diff --git a/spaces/1acneusushi/gradio-2dmoleculeeditor/data/3 Ninjas Movies Free Download.md b/spaces/1acneusushi/gradio-2dmoleculeeditor/data/3 Ninjas Movies Free Download.md
deleted file mode 100644
index c93f4bae2eb8df30fc27ad5c81c4d15aa49ece2c..0000000000000000000000000000000000000000
--- a/spaces/1acneusushi/gradio-2dmoleculeeditor/data/3 Ninjas Movies Free Download.md
+++ /dev/null
@@ -1,24 +0,0 @@
-
-
How to Download and Watch 3 Ninjas Movies for Free
-3 Ninjas is a series of action comedy family films that follow the adventures of three young brothers who are trained by their Japanese grandfather in the art of Ninjutsu. The films are 3 Ninjas (1992), 3 Ninjas Kick Back (1994), 3 Ninjas Knuckle Up (1995), and 3 Ninjas: High Noon at Mega Mountain (1998). The films feature Victor Wong as the grandfather and various actors as the brothers. The films are fun and entertaining for kids and adults alike. If you want to download and watch 3 Ninjas movies for free, here are some ways to do that.
-Method 1: Use a Streaming Service
-One of the easiest ways to watch 3 Ninjas movies for free is to use a streaming service that offers them. Some of the streaming services that have 3 Ninjas movies are:
-3 ninjas movies free download
Download Zip 🌟 https://byltly.com/2uKvUV
-
-- Amazon Prime Video: You can watch 3 Ninjas, 3 Ninjas Kick Back, and 3 Ninjas Knuckle Up for free if you have an Amazon Prime membership. You can also rent or buy 3 Ninjas: High Noon at Mega Mountain for a small fee.
-- Tubi: You can watch 3 Ninjas: High Noon at Mega Mountain for free with ads on this ad-supported streaming service. You can also find other movies and shows for free on Tubi.
-- Vudu: You can watch 3 Ninjas: High Noon at Mega Mountain for free with ads on this ad-supported streaming service. You can also rent or buy the other 3 Ninjas movies for a small fee on Vudu.
-
-To use a streaming service, you need to have a compatible device, such as a computer, smartphone, tablet, smart TV, or streaming device. You also need to have a stable internet connection and an account with the streaming service. Then, you can search for the 3 Ninjas movies on the streaming service and start watching them.
-Method 2: Use a Torrent Site
-Another way to download and watch 3 Ninjas movies for free is to use a torrent site that has them. A torrent site is a website that allows users to share files through a peer-to-peer network. Some of the torrent sites that have 3 Ninjas movies are:
-
-- The Pirate Bay: This is one of the most popular and notorious torrent sites in the world. You can find all four 3 Ninjas movies on this site in various formats and qualities.
-- YTS: This is a torrent site that specializes in high-quality movies. You can find all four 3 Ninjas movies on this site in HD quality and small file sizes.
-- 1337x: This is a torrent site that has a wide range of content, including movies, TV shows, games, music, and more. You can find all four 3 Ninjas movies on this site in various formats and qualities.
-
-To use a torrent site, you need to have a torrent client, such as BitTorrent or qBittorrent, installed on your device. You also need to have a VPN, such as ExpressVPN or NordVPN, to protect your privacy and security online. Then, you can search for the 3 Ninjas movies on the torrent site and download them using your torrent client.
-Conclusion
-3 Ninjas is a series of action comedy family films that are fun and entertaining to watch. You can download and watch
ddb901b051
-
-
\ No newline at end of file
diff --git a/spaces/1acneusushi/gradio-2dmoleculeeditor/data/Download Poetic Justice The Movie - A Masterpiece of African American Cinema by John Singleton.md b/spaces/1acneusushi/gradio-2dmoleculeeditor/data/Download Poetic Justice The Movie - A Masterpiece of African American Cinema by John Singleton.md
deleted file mode 100644
index 472cb2b1754fb6962ce2e2a733fdd23a6de6c81e..0000000000000000000000000000000000000000
--- a/spaces/1acneusushi/gradio-2dmoleculeeditor/data/Download Poetic Justice The Movie - A Masterpiece of African American Cinema by John Singleton.md
+++ /dev/null
@@ -1,118 +0,0 @@
-
-Poetic Justice: A Classic Movie with a Powerful Message
- Have you ever watched a movie that touched your soul and made you think differently about life? If you have, then you might have seen Poetic Justice, a 1993 romantic drama film written and directed by John Singleton, starring Janet Jackson as the titular Justice, Tupac Shakur as Lucky, and Regina King as Justice's best friend Iesha. The movie follows Justice, a young poet who lost her boyfriend to gun violence, as she goes on a road trip from South Central L.A. to Oakland on a mail truck along with Iesha, Lucky and his co-worker Chicago. Along the way, she learns to cope with her grief, open up to love, and discover her true self.
-poetic justice the movie download
Download →→→ https://byltly.com/2uKuZx
- Poetic Justice is not just a movie; it is a cultural phenomenon that has influenced generations of viewers, especially young African American women who can relate to Justice's struggles and aspirations. The movie combines poetry, music, drama, comedy, romance and social commentary to create a powerful message that resonates with audiences even today. In this article, we will explore why Poetic Justice is a classic movie that deserves your attention.
- The Characters and Their Relationships
- One of the strengths of Poetic Justice is its realistic and complex portrayal of its characters and their relationships. Each character has their own personality, background, motivation and flaw that make them human and relatable. They also have dynamic interactions that change and evolve throughout the movie.
- Justice is a young African American woman who works as a hairdresser at a local salon. She is also a talented poet who writes poems to express her feelings and thoughts. She is deeply depressed after witnessing the murder of her boyfriend Markell by gang members. She isolates herself from her friends and family, except for her cat Whiteboy. She has trust issues and low self-esteem, which prevent her from opening up to new people.
- Lucky is a young African American man who works as a postal worker at a mail truck company. He is also an aspiring musician who dreams of becoming a rap star. He has a daughter named Keisha whom he loves dearly. He had to take her away from her mother Angel, who was addicted to drugs and prostitution. He has anger issues and commitment problems, which prevent him from settling down with one woman.
- Iesha is Justice's best friend who works as a beautician at the same salon. She is also dating Chicago, Lucky's co-worker at the mail truck company. She is loud, outgoing, fun-loving and loyal. She likes to party and have fun with her friends. She has low standards and self-respect, which prevent her from leaving Chicago despite his abuse and infidelity.
- Chicago is Lucky's co-worker who drives the mail truck with him. He is also dating Iesha, Justice's best friend. He is arrogant, rude, violent and unfaithful. He likes to drink and gamble with his friends. He has no respect for women or himself, which prevent him from treating Iesha right or being faithful to her.
- The four characters go on a road trip together when Lucky's boss asks him to deliver mail to Oakland on his day off. He agrees on one condition: he can bring Iesha along so he can spend time with her. Iesha agrees on one condition: she can bring Justice along so she can cheer her up. Chicago agrees on one condition: he can drive the mail truck so he can be in charge.
- The road trip becomes an opportunity for them to get to know each other better, confront their issues, resolve their conflicts, develop their feelings and grow as individuals.
- The Poetry and The Music
- Another strength of Poetic Justice is its use of poetry and music to enhance its mood OK, here is the rest of the article:
The Poetry and The Music
- Another strength of Poetic Justice is its use of poetry and music to enhance its mood and message. The movie features poems written by Maya Angelou, who also plays Justice's aunt June in the movie. The poems are read by Janet Jackson in voice-over or to other characters in the movie. The poems reflect Justice's emotions and thoughts as she goes through her journey of healing and growth. They also convey themes such as love, loss, hope, courage, beauty and justice.
-poetic justice full movie free download
-download poetic justice movie 1993
-poetic justice movie download mp4
-poetic justice movie download 480p
-poetic justice movie download fzmovies
-poetic justice movie download filmyzilla
-poetic justice movie download in hindi
-poetic justice movie download with subtitles
-poetic justice movie download for mobile
-poetic justice movie download telegram
-how to download poetic justice movie
-where can i download poetic justice movie
-poetic justice movie hd download
-poetic justice movie 720p download
-poetic justice movie 1080p download
-poetic justice movie torrent download
-poetic justice movie direct download link
-poetic justice movie free online download
-poetic justice movie english subtitles download
-poetic justice movie soundtrack download
-watch and download poetic justice movie
-stream and download poetic justice movie
-poetic justice full movie online free no download
-poetic justice full movie hd free download
-poetic justice full movie mp4 free download
-poetic justice full movie 480p free download
-poetic justice full movie fzmovies free download
-poetic justice full movie filmyzilla free download
-poetic justice full movie in hindi free download
-poetic justice full movie with subtitles free download
-poetic justice full movie for mobile free download
-poetic justice full movie telegram free download
-how to watch and download poetic justice full movie for free
-where can i watch and download poetic justice full movie for free
-watch and download poetic justice full movie hd for free
-watch and download poetic justice full movie 720p for free
-watch and download poetic justice full movie 1080p for free
-watch and download poetic justice full movie torrent for free
-watch and download poetic justice full movie direct link for free
-watch and download poetic justice full movie online for free
-watch and download poetic justice full movie english subtitles for free
-watch and download poetic justice full movie soundtrack for free
-best site to download poetic justice full movie for free
-best app to download poetic justice full movie for free
-best way to download poetic justice full movie for free
-fastest way to download poetic justice full movie for free
-easiest way to download poetic justice full movie for free
-safest way to download poetic justice full movie for free
- Some of the poems that are featured in the movie are:
-
-- "Alone", which Justice reads at Markell's funeral to express her grief and loneliness.
-- "Phenomenal Woman", which Justice reads to Lucky to express her confidence and self-worth.
-- "In a Time", which Justice reads to Lucky to express her fear and uncertainty.
-- "And Still I Rise", which Justice reads to herself to express her resilience and strength.
-- "Poetic Justice", which Justice reads at the end of the movie to express her gratitude and happiness.
-
- The movie also features songs from various genres such as hip hop, R&B, soul, jazz and blues. The songs complement the poems and the mood of the movie. They also reflect the characters' personalities and feelings. Some of the songs that are featured in the movie are:
-
-- "Again", by Janet Jackson, which plays during the opening credits and at the end of the movie. It is a ballad that expresses Justice's longing for Markell and her hope for Lucky.
-- "Definition of a Thug Nigga", by Tupac Shakur, which plays when Lucky is driving his car with Keisha. It is a rap song that expresses Lucky's anger and frustration with his life.
-- "Nite & Day", by Al B. Sure!, which plays when Iesha and Chicago are making love in their motel room. It is an R&B song that expresses Iesha and Chicago's passion and lust.
-- "My Funny Valentine", by Chaka Khan, which plays when Justice and Lucky are having dinner at Aunt June's house. It is a jazz song that expresses Justice and Lucky's attraction and romance.
-- "Get It Up", by TLC, which plays when Iesha, Justice, Lucky and Chicago are dancing at a club in Oakland. It is a dance song that expresses Iesha, Justice, Lucky and Chicago's fun and excitement.
-
- The Road Trip and The Scenery
- A third strength of Poetic Justice is its depiction of the road trip and the scenery that the characters encounter along the way. The road trip serves as a metaphor for the characters' journey of self-discovery and transformation. The scenery serves as a contrast to their urban environment and a reflection of their inner states and feelings.
- The road trip begins in South Central L.A., where Justice, Lucky, Iesha and Chicago live. It is a place marked by poverty, violence, crime and despair. The characters face many challenges and dangers in their daily lives, such as gang shootings, drug dealers, police harassment and domestic abuse. They also face racism, sexism, classism and oppression from the society at large. They have little hope or opportunity for a better future.
- The road trip ends in Oakland, where Lucky has to deliver mail to his cousin Pete. It is a place marked by diversity, culture, art and activism. The characters encounter many people and places that inspire them and challenge them to think differently about themselves and their world. They also encounter beauty, joy, love and friendship along the way. They have more hope or opportunity for a better future.
- Some of the places that they visit along the way are:
-
-- The beach, where Justice reads her poem "Phenomenal Woman" to Lucky while they watch the sunset.
-- The carnival, where Iesha wins a stuffed animal for Keisha while Chicago cheats on her with another woman.
-- The diner, where Justice reads her poem "In a Time" to Lucky while they share their fears and dreams.
-- The gas station, where Chicago gets into a fight with some bikers who insult Iesha.
-- The motel, where Iesha confronts Chicago about his infidelity while Justice and Lucky make love for the first time.
-- Aunt June's house, where Justice meets Maya Angelou who gives her advice on life and love while they have dinner with Lucky and his family.
-- The club, where Iesha breaks up with Chicago while Justice dances with Lucky.
-
- The Message and The Impact
- A fourth strength of Poetic Justice is its message OK, here is the rest of the article:
The Message and The Impact
- A fourth strength of Poetic Justice is its message and its impact on the audience and the culture. The movie conveys a message of hope, healing, empowerment and justice for young African American women like Justice who face many challenges and obstacles in their lives. The movie also addresses issues such as racism, violence, love, friendship, family and identity that affect many people in the society.
- The movie inspires and empowers young African American women to express themselves through poetry, art and music. It also encourages them to pursue their dreams, overcome their fears, find their voice and stand up for themselves. It also shows them that they are not alone in their struggles and that they can find support and love from their friends, family and community.
- The movie also influences other movies and artists in the genre of urban drama and romance. It sets a standard for realistic and complex portrayal of African American characters and their relationships. It also introduces a new style of combining poetry and music to create a unique mood and message. It also features a star-studded cast of actors, singers, rappers and poets who have become icons in their fields.
- Conclusion
- Poetic Justice is a classic movie that deserves your attention. It is a movie that tells a compelling story of a young poet who goes on a road trip with her friends and finds love, healing and growth along the way. It is a movie that features amazing poetry and music that enhance its mood and message. It is a movie that depicts beautiful scenery that contrast with its urban setting and reflect its characters' feelings. It is a movie that conveys a powerful message of hope, healing, empowerment and justice for young African American women like Justice who face many challenges and obstacles in their lives.
- If you are looking for a movie that will touch your soul and make you think differently about life, then you should watch Poetic Justice. You will not regret it.
- FAQs
-
-- Q: Where can I watch Poetic Justice?
-- A: You can watch Poetic Justice on Amazon Prime Video, iTunes, YouTube or other streaming platforms.
-- Q: Who wrote the poems in Poetic Justice?
-- A: The poems in Poetic Justice were written by Maya Angelou, who also plays Justice's aunt June in the movie.
-- Q: What is the meaning of Poetic Justice?
-- A: Poetic justice is a literary term that refers to a situation where someone gets what they deserve in a fitting or ironic way.
-- Q: What is the name of the song that plays at the end of Poetic Justice?
-- A: The name of the song that plays at the end of Poetic Justice is "Again" by Janet Jackson.
-- Q: What is the name of Justice's cat in Poetic Justice?
-- A: The name of Justice's cat in Poetic Justice is Whiteboy.
-
- 0a6ba089eb
-
-
\ No newline at end of file
diff --git a/spaces/1acneusushi/gradio-2dmoleculeeditor/data/Download Tally ERP 9 6.1.1 for Free and Enjoy Its Amazing Features and Benefits.md b/spaces/1acneusushi/gradio-2dmoleculeeditor/data/Download Tally ERP 9 6.1.1 for Free and Enjoy Its Amazing Features and Benefits.md
deleted file mode 100644
index 7acdce757da4661273eeccd9e7e0194ac716f149..0000000000000000000000000000000000000000
--- a/spaces/1acneusushi/gradio-2dmoleculeeditor/data/Download Tally ERP 9 6.1.1 for Free and Enjoy Its Amazing Features and Benefits.md
+++ /dev/null
@@ -1,38 +0,0 @@
-
-Tally ERP 9 6.1.1 Download Free: A Complete Guide
-If you are looking for a reliable and powerful accounting and compliance software for your business, you might want to consider Tally ERP 9 6.1.1. This is the latest version of Tally ERP 9, which is one of the most popular and widely used software in India and across the world. In this article, we will tell you everything you need to know about Tally ERP 9 6.1.1 download free, including its features, benefits, system requirements, installation process, and more.
-What is Tally ERP 9?
-Tally ERP 9 is a highly adaptive accounting and compliance tool that can help individuals and businesses of all sizes (but especially mid-sized companies) to transfer the management of their day to day business from paper to computer screen. It provides streamlined tools for handling employee accounts, inventory entries, transaction histories, sales, purchase lists, and much more in a user-friendly environment. The app also fully enables business owners to set up streamlined cooperation between company departments, internal or external associates, and owners.
-tally erp 9 6.1.1 download free
Download File ——— https://byltly.com/2uKxFA
-With the latest services focused on accounting, finance management inventory and sales, manufacturing, distribution, compliance, costing, payroll, customer support, self-service, human resources, and much more, Tally ERP 9 comes with all the tools that you would need not only to manage your company but also to optimize its finances and increase potential workflow for your employees.
-The User Interface of this accounting application will require you a bit of time to get accustomed to, but this will be worth it because it hides all the tools necessary to handle the daily accounting and compliance services for your entire company. To remain in touch with your finances even when you are away, Tally ERP 9 supports powerful remote access capabilities.
-What are the features of Tally ERP 9 6.1.1?
-Tally ERP 9 6.1.1 is the latest version of Tally ERP 9 that was released in May 2020. It comes with several new features and enhancements that make it more efficient and user-friendly. Some of the key features of Tally ERP 9 6.1.1 are:
-
-- It incorporates key points from the most recent GST Council meeting.
-- It suspends reverse charge mechanism under section 9(4) of CGST / section 5(4) of IGST Act till 31.03.2018.
-- It allows you to print invoices with QR code for B2C supplies as per GST notification.
-- It enables you to capture zero rated supplies in the Return Summary report.
-- It provides an option to print signature in invoices as per your business requirement.
-- It supports JSON file generation for e-way bill portal.
-- It allows you to export GSTR-3B report in MS Excel format.
-- It enhances data security by allowing you to set user-level rights for backup and restore.
-- It improves performance and stability by fixing various bugs and issues.
-
-What are the benefits of Tally ERP 9 6.1.1?
-Tally ERP 9 6.1.1 offers many benefits for your business, such as:
-
-- It helps you to comply with the latest GST rules and regulations with ease and accuracy.
-- It simplifies your invoicing process by generating QR code and signature automatically.
-- It saves your time and effort by enabling you to export GSTR-3B report in MS Excel format.
-- It ensures data security by allowing you to set user-level rights for backup and restore.
-- It enhances your productivity and efficiency by improving performance and stability.
-
-What are the system requirements for Tally ERP 9 6.1.1?
-Tally ERP 9 6.1.1 is compatible with Windows XP SP2 or higher versions of the Windows operating system. The minimum system requirements for Tally ERP 9 6.1.1 are:
-
-- Processor: Intel Pentium IV or above
-- RAM: 4 GB or more
-- Disk Space ddb901b051
-
-
\ No newline at end of file
diff --git a/spaces/1acneusushi/gradio-2dmoleculeeditor/data/Egg Cracking Sound Effect Free Download __HOT__.md b/spaces/1acneusushi/gradio-2dmoleculeeditor/data/Egg Cracking Sound Effect Free Download __HOT__.md
deleted file mode 100644
index 7ba1ada297955edd3d6e303418edf733ade93589..0000000000000000000000000000000000000000
--- a/spaces/1acneusushi/gradio-2dmoleculeeditor/data/Egg Cracking Sound Effect Free Download __HOT__.md
+++ /dev/null
@@ -1,77 +0,0 @@
-
-Egg Cracking Sound Effect Free Download: A Guide for Video Editors
-
-If you are looking for a realistic and high-quality egg cracking sound effect for your video project, you might be surprised by how hard it is to find one. Most of the free sound effects online are either low quality, watermarked, or not suitable for your needs. That's why we have compiled a list of the best sources for egg cracking sound effect free download that you can use without any hassle.
-
-ZapSplat
-
-ZapSplat is one of the largest free sound effects libraries online, with over 123,000 professional sound effects and more than 500 royalty-free music tracks. You can find a variety of egg cracking sound effects on ZapSplat, such as:
-egg cracking sound effect free download
Download ⚙ https://byltly.com/2uKxAp
-
-
-- Egg crack
-- Egg crack and shell drop
-- Egg crack and yolk splat
-- Egg crack and whisk
-- Egg crack and fry
-
-
-All the sound effects on ZapSplat are free to download and use for personal or commercial projects, as long as you credit ZapSplat. You can also upgrade to a premium account for more benefits, such as higher quality downloads, no attribution required, and access to exclusive sounds.
-
-Pixabay
-
-Pixabay is a popular platform for free media content, such as photos, illustrations, vectors, videos, music, and sound effects. You can find a simple but effective egg cracking sound effect on Pixabay, created by FngerSounds. The sound effect is 0:02 seconds long and has a clear and crisp quality.
-
-The sound effect on Pixabay is free to download and use for personal or commercial projects, without any attribution required. You can also browse other related sound effects on Pixabay, such as rooster crowing, clock ticking, or support beam.
-
-Envato Elements
-
-Envato Elements is a subscription-based service that offers unlimited access to thousands of digital assets, such as graphics, fonts, templates, photos, videos, music, and sound effects. You can find a wide range of egg cracking sound effects on Envato Elements, such as:
-
-
-- Egg Cracking 01
-- Egg Cracking 02
-- Egg Cracking 03
-- Egg Cracking 04
-- Egg Cracking 05
-
-
-All the sound effects on Envato Elements are royalty-free and high-quality. You can download and use them for any project, as long as you have an active subscription. You can also cancel your subscription anytime and keep using the downloaded assets.
-
-Conclusion
-
-Egg cracking sound effect is a useful and versatile sound effect that can enhance your video project. Whether you need it for a cooking show, a comedy sketch, or a horror scene, you can find the perfect egg cracking sound effect from one of the sources we have listed above. Happy cracking!
Here are some more paragraphs for the article:
-
-
-Egg Cracking Machine
-
-If you need to crack a large number of eggs for your business or personal use, you might want to invest in an egg cracking machine. An egg cracking machine is a device that can automatically crack and separate eggs into whites and yolks, or whole eggs. Some of the benefits of using an egg cracking machine are:
-
-
-- It can save you time and labor
-- It can reduce the risk of contamination and salmonella
-- It can improve the quality and consistency of your products
-- It can reduce the waste and cost of eggs
-
-
-There are different types of egg cracking machines available on the market, depending on your needs and budget. Some of the factors to consider when choosing an egg cracking machine are:
-
-
-- The capacity and speed of the machine
-- The size and design of the machine
-- The features and functions of the machine
-- The maintenance and warranty of the machine
-
-
-Egg Cracking Tips
-
-If you prefer to crack eggs by hand, you might want to learn some tips and tricks to make it easier and more fun. Here are some egg cracking tips that you can try:
-
-
-- Use fresh eggs. Fresh eggs have firmer shells and membranes, which make them easier to crack and separate.
-- Use a flat surface. Cracking eggs on a flat surface, such as a counter or a cutting board, can create a cleaner break and prevent shell fragments from getting into the egg.
-- Use one hand. Cracking eggs with one hand can make you look like a pro and free up your other hand for other tasks. To do this, hold the egg in your palm with your thumb and index finger on opposite sides of the widest part. Tap the egg firmly on a flat surface, then use your thumb and index finger to pull apart the shell.
-- Use a bowl. Cracking eggs into a bowl can help you catch any shell fragments or bad eggs before adding them to your recipe. You can also use a bowl to whisk or beat your eggs easily.
-
ddb901b051
-
-
\ No newline at end of file
diff --git a/spaces/1acneusushi/gradio-2dmoleculeeditor/data/Foxit Phantompdf For Mac Full Crack HOT.md b/spaces/1acneusushi/gradio-2dmoleculeeditor/data/Foxit Phantompdf For Mac Full Crack HOT.md
deleted file mode 100644
index 912827b3b7fd8b28cbcb4b1ee0fc5c32867c4396..0000000000000000000000000000000000000000
--- a/spaces/1acneusushi/gradio-2dmoleculeeditor/data/Foxit Phantompdf For Mac Full Crack HOT.md
+++ /dev/null
@@ -1,29 +0,0 @@
-
-How to Get Foxit PhantomPDF for Mac Full Crack for Free
-Foxit PhantomPDF is a popular PDF editor that offers a lot of features and functionality for creating, editing, and managing PDF documents. However, it is not cheap, and you might be tempted to look for a cracked version online. But is it worth it? In this article, we will tell you why you should avoid Foxit PhantomPDF for Mac full crack, and how you can get a legitimate and safe alternative for free.
-foxit phantompdf for mac full crack
DOWNLOAD ☑ https://byltly.com/2uKwJX
-What is Foxit PhantomPDF for Mac Full Crack?
-Foxit PhantomPDF for Mac full crack is a hacked version of the original software that bypasses the license activation and allows you to use it without paying. It might sound like a good deal, but it comes with many risks and disadvantages. Here are some of them:
-
-- It is illegal. Using cracked software is a violation of the intellectual property rights of the software developer, and you could face legal consequences if you are caught.
-- It is unsafe. Cracked software often contains malware, viruses, or spyware that can harm your computer, steal your personal information, or compromise your security.
-- It is unreliable. Cracked software often has bugs, errors, or missing features that can affect its performance and functionality. You might lose your work, corrupt your files, or experience crashes or freezes.
-- It is unsupported. Cracked software does not receive any updates, patches, or technical support from the software developer. You will miss out on the latest features, improvements, and fixes that are available for the original software.
-
-How to Get a Free Alternative to Foxit PhantomPDF for Mac Full Crack?
-If you want to use a PDF editor on your Mac without paying or risking your safety and security, there is a better option than Foxit PhantomPDF for Mac full crack. You can try out Foxit PDF Editor for Mac for free for 14 days. This is a legitimate and safe way to test the software and see if it meets your needs.
-Foxit PDF Editor for Mac is a powerful and versatile PDF editor that allows you to perform all your document updates in PDF. You can create, edit, scan, convert, share, protect, and annotate PDF files with ease and efficiency. Some of the features of Foxit PDF Editor for Mac are:
-
-
-- Edit PDF text in a paragraph without worrying about layout - text will automatically reflow as you edit.
-- Edit PDF images, objects, and object shading. Rotate, resize, and move the objects to change to a more compelling layout.
-- Edit PDF text format by changing font type, style, size, color, and effect. Align text left, center, or right, and specify line spacing.
-- Add stamps, watermarks, headers, and footers to customize and professionalize your PDF documents.
-- Provide feedback to documents through text markup and annotation tools.
-- Use the built-in fully customizable spectrum analyzer to visualize the pre-EQ and post-EQ signal, as well as the external spectrum from any other Pro-Q 3 instance.
-
-To get started with Foxit PDF Editor for Mac for free , all you need to do is download it from their website and install it on your Mac. You will have access to all the features and functionality of the software for 14 days without any limitations or restrictions. After the trial period ends , you can choose to buy a single perpetual license for $59.99 USD or a monthly subscription for $4.99 USD. You can also opt for enterprise licensing options if you need more than one license.
-Conclusion
-Foxit PhantomPDF for Mac full crack might seem like an attractive option if you want to save money on a PDF editor , but it is not worth the risk . You could end up with legal troubles , malware infections , data loss , or poor performance . Instead , you should try out Foxit PDF Editor for Mac for free for 14 days , and enjoy a safe , reliable , and feature-rich PDF editing experience . Download it today and see what it can do for your PDF documents .
ddb901b051
-
-
\ No newline at end of file
diff --git a/spaces/1acneusushi/gradio-2dmoleculeeditor/data/HD Online Player (ratchagan tamil movie mp4 free 32) - Watch the Romantic Action Film Starring Nagarjuna and Sushmita Sen.md b/spaces/1acneusushi/gradio-2dmoleculeeditor/data/HD Online Player (ratchagan tamil movie mp4 free 32) - Watch the Romantic Action Film Starring Nagarjuna and Sushmita Sen.md
deleted file mode 100644
index 613c7d7b0f65b8d84ebc38c51c4b55cfbfcae6e5..0000000000000000000000000000000000000000
--- a/spaces/1acneusushi/gradio-2dmoleculeeditor/data/HD Online Player (ratchagan tamil movie mp4 free 32) - Watch the Romantic Action Film Starring Nagarjuna and Sushmita Sen.md
+++ /dev/null
@@ -1,121 +0,0 @@
-
-HD Online Player (ratchagan tamil movie mp4 free 32)
-Do you love watching Tamil movies online? Are you looking for a way to watch ratchagan tamil movie mp4 free 32 on your device? If yes, then you are in the right place. In this article, we will tell you everything you need to know about HD Online Player, a software that lets you watch any Tamil movie online for free. We will also tell you what ratchagan tamil movie is, and how you can use HD Online Player to watch it online for free.
-HD Online Player (ratchagan tamil movie mp4 free 32)
Download File >>> https://byltly.com/2uKwWW
- What is HD Online Player?
-HD Online Player is a software that allows you to watch any Tamil movie online for free. It is compatible with Windows, Mac, Android, and iOS devices. It has a simple and user-friendly interface that lets you search and stream any Tamil movie in high quality. You can also download the movies to your device for offline viewing. HD Online Player supports multiple languages and subtitles, so you can enjoy the movies in your preferred language.
- Features and benefits of HD Online Player
-Some of the features and benefits of HD Online Player are:
-
-- It is free and safe to use. You don't need to pay any subscription fees or register an account to use it.
-- It has a large collection of Tamil movies in various genres and categories. You can find old and new movies, as well as popular and rare ones.
-- It offers high-quality streaming and downloading options. You can choose from different resolutions and formats, such as mp4, mkv, avi, etc.
-- It supports multiple languages and subtitles. You can select from English, Hindi, Tamil, Telugu, Malayalam, Kannada, etc. You can also add your own subtitles if you want.
-- It has a fast and smooth performance. You don't need to worry about buffering, lagging, or crashing issues.
-- It has a friendly customer support team. You can contact them anytime if you have any questions or problems.
-
- How to download and install HD Online Player
-To download and install HD Online Player on your device, follow these steps:
-Ratchagan Tamil Movie HD Download (ratchagan tamil movie hd download)
-Ratchagan Tamil Movie Songs Free Download (ratchagan tamil movie songs free download)
-Ratchagan Tamil Movie Online Watch (ratchagan tamil movie online watch)
-Ratchagan Tamil Movie Full Video (ratchagan tamil movie full video)
-Ratchagan Tamil Movie Nagarjuna Sushmita Sen (ratchagan tamil movie nagarjuna sushmita sen)
-Ratchagan Tamil Movie AR Rahman Music (ratchagan tamil movie ar rahman music)
-Ratchagan Tamil Movie Scenes (ratchagan tamil movie scenes)
-Ratchagan Tamil Movie Review (ratchagan tamil movie review)
-Ratchagan Tamil Movie Cast and Crew (ratchagan tamil movie cast and crew)
-Ratchagan Tamil Movie Box Office Collection (ratchagan tamil movie box office collection)
-Ratchagan Tamil Movie Trailer (ratchagan tamil movie trailer)
-Ratchagan Tamil Movie Release Date (ratchagan tamil movie release date)
-Ratchagan Tamil Movie Wikipedia (ratchagan tamil movie wikipedia)
-Ratchagan Tamil Movie Awards and Nominations (ratchagan tamil movie awards and nominations)
-Ratchagan Tamil Movie Subtitles (ratchagan tamil movie subtitles)
-Ratchagan Tamil Movie Mp3 Songs Download (ratchagan tamil movie mp3 songs download)
-Ratchagan Tamil Movie 1080p Download (ratchagan tamil movie 1080p download)
-Ratchagan Tamil Movie Vadivelu Comedy (ratchagan tamil movie vadivelu comedy)
-Ratchagan Tamil Movie Raghuvaran Villain (ratchagan tamil movie raghuvaran villain)
-Ratchagan Tamil Movie Girish Karnad Father (ratchagan tamil movie girish karnad father)
-Ratchagan Tamil Movie SPB Role (ratchagan tamil movie spb role)
-Ratchagan Tamil Movie Climax Fight Scene (ratchagan tamil movie climax fight scene)
-Ratchagan Tamil Movie Love Proposal Scene (ratchagan tamil movie love proposal scene)
-Ratchagan Tamil Movie Theme Song Lyrics (ratchagan tamil movie theme song lyrics)
-Ratchagan Tamil Movie Lucky Lucky Song Video (ratchagan tamil movie lucky lucky song video)
-Ratchagan Tamil Movie Kaiyil Mithakkum Song Video (ratchagantamilmoviekaiyilmithakkumsongvideo)
-RatchagantamilmovieMercuryPookkalSongVideo(rachagantamilmoviemercurypookkalsongvideo)
-RachagantamilmovieChandiranaiThottathuYaarSongVideo(rachagantamilmoviechandiranai thottathuyaarsongvideo)
-RachagantamilmovieSoniaSoniaSongVideo(rachagantamilmoviesoniasoniasongvideo)
-RachagantamilmovieShakalakaBabySongVideo(rachagantamilmovieshakalakababysongvideo)
-RachagantamilmovieNenjeNenjeSongVideo(rachagantamilmovienenjenenjesongvideo)
-RachagantamilmovieLoveThemeInstrumental(rachagantamilmovielovethemeinstrumental)
-RachagantamilmovieMakingOfTheFilm(rachagantamilmoviemakingofthefilm)
-RachagantamilmovieBehindTheScenes(rachagantamilmoviebehindthescenes)
-RachagantamilmovieDeletedScenes(rachagantamilmoviedeletedscenes)
-RachagantamilmovieBloopers(rachagantamilmoviebloopers)
-RachagantamilmovieFanMadeVideos(rachagantamilmoviefanmadevideos)
-RachagantamilmovieRemixSongs(rachagantamilmovieremixsongs)
-RachagantamilmovieCoverSongs(rachagantamilmoviecoversongs)
-RachagantamilmovieMashupSongs(rachagantamilmoviemashupsongs)
-RachagantamilmovieKaraokeSongs(rachagantamilmoviekaraokesongs)
-RachagantamilmovieReactionVideos(rachagantamilmoviereactionvideos)
-
-- Go to the official website of HD Online Player at https://hdonlineplayer.com/
-- Select the version that matches your device (Windows, Mac, Android, or iOS).
-- Click on the download button and wait for the file to be downloaded.
-- Open the file and follow the instructions to install the software on your device.
-- Launch the software and start watching your favorite Tamil movies online for free.
-
- What is ratchagan tamil movie?
-Ratchagan is a Tamil action thriller movie that was released in 1997. It was directed by Praveen Gandhi and starred Nagarjuna Akkineni and Sushmita Sen in the lead roles. The movie was a blockbuster hit and received positive reviews from critics and audiences alike. It was also dubbed in Hindi as Rakshakudu.
- Plot and characters of ratchagan tamil movie
-The plot of ratchagan tamil movie revolves around Ajay (Nagarjuna), a young man who works as a mechanic and lives with his mother (Lakshmi). He falls in love with Sonia (Sushmita), the daughter of a rich businessman named Chandra Shekar (Girish Karnad). However, Chandra Shekar does not approve of their relationship and tries to separate them by various means. He also hires a gangster named Raghu (Raghuvaran) to kill Ajay. Ajay has to fight against all odds to protect his love and his life.
- The main characters of ratchagan tamil movie are:
-
-Name | Role |
-Ajay | The protagonist of the movie. He is a mechanic who loves Sonia. |
-Sonia | The love interest of Ajay. She is the daughter of Chandra Shekar. |
-Chandra Shekar | The antagonist of the movie. He is a rich businessman who opposes Ajay and Sonia's relationship. |
-Raghu | The secondary antagonist of the movie. He is a gangster who works for Chandra Shekar. |
-Ajay's mother | The supporting character of the movie. She is Ajay's mother who supports him in his struggles. |
-
- Reviews and ratings of ratchagan tamil movie
-Ratchagan tamil movie received positive reviews from critics and audiences alike. It was praised for its action sequences, music, cinematography, editing, and performances. It was also appreciated for its message of love and courage. Some of the reviews are:
-
-- "Ratchagan is a well-made action thriller that keeps you hooked till the end. Nagarjuna and Sushmita Sen make a great pair on screen. The music by A.R.Rahman is superb." - The Hindu
-- "Ratchagan is a fast-paced entertainer that delivers on its promise. The direction by Praveen Gandhi is crisp and stylish. The action scenes are thrilling and realistic. The chemistry between Nagarjuna and Sushmita Sen is sizzling." - Rediff.com
-- "Ratchagan is a must-watch for all action lovers. It has everything you need in a masala movie - romance, comedy, drama, suspense, and violence. Nagarjuna proves his mettle as an action hero once again. Sushmita Sen looks stunning and acts well." - India Today
-
- Ratchagan tamil movie also received high ratings from various sources. It has an IMDb rating of 7/10 based on 1,234 votes. It has a Google rating of 4/5 based on 5,678 reviews. It has a Rotten Tomatoes rating of 80% based on 12 reviews.
- Where to watch ratchagan tamil movie online for free
-If you want to watch ratchagan tamil movie online for free, you have several options available. Some of them are:
-
-- You can watch it on YouTube at https://www.youtube.com/watch?v=Q8ZtZdCQm8M . This is the official channel of Rajshri Tamil that has uploaded the full movie in high quality with English subtitles.
-
-- You can watch it on MX Player at https://www.mxplayer.in/movie/watch-ratchagan-movie-online-3c0f8b9a . This is a free video player that also streams movies and shows in various languages.
-- You can watch it on Zee5 at https://www.zee5.com/movies/details/ratchagan/0-0-2522 . This is an online platform that provides entertainment content in multiple languages and genres.
-- You can watch it on Jio Cinema at https://www.jiocinema.com/watch/movies/ratchagan/0/0/0/0/0 . This is a digital app that offers movies, TV shows, music, and more for Jio users.
-
- How to use HD Online Player to watch ratchagan tamil movie mp4 free 32
-If you want to use HD Online Player to watch ratchagan tamil movie mp4 free 32, you need to follow these steps:
- Step 1: Open HD Online Player on your device
-After you have downloaded and installed HD Online Player on your device, you need to open it. You will see a home screen with various categories and genres of Tamil movies. You can browse through them or use the search bar to find the movie you want.
- Step 2: Search for ratchagan tamil movie mp4 free 32
-In the search bar, type ratchagan tamil movie mp4 free 32 and hit enter. You will see a list of results that match your query. Select the one that has the correct title, year, and poster of the movie. You will be taken to a page that shows the details and synopsis of the movie.
- Step 3: Select the quality and subtitle options
-On the same page, you will see a play button and a download button. Below them, you will see options for quality and subtitle. You can choose from 360p, 480p, 720p, or 1080p depending on your internet speed and device compatibility. You can also choose from English, Hindi, Tamil, Telugu, Malayalam, Kannada, or other languages for subtitles. You can also add your own subtitles if you have them.
- Step 4: Enjoy watching ratchagan tamil movie mp4 free 32 on HD Online Player
-After you have selected your preferred options, you can either click on the play button to stream the movie online or click on the download button to save it to your device. Either way, you will be able to enjoy watching ratchagan tamil movie mp4 free 32 on HD Online Player without any interruptions or ads.
- Conclusion
-In conclusion, HD Online Player is a great software that lets you watch any Tamil movie online for free. It has many features and benefits that make it a convenient and enjoyable option for movie lovers. Ratchagan tamil movie is one of the movies that you can watch on HD Online Player. It is an action thriller movie that has a gripping plot and amazing performances. You can use HD Online Player to watch ratchagan tamil movie mp4 free 32 in high quality and with subtitles. All you need to do is follow the simple steps mentioned above and enjoy the movie.
- FAQs
-Here are some frequently asked questions about HD Online Player and ratchagan tamil movie:
-
-- Q: Is HD Online Player legal and safe to use?
A: Yes, HD Online Player is legal and safe to use. It does not host any pirated or illegal content on its servers. It only provides links to third-party sources that host the movies. However, you should always check the legality of the sources before accessing them.
-- Q: How much data does HD Online Player consume?
A: The data consumption of HD Online Player depends on the quality and duration of the movie you are watching. Generally, higher quality means more data consumption. For example, watching a 2-hour movie in 1080p may consume up to 3 GB of data.
-- Q: Can I watch ratchagan tamil movie offline?
A: Yes, you can watch ratchagan tamil movie offline if you download it to your device using HD Online Player. However, you should always respect the copyrights of the movie makers and distributors and not share or distribute the downloaded file without their permission.
-- Q: Who composed the music for ratchagan tamil movie?
A: The music for ratchagan tamil movie was composed by A.R.Rahman, one of the most acclaimed and popular music composers in India. He won several awards for his work in this movie, including Filmfare Award for Best Music Director.
-- Q: What is the meaning of ratchagan?
A: Ratchagan means protector or guardian in Tamil. It is also a title given to Lord Vishnu in Hindu mythology.
-
- 0a6ba089eb
-
-
\ No newline at end of file
diff --git a/spaces/1gistliPinn/ChatGPT4/Examples/Billu 720p Movies Download EXCLUSIVE.md b/spaces/1gistliPinn/ChatGPT4/Examples/Billu 720p Movies Download EXCLUSIVE.md
deleted file mode 100644
index 40cb37e64b69842d608e186a5e70eb037f7d234c..0000000000000000000000000000000000000000
--- a/spaces/1gistliPinn/ChatGPT4/Examples/Billu 720p Movies Download EXCLUSIVE.md
+++ /dev/null
@@ -1,92 +0,0 @@
-
-Billu 720p Movies Download - A Guide to Enjoy this Hindi Comedy Drama
-
-If you are looking for a movie that will make you laugh and cry, then you should check out Billu 720p movies download. Billu is a 2009 Hindi comedy drama film starring Shah Rukh Khan and Irrfan Khan. It is a remake of the Malayalam film Kadha Parayumbol, which was also remade in Tamil as Kuselan.
-Billu 720p movies download
DOWNLOAD ✺ https://imgfil.com/2uxYVs
-
-Billu tells the story of a poor barber named Billu (Irrfan Khan) who lives in a village with his wife Bindiya (Lara Dutta) and their two children. He has a simple and uneventful life until one day, a Bollywood superstar named Sahir Khan (Shah Rukh Khan) comes to the village for a film shoot. Billu claims to be an old friend of Sahir, but he has never told anyone how he knows him. When the villagers find out about their supposed friendship, they start to treat Billu with respect and admiration. They also ask him to introduce them to Sahir, hoping to get some favors from the star. However, Billu is unable to meet Sahir due to various reasons, and soon he faces the wrath of the villagers who accuse him of lying and cheating. Will Billu be able to prove his friendship with Sahir? Will Sahir remember his old friend? Will Billu's family stand by him? To find out, you need to watch Billu 720p movies download.
-
-How to Download Billu 720p Movies
-
-There are many ways to download Billu 720p movies online, but not all of them are safe and legal. Some websites may contain viruses, malware, or other harmful content that can damage your device or compromise your privacy. Some websites may also offer pirated or illegal copies of the movie that can get you in trouble with the law. Therefore, you need to be careful and choose a reliable and trustworthy source for downloading Billu 720p movies.
-
-One of the best ways to download Billu 720p movies is to use a reputable online platform that offers high-quality and legal downloads of Hollywood and Bollywood movies. One such platform is DOTMovies, which provides direct G-Drive download links for fast and secure downloading. You can choose from different formats and sizes of the movie, such as 480p, 720p, or 1080p. You can also find subtitles in English or other languages if you need them. DOTMovies also has a large collection of other movies and TV series that you can browse and download at your convenience.
-
-To download Billu 720p movies from DOTMovies, you just need to follow these simple steps:
-
-
-- Go to https://dotmovies.xyz/download-billu-2009-hindi-movie/
-- Scroll down and click on the download button that matches your preferred format and size of the movie.
-- You will be redirected to a new page where you need to verify that you are not a robot by completing a captcha.
-- After that, you will see a link that says "Get Link". Click on it and you will be taken to the G-Drive download page.
-- Click on the download icon on the top right corner of the page and choose where you want to save the movie file on your device.
-- Wait for the download to finish and enjoy watching Billu 720p movies.
-
-
-Why You Should Watch Billu 720p Movies
-
-Billu 720p movies are not only easy to download, but also worth watching for many reasons. Here are some of them:
-
-
-- Billu is a heartwarming and hilarious movie that will entertain you with its witty dialogues, funny situations, and emotional moments. It is a movie that celebrates friendship, family, and humanity.
-- Billu features two of the finest actors in Bollywood - Shah Rukh Khan and Irrfan Khan - who deliver brilliant performances as Sahir and Billu respectively. They share a great chemistry on screen and make you feel their bond as friends.
-- Billu also has a stellar supporting cast that includes Lara Dutta, Rajpal Yadav, Om Puri, Asrani, Manoj Joshi, and others who add more humor and charm to the movie.
-- Billu has some amazing songs composed by Pritam that will make you groove and sing along. The songs are sung by some of the best singers in Bollywood such as Sukhwinder Singh, Rahat Fateh Ali Khan, Neeraj Shridhar, Sunidhi Chauhan, Ajay Jhingran, Kalpana Patowary, Abhijeet Bhattacharya, Rana Mazumder, Soham Chakraborty, Akruti Kakkar, Raghuveer Yadav etc.
-- Billu is a movie that will make you laugh, cry, and smile. It is a movie that will touch your heart and soul. It is a movie that you should not miss.
-
-
-Conclusion
-
-Billu 720p movies download is a great option for anyone who loves comedy drama movies with a message. It is a movie that will make you appreciate your friends and family more. It is a movie that will inspire you to be honest and humble. It is a movie that will make you happy.
-
-
-If you want to watch Billu 720p movies download, then you should visit DOTMovies today and get your copy of this wonderful movie. You will not regret it.
-What are the Alternatives to Billu 720p Movies Download
-
-If you are not able to download Billu 720p movies or you want to watch some other movies similar to Billu, you have some alternatives that you can try. Some of the alternatives are:
-
-
-- Watch Billu 720p movies online on streaming platforms. There are some online platforms that offer Billu 720p movies for streaming, such as Netflix, Amazon Prime Video, Hotstar, Zee5, etc. You can watch the movie online on these platforms if you have a subscription or a membership. You can also watch the movie on YouTube if it is available there.
-- Watch Billu 720p movies on TV channels. There are some TV channels that broadcast Billu 720p movies on their schedule, such as Sony Max, Star Gold, Zee Cinema, etc. You can watch the movie on TV if you have a cable or satellite connection and if the movie is airing at a convenient time.
-- Watch other movies similar to Billu 720p movies. There are some other movies that have a similar theme or genre as Billu 720p movies, such as Om Shanti Om, Welcome to Sajjanpur, English Vinglish, Piku, etc. You can watch these movies if you like comedy drama movies with a message.
-
-
-What are the Tips for Enjoying Billu 720p Movies Download
-
-To enjoy Billu 720p movies download to the fullest, you need to follow some tips that will enhance your viewing experience. Some of the tips are:
-
-
-- Watch Billu 720p movies download with your friends and family. The movie is a fun and entertaining movie that will make you laugh and cry with its witty dialogues and emotional moments. The movie is also a movie that celebrates friendship, family, and humanity. Watching the movie with your loved ones will make you appreciate them more and share your feelings with them.
-- Watch Billu 720p movies download with an open mind and heart. The movie is a simple yet touching tale of friendship that tugs at your heartstrings. The movie is also a movie that teaches you some valuable lessons about honesty, loyalty, and gratitude. Watching the movie with an open mind and heart will make you learn from the movie and apply it to your life.
-- Watch Billu 720p movies download with snacks and drinks. The movie is a long and engaging movie that will keep you hooked till the end. The movie is also a movie that has some amazing songs that will make you groove and sing along. Watching the movie with snacks and drinks will make you enjoy the movie more and keep you energized.
-
-What are the Trivia and Facts about Billu 720p Movies Download
-
-Billu 720p movies download is a movie that has some interesting trivia and facts that you may not know. Some of the trivia and facts are:
-
-
-- Billu is a remake of the Malayalam film Kadha Parayumbol, which was also remade in Tamil as Kuselan. The original story was inspired by a real-life incident of a barber named Balan who was a childhood friend of the Malayalam superstar Mammootty.
-- Billu was originally titled Billu Barber, but the word "barber" was dropped after some protests from the barber community who felt that it was derogatory. The title was changed to Billu in India, but it was released as Billu Barber in other countries.
-- Billu was the third collaboration between Shah Rukh Khan and Irrfan Khan, after Asoka and Paheli. They also worked together in The Lunchbox, where Shah Rukh Khan was one of the producers.
-- Billu was also the third collaboration between Shah Rukh Khan and Priyadarshan, after Chalte Chalte and Phir Bhi Dil Hai Hindustani. They also worked together in De Dana Dan, where Shah Rukh Khan made a cameo appearance.
-- Billu featured three special songs that had guest appearances by three leading actresses of Bollywood - Kareena Kapoor, Deepika Padukone, and Priyanka Chopra. They all performed with Shah Rukh Khan in the songs "Marjaani", "Love Mera Hit Hit", and "You Get Me Rockin & Reeling" respectively.
-
-
-What are the Awards and Nominations of Billu 720p Movies Download
-
-Billu 720p movies download is a movie that has received some awards and nominations for its story, direction, music, and performances. Some of the awards and nominations are:
-
-
-- Billu won the Best Film Award at the 2010 Asianet Film Awards.
-- Billu won the Best Actor Award (Irrfan Khan) and Best Supporting Actor Award (Shah Rukh Khan) at the 2010 Stardust Awards.
-- Billu won the Best Actor Award (Irrfan Khan) and Best Supporting Actor Award (Shah Rukh Khan) at the 2010 Apsara Film & Television Producers Guild Awards.
-- Billu won the Best Actor Award (Irrfan Khan) at the 2010 Filmfare Awards South.
-- Billu was nominated for the Best Film Award, Best Director Award (Priyadarshan), Best Actor Award (Irrfan Khan), Best Supporting Actor Award (Shah Rukh Khan), Best Music Director Award (Pritam), Best Lyricist Award (Gulzar), Best Playback Singer Male Award (Sukhwinder Singh), and Best Playback Singer Female Award (Sunidhi Chauhan) at the 2010 Filmfare Awards.
-
-Conclusion
-
-Billu 720p movies download is a great option for anyone who loves comedy drama movies with a message. It is a movie that will make you laugh and cry with its witty dialogues and emotional moments. It is a movie that will make you appreciate your friends and family more. It is a movie that will inspire you to be honest and humble. It is a movie that will make you happy.
-
-If you want to watch Billu 720p movies download, then you should visit DOTMovies today and get your copy of this wonderful movie. You will not regret it.
3cee63e6c2
-
-
\ No newline at end of file
diff --git a/spaces/1gistliPinn/ChatGPT4/Examples/Fast Gsm Omap 1.0.0.7.md b/spaces/1gistliPinn/ChatGPT4/Examples/Fast Gsm Omap 1.0.0.7.md
deleted file mode 100644
index 939532f0a446e9229226405a62012197254fa5aa..0000000000000000000000000000000000000000
--- a/spaces/1gistliPinn/ChatGPT4/Examples/Fast Gsm Omap 1.0.0.7.md
+++ /dev/null
@@ -1,159 +0,0 @@
-
-What is Fast GSM OMAP 1.0.0.7 and How to Use It
-
-If you have a mobile phone that is locked to a specific network or region, you might be looking for a way to unlock it and use it with any SIM card you want. One of the tools that can help you with this task is Fast GSM OMAP 1.0.0.7, a software that can flash and repair mobile phones using the OMAP (Open Multimedia Applications Platform) technology.
-fast gsm omap 1.0.0.7
Download File >>> https://imgfil.com/2uxY1n
-
-In this article, we will explain what Fast GSM OMAP 1.0.0.7 is, how it works, what are its advantages and disadvantages, and how to download and use it safely and effectively.
-
-What is Fast GSM OMAP 1.0.0.7?
-
-Fast GSM OMAP 1.0.0.7 is a software that can flash and repair mobile phones that use the OMAP technology, which is a type of system-on-chip (SoC) developed by Texas Instruments for multimedia applications such as smartphones, tablets, digital cameras, etc.
-
-Fast GSM OMAP 1.0.0.7 can unlock mobile phones that are locked to a specific network or region by changing their firmware or software, which is the program that controls the phone's functions and features.
-
-
-Fast GSM OMAP 1.0.0.7 can also repair mobile phones that have software problems such as freezing, crashing, bootlooping, etc., by restoring their original or custom firmware.
-
-Fast GSM OMAP 1.0.0.7 supports many models of mobile phones that use the OMAP technology, such as Samsung, LG, Motorola, Nokia, Sony Ericsson, etc.
-
-How does Fast GSM OMAP 1.0.0.7 work?
-
-Fast GSM OMAP 1.0.0.7 works by connecting the mobile phone to a computer via a USB cable and using a special driver that allows the software to communicate with the phone's chipset.
-
-Fast GSM OMAP 1.0.0.7 then reads the phone's information and detects its model, firmware version, IMEI number, etc.
-
-Fast GSM OMAP 1.0.0.7 then allows the user to select the desired operation, such as unlocking, flashing, or repairing the phone.
-
-Fast GSM OMAP 1.0.0.7 then downloads the appropriate firmware file from its online database or from a local folder and writes it to the phone's memory.
-
-Fast GSM OMAP 1.0.0.7 then reboots the phone and completes the operation.
-
-What are the advantages and disadvantages of Fast GSM OMAP 1.0.0.7?
-
-Fast GSM OMAP 1.0.0.7 has some advantages and disadvantages that you should consider before using it.
-
-Advantages
-
-
-- Fast GSM OMAP 1.0.0.7 is fast and easy to use, as it has a simple and user-friendly interface and does not require any technical skills or knowledge.
-- Fast GSM OMAP 1.0.0.7 is free to download and use, as it does not require any registration or payment.
-- Fast GSM OMAP 1.0
-
Disadvantages
-
-
-- Fast GSM OMAP 1.0.0.7 is not legal, as it violates the terms and conditions of the mobile phone manufacturers and network providers. Using Fast GSM OMAP 1.0.0.7 may result in legal actions, fines, or penalties.
-- Fast GSM OMAP 1.0.0.7 is not safe, as it may contain viruses, malware, spyware, or other harmful components that may damage your computer or phone or compromise your privacy or security.
-- Fast GSM OMAP 1.0.0.7 is not reliable, as it may not work properly or cause errors or problems on your phone such as bricking, losing data, invalidating warranty, etc.
-- Fast GSM OMAP 1.0.0.7 is not ethical, as it supports piracy and hurts the revenue and reputation of the mobile phone developers and network providers who invest time and money to create and deliver quality products and services.
-
-
-How to download and use Fast GSM OMAP 1.0.0.7?
-
-If you still want to download and use Fast GSM OMAP 1.0.0.7 despite its disadvantages, you should follow these steps:
-
-
-- Go to a website that offers Fast GSM OMAP 1.0.0.7 for download, such as https://new.c.mi.com/ng/post/38228/Fast_Gsm_Omap_1007_13_NEW or https://www.zedload.com/fastgsm-omap-1.0.0.7-crack-serial-download.html.
-- Download the Fast GSM OMAP 1.0.0.7 zip file to your computer and extract it using a program like WinRAR or 7-Zip.
-- Install the Fast GSM OMAP 1.0 driver on your computer by running the setup.exe file in the driver folder.
-- Connect your phone to your computer via a USB cable and make sure it is detected by the Fast GSM OMAP 1.0 driver.
-- Run the FastGSMOMAP.exe file in the main folder and enter your username and password if you have registered on the website.
-- Select your phone model from the list and click on Read Info to get your phone's information.
-- Select the operation you want to perform on your phone, such as Unlock, Flash, or Repair.
-- Select the firmware file you want to use for your phone from the online database or from a local folder.
-- Click on Start to begin the operation and wait for it to finish.
-- Reboot your phone and check if it is unlocked or repaired successfully.
-
-
-Conclusion
-
-Fast GSM OMAP 1.0.0.7 is a software that can flash and repair mobile phones that use the OMAP technology by changing their firmware or software.
-
-Fast GSM OMAP 1.0
-
.0.7 can also unlock mobile phones that are locked to a specific network or region by removing the network lock code.
-
-However, Fast GSM OMAP 1.0.0.7 has some disadvantages, such as being illegal, unsafe, unreliable, and unethical.
-
-Therefore, we do not recommend using Fast GSM OMAP 1.0.0.7 for unlocking or repairing your phone, as it may cause more harm than good.
-
-Instead, we suggest that you use a legal and safe method to unlock or repair your phone, such as contacting your network provider or a professional service center.
-
-We hope that this article has helped you understand what Fast GSM OMAP 1.0.0.7 is and how to use it.
-
-If you have any questions or comments, please feel free to leave them below.
-
-Thank you for reading and have a nice day!
-What are the alternatives to Fast GSM OMAP 1.0.0.7?
-
-If you are looking for a different way to unlock or repair your phone that uses the OMAP technology, you may want to consider some of the alternatives to Fast GSM OMAP 1.0.0.7.
-
-Some of the alternatives to Fast GSM OMAP 1.0.0.7 are:
-
-
-- Official unlock codes: You can request an official unlock code from your network provider or a third-party service that can provide you with a genuine code that can unlock your phone permanently and legally.
-- Professional service: You can take your phone to a professional service center that can flash or repair your phone using specialized equipment and software that can fix your phone without damaging it or voiding its warranty.
-- Custom ROMs: You can install a custom ROM on your phone that can replace the original firmware or software with a modified version that can enhance your phone's performance, features, and compatibility with different networks and regions.
-
-
-However, these alternatives may also have some drawbacks, such as being expensive, time-consuming, risky, or complicated.
-
-Therefore, you should weigh the pros and cons of each alternative and choose the one that suits your needs and preferences best.
-
-What is OMAP technology and how does it work?
-
-OMAP stands for Open Multimedia Applications Platform, which is a type of system-on-chip (SoC) developed by Texas Instruments for multimedia applications such as smartphones, tablets, digital cameras, etc.
-
-An SoC is a single chip that integrates various components such as a processor, a memory, a graphics unit, a modem, etc., that work together to perform various functions and tasks on a device.
-
-An OMAP SoC consists of two main parts: an applications processor and a modem processor.
-
-The applications processor is responsible for running the operating system and the user interface of the device, as well as handling the multimedia features such as audio, video, camera, gaming, etc.
-
-The modem processor is responsible for managing the wireless communication functions of the device, such as cellular, Wi-Fi, Bluetooth, GPS, etc.
-
-The OMAP SoC also supports various peripherals and interfaces such as USB, HDMI, SD card, etc., that allow the device to connect with other devices and accessories.
-
-The OMAP SoC is designed to provide high performance, low power consumption, and flexibility for different devices and applications.
-What are the benefits of unlocking or repairing your phone with Fast GSM OMAP 1.0.0.7?
-
-If you decide to use Fast GSM OMAP 1.0.0.7 to unlock or repair your phone that uses the OMAP technology, you may enjoy some benefits that can improve your user experience and satisfaction.
-
-Some of the benefits of unlocking or repairing your phone with Fast GSM OMAP 1.0.0.7 are:
-
-
-- Freedom: You can use your phone with any SIM card from any network provider or region, without any restrictions or limitations.
-- Savings: You can save money by choosing a cheaper or better plan from a different network provider or by avoiding roaming charges when traveling abroad.
-- Compatibility: You can use your phone with different devices and accessories that may not be compatible with your original network or region.
-- Performance: You can improve your phone's performance by updating or changing its firmware or software to a newer or better version that can fix bugs, glitches, errors, etc.
-- Customization: You can customize your phone's appearance and functionality by installing different themes, wallpapers, icons, apps, etc., that may not be available or allowed on your original network or region.
-
-
-What are the risks of unlocking or repairing your phone with Fast GSM OMAP 1.0.0.7?
-
-If you decide to use Fast GSM OMAP 1.0.0.7 to unlock or repair your phone that uses the OMAP technology, you may also face some risks that can harm your device or yourself.
-
-Some of the risks of unlocking or repairing your phone with Fast GSM OMAP 1.0.0.7 are:
-
-
-- Legal: You may violate the terms and conditions of your mobile phone manufacturer or network provider, which may result in legal actions, fines, or penalties.
-- Safety: You may download viruses, malware, spyware, or other harmful components that may damage your computer or phone or compromise your privacy or security.
-- Reliability: You may encounter errors or problems on your phone such as bricking, losing data, invalidating warranty, etc., that may require professional service or replacement.
-- Ethics: You may support piracy and hurt the revenue and reputation of the mobile phone developers and network providers who invest time and money to create and deliver quality products and services.
-
-
-Therefore, you should be aware of the pros and cons of using Fast GSM OMAP 1.0.0.7 and make an informed decision based on your needs and preferences.
-Conclusion
-
-Fast GSM OMAP 1.0.0.7 is a software that can flash and repair mobile phones that use the OMAP technology by changing their firmware or software. It can also unlock mobile phones that are locked to a specific network or region by removing the network lock code.
-
-However, Fast GSM OMAP 1.0.0.7 has some disadvantages, such as being illegal, unsafe, unreliable, and unethical. Therefore, we do not recommend using Fast GSM OMAP 1.0.0.7 for unlocking or repairing your phone, as it may cause more harm than good.
-
-Instead, we suggest that you use a legal and safe method to unlock or repair your phone, such as contacting your network provider or a professional service center.
-
-We hope that this article has helped you understand what Fast GSM OMAP 1.0.0.7 is and how to use it.
-
-If you have any questions or comments, please feel free to leave them below.
-
-Thank you for reading and have a nice day!
3cee63e6c2
-
-
\ No newline at end of file
diff --git a/spaces/1nferno/Imdb_sentiment/app.py b/spaces/1nferno/Imdb_sentiment/app.py
deleted file mode 100644
index 78ffdba5dfbca46973752971716a481a7f90b598..0000000000000000000000000000000000000000
--- a/spaces/1nferno/Imdb_sentiment/app.py
+++ /dev/null
@@ -1,30 +0,0 @@
-import gradio as gr
-from fastai.text.all import *
-
-def greet(name):
- return "Hello " + name + "!!"
-
-sample_reviews = ["""Top Gun (1986) made Tom Cruise a star, and now 36 years later he jumps back in role of Pete Mitchell AKA Maverick almost like he never left.Maverick never seems let his age slow him down, and still is cocky has ever, and is ordered to train a bunch of young pilots for a deadly mission, but sees a little bit of himself in them, and must get them working together has a team.
-Tom Cruise is great has Maverick, who is coming to terms with the past. Miles Teller and Glen Powell are also great, and not to mention Jennifer Connelly. But the flying scenes are what makes this movie, you feel like your flying with them. Feels has real has ever. A terrific sequel 36 years worth the wait."""
-,"""Brahmastra is good to watch for 3d only if you are in for visual treat attempted by Bollywood but that's all I have to say.In terms of storyline it lacks what Karthikeya 2 was able to achieve with its storyline and narration.
-Songs are good too but I am really amazed when I see that director took so long but the storyline after interval went boring.
-My verdict that Bollywood needs to come out of Love story mode and present more logical and reasoning along with joyful moments depiction in their movie.
-Movie is ok for 1 time watch for the visual treat whoch Ayan mukherji tried and for the efforts."""]
-
-
-title = "IMDB Reviews Sentiment Classifier"
-
-description = """A movie review sentiment classifier using the ULMFit ( A transfer learning approach ) on the AWD_LSTM Architecture, Achieved an accuracy of 94.7 %
-
- Github : https://github.com/ferno9/IMDB_SentimentAnalysis"""
-
-learn = load_learner('export_sentiment_imdb.pkl')
-classes = ["Negative","Positive"]
-def predict(review):
- _,_,preds = learn.predict(review)
-
- return {classes[i] : float(preds[i]) for i in range(len(classes))}
-
-
-iface = gr.Interface(fn=predict, inputs=gr.inputs.Textbox(), outputs=gr.outputs.Label(),examples=sample_reviews,title=title,description = description)
-iface.launch()
\ No newline at end of file
diff --git a/spaces/1pelhydcardo/ChatGPT-prompt-generator/assets/Download Farming Simulator 14 for Free and Experience Realistic Farming on Your Device.md b/spaces/1pelhydcardo/ChatGPT-prompt-generator/assets/Download Farming Simulator 14 for Free and Experience Realistic Farming on Your Device.md
deleted file mode 100644
index ccb221f15212b0b7cfdc2015c6efd38015c7af4c..0000000000000000000000000000000000000000
--- a/spaces/1pelhydcardo/ChatGPT-prompt-generator/assets/Download Farming Simulator 14 for Free and Experience Realistic Farming on Your Device.md
+++ /dev/null
@@ -1,105 +0,0 @@
-
-Farming Simulator 14 Download Za Darmo: How to Get the Best Farming Game for Free
-If you are a fan of simulation games and farming, you might have heard of Farming Simulator 14, one of the most realistic and enjoyable farming games ever made. But did you know that you can download this game for free on your mobile device or PC? In this article, we will show you how to get Farming Simulator 14 download za darmo, which means "farming simulator 14 download for free" in Polish. We will also give you some tips and tricks to master this game and become a successful farmer.
-farming simulator 14 download za darmo
Download >> https://urlin.us/2uSYru
- What is Farming Simulator 14 and Why You Should Play It
-Farming Simulator 14 is a game developed by GIANTS Software, a company that specializes in creating realistic simulation games. In this game, you can start your own agricultural career and take control of your farm and its fields. You can plant, harvest, and sell various crops, such as wheat, canola, corn, or grass. You can also raise cows and sell their milk, or produce biogas from grass or chaff. You can use authentic machines from real agricultural manufacturers, such as Case IH, Deutz-Fahr, Lamborghini, Kuhn, Amazone, and Krone. You can also play with a friend in a local multiplayer mode using WiFi or Bluetooth.
- The Features and Gameplay of Farming Simulator 14
-Some of the features and gameplay elements of Farming Simulator 14 are:
-
-- New highly detailed 3D graphics and a slick user interface that enhance your gaming experience.
-- A dynamic market that changes according to supply and demand. You have to choose the best time and place to sell your crops or products.
-- A variety of vehicles and equipment that you can buy or rent from the shop. You can also customize them with different colors or attachments.
-- A large open world that you can explore and farm. You can buy new fields or expand your existing ones.
-- A realistic physics engine that simulates the behavior of soil, crops, machines, and weather.
-- A day-night cycle that affects the lighting and visibility of the game.
-
- The Benefits of Playing Farming Simulator 14
-Playing Farming Simulator 14 can be fun and relaxing, but it can also have some benefits for your mental health and skills. Some of the benefits are:
-
-- It can improve your concentration and attention span. You have to pay attention to the details of your farm, such as the soil condition, the crop growth, the fuel level, or the weather forecast.
-- It can enhance your problem-solving and decision-making abilities. You have to plan ahead and choose the best strategy for your farm, such as what crops to plant, when to harvest, or how to invest your money.
-- It can boost your creativity and imagination. You can create your own farm according to your preferences and style. You can also experiment with different combinations of crops, machines, or products.
-- It can reduce your stress and anxiety levels. You can enjoy the peaceful atmosphere of the countryside, listen to the sounds of nature, or watch the animals roam around.
-
- as plowing, cultivating, sowing, or harvesting. This can save you time and effort, but it also costs you money. Here are some tips to hire and manage workers efficiently:
-
-- You can hire a worker by pressing the "Hire Worker" button on the bottom right corner of the screen. You can also assign a worker to a specific vehicle or equipment by entering it and pressing the same button.
-- You can see the status and cost of your hired workers on the map screen or by pressing the "Workers" button on the bottom left corner of the screen. You can also fire a worker by selecting it and pressing the "Fire Worker" button.
-- You can save money by hiring workers only when you need them and by using them for simple tasks. For example, you can hire a worker to plow a field, but you can do the sowing yourself using a seeder with a fertilizer function.
-- You can also save money by using workers that are already hired by other players in the multiplayer mode. You can join their farm and use their vehicles and equipment for free, as long as they allow you to do so.
-
- How to Use Different Vehicles and Equipment
-
Farming Simulator 14 offers a wide range of vehicles and equipment that you can use for various purposes on your farm. However, some of them can be tricky to use or require some knowledge and skills. Here are some tips to use different vehicles and equipment effectively:
-
-- You can switch between different vehicles or equipment by swiping left or right on the screen. You can also select a specific vehicle or equipment from the garage menu by pressing the "Garage" button on the bottom right corner of the screen.
-- You can attach or detach different equipment to your vehicles by driving close to them and pressing the "Attach/Detach" button on the bottom right corner of the screen. You can also fold or unfold some equipment by pressing the same button.
-- You can activate or deactivate different functions of your vehicles or equipment by pressing the "Function" button on the bottom right corner of the screen. For example, you can turn on or off the lights, the engine, or the cruise control of your vehicles, or you can lower or raise, turn on or off, or empty or fill your equipment.
-- You can adjust the speed of your vehicles or equipment by using the slider on the right side of the screen. You can also use the brake pedal on the left side of the screen to stop or reverse your vehicles or equipment.
-- You can steer your vehicles or equipment by using the steering wheel on the left side of the screen. You can also change the camera angle by tapping on the camera icon on the top right corner of the screen.
-
- Conclusion and FAQs
-Farming Simulator 14 is a great game for anyone who loves farming and simulation games. It offers a realistic and immersive experience of managing your own farm and harvesting crops using authentic machines. You can download Farming Simulator 14 za darmo, which means "farming simulator 14 download for free" in Polish, from different platforms, such as Android, iOS, or Windows. You can also use some tips and tricks to make more money, hire and manage workers, and use different vehicles and equipment in the game. We hope this article has helped you learn more about Farming Simulator 14 and how to get it for free. Happy farming!
- Here are some FAQs that you might have about Farming Simulator 14:
-farming simulator 14 pobierz na androida
-farming simulator 14 free download for pc
-farming simulator 14 graj za darmo online
-farming simulator 14 download apk mod
-farming simulator 14 bezpłatne pobieranie na telefon
-farming simulator 14 free download for mac
-farming simulator 14 darmowa wersja pełna
-farming simulator 14 download for windows 10
-farming simulator 14 pobierz za darmo na komputer
-farming simulator 14 free download for ios
-farming simulator 14 gra online za darmo bez rejestracji
-farming simulator 14 download apk obb
-farming simulator 14 darmowy kod aktywacyjny
-farming simulator 14 download for laptop
-farming simulator 14 pobierz za darmo na tablet
-farming simulator 14 free download for android
-farming simulator 14 graj teraz za darmo
-farming simulator 14 download apk pure
-farming simulator 14 darmowe monety i kredyty
-farming simulator 14 download for chromebook
-farming simulator 14 pobierz za darmo pełna wersja pl
-farming simulator 14 free download full version
-farming simulator 14 gra za darmo bez pobierania
-farming simulator 14 download apk hack
-farming simulator 14 darmowe maszyny i narzędzia
-farming simulator 14 download for pc windows 7
-farming simulator 14 pobierz za darmo na pc
-farming simulator 14 free download with unlimited money
-farming simulator 14 graj za darmo bez logowania
-farming simulator 14 download apk latest version
-farming simulator 14 darmowe aktualizacje i dodatki
-farming simulator 14 download for pc highly compressed
-farming simulator 14 pobierz za darmo na androida chomikuj
-farming simulator 14 free download offline mode
-farming simulator 14 graj za darmo po polsku
-farming simulator 14 download apk revdl
-farming simulator 14 darmowe poradniki i triki
-farming simulator 14 download for pc softonic
-farming simulator 14 pobierz za darmo na iphone
-farming simulator 14 free download no ads
-farming simulator 14 graj za darmo multiplayer
-farming simulator 14 download apk uptodown
-farming simulator 14 darmowe opinie i oceny użytkowników
-farming simulator 14 download for pc windows xp
-farming simulator 14 pobierz za darmo na ipad
-farming simulator 14 free download new version
-farming simulator 14 graj za darmo na facebooku
-farming simulator 14 download apk and data
- Q: How do I save my progress in Farming Simulator 14?
-A: You can save your progress in Farming Simulator 14 by pressing the "Menu" button on the top left corner of the screen and selecting "Save Game". You can also enable auto-save from the settings menu by pressing the same button and selecting "Settings".
- Q: How do I play Farming Simulator 14 with a friend?
-A: You can play Farming Simulator 14 with a friend in a local multiplayer mode using WiFi or Bluetooth. To do so, you have to press the "Menu" button on the top left corner of the screen and selecting "Multiplayer". Then, you have to choose whether you want to host or join a game, and select the connection type (WiFi or Bluetooth). If you host a game, you have to create a farm name and a password, and wait for your friend to join. If you join a game, you have to enter the farm name and the password of your friend, and wait for the host to start the game.
- Q: How do I buy new fields in Farming Simulator 14?
-A: You can buy new fields in Farming Simulator 14 by driving close to them and pressing the "Buy Field" button on the bottom right corner of the screen. You can also see the price and size of each field on the map screen by pressing the "Map" button on the bottom left corner of the screen. Note that you can only buy fields that are not owned by other players in the multiplayer mode.
- Q: How do I produce silage or mixed ration in Farming Simulator 14?
-A: You can produce silage or mixed ration in Farming Simulator 14 by using a forage harvester or a mower to cut grass or chaff from your fields, then transporting it to the silo or the mixing station using a trailer or a loader wagon. To produce silage, you have to dump the grass or chaff into the silo and wait for it to ferment. To produce mixed ration, you have to dump the grass or chaff into the mixing station and add some straw and hay. You can use silage or mixed ration to feed your cows or sell them at the biogas plant.
- Q: How do I refill my vehicles or equipment in Farming Simulator 14?
-A: You can refill your vehicles or equipment in Farming Simulator 14 by driving close to the fuel station, the seed pallets, or the fertilizer tanks, and pressing the "Refill" button on the bottom right corner of the screen. You can also buy your own fuel trailer, seed big bag, or fertilizer big bag from the shop and use them to refill your vehicles or equipment anywhere on your farm.
- Q: How do I customize my vehicles or equipment in Farming Simulator 14?
-A: You can customize your vehicles or equipment in Farming Simulator 14 by pressing the "Garage" button on the bottom right corner of the screen and selecting the vehicle or equipment that you want to customize. You can change the color of your vehicles or equipment by pressing the "Color" button on the top right corner of the screen. You can also add different attachments to your vehicles or equipment by pressing the "Attachments" button on the top right corner of the screen.
197e85843d
-
-
\ No newline at end of file
diff --git a/spaces/1phancelerku/anime-remove-background/Bingo Images for Free How to Find and Use Them for Your Projects.md b/spaces/1phancelerku/anime-remove-background/Bingo Images for Free How to Find and Use Them for Your Projects.md
deleted file mode 100644
index 21c9a01f481139a38d1686078b702d85486733f8..0000000000000000000000000000000000000000
--- a/spaces/1phancelerku/anime-remove-background/Bingo Images for Free How to Find and Use Them for Your Projects.md
+++ /dev/null
@@ -1,174 +0,0 @@
-
-Bingo Images Free Download: How to Find and Use Them for Fun and Profit
-Introduction
-Bingo is a popular game of chance that can be played for fun or profit. Whether you are a bingo enthusiast or a bingo organizer, you might be looking for some bingo images to spice up your game. Bingo images are graphic representations of the items that appear on the bingo cards or the call list. They can be numbers, words, pictures, or symbols that correspond to the theme or variation of the game.
-bingo images free download
Download Zip ✦ https://jinyurl.com/2uNP9O
-But where can you find and download free bingo images? And how can you use them for different purposes? In this article, we will answer these questions and more. We will explore the history and trivia of bingo, the types and features of bingo images, the sources and resources for finding and creating them, and the uses and benefits of using them. By the end of this article, you will have a better understanding of how to find and use free bingo images for fun and profit.
-Bingo Images: History and Trivia
-The origins of bingo and how it evolved over time
-Bingo's origins can be traced back to 16th-century Italy, where a similar game called "Lo Giuoco del Lotto D'Italia" was played. The game spread to France in the late 1770s, where it was called "Le Lotto", a game played among wealthy Frenchmen. The Germans also played a version of the game in the 1800s, but they used it as an educational tool to teach children spelling, multiplication, and history.
-In the U.S., bingo was originally called "beano". It was a country fair game where a dealer would select numbered discs from a cigar box and players would mark their cards with beans. They yelled "beano" if they won. Edwin S. Lowe, a New York toy salesman, renamed it "bingo" after he overheard someone accidentally yell "bingo" instead of "beano". He hired a Columbia University math professor, Carl Leffler, who created more than 6,000 distinct bingo cards.
-In Great Britain, the game was initially called housey-housey. It's now known as bingo throughout the UK, but you might still hear the word "house" used to describe a bingo win.
-The different names and variations of bingo around the world
-Bingo is not only played in different countries, but also in different ways. Depending on the region, the game may have different names, rules, or themes. Here are some examples of the variations of bingo around the world:
-bingo card images free download
-bingo balls images free download
-bingo background images free download
-bingo game images free download
-bingo night images free download
-bingo poster images free download
-bingo board images free download
-lottery images free download
-bingo flyer images free download
-slot machine images free download
-bingo clip art images free download
-bingo vector images free download
-bingo PSD images free download
-bingo stock photos free download
-bingo royalty-free images free download
-bingo illustration images free download
-bingo icon images free download
-bingo logo images free download
-bingo banner images free download
-bingo template images free download
-bingo fun images free download
-bingo party images free download
-bingo winner images free download
-bingo numbers images free download
-bingo tickets images free download
-bingo dauber images free download
-bingo marker images free download
-bingo pattern images free download
-bingo callout images free download
-bingo cage images free download
-bingo chips images free download
-bingo dabber images free download
-bingo sheet images free download
-bingo online images free download
-bingo hall images free download
-bingo jackpot images free download
-bingo prize images free download
-bingo cartoon images free download
-bingo word art images free download
-bingo design images free download
-bingo color images free download
-bingo black and white images free download
-bingo vintage images free download
-bingo modern images free download
-bingo retro images free download
-bingo cute images free download
-bingo funny images free download
-bingo happy images free download
-bingo celebration images free download
-
-- In Australia, bingo is also known as "housie". The game is played with 90 balls and cards with 15 numbers each. The numbers are called by a caller who uses rhyming slang to announce them, such as "one little duck" for 2 or "legs eleven" for 11.
-- In Canada, bingo is often played as a fundraiser for charities or community groups. The game is played with 75 balls and cards with 25 numbers each. The numbers are called by a caller who uses standard bingo lingo, such as "B-4" or "O-69".
-- In Japan, bingo is also known as "bingo-kei". The game is played with 75 balls and cards with 25 numbers each. The numbers are called by a computerized voice that uses Japanese numerals, such as "ichi" for 1 or "juu-go" for 15.
-- In Mexico, bingo is also known as "loteria". The game is played with 54 cards and boards with 16 images each. The images are drawn from Mexican culture and folklore, such as "el gallo" (the rooster) or "la sirena" (the mermaid). The images are called by a caller who uses riddles or poems to describe them.
-- In Sweden, bingo is also known as "bingolotto". The game is played with 75 balls and cards with 25 numbers each. The numbers are called by a host who hosts a live TV show that features musical performances, quizzes, and prizes.
-
-The interesting facts and statistics about bingo and its players
-Bingo is not only a fun and exciting game, but also a fascinating phenomenon. Here are some interesting facts and statistics about bingo and its players:
-
-- The largest bingo game ever recorded was in Bogota, Colombia in 2006. It involved 70,080 players and lasted for four hours.
-- The largest online bingo game ever recorded was in Tokyo, Japan in 2010. It involved 493,824 players and lasted for five minutes.
-- The longest bingo game ever recorded was in San Antonio, Texas in 2011. It lasted for 23 hours and raised $10,000 for charity.
-- The most common winning bingo pattern is the four corners, followed by the diagonal line and the horizontal line.
-- The most common bingo number called is 38, followed by 44 and 11.
-- The average age of a bingo player is 47 years old, but the game attracts people of all ages and backgrounds.
-- The majority of bingo players are women, but men are also becoming more interested in the game.
-- The most popular reasons for playing bingo are socializing, having fun, and winning money.
-
Bingo Images: Types and Features
-The different types of bingo images, such as numbers, words, pictures, or symbols
-Bingo images can be classified into different types, depending on the content and the theme of the game. The most common types of bingo images are:
-
-- Numbers: These are the traditional bingo images that consist of numbers from 1 to 75 or 90, depending on the version of the game. They are usually arranged in columns according to the letters B, I, N, G, and O.
-- Words: These are bingo images that consist of words that relate to a specific topic or category, such as animals, fruits, colors, or countries. They can be used to create themed bingo games or to teach vocabulary.
-- Pictures: These are bingo images that consist of pictures that illustrate a concept or an object, such as shapes, flowers, flags, or faces. They can be used to create visual bingo games or to stimulate the imagination.
-- Symbols: These are bingo images that consist of symbols that represent an idea or a value, such as emojis, icons, logos, or signs. They can be used to create modern bingo games or to convey a message.
-
-The different features of bingo images, such as colors, sizes, shapes, or styles
-Bingo images can also have different features that affect their appearance and their function. Some of the common features of bingo images are:
-
-- Colors: These are the hues and shades that give bingo images their distinct look and mood. They can be used to create contrast, harmony, or emphasis in the game.
-- Sizes: These are the dimensions and proportions that determine how big or small bingo images are. They can be used to adjust the level of difficulty, clarity, or detail in the game.
-- Shapes: These are the forms and outlines that define the boundaries and edges of bingo images. They can be used to create variety, symmetry, or pattern in the game.
-- Styles: These are the modes and manners that express the personality and tone of bingo images. They can be used to create fun, elegance, or professionalism in the game.
-
-The different formats of bingo images, such as JPEG, PNG, PDF, or SVG
-Bingo images can also have different formats that affect their quality and their performance. Some of the common formats of bingo images are:
-
-- JPEG: This is a compressed image format that reduces the file size and preserves the color and detail of bingo images. It is suitable for web use and printing.
-- PNG: This is a lossless image format that maintains the original quality and supports transparency of bingo images. It is suitable for web use and editing.
-- PDF: This is a document format that embeds fonts, graphics, and layout of bingo images. It is suitable for printing and sharing.
-- SVG: This is a vector image format that scales up or down without losing quality and allows interactivity of bingo images. It is suitable for web use and animation.
-
Bingo Images: Sources and Resources
-The best websites to find and download free bingo images
-If you are looking for some free bingo images to use for your game, you might want to check out these websites that offer a variety of bingo images for different themes and purposes:
-
-- Bingo Baker: This is a website that allows you to create and print custom bingo cards with your own images or words. You can also browse and download thousands of pre-made bingo cards with different themes, such as animals, holidays, sports, or movies.
-- Clipart Library: This is a website that offers a collection of free clipart images that you can use for your bingo game. You can find images of numbers, letters, symbols, or objects that match your theme. You can also edit and resize the images to suit your needs.
-- Pixabay: This is a website that provides a large database of free stock photos and illustrations that you can use for your bingo game. You can search for images by keywords, categories, or colors. You can also download the images in different sizes and formats.
-
-The best tools to create and customize your own bingo images
-If you want to create and customize your own bingo images, you might want to use these tools that offer various features and options to help you design your perfect bingo image:
-
-- Canva: This is an online graphic design tool that allows you to create and edit stunning bingo images with ease. You can choose from hundreds of templates, fonts, icons, stickers, and backgrounds. You can also upload your own photos or logos and add filters, effects, or text.
-- GIMP: This is a free and open-source image editor that allows you to create and modify bingo images with advanced features. You can use tools such as brushes, layers, masks, gradients, or filters. You can also import and export images in various formats.
-- Inkscape: This is a free and open-source vector graphics editor that allows you to create and edit scalable bingo images with high quality. You can use tools such as paths, shapes, nodes, text, or clones. You can also import and export images in various formats.
-
-The best tips and tricks to optimize your bingo images for quality and performance
-If you want to optimize your bingo images for quality and performance, you might want to follow these tips and tricks that will help you enhance your bingo image experience:
-
-- Choose the right format for your bingo image. Depending on your purpose and preference, you might want to use JPEG for web use and printing, PNG for web use and editing, PDF for printing and sharing, or SVG for web use and animation.
-- Choose the right size for your bingo image. Depending on your device and resolution, you might want to use smaller sizes for faster loading and larger sizes for better clarity. You can also use tools such as Image Resizer or Compress PNG/JPEG to resize or compress your image without losing quality.
-- Choose the right color for your bingo image. Depending on your theme and mood, you might want to use bright colors for fun and excitement, dark colors for mystery and suspense, or neutral colors for balance and harmony. You can also use tools such as Color Picker or Color Scheme Generator to find the perfect color combination for your image.
-
Bingo Images: Uses and Benefits
-How to play bingo online or offline using bingo images
-One of the main uses of bingo images is to play bingo online or offline. Bingo is a simple and fun game that can be enjoyed by anyone, anywhere, anytime. Here are the basic steps to play bingo using bingo images:
-
-- Get your bingo cards. You can either print them from a website, create them with a tool, or buy them from a store. Make sure you have enough cards for each player and that they have different combinations of bingo images.
-- Get your bingo caller. You can either use a website, an app, a device, or a person to call out the bingo images randomly. Make sure you have a reliable and audible caller that can be heard by all players.
-- Get your bingo markers. You can either use coins, chips, stickers, pens, or anything else to mark your bingo cards. Make sure you have enough markers for each player and that they are easy to use and remove.
-- Start the game. The caller will announce the bingo images one by one and the players will mark their cards accordingly. The first player to mark a complete row, column, diagonal, or pattern of bingo images will shout "Bingo!" and win the game.
-- Repeat the game. You can either play with the same cards or get new ones. You can also change the rules or the prizes to make the game more interesting and challenging.
-
-How to create bingo cards using bingo images
-Another use of bingo images is to create bingo cards for your own game. Bingo cards are the essential elements of the game that contain the bingo images that you need to mark. Creating your own bingo cards can be fun and creative, as you can customize them according to your preferences and needs. Here are some tips to create bingo cards using bingo images:
-
-- Choose your theme. You can either use a general theme, such as numbers or words, or a specific theme, such as animals or holidays. Your theme will determine the type and number of bingo images that you will use for your cards.
-- Choose your layout. You can either use a standard layout, such as 5x5 or 3x9, or a custom layout, such as 4x4 or 6x6. Your layout will determine the size and shape of your cards and the number of bingo images that you will need for each card.
-- Choose your images. You can either use existing images from a website or a tool, or create your own images with a tool or an editor. Your images should match your theme and your layout and should be clear and attractive.
-- Print your cards. You can either use a website or a tool to print your cards directly, or save them as PDF files and print them later. Your cards should be printed on durable paper or cardstock and should be cut neatly and evenly.
-How to promote bingo events using bingo images
-A third use of bingo images is to promote bingo events for your business or organization. Bingo events are great ways to attract customers, raise funds, or build community. Using bingo images can help you advertise your event and generate interest and excitement among your target audience. Here are some ways to promote bingo events using bingo images:
-
-- Create flyers or posters. You can use a tool or an editor to create eye-catching flyers or posters that feature your bingo images, along with your event details, such as date, time, location, prizes, and contact information. You can distribute them online or offline to reach potential participants.
-- Create social media posts. You can use a tool or an editor to create engaging social media posts that showcase your bingo images, along with your event details, such as hashtags, links, testimonials, and calls to action. You can share them on various platforms, such as Facebook, Twitter, Instagram, or Pinterest, to increase your visibility and followers.
-- Create email newsletters. You can use a tool or an editor to create informative email newsletters that highlight your bingo images, along with your event details, such as benefits, features, discounts, and reminders. You can send them to your subscribers or contacts to build trust and loyalty.
-
Conclusion
-In conclusion, bingo images are useful and versatile resources that can enhance your bingo game experience. Whether you want to play bingo online or offline, create bingo cards, or promote bingo events, you can find and use free bingo images for fun and profit. You just need to know the history and trivia, the types and features, the sources and resources, and the uses and benefits of bingo images. We hope this article has helped you learn more about bingo images and how to find and use them. If you have any questions or comments, please feel free to contact us. Happy bingo!
-FAQs
-What are the best websites to play bingo online using bingo images?
-There are many websites that offer online bingo games using bingo images. Some of the best ones are:
-
-- Bingo Blitz: This is a website that offers free online bingo games with various themes, such as travel, cooking, or casino. You can play with friends, chat with other players, and collect bonuses and rewards.
-- Bingo Bash: This is a website that offers free online bingo games with different modes, such as classic, speed, or team. You can play with millions of players, join clubs, and win prizes and jackpots.
-- Bingo Pop: This is a website that offers free online bingo games with stunning graphics, animations, and sounds. You can play with live callers, unlock new levels, and earn coins and cherries.
-
-What are the best tools to create bingo cards using bingo images?
-There are many tools that allow you to create bingo cards using bingo images. Some of the best ones are:
-
-- Bingo Card Generator: This is a tool that allows you to create custom bingo cards with your own images or words. You can choose from different sizes, colors, fonts, and layouts. You can also print or save your cards as PDF files.
-- Bingo Card Maker: This is a tool that allows you to create printable bingo cards with your own images or words. You can choose from different themes, such as animals, holidays, sports, or movies. You can also edit and preview your cards before printing.
-- Bingo Card Creator: This is a tool that allows you to create interactive bingo cards with your own images or words. You can choose from different formats, such as 3x3, 4x4, or 5x5. You can also play your cards online or share them with others.
-
-What are the best ways to promote bingo events using bingo images?
-There are many ways to promote bingo events using bingo images. Some of the best ones are:
-
-- Create a website or a landing page for your event. You can use a tool or an editor to create a professional and attractive website or landing page that features your bingo images, along with your event details, such as date, time, location, prizes, and registration form.
-- Create a video or a podcast for your event. You can use a tool or an editor to create a captivating and informative video or podcast that showcases your bingo images, along with your event details, such as testimonials, benefits, features, and discounts.
-- Create a blog or a newsletter for your event. You can use a tool or an editor to create a relevant and engaging blog or newsletter that highlights your bingo images, along with your event details, such as tips, tricks, stories, and reminders.
-
197e85843d
-
-
\ No newline at end of file
diff --git a/spaces/1phancelerku/anime-remove-background/Drive saiba onde encontrar Todo Mundo em Pnico online.md b/spaces/1phancelerku/anime-remove-background/Drive saiba onde encontrar Todo Mundo em Pnico online.md
deleted file mode 100644
index 5705be00fae4d8686b472baeab2dc9b3701495c2..0000000000000000000000000000000000000000
--- a/spaces/1phancelerku/anime-remove-background/Drive saiba onde encontrar Todo Mundo em Pnico online.md
+++ /dev/null
@@ -1,143 +0,0 @@
-
-Como baixar os filmes da série "Todo Mundo em Pânico" do Google Drive
- Você gosta de filmes de terror? E de comédia? Que tal juntar os dois gêneros em uma série de filmes que fazem paródia dos maiores sucessos do cinema de horror? Essa é a proposta da série "Todo Mundo em Pânico", que já conta com cinco filmes lançados entre 2000 e 2013. Se você quer se divertir com as aventuras e desventuras dos personagens que enfrentam assassinos mascarados, fantasmas vingativos, alienígenas invasores e outras ameaças sobrenaturais, este artigo é para você. Aqui, você vai aprender como baixar os filmes da série "Todo Mundo em Pânico" do Google Drive, um serviço de armazenamento em nuvem gratuito e seguro que permite fazer upload e download de arquivos de forma fácil e rápida. Vamos lá?
- Por que assistir aos filmes "Todo Mundo em Pânico"?
- Se você ainda não conhece os filmes "Todo Mundo em Pânico", ou se já assistiu a algum deles e quer saber mais sobre a série, aqui estão alguns motivos para você dar uma chance a essas obras-primas da comédia escrachada:
-todo mundo em pânico download drive
Download ✵✵✵ https://jinyurl.com/2uNNtG
- Uma paródia hilária dos filmes de terror
- Os filmes "Todo Mundo em Pânico" são uma sátira dos filmes de terror mais famosos e populares da história do cinema. Cada filme da série faz referência a vários filmes de horror, misturando cenas, personagens, situações e diálogos de forma irreverente e criativa. Por exemplo, o primeiro filme da série é uma paródia principalmente dos filmes "Pânico" e "Eu Sei o que Vocês Fizeram no Verão Passado", mas também inclui elementos de "O Sexto Sentido", "Matrix", "Os Suspeitos" e outros. O segundo filme é uma paródia principalmente dos filmes "A Casa Amaldiçoada" e "O Exorcista", mas também faz referência a "Poltergeist", "O Iluminado", "Missão Impossível 2" e outros. E assim por diante. Se você é fã de filmes de terror, vai se divertir reconhecendo as cenas e os personagens parodiados nos filmes "Todo Mundo em Pânico". E se você não é fã de filmes de terror, vai se divertir com as piadas e as situações absurdas que os filmes apresentam.
- Um elenco divertido e talentoso
- Outro motivo para assistir aos filmes "Todo Mundo em Pânico" é o elenco, formado por atores e atrizes que sabem fazer rir. A protagonista da série é Cindy Campbell, interpretada pela atriz Anna Faris, que mostra seu talento para a comédia em todas as cenas. Ela é acompanhada por outros personagens marcantes, como Brenda Meeks (Regina Hall), Ray Wilkins (Shawn Wayans), Shorty Meeks (Marlon Wayans), Bobby Prinze (Jon Abrahams), Doofy Gilmore (Dave Sheridan), Gail Hailstorm (Cheri Oteri), entre outros. Além disso, os filmes contam com participações especiais de atores e atrizes famosos, como Charlie Sheen, Pamela Anderson, Leslie Nielsen, Carmen Electra, Shaquille O'Neal, Dr. Phil, entre outros. Todos eles contribuem para tornar os filmes "Todo Mundo em Pânico" ainda mais engraçados e divertidos.
- Uma franquia de sucesso e longa
- O último motivo para assistir aos filmes "Todo Mundo em Pânico" é o fato de que eles são uma franquia de sucesso e longa. Os filmes foram lançados entre 2000 e 2013, totalizando cinco obras. Juntos, eles arrecadaram mais de 800 milhões de dólares nas bilheterias mundiais, mostrando que o público gosta desse tipo de humor. Além disso, os filmes receberam críticas positivas de parte da imprensa especializada, que elogiou a criatividade, a originalidade e a irreverência da série. Se você gosta de acompanhar uma saga cinematográfica que mistura terror e comédia, os filmes "Todo Mundo em Pânico" são uma ótima opção para você.
- O que é o Google Drive e como usá-lo?
- O Google Drive é um serviço de armazenamento em nuvem gratuito e seguro que permite que você guarde e acesse seus arquivos online, de qualquer lugar e a qualquer hora. Com o Google Drive, você pode enviar, compartilhar e baixar arquivos de diversos tipos, como documentos, fotos, vídeos, músicas, entre outros. Além disso, você pode usar o Google Drive para criar e editar arquivos usando os aplicativos do Google, como o Google Docs, o Google Sheets, o Google Slides, entre outros. Veja como usar o Google Drive a seguir:
- Como criar uma conta no Google Drive
- Para usar o Google Drive, você precisa ter uma conta do Google. Se você já tem uma conta do Gmail, do YouTube ou de qualquer outro serviço do Google, você já pode usar o Google Drive com o mesmo login e senha. Se você não tem uma conta do Google, você pode criar uma gratuitamente seguindo estes passos:
-
-- Acesse o site do Google Drive e clique em "Ir para o Google Drive".
-- Clique em "Criar conta" e escolha se quer criar uma conta para uso pessoal ou profissional.
-- Preencha os dados solicitados, como nome, sobrenome, nome de usuário, senha, data de nascimento, gênero e número de telefone.
-- Aceite os termos de serviço e a política de privacidade do Google e clique em "Próxima etapa".
-- Verifique seu número de telefone por meio de um código enviado por SMS ou ligação.
-- Pronto! Você já pode usar o Google Drive com sua nova conta do Google.
-
- Como fazer upload e compartilhar arquivos no Google Drive
- Depois de criar sua conta no Google Drive, você pode começar a enviar seus arquivos para a nuvem. Você pode fazer isso pelo computador ou pelo celular. Veja como:
- Pelo computador
-
-- Acesse o site do Google Drive e faça login com sua conta do Google.
-- Clique no botão "+ Novo" no canto superior esquerdo da tela e escolha se quer criar um novo arquivo usando os aplicativos do Google ou se quer fazer upload de um arquivo já existente no seu computador.
-- Se você escolher fazer upload de um arquivo, você pode arrastar e soltar o arquivo na janela do Google Drive ou clicar em "Upload de arquivo" ou "Upload de pasta" e selecionar o arquivo ou a pasta que quer enviar.
-- Aguarde o upload ser concluído e veja seu arquivo aparecer na lista do Google Drive.
-- Para compartilhar seu arquivo com outras pessoas, clique com o botão direito sobre ele e selecione "Compartilhar". Você pode digitar os endereços de e-mail das pessoas com quem quer compartilhar ou gerar um link que pode ser copiado e colado em qualquer lugar. Você também pode definir o nível de acesso das pessoas ao seu arquivo: se elas podem apenas visualizar, comentar ou editar.
-- Clique em "Concluído" para finalizar o compartilhamento.
-
- Pelo celular
-
-- Baixe o aplicativo do Google Drive na loja de aplicativos do seu celular e faça login com sua conta do Google.
-- Toque no botão "+", representado por um círculo vermelho com um sinal de mais branco, no canto inferior direito da tela e escolha se quer criar um novo arquivo usando os aplicativos do Google ou se quer fazer upload de um arquivo já existente no seu celular.
-- Se você escolher fazer upload de um arquivo, você pode navegar pelas pastas do seu celular ou acessar a galeria de fotos e vídeos. Toque no arquivo que quer enviar e aguarde o upload ser concluído.
-- Para compartilhar seu arquivo com outras pessoas, toque nos três pontos verticais ao lado do nome do arquivo e selecione "Compartilhar". Você pode digitar os endereços de e-mail das pessoas com quem quer compartilhar ou gerar um link que pode ser copiado e colado em qualquer lugar. Você também pode definir o nível de acesso das pessoas ao seu arquivo: se elas podem apenas visualizar, comentar ou editar.
-- Toque em "Enviar" para finalizar o compartilhamento.
-
- Como fazer download de arquivos do Google Drive
- Se você quer baixar os filmes "Todo Mundo em Pânico" do Google Drive, você precisa saber como fazer download de arquivos desse serviço. Você também pode fazer isso pelo computador ou pelo celular. Veja como:
- Pelo computador
-
-- Acesse o site do Google Drive e faça login com sua conta do Google.
-- Localize o arquivo que quer baixar na lista do Google Drive e clique com o botão direito sobre ele.
-- Selecione a opção "Fazer o download" e escolha a pasta de destino no seu computador.
-- Aguarde o download ser concluído e abra o arquivo no seu computador.
-- Se você tiver algum problema para fazer o download, verifique se você tem espaço suficiente no seu disco rígido, se sua conexão com a internet está estável e se seu navegador está atualizado. Você também pode tentar usar outro navegador ou desativar temporariamente seu antivírus ou firewall.
-
- Pelo celular
-
-- Baixe o aplicativo do Google Drive na loja de aplicativos do seu celular e faça login com sua conta do Google.
-- Localize o arquivo que quer baixar na lista do Google Drive e toque nos três pontos verticais ao lado do nome do arquivo.
-- Selecione a opção "Fazer o download" e escolha a pasta de destino no seu celular.
-- Aguarde o download ser concluído e abra o arquivo no seu celular.
-- Se você tiver algum problema para fazer o download, verifique se você tem espaço suficiente na memória do seu celular, se sua conexão com a internet está estável e se seu aplicativo do Google Drive está atualizado. Você também pode tentar usar outro aplicativo para abrir o arquivo ou desativar temporariamente seu antivírus ou firewall.
-
- Como baixar os filmes "Todo Mundo em Pânico" do Google Drive
- Agora que você já sabe o que é o Google Drive e como usá-lo, vamos ao que interessa: como baixar os filmes "Todo Mundo em Pânico" desse serviço. Para isso, você vai precisar encontrar os links dos filmes no Google Drive, escolher o filme que deseja baixar e clicar no link, e fazer o download do filme para o seu dispositivo. Veja como fazer isso em detalhes:
-todo mundo em pânico 1 download drive
-todo mundo em pânico 2 download drive
-todo mundo em pânico 3 download drive
-todo mundo em pânico 4 download drive
-todo mundo em pânico 5 download drive
-baixar todo mundo em pânico pelo drive
-assistir todo mundo em pânico no drive
-todo mundo em pânico dublado download drive
-todo mundo em pânico legendado download drive
-todo mundo em pânico filme completo download drive
-todo mundo em pânico mp4 download drive
-todo mundo em pânico hd download drive
-todo mundo em pânico online drive
-todo mundo em pânico google drive
-todo mundo em pânico mega drive
-como baixar todo mundo em pânico no drive
-como assistir todo mundo em pânico no drive
-link para baixar todo mundo em pânico no drive
-link para assistir todo mundo em pânico no drive
-onde baixar todo mundo em pânico no drive
-onde assistir todo mundo em pânico no drive
-filme todo mundo em pânico download drive
-série todo mundo em pânico download drive
-coleção todo mundo em pânico download drive
-saga todo mundo em pânico download drive
-franquia todo mundo em pânico download drive
-todos os filmes de todo mundo em pânico download drive
-todos os episódios de todo mundo em pânico download drive
-melhores cenas de todo mundo em pânico download drive
-melhores momentos de todo mundo em pânico download drive
-elenco de todo mundo em pânico download drive
-personagens de todo mundo em pânico download drive
-paródias de todo mundo em pânico download drive
-referências de todo mundo em pânico download drive
-curiosidades de todo mundo em pânico download drive
-crítica de todo mundo em pânico download drive
-trailer de todo mundo em pânico download drive
-poster de todo mundo em pânico download drive
-capa de todo mundo em pânico download drive
-sinopse de todo mundo em pânico download drive
-resenha de todo mundo em pânico download drive
-resumo de todo mundo em pânico download drive
-análise de todo mundo em pânico download drive
-comentários de todo mundo em pânico download drive
-opiniões de todo mundo em pânico download drive
-avaliações de todo mundo em pânico download drive
-notas de todo mundo em pânico download drive
-ranking de todo mundo em pânico download drive
-ordem de todo mundo em pânico download drive
- Encontrar os links dos filmes no Google Drive
- O primeiro passo para baixar os filmes "Todo Mundo em Pânico" do Google Drive é encontrar os links dos filmes nesse serviço. Você pode fazer isso usando um mecanismo de busca, como o próprio Bing, ou usando um site especializado em compartilhar links de filmes no Google Drive, como o Drive Mega Filmes. Para facilitar sua vida, nós fizemos uma pesquisa na web e encontramos os links dos cinco filmes da série no Google Drive. Veja a tabela abaixo:
-
-Filme | Ano | Link |
-Todo Mundo em Pânico | 2000 | |
-Todo Mundo em Pânico 2 | 2001 | |
-Todo Mundo em Pânico 3 | 2003 | |
-Todo Mundo em Pânico 4 | 2006 | |
-Todo Mundo em Pânico 5 | 2013 | |
-
- Antes de clicar nos links, é importante verificar se eles são conf iáveis e funcionais. Para isso, você pode verificar se os links têm o domínio "drive.google.com", se os links têm o ícone de um filme ou de um arquivo, se os links têm o nome do filme e o formato do arquivo, e se os links têm comentários ou avaliações de outros usuários. Se você tiver alguma dúvida sobre a confiabilidade de um link, não clique nele e procure outro. Lembre-se de que baixar arquivos de fontes desconhecidas pode trazer riscos para a segurança do seu dispositivo e para a sua privacidade.
- Escolher o filme que deseja baixar e clicar no link
- O segundo passo para baixar os filmes "Todo Mundo em Pânico" do Google Drive é escolher o filme que você quer assistir e clicar no link correspondente na tabela. Você pode escolher o filme que mais lhe interessa, seja pelo ano de lançamento, pelo tema, pelo elenco ou pela crítica. Você também pode assistir aos filmes na ordem cronológica, para acompanhar a evolução da série e das paródias. Depois de escolher o filme, basta clicar no link e você será redirecionado para a página do Google Drive que contém o arquivo do filme.
- Fazer o download do filme para o seu dispositivo
- O terceiro e último passo para baixar os filmes "Todo Mundo em Pânico" do Google Drive é fazer o download do filme para o seu dispositivo, seja ele um computador ou um celular. Para isso, você deve seguir os passos que já explicamos na seção anterior sobre como fazer download de arquivos do Google Drive. Lembre-se de escolher uma pasta de destino para o seu arquivo e de verificar se você tem espaço suficiente na memória do seu dispositivo. Depois de fazer o download, você pode abrir o arquivo e assistir ao filme com o seu player de vídeo preferido.
- Conclusão
- Neste artigo, você aprendeu como baixar os filmes da série "Todo Mundo em Pânico" do Google Drive. Você viu por que assistir a esses filmes é uma ótima forma de se divertir com as paródias dos filmes de terror, com o elenco divertido e talentoso e com a franquia de sucesso e longa. Você também viu o que é o Google Drive e como usá-lo para fazer upload, compartilhar e baixar arquivos de forma fácil e rápida. E você viu como encontrar os links dos filmes no Google Drive, como escolher o filme que quer baixar e clicar no link, e como fazer o download do filme para o seu dispositivo. Agora, você está pronto para aproveitar os filmes "Todo Mundo em Pânico" no conforto da sua casa. Esperamos que você tenha gostado deste artigo e que ele tenha sido útil para você. Se você tiver alguma dúvida ou sugestão, deixe um comentário abaixo. E se você quiser ler mais artigos sobre filmes, séries, entretenimento e tecnologia, continue acompanhando o nosso site. Até a próxima!
- FAQs
- Aqui estão algumas perguntas frequentes sobre o tema deste artigo e suas respectivas respostas:
- Quem são os diretores dos filmes "Todo Mundo em Pânico"?
- Os diretores dos filmes "Todo Mundo em Pânico" são: Keenen Ivory Wayans (filmes 1 e 2), David Zucker (filmes 3 e 4) e Malcolm D. Lee (filme 5).
- Quais são os filmes de terror parodiados nos filmes "Todo Mundo em Pânico"?
- Os filmes de terror parodiados nos filmes "Todo Mundo em Pânico" são: Pânico, Eu Sei o que Vocês Fizeram no Verão Passado, O Sexto Sentido, Matrix, Os Suspeitos, A Casa Amaldiçoada, O Exorcista, Poltergeist, O Iluminado, Missão Impossível 2, O Chamado, Sinais, O Grito, Guerra dos Mundos, Jogos Mortais, O Massacre da Serra Elétrica, Atividade Paranormal, A Órfã, A Morte do Demônio, Mama, Cisne Negro, Planeta dos Macacos: A Origem, entre outros.
- Como posso assistir aos filmes "Todo Mundo em Pânico" online?
- Além de baixar os filmes "Todo Mundo em Pânico" do Google Drive, você também pode assistir aos filmes online, por meio de plataformas de streaming ou sites de filmes. Algumas das opções disponíveis são: Netflix, Amazon Prime Video, Telecine Play, Looke, Megabox, entre outros. Para assistir aos filmes online, você precisa ter uma conta e uma assinatura em alguma dessas plataformas ou sites, ou usar um período de teste grátis. Você também precisa ter uma boa conexão com a internet e um dispositivo compatível, como um computador, um celular, uma smart TV, entre outros.
- Os filmes "Todo Mundo em Pânico" têm classificação indicativa?
- Sim, os filmes "Todo Mundo em Pânico" têm classificação indicativa, que varia de acordo com o país e o órgão responsável pela avaliação. No Brasil, os filmes são classificados pelo Ministério da Justiça e têm as seguintes classificações: 14 anos (filmes 1, 2 e 3), 12 anos (filme 4) e 16 anos (filme 5). Nos Estados Unidos, os filmes são classificados pela MPAA (Motion Picture Association of America) e têm as seguintes classificações: R (filmes 1, 2 e 4), PG-13 (filme 3) e PG-13 (filme 5). As classificações indicam que os filmes contêm cenas de violência, sexo, drogas, linguagem imprópria e humor adulto.
- Existe algum livro ou quadrinho baseado nos filmes "Todo Mundo em Pânico"?
- Não, não existe nenhum livro ou quadrinho baseado nos filmes "Todo Mundo em Pânico". Os filmes são obras originais criadas pelos roteiristas e diretores da série. No entanto, existem alguns livros e quadrinhos que fazem paródia de filmes de terror, assim como os filmes "Todo Mundo em Pânico". Alguns exemplos são: "Scary Movie: A Novelization", de Michael Teitelbaum, baseado no primeiro filme da série; "The Walking Dead: The Official Cookbook and Survival Guide", de Lauren Wilson, baseado na série de TV e quadrinhos de zumbis; "The Simpsons Treehouse of Horror", uma série de quadrinhos que parodia vários filmes e histórias de terror; entre outros.
197e85843d
-
-
\ No newline at end of file
diff --git a/spaces/4Taps/SadTalker/Dockerfile b/spaces/4Taps/SadTalker/Dockerfile
deleted file mode 100644
index 5ddc6e3d8b246534a58f9612a88b309fa7e10795..0000000000000000000000000000000000000000
--- a/spaces/4Taps/SadTalker/Dockerfile
+++ /dev/null
@@ -1,59 +0,0 @@
-FROM nvidia/cuda:11.7.1-cudnn8-devel-ubuntu22.04
-ENV DEBIAN_FRONTEND=noninteractive
-RUN apt-get update && \
- apt-get upgrade -y && \
- apt-get install -y --no-install-recommends \
- git \
- zip \
- unzip \
- git-lfs \
- wget \
- curl \
- # ffmpeg \
- ffmpeg \
- x264 \
- # python build dependencies \
- build-essential \
- libssl-dev \
- zlib1g-dev \
- libbz2-dev \
- libreadline-dev \
- libsqlite3-dev \
- libncursesw5-dev \
- xz-utils \
- tk-dev \
- libxml2-dev \
- libxmlsec1-dev \
- libffi-dev \
- liblzma-dev && \
- apt-get clean && \
- rm -rf /var/lib/apt/lists/*
-
-RUN useradd -m -u 1000 user
-USER user
-ENV HOME=/home/user \
- PATH=/home/user/.local/bin:${PATH}
-WORKDIR ${HOME}/app
-
-RUN curl https://pyenv.run | bash
-ENV PATH=${HOME}/.pyenv/shims:${HOME}/.pyenv/bin:${PATH}
-ENV PYTHON_VERSION=3.10.9
-RUN pyenv install ${PYTHON_VERSION} && \
- pyenv global ${PYTHON_VERSION} && \
- pyenv rehash && \
- pip install --no-cache-dir -U pip setuptools wheel
-
-RUN pip install --no-cache-dir -U torch==1.12.1 torchvision==0.13.1
-COPY --chown=1000 requirements.txt /tmp/requirements.txt
-RUN pip install --no-cache-dir -U -r /tmp/requirements.txt
-
-COPY --chown=1000 . ${HOME}/app
-RUN ls -a
-ENV PYTHONPATH=${HOME}/app \
- PYTHONUNBUFFERED=1 \
- GRADIO_ALLOW_FLAGGING=never \
- GRADIO_NUM_PORTS=1 \
- GRADIO_SERVER_NAME=0.0.0.0 \
- GRADIO_THEME=huggingface \
- SYSTEM=spaces
-CMD ["python", "app.py"]
\ No newline at end of file
diff --git a/spaces/801artistry/RVC801/infer/lib/uvr5_pack/lib_v5/layers.py b/spaces/801artistry/RVC801/infer/lib/uvr5_pack/lib_v5/layers.py
deleted file mode 100644
index 4fc1b5cb85a3327f60cbb9f5deffbeeaaac516ad..0000000000000000000000000000000000000000
--- a/spaces/801artistry/RVC801/infer/lib/uvr5_pack/lib_v5/layers.py
+++ /dev/null
@@ -1,118 +0,0 @@
-import torch
-import torch.nn.functional as F
-from torch import nn
-
-from . import spec_utils
-
-
-class Conv2DBNActiv(nn.Module):
- def __init__(self, nin, nout, ksize=3, stride=1, pad=1, dilation=1, activ=nn.ReLU):
- super(Conv2DBNActiv, self).__init__()
- self.conv = nn.Sequential(
- nn.Conv2d(
- nin,
- nout,
- kernel_size=ksize,
- stride=stride,
- padding=pad,
- dilation=dilation,
- bias=False,
- ),
- nn.BatchNorm2d(nout),
- activ(),
- )
-
- def __call__(self, x):
- return self.conv(x)
-
-
-class SeperableConv2DBNActiv(nn.Module):
- def __init__(self, nin, nout, ksize=3, stride=1, pad=1, dilation=1, activ=nn.ReLU):
- super(SeperableConv2DBNActiv, self).__init__()
- self.conv = nn.Sequential(
- nn.Conv2d(
- nin,
- nin,
- kernel_size=ksize,
- stride=stride,
- padding=pad,
- dilation=dilation,
- groups=nin,
- bias=False,
- ),
- nn.Conv2d(nin, nout, kernel_size=1, bias=False),
- nn.BatchNorm2d(nout),
- activ(),
- )
-
- def __call__(self, x):
- return self.conv(x)
-
-
-class Encoder(nn.Module):
- def __init__(self, nin, nout, ksize=3, stride=1, pad=1, activ=nn.LeakyReLU):
- super(Encoder, self).__init__()
- self.conv1 = Conv2DBNActiv(nin, nout, ksize, 1, pad, activ=activ)
- self.conv2 = Conv2DBNActiv(nout, nout, ksize, stride, pad, activ=activ)
-
- def __call__(self, x):
- skip = self.conv1(x)
- h = self.conv2(skip)
-
- return h, skip
-
-
-class Decoder(nn.Module):
- def __init__(
- self, nin, nout, ksize=3, stride=1, pad=1, activ=nn.ReLU, dropout=False
- ):
- super(Decoder, self).__init__()
- self.conv = Conv2DBNActiv(nin, nout, ksize, 1, pad, activ=activ)
- self.dropout = nn.Dropout2d(0.1) if dropout else None
-
- def __call__(self, x, skip=None):
- x = F.interpolate(x, scale_factor=2, mode="bilinear", align_corners=True)
- if skip is not None:
- skip = spec_utils.crop_center(skip, x)
- x = torch.cat([x, skip], dim=1)
- h = self.conv(x)
-
- if self.dropout is not None:
- h = self.dropout(h)
-
- return h
-
-
-class ASPPModule(nn.Module):
- def __init__(self, nin, nout, dilations=(4, 8, 16), activ=nn.ReLU):
- super(ASPPModule, self).__init__()
- self.conv1 = nn.Sequential(
- nn.AdaptiveAvgPool2d((1, None)),
- Conv2DBNActiv(nin, nin, 1, 1, 0, activ=activ),
- )
- self.conv2 = Conv2DBNActiv(nin, nin, 1, 1, 0, activ=activ)
- self.conv3 = SeperableConv2DBNActiv(
- nin, nin, 3, 1, dilations[0], dilations[0], activ=activ
- )
- self.conv4 = SeperableConv2DBNActiv(
- nin, nin, 3, 1, dilations[1], dilations[1], activ=activ
- )
- self.conv5 = SeperableConv2DBNActiv(
- nin, nin, 3, 1, dilations[2], dilations[2], activ=activ
- )
- self.bottleneck = nn.Sequential(
- Conv2DBNActiv(nin * 5, nout, 1, 1, 0, activ=activ), nn.Dropout2d(0.1)
- )
-
- def forward(self, x):
- _, _, h, w = x.size()
- feat1 = F.interpolate(
- self.conv1(x), size=(h, w), mode="bilinear", align_corners=True
- )
- feat2 = self.conv2(x)
- feat3 = self.conv3(x)
- feat4 = self.conv4(x)
- feat5 = self.conv5(x)
- out = torch.cat((feat1, feat2, feat3, feat4, feat5), dim=1)
- bottle = self.bottleneck(out)
- return bottle
diff --git a/spaces/801artistry/RVC801/lib/uvr5_pack/lib_v5/nets_61968KB.py b/spaces/801artistry/RVC801/lib/uvr5_pack/lib_v5/nets_61968KB.py
deleted file mode 100644
index becbfae85683a13bbb19d3ea6c840da24e61e01e..0000000000000000000000000000000000000000
--- a/spaces/801artistry/RVC801/lib/uvr5_pack/lib_v5/nets_61968KB.py
+++ /dev/null
@@ -1,122 +0,0 @@
-import torch
-from torch import nn
-import torch.nn.functional as F
-
-from . import layers_123821KB as layers
-
-
-class BaseASPPNet(nn.Module):
- def __init__(self, nin, ch, dilations=(4, 8, 16)):
- super(BaseASPPNet, self).__init__()
- self.enc1 = layers.Encoder(nin, ch, 3, 2, 1)
- self.enc2 = layers.Encoder(ch, ch * 2, 3, 2, 1)
- self.enc3 = layers.Encoder(ch * 2, ch * 4, 3, 2, 1)
- self.enc4 = layers.Encoder(ch * 4, ch * 8, 3, 2, 1)
-
- self.aspp = layers.ASPPModule(ch * 8, ch * 16, dilations)
-
- self.dec4 = layers.Decoder(ch * (8 + 16), ch * 8, 3, 1, 1)
- self.dec3 = layers.Decoder(ch * (4 + 8), ch * 4, 3, 1, 1)
- self.dec2 = layers.Decoder(ch * (2 + 4), ch * 2, 3, 1, 1)
- self.dec1 = layers.Decoder(ch * (1 + 2), ch, 3, 1, 1)
-
- def __call__(self, x):
- h, e1 = self.enc1(x)
- h, e2 = self.enc2(h)
- h, e3 = self.enc3(h)
- h, e4 = self.enc4(h)
-
- h = self.aspp(h)
-
- h = self.dec4(h, e4)
- h = self.dec3(h, e3)
- h = self.dec2(h, e2)
- h = self.dec1(h, e1)
-
- return h
-
-
-class CascadedASPPNet(nn.Module):
- def __init__(self, n_fft):
- super(CascadedASPPNet, self).__init__()
- self.stg1_low_band_net = BaseASPPNet(2, 32)
- self.stg1_high_band_net = BaseASPPNet(2, 32)
-
- self.stg2_bridge = layers.Conv2DBNActiv(34, 16, 1, 1, 0)
- self.stg2_full_band_net = BaseASPPNet(16, 32)
-
- self.stg3_bridge = layers.Conv2DBNActiv(66, 32, 1, 1, 0)
- self.stg3_full_band_net = BaseASPPNet(32, 64)
-
- self.out = nn.Conv2d(64, 2, 1, bias=False)
- self.aux1_out = nn.Conv2d(32, 2, 1, bias=False)
- self.aux2_out = nn.Conv2d(32, 2, 1, bias=False)
-
- self.max_bin = n_fft // 2
- self.output_bin = n_fft // 2 + 1
-
- self.offset = 128
-
- def forward(self, x, aggressiveness=None):
- mix = x.detach()
- x = x.clone()
-
- x = x[:, :, : self.max_bin]
-
- bandw = x.size()[2] // 2
- aux1 = torch.cat(
- [
- self.stg1_low_band_net(x[:, :, :bandw]),
- self.stg1_high_band_net(x[:, :, bandw:]),
- ],
- dim=2,
- )
-
- h = torch.cat([x, aux1], dim=1)
- aux2 = self.stg2_full_band_net(self.stg2_bridge(h))
-
- h = torch.cat([x, aux1, aux2], dim=1)
- h = self.stg3_full_band_net(self.stg3_bridge(h))
-
- mask = torch.sigmoid(self.out(h))
- mask = F.pad(
- input=mask,
- pad=(0, 0, 0, self.output_bin - mask.size()[2]),
- mode="replicate",
- )
-
- if self.training:
- aux1 = torch.sigmoid(self.aux1_out(aux1))
- aux1 = F.pad(
- input=aux1,
- pad=(0, 0, 0, self.output_bin - aux1.size()[2]),
- mode="replicate",
- )
- aux2 = torch.sigmoid(self.aux2_out(aux2))
- aux2 = F.pad(
- input=aux2,
- pad=(0, 0, 0, self.output_bin - aux2.size()[2]),
- mode="replicate",
- )
- return mask * mix, aux1 * mix, aux2 * mix
- else:
- if aggressiveness:
- mask[:, :, : aggressiveness["split_bin"]] = torch.pow(
- mask[:, :, : aggressiveness["split_bin"]],
- 1 + aggressiveness["value"] / 3,
- )
- mask[:, :, aggressiveness["split_bin"] :] = torch.pow(
- mask[:, :, aggressiveness["split_bin"] :],
- 1 + aggressiveness["value"],
- )
-
- return mask * mix
-
- def predict(self, x_mag, aggressiveness=None):
- h = self.forward(x_mag, aggressiveness)
-
- if self.offset > 0:
- h = h[:, :, :, self.offset : -self.offset]
- assert h.size()[3] > 0
-
- return h
diff --git a/spaces/A-Celsius/Caption-Generator/README.md b/spaces/A-Celsius/Caption-Generator/README.md
deleted file mode 100644
index f77cf6d5a40ede0ac6a47e056c0df66f70f6a425..0000000000000000000000000000000000000000
--- a/spaces/A-Celsius/Caption-Generator/README.md
+++ /dev/null
@@ -1,12 +0,0 @@
----
-title: Caption Generator
-emoji: 🦀
-colorFrom: purple
-colorTo: yellow
-sdk: gradio
-sdk_version: 3.28.2
-app_file: app.py
-pinned: false
----
-
-Check out the configuration reference at https://huggingface.co/docs/hub/spaces-config-reference
diff --git a/spaces/AIConsultant/MusicGen/audiocraft/optim/inverse_sqrt_lr_scheduler.py b/spaces/AIConsultant/MusicGen/audiocraft/optim/inverse_sqrt_lr_scheduler.py
deleted file mode 100644
index 920192e8842c5635bf6f7f76618fa4a6f4b0114a..0000000000000000000000000000000000000000
--- a/spaces/AIConsultant/MusicGen/audiocraft/optim/inverse_sqrt_lr_scheduler.py
+++ /dev/null
@@ -1,38 +0,0 @@
-# Copyright (c) Meta Platforms, Inc. and affiliates.
-# All rights reserved.
-#
-# This source code is licensed under the license found in the
-# LICENSE file in the root directory of this source tree.
-
-import typing as tp
-
-from torch.optim import Optimizer
-from torch.optim.lr_scheduler import _LRScheduler
-
-
-class InverseSquareRootLRScheduler(_LRScheduler):
- """Inverse square root LR scheduler.
-
- Args:
- optimizer (Optimizer): Torch optimizer.
- warmup_steps (int): Number of warmup steps.
- warmup_init_lr (tp.Optional[float]): Initial learning rate
- during warmup phase. When not set, use the provided learning rate.
- """
- def __init__(self, optimizer: Optimizer, warmup_steps: int, warmup_init_lr: tp.Optional[float] = 0):
- self.warmup_steps = warmup_steps
- self.warmup_init_lr = warmup_init_lr
- super().__init__(optimizer)
-
- def _get_sched_lr(self, lr: float, step: int):
- if step < self.warmup_steps:
- warmup_init_lr = self.warmup_init_lr or 0
- lr_step = (lr - warmup_init_lr) / self.warmup_steps
- lr = warmup_init_lr + step * lr_step
- else:
- decay_factor = lr * self.warmup_steps**0.5
- lr = decay_factor * step**-0.5
- return lr
-
- def get_lr(self):
- return [self._get_sched_lr(base_lr, self._step_count) for base_lr in self.base_lrs]
diff --git a/spaces/AIZero2HeroBootcamp/AnimatedGifGallery/gifs/README.md b/spaces/AIZero2HeroBootcamp/AnimatedGifGallery/gifs/README.md
deleted file mode 100644
index e69de29bb2d1d6434b8b29ae775ad8c2e48c5391..0000000000000000000000000000000000000000
diff --git a/spaces/AP123/IllusionDiffusion/app.py b/spaces/AP123/IllusionDiffusion/app.py
deleted file mode 100644
index d82460d88330310696dff806bba6d7ba531e409e..0000000000000000000000000000000000000000
--- a/spaces/AP123/IllusionDiffusion/app.py
+++ /dev/null
@@ -1,281 +0,0 @@
-import torch
-import gradio as gr
-from gradio import processing_utils, utils
-from PIL import Image
-import random
-from diffusers import (
- DiffusionPipeline,
- AutoencoderKL,
- StableDiffusionControlNetPipeline,
- ControlNetModel,
- StableDiffusionLatentUpscalePipeline,
- StableDiffusionImg2ImgPipeline,
- StableDiffusionControlNetImg2ImgPipeline,
- DPMSolverMultistepScheduler, # <-- Added import
- EulerDiscreteScheduler # <-- Added import
-)
-import time
-from share_btn import community_icon_html, loading_icon_html, share_js
-import user_history
-from illusion_style import css
-
-BASE_MODEL = "SG161222/Realistic_Vision_V5.1_noVAE"
-
-# Initialize both pipelines
-vae = AutoencoderKL.from_pretrained("stabilityai/sd-vae-ft-mse", torch_dtype=torch.float16)
-#init_pipe = DiffusionPipeline.from_pretrained("SG161222/Realistic_Vision_V5.1_noVAE", torch_dtype=torch.float16)
-controlnet = ControlNetModel.from_pretrained("monster-labs/control_v1p_sd15_qrcode_monster", torch_dtype=torch.float16)#, torch_dtype=torch.float16)
-main_pipe = StableDiffusionControlNetPipeline.from_pretrained(
- BASE_MODEL,
- controlnet=controlnet,
- vae=vae,
- safety_checker=None,
- torch_dtype=torch.float16,
-).to("cuda")
-
-#main_pipe.unet = torch.compile(main_pipe.unet, mode="reduce-overhead", fullgraph=True)
-#main_pipe.unet.to(memory_format=torch.channels_last)
-#main_pipe.unet = torch.compile(main_pipe.unet, mode="reduce-overhead", fullgraph=True)
-#model_id = "stabilityai/sd-x2-latent-upscaler"
-image_pipe = StableDiffusionControlNetImg2ImgPipeline(**main_pipe.components)
-
-#image_pipe.unet = torch.compile(image_pipe.unet, mode="reduce-overhead", fullgraph=True)
-#upscaler = StableDiffusionLatentUpscalePipeline.from_pretrained(model_id, torch_dtype=torch.float16)
-#upscaler.to("cuda")
-
-
-# Sampler map
-SAMPLER_MAP = {
- "DPM++ Karras SDE": lambda config: DPMSolverMultistepScheduler.from_config(config, use_karras=True, algorithm_type="sde-dpmsolver++"),
- "Euler": lambda config: EulerDiscreteScheduler.from_config(config),
-}
-
-def center_crop_resize(img, output_size=(512, 512)):
- width, height = img.size
-
- # Calculate dimensions to crop to the center
- new_dimension = min(width, height)
- left = (width - new_dimension)/2
- top = (height - new_dimension)/2
- right = (width + new_dimension)/2
- bottom = (height + new_dimension)/2
-
- # Crop and resize
- img = img.crop((left, top, right, bottom))
- img = img.resize(output_size)
-
- return img
-
-def common_upscale(samples, width, height, upscale_method, crop=False):
- if crop == "center":
- old_width = samples.shape[3]
- old_height = samples.shape[2]
- old_aspect = old_width / old_height
- new_aspect = width / height
- x = 0
- y = 0
- if old_aspect > new_aspect:
- x = round((old_width - old_width * (new_aspect / old_aspect)) / 2)
- elif old_aspect < new_aspect:
- y = round((old_height - old_height * (old_aspect / new_aspect)) / 2)
- s = samples[:,:,y:old_height-y,x:old_width-x]
- else:
- s = samples
-
- return torch.nn.functional.interpolate(s, size=(height, width), mode=upscale_method)
-
-def upscale(samples, upscale_method, scale_by):
- #s = samples.copy()
- width = round(samples["images"].shape[3] * scale_by)
- height = round(samples["images"].shape[2] * scale_by)
- s = common_upscale(samples["images"], width, height, upscale_method, "disabled")
- return (s)
-
-def check_inputs(prompt: str, control_image: Image.Image):
- if control_image is None:
- raise gr.Error("Please select or upload an Input Illusion")
- if prompt is None or prompt == "":
- raise gr.Error("Prompt is required")
-
-def convert_to_pil(base64_image):
- pil_image = processing_utils.decode_base64_to_image(base64_image)
- return pil_image
-
-def convert_to_base64(pil_image):
- base64_image = processing_utils.encode_pil_to_base64(pil_image)
- return base64_image
-
-# Inference function
-def inference(
- control_image: Image.Image,
- prompt: str,
- negative_prompt: str,
- guidance_scale: float = 8.0,
- controlnet_conditioning_scale: float = 1,
- control_guidance_start: float = 1,
- control_guidance_end: float = 1,
- upscaler_strength: float = 0.5,
- seed: int = -1,
- sampler = "DPM++ Karras SDE",
- progress = gr.Progress(track_tqdm=True),
- profile: gr.OAuthProfile | None = None,
-):
- start_time = time.time()
- start_time_struct = time.localtime(start_time)
- start_time_formatted = time.strftime("%H:%M:%S", start_time_struct)
- print(f"Inference started at {start_time_formatted}")
-
- # Generate the initial image
- #init_image = init_pipe(prompt).images[0]
-
- # Rest of your existing code
- control_image_small = center_crop_resize(control_image)
- control_image_large = center_crop_resize(control_image, (1024, 1024))
-
- main_pipe.scheduler = SAMPLER_MAP[sampler](main_pipe.scheduler.config)
- my_seed = random.randint(0, 2**32 - 1) if seed == -1 else seed
- generator = torch.Generator(device="cuda").manual_seed(my_seed)
-
- out = main_pipe(
- prompt=prompt,
- negative_prompt=negative_prompt,
- image=control_image_small,
- guidance_scale=float(guidance_scale),
- controlnet_conditioning_scale=float(controlnet_conditioning_scale),
- generator=generator,
- control_guidance_start=float(control_guidance_start),
- control_guidance_end=float(control_guidance_end),
- num_inference_steps=15,
- output_type="latent"
- )
- upscaled_latents = upscale(out, "nearest-exact", 2)
- out_image = image_pipe(
- prompt=prompt,
- negative_prompt=negative_prompt,
- control_image=control_image_large,
- image=upscaled_latents,
- guidance_scale=float(guidance_scale),
- generator=generator,
- num_inference_steps=20,
- strength=upscaler_strength,
- control_guidance_start=float(control_guidance_start),
- control_guidance_end=float(control_guidance_end),
- controlnet_conditioning_scale=float(controlnet_conditioning_scale)
- )
- end_time = time.time()
- end_time_struct = time.localtime(end_time)
- end_time_formatted = time.strftime("%H:%M:%S", end_time_struct)
- print(f"Inference ended at {end_time_formatted}, taking {end_time-start_time}s")
-
- # Save image + metadata
- user_history.save_image(
- label=prompt,
- image=out_image["images"][0],
- profile=profile,
- metadata={
- "prompt": prompt,
- "negative_prompt": negative_prompt,
- "guidance_scale": guidance_scale,
- "controlnet_conditioning_scale": controlnet_conditioning_scale,
- "control_guidance_start": control_guidance_start,
- "control_guidance_end": control_guidance_end,
- "upscaler_strength": upscaler_strength,
- "seed": seed,
- "sampler": sampler,
- },
- )
-
- return out_image["images"][0], gr.update(visible=True), gr.update(visible=True), my_seed
-
-with gr.Blocks() as app:
- gr.Markdown(
- '''
- Illusion Diffusion HQ 🌀
- Generate stunning high quality illusion artwork with Stable Diffusion
-
-
- A space by AP [Follow me on Twitter](https://twitter.com/angrypenguinPNG) with big contributions from [multimodalart](https://twitter.com/multimodalart)
-
- This project works by using [Monster Labs QR Control Net](https://huggingface.co/monster-labs/control_v1p_sd15_qrcode_monster).
- Given a prompt and your pattern, we use a QR code conditioned controlnet to create a stunning illusion! Credit to: [MrUgleh](https://twitter.com/MrUgleh) for discovering the workflow :)
- '''
- )
- state_img_input = gr.State()
- state_img_output = gr.State()
- with gr.Row():
- with gr.Column():
- control_image = gr.Image(label="Input Illusion", type="pil", elem_id="control_image")
- controlnet_conditioning_scale = gr.Slider(minimum=0.0, maximum=5.0, step=0.01, value=0.8, label="Illusion strength", elem_id="illusion_strength", info="ControlNet conditioning scale")
- gr.Examples(examples=["checkers.png", "checkers_mid.jpg", "pattern.png", "ultra_checkers.png", "spiral.jpeg", "funky.jpeg" ], inputs=control_image)
- prompt = gr.Textbox(label="Prompt", elem_id="prompt", info="Type what you want to generate", placeholder="Medieval village scene with busy streets and castle in the distance")
- negative_prompt = gr.Textbox(label="Negative Prompt", info="Type what you don't want to see", value="low quality", elem_id="negative_prompt")
- with gr.Accordion(label="Advanced Options", open=False):
- guidance_scale = gr.Slider(minimum=0.0, maximum=50.0, step=0.25, value=7.5, label="Guidance Scale")
- sampler = gr.Dropdown(choices=list(SAMPLER_MAP.keys()), value="Euler")
- control_start = gr.Slider(minimum=0.0, maximum=1.0, step=0.1, value=0, label="Start of ControlNet")
- control_end = gr.Slider(minimum=0.0, maximum=1.0, step=0.1, value=1, label="End of ControlNet")
- strength = gr.Slider(minimum=0.0, maximum=1.0, step=0.1, value=1, label="Strength of the upscaler")
- seed = gr.Slider(minimum=-1, maximum=9999999999, step=1, value=-1, label="Seed", info="-1 means random seed")
- used_seed = gr.Number(label="Last seed used",interactive=False)
- run_btn = gr.Button("Run")
- with gr.Column():
- result_image = gr.Image(label="Illusion Diffusion Output", interactive=False, elem_id="output")
- with gr.Group(elem_id="share-btn-container", visible=False) as share_group:
- community_icon = gr.HTML(community_icon_html)
- loading_icon = gr.HTML(loading_icon_html)
- share_button = gr.Button("Share to community", elem_id="share-btn")
-
- prompt.submit(
- check_inputs,
- inputs=[prompt, control_image],
- queue=False
- ).success(
- convert_to_pil,
- inputs=[control_image],
- outputs=[state_img_input],
- queue=False,
- preprocess=False,
- ).success(
- inference,
- inputs=[state_img_input, prompt, negative_prompt, guidance_scale, controlnet_conditioning_scale, control_start, control_end, strength, seed, sampler],
- outputs=[state_img_output, result_image, share_group, used_seed]
- ).success(
- convert_to_base64,
- inputs=[state_img_output],
- outputs=[result_image],
- queue=False,
- postprocess=False
- )
- run_btn.click(
- check_inputs,
- inputs=[prompt, control_image],
- queue=False
- ).success(
- convert_to_pil,
- inputs=[control_image],
- outputs=[state_img_input],
- queue=False,
- preprocess=False,
- ).success(
- inference,
- inputs=[state_img_input, prompt, negative_prompt, guidance_scale, controlnet_conditioning_scale, control_start, control_end, strength, seed, sampler],
- outputs=[state_img_output, result_image, share_group, used_seed]
- ).success(
- convert_to_base64,
- inputs=[state_img_output],
- outputs=[result_image],
- queue=False,
- postprocess=False
- )
- share_button.click(None, [], [], _js=share_js)
-
-with gr.Blocks(css=css) as app_with_history:
- with gr.Tab("Demo"):
- app.render()
- with gr.Tab("Past generations"):
- user_history.render()
-
-app_with_history.queue(max_size=20,api_open=False )
-
-if __name__ == "__main__":
- app_with_history.launch(max_threads=400)
diff --git a/spaces/AchyuthGamer/OpenGPT-Chat-UI/src/routes/search/[id]/+server.ts b/spaces/AchyuthGamer/OpenGPT-Chat-UI/src/routes/search/[id]/+server.ts
deleted file mode 100644
index 240de4cd73a9d03090618a8d475313735ad0d96e..0000000000000000000000000000000000000000
--- a/spaces/AchyuthGamer/OpenGPT-Chat-UI/src/routes/search/[id]/+server.ts
+++ /dev/null
@@ -1,7 +0,0 @@
-import { collections } from "$lib/server/database";
-import { hashConv } from "$lib/utils/hashConv.js";
-import { error } from "@sveltejs/kit";
-
-export async function GET({ params, locals }) {
- return new Response(JSON.stringify(""), { headers: { "Content-Type": "application/json" } });
-}
diff --git a/spaces/AgentVerse/agentVerse/ui/src/phaser3-rex-plugins/templates/ui/menu/Menu.js b/spaces/AgentVerse/agentVerse/ui/src/phaser3-rex-plugins/templates/ui/menu/Menu.js
deleted file mode 100644
index 706ebe026aa53eeb5e7fc30432266363ff5ec251..0000000000000000000000000000000000000000
--- a/spaces/AgentVerse/agentVerse/ui/src/phaser3-rex-plugins/templates/ui/menu/Menu.js
+++ /dev/null
@@ -1,210 +0,0 @@
-import Buttons from '../buttons/Buttons.js';
-import Methods from './methods/Methods.js';
-import CreateBackground from './methods/CreateBackground.js';
-import CreateButtons from './methods/CreateButtons.js';
-import GetViewport from '../../../plugins/utils/system/GetViewport.js';
-import MenuSetInteractive from './methods/MenuSetInteractive.js';
-import ParseEaseConfig from './methods/ParseEaseConfig.js';
-import GetEaseConfig from './methods/GetEaseConfig.js';
-import Expand from './methods/Expand.js';
-
-const GetValue = Phaser.Utils.Objects.GetValue;
-
-class Menu extends Buttons {
- constructor(scene, config) {
- if (config === undefined) {
- config = {};
- }
-
- // Orientation
- if (!config.hasOwnProperty('orientation')) {
- config.orientation = 1; // y
- }
-
- // Parent
- var rootMenu = config._rootMenu;
- var parentMenu = config._parentMenu;
- var parentButton = config._parentButton;
- // Popup, root menu can be static, sub-menus are always popup.
- var popUp = GetValue(config, 'popup', true);
- // Items
- var items = GetValue(config, 'items', undefined);
- // Background
- var createBackgroundCallback = GetValue(config, 'createBackgroundCallback', undefined);
- var createBackgroundCallbackScope = GetValue(config, 'createBackgroundCallbackScope', undefined);
- config.background = CreateBackground(scene, items, createBackgroundCallback, createBackgroundCallbackScope);
- // Buttons
- var createButtonCallback = GetValue(config, 'createButtonCallback', undefined);
- var createButtonCallbackScope = GetValue(config, 'createButtonCallbackScope', undefined);
- config.buttons = CreateButtons(scene, items, createButtonCallback, createButtonCallbackScope);
-
- super(scene, config);
- this.type = 'rexMenu';
-
- this.items = items;
- this.root = (rootMenu === undefined) ? this : rootMenu;
- this.isRoot = (this.root === this);
- this.parentMenu = parentMenu;
- this.parentButton = parentButton;
- this.timer = undefined;
-
- // Root menu
- if (this.isRoot) {
- this.isPopUpMode = popUp;
- // Bounds
- var bounds = config.bounds;
- if (bounds === undefined) {
- bounds = GetViewport(scene);
- }
- this.bounds = bounds;
-
- // Side of submenu
- this.subMenuSide = [
- ((this.y < bounds.centerY) ? SUBMENU_DOWN : SUBMENU_UP),
- ((this.x < bounds.centerX) ? SUBMENU_RIGHT : SUBMENU_LEFT)
- ];
- // Overwrite subMenuSide value if given
- var subMenuSide = GetValue(config, 'subMenuSide', undefined);
- if (subMenuSide !== undefined) {
- if (typeof (subMenuSide) === 'string') {
- subMenuSide = SubMenuSideMode[subMenuSide];
- }
- this.subMenuSide[this.orientation] = subMenuSide;
- }
- // ToggleOrientation mode
- this.toggleOrientation = GetValue(config, 'toggleOrientation', false);
- // Expand mode
- this.expandEventName = GetValue(config, 'expandEvent', 'button.click');
- // Transition
- this.easeIn = ParseEaseConfig(this, GetValue(config, 'easeIn', 0));
- this.easeOut = ParseEaseConfig(this, GetValue(config, 'easeOut', 0));
- this.setTransitInCallback(GetValue(config, 'transitIn'));
- this.setTransitOutCallback(GetValue(config, 'transitOut'));
- // Callbacks
- this.createBackgroundCallback = createBackgroundCallback;
- this.createBackgroundCallbackScope = createBackgroundCallbackScope;
- this.createButtonCallback = createButtonCallback;
- this.createButtonCallbackScope = createButtonCallbackScope;
- // Children key
- this.childrenKey = GetValue(config, 'childrenKey', 'children');
- // Event flag
- this._isPassedEvent = false;
-
- // pointerdown-outside-collapse
- this.pointerDownOutsideCollapsing = GetValue(config, 'pointerDownOutsideCollapsing', true);
- if (this.pointerDownOutsideCollapsing) {
- scene.input.on('pointerdown', this.onPointerDownOutside, this);
- }
-
- } else { // Sub-menu
-
- }
-
- var originX = 0, originY = 0;
- if (!this.root.easeIn.sameOrientation) {
- var easeOrientation = GetEaseConfig(this.root.easeIn, this).orientation;
- var menuOrientation = (parentMenu) ? parentMenu.orientation : this.orientation;
- var subMenuSide = this.root.subMenuSide[menuOrientation];
- if ((easeOrientation === 0) && (subMenuSide === SUBMENU_LEFT)) {
- originX = 1;
- }
- if ((easeOrientation === 1) && (subMenuSide === SUBMENU_UP)) {
- originY = 1;
- }
- }
-
- if (popUp) {
- this.setOrigin(originX, originY).layout();
- }
-
- // Sub-menu:
- // - scale to root's scale value
- // - align to parent button
- if (!this.isRoot) {
- this.setScale(this.root.scaleX, this.root.scaleY);
- var subMenuSide = this.root.subMenuSide[parentMenu.orientation];
- switch (subMenuSide) {
- case SUBMENU_LEFT: //Put submene at left side
- this.alignTop(parentButton.top).alignRight(parentButton.left);
- break;
-
- case SUBMENU_RIGHT: //Put submene at right side
- this.alignTop(parentButton.top).alignLeft(parentButton.right);
- break;
-
- case SUBMENU_UP: //Put submene at up side
- this.alignLeft(parentButton.left).alignBottom(parentButton.top);
- break;
-
- case SUBMENU_DOWN: //Put submene at down side
- this.alignLeft(parentButton.left).alignTop(parentButton.bottom);
- break;
- }
- }
-
- MenuSetInteractive(this);
-
- if (popUp) {
- this.pushIntoBounds(this.root.bounds);
-
- // Expand this menu
- Expand.call(this);
- }
-
- }
-
- destroy(fromScene) {
- // This Game Object has already been destroyed
- if (!this.scene || this.ignoreDestroy) {
- return;
- }
-
- if (this.isRoot && this.pointerDownOutsideCollapsing) {
- this.scene.input.off('pointerdown', this.onPointerDownOutside, this);
- }
-
- super.destroy(fromScene);
- this.removeDelayCall();
- }
-
- isInTouching(pointer) {
- if (super.isInTouching(pointer)) {
- return true;
- } else if (this.childrenMap.subMenu) {
- return this.childrenMap.subMenu.isInTouching(pointer);
- } else {
- return false;
- }
- }
-
- onPointerDownOutside(pointer) {
- if (this.isInTouching(pointer)) {
- return;
- }
-
- if (this.isPopUpMode) {
- this.collapse();
- } else {
- this.collapseSubMenu();
- }
- }
-
-
-}
-
-const SUBMENU_LEFT = 2;
-const SUBMENU_RIGHT = 0;
-const SUBMENU_UP = 3;
-const SUBMENU_DOWN = 1;
-const SubMenuSideMode = {
- up: SUBMENU_UP,
- down: SUBMENU_DOWN,
- left: SUBMENU_LEFT,
- right: SUBMENU_RIGHT
-}
-
-Object.assign(
- Menu.prototype,
- Methods
-);
-export default Menu;
\ No newline at end of file
diff --git a/spaces/Agusbs98/automatic-ecg-diagnosis/tools/tools.py b/spaces/Agusbs98/automatic-ecg-diagnosis/tools/tools.py
deleted file mode 100644
index 3b7f7d52b9a7a488ed446d52e5ae79e400211bd9..0000000000000000000000000000000000000000
--- a/spaces/Agusbs98/automatic-ecg-diagnosis/tools/tools.py
+++ /dev/null
@@ -1,124 +0,0 @@
-from libs import *
-import configVars
-import ecg_plot
-def remove_baseline_filter(sample_rate):
- fc = 0.8 # [Hz], cutoff frequency
- fst = 0.2 # [Hz], rejection band
- rp = 0.5 # [dB], ripple in passband
- rs = 40 # [dB], attenuation in rejection band
- wn = fc / (sample_rate / 2)
- wst = fst / (sample_rate / 2)
-
- filterorder, aux = sgn.ellipord(wn, wst, rp, rs)
- sos = sgn.iirfilter(filterorder, wn, rp, rs, btype='high', ftype='ellip', output='sos')
-
- return sos
-
-reduced_leads = ['DI', 'DII', 'V1', 'V2', 'V3', 'V4', 'V5', 'V6']
-all_leads = ['DI', 'DII', 'DIII', 'AVR', 'AVL', 'AVF', 'V1', 'V2', 'V3', 'V4', 'V5', 'V6']
-
-def preprocess_ecg(ecg, sample_rate, leads, scale=1,
- use_all_leads=True, remove_baseline=False):
- # Remove baseline
- if remove_baseline:
- sos = remove_baseline_filter(sample_rate)
- ecg_nobaseline = sgn.sosfiltfilt(sos, ecg, padtype='constant', axis=-1)
- else:
- ecg_nobaseline = ecg
-
- # Rescale
- ecg_rescaled = scale * ecg_nobaseline
-
- # Resample
- if sample_rate != 500:
- ecg_resampled = sgn.resample_poly(ecg_rescaled, up=500, down=sample_rate, axis=-1)
- else:
- ecg_resampled = ecg_rescaled
- length = len(ecg_resampled[0])
-
- # Add leads if needed
- target_leads = all_leads if use_all_leads else reduced_leads
- n_leads_target = len(target_leads)
- l2p = dict(zip(target_leads, range(n_leads_target)))
- ecg_targetleads = np.zeros([n_leads_target, length])
- ecg_targetleads = ecg_rescaled
- if n_leads_target >= leads and use_all_leads:
- ecg_targetleads[l2p['DIII'], :] = ecg_targetleads[l2p['DII'], :] - ecg_targetleads[l2p['DI'], :]
- ecg_targetleads[l2p['AVR'], :] = -(ecg_targetleads[l2p['DI'], :] + ecg_targetleads[l2p['DII'], :]) / 2
- ecg_targetleads[l2p['AVL'], :] = (ecg_targetleads[l2p['DI'], :] - ecg_targetleads[l2p['DIII'], :]) / 2
- ecg_targetleads[l2p['AVF'], :] = (ecg_targetleads[l2p['DII'], :] + ecg_targetleads[l2p['DIII'], :]) / 2
-
- return ecg_targetleads
-
-
-def generateH5(input_file,out_file,new_freq=None,new_len=None,scale=1,sample_rate=None):
- n = len(input_file) # Get length
- try:
- h5f = h5py.File(f"{configVars.pathCasos}{out_file}", 'r+')
- h5f.clear()
- except:
- h5f = h5py.File(f"{configVars.pathCasos}{out_file}", 'w')
-
- # Resample
- if new_freq is not None:
- ecg_resampled = sgn.resample_poly(input_file, up=new_freq, down=sample_rate, axis=-1)
- else:
- ecg_resampled = input_file
- new_freq = sample_rate
- n_leads, length = ecg_resampled.shape
-
- # Rescale
- ecg_rescaled = scale * ecg_resampled
-
- # Reshape
- if new_len is None or new_len == length:
- ecg_reshaped = ecg_rescaled
- elif new_len > length:
- ecg_reshaped = np.zeros([n_leads, new_len])
- pad = (new_len - length) // 2
- ecg_reshaped[..., pad:length+pad] = ecg_rescaled
- else:
- extra = (length - new_len) // 2
- ecg_reshaped = ecg_rescaled[:, extra:new_len + extra]
-
- n_leads, n_samples = ecg_reshaped.shape
- x = h5f.create_dataset('tracings', (1, n_samples, n_leads), dtype='f8')
- x[0, :, :] = ecg_reshaped.T
- h5f.close()
-
-def LightX3ECG(
- train_loaders,
- config,
- save_ckp_dir,
-):
- model = torch.load(f"{save_ckp_dir}/best.ptl", map_location='cpu')
- #model = torch.load(f"{save_ckp_dir}/best.ptl", map_location = "cuda")
- model.to(torch.device('cpu'))
- with torch.no_grad():
- model.eval()
- running_preds = []
-
- for ecgs in train_loaders["pred"]:
- ecgs = ecgs.cpu()
- logits = model(ecgs)
- preds = list(torch.max(logits, 1)[1].detach().cpu().numpy()) if not config["is_multilabel"] else list(torch.sigmoid(logits).detach().cpu().numpy())
- running_preds.extend(preds)
-
- if config["is_multilabel"]:
- running_preds = np.array(running_preds)
- optimal_thresholds = pd.read_csv(f"{configVars.pathThresholds}CPSC-2018/optimal_thresholds_best.csv")
- preds = optimal_thresholds[optimal_thresholds["Threshold"]<=running_preds[0]]
- preds = preds['Pred'].values.tolist()
- else:
- enfermedades = ['AFIB','GSVT','SB','SR']
- running_preds = np.array(running_preds)
- #running_preds=np.reshape(running_preds, (len(running_preds),-1))
- preds = enfermedades[running_preds[0]]
- return preds
-
-def ecgPlot(source,sample):
- data = np.load(source)
- #print(data)
- xml_leads = ['DI', 'DII', 'DIII', 'AVR', 'AVL', 'AVF', 'V1', 'V2', 'V3', 'V4', 'V5', 'V6']
- ecg_plot.plot_12(data, sample_rate= sample,lead_index=xml_leads, title="Muestra")
- ecg_plot.save_as_png("ecg")
\ No newline at end of file
diff --git a/spaces/Alfasign/diffusers-gallery/Dockerfile b/spaces/Alfasign/diffusers-gallery/Dockerfile
deleted file mode 100644
index 0ba18d346de09532882673442ee72107556a887d..0000000000000000000000000000000000000000
--- a/spaces/Alfasign/diffusers-gallery/Dockerfile
+++ /dev/null
@@ -1,2 +0,0 @@
-FROM nginxinc/nginx-unprivileged:alpine
-COPY . /usr/share/nginx/html
\ No newline at end of file
diff --git a/spaces/Alpaca233/SadTalker/src/face3d/models/arcface_torch/backbones/iresnet.py b/spaces/Alpaca233/SadTalker/src/face3d/models/arcface_torch/backbones/iresnet.py
deleted file mode 100644
index c6d3b9c240c24687d432197f976ee01fbf423216..0000000000000000000000000000000000000000
--- a/spaces/Alpaca233/SadTalker/src/face3d/models/arcface_torch/backbones/iresnet.py
+++ /dev/null
@@ -1,187 +0,0 @@
-import torch
-from torch import nn
-
-__all__ = ['iresnet18', 'iresnet34', 'iresnet50', 'iresnet100', 'iresnet200']
-
-
-def conv3x3(in_planes, out_planes, stride=1, groups=1, dilation=1):
- """3x3 convolution with padding"""
- return nn.Conv2d(in_planes,
- out_planes,
- kernel_size=3,
- stride=stride,
- padding=dilation,
- groups=groups,
- bias=False,
- dilation=dilation)
-
-
-def conv1x1(in_planes, out_planes, stride=1):
- """1x1 convolution"""
- return nn.Conv2d(in_planes,
- out_planes,
- kernel_size=1,
- stride=stride,
- bias=False)
-
-
-class IBasicBlock(nn.Module):
- expansion = 1
- def __init__(self, inplanes, planes, stride=1, downsample=None,
- groups=1, base_width=64, dilation=1):
- super(IBasicBlock, self).__init__()
- if groups != 1 or base_width != 64:
- raise ValueError('BasicBlock only supports groups=1 and base_width=64')
- if dilation > 1:
- raise NotImplementedError("Dilation > 1 not supported in BasicBlock")
- self.bn1 = nn.BatchNorm2d(inplanes, eps=1e-05,)
- self.conv1 = conv3x3(inplanes, planes)
- self.bn2 = nn.BatchNorm2d(planes, eps=1e-05,)
- self.prelu = nn.PReLU(planes)
- self.conv2 = conv3x3(planes, planes, stride)
- self.bn3 = nn.BatchNorm2d(planes, eps=1e-05,)
- self.downsample = downsample
- self.stride = stride
-
- def forward(self, x):
- identity = x
- out = self.bn1(x)
- out = self.conv1(out)
- out = self.bn2(out)
- out = self.prelu(out)
- out = self.conv2(out)
- out = self.bn3(out)
- if self.downsample is not None:
- identity = self.downsample(x)
- out += identity
- return out
-
-
-class IResNet(nn.Module):
- fc_scale = 7 * 7
- def __init__(self,
- block, layers, dropout=0, num_features=512, zero_init_residual=False,
- groups=1, width_per_group=64, replace_stride_with_dilation=None, fp16=False):
- super(IResNet, self).__init__()
- self.fp16 = fp16
- self.inplanes = 64
- self.dilation = 1
- if replace_stride_with_dilation is None:
- replace_stride_with_dilation = [False, False, False]
- if len(replace_stride_with_dilation) != 3:
- raise ValueError("replace_stride_with_dilation should be None "
- "or a 3-element tuple, got {}".format(replace_stride_with_dilation))
- self.groups = groups
- self.base_width = width_per_group
- self.conv1 = nn.Conv2d(3, self.inplanes, kernel_size=3, stride=1, padding=1, bias=False)
- self.bn1 = nn.BatchNorm2d(self.inplanes, eps=1e-05)
- self.prelu = nn.PReLU(self.inplanes)
- self.layer1 = self._make_layer(block, 64, layers[0], stride=2)
- self.layer2 = self._make_layer(block,
- 128,
- layers[1],
- stride=2,
- dilate=replace_stride_with_dilation[0])
- self.layer3 = self._make_layer(block,
- 256,
- layers[2],
- stride=2,
- dilate=replace_stride_with_dilation[1])
- self.layer4 = self._make_layer(block,
- 512,
- layers[3],
- stride=2,
- dilate=replace_stride_with_dilation[2])
- self.bn2 = nn.BatchNorm2d(512 * block.expansion, eps=1e-05,)
- self.dropout = nn.Dropout(p=dropout, inplace=True)
- self.fc = nn.Linear(512 * block.expansion * self.fc_scale, num_features)
- self.features = nn.BatchNorm1d(num_features, eps=1e-05)
- nn.init.constant_(self.features.weight, 1.0)
- self.features.weight.requires_grad = False
-
- for m in self.modules():
- if isinstance(m, nn.Conv2d):
- nn.init.normal_(m.weight, 0, 0.1)
- elif isinstance(m, (nn.BatchNorm2d, nn.GroupNorm)):
- nn.init.constant_(m.weight, 1)
- nn.init.constant_(m.bias, 0)
-
- if zero_init_residual:
- for m in self.modules():
- if isinstance(m, IBasicBlock):
- nn.init.constant_(m.bn2.weight, 0)
-
- def _make_layer(self, block, planes, blocks, stride=1, dilate=False):
- downsample = None
- previous_dilation = self.dilation
- if dilate:
- self.dilation *= stride
- stride = 1
- if stride != 1 or self.inplanes != planes * block.expansion:
- downsample = nn.Sequential(
- conv1x1(self.inplanes, planes * block.expansion, stride),
- nn.BatchNorm2d(planes * block.expansion, eps=1e-05, ),
- )
- layers = []
- layers.append(
- block(self.inplanes, planes, stride, downsample, self.groups,
- self.base_width, previous_dilation))
- self.inplanes = planes * block.expansion
- for _ in range(1, blocks):
- layers.append(
- block(self.inplanes,
- planes,
- groups=self.groups,
- base_width=self.base_width,
- dilation=self.dilation))
-
- return nn.Sequential(*layers)
-
- def forward(self, x):
- with torch.cuda.amp.autocast(self.fp16):
- x = self.conv1(x)
- x = self.bn1(x)
- x = self.prelu(x)
- x = self.layer1(x)
- x = self.layer2(x)
- x = self.layer3(x)
- x = self.layer4(x)
- x = self.bn2(x)
- x = torch.flatten(x, 1)
- x = self.dropout(x)
- x = self.fc(x.float() if self.fp16 else x)
- x = self.features(x)
- return x
-
-
-def _iresnet(arch, block, layers, pretrained, progress, **kwargs):
- model = IResNet(block, layers, **kwargs)
- if pretrained:
- raise ValueError()
- return model
-
-
-def iresnet18(pretrained=False, progress=True, **kwargs):
- return _iresnet('iresnet18', IBasicBlock, [2, 2, 2, 2], pretrained,
- progress, **kwargs)
-
-
-def iresnet34(pretrained=False, progress=True, **kwargs):
- return _iresnet('iresnet34', IBasicBlock, [3, 4, 6, 3], pretrained,
- progress, **kwargs)
-
-
-def iresnet50(pretrained=False, progress=True, **kwargs):
- return _iresnet('iresnet50', IBasicBlock, [3, 4, 14, 3], pretrained,
- progress, **kwargs)
-
-
-def iresnet100(pretrained=False, progress=True, **kwargs):
- return _iresnet('iresnet100', IBasicBlock, [3, 13, 30, 3], pretrained,
- progress, **kwargs)
-
-
-def iresnet200(pretrained=False, progress=True, **kwargs):
- return _iresnet('iresnet200', IBasicBlock, [6, 26, 60, 6], pretrained,
- progress, **kwargs)
-
diff --git a/spaces/Amrrs/DragGan-Inversion/PTI/README.md b/spaces/Amrrs/DragGan-Inversion/PTI/README.md
deleted file mode 100644
index 19baf6bdb2e87aeeb87527be49969a579cc3f0e1..0000000000000000000000000000000000000000
--- a/spaces/Amrrs/DragGan-Inversion/PTI/README.md
+++ /dev/null
@@ -1,230 +0,0 @@
-# PTI: Pivotal Tuning for Latent-based editing of Real Images (ACM TOG 2022)
-
-
-
-
-
-Inference Notebook:
-
-
-
-
-Pivotal Tuning Inversion (PTI) enables employing off-the-shelf latent based
-semantic editing techniques on real images using StyleGAN.
-PTI excels in identity preserving edits, portrayed through recognizable figures —
-Serena Williams and Robert Downey Jr. (top), and in handling faces which
-are clearly out-of-domain, e.g., due to heavy makeup (bottom).
-
-
-
-## Description
-Official Implementation of our PTI paper + code for evaluation metrics. PTI introduces an optimization mechanizem for solving the StyleGAN inversion task.
-Providing near-perfect reconstruction results while maintaining the high editing abilitis of the native StyleGAN latent space W. For more details, see
-
-## Recent Updates
-**2021.07.01**: Fixed files download phase in the inference notebook. Which might caused the notebook not to run smoothly.
-
-**2021.06.29**: Added support for CPU. In order to run PTI on CPU please change `device` parameter under `configs/global_config.py` to "cpu" instead of "cuda".
-
-**2021.06.25** : Adding mohawk edit using StyleCLIP+PTI in inference notebook.
- Updating documentation in inference notebook due to Google Drive rate limit reached.
- Currently, Google Drive does not allow to download the pretrined models using Colab automatically. Manual intervention might be needed.
-
-## Getting Started
-### Prerequisites
-- Linux or macOS
-- NVIDIA GPU + CUDA CuDNN (Not mandatory bur recommended)
-- Python 3
-
-### Installation
-- Dependencies:
- 1. lpips
- 2. wandb
- 3. pytorch
- 4. torchvision
- 5. matplotlib
- 6. dlib
-- All dependencies can be installed using *pip install* and the package name
-
-## Pretrained Models
-Please download the pretrained models from the following links.
-
-### Auxiliary Models
-We provide various auxiliary models needed for PTI inversion task.
-This includes the StyleGAN generator and pre-trained models used for loss computation.
-| Path | Description
-| :--- | :----------
-|[FFHQ StyleGAN](https://nvlabs-fi-cdn.nvidia.com/stylegan2-ada-pytorch/pretrained/ffhq.pkl) | StyleGAN2-ada model trained on FFHQ with 1024x1024 output resolution.
-|[Dlib alignment](https://drive.google.com/file/d/1HKmjg6iXsWr4aFPuU0gBXPGR83wqMzq7/view?usp=sharing) | Dlib alignment used for images preproccessing.
-|[FFHQ e4e encoder](https://drive.google.com/file/d/1ALC5CLA89Ouw40TwvxcwebhzWXM5YSCm/view?usp=sharing) | Pretrained e4e encoder. Used for StyleCLIP editing.
-
-Note: The StyleGAN model is used directly from the official [stylegan2-ada-pytorch implementation](https://github.com/NVlabs/stylegan2-ada-pytorch).
-For StyleCLIP pretrained mappers, please see [StyleCLIP's official routes](https://github.com/orpatashnik/StyleCLIP/blob/main/utils.py)
-
-
-By default, we assume that all auxiliary models are downloaded and saved to the directory `pretrained_models`.
-However, you may use your own paths by changing the necessary values in `configs/path_configs.py`.
-
-
-## Inversion
-### Preparing your Data
-In order to invert a real image and edit it you should first align and crop it to the correct size. To do so you should perform *One* of the following steps:
-1. Run `notebooks/align_data.ipynb` and change the "images_path" variable to the raw images path
-2. Run `utils/align_data.py` and change the "images_path" variable to the raw images path
-
-
-### Weights And Biases
-The project supports [Weights And Biases](https://wandb.ai/home) framework for experiment tracking. For the inversion task it enables visualization of the losses progression and the generator intermediate results during the initial inversion and the *Pivotal Tuning*(PT) procedure.
-
-The log frequency can be adjusted using the parameters defined at `configs/global_config.py` under the "Logs" subsection.
-
-There is no no need to have an account. However, in order to use the features provided by Weights and Biases you first have to register on their site.
-
-
-### Running PTI
-The main training script is `scripts/run_pti.py`. The script receives aligned and cropped images from paths configured in the "Input info" subscetion in
- `configs/paths_config.py`.
-Results are saved to directories found at "Dirs for output files" under `configs/paths_config.py`. This includes inversion latent codes and tuned generators.
-The hyperparametrs for the inversion task can be found at `configs/hyperparameters.py`. They are intilized to the default values used in the paper.
-
-## Editing
-By default, we assume that all auxiliary edit directions are downloaded and saved to the directory `editings`.
-However, you may use your own paths by changing the necessary values in `configs/path_configs.py` under "Edit directions" subsection.
-
-Example of editing code can be found at `scripts/latent_editor_wrapper.py`
-
-## Inference Notebooks
-To help visualize the results of PTI we provide a Jupyter notebook found in `notebooks/inference_playground.ipynb`.
-The notebook will download the pretrained models and run inference on a sample image found online or
-on images of your choosing. It is recommended to run this in [Google Colab](https://colab.research.google.com/github/danielroich/PTI/blob/main/notebooks/inference_playground.ipynb).
-
-The notebook demonstrates how to:
-- Invert an image using PTI
-- Visualise the inversion and use the PTI output
-- Edit the image after PTI using InterfaceGAN and StyleCLIP
-- Compare to other inversion methods
-
-## Evaluation
-Currently the repository supports qualitative evaluation for reconstruction of: PTI, SG2 (*W Space*), e4e, SG2Plus (*W+ Space*).
-As well as editing using InterfaceGAN and GANSpace for the same inversion methods.
-To run the evaluation please see `evaluation/qualitative_edit_comparison.py`. Examples of the evaluation scripts are:
-
-
-
-
-Reconsturction comparison between different methods. The images order is: Original image, W+ inversion, e4e inversion, W inversion, PTI inversion
-
-
-
-
-
-
-InterfaceGAN pose edit comparison between different methods. The images order is: Original, W+, e4e, W, PTI
-
-
-
-
-
-
-
-Image per edit or several edits without comparison
-
-
-
-### Coming Soon - Quantitative evaluation and StyleCLIP qualitative evaluation
-
-## Repository structure
-| Path | Description
-| :--- | :---
-| ├ configs | Folder containing configs defining Hyperparameters, paths and logging
-| ├ criteria | Folder containing various loss and regularization criterias for the optimization
-| ├ dnnlib | Folder containing internal utils for StyleGAN2-ada
-| ├ docs | Folder containing the latent space edit directions
-| ├ editings | Folder containing images displayed in the README
-| ├ environment | Folder containing Anaconda environment used in our experiments
-| ├ licenses | Folder containing licenses of the open source projects used in this repository
-| ├ models | Folder containing models used in different editing techniques and first phase inversion
-| ├ notebooks | Folder with jupyter notebooks to demonstrate the usage of PTI end-to-end
-| ├ scripts | Folder with running scripts for inversion, editing and metric computations
-| ├ torch_utils | Folder containing internal utils for StyleGAN2-ada
-| ├ training | Folder containing the core training logic of PTI
-| ├ utils | Folder with various utility functions
-
-
-## Credits
-**StyleGAN2-ada model and implementation:**
-https://github.com/NVlabs/stylegan2-ada-pytorch
-Copyright © 2021, NVIDIA Corporation.
-Nvidia Source Code License https://nvlabs.github.io/stylegan2-ada-pytorch/license.html
-
-**LPIPS model and implementation:**
-https://github.com/richzhang/PerceptualSimilarity
-Copyright (c) 2020, Sou Uchida
-License (BSD 2-Clause) https://github.com/richzhang/PerceptualSimilarity/blob/master/LICENSE
-
-**e4e model and implementation:**
-https://github.com/omertov/encoder4editing
-Copyright (c) 2021 omertov
-License (MIT) https://github.com/omertov/encoder4editing/blob/main/LICENSE
-
-**StyleCLIP model and implementation:**
-https://github.com/orpatashnik/StyleCLIP
-Copyright (c) 2021 orpatashnik
-License (MIT) https://github.com/orpatashnik/StyleCLIP/blob/main/LICENSE
-
-**InterfaceGAN implementation:**
-https://github.com/genforce/interfacegan
-Copyright (c) 2020 genforce
-License (MIT) https://github.com/genforce/interfacegan/blob/master/LICENSE
-
-**GANSpace implementation:**
-https://github.com/harskish/ganspace
-Copyright (c) 2020 harkish
-License (Apache License 2.0) https://github.com/harskish/ganspace/blob/master/LICENSE
-
-
-## Acknowledgments
-This repository structure is based on [encoder4editing](https://github.com/omertov/encoder4editing) and [ReStyle](https://github.com/yuval-alaluf/restyle-encoder) repositories
-
-## Contact
-For any inquiry please contact us at our email addresses: danielroich@gmail.com or ron.mokady@gmail.com
-
-
-## Citation
-If you use this code for your research, please cite:
-```
-@article{roich2021pivotal,
- title={Pivotal Tuning for Latent-based Editing of Real Images},
- author={Roich, Daniel and Mokady, Ron and Bermano, Amit H and Cohen-Or, Daniel},
- publisher = {Association for Computing Machinery},
- journal={ACM Trans. Graph.},
- year={2021}
-}
-```
diff --git a/spaces/Amrrs/DragGan-Inversion/PTI/models/StyleCLIP/global_directions/dnnlib/tflib/ops/upfirdn_2d.py b/spaces/Amrrs/DragGan-Inversion/PTI/models/StyleCLIP/global_directions/dnnlib/tflib/ops/upfirdn_2d.py
deleted file mode 100644
index 55a31af7e146da7afeb964db018f14aca3134920..0000000000000000000000000000000000000000
--- a/spaces/Amrrs/DragGan-Inversion/PTI/models/StyleCLIP/global_directions/dnnlib/tflib/ops/upfirdn_2d.py
+++ /dev/null
@@ -1,418 +0,0 @@
-# Copyright (c) 2020, NVIDIA CORPORATION. All rights reserved.
-#
-# NVIDIA CORPORATION and its licensors retain all intellectual property
-# and proprietary rights in and to this software, related documentation
-# and any modifications thereto. Any use, reproduction, disclosure or
-# distribution of this software and related documentation without an express
-# license agreement from NVIDIA CORPORATION is strictly prohibited.
-
-"""Custom TensorFlow ops for efficient resampling of 2D images."""
-
-import os
-import numpy as np
-import tensorflow as tf
-from .. import custom_ops
-
-def _get_plugin():
- return custom_ops.get_plugin(os.path.splitext(__file__)[0] + '.cu')
-
-#----------------------------------------------------------------------------
-
-def upfirdn_2d(x, k, upx=1, upy=1, downx=1, downy=1, padx0=0, padx1=0, pady0=0, pady1=0, impl='cuda'):
- r"""Pad, upsample, FIR filter, and downsample a batch of 2D images.
-
- Accepts a batch of 2D images of the shape `[majorDim, inH, inW, minorDim]`
- and performs the following operations for each image, batched across
- `majorDim` and `minorDim`:
-
- 1. Upsample the image by inserting the zeros after each pixel (`upx`, `upy`).
-
- 2. Pad the image with zeros by the specified number of pixels on each side
- (`padx0`, `padx1`, `pady0`, `pady1`). Specifying a negative value
- corresponds to cropping the image.
-
- 3. Convolve the image with the specified 2D FIR filter (`k`), shrinking the
- image so that the footprint of all output pixels lies within the input image.
-
- 4. Downsample the image by throwing away pixels (`downx`, `downy`).
-
- This sequence of operations bears close resemblance to scipy.signal.upfirdn().
- The fused op is considerably more efficient than performing the same calculation
- using standard TensorFlow ops. It supports gradients of arbitrary order.
-
- Args:
- x: Input tensor of the shape `[majorDim, inH, inW, minorDim]`.
- k: 2D FIR filter of the shape `[firH, firW]`.
- upx: Integer upsampling factor along the X-axis (default: 1).
- upy: Integer upsampling factor along the Y-axis (default: 1).
- downx: Integer downsampling factor along the X-axis (default: 1).
- downy: Integer downsampling factor along the Y-axis (default: 1).
- padx0: Number of pixels to pad on the left side (default: 0).
- padx1: Number of pixels to pad on the right side (default: 0).
- pady0: Number of pixels to pad on the top side (default: 0).
- pady1: Number of pixels to pad on the bottom side (default: 0).
- impl: Name of the implementation to use. Can be `"ref"` or `"cuda"` (default).
-
- Returns:
- Tensor of the shape `[majorDim, outH, outW, minorDim]`, and same datatype as `x`.
- """
-
- impl_dict = {
- 'ref': _upfirdn_2d_ref,
- 'cuda': _upfirdn_2d_cuda,
- }
- return impl_dict[impl](x=x, k=k, upx=upx, upy=upy, downx=downx, downy=downy, padx0=padx0, padx1=padx1, pady0=pady0, pady1=pady1)
-
-#----------------------------------------------------------------------------
-
-def _upfirdn_2d_ref(x, k, upx, upy, downx, downy, padx0, padx1, pady0, pady1):
- """Slow reference implementation of `upfirdn_2d()` using standard TensorFlow ops."""
-
- x = tf.convert_to_tensor(x)
- k = np.asarray(k, dtype=np.float32)
- assert x.shape.rank == 4
- inH = x.shape[1].value
- inW = x.shape[2].value
- minorDim = _shape(x, 3)
- kernelH, kernelW = k.shape
- assert inW >= 1 and inH >= 1
- assert kernelW >= 1 and kernelH >= 1
- assert isinstance(upx, int) and isinstance(upy, int)
- assert isinstance(downx, int) and isinstance(downy, int)
- assert isinstance(padx0, int) and isinstance(padx1, int)
- assert isinstance(pady0, int) and isinstance(pady1, int)
-
- # Upsample (insert zeros).
- x = tf.reshape(x, [-1, inH, 1, inW, 1, minorDim])
- x = tf.pad(x, [[0, 0], [0, 0], [0, upy - 1], [0, 0], [0, upx - 1], [0, 0]])
- x = tf.reshape(x, [-1, inH * upy, inW * upx, minorDim])
-
- # Pad (crop if negative).
- x = tf.pad(x, [[0, 0], [max(pady0, 0), max(pady1, 0)], [max(padx0, 0), max(padx1, 0)], [0, 0]])
- x = x[:, max(-pady0, 0) : x.shape[1].value - max(-pady1, 0), max(-padx0, 0) : x.shape[2].value - max(-padx1, 0), :]
-
- # Convolve with filter.
- x = tf.transpose(x, [0, 3, 1, 2])
- x = tf.reshape(x, [-1, 1, inH * upy + pady0 + pady1, inW * upx + padx0 + padx1])
- w = tf.constant(k[::-1, ::-1, np.newaxis, np.newaxis], dtype=x.dtype)
- x = tf.nn.conv2d(x, w, strides=[1,1,1,1], padding='VALID', data_format='NCHW')
- x = tf.reshape(x, [-1, minorDim, inH * upy + pady0 + pady1 - kernelH + 1, inW * upx + padx0 + padx1 - kernelW + 1])
- x = tf.transpose(x, [0, 2, 3, 1])
-
- # Downsample (throw away pixels).
- return x[:, ::downy, ::downx, :]
-
-#----------------------------------------------------------------------------
-
-def _upfirdn_2d_cuda(x, k, upx, upy, downx, downy, padx0, padx1, pady0, pady1):
- """Fast CUDA implementation of `upfirdn_2d()` using custom ops."""
-
- x = tf.convert_to_tensor(x)
- k = np.asarray(k, dtype=np.float32)
- majorDim, inH, inW, minorDim = x.shape.as_list()
- kernelH, kernelW = k.shape
- assert inW >= 1 and inH >= 1
- assert kernelW >= 1 and kernelH >= 1
- assert isinstance(upx, int) and isinstance(upy, int)
- assert isinstance(downx, int) and isinstance(downy, int)
- assert isinstance(padx0, int) and isinstance(padx1, int)
- assert isinstance(pady0, int) and isinstance(pady1, int)
-
- outW = (inW * upx + padx0 + padx1 - kernelW) // downx + 1
- outH = (inH * upy + pady0 + pady1 - kernelH) // downy + 1
- assert outW >= 1 and outH >= 1
-
- cuda_op = _get_plugin().up_fir_dn2d
- kc = tf.constant(k, dtype=x.dtype)
- gkc = tf.constant(k[::-1, ::-1], dtype=x.dtype)
- gpadx0 = kernelW - padx0 - 1
- gpady0 = kernelH - pady0 - 1
- gpadx1 = inW * upx - outW * downx + padx0 - upx + 1
- gpady1 = inH * upy - outH * downy + pady0 - upy + 1
-
- @tf.custom_gradient
- def func(x):
- y = cuda_op(x=x, k=kc, upx=int(upx), upy=int(upy), downx=int(downx), downy=int(downy), padx0=int(padx0), padx1=int(padx1), pady0=int(pady0), pady1=int(pady1))
- y.set_shape([majorDim, outH, outW, minorDim])
- @tf.custom_gradient
- def grad(dy):
- dx = cuda_op(x=dy, k=gkc, upx=int(downx), upy=int(downy), downx=int(upx), downy=int(upy), padx0=int(gpadx0), padx1=int(gpadx1), pady0=int(gpady0), pady1=int(gpady1))
- dx.set_shape([majorDim, inH, inW, minorDim])
- return dx, func
- return y, grad
- return func(x)
-
-#----------------------------------------------------------------------------
-
-def filter_2d(x, k, gain=1, padding=0, data_format='NCHW', impl='cuda'):
- r"""Filter a batch of 2D images with the given FIR filter.
-
- Accepts a batch of 2D images of the shape `[N, C, H, W]` or `[N, H, W, C]`
- and filters each image with the given filter. The filter is normalized so that
- if the input pixels are constant, they will be scaled by the specified `gain`.
- Pixels outside the image are assumed to be zero.
-
- Args:
- x: Input tensor of the shape `[N, C, H, W]` or `[N, H, W, C]`.
- k: FIR filter of the shape `[firH, firW]` or `[firN]` (separable).
- gain: Scaling factor for signal magnitude (default: 1.0).
- padding: Number of pixels to pad or crop the output on each side (default: 0).
- data_format: `'NCHW'` or `'NHWC'` (default: `'NCHW'`).
- impl: Name of the implementation to use. Can be `"ref"` or `"cuda"` (default).
-
- Returns:
- Tensor of the same shape and datatype as `x`.
- """
-
- assert isinstance(padding, int)
- k = _FilterKernel(k=k, gain=gain)
- assert k.w == k.h
- pad0 = k.w // 2 + padding
- pad1 = (k.w - 1) // 2 + padding
- return _simple_upfirdn_2d(x, k, pad0=pad0, pad1=pad1, data_format=data_format, impl=impl)
-
-#----------------------------------------------------------------------------
-
-def upsample_2d(x, k=None, factor=2, gain=1, padding=0, data_format='NCHW', impl='cuda'):
- r"""Upsample a batch of 2D images with the given filter.
-
- Accepts a batch of 2D images of the shape `[N, C, H, W]` or `[N, H, W, C]`
- and upsamples each image with the given filter. The filter is normalized so that
- if the input pixels are constant, they will be scaled by the specified `gain`.
- Pixels outside the image are assumed to be zero, and the filter is padded with
- zeros so that its shape is a multiple of the upsampling factor.
-
- Args:
- x: Input tensor of the shape `[N, C, H, W]` or `[N, H, W, C]`.
- k: FIR filter of the shape `[firH, firW]` or `[firN]` (separable).
- The default is `[1] * factor`, which corresponds to nearest-neighbor
- upsampling.
- factor: Integer upsampling factor (default: 2).
- gain: Scaling factor for signal magnitude (default: 1.0).
- padding: Number of pixels to pad or crop the output on each side (default: 0).
- data_format: `'NCHW'` or `'NHWC'` (default: `'NCHW'`).
- impl: Name of the implementation to use. Can be `"ref"` or `"cuda"` (default).
-
- Returns:
- Tensor of the shape `[N, C, H * factor, W * factor]` or
- `[N, H * factor, W * factor, C]`, and same datatype as `x`.
- """
-
- assert isinstance(factor, int) and factor >= 1
- assert isinstance(padding, int)
- k = _FilterKernel(k if k is not None else [1] * factor, gain * (factor ** 2))
- assert k.w == k.h
- pad0 = (k.w + factor - 1) // 2 + padding
- pad1 = (k.w - factor) // 2 + padding
- return _simple_upfirdn_2d(x, k, up=factor, pad0=pad0, pad1=pad1, data_format=data_format, impl=impl)
-
-#----------------------------------------------------------------------------
-
-def downsample_2d(x, k=None, factor=2, gain=1, padding=0, data_format='NCHW', impl='cuda'):
- r"""Downsample a batch of 2D images with the given filter.
-
- Accepts a batch of 2D images of the shape `[N, C, H, W]` or `[N, H, W, C]`
- and downsamples each image with the given filter. The filter is normalized so that
- if the input pixels are constant, they will be scaled by the specified `gain`.
- Pixels outside the image are assumed to be zero, and the filter is padded with
- zeros so that its shape is a multiple of the downsampling factor.
-
- Args:
- x: Input tensor of the shape `[N, C, H, W]` or `[N, H, W, C]`.
- k: FIR filter of the shape `[firH, firW]` or `[firN]` (separable).
- The default is `[1] * factor`, which corresponds to average pooling.
- factor: Integer downsampling factor (default: 2).
- gain: Scaling factor for signal magnitude (default: 1.0).
- padding: Number of pixels to pad or crop the output on each side (default: 0).
- data_format: `'NCHW'` or `'NHWC'` (default: `'NCHW'`).
- impl: Name of the implementation to use. Can be `"ref"` or `"cuda"` (default).
-
- Returns:
- Tensor of the shape `[N, C, H // factor, W // factor]` or
- `[N, H // factor, W // factor, C]`, and same datatype as `x`.
- """
-
- assert isinstance(factor, int) and factor >= 1
- assert isinstance(padding, int)
- k = _FilterKernel(k if k is not None else [1] * factor, gain)
- assert k.w == k.h
- pad0 = (k.w - factor + 1) // 2 + padding * factor
- pad1 = (k.w - factor) // 2 + padding * factor
- return _simple_upfirdn_2d(x, k, down=factor, pad0=pad0, pad1=pad1, data_format=data_format, impl=impl)
-
-#----------------------------------------------------------------------------
-
-def upsample_conv_2d(x, w, k=None, factor=2, gain=1, padding=0, data_format='NCHW', impl='cuda'):
- r"""Fused `upsample_2d()` followed by `tf.nn.conv2d()`.
-
- Padding is performed only once at the beginning, not between the operations.
- The fused op is considerably more efficient than performing the same calculation
- using standard TensorFlow ops. It supports gradients of arbitrary order.
-
- Args:
- x: Input tensor of the shape `[N, C, H, W]` or `[N, H, W, C]`.
- w: Weight tensor of the shape `[filterH, filterW, inChannels, outChannels]`.
- Grouped convolution can be performed by `inChannels = x.shape[0] // numGroups`.
- k: FIR filter of the shape `[firH, firW]` or `[firN]` (separable).
- The default is `[1] * factor`, which corresponds to nearest-neighbor
- upsampling.
- factor: Integer upsampling factor (default: 2).
- gain: Scaling factor for signal magnitude (default: 1.0).
- padding: Number of pixels to pad or crop the output on each side (default: 0).
- data_format: `'NCHW'` or `'NHWC'` (default: `'NCHW'`).
- impl: Name of the implementation to use. Can be `"ref"` or `"cuda"` (default).
-
- Returns:
- Tensor of the shape `[N, C, H * factor, W * factor]` or
- `[N, H * factor, W * factor, C]`, and same datatype as `x`.
- """
-
- assert isinstance(factor, int) and factor >= 1
- assert isinstance(padding, int)
-
- # Check weight shape.
- w = tf.convert_to_tensor(w)
- ch, cw, _inC, _outC = w.shape.as_list()
- inC = _shape(w, 2)
- outC = _shape(w, 3)
- assert cw == ch
-
- # Fast path for 1x1 convolution.
- if cw == 1 and ch == 1:
- x = tf.nn.conv2d(x, w, data_format=data_format, strides=[1,1,1,1], padding='VALID')
- x = upsample_2d(x, k, factor=factor, gain=gain, padding=padding, data_format=data_format, impl=impl)
- return x
-
- # Setup filter kernel.
- k = _FilterKernel(k if k is not None else [1] * factor, gain * (factor ** 2))
- assert k.w == k.h
-
- # Determine data dimensions.
- if data_format == 'NCHW':
- stride = [1, 1, factor, factor]
- output_shape = [_shape(x, 0), outC, (_shape(x, 2) - 1) * factor + ch, (_shape(x, 3) - 1) * factor + cw]
- num_groups = _shape(x, 1) // inC
- else:
- stride = [1, factor, factor, 1]
- output_shape = [_shape(x, 0), (_shape(x, 1) - 1) * factor + ch, (_shape(x, 2) - 1) * factor + cw, outC]
- num_groups = _shape(x, 3) // inC
-
- # Transpose weights.
- w = tf.reshape(w, [ch, cw, inC, num_groups, -1])
- w = tf.transpose(w[::-1, ::-1], [0, 1, 4, 3, 2])
- w = tf.reshape(w, [ch, cw, -1, num_groups * inC])
-
- # Execute.
- x = tf.nn.conv2d_transpose(x, w, output_shape=output_shape, strides=stride, padding='VALID', data_format=data_format)
- pad0 = (k.w + factor - cw) // 2 + padding
- pad1 = (k.w - factor - cw + 3) // 2 + padding
- return _simple_upfirdn_2d(x, k, pad0=pad0, pad1=pad1, data_format=data_format, impl=impl)
-
-#----------------------------------------------------------------------------
-
-def conv_downsample_2d(x, w, k=None, factor=2, gain=1, padding=0, data_format='NCHW', impl='cuda'):
- r"""Fused `tf.nn.conv2d()` followed by `downsample_2d()`.
-
- Padding is performed only once at the beginning, not between the operations.
- The fused op is considerably more efficient than performing the same calculation
- using standard TensorFlow ops. It supports gradients of arbitrary order.
-
- Args:
- x: Input tensor of the shape `[N, C, H, W]` or `[N, H, W, C]`.
- w: Weight tensor of the shape `[filterH, filterW, inChannels, outChannels]`.
- Grouped convolution can be performed by `inChannels = x.shape[0] // numGroups`.
- k: FIR filter of the shape `[firH, firW]` or `[firN]` (separable).
- The default is `[1] * factor`, which corresponds to average pooling.
- factor: Integer downsampling factor (default: 2).
- gain: Scaling factor for signal magnitude (default: 1.0).
- padding: Number of pixels to pad or crop the output on each side (default: 0).
- data_format: `'NCHW'` or `'NHWC'` (default: `'NCHW'`).
- impl: Name of the implementation to use. Can be `"ref"` or `"cuda"` (default).
-
- Returns:
- Tensor of the shape `[N, C, H // factor, W // factor]` or
- `[N, H // factor, W // factor, C]`, and same datatype as `x`.
- """
-
- assert isinstance(factor, int) and factor >= 1
- assert isinstance(padding, int)
-
- # Check weight shape.
- w = tf.convert_to_tensor(w)
- ch, cw, _inC, _outC = w.shape.as_list()
- assert cw == ch
-
- # Fast path for 1x1 convolution.
- if cw == 1 and ch == 1:
- x = downsample_2d(x, k, factor=factor, gain=gain, padding=padding, data_format=data_format, impl=impl)
- x = tf.nn.conv2d(x, w, data_format=data_format, strides=[1,1,1,1], padding='VALID')
- return x
-
- # Setup filter kernel.
- k = _FilterKernel(k if k is not None else [1] * factor, gain)
- assert k.w == k.h
-
- # Determine stride.
- if data_format == 'NCHW':
- s = [1, 1, factor, factor]
- else:
- s = [1, factor, factor, 1]
-
- # Execute.
- pad0 = (k.w - factor + cw) // 2 + padding * factor
- pad1 = (k.w - factor + cw - 1) // 2 + padding * factor
- x = _simple_upfirdn_2d(x, k, pad0=pad0, pad1=pad1, data_format=data_format, impl=impl)
- return tf.nn.conv2d(x, w, strides=s, padding='VALID', data_format=data_format)
-
-#----------------------------------------------------------------------------
-# Internal helpers.
-
-class _FilterKernel:
- def __init__(self, k, gain=1):
- k = np.asarray(k, dtype=np.float32)
- k /= np.sum(k)
-
- # Separable.
- if k.ndim == 1 and k.size >= 8:
- self.w = k.size
- self.h = k.size
- self.kx = k[np.newaxis, :]
- self.ky = k[:, np.newaxis] * gain
- self.kxy = None
-
- # Non-separable.
- else:
- if k.ndim == 1:
- k = np.outer(k, k)
- assert k.ndim == 2
- self.w = k.shape[1]
- self.h = k.shape[0]
- self.kx = None
- self.ky = None
- self.kxy = k * gain
-
-def _simple_upfirdn_2d(x, k, up=1, down=1, pad0=0, pad1=0, data_format='NCHW', impl='cuda'):
- assert isinstance(k, _FilterKernel)
- assert data_format in ['NCHW', 'NHWC']
- assert x.shape.rank == 4
- y = x
- if data_format == 'NCHW':
- y = tf.reshape(y, [-1, _shape(y, 2), _shape(y, 3), 1])
- if k.kx is not None:
- y = upfirdn_2d(y, k.kx, upx=up, downx=down, padx0=pad0, padx1=pad1, impl=impl)
- if k.ky is not None:
- y = upfirdn_2d(y, k.ky, upy=up, downy=down, pady0=pad0, pady1=pad1, impl=impl)
- if k.kxy is not None:
- y = upfirdn_2d(y, k.kxy, upx=up, upy=up, downx=down, downy=down, padx0=pad0, padx1=pad1, pady0=pad0, pady1=pad1, impl=impl)
- if data_format == 'NCHW':
- y = tf.reshape(y, [-1, _shape(x, 1), _shape(y, 1), _shape(y, 2)])
- return y
-
-def _shape(tf_expr, dim_idx):
- if tf_expr.shape.rank is not None:
- dim = tf_expr.shape[dim_idx].value
- if dim is not None:
- return dim
- return tf.shape(tf_expr)[dim_idx]
-
-#----------------------------------------------------------------------------
diff --git a/spaces/Amrrs/DragGan-Inversion/torch_utils/ops/filtered_lrelu.py b/spaces/Amrrs/DragGan-Inversion/torch_utils/ops/filtered_lrelu.py
deleted file mode 100644
index 1a77f7e7a0ed0e951435cf6c7171d1baac8cf834..0000000000000000000000000000000000000000
--- a/spaces/Amrrs/DragGan-Inversion/torch_utils/ops/filtered_lrelu.py
+++ /dev/null
@@ -1,307 +0,0 @@
-# Copyright (c) 2021, NVIDIA CORPORATION & AFFILIATES. All rights reserved.
-#
-# NVIDIA CORPORATION and its licensors retain all intellectual property
-# and proprietary rights in and to this software, related documentation
-# and any modifications thereto. Any use, reproduction, disclosure or
-# distribution of this software and related documentation without an express
-# license agreement from NVIDIA CORPORATION is strictly prohibited.
-
-import os
-import numpy as np
-import torch
-import warnings
-
-from .. import custom_ops
-from .. import misc
-from . import upfirdn2d
-from . import bias_act
-
-# ----------------------------------------------------------------------------
-
-_plugin = None
-
-
-def _init():
- global _plugin
- if _plugin is None:
- _plugin = custom_ops.get_plugin(
- module_name='filtered_lrelu_plugin',
- sources=['filtered_lrelu.cpp', 'filtered_lrelu_wr.cu',
- 'filtered_lrelu_rd.cu', 'filtered_lrelu_ns.cu'],
- headers=['filtered_lrelu.h', 'filtered_lrelu.cu'],
- source_dir=os.path.dirname(__file__),
- extra_cuda_cflags=['--use_fast_math',
- '--allow-unsupported-compiler'],
- )
- return True
-
-
-def _get_filter_size(f):
- if f is None:
- return 1, 1
- assert isinstance(f, torch.Tensor)
- assert 1 <= f.ndim <= 2
- return f.shape[-1], f.shape[0] # width, height
-
-
-def _parse_padding(padding):
- if isinstance(padding, int):
- padding = [padding, padding]
- assert isinstance(padding, (list, tuple))
- assert all(isinstance(x, (int, np.integer)) for x in padding)
- padding = [int(x) for x in padding]
- if len(padding) == 2:
- px, py = padding
- padding = [px, px, py, py]
- px0, px1, py0, py1 = padding
- return px0, px1, py0, py1
-
-# ----------------------------------------------------------------------------
-
-
-def filtered_lrelu(x, fu=None, fd=None, b=None, up=1, down=1, padding=0, gain=np.sqrt(2), slope=0.2, clamp=None, flip_filter=False, impl='cuda'):
- r"""Filtered leaky ReLU for a batch of 2D images.
-
- Performs the following sequence of operations for each channel:
-
- 1. Add channel-specific bias if provided (`b`).
-
- 2. Upsample the image by inserting N-1 zeros after each pixel (`up`).
-
- 3. Pad the image with the specified number of zeros on each side (`padding`).
- Negative padding corresponds to cropping the image.
-
- 4. Convolve the image with the specified upsampling FIR filter (`fu`), shrinking it
- so that the footprint of all output pixels lies within the input image.
-
- 5. Multiply each value by the provided gain factor (`gain`).
-
- 6. Apply leaky ReLU activation function to each value.
-
- 7. Clamp each value between -clamp and +clamp, if `clamp` parameter is provided.
-
- 8. Convolve the image with the specified downsampling FIR filter (`fd`), shrinking
- it so that the footprint of all output pixels lies within the input image.
-
- 9. Downsample the image by keeping every Nth pixel (`down`).
-
- The fused op is considerably more efficient than performing the same calculation
- using standard PyTorch ops. It supports gradients of arbitrary order.
-
- Args:
- x: Float32/float16/float64 input tensor of the shape
- `[batch_size, num_channels, in_height, in_width]`.
- fu: Float32 upsampling FIR filter of the shape
- `[filter_height, filter_width]` (non-separable),
- `[filter_taps]` (separable), or
- `None` (identity).
- fd: Float32 downsampling FIR filter of the shape
- `[filter_height, filter_width]` (non-separable),
- `[filter_taps]` (separable), or
- `None` (identity).
- b: Bias vector, or `None` to disable. Must be a 1D tensor of the same type
- as `x`. The length of vector must must match the channel dimension of `x`.
- up: Integer upsampling factor (default: 1).
- down: Integer downsampling factor. (default: 1).
- padding: Padding with respect to the upsampled image. Can be a single number
- or a list/tuple `[x, y]` or `[x_before, x_after, y_before, y_after]`
- (default: 0).
- gain: Overall scaling factor for signal magnitude (default: sqrt(2)).
- slope: Slope on the negative side of leaky ReLU (default: 0.2).
- clamp: Maximum magnitude for leaky ReLU output (default: None).
- flip_filter: False = convolution, True = correlation (default: False).
- impl: Implementation to use. Can be `'ref'` or `'cuda'` (default: `'cuda'`).
-
- Returns:
- Tensor of the shape `[batch_size, num_channels, out_height, out_width]`.
- """
- assert isinstance(x, torch.Tensor)
- assert impl in ['ref', 'cuda']
- if impl == 'cuda' and x.device.type == 'cuda' and _init():
- return _filtered_lrelu_cuda(up=up, down=down, padding=padding, gain=gain, slope=slope, clamp=clamp, flip_filter=flip_filter).apply(x, fu, fd, b, None, 0, 0)
- return _filtered_lrelu_ref(x, fu=fu, fd=fd, b=b, up=up, down=down, padding=padding, gain=gain, slope=slope, clamp=clamp, flip_filter=flip_filter)
-
-# ----------------------------------------------------------------------------
-
-
-@misc.profiled_function
-def _filtered_lrelu_ref(x, fu=None, fd=None, b=None, up=1, down=1, padding=0, gain=np.sqrt(2), slope=0.2, clamp=None, flip_filter=False):
- """Slow and memory-inefficient reference implementation of `filtered_lrelu()` using
- existing `upfirdn2n()` and `bias_act()` ops.
- """
- assert isinstance(x, torch.Tensor) and x.ndim == 4
- fu_w, fu_h = _get_filter_size(fu)
- fd_w, fd_h = _get_filter_size(fd)
- if b is not None:
- assert isinstance(b, torch.Tensor) and b.dtype == x.dtype
- misc.assert_shape(b, [x.shape[1]])
- assert isinstance(up, int) and up >= 1
- assert isinstance(down, int) and down >= 1
- px0, px1, py0, py1 = _parse_padding(padding)
- assert gain == float(gain) and gain > 0
- assert slope == float(slope) and slope >= 0
- assert clamp is None or (clamp == float(clamp) and clamp >= 0)
-
- # Calculate output size.
- batch_size, channels, in_h, in_w = x.shape
- in_dtype = x.dtype
- out_w = (in_w * up + (px0 + px1) - (fu_w - 1) -
- (fd_w - 1) + (down - 1)) // down
- out_h = (in_h * up + (py0 + py1) - (fu_h - 1) -
- (fd_h - 1) + (down - 1)) // down
-
- # Compute using existing ops.
- x = bias_act.bias_act(x=x, b=b) # Apply bias.
- # Upsample.
- x = upfirdn2d.upfirdn2d(x=x, f=fu, up=up, padding=[
- px0, px1, py0, py1], gain=up**2, flip_filter=flip_filter)
- # Bias, leaky ReLU, clamp.
- x = bias_act.bias_act(x=x, act='lrelu', alpha=slope,
- gain=gain, clamp=clamp)
- # Downsample.
- x = upfirdn2d.upfirdn2d(x=x, f=fd, down=down, flip_filter=flip_filter)
-
- # Check output shape & dtype.
- misc.assert_shape(x, [batch_size, channels, out_h, out_w])
- assert x.dtype == in_dtype
- return x
-
-# ----------------------------------------------------------------------------
-
-
-_filtered_lrelu_cuda_cache = dict()
-
-
-def _filtered_lrelu_cuda(up=1, down=1, padding=0, gain=np.sqrt(2), slope=0.2, clamp=None, flip_filter=False):
- """Fast CUDA implementation of `filtered_lrelu()` using custom ops.
- """
- assert isinstance(up, int) and up >= 1
- assert isinstance(down, int) and down >= 1
- px0, px1, py0, py1 = _parse_padding(padding)
- assert gain == float(gain) and gain > 0
- gain = float(gain)
- assert slope == float(slope) and slope >= 0
- slope = float(slope)
- assert clamp is None or (clamp == float(clamp) and clamp >= 0)
- clamp = float(clamp if clamp is not None else 'inf')
-
- # Lookup from cache.
- key = (up, down, px0, px1, py0, py1, gain, slope, clamp, flip_filter)
- if key in _filtered_lrelu_cuda_cache:
- return _filtered_lrelu_cuda_cache[key]
-
- # Forward op.
- class FilteredLReluCuda(torch.autograd.Function):
- @staticmethod
- def forward(ctx, x, fu, fd, b, si, sx, sy): # pylint: disable=arguments-differ
- assert isinstance(x, torch.Tensor) and x.ndim == 4
-
- # Replace empty up/downsample kernels with full 1x1 kernels (faster than separable).
- if fu is None:
- fu = torch.ones([1, 1], dtype=torch.float32, device=x.device)
- if fd is None:
- fd = torch.ones([1, 1], dtype=torch.float32, device=x.device)
- assert 1 <= fu.ndim <= 2
- assert 1 <= fd.ndim <= 2
-
- # Replace separable 1x1 kernels with full 1x1 kernels when scale factor is 1.
- if up == 1 and fu.ndim == 1 and fu.shape[0] == 1:
- fu = fu.square()[None]
- if down == 1 and fd.ndim == 1 and fd.shape[0] == 1:
- fd = fd.square()[None]
-
- # Missing sign input tensor.
- if si is None:
- si = torch.empty([0])
-
- # Missing bias tensor.
- if b is None:
- b = torch.zeros([x.shape[1]], dtype=x.dtype, device=x.device)
-
- # Construct internal sign tensor only if gradients are needed.
- write_signs = (si.numel() == 0) and (
- x.requires_grad or b.requires_grad)
-
- # Warn if input storage strides are not in decreasing order due to e.g. channels-last layout.
- strides = [x.stride(i) for i in range(x.ndim) if x.size(i) > 1]
- if any(a < b for a, b in zip(strides[:-1], strides[1:])):
- warnings.warn(
- "low-performance memory layout detected in filtered_lrelu input", RuntimeWarning)
-
- # Call C++/Cuda plugin if datatype is supported.
- if x.dtype in [torch.float16, torch.float32]:
- if torch.cuda.current_stream(x.device) != torch.cuda.default_stream(x.device):
- warnings.warn(
- "filtered_lrelu called with non-default cuda stream but concurrent execution is not supported", RuntimeWarning)
- y, so, return_code = _plugin.filtered_lrelu(
- x, fu, fd, b, si, up, down, px0, px1, py0, py1, sx, sy, gain, slope, clamp, flip_filter, write_signs)
- else:
- return_code = -1
-
- # No Cuda kernel found? Fall back to generic implementation. Still more memory efficient than the reference implementation because
- # only the bit-packed sign tensor is retained for gradient computation.
- if return_code < 0:
- warnings.warn(
- "filtered_lrelu called with parameters that have no optimized CUDA kernel, using generic fallback", RuntimeWarning)
-
- y = x.add(b.unsqueeze(-1).unsqueeze(-1)) # Add bias.
- # Upsample.
- y = upfirdn2d.upfirdn2d(x=y, f=fu, up=up, padding=[
- px0, px1, py0, py1], gain=up**2, flip_filter=flip_filter)
- # Activation function and sign handling. Modifies y in-place.
- so = _plugin.filtered_lrelu_act_(
- y, si, sx, sy, gain, slope, clamp, write_signs)
- # Downsample.
- y = upfirdn2d.upfirdn2d(
- x=y, f=fd, down=down, flip_filter=flip_filter)
-
- # Prepare for gradient computation.
- ctx.save_for_backward(fu, fd, (si if si.numel() else so))
- ctx.x_shape = x.shape
- ctx.y_shape = y.shape
- ctx.s_ofs = sx, sy
- return y
-
- @staticmethod
- def backward(ctx, dy): # pylint: disable=arguments-differ
- fu, fd, si = ctx.saved_tensors
- _, _, xh, xw = ctx.x_shape
- _, _, yh, yw = ctx.y_shape
- sx, sy = ctx.s_ofs
- dx = None # 0
- dfu = None
- assert not ctx.needs_input_grad[1]
- dfd = None
- assert not ctx.needs_input_grad[2]
- db = None # 3
- dsi = None
- assert not ctx.needs_input_grad[4]
- dsx = None
- assert not ctx.needs_input_grad[5]
- dsy = None
- assert not ctx.needs_input_grad[6]
-
- if ctx.needs_input_grad[0] or ctx.needs_input_grad[3]:
- pp = [
- (fu.shape[-1] - 1) + (fd.shape[-1] - 1) - px0,
- xw * up - yw * down + px0 - (up - 1),
- (fu.shape[0] - 1) + (fd.shape[0] - 1) - py0,
- xh * up - yh * down + py0 - (up - 1),
- ]
- gg = gain * (up ** 2) / (down ** 2)
- ff = (not flip_filter)
- sx = sx - (fu.shape[-1] - 1) + px0
- sy = sy - (fu.shape[0] - 1) + py0
- dx = _filtered_lrelu_cuda(up=down, down=up, padding=pp, gain=gg, slope=slope,
- clamp=None, flip_filter=ff).apply(dy, fd, fu, None, si, sx, sy)
-
- if ctx.needs_input_grad[3]:
- db = dx.sum([0, 2, 3])
-
- return dx, dfu, dfd, db, dsi, dsx, dsy
-
- # Add to cache.
- _filtered_lrelu_cuda_cache[key] = FilteredLReluCuda
- return FilteredLReluCuda
-
-# ----------------------------------------------------------------------------
diff --git a/spaces/Anonymous-123/ImageNet-Editing/object_removal/TFill/model/stylegan_ops/fused_act.py b/spaces/Anonymous-123/ImageNet-Editing/object_removal/TFill/model/stylegan_ops/fused_act.py
deleted file mode 100644
index 973a84fffde53668d31397da5fb993bbc95f7be0..0000000000000000000000000000000000000000
--- a/spaces/Anonymous-123/ImageNet-Editing/object_removal/TFill/model/stylegan_ops/fused_act.py
+++ /dev/null
@@ -1,85 +0,0 @@
-import os
-
-import torch
-from torch import nn
-from torch.autograd import Function
-from torch.utils.cpp_extension import load
-
-module_path = os.path.dirname(__file__)
-fused = load(
- 'fused',
- sources=[
- os.path.join(module_path, 'fused_bias_act.cpp'),
- os.path.join(module_path, 'fused_bias_act_kernel.cu'),
- ],
-)
-
-
-class FusedLeakyReLUFunctionBackward(Function):
- @staticmethod
- def forward(ctx, grad_output, out, negative_slope, scale):
- ctx.save_for_backward(out)
- ctx.negative_slope = negative_slope
- ctx.scale = scale
-
- empty = grad_output.new_empty(0)
-
- grad_input = fused.fused_bias_act(
- grad_output, empty, out, 3, 1, negative_slope, scale
- )
-
- dim = [0]
-
- if grad_input.ndim > 2:
- dim += list(range(2, grad_input.ndim))
-
- grad_bias = grad_input.sum(dim).detach()
-
- return grad_input, grad_bias
-
- @staticmethod
- def backward(ctx, gradgrad_input, gradgrad_bias):
- out, = ctx.saved_tensors
- gradgrad_out = fused.fused_bias_act(
- gradgrad_input, gradgrad_bias, out, 3, 1, ctx.negative_slope, ctx.scale
- )
-
- return gradgrad_out, None, None, None
-
-
-class FusedLeakyReLUFunction(Function):
- @staticmethod
- def forward(ctx, input, bias, negative_slope, scale):
- empty = input.new_empty(0)
- out = fused.fused_bias_act(input, bias, empty, 3, 0, negative_slope, scale)
- ctx.save_for_backward(out)
- ctx.negative_slope = negative_slope
- ctx.scale = scale
-
- return out
-
- @staticmethod
- def backward(ctx, grad_output):
- out, = ctx.saved_tensors
-
- grad_input, grad_bias = FusedLeakyReLUFunctionBackward.apply(
- grad_output, out, ctx.negative_slope, ctx.scale
- )
-
- return grad_input, grad_bias, None, None
-
-
-class FusedLeakyReLU(nn.Module):
- def __init__(self, channel, negative_slope=0.2, scale=2 ** 0.5):
- super().__init__()
-
- self.bias = nn.Parameter(torch.zeros(channel))
- self.negative_slope = negative_slope
- self.scale = scale
-
- def forward(self, input):
- return fused_leaky_relu(input, self.bias, self.negative_slope, self.scale)
-
-
-def fused_leaky_relu(input, bias, negative_slope=0.2, scale=2 ** 0.5):
- return FusedLeakyReLUFunction.apply(input, bias, negative_slope, scale)
diff --git a/spaces/Anonymous-sub/Rerender/ControlNet/annotator/openpose/body.py b/spaces/Anonymous-sub/Rerender/ControlNet/annotator/openpose/body.py
deleted file mode 100644
index 7c3cf7a388b4ac81004524e64125e383bdd455bd..0000000000000000000000000000000000000000
--- a/spaces/Anonymous-sub/Rerender/ControlNet/annotator/openpose/body.py
+++ /dev/null
@@ -1,219 +0,0 @@
-import cv2
-import numpy as np
-import math
-import time
-from scipy.ndimage.filters import gaussian_filter
-import matplotlib.pyplot as plt
-import matplotlib
-import torch
-from torchvision import transforms
-
-from . import util
-from .model import bodypose_model
-
-class Body(object):
- def __init__(self, model_path):
- self.model = bodypose_model()
- if torch.cuda.is_available():
- self.model = self.model.cuda()
- print('cuda')
- model_dict = util.transfer(self.model, torch.load(model_path))
- self.model.load_state_dict(model_dict)
- self.model.eval()
-
- def __call__(self, oriImg):
- # scale_search = [0.5, 1.0, 1.5, 2.0]
- scale_search = [0.5]
- boxsize = 368
- stride = 8
- padValue = 128
- thre1 = 0.1
- thre2 = 0.05
- multiplier = [x * boxsize / oriImg.shape[0] for x in scale_search]
- heatmap_avg = np.zeros((oriImg.shape[0], oriImg.shape[1], 19))
- paf_avg = np.zeros((oriImg.shape[0], oriImg.shape[1], 38))
-
- for m in range(len(multiplier)):
- scale = multiplier[m]
- imageToTest = cv2.resize(oriImg, (0, 0), fx=scale, fy=scale, interpolation=cv2.INTER_CUBIC)
- imageToTest_padded, pad = util.padRightDownCorner(imageToTest, stride, padValue)
- im = np.transpose(np.float32(imageToTest_padded[:, :, :, np.newaxis]), (3, 2, 0, 1)) / 256 - 0.5
- im = np.ascontiguousarray(im)
-
- data = torch.from_numpy(im).float()
- if torch.cuda.is_available():
- data = data.cuda()
- # data = data.permute([2, 0, 1]).unsqueeze(0).float()
- with torch.no_grad():
- Mconv7_stage6_L1, Mconv7_stage6_L2 = self.model(data)
- Mconv7_stage6_L1 = Mconv7_stage6_L1.cpu().numpy()
- Mconv7_stage6_L2 = Mconv7_stage6_L2.cpu().numpy()
-
- # extract outputs, resize, and remove padding
- # heatmap = np.transpose(np.squeeze(net.blobs[output_blobs.keys()[1]].data), (1, 2, 0)) # output 1 is heatmaps
- heatmap = np.transpose(np.squeeze(Mconv7_stage6_L2), (1, 2, 0)) # output 1 is heatmaps
- heatmap = cv2.resize(heatmap, (0, 0), fx=stride, fy=stride, interpolation=cv2.INTER_CUBIC)
- heatmap = heatmap[:imageToTest_padded.shape[0] - pad[2], :imageToTest_padded.shape[1] - pad[3], :]
- heatmap = cv2.resize(heatmap, (oriImg.shape[1], oriImg.shape[0]), interpolation=cv2.INTER_CUBIC)
-
- # paf = np.transpose(np.squeeze(net.blobs[output_blobs.keys()[0]].data), (1, 2, 0)) # output 0 is PAFs
- paf = np.transpose(np.squeeze(Mconv7_stage6_L1), (1, 2, 0)) # output 0 is PAFs
- paf = cv2.resize(paf, (0, 0), fx=stride, fy=stride, interpolation=cv2.INTER_CUBIC)
- paf = paf[:imageToTest_padded.shape[0] - pad[2], :imageToTest_padded.shape[1] - pad[3], :]
- paf = cv2.resize(paf, (oriImg.shape[1], oriImg.shape[0]), interpolation=cv2.INTER_CUBIC)
-
- heatmap_avg += heatmap_avg + heatmap / len(multiplier)
- paf_avg += + paf / len(multiplier)
-
- all_peaks = []
- peak_counter = 0
-
- for part in range(18):
- map_ori = heatmap_avg[:, :, part]
- one_heatmap = gaussian_filter(map_ori, sigma=3)
-
- map_left = np.zeros(one_heatmap.shape)
- map_left[1:, :] = one_heatmap[:-1, :]
- map_right = np.zeros(one_heatmap.shape)
- map_right[:-1, :] = one_heatmap[1:, :]
- map_up = np.zeros(one_heatmap.shape)
- map_up[:, 1:] = one_heatmap[:, :-1]
- map_down = np.zeros(one_heatmap.shape)
- map_down[:, :-1] = one_heatmap[:, 1:]
-
- peaks_binary = np.logical_and.reduce(
- (one_heatmap >= map_left, one_heatmap >= map_right, one_heatmap >= map_up, one_heatmap >= map_down, one_heatmap > thre1))
- peaks = list(zip(np.nonzero(peaks_binary)[1], np.nonzero(peaks_binary)[0])) # note reverse
- peaks_with_score = [x + (map_ori[x[1], x[0]],) for x in peaks]
- peak_id = range(peak_counter, peak_counter + len(peaks))
- peaks_with_score_and_id = [peaks_with_score[i] + (peak_id[i],) for i in range(len(peak_id))]
-
- all_peaks.append(peaks_with_score_and_id)
- peak_counter += len(peaks)
-
- # find connection in the specified sequence, center 29 is in the position 15
- limbSeq = [[2, 3], [2, 6], [3, 4], [4, 5], [6, 7], [7, 8], [2, 9], [9, 10], \
- [10, 11], [2, 12], [12, 13], [13, 14], [2, 1], [1, 15], [15, 17], \
- [1, 16], [16, 18], [3, 17], [6, 18]]
- # the middle joints heatmap correpondence
- mapIdx = [[31, 32], [39, 40], [33, 34], [35, 36], [41, 42], [43, 44], [19, 20], [21, 22], \
- [23, 24], [25, 26], [27, 28], [29, 30], [47, 48], [49, 50], [53, 54], [51, 52], \
- [55, 56], [37, 38], [45, 46]]
-
- connection_all = []
- special_k = []
- mid_num = 10
-
- for k in range(len(mapIdx)):
- score_mid = paf_avg[:, :, [x - 19 for x in mapIdx[k]]]
- candA = all_peaks[limbSeq[k][0] - 1]
- candB = all_peaks[limbSeq[k][1] - 1]
- nA = len(candA)
- nB = len(candB)
- indexA, indexB = limbSeq[k]
- if (nA != 0 and nB != 0):
- connection_candidate = []
- for i in range(nA):
- for j in range(nB):
- vec = np.subtract(candB[j][:2], candA[i][:2])
- norm = math.sqrt(vec[0] * vec[0] + vec[1] * vec[1])
- norm = max(0.001, norm)
- vec = np.divide(vec, norm)
-
- startend = list(zip(np.linspace(candA[i][0], candB[j][0], num=mid_num), \
- np.linspace(candA[i][1], candB[j][1], num=mid_num)))
-
- vec_x = np.array([score_mid[int(round(startend[I][1])), int(round(startend[I][0])), 0] \
- for I in range(len(startend))])
- vec_y = np.array([score_mid[int(round(startend[I][1])), int(round(startend[I][0])), 1] \
- for I in range(len(startend))])
-
- score_midpts = np.multiply(vec_x, vec[0]) + np.multiply(vec_y, vec[1])
- score_with_dist_prior = sum(score_midpts) / len(score_midpts) + min(
- 0.5 * oriImg.shape[0] / norm - 1, 0)
- criterion1 = len(np.nonzero(score_midpts > thre2)[0]) > 0.8 * len(score_midpts)
- criterion2 = score_with_dist_prior > 0
- if criterion1 and criterion2:
- connection_candidate.append(
- [i, j, score_with_dist_prior, score_with_dist_prior + candA[i][2] + candB[j][2]])
-
- connection_candidate = sorted(connection_candidate, key=lambda x: x[2], reverse=True)
- connection = np.zeros((0, 5))
- for c in range(len(connection_candidate)):
- i, j, s = connection_candidate[c][0:3]
- if (i not in connection[:, 3] and j not in connection[:, 4]):
- connection = np.vstack([connection, [candA[i][3], candB[j][3], s, i, j]])
- if (len(connection) >= min(nA, nB)):
- break
-
- connection_all.append(connection)
- else:
- special_k.append(k)
- connection_all.append([])
-
- # last number in each row is the total parts number of that person
- # the second last number in each row is the score of the overall configuration
- subset = -1 * np.ones((0, 20))
- candidate = np.array([item for sublist in all_peaks for item in sublist])
-
- for k in range(len(mapIdx)):
- if k not in special_k:
- partAs = connection_all[k][:, 0]
- partBs = connection_all[k][:, 1]
- indexA, indexB = np.array(limbSeq[k]) - 1
-
- for i in range(len(connection_all[k])): # = 1:size(temp,1)
- found = 0
- subset_idx = [-1, -1]
- for j in range(len(subset)): # 1:size(subset,1):
- if subset[j][indexA] == partAs[i] or subset[j][indexB] == partBs[i]:
- subset_idx[found] = j
- found += 1
-
- if found == 1:
- j = subset_idx[0]
- if subset[j][indexB] != partBs[i]:
- subset[j][indexB] = partBs[i]
- subset[j][-1] += 1
- subset[j][-2] += candidate[partBs[i].astype(int), 2] + connection_all[k][i][2]
- elif found == 2: # if found 2 and disjoint, merge them
- j1, j2 = subset_idx
- membership = ((subset[j1] >= 0).astype(int) + (subset[j2] >= 0).astype(int))[:-2]
- if len(np.nonzero(membership == 2)[0]) == 0: # merge
- subset[j1][:-2] += (subset[j2][:-2] + 1)
- subset[j1][-2:] += subset[j2][-2:]
- subset[j1][-2] += connection_all[k][i][2]
- subset = np.delete(subset, j2, 0)
- else: # as like found == 1
- subset[j1][indexB] = partBs[i]
- subset[j1][-1] += 1
- subset[j1][-2] += candidate[partBs[i].astype(int), 2] + connection_all[k][i][2]
-
- # if find no partA in the subset, create a new subset
- elif not found and k < 17:
- row = -1 * np.ones(20)
- row[indexA] = partAs[i]
- row[indexB] = partBs[i]
- row[-1] = 2
- row[-2] = sum(candidate[connection_all[k][i, :2].astype(int), 2]) + connection_all[k][i][2]
- subset = np.vstack([subset, row])
- # delete some rows of subset which has few parts occur
- deleteIdx = []
- for i in range(len(subset)):
- if subset[i][-1] < 4 or subset[i][-2] / subset[i][-1] < 0.4:
- deleteIdx.append(i)
- subset = np.delete(subset, deleteIdx, axis=0)
-
- # subset: n*20 array, 0-17 is the index in candidate, 18 is the total score, 19 is the total parts
- # candidate: x, y, score, id
- return candidate, subset
-
-if __name__ == "__main__":
- body_estimation = Body('../model/body_pose_model.pth')
-
- test_image = '../images/ski.jpg'
- oriImg = cv2.imread(test_image) # B,G,R order
- candidate, subset = body_estimation(oriImg)
- canvas = util.draw_bodypose(oriImg, candidate, subset)
- plt.imshow(canvas[:, :, [2, 1, 0]])
- plt.show()
diff --git a/spaces/Anonymous-sub/Rerender/ControlNet/annotator/uniformer/mmcv/ops/iou3d.py b/spaces/Anonymous-sub/Rerender/ControlNet/annotator/uniformer/mmcv/ops/iou3d.py
deleted file mode 100644
index 6fc71979190323f44c09f8b7e1761cf49cd2d76b..0000000000000000000000000000000000000000
--- a/spaces/Anonymous-sub/Rerender/ControlNet/annotator/uniformer/mmcv/ops/iou3d.py
+++ /dev/null
@@ -1,85 +0,0 @@
-# Copyright (c) OpenMMLab. All rights reserved.
-import torch
-
-from ..utils import ext_loader
-
-ext_module = ext_loader.load_ext('_ext', [
- 'iou3d_boxes_iou_bev_forward', 'iou3d_nms_forward',
- 'iou3d_nms_normal_forward'
-])
-
-
-def boxes_iou_bev(boxes_a, boxes_b):
- """Calculate boxes IoU in the Bird's Eye View.
-
- Args:
- boxes_a (torch.Tensor): Input boxes a with shape (M, 5).
- boxes_b (torch.Tensor): Input boxes b with shape (N, 5).
-
- Returns:
- ans_iou (torch.Tensor): IoU result with shape (M, N).
- """
- ans_iou = boxes_a.new_zeros(
- torch.Size((boxes_a.shape[0], boxes_b.shape[0])))
-
- ext_module.iou3d_boxes_iou_bev_forward(boxes_a.contiguous(),
- boxes_b.contiguous(), ans_iou)
-
- return ans_iou
-
-
-def nms_bev(boxes, scores, thresh, pre_max_size=None, post_max_size=None):
- """NMS function GPU implementation (for BEV boxes). The overlap of two
- boxes for IoU calculation is defined as the exact overlapping area of the
- two boxes. In this function, one can also set ``pre_max_size`` and
- ``post_max_size``.
-
- Args:
- boxes (torch.Tensor): Input boxes with the shape of [N, 5]
- ([x1, y1, x2, y2, ry]).
- scores (torch.Tensor): Scores of boxes with the shape of [N].
- thresh (float): Overlap threshold of NMS.
- pre_max_size (int, optional): Max size of boxes before NMS.
- Default: None.
- post_max_size (int, optional): Max size of boxes after NMS.
- Default: None.
-
- Returns:
- torch.Tensor: Indexes after NMS.
- """
- assert boxes.size(1) == 5, 'Input boxes shape should be [N, 5]'
- order = scores.sort(0, descending=True)[1]
-
- if pre_max_size is not None:
- order = order[:pre_max_size]
- boxes = boxes[order].contiguous()
-
- keep = torch.zeros(boxes.size(0), dtype=torch.long)
- num_out = ext_module.iou3d_nms_forward(boxes, keep, thresh)
- keep = order[keep[:num_out].cuda(boxes.device)].contiguous()
- if post_max_size is not None:
- keep = keep[:post_max_size]
- return keep
-
-
-def nms_normal_bev(boxes, scores, thresh):
- """Normal NMS function GPU implementation (for BEV boxes). The overlap of
- two boxes for IoU calculation is defined as the exact overlapping area of
- the two boxes WITH their yaw angle set to 0.
-
- Args:
- boxes (torch.Tensor): Input boxes with shape (N, 5).
- scores (torch.Tensor): Scores of predicted boxes with shape (N).
- thresh (float): Overlap threshold of NMS.
-
- Returns:
- torch.Tensor: Remaining indices with scores in descending order.
- """
- assert boxes.shape[1] == 5, 'Input boxes shape should be [N, 5]'
- order = scores.sort(0, descending=True)[1]
-
- boxes = boxes[order].contiguous()
-
- keep = torch.zeros(boxes.size(0), dtype=torch.long)
- num_out = ext_module.iou3d_nms_normal_forward(boxes, keep, thresh)
- return order[keep[:num_out].cuda(boxes.device)].contiguous()
diff --git a/spaces/Anonymous-sub/Rerender/ControlNet/annotator/uniformer/mmcv/runner/hooks/logger/dvclive.py b/spaces/Anonymous-sub/Rerender/ControlNet/annotator/uniformer/mmcv/runner/hooks/logger/dvclive.py
deleted file mode 100644
index 687cdc58c0336c92b1e4f9a410ba67ebaab2bc7a..0000000000000000000000000000000000000000
--- a/spaces/Anonymous-sub/Rerender/ControlNet/annotator/uniformer/mmcv/runner/hooks/logger/dvclive.py
+++ /dev/null
@@ -1,58 +0,0 @@
-# Copyright (c) OpenMMLab. All rights reserved.
-from ...dist_utils import master_only
-from ..hook import HOOKS
-from .base import LoggerHook
-
-
-@HOOKS.register_module()
-class DvcliveLoggerHook(LoggerHook):
- """Class to log metrics with dvclive.
-
- It requires `dvclive`_ to be installed.
-
- Args:
- path (str): Directory where dvclive will write TSV log files.
- interval (int): Logging interval (every k iterations).
- Default 10.
- ignore_last (bool): Ignore the log of last iterations in each epoch
- if less than `interval`.
- Default: True.
- reset_flag (bool): Whether to clear the output buffer after logging.
- Default: True.
- by_epoch (bool): Whether EpochBasedRunner is used.
- Default: True.
-
- .. _dvclive:
- https://dvc.org/doc/dvclive
- """
-
- def __init__(self,
- path,
- interval=10,
- ignore_last=True,
- reset_flag=True,
- by_epoch=True):
-
- super(DvcliveLoggerHook, self).__init__(interval, ignore_last,
- reset_flag, by_epoch)
- self.path = path
- self.import_dvclive()
-
- def import_dvclive(self):
- try:
- import dvclive
- except ImportError:
- raise ImportError(
- 'Please run "pip install dvclive" to install dvclive')
- self.dvclive = dvclive
-
- @master_only
- def before_run(self, runner):
- self.dvclive.init(self.path)
-
- @master_only
- def log(self, runner):
- tags = self.get_loggable_tags(runner)
- if tags:
- for k, v in tags.items():
- self.dvclive.log(k, v, step=self.get_iter(runner))
diff --git a/spaces/AquaSuisei/ChatGPTXE/README.md b/spaces/AquaSuisei/ChatGPTXE/README.md
deleted file mode 100644
index 725a0fbb2151bb743de9c82948d2e850f66b7f4c..0000000000000000000000000000000000000000
--- a/spaces/AquaSuisei/ChatGPTXE/README.md
+++ /dev/null
@@ -1,14 +0,0 @@
----
-title: ChuanhuChatGPT
-emoji: 🐯
-colorFrom: green
-colorTo: red
-sdk: gradio
-sdk_version: 3.24.1
-app_file: ChuanhuChatbot.py
-pinned: false
-license: gpl-3.0
-duplicated_from: JohnSmith9982/ChuanhuChatGPT
----
-
-Check out the configuration reference at https://huggingface.co/docs/hub/spaces-config-reference
\ No newline at end of file
diff --git a/spaces/ArchitSharma/Digital-Photo-Color-Restoration/src/deoldify/_device.py b/spaces/ArchitSharma/Digital-Photo-Color-Restoration/src/deoldify/_device.py
deleted file mode 100644
index ed40ce131e3375a937c862fafa44e432f825f93b..0000000000000000000000000000000000000000
--- a/spaces/ArchitSharma/Digital-Photo-Color-Restoration/src/deoldify/_device.py
+++ /dev/null
@@ -1,30 +0,0 @@
-import os
-from enum import Enum
-from .device_id import DeviceId
-
-#NOTE: This must be called first before any torch imports in order to work properly!
-
-class DeviceException(Exception):
- pass
-
-class _Device:
- def __init__(self):
- self.set(DeviceId.CPU)
-
- def is_gpu(self):
- ''' Returns `True` if the current device is GPU, `False` otherwise. '''
- return self.current() is not DeviceId.CPU
-
- def current(self):
- return self._current_device
-
- def set(self, device:DeviceId):
- if device == DeviceId.CPU:
- os.environ['CUDA_VISIBLE_DEVICES']=''
- else:
- os.environ['CUDA_VISIBLE_DEVICES']=str(device.value)
- import torch
- torch.backends.cudnn.benchmark=False
-
- self._current_device = device
- return device
\ No newline at end of file
diff --git a/spaces/ArkanDash/rvc-models/README.md b/spaces/ArkanDash/rvc-models/README.md
deleted file mode 100644
index f077cd85340c26ebfcb0857816d0f1f511408242..0000000000000000000000000000000000000000
--- a/spaces/ArkanDash/rvc-models/README.md
+++ /dev/null
@@ -1,14 +0,0 @@
----
-title: Rvc Models
-emoji: 🎤
-colorFrom: red
-colorTo: blue
-sdk: gradio
-sdk_version: 3.27.0
-app_file: app.py
-pinned: false
-license: mit
-duplicated_from: ardha27/rvc-models
----
-
-Check out the configuration reference at https://huggingface.co/docs/hub/spaces-config-reference
diff --git a/spaces/Artrajz/vits-simple-api/vits/vits.py b/spaces/Artrajz/vits-simple-api/vits/vits.py
deleted file mode 100644
index 81d7d9cc7b13d6b3e39ae5ac0309cbed53d244f0..0000000000000000000000000000000000000000
--- a/spaces/Artrajz/vits-simple-api/vits/vits.py
+++ /dev/null
@@ -1,255 +0,0 @@
-import librosa
-import re
-import numpy as np
-import torch
-from torch import no_grad, LongTensor, inference_mode, FloatTensor
-import utils
-from contants import ModelType
-from utils import get_hparams_from_file, lang_dict
-from utils.sentence import sentence_split_and_markup
-from vits import commons
-from vits.mel_processing import spectrogram_torch
-from vits.text import text_to_sequence
-from vits.models import SynthesizerTrn
-
-
-class VITS:
- def __init__(self, model, config, additional_model=None, model_type=None, device=torch.device("cpu"), **kwargs):
- self.model_type = model_type
- self.hps_ms = get_hparams_from_file(config) if isinstance(config, str) else config
- self.n_speakers = getattr(self.hps_ms.data, 'n_speakers', 0)
- self.n_symbols = len(getattr(self.hps_ms, 'symbols', []))
- self.speakers = getattr(self.hps_ms, 'speakers', ['0'])
- if not isinstance(self.speakers, list):
- self.speakers = [item[0] for item in sorted(list(self.speakers.items()), key=lambda x: x[1])]
- self.use_f0 = getattr(self.hps_ms.data, 'use_f0', False)
- self.emotion_embedding = getattr(self.hps_ms.data, 'emotion_embedding',
- getattr(self.hps_ms.model, 'emotion_embedding', False))
- self.bert_embedding = getattr(self.hps_ms.data, 'bert_embedding',
- getattr(self.hps_ms.model, 'bert_embedding', False))
- self.hps_ms.model.emotion_embedding = self.emotion_embedding
- self.hps_ms.model.bert_embedding = self.bert_embedding
-
- self.net_g_ms = SynthesizerTrn(
- self.n_symbols,
- self.hps_ms.data.filter_length // 2 + 1,
- self.hps_ms.train.segment_size // self.hps_ms.data.hop_length,
- n_speakers=self.n_speakers,
- **self.hps_ms.model)
- _ = self.net_g_ms.eval()
- self.device = device
-
- key = getattr(self.hps_ms.data, "text_cleaners", ["none"])[0]
- self.lang = lang_dict.get(key, ["unknown"])
-
- # load model
- self.load_model(model, additional_model)
-
- def load_model(self, model, additional_model=None):
- utils.load_checkpoint(model, self.net_g_ms)
- self.net_g_ms.to(self.device)
- if self.model_type == ModelType.HUBERT_VITS:
- self.hubert = additional_model
- elif self.model_type == ModelType.W2V2_VITS:
- self.emotion_reference = additional_model
-
- def get_cleaned_text(self, text, hps, cleaned=False):
- if cleaned:
- text_norm = text_to_sequence(text, hps.symbols, [])
- else:
- if self.bert_embedding:
- text_norm, char_embed = text_to_sequence(text, hps.symbols, hps.data.text_cleaners,
- bert_embedding=self.bert_embedding)
- text_norm = LongTensor(text_norm)
- return text_norm, char_embed
- else:
- text_norm = text_to_sequence(text, hps.symbols, hps.data.text_cleaners)
- if hps.data.add_blank:
- text_norm = commons.intersperse(text_norm, 0)
- text_norm = LongTensor(text_norm)
- return text_norm
-
- def get_cleaner(self):
- return getattr(self.hps_ms.data, 'text_cleaners', [None])[0]
-
- def get_speakers(self, escape=False):
- return self.speakers
-
- @property
- def sampling_rate(self):
- return self.hps_ms.data.sampling_rate
-
- def infer(self, params):
- with no_grad():
- x_tst = params.get("stn_tst").unsqueeze(0).to(self.device)
- x_tst_lengths = LongTensor([params.get("stn_tst").size(0)]).to(self.device)
- x_tst_prosody = torch.FloatTensor(params.get("char_embeds")).unsqueeze(0).to(
- self.device) if self.bert_embedding else None
- sid = params.get("sid").to(self.device)
- emotion = params.get("emotion").to(self.device) if self.emotion_embedding else None
-
- audio = self.net_g_ms.infer(x=x_tst,
- x_lengths=x_tst_lengths,
- sid=sid,
- noise_scale=params.get("noise_scale"),
- noise_scale_w=params.get("noise_scale_w"),
- length_scale=params.get("length_scale"),
- emotion_embedding=emotion,
- bert=x_tst_prosody)[0][0, 0].data.float().cpu().numpy()
-
- torch.cuda.empty_cache()
-
- return audio
-
- def get_infer_param(self, length_scale, noise_scale, noise_scale_w, text=None, speaker_id=None, audio_path=None,
- emotion=None, cleaned=False, f0_scale=1):
- emo = None
- char_embeds = None
- if self.model_type != ModelType.HUBERT_VITS:
- if self.bert_embedding:
- stn_tst, char_embeds = self.get_cleaned_text(text, self.hps_ms, cleaned=cleaned)
- else:
- stn_tst = self.get_cleaned_text(text, self.hps_ms, cleaned=cleaned)
- sid = LongTensor([speaker_id])
-
- if self.model_type == ModelType.W2V2_VITS:
- # if emotion_reference.endswith('.npy'):
- # emotion = np.load(emotion_reference)
- # emotion = FloatTensor(emotion).unsqueeze(0)
- # else:
- # audio16000, sampling_rate = librosa.load(
- # emotion_reference, sr=16000, mono=True)
- # emotion = self.w2v2(audio16000, sampling_rate)[
- # 'hidden_states']
- # emotion_reference = re.sub(
- # r'\..*$', '', emotion_reference)
- # np.save(emotion_reference, emotion.squeeze(0))
- # emotion = FloatTensor(emotion)
- emo = torch.FloatTensor(self.emotion_reference[emotion]).unsqueeze(0)
-
-
- elif self.model_type == ModelType.HUBERT_VITS:
- if self.use_f0:
- audio, sampling_rate = librosa.load(audio_path, sr=self.hps_ms.data.sampling_rate, mono=True)
- audio16000 = librosa.resample(audio, orig_sr=sampling_rate, target_sr=16000)
- else:
- audio16000, sampling_rate = librosa.load(audio_path, sr=16000, mono=True)
-
- with inference_mode():
- units = self.hubert.units(FloatTensor(audio16000).unsqueeze(0).unsqueeze(0)).squeeze(0).numpy()
- if self.use_f0:
- f0 = librosa.pyin(audio,
- sr=sampling_rate,
- fmin=librosa.note_to_hz('C0'),
- fmax=librosa.note_to_hz('C7'),
- frame_length=1780)[0]
- target_length = len(units[:, 0])
- f0 = np.nan_to_num(np.interp(np.arange(0, len(f0) * target_length, len(f0)) / target_length,
- np.arange(0, len(f0)), f0)) * f0_scale
- units[:, 0] = f0 / 10
-
- stn_tst = FloatTensor(units)
- sid = LongTensor([speaker_id])
- params = {"length_scale": length_scale, "noise_scale": noise_scale,
- "noise_scale_w": noise_scale_w, "stn_tst": stn_tst,
- "sid": sid, "emotion": emo, "char_embeds": char_embeds}
-
- return params
-
- def get_tasks(self, voice):
- text = voice.get("text", None)
- speaker_id = voice.get("id", 0)
- length = voice.get("length", 1)
- noise = voice.get("noise", 0.667)
- noisew = voice.get("noisew", 0.8)
- max = voice.get("max", 50)
- lang = voice.get("lang", "auto")
- speaker_lang = voice.get("speaker_lang", None)
- audio_path = voice.get("audio_path", None)
- emotion = voice.get("emotion", 0)
-
- # 去除所有多余的空白字符
- if text is not None: text = re.sub(r'\s+', ' ', text).strip()
-
- tasks = []
- if self.model_type == ModelType.VITS:
- sentence_list = sentence_split_and_markup(text, max, lang, speaker_lang)
- for sentence in sentence_list:
- params = self.get_infer_param(text=sentence, speaker_id=speaker_id, length_scale=length,
- noise_scale=noise, noise_scale_w=noisew)
- tasks.append(params)
-
- elif self.model_type == ModelType.HUBERT_VITS:
- params = self.get_infer_param(speaker_id=speaker_id, length_scale=length, noise_scale=noise,
- noise_scale_w=noisew, audio_path=audio_path)
- tasks.append(params)
-
- elif self.model_type == ModelType.W2V2_VITS:
- sentence_list = sentence_split_and_markup(text, max, lang, speaker_lang)
- for sentence in sentence_list:
- params = self.get_infer_param(text=sentence, speaker_id=speaker_id, length_scale=length,
- noise_scale=noise, noise_scale_w=noisew, emotion=emotion)
- tasks.append(params)
- else:
- raise ValueError(f"Unsupported model type: {self.model_type}")
-
- return tasks
-
- def get_audio(self, voice, auto_break=False):
- tasks = self.get_tasks(voice)
- # 停顿0.75s,避免语音分段合成再拼接后的连接突兀
- brk = np.zeros(int(0.75 * self.sampling_rate), dtype=np.int16)
-
- audios = []
- num_tasks = len(tasks)
-
- for i, task in enumerate(tasks):
- if auto_break and i < num_tasks - 1:
- chunk = np.concatenate((self.infer(task), brk), axis=0)
- else:
- chunk = self.infer(task)
- audios.append(chunk)
-
- audio = np.concatenate(audios, axis=0)
- return audio
-
- def get_stream_audio(self, voice, auto_break=False):
- tasks = self.get_tasks(voice)
-
- brk = np.zeros(int(0.75 * 22050), dtype=np.int16)
-
- for task in tasks:
- if auto_break:
- chunk = np.concatenate((self.infer(task), brk), axis=0)
- else:
- chunk = self.infer(task)
-
- yield chunk
-
- def voice_conversion(self, voice):
- audio_path = voice.get("audio_path")
- original_id = voice.get("original_id")
- target_id = voice.get("target_id")
-
- audio = utils.load_audio_to_torch(
- audio_path, self.hps_ms.data.sampling_rate)
-
- y = audio.unsqueeze(0)
-
- spec = spectrogram_torch(y, self.hps_ms.data.filter_length,
- self.hps_ms.data.sampling_rate, self.hps_ms.data.hop_length,
- self.hps_ms.data.win_length,
- center=False)
- spec_lengths = LongTensor([spec.size(-1)])
- sid_src = LongTensor([original_id])
-
- with no_grad():
- sid_tgt = LongTensor([target_id])
- audio = self.net_g_ms.voice_conversion(spec.to(self.device),
- spec_lengths.to(self.device),
- sid_src=sid_src.to(self.device),
- sid_tgt=sid_tgt.to(self.device))[0][0, 0].data.cpu().float().numpy()
-
- torch.cuda.empty_cache()
-
- return audio
diff --git a/spaces/Awesimo/jojogan/README.md b/spaces/Awesimo/jojogan/README.md
deleted file mode 100644
index 98158fd9dbff2afc2f0d207cfbd825bf48a31844..0000000000000000000000000000000000000000
--- a/spaces/Awesimo/jojogan/README.md
+++ /dev/null
@@ -1,38 +0,0 @@
----
-title: JoJoGAN
-emoji: 🌍
-colorFrom: green
-colorTo: yellow
-sdk: gradio
-sdk_version: 3.1.1
-app_file: app.py
-pinned: false
----
-
-# Configuration
-
-`title`: _string_
-Display title for the Space
-
-`emoji`: _string_
-Space emoji (emoji-only character allowed)
-
-`colorFrom`: _string_
-Color for Thumbnail gradient (red, yellow, green, blue, indigo, purple, pink, gray)
-
-`colorTo`: _string_
-Color for Thumbnail gradient (red, yellow, green, blue, indigo, purple, pink, gray)
-
-`sdk`: _string_
-Can be either `gradio` or `streamlit`
-
-`sdk_version` : _string_
-Only applicable for `streamlit` SDK.
-See [doc](https://hf.co/docs/hub/spaces) for more info on supported versions.
-
-`app_file`: _string_
-Path to your main application file (which contains either `gradio` or `streamlit` Python code).
-Path is relative to the root of the repository.
-
-`pinned`: _boolean_
-Whether the Space stays on top of your list.
diff --git a/spaces/Awiny/Image2Paragraph/models/grit_src/third_party/CenterNet2/detectron2/layers/csrc/box_iou_rotated/box_iou_rotated.h b/spaces/Awiny/Image2Paragraph/models/grit_src/third_party/CenterNet2/detectron2/layers/csrc/box_iou_rotated/box_iou_rotated.h
deleted file mode 100644
index 3bf383b8ed9b358b5313d433a9682c294dfb77e4..0000000000000000000000000000000000000000
--- a/spaces/Awiny/Image2Paragraph/models/grit_src/third_party/CenterNet2/detectron2/layers/csrc/box_iou_rotated/box_iou_rotated.h
+++ /dev/null
@@ -1,35 +0,0 @@
-// Copyright (c) Facebook, Inc. and its affiliates.
-#pragma once
-#include
-
-namespace detectron2 {
-
-at::Tensor box_iou_rotated_cpu(
- const at::Tensor& boxes1,
- const at::Tensor& boxes2);
-
-#if defined(WITH_CUDA) || defined(WITH_HIP)
-at::Tensor box_iou_rotated_cuda(
- const at::Tensor& boxes1,
- const at::Tensor& boxes2);
-#endif
-
-// Interface for Python
-// inline is needed to prevent multiple function definitions when this header is
-// included by different cpps
-inline at::Tensor box_iou_rotated(
- const at::Tensor& boxes1,
- const at::Tensor& boxes2) {
- assert(boxes1.device().is_cuda() == boxes2.device().is_cuda());
- if (boxes1.device().is_cuda()) {
-#if defined(WITH_CUDA) || defined(WITH_HIP)
- return box_iou_rotated_cuda(boxes1.contiguous(), boxes2.contiguous());
-#else
- AT_ERROR("Detectron2 is not compiled with GPU support!");
-#endif
- }
-
- return box_iou_rotated_cpu(boxes1.contiguous(), boxes2.contiguous());
-}
-
-} // namespace detectron2
diff --git a/spaces/Awiny/Image2Paragraph/models/grit_src/third_party/CenterNet2/detectron2/modeling/meta_arch/panoptic_fpn.py b/spaces/Awiny/Image2Paragraph/models/grit_src/third_party/CenterNet2/detectron2/modeling/meta_arch/panoptic_fpn.py
deleted file mode 100644
index 13aeabce162f4114109efe2c7fb4770b89087ab0..0000000000000000000000000000000000000000
--- a/spaces/Awiny/Image2Paragraph/models/grit_src/third_party/CenterNet2/detectron2/modeling/meta_arch/panoptic_fpn.py
+++ /dev/null
@@ -1,266 +0,0 @@
-# -*- coding: utf-8 -*-
-# Copyright (c) Facebook, Inc. and its affiliates.
-
-import logging
-from typing import Dict, List
-import torch
-from torch import nn
-
-from detectron2.config import configurable
-from detectron2.structures import ImageList
-
-from ..postprocessing import detector_postprocess, sem_seg_postprocess
-from .build import META_ARCH_REGISTRY
-from .rcnn import GeneralizedRCNN
-from .semantic_seg import build_sem_seg_head
-
-__all__ = ["PanopticFPN"]
-
-
-@META_ARCH_REGISTRY.register()
-class PanopticFPN(GeneralizedRCNN):
- """
- Implement the paper :paper:`PanopticFPN`.
- """
-
- @configurable
- def __init__(
- self,
- *,
- sem_seg_head: nn.Module,
- combine_overlap_thresh: float = 0.5,
- combine_stuff_area_thresh: float = 4096,
- combine_instances_score_thresh: float = 0.5,
- **kwargs,
- ):
- """
- NOTE: this interface is experimental.
-
- Args:
- sem_seg_head: a module for the semantic segmentation head.
- combine_overlap_thresh: combine masks into one instances if
- they have enough overlap
- combine_stuff_area_thresh: ignore stuff areas smaller than this threshold
- combine_instances_score_thresh: ignore instances whose score is
- smaller than this threshold
-
- Other arguments are the same as :class:`GeneralizedRCNN`.
- """
- super().__init__(**kwargs)
- self.sem_seg_head = sem_seg_head
- # options when combining instance & semantic outputs
- self.combine_overlap_thresh = combine_overlap_thresh
- self.combine_stuff_area_thresh = combine_stuff_area_thresh
- self.combine_instances_score_thresh = combine_instances_score_thresh
-
- @classmethod
- def from_config(cls, cfg):
- ret = super().from_config(cfg)
- ret.update(
- {
- "combine_overlap_thresh": cfg.MODEL.PANOPTIC_FPN.COMBINE.OVERLAP_THRESH,
- "combine_stuff_area_thresh": cfg.MODEL.PANOPTIC_FPN.COMBINE.STUFF_AREA_LIMIT,
- "combine_instances_score_thresh": cfg.MODEL.PANOPTIC_FPN.COMBINE.INSTANCES_CONFIDENCE_THRESH, # noqa
- }
- )
- ret["sem_seg_head"] = build_sem_seg_head(cfg, ret["backbone"].output_shape())
- logger = logging.getLogger(__name__)
- if not cfg.MODEL.PANOPTIC_FPN.COMBINE.ENABLED:
- logger.warning(
- "PANOPTIC_FPN.COMBINED.ENABLED is no longer used. "
- " model.inference(do_postprocess=) should be used to toggle postprocessing."
- )
- if cfg.MODEL.PANOPTIC_FPN.INSTANCE_LOSS_WEIGHT != 1.0:
- w = cfg.MODEL.PANOPTIC_FPN.INSTANCE_LOSS_WEIGHT
- logger.warning(
- "PANOPTIC_FPN.INSTANCE_LOSS_WEIGHT should be replaced by weights on each ROI head."
- )
-
- def update_weight(x):
- if isinstance(x, dict):
- return {k: v * w for k, v in x.items()}
- else:
- return x * w
-
- roi_heads = ret["roi_heads"]
- roi_heads.box_predictor.loss_weight = update_weight(roi_heads.box_predictor.loss_weight)
- roi_heads.mask_head.loss_weight = update_weight(roi_heads.mask_head.loss_weight)
- return ret
-
- def forward(self, batched_inputs):
- """
- Args:
- batched_inputs: a list, batched outputs of :class:`DatasetMapper`.
- Each item in the list contains the inputs for one image.
-
- For now, each item in the list is a dict that contains:
-
- * "image": Tensor, image in (C, H, W) format.
- * "instances": Instances
- * "sem_seg": semantic segmentation ground truth.
- * Other information that's included in the original dicts, such as:
- "height", "width" (int): the output resolution of the model, used in inference.
- See :meth:`postprocess` for details.
-
- Returns:
- list[dict]:
- each dict has the results for one image. The dict contains the following keys:
-
- * "instances": see :meth:`GeneralizedRCNN.forward` for its format.
- * "sem_seg": see :meth:`SemanticSegmentor.forward` for its format.
- * "panoptic_seg": See the return value of
- :func:`combine_semantic_and_instance_outputs` for its format.
- """
- if not self.training:
- return self.inference(batched_inputs)
- images = self.preprocess_image(batched_inputs)
- features = self.backbone(images.tensor)
-
- assert "sem_seg" in batched_inputs[0]
- gt_sem_seg = [x["sem_seg"].to(self.device) for x in batched_inputs]
- gt_sem_seg = ImageList.from_tensors(
- gt_sem_seg, self.backbone.size_divisibility, self.sem_seg_head.ignore_value
- ).tensor
- sem_seg_results, sem_seg_losses = self.sem_seg_head(features, gt_sem_seg)
-
- gt_instances = [x["instances"].to(self.device) for x in batched_inputs]
- proposals, proposal_losses = self.proposal_generator(images, features, gt_instances)
- detector_results, detector_losses = self.roi_heads(
- images, features, proposals, gt_instances
- )
-
- losses = sem_seg_losses
- losses.update(proposal_losses)
- losses.update(detector_losses)
- return losses
-
- def inference(self, batched_inputs: List[Dict[str, torch.Tensor]], do_postprocess: bool = True):
- """
- Run inference on the given inputs.
-
- Args:
- batched_inputs (list[dict]): same as in :meth:`forward`
- do_postprocess (bool): whether to apply post-processing on the outputs.
-
- Returns:
- When do_postprocess=True, see docs in :meth:`forward`.
- Otherwise, returns a (list[Instances], list[Tensor]) that contains
- the raw detector outputs, and raw semantic segmentation outputs.
- """
- images = self.preprocess_image(batched_inputs)
- features = self.backbone(images.tensor)
- sem_seg_results, sem_seg_losses = self.sem_seg_head(features, None)
- proposals, _ = self.proposal_generator(images, features, None)
- detector_results, _ = self.roi_heads(images, features, proposals, None)
-
- if do_postprocess:
- processed_results = []
- for sem_seg_result, detector_result, input_per_image, image_size in zip(
- sem_seg_results, detector_results, batched_inputs, images.image_sizes
- ):
- height = input_per_image.get("height", image_size[0])
- width = input_per_image.get("width", image_size[1])
- sem_seg_r = sem_seg_postprocess(sem_seg_result, image_size, height, width)
- detector_r = detector_postprocess(detector_result, height, width)
-
- processed_results.append({"sem_seg": sem_seg_r, "instances": detector_r})
-
- panoptic_r = combine_semantic_and_instance_outputs(
- detector_r,
- sem_seg_r.argmax(dim=0),
- self.combine_overlap_thresh,
- self.combine_stuff_area_thresh,
- self.combine_instances_score_thresh,
- )
- processed_results[-1]["panoptic_seg"] = panoptic_r
- return processed_results
- else:
- return detector_results, sem_seg_results
-
-
-def combine_semantic_and_instance_outputs(
- instance_results,
- semantic_results,
- overlap_threshold,
- stuff_area_thresh,
- instances_score_thresh,
-):
- """
- Implement a simple combining logic following
- "combine_semantic_and_instance_predictions.py" in panopticapi
- to produce panoptic segmentation outputs.
-
- Args:
- instance_results: output of :func:`detector_postprocess`.
- semantic_results: an (H, W) tensor, each element is the contiguous semantic
- category id
-
- Returns:
- panoptic_seg (Tensor): of shape (height, width) where the values are ids for each segment.
- segments_info (list[dict]): Describe each segment in `panoptic_seg`.
- Each dict contains keys "id", "category_id", "isthing".
- """
- panoptic_seg = torch.zeros_like(semantic_results, dtype=torch.int32)
-
- # sort instance outputs by scores
- sorted_inds = torch.argsort(-instance_results.scores)
-
- current_segment_id = 0
- segments_info = []
-
- instance_masks = instance_results.pred_masks.to(dtype=torch.bool, device=panoptic_seg.device)
-
- # Add instances one-by-one, check for overlaps with existing ones
- for inst_id in sorted_inds:
- score = instance_results.scores[inst_id].item()
- if score < instances_score_thresh:
- break
- mask = instance_masks[inst_id] # H,W
- mask_area = mask.sum().item()
-
- if mask_area == 0:
- continue
-
- intersect = (mask > 0) & (panoptic_seg > 0)
- intersect_area = intersect.sum().item()
-
- if intersect_area * 1.0 / mask_area > overlap_threshold:
- continue
-
- if intersect_area > 0:
- mask = mask & (panoptic_seg == 0)
-
- current_segment_id += 1
- panoptic_seg[mask] = current_segment_id
- segments_info.append(
- {
- "id": current_segment_id,
- "isthing": True,
- "score": score,
- "category_id": instance_results.pred_classes[inst_id].item(),
- "instance_id": inst_id.item(),
- }
- )
-
- # Add semantic results to remaining empty areas
- semantic_labels = torch.unique(semantic_results).cpu().tolist()
- for semantic_label in semantic_labels:
- if semantic_label == 0: # 0 is a special "thing" class
- continue
- mask = (semantic_results == semantic_label) & (panoptic_seg == 0)
- mask_area = mask.sum().item()
- if mask_area < stuff_area_thresh:
- continue
-
- current_segment_id += 1
- panoptic_seg[mask] = current_segment_id
- segments_info.append(
- {
- "id": current_segment_id,
- "isthing": False,
- "category_id": semantic_label,
- "area": mask_area,
- }
- )
-
- return panoptic_seg, segments_info
diff --git a/spaces/Banbri/zcvzcv/src/lib/generateSeed.ts b/spaces/Banbri/zcvzcv/src/lib/generateSeed.ts
deleted file mode 100644
index 563e25ec894ab5af54c5025a15a9b7a5918325de..0000000000000000000000000000000000000000
--- a/spaces/Banbri/zcvzcv/src/lib/generateSeed.ts
+++ /dev/null
@@ -1,3 +0,0 @@
-export function generateSeed() {
- return Math.floor(Math.random() * Math.pow(2, 31));
-}
\ No newline at end of file
diff --git a/spaces/Benson/text-generation/Examples/Clash Royale Mod Apk Raja Apk.md b/spaces/Benson/text-generation/Examples/Clash Royale Mod Apk Raja Apk.md
deleted file mode 100644
index c726659e30a9455a14ecd5f4817f25cd1050f6aa..0000000000000000000000000000000000000000
--- a/spaces/Benson/text-generation/Examples/Clash Royale Mod Apk Raja Apk.md
+++ /dev/null
@@ -1,85 +0,0 @@
-
-Choque Royale Mod APK Raja APK: La guía definitiva
-Si eres un fan de los juegos de estrategia en tiempo real, debes haber oído hablar de Clash Royale, uno de los juegos más populares y adictivos en dispositivos móviles. ¿Pero sabías que hay una manera de disfrutar el juego aún más con recursos ilimitados, tarjetas personalizadas y otras características increíbles? Sí, estamos hablando de Clash Royale Mod APK Raja APK, una versión modificada del juego que le da una ventaja sobre sus oponentes. En este artículo, le diremos todo lo que necesita saber acerca de este apk mod, incluyendo sus beneficios, guía de instalación, y descargo de responsabilidad. ¡Sigue leyendo para saber más!
- ¿Qué es Clash Royale?
-Clash Royale es un juego multijugador en tiempo real desarrollado por Supercell, los creadores de Clash of Clans. Cuenta con tus personajes favoritos de Clash y mucho más. En este juego, tienes que recoger y actualizar las cartas que representan las tropas, hechizos y defensas del universo Clash. También tienes que construir tu propia baraja de batalla y usarla para luchar contra otros jugadores en partidas rápidas. El objetivo es destruir las torres de tu enemigo y ganar coronas que se pueden utilizar para desbloquear cofres con recompensas. También puedes unirte o formar un clan con otros jugadores para compartir cartas y participar en guerras de clanes para obtener premios más grandes.
-clash royale mod apk raja apk
Download File ✵✵✵ https://bltlly.com/2v6JaN
- Características de Clash Royale
-Clash Royale tiene muchas características que lo convierten en un juego emocionante y divertido para jugar. Algunas de ellas son:
-
-- Gana cofres para desbloquear recompensas, recoger nuevas cartas de gran alcance y actualizar los existentes.
-- Destruye las torres del oponente y gana coronas para ganar cofres épicos.
-- Construye y mejora tu colección de cartas con la familia Clash Royale junto con docenas de tus tropas, hechizos y defensas Clash favoritas.
-- Ábrete camino a la cima en diferentes arenas y ligas.
-- Compite en eventos estacionales y desafíos que ponen a prueba tus habilidades.
-
-
- Cómo jugar Clash Royale
-Jugar a Clash Royale es fácil y divertido. Estos son los pasos básicos a seguir:
-
-- Descargar e instalar el juego desde la Google Play Store o la App Store.
-- Cree o inicie sesión en su cuenta de Supercell.
-- Completa el tutorial para aprender los fundamentos del juego.
-- Iniciar un partido tocando el botón de batalla.
-- Selecciona cuatro cartas de tu mazo para usarlas en la batalla.
-- Arrastra y suelta las cartas en la arena para desplegar tus unidades.
-- Usa elixir sabiamente para administrar tus recursos.
-- Apunta a las torres del enemigo y trata de destruirlas antes de que destruyan la tuya.
-- Ganar coronas y cofres por cada victoria.
-- Abrir cofres para obtener recompensas como cartas, oro, gemas, etc.
-- Actualiza tus tarjetas con oro para hacerlas más fuertes.
-- Crea o únete a un clan para chatear, donar, solicitar e intercambiar cartas con otros jugadores.
-- Participa en guerras de clanes, torneos, eventos y desafíos para más diversión y recompensas.
-
- ¿Qué es Clash Royale Mod APK Raja APK?
-Clash Royale Mod APK Raja APK es una versión modificada del juego original que ofrece algunas características adicionales que no están disponibles en la versión oficial. También se conoce como CR mod apk o CR hack apk. Es desarrollado por Raja APK, un sitio web que proporciona varios apks mod para diferentes juegos y aplicaciones. Algunas de las características que ofrece este apk mod son:
- Beneficios Beneficios de choque Royale Mod APK Raja APK
-
Algunos de los beneficios que se pueden disfrutar mediante el uso de Clash Royale Mod APK Raja APK son:
-
-- Recursos ilimitados: Puede obtener oro ilimitado, gemas, elixir y elixir oscuro para actualizar sus tarjetas, comprar cofres y desbloquear nuevas funciones.
-- Tarjetas personalizadas: Puedes crear tus propias tarjetas con estadísticas, habilidades y diseños personalizados. También puedes usar cartas de otros juegos de Supercell como Brawl Stars, Boom Beach y Hay Day.
-
-- No hay anuncios: Puedes disfrutar del juego sin anuncios molestos o pop-ups.
-- No hay raíz: No es necesario rootear el dispositivo para utilizar este apk mod. Funciona tanto en dispositivos arraigados y no arraigados.
-
- Cómo descargar e instalar Clash Royale Mod APK Raja APK
-Para descargar e instalar Clash Royale Mod APK Raja APK, es necesario seguir estos sencillos pasos:
-
-- Ir a la página web oficial de Raja APK y encontrar el archivo Clash Royale Mod APK Raja APK. También puede utilizar este enlace: .
-- Descargue el archivo en su dispositivo. Asegúrese de tener suficiente espacio de almacenamiento y una conexión a Internet estable.
-- Habilita la instalación de fuentes desconocidas en tu dispositivo. Para hacer esto, ve a Configuración > Seguridad > Fuentes desconocidas y cámbiala.
-- Localice el archivo descargado en su dispositivo y toque en él para iniciar el proceso de instalación.
-- Siga las instrucciones en la pantalla y espere a que se complete la instalación.
-- Iniciar el juego y disfrutar!
-
- Descargo de responsabilidad y los riesgos de usar Clash Royale Mod APK Raja APK
-Aunque Clash Royale Mod APK Raja APK puede sonar tentador y divertido, también viene con algunos riesgos y desventajas que usted debe ser consciente de antes de usarlo. Estos son algunos de ellos:
-
- Cuestiones legales y prohibiciones
-Usando Clash Royale Mod APK Raja APK está en contra de los términos de servicio de Supercell, el desarrollador de Clash Royale. Esto significa que usted está violando sus reglas y reglamentos mediante el uso de una versión modificada de su juego. Esto puede dar lugar a acciones legales o prohibiciones de sus servidores. Si le pillan usando este mod apk, puede perder su cuenta, progreso y compras. También puede enfrentar consecuencias legales como multas o demandas.
- Malware y virus
-
- Pérdida de datos y corrupción
-Usando Clash Royale Mod APK Raja APK también puede causar pérdida de datos o corrupción en su dispositivo o juego. Algunos apks mod pueden no ser compatibles con su dispositivo o versión del juego, lo que puede conducir a errores, fallos o fallos. Esto puede resultar en la pérdida de los datos del juego o la corrupción de los archivos. Para evitar esto, siempre debe hacer una copia de seguridad de sus datos antes de usar cualquier apk mod. También debe actualizar su juego y mod apk regularmente para asegurar un rendimiento suave.
- Conclusión
-Clash Royale Mod APK Raja APK es una versión modificada de Clash Royale que ofrece recursos ilimitados, tarjetas personalizadas, servidor privado, sin anuncios, y sin características de raíz. Es una gran manera de disfrutar del juego con más libertad y diversión. Sin embargo, también viene con algunos riesgos y desventajas, como problemas legales, prohibiciones, malware, virus, pérdida de datos y corrupción. Por lo tanto, usted debe utilizar a su propio riesgo y discreción. Esperamos que este artículo le ha dado toda la información que necesita acerca de este apk mod. Si tiene alguna pregunta o comentario, háganoslo saber en los comentarios a continuación.
- Preguntas frecuentes
-Aquí hay algunas preguntas frecuentes sobre Clash Royale Mod APK Raja APK:
- Q: Es Clash Royale Mod APK Raja APK seguro de usar?
-A: Clash Royale Mod APK Raja APK es seguro de usar si lo descarga de una fuente de confianza como Raja APK. Sin embargo, siempre debe escanear el archivo en busca de virus antes de instalarlo en su dispositivo. También debe hacer una copia de seguridad de sus datos antes de usarlo para evitar cualquier pérdida de datos o corrupción.
Q: ¿Cómo puedo actualizar Clash Royale Mod APK Raja APK?
-A: Para actualizar Clash Royale Mod APK Raja APK, es necesario visitar el sitio web oficial de Raja APK y descargar la última versión del archivo. También puede utilizar este enlace: . Entonces, es necesario desinstalar la versión anterior de la apk mod e instalar el nuevo. Es posible que necesite habilitar la instalación de fuentes desconocidas de nuevo si se le solicita.
-
-A: Sí, puedes jugar Clash Royale Mod APK Raja APK con tus amigos que también utilizan el mismo mod apk. Puede unirse o crear un clan con ellos y chatear, donar, solicitar e intercambiar tarjetas. También puede luchar con ellos en el servidor privado. Sin embargo, no puedes jugar con tus amigos que usan la versión oficial del juego, ya que están en un servidor diferente.
- Q: ¿Puedo usar Clash Royale Mod APK Raja APK en dispositivos iOS?
-A: No, Clash Royale Mod APK Raja APK solo es compatible con dispositivos Android. No funciona en dispositivos iOS como iPhones o iPads. Si desea utilizar un apk mod en dispositivos iOS, es necesario encontrar uno diferente que está diseñado para iOS.
- Q: ¿Cuáles son algunas alternativas a Clash Royale Mod APK Raja APK?
-A: Algunas alternativas a Clash Royale Mod APK Raja APK son:
-
-- Choque Royale Mod APK Null’s Royale: Este es otro popular mod apk que ofrece recursos ilimitados, tarjetas personalizadas, servidor privado, y más.
-- Choque Royale Mod APK Master Royale: Este es un apk mod que ofrece gemas ilimitadas, monedas, cofres y tarjetas. También tiene un servidor privado y mods personalizados.
-- Clash Royale Mod APK PlenixRoyale: Este es un apk mod que ofrece gemas ilimitadas, oro, elixir y elixir oscuro. También tiene un servidor privado y tarjetas personalizadas.
-
- Q: ¿Dónde puedo encontrar más información sobre Clash Royale Mod APK Raja APK?
-A: Usted puede encontrar más información sobre Clash Royale Mod APK Raja APK en el sitio web oficial de Raja APK. También puede visitar sus páginas de redes sociales o contactarlos por correo electrónico o teléfono para cualquier consulta o retroalimentación.
64aa2da5cf
-
-
\ No newline at end of file
diff --git a/spaces/BetterAPI/BetterChat/PRIVACY.md b/spaces/BetterAPI/BetterChat/PRIVACY.md
deleted file mode 100644
index 583a08cb499cb95e426f80400d245446bb9a905d..0000000000000000000000000000000000000000
--- a/spaces/BetterAPI/BetterChat/PRIVACY.md
+++ /dev/null
@@ -1,35 +0,0 @@
-## Privacy
-
-> Last updated: May 2nd, 2023
-
-In this `v0.1` of BetterChat, users are not authenticated in any way, i.e. this app doesn't have access to your HF user account even if you're logged in to huggingface.co. The app is only using an anonymous session cookie. ❗️ Warning ❗️ this means if you switch browsers or clear cookies, you will currently lose your conversations.
-
-By default, your conversations are shared with the model's authors (for the `v0.1` model, to Open Assistant) to improve their training data and model over time. Model authors are the custodians of the data collected by their model, even if it's hosted on our platform.
-
-If you disable data sharing in your settings, your conversations will not be used for any downstream usage (including for research or model training purposes), and they will only be stored to let you access past conversations. You can click on the Delete icon to delete any past conversation at any moment.
-
-🗓 Please also consult huggingface.co's main privacy policy at https://huggingface.co/privacy. To exercise any of your legal privacy rights, please send an email to privacy@huggingface.co.
-
-## About available LLMs
-
-The goal of this app is to showcase that it is now (April 2023) possible to build an open source alternative to ChatGPT. 💪
-
-For now, it's running OpenAssistant's [latest LLaMA based model](https://huggingface.co/OpenAssistant/oasst-sft-6-llama-30b-xor) (which is one of the current best open source chat models), but the plan in the longer-term is to expose all good-quality chat models from the Hub.
-
-We are not affiliated with Open Assistant, but if you want to contribute to the training data for the next generation of open models, please consider contributing to https://open-assistant.io/ ❤️
-
-## Technical details
-
-This app is running in a [Space](https://huggingface.co/docs/hub/spaces-overview), which entails that the code for this UI is open source: https://huggingface.co/spaces/huggingchat/chat-ui/tree/main.
-The inference backend is running [text-generation-inference](https://github.com/huggingface/text-generation-inference) on HuggingFace's Inference API infrastructure.
-
-It is therefore possible to deploy a copy of this app to a Space and customize it (swap model, add some UI elements, or store user messages according to your own Terms and conditions)
-
-We welcome any feedback on this app: please participate to the public discussion at https://huggingface.co/spaces/huggingchat/chat-ui/discussions
-
-
-
-## Coming soon
-
-- LLM watermarking
-- User setting to share conversations with model authors (done ✅)
diff --git a/spaces/BetterAPI/BetterChat/src/routes/conversation/[id]/+page.server.ts b/spaces/BetterAPI/BetterChat/src/routes/conversation/[id]/+page.server.ts
deleted file mode 100644
index 3aced2cc895525e5bad8acd59546897df945f137..0000000000000000000000000000000000000000
--- a/spaces/BetterAPI/BetterChat/src/routes/conversation/[id]/+page.server.ts
+++ /dev/null
@@ -1,33 +0,0 @@
-import type { PageServerLoad } from "./$types";
-import { collections } from "$lib/server/database";
-import { ObjectId } from "mongodb";
-import { error } from "@sveltejs/kit";
-
-export const load: PageServerLoad = async (event) => {
- // todo: add validation on params.id
- const conversation = await collections.conversations.findOne({
- _id: new ObjectId(event.params.id),
- sessionId: event.locals.sessionId,
- });
-
- if (!conversation) {
- const conversationExists =
- (await collections.conversations.countDocuments({
- _id: new ObjectId(event.params.id),
- })) !== 0;
-
- if (conversationExists) {
- throw error(
- 403,
- "You don't have access to this conversation. If someone gave you this link, ask them to use the 'share' feature instead."
- );
- }
-
- throw error(404, "Conversation not found.");
- }
-
- return {
- messages: conversation.messages,
- title: conversation.title,
- };
-};
diff --git a/spaces/Big-Web/MMSD/env/Lib/site-packages/setuptools/_itertools.py b/spaces/Big-Web/MMSD/env/Lib/site-packages/setuptools/_itertools.py
deleted file mode 100644
index b8bf6d210aec669b6b948942eda1db953e8725fa..0000000000000000000000000000000000000000
--- a/spaces/Big-Web/MMSD/env/Lib/site-packages/setuptools/_itertools.py
+++ /dev/null
@@ -1,23 +0,0 @@
-from setuptools.extern.more_itertools import consume # noqa: F401
-
-
-# copied from jaraco.itertools 6.1
-def ensure_unique(iterable, key=lambda x: x):
- """
- Wrap an iterable to raise a ValueError if non-unique values are encountered.
-
- >>> list(ensure_unique('abc'))
- ['a', 'b', 'c']
- >>> consume(ensure_unique('abca'))
- Traceback (most recent call last):
- ...
- ValueError: Duplicate element 'a' encountered.
- """
- seen = set()
- seen_add = seen.add
- for element in iterable:
- k = key(element)
- if k in seen:
- raise ValueError(f"Duplicate element {element!r} encountered.")
- seen_add(k)
- yield element
diff --git a/spaces/Big-Web/MMSD/env/Lib/site-packages/urllib3/util/response.py b/spaces/Big-Web/MMSD/env/Lib/site-packages/urllib3/util/response.py
deleted file mode 100644
index 5ea609ccedf18eb4ab70f8fc6990448eb6407237..0000000000000000000000000000000000000000
--- a/spaces/Big-Web/MMSD/env/Lib/site-packages/urllib3/util/response.py
+++ /dev/null
@@ -1,107 +0,0 @@
-from __future__ import absolute_import
-
-from email.errors import MultipartInvariantViolationDefect, StartBoundaryNotFoundDefect
-
-from ..exceptions import HeaderParsingError
-from ..packages.six.moves import http_client as httplib
-
-
-def is_fp_closed(obj):
- """
- Checks whether a given file-like object is closed.
-
- :param obj:
- The file-like object to check.
- """
-
- try:
- # Check `isclosed()` first, in case Python3 doesn't set `closed`.
- # GH Issue #928
- return obj.isclosed()
- except AttributeError:
- pass
-
- try:
- # Check via the official file-like-object way.
- return obj.closed
- except AttributeError:
- pass
-
- try:
- # Check if the object is a container for another file-like object that
- # gets released on exhaustion (e.g. HTTPResponse).
- return obj.fp is None
- except AttributeError:
- pass
-
- raise ValueError("Unable to determine whether fp is closed.")
-
-
-def assert_header_parsing(headers):
- """
- Asserts whether all headers have been successfully parsed.
- Extracts encountered errors from the result of parsing headers.
-
- Only works on Python 3.
-
- :param http.client.HTTPMessage headers: Headers to verify.
-
- :raises urllib3.exceptions.HeaderParsingError:
- If parsing errors are found.
- """
-
- # This will fail silently if we pass in the wrong kind of parameter.
- # To make debugging easier add an explicit check.
- if not isinstance(headers, httplib.HTTPMessage):
- raise TypeError("expected httplib.Message, got {0}.".format(type(headers)))
-
- defects = getattr(headers, "defects", None)
- get_payload = getattr(headers, "get_payload", None)
-
- unparsed_data = None
- if get_payload:
- # get_payload is actually email.message.Message.get_payload;
- # we're only interested in the result if it's not a multipart message
- if not headers.is_multipart():
- payload = get_payload()
-
- if isinstance(payload, (bytes, str)):
- unparsed_data = payload
- if defects:
- # httplib is assuming a response body is available
- # when parsing headers even when httplib only sends
- # header data to parse_headers() This results in
- # defects on multipart responses in particular.
- # See: https://github.com/urllib3/urllib3/issues/800
-
- # So we ignore the following defects:
- # - StartBoundaryNotFoundDefect:
- # The claimed start boundary was never found.
- # - MultipartInvariantViolationDefect:
- # A message claimed to be a multipart but no subparts were found.
- defects = [
- defect
- for defect in defects
- if not isinstance(
- defect, (StartBoundaryNotFoundDefect, MultipartInvariantViolationDefect)
- )
- ]
-
- if defects or unparsed_data:
- raise HeaderParsingError(defects=defects, unparsed_data=unparsed_data)
-
-
-def is_response_to_head(response):
- """
- Checks whether the request of a response has been a HEAD-request.
- Handles the quirks of AppEngine.
-
- :param http.client.HTTPResponse response:
- Response to check if the originating request
- used 'HEAD' as a method.
- """
- # FIXME: Can we do this somehow without accessing private httplib _method?
- method = response._method
- if isinstance(method, int): # Platform-specific: Appengine
- return method == 3
- return method.upper() == "HEAD"
diff --git a/spaces/CVPR/LIVE/matrix.h b/spaces/CVPR/LIVE/matrix.h
deleted file mode 100644
index b53f484e2abf613c6d0c1b36890a332d778f24b5..0000000000000000000000000000000000000000
--- a/spaces/CVPR/LIVE/matrix.h
+++ /dev/null
@@ -1,544 +0,0 @@
-#pragma once
-
-#include "diffvg.h"
-#include "vector.h"
-#include
-
-template
-struct TMatrix3x3 {
- DEVICE
- TMatrix3x3() {
- for (int i = 0; i < 3; i++) {
- for (int j = 0; j < 3; j++) {
- data[i][j] = T(0);
- }
- }
- }
-
- template
- DEVICE
- TMatrix3x3(T2 *arr) {
- data[0][0] = arr[0];
- data[0][1] = arr[1];
- data[0][2] = arr[2];
- data[1][0] = arr[3];
- data[1][1] = arr[4];
- data[1][2] = arr[5];
- data[2][0] = arr[6];
- data[2][1] = arr[7];
- data[2][2] = arr[8];
- }
- DEVICE
- TMatrix3x3(T v00, T v01, T v02,
- T v10, T v11, T v12,
- T v20, T v21, T v22) {
- data[0][0] = v00;
- data[0][1] = v01;
- data[0][2] = v02;
- data[1][0] = v10;
- data[1][1] = v11;
- data[1][2] = v12;
- data[2][0] = v20;
- data[2][1] = v21;
- data[2][2] = v22;
- }
-
- DEVICE
- const T& operator()(int i, int j) const {
- return data[i][j];
- }
- DEVICE
- T& operator()(int i, int j) {
- return data[i][j];
- }
- DEVICE
- static TMatrix3x3 identity() {
- TMatrix3x3 m(1, 0, 0,
- 0, 1, 0,
- 0, 0, 1);
- return m;
- }
-
- T data[3][3];
-};
-
-using Matrix3x3 = TMatrix3x3;
-using Matrix3x3f = TMatrix3x3;
-
-template
-struct TMatrix4x4 {
- DEVICE TMatrix4x4() {
- for (int i = 0; i < 4; i++) {
- for (int j = 0; j < 4; j++) {
- data[i][j] = T(0);
- }
- }
- }
-
- template
- DEVICE TMatrix4x4(const T2 *arr) {
- for (int i = 0; i < 4; i++) {
- for (int j = 0; j < 4; j++) {
- data[i][j] = (T)arr[i * 4 + j];
- }
- }
- }
-
- template
- DEVICE TMatrix4x4(const TMatrix4x4 &m) {
- for (int i = 0; i < 4; i++) {
- for (int j = 0; j < 4; j++) {
- data[i][j] = T(m.data[i][j]);
- }
- }
- }
-
- template
- DEVICE TMatrix4x4(T2 v00, T2 v01, T2 v02, T2 v03,
- T2 v10, T2 v11, T2 v12, T2 v13,
- T2 v20, T2 v21, T2 v22, T2 v23,
- T2 v30, T2 v31, T2 v32, T2 v33) {
- data[0][0] = (T)v00;
- data[0][1] = (T)v01;
- data[0][2] = (T)v02;
- data[0][3] = (T)v03;
- data[1][0] = (T)v10;
- data[1][1] = (T)v11;
- data[1][2] = (T)v12;
- data[1][3] = (T)v13;
- data[2][0] = (T)v20;
- data[2][1] = (T)v21;
- data[2][2] = (T)v22;
- data[2][3] = (T)v23;
- data[3][0] = (T)v30;
- data[3][1] = (T)v31;
- data[3][2] = (T)v32;
- data[3][3] = (T)v33;
- }
-
- DEVICE
- const T& operator()(int i, int j) const {
- return data[i][j];
- }
-
- DEVICE
- T& operator()(int i, int j) {
- return data[i][j];
- }
-
- DEVICE
- static TMatrix4x4 identity() {
- TMatrix4x4 m(1, 0, 0, 0,
- 0, 1, 0, 0,
- 0, 0, 1, 0,
- 0, 0, 0, 1);
- return m;
- }
-
- T data[4][4];
-};
-
-using Matrix4x4 = TMatrix4x4;
-using Matrix4x4f = TMatrix4x4;
-
-template
-DEVICE
-inline auto operator+(const TMatrix3x3 &m0, const TMatrix3x3 &m1) -> TMatrix3x3 {
- TMatrix3x3 m;
- for (int i = 0; i < 3; i++) {
- for (int j = 0; j < 3; j++) {
- m(i, j) = m0(i, j) + m1(i, j);
- }
- }
- return m;
-}
-
-template
-DEVICE
-inline auto operator-(const TMatrix3x3 &m0, const TMatrix3x3 &m1) -> TMatrix3x3 {
- TMatrix3x3 m;
- for (int i = 0; i < 3; i++) {
- for (int j = 0; j < 3; j++) {
- m(i, j) = m0(i, j) - m1(i, j);
- }
- }
- return m;
-}
-
-template
-DEVICE
-inline auto operator*(const TMatrix3x3 &m0, const TMatrix3x3 &m1) -> TMatrix3x3 {
- TMatrix3x3 ret;
- for (int i = 0; i < 3; i++) {
- for (int j = 0; j < 3; j++) {
- ret(i, j) = T(0);
- for (int k = 0; k < 3; k++) {
- ret(i, j) += m0(i, k) * m1(k, j);
- }
- }
- }
- return ret;
-}
-
-template
-DEVICE
-inline auto operator*(const TVector3 &v, const TMatrix3x3 &m) -> TVector3 {
- TVector3 ret;
- for (int i = 0; i < 3; i++) {
- ret[i] = T(0);
- for (int j = 0; j < 3; j++) {
- ret[i] += v[j] * m(j, i);
- }
- }
- return ret;
-}
-
-template
-DEVICE
-inline auto operator*(const TMatrix3x3 &m, const TVector3 &v) -> TVector3 {
- TVector3 ret;
- for (int i = 0; i < 3; i++) {
- ret[i] = 0.f;
- for (int j = 0; j < 3; j++) {
- ret[i] += m(i, j) * v[j];
- }
- }
- return ret;
-}
-
-template
-DEVICE
-inline auto inverse(const TMatrix3x3 &m) -> TMatrix3x3 {
- // computes the inverse of a matrix m
- auto det = m(0, 0) * (m(1, 1) * m(2, 2) - m(2, 1) * m(1, 2)) -
- m(0, 1) * (m(1, 0) * m(2, 2) - m(1, 2) * m(2, 0)) +
- m(0, 2) * (m(1, 0) * m(2, 1) - m(1, 1) * m(2, 0));
-
- auto invdet = 1 / det;
-
- auto m_inv = TMatrix3x3{};
- m_inv(0, 0) = (m(1, 1) * m(2, 2) - m(2, 1) * m(1, 2)) * invdet;
- m_inv(0, 1) = (m(0, 2) * m(2, 1) - m(0, 1) * m(2, 2)) * invdet;
- m_inv(0, 2) = (m(0, 1) * m(1, 2) - m(0, 2) * m(1, 1)) * invdet;
- m_inv(1, 0) = (m(1, 2) * m(2, 0) - m(1, 0) * m(2, 2)) * invdet;
- m_inv(1, 1) = (m(0, 0) * m(2, 2) - m(0, 2) * m(2, 0)) * invdet;
- m_inv(1, 2) = (m(1, 0) * m(0, 2) - m(0, 0) * m(1, 2)) * invdet;
- m_inv(2, 0) = (m(1, 0) * m(2, 1) - m(2, 0) * m(1, 1)) * invdet;
- m_inv(2, 1) = (m(2, 0) * m(0, 1) - m(0, 0) * m(2, 1)) * invdet;
- m_inv(2, 2) = (m(0, 0) * m(1, 1) - m(1, 0) * m(0, 1)) * invdet;
- return m_inv;
-}
-
-template
-DEVICE
-inline auto operator+(const TMatrix4x4 &m0, const TMatrix4x4 &m1) -> TMatrix4x4 {
- TMatrix4x4 m;
- for (int i = 0; i < 4; i++) {
- for (int j = 0; j < 4; j++) {
- m(i, j) = m0(i, j) + m1(i, j);
- }
- }
- return m;
-}
-
-template
-DEVICE
-TMatrix3x3 transpose(const TMatrix3x3 &m) {
- return TMatrix3x3(m(0, 0), m(1, 0), m(2, 0),
- m(0, 1), m(1, 1), m(2, 1),
- m(0, 2), m(1, 2), m(2, 2));
-}
-
-template
-DEVICE
-TMatrix4x4 transpose(const TMatrix4x4 &m) {
- return TMatrix4x4(m(0, 0), m(1, 0), m(2, 0), m(3, 0),
- m(0, 1), m(1, 1), m(2, 1), m(3, 1),
- m(0, 2), m(1, 2), m(2, 2), m(3, 2),
- m(0, 3), m(1, 3), m(2, 3), m(3, 3));
-}
-
-template
-DEVICE
-inline TMatrix3x3 operator-(const TMatrix3x3 &m0) {
- TMatrix3x3 m;
- for (int i = 0; i < 3; i++) {
- for (int j = 0; j < 3; j++) {
- m(i, j) = -m0(i, j);
- }
- }
- return m;
-}
-
-template
-DEVICE
-inline TMatrix4x4 operator-(const TMatrix4x4 &m0) {
- TMatrix4x4 m;
- for (int i = 0; i < 4; i++) {
- for (int j = 0; j < 4; j++) {
- m(i, j) = -m0(i, j);
- }
- }
- return m;
-}
-
-template
-DEVICE
-inline TMatrix4x4 operator-(const TMatrix4x4 &m0, const TMatrix4x4 &m1) {
- TMatrix4x4 m;
- for (int i = 0; i < 4; i++) {
- for (int j = 0; j < 4; j++) {
- m(i, j) = m0(i, j) - m1(i, j);
- }
- }
- return m;
-}
-
-template
-DEVICE
-inline TMatrix3x3& operator+=(TMatrix3x3 &m0, const TMatrix3x3 &m1) {
- for (int i = 0; i < 3; i++) {
- for (int j = 0; j < 3; j++) {
- m0(i, j) += m1(i, j);
- }
- }
- return m0;
-}
-
-template
-DEVICE
-inline TMatrix4x4& operator+=(TMatrix4x4 &m0, const TMatrix4x4 &m1) {
- for (int i = 0; i < 4; i++) {
- for (int j = 0; j < 4; j++) {
- m0(i, j) += m1(i, j);
- }
- }
- return m0;
-}
-
-template
-DEVICE
-inline TMatrix4x4& operator-=(TMatrix4x4 &m0, const TMatrix4x4 &m1) {
- for (int i = 0; i < 4; i++) {
- for (int j = 0; j < 4; j++) {
- m0(i, j) -= m1(i, j);
- }
- }
- return m0;
-}
-
-template
-DEVICE
-inline TMatrix4x4 operator*(const TMatrix4x4 &m0, const TMatrix4x4 &m1) {
- TMatrix4x4 m;
- for (int i = 0; i < 4; i++) {
- for (int j = 0; j < 4; j++) {
- for (int k = 0; k < 4; k++) {
- m(i, j) += m0(i, k) * m1(k, j);
- }
- }
- }
- return m;
-}
-
-template
-DEVICE
-TMatrix4x4 inverse(const TMatrix4x4 &m) {
- // https://stackoverflow.com/questions/1148309/inverting-a-4x4-matrix
- TMatrix4x4 inv;
-
- inv(0, 0) = m(1, 1) * m(2, 2) * m(3, 3) -
- m(1, 1) * m(2, 3) * m(3, 2) -
- m(2, 1) * m(1, 2) * m(3, 3) +
- m(2, 1) * m(1, 3) * m(3, 2) +
- m(3, 1) * m(1, 2) * m(2, 3) -
- m(3, 1) * m(1, 3) * m(2, 2);
-
- inv(1, 0) = -m(1, 0) * m(2, 2) * m(3, 3) +
- m(1, 0) * m(2, 3) * m(3, 2) +
- m(2, 0) * m(1, 2) * m(3, 3) -
- m(2, 0) * m(1, 3) * m(3, 2) -
- m(3, 0) * m(1, 2) * m(2, 3) +
- m(3, 0) * m(1, 3) * m(2, 2);
-
- inv(2, 0) = m(1, 0) * m(2, 1) * m(3, 3) -
- m(1, 0) * m(2, 3) * m(3, 1) -
- m(2, 0) * m(1, 1) * m(3, 3) +
- m(2, 0) * m(1, 3) * m(3, 1) +
- m(3, 0) * m(1, 1) * m(2, 3) -
- m(3, 0) * m(1, 3) * m(2, 1);
-
- inv(3, 0) = -m(1, 0) * m(2, 1) * m(3, 2) +
- m(1, 0) * m(2, 2) * m(3, 1) +
- m(2, 0) * m(1, 1) * m(3, 2) -
- m(2, 0) * m(1, 2) * m(3, 1) -
- m(3, 0) * m(1, 1) * m(2, 2) +
- m(3, 0) * m(1, 2) * m(2, 1);
-
- inv(0, 1) = -m(0, 1) * m(2, 2) * m(3, 3) +
- m(0, 1) * m(2, 3) * m(3, 2) +
- m(2, 1) * m(0, 2) * m(3, 3) -
- m(2, 1) * m(0, 3) * m(3, 2) -
- m(3, 1) * m(0, 2) * m(2, 3) +
- m(3, 1) * m(0, 3) * m(2, 2);
-
- inv(1, 1) = m(0, 0) * m(2, 2) * m(3, 3) -
- m(0, 0) * m(2, 3) * m(3, 2) -
- m(2, 0) * m(0, 2) * m(3, 3) +
- m(2, 0) * m(0, 3) * m(3, 2) +
- m(3, 0) * m(0, 2) * m(2, 3) -
- m(3, 0) * m(0, 3) * m(2, 2);
-
- inv(2, 1) = -m(0, 0) * m(2, 1) * m(3, 3) +
- m(0, 0) * m(2, 3) * m(3, 1) +
- m(2, 0) * m(0, 1) * m(3, 3) -
- m(2, 0) * m(0, 3) * m(3, 1) -
- m(3, 0) * m(0, 1) * m(2, 3) +
- m(3, 0) * m(0, 3) * m(2, 1);
-
- inv(3, 1) = m(0, 0) * m(2, 1) * m(3, 2) -
- m(0, 0) * m(2, 2) * m(3, 1) -
- m(2, 0) * m(0, 1) * m(3, 2) +
- m(2, 0) * m(0, 2) * m(3, 1) +
- m(3, 0) * m(0, 1) * m(2, 2) -
- m(3, 0) * m(0, 2) * m(2, 1);
-
- inv(0, 2) = m(0, 1) * m(1, 2) * m(3, 3) -
- m(0, 1) * m(1, 3) * m(3, 2) -
- m(1, 1) * m(0, 2) * m(3, 3) +
- m(1, 1) * m(0, 3) * m(3, 2) +
- m(3, 1) * m(0, 2) * m(1, 3) -
- m(3, 1) * m(0, 3) * m(1, 2);
-
- inv(1, 2) = -m(0, 0) * m(1, 2) * m(3, 3) +
- m(0, 0) * m(1, 3) * m(3, 2) +
- m(1, 0) * m(0, 2) * m(3, 3) -
- m(1, 0) * m(0, 3) * m(3, 2) -
- m(3, 0) * m(0, 2) * m(1, 3) +
- m(3, 0) * m(0, 3) * m(1, 2);
-
- inv(2, 2) = m(0, 0) * m(1, 1) * m(3, 3) -
- m(0, 0) * m(1, 3) * m(3, 1) -
- m(1, 0) * m(0, 1) * m(3, 3) +
- m(1, 0) * m(0, 3) * m(3, 1) +
- m(3, 0) * m(0, 1) * m(1, 3) -
- m(3, 0) * m(0, 3) * m(1, 1);
-
- inv(3, 2) = -m(0, 0) * m(1, 1) * m(3, 2) +
- m(0, 0) * m(1, 2) * m(3, 1) +
- m(1, 0) * m(0, 1) * m(3, 2) -
- m(1, 0) * m(0, 2) * m(3, 1) -
- m(3, 0) * m(0, 1) * m(1, 2) +
- m(3, 0) * m(0, 2) * m(1, 1);
-
- inv(0, 3) = -m(0, 1) * m(1, 2) * m(2, 3) +
- m(0, 1) * m(1, 3) * m(2, 2) +
- m(1, 1) * m(0, 2) * m(2, 3) -
- m(1, 1) * m(0, 3) * m(2, 2) -
- m(2, 1) * m(0, 2) * m(1, 3) +
- m(2, 1) * m(0, 3) * m(1, 2);
-
- inv(1, 3) = m(0, 0) * m(1, 2) * m(2, 3) -
- m(0, 0) * m(1, 3) * m(2, 2) -
- m(1, 0) * m(0, 2) * m(2, 3) +
- m(1, 0) * m(0, 3) * m(2, 2) +
- m(2, 0) * m(0, 2) * m(1, 3) -
- m(2, 0) * m(0, 3) * m(1, 2);
-
- inv(2, 3) = -m(0, 0) * m(1, 1) * m(2, 3) +
- m(0, 0) * m(1, 3) * m(2, 1) +
- m(1, 0) * m(0, 1) * m(2, 3) -
- m(1, 0) * m(0, 3) * m(2, 1) -
- m(2, 0) * m(0, 1) * m(1, 3) +
- m(2, 0) * m(0, 3) * m(1, 1);
-
- inv(3, 3) = m(0, 0) * m(1, 1) * m(2, 2) -
- m(0, 0) * m(1, 2) * m(2, 1) -
- m(1, 0) * m(0, 1) * m(2, 2) +
- m(1, 0) * m(0, 2) * m(2, 1) +
- m(2, 0) * m(0, 1) * m(1, 2) -
- m(2, 0) * m(0, 2) * m(1, 1);
-
- auto det = m(0, 0) * inv(0, 0) +
- m(0, 1) * inv(1, 0) +
- m(0, 2) * inv(2, 0) +
- m(0, 3) * inv(3, 0);
-
- if (det == 0) {
- return TMatrix4x4{};
- }
-
- auto inv_det = 1.0 / det;
-
- for (int i = 0; i < 4; i++) {
- for (int j = 0; j < 4; j++) {
- inv(i, j) *= inv_det;
- }
- }
-
- return inv;
-}
-
-template
-inline std::ostream& operator<<(std::ostream &os, const TMatrix3x3 &m) {
- for (int i = 0; i < 3; i++) {
- for (int j = 0; j < 3; j++) {
- os << m(i, j) << " ";
- }
- os << std::endl;
- }
- return os;
-}
-
-template
-inline std::ostream& operator<<(std::ostream &os, const TMatrix4x4 &m) {
- for (int i = 0; i < 4; i++) {
- for (int j = 0; j < 4; j++) {
- os << m(i, j) << " ";
- }
- os << std::endl;
- }
- return os;
-}
-
-template
-DEVICE
-TVector2 xform_pt(const TMatrix3x3 &m, const TVector2 &pt) {
- TVector3 t{m(0, 0) * pt[0] + m(0, 1) * pt[1] + m(0, 2),
- m(1, 0) * pt[0] + m(1, 1) * pt[1] + m(1, 2),
- m(2, 0) * pt[0] + m(2, 1) * pt[1] + m(2, 2)};
- return TVector2{t[0] / t[2], t[1] / t[2]};
-}
-
-template
-DEVICE
-void d_xform_pt(const TMatrix3x3 &m, const TVector2 &pt,
- const TVector2 &d_out,
- TMatrix3x3 &d_m,
- TVector2 &d_pt) {
- TVector3 t{m(0, 0) * pt[0] + m(0, 1) * pt[1] + m(0, 2),
- m(1, 0) * pt[0] + m(1, 1) * pt[1] + m(1, 2),
- m(2, 0) * pt[0] + m(2, 1) * pt[1] + m(2, 2)};
- auto out = TVector2{t[0] / t[2], t[1] / t[2]};
- TVector3 d_t{d_out[0] / t[2],
- d_out[1] / t[2],
- -(d_out[0] * out[0] + d_out[1] * out[1]) / t[2]};
- d_m(0, 0) += d_t[0] * pt[0];
- d_m(0, 1) += d_t[0] * pt[1];
- d_m(0, 2) += d_t[0];
- d_m(1, 0) += d_t[1] * pt[0];
- d_m(1, 1) += d_t[1] * pt[1];
- d_m(1, 2) += d_t[1];
- d_m(2, 0) += d_t[2] * pt[0];
- d_m(2, 1) += d_t[2] * pt[1];
- d_m(2, 2) += d_t[2];
- d_pt[0] += d_t[0] * m(0, 0) + d_t[1] * m(1, 0) + d_t[2] * m(2, 0);
- d_pt[1] += d_t[0] * m(0, 1) + d_t[1] * m(1, 1) + d_t[2] * m(2, 1);
-}
-
-template
-DEVICE
-TVector2 xform_normal(const TMatrix3x3 &m_inv, const TVector2 &n) {
- return normalize(TVector2{m_inv(0, 0) * n[0] + m_inv(1, 0) * n[1],
- m_inv(0, 1) * n[0] + m_inv(1, 1) * n[1]});
-}
diff --git a/spaces/Callimethee/Imagine-CR/README.md b/spaces/Callimethee/Imagine-CR/README.md
deleted file mode 100644
index 40198ef611a7dc704d8c63466c61013c1cb3689b..0000000000000000000000000000000000000000
--- a/spaces/Callimethee/Imagine-CR/README.md
+++ /dev/null
@@ -1,13 +0,0 @@
----
-title: Imagine CR
-emoji: 🎲
-colorFrom: green
-colorTo: yellow
-sdk: gradio
-sdk_version: 3.4.1
-app_file: app.py
-pinned: false
-license: mit
----
-
-Generate your own Critical Role moments with this neural network!
diff --git a/spaces/CatNika/New_Cat_Proxy/README.md b/spaces/CatNika/New_Cat_Proxy/README.md
deleted file mode 100644
index b7940671089bddb21d6c2cbf2af3a63566c99709..0000000000000000000000000000000000000000
--- a/spaces/CatNika/New_Cat_Proxy/README.md
+++ /dev/null
@@ -1,10 +0,0 @@
----
-title: NikaProxy
-emoji: 🏆
-colorFrom: yellow
-colorTo: green
-sdk: docker
-pinned: false
----
-
-Check out the configuration reference at https://huggingface.co/docs/hub/spaces-config-reference
diff --git a/spaces/Chris4K/llms_compare/Arthashastra Book In Urdu Free [NEW] Download.md b/spaces/Chris4K/llms_compare/Arthashastra Book In Urdu Free [NEW] Download.md
deleted file mode 100644
index 361eeaac0e7b6d7154d20bcb4014c355d5266a6a..0000000000000000000000000000000000000000
--- a/spaces/Chris4K/llms_compare/Arthashastra Book In Urdu Free [NEW] Download.md
+++ /dev/null
@@ -1,80 +0,0 @@
-## Arthashastra Book In Urdu Free Download
-
-
-
-
-
- ![Arthashastra Book In Urdu Free \[NEW\] Download](https://cdn.shopify.com/s/files/1/0100/4001/6992/products/buy-chanakya-in-you-8184956606-urdu-bazaar-37264803037421.jpg?v\u003d1666102794)
-
-
-
-
-
-**Download File --->>> [https://urluso.com/2tBNCX](https://urluso.com/2tBNCX)**
-
-
-
-
-
-
-
-
-
-
-
-
-
-# How to Download Arthashastra Book in Urdu for Free
-
-
-
-Arthashastra is an ancient Indian treatise on statecraft, economic policy and military strategy, written by Chanakya, also known as Kautilya or Vishnugupta. It is considered one of the most influential works of political philosophy and practical wisdom in history.
-
-
-
-If you are interested in reading this classic book in Urdu language, you might be wondering how to get it for free. There are many websites that offer free ebooks, but not all of them are reliable or legal. Some might contain viruses, malware or spyware that can harm your device or compromise your privacy. Others might have poor quality translations, incomplete texts or broken links.
-
-
-
-To help you avoid these problems, we have compiled a list of three trustworthy sources where you can download Arthashastra book in Urdu for free. These are:
-
-
-
-- **Archive.org**: This is a non-profit library of millions of free books, movies, music and more. You can find Arthashastra book in Urdu along with other books by Chanakya and related topics. You can download the book in various formats, such as PDF, EPUB or MOBI. You can also read it online or borrow it for a limited time. To access the book, go to [this link](https://archive.org/details/Arthashastra_Of_Chanakya__Other_Books) [^1^] and select the format you prefer.
-
-- **SoundCloud**: This is a popular platform for streaming and sharing audio content. You can listen to Arthashastra book in Urdu as an audiobook or an excerpt on SoundCloud. You can also download the audio file for offline listening. To access the book, go to [this link](https://soundcloud.com/itembuhysko2/arthashastra-book-in-urdu-free-download) [^3^] and click on the download button.
-
-
-
-We hope you enjoy reading or listening to Arthashastra book in Urdu for free. If you do, please share this article with your friends and family who might also be interested in this topic. Thank you for your attention and happy reading!
-
-
-
-Arthashastra book in Urdu is not only a valuable source of historical and political knowledge, but also a guide for living a successful and ethical life. The book covers topics such as administration, diplomacy, law, taxation, welfare, trade, defense, agriculture, education and more. It also provides advice on personal conduct, leadership qualities, moral values and human psychology.
-
-
-
-The book is divided into 15 books and 180 chapters, each dealing with a specific aspect of statecraft or life. Some of the most famous concepts and quotes from the book are:
-
-
-
-- **Rajamandala**: This is a theory of foreign relations that divides the world into circles of friendly and hostile states. The king should seek to expand his influence by forming alliances with the friendly states and weakening the hostile ones.
-
-- **Mitrasamprapti**: This is a strategy of winning over enemies by using diplomacy, gifts, flattery and deception. The king should avoid direct confrontation and use subtle means to achieve his goals.
-
-- **Niti**: This is a term for ethical and prudent conduct that leads to happiness and prosperity. The king should follow the principles of niti in his personal and public life.
-
-- **Yuktivada**: This is a method of logical reasoning that helps to solve problems and make decisions. The king should use yuktivada to analyze situations and choose the best course of action.
-
-- **"The king shall consider as good, not what pleases himself but what pleases his subjects."**: This is one of the most famous quotes from the book that emphasizes the importance of benevolence and welfare of the people.
-
-
-
-By reading Arthashastra book in Urdu, you can learn a lot from the wisdom and experience of Chanakya, who was a master of statecraft and a genius of his time. You can apply his teachings to your own life and career, and improve your skills and abilities in various fields. You can also gain a deeper understanding of the ancient Indian culture and civilization, and appreciate its contributions to the world.
-
- 145887f19f
-
-
-
-
-
diff --git a/spaces/ClassCat/ViT-ImageNet-Classification/README.md b/spaces/ClassCat/ViT-ImageNet-Classification/README.md
deleted file mode 100644
index 2a9ee485fac7ecb96dd57a23dd133616b8385d22..0000000000000000000000000000000000000000
--- a/spaces/ClassCat/ViT-ImageNet-Classification/README.md
+++ /dev/null
@@ -1,12 +0,0 @@
----
-title: ViT ImageNet Classification
-emoji: 🔥
-colorFrom: pink
-colorTo: pink
-sdk: gradio
-sdk_version: 3.16.1
-app_file: app.py
-pinned: false
----
-
-Check out the configuration reference at https://huggingface.co/docs/hub/spaces-config-reference
diff --git a/spaces/CompVis/stable-diffusion-license/README.md b/spaces/CompVis/stable-diffusion-license/README.md
deleted file mode 100644
index 1c8a5bbad633babbf75e3c6811e742306e36c73d..0000000000000000000000000000000000000000
--- a/spaces/CompVis/stable-diffusion-license/README.md
+++ /dev/null
@@ -1,10 +0,0 @@
----
-title: License
-emoji: ⚖️
-colorFrom: red
-colorTo: indigo
-sdk: static
-pinned: false
----
-
-Check out the configuration reference at https://huggingface.co/docs/hub/spaces#reference
diff --git a/spaces/Cong723/gpt-academic-public/crazy_functions/test_project/latex/attention/background.tex b/spaces/Cong723/gpt-academic-public/crazy_functions/test_project/latex/attention/background.tex
deleted file mode 100644
index 785069dc0f9143bad24e640056dd1072d5c6e5b5..0000000000000000000000000000000000000000
--- a/spaces/Cong723/gpt-academic-public/crazy_functions/test_project/latex/attention/background.tex
+++ /dev/null
@@ -1,58 +0,0 @@
-The goal of reducing sequential computation also forms the foundation of the Extended Neural GPU \citep{extendedngpu}, ByteNet \citep{NalBytenet2017} and ConvS2S \citep{JonasFaceNet2017}, all of which use convolutional neural networks as basic building block, computing hidden representations in parallel for all input and output positions. In these models, the number of operations required to relate signals from two arbitrary input or output positions grows in the distance between positions, linearly for ConvS2S and logarithmically for ByteNet. This makes it more difficult to learn dependencies between distant positions \citep{hochreiter2001gradient}. In the Transformer this is reduced to a constant number of operations, albeit at the cost of reduced effective resolution due to averaging attention-weighted positions, an effect we counteract with Multi-Head Attention as described in section~\ref{sec:attention}.
-
-Self-attention, sometimes called intra-attention is an attention mechanism relating different positions of a single sequence in order to compute a representation of the sequence. Self-attention has been used successfully in a variety of tasks including reading comprehension, abstractive summarization, textual entailment and learning task-independent sentence representations \citep{cheng2016long, decomposableAttnModel, paulus2017deep, lin2017structured}.
-
-End-to-end memory networks are based on a recurrent attention mechanism instead of sequence-aligned recurrence and have been shown to perform well on simple-language question answering and language modeling tasks \citep{sukhbaatar2015}.
-
-To the best of our knowledge, however, the Transformer is the first transduction model relying entirely on self-attention to compute representations of its input and output without using sequence-aligned RNNs or convolution.
-In the following sections, we will describe the Transformer, motivate self-attention and discuss its advantages over models such as \citep{neural_gpu, NalBytenet2017} and \citep{JonasFaceNet2017}.
-
-
-%\citep{JonasFaceNet2017} report new SOTA on machine translation for English-to-German (EnDe), Enlish-to-French (EnFr) and English-to-Romanian language pairs.
-
-%For example,! in MT, we must draw information from both input and previous output words to translate an output word accurately. An attention layer \citep{bahdanau2014neural} can connect a very large number of positions at low computation cost, making it an essential ingredient in competitive recurrent models for machine translation.
-
-%A natural question to ask then is, "Could we replace recurrence with attention?". \marginpar{Don't know if it's the most natural question to ask given the previous statements. Also, need to say that the complexity table summarizes these statements} Such a model would be blessed with the computational efficiency of attention and the power of cross-positional communication. In this work, show that pure attention models work remarkably well for MT, achieving new SOTA results on EnDe and EnFr, and can be trained in under $2$ days on xyz architecture.
-
-%After the seminal models introduced in \citep{sutskever14, bahdanau2014neural, cho2014learning}, recurrent models have become the dominant solution for both sequence modeling and sequence-to-sequence transduction. Many efforts such as \citep{wu2016google,luong2015effective,jozefowicz2016exploring} have pushed the boundaries of machine translation (MT) and language modeling with recurrent endoder-decoder and recurrent language models. Recent effort \citep{shazeer2017outrageously} has successfully combined the power of conditional computation with sequence models to train very large models for MT, pushing SOTA at lower computational cost.
-
-%Recurrent models compute a vector of hidden states $h_t$, for each time step $t$ of computation. $h_t$ is a function of both the input at time $t$ and the previous hidden state $h_t$. This dependence on the previous hidden state precludes processing all timesteps at once, instead requiring long sequences of sequential operations. In practice, this results in greatly reduced computational efficiency, as on modern computing hardware, a single operation on a large batch is much faster than a large number of operations on small batches. The problem gets worse at longer sequence lengths. Although sequential computation is not a severe bottleneck at inference time, as autoregressively generating each output requires all previous outputs, the inability to compute scores at all output positions at once hinders us from rapidly training our models over large datasets. Although impressive work such as \citep{Kuchaiev2017Factorization} is able to significantly accelerate the training of LSTMs with factorization tricks, we are still bound by the linear dependence on sequence length.
-
-%If the model could compute hidden states at each time step using only the inputs and outputs, it would be liberated from the dependence on results from previous time steps during training. This line of thought is the foundation of recent efforts such as the Markovian neural GPU \citep{neural_gpu}, ByteNet \citep{NalBytenet2017} and ConvS2S \citep{JonasFaceNet2017}, all of which use convolutional neural networks as a building block to compute hidden representations simultaneously for all timesteps, resulting in $O(1)$ sequential time complexity. \citep{JonasFaceNet2017} report new SOTA on machine translation for English-to-German (EnDe), Enlish-to-French (EnFr) and English-to-Romanian language pairs.
-
-%A crucial component for accurate sequence prediction is modeling cross-positional communication. For example, in MT, we must draw information from both input and previous output words to translate an output word accurately. An attention layer \citep{bahdanau2014neural} can connect a very large number of positions at a low computation cost, also $O(1)$ sequential time complexity, making it an essential ingredient in recurrent encoder-decoder architectures for MT. A natural question to ask then is, "Could we replace recurrence with attention?". \marginpar{Don't know if it's the most natural question to ask given the previous statements. Also, need to say that the complexity table summarizes these statements} Such a model would be blessed with the computational efficiency of attention and the power of cross-positional communication. In this work, show that pure attention models work remarkably well for MT, achieving new SOTA results on EnDe and EnFr, and can be trained in under $2$ days on xyz architecture.
-
-
-
-%Note: Facebook model is no better than RNNs in this regard, since it requires a number of layers proportional to the distance you want to communicate. Bytenet is more promising, since it requires a logarithmnic number of layers (does bytenet have SOTA results)?
-
-%Note: An attention layer can connect a very large number of positions at a low computation cost in O(1) sequential operations. This is why encoder-decoder attention has been so successful in seq-to-seq models so far. It is only natural, then, to also use attention to connect the timesteps of the same sequence.
-
-%Note: I wouldn't say that long sequences are not a problem during inference. It would be great if we could infer with no long sequences. We could just say later on that, while our training graph is constant-depth, our model still requires sequential operations in the decoder part during inference due to the autoregressive nature of the model.
-
-%\begin{table}[h!]
-%\caption{Attention models are quite efficient for cross-positional communications when sequence length is smaller than channel depth. $n$ represents the sequence length and $d$ represents the channel depth.}
-%\label{tab:op_complexities}
-%\begin{center}
-%\vspace{-5pt}
-%\scalebox{0.75}{
-
-%\begin{tabular}{l|c|c|c}
-%\hline \hline
-%Layer Type & Receptive & Complexity & Sequential \\
-% & Field & & Operations \\
-%\hline
-%Pointwise Feed-Forward & $1$ & $O(n \cdot d^2)$ & $O(1)$ \\
-%\hline
-%Recurrent & $n$ & $O(n \cdot d^2)$ & $O(n)$ \\
-%\hline
-%Convolutional & $r$ & $O(r \cdot n \cdot d^2)$ & $O(1)$ \\
-%\hline
-%Convolutional (separable) & $r$ & $O(r \cdot n \cdot d + n %\cdot d^2)$ & $O(1)$ \\
-%\hline
-%Attention & $r$ & $O(r \cdot n \cdot d)$ & $O(1)$ \\
-%\hline \hline
-%\end{tabular}
-%}
-%\end{center}
-%\end{table}
\ No newline at end of file
diff --git a/spaces/Covert1107/sd-diffusers-webui/modules/model.py b/spaces/Covert1107/sd-diffusers-webui/modules/model.py
deleted file mode 100644
index 70fa00ee4b52f1c9ad7cc10c52c201b64ceb5fd8..0000000000000000000000000000000000000000
--- a/spaces/Covert1107/sd-diffusers-webui/modules/model.py
+++ /dev/null
@@ -1,897 +0,0 @@
-import importlib
-import inspect
-import math
-from pathlib import Path
-import re
-from collections import defaultdict
-from typing import List, Optional, Union
-
-import time
-import k_diffusion
-import numpy as np
-import PIL
-import torch
-import torch.nn as nn
-import torch.nn.functional as F
-from einops import rearrange
-from k_diffusion.external import CompVisDenoiser, CompVisVDenoiser
-from modules.prompt_parser import FrozenCLIPEmbedderWithCustomWords
-from torch import einsum
-from torch.autograd.function import Function
-
-from diffusers import DiffusionPipeline
-from diffusers.utils import PIL_INTERPOLATION, is_accelerate_available
-from diffusers.utils import logging, randn_tensor
-
-import modules.safe as _
-from safetensors.torch import load_file
-
-xformers_available = False
-try:
- import xformers
-
- xformers_available = True
-except ImportError:
- pass
-
-EPSILON = 1e-6
-exists = lambda val: val is not None
-default = lambda val, d: val if exists(val) else d
-logger = logging.get_logger(__name__) # pylint: disable=invalid-name
-
-
-def get_attention_scores(attn, query, key, attention_mask=None):
-
- if attn.upcast_attention:
- query = query.float()
- key = key.float()
-
- attention_scores = torch.baddbmm(
- torch.empty(
- query.shape[0],
- query.shape[1],
- key.shape[1],
- dtype=query.dtype,
- device=query.device,
- ),
- query,
- key.transpose(-1, -2),
- beta=0,
- alpha=attn.scale,
- )
-
- if attention_mask is not None:
- attention_scores = attention_scores + attention_mask
-
- if attn.upcast_softmax:
- attention_scores = attention_scores.float()
-
- return attention_scores
-
-
-class CrossAttnProcessor(nn.Module):
- def __call__(
- self,
- attn,
- hidden_states,
- encoder_hidden_states=None,
- attention_mask=None,
- ):
- batch_size, sequence_length, _ = hidden_states.shape
- attention_mask = attn.prepare_attention_mask(attention_mask, sequence_length)
-
- encoder_states = hidden_states
- is_xattn = False
- if encoder_hidden_states is not None:
- is_xattn = True
- img_state = encoder_hidden_states["img_state"]
- encoder_states = encoder_hidden_states["states"]
- weight_func = encoder_hidden_states["weight_func"]
- sigma = encoder_hidden_states["sigma"]
-
- query = attn.to_q(hidden_states)
- key = attn.to_k(encoder_states)
- value = attn.to_v(encoder_states)
-
- query = attn.head_to_batch_dim(query)
- key = attn.head_to_batch_dim(key)
- value = attn.head_to_batch_dim(value)
-
- if is_xattn and isinstance(img_state, dict):
- # use torch.baddbmm method (slow)
- attention_scores = get_attention_scores(attn, query, key, attention_mask)
- w = img_state[sequence_length].to(query.device)
- cross_attention_weight = weight_func(w, sigma, attention_scores)
- attention_scores += torch.repeat_interleave(
- cross_attention_weight, repeats=attn.heads, dim=0
- )
-
- # calc probs
- attention_probs = attention_scores.softmax(dim=-1)
- attention_probs = attention_probs.to(query.dtype)
- hidden_states = torch.bmm(attention_probs, value)
-
- elif xformers_available:
- hidden_states = xformers.ops.memory_efficient_attention(
- query.contiguous(),
- key.contiguous(),
- value.contiguous(),
- attn_bias=attention_mask,
- )
- hidden_states = hidden_states.to(query.dtype)
-
- else:
- q_bucket_size = 512
- k_bucket_size = 1024
-
- # use flash-attention
- hidden_states = FlashAttentionFunction.apply(
- query.contiguous(),
- key.contiguous(),
- value.contiguous(),
- attention_mask,
- False,
- q_bucket_size,
- k_bucket_size,
- )
- hidden_states = hidden_states.to(query.dtype)
-
- hidden_states = attn.batch_to_head_dim(hidden_states)
-
- # linear proj
- hidden_states = attn.to_out[0](hidden_states)
-
- # dropout
- hidden_states = attn.to_out[1](hidden_states)
-
- return hidden_states
-
-class ModelWrapper:
- def __init__(self, model, alphas_cumprod):
- self.model = model
- self.alphas_cumprod = alphas_cumprod
-
- def apply_model(self, *args, **kwargs):
- if len(args) == 3:
- encoder_hidden_states = args[-1]
- args = args[:2]
- if kwargs.get("cond", None) is not None:
- encoder_hidden_states = kwargs.pop("cond")
- return self.model(
- *args, encoder_hidden_states=encoder_hidden_states, **kwargs
- ).sample
-
-
-class StableDiffusionPipeline(DiffusionPipeline):
-
- _optional_components = ["safety_checker", "feature_extractor"]
-
- def __init__(
- self,
- vae,
- text_encoder,
- tokenizer,
- unet,
- scheduler,
- ):
- super().__init__()
-
- # get correct sigmas from LMS
- self.register_modules(
- vae=vae,
- text_encoder=text_encoder,
- tokenizer=tokenizer,
- unet=unet,
- scheduler=scheduler,
- )
- self.setup_unet(self.unet)
- self.setup_text_encoder()
-
- def setup_text_encoder(self, n=1, new_encoder=None):
- if new_encoder is not None:
- self.text_encoder = new_encoder
-
- self.prompt_parser = FrozenCLIPEmbedderWithCustomWords(self.tokenizer, self.text_encoder)
- self.prompt_parser.CLIP_stop_at_last_layers = n
-
- def setup_unet(self, unet):
- unet = unet.to(self.device)
- model = ModelWrapper(unet, self.scheduler.alphas_cumprod)
- if self.scheduler.prediction_type == "v_prediction":
- self.k_diffusion_model = CompVisVDenoiser(model)
- else:
- self.k_diffusion_model = CompVisDenoiser(model)
-
- def get_scheduler(self, scheduler_type: str):
- library = importlib.import_module("k_diffusion")
- sampling = getattr(library, "sampling")
- return getattr(sampling, scheduler_type)
-
- def encode_sketchs(self, state, scale_ratio=8, g_strength=1.0, text_ids=None):
- uncond, cond = text_ids[0], text_ids[1]
-
- img_state = []
- if state is None:
- return torch.FloatTensor(0)
-
- for k, v in state.items():
- if v["map"] is None:
- continue
-
- v_input = self.tokenizer(
- k,
- max_length=self.tokenizer.model_max_length,
- truncation=True,
- add_special_tokens=False,
- ).input_ids
-
- dotmap = v["map"] < 255
- out = dotmap.astype(float)
- if v["mask_outsides"]:
- out[out==0] = -1
-
- arr = torch.from_numpy(
- out * float(v["weight"]) * g_strength
- )
- img_state.append((v_input, arr))
-
- if len(img_state) == 0:
- return torch.FloatTensor(0)
-
- w_tensors = dict()
- cond = cond.tolist()
- uncond = uncond.tolist()
- for layer in self.unet.down_blocks:
- c = int(len(cond))
- w, h = img_state[0][1].shape
- w_r, h_r = w // scale_ratio, h // scale_ratio
-
- ret_cond_tensor = torch.zeros((1, int(w_r * h_r), c), dtype=torch.float32)
- ret_uncond_tensor = torch.zeros((1, int(w_r * h_r), c), dtype=torch.float32)
-
- for v_as_tokens, img_where_color in img_state:
- is_in = 0
-
- ret = (
- F.interpolate(
- img_where_color.unsqueeze(0).unsqueeze(1),
- scale_factor=1 / scale_ratio,
- mode="bilinear",
- align_corners=True,
- )
- .squeeze()
- .reshape(-1, 1)
- .repeat(1, len(v_as_tokens))
- )
-
- for idx, tok in enumerate(cond):
- if cond[idx : idx + len(v_as_tokens)] == v_as_tokens:
- is_in = 1
- ret_cond_tensor[0, :, idx : idx + len(v_as_tokens)] += ret
-
- for idx, tok in enumerate(uncond):
- if uncond[idx : idx + len(v_as_tokens)] == v_as_tokens:
- is_in = 1
- ret_uncond_tensor[0, :, idx : idx + len(v_as_tokens)] += ret
-
- if not is_in == 1:
- print(f"tokens {v_as_tokens} not found in text")
-
- w_tensors[w_r * h_r] = torch.cat([ret_uncond_tensor, ret_cond_tensor])
- scale_ratio *= 2
-
- return w_tensors
-
- def enable_attention_slicing(self, slice_size: Optional[Union[str, int]] = "auto"):
- r"""
- Enable sliced attention computation.
-
- When this option is enabled, the attention module will split the input tensor in slices, to compute attention
- in several steps. This is useful to save some memory in exchange for a small speed decrease.
-
- Args:
- slice_size (`str` or `int`, *optional*, defaults to `"auto"`):
- When `"auto"`, halves the input to the attention heads, so attention will be computed in two steps. If
- a number is provided, uses as many slices as `attention_head_dim // slice_size`. In this case,
- `attention_head_dim` must be a multiple of `slice_size`.
- """
- if slice_size == "auto":
- # half the attention head size is usually a good trade-off between
- # speed and memory
- slice_size = self.unet.config.attention_head_dim // 2
- self.unet.set_attention_slice(slice_size)
-
- def disable_attention_slicing(self):
- r"""
- Disable sliced attention computation. If `enable_attention_slicing` was previously invoked, this method will go
- back to computing attention in one step.
- """
- # set slice_size = `None` to disable `attention slicing`
- self.enable_attention_slicing(None)
-
- def enable_sequential_cpu_offload(self, gpu_id=0):
- r"""
- Offloads all models to CPU using accelerate, significantly reducing memory usage. When called, unet,
- text_encoder, vae and safety checker have their state dicts saved to CPU and then are moved to a
- `torch.device('meta') and loaded to GPU only when their specific submodule has its `forward` method called.
- """
- if is_accelerate_available():
- from accelerate import cpu_offload
- else:
- raise ImportError("Please install accelerate via `pip install accelerate`")
-
- device = torch.device(f"cuda:{gpu_id}")
-
- for cpu_offloaded_model in [
- self.unet,
- self.text_encoder,
- self.vae,
- self.safety_checker,
- ]:
- if cpu_offloaded_model is not None:
- cpu_offload(cpu_offloaded_model, device)
-
- @property
- def _execution_device(self):
- r"""
- Returns the device on which the pipeline's models will be executed. After calling
- `pipeline.enable_sequential_cpu_offload()` the execution device can only be inferred from Accelerate's module
- hooks.
- """
- if self.device != torch.device("meta") or not hasattr(self.unet, "_hf_hook"):
- return self.device
- for module in self.unet.modules():
- if (
- hasattr(module, "_hf_hook")
- and hasattr(module._hf_hook, "execution_device")
- and module._hf_hook.execution_device is not None
- ):
- return torch.device(module._hf_hook.execution_device)
- return self.device
-
- def decode_latents(self, latents):
- latents = latents.to(self.device, dtype=self.vae.dtype)
- latents = 1 / 0.18215 * latents
- image = self.vae.decode(latents).sample
- image = (image / 2 + 0.5).clamp(0, 1)
- # we always cast to float32 as this does not cause significant overhead and is compatible with bfloa16
- image = image.cpu().permute(0, 2, 3, 1).float().numpy()
- return image
-
- def check_inputs(self, prompt, height, width, callback_steps):
- if not isinstance(prompt, str) and not isinstance(prompt, list):
- raise ValueError(
- f"`prompt` has to be of type `str` or `list` but is {type(prompt)}"
- )
-
- if height % 8 != 0 or width % 8 != 0:
- raise ValueError(
- f"`height` and `width` have to be divisible by 8 but are {height} and {width}."
- )
-
- if (callback_steps is None) or (
- callback_steps is not None
- and (not isinstance(callback_steps, int) or callback_steps <= 0)
- ):
- raise ValueError(
- f"`callback_steps` has to be a positive integer but is {callback_steps} of type"
- f" {type(callback_steps)}."
- )
-
- def prepare_latents(
- self,
- batch_size,
- num_channels_latents,
- height,
- width,
- dtype,
- device,
- generator,
- latents=None,
- ):
- shape = (batch_size, num_channels_latents, height // 8, width // 8)
- if latents is None:
- if device.type == "mps":
- # randn does not work reproducibly on mps
- latents = torch.randn(
- shape, generator=generator, device="cpu", dtype=dtype
- ).to(device)
- else:
- latents = torch.randn(
- shape, generator=generator, device=device, dtype=dtype
- )
- else:
- # if latents.shape != shape:
- # raise ValueError(f"Unexpected latents shape, got {latents.shape}, expected {shape}")
- latents = latents.to(device)
-
- # scale the initial noise by the standard deviation required by the scheduler
- return latents
-
- def preprocess(self, image):
- if isinstance(image, torch.Tensor):
- return image
- elif isinstance(image, PIL.Image.Image):
- image = [image]
-
- if isinstance(image[0], PIL.Image.Image):
- w, h = image[0].size
- w, h = map(lambda x: x - x % 8, (w, h)) # resize to integer multiple of 8
-
- image = [
- np.array(i.resize((w, h), resample=PIL_INTERPOLATION["lanczos"]))[
- None, :
- ]
- for i in image
- ]
- image = np.concatenate(image, axis=0)
- image = np.array(image).astype(np.float32) / 255.0
- image = image.transpose(0, 3, 1, 2)
- image = 2.0 * image - 1.0
- image = torch.from_numpy(image)
- elif isinstance(image[0], torch.Tensor):
- image = torch.cat(image, dim=0)
- return image
-
- @torch.no_grad()
- def img2img(
- self,
- prompt: Union[str, List[str]],
- num_inference_steps: int = 50,
- guidance_scale: float = 7.5,
- negative_prompt: Optional[Union[str, List[str]]] = None,
- generator: Optional[torch.Generator] = None,
- image: Optional[torch.FloatTensor] = None,
- output_type: Optional[str] = "pil",
- latents=None,
- strength=1.0,
- pww_state=None,
- pww_attn_weight=1.0,
- sampler_name="",
- sampler_opt={},
- start_time=-1,
- timeout=180,
- scale_ratio=8.0,
- ):
- sampler = self.get_scheduler(sampler_name)
- if image is not None:
- image = self.preprocess(image)
- image = image.to(self.vae.device, dtype=self.vae.dtype)
-
- init_latents = self.vae.encode(image).latent_dist.sample(generator)
- latents = 0.18215 * init_latents
-
- # 2. Define call parameters
- batch_size = 1 if isinstance(prompt, str) else len(prompt)
- device = self._execution_device
- latents = latents.to(device, dtype=self.unet.dtype)
- # here `guidance_scale` is defined analog to the guidance weight `w` of equation (2)
- # of the Imagen paper: https://arxiv.org/pdf/2205.11487.pdf . `guidance_scale = 1`
- # corresponds to doing no classifier free guidance.
- do_classifier_free_guidance = True
- if guidance_scale <= 1.0:
- raise ValueError("has to use guidance_scale")
-
- # 3. Encode input prompt
- text_ids, text_embeddings = self.prompt_parser([negative_prompt, prompt])
- text_embeddings = text_embeddings.to(self.unet.dtype)
-
- init_timestep = (
- int(num_inference_steps / min(strength, 0.999)) if strength > 0 else 0
- )
- sigmas = self.get_sigmas(init_timestep, sampler_opt).to(
- text_embeddings.device, dtype=text_embeddings.dtype
- )
-
- t_start = max(init_timestep - num_inference_steps, 0)
- sigma_sched = sigmas[t_start:]
-
- noise = randn_tensor(
- latents.shape,
- generator=generator,
- device=device,
- dtype=text_embeddings.dtype,
- )
- latents = latents.to(device)
- latents = latents + noise * sigma_sched[0]
-
- # 5. Prepare latent variables
- self.k_diffusion_model.sigmas = self.k_diffusion_model.sigmas.to(latents.device)
- self.k_diffusion_model.log_sigmas = self.k_diffusion_model.log_sigmas.to(
- latents.device
- )
-
- img_state = self.encode_sketchs(
- pww_state,
- g_strength=pww_attn_weight,
- text_ids=text_ids,
- )
-
- def model_fn(x, sigma):
-
- if start_time > 0 and timeout > 0:
- assert (time.time() - start_time) < timeout, "inference process timed out"
-
- latent_model_input = torch.cat([x] * 2)
- weight_func = lambda w, sigma, qk: w * math.log(1 + sigma) * qk.max()
- encoder_state = {
- "img_state": img_state,
- "states": text_embeddings,
- "sigma": sigma[0],
- "weight_func": weight_func,
- }
-
- noise_pred = self.k_diffusion_model(
- latent_model_input, sigma, cond=encoder_state
- )
- noise_pred_uncond, noise_pred_text = noise_pred.chunk(2)
- noise_pred = noise_pred_uncond + guidance_scale * (
- noise_pred_text - noise_pred_uncond
- )
- return noise_pred
-
- sampler_args = self.get_sampler_extra_args_i2i(sigma_sched, sampler)
- latents = sampler(model_fn, latents, **sampler_args)
-
- # 8. Post-processing
- image = self.decode_latents(latents)
-
- # 10. Convert to PIL
- if output_type == "pil":
- image = self.numpy_to_pil(image)
-
- return (image,)
-
- def get_sigmas(self, steps, params):
- discard_next_to_last_sigma = params.get("discard_next_to_last_sigma", False)
- steps += 1 if discard_next_to_last_sigma else 0
-
- if params.get("scheduler", None) == "karras":
- sigma_min, sigma_max = (
- self.k_diffusion_model.sigmas[0].item(),
- self.k_diffusion_model.sigmas[-1].item(),
- )
- sigmas = k_diffusion.sampling.get_sigmas_karras(
- n=steps, sigma_min=sigma_min, sigma_max=sigma_max, device=self.device
- )
- else:
- sigmas = self.k_diffusion_model.get_sigmas(steps)
-
- if discard_next_to_last_sigma:
- sigmas = torch.cat([sigmas[:-2], sigmas[-1:]])
-
- return sigmas
-
- # https://github.com/AUTOMATIC1111/stable-diffusion-webui/blob/48a15821de768fea76e66f26df83df3fddf18f4b/modules/sd_samplers.py#L454
- def get_sampler_extra_args_t2i(self, sigmas, eta, steps, func):
- extra_params_kwargs = {}
-
- if "eta" in inspect.signature(func).parameters:
- extra_params_kwargs["eta"] = eta
-
- if "sigma_min" in inspect.signature(func).parameters:
- extra_params_kwargs["sigma_min"] = sigmas[0].item()
- extra_params_kwargs["sigma_max"] = sigmas[-1].item()
-
- if "n" in inspect.signature(func).parameters:
- extra_params_kwargs["n"] = steps
- else:
- extra_params_kwargs["sigmas"] = sigmas
-
- return extra_params_kwargs
-
- # https://github.com/AUTOMATIC1111/stable-diffusion-webui/blob/48a15821de768fea76e66f26df83df3fddf18f4b/modules/sd_samplers.py#L454
- def get_sampler_extra_args_i2i(self, sigmas, func):
- extra_params_kwargs = {}
-
- if "sigma_min" in inspect.signature(func).parameters:
- ## last sigma is zero which isn't allowed by DPM Fast & Adaptive so taking value before last
- extra_params_kwargs["sigma_min"] = sigmas[-2]
-
- if "sigma_max" in inspect.signature(func).parameters:
- extra_params_kwargs["sigma_max"] = sigmas[0]
-
- if "n" in inspect.signature(func).parameters:
- extra_params_kwargs["n"] = len(sigmas) - 1
-
- if "sigma_sched" in inspect.signature(func).parameters:
- extra_params_kwargs["sigma_sched"] = sigmas
-
- if "sigmas" in inspect.signature(func).parameters:
- extra_params_kwargs["sigmas"] = sigmas
-
- return extra_params_kwargs
-
- @torch.no_grad()
- def txt2img(
- self,
- prompt: Union[str, List[str]],
- height: int = 512,
- width: int = 512,
- num_inference_steps: int = 50,
- guidance_scale: float = 7.5,
- negative_prompt: Optional[Union[str, List[str]]] = None,
- eta: float = 0.0,
- generator: Optional[torch.Generator] = None,
- latents: Optional[torch.FloatTensor] = None,
- output_type: Optional[str] = "pil",
- callback_steps: Optional[int] = 1,
- upscale=False,
- upscale_x: float = 2.0,
- upscale_method: str = "bicubic",
- upscale_antialias: bool = False,
- upscale_denoising_strength: int = 0.7,
- pww_state=None,
- pww_attn_weight=1.0,
- sampler_name="",
- sampler_opt={},
- start_time=-1,
- timeout=180,
- ):
- sampler = self.get_scheduler(sampler_name)
- # 1. Check inputs. Raise error if not correct
- self.check_inputs(prompt, height, width, callback_steps)
-
- # 2. Define call parameters
- batch_size = 1 if isinstance(prompt, str) else len(prompt)
- device = self._execution_device
- # here `guidance_scale` is defined analog to the guidance weight `w` of equation (2)
- # of the Imagen paper: https://arxiv.org/pdf/2205.11487.pdf . `guidance_scale = 1`
- # corresponds to doing no classifier free guidance.
- do_classifier_free_guidance = True
- if guidance_scale <= 1.0:
- raise ValueError("has to use guidance_scale")
-
- # 3. Encode input prompt
- text_ids, text_embeddings = self.prompt_parser([negative_prompt, prompt])
- text_embeddings = text_embeddings.to(self.unet.dtype)
-
- # 4. Prepare timesteps
- sigmas = self.get_sigmas(num_inference_steps, sampler_opt).to(
- text_embeddings.device, dtype=text_embeddings.dtype
- )
-
- # 5. Prepare latent variables
- num_channels_latents = self.unet.in_channels
- latents = self.prepare_latents(
- batch_size,
- num_channels_latents,
- height,
- width,
- text_embeddings.dtype,
- device,
- generator,
- latents,
- )
- latents = latents * sigmas[0]
- self.k_diffusion_model.sigmas = self.k_diffusion_model.sigmas.to(latents.device)
- self.k_diffusion_model.log_sigmas = self.k_diffusion_model.log_sigmas.to(
- latents.device
- )
-
- img_state = self.encode_sketchs(
- pww_state,
- g_strength=pww_attn_weight,
- text_ids=text_ids,
- )
-
- def model_fn(x, sigma):
-
- if start_time > 0 and timeout > 0:
- assert (time.time() - start_time) < timeout, "inference process timed out"
-
- latent_model_input = torch.cat([x] * 2)
- weight_func = lambda w, sigma, qk: w * math.log(1 + sigma) * qk.max()
- encoder_state = {
- "img_state": img_state,
- "states": text_embeddings,
- "sigma": sigma[0],
- "weight_func": weight_func,
- }
-
- noise_pred = self.k_diffusion_model(
- latent_model_input, sigma, cond=encoder_state
- )
- noise_pred_uncond, noise_pred_text = noise_pred.chunk(2)
- noise_pred = noise_pred_uncond + guidance_scale * (
- noise_pred_text - noise_pred_uncond
- )
- return noise_pred
-
- extra_args = self.get_sampler_extra_args_t2i(
- sigmas, eta, num_inference_steps, sampler
- )
- latents = sampler(model_fn, latents, **extra_args)
-
- if upscale:
- target_height = height * upscale_x
- target_width = width * upscale_x
- vae_scale_factor = 2 ** (len(self.vae.config.block_out_channels) - 1)
- latents = torch.nn.functional.interpolate(
- latents,
- size=(
- int(target_height // vae_scale_factor),
- int(target_width // vae_scale_factor),
- ),
- mode=upscale_method,
- antialias=upscale_antialias,
- )
- return self.img2img(
- prompt=prompt,
- num_inference_steps=num_inference_steps,
- guidance_scale=guidance_scale,
- negative_prompt=negative_prompt,
- generator=generator,
- latents=latents,
- strength=upscale_denoising_strength,
- sampler_name=sampler_name,
- sampler_opt=sampler_opt,
- pww_state=None,
- pww_attn_weight=pww_attn_weight / 2,
- )
-
- # 8. Post-processing
- image = self.decode_latents(latents)
-
- # 10. Convert to PIL
- if output_type == "pil":
- image = self.numpy_to_pil(image)
-
- return (image,)
-
-
-class FlashAttentionFunction(Function):
- @staticmethod
- @torch.no_grad()
- def forward(ctx, q, k, v, mask, causal, q_bucket_size, k_bucket_size):
- """Algorithm 2 in the paper"""
-
- device = q.device
- max_neg_value = -torch.finfo(q.dtype).max
- qk_len_diff = max(k.shape[-2] - q.shape[-2], 0)
-
- o = torch.zeros_like(q)
- all_row_sums = torch.zeros((*q.shape[:-1], 1), device=device)
- all_row_maxes = torch.full((*q.shape[:-1], 1), max_neg_value, device=device)
-
- scale = q.shape[-1] ** -0.5
-
- if not exists(mask):
- mask = (None,) * math.ceil(q.shape[-2] / q_bucket_size)
- else:
- mask = rearrange(mask, "b n -> b 1 1 n")
- mask = mask.split(q_bucket_size, dim=-1)
-
- row_splits = zip(
- q.split(q_bucket_size, dim=-2),
- o.split(q_bucket_size, dim=-2),
- mask,
- all_row_sums.split(q_bucket_size, dim=-2),
- all_row_maxes.split(q_bucket_size, dim=-2),
- )
-
- for ind, (qc, oc, row_mask, row_sums, row_maxes) in enumerate(row_splits):
- q_start_index = ind * q_bucket_size - qk_len_diff
-
- col_splits = zip(
- k.split(k_bucket_size, dim=-2),
- v.split(k_bucket_size, dim=-2),
- )
-
- for k_ind, (kc, vc) in enumerate(col_splits):
- k_start_index = k_ind * k_bucket_size
-
- attn_weights = einsum("... i d, ... j d -> ... i j", qc, kc) * scale
-
- if exists(row_mask):
- attn_weights.masked_fill_(~row_mask, max_neg_value)
-
- if causal and q_start_index < (k_start_index + k_bucket_size - 1):
- causal_mask = torch.ones(
- (qc.shape[-2], kc.shape[-2]), dtype=torch.bool, device=device
- ).triu(q_start_index - k_start_index + 1)
- attn_weights.masked_fill_(causal_mask, max_neg_value)
-
- block_row_maxes = attn_weights.amax(dim=-1, keepdims=True)
- attn_weights -= block_row_maxes
- exp_weights = torch.exp(attn_weights)
-
- if exists(row_mask):
- exp_weights.masked_fill_(~row_mask, 0.0)
-
- block_row_sums = exp_weights.sum(dim=-1, keepdims=True).clamp(
- min=EPSILON
- )
-
- new_row_maxes = torch.maximum(block_row_maxes, row_maxes)
-
- exp_values = einsum("... i j, ... j d -> ... i d", exp_weights, vc)
-
- exp_row_max_diff = torch.exp(row_maxes - new_row_maxes)
- exp_block_row_max_diff = torch.exp(block_row_maxes - new_row_maxes)
-
- new_row_sums = (
- exp_row_max_diff * row_sums
- + exp_block_row_max_diff * block_row_sums
- )
-
- oc.mul_((row_sums / new_row_sums) * exp_row_max_diff).add_(
- (exp_block_row_max_diff / new_row_sums) * exp_values
- )
-
- row_maxes.copy_(new_row_maxes)
- row_sums.copy_(new_row_sums)
-
- lse = all_row_sums.log() + all_row_maxes
-
- ctx.args = (causal, scale, mask, q_bucket_size, k_bucket_size)
- ctx.save_for_backward(q, k, v, o, lse)
-
- return o
-
- @staticmethod
- @torch.no_grad()
- def backward(ctx, do):
- """Algorithm 4 in the paper"""
-
- causal, scale, mask, q_bucket_size, k_bucket_size = ctx.args
- q, k, v, o, lse = ctx.saved_tensors
-
- device = q.device
-
- max_neg_value = -torch.finfo(q.dtype).max
- qk_len_diff = max(k.shape[-2] - q.shape[-2], 0)
-
- dq = torch.zeros_like(q)
- dk = torch.zeros_like(k)
- dv = torch.zeros_like(v)
-
- row_splits = zip(
- q.split(q_bucket_size, dim=-2),
- o.split(q_bucket_size, dim=-2),
- do.split(q_bucket_size, dim=-2),
- mask,
- lse.split(q_bucket_size, dim=-2),
- dq.split(q_bucket_size, dim=-2),
- )
-
- for ind, (qc, oc, doc, row_mask, lsec, dqc) in enumerate(row_splits):
- q_start_index = ind * q_bucket_size - qk_len_diff
-
- col_splits = zip(
- k.split(k_bucket_size, dim=-2),
- v.split(k_bucket_size, dim=-2),
- dk.split(k_bucket_size, dim=-2),
- dv.split(k_bucket_size, dim=-2),
- )
-
- for k_ind, (kc, vc, dkc, dvc) in enumerate(col_splits):
- k_start_index = k_ind * k_bucket_size
-
- attn_weights = einsum("... i d, ... j d -> ... i j", qc, kc) * scale
-
- if causal and q_start_index < (k_start_index + k_bucket_size - 1):
- causal_mask = torch.ones(
- (qc.shape[-2], kc.shape[-2]), dtype=torch.bool, device=device
- ).triu(q_start_index - k_start_index + 1)
- attn_weights.masked_fill_(causal_mask, max_neg_value)
-
- p = torch.exp(attn_weights - lsec)
-
- if exists(row_mask):
- p.masked_fill_(~row_mask, 0.0)
-
- dv_chunk = einsum("... i j, ... i d -> ... j d", p, doc)
- dp = einsum("... i d, ... j d -> ... i j", doc, vc)
-
- D = (doc * oc).sum(dim=-1, keepdims=True)
- ds = p * scale * (dp - D)
-
- dq_chunk = einsum("... i j, ... j d -> ... i d", ds, kc)
- dk_chunk = einsum("... i j, ... i d -> ... j d", ds, qc)
-
- dqc.add_(dq_chunk)
- dkc.add_(dk_chunk)
- dvc.add_(dv_chunk)
-
- return dq, dk, dv, None, None, None, None
diff --git a/spaces/Cvandi/remake/inference_realesrgan_video.py b/spaces/Cvandi/remake/inference_realesrgan_video.py
deleted file mode 100644
index 639b848e6578a2480ee0784e664c7751e325c477..0000000000000000000000000000000000000000
--- a/spaces/Cvandi/remake/inference_realesrgan_video.py
+++ /dev/null
@@ -1,199 +0,0 @@
-import argparse
-import glob
-import mimetypes
-import os
-import queue
-import shutil
-import torch
-from basicsr.archs.rrdbnet_arch import RRDBNet
-from basicsr.utils.logger import AvgTimer
-from tqdm import tqdm
-
-from realesrgan import IOConsumer, PrefetchReader, RealESRGANer
-from realesrgan.archs.srvgg_arch import SRVGGNetCompact
-
-
-def main():
- """Inference demo for Real-ESRGAN.
- It mainly for restoring anime videos.
-
- """
- parser = argparse.ArgumentParser()
- parser.add_argument('-i', '--input', type=str, default='inputs', help='Input image or folder')
- parser.add_argument(
- '-n',
- '--model_name',
- type=str,
- default='RealESRGAN_x4plus',
- help=('Model names: RealESRGAN_x4plus | RealESRNet_x4plus | RealESRGAN_x4plus_anime_6B | RealESRGAN_x2plus'
- 'RealESRGANv2-anime-xsx2 | RealESRGANv2-animevideo-xsx2-nousm | RealESRGANv2-animevideo-xsx2'
- 'RealESRGANv2-anime-xsx4 | RealESRGANv2-animevideo-xsx4-nousm | RealESRGANv2-animevideo-xsx4'))
- parser.add_argument('-o', '--output', type=str, default='results', help='Output folder')
- parser.add_argument('-s', '--outscale', type=float, default=4, help='The final upsampling scale of the image')
- parser.add_argument('--suffix', type=str, default='out', help='Suffix of the restored video')
- parser.add_argument('-t', '--tile', type=int, default=0, help='Tile size, 0 for no tile during testing')
- parser.add_argument('--tile_pad', type=int, default=10, help='Tile padding')
- parser.add_argument('--pre_pad', type=int, default=0, help='Pre padding size at each border')
- parser.add_argument('--face_enhance', action='store_true', help='Use GFPGAN to enhance face')
- parser.add_argument('--half', action='store_true', help='Use half precision during inference')
- parser.add_argument('-v', '--video', action='store_true', help='Output a video using ffmpeg')
- parser.add_argument('-a', '--audio', action='store_true', help='Keep audio')
- parser.add_argument('--fps', type=float, default=None, help='FPS of the output video')
- parser.add_argument('--consumer', type=int, default=4, help='Number of IO consumers')
-
- parser.add_argument(
- '--alpha_upsampler',
- type=str,
- default='realesrgan',
- help='The upsampler for the alpha channels. Options: realesrgan | bicubic')
- parser.add_argument(
- '--ext',
- type=str,
- default='auto',
- help='Image extension. Options: auto | jpg | png, auto means using the same extension as inputs')
- args = parser.parse_args()
-
- # ---------------------- determine models according to model names ---------------------- #
- args.model_name = args.model_name.split('.')[0]
- if args.model_name in ['RealESRGAN_x4plus', 'RealESRNet_x4plus']: # x4 RRDBNet model
- model = RRDBNet(num_in_ch=3, num_out_ch=3, num_feat=64, num_block=23, num_grow_ch=32, scale=4)
- netscale = 4
- elif args.model_name in ['RealESRGAN_x4plus_anime_6B']: # x4 RRDBNet model with 6 blocks
- model = RRDBNet(num_in_ch=3, num_out_ch=3, num_feat=64, num_block=6, num_grow_ch=32, scale=4)
- netscale = 4
- elif args.model_name in ['RealESRGAN_x2plus']: # x2 RRDBNet model
- model = RRDBNet(num_in_ch=3, num_out_ch=3, num_feat=64, num_block=23, num_grow_ch=32, scale=2)
- netscale = 2
- elif args.model_name in [
- 'RealESRGANv2-anime-xsx2', 'RealESRGANv2-animevideo-xsx2-nousm', 'RealESRGANv2-animevideo-xsx2'
- ]: # x2 VGG-style model (XS size)
- model = SRVGGNetCompact(num_in_ch=3, num_out_ch=3, num_feat=64, num_conv=16, upscale=2, act_type='prelu')
- netscale = 2
- elif args.model_name in [
- 'RealESRGANv2-anime-xsx4', 'RealESRGANv2-animevideo-xsx4-nousm', 'RealESRGANv2-animevideo-xsx4'
- ]: # x4 VGG-style model (XS size)
- model = SRVGGNetCompact(num_in_ch=3, num_out_ch=3, num_feat=64, num_conv=16, upscale=4, act_type='prelu')
- netscale = 4
-
- # ---------------------- determine model paths ---------------------- #
- model_path = os.path.join('experiments/pretrained_models', args.model_name + '.pth')
- if not os.path.isfile(model_path):
- model_path = os.path.join('realesrgan/weights', args.model_name + '.pth')
- if not os.path.isfile(model_path):
- raise ValueError(f'Model {args.model_name} does not exist.')
-
- # restorer
- upsampler = RealESRGANer(
- scale=netscale,
- model_path=model_path,
- model=model,
- tile=args.tile,
- tile_pad=args.tile_pad,
- pre_pad=args.pre_pad,
- half=args.half)
-
- if args.face_enhance: # Use GFPGAN for face enhancement
- from gfpgan import GFPGANer
- face_enhancer = GFPGANer(
- model_path='https://github.com/TencentARC/GFPGAN/releases/download/v0.2.0/GFPGANCleanv1-NoCE-C2.pth',
- upscale=args.outscale,
- arch='clean',
- channel_multiplier=2,
- bg_upsampler=upsampler)
- os.makedirs(args.output, exist_ok=True)
- # for saving restored frames
- save_frame_folder = os.path.join(args.output, 'frames_tmpout')
- os.makedirs(save_frame_folder, exist_ok=True)
-
- if mimetypes.guess_type(args.input)[0].startswith('video'): # is a video file
- video_name = os.path.splitext(os.path.basename(args.input))[0]
- frame_folder = os.path.join('tmp_frames', video_name)
- os.makedirs(frame_folder, exist_ok=True)
- # use ffmpeg to extract frames
- os.system(f'ffmpeg -i {args.input} -qscale:v 1 -qmin 1 -qmax 1 -vsync 0 {frame_folder}/frame%08d.png')
- # get image path list
- paths = sorted(glob.glob(os.path.join(frame_folder, '*')))
- if args.video:
- if args.fps is None:
- # get input video fps
- import ffmpeg
- probe = ffmpeg.probe(args.input)
- video_streams = [stream for stream in probe['streams'] if stream['codec_type'] == 'video']
- args.fps = eval(video_streams[0]['avg_frame_rate'])
- elif mimetypes.guess_type(args.input)[0].startswith('image'): # is an image file
- paths = [args.input]
- video_name = 'video'
- else:
- paths = sorted(glob.glob(os.path.join(args.input, '*')))
- video_name = 'video'
-
- timer = AvgTimer()
- timer.start()
- pbar = tqdm(total=len(paths), unit='frame', desc='inference')
- # set up prefetch reader
- reader = PrefetchReader(paths, num_prefetch_queue=4)
- reader.start()
-
- que = queue.Queue()
- consumers = [IOConsumer(args, que, f'IO_{i}') for i in range(args.consumer)]
- for consumer in consumers:
- consumer.start()
-
- for idx, (path, img) in enumerate(zip(paths, reader)):
- imgname, extension = os.path.splitext(os.path.basename(path))
- if len(img.shape) == 3 and img.shape[2] == 4:
- img_mode = 'RGBA'
- else:
- img_mode = None
-
- try:
- if args.face_enhance:
- _, _, output = face_enhancer.enhance(img, has_aligned=False, only_center_face=False, paste_back=True)
- else:
- output, _ = upsampler.enhance(img, outscale=args.outscale)
- except RuntimeError as error:
- print('Error', error)
- print('If you encounter CUDA out of memory, try to set --tile with a smaller number.')
-
- else:
- if args.ext == 'auto':
- extension = extension[1:]
- else:
- extension = args.ext
- if img_mode == 'RGBA': # RGBA images should be saved in png format
- extension = 'png'
- save_path = os.path.join(save_frame_folder, f'{imgname}_out.{extension}')
-
- que.put({'output': output, 'save_path': save_path})
-
- pbar.update(1)
- torch.cuda.synchronize()
- timer.record()
- avg_fps = 1. / (timer.get_avg_time() + 1e-7)
- pbar.set_description(f'idx {idx}, fps {avg_fps:.2f}')
-
- for _ in range(args.consumer):
- que.put('quit')
- for consumer in consumers:
- consumer.join()
- pbar.close()
-
- # merge frames to video
- if args.video:
- video_save_path = os.path.join(args.output, f'{video_name}_{args.suffix}.mp4')
- if args.audio:
- os.system(
- f'ffmpeg -r {args.fps} -i {save_frame_folder}/frame%08d_out.{extension} -i {args.input}'
- f' -map 0:v:0 -map 1:a:0 -c:a copy -c:v libx264 -r {args.fps} -pix_fmt yuv420p {video_save_path}')
- else:
- os.system(f'ffmpeg -r {args.fps} -i {save_frame_folder}/frame%08d_out.{extension} '
- f'-c:v libx264 -r {args.fps} -pix_fmt yuv420p {video_save_path}')
-
- # delete tmp file
- shutil.rmtree(save_frame_folder)
- if os.path.isdir(frame_folder):
- shutil.rmtree(frame_folder)
-
-
-if __name__ == '__main__':
- main()
diff --git a/spaces/DQChoi/gpt-demo/venv/lib/python3.11/site-packages/aiohttp/test_utils.py b/spaces/DQChoi/gpt-demo/venv/lib/python3.11/site-packages/aiohttp/test_utils.py
deleted file mode 100644
index fcda2f3ddc045a381470012ba331c75299af4981..0000000000000000000000000000000000000000
--- a/spaces/DQChoi/gpt-demo/venv/lib/python3.11/site-packages/aiohttp/test_utils.py
+++ /dev/null
@@ -1,706 +0,0 @@
-"""Utilities shared by tests."""
-
-import asyncio
-import contextlib
-import gc
-import inspect
-import ipaddress
-import os
-import socket
-import sys
-import warnings
-from abc import ABC, abstractmethod
-from types import TracebackType
-from typing import (
- TYPE_CHECKING,
- Any,
- Callable,
- Iterator,
- List,
- Optional,
- Type,
- Union,
- cast,
-)
-from unittest import mock
-
-from aiosignal import Signal
-from multidict import CIMultiDict, CIMultiDictProxy
-from yarl import URL
-
-import aiohttp
-from aiohttp.client import _RequestContextManager, _WSRequestContextManager
-
-from . import ClientSession, hdrs
-from .abc import AbstractCookieJar
-from .client_reqrep import ClientResponse
-from .client_ws import ClientWebSocketResponse
-from .helpers import PY_38, sentinel
-from .http import HttpVersion, RawRequestMessage
-from .web import (
- Application,
- AppRunner,
- BaseRunner,
- Request,
- Server,
- ServerRunner,
- SockSite,
- UrlMappingMatchInfo,
-)
-from .web_protocol import _RequestHandler
-
-if TYPE_CHECKING: # pragma: no cover
- from ssl import SSLContext
-else:
- SSLContext = None
-
-if PY_38:
- from unittest import IsolatedAsyncioTestCase as TestCase
-else:
- from asynctest import TestCase # type: ignore[no-redef]
-
-REUSE_ADDRESS = os.name == "posix" and sys.platform != "cygwin"
-
-
-def get_unused_port_socket(
- host: str, family: socket.AddressFamily = socket.AF_INET
-) -> socket.socket:
- return get_port_socket(host, 0, family)
-
-
-def get_port_socket(
- host: str, port: int, family: socket.AddressFamily
-) -> socket.socket:
- s = socket.socket(family, socket.SOCK_STREAM)
- if REUSE_ADDRESS:
- # Windows has different semantics for SO_REUSEADDR,
- # so don't set it. Ref:
- # https://docs.microsoft.com/en-us/windows/win32/winsock/using-so-reuseaddr-and-so-exclusiveaddruse
- s.setsockopt(socket.SOL_SOCKET, socket.SO_REUSEADDR, 1)
- s.bind((host, port))
- return s
-
-
-def unused_port() -> int:
- """Return a port that is unused on the current host."""
- with socket.socket(socket.AF_INET, socket.SOCK_STREAM) as s:
- s.bind(("127.0.0.1", 0))
- return cast(int, s.getsockname()[1])
-
-
-class BaseTestServer(ABC):
- __test__ = False
-
- def __init__(
- self,
- *,
- scheme: Union[str, object] = sentinel,
- loop: Optional[asyncio.AbstractEventLoop] = None,
- host: str = "127.0.0.1",
- port: Optional[int] = None,
- skip_url_asserts: bool = False,
- socket_factory: Callable[
- [str, int, socket.AddressFamily], socket.socket
- ] = get_port_socket,
- **kwargs: Any,
- ) -> None:
- self._loop = loop
- self.runner: Optional[BaseRunner] = None
- self._root: Optional[URL] = None
- self.host = host
- self.port = port
- self._closed = False
- self.scheme = scheme
- self.skip_url_asserts = skip_url_asserts
- self.socket_factory = socket_factory
-
- async def start_server(
- self, loop: Optional[asyncio.AbstractEventLoop] = None, **kwargs: Any
- ) -> None:
- if self.runner:
- return
- self._loop = loop
- self._ssl = kwargs.pop("ssl", None)
- self.runner = await self._make_runner(**kwargs)
- await self.runner.setup()
- if not self.port:
- self.port = 0
- try:
- version = ipaddress.ip_address(self.host).version
- except ValueError:
- version = 4
- family = socket.AF_INET6 if version == 6 else socket.AF_INET
- _sock = self.socket_factory(self.host, self.port, family)
- self.host, self.port = _sock.getsockname()[:2]
- site = SockSite(self.runner, sock=_sock, ssl_context=self._ssl)
- await site.start()
- server = site._server
- assert server is not None
- sockets = server.sockets
- assert sockets is not None
- self.port = sockets[0].getsockname()[1]
- if self.scheme is sentinel:
- if self._ssl:
- scheme = "https"
- else:
- scheme = "http"
- self.scheme = scheme
- self._root = URL(f"{self.scheme}://{self.host}:{self.port}")
-
- @abstractmethod # pragma: no cover
- async def _make_runner(self, **kwargs: Any) -> BaseRunner:
- pass
-
- def make_url(self, path: str) -> URL:
- assert self._root is not None
- url = URL(path)
- if not self.skip_url_asserts:
- assert not url.is_absolute()
- return self._root.join(url)
- else:
- return URL(str(self._root) + path)
-
- @property
- def started(self) -> bool:
- return self.runner is not None
-
- @property
- def closed(self) -> bool:
- return self._closed
-
- @property
- def handler(self) -> Server:
- # for backward compatibility
- # web.Server instance
- runner = self.runner
- assert runner is not None
- assert runner.server is not None
- return runner.server
-
- async def close(self) -> None:
- """Close all fixtures created by the test client.
-
- After that point, the TestClient is no longer usable.
-
- This is an idempotent function: running close multiple times
- will not have any additional effects.
-
- close is also run when the object is garbage collected, and on
- exit when used as a context manager.
-
- """
- if self.started and not self.closed:
- assert self.runner is not None
- await self.runner.cleanup()
- self._root = None
- self.port = None
- self._closed = True
-
- def __enter__(self) -> None:
- raise TypeError("Use async with instead")
-
- def __exit__(
- self,
- exc_type: Optional[Type[BaseException]],
- exc_value: Optional[BaseException],
- traceback: Optional[TracebackType],
- ) -> None:
- # __exit__ should exist in pair with __enter__ but never executed
- pass # pragma: no cover
-
- async def __aenter__(self) -> "BaseTestServer":
- await self.start_server(loop=self._loop)
- return self
-
- async def __aexit__(
- self,
- exc_type: Optional[Type[BaseException]],
- exc_value: Optional[BaseException],
- traceback: Optional[TracebackType],
- ) -> None:
- await self.close()
-
-
-class TestServer(BaseTestServer):
- def __init__(
- self,
- app: Application,
- *,
- scheme: Union[str, object] = sentinel,
- host: str = "127.0.0.1",
- port: Optional[int] = None,
- **kwargs: Any,
- ):
- self.app = app
- super().__init__(scheme=scheme, host=host, port=port, **kwargs)
-
- async def _make_runner(self, **kwargs: Any) -> BaseRunner:
- return AppRunner(self.app, **kwargs)
-
-
-class RawTestServer(BaseTestServer):
- def __init__(
- self,
- handler: _RequestHandler,
- *,
- scheme: Union[str, object] = sentinel,
- host: str = "127.0.0.1",
- port: Optional[int] = None,
- **kwargs: Any,
- ) -> None:
- self._handler = handler
- super().__init__(scheme=scheme, host=host, port=port, **kwargs)
-
- async def _make_runner(self, debug: bool = True, **kwargs: Any) -> ServerRunner:
- srv = Server(self._handler, loop=self._loop, debug=debug, **kwargs)
- return ServerRunner(srv, debug=debug, **kwargs)
-
-
-class TestClient:
- """
- A test client implementation.
-
- To write functional tests for aiohttp based servers.
-
- """
-
- __test__ = False
-
- def __init__(
- self,
- server: BaseTestServer,
- *,
- cookie_jar: Optional[AbstractCookieJar] = None,
- loop: Optional[asyncio.AbstractEventLoop] = None,
- **kwargs: Any,
- ) -> None:
- if not isinstance(server, BaseTestServer):
- raise TypeError(
- "server must be TestServer " "instance, found type: %r" % type(server)
- )
- self._server = server
- self._loop = loop
- if cookie_jar is None:
- cookie_jar = aiohttp.CookieJar(unsafe=True, loop=loop)
- self._session = ClientSession(loop=loop, cookie_jar=cookie_jar, **kwargs)
- self._closed = False
- self._responses: List[ClientResponse] = []
- self._websockets: List[ClientWebSocketResponse] = []
-
- async def start_server(self) -> None:
- await self._server.start_server(loop=self._loop)
-
- @property
- def host(self) -> str:
- return self._server.host
-
- @property
- def port(self) -> Optional[int]:
- return self._server.port
-
- @property
- def server(self) -> BaseTestServer:
- return self._server
-
- @property
- def app(self) -> Optional[Application]:
- return cast(Optional[Application], getattr(self._server, "app", None))
-
- @property
- def session(self) -> ClientSession:
- """An internal aiohttp.ClientSession.
-
- Unlike the methods on the TestClient, client session requests
- do not automatically include the host in the url queried, and
- will require an absolute path to the resource.
-
- """
- return self._session
-
- def make_url(self, path: str) -> URL:
- return self._server.make_url(path)
-
- async def _request(self, method: str, path: str, **kwargs: Any) -> ClientResponse:
- resp = await self._session.request(method, self.make_url(path), **kwargs)
- # save it to close later
- self._responses.append(resp)
- return resp
-
- def request(self, method: str, path: str, **kwargs: Any) -> _RequestContextManager:
- """Routes a request to tested http server.
-
- The interface is identical to aiohttp.ClientSession.request,
- except the loop kwarg is overridden by the instance used by the
- test server.
-
- """
- return _RequestContextManager(self._request(method, path, **kwargs))
-
- def get(self, path: str, **kwargs: Any) -> _RequestContextManager:
- """Perform an HTTP GET request."""
- return _RequestContextManager(self._request(hdrs.METH_GET, path, **kwargs))
-
- def post(self, path: str, **kwargs: Any) -> _RequestContextManager:
- """Perform an HTTP POST request."""
- return _RequestContextManager(self._request(hdrs.METH_POST, path, **kwargs))
-
- def options(self, path: str, **kwargs: Any) -> _RequestContextManager:
- """Perform an HTTP OPTIONS request."""
- return _RequestContextManager(self._request(hdrs.METH_OPTIONS, path, **kwargs))
-
- def head(self, path: str, **kwargs: Any) -> _RequestContextManager:
- """Perform an HTTP HEAD request."""
- return _RequestContextManager(self._request(hdrs.METH_HEAD, path, **kwargs))
-
- def put(self, path: str, **kwargs: Any) -> _RequestContextManager:
- """Perform an HTTP PUT request."""
- return _RequestContextManager(self._request(hdrs.METH_PUT, path, **kwargs))
-
- def patch(self, path: str, **kwargs: Any) -> _RequestContextManager:
- """Perform an HTTP PATCH request."""
- return _RequestContextManager(self._request(hdrs.METH_PATCH, path, **kwargs))
-
- def delete(self, path: str, **kwargs: Any) -> _RequestContextManager:
- """Perform an HTTP PATCH request."""
- return _RequestContextManager(self._request(hdrs.METH_DELETE, path, **kwargs))
-
- def ws_connect(self, path: str, **kwargs: Any) -> _WSRequestContextManager:
- """Initiate websocket connection.
-
- The api corresponds to aiohttp.ClientSession.ws_connect.
-
- """
- return _WSRequestContextManager(self._ws_connect(path, **kwargs))
-
- async def _ws_connect(self, path: str, **kwargs: Any) -> ClientWebSocketResponse:
- ws = await self._session.ws_connect(self.make_url(path), **kwargs)
- self._websockets.append(ws)
- return ws
-
- async def close(self) -> None:
- """Close all fixtures created by the test client.
-
- After that point, the TestClient is no longer usable.
-
- This is an idempotent function: running close multiple times
- will not have any additional effects.
-
- close is also run on exit when used as a(n) (asynchronous)
- context manager.
-
- """
- if not self._closed:
- for resp in self._responses:
- resp.close()
- for ws in self._websockets:
- await ws.close()
- await self._session.close()
- await self._server.close()
- self._closed = True
-
- def __enter__(self) -> None:
- raise TypeError("Use async with instead")
-
- def __exit__(
- self,
- exc_type: Optional[Type[BaseException]],
- exc: Optional[BaseException],
- tb: Optional[TracebackType],
- ) -> None:
- # __exit__ should exist in pair with __enter__ but never executed
- pass # pragma: no cover
-
- async def __aenter__(self) -> "TestClient":
- await self.start_server()
- return self
-
- async def __aexit__(
- self,
- exc_type: Optional[Type[BaseException]],
- exc: Optional[BaseException],
- tb: Optional[TracebackType],
- ) -> None:
- await self.close()
-
-
-class AioHTTPTestCase(TestCase):
- """A base class to allow for unittest web applications using aiohttp.
-
- Provides the following:
-
- * self.client (aiohttp.test_utils.TestClient): an aiohttp test client.
- * self.loop (asyncio.BaseEventLoop): the event loop in which the
- application and server are running.
- * self.app (aiohttp.web.Application): the application returned by
- self.get_application()
-
- Note that the TestClient's methods are asynchronous: you have to
- execute function on the test client using asynchronous methods.
- """
-
- async def get_application(self) -> Application:
- """Get application.
-
- This method should be overridden
- to return the aiohttp.web.Application
- object to test.
- """
- return self.get_app()
-
- def get_app(self) -> Application:
- """Obsolete method used to constructing web application.
-
- Use .get_application() coroutine instead.
- """
- raise RuntimeError("Did you forget to define get_application()?")
-
- def setUp(self) -> None:
- if not PY_38:
- asyncio.get_event_loop().run_until_complete(self.asyncSetUp())
-
- async def asyncSetUp(self) -> None:
- try:
- self.loop = asyncio.get_running_loop()
- except (AttributeError, RuntimeError): # AttributeError->py36
- self.loop = asyncio.get_event_loop_policy().get_event_loop()
-
- return await self.setUpAsync()
-
- async def setUpAsync(self) -> None:
- self.app = await self.get_application()
- self.server = await self.get_server(self.app)
- self.client = await self.get_client(self.server)
-
- await self.client.start_server()
-
- def tearDown(self) -> None:
- if not PY_38:
- self.loop.run_until_complete(self.asyncTearDown())
-
- async def asyncTearDown(self) -> None:
- return await self.tearDownAsync()
-
- async def tearDownAsync(self) -> None:
- await self.client.close()
-
- async def get_server(self, app: Application) -> TestServer:
- """Return a TestServer instance."""
- return TestServer(app, loop=self.loop)
-
- async def get_client(self, server: TestServer) -> TestClient:
- """Return a TestClient instance."""
- return TestClient(server, loop=self.loop)
-
-
-def unittest_run_loop(func: Any, *args: Any, **kwargs: Any) -> Any:
- """
- A decorator dedicated to use with asynchronous AioHTTPTestCase test methods.
-
- In 3.8+, this does nothing.
- """
- warnings.warn(
- "Decorator `@unittest_run_loop` is no longer needed in aiohttp 3.8+",
- DeprecationWarning,
- stacklevel=2,
- )
- return func
-
-
-_LOOP_FACTORY = Callable[[], asyncio.AbstractEventLoop]
-
-
-@contextlib.contextmanager
-def loop_context(
- loop_factory: _LOOP_FACTORY = asyncio.new_event_loop, fast: bool = False
-) -> Iterator[asyncio.AbstractEventLoop]:
- """A contextmanager that creates an event_loop, for test purposes.
-
- Handles the creation and cleanup of a test loop.
- """
- loop = setup_test_loop(loop_factory)
- yield loop
- teardown_test_loop(loop, fast=fast)
-
-
-def setup_test_loop(
- loop_factory: _LOOP_FACTORY = asyncio.new_event_loop,
-) -> asyncio.AbstractEventLoop:
- """Create and return an asyncio.BaseEventLoop instance.
-
- The caller should also call teardown_test_loop,
- once they are done with the loop.
- """
- loop = loop_factory()
- try:
- module = loop.__class__.__module__
- skip_watcher = "uvloop" in module
- except AttributeError: # pragma: no cover
- # Just in case
- skip_watcher = True
- asyncio.set_event_loop(loop)
- if sys.platform != "win32" and not skip_watcher:
- policy = asyncio.get_event_loop_policy()
- watcher: asyncio.AbstractChildWatcher
- try: # Python >= 3.8
- # Refs:
- # * https://github.com/pytest-dev/pytest-xdist/issues/620
- # * https://stackoverflow.com/a/58614689/595220
- # * https://bugs.python.org/issue35621
- # * https://github.com/python/cpython/pull/14344
- watcher = asyncio.ThreadedChildWatcher()
- except AttributeError: # Python < 3.8
- watcher = asyncio.SafeChildWatcher()
- watcher.attach_loop(loop)
- with contextlib.suppress(NotImplementedError):
- policy.set_child_watcher(watcher)
- return loop
-
-
-def teardown_test_loop(loop: asyncio.AbstractEventLoop, fast: bool = False) -> None:
- """Teardown and cleanup an event_loop created by setup_test_loop."""
- closed = loop.is_closed()
- if not closed:
- loop.call_soon(loop.stop)
- loop.run_forever()
- loop.close()
-
- if not fast:
- gc.collect()
-
- asyncio.set_event_loop(None)
-
-
-def _create_app_mock() -> mock.MagicMock:
- def get_dict(app: Any, key: str) -> Any:
- return app.__app_dict[key]
-
- def set_dict(app: Any, key: str, value: Any) -> None:
- app.__app_dict[key] = value
-
- app = mock.MagicMock(spec=Application)
- app.__app_dict = {}
- app.__getitem__ = get_dict
- app.__setitem__ = set_dict
-
- app._debug = False
- app.on_response_prepare = Signal(app)
- app.on_response_prepare.freeze()
- return app
-
-
-def _create_transport(sslcontext: Optional[SSLContext] = None) -> mock.Mock:
- transport = mock.Mock()
-
- def get_extra_info(key: str) -> Optional[SSLContext]:
- if key == "sslcontext":
- return sslcontext
- else:
- return None
-
- transport.get_extra_info.side_effect = get_extra_info
- return transport
-
-
-def make_mocked_request(
- method: str,
- path: str,
- headers: Any = None,
- *,
- match_info: Any = sentinel,
- version: HttpVersion = HttpVersion(1, 1),
- closing: bool = False,
- app: Any = None,
- writer: Any = sentinel,
- protocol: Any = sentinel,
- transport: Any = sentinel,
- payload: Any = sentinel,
- sslcontext: Optional[SSLContext] = None,
- client_max_size: int = 1024**2,
- loop: Any = ...,
-) -> Request:
- """Creates mocked web.Request testing purposes.
-
- Useful in unit tests, when spinning full web server is overkill or
- specific conditions and errors are hard to trigger.
- """
- task = mock.Mock()
- if loop is ...:
- loop = mock.Mock()
- loop.create_future.return_value = ()
-
- if version < HttpVersion(1, 1):
- closing = True
-
- if headers:
- headers = CIMultiDictProxy(CIMultiDict(headers))
- raw_hdrs = tuple(
- (k.encode("utf-8"), v.encode("utf-8")) for k, v in headers.items()
- )
- else:
- headers = CIMultiDictProxy(CIMultiDict())
- raw_hdrs = ()
-
- chunked = "chunked" in headers.get(hdrs.TRANSFER_ENCODING, "").lower()
-
- message = RawRequestMessage(
- method,
- path,
- version,
- headers,
- raw_hdrs,
- closing,
- None,
- False,
- chunked,
- URL(path),
- )
- if app is None:
- app = _create_app_mock()
-
- if transport is sentinel:
- transport = _create_transport(sslcontext)
-
- if protocol is sentinel:
- protocol = mock.Mock()
- protocol.transport = transport
-
- if writer is sentinel:
- writer = mock.Mock()
- writer.write_headers = make_mocked_coro(None)
- writer.write = make_mocked_coro(None)
- writer.write_eof = make_mocked_coro(None)
- writer.drain = make_mocked_coro(None)
- writer.transport = transport
-
- protocol.transport = transport
- protocol.writer = writer
-
- if payload is sentinel:
- payload = mock.Mock()
-
- req = Request(
- message, payload, protocol, writer, task, loop, client_max_size=client_max_size
- )
-
- match_info = UrlMappingMatchInfo(
- {} if match_info is sentinel else match_info, mock.Mock()
- )
- match_info.add_app(app)
- req._match_info = match_info
-
- return req
-
-
-def make_mocked_coro(
- return_value: Any = sentinel, raise_exception: Any = sentinel
-) -> Any:
- """Creates a coroutine mock."""
-
- async def mock_coro(*args: Any, **kwargs: Any) -> Any:
- if raise_exception is not sentinel:
- raise raise_exception
- if not inspect.isawaitable(return_value):
- return return_value
- await return_value
-
- return mock.Mock(wraps=mock_coro)
diff --git a/spaces/DQChoi/gpt-demo/venv/lib/python3.11/site-packages/fsspec/implementations/http_sync.py b/spaces/DQChoi/gpt-demo/venv/lib/python3.11/site-packages/fsspec/implementations/http_sync.py
deleted file mode 100644
index 744b2bd315cb421b982af3c21254d22bacd77c16..0000000000000000000000000000000000000000
--- a/spaces/DQChoi/gpt-demo/venv/lib/python3.11/site-packages/fsspec/implementations/http_sync.py
+++ /dev/null
@@ -1,882 +0,0 @@
-from __future__ import absolute_import, division, print_function
-
-import io
-import logging
-import re
-import urllib.error
-import urllib.parse
-from copy import copy
-from json import dumps, loads
-from urllib.parse import urlparse
-
-try:
- import yarl
-except (ImportError, ModuleNotFoundError, OSError):
- yarl = False
-
-from fsspec.callbacks import _DEFAULT_CALLBACK
-from fsspec.registry import register_implementation
-from fsspec.spec import AbstractBufferedFile, AbstractFileSystem
-from fsspec.utils import DEFAULT_BLOCK_SIZE, isfilelike, nullcontext, tokenize
-
-from ..caching import AllBytes
-
-# https://stackoverflow.com/a/15926317/3821154
-ex = re.compile(r"""<(a|A)\s+(?:[^>]*?\s+)?(href|HREF)=["'](?P[^"']+)""")
-ex2 = re.compile(r"""(?Phttp[s]?://[-a-zA-Z0-9@:%_+.~#?&/=]+)""")
-logger = logging.getLogger("fsspec.http")
-
-
-class JsHttpException(urllib.error.HTTPError):
- ...
-
-
-class StreamIO(io.BytesIO):
- # fake class, so you can set attributes on it
- # will eventually actually stream
- ...
-
-
-class ResponseProxy:
- """Looks like a requests response"""
-
- def __init__(self, req, stream=False):
- self.request = req
- self.stream = stream
- self._data = None
- self._headers = None
-
- @property
- def raw(self):
- if self._data is None:
- b = self.request.response.to_bytes()
- if self.stream:
- self._data = StreamIO(b)
- else:
- self._data = b
- return self._data
-
- def close(self):
- if hasattr(self, "_data"):
- del self._data
-
- @property
- def headers(self):
- if self._headers is None:
- self._headers = dict(
- [
- _.split(": ")
- for _ in self.request.getAllResponseHeaders().strip().split("\r\n")
- ]
- )
- return self._headers
-
- @property
- def status_code(self):
- return int(self.request.status)
-
- def raise_for_status(self):
- if not self.ok:
- raise JsHttpException(
- self.url, self.status_code, self.reason, self.headers, None
- )
-
- @property
- def reason(self):
- return self.request.statusText
-
- @property
- def ok(self):
- return self.status_code < 400
-
- @property
- def url(self):
- return self.request.response.responseURL
-
- @property
- def text(self):
- # TODO: encoding from headers
- return self.content.decode()
-
- @property
- def content(self):
- self.stream = False
- return self.raw
-
- @property
- def json(self):
- return loads(self.text)
-
-
-class RequestsSessionShim:
- def __init__(self):
- self.headers = {}
-
- def request(
- self,
- method,
- url,
- params=None,
- data=None,
- headers=None,
- cookies=None,
- files=None,
- auth=None,
- timeout=None,
- allow_redirects=None,
- proxies=None,
- hooks=None,
- stream=None,
- verify=None,
- cert=None,
- json=None,
- ):
- import js
- from js import Blob, XMLHttpRequest
-
- if hasattr(js, "document"):
- raise RuntimeError("Filesystem can only be run from a worker, not main")
-
- logger.debug("JS request: %s %s", method, url)
-
- if cert or verify or proxies or files or cookies or hooks:
- raise NotImplementedError
- if data and json:
- raise ValueError("Use json= or data=, not both")
- req = XMLHttpRequest.new()
- extra = auth if auth else ()
- if params:
- url = f"{url}?{urllib.parse.urlencode(params)}"
- req.open(method, url, False, *extra)
- if timeout:
- req.timeout = timeout
- if headers:
- for k, v in headers.items():
- req.setRequestHeader(k, v)
-
- req.setRequestHeader("Accept", "application/octet-stream")
- req.responseType = "arraybuffer"
- if json:
- blob = Blob.new([dumps(data)], {type: "application/json"})
- req.send(blob)
- elif data:
- if isinstance(data, io.IOBase):
- data = data.read()
- blob = Blob.new([data], {type: "application/octet-stream"})
- req.send(blob)
- else:
- req.send(None)
- return ResponseProxy(req, stream=stream)
-
- def get(self, url, **kwargs):
- return self.request("GET", url, **kwargs)
-
- def head(self, url, **kwargs):
- return self.request("HEAD", url, **kwargs)
-
- def post(self, url, **kwargs):
- return self.request("POST}", url, **kwargs)
-
- def put(self, url, **kwargs):
- return self.request("PUT", url, **kwargs)
-
- def patch(self, url, **kwargs):
- return self.request("PATCH", url, **kwargs)
-
- def delete(self, url, **kwargs):
- return self.request("DELETE", url, **kwargs)
-
-
-class HTTPFileSystem(AbstractFileSystem):
- """
- Simple File-System for fetching data via HTTP(S)
-
- ``ls()`` is implemented by loading the parent page and doing a regex
- match on the result. If simple_link=True, anything of the form
- "http(s)://server.com/stuff?thing=other"; otherwise only links within
- HTML href tags will be used.
- """
-
- sep = "/"
-
- def __init__(
- self,
- simple_links=True,
- block_size=None,
- same_scheme=True,
- cache_type="readahead",
- cache_options=None,
- client_kwargs=None,
- encoded=False,
- **storage_options,
- ):
- """
-
- Parameters
- ----------
- block_size: int
- Blocks to read bytes; if 0, will default to raw requests file-like
- objects instead of HTTPFile instances
- simple_links: bool
- If True, will consider both HTML tags and anything that looks
- like a URL; if False, will consider only the former.
- same_scheme: True
- When doing ls/glob, if this is True, only consider paths that have
- http/https matching the input URLs.
- size_policy: this argument is deprecated
- client_kwargs: dict
- Passed to aiohttp.ClientSession, see
- https://docs.aiohttp.org/en/stable/client_reference.html
- For example, ``{'auth': aiohttp.BasicAuth('user', 'pass')}``
- storage_options: key-value
- Any other parameters passed on to requests
- cache_type, cache_options: defaults used in open
- """
- super().__init__(self, **storage_options)
- self.block_size = block_size if block_size is not None else DEFAULT_BLOCK_SIZE
- self.simple_links = simple_links
- self.same_schema = same_scheme
- self.cache_type = cache_type
- self.cache_options = cache_options
- self.client_kwargs = client_kwargs or {}
- self.encoded = encoded
- self.kwargs = storage_options
-
- try:
- import js # noqa: F401
-
- logger.debug("Starting JS session")
- self.session = RequestsSessionShim()
- self.js = True
- except Exception as e:
- import requests
-
- logger.debug("Starting cpython session because of: %s", e)
- self.session = requests.Session(**(client_kwargs or {}))
- self.js = False
-
- request_options = copy(storage_options)
- self.use_listings_cache = request_options.pop("use_listings_cache", False)
- request_options.pop("listings_expiry_time", None)
- request_options.pop("max_paths", None)
- request_options.pop("skip_instance_cache", None)
- self.kwargs = request_options
-
- @property
- def fsid(self):
- return "http"
-
- def encode_url(self, url):
- if yarl:
- return yarl.URL(url, encoded=self.encoded)
- return url
-
- @classmethod
- def _strip_protocol(cls, path):
- """For HTTP, we always want to keep the full URL"""
- return path
-
- @classmethod
- def _parent(cls, path):
- # override, since _strip_protocol is different for URLs
- par = super()._parent(path)
- if len(par) > 7: # "http://..."
- return par
- return ""
-
- def _ls_real(self, url, detail=True, **kwargs):
- # ignoring URL-encoded arguments
- kw = self.kwargs.copy()
- kw.update(kwargs)
- logger.debug(url)
- r = self.session.get(self.encode_url(url), **self.kwargs)
- self._raise_not_found_for_status(r, url)
- text = r.text
- if self.simple_links:
- links = ex2.findall(text) + [u[2] for u in ex.findall(text)]
- else:
- links = [u[2] for u in ex.findall(text)]
- out = set()
- parts = urlparse(url)
- for l in links:
- if isinstance(l, tuple):
- l = l[1]
- if l.startswith("/") and len(l) > 1:
- # absolute URL on this server
- l = parts.scheme + "://" + parts.netloc + l
- if l.startswith("http"):
- if self.same_schema and l.startswith(url.rstrip("/") + "/"):
- out.add(l)
- elif l.replace("https", "http").startswith(
- url.replace("https", "http").rstrip("/") + "/"
- ):
- # allowed to cross http <-> https
- out.add(l)
- else:
- if l not in ["..", "../"]:
- # Ignore FTP-like "parent"
- out.add("/".join([url.rstrip("/"), l.lstrip("/")]))
- if not out and url.endswith("/"):
- out = self._ls_real(url.rstrip("/"), detail=False)
- if detail:
- return [
- {
- "name": u,
- "size": None,
- "type": "directory" if u.endswith("/") else "file",
- }
- for u in out
- ]
- else:
- return list(sorted(out))
-
- def ls(self, url, detail=True, **kwargs):
-
- if self.use_listings_cache and url in self.dircache:
- out = self.dircache[url]
- else:
- out = self._ls_real(url, detail=detail, **kwargs)
- self.dircache[url] = out
- return out
-
- def _raise_not_found_for_status(self, response, url):
- """
- Raises FileNotFoundError for 404s, otherwise uses raise_for_status.
- """
- if response.status_code == 404:
- raise FileNotFoundError(url)
- response.raise_for_status()
-
- def cat_file(self, url, start=None, end=None, **kwargs):
- kw = self.kwargs.copy()
- kw.update(kwargs)
- logger.debug(url)
-
- if start is not None or end is not None:
- if start == end:
- return b""
- headers = kw.pop("headers", {}).copy()
-
- headers["Range"] = self._process_limits(url, start, end)
- kw["headers"] = headers
- r = self.session.get(self.encode_url(url), **kw)
- self._raise_not_found_for_status(r, url)
- return r.content
-
- def get_file(
- self, rpath, lpath, chunk_size=5 * 2**20, callback=_DEFAULT_CALLBACK, **kwargs
- ):
- kw = self.kwargs.copy()
- kw.update(kwargs)
- logger.debug(rpath)
- r = self.session.get(self.encode_url(rpath), **kw)
- try:
- size = int(r.headers["content-length"])
- except (ValueError, KeyError):
- size = None
-
- callback.set_size(size)
- self._raise_not_found_for_status(r, rpath)
- if not isfilelike(lpath):
- lpath = open(lpath, "wb")
- chunk = True
- while chunk:
- r.raw.decode_content = True
- chunk = r.raw.read(chunk_size)
- lpath.write(chunk)
- callback.relative_update(len(chunk))
-
- def put_file(
- self,
- lpath,
- rpath,
- chunk_size=5 * 2**20,
- callback=_DEFAULT_CALLBACK,
- method="post",
- **kwargs,
- ):
- def gen_chunks():
- # Support passing arbitrary file-like objects
- # and use them instead of streams.
- if isinstance(lpath, io.IOBase):
- context = nullcontext(lpath)
- use_seek = False # might not support seeking
- else:
- context = open(lpath, "rb")
- use_seek = True
-
- with context as f:
- if use_seek:
- callback.set_size(f.seek(0, 2))
- f.seek(0)
- else:
- callback.set_size(getattr(f, "size", None))
-
- chunk = f.read(chunk_size)
- while chunk:
- yield chunk
- callback.relative_update(len(chunk))
- chunk = f.read(chunk_size)
-
- kw = self.kwargs.copy()
- kw.update(kwargs)
-
- method = method.lower()
- if method not in ("post", "put"):
- raise ValueError(
- f"method has to be either 'post' or 'put', not: {method!r}"
- )
-
- meth = getattr(self.session, method)
- resp = meth(rpath, data=gen_chunks(), **kw)
- self._raise_not_found_for_status(resp, rpath)
-
- def exists(self, path, **kwargs):
- kw = self.kwargs.copy()
- kw.update(kwargs)
- try:
- logger.debug(path)
- r = self.session.get(self.encode_url(path), **kw)
- return r.status_code < 400
- except Exception:
- return False
-
- def isfile(self, path, **kwargs):
- return self.exists(path, **kwargs)
-
- def _open(
- self,
- path,
- mode="rb",
- block_size=None,
- autocommit=None, # XXX: This differs from the base class.
- cache_type=None,
- cache_options=None,
- size=None,
- **kwargs,
- ):
- """Make a file-like object
-
- Parameters
- ----------
- path: str
- Full URL with protocol
- mode: string
- must be "rb"
- block_size: int or None
- Bytes to download in one request; use instance value if None. If
- zero, will return a streaming Requests file-like instance.
- kwargs: key-value
- Any other parameters, passed to requests calls
- """
- if mode != "rb":
- raise NotImplementedError
- block_size = block_size if block_size is not None else self.block_size
- kw = self.kwargs.copy()
- kw.update(kwargs)
- size = size or self.info(path, **kwargs)["size"]
- if block_size and size:
- return HTTPFile(
- self,
- path,
- session=self.session,
- block_size=block_size,
- mode=mode,
- size=size,
- cache_type=cache_type or self.cache_type,
- cache_options=cache_options or self.cache_options,
- **kw,
- )
- else:
- return HTTPStreamFile(
- self,
- path,
- mode=mode,
- session=self.session,
- **kw,
- )
-
- def ukey(self, url):
- """Unique identifier; assume HTTP files are static, unchanging"""
- return tokenize(url, self.kwargs, self.protocol)
-
- def info(self, url, **kwargs):
- """Get info of URL
-
- Tries to access location via HEAD, and then GET methods, but does
- not fetch the data.
-
- It is possible that the server does not supply any size information, in
- which case size will be given as None (and certain operations on the
- corresponding file will not work).
- """
- info = {}
- for policy in ["head", "get"]:
- try:
- info.update(
- _file_info(
- self.encode_url(url),
- size_policy=policy,
- session=self.session,
- **self.kwargs,
- **kwargs,
- )
- )
- if info.get("size") is not None:
- break
- except Exception as exc:
- if policy == "get":
- # If get failed, then raise a FileNotFoundError
- raise FileNotFoundError(url) from exc
- logger.debug(str(exc))
-
- return {"name": url, "size": None, **info, "type": "file"}
-
- def glob(self, path, **kwargs):
- """
- Find files by glob-matching.
-
- This implementation is idntical to the one in AbstractFileSystem,
- but "?" is not considered as a character for globbing, because it is
- so common in URLs, often identifying the "query" part.
- """
- import re
-
- ends = path.endswith("/")
- path = self._strip_protocol(path)
- indstar = path.find("*") if path.find("*") >= 0 else len(path)
- indbrace = path.find("[") if path.find("[") >= 0 else len(path)
-
- ind = min(indstar, indbrace)
-
- detail = kwargs.pop("detail", False)
-
- if not has_magic(path):
- root = path
- depth = 1
- if ends:
- path += "/*"
- elif self.exists(path):
- if not detail:
- return [path]
- else:
- return {path: self.info(path)}
- else:
- if not detail:
- return [] # glob of non-existent returns empty
- else:
- return {}
- elif "/" in path[:ind]:
- ind2 = path[:ind].rindex("/")
- root = path[: ind2 + 1]
- depth = None if "**" in path else path[ind2 + 1 :].count("/") + 1
- else:
- root = ""
- depth = None if "**" in path else path[ind + 1 :].count("/") + 1
-
- allpaths = self.find(root, maxdepth=depth, withdirs=True, detail=True, **kwargs)
- # Escape characters special to python regex, leaving our supported
- # special characters in place.
- # See https://www.gnu.org/software/bash/manual/html_node/Pattern-Matching.html
- # for shell globbing details.
- pattern = (
- "^"
- + (
- path.replace("\\", r"\\")
- .replace(".", r"\.")
- .replace("+", r"\+")
- .replace("//", "/")
- .replace("(", r"\(")
- .replace(")", r"\)")
- .replace("|", r"\|")
- .replace("^", r"\^")
- .replace("$", r"\$")
- .replace("{", r"\{")
- .replace("}", r"\}")
- .rstrip("/")
- )
- + "$"
- )
- pattern = re.sub("[*]{2}", "=PLACEHOLDER=", pattern)
- pattern = re.sub("[*]", "[^/]*", pattern)
- pattern = re.compile(pattern.replace("=PLACEHOLDER=", ".*"))
- out = {
- p: allpaths[p]
- for p in sorted(allpaths)
- if pattern.match(p.replace("//", "/").rstrip("/"))
- }
- if detail:
- return out
- else:
- return list(out)
-
- def isdir(self, path):
- # override, since all URLs are (also) files
- try:
- return bool(self._ls(path))
- except (FileNotFoundError, ValueError):
- return False
-
-
-class HTTPFile(AbstractBufferedFile):
- """
- A file-like object pointing to a remove HTTP(S) resource
-
- Supports only reading, with read-ahead of a predermined block-size.
-
- In the case that the server does not supply the filesize, only reading of
- the complete file in one go is supported.
-
- Parameters
- ----------
- url: str
- Full URL of the remote resource, including the protocol
- session: requests.Session or None
- All calls will be made within this session, to avoid restarting
- connections where the server allows this
- block_size: int or None
- The amount of read-ahead to do, in bytes. Default is 5MB, or the value
- configured for the FileSystem creating this file
- size: None or int
- If given, this is the size of the file in bytes, and we don't attempt
- to call the server to find the value.
- kwargs: all other key-values are passed to requests calls.
- """
-
- def __init__(
- self,
- fs,
- url,
- session=None,
- block_size=None,
- mode="rb",
- cache_type="bytes",
- cache_options=None,
- size=None,
- **kwargs,
- ):
- if mode != "rb":
- raise NotImplementedError("File mode not supported")
- self.url = url
- self.session = session
- self.details = {"name": url, "size": size, "type": "file"}
- super().__init__(
- fs=fs,
- path=url,
- mode=mode,
- block_size=block_size,
- cache_type=cache_type,
- cache_options=cache_options,
- **kwargs,
- )
-
- def read(self, length=-1):
- """Read bytes from file
-
- Parameters
- ----------
- length: int
- Read up to this many bytes. If negative, read all content to end of
- file. If the server has not supplied the filesize, attempting to
- read only part of the data will raise a ValueError.
- """
- if (
- (length < 0 and self.loc == 0) # explicit read all
- # but not when the size is known and fits into a block anyways
- and not (self.size is not None and self.size <= self.blocksize)
- ):
- self._fetch_all()
- if self.size is None:
- if length < 0:
- self._fetch_all()
- else:
- length = min(self.size - self.loc, length)
- return super().read(length)
-
- def _fetch_all(self):
- """Read whole file in one shot, without caching
-
- This is only called when position is still at zero,
- and read() is called without a byte-count.
- """
- logger.debug(f"Fetch all for {self}")
- if not isinstance(self.cache, AllBytes):
- r = self.session.get(self.fs.encode_url(self.url), **self.kwargs)
- r.raise_for_status()
- out = r.content
- self.cache = AllBytes(size=len(out), fetcher=None, blocksize=None, data=out)
- self.size = len(out)
-
- def _parse_content_range(self, headers):
- """Parse the Content-Range header"""
- s = headers.get("Content-Range", "")
- m = re.match(r"bytes (\d+-\d+|\*)/(\d+|\*)", s)
- if not m:
- return None, None, None
-
- if m[1] == "*":
- start = end = None
- else:
- start, end = [int(x) for x in m[1].split("-")]
- total = None if m[2] == "*" else int(m[2])
- return start, end, total
-
- def _fetch_range(self, start, end):
- """Download a block of data
-
- The expectation is that the server returns only the requested bytes,
- with HTTP code 206. If this is not the case, we first check the headers,
- and then stream the output - if the data size is bigger than we
- requested, an exception is raised.
- """
- logger.debug(f"Fetch range for {self}: {start}-{end}")
- kwargs = self.kwargs.copy()
- headers = kwargs.pop("headers", {}).copy()
- headers["Range"] = "bytes=%i-%i" % (start, end - 1)
- logger.debug(str(self.url) + " : " + headers["Range"])
- r = self.session.get(self.fs.encode_url(self.url), headers=headers, **kwargs)
- if r.status_code == 416:
- # range request outside file
- return b""
- r.raise_for_status()
-
- # If the server has handled the range request, it should reply
- # with status 206 (partial content). But we'll guess that a suitable
- # Content-Range header or a Content-Length no more than the
- # requested range also mean we have got the desired range.
- cl = r.headers.get("Content-Length", r.headers.get("content-length", end + 1))
- response_is_range = (
- r.status_code == 206
- or self._parse_content_range(r.headers)[0] == start
- or int(cl) <= end - start
- )
-
- if response_is_range:
- # partial content, as expected
- out = r.content
- elif start > 0:
- raise ValueError(
- "The HTTP server doesn't appear to support range requests. "
- "Only reading this file from the beginning is supported. "
- "Open with block_size=0 for a streaming file interface."
- )
- else:
- # Response is not a range, but we want the start of the file,
- # so we can read the required amount anyway.
- cl = 0
- out = []
- while True:
- r.raw.decode_content = True
- chunk = r.raw.read(2**20)
- # data size unknown, let's read until we have enough
- if chunk:
- out.append(chunk)
- cl += len(chunk)
- if cl > end - start:
- break
- else:
- break
- r.raw.close()
- out = b"".join(out)[: end - start]
- return out
-
-
-magic_check = re.compile("([*[])")
-
-
-def has_magic(s):
- match = magic_check.search(s)
- return match is not None
-
-
-class HTTPStreamFile(AbstractBufferedFile):
- def __init__(self, fs, url, mode="rb", session=None, **kwargs):
- self.url = url
- self.session = session
- if mode != "rb":
- raise ValueError
- self.details = {"name": url, "size": None}
- super().__init__(fs=fs, path=url, mode=mode, cache_type="readahead", **kwargs)
-
- r = self.session.get(self.fs.encode_url(url), stream=True, **kwargs)
- r.raw.decode_content = True
- self.fs._raise_not_found_for_status(r, url)
-
- self.r = r
-
- def seek(self, *args, **kwargs):
- raise ValueError("Cannot seek streaming HTTP file")
-
- def read(self, num=-1):
- bufs = []
- leng = 0
- while not self.r.raw.closed and (leng < num or num < 0):
- out = self.r.raw.read(num)
- if out:
- bufs.append(out)
- else:
- break
- leng += len(out)
- self.loc += leng
- return b"".join(bufs)
-
- def close(self):
- self.r.close()
-
-
-def get_range(session, url, start, end, **kwargs):
- # explicit get a range when we know it must be safe
- kwargs = kwargs.copy()
- headers = kwargs.pop("headers", {}).copy()
- headers["Range"] = "bytes=%i-%i" % (start, end - 1)
- r = session.get(url, headers=headers, **kwargs)
- r.raise_for_status()
- return r.content
-
-
-def _file_info(url, session, size_policy="head", **kwargs):
- """Call HEAD on the server to get details about the file (size/checksum etc.)
-
- Default operation is to explicitly allow redirects and use encoding
- 'identity' (no compression) to get the true size of the target.
- """
- logger.debug("Retrieve file size for %s" % url)
- kwargs = kwargs.copy()
- ar = kwargs.pop("allow_redirects", True)
- head = kwargs.get("headers", {}).copy()
- # TODO: not allowed in JS
- # head["Accept-Encoding"] = "identity"
- kwargs["headers"] = head
-
- info = {}
- if size_policy == "head":
- r = session.head(url, allow_redirects=ar, **kwargs)
- elif size_policy == "get":
- r = session.get(url, allow_redirects=ar, **kwargs)
- else:
- raise TypeError('size_policy must be "head" or "get", got %s' "" % size_policy)
- r.raise_for_status()
-
- # TODO:
- # recognise lack of 'Accept-Ranges',
- # or 'Accept-Ranges': 'none' (not 'bytes')
- # to mean streaming only, no random access => return None
- if "Content-Length" in r.headers:
- info["size"] = int(r.headers["Content-Length"])
- elif "Content-Range" in r.headers:
- info["size"] = int(r.headers["Content-Range"].split("/")[1])
- if "content-length" in r.headers:
- info["size"] = int(r.headers["content-length"])
- elif "content-range" in r.headers:
- info["size"] = int(r.headers["content-range"].split("/")[1])
-
- for checksum_field in ["ETag", "Content-MD5", "Digest"]:
- if r.headers.get(checksum_field):
- info[checksum_field] = r.headers[checksum_field]
-
- return info
-
-
-# importing this is enough to register it
-register_implementation("http", HTTPFileSystem, clobber=True)
-register_implementation("https", HTTPFileSystem, clobber=True)
diff --git a/spaces/ECCV2022/PSG/README.md b/spaces/ECCV2022/PSG/README.md
deleted file mode 100644
index e98750a2dff055c52d540c2e20bd3b6b73f154a7..0000000000000000000000000000000000000000
--- a/spaces/ECCV2022/PSG/README.md
+++ /dev/null
@@ -1,13 +0,0 @@
----
-title: Panoptic Scene Graph Generation
-emoji: 🏞️🏙️🌄🌉
-colorFrom: yellow
-colorTo: blue
-sdk: gradio
-sdk_version: 3.1.4
-app_file: app.py
-pinned: false
-license: mit
----
-
-Check out the configuration reference at https://huggingface.co/docs/hub/spaces-config-reference
diff --git a/spaces/ECCV2022/bytetrack/tools/demo_track.py b/spaces/ECCV2022/bytetrack/tools/demo_track.py
deleted file mode 100644
index 9eb7eb0e27cc83822dcfa247a0a2dbde35f34ed6..0000000000000000000000000000000000000000
--- a/spaces/ECCV2022/bytetrack/tools/demo_track.py
+++ /dev/null
@@ -1,345 +0,0 @@
-from loguru import logger
-
-import cv2
-
-import torch
-
-from yolox.data.data_augment import preproc
-from yolox.exp import get_exp
-from yolox.utils import fuse_model, get_model_info, postprocess, vis
-from yolox.utils.visualize import plot_tracking
-from yolox.tracker.byte_tracker import BYTETracker
-from yolox.tracking_utils.timer import Timer
-
-import argparse
-import os
-import time
-
-IMAGE_EXT = [".jpg", ".jpeg", ".webp", ".bmp", ".png"]
-
-
-def make_parser():
- parser = argparse.ArgumentParser("ByteTrack Demo!")
- parser.add_argument(
- "demo", default="image", help="demo type, eg. image, video and webcam"
- )
- parser.add_argument("-expn", "--experiment-name", type=str, default=None)
- parser.add_argument("-n", "--name", type=str, default=None, help="model name")
-
- parser.add_argument(
- #"--path", default="./datasets/mot/train/MOT17-05-FRCNN/img1", help="path to images or video"
- "--path", default="./videos/palace.mp4", help="path to images or video"
- )
- parser.add_argument("--camid", type=int, default=0, help="webcam demo camera id")
- parser.add_argument(
- "--save_result",
- action="store_true",
- help="whether to save the inference result of image/video",
- )
-
- # exp file
- parser.add_argument(
- "-f",
- "--exp_file",
- default=None,
- type=str,
- help="pls input your expriment description file",
- )
- parser.add_argument("-c", "--ckpt", default=None, type=str, help="ckpt for eval")
- parser.add_argument(
- "--device",
- default="gpu",
- type=str,
- help="device to run our model, can either be cpu or gpu",
- )
- parser.add_argument("--conf", default=None, type=float, help="test conf")
- parser.add_argument("--nms", default=None, type=float, help="test nms threshold")
- parser.add_argument("--tsize", default=None, type=int, help="test img size")
- parser.add_argument(
- "--fp16",
- dest="fp16",
- default=False,
- action="store_true",
- help="Adopting mix precision evaluating.",
- )
- parser.add_argument(
- "--fuse",
- dest="fuse",
- default=False,
- action="store_true",
- help="Fuse conv and bn for testing.",
- )
- parser.add_argument(
- "--trt",
- dest="trt",
- default=False,
- action="store_true",
- help="Using TensorRT model for testing.",
- )
- # tracking args
- parser.add_argument("--track_thresh", type=float, default=0.5, help="tracking confidence threshold")
- parser.add_argument("--track_buffer", type=int, default=30, help="the frames for keep lost tracks")
- parser.add_argument("--match_thresh", type=int, default=0.8, help="matching threshold for tracking")
- parser.add_argument('--min-box-area', type=float, default=10, help='filter out tiny boxes')
- parser.add_argument("--mot20", dest="mot20", default=False, action="store_true", help="test mot20.")
- return parser
-
-
-def get_image_list(path):
- image_names = []
- for maindir, subdir, file_name_list in os.walk(path):
- for filename in file_name_list:
- apath = os.path.join(maindir, filename)
- ext = os.path.splitext(apath)[1]
- if ext in IMAGE_EXT:
- image_names.append(apath)
- return image_names
-
-
-def write_results(filename, results):
- save_format = '{frame},{id},{x1},{y1},{w},{h},{s},-1,-1,-1\n'
- with open(filename, 'w') as f:
- for frame_id, tlwhs, track_ids, scores in results:
- for tlwh, track_id, score in zip(tlwhs, track_ids, scores):
- if track_id < 0:
- continue
- x1, y1, w, h = tlwh
- line = save_format.format(frame=frame_id, id=track_id, x1=round(x1, 1), y1=round(y1, 1), w=round(w, 1), h=round(h, 1), s=round(score, 2))
- f.write(line)
- logger.info('save results to {}'.format(filename))
-
-
-class Predictor(object):
- def __init__(
- self,
- model,
- exp,
- trt_file=None,
- decoder=None,
- device="cpu",
- fp16=False
- ):
- self.model = model
- self.decoder = decoder
- self.num_classes = exp.num_classes
- self.confthre = exp.test_conf
- self.nmsthre = exp.nmsthre
- self.test_size = exp.test_size
- self.device = device
- self.fp16 = fp16
- if trt_file is not None:
- from torch2trt import TRTModule
-
- model_trt = TRTModule()
- model_trt.load_state_dict(torch.load(trt_file))
-
- x = torch.ones(1, 3, exp.test_size[0], exp.test_size[1]).cuda()
- self.model(x)
- self.model = model_trt
- self.rgb_means = (0.485, 0.456, 0.406)
- self.std = (0.229, 0.224, 0.225)
-
- def inference(self, img, timer):
- img_info = {"id": 0}
- if isinstance(img, str):
- img_info["file_name"] = os.path.basename(img)
- img = cv2.imread(img)
- else:
- img_info["file_name"] = None
-
- height, width = img.shape[:2]
- img_info["height"] = height
- img_info["width"] = width
- img_info["raw_img"] = img
-
- img, ratio = preproc(img, self.test_size, self.rgb_means, self.std)
- img_info["ratio"] = ratio
- img = torch.from_numpy(img).unsqueeze(0)
- img = img.float()
- if self.device == "gpu":
- img = img.cuda()
- if self.fp16:
- img = img.half() # to FP16
-
- with torch.no_grad():
- timer.tic()
- outputs = self.model(img)
- if self.decoder is not None:
- outputs = self.decoder(outputs, dtype=outputs.type())
- outputs = postprocess(
- outputs, self.num_classes, self.confthre, self.nmsthre
- )
- #logger.info("Infer time: {:.4f}s".format(time.time() - t0))
- return outputs, img_info
-
-
-def image_demo(predictor, vis_folder, path, current_time, save_result):
- if os.path.isdir(path):
- files = get_image_list(path)
- else:
- files = [path]
- files.sort()
- tracker = BYTETracker(args, frame_rate=30)
- timer = Timer()
- frame_id = 0
- results = []
- for image_name in files:
- if frame_id % 20 == 0:
- logger.info('Processing frame {} ({:.2f} fps)'.format(frame_id, 1. / max(1e-5, timer.average_time)))
- outputs, img_info = predictor.inference(image_name, timer)
- online_targets = tracker.update(outputs[0], [img_info['height'], img_info['width']], exp.test_size)
- online_tlwhs = []
- online_ids = []
- online_scores = []
- for t in online_targets:
- tlwh = t.tlwh
- tid = t.track_id
- vertical = tlwh[2] / tlwh[3] > 1.6
- if tlwh[2] * tlwh[3] > args.min_box_area and not vertical:
- online_tlwhs.append(tlwh)
- online_ids.append(tid)
- online_scores.append(t.score)
- timer.toc()
- # save results
- results.append((frame_id + 1, online_tlwhs, online_ids, online_scores))
- online_im = plot_tracking(img_info['raw_img'], online_tlwhs, online_ids, frame_id=frame_id + 1,
- fps=1. / timer.average_time)
-
- #result_image = predictor.visual(outputs[0], img_info, predictor.confthre)
- if save_result:
- save_file_name = "out.jpg"
- cv2.imwrite(save_file_name, online_im)
- ch = cv2.waitKey(0)
- frame_id += 1
- if ch == 27 or ch == ord("q") or ch == ord("Q"):
- break
- #write_results(result_filename, results)
-
-
-def imageflow_demo(predictor, vis_folder, current_time, args):
- cap = cv2.VideoCapture(args.path if args.demo == "video" else args.camid)
- width = cap.get(cv2.CAP_PROP_FRAME_WIDTH) # float
- height = cap.get(cv2.CAP_PROP_FRAME_HEIGHT) # float
- fps = cap.get(cv2.CAP_PROP_FPS)
- save_folder = os.path.join(
- vis_folder, time.strftime("%Y_%m_%d_%H_%M_%S", current_time)
- )
- os.makedirs(save_folder, exist_ok=True)
- if args.demo == "video":
- save_path = os.path.join(save_folder, args.path.split("/")[-1])
- else:
- save_path = os.path.join(save_folder, "camera.mp4")
- logger.info(f"video save_path is {save_path}")
- vid_writer = cv2.VideoWriter(
- save_path, cv2.VideoWriter_fourcc(*"mp4v"), fps, (int(width), int(height))
- )
- tracker = BYTETracker(args, frame_rate=30)
- timer = Timer()
- frame_id = 0
- results = []
- while True:
- if frame_id % 20 == 0:
- logger.info('Processing frame {} ({:.2f} fps)'.format(frame_id, 1. / max(1e-5, timer.average_time)))
- ret_val, frame = cap.read()
- if ret_val:
- outputs, img_info = predictor.inference(frame, timer)
- online_targets = tracker.update(outputs[0], [img_info['height'], img_info['width']], exp.test_size)
- online_tlwhs = []
- online_ids = []
- online_scores = []
- for t in online_targets:
- tlwh = t.tlwh
- tid = t.track_id
- vertical = tlwh[2] / tlwh[3] > 1.6
- if tlwh[2] * tlwh[3] > args.min_box_area and not vertical:
- online_tlwhs.append(tlwh)
- online_ids.append(tid)
- online_scores.append(t.score)
- timer.toc()
- results.append((frame_id + 1, online_tlwhs, online_ids, online_scores))
- online_im = plot_tracking(img_info['raw_img'], online_tlwhs, online_ids, frame_id=frame_id + 1,
- fps=1. / timer.average_time)
- if args.save_result:
- vid_writer.write(online_im)
- ch = cv2.waitKey(1)
- if ch == 27 or ch == ord("q") or ch == ord("Q"):
- break
- else:
- break
- frame_id += 1
-
-
-def main(exp, args):
- if not args.experiment_name:
- args.experiment_name = exp.exp_name
-
- file_name = os.path.join(exp.output_dir, args.experiment_name)
- os.makedirs(file_name, exist_ok=True)
-
- if args.save_result:
- vis_folder = os.path.join(file_name, "track_vis")
- os.makedirs(vis_folder, exist_ok=True)
-
- if args.trt:
- args.device = "gpu"
-
- logger.info("Args: {}".format(args))
-
- if args.conf is not None:
- exp.test_conf = args.conf
- if args.nms is not None:
- exp.nmsthre = args.nms
- if args.tsize is not None:
- exp.test_size = (args.tsize, args.tsize)
-
- model = exp.get_model()
- logger.info("Model Summary: {}".format(get_model_info(model, exp.test_size)))
-
- if args.device == "gpu":
- model.cuda()
- model.eval()
-
- if not args.trt:
- if args.ckpt is None:
- ckpt_file = os.path.join(file_name, "best_ckpt.pth.tar")
- else:
- ckpt_file = args.ckpt
- logger.info("loading checkpoint")
- ckpt = torch.load(ckpt_file, map_location="cpu")
- # load the model state dict
- model.load_state_dict(ckpt["model"])
- logger.info("loaded checkpoint done.")
-
- if args.fuse:
- logger.info("\tFusing model...")
- model = fuse_model(model)
-
- if args.fp16:
- model = model.half() # to FP16
-
- if args.trt:
- assert not args.fuse, "TensorRT model is not support model fusing!"
- trt_file = os.path.join(file_name, "model_trt.pth")
- assert os.path.exists(
- trt_file
- ), "TensorRT model is not found!\n Run python3 tools/trt.py first!"
- model.head.decode_in_inference = False
- decoder = model.head.decode_outputs
- logger.info("Using TensorRT to inference")
- else:
- trt_file = None
- decoder = None
-
- predictor = Predictor(model, exp, trt_file, decoder, args.device, args.fp16)
- current_time = time.localtime()
- if args.demo == "image":
- image_demo(predictor, vis_folder, args.path, current_time, args.save_result)
- elif args.demo == "video" or args.demo == "webcam":
- imageflow_demo(predictor, vis_folder, current_time, args)
-
-
-if __name__ == "__main__":
- args = make_parser().parse_args()
- exp = get_exp(args.exp_file, args.name)
-
- main(exp, args)
diff --git a/spaces/ECCV2022/bytetrack/tutorials/qdtrack/mot_online/kalman_filter.py b/spaces/ECCV2022/bytetrack/tutorials/qdtrack/mot_online/kalman_filter.py
deleted file mode 100644
index b4c4e9854d8abd2fea75ad6b1fe8cd6846c43680..0000000000000000000000000000000000000000
--- a/spaces/ECCV2022/bytetrack/tutorials/qdtrack/mot_online/kalman_filter.py
+++ /dev/null
@@ -1,269 +0,0 @@
-# vim: expandtab:ts=4:sw=4
-import numpy as np
-import scipy.linalg
-
-"""
-Table for the 0.95 quantile of the chi-square distribution with N degrees of
-freedom (contains values for N=1, ..., 9). Taken from MATLAB/Octave's chi2inv
-function and used as Mahalanobis gating threshold.
-"""
-chi2inv95 = {
- 1: 3.8415,
- 2: 5.9915,
- 3: 7.8147,
- 4: 9.4877,
- 5: 11.070,
- 6: 12.592,
- 7: 14.067,
- 8: 15.507,
- 9: 16.919}
-
-
-class KalmanFilter(object):
- """
- A simple Kalman filter for tracking bounding boxes in image space.
-
- The 8-dimensional state space
-
- x, y, a, h, vx, vy, va, vh
-
- contains the bounding box center position (x, y), aspect ratio a, height h,
- and their respective velocities.
-
- Object motion follows a constant velocity model. The bounding box location
- (x, y, a, h) is taken as direct observation of the state space (linear
- observation model).
-
- """
-
- def __init__(self):
- ndim, dt = 4, 1.
-
- # Create Kalman filter model matrices.
- self._motion_mat = np.eye(2 * ndim, 2 * ndim)
- for i in range(ndim):
- self._motion_mat[i, ndim + i] = dt
- self._update_mat = np.eye(ndim, 2 * ndim)
-
- # Motion and observation uncertainty are chosen relative to the current
- # state estimate. These weights control the amount of uncertainty in
- # the model. This is a bit hacky.
- self._std_weight_position = 1. / 20
- self._std_weight_velocity = 1. / 160
-
- def initiate(self, measurement):
- """Create track from unassociated measurement.
-
- Parameters
- ----------
- measurement : ndarray
- Bounding box coordinates (x, y, a, h) with center position (x, y),
- aspect ratio a, and height h.
-
- Returns
- -------
- (ndarray, ndarray)
- Returns the mean vector (8 dimensional) and covariance matrix (8x8
- dimensional) of the new track. Unobserved velocities are initialized
- to 0 mean.
-
- """
- mean_pos = measurement
- mean_vel = np.zeros_like(mean_pos)
- mean = np.r_[mean_pos, mean_vel]
-
- std = [
- 2 * self._std_weight_position * measurement[3],
- 2 * self._std_weight_position * measurement[3],
- 1e-2,
- 2 * self._std_weight_position * measurement[3],
- 10 * self._std_weight_velocity * measurement[3],
- 10 * self._std_weight_velocity * measurement[3],
- 1e-5,
- 10 * self._std_weight_velocity * measurement[3]]
- covariance = np.diag(np.square(std))
- return mean, covariance
-
- def predict(self, mean, covariance):
- """Run Kalman filter prediction step.
-
- Parameters
- ----------
- mean : ndarray
- The 8 dimensional mean vector of the object state at the previous
- time step.
- covariance : ndarray
- The 8x8 dimensional covariance matrix of the object state at the
- previous time step.
-
- Returns
- -------
- (ndarray, ndarray)
- Returns the mean vector and covariance matrix of the predicted
- state. Unobserved velocities are initialized to 0 mean.
-
- """
- std_pos = [
- self._std_weight_position * mean[3],
- self._std_weight_position * mean[3],
- 1e-2,
- self._std_weight_position * mean[3]]
- std_vel = [
- self._std_weight_velocity * mean[3],
- self._std_weight_velocity * mean[3],
- 1e-5,
- self._std_weight_velocity * mean[3]]
- motion_cov = np.diag(np.square(np.r_[std_pos, std_vel]))
-
- #mean = np.dot(self._motion_mat, mean)
- mean = np.dot(mean, self._motion_mat.T)
- covariance = np.linalg.multi_dot((
- self._motion_mat, covariance, self._motion_mat.T)) + motion_cov
-
- return mean, covariance
-
- def project(self, mean, covariance):
- """Project state distribution to measurement space.
-
- Parameters
- ----------
- mean : ndarray
- The state's mean vector (8 dimensional array).
- covariance : ndarray
- The state's covariance matrix (8x8 dimensional).
-
- Returns
- -------
- (ndarray, ndarray)
- Returns the projected mean and covariance matrix of the given state
- estimate.
-
- """
- std = [
- self._std_weight_position * mean[3],
- self._std_weight_position * mean[3],
- 1e-1,
- self._std_weight_position * mean[3]]
- innovation_cov = np.diag(np.square(std))
-
- mean = np.dot(self._update_mat, mean)
- covariance = np.linalg.multi_dot((
- self._update_mat, covariance, self._update_mat.T))
- return mean, covariance + innovation_cov
-
- def multi_predict(self, mean, covariance):
- """Run Kalman filter prediction step (Vectorized version).
- Parameters
- ----------
- mean : ndarray
- The Nx8 dimensional mean matrix of the object states at the previous
- time step.
- covariance : ndarray
- The Nx8x8 dimensional covariance matrics of the object states at the
- previous time step.
- Returns
- -------
- (ndarray, ndarray)
- Returns the mean vector and covariance matrix of the predicted
- state. Unobserved velocities are initialized to 0 mean.
- """
- std_pos = [
- self._std_weight_position * mean[:, 3],
- self._std_weight_position * mean[:, 3],
- 1e-2 * np.ones_like(mean[:, 3]),
- self._std_weight_position * mean[:, 3]]
- std_vel = [
- self._std_weight_velocity * mean[:, 3],
- self._std_weight_velocity * mean[:, 3],
- 1e-5 * np.ones_like(mean[:, 3]),
- self._std_weight_velocity * mean[:, 3]]
- sqr = np.square(np.r_[std_pos, std_vel]).T
-
- motion_cov = []
- for i in range(len(mean)):
- motion_cov.append(np.diag(sqr[i]))
- motion_cov = np.asarray(motion_cov)
-
- mean = np.dot(mean, self._motion_mat.T)
- left = np.dot(self._motion_mat, covariance).transpose((1, 0, 2))
- covariance = np.dot(left, self._motion_mat.T) + motion_cov
-
- return mean, covariance
-
- def update(self, mean, covariance, measurement):
- """Run Kalman filter correction step.
-
- Parameters
- ----------
- mean : ndarray
- The predicted state's mean vector (8 dimensional).
- covariance : ndarray
- The state's covariance matrix (8x8 dimensional).
- measurement : ndarray
- The 4 dimensional measurement vector (x, y, a, h), where (x, y)
- is the center position, a the aspect ratio, and h the height of the
- bounding box.
-
- Returns
- -------
- (ndarray, ndarray)
- Returns the measurement-corrected state distribution.
-
- """
- projected_mean, projected_cov = self.project(mean, covariance)
-
- chol_factor, lower = scipy.linalg.cho_factor(
- projected_cov, lower=True, check_finite=False)
- kalman_gain = scipy.linalg.cho_solve(
- (chol_factor, lower), np.dot(covariance, self._update_mat.T).T,
- check_finite=False).T
- innovation = measurement - projected_mean
-
- new_mean = mean + np.dot(innovation, kalman_gain.T)
- new_covariance = covariance - np.linalg.multi_dot((
- kalman_gain, projected_cov, kalman_gain.T))
- return new_mean, new_covariance
-
- def gating_distance(self, mean, covariance, measurements,
- only_position=False, metric='maha'):
- """Compute gating distance between state distribution and measurements.
- A suitable distance threshold can be obtained from `chi2inv95`. If
- `only_position` is False, the chi-square distribution has 4 degrees of
- freedom, otherwise 2.
- Parameters
- ----------
- mean : ndarray
- Mean vector over the state distribution (8 dimensional).
- covariance : ndarray
- Covariance of the state distribution (8x8 dimensional).
- measurements : ndarray
- An Nx4 dimensional matrix of N measurements, each in
- format (x, y, a, h) where (x, y) is the bounding box center
- position, a the aspect ratio, and h the height.
- only_position : Optional[bool]
- If True, distance computation is done with respect to the bounding
- box center position only.
- Returns
- -------
- ndarray
- Returns an array of length N, where the i-th element contains the
- squared Mahalanobis distance between (mean, covariance) and
- `measurements[i]`.
- """
- mean, covariance = self.project(mean, covariance)
- if only_position:
- mean, covariance = mean[:2], covariance[:2, :2]
- measurements = measurements[:, :2]
-
- d = measurements - mean
- if metric == 'gaussian':
- return np.sum(d * d, axis=1)
- elif metric == 'maha':
- cholesky_factor = np.linalg.cholesky(covariance)
- z = scipy.linalg.solve_triangular(
- cholesky_factor, d.T, lower=True, check_finite=False,
- overwrite_b=True)
- squared_maha = np.sum(z * z, axis=0)
- return squared_maha
- else:
- raise ValueError('invalid distance metric')
diff --git a/spaces/EPFL-VILAB/MultiMAE/mask2former/modeling/pixel_decoder/ops/test.py b/spaces/EPFL-VILAB/MultiMAE/mask2former/modeling/pixel_decoder/ops/test.py
deleted file mode 100644
index 6e1b545459f6fd3235767e721eb5a1090ae14bef..0000000000000000000000000000000000000000
--- a/spaces/EPFL-VILAB/MultiMAE/mask2former/modeling/pixel_decoder/ops/test.py
+++ /dev/null
@@ -1,92 +0,0 @@
-# ------------------------------------------------------------------------------------------------
-# Deformable DETR
-# Copyright (c) 2020 SenseTime. All Rights Reserved.
-# Licensed under the Apache License, Version 2.0 [see LICENSE for details]
-# ------------------------------------------------------------------------------------------------
-# Modified from https://github.com/chengdazhi/Deformable-Convolution-V2-PyTorch/tree/pytorch_1.0.0
-# ------------------------------------------------------------------------------------------------
-
-# Copyright (c) Facebook, Inc. and its affiliates.
-# Modified by Bowen Cheng from https://github.com/fundamentalvision/Deformable-DETR
-
-from __future__ import absolute_import
-from __future__ import print_function
-from __future__ import division
-
-import time
-import torch
-import torch.nn as nn
-from torch.autograd import gradcheck
-
-from functions.ms_deform_attn_func import MSDeformAttnFunction, ms_deform_attn_core_pytorch
-
-
-N, M, D = 1, 2, 2
-Lq, L, P = 2, 2, 2
-shapes = torch.as_tensor([(6, 4), (3, 2)], dtype=torch.long).cuda()
-level_start_index = torch.cat((shapes.new_zeros((1, )), shapes.prod(1).cumsum(0)[:-1]))
-S = sum([(H*W).item() for H, W in shapes])
-
-
-torch.manual_seed(3)
-
-
-@torch.no_grad()
-def check_forward_equal_with_pytorch_double():
- value = torch.rand(N, S, M, D).cuda() * 0.01
- sampling_locations = torch.rand(N, Lq, M, L, P, 2).cuda()
- attention_weights = torch.rand(N, Lq, M, L, P).cuda() + 1e-5
- attention_weights /= attention_weights.sum(-1, keepdim=True).sum(-2, keepdim=True)
- im2col_step = 2
- output_pytorch = ms_deform_attn_core_pytorch(value.double(), shapes, sampling_locations.double(), attention_weights.double()).detach().cpu()
- output_cuda = MSDeformAttnFunction.apply(value.double(), shapes, level_start_index, sampling_locations.double(), attention_weights.double(), im2col_step).detach().cpu()
- fwdok = torch.allclose(output_cuda, output_pytorch)
- max_abs_err = (output_cuda - output_pytorch).abs().max()
- max_rel_err = ((output_cuda - output_pytorch).abs() / output_pytorch.abs()).max()
-
- print(f'* {fwdok} check_forward_equal_with_pytorch_double: max_abs_err {max_abs_err:.2e} max_rel_err {max_rel_err:.2e}')
-
-
-@torch.no_grad()
-def check_forward_equal_with_pytorch_float():
- value = torch.rand(N, S, M, D).cuda() * 0.01
- sampling_locations = torch.rand(N, Lq, M, L, P, 2).cuda()
- attention_weights = torch.rand(N, Lq, M, L, P).cuda() + 1e-5
- attention_weights /= attention_weights.sum(-1, keepdim=True).sum(-2, keepdim=True)
- im2col_step = 2
- output_pytorch = ms_deform_attn_core_pytorch(value, shapes, sampling_locations, attention_weights).detach().cpu()
- output_cuda = MSDeformAttnFunction.apply(value, shapes, level_start_index, sampling_locations, attention_weights, im2col_step).detach().cpu()
- fwdok = torch.allclose(output_cuda, output_pytorch, rtol=1e-2, atol=1e-3)
- max_abs_err = (output_cuda - output_pytorch).abs().max()
- max_rel_err = ((output_cuda - output_pytorch).abs() / output_pytorch.abs()).max()
-
- print(f'* {fwdok} check_forward_equal_with_pytorch_float: max_abs_err {max_abs_err:.2e} max_rel_err {max_rel_err:.2e}')
-
-
-def check_gradient_numerical(channels=4, grad_value=True, grad_sampling_loc=True, grad_attn_weight=True):
-
- value = torch.rand(N, S, M, channels).cuda() * 0.01
- sampling_locations = torch.rand(N, Lq, M, L, P, 2).cuda()
- attention_weights = torch.rand(N, Lq, M, L, P).cuda() + 1e-5
- attention_weights /= attention_weights.sum(-1, keepdim=True).sum(-2, keepdim=True)
- im2col_step = 2
- func = MSDeformAttnFunction.apply
-
- value.requires_grad = grad_value
- sampling_locations.requires_grad = grad_sampling_loc
- attention_weights.requires_grad = grad_attn_weight
-
- gradok = gradcheck(func, (value.double(), shapes, level_start_index, sampling_locations.double(), attention_weights.double(), im2col_step))
-
- print(f'* {gradok} check_gradient_numerical(D={channels})')
-
-
-if __name__ == '__main__':
- check_forward_equal_with_pytorch_double()
- check_forward_equal_with_pytorch_float()
-
- for channels in [30, 32, 64, 71, 1025, 2048, 3096]:
- check_gradient_numerical(channels, True, True, True)
-
-
-
diff --git a/spaces/EddyCode/Portfolio/assets/bootstrap/css/bootstrap.min.css b/spaces/EddyCode/Portfolio/assets/bootstrap/css/bootstrap.min.css
deleted file mode 100644
index 4bf32529e91df3f6e9eaa11d51e59376b6a1d696..0000000000000000000000000000000000000000
--- a/spaces/EddyCode/Portfolio/assets/bootstrap/css/bootstrap.min.css
+++ /dev/null
@@ -1,5 +0,0 @@
-/*!
- * Bootstrap v5.3.0 (https://getbootstrap.com/)
- * Copyright 2011-2023 The Bootstrap Authors
- * Licensed under MIT (https://github.com/twbs/bootstrap/blob/main/LICENSE)
- */:root,[data-bs-theme=light]{--bs-blue: #0d6efd;--bs-indigo: #6610f2;--bs-purple: #6f42c1;--bs-pink: #d63384;--bs-red: #dc3545;--bs-orange: #fd7e14;--bs-yellow: #ffc107;--bs-green: #198754;--bs-teal: #20c997;--bs-cyan: #0dcaf0;--bs-black: #000;--bs-white: #fff;--bs-gray: #868e96;--bs-gray-dark: #343a40;--bs-gray-100: #f8f9fa;--bs-gray-200: #e9ecef;--bs-gray-300: #dee2e6;--bs-gray-400: #ced4da;--bs-gray-500: #adb5bd;--bs-gray-600: #868e96;--bs-gray-700: #495057;--bs-gray-800: #343a40;--bs-gray-900: #212529;--bs-primary: #1D809F;--bs-secondary: #ecb807;--bs-success: #198754;--bs-info: #0dcaf0;--bs-warning: #ffc107;--bs-danger: #dc3545;--bs-light: #f8f9fa;--bs-dark: #212529;--bs-primary-rgb: 29, 128, 159;--bs-secondary-rgb: 236, 184, 7;--bs-success-rgb: 25, 135, 84;--bs-info-rgb: 13, 202, 240;--bs-warning-rgb: 255, 193, 7;--bs-danger-rgb: 220, 53, 69;--bs-light-rgb: 248, 249, 250;--bs-dark-rgb: 33, 37, 41;--bs-primary-text-emphasis: #0c3340;--bs-secondary-text-emphasis: #5e4a03;--bs-success-text-emphasis: #0a3622;--bs-info-text-emphasis: #055160;--bs-warning-text-emphasis: #664d03;--bs-danger-text-emphasis: #58151c;--bs-light-text-emphasis: #495057;--bs-dark-text-emphasis: #495057;--bs-primary-bg-subtle: #d2e6ec;--bs-secondary-bg-subtle: #fbf1cd;--bs-success-bg-subtle: #d1e7dd;--bs-info-bg-subtle: #cff4fc;--bs-warning-bg-subtle: #fff3cd;--bs-danger-bg-subtle: #f8d7da;--bs-light-bg-subtle: #fcfcfd;--bs-dark-bg-subtle: #ced4da;--bs-primary-border-subtle: #a5ccd9;--bs-secondary-border-subtle: #f7e39c;--bs-success-border-subtle: #a3cfbb;--bs-info-border-subtle: #9eeaf9;--bs-warning-border-subtle: #ffe69c;--bs-danger-border-subtle: #f1aeb5;--bs-light-border-subtle: #e9ecef;--bs-dark-border-subtle: #adb5bd;--bs-white-rgb: 255, 255, 255;--bs-black-rgb: 0, 0, 0;--bs-font-sans-serif: system-ui, -apple-system, "Segoe UI", Roboto, "Helvetica Neue", "Noto Sans", "Liberation Sans", Arial, sans-serif, "Apple Color Emoji", "Segoe UI Emoji", "Segoe UI Symbol", "Noto Color Emoji";--bs-font-monospace: SFMono-Regular, Menlo, Monaco, Consolas, "Liberation Mono", "Courier New", monospace;--bs-gradient: linear-gradient(180deg, rgba(255, 255, 255, 0.15), rgba(255, 255, 255, 0));--bs-body-font-family: var(--bs-font-sans-serif);--bs-body-font-size:1rem;--bs-body-font-weight: 400;--bs-body-line-height: 1.5;--bs-body-color: #212529;--bs-body-color-rgb: 33, 37, 41;--bs-body-bg: #fff;--bs-body-bg-rgb: 255, 255, 255;--bs-emphasis-color: #000;--bs-emphasis-color-rgb: 0, 0, 0;--bs-secondary-color: rgba(33, 37, 41, 0.75);--bs-secondary-color-rgb: 33, 37, 41;--bs-secondary-bg: #e9ecef;--bs-secondary-bg-rgb: 233, 236, 239;--bs-tertiary-color: rgba(33, 37, 41, 0.5);--bs-tertiary-color-rgb: 33, 37, 41;--bs-tertiary-bg: #f8f9fa;--bs-tertiary-bg-rgb: 248, 249, 250;--bs-heading-color: inherit;--bs-link-color: #1D809F;--bs-link-color-rgb: 29, 128, 159;--bs-link-decoration: underline;--bs-link-hover-color: #17667f;--bs-link-hover-color-rgb: 23, 102, 127;--bs-code-color: #d63384;--bs-highlight-bg: #fff3cd;--bs-border-width: 1px;--bs-border-style: solid;--bs-border-color: #dee2e6;--bs-border-color-translucent: rgba(0, 0, 0, 0.175);--bs-border-radius: 0.375rem;--bs-border-radius-sm: 0.25rem;--bs-border-radius-lg: 0.5rem;--bs-border-radius-xl: 1rem;--bs-border-radius-xxl: 2rem;--bs-border-radius-2xl: var(--bs-border-radius-xxl);--bs-border-radius-pill: 50rem;--bs-box-shadow: 0 0.5rem 1rem rgba(0, 0, 0, 0.15);--bs-box-shadow-sm: 0 0.125rem 0.25rem rgba(0, 0, 0, 0.075);--bs-box-shadow-lg: 0 1rem 3rem rgba(0, 0, 0, 0.175);--bs-box-shadow-inset: inset 0 1px 2px rgba(0, 0, 0, 0.075);--bs-focus-ring-width: 0.25rem;--bs-focus-ring-opacity: 0.25;--bs-focus-ring-color: rgba(29, 128, 159, 0.25);--bs-form-valid-color: #198754;--bs-form-valid-border-color: #198754;--bs-form-invalid-color: #dc3545;--bs-form-invalid-border-color: #dc3545}[data-bs-theme=dark]{color-scheme:dark;--bs-body-color: #adb5bd;--bs-body-color-rgb: 173, 181, 189;--bs-body-bg: #212529;--bs-body-bg-rgb: 33, 37, 41;--bs-emphasis-color: #fff;--bs-emphasis-color-rgb: 255, 255, 255;--bs-secondary-color: rgba(173, 181, 189, 0.75);--bs-secondary-color-rgb: 173, 181, 189;--bs-secondary-bg: #343a40;--bs-secondary-bg-rgb: 52, 58, 64;--bs-tertiary-color: rgba(173, 181, 189, 0.5);--bs-tertiary-color-rgb: 173, 181, 189;--bs-tertiary-bg: #2b3035;--bs-tertiary-bg-rgb: 43, 48, 53;--bs-primary-text-emphasis: #77b3c5;--bs-secondary-text-emphasis: #f4d46a;--bs-success-text-emphasis: #75b798;--bs-info-text-emphasis: #6edff6;--bs-warning-text-emphasis: #ffda6a;--bs-danger-text-emphasis: #ea868f;--bs-light-text-emphasis: #f8f9fa;--bs-dark-text-emphasis: #dee2e6;--bs-primary-bg-subtle: #061a20;--bs-secondary-bg-subtle: #2f2501;--bs-success-bg-subtle: #051b11;--bs-info-bg-subtle: #032830;--bs-warning-bg-subtle: #332701;--bs-danger-bg-subtle: #2c0b0e;--bs-light-bg-subtle: #343a40;--bs-dark-bg-subtle: #1a1d20;--bs-primary-border-subtle: #114d5f;--bs-secondary-border-subtle: #8e6e04;--bs-success-border-subtle: #0f5132;--bs-info-border-subtle: #087990;--bs-warning-border-subtle: #997404;--bs-danger-border-subtle: #842029;--bs-light-border-subtle: #495057;--bs-dark-border-subtle: #343a40;--bs-heading-color: inherit;--bs-link-color: #77b3c5;--bs-link-hover-color: #92c2d1;--bs-link-color-rgb: 119, 179, 197;--bs-link-hover-color-rgb: 146, 194, 209;--bs-code-color: #e685b5;--bs-border-color: #495057;--bs-border-color-translucent: rgba(255, 255, 255, 0.15);--bs-form-valid-color: #75b798;--bs-form-valid-border-color: #75b798;--bs-form-invalid-color: #ea868f;--bs-form-invalid-border-color: #ea868f}*,*::before,*::after{box-sizing:border-box}@media(prefers-reduced-motion: no-preference){:root{scroll-behavior:smooth}}body{margin:0;font-family:var(--bs-body-font-family);font-size:var(--bs-body-font-size);font-weight:var(--bs-body-font-weight);line-height:var(--bs-body-line-height);color:var(--bs-body-color);text-align:var(--bs-body-text-align);background-color:var(--bs-body-bg);-webkit-text-size-adjust:100%;-webkit-tap-highlight-color:rgba(0,0,0,0)}hr{margin:1rem 0;color:inherit;border:0;border-top:var(--bs-border-width) solid;opacity:.25}h6,.h6,h5,.h5,h4,.h4,h3,.h3,h2,.h2,h1,.h1{margin-top:0;margin-bottom:.5rem;font-weight:500;line-height:1.2;color:var(--bs-heading-color)}h1,.h1{font-size:calc(1.375rem + 1.5vw)}@media(min-width: 1200px){h1,.h1{font-size:2.5rem}}h2,.h2{font-size:calc(1.325rem + 0.9vw)}@media(min-width: 1200px){h2,.h2{font-size:2rem}}h3,.h3{font-size:calc(1.3rem + 0.6vw)}@media(min-width: 1200px){h3,.h3{font-size:1.75rem}}h4,.h4{font-size:calc(1.275rem + 0.3vw)}@media(min-width: 1200px){h4,.h4{font-size:1.5rem}}h5,.h5{font-size:1.25rem}h6,.h6{font-size:1rem}p{margin-top:0;margin-bottom:1rem}abbr[title]{-webkit-text-decoration:underline dotted;text-decoration:underline dotted;cursor:help;-webkit-text-decoration-skip-ink:none;text-decoration-skip-ink:none}address{margin-bottom:1rem;font-style:normal;line-height:inherit}ol,ul{padding-left:2rem}ol,ul,dl{margin-top:0;margin-bottom:1rem}ol ol,ul ul,ol ul,ul ol{margin-bottom:0}dt{font-weight:700}dd{margin-bottom:.5rem;margin-left:0}blockquote{margin:0 0 1rem}b,strong{font-weight:bolder}small,.small{font-size:0.875em}mark,.mark{padding:.1875em;background-color:var(--bs-highlight-bg)}sub,sup{position:relative;font-size:0.75em;line-height:0;vertical-align:baseline}sub{bottom:-0.25em}sup{top:-0.5em}a{color:rgba(var(--bs-link-color-rgb), var(--bs-link-opacity, 1));text-decoration:underline}a:hover{--bs-link-color-rgb: var(--bs-link-hover-color-rgb)}a:not([href]):not([class]),a:not([href]):not([class]):hover{color:inherit;text-decoration:none}pre,code,kbd,samp{font-family:var(--bs-font-monospace);font-size:1em}pre{display:block;margin-top:0;margin-bottom:1rem;overflow:auto;font-size:0.875em}pre code{font-size:inherit;color:inherit;word-break:normal}code{font-size:0.875em;color:var(--bs-code-color);word-wrap:break-word}a>code{color:inherit}kbd{padding:.1875rem .375rem;font-size:0.875em;color:var(--bs-body-bg);background-color:var(--bs-body-color);border-radius:.25rem}kbd kbd{padding:0;font-size:1em}figure{margin:0 0 1rem}img,svg{vertical-align:middle}table{caption-side:bottom;border-collapse:collapse}caption{padding-top:.5rem;padding-bottom:.5rem;color:var(--bs-secondary-color);text-align:left}th{text-align:inherit;text-align:-webkit-match-parent}thead,tbody,tfoot,tr,td,th{border-color:inherit;border-style:solid;border-width:0}label{display:inline-block}button{border-radius:0}button:focus:not(:focus-visible){outline:0}input,button,select,optgroup,textarea{margin:0;font-family:inherit;font-size:inherit;line-height:inherit}button,select{text-transform:none}[role=button]{cursor:pointer}select{word-wrap:normal}select:disabled{opacity:1}[list]:not([type=date]):not([type=datetime-local]):not([type=month]):not([type=week]):not([type=time])::-webkit-calendar-picker-indicator{display:none !important}button,[type=button],[type=reset],[type=submit]{-webkit-appearance:button}button:not(:disabled),[type=button]:not(:disabled),[type=reset]:not(:disabled),[type=submit]:not(:disabled){cursor:pointer}::-moz-focus-inner{padding:0;border-style:none}textarea{resize:vertical}fieldset{min-width:0;padding:0;margin:0;border:0}legend{float:left;width:100%;padding:0;margin-bottom:.5rem;font-size:calc(1.275rem + 0.3vw);line-height:inherit}@media(min-width: 1200px){legend{font-size:1.5rem}}legend+*{clear:left}::-webkit-datetime-edit-fields-wrapper,::-webkit-datetime-edit-text,::-webkit-datetime-edit-minute,::-webkit-datetime-edit-hour-field,::-webkit-datetime-edit-day-field,::-webkit-datetime-edit-month-field,::-webkit-datetime-edit-year-field{padding:0}::-webkit-inner-spin-button{height:auto}[type=search]{outline-offset:-2px;-webkit-appearance:textfield}::-webkit-search-decoration{-webkit-appearance:none}::-webkit-color-swatch-wrapper{padding:0}::-webkit-file-upload-button{font:inherit;-webkit-appearance:button}::file-selector-button{font:inherit;-webkit-appearance:button}output{display:inline-block}iframe{border:0}summary{display:list-item;cursor:pointer}progress{vertical-align:baseline}[hidden]{display:none !important}.lead{font-size:1.25rem;font-weight:300}.display-1{font-size:calc(1.625rem + 4.5vw);font-weight:300;line-height:1.2}@media(min-width: 1200px){.display-1{font-size:5rem}}.display-2{font-size:calc(1.575rem + 3.9vw);font-weight:300;line-height:1.2}@media(min-width: 1200px){.display-2{font-size:4.5rem}}.display-3{font-size:calc(1.525rem + 3.3vw);font-weight:300;line-height:1.2}@media(min-width: 1200px){.display-3{font-size:4rem}}.display-4{font-size:calc(1.475rem + 2.7vw);font-weight:300;line-height:1.2}@media(min-width: 1200px){.display-4{font-size:3.5rem}}.display-5{font-size:calc(1.425rem + 2.1vw);font-weight:300;line-height:1.2}@media(min-width: 1200px){.display-5{font-size:3rem}}.display-6{font-size:calc(1.375rem + 1.5vw);font-weight:300;line-height:1.2}@media(min-width: 1200px){.display-6{font-size:2.5rem}}.list-unstyled{padding-left:0;list-style:none}.list-inline{padding-left:0;list-style:none}.list-inline-item{display:inline-block}.list-inline-item:not(:last-child){margin-right:.5rem}.initialism{font-size:0.875em;text-transform:uppercase}.blockquote{margin-bottom:1rem;font-size:1.25rem}.blockquote>:last-child{margin-bottom:0}.blockquote-footer{margin-top:-1rem;margin-bottom:1rem;font-size:0.875em;color:#868e96}.blockquote-footer::before{content:"— "}.img-fluid{max-width:100%;height:auto}.img-thumbnail{padding:.25rem;background-color:var(--bs-body-bg);border:var(--bs-border-width) solid var(--bs-border-color);border-radius:var(--bs-border-radius);max-width:100%;height:auto}.figure{display:inline-block}.figure-img{margin-bottom:.5rem;line-height:1}.figure-caption{font-size:0.875em;color:var(--bs-secondary-color)}.container,.container-fluid,.container-xxl,.container-xl,.container-lg,.container-md,.container-sm{--bs-gutter-x: 1.5rem;--bs-gutter-y: 0;width:100%;padding-right:calc(var(--bs-gutter-x) * .5);padding-left:calc(var(--bs-gutter-x) * .5);margin-right:auto;margin-left:auto}@media(min-width: 576px){.container-sm,.container{max-width:540px}}@media(min-width: 768px){.container-md,.container-sm,.container{max-width:720px}}@media(min-width: 992px){.container-lg,.container-md,.container-sm,.container{max-width:960px}}@media(min-width: 1200px){.container-xl,.container-lg,.container-md,.container-sm,.container{max-width:1140px}}@media(min-width: 1400px){.container-xxl,.container-xl,.container-lg,.container-md,.container-sm,.container{max-width:1320px}}:root{--bs-breakpoint-xs: 0;--bs-breakpoint-sm: 576px;--bs-breakpoint-md: 768px;--bs-breakpoint-lg: 992px;--bs-breakpoint-xl: 1200px;--bs-breakpoint-xxl: 1400px}.row{--bs-gutter-x: 1.5rem;--bs-gutter-y: 0;display:flex;flex-wrap:wrap;margin-top:calc(-1 * var(--bs-gutter-y));margin-right:calc(-.5 * var(--bs-gutter-x));margin-left:calc(-.5 * var(--bs-gutter-x))}.row>*{flex-shrink:0;width:100%;max-width:100%;padding-right:calc(var(--bs-gutter-x) * .5);padding-left:calc(var(--bs-gutter-x) * .5);margin-top:var(--bs-gutter-y)}.col{flex:1 0 0%}.row-cols-auto>*{flex:0 0 auto;width:auto}.row-cols-1>*{flex:0 0 auto;width:100%}.row-cols-2>*{flex:0 0 auto;width:50%}.row-cols-3>*{flex:0 0 auto;width:33.3333333333%}.row-cols-4>*{flex:0 0 auto;width:25%}.row-cols-5>*{flex:0 0 auto;width:20%}.row-cols-6>*{flex:0 0 auto;width:16.6666666667%}.col-auto{flex:0 0 auto;width:auto}.col-1{flex:0 0 auto;width:8.33333333%}.col-2{flex:0 0 auto;width:16.66666667%}.col-3{flex:0 0 auto;width:25%}.col-4{flex:0 0 auto;width:33.33333333%}.col-5{flex:0 0 auto;width:41.66666667%}.col-6{flex:0 0 auto;width:50%}.col-7{flex:0 0 auto;width:58.33333333%}.col-8{flex:0 0 auto;width:66.66666667%}.col-9{flex:0 0 auto;width:75%}.col-10{flex:0 0 auto;width:83.33333333%}.col-11{flex:0 0 auto;width:91.66666667%}.col-12{flex:0 0 auto;width:100%}.offset-1{margin-left:8.33333333%}.offset-2{margin-left:16.66666667%}.offset-3{margin-left:25%}.offset-4{margin-left:33.33333333%}.offset-5{margin-left:41.66666667%}.offset-6{margin-left:50%}.offset-7{margin-left:58.33333333%}.offset-8{margin-left:66.66666667%}.offset-9{margin-left:75%}.offset-10{margin-left:83.33333333%}.offset-11{margin-left:91.66666667%}.g-0,.gx-0{--bs-gutter-x: 0}.g-0,.gy-0{--bs-gutter-y: 0}.g-1,.gx-1{--bs-gutter-x: 0.25rem}.g-1,.gy-1{--bs-gutter-y: 0.25rem}.g-2,.gx-2{--bs-gutter-x: 0.5rem}.g-2,.gy-2{--bs-gutter-y: 0.5rem}.g-3,.gx-3{--bs-gutter-x: 1rem}.g-3,.gy-3{--bs-gutter-y: 1rem}.g-4,.gx-4{--bs-gutter-x: 1.5rem}.g-4,.gy-4{--bs-gutter-y: 1.5rem}.g-5,.gx-5{--bs-gutter-x: 3rem}.g-5,.gy-5{--bs-gutter-y: 3rem}@media(min-width: 576px){.col-sm{flex:1 0 0%}.row-cols-sm-auto>*{flex:0 0 auto;width:auto}.row-cols-sm-1>*{flex:0 0 auto;width:100%}.row-cols-sm-2>*{flex:0 0 auto;width:50%}.row-cols-sm-3>*{flex:0 0 auto;width:33.3333333333%}.row-cols-sm-4>*{flex:0 0 auto;width:25%}.row-cols-sm-5>*{flex:0 0 auto;width:20%}.row-cols-sm-6>*{flex:0 0 auto;width:16.6666666667%}.col-sm-auto{flex:0 0 auto;width:auto}.col-sm-1{flex:0 0 auto;width:8.33333333%}.col-sm-2{flex:0 0 auto;width:16.66666667%}.col-sm-3{flex:0 0 auto;width:25%}.col-sm-4{flex:0 0 auto;width:33.33333333%}.col-sm-5{flex:0 0 auto;width:41.66666667%}.col-sm-6{flex:0 0 auto;width:50%}.col-sm-7{flex:0 0 auto;width:58.33333333%}.col-sm-8{flex:0 0 auto;width:66.66666667%}.col-sm-9{flex:0 0 auto;width:75%}.col-sm-10{flex:0 0 auto;width:83.33333333%}.col-sm-11{flex:0 0 auto;width:91.66666667%}.col-sm-12{flex:0 0 auto;width:100%}.offset-sm-0{margin-left:0}.offset-sm-1{margin-left:8.33333333%}.offset-sm-2{margin-left:16.66666667%}.offset-sm-3{margin-left:25%}.offset-sm-4{margin-left:33.33333333%}.offset-sm-5{margin-left:41.66666667%}.offset-sm-6{margin-left:50%}.offset-sm-7{margin-left:58.33333333%}.offset-sm-8{margin-left:66.66666667%}.offset-sm-9{margin-left:75%}.offset-sm-10{margin-left:83.33333333%}.offset-sm-11{margin-left:91.66666667%}.g-sm-0,.gx-sm-0{--bs-gutter-x: 0}.g-sm-0,.gy-sm-0{--bs-gutter-y: 0}.g-sm-1,.gx-sm-1{--bs-gutter-x: 0.25rem}.g-sm-1,.gy-sm-1{--bs-gutter-y: 0.25rem}.g-sm-2,.gx-sm-2{--bs-gutter-x: 0.5rem}.g-sm-2,.gy-sm-2{--bs-gutter-y: 0.5rem}.g-sm-3,.gx-sm-3{--bs-gutter-x: 1rem}.g-sm-3,.gy-sm-3{--bs-gutter-y: 1rem}.g-sm-4,.gx-sm-4{--bs-gutter-x: 1.5rem}.g-sm-4,.gy-sm-4{--bs-gutter-y: 1.5rem}.g-sm-5,.gx-sm-5{--bs-gutter-x: 3rem}.g-sm-5,.gy-sm-5{--bs-gutter-y: 3rem}}@media(min-width: 768px){.col-md{flex:1 0 0%}.row-cols-md-auto>*{flex:0 0 auto;width:auto}.row-cols-md-1>*{flex:0 0 auto;width:100%}.row-cols-md-2>*{flex:0 0 auto;width:50%}.row-cols-md-3>*{flex:0 0 auto;width:33.3333333333%}.row-cols-md-4>*{flex:0 0 auto;width:25%}.row-cols-md-5>*{flex:0 0 auto;width:20%}.row-cols-md-6>*{flex:0 0 auto;width:16.6666666667%}.col-md-auto{flex:0 0 auto;width:auto}.col-md-1{flex:0 0 auto;width:8.33333333%}.col-md-2{flex:0 0 auto;width:16.66666667%}.col-md-3{flex:0 0 auto;width:25%}.col-md-4{flex:0 0 auto;width:33.33333333%}.col-md-5{flex:0 0 auto;width:41.66666667%}.col-md-6{flex:0 0 auto;width:50%}.col-md-7{flex:0 0 auto;width:58.33333333%}.col-md-8{flex:0 0 auto;width:66.66666667%}.col-md-9{flex:0 0 auto;width:75%}.col-md-10{flex:0 0 auto;width:83.33333333%}.col-md-11{flex:0 0 auto;width:91.66666667%}.col-md-12{flex:0 0 auto;width:100%}.offset-md-0{margin-left:0}.offset-md-1{margin-left:8.33333333%}.offset-md-2{margin-left:16.66666667%}.offset-md-3{margin-left:25%}.offset-md-4{margin-left:33.33333333%}.offset-md-5{margin-left:41.66666667%}.offset-md-6{margin-left:50%}.offset-md-7{margin-left:58.33333333%}.offset-md-8{margin-left:66.66666667%}.offset-md-9{margin-left:75%}.offset-md-10{margin-left:83.33333333%}.offset-md-11{margin-left:91.66666667%}.g-md-0,.gx-md-0{--bs-gutter-x: 0}.g-md-0,.gy-md-0{--bs-gutter-y: 0}.g-md-1,.gx-md-1{--bs-gutter-x: 0.25rem}.g-md-1,.gy-md-1{--bs-gutter-y: 0.25rem}.g-md-2,.gx-md-2{--bs-gutter-x: 0.5rem}.g-md-2,.gy-md-2{--bs-gutter-y: 0.5rem}.g-md-3,.gx-md-3{--bs-gutter-x: 1rem}.g-md-3,.gy-md-3{--bs-gutter-y: 1rem}.g-md-4,.gx-md-4{--bs-gutter-x: 1.5rem}.g-md-4,.gy-md-4{--bs-gutter-y: 1.5rem}.g-md-5,.gx-md-5{--bs-gutter-x: 3rem}.g-md-5,.gy-md-5{--bs-gutter-y: 3rem}}@media(min-width: 992px){.col-lg{flex:1 0 0%}.row-cols-lg-auto>*{flex:0 0 auto;width:auto}.row-cols-lg-1>*{flex:0 0 auto;width:100%}.row-cols-lg-2>*{flex:0 0 auto;width:50%}.row-cols-lg-3>*{flex:0 0 auto;width:33.3333333333%}.row-cols-lg-4>*{flex:0 0 auto;width:25%}.row-cols-lg-5>*{flex:0 0 auto;width:20%}.row-cols-lg-6>*{flex:0 0 auto;width:16.6666666667%}.col-lg-auto{flex:0 0 auto;width:auto}.col-lg-1{flex:0 0 auto;width:8.33333333%}.col-lg-2{flex:0 0 auto;width:16.66666667%}.col-lg-3{flex:0 0 auto;width:25%}.col-lg-4{flex:0 0 auto;width:33.33333333%}.col-lg-5{flex:0 0 auto;width:41.66666667%}.col-lg-6{flex:0 0 auto;width:50%}.col-lg-7{flex:0 0 auto;width:58.33333333%}.col-lg-8{flex:0 0 auto;width:66.66666667%}.col-lg-9{flex:0 0 auto;width:75%}.col-lg-10{flex:0 0 auto;width:83.33333333%}.col-lg-11{flex:0 0 auto;width:91.66666667%}.col-lg-12{flex:0 0 auto;width:100%}.offset-lg-0{margin-left:0}.offset-lg-1{margin-left:8.33333333%}.offset-lg-2{margin-left:16.66666667%}.offset-lg-3{margin-left:25%}.offset-lg-4{margin-left:33.33333333%}.offset-lg-5{margin-left:41.66666667%}.offset-lg-6{margin-left:50%}.offset-lg-7{margin-left:58.33333333%}.offset-lg-8{margin-left:66.66666667%}.offset-lg-9{margin-left:75%}.offset-lg-10{margin-left:83.33333333%}.offset-lg-11{margin-left:91.66666667%}.g-lg-0,.gx-lg-0{--bs-gutter-x: 0}.g-lg-0,.gy-lg-0{--bs-gutter-y: 0}.g-lg-1,.gx-lg-1{--bs-gutter-x: 0.25rem}.g-lg-1,.gy-lg-1{--bs-gutter-y: 0.25rem}.g-lg-2,.gx-lg-2{--bs-gutter-x: 0.5rem}.g-lg-2,.gy-lg-2{--bs-gutter-y: 0.5rem}.g-lg-3,.gx-lg-3{--bs-gutter-x: 1rem}.g-lg-3,.gy-lg-3{--bs-gutter-y: 1rem}.g-lg-4,.gx-lg-4{--bs-gutter-x: 1.5rem}.g-lg-4,.gy-lg-4{--bs-gutter-y: 1.5rem}.g-lg-5,.gx-lg-5{--bs-gutter-x: 3rem}.g-lg-5,.gy-lg-5{--bs-gutter-y: 3rem}}@media(min-width: 1200px){.col-xl{flex:1 0 0%}.row-cols-xl-auto>*{flex:0 0 auto;width:auto}.row-cols-xl-1>*{flex:0 0 auto;width:100%}.row-cols-xl-2>*{flex:0 0 auto;width:50%}.row-cols-xl-3>*{flex:0 0 auto;width:33.3333333333%}.row-cols-xl-4>*{flex:0 0 auto;width:25%}.row-cols-xl-5>*{flex:0 0 auto;width:20%}.row-cols-xl-6>*{flex:0 0 auto;width:16.6666666667%}.col-xl-auto{flex:0 0 auto;width:auto}.col-xl-1{flex:0 0 auto;width:8.33333333%}.col-xl-2{flex:0 0 auto;width:16.66666667%}.col-xl-3{flex:0 0 auto;width:25%}.col-xl-4{flex:0 0 auto;width:33.33333333%}.col-xl-5{flex:0 0 auto;width:41.66666667%}.col-xl-6{flex:0 0 auto;width:50%}.col-xl-7{flex:0 0 auto;width:58.33333333%}.col-xl-8{flex:0 0 auto;width:66.66666667%}.col-xl-9{flex:0 0 auto;width:75%}.col-xl-10{flex:0 0 auto;width:83.33333333%}.col-xl-11{flex:0 0 auto;width:91.66666667%}.col-xl-12{flex:0 0 auto;width:100%}.offset-xl-0{margin-left:0}.offset-xl-1{margin-left:8.33333333%}.offset-xl-2{margin-left:16.66666667%}.offset-xl-3{margin-left:25%}.offset-xl-4{margin-left:33.33333333%}.offset-xl-5{margin-left:41.66666667%}.offset-xl-6{margin-left:50%}.offset-xl-7{margin-left:58.33333333%}.offset-xl-8{margin-left:66.66666667%}.offset-xl-9{margin-left:75%}.offset-xl-10{margin-left:83.33333333%}.offset-xl-11{margin-left:91.66666667%}.g-xl-0,.gx-xl-0{--bs-gutter-x: 0}.g-xl-0,.gy-xl-0{--bs-gutter-y: 0}.g-xl-1,.gx-xl-1{--bs-gutter-x: 0.25rem}.g-xl-1,.gy-xl-1{--bs-gutter-y: 0.25rem}.g-xl-2,.gx-xl-2{--bs-gutter-x: 0.5rem}.g-xl-2,.gy-xl-2{--bs-gutter-y: 0.5rem}.g-xl-3,.gx-xl-3{--bs-gutter-x: 1rem}.g-xl-3,.gy-xl-3{--bs-gutter-y: 1rem}.g-xl-4,.gx-xl-4{--bs-gutter-x: 1.5rem}.g-xl-4,.gy-xl-4{--bs-gutter-y: 1.5rem}.g-xl-5,.gx-xl-5{--bs-gutter-x: 3rem}.g-xl-5,.gy-xl-5{--bs-gutter-y: 3rem}}@media(min-width: 1400px){.col-xxl{flex:1 0 0%}.row-cols-xxl-auto>*{flex:0 0 auto;width:auto}.row-cols-xxl-1>*{flex:0 0 auto;width:100%}.row-cols-xxl-2>*{flex:0 0 auto;width:50%}.row-cols-xxl-3>*{flex:0 0 auto;width:33.3333333333%}.row-cols-xxl-4>*{flex:0 0 auto;width:25%}.row-cols-xxl-5>*{flex:0 0 auto;width:20%}.row-cols-xxl-6>*{flex:0 0 auto;width:16.6666666667%}.col-xxl-auto{flex:0 0 auto;width:auto}.col-xxl-1{flex:0 0 auto;width:8.33333333%}.col-xxl-2{flex:0 0 auto;width:16.66666667%}.col-xxl-3{flex:0 0 auto;width:25%}.col-xxl-4{flex:0 0 auto;width:33.33333333%}.col-xxl-5{flex:0 0 auto;width:41.66666667%}.col-xxl-6{flex:0 0 auto;width:50%}.col-xxl-7{flex:0 0 auto;width:58.33333333%}.col-xxl-8{flex:0 0 auto;width:66.66666667%}.col-xxl-9{flex:0 0 auto;width:75%}.col-xxl-10{flex:0 0 auto;width:83.33333333%}.col-xxl-11{flex:0 0 auto;width:91.66666667%}.col-xxl-12{flex:0 0 auto;width:100%}.offset-xxl-0{margin-left:0}.offset-xxl-1{margin-left:8.33333333%}.offset-xxl-2{margin-left:16.66666667%}.offset-xxl-3{margin-left:25%}.offset-xxl-4{margin-left:33.33333333%}.offset-xxl-5{margin-left:41.66666667%}.offset-xxl-6{margin-left:50%}.offset-xxl-7{margin-left:58.33333333%}.offset-xxl-8{margin-left:66.66666667%}.offset-xxl-9{margin-left:75%}.offset-xxl-10{margin-left:83.33333333%}.offset-xxl-11{margin-left:91.66666667%}.g-xxl-0,.gx-xxl-0{--bs-gutter-x: 0}.g-xxl-0,.gy-xxl-0{--bs-gutter-y: 0}.g-xxl-1,.gx-xxl-1{--bs-gutter-x: 0.25rem}.g-xxl-1,.gy-xxl-1{--bs-gutter-y: 0.25rem}.g-xxl-2,.gx-xxl-2{--bs-gutter-x: 0.5rem}.g-xxl-2,.gy-xxl-2{--bs-gutter-y: 0.5rem}.g-xxl-3,.gx-xxl-3{--bs-gutter-x: 1rem}.g-xxl-3,.gy-xxl-3{--bs-gutter-y: 1rem}.g-xxl-4,.gx-xxl-4{--bs-gutter-x: 1.5rem}.g-xxl-4,.gy-xxl-4{--bs-gutter-y: 1.5rem}.g-xxl-5,.gx-xxl-5{--bs-gutter-x: 3rem}.g-xxl-5,.gy-xxl-5{--bs-gutter-y: 3rem}}.table{--bs-table-color-type: initial;--bs-table-bg-type: initial;--bs-table-color-state: initial;--bs-table-bg-state: initial;--bs-table-color: var(--bs-body-color);--bs-table-bg: var(--bs-body-bg);--bs-table-border-color: var(--bs-border-color);--bs-table-accent-bg: transparent;--bs-table-striped-color: var(--bs-body-color);--bs-table-striped-bg: rgba(0, 0, 0, 0.05);--bs-table-active-color: var(--bs-body-color);--bs-table-active-bg: rgba(0, 0, 0, 0.1);--bs-table-hover-color: var(--bs-body-color);--bs-table-hover-bg: rgba(0, 0, 0, 0.075);width:100%;margin-bottom:1rem;vertical-align:top;border-color:var(--bs-table-border-color)}.table>:not(caption)>*>*{padding:.5rem .5rem;color:var(--bs-table-color-state, var(--bs-table-color-type, var(--bs-table-color)));background-color:var(--bs-table-bg);border-bottom-width:var(--bs-border-width);box-shadow:inset 0 0 0 9999px var(--bs-table-bg-state, var(--bs-table-bg-type, var(--bs-table-accent-bg)))}.table>tbody{vertical-align:inherit}.table>thead{vertical-align:bottom}.table-group-divider{border-top:calc(var(--bs-border-width) * 2) solid currentcolor}.caption-top{caption-side:top}.table-sm>:not(caption)>*>*{padding:.25rem .25rem}.table-bordered>:not(caption)>*{border-width:var(--bs-border-width) 0}.table-bordered>:not(caption)>*>*{border-width:0 var(--bs-border-width)}.table-borderless>:not(caption)>*>*{border-bottom-width:0}.table-borderless>:not(:first-child){border-top-width:0}.table-striped>tbody>tr:nth-of-type(odd)>*{--bs-table-color-type: var(--bs-table-striped-color);--bs-table-bg-type: var(--bs-table-striped-bg)}.table-striped-columns>:not(caption)>tr>:nth-child(even){--bs-table-color-type: var(--bs-table-striped-color);--bs-table-bg-type: var(--bs-table-striped-bg)}.table-active{--bs-table-color-state: var(--bs-table-active-color);--bs-table-bg-state: var(--bs-table-active-bg)}.table-hover>tbody>tr:hover>*{--bs-table-color-state: var(--bs-table-hover-color);--bs-table-bg-state: var(--bs-table-hover-bg)}.table-primary{--bs-table-color: #000;--bs-table-bg: #d2e6ec;--bs-table-border-color: #bdcfd4;--bs-table-striped-bg: #c8dbe0;--bs-table-striped-color: #000;--bs-table-active-bg: #bdcfd4;--bs-table-active-color: #000;--bs-table-hover-bg: #c2d5da;--bs-table-hover-color: #000;color:var(--bs-table-color);border-color:var(--bs-table-border-color)}.table-secondary{--bs-table-color: #000;--bs-table-bg: #fbf1cd;--bs-table-border-color: #e2d9b9;--bs-table-striped-bg: #eee5c3;--bs-table-striped-color: #000;--bs-table-active-bg: #e2d9b9;--bs-table-active-color: #000;--bs-table-hover-bg: #e8dfbe;--bs-table-hover-color: #000;color:var(--bs-table-color);border-color:var(--bs-table-border-color)}.table-success{--bs-table-color: #000;--bs-table-bg: #d1e7dd;--bs-table-border-color: #bcd0c7;--bs-table-striped-bg: #c7dbd2;--bs-table-striped-color: #000;--bs-table-active-bg: #bcd0c7;--bs-table-active-color: #000;--bs-table-hover-bg: #c1d6cc;--bs-table-hover-color: #000;color:var(--bs-table-color);border-color:var(--bs-table-border-color)}.table-info{--bs-table-color: #000;--bs-table-bg: #cff4fc;--bs-table-border-color: #badce3;--bs-table-striped-bg: #c5e8ef;--bs-table-striped-color: #000;--bs-table-active-bg: #badce3;--bs-table-active-color: #000;--bs-table-hover-bg: #bfe2e9;--bs-table-hover-color: #000;color:var(--bs-table-color);border-color:var(--bs-table-border-color)}.table-warning{--bs-table-color: #000;--bs-table-bg: #fff3cd;--bs-table-border-color: #e6dbb9;--bs-table-striped-bg: #f2e7c3;--bs-table-striped-color: #000;--bs-table-active-bg: #e6dbb9;--bs-table-active-color: #000;--bs-table-hover-bg: #ece1be;--bs-table-hover-color: #000;color:var(--bs-table-color);border-color:var(--bs-table-border-color)}.table-danger{--bs-table-color: #000;--bs-table-bg: #f8d7da;--bs-table-border-color: #dfc2c4;--bs-table-striped-bg: #eccccf;--bs-table-striped-color: #000;--bs-table-active-bg: #dfc2c4;--bs-table-active-color: #000;--bs-table-hover-bg: #e5c7ca;--bs-table-hover-color: #000;color:var(--bs-table-color);border-color:var(--bs-table-border-color)}.table-light{--bs-table-color: #000;--bs-table-bg: #f8f9fa;--bs-table-border-color: #dfe0e1;--bs-table-striped-bg: #ecedee;--bs-table-striped-color: #000;--bs-table-active-bg: #dfe0e1;--bs-table-active-color: #000;--bs-table-hover-bg: #e5e6e7;--bs-table-hover-color: #000;color:var(--bs-table-color);border-color:var(--bs-table-border-color)}.table-dark{--bs-table-color: #fff;--bs-table-bg: #212529;--bs-table-border-color: #373b3e;--bs-table-striped-bg: #2c3034;--bs-table-striped-color: #fff;--bs-table-active-bg: #373b3e;--bs-table-active-color: #fff;--bs-table-hover-bg: #323539;--bs-table-hover-color: #fff;color:var(--bs-table-color);border-color:var(--bs-table-border-color)}.table-responsive{overflow-x:auto;-webkit-overflow-scrolling:touch}@media(max-width: 575.98px){.table-responsive-sm{overflow-x:auto;-webkit-overflow-scrolling:touch}}@media(max-width: 767.98px){.table-responsive-md{overflow-x:auto;-webkit-overflow-scrolling:touch}}@media(max-width: 991.98px){.table-responsive-lg{overflow-x:auto;-webkit-overflow-scrolling:touch}}@media(max-width: 1199.98px){.table-responsive-xl{overflow-x:auto;-webkit-overflow-scrolling:touch}}@media(max-width: 1399.98px){.table-responsive-xxl{overflow-x:auto;-webkit-overflow-scrolling:touch}}.form-label{margin-bottom:.5rem}.col-form-label{padding-top:calc(0.375rem + var(--bs-border-width));padding-bottom:calc(0.375rem + var(--bs-border-width));margin-bottom:0;font-size:inherit;line-height:1.5}.col-form-label-lg{padding-top:calc(0.5rem + var(--bs-border-width));padding-bottom:calc(0.5rem + var(--bs-border-width));font-size:1.25rem}.col-form-label-sm{padding-top:calc(0.25rem + var(--bs-border-width));padding-bottom:calc(0.25rem + var(--bs-border-width));font-size:0.875rem}.form-text{margin-top:.25rem;font-size:0.875em;color:var(--bs-secondary-color)}.form-control{display:block;width:100%;padding:.375rem .75rem;font-size:1rem;font-weight:400;line-height:1.5;color:var(--bs-body-color);background-color:var(--bs-body-bg);background-clip:padding-box;border:var(--bs-border-width) solid var(--bs-border-color);-webkit-appearance:none;-moz-appearance:none;appearance:none;border-radius:var(--bs-border-radius);transition:border-color .15s ease-in-out,box-shadow .15s ease-in-out}@media(prefers-reduced-motion: reduce){.form-control{transition:none}}.form-control[type=file]{overflow:hidden}.form-control[type=file]:not(:disabled):not([readonly]){cursor:pointer}.form-control:focus{color:var(--bs-body-color);background-color:var(--bs-body-bg);border-color:#8ec0cf;outline:0;box-shadow:0 0 0 .25rem rgba(29,128,159,.25)}.form-control::-webkit-date-and-time-value{min-width:85px;height:1.5em;margin:0}.form-control::-webkit-datetime-edit{display:block;padding:0}.form-control::-moz-placeholder{color:var(--bs-secondary-color);opacity:1}.form-control:-ms-input-placeholder{color:var(--bs-secondary-color);opacity:1}.form-control::placeholder{color:var(--bs-secondary-color);opacity:1}.form-control:disabled{background-color:var(--bs-secondary-bg);opacity:1}.form-control::-webkit-file-upload-button{padding:.375rem .75rem;margin:-0.375rem -0.75rem;-webkit-margin-end:.75rem;margin-inline-end:.75rem;color:var(--bs-body-color);background-color:var(--bs-tertiary-bg);pointer-events:none;border-color:inherit;border-style:solid;border-width:0;border-inline-end-width:var(--bs-border-width);border-radius:0;-webkit-transition:color .15s ease-in-out,background-color .15s ease-in-out,border-color .15s ease-in-out,box-shadow .15s ease-in-out;transition:color .15s ease-in-out,background-color .15s ease-in-out,border-color .15s ease-in-out,box-shadow .15s ease-in-out}.form-control::file-selector-button{padding:.375rem .75rem;margin:-0.375rem -0.75rem;-webkit-margin-end:.75rem;margin-inline-end:.75rem;color:var(--bs-body-color);background-color:var(--bs-tertiary-bg);pointer-events:none;border-color:inherit;border-style:solid;border-width:0;border-inline-end-width:var(--bs-border-width);border-radius:0;transition:color .15s ease-in-out,background-color .15s ease-in-out,border-color .15s ease-in-out,box-shadow .15s ease-in-out}@media(prefers-reduced-motion: reduce){.form-control::-webkit-file-upload-button{-webkit-transition:none;transition:none}.form-control::file-selector-button{transition:none}}.form-control:hover:not(:disabled):not([readonly])::-webkit-file-upload-button{background-color:var(--bs-secondary-bg)}.form-control:hover:not(:disabled):not([readonly])::file-selector-button{background-color:var(--bs-secondary-bg)}.form-control-plaintext{display:block;width:100%;padding:.375rem 0;margin-bottom:0;line-height:1.5;color:var(--bs-body-color);background-color:transparent;border:solid transparent;border-width:var(--bs-border-width) 0}.form-control-plaintext:focus{outline:0}.form-control-plaintext.form-control-sm,.form-control-plaintext.form-control-lg{padding-right:0;padding-left:0}.form-control-sm{min-height:calc(1.5em + 0.5rem + calc(var(--bs-border-width) * 2));padding:.25rem .5rem;font-size:0.875rem;border-radius:var(--bs-border-radius-sm)}.form-control-sm::-webkit-file-upload-button{padding:.25rem .5rem;margin:-0.25rem -0.5rem;-webkit-margin-end:.5rem;margin-inline-end:.5rem}.form-control-sm::file-selector-button{padding:.25rem .5rem;margin:-0.25rem -0.5rem;-webkit-margin-end:.5rem;margin-inline-end:.5rem}.form-control-lg{min-height:calc(1.5em + 1rem + calc(var(--bs-border-width) * 2));padding:.5rem 1rem;font-size:1.25rem;border-radius:var(--bs-border-radius-lg)}.form-control-lg::-webkit-file-upload-button{padding:.5rem 1rem;margin:-0.5rem -1rem;-webkit-margin-end:1rem;margin-inline-end:1rem}.form-control-lg::file-selector-button{padding:.5rem 1rem;margin:-0.5rem -1rem;-webkit-margin-end:1rem;margin-inline-end:1rem}textarea.form-control{min-height:calc(1.5em + 0.75rem + calc(var(--bs-border-width) * 2))}textarea.form-control-sm{min-height:calc(1.5em + 0.5rem + calc(var(--bs-border-width) * 2))}textarea.form-control-lg{min-height:calc(1.5em + 1rem + calc(var(--bs-border-width) * 2))}.form-control-color{width:3rem;height:calc(1.5em + 0.75rem + calc(var(--bs-border-width) * 2));padding:.375rem}.form-control-color:not(:disabled):not([readonly]){cursor:pointer}.form-control-color::-moz-color-swatch{border:0 !important;border-radius:var(--bs-border-radius)}.form-control-color::-webkit-color-swatch{border:0 !important;border-radius:var(--bs-border-radius)}.form-control-color.form-control-sm{height:calc(1.5em + 0.5rem + calc(var(--bs-border-width) * 2))}.form-control-color.form-control-lg{height:calc(1.5em + 1rem + calc(var(--bs-border-width) * 2))}.form-select{--bs-form-select-bg-img: url("data:image/svg+xml,%3csvg xmlns='http://www.w3.org/2000/svg' viewBox='0 0 16 16'%3e%3cpath fill='none' stroke='%23343a40' stroke-linecap='round' stroke-linejoin='round' stroke-width='2' d='m2 5 6 6 6-6'/%3e%3c/svg%3e");display:block;width:100%;padding:.375rem 2.25rem .375rem .75rem;font-size:1rem;font-weight:400;line-height:1.5;color:var(--bs-body-color);background-color:var(--bs-body-bg);background-image:var(--bs-form-select-bg-img),var(--bs-form-select-bg-icon, none);background-repeat:no-repeat;background-position:right .75rem center;background-size:16px 12px;border:var(--bs-border-width) solid var(--bs-border-color);border-radius:var(--bs-border-radius);transition:border-color .15s ease-in-out,box-shadow .15s ease-in-out;-webkit-appearance:none;-moz-appearance:none;appearance:none}@media(prefers-reduced-motion: reduce){.form-select{transition:none}}.form-select:focus{border-color:#8ec0cf;outline:0;box-shadow:0 0 0 .25rem rgba(29,128,159,.25)}.form-select[multiple],.form-select[size]:not([size="1"]){padding-right:.75rem;background-image:none}.form-select:disabled{background-color:var(--bs-secondary-bg)}.form-select:-moz-focusring{color:transparent;text-shadow:0 0 0 var(--bs-body-color)}.form-select-sm{padding-top:.25rem;padding-bottom:.25rem;padding-left:.5rem;font-size:0.875rem;border-radius:var(--bs-border-radius-sm)}.form-select-lg{padding-top:.5rem;padding-bottom:.5rem;padding-left:1rem;font-size:1.25rem;border-radius:var(--bs-border-radius-lg)}[data-bs-theme=dark] .form-select{--bs-form-select-bg-img: url("data:image/svg+xml,%3csvg xmlns='http://www.w3.org/2000/svg' viewBox='0 0 16 16'%3e%3cpath fill='none' stroke='%23adb5bd' stroke-linecap='round' stroke-linejoin='round' stroke-width='2' d='m2 5 6 6 6-6'/%3e%3c/svg%3e")}.form-check{display:block;min-height:1.5rem;padding-left:1.5em;margin-bottom:.125rem}.form-check .form-check-input{float:left;margin-left:-1.5em}.form-check-reverse{padding-right:1.5em;padding-left:0;text-align:right}.form-check-reverse .form-check-input{float:right;margin-right:-1.5em;margin-left:0}.form-check-input{--bs-form-check-bg: var(--bs-body-bg);width:1em;height:1em;margin-top:.25em;vertical-align:top;background-color:var(--bs-form-check-bg);background-image:var(--bs-form-check-bg-image);background-repeat:no-repeat;background-position:center;background-size:contain;border:var(--bs-border-width) solid var(--bs-border-color);-webkit-appearance:none;-moz-appearance:none;appearance:none;print-color-adjust:exact}.form-check-input[type=checkbox]{border-radius:.25em}.form-check-input[type=radio]{border-radius:50%}.form-check-input:active{filter:brightness(90%)}.form-check-input:focus{border-color:#8ec0cf;outline:0;box-shadow:0 0 0 .25rem rgba(29,128,159,.25)}.form-check-input:checked{background-color:#1d809f;border-color:#1d809f}.form-check-input:checked[type=checkbox]{--bs-form-check-bg-image: url("data:image/svg+xml,%3csvg xmlns='http://www.w3.org/2000/svg' viewBox='0 0 20 20'%3e%3cpath fill='none' stroke='%23fff' stroke-linecap='round' stroke-linejoin='round' stroke-width='3' d='m6 10 3 3 6-6'/%3e%3c/svg%3e")}.form-check-input:checked[type=radio]{--bs-form-check-bg-image: url("data:image/svg+xml,%3csvg xmlns='http://www.w3.org/2000/svg' viewBox='-4 -4 8 8'%3e%3ccircle r='2' fill='%23fff'/%3e%3c/svg%3e")}.form-check-input[type=checkbox]:indeterminate{background-color:#1d809f;border-color:#1d809f;--bs-form-check-bg-image: url("data:image/svg+xml,%3csvg xmlns='http://www.w3.org/2000/svg' viewBox='0 0 20 20'%3e%3cpath fill='none' stroke='%23fff' stroke-linecap='round' stroke-linejoin='round' stroke-width='3' d='M6 10h8'/%3e%3c/svg%3e")}.form-check-input:disabled{pointer-events:none;filter:none;opacity:.5}.form-check-input[disabled]~.form-check-label,.form-check-input:disabled~.form-check-label{cursor:default;opacity:.5}.form-switch{padding-left:2.5em}.form-switch .form-check-input{--bs-form-switch-bg: url("data:image/svg+xml,%3csvg xmlns='http://www.w3.org/2000/svg' viewBox='-4 -4 8 8'%3e%3ccircle r='3' fill='rgba%280, 0, 0, 0.25%29'/%3e%3c/svg%3e");width:2em;margin-left:-2.5em;background-image:var(--bs-form-switch-bg);background-position:left center;border-radius:2em;transition:background-position .15s ease-in-out}@media(prefers-reduced-motion: reduce){.form-switch .form-check-input{transition:none}}.form-switch .form-check-input:focus{--bs-form-switch-bg: url("data:image/svg+xml,%3csvg xmlns='http://www.w3.org/2000/svg' viewBox='-4 -4 8 8'%3e%3ccircle r='3' fill='%238ec0cf'/%3e%3c/svg%3e")}.form-switch .form-check-input:checked{background-position:right center;--bs-form-switch-bg: url("data:image/svg+xml,%3csvg xmlns='http://www.w3.org/2000/svg' viewBox='-4 -4 8 8'%3e%3ccircle r='3' fill='%23fff'/%3e%3c/svg%3e")}.form-switch.form-check-reverse{padding-right:2.5em;padding-left:0}.form-switch.form-check-reverse .form-check-input{margin-right:-2.5em;margin-left:0}.form-check-inline{display:inline-block;margin-right:1rem}.btn-check{position:absolute;clip:rect(0, 0, 0, 0);pointer-events:none}.btn-check[disabled]+.btn,.btn-check:disabled+.btn{pointer-events:none;filter:none;opacity:.65}[data-bs-theme=dark] .form-switch .form-check-input:not(:checked):not(:focus){--bs-form-switch-bg: url("data:image/svg+xml,%3csvg xmlns='http://www.w3.org/2000/svg' viewBox='-4 -4 8 8'%3e%3ccircle r='3' fill='rgba%28255, 255, 255, 0.25%29'/%3e%3c/svg%3e")}.form-range{width:100%;height:1.5rem;padding:0;background-color:transparent;-webkit-appearance:none;-moz-appearance:none;appearance:none}.form-range:focus{outline:0}.form-range:focus::-webkit-slider-thumb{box-shadow:0 0 0 1px #fff,0 0 0 .25rem rgba(29,128,159,.25)}.form-range:focus::-moz-range-thumb{box-shadow:0 0 0 1px #fff,0 0 0 .25rem rgba(29,128,159,.25)}.form-range::-moz-focus-outer{border:0}.form-range::-webkit-slider-thumb{width:1rem;height:1rem;margin-top:-0.25rem;background-color:#1d809f;border:0;border-radius:1rem;-webkit-transition:background-color .15s ease-in-out,border-color .15s ease-in-out,box-shadow .15s ease-in-out;transition:background-color .15s ease-in-out,border-color .15s ease-in-out,box-shadow .15s ease-in-out;-webkit-appearance:none;appearance:none}@media(prefers-reduced-motion: reduce){.form-range::-webkit-slider-thumb{-webkit-transition:none;transition:none}}.form-range::-webkit-slider-thumb:active{background-color:#bbd9e2}.form-range::-webkit-slider-runnable-track{width:100%;height:.5rem;color:transparent;cursor:pointer;background-color:var(--bs-tertiary-bg);border-color:transparent;border-radius:1rem}.form-range::-moz-range-thumb{width:1rem;height:1rem;background-color:#1d809f;border:0;border-radius:1rem;-moz-transition:background-color .15s ease-in-out,border-color .15s ease-in-out,box-shadow .15s ease-in-out;transition:background-color .15s ease-in-out,border-color .15s ease-in-out,box-shadow .15s ease-in-out;-moz-appearance:none;appearance:none}@media(prefers-reduced-motion: reduce){.form-range::-moz-range-thumb{-moz-transition:none;transition:none}}.form-range::-moz-range-thumb:active{background-color:#bbd9e2}.form-range::-moz-range-track{width:100%;height:.5rem;color:transparent;cursor:pointer;background-color:var(--bs-tertiary-bg);border-color:transparent;border-radius:1rem}.form-range:disabled{pointer-events:none}.form-range:disabled::-webkit-slider-thumb{background-color:var(--bs-secondary-color)}.form-range:disabled::-moz-range-thumb{background-color:var(--bs-secondary-color)}.form-floating{position:relative}.form-floating>.form-control,.form-floating>.form-control-plaintext,.form-floating>.form-select{height:calc(3.5rem + calc(var(--bs-border-width) * 2));min-height:calc(3.5rem + calc(var(--bs-border-width) * 2));line-height:1.25}.form-floating>label{position:absolute;top:0;left:0;z-index:2;height:100%;padding:1rem .75rem;overflow:hidden;text-align:start;text-overflow:ellipsis;white-space:nowrap;pointer-events:none;border:var(--bs-border-width) solid transparent;transform-origin:0 0;transition:opacity .1s ease-in-out,transform .1s ease-in-out}@media(prefers-reduced-motion: reduce){.form-floating>label{transition:none}}.form-floating>.form-control,.form-floating>.form-control-plaintext{padding:1rem .75rem}.form-floating>.form-control::-moz-placeholder, .form-floating>.form-control-plaintext::-moz-placeholder{color:transparent}.form-floating>.form-control:-ms-input-placeholder, .form-floating>.form-control-plaintext:-ms-input-placeholder{color:transparent}.form-floating>.form-control::placeholder,.form-floating>.form-control-plaintext::placeholder{color:transparent}.form-floating>.form-control:not(:-moz-placeholder-shown), .form-floating>.form-control-plaintext:not(:-moz-placeholder-shown){padding-top:1.625rem;padding-bottom:.625rem}.form-floating>.form-control:not(:-ms-input-placeholder), .form-floating>.form-control-plaintext:not(:-ms-input-placeholder){padding-top:1.625rem;padding-bottom:.625rem}.form-floating>.form-control:focus,.form-floating>.form-control:not(:placeholder-shown),.form-floating>.form-control-plaintext:focus,.form-floating>.form-control-plaintext:not(:placeholder-shown){padding-top:1.625rem;padding-bottom:.625rem}.form-floating>.form-control:-webkit-autofill,.form-floating>.form-control-plaintext:-webkit-autofill{padding-top:1.625rem;padding-bottom:.625rem}.form-floating>.form-select{padding-top:1.625rem;padding-bottom:.625rem}.form-floating>.form-control:not(:-moz-placeholder-shown)~label{color:rgba(var(--bs-body-color-rgb), 0.65);transform:scale(0.85) translateY(-0.5rem) translateX(0.15rem)}.form-floating>.form-control:not(:-ms-input-placeholder)~label{color:rgba(var(--bs-body-color-rgb), 0.65);transform:scale(0.85) translateY(-0.5rem) translateX(0.15rem)}.form-floating>.form-control:focus~label,.form-floating>.form-control:not(:placeholder-shown)~label,.form-floating>.form-control-plaintext~label,.form-floating>.form-select~label{color:rgba(var(--bs-body-color-rgb), 0.65);transform:scale(0.85) translateY(-0.5rem) translateX(0.15rem)}.form-floating>.form-control:not(:-moz-placeholder-shown)~label::after{position:absolute;inset:1rem .375rem;z-index:-1;height:1.5em;content:"";background-color:var(--bs-body-bg);border-radius:var(--bs-border-radius)}.form-floating>.form-control:not(:-ms-input-placeholder)~label::after{position:absolute;inset:1rem .375rem;z-index:-1;height:1.5em;content:"";background-color:var(--bs-body-bg);border-radius:var(--bs-border-radius)}.form-floating>.form-control:focus~label::after,.form-floating>.form-control:not(:placeholder-shown)~label::after,.form-floating>.form-control-plaintext~label::after,.form-floating>.form-select~label::after{position:absolute;inset:1rem .375rem;z-index:-1;height:1.5em;content:"";background-color:var(--bs-body-bg);border-radius:var(--bs-border-radius)}.form-floating>.form-control:-webkit-autofill~label{color:rgba(var(--bs-body-color-rgb), 0.65);transform:scale(0.85) translateY(-0.5rem) translateX(0.15rem)}.form-floating>.form-control-plaintext~label{border-width:var(--bs-border-width) 0}.form-floating>:disabled~label{color:#868e96}.form-floating>:disabled~label::after{background-color:var(--bs-secondary-bg)}.input-group{position:relative;display:flex;flex-wrap:wrap;align-items:stretch;width:100%}.input-group>.form-control,.input-group>.form-select,.input-group>.form-floating{position:relative;flex:1 1 auto;width:1%;min-width:0}.input-group>.form-control:focus,.input-group>.form-select:focus,.input-group>.form-floating:focus-within{z-index:5}.input-group .btn{position:relative;z-index:2}.input-group .btn:focus{z-index:5}.input-group-text{display:flex;align-items:center;padding:.375rem .75rem;font-size:1rem;font-weight:400;line-height:1.5;color:var(--bs-body-color);text-align:center;white-space:nowrap;background-color:var(--bs-tertiary-bg);border:var(--bs-border-width) solid var(--bs-border-color);border-radius:var(--bs-border-radius)}.input-group-lg>.form-control,.input-group-lg>.form-select,.input-group-lg>.input-group-text,.input-group-lg>.btn{padding:.5rem 1rem;font-size:1.25rem;border-radius:var(--bs-border-radius-lg)}.input-group-sm>.form-control,.input-group-sm>.form-select,.input-group-sm>.input-group-text,.input-group-sm>.btn{padding:.25rem .5rem;font-size:0.875rem;border-radius:var(--bs-border-radius-sm)}.input-group-lg>.form-select,.input-group-sm>.form-select{padding-right:3rem}.input-group:not(.has-validation)>:not(:last-child):not(.dropdown-toggle):not(.dropdown-menu):not(.form-floating),.input-group:not(.has-validation)>.dropdown-toggle:nth-last-child(n+3),.input-group:not(.has-validation)>.form-floating:not(:last-child)>.form-control,.input-group:not(.has-validation)>.form-floating:not(:last-child)>.form-select{border-top-right-radius:0;border-bottom-right-radius:0}.input-group.has-validation>:nth-last-child(n+3):not(.dropdown-toggle):not(.dropdown-menu):not(.form-floating),.input-group.has-validation>.dropdown-toggle:nth-last-child(n+4),.input-group.has-validation>.form-floating:nth-last-child(n+3)>.form-control,.input-group.has-validation>.form-floating:nth-last-child(n+3)>.form-select{border-top-right-radius:0;border-bottom-right-radius:0}.input-group>:not(:first-child):not(.dropdown-menu):not(.valid-tooltip):not(.valid-feedback):not(.invalid-tooltip):not(.invalid-feedback){margin-left:calc(var(--bs-border-width) * -1);border-top-left-radius:0;border-bottom-left-radius:0}.input-group>.form-floating:not(:first-child)>.form-control,.input-group>.form-floating:not(:first-child)>.form-select{border-top-left-radius:0;border-bottom-left-radius:0}.valid-feedback{display:none;width:100%;margin-top:.25rem;font-size:0.875em;color:var(--bs-form-valid-color)}.valid-tooltip{position:absolute;top:100%;z-index:5;display:none;max-width:100%;padding:.25rem .5rem;margin-top:.1rem;font-size:0.875rem;color:#fff;background-color:var(--bs-success);border-radius:var(--bs-border-radius)}.was-validated :valid~.valid-feedback,.was-validated :valid~.valid-tooltip,.is-valid~.valid-feedback,.is-valid~.valid-tooltip{display:block}.was-validated .form-control:valid,.form-control.is-valid{border-color:var(--bs-form-valid-border-color);padding-right:calc(1.5em + 0.75rem);background-image:url("data:image/svg+xml,%3csvg xmlns='http://www.w3.org/2000/svg' viewBox='0 0 8 8'%3e%3cpath fill='%23198754' d='M2.3 6.73.6 4.53c-.4-1.04.46-1.4 1.1-.8l1.1 1.4 3.4-3.8c.6-.63 1.6-.27 1.2.7l-4 4.6c-.43.5-.8.4-1.1.1z'/%3e%3c/svg%3e");background-repeat:no-repeat;background-position:right calc(0.375em + 0.1875rem) center;background-size:calc(0.75em + 0.375rem) calc(0.75em + 0.375rem)}.was-validated .form-control:valid:focus,.form-control.is-valid:focus{border-color:var(--bs-form-valid-border-color);box-shadow:0 0 0 .25rem rgba(var(--bs-success-rgb), 0.25)}.was-validated textarea.form-control:valid,textarea.form-control.is-valid{padding-right:calc(1.5em + 0.75rem);background-position:top calc(0.375em + 0.1875rem) right calc(0.375em + 0.1875rem)}.was-validated .form-select:valid,.form-select.is-valid{border-color:var(--bs-form-valid-border-color)}.was-validated .form-select:valid:not([multiple]):not([size]),.was-validated .form-select:valid:not([multiple])[size="1"],.form-select.is-valid:not([multiple]):not([size]),.form-select.is-valid:not([multiple])[size="1"]{--bs-form-select-bg-icon: url("data:image/svg+xml,%3csvg xmlns='http://www.w3.org/2000/svg' viewBox='0 0 8 8'%3e%3cpath fill='%23198754' d='M2.3 6.73.6 4.53c-.4-1.04.46-1.4 1.1-.8l1.1 1.4 3.4-3.8c.6-.63 1.6-.27 1.2.7l-4 4.6c-.43.5-.8.4-1.1.1z'/%3e%3c/svg%3e");padding-right:4.125rem;background-position:right .75rem center,center right 2.25rem;background-size:16px 12px,calc(0.75em + 0.375rem) calc(0.75em + 0.375rem)}.was-validated .form-select:valid:focus,.form-select.is-valid:focus{border-color:var(--bs-form-valid-border-color);box-shadow:0 0 0 .25rem rgba(var(--bs-success-rgb), 0.25)}.was-validated .form-control-color:valid,.form-control-color.is-valid{width:calc(3rem + calc(1.5em + 0.75rem))}.was-validated .form-check-input:valid,.form-check-input.is-valid{border-color:var(--bs-form-valid-border-color)}.was-validated .form-check-input:valid:checked,.form-check-input.is-valid:checked{background-color:var(--bs-form-valid-color)}.was-validated .form-check-input:valid:focus,.form-check-input.is-valid:focus{box-shadow:0 0 0 .25rem rgba(var(--bs-success-rgb), 0.25)}.was-validated .form-check-input:valid~.form-check-label,.form-check-input.is-valid~.form-check-label{color:var(--bs-form-valid-color)}.form-check-inline .form-check-input~.valid-feedback{margin-left:.5em}.was-validated .input-group>.form-control:not(:focus):valid,.input-group>.form-control:not(:focus).is-valid,.was-validated .input-group>.form-select:not(:focus):valid,.input-group>.form-select:not(:focus).is-valid,.was-validated .input-group>.form-floating:not(:focus-within):valid,.input-group>.form-floating:not(:focus-within).is-valid{z-index:3}.invalid-feedback{display:none;width:100%;margin-top:.25rem;font-size:0.875em;color:var(--bs-form-invalid-color)}.invalid-tooltip{position:absolute;top:100%;z-index:5;display:none;max-width:100%;padding:.25rem .5rem;margin-top:.1rem;font-size:0.875rem;color:#fff;background-color:var(--bs-danger);border-radius:var(--bs-border-radius)}.was-validated :invalid~.invalid-feedback,.was-validated :invalid~.invalid-tooltip,.is-invalid~.invalid-feedback,.is-invalid~.invalid-tooltip{display:block}.was-validated .form-control:invalid,.form-control.is-invalid{border-color:var(--bs-form-invalid-border-color);padding-right:calc(1.5em + 0.75rem);background-image:url("data:image/svg+xml,%3csvg xmlns='http://www.w3.org/2000/svg' viewBox='0 0 12 12' width='12' height='12' fill='none' stroke='%23dc3545'%3e%3ccircle cx='6' cy='6' r='4.5'/%3e%3cpath stroke-linejoin='round' d='M5.8 3.6h.4L6 6.5z'/%3e%3ccircle cx='6' cy='8.2' r='.6' fill='%23dc3545' stroke='none'/%3e%3c/svg%3e");background-repeat:no-repeat;background-position:right calc(0.375em + 0.1875rem) center;background-size:calc(0.75em + 0.375rem) calc(0.75em + 0.375rem)}.was-validated .form-control:invalid:focus,.form-control.is-invalid:focus{border-color:var(--bs-form-invalid-border-color);box-shadow:0 0 0 .25rem rgba(var(--bs-danger-rgb), 0.25)}.was-validated textarea.form-control:invalid,textarea.form-control.is-invalid{padding-right:calc(1.5em + 0.75rem);background-position:top calc(0.375em + 0.1875rem) right calc(0.375em + 0.1875rem)}.was-validated .form-select:invalid,.form-select.is-invalid{border-color:var(--bs-form-invalid-border-color)}.was-validated .form-select:invalid:not([multiple]):not([size]),.was-validated .form-select:invalid:not([multiple])[size="1"],.form-select.is-invalid:not([multiple]):not([size]),.form-select.is-invalid:not([multiple])[size="1"]{--bs-form-select-bg-icon: url("data:image/svg+xml,%3csvg xmlns='http://www.w3.org/2000/svg' viewBox='0 0 12 12' width='12' height='12' fill='none' stroke='%23dc3545'%3e%3ccircle cx='6' cy='6' r='4.5'/%3e%3cpath stroke-linejoin='round' d='M5.8 3.6h.4L6 6.5z'/%3e%3ccircle cx='6' cy='8.2' r='.6' fill='%23dc3545' stroke='none'/%3e%3c/svg%3e");padding-right:4.125rem;background-position:right .75rem center,center right 2.25rem;background-size:16px 12px,calc(0.75em + 0.375rem) calc(0.75em + 0.375rem)}.was-validated .form-select:invalid:focus,.form-select.is-invalid:focus{border-color:var(--bs-form-invalid-border-color);box-shadow:0 0 0 .25rem rgba(var(--bs-danger-rgb), 0.25)}.was-validated .form-control-color:invalid,.form-control-color.is-invalid{width:calc(3rem + calc(1.5em + 0.75rem))}.was-validated .form-check-input:invalid,.form-check-input.is-invalid{border-color:var(--bs-form-invalid-border-color)}.was-validated .form-check-input:invalid:checked,.form-check-input.is-invalid:checked{background-color:var(--bs-form-invalid-color)}.was-validated .form-check-input:invalid:focus,.form-check-input.is-invalid:focus{box-shadow:0 0 0 .25rem rgba(var(--bs-danger-rgb), 0.25)}.was-validated .form-check-input:invalid~.form-check-label,.form-check-input.is-invalid~.form-check-label{color:var(--bs-form-invalid-color)}.form-check-inline .form-check-input~.invalid-feedback{margin-left:.5em}.was-validated .input-group>.form-control:not(:focus):invalid,.input-group>.form-control:not(:focus).is-invalid,.was-validated .input-group>.form-select:not(:focus):invalid,.input-group>.form-select:not(:focus).is-invalid,.was-validated .input-group>.form-floating:not(:focus-within):invalid,.input-group>.form-floating:not(:focus-within).is-invalid{z-index:4}.btn{--bs-btn-padding-x: 0.75rem;--bs-btn-padding-y: 0.375rem;--bs-btn-font-family: ;--bs-btn-font-size:1rem;--bs-btn-font-weight: 400;--bs-btn-line-height: 1.5;--bs-btn-color: var(--bs-body-color);--bs-btn-bg: transparent;--bs-btn-border-width: var(--bs-border-width);--bs-btn-border-color: transparent;--bs-btn-border-radius: var(--bs-border-radius);--bs-btn-hover-border-color: transparent;--bs-btn-box-shadow: inset 0 1px 0 rgba(255, 255, 255, 0.15), 0 1px 1px rgba(0, 0, 0, 0.075);--bs-btn-disabled-opacity: 0.65;--bs-btn-focus-box-shadow: 0 0 0 0.25rem rgba(var(--bs-btn-focus-shadow-rgb), .5);display:inline-block;padding:var(--bs-btn-padding-y) var(--bs-btn-padding-x);font-family:var(--bs-btn-font-family);font-size:var(--bs-btn-font-size);font-weight:var(--bs-btn-font-weight);line-height:var(--bs-btn-line-height);color:var(--bs-btn-color);text-align:center;text-decoration:none;vertical-align:middle;cursor:pointer;-webkit-user-select:none;-moz-user-select:none;-ms-user-select:none;user-select:none;border:var(--bs-btn-border-width) solid var(--bs-btn-border-color);border-radius:var(--bs-btn-border-radius);background-color:var(--bs-btn-bg);transition:color .15s ease-in-out,background-color .15s ease-in-out,border-color .15s ease-in-out,box-shadow .15s ease-in-out}@media(prefers-reduced-motion: reduce){.btn{transition:none}}.btn:hover{color:var(--bs-btn-hover-color);background-color:var(--bs-btn-hover-bg);border-color:var(--bs-btn-hover-border-color)}.btn-check+.btn:hover{color:var(--bs-btn-color);background-color:var(--bs-btn-bg);border-color:var(--bs-btn-border-color)}.btn:focus-visible{color:var(--bs-btn-hover-color);background-color:var(--bs-btn-hover-bg);border-color:var(--bs-btn-hover-border-color);outline:0;box-shadow:var(--bs-btn-focus-box-shadow)}.btn-check:focus-visible+.btn{border-color:var(--bs-btn-hover-border-color);outline:0;box-shadow:var(--bs-btn-focus-box-shadow)}.btn-check:checked+.btn,:not(.btn-check)+.btn:active,.btn:first-child:active,.btn.active,.btn.show{color:var(--bs-btn-active-color);background-color:var(--bs-btn-active-bg);border-color:var(--bs-btn-active-border-color)}.btn-check:checked+.btn:focus-visible,:not(.btn-check)+.btn:active:focus-visible,.btn:first-child:active:focus-visible,.btn.active:focus-visible,.btn.show:focus-visible{box-shadow:var(--bs-btn-focus-box-shadow)}.btn:disabled,.btn.disabled,fieldset:disabled .btn{color:var(--bs-btn-disabled-color);pointer-events:none;background-color:var(--bs-btn-disabled-bg);border-color:var(--bs-btn-disabled-border-color);opacity:var(--bs-btn-disabled-opacity)}.btn-primary{--bs-btn-color: #fff;--bs-btn-bg: #1D809F;--bs-btn-border-color: #1D809F;--bs-btn-hover-color: #fff;--bs-btn-hover-bg: #196d87;--bs-btn-hover-border-color: #17667f;--bs-btn-focus-shadow-rgb: 63, 147, 173;--bs-btn-active-color: #fff;--bs-btn-active-bg: #17667f;--bs-btn-active-border-color: #166077;--bs-btn-active-shadow: inset 0 3px 5px rgba(0, 0, 0, 0.125);--bs-btn-disabled-color: #fff;--bs-btn-disabled-bg: #1D809F;--bs-btn-disabled-border-color: #1D809F}.btn-secondary{--bs-btn-color: #000;--bs-btn-bg: #ecb807;--bs-btn-border-color: #ecb807;--bs-btn-hover-color: #000;--bs-btn-hover-bg: #efc32c;--bs-btn-hover-border-color: #eebf20;--bs-btn-focus-shadow-rgb: 201, 156, 6;--bs-btn-active-color: #000;--bs-btn-active-bg: #f0c639;--bs-btn-active-border-color: #eebf20;--bs-btn-active-shadow: inset 0 3px 5px rgba(0, 0, 0, 0.125);--bs-btn-disabled-color: #000;--bs-btn-disabled-bg: #ecb807;--bs-btn-disabled-border-color: #ecb807}.btn-success{--bs-btn-color: #fff;--bs-btn-bg: #198754;--bs-btn-border-color: #198754;--bs-btn-hover-color: #fff;--bs-btn-hover-bg: #157347;--bs-btn-hover-border-color: #146c43;--bs-btn-focus-shadow-rgb: 60, 153, 110;--bs-btn-active-color: #fff;--bs-btn-active-bg: #146c43;--bs-btn-active-border-color: #13653f;--bs-btn-active-shadow: inset 0 3px 5px rgba(0, 0, 0, 0.125);--bs-btn-disabled-color: #fff;--bs-btn-disabled-bg: #198754;--bs-btn-disabled-border-color: #198754}.btn-info{--bs-btn-color: #000;--bs-btn-bg: #0dcaf0;--bs-btn-border-color: #0dcaf0;--bs-btn-hover-color: #000;--bs-btn-hover-bg: #31d2f2;--bs-btn-hover-border-color: #25cff2;--bs-btn-focus-shadow-rgb: 11, 172, 204;--bs-btn-active-color: #000;--bs-btn-active-bg: #3dd5f3;--bs-btn-active-border-color: #25cff2;--bs-btn-active-shadow: inset 0 3px 5px rgba(0, 0, 0, 0.125);--bs-btn-disabled-color: #000;--bs-btn-disabled-bg: #0dcaf0;--bs-btn-disabled-border-color: #0dcaf0}.btn-warning{--bs-btn-color: #000;--bs-btn-bg: #ffc107;--bs-btn-border-color: #ffc107;--bs-btn-hover-color: #000;--bs-btn-hover-bg: #ffca2c;--bs-btn-hover-border-color: #ffc720;--bs-btn-focus-shadow-rgb: 217, 164, 6;--bs-btn-active-color: #000;--bs-btn-active-bg: #ffcd39;--bs-btn-active-border-color: #ffc720;--bs-btn-active-shadow: inset 0 3px 5px rgba(0, 0, 0, 0.125);--bs-btn-disabled-color: #000;--bs-btn-disabled-bg: #ffc107;--bs-btn-disabled-border-color: #ffc107}.btn-danger{--bs-btn-color: #fff;--bs-btn-bg: #dc3545;--bs-btn-border-color: #dc3545;--bs-btn-hover-color: #fff;--bs-btn-hover-bg: #bb2d3b;--bs-btn-hover-border-color: #b02a37;--bs-btn-focus-shadow-rgb: 225, 83, 97;--bs-btn-active-color: #fff;--bs-btn-active-bg: #b02a37;--bs-btn-active-border-color: #a52834;--bs-btn-active-shadow: inset 0 3px 5px rgba(0, 0, 0, 0.125);--bs-btn-disabled-color: #fff;--bs-btn-disabled-bg: #dc3545;--bs-btn-disabled-border-color: #dc3545}.btn-light{--bs-btn-color: #000;--bs-btn-bg: #f8f9fa;--bs-btn-border-color: #f8f9fa;--bs-btn-hover-color: #000;--bs-btn-hover-bg: #d3d4d5;--bs-btn-hover-border-color: #c6c7c8;--bs-btn-focus-shadow-rgb: 211, 212, 213;--bs-btn-active-color: #000;--bs-btn-active-bg: #c6c7c8;--bs-btn-active-border-color: #babbbc;--bs-btn-active-shadow: inset 0 3px 5px rgba(0, 0, 0, 0.125);--bs-btn-disabled-color: #000;--bs-btn-disabled-bg: #f8f9fa;--bs-btn-disabled-border-color: #f8f9fa}.btn-dark{--bs-btn-color: #fff;--bs-btn-bg: #212529;--bs-btn-border-color: #212529;--bs-btn-hover-color: #fff;--bs-btn-hover-bg: #424649;--bs-btn-hover-border-color: #373b3e;--bs-btn-focus-shadow-rgb: 66, 70, 73;--bs-btn-active-color: #fff;--bs-btn-active-bg: #4d5154;--bs-btn-active-border-color: #373b3e;--bs-btn-active-shadow: inset 0 3px 5px rgba(0, 0, 0, 0.125);--bs-btn-disabled-color: #fff;--bs-btn-disabled-bg: #212529;--bs-btn-disabled-border-color: #212529}.btn-outline-primary{--bs-btn-color: #1D809F;--bs-btn-border-color: #1D809F;--bs-btn-hover-color: #fff;--bs-btn-hover-bg: #1D809F;--bs-btn-hover-border-color: #1D809F;--bs-btn-focus-shadow-rgb: 29, 128, 159;--bs-btn-active-color: #fff;--bs-btn-active-bg: #1D809F;--bs-btn-active-border-color: #1D809F;--bs-btn-active-shadow: inset 0 3px 5px rgba(0, 0, 0, 0.125);--bs-btn-disabled-color: #1D809F;--bs-btn-disabled-bg: transparent;--bs-btn-disabled-border-color: #1D809F;--bs-gradient: none}.btn-outline-secondary{--bs-btn-color: #ecb807;--bs-btn-border-color: #ecb807;--bs-btn-hover-color: #000;--bs-btn-hover-bg: #ecb807;--bs-btn-hover-border-color: #ecb807;--bs-btn-focus-shadow-rgb: 236, 184, 7;--bs-btn-active-color: #000;--bs-btn-active-bg: #ecb807;--bs-btn-active-border-color: #ecb807;--bs-btn-active-shadow: inset 0 3px 5px rgba(0, 0, 0, 0.125);--bs-btn-disabled-color: #ecb807;--bs-btn-disabled-bg: transparent;--bs-btn-disabled-border-color: #ecb807;--bs-gradient: none}.btn-outline-success{--bs-btn-color: #198754;--bs-btn-border-color: #198754;--bs-btn-hover-color: #fff;--bs-btn-hover-bg: #198754;--bs-btn-hover-border-color: #198754;--bs-btn-focus-shadow-rgb: 25, 135, 84;--bs-btn-active-color: #fff;--bs-btn-active-bg: #198754;--bs-btn-active-border-color: #198754;--bs-btn-active-shadow: inset 0 3px 5px rgba(0, 0, 0, 0.125);--bs-btn-disabled-color: #198754;--bs-btn-disabled-bg: transparent;--bs-btn-disabled-border-color: #198754;--bs-gradient: none}.btn-outline-info{--bs-btn-color: #0dcaf0;--bs-btn-border-color: #0dcaf0;--bs-btn-hover-color: #000;--bs-btn-hover-bg: #0dcaf0;--bs-btn-hover-border-color: #0dcaf0;--bs-btn-focus-shadow-rgb: 13, 202, 240;--bs-btn-active-color: #000;--bs-btn-active-bg: #0dcaf0;--bs-btn-active-border-color: #0dcaf0;--bs-btn-active-shadow: inset 0 3px 5px rgba(0, 0, 0, 0.125);--bs-btn-disabled-color: #0dcaf0;--bs-btn-disabled-bg: transparent;--bs-btn-disabled-border-color: #0dcaf0;--bs-gradient: none}.btn-outline-warning{--bs-btn-color: #ffc107;--bs-btn-border-color: #ffc107;--bs-btn-hover-color: #000;--bs-btn-hover-bg: #ffc107;--bs-btn-hover-border-color: #ffc107;--bs-btn-focus-shadow-rgb: 255, 193, 7;--bs-btn-active-color: #000;--bs-btn-active-bg: #ffc107;--bs-btn-active-border-color: #ffc107;--bs-btn-active-shadow: inset 0 3px 5px rgba(0, 0, 0, 0.125);--bs-btn-disabled-color: #ffc107;--bs-btn-disabled-bg: transparent;--bs-btn-disabled-border-color: #ffc107;--bs-gradient: none}.btn-outline-danger{--bs-btn-color: #dc3545;--bs-btn-border-color: #dc3545;--bs-btn-hover-color: #fff;--bs-btn-hover-bg: #dc3545;--bs-btn-hover-border-color: #dc3545;--bs-btn-focus-shadow-rgb: 220, 53, 69;--bs-btn-active-color: #fff;--bs-btn-active-bg: #dc3545;--bs-btn-active-border-color: #dc3545;--bs-btn-active-shadow: inset 0 3px 5px rgba(0, 0, 0, 0.125);--bs-btn-disabled-color: #dc3545;--bs-btn-disabled-bg: transparent;--bs-btn-disabled-border-color: #dc3545;--bs-gradient: none}.btn-outline-light{--bs-btn-color: #f8f9fa;--bs-btn-border-color: #f8f9fa;--bs-btn-hover-color: #000;--bs-btn-hover-bg: #f8f9fa;--bs-btn-hover-border-color: #f8f9fa;--bs-btn-focus-shadow-rgb: 248, 249, 250;--bs-btn-active-color: #000;--bs-btn-active-bg: #f8f9fa;--bs-btn-active-border-color: #f8f9fa;--bs-btn-active-shadow: inset 0 3px 5px rgba(0, 0, 0, 0.125);--bs-btn-disabled-color: #f8f9fa;--bs-btn-disabled-bg: transparent;--bs-btn-disabled-border-color: #f8f9fa;--bs-gradient: none}.btn-outline-dark{--bs-btn-color: #212529;--bs-btn-border-color: #212529;--bs-btn-hover-color: #fff;--bs-btn-hover-bg: #212529;--bs-btn-hover-border-color: #212529;--bs-btn-focus-shadow-rgb: 33, 37, 41;--bs-btn-active-color: #fff;--bs-btn-active-bg: #212529;--bs-btn-active-border-color: #212529;--bs-btn-active-shadow: inset 0 3px 5px rgba(0, 0, 0, 0.125);--bs-btn-disabled-color: #212529;--bs-btn-disabled-bg: transparent;--bs-btn-disabled-border-color: #212529;--bs-gradient: none}.btn-link{--bs-btn-font-weight: 400;--bs-btn-color: var(--bs-link-color);--bs-btn-bg: transparent;--bs-btn-border-color: transparent;--bs-btn-hover-color: var(--bs-link-hover-color);--bs-btn-hover-border-color: transparent;--bs-btn-active-color: var(--bs-link-hover-color);--bs-btn-active-border-color: transparent;--bs-btn-disabled-color: #868e96;--bs-btn-disabled-border-color: transparent;--bs-btn-box-shadow: 0 0 0 #000;--bs-btn-focus-shadow-rgb: 63, 147, 173;text-decoration:underline}.btn-link:focus-visible{color:var(--bs-btn-color)}.btn-link:hover{color:var(--bs-btn-hover-color)}.btn-lg,.btn-group-lg>.btn{--bs-btn-padding-y: 0.5rem;--bs-btn-padding-x: 1rem;--bs-btn-font-size:1.25rem;--bs-btn-border-radius: var(--bs-border-radius-lg)}.btn-sm,.btn-group-sm>.btn{--bs-btn-padding-y: 0.25rem;--bs-btn-padding-x: 0.5rem;--bs-btn-font-size:0.875rem;--bs-btn-border-radius: var(--bs-border-radius-sm)}.fade{transition:opacity .15s linear}@media(prefers-reduced-motion: reduce){.fade{transition:none}}.fade:not(.show){opacity:0}.collapse:not(.show){display:none}.collapsing{height:0;overflow:hidden;transition:height .35s ease}@media(prefers-reduced-motion: reduce){.collapsing{transition:none}}.collapsing.collapse-horizontal{width:0;height:auto;transition:width .35s ease}@media(prefers-reduced-motion: reduce){.collapsing.collapse-horizontal{transition:none}}.dropup,.dropend,.dropdown,.dropstart,.dropup-center,.dropdown-center{position:relative}.dropdown-toggle{white-space:nowrap}.dropdown-toggle::after{display:inline-block;margin-left:.255em;vertical-align:.255em;content:"";border-top:.3em solid;border-right:.3em solid transparent;border-bottom:0;border-left:.3em solid transparent}.dropdown-toggle:empty::after{margin-left:0}.dropdown-menu{--bs-dropdown-zindex: 1000;--bs-dropdown-min-width: 10rem;--bs-dropdown-padding-x: 0;--bs-dropdown-padding-y: 0.5rem;--bs-dropdown-spacer: 0.125rem;--bs-dropdown-font-size:1rem;--bs-dropdown-color: var(--bs-body-color);--bs-dropdown-bg: var(--bs-body-bg);--bs-dropdown-border-color: var(--bs-border-color-translucent);--bs-dropdown-border-radius: var(--bs-border-radius);--bs-dropdown-border-width: var(--bs-border-width);--bs-dropdown-inner-border-radius: calc(var(--bs-border-radius) - var(--bs-border-width));--bs-dropdown-divider-bg: var(--bs-border-color-translucent);--bs-dropdown-divider-margin-y: 0.5rem;--bs-dropdown-box-shadow: 0 0.5rem 1rem rgba(0, 0, 0, 0.15);--bs-dropdown-link-color: var(--bs-body-color);--bs-dropdown-link-hover-color: var(--bs-body-color);--bs-dropdown-link-hover-bg: var(--bs-tertiary-bg);--bs-dropdown-link-active-color: #fff;--bs-dropdown-link-active-bg: #1D809F;--bs-dropdown-link-disabled-color: var(--bs-tertiary-color);--bs-dropdown-item-padding-x: 1rem;--bs-dropdown-item-padding-y: 0.25rem;--bs-dropdown-header-color: #868e96;--bs-dropdown-header-padding-x: 1rem;--bs-dropdown-header-padding-y: 0.5rem;position:absolute;z-index:var(--bs-dropdown-zindex);display:none;min-width:var(--bs-dropdown-min-width);padding:var(--bs-dropdown-padding-y) var(--bs-dropdown-padding-x);margin:0;font-size:var(--bs-dropdown-font-size);color:var(--bs-dropdown-color);text-align:left;list-style:none;background-color:var(--bs-dropdown-bg);background-clip:padding-box;border:var(--bs-dropdown-border-width) solid var(--bs-dropdown-border-color);border-radius:var(--bs-dropdown-border-radius)}.dropdown-menu[data-bs-popper]{top:100%;left:0;margin-top:var(--bs-dropdown-spacer)}.dropdown-menu-start{--bs-position: start}.dropdown-menu-start[data-bs-popper]{right:auto;left:0}.dropdown-menu-end{--bs-position: end}.dropdown-menu-end[data-bs-popper]{right:0;left:auto}@media(min-width: 576px){.dropdown-menu-sm-start{--bs-position: start}.dropdown-menu-sm-start[data-bs-popper]{right:auto;left:0}.dropdown-menu-sm-end{--bs-position: end}.dropdown-menu-sm-end[data-bs-popper]{right:0;left:auto}}@media(min-width: 768px){.dropdown-menu-md-start{--bs-position: start}.dropdown-menu-md-start[data-bs-popper]{right:auto;left:0}.dropdown-menu-md-end{--bs-position: end}.dropdown-menu-md-end[data-bs-popper]{right:0;left:auto}}@media(min-width: 992px){.dropdown-menu-lg-start{--bs-position: start}.dropdown-menu-lg-start[data-bs-popper]{right:auto;left:0}.dropdown-menu-lg-end{--bs-position: end}.dropdown-menu-lg-end[data-bs-popper]{right:0;left:auto}}@media(min-width: 1200px){.dropdown-menu-xl-start{--bs-position: start}.dropdown-menu-xl-start[data-bs-popper]{right:auto;left:0}.dropdown-menu-xl-end{--bs-position: end}.dropdown-menu-xl-end[data-bs-popper]{right:0;left:auto}}@media(min-width: 1400px){.dropdown-menu-xxl-start{--bs-position: start}.dropdown-menu-xxl-start[data-bs-popper]{right:auto;left:0}.dropdown-menu-xxl-end{--bs-position: end}.dropdown-menu-xxl-end[data-bs-popper]{right:0;left:auto}}.dropup .dropdown-menu[data-bs-popper]{top:auto;bottom:100%;margin-top:0;margin-bottom:var(--bs-dropdown-spacer)}.dropup .dropdown-toggle::after{display:inline-block;margin-left:.255em;vertical-align:.255em;content:"";border-top:0;border-right:.3em solid transparent;border-bottom:.3em solid;border-left:.3em solid transparent}.dropup .dropdown-toggle:empty::after{margin-left:0}.dropend .dropdown-menu[data-bs-popper]{top:0;right:auto;left:100%;margin-top:0;margin-left:var(--bs-dropdown-spacer)}.dropend .dropdown-toggle::after{display:inline-block;margin-left:.255em;vertical-align:.255em;content:"";border-top:.3em solid transparent;border-right:0;border-bottom:.3em solid transparent;border-left:.3em solid}.dropend .dropdown-toggle:empty::after{margin-left:0}.dropend .dropdown-toggle::after{vertical-align:0}.dropstart .dropdown-menu[data-bs-popper]{top:0;right:100%;left:auto;margin-top:0;margin-right:var(--bs-dropdown-spacer)}.dropstart .dropdown-toggle::after{display:inline-block;margin-left:.255em;vertical-align:.255em;content:""}.dropstart .dropdown-toggle::after{display:none}.dropstart .dropdown-toggle::before{display:inline-block;margin-right:.255em;vertical-align:.255em;content:"";border-top:.3em solid transparent;border-right:.3em solid;border-bottom:.3em solid transparent}.dropstart .dropdown-toggle:empty::after{margin-left:0}.dropstart .dropdown-toggle::before{vertical-align:0}.dropdown-divider{height:0;margin:var(--bs-dropdown-divider-margin-y) 0;overflow:hidden;border-top:1px solid var(--bs-dropdown-divider-bg);opacity:1}.dropdown-item{display:block;width:100%;padding:var(--bs-dropdown-item-padding-y) var(--bs-dropdown-item-padding-x);clear:both;font-weight:400;color:var(--bs-dropdown-link-color);text-align:inherit;text-decoration:none;white-space:nowrap;background-color:transparent;border:0;border-radius:var(--bs-dropdown-item-border-radius, 0)}.dropdown-item:hover,.dropdown-item:focus{color:var(--bs-dropdown-link-hover-color);background-color:var(--bs-dropdown-link-hover-bg)}.dropdown-item.active,.dropdown-item:active{color:var(--bs-dropdown-link-active-color);text-decoration:none;background-color:var(--bs-dropdown-link-active-bg)}.dropdown-item.disabled,.dropdown-item:disabled{color:var(--bs-dropdown-link-disabled-color);pointer-events:none;background-color:transparent}.dropdown-menu.show{display:block}.dropdown-header{display:block;padding:var(--bs-dropdown-header-padding-y) var(--bs-dropdown-header-padding-x);margin-bottom:0;font-size:0.875rem;color:var(--bs-dropdown-header-color);white-space:nowrap}.dropdown-item-text{display:block;padding:var(--bs-dropdown-item-padding-y) var(--bs-dropdown-item-padding-x);color:var(--bs-dropdown-link-color)}.dropdown-menu-dark{--bs-dropdown-color: #dee2e6;--bs-dropdown-bg: #343a40;--bs-dropdown-border-color: var(--bs-border-color-translucent);--bs-dropdown-box-shadow: ;--bs-dropdown-link-color: #dee2e6;--bs-dropdown-link-hover-color: #fff;--bs-dropdown-divider-bg: var(--bs-border-color-translucent);--bs-dropdown-link-hover-bg: rgba(255, 255, 255, 0.15);--bs-dropdown-link-active-color: #fff;--bs-dropdown-link-active-bg: #1D809F;--bs-dropdown-link-disabled-color: #adb5bd;--bs-dropdown-header-color: #adb5bd}.btn-group,.btn-group-vertical{position:relative;display:inline-flex;vertical-align:middle}.btn-group>.btn,.btn-group-vertical>.btn{position:relative;flex:1 1 auto}.btn-group>.btn-check:checked+.btn,.btn-group>.btn-check:focus+.btn,.btn-group>.btn:hover,.btn-group>.btn:focus,.btn-group>.btn:active,.btn-group>.btn.active,.btn-group-vertical>.btn-check:checked+.btn,.btn-group-vertical>.btn-check:focus+.btn,.btn-group-vertical>.btn:hover,.btn-group-vertical>.btn:focus,.btn-group-vertical>.btn:active,.btn-group-vertical>.btn.active{z-index:1}.btn-toolbar{display:flex;flex-wrap:wrap;justify-content:flex-start}.btn-toolbar .input-group{width:auto}.btn-group{border-radius:var(--bs-border-radius)}.btn-group>:not(.btn-check:first-child)+.btn,.btn-group>.btn-group:not(:first-child){margin-left:calc(var(--bs-border-width) * -1)}.btn-group>.btn:not(:last-child):not(.dropdown-toggle),.btn-group>.btn.dropdown-toggle-split:first-child,.btn-group>.btn-group:not(:last-child)>.btn{border-top-right-radius:0;border-bottom-right-radius:0}.btn-group>.btn:nth-child(n+3),.btn-group>:not(.btn-check)+.btn,.btn-group>.btn-group:not(:first-child)>.btn{border-top-left-radius:0;border-bottom-left-radius:0}.dropdown-toggle-split{padding-right:.5625rem;padding-left:.5625rem}.dropdown-toggle-split::after,.dropup .dropdown-toggle-split::after,.dropend .dropdown-toggle-split::after{margin-left:0}.dropstart .dropdown-toggle-split::before{margin-right:0}.btn-sm+.dropdown-toggle-split,.btn-group-sm>.btn+.dropdown-toggle-split{padding-right:.375rem;padding-left:.375rem}.btn-lg+.dropdown-toggle-split,.btn-group-lg>.btn+.dropdown-toggle-split{padding-right:.75rem;padding-left:.75rem}.btn-group-vertical{flex-direction:column;align-items:flex-start;justify-content:center}.btn-group-vertical>.btn,.btn-group-vertical>.btn-group{width:100%}.btn-group-vertical>.btn:not(:first-child),.btn-group-vertical>.btn-group:not(:first-child){margin-top:calc(var(--bs-border-width) * -1)}.btn-group-vertical>.btn:not(:last-child):not(.dropdown-toggle),.btn-group-vertical>.btn-group:not(:last-child)>.btn{border-bottom-right-radius:0;border-bottom-left-radius:0}.btn-group-vertical>.btn~.btn,.btn-group-vertical>.btn-group:not(:first-child)>.btn{border-top-left-radius:0;border-top-right-radius:0}.nav{--bs-nav-link-padding-x: 1rem;--bs-nav-link-padding-y: 0.5rem;--bs-nav-link-font-weight: ;--bs-nav-link-color: var(--bs-link-color);--bs-nav-link-hover-color: var(--bs-link-hover-color);--bs-nav-link-disabled-color: var(--bs-secondary-color);display:flex;flex-wrap:wrap;padding-left:0;margin-bottom:0;list-style:none}.nav-link{display:block;padding:var(--bs-nav-link-padding-y) var(--bs-nav-link-padding-x);font-size:var(--bs-nav-link-font-size);font-weight:var(--bs-nav-link-font-weight);color:var(--bs-nav-link-color);text-decoration:none;background:none;border:0;transition:color .15s ease-in-out,background-color .15s ease-in-out,border-color .15s ease-in-out}@media(prefers-reduced-motion: reduce){.nav-link{transition:none}}.nav-link:hover,.nav-link:focus{color:var(--bs-nav-link-hover-color)}.nav-link:focus-visible{outline:0;box-shadow:0 0 0 .25rem rgba(29,128,159,.25)}.nav-link.disabled{color:var(--bs-nav-link-disabled-color);pointer-events:none;cursor:default}.nav-tabs{--bs-nav-tabs-border-width: var(--bs-border-width);--bs-nav-tabs-border-color: var(--bs-border-color);--bs-nav-tabs-border-radius: var(--bs-border-radius);--bs-nav-tabs-link-hover-border-color: var(--bs-secondary-bg) var(--bs-secondary-bg) var(--bs-border-color);--bs-nav-tabs-link-active-color: var(--bs-emphasis-color);--bs-nav-tabs-link-active-bg: var(--bs-body-bg);--bs-nav-tabs-link-active-border-color: var(--bs-border-color) var(--bs-border-color) var(--bs-body-bg);border-bottom:var(--bs-nav-tabs-border-width) solid var(--bs-nav-tabs-border-color)}.nav-tabs .nav-link{margin-bottom:calc(-1 * var(--bs-nav-tabs-border-width));border:var(--bs-nav-tabs-border-width) solid transparent;border-top-left-radius:var(--bs-nav-tabs-border-radius);border-top-right-radius:var(--bs-nav-tabs-border-radius)}.nav-tabs .nav-link:hover,.nav-tabs .nav-link:focus{isolation:isolate;border-color:var(--bs-nav-tabs-link-hover-border-color)}.nav-tabs .nav-link.disabled,.nav-tabs .nav-link:disabled{color:var(--bs-nav-link-disabled-color);background-color:transparent;border-color:transparent}.nav-tabs .nav-link.active,.nav-tabs .nav-item.show .nav-link{color:var(--bs-nav-tabs-link-active-color);background-color:var(--bs-nav-tabs-link-active-bg);border-color:var(--bs-nav-tabs-link-active-border-color)}.nav-tabs .dropdown-menu{margin-top:calc(-1 * var(--bs-nav-tabs-border-width));border-top-left-radius:0;border-top-right-radius:0}.nav-pills{--bs-nav-pills-border-radius: var(--bs-border-radius);--bs-nav-pills-link-active-color: #fff;--bs-nav-pills-link-active-bg: #1D809F}.nav-pills .nav-link{border-radius:var(--bs-nav-pills-border-radius)}.nav-pills .nav-link:disabled{color:var(--bs-nav-link-disabled-color);background-color:transparent;border-color:transparent}.nav-pills .nav-link.active,.nav-pills .show>.nav-link{color:var(--bs-nav-pills-link-active-color);background-color:var(--bs-nav-pills-link-active-bg)}.nav-underline{--bs-nav-underline-gap: 1rem;--bs-nav-underline-border-width: 0.125rem;--bs-nav-underline-link-active-color: var(--bs-emphasis-color);gap:var(--bs-nav-underline-gap)}.nav-underline .nav-link{padding-right:0;padding-left:0;border-bottom:var(--bs-nav-underline-border-width) solid transparent}.nav-underline .nav-link:hover,.nav-underline .nav-link:focus{border-bottom-color:currentcolor}.nav-underline .nav-link.active,.nav-underline .show>.nav-link{font-weight:700;color:var(--bs-nav-underline-link-active-color);border-bottom-color:currentcolor}.nav-fill>.nav-link,.nav-fill .nav-item{flex:1 1 auto;text-align:center}.nav-justified>.nav-link,.nav-justified .nav-item{flex-basis:0;flex-grow:1;text-align:center}.nav-fill .nav-item .nav-link,.nav-justified .nav-item .nav-link{width:100%}.tab-content>.tab-pane{display:none}.tab-content>.active{display:block}.navbar{--bs-navbar-padding-x: 0;--bs-navbar-padding-y: 0.5rem;--bs-navbar-color: rgba(var(--bs-emphasis-color-rgb), 0.65);--bs-navbar-hover-color: rgba(var(--bs-emphasis-color-rgb), 0.8);--bs-navbar-disabled-color: rgba(var(--bs-emphasis-color-rgb), 0.3);--bs-navbar-active-color: rgba(var(--bs-emphasis-color-rgb), 1);--bs-navbar-brand-padding-y: 0.3125rem;--bs-navbar-brand-margin-end: 1rem;--bs-navbar-brand-font-size: 1.25rem;--bs-navbar-brand-color: rgba(var(--bs-emphasis-color-rgb), 1);--bs-navbar-brand-hover-color: rgba(var(--bs-emphasis-color-rgb), 1);--bs-navbar-nav-link-padding-x: 0.5rem;--bs-navbar-toggler-padding-y: 0.25rem;--bs-navbar-toggler-padding-x: 0.75rem;--bs-navbar-toggler-font-size: 1.25rem;--bs-navbar-toggler-icon-bg: url("data:image/svg+xml,%3csvg xmlns='http://www.w3.org/2000/svg' viewBox='0 0 30 30'%3e%3cpath stroke='rgba%2833, 37, 41, 0.75%29' stroke-linecap='round' stroke-miterlimit='10' stroke-width='2' d='M4 7h22M4 15h22M4 23h22'/%3e%3c/svg%3e");--bs-navbar-toggler-border-color: rgba(var(--bs-emphasis-color-rgb), 0.15);--bs-navbar-toggler-border-radius: var(--bs-border-radius);--bs-navbar-toggler-focus-width: 0.25rem;--bs-navbar-toggler-transition: box-shadow 0.15s ease-in-out;position:relative;display:flex;flex-wrap:wrap;align-items:center;justify-content:space-between;padding:var(--bs-navbar-padding-y) var(--bs-navbar-padding-x)}.navbar>.container,.navbar>.container-fluid,.navbar>.container-sm,.navbar>.container-md,.navbar>.container-lg,.navbar>.container-xl,.navbar>.container-xxl{display:flex;flex-wrap:inherit;align-items:center;justify-content:space-between}.navbar-brand{padding-top:var(--bs-navbar-brand-padding-y);padding-bottom:var(--bs-navbar-brand-padding-y);margin-right:var(--bs-navbar-brand-margin-end);font-size:var(--bs-navbar-brand-font-size);color:var(--bs-navbar-brand-color);text-decoration:none;white-space:nowrap}.navbar-brand:hover,.navbar-brand:focus{color:var(--bs-navbar-brand-hover-color)}.navbar-nav{--bs-nav-link-padding-x: 0;--bs-nav-link-padding-y: 0.5rem;--bs-nav-link-font-weight: ;--bs-nav-link-color: var(--bs-navbar-color);--bs-nav-link-hover-color: var(--bs-navbar-hover-color);--bs-nav-link-disabled-color: var(--bs-navbar-disabled-color);display:flex;flex-direction:column;padding-left:0;margin-bottom:0;list-style:none}.navbar-nav .nav-link.active,.navbar-nav .nav-link.show{color:var(--bs-navbar-active-color)}.navbar-nav .dropdown-menu{position:static}.navbar-text{padding-top:.5rem;padding-bottom:.5rem;color:var(--bs-navbar-color)}.navbar-text a,.navbar-text a:hover,.navbar-text a:focus{color:var(--bs-navbar-active-color)}.navbar-collapse{flex-basis:100%;flex-grow:1;align-items:center}.navbar-toggler{padding:var(--bs-navbar-toggler-padding-y) var(--bs-navbar-toggler-padding-x);font-size:var(--bs-navbar-toggler-font-size);line-height:1;color:var(--bs-navbar-color);background-color:transparent;border:var(--bs-border-width) solid var(--bs-navbar-toggler-border-color);border-radius:var(--bs-navbar-toggler-border-radius);transition:var(--bs-navbar-toggler-transition)}@media(prefers-reduced-motion: reduce){.navbar-toggler{transition:none}}.navbar-toggler:hover{text-decoration:none}.navbar-toggler:focus{text-decoration:none;outline:0;box-shadow:0 0 0 var(--bs-navbar-toggler-focus-width)}.navbar-toggler-icon{display:inline-block;width:1.5em;height:1.5em;vertical-align:middle;background-image:var(--bs-navbar-toggler-icon-bg);background-repeat:no-repeat;background-position:center;background-size:100%}.navbar-nav-scroll{max-height:var(--bs-scroll-height, 75vh);overflow-y:auto}@media(min-width: 576px){.navbar-expand-sm{flex-wrap:nowrap;justify-content:flex-start}.navbar-expand-sm .navbar-nav{flex-direction:row}.navbar-expand-sm .navbar-nav .dropdown-menu{position:absolute}.navbar-expand-sm .navbar-nav .nav-link{padding-right:var(--bs-navbar-nav-link-padding-x);padding-left:var(--bs-navbar-nav-link-padding-x)}.navbar-expand-sm .navbar-nav-scroll{overflow:visible}.navbar-expand-sm .navbar-collapse{display:flex !important;flex-basis:auto}.navbar-expand-sm .navbar-toggler{display:none}.navbar-expand-sm .offcanvas{position:static;z-index:auto;flex-grow:1;width:auto !important;height:auto !important;visibility:visible !important;background-color:transparent !important;border:0 !important;transform:none !important;transition:none}.navbar-expand-sm .offcanvas .offcanvas-header{display:none}.navbar-expand-sm .offcanvas .offcanvas-body{display:flex;flex-grow:0;padding:0;overflow-y:visible}}@media(min-width: 768px){.navbar-expand-md{flex-wrap:nowrap;justify-content:flex-start}.navbar-expand-md .navbar-nav{flex-direction:row}.navbar-expand-md .navbar-nav .dropdown-menu{position:absolute}.navbar-expand-md .navbar-nav .nav-link{padding-right:var(--bs-navbar-nav-link-padding-x);padding-left:var(--bs-navbar-nav-link-padding-x)}.navbar-expand-md .navbar-nav-scroll{overflow:visible}.navbar-expand-md .navbar-collapse{display:flex !important;flex-basis:auto}.navbar-expand-md .navbar-toggler{display:none}.navbar-expand-md .offcanvas{position:static;z-index:auto;flex-grow:1;width:auto !important;height:auto !important;visibility:visible !important;background-color:transparent !important;border:0 !important;transform:none !important;transition:none}.navbar-expand-md .offcanvas .offcanvas-header{display:none}.navbar-expand-md .offcanvas .offcanvas-body{display:flex;flex-grow:0;padding:0;overflow-y:visible}}@media(min-width: 992px){.navbar-expand-lg{flex-wrap:nowrap;justify-content:flex-start}.navbar-expand-lg .navbar-nav{flex-direction:row}.navbar-expand-lg .navbar-nav .dropdown-menu{position:absolute}.navbar-expand-lg .navbar-nav .nav-link{padding-right:var(--bs-navbar-nav-link-padding-x);padding-left:var(--bs-navbar-nav-link-padding-x)}.navbar-expand-lg .navbar-nav-scroll{overflow:visible}.navbar-expand-lg .navbar-collapse{display:flex !important;flex-basis:auto}.navbar-expand-lg .navbar-toggler{display:none}.navbar-expand-lg .offcanvas{position:static;z-index:auto;flex-grow:1;width:auto !important;height:auto !important;visibility:visible !important;background-color:transparent !important;border:0 !important;transform:none !important;transition:none}.navbar-expand-lg .offcanvas .offcanvas-header{display:none}.navbar-expand-lg .offcanvas .offcanvas-body{display:flex;flex-grow:0;padding:0;overflow-y:visible}}@media(min-width: 1200px){.navbar-expand-xl{flex-wrap:nowrap;justify-content:flex-start}.navbar-expand-xl .navbar-nav{flex-direction:row}.navbar-expand-xl .navbar-nav .dropdown-menu{position:absolute}.navbar-expand-xl .navbar-nav .nav-link{padding-right:var(--bs-navbar-nav-link-padding-x);padding-left:var(--bs-navbar-nav-link-padding-x)}.navbar-expand-xl .navbar-nav-scroll{overflow:visible}.navbar-expand-xl .navbar-collapse{display:flex !important;flex-basis:auto}.navbar-expand-xl .navbar-toggler{display:none}.navbar-expand-xl .offcanvas{position:static;z-index:auto;flex-grow:1;width:auto !important;height:auto !important;visibility:visible !important;background-color:transparent !important;border:0 !important;transform:none !important;transition:none}.navbar-expand-xl .offcanvas .offcanvas-header{display:none}.navbar-expand-xl .offcanvas .offcanvas-body{display:flex;flex-grow:0;padding:0;overflow-y:visible}}@media(min-width: 1400px){.navbar-expand-xxl{flex-wrap:nowrap;justify-content:flex-start}.navbar-expand-xxl .navbar-nav{flex-direction:row}.navbar-expand-xxl .navbar-nav .dropdown-menu{position:absolute}.navbar-expand-xxl .navbar-nav .nav-link{padding-right:var(--bs-navbar-nav-link-padding-x);padding-left:var(--bs-navbar-nav-link-padding-x)}.navbar-expand-xxl .navbar-nav-scroll{overflow:visible}.navbar-expand-xxl .navbar-collapse{display:flex !important;flex-basis:auto}.navbar-expand-xxl .navbar-toggler{display:none}.navbar-expand-xxl .offcanvas{position:static;z-index:auto;flex-grow:1;width:auto !important;height:auto !important;visibility:visible !important;background-color:transparent !important;border:0 !important;transform:none !important;transition:none}.navbar-expand-xxl .offcanvas .offcanvas-header{display:none}.navbar-expand-xxl .offcanvas .offcanvas-body{display:flex;flex-grow:0;padding:0;overflow-y:visible}}.navbar-expand{flex-wrap:nowrap;justify-content:flex-start}.navbar-expand .navbar-nav{flex-direction:row}.navbar-expand .navbar-nav .dropdown-menu{position:absolute}.navbar-expand .navbar-nav .nav-link{padding-right:var(--bs-navbar-nav-link-padding-x);padding-left:var(--bs-navbar-nav-link-padding-x)}.navbar-expand .navbar-nav-scroll{overflow:visible}.navbar-expand .navbar-collapse{display:flex !important;flex-basis:auto}.navbar-expand .navbar-toggler{display:none}.navbar-expand .offcanvas{position:static;z-index:auto;flex-grow:1;width:auto !important;height:auto !important;visibility:visible !important;background-color:transparent !important;border:0 !important;transform:none !important;transition:none}.navbar-expand .offcanvas .offcanvas-header{display:none}.navbar-expand .offcanvas .offcanvas-body{display:flex;flex-grow:0;padding:0;overflow-y:visible}.navbar-dark,.navbar[data-bs-theme=dark]{--bs-navbar-color: rgba(255, 255, 255, 0.55);--bs-navbar-hover-color: rgba(255, 255, 255, 0.75);--bs-navbar-disabled-color: rgba(255, 255, 255, 0.25);--bs-navbar-active-color: #fff;--bs-navbar-brand-color: #fff;--bs-navbar-brand-hover-color: #fff;--bs-navbar-toggler-border-color: rgba(255, 255, 255, 0.1);--bs-navbar-toggler-icon-bg: url("data:image/svg+xml,%3csvg xmlns='http://www.w3.org/2000/svg' viewBox='0 0 30 30'%3e%3cpath stroke='rgba%28255, 255, 255, 0.55%29' stroke-linecap='round' stroke-miterlimit='10' stroke-width='2' d='M4 7h22M4 15h22M4 23h22'/%3e%3c/svg%3e")}[data-bs-theme=dark] .navbar-toggler-icon{--bs-navbar-toggler-icon-bg: url("data:image/svg+xml,%3csvg xmlns='http://www.w3.org/2000/svg' viewBox='0 0 30 30'%3e%3cpath stroke='rgba%28255, 255, 255, 0.55%29' stroke-linecap='round' stroke-miterlimit='10' stroke-width='2' d='M4 7h22M4 15h22M4 23h22'/%3e%3c/svg%3e")}.card{--bs-card-spacer-y: 1rem;--bs-card-spacer-x: 1rem;--bs-card-title-spacer-y: 0.5rem;--bs-card-title-color: ;--bs-card-subtitle-color: ;--bs-card-border-width: var(--bs-border-width);--bs-card-border-color: var(--bs-border-color-translucent);--bs-card-border-radius: var(--bs-border-radius);--bs-card-box-shadow: ;--bs-card-inner-border-radius: calc(var(--bs-border-radius) - (var(--bs-border-width)));--bs-card-cap-padding-y: 0.5rem;--bs-card-cap-padding-x: 1rem;--bs-card-cap-bg: rgba(var(--bs-body-color-rgb), 0.03);--bs-card-cap-color: ;--bs-card-height: ;--bs-card-color: ;--bs-card-bg: var(--bs-body-bg);--bs-card-img-overlay-padding: 1rem;--bs-card-group-margin: 0.75rem;position:relative;display:flex;flex-direction:column;min-width:0;height:var(--bs-card-height);color:var(--bs-body-color);word-wrap:break-word;background-color:var(--bs-card-bg);background-clip:border-box;border:var(--bs-card-border-width) solid var(--bs-card-border-color);border-radius:var(--bs-card-border-radius)}.card>hr{margin-right:0;margin-left:0}.card>.list-group{border-top:inherit;border-bottom:inherit}.card>.list-group:first-child{border-top-width:0;border-top-left-radius:var(--bs-card-inner-border-radius);border-top-right-radius:var(--bs-card-inner-border-radius)}.card>.list-group:last-child{border-bottom-width:0;border-bottom-right-radius:var(--bs-card-inner-border-radius);border-bottom-left-radius:var(--bs-card-inner-border-radius)}.card>.card-header+.list-group,.card>.list-group+.card-footer{border-top:0}.card-body{flex:1 1 auto;padding:var(--bs-card-spacer-y) var(--bs-card-spacer-x);color:var(--bs-card-color)}.card-title{margin-bottom:var(--bs-card-title-spacer-y);color:var(--bs-card-title-color)}.card-subtitle{margin-top:calc(-.5 * var(--bs-card-title-spacer-y));margin-bottom:0;color:var(--bs-card-subtitle-color)}.card-text:last-child{margin-bottom:0}.card-link+.card-link{margin-left:var(--bs-card-spacer-x)}.card-header{padding:var(--bs-card-cap-padding-y) var(--bs-card-cap-padding-x);margin-bottom:0;color:var(--bs-card-cap-color);background-color:var(--bs-card-cap-bg);border-bottom:var(--bs-card-border-width) solid var(--bs-card-border-color)}.card-header:first-child{border-radius:var(--bs-card-inner-border-radius) var(--bs-card-inner-border-radius) 0 0}.card-footer{padding:var(--bs-card-cap-padding-y) var(--bs-card-cap-padding-x);color:var(--bs-card-cap-color);background-color:var(--bs-card-cap-bg);border-top:var(--bs-card-border-width) solid var(--bs-card-border-color)}.card-footer:last-child{border-radius:0 0 var(--bs-card-inner-border-radius) var(--bs-card-inner-border-radius)}.card-header-tabs{margin-right:calc(-.5 * var(--bs-card-cap-padding-x));margin-bottom:calc(-1 * var(--bs-card-cap-padding-y));margin-left:calc(-.5 * var(--bs-card-cap-padding-x));border-bottom:0}.card-header-tabs .nav-link.active{background-color:var(--bs-card-bg);border-bottom-color:var(--bs-card-bg)}.card-header-pills{margin-right:calc(-.5 * var(--bs-card-cap-padding-x));margin-left:calc(-.5 * var(--bs-card-cap-padding-x))}.card-img-overlay{position:absolute;top:0;right:0;bottom:0;left:0;padding:var(--bs-card-img-overlay-padding);border-radius:var(--bs-card-inner-border-radius)}.card-img,.card-img-top,.card-img-bottom{width:100%}.card-img,.card-img-top{border-top-left-radius:var(--bs-card-inner-border-radius);border-top-right-radius:var(--bs-card-inner-border-radius)}.card-img,.card-img-bottom{border-bottom-right-radius:var(--bs-card-inner-border-radius);border-bottom-left-radius:var(--bs-card-inner-border-radius)}.card-group>.card{margin-bottom:var(--bs-card-group-margin)}@media(min-width: 576px){.card-group{display:flex;flex-flow:row wrap}.card-group>.card{flex:1 0 0%;margin-bottom:0}.card-group>.card+.card{margin-left:0;border-left:0}.card-group>.card:not(:last-child){border-top-right-radius:0;border-bottom-right-radius:0}.card-group>.card:not(:last-child) .card-img-top,.card-group>.card:not(:last-child) .card-header{border-top-right-radius:0}.card-group>.card:not(:last-child) .card-img-bottom,.card-group>.card:not(:last-child) .card-footer{border-bottom-right-radius:0}.card-group>.card:not(:first-child){border-top-left-radius:0;border-bottom-left-radius:0}.card-group>.card:not(:first-child) .card-img-top,.card-group>.card:not(:first-child) .card-header{border-top-left-radius:0}.card-group>.card:not(:first-child) .card-img-bottom,.card-group>.card:not(:first-child) .card-footer{border-bottom-left-radius:0}}.accordion{--bs-accordion-color: var(--bs-body-color);--bs-accordion-bg: var(--bs-body-bg);--bs-accordion-transition: color 0.15s ease-in-out, background-color 0.15s ease-in-out, border-color 0.15s ease-in-out, box-shadow 0.15s ease-in-out, border-radius 0.15s ease;--bs-accordion-border-color: var(--bs-border-color);--bs-accordion-border-width: var(--bs-border-width);--bs-accordion-border-radius: var(--bs-border-radius);--bs-accordion-inner-border-radius: calc(var(--bs-border-radius) - (var(--bs-border-width)));--bs-accordion-btn-padding-x: 1.25rem;--bs-accordion-btn-padding-y: 1rem;--bs-accordion-btn-color: var(--bs-body-color);--bs-accordion-btn-bg: var(--bs-accordion-bg);--bs-accordion-btn-icon: url("data:image/svg+xml,%3csvg xmlns='http://www.w3.org/2000/svg' viewBox='0 0 16 16' fill='%23212529'%3e%3cpath fill-rule='evenodd' d='M1.646 4.646a.5.5 0 0 1 .708 0L8 10.293l5.646-5.647a.5.5 0 0 1 .708.708l-6 6a.5.5 0 0 1-.708 0l-6-6a.5.5 0 0 1 0-.708z'/%3e%3c/svg%3e");--bs-accordion-btn-icon-width: 1.25rem;--bs-accordion-btn-icon-transform: rotate(-180deg);--bs-accordion-btn-icon-transition: transform 0.2s ease-in-out;--bs-accordion-btn-active-icon: url("data:image/svg+xml,%3csvg xmlns='http://www.w3.org/2000/svg' viewBox='0 0 16 16' fill='%230c3340'%3e%3cpath fill-rule='evenodd' d='M1.646 4.646a.5.5 0 0 1 .708 0L8 10.293l5.646-5.647a.5.5 0 0 1 .708.708l-6 6a.5.5 0 0 1-.708 0l-6-6a.5.5 0 0 1 0-.708z'/%3e%3c/svg%3e");--bs-accordion-btn-focus-border-color: #8ec0cf;--bs-accordion-btn-focus-box-shadow: 0 0 0 0.25rem rgba(29, 128, 159, 0.25);--bs-accordion-body-padding-x: 1.25rem;--bs-accordion-body-padding-y: 1rem;--bs-accordion-active-color: var(--bs-primary-text-emphasis);--bs-accordion-active-bg: var(--bs-primary-bg-subtle)}.accordion-button{position:relative;display:flex;align-items:center;width:100%;padding:var(--bs-accordion-btn-padding-y) var(--bs-accordion-btn-padding-x);font-size:1rem;color:var(--bs-accordion-btn-color);text-align:left;background-color:var(--bs-accordion-btn-bg);border:0;border-radius:0;overflow-anchor:none;transition:var(--bs-accordion-transition)}@media(prefers-reduced-motion: reduce){.accordion-button{transition:none}}.accordion-button:not(.collapsed){color:var(--bs-accordion-active-color);background-color:var(--bs-accordion-active-bg);box-shadow:inset 0 calc(-1 * var(--bs-accordion-border-width)) 0 var(--bs-accordion-border-color)}.accordion-button:not(.collapsed)::after{background-image:var(--bs-accordion-btn-active-icon);transform:var(--bs-accordion-btn-icon-transform)}.accordion-button::after{flex-shrink:0;width:var(--bs-accordion-btn-icon-width);height:var(--bs-accordion-btn-icon-width);margin-left:auto;content:"";background-image:var(--bs-accordion-btn-icon);background-repeat:no-repeat;background-size:var(--bs-accordion-btn-icon-width);transition:var(--bs-accordion-btn-icon-transition)}@media(prefers-reduced-motion: reduce){.accordion-button::after{transition:none}}.accordion-button:hover{z-index:2}.accordion-button:focus{z-index:3;border-color:var(--bs-accordion-btn-focus-border-color);outline:0;box-shadow:var(--bs-accordion-btn-focus-box-shadow)}.accordion-header{margin-bottom:0}.accordion-item{color:var(--bs-accordion-color);background-color:var(--bs-accordion-bg);border:var(--bs-accordion-border-width) solid var(--bs-accordion-border-color)}.accordion-item:first-of-type{border-top-left-radius:var(--bs-accordion-border-radius);border-top-right-radius:var(--bs-accordion-border-radius)}.accordion-item:first-of-type .accordion-button{border-top-left-radius:var(--bs-accordion-inner-border-radius);border-top-right-radius:var(--bs-accordion-inner-border-radius)}.accordion-item:not(:first-of-type){border-top:0}.accordion-item:last-of-type{border-bottom-right-radius:var(--bs-accordion-border-radius);border-bottom-left-radius:var(--bs-accordion-border-radius)}.accordion-item:last-of-type .accordion-button.collapsed{border-bottom-right-radius:var(--bs-accordion-inner-border-radius);border-bottom-left-radius:var(--bs-accordion-inner-border-radius)}.accordion-item:last-of-type .accordion-collapse{border-bottom-right-radius:var(--bs-accordion-border-radius);border-bottom-left-radius:var(--bs-accordion-border-radius)}.accordion-body{padding:var(--bs-accordion-body-padding-y) var(--bs-accordion-body-padding-x)}.accordion-flush .accordion-collapse{border-width:0}.accordion-flush .accordion-item{border-right:0;border-left:0;border-radius:0}.accordion-flush .accordion-item:first-child{border-top:0}.accordion-flush .accordion-item:last-child{border-bottom:0}.accordion-flush .accordion-item .accordion-button,.accordion-flush .accordion-item .accordion-button.collapsed{border-radius:0}[data-bs-theme=dark] .accordion-button::after{--bs-accordion-btn-icon: url("data:image/svg+xml,%3csvg xmlns='http://www.w3.org/2000/svg' viewBox='0 0 16 16' fill='%2377b3c5'%3e%3cpath fill-rule='evenodd' d='M1.646 4.646a.5.5 0 0 1 .708 0L8 10.293l5.646-5.647a.5.5 0 0 1 .708.708l-6 6a.5.5 0 0 1-.708 0l-6-6a.5.5 0 0 1 0-.708z'/%3e%3c/svg%3e");--bs-accordion-btn-active-icon: url("data:image/svg+xml,%3csvg xmlns='http://www.w3.org/2000/svg' viewBox='0 0 16 16' fill='%2377b3c5'%3e%3cpath fill-rule='evenodd' d='M1.646 4.646a.5.5 0 0 1 .708 0L8 10.293l5.646-5.647a.5.5 0 0 1 .708.708l-6 6a.5.5 0 0 1-.708 0l-6-6a.5.5 0 0 1 0-.708z'/%3e%3c/svg%3e")}.breadcrumb{--bs-breadcrumb-padding-x: 0;--bs-breadcrumb-padding-y: 0;--bs-breadcrumb-margin-bottom: 1rem;--bs-breadcrumb-bg: ;--bs-breadcrumb-border-radius: ;--bs-breadcrumb-divider-color: var(--bs-secondary-color);--bs-breadcrumb-item-padding-x: 0.5rem;--bs-breadcrumb-item-active-color: var(--bs-secondary-color);display:flex;flex-wrap:wrap;padding:var(--bs-breadcrumb-padding-y) var(--bs-breadcrumb-padding-x);margin-bottom:var(--bs-breadcrumb-margin-bottom);font-size:var(--bs-breadcrumb-font-size);list-style:none;background-color:var(--bs-breadcrumb-bg);border-radius:var(--bs-breadcrumb-border-radius)}.breadcrumb-item+.breadcrumb-item{padding-left:var(--bs-breadcrumb-item-padding-x)}.breadcrumb-item+.breadcrumb-item::before{float:left;padding-right:var(--bs-breadcrumb-item-padding-x);color:var(--bs-breadcrumb-divider-color);content:var(--bs-breadcrumb-divider, "/") /* rtl: var(--bs-breadcrumb-divider, "/") */}.breadcrumb-item.active{color:var(--bs-breadcrumb-item-active-color)}.pagination{--bs-pagination-padding-x: 0.75rem;--bs-pagination-padding-y: 0.375rem;--bs-pagination-font-size:1rem;--bs-pagination-color: var(--bs-link-color);--bs-pagination-bg: var(--bs-body-bg);--bs-pagination-border-width: var(--bs-border-width);--bs-pagination-border-color: var(--bs-border-color);--bs-pagination-border-radius: var(--bs-border-radius);--bs-pagination-hover-color: var(--bs-link-hover-color);--bs-pagination-hover-bg: var(--bs-tertiary-bg);--bs-pagination-hover-border-color: var(--bs-border-color);--bs-pagination-focus-color: var(--bs-link-hover-color);--bs-pagination-focus-bg: var(--bs-secondary-bg);--bs-pagination-focus-box-shadow: 0 0 0 0.25rem rgba(29, 128, 159, 0.25);--bs-pagination-active-color: #fff;--bs-pagination-active-bg: #1D809F;--bs-pagination-active-border-color: #1D809F;--bs-pagination-disabled-color: var(--bs-secondary-color);--bs-pagination-disabled-bg: var(--bs-secondary-bg);--bs-pagination-disabled-border-color: var(--bs-border-color);display:flex;padding-left:0;list-style:none}.page-link{position:relative;display:block;padding:var(--bs-pagination-padding-y) var(--bs-pagination-padding-x);font-size:var(--bs-pagination-font-size);color:var(--bs-pagination-color);text-decoration:none;background-color:var(--bs-pagination-bg);border:var(--bs-pagination-border-width) solid var(--bs-pagination-border-color);transition:color .15s ease-in-out,background-color .15s ease-in-out,border-color .15s ease-in-out,box-shadow .15s ease-in-out}@media(prefers-reduced-motion: reduce){.page-link{transition:none}}.page-link:hover{z-index:2;color:var(--bs-pagination-hover-color);background-color:var(--bs-pagination-hover-bg);border-color:var(--bs-pagination-hover-border-color)}.page-link:focus{z-index:3;color:var(--bs-pagination-focus-color);background-color:var(--bs-pagination-focus-bg);outline:0;box-shadow:var(--bs-pagination-focus-box-shadow)}.page-link.active,.active>.page-link{z-index:3;color:var(--bs-pagination-active-color);background-color:var(--bs-pagination-active-bg);border-color:var(--bs-pagination-active-border-color)}.page-link.disabled,.disabled>.page-link{color:var(--bs-pagination-disabled-color);pointer-events:none;background-color:var(--bs-pagination-disabled-bg);border-color:var(--bs-pagination-disabled-border-color)}.page-item:not(:first-child) .page-link{margin-left:calc(var(--bs-border-width) * -1)}.page-item:first-child .page-link{border-top-left-radius:var(--bs-pagination-border-radius);border-bottom-left-radius:var(--bs-pagination-border-radius)}.page-item:last-child .page-link{border-top-right-radius:var(--bs-pagination-border-radius);border-bottom-right-radius:var(--bs-pagination-border-radius)}.pagination-lg{--bs-pagination-padding-x: 1.5rem;--bs-pagination-padding-y: 0.75rem;--bs-pagination-font-size:1.25rem;--bs-pagination-border-radius: var(--bs-border-radius-lg)}.pagination-sm{--bs-pagination-padding-x: 0.5rem;--bs-pagination-padding-y: 0.25rem;--bs-pagination-font-size:0.875rem;--bs-pagination-border-radius: var(--bs-border-radius-sm)}.badge{--bs-badge-padding-x: 0.65em;--bs-badge-padding-y: 0.35em;--bs-badge-font-size:0.75em;--bs-badge-font-weight: 700;--bs-badge-color: #fff;--bs-badge-border-radius: var(--bs-border-radius);display:inline-block;padding:var(--bs-badge-padding-y) var(--bs-badge-padding-x);font-size:var(--bs-badge-font-size);font-weight:var(--bs-badge-font-weight);line-height:1;color:var(--bs-badge-color);text-align:center;white-space:nowrap;vertical-align:baseline;border-radius:var(--bs-badge-border-radius)}.badge:empty{display:none}.btn .badge{position:relative;top:-1px}.alert{--bs-alert-bg: transparent;--bs-alert-padding-x: 1rem;--bs-alert-padding-y: 1rem;--bs-alert-margin-bottom: 1rem;--bs-alert-color: inherit;--bs-alert-border-color: transparent;--bs-alert-border: var(--bs-border-width) solid var(--bs-alert-border-color);--bs-alert-border-radius: var(--bs-border-radius);--bs-alert-link-color: inherit;position:relative;padding:var(--bs-alert-padding-y) var(--bs-alert-padding-x);margin-bottom:var(--bs-alert-margin-bottom);color:var(--bs-alert-color);background-color:var(--bs-alert-bg);border:var(--bs-alert-border);border-radius:var(--bs-alert-border-radius)}.alert-heading{color:inherit}.alert-link{font-weight:700;color:var(--bs-alert-link-color)}.alert-dismissible{padding-right:3rem}.alert-dismissible .btn-close{position:absolute;top:0;right:0;z-index:2;padding:1.25rem 1rem}.alert-primary{--bs-alert-color: var(--bs-primary-text-emphasis);--bs-alert-bg: var(--bs-primary-bg-subtle);--bs-alert-border-color: var(--bs-primary-border-subtle);--bs-alert-link-color: var(--bs-primary-text-emphasis)}.alert-secondary{--bs-alert-color: var(--bs-secondary-text-emphasis);--bs-alert-bg: var(--bs-secondary-bg-subtle);--bs-alert-border-color: var(--bs-secondary-border-subtle);--bs-alert-link-color: var(--bs-secondary-text-emphasis)}.alert-success{--bs-alert-color: var(--bs-success-text-emphasis);--bs-alert-bg: var(--bs-success-bg-subtle);--bs-alert-border-color: var(--bs-success-border-subtle);--bs-alert-link-color: var(--bs-success-text-emphasis)}.alert-info{--bs-alert-color: var(--bs-info-text-emphasis);--bs-alert-bg: var(--bs-info-bg-subtle);--bs-alert-border-color: var(--bs-info-border-subtle);--bs-alert-link-color: var(--bs-info-text-emphasis)}.alert-warning{--bs-alert-color: var(--bs-warning-text-emphasis);--bs-alert-bg: var(--bs-warning-bg-subtle);--bs-alert-border-color: var(--bs-warning-border-subtle);--bs-alert-link-color: var(--bs-warning-text-emphasis)}.alert-danger{--bs-alert-color: var(--bs-danger-text-emphasis);--bs-alert-bg: var(--bs-danger-bg-subtle);--bs-alert-border-color: var(--bs-danger-border-subtle);--bs-alert-link-color: var(--bs-danger-text-emphasis)}.alert-light{--bs-alert-color: var(--bs-light-text-emphasis);--bs-alert-bg: var(--bs-light-bg-subtle);--bs-alert-border-color: var(--bs-light-border-subtle);--bs-alert-link-color: var(--bs-light-text-emphasis)}.alert-dark{--bs-alert-color: var(--bs-dark-text-emphasis);--bs-alert-bg: var(--bs-dark-bg-subtle);--bs-alert-border-color: var(--bs-dark-border-subtle);--bs-alert-link-color: var(--bs-dark-text-emphasis)}@-webkit-keyframes progress-bar-stripes{0%{background-position-x:1rem}}@keyframes progress-bar-stripes{0%{background-position-x:1rem}}.progress,.progress-stacked{--bs-progress-height: 1rem;--bs-progress-font-size:0.75rem;--bs-progress-bg: var(--bs-secondary-bg);--bs-progress-border-radius: var(--bs-border-radius);--bs-progress-box-shadow: var(--bs-box-shadow-inset);--bs-progress-bar-color: #fff;--bs-progress-bar-bg: #1D809F;--bs-progress-bar-transition: width 0.6s ease;display:flex;height:var(--bs-progress-height);overflow:hidden;font-size:var(--bs-progress-font-size);background-color:var(--bs-progress-bg);border-radius:var(--bs-progress-border-radius)}.progress-bar{display:flex;flex-direction:column;justify-content:center;overflow:hidden;color:var(--bs-progress-bar-color);text-align:center;white-space:nowrap;background-color:var(--bs-progress-bar-bg);transition:var(--bs-progress-bar-transition)}@media(prefers-reduced-motion: reduce){.progress-bar{transition:none}}.progress-bar-striped{background-image:linear-gradient(45deg, rgba(255, 255, 255, 0.15) 25%, transparent 25%, transparent 50%, rgba(255, 255, 255, 0.15) 50%, rgba(255, 255, 255, 0.15) 75%, transparent 75%, transparent);background-size:var(--bs-progress-height) var(--bs-progress-height)}.progress-stacked>.progress{overflow:visible}.progress-stacked>.progress>.progress-bar{width:100%}.progress-bar-animated{-webkit-animation:1s linear infinite progress-bar-stripes;animation:1s linear infinite progress-bar-stripes}@media(prefers-reduced-motion: reduce){.progress-bar-animated{-webkit-animation:none;animation:none}}.list-group{--bs-list-group-color: var(--bs-body-color);--bs-list-group-bg: var(--bs-body-bg);--bs-list-group-border-color: var(--bs-border-color);--bs-list-group-border-width: var(--bs-border-width);--bs-list-group-border-radius: var(--bs-border-radius);--bs-list-group-item-padding-x: 1rem;--bs-list-group-item-padding-y: 0.5rem;--bs-list-group-action-color: var(--bs-secondary-color);--bs-list-group-action-hover-color: var(--bs-emphasis-color);--bs-list-group-action-hover-bg: var(--bs-tertiary-bg);--bs-list-group-action-active-color: var(--bs-body-color);--bs-list-group-action-active-bg: var(--bs-secondary-bg);--bs-list-group-disabled-color: var(--bs-secondary-color);--bs-list-group-disabled-bg: var(--bs-body-bg);--bs-list-group-active-color: #fff;--bs-list-group-active-bg: #1D809F;--bs-list-group-active-border-color: #1D809F;display:flex;flex-direction:column;padding-left:0;margin-bottom:0;border-radius:var(--bs-list-group-border-radius)}.list-group-numbered{list-style-type:none;counter-reset:section}.list-group-numbered>.list-group-item::before{content:counters(section, ".") ". ";counter-increment:section}.list-group-item-action{width:100%;color:var(--bs-list-group-action-color);text-align:inherit}.list-group-item-action:hover,.list-group-item-action:focus{z-index:1;color:var(--bs-list-group-action-hover-color);text-decoration:none;background-color:var(--bs-list-group-action-hover-bg)}.list-group-item-action:active{color:var(--bs-list-group-action-active-color);background-color:var(--bs-list-group-action-active-bg)}.list-group-item{position:relative;display:block;padding:var(--bs-list-group-item-padding-y) var(--bs-list-group-item-padding-x);color:var(--bs-list-group-color);text-decoration:none;background-color:var(--bs-list-group-bg);border:var(--bs-list-group-border-width) solid var(--bs-list-group-border-color)}.list-group-item:first-child{border-top-left-radius:inherit;border-top-right-radius:inherit}.list-group-item:last-child{border-bottom-right-radius:inherit;border-bottom-left-radius:inherit}.list-group-item.disabled,.list-group-item:disabled{color:var(--bs-list-group-disabled-color);pointer-events:none;background-color:var(--bs-list-group-disabled-bg)}.list-group-item.active{z-index:2;color:var(--bs-list-group-active-color);background-color:var(--bs-list-group-active-bg);border-color:var(--bs-list-group-active-border-color)}.list-group-item+.list-group-item{border-top-width:0}.list-group-item+.list-group-item.active{margin-top:calc(-1 * var(--bs-list-group-border-width));border-top-width:var(--bs-list-group-border-width)}.list-group-horizontal{flex-direction:row}.list-group-horizontal>.list-group-item:first-child:not(:last-child){border-bottom-left-radius:var(--bs-list-group-border-radius);border-top-right-radius:0}.list-group-horizontal>.list-group-item:last-child:not(:first-child){border-top-right-radius:var(--bs-list-group-border-radius);border-bottom-left-radius:0}.list-group-horizontal>.list-group-item.active{margin-top:0}.list-group-horizontal>.list-group-item+.list-group-item{border-top-width:var(--bs-list-group-border-width);border-left-width:0}.list-group-horizontal>.list-group-item+.list-group-item.active{margin-left:calc(-1 * var(--bs-list-group-border-width));border-left-width:var(--bs-list-group-border-width)}@media(min-width: 576px){.list-group-horizontal-sm{flex-direction:row}.list-group-horizontal-sm>.list-group-item:first-child:not(:last-child){border-bottom-left-radius:var(--bs-list-group-border-radius);border-top-right-radius:0}.list-group-horizontal-sm>.list-group-item:last-child:not(:first-child){border-top-right-radius:var(--bs-list-group-border-radius);border-bottom-left-radius:0}.list-group-horizontal-sm>.list-group-item.active{margin-top:0}.list-group-horizontal-sm>.list-group-item+.list-group-item{border-top-width:var(--bs-list-group-border-width);border-left-width:0}.list-group-horizontal-sm>.list-group-item+.list-group-item.active{margin-left:calc(-1 * var(--bs-list-group-border-width));border-left-width:var(--bs-list-group-border-width)}}@media(min-width: 768px){.list-group-horizontal-md{flex-direction:row}.list-group-horizontal-md>.list-group-item:first-child:not(:last-child){border-bottom-left-radius:var(--bs-list-group-border-radius);border-top-right-radius:0}.list-group-horizontal-md>.list-group-item:last-child:not(:first-child){border-top-right-radius:var(--bs-list-group-border-radius);border-bottom-left-radius:0}.list-group-horizontal-md>.list-group-item.active{margin-top:0}.list-group-horizontal-md>.list-group-item+.list-group-item{border-top-width:var(--bs-list-group-border-width);border-left-width:0}.list-group-horizontal-md>.list-group-item+.list-group-item.active{margin-left:calc(-1 * var(--bs-list-group-border-width));border-left-width:var(--bs-list-group-border-width)}}@media(min-width: 992px){.list-group-horizontal-lg{flex-direction:row}.list-group-horizontal-lg>.list-group-item:first-child:not(:last-child){border-bottom-left-radius:var(--bs-list-group-border-radius);border-top-right-radius:0}.list-group-horizontal-lg>.list-group-item:last-child:not(:first-child){border-top-right-radius:var(--bs-list-group-border-radius);border-bottom-left-radius:0}.list-group-horizontal-lg>.list-group-item.active{margin-top:0}.list-group-horizontal-lg>.list-group-item+.list-group-item{border-top-width:var(--bs-list-group-border-width);border-left-width:0}.list-group-horizontal-lg>.list-group-item+.list-group-item.active{margin-left:calc(-1 * var(--bs-list-group-border-width));border-left-width:var(--bs-list-group-border-width)}}@media(min-width: 1200px){.list-group-horizontal-xl{flex-direction:row}.list-group-horizontal-xl>.list-group-item:first-child:not(:last-child){border-bottom-left-radius:var(--bs-list-group-border-radius);border-top-right-radius:0}.list-group-horizontal-xl>.list-group-item:last-child:not(:first-child){border-top-right-radius:var(--bs-list-group-border-radius);border-bottom-left-radius:0}.list-group-horizontal-xl>.list-group-item.active{margin-top:0}.list-group-horizontal-xl>.list-group-item+.list-group-item{border-top-width:var(--bs-list-group-border-width);border-left-width:0}.list-group-horizontal-xl>.list-group-item+.list-group-item.active{margin-left:calc(-1 * var(--bs-list-group-border-width));border-left-width:var(--bs-list-group-border-width)}}@media(min-width: 1400px){.list-group-horizontal-xxl{flex-direction:row}.list-group-horizontal-xxl>.list-group-item:first-child:not(:last-child){border-bottom-left-radius:var(--bs-list-group-border-radius);border-top-right-radius:0}.list-group-horizontal-xxl>.list-group-item:last-child:not(:first-child){border-top-right-radius:var(--bs-list-group-border-radius);border-bottom-left-radius:0}.list-group-horizontal-xxl>.list-group-item.active{margin-top:0}.list-group-horizontal-xxl>.list-group-item+.list-group-item{border-top-width:var(--bs-list-group-border-width);border-left-width:0}.list-group-horizontal-xxl>.list-group-item+.list-group-item.active{margin-left:calc(-1 * var(--bs-list-group-border-width));border-left-width:var(--bs-list-group-border-width)}}.list-group-flush{border-radius:0}.list-group-flush>.list-group-item{border-width:0 0 var(--bs-list-group-border-width)}.list-group-flush>.list-group-item:last-child{border-bottom-width:0}.list-group-item-primary{--bs-list-group-color: var(--bs-primary-text-emphasis);--bs-list-group-bg: var(--bs-primary-bg-subtle);--bs-list-group-border-color: var(--bs-primary-border-subtle);--bs-list-group-action-hover-color: var(--bs-emphasis-color);--bs-list-group-action-hover-bg: var(--bs-primary-border-subtle);--bs-list-group-action-active-color: var(--bs-emphasis-color);--bs-list-group-action-active-bg: var(--bs-primary-border-subtle);--bs-list-group-active-color: var(--bs-primary-bg-subtle);--bs-list-group-active-bg: var(--bs-primary-text-emphasis);--bs-list-group-active-border-color: var(--bs-primary-text-emphasis)}.list-group-item-secondary{--bs-list-group-color: var(--bs-secondary-text-emphasis);--bs-list-group-bg: var(--bs-secondary-bg-subtle);--bs-list-group-border-color: var(--bs-secondary-border-subtle);--bs-list-group-action-hover-color: var(--bs-emphasis-color);--bs-list-group-action-hover-bg: var(--bs-secondary-border-subtle);--bs-list-group-action-active-color: var(--bs-emphasis-color);--bs-list-group-action-active-bg: var(--bs-secondary-border-subtle);--bs-list-group-active-color: var(--bs-secondary-bg-subtle);--bs-list-group-active-bg: var(--bs-secondary-text-emphasis);--bs-list-group-active-border-color: var(--bs-secondary-text-emphasis)}.list-group-item-success{--bs-list-group-color: var(--bs-success-text-emphasis);--bs-list-group-bg: var(--bs-success-bg-subtle);--bs-list-group-border-color: var(--bs-success-border-subtle);--bs-list-group-action-hover-color: var(--bs-emphasis-color);--bs-list-group-action-hover-bg: var(--bs-success-border-subtle);--bs-list-group-action-active-color: var(--bs-emphasis-color);--bs-list-group-action-active-bg: var(--bs-success-border-subtle);--bs-list-group-active-color: var(--bs-success-bg-subtle);--bs-list-group-active-bg: var(--bs-success-text-emphasis);--bs-list-group-active-border-color: var(--bs-success-text-emphasis)}.list-group-item-info{--bs-list-group-color: var(--bs-info-text-emphasis);--bs-list-group-bg: var(--bs-info-bg-subtle);--bs-list-group-border-color: var(--bs-info-border-subtle);--bs-list-group-action-hover-color: var(--bs-emphasis-color);--bs-list-group-action-hover-bg: var(--bs-info-border-subtle);--bs-list-group-action-active-color: var(--bs-emphasis-color);--bs-list-group-action-active-bg: var(--bs-info-border-subtle);--bs-list-group-active-color: var(--bs-info-bg-subtle);--bs-list-group-active-bg: var(--bs-info-text-emphasis);--bs-list-group-active-border-color: var(--bs-info-text-emphasis)}.list-group-item-warning{--bs-list-group-color: var(--bs-warning-text-emphasis);--bs-list-group-bg: var(--bs-warning-bg-subtle);--bs-list-group-border-color: var(--bs-warning-border-subtle);--bs-list-group-action-hover-color: var(--bs-emphasis-color);--bs-list-group-action-hover-bg: var(--bs-warning-border-subtle);--bs-list-group-action-active-color: var(--bs-emphasis-color);--bs-list-group-action-active-bg: var(--bs-warning-border-subtle);--bs-list-group-active-color: var(--bs-warning-bg-subtle);--bs-list-group-active-bg: var(--bs-warning-text-emphasis);--bs-list-group-active-border-color: var(--bs-warning-text-emphasis)}.list-group-item-danger{--bs-list-group-color: var(--bs-danger-text-emphasis);--bs-list-group-bg: var(--bs-danger-bg-subtle);--bs-list-group-border-color: var(--bs-danger-border-subtle);--bs-list-group-action-hover-color: var(--bs-emphasis-color);--bs-list-group-action-hover-bg: var(--bs-danger-border-subtle);--bs-list-group-action-active-color: var(--bs-emphasis-color);--bs-list-group-action-active-bg: var(--bs-danger-border-subtle);--bs-list-group-active-color: var(--bs-danger-bg-subtle);--bs-list-group-active-bg: var(--bs-danger-text-emphasis);--bs-list-group-active-border-color: var(--bs-danger-text-emphasis)}.list-group-item-light{--bs-list-group-color: var(--bs-light-text-emphasis);--bs-list-group-bg: var(--bs-light-bg-subtle);--bs-list-group-border-color: var(--bs-light-border-subtle);--bs-list-group-action-hover-color: var(--bs-emphasis-color);--bs-list-group-action-hover-bg: var(--bs-light-border-subtle);--bs-list-group-action-active-color: var(--bs-emphasis-color);--bs-list-group-action-active-bg: var(--bs-light-border-subtle);--bs-list-group-active-color: var(--bs-light-bg-subtle);--bs-list-group-active-bg: var(--bs-light-text-emphasis);--bs-list-group-active-border-color: var(--bs-light-text-emphasis)}.list-group-item-dark{--bs-list-group-color: var(--bs-dark-text-emphasis);--bs-list-group-bg: var(--bs-dark-bg-subtle);--bs-list-group-border-color: var(--bs-dark-border-subtle);--bs-list-group-action-hover-color: var(--bs-emphasis-color);--bs-list-group-action-hover-bg: var(--bs-dark-border-subtle);--bs-list-group-action-active-color: var(--bs-emphasis-color);--bs-list-group-action-active-bg: var(--bs-dark-border-subtle);--bs-list-group-active-color: var(--bs-dark-bg-subtle);--bs-list-group-active-bg: var(--bs-dark-text-emphasis);--bs-list-group-active-border-color: var(--bs-dark-text-emphasis)}.btn-close{--bs-btn-close-color: #000;--bs-btn-close-bg: url("data:image/svg+xml,%3csvg xmlns='http://www.w3.org/2000/svg' viewBox='0 0 16 16' fill='%23000'%3e%3cpath d='M.293.293a1 1 0 0 1 1.414 0L8 6.586 14.293.293a1 1 0 1 1 1.414 1.414L9.414 8l6.293 6.293a1 1 0 0 1-1.414 1.414L8 9.414l-6.293 6.293a1 1 0 0 1-1.414-1.414L6.586 8 .293 1.707a1 1 0 0 1 0-1.414z'/%3e%3c/svg%3e");--bs-btn-close-opacity: 0.5;--bs-btn-close-hover-opacity: 0.75;--bs-btn-close-focus-shadow: 0 0 0 0.25rem rgba(29, 128, 159, 0.25);--bs-btn-close-focus-opacity: 1;--bs-btn-close-disabled-opacity: 0.25;--bs-btn-close-white-filter: invert(1) grayscale(100%) brightness(200%);box-sizing:content-box;width:1em;height:1em;padding:.25em .25em;color:var(--bs-btn-close-color);background:transparent var(--bs-btn-close-bg) center/1em auto no-repeat;border:0;border-radius:.375rem;opacity:var(--bs-btn-close-opacity)}.btn-close:hover{color:var(--bs-btn-close-color);text-decoration:none;opacity:var(--bs-btn-close-hover-opacity)}.btn-close:focus{outline:0;box-shadow:var(--bs-btn-close-focus-shadow);opacity:var(--bs-btn-close-focus-opacity)}.btn-close:disabled,.btn-close.disabled{pointer-events:none;-webkit-user-select:none;-moz-user-select:none;-ms-user-select:none;user-select:none;opacity:var(--bs-btn-close-disabled-opacity)}.btn-close-white{filter:var(--bs-btn-close-white-filter)}[data-bs-theme=dark] .btn-close{filter:var(--bs-btn-close-white-filter)}.toast{--bs-toast-zindex: 1090;--bs-toast-padding-x: 0.75rem;--bs-toast-padding-y: 0.5rem;--bs-toast-spacing: 1.5rem;--bs-toast-max-width: 350px;--bs-toast-font-size:0.875rem;--bs-toast-color: ;--bs-toast-bg: rgba(var(--bs-body-bg-rgb), 0.85);--bs-toast-border-width: var(--bs-border-width);--bs-toast-border-color: var(--bs-border-color-translucent);--bs-toast-border-radius: var(--bs-border-radius);--bs-toast-box-shadow: var(--bs-box-shadow);--bs-toast-header-color: var(--bs-secondary-color);--bs-toast-header-bg: rgba(var(--bs-body-bg-rgb), 0.85);--bs-toast-header-border-color: var(--bs-border-color-translucent);width:var(--bs-toast-max-width);max-width:100%;font-size:var(--bs-toast-font-size);color:var(--bs-toast-color);pointer-events:auto;background-color:var(--bs-toast-bg);background-clip:padding-box;border:var(--bs-toast-border-width) solid var(--bs-toast-border-color);box-shadow:var(--bs-toast-box-shadow);border-radius:var(--bs-toast-border-radius)}.toast.showing{opacity:0}.toast:not(.show){display:none}.toast-container{--bs-toast-zindex: 1090;position:absolute;z-index:var(--bs-toast-zindex);width:-webkit-max-content;width:-moz-max-content;width:max-content;max-width:100%;pointer-events:none}.toast-container>:not(:last-child){margin-bottom:var(--bs-toast-spacing)}.toast-header{display:flex;align-items:center;padding:var(--bs-toast-padding-y) var(--bs-toast-padding-x);color:var(--bs-toast-header-color);background-color:var(--bs-toast-header-bg);background-clip:padding-box;border-bottom:var(--bs-toast-border-width) solid var(--bs-toast-header-border-color);border-top-left-radius:calc(var(--bs-toast-border-radius) - var(--bs-toast-border-width));border-top-right-radius:calc(var(--bs-toast-border-radius) - var(--bs-toast-border-width))}.toast-header .btn-close{margin-right:calc(-.5 * var(--bs-toast-padding-x));margin-left:var(--bs-toast-padding-x)}.toast-body{padding:var(--bs-toast-padding-x);word-wrap:break-word}.modal{--bs-modal-zindex: 1055;--bs-modal-width: 500px;--bs-modal-padding: 1rem;--bs-modal-margin: 0.5rem;--bs-modal-color: ;--bs-modal-bg: var(--bs-body-bg);--bs-modal-border-color: var(--bs-border-color-translucent);--bs-modal-border-width: var(--bs-border-width);--bs-modal-border-radius: var(--bs-border-radius-lg);--bs-modal-box-shadow: 0 0.125rem 0.25rem rgba(0, 0, 0, 0.075);--bs-modal-inner-border-radius: calc(var(--bs-border-radius-lg) - (var(--bs-border-width)));--bs-modal-header-padding-x: 1rem;--bs-modal-header-padding-y: 1rem;--bs-modal-header-padding: 1rem 1rem;--bs-modal-header-border-color: var(--bs-border-color);--bs-modal-header-border-width: var(--bs-border-width);--bs-modal-title-line-height: 1.5;--bs-modal-footer-gap: 0.5rem;--bs-modal-footer-bg: ;--bs-modal-footer-border-color: var(--bs-border-color);--bs-modal-footer-border-width: var(--bs-border-width);position:fixed;top:0;left:0;z-index:var(--bs-modal-zindex);display:none;width:100%;height:100%;overflow-x:hidden;overflow-y:auto;outline:0}.modal-dialog{position:relative;width:auto;margin:var(--bs-modal-margin);pointer-events:none}.modal.fade .modal-dialog{transition:transform .3s ease-out;transform:translate(0, -50px)}@media(prefers-reduced-motion: reduce){.modal.fade .modal-dialog{transition:none}}.modal.show .modal-dialog{transform:none}.modal.modal-static .modal-dialog{transform:scale(1.02)}.modal-dialog-scrollable{height:calc(100% - var(--bs-modal-margin) * 2)}.modal-dialog-scrollable .modal-content{max-height:100%;overflow:hidden}.modal-dialog-scrollable .modal-body{overflow-y:auto}.modal-dialog-centered{display:flex;align-items:center;min-height:calc(100% - var(--bs-modal-margin) * 2)}.modal-content{position:relative;display:flex;flex-direction:column;width:100%;color:var(--bs-modal-color);pointer-events:auto;background-color:var(--bs-modal-bg);background-clip:padding-box;border:var(--bs-modal-border-width) solid var(--bs-modal-border-color);border-radius:var(--bs-modal-border-radius);outline:0}.modal-backdrop{--bs-backdrop-zindex: 1050;--bs-backdrop-bg: #000;--bs-backdrop-opacity: 0.5;position:fixed;top:0;left:0;z-index:var(--bs-backdrop-zindex);width:100vw;height:100vh;background-color:var(--bs-backdrop-bg)}.modal-backdrop.fade{opacity:0}.modal-backdrop.show{opacity:var(--bs-backdrop-opacity)}.modal-header{display:flex;flex-shrink:0;align-items:center;justify-content:space-between;padding:var(--bs-modal-header-padding);border-bottom:var(--bs-modal-header-border-width) solid var(--bs-modal-header-border-color);border-top-left-radius:var(--bs-modal-inner-border-radius);border-top-right-radius:var(--bs-modal-inner-border-radius)}.modal-header .btn-close{padding:calc(var(--bs-modal-header-padding-y) * .5) calc(var(--bs-modal-header-padding-x) * .5);margin:calc(-.5 * var(--bs-modal-header-padding-y)) calc(-.5 * var(--bs-modal-header-padding-x)) calc(-.5 * var(--bs-modal-header-padding-y)) auto}.modal-title{margin-bottom:0;line-height:var(--bs-modal-title-line-height)}.modal-body{position:relative;flex:1 1 auto;padding:var(--bs-modal-padding)}.modal-footer{display:flex;flex-shrink:0;flex-wrap:wrap;align-items:center;justify-content:flex-end;padding:calc(var(--bs-modal-padding) - var(--bs-modal-footer-gap) * .5);background-color:var(--bs-modal-footer-bg);border-top:var(--bs-modal-footer-border-width) solid var(--bs-modal-footer-border-color);border-bottom-right-radius:var(--bs-modal-inner-border-radius);border-bottom-left-radius:var(--bs-modal-inner-border-radius)}.modal-footer>*{margin:calc(var(--bs-modal-footer-gap) * .5)}@media(min-width: 576px){.modal{--bs-modal-margin: 1.75rem;--bs-modal-box-shadow: 0 0.5rem 1rem rgba(0, 0, 0, 0.15)}.modal-dialog{max-width:var(--bs-modal-width);margin-right:auto;margin-left:auto}.modal-sm{--bs-modal-width: 300px}}@media(min-width: 992px){.modal-lg,.modal-xl{--bs-modal-width: 800px}}@media(min-width: 1200px){.modal-xl{--bs-modal-width: 1140px}}.modal-fullscreen{width:100vw;max-width:none;height:100%;margin:0}.modal-fullscreen .modal-content{height:100%;border:0;border-radius:0}.modal-fullscreen .modal-header,.modal-fullscreen .modal-footer{border-radius:0}.modal-fullscreen .modal-body{overflow-y:auto}@media(max-width: 575.98px){.modal-fullscreen-sm-down{width:100vw;max-width:none;height:100%;margin:0}.modal-fullscreen-sm-down .modal-content{height:100%;border:0;border-radius:0}.modal-fullscreen-sm-down .modal-header,.modal-fullscreen-sm-down .modal-footer{border-radius:0}.modal-fullscreen-sm-down .modal-body{overflow-y:auto}}@media(max-width: 767.98px){.modal-fullscreen-md-down{width:100vw;max-width:none;height:100%;margin:0}.modal-fullscreen-md-down .modal-content{height:100%;border:0;border-radius:0}.modal-fullscreen-md-down .modal-header,.modal-fullscreen-md-down .modal-footer{border-radius:0}.modal-fullscreen-md-down .modal-body{overflow-y:auto}}@media(max-width: 991.98px){.modal-fullscreen-lg-down{width:100vw;max-width:none;height:100%;margin:0}.modal-fullscreen-lg-down .modal-content{height:100%;border:0;border-radius:0}.modal-fullscreen-lg-down .modal-header,.modal-fullscreen-lg-down .modal-footer{border-radius:0}.modal-fullscreen-lg-down .modal-body{overflow-y:auto}}@media(max-width: 1199.98px){.modal-fullscreen-xl-down{width:100vw;max-width:none;height:100%;margin:0}.modal-fullscreen-xl-down .modal-content{height:100%;border:0;border-radius:0}.modal-fullscreen-xl-down .modal-header,.modal-fullscreen-xl-down .modal-footer{border-radius:0}.modal-fullscreen-xl-down .modal-body{overflow-y:auto}}@media(max-width: 1399.98px){.modal-fullscreen-xxl-down{width:100vw;max-width:none;height:100%;margin:0}.modal-fullscreen-xxl-down .modal-content{height:100%;border:0;border-radius:0}.modal-fullscreen-xxl-down .modal-header,.modal-fullscreen-xxl-down .modal-footer{border-radius:0}.modal-fullscreen-xxl-down .modal-body{overflow-y:auto}}.tooltip{--bs-tooltip-zindex: 1080;--bs-tooltip-max-width: 200px;--bs-tooltip-padding-x: 0.5rem;--bs-tooltip-padding-y: 0.25rem;--bs-tooltip-margin: ;--bs-tooltip-font-size:0.875rem;--bs-tooltip-color: var(--bs-body-bg);--bs-tooltip-bg: var(--bs-emphasis-color);--bs-tooltip-border-radius: var(--bs-border-radius);--bs-tooltip-opacity: 0.9;--bs-tooltip-arrow-width: 0.8rem;--bs-tooltip-arrow-height: 0.4rem;z-index:var(--bs-tooltip-zindex);display:block;margin:var(--bs-tooltip-margin);font-family:var(--bs-font-sans-serif);font-style:normal;font-weight:400;line-height:1.5;text-align:left;text-align:start;text-decoration:none;text-shadow:none;text-transform:none;letter-spacing:normal;word-break:normal;white-space:normal;word-spacing:normal;line-break:auto;font-size:var(--bs-tooltip-font-size);word-wrap:break-word;opacity:0}.tooltip.show{opacity:var(--bs-tooltip-opacity)}.tooltip .tooltip-arrow{display:block;width:var(--bs-tooltip-arrow-width);height:var(--bs-tooltip-arrow-height)}.tooltip .tooltip-arrow::before{position:absolute;content:"";border-color:transparent;border-style:solid}.bs-tooltip-top .tooltip-arrow,.bs-tooltip-auto[data-popper-placement^=top] .tooltip-arrow{bottom:calc(-1 * var(--bs-tooltip-arrow-height))}.bs-tooltip-top .tooltip-arrow::before,.bs-tooltip-auto[data-popper-placement^=top] .tooltip-arrow::before{top:-1px;border-width:var(--bs-tooltip-arrow-height) calc(var(--bs-tooltip-arrow-width) * .5) 0;border-top-color:var(--bs-tooltip-bg)}.bs-tooltip-end .tooltip-arrow,.bs-tooltip-auto[data-popper-placement^=right] .tooltip-arrow{left:calc(-1 * var(--bs-tooltip-arrow-height));width:var(--bs-tooltip-arrow-height);height:var(--bs-tooltip-arrow-width)}.bs-tooltip-end .tooltip-arrow::before,.bs-tooltip-auto[data-popper-placement^=right] .tooltip-arrow::before{right:-1px;border-width:calc(var(--bs-tooltip-arrow-width) * .5) var(--bs-tooltip-arrow-height) calc(var(--bs-tooltip-arrow-width) * .5) 0;border-right-color:var(--bs-tooltip-bg)}.bs-tooltip-bottom .tooltip-arrow,.bs-tooltip-auto[data-popper-placement^=bottom] .tooltip-arrow{top:calc(-1 * var(--bs-tooltip-arrow-height))}.bs-tooltip-bottom .tooltip-arrow::before,.bs-tooltip-auto[data-popper-placement^=bottom] .tooltip-arrow::before{bottom:-1px;border-width:0 calc(var(--bs-tooltip-arrow-width) * .5) var(--bs-tooltip-arrow-height);border-bottom-color:var(--bs-tooltip-bg)}.bs-tooltip-start .tooltip-arrow,.bs-tooltip-auto[data-popper-placement^=left] .tooltip-arrow{right:calc(-1 * var(--bs-tooltip-arrow-height));width:var(--bs-tooltip-arrow-height);height:var(--bs-tooltip-arrow-width)}.bs-tooltip-start .tooltip-arrow::before,.bs-tooltip-auto[data-popper-placement^=left] .tooltip-arrow::before{left:-1px;border-width:calc(var(--bs-tooltip-arrow-width) * .5) 0 calc(var(--bs-tooltip-arrow-width) * .5) var(--bs-tooltip-arrow-height);border-left-color:var(--bs-tooltip-bg)}.tooltip-inner{max-width:var(--bs-tooltip-max-width);padding:var(--bs-tooltip-padding-y) var(--bs-tooltip-padding-x);color:var(--bs-tooltip-color);text-align:center;background-color:var(--bs-tooltip-bg);border-radius:var(--bs-tooltip-border-radius)}.popover{--bs-popover-zindex: 1070;--bs-popover-max-width: 276px;--bs-popover-font-size:0.875rem;--bs-popover-bg: var(--bs-body-bg);--bs-popover-border-width: var(--bs-border-width);--bs-popover-border-color: var(--bs-border-color-translucent);--bs-popover-border-radius: var(--bs-border-radius-lg);--bs-popover-inner-border-radius: calc(var(--bs-border-radius-lg) - var(--bs-border-width));--bs-popover-box-shadow: 0 0.5rem 1rem rgba(0, 0, 0, 0.15);--bs-popover-header-padding-x: 1rem;--bs-popover-header-padding-y: 0.5rem;--bs-popover-header-font-size:1rem;--bs-popover-header-color: inherit;--bs-popover-header-bg: var(--bs-secondary-bg);--bs-popover-body-padding-x: 1rem;--bs-popover-body-padding-y: 1rem;--bs-popover-body-color: var(--bs-body-color);--bs-popover-arrow-width: 1rem;--bs-popover-arrow-height: 0.5rem;--bs-popover-arrow-border: var(--bs-popover-border-color);z-index:var(--bs-popover-zindex);display:block;max-width:var(--bs-popover-max-width);font-family:var(--bs-font-sans-serif);font-style:normal;font-weight:400;line-height:1.5;text-align:left;text-align:start;text-decoration:none;text-shadow:none;text-transform:none;letter-spacing:normal;word-break:normal;white-space:normal;word-spacing:normal;line-break:auto;font-size:var(--bs-popover-font-size);word-wrap:break-word;background-color:var(--bs-popover-bg);background-clip:padding-box;border:var(--bs-popover-border-width) solid var(--bs-popover-border-color);border-radius:var(--bs-popover-border-radius)}.popover .popover-arrow{display:block;width:var(--bs-popover-arrow-width);height:var(--bs-popover-arrow-height)}.popover .popover-arrow::before,.popover .popover-arrow::after{position:absolute;display:block;content:"";border-color:transparent;border-style:solid;border-width:0}.bs-popover-top>.popover-arrow,.bs-popover-auto[data-popper-placement^=top]>.popover-arrow{bottom:calc(-1 * (var(--bs-popover-arrow-height)) - var(--bs-popover-border-width))}.bs-popover-top>.popover-arrow::before,.bs-popover-auto[data-popper-placement^=top]>.popover-arrow::before,.bs-popover-top>.popover-arrow::after,.bs-popover-auto[data-popper-placement^=top]>.popover-arrow::after{border-width:var(--bs-popover-arrow-height) calc(var(--bs-popover-arrow-width) * .5) 0}.bs-popover-top>.popover-arrow::before,.bs-popover-auto[data-popper-placement^=top]>.popover-arrow::before{bottom:0;border-top-color:var(--bs-popover-arrow-border)}.bs-popover-top>.popover-arrow::after,.bs-popover-auto[data-popper-placement^=top]>.popover-arrow::after{bottom:var(--bs-popover-border-width);border-top-color:var(--bs-popover-bg)}.bs-popover-end>.popover-arrow,.bs-popover-auto[data-popper-placement^=right]>.popover-arrow{left:calc(-1 * (var(--bs-popover-arrow-height)) - var(--bs-popover-border-width));width:var(--bs-popover-arrow-height);height:var(--bs-popover-arrow-width)}.bs-popover-end>.popover-arrow::before,.bs-popover-auto[data-popper-placement^=right]>.popover-arrow::before,.bs-popover-end>.popover-arrow::after,.bs-popover-auto[data-popper-placement^=right]>.popover-arrow::after{border-width:calc(var(--bs-popover-arrow-width) * .5) var(--bs-popover-arrow-height) calc(var(--bs-popover-arrow-width) * .5) 0}.bs-popover-end>.popover-arrow::before,.bs-popover-auto[data-popper-placement^=right]>.popover-arrow::before{left:0;border-right-color:var(--bs-popover-arrow-border)}.bs-popover-end>.popover-arrow::after,.bs-popover-auto[data-popper-placement^=right]>.popover-arrow::after{left:var(--bs-popover-border-width);border-right-color:var(--bs-popover-bg)}.bs-popover-bottom>.popover-arrow,.bs-popover-auto[data-popper-placement^=bottom]>.popover-arrow{top:calc(-1 * (var(--bs-popover-arrow-height)) - var(--bs-popover-border-width))}.bs-popover-bottom>.popover-arrow::before,.bs-popover-auto[data-popper-placement^=bottom]>.popover-arrow::before,.bs-popover-bottom>.popover-arrow::after,.bs-popover-auto[data-popper-placement^=bottom]>.popover-arrow::after{border-width:0 calc(var(--bs-popover-arrow-width) * .5) var(--bs-popover-arrow-height)}.bs-popover-bottom>.popover-arrow::before,.bs-popover-auto[data-popper-placement^=bottom]>.popover-arrow::before{top:0;border-bottom-color:var(--bs-popover-arrow-border)}.bs-popover-bottom>.popover-arrow::after,.bs-popover-auto[data-popper-placement^=bottom]>.popover-arrow::after{top:var(--bs-popover-border-width);border-bottom-color:var(--bs-popover-bg)}.bs-popover-bottom .popover-header::before,.bs-popover-auto[data-popper-placement^=bottom] .popover-header::before{position:absolute;top:0;left:50%;display:block;width:var(--bs-popover-arrow-width);margin-left:calc(-.5 * var(--bs-popover-arrow-width));content:"";border-bottom:var(--bs-popover-border-width) solid var(--bs-popover-header-bg)}.bs-popover-start>.popover-arrow,.bs-popover-auto[data-popper-placement^=left]>.popover-arrow{right:calc(-1 * (var(--bs-popover-arrow-height)) - var(--bs-popover-border-width));width:var(--bs-popover-arrow-height);height:var(--bs-popover-arrow-width)}.bs-popover-start>.popover-arrow::before,.bs-popover-auto[data-popper-placement^=left]>.popover-arrow::before,.bs-popover-start>.popover-arrow::after,.bs-popover-auto[data-popper-placement^=left]>.popover-arrow::after{border-width:calc(var(--bs-popover-arrow-width) * .5) 0 calc(var(--bs-popover-arrow-width) * .5) var(--bs-popover-arrow-height)}.bs-popover-start>.popover-arrow::before,.bs-popover-auto[data-popper-placement^=left]>.popover-arrow::before{right:0;border-left-color:var(--bs-popover-arrow-border)}.bs-popover-start>.popover-arrow::after,.bs-popover-auto[data-popper-placement^=left]>.popover-arrow::after{right:var(--bs-popover-border-width);border-left-color:var(--bs-popover-bg)}.popover-header{padding:var(--bs-popover-header-padding-y) var(--bs-popover-header-padding-x);margin-bottom:0;font-size:var(--bs-popover-header-font-size);color:var(--bs-popover-header-color);background-color:var(--bs-popover-header-bg);border-bottom:var(--bs-popover-border-width) solid var(--bs-popover-border-color);border-top-left-radius:var(--bs-popover-inner-border-radius);border-top-right-radius:var(--bs-popover-inner-border-radius)}.popover-header:empty{display:none}.popover-body{padding:var(--bs-popover-body-padding-y) var(--bs-popover-body-padding-x);color:var(--bs-popover-body-color)}.carousel{position:relative}.carousel.pointer-event{touch-action:pan-y}.carousel-inner{position:relative;width:100%;overflow:hidden}.carousel-inner::after{display:block;clear:both;content:""}.carousel-item{position:relative;display:none;float:left;width:100%;margin-right:-100%;-webkit-backface-visibility:hidden;backface-visibility:hidden;transition:transform .6s ease-in-out}@media(prefers-reduced-motion: reduce){.carousel-item{transition:none}}.carousel-item.active,.carousel-item-next,.carousel-item-prev{display:block}.carousel-item-next:not(.carousel-item-start),.active.carousel-item-end{transform:translateX(100%)}.carousel-item-prev:not(.carousel-item-end),.active.carousel-item-start{transform:translateX(-100%)}.carousel-fade .carousel-item{opacity:0;transition-property:opacity;transform:none}.carousel-fade .carousel-item.active,.carousel-fade .carousel-item-next.carousel-item-start,.carousel-fade .carousel-item-prev.carousel-item-end{z-index:1;opacity:1}.carousel-fade .active.carousel-item-start,.carousel-fade .active.carousel-item-end{z-index:0;opacity:0;transition:opacity 0s .6s}@media(prefers-reduced-motion: reduce){.carousel-fade .active.carousel-item-start,.carousel-fade .active.carousel-item-end{transition:none}}.carousel-control-prev,.carousel-control-next{position:absolute;top:0;bottom:0;z-index:1;display:flex;align-items:center;justify-content:center;width:15%;padding:0;color:#fff;text-align:center;background:none;border:0;opacity:.5;transition:opacity .15s ease}@media(prefers-reduced-motion: reduce){.carousel-control-prev,.carousel-control-next{transition:none}}.carousel-control-prev:hover,.carousel-control-prev:focus,.carousel-control-next:hover,.carousel-control-next:focus{color:#fff;text-decoration:none;outline:0;opacity:.9}.carousel-control-prev{left:0}.carousel-control-next{right:0}.carousel-control-prev-icon,.carousel-control-next-icon{display:inline-block;width:2rem;height:2rem;background-repeat:no-repeat;background-position:50%;background-size:100% 100%}.carousel-control-prev-icon{background-image:url("data:image/svg+xml,%3csvg xmlns='http://www.w3.org/2000/svg' viewBox='0 0 16 16' fill='%23fff'%3e%3cpath d='M11.354 1.646a.5.5 0 0 1 0 .708L5.707 8l5.647 5.646a.5.5 0 0 1-.708.708l-6-6a.5.5 0 0 1 0-.708l6-6a.5.5 0 0 1 .708 0z'/%3e%3c/svg%3e")}.carousel-control-next-icon{background-image:url("data:image/svg+xml,%3csvg xmlns='http://www.w3.org/2000/svg' viewBox='0 0 16 16' fill='%23fff'%3e%3cpath d='M4.646 1.646a.5.5 0 0 1 .708 0l6 6a.5.5 0 0 1 0 .708l-6 6a.5.5 0 0 1-.708-.708L10.293 8 4.646 2.354a.5.5 0 0 1 0-.708z'/%3e%3c/svg%3e")}.carousel-indicators{position:absolute;right:0;bottom:0;left:0;z-index:2;display:flex;justify-content:center;padding:0;margin-right:15%;margin-bottom:1rem;margin-left:15%}.carousel-indicators [data-bs-target]{box-sizing:content-box;flex:0 1 auto;width:30px;height:3px;padding:0;margin-right:3px;margin-left:3px;text-indent:-999px;cursor:pointer;background-color:#fff;background-clip:padding-box;border:0;border-top:10px solid transparent;border-bottom:10px solid transparent;opacity:.5;transition:opacity .6s ease}@media(prefers-reduced-motion: reduce){.carousel-indicators [data-bs-target]{transition:none}}.carousel-indicators .active{opacity:1}.carousel-caption{position:absolute;right:15%;bottom:1.25rem;left:15%;padding-top:1.25rem;padding-bottom:1.25rem;color:#fff;text-align:center}.carousel-dark .carousel-control-prev-icon,.carousel-dark .carousel-control-next-icon{filter:invert(1) grayscale(100)}.carousel-dark .carousel-indicators [data-bs-target]{background-color:#000}.carousel-dark .carousel-caption{color:#000}[data-bs-theme=dark] .carousel .carousel-control-prev-icon,[data-bs-theme=dark] .carousel .carousel-control-next-icon,[data-bs-theme=dark].carousel .carousel-control-prev-icon,[data-bs-theme=dark].carousel .carousel-control-next-icon{filter:invert(1) grayscale(100)}[data-bs-theme=dark] .carousel .carousel-indicators [data-bs-target],[data-bs-theme=dark].carousel .carousel-indicators [data-bs-target]{background-color:#000}[data-bs-theme=dark] .carousel .carousel-caption,[data-bs-theme=dark].carousel .carousel-caption{color:#000}.spinner-grow,.spinner-border{display:inline-block;width:var(--bs-spinner-width);height:var(--bs-spinner-height);vertical-align:var(--bs-spinner-vertical-align);border-radius:50%;-webkit-animation:var(--bs-spinner-animation-speed) linear infinite var(--bs-spinner-animation-name);animation:var(--bs-spinner-animation-speed) linear infinite var(--bs-spinner-animation-name)}@-webkit-keyframes spinner-border{to{transform:rotate(360deg) /* rtl:ignore */}}@keyframes spinner-border{to{transform:rotate(360deg) /* rtl:ignore */}}.spinner-border{--bs-spinner-width: 2rem;--bs-spinner-height: 2rem;--bs-spinner-vertical-align: -0.125em;--bs-spinner-border-width: 0.25em;--bs-spinner-animation-speed: 0.75s;--bs-spinner-animation-name: spinner-border;border:var(--bs-spinner-border-width) solid currentcolor;border-right-color:transparent}.spinner-border-sm{--bs-spinner-width: 1rem;--bs-spinner-height: 1rem;--bs-spinner-border-width: 0.2em}@-webkit-keyframes spinner-grow{0%{transform:scale(0)}50%{opacity:1;transform:none}}@keyframes spinner-grow{0%{transform:scale(0)}50%{opacity:1;transform:none}}.spinner-grow{--bs-spinner-width: 2rem;--bs-spinner-height: 2rem;--bs-spinner-vertical-align: -0.125em;--bs-spinner-animation-speed: 0.75s;--bs-spinner-animation-name: spinner-grow;background-color:currentcolor;opacity:0}.spinner-grow-sm{--bs-spinner-width: 1rem;--bs-spinner-height: 1rem}@media(prefers-reduced-motion: reduce){.spinner-border,.spinner-grow{--bs-spinner-animation-speed: 1.5s}}.offcanvas,.offcanvas-xxl,.offcanvas-xl,.offcanvas-lg,.offcanvas-md,.offcanvas-sm{--bs-offcanvas-zindex: 1045;--bs-offcanvas-width: 400px;--bs-offcanvas-height: 30vh;--bs-offcanvas-padding-x: 1rem;--bs-offcanvas-padding-y: 1rem;--bs-offcanvas-color: var(--bs-body-color);--bs-offcanvas-bg: var(--bs-body-bg);--bs-offcanvas-border-width: var(--bs-border-width);--bs-offcanvas-border-color: var(--bs-border-color-translucent);--bs-offcanvas-box-shadow: 0 0.125rem 0.25rem rgba(0, 0, 0, 0.075);--bs-offcanvas-transition: transform 0.3s ease-in-out;--bs-offcanvas-title-line-height: 1.5}@media(max-width: 575.98px){.offcanvas-sm{position:fixed;bottom:0;z-index:var(--bs-offcanvas-zindex);display:flex;flex-direction:column;max-width:100%;color:var(--bs-offcanvas-color);visibility:hidden;background-color:var(--bs-offcanvas-bg);background-clip:padding-box;outline:0;transition:var(--bs-offcanvas-transition)}}@media(max-width: 575.98px)and (prefers-reduced-motion: reduce){.offcanvas-sm{transition:none}}@media(max-width: 575.98px){.offcanvas-sm.offcanvas-start{top:0;left:0;width:var(--bs-offcanvas-width);border-right:var(--bs-offcanvas-border-width) solid var(--bs-offcanvas-border-color);transform:translateX(-100%)}}@media(max-width: 575.98px){.offcanvas-sm.offcanvas-end{top:0;right:0;width:var(--bs-offcanvas-width);border-left:var(--bs-offcanvas-border-width) solid var(--bs-offcanvas-border-color);transform:translateX(100%)}}@media(max-width: 575.98px){.offcanvas-sm.offcanvas-top{top:0;right:0;left:0;height:var(--bs-offcanvas-height);max-height:100%;border-bottom:var(--bs-offcanvas-border-width) solid var(--bs-offcanvas-border-color);transform:translateY(-100%)}}@media(max-width: 575.98px){.offcanvas-sm.offcanvas-bottom{right:0;left:0;height:var(--bs-offcanvas-height);max-height:100%;border-top:var(--bs-offcanvas-border-width) solid var(--bs-offcanvas-border-color);transform:translateY(100%)}}@media(max-width: 575.98px){.offcanvas-sm.showing,.offcanvas-sm.show:not(.hiding){transform:none}}@media(max-width: 575.98px){.offcanvas-sm.showing,.offcanvas-sm.hiding,.offcanvas-sm.show{visibility:visible}}@media(min-width: 576px){.offcanvas-sm{--bs-offcanvas-height: auto;--bs-offcanvas-border-width: 0;background-color:transparent !important}.offcanvas-sm .offcanvas-header{display:none}.offcanvas-sm .offcanvas-body{display:flex;flex-grow:0;padding:0;overflow-y:visible;background-color:transparent !important}}@media(max-width: 767.98px){.offcanvas-md{position:fixed;bottom:0;z-index:var(--bs-offcanvas-zindex);display:flex;flex-direction:column;max-width:100%;color:var(--bs-offcanvas-color);visibility:hidden;background-color:var(--bs-offcanvas-bg);background-clip:padding-box;outline:0;transition:var(--bs-offcanvas-transition)}}@media(max-width: 767.98px)and (prefers-reduced-motion: reduce){.offcanvas-md{transition:none}}@media(max-width: 767.98px){.offcanvas-md.offcanvas-start{top:0;left:0;width:var(--bs-offcanvas-width);border-right:var(--bs-offcanvas-border-width) solid var(--bs-offcanvas-border-color);transform:translateX(-100%)}}@media(max-width: 767.98px){.offcanvas-md.offcanvas-end{top:0;right:0;width:var(--bs-offcanvas-width);border-left:var(--bs-offcanvas-border-width) solid var(--bs-offcanvas-border-color);transform:translateX(100%)}}@media(max-width: 767.98px){.offcanvas-md.offcanvas-top{top:0;right:0;left:0;height:var(--bs-offcanvas-height);max-height:100%;border-bottom:var(--bs-offcanvas-border-width) solid var(--bs-offcanvas-border-color);transform:translateY(-100%)}}@media(max-width: 767.98px){.offcanvas-md.offcanvas-bottom{right:0;left:0;height:var(--bs-offcanvas-height);max-height:100%;border-top:var(--bs-offcanvas-border-width) solid var(--bs-offcanvas-border-color);transform:translateY(100%)}}@media(max-width: 767.98px){.offcanvas-md.showing,.offcanvas-md.show:not(.hiding){transform:none}}@media(max-width: 767.98px){.offcanvas-md.showing,.offcanvas-md.hiding,.offcanvas-md.show{visibility:visible}}@media(min-width: 768px){.offcanvas-md{--bs-offcanvas-height: auto;--bs-offcanvas-border-width: 0;background-color:transparent !important}.offcanvas-md .offcanvas-header{display:none}.offcanvas-md .offcanvas-body{display:flex;flex-grow:0;padding:0;overflow-y:visible;background-color:transparent !important}}@media(max-width: 991.98px){.offcanvas-lg{position:fixed;bottom:0;z-index:var(--bs-offcanvas-zindex);display:flex;flex-direction:column;max-width:100%;color:var(--bs-offcanvas-color);visibility:hidden;background-color:var(--bs-offcanvas-bg);background-clip:padding-box;outline:0;transition:var(--bs-offcanvas-transition)}}@media(max-width: 991.98px)and (prefers-reduced-motion: reduce){.offcanvas-lg{transition:none}}@media(max-width: 991.98px){.offcanvas-lg.offcanvas-start{top:0;left:0;width:var(--bs-offcanvas-width);border-right:var(--bs-offcanvas-border-width) solid var(--bs-offcanvas-border-color);transform:translateX(-100%)}}@media(max-width: 991.98px){.offcanvas-lg.offcanvas-end{top:0;right:0;width:var(--bs-offcanvas-width);border-left:var(--bs-offcanvas-border-width) solid var(--bs-offcanvas-border-color);transform:translateX(100%)}}@media(max-width: 991.98px){.offcanvas-lg.offcanvas-top{top:0;right:0;left:0;height:var(--bs-offcanvas-height);max-height:100%;border-bottom:var(--bs-offcanvas-border-width) solid var(--bs-offcanvas-border-color);transform:translateY(-100%)}}@media(max-width: 991.98px){.offcanvas-lg.offcanvas-bottom{right:0;left:0;height:var(--bs-offcanvas-height);max-height:100%;border-top:var(--bs-offcanvas-border-width) solid var(--bs-offcanvas-border-color);transform:translateY(100%)}}@media(max-width: 991.98px){.offcanvas-lg.showing,.offcanvas-lg.show:not(.hiding){transform:none}}@media(max-width: 991.98px){.offcanvas-lg.showing,.offcanvas-lg.hiding,.offcanvas-lg.show{visibility:visible}}@media(min-width: 992px){.offcanvas-lg{--bs-offcanvas-height: auto;--bs-offcanvas-border-width: 0;background-color:transparent !important}.offcanvas-lg .offcanvas-header{display:none}.offcanvas-lg .offcanvas-body{display:flex;flex-grow:0;padding:0;overflow-y:visible;background-color:transparent !important}}@media(max-width: 1199.98px){.offcanvas-xl{position:fixed;bottom:0;z-index:var(--bs-offcanvas-zindex);display:flex;flex-direction:column;max-width:100%;color:var(--bs-offcanvas-color);visibility:hidden;background-color:var(--bs-offcanvas-bg);background-clip:padding-box;outline:0;transition:var(--bs-offcanvas-transition)}}@media(max-width: 1199.98px)and (prefers-reduced-motion: reduce){.offcanvas-xl{transition:none}}@media(max-width: 1199.98px){.offcanvas-xl.offcanvas-start{top:0;left:0;width:var(--bs-offcanvas-width);border-right:var(--bs-offcanvas-border-width) solid var(--bs-offcanvas-border-color);transform:translateX(-100%)}}@media(max-width: 1199.98px){.offcanvas-xl.offcanvas-end{top:0;right:0;width:var(--bs-offcanvas-width);border-left:var(--bs-offcanvas-border-width) solid var(--bs-offcanvas-border-color);transform:translateX(100%)}}@media(max-width: 1199.98px){.offcanvas-xl.offcanvas-top{top:0;right:0;left:0;height:var(--bs-offcanvas-height);max-height:100%;border-bottom:var(--bs-offcanvas-border-width) solid var(--bs-offcanvas-border-color);transform:translateY(-100%)}}@media(max-width: 1199.98px){.offcanvas-xl.offcanvas-bottom{right:0;left:0;height:var(--bs-offcanvas-height);max-height:100%;border-top:var(--bs-offcanvas-border-width) solid var(--bs-offcanvas-border-color);transform:translateY(100%)}}@media(max-width: 1199.98px){.offcanvas-xl.showing,.offcanvas-xl.show:not(.hiding){transform:none}}@media(max-width: 1199.98px){.offcanvas-xl.showing,.offcanvas-xl.hiding,.offcanvas-xl.show{visibility:visible}}@media(min-width: 1200px){.offcanvas-xl{--bs-offcanvas-height: auto;--bs-offcanvas-border-width: 0;background-color:transparent !important}.offcanvas-xl .offcanvas-header{display:none}.offcanvas-xl .offcanvas-body{display:flex;flex-grow:0;padding:0;overflow-y:visible;background-color:transparent !important}}@media(max-width: 1399.98px){.offcanvas-xxl{position:fixed;bottom:0;z-index:var(--bs-offcanvas-zindex);display:flex;flex-direction:column;max-width:100%;color:var(--bs-offcanvas-color);visibility:hidden;background-color:var(--bs-offcanvas-bg);background-clip:padding-box;outline:0;transition:var(--bs-offcanvas-transition)}}@media(max-width: 1399.98px)and (prefers-reduced-motion: reduce){.offcanvas-xxl{transition:none}}@media(max-width: 1399.98px){.offcanvas-xxl.offcanvas-start{top:0;left:0;width:var(--bs-offcanvas-width);border-right:var(--bs-offcanvas-border-width) solid var(--bs-offcanvas-border-color);transform:translateX(-100%)}}@media(max-width: 1399.98px){.offcanvas-xxl.offcanvas-end{top:0;right:0;width:var(--bs-offcanvas-width);border-left:var(--bs-offcanvas-border-width) solid var(--bs-offcanvas-border-color);transform:translateX(100%)}}@media(max-width: 1399.98px){.offcanvas-xxl.offcanvas-top{top:0;right:0;left:0;height:var(--bs-offcanvas-height);max-height:100%;border-bottom:var(--bs-offcanvas-border-width) solid var(--bs-offcanvas-border-color);transform:translateY(-100%)}}@media(max-width: 1399.98px){.offcanvas-xxl.offcanvas-bottom{right:0;left:0;height:var(--bs-offcanvas-height);max-height:100%;border-top:var(--bs-offcanvas-border-width) solid var(--bs-offcanvas-border-color);transform:translateY(100%)}}@media(max-width: 1399.98px){.offcanvas-xxl.showing,.offcanvas-xxl.show:not(.hiding){transform:none}}@media(max-width: 1399.98px){.offcanvas-xxl.showing,.offcanvas-xxl.hiding,.offcanvas-xxl.show{visibility:visible}}@media(min-width: 1400px){.offcanvas-xxl{--bs-offcanvas-height: auto;--bs-offcanvas-border-width: 0;background-color:transparent !important}.offcanvas-xxl .offcanvas-header{display:none}.offcanvas-xxl .offcanvas-body{display:flex;flex-grow:0;padding:0;overflow-y:visible;background-color:transparent !important}}.offcanvas{position:fixed;bottom:0;z-index:var(--bs-offcanvas-zindex);display:flex;flex-direction:column;max-width:100%;color:var(--bs-offcanvas-color);visibility:hidden;background-color:var(--bs-offcanvas-bg);background-clip:padding-box;outline:0;transition:var(--bs-offcanvas-transition)}@media(prefers-reduced-motion: reduce){.offcanvas{transition:none}}.offcanvas.offcanvas-start{top:0;left:0;width:var(--bs-offcanvas-width);border-right:var(--bs-offcanvas-border-width) solid var(--bs-offcanvas-border-color);transform:translateX(-100%)}.offcanvas.offcanvas-end{top:0;right:0;width:var(--bs-offcanvas-width);border-left:var(--bs-offcanvas-border-width) solid var(--bs-offcanvas-border-color);transform:translateX(100%)}.offcanvas.offcanvas-top{top:0;right:0;left:0;height:var(--bs-offcanvas-height);max-height:100%;border-bottom:var(--bs-offcanvas-border-width) solid var(--bs-offcanvas-border-color);transform:translateY(-100%)}.offcanvas.offcanvas-bottom{right:0;left:0;height:var(--bs-offcanvas-height);max-height:100%;border-top:var(--bs-offcanvas-border-width) solid var(--bs-offcanvas-border-color);transform:translateY(100%)}.offcanvas.showing,.offcanvas.show:not(.hiding){transform:none}.offcanvas.showing,.offcanvas.hiding,.offcanvas.show{visibility:visible}.offcanvas-backdrop{position:fixed;top:0;left:0;z-index:1040;width:100vw;height:100vh;background-color:#000}.offcanvas-backdrop.fade{opacity:0}.offcanvas-backdrop.show{opacity:.5}.offcanvas-header{display:flex;align-items:center;justify-content:space-between;padding:var(--bs-offcanvas-padding-y) var(--bs-offcanvas-padding-x)}.offcanvas-header .btn-close{padding:calc(var(--bs-offcanvas-padding-y) * .5) calc(var(--bs-offcanvas-padding-x) * .5);margin-top:calc(-.5 * var(--bs-offcanvas-padding-y));margin-right:calc(-.5 * var(--bs-offcanvas-padding-x));margin-bottom:calc(-.5 * var(--bs-offcanvas-padding-y))}.offcanvas-title{margin-bottom:0;line-height:var(--bs-offcanvas-title-line-height)}.offcanvas-body{flex-grow:1;padding:var(--bs-offcanvas-padding-y) var(--bs-offcanvas-padding-x);overflow-y:auto}.placeholder{display:inline-block;min-height:1em;vertical-align:middle;cursor:wait;background-color:currentcolor;opacity:.5}.placeholder.btn::before{display:inline-block;content:""}.placeholder-xs{min-height:.6em}.placeholder-sm{min-height:.8em}.placeholder-lg{min-height:1.2em}.placeholder-glow .placeholder{-webkit-animation:placeholder-glow 2s ease-in-out infinite;animation:placeholder-glow 2s ease-in-out infinite}@-webkit-keyframes placeholder-glow{50%{opacity:.2}}@keyframes placeholder-glow{50%{opacity:.2}}.placeholder-wave{-webkit-mask-image:linear-gradient(130deg, #000 55%, rgba(0, 0, 0, 0.8) 75%, #000 95%);mask-image:linear-gradient(130deg, #000 55%, rgba(0, 0, 0, 0.8) 75%, #000 95%);-webkit-mask-size:200% 100%;mask-size:200% 100%;-webkit-animation:placeholder-wave 2s linear infinite;animation:placeholder-wave 2s linear infinite}@-webkit-keyframes placeholder-wave{100%{-webkit-mask-position:-200% 0%;mask-position:-200% 0%}}@keyframes placeholder-wave{100%{-webkit-mask-position:-200% 0%;mask-position:-200% 0%}}.clearfix::after{display:block;clear:both;content:""}.text-bg-primary{color:#fff !important;background-color:RGBA(29, 128, 159, var(--bs-bg-opacity, 1)) !important}.text-bg-secondary{color:#000 !important;background-color:RGBA(236, 184, 7, var(--bs-bg-opacity, 1)) !important}.text-bg-success{color:#fff !important;background-color:RGBA(25, 135, 84, var(--bs-bg-opacity, 1)) !important}.text-bg-info{color:#000 !important;background-color:RGBA(13, 202, 240, var(--bs-bg-opacity, 1)) !important}.text-bg-warning{color:#000 !important;background-color:RGBA(255, 193, 7, var(--bs-bg-opacity, 1)) !important}.text-bg-danger{color:#fff !important;background-color:RGBA(220, 53, 69, var(--bs-bg-opacity, 1)) !important}.text-bg-light{color:#000 !important;background-color:RGBA(248, 249, 250, var(--bs-bg-opacity, 1)) !important}.text-bg-dark{color:#fff !important;background-color:RGBA(33, 37, 41, var(--bs-bg-opacity, 1)) !important}.link-primary{color:RGBA(var(--bs-primary-rgb), var(--bs-link-opacity, 1)) !important;-webkit-text-decoration-color:RGBA(var(--bs-primary-rgb), var(--bs-link-underline-opacity, 1)) !important;text-decoration-color:RGBA(var(--bs-primary-rgb), var(--bs-link-underline-opacity, 1)) !important}.link-primary:hover,.link-primary:focus{color:RGBA(23, 102, 127, var(--bs-link-opacity, 1)) !important;-webkit-text-decoration-color:RGBA(23, 102, 127, var(--bs-link-underline-opacity, 1)) !important;text-decoration-color:RGBA(23, 102, 127, var(--bs-link-underline-opacity, 1)) !important}.link-secondary{color:RGBA(var(--bs-secondary-rgb), var(--bs-link-opacity, 1)) !important;-webkit-text-decoration-color:RGBA(var(--bs-secondary-rgb), var(--bs-link-underline-opacity, 1)) !important;text-decoration-color:RGBA(var(--bs-secondary-rgb), var(--bs-link-underline-opacity, 1)) !important}.link-secondary:hover,.link-secondary:focus{color:RGBA(240, 198, 57, var(--bs-link-opacity, 1)) !important;-webkit-text-decoration-color:RGBA(240, 198, 57, var(--bs-link-underline-opacity, 1)) !important;text-decoration-color:RGBA(240, 198, 57, var(--bs-link-underline-opacity, 1)) !important}.link-success{color:RGBA(var(--bs-success-rgb), var(--bs-link-opacity, 1)) !important;-webkit-text-decoration-color:RGBA(var(--bs-success-rgb), var(--bs-link-underline-opacity, 1)) !important;text-decoration-color:RGBA(var(--bs-success-rgb), var(--bs-link-underline-opacity, 1)) !important}.link-success:hover,.link-success:focus{color:RGBA(20, 108, 67, var(--bs-link-opacity, 1)) !important;-webkit-text-decoration-color:RGBA(20, 108, 67, var(--bs-link-underline-opacity, 1)) !important;text-decoration-color:RGBA(20, 108, 67, var(--bs-link-underline-opacity, 1)) !important}.link-info{color:RGBA(var(--bs-info-rgb), var(--bs-link-opacity, 1)) !important;-webkit-text-decoration-color:RGBA(var(--bs-info-rgb), var(--bs-link-underline-opacity, 1)) !important;text-decoration-color:RGBA(var(--bs-info-rgb), var(--bs-link-underline-opacity, 1)) !important}.link-info:hover,.link-info:focus{color:RGBA(61, 213, 243, var(--bs-link-opacity, 1)) !important;-webkit-text-decoration-color:RGBA(61, 213, 243, var(--bs-link-underline-opacity, 1)) !important;text-decoration-color:RGBA(61, 213, 243, var(--bs-link-underline-opacity, 1)) !important}.link-warning{color:RGBA(var(--bs-warning-rgb), var(--bs-link-opacity, 1)) !important;-webkit-text-decoration-color:RGBA(var(--bs-warning-rgb), var(--bs-link-underline-opacity, 1)) !important;text-decoration-color:RGBA(var(--bs-warning-rgb), var(--bs-link-underline-opacity, 1)) !important}.link-warning:hover,.link-warning:focus{color:RGBA(255, 205, 57, var(--bs-link-opacity, 1)) !important;-webkit-text-decoration-color:RGBA(255, 205, 57, var(--bs-link-underline-opacity, 1)) !important;text-decoration-color:RGBA(255, 205, 57, var(--bs-link-underline-opacity, 1)) !important}.link-danger{color:RGBA(var(--bs-danger-rgb), var(--bs-link-opacity, 1)) !important;-webkit-text-decoration-color:RGBA(var(--bs-danger-rgb), var(--bs-link-underline-opacity, 1)) !important;text-decoration-color:RGBA(var(--bs-danger-rgb), var(--bs-link-underline-opacity, 1)) !important}.link-danger:hover,.link-danger:focus{color:RGBA(176, 42, 55, var(--bs-link-opacity, 1)) !important;-webkit-text-decoration-color:RGBA(176, 42, 55, var(--bs-link-underline-opacity, 1)) !important;text-decoration-color:RGBA(176, 42, 55, var(--bs-link-underline-opacity, 1)) !important}.link-light{color:RGBA(var(--bs-light-rgb), var(--bs-link-opacity, 1)) !important;-webkit-text-decoration-color:RGBA(var(--bs-light-rgb), var(--bs-link-underline-opacity, 1)) !important;text-decoration-color:RGBA(var(--bs-light-rgb), var(--bs-link-underline-opacity, 1)) !important}.link-light:hover,.link-light:focus{color:RGBA(249, 250, 251, var(--bs-link-opacity, 1)) !important;-webkit-text-decoration-color:RGBA(249, 250, 251, var(--bs-link-underline-opacity, 1)) !important;text-decoration-color:RGBA(249, 250, 251, var(--bs-link-underline-opacity, 1)) !important}.link-dark{color:RGBA(var(--bs-dark-rgb), var(--bs-link-opacity, 1)) !important;-webkit-text-decoration-color:RGBA(var(--bs-dark-rgb), var(--bs-link-underline-opacity, 1)) !important;text-decoration-color:RGBA(var(--bs-dark-rgb), var(--bs-link-underline-opacity, 1)) !important}.link-dark:hover,.link-dark:focus{color:RGBA(26, 30, 33, var(--bs-link-opacity, 1)) !important;-webkit-text-decoration-color:RGBA(26, 30, 33, var(--bs-link-underline-opacity, 1)) !important;text-decoration-color:RGBA(26, 30, 33, var(--bs-link-underline-opacity, 1)) !important}.link-body-emphasis{color:RGBA(var(--bs-emphasis-color-rgb), var(--bs-link-opacity, 1)) !important;-webkit-text-decoration-color:RGBA(var(--bs-emphasis-color-rgb), var(--bs-link-underline-opacity, 1)) !important;text-decoration-color:RGBA(var(--bs-emphasis-color-rgb), var(--bs-link-underline-opacity, 1)) !important}.link-body-emphasis:hover,.link-body-emphasis:focus{color:RGBA(var(--bs-emphasis-color-rgb), var(--bs-link-opacity, 0.75)) !important;-webkit-text-decoration-color:RGBA(var(--bs-emphasis-color-rgb), var(--bs-link-underline-opacity, 0.75)) !important;text-decoration-color:RGBA(var(--bs-emphasis-color-rgb), var(--bs-link-underline-opacity, 0.75)) !important}.focus-ring:focus{outline:0;box-shadow:var(--bs-focus-ring-x, 0) var(--bs-focus-ring-y, 0) var(--bs-focus-ring-blur, 0) var(--bs-focus-ring-width) var(--bs-focus-ring-color)}.icon-link{display:inline-flex;gap:.375rem;align-items:center;-webkit-text-decoration-color:rgba(var(--bs-link-color-rgb), var(--bs-link-opacity, 0.5));text-decoration-color:rgba(var(--bs-link-color-rgb), var(--bs-link-opacity, 0.5));text-underline-offset:.25em;-webkit-backface-visibility:hidden;backface-visibility:hidden}.icon-link>.bi{flex-shrink:0;width:1em;height:1em;fill:currentcolor;transition:.2s ease-in-out transform}@media(prefers-reduced-motion: reduce){.icon-link>.bi{transition:none}}.icon-link-hover:hover>.bi,.icon-link-hover:focus-visible>.bi{transform:var(--bs-icon-link-transform, translate3d(0.25em, 0, 0))}.ratio{position:relative;width:100%}.ratio::before{display:block;padding-top:var(--bs-aspect-ratio);content:""}.ratio>*{position:absolute;top:0;left:0;width:100%;height:100%}.ratio-1x1{--bs-aspect-ratio: 100%}.ratio-4x3{--bs-aspect-ratio: calc(3 / 4 * 100%)}.ratio-16x9{--bs-aspect-ratio: calc(9 / 16 * 100%)}.ratio-21x9{--bs-aspect-ratio: calc(9 / 21 * 100%)}.fixed-top{position:fixed;top:0;right:0;left:0;z-index:1030}.fixed-bottom{position:fixed;right:0;bottom:0;left:0;z-index:1030}.sticky-top{position:sticky;top:0;z-index:1020}.sticky-bottom{position:sticky;bottom:0;z-index:1020}@media(min-width: 576px){.sticky-sm-top{position:sticky;top:0;z-index:1020}.sticky-sm-bottom{position:sticky;bottom:0;z-index:1020}}@media(min-width: 768px){.sticky-md-top{position:sticky;top:0;z-index:1020}.sticky-md-bottom{position:sticky;bottom:0;z-index:1020}}@media(min-width: 992px){.sticky-lg-top{position:sticky;top:0;z-index:1020}.sticky-lg-bottom{position:sticky;bottom:0;z-index:1020}}@media(min-width: 1200px){.sticky-xl-top{position:sticky;top:0;z-index:1020}.sticky-xl-bottom{position:sticky;bottom:0;z-index:1020}}@media(min-width: 1400px){.sticky-xxl-top{position:sticky;top:0;z-index:1020}.sticky-xxl-bottom{position:sticky;bottom:0;z-index:1020}}.hstack{display:flex;flex-direction:row;align-items:center;align-self:stretch}.vstack{display:flex;flex:1 1 auto;flex-direction:column;align-self:stretch}.visually-hidden,.visually-hidden-focusable:not(:focus):not(:focus-within){width:1px !important;height:1px !important;padding:0 !important;margin:-1px !important;overflow:hidden !important;clip:rect(0, 0, 0, 0) !important;white-space:nowrap !important;border:0 !important}.visually-hidden:not(caption),.visually-hidden-focusable:not(:focus):not(:focus-within):not(caption){position:absolute !important}.stretched-link::after{position:absolute;top:0;right:0;bottom:0;left:0;z-index:1;content:""}.text-truncate{overflow:hidden;text-overflow:ellipsis;white-space:nowrap}.vr{display:inline-block;align-self:stretch;width:1px;min-height:1em;background-color:currentcolor;opacity:.25}.align-baseline{vertical-align:baseline !important}.align-top{vertical-align:top !important}.align-middle{vertical-align:middle !important}.align-bottom{vertical-align:bottom !important}.align-text-bottom{vertical-align:text-bottom !important}.align-text-top{vertical-align:text-top !important}.float-start{float:left !important}.float-end{float:right !important}.float-none{float:none !important}.object-fit-contain{-o-object-fit:contain !important;object-fit:contain !important}.object-fit-cover{-o-object-fit:cover !important;object-fit:cover !important}.object-fit-fill{-o-object-fit:fill !important;object-fit:fill !important}.object-fit-scale{-o-object-fit:scale-down !important;object-fit:scale-down !important}.object-fit-none{-o-object-fit:none !important;object-fit:none !important}.opacity-0{opacity:0 !important}.opacity-25{opacity:.25 !important}.opacity-50{opacity:.5 !important}.opacity-75{opacity:.75 !important}.opacity-100{opacity:1 !important}.overflow-auto{overflow:auto !important}.overflow-hidden{overflow:hidden !important}.overflow-visible{overflow:visible !important}.overflow-scroll{overflow:scroll !important}.overflow-x-auto{overflow-x:auto !important}.overflow-x-hidden{overflow-x:hidden !important}.overflow-x-visible{overflow-x:visible !important}.overflow-x-scroll{overflow-x:scroll !important}.overflow-y-auto{overflow-y:auto !important}.overflow-y-hidden{overflow-y:hidden !important}.overflow-y-visible{overflow-y:visible !important}.overflow-y-scroll{overflow-y:scroll !important}.d-inline{display:inline !important}.d-inline-block{display:inline-block !important}.d-block{display:block !important}.d-grid{display:grid !important}.d-inline-grid{display:inline-grid !important}.d-table{display:table !important}.d-table-row{display:table-row !important}.d-table-cell{display:table-cell !important}.d-flex{display:flex !important}.d-inline-flex{display:inline-flex !important}.d-none{display:none !important}.shadow{box-shadow:0 .5rem 1rem rgba(0,0,0,.15) !important}.shadow-sm{box-shadow:0 .125rem .25rem rgba(0,0,0,.075) !important}.shadow-lg{box-shadow:0 1rem 3rem rgba(0,0,0,.175) !important}.shadow-none{box-shadow:none !important}.focus-ring-primary{--bs-focus-ring-color: rgba(var(--bs-primary-rgb), var(--bs-focus-ring-opacity))}.focus-ring-secondary{--bs-focus-ring-color: rgba(var(--bs-secondary-rgb), var(--bs-focus-ring-opacity))}.focus-ring-success{--bs-focus-ring-color: rgba(var(--bs-success-rgb), var(--bs-focus-ring-opacity))}.focus-ring-info{--bs-focus-ring-color: rgba(var(--bs-info-rgb), var(--bs-focus-ring-opacity))}.focus-ring-warning{--bs-focus-ring-color: rgba(var(--bs-warning-rgb), var(--bs-focus-ring-opacity))}.focus-ring-danger{--bs-focus-ring-color: rgba(var(--bs-danger-rgb), var(--bs-focus-ring-opacity))}.focus-ring-light{--bs-focus-ring-color: rgba(var(--bs-light-rgb), var(--bs-focus-ring-opacity))}.focus-ring-dark{--bs-focus-ring-color: rgba(var(--bs-dark-rgb), var(--bs-focus-ring-opacity))}.position-static{position:static !important}.position-relative{position:relative !important}.position-absolute{position:absolute !important}.position-fixed{position:fixed !important}.position-sticky{position:sticky !important}.top-0{top:0 !important}.top-50{top:50% !important}.top-100{top:100% !important}.bottom-0{bottom:0 !important}.bottom-50{bottom:50% !important}.bottom-100{bottom:100% !important}.start-0{left:0 !important}.start-50{left:50% !important}.start-100{left:100% !important}.end-0{right:0 !important}.end-50{right:50% !important}.end-100{right:100% !important}.translate-middle{transform:translate(-50%, -50%) !important}.translate-middle-x{transform:translateX(-50%) !important}.translate-middle-y{transform:translateY(-50%) !important}.border{border:var(--bs-border-width) var(--bs-border-style) var(--bs-border-color) !important}.border-0{border:0 !important}.border-top{border-top:var(--bs-border-width) var(--bs-border-style) var(--bs-border-color) !important}.border-top-0{border-top:0 !important}.border-end{border-right:var(--bs-border-width) var(--bs-border-style) var(--bs-border-color) !important}.border-end-0{border-right:0 !important}.border-bottom{border-bottom:var(--bs-border-width) var(--bs-border-style) var(--bs-border-color) !important}.border-bottom-0{border-bottom:0 !important}.border-start{border-left:var(--bs-border-width) var(--bs-border-style) var(--bs-border-color) !important}.border-start-0{border-left:0 !important}.border-primary{--bs-border-opacity: 1;border-color:rgba(var(--bs-primary-rgb), var(--bs-border-opacity)) !important}.border-secondary{--bs-border-opacity: 1;border-color:rgba(var(--bs-secondary-rgb), var(--bs-border-opacity)) !important}.border-success{--bs-border-opacity: 1;border-color:rgba(var(--bs-success-rgb), var(--bs-border-opacity)) !important}.border-info{--bs-border-opacity: 1;border-color:rgba(var(--bs-info-rgb), var(--bs-border-opacity)) !important}.border-warning{--bs-border-opacity: 1;border-color:rgba(var(--bs-warning-rgb), var(--bs-border-opacity)) !important}.border-danger{--bs-border-opacity: 1;border-color:rgba(var(--bs-danger-rgb), var(--bs-border-opacity)) !important}.border-light{--bs-border-opacity: 1;border-color:rgba(var(--bs-light-rgb), var(--bs-border-opacity)) !important}.border-dark{--bs-border-opacity: 1;border-color:rgba(var(--bs-dark-rgb), var(--bs-border-opacity)) !important}.border-black{--bs-border-opacity: 1;border-color:rgba(var(--bs-black-rgb), var(--bs-border-opacity)) !important}.border-white{--bs-border-opacity: 1;border-color:rgba(var(--bs-white-rgb), var(--bs-border-opacity)) !important}.border-primary-subtle{border-color:var(--bs-primary-border-subtle) !important}.border-secondary-subtle{border-color:var(--bs-secondary-border-subtle) !important}.border-success-subtle{border-color:var(--bs-success-border-subtle) !important}.border-info-subtle{border-color:var(--bs-info-border-subtle) !important}.border-warning-subtle{border-color:var(--bs-warning-border-subtle) !important}.border-danger-subtle{border-color:var(--bs-danger-border-subtle) !important}.border-light-subtle{border-color:var(--bs-light-border-subtle) !important}.border-dark-subtle{border-color:var(--bs-dark-border-subtle) !important}.border-1{border-width:1px !important}.border-2{border-width:2px !important}.border-3{border-width:3px !important}.border-4{border-width:4px !important}.border-5{border-width:5px !important}.border-opacity-10{--bs-border-opacity: 0.1}.border-opacity-25{--bs-border-opacity: 0.25}.border-opacity-50{--bs-border-opacity: 0.5}.border-opacity-75{--bs-border-opacity: 0.75}.border-opacity-100{--bs-border-opacity: 1}.w-25{width:25% !important}.w-50{width:50% !important}.w-75{width:75% !important}.w-100{width:100% !important}.w-auto{width:auto !important}.mw-100{max-width:100% !important}.vw-100{width:100vw !important}.min-vw-100{min-width:100vw !important}.h-25{height:25% !important}.h-50{height:50% !important}.h-75{height:75% !important}.h-100{height:100% !important}.h-auto{height:auto !important}.mh-100{max-height:100% !important}.vh-100{height:100vh !important}.min-vh-100{min-height:100vh !important}.flex-fill{flex:1 1 auto !important}.flex-row{flex-direction:row !important}.flex-column{flex-direction:column !important}.flex-row-reverse{flex-direction:row-reverse !important}.flex-column-reverse{flex-direction:column-reverse !important}.flex-grow-0{flex-grow:0 !important}.flex-grow-1{flex-grow:1 !important}.flex-shrink-0{flex-shrink:0 !important}.flex-shrink-1{flex-shrink:1 !important}.flex-wrap{flex-wrap:wrap !important}.flex-nowrap{flex-wrap:nowrap !important}.flex-wrap-reverse{flex-wrap:wrap-reverse !important}.justify-content-start{justify-content:flex-start !important}.justify-content-end{justify-content:flex-end !important}.justify-content-center{justify-content:center !important}.justify-content-between{justify-content:space-between !important}.justify-content-around{justify-content:space-around !important}.justify-content-evenly{justify-content:space-evenly !important}.align-items-start{align-items:flex-start !important}.align-items-end{align-items:flex-end !important}.align-items-center{align-items:center !important}.align-items-baseline{align-items:baseline !important}.align-items-stretch{align-items:stretch !important}.align-content-start{align-content:flex-start !important}.align-content-end{align-content:flex-end !important}.align-content-center{align-content:center !important}.align-content-between{align-content:space-between !important}.align-content-around{align-content:space-around !important}.align-content-stretch{align-content:stretch !important}.align-self-auto{align-self:auto !important}.align-self-start{align-self:flex-start !important}.align-self-end{align-self:flex-end !important}.align-self-center{align-self:center !important}.align-self-baseline{align-self:baseline !important}.align-self-stretch{align-self:stretch !important}.order-first{order:-1 !important}.order-0{order:0 !important}.order-1{order:1 !important}.order-2{order:2 !important}.order-3{order:3 !important}.order-4{order:4 !important}.order-5{order:5 !important}.order-last{order:6 !important}.m-0{margin:0 !important}.m-1{margin:.25rem !important}.m-2{margin:.5rem !important}.m-3{margin:1rem !important}.m-4{margin:1.5rem !important}.m-5{margin:3rem !important}.m-auto{margin:auto !important}.mx-0{margin-right:0 !important;margin-left:0 !important}.mx-1{margin-right:.25rem !important;margin-left:.25rem !important}.mx-2{margin-right:.5rem !important;margin-left:.5rem !important}.mx-3{margin-right:1rem !important;margin-left:1rem !important}.mx-4{margin-right:1.5rem !important;margin-left:1.5rem !important}.mx-5{margin-right:3rem !important;margin-left:3rem !important}.mx-auto{margin-right:auto !important;margin-left:auto !important}.my-0{margin-top:0 !important;margin-bottom:0 !important}.my-1{margin-top:.25rem !important;margin-bottom:.25rem !important}.my-2{margin-top:.5rem !important;margin-bottom:.5rem !important}.my-3{margin-top:1rem !important;margin-bottom:1rem !important}.my-4{margin-top:1.5rem !important;margin-bottom:1.5rem !important}.my-5{margin-top:3rem !important;margin-bottom:3rem !important}.my-auto{margin-top:auto !important;margin-bottom:auto !important}.mt-0{margin-top:0 !important}.mt-1{margin-top:.25rem !important}.mt-2{margin-top:.5rem !important}.mt-3{margin-top:1rem !important}.mt-4{margin-top:1.5rem !important}.mt-5{margin-top:3rem !important}.mt-auto{margin-top:auto !important}.me-0{margin-right:0 !important}.me-1{margin-right:.25rem !important}.me-2{margin-right:.5rem !important}.me-3{margin-right:1rem !important}.me-4{margin-right:1.5rem !important}.me-5{margin-right:3rem !important}.me-auto{margin-right:auto !important}.mb-0{margin-bottom:0 !important}.mb-1{margin-bottom:.25rem !important}.mb-2{margin-bottom:.5rem !important}.mb-3{margin-bottom:1rem !important}.mb-4{margin-bottom:1.5rem !important}.mb-5{margin-bottom:3rem !important}.mb-auto{margin-bottom:auto !important}.ms-0{margin-left:0 !important}.ms-1{margin-left:.25rem !important}.ms-2{margin-left:.5rem !important}.ms-3{margin-left:1rem !important}.ms-4{margin-left:1.5rem !important}.ms-5{margin-left:3rem !important}.ms-auto{margin-left:auto !important}.p-0{padding:0 !important}.p-1{padding:.25rem !important}.p-2{padding:.5rem !important}.p-3{padding:1rem !important}.p-4{padding:1.5rem !important}.p-5{padding:3rem !important}.px-0{padding-right:0 !important;padding-left:0 !important}.px-1{padding-right:.25rem !important;padding-left:.25rem !important}.px-2{padding-right:.5rem !important;padding-left:.5rem !important}.px-3{padding-right:1rem !important;padding-left:1rem !important}.px-4{padding-right:1.5rem !important;padding-left:1.5rem !important}.px-5{padding-right:3rem !important;padding-left:3rem !important}.py-0{padding-top:0 !important;padding-bottom:0 !important}.py-1{padding-top:.25rem !important;padding-bottom:.25rem !important}.py-2{padding-top:.5rem !important;padding-bottom:.5rem !important}.py-3{padding-top:1rem !important;padding-bottom:1rem !important}.py-4{padding-top:1.5rem !important;padding-bottom:1.5rem !important}.py-5{padding-top:3rem !important;padding-bottom:3rem !important}.pt-0{padding-top:0 !important}.pt-1{padding-top:.25rem !important}.pt-2{padding-top:.5rem !important}.pt-3{padding-top:1rem !important}.pt-4{padding-top:1.5rem !important}.pt-5{padding-top:3rem !important}.pe-0{padding-right:0 !important}.pe-1{padding-right:.25rem !important}.pe-2{padding-right:.5rem !important}.pe-3{padding-right:1rem !important}.pe-4{padding-right:1.5rem !important}.pe-5{padding-right:3rem !important}.pb-0{padding-bottom:0 !important}.pb-1{padding-bottom:.25rem !important}.pb-2{padding-bottom:.5rem !important}.pb-3{padding-bottom:1rem !important}.pb-4{padding-bottom:1.5rem !important}.pb-5{padding-bottom:3rem !important}.ps-0{padding-left:0 !important}.ps-1{padding-left:.25rem !important}.ps-2{padding-left:.5rem !important}.ps-3{padding-left:1rem !important}.ps-4{padding-left:1.5rem !important}.ps-5{padding-left:3rem !important}.gap-0{gap:0 !important}.gap-1{gap:.25rem !important}.gap-2{gap:.5rem !important}.gap-3{gap:1rem !important}.gap-4{gap:1.5rem !important}.gap-5{gap:3rem !important}.row-gap-0{row-gap:0 !important}.row-gap-1{row-gap:.25rem !important}.row-gap-2{row-gap:.5rem !important}.row-gap-3{row-gap:1rem !important}.row-gap-4{row-gap:1.5rem !important}.row-gap-5{row-gap:3rem !important}.column-gap-0{-moz-column-gap:0 !important;column-gap:0 !important}.column-gap-1{-moz-column-gap:.25rem !important;column-gap:.25rem !important}.column-gap-2{-moz-column-gap:.5rem !important;column-gap:.5rem !important}.column-gap-3{-moz-column-gap:1rem !important;column-gap:1rem !important}.column-gap-4{-moz-column-gap:1.5rem !important;column-gap:1.5rem !important}.column-gap-5{-moz-column-gap:3rem !important;column-gap:3rem !important}.font-monospace{font-family:var(--bs-font-monospace) !important}.fs-1{font-size:calc(1.375rem + 1.5vw) !important}.fs-2{font-size:calc(1.325rem + 0.9vw) !important}.fs-3{font-size:calc(1.3rem + 0.6vw) !important}.fs-4{font-size:calc(1.275rem + 0.3vw) !important}.fs-5{font-size:1.25rem !important}.fs-6{font-size:1rem !important}.fst-italic{font-style:italic !important}.fst-normal{font-style:normal !important}.fw-lighter{font-weight:lighter !important}.fw-light{font-weight:300 !important}.fw-normal{font-weight:400 !important}.fw-medium{font-weight:500 !important}.fw-semibold{font-weight:600 !important}.fw-bold{font-weight:700 !important}.fw-bolder{font-weight:bolder !important}.lh-1{line-height:1 !important}.lh-sm{line-height:1.25 !important}.lh-base{line-height:1.5 !important}.lh-lg{line-height:2 !important}.text-start{text-align:left !important}.text-end{text-align:right !important}.text-center{text-align:center !important}.text-decoration-none{text-decoration:none !important}.text-decoration-underline{text-decoration:underline !important}.text-decoration-line-through{text-decoration:line-through !important}.text-lowercase{text-transform:lowercase !important}.text-uppercase{text-transform:uppercase !important}.text-capitalize{text-transform:capitalize !important}.text-wrap{white-space:normal !important}.text-nowrap{white-space:nowrap !important}.text-break{word-wrap:break-word !important;word-break:break-word !important}.text-primary{--bs-text-opacity: 1;color:rgba(var(--bs-primary-rgb), var(--bs-text-opacity)) !important}.text-secondary{--bs-text-opacity: 1;color:rgba(var(--bs-secondary-rgb), var(--bs-text-opacity)) !important}.text-success{--bs-text-opacity: 1;color:rgba(var(--bs-success-rgb), var(--bs-text-opacity)) !important}.text-info{--bs-text-opacity: 1;color:rgba(var(--bs-info-rgb), var(--bs-text-opacity)) !important}.text-warning{--bs-text-opacity: 1;color:rgba(var(--bs-warning-rgb), var(--bs-text-opacity)) !important}.text-danger{--bs-text-opacity: 1;color:rgba(var(--bs-danger-rgb), var(--bs-text-opacity)) !important}.text-light{--bs-text-opacity: 1;color:rgba(var(--bs-light-rgb), var(--bs-text-opacity)) !important}.text-dark{--bs-text-opacity: 1;color:rgba(var(--bs-dark-rgb), var(--bs-text-opacity)) !important}.text-black{--bs-text-opacity: 1;color:rgba(var(--bs-black-rgb), var(--bs-text-opacity)) !important}.text-white{--bs-text-opacity: 1;color:rgba(var(--bs-white-rgb), var(--bs-text-opacity)) !important}.text-body{--bs-text-opacity: 1;color:rgba(var(--bs-body-color-rgb), var(--bs-text-opacity)) !important}.text-muted{--bs-text-opacity: 1;color:var(--bs-secondary-color) !important}.text-black-50{--bs-text-opacity: 1;color:rgba(0,0,0,.5) !important}.text-white-50{--bs-text-opacity: 1;color:rgba(255,255,255,.5) !important}.text-body-secondary{--bs-text-opacity: 1;color:var(--bs-secondary-color) !important}.text-body-tertiary{--bs-text-opacity: 1;color:var(--bs-tertiary-color) !important}.text-body-emphasis{--bs-text-opacity: 1;color:var(--bs-emphasis-color) !important}.text-reset{--bs-text-opacity: 1;color:inherit !important}.text-opacity-25{--bs-text-opacity: 0.25}.text-opacity-50{--bs-text-opacity: 0.5}.text-opacity-75{--bs-text-opacity: 0.75}.text-opacity-100{--bs-text-opacity: 1}.text-primary-emphasis{color:var(--bs-primary-text-emphasis) !important}.text-secondary-emphasis{color:var(--bs-secondary-text-emphasis) !important}.text-success-emphasis{color:var(--bs-success-text-emphasis) !important}.text-info-emphasis{color:var(--bs-info-text-emphasis) !important}.text-warning-emphasis{color:var(--bs-warning-text-emphasis) !important}.text-danger-emphasis{color:var(--bs-danger-text-emphasis) !important}.text-light-emphasis{color:var(--bs-light-text-emphasis) !important}.text-dark-emphasis{color:var(--bs-dark-text-emphasis) !important}.link-opacity-10{--bs-link-opacity: 0.1}.link-opacity-10-hover:hover{--bs-link-opacity: 0.1}.link-opacity-25{--bs-link-opacity: 0.25}.link-opacity-25-hover:hover{--bs-link-opacity: 0.25}.link-opacity-50{--bs-link-opacity: 0.5}.link-opacity-50-hover:hover{--bs-link-opacity: 0.5}.link-opacity-75{--bs-link-opacity: 0.75}.link-opacity-75-hover:hover{--bs-link-opacity: 0.75}.link-opacity-100{--bs-link-opacity: 1}.link-opacity-100-hover:hover{--bs-link-opacity: 1}.link-offset-1{text-underline-offset:.125em !important}.link-offset-1-hover:hover{text-underline-offset:.125em !important}.link-offset-2{text-underline-offset:.25em !important}.link-offset-2-hover:hover{text-underline-offset:.25em !important}.link-offset-3{text-underline-offset:.375em !important}.link-offset-3-hover:hover{text-underline-offset:.375em !important}.link-underline-primary{--bs-link-underline-opacity: 1;-webkit-text-decoration-color:rgba(var(--bs-primary-rgb), var(--bs-link-underline-opacity)) !important;text-decoration-color:rgba(var(--bs-primary-rgb), var(--bs-link-underline-opacity)) !important}.link-underline-secondary{--bs-link-underline-opacity: 1;-webkit-text-decoration-color:rgba(var(--bs-secondary-rgb), var(--bs-link-underline-opacity)) !important;text-decoration-color:rgba(var(--bs-secondary-rgb), var(--bs-link-underline-opacity)) !important}.link-underline-success{--bs-link-underline-opacity: 1;-webkit-text-decoration-color:rgba(var(--bs-success-rgb), var(--bs-link-underline-opacity)) !important;text-decoration-color:rgba(var(--bs-success-rgb), var(--bs-link-underline-opacity)) !important}.link-underline-info{--bs-link-underline-opacity: 1;-webkit-text-decoration-color:rgba(var(--bs-info-rgb), var(--bs-link-underline-opacity)) !important;text-decoration-color:rgba(var(--bs-info-rgb), var(--bs-link-underline-opacity)) !important}.link-underline-warning{--bs-link-underline-opacity: 1;-webkit-text-decoration-color:rgba(var(--bs-warning-rgb), var(--bs-link-underline-opacity)) !important;text-decoration-color:rgba(var(--bs-warning-rgb), var(--bs-link-underline-opacity)) !important}.link-underline-danger{--bs-link-underline-opacity: 1;-webkit-text-decoration-color:rgba(var(--bs-danger-rgb), var(--bs-link-underline-opacity)) !important;text-decoration-color:rgba(var(--bs-danger-rgb), var(--bs-link-underline-opacity)) !important}.link-underline-light{--bs-link-underline-opacity: 1;-webkit-text-decoration-color:rgba(var(--bs-light-rgb), var(--bs-link-underline-opacity)) !important;text-decoration-color:rgba(var(--bs-light-rgb), var(--bs-link-underline-opacity)) !important}.link-underline-dark{--bs-link-underline-opacity: 1;-webkit-text-decoration-color:rgba(var(--bs-dark-rgb), var(--bs-link-underline-opacity)) !important;text-decoration-color:rgba(var(--bs-dark-rgb), var(--bs-link-underline-opacity)) !important}.link-underline{--bs-link-underline-opacity: 1;-webkit-text-decoration-color:rgba(var(--bs-link-color-rgb), var(--bs-link-underline-opacity, 1)) !important;text-decoration-color:rgba(var(--bs-link-color-rgb), var(--bs-link-underline-opacity, 1)) !important}.link-underline-opacity-0{--bs-link-underline-opacity: 0}.link-underline-opacity-0-hover:hover{--bs-link-underline-opacity: 0}.link-underline-opacity-10{--bs-link-underline-opacity: 0.1}.link-underline-opacity-10-hover:hover{--bs-link-underline-opacity: 0.1}.link-underline-opacity-25{--bs-link-underline-opacity: 0.25}.link-underline-opacity-25-hover:hover{--bs-link-underline-opacity: 0.25}.link-underline-opacity-50{--bs-link-underline-opacity: 0.5}.link-underline-opacity-50-hover:hover{--bs-link-underline-opacity: 0.5}.link-underline-opacity-75{--bs-link-underline-opacity: 0.75}.link-underline-opacity-75-hover:hover{--bs-link-underline-opacity: 0.75}.link-underline-opacity-100{--bs-link-underline-opacity: 1}.link-underline-opacity-100-hover:hover{--bs-link-underline-opacity: 1}.bg-primary{--bs-bg-opacity: 1;background-color:rgba(var(--bs-primary-rgb), var(--bs-bg-opacity)) !important}.bg-secondary{--bs-bg-opacity: 1;background-color:rgba(var(--bs-secondary-rgb), var(--bs-bg-opacity)) !important}.bg-success{--bs-bg-opacity: 1;background-color:rgba(var(--bs-success-rgb), var(--bs-bg-opacity)) !important}.bg-info{--bs-bg-opacity: 1;background-color:rgba(var(--bs-info-rgb), var(--bs-bg-opacity)) !important}.bg-warning{--bs-bg-opacity: 1;background-color:rgba(var(--bs-warning-rgb), var(--bs-bg-opacity)) !important}.bg-danger{--bs-bg-opacity: 1;background-color:rgba(var(--bs-danger-rgb), var(--bs-bg-opacity)) !important}.bg-light{--bs-bg-opacity: 1;background-color:rgba(var(--bs-light-rgb), var(--bs-bg-opacity)) !important}.bg-dark{--bs-bg-opacity: 1;background-color:rgba(var(--bs-dark-rgb), var(--bs-bg-opacity)) !important}.bg-black{--bs-bg-opacity: 1;background-color:rgba(var(--bs-black-rgb), var(--bs-bg-opacity)) !important}.bg-white{--bs-bg-opacity: 1;background-color:rgba(var(--bs-white-rgb), var(--bs-bg-opacity)) !important}.bg-body{--bs-bg-opacity: 1;background-color:rgba(var(--bs-body-bg-rgb), var(--bs-bg-opacity)) !important}.bg-transparent{--bs-bg-opacity: 1;background-color:transparent !important}.bg-body-secondary{--bs-bg-opacity: 1;background-color:rgba(var(--bs-secondary-bg-rgb), var(--bs-bg-opacity)) !important}.bg-body-tertiary{--bs-bg-opacity: 1;background-color:rgba(var(--bs-tertiary-bg-rgb), var(--bs-bg-opacity)) !important}.bg-opacity-10{--bs-bg-opacity: 0.1}.bg-opacity-25{--bs-bg-opacity: 0.25}.bg-opacity-50{--bs-bg-opacity: 0.5}.bg-opacity-75{--bs-bg-opacity: 0.75}.bg-opacity-100{--bs-bg-opacity: 1}.bg-primary-subtle{background-color:var(--bs-primary-bg-subtle) !important}.bg-secondary-subtle{background-color:var(--bs-secondary-bg-subtle) !important}.bg-success-subtle{background-color:var(--bs-success-bg-subtle) !important}.bg-info-subtle{background-color:var(--bs-info-bg-subtle) !important}.bg-warning-subtle{background-color:var(--bs-warning-bg-subtle) !important}.bg-danger-subtle{background-color:var(--bs-danger-bg-subtle) !important}.bg-light-subtle{background-color:var(--bs-light-bg-subtle) !important}.bg-dark-subtle{background-color:var(--bs-dark-bg-subtle) !important}.bg-gradient{background-image:var(--bs-gradient) !important}.user-select-all{-webkit-user-select:all !important;-moz-user-select:all !important;user-select:all !important}.user-select-auto{-webkit-user-select:auto !important;-moz-user-select:auto !important;-ms-user-select:auto !important;user-select:auto !important}.user-select-none{-webkit-user-select:none !important;-moz-user-select:none !important;-ms-user-select:none !important;user-select:none !important}.pe-none{pointer-events:none !important}.pe-auto{pointer-events:auto !important}.rounded{border-radius:var(--bs-border-radius) !important}.rounded-0{border-radius:0 !important}.rounded-1{border-radius:var(--bs-border-radius-sm) !important}.rounded-2{border-radius:var(--bs-border-radius) !important}.rounded-3{border-radius:var(--bs-border-radius-lg) !important}.rounded-4{border-radius:var(--bs-border-radius-xl) !important}.rounded-5{border-radius:var(--bs-border-radius-xxl) !important}.rounded-circle{border-radius:50% !important}.rounded-pill{border-radius:var(--bs-border-radius-pill) !important}.rounded-top{border-top-left-radius:var(--bs-border-radius) !important;border-top-right-radius:var(--bs-border-radius) !important}.rounded-top-0{border-top-left-radius:0 !important;border-top-right-radius:0 !important}.rounded-top-1{border-top-left-radius:var(--bs-border-radius-sm) !important;border-top-right-radius:var(--bs-border-radius-sm) !important}.rounded-top-2{border-top-left-radius:var(--bs-border-radius) !important;border-top-right-radius:var(--bs-border-radius) !important}.rounded-top-3{border-top-left-radius:var(--bs-border-radius-lg) !important;border-top-right-radius:var(--bs-border-radius-lg) !important}.rounded-top-4{border-top-left-radius:var(--bs-border-radius-xl) !important;border-top-right-radius:var(--bs-border-radius-xl) !important}.rounded-top-5{border-top-left-radius:var(--bs-border-radius-xxl) !important;border-top-right-radius:var(--bs-border-radius-xxl) !important}.rounded-top-circle{border-top-left-radius:50% !important;border-top-right-radius:50% !important}.rounded-top-pill{border-top-left-radius:var(--bs-border-radius-pill) !important;border-top-right-radius:var(--bs-border-radius-pill) !important}.rounded-end{border-top-right-radius:var(--bs-border-radius) !important;border-bottom-right-radius:var(--bs-border-radius) !important}.rounded-end-0{border-top-right-radius:0 !important;border-bottom-right-radius:0 !important}.rounded-end-1{border-top-right-radius:var(--bs-border-radius-sm) !important;border-bottom-right-radius:var(--bs-border-radius-sm) !important}.rounded-end-2{border-top-right-radius:var(--bs-border-radius) !important;border-bottom-right-radius:var(--bs-border-radius) !important}.rounded-end-3{border-top-right-radius:var(--bs-border-radius-lg) !important;border-bottom-right-radius:var(--bs-border-radius-lg) !important}.rounded-end-4{border-top-right-radius:var(--bs-border-radius-xl) !important;border-bottom-right-radius:var(--bs-border-radius-xl) !important}.rounded-end-5{border-top-right-radius:var(--bs-border-radius-xxl) !important;border-bottom-right-radius:var(--bs-border-radius-xxl) !important}.rounded-end-circle{border-top-right-radius:50% !important;border-bottom-right-radius:50% !important}.rounded-end-pill{border-top-right-radius:var(--bs-border-radius-pill) !important;border-bottom-right-radius:var(--bs-border-radius-pill) !important}.rounded-bottom{border-bottom-right-radius:var(--bs-border-radius) !important;border-bottom-left-radius:var(--bs-border-radius) !important}.rounded-bottom-0{border-bottom-right-radius:0 !important;border-bottom-left-radius:0 !important}.rounded-bottom-1{border-bottom-right-radius:var(--bs-border-radius-sm) !important;border-bottom-left-radius:var(--bs-border-radius-sm) !important}.rounded-bottom-2{border-bottom-right-radius:var(--bs-border-radius) !important;border-bottom-left-radius:var(--bs-border-radius) !important}.rounded-bottom-3{border-bottom-right-radius:var(--bs-border-radius-lg) !important;border-bottom-left-radius:var(--bs-border-radius-lg) !important}.rounded-bottom-4{border-bottom-right-radius:var(--bs-border-radius-xl) !important;border-bottom-left-radius:var(--bs-border-radius-xl) !important}.rounded-bottom-5{border-bottom-right-radius:var(--bs-border-radius-xxl) !important;border-bottom-left-radius:var(--bs-border-radius-xxl) !important}.rounded-bottom-circle{border-bottom-right-radius:50% !important;border-bottom-left-radius:50% !important}.rounded-bottom-pill{border-bottom-right-radius:var(--bs-border-radius-pill) !important;border-bottom-left-radius:var(--bs-border-radius-pill) !important}.rounded-start{border-bottom-left-radius:var(--bs-border-radius) !important;border-top-left-radius:var(--bs-border-radius) !important}.rounded-start-0{border-bottom-left-radius:0 !important;border-top-left-radius:0 !important}.rounded-start-1{border-bottom-left-radius:var(--bs-border-radius-sm) !important;border-top-left-radius:var(--bs-border-radius-sm) !important}.rounded-start-2{border-bottom-left-radius:var(--bs-border-radius) !important;border-top-left-radius:var(--bs-border-radius) !important}.rounded-start-3{border-bottom-left-radius:var(--bs-border-radius-lg) !important;border-top-left-radius:var(--bs-border-radius-lg) !important}.rounded-start-4{border-bottom-left-radius:var(--bs-border-radius-xl) !important;border-top-left-radius:var(--bs-border-radius-xl) !important}.rounded-start-5{border-bottom-left-radius:var(--bs-border-radius-xxl) !important;border-top-left-radius:var(--bs-border-radius-xxl) !important}.rounded-start-circle{border-bottom-left-radius:50% !important;border-top-left-radius:50% !important}.rounded-start-pill{border-bottom-left-radius:var(--bs-border-radius-pill) !important;border-top-left-radius:var(--bs-border-radius-pill) !important}.visible{visibility:visible !important}.invisible{visibility:hidden !important}.z-n1{z-index:-1 !important}.z-0{z-index:0 !important}.z-1{z-index:1 !important}.z-2{z-index:2 !important}.z-3{z-index:3 !important}@media(min-width: 576px){.float-sm-start{float:left !important}.float-sm-end{float:right !important}.float-sm-none{float:none !important}.object-fit-sm-contain{-o-object-fit:contain !important;object-fit:contain !important}.object-fit-sm-cover{-o-object-fit:cover !important;object-fit:cover !important}.object-fit-sm-fill{-o-object-fit:fill !important;object-fit:fill !important}.object-fit-sm-scale{-o-object-fit:scale-down !important;object-fit:scale-down !important}.object-fit-sm-none{-o-object-fit:none !important;object-fit:none !important}.d-sm-inline{display:inline !important}.d-sm-inline-block{display:inline-block !important}.d-sm-block{display:block !important}.d-sm-grid{display:grid !important}.d-sm-inline-grid{display:inline-grid !important}.d-sm-table{display:table !important}.d-sm-table-row{display:table-row !important}.d-sm-table-cell{display:table-cell !important}.d-sm-flex{display:flex !important}.d-sm-inline-flex{display:inline-flex !important}.d-sm-none{display:none !important}.flex-sm-fill{flex:1 1 auto !important}.flex-sm-row{flex-direction:row !important}.flex-sm-column{flex-direction:column !important}.flex-sm-row-reverse{flex-direction:row-reverse !important}.flex-sm-column-reverse{flex-direction:column-reverse !important}.flex-sm-grow-0{flex-grow:0 !important}.flex-sm-grow-1{flex-grow:1 !important}.flex-sm-shrink-0{flex-shrink:0 !important}.flex-sm-shrink-1{flex-shrink:1 !important}.flex-sm-wrap{flex-wrap:wrap !important}.flex-sm-nowrap{flex-wrap:nowrap !important}.flex-sm-wrap-reverse{flex-wrap:wrap-reverse !important}.justify-content-sm-start{justify-content:flex-start !important}.justify-content-sm-end{justify-content:flex-end !important}.justify-content-sm-center{justify-content:center !important}.justify-content-sm-between{justify-content:space-between !important}.justify-content-sm-around{justify-content:space-around !important}.justify-content-sm-evenly{justify-content:space-evenly !important}.align-items-sm-start{align-items:flex-start !important}.align-items-sm-end{align-items:flex-end !important}.align-items-sm-center{align-items:center !important}.align-items-sm-baseline{align-items:baseline !important}.align-items-sm-stretch{align-items:stretch !important}.align-content-sm-start{align-content:flex-start !important}.align-content-sm-end{align-content:flex-end !important}.align-content-sm-center{align-content:center !important}.align-content-sm-between{align-content:space-between !important}.align-content-sm-around{align-content:space-around !important}.align-content-sm-stretch{align-content:stretch !important}.align-self-sm-auto{align-self:auto !important}.align-self-sm-start{align-self:flex-start !important}.align-self-sm-end{align-self:flex-end !important}.align-self-sm-center{align-self:center !important}.align-self-sm-baseline{align-self:baseline !important}.align-self-sm-stretch{align-self:stretch !important}.order-sm-first{order:-1 !important}.order-sm-0{order:0 !important}.order-sm-1{order:1 !important}.order-sm-2{order:2 !important}.order-sm-3{order:3 !important}.order-sm-4{order:4 !important}.order-sm-5{order:5 !important}.order-sm-last{order:6 !important}.m-sm-0{margin:0 !important}.m-sm-1{margin:.25rem !important}.m-sm-2{margin:.5rem !important}.m-sm-3{margin:1rem !important}.m-sm-4{margin:1.5rem !important}.m-sm-5{margin:3rem !important}.m-sm-auto{margin:auto !important}.mx-sm-0{margin-right:0 !important;margin-left:0 !important}.mx-sm-1{margin-right:.25rem !important;margin-left:.25rem !important}.mx-sm-2{margin-right:.5rem !important;margin-left:.5rem !important}.mx-sm-3{margin-right:1rem !important;margin-left:1rem !important}.mx-sm-4{margin-right:1.5rem !important;margin-left:1.5rem !important}.mx-sm-5{margin-right:3rem !important;margin-left:3rem !important}.mx-sm-auto{margin-right:auto !important;margin-left:auto !important}.my-sm-0{margin-top:0 !important;margin-bottom:0 !important}.my-sm-1{margin-top:.25rem !important;margin-bottom:.25rem !important}.my-sm-2{margin-top:.5rem !important;margin-bottom:.5rem !important}.my-sm-3{margin-top:1rem !important;margin-bottom:1rem !important}.my-sm-4{margin-top:1.5rem !important;margin-bottom:1.5rem !important}.my-sm-5{margin-top:3rem !important;margin-bottom:3rem !important}.my-sm-auto{margin-top:auto !important;margin-bottom:auto !important}.mt-sm-0{margin-top:0 !important}.mt-sm-1{margin-top:.25rem !important}.mt-sm-2{margin-top:.5rem !important}.mt-sm-3{margin-top:1rem !important}.mt-sm-4{margin-top:1.5rem !important}.mt-sm-5{margin-top:3rem !important}.mt-sm-auto{margin-top:auto !important}.me-sm-0{margin-right:0 !important}.me-sm-1{margin-right:.25rem !important}.me-sm-2{margin-right:.5rem !important}.me-sm-3{margin-right:1rem !important}.me-sm-4{margin-right:1.5rem !important}.me-sm-5{margin-right:3rem !important}.me-sm-auto{margin-right:auto !important}.mb-sm-0{margin-bottom:0 !important}.mb-sm-1{margin-bottom:.25rem !important}.mb-sm-2{margin-bottom:.5rem !important}.mb-sm-3{margin-bottom:1rem !important}.mb-sm-4{margin-bottom:1.5rem !important}.mb-sm-5{margin-bottom:3rem !important}.mb-sm-auto{margin-bottom:auto !important}.ms-sm-0{margin-left:0 !important}.ms-sm-1{margin-left:.25rem !important}.ms-sm-2{margin-left:.5rem !important}.ms-sm-3{margin-left:1rem !important}.ms-sm-4{margin-left:1.5rem !important}.ms-sm-5{margin-left:3rem !important}.ms-sm-auto{margin-left:auto !important}.p-sm-0{padding:0 !important}.p-sm-1{padding:.25rem !important}.p-sm-2{padding:.5rem !important}.p-sm-3{padding:1rem !important}.p-sm-4{padding:1.5rem !important}.p-sm-5{padding:3rem !important}.px-sm-0{padding-right:0 !important;padding-left:0 !important}.px-sm-1{padding-right:.25rem !important;padding-left:.25rem !important}.px-sm-2{padding-right:.5rem !important;padding-left:.5rem !important}.px-sm-3{padding-right:1rem !important;padding-left:1rem !important}.px-sm-4{padding-right:1.5rem !important;padding-left:1.5rem !important}.px-sm-5{padding-right:3rem !important;padding-left:3rem !important}.py-sm-0{padding-top:0 !important;padding-bottom:0 !important}.py-sm-1{padding-top:.25rem !important;padding-bottom:.25rem !important}.py-sm-2{padding-top:.5rem !important;padding-bottom:.5rem !important}.py-sm-3{padding-top:1rem !important;padding-bottom:1rem !important}.py-sm-4{padding-top:1.5rem !important;padding-bottom:1.5rem !important}.py-sm-5{padding-top:3rem !important;padding-bottom:3rem !important}.pt-sm-0{padding-top:0 !important}.pt-sm-1{padding-top:.25rem !important}.pt-sm-2{padding-top:.5rem !important}.pt-sm-3{padding-top:1rem !important}.pt-sm-4{padding-top:1.5rem !important}.pt-sm-5{padding-top:3rem !important}.pe-sm-0{padding-right:0 !important}.pe-sm-1{padding-right:.25rem !important}.pe-sm-2{padding-right:.5rem !important}.pe-sm-3{padding-right:1rem !important}.pe-sm-4{padding-right:1.5rem !important}.pe-sm-5{padding-right:3rem !important}.pb-sm-0{padding-bottom:0 !important}.pb-sm-1{padding-bottom:.25rem !important}.pb-sm-2{padding-bottom:.5rem !important}.pb-sm-3{padding-bottom:1rem !important}.pb-sm-4{padding-bottom:1.5rem !important}.pb-sm-5{padding-bottom:3rem !important}.ps-sm-0{padding-left:0 !important}.ps-sm-1{padding-left:.25rem !important}.ps-sm-2{padding-left:.5rem !important}.ps-sm-3{padding-left:1rem !important}.ps-sm-4{padding-left:1.5rem !important}.ps-sm-5{padding-left:3rem !important}.gap-sm-0{gap:0 !important}.gap-sm-1{gap:.25rem !important}.gap-sm-2{gap:.5rem !important}.gap-sm-3{gap:1rem !important}.gap-sm-4{gap:1.5rem !important}.gap-sm-5{gap:3rem !important}.row-gap-sm-0{row-gap:0 !important}.row-gap-sm-1{row-gap:.25rem !important}.row-gap-sm-2{row-gap:.5rem !important}.row-gap-sm-3{row-gap:1rem !important}.row-gap-sm-4{row-gap:1.5rem !important}.row-gap-sm-5{row-gap:3rem !important}.column-gap-sm-0{-moz-column-gap:0 !important;column-gap:0 !important}.column-gap-sm-1{-moz-column-gap:.25rem !important;column-gap:.25rem !important}.column-gap-sm-2{-moz-column-gap:.5rem !important;column-gap:.5rem !important}.column-gap-sm-3{-moz-column-gap:1rem !important;column-gap:1rem !important}.column-gap-sm-4{-moz-column-gap:1.5rem !important;column-gap:1.5rem !important}.column-gap-sm-5{-moz-column-gap:3rem !important;column-gap:3rem !important}.text-sm-start{text-align:left !important}.text-sm-end{text-align:right !important}.text-sm-center{text-align:center !important}}@media(min-width: 768px){.float-md-start{float:left !important}.float-md-end{float:right !important}.float-md-none{float:none !important}.object-fit-md-contain{-o-object-fit:contain !important;object-fit:contain !important}.object-fit-md-cover{-o-object-fit:cover !important;object-fit:cover !important}.object-fit-md-fill{-o-object-fit:fill !important;object-fit:fill !important}.object-fit-md-scale{-o-object-fit:scale-down !important;object-fit:scale-down !important}.object-fit-md-none{-o-object-fit:none !important;object-fit:none !important}.d-md-inline{display:inline !important}.d-md-inline-block{display:inline-block !important}.d-md-block{display:block !important}.d-md-grid{display:grid !important}.d-md-inline-grid{display:inline-grid !important}.d-md-table{display:table !important}.d-md-table-row{display:table-row !important}.d-md-table-cell{display:table-cell !important}.d-md-flex{display:flex !important}.d-md-inline-flex{display:inline-flex !important}.d-md-none{display:none !important}.flex-md-fill{flex:1 1 auto !important}.flex-md-row{flex-direction:row !important}.flex-md-column{flex-direction:column !important}.flex-md-row-reverse{flex-direction:row-reverse !important}.flex-md-column-reverse{flex-direction:column-reverse !important}.flex-md-grow-0{flex-grow:0 !important}.flex-md-grow-1{flex-grow:1 !important}.flex-md-shrink-0{flex-shrink:0 !important}.flex-md-shrink-1{flex-shrink:1 !important}.flex-md-wrap{flex-wrap:wrap !important}.flex-md-nowrap{flex-wrap:nowrap !important}.flex-md-wrap-reverse{flex-wrap:wrap-reverse !important}.justify-content-md-start{justify-content:flex-start !important}.justify-content-md-end{justify-content:flex-end !important}.justify-content-md-center{justify-content:center !important}.justify-content-md-between{justify-content:space-between !important}.justify-content-md-around{justify-content:space-around !important}.justify-content-md-evenly{justify-content:space-evenly !important}.align-items-md-start{align-items:flex-start !important}.align-items-md-end{align-items:flex-end !important}.align-items-md-center{align-items:center !important}.align-items-md-baseline{align-items:baseline !important}.align-items-md-stretch{align-items:stretch !important}.align-content-md-start{align-content:flex-start !important}.align-content-md-end{align-content:flex-end !important}.align-content-md-center{align-content:center !important}.align-content-md-between{align-content:space-between !important}.align-content-md-around{align-content:space-around !important}.align-content-md-stretch{align-content:stretch !important}.align-self-md-auto{align-self:auto !important}.align-self-md-start{align-self:flex-start !important}.align-self-md-end{align-self:flex-end !important}.align-self-md-center{align-self:center !important}.align-self-md-baseline{align-self:baseline !important}.align-self-md-stretch{align-self:stretch !important}.order-md-first{order:-1 !important}.order-md-0{order:0 !important}.order-md-1{order:1 !important}.order-md-2{order:2 !important}.order-md-3{order:3 !important}.order-md-4{order:4 !important}.order-md-5{order:5 !important}.order-md-last{order:6 !important}.m-md-0{margin:0 !important}.m-md-1{margin:.25rem !important}.m-md-2{margin:.5rem !important}.m-md-3{margin:1rem !important}.m-md-4{margin:1.5rem !important}.m-md-5{margin:3rem !important}.m-md-auto{margin:auto !important}.mx-md-0{margin-right:0 !important;margin-left:0 !important}.mx-md-1{margin-right:.25rem !important;margin-left:.25rem !important}.mx-md-2{margin-right:.5rem !important;margin-left:.5rem !important}.mx-md-3{margin-right:1rem !important;margin-left:1rem !important}.mx-md-4{margin-right:1.5rem !important;margin-left:1.5rem !important}.mx-md-5{margin-right:3rem !important;margin-left:3rem !important}.mx-md-auto{margin-right:auto !important;margin-left:auto !important}.my-md-0{margin-top:0 !important;margin-bottom:0 !important}.my-md-1{margin-top:.25rem !important;margin-bottom:.25rem !important}.my-md-2{margin-top:.5rem !important;margin-bottom:.5rem !important}.my-md-3{margin-top:1rem !important;margin-bottom:1rem !important}.my-md-4{margin-top:1.5rem !important;margin-bottom:1.5rem !important}.my-md-5{margin-top:3rem !important;margin-bottom:3rem !important}.my-md-auto{margin-top:auto !important;margin-bottom:auto !important}.mt-md-0{margin-top:0 !important}.mt-md-1{margin-top:.25rem !important}.mt-md-2{margin-top:.5rem !important}.mt-md-3{margin-top:1rem !important}.mt-md-4{margin-top:1.5rem !important}.mt-md-5{margin-top:3rem !important}.mt-md-auto{margin-top:auto !important}.me-md-0{margin-right:0 !important}.me-md-1{margin-right:.25rem !important}.me-md-2{margin-right:.5rem !important}.me-md-3{margin-right:1rem !important}.me-md-4{margin-right:1.5rem !important}.me-md-5{margin-right:3rem !important}.me-md-auto{margin-right:auto !important}.mb-md-0{margin-bottom:0 !important}.mb-md-1{margin-bottom:.25rem !important}.mb-md-2{margin-bottom:.5rem !important}.mb-md-3{margin-bottom:1rem !important}.mb-md-4{margin-bottom:1.5rem !important}.mb-md-5{margin-bottom:3rem !important}.mb-md-auto{margin-bottom:auto !important}.ms-md-0{margin-left:0 !important}.ms-md-1{margin-left:.25rem !important}.ms-md-2{margin-left:.5rem !important}.ms-md-3{margin-left:1rem !important}.ms-md-4{margin-left:1.5rem !important}.ms-md-5{margin-left:3rem !important}.ms-md-auto{margin-left:auto !important}.p-md-0{padding:0 !important}.p-md-1{padding:.25rem !important}.p-md-2{padding:.5rem !important}.p-md-3{padding:1rem !important}.p-md-4{padding:1.5rem !important}.p-md-5{padding:3rem !important}.px-md-0{padding-right:0 !important;padding-left:0 !important}.px-md-1{padding-right:.25rem !important;padding-left:.25rem !important}.px-md-2{padding-right:.5rem !important;padding-left:.5rem !important}.px-md-3{padding-right:1rem !important;padding-left:1rem !important}.px-md-4{padding-right:1.5rem !important;padding-left:1.5rem !important}.px-md-5{padding-right:3rem !important;padding-left:3rem !important}.py-md-0{padding-top:0 !important;padding-bottom:0 !important}.py-md-1{padding-top:.25rem !important;padding-bottom:.25rem !important}.py-md-2{padding-top:.5rem !important;padding-bottom:.5rem !important}.py-md-3{padding-top:1rem !important;padding-bottom:1rem !important}.py-md-4{padding-top:1.5rem !important;padding-bottom:1.5rem !important}.py-md-5{padding-top:3rem !important;padding-bottom:3rem !important}.pt-md-0{padding-top:0 !important}.pt-md-1{padding-top:.25rem !important}.pt-md-2{padding-top:.5rem !important}.pt-md-3{padding-top:1rem !important}.pt-md-4{padding-top:1.5rem !important}.pt-md-5{padding-top:3rem !important}.pe-md-0{padding-right:0 !important}.pe-md-1{padding-right:.25rem !important}.pe-md-2{padding-right:.5rem !important}.pe-md-3{padding-right:1rem !important}.pe-md-4{padding-right:1.5rem !important}.pe-md-5{padding-right:3rem !important}.pb-md-0{padding-bottom:0 !important}.pb-md-1{padding-bottom:.25rem !important}.pb-md-2{padding-bottom:.5rem !important}.pb-md-3{padding-bottom:1rem !important}.pb-md-4{padding-bottom:1.5rem !important}.pb-md-5{padding-bottom:3rem !important}.ps-md-0{padding-left:0 !important}.ps-md-1{padding-left:.25rem !important}.ps-md-2{padding-left:.5rem !important}.ps-md-3{padding-left:1rem !important}.ps-md-4{padding-left:1.5rem !important}.ps-md-5{padding-left:3rem !important}.gap-md-0{gap:0 !important}.gap-md-1{gap:.25rem !important}.gap-md-2{gap:.5rem !important}.gap-md-3{gap:1rem !important}.gap-md-4{gap:1.5rem !important}.gap-md-5{gap:3rem !important}.row-gap-md-0{row-gap:0 !important}.row-gap-md-1{row-gap:.25rem !important}.row-gap-md-2{row-gap:.5rem !important}.row-gap-md-3{row-gap:1rem !important}.row-gap-md-4{row-gap:1.5rem !important}.row-gap-md-5{row-gap:3rem !important}.column-gap-md-0{-moz-column-gap:0 !important;column-gap:0 !important}.column-gap-md-1{-moz-column-gap:.25rem !important;column-gap:.25rem !important}.column-gap-md-2{-moz-column-gap:.5rem !important;column-gap:.5rem !important}.column-gap-md-3{-moz-column-gap:1rem !important;column-gap:1rem !important}.column-gap-md-4{-moz-column-gap:1.5rem !important;column-gap:1.5rem !important}.column-gap-md-5{-moz-column-gap:3rem !important;column-gap:3rem !important}.text-md-start{text-align:left !important}.text-md-end{text-align:right !important}.text-md-center{text-align:center !important}}@media(min-width: 992px){.float-lg-start{float:left !important}.float-lg-end{float:right !important}.float-lg-none{float:none !important}.object-fit-lg-contain{-o-object-fit:contain !important;object-fit:contain !important}.object-fit-lg-cover{-o-object-fit:cover !important;object-fit:cover !important}.object-fit-lg-fill{-o-object-fit:fill !important;object-fit:fill !important}.object-fit-lg-scale{-o-object-fit:scale-down !important;object-fit:scale-down !important}.object-fit-lg-none{-o-object-fit:none !important;object-fit:none !important}.d-lg-inline{display:inline !important}.d-lg-inline-block{display:inline-block !important}.d-lg-block{display:block !important}.d-lg-grid{display:grid !important}.d-lg-inline-grid{display:inline-grid !important}.d-lg-table{display:table !important}.d-lg-table-row{display:table-row !important}.d-lg-table-cell{display:table-cell !important}.d-lg-flex{display:flex !important}.d-lg-inline-flex{display:inline-flex !important}.d-lg-none{display:none !important}.flex-lg-fill{flex:1 1 auto !important}.flex-lg-row{flex-direction:row !important}.flex-lg-column{flex-direction:column !important}.flex-lg-row-reverse{flex-direction:row-reverse !important}.flex-lg-column-reverse{flex-direction:column-reverse !important}.flex-lg-grow-0{flex-grow:0 !important}.flex-lg-grow-1{flex-grow:1 !important}.flex-lg-shrink-0{flex-shrink:0 !important}.flex-lg-shrink-1{flex-shrink:1 !important}.flex-lg-wrap{flex-wrap:wrap !important}.flex-lg-nowrap{flex-wrap:nowrap !important}.flex-lg-wrap-reverse{flex-wrap:wrap-reverse !important}.justify-content-lg-start{justify-content:flex-start !important}.justify-content-lg-end{justify-content:flex-end !important}.justify-content-lg-center{justify-content:center !important}.justify-content-lg-between{justify-content:space-between !important}.justify-content-lg-around{justify-content:space-around !important}.justify-content-lg-evenly{justify-content:space-evenly !important}.align-items-lg-start{align-items:flex-start !important}.align-items-lg-end{align-items:flex-end !important}.align-items-lg-center{align-items:center !important}.align-items-lg-baseline{align-items:baseline !important}.align-items-lg-stretch{align-items:stretch !important}.align-content-lg-start{align-content:flex-start !important}.align-content-lg-end{align-content:flex-end !important}.align-content-lg-center{align-content:center !important}.align-content-lg-between{align-content:space-between !important}.align-content-lg-around{align-content:space-around !important}.align-content-lg-stretch{align-content:stretch !important}.align-self-lg-auto{align-self:auto !important}.align-self-lg-start{align-self:flex-start !important}.align-self-lg-end{align-self:flex-end !important}.align-self-lg-center{align-self:center !important}.align-self-lg-baseline{align-self:baseline !important}.align-self-lg-stretch{align-self:stretch !important}.order-lg-first{order:-1 !important}.order-lg-0{order:0 !important}.order-lg-1{order:1 !important}.order-lg-2{order:2 !important}.order-lg-3{order:3 !important}.order-lg-4{order:4 !important}.order-lg-5{order:5 !important}.order-lg-last{order:6 !important}.m-lg-0{margin:0 !important}.m-lg-1{margin:.25rem !important}.m-lg-2{margin:.5rem !important}.m-lg-3{margin:1rem !important}.m-lg-4{margin:1.5rem !important}.m-lg-5{margin:3rem !important}.m-lg-auto{margin:auto !important}.mx-lg-0{margin-right:0 !important;margin-left:0 !important}.mx-lg-1{margin-right:.25rem !important;margin-left:.25rem !important}.mx-lg-2{margin-right:.5rem !important;margin-left:.5rem !important}.mx-lg-3{margin-right:1rem !important;margin-left:1rem !important}.mx-lg-4{margin-right:1.5rem !important;margin-left:1.5rem !important}.mx-lg-5{margin-right:3rem !important;margin-left:3rem !important}.mx-lg-auto{margin-right:auto !important;margin-left:auto !important}.my-lg-0{margin-top:0 !important;margin-bottom:0 !important}.my-lg-1{margin-top:.25rem !important;margin-bottom:.25rem !important}.my-lg-2{margin-top:.5rem !important;margin-bottom:.5rem !important}.my-lg-3{margin-top:1rem !important;margin-bottom:1rem !important}.my-lg-4{margin-top:1.5rem !important;margin-bottom:1.5rem !important}.my-lg-5{margin-top:3rem !important;margin-bottom:3rem !important}.my-lg-auto{margin-top:auto !important;margin-bottom:auto !important}.mt-lg-0{margin-top:0 !important}.mt-lg-1{margin-top:.25rem !important}.mt-lg-2{margin-top:.5rem !important}.mt-lg-3{margin-top:1rem !important}.mt-lg-4{margin-top:1.5rem !important}.mt-lg-5{margin-top:3rem !important}.mt-lg-auto{margin-top:auto !important}.me-lg-0{margin-right:0 !important}.me-lg-1{margin-right:.25rem !important}.me-lg-2{margin-right:.5rem !important}.me-lg-3{margin-right:1rem !important}.me-lg-4{margin-right:1.5rem !important}.me-lg-5{margin-right:3rem !important}.me-lg-auto{margin-right:auto !important}.mb-lg-0{margin-bottom:0 !important}.mb-lg-1{margin-bottom:.25rem !important}.mb-lg-2{margin-bottom:.5rem !important}.mb-lg-3{margin-bottom:1rem !important}.mb-lg-4{margin-bottom:1.5rem !important}.mb-lg-5{margin-bottom:3rem !important}.mb-lg-auto{margin-bottom:auto !important}.ms-lg-0{margin-left:0 !important}.ms-lg-1{margin-left:.25rem !important}.ms-lg-2{margin-left:.5rem !important}.ms-lg-3{margin-left:1rem !important}.ms-lg-4{margin-left:1.5rem !important}.ms-lg-5{margin-left:3rem !important}.ms-lg-auto{margin-left:auto !important}.p-lg-0{padding:0 !important}.p-lg-1{padding:.25rem !important}.p-lg-2{padding:.5rem !important}.p-lg-3{padding:1rem !important}.p-lg-4{padding:1.5rem !important}.p-lg-5{padding:3rem !important}.px-lg-0{padding-right:0 !important;padding-left:0 !important}.px-lg-1{padding-right:.25rem !important;padding-left:.25rem !important}.px-lg-2{padding-right:.5rem !important;padding-left:.5rem !important}.px-lg-3{padding-right:1rem !important;padding-left:1rem !important}.px-lg-4{padding-right:1.5rem !important;padding-left:1.5rem !important}.px-lg-5{padding-right:3rem !important;padding-left:3rem !important}.py-lg-0{padding-top:0 !important;padding-bottom:0 !important}.py-lg-1{padding-top:.25rem !important;padding-bottom:.25rem !important}.py-lg-2{padding-top:.5rem !important;padding-bottom:.5rem !important}.py-lg-3{padding-top:1rem !important;padding-bottom:1rem !important}.py-lg-4{padding-top:1.5rem !important;padding-bottom:1.5rem !important}.py-lg-5{padding-top:3rem !important;padding-bottom:3rem !important}.pt-lg-0{padding-top:0 !important}.pt-lg-1{padding-top:.25rem !important}.pt-lg-2{padding-top:.5rem !important}.pt-lg-3{padding-top:1rem !important}.pt-lg-4{padding-top:1.5rem !important}.pt-lg-5{padding-top:3rem !important}.pe-lg-0{padding-right:0 !important}.pe-lg-1{padding-right:.25rem !important}.pe-lg-2{padding-right:.5rem !important}.pe-lg-3{padding-right:1rem !important}.pe-lg-4{padding-right:1.5rem !important}.pe-lg-5{padding-right:3rem !important}.pb-lg-0{padding-bottom:0 !important}.pb-lg-1{padding-bottom:.25rem !important}.pb-lg-2{padding-bottom:.5rem !important}.pb-lg-3{padding-bottom:1rem !important}.pb-lg-4{padding-bottom:1.5rem !important}.pb-lg-5{padding-bottom:3rem !important}.ps-lg-0{padding-left:0 !important}.ps-lg-1{padding-left:.25rem !important}.ps-lg-2{padding-left:.5rem !important}.ps-lg-3{padding-left:1rem !important}.ps-lg-4{padding-left:1.5rem !important}.ps-lg-5{padding-left:3rem !important}.gap-lg-0{gap:0 !important}.gap-lg-1{gap:.25rem !important}.gap-lg-2{gap:.5rem !important}.gap-lg-3{gap:1rem !important}.gap-lg-4{gap:1.5rem !important}.gap-lg-5{gap:3rem !important}.row-gap-lg-0{row-gap:0 !important}.row-gap-lg-1{row-gap:.25rem !important}.row-gap-lg-2{row-gap:.5rem !important}.row-gap-lg-3{row-gap:1rem !important}.row-gap-lg-4{row-gap:1.5rem !important}.row-gap-lg-5{row-gap:3rem !important}.column-gap-lg-0{-moz-column-gap:0 !important;column-gap:0 !important}.column-gap-lg-1{-moz-column-gap:.25rem !important;column-gap:.25rem !important}.column-gap-lg-2{-moz-column-gap:.5rem !important;column-gap:.5rem !important}.column-gap-lg-3{-moz-column-gap:1rem !important;column-gap:1rem !important}.column-gap-lg-4{-moz-column-gap:1.5rem !important;column-gap:1.5rem !important}.column-gap-lg-5{-moz-column-gap:3rem !important;column-gap:3rem !important}.text-lg-start{text-align:left !important}.text-lg-end{text-align:right !important}.text-lg-center{text-align:center !important}}@media(min-width: 1200px){.float-xl-start{float:left !important}.float-xl-end{float:right !important}.float-xl-none{float:none !important}.object-fit-xl-contain{-o-object-fit:contain !important;object-fit:contain !important}.object-fit-xl-cover{-o-object-fit:cover !important;object-fit:cover !important}.object-fit-xl-fill{-o-object-fit:fill !important;object-fit:fill !important}.object-fit-xl-scale{-o-object-fit:scale-down !important;object-fit:scale-down !important}.object-fit-xl-none{-o-object-fit:none !important;object-fit:none !important}.d-xl-inline{display:inline !important}.d-xl-inline-block{display:inline-block !important}.d-xl-block{display:block !important}.d-xl-grid{display:grid !important}.d-xl-inline-grid{display:inline-grid !important}.d-xl-table{display:table !important}.d-xl-table-row{display:table-row !important}.d-xl-table-cell{display:table-cell !important}.d-xl-flex{display:flex !important}.d-xl-inline-flex{display:inline-flex !important}.d-xl-none{display:none !important}.flex-xl-fill{flex:1 1 auto !important}.flex-xl-row{flex-direction:row !important}.flex-xl-column{flex-direction:column !important}.flex-xl-row-reverse{flex-direction:row-reverse !important}.flex-xl-column-reverse{flex-direction:column-reverse !important}.flex-xl-grow-0{flex-grow:0 !important}.flex-xl-grow-1{flex-grow:1 !important}.flex-xl-shrink-0{flex-shrink:0 !important}.flex-xl-shrink-1{flex-shrink:1 !important}.flex-xl-wrap{flex-wrap:wrap !important}.flex-xl-nowrap{flex-wrap:nowrap !important}.flex-xl-wrap-reverse{flex-wrap:wrap-reverse !important}.justify-content-xl-start{justify-content:flex-start !important}.justify-content-xl-end{justify-content:flex-end !important}.justify-content-xl-center{justify-content:center !important}.justify-content-xl-between{justify-content:space-between !important}.justify-content-xl-around{justify-content:space-around !important}.justify-content-xl-evenly{justify-content:space-evenly !important}.align-items-xl-start{align-items:flex-start !important}.align-items-xl-end{align-items:flex-end !important}.align-items-xl-center{align-items:center !important}.align-items-xl-baseline{align-items:baseline !important}.align-items-xl-stretch{align-items:stretch !important}.align-content-xl-start{align-content:flex-start !important}.align-content-xl-end{align-content:flex-end !important}.align-content-xl-center{align-content:center !important}.align-content-xl-between{align-content:space-between !important}.align-content-xl-around{align-content:space-around !important}.align-content-xl-stretch{align-content:stretch !important}.align-self-xl-auto{align-self:auto !important}.align-self-xl-start{align-self:flex-start !important}.align-self-xl-end{align-self:flex-end !important}.align-self-xl-center{align-self:center !important}.align-self-xl-baseline{align-self:baseline !important}.align-self-xl-stretch{align-self:stretch !important}.order-xl-first{order:-1 !important}.order-xl-0{order:0 !important}.order-xl-1{order:1 !important}.order-xl-2{order:2 !important}.order-xl-3{order:3 !important}.order-xl-4{order:4 !important}.order-xl-5{order:5 !important}.order-xl-last{order:6 !important}.m-xl-0{margin:0 !important}.m-xl-1{margin:.25rem !important}.m-xl-2{margin:.5rem !important}.m-xl-3{margin:1rem !important}.m-xl-4{margin:1.5rem !important}.m-xl-5{margin:3rem !important}.m-xl-auto{margin:auto !important}.mx-xl-0{margin-right:0 !important;margin-left:0 !important}.mx-xl-1{margin-right:.25rem !important;margin-left:.25rem !important}.mx-xl-2{margin-right:.5rem !important;margin-left:.5rem !important}.mx-xl-3{margin-right:1rem !important;margin-left:1rem !important}.mx-xl-4{margin-right:1.5rem !important;margin-left:1.5rem !important}.mx-xl-5{margin-right:3rem !important;margin-left:3rem !important}.mx-xl-auto{margin-right:auto !important;margin-left:auto !important}.my-xl-0{margin-top:0 !important;margin-bottom:0 !important}.my-xl-1{margin-top:.25rem !important;margin-bottom:.25rem !important}.my-xl-2{margin-top:.5rem !important;margin-bottom:.5rem !important}.my-xl-3{margin-top:1rem !important;margin-bottom:1rem !important}.my-xl-4{margin-top:1.5rem !important;margin-bottom:1.5rem !important}.my-xl-5{margin-top:3rem !important;margin-bottom:3rem !important}.my-xl-auto{margin-top:auto !important;margin-bottom:auto !important}.mt-xl-0{margin-top:0 !important}.mt-xl-1{margin-top:.25rem !important}.mt-xl-2{margin-top:.5rem !important}.mt-xl-3{margin-top:1rem !important}.mt-xl-4{margin-top:1.5rem !important}.mt-xl-5{margin-top:3rem !important}.mt-xl-auto{margin-top:auto !important}.me-xl-0{margin-right:0 !important}.me-xl-1{margin-right:.25rem !important}.me-xl-2{margin-right:.5rem !important}.me-xl-3{margin-right:1rem !important}.me-xl-4{margin-right:1.5rem !important}.me-xl-5{margin-right:3rem !important}.me-xl-auto{margin-right:auto !important}.mb-xl-0{margin-bottom:0 !important}.mb-xl-1{margin-bottom:.25rem !important}.mb-xl-2{margin-bottom:.5rem !important}.mb-xl-3{margin-bottom:1rem !important}.mb-xl-4{margin-bottom:1.5rem !important}.mb-xl-5{margin-bottom:3rem !important}.mb-xl-auto{margin-bottom:auto !important}.ms-xl-0{margin-left:0 !important}.ms-xl-1{margin-left:.25rem !important}.ms-xl-2{margin-left:.5rem !important}.ms-xl-3{margin-left:1rem !important}.ms-xl-4{margin-left:1.5rem !important}.ms-xl-5{margin-left:3rem !important}.ms-xl-auto{margin-left:auto !important}.p-xl-0{padding:0 !important}.p-xl-1{padding:.25rem !important}.p-xl-2{padding:.5rem !important}.p-xl-3{padding:1rem !important}.p-xl-4{padding:1.5rem !important}.p-xl-5{padding:3rem !important}.px-xl-0{padding-right:0 !important;padding-left:0 !important}.px-xl-1{padding-right:.25rem !important;padding-left:.25rem !important}.px-xl-2{padding-right:.5rem !important;padding-left:.5rem !important}.px-xl-3{padding-right:1rem !important;padding-left:1rem !important}.px-xl-4{padding-right:1.5rem !important;padding-left:1.5rem !important}.px-xl-5{padding-right:3rem !important;padding-left:3rem !important}.py-xl-0{padding-top:0 !important;padding-bottom:0 !important}.py-xl-1{padding-top:.25rem !important;padding-bottom:.25rem !important}.py-xl-2{padding-top:.5rem !important;padding-bottom:.5rem !important}.py-xl-3{padding-top:1rem !important;padding-bottom:1rem !important}.py-xl-4{padding-top:1.5rem !important;padding-bottom:1.5rem !important}.py-xl-5{padding-top:3rem !important;padding-bottom:3rem !important}.pt-xl-0{padding-top:0 !important}.pt-xl-1{padding-top:.25rem !important}.pt-xl-2{padding-top:.5rem !important}.pt-xl-3{padding-top:1rem !important}.pt-xl-4{padding-top:1.5rem !important}.pt-xl-5{padding-top:3rem !important}.pe-xl-0{padding-right:0 !important}.pe-xl-1{padding-right:.25rem !important}.pe-xl-2{padding-right:.5rem !important}.pe-xl-3{padding-right:1rem !important}.pe-xl-4{padding-right:1.5rem !important}.pe-xl-5{padding-right:3rem !important}.pb-xl-0{padding-bottom:0 !important}.pb-xl-1{padding-bottom:.25rem !important}.pb-xl-2{padding-bottom:.5rem !important}.pb-xl-3{padding-bottom:1rem !important}.pb-xl-4{padding-bottom:1.5rem !important}.pb-xl-5{padding-bottom:3rem !important}.ps-xl-0{padding-left:0 !important}.ps-xl-1{padding-left:.25rem !important}.ps-xl-2{padding-left:.5rem !important}.ps-xl-3{padding-left:1rem !important}.ps-xl-4{padding-left:1.5rem !important}.ps-xl-5{padding-left:3rem !important}.gap-xl-0{gap:0 !important}.gap-xl-1{gap:.25rem !important}.gap-xl-2{gap:.5rem !important}.gap-xl-3{gap:1rem !important}.gap-xl-4{gap:1.5rem !important}.gap-xl-5{gap:3rem !important}.row-gap-xl-0{row-gap:0 !important}.row-gap-xl-1{row-gap:.25rem !important}.row-gap-xl-2{row-gap:.5rem !important}.row-gap-xl-3{row-gap:1rem !important}.row-gap-xl-4{row-gap:1.5rem !important}.row-gap-xl-5{row-gap:3rem !important}.column-gap-xl-0{-moz-column-gap:0 !important;column-gap:0 !important}.column-gap-xl-1{-moz-column-gap:.25rem !important;column-gap:.25rem !important}.column-gap-xl-2{-moz-column-gap:.5rem !important;column-gap:.5rem !important}.column-gap-xl-3{-moz-column-gap:1rem !important;column-gap:1rem !important}.column-gap-xl-4{-moz-column-gap:1.5rem !important;column-gap:1.5rem !important}.column-gap-xl-5{-moz-column-gap:3rem !important;column-gap:3rem !important}.text-xl-start{text-align:left !important}.text-xl-end{text-align:right !important}.text-xl-center{text-align:center !important}}@media(min-width: 1400px){.float-xxl-start{float:left !important}.float-xxl-end{float:right !important}.float-xxl-none{float:none !important}.object-fit-xxl-contain{-o-object-fit:contain !important;object-fit:contain !important}.object-fit-xxl-cover{-o-object-fit:cover !important;object-fit:cover !important}.object-fit-xxl-fill{-o-object-fit:fill !important;object-fit:fill !important}.object-fit-xxl-scale{-o-object-fit:scale-down !important;object-fit:scale-down !important}.object-fit-xxl-none{-o-object-fit:none !important;object-fit:none !important}.d-xxl-inline{display:inline !important}.d-xxl-inline-block{display:inline-block !important}.d-xxl-block{display:block !important}.d-xxl-grid{display:grid !important}.d-xxl-inline-grid{display:inline-grid !important}.d-xxl-table{display:table !important}.d-xxl-table-row{display:table-row !important}.d-xxl-table-cell{display:table-cell !important}.d-xxl-flex{display:flex !important}.d-xxl-inline-flex{display:inline-flex !important}.d-xxl-none{display:none !important}.flex-xxl-fill{flex:1 1 auto !important}.flex-xxl-row{flex-direction:row !important}.flex-xxl-column{flex-direction:column !important}.flex-xxl-row-reverse{flex-direction:row-reverse !important}.flex-xxl-column-reverse{flex-direction:column-reverse !important}.flex-xxl-grow-0{flex-grow:0 !important}.flex-xxl-grow-1{flex-grow:1 !important}.flex-xxl-shrink-0{flex-shrink:0 !important}.flex-xxl-shrink-1{flex-shrink:1 !important}.flex-xxl-wrap{flex-wrap:wrap !important}.flex-xxl-nowrap{flex-wrap:nowrap !important}.flex-xxl-wrap-reverse{flex-wrap:wrap-reverse !important}.justify-content-xxl-start{justify-content:flex-start !important}.justify-content-xxl-end{justify-content:flex-end !important}.justify-content-xxl-center{justify-content:center !important}.justify-content-xxl-between{justify-content:space-between !important}.justify-content-xxl-around{justify-content:space-around !important}.justify-content-xxl-evenly{justify-content:space-evenly !important}.align-items-xxl-start{align-items:flex-start !important}.align-items-xxl-end{align-items:flex-end !important}.align-items-xxl-center{align-items:center !important}.align-items-xxl-baseline{align-items:baseline !important}.align-items-xxl-stretch{align-items:stretch !important}.align-content-xxl-start{align-content:flex-start !important}.align-content-xxl-end{align-content:flex-end !important}.align-content-xxl-center{align-content:center !important}.align-content-xxl-between{align-content:space-between !important}.align-content-xxl-around{align-content:space-around !important}.align-content-xxl-stretch{align-content:stretch !important}.align-self-xxl-auto{align-self:auto !important}.align-self-xxl-start{align-self:flex-start !important}.align-self-xxl-end{align-self:flex-end !important}.align-self-xxl-center{align-self:center !important}.align-self-xxl-baseline{align-self:baseline !important}.align-self-xxl-stretch{align-self:stretch !important}.order-xxl-first{order:-1 !important}.order-xxl-0{order:0 !important}.order-xxl-1{order:1 !important}.order-xxl-2{order:2 !important}.order-xxl-3{order:3 !important}.order-xxl-4{order:4 !important}.order-xxl-5{order:5 !important}.order-xxl-last{order:6 !important}.m-xxl-0{margin:0 !important}.m-xxl-1{margin:.25rem !important}.m-xxl-2{margin:.5rem !important}.m-xxl-3{margin:1rem !important}.m-xxl-4{margin:1.5rem !important}.m-xxl-5{margin:3rem !important}.m-xxl-auto{margin:auto !important}.mx-xxl-0{margin-right:0 !important;margin-left:0 !important}.mx-xxl-1{margin-right:.25rem !important;margin-left:.25rem !important}.mx-xxl-2{margin-right:.5rem !important;margin-left:.5rem !important}.mx-xxl-3{margin-right:1rem !important;margin-left:1rem !important}.mx-xxl-4{margin-right:1.5rem !important;margin-left:1.5rem !important}.mx-xxl-5{margin-right:3rem !important;margin-left:3rem !important}.mx-xxl-auto{margin-right:auto !important;margin-left:auto !important}.my-xxl-0{margin-top:0 !important;margin-bottom:0 !important}.my-xxl-1{margin-top:.25rem !important;margin-bottom:.25rem !important}.my-xxl-2{margin-top:.5rem !important;margin-bottom:.5rem !important}.my-xxl-3{margin-top:1rem !important;margin-bottom:1rem !important}.my-xxl-4{margin-top:1.5rem !important;margin-bottom:1.5rem !important}.my-xxl-5{margin-top:3rem !important;margin-bottom:3rem !important}.my-xxl-auto{margin-top:auto !important;margin-bottom:auto !important}.mt-xxl-0{margin-top:0 !important}.mt-xxl-1{margin-top:.25rem !important}.mt-xxl-2{margin-top:.5rem !important}.mt-xxl-3{margin-top:1rem !important}.mt-xxl-4{margin-top:1.5rem !important}.mt-xxl-5{margin-top:3rem !important}.mt-xxl-auto{margin-top:auto !important}.me-xxl-0{margin-right:0 !important}.me-xxl-1{margin-right:.25rem !important}.me-xxl-2{margin-right:.5rem !important}.me-xxl-3{margin-right:1rem !important}.me-xxl-4{margin-right:1.5rem !important}.me-xxl-5{margin-right:3rem !important}.me-xxl-auto{margin-right:auto !important}.mb-xxl-0{margin-bottom:0 !important}.mb-xxl-1{margin-bottom:.25rem !important}.mb-xxl-2{margin-bottom:.5rem !important}.mb-xxl-3{margin-bottom:1rem !important}.mb-xxl-4{margin-bottom:1.5rem !important}.mb-xxl-5{margin-bottom:3rem !important}.mb-xxl-auto{margin-bottom:auto !important}.ms-xxl-0{margin-left:0 !important}.ms-xxl-1{margin-left:.25rem !important}.ms-xxl-2{margin-left:.5rem !important}.ms-xxl-3{margin-left:1rem !important}.ms-xxl-4{margin-left:1.5rem !important}.ms-xxl-5{margin-left:3rem !important}.ms-xxl-auto{margin-left:auto !important}.p-xxl-0{padding:0 !important}.p-xxl-1{padding:.25rem !important}.p-xxl-2{padding:.5rem !important}.p-xxl-3{padding:1rem !important}.p-xxl-4{padding:1.5rem !important}.p-xxl-5{padding:3rem !important}.px-xxl-0{padding-right:0 !important;padding-left:0 !important}.px-xxl-1{padding-right:.25rem !important;padding-left:.25rem !important}.px-xxl-2{padding-right:.5rem !important;padding-left:.5rem !important}.px-xxl-3{padding-right:1rem !important;padding-left:1rem !important}.px-xxl-4{padding-right:1.5rem !important;padding-left:1.5rem !important}.px-xxl-5{padding-right:3rem !important;padding-left:3rem !important}.py-xxl-0{padding-top:0 !important;padding-bottom:0 !important}.py-xxl-1{padding-top:.25rem !important;padding-bottom:.25rem !important}.py-xxl-2{padding-top:.5rem !important;padding-bottom:.5rem !important}.py-xxl-3{padding-top:1rem !important;padding-bottom:1rem !important}.py-xxl-4{padding-top:1.5rem !important;padding-bottom:1.5rem !important}.py-xxl-5{padding-top:3rem !important;padding-bottom:3rem !important}.pt-xxl-0{padding-top:0 !important}.pt-xxl-1{padding-top:.25rem !important}.pt-xxl-2{padding-top:.5rem !important}.pt-xxl-3{padding-top:1rem !important}.pt-xxl-4{padding-top:1.5rem !important}.pt-xxl-5{padding-top:3rem !important}.pe-xxl-0{padding-right:0 !important}.pe-xxl-1{padding-right:.25rem !important}.pe-xxl-2{padding-right:.5rem !important}.pe-xxl-3{padding-right:1rem !important}.pe-xxl-4{padding-right:1.5rem !important}.pe-xxl-5{padding-right:3rem !important}.pb-xxl-0{padding-bottom:0 !important}.pb-xxl-1{padding-bottom:.25rem !important}.pb-xxl-2{padding-bottom:.5rem !important}.pb-xxl-3{padding-bottom:1rem !important}.pb-xxl-4{padding-bottom:1.5rem !important}.pb-xxl-5{padding-bottom:3rem !important}.ps-xxl-0{padding-left:0 !important}.ps-xxl-1{padding-left:.25rem !important}.ps-xxl-2{padding-left:.5rem !important}.ps-xxl-3{padding-left:1rem !important}.ps-xxl-4{padding-left:1.5rem !important}.ps-xxl-5{padding-left:3rem !important}.gap-xxl-0{gap:0 !important}.gap-xxl-1{gap:.25rem !important}.gap-xxl-2{gap:.5rem !important}.gap-xxl-3{gap:1rem !important}.gap-xxl-4{gap:1.5rem !important}.gap-xxl-5{gap:3rem !important}.row-gap-xxl-0{row-gap:0 !important}.row-gap-xxl-1{row-gap:.25rem !important}.row-gap-xxl-2{row-gap:.5rem !important}.row-gap-xxl-3{row-gap:1rem !important}.row-gap-xxl-4{row-gap:1.5rem !important}.row-gap-xxl-5{row-gap:3rem !important}.column-gap-xxl-0{-moz-column-gap:0 !important;column-gap:0 !important}.column-gap-xxl-1{-moz-column-gap:.25rem !important;column-gap:.25rem !important}.column-gap-xxl-2{-moz-column-gap:.5rem !important;column-gap:.5rem !important}.column-gap-xxl-3{-moz-column-gap:1rem !important;column-gap:1rem !important}.column-gap-xxl-4{-moz-column-gap:1.5rem !important;column-gap:1.5rem !important}.column-gap-xxl-5{-moz-column-gap:3rem !important;column-gap:3rem !important}.text-xxl-start{text-align:left !important}.text-xxl-end{text-align:right !important}.text-xxl-center{text-align:center !important}}@media(min-width: 1200px){.fs-1{font-size:2.5rem !important}.fs-2{font-size:2rem !important}.fs-3{font-size:1.75rem !important}.fs-4{font-size:1.5rem !important}}@media print{.d-print-inline{display:inline !important}.d-print-inline-block{display:inline-block !important}.d-print-block{display:block !important}.d-print-grid{display:grid !important}.d-print-inline-grid{display:inline-grid !important}.d-print-table{display:table !important}.d-print-table-row{display:table-row !important}.d-print-table-cell{display:table-cell !important}.d-print-flex{display:flex !important}.d-print-inline-flex{display:inline-flex !important}.d-print-none{display:none !important}}body,html{width:100%;height:100%}body{font-family:"Source Sans Pro"}.btn-xl{padding:1.25rem 2.5rem}.content-section{padding-top:7.5rem;padding-bottom:7.5rem}.content-section-heading h2,.content-section-heading .h2{font-size:3rem}.content-section-heading h3,.content-section-heading .h3{font-size:1rem;text-transform:uppercase}h1,.h1,h2,.h2,h3,.h3,h4,.h4,h5,.h5,h6,.h6{font-weight:700}.text-faded{color:rgba(255,255,255,.7)}.map{height:30rem}@media(max-width: 992px){.map{height:75%}}.map iframe{pointer-events:none}.scroll-to-top{position:fixed;right:15px;bottom:15px;display:none;width:50px;height:50px;text-align:center;color:#fff;background:rgba(52,58,64,.5);line-height:45px}.scroll-to-top:focus,.scroll-to-top:hover{color:#fff}.scroll-to-top:hover{background:#343a40}.scroll-to-top i{font-weight:800}.masthead{min-height:30rem;position:relative;display:table;width:100%;height:auto;padding-top:8rem;padding-bottom:8rem;background:linear-gradient(90deg, rgba(255, 255, 255, 0.1) 0%, rgba(255, 255, 255, 0.1) 100%);background-position:center center;background-repeat:no-repeat;background-size:cover}.masthead h1,.masthead .h1{font-size:4rem;margin:0;padding:0}@media(min-width: 992px){.masthead{height:100vh}.masthead h1,.masthead .h1{font-size:5.5rem}}#sidebar-wrapper{position:fixed;z-index:2;right:0;width:250px;height:100%;transition:all .4s ease 0s;transform:translateX(250px);background:#1d809f;border-left:1px solid rgba(255,255,255,.1)}.sidebar-nav{position:absolute;top:0;left:0;width:250px;margin:0;padding:0;list-style:none}.sidebar-nav li.sidebar-nav-item a{display:block;text-decoration:none;color:#fff;padding:15px}.sidebar-nav li a:hover{text-decoration:none;color:#fff;background:rgba(255,255,255,.2)}.sidebar-nav li a:active,.sidebar-nav li a:focus{text-decoration:none}.sidebar-nav>.sidebar-brand{font-size:1.2rem;background:rgba(52,58,64,.1);height:80px;line-height:50px;padding-top:15px;padding-bottom:15px;padding-left:15px}.sidebar-nav>.sidebar-brand a{color:#fff}.sidebar-nav>.sidebar-brand a:hover{color:#fff;background:none}#sidebar-wrapper.active{right:250px;width:250px;transition:all .4s ease 0s}.menu-toggle{position:fixed;right:15px;top:15px;width:50px;height:50px;text-align:center;color:#fff;background:rgba(52,58,64,.5);line-height:50px;z-index:999}.menu-toggle:focus,.menu-toggle:hover{color:#fff}.menu-toggle:hover{background:#343a40}.service-icon{background-color:#fff;color:#1d809f;height:7rem;width:7rem;display:block;line-height:7.5rem;font-size:2.25rem;box-shadow:0 3px 3px 0 rgba(0,0,0,.1)}.callout{padding:15rem 0;background:linear-gradient(90deg, rgba(255, 255, 255, 0.1) 0%, rgba(255, 255, 255, 0.1) 100%);background-position:center center;background-repeat:no-repeat;background-size:cover}.callout h2,.callout .h2{font-size:3.5rem;font-weight:700;display:block;max-width:30rem}.portfolio-item{display:block;position:relative;overflow:hidden;max-width:530px;margin:auto auto 1rem}.portfolio-item .caption{display:flex;height:100%;width:100%;background-color:rgba(33,37,41,.2);position:absolute;top:0;bottom:0;z-index:1}.portfolio-item .caption .caption-content{color:#fff;margin:auto 2rem 2rem}.portfolio-item .caption .caption-content h2,.portfolio-item .caption .caption-content .h2{font-size:.8rem;text-transform:uppercase}.portfolio-item .caption .caption-content p{font-weight:300;font-size:1.2rem}@media(min-width: 992px){.portfolio-item{max-width:none;margin:0}.portfolio-item .caption{-webkit-transition:-webkit-clip-path .25s ease-out,background-color .7s;-webkit-clip-path:inset(0px);clip-path:inset(0px)}.portfolio-item .caption .caption-content{transition:opacity .25s;margin-left:5rem;margin-right:5rem;margin-bottom:5rem}.portfolio-item img{-webkit-transition:-webkit-clip-path .25s ease-out;-webkit-clip-path:inset(-1px);clip-path:inset(-1px)}.portfolio-item:hover img{-webkit-clip-path:inset(2rem);clip-path:inset(2rem)}.portfolio-item:hover .caption{background-color:rgba(29,128,159,.9);-webkit-clip-path:inset(2rem);clip-path:inset(2rem)}}#sidebar-nav{display:flex;flex-direction:column;justify-content:flex-start;color:#fff !important;padding:0px}#sidebar-nav a{color:#fff}#sidebar-wrapper,#sidebar-nav li{padding:0}footer.footer{padding-top:5rem;padding-bottom:5rem}footer.footer .social-link{display:block;height:4rem;width:4rem;line-height:4.3rem;font-size:1.5rem;background-color:#1d809f;transition:background-color .15s ease-in-out;box-shadow:0 3px 3px 0 rgba(0,0,0,.1);text-decoration:none}footer.footer .social-link:hover{background-color:#1a738f}
\ No newline at end of file
diff --git a/spaces/Fengbinbin/gpt-academic/crazy_functions/test_project/cpp/cppipc/prod_cons.h b/spaces/Fengbinbin/gpt-academic/crazy_functions/test_project/cpp/cppipc/prod_cons.h
deleted file mode 100644
index c9004bb8043a12e32814436baa6262a00c8ef68e..0000000000000000000000000000000000000000
--- a/spaces/Fengbinbin/gpt-academic/crazy_functions/test_project/cpp/cppipc/prod_cons.h
+++ /dev/null
@@ -1,433 +0,0 @@
-#pragma once
-
-#include
-#include
-#include
-#include
-#include
-
-#include "libipc/def.h"
-
-#include "libipc/platform/detail.h"
-#include "libipc/circ/elem_def.h"
-#include "libipc/utility/log.h"
-#include "libipc/utility/utility.h"
-
-namespace ipc {
-
-////////////////////////////////////////////////////////////////
-/// producer-consumer implementation
-////////////////////////////////////////////////////////////////
-
-template
-struct prod_cons_impl;
-
-template <>
-struct prod_cons_impl> {
-
- template
- struct elem_t {
- std::aligned_storage_t data_ {};
- };
-
- alignas(cache_line_size) std::atomic rd_; // read index
- alignas(cache_line_size) std::atomic wt_; // write index
-
- constexpr circ::u2_t cursor() const noexcept {
- return 0;
- }
-
- template
- bool push(W* /*wrapper*/, F&& f, E* elems) {
- auto cur_wt = circ::index_of(wt_.load(std::memory_order_relaxed));
- if (cur_wt == circ::index_of(rd_.load(std::memory_order_acquire) - 1)) {
- return false; // full
- }
- std::forward(f)(&(elems[cur_wt].data_));
- wt_.fetch_add(1, std::memory_order_release);
- return true;
- }
-
- /**
- * In single-single-unicast, 'force_push' means 'no reader' or 'the only one reader is dead'.
- * So we could just disconnect all connections of receiver, and return false.
- */
- template
- bool force_push(W* wrapper, F&&, E*) {
- wrapper->elems()->disconnect_receiver(~static_cast(0u));
- return false;
- }
-
- template
- bool pop(W* /*wrapper*/, circ::u2_t& /*cur*/, F&& f, R&& out, E* elems) {
- auto cur_rd = circ::index_of(rd_.load(std::memory_order_relaxed));
- if (cur_rd == circ::index_of(wt_.load(std::memory_order_acquire))) {
- return false; // empty
- }
- std::forward(f)(&(elems[cur_rd].data_));
- std::forward(out)(true);
- rd_.fetch_add(1, std::memory_order_release);
- return true;
- }
-};
-
-template <>
-struct prod_cons_impl>
- : prod_cons_impl> {
-
- template
- bool force_push(W* wrapper, F&&, E*) {
- wrapper->elems()->disconnect_receiver(1);
- return false;
- }
-
- template class E, std::size_t DS, std::size_t AS>
- bool pop(W* /*wrapper*/, circ::u2_t& /*cur*/, F&& f, R&& out, E* elems) {
- byte_t buff[DS];
- for (unsigned k = 0;;) {
- auto cur_rd = rd_.load(std::memory_order_relaxed);
- if (circ::index_of(cur_rd) ==
- circ::index_of(wt_.load(std::memory_order_acquire))) {
- return false; // empty
- }
- std::memcpy(buff, &(elems[circ::index_of(cur_rd)].data_), sizeof(buff));
- if (rd_.compare_exchange_weak(cur_rd, cur_rd + 1, std::memory_order_release)) {
- std::forward(f)(buff);
- std::forward(out)(true);
- return true;
- }
- ipc::yield(k);
- }
- }
-};
-
-template <>
-struct prod_cons_impl>
- : prod_cons_impl> {
-
- using flag_t = std::uint64_t;
-
- template
- struct elem_t {
- std::aligned_storage_t data_ {};
- std::atomic f_ct_ { 0 }; // commit flag
- };
-
- alignas(cache_line_size) std::atomic ct_; // commit index
-
- template
- bool push(W* /*wrapper*/, F&& f, E* elems) {
- circ::u2_t cur_ct, nxt_ct;
- for (unsigned k = 0;;) {
- cur_ct = ct_.load(std::memory_order_relaxed);
- if (circ::index_of(nxt_ct = cur_ct + 1) ==
- circ::index_of(rd_.load(std::memory_order_acquire))) {
- return false; // full
- }
- if (ct_.compare_exchange_weak(cur_ct, nxt_ct, std::memory_order_acq_rel)) {
- break;
- }
- ipc::yield(k);
- }
- auto* el = elems + circ::index_of(cur_ct);
- std::forward(f)(&(el->data_));
- // set flag & try update wt
- el->f_ct_.store(~static_cast(cur_ct), std::memory_order_release);
- while (1) {
- auto cac_ct = el->f_ct_.load(std::memory_order_acquire);
- if (cur_ct != wt_.load(std::memory_order_relaxed)) {
- return true;
- }
- if ((~cac_ct) != cur_ct) {
- return true;
- }
- if (!el->f_ct_.compare_exchange_strong(cac_ct, 0, std::memory_order_relaxed)) {
- return true;
- }
- wt_.store(nxt_ct, std::memory_order_release);
- cur_ct = nxt_ct;
- nxt_ct = cur_ct + 1;
- el = elems + circ::index_of(cur_ct);
- }
- return true;
- }
-
- template
- bool force_push(W* wrapper, F&&, E*) {
- wrapper->elems()->disconnect_receiver(1);
- return false;
- }
-
- template class E, std::size_t DS, std::size_t AS>
- bool pop(W* /*wrapper*/, circ::u2_t& /*cur*/, F&& f, R&& out, E* elems) {
- byte_t buff[DS];
- for (unsigned k = 0;;) {
- auto cur_rd = rd_.load(std::memory_order_relaxed);
- auto cur_wt = wt_.load(std::memory_order_acquire);
- auto id_rd = circ::index_of(cur_rd);
- auto id_wt = circ::index_of(cur_wt);
- if (id_rd == id_wt) {
- auto* el = elems + id_wt;
- auto cac_ct = el->f_ct_.load(std::memory_order_acquire);
- if ((~cac_ct) != cur_wt) {
- return false; // empty
- }
- if (el->f_ct_.compare_exchange_weak(cac_ct, 0, std::memory_order_relaxed)) {
- wt_.store(cur_wt + 1, std::memory_order_release);
- }
- k = 0;
- }
- else {
- std::memcpy(buff, &(elems[circ::index_of(cur_rd)].data_), sizeof(buff));
- if (rd_.compare_exchange_weak(cur_rd, cur_rd + 1, std::memory_order_release)) {
- std::forward(f)(buff);
- std::forward(out)(true);
- return true;
- }
- ipc::yield(k);
- }
- }
- }
-};
-
-template <>
-struct prod_cons_impl> {
-
- using rc_t = std::uint64_t;
-
- enum : rc_t {
- ep_mask = 0x00000000ffffffffull,
- ep_incr = 0x0000000100000000ull
- };
-
- template
- struct elem_t {
- std::aligned_storage_t data_ {};
- std::atomic rc_ { 0 }; // read-counter
- };
-
- alignas(cache_line_size) std::atomic wt_; // write index
- alignas(cache_line_size) rc_t epoch_ { 0 }; // only one writer
-
- circ::u2_t cursor() const noexcept {
- return wt_.load(std::memory_order_acquire);
- }
-
- template
- bool push(W* wrapper, F&& f, E* elems) {
- E* el;
- for (unsigned k = 0;;) {
- circ::cc_t cc = wrapper->elems()->connections(std::memory_order_relaxed);
- if (cc == 0) return false; // no reader
- el = elems + circ::index_of(wt_.load(std::memory_order_relaxed));
- // check all consumers have finished reading this element
- auto cur_rc = el->rc_.load(std::memory_order_acquire);
- circ::cc_t rem_cc = cur_rc & ep_mask;
- if ((cc & rem_cc) && ((cur_rc & ~ep_mask) == epoch_)) {
- return false; // has not finished yet
- }
- // consider rem_cc to be 0 here
- if (el->rc_.compare_exchange_weak(
- cur_rc, epoch_ | static_cast(cc), std::memory_order_release)) {
- break;
- }
- ipc::yield(k);
- }
- std::forward(f)(&(el->data_));
- wt_.fetch_add(1, std::memory_order_release);
- return true;
- }
-
- template
- bool force_push(W* wrapper, F&& f, E* elems) {
- E* el;
- epoch_ += ep_incr;
- for (unsigned k = 0;;) {
- circ::cc_t cc = wrapper->elems()->connections(std::memory_order_relaxed);
- if (cc == 0) return false; // no reader
- el = elems + circ::index_of(wt_.load(std::memory_order_relaxed));
- // check all consumers have finished reading this element
- auto cur_rc = el->rc_.load(std::memory_order_acquire);
- circ::cc_t rem_cc = cur_rc & ep_mask;
- if (cc & rem_cc) {
- ipc::log("force_push: k = %u, cc = %u, rem_cc = %u\n", k, cc, rem_cc);
- cc = wrapper->elems()->disconnect_receiver(rem_cc); // disconnect all invalid readers
- if (cc == 0) return false; // no reader
- }
- // just compare & exchange
- if (el->rc_.compare_exchange_weak(
- cur_rc, epoch_ | static_cast(cc), std::memory_order_release)) {
- break;
- }
- ipc::yield(k);
- }
- std::forward(f)(&(el->data_));
- wt_.fetch_add(1, std::memory_order_release);
- return true;
- }
-
- template
- bool pop(W* wrapper, circ::u2_t& cur, F&& f, R&& out, E* elems) {
- if (cur == cursor()) return false; // acquire
- auto* el = elems + circ::index_of(cur++);
- std::forward(f)(&(el->data_));
- for (unsigned k = 0;;) {
- auto cur_rc = el->rc_.load(std::memory_order_acquire);
- if ((cur_rc & ep_mask) == 0) {
- std::forward(out)(true);
- return true;
- }
- auto nxt_rc = cur_rc & ~static_cast(wrapper->connected_id());
- if (el->rc_.compare_exchange_weak(cur_rc, nxt_rc, std::memory_order_release)) {
- std::forward(out)((nxt_rc & ep_mask) == 0);
- return true;
- }
- ipc::yield(k);
- }
- }
-};
-
-template <>
-struct prod_cons_impl> {
-
- using rc_t = std::uint64_t;
- using flag_t = std::uint64_t;
-
- enum : rc_t {
- rc_mask = 0x00000000ffffffffull,
- ep_mask = 0x00ffffffffffffffull,
- ep_incr = 0x0100000000000000ull,
- ic_mask = 0xff000000ffffffffull,
- ic_incr = 0x0000000100000000ull
- };
-
- template
- struct elem_t {
- std::aligned_storage_t data_ {};
- std::atomic rc_ { 0 }; // read-counter
- std::atomic f_ct_ { 0 }; // commit flag
- };
-
- alignas(cache_line_size) std::atomic ct_; // commit index
- alignas(cache_line_size) std::atomic epoch_ { 0 };
-
- circ::u2_t cursor() const noexcept {
- return ct_.load(std::memory_order_acquire);
- }
-
- constexpr static rc_t inc_rc(rc_t rc) noexcept {
- return (rc & ic_mask) | ((rc + ic_incr) & ~ic_mask);
- }
-
- constexpr static rc_t inc_mask(rc_t rc) noexcept {
- return inc_rc(rc) & ~rc_mask;
- }
-
- template
- bool push(W* wrapper, F&& f, E* elems) {
- E* el;
- circ::u2_t cur_ct;
- rc_t epoch = epoch_.load(std::memory_order_acquire);
- for (unsigned k = 0;;) {
- circ::cc_t cc = wrapper->elems()->connections(std::memory_order_relaxed);
- if (cc == 0) return false; // no reader
- el = elems + circ::index_of(cur_ct = ct_.load(std::memory_order_relaxed));
- // check all consumers have finished reading this element
- auto cur_rc = el->rc_.load(std::memory_order_relaxed);
- circ::cc_t rem_cc = cur_rc & rc_mask;
- if ((cc & rem_cc) && ((cur_rc & ~ep_mask) == epoch)) {
- return false; // has not finished yet
- }
- else if (!rem_cc) {
- auto cur_fl = el->f_ct_.load(std::memory_order_acquire);
- if ((cur_fl != cur_ct) && cur_fl) {
- return false; // full
- }
- }
- // consider rem_cc to be 0 here
- if (el->rc_.compare_exchange_weak(
- cur_rc, inc_mask(epoch | (cur_rc & ep_mask)) | static_cast(cc), std::memory_order_relaxed) &&
- epoch_.compare_exchange_weak(epoch, epoch, std::memory_order_acq_rel)) {
- break;
- }
- ipc::yield(k);
- }
- // only one thread/process would touch here at one time
- ct_.store(cur_ct + 1, std::memory_order_release);
- std::forward(f)(&(el->data_));
- // set flag & try update wt
- el->f_ct_.store(~static_cast(cur_ct), std::memory_order_release);
- return true;
- }
-
- template
- bool force_push(W* wrapper, F&& f, E* elems) {
- E* el;
- circ::u2_t cur_ct;
- rc_t epoch = epoch_.fetch_add(ep_incr, std::memory_order_release) + ep_incr;
- for (unsigned k = 0;;) {
- circ::cc_t cc = wrapper->elems()->connections(std::memory_order_relaxed);
- if (cc == 0) return false; // no reader
- el = elems + circ::index_of(cur_ct = ct_.load(std::memory_order_relaxed));
- // check all consumers have finished reading this element
- auto cur_rc = el->rc_.load(std::memory_order_acquire);
- circ::cc_t rem_cc = cur_rc & rc_mask;
- if (cc & rem_cc) {
- ipc::log("force_push: k = %u, cc = %u, rem_cc = %u\n", k, cc, rem_cc);
- cc = wrapper->elems()->disconnect_receiver(rem_cc); // disconnect all invalid readers
- if (cc == 0) return false; // no reader
- }
- // just compare & exchange
- if (el->rc_.compare_exchange_weak(
- cur_rc, inc_mask(epoch | (cur_rc & ep_mask)) | static_cast(cc), std::memory_order_relaxed)) {
- if (epoch == epoch_.load(std::memory_order_acquire)) {
- break;
- }
- else if (push(wrapper, std::forward(f), elems)) {
- return true;
- }
- epoch = epoch_.fetch_add(ep_incr, std::memory_order_release) + ep_incr;
- }
- ipc::yield(k);
- }
- // only one thread/process would touch here at one time
- ct_.store(cur_ct + 1, std::memory_order_release);
- std::forward(f)(&(el->data_));
- // set flag & try update wt
- el->f_ct_.store(~static_cast(cur_ct), std::memory_order_release);
- return true;
- }
-
- template
- bool pop(W* wrapper, circ::u2_t& cur, F&& f, R&& out, E(& elems)[N]) {
- auto* el = elems + circ::index_of(cur);
- auto cur_fl = el->f_ct_.load(std::memory_order_acquire);
- if (cur_fl != ~static_cast(cur)) {
- return false; // empty
- }
- ++cur;
- std::forward(f)(&(el->data_));
- for (unsigned k = 0;;) {
- auto cur_rc = el->rc_.load(std::memory_order_acquire);
- if ((cur_rc & rc_mask) == 0) {
- std::forward(out)(true);
- el->f_ct_.store(cur + N - 1, std::memory_order_release);
- return true;
- }
- auto nxt_rc = inc_rc(cur_rc) & ~static_cast(wrapper->connected_id());
- bool last_one = false;
- if ((last_one = (nxt_rc & rc_mask) == 0)) {
- el->f_ct_.store(cur + N - 1, std::memory_order_release);
- }
- if (el->rc_.compare_exchange_weak(cur_rc, nxt_rc, std::memory_order_release)) {
- std::forward(out)(last_one);
- return true;
- }
- ipc::yield(k);
- }
- }
-};
-
-} // namespace ipc
diff --git a/spaces/FridaZuley/RVC_HFKawaii/extract_locale.py b/spaces/FridaZuley/RVC_HFKawaii/extract_locale.py
deleted file mode 100644
index a4ff5ea3ddd7c612c640544099ab98a861b8fe35..0000000000000000000000000000000000000000
--- a/spaces/FridaZuley/RVC_HFKawaii/extract_locale.py
+++ /dev/null
@@ -1,34 +0,0 @@
-import json
-import re
-
-# Define regular expression patterns
-pattern = r"""i18n\([\s\n\t]*(["'][^"']+["'])[\s\n\t]*\)"""
-
-# Initialize the dictionary to store key-value pairs
-data = {}
-
-
-def process(fn: str):
- global data
- with open(fn, "r", encoding="utf-8") as f:
- contents = f.read()
- matches = re.findall(pattern, contents)
- for key in matches:
- key = eval(key)
- print("extract:", key)
- data[key] = key
-
-
-print("processing infer-web.py")
-process("infer-web.py")
-
-print("processing gui_v0.py")
-process("gui_v0.py")
-
-print("processing gui_v1.py")
-process("gui_v1.py")
-
-# Save as a JSON file
-with open("./i18n/en_US.json", "w", encoding="utf-8") as f:
- json.dump(data, f, ensure_ascii=False, indent=4)
- f.write("\n")
diff --git a/spaces/FridaZuley/RVC_HFKawaii/infer/lib/train/data_utils.py b/spaces/FridaZuley/RVC_HFKawaii/infer/lib/train/data_utils.py
deleted file mode 100644
index 51a176cceba860acf79157ed0bad2b82c8e80406..0000000000000000000000000000000000000000
--- a/spaces/FridaZuley/RVC_HFKawaii/infer/lib/train/data_utils.py
+++ /dev/null
@@ -1,517 +0,0 @@
-import os
-import traceback
-import logging
-
-logger = logging.getLogger(__name__)
-
-import numpy as np
-import torch
-import torch.utils.data
-
-from infer.lib.train.mel_processing import spectrogram_torch
-from infer.lib.train.utils import load_filepaths_and_text, load_wav_to_torch
-
-
-class TextAudioLoaderMultiNSFsid(torch.utils.data.Dataset):
- """
- 1) loads audio, text pairs
- 2) normalizes text and converts them to sequences of integers
- 3) computes spectrograms from audio files.
- """
-
- def __init__(self, audiopaths_and_text, hparams):
- self.audiopaths_and_text = load_filepaths_and_text(audiopaths_and_text)
- self.max_wav_value = hparams.max_wav_value
- self.sampling_rate = hparams.sampling_rate
- self.filter_length = hparams.filter_length
- self.hop_length = hparams.hop_length
- self.win_length = hparams.win_length
- self.sampling_rate = hparams.sampling_rate
- self.min_text_len = getattr(hparams, "min_text_len", 1)
- self.max_text_len = getattr(hparams, "max_text_len", 5000)
- self._filter()
-
- def _filter(self):
- """
- Filter text & store spec lengths
- """
- # Store spectrogram lengths for Bucketing
- # wav_length ~= file_size / (wav_channels * Bytes per dim) = file_size / (1 * 2)
- # spec_length = wav_length // hop_length
- audiopaths_and_text_new = []
- lengths = []
- for audiopath, text, pitch, pitchf, dv in self.audiopaths_and_text:
- if self.min_text_len <= len(text) and len(text) <= self.max_text_len:
- audiopaths_and_text_new.append([audiopath, text, pitch, pitchf, dv])
- lengths.append(os.path.getsize(audiopath) // (3 * self.hop_length))
- self.audiopaths_and_text = audiopaths_and_text_new
- self.lengths = lengths
-
- def get_sid(self, sid):
- sid = torch.LongTensor([int(sid)])
- return sid
-
- def get_audio_text_pair(self, audiopath_and_text):
- # separate filename and text
- file = audiopath_and_text[0]
- phone = audiopath_and_text[1]
- pitch = audiopath_and_text[2]
- pitchf = audiopath_and_text[3]
- dv = audiopath_and_text[4]
-
- phone, pitch, pitchf = self.get_labels(phone, pitch, pitchf)
- spec, wav = self.get_audio(file)
- dv = self.get_sid(dv)
-
- len_phone = phone.size()[0]
- len_spec = spec.size()[-1]
- # print(123,phone.shape,pitch.shape,spec.shape)
- if len_phone != len_spec:
- len_min = min(len_phone, len_spec)
- # amor
- len_wav = len_min * self.hop_length
-
- spec = spec[:, :len_min]
- wav = wav[:, :len_wav]
-
- phone = phone[:len_min, :]
- pitch = pitch[:len_min]
- pitchf = pitchf[:len_min]
-
- return (spec, wav, phone, pitch, pitchf, dv)
-
- def get_labels(self, phone, pitch, pitchf):
- phone = np.load(phone)
- phone = np.repeat(phone, 2, axis=0)
- pitch = np.load(pitch)
- pitchf = np.load(pitchf)
- n_num = min(phone.shape[0], 900) # DistributedBucketSampler
- # print(234,phone.shape,pitch.shape)
- phone = phone[:n_num, :]
- pitch = pitch[:n_num]
- pitchf = pitchf[:n_num]
- phone = torch.FloatTensor(phone)
- pitch = torch.LongTensor(pitch)
- pitchf = torch.FloatTensor(pitchf)
- return phone, pitch, pitchf
-
- def get_audio(self, filename):
- audio, sampling_rate = load_wav_to_torch(filename)
- if sampling_rate != self.sampling_rate:
- raise ValueError(
- "{} SR doesn't match target {} SR".format(
- sampling_rate, self.sampling_rate
- )
- )
- audio_norm = audio
- # audio_norm = audio / self.max_wav_value
- # audio_norm = audio / np.abs(audio).max()
-
- audio_norm = audio_norm.unsqueeze(0)
- spec_filename = filename.replace(".wav", ".spec.pt")
- if os.path.exists(spec_filename):
- try:
- spec = torch.load(spec_filename)
- except:
- logger.warn("%s %s", spec_filename, traceback.format_exc())
- spec = spectrogram_torch(
- audio_norm,
- self.filter_length,
- self.sampling_rate,
- self.hop_length,
- self.win_length,
- center=False,
- )
- spec = torch.squeeze(spec, 0)
- torch.save(spec, spec_filename, _use_new_zipfile_serialization=False)
- else:
- spec = spectrogram_torch(
- audio_norm,
- self.filter_length,
- self.sampling_rate,
- self.hop_length,
- self.win_length,
- center=False,
- )
- spec = torch.squeeze(spec, 0)
- torch.save(spec, spec_filename, _use_new_zipfile_serialization=False)
- return spec, audio_norm
-
- def __getitem__(self, index):
- return self.get_audio_text_pair(self.audiopaths_and_text[index])
-
- def __len__(self):
- return len(self.audiopaths_and_text)
-
-
-class TextAudioCollateMultiNSFsid:
- """Zero-pads model inputs and targets"""
-
- def __init__(self, return_ids=False):
- self.return_ids = return_ids
-
- def __call__(self, batch):
- """Collate's training batch from normalized text and aduio
- PARAMS
- ------
- batch: [text_normalized, spec_normalized, wav_normalized]
- """
- # Right zero-pad all one-hot text sequences to max input length
- _, ids_sorted_decreasing = torch.sort(
- torch.LongTensor([x[0].size(1) for x in batch]), dim=0, descending=True
- )
-
- max_spec_len = max([x[0].size(1) for x in batch])
- max_wave_len = max([x[1].size(1) for x in batch])
- spec_lengths = torch.LongTensor(len(batch))
- wave_lengths = torch.LongTensor(len(batch))
- spec_padded = torch.FloatTensor(len(batch), batch[0][0].size(0), max_spec_len)
- wave_padded = torch.FloatTensor(len(batch), 1, max_wave_len)
- spec_padded.zero_()
- wave_padded.zero_()
-
- max_phone_len = max([x[2].size(0) for x in batch])
- phone_lengths = torch.LongTensor(len(batch))
- phone_padded = torch.FloatTensor(
- len(batch), max_phone_len, batch[0][2].shape[1]
- ) # (spec, wav, phone, pitch)
- pitch_padded = torch.LongTensor(len(batch), max_phone_len)
- pitchf_padded = torch.FloatTensor(len(batch), max_phone_len)
- phone_padded.zero_()
- pitch_padded.zero_()
- pitchf_padded.zero_()
- # dv = torch.FloatTensor(len(batch), 256)#gin=256
- sid = torch.LongTensor(len(batch))
-
- for i in range(len(ids_sorted_decreasing)):
- row = batch[ids_sorted_decreasing[i]]
-
- spec = row[0]
- spec_padded[i, :, : spec.size(1)] = spec
- spec_lengths[i] = spec.size(1)
-
- wave = row[1]
- wave_padded[i, :, : wave.size(1)] = wave
- wave_lengths[i] = wave.size(1)
-
- phone = row[2]
- phone_padded[i, : phone.size(0), :] = phone
- phone_lengths[i] = phone.size(0)
-
- pitch = row[3]
- pitch_padded[i, : pitch.size(0)] = pitch
- pitchf = row[4]
- pitchf_padded[i, : pitchf.size(0)] = pitchf
-
- # dv[i] = row[5]
- sid[i] = row[5]
-
- return (
- phone_padded,
- phone_lengths,
- pitch_padded,
- pitchf_padded,
- spec_padded,
- spec_lengths,
- wave_padded,
- wave_lengths,
- # dv
- sid,
- )
-
-
-class TextAudioLoader(torch.utils.data.Dataset):
- """
- 1) loads audio, text pairs
- 2) normalizes text and converts them to sequences of integers
- 3) computes spectrograms from audio files.
- """
-
- def __init__(self, audiopaths_and_text, hparams):
- self.audiopaths_and_text = load_filepaths_and_text(audiopaths_and_text)
- self.max_wav_value = hparams.max_wav_value
- self.sampling_rate = hparams.sampling_rate
- self.filter_length = hparams.filter_length
- self.hop_length = hparams.hop_length
- self.win_length = hparams.win_length
- self.sampling_rate = hparams.sampling_rate
- self.min_text_len = getattr(hparams, "min_text_len", 1)
- self.max_text_len = getattr(hparams, "max_text_len", 5000)
- self._filter()
-
- def _filter(self):
- """
- Filter text & store spec lengths
- """
- # Store spectrogram lengths for Bucketing
- # wav_length ~= file_size / (wav_channels * Bytes per dim) = file_size / (1 * 2)
- # spec_length = wav_length // hop_length
- audiopaths_and_text_new = []
- lengths = []
- for audiopath, text, dv in self.audiopaths_and_text:
- if self.min_text_len <= len(text) and len(text) <= self.max_text_len:
- audiopaths_and_text_new.append([audiopath, text, dv])
- lengths.append(os.path.getsize(audiopath) // (3 * self.hop_length))
- self.audiopaths_and_text = audiopaths_and_text_new
- self.lengths = lengths
-
- def get_sid(self, sid):
- sid = torch.LongTensor([int(sid)])
- return sid
-
- def get_audio_text_pair(self, audiopath_and_text):
- # separate filename and text
- file = audiopath_and_text[0]
- phone = audiopath_and_text[1]
- dv = audiopath_and_text[2]
-
- phone = self.get_labels(phone)
- spec, wav = self.get_audio(file)
- dv = self.get_sid(dv)
-
- len_phone = phone.size()[0]
- len_spec = spec.size()[-1]
- if len_phone != len_spec:
- len_min = min(len_phone, len_spec)
- len_wav = len_min * self.hop_length
- spec = spec[:, :len_min]
- wav = wav[:, :len_wav]
- phone = phone[:len_min, :]
- return (spec, wav, phone, dv)
-
- def get_labels(self, phone):
- phone = np.load(phone)
- phone = np.repeat(phone, 2, axis=0)
- n_num = min(phone.shape[0], 900) # DistributedBucketSampler
- phone = phone[:n_num, :]
- phone = torch.FloatTensor(phone)
- return phone
-
- def get_audio(self, filename):
- audio, sampling_rate = load_wav_to_torch(filename)
- if sampling_rate != self.sampling_rate:
- raise ValueError(
- "{} SR doesn't match target {} SR".format(
- sampling_rate, self.sampling_rate
- )
- )
- audio_norm = audio
- # audio_norm = audio / self.max_wav_value
- # audio_norm = audio / np.abs(audio).max()
-
- audio_norm = audio_norm.unsqueeze(0)
- spec_filename = filename.replace(".wav", ".spec.pt")
- if os.path.exists(spec_filename):
- try:
- spec = torch.load(spec_filename)
- except:
- logger.warn("%s %s", spec_filename, traceback.format_exc())
- spec = spectrogram_torch(
- audio_norm,
- self.filter_length,
- self.sampling_rate,
- self.hop_length,
- self.win_length,
- center=False,
- )
- spec = torch.squeeze(spec, 0)
- torch.save(spec, spec_filename, _use_new_zipfile_serialization=False)
- else:
- spec = spectrogram_torch(
- audio_norm,
- self.filter_length,
- self.sampling_rate,
- self.hop_length,
- self.win_length,
- center=False,
- )
- spec = torch.squeeze(spec, 0)
- torch.save(spec, spec_filename, _use_new_zipfile_serialization=False)
- return spec, audio_norm
-
- def __getitem__(self, index):
- return self.get_audio_text_pair(self.audiopaths_and_text[index])
-
- def __len__(self):
- return len(self.audiopaths_and_text)
-
-
-class TextAudioCollate:
- """Zero-pads model inputs and targets"""
-
- def __init__(self, return_ids=False):
- self.return_ids = return_ids
-
- def __call__(self, batch):
- """Collate's training batch from normalized text and aduio
- PARAMS
- ------
- batch: [text_normalized, spec_normalized, wav_normalized]
- """
- # Right zero-pad all one-hot text sequences to max input length
- _, ids_sorted_decreasing = torch.sort(
- torch.LongTensor([x[0].size(1) for x in batch]), dim=0, descending=True
- )
-
- max_spec_len = max([x[0].size(1) for x in batch])
- max_wave_len = max([x[1].size(1) for x in batch])
- spec_lengths = torch.LongTensor(len(batch))
- wave_lengths = torch.LongTensor(len(batch))
- spec_padded = torch.FloatTensor(len(batch), batch[0][0].size(0), max_spec_len)
- wave_padded = torch.FloatTensor(len(batch), 1, max_wave_len)
- spec_padded.zero_()
- wave_padded.zero_()
-
- max_phone_len = max([x[2].size(0) for x in batch])
- phone_lengths = torch.LongTensor(len(batch))
- phone_padded = torch.FloatTensor(
- len(batch), max_phone_len, batch[0][2].shape[1]
- )
- phone_padded.zero_()
- sid = torch.LongTensor(len(batch))
-
- for i in range(len(ids_sorted_decreasing)):
- row = batch[ids_sorted_decreasing[i]]
-
- spec = row[0]
- spec_padded[i, :, : spec.size(1)] = spec
- spec_lengths[i] = spec.size(1)
-
- wave = row[1]
- wave_padded[i, :, : wave.size(1)] = wave
- wave_lengths[i] = wave.size(1)
-
- phone = row[2]
- phone_padded[i, : phone.size(0), :] = phone
- phone_lengths[i] = phone.size(0)
-
- sid[i] = row[3]
-
- return (
- phone_padded,
- phone_lengths,
- spec_padded,
- spec_lengths,
- wave_padded,
- wave_lengths,
- sid,
- )
-
-
-class DistributedBucketSampler(torch.utils.data.distributed.DistributedSampler):
- """
- Maintain similar input lengths in a batch.
- Length groups are specified by boundaries.
- Ex) boundaries = [b1, b2, b3] -> any batch is included either {x | b1 < length(x) <=b2} or {x | b2 < length(x) <= b3}.
-
- It removes samples which are not included in the boundaries.
- Ex) boundaries = [b1, b2, b3] -> any x s.t. length(x) <= b1 or length(x) > b3 are discarded.
- """
-
- def __init__(
- self,
- dataset,
- batch_size,
- boundaries,
- num_replicas=None,
- rank=None,
- shuffle=True,
- ):
- super().__init__(dataset, num_replicas=num_replicas, rank=rank, shuffle=shuffle)
- self.lengths = dataset.lengths
- self.batch_size = batch_size
- self.boundaries = boundaries
-
- self.buckets, self.num_samples_per_bucket = self._create_buckets()
- self.total_size = sum(self.num_samples_per_bucket)
- self.num_samples = self.total_size // self.num_replicas
-
- def _create_buckets(self):
- buckets = [[] for _ in range(len(self.boundaries) - 1)]
- for i in range(len(self.lengths)):
- length = self.lengths[i]
- idx_bucket = self._bisect(length)
- if idx_bucket != -1:
- buckets[idx_bucket].append(i)
-
- for i in range(len(buckets) - 1, -1, -1): #
- if len(buckets[i]) == 0:
- buckets.pop(i)
- self.boundaries.pop(i + 1)
-
- num_samples_per_bucket = []
- for i in range(len(buckets)):
- len_bucket = len(buckets[i])
- total_batch_size = self.num_replicas * self.batch_size
- rem = (
- total_batch_size - (len_bucket % total_batch_size)
- ) % total_batch_size
- num_samples_per_bucket.append(len_bucket + rem)
- return buckets, num_samples_per_bucket
-
- def __iter__(self):
- # deterministically shuffle based on epoch
- g = torch.Generator()
- g.manual_seed(self.epoch)
-
- indices = []
- if self.shuffle:
- for bucket in self.buckets:
- indices.append(torch.randperm(len(bucket), generator=g).tolist())
- else:
- for bucket in self.buckets:
- indices.append(list(range(len(bucket))))
-
- batches = []
- for i in range(len(self.buckets)):
- bucket = self.buckets[i]
- len_bucket = len(bucket)
- ids_bucket = indices[i]
- num_samples_bucket = self.num_samples_per_bucket[i]
-
- # add extra samples to make it evenly divisible
- rem = num_samples_bucket - len_bucket
- ids_bucket = (
- ids_bucket
- + ids_bucket * (rem // len_bucket)
- + ids_bucket[: (rem % len_bucket)]
- )
-
- # subsample
- ids_bucket = ids_bucket[self.rank :: self.num_replicas]
-
- # batching
- for j in range(len(ids_bucket) // self.batch_size):
- batch = [
- bucket[idx]
- for idx in ids_bucket[
- j * self.batch_size : (j + 1) * self.batch_size
- ]
- ]
- batches.append(batch)
-
- if self.shuffle:
- batch_ids = torch.randperm(len(batches), generator=g).tolist()
- batches = [batches[i] for i in batch_ids]
- self.batches = batches
-
- assert len(self.batches) * self.batch_size == self.num_samples
- return iter(self.batches)
-
- def _bisect(self, x, lo=0, hi=None):
- if hi is None:
- hi = len(self.boundaries) - 1
-
- if hi > lo:
- mid = (hi + lo) // 2
- if self.boundaries[mid] < x and x <= self.boundaries[mid + 1]:
- return mid
- elif x <= self.boundaries[mid]:
- return self._bisect(x, lo, mid)
- else:
- return self._bisect(x, mid + 1, hi)
- else:
- return -1
-
- def __len__(self):
- return self.num_samples // self.batch_size
diff --git a/spaces/GIZ/SDSN-demo/appStore/sdg_analysis.py b/spaces/GIZ/SDSN-demo/appStore/sdg_analysis.py
deleted file mode 100644
index 3b45ddaf099fdff9307fd7f0b1f171fb5a2f730d..0000000000000000000000000000000000000000
--- a/spaces/GIZ/SDSN-demo/appStore/sdg_analysis.py
+++ /dev/null
@@ -1,179 +0,0 @@
-# set path
-import glob, os, sys;
-sys.path.append('../utils')
-
-#import needed libraries
-import seaborn as sns
-import matplotlib.pyplot as plt
-import numpy as np
-import pandas as pd
-import streamlit as st
-from st_aggrid import AgGrid
-from st_aggrid.shared import ColumnsAutoSizeMode
-from utils.sdg_classifier import sdg_classification
-from utils.sdg_classifier import runSDGPreprocessingPipeline, load_sdgClassifier
-from utils.keyword_extraction import textrank
-import logging
-logger = logging.getLogger(__name__)
-from utils.checkconfig import getconfig
-
-
-# Declare all the necessary variables
-config = getconfig('paramconfig.cfg')
-model_name = config.get('sdg','MODEL')
-split_by = config.get('sdg','SPLIT_BY')
-split_length = int(config.get('sdg','SPLIT_LENGTH'))
-split_overlap = int(config.get('sdg','SPLIT_OVERLAP'))
-remove_punc = bool(int(config.get('sdg','REMOVE_PUNC')))
-split_respect_sentence_boundary = bool(int(config.get('sdg','RESPECT_SENTENCE_BOUNDARY')))
-threshold = float(config.get('sdg','THRESHOLD'))
-top_n = int(config.get('sdg','TOP_KEY'))
-
-
-def app():
-
- #### APP INFO #####
- with st.container():
- st.markdown(" SDG Classification and Keyphrase Extraction
", unsafe_allow_html=True)
- st.write(' ')
- st.write(' ')
-
- with st.expander("ℹ️ - About this app", expanded=False):
-
- st.write(
- """
- The *SDG Analysis* app is an easy-to-use interface built \
- in Streamlit for analyzing policy documents with respect to SDG \
- Classification for the paragraphs/texts in the document and \
- extracting the keyphrase per SDG label - developed by GIZ Data \
- and the Sustainable Development Solution Network. \n
- """)
- st.write("""**Document Processing:** The Uploaded/Selected document is \
- automatically cleaned and split into paragraphs with a maximum \
- length of 120 words using a Haystack preprocessing pipeline. The \
- length of 120 is an empirical value which should reflect the length \
- of a “context” and should limit the paragraph length deviation. \
- However, since we want to respect the sentence boundary the limit \
- can breach and hence this limit of 120 is tentative. \n
- """)
- st.write("""**SDG cLassification:** The application assigns paragraphs \
- to 16 of the 17 United Nations Sustainable Development Goals (SDGs).\
- SDG 17 “Partnerships for the Goals” is excluded from the analysis due \
- to its broad nature which could potentially inflate the results. \
- Each paragraph is assigned to one SDG only. Again, the results are \
- displayed in a summary table including the number of the SDG, a \
- relevancy score highlighted through a green color shading, and the \
- respective text of the analyzed paragraph. Additionally, a pie \
- chart with a blue color shading is displayed which illustrates the \
- three most prominent SDGs in the document. The SDG classification \
- uses open-source training [data](https://zenodo.org/record/5550238#.Y25ICHbMJPY) \
- from [OSDG.ai](https://osdg.ai/) which is a global \
- partnerships and growing community of researchers and institutions \
- interested in the classification of research according to the \
- Sustainable Development Goals. The summary table only displays \
- paragraphs with a calculated relevancy score above 85%. \n""")
-
- st.write("""**Keyphrase Extraction:** The application extracts 15 \
- keyphrases from the document, for each SDG label and displays the \
- results in a summary table. The keyphrases are extracted using \
- using [Textrank](https://github.com/summanlp/textrank)\
- which is an easy-to-use computational less expensive \
- model leveraging combination of TFIDF and Graph networks.
- """)
- st.write("")
- st.write("")
- st.markdown("Some runtime metrics tested with cpu: Intel(R) Xeon(R) CPU @ 2.20GHz, memory: 13GB")
- col1,col2,col3,col4 = st.columns([2,2,4,4])
- with col1:
- st.caption("Loading Time Classifier")
- # st.markdown('12 sec
', unsafe_allow_html=True)
- st.write("12 sec")
- with col2:
- st.caption("OCR File processing")
- # st.markdown('50 sec
', unsafe_allow_html=True)
- st.write("50 sec")
- with col3:
- st.caption("SDG Classification of 200 paragraphs(~ 35 pages)")
- # st.markdown('120 sec
', unsafe_allow_html=True)
- st.write("120 sec")
- with col4:
- st.caption("Keyword extraction for 200 paragraphs(~ 35 pages)")
- # st.markdown('3 sec
', unsafe_allow_html=True)
- st.write("3 sec")
-
-
-
-
- ### Main app code ###
- with st.container():
- if st.button("RUN SDG Analysis"):
-
- if 'filepath' in st.session_state:
- file_name = st.session_state['filename']
- file_path = st.session_state['filepath']
- classifier = load_sdgClassifier(classifier_name=model_name)
- st.session_state['sdg_classifier'] = classifier
- all_documents = runSDGPreprocessingPipeline(file_name= file_name,
- file_path= file_path, split_by= split_by,
- split_length= split_length,
- split_respect_sentence_boundary= split_respect_sentence_boundary,
- split_overlap= split_overlap, remove_punc= remove_punc)
-
- if len(all_documents['documents']) > 100:
- warning_msg = ": This might take sometime, please sit back and relax."
- else:
- warning_msg = ""
-
- with st.spinner("Running SDG Classification{}".format(warning_msg)):
-
- df, x = sdg_classification(haystack_doc=all_documents['documents'],
- threshold= threshold)
- df = df.drop(['Relevancy'], axis = 1)
- sdg_labels = x.SDG.unique()
- textrank_keyword_list = []
- for label in sdg_labels:
- sdgdata = " ".join(df[df.SDG == label].text.to_list())
- textranklist_ = textrank(textdata=sdgdata, words= top_n)
- if len(textranklist_) > 0:
- textrank_keyword_list.append({'SDG':label, 'TextRank Keywords':",".join(textranklist_)})
- textrank_keywords_df = pd.DataFrame(textrank_keyword_list)
-
-
- plt.rcParams['font.size'] = 25
- colors = plt.get_cmap('Blues')(np.linspace(0.2, 0.7, len(x)))
- # plot
- fig, ax = plt.subplots()
- ax.pie(x['count'], colors=colors, radius=2, center=(4, 4),
- wedgeprops={"linewidth": 1, "edgecolor": "white"},
- textprops={'fontsize': 14},
- frame=False,labels =list(x.SDG_Num),
- labeldistance=1.2)
- # fig.savefig('temp.png', bbox_inches='tight',dpi= 100)
-
-
- st.markdown("#### Anything related to SDGs? ####")
-
- c4, c5, c6 = st.columns([1,2,2])
-
- with c5:
- st.pyplot(fig)
- with c6:
- labeldf = x['SDG_name'].values.tolist()
- labeldf = "
".join(labeldf)
- st.markdown(labeldf, unsafe_allow_html=True)
- st.write("")
- st.markdown("###### What keywords are present under SDG classified text? ######")
-
- AgGrid(textrank_keywords_df, reload_data = False,
- update_mode="value_changed",
- columns_auto_size_mode = ColumnsAutoSizeMode.FIT_CONTENTS)
- st.write("")
- st.markdown("###### Top few SDG Classified paragraph/text results ######")
-
- AgGrid(df, reload_data = False, update_mode="value_changed",
- columns_auto_size_mode = ColumnsAutoSizeMode.FIT_CONTENTS)
- else:
- st.info("🤔 No document found, please try to upload it at the sidebar!")
- logging.warning("Terminated as no document provided")
-
-
diff --git a/spaces/GIZ/vulnerability_analysis/utils/preprocessing.py b/spaces/GIZ/vulnerability_analysis/utils/preprocessing.py
deleted file mode 100644
index 0e0c44737cc37ededbd8d50f62407af2a16d5bc7..0000000000000000000000000000000000000000
--- a/spaces/GIZ/vulnerability_analysis/utils/preprocessing.py
+++ /dev/null
@@ -1,291 +0,0 @@
-from haystack.nodes.base import BaseComponent
-from haystack.schema import Document
-from haystack.nodes import PDFToTextOCRConverter, PDFToTextConverter
-from haystack.nodes import TextConverter, DocxToTextConverter, PreProcessor
-from typing import Callable, Dict, List, Optional, Text, Tuple, Union
-from typing_extensions import Literal
-import pandas as pd
-import logging
-import re
-import string
-from haystack.pipelines import Pipeline
-
-def useOCR(file_path: str)-> Text:
- """
- Converts image pdfs into text, Using the Farm-haystack[OCR]
-
- Params
- ----------
- file_path: file_path of uploade file, returned by add_upload function in
- uploadAndExample.py
-
- Returns the text file as string.
- """
-
-
- converter = PDFToTextOCRConverter(remove_numeric_tables=True,
- valid_languages=["eng"])
- docs = converter.convert(file_path=file_path, meta=None)
- return docs[0].content
-
-
-
-
-class FileConverter(BaseComponent):
- """
- Wrapper class to convert uploaded document into text by calling appropriate
- Converter class, will use internally haystack PDFToTextOCR in case of image
- pdf. Cannot use the FileClassifier from haystack as its doesnt has any
- label/output class for image.
-
- 1. https://haystack.deepset.ai/pipeline_nodes/custom-nodes
- 2. https://docs.haystack.deepset.ai/docs/file_converters
- 3. https://github.com/deepset-ai/haystack/tree/main/haystack/nodes/file_converter
- 4. https://docs.haystack.deepset.ai/reference/file-converters-api
-
-
- """
-
- outgoing_edges = 1
-
- def run(self, file_name: str , file_path: str, encoding: Optional[str]=None,
- id_hash_keys: Optional[List[str]] = None,
- ) -> Tuple[dict,str]:
- """ this is required method to invoke the component in
- the pipeline implementation.
-
- Params
- ----------
- file_name: name of file
- file_path: file_path of uploade file, returned by add_upload function in
- uploadAndExample.py
-
- See the links provided in Class docstring/description to see other params
-
- Return
- ---------
- output: dictionary, with key as identifier and value could be anything
- we need to return. In this case its the List of Hasyatck Document
-
- output_1: As there is only one outgoing edge, we pass 'output_1' string
- """
- try:
- if file_name.endswith('.pdf'):
- converter = PDFToTextConverter(remove_numeric_tables=True)
- if file_name.endswith('.txt'):
- converter = TextConverter(remove_numeric_tables=True)
- if file_name.endswith('.docx'):
- converter = DocxToTextConverter()
- except Exception as e:
- logging.error(e)
- return
-
-
-
- documents = []
-
-
-# encoding is empty, probably should be utf-8
- document = converter.convert(
- file_path=file_path, meta=None,
- encoding=encoding, id_hash_keys=id_hash_keys
- )[0]
-
- text = document.content
-
- # in case of scanned/images only PDF the content might contain only
- # the page separator (\f or \x0c). We check if is so and use
- # use the OCR to get the text.
- filtered = re.sub(r'\x0c', '', text)
-
- if filtered == "":
- logging.info("Using OCR")
- text = useOCR(file_path)
-
- documents.append(Document(content=text,
- meta={"name": file_name},
- id_hash_keys=id_hash_keys))
-
- logging.info('file conversion succesful')
- output = {'documents': documents}
- return output, 'output_1'
-
- def run_batch():
- """
- we dont have requirement to process the multiple files in one go
- therefore nothing here, however to use the custom node we need to have
- this method for the class.
- """
-
- return
-
-
-def basic(s:str, remove_punc:bool = False):
-
- """
- Performs basic cleaning of text.
-
- Params
- ----------
- s: string to be processed
- removePunc: to remove all Punctuation including ',' and '.' or not
-
- Returns: processed string: see comments in the source code for more info
- """
-
- # Remove URLs
- s = re.sub(r'^https?:\/\/.*[\r\n]*', ' ', s, flags=re.MULTILINE)
- s = re.sub(r"http\S+", " ", s)
-
- # Remove new line characters
- s = re.sub('\n', ' ', s)
-
- # Remove punctuations
- if remove_punc == True:
- translator = str.maketrans(' ', ' ', string.punctuation)
- s = s.translate(translator)
- # Remove distracting single quotes and dotted pattern
- s = re.sub("\'", " ", s)
- s = s.replace("..","")
-
- return s.strip()
-
-def paraLengthCheck(paraList, max_len = 100):
- """
- There are cases where preprocessor cannot respect word limit, when using
- respect sentence boundary flag due to missing sentence boundaries.
- Therefore we run one more round of split here for those paragraphs
-
- Params
- ---------------
- paraList : list of paragraphs/text
- max_len : max length to be respected by sentences which bypassed
- preprocessor strategy
-
- """
- new_para_list = []
- for passage in paraList:
- # check if para exceeds words limit
- if len(passage.content.split()) > max_len:
- # we might need few iterations example if para = 512 tokens
- # we need to iterate 5 times to reduce para to size limit of '100'
- iterations = int(len(passage.content.split())/max_len)
- for i in range(iterations):
- temp = " ".join(passage.content.split()[max_len*i:max_len*(i+1)])
- new_para_list.append((temp,passage.meta['page']))
- temp = " ".join(passage.content.split()[max_len*(i+1):])
- new_para_list.append((temp,passage.meta['page']))
- else:
- # paragraphs which dont need any splitting
- new_para_list.append((passage.content, passage.meta['page']))
-
- logging.info("New paragraphs length {}".format(len(new_para_list)))
- return new_para_list
-
-class UdfPreProcessor(BaseComponent):
- """
- class to preprocess the document returned by FileConverter. It will check
- for splitting strategy and splits the document by word or sentences and then
- synthetically create the paragraphs.
-
- 1. https://docs.haystack.deepset.ai/docs/preprocessor
- 2. https://docs.haystack.deepset.ai/reference/preprocessor-api
- 3. https://github.com/deepset-ai/haystack/tree/main/haystack/nodes/preprocessor
-
- """
- outgoing_edges = 1
-
- def run(self, documents:List[Document], remove_punc:bool=False,
- split_by: Literal["sentence", "word"] = 'sentence',
- split_length:int = 2, split_respect_sentence_boundary:bool = False,
- split_overlap:int = 0):
-
- """ this is required method to invoke the component in
- the pipeline implementation.
-
- Params
- ----------
- documents: documents from the output dictionary returned by Fileconverter
- remove_punc: to remove all Punctuation including ',' and '.' or not
- split_by: document splitting strategy either as word or sentence
- split_length: when synthetically creating the paragrpahs from document,
- it defines the length of paragraph.
- split_respect_sentence_boundary: Used when using 'word' strategy for
- splititng of text.
- split_overlap: Number of words or sentences that overlap when creating
- the paragraphs. This is done as one sentence or 'some words' make sense
- when read in together with others. Therefore the overlap is used.
-
- Return
- ---------
- output: dictionary, with key as identifier and value could be anything
- we need to return. In this case the output will contain 4 objects
- the paragraphs text list as List, Haystack document, Dataframe and
- one raw text file.
-
- output_1: As there is only one outgoing edge, we pass 'output_1' string
-
- """
-
- if split_by == 'sentence':
- split_respect_sentence_boundary = False
-
- else:
- split_respect_sentence_boundary = split_respect_sentence_boundary
-
- preprocessor = PreProcessor(
- clean_empty_lines=True,
- clean_whitespace=True,
- clean_header_footer=True,
- split_by=split_by,
- split_length=split_length,
- split_respect_sentence_boundary= split_respect_sentence_boundary,
- split_overlap=split_overlap,
-
- # will add page number only in case of PDF not for text/docx file.
- add_page_number=True
- )
-
- for i in documents:
- # # basic cleaning before passing it to preprocessor.
- # i = basic(i)
- docs_processed = preprocessor.process([i])
- for item in docs_processed:
- item.content = basic(item.content, remove_punc= remove_punc)
-
- df = pd.DataFrame(docs_processed)
- all_text = " ".join(df.content.to_list())
- para_list = df.content.to_list()
- logging.info('document split into {} paragraphs'.format(len(para_list)))
- output = {'documents': docs_processed,
- 'dataframe': df,
- 'text': all_text,
- 'paraList': para_list
- }
- return output, "output_1"
- def run_batch():
- """
- we dont have requirement to process the multiple files in one go
- therefore nothing here, however to use the custom node we need to have
- this method for the class.
- """
- return
-
-def processingpipeline():
- """
- Returns the preprocessing pipeline. Will use FileConverter and UdfPreProcesor
- from utils.preprocessing
-
- """
-
- preprocessing_pipeline = Pipeline()
- file_converter = FileConverter()
- custom_preprocessor = UdfPreProcessor()
-
- preprocessing_pipeline.add_node(component=file_converter,
- name="FileConverter", inputs=["File"])
- preprocessing_pipeline.add_node(component = custom_preprocessor,
- name ='UdfPreProcessor', inputs=["FileConverter"])
-
- return preprocessing_pipeline
-
diff --git a/spaces/Gen-Sim/Gen-Sim/cliport/generated_tasks/color_coordinated_sphere_and_cylinder_assembly.py b/spaces/Gen-Sim/Gen-Sim/cliport/generated_tasks/color_coordinated_sphere_and_cylinder_assembly.py
deleted file mode 100644
index 5bfbc2ac42a37fd5cc6b350ee893158e4e7e37bc..0000000000000000000000000000000000000000
--- a/spaces/Gen-Sim/Gen-Sim/cliport/generated_tasks/color_coordinated_sphere_and_cylinder_assembly.py
+++ /dev/null
@@ -1,46 +0,0 @@
-import numpy as np
-import os
-import pybullet as p
-import random
-from cliport.tasks import primitives
-from cliport.tasks.grippers import Spatula
-from cliport.tasks.task import Task
-from cliport.utils import utils
-import numpy as np
-from cliport.tasks.task import Task
-from cliport.utils import utils
-
-class ColorCoordinatedSphereAndCylinderAssembly(Task):
- """Pick up each sphere and place it on top of the cylinder of the same color, in a specific color sequence."""
-
- def __init__(self):
- super().__init__()
- self.max_steps = 20
- self.lang_template = "place the {color} sphere on the {color} cylinder"
- self.task_completed_desc = "done placing spheres on cylinders."
- self.colors = ['red', 'blue', 'green', 'yellow']
- self.color_sequence = ['red', 'blue', 'green', 'yellow']
-
- def reset(self, env):
- super().reset(env)
-
- # Add spheres and cylinders.
- sphere_size = (0.05, 0.05, 0.05)
- cylinder_size = (0.05, 0.05, 0.1)
- sphere_template = 'sphere/sphere-template.urdf'
- cylinder_template = 'cylinder/cylinder-template.urdf'
-
- # Add spheres and cylinders of each color.
- for color in self.colors:
- sphere_pose = self.get_random_pose(env, sphere_size)
- cylinder_pose = self.get_random_pose(env, cylinder_size)
- sphere_id = env.add_object(sphere_template, sphere_pose, color=color)
- cylinder_id = env.add_object(cylinder_template, cylinder_pose, color=color)
-
- # Goal: each sphere is on top of the cylinder of the same color.
- self.add_goal(objs=[sphere_id], matches=np.ones((1, 1)), targ_poses=[cylinder_pose], replace=False,
- rotations=True, metric='pose', params=None, step_max_reward=1/4,
- language_goal=self.lang_template.format(color=color))
-
- # The task is completed in a specific color sequence.
- self.color_sequence = ['red', 'blue', 'green', 'yellow']
\ No newline at end of file
diff --git a/spaces/GipAdonimus/PAIR-text2video-zero-controlnet-canny-gta5/app.py b/spaces/GipAdonimus/PAIR-text2video-zero-controlnet-canny-gta5/app.py
deleted file mode 100644
index 1ba557129fa222166fc98a42d73ab25d17ac0f20..0000000000000000000000000000000000000000
--- a/spaces/GipAdonimus/PAIR-text2video-zero-controlnet-canny-gta5/app.py
+++ /dev/null
@@ -1,3 +0,0 @@
-import gradio as gr
-
-gr.Interface.load("models/PAIR/text2video-zero-controlnet-canny-gta5").launch()
\ No newline at end of file
diff --git a/spaces/Gladiator/Text-Summarizer/extractive_summarizer/sentence_handler.py b/spaces/Gladiator/Text-Summarizer/extractive_summarizer/sentence_handler.py
deleted file mode 100644
index dfb94c71741375c028d5c30413258279b5a7ce43..0000000000000000000000000000000000000000
--- a/spaces/Gladiator/Text-Summarizer/extractive_summarizer/sentence_handler.py
+++ /dev/null
@@ -1,73 +0,0 @@
-from typing import List
-
-from spacy.lang.en import English
-
-
-class SentenceHandler(object):
-
- def __init__(self, language=English):
- """
- Base Sentence Handler with Spacy support.
-
- :param language: Determines the language to use with spacy.
- """
- self.nlp = language()
-
- try:
- # Supports spacy 2.0
- self.nlp.add_pipe(self.nlp.create_pipe('sentencizer'))
- self.is_spacy_3 = False
- except Exception:
- # Supports spacy 3.0
- self.nlp.add_pipe("sentencizer")
- self.is_spacy_3 = True
-
- def sentence_processor(self, doc,
- min_length: int = 40,
- max_length: int = 600) -> List[str]:
- """
- Processes a given spacy document and turns them into sentences.
-
- :param doc: The document to use from spacy.
- :param min_length: The minimum length a sentence should be to be considered.
- :param max_length: The maximum length a sentence should be to be considered.
- :return: Sentences.
- """
- to_return = []
-
- for c in doc.sents:
- if max_length > len(c.text.strip()) > min_length:
-
- if self.is_spacy_3:
- to_return.append(c.text.strip())
- else:
- to_return.append(c.string.strip())
-
- return to_return
-
- def process(self, body: str,
- min_length: int = 40,
- max_length: int = 600) -> List[str]:
- """
- Processes the content sentences.
-
- :param body: The raw string body to process
- :param min_length: Minimum length that the sentences must be
- :param max_length: Max length that the sentences mus fall under
- :return: Returns a list of sentences.
- """
- doc = self.nlp(body)
- return self.sentence_processor(doc, min_length, max_length)
-
- def __call__(self, body: str,
- min_length: int = 40,
- max_length: int = 600) -> List[str]:
- """
- Processes the content sentences.
-
- :param body: The raw string body to process
- :param min_length: Minimum length that the sentences must be
- :param max_length: Max length that the sentences mus fall under
- :return: Returns a list of sentences.
- """
- return self.process(body, min_length, max_length)
\ No newline at end of file
diff --git a/spaces/Gradio-Blocks/uniformer_image_detection/configs/foveabox/fovea_r50_fpn_4x4_2x_coco.py b/spaces/Gradio-Blocks/uniformer_image_detection/configs/foveabox/fovea_r50_fpn_4x4_2x_coco.py
deleted file mode 100644
index 68ce4d250ac673a274d1458963eb02614e4f5f98..0000000000000000000000000000000000000000
--- a/spaces/Gradio-Blocks/uniformer_image_detection/configs/foveabox/fovea_r50_fpn_4x4_2x_coco.py
+++ /dev/null
@@ -1,4 +0,0 @@
-_base_ = './fovea_r50_fpn_4x4_1x_coco.py'
-# learning policy
-lr_config = dict(step=[16, 22])
-runner = dict(type='EpochBasedRunner', max_epochs=24)
diff --git a/spaces/Gradio-Blocks/uniformer_image_detection/mmdet/models/backbones/__init__.py b/spaces/Gradio-Blocks/uniformer_image_detection/mmdet/models/backbones/__init__.py
deleted file mode 100644
index e54b088acf644d285ecbeb1440c414e722b9db58..0000000000000000000000000000000000000000
--- a/spaces/Gradio-Blocks/uniformer_image_detection/mmdet/models/backbones/__init__.py
+++ /dev/null
@@ -1,20 +0,0 @@
-from .darknet import Darknet
-from .detectors_resnet import DetectoRS_ResNet
-from .detectors_resnext import DetectoRS_ResNeXt
-from .hourglass import HourglassNet
-from .hrnet import HRNet
-from .regnet import RegNet
-from .res2net import Res2Net
-from .resnest import ResNeSt
-from .resnet import ResNet, ResNetV1d
-from .resnext import ResNeXt
-from .ssd_vgg import SSDVGG
-from .trident_resnet import TridentResNet
-from .swin_transformer import SwinTransformer
-from .uniformer import UniFormer
-
-__all__ = [
- 'RegNet', 'ResNet', 'ResNetV1d', 'ResNeXt', 'SSDVGG', 'HRNet', 'Res2Net',
- 'HourglassNet', 'DetectoRS_ResNet', 'DetectoRS_ResNeXt', 'Darknet',
- 'ResNeSt', 'TridentResNet', 'SwinTransformer', 'UniFormer'
-]
diff --git a/spaces/GrandaddyShmax/MusicGen_Plus_hfv2/tests/data/test_audio_utils.py b/spaces/GrandaddyShmax/MusicGen_Plus_hfv2/tests/data/test_audio_utils.py
deleted file mode 100644
index 0480671bb17281d61ce02bce6373a5ccec89fece..0000000000000000000000000000000000000000
--- a/spaces/GrandaddyShmax/MusicGen_Plus_hfv2/tests/data/test_audio_utils.py
+++ /dev/null
@@ -1,110 +0,0 @@
-# Copyright (c) Meta Platforms, Inc. and affiliates.
-# All rights reserved.
-#
-# This source code is licensed under the license found in the
-# LICENSE file in the root directory of this source tree.
-
-import julius
-import torch
-import pytest
-
-from audiocraft.data.audio_utils import (
- _clip_wav,
- convert_audio_channels,
- convert_audio,
- normalize_audio
-)
-from ..common_utils import get_batch_white_noise
-
-
-class TestConvertAudioChannels:
-
- def test_convert_audio_channels_downmix(self):
- b, c, t = 2, 3, 100
- audio = get_batch_white_noise(b, c, t)
- mixed = convert_audio_channels(audio, channels=2)
- assert list(mixed.shape) == [b, 2, t]
-
- def test_convert_audio_channels_nochange(self):
- b, c, t = 2, 3, 100
- audio = get_batch_white_noise(b, c, t)
- mixed = convert_audio_channels(audio, channels=c)
- assert list(mixed.shape) == list(audio.shape)
-
- def test_convert_audio_channels_upmix(self):
- b, c, t = 2, 1, 100
- audio = get_batch_white_noise(b, c, t)
- mixed = convert_audio_channels(audio, channels=3)
- assert list(mixed.shape) == [b, 3, t]
-
- def test_convert_audio_channels_upmix_error(self):
- b, c, t = 2, 2, 100
- audio = get_batch_white_noise(b, c, t)
- with pytest.raises(ValueError):
- convert_audio_channels(audio, channels=3)
-
-
-class TestConvertAudio:
-
- def test_convert_audio_channels_downmix(self):
- b, c, dur = 2, 3, 4.
- sr = 128
- audio = get_batch_white_noise(b, c, int(sr * dur))
- out = convert_audio(audio, from_rate=sr, to_rate=sr, to_channels=2)
- assert list(out.shape) == [audio.shape[0], 2, audio.shape[-1]]
-
- def test_convert_audio_channels_upmix(self):
- b, c, dur = 2, 1, 4.
- sr = 128
- audio = get_batch_white_noise(b, c, int(sr * dur))
- out = convert_audio(audio, from_rate=sr, to_rate=sr, to_channels=3)
- assert list(out.shape) == [audio.shape[0], 3, audio.shape[-1]]
-
- def test_convert_audio_upsample(self):
- b, c, dur = 2, 1, 4.
- sr = 2
- new_sr = 3
- audio = get_batch_white_noise(b, c, int(sr * dur))
- out = convert_audio(audio, from_rate=sr, to_rate=new_sr, to_channels=c)
- out_j = julius.resample.resample_frac(audio, old_sr=sr, new_sr=new_sr)
- assert torch.allclose(out, out_j)
-
- def test_convert_audio_resample(self):
- b, c, dur = 2, 1, 4.
- sr = 3
- new_sr = 2
- audio = get_batch_white_noise(b, c, int(sr * dur))
- out = convert_audio(audio, from_rate=sr, to_rate=new_sr, to_channels=c)
- out_j = julius.resample.resample_frac(audio, old_sr=sr, new_sr=new_sr)
- assert torch.allclose(out, out_j)
-
-
-class TestNormalizeAudio:
-
- def test_clip_wav(self):
- b, c, dur = 2, 1, 4.
- sr = 3
- audio = 10.0 * get_batch_white_noise(b, c, int(sr * dur))
- _clip_wav(audio)
- assert audio.abs().max() <= 1
-
- def test_normalize_audio_clip(self):
- b, c, dur = 2, 1, 4.
- sr = 3
- audio = 10.0 * get_batch_white_noise(b, c, int(sr * dur))
- norm_audio = normalize_audio(audio, strategy='clip')
- assert norm_audio.abs().max() <= 1
-
- def test_normalize_audio_rms(self):
- b, c, dur = 2, 1, 4.
- sr = 3
- audio = 10.0 * get_batch_white_noise(b, c, int(sr * dur))
- norm_audio = normalize_audio(audio, strategy='rms')
- assert norm_audio.abs().max() <= 1
-
- def test_normalize_audio_peak(self):
- b, c, dur = 2, 1, 4.
- sr = 3
- audio = 10.0 * get_batch_white_noise(b, c, int(sr * dur))
- norm_audio = normalize_audio(audio, strategy='peak')
- assert norm_audio.abs().max() <= 1
diff --git a/spaces/Grezz/generate_human_motion/VQ-Trans/options/option_vq.py b/spaces/Grezz/generate_human_motion/VQ-Trans/options/option_vq.py
deleted file mode 100644
index 08a53ff1270facc10ab44ec0647e673ed1336d0d..0000000000000000000000000000000000000000
--- a/spaces/Grezz/generate_human_motion/VQ-Trans/options/option_vq.py
+++ /dev/null
@@ -1,61 +0,0 @@
-import argparse
-
-def get_args_parser():
- parser = argparse.ArgumentParser(description='Optimal Transport AutoEncoder training for AIST',
- add_help=True,
- formatter_class=argparse.ArgumentDefaultsHelpFormatter)
-
- ## dataloader
- parser.add_argument('--dataname', type=str, default='kit', help='dataset directory')
- parser.add_argument('--batch-size', default=128, type=int, help='batch size')
- parser.add_argument('--window-size', type=int, default=64, help='training motion length')
-
- ## optimization
- parser.add_argument('--total-iter', default=200000, type=int, help='number of total iterations to run')
- parser.add_argument('--warm-up-iter', default=1000, type=int, help='number of total iterations for warmup')
- parser.add_argument('--lr', default=2e-4, type=float, help='max learning rate')
- parser.add_argument('--lr-scheduler', default=[50000, 400000], nargs="+", type=int, help="learning rate schedule (iterations)")
- parser.add_argument('--gamma', default=0.05, type=float, help="learning rate decay")
-
- parser.add_argument('--weight-decay', default=0.0, type=float, help='weight decay')
- parser.add_argument("--commit", type=float, default=0.02, help="hyper-parameter for the commitment loss")
- parser.add_argument('--loss-vel', type=float, default=0.1, help='hyper-parameter for the velocity loss')
- parser.add_argument('--recons-loss', type=str, default='l2', help='reconstruction loss')
-
- ## vqvae arch
- parser.add_argument("--code-dim", type=int, default=512, help="embedding dimension")
- parser.add_argument("--nb-code", type=int, default=512, help="nb of embedding")
- parser.add_argument("--mu", type=float, default=0.99, help="exponential moving average to update the codebook")
- parser.add_argument("--down-t", type=int, default=2, help="downsampling rate")
- parser.add_argument("--stride-t", type=int, default=2, help="stride size")
- parser.add_argument("--width", type=int, default=512, help="width of the network")
- parser.add_argument("--depth", type=int, default=3, help="depth of the network")
- parser.add_argument("--dilation-growth-rate", type=int, default=3, help="dilation growth rate")
- parser.add_argument("--output-emb-width", type=int, default=512, help="output embedding width")
- parser.add_argument('--vq-act', type=str, default='relu', choices = ['relu', 'silu', 'gelu'], help='dataset directory')
- parser.add_argument('--vq-norm', type=str, default=None, help='dataset directory')
-
- ## quantizer
- parser.add_argument("--quantizer", type=str, default='ema_reset', choices = ['ema', 'orig', 'ema_reset', 'reset'], help="eps for optimal transport")
- parser.add_argument('--beta', type=float, default=1.0, help='commitment loss in standard VQ')
-
- ## resume
- parser.add_argument("--resume-pth", type=str, default=None, help='resume pth for VQ')
- parser.add_argument("--resume-gpt", type=str, default=None, help='resume pth for GPT')
-
-
- ## output directory
- parser.add_argument('--out-dir', type=str, default='output_vqfinal/', help='output directory')
- parser.add_argument('--results-dir', type=str, default='visual_results/', help='output directory')
- parser.add_argument('--visual-name', type=str, default='baseline', help='output directory')
- parser.add_argument('--exp-name', type=str, default='exp_debug', help='name of the experiment, will create a file inside out-dir')
- ## other
- parser.add_argument('--print-iter', default=200, type=int, help='print frequency')
- parser.add_argument('--eval-iter', default=1000, type=int, help='evaluation frequency')
- parser.add_argument('--seed', default=123, type=int, help='seed for initializing training.')
-
- parser.add_argument('--vis-gt', action='store_true', help='whether visualize GT motions')
- parser.add_argument('--nb-vis', default=20, type=int, help='nb of visualizations')
-
-
- return parser.parse_args()
\ No newline at end of file
diff --git a/spaces/HarryLee/eCommerceImageCaptioning/fairseq/fairseq/optim/lr_scheduler/step_lr_scheduler.py b/spaces/HarryLee/eCommerceImageCaptioning/fairseq/fairseq/optim/lr_scheduler/step_lr_scheduler.py
deleted file mode 100644
index 8cb20068606a4afd2983430b794fa24647de2e7b..0000000000000000000000000000000000000000
--- a/spaces/HarryLee/eCommerceImageCaptioning/fairseq/fairseq/optim/lr_scheduler/step_lr_scheduler.py
+++ /dev/null
@@ -1,86 +0,0 @@
-# Copyright (c) Facebook, Inc. and its affiliates.
-#
-# This source code is licensed under the MIT license found in the
-# LICENSE file in the root directory of this source tree.
-
-from collections.abc import Collection
-from dataclasses import dataclass, field
-from typing import List
-
-from omegaconf import II
-
-from fairseq.dataclass import FairseqDataclass
-from fairseq.optim.lr_scheduler import FairseqLRScheduler, register_lr_scheduler
-
-
-@dataclass
-class StepLRScheduleConfig(FairseqDataclass):
- warmup_updates: int = field(
- default=0,
- metadata={"help": "warmup the learning rate linearly for the first N updates"},
- )
- warmup_init_lr: float = field(
- default=-1,
- metadata={
- "help": "initial learning rate during warmup phase; default is cfg.lr"
- },
- )
- lr: List[float] = field(
- default=II("optimization.lr"),
- metadata={"help": "max learning rate, must be more than cfg.min_lr"},
- )
- min_lr: float = field(default=0.0, metadata={"help": "min learning rate"})
- lr_deacy_period: int = field(default=25000, metadata={"help": "decay period"})
- lr_decay: float = field(default=0.5, metadata={"help": "decay factor"})
-
-
-@register_lr_scheduler("step", dataclass=StepLRScheduleConfig)
-class StepLRSchedule(FairseqLRScheduler):
- """Decay learning rate every k updates by a fixed factor
- """
-
- def __init__(self, cfg: StepLRScheduleConfig, fairseq_optimizer):
- super().__init__(cfg, fairseq_optimizer)
- self.max_lr = cfg.lr[0] if isinstance(cfg.lr, Collection) else cfg.lr
- self.min_lr = cfg.min_lr
- self.lr_deacy_period = cfg.lr_deacy_period
- self.lr_decay = cfg.lr_decay
- self.warmup_updates = cfg.warmup_updates
- self.warmup_init_lr = (
- cfg.warmup_init_lr if cfg.warmup_init_lr >= 0 else self.min_lr
- )
-
- assert(self.lr_deacy_period > 0)
- assert(self.lr_decay <= 1)
- assert(self.min_lr >= 0)
- assert(self.max_lr > self.min_lr)
-
- if cfg.warmup_updates > 0:
- # linearly warmup for the first cfg.warmup_updates
- self.warmup_lr_step = (
- (self.max_lr - self.warmup_init_lr) / self.warmup_updates
- )
- else:
- self.warmup_lr_step = 1
-
- # initial learning rate
- self.lr = self.warmup_init_lr
- self.optimizer.set_lr(self.lr)
-
- def step(self, epoch, val_loss=None):
- """Update the learning rate at the end of the given epoch."""
- super().step(epoch, val_loss)
- # we don't change the learning rate at epoch boundaries
- return self.optimizer.get_lr()
-
- def step_update(self, num_updates):
- """Update the learning rate after each update."""
- if num_updates < self.cfg.warmup_updates:
- self.lr = self.warmup_init_lr + num_updates * self.warmup_lr_step
- else:
- curr_updates = num_updates - self.cfg.warmup_updates
- lr_mult = self.lr_decay ** (curr_updates // self.lr_deacy_period)
- self.lr = max(self.max_lr * lr_mult, self.min_lr)
-
- self.optimizer.set_lr(self.lr)
- return self.lr
diff --git a/spaces/HighCWu/GFPGAN-1.3/inference_gfpgan.py b/spaces/HighCWu/GFPGAN-1.3/inference_gfpgan.py
deleted file mode 100644
index a426cfc7b9e67aef84e0f3c0666e09d875ebb222..0000000000000000000000000000000000000000
--- a/spaces/HighCWu/GFPGAN-1.3/inference_gfpgan.py
+++ /dev/null
@@ -1,116 +0,0 @@
-import argparse
-import cv2
-import glob
-import numpy as np
-import os
-import torch
-from basicsr.utils import imwrite
-
-from gfpgan import GFPGANer
-
-
-def main():
- """Inference demo for GFPGAN.
- """
- parser = argparse.ArgumentParser()
- parser.add_argument('--upscale', type=int, default=2, help='The final upsampling scale of the image')
- parser.add_argument('--arch', type=str, default='clean', help='The GFPGAN architecture. Option: clean | original')
- parser.add_argument('--channel', type=int, default=2, help='Channel multiplier for large networks of StyleGAN2')
- parser.add_argument('--model_path', type=str, default='experiments/pretrained_models/GFPGANCleanv1-NoCE-C2.pth')
- parser.add_argument('--bg_upsampler', type=str, default='realesrgan', help='background upsampler')
- parser.add_argument(
- '--bg_tile', type=int, default=400, help='Tile size for background sampler, 0 for no tile during testing')
- parser.add_argument('--test_path', type=str, default='inputs/whole_imgs', help='Input folder')
- parser.add_argument('--suffix', type=str, default=None, help='Suffix of the restored faces')
- parser.add_argument('--only_center_face', action='store_true', help='Only restore the center face')
- parser.add_argument('--aligned', action='store_true', help='Input are aligned faces')
- parser.add_argument('--paste_back', action='store_false', help='Paste the restored faces back to images')
- parser.add_argument('--save_root', type=str, default='results', help='Path to save root')
- parser.add_argument(
- '--ext',
- type=str,
- default='auto',
- help='Image extension. Options: auto | jpg | png, auto means using the same extension as inputs')
- args = parser.parse_args()
-
- args = parser.parse_args()
- if args.test_path.endswith('/'):
- args.test_path = args.test_path[:-1]
- os.makedirs(args.save_root, exist_ok=True)
-
- # background upsampler
- if args.bg_upsampler == 'realesrgan':
- if not torch.cuda.is_available(): # CPU
- import warnings
- warnings.warn('The unoptimized RealESRGAN is very slow on CPU. We do not use it. '
- 'If you really want to use it, please modify the corresponding codes.')
- bg_upsampler = None
- else:
- from basicsr.archs.rrdbnet_arch import RRDBNet
- from realesrgan import RealESRGANer
- model = RRDBNet(num_in_ch=3, num_out_ch=3, num_feat=64, num_block=23, num_grow_ch=32, scale=2)
- bg_upsampler = RealESRGANer(
- scale=2,
- model_path='https://github.com/xinntao/Real-ESRGAN/releases/download/v0.2.1/RealESRGAN_x2plus.pth',
- model=model,
- tile=args.bg_tile,
- tile_pad=10,
- pre_pad=0,
- half=True) # need to set False in CPU mode
- else:
- bg_upsampler = None
- # set up GFPGAN restorer
- restorer = GFPGANer(
- model_path=args.model_path,
- upscale=args.upscale,
- arch=args.arch,
- channel_multiplier=args.channel,
- bg_upsampler=bg_upsampler)
-
- img_list = sorted(glob.glob(os.path.join(args.test_path, '*')))
- for img_path in img_list:
- # read image
- img_name = os.path.basename(img_path)
- print(f'Processing {img_name} ...')
- basename, ext = os.path.splitext(img_name)
- input_img = cv2.imread(img_path, cv2.IMREAD_COLOR)
-
- # restore faces and background if necessary
- cropped_faces, restored_faces, restored_img = restorer.enhance(
- input_img, has_aligned=args.aligned, only_center_face=args.only_center_face, paste_back=args.paste_back)
-
- # save faces
- for idx, (cropped_face, restored_face) in enumerate(zip(cropped_faces, restored_faces)):
- # save cropped face
- save_crop_path = os.path.join(args.save_root, 'cropped_faces', f'{basename}_{idx:02d}.png')
- imwrite(cropped_face, save_crop_path)
- # save restored face
- if args.suffix is not None:
- save_face_name = f'{basename}_{idx:02d}_{args.suffix}.png'
- else:
- save_face_name = f'{basename}_{idx:02d}.png'
- save_restore_path = os.path.join(args.save_root, 'restored_faces', save_face_name)
- imwrite(restored_face, save_restore_path)
- # save comparison image
- cmp_img = np.concatenate((cropped_face, restored_face), axis=1)
- imwrite(cmp_img, os.path.join(args.save_root, 'cmp', f'{basename}_{idx:02d}.png'))
-
- # save restored img
- if restored_img is not None:
- if args.ext == 'auto':
- extension = ext[1:]
- else:
- extension = args.ext
-
- if args.suffix is not None:
- save_restore_path = os.path.join(args.save_root, 'restored_imgs',
- f'{basename}_{args.suffix}.{extension}')
- else:
- save_restore_path = os.path.join(args.save_root, 'restored_imgs', f'{basename}.{extension}')
- imwrite(restored_img, save_restore_path)
-
- print(f'Results are in the [{args.save_root}] folder.')
-
-
-if __name__ == '__main__':
- main()
diff --git a/spaces/Hina4867/bingo/src/pages/api/blob.ts b/spaces/Hina4867/bingo/src/pages/api/blob.ts
deleted file mode 100644
index fecd48031916b2284b8958892196e0a1ad420421..0000000000000000000000000000000000000000
--- a/spaces/Hina4867/bingo/src/pages/api/blob.ts
+++ /dev/null
@@ -1,40 +0,0 @@
-'use server'
-
-import { NextApiRequest, NextApiResponse } from 'next'
-import { Readable } from 'node:stream'
-import { fetch } from '@/lib/isomorphic'
-
-const API_DOMAIN = 'https://www.bing.com'
-
-export default async function handler(req: NextApiRequest, res: NextApiResponse) {
- try {
- const { bcid } = req.query
-
- const { headers, body } = await fetch(`${API_DOMAIN}/images/blob?bcid=${bcid}`,
- {
- method: 'GET',
- headers: {
- "sec-ch-ua": "\"Not/A)Brand\";v=\"99\", \"Google Chrome\";v=\"115\", \"Chromium\";v=\"115\"",
- "sec-ch-ua-mobile": "?0",
- "sec-ch-ua-platform": "\"Windows\"",
- "Referrer-Policy": "origin-when-cross-origin",
- },
- },
- )
-
- res.writeHead(200, {
- 'Content-Length': headers.get('content-length')!,
- 'Content-Type': headers.get('content-type')!,
- })
- // @ts-ignore
- return Readable.fromWeb(body!).pipe(res)
- } catch (e) {
- console.log('Error', e)
- return res.json({
- result: {
- value: 'UploadFailed',
- message: `${e}`
- }
- })
- }
-}
diff --git a/spaces/HoangHa/llama2-code/app.py b/spaces/HoangHa/llama2-code/app.py
deleted file mode 100644
index ffb42e42d106cc11515fa02f0acea004127a48b3..0000000000000000000000000000000000000000
--- a/spaces/HoangHa/llama2-code/app.py
+++ /dev/null
@@ -1,267 +0,0 @@
-from typing import Iterator
-
-import gradio as gr
-import torch
-
-from model import get_input_token_length, run
-
-DEFAULT_SYSTEM_PROMPT = """\
-You are a software engineer reporting to a senior software engineer. Reply with highest quality, PhD level, detailed, logical, precise, clean answers.
-"""
-MAX_MAX_NEW_TOKENS = 2048
-DEFAULT_MAX_NEW_TOKENS = 1024
-MAX_INPUT_TOKEN_LENGTH = 4000
-
-DESCRIPTION = """
-"""
-
-LICENSE = """
-
----
-"""
-
-if not torch.cuda.is_available():
- DESCRIPTION += '\nRunning on CPU.
'
-
-
-def clear_and_save_textbox(message: str) -> tuple[str, str]:
- return '', message
-
-
-def display_input(message: str,
- history: list[tuple[str, str]]) -> list[tuple[str, str]]:
- history.append((message, ''))
- return history
-
-
-def delete_prev_fn(
- history: list[tuple[str, str]]) -> tuple[list[tuple[str, str]], str]:
- try:
- message, _ = history.pop()
- except IndexError:
- message = ''
- return history, message or ''
-
-
-def generate(
- message: str,
- history_with_input: list[tuple[str, str]],
- system_prompt: str,
- max_new_tokens: int,
- temperature: float,
- top_p: float,
- top_k: int,
-) -> Iterator[list[tuple[str, str]]]:
- if max_new_tokens > MAX_MAX_NEW_TOKENS:
- raise ValueError
-
- history = history_with_input[:-1]
- generator = run(message, history, system_prompt, max_new_tokens, temperature, top_p, top_k)
- try:
- first_response = next(generator)
- yield history + [(message, first_response)]
- except StopIteration:
- yield history + [(message, '')]
- for response in generator:
- yield history + [(message, response)]
-
-
-def process_example(message: str) -> tuple[str, list[tuple[str, str]]]:
- generator = generate(message, [], DEFAULT_SYSTEM_PROMPT, 1024, 1, 0.95, 50)
- for x in generator:
- pass
- return '', x
-
-
-def check_input_token_length(message: str, chat_history: list[tuple[str, str]], system_prompt: str) -> None:
- input_token_length = get_input_token_length(message, chat_history, system_prompt)
- if input_token_length > MAX_INPUT_TOKEN_LENGTH:
- raise gr.Error(f'The accumulated input is too long ({input_token_length} > {MAX_INPUT_TOKEN_LENGTH}). Clear your chat history and try again.')
-
-
-with gr.Blocks(css='style.css') as demo:
- gr.Markdown(DESCRIPTION)
-
- with gr.Group():
- chatbot = gr.Chatbot(label='Chatbot')
- with gr.Row():
- textbox = gr.Textbox(
- container=False,
- show_label=False,
- placeholder='Type a message...',
- scale=10,
- )
- submit_button = gr.Button('Submit',
- variant='primary',
- scale=1,
- min_width=0)
- with gr.Row():
- retry_button = gr.Button('🔄 Retry', variant='secondary')
- undo_button = gr.Button('↩️ Undo', variant='secondary')
- clear_button = gr.Button('🗑️ Clear', variant='secondary')
-
- saved_input = gr.State()
-
- with gr.Accordion(label='Advanced options', open=False):
- system_prompt = gr.Textbox(label='System prompt',
- value=DEFAULT_SYSTEM_PROMPT,
- lines=6)
- max_new_tokens = gr.Slider(
- label='Max new tokens',
- minimum=1,
- maximum=MAX_MAX_NEW_TOKENS,
- step=1,
- value=DEFAULT_MAX_NEW_TOKENS,
- )
- temperature = gr.Slider(
- label='Temperature',
- minimum=0.1,
- maximum=4.0,
- step=0.1,
- value=1.0,
- )
- top_p = gr.Slider(
- label='Top-p (nucleus sampling)',
- minimum=0.05,
- maximum=1.0,
- step=0.05,
- value=0.95,
- )
- top_k = gr.Slider(
- label='Top-k',
- minimum=1,
- maximum=1000,
- step=1,
- value=50,
- )
-
- gr.Examples(
- examples=[
- "X_train, y_train, X_test, y_test = train_test_split(X, y, test_size=0.1)\n\n# Train a logistic regression model, predict the labels on the test set and compute the accuracy score",
- "// Returns every other value in the array as a new array.\nfunction everyOther(arr) {",
- "Poor English: She no went to the market. Corrected English:",
- "def alternating(list1, list2):\n results = []\n for i in range(min(len(list1), len(list2))):\n results.append(list1[i])\n results.append(list2[i])\n if len(list1) > len(list2):\n \n else:\n results.extend(list2[i+1:])\n return results",
- "def remove_non_ascii(s: str) -> str:\n \"\"\" \nprint(remove_non_ascii('afkdj$$('))",
- ],
- inputs=textbox,
- outputs=[textbox, chatbot],
- fn=process_example,
- cache_examples=True,
- )
-
- gr.Markdown(LICENSE)
-
- textbox.submit(
- fn=clear_and_save_textbox,
- inputs=textbox,
- outputs=[textbox, saved_input],
- api_name=False,
- queue=False,
- ).then(
- fn=display_input,
- inputs=[saved_input, chatbot],
- outputs=chatbot,
- api_name=False,
- queue=False,
- ).then(
- fn=check_input_token_length,
- inputs=[saved_input, chatbot, system_prompt],
- api_name=False,
- queue=False,
- ).success(
- fn=generate,
- inputs=[
- saved_input,
- chatbot,
- system_prompt,
- max_new_tokens,
- temperature,
- top_p,
- top_k,
- ],
- outputs=chatbot,
- api_name=False,
- )
-
- button_event_preprocess = submit_button.click(
- fn=clear_and_save_textbox,
- inputs=textbox,
- outputs=[textbox, saved_input],
- api_name=False,
- queue=False,
- ).then(
- fn=display_input,
- inputs=[saved_input, chatbot],
- outputs=chatbot,
- api_name=False,
- queue=False,
- ).then(
- fn=check_input_token_length,
- inputs=[saved_input, chatbot, system_prompt],
- api_name=False,
- queue=False,
- ).success(
- fn=generate,
- inputs=[
- saved_input,
- chatbot,
- system_prompt,
- max_new_tokens,
- temperature,
- top_p,
- top_k,
- ],
- outputs=chatbot,
- api_name=False,
- )
-
- retry_button.click(
- fn=delete_prev_fn,
- inputs=chatbot,
- outputs=[chatbot, saved_input],
- api_name=False,
- queue=False,
- ).then(
- fn=display_input,
- inputs=[saved_input, chatbot],
- outputs=chatbot,
- api_name=False,
- queue=False,
- ).then(
- fn=generate,
- inputs=[
- saved_input,
- chatbot,
- system_prompt,
- max_new_tokens,
- temperature,
- top_p,
- top_k,
- ],
- outputs=chatbot,
- api_name=False,
- )
-
- undo_button.click(
-
- fn=delete_prev_fn,
- inputs=chatbot,
- outputs=[chatbot, saved_input],
- api_name=False,
- queue=False,
- ).then(
- fn=lambda x: x,
- inputs=[saved_input],
- outputs=textbox,
- api_name=False,
- queue=False,
- )
-
- clear_button.click(
- fn=lambda: ([], ''),
- outputs=[chatbot, saved_input],
- queue=False,
- api_name=False,
- )
-
-demo.queue(max_size=20).launch()
\ No newline at end of file
diff --git a/spaces/ICML2023/ICML2023_papers/app.py b/spaces/ICML2023/ICML2023_papers/app.py
deleted file mode 100644
index c211890d1863cc537acb5e4357bf550acb661319..0000000000000000000000000000000000000000
--- a/spaces/ICML2023/ICML2023_papers/app.py
+++ /dev/null
@@ -1,72 +0,0 @@
-#!/usr/bin/env python
-
-from __future__ import annotations
-
-import gradio as gr
-
-from paper_list import PaperList
-
-DESCRIPTION = '# ICML 2023 Papers'
-
-paper_list = PaperList()
-
-with gr.Blocks(css='style.css') as demo:
- gr.Markdown(DESCRIPTION)
-
- search_box = gr.Textbox(
- label='Search Title',
- placeholder=
- 'You can search for titles with regular expressions. e.g. (? partial_frames:
- mel_slices = self.compute_partial_slices(mel_len, partial_frames, partial_hop)
- mels = list(mel[:,s] for s in mel_slices)
- mels.append(last_mel)
- mels = torch.stack(tuple(mels), 0).squeeze(1)
-
- with torch.no_grad():
- partial_embeds = self(mels)
- embed = torch.mean(partial_embeds, axis=0).unsqueeze(0)
- #embed = embed / torch.linalg.norm(embed, 2)
- else:
- with torch.no_grad():
- embed = self(last_mel)
-
- return embed
-
-
-class SynthesizerTrn(nn.Module):
- """
- Synthesizer for Training
- """
-
- def __init__(self,
- spec_channels,
- segment_size,
- inter_channels,
- hidden_channels,
- filter_channels,
- n_heads,
- n_layers,
- kernel_size,
- p_dropout,
- resblock,
- resblock_kernel_sizes,
- resblock_dilation_sizes,
- upsample_rates,
- upsample_initial_channel,
- upsample_kernel_sizes,
- gin_channels,
- ssl_dim,
- n_speakers,
- **kwargs):
-
- super().__init__()
- self.spec_channels = spec_channels
- self.inter_channels = inter_channels
- self.hidden_channels = hidden_channels
- self.filter_channels = filter_channels
- self.n_heads = n_heads
- self.n_layers = n_layers
- self.kernel_size = kernel_size
- self.p_dropout = p_dropout
- self.resblock = resblock
- self.resblock_kernel_sizes = resblock_kernel_sizes
- self.resblock_dilation_sizes = resblock_dilation_sizes
- self.upsample_rates = upsample_rates
- self.upsample_initial_channel = upsample_initial_channel
- self.upsample_kernel_sizes = upsample_kernel_sizes
- self.segment_size = segment_size
- self.gin_channels = gin_channels
- self.ssl_dim = ssl_dim
- self.emb_g = nn.Embedding(n_speakers, gin_channels)
-
- self.enc_p_ = TextEncoder(ssl_dim, inter_channels, hidden_channels, 5, 1, 16,0, filter_channels, n_heads, p_dropout)
- hps = {
- "sampling_rate": 48000,
- "inter_channels": 192,
- "resblock": "1",
- "resblock_kernel_sizes": [3, 7, 11],
- "resblock_dilation_sizes": [[1, 3, 5], [1, 3, 5], [1, 3, 5]],
- "upsample_rates": [10, 8, 2, 2],
- "upsample_initial_channel": 512,
- "upsample_kernel_sizes": [16, 16, 4, 4],
- "gin_channels": 256,
- }
- self.dec = Generator(h=hps)
- self.enc_q = Encoder(spec_channels, inter_channels, hidden_channels, 5, 1, 16, gin_channels=gin_channels)
- self.flow = ResidualCouplingBlock(inter_channels, hidden_channels, 5, 1, 4, gin_channels=gin_channels)
-
- def forward(self, c, c_lengths, f0, g=None):
- g = self.emb_g(g.unsqueeze(0)).transpose(1,2)
- z_p, m_p, logs_p, c_mask = self.enc_p_(c.transpose(1,2), c_lengths, f0=f0_to_coarse(f0))
- z = self.flow(z_p, c_mask, g=g, reverse=True)
- o = self.dec(z * c_mask, g=g, f0=f0.float())
- return o
-
diff --git a/spaces/InpaintAI/Inpaint-Anything/third_party/segment-anything/demo/configs/webpack/common.js b/spaces/InpaintAI/Inpaint-Anything/third_party/segment-anything/demo/configs/webpack/common.js
deleted file mode 100644
index 098f6686f063bf6c631df4f5f3b5921d48ed2d2a..0000000000000000000000000000000000000000
--- a/spaces/InpaintAI/Inpaint-Anything/third_party/segment-anything/demo/configs/webpack/common.js
+++ /dev/null
@@ -1,84 +0,0 @@
-// Copyright (c) Meta Platforms, Inc. and affiliates.
-// All rights reserved.
-
-// This source code is licensed under the license found in the
-// LICENSE file in the root directory of this source tree.
-
-const { resolve } = require("path");
-const HtmlWebpackPlugin = require("html-webpack-plugin");
-const FriendlyErrorsWebpackPlugin = require("friendly-errors-webpack-plugin");
-const CopyPlugin = require("copy-webpack-plugin");
-const webpack = require("webpack");
-
-module.exports = {
- entry: "./src/index.tsx",
- resolve: {
- extensions: [".js", ".jsx", ".ts", ".tsx"],
- },
- output: {
- path: resolve(__dirname, "dist"),
- },
- module: {
- rules: [
- {
- test: /\.mjs$/,
- include: /node_modules/,
- type: "javascript/auto",
- resolve: {
- fullySpecified: false,
- },
- },
- {
- test: [/\.jsx?$/, /\.tsx?$/],
- use: ["ts-loader"],
- exclude: /node_modules/,
- },
- {
- test: /\.css$/,
- use: ["style-loader", "css-loader"],
- },
- {
- test: /\.(scss|sass)$/,
- use: ["style-loader", "css-loader", "postcss-loader"],
- },
- {
- test: /\.(jpe?g|png|gif|svg)$/i,
- use: [
- "file-loader?hash=sha512&digest=hex&name=img/[contenthash].[ext]",
- "image-webpack-loader?bypassOnDebug&optipng.optimizationLevel=7&gifsicle.interlaced=false",
- ],
- },
- {
- test: /\.(woff|woff2|ttf)$/,
- use: {
- loader: "url-loader",
- },
- },
- ],
- },
- plugins: [
- new CopyPlugin({
- patterns: [
- {
- from: "node_modules/onnxruntime-web/dist/*.wasm",
- to: "[name][ext]",
- },
- {
- from: "model",
- to: "model",
- },
- {
- from: "src/assets",
- to: "assets",
- },
- ],
- }),
- new HtmlWebpackPlugin({
- template: "./src/assets/index.html",
- }),
- new FriendlyErrorsWebpackPlugin(),
- new webpack.ProvidePlugin({
- process: "process/browser",
- }),
- ],
-};
diff --git a/spaces/Intel/NeuralChat-ICX-INT4/fastchat/serve/huggingface_api.py b/spaces/Intel/NeuralChat-ICX-INT4/fastchat/serve/huggingface_api.py
deleted file mode 100644
index 9dd4ea466d72345670163c94874ae0a377e874b6..0000000000000000000000000000000000000000
--- a/spaces/Intel/NeuralChat-ICX-INT4/fastchat/serve/huggingface_api.py
+++ /dev/null
@@ -1,77 +0,0 @@
-"""
-Usage:
-python3 -m fastchat.serve.huggingface_api --model ~/model_weights/vicuna-7b/
-"""
-import argparse
-import json
-
-import torch
-from transformers import AutoTokenizer, AutoModelForCausalLM
-
-from fastchat.conversation import get_default_conv_template, compute_skip_echo_len
-from fastchat.serve.inference import load_model
-
-
-@torch.inference_mode()
-def main(args):
- model, tokenizer = load_model(
- args.model_path,
- args.device,
- args.num_gpus,
- args.max_gpu_memory,
- args.load_8bit,
- debug=args.debug,
- )
-
- msg = args.message
-
- conv = get_default_conv_template(args.model_path).copy()
- conv.append_message(conv.roles[0], msg)
- conv.append_message(conv.roles[1], None)
- prompt = conv.get_prompt()
-
- inputs = tokenizer([prompt])
- output_ids = model.generate(
- torch.as_tensor(inputs.input_ids).cuda(),
- do_sample=True,
- temperature=0.7,
- max_new_tokens=1024,
- )
- outputs = tokenizer.batch_decode(output_ids, skip_special_tokens=True)[0]
- skip_echo_len = compute_skip_echo_len(args.model_path, conv, prompt)
- outputs = outputs[skip_echo_len:]
-
- print(f"{conv.roles[0]}: {msg}")
- print(f"{conv.roles[1]}: {outputs}")
-
-
-if __name__ == "__main__":
- parser = argparse.ArgumentParser()
- parser.add_argument(
- "--model-path",
- type=str,
- default="facebook/opt-350m",
- help="The path to the weights",
- )
- parser.add_argument(
- "--device", type=str, choices=["cpu", "cuda", "mps"], default="cuda"
- )
- parser.add_argument("--num-gpus", type=str, default="1")
- parser.add_argument(
- "--max-gpu-memory",
- type=str,
- help="The maximum memory per gpu. Use a string like '13Gib'",
- )
- parser.add_argument(
- "--load-8bit", action="store_true", help="Use 8-bit quantization."
- )
- parser.add_argument(
- "--conv-template", type=str, default=None, help="Conversation prompt template."
- )
- parser.add_argument("--temperature", type=float, default=0.7)
- parser.add_argument("--max-new-tokens", type=int, default=512)
- parser.add_argument("--debug", action="store_true")
- parser.add_argument("--message", type=str, default="Hello! Who are you?")
- args = parser.parse_args()
-
- main(args)
diff --git a/spaces/JCTN/stable-diffusion-webui-cjtn/app.py b/spaces/JCTN/stable-diffusion-webui-cjtn/app.py
deleted file mode 100644
index 723fab1dcee0b8cade7795de3440be792b536048..0000000000000000000000000000000000000000
--- a/spaces/JCTN/stable-diffusion-webui-cjtn/app.py
+++ /dev/null
@@ -1,147 +0,0 @@
-import os
-from sys import executable as pyexecutable
-import subprocess
-import pathlib
-import gc
-
-def Gitclone(URI:str,ClonePath:str = "") -> int :
- if(ClonePath == "") :
- while True:
- i=subprocess.run([r"git",r"clone",URI])
- if(i.returncode == 0 ):
- del i
- gc.collect()
- return 0
- else :
- del i
- else:
- while True:
- i=subprocess.run([r"git",r"clone",URI,ClonePath])
- if(i.returncode == 0 ):
- del i
- gc.collect()
- return 0
- else :
- del i
-def DownLoad(URI:str,DownloadPath:str,DownLoadFileName:str ) -> int:
- while (True):
- i=subprocess.run([r"aria2c",r"-c",r"-x" ,r"16", r"-s",r"16", r"-k" ,r"1M" ,r"-m",r"0",r"--enable-mmap=false",r"--console-log-level=error",r"-d",DownloadPath,r"-o",DownLoadFileName,URI]);
- if(i.returncode == 0 ):
- del i
- gc.collect()
- return 0
- else :
- del i
-user_home =pathlib.Path.home().resolve()
-os.chdir(str(user_home))
-#clone stable-diffusion-webui repo
-print("cloning stable-diffusion-webui repo")
-Gitclone(r"https://github.com/AUTOMATIC1111/stable-diffusion-webui.git",str(user_home / r"stable-diffusion-webui"))
-os.chdir(str(user_home / r"stable-diffusion-webui"))
-os.system("git reset --hard 89f9faa63388756314e8a1d96cf86bf5e0663045")
-#
-
-#install extensions
-print("installing extensions")
-Gitclone(r"https://huggingface.co/embed/negative",str(user_home / r"stable-diffusion-webui" / r"embeddings" / r"negative"))
-Gitclone(r"https://huggingface.co/embed/lora",str(user_home / r"stable-diffusion-webui" / r"models" / r"Lora" / r"positive"))
-DownLoad(r"https://huggingface.co/embed/upscale/resolve/main/4x-UltraSharp.pth",str(user_home / r"stable-diffusion-webui" / r"models" / r"ESRGAN") ,r"4x-UltraSharp.pth")
-while True:
- if(subprocess.run([r"wget",r"https://raw.githubusercontent.com/camenduru/stable-diffusion-webui-scripts/main/run_n_times.py",r"-O",str(user_home / r"stable-diffusion-webui" / r"scripts" / r"run_n_times.py")]).returncode == 0):
- break
-Gitclone(r"https://github.com/deforum-art/deforum-for-automatic1111-webui",str(user_home / r"stable-diffusion-webui" / r"extensions" / r"deforum-for-automatic1111-webui" ))
-Gitclone(r"https://github.com/AlUlkesh/stable-diffusion-webui-images-browser",str(user_home / r"stable-diffusion-webui" / r"extensions"/ r"stable-diffusion-webui-images-browser"))
-Gitclone(r"https://github.com/camenduru/stable-diffusion-webui-huggingface",str(user_home / r"stable-diffusion-webui" / r"extensions" / r"stable-diffusion-webui-huggingface"))
-Gitclone(r"https://github.com/camenduru/sd-civitai-browser",str(user_home / r"stable-diffusion-webui" / r"extensions" / r"sd-civitai-browser"))
-Gitclone(r"https://github.com/kohya-ss/sd-webui-additional-networks",str(user_home / r"stable-diffusion-webui" / r"extensions" / r"sd-webui-additional-networks"))
-Gitclone(r"https://github.com/Mikubill/sd-webui-controlnet",str(user_home / r"stable-diffusion-webui" / r"extensions" / r"sd-webui-controlnet"))
-Gitclone(r"https://github.com/fkunn1326/openpose-editor",str(user_home / r"stable-diffusion-webui" / r"extensions" / r"openpose-editor"))
-Gitclone(r"https://github.com/jexom/sd-webui-depth-lib",str(user_home / r"stable-diffusion-webui" / r"extensions" / r"sd-webui-depth-lib"))
-Gitclone(r"https://github.com/hnmr293/posex",str(user_home / r"stable-diffusion-webui" / r"extensions" / r"posex"))
-Gitclone(r"https://github.com/nonnonstop/sd-webui-3d-open-pose-editor",str(user_home / r"stable-diffusion-webui" / r"extensions" / r"sd-webui-3d-open-pose-editor"))
-#中文本地化的请解除下一行的注释
-#Gitclone(r"https://github.com/dtlnor/stable-diffusion-webui-localization-zh_CN.git",str(user_home / r"stable-diffusion-webui" / r"extensions" / r"stable-diffusion-webui-localization-zh_CN"))
-Gitclone(r"https://github.com/DominikDoom/a1111-sd-webui-tagcomplete.git" , str(user_home / r"stable-diffusion-webui" / r"extensions" / r"a1111-sd-webui-tagcomplete"))
-Gitclone(r"https://github.com/camenduru/sd-webui-tunnels",str(user_home / r"stable-diffusion-webui" / r"extensions" / r"sd-webui-tunnels"))
-Gitclone(r"https://github.com/etherealxx/batchlinks-webui",str(user_home / r"stable-diffusion-webui" / r"extensions" / r"batchlinks-webui"))
-Gitclone(r"https://github.com/catppuccin/stable-diffusion-webui",str(user_home / r"stable-diffusion-webui" / r"extensions" / r"stable-diffusion-webui-catppuccin"))
-
-#Gitclone(r"https://github.com/KohakuBueleaf/a1111-sd-webui-locon",str(user_home / r"stable-diffusion-webui" / r"extensions" / r"a1111-sd-webui-locon" ))
-Gitclone(r"https://github.com/AUTOMATIC1111/stable-diffusion-webui-rembg",str(user_home / r"stable-diffusion-webui" / r"extensions" / r"stable-diffusion-webui-rembg"))
-Gitclone(r"https://github.com/ashen-sensored/stable-diffusion-webui-two-shot",str(user_home / r"stable-diffusion-webui" / r"extensions" / r"stable-diffusion-webui-two-shot"))
-Gitclone(r"https://github.com/camenduru/sd_webui_stealth_pnginfo",str(user_home / r"stable-diffusion-webui" / r"extensions" / r"sd_webui_stealth_pnginfo"))
-
-os.chdir(user_home / r"stable-diffusion-webui")
-
-#download ControlNet models
-print("extensions dolwnload done .\ndownloading ControlNet models")
-dList =[r"https://huggingface.co/ckpt/ControlNet-v1-1/resolve/main/control_v11e_sd15_ip2p_fp16.safetensors",
- r"https://huggingface.co/ckpt/ControlNet-v1-1/resolve/main/control_v11e_sd15_shuffle_fp16.safetensors",
- r"https://huggingface.co/ckpt/ControlNet-v1-1/resolve/main/control_v11p_sd15_canny_fp16.safetensors",
- r"https://huggingface.co/ckpt/ControlNet-v1-1/resolve/main/control_v11f1p_sd15_depth_fp16.safetensors",
- r"https://huggingface.co/ckpt/ControlNet-v1-1/resolve/main/control_v11p_sd15_inpaint_fp16.safetensors",
- r"https://huggingface.co/ckpt/ControlNet-v1-1/resolve/main/control_v11p_sd15_lineart_fp16.safetensors",
- r"https://huggingface.co/ckpt/ControlNet-v1-1/resolve/main/control_v11p_sd15_mlsd_fp16.safetensors",
- r"https://huggingface.co/ckpt/ControlNet-v1-1/resolve/main/control_v11p_sd15_normalbae_fp16.safetensors",
- r"https://huggingface.co/ckpt/ControlNet-v1-1/resolve/main/control_v11p_sd15_openpose_fp16.safetensors",
- r"https://huggingface.co/ckpt/ControlNet-v1-1/resolve/main/control_v11p_sd15_scribble_fp16.safetensors",
- r"https://huggingface.co/ckpt/ControlNet-v1-1/resolve/main/control_v11p_sd15_seg_fp16.safetensors",
- r"https://huggingface.co/ckpt/ControlNet-v1-1/resolve/main/control_v11p_sd15_softedge_fp16.safetensors",
- r"https://huggingface.co/ckpt/ControlNet-v1-1/resolve/main/control_v11p_sd15s2_lineart_anime_fp16.safetensors",
- r"https://huggingface.co/ckpt/ControlNet-v1-1/resolve/main/control_v11f1e_sd15_tile_fp16.safetensors",
- r"https://huggingface.co/ckpt/ControlNet-v1-1/raw/main/control_v11e_sd15_ip2p_fp16.yaml",
- r"https://huggingface.co/ckpt/ControlNet-v1-1/raw/main/control_v11e_sd15_shuffle_fp16.yaml",
- r"https://huggingface.co/ckpt/ControlNet-v1-1/raw/main/control_v11p_sd15_canny_fp16.yaml",
- r"https://huggingface.co/ckpt/ControlNet-v1-1/raw/main/control_v11f1p_sd15_depth_fp16.yaml",
- r"https://huggingface.co/ckpt/ControlNet-v1-1/raw/main/control_v11p_sd15_inpaint_fp16.yaml",
- r"https://huggingface.co/ckpt/ControlNet-v1-1/raw/main/control_v11p_sd15_lineart_fp16.yaml",
- r"https://huggingface.co/ckpt/ControlNet-v1-1/raw/main/control_v11p_sd15_mlsd_fp16.yaml",
- r"https://huggingface.co/ckpt/ControlNet-v1-1/raw/main/control_v11p_sd15_normalbae_fp16.yaml",
- r"https://huggingface.co/ckpt/ControlNet-v1-1/raw/main/control_v11p_sd15_openpose_fp16.yaml",
- r"https://huggingface.co/ckpt/ControlNet-v1-1/raw/main/control_v11p_sd15_scribble_fp16.yaml",
- r"https://huggingface.co/ckpt/ControlNet-v1-1/raw/main/control_v11p_sd15_seg_fp16.yaml",
- r"https://huggingface.co/ckpt/ControlNet-v1-1/raw/main/control_v11p_sd15_softedge_fp16.yaml",
- r"https://huggingface.co/ckpt/ControlNet-v1-1/raw/main/control_v11p_sd15s2_lineart_anime_fp16.yaml",
- r"https://huggingface.co/ckpt/ControlNet-v1-1/raw/main/control_v11f1e_sd15_tile_fp16.yaml",
- r"https://huggingface.co/ckpt/ControlNet-v1-1/resolve/main/t2iadapter_style_sd14v1.pth",
- r"https://huggingface.co/ckpt/ControlNet-v1-1/resolve/main/t2iadapter_sketch_sd14v1.pth",
- r"https://huggingface.co/ckpt/ControlNet-v1-1/resolve/main/t2iadapter_seg_sd14v1.pth",
- r"https://huggingface.co/ckpt/ControlNet-v1-1/resolve/main/t2iadapter_openpose_sd14v1.pth",
- r"https://huggingface.co/ckpt/ControlNet-v1-1/resolve/main/t2iadapter_keypose_sd14v1.pth",
- r"https://huggingface.co/ckpt/ControlNet-v1-1/resolve/main/t2iadapter_depth_sd14v1.pth",
- r"https://huggingface.co/ckpt/ControlNet-v1-1/resolve/main/t2iadapter_canny_sd14v1.pth",
- r"https://huggingface.co/ckpt/ControlNet-v1-1/resolve/main/t2iadapter_canny_sd15v2.pth",
- r"https://huggingface.co/ckpt/ControlNet-v1-1/resolve/main/t2iadapter_depth_sd15v2.pth",
- r"https://huggingface.co/ckpt/ControlNet-v1-1/resolve/main/t2iadapter_sketch_sd15v2.pth",
- r"https://huggingface.co/ckpt/ControlNet-v1-1/resolve/main/t2iadapter_zoedepth_sd15v1.pth"]
-for i in range(0,len(dList)): DownLoad(dList[i],str(user_home / "stable-diffusion-webui" / "extensions" / "sd-webui-controlnet" / "models"),pathlib.Path(dList[i]).name)
-del dList
-
-#download model
-#you can change model download address here
-print("ControlNet models download done.\ndownloading model")
-DownLoad(r"https://huggingface.co/ckpt/anything-v4.0/resolve/main/anything-v4.5-pruned.ckpt",str(user_home / r"stable-diffusion-webui" / r"models" / r"Stable-diffusion"),r"anything-v4.5-pruned.ckpt")
-DownLoad(r"https://huggingface.co/ckpt/anything-v4.0/resolve/main/anything-v4.0.vae.pt",str(user_home / r"stable-diffusion-webui" / r"models" / r"Stable-diffusion"),r"anything-v4.0.vae.pt")
-DownLoad(r"https://huggingface.co/gsdf/Counterfeit-V3.0/resolve/main/Counterfeit-V3.0_fp16.safetensors",str(user_home / r"stable-diffusion-webui" / r"models" / r"Stable-diffusion"),r"Counterfeit-V3.0_fp16.safetensors")
-DownLoad(r"https://huggingface.co/WarriorMama777/OrangeMixs/resolve/main/Models/AbyssOrangeMix3/AOM3A1B_orangemixs.safetensors",str(user_home / r"stable-diffusion-webui" / r"models" / r"Stable-diffusion"),r"AOM3A1B_orangemixs.safetensors")
-DownLoad(r"https://huggingface.co/WarriorMama777/OrangeMixs/resolve/main/VAEs/orangemix.vae.pt",str(user_home / r"stable-diffusion-webui" / r"models" / r"Stable-diffusion"),r"orangemix.vae.pt")
-DownLoad(r"https://huggingface.co/Meina/MeinaPastel/resolve/main/MeinaPastelV5%20-%20Baked%20VAE.safetensors",str(user_home / r"stable-diffusion-webui" / r"models" / r"Stable-diffusion"),r"MeinaPastelV5_BakedVAE.safetensors")
-DownLoad(r"https://huggingface.co/Meina/MeinaPastel/resolve/main/MeinaPastelV5%20-%20Without%20VAE.safetensors",str(user_home / r"stable-diffusion-webui" / r"models" / r"Stable-diffusion"),r"MeinaPastelV5_WithoutVAE.safetensors")
-DownLoad(r"https://civitai.com/api/download/models/9474",str(user_home / r"stable-diffusion-webui" / r"models" / r"Stable-diffusion"),r"chilloutmix_NiPrunedFp16.safetensors")
-
-DownLoad(r"https://civitai.com/api/download/models/39885",str(user_home / r"stable-diffusion-webui" / r"extensions" / r"sd-webui-additional-networks" / r"models"/ r"lora"),r"Better_light.safetensors")
-DownLoad(r"https://civitai.com/api/download/models/21065",str(user_home / r"stable-diffusion-webui" / r"extensions" / r"sd-webui-additional-networks" / r"models"/ r"lora"),r"LAS.safetensors")
-DownLoad(r"https://civitai.com/api/download/models/39164",str(user_home / r"stable-diffusion-webui" / r"extensions" / r"sd-webui-additional-networks" / r"models"/ r"lora"),r"backlighting.safetensors")
-#strt webui
-
-print("Done\nStarting Webui...")
-os.chdir(user_home / r"stable-diffusion-webui")
-while True:
- ret=subprocess.run([r"python3" ,r"launch.py",r"--precision",r"full",r"--no-half",r"--no-half-vae",r"--enable-insecure-extension-access",r"--medvram",r"--skip-torch-cuda-test",r"--enable-console-prompts",r"--ui-settings-file="+str(pathlib.Path(__file__).parent /r"config.json")])
- if(ret.returncode == 0 ):
- del ret
- gc.collect()
- else :
- del ret
-
-del os ,user_home ,pyexecutable ,subprocess
\ No newline at end of file
diff --git a/spaces/Jacob209/AUTOMATIC-promptgen-lexart/app.py b/spaces/Jacob209/AUTOMATIC-promptgen-lexart/app.py
deleted file mode 100644
index b06163218af29dd0948f34b428b860116493eb88..0000000000000000000000000000000000000000
--- a/spaces/Jacob209/AUTOMATIC-promptgen-lexart/app.py
+++ /dev/null
@@ -1,3 +0,0 @@
-import gradio as gr
-
-gr.Interface.load("models/AUTOMATIC/promptgen-lexart").launch()
\ No newline at end of file
diff --git a/spaces/JeffJing/ZookChatBot/revChatGPT/V1.py b/spaces/JeffJing/ZookChatBot/revChatGPT/V1.py
deleted file mode 100644
index c6357ec795319a0fca32bb84391bdd764b9e80f2..0000000000000000000000000000000000000000
--- a/spaces/JeffJing/ZookChatBot/revChatGPT/V1.py
+++ /dev/null
@@ -1,418 +0,0 @@
-"""
-Standard ChatGPT
-"""
-import json
-import logging
-import uuid
-from os import environ
-from os import getenv
-from os.path import exists
-from random import choice
-
-import requests
-from OpenAIAuth.OpenAIAuth import OpenAIAuth
-
-# Disable all logging
-logging.basicConfig(level=logging.ERROR)
-
-BASE_URL = environ.get("CHATGPT_BASE_URL") or choice(
- ["https://chatgpt-proxy.fly.dev/", "https://chatgpt-proxy2.fly.dev/"]
-)
-
-
-class Error(Exception):
- """Base class for exceptions in this module."""
-
- source: str
- message: str
- code: int
-
-
-class Chatbot:
- """
- Chatbot class for ChatGPT
- """
-
- def __init__(
- self,
- config,
- conversation_id=None,
- parent_id=None,
- ) -> None:
- self.config = config
- self.session = requests.Session()
- if "proxy" in config:
- if isinstance(config["proxy"], str) is False:
- raise Exception("Proxy must be a string!")
- proxies = {
- "http": config["proxy"],
- "https": config["proxy"],
- }
- self.session.proxies.update(proxies)
- if "verbose" in config:
- if type(config["verbose"]) != bool:
- raise Exception("Verbose must be a boolean!")
- self.verbose = config["verbose"]
- else:
- self.verbose = False
- self.conversation_id = conversation_id
- self.parent_id = parent_id
- self.conversation_mapping = {}
- self.conversation_id_prev_queue = []
- self.parent_id_prev_queue = []
- if "email" in config and "password" in config:
- pass
- elif "session_token" in config:
- pass
- elif "access_token" in config:
- self.__refresh_headers(config["access_token"])
- else:
- raise Exception("No login details provided!")
- if "access_token" not in config:
- self.__login()
-
- def __refresh_headers(self, access_token):
- self.session.headers.clear()
- self.session.headers.update(
- {
- "Accept": "text/event-stream",
- "Authorization": f"Bearer {access_token}",
- "Content-Type": "application/json",
- "X-Openai-Assistant-App-Id": "",
- "Connection": "close",
- "Accept-Language": "en-US,en;q=0.9",
- "Referer": "https://chat.openai.com/chat",
- },
- )
-
- def __login(self):
- if (
- "email" not in self.config or "password" not in self.config
- ) and "session_token" not in self.config:
- raise Exception("No login details provided!")
- auth = OpenAIAuth(
- email_address=self.config.get("email"),
- password=self.config.get("password"),
- proxy=self.config.get("proxy"),
- )
- if self.config.get("session_token"):
- auth.session_token = self.config["session_token"]
- auth.get_access_token()
- if auth.access_token is None:
- del self.config["session_token"]
- self.__login()
- return
- else:
- auth.begin()
- self.config["session_token"] = auth.session_token
- auth.get_access_token()
-
- self.__refresh_headers(auth.access_token)
-
- def ask(
- self,
- prompt,
- conversation_id=None,
- parent_id=None,
- # gen_title=True,
- ):
- """
- Ask a question to the chatbot
- :param prompt: String
- :param conversation_id: UUID
- :param parent_id: UUID
- :param gen_title: Boolean
- """
- if conversation_id is not None and parent_id is None:
- self.__map_conversations()
- if conversation_id is None:
- conversation_id = self.conversation_id
- if parent_id is None:
- parent_id = (
- self.parent_id
- if conversation_id == self.conversation_id
- else self.conversation_mapping[conversation_id]
- )
- # new_conv = conversation_id is None
- data = {
- "action": "next",
- "messages": [
- {
- "id": str(uuid.uuid4()),
- "role": "user",
- "content": {"content_type": "text", "parts": [prompt]},
- },
- ],
- "conversation_id": conversation_id,
- "parent_message_id": parent_id or str(uuid.uuid4()),
- "model": "text-davinci-002-render-sha",
- }
- # new_conv = data["conversation_id"] is None
- self.conversation_id_prev_queue.append(
- data["conversation_id"],
- ) # for rollback
- self.parent_id_prev_queue.append(data["parent_message_id"])
- response = self.session.post(
- url=BASE_URL + "api/conversation",
- data=json.dumps(data),
- timeout=360,
- stream=True,
- )
- self.__check_response(response)
- for line in response.iter_lines():
- line = str(line)[2:-1]
- if line == "" or line is None:
- continue
- if "data: " in line:
- line = line[6:]
- if line == "[DONE]":
- break
-
- # Replace accidentally escaped double quotes
- line = line.replace('\\"', '"')
- line = line.replace("\\'", "'")
- line = line.replace("\\\\", "\\")
- # Try parse JSON
- try:
- line = json.loads(line)
- except json.decoder.JSONDecodeError:
- continue
- if not self.__check_fields(line):
- print("Field missing")
- print(line)
- continue
- message = line["message"]["content"]["parts"][0]
- conversation_id = line["conversation_id"]
- parent_id = line["message"]["id"]
- yield {
- "message": message,
- "conversation_id": conversation_id,
- "parent_id": parent_id,
- }
- if parent_id is not None:
- self.parent_id = parent_id
- if conversation_id is not None:
- self.conversation_id = conversation_id
-
- def __check_fields(self, data: dict) -> bool:
- try:
- data["message"]["content"]
- except TypeError:
- return False
- except KeyError:
- return False
- return True
-
- def __check_response(self, response):
- if response.status_code != 200:
- print(response.text)
- error = Error()
- error.source = "OpenAI"
- error.code = response.status_code
- error.message = response.text
- raise error
-
- def get_conversations(self, offset=0, limit=20):
- """
- Get conversations
- :param offset: Integer
- :param limit: Integer
- """
- url = BASE_URL + f"api/conversations?offset={offset}&limit={limit}"
- response = self.session.get(url)
- self.__check_response(response)
- data = json.loads(response.text)
- return data["items"]
-
- def get_msg_history(self, convo_id):
- """
- Get message history
- :param id: UUID of conversation
- """
- url = BASE_URL + f"api/conversation/{convo_id}"
- response = self.session.get(url)
- self.__check_response(response)
- data = json.loads(response.text)
- return data
-
- # def __gen_title(self, convo_id, message_id):
- # """
- # Generate title for conversation
- # """
- # url = BASE_URL + f"api/conversation/gen_title/{convo_id}"
- # response = self.session.post(
- # url,
- # data=json.dumps(
- # {"message_id": message_id, "model": "text-davinci-002-render"},
- # ),
- # )
- # self.__check_response(response)
-
- def change_title(self, convo_id, title):
- """
- Change title of conversation
- :param id: UUID of conversation
- :param title: String
- """
- url = BASE_URL + f"api/conversation/{convo_id}"
- response = self.session.patch(url, data=f'{{"title": "{title}"}}')
- self.__check_response(response)
-
- def delete_conversation(self, convo_id):
- """
- Delete conversation
- :param id: UUID of conversation
- """
- url = BASE_URL + f"api/conversation/{convo_id}"
- response = self.session.patch(url, data='{"is_visible": false}')
- self.__check_response(response)
-
- def clear_conversations(self):
- """
- Delete all conversations
- """
- url = BASE_URL + "api/conversations"
- response = self.session.patch(url, data='{"is_visible": false}')
- self.__check_response(response)
-
- def __map_conversations(self):
- conversations = self.get_conversations()
- histories = [self.get_msg_history(x["id"]) for x in conversations]
- for x, y in zip(conversations, histories):
- self.conversation_mapping[x["id"]] = y["current_node"]
-
- def reset_chat(self) -> None:
- """
- Reset the conversation ID and parent ID.
-
- :return: None
- """
- self.conversation_id = None
- self.parent_id = str(uuid.uuid4())
-
- def rollback_conversation(self, num=1) -> None:
- """
- Rollback the conversation.
- :param num: The number of messages to rollback
- :return: None
- """
- for _ in range(num):
- self.conversation_id = self.conversation_id_prev_queue.pop()
- self.parent_id = self.parent_id_prev_queue.pop()
-
-
-def get_input(prompt):
- """
- Multiline input function.
- """
- # Display the prompt
- print(prompt, end="")
-
- # Initialize an empty list to store the input lines
- lines = []
-
- # Read lines of input until the user enters an empty line
- while True:
- line = input()
- if line == "":
- break
- lines.append(line)
-
- # Join the lines, separated by newlines, and store the result
- user_input = "\n".join(lines)
-
- # Return the input
- return user_input
-
-
-def configure():
- """
- Looks for a config file in the following locations:
- """
- config_files = ["config.json"]
- xdg_config_home = getenv("XDG_CONFIG_HOME")
- if xdg_config_home:
- config_files.append(f"{xdg_config_home}/revChatGPT/config.json")
- user_home = getenv("HOME")
- if user_home:
- config_files.append(f"{user_home}/.config/revChatGPT/config.json")
-
- config_file = next((f for f in config_files if exists(f)), None)
- if config_file:
- with open(config_file, encoding="utf-8") as f:
- config = json.load(f)
- else:
- print("No config file found.")
- raise Exception("No config file found.")
- return config
-
-
-def main(config):
- """
- Main function for the chatGPT program.
- """
- print("Logging in...")
- chatbot = Chatbot(config)
- while True:
- prompt = get_input("\nYou:\n")
- if prompt.startswith("!"):
- if prompt == "!help":
- print(
- """
- !help - Show this message
- !reset - Forget the current conversation
- !config - Show the current configuration
- !rollback x - Rollback the conversation (x being the number of messages to rollback)
- !exit - Exit this program
- """,
- )
- continue
- elif prompt == "!reset":
- chatbot.reset_chat()
- print("Chat session successfully reset.")
- continue
- elif prompt == "!config":
- print(json.dumps(chatbot.config, indent=4))
- continue
- elif prompt.startswith("!rollback"):
- # Default to 1 rollback if no number is specified
- try:
- rollback = int(prompt.split(" ")[1])
- except IndexError:
- rollback = 1
- chatbot.rollback_conversation(rollback)
- print(f"Rolled back {rollback} messages.")
- continue
- elif prompt.startswith("!setconversation"):
- try:
- chatbot.config["conversation"] = prompt.split(" ")[1]
- print("Conversation has been changed")
- except IndexError:
- print("Please include conversation UUID in command")
- continue
- elif prompt == "!exit":
- break
- print("Chatbot: ")
- prev_text = ""
- for data in chatbot.ask(
- prompt,
- conversation_id=chatbot.config.get("conversation"),
- parent_id=chatbot.config.get("parent_id"),
- ):
- message = data["message"][len(prev_text) :]
- print(message, end="", flush=True)
- prev_text = data["message"]
- print()
- # print(message["message"])
-
-
-if __name__ == "__main__":
- print(
- """
- ChatGPT - A command-line interface to OpenAI's ChatGPT (https://chat.openai.com/chat)
- Repo: github.com/acheong08/ChatGPT
- """,
- )
- print("Type '!help' to show a full list of commands")
- print("Press enter twice to submit your question.\n")
- main(configure())
diff --git a/spaces/KPCGD/bingo/src/pages/api/kblob.ts b/spaces/KPCGD/bingo/src/pages/api/kblob.ts
deleted file mode 100644
index 0ce7e6063cdc06838e76f1cff1d5982d34ef52de..0000000000000000000000000000000000000000
--- a/spaces/KPCGD/bingo/src/pages/api/kblob.ts
+++ /dev/null
@@ -1,56 +0,0 @@
-'use server'
-
-import { NextApiRequest, NextApiResponse } from 'next'
-import FormData from 'form-data'
-import { fetch } from '@/lib/isomorphic'
-import { KBlobRequest } from '@/lib/bots/bing/types'
-
-const API_DOMAIN = 'https://bing.vcanbb.top'
-
-export const config = {
- api: {
- bodyParser: {
- sizeLimit: '10mb' // Set desired value here
- }
- }
-}
-
-export default async function handler(req: NextApiRequest, res: NextApiResponse) {
- try {
- const { knowledgeRequest, imageBase64 } = req.body as KBlobRequest
-
- const formData = new FormData()
- formData.append('knowledgeRequest', JSON.stringify(knowledgeRequest))
- if (imageBase64) {
- formData.append('imageBase64', imageBase64)
- }
-
- const response = await fetch(`${API_DOMAIN}/images/kblob`,
- {
- method: 'POST',
- body: formData.getBuffer(),
- headers: {
- "sec-ch-ua": "\"Not/A)Brand\";v=\"99\", \"Google Chrome\";v=\"115\", \"Chromium\";v=\"115\"",
- "sec-ch-ua-mobile": "?0",
- "sec-ch-ua-platform": "\"Windows\"",
- "Referer": `${API_DOMAIN}/web/index.html`,
- "Referrer-Policy": "origin-when-cross-origin",
- 'x-ms-useragent': 'azsdk-js-api-client-factory/1.0.0-beta.1 core-rest-pipeline/1.10.0 OS/Win32',
- ...formData.getHeaders()
- }
- }
- ).then(res => res.text())
-
- res.writeHead(200, {
- 'Content-Type': 'application/json',
- })
- res.end(response || JSON.stringify({ result: { value: 'UploadFailed', message: '请更换 IP 或代理后重试' } }))
- } catch (e) {
- return res.json({
- result: {
- value: 'UploadFailed',
- message: `${e}`
- }
- })
- }
-}
diff --git a/spaces/Kangarroar/ApplioRVC-Inference/demucs/separate.py b/spaces/Kangarroar/ApplioRVC-Inference/demucs/separate.py
deleted file mode 100644
index 3fc7af9e711978b3e21398aa6f1deb9ae87dd370..0000000000000000000000000000000000000000
--- a/spaces/Kangarroar/ApplioRVC-Inference/demucs/separate.py
+++ /dev/null
@@ -1,185 +0,0 @@
-# Copyright (c) Facebook, Inc. and its affiliates.
-# All rights reserved.
-#
-# This source code is licensed under the license found in the
-# LICENSE file in the root directory of this source tree.
-
-import argparse
-import sys
-from pathlib import Path
-import subprocess
-
-import julius
-import torch as th
-import torchaudio as ta
-
-from .audio import AudioFile, convert_audio_channels
-from .pretrained import is_pretrained, load_pretrained
-from .utils import apply_model, load_model
-
-
-def load_track(track, device, audio_channels, samplerate):
- errors = {}
- wav = None
-
- try:
- wav = AudioFile(track).read(
- streams=0,
- samplerate=samplerate,
- channels=audio_channels).to(device)
- except FileNotFoundError:
- errors['ffmpeg'] = 'Ffmpeg is not installed.'
- except subprocess.CalledProcessError:
- errors['ffmpeg'] = 'FFmpeg could not read the file.'
-
- if wav is None:
- try:
- wav, sr = ta.load(str(track))
- except RuntimeError as err:
- errors['torchaudio'] = err.args[0]
- else:
- wav = convert_audio_channels(wav, audio_channels)
- wav = wav.to(device)
- wav = julius.resample_frac(wav, sr, samplerate)
-
- if wav is None:
- print(f"Could not load file {track}. "
- "Maybe it is not a supported file format? ")
- for backend, error in errors.items():
- print(f"When trying to load using {backend}, got the following error: {error}")
- sys.exit(1)
- return wav
-
-
-def encode_mp3(wav, path, bitrate=320, samplerate=44100, channels=2, verbose=False):
- try:
- import lameenc
- except ImportError:
- print("Failed to call lame encoder. Maybe it is not installed? "
- "On windows, run `python.exe -m pip install -U lameenc`, "
- "on OSX/Linux, run `python3 -m pip install -U lameenc`, "
- "then try again.", file=sys.stderr)
- sys.exit(1)
- encoder = lameenc.Encoder()
- encoder.set_bit_rate(bitrate)
- encoder.set_in_sample_rate(samplerate)
- encoder.set_channels(channels)
- encoder.set_quality(2) # 2-highest, 7-fastest
- if not verbose:
- encoder.silence()
- wav = wav.transpose(0, 1).numpy()
- mp3_data = encoder.encode(wav.tobytes())
- mp3_data += encoder.flush()
- with open(path, "wb") as f:
- f.write(mp3_data)
-
-
-def main():
- parser = argparse.ArgumentParser("demucs.separate",
- description="Separate the sources for the given tracks")
- parser.add_argument("tracks", nargs='+', type=Path, default=[], help='Path to tracks')
- parser.add_argument("-n",
- "--name",
- default="demucs_quantized",
- help="Model name. See README.md for the list of pretrained models. "
- "Default is demucs_quantized.")
- parser.add_argument("-v", "--verbose", action="store_true")
- parser.add_argument("-o",
- "--out",
- type=Path,
- default=Path("separated"),
- help="Folder where to put extracted tracks. A subfolder "
- "with the model name will be created.")
- parser.add_argument("--models",
- type=Path,
- default=Path("models"),
- help="Path to trained models. "
- "Also used to store downloaded pretrained models")
- parser.add_argument("-d",
- "--device",
- default="cuda" if th.cuda.is_available() else "cpu",
- help="Device to use, default is cuda if available else cpu")
- parser.add_argument("--shifts",
- default=0,
- type=int,
- help="Number of random shifts for equivariant stabilization."
- "Increase separation time but improves quality for Demucs. 10 was used "
- "in the original paper.")
- parser.add_argument("--overlap",
- default=0.25,
- type=float,
- help="Overlap between the splits.")
- parser.add_argument("--no-split",
- action="store_false",
- dest="split",
- default=True,
- help="Doesn't split audio in chunks. This can use large amounts of memory.")
- parser.add_argument("--float32",
- action="store_true",
- help="Convert the output wavefile to use pcm f32 format instead of s16. "
- "This should not make a difference if you just plan on listening to the "
- "audio but might be needed to compute exactly metrics like SDR etc.")
- parser.add_argument("--int16",
- action="store_false",
- dest="float32",
- help="Opposite of --float32, here for compatibility.")
- parser.add_argument("--mp3", action="store_true",
- help="Convert the output wavs to mp3.")
- parser.add_argument("--mp3-bitrate",
- default=320,
- type=int,
- help="Bitrate of converted mp3.")
-
- args = parser.parse_args()
- name = args.name + ".th"
- model_path = args.models / name
- if model_path.is_file():
- model = load_model(model_path)
- else:
- if is_pretrained(args.name):
- model = load_pretrained(args.name)
- else:
- print(f"No pre-trained model {args.name}", file=sys.stderr)
- sys.exit(1)
- model.to(args.device)
-
- out = args.out / args.name
- out.mkdir(parents=True, exist_ok=True)
- print(f"Separated tracks will be stored in {out.resolve()}")
- for track in args.tracks:
- if not track.exists():
- print(
- f"File {track} does not exist. If the path contains spaces, "
- "please try again after surrounding the entire path with quotes \"\".",
- file=sys.stderr)
- continue
- print(f"Separating track {track}")
- wav = load_track(track, args.device, model.audio_channels, model.samplerate)
-
- ref = wav.mean(0)
- wav = (wav - ref.mean()) / ref.std()
- sources = apply_model(model, wav, shifts=args.shifts, split=args.split,
- overlap=args.overlap, progress=True)
- sources = sources * ref.std() + ref.mean()
-
- track_folder = out / track.name.rsplit(".", 1)[0]
- track_folder.mkdir(exist_ok=True)
- for source, name in zip(sources, model.sources):
- source = source / max(1.01 * source.abs().max(), 1)
- if args.mp3 or not args.float32:
- source = (source * 2**15).clamp_(-2**15, 2**15 - 1).short()
- source = source.cpu()
- stem = str(track_folder / name)
- if args.mp3:
- encode_mp3(source, stem + ".mp3",
- bitrate=args.mp3_bitrate,
- samplerate=model.samplerate,
- channels=model.audio_channels,
- verbose=args.verbose)
- else:
- wavname = str(track_folder / f"{name}.wav")
- ta.save(wavname, source, sample_rate=model.samplerate)
-
-
-if __name__ == "__main__":
- main()
diff --git a/spaces/KaygNas/cut-it/README.md b/spaces/KaygNas/cut-it/README.md
deleted file mode 100644
index de1fa5cb6be2ebf874177a22e4e1b72816df124d..0000000000000000000000000000000000000000
--- a/spaces/KaygNas/cut-it/README.md
+++ /dev/null
@@ -1,10 +0,0 @@
----
-title: Cut it - A tiny robot cut anything you need from the picture.
-emoji: 👁
-colorFrom: gray
-colorTo: purple
-sdk: docker
-pinned: false
----
-
-Check out the configuration reference at https://huggingface.co/docs/hub/spaces-config-reference
diff --git a/spaces/Kelvinhjk/QnA_chatbot_for_Swinburne_cs_course/README.md b/spaces/Kelvinhjk/QnA_chatbot_for_Swinburne_cs_course/README.md
deleted file mode 100644
index 887fba4c9ed4daddefe5be0258ff55f93cd34244..0000000000000000000000000000000000000000
--- a/spaces/Kelvinhjk/QnA_chatbot_for_Swinburne_cs_course/README.md
+++ /dev/null
@@ -1,12 +0,0 @@
----
-title: QnA Chatbot For Swinburne Cs Course
-emoji: 🚀
-colorFrom: purple
-colorTo: indigo
-sdk: streamlit
-sdk_version: 1.21.0
-app_file: app.py
-pinned: false
----
-
-Check out the configuration reference at https://huggingface.co/docs/hub/spaces-config-reference
diff --git a/spaces/Keshav4/resume-data-extraction/ResumeParser.py b/spaces/Keshav4/resume-data-extraction/ResumeParser.py
deleted file mode 100644
index 1f26d650888670f04f0958dc4f415453a94c6a3f..0000000000000000000000000000000000000000
--- a/spaces/Keshav4/resume-data-extraction/ResumeParser.py
+++ /dev/null
@@ -1,258 +0,0 @@
-from Models import Models
-from ResumeSegmenter import ResumeSegmenter
-from datetime import datetime
-from dateutil import parser
-import re
-from string import punctuation
-
-class ResumeParser:
- def __init__(self, ner, ner_dates, zero_shot_classifier, tagger):
- self.models = Models()
- self.segmenter = ResumeSegmenter(zero_shot_classifier)
- self.ner, self.ner_dates, self.zero_shot_classifier, self.tagger = ner, ner_dates, zero_shot_classifier, tagger
- self.parsed_cv = {}
-
- def parse(self, resume_lines):
- resume_segments = self.segmenter.segment(resume_lines)
- print("***************************** Parsing the Resume...***************************** ")
- for segment_name in resume_segments:
- if segment_name == "work_and_employment":
- resume_segment = resume_segments[segment_name]
- self.parse_job_history(resume_segment)
- elif segment_name == "contact_info":
- contact_info = resume_segments[segment_name]
- self.parse_contact_info(contact_info)
- elif segment_name == "education_and_training":
- education_and_training = resume_segments[segment_name]
- self.parse_education(education_and_training)
- elif segment_name == "skills_header":
- skills_header = resume_segments[segment_name]
- self.parse_skills(skills_header)
- print("************************************** SKILLS HEADER *****************************
",skills_header)
- return self.parsed_cv
-
- def parse_education(self, education_and_training):
- print(education_and_training)
- self.parsed_cv['Education'] = education_and_training
-
- def parse_skills(self, skills_header):
- self.parsed_cv['Skills'] = skills_header
-
- def parse_contact_info(self, contact_info):
- contact_info_dict = {}
- name = self.find_person_name(contact_info)
- email = self.find_contact_email(contact_info)
- self.parsed_cv['Name'] = name
- contact_info_dict["Email"] = email
- self.parsed_cv['Contact Info'] = contact_info_dict
-
- def find_person_name(self, items):
- class_score = []
- splitter = re.compile(r'[{}]+'.format(re.escape(punctuation.replace("&", "") )))
- classes = ["person name", "address", "email", "title"]
- for item in items:
- elements = splitter.split(item)
- for element in elements:
- element = ''.join(i for i in element.strip() if not i.isdigit())
- if not len(element.strip().split()) > 1: continue
- out = self.zero_shot_classifier(element, classes)
- highest = sorted(zip(out["labels"], out["scores"]), key=lambda x: x[1])[-1]
- if highest[0] == "person name":
- class_score.append((element, highest[1]))
- if len(class_score):
- return sorted(class_score, key=lambda x: x[1], reverse=True)[0][0]
- return ""
-
- def find_contact_email(self, items):
- for item in items:
- match = re.search(r'[\w.+-]+@[\w-]+\.[\w.-]+', item)
- if match:
- return match.group(0)
- return ""
-
- def parse_job_history(self, resume_segment):
- idx_job_title = self.get_job_titles(resume_segment)
- current_and_below = False
- if not len(idx_job_title):
- self.parsed_cv["Job History"] = []
- return
- if idx_job_title[0][0] == 0: current_and_below = True
- job_history = []
- for ls_idx, (idx, job_title) in enumerate(idx_job_title):
- job_info = {}
- # print("
Job Title: ",job_title)
- job_info["Job Title"] = self.filter_job_title(job_title)
- # company
- if current_and_below: line1, line2 = idx, idx+1
- else: line1, line2 = idx, idx-1
- job_info["Company"] = self.get_job_company(line1, line2, resume_segment)
- if current_and_below: st_span = idx
- else: st_span = idx-1
- # Dates
- if ls_idx == len(idx_job_title) - 1: end_span = len(resume_segment)
- else: end_span = idx_job_title[ls_idx+1][0]
- start, end = self.get_job_dates(st_span, end_span, resume_segment)
- job_info["Start Date"] = start
- job_info["End Date"] = end
- # if(start != "" and end != ""):
- job_history.append(job_info)
- self.parsed_cv["Job History"] = job_history
-
- def get_job_titles(self, resume_segment):
- classes = ["organization", "institution", "company", "job title", "work details"]
- idx_line = []
- for idx, line in enumerate(resume_segment):
- has_verb = False
- line_modifed = ''.join(i for i in line if not i.isdigit())
- sentence = self.models.get_flair_sentence(line_modifed)
- self.tagger.predict(sentence)
- tags = []
- for entity in sentence.get_spans('pos'):
- tags.append(entity.tag)
- if entity.tag.startswith("V"):
- has_verb = True
-
- most_common_tag = max(set(tags), key=tags.count)
- if (most_common_tag == "NNP") or (most_common_tag == "NN"):
- # if most_common_tag == "NNP":
- if not has_verb:
- out = self.zero_shot_classifier(line, classes)
- class_score = zip(out["labels"], out["scores"])
- highest = sorted(class_score, key=lambda x: x[1])[-1]
-
- if (highest[0] == "job title") or (highest[0] == "organization"):
- # if highest[0] == "job title":
- idx_line.append((idx, line))
- return idx_line
-
- def get_job_dates(self, st, end, resume_segment):
- search_span = resume_segment[st:end]
- dates = []
- for line in search_span:
- for dt in self.get_ner_in_line(line, "DATE"):
- if self.isvalidyear(dt.strip()):
- dates.append(dt)
- if len(dates): first = dates[0]
- exists_second = False
- if len(dates) > 1:
- exists_second = True
- second = dates[1]
-
- if len(dates) > 0:
- if self.has_two_dates(first):
- d1, d2 = self.get_two_dates(first)
- return self.format_date(d1), self.format_date(d2)
- elif exists_second and self.has_two_dates(second):
- d1, d2 = self.get_two_dates(second)
- return self.format_date(d1), self.format_date(d2)
- else:
- if exists_second:
- st = self.format_date(first)
- end = self.format_date(second)
- return st, end
- else:
- return (self.format_date(first), "")
- else: return ("", "")
-
-
-
- def filter_job_title(self, job_title):
- job_title_splitter = re.compile(r'[{}]+'.format(re.escape(punctuation.replace("&", "") )))
- job_title = ''.join(i for i in job_title if not i.isdigit())
- tokens = job_title_splitter.split(job_title)
- tokens = [''.join([i for i in tok.strip() if (i.isalpha() or i.strip()=="")]) for tok in tokens if tok.strip()]
- classes = ["company", "organization", "institution", "job title", "responsibility", "details"]
- new_title = []
- for token in tokens:
- if not token: continue
- res = self.zero_shot_classifier(token, classes)
- class_score = zip(res["labels"], res["scores"])
- highest = sorted(class_score, key=lambda x: x[1])[-1]
- if (highest[0] == "job title") or (highest[0] == "organization"):
- # if highest[0] == "job title":
- new_title.append(token.strip())
- if len(new_title):
- return ', '.join(new_title)
- else: return ', '.join(tokens)
-
- def has_two_dates(self, date):
- years = self.get_valid_years()
- count = 0
- for year in years:
- if year in str(date):
- count+=1
- return count == 2
-
- def get_two_dates(self, date):
- years = self.get_valid_years()
- idxs = []
- for year in years:
- if year in date:
- idxs.append(date.index(year))
- min_idx = min(idxs)
- first = date[:min_idx+4]
- second = date[min_idx+4:]
- return first, second
- def get_valid_years(self):
- current_year = datetime.today().year
- years = [str(i) for i in range(current_year-100, current_year)]
- return years
-
- def format_date(self, date):
- out = self.parse_date(date)
- if out:
- return out
- else:
- date = self.clean_date(date)
- out = self.parse_date(date)
- if out:
- return out
- else:
- return date
-
- def clean_date(self, date):
- try:
- date = ''.join(i for i in date if i.isalnum() or i =='-' or i == '/')
- return date
- except:
- return date
-
- def parse_date(self, date):
- try:
- date = parser.parse(date)
- return date.strftime("%m-%Y")
- except:
- try:
- date = datetime(date)
- return date.strftime("%m-%Y")
- except:
- return 0
-
-
- def isvalidyear(self, date):
- current_year = datetime.today().year
- years = [str(i) for i in range(current_year-100, current_year)]
- for year in years:
- if year in str(date):
- return True
- return False
-
- def get_ner_in_line(self, line, entity_type):
- if entity_type == "DATE": ner = self.ner_dates
- else: ner = self.ner
- return [i['word'] for i in ner(line) if i['entity_group'] == entity_type]
-
-
- def get_job_company(self, idx, idx1, resume_segment):
- job_title = resume_segment[idx]
- if not idx1 <= len(resume_segment)-1: context = ""
- else:context = resume_segment[idx1]
- candidate_companies = self.get_ner_in_line(job_title, "ORG") + self.get_ner_in_line(context, "ORG")
- classes = ["organization", "company", "institution", "not organization", "not company", "not institution"]
- scores = []
- for comp in candidate_companies:
- res = self.zero_shot_classifier(comp, classes)['scores']
- scores.append(max(res[:3]))
- sorted_cmps = sorted(zip(candidate_companies, scores), key=lambda x: x[1], reverse=True)
- if len(sorted_cmps): return sorted_cmps[0][0]
- return context
\ No newline at end of file
diff --git a/spaces/KonradSzafer/HF-QA-Demo/discord_bot/client/client.py b/spaces/KonradSzafer/HF-QA-Demo/discord_bot/client/client.py
deleted file mode 100644
index e3d651e662a706733e0ea0ec614bc2e0ca543770..0000000000000000000000000000000000000000
--- a/spaces/KonradSzafer/HF-QA-Demo/discord_bot/client/client.py
+++ /dev/null
@@ -1,130 +0,0 @@
-import json
-import requests
-from urllib.parse import quote
-import discord
-from typing import List
-
-from qa_engine import logger, QAEngine
-from discord_bot.client.utils import split_text_into_chunks
-
-
-class DiscordClient(discord.Client):
- """
- Discord Client class, used for interacting with a Discord server.
-
- Args:
- qa_service_url (str): The URL of the question answering service.
- num_last_messages (int, optional): The number of previous messages to use as context for generating answers.
- Defaults to 5.
- use_names_in_context (bool, optional): Whether to include user names in the message context. Defaults to True.
- enable_commands (bool, optional): Whether to enable commands for the bot. Defaults to True.
-
- Attributes:
- qa_service_url (str): The URL of the question answering service.
- num_last_messages (int): The number of previous messages to use as context for generating answers.
- use_names_in_context (bool): Whether to include user names in the message context.
- enable_commands (bool): Whether to enable commands for the bot.
- max_message_len (int): The maximum length of a message.
- system_prompt (str): The system prompt to be used.
-
- """
- def __init__(
- self,
- qa_engine: QAEngine,
- num_last_messages: int = 5,
- use_names_in_context: bool = True,
- enable_commands: bool = True,
- debug: bool = False
- ):
- logger.info('Initializing Discord client...')
- intents = discord.Intents.all()
- intents.message_content = True
- super().__init__(intents=intents, command_prefix='!')
-
- assert num_last_messages >= 1, \
- 'The number of last messages in context should be at least 1'
-
- self.qa_engine: QAEngine = qa_engine
- self.num_last_messages: int = num_last_messages
- self.use_names_in_context: bool = use_names_in_context
- self.enable_commands: bool = enable_commands
- self.debug: bool = debug
- self.min_messgae_len: int = 1800
- self.max_message_len: int = 2000
-
-
- async def on_ready(self):
- """
- Callback function to be called when the client is ready.
- """
- logger.info('Successfully logged in as: {0.user}'.format(self))
- await self.change_presence(activity=discord.Game(name='Chatting...'))
-
-
- async def get_last_messages(self, message) -> List[str]:
- """
- Method to fetch recent messages from a message's channel.
-
- Args:
- message (Message): The discord Message object used to identify the channel.
-
- Returns:
- List[str]: Reversed list of recent messages from the channel,
- excluding the input message. Messages may be prefixed with the author's name
- if `self.use_names_in_context` is True.
- """
- last_messages: List[str] = []
- async for msg in message.channel.history(
- limit=self.num_last_messages):
- if self.use_names_in_context:
- last_messages.append(f'{msg.author}: {msg.content}')
- else:
- last_messages.append(msg.content)
- last_messages.reverse()
- last_messages.pop() # remove last message from context
- return last_messages
-
-
- async def send_message(self, message, answer: str, sources: str):
- chunks = split_text_into_chunks(
- text=answer,
- split_characters=['. ', ', ', '\n'],
- min_size=self.min_messgae_len,
- max_size=self.max_message_len
- )
- for chunk in chunks:
- await message.channel.send(chunk)
- await message.channel.send(sources)
-
-
- async def on_message(self, message):
- """
- Callback function to be called when a message is received.
-
- Args:
- message (discord.Message): The received message.
- """
- if message.author == self.user:
- return
- if self.enable_commands and message.content.startswith('!'):
- if message.content == '!clear':
- await message.channel.purge()
- return
-
- last_messages = await self.get_last_messages(message)
- context = '\n'.join(last_messages)
-
- logger.info('Received message: {0.content}'.format(message))
- response = self.qa_engine.get_response(
- question=message.content,
- messages_context=context
- )
- logger.info('Sending response: {0}'.format(response))
- try:
- await self.send_message(
- message,
- response.get_answer(),
- response.get_sources_as_text()
- )
- except Exception as e:
- logger.error('Failed to send response: {0}'.format(e))
diff --git a/spaces/Kreaols/ChuanhuChatGPT/modules/models/inspurai.py b/spaces/Kreaols/ChuanhuChatGPT/modules/models/inspurai.py
deleted file mode 100644
index c590859fa7717d032290ccc490d22f4494541576..0000000000000000000000000000000000000000
--- a/spaces/Kreaols/ChuanhuChatGPT/modules/models/inspurai.py
+++ /dev/null
@@ -1,345 +0,0 @@
-# 代码主要来源于 https://github.com/Shawn-Inspur/Yuan-1.0/blob/main/yuan_api/inspurai.py
-
-import hashlib
-import json
-import os
-import time
-import uuid
-from datetime import datetime
-
-import pytz
-import requests
-
-from modules.presets import NO_APIKEY_MSG
-from modules.models.base_model import BaseLLMModel
-
-
-class Example:
- """ store some examples(input, output pairs and formats) for few-shots to prime the model."""
-
- def __init__(self, inp, out):
- self.input = inp
- self.output = out
- self.id = uuid.uuid4().hex
-
- def get_input(self):
- """return the input of the example."""
- return self.input
-
- def get_output(self):
- """Return the output of the example."""
- return self.output
-
- def get_id(self):
- """Returns the unique ID of the example."""
- return self.id
-
- def as_dict(self):
- return {
- "input": self.get_input(),
- "output": self.get_output(),
- "id": self.get_id(),
- }
-
-
-class Yuan:
- """The main class for a user to interface with the Inspur Yuan API.
- A user can set account info and add examples of the API request.
- """
-
- def __init__(self,
- engine='base_10B',
- temperature=0.9,
- max_tokens=100,
- input_prefix='',
- input_suffix='\n',
- output_prefix='答:',
- output_suffix='\n\n',
- append_output_prefix_to_query=False,
- topK=1,
- topP=0.9,
- frequencyPenalty=1.2,
- responsePenalty=1.2,
- noRepeatNgramSize=2):
-
- self.examples = {}
- self.engine = engine
- self.temperature = temperature
- self.max_tokens = max_tokens
- self.topK = topK
- self.topP = topP
- self.frequencyPenalty = frequencyPenalty
- self.responsePenalty = responsePenalty
- self.noRepeatNgramSize = noRepeatNgramSize
- self.input_prefix = input_prefix
- self.input_suffix = input_suffix
- self.output_prefix = output_prefix
- self.output_suffix = output_suffix
- self.append_output_prefix_to_query = append_output_prefix_to_query
- self.stop = (output_suffix + input_prefix).strip()
- self.api = None
-
- # if self.engine not in ['base_10B','translate','dialog']:
- # raise Exception('engine must be one of [\'base_10B\',\'translate\',\'dialog\'] ')
- def set_account(self, api_key):
- account = api_key.split('||')
- self.api = YuanAPI(user=account[0], phone=account[1])
-
- def add_example(self, ex):
- """Add an example to the object.
- Example must be an instance of the Example class."""
- assert isinstance(ex, Example), "Please create an Example object."
- self.examples[ex.get_id()] = ex
-
- def delete_example(self, id):
- """Delete example with the specific id."""
- if id in self.examples:
- del self.examples[id]
-
- def get_example(self, id):
- """Get a single example."""
- return self.examples.get(id, None)
-
- def get_all_examples(self):
- """Returns all examples as a list of dicts."""
- return {k: v.as_dict() for k, v in self.examples.items()}
-
- def get_prime_text(self):
- """Formats all examples to prime the model."""
- return "".join(
- [self.format_example(ex) for ex in self.examples.values()])
-
- def get_engine(self):
- """Returns the engine specified for the API."""
- return self.engine
-
- def get_temperature(self):
- """Returns the temperature specified for the API."""
- return self.temperature
-
- def get_max_tokens(self):
- """Returns the max tokens specified for the API."""
- return self.max_tokens
-
- def craft_query(self, prompt):
- """Creates the query for the API request."""
- q = self.get_prime_text(
- ) + self.input_prefix + prompt + self.input_suffix
- if self.append_output_prefix_to_query:
- q = q + self.output_prefix
-
- return q
-
- def format_example(self, ex):
- """Formats the input, output pair."""
- return self.input_prefix + ex.get_input(
- ) + self.input_suffix + self.output_prefix + ex.get_output(
- ) + self.output_suffix
-
- def response(self,
- query,
- engine='base_10B',
- max_tokens=20,
- temperature=0.9,
- topP=0.1,
- topK=1,
- frequencyPenalty=1.0,
- responsePenalty=1.0,
- noRepeatNgramSize=0):
- """Obtains the original result returned by the API."""
-
- if self.api is None:
- return NO_APIKEY_MSG
- try:
- # requestId = submit_request(query,temperature,topP,topK,max_tokens, engine)
- requestId = self.api.submit_request(query, temperature, topP, topK, max_tokens, engine, frequencyPenalty,
- responsePenalty, noRepeatNgramSize)
- response_text = self.api.reply_request(requestId)
- except Exception as e:
- raise e
-
- return response_text
-
- def del_special_chars(self, msg):
- special_chars = ['', '', '#', '▃', '▁', '▂', ' ']
- for char in special_chars:
- msg = msg.replace(char, '')
- return msg
-
- def submit_API(self, prompt, trun=[]):
- """Submit prompt to yuan API interface and obtain an pure text reply.
- :prompt: Question or any content a user may input.
- :return: pure text response."""
- query = self.craft_query(prompt)
- res = self.response(query, engine=self.engine,
- max_tokens=self.max_tokens,
- temperature=self.temperature,
- topP=self.topP,
- topK=self.topK,
- frequencyPenalty=self.frequencyPenalty,
- responsePenalty=self.responsePenalty,
- noRepeatNgramSize=self.noRepeatNgramSize)
- if 'resData' in res and res['resData'] != None:
- txt = res['resData']
- else:
- txt = '模型返回为空,请尝试修改输入'
- # 单独针对翻译模型的后处理
- if self.engine == 'translate':
- txt = txt.replace(' ##', '').replace(' "', '"').replace(": ", ":").replace(" ,", ",") \
- .replace('英文:', '').replace('文:', '').replace("( ", "(").replace(" )", ")")
- else:
- txt = txt.replace(' ', '')
- txt = self.del_special_chars(txt)
-
- # trun多结束符截断模型输出
- if isinstance(trun, str):
- trun = [trun]
- try:
- if trun != None and isinstance(trun, list) and trun != []:
- for tr in trun:
- if tr in txt and tr != "":
- txt = txt[:txt.index(tr)]
- else:
- continue
- except:
- return txt
- return txt
-
-
-class YuanAPI:
- ACCOUNT = ''
- PHONE = ''
-
- SUBMIT_URL = "http://api.airyuan.cn:32102/v1/interface/api/infer/getRequestId?"
- REPLY_URL = "http://api.airyuan.cn:32102/v1/interface/api/result?"
-
- def __init__(self, user, phone):
- self.ACCOUNT = user
- self.PHONE = phone
-
- @staticmethod
- def code_md5(str):
- code = str.encode("utf-8")
- m = hashlib.md5()
- m.update(code)
- result = m.hexdigest()
- return result
-
- @staticmethod
- def rest_get(url, header, timeout, show_error=False):
- '''Call rest get method'''
- try:
- response = requests.get(url, headers=header, timeout=timeout, verify=False)
- return response
- except Exception as exception:
- if show_error:
- print(exception)
- return None
-
- def header_generation(self):
- """Generate header for API request."""
- t = datetime.now(pytz.timezone("Asia/Shanghai")).strftime("%Y-%m-%d")
- token = self.code_md5(self.ACCOUNT + self.PHONE + t)
- headers = {'token': token}
- return headers
-
- def submit_request(self, query, temperature, topP, topK, max_tokens, engine, frequencyPenalty, responsePenalty,
- noRepeatNgramSize):
- """Submit query to the backend server and get requestID."""
- headers = self.header_generation()
- # url=SUBMIT_URL + "account={0}&data={1}&temperature={2}&topP={3}&topK={4}&tokensToGenerate={5}&type={6}".format(ACCOUNT,query,temperature,topP,topK,max_tokens,"api")
- # url=SUBMIT_URL + "engine={0}&account={1}&data={2}&temperature={3}&topP={4}&topK={5}&tokensToGenerate={6}" \
- # "&type={7}".format(engine,ACCOUNT,query,temperature,topP,topK, max_tokens,"api")
- url = self.SUBMIT_URL + "engine={0}&account={1}&data={2}&temperature={3}&topP={4}&topK={5}&tokensToGenerate={6}" \
- "&type={7}&frequencyPenalty={8}&responsePenalty={9}&noRepeatNgramSize={10}". \
- format(engine, self.ACCOUNT, query, temperature, topP, topK, max_tokens, "api", frequencyPenalty,
- responsePenalty, noRepeatNgramSize)
- response = self.rest_get(url, headers, 30)
- response_text = json.loads(response.text)
- if response_text["flag"]:
- requestId = response_text["resData"]
- return requestId
- else:
- raise RuntimeWarning(response_text)
-
- def reply_request(self, requestId, cycle_count=5):
- """Check reply API to get the inference response."""
- url = self.REPLY_URL + "account={0}&requestId={1}".format(self.ACCOUNT, requestId)
- headers = self.header_generation()
- response_text = {"flag": True, "resData": None}
- for i in range(cycle_count):
- response = self.rest_get(url, headers, 30, show_error=True)
- response_text = json.loads(response.text)
- if response_text["resData"] is not None:
- return response_text
- if response_text["flag"] is False and i == cycle_count - 1:
- raise RuntimeWarning(response_text)
- time.sleep(3)
- return response_text
-
-
-class Yuan_Client(BaseLLMModel):
-
- def __init__(self, model_name, api_key, user_name="", system_prompt=None):
- super().__init__(model_name=model_name, user=user_name)
- self.history = []
- self.api_key = api_key
- self.system_prompt = system_prompt
-
- self.input_prefix = ""
- self.output_prefix = ""
-
- def set_text_prefix(self, option, value):
- if option == 'input_prefix':
- self.input_prefix = value
- elif option == 'output_prefix':
- self.output_prefix = value
-
- def get_answer_at_once(self):
- # yuan temperature is (0,1] and base model temperature is [0,2], and yuan 0.9 == base 1 so need to convert
- temperature = self.temperature if self.temperature <= 1 else 0.9 + (self.temperature - 1) / 10
- topP = self.top_p
- topK = self.n_choices
- # max_tokens should be in [1,200]
- max_tokens = self.max_generation_token if self.max_generation_token is not None else 50
- if max_tokens > 200:
- max_tokens = 200
- stop = self.stop_sequence if self.stop_sequence is not None else []
- examples = []
- system_prompt = self.system_prompt
- if system_prompt is not None:
- lines = system_prompt.splitlines()
- # TODO: support prefixes in system prompt or settings
- """
- if lines[0].startswith('-'):
- prefixes = lines.pop()[1:].split('|')
- self.input_prefix = prefixes[0]
- if len(prefixes) > 1:
- self.output_prefix = prefixes[1]
- if len(prefixes) > 2:
- stop = prefixes[2].split(',')
- """
- for i in range(0, len(lines), 2):
- in_line = lines[i]
- out_line = lines[i + 1] if i + 1 < len(lines) else ""
- examples.append((in_line, out_line))
- yuan = Yuan(engine=self.model_name.replace('yuanai-1.0-', ''),
- temperature=temperature,
- max_tokens=max_tokens,
- topK=topK,
- topP=topP,
- input_prefix=self.input_prefix,
- input_suffix="",
- output_prefix=self.output_prefix,
- output_suffix="".join(stop),
- )
- if not self.api_key:
- return NO_APIKEY_MSG, 0
- yuan.set_account(self.api_key)
-
- for in_line, out_line in examples:
- yuan.add_example(Example(inp=in_line, out=out_line))
-
- prompt = self.history[-1]["content"]
- answer = yuan.submit_API(prompt, trun=stop)
- return answer, len(answer)
diff --git a/spaces/KyanChen/RSPrompter/mmdet/models/roi_heads/pisa_roi_head.py b/spaces/KyanChen/RSPrompter/mmdet/models/roi_heads/pisa_roi_head.py
deleted file mode 100644
index 45d59879da73b48df790c55d40a4a88f1d099111..0000000000000000000000000000000000000000
--- a/spaces/KyanChen/RSPrompter/mmdet/models/roi_heads/pisa_roi_head.py
+++ /dev/null
@@ -1,148 +0,0 @@
-# Copyright (c) OpenMMLab. All rights reserved.
-from typing import List, Tuple
-
-from torch import Tensor
-
-from mmdet.models.task_modules import SamplingResult
-from mmdet.registry import MODELS
-from mmdet.structures import DetDataSample
-from mmdet.structures.bbox import bbox2roi
-from mmdet.utils import InstanceList
-from ..losses.pisa_loss import carl_loss, isr_p
-from ..utils import unpack_gt_instances
-from .standard_roi_head import StandardRoIHead
-
-
-@MODELS.register_module()
-class PISARoIHead(StandardRoIHead):
- r"""The RoI head for `Prime Sample Attention in Object Detection
- `_."""
-
- def loss(self, x: Tuple[Tensor], rpn_results_list: InstanceList,
- batch_data_samples: List[DetDataSample]) -> dict:
- """Perform forward propagation and loss calculation of the detection
- roi on the features of the upstream network.
-
- Args:
- x (tuple[Tensor]): List of multi-level img features.
- rpn_results_list (list[:obj:`InstanceData`]): List of region
- proposals.
- batch_data_samples (list[:obj:`DetDataSample`]): The batch
- data samples. It usually includes information such
- as `gt_instance` or `gt_panoptic_seg` or `gt_sem_seg`.
-
- Returns:
- dict[str, Tensor]: A dictionary of loss components
- """
- assert len(rpn_results_list) == len(batch_data_samples)
- outputs = unpack_gt_instances(batch_data_samples)
- batch_gt_instances, batch_gt_instances_ignore, _ = outputs
-
- # assign gts and sample proposals
- num_imgs = len(batch_data_samples)
- sampling_results = []
- neg_label_weights = []
- for i in range(num_imgs):
- # rename rpn_results.bboxes to rpn_results.priors
- rpn_results = rpn_results_list[i]
- rpn_results.priors = rpn_results.pop('bboxes')
-
- assign_result = self.bbox_assigner.assign(
- rpn_results, batch_gt_instances[i],
- batch_gt_instances_ignore[i])
- sampling_result = self.bbox_sampler.sample(
- assign_result,
- rpn_results,
- batch_gt_instances[i],
- feats=[lvl_feat[i][None] for lvl_feat in x])
- if isinstance(sampling_result, tuple):
- sampling_result, neg_label_weight = sampling_result
- sampling_results.append(sampling_result)
- neg_label_weights.append(neg_label_weight)
-
- losses = dict()
- # bbox head forward and loss
- if self.with_bbox:
- bbox_results = self.bbox_loss(
- x, sampling_results, neg_label_weights=neg_label_weights)
- losses.update(bbox_results['loss_bbox'])
-
- # mask head forward and loss
- if self.with_mask:
- mask_results = self.mask_loss(x, sampling_results,
- bbox_results['bbox_feats'],
- batch_gt_instances)
- losses.update(mask_results['loss_mask'])
-
- return losses
-
- def bbox_loss(self,
- x: Tuple[Tensor],
- sampling_results: List[SamplingResult],
- neg_label_weights: List[Tensor] = None) -> dict:
- """Perform forward propagation and loss calculation of the bbox head on
- the features of the upstream network.
-
- Args:
- x (tuple[Tensor]): List of multi-level img features.
- sampling_results (list["obj:`SamplingResult`]): Sampling results.
-
- Returns:
- dict[str, Tensor]: Usually returns a dictionary with keys:
-
- - `cls_score` (Tensor): Classification scores.
- - `bbox_pred` (Tensor): Box energies / deltas.
- - `bbox_feats` (Tensor): Extract bbox RoI features.
- - `loss_bbox` (dict): A dictionary of bbox loss components.
- """
- rois = bbox2roi([res.priors for res in sampling_results])
- bbox_results = self._bbox_forward(x, rois)
- bbox_targets = self.bbox_head.get_targets(sampling_results,
- self.train_cfg)
-
- # neg_label_weights obtained by sampler is image-wise, mapping back to
- # the corresponding location in label weights
- if neg_label_weights[0] is not None:
- label_weights = bbox_targets[1]
- cur_num_rois = 0
- for i in range(len(sampling_results)):
- num_pos = sampling_results[i].pos_inds.size(0)
- num_neg = sampling_results[i].neg_inds.size(0)
- label_weights[cur_num_rois + num_pos:cur_num_rois + num_pos +
- num_neg] = neg_label_weights[i]
- cur_num_rois += num_pos + num_neg
-
- cls_score = bbox_results['cls_score']
- bbox_pred = bbox_results['bbox_pred']
-
- # Apply ISR-P
- isr_cfg = self.train_cfg.get('isr', None)
- if isr_cfg is not None:
- bbox_targets = isr_p(
- cls_score,
- bbox_pred,
- bbox_targets,
- rois,
- sampling_results,
- self.bbox_head.loss_cls,
- self.bbox_head.bbox_coder,
- **isr_cfg,
- num_class=self.bbox_head.num_classes)
- loss_bbox = self.bbox_head.loss(cls_score, bbox_pred, rois,
- *bbox_targets)
-
- # Add CARL Loss
- carl_cfg = self.train_cfg.get('carl', None)
- if carl_cfg is not None:
- loss_carl = carl_loss(
- cls_score,
- bbox_targets[0],
- bbox_pred,
- bbox_targets[2],
- self.bbox_head.loss_bbox,
- **carl_cfg,
- num_class=self.bbox_head.num_classes)
- loss_bbox.update(loss_carl)
-
- bbox_results.update(loss_bbox=loss_bbox)
- return bbox_results
diff --git a/spaces/KyanChen/RSPrompter/mmdet/utils/contextmanagers.py b/spaces/KyanChen/RSPrompter/mmdet/utils/contextmanagers.py
deleted file mode 100644
index fa12bfcaff1e781b0a8cc7d7c8b839c2f2955a05..0000000000000000000000000000000000000000
--- a/spaces/KyanChen/RSPrompter/mmdet/utils/contextmanagers.py
+++ /dev/null
@@ -1,122 +0,0 @@
-# Copyright (c) OpenMMLab. All rights reserved.
-import asyncio
-import contextlib
-import logging
-import os
-import time
-from typing import List
-
-import torch
-
-logger = logging.getLogger(__name__)
-
-DEBUG_COMPLETED_TIME = bool(os.environ.get('DEBUG_COMPLETED_TIME', False))
-
-
-@contextlib.asynccontextmanager
-async def completed(trace_name='',
- name='',
- sleep_interval=0.05,
- streams: List[torch.cuda.Stream] = None):
- """Async context manager that waits for work to complete on given CUDA
- streams."""
- if not torch.cuda.is_available():
- yield
- return
-
- stream_before_context_switch = torch.cuda.current_stream()
- if not streams:
- streams = [stream_before_context_switch]
- else:
- streams = [s if s else stream_before_context_switch for s in streams]
-
- end_events = [
- torch.cuda.Event(enable_timing=DEBUG_COMPLETED_TIME) for _ in streams
- ]
-
- if DEBUG_COMPLETED_TIME:
- start = torch.cuda.Event(enable_timing=True)
- stream_before_context_switch.record_event(start)
-
- cpu_start = time.monotonic()
- logger.debug('%s %s starting, streams: %s', trace_name, name, streams)
- grad_enabled_before = torch.is_grad_enabled()
- try:
- yield
- finally:
- current_stream = torch.cuda.current_stream()
- assert current_stream == stream_before_context_switch
-
- if DEBUG_COMPLETED_TIME:
- cpu_end = time.monotonic()
- for i, stream in enumerate(streams):
- event = end_events[i]
- stream.record_event(event)
-
- grad_enabled_after = torch.is_grad_enabled()
-
- # observed change of torch.is_grad_enabled() during concurrent run of
- # async_test_bboxes code
- assert (grad_enabled_before == grad_enabled_after
- ), 'Unexpected is_grad_enabled() value change'
-
- are_done = [e.query() for e in end_events]
- logger.debug('%s %s completed: %s streams: %s', trace_name, name,
- are_done, streams)
- with torch.cuda.stream(stream_before_context_switch):
- while not all(are_done):
- await asyncio.sleep(sleep_interval)
- are_done = [e.query() for e in end_events]
- logger.debug(
- '%s %s completed: %s streams: %s',
- trace_name,
- name,
- are_done,
- streams,
- )
-
- current_stream = torch.cuda.current_stream()
- assert current_stream == stream_before_context_switch
-
- if DEBUG_COMPLETED_TIME:
- cpu_time = (cpu_end - cpu_start) * 1000
- stream_times_ms = ''
- for i, stream in enumerate(streams):
- elapsed_time = start.elapsed_time(end_events[i])
- stream_times_ms += f' {stream} {elapsed_time:.2f} ms'
- logger.info('%s %s %.2f ms %s', trace_name, name, cpu_time,
- stream_times_ms)
-
-
-@contextlib.asynccontextmanager
-async def concurrent(streamqueue: asyncio.Queue,
- trace_name='concurrent',
- name='stream'):
- """Run code concurrently in different streams.
-
- :param streamqueue: asyncio.Queue instance.
-
- Queue tasks define the pool of streams used for concurrent execution.
- """
- if not torch.cuda.is_available():
- yield
- return
-
- initial_stream = torch.cuda.current_stream()
-
- with torch.cuda.stream(initial_stream):
- stream = await streamqueue.get()
- assert isinstance(stream, torch.cuda.Stream)
-
- try:
- with torch.cuda.stream(stream):
- logger.debug('%s %s is starting, stream: %s', trace_name, name,
- stream)
- yield
- current = torch.cuda.current_stream()
- assert current == stream
- logger.debug('%s %s has finished, stream: %s', trace_name,
- name, stream)
- finally:
- streamqueue.task_done()
- streamqueue.put_nowait(stream)
diff --git a/spaces/LarissaHung/text_generator/README.md b/spaces/LarissaHung/text_generator/README.md
deleted file mode 100644
index c8ba7c9d0bed637d03d2afdd3cfbdb079ed34729..0000000000000000000000000000000000000000
--- a/spaces/LarissaHung/text_generator/README.md
+++ /dev/null
@@ -1,12 +0,0 @@
----
-title: Text Generator
-emoji: 🐠
-colorFrom: purple
-colorTo: gray
-sdk: gradio
-sdk_version: 3.18.0
-app_file: app.py
-pinned: false
----
-
-Check out the configuration reference at https://huggingface.co/docs/hub/spaces-config-reference
diff --git a/spaces/Loren/Streamlit_OCR_comparator/configs/textrecog/satrn/README.md b/spaces/Loren/Streamlit_OCR_comparator/configs/textrecog/satrn/README.md
deleted file mode 100644
index 4bb92f3fc9d37d0c1a9563769b645d20fc598eb2..0000000000000000000000000000000000000000
--- a/spaces/Loren/Streamlit_OCR_comparator/configs/textrecog/satrn/README.md
+++ /dev/null
@@ -1,51 +0,0 @@
-# SATRN
-
-> [On Recognizing Texts of Arbitrary Shapes with 2D Self-Attention](https://arxiv.org/abs/1910.04396)
-
-
-
-## Abstract
-
-Scene text recognition (STR) is the task of recognizing character sequences in natural scenes. While there have been great advances in STR methods, current methods still fail to recognize texts in arbitrary shapes, such as heavily curved or rotated texts, which are abundant in daily life (e.g. restaurant signs, product labels, company logos, etc). This paper introduces a novel architecture to recognizing texts of arbitrary shapes, named Self-Attention Text Recognition Network (SATRN), which is inspired by the Transformer. SATRN utilizes the self-attention mechanism to describe two-dimensional (2D) spatial dependencies of characters in a scene text image. Exploiting the full-graph propagation of self-attention, SATRN can recognize texts with arbitrary arrangements and large inter-character spacing. As a result, SATRN outperforms existing STR models by a large margin of 5.7 pp on average in "irregular text" benchmarks. We provide empirical analyses that illustrate the inner mechanisms and the extent to which the model is applicable (e.g. rotated and multi-line text). We will open-source the code.
-
-
-
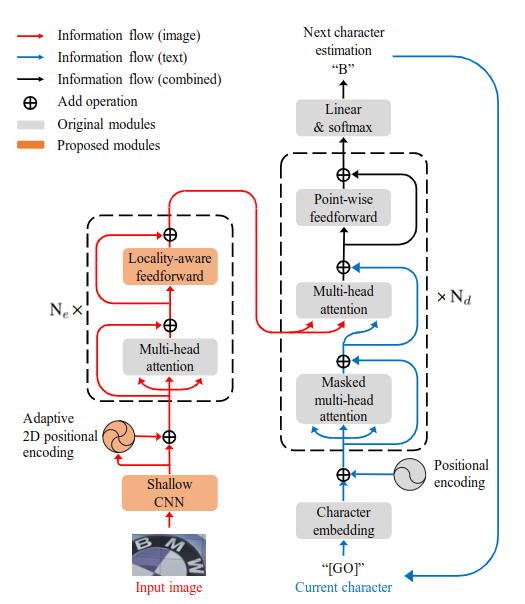
-
-
-## Dataset
-
-### Train Dataset
-
-| trainset | instance_num | repeat_num | source |
-| :-------: | :----------: | :--------: | :----: |
-| SynthText | 7266686 | 1 | synth |
-| Syn90k | 8919273 | 1 | synth |
-
-### Test Dataset
-
-| testset | instance_num | type |
-| :-----: | :----------: | :-------: |
-| IIIT5K | 3000 | regular |
-| SVT | 647 | regular |
-| IC13 | 1015 | regular |
-| IC15 | 2077 | irregular |
-| SVTP | 645 | irregular |
-| CT80 | 288 | irregular |
-
-## Results and Models
-
-| Methods | | Regular Text | | | | Irregular Text | | download |
-| :----------------------------------------------------: | :----: | :----------: | :--: | :-: | :--: | :------------: | :--: | :-------------------------------------------------------------------------------------------------: |
-| | IIIT5K | SVT | IC13 | | IC15 | SVTP | CT80 | |
-| [Satrn](/configs/textrecog/satrn/satrn_academic.py) | 96.1 | 93.5 | 95.7 | | 84.1 | 88.5 | 90.3 | [model](https://download.openmmlab.com/mmocr/textrecog/satrn/satrn_academic_20211009-cb8b1580.pth) \| [log](https://download.openmmlab.com/mmocr/textrecog/satrn/20210809_093244.log.json) |
-| [Satrn_small](/configs/textrecog/satrn/satrn_small.py) | 94.7 | 91.3 | 95.4 | | 81.9 | 85.9 | 86.5 | [model](https://download.openmmlab.com/mmocr/textrecog/satrn/satrn_small_20211009-2cf13355.pth) \| [log](https://download.openmmlab.com/mmocr/textrecog/satrn/20210811_053047.log.json) |
-
-## Citation
-
-```bibtex
-@article{junyeop2019recognizing,
- title={On Recognizing Texts of Arbitrary Shapes with 2D Self-Attention},
- author={Junyeop Lee, Sungrae Park, Jeonghun Baek, Seong Joon Oh, Seonghyeon Kim, Hwalsuk Lee},
- year={2019}
-}
-```
diff --git a/spaces/Maharani/stock_prediction/app.py b/spaces/Maharani/stock_prediction/app.py
deleted file mode 100644
index 2a5e16f0800dd3e416766d6e48fc379301d06dd1..0000000000000000000000000000000000000000
--- a/spaces/Maharani/stock_prediction/app.py
+++ /dev/null
@@ -1,83 +0,0 @@
-import yfinance as yf
-import streamlit as st
-import pandas as pd
-import datetime
-
-import numpy as np
-import matplotlib.pyplot as plt
-from keras.models import Sequential
-from keras.layers import LSTM
-from keras.layers import Dense
-from keras.layers import Bidirectional
-
-
-st.write("""
-# Simple Stock Price App
-
-Shown are the stock **closing price** and **volume**.
-""")
-
-def user_input_features() :
- stock_symbol = st.sidebar.selectbox('Symbol',('BMRI','APLN', 'MNCN', 'BFIN', 'CSAP'))
- date_start = st.sidebar.date_input("Start Date", datetime.date(2015, 5, 31))
- date_end = st.sidebar.date_input("End Date", datetime.date.today())
-
- tickerData = yf.Ticker(stock_symbol+'.JK')
- tickerDf = tickerData.history(period='1d', start=date_start, end=date_end)
- return tickerDf, stock_symbol
-
-input_df, stock_symbol = user_input_features()
-
-st.line_chart(input_df.Close)
-st.line_chart(input_df.Volume)
-
-st.write("""
-# Stock Price Prediction
-
-Shown are the stock prediction for next 20 days.
-""")
-
-n_steps = 100
-n_features = 1
-
-model = Sequential()
-model.add(Bidirectional(LSTM(300, activation='relu'), input_shape=(n_steps, n_features)))
-model.add(Dense(1))
-model.compile(optimizer='adam', loss='mse')
-
-model.load_weights(stock_symbol + ".h5")
-df = input_df.dropna(axis=0, how='any', thresh=None, subset=None, inplace=False)
-df = df[df.Volume > 0]
-
-close = df['Close'][-n_steps:].to_list()
-min_in = min(close)
-max_in = max(close)
-in_seq = []
-for i in close :
- in_seq.append((i - min_in) / (max_in - min_in))
-
-for i in range(20) :
- x_input = np.array(in_seq[-100:])
- x_input = x_input.reshape((1, n_steps, n_features))
- yhat = model.predict(x_input, verbose=0)
- in_seq.append(yhat[0][0])
-
-norm_res = in_seq[-20:]
-res = []
-for i in norm_res :
- res.append(i * (max_in - min_in) + min_in)
-
-closepred = close[-80:]
-for x in res :
- closepred.append(x)
-
-plt.figure(figsize = (20,10))
-plt.plot(closepred, label="Prediction")
-plt.plot(close[-80:], label="Previous")
-plt.ylabel('Price (Rp)', fontsize = 15 )
-plt.xlabel('Days', fontsize = 15 )
-plt.title(stock_symbol + " Stock Prediction", fontsize = 20)
-plt.legend()
-plt.grid()
-
-st.pyplot(plt)
\ No newline at end of file
diff --git a/spaces/Manjushri/MusicGen/tests/modules/test_codebooks_patterns.py b/spaces/Manjushri/MusicGen/tests/modules/test_codebooks_patterns.py
deleted file mode 100644
index b658f4779a369f9ec8dde692a61b7f0fe3485724..0000000000000000000000000000000000000000
--- a/spaces/Manjushri/MusicGen/tests/modules/test_codebooks_patterns.py
+++ /dev/null
@@ -1,246 +0,0 @@
-# Copyright (c) Meta Platforms, Inc. and affiliates.
-# All rights reserved.
-#
-# This source code is licensed under the license found in the
-# LICENSE file in the root directory of this source tree.
-
-import pytest
-import torch
-
-from audiocraft.modules.codebooks_patterns import (
- DelayedPatternProvider,
- ParallelPatternProvider,
- Pattern,
- UnrolledPatternProvider,
-)
-
-
-class TestParallelPatternProvider:
-
- @pytest.mark.parametrize("n_q", [1, 4, 32])
- @pytest.mark.parametrize("timesteps", [0, 1, 16, 100])
- def test_get_pattern(self, n_q: int, timesteps: int):
- provider = ParallelPatternProvider(n_q)
- pattern = provider.get_pattern(timesteps)
- # + 1 to account for 1st step
- assert len(pattern.layout) == timesteps + 1
-
- @pytest.mark.parametrize("n_q", [1, 4, 32])
- @pytest.mark.parametrize("timesteps", [8, 16, 100])
- def test_pattern_content(self, n_q: int, timesteps: int):
- provider = ParallelPatternProvider(n_q)
- pattern = provider.get_pattern(timesteps)
- for s, v in enumerate(pattern.layout):
- for i, code in enumerate(v):
- assert i == code.q
- assert code.t == s - 1 # account for the 1st empty step
-
- @pytest.mark.parametrize("n_q", [1, 4, 32])
- @pytest.mark.parametrize("timesteps", [8, 16, 100])
- def test_pattern_max_delay(self, n_q: int, timesteps: int):
- provider = ParallelPatternProvider(n_q)
- pattern = provider.get_pattern(timesteps)
- assert pattern.max_delay == 0
- assert len(pattern.valid_layout) == len(pattern.layout) - pattern.max_delay
-
-
-class TestDelayedPatternProvider:
-
- @pytest.mark.parametrize("n_q", [1, 4, 32])
- @pytest.mark.parametrize("timesteps", [0, 1, 16, 100])
- def test_get_pattern(self, n_q: int, timesteps: int):
- delays = [
- list(range(n_q)),
- [0] + [1] * (n_q - 1),
- [0] + [4] * (n_q - 1),
- ]
- for delay in delays:
- provider = DelayedPatternProvider(n_q, delay)
- pattern = provider.get_pattern(timesteps)
- # + 1 to account for 1st step
- assert len(pattern.layout) == timesteps + max(delay) + 1
-
- @pytest.mark.parametrize("n_q", [1, 4, 32])
- @pytest.mark.parametrize("timesteps", [8, 16, 100])
- def test_pattern_content(self, n_q: int, timesteps: int):
- provider = DelayedPatternProvider(n_q)
- pattern = provider.get_pattern(timesteps)
- for s, v in enumerate(pattern.layout):
- for i, code in enumerate(v):
- assert i == code.q
- assert code.t == max(0, s - code.q - 1)
-
- @pytest.mark.parametrize("timesteps", [8, 16, 100])
- @pytest.mark.parametrize("delay", [[0, 1, 2, 3], [0, 1, 1, 1], [0, 3, 3, 3], [0, 3]])
- def test_pattern_max_delay(self, timesteps: int, delay: list):
- provider = DelayedPatternProvider(len(delay), delay)
- pattern = provider.get_pattern(timesteps)
- assert pattern.max_delay == max(delay)
- assert len(pattern.valid_layout) == len(pattern.layout) - pattern.max_delay
-
-
-class TestUnrolledPatternProvider:
-
- @pytest.mark.parametrize("timesteps", [0, 1, 16])
- @pytest.mark.parametrize("flattening", [[0, 1, 2], [0, 1, 1]])
- @pytest.mark.parametrize("delays", [[0, 0, 0], [0, 5, 5]])
- def test_get_pattern(self, timesteps: int, flattening: list, delays: list):
- n_q = len(flattening)
- max_delay = max(delays)
- provider = UnrolledPatternProvider(n_q, flattening, delays)
- pattern = provider.get_pattern(timesteps)
- assert len(pattern.layout) == provider.num_virtual_steps(timesteps) + max_delay
-
- @pytest.mark.parametrize("timesteps", [0, 1, 16])
- @pytest.mark.parametrize("flattening", [[0, 1, 2], [0, 1, 1]])
- @pytest.mark.parametrize("delays", [[0, 0, 0], [0, 5, 5]])
- def test_pattern_max_delay(self, timesteps: int, flattening: list, delays: list):
- n_q = len(flattening)
- max_delay = max(delays)
- provider = UnrolledPatternProvider(n_q, flattening, delays)
- pattern = provider.get_pattern(timesteps)
- assert pattern.max_delay == max_delay
-
-
-class TestPattern:
-
- def ref_build_pattern_sequence(self, z: torch.Tensor, pattern: Pattern, special_token: int):
- """Reference method to build the sequence from the pattern without using fancy scatter."""
- bs, n_q, T = z.shape
- z = z.cpu().numpy()
- assert n_q == pattern.n_q
- assert T <= pattern.timesteps
- inp = torch.full((bs, n_q, len(pattern.layout)), special_token, dtype=torch.long).numpy()
- inp[:] = special_token
- for s, v in enumerate(pattern.layout):
- for (t, q) in v:
- if t < T:
- inp[:, q, s] = z[:, q, t]
- return torch.from_numpy(inp)
-
- def ref_revert_pattern_sequence(self, z: torch.Tensor, pattern: Pattern, special_token: int):
- """Reference method to revert the sequence from the pattern without using fancy scatter."""
- z = z.cpu().numpy()
- bs, n_q, S = z.shape
- assert pattern.n_q == n_q
- inp = torch.full((bs, pattern.n_q, pattern.timesteps), special_token, dtype=torch.long).numpy()
- inp[:] = special_token
- for s, v in enumerate(pattern.layout):
- for (t, q) in v:
- if t < pattern.timesteps:
- inp[:, q, t] = z[:, q, s]
- return torch.from_numpy(inp)
-
- def ref_revert_pattern_logits(self, z: torch.Tensor, pattern: Pattern, special_token: float):
- """Reference method to revert the logits from the pattern without using fancy scatter."""
- z = z.cpu().numpy()
- bs, card, n_q, S = z.shape
- assert pattern.n_q == n_q
- ref_layout = pattern.layout
- inp = torch.full((bs, card, pattern.n_q, pattern.timesteps), special_token, dtype=torch.float).numpy()
- inp[:] = special_token
- for s, v in enumerate(ref_layout[1:]):
- if s < S:
- for (t, q) in v:
- if t < pattern.timesteps:
- inp[:, :, q, t] = z[:, :, q, s]
- return torch.from_numpy(inp)
-
- def _get_pattern_providers(self, n_q: int):
- pattern_provider_1 = ParallelPatternProvider(n_q)
- pattern_provider_2 = DelayedPatternProvider(n_q, list(range(n_q)))
- pattern_provider_3 = DelayedPatternProvider(n_q, [0] + [1] * (n_q - 1))
- pattern_provider_4 = UnrolledPatternProvider(
- n_q, flattening=list(range(n_q)), delays=[0] * n_q
- )
- pattern_provider_5 = UnrolledPatternProvider(
- n_q, flattening=[0] + [1] * (n_q - 1), delays=[0] * n_q
- )
- pattern_provider_6 = UnrolledPatternProvider(
- n_q, flattening=[0] + [1] * (n_q - 1), delays=[0] + [5] * (n_q - 1)
- )
- return [
- pattern_provider_1,
- pattern_provider_2,
- pattern_provider_3,
- pattern_provider_4,
- pattern_provider_5,
- pattern_provider_6,
- ]
-
- @pytest.mark.parametrize("n_q", [1, 4, 32])
- @pytest.mark.parametrize("timesteps", [16, 72])
- def test_build_pattern_sequence(self, n_q: int, timesteps: int):
- bs = 2
- card = 256
- special_token = card
-
- pattern_providers = self._get_pattern_providers(n_q)
- for pattern_provider in pattern_providers:
- pattern = pattern_provider.get_pattern(timesteps)
- # we can correctly build the sequence from the pattern
- z = torch.randint(0, card, (bs, n_q, timesteps))
- ref_res = self.ref_build_pattern_sequence(z, pattern, special_token)
- res, indexes, mask = pattern.build_pattern_sequence(z, special_token)
- assert (res == ref_res).float().mean() == 1.0
-
- # expected assertion fails on the number of timesteps
- invalid_timesteps = [timesteps + 1]
- if pattern.num_sequence_steps != pattern.timesteps:
- invalid_timesteps.append(pattern.num_sequence_steps)
- for i_timesteps in invalid_timesteps:
- z2 = torch.randint(0, card, (bs, n_q, i_timesteps))
- with pytest.raises(AssertionError):
- pattern.build_pattern_sequence(z2, special_token)
-
- # expected assertion fails on the number of codebooks
- invalid_qs = [0, n_q - 1, n_q + 1]
- for i_q in invalid_qs:
- z3 = torch.randint(0, card, (bs, i_q, timesteps))
- with pytest.raises(AssertionError):
- pattern.build_pattern_sequence(z3, special_token)
-
- @pytest.mark.parametrize("n_q", [1, 4, 32])
- @pytest.mark.parametrize("timesteps", [16, 72])
- def test_revert_pattern_sequence(self, n_q: int, timesteps: int):
- bs = 2
- card = 256
- special_token = card
-
- pattern_providers = self._get_pattern_providers(n_q)
- for pattern_provider in pattern_providers:
- pattern = pattern_provider.get_pattern(timesteps)
- # this works assuming previous tests are successful
- z = torch.randint(0, card, (bs, n_q, timesteps))
- s = self.ref_build_pattern_sequence(z, pattern, special_token)
- ref_out = self.ref_revert_pattern_sequence(s, pattern, special_token)
- # ensure our reference script retrieve the original sequence
- assert z.shape == ref_out.shape
- assert (z == ref_out).float().mean() == 1.0
- # now we can test the scatter version
- out, indexes, mask = pattern.revert_pattern_sequence(s, special_token)
- assert out.shape == ref_out.shape
- assert (out == ref_out).float().mean() == 1.0
-
- @pytest.mark.parametrize("n_q", [1, 4, 32])
- @pytest.mark.parametrize("timesteps", [16, 72])
- @pytest.mark.parametrize("card", [1, 2, 256, 1024])
- def test_revert_pattern_logits(self, n_q: int, timesteps: int, card: int):
- bs = 2
- special_token = card
- logits_special_token = float('nan')
-
- pattern_providers = self._get_pattern_providers(n_q)
- for pattern_provider in pattern_providers:
- pattern = pattern_provider.get_pattern(timesteps)
- # this works assuming previous tests are successful
- z = torch.randint(0, card, (bs, n_q, timesteps))
- s = self.ref_build_pattern_sequence(z, pattern, special_token)
- logits = torch.randn((bs, card, n_q, s.shape[-1]))
- ref_out = self.ref_revert_pattern_logits(logits, pattern, logits_special_token)
- # ensure our reference script retrieve the original sequence
- assert ref_out.shape == torch.Size([bs, card, n_q, timesteps])
- # now we can test the scatter version
- out, indexes, mask = pattern.revert_pattern_logits(logits, logits_special_token)
- assert out.shape == ref_out.shape
- assert (out == ref_out).float().mean() == 1.0
diff --git a/spaces/Marshalls/testmtd/analysis/aistplusplus_api/__MACOSX/smpl/smpl_webuser/._lbs.py b/spaces/Marshalls/testmtd/analysis/aistplusplus_api/__MACOSX/smpl/smpl_webuser/._lbs.py
deleted file mode 100644
index c141f0b71b678ee836ef1b58733749b8aea579c9..0000000000000000000000000000000000000000
Binary files a/spaces/Marshalls/testmtd/analysis/aistplusplus_api/__MACOSX/smpl/smpl_webuser/._lbs.py and /dev/null differ
diff --git a/spaces/Mellow-ai/PhotoAI_Mellow/annotator/uniformer/mmcv/parallel/distributed_deprecated.py b/spaces/Mellow-ai/PhotoAI_Mellow/annotator/uniformer/mmcv/parallel/distributed_deprecated.py
deleted file mode 100644
index 676937a2085d4da20fa87923041a200fca6214eb..0000000000000000000000000000000000000000
--- a/spaces/Mellow-ai/PhotoAI_Mellow/annotator/uniformer/mmcv/parallel/distributed_deprecated.py
+++ /dev/null
@@ -1,70 +0,0 @@
-# Copyright (c) OpenMMLab. All rights reserved.
-import torch
-import torch.distributed as dist
-import torch.nn as nn
-from torch._utils import (_flatten_dense_tensors, _take_tensors,
- _unflatten_dense_tensors)
-
-from annotator.uniformer.mmcv.utils import TORCH_VERSION, digit_version
-from .registry import MODULE_WRAPPERS
-from .scatter_gather import scatter_kwargs
-
-
-@MODULE_WRAPPERS.register_module()
-class MMDistributedDataParallel(nn.Module):
-
- def __init__(self,
- module,
- dim=0,
- broadcast_buffers=True,
- bucket_cap_mb=25):
- super(MMDistributedDataParallel, self).__init__()
- self.module = module
- self.dim = dim
- self.broadcast_buffers = broadcast_buffers
-
- self.broadcast_bucket_size = bucket_cap_mb * 1024 * 1024
- self._sync_params()
-
- def _dist_broadcast_coalesced(self, tensors, buffer_size):
- for tensors in _take_tensors(tensors, buffer_size):
- flat_tensors = _flatten_dense_tensors(tensors)
- dist.broadcast(flat_tensors, 0)
- for tensor, synced in zip(
- tensors, _unflatten_dense_tensors(flat_tensors, tensors)):
- tensor.copy_(synced)
-
- def _sync_params(self):
- module_states = list(self.module.state_dict().values())
- if len(module_states) > 0:
- self._dist_broadcast_coalesced(module_states,
- self.broadcast_bucket_size)
- if self.broadcast_buffers:
- if (TORCH_VERSION != 'parrots'
- and digit_version(TORCH_VERSION) < digit_version('1.0')):
- buffers = [b.data for b in self.module._all_buffers()]
- else:
- buffers = [b.data for b in self.module.buffers()]
- if len(buffers) > 0:
- self._dist_broadcast_coalesced(buffers,
- self.broadcast_bucket_size)
-
- def scatter(self, inputs, kwargs, device_ids):
- return scatter_kwargs(inputs, kwargs, device_ids, dim=self.dim)
-
- def forward(self, *inputs, **kwargs):
- inputs, kwargs = self.scatter(inputs, kwargs,
- [torch.cuda.current_device()])
- return self.module(*inputs[0], **kwargs[0])
-
- def train_step(self, *inputs, **kwargs):
- inputs, kwargs = self.scatter(inputs, kwargs,
- [torch.cuda.current_device()])
- output = self.module.train_step(*inputs[0], **kwargs[0])
- return output
-
- def val_step(self, *inputs, **kwargs):
- inputs, kwargs = self.scatter(inputs, kwargs,
- [torch.cuda.current_device()])
- output = self.module.val_step(*inputs[0], **kwargs[0])
- return output
diff --git a/spaces/Mellow-ai/PhotoAI_Mellow/annotator/uniformer/mmcv/runner/hooks/logger/text.py b/spaces/Mellow-ai/PhotoAI_Mellow/annotator/uniformer/mmcv/runner/hooks/logger/text.py
deleted file mode 100644
index 87b1a3eca9595a130121526f8b4c29915387ab35..0000000000000000000000000000000000000000
--- a/spaces/Mellow-ai/PhotoAI_Mellow/annotator/uniformer/mmcv/runner/hooks/logger/text.py
+++ /dev/null
@@ -1,256 +0,0 @@
-# Copyright (c) OpenMMLab. All rights reserved.
-import datetime
-import os
-import os.path as osp
-from collections import OrderedDict
-
-import torch
-import torch.distributed as dist
-
-import annotator.uniformer.mmcv as mmcv
-from annotator.uniformer.mmcv.fileio.file_client import FileClient
-from annotator.uniformer.mmcv.utils import is_tuple_of, scandir
-from ..hook import HOOKS
-from .base import LoggerHook
-
-
-@HOOKS.register_module()
-class TextLoggerHook(LoggerHook):
- """Logger hook in text.
-
- In this logger hook, the information will be printed on terminal and
- saved in json file.
-
- Args:
- by_epoch (bool, optional): Whether EpochBasedRunner is used.
- Default: True.
- interval (int, optional): Logging interval (every k iterations).
- Default: 10.
- ignore_last (bool, optional): Ignore the log of last iterations in each
- epoch if less than :attr:`interval`. Default: True.
- reset_flag (bool, optional): Whether to clear the output buffer after
- logging. Default: False.
- interval_exp_name (int, optional): Logging interval for experiment
- name. This feature is to help users conveniently get the experiment
- information from screen or log file. Default: 1000.
- out_dir (str, optional): Logs are saved in ``runner.work_dir`` default.
- If ``out_dir`` is specified, logs will be copied to a new directory
- which is the concatenation of ``out_dir`` and the last level
- directory of ``runner.work_dir``. Default: None.
- `New in version 1.3.16.`
- out_suffix (str or tuple[str], optional): Those filenames ending with
- ``out_suffix`` will be copied to ``out_dir``.
- Default: ('.log.json', '.log', '.py').
- `New in version 1.3.16.`
- keep_local (bool, optional): Whether to keep local log when
- :attr:`out_dir` is specified. If False, the local log will be
- removed. Default: True.
- `New in version 1.3.16.`
- file_client_args (dict, optional): Arguments to instantiate a
- FileClient. See :class:`mmcv.fileio.FileClient` for details.
- Default: None.
- `New in version 1.3.16.`
- """
-
- def __init__(self,
- by_epoch=True,
- interval=10,
- ignore_last=True,
- reset_flag=False,
- interval_exp_name=1000,
- out_dir=None,
- out_suffix=('.log.json', '.log', '.py'),
- keep_local=True,
- file_client_args=None):
- super(TextLoggerHook, self).__init__(interval, ignore_last, reset_flag,
- by_epoch)
- self.by_epoch = by_epoch
- self.time_sec_tot = 0
- self.interval_exp_name = interval_exp_name
-
- if out_dir is None and file_client_args is not None:
- raise ValueError(
- 'file_client_args should be "None" when `out_dir` is not'
- 'specified.')
- self.out_dir = out_dir
-
- if not (out_dir is None or isinstance(out_dir, str)
- or is_tuple_of(out_dir, str)):
- raise TypeError('out_dir should be "None" or string or tuple of '
- 'string, but got {out_dir}')
- self.out_suffix = out_suffix
-
- self.keep_local = keep_local
- self.file_client_args = file_client_args
- if self.out_dir is not None:
- self.file_client = FileClient.infer_client(file_client_args,
- self.out_dir)
-
- def before_run(self, runner):
- super(TextLoggerHook, self).before_run(runner)
-
- if self.out_dir is not None:
- self.file_client = FileClient.infer_client(self.file_client_args,
- self.out_dir)
- # The final `self.out_dir` is the concatenation of `self.out_dir`
- # and the last level directory of `runner.work_dir`
- basename = osp.basename(runner.work_dir.rstrip(osp.sep))
- self.out_dir = self.file_client.join_path(self.out_dir, basename)
- runner.logger.info(
- (f'Text logs will be saved to {self.out_dir} by '
- f'{self.file_client.name} after the training process.'))
-
- self.start_iter = runner.iter
- self.json_log_path = osp.join(runner.work_dir,
- f'{runner.timestamp}.log.json')
- if runner.meta is not None:
- self._dump_log(runner.meta, runner)
-
- def _get_max_memory(self, runner):
- device = getattr(runner.model, 'output_device', None)
- mem = torch.cuda.max_memory_allocated(device=device)
- mem_mb = torch.tensor([mem / (1024 * 1024)],
- dtype=torch.int,
- device=device)
- if runner.world_size > 1:
- dist.reduce(mem_mb, 0, op=dist.ReduceOp.MAX)
- return mem_mb.item()
-
- def _log_info(self, log_dict, runner):
- # print exp name for users to distinguish experiments
- # at every ``interval_exp_name`` iterations and the end of each epoch
- if runner.meta is not None and 'exp_name' in runner.meta:
- if (self.every_n_iters(runner, self.interval_exp_name)) or (
- self.by_epoch and self.end_of_epoch(runner)):
- exp_info = f'Exp name: {runner.meta["exp_name"]}'
- runner.logger.info(exp_info)
-
- if log_dict['mode'] == 'train':
- if isinstance(log_dict['lr'], dict):
- lr_str = []
- for k, val in log_dict['lr'].items():
- lr_str.append(f'lr_{k}: {val:.3e}')
- lr_str = ' '.join(lr_str)
- else:
- lr_str = f'lr: {log_dict["lr"]:.3e}'
-
- # by epoch: Epoch [4][100/1000]
- # by iter: Iter [100/100000]
- if self.by_epoch:
- log_str = f'Epoch [{log_dict["epoch"]}]' \
- f'[{log_dict["iter"]}/{len(runner.data_loader)}]\t'
- else:
- log_str = f'Iter [{log_dict["iter"]}/{runner.max_iters}]\t'
- log_str += f'{lr_str}, '
-
- if 'time' in log_dict.keys():
- self.time_sec_tot += (log_dict['time'] * self.interval)
- time_sec_avg = self.time_sec_tot / (
- runner.iter - self.start_iter + 1)
- eta_sec = time_sec_avg * (runner.max_iters - runner.iter - 1)
- eta_str = str(datetime.timedelta(seconds=int(eta_sec)))
- log_str += f'eta: {eta_str}, '
- log_str += f'time: {log_dict["time"]:.3f}, ' \
- f'data_time: {log_dict["data_time"]:.3f}, '
- # statistic memory
- if torch.cuda.is_available():
- log_str += f'memory: {log_dict["memory"]}, '
- else:
- # val/test time
- # here 1000 is the length of the val dataloader
- # by epoch: Epoch[val] [4][1000]
- # by iter: Iter[val] [1000]
- if self.by_epoch:
- log_str = f'Epoch({log_dict["mode"]}) ' \
- f'[{log_dict["epoch"]}][{log_dict["iter"]}]\t'
- else:
- log_str = f'Iter({log_dict["mode"]}) [{log_dict["iter"]}]\t'
-
- log_items = []
- for name, val in log_dict.items():
- # TODO: resolve this hack
- # these items have been in log_str
- if name in [
- 'mode', 'Epoch', 'iter', 'lr', 'time', 'data_time',
- 'memory', 'epoch'
- ]:
- continue
- if isinstance(val, float):
- val = f'{val:.4f}'
- log_items.append(f'{name}: {val}')
- log_str += ', '.join(log_items)
-
- runner.logger.info(log_str)
-
- def _dump_log(self, log_dict, runner):
- # dump log in json format
- json_log = OrderedDict()
- for k, v in log_dict.items():
- json_log[k] = self._round_float(v)
- # only append log at last line
- if runner.rank == 0:
- with open(self.json_log_path, 'a+') as f:
- mmcv.dump(json_log, f, file_format='json')
- f.write('\n')
-
- def _round_float(self, items):
- if isinstance(items, list):
- return [self._round_float(item) for item in items]
- elif isinstance(items, float):
- return round(items, 5)
- else:
- return items
-
- def log(self, runner):
- if 'eval_iter_num' in runner.log_buffer.output:
- # this doesn't modify runner.iter and is regardless of by_epoch
- cur_iter = runner.log_buffer.output.pop('eval_iter_num')
- else:
- cur_iter = self.get_iter(runner, inner_iter=True)
-
- log_dict = OrderedDict(
- mode=self.get_mode(runner),
- epoch=self.get_epoch(runner),
- iter=cur_iter)
-
- # only record lr of the first param group
- cur_lr = runner.current_lr()
- if isinstance(cur_lr, list):
- log_dict['lr'] = cur_lr[0]
- else:
- assert isinstance(cur_lr, dict)
- log_dict['lr'] = {}
- for k, lr_ in cur_lr.items():
- assert isinstance(lr_, list)
- log_dict['lr'].update({k: lr_[0]})
-
- if 'time' in runner.log_buffer.output:
- # statistic memory
- if torch.cuda.is_available():
- log_dict['memory'] = self._get_max_memory(runner)
-
- log_dict = dict(log_dict, **runner.log_buffer.output)
-
- self._log_info(log_dict, runner)
- self._dump_log(log_dict, runner)
- return log_dict
-
- def after_run(self, runner):
- # copy or upload logs to self.out_dir
- if self.out_dir is not None:
- for filename in scandir(runner.work_dir, self.out_suffix, True):
- local_filepath = osp.join(runner.work_dir, filename)
- out_filepath = self.file_client.join_path(
- self.out_dir, filename)
- with open(local_filepath, 'r') as f:
- self.file_client.put_text(f.read(), out_filepath)
-
- runner.logger.info(
- (f'The file {local_filepath} has been uploaded to '
- f'{out_filepath}.'))
-
- if not self.keep_local:
- os.remove(local_filepath)
- runner.logger.info(
- (f'{local_filepath} was removed due to the '
- '`self.keep_local=False`'))
diff --git a/spaces/MingGatsby/Grounding_DINO_demo/groundingdino/util/slconfig.py b/spaces/MingGatsby/Grounding_DINO_demo/groundingdino/util/slconfig.py
deleted file mode 100644
index 0d84a4c24f3f3a2ce9ba60cb3f939bad89b35baa..0000000000000000000000000000000000000000
--- a/spaces/MingGatsby/Grounding_DINO_demo/groundingdino/util/slconfig.py
+++ /dev/null
@@ -1,424 +0,0 @@
-# ==========================================================
-# Modified from mmcv
-# ==========================================================
-import ast
-import os.path as osp
-import shutil
-import sys
-import tempfile
-from argparse import Action
-from importlib import import_module
-
-from addict import Dict
-from yapf.yapflib.yapf_api import FormatCode
-
-BASE_KEY = "_base_"
-DELETE_KEY = "_delete_"
-RESERVED_KEYS = ["filename", "text", "pretty_text", "get", "dump", "merge_from_dict"]
-
-
-def check_file_exist(filename, msg_tmpl='file "{}" does not exist'):
- if not osp.isfile(filename):
- raise FileNotFoundError(msg_tmpl.format(filename))
-
-
-class ConfigDict(Dict):
- def __missing__(self, name):
- raise KeyError(name)
-
- def __getattr__(self, name):
- try:
- value = super(ConfigDict, self).__getattr__(name)
- except KeyError:
- ex = AttributeError(f"'{self.__class__.__name__}' object has no " f"attribute '{name}'")
- except Exception as e:
- ex = e
- else:
- return value
- raise ex
-
-
-class SLConfig(object):
- """
- config files.
- only support .py file as config now.
-
- ref: mmcv.utils.config
-
- Example:
- >>> cfg = Config(dict(a=1, b=dict(b1=[0, 1])))
- >>> cfg.a
- 1
- >>> cfg.b
- {'b1': [0, 1]}
- >>> cfg.b.b1
- [0, 1]
- >>> cfg = Config.fromfile('tests/data/config/a.py')
- >>> cfg.filename
- "/home/kchen/projects/mmcv/tests/data/config/a.py"
- >>> cfg.item4
- 'test'
- >>> cfg
- "Config [path: /home/kchen/projects/mmcv/tests/data/config/a.py]: "
- "{'item1': [1, 2], 'item2': {'a': 0}, 'item3': True, 'item4': 'test'}"
- """
-
- @staticmethod
- def _validate_py_syntax(filename):
- with open(filename) as f:
- content = f.read()
- try:
- ast.parse(content)
- except SyntaxError:
- raise SyntaxError("There are syntax errors in config " f"file {filename}")
-
- @staticmethod
- def _file2dict(filename):
- filename = osp.abspath(osp.expanduser(filename))
- check_file_exist(filename)
- if filename.lower().endswith(".py"):
- with tempfile.TemporaryDirectory() as temp_config_dir:
- temp_config_file = tempfile.NamedTemporaryFile(dir=temp_config_dir, suffix=".py")
- temp_config_name = osp.basename(temp_config_file.name)
- shutil.copyfile(filename, osp.join(temp_config_dir, temp_config_name))
- temp_module_name = osp.splitext(temp_config_name)[0]
- sys.path.insert(0, temp_config_dir)
- SLConfig._validate_py_syntax(filename)
- mod = import_module(temp_module_name)
- sys.path.pop(0)
- cfg_dict = {
- name: value for name, value in mod.__dict__.items() if not name.startswith("__")
- }
- # delete imported module
- del sys.modules[temp_module_name]
- # close temp file
- temp_config_file.close()
- elif filename.lower().endswith((".yml", ".yaml", ".json")):
- from .slio import slload
-
- cfg_dict = slload(filename)
- else:
- raise IOError("Only py/yml/yaml/json type are supported now!")
-
- cfg_text = filename + "\n"
- with open(filename, "r") as f:
- cfg_text += f.read()
-
- # parse the base file
- if BASE_KEY in cfg_dict:
- cfg_dir = osp.dirname(filename)
- base_filename = cfg_dict.pop(BASE_KEY)
- base_filename = base_filename if isinstance(base_filename, list) else [base_filename]
-
- cfg_dict_list = list()
- cfg_text_list = list()
- for f in base_filename:
- _cfg_dict, _cfg_text = SLConfig._file2dict(osp.join(cfg_dir, f))
- cfg_dict_list.append(_cfg_dict)
- cfg_text_list.append(_cfg_text)
-
- base_cfg_dict = dict()
- for c in cfg_dict_list:
- if len(base_cfg_dict.keys() & c.keys()) > 0:
- raise KeyError("Duplicate key is not allowed among bases")
- # TODO Allow the duplicate key while warnning user
- base_cfg_dict.update(c)
-
- base_cfg_dict = SLConfig._merge_a_into_b(cfg_dict, base_cfg_dict)
- cfg_dict = base_cfg_dict
-
- # merge cfg_text
- cfg_text_list.append(cfg_text)
- cfg_text = "\n".join(cfg_text_list)
-
- return cfg_dict, cfg_text
-
- @staticmethod
- def _merge_a_into_b(a, b):
- """merge dict `a` into dict `b` (non-inplace).
- values in `a` will overwrite `b`.
- copy first to avoid inplace modification
-
- Args:
- a ([type]): [description]
- b ([type]): [description]
-
- Returns:
- [dict]: [description]
- """
- # import ipdb; ipdb.set_trace()
- if not isinstance(a, dict):
- return a
-
- b = b.copy()
- for k, v in a.items():
- if isinstance(v, dict) and k in b and not v.pop(DELETE_KEY, False):
-
- if not isinstance(b[k], dict) and not isinstance(b[k], list):
- # if :
- # import ipdb; ipdb.set_trace()
- raise TypeError(
- f"{k}={v} in child config cannot inherit from base "
- f"because {k} is a dict in the child config but is of "
- f"type {type(b[k])} in base config. You may set "
- f"`{DELETE_KEY}=True` to ignore the base config"
- )
- b[k] = SLConfig._merge_a_into_b(v, b[k])
- elif isinstance(b, list):
- try:
- _ = int(k)
- except:
- raise TypeError(
- f"b is a list, " f"index {k} should be an int when input but {type(k)}"
- )
- b[int(k)] = SLConfig._merge_a_into_b(v, b[int(k)])
- else:
- b[k] = v
-
- return b
-
- @staticmethod
- def fromfile(filename):
- cfg_dict, cfg_text = SLConfig._file2dict(filename)
- return SLConfig(cfg_dict, cfg_text=cfg_text, filename=filename)
-
- def __init__(self, cfg_dict=None, cfg_text=None, filename=None):
- if cfg_dict is None:
- cfg_dict = dict()
- elif not isinstance(cfg_dict, dict):
- raise TypeError("cfg_dict must be a dict, but " f"got {type(cfg_dict)}")
- for key in cfg_dict:
- if key in RESERVED_KEYS:
- raise KeyError(f"{key} is reserved for config file")
-
- super(SLConfig, self).__setattr__("_cfg_dict", ConfigDict(cfg_dict))
- super(SLConfig, self).__setattr__("_filename", filename)
- if cfg_text:
- text = cfg_text
- elif filename:
- with open(filename, "r") as f:
- text = f.read()
- else:
- text = ""
- super(SLConfig, self).__setattr__("_text", text)
-
- @property
- def filename(self):
- return self._filename
-
- @property
- def text(self):
- return self._text
-
- @property
- def pretty_text(self):
-
- indent = 4
-
- def _indent(s_, num_spaces):
- s = s_.split("\n")
- if len(s) == 1:
- return s_
- first = s.pop(0)
- s = [(num_spaces * " ") + line for line in s]
- s = "\n".join(s)
- s = first + "\n" + s
- return s
-
- def _format_basic_types(k, v, use_mapping=False):
- if isinstance(v, str):
- v_str = f"'{v}'"
- else:
- v_str = str(v)
-
- if use_mapping:
- k_str = f"'{k}'" if isinstance(k, str) else str(k)
- attr_str = f"{k_str}: {v_str}"
- else:
- attr_str = f"{str(k)}={v_str}"
- attr_str = _indent(attr_str, indent)
-
- return attr_str
-
- def _format_list(k, v, use_mapping=False):
- # check if all items in the list are dict
- if all(isinstance(_, dict) for _ in v):
- v_str = "[\n"
- v_str += "\n".join(
- f"dict({_indent(_format_dict(v_), indent)})," for v_ in v
- ).rstrip(",")
- if use_mapping:
- k_str = f"'{k}'" if isinstance(k, str) else str(k)
- attr_str = f"{k_str}: {v_str}"
- else:
- attr_str = f"{str(k)}={v_str}"
- attr_str = _indent(attr_str, indent) + "]"
- else:
- attr_str = _format_basic_types(k, v, use_mapping)
- return attr_str
-
- def _contain_invalid_identifier(dict_str):
- contain_invalid_identifier = False
- for key_name in dict_str:
- contain_invalid_identifier |= not str(key_name).isidentifier()
- return contain_invalid_identifier
-
- def _format_dict(input_dict, outest_level=False):
- r = ""
- s = []
-
- use_mapping = _contain_invalid_identifier(input_dict)
- if use_mapping:
- r += "{"
- for idx, (k, v) in enumerate(input_dict.items()):
- is_last = idx >= len(input_dict) - 1
- end = "" if outest_level or is_last else ","
- if isinstance(v, dict):
- v_str = "\n" + _format_dict(v)
- if use_mapping:
- k_str = f"'{k}'" if isinstance(k, str) else str(k)
- attr_str = f"{k_str}: dict({v_str}"
- else:
- attr_str = f"{str(k)}=dict({v_str}"
- attr_str = _indent(attr_str, indent) + ")" + end
- elif isinstance(v, list):
- attr_str = _format_list(k, v, use_mapping) + end
- else:
- attr_str = _format_basic_types(k, v, use_mapping) + end
-
- s.append(attr_str)
- r += "\n".join(s)
- if use_mapping:
- r += "}"
- return r
-
- cfg_dict = self._cfg_dict.to_dict()
- text = _format_dict(cfg_dict, outest_level=True)
- # copied from setup.cfg
- yapf_style = dict(
- based_on_style="pep8",
- blank_line_before_nested_class_or_def=True,
- split_before_expression_after_opening_paren=True,
- )
- text, _ = FormatCode(text, style_config=yapf_style, verify=True)
-
- return text
-
- def __repr__(self):
- return f"Config (path: {self.filename}): {self._cfg_dict.__repr__()}"
-
- def __len__(self):
- return len(self._cfg_dict)
-
- def __getattr__(self, name):
- # # debug
- # print('+'*15)
- # print('name=%s' % name)
- # print("addr:", id(self))
- # # print('type(self):', type(self))
- # print(self.__dict__)
- # print('+'*15)
- # if self.__dict__ == {}:
- # raise ValueError
-
- return getattr(self._cfg_dict, name)
-
- def __getitem__(self, name):
- return self._cfg_dict.__getitem__(name)
-
- def __setattr__(self, name, value):
- if isinstance(value, dict):
- value = ConfigDict(value)
- self._cfg_dict.__setattr__(name, value)
-
- def __setitem__(self, name, value):
- if isinstance(value, dict):
- value = ConfigDict(value)
- self._cfg_dict.__setitem__(name, value)
-
- def __iter__(self):
- return iter(self._cfg_dict)
-
- def dump(self, file=None):
- # import ipdb; ipdb.set_trace()
- if file is None:
- return self.pretty_text
- else:
- with open(file, "w") as f:
- f.write(self.pretty_text)
-
- def merge_from_dict(self, options):
- """Merge list into cfg_dict
-
- Merge the dict parsed by MultipleKVAction into this cfg.
-
- Examples:
- >>> options = {'model.backbone.depth': 50,
- ... 'model.backbone.with_cp':True}
- >>> cfg = Config(dict(model=dict(backbone=dict(type='ResNet'))))
- >>> cfg.merge_from_dict(options)
- >>> cfg_dict = super(Config, self).__getattribute__('_cfg_dict')
- >>> assert cfg_dict == dict(
- ... model=dict(backbone=dict(depth=50, with_cp=True)))
-
- Args:
- options (dict): dict of configs to merge from.
- """
- option_cfg_dict = {}
- for full_key, v in options.items():
- d = option_cfg_dict
- key_list = full_key.split(".")
- for subkey in key_list[:-1]:
- d.setdefault(subkey, ConfigDict())
- d = d[subkey]
- subkey = key_list[-1]
- d[subkey] = v
-
- cfg_dict = super(SLConfig, self).__getattribute__("_cfg_dict")
- super(SLConfig, self).__setattr__(
- "_cfg_dict", SLConfig._merge_a_into_b(option_cfg_dict, cfg_dict)
- )
-
- # for multiprocess
- def __setstate__(self, state):
- self.__init__(state)
-
- def copy(self):
- return SLConfig(self._cfg_dict.copy())
-
- def deepcopy(self):
- return SLConfig(self._cfg_dict.deepcopy())
-
-
-class DictAction(Action):
- """
- argparse action to split an argument into KEY=VALUE form
- on the first = and append to a dictionary. List options should
- be passed as comma separated values, i.e KEY=V1,V2,V3
- """
-
- @staticmethod
- def _parse_int_float_bool(val):
- try:
- return int(val)
- except ValueError:
- pass
- try:
- return float(val)
- except ValueError:
- pass
- if val.lower() in ["true", "false"]:
- return True if val.lower() == "true" else False
- if val.lower() in ["none", "null"]:
- return None
- return val
-
- def __call__(self, parser, namespace, values, option_string=None):
- options = {}
- for kv in values:
- key, val = kv.split("=", maxsplit=1)
- val = [self._parse_int_float_bool(v) for v in val.split(",")]
- if len(val) == 1:
- val = val[0]
- options[key] = val
- setattr(namespace, self.dest, options)
diff --git a/spaces/MirageML/sjc/my/utils/__init__.py b/spaces/MirageML/sjc/my/utils/__init__.py
deleted file mode 100644
index fc8cd6bb17eb8463e14845e0b4ecbbb86620ca0b..0000000000000000000000000000000000000000
--- a/spaces/MirageML/sjc/my/utils/__init__.py
+++ /dev/null
@@ -1,4 +0,0 @@
-from .event import EventStorage, get_event_storage, read_stats
-from .tqdm import tqdm
-from .heartbeat import HeartBeat, get_heartbeat
-from .debug import EarlyLoopBreak
diff --git a/spaces/NCTCMumbai/NCTC/models/official/vision/detection/ops/postprocess_ops.py b/spaces/NCTCMumbai/NCTC/models/official/vision/detection/ops/postprocess_ops.py
deleted file mode 100644
index 2cb06c34ab114d171f30cb52e69d8dc73996e302..0000000000000000000000000000000000000000
--- a/spaces/NCTCMumbai/NCTC/models/official/vision/detection/ops/postprocess_ops.py
+++ /dev/null
@@ -1,413 +0,0 @@
-# Copyright 2019 The TensorFlow Authors. All Rights Reserved.
-#
-# Licensed under the Apache License, Version 2.0 (the "License");
-# you may not use this file except in compliance with the License.
-# You may obtain a copy of the License at
-#
-# http://www.apache.org/licenses/LICENSE-2.0
-#
-# Unless required by applicable law or agreed to in writing, software
-# distributed under the License is distributed on an "AS IS" BASIS,
-# WITHOUT WARRANTIES OR CONDITIONS OF ANY KIND, either express or implied.
-# See the License for the specific language governing permissions and
-# limitations under the License.
-# ==============================================================================
-"""Post-processing model outputs to generate detection."""
-
-from __future__ import absolute_import
-from __future__ import division
-from __future__ import print_function
-
-import functools
-import tensorflow as tf
-
-from official.vision.detection.ops import nms
-from official.vision.detection.utils import box_utils
-
-
-def generate_detections_factory(params):
- """Factory to select function to generate detection."""
- if params.use_batched_nms:
- func = functools.partial(
- _generate_detections_batched,
- max_total_size=params.max_total_size,
- nms_iou_threshold=params.nms_iou_threshold,
- score_threshold=params.score_threshold)
- else:
- func = functools.partial(
- _generate_detections,
- max_total_size=params.max_total_size,
- nms_iou_threshold=params.nms_iou_threshold,
- score_threshold=params.score_threshold,
- pre_nms_num_boxes=params.pre_nms_num_boxes)
- return func
-
-
-def _select_top_k_scores(scores_in, pre_nms_num_detections):
- """Select top_k scores and indices for each class.
-
- Args:
- scores_in: a Tensor with shape [batch_size, N, num_classes], which stacks
- class logit outputs on all feature levels. The N is the number of total
- anchors on all levels. The num_classes is the number of classes predicted
- by the model.
- pre_nms_num_detections: Number of candidates before NMS.
-
- Returns:
- scores and indices: Tensors with shape [batch_size, pre_nms_num_detections,
- num_classes].
- """
- batch_size, num_anchors, num_class = scores_in.get_shape().as_list()
- scores_trans = tf.transpose(scores_in, perm=[0, 2, 1])
- scores_trans = tf.reshape(scores_trans, [-1, num_anchors])
-
- top_k_scores, top_k_indices = tf.nn.top_k(
- scores_trans, k=pre_nms_num_detections, sorted=True)
-
- top_k_scores = tf.reshape(top_k_scores,
- [batch_size, num_class, pre_nms_num_detections])
- top_k_indices = tf.reshape(top_k_indices,
- [batch_size, num_class, pre_nms_num_detections])
-
- return tf.transpose(top_k_scores,
- [0, 2, 1]), tf.transpose(top_k_indices, [0, 2, 1])
-
-
-def _generate_detections(boxes,
- scores,
- max_total_size=100,
- nms_iou_threshold=0.3,
- score_threshold=0.05,
- pre_nms_num_boxes=5000):
- """Generate the final detections given the model outputs.
-
- This uses classes unrolling with while loop based NMS, could be parralled
- at batch dimension.
-
- Args:
- boxes: a tensor with shape [batch_size, N, num_classes, 4] or [batch_size,
- N, 1, 4], which box predictions on all feature levels. The N is the number
- of total anchors on all levels.
- scores: a tensor with shape [batch_size, N, num_classes], which stacks class
- probability on all feature levels. The N is the number of total anchors on
- all levels. The num_classes is the number of classes predicted by the
- model. Note that the class_outputs here is the raw score.
- max_total_size: a scalar representing maximum number of boxes retained over
- all classes.
- nms_iou_threshold: a float representing the threshold for deciding whether
- boxes overlap too much with respect to IOU.
- score_threshold: a float representing the threshold for deciding when to
- remove boxes based on score.
- pre_nms_num_boxes: an int number of top candidate detections per class
- before NMS.
-
- Returns:
- nms_boxes: `float` Tensor of shape [batch_size, max_total_size, 4]
- representing top detected boxes in [y1, x1, y2, x2].
- nms_scores: `float` Tensor of shape [batch_size, max_total_size]
- representing sorted confidence scores for detected boxes. The values are
- between [0, 1].
- nms_classes: `int` Tensor of shape [batch_size, max_total_size] representing
- classes for detected boxes.
- valid_detections: `int` Tensor of shape [batch_size] only the top
- `valid_detections` boxes are valid detections.
- """
- with tf.name_scope('generate_detections'):
- nmsed_boxes = []
- nmsed_classes = []
- nmsed_scores = []
- valid_detections = []
- batch_size, _, num_classes_for_box, _ = boxes.get_shape().as_list()
- _, total_anchors, num_classes = scores.get_shape().as_list()
- # Selects top pre_nms_num scores and indices before NMS.
- scores, indices = _select_top_k_scores(
- scores, min(total_anchors, pre_nms_num_boxes))
- for i in range(num_classes):
- boxes_i = boxes[:, :, min(num_classes_for_box - 1, i), :]
- scores_i = scores[:, :, i]
- # Obtains pre_nms_num_boxes before running NMS.
- boxes_i = tf.gather(boxes_i, indices[:, :, i], batch_dims=1, axis=1)
-
- # Filter out scores.
- boxes_i, scores_i = box_utils.filter_boxes_by_scores(
- boxes_i, scores_i, min_score_threshold=score_threshold)
-
- (nmsed_scores_i, nmsed_boxes_i) = nms.sorted_non_max_suppression_padded(
- tf.cast(scores_i, tf.float32),
- tf.cast(boxes_i, tf.float32),
- max_total_size,
- iou_threshold=nms_iou_threshold)
- nmsed_classes_i = tf.fill([batch_size, max_total_size], i)
- nmsed_boxes.append(nmsed_boxes_i)
- nmsed_scores.append(nmsed_scores_i)
- nmsed_classes.append(nmsed_classes_i)
- nmsed_boxes = tf.concat(nmsed_boxes, axis=1)
- nmsed_scores = tf.concat(nmsed_scores, axis=1)
- nmsed_classes = tf.concat(nmsed_classes, axis=1)
- nmsed_scores, indices = tf.nn.top_k(
- nmsed_scores, k=max_total_size, sorted=True)
- nmsed_boxes = tf.gather(nmsed_boxes, indices, batch_dims=1, axis=1)
- nmsed_classes = tf.gather(nmsed_classes, indices, batch_dims=1)
- valid_detections = tf.reduce_sum(
- input_tensor=tf.cast(tf.greater(nmsed_scores, -1), tf.int32), axis=1)
- return nmsed_boxes, nmsed_scores, nmsed_classes, valid_detections
-
-
-def _generate_detections_per_image(boxes,
- scores,
- max_total_size=100,
- nms_iou_threshold=0.3,
- score_threshold=0.05,
- pre_nms_num_boxes=5000):
- """Generate the final detections per image given the model outputs.
-
- Args:
- boxes: a tensor with shape [N, num_classes, 4] or [N, 1, 4], which box
- predictions on all feature levels. The N is the number of total anchors on
- all levels.
- scores: a tensor with shape [N, num_classes], which stacks class probability
- on all feature levels. The N is the number of total anchors on all levels.
- The num_classes is the number of classes predicted by the model. Note that
- the class_outputs here is the raw score.
- max_total_size: a scalar representing maximum number of boxes retained over
- all classes.
- nms_iou_threshold: a float representing the threshold for deciding whether
- boxes overlap too much with respect to IOU.
- score_threshold: a float representing the threshold for deciding when to
- remove boxes based on score.
- pre_nms_num_boxes: an int number of top candidate detections per class
- before NMS.
-
- Returns:
- nms_boxes: `float` Tensor of shape [max_total_size, 4] representing top
- detected boxes in [y1, x1, y2, x2].
- nms_scores: `float` Tensor of shape [max_total_size] representing sorted
- confidence scores for detected boxes. The values are between [0, 1].
- nms_classes: `int` Tensor of shape [max_total_size] representing classes for
- detected boxes.
- valid_detections: `int` Tensor of shape [1] only the top `valid_detections`
- boxes are valid detections.
- """
- nmsed_boxes = []
- nmsed_scores = []
- nmsed_classes = []
- num_classes_for_box = boxes.get_shape().as_list()[1]
- num_classes = scores.get_shape().as_list()[1]
- for i in range(num_classes):
- boxes_i = boxes[:, min(num_classes_for_box - 1, i)]
- scores_i = scores[:, i]
-
- # Obtains pre_nms_num_boxes before running NMS.
- scores_i, indices = tf.nn.top_k(
- scores_i, k=tf.minimum(tf.shape(input=scores_i)[-1], pre_nms_num_boxes))
- boxes_i = tf.gather(boxes_i, indices)
-
- (nmsed_indices_i,
- nmsed_num_valid_i) = tf.image.non_max_suppression_padded(
- tf.cast(boxes_i, tf.float32),
- tf.cast(scores_i, tf.float32),
- max_total_size,
- iou_threshold=nms_iou_threshold,
- score_threshold=score_threshold,
- pad_to_max_output_size=True,
- name='nms_detections_' + str(i))
- nmsed_boxes_i = tf.gather(boxes_i, nmsed_indices_i)
- nmsed_scores_i = tf.gather(scores_i, nmsed_indices_i)
- # Sets scores of invalid boxes to -1.
- nmsed_scores_i = tf.where(
- tf.less(tf.range(max_total_size), [nmsed_num_valid_i]), nmsed_scores_i,
- -tf.ones_like(nmsed_scores_i))
- nmsed_classes_i = tf.fill([max_total_size], i)
- nmsed_boxes.append(nmsed_boxes_i)
- nmsed_scores.append(nmsed_scores_i)
- nmsed_classes.append(nmsed_classes_i)
-
- # Concats results from all classes and sort them.
- nmsed_boxes = tf.concat(nmsed_boxes, axis=0)
- nmsed_scores = tf.concat(nmsed_scores, axis=0)
- nmsed_classes = tf.concat(nmsed_classes, axis=0)
- nmsed_scores, indices = tf.nn.top_k(
- nmsed_scores, k=max_total_size, sorted=True)
- nmsed_boxes = tf.gather(nmsed_boxes, indices)
- nmsed_classes = tf.gather(nmsed_classes, indices)
- valid_detections = tf.reduce_sum(
- input_tensor=tf.cast(tf.greater(nmsed_scores, -1), tf.int32))
- return nmsed_boxes, nmsed_scores, nmsed_classes, valid_detections
-
-
-def _generate_detections_batched(boxes,
- scores,
- max_total_size,
- nms_iou_threshold,
- score_threshold):
- """Generates detected boxes with scores and classes for one-stage detector.
-
- The function takes output of multi-level ConvNets and anchor boxes and
- generates detected boxes. Note that this used batched nms, which is not
- supported on TPU currently.
-
- Args:
- boxes: a tensor with shape [batch_size, N, num_classes, 4] or
- [batch_size, N, 1, 4], which box predictions on all feature levels. The N
- is the number of total anchors on all levels.
- scores: a tensor with shape [batch_size, N, num_classes], which
- stacks class probability on all feature levels. The N is the number of
- total anchors on all levels. The num_classes is the number of classes
- predicted by the model. Note that the class_outputs here is the raw score.
- max_total_size: a scalar representing maximum number of boxes retained over
- all classes.
- nms_iou_threshold: a float representing the threshold for deciding whether
- boxes overlap too much with respect to IOU.
- score_threshold: a float representing the threshold for deciding when to
- remove boxes based on score.
- Returns:
- nms_boxes: `float` Tensor of shape [batch_size, max_total_size, 4]
- representing top detected boxes in [y1, x1, y2, x2].
- nms_scores: `float` Tensor of shape [batch_size, max_total_size]
- representing sorted confidence scores for detected boxes. The values are
- between [0, 1].
- nms_classes: `int` Tensor of shape [batch_size, max_total_size] representing
- classes for detected boxes.
- valid_detections: `int` Tensor of shape [batch_size] only the top
- `valid_detections` boxes are valid detections.
- """
- with tf.name_scope('generate_detections'):
- # TODO(tsungyi): Removes normalization/denomalization once the
- # tf.image.combined_non_max_suppression is coordinate system agnostic.
- # Normalizes maximum box cooridinates to 1.
- normalizer = tf.reduce_max(boxes)
- boxes /= normalizer
- (nmsed_boxes, nmsed_scores, nmsed_classes,
- valid_detections) = tf.image.combined_non_max_suppression(
- boxes,
- scores,
- max_output_size_per_class=max_total_size,
- max_total_size=max_total_size,
- iou_threshold=nms_iou_threshold,
- score_threshold=score_threshold,
- pad_per_class=False,)
- # De-normalizes box cooridinates.
- nmsed_boxes *= normalizer
- nmsed_classes = tf.cast(nmsed_classes, tf.int32)
- return nmsed_boxes, nmsed_scores, nmsed_classes, valid_detections
-
-
-class MultilevelDetectionGenerator(object):
- """Generates detected boxes with scores and classes for one-stage detector."""
-
- def __init__(self, min_level, max_level, params):
- self._min_level = min_level
- self._max_level = max_level
- self._generate_detections = generate_detections_factory(params)
-
- def __call__(self, box_outputs, class_outputs, anchor_boxes, image_shape):
- # Collects outputs from all levels into a list.
- boxes = []
- scores = []
- for i in range(self._min_level, self._max_level + 1):
- box_outputs_i_shape = tf.shape(box_outputs[i])
- batch_size = box_outputs_i_shape[0]
- num_anchors_per_locations = box_outputs_i_shape[-1] // 4
- num_classes = tf.shape(class_outputs[i])[-1] // num_anchors_per_locations
-
- # Applies score transformation and remove the implicit background class.
- scores_i = tf.sigmoid(
- tf.reshape(class_outputs[i], [batch_size, -1, num_classes]))
- scores_i = tf.slice(scores_i, [0, 0, 1], [-1, -1, -1])
-
- # Box decoding.
- # The anchor boxes are shared for all data in a batch.
- # One stage detector only supports class agnostic box regression.
- anchor_boxes_i = tf.reshape(anchor_boxes[i], [batch_size, -1, 4])
- box_outputs_i = tf.reshape(box_outputs[i], [batch_size, -1, 4])
- boxes_i = box_utils.decode_boxes(box_outputs_i, anchor_boxes_i)
-
- # Box clipping.
- boxes_i = box_utils.clip_boxes(boxes_i, image_shape)
-
- boxes.append(boxes_i)
- scores.append(scores_i)
- boxes = tf.concat(boxes, axis=1)
- scores = tf.concat(scores, axis=1)
-
- nmsed_boxes, nmsed_scores, nmsed_classes, valid_detections = (
- self._generate_detections(tf.expand_dims(boxes, axis=2), scores))
-
- # Adds 1 to offset the background class which has index 0.
- nmsed_classes += 1
- return nmsed_boxes, nmsed_scores, nmsed_classes, valid_detections
-
-
-class GenericDetectionGenerator(object):
- """Generates the final detected boxes with scores and classes."""
-
- def __init__(self, params):
- self._generate_detections = generate_detections_factory(params)
-
- def __call__(self, box_outputs, class_outputs, anchor_boxes, image_shape):
- """Generate final detections.
-
- Args:
- box_outputs: a tensor of shape of [batch_size, K, num_classes * 4]
- representing the class-specific box coordinates relative to anchors.
- class_outputs: a tensor of shape of [batch_size, K, num_classes]
- representing the class logits before applying score activiation.
- anchor_boxes: a tensor of shape of [batch_size, K, 4] representing the
- corresponding anchor boxes w.r.t `box_outputs`.
- image_shape: a tensor of shape of [batch_size, 2] storing the image height
- and width w.r.t. the scaled image, i.e. the same image space as
- `box_outputs` and `anchor_boxes`.
-
- Returns:
- nms_boxes: `float` Tensor of shape [batch_size, max_total_size, 4]
- representing top detected boxes in [y1, x1, y2, x2].
- nms_scores: `float` Tensor of shape [batch_size, max_total_size]
- representing sorted confidence scores for detected boxes. The values are
- between [0, 1].
- nms_classes: `int` Tensor of shape [batch_size, max_total_size]
- representing classes for detected boxes.
- valid_detections: `int` Tensor of shape [batch_size] only the top
- `valid_detections` boxes are valid detections.
- """
- class_outputs = tf.nn.softmax(class_outputs, axis=-1)
-
- # Removes the background class.
- class_outputs_shape = tf.shape(class_outputs)
- batch_size = class_outputs_shape[0]
- num_locations = class_outputs_shape[1]
- num_classes = class_outputs_shape[-1]
- num_detections = num_locations * (num_classes - 1)
-
- class_outputs = tf.slice(class_outputs, [0, 0, 1], [-1, -1, -1])
- box_outputs = tf.reshape(
- box_outputs,
- tf.stack([batch_size, num_locations, num_classes, 4], axis=-1))
- box_outputs = tf.slice(
- box_outputs, [0, 0, 1, 0], [-1, -1, -1, -1])
- anchor_boxes = tf.tile(
- tf.expand_dims(anchor_boxes, axis=2), [1, 1, num_classes - 1, 1])
- box_outputs = tf.reshape(
- box_outputs,
- tf.stack([batch_size, num_detections, 4], axis=-1))
- anchor_boxes = tf.reshape(
- anchor_boxes,
- tf.stack([batch_size, num_detections, 4], axis=-1))
-
- # Box decoding.
- decoded_boxes = box_utils.decode_boxes(
- box_outputs, anchor_boxes, weights=[10.0, 10.0, 5.0, 5.0])
-
- # Box clipping
- decoded_boxes = box_utils.clip_boxes(decoded_boxes, image_shape)
-
- decoded_boxes = tf.reshape(
- decoded_boxes,
- tf.stack([batch_size, num_locations, num_classes - 1, 4], axis=-1))
-
- nmsed_boxes, nmsed_scores, nmsed_classes, valid_detections = (
- self._generate_detections(decoded_boxes, class_outputs))
-
- # Adds 1 to offset the background class which has index 0.
- nmsed_classes += 1
-
- return nmsed_boxes, nmsed_scores, nmsed_classes, valid_detections
diff --git a/spaces/NCTCMumbai/NCTC/models/research/brain_coder/single_task/defaults.py b/spaces/NCTCMumbai/NCTC/models/research/brain_coder/single_task/defaults.py
deleted file mode 100644
index d9bd8b942532dfffcf06d90d331e58725c4d82a9..0000000000000000000000000000000000000000
--- a/spaces/NCTCMumbai/NCTC/models/research/brain_coder/single_task/defaults.py
+++ /dev/null
@@ -1,82 +0,0 @@
-from __future__ import absolute_import
-from __future__ import division
-from __future__ import print_function
-
-"""Default configuration for agent and environment."""
-
-from absl import logging
-
-from common import config_lib # brain coder
-
-
-def default_config():
- return config_lib.Config(
- agent=config_lib.OneOf(
- [config_lib.Config(
- algorithm='pg',
- policy_lstm_sizes=[35,35],
- # Set value_lstm_sizes to None to share weights with policy.
- value_lstm_sizes=[35,35],
- obs_embedding_size=10,
- grad_clip_threshold=10.0,
- param_init_factor=1.0,
- lr=5e-5,
- pi_loss_hparam=1.0,
- vf_loss_hparam=0.5,
- entropy_beta=1e-2,
- regularizer=0.0,
- softmax_tr=1.0, # Reciprocal temperature.
- optimizer='rmsprop', # 'adam', 'sgd', 'rmsprop'
- topk=0, # Top-k unique codes will be stored.
- topk_loss_hparam=0.0, # off policy loss multiplier.
- # Uniformly sample this many episodes from topk buffer per batch.
- # If topk is 0, this has no effect.
- topk_batch_size=1,
- # Exponential moving average baseline for REINFORCE.
- # If zero, A2C is used.
- # If non-zero, should be close to 1, like .99, .999, etc.
- ema_baseline_decay=0.99,
- # Whether agent can emit EOS token. If true, agent can emit EOS
- # token which ends the episode early (ends the sequence).
- # If false, agent must emit tokens until the timestep limit is
- # reached. e.g. True means variable length code, False means fixed
- # length code.
- # WARNING: Making this false slows things down.
- eos_token=False,
- replay_temperature=1.0,
- # Replay probability. 1 = always replay, 0 = always on policy.
- alpha=0.0,
- # Whether to normalize importance weights in each minibatch.
- iw_normalize=True),
- config_lib.Config(
- algorithm='ga',
- crossover_rate=0.99,
- mutation_rate=0.086),
- config_lib.Config(
- algorithm='rand')],
- algorithm='pg',
- ),
- env=config_lib.Config(
- # If True, task-specific settings are not needed.
- task='', # 'print', 'echo', 'reverse', 'remove', ...
- task_cycle=[], # If non-empty, reptitions will cycle through tasks.
- task_kwargs='{}', # Python dict literal.
- task_manager_config=config_lib.Config(
- # Reward recieved per test case. These bonuses will be scaled
- # based on how many test cases there are.
- correct_bonus=2.0, # Bonus for code getting correct answer.
- code_length_bonus=1.0), # Maximum bonus for short code.
- correct_syntax=False,
- ),
- batch_size=64,
- timestep_limit=32)
-
-
-def default_config_with_updates(config_string, do_logging=True):
- if do_logging:
- logging.info('Config string: "%s"', config_string)
- config = default_config()
- config.strict_update(config_lib.Config.parse(config_string))
- if do_logging:
- logging.info('Config:\n%s', config.pretty_str())
- return config
diff --git a/spaces/NSect/VALL-E-X/utils/symbol_table.py b/spaces/NSect/VALL-E-X/utils/symbol_table.py
deleted file mode 100644
index 7a86010a76280576f85490641623dbb27559aa99..0000000000000000000000000000000000000000
--- a/spaces/NSect/VALL-E-X/utils/symbol_table.py
+++ /dev/null
@@ -1,287 +0,0 @@
-# Copyright 2020 Mobvoi Inc. (authors: Fangjun Kuang)
-#
-# See ../../../LICENSE for clarification regarding multiple authors
-#
-# Licensed under the Apache License, Version 2.0 (the "License");
-# you may not use this file except in compliance with the License.
-# You may obtain a copy of the License at
-#
-# http://www.apache.org/licenses/LICENSE-2.0
-#
-# Unless required by applicable law or agreed to in writing, software
-# distributed under the License is distributed on an "AS IS" BASIS,
-# WITHOUT WARRANTIES OR CONDITIONS OF ANY KIND, either express or implied.
-# See the License for the specific language governing permissions and
-# limitations under the License.
-
-from dataclasses import dataclass
-from dataclasses import field
-from typing import Dict
-from typing import Generic
-from typing import List
-from typing import Optional
-from typing import TypeVar
-from typing import Union
-
-Symbol = TypeVar('Symbol')
-
-
-# Disable __repr__ otherwise it could freeze e.g. Jupyter.
-@dataclass(repr=False)
-class SymbolTable(Generic[Symbol]):
- '''SymbolTable that maps symbol IDs, found on the FSA arcs to
- actual objects. These objects can be arbitrary Python objects
- that can serve as keys in a dictionary (i.e. they need to be
- hashable and immutable).
-
- The SymbolTable can only be read to/written from disk if the
- symbols are strings.
- '''
- _id2sym: Dict[int, Symbol] = field(default_factory=dict)
- '''Map an integer to a symbol.
- '''
-
- _sym2id: Dict[Symbol, int] = field(default_factory=dict)
- '''Map a symbol to an integer.
- '''
-
- _next_available_id: int = 1
- '''A helper internal field that helps adding new symbols
- to the table efficiently.
- '''
-
- eps: Symbol = ''
- '''Null symbol, always mapped to index 0.
- '''
-
- def __post_init__(self):
- for idx, sym in self._id2sym.items():
- assert self._sym2id[sym] == idx
- assert idx >= 0
-
- for sym, idx in self._sym2id.items():
- assert idx >= 0
- assert self._id2sym[idx] == sym
-
- if 0 not in self._id2sym:
- self._id2sym[0] = self.eps
- self._sym2id[self.eps] = 0
- else:
- assert self._id2sym[0] == self.eps
- assert self._sym2id[self.eps] == 0
-
- self._next_available_id = max(self._id2sym) + 1
-
- @staticmethod
- def from_str(s: str) -> 'SymbolTable':
- '''Build a symbol table from a string.
-
- The string consists of lines. Every line has two fields separated
- by space(s), tab(s) or both. The first field is the symbol and the
- second the integer id of the symbol.
-
- Args:
- s:
- The input string with the format described above.
- Returns:
- An instance of :class:`SymbolTable`.
- '''
- id2sym: Dict[int, str] = dict()
- sym2id: Dict[str, int] = dict()
-
- for line in s.split('\n'):
- fields = line.split()
- if len(fields) == 0:
- continue # skip empty lines
- assert len(fields) == 2, \
- f'Expect a line with 2 fields. Given: {len(fields)}'
- sym, idx = fields[0], int(fields[1])
- assert sym not in sym2id, f'Duplicated symbol {sym}'
- assert idx not in id2sym, f'Duplicated id {idx}'
- id2sym[idx] = sym
- sym2id[sym] = idx
-
- eps = id2sym.get(0, '')
-
- return SymbolTable(_id2sym=id2sym, _sym2id=sym2id, eps=eps)
-
- @staticmethod
- def from_file(filename: str) -> 'SymbolTable':
- '''Build a symbol table from file.
-
- Every line in the symbol table file has two fields separated by
- space(s), tab(s) or both. The following is an example file:
-
- .. code-block::
-
- 0
- a 1
- b 2
- c 3
-
- Args:
- filename:
- Name of the symbol table file. Its format is documented above.
-
- Returns:
- An instance of :class:`SymbolTable`.
-
- '''
- with open(filename, 'r', encoding='utf-8') as f:
- return SymbolTable.from_str(f.read().strip())
-
- def to_str(self) -> str:
- '''
- Returns:
- Return a string representation of this object. You can pass
- it to the method ``from_str`` to recreate an identical object.
- '''
- s = ''
- for idx, symbol in sorted(self._id2sym.items()):
- s += f'{symbol} {idx}\n'
- return s
-
- def to_file(self, filename: str):
- '''Serialize the SymbolTable to a file.
-
- Every line in the symbol table file has two fields separated by
- space(s), tab(s) or both. The following is an example file:
-
- .. code-block::
-
- 0
- a 1
- b 2
- c 3
-
- Args:
- filename:
- Name of the symbol table file. Its format is documented above.
- '''
- with open(filename, 'w') as f:
- for idx, symbol in sorted(self._id2sym.items()):
- print(symbol, idx, file=f)
-
- def add(self, symbol: Symbol, index: Optional[int] = None) -> int:
- '''Add a new symbol to the SymbolTable.
-
- Args:
- symbol:
- The symbol to be added.
- index:
- Optional int id to which the symbol should be assigned.
- If it is not available, a ValueError will be raised.
-
- Returns:
- The int id to which the symbol has been assigned.
- '''
- # Already in the table? Return its ID.
- if symbol in self._sym2id:
- return self._sym2id[symbol]
- # Specific ID not provided - use next available.
- if index is None:
- index = self._next_available_id
- # Specific ID provided but not available.
- if index in self._id2sym:
- raise ValueError(f"Cannot assign id '{index}' to '{symbol}' - "
- f"already occupied by {self._id2sym[index]}")
- self._sym2id[symbol] = index
- self._id2sym[index] = symbol
-
- # Update next available ID if needed
- if self._next_available_id <= index:
- self._next_available_id = index + 1
-
- return index
-
- def get(self, k: Union[int, Symbol]) -> Union[Symbol, int]:
- '''Get a symbol for an id or get an id for a symbol
-
- Args:
- k:
- If it is an id, it tries to find the symbol corresponding
- to the id; if it is a symbol, it tries to find the id
- corresponding to the symbol.
-
- Returns:
- An id or a symbol depending on the given `k`.
- '''
- if isinstance(k, int):
- return self._id2sym[k]
- else:
- return self._sym2id[k]
-
- def merge(self, other: 'SymbolTable') -> 'SymbolTable':
- '''Create a union of two SymbolTables.
- Raises an AssertionError if the same IDs are occupied by
- different symbols.
-
- Args:
- other:
- A symbol table to merge with ``self``.
-
- Returns:
- A new symbol table.
- '''
- self._check_compatible(other)
-
- id2sym = {**self._id2sym, **other._id2sym}
- sym2id = {**self._sym2id, **other._sym2id}
-
- return SymbolTable(_id2sym=id2sym, _sym2id=sym2id, eps=self.eps)
-
- def _check_compatible(self, other: 'SymbolTable') -> None:
- # Epsilon compatibility
- assert self.eps == other.eps, f'Mismatched epsilon symbol: ' \
- f'{self.eps} != {other.eps}'
- # IDs compatibility
- common_ids = set(self._id2sym).intersection(other._id2sym)
- for idx in common_ids:
- assert self[idx] == other[idx], f'ID conflict for id: {idx}, ' \
- f'self[idx] = "{self[idx]}", ' \
- f'other[idx] = "{other[idx]}"'
- # Symbols compatibility
- common_symbols = set(self._sym2id).intersection(other._sym2id)
- for sym in common_symbols:
- assert self[sym] == other[sym], f'ID conflict for id: {sym}, ' \
- f'self[sym] = "{self[sym]}", ' \
- f'other[sym] = "{other[sym]}"'
-
- def __getitem__(self, item: Union[int, Symbol]) -> Union[Symbol, int]:
- return self.get(item)
-
- def __contains__(self, item: Union[int, Symbol]) -> bool:
- if isinstance(item, int):
- return item in self._id2sym
- else:
- return item in self._sym2id
-
- def __len__(self) -> int:
- return len(self._id2sym)
-
- def __eq__(self, other: 'SymbolTable') -> bool:
- if len(self) != len(other):
- return False
-
- for s in self.symbols:
- if self[s] != other[s]:
- return False
-
- return True
-
- @property
- def ids(self) -> List[int]:
- '''Returns a list of integer IDs corresponding to the symbols.
- '''
- ans = list(self._id2sym.keys())
- ans.sort()
- return ans
-
- @property
- def symbols(self) -> List[Symbol]:
- '''Returns a list of symbols (e.g., strings) corresponding to
- the integer IDs.
- '''
- ans = list(self._sym2id.keys())
- ans.sort()
- return ans
diff --git a/spaces/Navneet574/algerian-forest-fire-prediction/README.md b/spaces/Navneet574/algerian-forest-fire-prediction/README.md
deleted file mode 100644
index 1f3bf84a8e2d4fb50386837ab4671b16392bf7f9..0000000000000000000000000000000000000000
--- a/spaces/Navneet574/algerian-forest-fire-prediction/README.md
+++ /dev/null
@@ -1,13 +0,0 @@
----
-title: Algerian Forest Fire Prediction
-emoji: 🌍
-colorFrom: purple
-colorTo: purple
-sdk: streamlit
-sdk_version: 1.17.0
-app_file: app.py
-pinned: false
-license: cc-by-nc-4.0
----
-
-Check out the configuration reference at https://huggingface.co/docs/hub/spaces-config-reference
diff --git a/spaces/Nephele/bert-vits2-multi-voice/modules.py b/spaces/Nephele/bert-vits2-multi-voice/modules.py
deleted file mode 100644
index 92e0f32a51c472bfd1659a50a95a95d195281d2b..0000000000000000000000000000000000000000
--- a/spaces/Nephele/bert-vits2-multi-voice/modules.py
+++ /dev/null
@@ -1,452 +0,0 @@
-import copy
-import math
-import numpy as np
-import scipy
-import torch
-from torch import nn
-from torch.nn import functional as F
-
-from torch.nn import Conv1d, ConvTranspose1d, AvgPool1d, Conv2d
-from torch.nn.utils import weight_norm, remove_weight_norm
-
-import commons
-from commons import init_weights, get_padding
-from transforms import piecewise_rational_quadratic_transform
-from attentions import Encoder
-
-LRELU_SLOPE = 0.1
-
-class LayerNorm(nn.Module):
- def __init__(self, channels, eps=1e-5):
- super().__init__()
- self.channels = channels
- self.eps = eps
-
- self.gamma = nn.Parameter(torch.ones(channels))
- self.beta = nn.Parameter(torch.zeros(channels))
-
- def forward(self, x):
- x = x.transpose(1, -1)
- x = F.layer_norm(x, (self.channels,), self.gamma, self.beta, self.eps)
- return x.transpose(1, -1)
-
-class ConvReluNorm(nn.Module):
- def __init__(self, in_channels, hidden_channels, out_channels, kernel_size, n_layers, p_dropout):
- super().__init__()
- self.in_channels = in_channels
- self.hidden_channels = hidden_channels
- self.out_channels = out_channels
- self.kernel_size = kernel_size
- self.n_layers = n_layers
- self.p_dropout = p_dropout
- assert n_layers > 1, "Number of layers should be larger than 0."
-
- self.conv_layers = nn.ModuleList()
- self.norm_layers = nn.ModuleList()
- self.conv_layers.append(nn.Conv1d(in_channels, hidden_channels, kernel_size, padding=kernel_size//2))
- self.norm_layers.append(LayerNorm(hidden_channels))
- self.relu_drop = nn.Sequential(
- nn.ReLU(),
- nn.Dropout(p_dropout))
- for _ in range(n_layers-1):
- self.conv_layers.append(nn.Conv1d(hidden_channels, hidden_channels, kernel_size, padding=kernel_size//2))
- self.norm_layers.append(LayerNorm(hidden_channels))
- self.proj = nn.Conv1d(hidden_channels, out_channels, 1)
- self.proj.weight.data.zero_()
- self.proj.bias.data.zero_()
-
- def forward(self, x, x_mask):
- x_org = x
- for i in range(self.n_layers):
- x = self.conv_layers[i](x * x_mask)
- x = self.norm_layers[i](x)
- x = self.relu_drop(x)
- x = x_org + self.proj(x)
- return x * x_mask
-
-
-class DDSConv(nn.Module):
- """
- Dialted and Depth-Separable Convolution
- """
- def __init__(self, channels, kernel_size, n_layers, p_dropout=0.):
- super().__init__()
- self.channels = channels
- self.kernel_size = kernel_size
- self.n_layers = n_layers
- self.p_dropout = p_dropout
-
- self.drop = nn.Dropout(p_dropout)
- self.convs_sep = nn.ModuleList()
- self.convs_1x1 = nn.ModuleList()
- self.norms_1 = nn.ModuleList()
- self.norms_2 = nn.ModuleList()
- for i in range(n_layers):
- dilation = kernel_size ** i
- padding = (kernel_size * dilation - dilation) // 2
- self.convs_sep.append(nn.Conv1d(channels, channels, kernel_size,
- groups=channels, dilation=dilation, padding=padding
- ))
- self.convs_1x1.append(nn.Conv1d(channels, channels, 1))
- self.norms_1.append(LayerNorm(channels))
- self.norms_2.append(LayerNorm(channels))
-
- def forward(self, x, x_mask, g=None):
- if g is not None:
- x = x + g
- for i in range(self.n_layers):
- y = self.convs_sep[i](x * x_mask)
- y = self.norms_1[i](y)
- y = F.gelu(y)
- y = self.convs_1x1[i](y)
- y = self.norms_2[i](y)
- y = F.gelu(y)
- y = self.drop(y)
- x = x + y
- return x * x_mask
-
-
-class WN(torch.nn.Module):
- def __init__(self, hidden_channels, kernel_size, dilation_rate, n_layers, gin_channels=0, p_dropout=0):
- super(WN, self).__init__()
- assert(kernel_size % 2 == 1)
- self.hidden_channels =hidden_channels
- self.kernel_size = kernel_size,
- self.dilation_rate = dilation_rate
- self.n_layers = n_layers
- self.gin_channels = gin_channels
- self.p_dropout = p_dropout
-
- self.in_layers = torch.nn.ModuleList()
- self.res_skip_layers = torch.nn.ModuleList()
- self.drop = nn.Dropout(p_dropout)
-
- if gin_channels != 0:
- cond_layer = torch.nn.Conv1d(gin_channels, 2*hidden_channels*n_layers, 1)
- self.cond_layer = torch.nn.utils.weight_norm(cond_layer, name='weight')
-
- for i in range(n_layers):
- dilation = dilation_rate ** i
- padding = int((kernel_size * dilation - dilation) / 2)
- in_layer = torch.nn.Conv1d(hidden_channels, 2*hidden_channels, kernel_size,
- dilation=dilation, padding=padding)
- in_layer = torch.nn.utils.weight_norm(in_layer, name='weight')
- self.in_layers.append(in_layer)
-
- # last one is not necessary
- if i < n_layers - 1:
- res_skip_channels = 2 * hidden_channels
- else:
- res_skip_channels = hidden_channels
-
- res_skip_layer = torch.nn.Conv1d(hidden_channels, res_skip_channels, 1)
- res_skip_layer = torch.nn.utils.weight_norm(res_skip_layer, name='weight')
- self.res_skip_layers.append(res_skip_layer)
-
- def forward(self, x, x_mask, g=None, **kwargs):
- output = torch.zeros_like(x)
- n_channels_tensor = torch.IntTensor([self.hidden_channels])
-
- if g is not None:
- g = self.cond_layer(g)
-
- for i in range(self.n_layers):
- x_in = self.in_layers[i](x)
- if g is not None:
- cond_offset = i * 2 * self.hidden_channels
- g_l = g[:,cond_offset:cond_offset+2*self.hidden_channels,:]
- else:
- g_l = torch.zeros_like(x_in)
-
- acts = commons.fused_add_tanh_sigmoid_multiply(
- x_in,
- g_l,
- n_channels_tensor)
- acts = self.drop(acts)
-
- res_skip_acts = self.res_skip_layers[i](acts)
- if i < self.n_layers - 1:
- res_acts = res_skip_acts[:,:self.hidden_channels,:]
- x = (x + res_acts) * x_mask
- output = output + res_skip_acts[:,self.hidden_channels:,:]
- else:
- output = output + res_skip_acts
- return output * x_mask
-
- def remove_weight_norm(self):
- if self.gin_channels != 0:
- torch.nn.utils.remove_weight_norm(self.cond_layer)
- for l in self.in_layers:
- torch.nn.utils.remove_weight_norm(l)
- for l in self.res_skip_layers:
- torch.nn.utils.remove_weight_norm(l)
-
-
-class ResBlock1(torch.nn.Module):
- def __init__(self, channels, kernel_size=3, dilation=(1, 3, 5)):
- super(ResBlock1, self).__init__()
- self.convs1 = nn.ModuleList([
- weight_norm(Conv1d(channels, channels, kernel_size, 1, dilation=dilation[0],
- padding=get_padding(kernel_size, dilation[0]))),
- weight_norm(Conv1d(channels, channels, kernel_size, 1, dilation=dilation[1],
- padding=get_padding(kernel_size, dilation[1]))),
- weight_norm(Conv1d(channels, channels, kernel_size, 1, dilation=dilation[2],
- padding=get_padding(kernel_size, dilation[2])))
- ])
- self.convs1.apply(init_weights)
-
- self.convs2 = nn.ModuleList([
- weight_norm(Conv1d(channels, channels, kernel_size, 1, dilation=1,
- padding=get_padding(kernel_size, 1))),
- weight_norm(Conv1d(channels, channels, kernel_size, 1, dilation=1,
- padding=get_padding(kernel_size, 1))),
- weight_norm(Conv1d(channels, channels, kernel_size, 1, dilation=1,
- padding=get_padding(kernel_size, 1)))
- ])
- self.convs2.apply(init_weights)
-
- def forward(self, x, x_mask=None):
- for c1, c2 in zip(self.convs1, self.convs2):
- xt = F.leaky_relu(x, LRELU_SLOPE)
- if x_mask is not None:
- xt = xt * x_mask
- xt = c1(xt)
- xt = F.leaky_relu(xt, LRELU_SLOPE)
- if x_mask is not None:
- xt = xt * x_mask
- xt = c2(xt)
- x = xt + x
- if x_mask is not None:
- x = x * x_mask
- return x
-
- def remove_weight_norm(self):
- for l in self.convs1:
- remove_weight_norm(l)
- for l in self.convs2:
- remove_weight_norm(l)
-
-
-class ResBlock2(torch.nn.Module):
- def __init__(self, channels, kernel_size=3, dilation=(1, 3)):
- super(ResBlock2, self).__init__()
- self.convs = nn.ModuleList([
- weight_norm(Conv1d(channels, channels, kernel_size, 1, dilation=dilation[0],
- padding=get_padding(kernel_size, dilation[0]))),
- weight_norm(Conv1d(channels, channels, kernel_size, 1, dilation=dilation[1],
- padding=get_padding(kernel_size, dilation[1])))
- ])
- self.convs.apply(init_weights)
-
- def forward(self, x, x_mask=None):
- for c in self.convs:
- xt = F.leaky_relu(x, LRELU_SLOPE)
- if x_mask is not None:
- xt = xt * x_mask
- xt = c(xt)
- x = xt + x
- if x_mask is not None:
- x = x * x_mask
- return x
-
- def remove_weight_norm(self):
- for l in self.convs:
- remove_weight_norm(l)
-
-
-class Log(nn.Module):
- def forward(self, x, x_mask, reverse=False, **kwargs):
- if not reverse:
- y = torch.log(torch.clamp_min(x, 1e-5)) * x_mask
- logdet = torch.sum(-y, [1, 2])
- return y, logdet
- else:
- x = torch.exp(x) * x_mask
- return x
-
-
-class Flip(nn.Module):
- def forward(self, x, *args, reverse=False, **kwargs):
- x = torch.flip(x, [1])
- if not reverse:
- logdet = torch.zeros(x.size(0)).to(dtype=x.dtype, device=x.device)
- return x, logdet
- else:
- return x
-
-
-class ElementwiseAffine(nn.Module):
- def __init__(self, channels):
- super().__init__()
- self.channels = channels
- self.m = nn.Parameter(torch.zeros(channels,1))
- self.logs = nn.Parameter(torch.zeros(channels,1))
-
- def forward(self, x, x_mask, reverse=False, **kwargs):
- if not reverse:
- y = self.m + torch.exp(self.logs) * x
- y = y * x_mask
- logdet = torch.sum(self.logs * x_mask, [1,2])
- return y, logdet
- else:
- x = (x - self.m) * torch.exp(-self.logs) * x_mask
- return x
-
-
-class ResidualCouplingLayer(nn.Module):
- def __init__(self,
- channels,
- hidden_channels,
- kernel_size,
- dilation_rate,
- n_layers,
- p_dropout=0,
- gin_channels=0,
- mean_only=False):
- assert channels % 2 == 0, "channels should be divisible by 2"
- super().__init__()
- self.channels = channels
- self.hidden_channels = hidden_channels
- self.kernel_size = kernel_size
- self.dilation_rate = dilation_rate
- self.n_layers = n_layers
- self.half_channels = channels // 2
- self.mean_only = mean_only
-
- self.pre = nn.Conv1d(self.half_channels, hidden_channels, 1)
- self.enc = WN(hidden_channels, kernel_size, dilation_rate, n_layers, p_dropout=p_dropout, gin_channels=gin_channels)
- self.post = nn.Conv1d(hidden_channels, self.half_channels * (2 - mean_only), 1)
- self.post.weight.data.zero_()
- self.post.bias.data.zero_()
-
- def forward(self, x, x_mask, g=None, reverse=False):
- x0, x1 = torch.split(x, [self.half_channels]*2, 1)
- h = self.pre(x0) * x_mask
- h = self.enc(h, x_mask, g=g)
- stats = self.post(h) * x_mask
- if not self.mean_only:
- m, logs = torch.split(stats, [self.half_channels]*2, 1)
- else:
- m = stats
- logs = torch.zeros_like(m)
-
- if not reverse:
- x1 = m + x1 * torch.exp(logs) * x_mask
- x = torch.cat([x0, x1], 1)
- logdet = torch.sum(logs, [1,2])
- return x, logdet
- else:
- x1 = (x1 - m) * torch.exp(-logs) * x_mask
- x = torch.cat([x0, x1], 1)
- return x
-
-
-class ConvFlow(nn.Module):
- def __init__(self, in_channels, filter_channels, kernel_size, n_layers, num_bins=10, tail_bound=5.0):
- super().__init__()
- self.in_channels = in_channels
- self.filter_channels = filter_channels
- self.kernel_size = kernel_size
- self.n_layers = n_layers
- self.num_bins = num_bins
- self.tail_bound = tail_bound
- self.half_channels = in_channels // 2
-
- self.pre = nn.Conv1d(self.half_channels, filter_channels, 1)
- self.convs = DDSConv(filter_channels, kernel_size, n_layers, p_dropout=0.)
- self.proj = nn.Conv1d(filter_channels, self.half_channels * (num_bins * 3 - 1), 1)
- self.proj.weight.data.zero_()
- self.proj.bias.data.zero_()
-
- def forward(self, x, x_mask, g=None, reverse=False):
- x0, x1 = torch.split(x, [self.half_channels]*2, 1)
- h = self.pre(x0)
- h = self.convs(h, x_mask, g=g)
- h = self.proj(h) * x_mask
-
- b, c, t = x0.shape
- h = h.reshape(b, c, -1, t).permute(0, 1, 3, 2) # [b, cx?, t] -> [b, c, t, ?]
-
- unnormalized_widths = h[..., :self.num_bins] / math.sqrt(self.filter_channels)
- unnormalized_heights = h[..., self.num_bins:2*self.num_bins] / math.sqrt(self.filter_channels)
- unnormalized_derivatives = h[..., 2 * self.num_bins:]
-
- x1, logabsdet = piecewise_rational_quadratic_transform(x1,
- unnormalized_widths,
- unnormalized_heights,
- unnormalized_derivatives,
- inverse=reverse,
- tails='linear',
- tail_bound=self.tail_bound
- )
-
- x = torch.cat([x0, x1], 1) * x_mask
- logdet = torch.sum(logabsdet * x_mask, [1,2])
- if not reverse:
- return x, logdet
- else:
- return x
-class TransformerCouplingLayer(nn.Module):
- def __init__(self,
- channels,
- hidden_channels,
- kernel_size,
- n_layers,
- n_heads,
- p_dropout=0,
- filter_channels=0,
- mean_only=False,
- wn_sharing_parameter=None,
- gin_channels = 0
- ):
- assert channels % 2 == 0, "channels should be divisible by 2"
- super().__init__()
- self.channels = channels
- self.hidden_channels = hidden_channels
- self.kernel_size = kernel_size
- self.n_layers = n_layers
- self.half_channels = channels // 2
- self.mean_only = mean_only
-
- self.pre = nn.Conv1d(self.half_channels, hidden_channels, 1)
- self.enc = Encoder(hidden_channels, filter_channels, n_heads, n_layers, kernel_size, p_dropout, isflow = True, gin_channels = gin_channels) if wn_sharing_parameter is None else wn_sharing_parameter
- self.post = nn.Conv1d(hidden_channels, self.half_channels * (2 - mean_only), 1)
- self.post.weight.data.zero_()
- self.post.bias.data.zero_()
-
- def forward(self, x, x_mask, g=None, reverse=False):
- x0, x1 = torch.split(x, [self.half_channels]*2, 1)
- h = self.pre(x0) * x_mask
- h = self.enc(h, x_mask, g=g)
- stats = self.post(h) * x_mask
- if not self.mean_only:
- m, logs = torch.split(stats, [self.half_channels]*2, 1)
- else:
- m = stats
- logs = torch.zeros_like(m)
-
- if not reverse:
- x1 = m + x1 * torch.exp(logs) * x_mask
- x = torch.cat([x0, x1], 1)
- logdet = torch.sum(logs, [1,2])
- return x, logdet
- else:
- x1 = (x1 - m) * torch.exp(-logs) * x_mask
- x = torch.cat([x0, x1], 1)
- return x
-
- x1, logabsdet = piecewise_rational_quadratic_transform(x1,
- unnormalized_widths,
- unnormalized_heights,
- unnormalized_derivatives,
- inverse=reverse,
- tails='linear',
- tail_bound=self.tail_bound
- )
-
- x = torch.cat([x0, x1], 1) * x_mask
- logdet = torch.sum(logabsdet * x_mask, [1,2])
- if not reverse:
- return x, logdet
- else:
- return x
diff --git a/spaces/OFA-Sys/OFA-Generic_Interface/fairseq/examples/layerdrop/README.md b/spaces/OFA-Sys/OFA-Generic_Interface/fairseq/examples/layerdrop/README.md
deleted file mode 100644
index 4d48ee9615e1458e1e889635dc9938e427a7f64a..0000000000000000000000000000000000000000
--- a/spaces/OFA-Sys/OFA-Generic_Interface/fairseq/examples/layerdrop/README.md
+++ /dev/null
@@ -1,154 +0,0 @@
-# Reducing Transformer Depth on Demand with Structured Dropout (Fan et al., 2019)
-This page contains information for how to train models with LayerDrop, based on this [paper](https://arxiv.org/abs/1909.11556).
-
-## Citation:
-If you found this technique useful, please cite our paper:
-```bibtex
-@article{fan2019reducing,
- title={Reducing Transformer Depth on Demand with Structured Dropout},
- author={Fan, Angela and Grave, Edouard and Joulin, Armand},
- journal={arXiv preprint arXiv:1909.11556},
- year={2019}
-}
-```
-
-## Pre-trained models
-
-Model | Description | Download
----|---|---
-`layerdrop_wmt_en_de_12_6` | Transformer + LayerDrop 0.2 trained on WMT16 en-de with 12 encoder and 6 decoder layers | [layerdrop_wmt_en_de_12_6.tar.gz](https://dl.fbaipublicfiles.com/fairseq/models/layerdrop_wmt_en_de_12_6.tar.gz)
-`roberta_layerdrop.base` | RoBERTa Base + LayerDrop 0.2 | [roberta_layerdrop.base.tar.gz](https://dl.fbaipublicfiles.com/fairseq/models/roberta_layerdrop.base.qnli.tar.gz)
-`roberta_layerdrop.large` | RoBERTa Large + LayerDrop 0.2 | [roberta_layerdrop.large.tar.gz](https://dl.fbaipublicfiles.com/fairseq/models/roberta_layerdrop.large.tar.gz)
-`roberta_layerdrop.large.mnli` | `roberta_layerdrop.large` finetuned on [MNLI](http://www.nyu.edu/projects/bowman/multinli) | [roberta_layerdrop.large.mnli.tar.gz](https://dl.fbaipublicfiles.com/fairseq/models/roberta_layerdrop.large.mnli.tar.gz)
-`roberta_layerdrop.large.qnli` | `roberta_layerdrop.large` finetuned on [QNLI](https://arxiv.org/abs/1804.07461) | [roberta_layerdrop.large.mnli.tar.gz](https://dl.fbaipublicfiles.com/fairseq/models/roberta_layerdrop.large.qnli.tar.gz)
-
-
-Evaluate performance of these pre-trained models:
-```bash
-# Example for Machine Translation
-fairseq-generate /path/to/bped/wmt/data --path nmt_checkpoint.pt \
- --beam 8 --lenpen 0.4 \
- --batch-size 64 \
- --remove-bpe \
- --gen-subset test > wmt16_gen.txt
-bash scripts/compound_split_bleu.sh wmt16_gen.txt
-# prints BLEU4 = 30.17
-```
-
-```python
-# Example for RoBERTa + LayerDrop finetuned on MNLI:
-from fairseq.models.roberta import RobertaModel
-
-roberta_layerdrop = RobertaModel.from_pretrained(
- '/path/to/MNLI/model',
- checkpoint_file='mnli_checkpoint.pt',
- data_name_or_path='/path/to/MNLI/data/MNLI-bin'
-)
-label_map = {0: 'contradiction', 2: 'neutral', 1: 'entailment'}
-ncorrect, nsamples = 0, 0
-roberta_layerdrop.cuda()
-roberta_layerdrop.eval()
-with open('/path/to/MNLI/data/dev_matched.tsv') as fin:
- fin.readline()
- for index, line in enumerate(fin):
- tokens = line.strip().split('\t')
- sent1, sent2, target = tokens[8], tokens[9], tokens[-1]
- tokens = roberta_layerdrop.encode(sent1, sent2)
- prediction = roberta_layerdrop.predict('sentence_classification_head', tokens).argmax().item()
- prediction_label = label_map[prediction]
- ncorrect += int(prediction_label == target)
- nsamples += 1
-print('| Accuracy: ', float(ncorrect)/float(nsamples))
-# prints | Accuracy: 0.9026999490575649
-
-
-# Example for RoBERTa + LayerDrop finetuned on QNLI:
-roberta = RobertaModel.from_pretrained(
- '/path/to/QNLI/model',
- checkpoint_file='qnli_checkpoint.pt',
- data_name_or_path='/path/to/QNLI/data/QNLI-bin'
-)
-
-label_fn = lambda label: roberta.task.label_dictionary.string(
- [label + roberta.task.target_dictionary.nspecial]
-)
-ncorrect, nsamples = 0, 0
-roberta.cuda()
-roberta.eval()
-with open('/path/to/QNLI/data/dev.tsv') as fin:
- fin.readline()
- for index, line in enumerate(fin):
- tokens = line.strip().split('\t')
- sent1, sent2, target = tokens[1], tokens[2], tokens[3]
- tokens = roberta.encode(sent1, sent2)
- prediction = roberta.predict('sentence_classification_head', tokens).argmax().item()
- prediction_label = label_fn(prediction)
- ncorrect += int(prediction_label == target)
- nsamples += 1
-print('| Accuracy: ', float(ncorrect)/float(nsamples))
-# prints | Accuracy: 0.9480139117700896
-```
-
-
-## Example usage
-
-To train a model with LayerDrop, add the following flags. We recommend 0.2, a value that worked well in our experiments. For Language Models that are decoder-only, you need only the decoder flag. For RoBERTa, an encoder, you need only the encoder flag. The encoder and decoder LayerDrop values can be set differently.
-```
---encoder-layerdrop 0.2 --decoder-layerdrop 0.2
-```
-
-To prune a model that has been trained with LayerDrop, add the following flags followed by a comma separated list of which layers you would like to keep.
-```
---encoder-layers-to-keep 0,2,4,6,8,10,12,14 --decoder-layers-to-keep 0,2,4,6,8,10,12,14
-```
-Setting these flags should print a message such as:
-```
-| Pruning model to specified layer configuration
-```
-You should also see a smaller number of parameters in the model, for example the 16-Layer Transformer Language Model prints:
-```
-num. model params: 246933504
-```
-while a model pruned to 8 Layers prints:
-```
-num. model params: 146163712
-```
-
-If you would like to pick up training with a model that has been pruned, simply adding these flags is sufficient. If you would like to use a script that only does evaluation (no training), you may need to pass an override command. A specific example would be for language modeling:
-```bash
-fairseq-eval-lm /path/to/wikitext-103 \
- --path /path/to/model/checkpoint.pt \
- --model-overrides "{'decoder_layers_to_keep':'0,2,4,6,8,10,12,14'}"
-```
-This model override command overrides the training parameters and updates the model arguments so that the pruned model is run instead of the full model.
-
-## Reproduce Paper Results
-
-Looking to reproduce the results in the paper?
-
-1. For Translation on WMT16 en-de, we followed this setting [here](https://github.com/pytorch/fairseq/blob/main/examples/scaling_nmt/README.md)
-2. To train RoBERTa, we followed this setting [here](https://github.com/pytorch/fairseq/tree/main/examples/roberta)
-3. To train Language Models on Wikitext-103, we followed this setting [here](https://github.com/pytorch/fairseq/tree/main/examples/language_model)
-
-
-## Tips
-
-1. If you would like to train large models with better performance, LayerDrop should be set to a smaller value such as 0.1 or 0.2. Too much LayerDrop will mean the model has too much regularization, so may not reach the best performance. Since LayerDrop adds regularization, you may achieve the best performance by slightly reducing the amount of standard dropout (for example, reduce by 0.1).
-
-2. If you would like to train large models to be pruned and made smaller, LayerDrop should be set to a larger value such as 0.5 if you want to prune very aggressively (such as removing half the network or more). If you would like to prune fewer layers away, LayerDrop can be set to a smaller value such as 0.2. Our experiments were conducted with low values of LayerDrop (such as 0.1 and 0.2), for reference.
-
-3. When pruning layers at inference time, it is best to spread out the layers remaining so they are evenly spaced throughout the network. For example, if you want to remove 50% of the network, keeping every other layer is good.
-
-
-## FAQ
-
-1. How did the sharing layers experiment work? In an appendix (https://openreview.net/pdf?id=SylO2yStDr) we added an experiment on Wikitext-103 language modeling that combined LayerDrop with Weight Sharing. We shared chunks of 2 layers such that every other layer had shared weights. For example, if our network has layers 1 through 6, then layer 1 and 2 are shared, layer 3 and 4 are shared, and layer 5 and 6 are shared.
-
-2. LayerDrop hasn't been helping in my setting? During training time, LayerDrop can help regularize your network. This is most important if your network is already overfitting - if your network is underfitting, it is possible LayerDrop is adding too much regularization. We recommend using smaller values (such as 0.1 or 0.2) and also decreasing the quantity of standard dropout (for example, reduce by 0.1).
-
-3. Can you train a model without LayerDrop and finetune with LayerDrop (e.g. for BERT)? In our experiments, we did not see great performance. Models such as RoBERTa have trained for a long time in the pre-training setting, so only finetuning with LayerDrop for a few epochs on a downstream task such as MNLI does not achieve the robustness required for successful pruning.
-
-
-## Having an issue or have a question?
-
-Please open an issue in this repository with the details of your question. Thanks!
diff --git a/spaces/OFA-Sys/OFA-Image_Caption/fairseq/examples/speech_synthesis/__init__.py b/spaces/OFA-Sys/OFA-Image_Caption/fairseq/examples/speech_synthesis/__init__.py
deleted file mode 100644
index 6264236915a7269a4d920ee8213004374dd86a9a..0000000000000000000000000000000000000000
--- a/spaces/OFA-Sys/OFA-Image_Caption/fairseq/examples/speech_synthesis/__init__.py
+++ /dev/null
@@ -1,4 +0,0 @@
-# Copyright (c) Facebook, Inc. and its affiliates.
-#
-# This source code is licensed under the MIT license found in the
-# LICENSE file in the root directory of this source tree.
diff --git a/spaces/OFA-Sys/OFA-Image_Caption/fairseq/examples/speech_synthesis/evaluation/eval_sp.py b/spaces/OFA-Sys/OFA-Image_Caption/fairseq/examples/speech_synthesis/evaluation/eval_sp.py
deleted file mode 100644
index 702c4980389624f788abc0b42cdf54757a52512f..0000000000000000000000000000000000000000
--- a/spaces/OFA-Sys/OFA-Image_Caption/fairseq/examples/speech_synthesis/evaluation/eval_sp.py
+++ /dev/null
@@ -1,131 +0,0 @@
-# Copyright (c) Facebook, Inc. and its affiliates.
-#
-# This source code is licensed under the MIT license found in the
-# LICENSE file in the root directory of this source tree.
-
-
-"""
-Signal processing-based evaluation using waveforms
-"""
-
-import csv
-import numpy as np
-import os.path as op
-
-import torch
-import tqdm
-from tabulate import tabulate
-import torchaudio
-
-from examples.speech_synthesis.utils import batch_mel_spectral_distortion
-from fairseq.tasks.text_to_speech import batch_mel_cepstral_distortion
-
-
-def load_eval_spec(path):
- with open(path) as f:
- reader = csv.DictReader(f, delimiter='\t')
- samples = list(reader)
- return samples
-
-
-def eval_distortion(samples, distortion_fn, device="cuda"):
- nmiss = 0
- results = []
- for sample in tqdm.tqdm(samples):
- if not op.isfile(sample["ref"]) or not op.isfile(sample["syn"]):
- nmiss += 1
- results.append(None)
- continue
- # assume single channel
- yref, sr = torchaudio.load(sample["ref"])
- ysyn, _sr = torchaudio.load(sample["syn"])
- yref, ysyn = yref[0].to(device), ysyn[0].to(device)
- assert sr == _sr, f"{sr} != {_sr}"
-
- distortion, extra = distortion_fn([yref], [ysyn], sr, None)[0]
- _, _, _, _, _, pathmap = extra
- nins = torch.sum(pathmap.sum(dim=1) - 1) # extra frames in syn
- ndel = torch.sum(pathmap.sum(dim=0) - 1) # missing frames from syn
- results.append(
- (distortion.item(), # path distortion
- pathmap.size(0), # yref num frames
- pathmap.size(1), # ysyn num frames
- pathmap.sum().item(), # path length
- nins.item(), # insertion
- ndel.item(), # deletion
- )
- )
- return results
-
-
-def eval_mel_cepstral_distortion(samples, device="cuda"):
- return eval_distortion(samples, batch_mel_cepstral_distortion, device)
-
-
-def eval_mel_spectral_distortion(samples, device="cuda"):
- return eval_distortion(samples, batch_mel_spectral_distortion, device)
-
-
-def print_results(results, show_bin):
- results = np.array(list(filter(lambda x: x is not None, results)))
-
- np.set_printoptions(precision=3)
-
- def _print_result(results):
- dist, dur_ref, dur_syn, dur_ali, nins, ndel = results.sum(axis=0)
- res = {
- "nutt": len(results),
- "dist": dist,
- "dur_ref": int(dur_ref),
- "dur_syn": int(dur_syn),
- "dur_ali": int(dur_ali),
- "dist_per_ref_frm": dist/dur_ref,
- "dist_per_syn_frm": dist/dur_syn,
- "dist_per_ali_frm": dist/dur_ali,
- "ins": nins/dur_ref,
- "del": ndel/dur_ref,
- }
- print(tabulate(
- [res.values()],
- res.keys(),
- floatfmt=".4f"
- ))
-
- print(">>>> ALL")
- _print_result(results)
-
- if show_bin:
- edges = [0, 200, 400, 600, 800, 1000, 2000, 4000]
- for i in range(1, len(edges)):
- mask = np.logical_and(results[:, 1] >= edges[i-1],
- results[:, 1] < edges[i])
- if not mask.any():
- continue
- bin_results = results[mask]
- print(f">>>> ({edges[i-1]}, {edges[i]})")
- _print_result(bin_results)
-
-
-def main(eval_spec, mcd, msd, show_bin):
- samples = load_eval_spec(eval_spec)
- device = "cpu"
- if mcd:
- print("===== Evaluate Mean Cepstral Distortion =====")
- results = eval_mel_cepstral_distortion(samples, device)
- print_results(results, show_bin)
- if msd:
- print("===== Evaluate Mean Spectral Distortion =====")
- results = eval_mel_spectral_distortion(samples, device)
- print_results(results, show_bin)
-
-
-if __name__ == "__main__":
- import argparse
- parser = argparse.ArgumentParser()
- parser.add_argument("eval_spec")
- parser.add_argument("--mcd", action="store_true")
- parser.add_argument("--msd", action="store_true")
- parser.add_argument("--show-bin", action="store_true")
- args = parser.parse_args()
-
- main(args.eval_spec, args.mcd, args.msd, args.show_bin)
diff --git a/spaces/OFA-Sys/OFA-Image_Caption/fairseq/examples/wav2vec/unsupervised/data/random_input_dataset.py b/spaces/OFA-Sys/OFA-Image_Caption/fairseq/examples/wav2vec/unsupervised/data/random_input_dataset.py
deleted file mode 100644
index 886505616cc7f7a515ecebf34fae5c2bc541de03..0000000000000000000000000000000000000000
--- a/spaces/OFA-Sys/OFA-Image_Caption/fairseq/examples/wav2vec/unsupervised/data/random_input_dataset.py
+++ /dev/null
@@ -1,62 +0,0 @@
-# Copyright (c) Facebook, Inc. and its affiliates.
-#
-# This source code is licensed under the MIT license found in the
-# LICENSE file in the root directory of this source tree.
-
-import random
-from typing import List
-
-from fairseq.data import BaseWrapperDataset, data_utils
-
-
-class RandomInputDataset(BaseWrapperDataset):
- def __init__(
- self,
- dataset,
- random_input_dataset,
- input_key_path: List[str],
- add_to_input,
- pad_idx,
- ):
- super().__init__(dataset)
- self.random_input_dataset = random_input_dataset
- if isinstance(input_key_path, str):
- input_key_path = [input_key_path]
- assert len(input_key_path) > 0
- self.input_key_path = input_key_path
- self.add_to_input = add_to_input
- self.pad_idx = pad_idx
-
- def get_target(self, item):
- target_loc = item
- for p in self.input_key_path[:-1]:
- target_loc = target_loc[p]
- return self.input_key_path[-1], target_loc
-
- def get_target_value(self, item):
- k, target_loc = self.get_target(item)
- return target_loc[k]
-
- def __getitem__(self, index):
- item = self.dataset[index]
- k, target_loc = self.get_target(item)
- target_loc[k] = random.choice(self.random_input_dataset)
- return item
-
- def collater(self, samples):
- collated = self.dataset.collater(samples)
- if len(collated) == 0:
- return collated
- indices = set(collated["id"].tolist())
-
- random_inputs = data_utils.collate_tokens(
- [self.get_target_value(s) for s in samples if s["id"] in indices],
- pad_idx=self.pad_idx,
- left_pad=False,
- )
- k, target_loc = self.get_target(
- collated if not self.add_to_input else collated["net_input"]
- )
- target_loc[k] = random_inputs
-
- return collated
diff --git a/spaces/OFA-Sys/OFA-Image_Caption/fairseq/scripts/constraints/extract.py b/spaces/OFA-Sys/OFA-Image_Caption/fairseq/scripts/constraints/extract.py
deleted file mode 100644
index f6155d0a0538aadb46bf612256b6b949728de69e..0000000000000000000000000000000000000000
--- a/spaces/OFA-Sys/OFA-Image_Caption/fairseq/scripts/constraints/extract.py
+++ /dev/null
@@ -1,92 +0,0 @@
-#!/usr/bin/env python3
-#
-# Copyright (c) Facebook, Inc. and its affiliates.
-#
-# This source code is licensed under the MIT license found in the
-# LICENSE file in the root directory of this source tree.
-
-"""Extracts random constraints from reference files."""
-
-import argparse
-import random
-import sys
-
-from sacrebleu import extract_ngrams
-
-
-def get_phrase(words, index, length):
- assert index < len(words) - length + 1
- phr = " ".join(words[index : index + length])
- for i in range(index, index + length):
- words.pop(index)
- return phr
-
-
-def main(args):
-
- if args.seed:
- random.seed(args.seed)
-
- for line in sys.stdin:
- constraints = []
-
- def add_constraint(constraint):
- constraints.append(constraint)
-
- source = line.rstrip()
- if "\t" in line:
- source, target = line.split("\t")
- if args.add_sos:
- target = f" {target}"
- if args.add_eos:
- target = f"{target} "
-
- if len(target.split()) >= args.len:
- words = [target]
-
- num = args.number
-
- choices = {}
- for i in range(num):
- if len(words) == 0:
- break
- segmentno = random.choice(range(len(words)))
- segment = words.pop(segmentno)
- tokens = segment.split()
- phrase_index = random.choice(range(len(tokens)))
- choice = " ".join(
- tokens[phrase_index : min(len(tokens), phrase_index + args.len)]
- )
- for j in range(
- phrase_index, min(len(tokens), phrase_index + args.len)
- ):
- tokens.pop(phrase_index)
- if phrase_index > 0:
- words.append(" ".join(tokens[0:phrase_index]))
- if phrase_index + 1 < len(tokens):
- words.append(" ".join(tokens[phrase_index:]))
- choices[target.find(choice)] = choice
-
- # mask out with spaces
- target = target.replace(choice, " " * len(choice), 1)
-
- for key in sorted(choices.keys()):
- add_constraint(choices[key])
-
- print(source, *constraints, sep="\t")
-
-
-if __name__ == "__main__":
- parser = argparse.ArgumentParser()
- parser.add_argument("--number", "-n", type=int, default=1, help="number of phrases")
- parser.add_argument("--len", "-l", type=int, default=1, help="phrase length")
- parser.add_argument(
- "--add-sos", default=False, action="store_true", help="add token"
- )
- parser.add_argument(
- "--add-eos", default=False, action="store_true", help="add token"
- )
- parser.add_argument("--seed", "-s", default=0, type=int)
- args = parser.parse_args()
-
- main(args)
diff --git a/spaces/OFA-Sys/OFA-vqa/fairseq/fairseq/scoring/__init__.py b/spaces/OFA-Sys/OFA-vqa/fairseq/fairseq/scoring/__init__.py
deleted file mode 100644
index 58f2f563e493327394dff1265030d18f0814b5a2..0000000000000000000000000000000000000000
--- a/spaces/OFA-Sys/OFA-vqa/fairseq/fairseq/scoring/__init__.py
+++ /dev/null
@@ -1,55 +0,0 @@
-# Copyright (c) Facebook, Inc. and its affiliates.
-#
-# This source code is licensed under the MIT license found in the
-# LICENSE file in the root directory of this source tree.
-
-
-import importlib
-import os
-from abc import ABC, abstractmethod
-
-from fairseq import registry
-from omegaconf import DictConfig
-
-
-class BaseScorer(ABC):
- def __init__(self, cfg):
- self.cfg = cfg
- self.ref = []
- self.pred = []
-
- def add_string(self, ref, pred):
- self.ref.append(ref)
- self.pred.append(pred)
-
- @abstractmethod
- def score(self) -> float:
- pass
-
- @abstractmethod
- def result_string(self) -> str:
- pass
-
-
-_build_scorer, register_scorer, SCORER_REGISTRY, _ = registry.setup_registry(
- "--scoring", default="bleu"
-)
-
-
-def build_scorer(choice, tgt_dict):
- _choice = choice._name if isinstance(choice, DictConfig) else choice
-
- if _choice == "bleu":
- from fairseq.scoring import bleu
-
- return bleu.Scorer(
- bleu.BleuConfig(pad=tgt_dict.pad(), eos=tgt_dict.eos(), unk=tgt_dict.unk())
- )
- return _build_scorer(choice)
-
-
-# automatically import any Python files in the current directory
-for file in sorted(os.listdir(os.path.dirname(__file__))):
- if file.endswith(".py") and not file.startswith("_"):
- module = file[: file.find(".py")]
- importlib.import_module("fairseq.scoring." + module)
diff --git a/spaces/Omnibus/game-test/diff.py b/spaces/Omnibus/game-test/diff.py
deleted file mode 100644
index fdef4b8f81ea9fdf0b175b3bb1102c144d12d6ad..0000000000000000000000000000000000000000
--- a/spaces/Omnibus/game-test/diff.py
+++ /dev/null
@@ -1,65 +0,0 @@
-import gradio as gr
-class SendIt:
- def __init__(self):
-
- models =[
- "",
- "CompVis/stable-diffusion-v1-4",
- "runwayml/stable-diffusion-v1-5",
- "prompthero/openjourney",
- "stabilityai/stable-diffusion-2-1",
- "stabilityai/stable-diffusion-2-1-base",
- "SG161222/Realistic_Vision_V1.4",
- "Linaqruf/anything-v3.0",
- "eimiss/EimisAnimeDiffusion_1.0v",
- "nitrosocke/Nitro-Diffusion",
- "wavymulder/portraitplus",
- "22h/vintedois-diffusion-v0-1",
- "dreamlike-art/dreamlike-photoreal-2.0",
- "dreamlike-art/dreamlike-diffusion-1.0",
- "wavymulder/Analog-Diffusion",
- "nitrosocke/redshift-diffusion",
- "claudfuen/photorealistic-fuen-v1",
- "prompthero/openjourney-v2",
- "johnslegers/epic-diffusion",
- "nitrosocke/Arcane-Diffusion",
- "darkstorm2150/Protogen_x5.8_Official_Release",
-
- ]
-
- self.models2=[
- gr.Interface.load(f"models/{models[1]}",live=True,preprocess=True),
- gr.Interface.load(f"models/{models[2]}",live=True,preprocess=True),
- gr.Interface.load(f"models/{models[3]}",live=True,preprocess=True),
- gr.Interface.load(f"models/{models[4]}",live=True,preprocess=True),
- gr.Interface.load(f"models/{models[5]}",live=True,preprocess=True),
- gr.Interface.load(f"models/{models[6]}",live=True,preprocess=True),
- gr.Interface.load(f"models/{models[7]}",live=True,preprocess=True),
- gr.Interface.load(f"models/{models[8]}",live=True,preprocess=True),
- gr.Interface.load(f"models/{models[9]}",live=True,preprocess=True),
- gr.Interface.load(f"models/{models[10]}",live=True,preprocess=True),
- gr.Interface.load(f"models/{models[11]}",live=True,preprocess=True),
- gr.Interface.load(f"models/{models[12]}",live=True,preprocess=True),
- gr.Interface.load(f"models/{models[13]}",live=True,preprocess=True),
- gr.Interface.load(f"models/{models[14]}",live=True,preprocess=True),
- gr.Interface.load(f"models/{models[15]}",live=True,preprocess=True),
- gr.Interface.load(f"models/{models[16]}",live=True,preprocess=True),
- gr.Interface.load(f"models/{models[17]}",live=True,preprocess=True),
- gr.Interface.load(f"models/{models[18]}",live=True,preprocess=True),
- gr.Interface.load(f"models/{models[19]}",live=True,preprocess=True),
- gr.Interface.load(f"models/{models[20]}",live=True,preprocess=True),
-
-
- ]
-do_it=SendIt()
-
-def send_it(inputs,model_choice=5, count = 1):
- t=0
- output_list=[]
- while t`.
-
-## Usage
-
-ChatGLM3 Demo has three modes:
-
-- Chat: Dialogue mode, where you can interact with the model.
-- Tool: Tool mode, where the model, in addition to dialogue, can perform other operations through tools.
-- Code Interpreter: Code interpreter mode, where the model can execute code in a Jupyter environment and obtain results to complete complex tasks.
-
-### Dialogue Mode
-
-In dialogue mode, users can directly modify parameters such as top_p, temperature, System Prompt in the sidebar to adjust the behavior of the model. For example,
-
-
-
-### Tool Mode
-
-You can enhance the model's capabilities by registering new tools in `tool_registry.py`. Just use the `@register_tool` decorator to complete the registration. For tool declarations, the function name is the name of the tool, and the function docstring is the description of the tool; for tool parameters, use `Annotated[typ: type, description: str, required: bool]` to annotate the type, description, and whether it is necessary of the parameters.
-
-For example, the registration of the `get_weather` tool is as follows:
-
-```python
-@register_tool
-def get_weather(
- city_name: Annotated[str, 'The name of the city to be queried', True],
-) -> str:
- """
- Get the weather for `city_name` in the following week
- """
- ...
-```
-
-
-
-Additionally, you can enter the manual mode through `Manual mode` on the page. In this mode, you can directly specify the tool list through YAML, but you need to manually feed back the tool's output to the model.
-
-### Code Interpreter Mode
-
-Due to having a code execution environment, the model in this mode can perform more complex tasks, such as drawing charts, performing symbolic operations, etc. The model will automatically execute multiple code blocks in succession based on its understanding of the task completion status until the task is completed. Therefore, in this mode, you only need to specify the task you want the model to perform.
-
-For example, we can ask ChatGLM3 to draw a heart:
-
-
-
-### Additional Tips
-
-- While the model is generating text, it can be interrupted by the `Stop` button at the top right corner of the page.
-- Refreshing the page will clear the dialogue history.
-
-# Enjoy!
\ No newline at end of file
diff --git a/spaces/Osborn-bh/ChatGLM3-6B-Osborn/langchain_demo/ChatGLM3.py b/spaces/Osborn-bh/ChatGLM3-6B-Osborn/langchain_demo/ChatGLM3.py
deleted file mode 100644
index befe9f5d6bd3591d6e7644e15f7d21c5cb697458..0000000000000000000000000000000000000000
--- a/spaces/Osborn-bh/ChatGLM3-6B-Osborn/langchain_demo/ChatGLM3.py
+++ /dev/null
@@ -1,123 +0,0 @@
-import json
-from langchain.llms.base import LLM
-from transformers import AutoTokenizer, AutoModel, AutoConfig
-from typing import List, Optional
-from utils import tool_config_from_file
-
-
-class ChatGLM3(LLM):
- max_token: int = 8192
- do_sample: bool = False
- temperature: float = 0.8
- top_p = 0.8
- tokenizer: object = None
- model: object = None
- history: List = []
- tool_names: List = []
- has_search: bool = False
-
- def __init__(self):
- super().__init__()
-
- @property
- def _llm_type(self) -> str:
- return "ChatGLM3"
-
- def load_model(self, model_name_or_path=None):
- model_config = AutoConfig.from_pretrained(
- model_name_or_path,
- trust_remote_code=True
- )
- self.tokenizer = AutoTokenizer.from_pretrained(
- model_name_or_path,
- trust_remote_code=True
- )
- self.model = AutoModel.from_pretrained(
- model_name_or_path, config=model_config, trust_remote_code=True
- ).half().cuda()
-
- def _tool_history(self, prompt: str):
- ans = []
- tool_prompts = prompt.split(
- "You have access to the following tools:\n\n")[1].split("\n\nUse a json blob")[0].split("\n")
-
- tool_names = [tool.split(":")[0] for tool in tool_prompts]
- self.tool_names = tool_names
- tools_json = []
- for i, tool in enumerate(tool_names):
- tool_config = tool_config_from_file(tool)
- if tool_config:
- tools_json.append(tool_config)
- else:
- ValueError(
- f"Tool {tool} config not found! It's description is {tool_prompts[i]}"
- )
-
- ans.append({
- "role": "system",
- "content": "Answer the following questions as best as you can. You have access to the following tools:",
- "tools": tools_json
- })
- query = f"""{prompt.split("Human: ")[-1].strip()}"""
- return ans, query
-
- def _extract_observation(self, prompt: str):
- return_json = prompt.split("Observation: ")[-1].split("\nThought:")[0]
- self.history.append({
- "role": "observation",
- "content": return_json
- })
- return
-
- def _extract_tool(self):
- if len(self.history[-1]["metadata"]) > 0:
- metadata = self.history[-1]["metadata"]
- content = self.history[-1]["content"]
- if "tool_call" in content:
- for tool in self.tool_names:
- if tool in metadata:
- input_para = content.split("='")[-1].split("'")[0]
- action_json = {
- "action": tool,
- "action_input": input_para
- }
- self.has_search = True
- return f"""
-Action:
-```
-{json.dumps(action_json, ensure_ascii=False)}
-```"""
- final_answer_json = {
- "action": "Final Answer",
- "action_input": self.history[-1]["content"]
- }
- self.has_search = False
- return f"""
-Action:
-```
-{json.dumps(final_answer_json, ensure_ascii=False)}
-```"""
-
- def _call(self, prompt: str, history: List = [], stop: Optional[List[str]] = ["<|user|>"]):
- print("======")
- print(self.prompt)
- print("======")
- if not self.has_search:
- self.history, query = self._tool_history(prompt)
- else:
- self._extract_observation(prompt)
- query = ""
- # print("======")
- # print(self.history)
- # print("======")
- _, self.history = self.model.chat(
- self.tokenizer,
- query,
- history=self.history,
- do_sample=self.do_sample,
- max_length=self.max_token,
- temperature=self.temperature,
- )
- response = self._extract_tool()
- history.append((prompt, response))
- return response
diff --git a/spaces/PKUWilliamYang/VToonify/vtoonify/model/stylegan/dataset.py b/spaces/PKUWilliamYang/VToonify/vtoonify/model/stylegan/dataset.py
deleted file mode 100644
index 7713ea2f8bc94d202d2dfbe830af3cb96b1e803d..0000000000000000000000000000000000000000
--- a/spaces/PKUWilliamYang/VToonify/vtoonify/model/stylegan/dataset.py
+++ /dev/null
@@ -1,40 +0,0 @@
-from io import BytesIO
-
-import lmdb
-from PIL import Image
-from torch.utils.data import Dataset
-
-
-class MultiResolutionDataset(Dataset):
- def __init__(self, path, transform, resolution=256):
- self.env = lmdb.open(
- path,
- max_readers=32,
- readonly=True,
- lock=False,
- readahead=False,
- meminit=False,
- )
-
- if not self.env:
- raise IOError('Cannot open lmdb dataset', path)
-
- with self.env.begin(write=False) as txn:
- self.length = int(txn.get('length'.encode('utf-8')).decode('utf-8'))
-
- self.resolution = resolution
- self.transform = transform
-
- def __len__(self):
- return self.length
-
- def __getitem__(self, index):
- with self.env.begin(write=False) as txn:
- key = f'{self.resolution}-{str(index).zfill(5)}'.encode('utf-8')
- img_bytes = txn.get(key)
-
- buffer = BytesIO(img_bytes)
- img = Image.open(buffer)
- img = self.transform(img)
-
- return img
diff --git a/spaces/PSLD/PSLD/diffusion-posterior-sampling/bkse/models/dsd/dsd_stylegan2.py b/spaces/PSLD/PSLD/diffusion-posterior-sampling/bkse/models/dsd/dsd_stylegan2.py
deleted file mode 100644
index 32e99ac1eeab41908ebfe61ac99913587c6d7149..0000000000000000000000000000000000000000
--- a/spaces/PSLD/PSLD/diffusion-posterior-sampling/bkse/models/dsd/dsd_stylegan2.py
+++ /dev/null
@@ -1,78 +0,0 @@
-from pathlib import Path
-
-import torch
-from models.dsd.dsd import DSD
-from models.dsd.stylegan2 import Generator
-from models.losses.dsd_loss import LossBuilderStyleGAN2
-
-
-class DSDStyleGAN2(DSD):
- def __init__(self, opt, cache_dir):
- super(DSDStyleGAN2, self).__init__(opt, cache_dir)
-
- def load_synthesis_network(self):
- self.synthesis = Generator(size=256, style_dim=512, n_mlp=8).cuda()
- self.synthesis.load_state_dict(torch.load("experiments/pretrained/stylegan2.pt")["g_ema"], strict=False)
- for v in self.synthesis.parameters():
- v.requires_grad = False
-
- def initialize_mapping_network(self):
- if Path("experiments/pretrained/gaussian_fit_stylegan2.pt").exists():
- self.gaussian_fit = torch.load("experiments/pretrained/gaussian_fit_stylegan2.pt")
- else:
- if self.verbose:
- print("\tRunning Mapping Network")
- with torch.no_grad():
- torch.manual_seed(0)
- latent = torch.randn((1000000, 512), dtype=torch.float32, device="cuda")
- latent_out = torch.nn.LeakyReLU(5)(self.synthesis.get_latent(latent))
- self.gaussian_fit = {"mean": latent_out.mean(0), "std": latent_out.std(0)}
- torch.save(self.gaussian_fit, "experiments/pretrained/gaussian_fit_stylegan2.pt")
- if self.verbose:
- print('\tSaved "gaussian_fit_stylegan2.pt"')
-
- def initialize_latent_space(self):
- batch_size = self.opt["batch_size"]
-
- # Generate latent tensor
- if self.opt["tile_latent"]:
- self.latent = torch.randn((batch_size, 1, 512), dtype=torch.float, requires_grad=True, device="cuda")
- else:
- self.latent = torch.randn((batch_size, 14, 512), dtype=torch.float, requires_grad=True, device="cuda")
-
- # Generate list of noise tensors
- noise = [] # stores all of the noise tensors
- noise_vars = [] # stores the noise tensors that we want to optimize on
-
- for i in range(14):
- res = (i + 5) // 2
- res = [1, 1, 2 ** res, 2 ** res]
-
- noise_type = self.opt["noise_type"]
- bad_noise_layers = self.opt["bad_noise_layers"]
- if noise_type == "zero" or i in [int(layer) for layer in bad_noise_layers.split(".")]:
- new_noise = torch.zeros(res, dtype=torch.float, device="cuda")
- new_noise.requires_grad = False
- elif noise_type == "fixed":
- new_noise = torch.randn(res, dtype=torch.float, device="cuda")
- new_noise.requires_grad = False
- elif noise_type == "trainable":
- new_noise = torch.randn(res, dtype=torch.float, device="cuda")
- if i < self.opt["num_trainable_noise_layers"]:
- new_noise.requires_grad = True
- noise_vars.append(new_noise)
- else:
- new_noise.requires_grad = False
- else:
- raise Exception("unknown noise type")
-
- noise.append(new_noise)
-
- self.latent_x_var_list = [self.latent] + noise_vars
- self.noise = noise
-
- def initialize_loss(self, ref_im):
- self.loss_builder = LossBuilderStyleGAN2(ref_im, self.opt).cuda()
-
- def get_gen_im(self, latent_in):
- return (self.synthesis([latent_in], input_is_latent=True, noise=self.noise)[0] + 1) / 2
diff --git a/spaces/PaddlePaddle/UIE-X/footer.html b/spaces/PaddlePaddle/UIE-X/footer.html
deleted file mode 100644
index 2e2341539e0ed1d936da97de9255ad7b47795dba..0000000000000000000000000000000000000000
--- a/spaces/PaddlePaddle/UIE-X/footer.html
+++ /dev/null
@@ -1,4 +0,0 @@
-
\ No newline at end of file
diff --git a/spaces/PeepDaSlan9/HuggingFaceH4-starchat-alpha/README.md b/spaces/PeepDaSlan9/HuggingFaceH4-starchat-alpha/README.md
deleted file mode 100644
index 11c84a0a2456207f2bdb3c8cc54ca31199c21f61..0000000000000000000000000000000000000000
--- a/spaces/PeepDaSlan9/HuggingFaceH4-starchat-alpha/README.md
+++ /dev/null
@@ -1,13 +0,0 @@
----
-title: HuggingFaceH4 Starchat Alpha
-emoji: 👁
-colorFrom: indigo
-colorTo: pink
-sdk: gradio
-sdk_version: 3.34.0
-app_file: app.py
-pinned: false
-license: bigcode-openrail-m
----
-
-Check out the configuration reference at https://huggingface.co/docs/hub/spaces-config-reference
diff --git a/spaces/PeepDaSlan9/neon-tts-plugin-coqui/app.py b/spaces/PeepDaSlan9/neon-tts-plugin-coqui/app.py
deleted file mode 100644
index b5a0142ab4ea8d8a58100b085db43589aa41b8d5..0000000000000000000000000000000000000000
--- a/spaces/PeepDaSlan9/neon-tts-plugin-coqui/app.py
+++ /dev/null
@@ -1,67 +0,0 @@
-import tempfile
-
-import gradio as gr
-
-from neon_tts_plugin_coqui import CoquiTTS
-
-
-LANGUAGES = list(CoquiTTS.langs.keys())
-default_lang = "en"
-
-
-
-title = "🐸💬 - NeonAI Coqui AI TTS Plugin"
-description = "🐸💬 - a deep learning toolkit for Text-to-Speech, battle-tested in research and production"
-info = "more info at [Neon Coqui TTS Plugin](https://github.com/NeonGeckoCom/neon-tts-plugin-coqui), [Coqui TTS](https://github.com/coqui-ai/TTS)"
-badge = "https://visitor-badge-reloaded.herokuapp.com/badge?page_id=neongeckocom.neon-tts-plugin-coqui"
-
-
-
-coquiTTS = CoquiTTS()
-
-
-def tts(text: str, language: str):
- print(text, language)
- # return output
- with tempfile.NamedTemporaryFile(suffix=".wav", delete=False) as fp:
- coquiTTS.get_tts(text, fp, speaker = {"language" : language})
- return fp.name
-
-
-
-with gr.Blocks() as blocks:
- gr.Markdown(""
- + title
- + "
")
- gr.Markdown(description)
- with gr.Row():# equal_height=False
- with gr.Column():# variant="panel"
- textbox = gr.Textbox(
- label="Input",
- value=CoquiTTS.langs[default_lang]["sentence"],
- max_lines=3,
- )
- radio = gr.Radio(
- label="Language",
- choices=LANGUAGES,
- value=default_lang
- )
- with gr.Row():# mobile_collapse=False
- submit = gr.Button("Submit", variant="primary")
- audio = gr.Audio(label="Output", interactive=False)
- gr.Markdown(info)
- gr.Markdown(""
- +f'
'
- +"")
-
- # actions
- submit.click(
- tts,
- [textbox, radio],
- [audio],
- )
- radio.change(lambda lang: CoquiTTS.langs[lang]["sentence"], radio, textbox)
-
-
-
-blocks.launch()
diff --git a/spaces/Pie31415/control-animation/annotator/uniformer/mmcv/image/misc.py b/spaces/Pie31415/control-animation/annotator/uniformer/mmcv/image/misc.py
deleted file mode 100644
index 3e61f05e3b05e4c7b40de4eb6c8eb100e6da41d0..0000000000000000000000000000000000000000
--- a/spaces/Pie31415/control-animation/annotator/uniformer/mmcv/image/misc.py
+++ /dev/null
@@ -1,44 +0,0 @@
-# Copyright (c) OpenMMLab. All rights reserved.
-import numpy as np
-
-import annotator.uniformer.mmcv as mmcv
-
-try:
- import torch
-except ImportError:
- torch = None
-
-
-def tensor2imgs(tensor, mean=(0, 0, 0), std=(1, 1, 1), to_rgb=True):
- """Convert tensor to 3-channel images.
-
- Args:
- tensor (torch.Tensor): Tensor that contains multiple images, shape (
- N, C, H, W).
- mean (tuple[float], optional): Mean of images. Defaults to (0, 0, 0).
- std (tuple[float], optional): Standard deviation of images.
- Defaults to (1, 1, 1).
- to_rgb (bool, optional): Whether the tensor was converted to RGB
- format in the first place. If so, convert it back to BGR.
- Defaults to True.
-
- Returns:
- list[np.ndarray]: A list that contains multiple images.
- """
-
- if torch is None:
- raise RuntimeError('pytorch is not installed')
- assert torch.is_tensor(tensor) and tensor.ndim == 4
- assert len(mean) == 3
- assert len(std) == 3
-
- num_imgs = tensor.size(0)
- mean = np.array(mean, dtype=np.float32)
- std = np.array(std, dtype=np.float32)
- imgs = []
- for img_id in range(num_imgs):
- img = tensor[img_id, ...].cpu().numpy().transpose(1, 2, 0)
- img = mmcv.imdenormalize(
- img, mean, std, to_bgr=to_rgb).astype(np.uint8)
- imgs.append(np.ascontiguousarray(img))
- return imgs
diff --git a/spaces/Pinwheel/GLIP-BLIP-Object-Detection-VQA/maskrcnn_benchmark/data/datasets/box_label_loader.py b/spaces/Pinwheel/GLIP-BLIP-Object-Detection-VQA/maskrcnn_benchmark/data/datasets/box_label_loader.py
deleted file mode 100644
index fddd7aa2b5b859d7b410cacd9c61800fd7190c51..0000000000000000000000000000000000000000
--- a/spaces/Pinwheel/GLIP-BLIP-Object-Detection-VQA/maskrcnn_benchmark/data/datasets/box_label_loader.py
+++ /dev/null
@@ -1,251 +0,0 @@
-import torch
-import numpy as np
-import math
-import base64
-import collections
-import pycocotools.mask as mask_utils
-
-from maskrcnn_benchmark.structures.bounding_box import BoxList
-from maskrcnn_benchmark.structures.segmentation_mask import SegmentationMask
-
-
-class LabelLoader(object):
- def __init__(self, labelmap, extra_fields=(), filter_duplicate_relations=False, ignore_attr=None, ignore_rel=None,
- mask_mode="poly"):
- self.labelmap = labelmap
- self.extra_fields = extra_fields
- self.supported_fields = ["class", "conf", "attributes", 'scores_all', 'boxes_all', 'feature', "mask"]
- self.filter_duplicate_relations = filter_duplicate_relations
- self.ignore_attr = set(ignore_attr) if ignore_attr != None else set()
- self.ignore_rel = set(ignore_rel) if ignore_rel != None else set()
- assert mask_mode == "poly" or mask_mode == "mask"
- self.mask_mode = mask_mode
-
- def __call__(self, annotations, img_size, remove_empty=False, load_fields=None):
- boxes = [obj["rect"] for obj in annotations]
- boxes = torch.as_tensor(boxes).reshape(-1, 4)
- target = BoxList(boxes, img_size, mode="xyxy")
-
- if load_fields is None:
- load_fields = self.extra_fields
-
- for field in load_fields:
- assert field in self.supported_fields, "Unsupported field {}".format(field)
- if field == "class":
- classes = self.add_classes(annotations)
- target.add_field("labels", classes)
- elif field == "conf":
- confidences = self.add_confidences(annotations)
- target.add_field("scores", confidences)
- elif field == "attributes":
- attributes = self.add_attributes(annotations)
- target.add_field("attributes", attributes)
- elif field == "scores_all":
- scores_all = self.add_scores_all(annotations)
- target.add_field("scores_all", scores_all)
- elif field == "boxes_all":
- boxes_all = self.add_boxes_all(annotations)
- target.add_field("boxes_all", boxes_all)
- elif field == "feature":
- features = self.add_features(annotations)
- target.add_field("box_features", features)
- elif field == "mask":
- masks, is_box_mask = self.add_masks(annotations, img_size)
- target.add_field("masks", masks)
- target.add_field("is_box_mask", is_box_mask)
-
- target = target.clip_to_image(remove_empty=remove_empty)
- return target
-
- def get_box_mask(self, rect, img_size):
- x1, y1, x2, y2 = rect[0], rect[1], rect[2], rect[3]
- if self.mask_mode == "poly":
- return [[x1, y1, x1, y2, x2, y2, x2, y1]]
- elif self.mask_mode == "mask":
- # note the order of height/width order in mask is opposite to image
- mask = np.zeros([img_size[1], img_size[0]], dtype=np.uint8)
- mask[math.floor(y1):math.ceil(y2), math.floor(x1):math.ceil(x2)] = 255
- encoded_mask = mask_utils.encode(np.asfortranarray(mask))
- encoded_mask["counts"] = encoded_mask["counts"].decode("utf-8")
- return encoded_mask
-
- def add_masks(self, annotations, img_size):
- masks = []
- is_box_mask = []
- for obj in annotations:
- if "mask" in obj:
- masks.append(obj["mask"])
- is_box_mask.append(0)
- else:
- masks.append(self.get_box_mask(obj["rect"], img_size))
- is_box_mask.append(1)
- masks = SegmentationMask(masks, img_size, mode=self.mask_mode)
- is_box_mask = torch.tensor(is_box_mask)
- return masks, is_box_mask
-
- def add_classes(self, annotations):
- class_names = [obj["class"] for obj in annotations]
- classes = [None] * len(class_names)
- for i in range(len(class_names)):
- classes[i] = self.labelmap['class_to_ind'][class_names[i]]
- return torch.tensor(classes)
-
- def add_confidences(self, annotations):
- confidences = []
- for obj in annotations:
- if "conf" in obj:
- confidences.append(obj["conf"])
- else:
- confidences.append(1.0)
- return torch.tensor(confidences)
-
- def add_attributes(self, annotations):
- # the maximal number of attributes per object is 16
- attributes = [[0] * 16 for _ in range(len(annotations))]
- for i, obj in enumerate(annotations):
- for j, attr in enumerate(obj["attributes"]):
- attributes[i][j] = self.labelmap['attribute_to_ind'][attr]
- return torch.tensor(attributes)
-
- def add_features(self, annotations):
- features = []
- for obj in annotations:
- features.append(np.frombuffer(base64.b64decode(obj['feature']), np.float32))
- return torch.tensor(features)
-
- def add_scores_all(self, annotations):
- scores_all = []
- for obj in annotations:
- scores_all.append(np.frombuffer(base64.b64decode(obj['scores_all']), np.float32))
- return torch.tensor(scores_all)
-
- def add_boxes_all(self, annotations):
- boxes_all = []
- for obj in annotations:
- boxes_all.append(np.frombuffer(base64.b64decode(obj['boxes_all']), np.float32).reshape(-1, 4))
- return torch.tensor(boxes_all)
-
- def relation_loader(self, relation_annos, target):
- if self.filter_duplicate_relations:
- # Filter out dupes!
- all_rel_sets = collections.defaultdict(list)
- for triplet in relation_annos:
- all_rel_sets[(triplet['subj_id'], triplet['obj_id'])].append(triplet)
- relation_annos = [np.random.choice(v) for v in all_rel_sets.values()]
-
- # get M*M pred_labels
- relation_triplets = []
- relations = torch.zeros([len(target), len(target)], dtype=torch.int64)
- for i in range(len(relation_annos)):
- if len(self.ignore_rel) != 0 and relation_annos[i]['class'] in self.ignore_rel:
- continue
- subj_id = relation_annos[i]['subj_id']
- obj_id = relation_annos[i]['obj_id']
- predicate = self.labelmap['relation_to_ind'][relation_annos[i]['class']]
- relations[subj_id, obj_id] = predicate
- relation_triplets.append([subj_id, obj_id, predicate])
-
- relation_triplets = torch.tensor(relation_triplets)
- target.add_field("relation_labels", relation_triplets)
- target.add_field("pred_labels", relations)
- return target
-
-
-class BoxLabelLoader(object):
- def __init__(self, labelmap, extra_fields=(), ignore_attrs=(),
- mask_mode="poly"):
- self.labelmap = labelmap
- self.extra_fields = extra_fields
- self.ignore_attrs = ignore_attrs
- assert mask_mode == "poly" or mask_mode == "mask"
- self.mask_mode = mask_mode
- self.all_fields = ["class", "mask", "confidence",
- "attributes_encode", "IsGroupOf", "IsProposal"]
-
- def __call__(self, annotations, img_size, remove_empty=True):
- boxes = [obj["rect"] for obj in annotations]
- boxes = torch.as_tensor(boxes).reshape(-1, 4)
- target = BoxList(boxes, img_size, mode="xyxy")
-
- for field in self.extra_fields:
- assert field in self.all_fields, "Unsupported field {}".format(field)
- if field == "class":
- classes = self.add_classes_with_ignore(annotations)
- target.add_field("labels", classes)
- elif field == "mask":
- masks, is_box_mask = self.add_masks(annotations, img_size)
- target.add_field("masks", masks)
- target.add_field("is_box_mask", is_box_mask)
- elif field == "confidence":
- confidences = self.add_confidences(annotations)
- target.add_field("confidences", confidences)
- elif field == "attributes_encode":
- attributes = self.add_attributes(annotations)
- target.add_field("attributes", attributes)
- elif field == "IsGroupOf":
- is_group = [1 if 'IsGroupOf' in obj and obj['IsGroupOf'] == 1 else 0
- for obj in annotations]
- target.add_field("IsGroupOf", torch.tensor(is_group))
- elif field == "IsProposal":
- is_proposal = [1 if "IsProposal" in obj and obj['IsProposal'] == 1 else 0
- for obj in annotations]
- target.add_field("IsProposal", torch.tensor(is_proposal))
-
- target = target.clip_to_image(remove_empty=remove_empty)
- return target
-
- def add_classes_with_ignore(self, annotations):
- class_names = [obj["class"] for obj in annotations]
- classes = [None] * len(class_names)
- if self.ignore_attrs:
- for i, obj in enumerate(annotations):
- if any([obj[attr] for attr in self.ignore_attrs if attr in obj]):
- classes[i] = -1
- for i, cls in enumerate(classes):
- if cls != -1:
- classes[i] = self.labelmap[class_names[i]] + 1 # 0 is saved for background
- return torch.tensor(classes)
-
- def add_masks(self, annotations, img_size):
- masks = []
- is_box_mask = []
- for obj in annotations:
- if "mask" in obj:
- masks.append(obj["mask"])
- is_box_mask.append(0)
- else:
- masks.append(self.get_box_mask(obj["rect"], img_size))
- is_box_mask.append(1)
- masks = SegmentationMask(masks, img_size, mode=self.mask_mode)
- is_box_mask = torch.tensor(is_box_mask)
- return masks, is_box_mask
-
- def get_box_mask(self, rect, img_size):
- x1, y1, x2, y2 = rect[0], rect[1], rect[2], rect[3]
- if self.mask_mode == "poly":
- return [[x1, y1, x1, y2, x2, y2, x2, y1]]
- elif self.mask_mode == "mask":
- # note the order of height/width order in mask is opposite to image
- mask = np.zeros([img_size[1], img_size[0]], dtype=np.uint8)
- mask[math.floor(y1):math.ceil(y2), math.floor(x1):math.ceil(x2)] = 255
- encoded_mask = mask_utils.encode(np.asfortranarray(mask))
- encoded_mask["counts"] = encoded_mask["counts"].decode("utf-8")
- return encoded_mask
-
- def add_confidences(self, annotations):
- confidences = []
- for obj in annotations:
- if "confidence" in obj:
- confidences.append(obj["confidence"])
- elif "conf" in obj:
- confidences.append(obj["conf"])
- else:
- confidences.append(1.0)
- return torch.tensor(confidences)
-
- def add_attributes(self, annotations):
- # we know that the maximal number of attributes per object is 16
- attributes = [[0] * 16 for _ in range(len(annotations))]
- for i, obj in enumerate(annotations):
- attributes[i][:len(obj["attributes_encode"])] = obj["attributes_encode"]
- return torch.tensor(attributes)
diff --git a/spaces/Politrees/RVC_V2_Huggingface_Version/utils.py b/spaces/Politrees/RVC_V2_Huggingface_Version/utils.py
deleted file mode 100644
index 62be8d03a8e8b839f8747310ef0ec0e82fb8ff0a..0000000000000000000000000000000000000000
--- a/spaces/Politrees/RVC_V2_Huggingface_Version/utils.py
+++ /dev/null
@@ -1,151 +0,0 @@
-import ffmpeg
-import numpy as np
-
-# import praatio
-# import praatio.praat_scripts
-import os
-import sys
-
-import random
-
-import csv
-
-platform_stft_mapping = {
- "linux": "stftpitchshift",
- "darwin": "stftpitchshift",
- "win32": "stftpitchshift.exe",
-}
-
-stft = platform_stft_mapping.get(sys.platform)
-# praatEXE = join('.',os.path.abspath(os.getcwd()) + r"\Praat.exe")
-
-
-def CSVutil(file, rw, type, *args):
- if type == "formanting":
- if rw == "r":
- with open(file) as fileCSVread:
- csv_reader = list(csv.reader(fileCSVread))
- return (
- (csv_reader[0][0], csv_reader[0][1], csv_reader[0][2])
- if csv_reader is not None
- else (lambda: exec('raise ValueError("No data")'))()
- )
- else:
- if args:
- doformnt = args[0]
- else:
- doformnt = False
- qfr = args[1] if len(args) > 1 else 1.0
- tmb = args[2] if len(args) > 2 else 1.0
- with open(file, rw, newline="") as fileCSVwrite:
- csv_writer = csv.writer(fileCSVwrite, delimiter=",")
- csv_writer.writerow([doformnt, qfr, tmb])
- elif type == "stop":
- stop = args[0] if args else False
- with open(file, rw, newline="") as fileCSVwrite:
- csv_writer = csv.writer(fileCSVwrite, delimiter=",")
- csv_writer.writerow([stop])
-
-
-def load_audio(file, sr, DoFormant, Quefrency, Timbre):
- converted = False
- DoFormant, Quefrency, Timbre = CSVutil("csvdb/formanting.csv", "r", "formanting")
- try:
- # https://github.com/openai/whisper/blob/main/whisper/audio.py#L26
- # This launches a subprocess to decode audio while down-mixing and resampling as necessary.
- # Requires the ffmpeg CLI and `ffmpeg-python` package to be installed.
- file = (
- file.strip(" ").strip('"').strip("\n").strip('"').strip(" ")
- ) # 防止小白拷路径头尾带了空格和"和回车
- file_formanted = file.strip(" ").strip('"').strip("\n").strip('"').strip(" ")
-
- # print(f"dofor={bool(DoFormant)} timbr={Timbre} quef={Quefrency}\n")
-
- if (
- lambda DoFormant: True
- if DoFormant.lower() == "true"
- else (False if DoFormant.lower() == "false" else DoFormant)
- )(DoFormant):
- numerator = round(random.uniform(1, 4), 4)
- # os.system(f"stftpitchshift -i {file} -q {Quefrency} -t {Timbre} -o {file_formanted}")
- # print('stftpitchshift -i "%s" -p 1.0 --rms -w 128 -v 8 -q %s -t %s -o "%s"' % (file, Quefrency, Timbre, file_formanted))
-
- if not file.endswith(".wav"):
- if not os.path.isfile(f"{file_formanted}.wav"):
- converted = True
- # print(f"\nfile = {file}\n")
- # print(f"\nfile_formanted = {file_formanted}\n")
- converting = (
- ffmpeg.input(file_formanted, threads=0)
- .output(f"{file_formanted}.wav")
- .run(
- cmd=["ffmpeg", "-nostdin"],
- capture_stdout=True,
- capture_stderr=True,
- )
- )
- else:
- pass
-
- file_formanted = (
- f"{file_formanted}.wav"
- if not file_formanted.endswith(".wav")
- else file_formanted
- )
-
- print(f" · Formanting {file_formanted}...\n")
-
- os.system(
- '%s -i "%s" -q "%s" -t "%s" -o "%sFORMANTED_%s.wav"'
- % (
- stft,
- file_formanted,
- Quefrency,
- Timbre,
- file_formanted,
- str(numerator),
- )
- )
-
- print(f" · Formanted {file_formanted}!\n")
-
- # filepraat = (os.path.abspath(os.getcwd()) + '\\' + file).replace('/','\\')
- # file_formantedpraat = ('"' + os.path.abspath(os.getcwd()) + '/' + 'formanted'.join(file_formanted) + '"').replace('/','\\')
- # print("%sFORMANTED_%s.wav" % (file_formanted, str(numerator)))
-
- out, _ = (
- ffmpeg.input(
- "%sFORMANTED_%s.wav" % (file_formanted, str(numerator)), threads=0
- )
- .output("-", format="f32le", acodec="pcm_f32le", ac=1, ar=sr)
- .run(
- cmd=["ffmpeg", "-nostdin"], capture_stdout=True, capture_stderr=True
- )
- )
-
- try:
- os.remove("%sFORMANTED_%s.wav" % (file_formanted, str(numerator)))
- except Exception:
- pass
- print("couldn't remove formanted type of file")
-
- else:
- out, _ = (
- ffmpeg.input(file, threads=0)
- .output("-", format="f32le", acodec="pcm_f32le", ac=1, ar=sr)
- .run(
- cmd=["ffmpeg", "-nostdin"], capture_stdout=True, capture_stderr=True
- )
- )
- except Exception as e:
- raise RuntimeError(f"Failed to load audio: {e}")
-
- if converted:
- try:
- os.remove(file_formanted)
- except Exception:
- pass
- print("couldn't remove converted type of file")
- converted = False
-
- return np.frombuffer(out, np.float32).flatten()
diff --git a/spaces/Preetesh/VideoSummaryfromYouTubeVideo/app.py b/spaces/Preetesh/VideoSummaryfromYouTubeVideo/app.py
deleted file mode 100644
index ea0d92944bdf4e1fde3b7b46810816a97c6b4964..0000000000000000000000000000000000000000
--- a/spaces/Preetesh/VideoSummaryfromYouTubeVideo/app.py
+++ /dev/null
@@ -1,22 +0,0 @@
-import gradio as gr
-from summarize import Summarizer
-
-interface = gr.Interface(fn = Summarizer,
- inputs = [gr.inputs.Textbox(lines=2,
- placeholder="Enter your link...",
- label='YouTube Video Link'),
- gr.inputs.Radio(["mT5", "BART"], type="value", label='Model')],
- outputs = [gr.outputs.Textbox(
- label="Summary")],
-
- title = "Video Summary Generator",
- examples = [
- ['https://www.youtube.com/watch?v=OaeYUm06in0&list=PLHgX2IExbFouJoqEr8JMF5MbZSbyC91-L&t=5761s', 'BART'],
- ['https://www.youtube.com/watch?v=U5OD8MjYnOM', 'BART'],
- ['https://www.youtube.com/watch?v=Gfr50f6ZBvo', 'BART'],
- ['https://www.youtube.com/watch?v=G4hL5Om4IJ4&t=2680s', 'BART'],
- ['https://www.youtube.com/watch?v=0Jd7fJgFkPU&t=8776s', 'mT5']
- ],
- enable_queue=True)
-
-interface.launch(debug=True)
\ No newline at end of file
diff --git a/spaces/RMXK/RVC_HFF/tools/infer/train-index-v2.py b/spaces/RMXK/RVC_HFF/tools/infer/train-index-v2.py
deleted file mode 100644
index cbeed5d4fbf65fcb9a697a99d5f7b41c844e95d6..0000000000000000000000000000000000000000
--- a/spaces/RMXK/RVC_HFF/tools/infer/train-index-v2.py
+++ /dev/null
@@ -1,79 +0,0 @@
-"""
-格式:直接cid为自带的index位;aid放不下了,通过字典来查,反正就5w个
-"""
-import os
-import traceback
-import logging
-
-logger = logging.getLogger(__name__)
-
-from multiprocessing import cpu_count
-
-import faiss
-import numpy as np
-from sklearn.cluster import MiniBatchKMeans
-
-# ###########如果是原始特征要先写save
-n_cpu = 0
-if n_cpu == 0:
- n_cpu = cpu_count()
-inp_root = r"./logs/anz/3_feature768"
-npys = []
-listdir_res = list(os.listdir(inp_root))
-for name in sorted(listdir_res):
- phone = np.load("%s/%s" % (inp_root, name))
- npys.append(phone)
-big_npy = np.concatenate(npys, 0)
-big_npy_idx = np.arange(big_npy.shape[0])
-np.random.shuffle(big_npy_idx)
-big_npy = big_npy[big_npy_idx]
-logger.debug(big_npy.shape) # (6196072, 192)#fp32#4.43G
-if big_npy.shape[0] > 2e5:
- # if(1):
- info = "Trying doing kmeans %s shape to 10k centers." % big_npy.shape[0]
- logger.info(info)
- try:
- big_npy = (
- MiniBatchKMeans(
- n_clusters=10000,
- verbose=True,
- batch_size=256 * n_cpu,
- compute_labels=False,
- init="random",
- )
- .fit(big_npy)
- .cluster_centers_
- )
- except:
- info = traceback.format_exc()
- logger.warn(info)
-
-np.save("tools/infer/big_src_feature_mi.npy", big_npy)
-
-##################train+add
-# big_npy=np.load("/bili-coeus/jupyter/jupyterhub-liujing04/vits_ch/inference_f0/big_src_feature_mi.npy")
-n_ivf = min(int(16 * np.sqrt(big_npy.shape[0])), big_npy.shape[0] // 39)
-index = faiss.index_factory(768, "IVF%s,Flat" % n_ivf) # mi
-logger.info("Training...")
-index_ivf = faiss.extract_index_ivf(index) #
-index_ivf.nprobe = 1
-index.train(big_npy)
-faiss.write_index(
- index, "tools/infer/trained_IVF%s_Flat_baseline_src_feat_v2.index" % (n_ivf)
-)
-logger.info("Adding...")
-batch_size_add = 8192
-for i in range(0, big_npy.shape[0], batch_size_add):
- index.add(big_npy[i : i + batch_size_add])
-faiss.write_index(
- index, "tools/infer/added_IVF%s_Flat_mi_baseline_src_feat.index" % (n_ivf)
-)
-"""
-大小(都是FP32)
-big_src_feature 2.95G
- (3098036, 256)
-big_emb 4.43G
- (6196072, 192)
-big_emb双倍是因为求特征要repeat后再加pitch
-
-"""
diff --git a/spaces/Ramse/TTS_Hindi/modules/hifigan/model/mpd.py b/spaces/Ramse/TTS_Hindi/modules/hifigan/model/mpd.py
deleted file mode 100644
index a22b2d14b0b3dd6187ec64fecbdb25f966cd624e..0000000000000000000000000000000000000000
--- a/spaces/Ramse/TTS_Hindi/modules/hifigan/model/mpd.py
+++ /dev/null
@@ -1,24 +0,0 @@
-from torch import nn
-from model.period_discriminator import PeriodDiscriminator
-
-
-class MPD(nn.Module):
- def __init__(self, periods=[2, 3, 5, 7, 11]):
- super(MPD, self).__init__()
- self.discriminators = nn.ModuleList([ PeriodDiscriminator(periods[0]),
- PeriodDiscriminator(periods[1]),
- PeriodDiscriminator(periods[2]),
- PeriodDiscriminator(periods[3]),
- PeriodDiscriminator(periods[4]),
- ])
-
- def forward(self, x):
- scores = list()
- feats = list()
- for key, disc in enumerate(self.discriminators):
- score, feat = disc(x)
- scores.append(score)
- feats.append(feat)
- return scores, feats
-
-
diff --git a/spaces/Raspberry-ai/main/.env/lib/python3.11/site-packages/pip/_vendor/platformdirs/__init__.py b/spaces/Raspberry-ai/main/.env/lib/python3.11/site-packages/pip/_vendor/platformdirs/__init__.py
deleted file mode 100644
index 9d513dcf17767c6f80d721cb4191decc7633571c..0000000000000000000000000000000000000000
--- a/spaces/Raspberry-ai/main/.env/lib/python3.11/site-packages/pip/_vendor/platformdirs/__init__.py
+++ /dev/null
@@ -1,340 +0,0 @@
-"""
-Utilities for determining application-specific dirs. See for details and
-usage.
-"""
-from __future__ import annotations
-
-import os
-import sys
-from pathlib import Path
-from typing import TYPE_CHECKING
-
-if TYPE_CHECKING:
- from pip._vendor.typing_extensions import Literal # pragma: no cover
-
-from .api import PlatformDirsABC
-from .version import __version__, __version_info__
-
-
-def _set_platform_dir_class() -> type[PlatformDirsABC]:
- if sys.platform == "win32":
- from pip._vendor.platformdirs.windows import Windows as Result
- elif sys.platform == "darwin":
- from pip._vendor.platformdirs.macos import MacOS as Result
- else:
- from pip._vendor.platformdirs.unix import Unix as Result
-
- if os.getenv("ANDROID_DATA") == "/data" and os.getenv("ANDROID_ROOT") == "/system":
-
- if os.getenv("SHELL") is not None:
- return Result
-
- from pip._vendor.platformdirs.android import _android_folder
-
- if _android_folder() is not None:
- from pip._vendor.platformdirs.android import Android
-
- return Android # return to avoid redefinition of result
-
- return Result
-
-
-PlatformDirs = _set_platform_dir_class() #: Currently active platform
-AppDirs = PlatformDirs #: Backwards compatibility with appdirs
-
-
-def user_data_dir(
- appname: str | None = None,
- appauthor: str | None | Literal[False] = None,
- version: str | None = None,
- roaming: bool = False,
-) -> str:
- """
- :param appname: See `appname `.
- :param appauthor: See `appauthor `.
- :param version: See `version `.
- :param roaming: See `roaming `.
- :returns: data directory tied to the user
- """
- return PlatformDirs(appname=appname, appauthor=appauthor, version=version, roaming=roaming).user_data_dir
-
-
-def site_data_dir(
- appname: str | None = None,
- appauthor: str | None | Literal[False] = None,
- version: str | None = None,
- multipath: bool = False,
-) -> str:
- """
- :param appname: See `appname `.
- :param appauthor: See `appauthor `.
- :param version: See `version `.
- :param multipath: See `roaming `.
- :returns: data directory shared by users
- """
- return PlatformDirs(appname=appname, appauthor=appauthor, version=version, multipath=multipath).site_data_dir
-
-
-def user_config_dir(
- appname: str | None = None,
- appauthor: str | None | Literal[False] = None,
- version: str | None = None,
- roaming: bool = False,
-) -> str:
- """
- :param appname: See `appname `.
- :param appauthor: See `appauthor `.
- :param version: See `version `.
- :param roaming: See `roaming `.
- :returns: config directory tied to the user
- """
- return PlatformDirs(appname=appname, appauthor=appauthor, version=version, roaming=roaming).user_config_dir
-
-
-def site_config_dir(
- appname: str | None = None,
- appauthor: str | None | Literal[False] = None,
- version: str | None = None,
- multipath: bool = False,
-) -> str:
- """
- :param appname: See `appname `.
- :param appauthor: See `appauthor `.
- :param version: See `version `.
- :param multipath: See `roaming `.
- :returns: config directory shared by the users
- """
- return PlatformDirs(appname=appname, appauthor=appauthor, version=version, multipath=multipath).site_config_dir
-
-
-def user_cache_dir(
- appname: str | None = None,
- appauthor: str | None | Literal[False] = None,
- version: str | None = None,
- opinion: bool = True,
-) -> str:
- """
- :param appname: See `appname `.
- :param appauthor: See `appauthor `.
- :param version: See `version `.
- :param opinion: See `roaming `.
- :returns: cache directory tied to the user
- """
- return PlatformDirs(appname=appname, appauthor=appauthor, version=version, opinion=opinion).user_cache_dir
-
-
-def user_state_dir(
- appname: str | None = None,
- appauthor: str | None | Literal[False] = None,
- version: str | None = None,
- roaming: bool = False,
-) -> str:
- """
- :param appname: See `appname `.
- :param appauthor: See `appauthor `.
- :param version: See `version `.
- :param roaming: See `roaming `.
- :returns: state directory tied to the user
- """
- return PlatformDirs(appname=appname, appauthor=appauthor, version=version, roaming=roaming).user_state_dir
-
-
-def user_log_dir(
- appname: str | None = None,
- appauthor: str | None | Literal[False] = None,
- version: str | None = None,
- opinion: bool = True,
-) -> str:
- """
- :param appname: See `appname `.
- :param appauthor: See `appauthor `.
- :param version: See `version `.
- :param opinion: See `roaming `.
- :returns: log directory tied to the user
- """
- return PlatformDirs(appname=appname, appauthor=appauthor, version=version, opinion=opinion).user_log_dir
-
-
-def user_documents_dir() -> str:
- """
- :returns: documents directory tied to the user
- """
- return PlatformDirs().user_documents_dir
-
-
-def user_runtime_dir(
- appname: str | None = None,
- appauthor: str | None | Literal[False] = None,
- version: str | None = None,
- opinion: bool = True,
-) -> str:
- """
- :param appname: See `appname `.
- :param appauthor: See `appauthor `.
- :param version: See `version `.
- :param opinion: See `opinion `.
- :returns: runtime directory tied to the user
- """
- return PlatformDirs(appname=appname, appauthor=appauthor, version=version, opinion=opinion).user_runtime_dir
-
-
-def user_data_path(
- appname: str | None = None,
- appauthor: str | None | Literal[False] = None,
- version: str | None = None,
- roaming: bool = False,
-) -> Path:
- """
- :param appname: See `appname `.
- :param appauthor: See `appauthor `.
- :param version: See `version `.
- :param roaming: See `roaming `.
- :returns: data path tied to the user
- """
- return PlatformDirs(appname=appname, appauthor=appauthor, version=version, roaming=roaming).user_data_path
-
-
-def site_data_path(
- appname: str | None = None,
- appauthor: str | None | Literal[False] = None,
- version: str | None = None,
- multipath: bool = False,
-) -> Path:
- """
- :param appname: See `appname `.
- :param appauthor: See `appauthor