that lets you run your own supermarket chain and serve customers.
-
-
-
[Cash Register Games]
-
A game that lets you play as a cashier and count money.
-
-
-
[Idle Supermarket Tycoon]
-
A game that lets you build your own supermarket empire and make money.
-
-
-
Conclusion
-
Cashier 3D Mod APK is a game that combines supermarket simulation and cashier simulation in one. You can manage your own store and be a manager, count money quickly and correctly, upgrade your store and unlock new items, serve VIP customers and earn more money, stop robbers and protect your cash, and learn counting money and calculating change. You can also enjoy unlimited money and no ads with this mod apk. You can download and install Cashier 3D Mod APK from a trusted website and follow the steps we provided. You can also use our tips and tricks to play better and have more fun. You can also check out the reviews and alternatives for this game if you want to know more or try something different.
-
FAQs
-
Q: Is Cashier 3D Mod APK safe to download and install?
-
A: Yes, Cashier 3D Mod APK is safe to download and install if you get it from a trusted website that provides the mod apk file without viruses or malware. However, you should always be careful when downloading and installing apps from unknown sources.
-
Q: How do I update Cashier 3D Mod APK?
-
A: To update Cashier 3D Mod APK, you need to download and install the latest version of the mod apk file from the same website that you got it from. You may need to uninstall the previous version of the game before installing the new one.
-
Q: Can I play Cashier 3D Mod APK offline?
-
A: Yes, you can play Cashier 3D Mod APK offline without an internet connection. However, some features of the game may require an internet connection, such as watching videos or accessing online content.
-
Q: Can I play Cashier 3D Mod APK on PC?
-
A: Yes, you can play Cashier 3D Mod APK on PC using an Android emulator or a software that allows you to run Android apps on your PC, such as [BlueStacks] or [NoxPlayer]. You need to download and install the emulator and the mod apk file on your PC and follow the same steps as you would on your mobile device.
-
Q: Can I play Cashier 3D Mod APK with friends?
-
A: No, Cashier 3D Mod APK is not a multiplayer game. You can only play it solo and compete with yourself. However, you can share your progress and achievements with your friends on social media or other platforms.
401be4b1e0
-
-
\ No newline at end of file
diff --git a/spaces/congsaPfin/Manga-OCR/logs/Download 3 Real Racing Mod APK for Free and Experience the Ultimate Racing Simulation.md b/spaces/congsaPfin/Manga-OCR/logs/Download 3 Real Racing Mod APK for Free and Experience the Ultimate Racing Simulation.md
deleted file mode 100644
index c896218b679d2af45df17cf89e4728b3f555b192..0000000000000000000000000000000000000000
--- a/spaces/congsaPfin/Manga-OCR/logs/Download 3 Real Racing Mod APK for Free and Experience the Ultimate Racing Simulation.md
+++ /dev/null
@@ -1,92 +0,0 @@
-
-
Download 3 Real Racing Mod Apk: A Guide for Racing Game Lovers
-
If you are a fan of racing games, you might have heard of 3 Real Racing, one of the most popular and realistic racing games on Android. This game offers you the chance to experience the thrill of driving some of the most expensive and fastest sports cars in the world on stunning tracks. However, if you want to enjoy the game to the fullest, you might need to spend some real money to unlock all the cars, upgrades, and features. That's why many players opt for downloading 3 Real Racing mod apk, a modified version of the game that gives you unlimited money, gold, and access to everything in the game. In this article, we will tell you everything you need to know about 3 Real Racing mod apk, including its features, benefits, and how to download and install it on your device.
3 Real Racing is a racing game developed by Electronic Arts (EA) for Android and iOS devices. It is the third installment in the Real Racing series, which is known for its realistic graphics, physics, and gameplay. The game features over 250 licensed cars from 33 manufacturers, such as Ferrari, Lamborghini, Porsche, Bugatti, and more. You can customize your cars with various paint jobs, vinyls, rims, and performance parts. You can also race on 19 real-world tracks from locations like Silverstone, Le Mans, Dubai Autodrome, and more.
-
Features of 3 Real Racing
-
Realistic graphics and physics
-
One of the main attractions of 3 Real Racing is its stunning graphics and physics. The game uses the Mint 3 Engine, which delivers high-quality visuals and realistic lighting effects. The cars look detailed and authentic, with accurate reflections, shadows, and damage effects. The tracks also look amazing, with dynamic weather conditions, day and night cycles, and different camera angles. The game also simulates realistic car physics, such as traction, braking, steering, and suspension. You can feel the difference between different car models and driving modes.
-
Over 250 cars and 19 tracks
-
Another feature that makes 3 Real Racing stand out is its huge variety of cars and tracks. The game offers you over 250 cars from 33 manufacturers, ranging from classic muscle cars to modern supercars. You can choose from different classes of cars, such as production, sports, GT, formula, endurance, and more. You can also customize your cars with various paint jobs, vinyls, rims, and performance parts. The game also features 19 real-world tracks from locations like Silverstone, Le Mans, Dubai Autodrome, and more. You can race on different types of tracks, such as circuits, ovals, drag strips, and street courses.
-
Multiplayer and social modes
-
The game also offers you various multiplayer and social modes to challenge yourself and other players. You can compete in online events against players from around the world or join a team to participate in team events. You can also race against your friends or rivals in real-time or asynchronous modes. The game also integrates with Facebook and Google Play Games to let you share your achievements and progress with your friends.
-
Why download 3 Real Racing mod apk?
-
While 3 Real Racing is a free-to-play game, it also has some limitations that might affect your enjoyment and performance. For example, you might need to spend real money to buy more money and gold, which are the main currencies in the game. You might also need to wait for long periods of time to repair or service your cars, or to unlock new cars and upgrades. Moreover, you might have to deal with annoying ads that pop up every now and then. That's why many players prefer to download 3 Real Racing mod apk, a modified version of the game that removes all these limitations and gives you unlimited access to everything in the game.
-
Benefits of mod apk
-
Unlimited money and gold
-
One of the main benefits of downloading 3 Real Racing mod apk is that you get unlimited money and gold in the game. Money and gold are the main currencies in the game, which you can use to buy new cars, upgrades, paint jobs, vinyls, rims, and more. However, earning money and gold in the game can be quite slow and tedious, especially if you want to buy some of the most expensive and rare cars in the game. You might also need to spend real money to buy more money and gold with in-app purchases. But with 3 Real Racing mod apk, you don't have to worry about that. You can get unlimited money and gold for free, and buy anything you want in the game without any restrictions.
-
download real racing 3 mod apk unlimited money and gold
-download real racing 3 mod apk latest version
-download real racing 3 mod apk android 1
-download real racing 3 mod apk offline
-download real racing 3 mod apk data
-download real racing 3 mod apk revdl
-download real racing 3 mod apk rexdl
-download real racing 3 mod apk hack
-download real racing 3 mod apk obb
-download real racing 3 mod apk an1
-download real racing 3 mod apk for pc
-download real racing 3 mod apk highly compressed
-download real racing 3 mod apk all cars unlocked
-download real racing 3 mod apk no root
-download real racing 3 mod apk free shopping
-download real racing 3 mod apk full unlocked
-download real racing 3 mod apk unlimited everything
-download real racing 3 mod apk andropalace
-download real racing 3 mod apk anti ban
-download real racing 3 mod apk all tracks unlocked
-download real racing 3 mod apk android oyun club
-download real racing 3 mod apk blackmod
-download real racing 3 mod apk by axey
-download real racing 3 mod apk by lenov.ru
-download real racing 3 mod apk by ihackedit
-download real racing 3 mod apk by apkpure
-download real racing 3 mod apk by happymod
-download real racing 3 mod apk by mob.org
-download real racing 3 mod apk by techylist
-download real racing 3 mod apk by apkmody
-download real racing 3 mod apk cheat menu
-download real racing 3 mod apk cracked
-download real racing 3 mod apk car unlocked
-download real racing 3 mod apk custom decals unlocked
-download real racing 3 mod apk china version
-download real racing 3 mod apk club vip unlocked
-download real racing 3 mod apk coins and gold generator
-download real racing 3 mod apk compressed zip file
-download real racing 3 mod apk direct link
-download real racing 3 mod apk data file host
-download real racing 3 mod apk data obb offline latest version android game zone.com.zip (1.2 gb)
-download real racing 3 mod apk easy drive enabled
-download real racing 3 mod apk elite cars unlocked
-download real racing 3 mod apk everything unlocked and unlimited money/gold/coins/keys/fuel/energy/anti-ban/no ads/no root/no damage/no repair costs/no waiting time/no license verification/no internet connection required/offline mode enabled/updated/newest/latest/best/most popular/most downloaded/most rated/most reviewed/most recommended/most searched/most wanted/most played/most enjoyed/most fun/most addictive/most exciting/most thrilling/most realistic/most amazing/most awesome/most fantastic/most incredible/most spectacular/most stunning/most beautiful/most gorgeous/most breathtaking/most mind-blowing/most jaw-dropping/most eye-catching/most astonishing/most astounding/most marvelous/most wonderful/most fabulous/most magnificent/most glorious/most splendid/most brilliant/most excellent/most outstanding/most superb/most exceptional/most extraordinary/most phenomenal/mast sensational/
-
Unlocked cars and upgrades
-
Another benefit of downloading 3 Real Racing mod apk is that you get all the cars and upgrades unlocked in the game. The game features over 250 cars from 33 manufacturers, but not all of them are available from the start. You need to unlock them by completing certain events, reaching certain levels, or spending money and gold. Some of the cars are also exclusive to certain events or modes, which means you might miss out on them if you don't participate in those events or modes. Moreover, you need to upgrade your cars with various performance parts to improve their speed, acceleration, handling, and durability. But with 3 Real Racing mod apk, you don't have to worry about that either. You can get all the cars and upgrades unlocked for free, and choose any car you want from the garage without any limitations.
-
No ads and no root required
-
A final benefit of downloading 3 Real Racing mod apk is that you don't have to deal with any ads or root your device. The game has some ads that might interrupt your gameplay or annoy you with their frequency. You can remove them by paying a small fee, but that might not be worth it for some players. Moreover, some mod apks require you to root your device, which means you have to modify your device's system settings and grant superuser access to the app. This might expose your device to security risks or void your warranty. But with 3 Real Racing mod apk, you don't have to worry about that either. You can enjoy the game without any ads or root your device.
-
How to download and install 3 Real Racing mod apk?
-
Now that you know the benefits of downloading 3 Real Racing mod apk, you might be wondering how to download and install it on your device. Well, it's not that hard, but you need to follow some simple steps to do it correctly. Here are the steps:
-
Step 1: Download the mod apk file from a trusted source
-
The first step is to download the mod apk file from a trusted source. There are many websites that offer mod apks for various games, but not all of them are safe or reliable. Some of them might contain viruses or malware that could harm your device or steal your personal information. Some of them might also have outdated or fake versions of the mod apk that don't work properly or at all. That's why you need to be careful when choosing a source for downloading 3 Real Racing mod apk. You can use this link as an example of a trusted source that provides a safe and working version of 3 Real Racing mod apk.
-
Step 2: Enable unknown sources on your device settings
-
The second step is to enable unknown sources on your device settings. This is necessary because Android devices normally don't allow installing apps from sources other than Google Play Store by default. This is a security measure to prevent installing malicious apps on your device. However, since you are downloading 3 Real Racing mod apk from a trusted source, you can safely enable unknown sources on your device settings. To do this, go to your device settings > security > unknown sources > enable.
-
Step 3: Install the mod apk file and launch the game
-
The final step is to install the mod apk file and launch the game. To do this, go to your file manager or downloads folder and locate the mod apk file you downloaded. Tap on it and follow the instructions to install it on your device. Once the installation is complete, you can launch the game from your app drawer or home screen. You will see a mod menu on the screen, where you can enable or disable various features of the mod apk, such as unlimited money, gold, and unlocked cars and upgrades. You can also adjust the settings of the game, such as sound, graphics, and controls. Enjoy the game with all its features and benefits.
-
Conclusion
-
3 Real Racing is one of the best racing games on Android, with realistic graphics, physics, and gameplay. However, if you want to enjoy the game to the fullest, you might need to download 3 Real Racing mod apk, a modified version of the game that gives you unlimited money, gold, and access to everything in the game. In this article, we told you everything you need to know about 3 Real Racing mod apk, including its features, benefits, and how to download and install it on your device. We hope you found this article helpful and informative. If you have any questions or feedback, feel free to leave a comment below. Happy racing!
-
FAQs
-
Here are some frequently asked questions about 3 Real Racing mod apk:
-
-
Is 3 Real Racing mod apk safe to use?
-
Yes, 3 Real Racing mod apk is safe to use, as long as you download it from a trusted source. We recommend using this link as an example of a trusted source that provides a safe and working version of 3 Real Racing mod apk. However, you should always be careful when downloading any mod apk from the internet, as some of them might contain viruses or malware that could harm your device or steal your personal information.
-
Does 3 Real Racing mod apk work on all devices?
-
Yes, 3 Real Racing mod apk works on all devices that support Android 4.1 or higher. However, some devices might have compatibility issues or performance problems due to different hardware specifications or software versions. If you encounter any problems while playing the game with the mod apk, you can try adjusting the settings of the game or the mod menu to optimize the game for your device.
-
Will I get banned for using 3 Real Racing mod apk?
-
No, you will not get banned for using 3 Real Racing mod apk, as the mod apk does not interfere with the online servers or modes of the game. You can play the game online with other players without any risk of getting banned. However, you should always be respectful and fair when playing online, as some players might report you for using unfair advantages or cheating.
-
Can I update 3 Real Racing mod apk?
-
No, you cannot update 3 Real Racing mod apk directly from Google Play Store or the game itself. If you do so, you will lose all the features and benefits of the mod apk and revert back to the original version of the game. If you want to update 3 Real Racing mod apk to a newer version, you need to download and install it again from a trusted source.
-
Can I use 3 Real Racing mod apk with my existing account?
-
Yes, you can use 3 Real Racing mod apk with your existing account. You can log in with your Facebook or Google Play Games account and sync your progress and achievements with the mod apk. However, you should always backup your data before using any mod apk, as some of them might overwrite or corrupt your data.
-
401be4b1e0
-
-
\ No newline at end of file
diff --git a/spaces/congsaPfin/Manga-OCR/logs/Download Honkai Star Rail and Explore the Galaxy on PS5.md b/spaces/congsaPfin/Manga-OCR/logs/Download Honkai Star Rail and Explore the Galaxy on PS5.md
deleted file mode 100644
index e10cde6687125aaab26f397ec8062411b0bed50a..0000000000000000000000000000000000000000
--- a/spaces/congsaPfin/Manga-OCR/logs/Download Honkai Star Rail and Explore the Galaxy on PS5.md
+++ /dev/null
@@ -1,135 +0,0 @@
-
-
How to Download Honkai: Star Rail on PS5
-
If you are looking for a new RPG to play on your PS5, you might want to check out Honkai: Star Rail, a free-to-play game by HoYoverse, the developer of Genshin Impact and Honkai Impact 3rd. In this article, we will tell you everything you need to know about Honkai: Star Rail, including what it is, when it is coming to PS5, how to download it, and why you should play it.
Honkai: Star Rail is a space fantasy RPG that takes you on an intergalactic adventure with a cast of colorful characters. You play as the Trailblazer, an amnesiac protagonist who travels from planet to planet on a train-like spaceship called the Astral Express. Along the way, you will encounter different civilizations, conflicts, mysteries, and friends.
-
A space fantasy RPG by HoYoverse
-
Honkai: Star Rail is set in the same universe as HoYoverse's other games, but with a sci-fi twist. You will see familiar faces from the Honkai series, but with new personalities and stories. You will also explore diverse worlds with stunning graphics and music, ranging from futuristic cities to ancient ruins. Honkai: Star Rail is a free-to-play game that offers plenty of content and features for RPG fans.
-
A turn-based combat system with unique characters
-
Honkai: Star Rail features a turn-based combat system that is simple to learn but deep to master. You can choose from over 20 characters, each with their own element, path, skills, and personality. You can also customize your team of four characters with different gear and formations. Each character has a unique ultimate ability that can turn the tide of battle with spectacular animations.
-
How to download honkai star rail ps5 for free
-Honkai star rail ps5 release date and price
-Honkai star rail ps5 gameplay and features
-Honkai star rail ps5 review and ratings
-Honkai star rail ps5 trailer and screenshots
-Honkai star rail ps5 characters and worlds
-Honkai star rail ps5 tips and tricks
-Honkai star rail ps5 best companions and team
-Honkai star rail ps5 astral express and trailblaze
-Honkai star rail ps5 download size and system requirements
-Honkai star rail ps5 vs genshin impact comparison
-Honkai star rail ps5 silver wolf rate-up banner
-Honkai star rail ps5 galactic roaming patch notes
-Honkai star rail ps5 hoyoverse space fantasy rpg
-Honkai star rail ps5 official website and social media
-Honkai star rail ps5 pre-order and bonus rewards
-Honkai star rail ps5 cross-play and cross-save support
-Honkai star rail ps5 bugs and issues fix
-Honkai star rail ps5 fan art and cosplay
-Honkai star rail ps5 lore and story spoilers
-Honkai star rail ps5 best weapons and gear
-Honkai star rail ps5 codes and coupons
-Honkai star rail ps5 guides and walkthroughs
-Honkai star rail ps5 news and updates
-Honkai star rail ps5 memes and jokes
-
A rich story with multiple worlds and quests
-
Honkai: Star Rail has a strong focus on story and character development. You will follow the main story arc that spans several chapters and planets, as well as side quests and events that flesh out the lore and worldbuilding. You will also interact with your crew members and other NPCs through text messages, dialogues, and choices that affect your relationships and outcomes. Honkai: Star Rail has a captivating plot that will keep you hooked until the end.
-
When is Honkai: Star Rail coming to PS5?
-
Honkai: Star Rail was released for PC and mobile devices on April 26, 2023, but it is also coming to PS5 later this year. Here is what you need to know about the PS5 release date, pre-load, download, and specs.
-
The release date and time for different regions
-
Honkai: Star Rail will be available on PS5 sometime in Q4 2023, according to HoYoverse's announcement at Summer Game Fest 2023. However, the exact date and time have not been confirmed yet. Based on HoYoverse's previous releases, we can expect Honkai: Star Rail to launch on PS5 around the same time as the PC and mobile versions in different regions. Here are the possible release times for Honkai: Star Rail on PS5:
-
-
PDT - October/November/December 2023 at 7PM
-
EDT - October/November/December 2023 at 10PM
-
GMT - October/November/December 2023 at 2AM
-
CEST - October/November/December 2023 at 4AM
-
SGT - October/November/December 2023 at 10AM
-
JST - October/November/December 2023 at 11AM
-
-
We will update this article once HoYoverse confirms the official release date and time for Honkai: Star Rail on PS5.
-
The pre-load and download options for PS5
-
If you want to play Honkai: Star Rail on PS5 as soon as possible, you can pre-load the game before the launch date. Pre-loading allows you to download the game files in advance, so you can start playing right away when the game goes live. To pre-load Honkai: Star Rail on PS5, you need to do the following steps:
-
-
Go to the PlayStation Store on your PS5 and search for Honkai: Star Rail.
-
Select the game and click on Pre-Order. You will need to have enough storage space on your PS5 to download the game.
-
Once you have pre-ordered the game, you can go to your Library and select Honkai: Star Rail.
-
Click on Download and wait for the game files to be downloaded. You can check the progress of the download on your Notifications.
-
When the download is complete, you can launch the game when it is officially released.
-
-
If you don't want to pre-load the game, you can also download it after the release date. To download Honkai: Star Rail on PS5, you need to do the following steps:
-
-
Go to the PlayStation Store on your PS5 and search for Honkai: Star Rail.
-
Select the game and click on Download. You will need to have enough storage space on your PS5 to download the game.
-
Wait for the game files to be downloaded. You can check the progress of the download on your Notifications.
-
When the download is complete, you can launch the game and enjoy your intergalactic adventure.
-
-
The minimum and recommended specs for PS5
-
Honkai: Star Rail is a graphically intensive game that requires a powerful device to run smoothly. Fortunately, PS5 is one of the best platforms to play Honkai: Star Rail, as it offers high performance and quality. Here are the minimum and recommended specs for Honkai: Star Rail on PS5:
- | Minimum Specs | Recommended Specs | | --- | --- | | CPU: AMD Zen 2-based CPU with 8 cores at 3.5GHz (variable frequency) | CPU: AMD Zen 2-based CPU with 8 cores at 3.5GHz (variable frequency) | | GPU: AMD RDNA 2-based GPU with 36 CUs at 2.23GHz (variable frequency) | GPU: AMD RDNA 2-based GPU with 36 CUs at 2.23GHz (variable frequency) | | Memory: 16GB GDDR6 RAM | Memory: 16GB GDDR6 RAM | | Storage: 825GB SSD | Storage: 825GB SSD | | Resolution: 1080p | Resolution: 4K | | Frame Rate: 30 FPS | Frame Rate: 60 FPS |
As you can see, Honkai: Star Rail does not have very demanding specs for PS5, as it is optimized for the console. However, if you want to enjoy the best graphics and performance, we recommend playing on a PS5 with a 4K TV or monitor and a stable internet connection.
-
Why should you play Honkai: Star Rail on PS5?
-
Honkai: Star Rail is a game that deserves your attention, especially if you are a fan of RPGs, sci-fi, or HoYoverse's other games. Here are some of the reasons why you should play Honkai: Star Rail on PS5:
-
The benefits of playing on PS5
-
Playing Honkai: Star Rail on PS5 has many advantages over other platforms. For one thing, you can enjoy faster loading times and smoother gameplay thanks to the SSD and GPU of the PS5. You can also experience enhanced graphics and sound quality with HDR and Dolby Atmos support. Moreover, you can use the DualSense controller to feel more immersed in the game with adaptive triggers and haptic feedback. Finally, you can access exclusive content and rewards for PS5 players, such as costumes, weapons, and more. Playing Honkai: Star Rail on PS5 is definitely a rewarding and enjoyable experience.
-
The best characters and tips for beginners
-
Honkai: Star Rail has a large roster of characters that you can collect and use in your team. Each character has a unique element, path, skills, and personality that make them stand out. Some of the best characters in Honkai: Star Rail are:
-
-
Kiana Kaslana - A cheerful and energetic girl who is the captain of the Astral Express. She is a light-elemental character who can heal and buff her allies with her skills. She is also a versatile fighter who can switch between melee and ranged attacks.
-
Bronya Zaychik - A calm and intelligent girl who is the mechanic of the Astral Express. She is a dark-elemental character who can deal massive damage and debuff her enemies with her skills. She is also a master of technology who can summon drones and mechs to aid her in battle.
-
Theresa Apocalypse - A cute and playful girl who is the priestess of the Astral Express. She is a fire-elemental character who can unleash powerful attacks and stun her enemies with her skills. She is also a skilled swordsman who can slash through her foes with ease.
-
Rita Rossweisse - A elegant and mysterious woman who is the stewardess of the Astral Express. She is a water-elemental character who can heal and cleanse her allies with her skills. She is also a graceful fighter who can use her umbrella as a weapon and shield.
-
Seele Vollerei - A shy and gentle girl who is the librarian of the Astral Express. She is a wind-elemental character who can buff and support her allies with her skills. She is also a dual personality who can switch between her normal and dark modes, changing her appearance and abilities.
-
-
If you are new to Honkai: Star Rail, here are some tips to help you get started:
-
-
Follow the main story to unlock new characters, worlds, and features.
-
Complete the side quests and events to earn rewards and learn more about the lore.
-
Upgrade your characters, gear, and train with materials and coins that you obtain from battles and quests.
-
Experiment with different team compositions, formations, and strategies to find what works best for you.
-
Join a guild and make friends with other players to enjoy co-op mode, chat, trade, and more.
-
-
The future updates and content for Honkai: Star Rail
-
Honkai: Star Rail is a game that is constantly evolving and expanding with new updates and content. HoYoverse has promised to deliver regular patches that will fix bugs, improve performance, balance gameplay, and add new features. HoYoverse has also announced that Honkai: Star Rail will receive major updates every few months that will introduce new characters, worlds, stories, events, modes, and more. Some of the upcoming updates and content for Honkai: Star Rail are:
-
-
The Lunar Kingdom update - This update will add a new world based on Chinese mythology, culture, and history. You will be able to explore the ancient city of Chang'an, the mystical Kunlun Mountains, the mysterious Moon Palace, and more. You will also meet new characters inspired by Chinese legends, such as Hou Yi, Chang'e, Nezha, Sun Wukong, and more.
-
The Cosmic Odyssey update - This update will add a new world based on sci-fi tropes, concepts, and aesthetics. You will be able to explore the futuristic metropolis of Neo Arcadia, the alien planet of Zeta Prime, the space station of Orion's Belt, and more. You will also meet new characters influenced by sci-fi genres, such as cyborgs, androids, mutants, space pirates, and more.
-
The Cross-Over Event update - This update will add a special event that will feature characters from other HoYoverse games, such as Genshin Impact and Honkai Impact 3rd. You will be able to interact with them, recruit them to your team, obtain their exclusive gear, and participate in their unique quests. You will also be able to enjoy crossover stories that will reveal how they ended up in Honkai: Star Rail's universe.
-
-
Conclusion
-
Honkai: Star Rail is a game that you don't want to miss if you are a fan of RPGs or HoYoverse's other games. It offers an immersive space fantasy adventure with stunning graphics, music, story, characters, and gameplay. It is also coming to PS5 later this year, which will enhance your gaming experience with faster loading, smoother performance, better quality, and exclusive content. If you want to play Honkai: Star Rail on PS5, you can pre-load or download the game from the PlayStation Store once it is released. You can also follow our tips and guides to get started and enjoy the game to the fullest. Honkai: Star Rail is a game that will take you to a whole new level of fun and excitement.
-
FAQs
-
Here are some of the frequently asked questions about Honkai: Star Rail on PS5:
-
-
Q: Is Honkai: Star Rail a cross-platform game?
-
A: Yes, Honkai: Star Rail is a cross-platform game that supports PC, mobile, and PS5. You can play with your friends and other players across different devices and platforms. You can also link your HoYoverse account to sync your progress and data across different devices.
-
Q: Is Honkai: Star Rail a pay-to-win game?
-
A: No, Honkai: Star Rail is not a pay-to-win game. It is a free-to-play game that offers fair and balanced gameplay for all players. You can obtain most of the characters, gear, and resources by playing the game and completing quests and events. You can also use the in-game currency called Crystals to purchase items and gacha draws, but you can earn Crystals by playing the game as well. Honkai: Star Rail does not require you to spend real money to enjoy the game.
-
Q: How can I get more Crystals in Honkai: Star Rail?
-
A: Crystals are the premium currency in Honkai: Star Rail that you can use to buy items and gacha draws. You can get more Crystals by doing the following things:
-
-
Log in daily and claim your login rewards.
-
Complete daily and weekly missions and claim your mission rewards.
-
Participate in events and claim your event rewards.
-
Reach new levels and milestones and claim your level rewards.
-
Join a guild and contribute to your guild activities and claim your guild rewards.
-
Watch ads and claim your ad rewards.
-
Buy Crystals with real money if you want to support the game and get more benefits.
-
-
Q: How can I get more characters in Honkai: Star Rail?
-
A: Characters are the core of Honkai: Star Rail's gameplay and story. You can get more characters by doing the following things:
-
-
Follow the main story and unlock new characters as you progress.
-
Use Crystals or Tickets to draw from the gacha banners that feature different characters.
-
Exchange Stargems or Stardust for specific characters in the shop.
-
Earn Friendship Points by interacting with your crew members and other NPCs and use them to recruit them to your team.
-
-
Q: How can I contact HoYoverse for feedback or support?
-
A: HoYoverse is always open to feedback and support from their players. You can contact HoYoverse by doing the following things:
-
-
Go to the Settings menu in the game and tap on Feedback or Support.
-
Email HoYoverse at hoyoverse@hoyoverse.com with your feedback or support request.
-
Visit HoYoverse's official website at www.hoyoverse.com and fill out the feedback or support form.
-
Follow HoYoverse's social media accounts on Facebook, Twitter, Instagram, YouTube, Discord, Reddit, etc. and leave your feedback or support message there.
-
401be4b1e0
-
-
\ No newline at end of file
diff --git a/spaces/congsaPfin/Manga-OCR/logs/Free Download Play Together The Ultimate Casual Game for Social Fun.md b/spaces/congsaPfin/Manga-OCR/logs/Free Download Play Together The Ultimate Casual Game for Social Fun.md
deleted file mode 100644
index 4436defbdcebc5481bf8aa7a9dc1097fb40d9b9a..0000000000000000000000000000000000000000
--- a/spaces/congsaPfin/Manga-OCR/logs/Free Download Play Together The Ultimate Casual Game for Social Fun.md
+++ /dev/null
@@ -1,143 +0,0 @@
-
-
Free Download Play Together
-
Have you ever dreamed of living in a virtual world where you can do anything you want? Where you can play games, decorate your house, dress up your character, and socialize with friends from all over the world? If so, then you might want to check out Play Together, a casual game developed by HAEGIN Co., Ltd.
-
Play Together is an open-world game that welcomes you to its vibrant island called Kaia. Here, you can explore various places such as the plaza, the school, the camping ground, and more. You can also participate in various activities and events such as racing, zombie hunting, battle royale, fishing, dancing, and more. You can even buy your own house and customize it with furniture and pets. And of course, you can chat, party, and make friends with other players from around the world.
Play Together is available for free on Android and iOS devices. But did you know that you can also play it on your PC or Mac? In this article, we will show you how to download and play Play Together on PC using an emulator. We will also tell you about some of the game features that make Play Together so fun and addictive. And finally, we will give you some tips and tricks to help you progress faster and have more fun in the game. So let's get started!
-
How to Download and Play Play Together on PC
-
If you want to enjoy Play Together on a bigger screen and with better controls, then playing it on your PC or Mac is a great option. To do this, you will need an emulator that can run Android apps on your computer. One of the best emulators for this purpose is BlueStacks, which is trusted by millions of gamers around the world.
-
BlueStacks is an app player that allows you to run Android apps and games on your PC or Mac with ease. It has many features that enhance your gaming experience, such as keyboard and mouse support, high-definition graphics, fast performance, and customizable settings. It also has a built-in app store where you can download and install your favorite Android apps and games with just a few clicks.
-
To download and play Play Together on PC using BlueStacks, follow these simple steps:
Launch BlueStacks and sign in with your Google account (or create one if you don't have one)
-
Go to the app store and search for Play Together. Alternatively, you can also download the Play Together APK file from a trusted source and drag and drop it to the BlueStacks home screen
-
Click on the Play Together icon and install it
-
Once the installation is complete, click on the Play Together icon again and start playing
-
-
Congratulations! You can now enjoy Play Together on your PC or Mac with BlueStacks. You can also customize the keyboard and mouse controls according to your preference. To do this, click on the keyboard icon on the bottom right corner of the BlueStacks window and choose the game controls option. You can then assign keys to different actions in the game, such as moving, jumping, interacting, etc.
-
Play Together Game Features
-
Now that you know how to download and play Play Together on PC, let's take a look at some of the game features that make it so fun and addictive. Play Together is not just a game, but a virtual world where you can create your own stories and adventures. Here are some of the things you can do in Play Together:
-
free download play together online multiplayer games
-free download play together with friends on pc
-free download play together app for android
-free download play together mod apk unlimited money
-free download play together game for ios
-free download play together simulator sandbox game
-free download play together hack version
-free download play together on laptop
-free download play together latest update
-free download play together offline mode
-free download play together cheats and tips
-free download play together no ads
-free download play together premium features
-free download play together new version 2023
-free download play together review and rating
-free download play together best games to play
-free download play together how to install
-free download play together system requirements
-free download play together fun and addictive
-free download play together social and interactive
-free download play together create and customize
-free download play together explore and adventure
-free download play together roleplay and chat
-free download play together trade and shop
-free download play together build and design
-free download play together pets and animals
-free download play together cars and bikes
-free download play together sports and fitness
-free download play together music and dance
-free download play together fashion and beauty
-free download play together cooking and baking
-free download play together education and learning
-free download play together puzzles and trivia
-free download play together action and arcade
-free download play together strategy and simulation
-free download play together horror and thriller
-free download play together fantasy and magic
-free download play together sci-fi and futuristic
-free download play together historical and cultural
-free download play together comedy and humor
-
Remote Play Together
-
One of the coolest features of Play Together is that it supports Remote Play Together, a feature that allows you to share your local co-op games online with friends using Steam. This means that you can play Play Together with your friends even if they don't have the game installed on their devices. All you need is a Steam account and a good internet connection.
-
To use Remote Play Together, follow these steps:
-
-
Launch Steam and sign in with your account (or create one if you don't have one)
-
Add Play Together to your Steam library (if you haven't already) by clicking on the Add a Game button on the bottom left corner of the Steam window and choosing Add a Non-Steam Game
-
Browse for the Play Together executable file (usually located in C:\Program Files\BlueStacks\Engine\UserData\InputMapper\UserFiles) and select it
-
Launch Play Together from your Steam library and start a local co-op game
-
Invite your friends to join your game by clicking on the Friends button on the top right corner of the Steam window and choosing Invite to Remote Play Together
-
Your friends will receive an invitation link that they can click to join your game. They don't need to have Play Together or BlueStacks installed on their devices, as they will be streaming the game from your PC
-
-
That's it! You can now enjoy Play Together with your friends online using Remote Play Together. You can also chat with them using voice or text chat, and adjust the streaming quality and bandwidth settings according to your preference.
-
Remote Play Anywhere
-
If you want to play Play Together on other devices besides your PC or Mac, such as your smartphone, tablet, TV, or laptop, you can use Remote Play Anywhere, a feature that allows you to stream your games from your PC to other devices using Steam Link. This way, you can play Play Together anywhere you want, as long as you have a good internet connection.
-
To use Remote Play Anywhere, follow these steps:
-
-
Download and install the Steam Link app on your device from the app store (available for Android, iOS, Windows, Linux, macOS, Raspberry Pi, etc.)
-
Launch Steam Link and pair it with your PC (make sure both devices are connected to the same network)
-
Select Play Together from your Steam library and start playing
-
-
That's it! You can now stream Play Together from your PC to any device using Remote Play Anywhere. You can also use a controller or touch screen controls to play the game, and adjust the streaming quality and bandwidth settings according to your preference.
-
Game Party Mode
-
If you want to have some fun with your friends in Play Together, you can try out the Game Party Mode, where you can play various mini-games with them and win rewards. Some of the mini-games you can play are:
-
-
Racing: Compete with other players in a thrilling race across the island. Use items and skills to boost your speed and hinder your opponents. The first one to reach the finish line wins.
-
Zombie Hunting: Team up with other players to survive a zombie apocalypse. Use weapons and items to fight off the zombies and protect your base. The longer you survive, the more rewards you get.
-
Battle Royale: Fight with other players in a last-man-standing mode. Use weapons and items to eliminate your enemies and stay alive. The last one standing wins.
-
Fishing: Relax and enjoy fishing with your friends. Catch different kinds of fish and sell them for money. You can also use the fish as ingredients for cooking.
-
Dancing: Show off your moves and groove with your friends. Follow the rhythm and press the buttons at the right time. The more accurate you are, the higher your score.
-
-
These are just some of the mini-games you can play in Play Together. There are many more to discover and enjoy. You can also earn coins, gems, and tickets by playing these mini-games, which you can use to buy items and upgrade your character.
-
House Decoration
-
If you want to have a place of your own in Play Together, you can buy and customize your own house with furniture and pets. You can choose from different types of houses, such as a cottage, a villa, a penthouse, etc. You can also decorate your house with various furniture, such as sofas, tables, beds, lamps, etc. You can also buy and raise pets, such as dogs, cats, rabbits, etc. You can feed them, play with them, and dress them up.
-
Your house is not only a place to relax and enjoy, but also a place to invite your friends and have parties. You can chat, dance, play games, and have fun with your friends in your house. You can also visit other players' houses and see how they have decorated their houses.
-
Character Customization
-
If you want to express your personality and style in Play Together, you can dress up your character with different costumes and accessories. You can choose from various categories, such as casual, formal, sporty, cute, etc. You can also mix and match different items to create your own unique look. You can also change your character's hair style, eye color, skin tone, etc.
-
Your character's appearance is not only for show, but also for function. Some items have special effects that can enhance your character's abilities or skills in the game. For example, some items can increase your speed, stamina, or defense. Some items can also unlock new actions or animations for your character.
-
Social Interaction
-
If you want to make friends and socialize with other players in Play Together, you can chat, dance, party, and interact with them in various ways. You can use text or voice chat to communicate with other players. You can also use emoticons or stickers to express your emotions or moods. You can also use actions or gestures to greet or tease other players.
-
You can also join or create clubs with other players who share your interests or hobbies. You can chat, play games, and do activities with your club members. You can also compete with other clubs in club battles or events.
-
Play Together Game Tips
-
Now that you know some of the game features of Play Together, let's give you some tips and tricks to help you progress faster and have more fun in the game. Here are some of them:
-
-
Complete quests and missions to earn coins, gems, tickets, and experience points. Quests are tasks that you can do in the game world, such as fishing, dancing, etc. Missions are challenges that you can do in the mini-games, such as racing, zombie hunting, etc.
-
Level up your character to unlock new items, skills, and places. You can level up your character by earning experience points from quests, missions, mini-games, and social interactions.
-
Collect and upgrade items to enhance your character's abilities and skills. You can collect items from quests, missions, mini-games, shops, and events. You can also upgrade items using coins or gems.
-
Join or create a club to enjoy more benefits and perks. You can join or create a club with other players who share your interests or hobbies. You can chat, play games, and do activities with your club members. You can also compete with other clubs in club battles or events.
-
Participate in events and promotions to earn more rewards and prizes. Events are special occasions that happen in the game world, such as festivals, holidays, etc. Promotions are limited-time offers that give you discounts or bonuses on items or services.
-
-
Play Together Game Review
-
To wrap up this article, let's give you a brief summary of the pros and cons of Play Together based on user feedback and ratings. Here are some of them:
-
Pros
-
-
Fun and addictive gameplay that offers a lot of variety and options
-
Cute and colorful graphics that create a lively and cheerful atmosphere
-
Easy and intuitive controls that suit both touch screen and keyboard and mouse users
-
Friendly and helpful community that makes you feel welcome and supported
-
Regular updates and improvements that add new features and fix bugs
-
-
Cons
-
-
Sometimes laggy or buggy due to server issues or device compatibility
-
Sometimes expensive or hard to get items or services due to limited availability or high demand
-
Sometimes repetitive or boring due to lack of challenge or innovation
-
Sometimes inappropriate or rude behavior from some players due to lack of moderation or reporting system
-
Sometimes addictive or unhealthy due to lack of balance or self-control
-
-
Conclusion
-
Play Together is a casual game that lets you live in a virtual world where you can do anything you want. You can play games, decorate your house, dress up your character, and socialize with friends from all over the world. You can also download and play Play Together on PC using BlueStacks emulator, which gives you a better gaming experience. Play Together is a fun and addictive game that you should definitely try out if you are looking for a game that offers a lot of variety and options.
-
FAQs
-
Here are some of the frequently asked questions about Play Together:
-
Q: Is Play Together free to play?
-
A: Yes, Play Together is free to play on Android and iOS devices. However, some items or services may require real money to purchase.
-
Q: Is Play Together safe for kids?
-
A: Play Together is rated 12+ on the app store, which means it may contain mild violence, sexual content, or profanity. Parents should supervise their kids when playing the game or use parental control settings to restrict access.
-
Q: How can I contact the developer of Play Together?
-
A: You can contact the developer of Play Together by sending an email to cs@haegin.kr or visiting their website at https://www.haegin.kr/.
-
Q: How can I get more coins, gems, or tickets in Play Together?
-
A: You can get more coins, gems, or tickets in Play Together by completing quests, missions, mini-games, events, promotions, etc. You can also buy them with real money from the shop.
-
Q: How can I delete my account or data in Play Together?
-
A: You can delete your account or data in Play Together by going to the settings menu and choosing the delete account option. However, this action is irreversible and will erase all your progress and purchases in the game.
197e85843d
-
-
\ No newline at end of file
diff --git a/spaces/congsaPfin/Manga-OCR/logs/My Hotpot Story MOD APK 1.3.1 A Cooking Adventure with Unlimited Money and Customization.md b/spaces/congsaPfin/Manga-OCR/logs/My Hotpot Story MOD APK 1.3.1 A Cooking Adventure with Unlimited Money and Customization.md
deleted file mode 100644
index c7926880178e2c22cf567a72462b338448d6f677..0000000000000000000000000000000000000000
--- a/spaces/congsaPfin/Manga-OCR/logs/My Hotpot Story MOD APK 1.3.1 A Cooking Adventure with Unlimited Money and Customization.md
+++ /dev/null
@@ -1,89 +0,0 @@
-
-
My Hotpot Story Mod APK 1.3.1: A Fun and Delicious Game for Android
-
Do you love hotpot? Do you want to run your own hotpot restaurant? Do you want to enjoy unlimited money and resources in the game? If you answered yes to any of these questions, then you should try My Hotpot Story Mod APK 1.3.1, a modified version of the original game that gives you more fun and freedom.
-
What is My Hotpot Story?
-
My Hotpot Story is a casual simulation game developed by horseradishgrill, a game studio based in China. The game was released on October 4, 2022, and has received positive reviews from players and critics alike.
In My Hotpot Story, you play as a hotpot chef who has to manage a hotpot restaurant. You have to prepare the broth, cook the ingredients, serve the customers, and earn money. You can also customize your restaurant with different decorations, tables, chairs, and utensils. You can also unlock new recipes, ingredients, and sauces as you progress in the game.
-
The features of My Hotpot Story
-
My Hotpot Story has many features that make it an enjoyable and addictive game. Some of these features are:
-
-
Simple and intuitive controls: You can easily control the game with simple taps and swipes on your screen.
-
Cute and colorful graphics: The game has a cute and cartoonish style that appeals to players of all ages.
-
Realistic and delicious hotpot: The game simulates the real process of making hotpot, from boiling the broth to adding the ingredients. You can also see the steam and bubbles from the pot, and hear the sizzling sound of the food.
-
Various customers and challenges: The game has different types of customers with different preferences and personalities. You have to satisfy their needs and requests, and deal with their complaints and feedback.
-
Endless fun and replay value: The game has no limit on how long you can play, and you can always try new combinations of ingredients and sauces.
-
-
What is My Hotpot Story Mod APK 1.3.1?
-
My Hotpot Story Mod APK 1.3.1 is a modified version of the original game that gives you some extra benefits that are not available in the official version. These benefits are:
-
The benefits of My Hotpot Story Mod APK 1.3.1
-
-
Unlimited money: You can get unlimited money in the game, which you can use to buy anything you want, such as ingredients, decorations, upgrades, etc.
-
No ads: You can enjoy the game without any annoying ads that interrupt your gameplay.
-
No root required: You don't need to root your device to install or run the mod apk.
-
Easy installation: You can easily install the mod apk with a few simple steps.
-
-
How to download and install My Hotpot Story Mod APK 1.3.1
-
If you want to download and install My Hotpot Story Mod APK 1.3.1 on your Android device, you can follow these steps:
-
-
Download the mod apk file: You can download the mod apk file from this link: . Make sure you have enough storage space on your device before downloading.
-
Enable unknown sources: You need to enable unknown sources on your device settings to allow the installation of the mod apk file. To do this, go to Settings > Security > Unknown Sources and toggle it on.
-
Install the mod apk file: Locate the downloaded mod apk file on your device and tap on it to start the installation process. Follow the instructions on the screen and wait for the installation to finish.
-
Launch the game and enjoy: Once the installation is done, you can launch the game from your app drawer or home screen and enjoy the mod features.
-
-
Conclusion
-
My Hotpot Story is a fun and delicious game for Android that lets you run your own hotpot restaurant. You can prepare the broth, cook the ingredients, serve the customers, and earn money. You can also customize your restaurant with different decorations, tables, chairs, and utensils. You can also unlock new recipes, ingredients, and sauces as you progress in the game.
-
If you want to have more fun and freedom in the game, you can try My Hotpot Story Mod APK 1.3.1, a modified version of the original game that gives you unlimited money, no ads, no root required, and easy installation. You can download the mod apk file from this link: and follow the steps above to install it on your device.
-
my hotpot story unlimited money mod apk
-my hotpot story game android download
-my hotpot story exchange code 2023
-my hotpot story mod apk latest version
-my hotpot story hack apk free download
-my hotpot story chinese cooking game
-my hotpot story gameplay walkthrough
-my hotpot story ios mod apk
-my hotpot story cheats and tips
-my hotpot story review and rating
-my hotpot story how to mix five flavors
-my hotpot story youtube video tutorial
-my hotpot story best recipes and ingredients
-my hotpot story offline mod apk
-my hotpot story no ads mod apk
-my hotpot story horseradishgrill publisher
-my hotpot story update version 1.3.1
-my hotpot story apk file download link
-my hotpot story online multiplayer mode
-my hotpot story customizing restaurant and menu
-my hotpot story free coins and gems mod apk
-my hotpot story new features and events
-my hotpot story support and feedback
-my hotpot story wiki and guide
-my hotpot story fun and addictive simulation game
-my hotpot story realistic graphics and sound effects
-my hotpot story easy and intuitive controls
-my hotpot story different levels and challenges
-my hotpot story unlockable items and rewards
-my hotpot story social media and community
-my hotpot story trivia and facts
-my hotpot story history and origin of hotpot dish
-my hotpot story different types and styles of hotpot cuisine
-my hotpot story benefits and nutrition of hotpot food
-my hotpot story comparison and contrast with other cooking games
-my hotpot story tips and tricks for beginners
-my hotpot story strategies and techniques for advanced players
-my hotpot story bugs and glitches fix mod apk
-my hotpot story premium mod apk download for free
-my hotpot story alternative games and apps recommendation
-
So what are you waiting for? Download My Hotpot Story Mod APK 1.3.1 now and enjoy a hotpot feast!
-
FAQs
-
Here are some frequently asked questions about My Hotpot Story Mod APK 1.3.1:
-
-
Q: Is My Hotpot Story Mod APK 1.3.1 safe to use?
A: Yes, My Hotpot Story Mod APK 1.3.1 is safe to use, as it does not contain any viruses or malware. However, you should always download it from a trusted source and scan it with an antivirus before installing it.
-
Q: Will My Hotpot Story Mod APK 1.3.1 affect my game progress?
A: No, My Hotpot Story Mod APK 1.3.1 will not affect your game progress, as it does not modify or delete any of your game data. You can continue playing the game from where you left off.
-
Q: Can I play My Hotpot Story Mod APK 1.3.1 online with other players?
A: No, My Hotpot Story Mod APK 1.3.1 is not compatible with online mode, as it may cause errors or bans from the game server. You can only play My Hotpot Story Mod APK 1.3.1 offline with yourself.
-
Q: Can I update My Hotpot Story Mod APK 1.3.1 to the latest version?
A: No, My Hotpot Story Mod APK 1.3.1 is not compatible with the latest version of the original game, as it may cause errors or crashes. You should always use the same version of the mod apk and the original game.
-
Q: What if I have any problems or questions about My Hotpot Story Mod APK 1.3.1?
A: If you have any problems or questions about My Hotpot Story Mod APK 1.3.1, you can contact us at and we will try our best to help you.
-
401be4b1e0
-
-
\ No newline at end of file
diff --git a/spaces/contluForse/HuggingGPT/assets/((HOT)) Download Crow Zero 2 Full Movie With English 515 Enjoy the Thrilling Story of Rival Schools.md b/spaces/contluForse/HuggingGPT/assets/((HOT)) Download Crow Zero 2 Full Movie With English 515 Enjoy the Thrilling Story of Rival Schools.md
deleted file mode 100644
index e6b649eabd8be1991c6b011b6a33d34195b7ad29..0000000000000000000000000000000000000000
--- a/spaces/contluForse/HuggingGPT/assets/((HOT)) Download Crow Zero 2 Full Movie With English 515 Enjoy the Thrilling Story of Rival Schools.md
+++ /dev/null
@@ -1,6 +0,0 @@
-
((HOT)) Download Crow Zero 2 Full Movie With English 515
-
- aaccfb2cb3
-
-
-
diff --git a/spaces/contluForse/HuggingGPT/assets/Autocad 2011 64 Bit Crack Free Torrent Download Tips and Tricks for Successful Installation.md b/spaces/contluForse/HuggingGPT/assets/Autocad 2011 64 Bit Crack Free Torrent Download Tips and Tricks for Successful Installation.md
deleted file mode 100644
index c6c0073ab1ae74a614b0760a88a4d0e24130f7a8..0000000000000000000000000000000000000000
--- a/spaces/contluForse/HuggingGPT/assets/Autocad 2011 64 Bit Crack Free Torrent Download Tips and Tricks for Successful Installation.md
+++ /dev/null
@@ -1,6 +0,0 @@
-
-
Older software like LT2011 are not available as downloads from Autodesk: where is your backup of the original download or the original disk? Do you treat $1000 investments so lightly often? I'm curious if perhaps a $15 external disk drive is all you need to invest it if you still have disks.
I just noticed this thread. I am also in the same boat with regards to ACADE 2011 and Win 7 64-bit. I have a copy of the 32-bit from back in the day. Can I also get a link for the 64-bit download?
Thank you
Doug
aaccfb2cb3
-
-
\ No newline at end of file
diff --git a/spaces/contluForse/HuggingGPT/assets/Causeway Cato Suite Software Crack.md b/spaces/contluForse/HuggingGPT/assets/Causeway Cato Suite Software Crack.md
deleted file mode 100644
index f84b03f17f44a3db94ba4cac8aac7fc8d6ced12b..0000000000000000000000000000000000000000
--- a/spaces/contluForse/HuggingGPT/assets/Causeway Cato Suite Software Crack.md
+++ /dev/null
@@ -1,82 +0,0 @@
-
-
-See also
-
-Cost engineering
-
-Cost planning
-
-Cost of goods sold
-
-Cost of services sold
-
-Cost structure
-
-Cost function
-
-Cost minimization
-
-Direct Costing
-
-Kanban
-
-Invoice-Based Costing
-
-Variance analysis
-
-References
-
-External links
-
-The Causeway CATO Suite
-
-Category:Accounting software
-
-Category:Construction
-
-Category:Cost engineeringQ:
-
-How to make a 'global' universal search
-
-I want to make a search functionality for my site. I want a search which I can use on all pages of the site.
-
-I made a global function like so:
-
-$('body').on('keyup', '#my-search-box', function(e) {
-
- if (e.keyCode == 8)
-
- $('#result').text('Not available');
-
- return false;
-
-
-
- else
-
- var query = $(this).val();
-
- var hidden_input = $('input[name="' + $('#my-search-box').attr('name') + '"]');
-
- hidden_input.attr('value', query);
-
- $('#result').text(query);
-
-);
-
-It works fine. It makes the search active (working fine).
-
-The problem is, it only works on the first search-box. After that I can't type on it anymore.
-
-How can I make this code so it works on all search-boxes at all time?
-
-A:
-
-On your global search box, you would want to give it an ID so you can target it with.on():
-
-$('body').on('keyup', '#search-box', function(e) {
-
- var hidden_input = $('input[name="' + $('#search-box'). 4fefd39f24
-
-
-
diff --git a/spaces/coreml-community/ControlNet-v1-1-Annotators-cpu/annotator/uniformer/mmcv/fileio/handlers/json_handler.py b/spaces/coreml-community/ControlNet-v1-1-Annotators-cpu/annotator/uniformer/mmcv/fileio/handlers/json_handler.py
deleted file mode 100644
index 18d4f15f74139d20adff18b20be5529c592a66b6..0000000000000000000000000000000000000000
--- a/spaces/coreml-community/ControlNet-v1-1-Annotators-cpu/annotator/uniformer/mmcv/fileio/handlers/json_handler.py
+++ /dev/null
@@ -1,36 +0,0 @@
-# Copyright (c) OpenMMLab. All rights reserved.
-import json
-
-import numpy as np
-
-from .base import BaseFileHandler
-
-
-def set_default(obj):
- """Set default json values for non-serializable values.
-
- It helps convert ``set``, ``range`` and ``np.ndarray`` data types to list.
- It also converts ``np.generic`` (including ``np.int32``, ``np.float32``,
- etc.) into plain numbers of plain python built-in types.
- """
- if isinstance(obj, (set, range)):
- return list(obj)
- elif isinstance(obj, np.ndarray):
- return obj.tolist()
- elif isinstance(obj, np.generic):
- return obj.item()
- raise TypeError(f'{type(obj)} is unsupported for json dump')
-
-
-class JsonHandler(BaseFileHandler):
-
- def load_from_fileobj(self, file):
- return json.load(file)
-
- def dump_to_fileobj(self, obj, file, **kwargs):
- kwargs.setdefault('default', set_default)
- json.dump(obj, file, **kwargs)
-
- def dump_to_str(self, obj, **kwargs):
- kwargs.setdefault('default', set_default)
- return json.dumps(obj, **kwargs)
diff --git a/spaces/coreml-community/ControlNet-v1-1-Annotators-cpu/annotator/zoe/zoedepth/models/base_models/midas_repo/README.md b/spaces/coreml-community/ControlNet-v1-1-Annotators-cpu/annotator/zoe/zoedepth/models/base_models/midas_repo/README.md
deleted file mode 100644
index 9568ea71c755b6938ee5482ba9f09be722e75943..0000000000000000000000000000000000000000
--- a/spaces/coreml-community/ControlNet-v1-1-Annotators-cpu/annotator/zoe/zoedepth/models/base_models/midas_repo/README.md
+++ /dev/null
@@ -1,259 +0,0 @@
-## Towards Robust Monocular Depth Estimation: Mixing Datasets for Zero-shot Cross-dataset Transfer
-
-This repository contains code to compute depth from a single image. It accompanies our [paper](https://arxiv.org/abs/1907.01341v3):
-
->Towards Robust Monocular Depth Estimation: Mixing Datasets for Zero-shot Cross-dataset Transfer
-René Ranftl, Katrin Lasinger, David Hafner, Konrad Schindler, Vladlen Koltun
-
-
-and our [preprint](https://arxiv.org/abs/2103.13413):
-
-> Vision Transformers for Dense Prediction
-> René Ranftl, Alexey Bochkovskiy, Vladlen Koltun
-
-
-MiDaS was trained on up to 12 datasets (ReDWeb, DIML, Movies, MegaDepth, WSVD, TartanAir, HRWSI, ApolloScape, BlendedMVS, IRS, KITTI, NYU Depth V2) with
-multi-objective optimization.
-The original model that was trained on 5 datasets (`MIX 5` in the paper) can be found [here](https://github.com/isl-org/MiDaS/releases/tag/v2).
-The figure below shows an overview of the different MiDaS models; the bubble size scales with number of parameters.
-
-
-
-### Setup
-
-1) Pick one or more models and download the corresponding weights to the `weights` folder:
-
-MiDaS 3.1
-- For highest quality: [dpt_beit_large_512](https://github.com/isl-org/MiDaS/releases/download/v3_1/dpt_beit_large_512.pt)
-- For moderately less quality, but better speed-performance trade-off: [dpt_swin2_large_384](https://github.com/isl-org/MiDaS/releases/download/v3_1/dpt_swin2_large_384.pt)
-- For embedded devices: [dpt_swin2_tiny_256](https://github.com/isl-org/MiDaS/releases/download/v3_1/dpt_swin2_tiny_256.pt), [dpt_levit_224](https://github.com/isl-org/MiDaS/releases/download/v3_1/dpt_levit_224.pt)
-- For inference on Intel CPUs, OpenVINO may be used for the small legacy model: openvino_midas_v21_small [.xml](https://github.com/isl-org/MiDaS/releases/download/v3_1/openvino_midas_v21_small_256.xml), [.bin](https://github.com/isl-org/MiDaS/releases/download/v3_1/openvino_midas_v21_small_256.bin)
-
-MiDaS 3.0: Legacy transformer models [dpt_large_384](https://github.com/isl-org/MiDaS/releases/download/v3/dpt_large_384.pt) and [dpt_hybrid_384](https://github.com/isl-org/MiDaS/releases/download/v3/dpt_hybrid_384.pt)
-
-MiDaS 2.1: Legacy convolutional models [midas_v21_384](https://github.com/isl-org/MiDaS/releases/download/v2_1/midas_v21_384.pt) and [midas_v21_small_256](https://github.com/isl-org/MiDaS/releases/download/v2_1/midas_v21_small_256.pt)
-
-1) Set up dependencies:
-
- ```shell
- conda env create -f environment.yaml
- conda activate midas-py310
- ```
-
-#### optional
-
-For the Next-ViT model, execute
-
-```shell
-git submodule add https://github.com/isl-org/Next-ViT midas/external/next_vit
-```
-
-For the OpenVINO model, install
-
-```shell
-pip install openvino
-```
-
-### Usage
-
-1) Place one or more input images in the folder `input`.
-
-2) Run the model with
-
- ```shell
- python run.py --model_type --input_path input --output_path output
- ```
- where `````` is chosen from [dpt_beit_large_512](#model_type), [dpt_beit_large_384](#model_type),
- [dpt_beit_base_384](#model_type), [dpt_swin2_large_384](#model_type), [dpt_swin2_base_384](#model_type),
- [dpt_swin2_tiny_256](#model_type), [dpt_swin_large_384](#model_type), [dpt_next_vit_large_384](#model_type),
- [dpt_levit_224](#model_type), [dpt_large_384](#model_type), [dpt_hybrid_384](#model_type),
- [midas_v21_384](#model_type), [midas_v21_small_256](#model_type), [openvino_midas_v21_small_256](#model_type).
-
-3) The resulting depth maps are written to the `output` folder.
-
-#### optional
-
-1) By default, the inference resizes the height of input images to the size of a model to fit into the encoder. This
- size is given by the numbers in the model names of the [accuracy table](#accuracy). Some models do not only support a single
- inference height but a range of different heights. Feel free to explore different heights by appending the extra
- command line argument `--height`. Unsupported height values will throw an error. Note that using this argument may
- decrease the model accuracy.
-2) By default, the inference keeps the aspect ratio of input images when feeding them into the encoder if this is
- supported by a model (all models except for Swin, Swin2, LeViT). In order to resize to a square resolution,
- disregarding the aspect ratio while preserving the height, use the command line argument `--square`.
-
-#### via Camera
-
- If you want the input images to be grabbed from the camera and shown in a window, leave the input and output paths
- away and choose a model type as shown above:
-
- ```shell
- python run.py --model_type --side
- ```
-
- The argument `--side` is optional and causes both the input RGB image and the output depth map to be shown
- side-by-side for comparison.
-
-#### via Docker
-
-1) Make sure you have installed Docker and the
- [NVIDIA Docker runtime](https://github.com/NVIDIA/nvidia-docker/wiki/Installation-\(Native-GPU-Support\)).
-
-2) Build the Docker image:
-
- ```shell
- docker build -t midas .
- ```
-
-3) Run inference:
-
- ```shell
- docker run --rm --gpus all -v $PWD/input:/opt/MiDaS/input -v $PWD/output:/opt/MiDaS/output -v $PWD/weights:/opt/MiDaS/weights midas
- ```
-
- This command passes through all of your NVIDIA GPUs to the container, mounts the
- `input` and `output` directories and then runs the inference.
-
-#### via PyTorch Hub
-
-The pretrained model is also available on [PyTorch Hub](https://pytorch.org/hub/intelisl_midas_v2/)
-
-#### via TensorFlow or ONNX
-
-See [README](https://github.com/isl-org/MiDaS/tree/master/tf) in the `tf` subdirectory.
-
-Currently only supports MiDaS v2.1.
-
-
-#### via Mobile (iOS / Android)
-
-See [README](https://github.com/isl-org/MiDaS/tree/master/mobile) in the `mobile` subdirectory.
-
-#### via ROS1 (Robot Operating System)
-
-See [README](https://github.com/isl-org/MiDaS/tree/master/ros) in the `ros` subdirectory.
-
-Currently only supports MiDaS v2.1. DPT-based models to be added.
-
-
-### Accuracy
-
-We provide a **zero-shot error** $\epsilon_d$ which is evaluated for 6 different datasets
-(see [paper](https://arxiv.org/abs/1907.01341v3)). **Lower error values are better**.
-$\color{green}{\textsf{Overall model quality is represented by the improvement}}$ ([Imp.](#improvement)) with respect to
-MiDaS 3.0 DPTL-384. The models are grouped by the height used for inference, whereas the square training resolution is given by
-the numbers in the model names. The table also shows the **number of parameters** (in millions) and the
-**frames per second** for inference at the training resolution (for GPU RTX 3090):
-
-| MiDaS Model | DIW WHDR | Eth3d AbsRel | Sintel AbsRel | TUM δ1 | KITTI δ1 | NYUv2 δ1 | $\color{green}{\textsf{Imp.}}$ % | Par.M | FPS |
-|-----------------------------------------------------------------------------------------------------------------------|-------------------------:|-----------------------------:|------------------------------:|-------------------------:|-------------------------:|-------------------------:|-------------------------------------------------:|----------------------:|--------------------------:|
-| **Inference height 512** | | | | | | | | | |
-| [v3.1 BEiTL-512](https://github.com/isl-org/MiDaS/releases/download/v3_1/dpt_beit_large_512.pt) | 0.1137 | 0.0659 | 0.2366 | **6.13** | 11.56* | **1.86*** | $\color{green}{\textsf{19}}$ | **345** | **5.7** |
-| [v3.1 BEiTL-512](https://github.com/isl-org/MiDaS/releases/download/v3_1/dpt_beit_large_512.pt)$\tiny{\square}$ | **0.1121** | **0.0614** | **0.2090** | 6.46 | **5.00*** | 1.90* | $\color{green}{\textsf{34}}$ | **345** | **5.7** |
-| | | | | | | | | | |
-| **Inference height 384** | | | | | | | | | |
-| [v3.1 BEiTL-512](https://github.com/isl-org/MiDaS/releases/download/v3_1/dpt_beit_large_512.pt) | 0.1245 | 0.0681 | **0.2176** | **6.13** | 6.28* | **2.16*** | $\color{green}{\textsf{28}}$ | 345 | 12 |
-| [v3.1 Swin2L-384](https://github.com/isl-org/MiDaS/releases/download/v3_1/dpt_swin2_large_384.pt)$\tiny{\square}$ | 0.1106 | 0.0732 | 0.2442 | 8.87 | **5.84*** | 2.92* | $\color{green}{\textsf{22}}$ | 213 | 41 |
-| [v3.1 Swin2B-384](https://github.com/isl-org/MiDaS/releases/download/v3_1/dpt_swin2_base_384.pt)$\tiny{\square}$ | 0.1095 | 0.0790 | 0.2404 | 8.93 | 5.97* | 3.28* | $\color{green}{\textsf{22}}$ | 102 | 39 |
-| [v3.1 SwinL-384](https://github.com/isl-org/MiDaS/releases/download/v3_1/dpt_swin_large_384.pt)$\tiny{\square}$ | 0.1126 | 0.0853 | 0.2428 | 8.74 | 6.60* | 3.34* | $\color{green}{\textsf{17}}$ | 213 | 49 |
-| [v3.1 BEiTL-384](https://github.com/isl-org/MiDaS/releases/download/v3_1/dpt_beit_large_384.pt) | 0.1239 | **0.0667** | 0.2545 | 7.17 | 9.84* | 2.21* | $\color{green}{\textsf{17}}$ | 344 | 13 |
-| [v3.1 Next-ViTL-384](https://github.com/isl-org/MiDaS/releases/download/v3_1/dpt_next_vit_large_384.pt) | **0.1031** | 0.0954 | 0.2295 | 9.21 | 6.89* | 3.47* | $\color{green}{\textsf{16}}$ | **72** | 30 |
-| [v3.1 BEiTB-384](https://github.com/isl-org/MiDaS/releases/download/v3_1/dpt_beit_base_384.pt) | 0.1159 | 0.0967 | 0.2901 | 9.88 | 26.60* | 3.91* | $\color{green}{\textsf{-31}}$ | 112 | 31 |
-| [v3.0 DPTL-384](https://github.com/isl-org/MiDaS/releases/download/v3/dpt_large_384.pt) | 0.1082 | 0.0888 | 0.2697 | 9.97 | 8.46 | 8.32 | $\color{green}{\textsf{0}}$ | 344 | **61** |
-| [v3.0 DPTH-384](https://github.com/isl-org/MiDaS/releases/download/v3/dpt_hybrid_384.pt) | 0.1106 | 0.0934 | 0.2741 | 10.89 | 11.56 | 8.69 | $\color{green}{\textsf{-10}}$ | 123 | 50 |
-| [v2.1 Large384](https://github.com/isl-org/MiDaS/releases/download/v2_1/midas_v21_384.pt) | 0.1295 | 0.1155 | 0.3285 | 12.51 | 16.08 | 8.71 | $\color{green}{\textsf{-32}}$ | 105 | 47 |
-| | | | | | | | | | |
-| **Inference height 256** | | | | | | | | | |
-| [v3.1 Swin2T-256](https://github.com/isl-org/MiDaS/releases/download/v3_1/dpt_swin2_tiny_256.pt)$\tiny{\square}$ | **0.1211** | **0.1106** | **0.2868** | **13.43** | **10.13*** | **5.55*** | $\color{green}{\textsf{-11}}$ | 42 | 64 |
-| [v2.1 Small256](https://github.com/isl-org/MiDaS/releases/download/v2_1/midas_v21_small_256.pt) | 0.1344 | 0.1344 | 0.3370 | 14.53 | 29.27 | 13.43 | $\color{green}{\textsf{-76}}$ | **21** | **90** |
-| | | | | | | | | | |
-| **Inference height 224** | | | | | | | | | |
-| [v3.1 LeViT224](https://github.com/isl-org/MiDaS/releases/download/v3_1/dpt_levit_224.pt)$\tiny{\square}$ | **0.1314** | **0.1206** | **0.3148** | **18.21** | **15.27*** | **8.64*** | $\color{green}{\textsf{-40}}$ | **51** | **73** |
-
-* No zero-shot error, because models are also trained on KITTI and NYU Depth V2\
-$\square$ Validation performed at **square resolution**, either because the transformer encoder backbone of a model
-does not support non-square resolutions (Swin, Swin2, LeViT) or for comparison with these models. All other
-validations keep the aspect ratio. A difference in resolution limits the comparability of the zero-shot error and the
-improvement, because these quantities are averages over the pixels of an image and do not take into account the
-advantage of more details due to a higher resolution.\
-Best values per column and same validation height in bold
-
-#### Improvement
-
-The improvement in the above table is defined as the relative zero-shot error with respect to MiDaS v3.0
-DPTL-384 and averaging over the datasets. So, if $\epsilon_d$ is the zero-shot error for dataset $d$, then
-the $\color{green}{\textsf{improvement}}$ is given by $100(1-(1/6)\sum_d\epsilon_d/\epsilon_{d,\rm{DPT_{L-384}}})$%.
-
-Note that the improvements of 10% for MiDaS v2.0 → v2.1 and 21% for MiDaS v2.1 → v3.0 are not visible from the
-improvement column (Imp.) in the table but would require an evaluation with respect to MiDaS v2.1 Large384
-and v2.0 Large384 respectively instead of v3.0 DPTL-384.
-
-### Depth map comparison
-
-Zoom in for better visibility
-
-
-### Speed on Camera Feed
-
-Test configuration
-- Windows 10
-- 11th Gen Intel Core i7-1185G7 3.00GHz
-- 16GB RAM
-- Camera resolution 640x480
-- openvino_midas_v21_small_256
-
-Speed: 22 FPS
-
-### Changelog
-
-* [Dec 2022] Released MiDaS v3.1:
- - New models based on 5 different types of transformers ([BEiT](https://arxiv.org/pdf/2106.08254.pdf), [Swin2](https://arxiv.org/pdf/2111.09883.pdf), [Swin](https://arxiv.org/pdf/2103.14030.pdf), [Next-ViT](https://arxiv.org/pdf/2207.05501.pdf), [LeViT](https://arxiv.org/pdf/2104.01136.pdf))
- - Training datasets extended from 10 to 12, including also KITTI and NYU Depth V2 using [BTS](https://github.com/cleinc/bts) split
- - Best model, BEiTLarge 512, with resolution 512x512, is on average about [28% more accurate](#Accuracy) than MiDaS v3.0
- - Integrated live depth estimation from camera feed
-* [Sep 2021] Integrated to [Huggingface Spaces](https://huggingface.co/spaces) with [Gradio](https://github.com/gradio-app/gradio). See [Gradio Web Demo](https://huggingface.co/spaces/akhaliq/DPT-Large).
-* [Apr 2021] Released MiDaS v3.0:
- - New models based on [Dense Prediction Transformers](https://arxiv.org/abs/2103.13413) are on average [21% more accurate](#Accuracy) than MiDaS v2.1
- - Additional models can be found [here](https://github.com/isl-org/DPT)
-* [Nov 2020] Released MiDaS v2.1:
- - New model that was trained on 10 datasets and is on average about [10% more accurate](#Accuracy) than [MiDaS v2.0](https://github.com/isl-org/MiDaS/releases/tag/v2)
- - New light-weight model that achieves [real-time performance](https://github.com/isl-org/MiDaS/tree/master/mobile) on mobile platforms.
- - Sample applications for [iOS](https://github.com/isl-org/MiDaS/tree/master/mobile/ios) and [Android](https://github.com/isl-org/MiDaS/tree/master/mobile/android)
- - [ROS package](https://github.com/isl-org/MiDaS/tree/master/ros) for easy deployment on robots
-* [Jul 2020] Added TensorFlow and ONNX code. Added [online demo](http://35.202.76.57/).
-* [Dec 2019] Released new version of MiDaS - the new model is significantly more accurate and robust
-* [Jul 2019] Initial release of MiDaS ([Link](https://github.com/isl-org/MiDaS/releases/tag/v1))
-
-### Citation
-
-Please cite our paper if you use this code or any of the models:
-```
-@ARTICLE {Ranftl2022,
- author = "Ren\'{e} Ranftl and Katrin Lasinger and David Hafner and Konrad Schindler and Vladlen Koltun",
- title = "Towards Robust Monocular Depth Estimation: Mixing Datasets for Zero-Shot Cross-Dataset Transfer",
- journal = "IEEE Transactions on Pattern Analysis and Machine Intelligence",
- year = "2022",
- volume = "44",
- number = "3"
-}
-```
-
-If you use a DPT-based model, please also cite:
-
-```
-@article{Ranftl2021,
- author = {Ren\'{e} Ranftl and Alexey Bochkovskiy and Vladlen Koltun},
- title = {Vision Transformers for Dense Prediction},
- journal = {ICCV},
- year = {2021},
-}
-```
-
-### Acknowledgements
-
-Our work builds on and uses code from [timm](https://github.com/rwightman/pytorch-image-models) and [Next-ViT](https://github.com/bytedance/Next-ViT).
-We'd like to thank the authors for making these libraries available.
-
-### License
-
-MIT License
diff --git a/spaces/coreml-community/ControlNet-v1-1-Annotators-cpu/annotator/zoe/zoedepth/models/base_models/midas_repo/mobile/android/lib_task_api/src/main/java/org/tensorflow/lite/examples/classification/tflite/Classifier.java b/spaces/coreml-community/ControlNet-v1-1-Annotators-cpu/annotator/zoe/zoedepth/models/base_models/midas_repo/mobile/android/lib_task_api/src/main/java/org/tensorflow/lite/examples/classification/tflite/Classifier.java
deleted file mode 100644
index 45da52a0d0dfa203255e0f2d44901ee0618e739f..0000000000000000000000000000000000000000
--- a/spaces/coreml-community/ControlNet-v1-1-Annotators-cpu/annotator/zoe/zoedepth/models/base_models/midas_repo/mobile/android/lib_task_api/src/main/java/org/tensorflow/lite/examples/classification/tflite/Classifier.java
+++ /dev/null
@@ -1,278 +0,0 @@
-/* Copyright 2019 The TensorFlow Authors. All Rights Reserved.
-
-Licensed under the Apache License, Version 2.0 (the "License");
-you may not use this file except in compliance with the License.
-You may obtain a copy of the License at
-
- http://www.apache.org/licenses/LICENSE-2.0
-
-Unless required by applicable law or agreed to in writing, software
-distributed under the License is distributed on an "AS IS" BASIS,
-WITHOUT WARRANTIES OR CONDITIONS OF ANY KIND, either express or implied.
-See the License for the specific language governing permissions and
-limitations under the License.
-==============================================================================*/
-
-package org.tensorflow.lite.examples.classification.tflite;
-
-import static java.lang.Math.min;
-
-import android.app.Activity;
-import android.graphics.Bitmap;
-import android.graphics.Rect;
-import android.graphics.RectF;
-import android.os.SystemClock;
-import android.os.Trace;
-import android.util.Log;
-import java.io.IOException;
-import java.nio.MappedByteBuffer;
-import java.util.ArrayList;
-import java.util.List;
-import org.tensorflow.lite.examples.classification.tflite.Classifier.Device;
-import org.tensorflow.lite.support.common.FileUtil;
-import org.tensorflow.lite.support.image.TensorImage;
-import org.tensorflow.lite.support.label.Category;
-import org.tensorflow.lite.support.metadata.MetadataExtractor;
-import org.tensorflow.lite.task.core.vision.ImageProcessingOptions;
-import org.tensorflow.lite.task.core.vision.ImageProcessingOptions.Orientation;
-import org.tensorflow.lite.task.vision.classifier.Classifications;
-import org.tensorflow.lite.task.vision.classifier.ImageClassifier;
-import org.tensorflow.lite.task.vision.classifier.ImageClassifier.ImageClassifierOptions;
-
-/** A classifier specialized to label images using TensorFlow Lite. */
-public abstract class Classifier {
- public static final String TAG = "ClassifierWithTaskApi";
-
- /** The model type used for classification. */
- public enum Model {
- FLOAT_MOBILENET,
- QUANTIZED_MOBILENET,
- FLOAT_EFFICIENTNET,
- QUANTIZED_EFFICIENTNET
- }
-
- /** The runtime device type used for executing classification. */
- public enum Device {
- CPU,
- NNAPI,
- GPU
- }
-
- /** Number of results to show in the UI. */
- private static final int MAX_RESULTS = 3;
-
- /** Image size along the x axis. */
- private final int imageSizeX;
-
- /** Image size along the y axis. */
- private final int imageSizeY;
- /** An instance of the driver class to run model inference with Tensorflow Lite. */
- protected final ImageClassifier imageClassifier;
-
- /**
- * Creates a classifier with the provided configuration.
- *
- * @param activity The current Activity.
- * @param model The model to use for classification.
- * @param device The device to use for classification.
- * @param numThreads The number of threads to use for classification.
- * @return A classifier with the desired configuration.
- */
- public static Classifier create(Activity activity, Model model, Device device, int numThreads)
- throws IOException {
- if (model == Model.QUANTIZED_MOBILENET) {
- return new ClassifierQuantizedMobileNet(activity, device, numThreads);
- } else if (model == Model.FLOAT_MOBILENET) {
- return new ClassifierFloatMobileNet(activity, device, numThreads);
- } else if (model == Model.FLOAT_EFFICIENTNET) {
- return new ClassifierFloatEfficientNet(activity, device, numThreads);
- } else if (model == Model.QUANTIZED_EFFICIENTNET) {
- return new ClassifierQuantizedEfficientNet(activity, device, numThreads);
- } else {
- throw new UnsupportedOperationException();
- }
- }
-
- /** An immutable result returned by a Classifier describing what was recognized. */
- public static class Recognition {
- /**
- * A unique identifier for what has been recognized. Specific to the class, not the instance of
- * the object.
- */
- private final String id;
-
- /** Display name for the recognition. */
- private final String title;
-
- /**
- * A sortable score for how good the recognition is relative to others. Higher should be better.
- */
- private final Float confidence;
-
- /** Optional location within the source image for the location of the recognized object. */
- private RectF location;
-
- public Recognition(
- final String id, final String title, final Float confidence, final RectF location) {
- this.id = id;
- this.title = title;
- this.confidence = confidence;
- this.location = location;
- }
-
- public String getId() {
- return id;
- }
-
- public String getTitle() {
- return title;
- }
-
- public Float getConfidence() {
- return confidence;
- }
-
- public RectF getLocation() {
- return new RectF(location);
- }
-
- public void setLocation(RectF location) {
- this.location = location;
- }
-
- @Override
- public String toString() {
- String resultString = "";
- if (id != null) {
- resultString += "[" + id + "] ";
- }
-
- if (title != null) {
- resultString += title + " ";
- }
-
- if (confidence != null) {
- resultString += String.format("(%.1f%%) ", confidence * 100.0f);
- }
-
- if (location != null) {
- resultString += location + " ";
- }
-
- return resultString.trim();
- }
- }
-
- /** Initializes a {@code Classifier}. */
- protected Classifier(Activity activity, Device device, int numThreads) throws IOException {
- if (device != Device.CPU || numThreads != 1) {
- throw new IllegalArgumentException(
- "Manipulating the hardware accelerators and numbers of threads is not allowed in the Task"
- + " library currently. Only CPU + single thread is allowed.");
- }
-
- // Create the ImageClassifier instance.
- ImageClassifierOptions options =
- ImageClassifierOptions.builder().setMaxResults(MAX_RESULTS).build();
- imageClassifier = ImageClassifier.createFromFileAndOptions(activity, getModelPath(), options);
- Log.d(TAG, "Created a Tensorflow Lite Image Classifier.");
-
- // Get the input image size information of the underlying tflite model.
- MappedByteBuffer tfliteModel = FileUtil.loadMappedFile(activity, getModelPath());
- MetadataExtractor metadataExtractor = new MetadataExtractor(tfliteModel);
- // Image shape is in the format of {1, height, width, 3}.
- int[] imageShape = metadataExtractor.getInputTensorShape(/*inputIndex=*/ 0);
- imageSizeY = imageShape[1];
- imageSizeX = imageShape[2];
- }
-
- /** Runs inference and returns the classification results. */
- public List recognizeImage(final Bitmap bitmap, int sensorOrientation) {
- // Logs this method so that it can be analyzed with systrace.
- Trace.beginSection("recognizeImage");
-
- TensorImage inputImage = TensorImage.fromBitmap(bitmap);
- int width = bitmap.getWidth();
- int height = bitmap.getHeight();
- int cropSize = min(width, height);
- // TODO(b/169379396): investigate the impact of the resize algorithm on accuracy.
- // Task Library resize the images using bilinear interpolation, which is slightly different from
- // the nearest neighbor sampling algorithm used in lib_support. See
- // https://github.com/tensorflow/examples/blob/0ef3d93e2af95d325c70ef3bcbbd6844d0631e07/lite/examples/image_classification/android/lib_support/src/main/java/org/tensorflow/lite/examples/classification/tflite/Classifier.java#L310.
- ImageProcessingOptions imageOptions =
- ImageProcessingOptions.builder()
- .setOrientation(getOrientation(sensorOrientation))
- // Set the ROI to the center of the image.
- .setRoi(
- new Rect(
- /*left=*/ (width - cropSize) / 2,
- /*top=*/ (height - cropSize) / 2,
- /*right=*/ (width + cropSize) / 2,
- /*bottom=*/ (height + cropSize) / 2))
- .build();
-
- // Runs the inference call.
- Trace.beginSection("runInference");
- long startTimeForReference = SystemClock.uptimeMillis();
- List results = imageClassifier.classify(inputImage, imageOptions);
- long endTimeForReference = SystemClock.uptimeMillis();
- Trace.endSection();
- Log.v(TAG, "Timecost to run model inference: " + (endTimeForReference - startTimeForReference));
-
- Trace.endSection();
-
- return getRecognitions(results);
- }
-
- /** Closes the interpreter and model to release resources. */
- public void close() {
- if (imageClassifier != null) {
- imageClassifier.close();
- }
- }
-
- /** Get the image size along the x axis. */
- public int getImageSizeX() {
- return imageSizeX;
- }
-
- /** Get the image size along the y axis. */
- public int getImageSizeY() {
- return imageSizeY;
- }
-
- /**
- * Converts a list of {@link Classifications} objects into a list of {@link Recognition} objects
- * to match the interface of other inference method, such as using the TFLite
- * Support Library..
- */
- private static List getRecognitions(List classifications) {
-
- final ArrayList recognitions = new ArrayList<>();
- // All the demo models are single head models. Get the first Classifications in the results.
- for (Category category : classifications.get(0).getCategories()) {
- recognitions.add(
- new Recognition(
- "" + category.getLabel(), category.getLabel(), category.getScore(), null));
- }
- return recognitions;
- }
-
- /* Convert the camera orientation in degree into {@link ImageProcessingOptions#Orientation}.*/
- private static Orientation getOrientation(int cameraOrientation) {
- switch (cameraOrientation / 90) {
- case 3:
- return Orientation.BOTTOM_LEFT;
- case 2:
- return Orientation.BOTTOM_RIGHT;
- case 1:
- return Orientation.TOP_RIGHT;
- default:
- return Orientation.TOP_LEFT;
- }
- }
-
- /** Gets the name of the model file stored in Assets. */
- protected abstract String getModelPath();
-}
diff --git a/spaces/cymic/Talking_Head_Anime_3/tha3/nn/common/__init__.py b/spaces/cymic/Talking_Head_Anime_3/tha3/nn/common/__init__.py
deleted file mode 100644
index e69de29bb2d1d6434b8b29ae775ad8c2e48c5391..0000000000000000000000000000000000000000
diff --git a/spaces/daddyjin/TalkingFaceGeneration/FONT/modules/keypoint_detector.py b/spaces/daddyjin/TalkingFaceGeneration/FONT/modules/keypoint_detector.py
deleted file mode 100644
index c92b0bb77bd08b6612ff11c8820afe5e5ee115eb..0000000000000000000000000000000000000000
--- a/spaces/daddyjin/TalkingFaceGeneration/FONT/modules/keypoint_detector.py
+++ /dev/null
@@ -1,260 +0,0 @@
-from torch import nn
-import torch
-import torch.nn.functional as F
-from .util import Hourglass, make_coordinate_grid, AntiAliasInterpolation2d, Ct_encoder, EmotionNet, AF2F, AF2F_s, draw_heatmap
-
-
-class KPDetector(nn.Module):
- """
- Detecting a keypoints. Return keypoint position and jacobian near each keypoint.
- """
-
- def __init__(self, block_expansion, num_kp, num_channels, max_features,
- num_blocks, temperature, estimate_jacobian=False, scale_factor=1,
- single_jacobian_map=False, pad=0):
- super(KPDetector, self).__init__()
-
- self.predictor = Hourglass(block_expansion, in_features=num_channels,
- max_features=max_features, num_blocks=num_blocks)
-
- self.kp = nn.Conv2d(in_channels=self.predictor.out_filters, out_channels=num_kp, kernel_size=(7, 7),
- padding=pad)
-
- if estimate_jacobian:
- self.num_jacobian_maps = 1 if single_jacobian_map else num_kp
- self.jacobian = nn.Conv2d(in_channels=self.predictor.out_filters,
- out_channels=4 * self.num_jacobian_maps, kernel_size=(7, 7), padding=pad)
- self.jacobian.weight.data.zero_()
- self.jacobian.bias.data.copy_(torch.tensor([1, 0, 0, 1] * self.num_jacobian_maps, dtype=torch.float))
- else:
- self.jacobian = None
-
- self.temperature = temperature
- self.scale_factor = scale_factor
- if self.scale_factor != 1:
- self.down = AntiAliasInterpolation2d(num_channels, self.scale_factor)
-
-
-
-
- def gaussian2kp(self, heatmap):
- """
- Extract the mean and from a heatmap
- """
- shape = heatmap.shape
- heatmap = heatmap.unsqueeze(-1) #[4,10,58,58,1]
- grid = make_coordinate_grid(shape[2:], heatmap.type()).unsqueeze_(0).unsqueeze_(0) #[1,1,58,58,2]
- value = (heatmap * grid).sum(dim=(2, 3)) #[4,10,2]
- kp = {'value': value}
-
- return kp
-
- def audio_feature(self, x, heatmap):
-
- # prediction = self.kp(x) #[4,10,H/4-6, W/4-6]
-
- # final_shape = prediction.shape
- # heatmap = prediction.view(final_shape[0], final_shape[1], -1) #[4, 10, 58*58]
- # heatmap = F.softmax(heatmap / self.temperature, dim=2)
- # heatmap = heatmap.view(*final_shape) #[4,10,58,58]
-
- # out = self.gaussian2kp(heatmap)
- final_shape = heatmap.squeeze(2).shape
-
- if self.jacobian is not None:
- jacobian_map = self.jacobian(x) ##[4,40,H/4-6, W/4-6]
- jacobian_map = jacobian_map.reshape(final_shape[0], self.num_jacobian_maps, 4, final_shape[2],
- final_shape[3])
- heatmap = heatmap.unsqueeze(2)
-
- jacobian = heatmap * jacobian_map #[4,10,4,H/4-6, W/4-6]
- jacobian = jacobian.view(final_shape[0], final_shape[1], 4, -1)
- jacobian = jacobian.sum(dim=-1) #[4,10,4]
- jacobian = jacobian.view(jacobian.shape[0], jacobian.shape[1], 2, 2) #[4,10,2,2]
-
- return jacobian
-
- def forward(self, x): #torch.Size([4, 3, H, W])
- if self.scale_factor != 1:
- x = self.down(x) # 0.25 [4, 3, H/4, W/4]
-
- feature_map = self.predictor(x) #[4,3+32,H/4, W/4]
- prediction = self.kp(feature_map) #[4,10,H/4-6, W/4-6]
-
- final_shape = prediction.shape
-
- heatmap = prediction.view(final_shape[0], final_shape[1], -1) #[4, 10, 58*58]
- heatmap = F.softmax(heatmap / self.temperature, dim=2)
- heatmap = heatmap.view(*final_shape) #[4,10,58,58]
-
- out = self.gaussian2kp(heatmap)
- out['heatmap'] = heatmap
-
- if self.jacobian is not None:
- jacobian_map = self.jacobian(feature_map) ##[4,40,H/4-6, W/4-6]
- jacobian_map = jacobian_map.reshape(final_shape[0], self.num_jacobian_maps, 4, final_shape[2],
- final_shape[3])
- heatmap = heatmap.unsqueeze(2)
-
- jacobian = heatmap * jacobian_map #[4,10,4,H/4-6, W/4-6]
- jacobian = jacobian.view(final_shape[0], final_shape[1], 4, -1)
- jacobian = jacobian.sum(dim=-1) #[4,10,4]
- jacobian = jacobian.view(jacobian.shape[0], jacobian.shape[1], 2, 2) #[4,10,2,2]
- out['jacobian'] = jacobian
-
- return out
-
-
-
-
-class KPDetector_a(nn.Module):
- """
- Detecting a keypoints. Return keypoint position and jacobian near each keypoint.
- """
-
- def __init__(self, block_expansion, num_kp, num_channels,num_channels_a, max_features,
- num_blocks, temperature, estimate_jacobian=False, scale_factor=1,
- single_jacobian_map=False, pad=0):
- super(KPDetector_a, self).__init__()
-
- self.predictor = Hourglass(block_expansion, in_features=num_channels_a,
- max_features=max_features, num_blocks=num_blocks)
-
- self.kp = nn.Conv2d(in_channels=self.predictor.out_filters, out_channels=num_kp, kernel_size=(7, 7),
- padding=pad)
-
- if estimate_jacobian:
- self.num_jacobian_maps = 1 if single_jacobian_map else num_kp
- self.jacobian = nn.Conv2d(in_channels=self.predictor.out_filters,
- out_channels=4 * self.num_jacobian_maps, kernel_size=(7, 7), padding=pad)
- self.jacobian.weight.data.zero_()
- self.jacobian.bias.data.copy_(torch.tensor([1, 0, 0, 1] * self.num_jacobian_maps, dtype=torch.float))
- else:
- self.jacobian = None
-
- self.temperature = temperature
- self.scale_factor = scale_factor
- if self.scale_factor != 1:
- self.down = AntiAliasInterpolation2d(num_channels, self.scale_factor)
-
-
-
-
- def gaussian2kp(self, heatmap):
- """
- Extract the mean and from a heatmap
- """
- shape = heatmap.shape
- heatmap = heatmap.unsqueeze(-1) #[4,10,58,58,1]
- grid = make_coordinate_grid(shape[2:], heatmap.type()).unsqueeze_(0).unsqueeze_(0) #[1,1,58,58,2]
- value = (heatmap * grid).sum(dim=(2, 3)) #[4,10,2]
- kp = {'value': value}
-
- return kp
-
- def audio_feature(self, x, heatmap):
-
- # prediction = self.kp(x) #[4,10,H/4-6, W/4-6]
-
- # final_shape = prediction.shape
- # heatmap = prediction.view(final_shape[0], final_shape[1], -1) #[4, 10, 58*58]
- # heatmap = F.softmax(heatmap / self.temperature, dim=2)
- # heatmap = heatmap.view(*final_shape) #[4,10,58,58]
-
- # out = self.gaussian2kp(heatmap)
- final_shape = heatmap.squeeze(2).shape
-
- if self.jacobian is not None:
- jacobian_map = self.jacobian(x) ##[4,40,H/4-6, W/4-6]
- jacobian_map = jacobian_map.reshape(final_shape[0], self.num_jacobian_maps, 4, final_shape[2],
- final_shape[3])
- heatmap = heatmap.unsqueeze(2)
-
- jacobian = heatmap * jacobian_map #[4,10,4,H/4-6, W/4-6]
- jacobian = jacobian.view(final_shape[0], final_shape[1], 4, -1)
- jacobian = jacobian.sum(dim=-1) #[4,10,4]
- jacobian = jacobian.view(jacobian.shape[0], jacobian.shape[1], 2, 2) #[4,10,2,2]
-
- return jacobian
-
- def forward(self, feature_map): #torch.Size([4, 3, H, W])
-
-
- prediction = self.kp(feature_map) #[4,10,H/4-6, W/4-6]
-
- final_shape = prediction.shape
-
- heatmap = prediction.view(final_shape[0], final_shape[1], -1) #[4, 10, 58*58]
- heatmap = F.softmax(heatmap / self.temperature, dim=2)
- heatmap = heatmap.view(*final_shape) #[4,10,58,58]
-
- out = self.gaussian2kp(heatmap)
- out['heatmap'] = heatmap #B,10,58,58
-
- if self.jacobian is not None:
- jacobian_map = self.jacobian(feature_map) ##[4,40,H/4-6, W/4-6]
- jacobian_map = jacobian_map.reshape(final_shape[0], self.num_jacobian_maps, 4, final_shape[2],
- final_shape[3])
- heatmap = heatmap.unsqueeze(2)
-
- jacobian = heatmap * jacobian_map #[4,10,4,H/4-6, W/4-6]
- jacobian = jacobian.view(final_shape[0], final_shape[1], 4, -1)
- jacobian = jacobian.sum(dim=-1) #[4,10,4]
- jacobian = jacobian.view(jacobian.shape[0], jacobian.shape[1], 2, 2) #[4,10,2,2]
- out['jacobian'] = jacobian #B,10,2,2
-
- return out
-
-
-class Audio_Feature(nn.Module):
- def __init__(self):
- super(Audio_Feature, self).__init__()
-
- self.con_encoder = Ct_encoder()
- self.emo_encoder = EmotionNet()
- self.decoder = AF2F_s()
-
-
-
- def forward(self, x):
- x = x.unsqueeze(1)
-
- c = self.con_encoder(x)
- e = self.emo_encoder(x)
-
- # d = torch.cat([c, e], dim=1)
- d = self.decoder(c)
-
-
- return d
-'''
-def forward(self, x, cube, audio): #torch.Size([4, 3, H, W])
- if self.scale_factor != 1:
- x = self.down(x) # 0.25 [4, 3, H/4, W/4]
-
- cube = cube.unsqueeze(1)
- feature = torch.cat([x,cube,audio],dim=1)
- feature_map = self.predictor(feature) #[4,3+32,H/4, W/4]
- prediction = self.kp(feature_map) #[4,10,H/4-6, W/4-6]
-
- final_shape = prediction.shape
- heatmap = prediction.view(final_shape[0], final_shape[1], -1) #[4, 10, 58*58]
- heatmap = F.softmax(heatmap / self.temperature, dim=2)
- heatmap = heatmap.view(*final_shape) #[4,10,58,58]
-
- out = self.gaussian2kp(heatmap)
- out['heatmap'] = heatmap
- if self.jacobian is not None:
- jacobian_map = self.jacobian(feature_map) ##[4,40,H/4-6, W/4-6]
- jacobian_map = jacobian_map.reshape(final_shape[0], self.num_jacobian_maps, 4, final_shape[2],
- final_shape[3])
- heatmap = heatmap.unsqueeze(2)
-
- jacobian = heatmap * jacobian_map #[4,10,4,H/4-6, W/4-6]
- jacobian = jacobian.view(final_shape[0], final_shape[1], 4, -1)
- jacobian = jacobian.sum(dim=-1) #[4,10,4]
- jacobian = jacobian.view(jacobian.shape[0], jacobian.shape[1], 2, 2) #[4,10,2,2]
- out['jacobian'] = jacobian
-
- return out
-'''
diff --git a/spaces/devthedeveloper/Bark-with-Voice-Cloning/bark/settings.py b/spaces/devthedeveloper/Bark-with-Voice-Cloning/bark/settings.py
deleted file mode 100644
index 81c660f3d2e33b21821583cb34c872c2ca23928b..0000000000000000000000000000000000000000
--- a/spaces/devthedeveloper/Bark-with-Voice-Cloning/bark/settings.py
+++ /dev/null
@@ -1,7 +0,0 @@
-import os
-
-def initenv(args):
- os.environ['SUNO_USE_SMALL_MODELS'] = str("-smallmodels" in args)
- os.environ['BARK_FORCE_CPU'] = str("-forcecpu" in args)
- os.environ['SUNO_ENABLE_MPS'] = str("-enablemps" in args)
- os.environ['SUNO_OFFLOAD_CPU'] = str("-offloadcpu" in args)
diff --git a/spaces/diacanFperku/AutoGPT/Avenged Sevenfold Amplitube Presetl.md b/spaces/diacanFperku/AutoGPT/Avenged Sevenfold Amplitube Presetl.md
deleted file mode 100644
index d4eb401e5371f8426894b854a182691dd65c4628..0000000000000000000000000000000000000000
--- a/spaces/diacanFperku/AutoGPT/Avenged Sevenfold Amplitube Presetl.md
+++ /dev/null
@@ -1,29 +0,0 @@
-
-
How to Get the Avenged Sevenfold Tone with Amplitube 3
-
Avenged Sevenfold is one of the most popular metal bands of the 21st century, known for their melodic and aggressive sound. Their guitar tone is a blend of high-gain distortion, tight low-end, and rich harmonies. If you want to emulate their tone with Amplitube 3, here are some tips and tricks to help you out.
First, you need to choose the right amp model and cabinet. Avenged Sevenfold's guitarists, Synyster Gates and Zacky Vengeance, use custom Schecter guitars with active pickups, which have a lot of output and clarity. They also use Mesa Boogie Dual Rectifier amps, which have a lot of gain and punch. In Amplitube 3, you can use the Metal Lead V amp model, which is based on the Mesa Boogie Mark V. For the cabinet, you can use the 4x12 Metal T1 cab, which is based on the Mesa Boogie Rectifier cabinet.
-
Next, you need to tweak the amp settings to get the right balance of distortion, EQ, and dynamics. Here are some suggested settings:
-
-
Gain: 7
-
Bass: 6
-
Middle: 4
-
Treble: 6
-
Presence: 7
-
Master: 5
-
-
You can adjust these settings according to your taste and guitar. The key is to have enough gain to get a saturated tone, but not too much to lose definition and clarity. You also want to have a scooped midrange to get a heavy sound, but not too much to lose body and warmth. You also want to have a bright presence to cut through the mix, but not too much to sound harsh or fizzy.
-
Finally, you need to add some effects to enhance your tone and create some ambience. Avenged Sevenfold uses a variety of effects, such as chorus, delay, reverb, flanger, phaser, wah, and more. In Amplitube 3, you can use the built-in effects or add your own pedals from the Custom Shop. Here are some suggested effects:
-
-
Chorus: Use a subtle chorus effect to add some width and depth to your tone. You can use the Chorus pedal from the Custom Shop or the Chorus effect from the Rack section. Set the rate and depth to low values and adjust the mix to your liking.
-
Delay: Use a short delay effect to add some space and dimension to your tone. You can use the Digital Delay pedal from the Custom Shop or the Delay effect from the Rack section. Set the time to around 300 ms and adjust the feedback and mix to your liking.
-
Reverb: Use a medium reverb effect to add some ambience and realism to your tone. You can use the Spring Reverb pedal from the Custom Shop or the Reverb effect from the Rack section. Set the type to Hall or Plate and adjust the decay and mix to your liking.
-
-
With these settings, you should be able to get a close approximation of the Avenged Sevenfold tone with Amplitube 3. Of course, you can experiment with different settings and effects to find your own sound. The most important thing is to have fun and rock on!
-
-
-
Now that you have the basic tone of Avenged Sevenfold, you can try to play some of their songs and riffs. Avenged Sevenfold has a diverse and eclectic musical style, ranging from metalcore to hard rock to progressive metal. They are known for their complex and catchy melodies, intricate and fast solos, and dynamic and syncopated rhythms. Some of their most popular songs include "Nightmare", "Hail to the King", "Bat Country", "Afterlife", "The Stage", and "Almost Easy".
-
To play these songs, you need to have a good grasp of guitar techniques, such as alternate picking, sweep picking, tapping, hammer-ons, pull-offs, slides, bends, vibrato, palm muting, and more. You also need to have a good ear for music theory, such as scales, modes, chords, arpeggios, intervals, harmonies, and more. You can find tabs and lessons for these songs online or in books and magazines. You can also watch videos of Avenged Sevenfold's guitarists playing these songs on their official YouTube channel[^1^].
-
One of the best ways to learn these songs is to practice them slowly and accurately at first, then gradually increase the speed and difficulty. You can use a metronome or a backing track to help you keep time and groove. You can also use a looper pedal or a recording software to record yourself playing these songs and listen back to your performance. This way, you can identify your strengths and weaknesses and improve your skills.
d5da3c52bf
-
-
\ No newline at end of file
diff --git a/spaces/diacanFperku/AutoGPT/Gifs Gay Mia Khalifa Porn Movies..md b/spaces/diacanFperku/AutoGPT/Gifs Gay Mia Khalifa Porn Movies..md
deleted file mode 100644
index 8087f67f7e92a3a533052d331391e46cc1881c91..0000000000000000000000000000000000000000
--- a/spaces/diacanFperku/AutoGPT/Gifs Gay Mia Khalifa Porn Movies..md
+++ /dev/null
@@ -1,6 +0,0 @@
-
-
- 1fdad05405
-
-
-
diff --git a/spaces/diacanFperku/AutoGPT/Luvi Night Out Official Video [PATCHED].md b/spaces/diacanFperku/AutoGPT/Luvi Night Out Official Video [PATCHED].md
deleted file mode 100644
index 44a2c295a0c9e86dd1f919a5d05aaadec5e8a564..0000000000000000000000000000000000000000
--- a/spaces/diacanFperku/AutoGPT/Luvi Night Out Official Video [PATCHED].md
+++ /dev/null
@@ -1,19 +0,0 @@
-
-
Luvi Releases New Music Video for Night Out
-
Luvi, a rising pop star, has just dropped a new music video for his song Night Out, which is part of his debut album Love Is Blind. The video, directed by Alex Nazari, features Luvi and his friends having fun in various locations, such as a bowling alley, a rooftop pool, and a nightclub. The video also showcases Luvi's dance moves and charismatic personality.
Night Out is a catchy and upbeat song that celebrates living in the moment and enjoying life. Luvi said that he wanted to make a song that would make people feel good and want to dance. He also said that he was inspired by his own experiences of going out with his friends and having a blast.
-
Luvi is a talented singer, songwriter, and dancer who started his musical journey at a young age. He has been influenced by artists such as Michael Jackson, Bruno Mars, and Justin Timberlake. He has released several singles before his album, such as Crazy For You, All I Need, and Let Me Love You. He has also collaborated with other artists, such as DJ Snake, Zedd, and Selena Gomez.
-
Luvi's fans can watch his new music video for Night Out on YouTube[^1^] or on his official website. They can also follow him on social media platforms such as Facebook, Twitter, and SoundCloud to stay updated on his latest news and projects.
-
-
-
Luvi said that he is very proud of his album Love Is Blind, which he described as a journey of love, heartbreak, and self-discovery. He said that he poured his heart and soul into every song and that he hopes his fans can relate to his stories and emotions. He also said that he worked with some amazing producers and writers who helped him bring his vision to life.
-
Luvi also revealed that he is planning to go on tour soon to perform his songs live for his fans. He said that he is very excited to meet his fans and to share his music with them. He said that he wants to create a memorable and interactive show that will make his fans feel happy and inspired.
-
Luvi thanked his fans for their support and love and said that they are the reason why he does what he does. He said that he hopes his music can make a positive impact on their lives and that he can't wait to see them on the road.
-
-
Luvi's fans have been showing their love and appreciation for his new music video and album on social media. They have been posting comments, tweets, and videos expressing their admiration and excitement for Luvi and his music. Some of them have also been creating fan art, covers, and remixes of his songs.
-
One fan commented on YouTube: "Luvi is amazing! He has such a great voice and style. I love his new video and song. It makes me want to go out and have fun with my friends. He is such an inspiration to me."
-
Another fan tweeted: "Luvi's album is a masterpiece. Every song is a bop and a vibe. He sings with so much passion and emotion. He is the best thing that ever happened to pop music."
-
A third fan posted a video on TikTok: "I'm obsessed with Luvi's music. He is so talented and handsome. I made this dance routine to his song Night Out. I hope he sees it and likes it."
d5da3c52bf
-
-
\ No newline at end of file
diff --git a/spaces/diacanFperku/AutoGPT/Mapinfo 105 Portable Download NEW!.md b/spaces/diacanFperku/AutoGPT/Mapinfo 105 Portable Download NEW!.md
deleted file mode 100644
index 172c0b82b9a5fcb36972a3d84a6570d9d140b28f..0000000000000000000000000000000000000000
--- a/spaces/diacanFperku/AutoGPT/Mapinfo 105 Portable Download NEW!.md
+++ /dev/null
@@ -1,26 +0,0 @@
-
-
-When i try it using dns query from a windows 10 machine it works fine.
-
-I need to run this in azure.
-
-Any help is much appreciated.
-
-A:
-
-Currently Azure Maps support the Google Geocoding API and the Bing Address Search API.
-
-The Geocoding API is for getting latitude, longitude coordinates, while the Address Search API provides a textual representation of an address.
-
-In general, the Address Search API is a good choice when you want to get the street name, city, state, postal code, country etc of a given address, and the Geocoding API is the best choice when you want to get the location information, such as latitude, longitude, address etc, of a given location.
-
-The service type determines the service data type that the Geocoding API returns. The service type can be POI, Location or Address.
-
-For more information, you could check this official document: Azure Maps Geocoding API.
-
-En medio de una sesión conjunta de la Cámara de Diputados y la Comisión Permanente de la Cámara de Diputados, la presentación de la iniciativa conocida como “Eliminación de la discriminación en la Cámara de Diputados” tuvo uno de sus mayores éxitos. Para que el proyecto pase a segunda lectura, tanto el PRI como el PAN también votaron a favor, pues este tema, desde que fue presentado por el diputado Oaxaca Francisco Agrón, es el tema que los priistas más querían, en efecto, tener resuelto para un punto que presentaron a la Mesa de la Cámara de Diputados.
-
-Aclarado, el PRI votó a favor, tal y como lo dijo su líder de las Cortes de la República, Francisco Gómez Morín, quien señaló que en el Senado ya se han discutido muchos temas, pero que es importante que los que votaron a favor entiendan que en este caso se trata de aprobar una propuesta en el recinto legislat 4fefd39f24
-
-
-
diff --git a/spaces/diacanFperku/AutoGPT/Sa-mp-0.3.7-install Money Hack.md b/spaces/diacanFperku/AutoGPT/Sa-mp-0.3.7-install Money Hack.md
deleted file mode 100644
index c1289b89aae672efa51642e776f29bb6948a80cc..0000000000000000000000000000000000000000
--- a/spaces/diacanFperku/AutoGPT/Sa-mp-0.3.7-install Money Hack.md
+++ /dev/null
@@ -1,6 +0,0 @@
-
-
- 4fefd39f24
-
-
-
diff --git a/spaces/duycse1603/math2tex/utils/p2l_utils.py b/spaces/duycse1603/math2tex/utils/p2l_utils.py
deleted file mode 100644
index ffed8567c8dce90b5d8b742fe44917b6e0c2c8af..0000000000000000000000000000000000000000
--- a/spaces/duycse1603/math2tex/utils/p2l_utils.py
+++ /dev/null
@@ -1,71 +0,0 @@
-import math
-import numpy as np
-
-def get_rolling_crops(image, stride = [128, 128], window_size = 512):
- # as of now stride is not implemented
- image_height, image_width, channels = image.shape
-
-
- # Compute the number of rolling windows
- # nwindows_vertical = math.ceil(image_height / window_size)
- # nwindows_horizontal = math.ceil(image_width / window_size)
- nwindows_vertical = math.ceil((image_height - window_size) / stride[0]) + 1
- nwindows_horizontal = math.ceil((image_width - window_size) / stride[1]) + 1
-
- print(f"Number of windows: {nwindows_vertical} x {nwindows_horizontal}")
- crops_list = []
- padded_crops_list = []
- crops_info_list = []
-
- for i in range(nwindows_vertical):
- for j in range(nwindows_horizontal):
- # window_x_start = j * window_size
- window_x_start = j * stride[1]
- window_x_end = min(window_x_start + window_size, image_width)
- # window_y_start = i * window_size
- window_y_start = i * stride[0]
- window_y_end = min(window_y_start + window_size, image_height)
- window_width = window_x_end - window_x_start
- window_height = window_y_end - window_y_start
-
- rolling_window = image[window_y_start:window_y_end, window_x_start:window_x_end]
-
- # create new image of desired size with white background
- color = (255,255,255)
- padded_window = np.full((window_size,window_size, channels), color, dtype=np.uint8)
-
- # compute center offset
- x_center = (window_size - window_width) // 2
- y_center = (window_size - window_height) // 2
-
- # Copy the window to the center of the white square
- padded_window[y_center:y_center+window_height, x_center:x_center+window_width] = rolling_window
-
- crops_list.append(rolling_window)
- padded_crops_list.append(padded_window)
-
- crops_info_list.append((window_x_start, window_y_start, window_width, window_height))
- return crops_list, padded_crops_list, crops_info_list
-
-
-def postprocess(window_borders, scores, crops_info_list, window_size=512):
- bb_list = []
- scores_list = []
-
- for i in range(len(window_borders)):
- window_border = window_borders[i]
- score = scores[i]
- window_x_start, window_y_start, window_width, window_height = crops_info_list[i]
- for k in range(len(window_border)):
-
- x0 = window_x_start+(window_border[k][0]-(window_size-window_width)//2)
- y0 = window_y_start+(window_border[k][1]-(window_size-window_height)//2)
- x1 = window_x_start+(window_border[k][2]-(window_size-window_width)//2)
- y1 = window_y_start+(window_border[k][3]-(window_size-window_height)//2)
-
- bb_list.append([x0, y0, x1, y1])
- scores_list.append(score[k])
- return bb_list, scores_list
-
-if __name__ == "__main__":
- print("hello world")
\ No newline at end of file
diff --git a/spaces/elkraken/Video-Object-Detection/LICENSE.md b/spaces/elkraken/Video-Object-Detection/LICENSE.md
deleted file mode 100644
index f288702d2fa16d3cdf0035b15a9fcbc552cd88e7..0000000000000000000000000000000000000000
--- a/spaces/elkraken/Video-Object-Detection/LICENSE.md
+++ /dev/null
@@ -1,674 +0,0 @@
- GNU GENERAL PUBLIC LICENSE
- Version 3, 29 June 2007
-
- Copyright (C) 2007 Free Software Foundation, Inc.
- Everyone is permitted to copy and distribute verbatim copies
- of this license document, but changing it is not allowed.
-
- Preamble
-
- The GNU General Public License is a free, copyleft license for
-software and other kinds of works.
-
- The licenses for most software and other practical works are designed
-to take away your freedom to share and change the works. By contrast,
-the GNU General Public License is intended to guarantee your freedom to
-share and change all versions of a program--to make sure it remains free
-software for all its users. We, the Free Software Foundation, use the
-GNU General Public License for most of our software; it applies also to
-any other work released this way by its authors. You can apply it to
-your programs, too.
-
- When we speak of free software, we are referring to freedom, not
-price. Our General Public Licenses are designed to make sure that you
-have the freedom to distribute copies of free software (and charge for
-them if you wish), that you receive source code or can get it if you
-want it, that you can change the software or use pieces of it in new
-free programs, and that you know you can do these things.
-
- To protect your rights, we need to prevent others from denying you
-these rights or asking you to surrender the rights. Therefore, you have
-certain responsibilities if you distribute copies of the software, or if
-you modify it: responsibilities to respect the freedom of others.
-
- For example, if you distribute copies of such a program, whether
-gratis or for a fee, you must pass on to the recipients the same
-freedoms that you received. You must make sure that they, too, receive
-or can get the source code. And you must show them these terms so they
-know their rights.
-
- Developers that use the GNU GPL protect your rights with two steps:
-(1) assert copyright on the software, and (2) offer you this License
-giving you legal permission to copy, distribute and/or modify it.
-
- For the developers' and authors' protection, the GPL clearly explains
-that there is no warranty for this free software. For both users' and
-authors' sake, the GPL requires that modified versions be marked as
-changed, so that their problems will not be attributed erroneously to
-authors of previous versions.
-
- Some devices are designed to deny users access to install or run
-modified versions of the software inside them, although the manufacturer
-can do so. This is fundamentally incompatible with the aim of
-protecting users' freedom to change the software. The systematic
-pattern of such abuse occurs in the area of products for individuals to
-use, which is precisely where it is most unacceptable. Therefore, we
-have designed this version of the GPL to prohibit the practice for those
-products. If such problems arise substantially in other domains, we
-stand ready to extend this provision to those domains in future versions
-of the GPL, as needed to protect the freedom of users.
-
- Finally, every program is threatened constantly by software patents.
-States should not allow patents to restrict development and use of
-software on general-purpose computers, but in those that do, we wish to
-avoid the special danger that patents applied to a free program could
-make it effectively proprietary. To prevent this, the GPL assures that
-patents cannot be used to render the program non-free.
-
- The precise terms and conditions for copying, distribution and
-modification follow.
-
- TERMS AND CONDITIONS
-
- 0. Definitions.
-
- "This License" refers to version 3 of the GNU General Public License.
-
- "Copyright" also means copyright-like laws that apply to other kinds of
-works, such as semiconductor masks.
-
- "The Program" refers to any copyrightable work licensed under this
-License. Each licensee is addressed as "you". "Licensees" and
-"recipients" may be individuals or organizations.
-
- To "modify" a work means to copy from or adapt all or part of the work
-in a fashion requiring copyright permission, other than the making of an
-exact copy. The resulting work is called a "modified version" of the
-earlier work or a work "based on" the earlier work.
-
- A "covered work" means either the unmodified Program or a work based
-on the Program.
-
- To "propagate" a work means to do anything with it that, without
-permission, would make you directly or secondarily liable for
-infringement under applicable copyright law, except executing it on a
-computer or modifying a private copy. Propagation includes copying,
-distribution (with or without modification), making available to the
-public, and in some countries other activities as well.
-
- To "convey" a work means any kind of propagation that enables other
-parties to make or receive copies. Mere interaction with a user through
-a computer network, with no transfer of a copy, is not conveying.
-
- An interactive user interface displays "Appropriate Legal Notices"
-to the extent that it includes a convenient and prominently visible
-feature that (1) displays an appropriate copyright notice, and (2)
-tells the user that there is no warranty for the work (except to the
-extent that warranties are provided), that licensees may convey the
-work under this License, and how to view a copy of this License. If
-the interface presents a list of user commands or options, such as a
-menu, a prominent item in the list meets this criterion.
-
- 1. Source Code.
-
- The "source code" for a work means the preferred form of the work
-for making modifications to it. "Object code" means any non-source
-form of a work.
-
- A "Standard Interface" means an interface that either is an official
-standard defined by a recognized standards body, or, in the case of
-interfaces specified for a particular programming language, one that
-is widely used among developers working in that language.
-
- The "System Libraries" of an executable work include anything, other
-than the work as a whole, that (a) is included in the normal form of
-packaging a Major Component, but which is not part of that Major
-Component, and (b) serves only to enable use of the work with that
-Major Component, or to implement a Standard Interface for which an
-implementation is available to the public in source code form. A
-"Major Component", in this context, means a major essential component
-(kernel, window system, and so on) of the specific operating system
-(if any) on which the executable work runs, or a compiler used to
-produce the work, or an object code interpreter used to run it.
-
- The "Corresponding Source" for a work in object code form means all
-the source code needed to generate, install, and (for an executable
-work) run the object code and to modify the work, including scripts to
-control those activities. However, it does not include the work's
-System Libraries, or general-purpose tools or generally available free
-programs which are used unmodified in performing those activities but
-which are not part of the work. For example, Corresponding Source
-includes interface definition files associated with source files for
-the work, and the source code for shared libraries and dynamically
-linked subprograms that the work is specifically designed to require,
-such as by intimate data communication or control flow between those
-subprograms and other parts of the work.
-
- The Corresponding Source need not include anything that users
-can regenerate automatically from other parts of the Corresponding
-Source.
-
- The Corresponding Source for a work in source code form is that
-same work.
-
- 2. Basic Permissions.
-
- All rights granted under this License are granted for the term of
-copyright on the Program, and are irrevocable provided the stated
-conditions are met. This License explicitly affirms your unlimited
-permission to run the unmodified Program. The output from running a
-covered work is covered by this License only if the output, given its
-content, constitutes a covered work. This License acknowledges your
-rights of fair use or other equivalent, as provided by copyright law.
-
- You may make, run and propagate covered works that you do not
-convey, without conditions so long as your license otherwise remains
-in force. You may convey covered works to others for the sole purpose
-of having them make modifications exclusively for you, or provide you
-with facilities for running those works, provided that you comply with
-the terms of this License in conveying all material for which you do
-not control copyright. Those thus making or running the covered works
-for you must do so exclusively on your behalf, under your direction
-and control, on terms that prohibit them from making any copies of
-your copyrighted material outside their relationship with you.
-
- Conveying under any other circumstances is permitted solely under
-the conditions stated below. Sublicensing is not allowed; section 10
-makes it unnecessary.
-
- 3. Protecting Users' Legal Rights From Anti-Circumvention Law.
-
- No covered work shall be deemed part of an effective technological
-measure under any applicable law fulfilling obligations under article
-11 of the WIPO copyright treaty adopted on 20 December 1996, or
-similar laws prohibiting or restricting circumvention of such
-measures.
-
- When you convey a covered work, you waive any legal power to forbid
-circumvention of technological measures to the extent such circumvention
-is effected by exercising rights under this License with respect to
-the covered work, and you disclaim any intention to limit operation or
-modification of the work as a means of enforcing, against the work's
-users, your or third parties' legal rights to forbid circumvention of
-technological measures.
-
- 4. Conveying Verbatim Copies.
-
- You may convey verbatim copies of the Program's source code as you
-receive it, in any medium, provided that you conspicuously and
-appropriately publish on each copy an appropriate copyright notice;
-keep intact all notices stating that this License and any
-non-permissive terms added in accord with section 7 apply to the code;
-keep intact all notices of the absence of any warranty; and give all
-recipients a copy of this License along with the Program.
-
- You may charge any price or no price for each copy that you convey,
-and you may offer support or warranty protection for a fee.
-
- 5. Conveying Modified Source Versions.
-
- You may convey a work based on the Program, or the modifications to
-produce it from the Program, in the form of source code under the
-terms of section 4, provided that you also meet all of these conditions:
-
- a) The work must carry prominent notices stating that you modified
- it, and giving a relevant date.
-
- b) The work must carry prominent notices stating that it is
- released under this License and any conditions added under section
- 7. This requirement modifies the requirement in section 4 to
- "keep intact all notices".
-
- c) You must license the entire work, as a whole, under this
- License to anyone who comes into possession of a copy. This
- License will therefore apply, along with any applicable section 7
- additional terms, to the whole of the work, and all its parts,
- regardless of how they are packaged. This License gives no
- permission to license the work in any other way, but it does not
- invalidate such permission if you have separately received it.
-
- d) If the work has interactive user interfaces, each must display
- Appropriate Legal Notices; however, if the Program has interactive
- interfaces that do not display Appropriate Legal Notices, your
- work need not make them do so.
-
- A compilation of a covered work with other separate and independent
-works, which are not by their nature extensions of the covered work,
-and which are not combined with it such as to form a larger program,
-in or on a volume of a storage or distribution medium, is called an
-"aggregate" if the compilation and its resulting copyright are not
-used to limit the access or legal rights of the compilation's users
-beyond what the individual works permit. Inclusion of a covered work
-in an aggregate does not cause this License to apply to the other
-parts of the aggregate.
-
- 6. Conveying Non-Source Forms.
-
- You may convey a covered work in object code form under the terms
-of sections 4 and 5, provided that you also convey the
-machine-readable Corresponding Source under the terms of this License,
-in one of these ways:
-
- a) Convey the object code in, or embodied in, a physical product
- (including a physical distribution medium), accompanied by the
- Corresponding Source fixed on a durable physical medium
- customarily used for software interchange.
-
- b) Convey the object code in, or embodied in, a physical product
- (including a physical distribution medium), accompanied by a
- written offer, valid for at least three years and valid for as
- long as you offer spare parts or customer support for that product
- model, to give anyone who possesses the object code either (1) a
- copy of the Corresponding Source for all the software in the
- product that is covered by this License, on a durable physical
- medium customarily used for software interchange, for a price no
- more than your reasonable cost of physically performing this
- conveying of source, or (2) access to copy the
- Corresponding Source from a network server at no charge.
-
- c) Convey individual copies of the object code with a copy of the
- written offer to provide the Corresponding Source. This
- alternative is allowed only occasionally and noncommercially, and
- only if you received the object code with such an offer, in accord
- with subsection 6b.
-
- d) Convey the object code by offering access from a designated
- place (gratis or for a charge), and offer equivalent access to the
- Corresponding Source in the same way through the same place at no
- further charge. You need not require recipients to copy the
- Corresponding Source along with the object code. If the place to
- copy the object code is a network server, the Corresponding Source
- may be on a different server (operated by you or a third party)
- that supports equivalent copying facilities, provided you maintain
- clear directions next to the object code saying where to find the
- Corresponding Source. Regardless of what server hosts the
- Corresponding Source, you remain obligated to ensure that it is
- available for as long as needed to satisfy these requirements.
-
- e) Convey the object code using peer-to-peer transmission, provided
- you inform other peers where the object code and Corresponding
- Source of the work are being offered to the general public at no
- charge under subsection 6d.
-
- A separable portion of the object code, whose source code is excluded
-from the Corresponding Source as a System Library, need not be
-included in conveying the object code work.
-
- A "User Product" is either (1) a "consumer product", which means any
-tangible personal property which is normally used for personal, family,
-or household purposes, or (2) anything designed or sold for incorporation
-into a dwelling. In determining whether a product is a consumer product,
-doubtful cases shall be resolved in favor of coverage. For a particular
-product received by a particular user, "normally used" refers to a
-typical or common use of that class of product, regardless of the status
-of the particular user or of the way in which the particular user
-actually uses, or expects or is expected to use, the product. A product
-is a consumer product regardless of whether the product has substantial
-commercial, industrial or non-consumer uses, unless such uses represent
-the only significant mode of use of the product.
-
- "Installation Information" for a User Product means any methods,
-procedures, authorization keys, or other information required to install
-and execute modified versions of a covered work in that User Product from
-a modified version of its Corresponding Source. The information must
-suffice to ensure that the continued functioning of the modified object
-code is in no case prevented or interfered with solely because
-modification has been made.
-
- If you convey an object code work under this section in, or with, or
-specifically for use in, a User Product, and the conveying occurs as
-part of a transaction in which the right of possession and use of the
-User Product is transferred to the recipient in perpetuity or for a
-fixed term (regardless of how the transaction is characterized), the
-Corresponding Source conveyed under this section must be accompanied
-by the Installation Information. But this requirement does not apply
-if neither you nor any third party retains the ability to install
-modified object code on the User Product (for example, the work has
-been installed in ROM).
-
- The requirement to provide Installation Information does not include a
-requirement to continue to provide support service, warranty, or updates
-for a work that has been modified or installed by the recipient, or for
-the User Product in which it has been modified or installed. Access to a
-network may be denied when the modification itself materially and
-adversely affects the operation of the network or violates the rules and
-protocols for communication across the network.
-
- Corresponding Source conveyed, and Installation Information provided,
-in accord with this section must be in a format that is publicly
-documented (and with an implementation available to the public in
-source code form), and must require no special password or key for
-unpacking, reading or copying.
-
- 7. Additional Terms.
-
- "Additional permissions" are terms that supplement the terms of this
-License by making exceptions from one or more of its conditions.
-Additional permissions that are applicable to the entire Program shall
-be treated as though they were included in this License, to the extent
-that they are valid under applicable law. If additional permissions
-apply only to part of the Program, that part may be used separately
-under those permissions, but the entire Program remains governed by
-this License without regard to the additional permissions.
-
- When you convey a copy of a covered work, you may at your option
-remove any additional permissions from that copy, or from any part of
-it. (Additional permissions may be written to require their own
-removal in certain cases when you modify the work.) You may place
-additional permissions on material, added by you to a covered work,
-for which you have or can give appropriate copyright permission.
-
- Notwithstanding any other provision of this License, for material you
-add to a covered work, you may (if authorized by the copyright holders of
-that material) supplement the terms of this License with terms:
-
- a) Disclaiming warranty or limiting liability differently from the
- terms of sections 15 and 16 of this License; or
-
- b) Requiring preservation of specified reasonable legal notices or
- author attributions in that material or in the Appropriate Legal
- Notices displayed by works containing it; or
-
- c) Prohibiting misrepresentation of the origin of that material, or
- requiring that modified versions of such material be marked in
- reasonable ways as different from the original version; or
-
- d) Limiting the use for publicity purposes of names of licensors or
- authors of the material; or
-
- e) Declining to grant rights under trademark law for use of some
- trade names, trademarks, or service marks; or
-
- f) Requiring indemnification of licensors and authors of that
- material by anyone who conveys the material (or modified versions of
- it) with contractual assumptions of liability to the recipient, for
- any liability that these contractual assumptions directly impose on
- those licensors and authors.
-
- All other non-permissive additional terms are considered "further
-restrictions" within the meaning of section 10. If the Program as you
-received it, or any part of it, contains a notice stating that it is
-governed by this License along with a term that is a further
-restriction, you may remove that term. If a license document contains
-a further restriction but permits relicensing or conveying under this
-License, you may add to a covered work material governed by the terms
-of that license document, provided that the further restriction does
-not survive such relicensing or conveying.
-
- If you add terms to a covered work in accord with this section, you
-must place, in the relevant source files, a statement of the
-additional terms that apply to those files, or a notice indicating
-where to find the applicable terms.
-
- Additional terms, permissive or non-permissive, may be stated in the
-form of a separately written license, or stated as exceptions;
-the above requirements apply either way.
-
- 8. Termination.
-
- You may not propagate or modify a covered work except as expressly
-provided under this License. Any attempt otherwise to propagate or
-modify it is void, and will automatically terminate your rights under
-this License (including any patent licenses granted under the third
-paragraph of section 11).
-
- However, if you cease all violation of this License, then your
-license from a particular copyright holder is reinstated (a)
-provisionally, unless and until the copyright holder explicitly and
-finally terminates your license, and (b) permanently, if the copyright
-holder fails to notify you of the violation by some reasonable means
-prior to 60 days after the cessation.
-
- Moreover, your license from a particular copyright holder is
-reinstated permanently if the copyright holder notifies you of the
-violation by some reasonable means, this is the first time you have
-received notice of violation of this License (for any work) from that
-copyright holder, and you cure the violation prior to 30 days after
-your receipt of the notice.
-
- Termination of your rights under this section does not terminate the
-licenses of parties who have received copies or rights from you under
-this License. If your rights have been terminated and not permanently
-reinstated, you do not qualify to receive new licenses for the same
-material under section 10.
-
- 9. Acceptance Not Required for Having Copies.
-
- You are not required to accept this License in order to receive or
-run a copy of the Program. Ancillary propagation of a covered work
-occurring solely as a consequence of using peer-to-peer transmission
-to receive a copy likewise does not require acceptance. However,
-nothing other than this License grants you permission to propagate or
-modify any covered work. These actions infringe copyright if you do
-not accept this License. Therefore, by modifying or propagating a
-covered work, you indicate your acceptance of this License to do so.
-
- 10. Automatic Licensing of Downstream Recipients.
-
- Each time you convey a covered work, the recipient automatically
-receives a license from the original licensors, to run, modify and
-propagate that work, subject to this License. You are not responsible
-for enforcing compliance by third parties with this License.
-
- An "entity transaction" is a transaction transferring control of an
-organization, or substantially all assets of one, or subdividing an
-organization, or merging organizations. If propagation of a covered
-work results from an entity transaction, each party to that
-transaction who receives a copy of the work also receives whatever
-licenses to the work the party's predecessor in interest had or could
-give under the previous paragraph, plus a right to possession of the
-Corresponding Source of the work from the predecessor in interest, if
-the predecessor has it or can get it with reasonable efforts.
-
- You may not impose any further restrictions on the exercise of the
-rights granted or affirmed under this License. For example, you may
-not impose a license fee, royalty, or other charge for exercise of
-rights granted under this License, and you may not initiate litigation
-(including a cross-claim or counterclaim in a lawsuit) alleging that
-any patent claim is infringed by making, using, selling, offering for
-sale, or importing the Program or any portion of it.
-
- 11. Patents.
-
- A "contributor" is a copyright holder who authorizes use under this
-License of the Program or a work on which the Program is based. The
-work thus licensed is called the contributor's "contributor version".
-
- A contributor's "essential patent claims" are all patent claims
-owned or controlled by the contributor, whether already acquired or
-hereafter acquired, that would be infringed by some manner, permitted
-by this License, of making, using, or selling its contributor version,
-but do not include claims that would be infringed only as a
-consequence of further modification of the contributor version. For
-purposes of this definition, "control" includes the right to grant
-patent sublicenses in a manner consistent with the requirements of
-this License.
-
- Each contributor grants you a non-exclusive, worldwide, royalty-free
-patent license under the contributor's essential patent claims, to
-make, use, sell, offer for sale, import and otherwise run, modify and
-propagate the contents of its contributor version.
-
- In the following three paragraphs, a "patent license" is any express
-agreement or commitment, however denominated, not to enforce a patent
-(such as an express permission to practice a patent or covenant not to
-sue for patent infringement). To "grant" such a patent license to a
-party means to make such an agreement or commitment not to enforce a
-patent against the party.
-
- If you convey a covered work, knowingly relying on a patent license,
-and the Corresponding Source of the work is not available for anyone
-to copy, free of charge and under the terms of this License, through a
-publicly available network server or other readily accessible means,
-then you must either (1) cause the Corresponding Source to be so
-available, or (2) arrange to deprive yourself of the benefit of the
-patent license for this particular work, or (3) arrange, in a manner
-consistent with the requirements of this License, to extend the patent
-license to downstream recipients. "Knowingly relying" means you have
-actual knowledge that, but for the patent license, your conveying the
-covered work in a country, or your recipient's use of the covered work
-in a country, would infringe one or more identifiable patents in that
-country that you have reason to believe are valid.
-
- If, pursuant to or in connection with a single transaction or
-arrangement, you convey, or propagate by procuring conveyance of, a
-covered work, and grant a patent license to some of the parties
-receiving the covered work authorizing them to use, propagate, modify
-or convey a specific copy of the covered work, then the patent license
-you grant is automatically extended to all recipients of the covered
-work and works based on it.
-
- A patent license is "discriminatory" if it does not include within
-the scope of its coverage, prohibits the exercise of, or is
-conditioned on the non-exercise of one or more of the rights that are
-specifically granted under this License. You may not convey a covered
-work if you are a party to an arrangement with a third party that is
-in the business of distributing software, under which you make payment
-to the third party based on the extent of your activity of conveying
-the work, and under which the third party grants, to any of the
-parties who would receive the covered work from you, a discriminatory
-patent license (a) in connection with copies of the covered work
-conveyed by you (or copies made from those copies), or (b) primarily
-for and in connection with specific products or compilations that
-contain the covered work, unless you entered into that arrangement,
-or that patent license was granted, prior to 28 March 2007.
-
- Nothing in this License shall be construed as excluding or limiting
-any implied license or other defenses to infringement that may
-otherwise be available to you under applicable patent law.
-
- 12. No Surrender of Others' Freedom.
-
- If conditions are imposed on you (whether by court order, agreement or
-otherwise) that contradict the conditions of this License, they do not
-excuse you from the conditions of this License. If you cannot convey a
-covered work so as to satisfy simultaneously your obligations under this
-License and any other pertinent obligations, then as a consequence you may
-not convey it at all. For example, if you agree to terms that obligate you
-to collect a royalty for further conveying from those to whom you convey
-the Program, the only way you could satisfy both those terms and this
-License would be to refrain entirely from conveying the Program.
-
- 13. Use with the GNU Affero General Public License.
-
- Notwithstanding any other provision of this License, you have
-permission to link or combine any covered work with a work licensed
-under version 3 of the GNU Affero General Public License into a single
-combined work, and to convey the resulting work. The terms of this
-License will continue to apply to the part which is the covered work,
-but the special requirements of the GNU Affero General Public License,
-section 13, concerning interaction through a network will apply to the
-combination as such.
-
- 14. Revised Versions of this License.
-
- The Free Software Foundation may publish revised and/or new versions of
-the GNU General Public License from time to time. Such new versions will
-be similar in spirit to the present version, but may differ in detail to
-address new problems or concerns.
-
- Each version is given a distinguishing version number. If the
-Program specifies that a certain numbered version of the GNU General
-Public License "or any later version" applies to it, you have the
-option of following the terms and conditions either of that numbered
-version or of any later version published by the Free Software
-Foundation. If the Program does not specify a version number of the
-GNU General Public License, you may choose any version ever published
-by the Free Software Foundation.
-
- If the Program specifies that a proxy can decide which future
-versions of the GNU General Public License can be used, that proxy's
-public statement of acceptance of a version permanently authorizes you
-to choose that version for the Program.
-
- Later license versions may give you additional or different
-permissions. However, no additional obligations are imposed on any
-author or copyright holder as a result of your choosing to follow a
-later version.
-
- 15. Disclaimer of Warranty.
-
- THERE IS NO WARRANTY FOR THE PROGRAM, TO THE EXTENT PERMITTED BY
-APPLICABLE LAW. EXCEPT WHEN OTHERWISE STATED IN WRITING THE COPYRIGHT
-HOLDERS AND/OR OTHER PARTIES PROVIDE THE PROGRAM "AS IS" WITHOUT WARRANTY
-OF ANY KIND, EITHER EXPRESSED OR IMPLIED, INCLUDING, BUT NOT LIMITED TO,
-THE IMPLIED WARRANTIES OF MERCHANTABILITY AND FITNESS FOR A PARTICULAR
-PURPOSE. THE ENTIRE RISK AS TO THE QUALITY AND PERFORMANCE OF THE PROGRAM
-IS WITH YOU. SHOULD THE PROGRAM PROVE DEFECTIVE, YOU ASSUME THE COST OF
-ALL NECESSARY SERVICING, REPAIR OR CORRECTION.
-
- 16. Limitation of Liability.
-
- IN NO EVENT UNLESS REQUIRED BY APPLICABLE LAW OR AGREED TO IN WRITING
-WILL ANY COPYRIGHT HOLDER, OR ANY OTHER PARTY WHO MODIFIES AND/OR CONVEYS
-THE PROGRAM AS PERMITTED ABOVE, BE LIABLE TO YOU FOR DAMAGES, INCLUDING ANY
-GENERAL, SPECIAL, INCIDENTAL OR CONSEQUENTIAL DAMAGES ARISING OUT OF THE
-USE OR INABILITY TO USE THE PROGRAM (INCLUDING BUT NOT LIMITED TO LOSS OF
-DATA OR DATA BEING RENDERED INACCURATE OR LOSSES SUSTAINED BY YOU OR THIRD
-PARTIES OR A FAILURE OF THE PROGRAM TO OPERATE WITH ANY OTHER PROGRAMS),
-EVEN IF SUCH HOLDER OR OTHER PARTY HAS BEEN ADVISED OF THE POSSIBILITY OF
-SUCH DAMAGES.
-
- 17. Interpretation of Sections 15 and 16.
-
- If the disclaimer of warranty and limitation of liability provided
-above cannot be given local legal effect according to their terms,
-reviewing courts shall apply local law that most closely approximates
-an absolute waiver of all civil liability in connection with the
-Program, unless a warranty or assumption of liability accompanies a
-copy of the Program in return for a fee.
-
- END OF TERMS AND CONDITIONS
-
- How to Apply These Terms to Your New Programs
-
- If you develop a new program, and you want it to be of the greatest
-possible use to the public, the best way to achieve this is to make it
-free software which everyone can redistribute and change under these terms.
-
- To do so, attach the following notices to the program. It is safest
-to attach them to the start of each source file to most effectively
-state the exclusion of warranty; and each file should have at least
-the "copyright" line and a pointer to where the full notice is found.
-
-
- Copyright (C)
-
- This program is free software: you can redistribute it and/or modify
- it under the terms of the GNU General Public License as published by
- the Free Software Foundation, either version 3 of the License, or
- (at your option) any later version.
-
- This program is distributed in the hope that it will be useful,
- but WITHOUT ANY WARRANTY; without even the implied warranty of
- MERCHANTABILITY or FITNESS FOR A PARTICULAR PURPOSE. See the
- GNU General Public License for more details.
-
- You should have received a copy of the GNU General Public License
- along with this program. If not, see .
-
-Also add information on how to contact you by electronic and paper mail.
-
- If the program does terminal interaction, make it output a short
-notice like this when it starts in an interactive mode:
-
- Copyright (C)
- This program comes with ABSOLUTELY NO WARRANTY; for details type `show w'.
- This is free software, and you are welcome to redistribute it
- under certain conditions; type `show c' for details.
-
-The hypothetical commands `show w' and `show c' should show the appropriate
-parts of the General Public License. Of course, your program's commands
-might be different; for a GUI interface, you would use an "about box".
-
- You should also get your employer (if you work as a programmer) or school,
-if any, to sign a "copyright disclaimer" for the program, if necessary.
-For more information on this, and how to apply and follow the GNU GPL, see
-.
-
- The GNU General Public License does not permit incorporating your program
-into proprietary programs. If your program is a subroutine library, you
-may consider it more useful to permit linking proprietary applications with
-the library. If this is what you want to do, use the GNU Lesser General
-Public License instead of this License. But first, please read
-.
diff --git a/spaces/elonmuskceo/shiny-cpu-info/app.py b/spaces/elonmuskceo/shiny-cpu-info/app.py
deleted file mode 100644
index ccc87d46d9990538f7ec82191b4d911725c6ceff..0000000000000000000000000000000000000000
--- a/spaces/elonmuskceo/shiny-cpu-info/app.py
+++ /dev/null
@@ -1,232 +0,0 @@
-import sys
-
-from psutil import cpu_count, cpu_percent
-
-from math import ceil
-
-import matplotlib
-import matplotlib.pyplot as plt
-import numpy as np
-import pandas as pd
-from shiny import App, Inputs, Outputs, Session, reactive, render, ui
-
-shinylive_message = ""
-
-# The agg matplotlib backend seems to be a little more efficient than the default when
-# running on macOS, and also gives more consistent results across operating systems
-matplotlib.use("agg")
-
-# max number of samples to retain
-MAX_SAMPLES = 1000
-# secs between samples
-SAMPLE_PERIOD = 1
-
-
-ncpu = cpu_count(logical=True)
-
-app_ui = ui.page_fluid(
- ui.tags.style(
- """
- /* Don't apply fade effect, it's constantly recalculating */
- .recalculating {
- opacity: 1;
- }
- tbody > tr:last-child {
- /*border: 3px solid var(--bs-dark);*/
- box-shadow:
- 0 0 2px 1px #fff, /* inner white */
- 0 0 4px 2px #0ff, /* middle cyan */
- 0 0 5px 3px #00f; /* outer blue */
- }
- #table table {
- table-layout: fixed;
- width: %s;
- font-size: 0.8em;
- }
- th, td {
- text-align: center;
- }
- """
- % f"{ncpu*4}em"
- ),
- ui.h3("CPU Usage %", class_="mt-2"),
- ui.layout_sidebar(
- ui.panel_sidebar(
- ui.input_select(
- "cmap",
- "Colormap",
- {
- "inferno": "inferno",
- "viridis": "viridis",
- "copper": "copper",
- "prism": "prism (not recommended)",
- },
- ),
- ui.p(ui.input_action_button("reset", "Clear history", class_="btn-sm")),
- ui.input_switch("hold", "Freeze output", value=False),
- shinylive_message,
- class_="mb-3",
- ),
- ui.panel_main(
- ui.div(
- {"class": "card mb-3"},
- ui.div(
- {"class": "card-body"},
- ui.h5({"class": "card-title mt-0"}, "Graphs"),
- ui.output_plot("plot", height=f"{ncpu * 40}px"),
- ),
- ui.div(
- {"class": "card-footer"},
- ui.input_numeric("sample_count", "Number of samples per graph", 50),
- ),
- ),
- ui.div(
- {"class": "card"},
- ui.div(
- {"class": "card-body"},
- ui.h5({"class": "card-title m-0"}, "Heatmap"),
- ),
- ui.div(
- {"class": "card-body overflow-auto pt-0"},
- ui.output_table("table"),
- ),
- ui.div(
- {"class": "card-footer"},
- ui.input_numeric("table_rows", "Rows to display", 5),
- ),
- ),
- ),
- ),
-)
-
-
-@reactive.Calc
-def cpu_current():
- reactive.invalidate_later(SAMPLE_PERIOD)
- return cpu_percent(percpu=True)
-
-
-def server(input: Inputs, output: Outputs, session: Session):
- cpu_history = reactive.Value(None)
-
- @reactive.Calc
- def cpu_history_with_hold():
- # If "hold" is on, grab an isolated snapshot of cpu_history; if not, then do a
- # regular read
- if not input.hold():
- return cpu_history()
- else:
- # Even if frozen, we still want to respond to input.reset()
- input.reset()
- with reactive.isolate():
- return cpu_history()
-
- @reactive.Effect
- def collect_cpu_samples():
- """cpu_percent() reports just the current CPU usage sample; this Effect gathers
- them up and stores them in the cpu_history reactive value, in a numpy 2D array
- (rows are CPUs, columns are time)."""
-
- new_data = np.vstack(cpu_current())
- with reactive.isolate():
- if cpu_history() is None:
- cpu_history.set(new_data)
- else:
- combined_data = np.hstack([cpu_history(), new_data])
- # Throw away extra data so we don't consume unbounded amounts of memory
- if combined_data.shape[1] > MAX_SAMPLES:
- combined_data = combined_data[:, -MAX_SAMPLES:]
- cpu_history.set(combined_data)
-
- @reactive.Effect(priority=100)
- @reactive.event(input.reset)
- def reset_history():
- cpu_history.set(None)
-
- @output
- @render.plot
- def plot():
- history = cpu_history_with_hold()
-
- if history is None:
- history = np.array([])
- history.shape = (ncpu, 0)
-
- nsamples = input.sample_count()
-
- # Throw away samples too old to fit on the plot
- if history.shape[1] > nsamples:
- history = history[:, -nsamples:]
-
- ncols = 2
- nrows = int(ceil(ncpu / ncols))
- fig, axeses = plt.subplots(
- nrows=nrows,
- ncols=ncols,
- squeeze=False,
- )
- for i in range(0, ncols * nrows):
- row = i // ncols
- col = i % ncols
- axes = axeses[row, col]
- if i >= len(history):
- axes.set_visible(False)
- continue
- data = history[i]
- axes.yaxis.set_label_position("right")
- axes.yaxis.tick_right()
- axes.set_xlim(-(nsamples - 1), 0)
- axes.set_ylim(0, 100)
-
- assert len(data) <= nsamples
-
- # Set up an array of x-values that will right-align the data relative to the
- # plotting area
- x = np.arange(0, len(data))
- x = np.flip(-x)
-
- # Color bars by cmap
- color = plt.get_cmap(input.cmap())(data / 100)
- axes.bar(x, data, color=color, linewidth=0, width=1.0)
-
- axes.set_yticks([25, 50, 75])
- for ytl in axes.get_yticklabels():
- if col == ncols - 1 or i == ncpu - 1 or True:
- ytl.set_fontsize(7)
- else:
- ytl.set_visible(False)
- hide_ticks(axes.yaxis)
- for xtl in axes.get_xticklabels():
- xtl.set_visible(False)
- hide_ticks(axes.xaxis)
- axes.grid(True, linewidth=0.25)
-
- return fig
-
- @output
- @render.table
- def table():
- history = cpu_history_with_hold()
- latest = pd.DataFrame(history).transpose().tail(input.table_rows())
- if latest.shape[0] == 0:
- return latest
- return (
- latest.style.format(precision=0)
- .hide(axis="index")
- .set_table_attributes(
- 'class="dataframe shiny-table table table-borderless font-monospace"'
- )
- .background_gradient(cmap=input.cmap(), vmin=0, vmax=100)
- )
-
-
-def hide_ticks(axis):
- for ticks in [axis.get_major_ticks(), axis.get_minor_ticks()]:
- for tick in ticks:
- tick.tick1line.set_visible(False)
- tick.tick2line.set_visible(False)
- tick.label1.set_visible(False)
- tick.label2.set_visible(False)
-
-
-app = App(app_ui, server)
diff --git a/spaces/elplaguister/Yuuka_TTS/src/attentions.py b/spaces/elplaguister/Yuuka_TTS/src/attentions.py
deleted file mode 100644
index 8406aed31636b37f017a11b84aca1d8175e58b8d..0000000000000000000000000000000000000000
--- a/spaces/elplaguister/Yuuka_TTS/src/attentions.py
+++ /dev/null
@@ -1,300 +0,0 @@
-import math
-import torch
-from torch import nn
-from torch.nn import functional as F
-
-from src import commons
-from src.modules import LayerNorm
-
-
-class Encoder(nn.Module):
- def __init__(self, hidden_channels, filter_channels, n_heads, n_layers, kernel_size=1, p_dropout=0., window_size=4, **kwargs):
- super().__init__()
- self.hidden_channels = hidden_channels
- self.filter_channels = filter_channels
- self.n_heads = n_heads
- self.n_layers = n_layers
- self.kernel_size = kernel_size
- self.p_dropout = p_dropout
- self.window_size = window_size
-
- self.drop = nn.Dropout(p_dropout)
- self.attn_layers = nn.ModuleList()
- self.norm_layers_1 = nn.ModuleList()
- self.ffn_layers = nn.ModuleList()
- self.norm_layers_2 = nn.ModuleList()
- for i in range(self.n_layers):
- self.attn_layers.append(MultiHeadAttention(hidden_channels, hidden_channels, n_heads, p_dropout=p_dropout, window_size=window_size))
- self.norm_layers_1.append(LayerNorm(hidden_channels))
- self.ffn_layers.append(FFN(hidden_channels, hidden_channels, filter_channels, kernel_size, p_dropout=p_dropout))
- self.norm_layers_2.append(LayerNorm(hidden_channels))
-
- def forward(self, x, x_mask):
- attn_mask = x_mask.unsqueeze(2) * x_mask.unsqueeze(-1)
- x = x * x_mask
- for i in range(self.n_layers):
- y = self.attn_layers[i](x, x, attn_mask)
- y = self.drop(y)
- x = self.norm_layers_1[i](x + y)
-
- y = self.ffn_layers[i](x, x_mask)
- y = self.drop(y)
- x = self.norm_layers_2[i](x + y)
- x = x * x_mask
- return x
-
-
-class Decoder(nn.Module):
- def __init__(self, hidden_channels, filter_channels, n_heads, n_layers, kernel_size=1, p_dropout=0., proximal_bias=False, proximal_init=True, **kwargs):
- super().__init__()
- self.hidden_channels = hidden_channels
- self.filter_channels = filter_channels
- self.n_heads = n_heads
- self.n_layers = n_layers
- self.kernel_size = kernel_size
- self.p_dropout = p_dropout
- self.proximal_bias = proximal_bias
- self.proximal_init = proximal_init
-
- self.drop = nn.Dropout(p_dropout)
- self.self_attn_layers = nn.ModuleList()
- self.norm_layers_0 = nn.ModuleList()
- self.encdec_attn_layers = nn.ModuleList()
- self.norm_layers_1 = nn.ModuleList()
- self.ffn_layers = nn.ModuleList()
- self.norm_layers_2 = nn.ModuleList()
- for i in range(self.n_layers):
- self.self_attn_layers.append(MultiHeadAttention(hidden_channels, hidden_channels, n_heads, p_dropout=p_dropout, proximal_bias=proximal_bias, proximal_init=proximal_init))
- self.norm_layers_0.append(LayerNorm(hidden_channels))
- self.encdec_attn_layers.append(MultiHeadAttention(hidden_channels, hidden_channels, n_heads, p_dropout=p_dropout))
- self.norm_layers_1.append(LayerNorm(hidden_channels))
- self.ffn_layers.append(FFN(hidden_channels, hidden_channels, filter_channels, kernel_size, p_dropout=p_dropout, causal=True))
- self.norm_layers_2.append(LayerNorm(hidden_channels))
-
- def forward(self, x, x_mask, h, h_mask):
- """
- x: decoder input
- h: encoder output
- """
- self_attn_mask = commons.subsequent_mask(x_mask.size(2)).to(device=x.device, dtype=x.dtype)
- encdec_attn_mask = h_mask.unsqueeze(2) * x_mask.unsqueeze(-1)
- x = x * x_mask
- for i in range(self.n_layers):
- y = self.self_attn_layers[i](x, x, self_attn_mask)
- y = self.drop(y)
- x = self.norm_layers_0[i](x + y)
-
- y = self.encdec_attn_layers[i](x, h, encdec_attn_mask)
- y = self.drop(y)
- x = self.norm_layers_1[i](x + y)
-
- y = self.ffn_layers[i](x, x_mask)
- y = self.drop(y)
- x = self.norm_layers_2[i](x + y)
- x = x * x_mask
- return x
-
-
-class MultiHeadAttention(nn.Module):
- def __init__(self, channels, out_channels, n_heads, p_dropout=0., window_size=None, heads_share=True, block_length=None, proximal_bias=False, proximal_init=False):
- super().__init__()
- assert channels % n_heads == 0
-
- self.channels = channels
- self.out_channels = out_channels
- self.n_heads = n_heads
- self.p_dropout = p_dropout
- self.window_size = window_size
- self.heads_share = heads_share
- self.block_length = block_length
- self.proximal_bias = proximal_bias
- self.proximal_init = proximal_init
- self.attn = None
-
- self.k_channels = channels // n_heads
- self.conv_q = nn.Conv1d(channels, channels, 1)
- self.conv_k = nn.Conv1d(channels, channels, 1)
- self.conv_v = nn.Conv1d(channels, channels, 1)
- self.conv_o = nn.Conv1d(channels, out_channels, 1)
- self.drop = nn.Dropout(p_dropout)
-
- if window_size is not None:
- n_heads_rel = 1 if heads_share else n_heads
- rel_stddev = self.k_channels**-0.5
- self.emb_rel_k = nn.Parameter(torch.randn(n_heads_rel, window_size * 2 + 1, self.k_channels) * rel_stddev)
- self.emb_rel_v = nn.Parameter(torch.randn(n_heads_rel, window_size * 2 + 1, self.k_channels) * rel_stddev)
-
- nn.init.xavier_uniform_(self.conv_q.weight)
- nn.init.xavier_uniform_(self.conv_k.weight)
- nn.init.xavier_uniform_(self.conv_v.weight)
- if proximal_init:
- with torch.no_grad():
- self.conv_k.weight.copy_(self.conv_q.weight)
- self.conv_k.bias.copy_(self.conv_q.bias)
-
- def forward(self, x, c, attn_mask=None):
- q = self.conv_q(x)
- k = self.conv_k(c)
- v = self.conv_v(c)
-
- x, self.attn = self.attention(q, k, v, mask=attn_mask)
-
- x = self.conv_o(x)
- return x
-
- def attention(self, query, key, value, mask=None):
- # reshape [b, d, t] -> [b, n_h, t, d_k]
- b, d, t_s, t_t = (*key.size(), query.size(2))
- query = query.view(b, self.n_heads, self.k_channels, t_t).transpose(2, 3)
- key = key.view(b, self.n_heads, self.k_channels, t_s).transpose(2, 3)
- value = value.view(b, self.n_heads, self.k_channels, t_s).transpose(2, 3)
-
- scores = torch.matmul(query / math.sqrt(self.k_channels), key.transpose(-2, -1))
- if self.window_size is not None:
- assert t_s == t_t, "Relative attention is only available for self-attention."
- key_relative_embeddings = self._get_relative_embeddings(self.emb_rel_k, t_s)
- rel_logits = self._matmul_with_relative_keys(query /math.sqrt(self.k_channels), key_relative_embeddings)
- scores_local = self._relative_position_to_absolute_position(rel_logits)
- scores = scores + scores_local
- if self.proximal_bias:
- assert t_s == t_t, "Proximal bias is only available for self-attention."
- scores = scores + self._attention_bias_proximal(t_s).to(device=scores.device, dtype=scores.dtype)
- if mask is not None:
- scores = scores.masked_fill(mask == 0, -1e4)
- if self.block_length is not None:
- assert t_s == t_t, "Local attention is only available for self-attention."
- block_mask = torch.ones_like(scores).triu(-self.block_length).tril(self.block_length)
- scores = scores.masked_fill(block_mask == 0, -1e4)
- p_attn = F.softmax(scores, dim=-1) # [b, n_h, t_t, t_s]
- p_attn = self.drop(p_attn)
- output = torch.matmul(p_attn, value)
- if self.window_size is not None:
- relative_weights = self._absolute_position_to_relative_position(p_attn)
- value_relative_embeddings = self._get_relative_embeddings(self.emb_rel_v, t_s)
- output = output + self._matmul_with_relative_values(relative_weights, value_relative_embeddings)
- output = output.transpose(2, 3).contiguous().view(b, d, t_t) # [b, n_h, t_t, d_k] -> [b, d, t_t]
- return output, p_attn
-
- def _matmul_with_relative_values(self, x, y):
- """
- x: [b, h, l, m]
- y: [h or 1, m, d]
- ret: [b, h, l, d]
- """
- ret = torch.matmul(x, y.unsqueeze(0))
- return ret
-
- def _matmul_with_relative_keys(self, x, y):
- """
- x: [b, h, l, d]
- y: [h or 1, m, d]
- ret: [b, h, l, m]
- """
- ret = torch.matmul(x, y.unsqueeze(0).transpose(-2, -1))
- return ret
-
- def _get_relative_embeddings(self, relative_embeddings, length):
- max_relative_position = 2 * self.window_size + 1
- # Pad first before slice to avoid using cond ops.
- pad_length = max(length - (self.window_size + 1), 0)
- slice_start_position = max((self.window_size + 1) - length, 0)
- slice_end_position = slice_start_position + 2 * length - 1
- if pad_length > 0:
- padded_relative_embeddings = F.pad(
- relative_embeddings,
- commons.convert_pad_shape([[0, 0], [pad_length, pad_length], [0, 0]]))
- else:
- padded_relative_embeddings = relative_embeddings
- used_relative_embeddings = padded_relative_embeddings[:,slice_start_position:slice_end_position]
- return used_relative_embeddings
-
- def _relative_position_to_absolute_position(self, x):
- """
- x: [b, h, l, 2*l-1]
- ret: [b, h, l, l]
- """
- batch, heads, length, _ = x.size()
- # Concat columns of pad to shift from relative to absolute indexing.
- x = F.pad(x, commons.convert_pad_shape([[0,0],[0,0],[0,0],[0,1]]))
-
- # Concat extra elements so to add up to shape (len+1, 2*len-1).
- x_flat = x.view([batch, heads, length * 2 * length])
- x_flat = F.pad(x_flat, commons.convert_pad_shape([[0,0],[0,0],[0,length-1]]))
-
- # Reshape and slice out the padded elements.
- x_final = x_flat.view([batch, heads, length+1, 2*length-1])[:, :, :length, length-1:]
- return x_final
-
- def _absolute_position_to_relative_position(self, x):
- """
- x: [b, h, l, l]
- ret: [b, h, l, 2*l-1]
- """
- batch, heads, length, _ = x.size()
- # padd along column
- x = F.pad(x, commons.convert_pad_shape([[0, 0], [0, 0], [0, 0], [0, length-1]]))
- x_flat = x.view([batch, heads, length**2 + length*(length -1)])
- # add 0's in the beginning that will skew the elements after reshape
- x_flat = F.pad(x_flat, commons.convert_pad_shape([[0, 0], [0, 0], [length, 0]]))
- x_final = x_flat.view([batch, heads, length, 2*length])[:,:,:,1:]
- return x_final
-
- def _attention_bias_proximal(self, length):
- """Bias for self-attention to encourage attention to close positions.
- Args:
- length: an integer scalar.
- Returns:
- a Tensor with shape [1, 1, length, length]
- """
- r = torch.arange(length, dtype=torch.float32)
- diff = torch.unsqueeze(r, 0) - torch.unsqueeze(r, 1)
- return torch.unsqueeze(torch.unsqueeze(-torch.log1p(torch.abs(diff)), 0), 0)
-
-
-class FFN(nn.Module):
- def __init__(self, in_channels, out_channels, filter_channels, kernel_size, p_dropout=0., activation=None, causal=False):
- super().__init__()
- self.in_channels = in_channels
- self.out_channels = out_channels
- self.filter_channels = filter_channels
- self.kernel_size = kernel_size
- self.p_dropout = p_dropout
- self.activation = activation
- self.causal = causal
-
- if causal:
- self.padding = self._causal_padding
- else:
- self.padding = self._same_padding
-
- self.conv_1 = nn.Conv1d(in_channels, filter_channels, kernel_size)
- self.conv_2 = nn.Conv1d(filter_channels, out_channels, kernel_size)
- self.drop = nn.Dropout(p_dropout)
-
- def forward(self, x, x_mask):
- x = self.conv_1(self.padding(x * x_mask))
- if self.activation == "gelu":
- x = x * torch.sigmoid(1.702 * x)
- else:
- x = torch.relu(x)
- x = self.drop(x)
- x = self.conv_2(self.padding(x * x_mask))
- return x * x_mask
-
- def _causal_padding(self, x):
- if self.kernel_size == 1:
- return x
- pad_l = self.kernel_size - 1
- pad_r = 0
- padding = [[0, 0], [0, 0], [pad_l, pad_r]]
- x = F.pad(x, commons.convert_pad_shape(padding))
- return x
-
- def _same_padding(self, x):
- if self.kernel_size == 1:
- return x
- pad_l = (self.kernel_size - 1) // 2
- pad_r = self.kernel_size // 2
- padding = [[0, 0], [0, 0], [pad_l, pad_r]]
- x = F.pad(x, commons.convert_pad_shape(padding))
- return x
diff --git a/spaces/epexVfeibi/Imagedeblurr/Account Hitman V0.98l.md b/spaces/epexVfeibi/Imagedeblurr/Account Hitman V0.98l.md
deleted file mode 100644
index 1144fb4650ea1bac40b8042e3bca566d8c14d92c..0000000000000000000000000000000000000000
--- a/spaces/epexVfeibi/Imagedeblurr/Account Hitman V0.98l.md
+++ /dev/null
@@ -1,19 +0,0 @@
-
-
Account Hitman V0.98l: A Dangerous Tool for Credential Stuffing
-
Account Hitman V0.98l is a software tool that can be used to automate the process of testing leaked username and password combinations on various websites and services. It is one of the popular tools for credential stuffing, a cyberattack technique that exploits the use of weak passwords and password reuse by users.
-
Credential stuffing can lead to account takeover, identity theft, fraud, and other malicious activities. According to a report by Digital Shadows, credential leaks such as the Anti Public Combo List and others have fueled the market for credential stuffing and made it a lucrative part of the black market economy[^5^].
Account Hitman V0.98l is available for download on various websites and forums, some of which claim to offer it for free or with a premium subscription. However, downloading and using this tool can be risky, as it may contain malware, viruses, or backdoors that can compromise the user's system or data. Moreover, using this tool for illegal purposes can result in legal consequences, as it violates the terms of service and privacy policies of the websites and services that it targets.
-
Therefore, users are advised to avoid downloading or using Account Hitman V0.98l or any similar tools for credential stuffing. Instead, users should follow good password hygiene practices, such as using strong and unique passwords for each account, changing passwords regularly, enabling two-factor authentication, and using a reputable password manager.
Here are some additional paragraphs for the article:
-
3. Research facts that reinforce your story
-
Once you have chosen a topic and identified your target audience, you need to do some research to find reliable sources that support your main points. You can use online databases, libraries, newspapers, magazines, journals, books, or interviews to gather relevant information for your article. Make sure to cite your sources properly and avoid plagiarism. You can also use tools like Grammarly or Copyscape to check your article for originality and accuracy.
-
4. Come up with an outline of your article
-
An outline is a helpful tool that can help you organize your ideas and structure your article. You can use bullet points, headings, subheadings, or numbers to list the main sections of your article and the key points you want to cover in each section. An outline can also help you identify any gaps or weaknesses in your argument and make necessary adjustments before you start writing. A typical outline for an article may look something like this:
-
-
-
Introduction: Hook the reader's attention with a catchy opening sentence, provide some background information on the topic, and state your main purpose or thesis statement.
-
Body: Develop your main points with supporting evidence, examples, statistics, quotes, or anecdotes. Use transitions to connect your paragraphs and maintain a logical flow of information.
-
Conclusion: Summarize your main points and restate your thesis statement. Provide a call to action or a recommendation for further action or research.
-
d5da3c52bf
-
-
\ No newline at end of file
diff --git a/spaces/eson/tokenizer-arena/vocab/gpt2/test_fairseq_gpt2.py b/spaces/eson/tokenizer-arena/vocab/gpt2/test_fairseq_gpt2.py
deleted file mode 100644
index 061cbaad64d083437818be193f467b9ffa90dd9b..0000000000000000000000000000000000000000
--- a/spaces/eson/tokenizer-arena/vocab/gpt2/test_fairseq_gpt2.py
+++ /dev/null
@@ -1,18 +0,0 @@
-
-
-from fairseq.data.encoders.gpt2_bpe import get_encoder
-bpe = get_encoder('/workspace/fairseq-models/data/vocab/gpt2/encoder.json', '/workspace/fairseq-models/data/vocab/gpt2/vocab.bpe')
-
-codes = bpe.encode('Hello world')
-print(codes)
-print(bpe.decode(codes))
-
-
-test_str = 'Leonardo DiCaprio was born in Los Angeles'
-print(bpe.bpe(test_str))
-codes = bpe.encode(test_str)
-print(codes)
-print(bpe.decode(codes))
-
-
-
diff --git a/spaces/facebook/XLS-R-1B-EN-15/app.py b/spaces/facebook/XLS-R-1B-EN-15/app.py
deleted file mode 100644
index 03880f8aa3a104bda398dbbf0145ede060902605..0000000000000000000000000000000000000000
--- a/spaces/facebook/XLS-R-1B-EN-15/app.py
+++ /dev/null
@@ -1,98 +0,0 @@
-import os
-os.system("pip install gradio==2.8.0b2")
-import gradio as gr
-import librosa
-from transformers import AutoFeatureExtractor, AutoTokenizer, SpeechEncoderDecoderModel
-
-model_name = "facebook/wav2vec2-xls-r-1b-en-to-15"
-
-feature_extractor = AutoFeatureExtractor.from_pretrained(model_name)
-tokenizer = AutoTokenizer.from_pretrained(model_name, use_fast=False)
-model = SpeechEncoderDecoderModel.from_pretrained(model_name)
-
-def process_audio_file(file):
- data, sr = librosa.load(file)
- if sr != 16000:
- data = librosa.resample(data, sr, 16000)
- print(data.shape)
- input_values = feature_extractor(data, return_tensors="pt").input_values
- return input_values
-
-def transcribe(file_mic, file_upload, target_language):
-
- target_code = target_language.split("(")[-1].split(")")[0]
- forced_bos_token_id = MAPPING[target_code]
-
- warn_output = ""
- if (file_mic is not None) and (file_upload is not None):
- warn_output = "WARNING: You've uploaded an audio file and used the microphone. The recorded file from the microphone will be used and the uploaded audio will be discarded.\n"
- file = file_mic
- elif (file_mic is None) and (file_upload is None):
- return "ERROR: You have to either use the microphone or upload an audio file"
- elif file_mic is not None:
- file = file_mic
- else:
- file = file_upload
-
- input_values = process_audio_file(file)
-
- sequences = model.generate(input_values, forced_bos_token_id=forced_bos_token_id, num_beams=1, max_length=30)
-
- transcription = tokenizer.batch_decode(sequences, skip_special_tokens=True)
- return warn_output + transcription[0]
-
-target_language = [
- "English (en)",
- "German (de)",
- "Turkish (tr)",
- "Persian (fa)",
- "Swedish (sv)",
- "Mongolian (mn)",
- "Chinese (zh)",
- "Welsh (cy)",
- "Catalan (ca)",
- "Slovenian (sl)",
- "Estonian (et)",
- "Indonesian (id)",
- "Arabic (ar)",
- "Tamil (ta)",
- "Latvian (lv)",
- "Japanese (ja)",
-]
-
-MAPPING = {
- "en": 250004,
- "de": 250003,
- "tr": 250023,
- "fa": 250029,
- "sv": 250042,
- "mn": 250037,
- "zh": 250025,
- "cy": 250007,
- "ca": 250005,
- "sl": 250052,
- "et": 250006,
- "id": 250032,
- "ar": 250001,
- "ta": 250044,
- "lv": 250017,
- "ja": 250012,
-}
-
-iface = gr.Interface(
- fn=transcribe,
- inputs=[
- gr.inputs.Audio(source="microphone", type='filepath', optional=True),
- gr.inputs.Audio(source="upload", type='filepath', optional=True),
- gr.inputs.Dropdown(target_language),
- ],
- outputs="text",
- layout="horizontal",
- theme="huggingface",
- title="XLS-R 1B EN-to-15 Speech Translation",
- description="A simple interface to translate from spoken English to 15 written languages.",
- article = "
",
- enable_queue=True,
- allow_flagging=False,
-)
-iface.launch()
diff --git a/spaces/falterWliame/Face_Mask_Detection/AVG PC TuneUp 2020 Crack Product Key Full Torrent (New).md b/spaces/falterWliame/Face_Mask_Detection/AVG PC TuneUp 2020 Crack Product Key Full Torrent (New).md
deleted file mode 100644
index ef1ae40ded57dd11029347488ebb8200f26424bd..0000000000000000000000000000000000000000
--- a/spaces/falterWliame/Face_Mask_Detection/AVG PC TuneUp 2020 Crack Product Key Full Torrent (New).md
+++ /dev/null
@@ -1,22 +0,0 @@
-
-Here is what I created:
-
-
AVG PC TuneUp 2020 Crack Product Key Full Torrent (New)
-
AVG PC TuneUp 2020 Crack is a powerful software that helps you optimize your PC performance and speed. It can scan your system for junk files, registry errors, outdated drivers, and other issues that can slow down your PC. It can also fix these problems with just one click, and provide you with various tools to customize your PC settings, manage startup programs, uninstall unwanted applications, and more.
-
AVG PC TuneUp 2020 Product Key is the license key that you need to activate the full version of the software. It can unlock all the premium features and functions that can enhance your PC experience. With AVG PC TuneUp 2020 Product Key, you can enjoy faster boot times, smoother gaming, longer battery life, and more disk space. You can also get automatic updates and priority support from AVG.
-
AVG PC TuneUp 2020 Crack Product Key Full Torrent (New)
AVG PC TuneUp 2020 Crack Product Key Full Torrent (New) is the best way to get the latest version of the software for free. You can download the torrent file from the link below and use the crack to generate a valid product key. You can then use the product key to activate AVG PC TuneUp 2020 and enjoy its benefits. However, this method is not recommended as it may be illegal and unsafe. You may risk getting viruses, malware, or legal issues by using cracked software. Therefore, it is better to buy the official product key from AVG website or authorized resellers.
-Here is what I continued:
-
-
AVG PC TuneUp 2020 has many features and functions that can help you optimize your PC performance and speed. Some of the main features are:
-
-
Cleaner: This feature can remove junk files, temporary files, browser cache, and other unnecessary data that can clutter your disk space and slow down your PC. It can also clean your registry and fix any errors that can cause stability issues.
-
Speed Up: This feature can boost your PC speed by managing your startup programs, disabling unnecessary background processes, and optimizing your system settings. It can also update your drivers and software to ensure compatibility and security.
-
Free Up Space: This feature can free up more disk space by deleting duplicate files, old downloads, unused applications, and other data that you don't need. It can also help you find and remove large files that take up a lot of space.
-
Battery Saver: This feature can extend your battery life by reducing the power consumption of your PC. It can also switch your PC to a low-power mode when it is not in use or when you are on the go.
-
Game Mode: This feature can improve your gaming performance by optimizing your PC resources for gaming. It can also disable notifications, pop-ups, and other distractions that can interrupt your gaming experience.
-
-
AVG PC TuneUp 2020 is compatible with Windows 10, 8.1, 8, and 7. It requires a minimum of 1 GB of RAM and 2 GB of disk space. It also supports over 35 languages. You can download AVG PC TuneUp 2020 from the official website or from the torrent link below. However, as mentioned before, using the torrent link may be risky and illegal. Therefore, it is better to buy the official product key from AVG website or authorized resellers.
- d5da3c52bf
-
-
\ No newline at end of file
diff --git a/spaces/farandclose/AudioChatGPT/README.md b/spaces/farandclose/AudioChatGPT/README.md
deleted file mode 100644
index 2668c63a51c63749210400ed6e335e8ee10918e9..0000000000000000000000000000000000000000
--- a/spaces/farandclose/AudioChatGPT/README.md
+++ /dev/null
@@ -1,12 +0,0 @@
----
-title: AudioChatGPT
-emoji: 🏃
-colorFrom: blue
-colorTo: gray
-sdk: gradio
-sdk_version: 3.20.1
-app_file: app.py
-pinned: false
----
-
-Check out the configuration reference at https://huggingface.co/docs/hub/spaces-config-reference
diff --git a/spaces/feng2022/Time-TravelRephotography/Time_TravelRephotography/op/upfirdn2d.cpp b/spaces/feng2022/Time-TravelRephotography/Time_TravelRephotography/op/upfirdn2d.cpp
deleted file mode 100644
index d2e633dc896433c205e18bc3e455539192ff968e..0000000000000000000000000000000000000000
--- a/spaces/feng2022/Time-TravelRephotography/Time_TravelRephotography/op/upfirdn2d.cpp
+++ /dev/null
@@ -1,23 +0,0 @@
-#include
-
-
-torch::Tensor upfirdn2d_op(const torch::Tensor& input, const torch::Tensor& kernel,
- int up_x, int up_y, int down_x, int down_y,
- int pad_x0, int pad_x1, int pad_y0, int pad_y1);
-
-#define CHECK_CUDA(x) TORCH_CHECK(x.type().is_cuda(), #x " must be a CUDA tensor")
-#define CHECK_CONTIGUOUS(x) TORCH_CHECK(x.is_contiguous(), #x " must be contiguous")
-#define CHECK_INPUT(x) CHECK_CUDA(x); CHECK_CONTIGUOUS(x)
-
-torch::Tensor upfirdn2d(const torch::Tensor& input, const torch::Tensor& kernel,
- int up_x, int up_y, int down_x, int down_y,
- int pad_x0, int pad_x1, int pad_y0, int pad_y1) {
- CHECK_CUDA(input);
- CHECK_CUDA(kernel);
-
- return upfirdn2d_op(input, kernel, up_x, up_y, down_x, down_y, pad_x0, pad_x1, pad_y0, pad_y1);
-}
-
-PYBIND11_MODULE(TORCH_EXTENSION_NAME, m) {
- m.def("upfirdn2d", &upfirdn2d, "upfirdn2d (CUDA)");
-}
\ No newline at end of file
diff --git a/spaces/feng2022/Time-TravelRephotography/Time_TravelRephotography/utils/projector_arguments.py b/spaces/feng2022/Time-TravelRephotography/Time_TravelRephotography/utils/projector_arguments.py
deleted file mode 100644
index 5fdf92897177fab9040abf666cbf6f4c7153ad78..0000000000000000000000000000000000000000
--- a/spaces/feng2022/Time-TravelRephotography/Time_TravelRephotography/utils/projector_arguments.py
+++ /dev/null
@@ -1,76 +0,0 @@
-import os
-from argparse import (
- ArgumentParser,
- Namespace,
-)
-
-from models.degrade import DegradeArguments
-from tools.initialize import InitializerArguments
-from losses.joint_loss import LossArguments
-from utils.optimize import OptimizerArguments
-from .misc import (
- optional_string,
- iterable_to_str,
-)
-
-
-class ProjectorArguments:
- def __init__(self):
- parser = ArgumentParser("Project image into stylegan2")
- self.add_arguments(parser)
- self.parser = parser
-
- @classmethod
- def add_arguments(cls, parser: ArgumentParser):
- parser.add_argument('--rand_seed', type=int, default=None,
- help="random seed")
- cls.add_io_args(parser)
- cls.add_preprocess_args(parser)
- cls.add_stylegan_args(parser)
-
- InitializerArguments.add_arguments(parser)
- LossArguments.add_arguments(parser)
- OptimizerArguments.add_arguments(parser)
- DegradeArguments.add_arguments(parser)
-
- @staticmethod
- def add_stylegan_args(parser: ArgumentParser):
- parser.add_argument('--ckpt', type=str, default="checkpoint/stylegan2-ffhq-config-f.pt",
- help="stylegan2 checkpoint")
- parser.add_argument('--generator_size', type=int, default=1024,
- help="output size of the generator")
-
- @staticmethod
- def add_io_args(parser: ArgumentParser) -> ArgumentParser:
- parser.add_argument('input', type=str, help="input image path")
- parser.add_argument('--results_dir', default="results/projector", help="directory to save results.")
-
- @staticmethod
- def add_preprocess_args(parser: ArgumentParser):
- # parser.add_argument("--match_histogram", action='store_true', help="match the histogram of the input image to the sibling")
- pass
-
- def parse(self, args=None, namespace=None) -> Namespace:
- args = self.parser.parse_args(args, namespace=namespace)
- self.print(args)
- return args
-
- @staticmethod
- def print(args: Namespace):
- print("------------ Parameters -------------")
- args = vars(args)
- for k, v in sorted(args.items()):
- print(f"{k}: {v}")
- print("-------------------------------------")
-
- @staticmethod
- def to_string(args: Namespace) -> str:
- return "-".join([
- #+ optional_string(args.no_camera_response, "-noCR")
- #+ optional_string(args.match_histogram, "-MH")
- DegradeArguments.to_string(args),
- InitializerArguments.to_string(args),
- LossArguments.to_string(args),
- OptimizerArguments.to_string(args),
- ]) + optional_string(args.rand_seed is not None, f"-S{args.rand_seed}")
-
diff --git a/spaces/feregVcuzo/sanity-test-midi/checkpoint/Benefits of Downloading Uplay Game Launcher for PC Gamers.md b/spaces/feregVcuzo/sanity-test-midi/checkpoint/Benefits of Downloading Uplay Game Launcher for PC Gamers.md
deleted file mode 100644
index eca7e6e5c0b45e050f0287324505eeee65d5aa96..0000000000000000000000000000000000000000
--- a/spaces/feregVcuzo/sanity-test-midi/checkpoint/Benefits of Downloading Uplay Game Launcher for PC Gamers.md
+++ /dev/null
@@ -1,87 +0,0 @@
-
-
How to Download Uplay Game Launcher
-
If you are a fan of Ubisoft games, you might have heard of Uplay Game Launcher. It is a free service that allows you to access, manage, and play your Ubisoft games on PC. But what exactly is Uplay Game Launcher and why should you download it? In this article, we will answer these questions and show you how to download Uplay Game Launcher on your PC in four easy steps.
-
What is Uplay Game Launcher?
-
Uplay Game Launcher is part of Ubisoft Connect, which is the ecosystem of player services for Ubisoft games across all platforms. It aims at giving the best environment for all players to enjoy their games and connect with each other whatever the device. Here are some of the features of Uplay Game Launcher:
Uplay Game Launcher is a free service that you can access on your PC, through a mobile app, or on consoles (directly from your games). All you need to log in is a Ubisoft account. You can use Uplay Game Launcher to launch and update your Ubisoft games, as well as access additional content and DLCs.
-
A cross-platform network for players
-
Uplay Game Launcher allows you to connect with players across all platforms, for all games. Whether you play on PC or console, you'll be part of a global network of Ubisoft players with access to all the same services. You can find friends on all platforms, see what they're playing, and check their achievements. You can also keep your progression on all devices for the newest releases, such as Assassin's Creed Valhalla, Immortals Fenyx Rising, and Riders Republic.
-
A loyalty program with rewards
-
Uplay Game Launcher also offers a loyalty program that rewards you for playing your games. You can win over 1000 free rewards across the back catalogue of games, such as legendary weapons, character outfits, emotes, and consumables. Every time you level up in Ubisoft Connect, you'll earn Units that you can spend on unique rewards. You can also redeem 100 Units to get 20% off your next purchase in the Ubisoft Store.
-
A desktop app with features
-
Uplay Game Launcher also has a desktop app that enhances your experience on PC. You can use it to manage and start your games through the library, discover new content and download it with just a click. You can also access your stats, progression, and performance in your favorite games, as well as check your feed for the latest news, events, and challenges. You can also join multiplayer sessions, create new chats and group chats, and enjoy the Ubisoft+ subscription service that gives you access to over 100 games on PC.
-
Why Download Uplay Game Launcher?
-
Now that you know what Uplay Game Launcher is and what it offers, you might be wondering why you should download it. Here are some of the reasons why downloading Uplay Game Launcher is a good idea:
-
To access your Ubisoft games on PC
-
If you want to play your Ubisoft games on PC, you will need Uplay Game Launcher to launch them. Uplay Game Launcher will also keep your games updated and let you download additional content and DLCs. You can also use Uplay Game Launcher to browse and buy new Ubisoft games from the store.
-
To enjoy the benefits of Ubisoft Connect
-
By downloading Uplay Game Launcher, you will also be able to enjoy all the benefits of Ubisoft Connect, such as connecting with other players, keeping your progression on all devices, winning rewards, accessing your stats and tips, and more. You will also be able to
To participate in events and giveaways
-
Another reason to download Uplay Game Launcher is to participate in events and giveaways that Ubisoft organizes regularly. You can join community challenges, live streams, contests, and more to win exclusive rewards, such as in-game items, beta access, or even physical goodies. You can also get free games and trials from time to time, so don't miss out on these opportunities.
-
How to Download Uplay Game Launcher on PC?
-
Now that you have decided to download Uplay Game Launcher, you might be wondering how to do it. Don't worry, it's very simple and fast. Just follow these four steps and you'll be ready to play your Ubisoft games on PC in no time:
-
How to download uplay game launcher on PC
-Download uplay game launcher for free
-Download uplay game launcher and enjoy 100+ games on PC
-Download uplay game launcher and get 20% off on Ubisoft Store
-Download uplay game launcher and join Ubisoft Connect community
-Download uplay game launcher and access Ubisoft+ subscription service
-Download uplay game launcher and play Ubisoft games across all platforms
-Download uplay game launcher and unlock free rewards
-Download uplay game launcher and check your stats and achievements
-Download uplay game launcher and participate in free week-ends and giveaways
-Download uplay game launcher and register for beta events and test servers
-Download uplay game launcher and manage your games library
-Download uplay game launcher and discover new content
-Download uplay game launcher and connect with your friends
-Download uplay game launcher and get the best gaming experience on PC
-How to install or re-install uplay game launcher on PC
-Uplay game launcher download error: how to fix it
-Uplay game launcher download speed: how to improve it
-Uplay game launcher download size: how much space do you need
-Uplay game launcher download location: where to find it on your PC
-How to update uplay game launcher on PC
-How to uninstall uplay game launcher on PC
-How to troubleshoot uplay game launcher issues on PC
-How to contact Ubisoft support for uplay game launcher problems on PC
-How to change your language settings on uplay game launcher on PC
-Uplay game launcher vs Steam: which one is better for PC gaming
-Uplay game launcher vs Epic Games Store: which one is better for PC gaming
-Uplay game launcher vs Origin: which one is better for PC gaming
-Uplay game launcher vs GOG Galaxy: which one is better for PC gaming
-Uplay game launcher vs Microsoft Store: which one is better for PC gaming
-How to link your Steam account to your uplay game launcher account on PC
-How to link your Epic Games Store account to your uplay game launcher account on PC
-How to link your Origin account to your uplay game launcher account on PC
-How to link your GOG Galaxy account to your uplay game launcher account on PC
-How to link your Microsoft Store account to your uplay game launcher account on PC
-How to play Steam games on uplay game launcher on PC
-How to play Epic Games Store games on uplay game launcher on PC
-How to play Origin games on uplay game launcher on PC
-How to play GOG Galaxy games on uplay game launcher on PC
-How to play Microsoft Store games on uplay game launcher on PC
-
Step 1: Visit the Ubisoft Connect website
-
The first thing you need to do is to visit the Ubisoft Connect website. You can do this by clicking on this link: https://ubisoftconnect.com/en-US/. This will take you to the official website of Ubisoft Connect, where you can learn more about the service and its features.
-
Step 2: Click on the download button
-
The next thing you need to do is to click on the download button that you will see on the top right corner of the website. This will start the download of the Uplay Game Launcher installer on your PC. The file size is about 100 MB, so it shouldn't take too long to download.
-
Step 3: Run the installer and follow the instructions
-
Once the download is complete, you need to run the installer and follow the instructions that will appear on your screen. The installation process is very easy and straightforward. You just need to agree to the terms and conditions, choose a destination folder, and wait for the installation to finish.
-
Step 4: Log in with your Ubisoft account or create one
-
The last step is to log in with your Ubisoft account or create one if you don't have one already. You can use your email address, Facebook account, or Google account to log in or sign up. Once you do that, you will be able to access Uplay Game Launcher and start playing your Ubisoft games on PC.
-
Conclusion
-
Uplay Game Launcher is a free service that allows you to access, manage, and play your Ubisoft games on PC. It is part of Ubisoft Connect, which is the ecosystem of player services for Ubisoft games across all platforms. By downloading Uplay Game Launcher, you will be able to enjoy all the benefits of Ubisoft Connect, such as connecting with other players, keeping your progression on all devices, winning rewards, accessing your stats and tips, and more. You will also be able to participate in events and giveaways that Ubisoft organizes regularly. To download Uplay Game Launcher on PC, you just need to follow four simple steps: visit the Ubisoft Connect website, click on the download button, run the installer and follow the instructions, and log in with your Ubisoft account or create one. We hope this article was helpful and informative for you. If you have any questions or feedback, feel free to leave a comment below.
-
FAQs
-
Here are some of the frequently asked questions about Uplay Game Launcher:
-
Q: Is Uplay Game Launcher safe?
-
A: Yes, Uplay Game Launcher is safe and secure. It is developed by Ubisoft, which is a reputable company in the gaming industry. It does not contain any viruses or malware that could harm your PC or compromise your data.
-
Q: Is Uplay Game Launcher free?
-
A: Yes, Uplay Game Launcher is free to download and use. You don't need to pay anything to access it or play your Ubisoft games on PC.
-
Q: Can I play my Ubisoft games without Uplay Game Launcher?
-
A: No, you cannot play your Ubisoft games without Uplay Game Launcher on PC. You need Uplay Game Launcher to launch and update your games, as well as access additional content and DLCs.
-
Q: Can I uninstall Uplay Game Launcher?
-
A: Yes, you can uninstall Uplay Game Launcher if you want to. However, this will also remove all your Ubisoft games from your PC. If you want to reinstall them later, you will need to download Uplay Game Launcher again.
-
Q: How can I contact Ubisoft support if I have any issues with Uplay Game Launcher?
-
A: If you have any issues with Uplay Game Launcher or your Ubisoft games, you can contact Ubisoft support through this link: < a href="https://support.ubisoft.com/">https://support.ubisoft.com/. You can also check the FAQ section or the forums for more information and help from other players.
The 1991 Lokhandwala Complex shootout was a gunbattle that occurred on 16 November 1991 at the Lokhandwala Complex, Bombay (now Mumbai), between seven gangsters led by Maya Dolas and members of the Mumbai Police and the Anti-Terrorism Squad (ATS) led by the then Additional Commissioner of Police, A. A. Khan. The four-hour-long shootout was termed as India's "first daylight encounter" and was videographed and conducted in full view of the public.[1][2][3] It ended in the deaths of all seven gangsters, including Maya Dolas, Dilip Buwa and Anil Pawar.
download Bollywood Crime Movies unlimited Movies and videos Download Here.Bollywood Crime Movies Hd,3gp. mp4 320p and More Videos You Can Download Easyly. tamilrockers and movierulz, tamilgun, filmywap, and pagalworld videos and Movies download.
-
The leading Premium South Asian streaming content provider of full-length feature films in on-demand superior HD. We're expanding to bring you high-bitrate audio albums, movie clips, and music videos.
aaccfb2cb3
-
-
\ No newline at end of file
diff --git a/spaces/inigosarralde/mushroom_edibility_classifier/app.py b/spaces/inigosarralde/mushroom_edibility_classifier/app.py
deleted file mode 100644
index b8dfaea5fd07d37ec2f94feb0bf5f76fadac4091..0000000000000000000000000000000000000000
--- a/spaces/inigosarralde/mushroom_edibility_classifier/app.py
+++ /dev/null
@@ -1,125 +0,0 @@
-import gradio as gr
-import matplotlib.pyplot as plt
-import numpy as np
-import PIL
-import tensorflow as tf
-
-
-model = tf.keras.models.load_model('model.h5')
-class_name_list = ['Edible', 'Inedible', 'Poisonous']
-
-def predict_image(img):
- # Reescalamos la imagen en 4 dimensiones
- img_4d = img.reshape(-1,224,224,3)
- # Predicción del modelo
- prediction = model.predict(img_4d)[0]
- # Diccionario con todas las clases y las probabilidades correspondientes
- return {class_name_list[i]: float(prediction[i]) for i in range(3)}
-image = gr.inputs.Image(shape=(224,224))
-label = gr.outputs.Label(num_top_classes=3)
-title = 'Mushroom Edibility Classifier'
-description = 'Get the edibility classification for the input mushroom image'
-examples=[['app_interface/Boletus edulis 15 wf.jpg'],
- ['app_interface/Cantharelluscibarius5 mw.jpg'],
- ['app_interface/Agaricus augustus 2 wf.jpg'],
- ['app_interface/Coprinellus micaceus 8 wf.jpg'],
- ['app_interface/Clavulinopsis fusiformis 2 fp.jpg'],
- ['app_interface/Amanita torrendii 8 fp.jpg'],
- ['app_interface/Russula sanguinea 5 fp.jpg'],
- ['app_interface/Caloceraviscosa1 mw.jpg'],
- ['app_interface/Amanita muscaria 1 wf.jpg'],
- ['app_interface/Amanita pantherina 11 wf.jpg'],
- ['app_interface/Lactarius torminosus 6 fp.jpg'],
- ['app_interface/Amanitaphalloides1 mw.jpg']]
-thumbnail = 'app_interface/thumbnail.png'
-article = '''
-
-
-
-
The Mushroom Edibility Classifier is an MVP for CNN multiclass classification model.
-It has been trained after gathering 5500 mushroom images through Web Scraping techniques from the following web sites:
Note: model created solely and exclusively for academic purposes. The results provided by the model should never be considered definitive as the accuracy of the model is not guaranteed.
-
-
-
MODEL METRICS:
-
-
-
-
precision
-
recall
-
f1-score
-
support
-
-
-
Edible
-
0.61
-
0.70
-
0.65
-
481
-
-
-
Inedible
-
0.67
-
0.69
-
0.68
-
439
-
-
-
Poisonous
-
0.52
-
0.28
-
0.36
-
192
-
-
-
-
-
-
Global Accuracy
-
-
-
0.63
-
1112
-
-
-
Macro Average
-
0.60
-
0.56
-
0.57
-
1112
-
-
-
Weighted Average
-
0.62
-
0.63
-
0.61
-
1112
-
-
-
-
Author: Íñigo Sarralde Alzórriz
-
-
-'''
-
-iface = gr.Interface(fn=predict_image,
- inputs=image,
- outputs=label,
- interpretation='default',
- title = title,
- description = description,
- theme = 'darkpeach',
- examples = examples,
- thumbnail = thumbnail,
- article = article,
- allow_flagging = False,
- allow_screenshot = False,
- )
-iface.launch()
\ No newline at end of file
diff --git a/spaces/innnky/visinger2-nomidi/modules/stft.py b/spaces/innnky/visinger2-nomidi/modules/stft.py
deleted file mode 100644
index 4bec9a72788902b38d104b7307ba415408b2477d..0000000000000000000000000000000000000000
--- a/spaces/innnky/visinger2-nomidi/modules/stft.py
+++ /dev/null
@@ -1,512 +0,0 @@
-from librosa.util import pad_center, tiny
-from scipy.signal import get_window
-from torch import Tensor
-from torch.autograd import Variable
-from typing import Optional, Tuple
-
-import librosa
-import librosa.util as librosa_util
-import math
-import numpy as np
-import scipy
-import torch
-import torch.nn.functional as F
-import warnings
-
-
-def create_fb_matrix(
- n_freqs: int,
- f_min: float,
- f_max: float,
- n_mels: int,
- sample_rate: int,
- norm: Optional[str] = None
-) -> Tensor:
- r"""Create a frequency bin conversion matrix.
-
- Args:
- n_freqs (int): Number of frequencies to highlight/apply
- f_min (float): Minimum frequency (Hz)
- f_max (float): Maximum frequency (Hz)
- n_mels (int): Number of mel filterbanks
- sample_rate (int): Sample rate of the audio waveform
- norm (Optional[str]): If 'slaney', divide the triangular mel weights by the width of the mel band
- (area normalization). (Default: ``None``)
-
- Returns:
- Tensor: Triangular filter banks (fb matrix) of size (``n_freqs``, ``n_mels``)
- meaning number of frequencies to highlight/apply to x the number of filterbanks.
- Each column is a filterbank so that assuming there is a matrix A of
- size (..., ``n_freqs``), the applied result would be
- ``A * create_fb_matrix(A.size(-1), ...)``.
- """
-
- if norm is not None and norm != "slaney":
- raise ValueError("norm must be one of None or 'slaney'")
-
- # freq bins
- # Equivalent filterbank construction by Librosa
- all_freqs = torch.linspace(0, sample_rate // 2, n_freqs)
-
- # calculate mel freq bins
- # hertz to mel(f) is 2595. * math.log10(1. + (f / 700.))
- m_min = 2595.0 * math.log10(1.0 + (f_min / 700.0))
- m_max = 2595.0 * math.log10(1.0 + (f_max / 700.0))
- m_pts = torch.linspace(m_min, m_max, n_mels + 2)
- # mel to hertz(mel) is 700. * (10**(mel / 2595.) - 1.)
- f_pts = 700.0 * (10 ** (m_pts / 2595.0) - 1.0)
- # calculate the difference between each mel point and each stft freq point in hertz
- f_diff = f_pts[1:] - f_pts[:-1] # (n_mels + 1)
- slopes = f_pts.unsqueeze(0) - all_freqs.unsqueeze(1) # (n_freqs, n_mels + 2)
- # create overlapping triangles
- down_slopes = (-1.0 * slopes[:, :-2]) / f_diff[:-1] # (n_freqs, n_mels)
- up_slopes = slopes[:, 2:] / f_diff[1:] # (n_freqs, n_mels)
- fb = torch.min(down_slopes, up_slopes)
- fb = torch.clamp(fb, 1e-6, 1)
-
- if norm is not None and norm == "slaney":
- # Slaney-style mel is scaled to be approx constant energy per channel
- enorm = 2.0 / (f_pts[2:n_mels + 2] - f_pts[:n_mels])
- fb *= enorm.unsqueeze(0)
- return fb
-
-
-def lfilter(
- waveform: Tensor,
- a_coeffs: Tensor,
- b_coeffs: Tensor,
- clamp: bool = True,
-) -> Tensor:
- r"""Perform an IIR filter by evaluating difference equation.
-
- Args:
- waveform (Tensor): audio waveform of dimension of ``(..., time)``. Must be normalized to -1 to 1.
- a_coeffs (Tensor): denominator coefficients of difference equation of dimension of ``(n_order + 1)``.
- Lower delays coefficients are first, e.g. ``[a0, a1, a2, ...]``.
- Must be same size as b_coeffs (pad with 0's as necessary).
- b_coeffs (Tensor): numerator coefficients of difference equation of dimension of ``(n_order + 1)``.
- Lower delays coefficients are first, e.g. ``[b0, b1, b2, ...]``.
- Must be same size as a_coeffs (pad with 0's as necessary).
- clamp (bool, optional): If ``True``, clamp the output signal to be in the range [-1, 1] (Default: ``True``)
-
- Returns:
- Tensor: Waveform with dimension of ``(..., time)``.
- """
- # pack batch
- shape = waveform.size()
- waveform = waveform.reshape(-1, shape[-1])
-
- assert (a_coeffs.size(0) == b_coeffs.size(0))
- assert (len(waveform.size()) == 2)
- assert (waveform.device == a_coeffs.device)
- assert (b_coeffs.device == a_coeffs.device)
-
- device = waveform.device
- dtype = waveform.dtype
- n_channel, n_sample = waveform.size()
- n_order = a_coeffs.size(0)
- n_sample_padded = n_sample + n_order - 1
- assert (n_order > 0)
-
- # Pad the input and create output
- padded_waveform = torch.zeros(n_channel, n_sample_padded, dtype=dtype, device=device)
- padded_waveform[:, (n_order - 1):] = waveform
- padded_output_waveform = torch.zeros(n_channel, n_sample_padded, dtype=dtype, device=device)
-
- # Set up the coefficients matrix
- # Flip coefficients' order
- a_coeffs_flipped = a_coeffs.flip(0)
- b_coeffs_flipped = b_coeffs.flip(0)
-
- # calculate windowed_input_signal in parallel
- # create indices of original with shape (n_channel, n_order, n_sample)
- window_idxs = torch.arange(n_sample, device=device).unsqueeze(0) + torch.arange(n_order, device=device).unsqueeze(1)
- window_idxs = window_idxs.repeat(n_channel, 1, 1)
- window_idxs += (torch.arange(n_channel, device=device).unsqueeze(-1).unsqueeze(-1) * n_sample_padded)
- window_idxs = window_idxs.long()
- # (n_order, ) matmul (n_channel, n_order, n_sample) -> (n_channel, n_sample)
- input_signal_windows = torch.matmul(b_coeffs_flipped, torch.take(padded_waveform, window_idxs))
-
- input_signal_windows.div_(a_coeffs[0])
- a_coeffs_flipped.div_(a_coeffs[0])
- for i_sample, o0 in enumerate(input_signal_windows.t()):
- windowed_output_signal = padded_output_waveform[:, i_sample:(i_sample + n_order)]
- o0.addmv_(windowed_output_signal, a_coeffs_flipped, alpha=-1)
- padded_output_waveform[:, i_sample + n_order - 1] = o0
-
- output = padded_output_waveform[:, (n_order - 1):]
-
- if clamp:
- output = torch.clamp(output, min=-1., max=1.)
-
- # unpack batch
- output = output.reshape(shape[:-1] + output.shape[-1:])
-
- return output
-
-
-
-def biquad(
- waveform: Tensor,
- b0: float,
- b1: float,
- b2: float,
- a0: float,
- a1: float,
- a2: float
-) -> Tensor:
- r"""Perform a biquad filter of input tensor. Initial conditions set to 0.
- https://en.wikipedia.org/wiki/Digital_biquad_filter
-
- Args:
- waveform (Tensor): audio waveform of dimension of `(..., time)`
- b0 (float): numerator coefficient of current input, x[n]
- b1 (float): numerator coefficient of input one time step ago x[n-1]
- b2 (float): numerator coefficient of input two time steps ago x[n-2]
- a0 (float): denominator coefficient of current output y[n], typically 1
- a1 (float): denominator coefficient of current output y[n-1]
- a2 (float): denominator coefficient of current output y[n-2]
-
- Returns:
- Tensor: Waveform with dimension of `(..., time)`
- """
-
- device = waveform.device
- dtype = waveform.dtype
-
- output_waveform = lfilter(
- waveform,
- torch.tensor([a0, a1, a2], dtype=dtype, device=device),
- torch.tensor([b0, b1, b2], dtype=dtype, device=device)
- )
- return output_waveform
-
-
-
-def _dB2Linear(x: float) -> float:
- return math.exp(x * math.log(10) / 20.0)
-
-
-def highpass_biquad(
- waveform: Tensor,
- sample_rate: int,
- cutoff_freq: float,
- Q: float = 0.707
-) -> Tensor:
- r"""Design biquad highpass filter and perform filtering. Similar to SoX implementation.
-
- Args:
- waveform (Tensor): audio waveform of dimension of `(..., time)`
- sample_rate (int): sampling rate of the waveform, e.g. 44100 (Hz)
- cutoff_freq (float): filter cutoff frequency
- Q (float, optional): https://en.wikipedia.org/wiki/Q_factor (Default: ``0.707``)
-
- Returns:
- Tensor: Waveform dimension of `(..., time)`
- """
- w0 = 2 * math.pi * cutoff_freq / sample_rate
- alpha = math.sin(w0) / 2. / Q
-
- b0 = (1 + math.cos(w0)) / 2
- b1 = -1 - math.cos(w0)
- b2 = b0
- a0 = 1 + alpha
- a1 = -2 * math.cos(w0)
- a2 = 1 - alpha
- return biquad(waveform, b0, b1, b2, a0, a1, a2)
-
-
-
-def lowpass_biquad(
- waveform: Tensor,
- sample_rate: int,
- cutoff_freq: float,
- Q: float = 0.707
-) -> Tensor:
- r"""Design biquad lowpass filter and perform filtering. Similar to SoX implementation.
-
- Args:
- waveform (torch.Tensor): audio waveform of dimension of `(..., time)`
- sample_rate (int): sampling rate of the waveform, e.g. 44100 (Hz)
- cutoff_freq (float): filter cutoff frequency
- Q (float, optional): https://en.wikipedia.org/wiki/Q_factor (Default: ``0.707``)
-
- Returns:
- Tensor: Waveform of dimension of `(..., time)`
- """
- w0 = 2 * math.pi * cutoff_freq / sample_rate
- alpha = math.sin(w0) / 2 / Q
-
- b0 = (1 - math.cos(w0)) / 2
- b1 = 1 - math.cos(w0)
- b2 = b0
- a0 = 1 + alpha
- a1 = -2 * math.cos(w0)
- a2 = 1 - alpha
- return biquad(waveform, b0, b1, b2, a0, a1, a2)
-
-
-def window_sumsquare(window, n_frames, hop_length=200, win_length=800,
- n_fft=800, dtype=np.float32, norm=None):
- """
- # from librosa 0.6
- Compute the sum-square envelope of a window function at a given hop length.
-
- This is used to estimate modulation effects induced by windowing
- observations in short-time fourier transforms.
-
- Parameters
- ----------
- window : string, tuple, number, callable, or list-like
- Window specification, as in `get_window`
-
- n_frames : int > 0
- The number of analysis frames
-
- hop_length : int > 0
- The number of samples to advance between frames
-
- win_length : [optional]
- The length of the window function. By default, this matches `n_fft`.
-
- n_fft : int > 0
- The length of each analysis frame.
-
- dtype : np.dtype
- The data type of the output
-
- Returns
- -------
- wss : np.ndarray, shape=`(n_fft + hop_length * (n_frames - 1))`
- The sum-squared envelope of the window function
- """
- if win_length is None:
- win_length = n_fft
-
- n = n_fft + hop_length * (n_frames - 1)
- x = np.zeros(n, dtype=dtype)
-
- # Compute the squared window at the desired length
- win_sq = get_window(window, win_length, fftbins=True)
- win_sq = librosa_util.normalize(win_sq, norm=norm)**2
- win_sq = librosa_util.pad_center(win_sq, n_fft)
-
- # Fill the envelope
- for i in range(n_frames):
- sample = i * hop_length
- x[sample:min(n, sample + n_fft)] += win_sq[:max(0, min(n_fft, n - sample))]
- return x
-
-
-class MelScale(torch.nn.Module):
- r"""Turn a normal STFT into a mel frequency STFT, using a conversion
- matrix. This uses triangular filter banks.
-
- User can control which device the filter bank (`fb`) is (e.g. fb.to(spec_f.device)).
-
- Args:
- n_mels (int, optional): Number of mel filterbanks. (Default: ``128``)
- sample_rate (int, optional): Sample rate of audio signal. (Default: ``16000``)
- f_min (float, optional): Minimum frequency. (Default: ``0.``)
- f_max (float or None, optional): Maximum frequency. (Default: ``sample_rate // 2``)
- n_stft (int, optional): Number of bins in STFT. Calculated from first input
- if None is given. See ``n_fft`` in :class:`Spectrogram`. (Default: ``None``)
- """
- __constants__ = ['n_mels', 'sample_rate', 'f_min', 'f_max']
-
- def __init__(self,
- n_mels: int = 128,
- sample_rate: int = 24000,
- f_min: float = 0.,
- f_max: Optional[float] = None,
- n_stft: Optional[int] = None) -> None:
- super(MelScale, self).__init__()
- self.n_mels = n_mels
- self.sample_rate = sample_rate
- self.f_max = f_max if f_max is not None else float(sample_rate // 2)
- self.f_min = f_min
-
- assert f_min <= self.f_max, 'Require f_min: %f < f_max: %f' % (f_min, self.f_max)
-
- fb = torch.empty(0) if n_stft is None else create_fb_matrix(
- n_stft, self.f_min, self.f_max, self.n_mels, self.sample_rate)
- self.register_buffer('fb', fb)
-
- def forward(self, specgram: Tensor) -> Tensor:
- r"""
- Args:
- specgram (Tensor): A spectrogram STFT of dimension (..., freq, time).
-
- Returns:
- Tensor: Mel frequency spectrogram of size (..., ``n_mels``, time).
- """
-
- # pack batch
- shape = specgram.size()
- specgram = specgram.reshape(-1, shape[-2], shape[-1])
-
- if self.fb.numel() == 0:
- tmp_fb = create_fb_matrix(specgram.size(1), self.f_min, self.f_max, self.n_mels, self.sample_rate)
- # Attributes cannot be reassigned outside __init__ so workaround
- self.fb.resize_(tmp_fb.size())
- self.fb.copy_(tmp_fb)
-
- # (channel, frequency, time).transpose(...) dot (frequency, n_mels)
- # -> (channel, time, n_mels).transpose(...)
- mel_specgram = torch.matmul(specgram.transpose(1, 2), self.fb).transpose(1, 2)
-
- # unpack batch
- mel_specgram = mel_specgram.reshape(shape[:-2] + mel_specgram.shape[-2:])
-
- return mel_specgram
-
-
-class TorchSTFT(torch.nn.Module):
- def __init__(self, fft_size, hop_size, win_size,
- normalized=False, domain='linear',
- mel_scale=False, ref_level_db=20, min_level_db=-100):
- super().__init__()
- self.fft_size = fft_size
- self.hop_size = hop_size
- self.win_size = win_size
- self.ref_level_db = ref_level_db
- self.min_level_db = min_level_db
- self.window = torch.hann_window(win_size)
- self.normalized = normalized
- self.domain = domain
- self.mel_scale = MelScale(n_mels=(fft_size // 2 + 1),
- n_stft=(fft_size // 2 + 1)) if mel_scale else None
-
- def transform(self, x):
- x_stft = torch.stft(x, self.fft_size, self.hop_size, self.win_size,
- self.window.type_as(x), normalized=self.normalized)
- real = x_stft[..., 0]
- imag = x_stft[..., 1]
- mag = torch.clamp(real ** 2 + imag ** 2, min=1e-7)
- mag = torch.sqrt(mag)
- phase = torch.atan2(imag, real)
-
- if self.mel_scale is not None:
- mag = self.mel_scale(mag)
-
- if self.domain == 'log':
- mag = 20 * torch.log10(mag) - self.ref_level_db
- mag = torch.clamp((mag - self.min_level_db) / -self.min_level_db, 0, 1)
- return mag, phase
- elif self.domain == 'linear':
- return mag, phase
- elif self.domain == 'double':
- log_mag = 20 * torch.log10(mag) - self.ref_level_db
- log_mag = torch.clamp((log_mag - self.min_level_db) / -self.min_level_db, 0, 1)
- return torch.cat((mag, log_mag), dim=1), phase
-
- def complex(self, x):
- x_stft = torch.stft(x, self.fft_size, self.hop_size, self.win_size,
- self.window.type_as(x), normalized=self.normalized)
- real = x_stft[..., 0]
- imag = x_stft[..., 1]
- return real, imag
-
-
-
-class STFT(torch.nn.Module):
- """adapted from Prem Seetharaman's https://github.com/pseeth/pytorch-stft"""
- def __init__(self, filter_length=800, hop_length=200, win_length=800,
- window='hann'):
- super(STFT, self).__init__()
- self.filter_length = filter_length
- self.hop_length = hop_length
- self.win_length = win_length
- self.window = window
- self.forward_transform = None
- scale = self.filter_length / self.hop_length
- fourier_basis = np.fft.fft(np.eye(self.filter_length))
-
- cutoff = int((self.filter_length / 2 + 1))
- fourier_basis = np.vstack([np.real(fourier_basis[:cutoff, :]),
- np.imag(fourier_basis[:cutoff, :])])
-
- forward_basis = torch.FloatTensor(fourier_basis[:, None, :])
- inverse_basis = torch.FloatTensor(
- np.linalg.pinv(scale * fourier_basis).T[:, None, :])
-
- if window is not None:
- assert(filter_length >= win_length)
- # get window and zero center pad it to filter_length
- fft_window = get_window(window, win_length, fftbins=True)
- fft_window = pad_center(fft_window, filter_length)
- fft_window = torch.from_numpy(fft_window).float()
-
- # window the bases
- forward_basis *= fft_window
- inverse_basis *= fft_window
-
- self.register_buffer('forward_basis', forward_basis.float())
- self.register_buffer('inverse_basis', inverse_basis.float())
-
- def transform(self, input_data):
- num_batches = input_data.size(0)
- num_samples = input_data.size(1)
-
- self.num_samples = num_samples
-
- # similar to librosa, reflect-pad the input
- input_data = input_data.view(num_batches, 1, num_samples)
- input_data = F.pad(
- input_data.unsqueeze(1),
- (int(self.filter_length / 2), int(self.filter_length / 2), 0, 0),
- mode='reflect')
- input_data = input_data.squeeze(1)
-
- forward_transform = F.conv1d(
- input_data,
- Variable(self.forward_basis, requires_grad=False),
- stride=self.hop_length,
- padding=0)
-
- cutoff = int((self.filter_length / 2) + 1)
- real_part = forward_transform[:, :cutoff, :]
- imag_part = forward_transform[:, cutoff:, :]
-
- magnitude = torch.sqrt(real_part**2 + imag_part**2)
- phase = torch.autograd.Variable(
- torch.atan2(imag_part.data, real_part.data))
-
- return magnitude, phase
-
- def inverse(self, magnitude, phase):
- recombine_magnitude_phase = torch.cat(
- [magnitude*torch.cos(phase), magnitude*torch.sin(phase)], dim=1)
-
- inverse_transform = F.conv_transpose1d(
- recombine_magnitude_phase,
- Variable(self.inverse_basis, requires_grad=False),
- stride=self.hop_length,
- padding=0)
-
- if self.window is not None:
- window_sum = window_sumsquare(
- self.window, magnitude.size(-1), hop_length=self.hop_length,
- win_length=self.win_length, n_fft=self.filter_length,
- dtype=np.float32)
- # remove modulation effects
- approx_nonzero_indices = torch.from_numpy(
- np.where(window_sum > tiny(window_sum))[0])
- window_sum = torch.autograd.Variable(
- torch.from_numpy(window_sum), requires_grad=False)
- window_sum = window_sum.cuda() if magnitude.is_cuda else window_sum
- inverse_transform[:, :, approx_nonzero_indices] /= window_sum[approx_nonzero_indices]
-
- # scale by hop ratio
- inverse_transform *= float(self.filter_length) / self.hop_length
-
- inverse_transform = inverse_transform[:, :, int(self.filter_length/2):]
- inverse_transform = inverse_transform[:, :, :-int(self.filter_length/2):]
-
- return inverse_transform
-
- def forward(self, input_data):
- self.magnitude, self.phase = self.transform(input_data)
- reconstruction = self.inverse(self.magnitude, self.phase)
- return reconstruction
-
diff --git a/spaces/inplisQlawa/anything-midjourney-v4-1/Download __TOP__ Password.txt 0.01 Kb.md b/spaces/inplisQlawa/anything-midjourney-v4-1/Download __TOP__ Password.txt 0.01 Kb.md
deleted file mode 100644
index 9cfe8868c8aa4e49adbb882d2469b12f9b435089..0000000000000000000000000000000000000000
--- a/spaces/inplisQlawa/anything-midjourney-v4-1/Download __TOP__ Password.txt 0.01 Kb.md
+++ /dev/null
@@ -1,118 +0,0 @@
-
-
How to Download Password.txt (0.01 KB) Without Survey
-
-
If you are looking for a way to download password.txt (0.01 KB) without survey, you are not alone. Many people are frustrated by the fake surveys and scams that prevent them from accessing the files they need. Password.txt (0.01 KB) is a common file that is used to unlock RAR archives that contain games, movies, software, or other files. However, finding a reliable source to download password.txt (0.01 KB) can be challenging.
-
-
In this article, we will show you how to download password.txt (0.01 KB) without survey, using some simple and effective methods. We will also explain what password.txt (0.01 KB) is, why it is used, and how to use it to open RAR files.
Password.txt (0.01 KB) is a text file that contains a password or a list of passwords that can be used to unlock RAR archives. RAR archives are compressed files that can store multiple files or folders in a single file. They are often used to reduce the size of large files and make them easier to download or share.
-
-
However, some RAR archives are protected by a password that prevents unauthorized users from opening them. The password can be set by the creator of the archive or by a third-party website that hosts the archive. In order to open a password-protected RAR archive, you need to enter the correct password or use a tool that can crack the password.
-
-
Some websites claim to provide the password for certain RAR archives, but they require you to complete a survey or an offer before they give you the password. These surveys or offers are usually fake and designed to trick you into giving away your personal information, money, or downloading malware. They are also very time-consuming and annoying.
-
-
That's why many people look for alternative ways to download password.txt (0.01 KB) without survey. Password.txt (0.01 KB) can help you bypass the survey and access the RAR archive directly.
-
-
How to Download Password.txt (0.01 KB) Without Survey?
-
-
There are several methods that can help you download password.txt (0.01 KB) without survey. Here are some of them:
-
-
-
Method 1: Use a Search Engine
-
One of the easiest ways to find password.txt (0.01 KB) is to use a search engine like Google or Bing. You can simply type in the name of the RAR archive or the website that hosts it, followed by "password.txt" or "password". For example, if you want to download password.txt for GTA 5 RAR archive from uploadsnack.com, you can search for "GTA 5 uploadsnack.com password.txt" or "GTA 5 uploadsnack.com password".
-
-
This will show you some results that may contain the password or a link to download password.txt (0.01 KB). However, you need to be careful and check the credibility of the source before you click on any link or download any file. Some sources may be fake or malicious and may harm your computer or steal your data.
-
-
Method 2: Use a Password Cracker
-
Another way to download password.txt (0.01 KB) without survey is to use a password cracker tool that can try to guess or break the password of the RAR archive. There are many tools available online that can do this, such as RAR Password Cracker, RAR Password Unlocker, WinRAR Password Remover, etc.
-
-
-
These tools work by using different techniques such as brute force, dictionary attack, mask attack, etc., to try different combinations of characters until they find the correct password or a close match. However, these tools may take a long time and consume a lot of CPU and memory resources depending on the complexity and length of the password.
-
-
Method 3: Use a Password Database
-
A third way to download password.txt (0.01 KB) without survey is to use a password database that contains a list of passwords for various RAR archives. These databases are usually created by users who have successfully cracked or obtained the passwords for different RAR archives and shared them online.
-
-
You can find these databases by searching for "password database" or "password list" on Google or Bing. You may also find some websites that specialize in providing passwords for certain RAR archives, such as uploadsnackpassword.com, memory1gigabyte.blogspot.com, etc.
-
-
However, these databases may not be updated regularly and may not contain the password for the RAR archive you are looking for. They may also contain some fake or incorrect passwords that may not work.
-
-
-
How to Use Password.txt (0.01 KB) to Open RAR Files?
-
-
Once you have downloaded password.txt (0.01 KB), you can use it to open the RAR archive you want. Here are the steps:
-
-
-
Download and install WinRAR or any other software that can open RAR files.
-
Right-click on the RAR file and select "Extract Here" or "Extract Files".
-
A window will pop up asking you to enter the password.
-
Open password.txt (0.01 KB) with Notepad or any other text editor.
-
Copy and paste one of the passwords from password.txt (0.01 KB) into the window and click "OK".
-
If the password is correct, the RAR file will be extracted and you can access its contents.
-
If the password is wrong, try another one from password.txt (0.01 KB) until you find the right one.
-
-
-
Conclusion
-
-
Password.txt (0.01 KB) is a useful file that can help you unlock RAR archives without completing surveys or offers. However, finding a reliable source to download password.txt (0.01 KB) can be difficult and risky.
-
-
In this article, we have shown you some methods that can help you download password.txt (0.01 KB) without survey, such as using a search engine, a password cracker tool, or a password database. We have also explained how to use password.txt (0.01 KB) to open RAR files.
-
-
We hope this article has been helpful and informative for you. If you have any questions or suggestions, please feel free to leave a comment below.
-
What are the Benefits of Downloading Password.txt (0.01 KB) Without Survey?
-
-
Downloading password.txt (0.01 KB) without survey has many benefits for you as a user. Here are some of them:
-
-
-
Save Time and Effort
-
By downloading password.txt (0.01 KB) without survey, you can save a lot of time and effort that you would otherwise spend on completing surveys or offers that may not even work. You can also avoid wasting your bandwidth and data on downloading unnecessary files or malware.
-
-
Protect Your Privacy and Security
-
By downloading password.txt (0.01 KB) without survey, you can protect your privacy and security from potential threats. Some surveys or offers may ask you to provide your personal information, such as your name, email, phone number, address, credit card details, etc. This information can be used by hackers or scammers to steal your identity, money, or data. Some surveys or offers may also require you to download files or software that may contain viruses, spyware, ransomware, or other malware that can harm your computer or device.
-
-
Access the Files You Want
-
By downloading password.txt (0.01 KB) without survey, you can access the files you want without any hassle. You can enjoy the games, movies, software, or other files that are stored in the RAR archive without any restrictions or limitations.
-
-
-
What are the Risks of Downloading Password.txt (0.01 KB) Without Survey?
-
-
While downloading password.txt (0.01 KB) without survey has many benefits, it also has some risks that you need to be aware of. Here are some of them:
-
-
-
Possibility of Fake or Incorrect Passwords
-
Not all sources that claim to provide password.txt (0.01 KB) are reliable or trustworthy. Some sources may provide fake or incorrect passwords that may not work for the RAR archive you want to open. This can be frustrating and disappointing for you as a user.
-
-
Possibility of Legal Issues
-
Some RAR archives may contain copyrighted or illegal content that you are not allowed to access or use without permission from the owner or the law. By downloading password.txt (0.01 KB) and opening these RAR archives, you may be violating the terms of service of the website that hosts them or the laws of your country. This can result in legal issues such as fines, lawsuits, or even jail time.
-
-
Possibility of Ethical Issues
-
Some RAR archives may contain content that is unethical or immoral, such as pornography, violence, hate speech, etc. By downloading password.txt (0.01 KB) and opening these RAR archives, you may be exposing yourself to content that may offend you or harm your mental health.
-
-
-
How to Download Password.txt (0.01 KB) Without Survey Safely?
-
-
To download password.txt (0.01 KB) without survey safely, you need to follow some precautions and tips. Here are some of them:
-
-
-
Use a Trusted Source
-
The best way to download password.txt (0.01 KB) without survey safely is to use a trusted source that has a good reputation and positive feedback from other users. You can check the credibility of the source by looking at its domain name, design, content quality, reviews, ratings, comments, etc. You can also use tools such as VirusTotal or URLVoid to scan the source for any malware or phishing signs.
-
-
Use a VPN Service
-
A VPN service is a tool that can help you hide your IP address and encrypt your online traffic when you download password.txt (0.01 KB) without survey. This can help you protect your privacy and security from hackers or trackers who may try to monitor your online activity or steal your data. It can also help you bypass geo-restrictions or censorship that may prevent you from accessing certain sources.
-
-
Use an Antivirus Software
-
An antivirus software is a tool that can help you detect and remove any malware that may infect your computer or device when you download password.txt (0.01 KB) without survey. This can help you prevent any damage or loss of data that may occur due to viruses, spyware, ransomware, or other malware.
-
-
Conclusion
-
-
Downloading password.txt (0.01 KB) without survey is a common and useful way to unlock RAR archives that contain games, movies, software, or other files. However, finding a reliable source to download password.txt (0.01 KB) can be difficult and risky.
-
-
In this article, we have shown you some methods that can help you download password.txt (0.01 KB) without survey, such as using a search engine, a password cracker tool, or a password database. We have also explained how to use password.txt (0.01 KB) to open RAR files.
-
-
We have also discussed the benefits and risks of downloading password.txt (0.01 KB) without survey, and how to download password.txt (0.01 KB) without survey safely.
-
-
We hope this article has been helpful and informative for you. If you have any questions or suggestions, please feel free to leave a comment below.
3cee63e6c2
-
-
\ No newline at end of file
diff --git a/spaces/inplisQlawa/anything-midjourney-v4-1/Lantek Expert Cut Rapidshare.md b/spaces/inplisQlawa/anything-midjourney-v4-1/Lantek Expert Cut Rapidshare.md
deleted file mode 100644
index 6e41f015e11d73da3986cfff32c1686e531e4d41..0000000000000000000000000000000000000000
--- a/spaces/inplisQlawa/anything-midjourney-v4-1/Lantek Expert Cut Rapidshare.md
+++ /dev/null
@@ -1,22 +0,0 @@
-
-
-Alberts Easy Activator v6.15.. For updating the software, you have to have a registration code for Alberts Easy Activator for you.. ABT can be uninstalled using the Windows Control Panel. Remove and then search and delete the.apk file from your computer.
-
-Alberts Easy Activator v6.15.. 7 Best GPS Navigation Apps for Android in 2019. this system has an interface like a desktop software, while there are.
-
-Alberts Easy Activator v6.15.. albert's easy activator for android: alberts easy activator.. download.
-
-Alberts Easy Activator v6.15.. Alberts Easy Activator is a fully-featured GPS navigation app for your Android phone. With 2D and 3D maps, voice-guided navigation, free lifetime updates, and 24-hour service..
-
-Alberts Easy Activator 6.15.. albert's easy activator v6.15 download, albert easy activator version v0.57.21.
-
-Alberts Easy Activator 6.15.. albert's easy activator v6.15 download, albert easy activator tomtom, albert easy activator v0.57.21.
-
-Alberts Easy Activator v6.15.. albert's easy activator version v0.57.21 (1) download, albert easy activator version v0.57.21.
-
-Alberts Easy Activator v6.15.. albert's easy activator version v0.57.21 (2) download, albert easy activator version v0.57.21.
-
-Alberts Easy Activator v6.15.. albert's easy activator version v0.57.21 (3) download, albert easy activator version v0.57.21.
-
-Alberts Easy Activator v6.15.. albert's easy activator version v0.57. 4fefd39f24
-
-
-
diff --git a/spaces/inreVtussa/clothingai/Examples/Bhopal A Prayer For Rain Full Movie Download In 720p Hd.md b/spaces/inreVtussa/clothingai/Examples/Bhopal A Prayer For Rain Full Movie Download In 720p Hd.md
deleted file mode 100644
index c0a94d1a38481f275562f9d73a1e35adbabbff24..0000000000000000000000000000000000000000
--- a/spaces/inreVtussa/clothingai/Examples/Bhopal A Prayer For Rain Full Movie Download In 720p Hd.md
+++ /dev/null
@@ -1,76 +0,0 @@
-
-
Bhopal: A Prayer For Rain Full Movie Download In 720p Hd - A Gripping Drama Based On A True Story
-
Bhopal: A Prayer For Rain is a 2014 drama film that depicts the events leading up to and following the Bhopal disaster, one of the worst industrial accidents in history that killed thousands of people and injured many more in India in 1984. The film is directed by Ravi Kumar and stars Mischa Barton, Martin Sheen, Kal Penn, David Brooks and others. The film is inspired by real events and features a mix of fictional and real characters.
-
Bhopal A Prayer For Rain Full Movie Download In 720p Hd
The film follows the lives of several people in Bhopal, a city in central India, where a pesticide plant owned by an American corporation called Union Carbide operates. The plant is notorious for its poor safety standards and environmental violations, but it provides jobs and income for many locals. Among them are Kuugo (Rajpal Yadav), a rickshaw driver who gets hired as a worker at the plant; Motwani (Kal Penn), a journalist who exposes the dangers of the plant; Eva Gascon (Mischa Barton), an American journalist who visits Bhopal to cover the story; Warren Anderson (Martin Sheen), the CEO of Union Carbide who tries to avoid responsibility; and Dilip (Vineet Kumar), Kuugo's friend who also works at the plant.
-
The film shows how the lives of these characters are affected by the disaster that occurs on the night of December 2nd, 1984, when a gas leak from the plant releases a toxic cloud of methyl isocyanate (MIC) that engulfs the city. The film portrays the horror and tragedy of the disaster, as well as the aftermath and the struggle for justice.
-
Why You Should Watch Bhopal: A Prayer For Rain Full Movie Download In 720p Hd
-
Bhopal: A Prayer For Rain is a film that will make you think and feel. It is a film that tells a powerful and important story that deserves to be seen and heard. Here are some reasons why you should watch Bhopal: A Prayer For Rain Full Movie Download In 720p Hd:
-
-
-
The film is based on a true story that has global relevance and impact. The Bhopal disaster is not only a tragedy that happened in India, but also a symbol of corporate greed, negligence and injustice that affects many people around the world. The film raises awareness and questions about the ethical and social responsibility of corporations, governments and individuals.
-
The film is well-made and well-acted. The film has a realistic and authentic look and feel, thanks to the use of real locations, costumes and props. The film also has a talented cast that delivers convincing and emotional performances. Mischa Barton, Martin Sheen, Kal Penn and Rajpal Yadav are especially noteworthy for their roles.
-
The film is gripping and moving. The film has a compelling narrative that keeps you engaged and invested in the characters and their fate. The film also has moments of suspense, drama and humor that balance the tone and mood. The film does not shy away from showing the harsh reality of the disaster, but also shows the resilience and courage of the survivors.
-
-
How To Download Bhopal: A Prayer For Rain Full Movie In 720p Hd
-
If you want to watch Bhopal: A Prayer For Rain Full Movie Download In 720p Hd, you have several options to choose from. You can either stream it online or download it offline. Here are some ways to do so:
-
-
Stream it online. You can watch Bhopal: A Prayer For Rain online on various platforms such as Amazon Prime Video, YouTube, Google Play Movies & TV, iTunes and others. You can rent or buy the movie depending on your preference and availability.
-
Download it offline. You can also download Bhopal: A Prayer For Rain offline on your device or computer. You can use various websites or apps that offer movie downloads such as uTorrent, BitTorrent, Vidmate, Tubemate and others. However, you should be careful about the legality and safety of these sources, as some of them might contain viruses or malware.
-
-
Whichever option you choose, make sure you have a good internet connection and enough storage space on your device or computer. Also, make sure you respect the copyright laws and do not share or distribute the movie illegally.
-
Conclusion
-
Bhopal: A Prayer For Rain is a film that will touch your heart and mind. It is a film that tells a true story that needs to be remembered and learned from. It is a film that showcases the best and worst of humanity in times of crisis. It is a film that you should not miss.
-
If you want to watch Bhopal: A Prayer For Rain Full Movie Download In 720p Hd, you can stream it online or download it offline from various sources. However you choose to watch it, make sure you enjoy it and appreciate it.
-
What are the reviews and ratings of Bhopal: A Prayer For Rain Full Movie Download In 720p Hd?
-
Bhopal: A Prayer For Rain has received mostly positive reviews and ratings from critics and audiences alike. The film has a rating of 7.1 out of 10 on IMDb, based on over 2,000 user votes. The film also has a metascore of 50 out of 100 on Metacritic, based on 18 critic reviews. The film has been praised for its realistic portrayal of the disaster, its powerful performances and its social message.
-
Some of the positive reviews of the film are as follows:
-
-
"Bhopal: A Prayer for Rain is a harrowing account of one of the worst industrial disasters in history, told from multiple perspectives and with a keen eye for detail." - Mark Adams, Screen International
-
"Bhopal: A Prayer for Rain is a gripping drama that exposes the human cost of corporate negligence and corruption." - Frank Scheck, The Hollywood Reporter
-
"Bhopal: A Prayer for Rain is a compelling and compassionate film that honors the victims and survivors of the tragedy, while also holding the perpetrators accountable." - Anupama Chopra, Hindustan Times
-
-
What are the awards and nominations of Bhopal: A Prayer For Rain Full Movie Download In 720p Hd?
-
Bhopal: A Prayer For Rain has also received some awards and nominations for its quality and impact. The film has won one award and received one nomination so far. The film has won the Best Feature Film Award at the Indian Film Festival of Los Angeles in 2015. The film has also been nominated for the Best International Feature Film Award at the Edinburgh International Film Festival in 2014.
-
The film has also been screened at various other film festivals and events around the world, such as the Palm Springs International Film Festival, the Tokyo International Film Festival, the London Indian Film Festival, the Mumbai Film Festival and others.
-
What are the themes and messages of Bhopal: A Prayer For Rain Full Movie Download In 720p Hd?
-
Bhopal: A Prayer For Rain is not just a film that tells a story, but also a film that conveys some themes and messages that are relevant and meaningful. The film explores some of the following themes and messages:
-
-
The human cost of industrialization and globalization. The film shows how the pursuit of profit and growth by multinational corporations can have devastating consequences for the environment and the people who live in it. The film also shows how the local authorities and the media can be complicit or indifferent to the plight of the victims.
-
The struggle for justice and accountability. The film shows how the survivors of the disaster have to fight for their rights and compensation, as well as for the recognition and apology from the perpetrators. The film also shows how the legal system and the international community can fail to deliver justice and accountability.
-
The resilience and courage of the human spirit. The film shows how the people of Bhopal cope with the tragedy and its aftermath, as well as how they support each other and find hope and meaning in their lives. The film also shows how some of the characters overcome their fears and doubts and stand up for their beliefs and values.
-
-
What are some interesting facts about Bhopal: A Prayer For Rain Full Movie Download In 720p Hd?
-
Bhopal: A Prayer For Rain is a film that has some interesting facts behind its making and its impact. Here are some of them:
-
-
The film was shot in Hyderabad, India, using real locations, costumes and props that resembled Bhopal in 1984. The film also used some archival footage and photos from the actual disaster.
-
The film was released in India on December 5th, 2014, coinciding with the 30th anniversary of the disaster. The film was also released in other countries such as the UK, the US, Canada, Australia and others.
-
The film received a positive response from some of the survivors and activists of the disaster, who praised its accuracy and sensitivity. The film also received support from some celebrities such as Amitabh Bachchan, Shabana Azmi, Anil Kapoor and others.
-
-
Who are the cast and crew of Bhopal: A Prayer For Rain Full Movie Download In 720p Hd?
-
Bhopal: A Prayer For Rain has a talented and diverse cast and crew that bring the film to life. The film is directed by Ravi Kumar, who is also one of the writers along with David Brooks. The film is produced by Ravi Walia, Ravi Kumar, Seemanto Roy and others. The film has a music score by Benjamin Wallfisch and a cinematography by Charlie Wuppermann and Anil Chandel.
-
The film has a star-studded cast that includes some of the following actors:
-
-
Mischa Barton as Eva Gascon, an American journalist who visits Bhopal to cover the story of the plant and the disaster.
-
Martin Sheen as Warren Anderson, the CEO of Union Carbide who tries to avoid responsibility and accountability for the disaster.
-
Kal Penn as Motwani, a local journalist who exposes the dangers of the plant and helps Eva with her investigation.
-
Rajpal Yadav as Dilip, a rickshaw driver who gets hired as a worker at the plant and becomes a victim of the disaster.
-
Tannishtha Chatterjee as Leela, Dilip's wife who is pregnant and also suffers from the disaster.
-
Vineet Kumar as Kuugo, Dilip's friend who also works at the plant and tries to warn him about the risks.
-
Manoj Joshi as Dr. Chandra, a doctor who treats the victims of the disaster and advocates for their rights.
-
Satish Kaushik as Chief Minister, the political leader of Madhya Pradesh who is in charge of handling the crisis.
-
-
What are some related movies to Bhopal: A Prayer For Rain Full Movie Download In 720p Hd?
-
If you liked Bhopal: A Prayer For Rain Full Movie Download In 720p Hd, you might also like some of these related movies that deal with similar themes and topics:
-
-
Erin Brockovich (2000), a biographical drama film that tells the story of a legal clerk who fights against a California power company that polluted a city's water supply.
-
Chernobyl (2019), a historical drama miniseries that depicts the 1986 Chernobyl nuclear disaster and its aftermath.
-
Dark Waters (2019), a legal thriller film that follows a corporate lawyer who takes on an environmental lawsuit against a chemical company that contaminated a town's water.
-
Silkwood (1983), a biographical drama film that portrays the life and death of a nuclear whistleblower who exposed safety violations at a plutonium plant.
-
The China Syndrome (1979), a thriller film that revolves around a reporter and a cameraman who witness a near-meltdown at a nuclear power plant.
-
-
Conclusion
-
Bhopal: A Prayer For Rain is a film that will touch your heart and mind. It is a film that tells a true story that needs to be remembered and learned from. It is a film that showcases the best and worst of humanity in times of crisis. It is a film that you should not miss.
-
If you want to watch Bhopal: A Prayer For Rain Full Movie Download In 720p Hd, you can stream it online or download it offline from various sources. However you choose to watch it, make sure you enjoy it and appreciate it.
3cee63e6c2
-
-
\ No newline at end of file
diff --git a/spaces/ivotai/VITS-Umamusume-voice-synthesizer/monotonic_align/__init__.py b/spaces/ivotai/VITS-Umamusume-voice-synthesizer/monotonic_align/__init__.py
deleted file mode 100644
index 3d7009c40fea3a98168e3e3bc9ae061e91327422..0000000000000000000000000000000000000000
--- a/spaces/ivotai/VITS-Umamusume-voice-synthesizer/monotonic_align/__init__.py
+++ /dev/null
@@ -1,19 +0,0 @@
-import numpy as np
-import torch
-from .monotonic_align.core import maximum_path_c
-
-
-def maximum_path(neg_cent, mask):
- """ Cython optimized version.
- neg_cent: [b, t_t, t_s]
- mask: [b, t_t, t_s]
- """
- device = neg_cent.device
- dtype = neg_cent.dtype
- neg_cent = neg_cent.data.cpu().numpy().astype(np.float32)
- path = np.zeros(neg_cent.shape, dtype=np.int32)
-
- t_t_max = mask.sum(1)[:, 0].data.cpu().numpy().astype(np.int32)
- t_s_max = mask.sum(2)[:, 0].data.cpu().numpy().astype(np.int32)
- maximum_path_c(path, neg_cent, t_t_max, t_s_max)
- return torch.from_numpy(path).to(device=device, dtype=dtype)
diff --git a/spaces/james-oldfield/PandA/networks/biggan/convert.sh b/spaces/james-oldfield/PandA/networks/biggan/convert.sh
deleted file mode 100644
index 09e4d91ebd11e804b0f937dfe968cdc18bcee0f1..0000000000000000000000000000000000000000
--- a/spaces/james-oldfield/PandA/networks/biggan/convert.sh
+++ /dev/null
@@ -1,21 +0,0 @@
-# Copyright (c) 2019-present, Thomas Wolf, Huggingface Inc.
-# All rights reserved.
-#
-# This source code is licensed under the license found in the
-# LICENSE file in the root directory of this source tree.
-#
-
-set -e
-set -x
-
-models="128 256 512"
-
-mkdir -p models/model_128
-mkdir -p models/model_256
-mkdir -p models/model_512
-
-# Convert TF Hub models.
-for model in $models
-do
- pytorch_pretrained_biggan --model_type $model --tf_model_path models/model_$model --pt_save_path models/model_$model
-done
\ No newline at end of file
diff --git a/spaces/jbilcke-hf/ai-comic-factory/src/app/interface/settings-dialog/getSettings.ts b/spaces/jbilcke-hf/ai-comic-factory/src/app/interface/settings-dialog/getSettings.ts
deleted file mode 100644
index 655b6da9a8d2f130cb79959cd8acb6529227a26b..0000000000000000000000000000000000000000
--- a/spaces/jbilcke-hf/ai-comic-factory/src/app/interface/settings-dialog/getSettings.ts
+++ /dev/null
@@ -1,26 +0,0 @@
-import { RenderingModelVendor, Settings } from "@/types"
-
-import { getValidString } from "@/lib/getValidString"
-import { localStorageKeys } from "./localStorageKeys"
-import { defaultSettings } from "./defaultSettings"
-
-export function getSettings(): Settings {
- try {
- return {
- renderingModelVendor: getValidString(localStorage?.getItem?.(localStorageKeys.renderingModelVendor), defaultSettings.renderingModelVendor) as RenderingModelVendor,
- huggingfaceApiKey: getValidString(localStorage?.getItem?.(localStorageKeys.huggingfaceApiKey), defaultSettings.huggingfaceApiKey),
- huggingfaceInferenceApiModel: getValidString(localStorage?.getItem?.(localStorageKeys.huggingfaceInferenceApiModel), defaultSettings.huggingfaceInferenceApiModel),
- huggingfaceInferenceApiModelTrigger: getValidString(localStorage?.getItem?.(localStorageKeys.huggingfaceInferenceApiModelTrigger), defaultSettings.huggingfaceInferenceApiModelTrigger),
- replicateApiKey: getValidString(localStorage?.getItem?.(localStorageKeys.replicateApiKey), defaultSettings.replicateApiKey),
- replicateApiModel: getValidString(localStorage?.getItem?.(localStorageKeys.replicateApiModel), defaultSettings.replicateApiModel),
- replicateApiModelVersion: getValidString(localStorage?.getItem?.(localStorageKeys.replicateApiModelVersion), defaultSettings.replicateApiModelVersion),
- replicateApiModelTrigger: getValidString(localStorage?.getItem?.(localStorageKeys.replicateApiModelTrigger), defaultSettings.replicateApiModelTrigger),
- openaiApiKey: getValidString(localStorage?.getItem?.(localStorageKeys.openaiApiKey), defaultSettings.openaiApiKey),
- openaiApiModel: getValidString(localStorage?.getItem?.(localStorageKeys.openaiApiModel), defaultSettings.openaiApiModel),
- }
- } catch (err) {
- return {
- ...defaultSettings
- }
- }
-}
\ No newline at end of file
diff --git a/spaces/jbilcke-hf/media-server/media-server.js b/spaces/jbilcke-hf/media-server/media-server.js
deleted file mode 100644
index 6139d112f633d4600c34c29fd3ae1f6786711d0c..0000000000000000000000000000000000000000
--- a/spaces/jbilcke-hf/media-server/media-server.js
+++ /dev/null
@@ -1,26 +0,0 @@
-const NodeMediaServer = require('node-media-server')
-
-const config = {
- /*
- auth: {
- api: true,
- api_user: process.env.WEBTV_MEDIA_SERVER_USER,
- api_pass: process.env.WEBTV_MEDIA_SERVER_PASSWORD
- },
- */
- rtmp: {
- port: 1935,
- chunk_size: 60000,
- gop_cache: true,
- ping: 30,
- ping_timeout: 60
- },
- http: {
- port: 8000,
- allow_origin: '*'
- }
-};
-
-console.log("starting the RTMP server..")
-var nms = new NodeMediaServer(config)
-nms.run()
\ No newline at end of file
diff --git a/spaces/jesuspj/jp/README.md b/spaces/jesuspj/jp/README.md
deleted file mode 100644
index 1111a5cd6f34b1ce8e68483fe2c1e4d45f87614f..0000000000000000000000000000000000000000
--- a/spaces/jesuspj/jp/README.md
+++ /dev/null
@@ -1,12 +0,0 @@
----
-title: AutoTrain Advanced
-emoji: 🚀
-colorFrom: blue
-colorTo: green
-sdk: docker
-pinned: false
-duplicated_from: autotrain-projects/autotrain-advanced
-license: apache-2.0
----
-
-Check out the configuration reference at https://huggingface.co/docs/hub/spaces-config-reference
diff --git a/spaces/joaopereirajp/livvieChatBot/venv/lib/python3.9/site-packages/bs4/tests/test_builder_registry.py b/spaces/joaopereirajp/livvieChatBot/venv/lib/python3.9/site-packages/bs4/tests/test_builder_registry.py
deleted file mode 100644
index 9327174f3775a763f5379eeaafddbd6cf1c69c52..0000000000000000000000000000000000000000
--- a/spaces/joaopereirajp/livvieChatBot/venv/lib/python3.9/site-packages/bs4/tests/test_builder_registry.py
+++ /dev/null
@@ -1,137 +0,0 @@
-"""Tests of the builder registry."""
-
-import pytest
-import warnings
-
-from bs4 import BeautifulSoup
-from bs4.builder import (
- builder_registry as registry,
- HTMLParserTreeBuilder,
- TreeBuilderRegistry,
-)
-
-from . import (
- HTML5LIB_PRESENT,
- LXML_PRESENT,
-)
-
-if HTML5LIB_PRESENT:
- from bs4.builder import HTML5TreeBuilder
-
-if LXML_PRESENT:
- from bs4.builder import (
- LXMLTreeBuilderForXML,
- LXMLTreeBuilder,
- )
-
-
-# TODO: Split out the lxml and html5lib tests into their own classes
-# and gate with pytest.mark.skipIf.
-class TestBuiltInRegistry(object):
- """Test the built-in registry with the default builders registered."""
-
- def test_combination(self):
- assert registry.lookup('strict', 'html') == HTMLParserTreeBuilder
- if LXML_PRESENT:
- assert registry.lookup('fast', 'html') == LXMLTreeBuilder
- assert registry.lookup('permissive', 'xml') == LXMLTreeBuilderForXML
- if HTML5LIB_PRESENT:
- assert registry.lookup('html5lib', 'html') == HTML5TreeBuilder
-
- def test_lookup_by_markup_type(self):
- if LXML_PRESENT:
- assert registry.lookup('html') == LXMLTreeBuilder
- assert registry.lookup('xml') == LXMLTreeBuilderForXML
- else:
- assert registry.lookup('xml') == None
- if HTML5LIB_PRESENT:
- assert registry.lookup('html') == HTML5TreeBuilder
- else:
- assert registry.lookup('html') == HTMLParserTreeBuilder
-
- def test_named_library(self):
- if LXML_PRESENT:
- assert registry.lookup('lxml', 'xml') == LXMLTreeBuilderForXML
- assert registry.lookup('lxml', 'html') == LXMLTreeBuilder
- if HTML5LIB_PRESENT:
- assert registry.lookup('html5lib') == HTML5TreeBuilder
-
- assert registry.lookup('html.parser') == HTMLParserTreeBuilder
-
- def test_beautifulsoup_constructor_does_lookup(self):
-
- with warnings.catch_warnings(record=True) as w:
- # This will create a warning about not explicitly
- # specifying a parser, but we'll ignore it.
-
- # You can pass in a string.
- BeautifulSoup("", features="html")
- # Or a list of strings.
- BeautifulSoup("", features=["html", "fast"])
- pass
-
- # You'll get an exception if BS can't find an appropriate
- # builder.
- with pytest.raises(ValueError):
- BeautifulSoup("", features="no-such-feature")
-
-class TestRegistry(object):
- """Test the TreeBuilderRegistry class in general."""
-
- def setup_method(self):
- self.registry = TreeBuilderRegistry()
-
- def builder_for_features(self, *feature_list):
- cls = type('Builder_' + '_'.join(feature_list),
- (object,), {'features' : feature_list})
-
- self.registry.register(cls)
- return cls
-
- def test_register_with_no_features(self):
- builder = self.builder_for_features()
-
- # Since the builder advertises no features, you can't find it
- # by looking up features.
- assert self.registry.lookup('foo') is None
-
- # But you can find it by doing a lookup with no features, if
- # this happens to be the only registered builder.
- assert self.registry.lookup() == builder
-
- def test_register_with_features_makes_lookup_succeed(self):
- builder = self.builder_for_features('foo', 'bar')
- assert self.registry.lookup('foo') is builder
- assert self.registry.lookup('bar') is builder
-
- def test_lookup_fails_when_no_builder_implements_feature(self):
- builder = self.builder_for_features('foo', 'bar')
- assert self.registry.lookup('baz') is None
-
- def test_lookup_gets_most_recent_registration_when_no_feature_specified(self):
- builder1 = self.builder_for_features('foo')
- builder2 = self.builder_for_features('bar')
- assert self.registry.lookup() == builder2
-
- def test_lookup_fails_when_no_tree_builders_registered(self):
- assert self.registry.lookup() is None
-
- def test_lookup_gets_most_recent_builder_supporting_all_features(self):
- has_one = self.builder_for_features('foo')
- has_the_other = self.builder_for_features('bar')
- has_both_early = self.builder_for_features('foo', 'bar', 'baz')
- has_both_late = self.builder_for_features('foo', 'bar', 'quux')
- lacks_one = self.builder_for_features('bar')
- has_the_other = self.builder_for_features('foo')
-
- # There are two builders featuring 'foo' and 'bar', but
- # the one that also features 'quux' was registered later.
- assert self.registry.lookup('foo', 'bar') == has_both_late
-
- # There is only one builder featuring 'foo', 'bar', and 'baz'.
- assert self.registry.lookup('foo', 'bar', 'baz') == has_both_early
-
- def test_lookup_fails_when_cannot_reconcile_requested_features(self):
- builder1 = self.builder_for_features('foo', 'bar')
- builder2 = self.builder_for_features('foo', 'baz')
- assert self.registry.lookup('bar', 'baz') is None
diff --git a/spaces/joaopereirajp/livvieChatBot/venv/lib/python3.9/site-packages/bson/datetime_ms.py b/spaces/joaopereirajp/livvieChatBot/venv/lib/python3.9/site-packages/bson/datetime_ms.py
deleted file mode 100644
index 6b9472b5b6b53238ef4c91109a45de0b726fdaf5..0000000000000000000000000000000000000000
--- a/spaces/joaopereirajp/livvieChatBot/venv/lib/python3.9/site-packages/bson/datetime_ms.py
+++ /dev/null
@@ -1,158 +0,0 @@
-# Copyright 2022-present MongoDB, Inc.
-#
-# Licensed under the Apache License, Version 2.0 (the "License"); you
-# may not use this file except in compliance with the License. You
-# may obtain a copy of the License at
-#
-# http://www.apache.org/licenses/LICENSE-2.0
-#
-# Unless required by applicable law or agreed to in writing, software
-# distributed under the License is distributed on an "AS IS" BASIS,
-# WITHOUT WARRANTIES OR CONDITIONS OF ANY KIND, either express or
-# implied. See the License for the specific language governing
-# permissions and limitations under the License.
-
-"""Tools for representing the BSON datetime type.
-
-.. versionadded:: 4.3
-"""
-
-import calendar
-import datetime
-import functools
-from typing import Any, Union, cast
-
-from bson.codec_options import DEFAULT_CODEC_OPTIONS, CodecOptions, DatetimeConversion
-from bson.tz_util import utc
-
-EPOCH_AWARE = datetime.datetime.fromtimestamp(0, utc)
-EPOCH_NAIVE = EPOCH_AWARE.replace(tzinfo=None)
-
-
-class DatetimeMS:
- """Represents a BSON UTC datetime."""
-
- __slots__ = ("_value",)
-
- def __init__(self, value: Union[int, datetime.datetime]):
- """Represents a BSON UTC datetime.
-
- BSON UTC datetimes are defined as an int64 of milliseconds since the
- Unix epoch. The principal use of DatetimeMS is to represent
- datetimes outside the range of the Python builtin
- :class:`~datetime.datetime` class when
- encoding/decoding BSON.
-
- To decode UTC datetimes as a ``DatetimeMS``, `datetime_conversion` in
- :class:`~bson.CodecOptions` must be set to 'datetime_ms' or
- 'datetime_auto'. See :ref:`handling-out-of-range-datetimes` for
- details.
-
- :Parameters:
- - `value`: An instance of :class:`datetime.datetime` to be
- represented as milliseconds since the Unix epoch, or int of
- milliseconds since the Unix epoch.
- """
- if isinstance(value, int):
- if not (-(2**63) <= value <= 2**63 - 1):
- raise OverflowError("Must be a 64-bit integer of milliseconds")
- self._value = value
- elif isinstance(value, datetime.datetime):
- self._value = _datetime_to_millis(value)
- else:
- raise TypeError(f"{type(value)} is not a valid type for DatetimeMS")
-
- def __hash__(self) -> int:
- return hash(self._value)
-
- def __repr__(self) -> str:
- return type(self).__name__ + "(" + str(self._value) + ")"
-
- def __lt__(self, other: Union["DatetimeMS", int]) -> bool:
- return self._value < other
-
- def __le__(self, other: Union["DatetimeMS", int]) -> bool:
- return self._value <= other
-
- def __eq__(self, other: Any) -> bool:
- if isinstance(other, DatetimeMS):
- return self._value == other._value
- return False
-
- def __ne__(self, other: Any) -> bool:
- if isinstance(other, DatetimeMS):
- return self._value != other._value
- return True
-
- def __gt__(self, other: Union["DatetimeMS", int]) -> bool:
- return self._value > other
-
- def __ge__(self, other: Union["DatetimeMS", int]) -> bool:
- return self._value >= other
-
- _type_marker = 9
-
- def as_datetime(self, codec_options: CodecOptions = DEFAULT_CODEC_OPTIONS) -> datetime.datetime:
- """Create a Python :class:`~datetime.datetime` from this DatetimeMS object.
-
- :Parameters:
- - `codec_options`: A CodecOptions instance for specifying how the
- resulting DatetimeMS object will be formatted using ``tz_aware``
- and ``tz_info``. Defaults to
- :const:`~bson.codec_options.DEFAULT_CODEC_OPTIONS`.
- """
- return cast(datetime.datetime, _millis_to_datetime(self._value, codec_options))
-
- def __int__(self) -> int:
- return self._value
-
-
-# Inclusive and exclusive min and max for timezones.
-# Timezones are hashed by their offset, which is a timedelta
-# and therefore there are more than 24 possible timezones.
-@functools.lru_cache(maxsize=None)
-def _min_datetime_ms(tz: datetime.timezone = datetime.timezone.utc) -> int:
- return _datetime_to_millis(datetime.datetime.min.replace(tzinfo=tz))
-
-
-@functools.lru_cache(maxsize=None)
-def _max_datetime_ms(tz: datetime.timezone = datetime.timezone.utc) -> int:
- return _datetime_to_millis(datetime.datetime.max.replace(tzinfo=tz))
-
-
-def _millis_to_datetime(millis: int, opts: CodecOptions) -> Union[datetime.datetime, DatetimeMS]:
- """Convert milliseconds since epoch UTC to datetime."""
- if (
- opts.datetime_conversion == DatetimeConversion.DATETIME
- or opts.datetime_conversion == DatetimeConversion.DATETIME_CLAMP
- or opts.datetime_conversion == DatetimeConversion.DATETIME_AUTO
- ):
- tz = opts.tzinfo or datetime.timezone.utc
- if opts.datetime_conversion == DatetimeConversion.DATETIME_CLAMP:
- millis = max(_min_datetime_ms(tz), min(millis, _max_datetime_ms(tz)))
- elif opts.datetime_conversion == DatetimeConversion.DATETIME_AUTO:
- if not (_min_datetime_ms(tz) <= millis <= _max_datetime_ms(tz)):
- return DatetimeMS(millis)
-
- diff = ((millis % 1000) + 1000) % 1000
- seconds = (millis - diff) // 1000
- micros = diff * 1000
-
- if opts.tz_aware:
- dt = EPOCH_AWARE + datetime.timedelta(seconds=seconds, microseconds=micros)
- if opts.tzinfo:
- dt = dt.astimezone(tz)
- return dt
- else:
- return EPOCH_NAIVE + datetime.timedelta(seconds=seconds, microseconds=micros)
- elif opts.datetime_conversion == DatetimeConversion.DATETIME_MS:
- return DatetimeMS(millis)
- else:
- raise ValueError("datetime_conversion must be an element of DatetimeConversion")
-
-
-def _datetime_to_millis(dtm: datetime.datetime) -> int:
- """Convert datetime to milliseconds since epoch UTC."""
- if dtm.utcoffset() is not None:
- dtm = dtm - dtm.utcoffset() # type: ignore
- return int(calendar.timegm(dtm.timetuple()) * 1000 + dtm.microsecond // 1000)
diff --git a/spaces/joaopereirajp/livvieChatBot/venv/lib/python3.9/site-packages/click/_winconsole.py b/spaces/joaopereirajp/livvieChatBot/venv/lib/python3.9/site-packages/click/_winconsole.py
deleted file mode 100644
index 6b20df315b23ecd1e3d0ec32c11c0b5ced577efe..0000000000000000000000000000000000000000
--- a/spaces/joaopereirajp/livvieChatBot/venv/lib/python3.9/site-packages/click/_winconsole.py
+++ /dev/null
@@ -1,279 +0,0 @@
-# This module is based on the excellent work by Adam Bartoš who
-# provided a lot of what went into the implementation here in
-# the discussion to issue1602 in the Python bug tracker.
-#
-# There are some general differences in regards to how this works
-# compared to the original patches as we do not need to patch
-# the entire interpreter but just work in our little world of
-# echo and prompt.
-import io
-import sys
-import time
-import typing as t
-from ctypes import byref
-from ctypes import c_char
-from ctypes import c_char_p
-from ctypes import c_int
-from ctypes import c_ssize_t
-from ctypes import c_ulong
-from ctypes import c_void_p
-from ctypes import POINTER
-from ctypes import py_object
-from ctypes import Structure
-from ctypes.wintypes import DWORD
-from ctypes.wintypes import HANDLE
-from ctypes.wintypes import LPCWSTR
-from ctypes.wintypes import LPWSTR
-
-from ._compat import _NonClosingTextIOWrapper
-
-assert sys.platform == "win32"
-import msvcrt # noqa: E402
-from ctypes import windll # noqa: E402
-from ctypes import WINFUNCTYPE # noqa: E402
-
-c_ssize_p = POINTER(c_ssize_t)
-
-kernel32 = windll.kernel32
-GetStdHandle = kernel32.GetStdHandle
-ReadConsoleW = kernel32.ReadConsoleW
-WriteConsoleW = kernel32.WriteConsoleW
-GetConsoleMode = kernel32.GetConsoleMode
-GetLastError = kernel32.GetLastError
-GetCommandLineW = WINFUNCTYPE(LPWSTR)(("GetCommandLineW", windll.kernel32))
-CommandLineToArgvW = WINFUNCTYPE(POINTER(LPWSTR), LPCWSTR, POINTER(c_int))(
- ("CommandLineToArgvW", windll.shell32)
-)
-LocalFree = WINFUNCTYPE(c_void_p, c_void_p)(("LocalFree", windll.kernel32))
-
-STDIN_HANDLE = GetStdHandle(-10)
-STDOUT_HANDLE = GetStdHandle(-11)
-STDERR_HANDLE = GetStdHandle(-12)
-
-PyBUF_SIMPLE = 0
-PyBUF_WRITABLE = 1
-
-ERROR_SUCCESS = 0
-ERROR_NOT_ENOUGH_MEMORY = 8
-ERROR_OPERATION_ABORTED = 995
-
-STDIN_FILENO = 0
-STDOUT_FILENO = 1
-STDERR_FILENO = 2
-
-EOF = b"\x1a"
-MAX_BYTES_WRITTEN = 32767
-
-try:
- from ctypes import pythonapi
-except ImportError:
- # On PyPy we cannot get buffers so our ability to operate here is
- # severely limited.
- get_buffer = None
-else:
-
- class Py_buffer(Structure):
- _fields_ = [
- ("buf", c_void_p),
- ("obj", py_object),
- ("len", c_ssize_t),
- ("itemsize", c_ssize_t),
- ("readonly", c_int),
- ("ndim", c_int),
- ("format", c_char_p),
- ("shape", c_ssize_p),
- ("strides", c_ssize_p),
- ("suboffsets", c_ssize_p),
- ("internal", c_void_p),
- ]
-
- PyObject_GetBuffer = pythonapi.PyObject_GetBuffer
- PyBuffer_Release = pythonapi.PyBuffer_Release
-
- def get_buffer(obj, writable=False):
- buf = Py_buffer()
- flags = PyBUF_WRITABLE if writable else PyBUF_SIMPLE
- PyObject_GetBuffer(py_object(obj), byref(buf), flags)
-
- try:
- buffer_type = c_char * buf.len
- return buffer_type.from_address(buf.buf)
- finally:
- PyBuffer_Release(byref(buf))
-
-
-class _WindowsConsoleRawIOBase(io.RawIOBase):
- def __init__(self, handle):
- self.handle = handle
-
- def isatty(self):
- super().isatty()
- return True
-
-
-class _WindowsConsoleReader(_WindowsConsoleRawIOBase):
- def readable(self):
- return True
-
- def readinto(self, b):
- bytes_to_be_read = len(b)
- if not bytes_to_be_read:
- return 0
- elif bytes_to_be_read % 2:
- raise ValueError(
- "cannot read odd number of bytes from UTF-16-LE encoded console"
- )
-
- buffer = get_buffer(b, writable=True)
- code_units_to_be_read = bytes_to_be_read // 2
- code_units_read = c_ulong()
-
- rv = ReadConsoleW(
- HANDLE(self.handle),
- buffer,
- code_units_to_be_read,
- byref(code_units_read),
- None,
- )
- if GetLastError() == ERROR_OPERATION_ABORTED:
- # wait for KeyboardInterrupt
- time.sleep(0.1)
- if not rv:
- raise OSError(f"Windows error: {GetLastError()}")
-
- if buffer[0] == EOF:
- return 0
- return 2 * code_units_read.value
-
-
-class _WindowsConsoleWriter(_WindowsConsoleRawIOBase):
- def writable(self):
- return True
-
- @staticmethod
- def _get_error_message(errno):
- if errno == ERROR_SUCCESS:
- return "ERROR_SUCCESS"
- elif errno == ERROR_NOT_ENOUGH_MEMORY:
- return "ERROR_NOT_ENOUGH_MEMORY"
- return f"Windows error {errno}"
-
- def write(self, b):
- bytes_to_be_written = len(b)
- buf = get_buffer(b)
- code_units_to_be_written = min(bytes_to_be_written, MAX_BYTES_WRITTEN) // 2
- code_units_written = c_ulong()
-
- WriteConsoleW(
- HANDLE(self.handle),
- buf,
- code_units_to_be_written,
- byref(code_units_written),
- None,
- )
- bytes_written = 2 * code_units_written.value
-
- if bytes_written == 0 and bytes_to_be_written > 0:
- raise OSError(self._get_error_message(GetLastError()))
- return bytes_written
-
-
-class ConsoleStream:
- def __init__(self, text_stream: t.TextIO, byte_stream: t.BinaryIO) -> None:
- self._text_stream = text_stream
- self.buffer = byte_stream
-
- @property
- def name(self) -> str:
- return self.buffer.name
-
- def write(self, x: t.AnyStr) -> int:
- if isinstance(x, str):
- return self._text_stream.write(x)
- try:
- self.flush()
- except Exception:
- pass
- return self.buffer.write(x)
-
- def writelines(self, lines: t.Iterable[t.AnyStr]) -> None:
- for line in lines:
- self.write(line)
-
- def __getattr__(self, name: str) -> t.Any:
- return getattr(self._text_stream, name)
-
- def isatty(self) -> bool:
- return self.buffer.isatty()
-
- def __repr__(self):
- return f""
-
-
-def _get_text_stdin(buffer_stream: t.BinaryIO) -> t.TextIO:
- text_stream = _NonClosingTextIOWrapper(
- io.BufferedReader(_WindowsConsoleReader(STDIN_HANDLE)),
- "utf-16-le",
- "strict",
- line_buffering=True,
- )
- return t.cast(t.TextIO, ConsoleStream(text_stream, buffer_stream))
-
-
-def _get_text_stdout(buffer_stream: t.BinaryIO) -> t.TextIO:
- text_stream = _NonClosingTextIOWrapper(
- io.BufferedWriter(_WindowsConsoleWriter(STDOUT_HANDLE)),
- "utf-16-le",
- "strict",
- line_buffering=True,
- )
- return t.cast(t.TextIO, ConsoleStream(text_stream, buffer_stream))
-
-
-def _get_text_stderr(buffer_stream: t.BinaryIO) -> t.TextIO:
- text_stream = _NonClosingTextIOWrapper(
- io.BufferedWriter(_WindowsConsoleWriter(STDERR_HANDLE)),
- "utf-16-le",
- "strict",
- line_buffering=True,
- )
- return t.cast(t.TextIO, ConsoleStream(text_stream, buffer_stream))
-
-
-_stream_factories: t.Mapping[int, t.Callable[[t.BinaryIO], t.TextIO]] = {
- 0: _get_text_stdin,
- 1: _get_text_stdout,
- 2: _get_text_stderr,
-}
-
-
-def _is_console(f: t.TextIO) -> bool:
- if not hasattr(f, "fileno"):
- return False
-
- try:
- fileno = f.fileno()
- except (OSError, io.UnsupportedOperation):
- return False
-
- handle = msvcrt.get_osfhandle(fileno)
- return bool(GetConsoleMode(handle, byref(DWORD())))
-
-
-def _get_windows_console_stream(
- f: t.TextIO, encoding: t.Optional[str], errors: t.Optional[str]
-) -> t.Optional[t.TextIO]:
- if (
- get_buffer is not None
- and encoding in {"utf-16-le", None}
- and errors in {"strict", None}
- and _is_console(f)
- ):
- func = _stream_factories.get(f.fileno())
- if func is not None:
- b = getattr(f, "buffer", None)
-
- if b is None:
- return None
-
- return func(b)
diff --git a/spaces/joaopereirajp/livvieChatBot/venv/lib/python3.9/site-packages/dns/_immutable_ctx.py b/spaces/joaopereirajp/livvieChatBot/venv/lib/python3.9/site-packages/dns/_immutable_ctx.py
deleted file mode 100644
index ae7a33bf3a5f92252a5191b23086fd62e431e785..0000000000000000000000000000000000000000
--- a/spaces/joaopereirajp/livvieChatBot/venv/lib/python3.9/site-packages/dns/_immutable_ctx.py
+++ /dev/null
@@ -1,76 +0,0 @@
-# Copyright (C) Dnspython Contributors, see LICENSE for text of ISC license
-
-# This implementation of the immutable decorator requires python >=
-# 3.7, and is significantly more storage efficient when making classes
-# with slots immutable. It's also faster.
-
-import contextvars
-import inspect
-
-_in__init__ = contextvars.ContextVar("_immutable_in__init__", default=False)
-
-
-class _Immutable:
- """Immutable mixin class"""
-
- # We set slots to the empty list to say "we don't have any attributes".
- # We do this so that if we're mixed in with a class with __slots__, we
- # don't cause a __dict__ to be added which would waste space.
-
- __slots__ = ()
-
- def __setattr__(self, name, value):
- if _in__init__.get() is not self:
- raise TypeError("object doesn't support attribute assignment")
- else:
- super().__setattr__(name, value)
-
- def __delattr__(self, name):
- if _in__init__.get() is not self:
- raise TypeError("object doesn't support attribute assignment")
- else:
- super().__delattr__(name)
-
-
-def _immutable_init(f):
- def nf(*args, **kwargs):
- previous = _in__init__.set(args[0])
- try:
- # call the actual __init__
- f(*args, **kwargs)
- finally:
- _in__init__.reset(previous)
-
- nf.__signature__ = inspect.signature(f)
- return nf
-
-
-def immutable(cls):
- if _Immutable in cls.__mro__:
- # Some ancestor already has the mixin, so just make sure we keep
- # following the __init__ protocol.
- cls.__init__ = _immutable_init(cls.__init__)
- if hasattr(cls, "__setstate__"):
- cls.__setstate__ = _immutable_init(cls.__setstate__)
- ncls = cls
- else:
- # Mixin the Immutable class and follow the __init__ protocol.
- class ncls(_Immutable, cls):
- # We have to do the __slots__ declaration here too!
- __slots__ = ()
-
- @_immutable_init
- def __init__(self, *args, **kwargs):
- super().__init__(*args, **kwargs)
-
- if hasattr(cls, "__setstate__"):
-
- @_immutable_init
- def __setstate__(self, *args, **kwargs):
- super().__setstate__(*args, **kwargs)
-
- # make ncls have the same name and module as cls
- ncls.__name__ = cls.__name__
- ncls.__qualname__ = cls.__qualname__
- ncls.__module__ = cls.__module__
- return ncls
diff --git a/spaces/joaopereirajp/livvieChatBot/venv/lib/python3.9/site-packages/fastapi/__init__.py b/spaces/joaopereirajp/livvieChatBot/venv/lib/python3.9/site-packages/fastapi/__init__.py
deleted file mode 100644
index 981ca49455a34e34f1414b0e04b8d9874135c94b..0000000000000000000000000000000000000000
--- a/spaces/joaopereirajp/livvieChatBot/venv/lib/python3.9/site-packages/fastapi/__init__.py
+++ /dev/null
@@ -1,25 +0,0 @@
-"""FastAPI framework, high performance, easy to learn, fast to code, ready for production"""
-
-__version__ = "0.103.2"
-
-from starlette import status as status
-
-from .applications import FastAPI as FastAPI
-from .background import BackgroundTasks as BackgroundTasks
-from .datastructures import UploadFile as UploadFile
-from .exceptions import HTTPException as HTTPException
-from .exceptions import WebSocketException as WebSocketException
-from .param_functions import Body as Body
-from .param_functions import Cookie as Cookie
-from .param_functions import Depends as Depends
-from .param_functions import File as File
-from .param_functions import Form as Form
-from .param_functions import Header as Header
-from .param_functions import Path as Path
-from .param_functions import Query as Query
-from .param_functions import Security as Security
-from .requests import Request as Request
-from .responses import Response as Response
-from .routing import APIRouter as APIRouter
-from .websockets import WebSocket as WebSocket
-from .websockets import WebSocketDisconnect as WebSocketDisconnect
diff --git a/spaces/jordyvl/ece/test_resnet-cifar_logits.py b/spaces/jordyvl/ece/test_resnet-cifar_logits.py
deleted file mode 100644
index b07aae24dcf15c7d1d24c83ad2ae9609baf2001b..0000000000000000000000000000000000000000
--- a/spaces/jordyvl/ece/test_resnet-cifar_logits.py
+++ /dev/null
@@ -1,164 +0,0 @@
-"""
-This testing script loads actual probabilisitic predictions from a resnet finetuned on CIFAR
-
-There are a number of logits-groundtruth pickles available @ https://github.com/markus93/NN_calibration/tree/master/logits
-[Seems to have moved from Git-LFS to sharepoint]
-https://tartuulikool-my.sharepoint.com/:f:/g/personal/markus93_ut_ee/EmW0xbhcic5Ou0lRbTrySOUBF2ccSsN7lo6lvSfuG1djew?e=l0TErb
-
-See https://github.com/markus93/NN_calibration/blob/master/logits/Readme.txt to decode the [model_dataset] filenames
-
-As a bonus, one could consider temperature scaling and measuring after calibration.
-"""
-import sys
-import numpy as np
-import scipy.stats as stats
-from scipy.special import softmax
-import pickle
-from sklearn.model_selection import train_test_split
-
-from matplotlib import pyplot as plt
-
-from ece import create_bins, discretize_into_bins, ECE
-
-
-# Open file with pickled variables
-def unpickle_probs(file, verbose=0, normalize=True):
- with open(file, "rb") as f: # Python 3: open(..., 'rb')
- y1, y2 = pickle.load(f) # unpickle the content
-
- if isinstance(y1, tuple):
- y_probs_val, y_val = y1
- y_probs_test, y_test = y2
- else:
- y_probs_val, y_probs_test, y_val, y_test = train_test_split(
- y1, y2.reshape(-1, 1), test_size=len(y2) - 5000, random_state=15
- ) # Splits the data in the case of pretrained models
-
- if normalize:
- y_probs_val = softmax(y_probs_val, -1)
- y_probs_test = softmax(y_probs_test, -1)
-
- if verbose:
- print(
- "y_probs_val:", y_probs_val.shape
- ) # (5000, 10); Validation set probabilities of predictions
- print("y_true_val:", y_val.shape) # (5000, 1); Validation set true labels
- print("y_probs_test:", y_probs_test.shape) # (10000, 10); Test set probabilities
- print("y_true_test:", y_test.shape) # (10000, 1); Test set true labels
-
- return ((y_probs_val, y_val.ravel()), (y_probs_test, y_test.ravel()))
-
-
-def unpickle_structured_probs(valpath=None, testpath=None):
- valpath = "/home/jordy/code/gordon/arkham/arkham/StructuredCalibration/models/jordyvl/bert-base-cased_conll2003-sm-first-ner_validation_UTY.pickle"
- testpath = "/home/jordy/code/gordon/arkham/arkham/StructuredCalibration/models/jordyvl/bert-base-cased_conll2003-sm-first-ner_test_UTY.pickle"
-
- with open(valpath, "rb") as f:
- X_val, _, y_val, _ = pickle.load(f)
-
- with open(testpath, "rb") as f:
- X_test, _, y_test, _ = pickle.load(f)
-
- X_val = np.log(X_val) # originally exponentiated [different purposes]
- X_test = np.log(X_test) # originally exponentiated [different purposes]
- # structured logits
-
-
-"""
-ALTERNATE equal mass binning
-"""
-# Define data types.
-from typing import List, Tuple, NewType, TypeVar
-Data = List[Tuple[float, float]] # List of (predicted_probability, true_label).
-Bins = List[float] # List of bin boundaries, excluding 0.0, but including 1.0.
-BinnedData = List[Data] # binned_data[i] contains the data in bin i.
-T = TypeVar('T')
-
-eps = 1e-6
-
-def split(sequence: List[T], parts: int) -> List[List[T]]:
- assert parts <= len(sequence), "more bins than probabilities"
- part_size = int(np.ceil(len(sequence) * 1.0 / parts))
- assert part_size * parts >= len(sequence), "no missing instances when partitioning"
- assert (part_size - 1) * parts < len(sequence), "dropping 1 does not make for missing"
- return [sequence[i:i + part_size] for i in range(0, len(sequence), part_size)]
-
-
-def get_equal_bins(probs: List[float], n_bins: int=10) -> Bins:
- """Get bins that contain approximately an equal number of data points."""
- sorted_probs = sorted(probs)
- binned_data = split(sorted_probs, n_bins)
- bins: Bins = []
- for i in range(len(binned_data) - 1):
- last_prob = binned_data[i][-1]
- next_first_prob = binned_data[i + 1][0]
- bins.append((last_prob + next_first_prob) / 2.0)
- bins.append(1.0)
- bins = sorted(list(set(bins))) #this is the special thing!
- return bins
-
-def histedges_equalN(x, nbin):
- npt = len(x)
- return np.interp(np.linspace(0, npt, nbin + 1),
- np.arange(npt),
- np.sort(x))
-
- '''
- bin_upper_edges = histedges_equalN(P, n_bins)
- #n, bins, patches = plt.hist(x, histedges_equalN(x, 10))
- '''
-
-
-def test_equalmass_binning(P, Y):
- #probs = np.array([0.63, 0.2, 0.2, 0, 0.95, 0.05, 0.72, 0.1, 0.2])
-
- kwargs = dict(
- n_bins= 10,
- scheme="equal-mass",
- bin_range=None,
- proxy="upper-edge",
- #proxy="center",
- p=1,
- detail=True,
- )
-
- if P.ndim == 2: #can assume ECE
- p_max = np.max(P, -1) # create p̂ as top-1 softmax probability € [0,1]
-
- eqr_bins = create_bins(n_bins=kwargs["n_bins"], scheme="equal-range", bin_range=kwargs["bin_range"], P=p_max)
- eqm_bins = create_bins(n_bins=kwargs["n_bins"], scheme=kwargs["scheme"], bin_range=kwargs["bin_range"], P=p_max)
- #alternate_eqm_bins = get_equal_bins(p_max, kwargs["n_bins"])
-
-
- eqr_hist = np.digitize(p_max, eqr_bins, right=True)
- eqm_hist = np.digitize(p_max, eqm_bins, right=True)
- eqml_hist = np.digitize(p_max, eqm_bins, right=False)
-
- #eqm_bins = [0] + eqm_bins
-
- other_hist = discretize_into_bins(np.expand_dims(p_max, 0), eqm_bins)
- hist_difference = stats.power_divergence(eqr_hist, eqm_hist, lambda_="pearson") #chisquare
-
- #plt.hist(eqr_hist, color="green", label="equal-range")
- plt.hist(eqm_hist, color="blue", label="equal-mass")
- plt.legend()
- #plt.show()
-
-
- res = ECE()._compute(P, Y, **kwargs)
- print(f"eqm ECE: {res['ECE']}")
-
- kwargs["scheme"] = "equal-range"
- res = ECE()._compute(P, Y, **kwargs)
- print(f"eqr ECE: {res['ECE']}")
-
- # res = ECE()._compute(predictions, references, detail=True)
- # print(f"ECE: {res['ECE']}")
-
-
-
-if __name__ == "__main__":
- FILE_PATH = sys.argv[1] if len(sys.argv) > 1 else "resnet110_c10_logits.p"
- (p_val, y_val), (p_test, y_test) = unpickle_probs(FILE_PATH, False, True)
- test_equalmass_binning(p_val, y_val)
- # do on val
diff --git a/spaces/josuelmet/Metal_Music_Interpolator/data/README.md b/spaces/josuelmet/Metal_Music_Interpolator/data/README.md
deleted file mode 100644
index 9b1b56e0f62bdcef5b816b90e0297663abc28028..0000000000000000000000000000000000000000
--- a/spaces/josuelmet/Metal_Music_Interpolator/data/README.md
+++ /dev/null
@@ -1 +0,0 @@
-Pre-processed data.
\ No newline at end of file
diff --git a/spaces/juanhuggingface/ChuanhuChatGPT_Beta/run_Linux.sh b/spaces/juanhuggingface/ChuanhuChatGPT_Beta/run_Linux.sh
deleted file mode 100644
index 2d26597ae47519f42336ccffc16646713a192ae1..0000000000000000000000000000000000000000
--- a/spaces/juanhuggingface/ChuanhuChatGPT_Beta/run_Linux.sh
+++ /dev/null
@@ -1,31 +0,0 @@
-#!/bin/bash
-
-# 获取脚本所在目录
-script_dir=$(dirname "$(readlink -f "$0")")
-
-# 将工作目录更改为脚本所在目录
-cd "$script_dir" || exit
-
-# 检查Git仓库是否有更新
-git remote update
-pwd
-
-if ! git status -uno | grep 'up to date' > /dev/null; then
- # 如果有更新,关闭当前运行的服务器
- pkill -f ChuanhuChatbot.py
-
- # 拉取最新更改
- git pull
-
- # 安装依赖
- pip3 install -r requirements.txt
-
- # 重新启动服务器
- nohup python3 ChuanhuChatbot.py &
-fi
-
-# 检查ChuanhuChatbot.py是否在运行
-if ! pgrep -f ChuanhuChatbot.py > /dev/null; then
- # 如果没有运行,启动服务器
- nohup python3 ChuanhuChatbot.py &
-fi
diff --git a/spaces/juliensimon/imdb-demo-space/app.py b/spaces/juliensimon/imdb-demo-space/app.py
deleted file mode 100644
index e0d84e2c298292d2db9ffc60fe42a26d27cb4ca3..0000000000000000000000000000000000000000
--- a/spaces/juliensimon/imdb-demo-space/app.py
+++ /dev/null
@@ -1,19 +0,0 @@
-import torch
-import numpy as np
-import gradio as gr
-from transformers import AutoTokenizer, AutoModelForSequenceClassification
-
-tokenizer = AutoTokenizer.from_pretrained("juliensimon/autonlp-imdb-demo-hf-16622775")
-model = AutoModelForSequenceClassification.from_pretrained("juliensimon/autonlp-imdb-demo-hf-16622775")
-
-def predict(review):
- inputs = tokenizer(review, padding=True, truncation=True, return_tensors="pt")
- outputs = model(**inputs)
- predictions = torch.nn.functional.softmax(outputs.logits, dim=-1)
- predictions = predictions.detach().numpy()[0]
- index = np.argmax(predictions)
- score = predictions[index]
- return "This review is {:.2f}% {}".format(100*score, "negative" if index==0 else "positive")
-
-iface = gr.Interface(fn=predict, inputs="text", outputs="text")
-iface.launch()
diff --git a/spaces/juuxn/SimpleRVC/utils/model.py b/spaces/juuxn/SimpleRVC/utils/model.py
deleted file mode 100644
index 5d97d86fb8adff1cfe65b8130b3d931768556c35..0000000000000000000000000000000000000000
--- a/spaces/juuxn/SimpleRVC/utils/model.py
+++ /dev/null
@@ -1,111 +0,0 @@
-import os
-import shutil
-from mega import Mega
-import gdown
-import re
-import wget
-import sys
-import uuid
-import zipfile
-
-
-class InvalidDriveId(Exception):
- def __init__(self, message="Error de la url"):
- self.message = message
- super().__init__(self.message)
-
-
-def model_downloader(url, zip_path, dest_path):
- """Download and unzip a file from Google Drive or Mega."""
-
- def drive_download(url, dest_folder):
- print(f"Descargando desde drive...")
- try:
- filename = gdown.download(url, os.path.join(dest_folder, f"{uuid.uuid4()}.zip"), fuzzy=True)
- return os.path.basename(filename)
- except:
- print("El intento de descargar con drive no funcionó")
- return None
-
- def mega_download(url, dest_folder):
- try:
- file_id = None
- if "#!" in url:
- file_id = url.split("#!")[1].split("!")[0]
- elif "file/" in url:
- file_id = url.split("file/")[1].split("/")[0]
- else:
- file_id = None
-
- print(f"Descargando desde mega...")
- if file_id:
- mega = Mega()
- m = mega.login()
- filename = m.download_url(url, dest_path=dest_folder, dest_filename=f"{uuid.uuid4()}.zip")
-
- return os.path.basename(filename)
- else:
- return None
-
- except Exception as e:
- print("Ocurrio un error**")
- print(e)
- return None
-
- def download(url, dest_folder):
- try:
- print(f"Descargando desde url generica...")
- dest_path = wget.download(url=url, out=os.path.join(dest_folder, f"{uuid.uuid4()}.zip"))
-
- return os.path.basename(dest_path)
- except Exception as e:
- print(f"Error al descargar el archivo: {str(e)}")
-
- filename = ""
-
- if not os.path.exists(zip_path):
- os.mkdir(zip_path)
-
- if url and 'drive.google.com' in url:
- # Descargar el elemento si la URL es de Google Drive
- filename = drive_download(url, zip_path)
- elif url and 'mega.nz' in url:
- filename = mega_download(url, zip_path)
- elif url and 'pixeldrain' in url:
- print("No se puede descargar de pixeldrain")
- sys.exit()
- else:
- filename = download(url, zip_path)
-
- if filename:
- print(f"Descomprimiendo {filename}...")
- modelname = str(filename).replace(".zip", "")
- zip_file_path = os.path.join(zip_path, filename)
-
- try:
- shutil.unpack_archive(zip_file_path, os.path.join(dest_path, modelname))
- except Exception as e:
- try:
- with zipfile.ZipFile(zip_file_path, 'r') as zip_ref:
- zip_ref.extractall(dest_path)
- except zipfile.BadZipFile as e:
- print(f"Error: El archivo ZIP no es válido - {e}")
- except Exception as e:
- print(f"Error inesperado: {e}")
-
- if os.path.exists(zip_file_path):
- os.remove(zip_file_path)
-
- return modelname
- else:
- return None
-
-def get_model(weight_path, modelname):
- resources = {}
- for root, dirs, files in os.walk(os.path.join(weight_path, modelname)):
- for file in files:
- if file.endswith('.index'):
- resources['index'] = os.path.relpath(os.path.join(root, file))
- if file.endswith('.pth') and not 'G_' in file and not 'D_' in file:
- resources['pth'] = os.path.relpath(os.path.join(root, file), start=weight_path)
- return resources
\ No newline at end of file
diff --git a/spaces/kernel982/Youtube-Transcriber/utils.py b/spaces/kernel982/Youtube-Transcriber/utils.py
deleted file mode 100644
index 17252fa2ac5ac9e64887184574561fb0f340545a..0000000000000000000000000000000000000000
--- a/spaces/kernel982/Youtube-Transcriber/utils.py
+++ /dev/null
@@ -1,115 +0,0 @@
-import textwrap
-import unicodedata
-import re
-
-import zlib
-from typing import Iterator, TextIO
-
-
-def exact_div(x, y):
- assert x % y == 0
- return x // y
-
-
-def str2bool(string):
- str2val = {"True": True, "False": False}
- if string in str2val:
- return str2val[string]
- else:
- raise ValueError(f"Expected one of {set(str2val.keys())}, got {string}")
-
-
-def optional_int(string):
- return None if string == "None" else int(string)
-
-
-def optional_float(string):
- return None if string == "None" else float(string)
-
-
-def compression_ratio(text) -> float:
- return len(text) / len(zlib.compress(text.encode("utf-8")))
-
-
-def format_timestamp(seconds: float, always_include_hours: bool = False, fractionalSeperator: str = '.'):
- assert seconds >= 0, "non-negative timestamp expected"
- milliseconds = round(seconds * 1000.0)
-
- hours = milliseconds // 3_600_000
- milliseconds -= hours * 3_600_000
-
- minutes = milliseconds // 60_000
- milliseconds -= minutes * 60_000
-
- seconds = milliseconds // 1_000
- milliseconds -= seconds * 1_000
-
- hours_marker = f"{hours:02d}:" if always_include_hours or hours > 0 else ""
- return f"{hours_marker}{minutes:02d}:{seconds:02d}{fractionalSeperator}{milliseconds:03d}"
-
-
-def write_txt(transcript: Iterator[dict], file: TextIO):
- for segment in transcript:
- print(segment['text'].strip(), file=file, flush=True)
-
-
-def write_vtt(transcript: Iterator[dict], file: TextIO, maxLineWidth=None):
- print("WEBVTT\n", file=file)
- for segment in transcript:
- text = processText(segment['text'], maxLineWidth).replace('-->', '->')
-
- print(
- f"{format_timestamp(segment['start'])} --> {format_timestamp(segment['end'])}\n"
- f"{text}\n",
- file=file,
- flush=True,
- )
-
-
-def write_srt(transcript: Iterator[dict], file: TextIO, maxLineWidth=None):
- """
- Write a transcript to a file in SRT format.
- Example usage:
- from pathlib import Path
- from whisper.utils import write_srt
- result = transcribe(model, audio_path, temperature=temperature, **args)
- # save SRT
- audio_basename = Path(audio_path).stem
- with open(Path(output_dir) / (audio_basename + ".srt"), "w", encoding="utf-8") as srt:
- write_srt(result["segments"], file=srt)
- """
- for i, segment in enumerate(transcript, start=1):
- text = processText(segment['text'].strip(), maxLineWidth).replace('-->', '->')
-
- # write srt lines
- print(
- f"{i}\n"
- f"{format_timestamp(segment['start'], always_include_hours=True, fractionalSeperator=',')} --> "
- f"{format_timestamp(segment['end'], always_include_hours=True, fractionalSeperator=',')}\n"
- f"{text}\n",
- file=file,
- flush=True,
- )
-
-def processText(text: str, maxLineWidth=None):
- if (maxLineWidth is None or maxLineWidth < 0):
- return text
-
- lines = textwrap.wrap(text, width=maxLineWidth, tabsize=4)
- return '\n'.join(lines)
-
-def slugify(value, allow_unicode=False):
- """
- Taken from https://github.com/django/django/blob/master/django/utils/text.py
- Convert to ASCII if 'allow_unicode' is False. Convert spaces or repeated
- dashes to single dashes. Remove characters that aren't alphanumerics,
- underscores, or hyphens. Convert to lowercase. Also strip leading and
- trailing whitespace, dashes, and underscores.
- """
- value = str(value)
- if allow_unicode:
- value = unicodedata.normalize('NFKC', value)
- else:
- value = unicodedata.normalize('NFKD', value).encode('ascii', 'ignore').decode('ascii')
- value = re.sub(r'[^\w\s-]', '', value.lower())
- return re.sub(r'[-\s]+', '-', value).strip('-_')
\ No newline at end of file
diff --git a/spaces/kevinwang676/Bark-with-Voice-Cloning/util/__init__.py b/spaces/kevinwang676/Bark-with-Voice-Cloning/util/__init__.py
deleted file mode 100644
index e69de29bb2d1d6434b8b29ae775ad8c2e48c5391..0000000000000000000000000000000000000000
diff --git a/spaces/kevinwang676/M4Singer/usr/diff/shallow_diffusion_tts.py b/spaces/kevinwang676/M4Singer/usr/diff/shallow_diffusion_tts.py
deleted file mode 100644
index 835c57efffae63df1a70165d8fb10e507070435a..0000000000000000000000000000000000000000
--- a/spaces/kevinwang676/M4Singer/usr/diff/shallow_diffusion_tts.py
+++ /dev/null
@@ -1,320 +0,0 @@
-import math
-import random
-from collections import deque
-from functools import partial
-from inspect import isfunction
-from pathlib import Path
-import numpy as np
-import torch
-import torch.nn.functional as F
-from torch import nn
-from tqdm import tqdm
-from einops import rearrange
-
-from modules.fastspeech.fs2 import FastSpeech2
-from modules.diffsinger_midi.fs2 import FastSpeech2MIDI
-from utils.hparams import hparams
-
-
-
-def exists(x):
- return x is not None
-
-
-def default(val, d):
- if exists(val):
- return val
- return d() if isfunction(d) else d
-
-
-# gaussian diffusion trainer class
-
-def extract(a, t, x_shape):
- b, *_ = t.shape
- out = a.gather(-1, t)
- return out.reshape(b, *((1,) * (len(x_shape) - 1)))
-
-
-def noise_like(shape, device, repeat=False):
- repeat_noise = lambda: torch.randn((1, *shape[1:]), device=device).repeat(shape[0], *((1,) * (len(shape) - 1)))
- noise = lambda: torch.randn(shape, device=device)
- return repeat_noise() if repeat else noise()
-
-
-def linear_beta_schedule(timesteps, max_beta=hparams.get('max_beta', 0.01)):
- """
- linear schedule
- """
- betas = np.linspace(1e-4, max_beta, timesteps)
- return betas
-
-
-def cosine_beta_schedule(timesteps, s=0.008):
- """
- cosine schedule
- as proposed in https://openreview.net/forum?id=-NEXDKk8gZ
- """
- steps = timesteps + 1
- x = np.linspace(0, steps, steps)
- alphas_cumprod = np.cos(((x / steps) + s) / (1 + s) * np.pi * 0.5) ** 2
- alphas_cumprod = alphas_cumprod / alphas_cumprod[0]
- betas = 1 - (alphas_cumprod[1:] / alphas_cumprod[:-1])
- return np.clip(betas, a_min=0, a_max=0.999)
-
-
-beta_schedule = {
- "cosine": cosine_beta_schedule,
- "linear": linear_beta_schedule,
-}
-
-
-class GaussianDiffusion(nn.Module):
- def __init__(self, phone_encoder, out_dims, denoise_fn,
- timesteps=1000, K_step=1000, loss_type=hparams.get('diff_loss_type', 'l1'), betas=None, spec_min=None, spec_max=None):
- super().__init__()
- self.denoise_fn = denoise_fn
- if hparams.get('use_midi') is not None and hparams['use_midi']:
- self.fs2 = FastSpeech2MIDI(phone_encoder, out_dims)
- else:
- self.fs2 = FastSpeech2(phone_encoder, out_dims)
- self.mel_bins = out_dims
-
- if exists(betas):
- betas = betas.detach().cpu().numpy() if isinstance(betas, torch.Tensor) else betas
- else:
- if 'schedule_type' in hparams.keys():
- betas = beta_schedule[hparams['schedule_type']](timesteps)
- else:
- betas = cosine_beta_schedule(timesteps)
-
- alphas = 1. - betas
- alphas_cumprod = np.cumprod(alphas, axis=0)
- alphas_cumprod_prev = np.append(1., alphas_cumprod[:-1])
-
- timesteps, = betas.shape
- self.num_timesteps = int(timesteps)
- self.K_step = K_step
- self.loss_type = loss_type
-
- self.noise_list = deque(maxlen=4)
-
- to_torch = partial(torch.tensor, dtype=torch.float32)
-
- self.register_buffer('betas', to_torch(betas))
- self.register_buffer('alphas_cumprod', to_torch(alphas_cumprod))
- self.register_buffer('alphas_cumprod_prev', to_torch(alphas_cumprod_prev))
-
- # calculations for diffusion q(x_t | x_{t-1}) and others
- self.register_buffer('sqrt_alphas_cumprod', to_torch(np.sqrt(alphas_cumprod)))
- self.register_buffer('sqrt_one_minus_alphas_cumprod', to_torch(np.sqrt(1. - alphas_cumprod)))
- self.register_buffer('log_one_minus_alphas_cumprod', to_torch(np.log(1. - alphas_cumprod)))
- self.register_buffer('sqrt_recip_alphas_cumprod', to_torch(np.sqrt(1. / alphas_cumprod)))
- self.register_buffer('sqrt_recipm1_alphas_cumprod', to_torch(np.sqrt(1. / alphas_cumprod - 1)))
-
- # calculations for posterior q(x_{t-1} | x_t, x_0)
- posterior_variance = betas * (1. - alphas_cumprod_prev) / (1. - alphas_cumprod)
- # above: equal to 1. / (1. / (1. - alpha_cumprod_tm1) + alpha_t / beta_t)
- self.register_buffer('posterior_variance', to_torch(posterior_variance))
- # below: log calculation clipped because the posterior variance is 0 at the beginning of the diffusion chain
- self.register_buffer('posterior_log_variance_clipped', to_torch(np.log(np.maximum(posterior_variance, 1e-20))))
- self.register_buffer('posterior_mean_coef1', to_torch(
- betas * np.sqrt(alphas_cumprod_prev) / (1. - alphas_cumprod)))
- self.register_buffer('posterior_mean_coef2', to_torch(
- (1. - alphas_cumprod_prev) * np.sqrt(alphas) / (1. - alphas_cumprod)))
-
- self.register_buffer('spec_min', torch.FloatTensor(spec_min)[None, None, :hparams['keep_bins']])
- self.register_buffer('spec_max', torch.FloatTensor(spec_max)[None, None, :hparams['keep_bins']])
-
- def q_mean_variance(self, x_start, t):
- mean = extract(self.sqrt_alphas_cumprod, t, x_start.shape) * x_start
- variance = extract(1. - self.alphas_cumprod, t, x_start.shape)
- log_variance = extract(self.log_one_minus_alphas_cumprod, t, x_start.shape)
- return mean, variance, log_variance
-
- def predict_start_from_noise(self, x_t, t, noise):
- return (
- extract(self.sqrt_recip_alphas_cumprod, t, x_t.shape) * x_t -
- extract(self.sqrt_recipm1_alphas_cumprod, t, x_t.shape) * noise
- )
-
- def q_posterior(self, x_start, x_t, t):
- posterior_mean = (
- extract(self.posterior_mean_coef1, t, x_t.shape) * x_start +
- extract(self.posterior_mean_coef2, t, x_t.shape) * x_t
- )
- posterior_variance = extract(self.posterior_variance, t, x_t.shape)
- posterior_log_variance_clipped = extract(self.posterior_log_variance_clipped, t, x_t.shape)
- return posterior_mean, posterior_variance, posterior_log_variance_clipped
-
- def p_mean_variance(self, x, t, cond, clip_denoised: bool):
- noise_pred = self.denoise_fn(x, t, cond=cond)
- x_recon = self.predict_start_from_noise(x, t=t, noise=noise_pred)
-
- if clip_denoised:
- x_recon.clamp_(-1., 1.)
-
- model_mean, posterior_variance, posterior_log_variance = self.q_posterior(x_start=x_recon, x_t=x, t=t)
- return model_mean, posterior_variance, posterior_log_variance
-
- @torch.no_grad()
- def p_sample(self, x, t, cond, clip_denoised=True, repeat_noise=False):
- b, *_, device = *x.shape, x.device
- model_mean, _, model_log_variance = self.p_mean_variance(x=x, t=t, cond=cond, clip_denoised=clip_denoised)
- noise = noise_like(x.shape, device, repeat_noise)
- # no noise when t == 0
- nonzero_mask = (1 - (t == 0).float()).reshape(b, *((1,) * (len(x.shape) - 1)))
- return model_mean + nonzero_mask * (0.5 * model_log_variance).exp() * noise
-
- @torch.no_grad()
- def p_sample_plms(self, x, t, interval, cond, clip_denoised=True, repeat_noise=False):
- """
- Use the PLMS method from [Pseudo Numerical Methods for Diffusion Models on Manifolds](https://arxiv.org/abs/2202.09778).
- """
-
- def get_x_pred(x, noise_t, t):
- a_t = extract(self.alphas_cumprod, t, x.shape)
- a_prev = extract(self.alphas_cumprod, torch.max(t-interval, torch.zeros_like(t)), x.shape)
- a_t_sq, a_prev_sq = a_t.sqrt(), a_prev.sqrt()
-
- x_delta = (a_prev - a_t) * ((1 / (a_t_sq * (a_t_sq + a_prev_sq))) * x - 1 / (a_t_sq * (((1 - a_prev) * a_t).sqrt() + ((1 - a_t) * a_prev).sqrt())) * noise_t)
- x_pred = x + x_delta
-
- return x_pred
-
- noise_list = self.noise_list
- noise_pred = self.denoise_fn(x, t, cond=cond)
-
- if len(noise_list) == 0:
- x_pred = get_x_pred(x, noise_pred, t)
- noise_pred_prev = self.denoise_fn(x_pred, max(t-interval, 0), cond=cond)
- noise_pred_prime = (noise_pred + noise_pred_prev) / 2
- elif len(noise_list) == 1:
- noise_pred_prime = (3 * noise_pred - noise_list[-1]) / 2
- elif len(noise_list) == 2:
- noise_pred_prime = (23 * noise_pred - 16 * noise_list[-1] + 5 * noise_list[-2]) / 12
- elif len(noise_list) >= 3:
- noise_pred_prime = (55 * noise_pred - 59 * noise_list[-1] + 37 * noise_list[-2] - 9 * noise_list[-3]) / 24
-
- x_prev = get_x_pred(x, noise_pred_prime, t)
- noise_list.append(noise_pred)
-
- return x_prev
-
- def q_sample(self, x_start, t, noise=None):
- noise = default(noise, lambda: torch.randn_like(x_start))
- return (
- extract(self.sqrt_alphas_cumprod, t, x_start.shape) * x_start +
- extract(self.sqrt_one_minus_alphas_cumprod, t, x_start.shape) * noise
- )
-
- def p_losses(self, x_start, t, cond, noise=None, nonpadding=None):
- noise = default(noise, lambda: torch.randn_like(x_start))
-
- x_noisy = self.q_sample(x_start=x_start, t=t, noise=noise)
- x_recon = self.denoise_fn(x_noisy, t, cond)
-
- if self.loss_type == 'l1':
- if nonpadding is not None:
- loss = ((noise - x_recon).abs() * nonpadding.unsqueeze(1)).mean()
- else:
- # print('are you sure w/o nonpadding?')
- loss = (noise - x_recon).abs().mean()
-
- elif self.loss_type == 'l2':
- loss = F.mse_loss(noise, x_recon)
- else:
- raise NotImplementedError()
-
- return loss
-
- def forward(self, txt_tokens, mel2ph=None, spk_embed=None,
- ref_mels=None, f0=None, uv=None, energy=None, infer=False, **kwargs):
- b, *_, device = *txt_tokens.shape, txt_tokens.device
- ret = self.fs2(txt_tokens, mel2ph, spk_embed, ref_mels, f0, uv, energy,
- skip_decoder=(not infer), infer=infer, **kwargs)
- cond = ret['decoder_inp'].transpose(1, 2)
-
- if not infer:
- t = torch.randint(0, self.K_step, (b,), device=device).long()
- x = ref_mels
- x = self.norm_spec(x)
- x = x.transpose(1, 2)[:, None, :, :] # [B, 1, M, T]
- ret['diff_loss'] = self.p_losses(x, t, cond)
- # nonpadding = (mel2ph != 0).float()
- # ret['diff_loss'] = self.p_losses(x, t, cond, nonpadding=nonpadding)
- else:
- ret['fs2_mel'] = ret['mel_out']
- fs2_mels = ret['mel_out']
- t = self.K_step
- fs2_mels = self.norm_spec(fs2_mels)
- fs2_mels = fs2_mels.transpose(1, 2)[:, None, :, :]
-
- x = self.q_sample(x_start=fs2_mels, t=torch.tensor([t - 1], device=device).long())
- if hparams.get('gaussian_start') is not None and hparams['gaussian_start']:
- print('===> gaussion start.')
- shape = (cond.shape[0], 1, self.mel_bins, cond.shape[2])
- x = torch.randn(shape, device=device)
-
- if hparams.get('pndm_speedup'):
- self.noise_list = deque(maxlen=4)
- iteration_interval = hparams['pndm_speedup']
- for i in tqdm(reversed(range(0, t, iteration_interval)), desc='sample time step',
- total=t // iteration_interval):
- x = self.p_sample_plms(x, torch.full((b,), i, device=device, dtype=torch.long), iteration_interval,
- cond)
- else:
- for i in tqdm(reversed(range(0, t)), desc='sample time step', total=t):
- x = self.p_sample(x, torch.full((b,), i, device=device, dtype=torch.long), cond)
- x = x[:, 0].transpose(1, 2)
- if mel2ph is not None: # for singing
- ret['mel_out'] = self.denorm_spec(x) * ((mel2ph > 0).float()[:, :, None])
- else:
- ret['mel_out'] = self.denorm_spec(x)
- return ret
-
- def norm_spec(self, x):
- return (x - self.spec_min) / (self.spec_max - self.spec_min) * 2 - 1
-
- def denorm_spec(self, x):
- return (x + 1) / 2 * (self.spec_max - self.spec_min) + self.spec_min
-
- def cwt2f0_norm(self, cwt_spec, mean, std, mel2ph):
- return self.fs2.cwt2f0_norm(cwt_spec, mean, std, mel2ph)
-
- def out2mel(self, x):
- return x
-
-
-class OfflineGaussianDiffusion(GaussianDiffusion):
- def forward(self, txt_tokens, mel2ph=None, spk_embed=None,
- ref_mels=None, f0=None, uv=None, energy=None, infer=False, **kwargs):
- b, *_, device = *txt_tokens.shape, txt_tokens.device
-
- ret = self.fs2(txt_tokens, mel2ph, spk_embed, ref_mels, f0, uv, energy,
- skip_decoder=True, infer=True, **kwargs)
- cond = ret['decoder_inp'].transpose(1, 2)
- fs2_mels = ref_mels[1]
- ref_mels = ref_mels[0]
-
- if not infer:
- t = torch.randint(0, self.K_step, (b,), device=device).long()
- x = ref_mels
- x = self.norm_spec(x)
- x = x.transpose(1, 2)[:, None, :, :] # [B, 1, M, T]
- ret['diff_loss'] = self.p_losses(x, t, cond)
- else:
- t = self.K_step
- fs2_mels = self.norm_spec(fs2_mels)
- fs2_mels = fs2_mels.transpose(1, 2)[:, None, :, :]
-
- x = self.q_sample(x_start=fs2_mels, t=torch.tensor([t - 1], device=device).long())
-
- if hparams.get('gaussian_start') is not None and hparams['gaussian_start']:
- print('===> gaussion start.')
- shape = (cond.shape[0], 1, self.mel_bins, cond.shape[2])
- x = torch.randn(shape, device=device)
- for i in tqdm(reversed(range(0, t)), desc='sample time step', total=t):
- x = self.p_sample(x, torch.full((b,), i, device=device, dtype=torch.long), cond)
- x = x[:, 0].transpose(1, 2)
- ret['mel_out'] = self.denorm_spec(x)
- return ret
diff --git a/spaces/kira4424/Tacotron-zero-short-voice-clone/ppg2mel/utils/basic_layers.py b/spaces/kira4424/Tacotron-zero-short-voice-clone/ppg2mel/utils/basic_layers.py
deleted file mode 100644
index 45d80f1ef9e459a6e2d8494cf8d4ca1e599f772f..0000000000000000000000000000000000000000
--- a/spaces/kira4424/Tacotron-zero-short-voice-clone/ppg2mel/utils/basic_layers.py
+++ /dev/null
@@ -1,79 +0,0 @@
-import torch
-from torch import nn
-from torch.nn import functional as F
-from torch.autograd import Function
-
-def tile(x, count, dim=0):
- """
- Tiles x on dimension dim count times.
- """
- perm = list(range(len(x.size())))
- if dim != 0:
- perm[0], perm[dim] = perm[dim], perm[0]
- x = x.permute(perm).contiguous()
- out_size = list(x.size())
- out_size[0] *= count
- batch = x.size(0)
- x = x.view(batch, -1) \
- .transpose(0, 1) \
- .repeat(count, 1) \
- .transpose(0, 1) \
- .contiguous() \
- .view(*out_size)
- if dim != 0:
- x = x.permute(perm).contiguous()
- return x
-
-class Linear(torch.nn.Module):
- def __init__(self, in_dim, out_dim, bias=True, w_init_gain='linear'):
- super(Linear, self).__init__()
- self.linear_layer = torch.nn.Linear(in_dim, out_dim, bias=bias)
-
- torch.nn.init.xavier_uniform_(
- self.linear_layer.weight,
- gain=torch.nn.init.calculate_gain(w_init_gain))
-
- def forward(self, x):
- return self.linear_layer(x)
-
-class Conv1d(torch.nn.Module):
- def __init__(self, in_channels, out_channels, kernel_size=1, stride=1,
- padding=None, dilation=1, bias=True, w_init_gain='linear', param=None):
- super(Conv1d, self).__init__()
- if padding is None:
- assert(kernel_size % 2 == 1)
- padding = int(dilation * (kernel_size - 1)/2)
-
- self.conv = torch.nn.Conv1d(in_channels, out_channels,
- kernel_size=kernel_size, stride=stride,
- padding=padding, dilation=dilation,
- bias=bias)
- torch.nn.init.xavier_uniform_(
- self.conv.weight, gain=torch.nn.init.calculate_gain(w_init_gain, param=param))
-
- def forward(self, x):
- # x: BxDxT
- return self.conv(x)
-
-
-
-def tile(x, count, dim=0):
- """
- Tiles x on dimension dim count times.
- """
- perm = list(range(len(x.size())))
- if dim != 0:
- perm[0], perm[dim] = perm[dim], perm[0]
- x = x.permute(perm).contiguous()
- out_size = list(x.size())
- out_size[0] *= count
- batch = x.size(0)
- x = x.view(batch, -1) \
- .transpose(0, 1) \
- .repeat(count, 1) \
- .transpose(0, 1) \
- .contiguous() \
- .view(*out_size)
- if dim != 0:
- x = x.permute(perm).contiguous()
- return x
diff --git a/spaces/kirch/Text2Video-Zero/annotator/uniformer/configs/_base_/models/fcn_r50-d8.py b/spaces/kirch/Text2Video-Zero/annotator/uniformer/configs/_base_/models/fcn_r50-d8.py
deleted file mode 100644
index 5e98f6cc918b6146fc6d613c6918e825ef1355c3..0000000000000000000000000000000000000000
--- a/spaces/kirch/Text2Video-Zero/annotator/uniformer/configs/_base_/models/fcn_r50-d8.py
+++ /dev/null
@@ -1,45 +0,0 @@
-# model settings
-norm_cfg = dict(type='SyncBN', requires_grad=True)
-model = dict(
- type='EncoderDecoder',
- pretrained='open-mmlab://resnet50_v1c',
- backbone=dict(
- type='ResNetV1c',
- depth=50,
- num_stages=4,
- out_indices=(0, 1, 2, 3),
- dilations=(1, 1, 2, 4),
- strides=(1, 2, 1, 1),
- norm_cfg=norm_cfg,
- norm_eval=False,
- style='pytorch',
- contract_dilation=True),
- decode_head=dict(
- type='FCNHead',
- in_channels=2048,
- in_index=3,
- channels=512,
- num_convs=2,
- concat_input=True,
- dropout_ratio=0.1,
- num_classes=19,
- norm_cfg=norm_cfg,
- align_corners=False,
- loss_decode=dict(
- type='CrossEntropyLoss', use_sigmoid=False, loss_weight=1.0)),
- auxiliary_head=dict(
- type='FCNHead',
- in_channels=1024,
- in_index=2,
- channels=256,
- num_convs=1,
- concat_input=False,
- dropout_ratio=0.1,
- num_classes=19,
- norm_cfg=norm_cfg,
- align_corners=False,
- loss_decode=dict(
- type='CrossEntropyLoss', use_sigmoid=False, loss_weight=0.4)),
- # model training and testing settings
- train_cfg=dict(),
- test_cfg=dict(mode='whole'))
diff --git a/spaces/koajoel/PolyFormer/fairseq/examples/speech_recognition/data/replabels.py b/spaces/koajoel/PolyFormer/fairseq/examples/speech_recognition/data/replabels.py
deleted file mode 100644
index 441f1bd432b95865fc981c6c695cee299b07ed62..0000000000000000000000000000000000000000
--- a/spaces/koajoel/PolyFormer/fairseq/examples/speech_recognition/data/replabels.py
+++ /dev/null
@@ -1,70 +0,0 @@
-#!/usr/bin/env python3
-
-# Copyright (c) Facebook, Inc. and its affiliates.
-#
-# This source code is licensed under the MIT license found in the
-# LICENSE file in the root directory of this source tree.
-
-"""
-Replabel transforms for use with flashlight's ASG criterion.
-"""
-
-
-def replabel_symbol(i):
- """
- Replabel symbols used in flashlight, currently just "1", "2", ...
- This prevents training with numeral tokens, so this might change in the future
- """
- return str(i)
-
-
-def pack_replabels(tokens, dictionary, max_reps):
- """
- Pack a token sequence so that repeated symbols are replaced by replabels
- """
- if len(tokens) == 0 or max_reps <= 0:
- return tokens
-
- replabel_value_to_idx = [0] * (max_reps + 1)
- for i in range(1, max_reps + 1):
- replabel_value_to_idx[i] = dictionary.index(replabel_symbol(i))
-
- result = []
- prev_token = -1
- num_reps = 0
- for token in tokens:
- if token == prev_token and num_reps < max_reps:
- num_reps += 1
- else:
- if num_reps > 0:
- result.append(replabel_value_to_idx[num_reps])
- num_reps = 0
- result.append(token)
- prev_token = token
- if num_reps > 0:
- result.append(replabel_value_to_idx[num_reps])
- return result
-
-
-def unpack_replabels(tokens, dictionary, max_reps):
- """
- Unpack a token sequence so that replabels are replaced by repeated symbols
- """
- if len(tokens) == 0 or max_reps <= 0:
- return tokens
-
- replabel_idx_to_value = {}
- for i in range(1, max_reps + 1):
- replabel_idx_to_value[dictionary.index(replabel_symbol(i))] = i
-
- result = []
- prev_token = -1
- for token in tokens:
- try:
- for _ in range(replabel_idx_to_value[token]):
- result.append(prev_token)
- prev_token = -1
- except KeyError:
- result.append(token)
- prev_token = token
- return result
diff --git a/spaces/koajoel/PolyFormer/fairseq/examples/speech_synthesis/preprocessing/denoiser/pretrained.py b/spaces/koajoel/PolyFormer/fairseq/examples/speech_synthesis/preprocessing/denoiser/pretrained.py
deleted file mode 100644
index 2fa846075b6872cdcc0baebca0b9acbb9ffcd287..0000000000000000000000000000000000000000
--- a/spaces/koajoel/PolyFormer/fairseq/examples/speech_synthesis/preprocessing/denoiser/pretrained.py
+++ /dev/null
@@ -1,81 +0,0 @@
-# Copyright (c) Facebook, Inc. and its affiliates.
-# All rights reserved.
-#
-# This source code is licensed under the license found in the
-# LICENSE file in the root directory of this source tree.
-# author: adefossez
-
-import logging
-
-import torch.hub
-
-from .demucs import Demucs
-from .utils import deserialize_model
-
-logger = logging.getLogger(__name__)
-ROOT = "https://dl.fbaipublicfiles.com/adiyoss/denoiser/"
-DNS_48_URL = ROOT + "dns48-11decc9d8e3f0998.th"
-DNS_64_URL = ROOT + "dns64-a7761ff99a7d5bb6.th"
-MASTER_64_URL = ROOT + "master64-8a5dfb4bb92753dd.th"
-
-
-def _demucs(pretrained, url, **kwargs):
- model = Demucs(**kwargs)
- if pretrained:
- state_dict = torch.hub.load_state_dict_from_url(url, map_location='cpu')
- model.load_state_dict(state_dict)
- return model
-
-
-def dns48(pretrained=True):
- return _demucs(pretrained, DNS_48_URL, hidden=48)
-
-
-def dns64(pretrained=True):
- return _demucs(pretrained, DNS_64_URL, hidden=64)
-
-
-def master64(pretrained=True):
- return _demucs(pretrained, MASTER_64_URL, hidden=64)
-
-
-def add_model_flags(parser):
- group = parser.add_mutually_exclusive_group(required=False)
- group.add_argument(
- "-m", "--model_path", help="Path to local trained model."
- )
- group.add_argument(
- "--dns48", action="store_true",
- help="Use pre-trained real time H=48 model trained on DNS."
- )
- group.add_argument(
- "--dns64", action="store_true",
- help="Use pre-trained real time H=64 model trained on DNS."
- )
- group.add_argument(
- "--master64", action="store_true",
- help="Use pre-trained real time H=64 model trained on DNS and Valentini."
- )
-
-
-def get_model(args):
- """
- Load local model package or torchhub pre-trained model.
- """
- if args.model_path:
- logger.info("Loading model from %s", args.model_path)
- pkg = torch.load(args.model_path)
- model = deserialize_model(pkg)
- elif args.dns64:
- logger.info("Loading pre-trained real time H=64 model trained on DNS.")
- model = dns64()
- elif args.master64:
- logger.info(
- "Loading pre-trained real time H=64 model trained on DNS and Valentini."
- )
- model = master64()
- else:
- logger.info("Loading pre-trained real time H=48 model trained on DNS.")
- model = dns48()
- logger.debug(model)
- return model
diff --git a/spaces/kony1337/frame-interpolation-fix/app.py b/spaces/kony1337/frame-interpolation-fix/app.py
deleted file mode 100644
index 9a12e7ea8a1a07e91ccedd5a880cafb0da9e4d1e..0000000000000000000000000000000000000000
--- a/spaces/kony1337/frame-interpolation-fix/app.py
+++ /dev/null
@@ -1,97 +0,0 @@
-import os
-
-os.system("git clone https://github.com/google-research/frame-interpolation")
-import sys
-
-sys.path.append("frame-interpolation")
-import numpy as np
-import tensorflow as tf
-import mediapy
-from PIL import Image
-from eval import interpolator, util
-import gradio as gr
-
-from huggingface_hub import snapshot_download
-
-from image_tools.sizes import resize_and_crop
-
-
-def load_model(model_name):
- model = interpolator.Interpolator(snapshot_download(repo_id=model_name), None)
-
- return model
-
-
-model_names = [
- "akhaliq/frame-interpolation-film-style",
- "NimaBoscarino/frame-interpolation_film_l1",
- "NimaBoscarino/frame_interpolation_film_vgg",
-]
-
-models = {model_name: load_model(model_name) for model_name in model_names}
-
-ffmpeg_path = util.get_ffmpeg_path()
-mediapy.set_ffmpeg(ffmpeg_path)
-
-
-def resize(width, img):
- basewidth = width
- img = Image.open(img)
- wpercent = (basewidth / float(img.size[0]))
- hsize = int((float(img.size[1]) * float(wpercent)))
- img = img.resize((basewidth, hsize), Image.ANTIALIAS)
- return img
-
-
-def resize_img(img1, img2):
- img_target_size = Image.open(img1)
- img_to_resize = resize_and_crop(
- img2,
- (img_target_size.size[0], img_target_size.size[1]), # set width and height to match img1
- crop_origin="middle"
- )
- img_to_resize.save('resized_img2.png')
-
-
-def predict(frame1, frame2, times_to_interpolate, model_name):
- model = models[model_name]
-
- frame1 = resize(256, frame1)
- frame2 = resize(256, frame2)
-
- frame1.save("test1.png")
- frame2.save("test2.png")
-
- resize_img("test1.png", "test2.png")
- input_frames = ["test1.png", "resized_img2.png"]
-
- frames = list(
- util.interpolate_recursively_from_files(
- input_frames, times_to_interpolate, model))
-
- mediapy.write_video("out.mp4", frames, fps=30)
- return "out.mp4"
-
-
-title = "frame-interpolation"
-description = "Gradio demo for FILM: Frame Interpolation for Large Scene Motion. To use it, simply upload your images and add the times to interpolate number or click on one of the examples to load them. Read more at the links below."
-article = "
-
-download and read online AutoCADMEP201764bitactivationcodezipfile - Download and Read Online AutoCADMEP201764bitactivationcodezipfile. AutoCADMEP201764bitactivationcodezipfile - Download and Read Online ] We advise customers that they have 30 days after receiving the product to make sure that they are satisfied with it (ie: If they are not, they may return it for full refund). [ Download: Download and Read Online ] AutoCAD2017Map - Download and Read Online ] Download and Read Online AutoCAD2017Map - Download and Read Online ] We advise customers that they have 30 days after receiving the product to make sure that they are satisfied with it (ie: If they are not, they may return it for full refund). [ Download: Download and Read Online ] What's new for AutoCAD 2017? - Download and Read Online ] Download and Read Online AutoCAD2017Map - Download and Read Online ] Download and Read Online AutoCAD2017Map - Download and Read Online ] We advise customers that they have 30 days after receiving the product to make sure that they are satisfied with it (ie: If they are not, they may return it for full refund). [ Download: Download and Read Online ] Autocad 2017 XED Version The latest version of the popular Autocad 2017 software has been upgraded for the first time in years! With XED, you can save time and automate many routine tasks, and enjoy improved reliability and error prevention. Support for concurrent AutoCAD users, enhancements to the interface, and performance improvements are just a few of the new features of XED! This edition comes with a free 30-day trial. [ Download: Download and Read Online ] Autocad2017Map - Download and Read Online ] AutoCADMEP201764bitactivationcodezipfile - Download and Read Online ] We advise customers that they have 30 days after receiving the product to make sure that they are satisfied with it (ie: If they are not, they may return it for full refund). [ Download: Download and Read Online ] What's new for AutoCAD 2017? - Download and Read Online ] Download and Read Online AutoCAD2017Map - Download and Read Online ] Download and Read Online AutoCAD2017Map - Download and Read Online ] We advise customers that they have 30 days after receiving the product to make sure that they are satisfied with it (ie: If they are not, they may return it 4fefd39f24
-
-
-
diff --git a/spaces/lincquiQcaudo/Top-20-Diffusion/DellMih61rMbManual [UPDATED].md b/spaces/lincquiQcaudo/Top-20-Diffusion/DellMih61rMbManual [UPDATED].md
deleted file mode 100644
index 8b3db36c677b40277cb12bca991c4080f7deec8a..0000000000000000000000000000000000000000
--- a/spaces/lincquiQcaudo/Top-20-Diffusion/DellMih61rMbManual [UPDATED].md
+++ /dev/null
@@ -1,6 +0,0 @@
-
-
- Fundamentos De Radiologia Novelline Pdf 27. Fundamentos De Radiologia Novelline Pdf 27. Fundamentos De Radiologia Novelline Pdf 27. Fundamentos De Radiologia Novelline Pdf 27. Fundamentos De Radiologia Novelline Pdf 27. Fundamentos De Radiologia Novelline Pdf 27. Fundamentos De Radiologia Novelline Pdf 27. Fundamentos De Radiologia Novelline Pdf 27. Fundamentos De Radiologia Novelline Pdf 27. Fundamentos De Radiologia Novelline Pdf 27. Fundamentos De Radiologia Novelline Pdf 27. Fundamentos De Radiologia Novelline Pdf 27. Fundamentos De Radiologia Novelline Pdf 27. Fundamentos De Radiologia Novelline Pdf 27. Fundamentos De Radiologia Novelline Pdf 27. Fundamentos De Radiologia Novelline Pdf 27. Fundamentos De Radiologia Novelline Pdf 27. Fundamentos De Radiologia Novelline Pdf 27. Fundamentos De Radiologia Novelline Pdf 27. Fundamentos De Radiologia Novelline Pdf 27. Fundamentos De Radiologia Novelline Pdf 27. Fundamentos De Radiologia Novelline Pdf 27. Fundamentos De Radiologia Novelline Pdf 27. Fundamentos De Radiologia Novelline Pdf 27. Fundamentos De Radiologia Novelline Pdf 27. Fundamentos De Radiologia Novelline Pdf 27. Fundamentos De Radiologia Novelline Pdf 27. Fundamentos De Radiologia Novelline Pdf 27. Fundamentos De Radiologia Novelline Pdf 27. Fundamentos De Radiologia Novelline Pdf 27. Fund 4fefd39f24
-
-
-
diff --git a/spaces/lincquiQcaudo/Top-20-Diffusion/Jopieksstrongholdcrusadertrainerv1001.md b/spaces/lincquiQcaudo/Top-20-Diffusion/Jopieksstrongholdcrusadertrainerv1001.md
deleted file mode 100644
index ae37e3f7e76be6c88c0d7e8a2bc1b7af2cdb7ff0..0000000000000000000000000000000000000000
--- a/spaces/lincquiQcaudo/Top-20-Diffusion/Jopieksstrongholdcrusadertrainerv1001.md
+++ /dev/null
@@ -1,6 +0,0 @@
-