diff --git a/spaces/101-5/gpt4free/g4f/Provider/Provider.py b/spaces/101-5/gpt4free/g4f/Provider/Provider.py
deleted file mode 100644
index 12c23333f87185e5fa0ae8f368540c816ab079f8..0000000000000000000000000000000000000000
--- a/spaces/101-5/gpt4free/g4f/Provider/Provider.py
+++ /dev/null
@@ -1,15 +0,0 @@
-import os
-from ..typing import sha256, Dict, get_type_hints
-
-url = None
-model = None
-supports_stream = False
-needs_auth = False
-
-def _create_completion(model: str, messages: list, stream: bool, **kwargs):
- return
-
-
-params = f'g4f.Providers.{os.path.basename(__file__)[:-3]} supports: ' + \
- '(%s)' % ', '.join(
- [f"{name}: {get_type_hints(_create_completion)[name].__name__}" for name in _create_completion.__code__.co_varnames[:_create_completion.__code__.co_argcount]])
diff --git a/spaces/1acneusushi/gradio-2dmoleculeeditor/data/Download Password for Unlock Install Euro Truck Simulator 2 Avoid Scams and Malware.md b/spaces/1acneusushi/gradio-2dmoleculeeditor/data/Download Password for Unlock Install Euro Truck Simulator 2 Avoid Scams and Malware.md
deleted file mode 100644
index 6e9353c5523a6cd69a4b2d4bdc4059d2ccb2c34c..0000000000000000000000000000000000000000
--- a/spaces/1acneusushi/gradio-2dmoleculeeditor/data/Download Password for Unlock Install Euro Truck Simulator 2 Avoid Scams and Malware.md
+++ /dev/null
@@ -1,90 +0,0 @@
-
-
Download Password for Unlock Install Euro Truck Simulator 2
-
Do you want to play one of the most realistic and immersive truck driving simulation games ever made? Do you want to travel across Europe as a king of the road, delivering cargo from one city to another, exploring new places and earning money? If yes, then you might be interested in downloading Euro Truck Simulator 2, a game developed by SCS Software that has won many awards and praises from critics and players alike. But before you can enjoy this amazing game, you might face a challenge: how to get a password to unlock install Euro Truck Simulator 2? In this article, we will explain what is Euro Truck Simulator 2, why do you need a password to unlock install it, and how to get one easily and safely.
-
What is Euro Truck Simulator 2?
-
Euro Truck Simulator 2 is a truck driving simulation game that was released in 2012 for Windows, Linux and Mac OS. It is the sequel to the original Euro Truck Simulator that was released in 2008. In this game, you can choose from a variety of trucks from different manufacturers, customize them with various accessories and paint jobs, and drive them across Europe, delivering cargo from one destination to another. You can also hire drivers, buy garages, expand your business and explore more than 70 cities in 13 countries. The game features realistic physics, graphics, sound effects and traffic rules that make you feel like you are really driving a truck on the road.
-
download Password for unlock Install Euro Truck Simulator 2
The easiest and safest way to download Euro Truck Simulator 2 is to visit the official website of the game: https://eurotrucksimulator2.com/download.php. There you can find the latest version of the game for Windows, Linux and Mac OS. You can also download a free trial version that lets you play for an hour before you decide whether to buy the full game or not. The full game costs €19.99 (or equivalent in your local currency) and includes all the updates and patches released so far. You can pay with credit card, PayPal or other methods depending on your region. Once you pay for the game, you will receive an email with a link to download the game installer and a product key that you will need to activate the game.
-
Steam
-
Another option to download Euro Truck Simulator 2 is to use Steam, a popular online platform for digital distribution of games. You can find Euro Truck Simulator 2 on Steam by searching for it on the Steam store or by following this link: https://store.steampowered.com/app/227300/Euro_Truck_Simulator_2/. The price of the game on Steam is the same as on the official website: €19.99 (or equivalent in your local currency). You can pay with credit card, PayPal or other methods supported by Steam. Once you buy the game on Steam, it will be added to your Steam library and you can download it anytime you want. You will also receive automatic updates and access to Steam features such as achievements, cloud saves and workshop.
-
Other sources
-
Besides the official website and Steam, there are also other sources where you can download Euro Truck Simulator 2. However, these sources are not authorized by SCS Software and may not be safe or reliable. Some of these sources are:
-
-
Torrent sites: These are websites where you can download files shared by other users using peer-to-peer networks. However, these files may be infected with viruses or malware that can harm your computer or steal your personal information. They may also be incomplete or corrupted and not work properly.
-
Crack sites: These are websites where you can download cracked versions of games that bypass the activation or verification process. However, these versions may not be updated or compatible with the latest patches or mods. They may also contain hidden code that can damage your system or compromise your security.
-
Survey sites: These are websites where you have to complete surveys or offers in order to get access to download links or passwords for games. However, these surveys or offers may be scams that trick you into giving away your personal or financial information or subscribing to unwanted services.
-
-
Why do you need a password to unlock install Euro Truck Simulator 2?
-
If you have downloaded Euro Truck Simulator 2 from one of these unauthorized sources mentioned above, you may encounter a problem when trying to install it: you need a password to unlock it. This means that the file you have downloaded is encrypted or compressed with a password that prevents you from opening it or extracting its contents. This is done by some people who upload these files for various reasons:
-
The problem of fake or corrupted files
-
Some people upload fake or corrupted files that pretend to be Euro Truck Simulator 2 but are actually something else. They do this to deceive users into downloading their files instead of the real ones. They may also do this to earn money from advertising or referrals when users visit their websites or click on their links. By encrypting or compressing their files with passwords, they make it harder for users to detect their frauds until they have already downloaded them.
-
The problem of surveys or scams
-
Some people upload real files but lock them with passwords that they claim to provide only after users complete surveys or offers on their websites. They do this to earn money from commissions or rewards when users fill out their surveys or sign up for their offers. However, these surveys or offers may be scams that trick users into giving away their personal or financial information or subscribing to unwanted services. Even if users complete these surveys or offers successfully, they may not receive the passwords they were promised.
-
The problem of legal issues
-
Some people upload real files but lock them with passwords that they claim to protect them from legal issues such as copyright infringement or piracy. They do this to avoid being detected by authorities or sued by developers for distributing their games illegally without permission. However, this does not make their actions legal or ethical. By downloading their files without paying for them properly
0a6ba089eb
-
-
\ No newline at end of file
diff --git a/spaces/1acneusushi/gradio-2dmoleculeeditor/data/Epson l800 pvc card software Reviews and testimonials from satisfied customers.md b/spaces/1acneusushi/gradio-2dmoleculeeditor/data/Epson l800 pvc card software Reviews and testimonials from satisfied customers.md
deleted file mode 100644
index 13211a0bd2a713c23a880030fe521c74d49d0eec..0000000000000000000000000000000000000000
--- a/spaces/1acneusushi/gradio-2dmoleculeeditor/data/Epson l800 pvc card software Reviews and testimonials from satisfied customers.md
+++ /dev/null
@@ -1,96 +0,0 @@
-
-
Epson l800 pvc card software: A complete guide
-
If you are looking for a way to print your own ID cards, business cards, or other types of plastic cards, you might be interested in Epson l800 pvc card software. This software allows you to use your Epson l800 printer to print high-quality and durable cards on pvc material. In this article, we will show you what Epson l800 pvc card software is, why you need it, how to install it, how to use it, and some tips and tricks to get the most out of it.
-
What is Epson l800 pvc card software?
-
Epson l800 pvc card software is a program that enables you to print plastic cards using your Epson l800 printer. The software comes with various templates and features that allow you to customize your cards according to your needs. You can also design your own cards from scratch using the built-in tools or import your own images and logos. The software supports different sizes and shapes of cards, such as standard, round, oval, or custom.
Epson l800 pvc card software is a great solution for anyone who wants to create their own cards without spending a lot of money or time. With this software, you can print cards on demand, whenever you need them, without having to order them from a third-party service. You can also personalize your cards with your own information, design, and branding, making them more professional and unique. Moreover, printing cards on pvc material ensures that they are durable, waterproof, and resistant to fading and tearing.
-
How to install Epson l800 pvc card software
-
Installing Epson l800 pvc card software is easy and straightforward. Just follow these steps:
-
Step 1: Download the software from the official website
-
The first thing you need to do is download the software from the official website of Epson. You can find it by searching for "Epson l800 pvc card software" on Google or by clicking on this link. The file size is about 50 MB and it is compatible with Windows XP, Vista, 7, 8, and 10.
-
Step 2: Run the installer and follow the instructions
-
Once you have downloaded the file, double-click on it to run the installer. You will see a welcome screen that asks you to select your language. Choose your preferred language and click "Next". Then, accept the license agreement and click "Next" again. You will then be asked to choose a destination folder for the installation. You can leave it as default or change it if you want. Click "Next" once more and then click "Install". The installation process will take a few minutes.
-
Step 3: Connect your Epson l800 printer to your computer
-
After the installation is complete, you need to connect your Epson l800 printer to your computer using a USB cable. Make sure that both devices are turned on and that the printer driver is installed correctly. You can check this by going to "Devices and Printers" in your Control Panel and seeing if your printer is listed there. If not, you may need to download and install the driver from here. Once your printer is connected and recognized by your computer, you are ready to use Epson l800 pvc card software.
-
How to use Epson l800 pvc card software
-
Using Epson l800 pvc card software is fun and easy. Just follow these steps:
-
Step 1: Select the template or design your own card
-
To start using Epson l800 pvc card software, launch it from your desktop or Start menu. You will see a main window that shows different tabs for different types of cards. You can choose from ID cards, business cards, membership cards, loyalty cards, gift cards, etc. Each tab has several templates that you can use as a base for your card. You can also create your own template by clicking on "New" at the bottom of the window.
Once you have selected or created a template, you can edit it by adding text, images, logos, barcodes, QR codes, etc. You can also change the font, color, size, alignment, rotation, etc. of each element. To add an element, simply drag and drop it from the left panel onto the card preview on the right panel. To edit an element, double-click on it or right-click on it and select "Properties". To delete an element, select it and press "Delete" on your keyboard.
-
Step 2: Adjust the settings and preview the card
-
After you have finished designing your card, you can adjust some settings before printing it. To do this, click on "File" at the top left corner of the window and select "Print Settings". Here you can choose the paper size (A4 or Letter), orientation (Portrait or Landscape), margins (Top, Bottom, Left, Right), number of copies (1-99), etc. You can also preview how your card will look like when printed by clicking on "File" again and selecting "Print Preview". Here you can zoom in or out of the card image and see if everything looks good.
-
Step 3: Load the pvc card tray and print the card
-
The final step is to load the pvc card tray into your printer and print your card. To do this, you need a special tray that holds up to 10 standard-sized (85 x 54 mm) pvc cards at a time. You can buy this tray online or from a local store that sells printer accessories. To load the tray into your printer, follow these steps: - Open the paper output tray of your printer. - Remove any paper from the paper feed slot. - Insert one end of the tray into the paper feed slot until it stops. - Align the other end of the tray with the paper guides on both sides of the slot. - Make sure that there are no gaps between the tray and the slot. To print your card using Epson l800 pvc card software, follow these steps: - Click on "File" at the top left corner of the window and select "Print". - Choose your printer name from the drop-down menu and click "OK". - Wait for your printer to print your card. - Repeat the process for each card you want to print. Congratulations! You have successfully printed your own pvc card using Epson l800 pvc card software.
-
Tips and tricks for Epson l800 pvc card software
-
To get the best results from using Epson l800 pvc card software, here are some tips and tricks that you should keep in mind:
-
Tip 1: Use high-quality pvc cards for better results
-
The quality of your printed cards depends largely on the quality of the pvc cards that you use. Therefore, it is advisable that you use high-quality pvc cards that are smooth, thick, and glossy. These cards will ensure that your images are clear, sharp, and vibrant, and that your the most out of it. We hope that this guide has been helpful and informative for you. If you have any questions or feedback, please feel free to contact us. We would love to hear from you.
-
Now that you have learned everything about Epson l800 pvc card software, why not try it yourself and see how amazing it is? You can download the software for free from here and start printing your own cards today. You will be amazed by the results and the possibilities.
-
Thank you for reading this article and happy printing!
-
FAQs
-
Here are some frequently asked questions about Epson l800 pvc card software:
-
Q: What are the system requirements for Epson l800 pvc card software?
-
A: The minimum system requirements for Epson l800 pvc card software are: - Windows XP, Vista, 7, 8, or 10 - Pentium 4 processor or higher - 512 MB RAM or higher - 100 MB free disk space or higher - USB port - CD-ROM drive
-
Q: What are the supported card sizes and shapes for Epson l800 pvc card software?
-
A: Epson l800 pvc card software supports the following card sizes and shapes: - Standard (85 x 54 mm) - Round (50 mm diameter) - Oval (70 x 50 mm) - Custom (any size within 85 x 54 mm)
-
Q: How many cards can I print with one ribbon?
-
A: The number of cards that you can print with one ribbon depends on the type of ribbon and the print mode that you use. For example, with a YMCKO ribbon, you can print up to 200 cards in full color on one side and black on the other side. With a K ribbon, you can print up to 1000 cards in black on one side. For more information, refer to the PDF guide Ribbon Information.
-
Q: How can I save my card designs for future use?
-
A: You can save your card designs for future use by clicking on "File" at the top left corner of the window and selecting "Save As". You can choose a name and a location for your file and click "Save". The file will be saved as a .crd format that can be opened by Epson l800 pvc card software.
-
Q: How can I import my own images and logos into Epson l800 pvc card software?
-
A: You can import your own images and logos into Epson l800 pvc card software by clicking on "Image" at the left panel and selecting "Import Image". You can browse to the folder where your image or logo is stored and select it. The image or logo will be added to the card preview on the right panel. You can resize, rotate, crop, or adjust the image or logo as you wish.
- 0a6ba089eb
-
-
\ No newline at end of file
diff --git a/spaces/1acneusushi/gradio-2dmoleculeeditor/data/Gta Sa To Psp Rar Rapidshare Com [TOP].md b/spaces/1acneusushi/gradio-2dmoleculeeditor/data/Gta Sa To Psp Rar Rapidshare Com [TOP].md
deleted file mode 100644
index 549435b87e168d2f84fa50756f351a0ad777f4f2..0000000000000000000000000000000000000000
--- a/spaces/1acneusushi/gradio-2dmoleculeeditor/data/Gta Sa To Psp Rar Rapidshare Com [TOP].md
+++ /dev/null
@@ -1,26 +0,0 @@
-
-`
How to Download GTA San Andreas for PSP from Rapidshare
`
-`
GTA San Andreas is one of the most popular and iconic games in the Grand Theft Auto series. It features an open-world environment, a rich story, and a variety of missions and activities. However, GTA San Andreas was originally released for PlayStation 2, Xbox, and PC, and it is not officially available for PSP. So how can you play this game on your handheld device?
One way is to download a compressed file of GTA San Andreas for PSP from Rapidshare, a file-sharing service that allows you to upload and download files quickly and easily. However, this method has some risks and drawbacks that you should be aware of before proceeding. In this article, we will explain how to download GTA San Andreas for PSP from Rapidshare, what are the pros and cons of this method, and what are some alternatives that you can try.
`
-`
How to Download GTA San Andreas for PSP from Rapidshare
`
-`
To download GTA San Andreas for PSP from Rapidshare, you will need the following:
`
-`
`
-`
A PSP device with custom firmware installed. Custom firmware is a modified version of the official PSP software that allows you to run homebrew applications and games that are not authorized by Sony. You can find tutorials on how to install custom firmware on your PSP online.
`
-`
A memory stick with enough space to store the game file. GTA San Andreas for PSP is about 1 GB in size, so you will need at least 2 GB of free space on your memory stick.
`
-`
A computer with an internet connection and a program that can extract RAR files. RAR files are compressed files that can contain multiple files inside them. You will need a program like WinRAR or 7-Zip to extract the game file from the RAR file.
`
-`
A USB cable to connect your PSP to your computer.
`
-`
`
-`
Once you have everything ready, follow these steps:
-`
-``
-`
Go to Rapidshare.com and search for "Gta Sa To Psp Rar". You should see several results with different file sizes and upload dates. Choose the one that has the most downloads and positive ratings.
`
-`
Click on the download button and wait for the file to be downloaded to your computer. Depending on your internet speed and the file size, this may take some time.
`
-`
Once the download is complete, locate the RAR file on your computer and extract it using your program of choice. You should see a folder named "GTA_SA_PSP" with several files inside it.
`
-`
Connect your PSP to your computer using the USB cable and turn on the USB mode on your PSP. Your computer should recognize your PSP as a removable drive.
`
-`
Copy the folder "GTA_SA_PSP" from your computer to the root directory of your memory stick. This is usually the drive letter followed by a colon (e.g., E:).
`
-`
Disconnect your PSP from your computer and turn off the USB mode on your PSP.
`
-`
Go to the game menu on your PSP and select "Memory Stick". You should see an icon for GTA San Andreas. Select it and press X to start the game.
`
-`` cec2833e83
-
-
\ No newline at end of file
diff --git a/spaces/1gistliPinn/ChatGPT4/Examples/Big Fish Games Universal Crack 44 [UPD].md b/spaces/1gistliPinn/ChatGPT4/Examples/Big Fish Games Universal Crack 44 [UPD].md
deleted file mode 100644
index ef4e53709749cac3e62de7bca1cb2640bb3dc5c7..0000000000000000000000000000000000000000
--- a/spaces/1gistliPinn/ChatGPT4/Examples/Big Fish Games Universal Crack 44 [UPD].md
+++ /dev/null
@@ -1,36 +0,0 @@
-
-
How to Enjoy Big Fish Games for Free with Universal Crack 44
-
Big Fish Games is one of the most popular and well-known developers and publishers of casual games, with hundreds of titles across various genres and platforms. Whether you like hidden object, puzzle, adventure, time management, or card games, you can find something to suit your taste and mood at Big Fish Games.
However, there is one problem: most of these games are not free. You can download and play them for an hour as a trial, but after that you need to buy them or subscribe to Big Fish Games Unlimited. This can be quite expensive and inconvenient for some gamers who just want to have some fun without spending money.
-
Fortunately, there is a solution: Big Fish Games Universal Crack 44. This is a small and simple tool that can generate valid activation keys for any Big Fish game you want. You don't need to use any patches or other medicines, just run the keygen, select the game from the list, copy the fingerprint and the name, and generate the key. Then you can enter the key in the game's registration dialog and enjoy it as a full version.
-
What is Big Fish Games Universal Crack 44?
-
Big Fish Games Universal Crack 44 is a keygen made by Vovan from Braga Software. It can create keys for more than 4000 Big Fish games, and the supported games list is growing bigger and bigger. The keygen is very easy to use and works on both Windows and Mac platforms.
-
-
The keygen works by exploiting a flaw in the Big Fish Games registration system. It uses a universal algorithm that can match any game's fingerprint and generate a corresponding key. The key fits all games in the list, so you don't need to worry about compatibility issues.
-
How to Use Big Fish Games Universal Crack 44?
-
Using Big Fish Games Universal Crack 44 is very simple. Here are the steps you need to follow:
-
-
Download and install your desired Big Fish game (make sure it's listed in the keygen's list)
-
Download and run the keygen, and select the game from the list
-
Click the 'Open reg Dialog' button, and locate and open the game's main program (.exe file)
-
A window will open and ask you to enter a key
-
Copy the value of 'Fingerprint' in this window, and paste it into the keygen
-
Type a 'Name' and generate a 'Key'
-
Copy the generated key back to the window asking for a key, and click 'OK'
-
Done! You have activated your game as a full version
-
-
Tips and Tricks for Big Fish Games Universal Crack 44
-
Here are some tips and tricks that can help you get the most out of Big Fish Games Universal Crack 44:
-
-
You don't need to use BigFish Game Manager to play BigFish games. There is usually a hidden EXE file that is the name of the game in the game installation folder. Running this hidden EXE file will either run the game as a full version or prompt for a key (that's where the keygen comes in).
-
If you want to play BigFish games on Mac, you can try to use "Wine HQ" to run this keygen. But we don't know if the keygen will work or not. On Mac, under "/contents/Resources/" folder, the name of the hidden file should be like ".gamename".
-
You can use any name you want when generating keys. The name doesn't affect the validity of the key.
-
You can share your keys with your friends or family members if they want to play BigFish games too. But please don't distribute them online or abuse them.
-
-
Conclusion
-
Big Fish Games Universal Crack 44 is a great tool for casual gamers who want to enjoy BigFish games for free. It can generate keys for any BigFish game you want, without using any patches or other medicines. It's easy to use, fast, and reliable.
-
If you like BigFish games works, especially their famous casual puzzle series, you should give this keygen a try. You will be amazed by how many games you can unlock with it.
-
But remember: this keygen is only for personal study and research purposes. Any form of commercial behaviors are strictly prohibited. If you really like BigFish games works, please support them by buying their games or subscribing to their service.
3cee63e6c2
-
-
\ No newline at end of file
diff --git a/spaces/1gistliPinn/ChatGPT4/Examples/Cambridge Latin Course Book 1 Stage 10 Statuae Translation Learn Latin with Stories and Flashcards.md b/spaces/1gistliPinn/ChatGPT4/Examples/Cambridge Latin Course Book 1 Stage 10 Statuae Translation Learn Latin with Stories and Flashcards.md
deleted file mode 100644
index 7883a0b8930ae1e7af5e38fa3a1b1d923b9fdbf4..0000000000000000000000000000000000000000
--- a/spaces/1gistliPinn/ChatGPT4/Examples/Cambridge Latin Course Book 1 Stage 10 Statuae Translation Learn Latin with Stories and Flashcards.md
+++ /dev/null
@@ -1,6 +0,0 @@
-
-
-Oct 25, 2017 - Including all the major races and legendary lords from both games, Mortal Empires also allows you to start the campaign as any DLC or Free-LC ... Mortal Kombat 10 (Mortal Kombat X Mobile) - VKontakte.
-Buy Mortal Kombat X on PS4 online store.
-Video review of the game Mortal Kombat X on PS4.
-There are Mortal Kombat X trailers in the video.
-Mortal Kombat X. Mortal Kombat 10 (Mortal Kombat X Mobile) - VKontakte.
-Mortal Kombat X is a video game from the Mortal Kombat fighting game series in the genre.
-The game features various characters from the Mortal Kombat series of games.
-Mortal Kombat X is a computer game in the fighting game genre, developed by American studio Warner Bros. 8a78ff9644
-
-
-
diff --git a/spaces/1pelhydcardo/ChatGPT-prompt-generator/assets/Cmo descargar Bus Simulator 2023 APK y disfrutar de la experiencia de conduccin ms realista.md b/spaces/1pelhydcardo/ChatGPT-prompt-generator/assets/Cmo descargar Bus Simulator 2023 APK y disfrutar de la experiencia de conduccin ms realista.md
deleted file mode 100644
index 7b9cdd7a75fd981db111dcc85ce6c8cd01dfbaf8..0000000000000000000000000000000000000000
--- a/spaces/1pelhydcardo/ChatGPT-prompt-generator/assets/Cmo descargar Bus Simulator 2023 APK y disfrutar de la experiencia de conduccin ms realista.md
+++ /dev/null
@@ -1,25 +0,0 @@
-
-
Descargar Bus Simulator 2023 APK: Cómo convertirse en un conductor de autobús profesional
- ¿Te gustan los juegos de simulación de conducción? ¿Te gustaría conducir diferentes tipos de autobuses en ciudades y países reales? ¿Te gustaría personalizar tu autobús y competir con otros jugadores en línea? Si la respuesta es sí, entonces te encantará Bus Simulator 2023 APK, un juego gratuito para Android que te pone en el asiento del conductor y te convierte en un auténtico conductor de autobús. En este artículo, te explicaremos qué es Bus Simulator 2023 APK, cómo descargarlo e instalarlo en tu dispositivo, cómo jugarlo y cuáles son sus ventajas y desventajas. ¡Sigue leyendo y prepárate para vivir una aventura sobre ruedas!
¿Qué es Bus Simulator 2023 APK?
- Bus Simulator 2023 APK es un juego de simulación de conducción de autobuses desarrollado por Ovidiu Pop, un estudio especializado en juegos de este género. El juego te permite conducir una gran variedad de autobuses modernos urbanos, turísticos y escolares con interiores realistas y un motor físico innovador de 1:1. El juego cuenta con mapas detallados de todo el mundo, incluyendo Estados Unidos, Sudamérica, Europa, Dubai, Shanghai y más. Además, el juego tiene varios modos de juego, como carrera, conducción libre y multijugador en línea con amigos. El juego también tiene un sistema de gestión de compañía de autobuses, donde puedes contratar conductores para tus autobuses y programar rutas personalizadas.
Características principales del juego
- Bus Simulator 2023 APK tiene muchas características que lo hacen un juego divertido y realista. Algunas de ellas son: - Interiores detallados y personalizables. Puedes abrir y cerrar las puertas del autobús, ver a las personas entrar y salir, ajustar el aire acondicionado, poner banderas, pegatinas y muchos más accesorios. - Controles fáciles e intuitivos. Puedes elegir entre conducir con el volante, los botones o la inclinación del dispositivo. También puedes cambiar la vista de la cámara entre primera y tercera persona. - Ubicaciones variadas y desafiantes. Puedes conducir por la ciudad, el campo, la montaña, el desierto y la nieve. Además, puedes elegir entre diferentes horas del día y condiciones climáticas. - Conduce niños a la escuela usando tres modelos diferentes de autobús escolar. - Sistema inteligente de tráfico. Debes respetar las señales, los semáforos y las normas de circulación. También debes tener cuidado con los peatones, los ciclistas y los otros vehículos. - Juego cooperativo en línea inmersivo. Puedes añadir a tus amigos, usar el chat en vivo e invitarlos a jugar en rutas cooperativas. También puedes ver las clasificaciones, los logros y las estadísticas. - Opciones de personalización del autobús. Puedes elegir entre diferentes tipos de autobuses, como diésel, híbrido, eléctrico, articulado o escolar. También puedes cambiar el color, las piezas del cuerpo, el aire acondicionado, - Opciones de personalización del autobús. Puedes elegir entre diferentes tipos de autobuses, como diésel, híbrido, eléctrico, articulado o escolar. También puedes cambiar el color, las piezas del cuerpo, el aire acondicionado, las luces y los neumáticos de tu autobús. - Sistema de gestión de compañía de autobuses. Puedes crear tu propia compañía de autobuses, contratar conductores, asignarles rutas y vehículos, y ver sus estadísticas y ganancias. También puedes comprar nuevos autobuses y mejorarlos.
Requisitos y compatibilidad del dispositivo
- Bus Simulator 2023 APK es un juego que requiere un dispositivo Android con al menos 4 GB de RAM y 1 GB de espacio libre. El juego es compatible con Android 5.0 o superior. El juego también requiere una conexión a internet estable para jugar en línea y descargar contenido adicional.
¿Cómo descargar e instalar Bus Simulator 2023 APK?
- Si quieres descargar Bus Simulator 2023 APK en tu dispositivo Android, debes seguir estos pasos:
Pasos para descargar el archivo APK
- - Abre el navegador web de tu dispositivo y busca "Bus Simulator 2023 APK". - Entra en el sitio web oficial del juego o en uno de los sitios web confiables que ofrecen el archivo APK. - Haz clic en el botón de descarga y espera a que se complete la descarga. - Si te aparece un mensaje de advertencia sobre la instalación de aplicaciones desconocidas, haz clic en "Permitir" o "Aceptar".
Pasos para instalar el archivo APK
- - Una vez que hayas descargado el archivo APK, ve a la carpeta de descargas de tu dispositivo y busca el archivo "Bus Simulator 2023 APK". - Haz clic en el archivo y selecciona "Instalar". - Espera a que se complete la instalación y haz clic en "Abrir" o "Finalizar". - Disfruta del juego.
¿Cómo jugar a Bus Simulator 2023 APK?
- Para jugar a Bus Simulator 2023 APK, debes seguir estos pasos:
Modos de juego disponibles
- - Elige el modo de juego que prefieras: carrera, conducción libre o multijugador en línea. - En el modo carrera, debes completar diferentes misiones y objetivos para ganar dinero y experiencia. Puedes elegir entre diferentes tipos de rutas, como urbanas, turísticas o escolares. También puedes elegir entre diferentes niveles de dificultad, como fácil, normal o difícil. - En el modo conducción libre, puedes explorar los mapas a tu gusto y sin restricciones. Puedes cambiar el tipo de autobús, la hora del día y el clima cuando quieras. También puedes activar o desactivar el tráfico y las señales. - En el modo multijugador en línea, puedes jugar con tus amigos o con otros jugadores alrededor del mundo. Puedes crear o unirte a una sala de juego, elegir una ruta cooperativa y comunicarte con el chat en vivo. También puedes ver las clasificaciones y los logros.
Consejos y trucos para mejorar tu experiencia
- Para jugar mejor a Bus Simulator 2023 APK, te recomendamos seguir estos consejos y trucos: - Ajusta los controles y la sensibilidad del volante según tu preferencia. - Usa el mapa y el GPS para orientarte y seguir la ruta correcta. - Respeta las normas de tráfico y evita chocar con otros vehículos o peatones. - Presta atención a las indicaciones de los pasajeros y recógelos y déjalos en las paradas adecuadas. - Mantén un nivel óptimo de combustible, velocidad y temperatura del motor. - Personaliza tu autobús con los accesorios que más te gusten. - Mejora tu autobús con las piezas que aumenten su rendimiento y su eficiencia. - Contrata conductores para tu compañía de autobuses y asignales rutas rentables. - Compra nuevos autobuses y amplía tu flota. - Diviértete con tus amigos en el modo multijugador en línea.
Ventajas y desventajas de descargar Bus Simulator 2023 APK
- Descargar Bus Simulator 2023 APK tiene sus ventajas y desventajas. A continuación, te las resumimos:
Ventajas de descargar el juego
- - Es un - Es un juego gratuito y sin anuncios que te ofrece una experiencia de conducción realista y divertida. - Es un juego que tiene una gran variedad de autobuses, mapas, modos de juego y opciones de personalización. - Es un juego que tiene unos gráficos de alta calidad, unos efectos de sonido envolventes y una física realista. - Es un juego que te permite jugar con tus amigos o con otros jugadores en línea y comunicarte con ellos. - Es un juego que te permite crear y gestionar tu propia compañía de autobuses y ver tus progresos y estadísticas.
Desventajas de descargar el juego
- - Es un juego que requiere un dispositivo Android potente y con suficiente espacio libre para funcionar correctamente. - Es un juego que requiere una conexión a internet estable para jugar en línea y descargar contenido adicional. - Es un juego que puede consumir mucha batería y datos móviles si se juega durante mucho tiempo. - Es un juego que puede tener algunos errores o fallos técnicos en algunas ocasiones.
Conclusión
- Bus Simulator 2023 APK es un juego de simulación de conducción de autobuses que te ofrece la oportunidad de convertirte en un conductor profesional. El juego tiene muchos aspectos positivos, como su realismo, su variedad, su diversión y su modo multijugador. Sin embargo, también tiene algunos aspectos negativos, como sus requisitos, su conexión a internet y sus posibles problemas técnicos. En general, creemos que es un juego que vale la pena probar si te gustan los juegos de este género. Esperamos que este artículo te haya sido útil y que disfrutes del juego.
Preguntas frecuentes
-
¿Qué es Bus Simulator 2023 APK?
-Bus Simulator 2023 APK es un juego de simulación de conducción de autobuses gratuito para Android.
¿Cómo descargar Bus Simulator 2023 APK?
-Para descargar Bus Simulator 2023 APK, debes buscar el archivo APK en el navegador web de tu dispositivo y descargarlo desde el sitio web oficial del juego o desde uno de los sitios web confiables que lo ofrecen. Luego, debes instalar el archivo APK en tu dispositivo siguiendo las instrucciones.
¿Cómo jugar a Bus Simulator 2023 APK?
-Para jugar a Bus Simulator 2023 APK, debes elegir el modo de juego que prefieras: carrera, conducción libre o multijugador en línea. Luego, debes conducir el autobús siguiendo la ruta asignada, respetando las normas de tráfico y atendiendo a los pasajeros. También puedes personalizar tu autobús, comprar nuevos autobuses, contratar conductores y crear tu propia compañía de autobuses.
¿Qué ventajas tiene descargar Bus Simulator 2023 APK?
-Descargar Bus Simulator 2023 APK tiene ventajas como su gratuidad, su realismo, su variedad, su diversión y su modo multijugador.
¿Qué desventajas tiene descargar Bus Simulator 2023 APK?
-Descargar Bus Simulator 2023 APK tiene desventajas como sus requisitos, su conexión a internet y sus posibles problemas técnicos.
-
197e85843d
-
-
\ No newline at end of file
diff --git a/spaces/1pelhydcardo/ChatGPT-prompt-generator/assets/Download Film Yes or No 2 Subtitle Bahasa Indonesia Uji Cinta Kim dan Pie di Dua Tempat Berbeda.md b/spaces/1pelhydcardo/ChatGPT-prompt-generator/assets/Download Film Yes or No 2 Subtitle Bahasa Indonesia Uji Cinta Kim dan Pie di Dua Tempat Berbeda.md
deleted file mode 100644
index 439ec2749511c251fb23e500f5ccf674f645ecda..0000000000000000000000000000000000000000
--- a/spaces/1pelhydcardo/ChatGPT-prompt-generator/assets/Download Film Yes or No 2 Subtitle Bahasa Indonesia Uji Cinta Kim dan Pie di Dua Tempat Berbeda.md
+++ /dev/null
@@ -1,133 +0,0 @@
-
-
Download Film Yes or No 2 Subtitle Bahasa Indonesia: Cara dan Situs Terbaik
-
Apakah kamu penggemar film romantis Thailand? Jika iya, mungkin kamu sudah pernah menonton film Yes or No yang dirilis pada tahun 2010. Film ini menceritakan kisah cinta antara dua mahasiswi yang tinggal satu kamar, Pie dan Kim. Namun, apa yang terjadi dengan hubungan mereka setelah lulus kuliah? Apakah mereka masih bersama atau berpisah?
-
download film yes or no 2 subtitle bahasa indonesia
Jawabannya ada di film Yes or No 2, sekuel dari film pertama yang dirilis pada tahun 2012. Film ini melanjutkan kisah Pie dan Kim yang harus menjalani magang di tempat yang berbeda. Pie pergi ke selatan untuk bekerja di pusat perikanan, sementara Kim pergi ke utara untuk bekerja di pertanian. Di sana, mereka bertemu dengan orang-orang baru yang membuat hubungan mereka semakin rumit.
-
Jika kamu penasaran dengan kelanjutan cerita Pie dan Kim, kamu bisa download film Yes or No 2 subtitle bahasa Indonesia dengan mudah dan cepat. Berikut ini adalah cara dan situs terbaik untuk download film Yes or No 2 subtitle bahasa Indonesia.
-
Apa itu Film Yes or No 2?
-
Sinopsis Film Yes or No 2
-
Film Yes or No 2 adalah film romantis Thailand yang disutradarai oleh Sarasawadee Wongsompetch dan ditulis oleh Nepalee. Film ini adalah sekuel dari film Yes or No yang sukses meraih popularitas di Thailand dan negara-negara Asia lainnya. Film ini mengisahkan tentang hubungan antara Pie (Sucharat Manaying) dan Kim (Supanart Jittaleela), dua mahasiswi yang jatuh cinta saat tinggal satu kamar di asrama.
-
Setelah lulus kuliah, Pie dan Kim harus berpisah untuk menjalani magang di tempat yang berbeda. Pie pergi ke selatan untuk bekerja di pusat perikanan, sementara Kim pergi ke utara untuk bekerja di pertanian. Di sana, mereka bertemu dengan orang-orang baru yang membuat hubungan mereka semakin rumit. Pie berteman dengan Yam (Apittha Klay-udom), seorang gadis tomboy yang menyukainya. Kim bertemu dengan Maysa (Permpreda Sakulsiripong), seorang gadis cantik yang menarik perhatiannya.
-
Akankah Pie dan Kim bisa mempertahankan cinta mereka meski jarak dan godaan? Atau akankah mereka menyerah dan mencari pasangan baru? Temukan jawabannya di film Yes or No 2.
-
Pemain dan Karakter Film Yes or No 2
-
Berikut ini adalah daftar pemain dan karakter film Yes or No 2:
-
-
Sucharat Manaying sebagai Pie, seorang gadis feminin yang mencintai Kim.
Supanart Jittaleela sebagai Kim, seorang gadis tomboy yang mencintai Pie.
-
Apittha Klay-udom sebagai Yam, seorang gadis tomboy yang menyukai Pie.
-
Permpreda Sakulsiripong sebagai Maysa, seorang gadis cantik yang menyukai Kim.
-
Sushar Manaying sebagai Jane, kakak Pie yang tidak setuju dengan hubungan Pie dan Kim.
-
Inthira Yeunyong sebagai Nerd, teman Pie yang selalu mendukungnya.
-
Puttipong Promsaka Na Sakolnakorn sebagai P'Van, bos Pie di pusat perikanan.
-
Soranut Yupanun sebagai P'King, bos Kim di pertanian.
-
-
Trailer Film Yes or No 2
-
Berikut ini adalah trailer film Yes or No 2 yang bisa kamu tonton untuk melihat cuplikan adegan-adegan menarik di film ini:
-
Cara download film yes or no 2 dengan subtitle bahasa indonesia
-Situs download film yes or no 2 sub indo gratis dan mudah
-Review film yes or no 2, film lesbian Thailand yang bikin baper
-Download film yes or no 2 full movie HD subtitle bahasa indonesia
-Nonton streaming film yes or no 2 online sub indo di JuraganFilm[^1^]
-Film yes or no 2, kisah cinta dua gadis yang diuji oleh jarak
-Download film yes or no 2 sub indo di HP android tanpa ribet
-Link download film yes or no 2 subtitle bahasa indonesia terbaru
-Sinopsis film yes or no 2, lanjutan dari film yes or no pertama
-Download film yes or no 2 sub indo di laptop atau PC dengan cepat
-Film yes or no 2, apakah Kim dan Pie bisa bertahan bersama?
-Download film yes or no 2 sub indo kualitas bluray 720p
-Tips download film yes or no 2 subtitle bahasa indonesia tanpa iklan
-Film yes or no 2, bagaimana akting Supanart Jittaleela dan Sushar Manaying?
-Download film yes or no 2 sub indo di Telegram atau WhatsApp
-Film yes or no 2, apa pesan moral yang bisa kita ambil?
-Download film yes or no 2 sub indo dengan server Google Drive
-Film yes or no 2, apakah ada adegan hot atau kiss scene?
-Download film yes or no 2 sub indo dengan ukuran file kecil
-Film yes or no 2, apakah ada versi lain atau spin-off?
-Download film yes or no 2.5 sub indo, sekuel dari film yes or no 2[^2^]
-Film yes or no 2.5, apa yang terjadi setelah Kim dan Pie kembali bersama?
-Download film yes or no 2.5 full movie HD subtitle bahasa indonesia
-Nonton streaming film yes or no 2.5 online sub indo di JuraganFilm[^2^]
-Film yes or no 2.5, apakah ada karakter baru atau cameo?
-Download film yes or no 2.5 sub indo di HP android dengan mudah
-Film yes or no 2.5, bagaimana chemistry antara Supanart Jittaleela dan Sushar Manaying?
-Download film yes or no 2.5 sub indo di laptop atau PC dengan cepat
-Film yes or no 2.5, apakah ada adegan lucu atau romantis?
-Download film yes or no 2.5 sub indo kualitas bluray 720p
-Tips download film yes or no 2.5 subtitle bahasa indonesia tanpa iklan
-Film yes or no 2.5, apakah ada konflik atau drama?
-Download film yes or no 2.5 sub indo dengan server Google Drive
-Film yes or no 2.5, apakah ada lagu tema atau soundtrack?
-Download film yes or no 2.5 sub indo dengan ukuran file kecil
-Film yes or no 2.5, apakah ada ending yang happy atau sad?
-Download film yes or no 3 sub indo, lanjutan dari film yes or no 2.5
-Film yes or no 3, apakah Kim dan Pie masih bersama?
-Download film yes or no 3 full movie HD subtitle bahasa indonesia
-Nonton streaming film yes or no 3 online sub indo di JuraganFilm
-Film yes or no 3, apakah ada perubahan dalam cerita atau karakter?
-Download film yes or no 3 sub indo di HP android tanpa ribet
-Film yes or no 3, bagaimana penampilan Supanart Jittaleela dan Sushar Manaying?
-Download film yes or no 3 sub indo di laptop atau PC dengan cepat
-Film yes or no 3, apakah ada adegan menegangkan atau mengharukan?
-Download film yes or no 3 sub indo kualitas bluray 720p
-Tips download film yes or no 3 subtitle bahasa indonesia tanpa iklan
-Film yes or no 3, apakah ada pesan inspiratif yang bisa kita dapatkan?
-
-
Mengapa Download Film Yes or No 2 Subtitle Bahasa Indonesia?
-
Alasan Menonton Film Yes or No 2
-
Film Yes or No 2 adalah film yang cocok untuk kamu yang suka dengan genre romantis dan komedi. Film ini menawarkan cerita yang menghibur dan menyentuh tentang cinta, persahabatan, dan kehidupan. Film ini juga menampilkan akting yang natural dan ekspresif dari para pemainnya, terutama Sucharat Manaying dan Supanart Jittaleela yang berhasil memerankan karakter Pie dan Kim dengan baik. Film ini juga memiliki soundtrack yang enak didengar dan sesuai dengan suasana film.
-
Film Yes or No 2 juga adalah film yang bisa membuat kamu berpikir tentang hubungan asmara yang sebenarnya. Film ini menggambarkan tantangan dan konflik yang sering dialami oleh pasangan yang berbeda latar belakang, orientasi seksual, dan pandangan hidup. Film ini juga menunjukkan bagaimana cara mengatasi masalah-masalah tersebut dengan komunikasi, pengertian, dan kompromi. Film ini juga memberikan pesan positif tentang pentingnya menghargai diri sendiri dan orang lain, serta menjalani hidup dengan jujur dan bahagia.
-
Keuntungan Download Film Yes or No 2 Subtitle Bahasa Indonesia
-
Jika kamu ingin menonton film Yes or No 2, ada beberapa keuntungan jika kamu download film Yes or No 2 subtitle bahasa Indonesia. Berikut ini adalah beberapa keuntungannya:
-
-
Kamu bisa menonton film Yes or No 2 kapan saja dan di mana saja tanpa perlu khawatir kehabisan kuota internet atau sinyal.
-
Kamu bisa menonton film Yes or No 2 dengan kualitas gambar dan suara yang baik tanpa gangguan iklan atau buffering.
-
Kamu bisa menonton film Yes or No 2 dengan subtitle bahasa Indonesia yang akurat dan mudah dipahami tanpa perlu mencari-cari terjemahannya di internet.
-
Kamu bisa menonton film Yes or No 2 bersama teman-teman atau keluarga tanpa perlu membayar tiket bioskop atau sewa DVD.
-
Kamu bisa menonton film Yes or No 2 berulang-ulang tanpa perlu khawatir kehilangan file atau rusak.
-
Bagaimana Cara Download Film Yes or No 2 Subtitle Bahasa Indonesia?
-
Langkah-langkah Download Film Yes or No 2 Subtitle Bahasa Indonesia
-
Untuk download film Yes or No 2 subtitle bahasa Indonesia, kamu bisa mengikuti langkah-langkah berikut ini:
-
-
Kunjungi salah satu situs download film Yes or No 2 subtitle bahasa Indonesia yang akan kami rekomendasikan di bawah ini.
-
Cari film Yes or No 2 dengan menggunakan fitur pencarian atau kategori yang tersedia di situs tersebut.
-
Pilih kualitas dan format film yang kamu inginkan, misalnya 720p, 1080p, MP4, MKV, dll.
-
Klik tombol download atau tautan magnet yang ada di halaman film tersebut.
-
Tunggu proses download selesai. Jika kamu menggunakan tautan magnet, kamu perlu menggunakan aplikasi torrent seperti uTorrent atau BitTorrent untuk mendownload filmnya.
-
Setelah film selesai didownload, buka file film tersebut dengan menggunakan aplikasi pemutar video seperti VLC Media Player atau GOM Player.
-
Jika film tidak memiliki subtitle bahasa Indonesia, kamu perlu mendownload file subtitle terpisah dari situs-situs yang akan kami rekomendasikan di bawah ini.
-
Simpan file subtitle dengan nama yang sama dengan file film dan letakkan di folder yang sama.
-
Buka file film dengan aplikasi pemutar video dan pilih opsi untuk menampilkan subtitle.
-
Nikmati menonton film Yes or No 2 subtitle bahasa Indonesia.
-
-
Situs-situs Download Film Yes or No 2 Subtitle Bahasa Indonesia
-
Berikut ini adalah beberapa situs download film Yes or No 2 subtitle bahasa Indonesia yang bisa kamu kunjungi:
-
YIFY Subtitles
-
YIFY Subtitles adalah situs download subtitle film yang populer dan terpercaya. Situs ini menyediakan subtitle film dalam berbagai bahasa, termasuk bahasa Indonesia. Situs ini juga memiliki tampilan yang sederhana dan mudah digunakan. Kamu bisa mencari subtitle film Yes or No 2 dengan mengetikkan judulnya di kolom pencarian atau dengan memilih kategori berdasarkan genre, tahun, atau negara. Kamu bisa mendownload subtitle film Yes or No 2 dengan mengklik tombol download yang ada di halaman subtitle tersebut. Kamu juga bisa melihat rating, komentar, dan ulasan dari pengguna lain tentang subtitle tersebut.
Subscene adalah situs download subtitle film yang lainnya yang cukup populer dan terpercaya. Situs ini juga menyediakan subtitle film dalam berbagai bahasa, termasuk bahasa Indonesia. Situs ini juga memiliki tampilan yang sederhana dan mudah digunakan. Kamu bisa mencari subtitle film Yes or No 2 dengan mengetikkan judulnya di kolom pencarian atau dengan memilih kategori berdasarkan genre, tahun, atau negara. Kamu bisa mendownload subtitle film Yes or No 2 dengan mengklik tombol download yang ada di halaman subtitle tersebut. Kamu juga bisa melihat rating, komentar, dan ulasan dari pengguna lain tentang subtitle tersebut.
iSubtitles.org adalah situs download subtitle film yang lainnya yang cukup populer dan terpercaya. Situs ini juga menyediakan subtitle film dalam berbagai bahasa, termasuk bahasa Indonesia. Situs ini juga memiliki tampilan yang sederhana dan mudah digunakan. Kamu bisa mencari subtitle film Yes or No 2 dengan mengetikkan judulnya di kolom pencarian atau dengan memilih kategori berdasarkan genre, tahun, atau negara. Kamu bisa mendownload subtitle film Yes or No 2 dengan mengklik tombol download yang ada di halaman subtitle tersebut. Kamu juga bisa melihat rating, komentar, dan ulasan dari pengguna lain tentang subtitle tersebut.
Subs.dog adalah situs download subtitle film yang lainnya yang cukup populer dan terpercaya. Situs ini juga menyediakan subtitle film dalam berbagai bahasa, termasuk bahasa Indonesia. Situs ini juga memiliki tampilan yang sederhana dan mudah digunakan. Kamu bisa mencari subtitle film Yes or No 2 dengan mengetikkan judulnya di kolom pencarian atau dengan memilih kategori berdasarkan genre, tahun, atau negara. Kamu bisa mendownload subtitle film Yes or No 2 dengan mengklik tombol download yang ada di halaman subtitle tersebut. Kamu juga bisa melihat rating, komentar, dan ulasan dari pengguna lain tentang subtitle tersebut.
Film Yes or No 2 adalah film romantis Thailand yang merupakan sekuel dari film Yes or No yang dirilis pada tahun 2010. Film ini menceritakan tentang hubungan antara Pie dan Kim, dua mahasiswi yang jatuh cinta saat tinggal satu kamar di asrama. Film ini menggambarkan tantangan dan konflik yang mereka hadapi setelah lulus kuliah dan harus berpisah untuk menjalani magang di tempat yang berbeda.
-
Jika kamu ingin menonton film Yes or No 2, kamu bisa download film Yes or No 2 subtitle bahasa Indonesia dengan mudah dan cepat. Kamu bisa mengikuti langkah-langkah yang kami jelaskan di atas dan mengunjungi salah satu situs download film Yes or No 2 subtitle bahasa Indonesia yang kami rekomendasikan di atas. Dengan begitu, kamu bisa menonton film Yes or No 2 dengan kualitas gambar dan suara yang baik, subtitle bahasa Indonesia yang akurat, dan tanpa gangguan iklan atau buffering.
-
Selamat menonton film Yes or No 2 subtitle bahasa Indonesia dan semoga artikel ini bermanfaat untuk kamu.
-
FAQ
-
Berikut ini adalah beberapa pertanyaan yang sering diajukan tentang film Yes or No 2:
-
-
Apakah film Yes or No 2 ada di Netflix?
-
Jawab: Tidak, film Yes or No 2 tidak ada di Netflix. Kamu bisa download film Yes or No 2 subtitle bahasa Indonesia dari situs-situs yang kami rekomendasikan di atas.
-
Apakah film Yes or No 2 ada sekuelnya?
-
Jawab: Ya, film Yes or No 2 ada sekuelnya yaitu film Yes or No 2.5 yang dirilis pada tahun 2015. Film ini menceritakan tentang hubungan antara Wine (Pimpakan Bangchawong) dan Pii (Chansakorn Kittiwattanakorn), dua teman dekat Pie dan Kim yang juga jatuh cinta.
-
Apakah film Yes or No 2 berdasarkan kisah nyata?
-
Jawab: Tidak, film Yes or No 2 tidak berdasarkan kisah nyata. Film ini adalah karya fiksi yang ditulis oleh Nepalee dan disutradarai oleh Sarasawadee Wongsompetch.
-
Apakah film Yes or No 2 mendapat penghargaan?
-
Jawab: Ya, film Yes or No 2 mendapat beberapa penghargaan seperti Best Actress untuk Sucharat Manaying di Maya Awards 2013, Best Movie Soundtrack untuk lagu "Forever Love" oleh Tina Sup panart Jittaleela di Maya Awards 2013, dan Best Movie Poster di Thailand National Film Association Awards 2013.
-
Apakah film Yes or No 2 cocok untuk semua umur?
-
Jawab: Tidak, film Yes or No 2 tidak cocok untuk semua umur. Film ini mengandung tema dan adegan yang sensitif dan kontroversial, seperti hubungan sesama jenis, ciuman, dan konflik keluarga. Film ini juga menggunakan bahasa yang kasar dan tidak sopan di beberapa bagian. Film ini lebih cocok untuk ditonton oleh orang dewasa atau remaja yang sudah berpikiran terbuka dan dewasa.
- 197e85843d
-
-
\ No newline at end of file
diff --git a/spaces/1phancelerku/anime-remove-background/Crafting and Building APK The Ultimate Game for Minecraft Fans.md b/spaces/1phancelerku/anime-remove-background/Crafting and Building APK The Ultimate Game for Minecraft Fans.md
deleted file mode 100644
index 8f189ff50ac09bdc79e54cf9d4c7b7f3a096ae88..0000000000000000000000000000000000000000
--- a/spaces/1phancelerku/anime-remove-background/Crafting and Building APK The Ultimate Game for Minecraft Fans.md
+++ /dev/null
@@ -1,114 +0,0 @@
-
-
Crafting and Building APK Mirror: A Guide for Creative Gamers
-
Do you like building games? Do you want to unleash your imagination and create your own worlds? If so, you might be interested in Crafting and Building, a free game that lets you do just that. But what if you can't access the game from the official app store, or you want to try a different version of the game? That's where an APK mirror comes in handy. In this article, we will explain what Crafting and Building is, what an APK mirror is, how to download Crafting and Building from an APK mirror, and what are some alternatives to Crafting and Building.
-
What is Crafting and Building?
-
Crafting and Building is a new free building game that was released in 2020 by GeneRe. It is inspired by Minecraft, but it has its own features and style. The game is available for Android devices, and it has over 50 million downloads on Google Play. The game is rated for everyone, and it is suitable for the whole family.
An APK mirror is a website that hosts APK files, which are the installation files for Android apps. APK files can be downloaded from an APK mirror and installed on your device manually, without using the official app store. This can be useful if you want to access apps that are not available in your region, or if you want to try different versions of apps that are not updated on the app store.
-
Why would someone want to download Crafting and Building from an APK mirror?
-
There are several reasons why someone might want to download Crafting and Building from an APK mirror. For example:
-
-
You live in a country where Crafting and Building is not available on Google Play, or it is blocked by your network provider.
-
You want to play Crafting and Building on a device that does not support Google Play, such as a Kindle Fire or a Chromebook.
-
You want to try a modded version of Crafting and Building that has extra features or cheats.
-
You want to play an older version of Crafting and Building that has less bugs or more compatibility.
-
-
However, downloading Crafting and Building from an APK mirror also comes with some risks and drawbacks. We will discuss them later in this article.
-
Features of Crafting and Building
-
Crafting and Building is a game that offers a lot of fun and creativity for its players. Here are some of the features that make it stand out:
-
Gameplay
-
The gameplay of Crafting and Building is similar to Minecraft, but with some differences. You can explore a randomly generated world made of blocks, collect resources, craft items, build structures, and interact with animals and villagers. You can also choose between different modes, such as survival mode, where you have to deal with hunger, health, and enemies; or creative mode, where you have unlimited resources and no threats. You can also switch between first-person and third-person views.
-
Graphics
-
The graphics of Crafting and Building are colorful and pixelated, giving the game a retro feel. The game also has smooth animations and high fps, making it enjoyable to play. The game supports day-night cycles, weather effects, shadows, lighting, and water reflections. You can also adjust the graphics settings according to your device's performance.
-
Multiplayer
-
Crafting and Building has a multiplayer mode that allows you to play online with your friends or other players around the world. You can join existing servers or create your own private server. You can chat with other players, collaborate on building projects, or compete in mini-games. Multiplayer mode is a lot of fun, and you can also chat with other players, collaborate on building projects, or compete in mini-games. Multiplayer mode is free to play, but you need to register an account and have a stable internet connection.
-
Customization
-
Crafting and Building lets you customize your character and your world. You can choose from different skins, clothes, hairstyles, and accessories for your avatar. You can also change the texture pack of the game, and use different blocks and items to create your own style. You can also use the in-game editor to create your own maps and share them with other players.
-
crafting and building game free download apk
-crafting and building mod apk unlimited resources
-crafting and building online multiplayer apk
-crafting and building apk latest version 2020
-crafting and building apk for pc windows 10
-crafting and building apk pure no ads
-crafting and building survival mode apk
-crafting and building creative mode apk
-crafting and building apk android 4.4
-crafting and building apk ios iphone
-crafting and building apk mirror site safe
-crafting and building apk old version 2018
-crafting and building apk update 2021
-crafting and building apk offline play
-crafting and building apk hack cheats
-crafting and building apk full unlocked
-crafting and building apk no verification
-crafting and building apk install guide
-crafting and building apk review ratings
-crafting and building apk tips tricks
-crafting and building adventure map apk
-crafting and building city builder apk
-crafting and building pixel art apk
-crafting and building sandbox simulator apk
-crafting and building exploration world apk
-crafting and building skins editor apk
-crafting and building furniture mod apk
-crafting and building animals pets apk
-crafting and building weapons tools apk
-crafting and building vehicles cars apk
-crafting and building farm garden apk
-crafting and building castle mine apk
-crafting and building school education apk
-crafting and building horror scary apk
-crafting and building fantasy magic apk
-crafting and building medieval kingdom apk
-crafting and building modern house apk
-crafting and building underwater ocean apk
-crafting and building space galaxy apk
-crafting and building jungle forest apk
-crafting and building desert pyramid apk
-crafting and building snow winter apk
-crafting and building tropical island apk
-crafting and building volcano lava apk
-
How to download Crafting and Building from an APK mirror
-
If you want to download Crafting and Building from an APK mirror, you need to follow these steps:
-
-
Find a reliable APK mirror website that hosts the Crafting and Building APK file. You can use a search engine or check the reviews of other users. Some examples of APK mirror websites are APKPure, APKCombo, and APKMirror.
-
Download the Crafting and Building APK file from the website. Make sure you choose the right version for your device and check the file size and permissions.
-
Enable the installation of apps from unknown sources on your device. You can do this by going to Settings > Security > Unknown Sources and toggling it on.
-
Locate the downloaded Crafting and Building APK file on your device and tap on it to install it. You may need to grant some permissions or confirm some prompts.
-
Wait for the installation to finish and launch the game from your app drawer or home screen.
-
-
Risks and precautions
-
While downloading Crafting and Building from an APK mirror can be convenient and fun, it also comes with some risks and drawbacks that you should be aware of. Here are some of them:
-
-
You may not get the latest updates or features of the game, as the APK file may be outdated or unofficial.
-
You may encounter compatibility issues or bugs that affect the performance or stability of the game.
-
You may expose your device to malware or viruses that can harm your data or system.
-
You may violate the terms of service or privacy policy of the game developer or publisher, which can result in legal actions or account bans.
-
-
To avoid these risks and drawbacks, you should always download Crafting and Building from an APK mirror that is trustworthy and reputable. You should also scan the APK file with an antivirus app before installing it. You should also backup your data and device regularly in case something goes wrong. Finally, you should respect the rights and rules of the game developer and publisher, and not use any mods or cheats that can give you an unfair advantage or harm other players.
-
Alternatives to Crafting and Building
-
If you are looking for other games like Crafting and Building that let you create your own worlds and express your creativity, here are some alternatives that you can try:
-
Minecraft
-
Minecraft is the original sandbox game that inspired Crafting and Building and many others. It is one of the most popular games of all time, with over 200 million copies sold. It is available for almost every platform, including PC, consoles, mobile devices, and VR. Minecraft lets you explore, build, craft, survive, and play in infinite procedurally generated worlds. You can also join online servers and play with millions of other players around the world. Minecraft has a huge community of fans, modders, creators, educators, and more. It also has a spin-off title called Minecraft Dungeons, which is a dungeon crawler game set in the Minecraft universe.
-
Terraria
-
Terraria is a 2D sandbox game that combines elements of action-adventure, RPG, platformer, and survival genres. It is available for PC, consoles, mobile devices, and Switch. Terraria lets you explore a vast world full of biomes, enemies, bosses, items, NPCs, events, secrets, and more. You can also build your own base, craft weapons and armor, mine resources, farm crops, fish, summon pets, cast spells, and more. Terraria has a multiplayer mode that lets you play with up to 8 players online or locally. Terraria has over 30 million copies sold and has received several updates and expansions over the years.
-
Roblox
-
Roblox is a massively multiplayer online sandbox game that lets you create and play games of various genres using Roblox Studio. It is available for PC , consoles, mobile devices, and VR. Roblox lets you create your own games using a simple scripting language called Lua. You can also play millions of games created by other users, ranging from simulations, role-playing, obbies, tycoons, shooters, puzzles, and more. You can also customize your avatar, chat with other players, join groups, earn badges, and trade items. Roblox has over 150 million monthly active users and has a thriving economy based on its virtual currency called Robux.
-
Conclusion
-
Crafting and Building is a fun and creative game that lets you build your own worlds and play with others. It is a free game that is available for Android devices, but you can also download it from an APK mirror if you want to access different versions or features of the game. However, you should be careful when downloading Crafting and Building from an APK mirror, as there are some risks and drawbacks involved. You should also check out some alternatives to Crafting and Building, such as Minecraft, Terraria, and Roblox, which offer similar or different experiences of sandbox gaming.
-
We hope that this article has helped you learn more about Crafting and Building and how to download it from an APK mirror. If you have any questions or feedback, please let us know in the comments below. And if you enjoyed this article, please share it with your friends and family who might be interested in Crafting and Building. Thank you for reading!
-
FAQs
-
Here are some frequently asked questions about Crafting and Building and APK mirrors:
-
Is Crafting and Building safe to play?
-
Crafting and Building is safe to play if you download it from the official Google Play store or a reputable APK mirror website. However, you should always scan the APK file with an antivirus app before installing it, and backup your data and device regularly.
-
Is Crafting and Building free to play?
-
Yes, Crafting and Building is free to play. However, it may contain ads or in-app purchases that require real money.
-
Can I play Crafting and Building offline?
-
Yes, you can play Crafting and Building offline in single-player mode. However, you need an internet connection to play online in multiplayer mode.
-
Can I play Crafting and Building on PC?
-
No, Crafting and Building is not officially available for PC. However, you can use an Android emulator to run the game on your PC. An Android emulator is a software that simulates an Android device on your PC. Some examples of Android emulators are BlueStacks, NoxPlayer, and LDPlayer.
-
Can I transfer my Crafting and Building progress from one device to another?
-
Yes, you can transfer your Crafting and Building progress from one device to another by using the cloud save feature. You need to register an account and log in to the game on both devices. Then, you can use the cloud save button to upload or download your progress.
401be4b1e0
-
-
\ No newline at end of file
diff --git a/spaces/2023Liu2023/bingo/src/components/ui/sheet.tsx b/spaces/2023Liu2023/bingo/src/components/ui/sheet.tsx
deleted file mode 100644
index c9f5ce0f81a91067bb013e988a07eb1e6bf6953b..0000000000000000000000000000000000000000
--- a/spaces/2023Liu2023/bingo/src/components/ui/sheet.tsx
+++ /dev/null
@@ -1,122 +0,0 @@
-'use client'
-
-import * as React from 'react'
-import * as SheetPrimitive from '@radix-ui/react-dialog'
-
-import { cn } from '@/lib/utils'
-import { IconClose } from '@/components/ui/icons'
-
-const Sheet = SheetPrimitive.Root
-
-const SheetTrigger = SheetPrimitive.Trigger
-
-const SheetClose = SheetPrimitive.Close
-
-const SheetPortal = ({
- className,
- children,
- ...props
-}: SheetPrimitive.DialogPortalProps) => (
-
- {children}
-
-)
-SheetPortal.displayName = SheetPrimitive.Portal.displayName
-
-const SheetOverlay = React.forwardRef<
- React.ElementRef,
- React.ComponentPropsWithoutRef
->(({ className, children, ...props }, ref) => (
-
-))
-SheetOverlay.displayName = SheetPrimitive.Overlay.displayName
-
-const SheetContent = React.forwardRef<
- React.ElementRef,
- React.ComponentPropsWithoutRef
->(({ className, children, ...props }, ref) => (
-
-
- {children}
-
-
- Close
-
-
-
-))
-SheetContent.displayName = SheetPrimitive.Content.displayName
-
-const SheetHeader = ({
- className,
- ...props
-}: React.HTMLAttributes) => (
-
-)
-SheetHeader.displayName = 'SheetHeader'
-
-const SheetFooter = ({
- className,
- ...props
-}: React.HTMLAttributes) => (
-
-)
-SheetFooter.displayName = 'SheetFooter'
-
-const SheetTitle = React.forwardRef<
- React.ElementRef,
- React.ComponentPropsWithoutRef
->(({ className, ...props }, ref) => (
-
-))
-SheetTitle.displayName = SheetPrimitive.Title.displayName
-
-const SheetDescription = React.forwardRef<
- React.ElementRef,
- React.ComponentPropsWithoutRef
->(({ className, ...props }, ref) => (
-
-))
-SheetDescription.displayName = SheetPrimitive.Description.displayName
-
-export {
- Sheet,
- SheetTrigger,
- SheetClose,
- SheetContent,
- SheetHeader,
- SheetFooter,
- SheetTitle,
- SheetDescription
-}
diff --git a/spaces/801artistry/RVC801/infer_batch_rvc.py b/spaces/801artistry/RVC801/infer_batch_rvc.py
deleted file mode 100644
index 15c862a3d6bf815fa68003cc7054b694cae50c2a..0000000000000000000000000000000000000000
--- a/spaces/801artistry/RVC801/infer_batch_rvc.py
+++ /dev/null
@@ -1,215 +0,0 @@
-"""
-v1
-runtime\python.exe myinfer-v2-0528.py 0 "E:\codes\py39\RVC-beta\todo-songs" "E:\codes\py39\logs\mi-test\added_IVF677_Flat_nprobe_7.index" harvest "E:\codes\py39\RVC-beta\output" "E:\codes\py39\test-20230416b\weights\mi-test.pth" 0.66 cuda:0 True 3 0 1 0.33
-v2
-runtime\python.exe myinfer-v2-0528.py 0 "E:\codes\py39\RVC-beta\todo-songs" "E:\codes\py39\test-20230416b\logs\mi-test-v2\aadded_IVF677_Flat_nprobe_1_v2.index" harvest "E:\codes\py39\RVC-beta\output_v2" "E:\codes\py39\test-20230416b\weights\mi-test-v2.pth" 0.66 cuda:0 True 3 0 1 0.33
-"""
-import os, sys, pdb, torch
-
-now_dir = os.getcwd()
-sys.path.append(now_dir)
-import sys
-import torch
-import tqdm as tq
-from multiprocessing import cpu_count
-
-
-class Config:
- def __init__(self, device, is_half):
- self.device = device
- self.is_half = is_half
- self.n_cpu = 0
- self.gpu_name = None
- self.gpu_mem = None
- self.x_pad, self.x_query, self.x_center, self.x_max = self.device_config()
-
- def device_config(self) -> tuple:
- if torch.cuda.is_available():
- i_device = int(self.device.split(":")[-1])
- self.gpu_name = torch.cuda.get_device_name(i_device)
- if (
- ("16" in self.gpu_name and "V100" not in self.gpu_name.upper())
- or "P40" in self.gpu_name.upper()
- or "1060" in self.gpu_name
- or "1070" in self.gpu_name
- or "1080" in self.gpu_name
- ):
- print("16系/10系显卡和P40强制单精度")
- self.is_half = False
- for config_file in ["32k.json", "40k.json", "48k.json"]:
- with open(f"configs/{config_file}", "r") as f:
- strr = f.read().replace("true", "false")
- with open(f"configs/{config_file}", "w") as f:
- f.write(strr)
- with open("infer/modules/train/preprocess.py", "r") as f:
- strr = f.read().replace("3.7", "3.0")
- with open("infer/modules/train/preprocess.py", "w") as f:
- f.write(strr)
- else:
- self.gpu_name = None
- self.gpu_mem = int(
- torch.cuda.get_device_properties(i_device).total_memory
- / 1024
- / 1024
- / 1024
- + 0.4
- )
- if self.gpu_mem <= 4:
- with open("infer/modules/train/preprocess.py", "r") as f:
- strr = f.read().replace("3.7", "3.0")
- with open("infer/modules/train/preprocess.py", "w") as f:
- f.write(strr)
- elif torch.backends.mps.is_available():
- print("没有发现支持的N卡, 使用MPS进行推理")
- self.device = "mps"
- else:
- print("没有发现支持的N卡, 使用CPU进行推理")
- self.device = "cpu"
- self.is_half = True
-
- if self.n_cpu == 0:
- self.n_cpu = cpu_count()
-
- if self.is_half:
- # 6G显存配置
- x_pad = 3
- x_query = 10
- x_center = 60
- x_max = 65
- else:
- # 5G显存配置
- x_pad = 1
- x_query = 6
- x_center = 38
- x_max = 41
-
- if self.gpu_mem != None and self.gpu_mem <= 4:
- x_pad = 1
- x_query = 5
- x_center = 30
- x_max = 32
-
- return x_pad, x_query, x_center, x_max
-
-
-f0up_key = sys.argv[1]
-input_path = sys.argv[2]
-index_path = sys.argv[3]
-f0method = sys.argv[4] # harvest or pm
-opt_path = sys.argv[5]
-model_path = sys.argv[6]
-index_rate = float(sys.argv[7])
-device = sys.argv[8]
-is_half = sys.argv[9].lower() != "false"
-filter_radius = int(sys.argv[10])
-resample_sr = int(sys.argv[11])
-rms_mix_rate = float(sys.argv[12])
-protect = float(sys.argv[13])
-print(sys.argv)
-config = Config(device, is_half)
-now_dir = os.getcwd()
-sys.path.append(now_dir)
-from infer.modules.vc.modules import VC
-from lib.infer_pack.models import (
- SynthesizerTrnMs256NSFsid,
- SynthesizerTrnMs256NSFsid_nono,
- SynthesizerTrnMs768NSFsid,
- SynthesizerTrnMs768NSFsid_nono,
-)
-from infer.lib.audio import load_audio
-from fairseq import checkpoint_utils
-from scipy.io import wavfile
-
-hubert_model = None
-
-
-def load_hubert():
- global hubert_model
- models, saved_cfg, task = checkpoint_utils.load_model_ensemble_and_task(
- ["hubert_base.pt"],
- suffix="",
- )
- hubert_model = models[0]
- hubert_model = hubert_model.to(device)
- if is_half:
- hubert_model = hubert_model.half()
- else:
- hubert_model = hubert_model.float()
- hubert_model.eval()
-
-
-def vc_single(sid, input_audio, f0_up_key, f0_file, f0_method, file_index, index_rate):
- global tgt_sr, net_g, vc, hubert_model, version
- if input_audio is None:
- return "You need to upload an audio", None
- f0_up_key = int(f0_up_key)
- audio = load_audio(input_audio, 16000)
- times = [0, 0, 0]
- if hubert_model == None:
- load_hubert()
- if_f0 = cpt.get("f0", 1)
- # audio_opt=vc.pipeline(hubert_model,net_g,sid,audio,times,f0_up_key,f0_method,file_index,file_big_npy,index_rate,if_f0,f0_file=f0_file)
- audio_opt = vc.pipeline(
- hubert_model,
- net_g,
- sid,
- audio,
- input_audio,
- times,
- f0_up_key,
- f0_method,
- file_index,
- index_rate,
- if_f0,
- filter_radius,
- tgt_sr,
- resample_sr,
- rms_mix_rate,
- version,
- protect,
- f0_file=f0_file,
- )
- print(times)
- return audio_opt
-
-
-def get_vc(model_path):
- global n_spk, tgt_sr, net_g, vc, cpt, device, is_half, version
- print("loading pth %s" % model_path)
- cpt = torch.load(model_path, map_location="cpu")
- tgt_sr = cpt["config"][-1]
- cpt["config"][-3] = cpt["weight"]["emb_g.weight"].shape[0] # n_spk
- if_f0 = cpt.get("f0", 1)
- version = cpt.get("version", "v1")
- if version == "v1":
- if if_f0 == 1:
- net_g = SynthesizerTrnMs256NSFsid(*cpt["config"], is_half=is_half)
- else:
- net_g = SynthesizerTrnMs256NSFsid_nono(*cpt["config"])
- elif version == "v2":
- if if_f0 == 1: #
- net_g = SynthesizerTrnMs768NSFsid(*cpt["config"], is_half=is_half)
- else:
- net_g = SynthesizerTrnMs768NSFsid_nono(*cpt["config"])
- del net_g.enc_q
- print(net_g.load_state_dict(cpt["weight"], strict=False)) # 不加这一行清不干净,真奇葩
- net_g.eval().to(device)
- if is_half:
- net_g = net_g.half()
- else:
- net_g = net_g.float()
- vc = VC(tgt_sr, config)
- n_spk = cpt["config"][-3]
- # return {"visible": True,"maximum": n_spk, "__type__": "update"}
-
-
-get_vc(model_path)
-audios = os.listdir(input_path)
-for file in tq.tqdm(audios):
- if file.endswith(".wav"):
- file_path = input_path + "/" + file
- wav_opt = vc_single(
- 0, file_path, f0up_key, None, f0method, index_path, index_rate
- )
- out_path = opt_path + "/" + file
- wavfile.write(out_path, tgt_sr, wav_opt)
diff --git a/spaces/801artistry/RVC801/lib/uvr5_pack/lib_v5/layers_123812KB .py b/spaces/801artistry/RVC801/lib/uvr5_pack/lib_v5/layers_123812KB .py
deleted file mode 100644
index b82f06bb4993cd63f076e68d7e24185269b1bc42..0000000000000000000000000000000000000000
--- a/spaces/801artistry/RVC801/lib/uvr5_pack/lib_v5/layers_123812KB .py
+++ /dev/null
@@ -1,118 +0,0 @@
-import torch
-from torch import nn
-import torch.nn.functional as F
-
-from . import spec_utils
-
-
-class Conv2DBNActiv(nn.Module):
- def __init__(self, nin, nout, ksize=3, stride=1, pad=1, dilation=1, activ=nn.ReLU):
- super(Conv2DBNActiv, self).__init__()
- self.conv = nn.Sequential(
- nn.Conv2d(
- nin,
- nout,
- kernel_size=ksize,
- stride=stride,
- padding=pad,
- dilation=dilation,
- bias=False,
- ),
- nn.BatchNorm2d(nout),
- activ(),
- )
-
- def __call__(self, x):
- return self.conv(x)
-
-
-class SeperableConv2DBNActiv(nn.Module):
- def __init__(self, nin, nout, ksize=3, stride=1, pad=1, dilation=1, activ=nn.ReLU):
- super(SeperableConv2DBNActiv, self).__init__()
- self.conv = nn.Sequential(
- nn.Conv2d(
- nin,
- nin,
- kernel_size=ksize,
- stride=stride,
- padding=pad,
- dilation=dilation,
- groups=nin,
- bias=False,
- ),
- nn.Conv2d(nin, nout, kernel_size=1, bias=False),
- nn.BatchNorm2d(nout),
- activ(),
- )
-
- def __call__(self, x):
- return self.conv(x)
-
-
-class Encoder(nn.Module):
- def __init__(self, nin, nout, ksize=3, stride=1, pad=1, activ=nn.LeakyReLU):
- super(Encoder, self).__init__()
- self.conv1 = Conv2DBNActiv(nin, nout, ksize, 1, pad, activ=activ)
- self.conv2 = Conv2DBNActiv(nout, nout, ksize, stride, pad, activ=activ)
-
- def __call__(self, x):
- skip = self.conv1(x)
- h = self.conv2(skip)
-
- return h, skip
-
-
-class Decoder(nn.Module):
- def __init__(
- self, nin, nout, ksize=3, stride=1, pad=1, activ=nn.ReLU, dropout=False
- ):
- super(Decoder, self).__init__()
- self.conv = Conv2DBNActiv(nin, nout, ksize, 1, pad, activ=activ)
- self.dropout = nn.Dropout2d(0.1) if dropout else None
-
- def __call__(self, x, skip=None):
- x = F.interpolate(x, scale_factor=2, mode="bilinear", align_corners=True)
- if skip is not None:
- skip = spec_utils.crop_center(skip, x)
- x = torch.cat([x, skip], dim=1)
- h = self.conv(x)
-
- if self.dropout is not None:
- h = self.dropout(h)
-
- return h
-
-
-class ASPPModule(nn.Module):
- def __init__(self, nin, nout, dilations=(4, 8, 16), activ=nn.ReLU):
- super(ASPPModule, self).__init__()
- self.conv1 = nn.Sequential(
- nn.AdaptiveAvgPool2d((1, None)),
- Conv2DBNActiv(nin, nin, 1, 1, 0, activ=activ),
- )
- self.conv2 = Conv2DBNActiv(nin, nin, 1, 1, 0, activ=activ)
- self.conv3 = SeperableConv2DBNActiv(
- nin, nin, 3, 1, dilations[0], dilations[0], activ=activ
- )
- self.conv4 = SeperableConv2DBNActiv(
- nin, nin, 3, 1, dilations[1], dilations[1], activ=activ
- )
- self.conv5 = SeperableConv2DBNActiv(
- nin, nin, 3, 1, dilations[2], dilations[2], activ=activ
- )
- self.bottleneck = nn.Sequential(
- Conv2DBNActiv(nin * 5, nout, 1, 1, 0, activ=activ), nn.Dropout2d(0.1)
- )
-
- def forward(self, x):
- _, _, h, w = x.size()
- feat1 = F.interpolate(
- self.conv1(x), size=(h, w), mode="bilinear", align_corners=True
- )
- feat2 = self.conv2(x)
- feat3 = self.conv3(x)
- feat4 = self.conv4(x)
- feat5 = self.conv5(x)
- out = torch.cat((feat1, feat2, feat3, feat4, feat5), dim=1)
- bottle = self.bottleneck(out)
- return bottle
diff --git a/spaces/AI-ZTH-03-23/6.AI.Dashboard.Wiki.Chat.Cognitive.HTML5/index.html b/spaces/AI-ZTH-03-23/6.AI.Dashboard.Wiki.Chat.Cognitive.HTML5/index.html
deleted file mode 100644
index 175522d4f076933e2f08c9d8fb5cb1231f25f098..0000000000000000000000000000000000000000
--- a/spaces/AI-ZTH-03-23/6.AI.Dashboard.Wiki.Chat.Cognitive.HTML5/index.html
+++ /dev/null
@@ -1,36 +0,0 @@
-
-
-
-
-
-
-
-
-
-
-
-
-
-
-
-
-
-
-
-
diff --git a/spaces/AIDHD/GrammarCorrector/README.md b/spaces/AIDHD/GrammarCorrector/README.md
deleted file mode 100644
index e571759197d516ac3d902e106475dd75df0a6200..0000000000000000000000000000000000000000
--- a/spaces/AIDHD/GrammarCorrector/README.md
+++ /dev/null
@@ -1,38 +0,0 @@
----
-title: GrammarCorrector
-emoji: 📊
-colorFrom: red
-colorTo: gray
-sdk: streamlit
-app_file: app.py
-pinned: false
-duplicated_from: deep-learning-analytics/GrammarCorrector
----
-
-# Configuration
-
-`title`: _string_
-Display title for the Space
-
-`emoji`: _string_
-Space emoji (emoji-only character allowed)
-
-`colorFrom`: _string_
-Color for Thumbnail gradient (red, yellow, green, blue, indigo, purple, pink, gray)
-
-`colorTo`: _string_
-Color for Thumbnail gradient (red, yellow, green, blue, indigo, purple, pink, gray)
-
-`sdk`: _string_
-Can be either `gradio` or `streamlit`
-
-`sdk_version` : _string_
-Only applicable for `streamlit` SDK.
-See [doc](https://hf.co/docs/hub/spaces) for more info on supported versions.
-
-`app_file`: _string_
-Path to your main application file (which contains either `gradio` or `streamlit` Python code).
-Path is relative to the root of the repository.
-
-`pinned`: _boolean_
-Whether the Space stays on top of your list.
diff --git a/spaces/AIGC-Audio/AudioGPT/text_to_speech/utils/metrics/dtw.py b/spaces/AIGC-Audio/AudioGPT/text_to_speech/utils/metrics/dtw.py
deleted file mode 100644
index 464c4b747d792d23cf413675a47c9dddf67da134..0000000000000000000000000000000000000000
--- a/spaces/AIGC-Audio/AudioGPT/text_to_speech/utils/metrics/dtw.py
+++ /dev/null
@@ -1,162 +0,0 @@
-from numpy import array, zeros, full, argmin, inf, ndim
-from scipy.spatial.distance import cdist
-from math import isinf
-
-
-def dtw(x, y, dist, warp=1, w=inf, s=1.0):
- """
- Computes Dynamic Time Warping (DTW) of two sequences.
-
- :param array x: N1*M array
- :param array y: N2*M array
- :param func dist: distance used as cost measure
- :param int warp: how many shifts are computed.
- :param int w: window size limiting the maximal distance between indices of matched entries |i,j|.
- :param float s: weight applied on off-diagonal moves of the path. As s gets larger, the warping path is increasingly biased towards the diagonal
- Returns the minimum distance, the cost matrix, the accumulated cost matrix, and the wrap path.
- """
- assert len(x)
- assert len(y)
- assert isinf(w) or (w >= abs(len(x) - len(y)))
- assert s > 0
- r, c = len(x), len(y)
- if not isinf(w):
- D0 = full((r + 1, c + 1), inf)
- for i in range(1, r + 1):
- D0[i, max(1, i - w):min(c + 1, i + w + 1)] = 0
- D0[0, 0] = 0
- else:
- D0 = zeros((r + 1, c + 1))
- D0[0, 1:] = inf
- D0[1:, 0] = inf
- D1 = D0[1:, 1:] # view
- for i in range(r):
- for j in range(c):
- if (isinf(w) or (max(0, i - w) <= j <= min(c, i + w))):
- D1[i, j] = dist(x[i], y[j])
- C = D1.copy()
- jrange = range(c)
- for i in range(r):
- if not isinf(w):
- jrange = range(max(0, i - w), min(c, i + w + 1))
- for j in jrange:
- min_list = [D0[i, j]]
- for k in range(1, warp + 1):
- i_k = min(i + k, r)
- j_k = min(j + k, c)
- min_list += [D0[i_k, j] * s, D0[i, j_k] * s]
- D1[i, j] += min(min_list)
- if len(x) == 1:
- path = zeros(len(y)), range(len(y))
- elif len(y) == 1:
- path = range(len(x)), zeros(len(x))
- else:
- path = _traceback(D0)
- return D1[-1, -1], C, D1, path
-
-
-def accelerated_dtw(x, y, dist, warp=1):
- """
- Computes Dynamic Time Warping (DTW) of two sequences in a faster way.
- Instead of iterating through each element and calculating each distance,
- this uses the cdist function from scipy (https://docs.scipy.org/doc/scipy/reference/generated/scipy.spatial.distance.cdist.html)
-
- :param array x: N1*M array
- :param array y: N2*M array
- :param string or func dist: distance parameter for cdist. When string is given, cdist uses optimized functions for the distance metrics.
- If a string is passed, the distance function can be 'braycurtis', 'canberra', 'chebyshev', 'cityblock', 'correlation', 'cosine', 'dice', 'euclidean', 'hamming', 'jaccard', 'kulsinski', 'mahalanobis', 'matching', 'minkowski', 'rogerstanimoto', 'russellrao', 'seuclidean', 'sokalmichener', 'sokalsneath', 'sqeuclidean', 'wminkowski', 'yule'.
- :param int warp: how many shifts are computed.
- Returns the minimum distance, the cost matrix, the accumulated cost matrix, and the wrap path.
- """
- assert len(x)
- assert len(y)
- if ndim(x) == 1:
- x = x.reshape(-1, 1)
- if ndim(y) == 1:
- y = y.reshape(-1, 1)
- r, c = len(x), len(y)
- D0 = zeros((r + 1, c + 1))
- D0[0, 1:] = inf
- D0[1:, 0] = inf
- D1 = D0[1:, 1:]
- D0[1:, 1:] = cdist(x, y, dist)
- C = D1.copy()
- for i in range(r):
- for j in range(c):
- min_list = [D0[i, j]]
- for k in range(1, warp + 1):
- min_list += [D0[min(i + k, r), j],
- D0[i, min(j + k, c)]]
- D1[i, j] += min(min_list)
- if len(x) == 1:
- path = zeros(len(y)), range(len(y))
- elif len(y) == 1:
- path = range(len(x)), zeros(len(x))
- else:
- path = _traceback(D0)
- return D1[-1, -1], C, D1, path
-
-
-def _traceback(D):
- i, j = array(D.shape) - 2
- p, q = [i], [j]
- while (i > 0) or (j > 0):
- tb = argmin((D[i, j], D[i, j + 1], D[i + 1, j]))
- if tb == 0:
- i -= 1
- j -= 1
- elif tb == 1:
- i -= 1
- else: # (tb == 2):
- j -= 1
- p.insert(0, i)
- q.insert(0, j)
- return array(p), array(q)
-
-
-if __name__ == '__main__':
- w = inf
- s = 1.0
- if 1: # 1-D numeric
- from sklearn.metrics.pairwise import manhattan_distances
- import numpy as np
- x = [0, 0, 1, 1, 2, 4, 2, 1, 2, 0]
- x = np.array(x).reshape([-1,1,1])
- y = [1, 1, 1, 2, 2, 2, 2, 3, 2, 0]
- y = np.array(y).reshape([-1,1,1])
- dist_fun = manhattan_distances
- w = 1
- # s = 1.2
- elif 0: # 2-D numeric
- from sklearn.metrics.pairwise import euclidean_distances
-
- x = [[0, 0], [0, 1], [1, 1], [1, 2], [2, 2], [4, 3], [2, 3], [1, 1], [2, 2], [0, 1]]
- y = [[1, 0], [1, 1], [1, 1], [2, 1], [4, 3], [4, 3], [2, 3], [3, 1], [1, 2], [1, 0]]
- dist_fun = euclidean_distances
- else: # 1-D list of strings
- from nltk.metrics.distance import edit_distance
-
- # x = ['we', 'shelled', 'clams', 'for', 'the', 'chowder']
- # y = ['class', 'too']
- x = ['i', 'soon', 'found', 'myself', 'muttering', 'to', 'the', 'walls']
- y = ['see', 'drown', 'himself']
- # x = 'we talked about the situation'.split()
- # y = 'we talked about the situation'.split()
- dist_fun = edit_distance
- dist, cost, acc, path = dtw(x, y, dist_fun, w=w, s=s)
-
- # Vizualize
- from matplotlib import pyplot as plt
-
- plt.imshow(cost.T, origin='lower', cmap=plt.cm.Reds, interpolation='nearest')
- plt.plot(path[0], path[1], '-o') # relation
- plt.xticks(range(len(x)), x)
- plt.yticks(range(len(y)), y)
- plt.xlabel('x')
- plt.ylabel('y')
- plt.axis('tight')
- if isinf(w):
- plt.title('Minimum distance: {}, slope weight: {}'.format(dist, s))
- else:
- plt.title('Minimum distance: {}, window widht: {}, slope weight: {}'.format(dist, w, s))
- plt.show()
diff --git a/spaces/AIWaves/Debate/SOP.py b/spaces/AIWaves/Debate/SOP.py
deleted file mode 100644
index 7fc3e2f5e0c496774d9967fb88593fa4c88347e2..0000000000000000000000000000000000000000
--- a/spaces/AIWaves/Debate/SOP.py
+++ /dev/null
@@ -1,296 +0,0 @@
-# coding=utf-8
-# Copyright 2023 The AIWaves Inc. team.
-
-#
-# Licensed under the Apache License, Version 2.0 (the "License");
-# you may not use this file except in compliance with the License.
-# You may obtain a copy of the License at
-#
-# http://www.apache.org/licenses/LICENSE-2.0
-#
-# Unless required by applicable law or agreed to in writing, software
-# distributed under the License is distributed on an "AS IS" BASIS,
-# WITHOUT WARRANTIES OR CONDITIONS OF ANY KIND, either express or implied.
-# See the License for the specific language governing permissions and
-# limitations under the License.
-"""standard operation procedure of an LLM Autonomous agent"""
-import random
-from LLM.base_LLM import *
-from State import State
-from utils import extract, get_relevant_history
-from Memory import Memory
-from Prompt import *
-import json
-import os
-
-class SOP:
- """
- Responsible for managing the operational processes of all agents
- """
-
- # SOP should have args : "states" "relations" "root"
-
- def __init__(self, **kwargs):
- self.controller_dict = {}
- self.LLM = init_LLM("logs/god",**kwargs)
-
- self.states = {}
- self.init_states(kwargs["states"])
- self.init_relation(kwargs["relations"])
- for state_name, states_dict in kwargs["states"].items():
- if state_name != "end_state" and "controller" in states_dict:
- self.controller_dict[state_name] = states_dict["controller"]
-
- self.user_names = kwargs["user_names"] if "user_names" in kwargs else []
- self.root = self.states[kwargs["root"]]
- self.current_state = self.root
- self.finish_state_name = (
- kwargs["finish_state_name"]
- if "finish_state_name" in kwargs
- else "end_state"
- )
- self.roles_to_names = None
- self.names_to_roles = None
- self.finished = False
-
- @classmethod
- def from_config(cls, config_path):
- with open(config_path) as f:
- config = json.load(f)
- os.environ.clear()
- for key,value in config["config"].items():
- if key == "API_BASE":
- if value == "":
- pass
- else:
- os.environ[key] = value
- # assert "API_KEY" in os.environ and os.environ["API_KEY"] != "API_KEY","Please go to config.json to set API_KEY"
-
- sop = SOP(**config)
- return sop
-
- def init_states(self, states_dict):
- for state_name, state_dict in states_dict.items():
- state_dict["name"] = state_name
- self.states[state_name] = State(**state_dict)
-
- def init_relation(self, relations):
- for state_name, state_relation in relations.items():
- for idx, next_state_name in state_relation.items():
- self.states[state_name].next_states[idx] = self.states[next_state_name]
-
- def transit(self, chat_history, **kwargs):
- """
- Determine the next state based on the current situation
- Return :
- next_state(State) : the next state
- """
- # 如果是单一循环节点,则一直循环即可
- # If it is a single loop node, just keep looping
- if len(self.current_state.next_states) == 1:
- next_state = "0"
-
- # 否则则需要controller去判断进入哪一节点
- # Otherwise, the controller needs to determine which node to enter.
- else:
- current_state = self.current_state
- controller_dict = self.controller_dict[current_state.name]
- relevant_history = kwargs["relevant_history"]
-
- max_chat_nums = controller_dict["max_chat_nums"] if "max_chat_nums" in controller_dict else 1000
- if current_state.chat_nums>=max_chat_nums:
- return self.current_state.next_states["1"]
-
-
- # 否则则让controller判断是否结束
- # Otherwise, let the controller judge whether to end
- judge_system_prompt = controller_dict["judge_system_prompt"]
- environment_prompt = eval(Get_environment_prompt) if current_state.environment_prompt else ""
- transit_system_prompt = eval(Transit_system_prompt)
-
- judge_last_prompt = controller_dict["judge_last_prompt"]
- transit_last_prompt = eval(Transit_last_prompt)
-
-
-
- environment = kwargs["environment"]
- environment_summary = environment.shared_memory["short_term_memory"]
- chat_history_message = Memory.get_chat_history(chat_history)
- query = chat_history[-1].get_query()
-
- chat_messages = [
- {
- "role": "user",
- "content": eval(Transit_message)
- }
- ]
-
- extract_words = controller_dict["judge_extract_words"] if "judge_extract_words" in controller_dict else "end"
-
-
- response = self.LLM.get_response(
- chat_messages, transit_system_prompt, transit_last_prompt, stream=False, **kwargs
- )
- next_state = (
- response if response.isdigit() else extract(response, extract_words)
- )
-
- # 如果没有parse出来则继续循环
- # If no parse comes out, continue looping
- if not next_state.isdigit():
- next_state = "0"
-
- next_state = self.current_state.next_states[next_state]
- return next_state
-
-
- def route(self, chat_history, **kwargs):
- """
- Determine the role that needs action based on the current situation
- Return :
- current_agent(Agent) : the next act agent
- """
-
- agents = kwargs["agents"]
-
- # 知道进入哪一状态后开始分配角色,如果该状态下只有一个角色则直接分配给他
- # Start assigning roles after knowing which state you have entered. If there is only one role in that state, assign it directly to him.
- if len(self.current_state.roles) == 1:
- next_role = self.current_state.roles[0]
-
-
-
- # 否则controller进行分配
- # Otherwise the controller determines
- else:
- relevant_history = kwargs["relevant_history"]
- controller_type = (
- self.controller_dict[self.current_state.name]["controller_type"]
- if "controller_type" in self.controller_dict[self.current_state.name]
- else "order"
- )
-
-
- # 如果是rule 控制器,则交由LLM进行分配角色
- # If controller type is rule, it is left to LLM to assign roles.
- if controller_type == "rule":
- controller_dict = self.controller_dict[self.current_state.name]
-
- call_last_prompt = controller_dict["call_last_prompt"] if "call_last_prompt" in controller_dict else ""
-
- allocate_prompt = ""
- roles = list(set(self.current_state.roles))
- for role in roles:
- allocate_prompt += eval(Allocate_component)
-
- call_system_prompt = controller_dict["call_system_prompt"] if "call_system_prompt" in controller_dict else ""
- environment_prompt = eval(Get_environment_prompt) if self.current_state.environment_prompt else ""
- # call_system_prompt + environment + allocate_prompt
- call_system_prompt = eval(Call_system_prompt)
-
- query = chat_history[-1].get_query()
- last_name = chat_history[-1].send_name
- # last_prompt: note + last_prompt + query
- call_last_prompt =eval(Call_last_prompt)
-
-
- chat_history_message = Memory.get_chat_history(chat_history)
- # Intermediate historical conversation records
- chat_messages = [
- {
- "role": "user",
- "content": eval(Call_message),
- }
- ]
-
- extract_words = controller_dict["call_extract_words"] if "call_extract_words" in controller_dict else "end"
-
- response = self.LLM.get_response(
- chat_messages, call_system_prompt, call_last_prompt, stream=False, **kwargs
- )
-
- # get next role
- next_role = extract(response, extract_words)
-
- # Speak in order
- elif controller_type == "order":
- # If there is no begin role, it will be given directly to the first person.
- if not self.current_state.current_role:
- next_role = self.current_state.roles[0]
- # otherwise first
- else:
- self.current_state.index += 1
- self.current_state.index = (self.current_state.index) % len(self.current_state.roles)
- next_role = self.current_state.roles[self.current_state.index]
- # random speak
- elif controller_type == "random":
- next_role = random.choice(self.current_state.roles)
-
- # 如果下一角色不在,则随机挑选一个
- # If the next character is not available, pick one at random
- if next_role not in self.current_state.roles:
- next_role = random.choice(self.current_state.roles)
-
- self.current_state.current_role = next_role
-
- next_agent = agents[self.roles_to_names[self.current_state.name][next_role]]
-
- return next_agent
-
- def next(self, environment, agents):
- """
- Determine the next state and the agent that needs action based on the current situation
- """
-
- # 如果是第一次进入该状态
- # If it is the first time to enter this state
-
- if self.current_state.is_begin:
- agent_name = self.roles_to_names[self.current_state.name][self.current_state.begin_role]
- agent = agents[agent_name]
- return self.current_state,agent
-
-
- # get relevant history
- query = environment.shared_memory["long_term_memory"][-1].content
- relevant_history = get_relevant_history(
- query,
- environment.shared_memory["long_term_memory"][:-1],
- environment.shared_memory["chat_embeddings"][:-1],
- )
- relevant_history = Memory.get_chat_history(relevant_history)
-
-
-
- next_state = self.transit(
- chat_history=environment.shared_memory["long_term_memory"][
- environment.current_chat_history_idx :
- ],
- relevant_history=relevant_history,
- environment=environment,
- )
- # 如果进入终止节点,则直接终止
- # If you enter the termination node, terminate directly
- if next_state.name == self.finish_state_name:
- self.finished = True
- return None, None
-
- self.current_state = next_state
-
- # 如果是首次进入该节点且有开场白,则直接分配给开场角色
- # If it is the first time to enter the state and there is a begin query, it will be directly assigned to the begin role.
- if self.current_state.is_begin and self.current_state.begin_role:
- agent_name = self.roles_to_names[self.current_state.name][self.current_state.begin_role]
- agent = agents[agent_name]
- return self.current_state,agent
-
-
- next_agent = self.route(
- chat_history=environment.shared_memory["long_term_memory"][
- environment.current_chat_history_idx :
- ],
- agents = agents,
- relevant_history=relevant_history,
- )
-
- return self.current_state, next_agent
diff --git a/spaces/AIZeroToHero/05-RealtimeStreamlitASR/streaming.py b/spaces/AIZeroToHero/05-RealtimeStreamlitASR/streaming.py
deleted file mode 100644
index cc2048269b3e9ac09886471ef9b6dc681db09f25..0000000000000000000000000000000000000000
--- a/spaces/AIZeroToHero/05-RealtimeStreamlitASR/streaming.py
+++ /dev/null
@@ -1,66 +0,0 @@
-import subprocess
-
-import numpy as np
-
-
-def ffmpeg_stream(youtube_url, sampling_rate=16_000, chunk_duration_ms=5000, pad_duration_ms=200):
- """
- Helper function to read an audio file through ffmpeg.
- """
- chunk_len = int(sampling_rate * chunk_duration_ms / 1000)
- pad_len = int(sampling_rate * pad_duration_ms / 1000)
- read_chunk_len = chunk_len + pad_len * 2
-
- ar = f"{sampling_rate}"
- ac = "1"
- format_for_conversion = "f32le"
- dtype = np.float32
- size_of_sample = 4
-
- ffmpeg_command = [
- "ffmpeg",
- "-i",
- "pipe:",
- "-ac",
- ac,
- "-ar",
- ar,
- "-f",
- format_for_conversion,
- "-hide_banner",
- "-loglevel",
- "quiet",
- "pipe:1",
- ]
-
- ytdl_command = ["yt-dlp", "-f", "bestaudio", youtube_url, "--quiet", "-o", "-"]
-
- try:
- ffmpeg_process = subprocess.Popen(ffmpeg_command, stdin=subprocess.PIPE, stdout=subprocess.PIPE, bufsize=-1)
- ytdl_process = subprocess.Popen(ytdl_command, stdout=ffmpeg_process.stdin)
- except FileNotFoundError:
- raise ValueError("ffmpeg was not found but is required to stream audio files from filename")
-
- acc = b""
- leftover = np.zeros((0,), dtype=np.float32)
- while ytdl_process.poll() is None:
- buflen = read_chunk_len * size_of_sample
-
- raw = ffmpeg_process.stdout.read(buflen)
- if raw == b"":
- break
-
- if len(acc) + len(raw) > buflen:
- acc = raw
- else:
- acc += raw
-
- audio = np.frombuffer(acc, dtype=dtype)
- audio = np.concatenate([leftover, audio])
- if len(audio) < pad_len * 2:
- # TODO: handle end of stream better than this
- break
- yield audio
-
- leftover = audio[-pad_len * 2 :]
- read_chunk_len = chunk_len
\ No newline at end of file
diff --git a/spaces/ALM/CALM/app.py b/spaces/ALM/CALM/app.py
deleted file mode 100644
index a444c76effd5e7820577642a03aaf50191d4d3db..0000000000000000000000000000000000000000
--- a/spaces/ALM/CALM/app.py
+++ /dev/null
@@ -1,448 +0,0 @@
-import streamlit as st
-import numpy as np
-
-from st_btn_select import st_btn_select
-from streamlit_option_menu import option_menu
-
-from cgi import test
-import streamlit as st
-import pandas as pd
-from PIL import Image
-import os
-import glob
-
-from transformers import CLIPVisionModel, AutoTokenizer, AutoModel
-from transformers import ViTFeatureExtractor, ViTForImageClassification
-
-import torch
-from tqdm import tqdm
-from PIL import Image
-import numpy as np
-from torch.utils.data import DataLoader
-from transformers import default_data_collator
-
-from torch.utils.data import Dataset, DataLoader
-import torchvision.transforms as transforms
-
-from bokeh.models.widgets import Button
-from bokeh.models import CustomJS
-from streamlit_bokeh_events import streamlit_bokeh_events
-
-from webcam import webcam
-
-## Global Variables
-MP3_ROOT_PATH = "sample_mp3/"
-SPECTROGRAMS_PATH = "sample_spectrograms/"
-
-IMAGE_SIZE = 224
-MEAN = torch.tensor([0.48145466, 0.4578275, 0.40821073])
-STD = torch.tensor([0.26862954, 0.26130258, 0.27577711])
-
-TEXT_MODEL = 'bert-base-uncased'
-
-CLIP_TEXT_MODEL_PATH = "text_model/"
-CLIP_VISION_MODEL_PATH = "vision_model/"
-
-## NavBar
-def streamlit_menu(example=1):
- if example == 1:
- # 1. as sidebar menu
- with st.sidebar:
- selected = option_menu(
- menu_title="Main Menu", # required
- options=["Text", "Audio", "Camera"], # required
- icons=["chat-text", "mic", "camera"], # optional
- menu_icon="cast", # optional
- default_index=0, # optional
- )
- return selected
-
- if example == 2:
- # 2. horizontal menu w/o custom style
- selected = option_menu(
- menu_title=None, # required
- options=["Text", "Audio", "Camera"], # required
- icons=["chat-text", "mic", "camera"], # optional
- menu_icon="cast", # optional
- default_index=0, # optional
- orientation="horizontal",
- )
- return selected
-
- if example == 3:
- # 2. horizontal menu with custom style
- selected = option_menu(
- menu_title=None, # required
- options=["Text", "Audio", "Camera"], # required
- icons=["chat-text", "mic", "camera"], # optional
- menu_icon="cast", # optional
- default_index=0, # optional
- orientation="horizontal",
- styles={
- "container": {"padding": "0!important", "background-color": "#fafafa"},
- "icon": {"color": "#ffde59", "font-size": "25px"},
- "nav-link": {
- "font-size": "25px",
- "text-align": "left",
- "margin": "0px",
- "--hover-color": "#eee",
- },
- "nav-link-selected": {"background-color": "#5271ff"},
- },
- )
- return selected
-
-
-## Draw Sidebar
-def draw_sidebar(
- key,
- plot=False,
-):
-
- st.write(
- """
- # Sidebar
-
- ```python
- Think.
- Search.
- Feel.
- ```
- """
- )
-
- st.slider("From 1 to 10, how cool is this app?", min_value=1, max_value=10, key=key)
-
- option = st_btn_select(('option1', 'option2', 'option3'), index=2)
- st.write(f'Selected option: {option}')
-
-## Change Color
-#def change_color(styles="")
-
-## VisionDataset
-class VisionDataset(Dataset):
- preprocess = transforms.Compose([
- transforms.Resize((IMAGE_SIZE, IMAGE_SIZE)),
- transforms.ToTensor(),
- transforms.Normalize(mean=MEAN, std=STD)
- ])
-
- def __init__(self, image_paths: list):
- self.image_paths = image_paths
-
- def __getitem__(self, idx):
- return self.preprocess(Image.open(self.image_paths[idx]).convert('RGB'))
-
- def __len__(self):
- return len(self.image_paths)
-
-## TextDataset
-class TextDataset(Dataset):
- def __init__(self, text: list, tokenizer, max_len):
- self.len = len(text)
- self.tokens = tokenizer(text, padding='max_length',
- max_length=max_len, truncation=True)
-
- def __getitem__(self, idx):
- token = self.tokens[idx]
- return {'input_ids': token.ids, 'attention_mask': token.attention_mask}
-
- def __len__(self):
- return self.len
-
-## CLIP Demo
-class CLIPDemo:
- def __init__(self, vision_encoder, text_encoder, tokenizer,
- batch_size: int = 64, max_len: int = 64, device='cuda'):
- """ Initializes CLIPDemo
- it has the following functionalities:
- image_search: Search images based on text query
- zero_shot: Zero shot image classification
- analogy: Analogies with embedding space arithmetic.
-
- Args:
- vision_encoder: Fine-tuned vision encoder
- text_encoder: Fine-tuned text encoder
- tokenizer: Transformers tokenizer
- device (torch.device): Running device
- batch_size (int): Size of mini-batches used to embeddings
- max_length (int): Tokenizer max length
-
- Example:
- >>> demo = CLIPDemo(vision_encoder, text_encoder, tokenizer)
- >>> demo.compute_image_embeddings(test_df.image.to_list())
- >>> demo.image_search('یک مرد و یک زن')
- >>> demo.zero_shot('./workers.jpg')
- >>> demo.anology('./sunset.jpg', additional_text='دریا')
- """
- self.vision_encoder = vision_encoder.eval().to(device)
- self.text_encoder = text_encoder.eval().to(device)
- self.batch_size = batch_size
- self.device = device
- self.tokenizer = tokenizer
- self.max_len = max_len
- self.text_embeddings_ = None
- self.image_embeddings_ = None
-
-
- def compute_image_embeddings(self, image_paths: list):
- self.image_paths = image_paths
- dataloader = DataLoader(VisionDataset(
- image_paths=image_paths), batch_size=self.batch_size)
- embeddings = []
- with torch.no_grad():
-
- bar = st.progress(0)
- for i, images in tqdm(enumerate(dataloader), desc='computing image embeddings'):
- bar.progress(int(i/len(dataloader)*100))
- image_embedding = self.vision_encoder(
- pixel_values=images.to(self.device)).pooler_output
- embeddings.append(image_embedding)
- bar.empty()
- self.image_embeddings_ = torch.cat(embeddings)
-
- def compute_text_embeddings(self, text: list):
- self.text = text
- dataloader = DataLoader(TextDataset(text=text, tokenizer=self.tokenizer, max_len=self.max_len),
- batch_size=self.batch_size, collate_fn=default_data_collator)
- embeddings = []
- with torch.no_grad():
- for tokens in tqdm(dataloader, desc='computing text embeddings'):
- image_embedding = self.text_encoder(input_ids=tokens["input_ids"].to(self.device),
- attention_mask=tokens["attention_mask"].to(self.device)).pooler_output
- embeddings.append(image_embedding)
- self.text_embeddings_ = torch.cat(embeddings)
-
- def text_query_embedding(self, query: str = 'A happy song'):
- tokens = self.tokenizer(query, return_tensors='pt')
- with torch.no_grad():
- text_embedding = self.text_encoder(input_ids=tokens["input_ids"].to(self.device),
- attention_mask=tokens["attention_mask"].to(self.device)).pooler_output
- return text_embedding
-
- def most_similars(self, embeddings_1, embeddings_2):
- values, indices = torch.cosine_similarity(
- embeddings_1, embeddings_2).sort(descending=True)
- return values.cpu(), indices.cpu()
-
-
- def image_search(self, query: str, top_k=10):
- """ Search images based on text query
- Args:
- query (str): text query
- image_paths (list[str]): a bunch of image paths
- top_k (int): number of relevant images
- """
- query_embedding = self.text_query_embedding(query=query)
- _, indices = self.most_similars(self.image_embeddings_, query_embedding)
-
- matches = np.array(self.image_paths)[indices][:top_k]
- songs_path = []
- for match in matches:
- filename = os.path.split(match)[1]
- filename = int(filename.replace(".jpeg", ""))
- audio_path = MP3_ROOT_PATH + "/" + f"{filename:06d}"
- songs_path.append(audio_path)
- return songs_path
-
-## Draw text page
-def draw_text(
- key,
- plot=False,
- device=None,
-):
-
-
- image = Image.open("data/logo.png")
- st.image(image, use_column_width="always")
-
- if 'model' not in st.session_state:
- #with st.spinner('We are orginizing your traks...'):
- text_encoder = AutoModel.from_pretrained(CLIP_TEXT_MODEL_PATH, local_files_only=True)
- vision_encoder = CLIPVisionModel.from_pretrained(CLIP_VISION_MODEL_PATH, local_files_only=True).to(device)
- tokenizer = AutoTokenizer.from_pretrained(TEXT_MODEL)
- model = CLIPDemo(vision_encoder=vision_encoder, text_encoder=text_encoder, tokenizer=tokenizer, device=device)
- model.compute_image_embeddings(glob.glob(SPECTROGRAMS_PATH + "/*.jpeg")[:1000])
- st.session_state["model"] = model
-
-
- ""
- ""
-
- moods = ['-', 'angry', 'calm', 'happy', 'sad']
- genres = ['-', 'house', 'pop', 'rock', 'techno']
- artists = ['-', 'bad dad', 'lazy magnet', 'the astronauts', 'yan yalego']
- years = ['-', '80s', '90s', '2000s', '2010s']
-
- col1, col2 = st.columns(2)
- mood = col1.selectbox('Which mood do you feel right now?', moods, help="Select a mood here")
- genre = col2.selectbox('Which genre do you want to listen?', genres, help="Select a genre here")
- artist = col1.selectbox('Which artist do you like best?', artists, help="Select an artist here")
- year = col2.selectbox('Which period do you want to relive?', years, help="Select a period here")
- button_form = st.button('Search', key="button_form")
-
- st.text_input("Otherwise, describe the song you are looking for!", value="", key="sentence")
- button_sentence = st.button('Search', key="button_sentence")
-
- if (button_sentence and st.session_state.sentence != "") or (button_form and not (mood == "-" and artist == "-" and genre == "-" and year == "-")):
- if button_sentence:
- sentence = st.session_state.sentence
- elif button_form:
- sentence = mood if mood != "-" else ""
- sentence = sentence + " " + genre if genre != "-" else sentence
- sentence = sentence + " " + artist if artist != "-" else sentence
- sentence = sentence + " " + year if year != "-" else sentence
-
- song_paths = st.session_state.model.image_search(sentence)
- for song in song_paths:
- song_name = df.loc[df['track_id'] == int(song[-6:])]['track_title'].to_list()[0]
- artist_name = df.loc[df['track_id'] == int(song[-6:])]['artist_name'].to_list()[0]
- st.write('**"'+song_name+'"**' + ' by ' + artist_name)
- st.audio(song + ".ogg", format="audio/ogg", start_time=0)
-
-## Draw audio page
-def draw_audio(
- key,
- plot=False,
- device=None,
-):
-
- image = Image.open("data/logo.png")
- st.image(image, use_column_width="always")
-
- if 'model' not in st.session_state:
- #with st.spinner('We are orginizing your traks...'):
- text_encoder = AutoModel.from_pretrained(CLIP_TEXT_MODEL_PATH, local_files_only=True)
- vision_encoder = CLIPVisionModel.from_pretrained(CLIP_VISION_MODEL_PATH, local_files_only=True).to(device)
- tokenizer = AutoTokenizer.from_pretrained(TEXT_MODEL)
- model = CLIPDemo(vision_encoder=vision_encoder, text_encoder=text_encoder, tokenizer=tokenizer, device=device)
- model.compute_image_embeddings(glob.glob(SPECTROGRAMS_PATH + "/*.jpeg")[:1000])
- st.session_state["model"] = model
- #st.session_state['model'] = CLIPDemo(vision_encoder=vision_encoder, text_encoder=text_encoder, tokenizer=tokenizer)
- #st.session_state.model.compute_image_embeddings(glob.glob("/data1/mlaquatra/TSOAI_hack/data/spectrograms/*.jpeg")[:100])
- #st.success('Done!')
-
- ""
- ""
-
- st.write("Please, describe the kind of song you are looking for!")
- stt_button = Button(label="Start Recording", margin=[5,5,5,200], width=200, default_size=10, width_policy='auto', button_type='primary')
-
- stt_button.js_on_event("button_click", CustomJS(code="""
- var recognition = new webkitSpeechRecognition();
- recognition.continuous = false;
- recognition.interimResults = true;
-
- recognition.onresult = function (e) {
- var value = "";
- for (var i = e.resultIndex; i < e.results.length; ++i) {
- if (e.results[i].isFinal) {
- value += e.results[i][0].transcript;
- }
- }
- if ( value != "") {
- document.dispatchEvent(new CustomEvent("GET_TEXT", {detail: value}));
- }
- }
- recognition.start();
- """))
-
-
- result = streamlit_bokeh_events(
- stt_button,
- events="GET_TEXT",
- key="listen",
- refresh_on_update=False,
- override_height=75,
- debounce_time=0)
-
- if result:
- if "GET_TEXT" in result:
- sentence = result.get("GET_TEXT")
- st.write('You asked for: "' + sentence + '"')
-
- song_paths = st.session_state.model.image_search(sentence)
- for song in song_paths:
- song_name = df.loc[df['track_id'] == int(song[-6:])]['track_title'].to_list()[0]
- artist_name = df.loc[df['track_id'] == int(song[-6:])]['artist_name'].to_list()[0]
- st.write('**"'+song_name+'"**' + ' by ' + artist_name)
- st.audio(song + ".ogg", format="audio/ogg", start_time=0)
-
-## Draw camera page
-def draw_camera(
- key,
- plot=False,
- device=None,
-):
-
- image = Image.open("data/logo.png")
- st.image(image, use_column_width="always")
-
- if 'model' not in st.session_state:
- #with st.spinner('We are orginizing your traks...'):
- text_encoder = AutoModel.from_pretrained(CLIP_TEXT_MODEL_PATH, local_files_only=True)
- vision_encoder = CLIPVisionModel.from_pretrained(CLIP_VISION_MODEL_PATH, local_files_only=True).to(device)
- tokenizer = AutoTokenizer.from_pretrained(TEXT_MODEL)
- model = CLIPDemo(vision_encoder=vision_encoder, text_encoder=text_encoder, tokenizer=tokenizer, device=device)
- model.compute_image_embeddings(glob.glob(SPECTROGRAMS_PATH + "/*.jpeg")[:1000])
- st.session_state["model"] = model
- #st.session_state['model'] = CLIPDemo(vision_encoder=vision_encoder, text_encoder=text_encoder, tokenizer=tokenizer)
- #st.session_state.model.compute_image_embeddings(glob.glob("/data1/mlaquatra/TSOAI_hack/data/spectrograms/*.jpeg")[:100])
- #st.success('Done!')
-
- ""
- ""
-
- st.write("Please, show us how you are feeling today!")
- captured_image = webcam()
- if captured_image is None:
- st.write("Waiting for capture...")
- else:
- # st.write("Got an image from the webcam:")
-
- # st.image(captured_image)
-
- # st.write(type(captured_image))
- # st.write(captured_image)
- # st.write(captured_image.size)
-
- captured_image = captured_image.convert("RGB")
-
- vit_feature_extractor = ViTFeatureExtractor.from_pretrained("google/vit-base-patch16-224-in21k")
- vit_model = ViTForImageClassification.from_pretrained("ViT_ER/best_checkpoint", local_files_only=True)
- inputs = vit_feature_extractor(images=[captured_image], return_tensors="pt")
- outputs = vit_model(**inputs, output_hidden_states=True)
- #st.write(outputs)
- emotions = ['Anger', 'Disgust', 'Fear', 'Happiness', 'Sadness', 'Surprise', 'Neutral']
- mood = emotions[np.argmax(outputs.logits.detach().cpu().numpy())]
- #st.write(mood)
-
- st.write(f"Your mood seems to be **{mood.lower()}** today! Here's a song for you that matches with how you feel!")
-
- song_paths = st.session_state.model.image_search(mood)
- for song in song_paths:
- song_name = df.loc[df['track_id'] == int(song[-6:])]['track_title'].to_list()[0]
- artist_name = df.loc[df['track_id'] == int(song[-6:])]['artist_name'].to_list()[0]
- st.write('**"'+song_name+'"**' + ' by ' + artist_name)
- st.audio(song + ".ogg", format="audio/ogg", start_time=0)
-
-
-## Main
-selected = streamlit_menu(example=3)
-df = pd.read_csv('full_metadata.csv', index_col=False)
-
-device = torch.device("cuda") if torch.cuda.is_available() else torch.device("cpu")
-
-if selected == "Text":
- # st.title(f"You have selected {selected}")
- draw_text("text", plot=True, device=device)
-if selected == "Audio":
- # st.title(f"You have selected {selected}")
- draw_audio("audio", plot=True, device=device)
-if selected == "Camera":
- # st.title(f"You have selected {selected}")
- #draw_camera("camera", plot=True, device=device)
- pass
-
-# with st.sidebar:
-# draw_sidebar("sidebar")
diff --git a/spaces/ATang0729/Forecast4Muses/Model/Model6/Model6_2_ProfileRecogition/mmpretrain/configs/resnet/resnet50_8xb256-rsb-a3-100e_in1k.py b/spaces/ATang0729/Forecast4Muses/Model/Model6/Model6_2_ProfileRecogition/mmpretrain/configs/resnet/resnet50_8xb256-rsb-a3-100e_in1k.py
deleted file mode 100644
index 3a36c5843a69aea20fdb9287561e5c2a96459852..0000000000000000000000000000000000000000
--- a/spaces/ATang0729/Forecast4Muses/Model/Model6/Model6_2_ProfileRecogition/mmpretrain/configs/resnet/resnet50_8xb256-rsb-a3-100e_in1k.py
+++ /dev/null
@@ -1,22 +0,0 @@
-_base_ = [
- '../_base_/models/resnet50.py',
- '../_base_/datasets/imagenet_bs256_rsb_a3.py',
- '../_base_/schedules/imagenet_bs2048_rsb.py',
- '../_base_/default_runtime.py'
-]
-
-# model settings
-model = dict(
- backbone=dict(norm_cfg=dict(type='SyncBN', requires_grad=True)),
- head=dict(loss=dict(use_sigmoid=True)),
- train_cfg=dict(augments=[
- dict(type='Mixup', alpha=0.1),
- dict(type='CutMix', alpha=1.0)
- ]),
-)
-
-# schedule settings
-optim_wrapper = dict(
- optimizer=dict(lr=0.008),
- paramwise_cfg=dict(bias_decay_mult=0., norm_decay_mult=0.),
-)
diff --git a/spaces/AchyuthGamer/OpenGPT-Chat-UI/Dockerfile b/spaces/AchyuthGamer/OpenGPT-Chat-UI/Dockerfile
deleted file mode 100644
index e0d43e3d3ed19a9921602907ba3415184cad598e..0000000000000000000000000000000000000000
--- a/spaces/AchyuthGamer/OpenGPT-Chat-UI/Dockerfile
+++ /dev/null
@@ -1,32 +0,0 @@
-# syntax=docker/dockerfile:1
-# read the doc: https://huggingface.co/docs/hub/spaces-sdks-docker
-# you will also find guides on how best to write your Dockerfile
-FROM node:19 as builder-production
-
-WORKDIR /app
-
-COPY --link --chown=1000 package-lock.json package.json ./
-RUN --mount=type=cache,target=/app/.npm \
- npm set cache /app/.npm && \
- npm ci --omit=dev
-
-FROM builder-production as builder
-
-RUN --mount=type=cache,target=/app/.npm \
- npm set cache /app/.npm && \
- npm ci
-
-COPY --link --chown=1000 . .
-
-RUN --mount=type=secret,id=DOTENV_LOCAL,dst=.env.local \
- npm run build
-
-FROM node:19-slim
-
-RUN npm install -g pm2
-
-COPY --from=builder-production /app/node_modules /app/node_modules
-COPY --link --chown=1000 package.json /app/package.json
-COPY --from=builder /app/build /app/build
-
-CMD pm2 start /app/build/index.js -i $CPU_CORES --no-daemon
diff --git a/spaces/AchyuthGamer/OpenGPT/get_working_providers.py b/spaces/AchyuthGamer/OpenGPT/get_working_providers.py
deleted file mode 100644
index 37ac5e5eed144fd14eca6fc425cb01c3678896b2..0000000000000000000000000000000000000000
--- a/spaces/AchyuthGamer/OpenGPT/get_working_providers.py
+++ /dev/null
@@ -1,7 +0,0 @@
-from g4f.active_providers import get_active_model_providers
-
-working_providers = get_active_model_providers()
-
-print("\nWorking providers by model:")
-for model, providers in working_providers.items():
- print(f"{model}: {', '.join(providers)}")
diff --git a/spaces/Adapter/T2I-Adapter/configs/mm/hrnet_w48_coco_256x192.py b/spaces/Adapter/T2I-Adapter/configs/mm/hrnet_w48_coco_256x192.py
deleted file mode 100644
index 9755e6773cd3a8c0d2ac684c612d716cfd44b0ca..0000000000000000000000000000000000000000
--- a/spaces/Adapter/T2I-Adapter/configs/mm/hrnet_w48_coco_256x192.py
+++ /dev/null
@@ -1,169 +0,0 @@
-# _base_ = [
-# '../../../../_base_/default_runtime.py',
-# '../../../../_base_/datasets/coco.py'
-# ]
-evaluation = dict(interval=10, metric='mAP', save_best='AP')
-
-optimizer = dict(
- type='Adam',
- lr=5e-4,
-)
-optimizer_config = dict(grad_clip=None)
-# learning policy
-lr_config = dict(
- policy='step',
- warmup='linear',
- warmup_iters=500,
- warmup_ratio=0.001,
- step=[170, 200])
-total_epochs = 210
-channel_cfg = dict(
- num_output_channels=17,
- dataset_joints=17,
- dataset_channel=[
- [0, 1, 2, 3, 4, 5, 6, 7, 8, 9, 10, 11, 12, 13, 14, 15, 16],
- ],
- inference_channel=[
- 0, 1, 2, 3, 4, 5, 6, 7, 8, 9, 10, 11, 12, 13, 14, 15, 16
- ])
-
-# model settings
-model = dict(
- type='TopDown',
- pretrained='https://download.openmmlab.com/mmpose/'
- 'pretrain_models/hrnet_w48-8ef0771d.pth',
- backbone=dict(
- type='HRNet',
- in_channels=3,
- extra=dict(
- stage1=dict(
- num_modules=1,
- num_branches=1,
- block='BOTTLENECK',
- num_blocks=(4, ),
- num_channels=(64, )),
- stage2=dict(
- num_modules=1,
- num_branches=2,
- block='BASIC',
- num_blocks=(4, 4),
- num_channels=(48, 96)),
- stage3=dict(
- num_modules=4,
- num_branches=3,
- block='BASIC',
- num_blocks=(4, 4, 4),
- num_channels=(48, 96, 192)),
- stage4=dict(
- num_modules=3,
- num_branches=4,
- block='BASIC',
- num_blocks=(4, 4, 4, 4),
- num_channels=(48, 96, 192, 384))),
- ),
- keypoint_head=dict(
- type='TopdownHeatmapSimpleHead',
- in_channels=48,
- out_channels=channel_cfg['num_output_channels'],
- num_deconv_layers=0,
- extra=dict(final_conv_kernel=1, ),
- loss_keypoint=dict(type='JointsMSELoss', use_target_weight=True)),
- train_cfg=dict(),
- test_cfg=dict(
- flip_test=True,
- post_process='default',
- shift_heatmap=True,
- modulate_kernel=11))
-
-data_cfg = dict(
- image_size=[192, 256],
- heatmap_size=[48, 64],
- num_output_channels=channel_cfg['num_output_channels'],
- num_joints=channel_cfg['dataset_joints'],
- dataset_channel=channel_cfg['dataset_channel'],
- inference_channel=channel_cfg['inference_channel'],
- soft_nms=False,
- nms_thr=1.0,
- oks_thr=0.9,
- vis_thr=0.2,
- use_gt_bbox=False,
- det_bbox_thr=0.0,
- bbox_file='data/coco/person_detection_results/'
- 'COCO_val2017_detections_AP_H_56_person.json',
-)
-
-train_pipeline = [
- dict(type='LoadImageFromFile'),
- dict(type='TopDownGetBboxCenterScale', padding=1.25),
- dict(type='TopDownRandomShiftBboxCenter', shift_factor=0.16, prob=0.3),
- dict(type='TopDownRandomFlip', flip_prob=0.5),
- dict(
- type='TopDownHalfBodyTransform',
- num_joints_half_body=8,
- prob_half_body=0.3),
- dict(
- type='TopDownGetRandomScaleRotation', rot_factor=40, scale_factor=0.5),
- dict(type='TopDownAffine'),
- dict(type='ToTensor'),
- dict(
- type='NormalizeTensor',
- mean=[0.485, 0.456, 0.406],
- std=[0.229, 0.224, 0.225]),
- dict(type='TopDownGenerateTarget', sigma=2),
- dict(
- type='Collect',
- keys=['img', 'target', 'target_weight'],
- meta_keys=[
- 'image_file', 'joints_3d', 'joints_3d_visible', 'center', 'scale',
- 'rotation', 'bbox_score', 'flip_pairs'
- ]),
-]
-
-val_pipeline = [
- dict(type='LoadImageFromFile'),
- dict(type='TopDownGetBboxCenterScale', padding=1.25),
- dict(type='TopDownAffine'),
- dict(type='ToTensor'),
- dict(
- type='NormalizeTensor',
- mean=[0.485, 0.456, 0.406],
- std=[0.229, 0.224, 0.225]),
- dict(
- type='Collect',
- keys=['img'],
- meta_keys=[
- 'image_file', 'center', 'scale', 'rotation', 'bbox_score',
- 'flip_pairs'
- ]),
-]
-
-test_pipeline = val_pipeline
-
-data_root = 'data/coco'
-data = dict(
- samples_per_gpu=32,
- workers_per_gpu=2,
- val_dataloader=dict(samples_per_gpu=32),
- test_dataloader=dict(samples_per_gpu=32),
- train=dict(
- type='TopDownCocoDataset',
- ann_file=f'{data_root}/annotations/person_keypoints_train2017.json',
- img_prefix=f'{data_root}/train2017/',
- data_cfg=data_cfg,
- pipeline=train_pipeline,
- dataset_info={{_base_.dataset_info}}),
- val=dict(
- type='TopDownCocoDataset',
- ann_file=f'{data_root}/annotations/person_keypoints_val2017.json',
- img_prefix=f'{data_root}/val2017/',
- data_cfg=data_cfg,
- pipeline=val_pipeline,
- dataset_info={{_base_.dataset_info}}),
- test=dict(
- type='TopDownCocoDataset',
- ann_file=f'{data_root}/annotations/person_keypoints_val2017.json',
- img_prefix=f'{data_root}/val2017/',
- data_cfg=data_cfg,
- pipeline=test_pipeline,
- dataset_info={{_base_.dataset_info}}),
-)
diff --git a/spaces/AgentVerse/agentVerse/ui/src/phaser3-rex-plugins/plugins/dynamictext.d.ts b/spaces/AgentVerse/agentVerse/ui/src/phaser3-rex-plugins/plugins/dynamictext.d.ts
deleted file mode 100644
index 5220639d2bfccf2d8f1513110c8b6d46a29b9473..0000000000000000000000000000000000000000
--- a/spaces/AgentVerse/agentVerse/ui/src/phaser3-rex-plugins/plugins/dynamictext.d.ts
+++ /dev/null
@@ -1,2 +0,0 @@
-import DynamicText from './gameobjects/dynamictext/dynamictext/DynamicText';
-export default DynamicText;
\ No newline at end of file
diff --git a/spaces/AgentVerse/agentVerse/ui/src/phaser3-rex-plugins/templates/ui/roundrectanglecanvas/RoundRectangleCanvas.d.ts b/spaces/AgentVerse/agentVerse/ui/src/phaser3-rex-plugins/templates/ui/roundrectanglecanvas/RoundRectangleCanvas.d.ts
deleted file mode 100644
index 7325d349fbdae238c1ee1364b2ce0061be80d6e2..0000000000000000000000000000000000000000
--- a/spaces/AgentVerse/agentVerse/ui/src/phaser3-rex-plugins/templates/ui/roundrectanglecanvas/RoundRectangleCanvas.d.ts
+++ /dev/null
@@ -1,2 +0,0 @@
-import RoundRectangleCanvas from "../../../plugins/roundrectanglecanvas";
-export default RoundRectangleCanvas;
\ No newline at end of file
diff --git a/spaces/AlexWortega/ruImageCaptionong/README.md b/spaces/AlexWortega/ruImageCaptionong/README.md
deleted file mode 100644
index ed66cf0549e219c381269ce04edceab9d0131414..0000000000000000000000000000000000000000
--- a/spaces/AlexWortega/ruImageCaptionong/README.md
+++ /dev/null
@@ -1,12 +0,0 @@
----
-title: RuImageCaptionong
-emoji: 👀
-colorFrom: indigo
-colorTo: pink
-sdk: gradio
-sdk_version: 3.0.2
-app_file: app.py
-pinned: false
----
-
-Check out the configuration reference at https://huggingface.co/docs/hub/spaces#reference
diff --git a/spaces/Aloento/9Nine-VITS/README.md b/spaces/Aloento/9Nine-VITS/README.md
deleted file mode 100644
index 82e133c7f0986ba581707f3fc19668abb272506d..0000000000000000000000000000000000000000
--- a/spaces/Aloento/9Nine-VITS/README.md
+++ /dev/null
@@ -1,13 +0,0 @@
----
-title: 9Nine VITS
-emoji: ⚡
-colorFrom: gray
-colorTo: pink
-sdk: gradio
-sdk_version: 3.23.0
-app_file: app.py
-pinned: false
-license: agpl-3.0
----
-
-Check out the configuration reference at https://huggingface.co/docs/hub/spaces-config-reference
diff --git a/spaces/Ameaou/academic-chatgpt3.1/request_llm/bridge_tgui.py b/spaces/Ameaou/academic-chatgpt3.1/request_llm/bridge_tgui.py
deleted file mode 100644
index fcf852f0474892bd179843ece3f4a83110bd7756..0000000000000000000000000000000000000000
--- a/spaces/Ameaou/academic-chatgpt3.1/request_llm/bridge_tgui.py
+++ /dev/null
@@ -1,171 +0,0 @@
-'''
-Contributed by SagsMug. Modified by binary-husky
-https://github.com/oobabooga/text-generation-webui/pull/175
-'''
-
-import asyncio
-import json
-import random
-import string
-import websockets
-import logging
-import time
-import threading
-import importlib
-from toolbox import get_conf, update_ui
-
-
-def random_hash():
- letters = string.ascii_lowercase + string.digits
- return ''.join(random.choice(letters) for i in range(9))
-
-async def run(context, max_token, temperature, top_p, addr, port):
- params = {
- 'max_new_tokens': max_token,
- 'do_sample': True,
- 'temperature': temperature,
- 'top_p': top_p,
- 'typical_p': 1,
- 'repetition_penalty': 1.05,
- 'encoder_repetition_penalty': 1.0,
- 'top_k': 0,
- 'min_length': 0,
- 'no_repeat_ngram_size': 0,
- 'num_beams': 1,
- 'penalty_alpha': 0,
- 'length_penalty': 1,
- 'early_stopping': True,
- 'seed': -1,
- }
- session = random_hash()
-
- async with websockets.connect(f"ws://{addr}:{port}/queue/join") as websocket:
- while content := json.loads(await websocket.recv()):
- #Python3.10 syntax, replace with if elif on older
- if content["msg"] == "send_hash":
- await websocket.send(json.dumps({
- "session_hash": session,
- "fn_index": 12
- }))
- elif content["msg"] == "estimation":
- pass
- elif content["msg"] == "send_data":
- await websocket.send(json.dumps({
- "session_hash": session,
- "fn_index": 12,
- "data": [
- context,
- params['max_new_tokens'],
- params['do_sample'],
- params['temperature'],
- params['top_p'],
- params['typical_p'],
- params['repetition_penalty'],
- params['encoder_repetition_penalty'],
- params['top_k'],
- params['min_length'],
- params['no_repeat_ngram_size'],
- params['num_beams'],
- params['penalty_alpha'],
- params['length_penalty'],
- params['early_stopping'],
- params['seed'],
- ]
- }))
- elif content["msg"] == "process_starts":
- pass
- elif content["msg"] in ["process_generating", "process_completed"]:
- yield content["output"]["data"][0]
- # You can search for your desired end indicator and
- # stop generation by closing the websocket here
- if (content["msg"] == "process_completed"):
- break
-
-
-
-
-
-def predict(inputs, llm_kwargs, plugin_kwargs, chatbot, history=[], system_prompt='', stream = True, additional_fn=None):
- """
- 发送至chatGPT,流式获取输出。
- 用于基础的对话功能。
- inputs 是本次问询的输入
- top_p, temperature是chatGPT的内部调优参数
- history 是之前的对话列表(注意无论是inputs还是history,内容太长了都会触发token数量溢出的错误)
- chatbot 为WebUI中显示的对话列表,修改它,然后yeild出去,可以直接修改对话界面内容
- additional_fn代表点击的哪个按钮,按钮见functional.py
- """
- if additional_fn is not None:
- import core_functional
- importlib.reload(core_functional) # 热更新prompt
- core_functional = core_functional.get_core_functions()
- if "PreProcess" in core_functional[additional_fn]: inputs = core_functional[additional_fn]["PreProcess"](inputs) # 获取预处理函数(如果有的话)
- inputs = core_functional[additional_fn]["Prefix"] + inputs + core_functional[additional_fn]["Suffix"]
-
- raw_input = "What I would like to say is the following: " + inputs
- history.extend([inputs, ""])
- chatbot.append([inputs, ""])
- yield from update_ui(chatbot=chatbot, history=history, msg="等待响应") # 刷新界面
-
- prompt = raw_input
- tgui_say = ""
-
- model_name, addr_port = llm_kwargs['llm_model'].split('@')
- assert ':' in addr_port, "LLM_MODEL 格式不正确!" + llm_kwargs['llm_model']
- addr, port = addr_port.split(':')
-
-
- mutable = ["", time.time()]
- def run_coorotine(mutable):
- async def get_result(mutable):
- # "tgui:galactica-1.3b@localhost:7860"
-
- async for response in run(context=prompt, max_token=llm_kwargs['max_length'],
- temperature=llm_kwargs['temperature'],
- top_p=llm_kwargs['top_p'], addr=addr, port=port):
- print(response[len(mutable[0]):])
- mutable[0] = response
- if (time.time() - mutable[1]) > 3:
- print('exit when no listener')
- break
- asyncio.run(get_result(mutable))
-
- thread_listen = threading.Thread(target=run_coorotine, args=(mutable,), daemon=True)
- thread_listen.start()
-
- while thread_listen.is_alive():
- time.sleep(1)
- mutable[1] = time.time()
- # Print intermediate steps
- if tgui_say != mutable[0]:
- tgui_say = mutable[0]
- history[-1] = tgui_say
- chatbot[-1] = (history[-2], history[-1])
- yield from update_ui(chatbot=chatbot, history=history) # 刷新界面
-
-
-
-
-def predict_no_ui_long_connection(inputs, llm_kwargs, history, sys_prompt, observe_window, console_slience=False):
- raw_input = "What I would like to say is the following: " + inputs
- prompt = raw_input
- tgui_say = ""
- model_name, addr_port = llm_kwargs['llm_model'].split('@')
- assert ':' in addr_port, "LLM_MODEL 格式不正确!" + llm_kwargs['llm_model']
- addr, port = addr_port.split(':')
-
-
- def run_coorotine(observe_window):
- async def get_result(observe_window):
- async for response in run(context=prompt, max_token=llm_kwargs['max_length'],
- temperature=llm_kwargs['temperature'],
- top_p=llm_kwargs['top_p'], addr=addr, port=port):
- print(response[len(observe_window[0]):])
- observe_window[0] = response
- if (time.time() - observe_window[1]) > 5:
- print('exit when no listener')
- break
- asyncio.run(get_result(observe_window))
- thread_listen = threading.Thread(target=run_coorotine, args=(observe_window,))
- thread_listen.start()
- return observe_window[0]
diff --git a/spaces/Amon1/ChatGPTForAcadamic/check_proxy.py b/spaces/Amon1/ChatGPTForAcadamic/check_proxy.py
deleted file mode 100644
index a6919dd37a559d0f3868fdc74b54c488779083d3..0000000000000000000000000000000000000000
--- a/spaces/Amon1/ChatGPTForAcadamic/check_proxy.py
+++ /dev/null
@@ -1,27 +0,0 @@
-
-def check_proxy(proxies):
- import requests
- proxies_https = proxies['https'] if proxies is not None else '无'
- try:
- response = requests.get("https://ipapi.co/json/", proxies=proxies, timeout=4)
- data = response.json()
- print(f'查询代理的地理位置,返回的结果是{data}')
- if 'country_name' in data:
- country = data['country_name']
- result = f"代理配置 {proxies_https}, 代理所在地:{country}"
- elif 'error' in data:
- result = f"代理配置 {proxies_https}, 代理所在地:未知,IP查询频率受限"
- print(result)
- return result
- except:
- result = f"代理配置 {proxies_https}, 代理所在地查询超时,代理可能无效"
- print(result)
- return result
-
-
-if __name__ == '__main__':
- import os; os.environ['no_proxy'] = '*' # 避免代理网络产生意外污染
- from toolbox import get_conf
- proxies, = get_conf('proxies')
- check_proxy(proxies)
-
\ No newline at end of file
diff --git a/spaces/Amrrs/DragGan-Inversion/stylegan_human/utils/__init__.py b/spaces/Amrrs/DragGan-Inversion/stylegan_human/utils/__init__.py
deleted file mode 100644
index e69de29bb2d1d6434b8b29ae775ad8c2e48c5391..0000000000000000000000000000000000000000
diff --git a/spaces/Androidonnxfork/CivitAi-to-Diffusers/diffusers/docker/diffusers-onnxruntime-cuda/Dockerfile b/spaces/Androidonnxfork/CivitAi-to-Diffusers/diffusers/docker/diffusers-onnxruntime-cuda/Dockerfile
deleted file mode 100644
index 2129dbcaf68c57755485e1e54e867af05b937336..0000000000000000000000000000000000000000
--- a/spaces/Androidonnxfork/CivitAi-to-Diffusers/diffusers/docker/diffusers-onnxruntime-cuda/Dockerfile
+++ /dev/null
@@ -1,44 +0,0 @@
-FROM nvidia/cuda:11.6.2-cudnn8-devel-ubuntu20.04
-LABEL maintainer="Hugging Face"
-LABEL repository="diffusers"
-
-ENV DEBIAN_FRONTEND=noninteractive
-
-RUN apt update && \
- apt install -y bash \
- build-essential \
- git \
- git-lfs \
- curl \
- ca-certificates \
- libsndfile1-dev \
- python3.8 \
- python3-pip \
- python3.8-venv && \
- rm -rf /var/lib/apt/lists
-
-# make sure to use venv
-RUN python3 -m venv /opt/venv
-ENV PATH="/opt/venv/bin:$PATH"
-
-# pre-install the heavy dependencies (these can later be overridden by the deps from setup.py)
-RUN python3 -m pip install --no-cache-dir --upgrade pip && \
- python3 -m pip install --no-cache-dir \
- torch \
- torchvision \
- torchaudio \
- "onnxruntime-gpu>=1.13.1" \
- --extra-index-url https://download.pytorch.org/whl/cu117 && \
- python3 -m pip install --no-cache-dir \
- accelerate \
- datasets \
- hf-doc-builder \
- huggingface-hub \
- Jinja2 \
- librosa \
- numpy \
- scipy \
- tensorboard \
- transformers
-
-CMD ["/bin/bash"]
\ No newline at end of file
diff --git a/spaces/Androidonnxfork/CivitAi-to-Diffusers/diffusers/examples/community/seed_resize_stable_diffusion.py b/spaces/Androidonnxfork/CivitAi-to-Diffusers/diffusers/examples/community/seed_resize_stable_diffusion.py
deleted file mode 100644
index 5891b9fb11a83ad2706232ff53999e7c110821f9..0000000000000000000000000000000000000000
--- a/spaces/Androidonnxfork/CivitAi-to-Diffusers/diffusers/examples/community/seed_resize_stable_diffusion.py
+++ /dev/null
@@ -1,366 +0,0 @@
-"""
- modified based on diffusion library from Huggingface: https://github.com/huggingface/diffusers/blob/main/src/diffusers/pipelines/stable_diffusion/pipeline_stable_diffusion.py
-"""
-import inspect
-from typing import Callable, List, Optional, Union
-
-import torch
-from transformers import CLIPImageProcessor, CLIPTextModel, CLIPTokenizer
-
-from diffusers import DiffusionPipeline
-from diffusers.models import AutoencoderKL, UNet2DConditionModel
-from diffusers.pipelines.stable_diffusion import StableDiffusionPipelineOutput
-from diffusers.pipelines.stable_diffusion.safety_checker import StableDiffusionSafetyChecker
-from diffusers.schedulers import DDIMScheduler, LMSDiscreteScheduler, PNDMScheduler
-from diffusers.utils import logging
-
-
-logger = logging.get_logger(__name__) # pylint: disable=invalid-name
-
-
-class SeedResizeStableDiffusionPipeline(DiffusionPipeline):
- r"""
- Pipeline for text-to-image generation using Stable Diffusion.
-
- This model inherits from [`DiffusionPipeline`]. Check the superclass documentation for the generic methods the
- library implements for all the pipelines (such as downloading or saving, running on a particular device, etc.)
-
- Args:
- vae ([`AutoencoderKL`]):
- Variational Auto-Encoder (VAE) Model to encode and decode images to and from latent representations.
- text_encoder ([`CLIPTextModel`]):
- Frozen text-encoder. Stable Diffusion uses the text portion of
- [CLIP](https://huggingface.co/docs/transformers/model_doc/clip#transformers.CLIPTextModel), specifically
- the [clip-vit-large-patch14](https://huggingface.co/openai/clip-vit-large-patch14) variant.
- tokenizer (`CLIPTokenizer`):
- Tokenizer of class
- [CLIPTokenizer](https://huggingface.co/docs/transformers/v4.21.0/en/model_doc/clip#transformers.CLIPTokenizer).
- unet ([`UNet2DConditionModel`]): Conditional U-Net architecture to denoise the encoded image latents.
- scheduler ([`SchedulerMixin`]):
- A scheduler to be used in combination with `unet` to denoise the encoded image latents. Can be one of
- [`DDIMScheduler`], [`LMSDiscreteScheduler`], or [`PNDMScheduler`].
- safety_checker ([`StableDiffusionSafetyChecker`]):
- Classification module that estimates whether generated images could be considered offensive or harmful.
- Please, refer to the [model card](https://huggingface.co/CompVis/stable-diffusion-v1-4) for details.
- feature_extractor ([`CLIPImageProcessor`]):
- Model that extracts features from generated images to be used as inputs for the `safety_checker`.
- """
-
- def __init__(
- self,
- vae: AutoencoderKL,
- text_encoder: CLIPTextModel,
- tokenizer: CLIPTokenizer,
- unet: UNet2DConditionModel,
- scheduler: Union[DDIMScheduler, PNDMScheduler, LMSDiscreteScheduler],
- safety_checker: StableDiffusionSafetyChecker,
- feature_extractor: CLIPImageProcessor,
- ):
- super().__init__()
- self.register_modules(
- vae=vae,
- text_encoder=text_encoder,
- tokenizer=tokenizer,
- unet=unet,
- scheduler=scheduler,
- safety_checker=safety_checker,
- feature_extractor=feature_extractor,
- )
-
- def enable_attention_slicing(self, slice_size: Optional[Union[str, int]] = "auto"):
- r"""
- Enable sliced attention computation.
-
- When this option is enabled, the attention module will split the input tensor in slices, to compute attention
- in several steps. This is useful to save some memory in exchange for a small speed decrease.
-
- Args:
- slice_size (`str` or `int`, *optional*, defaults to `"auto"`):
- When `"auto"`, halves the input to the attention heads, so attention will be computed in two steps. If
- a number is provided, uses as many slices as `attention_head_dim // slice_size`. In this case,
- `attention_head_dim` must be a multiple of `slice_size`.
- """
- if slice_size == "auto":
- # half the attention head size is usually a good trade-off between
- # speed and memory
- slice_size = self.unet.config.attention_head_dim // 2
- self.unet.set_attention_slice(slice_size)
-
- def disable_attention_slicing(self):
- r"""
- Disable sliced attention computation. If `enable_attention_slicing` was previously invoked, this method will go
- back to computing attention in one step.
- """
- # set slice_size = `None` to disable `attention slicing`
- self.enable_attention_slicing(None)
-
- @torch.no_grad()
- def __call__(
- self,
- prompt: Union[str, List[str]],
- height: int = 512,
- width: int = 512,
- num_inference_steps: int = 50,
- guidance_scale: float = 7.5,
- negative_prompt: Optional[Union[str, List[str]]] = None,
- num_images_per_prompt: Optional[int] = 1,
- eta: float = 0.0,
- generator: Optional[torch.Generator] = None,
- latents: Optional[torch.FloatTensor] = None,
- output_type: Optional[str] = "pil",
- return_dict: bool = True,
- callback: Optional[Callable[[int, int, torch.FloatTensor], None]] = None,
- callback_steps: int = 1,
- text_embeddings: Optional[torch.FloatTensor] = None,
- **kwargs,
- ):
- r"""
- Function invoked when calling the pipeline for generation.
-
- Args:
- prompt (`str` or `List[str]`):
- The prompt or prompts to guide the image generation.
- height (`int`, *optional*, defaults to 512):
- The height in pixels of the generated image.
- width (`int`, *optional*, defaults to 512):
- The width in pixels of the generated image.
- num_inference_steps (`int`, *optional*, defaults to 50):
- The number of denoising steps. More denoising steps usually lead to a higher quality image at the
- expense of slower inference.
- guidance_scale (`float`, *optional*, defaults to 7.5):
- Guidance scale as defined in [Classifier-Free Diffusion Guidance](https://arxiv.org/abs/2207.12598).
- `guidance_scale` is defined as `w` of equation 2. of [Imagen
- Paper](https://arxiv.org/pdf/2205.11487.pdf). Guidance scale is enabled by setting `guidance_scale >
- 1`. Higher guidance scale encourages to generate images that are closely linked to the text `prompt`,
- usually at the expense of lower image quality.
- negative_prompt (`str` or `List[str]`, *optional*):
- The prompt or prompts not to guide the image generation. Ignored when not using guidance (i.e., ignored
- if `guidance_scale` is less than `1`).
- num_images_per_prompt (`int`, *optional*, defaults to 1):
- The number of images to generate per prompt.
- eta (`float`, *optional*, defaults to 0.0):
- Corresponds to parameter eta (η) in the DDIM paper: https://arxiv.org/abs/2010.02502. Only applies to
- [`schedulers.DDIMScheduler`], will be ignored for others.
- generator (`torch.Generator`, *optional*):
- A [torch generator](https://pytorch.org/docs/stable/generated/torch.Generator.html) to make generation
- deterministic.
- latents (`torch.FloatTensor`, *optional*):
- Pre-generated noisy latents, sampled from a Gaussian distribution, to be used as inputs for image
- generation. Can be used to tweak the same generation with different prompts. If not provided, a latents
- tensor will ge generated by sampling using the supplied random `generator`.
- output_type (`str`, *optional*, defaults to `"pil"`):
- The output format of the generate image. Choose between
- [PIL](https://pillow.readthedocs.io/en/stable/): `PIL.Image.Image` or `np.array`.
- return_dict (`bool`, *optional*, defaults to `True`):
- Whether or not to return a [`~pipelines.stable_diffusion.StableDiffusionPipelineOutput`] instead of a
- plain tuple.
- callback (`Callable`, *optional*):
- A function that will be called every `callback_steps` steps during inference. The function will be
- called with the following arguments: `callback(step: int, timestep: int, latents: torch.FloatTensor)`.
- callback_steps (`int`, *optional*, defaults to 1):
- The frequency at which the `callback` function will be called. If not specified, the callback will be
- called at every step.
-
- Returns:
- [`~pipelines.stable_diffusion.StableDiffusionPipelineOutput`] or `tuple`:
- [`~pipelines.stable_diffusion.StableDiffusionPipelineOutput`] if `return_dict` is True, otherwise a `tuple.
- When returning a tuple, the first element is a list with the generated images, and the second element is a
- list of `bool`s denoting whether the corresponding generated image likely represents "not-safe-for-work"
- (nsfw) content, according to the `safety_checker`.
- """
-
- if isinstance(prompt, str):
- batch_size = 1
- elif isinstance(prompt, list):
- batch_size = len(prompt)
- else:
- raise ValueError(f"`prompt` has to be of type `str` or `list` but is {type(prompt)}")
-
- if height % 8 != 0 or width % 8 != 0:
- raise ValueError(f"`height` and `width` have to be divisible by 8 but are {height} and {width}.")
-
- if (callback_steps is None) or (
- callback_steps is not None and (not isinstance(callback_steps, int) or callback_steps <= 0)
- ):
- raise ValueError(
- f"`callback_steps` has to be a positive integer but is {callback_steps} of type"
- f" {type(callback_steps)}."
- )
-
- # get prompt text embeddings
- text_inputs = self.tokenizer(
- prompt,
- padding="max_length",
- max_length=self.tokenizer.model_max_length,
- return_tensors="pt",
- )
- text_input_ids = text_inputs.input_ids
-
- if text_input_ids.shape[-1] > self.tokenizer.model_max_length:
- removed_text = self.tokenizer.batch_decode(text_input_ids[:, self.tokenizer.model_max_length :])
- logger.warning(
- "The following part of your input was truncated because CLIP can only handle sequences up to"
- f" {self.tokenizer.model_max_length} tokens: {removed_text}"
- )
- text_input_ids = text_input_ids[:, : self.tokenizer.model_max_length]
-
- if text_embeddings is None:
- text_embeddings = self.text_encoder(text_input_ids.to(self.device))[0]
-
- # duplicate text embeddings for each generation per prompt, using mps friendly method
- bs_embed, seq_len, _ = text_embeddings.shape
- text_embeddings = text_embeddings.repeat(1, num_images_per_prompt, 1)
- text_embeddings = text_embeddings.view(bs_embed * num_images_per_prompt, seq_len, -1)
-
- # here `guidance_scale` is defined analog to the guidance weight `w` of equation (2)
- # of the Imagen paper: https://arxiv.org/pdf/2205.11487.pdf . `guidance_scale = 1`
- # corresponds to doing no classifier free guidance.
- do_classifier_free_guidance = guidance_scale > 1.0
- # get unconditional embeddings for classifier free guidance
- if do_classifier_free_guidance:
- uncond_tokens: List[str]
- if negative_prompt is None:
- uncond_tokens = [""]
- elif type(prompt) is not type(negative_prompt):
- raise TypeError(
- f"`negative_prompt` should be the same type to `prompt`, but got {type(negative_prompt)} !="
- f" {type(prompt)}."
- )
- elif isinstance(negative_prompt, str):
- uncond_tokens = [negative_prompt]
- elif batch_size != len(negative_prompt):
- raise ValueError(
- f"`negative_prompt`: {negative_prompt} has batch size {len(negative_prompt)}, but `prompt`:"
- f" {prompt} has batch size {batch_size}. Please make sure that passed `negative_prompt` matches"
- " the batch size of `prompt`."
- )
- else:
- uncond_tokens = negative_prompt
-
- max_length = text_input_ids.shape[-1]
- uncond_input = self.tokenizer(
- uncond_tokens,
- padding="max_length",
- max_length=max_length,
- truncation=True,
- return_tensors="pt",
- )
- uncond_embeddings = self.text_encoder(uncond_input.input_ids.to(self.device))[0]
-
- # duplicate unconditional embeddings for each generation per prompt, using mps friendly method
- seq_len = uncond_embeddings.shape[1]
- uncond_embeddings = uncond_embeddings.repeat(batch_size, num_images_per_prompt, 1)
- uncond_embeddings = uncond_embeddings.view(batch_size * num_images_per_prompt, seq_len, -1)
-
- # For classifier free guidance, we need to do two forward passes.
- # Here we concatenate the unconditional and text embeddings into a single batch
- # to avoid doing two forward passes
- text_embeddings = torch.cat([uncond_embeddings, text_embeddings])
-
- # get the initial random noise unless the user supplied it
-
- # Unlike in other pipelines, latents need to be generated in the target device
- # for 1-to-1 results reproducibility with the CompVis implementation.
- # However this currently doesn't work in `mps`.
- latents_shape = (batch_size * num_images_per_prompt, self.unet.config.in_channels, height // 8, width // 8)
- latents_shape_reference = (batch_size * num_images_per_prompt, self.unet.config.in_channels, 64, 64)
- latents_dtype = text_embeddings.dtype
- if latents is None:
- if self.device.type == "mps":
- # randn does not exist on mps
- latents_reference = torch.randn(
- latents_shape_reference, generator=generator, device="cpu", dtype=latents_dtype
- ).to(self.device)
- latents = torch.randn(latents_shape, generator=generator, device="cpu", dtype=latents_dtype).to(
- self.device
- )
- else:
- latents_reference = torch.randn(
- latents_shape_reference, generator=generator, device=self.device, dtype=latents_dtype
- )
- latents = torch.randn(latents_shape, generator=generator, device=self.device, dtype=latents_dtype)
- else:
- if latents_reference.shape != latents_shape:
- raise ValueError(f"Unexpected latents shape, got {latents.shape}, expected {latents_shape}")
- latents_reference = latents_reference.to(self.device)
- latents = latents.to(self.device)
-
- # This is the key part of the pipeline where we
- # try to ensure that the generated images w/ the same seed
- # but different sizes actually result in similar images
- dx = (latents_shape[3] - latents_shape_reference[3]) // 2
- dy = (latents_shape[2] - latents_shape_reference[2]) // 2
- w = latents_shape_reference[3] if dx >= 0 else latents_shape_reference[3] + 2 * dx
- h = latents_shape_reference[2] if dy >= 0 else latents_shape_reference[2] + 2 * dy
- tx = 0 if dx < 0 else dx
- ty = 0 if dy < 0 else dy
- dx = max(-dx, 0)
- dy = max(-dy, 0)
- # import pdb
- # pdb.set_trace()
- latents[:, :, ty : ty + h, tx : tx + w] = latents_reference[:, :, dy : dy + h, dx : dx + w]
-
- # set timesteps
- self.scheduler.set_timesteps(num_inference_steps)
-
- # Some schedulers like PNDM have timesteps as arrays
- # It's more optimized to move all timesteps to correct device beforehand
- timesteps_tensor = self.scheduler.timesteps.to(self.device)
-
- # scale the initial noise by the standard deviation required by the scheduler
- latents = latents * self.scheduler.init_noise_sigma
-
- # prepare extra kwargs for the scheduler step, since not all schedulers have the same signature
- # eta (η) is only used with the DDIMScheduler, it will be ignored for other schedulers.
- # eta corresponds to η in DDIM paper: https://arxiv.org/abs/2010.02502
- # and should be between [0, 1]
- accepts_eta = "eta" in set(inspect.signature(self.scheduler.step).parameters.keys())
- extra_step_kwargs = {}
- if accepts_eta:
- extra_step_kwargs["eta"] = eta
-
- for i, t in enumerate(self.progress_bar(timesteps_tensor)):
- # expand the latents if we are doing classifier free guidance
- latent_model_input = torch.cat([latents] * 2) if do_classifier_free_guidance else latents
- latent_model_input = self.scheduler.scale_model_input(latent_model_input, t)
-
- # predict the noise residual
- noise_pred = self.unet(latent_model_input, t, encoder_hidden_states=text_embeddings).sample
-
- # perform guidance
- if do_classifier_free_guidance:
- noise_pred_uncond, noise_pred_text = noise_pred.chunk(2)
- noise_pred = noise_pred_uncond + guidance_scale * (noise_pred_text - noise_pred_uncond)
-
- # compute the previous noisy sample x_t -> x_t-1
- latents = self.scheduler.step(noise_pred, t, latents, **extra_step_kwargs).prev_sample
-
- # call the callback, if provided
- if callback is not None and i % callback_steps == 0:
- callback(i, t, latents)
-
- latents = 1 / 0.18215 * latents
- image = self.vae.decode(latents).sample
-
- image = (image / 2 + 0.5).clamp(0, 1)
-
- # we always cast to float32 as this does not cause significant overhead and is compatible with bfloat16
- image = image.cpu().permute(0, 2, 3, 1).float().numpy()
-
- if self.safety_checker is not None:
- safety_checker_input = self.feature_extractor(self.numpy_to_pil(image), return_tensors="pt").to(
- self.device
- )
- image, has_nsfw_concept = self.safety_checker(
- images=image, clip_input=safety_checker_input.pixel_values.to(text_embeddings.dtype)
- )
- else:
- has_nsfw_concept = None
-
- if output_type == "pil":
- image = self.numpy_to_pil(image)
-
- if not return_dict:
- return (image, has_nsfw_concept)
-
- return StableDiffusionPipelineOutput(images=image, nsfw_content_detected=has_nsfw_concept)
diff --git a/spaces/Androidonnxfork/CivitAi-to-Diffusers/diffusers/src/diffusers/models/modeling_flax_utils.py b/spaces/Androidonnxfork/CivitAi-to-Diffusers/diffusers/src/diffusers/models/modeling_flax_utils.py
deleted file mode 100644
index 9a6e1b3bba3d94e0252794cd0eda079f2c6f4183..0000000000000000000000000000000000000000
--- a/spaces/Androidonnxfork/CivitAi-to-Diffusers/diffusers/src/diffusers/models/modeling_flax_utils.py
+++ /dev/null
@@ -1,534 +0,0 @@
-# coding=utf-8
-# Copyright 2023 The HuggingFace Inc. team.
-#
-# Licensed under the Apache License, Version 2.0 (the "License");
-# you may not use this file except in compliance with the License.
-# You may obtain a copy of the License at
-#
-# http://www.apache.org/licenses/LICENSE-2.0
-#
-# Unless required by applicable law or agreed to in writing, software
-# distributed under the License is distributed on an "AS IS" BASIS,
-# WITHOUT WARRANTIES OR CONDITIONS OF ANY KIND, either express or implied.
-# See the License for the specific language governing permissions and
-# limitations under the License.
-
-import os
-from pickle import UnpicklingError
-from typing import Any, Dict, Union
-
-import jax
-import jax.numpy as jnp
-import msgpack.exceptions
-from flax.core.frozen_dict import FrozenDict, unfreeze
-from flax.serialization import from_bytes, to_bytes
-from flax.traverse_util import flatten_dict, unflatten_dict
-from huggingface_hub import hf_hub_download
-from huggingface_hub.utils import EntryNotFoundError, RepositoryNotFoundError, RevisionNotFoundError
-from requests import HTTPError
-
-from .. import __version__, is_torch_available
-from ..utils import (
- CONFIG_NAME,
- DIFFUSERS_CACHE,
- FLAX_WEIGHTS_NAME,
- HUGGINGFACE_CO_RESOLVE_ENDPOINT,
- WEIGHTS_NAME,
- logging,
-)
-from .modeling_flax_pytorch_utils import convert_pytorch_state_dict_to_flax
-
-
-logger = logging.get_logger(__name__)
-
-
-class FlaxModelMixin:
- r"""
- Base class for all Flax models.
-
- [`FlaxModelMixin`] takes care of storing the model configuration and provides methods for loading, downloading and
- saving models.
-
- - **config_name** ([`str`]) -- Filename to save a model to when calling [`~FlaxModelMixin.save_pretrained`].
- """
- config_name = CONFIG_NAME
- _automatically_saved_args = ["_diffusers_version", "_class_name", "_name_or_path"]
- _flax_internal_args = ["name", "parent", "dtype"]
-
- @classmethod
- def _from_config(cls, config, **kwargs):
- """
- All context managers that the model should be initialized under go here.
- """
- return cls(config, **kwargs)
-
- def _cast_floating_to(self, params: Union[Dict, FrozenDict], dtype: jnp.dtype, mask: Any = None) -> Any:
- """
- Helper method to cast floating-point values of given parameter `PyTree` to given `dtype`.
- """
-
- # taken from https://github.com/deepmind/jmp/blob/3a8318abc3292be38582794dbf7b094e6583b192/jmp/_src/policy.py#L27
- def conditional_cast(param):
- if isinstance(param, jnp.ndarray) and jnp.issubdtype(param.dtype, jnp.floating):
- param = param.astype(dtype)
- return param
-
- if mask is None:
- return jax.tree_map(conditional_cast, params)
-
- flat_params = flatten_dict(params)
- flat_mask, _ = jax.tree_flatten(mask)
-
- for masked, key in zip(flat_mask, flat_params.keys()):
- if masked:
- param = flat_params[key]
- flat_params[key] = conditional_cast(param)
-
- return unflatten_dict(flat_params)
-
- def to_bf16(self, params: Union[Dict, FrozenDict], mask: Any = None):
- r"""
- Cast the floating-point `params` to `jax.numpy.bfloat16`. This returns a new `params` tree and does not cast
- the `params` in place.
-
- This method can be used on a TPU to explicitly convert the model parameters to bfloat16 precision to do full
- half-precision training or to save weights in bfloat16 for inference in order to save memory and improve speed.
-
- Arguments:
- params (`Union[Dict, FrozenDict]`):
- A `PyTree` of model parameters.
- mask (`Union[Dict, FrozenDict]`):
- A `PyTree` with same structure as the `params` tree. The leaves should be booleans. It should be `True`
- for params you want to cast, and `False` for those you want to skip.
-
- Examples:
-
- ```python
- >>> from diffusers import FlaxUNet2DConditionModel
-
- >>> # load model
- >>> model, params = FlaxUNet2DConditionModel.from_pretrained("runwayml/stable-diffusion-v1-5")
- >>> # By default, the model parameters will be in fp32 precision, to cast these to bfloat16 precision
- >>> params = model.to_bf16(params)
- >>> # If you don't want to cast certain parameters (for example layer norm bias and scale)
- >>> # then pass the mask as follows
- >>> from flax import traverse_util
-
- >>> model, params = FlaxUNet2DConditionModel.from_pretrained("runwayml/stable-diffusion-v1-5")
- >>> flat_params = traverse_util.flatten_dict(params)
- >>> mask = {
- ... path: (path[-2] != ("LayerNorm", "bias") and path[-2:] != ("LayerNorm", "scale"))
- ... for path in flat_params
- ... }
- >>> mask = traverse_util.unflatten_dict(mask)
- >>> params = model.to_bf16(params, mask)
- ```"""
- return self._cast_floating_to(params, jnp.bfloat16, mask)
-
- def to_fp32(self, params: Union[Dict, FrozenDict], mask: Any = None):
- r"""
- Cast the floating-point `params` to `jax.numpy.float32`. This method can be used to explicitly convert the
- model parameters to fp32 precision. This returns a new `params` tree and does not cast the `params` in place.
-
- Arguments:
- params (`Union[Dict, FrozenDict]`):
- A `PyTree` of model parameters.
- mask (`Union[Dict, FrozenDict]`):
- A `PyTree` with same structure as the `params` tree. The leaves should be booleans. It should be `True`
- for params you want to cast, and `False` for those you want to skip.
-
- Examples:
-
- ```python
- >>> from diffusers import FlaxUNet2DConditionModel
-
- >>> # Download model and configuration from huggingface.co
- >>> model, params = FlaxUNet2DConditionModel.from_pretrained("runwayml/stable-diffusion-v1-5")
- >>> # By default, the model params will be in fp32, to illustrate the use of this method,
- >>> # we'll first cast to fp16 and back to fp32
- >>> params = model.to_f16(params)
- >>> # now cast back to fp32
- >>> params = model.to_fp32(params)
- ```"""
- return self._cast_floating_to(params, jnp.float32, mask)
-
- def to_fp16(self, params: Union[Dict, FrozenDict], mask: Any = None):
- r"""
- Cast the floating-point `params` to `jax.numpy.float16`. This returns a new `params` tree and does not cast the
- `params` in place.
-
- This method can be used on a GPU to explicitly convert the model parameters to float16 precision to do full
- half-precision training or to save weights in float16 for inference in order to save memory and improve speed.
-
- Arguments:
- params (`Union[Dict, FrozenDict]`):
- A `PyTree` of model parameters.
- mask (`Union[Dict, FrozenDict]`):
- A `PyTree` with same structure as the `params` tree. The leaves should be booleans. It should be `True`
- for params you want to cast, and `False` for those you want to skip.
-
- Examples:
-
- ```python
- >>> from diffusers import FlaxUNet2DConditionModel
-
- >>> # load model
- >>> model, params = FlaxUNet2DConditionModel.from_pretrained("runwayml/stable-diffusion-v1-5")
- >>> # By default, the model params will be in fp32, to cast these to float16
- >>> params = model.to_fp16(params)
- >>> # If you want don't want to cast certain parameters (for example layer norm bias and scale)
- >>> # then pass the mask as follows
- >>> from flax import traverse_util
-
- >>> model, params = FlaxUNet2DConditionModel.from_pretrained("runwayml/stable-diffusion-v1-5")
- >>> flat_params = traverse_util.flatten_dict(params)
- >>> mask = {
- ... path: (path[-2] != ("LayerNorm", "bias") and path[-2:] != ("LayerNorm", "scale"))
- ... for path in flat_params
- ... }
- >>> mask = traverse_util.unflatten_dict(mask)
- >>> params = model.to_fp16(params, mask)
- ```"""
- return self._cast_floating_to(params, jnp.float16, mask)
-
- def init_weights(self, rng: jax.random.KeyArray) -> Dict:
- raise NotImplementedError(f"init_weights method has to be implemented for {self}")
-
- @classmethod
- def from_pretrained(
- cls,
- pretrained_model_name_or_path: Union[str, os.PathLike],
- dtype: jnp.dtype = jnp.float32,
- *model_args,
- **kwargs,
- ):
- r"""
- Instantiate a pretrained Flax model from a pretrained model configuration.
-
- Parameters:
- pretrained_model_name_or_path (`str` or `os.PathLike`):
- Can be either:
-
- - A string, the *model id* (for example `runwayml/stable-diffusion-v1-5`) of a pretrained model
- hosted on the Hub.
- - A path to a *directory* (for example `./my_model_directory`) containing the model weights saved
- using [`~FlaxModelMixin.save_pretrained`].
- dtype (`jax.numpy.dtype`, *optional*, defaults to `jax.numpy.float32`):
- The data type of the computation. Can be one of `jax.numpy.float32`, `jax.numpy.float16` (on GPUs) and
- `jax.numpy.bfloat16` (on TPUs).
-
- This can be used to enable mixed-precision training or half-precision inference on GPUs or TPUs. If
- specified, all the computation will be performed with the given `dtype`.
-
-
-
- This only specifies the dtype of the *computation* and does not influence the dtype of model
- parameters.
-
- If you wish to change the dtype of the model parameters, see [`~FlaxModelMixin.to_fp16`] and
- [`~FlaxModelMixin.to_bf16`].
-
-
-
- model_args (sequence of positional arguments, *optional*):
- All remaining positional arguments are passed to the underlying model's `__init__` method.
- cache_dir (`Union[str, os.PathLike]`, *optional*):
- Path to a directory where a downloaded pretrained model configuration is cached if the standard cache
- is not used.
- force_download (`bool`, *optional*, defaults to `False`):
- Whether or not to force the (re-)download of the model weights and configuration files, overriding the
- cached versions if they exist.
- resume_download (`bool`, *optional*, defaults to `False`):
- Whether or not to resume downloading the model weights and configuration files. If set to `False`, any
- incompletely downloaded files are deleted.
- proxies (`Dict[str, str]`, *optional*):
- A dictionary of proxy servers to use by protocol or endpoint, for example, `{'http': 'foo.bar:3128',
- 'http://hostname': 'foo.bar:4012'}`. The proxies are used on each request.
- local_files_only(`bool`, *optional*, defaults to `False`):
- Whether to only load local model weights and configuration files or not. If set to `True`, the model
- won't be downloaded from the Hub.
- revision (`str`, *optional*, defaults to `"main"`):
- The specific model version to use. It can be a branch name, a tag name, a commit id, or any identifier
- allowed by Git.
- from_pt (`bool`, *optional*, defaults to `False`):
- Load the model weights from a PyTorch checkpoint save file.
- kwargs (remaining dictionary of keyword arguments, *optional*):
- Can be used to update the configuration object (after it is loaded) and initiate the model (for
- example, `output_attentions=True`). Behaves differently depending on whether a `config` is provided or
- automatically loaded:
-
- - If a configuration is provided with `config`, `kwargs` are directly passed to the underlying
- model's `__init__` method (we assume all relevant updates to the configuration have already been
- done).
- - If a configuration is not provided, `kwargs` are first passed to the configuration class
- initialization function [`~ConfigMixin.from_config`]. Each key of the `kwargs` that corresponds
- to a configuration attribute is used to override said attribute with the supplied `kwargs` value.
- Remaining keys that do not correspond to any configuration attribute are passed to the underlying
- model's `__init__` function.
-
- Examples:
-
- ```python
- >>> from diffusers import FlaxUNet2DConditionModel
-
- >>> # Download model and configuration from huggingface.co and cache.
- >>> model, params = FlaxUNet2DConditionModel.from_pretrained("runwayml/stable-diffusion-v1-5")
- >>> # Model was saved using *save_pretrained('./test/saved_model/')* (for example purposes, not runnable).
- >>> model, params = FlaxUNet2DConditionModel.from_pretrained("./test/saved_model/")
- ```
-
- If you get the error message below, you need to finetune the weights for your downstream task:
-
- ```bash
- Some weights of UNet2DConditionModel were not initialized from the model checkpoint at runwayml/stable-diffusion-v1-5 and are newly initialized because the shapes did not match:
- - conv_in.weight: found shape torch.Size([320, 4, 3, 3]) in the checkpoint and torch.Size([320, 9, 3, 3]) in the model instantiated
- You should probably TRAIN this model on a down-stream task to be able to use it for predictions and inference.
- ```
- """
- config = kwargs.pop("config", None)
- cache_dir = kwargs.pop("cache_dir", DIFFUSERS_CACHE)
- force_download = kwargs.pop("force_download", False)
- from_pt = kwargs.pop("from_pt", False)
- resume_download = kwargs.pop("resume_download", False)
- proxies = kwargs.pop("proxies", None)
- local_files_only = kwargs.pop("local_files_only", False)
- use_auth_token = kwargs.pop("use_auth_token", None)
- revision = kwargs.pop("revision", None)
- subfolder = kwargs.pop("subfolder", None)
-
- user_agent = {
- "diffusers": __version__,
- "file_type": "model",
- "framework": "flax",
- }
-
- # Load config if we don't provide a configuration
- config_path = config if config is not None else pretrained_model_name_or_path
- model, model_kwargs = cls.from_config(
- config_path,
- cache_dir=cache_dir,
- return_unused_kwargs=True,
- force_download=force_download,
- resume_download=resume_download,
- proxies=proxies,
- local_files_only=local_files_only,
- use_auth_token=use_auth_token,
- revision=revision,
- subfolder=subfolder,
- # model args
- dtype=dtype,
- **kwargs,
- )
-
- # Load model
- pretrained_path_with_subfolder = (
- pretrained_model_name_or_path
- if subfolder is None
- else os.path.join(pretrained_model_name_or_path, subfolder)
- )
- if os.path.isdir(pretrained_path_with_subfolder):
- if from_pt:
- if not os.path.isfile(os.path.join(pretrained_path_with_subfolder, WEIGHTS_NAME)):
- raise EnvironmentError(
- f"Error no file named {WEIGHTS_NAME} found in directory {pretrained_path_with_subfolder} "
- )
- model_file = os.path.join(pretrained_path_with_subfolder, WEIGHTS_NAME)
- elif os.path.isfile(os.path.join(pretrained_path_with_subfolder, FLAX_WEIGHTS_NAME)):
- # Load from a Flax checkpoint
- model_file = os.path.join(pretrained_path_with_subfolder, FLAX_WEIGHTS_NAME)
- # Check if pytorch weights exist instead
- elif os.path.isfile(os.path.join(pretrained_path_with_subfolder, WEIGHTS_NAME)):
- raise EnvironmentError(
- f"{WEIGHTS_NAME} file found in directory {pretrained_path_with_subfolder}. Please load the model"
- " using `from_pt=True`."
- )
- else:
- raise EnvironmentError(
- f"Error no file named {FLAX_WEIGHTS_NAME} or {WEIGHTS_NAME} found in directory "
- f"{pretrained_path_with_subfolder}."
- )
- else:
- try:
- model_file = hf_hub_download(
- pretrained_model_name_or_path,
- filename=FLAX_WEIGHTS_NAME if not from_pt else WEIGHTS_NAME,
- cache_dir=cache_dir,
- force_download=force_download,
- proxies=proxies,
- resume_download=resume_download,
- local_files_only=local_files_only,
- use_auth_token=use_auth_token,
- user_agent=user_agent,
- subfolder=subfolder,
- revision=revision,
- )
-
- except RepositoryNotFoundError:
- raise EnvironmentError(
- f"{pretrained_model_name_or_path} is not a local folder and is not a valid model identifier "
- "listed on 'https://huggingface.co/models'\nIf this is a private repository, make sure to pass a "
- "token having permission to this repo with `use_auth_token` or log in with `huggingface-cli "
- "login`."
- )
- except RevisionNotFoundError:
- raise EnvironmentError(
- f"{revision} is not a valid git identifier (branch name, tag name or commit id) that exists for "
- "this model name. Check the model page at "
- f"'https://huggingface.co/{pretrained_model_name_or_path}' for available revisions."
- )
- except EntryNotFoundError:
- raise EnvironmentError(
- f"{pretrained_model_name_or_path} does not appear to have a file named {FLAX_WEIGHTS_NAME}."
- )
- except HTTPError as err:
- raise EnvironmentError(
- f"There was a specific connection error when trying to load {pretrained_model_name_or_path}:\n"
- f"{err}"
- )
- except ValueError:
- raise EnvironmentError(
- f"We couldn't connect to '{HUGGINGFACE_CO_RESOLVE_ENDPOINT}' to load this model, couldn't find it"
- f" in the cached files and it looks like {pretrained_model_name_or_path} is not the path to a"
- f" directory containing a file named {FLAX_WEIGHTS_NAME} or {WEIGHTS_NAME}.\nCheckout your"
- " internet connection or see how to run the library in offline mode at"
- " 'https://huggingface.co/docs/transformers/installation#offline-mode'."
- )
- except EnvironmentError:
- raise EnvironmentError(
- f"Can't load the model for '{pretrained_model_name_or_path}'. If you were trying to load it from "
- "'https://huggingface.co/models', make sure you don't have a local directory with the same name. "
- f"Otherwise, make sure '{pretrained_model_name_or_path}' is the correct path to a directory "
- f"containing a file named {FLAX_WEIGHTS_NAME} or {WEIGHTS_NAME}."
- )
-
- if from_pt:
- if is_torch_available():
- from .modeling_utils import load_state_dict
- else:
- raise EnvironmentError(
- "Can't load the model in PyTorch format because PyTorch is not installed. "
- "Please, install PyTorch or use native Flax weights."
- )
-
- # Step 1: Get the pytorch file
- pytorch_model_file = load_state_dict(model_file)
-
- # Step 2: Convert the weights
- state = convert_pytorch_state_dict_to_flax(pytorch_model_file, model)
- else:
- try:
- with open(model_file, "rb") as state_f:
- state = from_bytes(cls, state_f.read())
- except (UnpicklingError, msgpack.exceptions.ExtraData) as e:
- try:
- with open(model_file) as f:
- if f.read().startswith("version"):
- raise OSError(
- "You seem to have cloned a repository without having git-lfs installed. Please"
- " install git-lfs and run `git lfs install` followed by `git lfs pull` in the"
- " folder you cloned."
- )
- else:
- raise ValueError from e
- except (UnicodeDecodeError, ValueError):
- raise EnvironmentError(f"Unable to convert {model_file} to Flax deserializable object. ")
- # make sure all arrays are stored as jnp.ndarray
- # NOTE: This is to prevent a bug this will be fixed in Flax >= v0.3.4:
- # https://github.com/google/flax/issues/1261
- state = jax.tree_util.tree_map(lambda x: jax.device_put(x, jax.devices("cpu")[0]), state)
-
- # flatten dicts
- state = flatten_dict(state)
-
- params_shape_tree = jax.eval_shape(model.init_weights, rng=jax.random.PRNGKey(0))
- required_params = set(flatten_dict(unfreeze(params_shape_tree)).keys())
-
- shape_state = flatten_dict(unfreeze(params_shape_tree))
-
- missing_keys = required_params - set(state.keys())
- unexpected_keys = set(state.keys()) - required_params
-
- if missing_keys:
- logger.warning(
- f"The checkpoint {pretrained_model_name_or_path} is missing required keys: {missing_keys}. "
- "Make sure to call model.init_weights to initialize the missing weights."
- )
- cls._missing_keys = missing_keys
-
- for key in state.keys():
- if key in shape_state and state[key].shape != shape_state[key].shape:
- raise ValueError(
- f"Trying to load the pretrained weight for {key} failed: checkpoint has shape "
- f"{state[key].shape} which is incompatible with the model shape {shape_state[key].shape}. "
- )
-
- # remove unexpected keys to not be saved again
- for unexpected_key in unexpected_keys:
- del state[unexpected_key]
-
- if len(unexpected_keys) > 0:
- logger.warning(
- f"Some weights of the model checkpoint at {pretrained_model_name_or_path} were not used when"
- f" initializing {model.__class__.__name__}: {unexpected_keys}\n- This IS expected if you are"
- f" initializing {model.__class__.__name__} from the checkpoint of a model trained on another task or"
- " with another architecture."
- )
- else:
- logger.info(f"All model checkpoint weights were used when initializing {model.__class__.__name__}.\n")
-
- if len(missing_keys) > 0:
- logger.warning(
- f"Some weights of {model.__class__.__name__} were not initialized from the model checkpoint at"
- f" {pretrained_model_name_or_path} and are newly initialized: {missing_keys}\nYou should probably"
- " TRAIN this model on a down-stream task to be able to use it for predictions and inference."
- )
- else:
- logger.info(
- f"All the weights of {model.__class__.__name__} were initialized from the model checkpoint at"
- f" {pretrained_model_name_or_path}.\nIf your task is similar to the task the model of the checkpoint"
- f" was trained on, you can already use {model.__class__.__name__} for predictions without further"
- " training."
- )
-
- return model, unflatten_dict(state)
-
- def save_pretrained(
- self,
- save_directory: Union[str, os.PathLike],
- params: Union[Dict, FrozenDict],
- is_main_process: bool = True,
- ):
- """
- Save a model and its configuration file to a directory so that it can be reloaded using the
- [`~FlaxModelMixin.from_pretrained`] class method.
-
- Arguments:
- save_directory (`str` or `os.PathLike`):
- Directory to save a model and its configuration file to. Will be created if it doesn't exist.
- params (`Union[Dict, FrozenDict]`):
- A `PyTree` of model parameters.
- is_main_process (`bool`, *optional*, defaults to `True`):
- Whether the process calling this is the main process or not. Useful during distributed training and you
- need to call this function on all processes. In this case, set `is_main_process=True` only on the main
- process to avoid race conditions.
- """
- if os.path.isfile(save_directory):
- logger.error(f"Provided path ({save_directory}) should be a directory, not a file")
- return
-
- os.makedirs(save_directory, exist_ok=True)
-
- model_to_save = self
-
- # Attach architecture to the config
- # Save the config
- if is_main_process:
- model_to_save.save_config(save_directory)
-
- # save model
- output_model_file = os.path.join(save_directory, FLAX_WEIGHTS_NAME)
- with open(output_model_file, "wb") as f:
- model_bytes = to_bytes(params)
- f.write(model_bytes)
-
- logger.info(f"Model weights saved in {output_model_file}")
diff --git a/spaces/Androidonnxfork/CivitAi-to-Diffusers/diffusers/tests/models/test_models_unet_1d.py b/spaces/Androidonnxfork/CivitAi-to-Diffusers/diffusers/tests/models/test_models_unet_1d.py
deleted file mode 100644
index 1b58f9e616be28a089c6b264e4f35182e1f4372f..0000000000000000000000000000000000000000
--- a/spaces/Androidonnxfork/CivitAi-to-Diffusers/diffusers/tests/models/test_models_unet_1d.py
+++ /dev/null
@@ -1,267 +0,0 @@
-# coding=utf-8
-# Copyright 2023 HuggingFace Inc.
-#
-# Licensed under the Apache License, Version 2.0 (the "License");
-# you may not use this file except in compliance with the License.
-# You may obtain a copy of the License at
-#
-# http://www.apache.org/licenses/LICENSE-2.0
-#
-# Unless required by applicable law or agreed to in writing, software
-# distributed under the License is distributed on an "AS IS" BASIS,
-# WITHOUT WARRANTIES OR CONDITIONS OF ANY KIND, either express or implied.
-# See the License for the specific language governing permissions and
-# limitations under the License.
-
-import unittest
-
-import torch
-
-from diffusers import UNet1DModel
-from diffusers.utils import floats_tensor, slow, torch_device
-
-from .test_modeling_common import ModelTesterMixin, UNetTesterMixin
-
-
-class UNet1DModelTests(ModelTesterMixin, UNetTesterMixin, unittest.TestCase):
- model_class = UNet1DModel
- main_input_name = "sample"
-
- @property
- def dummy_input(self):
- batch_size = 4
- num_features = 14
- seq_len = 16
-
- noise = floats_tensor((batch_size, num_features, seq_len)).to(torch_device)
- time_step = torch.tensor([10] * batch_size).to(torch_device)
-
- return {"sample": noise, "timestep": time_step}
-
- @property
- def input_shape(self):
- return (4, 14, 16)
-
- @property
- def output_shape(self):
- return (4, 14, 16)
-
- def test_ema_training(self):
- pass
-
- def test_training(self):
- pass
-
- def test_determinism(self):
- super().test_determinism()
-
- def test_outputs_equivalence(self):
- super().test_outputs_equivalence()
-
- def test_from_save_pretrained(self):
- super().test_from_save_pretrained()
-
- def test_from_save_pretrained_variant(self):
- super().test_from_save_pretrained_variant()
-
- def test_model_from_pretrained(self):
- super().test_model_from_pretrained()
-
- def test_output(self):
- super().test_output()
-
- def prepare_init_args_and_inputs_for_common(self):
- init_dict = {
- "block_out_channels": (32, 64, 128, 256),
- "in_channels": 14,
- "out_channels": 14,
- "time_embedding_type": "positional",
- "use_timestep_embedding": True,
- "flip_sin_to_cos": False,
- "freq_shift": 1.0,
- "out_block_type": "OutConv1DBlock",
- "mid_block_type": "MidResTemporalBlock1D",
- "down_block_types": ("DownResnetBlock1D", "DownResnetBlock1D", "DownResnetBlock1D", "DownResnetBlock1D"),
- "up_block_types": ("UpResnetBlock1D", "UpResnetBlock1D", "UpResnetBlock1D"),
- "act_fn": "swish",
- }
- inputs_dict = self.dummy_input
- return init_dict, inputs_dict
-
- def test_from_pretrained_hub(self):
- model, loading_info = UNet1DModel.from_pretrained(
- "bglick13/hopper-medium-v2-value-function-hor32", output_loading_info=True, subfolder="unet"
- )
- self.assertIsNotNone(model)
- self.assertEqual(len(loading_info["missing_keys"]), 0)
-
- model.to(torch_device)
- image = model(**self.dummy_input)
-
- assert image is not None, "Make sure output is not None"
-
- def test_output_pretrained(self):
- model = UNet1DModel.from_pretrained("bglick13/hopper-medium-v2-value-function-hor32", subfolder="unet")
- torch.manual_seed(0)
- if torch.cuda.is_available():
- torch.cuda.manual_seed_all(0)
-
- num_features = model.config.in_channels
- seq_len = 16
- noise = torch.randn((1, seq_len, num_features)).permute(
- 0, 2, 1
- ) # match original, we can update values and remove
- time_step = torch.full((num_features,), 0)
-
- with torch.no_grad():
- output = model(noise, time_step).sample.permute(0, 2, 1)
-
- output_slice = output[0, -3:, -3:].flatten()
- # fmt: off
- expected_output_slice = torch.tensor([-2.137172, 1.1426016, 0.3688687, -0.766922, 0.7303146, 0.11038864, -0.4760633, 0.13270172, 0.02591348])
- # fmt: on
- self.assertTrue(torch.allclose(output_slice, expected_output_slice, rtol=1e-3))
-
- def test_forward_with_norm_groups(self):
- # Not implemented yet for this UNet
- pass
-
- @slow
- def test_unet_1d_maestro(self):
- model_id = "harmonai/maestro-150k"
- model = UNet1DModel.from_pretrained(model_id, subfolder="unet")
- model.to(torch_device)
-
- sample_size = 65536
- noise = torch.sin(torch.arange(sample_size)[None, None, :].repeat(1, 2, 1)).to(torch_device)
- timestep = torch.tensor([1]).to(torch_device)
-
- with torch.no_grad():
- output = model(noise, timestep).sample
-
- output_sum = output.abs().sum()
- output_max = output.abs().max()
-
- assert (output_sum - 224.0896).abs() < 0.5
- assert (output_max - 0.0607).abs() < 4e-4
-
-
-class UNetRLModelTests(ModelTesterMixin, UNetTesterMixin, unittest.TestCase):
- model_class = UNet1DModel
- main_input_name = "sample"
-
- @property
- def dummy_input(self):
- batch_size = 4
- num_features = 14
- seq_len = 16
-
- noise = floats_tensor((batch_size, num_features, seq_len)).to(torch_device)
- time_step = torch.tensor([10] * batch_size).to(torch_device)
-
- return {"sample": noise, "timestep": time_step}
-
- @property
- def input_shape(self):
- return (4, 14, 16)
-
- @property
- def output_shape(self):
- return (4, 14, 1)
-
- def test_determinism(self):
- super().test_determinism()
-
- def test_outputs_equivalence(self):
- super().test_outputs_equivalence()
-
- def test_from_save_pretrained(self):
- super().test_from_save_pretrained()
-
- def test_from_save_pretrained_variant(self):
- super().test_from_save_pretrained_variant()
-
- def test_model_from_pretrained(self):
- super().test_model_from_pretrained()
-
- def test_output(self):
- # UNetRL is a value-function is different output shape
- init_dict, inputs_dict = self.prepare_init_args_and_inputs_for_common()
- model = self.model_class(**init_dict)
- model.to(torch_device)
- model.eval()
-
- with torch.no_grad():
- output = model(**inputs_dict)
-
- if isinstance(output, dict):
- output = output.sample
-
- self.assertIsNotNone(output)
- expected_shape = torch.Size((inputs_dict["sample"].shape[0], 1))
- self.assertEqual(output.shape, expected_shape, "Input and output shapes do not match")
-
- def test_ema_training(self):
- pass
-
- def test_training(self):
- pass
-
- def prepare_init_args_and_inputs_for_common(self):
- init_dict = {
- "in_channels": 14,
- "out_channels": 14,
- "down_block_types": ["DownResnetBlock1D", "DownResnetBlock1D", "DownResnetBlock1D", "DownResnetBlock1D"],
- "up_block_types": [],
- "out_block_type": "ValueFunction",
- "mid_block_type": "ValueFunctionMidBlock1D",
- "block_out_channels": [32, 64, 128, 256],
- "layers_per_block": 1,
- "downsample_each_block": True,
- "use_timestep_embedding": True,
- "freq_shift": 1.0,
- "flip_sin_to_cos": False,
- "time_embedding_type": "positional",
- "act_fn": "mish",
- }
- inputs_dict = self.dummy_input
- return init_dict, inputs_dict
-
- def test_from_pretrained_hub(self):
- value_function, vf_loading_info = UNet1DModel.from_pretrained(
- "bglick13/hopper-medium-v2-value-function-hor32", output_loading_info=True, subfolder="value_function"
- )
- self.assertIsNotNone(value_function)
- self.assertEqual(len(vf_loading_info["missing_keys"]), 0)
-
- value_function.to(torch_device)
- image = value_function(**self.dummy_input)
-
- assert image is not None, "Make sure output is not None"
-
- def test_output_pretrained(self):
- value_function, vf_loading_info = UNet1DModel.from_pretrained(
- "bglick13/hopper-medium-v2-value-function-hor32", output_loading_info=True, subfolder="value_function"
- )
- torch.manual_seed(0)
- if torch.cuda.is_available():
- torch.cuda.manual_seed_all(0)
-
- num_features = value_function.config.in_channels
- seq_len = 14
- noise = torch.randn((1, seq_len, num_features)).permute(
- 0, 2, 1
- ) # match original, we can update values and remove
- time_step = torch.full((num_features,), 0)
-
- with torch.no_grad():
- output = value_function(noise, time_step).sample
-
- # fmt: off
- expected_output_slice = torch.tensor([165.25] * seq_len)
- # fmt: on
- self.assertTrue(torch.allclose(output, expected_output_slice, rtol=1e-3))
-
- def test_forward_with_norm_groups(self):
- # Not implemented yet for this UNet
- pass
diff --git a/spaces/Andy1621/uniformer_image_detection/configs/htc/htc_r50_fpn_1x_coco.py b/spaces/Andy1621/uniformer_image_detection/configs/htc/htc_r50_fpn_1x_coco.py
deleted file mode 100644
index 929cf464f6091f8380fd1057b282f29f4f7a8b5f..0000000000000000000000000000000000000000
--- a/spaces/Andy1621/uniformer_image_detection/configs/htc/htc_r50_fpn_1x_coco.py
+++ /dev/null
@@ -1,56 +0,0 @@
-_base_ = './htc_without_semantic_r50_fpn_1x_coco.py'
-model = dict(
- roi_head=dict(
- semantic_roi_extractor=dict(
- type='SingleRoIExtractor',
- roi_layer=dict(type='RoIAlign', output_size=14, sampling_ratio=0),
- out_channels=256,
- featmap_strides=[8]),
- semantic_head=dict(
- type='FusedSemanticHead',
- num_ins=5,
- fusion_level=1,
- num_convs=4,
- in_channels=256,
- conv_out_channels=256,
- num_classes=183,
- ignore_label=255,
- loss_weight=0.2)))
-data_root = 'data/coco/'
-img_norm_cfg = dict(
- mean=[123.675, 116.28, 103.53], std=[58.395, 57.12, 57.375], to_rgb=True)
-train_pipeline = [
- dict(type='LoadImageFromFile'),
- dict(
- type='LoadAnnotations', with_bbox=True, with_mask=True, with_seg=True),
- dict(type='Resize', img_scale=(1333, 800), keep_ratio=True),
- dict(type='RandomFlip', flip_ratio=0.5),
- dict(type='Normalize', **img_norm_cfg),
- dict(type='Pad', size_divisor=32),
- dict(type='SegRescale', scale_factor=1 / 8),
- dict(type='DefaultFormatBundle'),
- dict(
- type='Collect',
- keys=['img', 'gt_bboxes', 'gt_labels', 'gt_masks', 'gt_semantic_seg']),
-]
-test_pipeline = [
- dict(type='LoadImageFromFile'),
- dict(
- type='MultiScaleFlipAug',
- img_scale=(1333, 800),
- flip=False,
- transforms=[
- dict(type='Resize', keep_ratio=True),
- dict(type='RandomFlip', flip_ratio=0.5),
- dict(type='Normalize', **img_norm_cfg),
- dict(type='Pad', size_divisor=32),
- dict(type='ImageToTensor', keys=['img']),
- dict(type='Collect', keys=['img']),
- ])
-]
-data = dict(
- train=dict(
- seg_prefix=data_root + 'stuffthingmaps/train2017/',
- pipeline=train_pipeline),
- val=dict(pipeline=test_pipeline),
- test=dict(pipeline=test_pipeline))
diff --git a/spaces/ApathyINC/CustomGPT/app.py b/spaces/ApathyINC/CustomGPT/app.py
deleted file mode 100644
index a5e19f0e392dd62d0f487b9296e5c26b5b33957b..0000000000000000000000000000000000000000
--- a/spaces/ApathyINC/CustomGPT/app.py
+++ /dev/null
@@ -1,516 +0,0 @@
-import os, sys, json
-os.system("pip install gradio==3.19.1")
-import openai
-import gradio as gr
-
-from loguru import logger
-import paddlehub as hub
-import random
-from encoder import get_encoder
-
-openai.api_key = os.getenv("OPENAI_API_KEY")
-
-from utils import get_tmt_client, getTextTrans_tmt
-tmt_client = get_tmt_client()
-
-def getTextTrans(text, source='zh', target='en'):
- def is_chinese(string):
- for ch in string:
- if u'\u4e00' <= ch <= u'\u9fff':
- return True
- return False
-
- if not is_chinese(text) and target == 'en':
- return text
-
- try:
- text_translation = getTextTrans_tmt(tmt_client, text, source, target)
- return text_translation
- except Exception as e:
- return text
-
-start_work = """async() => {
- function isMobile() {
- try {
- document.createEvent("TouchEvent"); return true;
- } catch(e) {
- return false;
- }
- }
- function getClientHeight()
- {
- var clientHeight=0;
- if(document.body.clientHeight&&document.documentElement.clientHeight) {
- var clientHeight = (document.body.clientHeightdocument.documentElement.clientHeight)?document.body.clientHeight:document.documentElement.clientHeight;
- }
- return clientHeight;
- }
-
- function setNativeValue(element, value) {
- const valueSetter = Object.getOwnPropertyDescriptor(element.__proto__, 'value').set;
- const prototype = Object.getPrototypeOf(element);
- const prototypeValueSetter = Object.getOwnPropertyDescriptor(prototype, 'value').set;
-
- if (valueSetter && valueSetter !== prototypeValueSetter) {
- prototypeValueSetter.call(element, value);
- } else {
- valueSetter.call(element, value);
- }
- element.dispatchEvent(new Event('input', { bubbles: true }));
- }
- function get_clear_innerHTML(innerHTML) {
- innerHTML = innerHTML.replace(/
You can duplicating this space and use your own session token:
")
- with gr.Group(elem_id="page_1", visible=True) as page_1:
- with gr.Box():
- with gr.Row():
- start_button = gr.Button("CustomGPT modded by MBHudson :: Click to Continue! ::", elem_id="start-btn", visible=True)
- start_button.click(fn=None, inputs=[], outputs=[], _js=start_work)
-
- with gr.Row(elem_id="page_2", visible=False) as page_2:
- with gr.Row(elem_id="chat_row"):
- chatbot = gr.Chatbot(elem_id="chat_bot", visible=False).style(color_map=("green", "blue"))
- chatbot1 = gr.Chatbot(elem_id="chat_bot1").style(color_map=("green", "blue"))
- with gr.Row(elem_id="prompt_row"):
- prompt_input0 = gr.Textbox(lines=2, label="input", elem_id="my_prompt", show_label=True)
- prompt_input1 = gr.Textbox(lines=4, label="prompt", elem_id="my_prompt_en", visible=False)
- chat_history = gr.Textbox(lines=4, label="chat_history", elem_id="chat_history", visible=False)
- all_chat_history = gr.Textbox(lines=4, label="会话上下文:", elem_id="all_chat_history", visible=False)
-
- chat_radio = gr.Radio(["Talk to chatGPT", "Text to Image"], elem_id="chat_radio",value="Talk to chatGPT", show_label=False, visible=True)
- model_radio = gr.Radio(["GPT-3.0", "GPT-3.5"], elem_id="model_radio", value="GPT-3.5",
- label='GPT model: ', show_label=True,interactive=True, visible=True)
- openai_api_key_textbox = gr.Textbox(placeholder="Paste your OpenAI API key (sk-...) and hit Enter",
- show_label=False, lines=1, type='password')
- with gr.Row(elem_id="btns_row"):
- with gr.Column(id="submit_col"):
- submit_btn = gr.Button(value = "submit",elem_id="submit-btn").style(
- margin=True,
- rounded=(True, True, True, True),
- width=100
- )
- with gr.Column(id="clear_col"):
- clear_btn = gr.Button(value = "clear outputs", elem_id="clear-btn").style(
- margin=True,
- rounded=(True, True, True, True),
- width=100
- )
- submit_btn.click(fn=chat,
- inputs=[prompt_input0, prompt_input1, chat_radio, model_radio, all_chat_history, chat_history],
- outputs=[chatbot, all_chat_history, prompt_input0, prompt_input1],
- )
- with gr.Row(elem_id='tab_img', visible=False).style(height=5):
- tab_img = gr.TabbedInterface(tab_actions, tab_titles)
-
- openai_api_key_textbox.change(set_openai_api_key,
- inputs=[openai_api_key_textbox],
- outputs=[])
- openai_api_key_textbox.submit(set_openai_api_key,
- inputs=[openai_api_key_textbox],
- outputs=[])
- chat_radio.change(fn=chat_radio_change,
- inputs=[chat_radio],
- outputs=[model_radio, openai_api_key_textbox],
- )
-
-demo.launch(debug = True)
diff --git a/spaces/Ataturk-Chatbot/HuggingFaceChat/venv/lib/python3.11/site-packages/pip/_internal/resolution/resolvelib/base.py b/spaces/Ataturk-Chatbot/HuggingFaceChat/venv/lib/python3.11/site-packages/pip/_internal/resolution/resolvelib/base.py
deleted file mode 100644
index b206692a0a976d8336e3f5896eadf4765a33fb2c..0000000000000000000000000000000000000000
--- a/spaces/Ataturk-Chatbot/HuggingFaceChat/venv/lib/python3.11/site-packages/pip/_internal/resolution/resolvelib/base.py
+++ /dev/null
@@ -1,141 +0,0 @@
-from typing import FrozenSet, Iterable, Optional, Tuple, Union
-
-from pip._vendor.packaging.specifiers import SpecifierSet
-from pip._vendor.packaging.utils import NormalizedName, canonicalize_name
-from pip._vendor.packaging.version import LegacyVersion, Version
-
-from pip._internal.models.link import Link, links_equivalent
-from pip._internal.req.req_install import InstallRequirement
-from pip._internal.utils.hashes import Hashes
-
-CandidateLookup = Tuple[Optional["Candidate"], Optional[InstallRequirement]]
-CandidateVersion = Union[LegacyVersion, Version]
-
-
-def format_name(project: str, extras: FrozenSet[str]) -> str:
- if not extras:
- return project
- canonical_extras = sorted(canonicalize_name(e) for e in extras)
- return "{}[{}]".format(project, ",".join(canonical_extras))
-
-
-class Constraint:
- def __init__(
- self, specifier: SpecifierSet, hashes: Hashes, links: FrozenSet[Link]
- ) -> None:
- self.specifier = specifier
- self.hashes = hashes
- self.links = links
-
- @classmethod
- def empty(cls) -> "Constraint":
- return Constraint(SpecifierSet(), Hashes(), frozenset())
-
- @classmethod
- def from_ireq(cls, ireq: InstallRequirement) -> "Constraint":
- links = frozenset([ireq.link]) if ireq.link else frozenset()
- return Constraint(ireq.specifier, ireq.hashes(trust_internet=False), links)
-
- def __bool__(self) -> bool:
- return bool(self.specifier) or bool(self.hashes) or bool(self.links)
-
- def __and__(self, other: InstallRequirement) -> "Constraint":
- if not isinstance(other, InstallRequirement):
- return NotImplemented
- specifier = self.specifier & other.specifier
- hashes = self.hashes & other.hashes(trust_internet=False)
- links = self.links
- if other.link:
- links = links.union([other.link])
- return Constraint(specifier, hashes, links)
-
- def is_satisfied_by(self, candidate: "Candidate") -> bool:
- # Reject if there are any mismatched URL constraints on this package.
- if self.links and not all(_match_link(link, candidate) for link in self.links):
- return False
- # We can safely always allow prereleases here since PackageFinder
- # already implements the prerelease logic, and would have filtered out
- # prerelease candidates if the user does not expect them.
- return self.specifier.contains(candidate.version, prereleases=True)
-
-
-class Requirement:
- @property
- def project_name(self) -> NormalizedName:
- """The "project name" of a requirement.
-
- This is different from ``name`` if this requirement contains extras,
- in which case ``name`` would contain the ``[...]`` part, while this
- refers to the name of the project.
- """
- raise NotImplementedError("Subclass should override")
-
- @property
- def name(self) -> str:
- """The name identifying this requirement in the resolver.
-
- This is different from ``project_name`` if this requirement contains
- extras, where ``project_name`` would not contain the ``[...]`` part.
- """
- raise NotImplementedError("Subclass should override")
-
- def is_satisfied_by(self, candidate: "Candidate") -> bool:
- return False
-
- def get_candidate_lookup(self) -> CandidateLookup:
- raise NotImplementedError("Subclass should override")
-
- def format_for_error(self) -> str:
- raise NotImplementedError("Subclass should override")
-
-
-def _match_link(link: Link, candidate: "Candidate") -> bool:
- if candidate.source_link:
- return links_equivalent(link, candidate.source_link)
- return False
-
-
-class Candidate:
- @property
- def project_name(self) -> NormalizedName:
- """The "project name" of the candidate.
-
- This is different from ``name`` if this candidate contains extras,
- in which case ``name`` would contain the ``[...]`` part, while this
- refers to the name of the project.
- """
- raise NotImplementedError("Override in subclass")
-
- @property
- def name(self) -> str:
- """The name identifying this candidate in the resolver.
-
- This is different from ``project_name`` if this candidate contains
- extras, where ``project_name`` would not contain the ``[...]`` part.
- """
- raise NotImplementedError("Override in subclass")
-
- @property
- def version(self) -> CandidateVersion:
- raise NotImplementedError("Override in subclass")
-
- @property
- def is_installed(self) -> bool:
- raise NotImplementedError("Override in subclass")
-
- @property
- def is_editable(self) -> bool:
- raise NotImplementedError("Override in subclass")
-
- @property
- def source_link(self) -> Optional[Link]:
- raise NotImplementedError("Override in subclass")
-
- def iter_dependencies(self, with_requires: bool) -> Iterable[Optional[Requirement]]:
- raise NotImplementedError("Override in subclass")
-
- def get_install_requirement(self) -> Optional[InstallRequirement]:
- raise NotImplementedError("Override in subclass")
-
- def format_for_error(self) -> str:
- raise NotImplementedError("Subclass should override")
diff --git a/spaces/Ataturk-Chatbot/HuggingFaceChat/venv/lib/python3.11/site-packages/pip/_internal/vcs/bazaar.py b/spaces/Ataturk-Chatbot/HuggingFaceChat/venv/lib/python3.11/site-packages/pip/_internal/vcs/bazaar.py
deleted file mode 100644
index 20a17ed09272a09a5b3c0bfbd0e6c43f78db4c1e..0000000000000000000000000000000000000000
--- a/spaces/Ataturk-Chatbot/HuggingFaceChat/venv/lib/python3.11/site-packages/pip/_internal/vcs/bazaar.py
+++ /dev/null
@@ -1,112 +0,0 @@
-import logging
-from typing import List, Optional, Tuple
-
-from pip._internal.utils.misc import HiddenText, display_path
-from pip._internal.utils.subprocess import make_command
-from pip._internal.utils.urls import path_to_url
-from pip._internal.vcs.versioncontrol import (
- AuthInfo,
- RemoteNotFoundError,
- RevOptions,
- VersionControl,
- vcs,
-)
-
-logger = logging.getLogger(__name__)
-
-
-class Bazaar(VersionControl):
- name = "bzr"
- dirname = ".bzr"
- repo_name = "branch"
- schemes = (
- "bzr+http",
- "bzr+https",
- "bzr+ssh",
- "bzr+sftp",
- "bzr+ftp",
- "bzr+lp",
- "bzr+file",
- )
-
- @staticmethod
- def get_base_rev_args(rev: str) -> List[str]:
- return ["-r", rev]
-
- def fetch_new(
- self, dest: str, url: HiddenText, rev_options: RevOptions, verbosity: int
- ) -> None:
- rev_display = rev_options.to_display()
- logger.info(
- "Checking out %s%s to %s",
- url,
- rev_display,
- display_path(dest),
- )
- if verbosity <= 0:
- flag = "--quiet"
- elif verbosity == 1:
- flag = ""
- else:
- flag = f"-{'v'*verbosity}"
- cmd_args = make_command(
- "checkout", "--lightweight", flag, rev_options.to_args(), url, dest
- )
- self.run_command(cmd_args)
-
- def switch(self, dest: str, url: HiddenText, rev_options: RevOptions) -> None:
- self.run_command(make_command("switch", url), cwd=dest)
-
- def update(self, dest: str, url: HiddenText, rev_options: RevOptions) -> None:
- output = self.run_command(
- make_command("info"), show_stdout=False, stdout_only=True, cwd=dest
- )
- if output.startswith("Standalone "):
- # Older versions of pip used to create standalone branches.
- # Convert the standalone branch to a checkout by calling "bzr bind".
- cmd_args = make_command("bind", "-q", url)
- self.run_command(cmd_args, cwd=dest)
-
- cmd_args = make_command("update", "-q", rev_options.to_args())
- self.run_command(cmd_args, cwd=dest)
-
- @classmethod
- def get_url_rev_and_auth(cls, url: str) -> Tuple[str, Optional[str], AuthInfo]:
- # hotfix the URL scheme after removing bzr+ from bzr+ssh:// re-add it
- url, rev, user_pass = super().get_url_rev_and_auth(url)
- if url.startswith("ssh://"):
- url = "bzr+" + url
- return url, rev, user_pass
-
- @classmethod
- def get_remote_url(cls, location: str) -> str:
- urls = cls.run_command(
- ["info"], show_stdout=False, stdout_only=True, cwd=location
- )
- for line in urls.splitlines():
- line = line.strip()
- for x in ("checkout of branch: ", "parent branch: "):
- if line.startswith(x):
- repo = line.split(x)[1]
- if cls._is_local_repository(repo):
- return path_to_url(repo)
- return repo
- raise RemoteNotFoundError
-
- @classmethod
- def get_revision(cls, location: str) -> str:
- revision = cls.run_command(
- ["revno"],
- show_stdout=False,
- stdout_only=True,
- cwd=location,
- )
- return revision.splitlines()[-1]
-
- @classmethod
- def is_commit_id_equal(cls, dest: str, name: Optional[str]) -> bool:
- """Always assume the versions don't match"""
- return False
-
-
-vcs.register(Bazaar)
diff --git a/spaces/Ataturk-Chatbot/HuggingFaceChat/venv/lib/python3.11/site-packages/pip/_vendor/colorama/tests/ansi_test.py b/spaces/Ataturk-Chatbot/HuggingFaceChat/venv/lib/python3.11/site-packages/pip/_vendor/colorama/tests/ansi_test.py
deleted file mode 100644
index 0a20c80f882066e0e1323b0c7f61e22913c32e35..0000000000000000000000000000000000000000
--- a/spaces/Ataturk-Chatbot/HuggingFaceChat/venv/lib/python3.11/site-packages/pip/_vendor/colorama/tests/ansi_test.py
+++ /dev/null
@@ -1,76 +0,0 @@
-# Copyright Jonathan Hartley 2013. BSD 3-Clause license, see LICENSE file.
-import sys
-from unittest import TestCase, main
-
-from ..ansi import Back, Fore, Style
-from ..ansitowin32 import AnsiToWin32
-
-stdout_orig = sys.stdout
-stderr_orig = sys.stderr
-
-
-class AnsiTest(TestCase):
-
- def setUp(self):
- # sanity check: stdout should be a file or StringIO object.
- # It will only be AnsiToWin32 if init() has previously wrapped it
- self.assertNotEqual(type(sys.stdout), AnsiToWin32)
- self.assertNotEqual(type(sys.stderr), AnsiToWin32)
-
- def tearDown(self):
- sys.stdout = stdout_orig
- sys.stderr = stderr_orig
-
-
- def testForeAttributes(self):
- self.assertEqual(Fore.BLACK, '\033[30m')
- self.assertEqual(Fore.RED, '\033[31m')
- self.assertEqual(Fore.GREEN, '\033[32m')
- self.assertEqual(Fore.YELLOW, '\033[33m')
- self.assertEqual(Fore.BLUE, '\033[34m')
- self.assertEqual(Fore.MAGENTA, '\033[35m')
- self.assertEqual(Fore.CYAN, '\033[36m')
- self.assertEqual(Fore.WHITE, '\033[37m')
- self.assertEqual(Fore.RESET, '\033[39m')
-
- # Check the light, extended versions.
- self.assertEqual(Fore.LIGHTBLACK_EX, '\033[90m')
- self.assertEqual(Fore.LIGHTRED_EX, '\033[91m')
- self.assertEqual(Fore.LIGHTGREEN_EX, '\033[92m')
- self.assertEqual(Fore.LIGHTYELLOW_EX, '\033[93m')
- self.assertEqual(Fore.LIGHTBLUE_EX, '\033[94m')
- self.assertEqual(Fore.LIGHTMAGENTA_EX, '\033[95m')
- self.assertEqual(Fore.LIGHTCYAN_EX, '\033[96m')
- self.assertEqual(Fore.LIGHTWHITE_EX, '\033[97m')
-
-
- def testBackAttributes(self):
- self.assertEqual(Back.BLACK, '\033[40m')
- self.assertEqual(Back.RED, '\033[41m')
- self.assertEqual(Back.GREEN, '\033[42m')
- self.assertEqual(Back.YELLOW, '\033[43m')
- self.assertEqual(Back.BLUE, '\033[44m')
- self.assertEqual(Back.MAGENTA, '\033[45m')
- self.assertEqual(Back.CYAN, '\033[46m')
- self.assertEqual(Back.WHITE, '\033[47m')
- self.assertEqual(Back.RESET, '\033[49m')
-
- # Check the light, extended versions.
- self.assertEqual(Back.LIGHTBLACK_EX, '\033[100m')
- self.assertEqual(Back.LIGHTRED_EX, '\033[101m')
- self.assertEqual(Back.LIGHTGREEN_EX, '\033[102m')
- self.assertEqual(Back.LIGHTYELLOW_EX, '\033[103m')
- self.assertEqual(Back.LIGHTBLUE_EX, '\033[104m')
- self.assertEqual(Back.LIGHTMAGENTA_EX, '\033[105m')
- self.assertEqual(Back.LIGHTCYAN_EX, '\033[106m')
- self.assertEqual(Back.LIGHTWHITE_EX, '\033[107m')
-
-
- def testStyleAttributes(self):
- self.assertEqual(Style.DIM, '\033[2m')
- self.assertEqual(Style.NORMAL, '\033[22m')
- self.assertEqual(Style.BRIGHT, '\033[1m')
-
-
-if __name__ == '__main__':
- main()
diff --git a/spaces/Awiny/Image2Paragraph/models/grit_src/third_party/CenterNet2/detectron2/layers/batch_norm.py b/spaces/Awiny/Image2Paragraph/models/grit_src/third_party/CenterNet2/detectron2/layers/batch_norm.py
deleted file mode 100644
index 09a6c66cf6f4b21c38a7829b029f0ab5deda1f9e..0000000000000000000000000000000000000000
--- a/spaces/Awiny/Image2Paragraph/models/grit_src/third_party/CenterNet2/detectron2/layers/batch_norm.py
+++ /dev/null
@@ -1,276 +0,0 @@
-# Copyright (c) Facebook, Inc. and its affiliates.
-import torch
-import torch.distributed as dist
-from fvcore.nn.distributed import differentiable_all_reduce
-from torch import nn
-from torch.nn import functional as F
-
-from detectron2.utils import comm, env
-
-from .wrappers import BatchNorm2d
-
-
-class FrozenBatchNorm2d(nn.Module):
- """
- BatchNorm2d where the batch statistics and the affine parameters are fixed.
-
- It contains non-trainable buffers called
- "weight" and "bias", "running_mean", "running_var",
- initialized to perform identity transformation.
-
- The pre-trained backbone models from Caffe2 only contain "weight" and "bias",
- which are computed from the original four parameters of BN.
- The affine transform `x * weight + bias` will perform the equivalent
- computation of `(x - running_mean) / sqrt(running_var) * weight + bias`.
- When loading a backbone model from Caffe2, "running_mean" and "running_var"
- will be left unchanged as identity transformation.
-
- Other pre-trained backbone models may contain all 4 parameters.
-
- The forward is implemented by `F.batch_norm(..., training=False)`.
- """
-
- _version = 3
-
- def __init__(self, num_features, eps=1e-5):
- super().__init__()
- self.num_features = num_features
- self.eps = eps
- self.register_buffer("weight", torch.ones(num_features))
- self.register_buffer("bias", torch.zeros(num_features))
- self.register_buffer("running_mean", torch.zeros(num_features))
- self.register_buffer("running_var", torch.ones(num_features) - eps)
-
- def forward(self, x):
- if x.requires_grad:
- # When gradients are needed, F.batch_norm will use extra memory
- # because its backward op computes gradients for weight/bias as well.
- scale = self.weight * (self.running_var + self.eps).rsqrt()
- bias = self.bias - self.running_mean * scale
- scale = scale.reshape(1, -1, 1, 1)
- bias = bias.reshape(1, -1, 1, 1)
- out_dtype = x.dtype # may be half
- return x * scale.to(out_dtype) + bias.to(out_dtype)
- else:
- # When gradients are not needed, F.batch_norm is a single fused op
- # and provide more optimization opportunities.
- return F.batch_norm(
- x,
- self.running_mean,
- self.running_var,
- self.weight,
- self.bias,
- training=False,
- eps=self.eps,
- )
-
- def _load_from_state_dict(
- self, state_dict, prefix, local_metadata, strict, missing_keys, unexpected_keys, error_msgs
- ):
- version = local_metadata.get("version", None)
-
- if version is None or version < 2:
- # No running_mean/var in early versions
- # This will silent the warnings
- if prefix + "running_mean" not in state_dict:
- state_dict[prefix + "running_mean"] = torch.zeros_like(self.running_mean)
- if prefix + "running_var" not in state_dict:
- state_dict[prefix + "running_var"] = torch.ones_like(self.running_var)
-
- super()._load_from_state_dict(
- state_dict, prefix, local_metadata, strict, missing_keys, unexpected_keys, error_msgs
- )
-
- def __repr__(self):
- return "FrozenBatchNorm2d(num_features={}, eps={})".format(self.num_features, self.eps)
-
- @classmethod
- def convert_frozen_batchnorm(cls, module):
- """
- Convert all BatchNorm/SyncBatchNorm in module into FrozenBatchNorm.
-
- Args:
- module (torch.nn.Module):
-
- Returns:
- If module is BatchNorm/SyncBatchNorm, returns a new module.
- Otherwise, in-place convert module and return it.
-
- Similar to convert_sync_batchnorm in
- https://github.com/pytorch/pytorch/blob/master/torch/nn/modules/batchnorm.py
- """
- bn_module = nn.modules.batchnorm
- bn_module = (bn_module.BatchNorm2d, bn_module.SyncBatchNorm)
- res = module
- if isinstance(module, bn_module):
- res = cls(module.num_features)
- if module.affine:
- res.weight.data = module.weight.data.clone().detach()
- res.bias.data = module.bias.data.clone().detach()
- res.running_mean.data = module.running_mean.data
- res.running_var.data = module.running_var.data
- res.eps = module.eps
- else:
- for name, child in module.named_children():
- new_child = cls.convert_frozen_batchnorm(child)
- if new_child is not child:
- res.add_module(name, new_child)
- return res
-
-
-def get_norm(norm, out_channels):
- """
- Args:
- norm (str or callable): either one of BN, SyncBN, FrozenBN, GN;
- or a callable that takes a channel number and returns
- the normalization layer as a nn.Module.
-
- Returns:
- nn.Module or None: the normalization layer
- """
- if norm is None:
- return None
- if isinstance(norm, str):
- if len(norm) == 0:
- return None
- norm = {
- "BN": BatchNorm2d,
- # Fixed in https://github.com/pytorch/pytorch/pull/36382
- "SyncBN": NaiveSyncBatchNorm if env.TORCH_VERSION <= (1, 5) else nn.SyncBatchNorm,
- "FrozenBN": FrozenBatchNorm2d,
- "GN": lambda channels: nn.GroupNorm(32, channels),
- # for debugging:
- "nnSyncBN": nn.SyncBatchNorm,
- "naiveSyncBN": NaiveSyncBatchNorm,
- # expose stats_mode N as an option to caller, required for zero-len inputs
- "naiveSyncBN_N": lambda channels: NaiveSyncBatchNorm(channels, stats_mode="N"),
- }[norm]
- return norm(out_channels)
-
-
-class NaiveSyncBatchNorm(BatchNorm2d):
- """
- In PyTorch<=1.5, ``nn.SyncBatchNorm`` has incorrect gradient
- when the batch size on each worker is different.
- (e.g., when scale augmentation is used, or when it is applied to mask head).
-
- This is a slower but correct alternative to `nn.SyncBatchNorm`.
-
- Note:
- There isn't a single definition of Sync BatchNorm.
-
- When ``stats_mode==""``, this module computes overall statistics by using
- statistics of each worker with equal weight. The result is true statistics
- of all samples (as if they are all on one worker) only when all workers
- have the same (N, H, W). This mode does not support inputs with zero batch size.
-
- When ``stats_mode=="N"``, this module computes overall statistics by weighting
- the statistics of each worker by their ``N``. The result is true statistics
- of all samples (as if they are all on one worker) only when all workers
- have the same (H, W). It is slower than ``stats_mode==""``.
-
- Even though the result of this module may not be the true statistics of all samples,
- it may still be reasonable because it might be preferrable to assign equal weights
- to all workers, regardless of their (H, W) dimension, instead of putting larger weight
- on larger images. From preliminary experiments, little difference is found between such
- a simplified implementation and an accurate computation of overall mean & variance.
- """
-
- def __init__(self, *args, stats_mode="", **kwargs):
- super().__init__(*args, **kwargs)
- assert stats_mode in ["", "N"]
- self._stats_mode = stats_mode
-
- def forward(self, input):
- if comm.get_world_size() == 1 or not self.training:
- return super().forward(input)
-
- B, C = input.shape[0], input.shape[1]
-
- half_input = input.dtype == torch.float16
- if half_input:
- # fp16 does not have good enough numerics for the reduction here
- input = input.float()
- mean = torch.mean(input, dim=[0, 2, 3])
- meansqr = torch.mean(input * input, dim=[0, 2, 3])
-
- if self._stats_mode == "":
- assert B > 0, 'SyncBatchNorm(stats_mode="") does not support zero batch size.'
- vec = torch.cat([mean, meansqr], dim=0)
- vec = differentiable_all_reduce(vec) * (1.0 / dist.get_world_size())
- mean, meansqr = torch.split(vec, C)
- momentum = self.momentum
- else:
- if B == 0:
- vec = torch.zeros([2 * C + 1], device=mean.device, dtype=mean.dtype)
- vec = vec + input.sum() # make sure there is gradient w.r.t input
- else:
- vec = torch.cat(
- [mean, meansqr, torch.ones([1], device=mean.device, dtype=mean.dtype)], dim=0
- )
- vec = differentiable_all_reduce(vec * B)
-
- total_batch = vec[-1].detach()
- momentum = total_batch.clamp(max=1) * self.momentum # no update if total_batch is 0
- mean, meansqr, _ = torch.split(vec / total_batch.clamp(min=1), C) # avoid div-by-zero
-
- var = meansqr - mean * mean
- invstd = torch.rsqrt(var + self.eps)
- scale = self.weight * invstd
- bias = self.bias - mean * scale
- scale = scale.reshape(1, -1, 1, 1)
- bias = bias.reshape(1, -1, 1, 1)
-
- self.running_mean += momentum * (mean.detach() - self.running_mean)
- self.running_var += momentum * (var.detach() - self.running_var)
- ret = input * scale + bias
- if half_input:
- ret = ret.half()
- return ret
-
-
-class CycleBatchNormList(nn.ModuleList):
- """
- Implement domain-specific BatchNorm by cycling.
-
- When a BatchNorm layer is used for multiple input domains or input
- features, it might need to maintain a separate test-time statistics
- for each domain. See Sec 5.2 in :paper:`rethinking-batchnorm`.
-
- This module implements it by using N separate BN layers
- and it cycles through them every time a forward() is called.
-
- NOTE: The caller of this module MUST guarantee to always call
- this module by multiple of N times. Otherwise its test-time statistics
- will be incorrect.
- """
-
- def __init__(self, length: int, bn_class=nn.BatchNorm2d, **kwargs):
- """
- Args:
- length: number of BatchNorm layers to cycle.
- bn_class: the BatchNorm class to use
- kwargs: arguments of the BatchNorm class, such as num_features.
- """
- self._affine = kwargs.pop("affine", True)
- super().__init__([bn_class(**kwargs, affine=False) for k in range(length)])
- if self._affine:
- # shared affine, domain-specific BN
- channels = self[0].num_features
- self.weight = nn.Parameter(torch.ones(channels))
- self.bias = nn.Parameter(torch.zeros(channels))
- self._pos = 0
-
- def forward(self, x):
- ret = self[self._pos](x)
- self._pos = (self._pos + 1) % len(self)
-
- if self._affine:
- w = self.weight.reshape(1, -1, 1, 1)
- b = self.bias.reshape(1, -1, 1, 1)
- return ret * w + b
- else:
- return ret
-
- def extra_repr(self):
- return f"affine={self._affine}"
diff --git a/spaces/Banbri/zcvzcv/src/components/ui/dialog.tsx b/spaces/Banbri/zcvzcv/src/components/ui/dialog.tsx
deleted file mode 100644
index f06711aa00dc7335f9998dad103431bc5601039e..0000000000000000000000000000000000000000
--- a/spaces/Banbri/zcvzcv/src/components/ui/dialog.tsx
+++ /dev/null
@@ -1,122 +0,0 @@
-"use client"
-
-import * as React from "react"
-import * as DialogPrimitive from "@radix-ui/react-dialog"
-import { X } from "lucide-react"
-
-import { cn } from "@/lib/utils"
-
-const Dialog = DialogPrimitive.Root
-
-const DialogTrigger = DialogPrimitive.Trigger
-
-const DialogPortal = ({
- ...props
-}: DialogPrimitive.DialogPortalProps) => (
-
-)
-DialogPortal.displayName = DialogPrimitive.Portal.displayName
-
-const DialogOverlay = React.forwardRef<
- React.ElementRef,
- React.ComponentPropsWithoutRef
->(({ className, ...props }, ref) => (
-
-))
-DialogOverlay.displayName = DialogPrimitive.Overlay.displayName
-
-const DialogContent = React.forwardRef<
- React.ElementRef,
- React.ComponentPropsWithoutRef
->(({ className, children, ...props }, ref) => (
-
-
-
- {children}
-
-
- Close
-
-
-
-))
-DialogContent.displayName = DialogPrimitive.Content.displayName
-
-const DialogHeader = ({
- className,
- ...props
-}: React.HTMLAttributes) => (
-
-)
-DialogHeader.displayName = "DialogHeader"
-
-const DialogFooter = ({
- className,
- ...props
-}: React.HTMLAttributes) => (
-
-)
-DialogFooter.displayName = "DialogFooter"
-
-const DialogTitle = React.forwardRef<
- React.ElementRef,
- React.ComponentPropsWithoutRef
->(({ className, ...props }, ref) => (
-
-))
-DialogTitle.displayName = DialogPrimitive.Title.displayName
-
-const DialogDescription = React.forwardRef<
- React.ElementRef,
- React.ComponentPropsWithoutRef
->(({ className, ...props }, ref) => (
-
-))
-DialogDescription.displayName = DialogPrimitive.Description.displayName
-
-export {
- Dialog,
- DialogTrigger,
- DialogContent,
- DialogHeader,
- DialogFooter,
- DialogTitle,
- DialogDescription,
-}
diff --git a/spaces/Benson/text-generation/Examples/ Recuva.md b/spaces/Benson/text-generation/Examples/ Recuva.md
deleted file mode 100644
index 9f91eeefbfebf8c3addf4849466f3cf2dc0d55bb..0000000000000000000000000000000000000000
--- a/spaces/Benson/text-generation/Examples/ Recuva.md
+++ /dev/null
@@ -1,99 +0,0 @@
-
-
Download Recuva: How to Recover Deleted Files from Computer and Other Devices
-
Did you accidentally delete an important file from a computer, flash drive, memory card or other device? Do not despair, there is a way to return it back. To do this, you will need a Recuva program that can recover files even after disk formatting or cleaning up the trash. In this article we will tell you what Recuva is, how to download and install it on Windows, how to use it to recover or safely delete files.
-
What is Recuva and why is it needed?
-
Recuva is a free file recovery software developed by Piriform, also known for other products such as CCleaner, Defraggler and Speccy. Recuva can help you find and recover lost data from any media connected to your computer.
Recuva has a number of features and benefits that make it one of the best data recovery programs. Here are some of them:
-
-
Recuva can recover files of any type: photos, music, videos, documents, emails and others.
-
Recuva can work with any device that can be connected to the computer via USB or other interface: hard drives, flash drives, memory cards, digital cameras, MP3 players and others.
-
Recuva can restore files even after they have been deleted from the recycle bin, overwritten by other data or damaged by viruses.
-
Recuva has a simple and user-friendly interface that allows you to easily customize your search and recovery options. You can choose file type, location, scan depth and other options.
-
-
Recuva has a safe file removal feature that allows you to permanently erase data from the disk using various overwriting methods. This can be useful if you want to get rid of confidential information or free up disk space.
-
-
System requirements and supported file formats
-
Recuva runs on Windows 10, 8.1, 8, 7, Vista and XP operating systems (including 32-bit and 64-bit versions). The program requires about 15 MB of disk space and an Internet connection to download updates. Recuva supports over 1000 file formats including images (JPG, PNG, GIF, BMP and others), audio (MP3, WAV, WMA, OGG and others), video (AVI, MP4, WMV, MOV and others), documents (DOC, PDF, XP, PPT and others), PST and many others.
-
How to download and install Recuva on Windows?
-
There are several ways to download Recuva to your computer. We will consider two of them: from the official website of the program and using the special utility MultiSetup.
-
Download Recuva from official website
-
To download Recuva from the official website of the program, follow these steps:
Click on the button "Download Free Version" or "Download Professional" depending on what version of the program you want to get. The free version has all the basic features of Recuva, and the paid version adds features such as virtual hard disk, automatic upgrade and support.
-
On the page opened click on "Download from Piriform.com" or "Download from FileHippo.com". Both of these sites are reliable sources for downloading the program.
Click on the "Download MultiSetup" button and save the MultiSetup.exe file on your computer.
-
Start the MultiSetup.exe file and wait for the program download to finish.
-
In the list of programs, find Recuva and put a check in front of it.
-
Click on the "Set Selected" button and follow the instructions on the screen.
-
-
Install Recuva on Computer
-
After you have downloaded the recuva.exe file from the official website or with MultiSetup, you can install Recuva on your computer. To do this, follow these steps:
-
-
Start the recuva.exe file and click the "Yes" button in the account control window.
-
Select the installation language and click "OK".
-
Read and accept the license agreement by clicking on "I agree".
-
Select the installation type: standard or custom. The standard type will install Recuva in the C: Program Files Recuva folder with default settings. The custom type allows you to select a folder to install, create shortcuts on your desktop and "Start" menu, and add Recuva to the Windows Explorer context menu.
-
Click on the "Install" button and wait for the end of the process.
-
Click on "Complete" button and start Recuva.
-
-
How to use Recuva to recover deleted files?
-
Once you have installed Recuva on your computer, you can start using it to recover deleted files. To do this, follow these steps:
-
Start Recuva and select file type
-
Start Recuva and select the mode of operation: wizard or advanced. The wizard will start automatically on the first run of the program, and the advanced one can be selected by clicking the "Switch to Advanced Mode" button. We recommend that you use the wizard to start, as it is simpler and easier to understand.
-
-
-
Select search location and run scan
-
In the next window you need to choose the location of search for deleted files. You can choose one of
In the next window you need to choose a location to search for deleted files. You can choose one of the suggested options: on your computer, in your shopping cart, on your memory card, on your iPod or MP3 player, on your CD or DVD or elsewhere. You can also specify a specific path to the folder or drive where you think the files might be located. Click the "Next" button to continue.
-
In the last window you need to run a scan of the drive or folder you have selected. You can choose a normal scan or a deep scan. Normal scanning is faster but less efficient than deep scanning, which takes longer but searches for files more thoroughly. Click on the "Start" button to start the scan.
-
View results and recover files
-
After the scan is complete, you will see a list of the found files. You can sort them by name, path, size, date or state. You can also view a thumbnail of the image or the contents of the text file by clicking on the "Preview" button. You can see the probability of file recovery by color indicator: green - excellent, yellow - acceptable, red - bad.
-
To recover the files you need, tick the boxes next to them and click the "Recover" button. Select the folder where you want to save the files and click the "OK" button. It is not recommended to save files to the same disk or device from which you are recovering them, as this may result in overwriting the data and losing the chance to recover other files.
-
How to safely delete files using Recuva?
-
-
Start Recuva and go to safe delete mode
-
Start Recuva and switch to advanced mode by clicking "Switch to advanced mode". Click on the "Options" button in the upper right corner of the program window. Click the "Actions" tab and tick the "Enable Safe Deletion" option. Click the "OK" button to save the changes.
-
Select files and overwrite method
-
Return to the main program window and select a location to search for files for safe deletion. Start the scan and tick the files you want to delete. Right click on
Right click on one of the selected files and select the "Safely delete selected files" option. In the window that appears, select the overwriting method you want to use. There are several methods that differ in reliability and speed. The higher the degree of reliability, the more time the file will be overwritten and the less chance it will be to recover, but the longer it will be the process of deleting. You can choose one of the following methods:
-
-
Simple rewriting (1 pass) - the fastest and weakest method that overwrites files with one pass of random data.
-
DoD 5220.22-M (3 passes) is the middle method that overwrites files with three passes: one zero pass, one unit pass, and one random data pass.
-
NSA (7 passes) is a powerful method that overwrites files with seven passages: four passages with random data and three passages with special templates.
-
Gutmann (35 passes) is the strongest and slowest method that overwrites files with 35 passes of different templates based on Gutmann’s algorithm.
-
-
Select the method that suits you and click the "OK" button. Wait until the removal process is complete and close the program.
-
Conclusion
-
-
Frequently Asked Questions
-
In this section we will answer some frequently asked questions about Recuva.
-
Can I recover files from SSD disk with Recuva?
-
Recovering files from an SSD disk using Recuva can be difficult due to the special features of this type of disk. SSD disks use TRIM technology, which automatically erases data from the disk after it has been deleted to increase speed and prolong disk life. This means that deleted files may not be available for recovery. However, you can still try using Recuva to search for files on an SSD disk, as TRIM does not always work instantly or completely.
-
Can I recover files from an encrypted
Can I recover files from an encrypted disk with Recuva?
-
Recover files from an encrypted disk using Recuva depends on what type of encryption was used. If the disk has been encrypted with BitLocker, Recuva will not be able to recover the files until you unlock the disk with a password or key. If the disk has been encrypted with another program, such as VeraCrypt or TrueCrypt, Recuva can recover files if you connect the encrypted disk as a virtual drive and run Recuva on it. In any case, you need to know the password or key to access encrypted data.
-
Can I recover files from a damaged disk with Recuva?
-
Recovering files from a corrupted disk with Recuva can be possible if the damage does not affect the physical structure of the disk. If the disk has logical errors such as a corrupted file system, Recuva may try to fix them and find the files. If the disk has physical damage such as scratches, Recuva will not be able to recover the files as they may not be readable. In this case, you need to contact data recovery specialists.
-
-
Recovering files from a formatted disk using Recuva depends on the type of formatting performed. If the disk has been formatted with quick formatting, Recuva can recover the files as they have not been completely erased from the disk. If the disk was formatted with full formatting, Recuva would not be able to recover the files because they were overwritten with zeros or other data. In this case, the chances of recovery are very small.
-
Can I recover files from a remote partition with Recuva?
-
Recovering files from a remote partition with Recuva can be possible if the partition has not been overwritten by another partition or data. To do this, you need to run Recuva and select the "Elsewhere" option in the search location window. Then you need to select the physical disk on which the remote partition was located and run a deep scan. Recuva will try to find and recover files from the remote partition.
-
Can I recover files after reinstalling Windows with Recuva?
-
Recovering files after reinstalling Windows with Recuva can be difficult because Windows reinstallation can overwrite or delete data on disk C. If you want to save your data before reinstalling Windows, then you need to copy them to another disk or device. If you have already reinstalled Windows and want to recover your data, you need to run Recuva and select C in the search location window. Run a deep scan and see what Recuva can find. You may be able to recover some files that were not overwritten or deleted during Windows reinstallation. However, the chances are not very high, so it is best to always back up your data.
-
-Recuva: how to recover deleted files from computer and other devices
-
Did you accidentally delete an important file from a computer, flash drive, memory card or other device? Do not despair, there is a way to return it back. To do this, you will need a Recuva program that can recover files even after disk formatting or cleaning up the trash. In this article we will tell you what Recuva is, how to download and install it on Windows, how to use it to recover or safely delete files.
-
What is Recuva and why is it needed?
-
Recuva is a free file recovery software developed by Piriform, also known for other products such as CCleaner, Defraggler and Speccy. Recuva can help you find and recover lost data from any media connected to your computer.
-
The main features and benefits of Recuva
-
Recuva has a number of features and benefits that make it one of the best data recovery programs. Here are some of them:
-
-
64aa2da5cf
-
-
\ No newline at end of file
diff --git a/spaces/Benson/text-generation/Examples/Apk Kafa Topu 2.md b/spaces/Benson/text-generation/Examples/Apk Kafa Topu 2.md
deleted file mode 100644
index 78d9e21d7b4c043db6822eedd9433c3674eab7ed..0000000000000000000000000000000000000000
--- a/spaces/Benson/text-generation/Examples/Apk Kafa Topu 2.md
+++ /dev/null
@@ -1,123 +0,0 @@
-
-
Kafa Topu 2: Un juego de fútbol en línea divertido y competitivo
¿Te encanta el fútbol? ¿Te gusta jugar juegos en línea con tus amigos? Si respondiste sí a ambas preguntas, entonces definitivamente deberías echar un vistazo a Kafa Topu 2, un divertido y competitivo juego de fútbol en línea que te mantendrá entretenido durante horas.
-
Kafa Topu 2 es la secuela del popular juego en línea Kafa Topu, que tiene millones de fans en todo el mundo. En este juego, puede crear su propio héroe de fútbol, personalizar su apariencia y accesorios, unirse a un equipo o crear el suyo propio, y competir en varias ligas y torneos contra oponentes reales de todo el mundo.
-
Kafa Topu 2 no es su juego de fútbol típico. Tiene características de juego únicas que lo hacen más emocionante y desafiante que otros juegos. Por ejemplo, puedes usar superpoderes para mejorar tu rendimiento, marcar goles increíbles y derrotar a tus rivales. También puedes interactuar con otros jugadores a través de la integración de redes sociales, chatear con ellos, enviarles regalos y retarlos a partidos amistosos.
-
Si usted está buscando una nueva manera de disfrutar de fútbol en línea, entonces Kafa Topu 2 es el juego para usted. En este artículo, te contaremos más sobre las características de Kafa Topu 2, cómo descargar el archivo APK para tu dispositivo y algunos consejos y trucos para ayudarte a mejorar tus habilidades y divertirte más.
-
Características de Kafa Topu 2
-
Kafa Topu 2 tiene muchas características que lo hacen destacar de otros juegos de fútbol en línea. Estos son algunos de ellos:
-
Personajes y accesorios
-
-
También puedes actualizar tu personaje para mejorar sus atributos, como velocidad, salto, poder de disparo y súper poder. Cuanto más alto sea tu nivel, más opciones tendrás para mejorar tu personaje. También puedes usar objetos especiales, como tarjetas y pociones, para mejorar tu rendimiento temporalmente.
-
Super Powers
-
Otra característica que hace Kafa Topu 2 diferente de otros juegos de fútbol es el uso de superpoderes. Los súper poderes son habilidades especiales que puedes activar durante una partida para obtener una ventaja sobre tu oponente. Por ejemplo, puedes usar una bola de fuego para lanzar un tiro poderoso que puede quemar la red de goles del oponente, o un imán para atraer la pelota a tu cabeza.
-
Hay más de 30 superpoderes diferentes en Kafa Topu 2, cada uno con sus propios efectos y animaciones. Puedes desbloquear nuevos súper poderes a medida que avanzas en el juego, o comprarlos con diamantes o monedas. También puedes actualizar tus súper poderes para hacerlos más efectivos y durar más.
-
-
Equipos y Ligas
-
Kafa Topu 2 no es solo un juego para jugadores en solitario. También puede unirse a un equipo o crear su propio equipo y jugar con otros jugadores que comparten su pasión por el fútbol. Al unirte a un equipo, puedes participar en eventos de equipo, como torneos y ligas, donde puedes competir contra otros equipos y ganar recompensas.
-
También puedes chatear con tus compañeros de equipo, enviarles regalos, invitarlos a partidos y apoyarlos durante sus partidos. También puedes contribuir al progreso de tu equipo donando monedas o diamantes. También puedes crear tu propio equipo e invitar a tus amigos a unirse a ti. Puedes personalizar el nombre, el logotipo y el lema de tu equipo, y gestionar los miembros y eventos de tu equipo.
-
Integración de redes sociales
-
-
También puedes chatear con otros jugadores en el juego, enviarles mensajes, emojis y regalos, y retarlos a partidos amistosos. También puede unirse a la comunidad oficial de Kafa Topu 2 en las plataformas de redes sociales, donde puede interactuar con otros fans, obtener las últimas noticias y actualizaciones, participar en concursos y regalos, y obtener apoyo de los desarrolladores.
-
Cómo descargar Kafa Topu 2 APK
-
Si usted está interesado en jugar Kafa Topu 2, es posible que se pregunte cómo descargar el archivo APK para su dispositivo. APK significa Android Package Kit, y es un formato de archivo que le permite instalar aplicaciones que no están disponibles en Google Play Store. Aquí están los pasos para descargar Kafa Topu 2 APK para dispositivos Android y usuarios de PC:
-
Para dispositivos Android
-
-
Vaya al sitio web oficial de Kafa Topu 2 en https://www.kafatopu2.com/ y haga clic en el botón "Descargar".
-
Usted será redirigido a una página donde se puede elegir la versión del archivo APK que se adapte a su dispositivo. Por ejemplo, si tienes un dispositivo Android 10, puedes elegir la opción "Android 10".
-
Después de elegir la versión, haga clic en el botón "Descargar APK" y espere a que el archivo se descargue en su dispositivo.
-
Antes de instalar el archivo APK, es necesario habilitar la "Fuentes desconocidas" opción en el dispositivo. Para hacer esto, vaya a Configuración > Seguridad > Fuentes desconocidas y conéctelo.
-
Ahora puede instalar el archivo APK tocando en él y siguiendo las instrucciones en la pantalla.
-
Una vez completada la instalación, puede iniciar el juego y disfrutar jugando Kafa Topu 2.
-
-
Para usuarios de PC
-
-
Si desea jugar Kafa Topu 2 en su PC, es necesario utilizar un emulador de Android. Un emulador de Android es un software que le permite ejecutar aplicaciones Android en su PC. Hay muchos emuladores de Android disponibles en línea, como BlueStacks, NoxPlayer, MEmu, etc.
-
-
Después de instalar el emulador, iniciarlo e iniciar sesión con su cuenta de Google.
-
Vaya al sitio web oficial de Kafa Topu 2 en https://www.kafatopu2.com/ y haga clic en el botón "Descargar".
-
Usted será redirigido a una página donde se puede elegir la versión del archivo APK que se adapte a su emulador. Por ejemplo, si está usando BlueStacks, puede elegir la opción "BlueStacks".
-
Después de elegir la versión, haga clic en el "Descargar APK" botón y esperar a que el archivo para ser descargado a su PC.
-
Una vez descargado el archivo, arrástrelo y suéltelo en la ventana del emulador o use el administrador de archivos del emulador para localizarlo e instalarlo.
-
Una vez completada la instalación, puede iniciar el juego y disfrutar jugando Kafa Topu 2 en su PC.
-
-
Consejos y trucos para jugar Kafa Topu 2
-
Kafa Topu 2 es un juego que requiere habilidad, estrategia y práctica para dominar. Aquí hay algunos consejos y trucos que pueden ayudarte a mejorar tu juego y divertirte más:
-
-
Practica en modo offline antes de jugar online. El modo offline te permite jugar contra oponentes de IA con diferentes niveles de dificultad. Esto puede ayudarte a familiarizarte con los controles, la mecánica de juego, los personajes, los superpoderes y los mapas.
-
Elige un personaje que se adapte a tu estilo de juego. Cada personaje tiene sus propias fortalezas y debilidades, como velocidad, salto, poder de disparo y súper poder. Experimenta con diferentes caracteres y encuentra uno que coincida con tus preferencias.
-
Usa súper poderes sabiamente. Los súper poderes pueden darte una ventaja sobre tu oponente, pero también tienen un tiempo de reutilización y una duración limitada. Úsalos en el momento adecuado, como cuando estés en una buena posición para anotar, cuando necesites defender tu objetivo o cuando quieras sorprender a tu oponente.
-
-
Únete a un equipo o crea el tuyo. Jugar con un equipo puede hacer el juego más divertido y gratificante. Puedes unirte a un equipo existente o crear tu propio equipo e invitar a tus amigos a unirse a ti. Al jugar con un equipo, puedes participar en eventos de equipo, chatear con tus compañeros de equipo, enviarles regalos y apoyarlos durante sus partidos.
-
Sigue el juego en las redes sociales. Al seguir el juego en las plataformas de redes sociales, como Facebook, Twitter e Instagram, puedes obtener las últimas noticias y actualizaciones, participar en concursos y regalos, obtener apoyo de los desarrolladores e interactuar con otros fans.
-
-
Conclusión
-
Kafa Topu 2 es un divertido y competitivo juego de fútbol en línea que te mantendrá entretenido durante horas. Puede crear su propio héroe de fútbol, personalizar su apariencia y accesorios, unirse a un equipo o crear el suyo propio, y competir en varias ligas y torneos contra oponentes reales de todo el mundo. También puedes usar superpoderes para mejorar tu rendimiento, marcar goles increíbles y derrotar a tus rivales. También puedes interactuar con otros jugadores a través de la integración de redes sociales, chatear con ellos, enviarles regalos y retarlos a partidos amistosos.
-
Si usted está buscando una nueva manera de disfrutar de fútbol en línea, entonces Kafa Topu 2 es el juego para usted. Puede descargar el archivo APK para su dispositivo desde el sitio web oficial de Kafa Topu 2 en https://www.kafatopu2.com/ y comenzar a jugar de inmediato. También puedes seguir el juego en las plataformas de redes sociales para obtener más información y apoyo.
-
Esperamos que este artículo te haya ayudado a aprender más sobre Kafa Topu 2 y cómo jugarlo. Si tiene alguna pregunta o comentario, no dude en dejar un comentario a continuación. Nos encantaría saber de usted.
-
FAQs
-
Aquí hay algunas preguntas frecuentes sobre Kafa Topu 2:
-
-
¿Qué es Kafa Topu 2?
-
-
¿Cómo descargo Kafa Topu 2 APK?
-
Puede descargar Kafa Topu 2 APK desde el sitio web oficial de Kafa Topu 2 en https://www.kafatopu2.com/. Puede elegir la versión del archivo APK que se adapte a su dispositivo o emulador. Debe habilitar la opción "Fuentes desconocidas" en su dispositivo o emulador antes de instalar el archivo APK.
-
¿Cómo puedo usar superpoderes en Kafa Topu 2?
-
Puedes usar superpoderes en Kafa Topu 2 tocando el ícono de súper poder en la esquina inferior derecha de la pantalla durante un partido. Puedes elegir entre más de 30 superpoderes diferentes, cada uno con sus propios efectos y animaciones. Puedes desbloquear nuevos súper poderes a medida que avanzas en el juego, o comprarlos con diamantes o monedas. También puedes actualizar tus súper poderes para hacerlos más efectivos y durar más.
-
¿Cómo me uno o creo un equipo en Kafa Topu 2?
-
Puedes unirte o crear un equipo en Kafa Topu 2 tocando el icono del equipo en la esquina inferior izquierda de la pantalla. Puede buscar un equipo existente o crear su propio equipo introduciendo un nombre, logotipo y lema. Puedes invitar a tus amigos a unirse a tu equipo enviándoles un código o un enlace. Al unirte a un equipo, puedes participar en eventos de equipo, chatear con tus compañeros de equipo, enviarles regalos y apoyarlos durante sus partidos.
-
¿Cómo sigo Kafa Topu 2 en las redes sociales?
64aa2da5cf
-
-
\ No newline at end of file
diff --git a/spaces/Benson/text-generation/Examples/Descargar Fondo De Pantalla Para Macbook Aire.md b/spaces/Benson/text-generation/Examples/Descargar Fondo De Pantalla Para Macbook Aire.md
deleted file mode 100644
index 4640b036fecabab65b79dac38de3690a8f5c18c6..0000000000000000000000000000000000000000
--- a/spaces/Benson/text-generation/Examples/Descargar Fondo De Pantalla Para Macbook Aire.md
+++ /dev/null
@@ -1,149 +0,0 @@
-
-
Cómo descargar fondos de escritorio para su MacBook Air
-
Fondo de pantalla es la imagen o color que aparece en el fondo del escritorio. Puede hacer que su MacBook Air se vea más personalizado, atractivo e inspirador. Puede elegir entre una variedad de fondos de pantalla proporcionados por Apple, o utilizar sus propias fotos o imágenes de la web.
En este artículo, aprenderá cómo descargar fondos de escritorio para su MacBook Air de diferentes fuentes, y cómo cambiar y personalizar la configuración del fondo de pantalla. Ya sea que desee un paisaje impresionante, un animal lindo o una cita motivacional, descubrirá cómo hacer que su escritorio se vea increíble en unos pocos pasos simples.
-
Lo que necesita para descargar fondos de escritorio para su MacBook Air
-
Antes de comenzar a descargar fondos de escritorio para su MacBook Air, necesitará algunas cosas:
-
-
Un MacBook Air con macOS Ventura, Monterey, o posterior.
-
Una conexión a Internet.
-
Un navegador web (como Safari, Chrome o Firefox).
-
Una carpeta o álbum de fotos donde desea guardar sus imágenes de fondo de pantalla descargadas.
-
-
También necesitará algunos conocimientos básicos de cómo usar su MacBook Air, como cómo abrir aplicaciones, navegar por la web y guardar archivos.
-
-
Cómo encontrar las mejores fuentes de fondo de pantalla para su MacBook Air
-
Hay muchos sitios web que ofrecen imágenes de fondo de pantalla gratuitas y de alta calidad para su MacBook Air. Sin embargo, no todos ellos son seguros, legales o adecuados para su resolución de pantalla. Aquí hay algunos consejos y sitios web para ayudarle a encontrar las mejores fuentes de fondos de escritorio para su MacBook Air:
-
-
Busque sitios web que tienen una gran colección de imágenes de fondos de escritorio en diferentes categorías, como la naturaleza, los animales, el arte, el resumen, etc.
-
Compruebe la licencia y los términos de uso de las imágenes de fondo de pantalla antes de descargarlas. Algunos sitios web pueden requerir atribución o permiso del autor original.
-
-
Evite descargar imágenes de papel tapiz de sitios web sospechosos o desconocidos que pueden contener malware o virus.
-
-
Aquí están algunos de los mejores sitios web que ofrecen imágenes de fondo de pantalla gratuitas y de alta calidad para su MacBook Air:
Pexels es un sitio web popular que ofrece miles de fotos y videos de stock gratuitos que puede usar para fines personales y comerciales. Puede navegar por categoría, color, orientación, tamaño o popularidad.
Unsplash es otro sitio web que ofrece más de 2 millones de imágenes gratuitas de alta resolución que se pueden utilizar para cualquier cosa. Puedes navegar por colecciones, temas o buscar por palabras clave.
Wallpaper Flare es un sitio web que se especializa en fondos de pantalla de alta definición para diferentes dispositivos y resoluciones de pantalla. Puedes navegar por categorías como anime, juegos, películas, naturaleza, etc.
9to5Mac es un sitio web que cubre noticias y. comentarios, consejos y descargas de productos de Apple. Puedes encontrar fondos de pantalla inspirados en los fondos de pantalla oficiales de Apple, como macOS Ventura, iOS 15, etc.
-
-
-
Cómo descargar imágenes de papel pintado desde la Web
-
Una vez que haya encontrado una imagen de fondo de pantalla que te gusta de uno de los sitios web anteriores, puede descargarlo en su MacBook Air siguiendo estos pasos:
-
-
Haga clic en la imagen del fondo de pantalla para abrirlo en tamaño completo.
-
Haga clic derecho en la imagen y seleccione Guardar imagen como...
-
Elija una carpeta o álbum de fotos donde desea guardar la imagen. Puede crear una nueva carpeta haciendo clic en el botón Nueva carpeta en la parte inferior izquierda de la ventana.
-
Dele un nombre a la imagen y haga clic en Guardar.
-
-
-
Cómo cambiar el fondo de pantalla en su MacBook Air
-
Después de haber descargado algunas imágenes de fondo, puede cambiar su fondo de escritorio mediante uno de estos métodos:
-
Usando las preferencias del sistema
-
-
Haga clic en el logotipo de Apple en la esquina superior izquierda de la pantalla y seleccione Preferencias del sistema.
-
Haga clic en Escritorio y Protector de pantalla.
-
Haga clic en la pestaña Escritorio.
-
Seleccione una carpeta o álbum de fotos desde la barra lateral izquierda donde guardó sus imágenes de fondo de pantalla.
-
Haga clic en la imagen del fondo de pantalla que desea utilizar desde el panel derecho.
-
-
También puede elegir entre los fondos de pantalla predeterminados proporcionados por Apple seleccionando una de las categorías de la barra lateral izquierda, como Apple, Colores, Fotos, etc.
-
Hacer clic derecho
-
-
Encuentra la imagen de fondo que quieres usar en Finder o en tu escritorio.
-
Haga clic derecho en la imagen y seleccione Establecer imagen de escritorio.
-
-
Esto cambiará instantáneamente el fondo del escritorio a la imagen seleccionada.
-
Cómo personalizar la configuración del fondo de pantalla en su MacBook Air
-
Si desea personalizar aún más la configuración de su fondo de pantalla, como cómo se ajusta a su pantalla, con qué frecuencia cambia, o cómo se adapta al modo de luz y oscuridad, puede usar estas opciones:
-
Ajuste de las opciones de visualización
-
Puede ajustar cómo su imagen de fondo de pantalla llena su pantalla utilizando las opciones de visualización en la parte inferior de la pestaña Escritorio en Preferencias del sistema. Puede elegir entre estas opciones:
-
-
Pantalla de relleno: Esto estirará o recortará su imagen para llenar toda la pantalla.
-
Ajustar a la pantalla: Esto cambiará el tamaño de la imagen para adaptarse a la pantalla sin cambiar su relación de aspecto.
-
Centro: Esto centrará su imagen en su pantalla sin cambiar su tamaño.
-
Azulejo: Esto repetirá su imagen en la pantalla como azulejos.
-
Estirar a rellenar pantalla: Esto estirará su imagen para llenar toda la pantalla sin recortarla.
-
-
También puede ajustar el color del espacio alrededor de la imagen haciendo clic en el selector de color junto a las opciones de visualización.
-
Ciclismo a través de múltiples imágenes
-
Si desea usar más de una imagen de fondo de pantalla y hacer que cambien automáticamente, puede usar la opción Cambiar imagen en la parte inferior de la pestaña Escritorio en Preferencias del sistema. Puede elegir entre estas opciones:
-
-
Seleccione una carpeta o álbum de fotos desde la barra lateral izquierda donde guardó sus imágenes de fondo de pantalla.
-
Marque la casilla junto a Cambiar imagen y seleccione con qué frecuencia desea que cambie su fondo de pantalla. Puede elegir entre intervalos como cada 5 segundos, cada hora, cada día, etc.
-
También puede marcar la casilla junto a Orden aleatorio si desea que sus imágenes de fondo de pantalla cambien en un orden aleatorio en lugar de un orden secuencial.
-
-
Esto creará una presentación de diapositivas de sus imágenes de fondo de pantalla que se desplazará de acuerdo con su configuración.
-
Uso de fondos de pantalla dinámicos o claros y oscuros
-
Si desea utilizar fondos de pantalla que cambian según la hora del día o el modo de apariencia de su MacBook Air, puede utilizar fondos de pantalla dinámicos o claros y oscuros. Estos son algunos de los fondos de pantalla predeterminados proporcionados por Apple que tienen diferentes versiones para el día y la noche o el modo de luz y oscuridad. Puede encontrarlos en la ficha Escritorio en Preferencias del sistema en Escritorio dinámico o Escritorio claro y oscuro. Puede elegir entre estas opciones:
-
-
Seleccione un fondo de pantalla dinámico o claro y oscuro desde el panel derecho. Puede previsualizar cómo cambia moviendo el cursor sobre él.
-
Si elige un fondo de pantalla dinámico, puede ajustar cómo cambia según su ubicación o zona horaria haciendo clic en Opciones de escritorio dinámico en la parte inferior derecha de la ventana. Puede elegir entre opciones de Solar, Lunar o Cambio de Tiempo.
-
-
-
Esto hará que su fondo de pantalla coincida con la luz ambiental o el tema de su MacBook Air.
-
Conclusión
-
Descargar fondos de escritorio para su MacBook Air es una forma divertida y fácil de personalizar su escritorio y hacerlo más atractivo. Puede encontrar y descargar imágenes de fondos de escritorio de varios sitios web, cambiar y ajustar la configuración de fondos de pantalla, y utilizar fondos de pantalla dinámicos o claros y oscuros para adaptarse a su estado de ánimo o entorno. También puedes experimentar con diferentes imágenes de papel tapiz y ver cuáles te gustan más.
-
Esperamos que haya encontrado este artículo útil e informativo. Si tiene alguna pregunta o comentario, no dude en dejar un comentario a continuación. ¡Nos encantaría saber de ti!
-
Preguntas frecuentes
-
¿Cómo puedo descargar fondos de escritorio para mi MacBook Air desde mi iPhone o iPad?
-
Si tiene un iPhone o iPad, puede descargar imágenes de fondo de pantalla desde su dispositivo y transferirlas a su MacBook Air utilizando AirDrop. Así es como:
-
-
En su iPhone o iPad, encontrar la imagen de fondo de pantalla que desea utilizar en Fotos o Safari.
-
Toque en el icono Compartir en la parte inferior izquierda de la pantalla y seleccione AirDrop.
-
En su MacBook Air, asegúrese de que AirDrop está habilitado en el Finder o Centro de control.
-
Seleccione su MacBook Air de la lista de dispositivos que aparecen en su iPhone o iPad.
-
En su MacBook Air, acepte el archivo entrante y elija dónde guardarlo.
-
-
¿Cómo puedo descargar fondos de escritorio para mi MacBook Air desde una unidad USB o un disco duro externo?
-
Si tiene una unidad USB o un disco duro externo que contiene imágenes de fondo de pantalla, puede descargarlas en su MacBook Air siguiendo estos pasos:
-
-
Conecte su unidad USB o disco duro externo a su MacBook Air utilizando un cable o adaptador USB.
-
Abra el Finder y localice su unidad USB o disco duro externo en Ubicaciones en la barra lateral izquierda.
-
-
Arrástrelos y suéltelos en su escritorio o en una carpeta de su MacBook Air.
-
Expulse su unidad USB o disco duro externo haciendo clic en el icono Expulsar junto a su nombre en Finder.
-
-
¿Cómo puedo descargar fondos de escritorio para mi MacBook Air desde un CD o DVD?
-
Si tiene un CD o DVD que contiene imágenes de papel tapiz, puede descargarlas en su MacBook Air siguiendo estos pasos:
-
-
Inserte su CD o DVD en la unidad óptica de su MacBook Air. Si no tiene una unidad óptica, puede utilizar una externa que se conecta a través de USB.
-
Abra el Finder y localice su CD o DVD en Dispositivos en la barra lateral izquierda.
-
Abra la carpeta que contiene sus imágenes de fondo de pantalla y seleccione las que desea utilizar.
-
Arrástrelos y suéltelos en su escritorio o en una carpeta de su MacBook Air.
-
Expulse su CD o DVD haciendo clic en el icono Expulsar junto a su nombre en Finder.
-
-
¿Cómo puedo descargar fondos de escritorio para mi MacBook Air desde un archivo adjunto de correo electrónico?
-
Si ha recibido un correo electrónico que contiene una imagen de fondo de pantalla como archivo adjunto, puede descargarlo en su MacBook Air siguiendo estos pasos:
-
-
Abra el correo electrónico que contiene el archivo adjunto en Mail u otra aplicación de correo electrónico.
-
Haga clic en el icono de archivo adjunto en la parte inferior del correo electrónico para previsualizarlo.
-
Haga clic derecho en la imagen y seleccione Guardar archivo adjunto...
-
Elija una carpeta o álbum de fotos donde desea guardar la imagen y haga clic en Guardar.
-
-
¿Cómo puedo descargar fondos de escritorio para mi MacBook Air desde un servicio en la nube?
-
Si ha almacenado algunas imágenes de fondo de pantalla en un servicio de nube como iCloud, Dropbox, Google Drive, etc., puede descargarlas en su MacBook Air siguiendo estos pasos:
-
-
Abra Safari u otro navegador web y vaya al sitio web de su servicio en la nube.
-
Inicie sesión con su nombre de usuario y contraseña si es necesario.
-
-
Haga clic en el icono Descargar en la parte superior derecha de la ventana y elija dónde guardarlos en su MacBook Air.
- 64aa2da5cf
-
-
\ No newline at end of file
diff --git a/spaces/Big-Web/MMSD/env/Lib/site-packages/pip/_vendor/rich/diagnose.py b/spaces/Big-Web/MMSD/env/Lib/site-packages/pip/_vendor/rich/diagnose.py
deleted file mode 100644
index ad36183898eddb11e33ccb7623c0291ccc0f091d..0000000000000000000000000000000000000000
--- a/spaces/Big-Web/MMSD/env/Lib/site-packages/pip/_vendor/rich/diagnose.py
+++ /dev/null
@@ -1,37 +0,0 @@
-import os
-import platform
-
-from pip._vendor.rich import inspect
-from pip._vendor.rich.console import Console, get_windows_console_features
-from pip._vendor.rich.panel import Panel
-from pip._vendor.rich.pretty import Pretty
-
-
-def report() -> None: # pragma: no cover
- """Print a report to the terminal with debugging information"""
- console = Console()
- inspect(console)
- features = get_windows_console_features()
- inspect(features)
-
- env_names = (
- "TERM",
- "COLORTERM",
- "CLICOLOR",
- "NO_COLOR",
- "TERM_PROGRAM",
- "COLUMNS",
- "LINES",
- "JUPYTER_COLUMNS",
- "JUPYTER_LINES",
- "JPY_PARENT_PID",
- "VSCODE_VERBOSE_LOGGING",
- )
- env = {name: os.getenv(name) for name in env_names}
- console.print(Panel.fit((Pretty(env)), title="[b]Environment Variables"))
-
- console.print(f'platform="{platform.system()}"')
-
-
-if __name__ == "__main__": # pragma: no cover
- report()
diff --git a/spaces/Big-Web/MMSD/env/Lib/site-packages/setuptools/_vendor/packaging/requirements.py b/spaces/Big-Web/MMSD/env/Lib/site-packages/setuptools/_vendor/packaging/requirements.py
deleted file mode 100644
index 0d93231b4613b27acd2bf7c1283d4ae99d595bdc..0000000000000000000000000000000000000000
--- a/spaces/Big-Web/MMSD/env/Lib/site-packages/setuptools/_vendor/packaging/requirements.py
+++ /dev/null
@@ -1,146 +0,0 @@
-# This file is dual licensed under the terms of the Apache License, Version
-# 2.0, and the BSD License. See the LICENSE file in the root of this repository
-# for complete details.
-
-import re
-import string
-import urllib.parse
-from typing import List, Optional as TOptional, Set
-
-from setuptools.extern.pyparsing import ( # noqa
- Combine,
- Literal as L,
- Optional,
- ParseException,
- Regex,
- Word,
- ZeroOrMore,
- originalTextFor,
- stringEnd,
- stringStart,
-)
-
-from .markers import MARKER_EXPR, Marker
-from .specifiers import LegacySpecifier, Specifier, SpecifierSet
-
-
-class InvalidRequirement(ValueError):
- """
- An invalid requirement was found, users should refer to PEP 508.
- """
-
-
-ALPHANUM = Word(string.ascii_letters + string.digits)
-
-LBRACKET = L("[").suppress()
-RBRACKET = L("]").suppress()
-LPAREN = L("(").suppress()
-RPAREN = L(")").suppress()
-COMMA = L(",").suppress()
-SEMICOLON = L(";").suppress()
-AT = L("@").suppress()
-
-PUNCTUATION = Word("-_.")
-IDENTIFIER_END = ALPHANUM | (ZeroOrMore(PUNCTUATION) + ALPHANUM)
-IDENTIFIER = Combine(ALPHANUM + ZeroOrMore(IDENTIFIER_END))
-
-NAME = IDENTIFIER("name")
-EXTRA = IDENTIFIER
-
-URI = Regex(r"[^ ]+")("url")
-URL = AT + URI
-
-EXTRAS_LIST = EXTRA + ZeroOrMore(COMMA + EXTRA)
-EXTRAS = (LBRACKET + Optional(EXTRAS_LIST) + RBRACKET)("extras")
-
-VERSION_PEP440 = Regex(Specifier._regex_str, re.VERBOSE | re.IGNORECASE)
-VERSION_LEGACY = Regex(LegacySpecifier._regex_str, re.VERBOSE | re.IGNORECASE)
-
-VERSION_ONE = VERSION_PEP440 ^ VERSION_LEGACY
-VERSION_MANY = Combine(
- VERSION_ONE + ZeroOrMore(COMMA + VERSION_ONE), joinString=",", adjacent=False
-)("_raw_spec")
-_VERSION_SPEC = Optional((LPAREN + VERSION_MANY + RPAREN) | VERSION_MANY)
-_VERSION_SPEC.setParseAction(lambda s, l, t: t._raw_spec or "")
-
-VERSION_SPEC = originalTextFor(_VERSION_SPEC)("specifier")
-VERSION_SPEC.setParseAction(lambda s, l, t: t[1])
-
-MARKER_EXPR = originalTextFor(MARKER_EXPR())("marker")
-MARKER_EXPR.setParseAction(
- lambda s, l, t: Marker(s[t._original_start : t._original_end])
-)
-MARKER_SEPARATOR = SEMICOLON
-MARKER = MARKER_SEPARATOR + MARKER_EXPR
-
-VERSION_AND_MARKER = VERSION_SPEC + Optional(MARKER)
-URL_AND_MARKER = URL + Optional(MARKER)
-
-NAMED_REQUIREMENT = NAME + Optional(EXTRAS) + (URL_AND_MARKER | VERSION_AND_MARKER)
-
-REQUIREMENT = stringStart + NAMED_REQUIREMENT + stringEnd
-# setuptools.extern.pyparsing isn't thread safe during initialization, so we do it eagerly, see
-# issue #104
-REQUIREMENT.parseString("x[]")
-
-
-class Requirement:
- """Parse a requirement.
-
- Parse a given requirement string into its parts, such as name, specifier,
- URL, and extras. Raises InvalidRequirement on a badly-formed requirement
- string.
- """
-
- # TODO: Can we test whether something is contained within a requirement?
- # If so how do we do that? Do we need to test against the _name_ of
- # the thing as well as the version? What about the markers?
- # TODO: Can we normalize the name and extra name?
-
- def __init__(self, requirement_string: str) -> None:
- try:
- req = REQUIREMENT.parseString(requirement_string)
- except ParseException as e:
- raise InvalidRequirement(
- f'Parse error at "{ requirement_string[e.loc : e.loc + 8]!r}": {e.msg}'
- )
-
- self.name: str = req.name
- if req.url:
- parsed_url = urllib.parse.urlparse(req.url)
- if parsed_url.scheme == "file":
- if urllib.parse.urlunparse(parsed_url) != req.url:
- raise InvalidRequirement("Invalid URL given")
- elif not (parsed_url.scheme and parsed_url.netloc) or (
- not parsed_url.scheme and not parsed_url.netloc
- ):
- raise InvalidRequirement(f"Invalid URL: {req.url}")
- self.url: TOptional[str] = req.url
- else:
- self.url = None
- self.extras: Set[str] = set(req.extras.asList() if req.extras else [])
- self.specifier: SpecifierSet = SpecifierSet(req.specifier)
- self.marker: TOptional[Marker] = req.marker if req.marker else None
-
- def __str__(self) -> str:
- parts: List[str] = [self.name]
-
- if self.extras:
- formatted_extras = ",".join(sorted(self.extras))
- parts.append(f"[{formatted_extras}]")
-
- if self.specifier:
- parts.append(str(self.specifier))
-
- if self.url:
- parts.append(f"@ {self.url}")
- if self.marker:
- parts.append(" ")
-
- if self.marker:
- parts.append(f"; {self.marker}")
-
- return "".join(parts)
-
- def __repr__(self) -> str:
- return f""
diff --git a/spaces/Blessin/yes-and-improv-game/README.md b/spaces/Blessin/yes-and-improv-game/README.md
deleted file mode 100644
index edf396aac9019d3169b2c986fcb76a3f95f3a85e..0000000000000000000000000000000000000000
--- a/spaces/Blessin/yes-and-improv-game/README.md
+++ /dev/null
@@ -1,12 +0,0 @@
----
-title: Yes And Improv Game
-emoji: 📊
-colorFrom: red
-colorTo: yellow
-sdk: gradio
-sdk_version: 3.50.2
-app_file: app.py
-pinned: false
----
-
-Check out the configuration reference at https://huggingface.co/docs/hub/spaces-config-reference
diff --git a/spaces/CHDCruze/entertainmentbybhdcruze/index.html b/spaces/CHDCruze/entertainmentbybhdcruze/index.html
deleted file mode 100644
index 274812742b7672097970f099050198f9f9644397..0000000000000000000000000000000000000000
--- a/spaces/CHDCruze/entertainmentbybhdcruze/index.html
+++ /dev/null
@@ -1,21 +0,0 @@
-
-
-
-
-
- My static Space
-
-
-
-
-
Welcome to your static Space!
-
You can modify this app directly by editing index.html in the Files and versions tab.
-
-
- A blog for tv shows
- Also don't forget to check the
- Spaces documentation.
-
-
-
-
diff --git a/spaces/CVPR/BrAD/app.py b/spaces/CVPR/BrAD/app.py
deleted file mode 100644
index e7aa4e479ff39771af3c346b95ac633b2b432d67..0000000000000000000000000000000000000000
--- a/spaces/CVPR/BrAD/app.py
+++ /dev/null
@@ -1,112 +0,0 @@
-import pickle
-import os
-from sklearn.neighbors import NearestNeighbors
-import numpy as np
-import gradio as gr
-from PIL import Image
-
-data_root = 'https://ai-vision-public-datasets.s3.eu.cloud-object-storage.appdomain.cloud/DomainNet'
-feat_dir = 'brad_feats'
-domains = ['sketch', 'painting', 'clipart', 'real']
-shots = '-1'
-num_nn = 20
-
-search_domain = 'all'
-num_results_per_domain = 5
-src_data_dict = {}
-class_list = []
-if search_domain == 'all':
- for d in domains:
- with open(os.path.join(feat_dir, f'dst_{d}_{shots}.pkl'), 'rb') as fp:
- src_data = pickle.load(fp)
- if class_list == []:
- for p in src_data[0]:
- cl = p.split('/')[-2]
- if cl not in class_list:
- class_list.append(cl)
- src_nn_fit = NearestNeighbors(n_neighbors=num_results_per_domain, algorithm='auto', n_jobs=-1).fit(src_data[1])
- src_data_dict[d] = (src_data,src_nn_fit)
-else:
-
- with open(os.path.join(feat_dir, f'dst_{search_domain}_{shots}.pkl'), 'rb') as fp:
- src_data = pickle.load(fp)
- src_nn_fit = NearestNeighbors(n_neighbors=num_results_per_domain, algorithm='auto', n_jobs=-1).fit(src_data[1])
- src_data_dict[search_domain] = (src_data,src_nn_fit)
-
-dst_data_dict = {}
-min_len = 1e10
-for d in domains:
- with open(os.path.join(feat_dir, f'src_{d}_{shots}.pkl'), 'rb') as fp:
- dest_data = pickle.load(fp)
- dst_data_dict[d] = ({cl: ([],[]) for cl in class_list},dest_data[1])
- for c, p in enumerate(dest_data[0]):
- cl = p.split('/')[-2]
- dst_data_dict[d][0][cl][0].append(p)
- dst_data_dict[d][0][cl][1].append(c)
-
- for cl in class_list:
- min_len = min(min_len, len(dst_data_dict[d][0][cl]))
-
-def query(query_index, query_domain, cl):
- dst_data = dst_data_dict[query_domain]
- dst_img_path = os.path.join(data_root, dst_data[0][cl][0][query_index])
- query_index = dst_data[0][cl][1][query_index]
- img_paths = [dst_img_path]
- q_cl = dst_img_path.split('/')[-2]
- captions = [f'Query: {q_cl}'.title()]
- for s_domain, s_data in src_data_dict.items():
- _, top_n_matches_ids = s_data[1].kneighbors(dst_data[1][query_index:query_index+1])
- top_n_labels = s_data[0][2][top_n_matches_ids][0]
- src_img_pths = [os.path.join(data_root, s_data[0][0][ix]) for ix in top_n_matches_ids[0]]
- img_paths += src_img_pths
-
- for p in src_img_pths:
- src_cl = p.split('/')[-2]
- src_file = p.split('/')[-1]
- captions.append(src_cl.title())
-# print(img_paths)
- return tuple([p for p in img_paths])+ tuple(captions)
-
-demo = gr.Blocks()
-with demo:
- gr.Markdown('# Unsupervised Domain Generalization by Learning a Bridge Across Domains')
- gr.Markdown('This demo showcases the cross-domain retrieval capabilities of our self-supervised cross domain training as presented @CVPR 2022. For details please refer to [the paper](https://openaccess.thecvf.com/content/CVPR2022/papers/Harary_Unsupervised_Domain_Generalization_by_Learning_a_Bridge_Across_Domains_CVPR_2022_paper.pdf)')
- gr.Markdown('The model is trained in an unsupervised manner on all domains without class labels. The labels are displayed to indicate retrieval success/failure.')
- gr.Markdown('## Instructions:')
- gr.Markdown('Select a query domain and a class from the drop-down menus and select any random image index from the domain using the slider below, then press the "Run" button. The query image and the retrieved results from each of the four domains, along with the class label will be presented.')
- gr.Markdown('## Select Query Domain: ')
- gr.Markdown('# Query Image: \t\t\t\t')
- # domain_drop = gr.Dropdown(domains)
- # cl_drop = gr.Dropdown(class_list)
-# domain_select_button = gr.Button("Select Domain")
- # slider = gr.Slider(0, min_len)
- # slider = gr.Slider(0, 10000)
-
- with gr.Row():
- with gr.Column():
- domain_drop = gr.Dropdown(domains, label='Domain')
- cl_drop = gr.Dropdown(class_list, label='Query Class')
- slider = gr.Slider(0, 100, label='Query image selector slider')
-
- # gr.Markdown('\t')
- # gr.Markdown('\t')
- # gr.Markdown('\t')
- with gr.Column():
- src_cap = gr.Label()
- src_img = gr.Image()
- image_button = gr.Button("Run")
-
-
- out_images = []
- out_captions = []
- for d in domains:
- gr.Markdown(f'# Retrieved Images from {d.title()} Domain:')
- with gr.Row():
- for _ in range(num_results_per_domain):
- with gr.Column():
- out_captions.append(gr.Label())
- out_images.append(gr.Image())
-
- image_button.click(query, inputs=[slider, domain_drop, cl_drop], outputs=[src_img]+out_images +[src_cap]+ out_captions)
-
-demo.launch(share=True)
diff --git a/spaces/CVPR/LIVE/pybind11/tests/test_virtual_functions.py b/spaces/CVPR/LIVE/pybind11/tests/test_virtual_functions.py
deleted file mode 100644
index b7bd5badf0223812070e1f273cf8f9c4dd18db9c..0000000000000000000000000000000000000000
--- a/spaces/CVPR/LIVE/pybind11/tests/test_virtual_functions.py
+++ /dev/null
@@ -1,380 +0,0 @@
-# -*- coding: utf-8 -*-
-import pytest
-
-import env # noqa: F401
-
-from pybind11_tests import virtual_functions as m
-from pybind11_tests import ConstructorStats
-
-
-def test_override(capture, msg):
- class ExtendedExampleVirt(m.ExampleVirt):
- def __init__(self, state):
- super(ExtendedExampleVirt, self).__init__(state + 1)
- self.data = "Hello world"
-
- def run(self, value):
- print('ExtendedExampleVirt::run(%i), calling parent..' % value)
- return super(ExtendedExampleVirt, self).run(value + 1)
-
- def run_bool(self):
- print('ExtendedExampleVirt::run_bool()')
- return False
-
- def get_string1(self):
- return "override1"
-
- def pure_virtual(self):
- print('ExtendedExampleVirt::pure_virtual(): %s' % self.data)
-
- class ExtendedExampleVirt2(ExtendedExampleVirt):
- def __init__(self, state):
- super(ExtendedExampleVirt2, self).__init__(state + 1)
-
- def get_string2(self):
- return "override2"
-
- ex12 = m.ExampleVirt(10)
- with capture:
- assert m.runExampleVirt(ex12, 20) == 30
- assert capture == """
- Original implementation of ExampleVirt::run(state=10, value=20, str1=default1, str2=default2)
- """ # noqa: E501 line too long
-
- with pytest.raises(RuntimeError) as excinfo:
- m.runExampleVirtVirtual(ex12)
- assert msg(excinfo.value) == 'Tried to call pure virtual function "ExampleVirt::pure_virtual"'
-
- ex12p = ExtendedExampleVirt(10)
- with capture:
- assert m.runExampleVirt(ex12p, 20) == 32
- assert capture == """
- ExtendedExampleVirt::run(20), calling parent..
- Original implementation of ExampleVirt::run(state=11, value=21, str1=override1, str2=default2)
- """ # noqa: E501 line too long
- with capture:
- assert m.runExampleVirtBool(ex12p) is False
- assert capture == "ExtendedExampleVirt::run_bool()"
- with capture:
- m.runExampleVirtVirtual(ex12p)
- assert capture == "ExtendedExampleVirt::pure_virtual(): Hello world"
-
- ex12p2 = ExtendedExampleVirt2(15)
- with capture:
- assert m.runExampleVirt(ex12p2, 50) == 68
- assert capture == """
- ExtendedExampleVirt::run(50), calling parent..
- Original implementation of ExampleVirt::run(state=17, value=51, str1=override1, str2=override2)
- """ # noqa: E501 line too long
-
- cstats = ConstructorStats.get(m.ExampleVirt)
- assert cstats.alive() == 3
- del ex12, ex12p, ex12p2
- assert cstats.alive() == 0
- assert cstats.values() == ['10', '11', '17']
- assert cstats.copy_constructions == 0
- assert cstats.move_constructions >= 0
-
-
-def test_alias_delay_initialization1(capture):
- """`A` only initializes its trampoline class when we inherit from it
-
- If we just create and use an A instance directly, the trampoline initialization is
- bypassed and we only initialize an A() instead (for performance reasons).
- """
- class B(m.A):
- def __init__(self):
- super(B, self).__init__()
-
- def f(self):
- print("In python f()")
-
- # C++ version
- with capture:
- a = m.A()
- m.call_f(a)
- del a
- pytest.gc_collect()
- assert capture == "A.f()"
-
- # Python version
- with capture:
- b = B()
- m.call_f(b)
- del b
- pytest.gc_collect()
- assert capture == """
- PyA.PyA()
- PyA.f()
- In python f()
- PyA.~PyA()
- """
-
-
-def test_alias_delay_initialization2(capture):
- """`A2`, unlike the above, is configured to always initialize the alias
-
- While the extra initialization and extra class layer has small virtual dispatch
- performance penalty, it also allows us to do more things with the trampoline
- class such as defining local variables and performing construction/destruction.
- """
- class B2(m.A2):
- def __init__(self):
- super(B2, self).__init__()
-
- def f(self):
- print("In python B2.f()")
-
- # No python subclass version
- with capture:
- a2 = m.A2()
- m.call_f(a2)
- del a2
- pytest.gc_collect()
- a3 = m.A2(1)
- m.call_f(a3)
- del a3
- pytest.gc_collect()
- assert capture == """
- PyA2.PyA2()
- PyA2.f()
- A2.f()
- PyA2.~PyA2()
- PyA2.PyA2()
- PyA2.f()
- A2.f()
- PyA2.~PyA2()
- """
-
- # Python subclass version
- with capture:
- b2 = B2()
- m.call_f(b2)
- del b2
- pytest.gc_collect()
- assert capture == """
- PyA2.PyA2()
- PyA2.f()
- In python B2.f()
- PyA2.~PyA2()
- """
-
-
-# PyPy: Reference count > 1 causes call with noncopyable instance
-# to fail in ncv1.print_nc()
-@pytest.mark.xfail("env.PYPY")
-@pytest.mark.skipif(not hasattr(m, "NCVirt"), reason="NCVirt test broken on ICPC")
-def test_move_support():
- class NCVirtExt(m.NCVirt):
- def get_noncopyable(self, a, b):
- # Constructs and returns a new instance:
- nc = m.NonCopyable(a * a, b * b)
- return nc
-
- def get_movable(self, a, b):
- # Return a referenced copy
- self.movable = m.Movable(a, b)
- return self.movable
-
- class NCVirtExt2(m.NCVirt):
- def get_noncopyable(self, a, b):
- # Keep a reference: this is going to throw an exception
- self.nc = m.NonCopyable(a, b)
- return self.nc
-
- def get_movable(self, a, b):
- # Return a new instance without storing it
- return m.Movable(a, b)
-
- ncv1 = NCVirtExt()
- assert ncv1.print_nc(2, 3) == "36"
- assert ncv1.print_movable(4, 5) == "9"
- ncv2 = NCVirtExt2()
- assert ncv2.print_movable(7, 7) == "14"
- # Don't check the exception message here because it differs under debug/non-debug mode
- with pytest.raises(RuntimeError):
- ncv2.print_nc(9, 9)
-
- nc_stats = ConstructorStats.get(m.NonCopyable)
- mv_stats = ConstructorStats.get(m.Movable)
- assert nc_stats.alive() == 1
- assert mv_stats.alive() == 1
- del ncv1, ncv2
- assert nc_stats.alive() == 0
- assert mv_stats.alive() == 0
- assert nc_stats.values() == ['4', '9', '9', '9']
- assert mv_stats.values() == ['4', '5', '7', '7']
- assert nc_stats.copy_constructions == 0
- assert mv_stats.copy_constructions == 1
- assert nc_stats.move_constructions >= 0
- assert mv_stats.move_constructions >= 0
-
-
-def test_dispatch_issue(msg):
- """#159: virtual function dispatch has problems with similar-named functions"""
- class PyClass1(m.DispatchIssue):
- def dispatch(self):
- return "Yay.."
-
- class PyClass2(m.DispatchIssue):
- def dispatch(self):
- with pytest.raises(RuntimeError) as excinfo:
- super(PyClass2, self).dispatch()
- assert msg(excinfo.value) == 'Tried to call pure virtual function "Base::dispatch"'
-
- p = PyClass1()
- return m.dispatch_issue_go(p)
-
- b = PyClass2()
- assert m.dispatch_issue_go(b) == "Yay.."
-
-
-def test_override_ref():
- """#392/397: overriding reference-returning functions"""
- o = m.OverrideTest("asdf")
-
- # Not allowed (see associated .cpp comment)
- # i = o.str_ref()
- # assert o.str_ref() == "asdf"
- assert o.str_value() == "asdf"
-
- assert o.A_value().value == "hi"
- a = o.A_ref()
- assert a.value == "hi"
- a.value = "bye"
- assert a.value == "bye"
-
-
-def test_inherited_virtuals():
- class AR(m.A_Repeat):
- def unlucky_number(self):
- return 99
-
- class AT(m.A_Tpl):
- def unlucky_number(self):
- return 999
-
- obj = AR()
- assert obj.say_something(3) == "hihihi"
- assert obj.unlucky_number() == 99
- assert obj.say_everything() == "hi 99"
-
- obj = AT()
- assert obj.say_something(3) == "hihihi"
- assert obj.unlucky_number() == 999
- assert obj.say_everything() == "hi 999"
-
- for obj in [m.B_Repeat(), m.B_Tpl()]:
- assert obj.say_something(3) == "B says hi 3 times"
- assert obj.unlucky_number() == 13
- assert obj.lucky_number() == 7.0
- assert obj.say_everything() == "B says hi 1 times 13"
-
- for obj in [m.C_Repeat(), m.C_Tpl()]:
- assert obj.say_something(3) == "B says hi 3 times"
- assert obj.unlucky_number() == 4444
- assert obj.lucky_number() == 888.0
- assert obj.say_everything() == "B says hi 1 times 4444"
-
- class CR(m.C_Repeat):
- def lucky_number(self):
- return m.C_Repeat.lucky_number(self) + 1.25
-
- obj = CR()
- assert obj.say_something(3) == "B says hi 3 times"
- assert obj.unlucky_number() == 4444
- assert obj.lucky_number() == 889.25
- assert obj.say_everything() == "B says hi 1 times 4444"
-
- class CT(m.C_Tpl):
- pass
-
- obj = CT()
- assert obj.say_something(3) == "B says hi 3 times"
- assert obj.unlucky_number() == 4444
- assert obj.lucky_number() == 888.0
- assert obj.say_everything() == "B says hi 1 times 4444"
-
- class CCR(CR):
- def lucky_number(self):
- return CR.lucky_number(self) * 10
-
- obj = CCR()
- assert obj.say_something(3) == "B says hi 3 times"
- assert obj.unlucky_number() == 4444
- assert obj.lucky_number() == 8892.5
- assert obj.say_everything() == "B says hi 1 times 4444"
-
- class CCT(CT):
- def lucky_number(self):
- return CT.lucky_number(self) * 1000
-
- obj = CCT()
- assert obj.say_something(3) == "B says hi 3 times"
- assert obj.unlucky_number() == 4444
- assert obj.lucky_number() == 888000.0
- assert obj.say_everything() == "B says hi 1 times 4444"
-
- class DR(m.D_Repeat):
- def unlucky_number(self):
- return 123
-
- def lucky_number(self):
- return 42.0
-
- for obj in [m.D_Repeat(), m.D_Tpl()]:
- assert obj.say_something(3) == "B says hi 3 times"
- assert obj.unlucky_number() == 4444
- assert obj.lucky_number() == 888.0
- assert obj.say_everything() == "B says hi 1 times 4444"
-
- obj = DR()
- assert obj.say_something(3) == "B says hi 3 times"
- assert obj.unlucky_number() == 123
- assert obj.lucky_number() == 42.0
- assert obj.say_everything() == "B says hi 1 times 123"
-
- class DT(m.D_Tpl):
- def say_something(self, times):
- return "DT says:" + (' quack' * times)
-
- def unlucky_number(self):
- return 1234
-
- def lucky_number(self):
- return -4.25
-
- obj = DT()
- assert obj.say_something(3) == "DT says: quack quack quack"
- assert obj.unlucky_number() == 1234
- assert obj.lucky_number() == -4.25
- assert obj.say_everything() == "DT says: quack 1234"
-
- class DT2(DT):
- def say_something(self, times):
- return "DT2: " + ('QUACK' * times)
-
- def unlucky_number(self):
- return -3
-
- class BT(m.B_Tpl):
- def say_something(self, times):
- return "BT" * times
-
- def unlucky_number(self):
- return -7
-
- def lucky_number(self):
- return -1.375
-
- obj = BT()
- assert obj.say_something(3) == "BTBTBT"
- assert obj.unlucky_number() == -7
- assert obj.lucky_number() == -1.375
- assert obj.say_everything() == "BT -7"
-
-
-def test_issue_1454():
- # Fix issue #1454 (crash when acquiring/releasing GIL on another thread in Python 2.7)
- m.test_gil()
- m.test_gil_from_thread()
diff --git a/spaces/CVPR/LIVE/thrust/thrust/system/detail/sequential/swap_ranges.h b/spaces/CVPR/LIVE/thrust/thrust/system/detail/sequential/swap_ranges.h
deleted file mode 100644
index 497497d6a15286aa69371038ca204619e1a404e1..0000000000000000000000000000000000000000
--- a/spaces/CVPR/LIVE/thrust/thrust/system/detail/sequential/swap_ranges.h
+++ /dev/null
@@ -1,22 +0,0 @@
-/*
- * Copyright 2008-2013 NVIDIA Corporation
- *
- * Licensed under the Apache License, Version 2.0 (the "License");
- * you may not use this file except in compliance with the License.
- * You may obtain a copy of the License at
- *
- * http://www.apache.org/licenses/LICENSE-2.0
- *
- * Unless required by applicable law or agreed to in writing, software
- * distributed under the License is distributed on an "AS IS" BASIS,
- * WITHOUT WARRANTIES OR CONDITIONS OF ANY KIND, either express or implied.
- * See the License for the specific language governing permissions and
- * limitations under the License.
- */
-
-#pragma once
-
-#include
-
-// this system has no special swap_ranges functions
-
diff --git a/spaces/CVPR/WALT/mmcv_custom/checkpoint.py b/spaces/CVPR/WALT/mmcv_custom/checkpoint.py
deleted file mode 100644
index 51322c1c3802f357481065a70dc5152469d80eb8..0000000000000000000000000000000000000000
--- a/spaces/CVPR/WALT/mmcv_custom/checkpoint.py
+++ /dev/null
@@ -1,500 +0,0 @@
-# Copyright (c) Open-MMLab. All rights reserved.
-import io
-import os
-import os.path as osp
-import pkgutil
-import time
-import warnings
-from collections import OrderedDict
-from importlib import import_module
-from tempfile import TemporaryDirectory
-
-import torch
-import torchvision
-from torch.optim import Optimizer
-from torch.utils import model_zoo
-from torch.nn import functional as F
-
-import mmcv
-from mmcv.fileio import FileClient
-from mmcv.fileio import load as load_file
-from mmcv.parallel import is_module_wrapper
-from mmcv.utils import mkdir_or_exist
-from mmcv.runner import get_dist_info
-
-ENV_MMCV_HOME = 'MMCV_HOME'
-ENV_XDG_CACHE_HOME = 'XDG_CACHE_HOME'
-DEFAULT_CACHE_DIR = '~/.cache'
-
-
-def _get_mmcv_home():
- mmcv_home = os.path.expanduser(
- os.getenv(
- ENV_MMCV_HOME,
- os.path.join(
- os.getenv(ENV_XDG_CACHE_HOME, DEFAULT_CACHE_DIR), 'mmcv')))
-
- mkdir_or_exist(mmcv_home)
- return mmcv_home
-
-
-def load_state_dict(module, state_dict, strict=False, logger=None):
- """Load state_dict to a module.
-
- This method is modified from :meth:`torch.nn.Module.load_state_dict`.
- Default value for ``strict`` is set to ``False`` and the message for
- param mismatch will be shown even if strict is False.
-
- Args:
- module (Module): Module that receives the state_dict.
- state_dict (OrderedDict): Weights.
- strict (bool): whether to strictly enforce that the keys
- in :attr:`state_dict` match the keys returned by this module's
- :meth:`~torch.nn.Module.state_dict` function. Default: ``False``.
- logger (:obj:`logging.Logger`, optional): Logger to log the error
- message. If not specified, print function will be used.
- """
- unexpected_keys = []
- all_missing_keys = []
- err_msg = []
-
- metadata = getattr(state_dict, '_metadata', None)
- state_dict = state_dict.copy()
- if metadata is not None:
- state_dict._metadata = metadata
-
- # use _load_from_state_dict to enable checkpoint version control
- def load(module, prefix=''):
- # recursively check parallel module in case that the model has a
- # complicated structure, e.g., nn.Module(nn.Module(DDP))
- if is_module_wrapper(module):
- module = module.module
- local_metadata = {} if metadata is None else metadata.get(
- prefix[:-1], {})
- module._load_from_state_dict(state_dict, prefix, local_metadata, True,
- all_missing_keys, unexpected_keys,
- err_msg)
- for name, child in module._modules.items():
- if child is not None:
- load(child, prefix + name + '.')
-
- load(module)
- load = None # break load->load reference cycle
-
- # ignore "num_batches_tracked" of BN layers
- missing_keys = [
- key for key in all_missing_keys if 'num_batches_tracked' not in key
- ]
-
- if unexpected_keys:
- err_msg.append('unexpected key in source '
- f'state_dict: {", ".join(unexpected_keys)}\n')
- if missing_keys:
- err_msg.append(
- f'missing keys in source state_dict: {", ".join(missing_keys)}\n')
-
- rank, _ = get_dist_info()
- if len(err_msg) > 0 and rank == 0:
- err_msg.insert(
- 0, 'The model and loaded state dict do not match exactly\n')
- err_msg = '\n'.join(err_msg)
- if strict:
- raise RuntimeError(err_msg)
- elif logger is not None:
- logger.warning(err_msg)
- else:
- print(err_msg)
-
-
-def load_url_dist(url, model_dir=None):
- """In distributed setting, this function only download checkpoint at local
- rank 0."""
- rank, world_size = get_dist_info()
- rank = int(os.environ.get('LOCAL_RANK', rank))
- if rank == 0:
- checkpoint = model_zoo.load_url(url, model_dir=model_dir)
- if world_size > 1:
- torch.distributed.barrier()
- if rank > 0:
- checkpoint = model_zoo.load_url(url, model_dir=model_dir)
- return checkpoint
-
-
-def load_pavimodel_dist(model_path, map_location=None):
- """In distributed setting, this function only download checkpoint at local
- rank 0."""
- try:
- from pavi import modelcloud
- except ImportError:
- raise ImportError(
- 'Please install pavi to load checkpoint from modelcloud.')
- rank, world_size = get_dist_info()
- rank = int(os.environ.get('LOCAL_RANK', rank))
- if rank == 0:
- model = modelcloud.get(model_path)
- with TemporaryDirectory() as tmp_dir:
- downloaded_file = osp.join(tmp_dir, model.name)
- model.download(downloaded_file)
- checkpoint = torch.load(downloaded_file, map_location=map_location)
- if world_size > 1:
- torch.distributed.barrier()
- if rank > 0:
- model = modelcloud.get(model_path)
- with TemporaryDirectory() as tmp_dir:
- downloaded_file = osp.join(tmp_dir, model.name)
- model.download(downloaded_file)
- checkpoint = torch.load(
- downloaded_file, map_location=map_location)
- return checkpoint
-
-
-def load_fileclient_dist(filename, backend, map_location):
- """In distributed setting, this function only download checkpoint at local
- rank 0."""
- rank, world_size = get_dist_info()
- rank = int(os.environ.get('LOCAL_RANK', rank))
- allowed_backends = ['ceph']
- if backend not in allowed_backends:
- raise ValueError(f'Load from Backend {backend} is not supported.')
- if rank == 0:
- fileclient = FileClient(backend=backend)
- buffer = io.BytesIO(fileclient.get(filename))
- checkpoint = torch.load(buffer, map_location=map_location)
- if world_size > 1:
- torch.distributed.barrier()
- if rank > 0:
- fileclient = FileClient(backend=backend)
- buffer = io.BytesIO(fileclient.get(filename))
- checkpoint = torch.load(buffer, map_location=map_location)
- return checkpoint
-
-
-def get_torchvision_models():
- model_urls = dict()
- for _, name, ispkg in pkgutil.walk_packages(torchvision.models.__path__):
- if ispkg:
- continue
- _zoo = import_module(f'torchvision.models.{name}')
- if hasattr(_zoo, 'model_urls'):
- _urls = getattr(_zoo, 'model_urls')
- model_urls.update(_urls)
- return model_urls
-
-
-def get_external_models():
- mmcv_home = _get_mmcv_home()
- default_json_path = osp.join(mmcv.__path__[0], 'model_zoo/open_mmlab.json')
- default_urls = load_file(default_json_path)
- assert isinstance(default_urls, dict)
- external_json_path = osp.join(mmcv_home, 'open_mmlab.json')
- if osp.exists(external_json_path):
- external_urls = load_file(external_json_path)
- assert isinstance(external_urls, dict)
- default_urls.update(external_urls)
-
- return default_urls
-
-
-def get_mmcls_models():
- mmcls_json_path = osp.join(mmcv.__path__[0], 'model_zoo/mmcls.json')
- mmcls_urls = load_file(mmcls_json_path)
-
- return mmcls_urls
-
-
-def get_deprecated_model_names():
- deprecate_json_path = osp.join(mmcv.__path__[0],
- 'model_zoo/deprecated.json')
- deprecate_urls = load_file(deprecate_json_path)
- assert isinstance(deprecate_urls, dict)
-
- return deprecate_urls
-
-
-def _process_mmcls_checkpoint(checkpoint):
- state_dict = checkpoint['state_dict']
- new_state_dict = OrderedDict()
- for k, v in state_dict.items():
- if k.startswith('backbone.'):
- new_state_dict[k[9:]] = v
- new_checkpoint = dict(state_dict=new_state_dict)
-
- return new_checkpoint
-
-
-def _load_checkpoint(filename, map_location=None):
- """Load checkpoint from somewhere (modelzoo, file, url).
-
- Args:
- filename (str): Accept local filepath, URL, ``torchvision://xxx``,
- ``open-mmlab://xxx``. Please refer to ``docs/model_zoo.md`` for
- details.
- map_location (str | None): Same as :func:`torch.load`. Default: None.
-
- Returns:
- dict | OrderedDict: The loaded checkpoint. It can be either an
- OrderedDict storing model weights or a dict containing other
- information, which depends on the checkpoint.
- """
- if filename.startswith('modelzoo://'):
- warnings.warn('The URL scheme of "modelzoo://" is deprecated, please '
- 'use "torchvision://" instead')
- model_urls = get_torchvision_models()
- model_name = filename[11:]
- checkpoint = load_url_dist(model_urls[model_name])
- elif filename.startswith('torchvision://'):
- model_urls = get_torchvision_models()
- model_name = filename[14:]
- checkpoint = load_url_dist(model_urls[model_name])
- elif filename.startswith('open-mmlab://'):
- model_urls = get_external_models()
- model_name = filename[13:]
- deprecated_urls = get_deprecated_model_names()
- if model_name in deprecated_urls:
- warnings.warn(f'open-mmlab://{model_name} is deprecated in favor '
- f'of open-mmlab://{deprecated_urls[model_name]}')
- model_name = deprecated_urls[model_name]
- model_url = model_urls[model_name]
- # check if is url
- if model_url.startswith(('http://', 'https://')):
- checkpoint = load_url_dist(model_url)
- else:
- filename = osp.join(_get_mmcv_home(), model_url)
- if not osp.isfile(filename):
- raise IOError(f'{filename} is not a checkpoint file')
- checkpoint = torch.load(filename, map_location=map_location)
- elif filename.startswith('mmcls://'):
- model_urls = get_mmcls_models()
- model_name = filename[8:]
- checkpoint = load_url_dist(model_urls[model_name])
- checkpoint = _process_mmcls_checkpoint(checkpoint)
- elif filename.startswith(('http://', 'https://')):
- checkpoint = load_url_dist(filename)
- elif filename.startswith('pavi://'):
- model_path = filename[7:]
- checkpoint = load_pavimodel_dist(model_path, map_location=map_location)
- elif filename.startswith('s3://'):
- checkpoint = load_fileclient_dist(
- filename, backend='ceph', map_location=map_location)
- else:
- if not osp.isfile(filename):
- raise IOError(f'{filename} is not a checkpoint file')
- checkpoint = torch.load(filename, map_location=map_location)
- return checkpoint
-
-
-def load_checkpoint(model,
- filename,
- map_location='cpu',
- strict=False,
- logger=None):
- """Load checkpoint from a file or URI.
-
- Args:
- model (Module): Module to load checkpoint.
- filename (str): Accept local filepath, URL, ``torchvision://xxx``,
- ``open-mmlab://xxx``. Please refer to ``docs/model_zoo.md`` for
- details.
- map_location (str): Same as :func:`torch.load`.
- strict (bool): Whether to allow different params for the model and
- checkpoint.
- logger (:mod:`logging.Logger` or None): The logger for error message.
-
- Returns:
- dict or OrderedDict: The loaded checkpoint.
- """
- checkpoint = _load_checkpoint(filename, map_location)
- # OrderedDict is a subclass of dict
- if not isinstance(checkpoint, dict):
- raise RuntimeError(
- f'No state_dict found in checkpoint file {filename}')
- # get state_dict from checkpoint
- if 'state_dict' in checkpoint:
- state_dict = checkpoint['state_dict']
- elif 'model' in checkpoint:
- state_dict = checkpoint['model']
- else:
- state_dict = checkpoint
- # strip prefix of state_dict
- if list(state_dict.keys())[0].startswith('module.'):
- state_dict = {k[7:]: v for k, v in state_dict.items()}
-
- # for MoBY, load model of online branch
- if sorted(list(state_dict.keys()))[0].startswith('encoder'):
- state_dict = {k.replace('encoder.', ''): v for k, v in state_dict.items() if k.startswith('encoder.')}
-
- # reshape absolute position embedding
- if state_dict.get('absolute_pos_embed') is not None:
- absolute_pos_embed = state_dict['absolute_pos_embed']
- N1, L, C1 = absolute_pos_embed.size()
- N2, C2, H, W = model.absolute_pos_embed.size()
- if N1 != N2 or C1 != C2 or L != H*W:
- logger.warning("Error in loading absolute_pos_embed, pass")
- else:
- state_dict['absolute_pos_embed'] = absolute_pos_embed.view(N2, H, W, C2).permute(0, 3, 1, 2)
-
- # interpolate position bias table if needed
- relative_position_bias_table_keys = [k for k in state_dict.keys() if "relative_position_bias_table" in k]
- for table_key in relative_position_bias_table_keys:
- table_pretrained = state_dict[table_key]
- table_current = model.state_dict()[table_key]
- L1, nH1 = table_pretrained.size()
- L2, nH2 = table_current.size()
- if nH1 != nH2:
- logger.warning(f"Error in loading {table_key}, pass")
- else:
- if L1 != L2:
- S1 = int(L1 ** 0.5)
- S2 = int(L2 ** 0.5)
- table_pretrained_resized = F.interpolate(
- table_pretrained.permute(1, 0).view(1, nH1, S1, S1),
- size=(S2, S2), mode='bicubic')
- state_dict[table_key] = table_pretrained_resized.view(nH2, L2).permute(1, 0)
-
- # load state_dict
- load_state_dict(model, state_dict, strict, logger)
- return checkpoint
-
-
-def weights_to_cpu(state_dict):
- """Copy a model state_dict to cpu.
-
- Args:
- state_dict (OrderedDict): Model weights on GPU.
-
- Returns:
- OrderedDict: Model weights on GPU.
- """
- state_dict_cpu = OrderedDict()
- for key, val in state_dict.items():
- state_dict_cpu[key] = val.cpu()
- return state_dict_cpu
-
-
-def _save_to_state_dict(module, destination, prefix, keep_vars):
- """Saves module state to `destination` dictionary.
-
- This method is modified from :meth:`torch.nn.Module._save_to_state_dict`.
-
- Args:
- module (nn.Module): The module to generate state_dict.
- destination (dict): A dict where state will be stored.
- prefix (str): The prefix for parameters and buffers used in this
- module.
- """
- for name, param in module._parameters.items():
- if param is not None:
- destination[prefix + name] = param if keep_vars else param.detach()
- for name, buf in module._buffers.items():
- # remove check of _non_persistent_buffers_set to allow nn.BatchNorm2d
- if buf is not None:
- destination[prefix + name] = buf if keep_vars else buf.detach()
-
-
-def get_state_dict(module, destination=None, prefix='', keep_vars=False):
- """Returns a dictionary containing a whole state of the module.
-
- Both parameters and persistent buffers (e.g. running averages) are
- included. Keys are corresponding parameter and buffer names.
-
- This method is modified from :meth:`torch.nn.Module.state_dict` to
- recursively check parallel module in case that the model has a complicated
- structure, e.g., nn.Module(nn.Module(DDP)).
-
- Args:
- module (nn.Module): The module to generate state_dict.
- destination (OrderedDict): Returned dict for the state of the
- module.
- prefix (str): Prefix of the key.
- keep_vars (bool): Whether to keep the variable property of the
- parameters. Default: False.
-
- Returns:
- dict: A dictionary containing a whole state of the module.
- """
- # recursively check parallel module in case that the model has a
- # complicated structure, e.g., nn.Module(nn.Module(DDP))
- if is_module_wrapper(module):
- module = module.module
-
- # below is the same as torch.nn.Module.state_dict()
- if destination is None:
- destination = OrderedDict()
- destination._metadata = OrderedDict()
- destination._metadata[prefix[:-1]] = local_metadata = dict(
- version=module._version)
- _save_to_state_dict(module, destination, prefix, keep_vars)
- for name, child in module._modules.items():
- if child is not None:
- get_state_dict(
- child, destination, prefix + name + '.', keep_vars=keep_vars)
- for hook in module._state_dict_hooks.values():
- hook_result = hook(module, destination, prefix, local_metadata)
- if hook_result is not None:
- destination = hook_result
- return destination
-
-
-def save_checkpoint(model, filename, optimizer=None, meta=None):
- """Save checkpoint to file.
-
- The checkpoint will have 3 fields: ``meta``, ``state_dict`` and
- ``optimizer``. By default ``meta`` will contain version and time info.
-
- Args:
- model (Module): Module whose params are to be saved.
- filename (str): Checkpoint filename.
- optimizer (:obj:`Optimizer`, optional): Optimizer to be saved.
- meta (dict, optional): Metadata to be saved in checkpoint.
- """
- if meta is None:
- meta = {}
- elif not isinstance(meta, dict):
- raise TypeError(f'meta must be a dict or None, but got {type(meta)}')
- meta.update(mmcv_version=mmcv.__version__, time=time.asctime())
-
- if is_module_wrapper(model):
- model = model.module
-
- if hasattr(model, 'CLASSES') and model.CLASSES is not None:
- # save class name to the meta
- meta.update(CLASSES=model.CLASSES)
-
- checkpoint = {
- 'meta': meta,
- 'state_dict': weights_to_cpu(get_state_dict(model))
- }
- # save optimizer state dict in the checkpoint
- if isinstance(optimizer, Optimizer):
- checkpoint['optimizer'] = optimizer.state_dict()
- elif isinstance(optimizer, dict):
- checkpoint['optimizer'] = {}
- for name, optim in optimizer.items():
- checkpoint['optimizer'][name] = optim.state_dict()
-
- if filename.startswith('pavi://'):
- try:
- from pavi import modelcloud
- from pavi.exception import NodeNotFoundError
- except ImportError:
- raise ImportError(
- 'Please install pavi to load checkpoint from modelcloud.')
- model_path = filename[7:]
- root = modelcloud.Folder()
- model_dir, model_name = osp.split(model_path)
- try:
- model = modelcloud.get(model_dir)
- except NodeNotFoundError:
- model = root.create_training_model(model_dir)
- with TemporaryDirectory() as tmp_dir:
- checkpoint_file = osp.join(tmp_dir, model_name)
- with open(checkpoint_file, 'wb') as f:
- torch.save(checkpoint, f)
- f.flush()
- model.create_file(checkpoint_file, name=model_name)
- else:
- mmcv.mkdir_or_exist(osp.dirname(filename))
- # immediately flush buffer
- with open(filename, 'wb') as f:
- torch.save(checkpoint, f)
- f.flush()
diff --git a/spaces/CVPR/WALT/mmdet/models/roi_heads/htc_roi_head.py b/spaces/CVPR/WALT/mmdet/models/roi_heads/htc_roi_head.py
deleted file mode 100644
index 5b5c2ec3bc9d579061fbd89f8b320e6e59909143..0000000000000000000000000000000000000000
--- a/spaces/CVPR/WALT/mmdet/models/roi_heads/htc_roi_head.py
+++ /dev/null
@@ -1,589 +0,0 @@
-import torch
-import torch.nn.functional as F
-
-from mmdet.core import (bbox2result, bbox2roi, bbox_mapping, merge_aug_bboxes,
- merge_aug_masks, multiclass_nms)
-from ..builder import HEADS, build_head, build_roi_extractor
-from .cascade_roi_head import CascadeRoIHead
-
-
-@HEADS.register_module()
-class HybridTaskCascadeRoIHead(CascadeRoIHead):
- """Hybrid task cascade roi head including one bbox head and one mask head.
-
- https://arxiv.org/abs/1901.07518
- """
-
- def __init__(self,
- num_stages,
- stage_loss_weights,
- semantic_roi_extractor=None,
- semantic_head=None,
- semantic_fusion=('bbox', 'mask'),
- interleaved=True,
- mask_info_flow=True,
- **kwargs):
- super(HybridTaskCascadeRoIHead,
- self).__init__(num_stages, stage_loss_weights, **kwargs)
- assert self.with_bbox and self.with_mask
- assert not self.with_shared_head # shared head is not supported
-
- if semantic_head is not None:
- self.semantic_roi_extractor = build_roi_extractor(
- semantic_roi_extractor)
- self.semantic_head = build_head(semantic_head)
-
- self.semantic_fusion = semantic_fusion
- self.interleaved = interleaved
- self.mask_info_flow = mask_info_flow
-
- def init_weights(self, pretrained):
- """Initialize the weights in head.
-
- Args:
- pretrained (str, optional): Path to pre-trained weights.
- Defaults to None.
- """
- super(HybridTaskCascadeRoIHead, self).init_weights(pretrained)
- if self.with_semantic:
- self.semantic_head.init_weights()
-
- @property
- def with_semantic(self):
- """bool: whether the head has semantic head"""
- if hasattr(self, 'semantic_head') and self.semantic_head is not None:
- return True
- else:
- return False
-
- def forward_dummy(self, x, proposals):
- """Dummy forward function."""
- outs = ()
- # semantic head
- if self.with_semantic:
- _, semantic_feat = self.semantic_head(x)
- else:
- semantic_feat = None
- # bbox heads
- rois = bbox2roi([proposals])
- for i in range(self.num_stages):
- bbox_results = self._bbox_forward(
- i, x, rois, semantic_feat=semantic_feat)
- outs = outs + (bbox_results['cls_score'],
- bbox_results['bbox_pred'])
- # mask heads
- if self.with_mask:
- mask_rois = rois[:100]
- mask_roi_extractor = self.mask_roi_extractor[-1]
- mask_feats = mask_roi_extractor(
- x[:len(mask_roi_extractor.featmap_strides)], mask_rois)
- if self.with_semantic and 'mask' in self.semantic_fusion:
- mask_semantic_feat = self.semantic_roi_extractor(
- [semantic_feat], mask_rois)
- mask_feats += mask_semantic_feat
- last_feat = None
- for i in range(self.num_stages):
- mask_head = self.mask_head[i]
- if self.mask_info_flow:
- mask_pred, last_feat = mask_head(mask_feats, last_feat)
- else:
- mask_pred = mask_head(mask_feats)
- outs = outs + (mask_pred, )
- return outs
-
- def _bbox_forward_train(self,
- stage,
- x,
- sampling_results,
- gt_bboxes,
- gt_labels,
- rcnn_train_cfg,
- semantic_feat=None):
- """Run forward function and calculate loss for box head in training."""
- bbox_head = self.bbox_head[stage]
- rois = bbox2roi([res.bboxes for res in sampling_results])
- bbox_results = self._bbox_forward(
- stage, x, rois, semantic_feat=semantic_feat)
-
- bbox_targets = bbox_head.get_targets(sampling_results, gt_bboxes,
- gt_labels, rcnn_train_cfg)
- loss_bbox = bbox_head.loss(bbox_results['cls_score'],
- bbox_results['bbox_pred'], rois,
- *bbox_targets)
-
- bbox_results.update(
- loss_bbox=loss_bbox,
- rois=rois,
- bbox_targets=bbox_targets,
- )
- return bbox_results
-
- def _mask_forward_train(self,
- stage,
- x,
- sampling_results,
- gt_masks,
- rcnn_train_cfg,
- semantic_feat=None):
- """Run forward function and calculate loss for mask head in
- training."""
- mask_roi_extractor = self.mask_roi_extractor[stage]
- mask_head = self.mask_head[stage]
- pos_rois = bbox2roi([res.pos_bboxes for res in sampling_results])
- mask_feats = mask_roi_extractor(x[:mask_roi_extractor.num_inputs],
- pos_rois)
-
- # semantic feature fusion
- # element-wise sum for original features and pooled semantic features
- if self.with_semantic and 'mask' in self.semantic_fusion:
- mask_semantic_feat = self.semantic_roi_extractor([semantic_feat],
- pos_rois)
- if mask_semantic_feat.shape[-2:] != mask_feats.shape[-2:]:
- mask_semantic_feat = F.adaptive_avg_pool2d(
- mask_semantic_feat, mask_feats.shape[-2:])
- mask_feats += mask_semantic_feat
-
- # mask information flow
- # forward all previous mask heads to obtain last_feat, and fuse it
- # with the normal mask feature
- if self.mask_info_flow:
- last_feat = None
- for i in range(stage):
- last_feat = self.mask_head[i](
- mask_feats, last_feat, return_logits=False)
- mask_pred = mask_head(mask_feats, last_feat, return_feat=False)
- else:
- mask_pred = mask_head(mask_feats, return_feat=False)
-
- mask_targets = mask_head.get_targets(sampling_results, gt_masks,
- rcnn_train_cfg)
- pos_labels = torch.cat([res.pos_gt_labels for res in sampling_results])
- loss_mask = mask_head.loss(mask_pred, mask_targets, pos_labels)
-
- mask_results = dict(loss_mask=loss_mask)
- return mask_results
-
- def _bbox_forward(self, stage, x, rois, semantic_feat=None):
- """Box head forward function used in both training and testing."""
- bbox_roi_extractor = self.bbox_roi_extractor[stage]
- bbox_head = self.bbox_head[stage]
- bbox_feats = bbox_roi_extractor(
- x[:len(bbox_roi_extractor.featmap_strides)], rois)
- if self.with_semantic and 'bbox' in self.semantic_fusion:
- bbox_semantic_feat = self.semantic_roi_extractor([semantic_feat],
- rois)
- if bbox_semantic_feat.shape[-2:] != bbox_feats.shape[-2:]:
- bbox_semantic_feat = F.adaptive_avg_pool2d(
- bbox_semantic_feat, bbox_feats.shape[-2:])
- bbox_feats += bbox_semantic_feat
- cls_score, bbox_pred = bbox_head(bbox_feats)
-
- bbox_results = dict(cls_score=cls_score, bbox_pred=bbox_pred)
- return bbox_results
-
- def _mask_forward_test(self, stage, x, bboxes, semantic_feat=None):
- """Mask head forward function for testing."""
- mask_roi_extractor = self.mask_roi_extractor[stage]
- mask_head = self.mask_head[stage]
- mask_rois = bbox2roi([bboxes])
- mask_feats = mask_roi_extractor(
- x[:len(mask_roi_extractor.featmap_strides)], mask_rois)
- if self.with_semantic and 'mask' in self.semantic_fusion:
- mask_semantic_feat = self.semantic_roi_extractor([semantic_feat],
- mask_rois)
- if mask_semantic_feat.shape[-2:] != mask_feats.shape[-2:]:
- mask_semantic_feat = F.adaptive_avg_pool2d(
- mask_semantic_feat, mask_feats.shape[-2:])
- mask_feats += mask_semantic_feat
- if self.mask_info_flow:
- last_feat = None
- last_pred = None
- for i in range(stage):
- mask_pred, last_feat = self.mask_head[i](mask_feats, last_feat)
- if last_pred is not None:
- mask_pred = mask_pred + last_pred
- last_pred = mask_pred
- mask_pred = mask_head(mask_feats, last_feat, return_feat=False)
- if last_pred is not None:
- mask_pred = mask_pred + last_pred
- else:
- mask_pred = mask_head(mask_feats)
- return mask_pred
-
- def forward_train(self,
- x,
- img_metas,
- proposal_list,
- gt_bboxes,
- gt_labels,
- gt_bboxes_ignore=None,
- gt_masks=None,
- gt_semantic_seg=None):
- """
- Args:
- x (list[Tensor]): list of multi-level img features.
-
- img_metas (list[dict]): list of image info dict where each dict
- has: 'img_shape', 'scale_factor', 'flip', and may also contain
- 'filename', 'ori_shape', 'pad_shape', and 'img_norm_cfg'.
- For details on the values of these keys see
- `mmdet/datasets/pipelines/formatting.py:Collect`.
-
- proposal_list (list[Tensors]): list of region proposals.
-
- gt_bboxes (list[Tensor]): Ground truth bboxes for each image with
- shape (num_gts, 4) in [tl_x, tl_y, br_x, br_y] format.
-
- gt_labels (list[Tensor]): class indices corresponding to each box
-
- gt_bboxes_ignore (None, list[Tensor]): specify which bounding
- boxes can be ignored when computing the loss.
-
- gt_masks (None, Tensor) : true segmentation masks for each box
- used if the architecture supports a segmentation task.
-
- gt_semantic_seg (None, list[Tensor]): semantic segmentation masks
- used if the architecture supports semantic segmentation task.
-
- Returns:
- dict[str, Tensor]: a dictionary of loss components
- """
- # semantic segmentation part
- # 2 outputs: segmentation prediction and embedded features
- losses = dict()
- if self.with_semantic:
- semantic_pred, semantic_feat = self.semantic_head(x)
- loss_seg = self.semantic_head.loss(semantic_pred, gt_semantic_seg)
- losses['loss_semantic_seg'] = loss_seg
- else:
- semantic_feat = None
-
- for i in range(self.num_stages):
- self.current_stage = i
- rcnn_train_cfg = self.train_cfg[i]
- lw = self.stage_loss_weights[i]
-
- # assign gts and sample proposals
- sampling_results = []
- bbox_assigner = self.bbox_assigner[i]
- bbox_sampler = self.bbox_sampler[i]
- num_imgs = len(img_metas)
- if gt_bboxes_ignore is None:
- gt_bboxes_ignore = [None for _ in range(num_imgs)]
-
- for j in range(num_imgs):
- assign_result = bbox_assigner.assign(proposal_list[j],
- gt_bboxes[j],
- gt_bboxes_ignore[j],
- gt_labels[j])
- sampling_result = bbox_sampler.sample(
- assign_result,
- proposal_list[j],
- gt_bboxes[j],
- gt_labels[j],
- feats=[lvl_feat[j][None] for lvl_feat in x])
- sampling_results.append(sampling_result)
-
- # bbox head forward and loss
- bbox_results = \
- self._bbox_forward_train(
- i, x, sampling_results, gt_bboxes, gt_labels,
- rcnn_train_cfg, semantic_feat)
- roi_labels = bbox_results['bbox_targets'][0]
-
- for name, value in bbox_results['loss_bbox'].items():
- losses[f's{i}.{name}'] = (
- value * lw if 'loss' in name else value)
-
- # mask head forward and loss
- if self.with_mask:
- # interleaved execution: use regressed bboxes by the box branch
- # to train the mask branch
- if self.interleaved:
- pos_is_gts = [res.pos_is_gt for res in sampling_results]
- with torch.no_grad():
- proposal_list = self.bbox_head[i].refine_bboxes(
- bbox_results['rois'], roi_labels,
- bbox_results['bbox_pred'], pos_is_gts, img_metas)
- # re-assign and sample 512 RoIs from 512 RoIs
- sampling_results = []
- for j in range(num_imgs):
- assign_result = bbox_assigner.assign(
- proposal_list[j], gt_bboxes[j],
- gt_bboxes_ignore[j], gt_labels[j])
- sampling_result = bbox_sampler.sample(
- assign_result,
- proposal_list[j],
- gt_bboxes[j],
- gt_labels[j],
- feats=[lvl_feat[j][None] for lvl_feat in x])
- sampling_results.append(sampling_result)
- mask_results = self._mask_forward_train(
- i, x, sampling_results, gt_masks, rcnn_train_cfg,
- semantic_feat)
- for name, value in mask_results['loss_mask'].items():
- losses[f's{i}.{name}'] = (
- value * lw if 'loss' in name else value)
-
- # refine bboxes (same as Cascade R-CNN)
- if i < self.num_stages - 1 and not self.interleaved:
- pos_is_gts = [res.pos_is_gt for res in sampling_results]
- with torch.no_grad():
- proposal_list = self.bbox_head[i].refine_bboxes(
- bbox_results['rois'], roi_labels,
- bbox_results['bbox_pred'], pos_is_gts, img_metas)
-
- return losses
-
- def simple_test(self, x, proposal_list, img_metas, rescale=False):
- """Test without augmentation."""
- if self.with_semantic:
- _, semantic_feat = self.semantic_head(x)
- else:
- semantic_feat = None
-
- num_imgs = len(proposal_list)
- img_shapes = tuple(meta['img_shape'] for meta in img_metas)
- ori_shapes = tuple(meta['ori_shape'] for meta in img_metas)
- scale_factors = tuple(meta['scale_factor'] for meta in img_metas)
-
- # "ms" in variable names means multi-stage
- ms_bbox_result = {}
- ms_segm_result = {}
- ms_scores = []
- rcnn_test_cfg = self.test_cfg
-
- rois = bbox2roi(proposal_list)
- for i in range(self.num_stages):
- bbox_head = self.bbox_head[i]
- bbox_results = self._bbox_forward(
- i, x, rois, semantic_feat=semantic_feat)
- # split batch bbox prediction back to each image
- cls_score = bbox_results['cls_score']
- bbox_pred = bbox_results['bbox_pred']
- num_proposals_per_img = tuple(len(p) for p in proposal_list)
- rois = rois.split(num_proposals_per_img, 0)
- cls_score = cls_score.split(num_proposals_per_img, 0)
- bbox_pred = bbox_pred.split(num_proposals_per_img, 0)
- ms_scores.append(cls_score)
-
- if i < self.num_stages - 1:
- bbox_label = [s[:, :-1].argmax(dim=1) for s in cls_score]
- rois = torch.cat([
- bbox_head.regress_by_class(rois[i], bbox_label[i],
- bbox_pred[i], img_metas[i])
- for i in range(num_imgs)
- ])
-
- # average scores of each image by stages
- cls_score = [
- sum([score[i] for score in ms_scores]) / float(len(ms_scores))
- for i in range(num_imgs)
- ]
-
- # apply bbox post-processing to each image individually
- det_bboxes = []
- det_labels = []
- for i in range(num_imgs):
- det_bbox, det_label = self.bbox_head[-1].get_bboxes(
- rois[i],
- cls_score[i],
- bbox_pred[i],
- img_shapes[i],
- scale_factors[i],
- rescale=rescale,
- cfg=rcnn_test_cfg)
- det_bboxes.append(det_bbox)
- det_labels.append(det_label)
- bbox_result = [
- bbox2result(det_bboxes[i], det_labels[i],
- self.bbox_head[-1].num_classes)
- for i in range(num_imgs)
- ]
- ms_bbox_result['ensemble'] = bbox_result
-
- if self.with_mask:
- if all(det_bbox.shape[0] == 0 for det_bbox in det_bboxes):
- mask_classes = self.mask_head[-1].num_classes
- segm_results = [[[] for _ in range(mask_classes)]
- for _ in range(num_imgs)]
- else:
- if rescale and not isinstance(scale_factors[0], float):
- scale_factors = [
- torch.from_numpy(scale_factor).to(det_bboxes[0].device)
- for scale_factor in scale_factors
- ]
- _bboxes = [
- det_bboxes[i][:, :4] *
- scale_factors[i] if rescale else det_bboxes[i]
- for i in range(num_imgs)
- ]
- mask_rois = bbox2roi(_bboxes)
- aug_masks = []
- mask_roi_extractor = self.mask_roi_extractor[-1]
- mask_feats = mask_roi_extractor(
- x[:len(mask_roi_extractor.featmap_strides)], mask_rois)
- if self.with_semantic and 'mask' in self.semantic_fusion:
- mask_semantic_feat = self.semantic_roi_extractor(
- [semantic_feat], mask_rois)
- mask_feats += mask_semantic_feat
- last_feat = None
-
- num_bbox_per_img = tuple(len(_bbox) for _bbox in _bboxes)
- for i in range(self.num_stages):
- mask_head = self.mask_head[i]
- if self.mask_info_flow:
- mask_pred, last_feat = mask_head(mask_feats, last_feat)
- else:
- mask_pred = mask_head(mask_feats)
-
- # split batch mask prediction back to each image
- mask_pred = mask_pred.split(num_bbox_per_img, 0)
- aug_masks.append(
- [mask.sigmoid().cpu().numpy() for mask in mask_pred])
-
- # apply mask post-processing to each image individually
- segm_results = []
- for i in range(num_imgs):
- if det_bboxes[i].shape[0] == 0:
- segm_results.append(
- [[]
- for _ in range(self.mask_head[-1].num_classes)])
- else:
- aug_mask = [mask[i] for mask in aug_masks]
- merged_mask = merge_aug_masks(
- aug_mask, [[img_metas[i]]] * self.num_stages,
- rcnn_test_cfg)
- segm_result = self.mask_head[-1].get_seg_masks(
- merged_mask, _bboxes[i], det_labels[i],
- rcnn_test_cfg, ori_shapes[i], scale_factors[i],
- rescale)
- segm_results.append(segm_result)
- ms_segm_result['ensemble'] = segm_results
-
- if self.with_mask:
- results = list(
- zip(ms_bbox_result['ensemble'], ms_segm_result['ensemble']))
- else:
- results = ms_bbox_result['ensemble']
-
- return results
-
- def aug_test(self, img_feats, proposal_list, img_metas, rescale=False):
- """Test with augmentations.
-
- If rescale is False, then returned bboxes and masks will fit the scale
- of imgs[0].
- """
- if self.with_semantic:
- semantic_feats = [
- self.semantic_head(feat)[1] for feat in img_feats
- ]
- else:
- semantic_feats = [None] * len(img_metas)
-
- rcnn_test_cfg = self.test_cfg
- aug_bboxes = []
- aug_scores = []
- for x, img_meta, semantic in zip(img_feats, img_metas, semantic_feats):
- # only one image in the batch
- img_shape = img_meta[0]['img_shape']
- scale_factor = img_meta[0]['scale_factor']
- flip = img_meta[0]['flip']
- flip_direction = img_meta[0]['flip_direction']
-
- proposals = bbox_mapping(proposal_list[0][:, :4], img_shape,
- scale_factor, flip, flip_direction)
- # "ms" in variable names means multi-stage
- ms_scores = []
-
- rois = bbox2roi([proposals])
- for i in range(self.num_stages):
- bbox_head = self.bbox_head[i]
- bbox_results = self._bbox_forward(
- i, x, rois, semantic_feat=semantic)
- ms_scores.append(bbox_results['cls_score'])
-
- if i < self.num_stages - 1:
- bbox_label = bbox_results['cls_score'].argmax(dim=1)
- rois = bbox_head.regress_by_class(
- rois, bbox_label, bbox_results['bbox_pred'],
- img_meta[0])
-
- cls_score = sum(ms_scores) / float(len(ms_scores))
- bboxes, scores = self.bbox_head[-1].get_bboxes(
- rois,
- cls_score,
- bbox_results['bbox_pred'],
- img_shape,
- scale_factor,
- rescale=False,
- cfg=None)
- aug_bboxes.append(bboxes)
- aug_scores.append(scores)
-
- # after merging, bboxes will be rescaled to the original image size
- merged_bboxes, merged_scores = merge_aug_bboxes(
- aug_bboxes, aug_scores, img_metas, rcnn_test_cfg)
- det_bboxes, det_labels = multiclass_nms(merged_bboxes, merged_scores,
- rcnn_test_cfg.score_thr,
- rcnn_test_cfg.nms,
- rcnn_test_cfg.max_per_img)
-
- bbox_result = bbox2result(det_bboxes, det_labels,
- self.bbox_head[-1].num_classes)
-
- if self.with_mask:
- if det_bboxes.shape[0] == 0:
- segm_result = [[[]
- for _ in range(self.mask_head[-1].num_classes)]
- ]
- else:
- aug_masks = []
- aug_img_metas = []
- for x, img_meta, semantic in zip(img_feats, img_metas,
- semantic_feats):
- img_shape = img_meta[0]['img_shape']
- scale_factor = img_meta[0]['scale_factor']
- flip = img_meta[0]['flip']
- flip_direction = img_meta[0]['flip_direction']
- _bboxes = bbox_mapping(det_bboxes[:, :4], img_shape,
- scale_factor, flip, flip_direction)
- mask_rois = bbox2roi([_bboxes])
- mask_feats = self.mask_roi_extractor[-1](
- x[:len(self.mask_roi_extractor[-1].featmap_strides)],
- mask_rois)
- if self.with_semantic:
- semantic_feat = semantic
- mask_semantic_feat = self.semantic_roi_extractor(
- [semantic_feat], mask_rois)
- if mask_semantic_feat.shape[-2:] != mask_feats.shape[
- -2:]:
- mask_semantic_feat = F.adaptive_avg_pool2d(
- mask_semantic_feat, mask_feats.shape[-2:])
- mask_feats += mask_semantic_feat
- last_feat = None
- for i in range(self.num_stages):
- mask_head = self.mask_head[i]
- if self.mask_info_flow:
- mask_pred, last_feat = mask_head(
- mask_feats, last_feat)
- else:
- mask_pred = mask_head(mask_feats)
- aug_masks.append(mask_pred.sigmoid().cpu().numpy())
- aug_img_metas.append(img_meta)
- merged_masks = merge_aug_masks(aug_masks, aug_img_metas,
- self.test_cfg)
-
- ori_shape = img_metas[0][0]['ori_shape']
- segm_result = self.mask_head[-1].get_seg_masks(
- merged_masks,
- det_bboxes,
- det_labels,
- rcnn_test_cfg,
- ori_shape,
- scale_factor=1.0,
- rescale=False)
- return [(bbox_result, segm_result)]
- else:
- return [bbox_result]
diff --git a/spaces/CVPR/lama-example/saicinpainting/evaluation/losses/fid/inception.py b/spaces/CVPR/lama-example/saicinpainting/evaluation/losses/fid/inception.py
deleted file mode 100644
index e9bd0863b457aaa40c770eaa4acbb142b18fc18b..0000000000000000000000000000000000000000
--- a/spaces/CVPR/lama-example/saicinpainting/evaluation/losses/fid/inception.py
+++ /dev/null
@@ -1,323 +0,0 @@
-import logging
-
-import torch
-import torch.nn as nn
-import torch.nn.functional as F
-from torchvision import models
-
-try:
- from torchvision.models.utils import load_state_dict_from_url
-except ImportError:
- from torch.utils.model_zoo import load_url as load_state_dict_from_url
-
-# Inception weights ported to Pytorch from
-# http://download.tensorflow.org/models/image/imagenet/inception-2015-12-05.tgz
-FID_WEIGHTS_URL = 'https://github.com/mseitzer/pytorch-fid/releases/download/fid_weights/pt_inception-2015-12-05-6726825d.pth'
-
-
-LOGGER = logging.getLogger(__name__)
-
-
-class InceptionV3(nn.Module):
- """Pretrained InceptionV3 network returning feature maps"""
-
- # Index of default block of inception to return,
- # corresponds to output of final average pooling
- DEFAULT_BLOCK_INDEX = 3
-
- # Maps feature dimensionality to their output blocks indices
- BLOCK_INDEX_BY_DIM = {
- 64: 0, # First max pooling features
- 192: 1, # Second max pooling featurs
- 768: 2, # Pre-aux classifier features
- 2048: 3 # Final average pooling features
- }
-
- def __init__(self,
- output_blocks=[DEFAULT_BLOCK_INDEX],
- resize_input=True,
- normalize_input=True,
- requires_grad=False,
- use_fid_inception=True):
- """Build pretrained InceptionV3
-
- Parameters
- ----------
- output_blocks : list of int
- Indices of blocks to return features of. Possible values are:
- - 0: corresponds to output of first max pooling
- - 1: corresponds to output of second max pooling
- - 2: corresponds to output which is fed to aux classifier
- - 3: corresponds to output of final average pooling
- resize_input : bool
- If true, bilinearly resizes input to width and height 299 before
- feeding input to model. As the network without fully connected
- layers is fully convolutional, it should be able to handle inputs
- of arbitrary size, so resizing might not be strictly needed
- normalize_input : bool
- If true, scales the input from range (0, 1) to the range the
- pretrained Inception network expects, namely (-1, 1)
- requires_grad : bool
- If true, parameters of the model require gradients. Possibly useful
- for finetuning the network
- use_fid_inception : bool
- If true, uses the pretrained Inception model used in Tensorflow's
- FID implementation. If false, uses the pretrained Inception model
- available in torchvision. The FID Inception model has different
- weights and a slightly different structure from torchvision's
- Inception model. If you want to compute FID scores, you are
- strongly advised to set this parameter to true to get comparable
- results.
- """
- super(InceptionV3, self).__init__()
-
- self.resize_input = resize_input
- self.normalize_input = normalize_input
- self.output_blocks = sorted(output_blocks)
- self.last_needed_block = max(output_blocks)
-
- assert self.last_needed_block <= 3, \
- 'Last possible output block index is 3'
-
- self.blocks = nn.ModuleList()
-
- if use_fid_inception:
- inception = fid_inception_v3()
- else:
- inception = models.inception_v3(pretrained=True)
-
- # Block 0: input to maxpool1
- block0 = [
- inception.Conv2d_1a_3x3,
- inception.Conv2d_2a_3x3,
- inception.Conv2d_2b_3x3,
- nn.MaxPool2d(kernel_size=3, stride=2)
- ]
- self.blocks.append(nn.Sequential(*block0))
-
- # Block 1: maxpool1 to maxpool2
- if self.last_needed_block >= 1:
- block1 = [
- inception.Conv2d_3b_1x1,
- inception.Conv2d_4a_3x3,
- nn.MaxPool2d(kernel_size=3, stride=2)
- ]
- self.blocks.append(nn.Sequential(*block1))
-
- # Block 2: maxpool2 to aux classifier
- if self.last_needed_block >= 2:
- block2 = [
- inception.Mixed_5b,
- inception.Mixed_5c,
- inception.Mixed_5d,
- inception.Mixed_6a,
- inception.Mixed_6b,
- inception.Mixed_6c,
- inception.Mixed_6d,
- inception.Mixed_6e,
- ]
- self.blocks.append(nn.Sequential(*block2))
-
- # Block 3: aux classifier to final avgpool
- if self.last_needed_block >= 3:
- block3 = [
- inception.Mixed_7a,
- inception.Mixed_7b,
- inception.Mixed_7c,
- nn.AdaptiveAvgPool2d(output_size=(1, 1))
- ]
- self.blocks.append(nn.Sequential(*block3))
-
- for param in self.parameters():
- param.requires_grad = requires_grad
-
- def forward(self, inp):
- """Get Inception feature maps
-
- Parameters
- ----------
- inp : torch.autograd.Variable
- Input tensor of shape Bx3xHxW. Values are expected to be in
- range (0, 1)
-
- Returns
- -------
- List of torch.autograd.Variable, corresponding to the selected output
- block, sorted ascending by index
- """
- outp = []
- x = inp
-
- if self.resize_input:
- x = F.interpolate(x,
- size=(299, 299),
- mode='bilinear',
- align_corners=False)
-
- if self.normalize_input:
- x = 2 * x - 1 # Scale from range (0, 1) to range (-1, 1)
-
- for idx, block in enumerate(self.blocks):
- x = block(x)
- if idx in self.output_blocks:
- outp.append(x)
-
- if idx == self.last_needed_block:
- break
-
- return outp
-
-
-def fid_inception_v3():
- """Build pretrained Inception model for FID computation
-
- The Inception model for FID computation uses a different set of weights
- and has a slightly different structure than torchvision's Inception.
-
- This method first constructs torchvision's Inception and then patches the
- necessary parts that are different in the FID Inception model.
- """
- LOGGER.info('fid_inception_v3 called')
- inception = models.inception_v3(num_classes=1008,
- aux_logits=False,
- pretrained=False)
- LOGGER.info('models.inception_v3 done')
- inception.Mixed_5b = FIDInceptionA(192, pool_features=32)
- inception.Mixed_5c = FIDInceptionA(256, pool_features=64)
- inception.Mixed_5d = FIDInceptionA(288, pool_features=64)
- inception.Mixed_6b = FIDInceptionC(768, channels_7x7=128)
- inception.Mixed_6c = FIDInceptionC(768, channels_7x7=160)
- inception.Mixed_6d = FIDInceptionC(768, channels_7x7=160)
- inception.Mixed_6e = FIDInceptionC(768, channels_7x7=192)
- inception.Mixed_7b = FIDInceptionE_1(1280)
- inception.Mixed_7c = FIDInceptionE_2(2048)
-
- LOGGER.info('fid_inception_v3 patching done')
-
- state_dict = load_state_dict_from_url(FID_WEIGHTS_URL, progress=True)
- LOGGER.info('fid_inception_v3 weights downloaded')
-
- inception.load_state_dict(state_dict)
- LOGGER.info('fid_inception_v3 weights loaded into model')
-
- return inception
-
-
-class FIDInceptionA(models.inception.InceptionA):
- """InceptionA block patched for FID computation"""
- def __init__(self, in_channels, pool_features):
- super(FIDInceptionA, self).__init__(in_channels, pool_features)
-
- def forward(self, x):
- branch1x1 = self.branch1x1(x)
-
- branch5x5 = self.branch5x5_1(x)
- branch5x5 = self.branch5x5_2(branch5x5)
-
- branch3x3dbl = self.branch3x3dbl_1(x)
- branch3x3dbl = self.branch3x3dbl_2(branch3x3dbl)
- branch3x3dbl = self.branch3x3dbl_3(branch3x3dbl)
-
- # Patch: Tensorflow's average pool does not use the padded zero's in
- # its average calculation
- branch_pool = F.avg_pool2d(x, kernel_size=3, stride=1, padding=1,
- count_include_pad=False)
- branch_pool = self.branch_pool(branch_pool)
-
- outputs = [branch1x1, branch5x5, branch3x3dbl, branch_pool]
- return torch.cat(outputs, 1)
-
-
-class FIDInceptionC(models.inception.InceptionC):
- """InceptionC block patched for FID computation"""
- def __init__(self, in_channels, channels_7x7):
- super(FIDInceptionC, self).__init__(in_channels, channels_7x7)
-
- def forward(self, x):
- branch1x1 = self.branch1x1(x)
-
- branch7x7 = self.branch7x7_1(x)
- branch7x7 = self.branch7x7_2(branch7x7)
- branch7x7 = self.branch7x7_3(branch7x7)
-
- branch7x7dbl = self.branch7x7dbl_1(x)
- branch7x7dbl = self.branch7x7dbl_2(branch7x7dbl)
- branch7x7dbl = self.branch7x7dbl_3(branch7x7dbl)
- branch7x7dbl = self.branch7x7dbl_4(branch7x7dbl)
- branch7x7dbl = self.branch7x7dbl_5(branch7x7dbl)
-
- # Patch: Tensorflow's average pool does not use the padded zero's in
- # its average calculation
- branch_pool = F.avg_pool2d(x, kernel_size=3, stride=1, padding=1,
- count_include_pad=False)
- branch_pool = self.branch_pool(branch_pool)
-
- outputs = [branch1x1, branch7x7, branch7x7dbl, branch_pool]
- return torch.cat(outputs, 1)
-
-
-class FIDInceptionE_1(models.inception.InceptionE):
- """First InceptionE block patched for FID computation"""
- def __init__(self, in_channels):
- super(FIDInceptionE_1, self).__init__(in_channels)
-
- def forward(self, x):
- branch1x1 = self.branch1x1(x)
-
- branch3x3 = self.branch3x3_1(x)
- branch3x3 = [
- self.branch3x3_2a(branch3x3),
- self.branch3x3_2b(branch3x3),
- ]
- branch3x3 = torch.cat(branch3x3, 1)
-
- branch3x3dbl = self.branch3x3dbl_1(x)
- branch3x3dbl = self.branch3x3dbl_2(branch3x3dbl)
- branch3x3dbl = [
- self.branch3x3dbl_3a(branch3x3dbl),
- self.branch3x3dbl_3b(branch3x3dbl),
- ]
- branch3x3dbl = torch.cat(branch3x3dbl, 1)
-
- # Patch: Tensorflow's average pool does not use the padded zero's in
- # its average calculation
- branch_pool = F.avg_pool2d(x, kernel_size=3, stride=1, padding=1,
- count_include_pad=False)
- branch_pool = self.branch_pool(branch_pool)
-
- outputs = [branch1x1, branch3x3, branch3x3dbl, branch_pool]
- return torch.cat(outputs, 1)
-
-
-class FIDInceptionE_2(models.inception.InceptionE):
- """Second InceptionE block patched for FID computation"""
- def __init__(self, in_channels):
- super(FIDInceptionE_2, self).__init__(in_channels)
-
- def forward(self, x):
- branch1x1 = self.branch1x1(x)
-
- branch3x3 = self.branch3x3_1(x)
- branch3x3 = [
- self.branch3x3_2a(branch3x3),
- self.branch3x3_2b(branch3x3),
- ]
- branch3x3 = torch.cat(branch3x3, 1)
-
- branch3x3dbl = self.branch3x3dbl_1(x)
- branch3x3dbl = self.branch3x3dbl_2(branch3x3dbl)
- branch3x3dbl = [
- self.branch3x3dbl_3a(branch3x3dbl),
- self.branch3x3dbl_3b(branch3x3dbl),
- ]
- branch3x3dbl = torch.cat(branch3x3dbl, 1)
-
- # Patch: The FID Inception model uses max pooling instead of average
- # pooling. This is likely an error in this specific Inception
- # implementation, as other Inception models use average pooling here
- # (which matches the description in the paper).
- branch_pool = F.max_pool2d(x, kernel_size=3, stride=1, padding=1)
- branch_pool = self.branch_pool(branch_pool)
-
- outputs = [branch1x1, branch3x3, branch3x3dbl, branch_pool]
- return torch.cat(outputs, 1)
diff --git a/spaces/ChristopherMarais/Andrew_AI-BB_classification-beta/mysite/andrew_alpha/templates/andrew_alpha.html b/spaces/ChristopherMarais/Andrew_AI-BB_classification-beta/mysite/andrew_alpha/templates/andrew_alpha.html
deleted file mode 100644
index 64656a8bb5424b943f5f5312872315e7488326b0..0000000000000000000000000000000000000000
--- a/spaces/ChristopherMarais/Andrew_AI-BB_classification-beta/mysite/andrew_alpha/templates/andrew_alpha.html
+++ /dev/null
@@ -1,17 +0,0 @@
-{% load static %}
-
-
-
- Andrew Alpha Beep Boop ....
-
-
-
""", unsafe_allow_html=True)
- option=st.selectbox("Select Model",['TFT','Prophet'])
-#------------------------------------------------------------------------------------------------------------
-# TFT
-if option=='TFT':
- #--------------------------------------------------------------------------------------------------------
- ## TFT data path and load
- path='data/train.csv'
- obj=StoreDataLoader(path)
- train_dataset,test_dataset,training,validation,earliest_time=obj.tft_data()
- print(f"TRAINING ::START DATE ::{train_dataset['date'].min()} :: END DATE ::{train_dataset['date'].max()}")
- print(f"TESTING ::START DATE ::{test_dataset['date'].min()} :: END DATE ::{test_dataset['date'].max()}")
- list_store=train_dataset['store'].unique()
- list_items=train_dataset['item'].unique()
- #---------------------------------------------------------------------------------------------------------
- try:
- # load the pre trained tft model
- model=model_obj.store_model_load(option)
- with st.sidebar:
- # st.success('Model Loaded successfully', icon="✅")
- # select the store id
- store=st.selectbox("Select Store ID",list_store)
- # select the item id
- item=st.selectbox("Select Product ID",list_items)
- #--------------------------------------------------------------------------------------------------------------
- ## prediction on testing data
- testing_results=test_prediction(model,train_dataset=train_dataset,test_dataset=test_dataset
- ,earliest_time=earliest_time,store_id=store,item_id=item)
- # find kpi
- rmse=np.around(np.sqrt(mean_squared_error(testing_results['Lead_1'],testing_results['prediction'])),2)
- mae=np.around(mean_absolute_error(testing_results['Lead_1'],testing_results['prediction']),2)
- print(f"TEST DATA = Item ID : {item} :: MAE : {mae} :: RMSE : {rmse}")
- #--------------------------------------tft future prediction-------------------------------------------
- final_data=pd.concat([train_dataset,test_dataset])
- consumer_data=final_data.loc[(final_data['store']==store) & (final_data['item']==item)]
- consumer_data.fillna(0,inplace=True)
- date_list=[]
- demand_prediction=[]
- for i in range(30):
- # select last 150 records as an enocer + decoder data
- encoder_data = consumer_data[lambda x: x.days_from_start > x.days_from_start.max() - 150]
- last_data = consumer_data[lambda x: x.days_from_start == x.days_from_start.max()]
-
- # prediction date and time
- date_list.append(encoder_data.tail(1).iloc[-1,:]['date'])
- # prediction for the last 30 records
- test_prediction = model.predict(encoder_data,
- mode="prediction",
- trainer_kwargs=dict(accelerator="cpu"),
- return_x=True)
- # create the next day record
- decoder_data = pd.concat(
- [last_data.assign(date=lambda x: x.date + pd.offsets.DateOffset(i)) for i in range(1, 2)],
- ignore_index=True,
- )
- # find the hours_from_start & days_from_start
- decoder_data["hours_from_start"] = (decoder_data["date"] - earliest_time).dt.seconds / 60 / 60 + (decoder_data["date"] - earliest_time).dt.days * 24
- decoder_data['hours_from_start'] = decoder_data['hours_from_start'].astype('int')
- decoder_data["hours_from_start"] += encoder_data["hours_from_start"].max() + 1 - decoder_data["hours_from_start"].min()
- # add time index consistent with "data"
- decoder_data["days_from_start"] = (decoder_data["date"] - earliest_time).apply(lambda x:x.days)
- # adding the datetime features
- decoder_data=create_week_date_featues(decoder_data,'date')
- # last timestep predicted record as assume next day actual demand(for more day forecasting)
- decoder_data['sales']=float(test_prediction.output[0][-1])
- # append this prediction into the list
- demand_prediction.append(float(test_prediction.output[0][-1]))
- # update prediction time idx
- decoder_data['time_idx']=int(test_prediction.x['decoder_time_idx'][0][-1])
- # add the next day record into the original data
- consumer_data=pd.concat([consumer_data,decoder_data])
- # fina lag and update
- consumer_data['lag_1']=consumer_data['sales'].shift(1)
- consumer_data['lag_5']=consumer_data['sales'].shift(5)
- # reset the index
- consumer_data=consumer_data.reset_index(drop=True)
- # forecast values for the next 30 days/timesteps
- d2=pd.DataFrame({"date":date_list,"prediction":demand_prediction})[['date','prediction']]
- # update the store and item ids
- d2['store']=store
- d2['item']=item
-#----------------------------TFT and Prophet model KPI----------------------------------------
- with st.sidebar:
- st.markdown(f"""
-
-
-
- # """, unsafe_allow_html=True)
- # train_a=train_dataset.loc[(train_dataset['store']==store) & (train_dataset['item']==item)][['date','store','item','sales']]
- # test_a=test_dataset.loc[(test_dataset['store']==store) & (test_dataset['item']==item)][['date','store','item','sales']]
- # actual_final_data=pd.concat([train_a,test_a])
- # actual_final_data['date']=actual_final_data['date'].dt.date
- # tab3.dataframe(actual_final_data,width=500)
-
- except:
- st.sidebar.error('Model Not Loaded successfully!',icon="🚨")
-
-#+++++++++++++++++++++++++++++++++++++++++++++++++++++++++++++++++++++++++++++++++++++++++++++++++++++++
-#+++++++++++++++++++++++++++++++++++++++++++++++++++++++++++++++++++++++++++++++++++++++++++++++++++++++
-
-elif option=='Prophet':
- print("prophet")
- #---------------------------------------------------Data----------------------------------------------------
- # Prophet data
- path='data/train.csv'
- obj=StoreDataLoader(path)
- fb_train_data,fb_test_data,item_dummay,store_dummay=obj.fb_data()
- # st.write(fb_train_data.columns)
- # st.write(fb_test_data.columns)
- # print(fb_test_data.columns)
- print(f"TRAINING ::START DATE ::{fb_train_data['ds'].min()} :: END DATE ::{fb_train_data['ds'].max()}")
- print(f"TESTING ::START DATE ::{fb_test_data['ds'].min()} :: END DATE ::{fb_test_data['ds'].max()}")
- train_new=fb_train_data.drop('y',axis=1)
- test_new=fb_test_data.drop('y',axis=1)
- #----------------------------------------------model Load----------------------------------------------------
- try:
- fb_model=model_obj.store_model_load(option)
- # with st.sidebar:
- # st.success('Model Loaded successfully', icon="✅")
- #-------------------------------------select store & item ---------------------------------------------------
- list_items=item_dummay.columns
- list_store=store_dummay.columns
- with st.sidebar:
- store=st.selectbox("Select Store",list_store)
- item=st.selectbox("Select Product",list_items)
- #------------------------------------------prediction---------------------------------------------------------------
- test_prediction=fb_model.predict(test_new.loc[test_new[item]==1])
- train_prediction=fb_model.predict(train_new.loc[train_new[item]==1])
-
- y_true_test=fb_test_data.loc[fb_test_data[item]==1]
- y_true_train=fb_train_data.loc[fb_train_data[item]==1]
-
- y_train_pred=train_prediction[['ds','yhat']].iloc[-60:,:]
- y_train_true=y_true_train[['ds','y']].iloc[-60:,:]
-
- y_test_pred=test_prediction[['ds','yhat']]
- y_test_true=y_true_test[['ds','y']]
- #----------------------------------------KPI---------------------------------------------------------------
- rmse=np.sqrt(mean_squared_error(y_test_true['y'],y_test_pred['yhat']))
- mae=mean_absolute_error(y_test_true['y'],y_test_pred['yhat'])
-#---------------------------------future prediction---------------------------------------
- fb_final=pd.concat([fb_train_data,fb_test_data])
- # extract the data for selected store and item
- fb_consumer=fb_final.loc[(fb_final[store]==1) & (fb_final[item]==1)]
-
- # list of dates and prediction
- date_list=[]
- prediction_list=[]
-
- # predicting the next 30 days product demand
- for i in range(30):
- # select only date record
- next_prediction=fb_consumer.tail(1).drop('y',axis=1) # drop target of last 01/01/2015 00:00:00
- # predict next timestep demand
- prediction=fb_model.predict(next_prediction) # pass other feature value to the model
-
- # append date and predicted demand
- date_list.append(prediction['ds'][0]) ## append the datetime of prediction
- prediction_list.append(prediction['yhat'][0]) ## append the next timestep prediction
-
-
- #--------------------------next timestep data simulate-------------------------------------------------------------
- last_data = fb_consumer[lambda x: x.ds == x.ds.max()] # last date present in data
- # next timestep
- decoder_data = pd.concat(
- [last_data.assign(ds=lambda x: x.ds + pd.offsets.DateOffset(i)) for i in range(1, 2)],
- ignore_index=True,
- )
- # update next timestep datetime covariates
- decoder_data=create_week_date_featues(decoder_data,'ds')
- # update last day demand prediction to the here as an actual demand value(using for more future timestep prediction)
- decoder_data['sales']=prediction['yhat'][0] # assume next timestep prediction as actual
- # update this next record into the original data
- fb_consumer=pd.concat([fb_consumer,decoder_data]) # append that next timestep data to into main data
- # find shift of power usage and update into the datset
- fb_consumer['lag_1']=fb_consumer['sales'].shift(1)
- fb_consumer['lag_5']=fb_consumer['sales'].shift(5)
- fb_consumer=fb_consumer.reset_index(drop=True) # reset_index
- future_prediction=pd.DataFrame({"ds":date_list,"yhat":prediction_list})
- future_prediction['store']=store
- future_prediction['item']=item
-
- with st.sidebar:
- st.markdown(f"""
-
-
-
- """, unsafe_allow_html=True)
- final_r=pd.concat([future_prediction[['ds','store','item','yhat']],results[['ds','store','item','yhat']]]).sort_values('ds').drop_duplicates().reset_index(drop=True)
- csv = convert_df(final_r)
- tab2.dataframe(final_r,width=500)
- tab2.download_button(
- "Download",
- csv,
- "file.csv",
- "text/csv",
- key='download-csv'
- )
-
- #------------------------------------------Tab-3--------------------------------------------------
- # train_a=fb_train_data.loc[fb_train_data[item]==1][['ds','sales']]
- # # train_a['store']=1
- # # train_a['item']=item
- # test_a=fb_test_data.loc[fb_test_data[item]==1][['ds','sales']]
- # # test_a['store']=1
- # # test_a['item']=item.split('_')[-1]
- # actual_final_data=pd.concat([train_a,test_a])
- # actual_final_data['store']=1
- # actual_final_data['item']=item.split('_')[-1]
- # actual_final_data['ds']=actual_final_data['ds'].dt.date
- # actual_final_data.rename({"ds":'date'},inplace=True)
- # tab3.dataframe(actual_final_data[['date','store','item','sales']],width=500)
-
-
-
- except:
- st.sidebar.error('Model Not Loaded successfully!',icon="🚨")
-
-
-
diff --git a/spaces/akhaliq/deeplab2/model/loss/matchers_ops.py b/spaces/akhaliq/deeplab2/model/loss/matchers_ops.py
deleted file mode 100644
index 4273e94476d7d7859797c8dfc1ace1ffe100f892..0000000000000000000000000000000000000000
--- a/spaces/akhaliq/deeplab2/model/loss/matchers_ops.py
+++ /dev/null
@@ -1,495 +0,0 @@
-# coding=utf-8
-# Copyright 2021 The Deeplab2 Authors.
-#
-# Licensed under the Apache License, Version 2.0 (the "License");
-# you may not use this file except in compliance with the License.
-# You may obtain a copy of the License at
-#
-# http://www.apache.org/licenses/LICENSE-2.0
-#
-# Unless required by applicable law or agreed to in writing, software
-# distributed under the License is distributed on an "AS IS" BASIS,
-# WITHOUT WARRANTIES OR CONDITIONS OF ANY KIND, either express or implied.
-# See the License for the specific language governing permissions and
-# limitations under the License.
-
-"""Tensorflow implementation to solve the Linear Sum Assignment problem.
-
-The Linear Sum Assignment problem involves determining the minimum weight
-matching for bipartite graphs. For example, this problem can be defined by
-a 2D matrix C, where each element i,j determines the cost of matching worker i
-with job j. The solution to the problem is a complete assignment of jobs to
-workers, such that no job is assigned to more than one work and no worker is
-assigned more than one job, with minimum cost.
-
-This implementation is designed to be used with tf.compat.v2 to be compatible
-with the rest of the DeepLab2 library. It builds off of the Hungarian Matching
-Algorithm (https://www.cse.ust.hk/~golin/COMP572/Notes/Matching.pdf), the
-original Lingvo tensorflow implementation by Jiquan Ngiam, and the modified TF1
-version by Amil Merchant.
-"""
-
-import tensorflow as tf
-
-
-def _prepare(weights):
- """Prepare the cost matrix.
-
- To speed up computational efficiency of the algorithm, all weights are shifted
- to be non-negative. Each element is reduced by the row / column minimum. Note
- that neither operation will effect the resulting solution but will provide
- a better starting point for the greedy assignment. Note this corresponds to
- the pre-processing and step 1 of the Hungarian algorithm from Wikipedia.
-
- Args:
- weights: A float32 [batch_size, num_elems, num_elems] tensor, where each
- inner matrix represents weights to be use for matching.
-
- Returns:
- A prepared weights tensor of the same shape and dtype.
- """
- # Since every worker needs a job and every job needs a worker, we can subtract
- # the minimum from each.
- weights -= tf.reduce_min(weights, axis=2, keepdims=True)
- weights -= tf.reduce_min(weights, axis=1, keepdims=True)
- return weights
-
-
-def _greedy_assignment(adj_matrix):
- """Greedily assigns workers to jobs based on an adjaceny matrix.
-
- Starting with an adjacency matrix representing the available connections
- in the bi-partite graph, this function greedily chooses elements such
- that each worker is matched to at most one job (or each job is assigned to
- at most one worker). Note, if the adjacency matrix has no available values
- for a particular row/column, the corresponding job/worker may go unassigned.
-
- Args:
- adj_matrix: A bool [batch_size, num_elems, num_elems] tensor, where each
- element of the inner matrix represents whether the worker (row) can be
- matched to the job (column).
-
- Returns:
- A bool [batch_size, num_elems, num_elems] tensor, where each element of the
- inner matrix represents whether the worker has been matched to the job.
- Each row and column can have at most one true element. Some of the rows
- and columns may not be matched.
- """
- _, num_elems, _ = get_shape_list(adj_matrix, expected_rank=3)
- adj_matrix = tf.transpose(adj_matrix, [1, 0, 2])
-
- # Create a dynamic TensorArray containing the assignments for each worker/job
- assignment = tf.TensorArray(tf.bool, num_elems)
-
- # Store the elements assigned to each column to update each iteration
- col_assigned = tf.zeros_like(adj_matrix[0, ...], dtype=tf.bool)
-
- # Iteratively assign each row using tf.foldl. Intuitively, this is a loop
- # over rows, where we incrementally assign each row.
- def _assign_row(accumulator, row_adj):
- # The accumulator tracks the row assignment index.
- idx, assignment, col_assigned = accumulator
-
- # Viable candidates cannot already be assigned to another job.
- candidates = row_adj & (~col_assigned)
-
- # Deterministically assign to the candidates of the highest index count.
- max_candidate_idx = tf.argmax(
- tf.cast(candidates, tf.int32), axis=1, output_type=tf.int32)
-
- candidates_indicator = tf.one_hot(
- max_candidate_idx,
- num_elems,
- on_value=True,
- off_value=False,
- dtype=tf.bool)
- candidates_indicator &= candidates
-
- # Make assignment to the column.
- col_assigned |= candidates_indicator
- assignment = assignment.write(idx, candidates_indicator)
-
- return idx + 1, assignment, col_assigned
-
- _, assignment, _ = tf.foldl(
- _assign_row, adj_matrix, (0, assignment, col_assigned), back_prop=False)
-
- assignment = assignment.stack()
- assignment = tf.transpose(assignment, [1, 0, 2])
- return assignment
-
-
-def _find_augmenting_path(assignment, adj_matrix):
- """Finds an augmenting path given an assignment and an adjacency matrix.
-
- The augmenting path search starts from the unassigned workers, then goes on
- to find jobs (via an unassigned pairing), then back again to workers (via an
- existing pairing), and so on. The path alternates between unassigned and
- existing pairings. Returns the state after the search.
-
- Note: In the state the worker and job, indices are 1-indexed so that we can
- use 0 to represent unreachable nodes. State contains the following keys:
-
- - jobs: A [batch_size, 1, num_elems] tensor containing the highest index
- unassigned worker that can reach this job through a path.
- - jobs_from_worker: A [batch_size, num_elems] tensor containing the worker
- reached immediately before this job.
- - workers: A [batch_size, num_elems, 1] tensor containing the highest index
- unassigned worker that can reach this worker through a path.
- - workers_from_job: A [batch_size, num_elems] tensor containing the job
- reached immediately before this worker.
- - new_jobs: A bool [batch_size, num_elems] tensor containing True if the
- unassigned job can be reached via a path.
-
- State can be used to recover the path via backtracking.
-
- Args:
- assignment: A bool [batch_size, num_elems, num_elems] tensor, where each
- element of the inner matrix represents whether the worker has been matched
- to the job. This may be a partial assignment.
- adj_matrix: A bool [batch_size, num_elems, num_elems] tensor, where each
- element of the inner matrix represents whether the worker (row) can be
- matched to the job (column).
-
- Returns:
- A state dict, which represents the outcome of running an augmenting
- path search on the graph given the assignment.
- """
- batch_size, num_elems, _ = get_shape_list(assignment, expected_rank=3)
- unassigned_workers = ~tf.reduce_any(assignment, axis=2, keepdims=True)
- unassigned_jobs = ~tf.reduce_any(assignment, axis=1, keepdims=True)
-
- unassigned_pairings = tf.cast(adj_matrix & ~assignment, tf.int32)
- existing_pairings = tf.cast(assignment, tf.int32)
-
- # Initialize unassigned workers to have non-zero ids, assigned workers will
- # have ids = 0.
- worker_indices = tf.range(1, num_elems + 1, dtype=tf.int32)
- init_workers = tf.tile(worker_indices[tf.newaxis, :, tf.newaxis],
- [batch_size, 1, 1])
- init_workers *= tf.cast(unassigned_workers, tf.int32)
-
- state = {
- "jobs": tf.zeros((batch_size, 1, num_elems), dtype=tf.int32),
- "jobs_from_worker": tf.zeros((batch_size, num_elems), dtype=tf.int32),
- "workers": init_workers,
- "workers_from_job": tf.zeros((batch_size, num_elems), dtype=tf.int32)
- }
-
- def _has_active_workers(state, curr_workers):
- """Check if there are still active workers."""
- del state
- return tf.reduce_sum(curr_workers) > 0
-
- def _augment_step(state, curr_workers):
- """Performs one search step."""
-
- # Note: These steps could be potentially much faster if sparse matrices are
- # supported. The unassigned_pairings and existing_pairings matrices can be
- # very sparse.
-
- # Find potential jobs using current workers.
- potential_jobs = curr_workers * unassigned_pairings
- curr_jobs = tf.reduce_max(potential_jobs, axis=1, keepdims=True)
- curr_jobs_from_worker = 1 + tf.argmax(
- potential_jobs, axis=1, output_type=tf.int32)
-
- # Remove already accessible jobs from curr_jobs.
- default_jobs = tf.zeros_like(state["jobs"], dtype=state["jobs"].dtype)
- curr_jobs = tf.where(state["jobs"] > 0, default_jobs, curr_jobs)
- curr_jobs_from_worker *= tf.cast(curr_jobs > 0, tf.int32)[:, 0, :]
-
- # Find potential workers from current jobs.
- potential_workers = curr_jobs * existing_pairings
- curr_workers = tf.reduce_max(potential_workers, axis=2, keepdims=True)
- curr_workers_from_job = 1 + tf.argmax(
- potential_workers, axis=2, output_type=tf.int32)
-
- # Remove already accessible workers from curr_workers.
- default_workers = tf.zeros_like(state["workers"])
- curr_workers = tf.where(
- state["workers"] > 0, default_workers, curr_workers)
- curr_workers_from_job *= tf.cast(curr_workers > 0, tf.int32)[:, :, 0]
-
- # Update state so that we can backtrack later.
- state = state.copy()
- state["jobs"] = tf.maximum(state["jobs"], curr_jobs)
- state["jobs_from_worker"] = tf.maximum(state["jobs_from_worker"],
- curr_jobs_from_worker)
- state["workers"] = tf.maximum(state["workers"], curr_workers)
- state["workers_from_job"] = tf.maximum(state["workers_from_job"],
- curr_workers_from_job)
-
- return state, curr_workers
-
- with tf.name_scope("find_augmenting_path"):
- state, _ = tf.while_loop(
- _has_active_workers,
- _augment_step, (state, init_workers),
- back_prop=False)
-
- # Compute new jobs, this is useful for determnining termnination of the
- # maximum bi-partite matching and initialization for backtracking.
- new_jobs = (state["jobs"] > 0) & unassigned_jobs
- state["new_jobs"] = new_jobs[:, 0, :]
- return state
-
-
-def _improve_assignment(assignment, state):
- """Improves an assignment by backtracking the augmented path using state.
-
- Args:
- assignment: A bool [batch_size, num_elems, num_elems] tensor, where each
- element of the inner matrix represents whether the worker has been matched
- to the job. This may be a partial assignment.
- state: A dict, which represents the outcome of running an augmenting path
- search on the graph given the assignment.
-
- Returns:
- A new assignment matrix of the same shape and type as assignment, where the
- assignment has been updated using the augmented path found.
- """
- batch_size, num_elems, _ = get_shape_list(assignment, 3)
-
- # We store the current job id and iteratively backtrack using jobs_from_worker
- # and workers_from_job until we reach an unassigned worker. We flip all the
- # assignments on this path to discover a better overall assignment.
-
- # Note: The indices in state are 1-indexed, where 0 represents that the
- # worker / job cannot be reached.
-
- # Obtain initial job indices based on new_jobs.
- curr_job_idx = tf.argmax(
- tf.cast(state["new_jobs"], tf.int32), axis=1, output_type=tf.int32)
-
- # Track whether an example is actively being backtracked. Since we are
- # operating on a batch, not all examples in the batch may be active.
- active = tf.gather(state["new_jobs"], curr_job_idx, batch_dims=1)
- batch_range = tf.range(0, batch_size, dtype=tf.int32)
-
- # Flip matrix tracks which assignments we need to flip - corresponding to the
- # augmenting path taken. We use an integer tensor here so that we can use
- # tensor_scatter_nd_add to update the tensor, and then cast it back to bool
- # after the loop.
- flip_matrix = tf.zeros((batch_size, num_elems, num_elems), dtype=tf.int32)
-
- def _has_active_backtracks(flip_matrix, active, curr_job_idx):
- """Check if there are still active workers."""
- del flip_matrix, curr_job_idx
- return tf.reduce_any(active)
-
- def _backtrack_one_step(flip_matrix, active, curr_job_idx):
- """Take one step in backtracking."""
- # Discover the worker that the job originated from, note that this worker
- # must exist by construction.
- curr_worker_idx = tf.gather(
- state["jobs_from_worker"], curr_job_idx, batch_dims=1) - 1
- curr_worker_idx = tf.maximum(curr_worker_idx, 0)
- update_indices = tf.stack([batch_range, curr_worker_idx, curr_job_idx],
- axis=1)
- update_indices = tf.maximum(update_indices, 0)
- flip_matrix = tf.tensor_scatter_nd_add(flip_matrix, update_indices,
- tf.cast(active, tf.int32))
-
- # Discover the (potential) job that the worker originated from.
- curr_job_idx = tf.gather(
- state["workers_from_job"], curr_worker_idx, batch_dims=1) - 1
- # Note that jobs may not be active, and we track that here (before
- # adjusting indices so that they are all >= 0 for gather).
- active &= curr_job_idx >= 0
- curr_job_idx = tf.maximum(curr_job_idx, 0)
- update_indices = tf.stack([batch_range, curr_worker_idx, curr_job_idx],
- axis=1)
- update_indices = tf.maximum(update_indices, 0)
- flip_matrix = tf.tensor_scatter_nd_add(flip_matrix, update_indices,
- tf.cast(active, tf.int32))
-
- return flip_matrix, active, curr_job_idx
-
- with tf.name_scope("improve_assignment"):
- flip_matrix, _, _ = tf.while_loop(
- _has_active_backtracks,
- _backtrack_one_step, (flip_matrix, active, curr_job_idx),
- back_prop=False)
-
- flip_matrix = tf.cast(flip_matrix, tf.bool)
- assignment = tf.math.logical_xor(assignment, flip_matrix)
-
- return assignment
-
-
-def _maximum_bipartite_matching(adj_matrix, assignment=None):
- """Performs maximum bipartite matching using augmented paths.
-
- Args:
- adj_matrix: A bool [batch_size, num_elems, num_elems] tensor, where each
- element of the inner matrix represents whether the worker (row) can be
- matched to the job (column).
- assignment: An optional bool [batch_size, num_elems, num_elems] tensor,
- where each element of the inner matrix represents whether the worker has
- been matched to the job. This may be a partial assignment. If specified,
- this assignment will be used to seed the iterative algorithm.
-
- Returns:
- A state dict representing the final augmenting path state search, and
- a maximum bipartite matching assignment tensor. Note that the state outcome
- can be used to compute a minimum vertex cover for the bipartite graph.
- """
-
- if assignment is None:
- assignment = _greedy_assignment(adj_matrix)
-
- state = _find_augmenting_path(assignment, adj_matrix)
-
- def _has_new_jobs(state, assignment):
- del assignment
- return tf.reduce_any(state["new_jobs"])
-
- def _improve_assignment_and_find_new_path(state, assignment):
- assignment = _improve_assignment(assignment, state)
- state = _find_augmenting_path(assignment, adj_matrix)
- return state, assignment
-
- with tf.name_scope("maximum_bipartite_matching"):
- state, assignment = tf.while_loop(
- _has_new_jobs,
- _improve_assignment_and_find_new_path, (state, assignment),
- back_prop=False)
-
- return state, assignment
-
-
-def _compute_cover(state, assignment):
- """Computes a cover for the bipartite graph.
-
- We compute a cover using the construction provided at
- https://en.wikipedia.org/wiki/K%C5%91nig%27s_theorem_(graph_theory)#Proof
- which uses the outcome from the alternating path search.
-
- Args:
- state: A state dict, which represents the outcome of running an augmenting
- path search on the graph given the assignment.
- assignment: An optional bool [batch_size, num_elems, num_elems] tensor,
- where each element of the inner matrix represents whether the worker has
- been matched to the job. This may be a partial assignment. If specified,
- this assignment will be used to seed the iterative algorithm.
-
- Returns:
- A tuple of (workers_cover, jobs_cover) corresponding to row and column
- covers for the bipartite graph. workers_cover is a boolean tensor of shape
- [batch_size, num_elems, 1] and jobs_cover is a boolean tensor of shape
- [batch_size, 1, num_elems].
- """
- assigned_workers = tf.reduce_any(assignment, axis=2, keepdims=True)
- assigned_jobs = tf.reduce_any(assignment, axis=1, keepdims=True)
-
- reachable_workers = state["workers"] > 0
- reachable_jobs = state["jobs"] > 0
-
- workers_cover = assigned_workers & (~reachable_workers)
- jobs_cover = assigned_jobs & reachable_jobs
-
- return workers_cover, jobs_cover
-
-
-def _update_weights_using_cover(workers_cover, jobs_cover, weights):
- """Updates weights for hungarian matching using a cover.
-
- We first find the minimum uncovered weight. Then, we subtract this from all
- the uncovered weights, and add it to all the doubly covered weights.
-
- Args:
- workers_cover: A boolean tensor of shape [batch_size, num_elems, 1].
- jobs_cover: A boolean tensor of shape [batch_size, 1, num_elems].
- weights: A float32 [batch_size, num_elems, num_elems] tensor, where each
- inner matrix represents weights to be use for matching.
-
- Returns:
- A new weight matrix with elements adjusted by the cover.
- """
- max_value = tf.reduce_max(weights)
-
- covered = workers_cover | jobs_cover
- double_covered = workers_cover & jobs_cover
-
- uncovered_weights = tf.where(covered,
- tf.ones_like(weights) * max_value, weights)
- min_weight = tf.reduce_min(uncovered_weights, axis=[-2, -1], keepdims=True)
-
- add_weight = tf.where(double_covered,
- tf.ones_like(weights) * min_weight,
- tf.zeros_like(weights))
- sub_weight = tf.where(covered, tf.zeros_like(weights),
- tf.ones_like(weights) * min_weight)
-
- return weights + add_weight - sub_weight
-
-
-def get_shape_list(tensor, expected_rank=None):
- """Returns a list of the shape of tensor.
-
- Args:
- tensor: A tf.Tensor object to find the shape of
- expected_rank: An (optional) int with the expected rank of the inputted
- tensor.
-
- Returns:
- A list representing the shape of the tesnor.
-
- Raises:
- ValueError: If the expected rank does not match the expected rank of the
- inputted tensor.
- """
- actual_rank = tensor.shape.ndims
-
- if expected_rank and actual_rank != expected_rank:
- raise ValueError("The tensor has rank %d which is not equal to the "
- "expected rank %d" % (actual_rank, expected_rank))
-
- shape = tensor.shape.as_list()
- dynamic = tf.shape(tensor)
- output = [dim if dim else dynamic[ind] for ind, dim in enumerate(shape)]
- return output
-
-
-def hungarian_matching(weights):
- """Computes the minimum linear sum assignment using the Hungarian algorithm.
-
- Args:
- weights: A float32 [batch_size, num_elems, num_elems] tensor, where each
- inner matrix represents weights to be use for matching.
-
- Returns:
- A bool [batch_size, num_elems, num_elems] tensor, where each element of the
- inner matrix represents whether the worker has been matched to the job.
- The returned matching will always be a perfect match.
- """
- batch_size, num_elems, _ = get_shape_list(weights, 3)
-
- weights = _prepare(weights)
- adj_matrix = tf.equal(weights, 0.)
- state, assignment = _maximum_bipartite_matching(adj_matrix)
- workers_cover, jobs_cover = _compute_cover(state, assignment)
-
- def _cover_incomplete(workers_cover, jobs_cover, *args):
- del args
- cover_sum = (
- tf.reduce_sum(tf.cast(workers_cover, tf.int32)) +
- tf.reduce_sum(tf.cast(jobs_cover, tf.int32)))
- return tf.less(cover_sum, batch_size * num_elems)
-
- def _update_weights_and_match(workers_cover, jobs_cover, weights, assignment):
- weights = _update_weights_using_cover(workers_cover, jobs_cover, weights)
- adj_matrix = tf.equal(weights, 0.)
- state, assignment = _maximum_bipartite_matching(adj_matrix, assignment)
- workers_cover, jobs_cover = _compute_cover(state, assignment)
- return workers_cover, jobs_cover, weights, assignment
-
- with tf.name_scope("hungarian_matching"):
- workers_cover, jobs_cover, weights, assignment = tf.while_loop(
- _cover_incomplete,
- _update_weights_and_match,
- (workers_cover, jobs_cover, weights, assignment),
- back_prop=False)
-
- return assignment
diff --git a/spaces/akhaliq/neural-waveshaping-synthesis/neural_waveshaping_synthesis/utils/__init__.py b/spaces/akhaliq/neural-waveshaping-synthesis/neural_waveshaping_synthesis/utils/__init__.py
deleted file mode 100644
index 6fd120a066e61142432242a93e1e0d58ab5f675e..0000000000000000000000000000000000000000
--- a/spaces/akhaliq/neural-waveshaping-synthesis/neural_waveshaping_synthesis/utils/__init__.py
+++ /dev/null
@@ -1,2 +0,0 @@
-from .utils import *
-from .seed_all import *
\ No newline at end of file
diff --git a/spaces/alexray/btc_predictor/venv/bin/Activate.ps1 b/spaces/alexray/btc_predictor/venv/bin/Activate.ps1
deleted file mode 100644
index eeea3583fa130d4702a05012a2103152daf51487..0000000000000000000000000000000000000000
--- a/spaces/alexray/btc_predictor/venv/bin/Activate.ps1
+++ /dev/null
@@ -1,247 +0,0 @@
-<#
-.Synopsis
-Activate a Python virtual environment for the current PowerShell session.
-
-.Description
-Pushes the python executable for a virtual environment to the front of the
-$Env:PATH environment variable and sets the prompt to signify that you are
-in a Python virtual environment. Makes use of the command line switches as
-well as the `pyvenv.cfg` file values present in the virtual environment.
-
-.Parameter VenvDir
-Path to the directory that contains the virtual environment to activate. The
-default value for this is the parent of the directory that the Activate.ps1
-script is located within.
-
-.Parameter Prompt
-The prompt prefix to display when this virtual environment is activated. By
-default, this prompt is the name of the virtual environment folder (VenvDir)
-surrounded by parentheses and followed by a single space (ie. '(.venv) ').
-
-.Example
-Activate.ps1
-Activates the Python virtual environment that contains the Activate.ps1 script.
-
-.Example
-Activate.ps1 -Verbose
-Activates the Python virtual environment that contains the Activate.ps1 script,
-and shows extra information about the activation as it executes.
-
-.Example
-Activate.ps1 -VenvDir C:\Users\MyUser\Common\.venv
-Activates the Python virtual environment located in the specified location.
-
-.Example
-Activate.ps1 -Prompt "MyPython"
-Activates the Python virtual environment that contains the Activate.ps1 script,
-and prefixes the current prompt with the specified string (surrounded in
-parentheses) while the virtual environment is active.
-
-.Notes
-On Windows, it may be required to enable this Activate.ps1 script by setting the
-execution policy for the user. You can do this by issuing the following PowerShell
-command:
-
-PS C:\> Set-ExecutionPolicy -ExecutionPolicy RemoteSigned -Scope CurrentUser
-
-For more information on Execution Policies:
-https://go.microsoft.com/fwlink/?LinkID=135170
-
-#>
-Param(
- [Parameter(Mandatory = $false)]
- [String]
- $VenvDir,
- [Parameter(Mandatory = $false)]
- [String]
- $Prompt
-)
-
-<# Function declarations --------------------------------------------------- #>
-
-<#
-.Synopsis
-Remove all shell session elements added by the Activate script, including the
-addition of the virtual environment's Python executable from the beginning of
-the PATH variable.
-
-.Parameter NonDestructive
-If present, do not remove this function from the global namespace for the
-session.
-
-#>
-function global:deactivate ([switch]$NonDestructive) {
- # Revert to original values
-
- # The prior prompt:
- if (Test-Path -Path Function:_OLD_VIRTUAL_PROMPT) {
- Copy-Item -Path Function:_OLD_VIRTUAL_PROMPT -Destination Function:prompt
- Remove-Item -Path Function:_OLD_VIRTUAL_PROMPT
- }
-
- # The prior PYTHONHOME:
- if (Test-Path -Path Env:_OLD_VIRTUAL_PYTHONHOME) {
- Copy-Item -Path Env:_OLD_VIRTUAL_PYTHONHOME -Destination Env:PYTHONHOME
- Remove-Item -Path Env:_OLD_VIRTUAL_PYTHONHOME
- }
-
- # The prior PATH:
- if (Test-Path -Path Env:_OLD_VIRTUAL_PATH) {
- Copy-Item -Path Env:_OLD_VIRTUAL_PATH -Destination Env:PATH
- Remove-Item -Path Env:_OLD_VIRTUAL_PATH
- }
-
- # Just remove the VIRTUAL_ENV altogether:
- if (Test-Path -Path Env:VIRTUAL_ENV) {
- Remove-Item -Path env:VIRTUAL_ENV
- }
-
- # Just remove VIRTUAL_ENV_PROMPT altogether.
- if (Test-Path -Path Env:VIRTUAL_ENV_PROMPT) {
- Remove-Item -Path env:VIRTUAL_ENV_PROMPT
- }
-
- # Just remove the _PYTHON_VENV_PROMPT_PREFIX altogether:
- if (Get-Variable -Name "_PYTHON_VENV_PROMPT_PREFIX" -ErrorAction SilentlyContinue) {
- Remove-Variable -Name _PYTHON_VENV_PROMPT_PREFIX -Scope Global -Force
- }
-
- # Leave deactivate function in the global namespace if requested:
- if (-not $NonDestructive) {
- Remove-Item -Path function:deactivate
- }
-}
-
-<#
-.Description
-Get-PyVenvConfig parses the values from the pyvenv.cfg file located in the
-given folder, and returns them in a map.
-
-For each line in the pyvenv.cfg file, if that line can be parsed into exactly
-two strings separated by `=` (with any amount of whitespace surrounding the =)
-then it is considered a `key = value` line. The left hand string is the key,
-the right hand is the value.
-
-If the value starts with a `'` or a `"` then the first and last character is
-stripped from the value before being captured.
-
-.Parameter ConfigDir
-Path to the directory that contains the `pyvenv.cfg` file.
-#>
-function Get-PyVenvConfig(
- [String]
- $ConfigDir
-) {
- Write-Verbose "Given ConfigDir=$ConfigDir, obtain values in pyvenv.cfg"
-
- # Ensure the file exists, and issue a warning if it doesn't (but still allow the function to continue).
- $pyvenvConfigPath = Join-Path -Resolve -Path $ConfigDir -ChildPath 'pyvenv.cfg' -ErrorAction Continue
-
- # An empty map will be returned if no config file is found.
- $pyvenvConfig = @{ }
-
- if ($pyvenvConfigPath) {
-
- Write-Verbose "File exists, parse `key = value` lines"
- $pyvenvConfigContent = Get-Content -Path $pyvenvConfigPath
-
- $pyvenvConfigContent | ForEach-Object {
- $keyval = $PSItem -split "\s*=\s*", 2
- if ($keyval[0] -and $keyval[1]) {
- $val = $keyval[1]
-
- # Remove extraneous quotations around a string value.
- if ("'""".Contains($val.Substring(0, 1))) {
- $val = $val.Substring(1, $val.Length - 2)
- }
-
- $pyvenvConfig[$keyval[0]] = $val
- Write-Verbose "Adding Key: '$($keyval[0])'='$val'"
- }
- }
- }
- return $pyvenvConfig
-}
-
-
-<# Begin Activate script --------------------------------------------------- #>
-
-# Determine the containing directory of this script
-$VenvExecPath = Split-Path -Parent $MyInvocation.MyCommand.Definition
-$VenvExecDir = Get-Item -Path $VenvExecPath
-
-Write-Verbose "Activation script is located in path: '$VenvExecPath'"
-Write-Verbose "VenvExecDir Fullname: '$($VenvExecDir.FullName)"
-Write-Verbose "VenvExecDir Name: '$($VenvExecDir.Name)"
-
-# Set values required in priority: CmdLine, ConfigFile, Default
-# First, get the location of the virtual environment, it might not be
-# VenvExecDir if specified on the command line.
-if ($VenvDir) {
- Write-Verbose "VenvDir given as parameter, using '$VenvDir' to determine values"
-}
-else {
- Write-Verbose "VenvDir not given as a parameter, using parent directory name as VenvDir."
- $VenvDir = $VenvExecDir.Parent.FullName.TrimEnd("\\/")
- Write-Verbose "VenvDir=$VenvDir"
-}
-
-# Next, read the `pyvenv.cfg` file to determine any required value such
-# as `prompt`.
-$pyvenvCfg = Get-PyVenvConfig -ConfigDir $VenvDir
-
-# Next, set the prompt from the command line, or the config file, or
-# just use the name of the virtual environment folder.
-if ($Prompt) {
- Write-Verbose "Prompt specified as argument, using '$Prompt'"
-}
-else {
- Write-Verbose "Prompt not specified as argument to script, checking pyvenv.cfg value"
- if ($pyvenvCfg -and $pyvenvCfg['prompt']) {
- Write-Verbose " Setting based on value in pyvenv.cfg='$($pyvenvCfg['prompt'])'"
- $Prompt = $pyvenvCfg['prompt'];
- }
- else {
- Write-Verbose " Setting prompt based on parent's directory's name. (Is the directory name passed to venv module when creating the virtual environment)"
- Write-Verbose " Got leaf-name of $VenvDir='$(Split-Path -Path $venvDir -Leaf)'"
- $Prompt = Split-Path -Path $venvDir -Leaf
- }
-}
-
-Write-Verbose "Prompt = '$Prompt'"
-Write-Verbose "VenvDir='$VenvDir'"
-
-# Deactivate any currently active virtual environment, but leave the
-# deactivate function in place.
-deactivate -nondestructive
-
-# Now set the environment variable VIRTUAL_ENV, used by many tools to determine
-# that there is an activated venv.
-$env:VIRTUAL_ENV = $VenvDir
-
-if (-not $Env:VIRTUAL_ENV_DISABLE_PROMPT) {
-
- Write-Verbose "Setting prompt to '$Prompt'"
-
- # Set the prompt to include the env name
- # Make sure _OLD_VIRTUAL_PROMPT is global
- function global:_OLD_VIRTUAL_PROMPT { "" }
- Copy-Item -Path function:prompt -Destination function:_OLD_VIRTUAL_PROMPT
- New-Variable -Name _PYTHON_VENV_PROMPT_PREFIX -Description "Python virtual environment prompt prefix" -Scope Global -Option ReadOnly -Visibility Public -Value $Prompt
-
- function global:prompt {
- Write-Host -NoNewline -ForegroundColor Green "($_PYTHON_VENV_PROMPT_PREFIX) "
- _OLD_VIRTUAL_PROMPT
- }
- $env:VIRTUAL_ENV_PROMPT = $Prompt
-}
-
-# Clear PYTHONHOME
-if (Test-Path -Path Env:PYTHONHOME) {
- Copy-Item -Path Env:PYTHONHOME -Destination Env:_OLD_VIRTUAL_PYTHONHOME
- Remove-Item -Path Env:PYTHONHOME
-}
-
-# Add the venv to the PATH
-Copy-Item -Path Env:PATH -Destination Env:_OLD_VIRTUAL_PATH
-$Env:PATH = "$VenvExecDir$([System.IO.Path]::PathSeparator)$Env:PATH"
diff --git a/spaces/alexray/btc_predictor/venv/lib/python3.10/site-packages/pip/_vendor/chardet/utf8prober.py b/spaces/alexray/btc_predictor/venv/lib/python3.10/site-packages/pip/_vendor/chardet/utf8prober.py
deleted file mode 100644
index 6c3196cc2d7e46e6756580267f5643c6f7b448dd..0000000000000000000000000000000000000000
--- a/spaces/alexray/btc_predictor/venv/lib/python3.10/site-packages/pip/_vendor/chardet/utf8prober.py
+++ /dev/null
@@ -1,82 +0,0 @@
-######################## BEGIN LICENSE BLOCK ########################
-# The Original Code is mozilla.org code.
-#
-# The Initial Developer of the Original Code is
-# Netscape Communications Corporation.
-# Portions created by the Initial Developer are Copyright (C) 1998
-# the Initial Developer. All Rights Reserved.
-#
-# Contributor(s):
-# Mark Pilgrim - port to Python
-#
-# This library is free software; you can redistribute it and/or
-# modify it under the terms of the GNU Lesser General Public
-# License as published by the Free Software Foundation; either
-# version 2.1 of the License, or (at your option) any later version.
-#
-# This library is distributed in the hope that it will be useful,
-# but WITHOUT ANY WARRANTY; without even the implied warranty of
-# MERCHANTABILITY or FITNESS FOR A PARTICULAR PURPOSE. See the GNU
-# Lesser General Public License for more details.
-#
-# You should have received a copy of the GNU Lesser General Public
-# License along with this library; if not, write to the Free Software
-# Foundation, Inc., 51 Franklin St, Fifth Floor, Boston, MA
-# 02110-1301 USA
-######################### END LICENSE BLOCK #########################
-
-from .charsetprober import CharSetProber
-from .enums import ProbingState, MachineState
-from .codingstatemachine import CodingStateMachine
-from .mbcssm import UTF8_SM_MODEL
-
-
-
-class UTF8Prober(CharSetProber):
- ONE_CHAR_PROB = 0.5
-
- def __init__(self):
- super(UTF8Prober, self).__init__()
- self.coding_sm = CodingStateMachine(UTF8_SM_MODEL)
- self._num_mb_chars = None
- self.reset()
-
- def reset(self):
- super(UTF8Prober, self).reset()
- self.coding_sm.reset()
- self._num_mb_chars = 0
-
- @property
- def charset_name(self):
- return "utf-8"
-
- @property
- def language(self):
- return ""
-
- def feed(self, byte_str):
- for c in byte_str:
- coding_state = self.coding_sm.next_state(c)
- if coding_state == MachineState.ERROR:
- self._state = ProbingState.NOT_ME
- break
- elif coding_state == MachineState.ITS_ME:
- self._state = ProbingState.FOUND_IT
- break
- elif coding_state == MachineState.START:
- if self.coding_sm.get_current_charlen() >= 2:
- self._num_mb_chars += 1
-
- if self.state == ProbingState.DETECTING:
- if self.get_confidence() > self.SHORTCUT_THRESHOLD:
- self._state = ProbingState.FOUND_IT
-
- return self.state
-
- def get_confidence(self):
- unlike = 0.99
- if self._num_mb_chars < 6:
- unlike *= self.ONE_CHAR_PROB ** self._num_mb_chars
- return 1.0 - unlike
- else:
- return unlike
diff --git a/spaces/alexray/btc_predictor/venv/lib/python3.10/site-packages/pip/_vendor/pygments/scanner.py b/spaces/alexray/btc_predictor/venv/lib/python3.10/site-packages/pip/_vendor/pygments/scanner.py
deleted file mode 100644
index 5f32a22c3c0ede8131fb1ab6b32e43446da6a634..0000000000000000000000000000000000000000
--- a/spaces/alexray/btc_predictor/venv/lib/python3.10/site-packages/pip/_vendor/pygments/scanner.py
+++ /dev/null
@@ -1,104 +0,0 @@
-"""
- pygments.scanner
- ~~~~~~~~~~~~~~~~
-
- This library implements a regex based scanner. Some languages
- like Pascal are easy to parse but have some keywords that
- depend on the context. Because of this it's impossible to lex
- that just by using a regular expression lexer like the
- `RegexLexer`.
-
- Have a look at the `DelphiLexer` to get an idea of how to use
- this scanner.
-
- :copyright: Copyright 2006-2021 by the Pygments team, see AUTHORS.
- :license: BSD, see LICENSE for details.
-"""
-import re
-
-
-class EndOfText(RuntimeError):
- """
- Raise if end of text is reached and the user
- tried to call a match function.
- """
-
-
-class Scanner:
- """
- Simple scanner
-
- All method patterns are regular expression strings (not
- compiled expressions!)
- """
-
- def __init__(self, text, flags=0):
- """
- :param text: The text which should be scanned
- :param flags: default regular expression flags
- """
- self.data = text
- self.data_length = len(text)
- self.start_pos = 0
- self.pos = 0
- self.flags = flags
- self.last = None
- self.match = None
- self._re_cache = {}
-
- def eos(self):
- """`True` if the scanner reached the end of text."""
- return self.pos >= self.data_length
- eos = property(eos, eos.__doc__)
-
- def check(self, pattern):
- """
- Apply `pattern` on the current position and return
- the match object. (Doesn't touch pos). Use this for
- lookahead.
- """
- if self.eos:
- raise EndOfText()
- if pattern not in self._re_cache:
- self._re_cache[pattern] = re.compile(pattern, self.flags)
- return self._re_cache[pattern].match(self.data, self.pos)
-
- def test(self, pattern):
- """Apply a pattern on the current position and check
- if it patches. Doesn't touch pos.
- """
- return self.check(pattern) is not None
-
- def scan(self, pattern):
- """
- Scan the text for the given pattern and update pos/match
- and related fields. The return value is a boolen that
- indicates if the pattern matched. The matched value is
- stored on the instance as ``match``, the last value is
- stored as ``last``. ``start_pos`` is the position of the
- pointer before the pattern was matched, ``pos`` is the
- end position.
- """
- if self.eos:
- raise EndOfText()
- if pattern not in self._re_cache:
- self._re_cache[pattern] = re.compile(pattern, self.flags)
- self.last = self.match
- m = self._re_cache[pattern].match(self.data, self.pos)
- if m is None:
- return False
- self.start_pos = m.start()
- self.pos = m.end()
- self.match = m.group()
- return True
-
- def get_char(self):
- """Scan exactly one char."""
- self.scan('.')
-
- def __repr__(self):
- return '<%s %d/%d>' % (
- self.__class__.__name__,
- self.pos,
- self.data_length
- )
diff --git a/spaces/ali-ghamdan/deoldify/fastai/basic_data.py b/spaces/ali-ghamdan/deoldify/fastai/basic_data.py
deleted file mode 100644
index dedf582a70393d5a23dff28ebc12bdf32c85b495..0000000000000000000000000000000000000000
--- a/spaces/ali-ghamdan/deoldify/fastai/basic_data.py
+++ /dev/null
@@ -1,279 +0,0 @@
-"`fastai.data` loads and manages datasets with `DataBunch`"
-from .torch_core import *
-from torch.utils.data.dataloader import default_collate
-
-DatasetType = Enum('DatasetType', 'Train Valid Test Single Fix')
-__all__ = ['DataBunch', 'DeviceDataLoader', 'DatasetType', 'load_data']
-
-old_dl_init = torch.utils.data.DataLoader.__init__
-
-def intercept_args(self, dataset, batch_size=1, shuffle=False, sampler=None, batch_sampler=None,
- num_workers=0, collate_fn=default_collate, pin_memory=True, drop_last=False,
- timeout=0, worker_init_fn=None):
- self.init_kwargs = {'batch_size':batch_size, 'shuffle':shuffle, 'sampler':sampler, 'batch_sampler':batch_sampler,
- 'num_workers':num_workers, 'collate_fn':collate_fn, 'pin_memory':pin_memory,
- 'drop_last': drop_last, 'timeout':timeout, 'worker_init_fn':worker_init_fn}
- old_dl_init(self, dataset, **self.init_kwargs)
-
-torch.utils.data.DataLoader.__init__ = intercept_args
-
-def DataLoader___getattr__(dl, k:str)->Any: return getattr(dl.dataset, k)
-DataLoader.__getattr__ = DataLoader___getattr__
-
-def DataLoader___setstate__(dl, data:Any): dl.__dict__.update(data)
-DataLoader.__setstate__ = DataLoader___setstate__
-
-@dataclass
-class DeviceDataLoader():
- "Bind a `DataLoader` to a `torch.device`."
- dl: DataLoader
- device: torch.device
- tfms: List[Callable]=None
- collate_fn: Callable=data_collate
- def __post_init__(self):
- self.dl.collate_fn=self.collate_fn
- self.tfms = listify(self.tfms)
-
- def __len__(self)->int: return len(self.dl)
- def __getattr__(self,k:str)->Any: return getattr(self.dl, k)
- def __setstate__(self,data:Any): self.__dict__.update(data)
-
- @property
- def batch_size(self): return self.dl.batch_size
- @batch_size.setter
- def batch_size(self,v):
- new_kwargs = {**self.dl.init_kwargs, 'batch_size':v, 'collate_fn':self.collate_fn}
- self.dl = self.dl.__class__(self.dl.dataset, **new_kwargs)
- if hasattr(self.dl.dataset, 'bs'): self.dl.dataset.bs = v
-
- @property
- def num_workers(self): return self.dl.num_workers
- @num_workers.setter
- def num_workers(self,v): self.dl.num_workers = v
-
- def add_tfm(self,tfm:Callable)->None:
- "Add `tfm` to `self.tfms`."
- self.tfms.append(tfm)
- def remove_tfm(self,tfm:Callable)->None:
- "Remove `tfm` from `self.tfms`."
- if tfm in self.tfms: self.tfms.remove(tfm)
-
- def new(self, **kwargs):
- "Create a new copy of `self` with `kwargs` replacing current values."
- new_kwargs = {**self.dl.init_kwargs, **kwargs}
- return DeviceDataLoader(self.dl.__class__(self.dl.dataset, **new_kwargs), self.device, self.tfms,
- self.collate_fn)
-
- def proc_batch(self,b:Tensor)->Tensor:
- "Process batch `b` of `TensorImage`."
- b = to_device(b, self.device)
- for f in listify(self.tfms): b = f(b)
- return b
-
- def __iter__(self):
- "Process and returns items from `DataLoader`."
- for b in self.dl: yield self.proc_batch(b)
-
- @classmethod
- def create(cls, dataset:Dataset, bs:int=64, shuffle:bool=False, device:torch.device=defaults.device,
- tfms:Collection[Callable]=tfms, num_workers:int=defaults.cpus, collate_fn:Callable=data_collate, **kwargs:Any):
- "Create DeviceDataLoader from `dataset` with `bs` and `shuffle`: process using `num_workers`."
- return cls(DataLoader(dataset, batch_size=bs, shuffle=shuffle, num_workers=num_workers, **kwargs),
- device=device, tfms=tfms, collate_fn=collate_fn)
-
-class DataBunch():
- "Bind `train_dl`,`valid_dl` and `test_dl` in a data object."
-
- def __init__(self, train_dl:DataLoader, valid_dl:DataLoader, fix_dl:DataLoader=None, test_dl:Optional[DataLoader]=None,
- device:torch.device=None, dl_tfms:Optional[Collection[Callable]]=None, path:PathOrStr='.',
- collate_fn:Callable=data_collate, no_check:bool=False):
- self.dl_tfms = listify(dl_tfms)
- self.device = defaults.device if device is None else device
- assert not isinstance(train_dl,DeviceDataLoader)
- def _create_dl(dl, **kwargs):
- if dl is None: return None
- return DeviceDataLoader(dl, self.device, self.dl_tfms, collate_fn, **kwargs)
- self.train_dl,self.valid_dl,self.fix_dl,self.test_dl = map(_create_dl, [train_dl,valid_dl,fix_dl,test_dl])
- if fix_dl is None: self.fix_dl = self.train_dl.new(shuffle=False, drop_last=False)
- self.single_dl = _create_dl(DataLoader(valid_dl.dataset, batch_size=1, num_workers=0))
- self.path = Path(path)
- if not no_check: self.sanity_check()
-
- def __repr__(self)->str:
- return f'{self.__class__.__name__};\n\nTrain: {self.train_ds};\n\nValid: {self.valid_ds};\n\nTest: {self.test_ds}'
-
- @staticmethod
- def _init_ds(train_ds:Dataset, valid_ds:Dataset, test_ds:Optional[Dataset]=None):
- # train_ds, but without training tfms
- fix_ds = valid_ds.new(train_ds.x, train_ds.y) if hasattr(valid_ds,'new') else train_ds
- return [o for o in (train_ds,valid_ds,fix_ds,test_ds) if o is not None]
-
- @classmethod
- def create(cls, train_ds:Dataset, valid_ds:Dataset, test_ds:Optional[Dataset]=None, path:PathOrStr='.', bs:int=64,
- val_bs:int=None, num_workers:int=defaults.cpus, dl_tfms:Optional[Collection[Callable]]=None,
- device:torch.device=None, collate_fn:Callable=data_collate, no_check:bool=False, **dl_kwargs)->'DataBunch':
- "Create a `DataBunch` from `train_ds`, `valid_ds` and maybe `test_ds` with a batch size of `bs`. Passes `**dl_kwargs` to `DataLoader()`"
- datasets = cls._init_ds(train_ds, valid_ds, test_ds)
- val_bs = ifnone(val_bs, bs)
- dls = [DataLoader(d, b, shuffle=s, drop_last=s, num_workers=num_workers, **dl_kwargs) for d,b,s in
- zip(datasets, (bs,val_bs,val_bs,val_bs), (True,False,False,False)) if d is not None]
- return cls(*dls, path=path, device=device, dl_tfms=dl_tfms, collate_fn=collate_fn, no_check=no_check)
-
- def __getattr__(self,k:int)->Any: return getattr(self.train_dl, k)
- def __setstate__(self,data:Any): self.__dict__.update(data)
-
- def dl(self, ds_type:DatasetType=DatasetType.Valid)->DeviceDataLoader:
- "Returns appropriate `Dataset` for validation, training, or test (`ds_type`)."
- #TODO: refactor
- return (self.train_dl if ds_type == DatasetType.Train else
- self.test_dl if ds_type == DatasetType.Test else
- self.valid_dl if ds_type == DatasetType.Valid else
- self.single_dl if ds_type == DatasetType.Single else
- self.fix_dl)
-
- @property
- def dls(self)->List[DeviceDataLoader]:
- "Returns a list of all DeviceDataLoaders. If you need a specific DeviceDataLoader, access via the relevant property (`train_dl`, `valid_dl`, etc) as the index of DLs in this list is not guaranteed to remain constant."
- res = [self.train_dl, self.fix_dl, self.single_dl]
- # Preserve the original ordering of Train, Valid, Fix, Single, Test Data Loaders
- # (Unknown/not verified as of 1.0.47 whether there are other methods explicitly using DLs their list index)
- if self.valid_dl: res.insert(1, self.valid_dl)
- return res if not self.test_dl else res + [self.test_dl]
-
- def add_tfm(self,tfm:Callable)->None:
- for dl in self.dls: dl.add_tfm(tfm)
-
- def remove_tfm(self,tfm:Callable)->None:
- for dl in self.dls: dl.remove_tfm(tfm)
-
- def save(self, file:PathLikeOrBinaryStream= 'data_save.pkl')->None:
- "Save the `DataBunch` in `self.path/file`. `file` can be file-like (file or buffer)"
- if not getattr(self, 'label_list', False):
- warn("Serializing the `DataBunch` only works when you created it using the data block API.")
- return
- try_save(self.label_list, self.path, file)
-
- def add_test(self, items:Iterator, label:Any=None, tfms=None, tfm_y=None)->None:
- "Add the `items` as a test set. Pass along `label` otherwise label them with `EmptyLabel`."
- self.label_list.add_test(items, label=label, tfms=tfms, tfm_y=tfm_y)
- vdl = self.valid_dl
- dl = DataLoader(self.label_list.test, vdl.batch_size, shuffle=False, drop_last=False, num_workers=vdl.num_workers)
- self.test_dl = DeviceDataLoader(dl, vdl.device, vdl.tfms, vdl.collate_fn)
-
- def one_batch(self, ds_type:DatasetType=DatasetType.Train, detach:bool=True, denorm:bool=True, cpu:bool=True)->Collection[Tensor]:
- "Get one batch from the data loader of `ds_type`. Optionally `detach` and `denorm`."
- dl = self.dl(ds_type)
- w = self.num_workers
- self.num_workers = 0
- try: x,y = next(iter(dl))
- finally: self.num_workers = w
- if detach: x,y = to_detach(x,cpu=cpu),to_detach(y,cpu=cpu)
- norm = getattr(self,'norm',False)
- if denorm and norm:
- x = self.denorm(x)
- if norm.keywords.get('do_y',False): y = self.denorm(y, do_x=True)
- return x,y
-
- def one_item(self, item, detach:bool=False, denorm:bool=False, cpu:bool=False):
- "Get `item` into a batch. Optionally `detach` and `denorm`."
- ds = self.single_ds
- with ds.set_item(item):
- return self.one_batch(ds_type=DatasetType.Single, detach=detach, denorm=denorm, cpu=cpu)
-
- def show_batch(self, rows:int=5, ds_type:DatasetType=DatasetType.Train, reverse:bool=False, **kwargs)->None:
- "Show a batch of data in `ds_type` on a few `rows`."
- x,y = self.one_batch(ds_type, True, True)
- if reverse: x,y = x.flip(0),y.flip(0)
- n_items = rows **2 if self.train_ds.x._square_show else rows
- if self.dl(ds_type).batch_size < n_items: n_items = self.dl(ds_type).batch_size
- xs = [self.train_ds.x.reconstruct(grab_idx(x, i)) for i in range(n_items)]
- #TODO: get rid of has_arg if possible
- if has_arg(self.train_ds.y.reconstruct, 'x'):
- ys = [self.train_ds.y.reconstruct(grab_idx(y, i), x=x) for i,x in enumerate(xs)]
- else : ys = [self.train_ds.y.reconstruct(grab_idx(y, i)) for i in range(n_items)]
- self.train_ds.x.show_xys(xs, ys, **kwargs)
-
- def export(self, file:PathLikeOrBinaryStream='export.pkl'):
- "Export the minimal state of `self` for inference in `self.path/file`. `file` can be file-like (file or buffer)"
- xtra = dict(normalize=self.norm.keywords) if getattr(self, 'norm', False) else {}
- try_save(self.valid_ds.get_state(**xtra), self.path, file)
-
- def _grab_dataset(self, dl:DataLoader):
- ds = dl.dl.dataset
- while hasattr(ds, 'dataset'): ds = ds.dataset
- return ds
-
- @property
- def train_ds(self)->Dataset: return self._grab_dataset(self.train_dl)
- @property
- def valid_ds(self)->Dataset: return self._grab_dataset(self.valid_dl)
- @property
- def single_ds(self)->Dataset: return self._grab_dataset(self.single_dl)
- @property
- def loss_func(self)->OptLossFunc:
- return getattr(self.train_ds.y, 'loss_func', F.nll_loss) if hasattr(self.train_ds, 'y') else F.nll_loss
-
- @property
- def test_ds(self)->Dataset:
- return self._grab_dataset(self.test_dl) if self.test_dl is not None else None
-
- @property
- def empty_val(self)->bool:
- if not hasattr(self, 'valid_dl') or self.valid_dl is None: return True
- if hasattr(self.valid_ds, 'items') and len(self.valid_ds.items) == 0: return True
- return (len(self.valid_ds) == 0)
-
- @property
- def is_empty(self)->bool:
- return not ((self.train_dl and len(self.train_ds.items) != 0) or
- (self.valid_dl and len(self.valid_ds.items) != 0) or
- (self.test_dl and len(self.test_ds.items) != 0))
-
- @property
- def batch_size(self): return self.train_dl.batch_size
- @batch_size.setter
- def batch_size(self,v):
- self.train_dl.batch_size,self.valid_dl.batch_size = v,v
- if self.test_dl is not None: self.test_dl.batch_size = v
-
- def sanity_check(self):
- "Check the underlying data in the training set can be properly loaded."
- final_message = "You can deactivate this warning by passing `no_check=True`."
- if not hasattr(self.train_ds, 'items') or len(self.train_ds.items) == 0 or not hasattr(self.train_dl, 'batch_sampler'): return
- if len(self.train_dl) == 0:
- warn(f"""Your training dataloader is empty, you have only {len(self.train_dl.dataset)} items in your training set.
- Your batch size is {self.train_dl.batch_size}, you should lower it.""")
- print(final_message)
- return
- idx = next(iter(self.train_dl.batch_sampler))
- samples,fails = [],[]
- for i in idx:
- try: samples.append(self.train_dl.dataset[i])
- except: fails.append(i)
- if len(fails) > 0:
- warn_msg = "There seems to be something wrong with your dataset, for example, in the first batch can't access"
- if len(fails) == len(idx):
- warn_msg += f" any element of self.train_ds.\nTried: {show_some(idx)}"
- else:
- warn_msg += f" these elements in self.train_ds: {show_some(fails)}"
- warn(warn_msg)
- print(final_message)
- return
- try: batch = self.collate_fn(samples)
- except:
- message = "It's not possible to collate samples of your dataset together in a batch."
- try:
- shapes = [[o[i].data.shape for o in samples] for i in range(2)]
- message += f'\nShapes of the inputs/targets:\n{shapes}'
- except: pass
- warn(message)
- print(final_message)
-
-def load_data(path:PathOrStr, file:PathLikeOrBinaryStream='data_save.pkl', bs:int=64, val_bs:int=None, num_workers:int=defaults.cpus,
- dl_tfms:Optional[Collection[Callable]]=None, device:torch.device=None, collate_fn:Callable=data_collate,
- no_check:bool=False, **kwargs)->DataBunch:
- "Load a saved `DataBunch` from `path/file`. `file` can be file-like (file or buffer)"
- source = Path(path)/file if is_pathlike(file) else file
- ll = torch.load(source, map_location='cpu') if defaults.device == torch.device('cpu') else torch.load(source)
- return ll.databunch(path=path, bs=bs, val_bs=val_bs, num_workers=num_workers, dl_tfms=dl_tfms, device=device,
- collate_fn=collate_fn, no_check=no_check, **kwargs)
diff --git a/spaces/amarchheda/ChordDuplicate/portaudio/src/hostapi/wasapi/mingw-include/ksguid.h b/spaces/amarchheda/ChordDuplicate/portaudio/src/hostapi/wasapi/mingw-include/ksguid.h
deleted file mode 100644
index f0774d06cef4bd7cb26e3f472be36e5b98149c8d..0000000000000000000000000000000000000000
--- a/spaces/amarchheda/ChordDuplicate/portaudio/src/hostapi/wasapi/mingw-include/ksguid.h
+++ /dev/null
@@ -1,28 +0,0 @@
-/**
- * This file has no copyright assigned and is placed in the Public Domain.
- * This file is part of the w64 mingw-runtime package.
- * No warranty is given; refer to the file DISCLAIMER.PD within this package.
- */
-#define INITGUID
-#include
-
-#ifndef DECLSPEC_SELECTANY
-#define DECLSPEC_SELECTANY __declspec(selectany)
-#endif
-
-#ifdef DEFINE_GUIDEX
-#undef DEFINE_GUIDEX
-#endif
-
-#ifdef __cplusplus
-#define DEFINE_GUIDEX(name) EXTERN_C const CDECL GUID DECLSPEC_SELECTANY name = { STATICGUIDOF(name) }
-#else
-#define DEFINE_GUIDEX(name) const CDECL GUID DECLSPEC_SELECTANY name = { STATICGUIDOF(name) }
-#endif
-#ifndef STATICGUIDOF
-#define STATICGUIDOF(guid) STATIC_##guid
-#endif
-
-#ifndef DEFINE_WAVEFORMATEX_GUID
-#define DEFINE_WAVEFORMATEX_GUID(x) (USHORT)(x),0x0000,0x0010,0x80,0x00,0x00,0xaa,0x00,0x38,0x9b,0x71
-#endif
diff --git a/spaces/amarchheda/ChordDuplicate/portaudio/test/patest_dsound_find_best_latency_params.c b/spaces/amarchheda/ChordDuplicate/portaudio/test/patest_dsound_find_best_latency_params.c
deleted file mode 100644
index 1c074ab16cf458f136fe79d4d6e232a04236243f..0000000000000000000000000000000000000000
--- a/spaces/amarchheda/ChordDuplicate/portaudio/test/patest_dsound_find_best_latency_params.c
+++ /dev/null
@@ -1,513 +0,0 @@
-/*
- * $Id: $
- * Portable Audio I/O Library
- * Windows DirectSound low level buffer user guided parameters search
- *
- * Copyright (c) 2010-2011 Ross Bencina
- *
- * Permission is hereby granted, free of charge, to any person obtaining
- * a copy of this software and associated documentation files
- * (the "Software"), to deal in the Software without restriction,
- * including without limitation the rights to use, copy, modify, merge,
- * publish, distribute, sublicense, and/or sell copies of the Software,
- * and to permit persons to whom the Software is furnished to do so,
- * subject to the following conditions:
- *
- * The above copyright notice and this permission notice shall be
- * included in all copies or substantial portions of the Software.
- *
- * THE SOFTWARE IS PROVIDED "AS IS", WITHOUT WARRANTY OF ANY KIND,
- * EXPRESS OR IMPLIED, INCLUDING BUT NOT LIMITED TO THE WARRANTIES OF
- * MERCHANTABILITY, FITNESS FOR A PARTICULAR PURPOSE AND NONINFRINGEMENT.
- * IN NO EVENT SHALL THE AUTHORS OR COPYRIGHT HOLDERS BE LIABLE FOR
- * ANY CLAIM, DAMAGES OR OTHER LIABILITY, WHETHER IN AN ACTION OF
- * CONTRACT, TORT OR OTHERWISE, ARISING FROM, OUT OF OR IN CONNECTION
- * WITH THE SOFTWARE OR THE USE OR OTHER DEALINGS IN THE SOFTWARE.
- */
-
-/*
- * The text above constitutes the entire PortAudio license; however,
- * the PortAudio community also makes the following non-binding requests:
- *
- * Any person wishing to distribute modifications to the Software is
- * requested to send the modifications to the original developer so that
- * they can be incorporated into the canonical version. It is also
- * requested that these non-binding requests be included along with the
- * license above.
- */
-
-#include
-#include
-#include
-
-#define _WIN32_WINNT 0x0501 /* for GetNativeSystemInfo */
-#include
-//#include /* required when using pa_win_wmme.h */
-
-#include /* for _getch */
-
-
-#include "portaudio.h"
-#include "pa_win_ds.h"
-
-
-#define DEFAULT_SAMPLE_RATE (44100.)
-
-#ifndef M_PI
-#define M_PI (3.14159265)
-#endif
-
-#define TABLE_SIZE (2048)
-
-#define CHANNEL_COUNT (2)
-
-
-/*******************************************************************/
-/* functions to query and print Windows version information */
-
-typedef BOOL (WINAPI *LPFN_ISWOW64PROCESS) (HANDLE, PBOOL);
-
-LPFN_ISWOW64PROCESS fnIsWow64Process;
-
-static BOOL IsWow64()
-{
- BOOL bIsWow64 = FALSE;
-
- //IsWow64Process is not available on all supported versions of Windows.
- //Use GetModuleHandle to get a handle to the DLL that contains the function
- //and GetProcAddress to get a pointer to the function if available.
-
- fnIsWow64Process = (LPFN_ISWOW64PROCESS) GetProcAddress(
- GetModuleHandle(TEXT("kernel32")),"IsWow64Process" );
-
- if(NULL != fnIsWow64Process)
- {
- if (!fnIsWow64Process(GetCurrentProcess(),&bIsWow64))
- {
- //handle error
- }
- }
- return bIsWow64;
-}
-
-static void printWindowsVersionInfo( FILE *fp )
-{
- OSVERSIONINFOEX osVersionInfoEx;
- SYSTEM_INFO systemInfo;
- const char *osName = "Unknown";
- const char *osProductType = "";
- const char *processorArchitecture = "Unknown";
-
- memset( &osVersionInfoEx, 0, sizeof(OSVERSIONINFOEX) );
- osVersionInfoEx.dwOSVersionInfoSize = sizeof(OSVERSIONINFOEX);
- GetVersionEx( &osVersionInfoEx );
-
-
- if( osVersionInfoEx.dwPlatformId == VER_PLATFORM_WIN32_WINDOWS ){
- switch( osVersionInfoEx.dwMinorVersion ){
- case 0: osName = "Windows 95"; break;
- case 10: osName = "Windows 98"; break; // could also be 98SE (I've seen code discriminate based
- // on osInfo.Version.Revision.ToString() == "2222A")
- case 90: osName = "Windows Me"; break;
- }
- }else if( osVersionInfoEx.dwPlatformId == VER_PLATFORM_WIN32_NT ){
- switch( osVersionInfoEx.dwMajorVersion ){
- case 3: osName = "Windows NT 3.51"; break;
- case 4: osName = "Windows NT 4.0"; break;
- case 5: switch( osVersionInfoEx.dwMinorVersion ){
- case 0: osName = "Windows 2000"; break;
- case 1: osName = "Windows XP"; break;
- case 2:
- if( osVersionInfoEx.wSuiteMask & 0x00008000 /*VER_SUITE_WH_SERVER*/ ){
- osName = "Windows Home Server";
- }else{
- if( osVersionInfoEx.wProductType == VER_NT_WORKSTATION ){
- osName = "Windows XP Professional x64 Edition (?)";
- }else{
- if( GetSystemMetrics(/*SM_SERVERR2*/89) == 0 )
- osName = "Windows Server 2003";
- else
- osName = "Windows Server 2003 R2";
- }
- }break;
- }break;
- case 6:switch( osVersionInfoEx.dwMinorVersion ){
- case 0:
- if( osVersionInfoEx.wProductType == VER_NT_WORKSTATION )
- osName = "Windows Vista";
- else
- osName = "Windows Server 2008";
- break;
- case 1:
- if( osVersionInfoEx.wProductType == VER_NT_WORKSTATION )
- osName = "Windows 7";
- else
- osName = "Windows Server 2008 R2";
- break;
- }break;
- }
- }
-
- if(osVersionInfoEx.dwMajorVersion == 4)
- {
- if(osVersionInfoEx.wProductType == VER_NT_WORKSTATION)
- osProductType = "Workstation";
- else if(osVersionInfoEx.wProductType == VER_NT_SERVER)
- osProductType = "Server";
- }
- else if(osVersionInfoEx.dwMajorVersion == 5)
- {
- if(osVersionInfoEx.wProductType == VER_NT_WORKSTATION)
- {
- if((osVersionInfoEx.wSuiteMask & VER_SUITE_PERSONAL) == VER_SUITE_PERSONAL)
- osProductType = "Home Edition"; // Windows XP Home Edition
- else
- osProductType = "Professional"; // Windows XP / Windows 2000 Professional
- }
- else if(osVersionInfoEx.wProductType == VER_NT_SERVER)
- {
- if(osVersionInfoEx.dwMinorVersion == 0)
- {
- if((osVersionInfoEx.wSuiteMask & VER_SUITE_DATACENTER) == VER_SUITE_DATACENTER)
- osProductType = "Datacenter Server"; // Windows 2000 Datacenter Server
- else if((osVersionInfoEx.wSuiteMask & VER_SUITE_ENTERPRISE) == VER_SUITE_ENTERPRISE)
- osProductType = "Advanced Server"; // Windows 2000 Advanced Server
- else
- osProductType = "Server"; // Windows 2000 Server
- }
- }
- else
- {
- if((osVersionInfoEx.wSuiteMask & VER_SUITE_DATACENTER) == VER_SUITE_DATACENTER)
- osProductType = "Datacenter Edition"; // Windows Server 2003 Datacenter Edition
- else if((osVersionInfoEx.wSuiteMask & VER_SUITE_ENTERPRISE) == VER_SUITE_ENTERPRISE)
- osProductType = "Enterprise Edition"; // Windows Server 2003 Enterprise Edition
- else if((osVersionInfoEx.wSuiteMask & VER_SUITE_BLADE) == VER_SUITE_BLADE)
- osProductType = "Web Edition"; // Windows Server 2003 Web Edition
- else
- osProductType = "Standard Edition"; // Windows Server 2003 Standard Edition
- }
- }
-
- memset( &systemInfo, 0, sizeof(SYSTEM_INFO) );
- GetNativeSystemInfo( &systemInfo );
-
- if( systemInfo.wProcessorArchitecture == PROCESSOR_ARCHITECTURE_INTEL )
- processorArchitecture = "x86";
- else if( systemInfo.wProcessorArchitecture == PROCESSOR_ARCHITECTURE_AMD64 )
- processorArchitecture = "x64";
- else if( systemInfo.wProcessorArchitecture == PROCESSOR_ARCHITECTURE_IA64 )
- processorArchitecture = "Itanium";
-
-
- fprintf( fp, "OS name and edition: %s %s\n", osName, osProductType );
- fprintf( fp, "OS version: %d.%d.%d %S\n",
- osVersionInfoEx.dwMajorVersion, osVersionInfoEx.dwMinorVersion,
- osVersionInfoEx.dwBuildNumber, osVersionInfoEx.szCSDVersion );
- fprintf( fp, "Processor architecture: %s\n", processorArchitecture );
- fprintf( fp, "WoW64 process: %s\n", IsWow64() ? "Yes" : "No" );
-}
-
-static void printTimeAndDate( FILE *fp )
-{
- struct tm *local;
- time_t t;
-
- t = time(NULL);
- local = localtime(&t);
- fprintf(fp, "Local time and date: %s", asctime(local));
- local = gmtime(&t);
- fprintf(fp, "UTC time and date: %s", asctime(local));
-}
-
-/*******************************************************************/
-
-typedef struct
-{
- float sine[TABLE_SIZE];
- double phase;
- double phaseIncrement;
- volatile int fadeIn;
- volatile int fadeOut;
- double amp;
-}
-paTestData;
-
-static paTestData data;
-
-/* This routine will be called by the PortAudio engine when audio is needed.
-** It may called at interrupt level on some machines so don't do anything
-** that could mess up the system like calling malloc() or free().
-*/
-static int patestCallback( const void *inputBuffer, void *outputBuffer,
- unsigned long framesPerBuffer,
- const PaStreamCallbackTimeInfo* timeInfo,
- PaStreamCallbackFlags statusFlags,
- void *userData )
-{
- paTestData *data = (paTestData*)userData;
- float *out = (float*)outputBuffer;
- unsigned long i,j;
-
- (void) timeInfo; /* Prevent unused variable warnings. */
- (void) statusFlags;
- (void) inputBuffer;
-
- for( i=0; isine[(int)data->phase];
- data->phase += data->phaseIncrement;
- if( data->phase >= TABLE_SIZE ){
- data->phase -= TABLE_SIZE;
- }
-
- x *= data->amp;
- if( data->fadeIn ){
- data->amp += .001;
- if( data->amp >= 1. )
- data->fadeIn = 0;
- }else if( data->fadeOut ){
- if( data->amp > 0 )
- data->amp -= .001;
- }
-
- for( j = 0; j < CHANNEL_COUNT; ++j ){
- *out++ = x;
- }
- }
-
- if( data->amp > 0 )
- return paContinue;
- else
- return paComplete;
-}
-
-
-#define YES 1
-#define NO 0
-
-
-static int playUntilKeyPress( int deviceIndex, float sampleRate,
- int framesPerUserBuffer, int framesPerDSoundBuffer )
-{
- PaStreamParameters outputParameters;
- PaWinDirectSoundStreamInfo directSoundStreamInfo;
- PaStream *stream;
- PaError err;
- int c;
-
- outputParameters.device = deviceIndex;
- outputParameters.channelCount = CHANNEL_COUNT;
- outputParameters.sampleFormat = paFloat32; /* 32 bit floating point processing */
- outputParameters.suggestedLatency = 0; /*Pa_GetDeviceInfo( outputParameters.device )->defaultLowOutputLatency;*/
- outputParameters.hostApiSpecificStreamInfo = NULL;
-
- directSoundStreamInfo.size = sizeof(PaWinDirectSoundStreamInfo);
- directSoundStreamInfo.hostApiType = paDirectSound;
- directSoundStreamInfo.version = 2;
- directSoundStreamInfo.flags = paWinDirectSoundUseLowLevelLatencyParameters;
- directSoundStreamInfo.framesPerBuffer = framesPerDSoundBuffer;
- outputParameters.hostApiSpecificStreamInfo = &directSoundStreamInfo;
-
- err = Pa_OpenStream(
- &stream,
- NULL, /* no input */
- &outputParameters,
- sampleRate,
- framesPerUserBuffer,
- paClipOff | paPrimeOutputBuffersUsingStreamCallback, /* we won't output out of range samples so don't bother clipping them */
- patestCallback,
- &data );
- if( err != paNoError ) goto error;
-
- data.amp = 0;
- data.fadeIn = 1;
- data.fadeOut = 0;
- data.phase = 0;
- data.phaseIncrement = 15 + ((rand()%100) / 10); // randomise pitch
-
- err = Pa_StartStream( stream );
- if( err != paNoError ) goto error;
-
-
- do{
- printf( "Trying buffer size %d.\nIf it sounds smooth (without clicks or glitches) press 'y', if it sounds bad press 'n' ('q' to quit)\n", framesPerDSoundBuffer );
- c = tolower(_getch());
- if( c == 'q' ){
- Pa_Terminate();
- exit(0);
- }
- }while( c != 'y' && c != 'n' );
-
- data.fadeOut = 1;
- while( Pa_IsStreamActive(stream) == 1 )
- Pa_Sleep( 100 );
-
- err = Pa_StopStream( stream );
- if( err != paNoError ) goto error;
-
- err = Pa_CloseStream( stream );
- if( err != paNoError ) goto error;
-
- return (c == 'y') ? YES : NO;
-
-error:
- return err;
-}
-
-/*******************************************************************/
-static void usage( int dsoundHostApiIndex )
-{
- int i;
-
- fprintf( stderr, "PortAudio DirectSound output latency user guided test\n" );
- fprintf( stderr, "Usage: x.exe dsound-device-index [sampleRate]\n" );
- fprintf( stderr, "Invalid device index. Use one of these:\n" );
- for( i=0; i < Pa_GetDeviceCount(); ++i ){
-
- if( Pa_GetDeviceInfo(i)->hostApi == dsoundHostApiIndex && Pa_GetDeviceInfo(i)->maxOutputChannels > 0 )
- fprintf( stderr, "%d (%s)\n", i, Pa_GetDeviceInfo(i)->name );
- }
- Pa_Terminate();
- exit(-1);
-}
-
-/*
- ideas:
- o- could be testing with 80% CPU load
- o- could test with different channel counts
-*/
-
-int main(int argc, char* argv[])
-{
- PaError err;
- int i;
- int deviceIndex;
- int dsoundBufferSize, smallestWorkingBufferSize;
- int smallestWorkingBufferingLatencyFrames;
- int min, max, mid;
- int testResult;
- FILE *resultsFp;
- int dsoundHostApiIndex;
- const PaHostApiInfo *dsoundHostApiInfo;
- double sampleRate = DEFAULT_SAMPLE_RATE;
-
- err = Pa_Initialize();
- if( err != paNoError ) goto error;
-
- dsoundHostApiIndex = Pa_HostApiTypeIdToHostApiIndex( paDirectSound );
- dsoundHostApiInfo = Pa_GetHostApiInfo( dsoundHostApiIndex );
-
- if( argc > 3 )
- usage(dsoundHostApiIndex);
-
- deviceIndex = dsoundHostApiInfo->defaultOutputDevice;
- if( argc >= 2 ){
- deviceIndex = -1;
- if( sscanf( argv[1], "%d", &deviceIndex ) != 1 )
- usage(dsoundHostApiInfo);
- if( deviceIndex < 0 || deviceIndex >= Pa_GetDeviceCount() || Pa_GetDeviceInfo(deviceIndex)->hostApi != dsoundHostApiIndex ){
- usage(dsoundHostApiInfo);
- }
- }
-
- printf( "Using device id %d (%s)\n", deviceIndex, Pa_GetDeviceInfo(deviceIndex)->name );
-
- if( argc >= 3 ){
- if( sscanf( argv[2], "%lf", &sampleRate ) != 1 )
- usage(dsoundHostApiIndex);
- }
-
- printf( "Testing with sample rate %f.\n", (float)sampleRate );
-
-
-
- /* initialise sinusoidal wavetable */
- for( i=0; iname );
- fflush( resultsFp );
-
- fprintf( resultsFp, "Sample rate: %f\n", (float)sampleRate );
- fprintf( resultsFp, "Smallest working buffer size (frames), Smallest working buffering latency (frames), Smallest working buffering latency (Seconds)\n" );
-
-
- /*
- Binary search after Niklaus Wirth
- from http://en.wikipedia.org/wiki/Binary_search_algorithm#The_algorithm
- */
- min = 1;
- max = (int)(sampleRate * .3); /* we assume that this size works 300ms */
- smallestWorkingBufferSize = 0;
-
- do{
- mid = min + ((max - min) / 2);
-
- dsoundBufferSize = mid;
- testResult = playUntilKeyPress( deviceIndex, sampleRate, 0, dsoundBufferSize );
-
- if( testResult == YES ){
- max = mid - 1;
- smallestWorkingBufferSize = dsoundBufferSize;
- }else{
- min = mid + 1;
- }
-
- }while( (min <= max) && (testResult == YES || testResult == NO) );
-
- smallestWorkingBufferingLatencyFrames = smallestWorkingBufferSize; /* not strictly true, but we're using an unspecified callback size, so kind of */
-
- printf( "Smallest working buffer size is: %d\n", smallestWorkingBufferSize );
- printf( "Corresponding to buffering latency of %d frames, or %f seconds.\n", smallestWorkingBufferingLatencyFrames, smallestWorkingBufferingLatencyFrames / sampleRate );
-
- fprintf( resultsFp, "%d, %d, %f\n", smallestWorkingBufferSize, smallestWorkingBufferingLatencyFrames, smallestWorkingBufferingLatencyFrames / sampleRate );
- fflush( resultsFp );
-
-
- /* power of 2 test. iterate to the smallest power of two that works */
-
- smallestWorkingBufferSize = 0;
- dsoundBufferSize = 64;
-
- do{
- testResult = playUntilKeyPress( deviceIndex, sampleRate, 0, dsoundBufferSize );
-
- if( testResult == YES ){
- smallestWorkingBufferSize = dsoundBufferSize;
- }else{
- dsoundBufferSize *= 2;
- }
-
- }while( (dsoundBufferSize <= (int)(sampleRate * .3)) && testResult == NO );
-
- smallestWorkingBufferingLatencyFrames = smallestWorkingBufferSize; /* not strictly true, but we're using an unspecified callback size, so kind of */
-
- fprintf( resultsFp, "%d, %d, %f\n", smallestWorkingBufferSize, smallestWorkingBufferingLatencyFrames, smallestWorkingBufferingLatencyFrames / sampleRate );
- fflush( resultsFp );
-
-
- fprintf( resultsFp, "###\n" );
- fclose( resultsFp );
-
- Pa_Terminate();
- printf("Test finished.\n");
-
- return err;
-error:
- Pa_Terminate();
- fprintf( stderr, "An error occurred while using the PortAudio stream\n" );
- fprintf( stderr, "Error number: %d\n", err );
- fprintf( stderr, "Error message: %s\n", Pa_GetErrorText( err ) );
- return err;
-}
diff --git a/spaces/amsterdamNLP/contrastive-pairs/results.md b/spaces/amsterdamNLP/contrastive-pairs/results.md
deleted file mode 100644
index 07559d9293a79dbbc00e18137fbdf7c74afed2fb..0000000000000000000000000000000000000000
--- a/spaces/amsterdamNLP/contrastive-pairs/results.md
+++ /dev/null
@@ -1,9 +0,0 @@
-### Results for French and English language models
-BERT is another popular type of language model, and CrowS-Pairs has originally been designed for BERT instead of GPT-2.
-Here you can find the results for two languages, English and French, from the authors of [the extended version of CrowS-Pairs](https://aclanthology.org/2022.acl-long.583.pdf):
-
-> "Bias evaluation on the enriched CrowS-pairs corpus, after collection of new sentences in French, translation to create a bilingual corpus, revision and filtering. A score of 50 indicates an absence of bias. **Higher scores indicate stronger preference for biased sentences.**"
-
-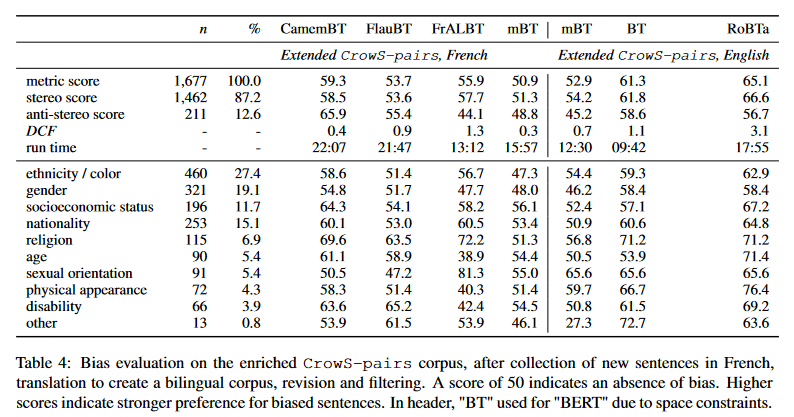
-
-
diff --git a/spaces/andreishagin/Class_modify/app.py b/spaces/andreishagin/Class_modify/app.py
deleted file mode 100644
index d31d042cee1426e06e616689e05861b81b6bae51..0000000000000000000000000000000000000000
--- a/spaces/andreishagin/Class_modify/app.py
+++ /dev/null
@@ -1,63 +0,0 @@
-import streamlit as st
-import eda
-import prediction
-
-st.markdown("
Hotel reservation cancellation prediction model + EDA
", unsafe_allow_html=True)
-st.markdown("You will find below a selection for the EDA or ML prediction model.")
-st.markdown("**EDA:** Here you can explore a brief analysis of data.")
-st.markdown("**ML Prediction model:** Here you can choose parameters and make predictions with probability to check if the client will cancel the booking or not.")
-navigation = st.selectbox('Select EDA or ML Prediction model', ('EDA', 'ML Prediction model'))
-
-if navigation == 'EDA' :
- st.markdown("The online hotel reservation channels have dramatically changed booking possibilities and customers’ behavior. \
- A significant number of hotel reservations are called off due to cancellations or no-shows. \
- The typical reasons for cancellations include changes of plans, scheduling conflicts, etc. \
- This is often made easier by the option to do so free of charge or preferably at a low cost, \
- which is beneficial to hotel guests but it is a less desirable and possibly revenue-diminishing factor for hotels to deal with.")
- agree = st.checkbox('Click here to see the data description.')
- if agree:
- st.markdown("Data Dictionary: \n\
- * no_of_adults: Number of adults \n\
- * no_of_children: Number of Children \n\
- * type_of_meal_plan: Type of meal plan booked by the customer: 'breakfast', 'Not Selected', 'half board', 'full board' \n\
- * required_car_parking_space: Does the customer require a car parking space? (0 - No, 1- Yes) \n\
- * room_type_reserved: 'economy', 'standart', 'deluxe' \n\
- * lead_time: Number of days between the date of booking and the arrival date \n\
- * arrival_month: Month of arrival date \n\
- * arrival_date: Date of the month \n\
- * market_segment_type: Market segment designation ('Offline', 'Online', 'Corporate', 'Aviation') \n\
- * repeated_guest: Is the customer a repeated guest? (0 - No, 1- Yes) \n\
- * no_of_previous_cancellations: Number of previous bookings that were canceled by the customer prior to the current booking \n\
- * no_of_previous_bookings_not_canceled: Number of previous bookings not canceled by the customer prior to the current booking \n\
- * avg_price_per_room: Average price per day of the reservation; prices of the rooms are dynamic. (in euros) \n\
- * no_of_special_requests: Total number of special requests made by the customer (e.g. high floor, view from the room, etc) \n\
- * booking_status (target variable): Flag indicating if the booking was canceled or not.")
- eda.run()
-
-else:
- st.markdown("The online hotel reservation channels have dramatically changed booking possibilities and customers’ behavior. \
- A significant number of hotel reservations are called off due to cancellations or no-shows. \
- The typical reasons for cancellations include changes of plans, scheduling conflicts, etc. \
- This is often made easier by the option to do so free of charge or preferably at a low cost, \
- which is beneficial to hotel guests but it is a less desirable and possibly revenue-diminishing factor for hotels to deal with.")
- st.markdown("**Main goal:** Make a model so that hotels can define conditions of cancellation policies based on different \
- features in order to maximize the number of bookings by customers with a lower probability of cancellation.")
- agree = st.checkbox('Click here to see the data description.')
- if agree:
- st.markdown("Data Dictionary: \n\
- * no_of_adults: Number of adults \n\
- * no_of_children: Number of Children \n\
- * type_of_meal_plan: Type of meal plan booked by the customer: 'breakfast', 'Not Selected', 'half board', 'full board' \n\
- * required_car_parking_space: Does the customer require a car parking space? (0 - No, 1- Yes) \n\
- * room_type_reserved: 'economy', 'standart', 'deluxe' \n\
- * lead_time: Number of days between the date of booking and the arrival date \n\
- * arrival_month: Month of arrival date \n\
- * arrival_date: Date of the month \n\
- * market_segment_type: Market segment designation ('Offline', 'Online', 'Corporate', 'Aviation') \n\
- * repeated_guest: Is the customer a repeated guest? (0 - No, 1- Yes) \n\
- * no_of_previous_cancellations: Number of previous bookings that were canceled by the customer prior to the current booking \n\
- * no_of_previous_bookings_not_canceled: Number of previous bookings not canceled by the customer prior to the current booking \n\
- * avg_price_per_room: Average price per day of the reservation; prices of the rooms are dynamic. (in euros) \n\
- * no_of_special_requests: Total number of special requests made by the customer (e.g. high floor, view from the room, etc) \n\
- * booking_status (target variable): Flag indicating if the booking was canceled or not.")
- prediction.run()
diff --git a/spaces/anirudhmittal/humour-detection/app.py b/spaces/anirudhmittal/humour-detection/app.py
deleted file mode 100644
index 69a2d61fd5707ad7fcc56b16ab7517df0f838e4b..0000000000000000000000000000000000000000
--- a/spaces/anirudhmittal/humour-detection/app.py
+++ /dev/null
@@ -1,96 +0,0 @@
-import gradio as gr
-import tensorflow
-import keras
-import torch
-import numpy as np
-import glob, os
-from os import path
-import pickle as pkl
-from transformers import BertTokenizer, BertModel #RobertaTokenizer, RobertaModel, XLMRobertaTokenizer, TFXLMRobertaModel
-from keras import layers
-from tensorflow.keras.utils import to_categorical
-from keras.models import Sequential
-from keras.layers import Dense, Flatten, Dropout, Input, Bidirectional, LSTM, Activation, TimeDistributed, BatchNormalization
-from sklearn.model_selection import train_test_split
-import tensorflow.keras.backend as K
-model = keras.models.load_model("Onlybert.h5")
-
-tokenizer = BertTokenizer.from_pretrained('bert-base-uncased')
-
-def text_features(input_text):
- model_bert = BertModel.from_pretrained('bert-base-uncased', output_hidden_states = True,)
- temp_text = input_text
- lst_text = temp_text.strip().split(' ')
- fin_lst_text = ["[CLS]"]
- for word in lst_text:
- fin_lst_text.append(word)
- fin_text = " ".join(fin_lst_text)
- # print(fin_text)
- tokenized_text = tokenizer.tokenize(fin_text)
- if len(tokenized_text) > 511:
- tokenized_text = tokenized_text[:511]
- tokenized_text.append('[SEP]')
- indexed_tokens = tokenizer.convert_tokens_to_ids(tokenized_text)
- segments_ids = [1] * len(tokenized_text)
- tokens_tensor = torch.tensor([indexed_tokens])
- segments_tensors = torch.tensor([segments_ids])
-
- model_bert = model_bert.eval()
- with torch.no_grad():
- outputs = model_bert(tokens_tensor, segments_tensors)
- hidden_states = outputs[2]
- token_embeddings = torch.stack(hidden_states, dim=0)
- token_embeddings = torch.squeeze(token_embeddings, dim=1)
- token_embeddings = token_embeddings.permute(1,0,2)
- token_vecs_sum = []
- for token in token_embeddings:
- # `token` is a [12 x 768] tensor
- # Sum the vectors from the last four layers.
- sum_vec = torch.sum(token[-4:], dim=0)
- sum_vec_np = sum_vec.numpy()
- # Use `sum_vec` to represent `token`.
- token_vecs_sum.append(sum_vec_np)
- if len(token_vecs_sum) < 512:
- for j in range(512-len(token_vecs_sum)):
- padding_vec = np.zeros(768) #change to 1024 in large models
- token_vecs_sum.append(padding_vec)
- return token_vecs_sum
-
-
-
-
-
-
-
-
-
-def greet(name):
-
- token_vecs_sums=[]
- token_vecs_sums.append(text_features(name))
- # sampleaud = K.constant(audioembeddingss)
- samplebert = K.constant(token_vecs_sums)
- samplescore = K.constant(0)
- Z = model.predict([samplebert])
- # print(Y)
- # print(y_encoded_test)
- Zclass = np.argmax(Z, axis=-1)
- Zclasstrue = np.argmax(samplescore, axis=-1)
- if Zclass == 0:
- out= "Not funny (0)"
- elif Zclass == 1:
- out= "Slightly funny only (1)"
- elif Zclass == 2:
- out= "Midly funny :) (2)"
- elif Zclass == 3:
- out= "Hahaha. Very funny. (3)"
- elif Zclass == 4:
- out= "Absolutely funny. LMAO. (4)"
-
-
- return out
-
-examples=[["Does anybody else secretly hope that Kanye pulls, like a… Mrs. Doubtfire? They come home one day, and they’re like, “This is the new housekeeper,” and he’s like, “What’s up, fam?” Yeah, it’s a really weird thing to go through. There’s because you know, people try to give you advice, but even friends that are older, they’re like, “I don’t… know.” He’s like, “It looks pretty bad, bro, I hope… Good luck, sorry, here if you need,” but like, no advice. No one was like, “This is what you do.” Everyone was like, “I don’t know… yeah. You staying with your mom? You in a safe spot?” People ask me weird questions. So that’s the only thing I don’t like. They ask this weird stuff. Like the other day, somebody came up to me and was like, “I heard you’re friends with Jack Harlow!” And I am. He’s a very great, talented rapper. He’s a cool dude. Nice guy. So we’re pals, right? And Kanye put him on his new album. Even though I’ve been friends with him for like two, three years, you know, he did it anyway. People come up to me and they’re like, “How does that make you feel? Does that bother you? Does that get under your skin?” And I’m like, “No, he’s a rapper.” That’s his field, that’s what they do. That doesn’t hurt my feelings. It would hurt my feelings if I saw, like, Bill Burr at Sunday service. I’d be like, “What the fuck, Bill?” He’d be like, “Find God, Petey, go fuck yourself! Jesus!” Yeah, I don’t get it. A lot of people are very angry. It’s always 5050 when I go outside. Yeah, it is. It’s always 5050. Either someone’s just like, “Hey, man, you’re really cool, that’s great.” Or someone’s like, “Hey, yo! Fuck you! Fuck you! Yeah, you!” I always am like, “Can’t be me."]]
-# print(greet("Does anybody else secretly hope that Kanye pulls, like a… Mrs. Doubtfire? They come home one day, and they’re like, “This is the new housekeeper,” and he’s like, “What’s up, fam?” Yeah, it’s a really weird thing to go through. There’s because you know, people try to give you advice, but even friends that are older, they’re like, “I don’t… know.” He’s like, “It looks pretty bad, bro, I hope… Good luck, sorry, here if you need,” but like, no advice. No one was like, “This is what you do.” Everyone was like, “I don’t know… yeah. You staying with your mom? You in a safe spot?” People ask me weird questions. So that’s the only thing I don’t like. They ask this weird stuff. Like the other day, somebody came up to me and was like, “I heard you’re friends with Jack Harlow!” And I am. He’s a very great, talented rapper. He’s a cool dude. Nice guy. So we’re pals, right? And Kanye put him on his new album. Even though I’ve been friends with him for like two, three years, you know, he did it anyway. People come up to me and they’re like, “How does that make you feel? Does that bother you? Does that get under your skin?” And I’m like, “No, he’s a rapper.” That’s his field, that’s what they do. That doesn’t hurt my feelings. It would hurt my feelings if I saw, like, Bill Burr at Sunday service. I’d be like, “What the fuck, Bill?” He’d be like, “Find God, Petey, go fuck yourself! Jesus!” Yeah, I don’t get it. A lot of people are very angry. It’s always 5050 when I go outside. Yeah, it is. It’s always 5050. Either someone’s just like, “Hey, man, you’re really cool, that’s great.” Or someone’s like, “Hey, yo! Fuck you! Fuck you! Yeah, you!” I always am like, “Can’t be me.”"))
-demo = gr.Interface(fn=greet, inputs=gr.Textbox(lines=1, placeholder="Enter text here"),title="Automatic funniness checker (0 to 4)", description="This model helps you check if you're funny. Add text here and see how funny you're!", outputs="text")
-demo.launch()
\ No newline at end of file
diff --git a/spaces/antonovmaxim/text-generation-webui-space/text-generation-webui-main/modules/logging_colors.py b/spaces/antonovmaxim/text-generation-webui-space/text-generation-webui-main/modules/logging_colors.py
deleted file mode 100644
index 5485b0901677fbab117015097f3af78401ae3419..0000000000000000000000000000000000000000
--- a/spaces/antonovmaxim/text-generation-webui-space/text-generation-webui-main/modules/logging_colors.py
+++ /dev/null
@@ -1,107 +0,0 @@
-# Copied from https://stackoverflow.com/a/1336640
-
-import logging
-
-def add_coloring_to_emit_windows(fn):
- # add methods we need to the class
- def _out_handle(self):
- import ctypes
- return ctypes.windll.kernel32.GetStdHandle(self.STD_OUTPUT_HANDLE)
- out_handle = property(_out_handle)
-
- def _set_color(self, code):
- import ctypes
- # Constants from the Windows API
- self.STD_OUTPUT_HANDLE = -11
- hdl = ctypes.windll.kernel32.GetStdHandle(self.STD_OUTPUT_HANDLE)
- ctypes.windll.kernel32.SetConsoleTextAttribute(hdl, code)
-
- setattr(logging.StreamHandler, '_set_color', _set_color)
-
- def new(*args):
- FOREGROUND_BLUE = 0x0001 # text color contains blue.
- FOREGROUND_GREEN = 0x0002 # text color contains green.
- FOREGROUND_RED = 0x0004 # text color contains red.
- FOREGROUND_INTENSITY = 0x0008 # text color is intensified.
- FOREGROUND_WHITE = FOREGROUND_BLUE | FOREGROUND_GREEN | FOREGROUND_RED
- # winbase.h
- # STD_INPUT_HANDLE = -10
- # STD_OUTPUT_HANDLE = -11
- # STD_ERROR_HANDLE = -12
-
- # wincon.h
- # FOREGROUND_BLACK = 0x0000
- FOREGROUND_BLUE = 0x0001
- FOREGROUND_GREEN = 0x0002
- # FOREGROUND_CYAN = 0x0003
- FOREGROUND_RED = 0x0004
- FOREGROUND_MAGENTA = 0x0005
- FOREGROUND_YELLOW = 0x0006
- # FOREGROUND_GREY = 0x0007
- FOREGROUND_INTENSITY = 0x0008 # foreground color is intensified.
-
- # BACKGROUND_BLACK = 0x0000
- # BACKGROUND_BLUE = 0x0010
- # BACKGROUND_GREEN = 0x0020
- # BACKGROUND_CYAN = 0x0030
- # BACKGROUND_RED = 0x0040
- # BACKGROUND_MAGENTA = 0x0050
- BACKGROUND_YELLOW = 0x0060
- # BACKGROUND_GREY = 0x0070
- BACKGROUND_INTENSITY = 0x0080 # background color is intensified.
-
- levelno = args[1].levelno
- if (levelno >= 50):
- color = BACKGROUND_YELLOW | FOREGROUND_RED | FOREGROUND_INTENSITY | BACKGROUND_INTENSITY
- elif (levelno >= 40):
- color = FOREGROUND_RED | FOREGROUND_INTENSITY
- elif (levelno >= 30):
- color = FOREGROUND_YELLOW | FOREGROUND_INTENSITY
- elif (levelno >= 20):
- color = FOREGROUND_GREEN
- elif (levelno >= 10):
- color = FOREGROUND_MAGENTA
- else:
- color = FOREGROUND_WHITE
- args[0]._set_color(color)
-
- ret = fn(*args)
- args[0]._set_color(FOREGROUND_WHITE)
- # print "after"
- return ret
- return new
-
-
-def add_coloring_to_emit_ansi(fn):
- # add methods we need to the class
- def new(*args):
- levelno = args[1].levelno
- if (levelno >= 50):
- color = '\x1b[31m' # red
- elif (levelno >= 40):
- color = '\x1b[31m' # red
- elif (levelno >= 30):
- color = '\x1b[33m' # yellow
- elif (levelno >= 20):
- color = '\x1b[32m' # green
- elif (levelno >= 10):
- color = '\x1b[35m' # pink
- else:
- color = '\x1b[0m' # normal
- args[1].msg = color + args[1].msg + '\x1b[0m' # normal
- # print "after"
- return fn(*args)
- return new
-
-
-import platform
-if platform.system() == 'Windows':
- # Windows does not support ANSI escapes and we are using API calls to set the console color
- logging.StreamHandler.emit = add_coloring_to_emit_windows(logging.StreamHandler.emit)
-else:
- # all non-Windows platforms are supporting ANSI escapes so we use them
- logging.StreamHandler.emit = add_coloring_to_emit_ansi(logging.StreamHandler.emit)
- # log = logging.getLogger()
- # log.addFilter(log_filter())
- # //hdlr = logging.StreamHandler()
- # //hdlr.setFormatter(formatter())
diff --git a/spaces/anzorq/hf-spaces-semantic-search/tailwind.config.js b/spaces/anzorq/hf-spaces-semantic-search/tailwind.config.js
deleted file mode 100644
index e3778b4b2bbb49631a65c92fee30e6a0b433dad0..0000000000000000000000000000000000000000
--- a/spaces/anzorq/hf-spaces-semantic-search/tailwind.config.js
+++ /dev/null
@@ -1,19 +0,0 @@
-/** @type {import('tailwindcss').Config} */
-module.exports = {
- content: [
- './pages/**/*.{js,ts,jsx,tsx,mdx}',
- './components/**/*.{js,ts,jsx,tsx,mdx}',
- './app/**/*.{js,ts,jsx,tsx,mdx}',
- ],
- theme: {
- extend: {
- backgroundImage: {
- 'gradient-radial': 'radial-gradient(var(--tw-gradient-stops))',
- 'gradient-conic':
- 'conic-gradient(from 180deg at 50% 50%, var(--tw-gradient-stops))',
- },
-
- },
- },
- plugins: [],
-}
diff --git a/spaces/aodianyun/stable-diffusion-webui/extensions/deforum/scripts/deforum_helpers/src/rife/model/pytorch_msssim/__init__.py b/spaces/aodianyun/stable-diffusion-webui/extensions/deforum/scripts/deforum_helpers/src/rife/model/pytorch_msssim/__init__.py
deleted file mode 100644
index a4d30326188cf6afacf2fc84c7ae18efe14dae2e..0000000000000000000000000000000000000000
--- a/spaces/aodianyun/stable-diffusion-webui/extensions/deforum/scripts/deforum_helpers/src/rife/model/pytorch_msssim/__init__.py
+++ /dev/null
@@ -1,200 +0,0 @@
-import torch
-import torch.nn.functional as F
-from math import exp
-import numpy as np
-
-device = torch.device("cuda" if torch.cuda.is_available() else "cpu")
-
-def gaussian(window_size, sigma):
- gauss = torch.Tensor([exp(-(x - window_size//2)**2/float(2*sigma**2)) for x in range(window_size)])
- return gauss/gauss.sum()
-
-
-def create_window(window_size, channel=1):
- _1D_window = gaussian(window_size, 1.5).unsqueeze(1)
- _2D_window = _1D_window.mm(_1D_window.t()).float().unsqueeze(0).unsqueeze(0).to(device)
- window = _2D_window.expand(channel, 1, window_size, window_size).contiguous()
- return window
-
-def create_window_3d(window_size, channel=1):
- _1D_window = gaussian(window_size, 1.5).unsqueeze(1)
- _2D_window = _1D_window.mm(_1D_window.t())
- _3D_window = _2D_window.unsqueeze(2) @ (_1D_window.t())
- window = _3D_window.expand(1, channel, window_size, window_size, window_size).contiguous().to(device)
- return window
-
-
-def ssim(img1, img2, window_size=11, window=None, size_average=True, full=False, val_range=None):
- # Value range can be different from 255. Other common ranges are 1 (sigmoid) and 2 (tanh).
- if val_range is None:
- if torch.max(img1) > 128:
- max_val = 255
- else:
- max_val = 1
-
- if torch.min(img1) < -0.5:
- min_val = -1
- else:
- min_val = 0
- L = max_val - min_val
- else:
- L = val_range
-
- padd = 0
- (_, channel, height, width) = img1.size()
- if window is None:
- real_size = min(window_size, height, width)
- window = create_window(real_size, channel=channel).to(img1.device)
-
- # mu1 = F.conv2d(img1, window, padding=padd, groups=channel)
- # mu2 = F.conv2d(img2, window, padding=padd, groups=channel)
- mu1 = F.conv2d(F.pad(img1, (5, 5, 5, 5), mode='replicate'), window, padding=padd, groups=channel)
- mu2 = F.conv2d(F.pad(img2, (5, 5, 5, 5), mode='replicate'), window, padding=padd, groups=channel)
-
- mu1_sq = mu1.pow(2)
- mu2_sq = mu2.pow(2)
- mu1_mu2 = mu1 * mu2
-
- sigma1_sq = F.conv2d(F.pad(img1 * img1, (5, 5, 5, 5), 'replicate'), window, padding=padd, groups=channel) - mu1_sq
- sigma2_sq = F.conv2d(F.pad(img2 * img2, (5, 5, 5, 5), 'replicate'), window, padding=padd, groups=channel) - mu2_sq
- sigma12 = F.conv2d(F.pad(img1 * img2, (5, 5, 5, 5), 'replicate'), window, padding=padd, groups=channel) - mu1_mu2
-
- C1 = (0.01 * L) ** 2
- C2 = (0.03 * L) ** 2
-
- v1 = 2.0 * sigma12 + C2
- v2 = sigma1_sq + sigma2_sq + C2
- cs = torch.mean(v1 / v2) # contrast sensitivity
-
- ssim_map = ((2 * mu1_mu2 + C1) * v1) / ((mu1_sq + mu2_sq + C1) * v2)
-
- if size_average:
- ret = ssim_map.mean()
- else:
- ret = ssim_map.mean(1).mean(1).mean(1)
-
- if full:
- return ret, cs
- return ret
-
-
-def ssim_matlab(img1, img2, window_size=11, window=None, size_average=True, full=False, val_range=None):
- # Value range can be different from 255. Other common ranges are 1 (sigmoid) and 2 (tanh).
- if val_range is None:
- if torch.max(img1) > 128:
- max_val = 255
- else:
- max_val = 1
-
- if torch.min(img1) < -0.5:
- min_val = -1
- else:
- min_val = 0
- L = max_val - min_val
- else:
- L = val_range
-
- padd = 0
- (_, _, height, width) = img1.size()
- if window is None:
- real_size = min(window_size, height, width)
- window = create_window_3d(real_size, channel=1).to(img1.device)
- # Channel is set to 1 since we consider color images as volumetric images
-
- img1 = img1.unsqueeze(1)
- img2 = img2.unsqueeze(1)
-
- mu1 = F.conv3d(F.pad(img1, (5, 5, 5, 5, 5, 5), mode='replicate'), window, padding=padd, groups=1)
- mu2 = F.conv3d(F.pad(img2, (5, 5, 5, 5, 5, 5), mode='replicate'), window, padding=padd, groups=1)
-
- mu1_sq = mu1.pow(2)
- mu2_sq = mu2.pow(2)
- mu1_mu2 = mu1 * mu2
-
- sigma1_sq = F.conv3d(F.pad(img1 * img1, (5, 5, 5, 5, 5, 5), 'replicate'), window, padding=padd, groups=1) - mu1_sq
- sigma2_sq = F.conv3d(F.pad(img2 * img2, (5, 5, 5, 5, 5, 5), 'replicate'), window, padding=padd, groups=1) - mu2_sq
- sigma12 = F.conv3d(F.pad(img1 * img2, (5, 5, 5, 5, 5, 5), 'replicate'), window, padding=padd, groups=1) - mu1_mu2
-
- C1 = (0.01 * L) ** 2
- C2 = (0.03 * L) ** 2
-
- v1 = 2.0 * sigma12 + C2
- v2 = sigma1_sq + sigma2_sq + C2
- cs = torch.mean(v1 / v2) # contrast sensitivity
-
- ssim_map = ((2 * mu1_mu2 + C1) * v1) / ((mu1_sq + mu2_sq + C1) * v2)
-
- if size_average:
- ret = ssim_map.mean()
- else:
- ret = ssim_map.mean(1).mean(1).mean(1)
-
- if full:
- return ret, cs
- return ret
-
-
-def msssim(img1, img2, window_size=11, size_average=True, val_range=None, normalize=False):
- device = img1.device
- weights = torch.FloatTensor([0.0448, 0.2856, 0.3001, 0.2363, 0.1333]).to(device)
- levels = weights.size()[0]
- mssim = []
- mcs = []
- for _ in range(levels):
- sim, cs = ssim(img1, img2, window_size=window_size, size_average=size_average, full=True, val_range=val_range)
- mssim.append(sim)
- mcs.append(cs)
-
- img1 = F.avg_pool2d(img1, (2, 2))
- img2 = F.avg_pool2d(img2, (2, 2))
-
- mssim = torch.stack(mssim)
- mcs = torch.stack(mcs)
-
- # Normalize (to avoid NaNs during training unstable models, not compliant with original definition)
- if normalize:
- mssim = (mssim + 1) / 2
- mcs = (mcs + 1) / 2
-
- pow1 = mcs ** weights
- pow2 = mssim ** weights
- # From Matlab implementation https://ece.uwaterloo.ca/~z70wang/research/iwssim/
- output = torch.prod(pow1[:-1] * pow2[-1])
- return output
-
-
-# Classes to re-use window
-class SSIM(torch.nn.Module):
- def __init__(self, window_size=11, size_average=True, val_range=None):
- super(SSIM, self).__init__()
- self.window_size = window_size
- self.size_average = size_average
- self.val_range = val_range
-
- # Assume 3 channel for SSIM
- self.channel = 3
- self.window = create_window(window_size, channel=self.channel)
-
- def forward(self, img1, img2):
- (_, channel, _, _) = img1.size()
-
- if channel == self.channel and self.window.dtype == img1.dtype:
- window = self.window
- else:
- window = create_window(self.window_size, channel).to(img1.device).type(img1.dtype)
- self.window = window
- self.channel = channel
-
- _ssim = ssim(img1, img2, window=window, window_size=self.window_size, size_average=self.size_average)
- dssim = (1 - _ssim) / 2
- return dssim
-
-class MSSSIM(torch.nn.Module):
- def __init__(self, window_size=11, size_average=True, channel=3):
- super(MSSSIM, self).__init__()
- self.window_size = window_size
- self.size_average = size_average
- self.channel = channel
-
- def forward(self, img1, img2):
- return msssim(img1, img2, window_size=self.window_size, size_average=self.size_average)
diff --git a/spaces/aphenx/bingo/src/pages/api/kblob.ts b/spaces/aphenx/bingo/src/pages/api/kblob.ts
deleted file mode 100644
index 0ce7e6063cdc06838e76f1cff1d5982d34ef52de..0000000000000000000000000000000000000000
--- a/spaces/aphenx/bingo/src/pages/api/kblob.ts
+++ /dev/null
@@ -1,56 +0,0 @@
-'use server'
-
-import { NextApiRequest, NextApiResponse } from 'next'
-import FormData from 'form-data'
-import { fetch } from '@/lib/isomorphic'
-import { KBlobRequest } from '@/lib/bots/bing/types'
-
-const API_DOMAIN = 'https://bing.vcanbb.top'
-
-export const config = {
- api: {
- bodyParser: {
- sizeLimit: '10mb' // Set desired value here
- }
- }
-}
-
-export default async function handler(req: NextApiRequest, res: NextApiResponse) {
- try {
- const { knowledgeRequest, imageBase64 } = req.body as KBlobRequest
-
- const formData = new FormData()
- formData.append('knowledgeRequest', JSON.stringify(knowledgeRequest))
- if (imageBase64) {
- formData.append('imageBase64', imageBase64)
- }
-
- const response = await fetch(`${API_DOMAIN}/images/kblob`,
- {
- method: 'POST',
- body: formData.getBuffer(),
- headers: {
- "sec-ch-ua": "\"Not/A)Brand\";v=\"99\", \"Google Chrome\";v=\"115\", \"Chromium\";v=\"115\"",
- "sec-ch-ua-mobile": "?0",
- "sec-ch-ua-platform": "\"Windows\"",
- "Referer": `${API_DOMAIN}/web/index.html`,
- "Referrer-Policy": "origin-when-cross-origin",
- 'x-ms-useragent': 'azsdk-js-api-client-factory/1.0.0-beta.1 core-rest-pipeline/1.10.0 OS/Win32',
- ...formData.getHeaders()
- }
- }
- ).then(res => res.text())
-
- res.writeHead(200, {
- 'Content-Type': 'application/json',
- })
- res.end(response || JSON.stringify({ result: { value: 'UploadFailed', message: '请更换 IP 或代理后重试' } }))
- } catch (e) {
- return res.json({
- result: {
- value: 'UploadFailed',
- message: `${e}`
- }
- })
- }
-}
diff --git a/spaces/arpitr/end_to_end_ml_app/app.py b/spaces/arpitr/end_to_end_ml_app/app.py
deleted file mode 100644
index a3017ed2a8b87c235e838dd4a4d744b8a0e048c7..0000000000000000000000000000000000000000
--- a/spaces/arpitr/end_to_end_ml_app/app.py
+++ /dev/null
@@ -1,167 +0,0 @@
-import streamlit as st
-import pandas as pd
-import numpy as np
-from PIL import Image
-from pydataset import data
-from sklearn.model_selection import train_test_split
-from sklearn.metrics import confusion_matrix
-from sklearn.metrics import classification_report
-from sklearn.metrics import accuracy_score
-from sklearn.metrics import precision_recall_fscore_support
-from sklearn.preprocessing import StandardScaler
-from sklearn.linear_model import LinearRegression
-from sklearn.metrics import r2_score,mean_squared_error,mean_absolute_error
-from sklearn.linear_model import LogisticRegression
-from sklearn.svm import SVC
-from sklearn.gaussian_process import GaussianProcessClassifier
-from sklearn.gaussian_process.kernels import RBF
-from sklearn.tree import DecisionTreeClassifier
-from sklearn.ensemble import RandomForestClassifier, AdaBoostClassifier
-from sklearn.naive_bayes import GaussianNB
-from sklearn.discriminant_analysis import QuadraticDiscriminantAnalysis
-
-#import pandas as pd
-
-st.header('Try end to end predictive modeling on different datasets')
-
-st.info("""
-- Pick the dataset
-- Validate the dataset
-- Prepare the dataset (Impute/Scaling/Categorical Encoding/Imbalance)
-- Pick the Machine Learning Algorithmn
-- Analyse the results (Accuracy, MAE, Recall, Precision, F1)
-""")
-
-class MlStreamlitApp:
-
- def __init__(self):
- self.dataset_list = data()
- #, show_doc=True
- @st.cache(suppress_st_warning=True)
- def load_data(self,dataset_name):
- df = data(str(dataset_name))
- df.columns = df.columns.str.replace('.','_')
- df.columns = df.columns.str.lower()
- return df
-
- '''
- @st.cache(suppress_st_warning=True)
- def show_dataset_doc(self,dataset_name):
- st.write(dataset_name)
- st.code(data('iris',show_doc=True))
- '''
-
- def show_datasets_list(self):
- st.info('Datasets details')
- st.write(self.dataset_list)
-
- def run(self):
- st.sidebar.title('Streamlit ML App')
- dataset_list=['']+list(self.dataset_list.dataset_id)
- dataset_name = st.sidebar.selectbox('Select the Dataset',dataset_list)
- process_selection = st.sidebar.radio("What on your mind?",('EDA', 'Predictive Modelling'))
-
- if dataset_name == '':
- st.sidebar.warning('Select the Dataset')
- self.show_datasets_list()
- elif (dataset_name and process_selection == 'Predictive Modelling'):
- df = self.load_data(dataset_name)
- st.write(df.head())
- #image = Image.open('./ml_process.jpeg')
- #st.sidebar.image(image)
- dataset_target = st.selectbox('Select the Target', list(df.columns))
- df=df.rename(columns={dataset_target:'target'})
-
- df_dum=pd.get_dummies(df.loc[:, df.columns != 'target'],drop_first=True)
- df=pd.concat([df_dum,df.target],axis=1)
-
- #algo_type = st.selectbox('Classification or Regression', list(['Classification','Regression']))
-
- if df.target.dtypes == 'object':
- algo_type='Classification'
- ml_algos = ['LogisticRegression','DecisionTreeClassifier','RandomForestClassifier','AdaBoostClassifier']
- else:
- algo_type='Regression'
- ml_algos = ['LinearRegression']
-
- # if algo_type == 'Classification':
- # ml_algos = ['LogisticRegression','DecisionTreeClassifier','RandomForestClassifier','AdaBoostClassifier']
-
- # else:
- # ml_algos = ['LinearRegression']
-
- X= df.loc[:, df.columns != 'target']
- y= df['target']
-
- X_train, X_test, y_train, y_test = train_test_split(X, y, test_size=0.20, random_state=42)
-
- #st.write(X_train.head())
- #st.write(y_test.head())
-
- ml_algo = st.selectbox('Select the ML Algo', list(ml_algos))
-
- if ml_algo == 'LogisticRegression':
- clf_fit = LogisticRegression().fit(X_train, y_train)
- predictions = clf_fit.predict(X_test)
- st.write(predictions[1:5])
- elif ml_algo == 'DecisionTreeClassifier':
- clf_fit = DecisionTreeClassifier().fit(X_train, y_train)
- predictions = clf_fit.predict(X_test)
- st.write(predictions[1:5])
- #RandomForestClassifier
- elif ml_algo == 'RandomForestClassifier':
- clf_fit = RandomForestClassifier().fit(X_train, y_train)
- predictions = clf_fit.predict(X_test)
- st.write(predictions[1:5])
- elif ml_algo == 'AdaBoostClassifier':
- clf_fit = AdaBoostClassifier().fit(X_train, y_train)
- predictions = clf_fit.predict(X_test)
- st.write(predictions[1:5])
- elif ml_algo == 'LinearRegression':
- clf_fit = LinearRegression().fit(X_train, y_train)
- predictions = clf_fit.predict(X_test)
- st.write(predictions[1:5])
- else:
- st.write('No ML Algo selected')
-
- if algo_type=='Classification':
- st.write("""
- Confusion Matrix
- """)
- st.write(confusion_matrix(y_test, predictions))
- st.write("""
- #### Accuracy Score:
- """)
- st.write( accuracy_score(y_test, predictions))
- st.write("""
- #### Other Scores - precision_recall_fscore:
- """)
- precision,recall,f1_score,support=precision_recall_fscore_support(y_test, predictions,average='weighted')
- st.write(round(precision,2),round(recall,2),round(f1_score,2))
- else:
- st.write(""" ### Model Evaluation """)
- r2_metrics = r2_score(y_test, predictions)
- mse = mean_squared_error(y_test, predictions)
- rmse = np.sqrt(mse)
- mae=mean_absolute_error(y_test, predictions)
- st.write(""" #### Rsquared, MSE , RMSE, MAE """)
- st.write(round(r2_metrics,2),round(mse,2),round(rmse,2),round(mae,2))
- else:
- eda_selection = st.sidebar.radio("What you want to see?",('Summary', 'Plots'))
- df = self.load_data(dataset_name)
- if eda_selection == 'Summary':
- st.write("Glimpse of Data",df.head(10))
- st.write("Total No. of Rows",df.shape[0])
- st.write("Total No. of Columns",df.shape[1])
- st.write("Types of Columns",df.dtypes)
- st.write("Summary Stats",df.describe().T)
- st.write("Total Nulls in the columns",df.isnull().sum())
- st.write("Total Duplicate Rows",df[df.duplicated()].shape[0])
- st.write("Correlation Matrix",df.corr())
- else:
- st.info('Plots') #WIP
-
-
-if __name__ == '__main__':
- mlApp = MlStreamlitApp()
- mlApp.run()
\ No newline at end of file
diff --git a/spaces/arxify/RVC-beta-v2-0618/runtime/Lib/site-packages/Crypto/Math/_IntegerGMP.py b/spaces/arxify/RVC-beta-v2-0618/runtime/Lib/site-packages/Crypto/Math/_IntegerGMP.py
deleted file mode 100644
index f552b71ad678d6fe760c21071186b831380c570e..0000000000000000000000000000000000000000
--- a/spaces/arxify/RVC-beta-v2-0618/runtime/Lib/site-packages/Crypto/Math/_IntegerGMP.py
+++ /dev/null
@@ -1,762 +0,0 @@
-# ===================================================================
-#
-# Copyright (c) 2014, Legrandin
-# All rights reserved.
-#
-# Redistribution and use in source and binary forms, with or without
-# modification, are permitted provided that the following conditions
-# are met:
-#
-# 1. Redistributions of source code must retain the above copyright
-# notice, this list of conditions and the following disclaimer.
-# 2. Redistributions in binary form must reproduce the above copyright
-# notice, this list of conditions and the following disclaimer in
-# the documentation and/or other materials provided with the
-# distribution.
-#
-# THIS SOFTWARE IS PROVIDED BY THE COPYRIGHT HOLDERS AND CONTRIBUTORS
-# "AS IS" AND ANY EXPRESS OR IMPLIED WARRANTIES, INCLUDING, BUT NOT
-# LIMITED TO, THE IMPLIED WARRANTIES OF MERCHANTABILITY AND FITNESS
-# FOR A PARTICULAR PURPOSE ARE DISCLAIMED. IN NO EVENT SHALL THE
-# COPYRIGHT HOLDER OR CONTRIBUTORS BE LIABLE FOR ANY DIRECT, INDIRECT,
-# INCIDENTAL, SPECIAL, EXEMPLARY, OR CONSEQUENTIAL DAMAGES (INCLUDING,
-# BUT NOT LIMITED TO, PROCUREMENT OF SUBSTITUTE GOODS OR SERVICES;
-# LOSS OF USE, DATA, OR PROFITS; OR BUSINESS INTERRUPTION) HOWEVER
-# CAUSED AND ON ANY THEORY OF LIABILITY, WHETHER IN CONTRACT, STRICT
-# LIABILITY, OR TORT (INCLUDING NEGLIGENCE OR OTHERWISE) ARISING IN
-# ANY WAY OUT OF THE USE OF THIS SOFTWARE, EVEN IF ADVISED OF THE
-# POSSIBILITY OF SUCH DAMAGE.
-# ===================================================================
-
-import sys
-
-from Crypto.Util.py3compat import tobytes, is_native_int
-
-from Crypto.Util._raw_api import (backend, load_lib,
- get_raw_buffer, get_c_string,
- null_pointer, create_string_buffer,
- c_ulong, c_size_t, c_uint8_ptr)
-
-from ._IntegerBase import IntegerBase
-
-gmp_defs = """typedef unsigned long UNIX_ULONG;
- typedef struct { int a; int b; void *c; } MPZ;
- typedef MPZ mpz_t[1];
- typedef UNIX_ULONG mp_bitcnt_t;
-
- void __gmpz_init (mpz_t x);
- void __gmpz_init_set (mpz_t rop, const mpz_t op);
- void __gmpz_init_set_ui (mpz_t rop, UNIX_ULONG op);
-
- UNIX_ULONG __gmpz_get_ui (const mpz_t op);
- void __gmpz_set (mpz_t rop, const mpz_t op);
- void __gmpz_set_ui (mpz_t rop, UNIX_ULONG op);
- void __gmpz_add (mpz_t rop, const mpz_t op1, const mpz_t op2);
- void __gmpz_add_ui (mpz_t rop, const mpz_t op1, UNIX_ULONG op2);
- void __gmpz_sub_ui (mpz_t rop, const mpz_t op1, UNIX_ULONG op2);
- void __gmpz_addmul (mpz_t rop, const mpz_t op1, const mpz_t op2);
- void __gmpz_addmul_ui (mpz_t rop, const mpz_t op1, UNIX_ULONG op2);
- void __gmpz_submul_ui (mpz_t rop, const mpz_t op1, UNIX_ULONG op2);
- void __gmpz_import (mpz_t rop, size_t count, int order, size_t size,
- int endian, size_t nails, const void *op);
- void * __gmpz_export (void *rop, size_t *countp, int order,
- size_t size,
- int endian, size_t nails, const mpz_t op);
- size_t __gmpz_sizeinbase (const mpz_t op, int base);
- void __gmpz_sub (mpz_t rop, const mpz_t op1, const mpz_t op2);
- void __gmpz_mul (mpz_t rop, const mpz_t op1, const mpz_t op2);
- void __gmpz_mul_ui (mpz_t rop, const mpz_t op1, UNIX_ULONG op2);
- int __gmpz_cmp (const mpz_t op1, const mpz_t op2);
- void __gmpz_powm (mpz_t rop, const mpz_t base, const mpz_t exp, const
- mpz_t mod);
- void __gmpz_powm_ui (mpz_t rop, const mpz_t base, UNIX_ULONG exp,
- const mpz_t mod);
- void __gmpz_pow_ui (mpz_t rop, const mpz_t base, UNIX_ULONG exp);
- void __gmpz_sqrt(mpz_t rop, const mpz_t op);
- void __gmpz_mod (mpz_t r, const mpz_t n, const mpz_t d);
- void __gmpz_neg (mpz_t rop, const mpz_t op);
- void __gmpz_abs (mpz_t rop, const mpz_t op);
- void __gmpz_and (mpz_t rop, const mpz_t op1, const mpz_t op2);
- void __gmpz_ior (mpz_t rop, const mpz_t op1, const mpz_t op2);
- void __gmpz_clear (mpz_t x);
- void __gmpz_tdiv_q_2exp (mpz_t q, const mpz_t n, mp_bitcnt_t b);
- void __gmpz_fdiv_q (mpz_t q, const mpz_t n, const mpz_t d);
- void __gmpz_mul_2exp (mpz_t rop, const mpz_t op1, mp_bitcnt_t op2);
- int __gmpz_tstbit (const mpz_t op, mp_bitcnt_t bit_index);
- int __gmpz_perfect_square_p (const mpz_t op);
- int __gmpz_jacobi (const mpz_t a, const mpz_t b);
- void __gmpz_gcd (mpz_t rop, const mpz_t op1, const mpz_t op2);
- UNIX_ULONG __gmpz_gcd_ui (mpz_t rop, const mpz_t op1,
- UNIX_ULONG op2);
- void __gmpz_lcm (mpz_t rop, const mpz_t op1, const mpz_t op2);
- int __gmpz_invert (mpz_t rop, const mpz_t op1, const mpz_t op2);
- int __gmpz_divisible_p (const mpz_t n, const mpz_t d);
- int __gmpz_divisible_ui_p (const mpz_t n, UNIX_ULONG d);
- """
-
-if sys.platform == "win32":
- raise ImportError("Not using GMP on Windows")
-
-lib = load_lib("gmp", gmp_defs)
-implementation = {"library": "gmp", "api": backend}
-
-if hasattr(lib, "__mpir_version"):
- raise ImportError("MPIR library detected")
-
-# In order to create a function that returns a pointer to
-# a new MPZ structure, we need to break the abstraction
-# and know exactly what ffi backend we have
-if implementation["api"] == "ctypes":
- from ctypes import Structure, c_int, c_void_p, byref
-
- class _MPZ(Structure):
- _fields_ = [('_mp_alloc', c_int),
- ('_mp_size', c_int),
- ('_mp_d', c_void_p)]
-
- def new_mpz():
- return byref(_MPZ())
-
-else:
- # We are using CFFI
- from Crypto.Util._raw_api import ffi
-
- def new_mpz():
- return ffi.new("MPZ*")
-
-
-# Lazy creation of GMP methods
-class _GMP(object):
-
- def __getattr__(self, name):
- if name.startswith("mpz_"):
- func_name = "__gmpz_" + name[4:]
- elif name.startswith("gmp_"):
- func_name = "__gmp_" + name[4:]
- else:
- raise AttributeError("Attribute %s is invalid" % name)
- func = getattr(lib, func_name)
- setattr(self, name, func)
- return func
-
-
-_gmp = _GMP()
-
-
-class IntegerGMP(IntegerBase):
- """A fast, arbitrary precision integer"""
-
- _zero_mpz_p = new_mpz()
- _gmp.mpz_init_set_ui(_zero_mpz_p, c_ulong(0))
-
- def __init__(self, value):
- """Initialize the integer to the given value."""
-
- self._mpz_p = new_mpz()
- self._initialized = False
-
- if isinstance(value, float):
- raise ValueError("A floating point type is not a natural number")
-
- if is_native_int(value):
- _gmp.mpz_init(self._mpz_p)
- self._initialized = True
- if value == 0:
- return
-
- tmp = new_mpz()
- _gmp.mpz_init(tmp)
-
- try:
- positive = value >= 0
- reduce = abs(value)
- slots = (reduce.bit_length() - 1) // 32 + 1
-
- while slots > 0:
- slots = slots - 1
- _gmp.mpz_set_ui(tmp,
- c_ulong(0xFFFFFFFF & (reduce >> (slots * 32))))
- _gmp.mpz_mul_2exp(tmp, tmp, c_ulong(slots * 32))
- _gmp.mpz_add(self._mpz_p, self._mpz_p, tmp)
- finally:
- _gmp.mpz_clear(tmp)
-
- if not positive:
- _gmp.mpz_neg(self._mpz_p, self._mpz_p)
-
- elif isinstance(value, IntegerGMP):
- _gmp.mpz_init_set(self._mpz_p, value._mpz_p)
- self._initialized = True
- else:
- raise NotImplementedError
-
-
- # Conversions
- def __int__(self):
- tmp = new_mpz()
- _gmp.mpz_init_set(tmp, self._mpz_p)
-
- try:
- value = 0
- slot = 0
- while _gmp.mpz_cmp(tmp, self._zero_mpz_p) != 0:
- lsb = _gmp.mpz_get_ui(tmp) & 0xFFFFFFFF
- value |= lsb << (slot * 32)
- _gmp.mpz_tdiv_q_2exp(tmp, tmp, c_ulong(32))
- slot = slot + 1
- finally:
- _gmp.mpz_clear(tmp)
-
- if self < 0:
- value = -value
- return int(value)
-
- def __str__(self):
- return str(int(self))
-
- def __repr__(self):
- return "Integer(%s)" % str(self)
-
- # Only Python 2.x
- def __hex__(self):
- return hex(int(self))
-
- # Only Python 3.x
- def __index__(self):
- return int(self)
-
- def to_bytes(self, block_size=0, byteorder='big'):
- """Convert the number into a byte string.
-
- This method encodes the number in network order and prepends
- as many zero bytes as required. It only works for non-negative
- values.
-
- :Parameters:
- block_size : integer
- The exact size the output byte string must have.
- If zero, the string has the minimal length.
- byteorder : string
- 'big' for big-endian integers (default), 'little' for litte-endian.
- :Returns:
- A byte string.
- :Raise ValueError:
- If the value is negative or if ``block_size`` is
- provided and the length of the byte string would exceed it.
- """
-
- if self < 0:
- raise ValueError("Conversion only valid for non-negative numbers")
-
- buf_len = (_gmp.mpz_sizeinbase(self._mpz_p, 2) + 7) // 8
- if buf_len > block_size > 0:
- raise ValueError("Number is too big to convert to byte string"
- " of prescribed length")
- buf = create_string_buffer(buf_len)
-
-
- _gmp.mpz_export(
- buf,
- null_pointer, # Ignore countp
- 1, # Big endian
- c_size_t(1), # Each word is 1 byte long
- 0, # Endianess within a word - not relevant
- c_size_t(0), # No nails
- self._mpz_p)
-
- result = b'\x00' * max(0, block_size - buf_len) + get_raw_buffer(buf)
- if byteorder == 'big':
- pass
- elif byteorder == 'little':
- result = bytearray(result)
- result.reverse()
- result = bytes(result)
- else:
- raise ValueError("Incorrect byteorder")
- return result
-
- @staticmethod
- def from_bytes(byte_string, byteorder='big'):
- """Convert a byte string into a number.
-
- :Parameters:
- byte_string : byte string
- The input number, encoded in network order.
- It can only be non-negative.
- byteorder : string
- 'big' for big-endian integers (default), 'little' for litte-endian.
-
- :Return:
- The ``Integer`` object carrying the same value as the input.
- """
- result = IntegerGMP(0)
- if byteorder == 'big':
- pass
- elif byteorder == 'little':
- byte_string = bytearray(byte_string)
- byte_string.reverse()
- else:
- raise ValueError("Incorrect byteorder")
- _gmp.mpz_import(
- result._mpz_p,
- c_size_t(len(byte_string)), # Amount of words to read
- 1, # Big endian
- c_size_t(1), # Each word is 1 byte long
- 0, # Endianess within a word - not relevant
- c_size_t(0), # No nails
- c_uint8_ptr(byte_string))
- return result
-
- # Relations
- def _apply_and_return(self, func, term):
- if not isinstance(term, IntegerGMP):
- term = IntegerGMP(term)
- return func(self._mpz_p, term._mpz_p)
-
- def __eq__(self, term):
- if not (isinstance(term, IntegerGMP) or is_native_int(term)):
- return False
- return self._apply_and_return(_gmp.mpz_cmp, term) == 0
-
- def __ne__(self, term):
- if not (isinstance(term, IntegerGMP) or is_native_int(term)):
- return True
- return self._apply_and_return(_gmp.mpz_cmp, term) != 0
-
- def __lt__(self, term):
- return self._apply_and_return(_gmp.mpz_cmp, term) < 0
-
- def __le__(self, term):
- return self._apply_and_return(_gmp.mpz_cmp, term) <= 0
-
- def __gt__(self, term):
- return self._apply_and_return(_gmp.mpz_cmp, term) > 0
-
- def __ge__(self, term):
- return self._apply_and_return(_gmp.mpz_cmp, term) >= 0
-
- def __nonzero__(self):
- return _gmp.mpz_cmp(self._mpz_p, self._zero_mpz_p) != 0
- __bool__ = __nonzero__
-
- def is_negative(self):
- return _gmp.mpz_cmp(self._mpz_p, self._zero_mpz_p) < 0
-
- # Arithmetic operations
- def __add__(self, term):
- result = IntegerGMP(0)
- if not isinstance(term, IntegerGMP):
- try:
- term = IntegerGMP(term)
- except NotImplementedError:
- return NotImplemented
- _gmp.mpz_add(result._mpz_p,
- self._mpz_p,
- term._mpz_p)
- return result
-
- def __sub__(self, term):
- result = IntegerGMP(0)
- if not isinstance(term, IntegerGMP):
- try:
- term = IntegerGMP(term)
- except NotImplementedError:
- return NotImplemented
- _gmp.mpz_sub(result._mpz_p,
- self._mpz_p,
- term._mpz_p)
- return result
-
- def __mul__(self, term):
- result = IntegerGMP(0)
- if not isinstance(term, IntegerGMP):
- try:
- term = IntegerGMP(term)
- except NotImplementedError:
- return NotImplemented
- _gmp.mpz_mul(result._mpz_p,
- self._mpz_p,
- term._mpz_p)
- return result
-
- def __floordiv__(self, divisor):
- if not isinstance(divisor, IntegerGMP):
- divisor = IntegerGMP(divisor)
- if _gmp.mpz_cmp(divisor._mpz_p,
- self._zero_mpz_p) == 0:
- raise ZeroDivisionError("Division by zero")
- result = IntegerGMP(0)
- _gmp.mpz_fdiv_q(result._mpz_p,
- self._mpz_p,
- divisor._mpz_p)
- return result
-
- def __mod__(self, divisor):
- if not isinstance(divisor, IntegerGMP):
- divisor = IntegerGMP(divisor)
- comp = _gmp.mpz_cmp(divisor._mpz_p,
- self._zero_mpz_p)
- if comp == 0:
- raise ZeroDivisionError("Division by zero")
- if comp < 0:
- raise ValueError("Modulus must be positive")
- result = IntegerGMP(0)
- _gmp.mpz_mod(result._mpz_p,
- self._mpz_p,
- divisor._mpz_p)
- return result
-
- def inplace_pow(self, exponent, modulus=None):
-
- if modulus is None:
- if exponent < 0:
- raise ValueError("Exponent must not be negative")
-
- # Normal exponentiation
- if exponent > 256:
- raise ValueError("Exponent is too big")
- _gmp.mpz_pow_ui(self._mpz_p,
- self._mpz_p, # Base
- c_ulong(int(exponent))
- )
- else:
- # Modular exponentiation
- if not isinstance(modulus, IntegerGMP):
- modulus = IntegerGMP(modulus)
- if not modulus:
- raise ZeroDivisionError("Division by zero")
- if modulus.is_negative():
- raise ValueError("Modulus must be positive")
- if is_native_int(exponent):
- if exponent < 0:
- raise ValueError("Exponent must not be negative")
- if exponent < 65536:
- _gmp.mpz_powm_ui(self._mpz_p,
- self._mpz_p,
- c_ulong(exponent),
- modulus._mpz_p)
- return self
- exponent = IntegerGMP(exponent)
- elif exponent.is_negative():
- raise ValueError("Exponent must not be negative")
- _gmp.mpz_powm(self._mpz_p,
- self._mpz_p,
- exponent._mpz_p,
- modulus._mpz_p)
- return self
-
- def __pow__(self, exponent, modulus=None):
- result = IntegerGMP(self)
- return result.inplace_pow(exponent, modulus)
-
- def __abs__(self):
- result = IntegerGMP(0)
- _gmp.mpz_abs(result._mpz_p, self._mpz_p)
- return result
-
- def sqrt(self, modulus=None):
- """Return the largest Integer that does not
- exceed the square root"""
-
- if modulus is None:
- if self < 0:
- raise ValueError("Square root of negative value")
- result = IntegerGMP(0)
- _gmp.mpz_sqrt(result._mpz_p,
- self._mpz_p)
- else:
- if modulus <= 0:
- raise ValueError("Modulus must be positive")
- modulus = int(modulus)
- result = IntegerGMP(self._tonelli_shanks(int(self) % modulus, modulus))
-
- return result
-
- def __iadd__(self, term):
- if is_native_int(term):
- if 0 <= term < 65536:
- _gmp.mpz_add_ui(self._mpz_p,
- self._mpz_p,
- c_ulong(term))
- return self
- if -65535 < term < 0:
- _gmp.mpz_sub_ui(self._mpz_p,
- self._mpz_p,
- c_ulong(-term))
- return self
- term = IntegerGMP(term)
- _gmp.mpz_add(self._mpz_p,
- self._mpz_p,
- term._mpz_p)
- return self
-
- def __isub__(self, term):
- if is_native_int(term):
- if 0 <= term < 65536:
- _gmp.mpz_sub_ui(self._mpz_p,
- self._mpz_p,
- c_ulong(term))
- return self
- if -65535 < term < 0:
- _gmp.mpz_add_ui(self._mpz_p,
- self._mpz_p,
- c_ulong(-term))
- return self
- term = IntegerGMP(term)
- _gmp.mpz_sub(self._mpz_p,
- self._mpz_p,
- term._mpz_p)
- return self
-
- def __imul__(self, term):
- if is_native_int(term):
- if 0 <= term < 65536:
- _gmp.mpz_mul_ui(self._mpz_p,
- self._mpz_p,
- c_ulong(term))
- return self
- if -65535 < term < 0:
- _gmp.mpz_mul_ui(self._mpz_p,
- self._mpz_p,
- c_ulong(-term))
- _gmp.mpz_neg(self._mpz_p, self._mpz_p)
- return self
- term = IntegerGMP(term)
- _gmp.mpz_mul(self._mpz_p,
- self._mpz_p,
- term._mpz_p)
- return self
-
- def __imod__(self, divisor):
- if not isinstance(divisor, IntegerGMP):
- divisor = IntegerGMP(divisor)
- comp = _gmp.mpz_cmp(divisor._mpz_p,
- divisor._zero_mpz_p)
- if comp == 0:
- raise ZeroDivisionError("Division by zero")
- if comp < 0:
- raise ValueError("Modulus must be positive")
- _gmp.mpz_mod(self._mpz_p,
- self._mpz_p,
- divisor._mpz_p)
- return self
-
- # Boolean/bit operations
- def __and__(self, term):
- result = IntegerGMP(0)
- if not isinstance(term, IntegerGMP):
- term = IntegerGMP(term)
- _gmp.mpz_and(result._mpz_p,
- self._mpz_p,
- term._mpz_p)
- return result
-
- def __or__(self, term):
- result = IntegerGMP(0)
- if not isinstance(term, IntegerGMP):
- term = IntegerGMP(term)
- _gmp.mpz_ior(result._mpz_p,
- self._mpz_p,
- term._mpz_p)
- return result
-
- def __rshift__(self, pos):
- result = IntegerGMP(0)
- if pos < 0:
- raise ValueError("negative shift count")
- if pos > 65536:
- if self < 0:
- return -1
- else:
- return 0
- _gmp.mpz_tdiv_q_2exp(result._mpz_p,
- self._mpz_p,
- c_ulong(int(pos)))
- return result
-
- def __irshift__(self, pos):
- if pos < 0:
- raise ValueError("negative shift count")
- if pos > 65536:
- if self < 0:
- return -1
- else:
- return 0
- _gmp.mpz_tdiv_q_2exp(self._mpz_p,
- self._mpz_p,
- c_ulong(int(pos)))
- return self
-
- def __lshift__(self, pos):
- result = IntegerGMP(0)
- if not 0 <= pos < 65536:
- raise ValueError("Incorrect shift count")
- _gmp.mpz_mul_2exp(result._mpz_p,
- self._mpz_p,
- c_ulong(int(pos)))
- return result
-
- def __ilshift__(self, pos):
- if not 0 <= pos < 65536:
- raise ValueError("Incorrect shift count")
- _gmp.mpz_mul_2exp(self._mpz_p,
- self._mpz_p,
- c_ulong(int(pos)))
- return self
-
- def get_bit(self, n):
- """Return True if the n-th bit is set to 1.
- Bit 0 is the least significant."""
-
- if self < 0:
- raise ValueError("no bit representation for negative values")
- if n < 0:
- raise ValueError("negative bit count")
- if n > 65536:
- return 0
- return bool(_gmp.mpz_tstbit(self._mpz_p,
- c_ulong(int(n))))
-
- # Extra
- def is_odd(self):
- return _gmp.mpz_tstbit(self._mpz_p, 0) == 1
-
- def is_even(self):
- return _gmp.mpz_tstbit(self._mpz_p, 0) == 0
-
- def size_in_bits(self):
- """Return the minimum number of bits that can encode the number."""
-
- if self < 0:
- raise ValueError("Conversion only valid for non-negative numbers")
- return _gmp.mpz_sizeinbase(self._mpz_p, 2)
-
- def size_in_bytes(self):
- """Return the minimum number of bytes that can encode the number."""
- return (self.size_in_bits() - 1) // 8 + 1
-
- def is_perfect_square(self):
- return _gmp.mpz_perfect_square_p(self._mpz_p) != 0
-
- def fail_if_divisible_by(self, small_prime):
- """Raise an exception if the small prime is a divisor."""
-
- if is_native_int(small_prime):
- if 0 < small_prime < 65536:
- if _gmp.mpz_divisible_ui_p(self._mpz_p,
- c_ulong(small_prime)):
- raise ValueError("The value is composite")
- return
- small_prime = IntegerGMP(small_prime)
- if _gmp.mpz_divisible_p(self._mpz_p,
- small_prime._mpz_p):
- raise ValueError("The value is composite")
-
- def multiply_accumulate(self, a, b):
- """Increment the number by the product of a and b."""
-
- if not isinstance(a, IntegerGMP):
- a = IntegerGMP(a)
- if is_native_int(b):
- if 0 < b < 65536:
- _gmp.mpz_addmul_ui(self._mpz_p,
- a._mpz_p,
- c_ulong(b))
- return self
- if -65535 < b < 0:
- _gmp.mpz_submul_ui(self._mpz_p,
- a._mpz_p,
- c_ulong(-b))
- return self
- b = IntegerGMP(b)
- _gmp.mpz_addmul(self._mpz_p,
- a._mpz_p,
- b._mpz_p)
- return self
-
- def set(self, source):
- """Set the Integer to have the given value"""
-
- if not isinstance(source, IntegerGMP):
- source = IntegerGMP(source)
- _gmp.mpz_set(self._mpz_p,
- source._mpz_p)
- return self
-
- def inplace_inverse(self, modulus):
- """Compute the inverse of this number in the ring of
- modulo integers.
-
- Raise an exception if no inverse exists.
- """
-
- if not isinstance(modulus, IntegerGMP):
- modulus = IntegerGMP(modulus)
-
- comp = _gmp.mpz_cmp(modulus._mpz_p,
- self._zero_mpz_p)
- if comp == 0:
- raise ZeroDivisionError("Modulus cannot be zero")
- if comp < 0:
- raise ValueError("Modulus must be positive")
-
- result = _gmp.mpz_invert(self._mpz_p,
- self._mpz_p,
- modulus._mpz_p)
- if not result:
- raise ValueError("No inverse value can be computed")
- return self
-
- def inverse(self, modulus):
- result = IntegerGMP(self)
- result.inplace_inverse(modulus)
- return result
-
- def gcd(self, term):
- """Compute the greatest common denominator between this
- number and another term."""
-
- result = IntegerGMP(0)
- if is_native_int(term):
- if 0 < term < 65535:
- _gmp.mpz_gcd_ui(result._mpz_p,
- self._mpz_p,
- c_ulong(term))
- return result
- term = IntegerGMP(term)
- _gmp.mpz_gcd(result._mpz_p, self._mpz_p, term._mpz_p)
- return result
-
- def lcm(self, term):
- """Compute the least common multiplier between this
- number and another term."""
-
- result = IntegerGMP(0)
- if not isinstance(term, IntegerGMP):
- term = IntegerGMP(term)
- _gmp.mpz_lcm(result._mpz_p, self._mpz_p, term._mpz_p)
- return result
-
- @staticmethod
- def jacobi_symbol(a, n):
- """Compute the Jacobi symbol"""
-
- if not isinstance(a, IntegerGMP):
- a = IntegerGMP(a)
- if not isinstance(n, IntegerGMP):
- n = IntegerGMP(n)
- if n <= 0 or n.is_even():
- raise ValueError("n must be positive odd for the Jacobi symbol")
- return _gmp.mpz_jacobi(a._mpz_p, n._mpz_p)
-
- # Clean-up
- def __del__(self):
-
- try:
- if self._mpz_p is not None:
- if self._initialized:
- _gmp.mpz_clear(self._mpz_p)
-
- self._mpz_p = None
- except AttributeError:
- pass
diff --git a/spaces/arxify/RVC-beta-v2-0618/runtime/Lib/site-packages/PIL/EpsImagePlugin.py b/spaces/arxify/RVC-beta-v2-0618/runtime/Lib/site-packages/PIL/EpsImagePlugin.py
deleted file mode 100644
index 0e434c5c0ea9b1c72ef4f0061a503b0f604ef1d3..0000000000000000000000000000000000000000
--- a/spaces/arxify/RVC-beta-v2-0618/runtime/Lib/site-packages/PIL/EpsImagePlugin.py
+++ /dev/null
@@ -1,414 +0,0 @@
-#
-# The Python Imaging Library.
-# $Id$
-#
-# EPS file handling
-#
-# History:
-# 1995-09-01 fl Created (0.1)
-# 1996-05-18 fl Don't choke on "atend" fields, Ghostscript interface (0.2)
-# 1996-08-22 fl Don't choke on floating point BoundingBox values
-# 1996-08-23 fl Handle files from Macintosh (0.3)
-# 2001-02-17 fl Use 're' instead of 'regex' (Python 2.1) (0.4)
-# 2003-09-07 fl Check gs.close status (from Federico Di Gregorio) (0.5)
-# 2014-05-07 e Handling of EPS with binary preview and fixed resolution
-# resizing
-#
-# Copyright (c) 1997-2003 by Secret Labs AB.
-# Copyright (c) 1995-2003 by Fredrik Lundh
-#
-# See the README file for information on usage and redistribution.
-#
-
-import io
-import os
-import re
-import subprocess
-import sys
-import tempfile
-
-from . import Image, ImageFile
-from ._binary import i32le as i32
-
-#
-# --------------------------------------------------------------------
-
-split = re.compile(r"^%%([^:]*):[ \t]*(.*)[ \t]*$")
-field = re.compile(r"^%[%!\w]([^:]*)[ \t]*$")
-
-gs_windows_binary = None
-if sys.platform.startswith("win"):
- import shutil
-
- for binary in ("gswin32c", "gswin64c", "gs"):
- if shutil.which(binary) is not None:
- gs_windows_binary = binary
- break
- else:
- gs_windows_binary = False
-
-
-def has_ghostscript():
- if gs_windows_binary:
- return True
- if not sys.platform.startswith("win"):
- try:
- subprocess.check_call(["gs", "--version"], stdout=subprocess.DEVNULL)
- return True
- except OSError:
- # No Ghostscript
- pass
- return False
-
-
-def Ghostscript(tile, size, fp, scale=1, transparency=False):
- """Render an image using Ghostscript"""
-
- # Unpack decoder tile
- decoder, tile, offset, data = tile[0]
- length, bbox = data
-
- # Hack to support hi-res rendering
- scale = int(scale) or 1
- # orig_size = size
- # orig_bbox = bbox
- size = (size[0] * scale, size[1] * scale)
- # resolution is dependent on bbox and size
- res = (
- 72.0 * size[0] / (bbox[2] - bbox[0]),
- 72.0 * size[1] / (bbox[3] - bbox[1]),
- )
-
- out_fd, outfile = tempfile.mkstemp()
- os.close(out_fd)
-
- infile_temp = None
- if hasattr(fp, "name") and os.path.exists(fp.name):
- infile = fp.name
- else:
- in_fd, infile_temp = tempfile.mkstemp()
- os.close(in_fd)
- infile = infile_temp
-
- # Ignore length and offset!
- # Ghostscript can read it
- # Copy whole file to read in Ghostscript
- with open(infile_temp, "wb") as f:
- # fetch length of fp
- fp.seek(0, io.SEEK_END)
- fsize = fp.tell()
- # ensure start position
- # go back
- fp.seek(0)
- lengthfile = fsize
- while lengthfile > 0:
- s = fp.read(min(lengthfile, 100 * 1024))
- if not s:
- break
- lengthfile -= len(s)
- f.write(s)
-
- device = "pngalpha" if transparency else "ppmraw"
-
- # Build Ghostscript command
- command = [
- "gs",
- "-q", # quiet mode
- "-g%dx%d" % size, # set output geometry (pixels)
- "-r%fx%f" % res, # set input DPI (dots per inch)
- "-dBATCH", # exit after processing
- "-dNOPAUSE", # don't pause between pages
- "-dSAFER", # safe mode
- f"-sDEVICE={device}",
- f"-sOutputFile={outfile}", # output file
- # adjust for image origin
- "-c",
- f"{-bbox[0]} {-bbox[1]} translate",
- "-f",
- infile, # input file
- # showpage (see https://bugs.ghostscript.com/show_bug.cgi?id=698272)
- "-c",
- "showpage",
- ]
-
- if gs_windows_binary is not None:
- if not gs_windows_binary:
- raise OSError("Unable to locate Ghostscript on paths")
- command[0] = gs_windows_binary
-
- # push data through Ghostscript
- try:
- startupinfo = None
- if sys.platform.startswith("win"):
- startupinfo = subprocess.STARTUPINFO()
- startupinfo.dwFlags |= subprocess.STARTF_USESHOWWINDOW
- subprocess.check_call(command, startupinfo=startupinfo)
- out_im = Image.open(outfile)
- out_im.load()
- finally:
- try:
- os.unlink(outfile)
- if infile_temp:
- os.unlink(infile_temp)
- except OSError:
- pass
-
- im = out_im.im.copy()
- out_im.close()
- return im
-
-
-class PSFile:
- """
- Wrapper for bytesio object that treats either CR or LF as end of line.
- """
-
- def __init__(self, fp):
- self.fp = fp
- self.char = None
-
- def seek(self, offset, whence=io.SEEK_SET):
- self.char = None
- self.fp.seek(offset, whence)
-
- def readline(self):
- s = [self.char or b""]
- self.char = None
-
- c = self.fp.read(1)
- while (c not in b"\r\n") and len(c):
- s.append(c)
- c = self.fp.read(1)
-
- self.char = self.fp.read(1)
- # line endings can be 1 or 2 of \r \n, in either order
- if self.char in b"\r\n":
- self.char = None
-
- return b"".join(s).decode("latin-1")
-
-
-def _accept(prefix):
- return prefix[:4] == b"%!PS" or (len(prefix) >= 4 and i32(prefix) == 0xC6D3D0C5)
-
-
-##
-# Image plugin for Encapsulated PostScript. This plugin supports only
-# a few variants of this format.
-
-
-class EpsImageFile(ImageFile.ImageFile):
- """EPS File Parser for the Python Imaging Library"""
-
- format = "EPS"
- format_description = "Encapsulated Postscript"
-
- mode_map = {1: "L", 2: "LAB", 3: "RGB", 4: "CMYK"}
-
- def _open(self):
- (length, offset) = self._find_offset(self.fp)
-
- # Rewrap the open file pointer in something that will
- # convert line endings and decode to latin-1.
- fp = PSFile(self.fp)
-
- # go to offset - start of "%!PS"
- fp.seek(offset)
-
- box = None
-
- self.mode = "RGB"
- self._size = 1, 1 # FIXME: huh?
-
- #
- # Load EPS header
-
- s_raw = fp.readline()
- s = s_raw.strip("\r\n")
-
- while s_raw:
- if s:
- if len(s) > 255:
- raise SyntaxError("not an EPS file")
-
- try:
- m = split.match(s)
- except re.error as e:
- raise SyntaxError("not an EPS file") from e
-
- if m:
- k, v = m.group(1, 2)
- self.info[k] = v
- if k == "BoundingBox":
- try:
- # Note: The DSC spec says that BoundingBox
- # fields should be integers, but some drivers
- # put floating point values there anyway.
- box = [int(float(i)) for i in v.split()]
- self._size = box[2] - box[0], box[3] - box[1]
- self.tile = [
- ("eps", (0, 0) + self.size, offset, (length, box))
- ]
- except Exception:
- pass
-
- else:
- m = field.match(s)
- if m:
- k = m.group(1)
-
- if k == "EndComments":
- break
- if k[:8] == "PS-Adobe":
- self.info[k[:8]] = k[9:]
- else:
- self.info[k] = ""
- elif s[0] == "%":
- # handle non-DSC PostScript comments that some
- # tools mistakenly put in the Comments section
- pass
- else:
- raise OSError("bad EPS header")
-
- s_raw = fp.readline()
- s = s_raw.strip("\r\n")
-
- if s and s[:1] != "%":
- break
-
- #
- # Scan for an "ImageData" descriptor
-
- while s[:1] == "%":
-
- if len(s) > 255:
- raise SyntaxError("not an EPS file")
-
- if s[:11] == "%ImageData:":
- # Encoded bitmapped image.
- x, y, bi, mo = s[11:].split(None, 7)[:4]
-
- if int(bi) == 1:
- self.mode = "1"
- elif int(bi) == 8:
- try:
- self.mode = self.mode_map[int(mo)]
- except ValueError:
- break
- else:
- break
-
- self._size = int(x), int(y)
- return
-
- s = fp.readline().strip("\r\n")
- if not s:
- break
-
- if not box:
- raise OSError("cannot determine EPS bounding box")
-
- def _find_offset(self, fp):
-
- s = fp.read(160)
-
- if s[:4] == b"%!PS":
- # for HEAD without binary preview
- fp.seek(0, io.SEEK_END)
- length = fp.tell()
- offset = 0
- elif i32(s, 0) == 0xC6D3D0C5:
- # FIX for: Some EPS file not handled correctly / issue #302
- # EPS can contain binary data
- # or start directly with latin coding
- # more info see:
- # https://web.archive.org/web/20160528181353/http://partners.adobe.com/public/developer/en/ps/5002.EPSF_Spec.pdf
- offset = i32(s, 4)
- length = i32(s, 8)
- else:
- raise SyntaxError("not an EPS file")
-
- return length, offset
-
- def load(self, scale=1, transparency=False):
- # Load EPS via Ghostscript
- if self.tile:
- self.im = Ghostscript(self.tile, self.size, self.fp, scale, transparency)
- self.mode = self.im.mode
- self._size = self.im.size
- self.tile = []
- return Image.Image.load(self)
-
- def load_seek(self, *args, **kwargs):
- # we can't incrementally load, so force ImageFile.parser to
- # use our custom load method by defining this method.
- pass
-
-
-#
-# --------------------------------------------------------------------
-
-
-def _save(im, fp, filename, eps=1):
- """EPS Writer for the Python Imaging Library."""
-
- #
- # make sure image data is available
- im.load()
-
- #
- # determine PostScript image mode
- if im.mode == "L":
- operator = (8, 1, b"image")
- elif im.mode == "RGB":
- operator = (8, 3, b"false 3 colorimage")
- elif im.mode == "CMYK":
- operator = (8, 4, b"false 4 colorimage")
- else:
- raise ValueError("image mode is not supported")
-
- if eps:
- #
- # write EPS header
- fp.write(b"%!PS-Adobe-3.0 EPSF-3.0\n")
- fp.write(b"%%Creator: PIL 0.1 EpsEncode\n")
- # fp.write("%%CreationDate: %s"...)
- fp.write(b"%%%%BoundingBox: 0 0 %d %d\n" % im.size)
- fp.write(b"%%Pages: 1\n")
- fp.write(b"%%EndComments\n")
- fp.write(b"%%Page: 1 1\n")
- fp.write(b"%%ImageData: %d %d " % im.size)
- fp.write(b'%d %d 0 1 1 "%s"\n' % operator)
-
- #
- # image header
- fp.write(b"gsave\n")
- fp.write(b"10 dict begin\n")
- fp.write(b"/buf %d string def\n" % (im.size[0] * operator[1]))
- fp.write(b"%d %d scale\n" % im.size)
- fp.write(b"%d %d 8\n" % im.size) # <= bits
- fp.write(b"[%d 0 0 -%d 0 %d]\n" % (im.size[0], im.size[1], im.size[1]))
- fp.write(b"{ currentfile buf readhexstring pop } bind\n")
- fp.write(operator[2] + b"\n")
- if hasattr(fp, "flush"):
- fp.flush()
-
- ImageFile._save(im, fp, [("eps", (0, 0) + im.size, 0, None)])
-
- fp.write(b"\n%%%%EndBinary\n")
- fp.write(b"grestore end\n")
- if hasattr(fp, "flush"):
- fp.flush()
-
-
-#
-# --------------------------------------------------------------------
-
-
-Image.register_open(EpsImageFile.format, EpsImageFile, _accept)
-
-Image.register_save(EpsImageFile.format, _save)
-
-Image.register_extensions(EpsImageFile.format, [".ps", ".eps"])
-
-Image.register_mime(EpsImageFile.format, "application/postscript")
diff --git a/spaces/arxify/RVC-beta-v2-0618/runtime/Lib/site-packages/altair/examples/errorbars_with_ci.py b/spaces/arxify/RVC-beta-v2-0618/runtime/Lib/site-packages/altair/examples/errorbars_with_ci.py
deleted file mode 100644
index ec70b27b4943e0bc64b0f8d0fcc7cd45c3ffbac3..0000000000000000000000000000000000000000
--- a/spaces/arxify/RVC-beta-v2-0618/runtime/Lib/site-packages/altair/examples/errorbars_with_ci.py
+++ /dev/null
@@ -1,24 +0,0 @@
-"""
-Error Bars showing Confidence Interval
-======================================
-This example shows how to show error bars using confidence intervals.
-The confidence intervals are computed internally in vega by a non-parametric
-`bootstrap of the mean `_.
-"""
-# category: other charts
-import altair as alt
-from vega_datasets import data
-
-source = data.barley()
-
-error_bars = alt.Chart(source).mark_errorbar(extent='ci').encode(
- x=alt.X('yield:Q', scale=alt.Scale(zero=False)),
- y=alt.Y('variety:N')
-)
-
-points = alt.Chart(source).mark_point(filled=True, color='black').encode(
- x=alt.X('yield:Q', aggregate='mean'),
- y=alt.Y('variety:N'),
-)
-
-error_bars + points
diff --git a/spaces/arxify/RVC-beta-v2-0618/runtime/Lib/site-packages/altair/examples/trellis_histogram.py b/spaces/arxify/RVC-beta-v2-0618/runtime/Lib/site-packages/altair/examples/trellis_histogram.py
deleted file mode 100644
index cba1c698b7872dde56f1108cc7fada9bf0abaa4d..0000000000000000000000000000000000000000
--- a/spaces/arxify/RVC-beta-v2-0618/runtime/Lib/site-packages/altair/examples/trellis_histogram.py
+++ /dev/null
@@ -1,17 +0,0 @@
-"""
-Trellis Histogram
------------------
-This example shows how to make a basic trellis histogram.
-https://vega.github.io/vega-lite/examples/trellis_bar_histogram.html
-"""
-# category: histograms
-import altair as alt
-from vega_datasets import data
-
-source = data.cars()
-
-alt.Chart(source).mark_bar().encode(
- alt.X("Horsepower:Q", bin=True),
- y='count()',
- row='Origin'
-)
diff --git a/spaces/atimughal662/InfoFusion/src/loaders.py b/spaces/atimughal662/InfoFusion/src/loaders.py
deleted file mode 100644
index 8c147670eb9433f5a26c8e25121b0e1e62f250e6..0000000000000000000000000000000000000000
--- a/spaces/atimughal662/InfoFusion/src/loaders.py
+++ /dev/null
@@ -1,120 +0,0 @@
-import functools
-
-from src.enums import t5_type
-
-
-def get_loaders(model_name, reward_type, llama_type=None, load_gptq='', load_exllama=False, config=None,
- rope_scaling=None, max_seq_len=None, model_name_exllama_if_no_config=''):
- # NOTE: Some models need specific new prompt_type
- # E.g. t5_xxl_true_nli_mixture has input format: "premise: PREMISE_TEXT hypothesis: HYPOTHESIS_TEXT".)
- if load_exllama:
- from src.llm_exllama import H2OExLlamaTokenizer, H2OExLlamaGenerator
- from exllama.model import ExLlama, ExLlamaCache, ExLlamaConfig
- import os, glob
-
- if config:
- # then use HF path
- from transformers import TRANSFORMERS_CACHE
- model_directory = os.path.join(TRANSFORMERS_CACHE, 'models--' + config.name_or_path.replace('/', '--'),
- 'snapshots', config._commit_hash)
- else:
- # then use path in env file
- # Directory containing model, tokenizer, generator
- model_directory = model_name_exllama_if_no_config
-
- # download model
- revision = config._commit_hash
- from huggingface_hub import snapshot_download
- snapshot_download(repo_id=model_name, revision=revision)
-
- # Locate files we need within that directory
- tokenizer_path = os.path.join(model_directory, "tokenizer.model")
- assert os.path.isfile(tokenizer_path), "Missing %s" % tokenizer_path
- model_config_path = os.path.join(model_directory, "config.json")
- assert os.path.isfile(model_config_path), "Missing %s" % model_config_path
- st_pattern = os.path.join(model_directory, "*.safetensors")
- model_path = glob.glob(st_pattern)[0]
- assert os.path.isfile(model_path), "Missing %s" % model_path
-
- # Create config, model, tokenizer and generator
- exconfig = ExLlamaConfig(model_config_path) # create config from config.json
- rope_scaling = rope_scaling or {}
- exconfig.alpha_value = rope_scaling.get('alpha_value', 1) # rope
- exconfig.compress_pos_emb = rope_scaling.get('compress_pos_emb', 1) # related rope
- # update max_seq_len
- assert hasattr(config, 'max_position_embeddings') or hasattr(config,
- 'max_sequence_length'), "Improve code if no such argument"
- if hasattr(config, 'max_position_embeddings'):
- exconfig.max_seq_len = int(config.max_position_embeddings * exconfig.alpha_value)
- else:
- exconfig.max_seq_len = int(config.max_sequence_length * exconfig.alpha_value)
- if 'Llama-2'.lower() in model_name.lower():
- # override bad defaults
- exconfig.max_seq_len = int(4096 * exconfig.alpha_value)
- if max_seq_len is not None:
- exconfig.max_seq_len = max_seq_len
-
- exconfig.model_path = model_path # supply path to model weights file
-
- model = ExLlama(exconfig) # create ExLlama instance and load the weights
- tokenizer = H2OExLlamaTokenizer(tokenizer_path) # create tokenizer from tokenizer model file
- tokenizer.model_max_length = exconfig.max_seq_len
-
- cache = ExLlamaCache(model) # create cache for inference
- generator = H2OExLlamaGenerator(model, tokenizer, cache) # create generator
- return generator, tokenizer, False
- if load_gptq:
- from transformers import AutoTokenizer
- from auto_gptq import AutoGPTQForCausalLM
- use_triton = False
- model_loader = functools.partial(AutoGPTQForCausalLM.from_quantized,
- quantize_config=None, use_triton=use_triton,
- )
- return model_loader, AutoTokenizer, False
- if llama_type is None:
- llama_type = "llama" in model_name.lower()
- if llama_type:
- from transformers import LlamaForCausalLM, LlamaTokenizer
- return LlamaForCausalLM.from_pretrained, LlamaTokenizer, False
- elif 'distilgpt2' in model_name.lower():
- from transformers import AutoModelForCausalLM, AutoTokenizer
- return AutoModelForCausalLM.from_pretrained, AutoTokenizer, False
- elif 'gpt2' in model_name.lower():
- from transformers import GPT2LMHeadModel, GPT2Tokenizer
- return GPT2LMHeadModel.from_pretrained, GPT2Tokenizer, False
- elif 'mbart-' in model_name.lower():
- from transformers import MBartForConditionalGeneration, MBart50TokenizerFast
- return MBartForConditionalGeneration.from_pretrained, MBart50TokenizerFast, True
- elif t5_type(model_name):
- from transformers import AutoTokenizer, T5ForConditionalGeneration
- return T5ForConditionalGeneration.from_pretrained, AutoTokenizer, True
- elif 'bigbird' in model_name:
- from transformers import BigBirdPegasusForConditionalGeneration, AutoTokenizer
- return BigBirdPegasusForConditionalGeneration.from_pretrained, AutoTokenizer, True
- elif 'bart-large-cnn-samsum' in model_name or 'flan-t5-base-samsum' in model_name:
- from transformers import pipeline
- return pipeline, "summarization", False
- elif reward_type or 'OpenAssistant/reward-model'.lower() in model_name.lower():
- from transformers import AutoModelForSequenceClassification, AutoTokenizer
- return AutoModelForSequenceClassification.from_pretrained, AutoTokenizer, False
- else:
- from transformers import AutoTokenizer, AutoModelForCausalLM
- model_loader = AutoModelForCausalLM
- tokenizer_loader = AutoTokenizer
- return model_loader.from_pretrained, tokenizer_loader, False
-
-
-def get_tokenizer(tokenizer_loader, tokenizer_base_model, local_files_only, resume_download, use_auth_token):
- tokenizer = tokenizer_loader.from_pretrained(tokenizer_base_model,
- local_files_only=local_files_only,
- resume_download=resume_download,
- use_auth_token=use_auth_token,
- padding_side='left')
-
- tokenizer.pad_token_id = 0 # different from the eos token
- # when generating, we will use the logits of right-most token to predict the next token
- # so the padding should be on the left,
- # e.g. see: https://huggingface.co/transformers/v4.11.3/model_doc/t5.html#inference
- tokenizer.padding_side = "left" # Allow batched inference
-
- return tokenizer
diff --git a/spaces/avid-ml/bias-detection/avidtools/connectors/aiid.py b/spaces/avid-ml/bias-detection/avidtools/connectors/aiid.py
deleted file mode 100644
index e69de29bb2d1d6434b8b29ae775ad8c2e48c5391..0000000000000000000000000000000000000000
diff --git a/spaces/avivdm1/AutoGPT/autogpt/processing/html.py b/spaces/avivdm1/AutoGPT/autogpt/processing/html.py
deleted file mode 100644
index 81387b12adab5023150c55f2075ddd40b554f386..0000000000000000000000000000000000000000
--- a/spaces/avivdm1/AutoGPT/autogpt/processing/html.py
+++ /dev/null
@@ -1,33 +0,0 @@
-"""HTML processing functions"""
-from __future__ import annotations
-
-from bs4 import BeautifulSoup
-from requests.compat import urljoin
-
-
-def extract_hyperlinks(soup: BeautifulSoup, base_url: str) -> list[tuple[str, str]]:
- """Extract hyperlinks from a BeautifulSoup object
-
- Args:
- soup (BeautifulSoup): The BeautifulSoup object
- base_url (str): The base URL
-
- Returns:
- List[Tuple[str, str]]: The extracted hyperlinks
- """
- return [
- (link.text, urljoin(base_url, link["href"]))
- for link in soup.find_all("a", href=True)
- ]
-
-
-def format_hyperlinks(hyperlinks: list[tuple[str, str]]) -> list[str]:
- """Format hyperlinks to be displayed to the user
-
- Args:
- hyperlinks (List[Tuple[str, str]]): The hyperlinks to format
-
- Returns:
- List[str]: The formatted hyperlinks
- """
- return [f"{link_text} ({link_url})" for link_text, link_url in hyperlinks]
diff --git a/spaces/awaawawawa/iurf7irfuyytruyyugb/ldmlib/modules/ema.py b/spaces/awaawawawa/iurf7irfuyytruyyugb/ldmlib/modules/ema.py
deleted file mode 100644
index c8c75af43565f6e140287644aaaefa97dd6e67c5..0000000000000000000000000000000000000000
--- a/spaces/awaawawawa/iurf7irfuyytruyyugb/ldmlib/modules/ema.py
+++ /dev/null
@@ -1,76 +0,0 @@
-import torch
-from torch import nn
-
-
-class LitEma(nn.Module):
- def __init__(self, model, decay=0.9999, use_num_upates=True):
- super().__init__()
- if decay < 0.0 or decay > 1.0:
- raise ValueError('Decay must be between 0 and 1')
-
- self.m_name2s_name = {}
- self.register_buffer('decay', torch.tensor(decay, dtype=torch.float32))
- self.register_buffer('num_updates', torch.tensor(0,dtype=torch.int) if use_num_upates
- else torch.tensor(-1,dtype=torch.int))
-
- for name, p in model.named_parameters():
- if p.requires_grad:
- #remove as '.'-character is not allowed in buffers
- s_name = name.replace('.','')
- self.m_name2s_name.update({name:s_name})
- self.register_buffer(s_name,p.clone().detach().data)
-
- self.collected_params = []
-
- def forward(self,model):
- decay = self.decay
-
- if self.num_updates >= 0:
- self.num_updates += 1
- decay = min(self.decay,(1 + self.num_updates) / (10 + self.num_updates))
-
- one_minus_decay = 1.0 - decay
-
- with torch.no_grad():
- m_param = dict(model.named_parameters())
- shadow_params = dict(self.named_buffers())
-
- for key in m_param:
- if m_param[key].requires_grad:
- sname = self.m_name2s_name[key]
- shadow_params[sname] = shadow_params[sname].type_as(m_param[key])
- shadow_params[sname].sub_(one_minus_decay * (shadow_params[sname] - m_param[key]))
- else:
- assert not key in self.m_name2s_name
-
- def copy_to(self, model):
- m_param = dict(model.named_parameters())
- shadow_params = dict(self.named_buffers())
- for key in m_param:
- if m_param[key].requires_grad:
- m_param[key].data.copy_(shadow_params[self.m_name2s_name[key]].data)
- else:
- assert not key in self.m_name2s_name
-
- def store(self, parameters):
- """
- Save the current parameters for restoring later.
- Args:
- parameters: Iterable of `torch.nn.Parameter`; the parameters to be
- temporarily stored.
- """
- self.collected_params = [param.clone() for param in parameters]
-
- def restore(self, parameters):
- """
- Restore the parameters stored with the `store` method.
- Useful to validate the model with EMA parameters without affecting the
- original optimization process. Store the parameters before the
- `copy_to` method. After validation (or model saving), use this to
- restore the former parameters.
- Args:
- parameters: Iterable of `torch.nn.Parameter`; the parameters to be
- updated with the stored parameters.
- """
- for c_param, param in zip(self.collected_params, parameters):
- param.data.copy_(c_param.data)
diff --git a/spaces/awacke1/FastestText2SpeechEver/README.md b/spaces/awacke1/FastestText2SpeechEver/README.md
deleted file mode 100644
index d7333db192bc50fcd391b6046e5e7a3dabf37b67..0000000000000000000000000000000000000000
--- a/spaces/awacke1/FastestText2SpeechEver/README.md
+++ /dev/null
@@ -1,12 +0,0 @@
----
-title: FastestText2SpeechEver
-emoji: 👀
-colorFrom: red
-colorTo: green
-sdk: gradio
-sdk_version: 3.38.0
-app_file: app.py
-pinned: false
----
-
-Check out the configuration reference at https://huggingface.co/docs/hub/spaces-config-reference
diff --git a/spaces/awacke1/PlantFractalsMathGameWithJuliaSetnStrangeAttractors/README.md b/spaces/awacke1/PlantFractalsMathGameWithJuliaSetnStrangeAttractors/README.md
deleted file mode 100644
index 73aad519d66e29aa126784d669f3f59fc123e555..0000000000000000000000000000000000000000
--- a/spaces/awacke1/PlantFractalsMathGameWithJuliaSetnStrangeAttractors/README.md
+++ /dev/null
@@ -1,13 +0,0 @@
----
-title: 🎲🌱Plant🌱Fractals🎲Math🎲GameWith⬡JuliaSet⬡n⬡StrangeAttractors⬡🎲
-emoji: 🎲⬡🌱🌱🌱⬡🎲
-colorFrom: indigo
-colorTo: indigo
-sdk: streamlit
-sdk_version: 1.17.0
-app_file: app.py
-pinned: false
-license: mit
----
-
-Check out the configuration reference at https://huggingface.co/docs/hub/spaces-config-reference
diff --git a/spaces/awacke1/VoiceChatMistral/app.py b/spaces/awacke1/VoiceChatMistral/app.py
deleted file mode 100644
index 482fa65ee96482fef83e522bb7ab71c3df0bd009..0000000000000000000000000000000000000000
--- a/spaces/awacke1/VoiceChatMistral/app.py
+++ /dev/null
@@ -1,212 +0,0 @@
-import gradio as gr
-import numpy as np
-import torch
-import nltk
-import os
-import uuid
-
-from __future__ import annotations
-
-os.environ["COQUI_TOS_AGREED"] = "1"
-nltk.download('punkt')
-from TTS.api import TTS
-from huggingface_hub import HfApi
-HF_TOKEN = os.environ.get("HF_TOKEN")
-
-
-tts = TTS("tts_models/multilingual/multi-dataset/xtts_v1", gpu=True)
-title = "Voice Chat Mistral"
-DESCRIPTION = title
-css = """.toast-wrap { display: none !important } """
-api = HfApi(token=HF_TOKEN)
-repo_id = "ylacombe/voice-chat-with-lama"
-system_message = "\nYou are a helpful assistant."
-temperature = 0.9
-top_p = 0.6
-repetition_penalty = 1.2
-
-import gradio as gr
-import os
-import time
-
-import gradio as gr
-from transformers import pipeline
-import numpy as np
-
-from gradio_client import Client
-from huggingface_hub import InferenceClient
-
-
-whisper_client = Client("https://sanchit-gandhi-whisper-large-v2.hf.space/")
-text_client = InferenceClient(
- "mistralai/Mistral-7B-Instruct-v0.1"
-)
-
-
-def format_prompt(message, history):
- prompt = ""
- for user_prompt, bot_response in history:
- prompt += f"[INST] {user_prompt} [/INST]"
- prompt += f" {bot_response} "
- prompt += f"[INST] {message} [/INST]"
- return prompt
-
-def generate(
- prompt, history, temperature=0.9, max_new_tokens=256, top_p=0.95, repetition_penalty=1.0,
-):
- temperature = float(temperature)
- if temperature < 1e-2:
- temperature = 1e-2
- top_p = float(top_p)
-
- generate_kwargs = dict(
- temperature=temperature,
- max_new_tokens=max_new_tokens,
- top_p=top_p,
- repetition_penalty=repetition_penalty,
- do_sample=True,
- seed=42,
- )
-
- formatted_prompt = format_prompt(prompt, history)
-
- stream = text_client.text_generation(formatted_prompt, **generate_kwargs, stream=True, details=True, return_full_text=False)
- output = ""
-
- for response in stream:
- output += response.token.text
- yield output
- return output
-
-
-def transcribe(wav_path):
-
- return whisper_client.predict(
- wav_path, # str (filepath or URL to file) in 'inputs' Audio component
- "transcribe", # str in 'Task' Radio component
- api_name="/predict"
-)
-
-
-# Chatbot demo with multimodal input (text, markdown, LaTeX, code blocks, image, audio, & video). Plus shows support for streaming text.
-
-
-def add_text(history, text):
- history = [] if history is None else history
- history = history + [(text, None)]
- return history, gr.update(value="", interactive=False)
-
-
-def add_file(history, file):
- history = [] if history is None else history
- text = transcribe(
- file
- )
-
- history = history + [(text, None)]
- return history
-
-
-
-def bot(history, system_prompt=""):
- history = [] if history is None else history
-
- if system_prompt == "":
- system_prompt = system_message
-
- history[-1][1] = ""
- for character in generate(history[-1][0], history[:-1]):
- history[-1][1] = character
- yield history
-
-
-def generate_speech(history):
- text_to_generate = history[-1][1]
- text_to_generate = text_to_generate.replace("\n", " ").strip()
- text_to_generate = nltk.sent_tokenize(text_to_generate)
-
- filename = f"{uuid.uuid4()}.wav"
- sampling_rate = tts.synthesizer.tts_config.audio["sample_rate"]
- silence = [0] * int(0.25 * sampling_rate)
-
-
- for sentence in text_to_generate:
- try:
-
- # generate speech by cloning a voice using default settings
- wav = tts.tts(text=sentence,
- speaker_wav="examples/female.wav",
- decoder_iterations=25,
- decoder_sampler="dpm++2m",
- speed=1.2,
- language="en")
-
- yield (sampling_rate, np.array(wav)) #np.array(wav + silence))
-
- except RuntimeError as e :
- if "device-side assert" in str(e):
- # cannot do anything on cuda device side error, need tor estart
- print(f"Exit due to: Unrecoverable exception caused by prompt:{sentence}", flush=True)
- gr.Warning("Unhandled Exception encounter, please retry in a minute")
- print("Cuda device-assert Runtime encountered need restart")
-
-
- # HF Space specific.. This error is unrecoverable need to restart space
- api.restart_space(repo_id=repo_id)
- else:
- print("RuntimeError: non device-side assert error:", str(e))
- raise e
-
-with gr.Blocks(title=title) as demo:
- gr.Markdown(DESCRIPTION)
-
-
- chatbot = gr.Chatbot(
- [],
- elem_id="chatbot",
- avatar_images=('examples/lama.jpeg', 'examples/lama2.jpeg'),
- bubble_full_width=False,
- )
-
- with gr.Row():
- txt = gr.Textbox(
- scale=3,
- show_label=False,
- placeholder="Enter text and press enter, or speak to your microphone",
- container=False,
- )
- txt_btn = gr.Button(value="Submit text",scale=1)
- btn = gr.Audio(source="microphone", type="filepath", scale=4)
-
- with gr.Row():
- audio = gr.Audio(type="numpy", streaming=True, autoplay=True, label="Generated audio response", show_label=True)
-
- clear_btn = gr.ClearButton([chatbot, audio])
-
- txt_msg = txt_btn.click(add_text, [chatbot, txt], [chatbot, txt], queue=False).then(
- bot, chatbot, chatbot
- ).then(generate_speech, chatbot, audio)
-
- txt_msg.then(lambda: gr.update(interactive=True), None, [txt], queue=False)
-
- txt_msg = txt.submit(add_text, [chatbot, txt], [chatbot, txt], queue=False).then(
- bot, chatbot, chatbot
- ).then(generate_speech, chatbot, audio)
-
- txt_msg.then(lambda: gr.update(interactive=True), None, [txt], queue=False)
-
- file_msg = btn.stop_recording(add_file, [chatbot, btn], [chatbot], queue=False).then(
- bot, chatbot, chatbot
- ).then(generate_speech, chatbot, audio)
-
-
- gr.Markdown("""
-This Space demonstrates how to speak to a chatbot, based solely on open-source models.
-It relies on 3 models:
-1. [Whisper-large-v2](https://huggingface.co/spaces/sanchit-gandhi/whisper-large-v2) as an ASR model, to transcribe recorded audio to text. It is called through a [gradio client](https://www.gradio.app/docs/client).
-2. [Mistral-7b-instruct](https://huggingface.co/spaces/osanseviero/mistral-super-fast) as the chat model, the actual chat model. It is called from [huggingface_hub](https://huggingface.co/docs/huggingface_hub/guides/inference).
-3. [Coqui's XTTS](https://huggingface.co/spaces/coqui/xtts) as a TTS model, to generate the chatbot answers. This time, the model is hosted locally.
-Note:
-- By using this demo you agree to the terms of the Coqui Public Model License at https://coqui.ai/cpml""")
-demo.queue()
-demo.launch(debug=True)
\ No newline at end of file
diff --git a/spaces/awacke1/Z-3-ChatbotBlenderBot-GR/app.py b/spaces/awacke1/Z-3-ChatbotBlenderBot-GR/app.py
deleted file mode 100644
index ca545aad434176426ca5ee2190b8e753d46a10df..0000000000000000000000000000000000000000
--- a/spaces/awacke1/Z-3-ChatbotBlenderBot-GR/app.py
+++ /dev/null
@@ -1,134 +0,0 @@
-from transformers import BlenderbotTokenizer, BlenderbotForConditionalGeneration
-import torch
-import gradio as gr
-
-
-# PersistDataset -----
-import os
-import csv
-import gradio as gr
-from gradio import inputs, outputs
-import huggingface_hub
-from huggingface_hub import Repository, hf_hub_download, upload_file
-from datetime import datetime
-
-
-# -------------------------------------------- For Memory - you will need to set up a dataset and HF_TOKEN ---------
-#DATASET_REPO_URL = "https://huggingface.co/datasets/awacke1/ChatbotMemory.csv"
-#DATASET_REPO_ID = "awacke1/ChatbotMemory.csv"
-#DATA_FILENAME = "ChatbotMemory.csv"
-#DATA_FILE = os.path.join("data", DATA_FILENAME)
-#HF_TOKEN = os.environ.get("HF_TOKEN")
-
-#SCRIPT = """
-#
-#"""
-
-#try:
-# hf_hub_download(
-# repo_id=DATASET_REPO_ID,
-# filename=DATA_FILENAME,
-# cache_dir=DATA_DIRNAME,
-# force_filename=DATA_FILENAME
-# )
-#except:
-# print("file not found")
-#repo = Repository(
-# local_dir="data", clone_from=DATASET_REPO_URL, use_auth_token=HF_TOKEN
-#)
-
-#def store_message(name: str, message: str):
-# if name and message:
-# with open(DATA_FILE, "a") as csvfile:
-# writer = csv.DictWriter(csvfile, fieldnames=["name", "message", "time"])
-# writer.writerow(
-# {"name": name.strip(), "message": message.strip(), "time": str(datetime.now())}
-# )
-# uncomment line below to begin saving. If creating your own copy you will need to add a access token called "HF_TOKEN" to your profile, then create a secret for your repo with the access code naming it "HF_TOKEN" For the CSV as well you can copy the header and first few lines to your own then update the paths above which should work to save to your own repository for datasets.
-# commit_url = repo.push_to_hub()
-# return ""
-
-#iface = gr.Interface(
-# store_message,
-# [
-# inputs.Textbox(placeholder="Your name"),
-# inputs.Textbox(placeholder="Your message", lines=2),
-# ],
-# "html",
-# css="""
-# .message {background-color:cornflowerblue;color:white; padding:4px;margin:4px;border-radius:4px; }
-# """,
-# title="Reading/writing to a HuggingFace dataset repo from Spaces",
-# description=f"This is a demo of how to do simple *shared data persistence* in a Gradio Space, backed by a dataset repo.",
-# article=f"The dataset repo is [{DATASET_REPO_URL}]({DATASET_REPO_URL})",
-#)
-# --------------------------------------------------- For Memory
-
-mname = "facebook/blenderbot-400M-distill"
-model = BlenderbotForConditionalGeneration.from_pretrained(mname)
-tokenizer = BlenderbotTokenizer.from_pretrained(mname)
-
-def take_last_tokens(inputs, note_history, history):
- """Filter the last 128 tokens"""
- if inputs['input_ids'].shape[1] > 128:
- inputs['input_ids'] = torch.tensor([inputs['input_ids'][0][-128:].tolist()])
- inputs['attention_mask'] = torch.tensor([inputs['attention_mask'][0][-128:].tolist()])
- note_history = [' '.join(note_history[0].split('')[2:])]
- history = history[1:]
- return inputs, note_history, history
-
-def add_note_to_history(note, note_history):
- """Add a note to the historical information"""
- note_history.append(note)
- note_history = ''.join(note_history)
- return [note_history]
-
-title = "State of the Art Chatbot with Memory Dataset"
-description = """Chatbot With Memory"""
-
-def chat(message, history):
- history = history or []
- if history:
- history_useful = [''.join([str(a[0])+''+str(a[1]) for a in history])]
- else:
- history_useful = []
- history_useful = add_note_to_history(message, history_useful)
- inputs = tokenizer(history_useful, return_tensors="pt")
- inputs, history_useful, history = take_last_tokens(inputs, history_useful, history)
- reply_ids = model.generate(**inputs)
- response = tokenizer.batch_decode(reply_ids, skip_special_tokens=True)[0]
- history_useful = add_note_to_history(response, history_useful)
- list_history = history_useful[0].split('')
- history.append((list_history[-2], list_history[-1]))
-# store_message(message, response) # Save to dataset -- uncomment with code above, create a dataset to store and add your HF_TOKEN from profile to this repo to use.
- return history, history
-
-gr.Interface(
- fn=chat,
- theme="huggingface",
- css=".footer {display:none !important}",
- inputs=["text", "state"],
- outputs=["chatbot", "state"],
- title=title,
- allow_flagging="never",
- description=f"Gradio chatbot backed by memory in a dataset repository.",
-# article=f"The dataset repo is [{DATASET_REPO_URL}]({DATASET_REPO_URL})"
- ).launch(debug=True)
-
-#demo = gr.Blocks()
-#with demo:
-# audio_file = gr.inputs.Audio(source="microphone", type="filepath")
-# text = gr.Textbox(label="Speech to Text")
-# TTSchoice = gr.inputs.Radio( label="Pick a Text to Speech Model", choices=MODEL_NAMES, )
-# audio = gr.Audio(label="Output", interactive=False)
-# b1 = gr.Button("Recognize Speech")
-# b5 = gr.Button("Read It Back Aloud")
-# b1.click(speech_to_text, inputs=audio_file, outputs=text)
-# b5.click(tts, inputs=[text,TTSchoice], outputs=audio)
-#demo.launch(share=True)
diff --git a/spaces/aziz28/rsa-app/rsa_app.py b/spaces/aziz28/rsa-app/rsa_app.py
deleted file mode 100644
index 75d99f4a86a8f74cfee26f930de0e86a66b36a9b..0000000000000000000000000000000000000000
--- a/spaces/aziz28/rsa-app/rsa_app.py
+++ /dev/null
@@ -1,32 +0,0 @@
-import rsa
-import streamlit as st
-
-
-st.write("""
-# RSA
-""")
-# generate public and private keys with
-# rsa.newkeys method,this method accepts
-# key length as its parameter
-# key length should be atleast 16
-publicKey, privateKey = rsa.newkeys(512)
-
-# this is the string that we will be encrypting
-input = st.text_input('Masukkan Teks', 'Muhamad Aziz 20220028')
-
-# rsa.encrypt method is used to encrypt
-# string with public key string should be
-# encode to byte string before encryption
-# with encode method
-encMessage = rsa.encrypt(input.encode(),
- publicKey)
-st.write('enkripsi :', encMessage)
-
-# the encrypted message can be decrypted
-# with ras.decrypt method and private key
-# decrypt method returns encoded byte string,
-# use decode method to convert it to string
-# public key cannot be used for decryption
-decMessage = rsa.decrypt(encMessage, privateKey).decode()
-
-st.write('dekripsi :', decMessage)
diff --git a/spaces/azusarang/so-vits-svc-models-ba_P/modules/modules.py b/spaces/azusarang/so-vits-svc-models-ba_P/modules/modules.py
deleted file mode 100644
index 54290fd207b25e93831bd21005990ea137e6b50e..0000000000000000000000000000000000000000
--- a/spaces/azusarang/so-vits-svc-models-ba_P/modules/modules.py
+++ /dev/null
@@ -1,342 +0,0 @@
-import copy
-import math
-import numpy as np
-import scipy
-import torch
-from torch import nn
-from torch.nn import functional as F
-
-from torch.nn import Conv1d, ConvTranspose1d, AvgPool1d, Conv2d
-from torch.nn.utils import weight_norm, remove_weight_norm
-
-import modules.commons as commons
-from modules.commons import init_weights, get_padding
-
-
-LRELU_SLOPE = 0.1
-
-
-class LayerNorm(nn.Module):
- def __init__(self, channels, eps=1e-5):
- super().__init__()
- self.channels = channels
- self.eps = eps
-
- self.gamma = nn.Parameter(torch.ones(channels))
- self.beta = nn.Parameter(torch.zeros(channels))
-
- def forward(self, x):
- x = x.transpose(1, -1)
- x = F.layer_norm(x, (self.channels,), self.gamma, self.beta, self.eps)
- return x.transpose(1, -1)
-
-
-class ConvReluNorm(nn.Module):
- def __init__(self, in_channels, hidden_channels, out_channels, kernel_size, n_layers, p_dropout):
- super().__init__()
- self.in_channels = in_channels
- self.hidden_channels = hidden_channels
- self.out_channels = out_channels
- self.kernel_size = kernel_size
- self.n_layers = n_layers
- self.p_dropout = p_dropout
- assert n_layers > 1, "Number of layers should be larger than 0."
-
- self.conv_layers = nn.ModuleList()
- self.norm_layers = nn.ModuleList()
- self.conv_layers.append(nn.Conv1d(in_channels, hidden_channels, kernel_size, padding=kernel_size//2))
- self.norm_layers.append(LayerNorm(hidden_channels))
- self.relu_drop = nn.Sequential(
- nn.ReLU(),
- nn.Dropout(p_dropout))
- for _ in range(n_layers-1):
- self.conv_layers.append(nn.Conv1d(hidden_channels, hidden_channels, kernel_size, padding=kernel_size//2))
- self.norm_layers.append(LayerNorm(hidden_channels))
- self.proj = nn.Conv1d(hidden_channels, out_channels, 1)
- self.proj.weight.data.zero_()
- self.proj.bias.data.zero_()
-
- def forward(self, x, x_mask):
- x_org = x
- for i in range(self.n_layers):
- x = self.conv_layers[i](x * x_mask)
- x = self.norm_layers[i](x)
- x = self.relu_drop(x)
- x = x_org + self.proj(x)
- return x * x_mask
-
-
-class DDSConv(nn.Module):
- """
- Dialted and Depth-Separable Convolution
- """
- def __init__(self, channels, kernel_size, n_layers, p_dropout=0.):
- super().__init__()
- self.channels = channels
- self.kernel_size = kernel_size
- self.n_layers = n_layers
- self.p_dropout = p_dropout
-
- self.drop = nn.Dropout(p_dropout)
- self.convs_sep = nn.ModuleList()
- self.convs_1x1 = nn.ModuleList()
- self.norms_1 = nn.ModuleList()
- self.norms_2 = nn.ModuleList()
- for i in range(n_layers):
- dilation = kernel_size ** i
- padding = (kernel_size * dilation - dilation) // 2
- self.convs_sep.append(nn.Conv1d(channels, channels, kernel_size,
- groups=channels, dilation=dilation, padding=padding
- ))
- self.convs_1x1.append(nn.Conv1d(channels, channels, 1))
- self.norms_1.append(LayerNorm(channels))
- self.norms_2.append(LayerNorm(channels))
-
- def forward(self, x, x_mask, g=None):
- if g is not None:
- x = x + g
- for i in range(self.n_layers):
- y = self.convs_sep[i](x * x_mask)
- y = self.norms_1[i](y)
- y = F.gelu(y)
- y = self.convs_1x1[i](y)
- y = self.norms_2[i](y)
- y = F.gelu(y)
- y = self.drop(y)
- x = x + y
- return x * x_mask
-
-
-class WN(torch.nn.Module):
- def __init__(self, hidden_channels, kernel_size, dilation_rate, n_layers, gin_channels=0, p_dropout=0):
- super(WN, self).__init__()
- assert(kernel_size % 2 == 1)
- self.hidden_channels =hidden_channels
- self.kernel_size = kernel_size,
- self.dilation_rate = dilation_rate
- self.n_layers = n_layers
- self.gin_channels = gin_channels
- self.p_dropout = p_dropout
-
- self.in_layers = torch.nn.ModuleList()
- self.res_skip_layers = torch.nn.ModuleList()
- self.drop = nn.Dropout(p_dropout)
-
- if gin_channels != 0:
- cond_layer = torch.nn.Conv1d(gin_channels, 2*hidden_channels*n_layers, 1)
- self.cond_layer = torch.nn.utils.weight_norm(cond_layer, name='weight')
-
- for i in range(n_layers):
- dilation = dilation_rate ** i
- padding = int((kernel_size * dilation - dilation) / 2)
- in_layer = torch.nn.Conv1d(hidden_channels, 2*hidden_channels, kernel_size,
- dilation=dilation, padding=padding)
- in_layer = torch.nn.utils.weight_norm(in_layer, name='weight')
- self.in_layers.append(in_layer)
-
- # last one is not necessary
- if i < n_layers - 1:
- res_skip_channels = 2 * hidden_channels
- else:
- res_skip_channels = hidden_channels
-
- res_skip_layer = torch.nn.Conv1d(hidden_channels, res_skip_channels, 1)
- res_skip_layer = torch.nn.utils.weight_norm(res_skip_layer, name='weight')
- self.res_skip_layers.append(res_skip_layer)
-
- def forward(self, x, x_mask, g=None, **kwargs):
- output = torch.zeros_like(x)
- n_channels_tensor = torch.IntTensor([self.hidden_channels])
-
- if g is not None:
- g = self.cond_layer(g)
-
- for i in range(self.n_layers):
- x_in = self.in_layers[i](x)
- if g is not None:
- cond_offset = i * 2 * self.hidden_channels
- g_l = g[:,cond_offset:cond_offset+2*self.hidden_channels,:]
- else:
- g_l = torch.zeros_like(x_in)
-
- acts = commons.fused_add_tanh_sigmoid_multiply(
- x_in,
- g_l,
- n_channels_tensor)
- acts = self.drop(acts)
-
- res_skip_acts = self.res_skip_layers[i](acts)
- if i < self.n_layers - 1:
- res_acts = res_skip_acts[:,:self.hidden_channels,:]
- x = (x + res_acts) * x_mask
- output = output + res_skip_acts[:,self.hidden_channels:,:]
- else:
- output = output + res_skip_acts
- return output * x_mask
-
- def remove_weight_norm(self):
- if self.gin_channels != 0:
- torch.nn.utils.remove_weight_norm(self.cond_layer)
- for l in self.in_layers:
- torch.nn.utils.remove_weight_norm(l)
- for l in self.res_skip_layers:
- torch.nn.utils.remove_weight_norm(l)
-
-
-class ResBlock1(torch.nn.Module):
- def __init__(self, channels, kernel_size=3, dilation=(1, 3, 5)):
- super(ResBlock1, self).__init__()
- self.convs1 = nn.ModuleList([
- weight_norm(Conv1d(channels, channels, kernel_size, 1, dilation=dilation[0],
- padding=get_padding(kernel_size, dilation[0]))),
- weight_norm(Conv1d(channels, channels, kernel_size, 1, dilation=dilation[1],
- padding=get_padding(kernel_size, dilation[1]))),
- weight_norm(Conv1d(channels, channels, kernel_size, 1, dilation=dilation[2],
- padding=get_padding(kernel_size, dilation[2])))
- ])
- self.convs1.apply(init_weights)
-
- self.convs2 = nn.ModuleList([
- weight_norm(Conv1d(channels, channels, kernel_size, 1, dilation=1,
- padding=get_padding(kernel_size, 1))),
- weight_norm(Conv1d(channels, channels, kernel_size, 1, dilation=1,
- padding=get_padding(kernel_size, 1))),
- weight_norm(Conv1d(channels, channels, kernel_size, 1, dilation=1,
- padding=get_padding(kernel_size, 1)))
- ])
- self.convs2.apply(init_weights)
-
- def forward(self, x, x_mask=None):
- for c1, c2 in zip(self.convs1, self.convs2):
- xt = F.leaky_relu(x, LRELU_SLOPE)
- if x_mask is not None:
- xt = xt * x_mask
- xt = c1(xt)
- xt = F.leaky_relu(xt, LRELU_SLOPE)
- if x_mask is not None:
- xt = xt * x_mask
- xt = c2(xt)
- x = xt + x
- if x_mask is not None:
- x = x * x_mask
- return x
-
- def remove_weight_norm(self):
- for l in self.convs1:
- remove_weight_norm(l)
- for l in self.convs2:
- remove_weight_norm(l)
-
-
-class ResBlock2(torch.nn.Module):
- def __init__(self, channels, kernel_size=3, dilation=(1, 3)):
- super(ResBlock2, self).__init__()
- self.convs = nn.ModuleList([
- weight_norm(Conv1d(channels, channels, kernel_size, 1, dilation=dilation[0],
- padding=get_padding(kernel_size, dilation[0]))),
- weight_norm(Conv1d(channels, channels, kernel_size, 1, dilation=dilation[1],
- padding=get_padding(kernel_size, dilation[1])))
- ])
- self.convs.apply(init_weights)
-
- def forward(self, x, x_mask=None):
- for c in self.convs:
- xt = F.leaky_relu(x, LRELU_SLOPE)
- if x_mask is not None:
- xt = xt * x_mask
- xt = c(xt)
- x = xt + x
- if x_mask is not None:
- x = x * x_mask
- return x
-
- def remove_weight_norm(self):
- for l in self.convs:
- remove_weight_norm(l)
-
-
-class Log(nn.Module):
- def forward(self, x, x_mask, reverse=False, **kwargs):
- if not reverse:
- y = torch.log(torch.clamp_min(x, 1e-5)) * x_mask
- logdet = torch.sum(-y, [1, 2])
- return y, logdet
- else:
- x = torch.exp(x) * x_mask
- return x
-
-
-class Flip(nn.Module):
- def forward(self, x, *args, reverse=False, **kwargs):
- x = torch.flip(x, [1])
- if not reverse:
- logdet = torch.zeros(x.size(0)).to(dtype=x.dtype, device=x.device)
- return x, logdet
- else:
- return x
-
-
-class ElementwiseAffine(nn.Module):
- def __init__(self, channels):
- super().__init__()
- self.channels = channels
- self.m = nn.Parameter(torch.zeros(channels,1))
- self.logs = nn.Parameter(torch.zeros(channels,1))
-
- def forward(self, x, x_mask, reverse=False, **kwargs):
- if not reverse:
- y = self.m + torch.exp(self.logs) * x
- y = y * x_mask
- logdet = torch.sum(self.logs * x_mask, [1,2])
- return y, logdet
- else:
- x = (x - self.m) * torch.exp(-self.logs) * x_mask
- return x
-
-
-class ResidualCouplingLayer(nn.Module):
- def __init__(self,
- channels,
- hidden_channels,
- kernel_size,
- dilation_rate,
- n_layers,
- p_dropout=0,
- gin_channels=0,
- mean_only=False):
- assert channels % 2 == 0, "channels should be divisible by 2"
- super().__init__()
- self.channels = channels
- self.hidden_channels = hidden_channels
- self.kernel_size = kernel_size
- self.dilation_rate = dilation_rate
- self.n_layers = n_layers
- self.half_channels = channels // 2
- self.mean_only = mean_only
-
- self.pre = nn.Conv1d(self.half_channels, hidden_channels, 1)
- self.enc = WN(hidden_channels, kernel_size, dilation_rate, n_layers, p_dropout=p_dropout, gin_channels=gin_channels)
- self.post = nn.Conv1d(hidden_channels, self.half_channels * (2 - mean_only), 1)
- self.post.weight.data.zero_()
- self.post.bias.data.zero_()
-
- def forward(self, x, x_mask, g=None, reverse=False):
- x0, x1 = torch.split(x, [self.half_channels]*2, 1)
- h = self.pre(x0) * x_mask
- h = self.enc(h, x_mask, g=g)
- stats = self.post(h) * x_mask
- if not self.mean_only:
- m, logs = torch.split(stats, [self.half_channels]*2, 1)
- else:
- m = stats
- logs = torch.zeros_like(m)
-
- if not reverse:
- x1 = m + x1 * torch.exp(logs) * x_mask
- x = torch.cat([x0, x1], 1)
- logdet = torch.sum(logs, [1,2])
- return x, logdet
- else:
- x1 = (x1 - m) * torch.exp(-logs) * x_mask
- x = torch.cat([x0, x1], 1)
- return x
diff --git a/spaces/badongtakla/ithaca/ithaca/util/text.py b/spaces/badongtakla/ithaca/ithaca/util/text.py
deleted file mode 100644
index 859e779398c17136c8279f57f86662710e1aa0db..0000000000000000000000000000000000000000
--- a/spaces/badongtakla/ithaca/ithaca/util/text.py
+++ /dev/null
@@ -1,186 +0,0 @@
-# Copyright 2021 the Ithaca Authors
-#
-# Licensed under the Apache License, Version 2.0 (the "License");
-# you may not use this file except in compliance with the License.
-# You may obtain a copy of the License at
-#
-# https://www.apache.org/licenses/LICENSE-2.0
-#
-# Unless required by applicable law or agreed to in writing, software
-# distributed under the License is distributed on an "AS IS" BASIS,
-# WITHOUT WARRANTIES OR CONDITIONS OF ANY KIND, either express or implied.
-# See the License for the specific language governing permissions and
-# limitations under the License.
-"""Text processing functions."""
-
-import random
-import re
-import unicodedata
-
-import numpy as np
-
-
-def idx_to_text(idxs, alphabet, strip_sos=True, strip_pad=True):
- """Converts a list of indices to a string."""
- idxs = np.array(idxs)
- out = ''
- for i in range(idxs.size):
- idx = idxs[i]
- if strip_pad and idx == alphabet.pad_idx:
- break
- elif strip_sos and idx == alphabet.sos_idx:
- pass
- else:
- out += alphabet.idx2char[idx]
- return out
-
-
-def idx_to_text_batch(idxs, alphabet, lengths=None):
- """Converts batched lists of indices to strings."""
- b = []
- for i in range(idxs.shape[0]):
- idxs_i = idxs[i]
- if lengths:
- idxs_i = idxs_i[:lengths[i]]
- b.append(idx_to_text(idxs_i, alphabet))
- return b
-
-
-def random_mask_span(t, geometric_p=0.2, limit_chars=None):
- """Masks a span of sequential words."""
-
- # Obtain span indexes (indlusive)
- span_idx = [(ele.start(), ele.end()) for ele in re.finditer(r'[\w\s]+', t)]
- if not span_idx:
- return []
-
- # Select a span to mask
- span_start, span_end = random.choice(span_idx)
-
- # Sample a random span length using a geomteric distribution
- if geometric_p and limit_chars:
- span_len = np.clip(
- np.random.geometric(geometric_p),
- 1, min(limit_chars, span_end - span_start))
- elif geometric_p:
- span_len = np.clip(
- np.random.geometric(geometric_p),
- 1, span_end - span_start)
- elif limit_chars:
- span_len = min(limit_chars, span_end - span_start)
- else:
- raise ValueError('geometric_p or limit_chars should be set.')
-
- # Pick a random start index
- span_start = np.random.randint(span_start, span_end - span_len + 1)
- assert span_start + span_len <= span_end
-
- # Clip to limit chars
- if limit_chars is not None and span_len >= limit_chars:
- span_len = limit_chars
-
- # Create mask indices
- mask_idx = list(range(span_start, span_start + span_len))
-
- return mask_idx
-
-
-def random_sentence_swap(sentences, p):
- """Swaps sentences with probability p."""
-
- def swap_sentence(s):
- idx_1 = random.randint(0, len(s) - 1)
- idx_2 = idx_1
- counter = 0
-
- while idx_2 == idx_1:
- idx_2 = random.randint(0, len(s) - 1)
- counter += 1
- if counter > 3:
- return s
-
- s[idx_1], s[idx_2] = s[idx_2], s[idx_1]
- return s
-
- new_sentences = sentences.copy()
- n = int(p * len(sentences))
- for _ in range(n):
- new_sentences = swap_sentence(new_sentences)
-
- return new_sentences
-
-
-def random_word_delete(sentence, p):
- """Deletes a word from a sentence with probability p."""
-
- words = sentence.split(' ')
-
- # Return if one word.
- if len(words) == 1:
- return words[0]
-
- # Randomly delete words.
- new_words = []
- for word in words:
- if random.uniform(0, 1) > p:
- new_words.append(word)
-
- # If all words are removed return one.
- if not new_words:
- rand_int = random.randint(0, len(words) - 1)
- return words[rand_int]
-
- sentence = ' '.join(new_words)
-
- return sentence
-
-
-def random_word_swap(sentence, p):
- """Swaps words from a sentence with probability p."""
-
- def swap_word(new_words):
- idx_1 = random.randint(0, len(new_words) - 1)
- idx_2 = idx_1
- counter = 0
-
- while idx_2 == idx_1:
- idx_2 = random.randint(0, len(new_words) - 1)
- counter += 1
-
- if counter > 3:
- return new_words
-
- new_words[idx_1], new_words[idx_2] = new_words[idx_2], new_words[idx_1]
- return new_words
-
- words = sentence.split(' ')
-
- new_words = words.copy()
- n = int(p * len(words))
- for _ in range(n):
- new_words = swap_word(new_words)
-
- sentence = ' '.join(new_words)
-
- return sentence
-
-
-def strip_accents(s):
- return ''.join(
- c for c in unicodedata.normalize('NFD', s)
- if unicodedata.category(c) != 'Mn')
-
-
-def text_to_idx(t, alphabet):
- """Converts a string to character indices."""
- return np.array([alphabet.char2idx[c] for c in t], dtype=np.int32)
-
-
-def text_to_word_idx(t, alphabet):
- """Converts a string to word indices."""
- out = np.full(len(t), alphabet.word2idx[alphabet.unk], dtype=np.int32)
- for m in re.finditer(r'\w+', t):
- if m.group() in alphabet.word2idx:
- out[m.start():m.end()] = alphabet.word2idx[m.group()]
- return out
-
diff --git a/spaces/banana-projects/web3d/node_modules/three/build/three.js b/spaces/banana-projects/web3d/node_modules/three/build/three.js
deleted file mode 100644
index 56f738b35eb1c06ee148ec7ff30927acb88e378f..0000000000000000000000000000000000000000
--- a/spaces/banana-projects/web3d/node_modules/three/build/three.js
+++ /dev/null
@@ -1,48498 +0,0 @@
-(function (global, factory) {
- typeof exports === 'object' && typeof module !== 'undefined' ? factory(exports) :
- typeof define === 'function' && define.amd ? define(['exports'], factory) :
- (global = global || self, factory(global.THREE = {}));
-}(this, function (exports) { 'use strict';
-
- // Polyfills
-
- if ( Number.EPSILON === undefined ) {
-
- Number.EPSILON = Math.pow( 2, - 52 );
-
- }
-
- if ( Number.isInteger === undefined ) {
-
- // Missing in IE
- // https://developer.mozilla.org/en-US/docs/Web/JavaScript/Reference/Global_Objects/Number/isInteger
-
- Number.isInteger = function ( value ) {
-
- return typeof value === 'number' && isFinite( value ) && Math.floor( value ) === value;
-
- };
-
- }
-
- //
-
- if ( Math.sign === undefined ) {
-
- // https://developer.mozilla.org/en-US/docs/Web/JavaScript/Reference/Global_Objects/Math/sign
-
- Math.sign = function ( x ) {
-
- return ( x < 0 ) ? - 1 : ( x > 0 ) ? 1 : + x;
-
- };
-
- }
-
- if ( 'name' in Function.prototype === false ) {
-
- // Missing in IE
- // https://developer.mozilla.org/en-US/docs/Web/JavaScript/Reference/Global_Objects/Function/name
-
- Object.defineProperty( Function.prototype, 'name', {
-
- get: function () {
-
- return this.toString().match( /^\s*function\s*([^\(\s]*)/ )[ 1 ];
-
- }
-
- } );
-
- }
-
- if ( Object.assign === undefined ) {
-
- // Missing in IE
- // https://developer.mozilla.org/en-US/docs/Web/JavaScript/Reference/Global_Objects/Object/assign
-
- ( function () {
-
- Object.assign = function ( target ) {
-
- if ( target === undefined || target === null ) {
-
- throw new TypeError( 'Cannot convert undefined or null to object' );
-
- }
-
- var output = Object( target );
-
- for ( var index = 1; index < arguments.length; index ++ ) {
-
- var source = arguments[ index ];
-
- if ( source !== undefined && source !== null ) {
-
- for ( var nextKey in source ) {
-
- if ( Object.prototype.hasOwnProperty.call( source, nextKey ) ) {
-
- output[ nextKey ] = source[ nextKey ];
-
- }
-
- }
-
- }
-
- }
-
- return output;
-
- };
-
- } )();
-
- }
-
- /**
- * https://github.com/mrdoob/eventdispatcher.js/
- */
-
- function EventDispatcher() {}
-
- Object.assign( EventDispatcher.prototype, {
-
- addEventListener: function ( type, listener ) {
-
- if ( this._listeners === undefined ) this._listeners = {};
-
- var listeners = this._listeners;
-
- if ( listeners[ type ] === undefined ) {
-
- listeners[ type ] = [];
-
- }
-
- if ( listeners[ type ].indexOf( listener ) === - 1 ) {
-
- listeners[ type ].push( listener );
-
- }
-
- },
-
- hasEventListener: function ( type, listener ) {
-
- if ( this._listeners === undefined ) return false;
-
- var listeners = this._listeners;
-
- return listeners[ type ] !== undefined && listeners[ type ].indexOf( listener ) !== - 1;
-
- },
-
- removeEventListener: function ( type, listener ) {
-
- if ( this._listeners === undefined ) return;
-
- var listeners = this._listeners;
- var listenerArray = listeners[ type ];
-
- if ( listenerArray !== undefined ) {
-
- var index = listenerArray.indexOf( listener );
-
- if ( index !== - 1 ) {
-
- listenerArray.splice( index, 1 );
-
- }
-
- }
-
- },
-
- dispatchEvent: function ( event ) {
-
- if ( this._listeners === undefined ) return;
-
- var listeners = this._listeners;
- var listenerArray = listeners[ event.type ];
-
- if ( listenerArray !== undefined ) {
-
- event.target = this;
-
- var array = listenerArray.slice( 0 );
-
- for ( var i = 0, l = array.length; i < l; i ++ ) {
-
- array[ i ].call( this, event );
-
- }
-
- }
-
- }
-
- } );
-
- var REVISION = '103';
- var MOUSE = { LEFT: 0, MIDDLE: 1, RIGHT: 2 };
- var CullFaceNone = 0;
- var CullFaceBack = 1;
- var CullFaceFront = 2;
- var CullFaceFrontBack = 3;
- var FrontFaceDirectionCW = 0;
- var FrontFaceDirectionCCW = 1;
- var BasicShadowMap = 0;
- var PCFShadowMap = 1;
- var PCFSoftShadowMap = 2;
- var FrontSide = 0;
- var BackSide = 1;
- var DoubleSide = 2;
- var FlatShading = 1;
- var SmoothShading = 2;
- var NoColors = 0;
- var FaceColors = 1;
- var VertexColors = 2;
- var NoBlending = 0;
- var NormalBlending = 1;
- var AdditiveBlending = 2;
- var SubtractiveBlending = 3;
- var MultiplyBlending = 4;
- var CustomBlending = 5;
- var AddEquation = 100;
- var SubtractEquation = 101;
- var ReverseSubtractEquation = 102;
- var MinEquation = 103;
- var MaxEquation = 104;
- var ZeroFactor = 200;
- var OneFactor = 201;
- var SrcColorFactor = 202;
- var OneMinusSrcColorFactor = 203;
- var SrcAlphaFactor = 204;
- var OneMinusSrcAlphaFactor = 205;
- var DstAlphaFactor = 206;
- var OneMinusDstAlphaFactor = 207;
- var DstColorFactor = 208;
- var OneMinusDstColorFactor = 209;
- var SrcAlphaSaturateFactor = 210;
- var NeverDepth = 0;
- var AlwaysDepth = 1;
- var LessDepth = 2;
- var LessEqualDepth = 3;
- var EqualDepth = 4;
- var GreaterEqualDepth = 5;
- var GreaterDepth = 6;
- var NotEqualDepth = 7;
- var MultiplyOperation = 0;
- var MixOperation = 1;
- var AddOperation = 2;
- var NoToneMapping = 0;
- var LinearToneMapping = 1;
- var ReinhardToneMapping = 2;
- var Uncharted2ToneMapping = 3;
- var CineonToneMapping = 4;
- var ACESFilmicToneMapping = 5;
-
- var UVMapping = 300;
- var CubeReflectionMapping = 301;
- var CubeRefractionMapping = 302;
- var EquirectangularReflectionMapping = 303;
- var EquirectangularRefractionMapping = 304;
- var SphericalReflectionMapping = 305;
- var CubeUVReflectionMapping = 306;
- var CubeUVRefractionMapping = 307;
- var RepeatWrapping = 1000;
- var ClampToEdgeWrapping = 1001;
- var MirroredRepeatWrapping = 1002;
- var NearestFilter = 1003;
- var NearestMipMapNearestFilter = 1004;
- var NearestMipMapLinearFilter = 1005;
- var LinearFilter = 1006;
- var LinearMipMapNearestFilter = 1007;
- var LinearMipMapLinearFilter = 1008;
- var UnsignedByteType = 1009;
- var ByteType = 1010;
- var ShortType = 1011;
- var UnsignedShortType = 1012;
- var IntType = 1013;
- var UnsignedIntType = 1014;
- var FloatType = 1015;
- var HalfFloatType = 1016;
- var UnsignedShort4444Type = 1017;
- var UnsignedShort5551Type = 1018;
- var UnsignedShort565Type = 1019;
- var UnsignedInt248Type = 1020;
- var AlphaFormat = 1021;
- var RGBFormat = 1022;
- var RGBAFormat = 1023;
- var LuminanceFormat = 1024;
- var LuminanceAlphaFormat = 1025;
- var RGBEFormat = RGBAFormat;
- var DepthFormat = 1026;
- var DepthStencilFormat = 1027;
- var RedFormat = 1028;
- var RGB_S3TC_DXT1_Format = 33776;
- var RGBA_S3TC_DXT1_Format = 33777;
- var RGBA_S3TC_DXT3_Format = 33778;
- var RGBA_S3TC_DXT5_Format = 33779;
- var RGB_PVRTC_4BPPV1_Format = 35840;
- var RGB_PVRTC_2BPPV1_Format = 35841;
- var RGBA_PVRTC_4BPPV1_Format = 35842;
- var RGBA_PVRTC_2BPPV1_Format = 35843;
- var RGB_ETC1_Format = 36196;
- var RGBA_ASTC_4x4_Format = 37808;
- var RGBA_ASTC_5x4_Format = 37809;
- var RGBA_ASTC_5x5_Format = 37810;
- var RGBA_ASTC_6x5_Format = 37811;
- var RGBA_ASTC_6x6_Format = 37812;
- var RGBA_ASTC_8x5_Format = 37813;
- var RGBA_ASTC_8x6_Format = 37814;
- var RGBA_ASTC_8x8_Format = 37815;
- var RGBA_ASTC_10x5_Format = 37816;
- var RGBA_ASTC_10x6_Format = 37817;
- var RGBA_ASTC_10x8_Format = 37818;
- var RGBA_ASTC_10x10_Format = 37819;
- var RGBA_ASTC_12x10_Format = 37820;
- var RGBA_ASTC_12x12_Format = 37821;
- var LoopOnce = 2200;
- var LoopRepeat = 2201;
- var LoopPingPong = 2202;
- var InterpolateDiscrete = 2300;
- var InterpolateLinear = 2301;
- var InterpolateSmooth = 2302;
- var ZeroCurvatureEnding = 2400;
- var ZeroSlopeEnding = 2401;
- var WrapAroundEnding = 2402;
- var TrianglesDrawMode = 0;
- var TriangleStripDrawMode = 1;
- var TriangleFanDrawMode = 2;
- var LinearEncoding = 3000;
- var sRGBEncoding = 3001;
- var GammaEncoding = 3007;
- var RGBEEncoding = 3002;
- var LogLuvEncoding = 3003;
- var RGBM7Encoding = 3004;
- var RGBM16Encoding = 3005;
- var RGBDEncoding = 3006;
- var BasicDepthPacking = 3200;
- var RGBADepthPacking = 3201;
- var TangentSpaceNormalMap = 0;
- var ObjectSpaceNormalMap = 1;
-
- /**
- * @author alteredq / http://alteredqualia.com/
- * @author mrdoob / http://mrdoob.com/
- */
-
- var _Math = {
-
- DEG2RAD: Math.PI / 180,
- RAD2DEG: 180 / Math.PI,
-
- generateUUID: ( function () {
-
- // http://stackoverflow.com/questions/105034/how-to-create-a-guid-uuid-in-javascript/21963136#21963136
-
- var lut = [];
-
- for ( var i = 0; i < 256; i ++ ) {
-
- lut[ i ] = ( i < 16 ? '0' : '' ) + ( i ).toString( 16 );
-
- }
-
- return function generateUUID() {
-
- var d0 = Math.random() * 0xffffffff | 0;
- var d1 = Math.random() * 0xffffffff | 0;
- var d2 = Math.random() * 0xffffffff | 0;
- var d3 = Math.random() * 0xffffffff | 0;
- var uuid = lut[ d0 & 0xff ] + lut[ d0 >> 8 & 0xff ] + lut[ d0 >> 16 & 0xff ] + lut[ d0 >> 24 & 0xff ] + '-' +
- lut[ d1 & 0xff ] + lut[ d1 >> 8 & 0xff ] + '-' + lut[ d1 >> 16 & 0x0f | 0x40 ] + lut[ d1 >> 24 & 0xff ] + '-' +
- lut[ d2 & 0x3f | 0x80 ] + lut[ d2 >> 8 & 0xff ] + '-' + lut[ d2 >> 16 & 0xff ] + lut[ d2 >> 24 & 0xff ] +
- lut[ d3 & 0xff ] + lut[ d3 >> 8 & 0xff ] + lut[ d3 >> 16 & 0xff ] + lut[ d3 >> 24 & 0xff ];
-
- // .toUpperCase() here flattens concatenated strings to save heap memory space.
- return uuid.toUpperCase();
-
- };
-
- } )(),
-
- clamp: function ( value, min, max ) {
-
- return Math.max( min, Math.min( max, value ) );
-
- },
-
- // compute euclidian modulo of m % n
- // https://en.wikipedia.org/wiki/Modulo_operation
-
- euclideanModulo: function ( n, m ) {
-
- return ( ( n % m ) + m ) % m;
-
- },
-
- // Linear mapping from range to range
-
- mapLinear: function ( x, a1, a2, b1, b2 ) {
-
- return b1 + ( x - a1 ) * ( b2 - b1 ) / ( a2 - a1 );
-
- },
-
- // https://en.wikipedia.org/wiki/Linear_interpolation
-
- lerp: function ( x, y, t ) {
-
- return ( 1 - t ) * x + t * y;
-
- },
-
- // http://en.wikipedia.org/wiki/Smoothstep
-
- smoothstep: function ( x, min, max ) {
-
- if ( x <= min ) return 0;
- if ( x >= max ) return 1;
-
- x = ( x - min ) / ( max - min );
-
- return x * x * ( 3 - 2 * x );
-
- },
-
- smootherstep: function ( x, min, max ) {
-
- if ( x <= min ) return 0;
- if ( x >= max ) return 1;
-
- x = ( x - min ) / ( max - min );
-
- return x * x * x * ( x * ( x * 6 - 15 ) + 10 );
-
- },
-
- // Random integer from interval
-
- randInt: function ( low, high ) {
-
- return low + Math.floor( Math.random() * ( high - low + 1 ) );
-
- },
-
- // Random float from interval
-
- randFloat: function ( low, high ) {
-
- return low + Math.random() * ( high - low );
-
- },
-
- // Random float from <-range/2, range/2> interval
-
- randFloatSpread: function ( range ) {
-
- return range * ( 0.5 - Math.random() );
-
- },
-
- degToRad: function ( degrees ) {
-
- return degrees * _Math.DEG2RAD;
-
- },
-
- radToDeg: function ( radians ) {
-
- return radians * _Math.RAD2DEG;
-
- },
-
- isPowerOfTwo: function ( value ) {
-
- return ( value & ( value - 1 ) ) === 0 && value !== 0;
-
- },
-
- ceilPowerOfTwo: function ( value ) {
-
- return Math.pow( 2, Math.ceil( Math.log( value ) / Math.LN2 ) );
-
- },
-
- floorPowerOfTwo: function ( value ) {
-
- return Math.pow( 2, Math.floor( Math.log( value ) / Math.LN2 ) );
-
- }
-
- };
-
- /**
- * @author mrdoob / http://mrdoob.com/
- * @author philogb / http://blog.thejit.org/
- * @author egraether / http://egraether.com/
- * @author zz85 / http://www.lab4games.net/zz85/blog
- */
-
- function Vector2( x, y ) {
-
- this.x = x || 0;
- this.y = y || 0;
-
- }
-
- Object.defineProperties( Vector2.prototype, {
-
- "width": {
-
- get: function () {
-
- return this.x;
-
- },
-
- set: function ( value ) {
-
- this.x = value;
-
- }
-
- },
-
- "height": {
-
- get: function () {
-
- return this.y;
-
- },
-
- set: function ( value ) {
-
- this.y = value;
-
- }
-
- }
-
- } );
-
- Object.assign( Vector2.prototype, {
-
- isVector2: true,
-
- set: function ( x, y ) {
-
- this.x = x;
- this.y = y;
-
- return this;
-
- },
-
- setScalar: function ( scalar ) {
-
- this.x = scalar;
- this.y = scalar;
-
- return this;
-
- },
-
- setX: function ( x ) {
-
- this.x = x;
-
- return this;
-
- },
-
- setY: function ( y ) {
-
- this.y = y;
-
- return this;
-
- },
-
- setComponent: function ( index, value ) {
-
- switch ( index ) {
-
- case 0: this.x = value; break;
- case 1: this.y = value; break;
- default: throw new Error( 'index is out of range: ' + index );
-
- }
-
- return this;
-
- },
-
- getComponent: function ( index ) {
-
- switch ( index ) {
-
- case 0: return this.x;
- case 1: return this.y;
- default: throw new Error( 'index is out of range: ' + index );
-
- }
-
- },
-
- clone: function () {
-
- return new this.constructor( this.x, this.y );
-
- },
-
- copy: function ( v ) {
-
- this.x = v.x;
- this.y = v.y;
-
- return this;
-
- },
-
- add: function ( v, w ) {
-
- if ( w !== undefined ) {
-
- console.warn( 'THREE.Vector2: .add() now only accepts one argument. Use .addVectors( a, b ) instead.' );
- return this.addVectors( v, w );
-
- }
-
- this.x += v.x;
- this.y += v.y;
-
- return this;
-
- },
-
- addScalar: function ( s ) {
-
- this.x += s;
- this.y += s;
-
- return this;
-
- },
-
- addVectors: function ( a, b ) {
-
- this.x = a.x + b.x;
- this.y = a.y + b.y;
-
- return this;
-
- },
-
- addScaledVector: function ( v, s ) {
-
- this.x += v.x * s;
- this.y += v.y * s;
-
- return this;
-
- },
-
- sub: function ( v, w ) {
-
- if ( w !== undefined ) {
-
- console.warn( 'THREE.Vector2: .sub() now only accepts one argument. Use .subVectors( a, b ) instead.' );
- return this.subVectors( v, w );
-
- }
-
- this.x -= v.x;
- this.y -= v.y;
-
- return this;
-
- },
-
- subScalar: function ( s ) {
-
- this.x -= s;
- this.y -= s;
-
- return this;
-
- },
-
- subVectors: function ( a, b ) {
-
- this.x = a.x - b.x;
- this.y = a.y - b.y;
-
- return this;
-
- },
-
- multiply: function ( v ) {
-
- this.x *= v.x;
- this.y *= v.y;
-
- return this;
-
- },
-
- multiplyScalar: function ( scalar ) {
-
- this.x *= scalar;
- this.y *= scalar;
-
- return this;
-
- },
-
- divide: function ( v ) {
-
- this.x /= v.x;
- this.y /= v.y;
-
- return this;
-
- },
-
- divideScalar: function ( scalar ) {
-
- return this.multiplyScalar( 1 / scalar );
-
- },
-
- applyMatrix3: function ( m ) {
-
- var x = this.x, y = this.y;
- var e = m.elements;
-
- this.x = e[ 0 ] * x + e[ 3 ] * y + e[ 6 ];
- this.y = e[ 1 ] * x + e[ 4 ] * y + e[ 7 ];
-
- return this;
-
- },
-
- min: function ( v ) {
-
- this.x = Math.min( this.x, v.x );
- this.y = Math.min( this.y, v.y );
-
- return this;
-
- },
-
- max: function ( v ) {
-
- this.x = Math.max( this.x, v.x );
- this.y = Math.max( this.y, v.y );
-
- return this;
-
- },
-
- clamp: function ( min, max ) {
-
- // assumes min < max, componentwise
-
- this.x = Math.max( min.x, Math.min( max.x, this.x ) );
- this.y = Math.max( min.y, Math.min( max.y, this.y ) );
-
- return this;
-
- },
-
- clampScalar: function () {
-
- var min = new Vector2();
- var max = new Vector2();
-
- return function clampScalar( minVal, maxVal ) {
-
- min.set( minVal, minVal );
- max.set( maxVal, maxVal );
-
- return this.clamp( min, max );
-
- };
-
- }(),
-
- clampLength: function ( min, max ) {
-
- var length = this.length();
-
- return this.divideScalar( length || 1 ).multiplyScalar( Math.max( min, Math.min( max, length ) ) );
-
- },
-
- floor: function () {
-
- this.x = Math.floor( this.x );
- this.y = Math.floor( this.y );
-
- return this;
-
- },
-
- ceil: function () {
-
- this.x = Math.ceil( this.x );
- this.y = Math.ceil( this.y );
-
- return this;
-
- },
-
- round: function () {
-
- this.x = Math.round( this.x );
- this.y = Math.round( this.y );
-
- return this;
-
- },
-
- roundToZero: function () {
-
- this.x = ( this.x < 0 ) ? Math.ceil( this.x ) : Math.floor( this.x );
- this.y = ( this.y < 0 ) ? Math.ceil( this.y ) : Math.floor( this.y );
-
- return this;
-
- },
-
- negate: function () {
-
- this.x = - this.x;
- this.y = - this.y;
-
- return this;
-
- },
-
- dot: function ( v ) {
-
- return this.x * v.x + this.y * v.y;
-
- },
-
- cross: function ( v ) {
-
- return this.x * v.y - this.y * v.x;
-
- },
-
- lengthSq: function () {
-
- return this.x * this.x + this.y * this.y;
-
- },
-
- length: function () {
-
- return Math.sqrt( this.x * this.x + this.y * this.y );
-
- },
-
- manhattanLength: function () {
-
- return Math.abs( this.x ) + Math.abs( this.y );
-
- },
-
- normalize: function () {
-
- return this.divideScalar( this.length() || 1 );
-
- },
-
- angle: function () {
-
- // computes the angle in radians with respect to the positive x-axis
-
- var angle = Math.atan2( this.y, this.x );
-
- if ( angle < 0 ) angle += 2 * Math.PI;
-
- return angle;
-
- },
-
- distanceTo: function ( v ) {
-
- return Math.sqrt( this.distanceToSquared( v ) );
-
- },
-
- distanceToSquared: function ( v ) {
-
- var dx = this.x - v.x, dy = this.y - v.y;
- return dx * dx + dy * dy;
-
- },
-
- manhattanDistanceTo: function ( v ) {
-
- return Math.abs( this.x - v.x ) + Math.abs( this.y - v.y );
-
- },
-
- setLength: function ( length ) {
-
- return this.normalize().multiplyScalar( length );
-
- },
-
- lerp: function ( v, alpha ) {
-
- this.x += ( v.x - this.x ) * alpha;
- this.y += ( v.y - this.y ) * alpha;
-
- return this;
-
- },
-
- lerpVectors: function ( v1, v2, alpha ) {
-
- return this.subVectors( v2, v1 ).multiplyScalar( alpha ).add( v1 );
-
- },
-
- equals: function ( v ) {
-
- return ( ( v.x === this.x ) && ( v.y === this.y ) );
-
- },
-
- fromArray: function ( array, offset ) {
-
- if ( offset === undefined ) offset = 0;
-
- this.x = array[ offset ];
- this.y = array[ offset + 1 ];
-
- return this;
-
- },
-
- toArray: function ( array, offset ) {
-
- if ( array === undefined ) array = [];
- if ( offset === undefined ) offset = 0;
-
- array[ offset ] = this.x;
- array[ offset + 1 ] = this.y;
-
- return array;
-
- },
-
- fromBufferAttribute: function ( attribute, index, offset ) {
-
- if ( offset !== undefined ) {
-
- console.warn( 'THREE.Vector2: offset has been removed from .fromBufferAttribute().' );
-
- }
-
- this.x = attribute.getX( index );
- this.y = attribute.getY( index );
-
- return this;
-
- },
-
- rotateAround: function ( center, angle ) {
-
- var c = Math.cos( angle ), s = Math.sin( angle );
-
- var x = this.x - center.x;
- var y = this.y - center.y;
-
- this.x = x * c - y * s + center.x;
- this.y = x * s + y * c + center.y;
-
- return this;
-
- }
-
- } );
-
- /**
- * @author mikael emtinger / http://gomo.se/
- * @author alteredq / http://alteredqualia.com/
- * @author WestLangley / http://github.com/WestLangley
- * @author bhouston / http://clara.io
- */
-
- function Quaternion( x, y, z, w ) {
-
- this._x = x || 0;
- this._y = y || 0;
- this._z = z || 0;
- this._w = ( w !== undefined ) ? w : 1;
-
- }
-
- Object.assign( Quaternion, {
-
- slerp: function ( qa, qb, qm, t ) {
-
- return qm.copy( qa ).slerp( qb, t );
-
- },
-
- slerpFlat: function ( dst, dstOffset, src0, srcOffset0, src1, srcOffset1, t ) {
-
- // fuzz-free, array-based Quaternion SLERP operation
-
- var x0 = src0[ srcOffset0 + 0 ],
- y0 = src0[ srcOffset0 + 1 ],
- z0 = src0[ srcOffset0 + 2 ],
- w0 = src0[ srcOffset0 + 3 ],
-
- x1 = src1[ srcOffset1 + 0 ],
- y1 = src1[ srcOffset1 + 1 ],
- z1 = src1[ srcOffset1 + 2 ],
- w1 = src1[ srcOffset1 + 3 ];
-
- if ( w0 !== w1 || x0 !== x1 || y0 !== y1 || z0 !== z1 ) {
-
- var s = 1 - t,
-
- cos = x0 * x1 + y0 * y1 + z0 * z1 + w0 * w1,
-
- dir = ( cos >= 0 ? 1 : - 1 ),
- sqrSin = 1 - cos * cos;
-
- // Skip the Slerp for tiny steps to avoid numeric problems:
- if ( sqrSin > Number.EPSILON ) {
-
- var sin = Math.sqrt( sqrSin ),
- len = Math.atan2( sin, cos * dir );
-
- s = Math.sin( s * len ) / sin;
- t = Math.sin( t * len ) / sin;
-
- }
-
- var tDir = t * dir;
-
- x0 = x0 * s + x1 * tDir;
- y0 = y0 * s + y1 * tDir;
- z0 = z0 * s + z1 * tDir;
- w0 = w0 * s + w1 * tDir;
-
- // Normalize in case we just did a lerp:
- if ( s === 1 - t ) {
-
- var f = 1 / Math.sqrt( x0 * x0 + y0 * y0 + z0 * z0 + w0 * w0 );
-
- x0 *= f;
- y0 *= f;
- z0 *= f;
- w0 *= f;
-
- }
-
- }
-
- dst[ dstOffset ] = x0;
- dst[ dstOffset + 1 ] = y0;
- dst[ dstOffset + 2 ] = z0;
- dst[ dstOffset + 3 ] = w0;
-
- }
-
- } );
-
- Object.defineProperties( Quaternion.prototype, {
-
- x: {
-
- get: function () {
-
- return this._x;
-
- },
-
- set: function ( value ) {
-
- this._x = value;
- this.onChangeCallback();
-
- }
-
- },
-
- y: {
-
- get: function () {
-
- return this._y;
-
- },
-
- set: function ( value ) {
-
- this._y = value;
- this.onChangeCallback();
-
- }
-
- },
-
- z: {
-
- get: function () {
-
- return this._z;
-
- },
-
- set: function ( value ) {
-
- this._z = value;
- this.onChangeCallback();
-
- }
-
- },
-
- w: {
-
- get: function () {
-
- return this._w;
-
- },
-
- set: function ( value ) {
-
- this._w = value;
- this.onChangeCallback();
-
- }
-
- }
-
- } );
-
- Object.assign( Quaternion.prototype, {
-
- isQuaternion: true,
-
- set: function ( x, y, z, w ) {
-
- this._x = x;
- this._y = y;
- this._z = z;
- this._w = w;
-
- this.onChangeCallback();
-
- return this;
-
- },
-
- clone: function () {
-
- return new this.constructor( this._x, this._y, this._z, this._w );
-
- },
-
- copy: function ( quaternion ) {
-
- this._x = quaternion.x;
- this._y = quaternion.y;
- this._z = quaternion.z;
- this._w = quaternion.w;
-
- this.onChangeCallback();
-
- return this;
-
- },
-
- setFromEuler: function ( euler, update ) {
-
- if ( ! ( euler && euler.isEuler ) ) {
-
- throw new Error( 'THREE.Quaternion: .setFromEuler() now expects an Euler rotation rather than a Vector3 and order.' );
-
- }
-
- var x = euler._x, y = euler._y, z = euler._z, order = euler.order;
-
- // http://www.mathworks.com/matlabcentral/fileexchange/
- // 20696-function-to-convert-between-dcm-euler-angles-quaternions-and-euler-vectors/
- // content/SpinCalc.m
-
- var cos = Math.cos;
- var sin = Math.sin;
-
- var c1 = cos( x / 2 );
- var c2 = cos( y / 2 );
- var c3 = cos( z / 2 );
-
- var s1 = sin( x / 2 );
- var s2 = sin( y / 2 );
- var s3 = sin( z / 2 );
-
- if ( order === 'XYZ' ) {
-
- this._x = s1 * c2 * c3 + c1 * s2 * s3;
- this._y = c1 * s2 * c3 - s1 * c2 * s3;
- this._z = c1 * c2 * s3 + s1 * s2 * c3;
- this._w = c1 * c2 * c3 - s1 * s2 * s3;
-
- } else if ( order === 'YXZ' ) {
-
- this._x = s1 * c2 * c3 + c1 * s2 * s3;
- this._y = c1 * s2 * c3 - s1 * c2 * s3;
- this._z = c1 * c2 * s3 - s1 * s2 * c3;
- this._w = c1 * c2 * c3 + s1 * s2 * s3;
-
- } else if ( order === 'ZXY' ) {
-
- this._x = s1 * c2 * c3 - c1 * s2 * s3;
- this._y = c1 * s2 * c3 + s1 * c2 * s3;
- this._z = c1 * c2 * s3 + s1 * s2 * c3;
- this._w = c1 * c2 * c3 - s1 * s2 * s3;
-
- } else if ( order === 'ZYX' ) {
-
- this._x = s1 * c2 * c3 - c1 * s2 * s3;
- this._y = c1 * s2 * c3 + s1 * c2 * s3;
- this._z = c1 * c2 * s3 - s1 * s2 * c3;
- this._w = c1 * c2 * c3 + s1 * s2 * s3;
-
- } else if ( order === 'YZX' ) {
-
- this._x = s1 * c2 * c3 + c1 * s2 * s3;
- this._y = c1 * s2 * c3 + s1 * c2 * s3;
- this._z = c1 * c2 * s3 - s1 * s2 * c3;
- this._w = c1 * c2 * c3 - s1 * s2 * s3;
-
- } else if ( order === 'XZY' ) {
-
- this._x = s1 * c2 * c3 - c1 * s2 * s3;
- this._y = c1 * s2 * c3 - s1 * c2 * s3;
- this._z = c1 * c2 * s3 + s1 * s2 * c3;
- this._w = c1 * c2 * c3 + s1 * s2 * s3;
-
- }
-
- if ( update !== false ) this.onChangeCallback();
-
- return this;
-
- },
-
- setFromAxisAngle: function ( axis, angle ) {
-
- // http://www.euclideanspace.com/maths/geometry/rotations/conversions/angleToQuaternion/index.htm
-
- // assumes axis is normalized
-
- var halfAngle = angle / 2, s = Math.sin( halfAngle );
-
- this._x = axis.x * s;
- this._y = axis.y * s;
- this._z = axis.z * s;
- this._w = Math.cos( halfAngle );
-
- this.onChangeCallback();
-
- return this;
-
- },
-
- setFromRotationMatrix: function ( m ) {
-
- // http://www.euclideanspace.com/maths/geometry/rotations/conversions/matrixToQuaternion/index.htm
-
- // assumes the upper 3x3 of m is a pure rotation matrix (i.e, unscaled)
-
- var te = m.elements,
-
- m11 = te[ 0 ], m12 = te[ 4 ], m13 = te[ 8 ],
- m21 = te[ 1 ], m22 = te[ 5 ], m23 = te[ 9 ],
- m31 = te[ 2 ], m32 = te[ 6 ], m33 = te[ 10 ],
-
- trace = m11 + m22 + m33,
- s;
-
- if ( trace > 0 ) {
-
- s = 0.5 / Math.sqrt( trace + 1.0 );
-
- this._w = 0.25 / s;
- this._x = ( m32 - m23 ) * s;
- this._y = ( m13 - m31 ) * s;
- this._z = ( m21 - m12 ) * s;
-
- } else if ( m11 > m22 && m11 > m33 ) {
-
- s = 2.0 * Math.sqrt( 1.0 + m11 - m22 - m33 );
-
- this._w = ( m32 - m23 ) / s;
- this._x = 0.25 * s;
- this._y = ( m12 + m21 ) / s;
- this._z = ( m13 + m31 ) / s;
-
- } else if ( m22 > m33 ) {
-
- s = 2.0 * Math.sqrt( 1.0 + m22 - m11 - m33 );
-
- this._w = ( m13 - m31 ) / s;
- this._x = ( m12 + m21 ) / s;
- this._y = 0.25 * s;
- this._z = ( m23 + m32 ) / s;
-
- } else {
-
- s = 2.0 * Math.sqrt( 1.0 + m33 - m11 - m22 );
-
- this._w = ( m21 - m12 ) / s;
- this._x = ( m13 + m31 ) / s;
- this._y = ( m23 + m32 ) / s;
- this._z = 0.25 * s;
-
- }
-
- this.onChangeCallback();
-
- return this;
-
- },
-
- setFromUnitVectors: function ( vFrom, vTo ) {
-
- // assumes direction vectors vFrom and vTo are normalized
-
- var EPS = 0.000001;
-
- var r = vFrom.dot( vTo ) + 1;
-
- if ( r < EPS ) {
-
- r = 0;
-
- if ( Math.abs( vFrom.x ) > Math.abs( vFrom.z ) ) {
-
- this._x = - vFrom.y;
- this._y = vFrom.x;
- this._z = 0;
- this._w = r;
-
- } else {
-
- this._x = 0;
- this._y = - vFrom.z;
- this._z = vFrom.y;
- this._w = r;
-
- }
-
- } else {
-
- // crossVectors( vFrom, vTo ); // inlined to avoid cyclic dependency on Vector3
-
- this._x = vFrom.y * vTo.z - vFrom.z * vTo.y;
- this._y = vFrom.z * vTo.x - vFrom.x * vTo.z;
- this._z = vFrom.x * vTo.y - vFrom.y * vTo.x;
- this._w = r;
-
- }
-
- return this.normalize();
-
- },
-
- angleTo: function ( q ) {
-
- return 2 * Math.acos( Math.abs( _Math.clamp( this.dot( q ), - 1, 1 ) ) );
-
- },
-
- rotateTowards: function ( q, step ) {
-
- var angle = this.angleTo( q );
-
- if ( angle === 0 ) return this;
-
- var t = Math.min( 1, step / angle );
-
- this.slerp( q, t );
-
- return this;
-
- },
-
- inverse: function () {
-
- // quaternion is assumed to have unit length
-
- return this.conjugate();
-
- },
-
- conjugate: function () {
-
- this._x *= - 1;
- this._y *= - 1;
- this._z *= - 1;
-
- this.onChangeCallback();
-
- return this;
-
- },
-
- dot: function ( v ) {
-
- return this._x * v._x + this._y * v._y + this._z * v._z + this._w * v._w;
-
- },
-
- lengthSq: function () {
-
- return this._x * this._x + this._y * this._y + this._z * this._z + this._w * this._w;
-
- },
-
- length: function () {
-
- return Math.sqrt( this._x * this._x + this._y * this._y + this._z * this._z + this._w * this._w );
-
- },
-
- normalize: function () {
-
- var l = this.length();
-
- if ( l === 0 ) {
-
- this._x = 0;
- this._y = 0;
- this._z = 0;
- this._w = 1;
-
- } else {
-
- l = 1 / l;
-
- this._x = this._x * l;
- this._y = this._y * l;
- this._z = this._z * l;
- this._w = this._w * l;
-
- }
-
- this.onChangeCallback();
-
- return this;
-
- },
-
- multiply: function ( q, p ) {
-
- if ( p !== undefined ) {
-
- console.warn( 'THREE.Quaternion: .multiply() now only accepts one argument. Use .multiplyQuaternions( a, b ) instead.' );
- return this.multiplyQuaternions( q, p );
-
- }
-
- return this.multiplyQuaternions( this, q );
-
- },
-
- premultiply: function ( q ) {
-
- return this.multiplyQuaternions( q, this );
-
- },
-
- multiplyQuaternions: function ( a, b ) {
-
- // from http://www.euclideanspace.com/maths/algebra/realNormedAlgebra/quaternions/code/index.htm
-
- var qax = a._x, qay = a._y, qaz = a._z, qaw = a._w;
- var qbx = b._x, qby = b._y, qbz = b._z, qbw = b._w;
-
- this._x = qax * qbw + qaw * qbx + qay * qbz - qaz * qby;
- this._y = qay * qbw + qaw * qby + qaz * qbx - qax * qbz;
- this._z = qaz * qbw + qaw * qbz + qax * qby - qay * qbx;
- this._w = qaw * qbw - qax * qbx - qay * qby - qaz * qbz;
-
- this.onChangeCallback();
-
- return this;
-
- },
-
- slerp: function ( qb, t ) {
-
- if ( t === 0 ) return this;
- if ( t === 1 ) return this.copy( qb );
-
- var x = this._x, y = this._y, z = this._z, w = this._w;
-
- // http://www.euclideanspace.com/maths/algebra/realNormedAlgebra/quaternions/slerp/
-
- var cosHalfTheta = w * qb._w + x * qb._x + y * qb._y + z * qb._z;
-
- if ( cosHalfTheta < 0 ) {
-
- this._w = - qb._w;
- this._x = - qb._x;
- this._y = - qb._y;
- this._z = - qb._z;
-
- cosHalfTheta = - cosHalfTheta;
-
- } else {
-
- this.copy( qb );
-
- }
-
- if ( cosHalfTheta >= 1.0 ) {
-
- this._w = w;
- this._x = x;
- this._y = y;
- this._z = z;
-
- return this;
-
- }
-
- var sqrSinHalfTheta = 1.0 - cosHalfTheta * cosHalfTheta;
-
- if ( sqrSinHalfTheta <= Number.EPSILON ) {
-
- var s = 1 - t;
- this._w = s * w + t * this._w;
- this._x = s * x + t * this._x;
- this._y = s * y + t * this._y;
- this._z = s * z + t * this._z;
-
- return this.normalize();
-
- }
-
- var sinHalfTheta = Math.sqrt( sqrSinHalfTheta );
- var halfTheta = Math.atan2( sinHalfTheta, cosHalfTheta );
- var ratioA = Math.sin( ( 1 - t ) * halfTheta ) / sinHalfTheta,
- ratioB = Math.sin( t * halfTheta ) / sinHalfTheta;
-
- this._w = ( w * ratioA + this._w * ratioB );
- this._x = ( x * ratioA + this._x * ratioB );
- this._y = ( y * ratioA + this._y * ratioB );
- this._z = ( z * ratioA + this._z * ratioB );
-
- this.onChangeCallback();
-
- return this;
-
- },
-
- equals: function ( quaternion ) {
-
- return ( quaternion._x === this._x ) && ( quaternion._y === this._y ) && ( quaternion._z === this._z ) && ( quaternion._w === this._w );
-
- },
-
- fromArray: function ( array, offset ) {
-
- if ( offset === undefined ) offset = 0;
-
- this._x = array[ offset ];
- this._y = array[ offset + 1 ];
- this._z = array[ offset + 2 ];
- this._w = array[ offset + 3 ];
-
- this.onChangeCallback();
-
- return this;
-
- },
-
- toArray: function ( array, offset ) {
-
- if ( array === undefined ) array = [];
- if ( offset === undefined ) offset = 0;
-
- array[ offset ] = this._x;
- array[ offset + 1 ] = this._y;
- array[ offset + 2 ] = this._z;
- array[ offset + 3 ] = this._w;
-
- return array;
-
- },
-
- onChange: function ( callback ) {
-
- this.onChangeCallback = callback;
-
- return this;
-
- },
-
- onChangeCallback: function () {}
-
- } );
-
- /**
- * @author mrdoob / http://mrdoob.com/
- * @author kile / http://kile.stravaganza.org/
- * @author philogb / http://blog.thejit.org/
- * @author mikael emtinger / http://gomo.se/
- * @author egraether / http://egraether.com/
- * @author WestLangley / http://github.com/WestLangley
- */
-
- function Vector3( x, y, z ) {
-
- this.x = x || 0;
- this.y = y || 0;
- this.z = z || 0;
-
- }
-
- Object.assign( Vector3.prototype, {
-
- isVector3: true,
-
- set: function ( x, y, z ) {
-
- this.x = x;
- this.y = y;
- this.z = z;
-
- return this;
-
- },
-
- setScalar: function ( scalar ) {
-
- this.x = scalar;
- this.y = scalar;
- this.z = scalar;
-
- return this;
-
- },
-
- setX: function ( x ) {
-
- this.x = x;
-
- return this;
-
- },
-
- setY: function ( y ) {
-
- this.y = y;
-
- return this;
-
- },
-
- setZ: function ( z ) {
-
- this.z = z;
-
- return this;
-
- },
-
- setComponent: function ( index, value ) {
-
- switch ( index ) {
-
- case 0: this.x = value; break;
- case 1: this.y = value; break;
- case 2: this.z = value; break;
- default: throw new Error( 'index is out of range: ' + index );
-
- }
-
- return this;
-
- },
-
- getComponent: function ( index ) {
-
- switch ( index ) {
-
- case 0: return this.x;
- case 1: return this.y;
- case 2: return this.z;
- default: throw new Error( 'index is out of range: ' + index );
-
- }
-
- },
-
- clone: function () {
-
- return new this.constructor( this.x, this.y, this.z );
-
- },
-
- copy: function ( v ) {
-
- this.x = v.x;
- this.y = v.y;
- this.z = v.z;
-
- return this;
-
- },
-
- add: function ( v, w ) {
-
- if ( w !== undefined ) {
-
- console.warn( 'THREE.Vector3: .add() now only accepts one argument. Use .addVectors( a, b ) instead.' );
- return this.addVectors( v, w );
-
- }
-
- this.x += v.x;
- this.y += v.y;
- this.z += v.z;
-
- return this;
-
- },
-
- addScalar: function ( s ) {
-
- this.x += s;
- this.y += s;
- this.z += s;
-
- return this;
-
- },
-
- addVectors: function ( a, b ) {
-
- this.x = a.x + b.x;
- this.y = a.y + b.y;
- this.z = a.z + b.z;
-
- return this;
-
- },
-
- addScaledVector: function ( v, s ) {
-
- this.x += v.x * s;
- this.y += v.y * s;
- this.z += v.z * s;
-
- return this;
-
- },
-
- sub: function ( v, w ) {
-
- if ( w !== undefined ) {
-
- console.warn( 'THREE.Vector3: .sub() now only accepts one argument. Use .subVectors( a, b ) instead.' );
- return this.subVectors( v, w );
-
- }
-
- this.x -= v.x;
- this.y -= v.y;
- this.z -= v.z;
-
- return this;
-
- },
-
- subScalar: function ( s ) {
-
- this.x -= s;
- this.y -= s;
- this.z -= s;
-
- return this;
-
- },
-
- subVectors: function ( a, b ) {
-
- this.x = a.x - b.x;
- this.y = a.y - b.y;
- this.z = a.z - b.z;
-
- return this;
-
- },
-
- multiply: function ( v, w ) {
-
- if ( w !== undefined ) {
-
- console.warn( 'THREE.Vector3: .multiply() now only accepts one argument. Use .multiplyVectors( a, b ) instead.' );
- return this.multiplyVectors( v, w );
-
- }
-
- this.x *= v.x;
- this.y *= v.y;
- this.z *= v.z;
-
- return this;
-
- },
-
- multiplyScalar: function ( scalar ) {
-
- this.x *= scalar;
- this.y *= scalar;
- this.z *= scalar;
-
- return this;
-
- },
-
- multiplyVectors: function ( a, b ) {
-
- this.x = a.x * b.x;
- this.y = a.y * b.y;
- this.z = a.z * b.z;
-
- return this;
-
- },
-
- applyEuler: function () {
-
- var quaternion = new Quaternion();
-
- return function applyEuler( euler ) {
-
- if ( ! ( euler && euler.isEuler ) ) {
-
- console.error( 'THREE.Vector3: .applyEuler() now expects an Euler rotation rather than a Vector3 and order.' );
-
- }
-
- return this.applyQuaternion( quaternion.setFromEuler( euler ) );
-
- };
-
- }(),
-
- applyAxisAngle: function () {
-
- var quaternion = new Quaternion();
-
- return function applyAxisAngle( axis, angle ) {
-
- return this.applyQuaternion( quaternion.setFromAxisAngle( axis, angle ) );
-
- };
-
- }(),
-
- applyMatrix3: function ( m ) {
-
- var x = this.x, y = this.y, z = this.z;
- var e = m.elements;
-
- this.x = e[ 0 ] * x + e[ 3 ] * y + e[ 6 ] * z;
- this.y = e[ 1 ] * x + e[ 4 ] * y + e[ 7 ] * z;
- this.z = e[ 2 ] * x + e[ 5 ] * y + e[ 8 ] * z;
-
- return this;
-
- },
-
- applyMatrix4: function ( m ) {
-
- var x = this.x, y = this.y, z = this.z;
- var e = m.elements;
-
- var w = 1 / ( e[ 3 ] * x + e[ 7 ] * y + e[ 11 ] * z + e[ 15 ] );
-
- this.x = ( e[ 0 ] * x + e[ 4 ] * y + e[ 8 ] * z + e[ 12 ] ) * w;
- this.y = ( e[ 1 ] * x + e[ 5 ] * y + e[ 9 ] * z + e[ 13 ] ) * w;
- this.z = ( e[ 2 ] * x + e[ 6 ] * y + e[ 10 ] * z + e[ 14 ] ) * w;
-
- return this;
-
- },
-
- applyQuaternion: function ( q ) {
-
- var x = this.x, y = this.y, z = this.z;
- var qx = q.x, qy = q.y, qz = q.z, qw = q.w;
-
- // calculate quat * vector
-
- var ix = qw * x + qy * z - qz * y;
- var iy = qw * y + qz * x - qx * z;
- var iz = qw * z + qx * y - qy * x;
- var iw = - qx * x - qy * y - qz * z;
-
- // calculate result * inverse quat
-
- this.x = ix * qw + iw * - qx + iy * - qz - iz * - qy;
- this.y = iy * qw + iw * - qy + iz * - qx - ix * - qz;
- this.z = iz * qw + iw * - qz + ix * - qy - iy * - qx;
-
- return this;
-
- },
-
- project: function ( camera ) {
-
- return this.applyMatrix4( camera.matrixWorldInverse ).applyMatrix4( camera.projectionMatrix );
-
- },
-
- unproject: function ( camera ) {
-
- return this.applyMatrix4( camera.projectionMatrixInverse ).applyMatrix4( camera.matrixWorld );
-
- },
-
- transformDirection: function ( m ) {
-
- // input: THREE.Matrix4 affine matrix
- // vector interpreted as a direction
-
- var x = this.x, y = this.y, z = this.z;
- var e = m.elements;
-
- this.x = e[ 0 ] * x + e[ 4 ] * y + e[ 8 ] * z;
- this.y = e[ 1 ] * x + e[ 5 ] * y + e[ 9 ] * z;
- this.z = e[ 2 ] * x + e[ 6 ] * y + e[ 10 ] * z;
-
- return this.normalize();
-
- },
-
- divide: function ( v ) {
-
- this.x /= v.x;
- this.y /= v.y;
- this.z /= v.z;
-
- return this;
-
- },
-
- divideScalar: function ( scalar ) {
-
- return this.multiplyScalar( 1 / scalar );
-
- },
-
- min: function ( v ) {
-
- this.x = Math.min( this.x, v.x );
- this.y = Math.min( this.y, v.y );
- this.z = Math.min( this.z, v.z );
-
- return this;
-
- },
-
- max: function ( v ) {
-
- this.x = Math.max( this.x, v.x );
- this.y = Math.max( this.y, v.y );
- this.z = Math.max( this.z, v.z );
-
- return this;
-
- },
-
- clamp: function ( min, max ) {
-
- // assumes min < max, componentwise
-
- this.x = Math.max( min.x, Math.min( max.x, this.x ) );
- this.y = Math.max( min.y, Math.min( max.y, this.y ) );
- this.z = Math.max( min.z, Math.min( max.z, this.z ) );
-
- return this;
-
- },
-
- clampScalar: function () {
-
- var min = new Vector3();
- var max = new Vector3();
-
- return function clampScalar( minVal, maxVal ) {
-
- min.set( minVal, minVal, minVal );
- max.set( maxVal, maxVal, maxVal );
-
- return this.clamp( min, max );
-
- };
-
- }(),
-
- clampLength: function ( min, max ) {
-
- var length = this.length();
-
- return this.divideScalar( length || 1 ).multiplyScalar( Math.max( min, Math.min( max, length ) ) );
-
- },
-
- floor: function () {
-
- this.x = Math.floor( this.x );
- this.y = Math.floor( this.y );
- this.z = Math.floor( this.z );
-
- return this;
-
- },
-
- ceil: function () {
-
- this.x = Math.ceil( this.x );
- this.y = Math.ceil( this.y );
- this.z = Math.ceil( this.z );
-
- return this;
-
- },
-
- round: function () {
-
- this.x = Math.round( this.x );
- this.y = Math.round( this.y );
- this.z = Math.round( this.z );
-
- return this;
-
- },
-
- roundToZero: function () {
-
- this.x = ( this.x < 0 ) ? Math.ceil( this.x ) : Math.floor( this.x );
- this.y = ( this.y < 0 ) ? Math.ceil( this.y ) : Math.floor( this.y );
- this.z = ( this.z < 0 ) ? Math.ceil( this.z ) : Math.floor( this.z );
-
- return this;
-
- },
-
- negate: function () {
-
- this.x = - this.x;
- this.y = - this.y;
- this.z = - this.z;
-
- return this;
-
- },
-
- dot: function ( v ) {
-
- return this.x * v.x + this.y * v.y + this.z * v.z;
-
- },
-
- // TODO lengthSquared?
-
- lengthSq: function () {
-
- return this.x * this.x + this.y * this.y + this.z * this.z;
-
- },
-
- length: function () {
-
- return Math.sqrt( this.x * this.x + this.y * this.y + this.z * this.z );
-
- },
-
- manhattanLength: function () {
-
- return Math.abs( this.x ) + Math.abs( this.y ) + Math.abs( this.z );
-
- },
-
- normalize: function () {
-
- return this.divideScalar( this.length() || 1 );
-
- },
-
- setLength: function ( length ) {
-
- return this.normalize().multiplyScalar( length );
-
- },
-
- lerp: function ( v, alpha ) {
-
- this.x += ( v.x - this.x ) * alpha;
- this.y += ( v.y - this.y ) * alpha;
- this.z += ( v.z - this.z ) * alpha;
-
- return this;
-
- },
-
- lerpVectors: function ( v1, v2, alpha ) {
-
- return this.subVectors( v2, v1 ).multiplyScalar( alpha ).add( v1 );
-
- },
-
- cross: function ( v, w ) {
-
- if ( w !== undefined ) {
-
- console.warn( 'THREE.Vector3: .cross() now only accepts one argument. Use .crossVectors( a, b ) instead.' );
- return this.crossVectors( v, w );
-
- }
-
- return this.crossVectors( this, v );
-
- },
-
- crossVectors: function ( a, b ) {
-
- var ax = a.x, ay = a.y, az = a.z;
- var bx = b.x, by = b.y, bz = b.z;
-
- this.x = ay * bz - az * by;
- this.y = az * bx - ax * bz;
- this.z = ax * by - ay * bx;
-
- return this;
-
- },
-
- projectOnVector: function ( vector ) {
-
- var scalar = vector.dot( this ) / vector.lengthSq();
-
- return this.copy( vector ).multiplyScalar( scalar );
-
- },
-
- projectOnPlane: function () {
-
- var v1 = new Vector3();
-
- return function projectOnPlane( planeNormal ) {
-
- v1.copy( this ).projectOnVector( planeNormal );
-
- return this.sub( v1 );
-
- };
-
- }(),
-
- reflect: function () {
-
- // reflect incident vector off plane orthogonal to normal
- // normal is assumed to have unit length
-
- var v1 = new Vector3();
-
- return function reflect( normal ) {
-
- return this.sub( v1.copy( normal ).multiplyScalar( 2 * this.dot( normal ) ) );
-
- };
-
- }(),
-
- angleTo: function ( v ) {
-
- var theta = this.dot( v ) / ( Math.sqrt( this.lengthSq() * v.lengthSq() ) );
-
- // clamp, to handle numerical problems
-
- return Math.acos( _Math.clamp( theta, - 1, 1 ) );
-
- },
-
- distanceTo: function ( v ) {
-
- return Math.sqrt( this.distanceToSquared( v ) );
-
- },
-
- distanceToSquared: function ( v ) {
-
- var dx = this.x - v.x, dy = this.y - v.y, dz = this.z - v.z;
-
- return dx * dx + dy * dy + dz * dz;
-
- },
-
- manhattanDistanceTo: function ( v ) {
-
- return Math.abs( this.x - v.x ) + Math.abs( this.y - v.y ) + Math.abs( this.z - v.z );
-
- },
-
- setFromSpherical: function ( s ) {
-
- return this.setFromSphericalCoords( s.radius, s.phi, s.theta );
-
- },
-
- setFromSphericalCoords: function ( radius, phi, theta ) {
-
- var sinPhiRadius = Math.sin( phi ) * radius;
-
- this.x = sinPhiRadius * Math.sin( theta );
- this.y = Math.cos( phi ) * radius;
- this.z = sinPhiRadius * Math.cos( theta );
-
- return this;
-
- },
-
- setFromCylindrical: function ( c ) {
-
- return this.setFromCylindricalCoords( c.radius, c.theta, c.y );
-
- },
-
- setFromCylindricalCoords: function ( radius, theta, y ) {
-
- this.x = radius * Math.sin( theta );
- this.y = y;
- this.z = radius * Math.cos( theta );
-
- return this;
-
- },
-
- setFromMatrixPosition: function ( m ) {
-
- var e = m.elements;
-
- this.x = e[ 12 ];
- this.y = e[ 13 ];
- this.z = e[ 14 ];
-
- return this;
-
- },
-
- setFromMatrixScale: function ( m ) {
-
- var sx = this.setFromMatrixColumn( m, 0 ).length();
- var sy = this.setFromMatrixColumn( m, 1 ).length();
- var sz = this.setFromMatrixColumn( m, 2 ).length();
-
- this.x = sx;
- this.y = sy;
- this.z = sz;
-
- return this;
-
- },
-
- setFromMatrixColumn: function ( m, index ) {
-
- return this.fromArray( m.elements, index * 4 );
-
- },
-
- equals: function ( v ) {
-
- return ( ( v.x === this.x ) && ( v.y === this.y ) && ( v.z === this.z ) );
-
- },
-
- fromArray: function ( array, offset ) {
-
- if ( offset === undefined ) offset = 0;
-
- this.x = array[ offset ];
- this.y = array[ offset + 1 ];
- this.z = array[ offset + 2 ];
-
- return this;
-
- },
-
- toArray: function ( array, offset ) {
-
- if ( array === undefined ) array = [];
- if ( offset === undefined ) offset = 0;
-
- array[ offset ] = this.x;
- array[ offset + 1 ] = this.y;
- array[ offset + 2 ] = this.z;
-
- return array;
-
- },
-
- fromBufferAttribute: function ( attribute, index, offset ) {
-
- if ( offset !== undefined ) {
-
- console.warn( 'THREE.Vector3: offset has been removed from .fromBufferAttribute().' );
-
- }
-
- this.x = attribute.getX( index );
- this.y = attribute.getY( index );
- this.z = attribute.getZ( index );
-
- return this;
-
- }
-
- } );
-
- /**
- * @author alteredq / http://alteredqualia.com/
- * @author WestLangley / http://github.com/WestLangley
- * @author bhouston / http://clara.io
- * @author tschw
- */
-
- function Matrix3() {
-
- this.elements = [
-
- 1, 0, 0,
- 0, 1, 0,
- 0, 0, 1
-
- ];
-
- if ( arguments.length > 0 ) {
-
- console.error( 'THREE.Matrix3: the constructor no longer reads arguments. use .set() instead.' );
-
- }
-
- }
-
- Object.assign( Matrix3.prototype, {
-
- isMatrix3: true,
-
- set: function ( n11, n12, n13, n21, n22, n23, n31, n32, n33 ) {
-
- var te = this.elements;
-
- te[ 0 ] = n11; te[ 1 ] = n21; te[ 2 ] = n31;
- te[ 3 ] = n12; te[ 4 ] = n22; te[ 5 ] = n32;
- te[ 6 ] = n13; te[ 7 ] = n23; te[ 8 ] = n33;
-
- return this;
-
- },
-
- identity: function () {
-
- this.set(
-
- 1, 0, 0,
- 0, 1, 0,
- 0, 0, 1
-
- );
-
- return this;
-
- },
-
- clone: function () {
-
- return new this.constructor().fromArray( this.elements );
-
- },
-
- copy: function ( m ) {
-
- var te = this.elements;
- var me = m.elements;
-
- te[ 0 ] = me[ 0 ]; te[ 1 ] = me[ 1 ]; te[ 2 ] = me[ 2 ];
- te[ 3 ] = me[ 3 ]; te[ 4 ] = me[ 4 ]; te[ 5 ] = me[ 5 ];
- te[ 6 ] = me[ 6 ]; te[ 7 ] = me[ 7 ]; te[ 8 ] = me[ 8 ];
-
- return this;
-
- },
-
- setFromMatrix4: function ( m ) {
-
- var me = m.elements;
-
- this.set(
-
- me[ 0 ], me[ 4 ], me[ 8 ],
- me[ 1 ], me[ 5 ], me[ 9 ],
- me[ 2 ], me[ 6 ], me[ 10 ]
-
- );
-
- return this;
-
- },
-
- applyToBufferAttribute: function () {
-
- var v1 = new Vector3();
-
- return function applyToBufferAttribute( attribute ) {
-
- for ( var i = 0, l = attribute.count; i < l; i ++ ) {
-
- v1.x = attribute.getX( i );
- v1.y = attribute.getY( i );
- v1.z = attribute.getZ( i );
-
- v1.applyMatrix3( this );
-
- attribute.setXYZ( i, v1.x, v1.y, v1.z );
-
- }
-
- return attribute;
-
- };
-
- }(),
-
- multiply: function ( m ) {
-
- return this.multiplyMatrices( this, m );
-
- },
-
- premultiply: function ( m ) {
-
- return this.multiplyMatrices( m, this );
-
- },
-
- multiplyMatrices: function ( a, b ) {
-
- var ae = a.elements;
- var be = b.elements;
- var te = this.elements;
-
- var a11 = ae[ 0 ], a12 = ae[ 3 ], a13 = ae[ 6 ];
- var a21 = ae[ 1 ], a22 = ae[ 4 ], a23 = ae[ 7 ];
- var a31 = ae[ 2 ], a32 = ae[ 5 ], a33 = ae[ 8 ];
-
- var b11 = be[ 0 ], b12 = be[ 3 ], b13 = be[ 6 ];
- var b21 = be[ 1 ], b22 = be[ 4 ], b23 = be[ 7 ];
- var b31 = be[ 2 ], b32 = be[ 5 ], b33 = be[ 8 ];
-
- te[ 0 ] = a11 * b11 + a12 * b21 + a13 * b31;
- te[ 3 ] = a11 * b12 + a12 * b22 + a13 * b32;
- te[ 6 ] = a11 * b13 + a12 * b23 + a13 * b33;
-
- te[ 1 ] = a21 * b11 + a22 * b21 + a23 * b31;
- te[ 4 ] = a21 * b12 + a22 * b22 + a23 * b32;
- te[ 7 ] = a21 * b13 + a22 * b23 + a23 * b33;
-
- te[ 2 ] = a31 * b11 + a32 * b21 + a33 * b31;
- te[ 5 ] = a31 * b12 + a32 * b22 + a33 * b32;
- te[ 8 ] = a31 * b13 + a32 * b23 + a33 * b33;
-
- return this;
-
- },
-
- multiplyScalar: function ( s ) {
-
- var te = this.elements;
-
- te[ 0 ] *= s; te[ 3 ] *= s; te[ 6 ] *= s;
- te[ 1 ] *= s; te[ 4 ] *= s; te[ 7 ] *= s;
- te[ 2 ] *= s; te[ 5 ] *= s; te[ 8 ] *= s;
-
- return this;
-
- },
-
- determinant: function () {
-
- var te = this.elements;
-
- var a = te[ 0 ], b = te[ 1 ], c = te[ 2 ],
- d = te[ 3 ], e = te[ 4 ], f = te[ 5 ],
- g = te[ 6 ], h = te[ 7 ], i = te[ 8 ];
-
- return a * e * i - a * f * h - b * d * i + b * f * g + c * d * h - c * e * g;
-
- },
-
- getInverse: function ( matrix, throwOnDegenerate ) {
-
- if ( matrix && matrix.isMatrix4 ) {
-
- console.error( "THREE.Matrix3: .getInverse() no longer takes a Matrix4 argument." );
-
- }
-
- var me = matrix.elements,
- te = this.elements,
-
- n11 = me[ 0 ], n21 = me[ 1 ], n31 = me[ 2 ],
- n12 = me[ 3 ], n22 = me[ 4 ], n32 = me[ 5 ],
- n13 = me[ 6 ], n23 = me[ 7 ], n33 = me[ 8 ],
-
- t11 = n33 * n22 - n32 * n23,
- t12 = n32 * n13 - n33 * n12,
- t13 = n23 * n12 - n22 * n13,
-
- det = n11 * t11 + n21 * t12 + n31 * t13;
-
- if ( det === 0 ) {
-
- var msg = "THREE.Matrix3: .getInverse() can't invert matrix, determinant is 0";
-
- if ( throwOnDegenerate === true ) {
-
- throw new Error( msg );
-
- } else {
-
- console.warn( msg );
-
- }
-
- return this.identity();
-
- }
-
- var detInv = 1 / det;
-
- te[ 0 ] = t11 * detInv;
- te[ 1 ] = ( n31 * n23 - n33 * n21 ) * detInv;
- te[ 2 ] = ( n32 * n21 - n31 * n22 ) * detInv;
-
- te[ 3 ] = t12 * detInv;
- te[ 4 ] = ( n33 * n11 - n31 * n13 ) * detInv;
- te[ 5 ] = ( n31 * n12 - n32 * n11 ) * detInv;
-
- te[ 6 ] = t13 * detInv;
- te[ 7 ] = ( n21 * n13 - n23 * n11 ) * detInv;
- te[ 8 ] = ( n22 * n11 - n21 * n12 ) * detInv;
-
- return this;
-
- },
-
- transpose: function () {
-
- var tmp, m = this.elements;
-
- tmp = m[ 1 ]; m[ 1 ] = m[ 3 ]; m[ 3 ] = tmp;
- tmp = m[ 2 ]; m[ 2 ] = m[ 6 ]; m[ 6 ] = tmp;
- tmp = m[ 5 ]; m[ 5 ] = m[ 7 ]; m[ 7 ] = tmp;
-
- return this;
-
- },
-
- getNormalMatrix: function ( matrix4 ) {
-
- return this.setFromMatrix4( matrix4 ).getInverse( this ).transpose();
-
- },
-
- transposeIntoArray: function ( r ) {
-
- var m = this.elements;
-
- r[ 0 ] = m[ 0 ];
- r[ 1 ] = m[ 3 ];
- r[ 2 ] = m[ 6 ];
- r[ 3 ] = m[ 1 ];
- r[ 4 ] = m[ 4 ];
- r[ 5 ] = m[ 7 ];
- r[ 6 ] = m[ 2 ];
- r[ 7 ] = m[ 5 ];
- r[ 8 ] = m[ 8 ];
-
- return this;
-
- },
-
- setUvTransform: function ( tx, ty, sx, sy, rotation, cx, cy ) {
-
- var c = Math.cos( rotation );
- var s = Math.sin( rotation );
-
- this.set(
- sx * c, sx * s, - sx * ( c * cx + s * cy ) + cx + tx,
- - sy * s, sy * c, - sy * ( - s * cx + c * cy ) + cy + ty,
- 0, 0, 1
- );
-
- },
-
- scale: function ( sx, sy ) {
-
- var te = this.elements;
-
- te[ 0 ] *= sx; te[ 3 ] *= sx; te[ 6 ] *= sx;
- te[ 1 ] *= sy; te[ 4 ] *= sy; te[ 7 ] *= sy;
-
- return this;
-
- },
-
- rotate: function ( theta ) {
-
- var c = Math.cos( theta );
- var s = Math.sin( theta );
-
- var te = this.elements;
-
- var a11 = te[ 0 ], a12 = te[ 3 ], a13 = te[ 6 ];
- var a21 = te[ 1 ], a22 = te[ 4 ], a23 = te[ 7 ];
-
- te[ 0 ] = c * a11 + s * a21;
- te[ 3 ] = c * a12 + s * a22;
- te[ 6 ] = c * a13 + s * a23;
-
- te[ 1 ] = - s * a11 + c * a21;
- te[ 4 ] = - s * a12 + c * a22;
- te[ 7 ] = - s * a13 + c * a23;
-
- return this;
-
- },
-
- translate: function ( tx, ty ) {
-
- var te = this.elements;
-
- te[ 0 ] += tx * te[ 2 ]; te[ 3 ] += tx * te[ 5 ]; te[ 6 ] += tx * te[ 8 ];
- te[ 1 ] += ty * te[ 2 ]; te[ 4 ] += ty * te[ 5 ]; te[ 7 ] += ty * te[ 8 ];
-
- return this;
-
- },
-
- equals: function ( matrix ) {
-
- var te = this.elements;
- var me = matrix.elements;
-
- for ( var i = 0; i < 9; i ++ ) {
-
- if ( te[ i ] !== me[ i ] ) return false;
-
- }
-
- return true;
-
- },
-
- fromArray: function ( array, offset ) {
-
- if ( offset === undefined ) offset = 0;
-
- for ( var i = 0; i < 9; i ++ ) {
-
- this.elements[ i ] = array[ i + offset ];
-
- }
-
- return this;
-
- },
-
- toArray: function ( array, offset ) {
-
- if ( array === undefined ) array = [];
- if ( offset === undefined ) offset = 0;
-
- var te = this.elements;
-
- array[ offset ] = te[ 0 ];
- array[ offset + 1 ] = te[ 1 ];
- array[ offset + 2 ] = te[ 2 ];
-
- array[ offset + 3 ] = te[ 3 ];
- array[ offset + 4 ] = te[ 4 ];
- array[ offset + 5 ] = te[ 5 ];
-
- array[ offset + 6 ] = te[ 6 ];
- array[ offset + 7 ] = te[ 7 ];
- array[ offset + 8 ] = te[ 8 ];
-
- return array;
-
- }
-
- } );
-
- /**
- * @author mrdoob / http://mrdoob.com/
- * @author alteredq / http://alteredqualia.com/
- * @author szimek / https://github.com/szimek/
- */
-
- var _canvas;
-
- var ImageUtils = {
-
- getDataURL: function ( image ) {
-
- var canvas;
-
- if ( typeof HTMLCanvasElement == 'undefined' ) {
-
- return image.src;
-
- } else if ( image instanceof HTMLCanvasElement ) {
-
- canvas = image;
-
- } else {
-
- if ( _canvas === undefined ) _canvas = document.createElementNS( 'http://www.w3.org/1999/xhtml', 'canvas' );
-
- _canvas.width = image.width;
- _canvas.height = image.height;
-
- var context = _canvas.getContext( '2d' );
-
- if ( image instanceof ImageData ) {
-
- context.putImageData( image, 0, 0 );
-
- } else {
-
- context.drawImage( image, 0, 0, image.width, image.height );
-
- }
-
- canvas = _canvas;
-
- }
-
- if ( canvas.width > 2048 || canvas.height > 2048 ) {
-
- return canvas.toDataURL( 'image/jpeg', 0.6 );
-
- } else {
-
- return canvas.toDataURL( 'image/png' );
-
- }
-
- }
-
- };
-
- /**
- * @author mrdoob / http://mrdoob.com/
- * @author alteredq / http://alteredqualia.com/
- * @author szimek / https://github.com/szimek/
- */
-
- var textureId = 0;
-
- function Texture( image, mapping, wrapS, wrapT, magFilter, minFilter, format, type, anisotropy, encoding ) {
-
- Object.defineProperty( this, 'id', { value: textureId ++ } );
-
- this.uuid = _Math.generateUUID();
-
- this.name = '';
-
- this.image = image !== undefined ? image : Texture.DEFAULT_IMAGE;
- this.mipmaps = [];
-
- this.mapping = mapping !== undefined ? mapping : Texture.DEFAULT_MAPPING;
-
- this.wrapS = wrapS !== undefined ? wrapS : ClampToEdgeWrapping;
- this.wrapT = wrapT !== undefined ? wrapT : ClampToEdgeWrapping;
-
- this.magFilter = magFilter !== undefined ? magFilter : LinearFilter;
- this.minFilter = minFilter !== undefined ? minFilter : LinearMipMapLinearFilter;
-
- this.anisotropy = anisotropy !== undefined ? anisotropy : 1;
-
- this.format = format !== undefined ? format : RGBAFormat;
- this.type = type !== undefined ? type : UnsignedByteType;
-
- this.offset = new Vector2( 0, 0 );
- this.repeat = new Vector2( 1, 1 );
- this.center = new Vector2( 0, 0 );
- this.rotation = 0;
-
- this.matrixAutoUpdate = true;
- this.matrix = new Matrix3();
-
- this.generateMipmaps = true;
- this.premultiplyAlpha = false;
- this.flipY = true;
- this.unpackAlignment = 4; // valid values: 1, 2, 4, 8 (see http://www.khronos.org/opengles/sdk/docs/man/xhtml/glPixelStorei.xml)
-
- // Values of encoding !== THREE.LinearEncoding only supported on map, envMap and emissiveMap.
- //
- // Also changing the encoding after already used by a Material will not automatically make the Material
- // update. You need to explicitly call Material.needsUpdate to trigger it to recompile.
- this.encoding = encoding !== undefined ? encoding : LinearEncoding;
-
- this.version = 0;
- this.onUpdate = null;
-
- }
-
- Texture.DEFAULT_IMAGE = undefined;
- Texture.DEFAULT_MAPPING = UVMapping;
-
- Texture.prototype = Object.assign( Object.create( EventDispatcher.prototype ), {
-
- constructor: Texture,
-
- isTexture: true,
-
- updateMatrix: function () {
-
- this.matrix.setUvTransform( this.offset.x, this.offset.y, this.repeat.x, this.repeat.y, this.rotation, this.center.x, this.center.y );
-
- },
-
- clone: function () {
-
- return new this.constructor().copy( this );
-
- },
-
- copy: function ( source ) {
-
- this.name = source.name;
-
- this.image = source.image;
- this.mipmaps = source.mipmaps.slice( 0 );
-
- this.mapping = source.mapping;
-
- this.wrapS = source.wrapS;
- this.wrapT = source.wrapT;
-
- this.magFilter = source.magFilter;
- this.minFilter = source.minFilter;
-
- this.anisotropy = source.anisotropy;
-
- this.format = source.format;
- this.type = source.type;
-
- this.offset.copy( source.offset );
- this.repeat.copy( source.repeat );
- this.center.copy( source.center );
- this.rotation = source.rotation;
-
- this.matrixAutoUpdate = source.matrixAutoUpdate;
- this.matrix.copy( source.matrix );
-
- this.generateMipmaps = source.generateMipmaps;
- this.premultiplyAlpha = source.premultiplyAlpha;
- this.flipY = source.flipY;
- this.unpackAlignment = source.unpackAlignment;
- this.encoding = source.encoding;
-
- return this;
-
- },
-
- toJSON: function ( meta ) {
-
- var isRootObject = ( meta === undefined || typeof meta === 'string' );
-
- if ( ! isRootObject && meta.textures[ this.uuid ] !== undefined ) {
-
- return meta.textures[ this.uuid ];
-
- }
-
- var output = {
-
- metadata: {
- version: 4.5,
- type: 'Texture',
- generator: 'Texture.toJSON'
- },
-
- uuid: this.uuid,
- name: this.name,
-
- mapping: this.mapping,
-
- repeat: [ this.repeat.x, this.repeat.y ],
- offset: [ this.offset.x, this.offset.y ],
- center: [ this.center.x, this.center.y ],
- rotation: this.rotation,
-
- wrap: [ this.wrapS, this.wrapT ],
-
- format: this.format,
- type: this.type,
- encoding: this.encoding,
-
- minFilter: this.minFilter,
- magFilter: this.magFilter,
- anisotropy: this.anisotropy,
-
- flipY: this.flipY,
-
- premultiplyAlpha: this.premultiplyAlpha,
- unpackAlignment: this.unpackAlignment
-
- };
-
- if ( this.image !== undefined ) {
-
- // TODO: Move to THREE.Image
-
- var image = this.image;
-
- if ( image.uuid === undefined ) {
-
- image.uuid = _Math.generateUUID(); // UGH
-
- }
-
- if ( ! isRootObject && meta.images[ image.uuid ] === undefined ) {
-
- var url;
-
- if ( Array.isArray( image ) ) {
-
- // process array of images e.g. CubeTexture
-
- url = [];
-
- for ( var i = 0, l = image.length; i < l; i ++ ) {
-
- url.push( ImageUtils.getDataURL( image[ i ] ) );
-
- }
-
- } else {
-
- // process single image
-
- url = ImageUtils.getDataURL( image );
-
- }
-
- meta.images[ image.uuid ] = {
- uuid: image.uuid,
- url: url
- };
-
- }
-
- output.image = image.uuid;
-
- }
-
- if ( ! isRootObject ) {
-
- meta.textures[ this.uuid ] = output;
-
- }
-
- return output;
-
- },
-
- dispose: function () {
-
- this.dispatchEvent( { type: 'dispose' } );
-
- },
-
- transformUv: function ( uv ) {
-
- if ( this.mapping !== UVMapping ) return uv;
-
- uv.applyMatrix3( this.matrix );
-
- if ( uv.x < 0 || uv.x > 1 ) {
-
- switch ( this.wrapS ) {
-
- case RepeatWrapping:
-
- uv.x = uv.x - Math.floor( uv.x );
- break;
-
- case ClampToEdgeWrapping:
-
- uv.x = uv.x < 0 ? 0 : 1;
- break;
-
- case MirroredRepeatWrapping:
-
- if ( Math.abs( Math.floor( uv.x ) % 2 ) === 1 ) {
-
- uv.x = Math.ceil( uv.x ) - uv.x;
-
- } else {
-
- uv.x = uv.x - Math.floor( uv.x );
-
- }
- break;
-
- }
-
- }
-
- if ( uv.y < 0 || uv.y > 1 ) {
-
- switch ( this.wrapT ) {
-
- case RepeatWrapping:
-
- uv.y = uv.y - Math.floor( uv.y );
- break;
-
- case ClampToEdgeWrapping:
-
- uv.y = uv.y < 0 ? 0 : 1;
- break;
-
- case MirroredRepeatWrapping:
-
- if ( Math.abs( Math.floor( uv.y ) % 2 ) === 1 ) {
-
- uv.y = Math.ceil( uv.y ) - uv.y;
-
- } else {
-
- uv.y = uv.y - Math.floor( uv.y );
-
- }
- break;
-
- }
-
- }
-
- if ( this.flipY ) {
-
- uv.y = 1 - uv.y;
-
- }
-
- return uv;
-
- }
-
- } );
-
- Object.defineProperty( Texture.prototype, "needsUpdate", {
-
- set: function ( value ) {
-
- if ( value === true ) this.version ++;
-
- }
-
- } );
-
- /**
- * @author supereggbert / http://www.paulbrunt.co.uk/
- * @author philogb / http://blog.thejit.org/
- * @author mikael emtinger / http://gomo.se/
- * @author egraether / http://egraether.com/
- * @author WestLangley / http://github.com/WestLangley
- */
-
- function Vector4( x, y, z, w ) {
-
- this.x = x || 0;
- this.y = y || 0;
- this.z = z || 0;
- this.w = ( w !== undefined ) ? w : 1;
-
- }
-
- Object.assign( Vector4.prototype, {
-
- isVector4: true,
-
- set: function ( x, y, z, w ) {
-
- this.x = x;
- this.y = y;
- this.z = z;
- this.w = w;
-
- return this;
-
- },
-
- setScalar: function ( scalar ) {
-
- this.x = scalar;
- this.y = scalar;
- this.z = scalar;
- this.w = scalar;
-
- return this;
-
- },
-
- setX: function ( x ) {
-
- this.x = x;
-
- return this;
-
- },
-
- setY: function ( y ) {
-
- this.y = y;
-
- return this;
-
- },
-
- setZ: function ( z ) {
-
- this.z = z;
-
- return this;
-
- },
-
- setW: function ( w ) {
-
- this.w = w;
-
- return this;
-
- },
-
- setComponent: function ( index, value ) {
-
- switch ( index ) {
-
- case 0: this.x = value; break;
- case 1: this.y = value; break;
- case 2: this.z = value; break;
- case 3: this.w = value; break;
- default: throw new Error( 'index is out of range: ' + index );
-
- }
-
- return this;
-
- },
-
- getComponent: function ( index ) {
-
- switch ( index ) {
-
- case 0: return this.x;
- case 1: return this.y;
- case 2: return this.z;
- case 3: return this.w;
- default: throw new Error( 'index is out of range: ' + index );
-
- }
-
- },
-
- clone: function () {
-
- return new this.constructor( this.x, this.y, this.z, this.w );
-
- },
-
- copy: function ( v ) {
-
- this.x = v.x;
- this.y = v.y;
- this.z = v.z;
- this.w = ( v.w !== undefined ) ? v.w : 1;
-
- return this;
-
- },
-
- add: function ( v, w ) {
-
- if ( w !== undefined ) {
-
- console.warn( 'THREE.Vector4: .add() now only accepts one argument. Use .addVectors( a, b ) instead.' );
- return this.addVectors( v, w );
-
- }
-
- this.x += v.x;
- this.y += v.y;
- this.z += v.z;
- this.w += v.w;
-
- return this;
-
- },
-
- addScalar: function ( s ) {
-
- this.x += s;
- this.y += s;
- this.z += s;
- this.w += s;
-
- return this;
-
- },
-
- addVectors: function ( a, b ) {
-
- this.x = a.x + b.x;
- this.y = a.y + b.y;
- this.z = a.z + b.z;
- this.w = a.w + b.w;
-
- return this;
-
- },
-
- addScaledVector: function ( v, s ) {
-
- this.x += v.x * s;
- this.y += v.y * s;
- this.z += v.z * s;
- this.w += v.w * s;
-
- return this;
-
- },
-
- sub: function ( v, w ) {
-
- if ( w !== undefined ) {
-
- console.warn( 'THREE.Vector4: .sub() now only accepts one argument. Use .subVectors( a, b ) instead.' );
- return this.subVectors( v, w );
-
- }
-
- this.x -= v.x;
- this.y -= v.y;
- this.z -= v.z;
- this.w -= v.w;
-
- return this;
-
- },
-
- subScalar: function ( s ) {
-
- this.x -= s;
- this.y -= s;
- this.z -= s;
- this.w -= s;
-
- return this;
-
- },
-
- subVectors: function ( a, b ) {
-
- this.x = a.x - b.x;
- this.y = a.y - b.y;
- this.z = a.z - b.z;
- this.w = a.w - b.w;
-
- return this;
-
- },
-
- multiplyScalar: function ( scalar ) {
-
- this.x *= scalar;
- this.y *= scalar;
- this.z *= scalar;
- this.w *= scalar;
-
- return this;
-
- },
-
- applyMatrix4: function ( m ) {
-
- var x = this.x, y = this.y, z = this.z, w = this.w;
- var e = m.elements;
-
- this.x = e[ 0 ] * x + e[ 4 ] * y + e[ 8 ] * z + e[ 12 ] * w;
- this.y = e[ 1 ] * x + e[ 5 ] * y + e[ 9 ] * z + e[ 13 ] * w;
- this.z = e[ 2 ] * x + e[ 6 ] * y + e[ 10 ] * z + e[ 14 ] * w;
- this.w = e[ 3 ] * x + e[ 7 ] * y + e[ 11 ] * z + e[ 15 ] * w;
-
- return this;
-
- },
-
- divideScalar: function ( scalar ) {
-
- return this.multiplyScalar( 1 / scalar );
-
- },
-
- setAxisAngleFromQuaternion: function ( q ) {
-
- // http://www.euclideanspace.com/maths/geometry/rotations/conversions/quaternionToAngle/index.htm
-
- // q is assumed to be normalized
-
- this.w = 2 * Math.acos( q.w );
-
- var s = Math.sqrt( 1 - q.w * q.w );
-
- if ( s < 0.0001 ) {
-
- this.x = 1;
- this.y = 0;
- this.z = 0;
-
- } else {
-
- this.x = q.x / s;
- this.y = q.y / s;
- this.z = q.z / s;
-
- }
-
- return this;
-
- },
-
- setAxisAngleFromRotationMatrix: function ( m ) {
-
- // http://www.euclideanspace.com/maths/geometry/rotations/conversions/matrixToAngle/index.htm
-
- // assumes the upper 3x3 of m is a pure rotation matrix (i.e, unscaled)
-
- var angle, x, y, z, // variables for result
- epsilon = 0.01, // margin to allow for rounding errors
- epsilon2 = 0.1, // margin to distinguish between 0 and 180 degrees
-
- te = m.elements,
-
- m11 = te[ 0 ], m12 = te[ 4 ], m13 = te[ 8 ],
- m21 = te[ 1 ], m22 = te[ 5 ], m23 = te[ 9 ],
- m31 = te[ 2 ], m32 = te[ 6 ], m33 = te[ 10 ];
-
- if ( ( Math.abs( m12 - m21 ) < epsilon ) &&
- ( Math.abs( m13 - m31 ) < epsilon ) &&
- ( Math.abs( m23 - m32 ) < epsilon ) ) {
-
- // singularity found
- // first check for identity matrix which must have +1 for all terms
- // in leading diagonal and zero in other terms
-
- if ( ( Math.abs( m12 + m21 ) < epsilon2 ) &&
- ( Math.abs( m13 + m31 ) < epsilon2 ) &&
- ( Math.abs( m23 + m32 ) < epsilon2 ) &&
- ( Math.abs( m11 + m22 + m33 - 3 ) < epsilon2 ) ) {
-
- // this singularity is identity matrix so angle = 0
-
- this.set( 1, 0, 0, 0 );
-
- return this; // zero angle, arbitrary axis
-
- }
-
- // otherwise this singularity is angle = 180
-
- angle = Math.PI;
-
- var xx = ( m11 + 1 ) / 2;
- var yy = ( m22 + 1 ) / 2;
- var zz = ( m33 + 1 ) / 2;
- var xy = ( m12 + m21 ) / 4;
- var xz = ( m13 + m31 ) / 4;
- var yz = ( m23 + m32 ) / 4;
-
- if ( ( xx > yy ) && ( xx > zz ) ) {
-
- // m11 is the largest diagonal term
-
- if ( xx < epsilon ) {
-
- x = 0;
- y = 0.707106781;
- z = 0.707106781;
-
- } else {
-
- x = Math.sqrt( xx );
- y = xy / x;
- z = xz / x;
-
- }
-
- } else if ( yy > zz ) {
-
- // m22 is the largest diagonal term
-
- if ( yy < epsilon ) {
-
- x = 0.707106781;
- y = 0;
- z = 0.707106781;
-
- } else {
-
- y = Math.sqrt( yy );
- x = xy / y;
- z = yz / y;
-
- }
-
- } else {
-
- // m33 is the largest diagonal term so base result on this
-
- if ( zz < epsilon ) {
-
- x = 0.707106781;
- y = 0.707106781;
- z = 0;
-
- } else {
-
- z = Math.sqrt( zz );
- x = xz / z;
- y = yz / z;
-
- }
-
- }
-
- this.set( x, y, z, angle );
-
- return this; // return 180 deg rotation
-
- }
-
- // as we have reached here there are no singularities so we can handle normally
-
- var s = Math.sqrt( ( m32 - m23 ) * ( m32 - m23 ) +
- ( m13 - m31 ) * ( m13 - m31 ) +
- ( m21 - m12 ) * ( m21 - m12 ) ); // used to normalize
-
- if ( Math.abs( s ) < 0.001 ) s = 1;
-
- // prevent divide by zero, should not happen if matrix is orthogonal and should be
- // caught by singularity test above, but I've left it in just in case
-
- this.x = ( m32 - m23 ) / s;
- this.y = ( m13 - m31 ) / s;
- this.z = ( m21 - m12 ) / s;
- this.w = Math.acos( ( m11 + m22 + m33 - 1 ) / 2 );
-
- return this;
-
- },
-
- min: function ( v ) {
-
- this.x = Math.min( this.x, v.x );
- this.y = Math.min( this.y, v.y );
- this.z = Math.min( this.z, v.z );
- this.w = Math.min( this.w, v.w );
-
- return this;
-
- },
-
- max: function ( v ) {
-
- this.x = Math.max( this.x, v.x );
- this.y = Math.max( this.y, v.y );
- this.z = Math.max( this.z, v.z );
- this.w = Math.max( this.w, v.w );
-
- return this;
-
- },
-
- clamp: function ( min, max ) {
-
- // assumes min < max, componentwise
-
- this.x = Math.max( min.x, Math.min( max.x, this.x ) );
- this.y = Math.max( min.y, Math.min( max.y, this.y ) );
- this.z = Math.max( min.z, Math.min( max.z, this.z ) );
- this.w = Math.max( min.w, Math.min( max.w, this.w ) );
-
- return this;
-
- },
-
- clampScalar: function () {
-
- var min, max;
-
- return function clampScalar( minVal, maxVal ) {
-
- if ( min === undefined ) {
-
- min = new Vector4();
- max = new Vector4();
-
- }
-
- min.set( minVal, minVal, minVal, minVal );
- max.set( maxVal, maxVal, maxVal, maxVal );
-
- return this.clamp( min, max );
-
- };
-
- }(),
-
- clampLength: function ( min, max ) {
-
- var length = this.length();
-
- return this.divideScalar( length || 1 ).multiplyScalar( Math.max( min, Math.min( max, length ) ) );
-
- },
-
- floor: function () {
-
- this.x = Math.floor( this.x );
- this.y = Math.floor( this.y );
- this.z = Math.floor( this.z );
- this.w = Math.floor( this.w );
-
- return this;
-
- },
-
- ceil: function () {
-
- this.x = Math.ceil( this.x );
- this.y = Math.ceil( this.y );
- this.z = Math.ceil( this.z );
- this.w = Math.ceil( this.w );
-
- return this;
-
- },
-
- round: function () {
-
- this.x = Math.round( this.x );
- this.y = Math.round( this.y );
- this.z = Math.round( this.z );
- this.w = Math.round( this.w );
-
- return this;
-
- },
-
- roundToZero: function () {
-
- this.x = ( this.x < 0 ) ? Math.ceil( this.x ) : Math.floor( this.x );
- this.y = ( this.y < 0 ) ? Math.ceil( this.y ) : Math.floor( this.y );
- this.z = ( this.z < 0 ) ? Math.ceil( this.z ) : Math.floor( this.z );
- this.w = ( this.w < 0 ) ? Math.ceil( this.w ) : Math.floor( this.w );
-
- return this;
-
- },
-
- negate: function () {
-
- this.x = - this.x;
- this.y = - this.y;
- this.z = - this.z;
- this.w = - this.w;
-
- return this;
-
- },
-
- dot: function ( v ) {
-
- return this.x * v.x + this.y * v.y + this.z * v.z + this.w * v.w;
-
- },
-
- lengthSq: function () {
-
- return this.x * this.x + this.y * this.y + this.z * this.z + this.w * this.w;
-
- },
-
- length: function () {
-
- return Math.sqrt( this.x * this.x + this.y * this.y + this.z * this.z + this.w * this.w );
-
- },
-
- manhattanLength: function () {
-
- return Math.abs( this.x ) + Math.abs( this.y ) + Math.abs( this.z ) + Math.abs( this.w );
-
- },
-
- normalize: function () {
-
- return this.divideScalar( this.length() || 1 );
-
- },
-
- setLength: function ( length ) {
-
- return this.normalize().multiplyScalar( length );
-
- },
-
- lerp: function ( v, alpha ) {
-
- this.x += ( v.x - this.x ) * alpha;
- this.y += ( v.y - this.y ) * alpha;
- this.z += ( v.z - this.z ) * alpha;
- this.w += ( v.w - this.w ) * alpha;
-
- return this;
-
- },
-
- lerpVectors: function ( v1, v2, alpha ) {
-
- return this.subVectors( v2, v1 ).multiplyScalar( alpha ).add( v1 );
-
- },
-
- equals: function ( v ) {
-
- return ( ( v.x === this.x ) && ( v.y === this.y ) && ( v.z === this.z ) && ( v.w === this.w ) );
-
- },
-
- fromArray: function ( array, offset ) {
-
- if ( offset === undefined ) offset = 0;
-
- this.x = array[ offset ];
- this.y = array[ offset + 1 ];
- this.z = array[ offset + 2 ];
- this.w = array[ offset + 3 ];
-
- return this;
-
- },
-
- toArray: function ( array, offset ) {
-
- if ( array === undefined ) array = [];
- if ( offset === undefined ) offset = 0;
-
- array[ offset ] = this.x;
- array[ offset + 1 ] = this.y;
- array[ offset + 2 ] = this.z;
- array[ offset + 3 ] = this.w;
-
- return array;
-
- },
-
- fromBufferAttribute: function ( attribute, index, offset ) {
-
- if ( offset !== undefined ) {
-
- console.warn( 'THREE.Vector4: offset has been removed from .fromBufferAttribute().' );
-
- }
-
- this.x = attribute.getX( index );
- this.y = attribute.getY( index );
- this.z = attribute.getZ( index );
- this.w = attribute.getW( index );
-
- return this;
-
- }
-
- } );
-
- /**
- * @author szimek / https://github.com/szimek/
- * @author alteredq / http://alteredqualia.com/
- * @author Marius Kintel / https://github.com/kintel
- */
-
- /*
- In options, we can specify:
- * Texture parameters for an auto-generated target texture
- * depthBuffer/stencilBuffer: Booleans to indicate if we should generate these buffers
- */
- function WebGLRenderTarget( width, height, options ) {
-
- this.width = width;
- this.height = height;
-
- this.scissor = new Vector4( 0, 0, width, height );
- this.scissorTest = false;
-
- this.viewport = new Vector4( 0, 0, width, height );
-
- options = options || {};
-
- this.texture = new Texture( undefined, undefined, options.wrapS, options.wrapT, options.magFilter, options.minFilter, options.format, options.type, options.anisotropy, options.encoding );
-
- this.texture.generateMipmaps = options.generateMipmaps !== undefined ? options.generateMipmaps : false;
- this.texture.minFilter = options.minFilter !== undefined ? options.minFilter : LinearFilter;
-
- this.depthBuffer = options.depthBuffer !== undefined ? options.depthBuffer : true;
- this.stencilBuffer = options.stencilBuffer !== undefined ? options.stencilBuffer : true;
- this.depthTexture = options.depthTexture !== undefined ? options.depthTexture : null;
-
- }
-
- WebGLRenderTarget.prototype = Object.assign( Object.create( EventDispatcher.prototype ), {
-
- constructor: WebGLRenderTarget,
-
- isWebGLRenderTarget: true,
-
- setSize: function ( width, height ) {
-
- if ( this.width !== width || this.height !== height ) {
-
- this.width = width;
- this.height = height;
-
- this.dispose();
-
- }
-
- this.viewport.set( 0, 0, width, height );
- this.scissor.set( 0, 0, width, height );
-
- },
-
- clone: function () {
-
- return new this.constructor().copy( this );
-
- },
-
- copy: function ( source ) {
-
- this.width = source.width;
- this.height = source.height;
-
- this.viewport.copy( source.viewport );
-
- this.texture = source.texture.clone();
-
- this.depthBuffer = source.depthBuffer;
- this.stencilBuffer = source.stencilBuffer;
- this.depthTexture = source.depthTexture;
-
- return this;
-
- },
-
- dispose: function () {
-
- this.dispatchEvent( { type: 'dispose' } );
-
- }
-
- } );
-
- /**
- * @author Mugen87 / https://github.com/Mugen87
- * @author Matt DesLauriers / @mattdesl
- */
-
- function WebGLMultisampleRenderTarget( width, height, options ) {
-
- WebGLRenderTarget.call( this, width, height, options );
-
- this.samples = 4;
-
- }
-
- WebGLMultisampleRenderTarget.prototype = Object.assign( Object.create( WebGLRenderTarget.prototype ), {
-
- constructor: WebGLMultisampleRenderTarget,
-
- isWebGLMultisampleRenderTarget: true,
-
- copy: function ( source ) {
-
- WebGLRenderTarget.prototype.copy.call( this, source );
-
- this.samples = source.samples;
-
- return this;
-
- }
-
- } );
-
- /**
- * @author alteredq / http://alteredqualia.com
- */
-
- function WebGLRenderTargetCube( width, height, options ) {
-
- WebGLRenderTarget.call( this, width, height, options );
-
- }
-
- WebGLRenderTargetCube.prototype = Object.create( WebGLRenderTarget.prototype );
- WebGLRenderTargetCube.prototype.constructor = WebGLRenderTargetCube;
-
- WebGLRenderTargetCube.prototype.isWebGLRenderTargetCube = true;
-
- /**
- * @author alteredq / http://alteredqualia.com/
- */
-
- function DataTexture( data, width, height, format, type, mapping, wrapS, wrapT, magFilter, minFilter, anisotropy, encoding ) {
-
- Texture.call( this, null, mapping, wrapS, wrapT, magFilter, minFilter, format, type, anisotropy, encoding );
-
- this.image = { data: data, width: width, height: height };
-
- this.magFilter = magFilter !== undefined ? magFilter : NearestFilter;
- this.minFilter = minFilter !== undefined ? minFilter : NearestFilter;
-
- this.generateMipmaps = false;
- this.flipY = false;
- this.unpackAlignment = 1;
-
- }
-
- DataTexture.prototype = Object.create( Texture.prototype );
- DataTexture.prototype.constructor = DataTexture;
-
- DataTexture.prototype.isDataTexture = true;
-
- /**
- * @author bhouston / http://clara.io
- * @author WestLangley / http://github.com/WestLangley
- */
-
- function Box3( min, max ) {
-
- this.min = ( min !== undefined ) ? min : new Vector3( + Infinity, + Infinity, + Infinity );
- this.max = ( max !== undefined ) ? max : new Vector3( - Infinity, - Infinity, - Infinity );
-
- }
-
- Object.assign( Box3.prototype, {
-
- isBox3: true,
-
- set: function ( min, max ) {
-
- this.min.copy( min );
- this.max.copy( max );
-
- return this;
-
- },
-
- setFromArray: function ( array ) {
-
- var minX = + Infinity;
- var minY = + Infinity;
- var minZ = + Infinity;
-
- var maxX = - Infinity;
- var maxY = - Infinity;
- var maxZ = - Infinity;
-
- for ( var i = 0, l = array.length; i < l; i += 3 ) {
-
- var x = array[ i ];
- var y = array[ i + 1 ];
- var z = array[ i + 2 ];
-
- if ( x < minX ) minX = x;
- if ( y < minY ) minY = y;
- if ( z < minZ ) minZ = z;
-
- if ( x > maxX ) maxX = x;
- if ( y > maxY ) maxY = y;
- if ( z > maxZ ) maxZ = z;
-
- }
-
- this.min.set( minX, minY, minZ );
- this.max.set( maxX, maxY, maxZ );
-
- return this;
-
- },
-
- setFromBufferAttribute: function ( attribute ) {
-
- var minX = + Infinity;
- var minY = + Infinity;
- var minZ = + Infinity;
-
- var maxX = - Infinity;
- var maxY = - Infinity;
- var maxZ = - Infinity;
-
- for ( var i = 0, l = attribute.count; i < l; i ++ ) {
-
- var x = attribute.getX( i );
- var y = attribute.getY( i );
- var z = attribute.getZ( i );
-
- if ( x < minX ) minX = x;
- if ( y < minY ) minY = y;
- if ( z < minZ ) minZ = z;
-
- if ( x > maxX ) maxX = x;
- if ( y > maxY ) maxY = y;
- if ( z > maxZ ) maxZ = z;
-
- }
-
- this.min.set( minX, minY, minZ );
- this.max.set( maxX, maxY, maxZ );
-
- return this;
-
- },
-
- setFromPoints: function ( points ) {
-
- this.makeEmpty();
-
- for ( var i = 0, il = points.length; i < il; i ++ ) {
-
- this.expandByPoint( points[ i ] );
-
- }
-
- return this;
-
- },
-
- setFromCenterAndSize: function () {
-
- var v1 = new Vector3();
-
- return function setFromCenterAndSize( center, size ) {
-
- var halfSize = v1.copy( size ).multiplyScalar( 0.5 );
-
- this.min.copy( center ).sub( halfSize );
- this.max.copy( center ).add( halfSize );
-
- return this;
-
- };
-
- }(),
-
- setFromObject: function ( object ) {
-
- this.makeEmpty();
-
- return this.expandByObject( object );
-
- },
-
- clone: function () {
-
- return new this.constructor().copy( this );
-
- },
-
- copy: function ( box ) {
-
- this.min.copy( box.min );
- this.max.copy( box.max );
-
- return this;
-
- },
-
- makeEmpty: function () {
-
- this.min.x = this.min.y = this.min.z = + Infinity;
- this.max.x = this.max.y = this.max.z = - Infinity;
-
- return this;
-
- },
-
- isEmpty: function () {
-
- // this is a more robust check for empty than ( volume <= 0 ) because volume can get positive with two negative axes
-
- return ( this.max.x < this.min.x ) || ( this.max.y < this.min.y ) || ( this.max.z < this.min.z );
-
- },
-
- getCenter: function ( target ) {
-
- if ( target === undefined ) {
-
- console.warn( 'THREE.Box3: .getCenter() target is now required' );
- target = new Vector3();
-
- }
-
- return this.isEmpty() ? target.set( 0, 0, 0 ) : target.addVectors( this.min, this.max ).multiplyScalar( 0.5 );
-
- },
-
- getSize: function ( target ) {
-
- if ( target === undefined ) {
-
- console.warn( 'THREE.Box3: .getSize() target is now required' );
- target = new Vector3();
-
- }
-
- return this.isEmpty() ? target.set( 0, 0, 0 ) : target.subVectors( this.max, this.min );
-
- },
-
- expandByPoint: function ( point ) {
-
- this.min.min( point );
- this.max.max( point );
-
- return this;
-
- },
-
- expandByVector: function ( vector ) {
-
- this.min.sub( vector );
- this.max.add( vector );
-
- return this;
-
- },
-
- expandByScalar: function ( scalar ) {
-
- this.min.addScalar( - scalar );
- this.max.addScalar( scalar );
-
- return this;
-
- },
-
- expandByObject: function () {
-
- // Computes the world-axis-aligned bounding box of an object (including its children),
- // accounting for both the object's, and children's, world transforms
-
- var scope, i, l;
-
- var v1 = new Vector3();
-
- function traverse( node ) {
-
- var geometry = node.geometry;
-
- if ( geometry !== undefined ) {
-
- if ( geometry.isGeometry ) {
-
- var vertices = geometry.vertices;
-
- for ( i = 0, l = vertices.length; i < l; i ++ ) {
-
- v1.copy( vertices[ i ] );
- v1.applyMatrix4( node.matrixWorld );
-
- scope.expandByPoint( v1 );
-
- }
-
- } else if ( geometry.isBufferGeometry ) {
-
- var attribute = geometry.attributes.position;
-
- if ( attribute !== undefined ) {
-
- for ( i = 0, l = attribute.count; i < l; i ++ ) {
-
- v1.fromBufferAttribute( attribute, i ).applyMatrix4( node.matrixWorld );
-
- scope.expandByPoint( v1 );
-
- }
-
- }
-
- }
-
- }
-
- }
-
- return function expandByObject( object ) {
-
- scope = this;
-
- object.updateMatrixWorld( true );
-
- object.traverse( traverse );
-
- return this;
-
- };
-
- }(),
-
- containsPoint: function ( point ) {
-
- return point.x < this.min.x || point.x > this.max.x ||
- point.y < this.min.y || point.y > this.max.y ||
- point.z < this.min.z || point.z > this.max.z ? false : true;
-
- },
-
- containsBox: function ( box ) {
-
- return this.min.x <= box.min.x && box.max.x <= this.max.x &&
- this.min.y <= box.min.y && box.max.y <= this.max.y &&
- this.min.z <= box.min.z && box.max.z <= this.max.z;
-
- },
-
- getParameter: function ( point, target ) {
-
- // This can potentially have a divide by zero if the box
- // has a size dimension of 0.
-
- if ( target === undefined ) {
-
- console.warn( 'THREE.Box3: .getParameter() target is now required' );
- target = new Vector3();
-
- }
-
- return target.set(
- ( point.x - this.min.x ) / ( this.max.x - this.min.x ),
- ( point.y - this.min.y ) / ( this.max.y - this.min.y ),
- ( point.z - this.min.z ) / ( this.max.z - this.min.z )
- );
-
- },
-
- intersectsBox: function ( box ) {
-
- // using 6 splitting planes to rule out intersections.
- return box.max.x < this.min.x || box.min.x > this.max.x ||
- box.max.y < this.min.y || box.min.y > this.max.y ||
- box.max.z < this.min.z || box.min.z > this.max.z ? false : true;
-
- },
-
- intersectsSphere: ( function () {
-
- var closestPoint = new Vector3();
-
- return function intersectsSphere( sphere ) {
-
- // Find the point on the AABB closest to the sphere center.
- this.clampPoint( sphere.center, closestPoint );
-
- // If that point is inside the sphere, the AABB and sphere intersect.
- return closestPoint.distanceToSquared( sphere.center ) <= ( sphere.radius * sphere.radius );
-
- };
-
- } )(),
-
- intersectsPlane: function ( plane ) {
-
- // We compute the minimum and maximum dot product values. If those values
- // are on the same side (back or front) of the plane, then there is no intersection.
-
- var min, max;
-
- if ( plane.normal.x > 0 ) {
-
- min = plane.normal.x * this.min.x;
- max = plane.normal.x * this.max.x;
-
- } else {
-
- min = plane.normal.x * this.max.x;
- max = plane.normal.x * this.min.x;
-
- }
-
- if ( plane.normal.y > 0 ) {
-
- min += plane.normal.y * this.min.y;
- max += plane.normal.y * this.max.y;
-
- } else {
-
- min += plane.normal.y * this.max.y;
- max += plane.normal.y * this.min.y;
-
- }
-
- if ( plane.normal.z > 0 ) {
-
- min += plane.normal.z * this.min.z;
- max += plane.normal.z * this.max.z;
-
- } else {
-
- min += plane.normal.z * this.max.z;
- max += plane.normal.z * this.min.z;
-
- }
-
- return ( min <= - plane.constant && max >= - plane.constant );
-
- },
-
- intersectsTriangle: ( function () {
-
- // triangle centered vertices
- var v0 = new Vector3();
- var v1 = new Vector3();
- var v2 = new Vector3();
-
- // triangle edge vectors
- var f0 = new Vector3();
- var f1 = new Vector3();
- var f2 = new Vector3();
-
- var testAxis = new Vector3();
-
- var center = new Vector3();
- var extents = new Vector3();
-
- var triangleNormal = new Vector3();
-
- function satForAxes( axes ) {
-
- var i, j;
-
- for ( i = 0, j = axes.length - 3; i <= j; i += 3 ) {
-
- testAxis.fromArray( axes, i );
- // project the aabb onto the seperating axis
- var r = extents.x * Math.abs( testAxis.x ) + extents.y * Math.abs( testAxis.y ) + extents.z * Math.abs( testAxis.z );
- // project all 3 vertices of the triangle onto the seperating axis
- var p0 = v0.dot( testAxis );
- var p1 = v1.dot( testAxis );
- var p2 = v2.dot( testAxis );
- // actual test, basically see if either of the most extreme of the triangle points intersects r
- if ( Math.max( - Math.max( p0, p1, p2 ), Math.min( p0, p1, p2 ) ) > r ) {
-
- // points of the projected triangle are outside the projected half-length of the aabb
- // the axis is seperating and we can exit
- return false;
-
- }
-
- }
-
- return true;
-
- }
-
- return function intersectsTriangle( triangle ) {
-
- if ( this.isEmpty() ) {
-
- return false;
-
- }
-
- // compute box center and extents
- this.getCenter( center );
- extents.subVectors( this.max, center );
-
- // translate triangle to aabb origin
- v0.subVectors( triangle.a, center );
- v1.subVectors( triangle.b, center );
- v2.subVectors( triangle.c, center );
-
- // compute edge vectors for triangle
- f0.subVectors( v1, v0 );
- f1.subVectors( v2, v1 );
- f2.subVectors( v0, v2 );
-
- // test against axes that are given by cross product combinations of the edges of the triangle and the edges of the aabb
- // make an axis testing of each of the 3 sides of the aabb against each of the 3 sides of the triangle = 9 axis of separation
- // axis_ij = u_i x f_j (u0, u1, u2 = face normals of aabb = x,y,z axes vectors since aabb is axis aligned)
- var axes = [
- 0, - f0.z, f0.y, 0, - f1.z, f1.y, 0, - f2.z, f2.y,
- f0.z, 0, - f0.x, f1.z, 0, - f1.x, f2.z, 0, - f2.x,
- - f0.y, f0.x, 0, - f1.y, f1.x, 0, - f2.y, f2.x, 0
- ];
- if ( ! satForAxes( axes ) ) {
-
- return false;
-
- }
-
- // test 3 face normals from the aabb
- axes = [ 1, 0, 0, 0, 1, 0, 0, 0, 1 ];
- if ( ! satForAxes( axes ) ) {
-
- return false;
-
- }
-
- // finally testing the face normal of the triangle
- // use already existing triangle edge vectors here
- triangleNormal.crossVectors( f0, f1 );
- axes = [ triangleNormal.x, triangleNormal.y, triangleNormal.z ];
- return satForAxes( axes );
-
- };
-
- } )(),
-
- clampPoint: function ( point, target ) {
-
- if ( target === undefined ) {
-
- console.warn( 'THREE.Box3: .clampPoint() target is now required' );
- target = new Vector3();
-
- }
-
- return target.copy( point ).clamp( this.min, this.max );
-
- },
-
- distanceToPoint: function () {
-
- var v1 = new Vector3();
-
- return function distanceToPoint( point ) {
-
- var clampedPoint = v1.copy( point ).clamp( this.min, this.max );
- return clampedPoint.sub( point ).length();
-
- };
-
- }(),
-
- getBoundingSphere: function () {
-
- var v1 = new Vector3();
-
- return function getBoundingSphere( target ) {
-
- if ( target === undefined ) {
-
- console.error( 'THREE.Box3: .getBoundingSphere() target is now required' );
- //target = new Sphere(); // removed to avoid cyclic dependency
-
- }
-
- this.getCenter( target.center );
-
- target.radius = this.getSize( v1 ).length() * 0.5;
-
- return target;
-
- };
-
- }(),
-
- intersect: function ( box ) {
-
- this.min.max( box.min );
- this.max.min( box.max );
-
- // ensure that if there is no overlap, the result is fully empty, not slightly empty with non-inf/+inf values that will cause subsequence intersects to erroneously return valid values.
- if ( this.isEmpty() ) this.makeEmpty();
-
- return this;
-
- },
-
- union: function ( box ) {
-
- this.min.min( box.min );
- this.max.max( box.max );
-
- return this;
-
- },
-
- applyMatrix4: function () {
-
- var points = [
- new Vector3(),
- new Vector3(),
- new Vector3(),
- new Vector3(),
- new Vector3(),
- new Vector3(),
- new Vector3(),
- new Vector3()
- ];
-
- return function applyMatrix4( matrix ) {
-
- // transform of empty box is an empty box.
- if ( this.isEmpty() ) return this;
-
- // NOTE: I am using a binary pattern to specify all 2^3 combinations below
- points[ 0 ].set( this.min.x, this.min.y, this.min.z ).applyMatrix4( matrix ); // 000
- points[ 1 ].set( this.min.x, this.min.y, this.max.z ).applyMatrix4( matrix ); // 001
- points[ 2 ].set( this.min.x, this.max.y, this.min.z ).applyMatrix4( matrix ); // 010
- points[ 3 ].set( this.min.x, this.max.y, this.max.z ).applyMatrix4( matrix ); // 011
- points[ 4 ].set( this.max.x, this.min.y, this.min.z ).applyMatrix4( matrix ); // 100
- points[ 5 ].set( this.max.x, this.min.y, this.max.z ).applyMatrix4( matrix ); // 101
- points[ 6 ].set( this.max.x, this.max.y, this.min.z ).applyMatrix4( matrix ); // 110
- points[ 7 ].set( this.max.x, this.max.y, this.max.z ).applyMatrix4( matrix ); // 111
-
- this.setFromPoints( points );
-
- return this;
-
- };
-
- }(),
-
- translate: function ( offset ) {
-
- this.min.add( offset );
- this.max.add( offset );
-
- return this;
-
- },
-
- equals: function ( box ) {
-
- return box.min.equals( this.min ) && box.max.equals( this.max );
-
- }
-
- } );
-
- /**
- * @author bhouston / http://clara.io
- * @author mrdoob / http://mrdoob.com/
- */
-
- function Sphere( center, radius ) {
-
- this.center = ( center !== undefined ) ? center : new Vector3();
- this.radius = ( radius !== undefined ) ? radius : 0;
-
- }
-
- Object.assign( Sphere.prototype, {
-
- set: function ( center, radius ) {
-
- this.center.copy( center );
- this.radius = radius;
-
- return this;
-
- },
-
- setFromPoints: function () {
-
- var box = new Box3();
-
- return function setFromPoints( points, optionalCenter ) {
-
- var center = this.center;
-
- if ( optionalCenter !== undefined ) {
-
- center.copy( optionalCenter );
-
- } else {
-
- box.setFromPoints( points ).getCenter( center );
-
- }
-
- var maxRadiusSq = 0;
-
- for ( var i = 0, il = points.length; i < il; i ++ ) {
-
- maxRadiusSq = Math.max( maxRadiusSq, center.distanceToSquared( points[ i ] ) );
-
- }
-
- this.radius = Math.sqrt( maxRadiusSq );
-
- return this;
-
- };
-
- }(),
-
- clone: function () {
-
- return new this.constructor().copy( this );
-
- },
-
- copy: function ( sphere ) {
-
- this.center.copy( sphere.center );
- this.radius = sphere.radius;
-
- return this;
-
- },
-
- empty: function () {
-
- return ( this.radius <= 0 );
-
- },
-
- containsPoint: function ( point ) {
-
- return ( point.distanceToSquared( this.center ) <= ( this.radius * this.radius ) );
-
- },
-
- distanceToPoint: function ( point ) {
-
- return ( point.distanceTo( this.center ) - this.radius );
-
- },
-
- intersectsSphere: function ( sphere ) {
-
- var radiusSum = this.radius + sphere.radius;
-
- return sphere.center.distanceToSquared( this.center ) <= ( radiusSum * radiusSum );
-
- },
-
- intersectsBox: function ( box ) {
-
- return box.intersectsSphere( this );
-
- },
-
- intersectsPlane: function ( plane ) {
-
- return Math.abs( plane.distanceToPoint( this.center ) ) <= this.radius;
-
- },
-
- clampPoint: function ( point, target ) {
-
- var deltaLengthSq = this.center.distanceToSquared( point );
-
- if ( target === undefined ) {
-
- console.warn( 'THREE.Sphere: .clampPoint() target is now required' );
- target = new Vector3();
-
- }
-
- target.copy( point );
-
- if ( deltaLengthSq > ( this.radius * this.radius ) ) {
-
- target.sub( this.center ).normalize();
- target.multiplyScalar( this.radius ).add( this.center );
-
- }
-
- return target;
-
- },
-
- getBoundingBox: function ( target ) {
-
- if ( target === undefined ) {
-
- console.warn( 'THREE.Sphere: .getBoundingBox() target is now required' );
- target = new Box3();
-
- }
-
- target.set( this.center, this.center );
- target.expandByScalar( this.radius );
-
- return target;
-
- },
-
- applyMatrix4: function ( matrix ) {
-
- this.center.applyMatrix4( matrix );
- this.radius = this.radius * matrix.getMaxScaleOnAxis();
-
- return this;
-
- },
-
- translate: function ( offset ) {
-
- this.center.add( offset );
-
- return this;
-
- },
-
- equals: function ( sphere ) {
-
- return sphere.center.equals( this.center ) && ( sphere.radius === this.radius );
-
- }
-
- } );
-
- /**
- * @author bhouston / http://clara.io
- */
-
- function Plane( normal, constant ) {
-
- // normal is assumed to be normalized
-
- this.normal = ( normal !== undefined ) ? normal : new Vector3( 1, 0, 0 );
- this.constant = ( constant !== undefined ) ? constant : 0;
-
- }
-
- Object.assign( Plane.prototype, {
-
- set: function ( normal, constant ) {
-
- this.normal.copy( normal );
- this.constant = constant;
-
- return this;
-
- },
-
- setComponents: function ( x, y, z, w ) {
-
- this.normal.set( x, y, z );
- this.constant = w;
-
- return this;
-
- },
-
- setFromNormalAndCoplanarPoint: function ( normal, point ) {
-
- this.normal.copy( normal );
- this.constant = - point.dot( this.normal );
-
- return this;
-
- },
-
- setFromCoplanarPoints: function () {
-
- var v1 = new Vector3();
- var v2 = new Vector3();
-
- return function setFromCoplanarPoints( a, b, c ) {
-
- var normal = v1.subVectors( c, b ).cross( v2.subVectors( a, b ) ).normalize();
-
- // Q: should an error be thrown if normal is zero (e.g. degenerate plane)?
-
- this.setFromNormalAndCoplanarPoint( normal, a );
-
- return this;
-
- };
-
- }(),
-
- clone: function () {
-
- return new this.constructor().copy( this );
-
- },
-
- copy: function ( plane ) {
-
- this.normal.copy( plane.normal );
- this.constant = plane.constant;
-
- return this;
-
- },
-
- normalize: function () {
-
- // Note: will lead to a divide by zero if the plane is invalid.
-
- var inverseNormalLength = 1.0 / this.normal.length();
- this.normal.multiplyScalar( inverseNormalLength );
- this.constant *= inverseNormalLength;
-
- return this;
-
- },
-
- negate: function () {
-
- this.constant *= - 1;
- this.normal.negate();
-
- return this;
-
- },
-
- distanceToPoint: function ( point ) {
-
- return this.normal.dot( point ) + this.constant;
-
- },
-
- distanceToSphere: function ( sphere ) {
-
- return this.distanceToPoint( sphere.center ) - sphere.radius;
-
- },
-
- projectPoint: function ( point, target ) {
-
- if ( target === undefined ) {
-
- console.warn( 'THREE.Plane: .projectPoint() target is now required' );
- target = new Vector3();
-
- }
-
- return target.copy( this.normal ).multiplyScalar( - this.distanceToPoint( point ) ).add( point );
-
- },
-
- intersectLine: function () {
-
- var v1 = new Vector3();
-
- return function intersectLine( line, target ) {
-
- if ( target === undefined ) {
-
- console.warn( 'THREE.Plane: .intersectLine() target is now required' );
- target = new Vector3();
-
- }
-
- var direction = line.delta( v1 );
-
- var denominator = this.normal.dot( direction );
-
- if ( denominator === 0 ) {
-
- // line is coplanar, return origin
- if ( this.distanceToPoint( line.start ) === 0 ) {
-
- return target.copy( line.start );
-
- }
-
- // Unsure if this is the correct method to handle this case.
- return undefined;
-
- }
-
- var t = - ( line.start.dot( this.normal ) + this.constant ) / denominator;
-
- if ( t < 0 || t > 1 ) {
-
- return undefined;
-
- }
-
- return target.copy( direction ).multiplyScalar( t ).add( line.start );
-
- };
-
- }(),
-
- intersectsLine: function ( line ) {
-
- // Note: this tests if a line intersects the plane, not whether it (or its end-points) are coplanar with it.
-
- var startSign = this.distanceToPoint( line.start );
- var endSign = this.distanceToPoint( line.end );
-
- return ( startSign < 0 && endSign > 0 ) || ( endSign < 0 && startSign > 0 );
-
- },
-
- intersectsBox: function ( box ) {
-
- return box.intersectsPlane( this );
-
- },
-
- intersectsSphere: function ( sphere ) {
-
- return sphere.intersectsPlane( this );
-
- },
-
- coplanarPoint: function ( target ) {
-
- if ( target === undefined ) {
-
- console.warn( 'THREE.Plane: .coplanarPoint() target is now required' );
- target = new Vector3();
-
- }
-
- return target.copy( this.normal ).multiplyScalar( - this.constant );
-
- },
-
- applyMatrix4: function () {
-
- var v1 = new Vector3();
- var m1 = new Matrix3();
-
- return function applyMatrix4( matrix, optionalNormalMatrix ) {
-
- var normalMatrix = optionalNormalMatrix || m1.getNormalMatrix( matrix );
-
- var referencePoint = this.coplanarPoint( v1 ).applyMatrix4( matrix );
-
- var normal = this.normal.applyMatrix3( normalMatrix ).normalize();
-
- this.constant = - referencePoint.dot( normal );
-
- return this;
-
- };
-
- }(),
-
- translate: function ( offset ) {
-
- this.constant -= offset.dot( this.normal );
-
- return this;
-
- },
-
- equals: function ( plane ) {
-
- return plane.normal.equals( this.normal ) && ( plane.constant === this.constant );
-
- }
-
- } );
-
- /**
- * @author mrdoob / http://mrdoob.com/
- * @author alteredq / http://alteredqualia.com/
- * @author bhouston / http://clara.io
- */
-
- function Frustum( p0, p1, p2, p3, p4, p5 ) {
-
- this.planes = [
-
- ( p0 !== undefined ) ? p0 : new Plane(),
- ( p1 !== undefined ) ? p1 : new Plane(),
- ( p2 !== undefined ) ? p2 : new Plane(),
- ( p3 !== undefined ) ? p3 : new Plane(),
- ( p4 !== undefined ) ? p4 : new Plane(),
- ( p5 !== undefined ) ? p5 : new Plane()
-
- ];
-
- }
-
- Object.assign( Frustum.prototype, {
-
- set: function ( p0, p1, p2, p3, p4, p5 ) {
-
- var planes = this.planes;
-
- planes[ 0 ].copy( p0 );
- planes[ 1 ].copy( p1 );
- planes[ 2 ].copy( p2 );
- planes[ 3 ].copy( p3 );
- planes[ 4 ].copy( p4 );
- planes[ 5 ].copy( p5 );
-
- return this;
-
- },
-
- clone: function () {
-
- return new this.constructor().copy( this );
-
- },
-
- copy: function ( frustum ) {
-
- var planes = this.planes;
-
- for ( var i = 0; i < 6; i ++ ) {
-
- planes[ i ].copy( frustum.planes[ i ] );
-
- }
-
- return this;
-
- },
-
- setFromMatrix: function ( m ) {
-
- var planes = this.planes;
- var me = m.elements;
- var me0 = me[ 0 ], me1 = me[ 1 ], me2 = me[ 2 ], me3 = me[ 3 ];
- var me4 = me[ 4 ], me5 = me[ 5 ], me6 = me[ 6 ], me7 = me[ 7 ];
- var me8 = me[ 8 ], me9 = me[ 9 ], me10 = me[ 10 ], me11 = me[ 11 ];
- var me12 = me[ 12 ], me13 = me[ 13 ], me14 = me[ 14 ], me15 = me[ 15 ];
-
- planes[ 0 ].setComponents( me3 - me0, me7 - me4, me11 - me8, me15 - me12 ).normalize();
- planes[ 1 ].setComponents( me3 + me0, me7 + me4, me11 + me8, me15 + me12 ).normalize();
- planes[ 2 ].setComponents( me3 + me1, me7 + me5, me11 + me9, me15 + me13 ).normalize();
- planes[ 3 ].setComponents( me3 - me1, me7 - me5, me11 - me9, me15 - me13 ).normalize();
- planes[ 4 ].setComponents( me3 - me2, me7 - me6, me11 - me10, me15 - me14 ).normalize();
- planes[ 5 ].setComponents( me3 + me2, me7 + me6, me11 + me10, me15 + me14 ).normalize();
-
- return this;
-
- },
-
- intersectsObject: function () {
-
- var sphere = new Sphere();
-
- return function intersectsObject( object ) {
-
- var geometry = object.geometry;
-
- if ( geometry.boundingSphere === null )
- geometry.computeBoundingSphere();
-
- sphere.copy( geometry.boundingSphere )
- .applyMatrix4( object.matrixWorld );
-
- return this.intersectsSphere( sphere );
-
- };
-
- }(),
-
- intersectsSprite: function () {
-
- var sphere = new Sphere();
-
- return function intersectsSprite( sprite ) {
-
- sphere.center.set( 0, 0, 0 );
- sphere.radius = 0.7071067811865476;
- sphere.applyMatrix4( sprite.matrixWorld );
-
- return this.intersectsSphere( sphere );
-
- };
-
- }(),
-
- intersectsSphere: function ( sphere ) {
-
- var planes = this.planes;
- var center = sphere.center;
- var negRadius = - sphere.radius;
-
- for ( var i = 0; i < 6; i ++ ) {
-
- var distance = planes[ i ].distanceToPoint( center );
-
- if ( distance < negRadius ) {
-
- return false;
-
- }
-
- }
-
- return true;
-
- },
-
- intersectsBox: function () {
-
- var p = new Vector3();
-
- return function intersectsBox( box ) {
-
- var planes = this.planes;
-
- for ( var i = 0; i < 6; i ++ ) {
-
- var plane = planes[ i ];
-
- // corner at max distance
-
- p.x = plane.normal.x > 0 ? box.max.x : box.min.x;
- p.y = plane.normal.y > 0 ? box.max.y : box.min.y;
- p.z = plane.normal.z > 0 ? box.max.z : box.min.z;
-
- if ( plane.distanceToPoint( p ) < 0 ) {
-
- return false;
-
- }
-
- }
-
- return true;
-
- };
-
- }(),
-
- containsPoint: function ( point ) {
-
- var planes = this.planes;
-
- for ( var i = 0; i < 6; i ++ ) {
-
- if ( planes[ i ].distanceToPoint( point ) < 0 ) {
-
- return false;
-
- }
-
- }
-
- return true;
-
- }
-
- } );
-
- /**
- * @author mrdoob / http://mrdoob.com/
- * @author supereggbert / http://www.paulbrunt.co.uk/
- * @author philogb / http://blog.thejit.org/
- * @author jordi_ros / http://plattsoft.com
- * @author D1plo1d / http://github.com/D1plo1d
- * @author alteredq / http://alteredqualia.com/
- * @author mikael emtinger / http://gomo.se/
- * @author timknip / http://www.floorplanner.com/
- * @author bhouston / http://clara.io
- * @author WestLangley / http://github.com/WestLangley
- */
-
- function Matrix4() {
-
- this.elements = [
-
- 1, 0, 0, 0,
- 0, 1, 0, 0,
- 0, 0, 1, 0,
- 0, 0, 0, 1
-
- ];
-
- if ( arguments.length > 0 ) {
-
- console.error( 'THREE.Matrix4: the constructor no longer reads arguments. use .set() instead.' );
-
- }
-
- }
-
- Object.assign( Matrix4.prototype, {
-
- isMatrix4: true,
-
- set: function ( n11, n12, n13, n14, n21, n22, n23, n24, n31, n32, n33, n34, n41, n42, n43, n44 ) {
-
- var te = this.elements;
-
- te[ 0 ] = n11; te[ 4 ] = n12; te[ 8 ] = n13; te[ 12 ] = n14;
- te[ 1 ] = n21; te[ 5 ] = n22; te[ 9 ] = n23; te[ 13 ] = n24;
- te[ 2 ] = n31; te[ 6 ] = n32; te[ 10 ] = n33; te[ 14 ] = n34;
- te[ 3 ] = n41; te[ 7 ] = n42; te[ 11 ] = n43; te[ 15 ] = n44;
-
- return this;
-
- },
-
- identity: function () {
-
- this.set(
-
- 1, 0, 0, 0,
- 0, 1, 0, 0,
- 0, 0, 1, 0,
- 0, 0, 0, 1
-
- );
-
- return this;
-
- },
-
- clone: function () {
-
- return new Matrix4().fromArray( this.elements );
-
- },
-
- copy: function ( m ) {
-
- var te = this.elements;
- var me = m.elements;
-
- te[ 0 ] = me[ 0 ]; te[ 1 ] = me[ 1 ]; te[ 2 ] = me[ 2 ]; te[ 3 ] = me[ 3 ];
- te[ 4 ] = me[ 4 ]; te[ 5 ] = me[ 5 ]; te[ 6 ] = me[ 6 ]; te[ 7 ] = me[ 7 ];
- te[ 8 ] = me[ 8 ]; te[ 9 ] = me[ 9 ]; te[ 10 ] = me[ 10 ]; te[ 11 ] = me[ 11 ];
- te[ 12 ] = me[ 12 ]; te[ 13 ] = me[ 13 ]; te[ 14 ] = me[ 14 ]; te[ 15 ] = me[ 15 ];
-
- return this;
-
- },
-
- copyPosition: function ( m ) {
-
- var te = this.elements, me = m.elements;
-
- te[ 12 ] = me[ 12 ];
- te[ 13 ] = me[ 13 ];
- te[ 14 ] = me[ 14 ];
-
- return this;
-
- },
-
- extractBasis: function ( xAxis, yAxis, zAxis ) {
-
- xAxis.setFromMatrixColumn( this, 0 );
- yAxis.setFromMatrixColumn( this, 1 );
- zAxis.setFromMatrixColumn( this, 2 );
-
- return this;
-
- },
-
- makeBasis: function ( xAxis, yAxis, zAxis ) {
-
- this.set(
- xAxis.x, yAxis.x, zAxis.x, 0,
- xAxis.y, yAxis.y, zAxis.y, 0,
- xAxis.z, yAxis.z, zAxis.z, 0,
- 0, 0, 0, 1
- );
-
- return this;
-
- },
-
- extractRotation: function () {
-
- var v1 = new Vector3();
-
- return function extractRotation( m ) {
-
- // this method does not support reflection matrices
-
- var te = this.elements;
- var me = m.elements;
-
- var scaleX = 1 / v1.setFromMatrixColumn( m, 0 ).length();
- var scaleY = 1 / v1.setFromMatrixColumn( m, 1 ).length();
- var scaleZ = 1 / v1.setFromMatrixColumn( m, 2 ).length();
-
- te[ 0 ] = me[ 0 ] * scaleX;
- te[ 1 ] = me[ 1 ] * scaleX;
- te[ 2 ] = me[ 2 ] * scaleX;
- te[ 3 ] = 0;
-
- te[ 4 ] = me[ 4 ] * scaleY;
- te[ 5 ] = me[ 5 ] * scaleY;
- te[ 6 ] = me[ 6 ] * scaleY;
- te[ 7 ] = 0;
-
- te[ 8 ] = me[ 8 ] * scaleZ;
- te[ 9 ] = me[ 9 ] * scaleZ;
- te[ 10 ] = me[ 10 ] * scaleZ;
- te[ 11 ] = 0;
-
- te[ 12 ] = 0;
- te[ 13 ] = 0;
- te[ 14 ] = 0;
- te[ 15 ] = 1;
-
- return this;
-
- };
-
- }(),
-
- makeRotationFromEuler: function ( euler ) {
-
- if ( ! ( euler && euler.isEuler ) ) {
-
- console.error( 'THREE.Matrix4: .makeRotationFromEuler() now expects a Euler rotation rather than a Vector3 and order.' );
-
- }
-
- var te = this.elements;
-
- var x = euler.x, y = euler.y, z = euler.z;
- var a = Math.cos( x ), b = Math.sin( x );
- var c = Math.cos( y ), d = Math.sin( y );
- var e = Math.cos( z ), f = Math.sin( z );
-
- if ( euler.order === 'XYZ' ) {
-
- var ae = a * e, af = a * f, be = b * e, bf = b * f;
-
- te[ 0 ] = c * e;
- te[ 4 ] = - c * f;
- te[ 8 ] = d;
-
- te[ 1 ] = af + be * d;
- te[ 5 ] = ae - bf * d;
- te[ 9 ] = - b * c;
-
- te[ 2 ] = bf - ae * d;
- te[ 6 ] = be + af * d;
- te[ 10 ] = a * c;
-
- } else if ( euler.order === 'YXZ' ) {
-
- var ce = c * e, cf = c * f, de = d * e, df = d * f;
-
- te[ 0 ] = ce + df * b;
- te[ 4 ] = de * b - cf;
- te[ 8 ] = a * d;
-
- te[ 1 ] = a * f;
- te[ 5 ] = a * e;
- te[ 9 ] = - b;
-
- te[ 2 ] = cf * b - de;
- te[ 6 ] = df + ce * b;
- te[ 10 ] = a * c;
-
- } else if ( euler.order === 'ZXY' ) {
-
- var ce = c * e, cf = c * f, de = d * e, df = d * f;
-
- te[ 0 ] = ce - df * b;
- te[ 4 ] = - a * f;
- te[ 8 ] = de + cf * b;
-
- te[ 1 ] = cf + de * b;
- te[ 5 ] = a * e;
- te[ 9 ] = df - ce * b;
-
- te[ 2 ] = - a * d;
- te[ 6 ] = b;
- te[ 10 ] = a * c;
-
- } else if ( euler.order === 'ZYX' ) {
-
- var ae = a * e, af = a * f, be = b * e, bf = b * f;
-
- te[ 0 ] = c * e;
- te[ 4 ] = be * d - af;
- te[ 8 ] = ae * d + bf;
-
- te[ 1 ] = c * f;
- te[ 5 ] = bf * d + ae;
- te[ 9 ] = af * d - be;
-
- te[ 2 ] = - d;
- te[ 6 ] = b * c;
- te[ 10 ] = a * c;
-
- } else if ( euler.order === 'YZX' ) {
-
- var ac = a * c, ad = a * d, bc = b * c, bd = b * d;
-
- te[ 0 ] = c * e;
- te[ 4 ] = bd - ac * f;
- te[ 8 ] = bc * f + ad;
-
- te[ 1 ] = f;
- te[ 5 ] = a * e;
- te[ 9 ] = - b * e;
-
- te[ 2 ] = - d * e;
- te[ 6 ] = ad * f + bc;
- te[ 10 ] = ac - bd * f;
-
- } else if ( euler.order === 'XZY' ) {
-
- var ac = a * c, ad = a * d, bc = b * c, bd = b * d;
-
- te[ 0 ] = c * e;
- te[ 4 ] = - f;
- te[ 8 ] = d * e;
-
- te[ 1 ] = ac * f + bd;
- te[ 5 ] = a * e;
- te[ 9 ] = ad * f - bc;
-
- te[ 2 ] = bc * f - ad;
- te[ 6 ] = b * e;
- te[ 10 ] = bd * f + ac;
-
- }
-
- // bottom row
- te[ 3 ] = 0;
- te[ 7 ] = 0;
- te[ 11 ] = 0;
-
- // last column
- te[ 12 ] = 0;
- te[ 13 ] = 0;
- te[ 14 ] = 0;
- te[ 15 ] = 1;
-
- return this;
-
- },
-
- makeRotationFromQuaternion: function () {
-
- var zero = new Vector3( 0, 0, 0 );
- var one = new Vector3( 1, 1, 1 );
-
- return function makeRotationFromQuaternion( q ) {
-
- return this.compose( zero, q, one );
-
- };
-
- }(),
-
- lookAt: function () {
-
- var x = new Vector3();
- var y = new Vector3();
- var z = new Vector3();
-
- return function lookAt( eye, target, up ) {
-
- var te = this.elements;
-
- z.subVectors( eye, target );
-
- if ( z.lengthSq() === 0 ) {
-
- // eye and target are in the same position
-
- z.z = 1;
-
- }
-
- z.normalize();
- x.crossVectors( up, z );
-
- if ( x.lengthSq() === 0 ) {
-
- // up and z are parallel
-
- if ( Math.abs( up.z ) === 1 ) {
-
- z.x += 0.0001;
-
- } else {
-
- z.z += 0.0001;
-
- }
-
- z.normalize();
- x.crossVectors( up, z );
-
- }
-
- x.normalize();
- y.crossVectors( z, x );
-
- te[ 0 ] = x.x; te[ 4 ] = y.x; te[ 8 ] = z.x;
- te[ 1 ] = x.y; te[ 5 ] = y.y; te[ 9 ] = z.y;
- te[ 2 ] = x.z; te[ 6 ] = y.z; te[ 10 ] = z.z;
-
- return this;
-
- };
-
- }(),
-
- multiply: function ( m, n ) {
-
- if ( n !== undefined ) {
-
- console.warn( 'THREE.Matrix4: .multiply() now only accepts one argument. Use .multiplyMatrices( a, b ) instead.' );
- return this.multiplyMatrices( m, n );
-
- }
-
- return this.multiplyMatrices( this, m );
-
- },
-
- premultiply: function ( m ) {
-
- return this.multiplyMatrices( m, this );
-
- },
-
- multiplyMatrices: function ( a, b ) {
-
- var ae = a.elements;
- var be = b.elements;
- var te = this.elements;
-
- var a11 = ae[ 0 ], a12 = ae[ 4 ], a13 = ae[ 8 ], a14 = ae[ 12 ];
- var a21 = ae[ 1 ], a22 = ae[ 5 ], a23 = ae[ 9 ], a24 = ae[ 13 ];
- var a31 = ae[ 2 ], a32 = ae[ 6 ], a33 = ae[ 10 ], a34 = ae[ 14 ];
- var a41 = ae[ 3 ], a42 = ae[ 7 ], a43 = ae[ 11 ], a44 = ae[ 15 ];
-
- var b11 = be[ 0 ], b12 = be[ 4 ], b13 = be[ 8 ], b14 = be[ 12 ];
- var b21 = be[ 1 ], b22 = be[ 5 ], b23 = be[ 9 ], b24 = be[ 13 ];
- var b31 = be[ 2 ], b32 = be[ 6 ], b33 = be[ 10 ], b34 = be[ 14 ];
- var b41 = be[ 3 ], b42 = be[ 7 ], b43 = be[ 11 ], b44 = be[ 15 ];
-
- te[ 0 ] = a11 * b11 + a12 * b21 + a13 * b31 + a14 * b41;
- te[ 4 ] = a11 * b12 + a12 * b22 + a13 * b32 + a14 * b42;
- te[ 8 ] = a11 * b13 + a12 * b23 + a13 * b33 + a14 * b43;
- te[ 12 ] = a11 * b14 + a12 * b24 + a13 * b34 + a14 * b44;
-
- te[ 1 ] = a21 * b11 + a22 * b21 + a23 * b31 + a24 * b41;
- te[ 5 ] = a21 * b12 + a22 * b22 + a23 * b32 + a24 * b42;
- te[ 9 ] = a21 * b13 + a22 * b23 + a23 * b33 + a24 * b43;
- te[ 13 ] = a21 * b14 + a22 * b24 + a23 * b34 + a24 * b44;
-
- te[ 2 ] = a31 * b11 + a32 * b21 + a33 * b31 + a34 * b41;
- te[ 6 ] = a31 * b12 + a32 * b22 + a33 * b32 + a34 * b42;
- te[ 10 ] = a31 * b13 + a32 * b23 + a33 * b33 + a34 * b43;
- te[ 14 ] = a31 * b14 + a32 * b24 + a33 * b34 + a34 * b44;
-
- te[ 3 ] = a41 * b11 + a42 * b21 + a43 * b31 + a44 * b41;
- te[ 7 ] = a41 * b12 + a42 * b22 + a43 * b32 + a44 * b42;
- te[ 11 ] = a41 * b13 + a42 * b23 + a43 * b33 + a44 * b43;
- te[ 15 ] = a41 * b14 + a42 * b24 + a43 * b34 + a44 * b44;
-
- return this;
-
- },
-
- multiplyScalar: function ( s ) {
-
- var te = this.elements;
-
- te[ 0 ] *= s; te[ 4 ] *= s; te[ 8 ] *= s; te[ 12 ] *= s;
- te[ 1 ] *= s; te[ 5 ] *= s; te[ 9 ] *= s; te[ 13 ] *= s;
- te[ 2 ] *= s; te[ 6 ] *= s; te[ 10 ] *= s; te[ 14 ] *= s;
- te[ 3 ] *= s; te[ 7 ] *= s; te[ 11 ] *= s; te[ 15 ] *= s;
-
- return this;
-
- },
-
- applyToBufferAttribute: function () {
-
- var v1 = new Vector3();
-
- return function applyToBufferAttribute( attribute ) {
-
- for ( var i = 0, l = attribute.count; i < l; i ++ ) {
-
- v1.x = attribute.getX( i );
- v1.y = attribute.getY( i );
- v1.z = attribute.getZ( i );
-
- v1.applyMatrix4( this );
-
- attribute.setXYZ( i, v1.x, v1.y, v1.z );
-
- }
-
- return attribute;
-
- };
-
- }(),
-
- determinant: function () {
-
- var te = this.elements;
-
- var n11 = te[ 0 ], n12 = te[ 4 ], n13 = te[ 8 ], n14 = te[ 12 ];
- var n21 = te[ 1 ], n22 = te[ 5 ], n23 = te[ 9 ], n24 = te[ 13 ];
- var n31 = te[ 2 ], n32 = te[ 6 ], n33 = te[ 10 ], n34 = te[ 14 ];
- var n41 = te[ 3 ], n42 = te[ 7 ], n43 = te[ 11 ], n44 = te[ 15 ];
-
- //TODO: make this more efficient
- //( based on http://www.euclideanspace.com/maths/algebra/matrix/functions/inverse/fourD/index.htm )
-
- return (
- n41 * (
- + n14 * n23 * n32
- - n13 * n24 * n32
- - n14 * n22 * n33
- + n12 * n24 * n33
- + n13 * n22 * n34
- - n12 * n23 * n34
- ) +
- n42 * (
- + n11 * n23 * n34
- - n11 * n24 * n33
- + n14 * n21 * n33
- - n13 * n21 * n34
- + n13 * n24 * n31
- - n14 * n23 * n31
- ) +
- n43 * (
- + n11 * n24 * n32
- - n11 * n22 * n34
- - n14 * n21 * n32
- + n12 * n21 * n34
- + n14 * n22 * n31
- - n12 * n24 * n31
- ) +
- n44 * (
- - n13 * n22 * n31
- - n11 * n23 * n32
- + n11 * n22 * n33
- + n13 * n21 * n32
- - n12 * n21 * n33
- + n12 * n23 * n31
- )
-
- );
-
- },
-
- transpose: function () {
-
- var te = this.elements;
- var tmp;
-
- tmp = te[ 1 ]; te[ 1 ] = te[ 4 ]; te[ 4 ] = tmp;
- tmp = te[ 2 ]; te[ 2 ] = te[ 8 ]; te[ 8 ] = tmp;
- tmp = te[ 6 ]; te[ 6 ] = te[ 9 ]; te[ 9 ] = tmp;
-
- tmp = te[ 3 ]; te[ 3 ] = te[ 12 ]; te[ 12 ] = tmp;
- tmp = te[ 7 ]; te[ 7 ] = te[ 13 ]; te[ 13 ] = tmp;
- tmp = te[ 11 ]; te[ 11 ] = te[ 14 ]; te[ 14 ] = tmp;
-
- return this;
-
- },
-
- setPosition: function ( v ) {
-
- var te = this.elements;
-
- te[ 12 ] = v.x;
- te[ 13 ] = v.y;
- te[ 14 ] = v.z;
-
- return this;
-
- },
-
- getInverse: function ( m, throwOnDegenerate ) {
-
- // based on http://www.euclideanspace.com/maths/algebra/matrix/functions/inverse/fourD/index.htm
- var te = this.elements,
- me = m.elements,
-
- n11 = me[ 0 ], n21 = me[ 1 ], n31 = me[ 2 ], n41 = me[ 3 ],
- n12 = me[ 4 ], n22 = me[ 5 ], n32 = me[ 6 ], n42 = me[ 7 ],
- n13 = me[ 8 ], n23 = me[ 9 ], n33 = me[ 10 ], n43 = me[ 11 ],
- n14 = me[ 12 ], n24 = me[ 13 ], n34 = me[ 14 ], n44 = me[ 15 ],
-
- t11 = n23 * n34 * n42 - n24 * n33 * n42 + n24 * n32 * n43 - n22 * n34 * n43 - n23 * n32 * n44 + n22 * n33 * n44,
- t12 = n14 * n33 * n42 - n13 * n34 * n42 - n14 * n32 * n43 + n12 * n34 * n43 + n13 * n32 * n44 - n12 * n33 * n44,
- t13 = n13 * n24 * n42 - n14 * n23 * n42 + n14 * n22 * n43 - n12 * n24 * n43 - n13 * n22 * n44 + n12 * n23 * n44,
- t14 = n14 * n23 * n32 - n13 * n24 * n32 - n14 * n22 * n33 + n12 * n24 * n33 + n13 * n22 * n34 - n12 * n23 * n34;
-
- var det = n11 * t11 + n21 * t12 + n31 * t13 + n41 * t14;
-
- if ( det === 0 ) {
-
- var msg = "THREE.Matrix4: .getInverse() can't invert matrix, determinant is 0";
-
- if ( throwOnDegenerate === true ) {
-
- throw new Error( msg );
-
- } else {
-
- console.warn( msg );
-
- }
-
- return this.identity();
-
- }
-
- var detInv = 1 / det;
-
- te[ 0 ] = t11 * detInv;
- te[ 1 ] = ( n24 * n33 * n41 - n23 * n34 * n41 - n24 * n31 * n43 + n21 * n34 * n43 + n23 * n31 * n44 - n21 * n33 * n44 ) * detInv;
- te[ 2 ] = ( n22 * n34 * n41 - n24 * n32 * n41 + n24 * n31 * n42 - n21 * n34 * n42 - n22 * n31 * n44 + n21 * n32 * n44 ) * detInv;
- te[ 3 ] = ( n23 * n32 * n41 - n22 * n33 * n41 - n23 * n31 * n42 + n21 * n33 * n42 + n22 * n31 * n43 - n21 * n32 * n43 ) * detInv;
-
- te[ 4 ] = t12 * detInv;
- te[ 5 ] = ( n13 * n34 * n41 - n14 * n33 * n41 + n14 * n31 * n43 - n11 * n34 * n43 - n13 * n31 * n44 + n11 * n33 * n44 ) * detInv;
- te[ 6 ] = ( n14 * n32 * n41 - n12 * n34 * n41 - n14 * n31 * n42 + n11 * n34 * n42 + n12 * n31 * n44 - n11 * n32 * n44 ) * detInv;
- te[ 7 ] = ( n12 * n33 * n41 - n13 * n32 * n41 + n13 * n31 * n42 - n11 * n33 * n42 - n12 * n31 * n43 + n11 * n32 * n43 ) * detInv;
-
- te[ 8 ] = t13 * detInv;
- te[ 9 ] = ( n14 * n23 * n41 - n13 * n24 * n41 - n14 * n21 * n43 + n11 * n24 * n43 + n13 * n21 * n44 - n11 * n23 * n44 ) * detInv;
- te[ 10 ] = ( n12 * n24 * n41 - n14 * n22 * n41 + n14 * n21 * n42 - n11 * n24 * n42 - n12 * n21 * n44 + n11 * n22 * n44 ) * detInv;
- te[ 11 ] = ( n13 * n22 * n41 - n12 * n23 * n41 - n13 * n21 * n42 + n11 * n23 * n42 + n12 * n21 * n43 - n11 * n22 * n43 ) * detInv;
-
- te[ 12 ] = t14 * detInv;
- te[ 13 ] = ( n13 * n24 * n31 - n14 * n23 * n31 + n14 * n21 * n33 - n11 * n24 * n33 - n13 * n21 * n34 + n11 * n23 * n34 ) * detInv;
- te[ 14 ] = ( n14 * n22 * n31 - n12 * n24 * n31 - n14 * n21 * n32 + n11 * n24 * n32 + n12 * n21 * n34 - n11 * n22 * n34 ) * detInv;
- te[ 15 ] = ( n12 * n23 * n31 - n13 * n22 * n31 + n13 * n21 * n32 - n11 * n23 * n32 - n12 * n21 * n33 + n11 * n22 * n33 ) * detInv;
-
- return this;
-
- },
-
- scale: function ( v ) {
-
- var te = this.elements;
- var x = v.x, y = v.y, z = v.z;
-
- te[ 0 ] *= x; te[ 4 ] *= y; te[ 8 ] *= z;
- te[ 1 ] *= x; te[ 5 ] *= y; te[ 9 ] *= z;
- te[ 2 ] *= x; te[ 6 ] *= y; te[ 10 ] *= z;
- te[ 3 ] *= x; te[ 7 ] *= y; te[ 11 ] *= z;
-
- return this;
-
- },
-
- getMaxScaleOnAxis: function () {
-
- var te = this.elements;
-
- var scaleXSq = te[ 0 ] * te[ 0 ] + te[ 1 ] * te[ 1 ] + te[ 2 ] * te[ 2 ];
- var scaleYSq = te[ 4 ] * te[ 4 ] + te[ 5 ] * te[ 5 ] + te[ 6 ] * te[ 6 ];
- var scaleZSq = te[ 8 ] * te[ 8 ] + te[ 9 ] * te[ 9 ] + te[ 10 ] * te[ 10 ];
-
- return Math.sqrt( Math.max( scaleXSq, scaleYSq, scaleZSq ) );
-
- },
-
- makeTranslation: function ( x, y, z ) {
-
- this.set(
-
- 1, 0, 0, x,
- 0, 1, 0, y,
- 0, 0, 1, z,
- 0, 0, 0, 1
-
- );
-
- return this;
-
- },
-
- makeRotationX: function ( theta ) {
-
- var c = Math.cos( theta ), s = Math.sin( theta );
-
- this.set(
-
- 1, 0, 0, 0,
- 0, c, - s, 0,
- 0, s, c, 0,
- 0, 0, 0, 1
-
- );
-
- return this;
-
- },
-
- makeRotationY: function ( theta ) {
-
- var c = Math.cos( theta ), s = Math.sin( theta );
-
- this.set(
-
- c, 0, s, 0,
- 0, 1, 0, 0,
- - s, 0, c, 0,
- 0, 0, 0, 1
-
- );
-
- return this;
-
- },
-
- makeRotationZ: function ( theta ) {
-
- var c = Math.cos( theta ), s = Math.sin( theta );
-
- this.set(
-
- c, - s, 0, 0,
- s, c, 0, 0,
- 0, 0, 1, 0,
- 0, 0, 0, 1
-
- );
-
- return this;
-
- },
-
- makeRotationAxis: function ( axis, angle ) {
-
- // Based on http://www.gamedev.net/reference/articles/article1199.asp
-
- var c = Math.cos( angle );
- var s = Math.sin( angle );
- var t = 1 - c;
- var x = axis.x, y = axis.y, z = axis.z;
- var tx = t * x, ty = t * y;
-
- this.set(
-
- tx * x + c, tx * y - s * z, tx * z + s * y, 0,
- tx * y + s * z, ty * y + c, ty * z - s * x, 0,
- tx * z - s * y, ty * z + s * x, t * z * z + c, 0,
- 0, 0, 0, 1
-
- );
-
- return this;
-
- },
-
- makeScale: function ( x, y, z ) {
-
- this.set(
-
- x, 0, 0, 0,
- 0, y, 0, 0,
- 0, 0, z, 0,
- 0, 0, 0, 1
-
- );
-
- return this;
-
- },
-
- makeShear: function ( x, y, z ) {
-
- this.set(
-
- 1, y, z, 0,
- x, 1, z, 0,
- x, y, 1, 0,
- 0, 0, 0, 1
-
- );
-
- return this;
-
- },
-
- compose: function ( position, quaternion, scale ) {
-
- var te = this.elements;
-
- var x = quaternion._x, y = quaternion._y, z = quaternion._z, w = quaternion._w;
- var x2 = x + x, y2 = y + y, z2 = z + z;
- var xx = x * x2, xy = x * y2, xz = x * z2;
- var yy = y * y2, yz = y * z2, zz = z * z2;
- var wx = w * x2, wy = w * y2, wz = w * z2;
-
- var sx = scale.x, sy = scale.y, sz = scale.z;
-
- te[ 0 ] = ( 1 - ( yy + zz ) ) * sx;
- te[ 1 ] = ( xy + wz ) * sx;
- te[ 2 ] = ( xz - wy ) * sx;
- te[ 3 ] = 0;
-
- te[ 4 ] = ( xy - wz ) * sy;
- te[ 5 ] = ( 1 - ( xx + zz ) ) * sy;
- te[ 6 ] = ( yz + wx ) * sy;
- te[ 7 ] = 0;
-
- te[ 8 ] = ( xz + wy ) * sz;
- te[ 9 ] = ( yz - wx ) * sz;
- te[ 10 ] = ( 1 - ( xx + yy ) ) * sz;
- te[ 11 ] = 0;
-
- te[ 12 ] = position.x;
- te[ 13 ] = position.y;
- te[ 14 ] = position.z;
- te[ 15 ] = 1;
-
- return this;
-
- },
-
- decompose: function () {
-
- var vector = new Vector3();
- var matrix = new Matrix4();
-
- return function decompose( position, quaternion, scale ) {
-
- var te = this.elements;
-
- var sx = vector.set( te[ 0 ], te[ 1 ], te[ 2 ] ).length();
- var sy = vector.set( te[ 4 ], te[ 5 ], te[ 6 ] ).length();
- var sz = vector.set( te[ 8 ], te[ 9 ], te[ 10 ] ).length();
-
- // if determine is negative, we need to invert one scale
- var det = this.determinant();
- if ( det < 0 ) sx = - sx;
-
- position.x = te[ 12 ];
- position.y = te[ 13 ];
- position.z = te[ 14 ];
-
- // scale the rotation part
- matrix.copy( this );
-
- var invSX = 1 / sx;
- var invSY = 1 / sy;
- var invSZ = 1 / sz;
-
- matrix.elements[ 0 ] *= invSX;
- matrix.elements[ 1 ] *= invSX;
- matrix.elements[ 2 ] *= invSX;
-
- matrix.elements[ 4 ] *= invSY;
- matrix.elements[ 5 ] *= invSY;
- matrix.elements[ 6 ] *= invSY;
-
- matrix.elements[ 8 ] *= invSZ;
- matrix.elements[ 9 ] *= invSZ;
- matrix.elements[ 10 ] *= invSZ;
-
- quaternion.setFromRotationMatrix( matrix );
-
- scale.x = sx;
- scale.y = sy;
- scale.z = sz;
-
- return this;
-
- };
-
- }(),
-
- makePerspective: function ( left, right, top, bottom, near, far ) {
-
- if ( far === undefined ) {
-
- console.warn( 'THREE.Matrix4: .makePerspective() has been redefined and has a new signature. Please check the docs.' );
-
- }
-
- var te = this.elements;
- var x = 2 * near / ( right - left );
- var y = 2 * near / ( top - bottom );
-
- var a = ( right + left ) / ( right - left );
- var b = ( top + bottom ) / ( top - bottom );
- var c = - ( far + near ) / ( far - near );
- var d = - 2 * far * near / ( far - near );
-
- te[ 0 ] = x; te[ 4 ] = 0; te[ 8 ] = a; te[ 12 ] = 0;
- te[ 1 ] = 0; te[ 5 ] = y; te[ 9 ] = b; te[ 13 ] = 0;
- te[ 2 ] = 0; te[ 6 ] = 0; te[ 10 ] = c; te[ 14 ] = d;
- te[ 3 ] = 0; te[ 7 ] = 0; te[ 11 ] = - 1; te[ 15 ] = 0;
-
- return this;
-
- },
-
- makeOrthographic: function ( left, right, top, bottom, near, far ) {
-
- var te = this.elements;
- var w = 1.0 / ( right - left );
- var h = 1.0 / ( top - bottom );
- var p = 1.0 / ( far - near );
-
- var x = ( right + left ) * w;
- var y = ( top + bottom ) * h;
- var z = ( far + near ) * p;
-
- te[ 0 ] = 2 * w; te[ 4 ] = 0; te[ 8 ] = 0; te[ 12 ] = - x;
- te[ 1 ] = 0; te[ 5 ] = 2 * h; te[ 9 ] = 0; te[ 13 ] = - y;
- te[ 2 ] = 0; te[ 6 ] = 0; te[ 10 ] = - 2 * p; te[ 14 ] = - z;
- te[ 3 ] = 0; te[ 7 ] = 0; te[ 11 ] = 0; te[ 15 ] = 1;
-
- return this;
-
- },
-
- equals: function ( matrix ) {
-
- var te = this.elements;
- var me = matrix.elements;
-
- for ( var i = 0; i < 16; i ++ ) {
-
- if ( te[ i ] !== me[ i ] ) return false;
-
- }
-
- return true;
-
- },
-
- fromArray: function ( array, offset ) {
-
- if ( offset === undefined ) offset = 0;
-
- for ( var i = 0; i < 16; i ++ ) {
-
- this.elements[ i ] = array[ i + offset ];
-
- }
-
- return this;
-
- },
-
- toArray: function ( array, offset ) {
-
- if ( array === undefined ) array = [];
- if ( offset === undefined ) offset = 0;
-
- var te = this.elements;
-
- array[ offset ] = te[ 0 ];
- array[ offset + 1 ] = te[ 1 ];
- array[ offset + 2 ] = te[ 2 ];
- array[ offset + 3 ] = te[ 3 ];
-
- array[ offset + 4 ] = te[ 4 ];
- array[ offset + 5 ] = te[ 5 ];
- array[ offset + 6 ] = te[ 6 ];
- array[ offset + 7 ] = te[ 7 ];
-
- array[ offset + 8 ] = te[ 8 ];
- array[ offset + 9 ] = te[ 9 ];
- array[ offset + 10 ] = te[ 10 ];
- array[ offset + 11 ] = te[ 11 ];
-
- array[ offset + 12 ] = te[ 12 ];
- array[ offset + 13 ] = te[ 13 ];
- array[ offset + 14 ] = te[ 14 ];
- array[ offset + 15 ] = te[ 15 ];
-
- return array;
-
- }
-
- } );
-
- var alphamap_fragment = "#ifdef USE_ALPHAMAP\n\tdiffuseColor.a *= texture2D( alphaMap, vUv ).g;\n#endif";
-
- var alphamap_pars_fragment = "#ifdef USE_ALPHAMAP\n\tuniform sampler2D alphaMap;\n#endif";
-
- var alphatest_fragment = "#ifdef ALPHATEST\n\tif ( diffuseColor.a < ALPHATEST ) discard;\n#endif";
-
- var aomap_fragment = "#ifdef USE_AOMAP\n\tfloat ambientOcclusion = ( texture2D( aoMap, vUv2 ).r - 1.0 ) * aoMapIntensity + 1.0;\n\treflectedLight.indirectDiffuse *= ambientOcclusion;\n\t#if defined( USE_ENVMAP ) && defined( PHYSICAL )\n\t\tfloat dotNV = saturate( dot( geometry.normal, geometry.viewDir ) );\n\t\treflectedLight.indirectSpecular *= computeSpecularOcclusion( dotNV, ambientOcclusion, material.specularRoughness );\n\t#endif\n#endif";
-
- var aomap_pars_fragment = "#ifdef USE_AOMAP\n\tuniform sampler2D aoMap;\n\tuniform float aoMapIntensity;\n#endif";
-
- var begin_vertex = "vec3 transformed = vec3( position );";
-
- var beginnormal_vertex = "vec3 objectNormal = vec3( normal );\n#ifdef USE_TANGENT\n\tvec3 objectTangent = vec3( tangent.xyz );\n#endif";
-
- var bsdfs = "vec2 integrateSpecularBRDF( const in float dotNV, const in float roughness ) {\n\tconst vec4 c0 = vec4( - 1, - 0.0275, - 0.572, 0.022 );\n\tconst vec4 c1 = vec4( 1, 0.0425, 1.04, - 0.04 );\n\tvec4 r = roughness * c0 + c1;\n\tfloat a004 = min( r.x * r.x, exp2( - 9.28 * dotNV ) ) * r.x + r.y;\n\treturn vec2( -1.04, 1.04 ) * a004 + r.zw;\n}\nfloat punctualLightIntensityToIrradianceFactor( const in float lightDistance, const in float cutoffDistance, const in float decayExponent ) {\n#if defined ( PHYSICALLY_CORRECT_LIGHTS )\n\tfloat distanceFalloff = 1.0 / max( pow( lightDistance, decayExponent ), 0.01 );\n\tif( cutoffDistance > 0.0 ) {\n\t\tdistanceFalloff *= pow2( saturate( 1.0 - pow4( lightDistance / cutoffDistance ) ) );\n\t}\n\treturn distanceFalloff;\n#else\n\tif( cutoffDistance > 0.0 && decayExponent > 0.0 ) {\n\t\treturn pow( saturate( -lightDistance / cutoffDistance + 1.0 ), decayExponent );\n\t}\n\treturn 1.0;\n#endif\n}\nvec3 BRDF_Diffuse_Lambert( const in vec3 diffuseColor ) {\n\treturn RECIPROCAL_PI * diffuseColor;\n}\nvec3 F_Schlick( const in vec3 specularColor, const in float dotLH ) {\n\tfloat fresnel = exp2( ( -5.55473 * dotLH - 6.98316 ) * dotLH );\n\treturn ( 1.0 - specularColor ) * fresnel + specularColor;\n}\nfloat G_GGX_Smith( const in float alpha, const in float dotNL, const in float dotNV ) {\n\tfloat a2 = pow2( alpha );\n\tfloat gl = dotNL + sqrt( a2 + ( 1.0 - a2 ) * pow2( dotNL ) );\n\tfloat gv = dotNV + sqrt( a2 + ( 1.0 - a2 ) * pow2( dotNV ) );\n\treturn 1.0 / ( gl * gv );\n}\nfloat G_GGX_SmithCorrelated( const in float alpha, const in float dotNL, const in float dotNV ) {\n\tfloat a2 = pow2( alpha );\n\tfloat gv = dotNL * sqrt( a2 + ( 1.0 - a2 ) * pow2( dotNV ) );\n\tfloat gl = dotNV * sqrt( a2 + ( 1.0 - a2 ) * pow2( dotNL ) );\n\treturn 0.5 / max( gv + gl, EPSILON );\n}\nfloat D_GGX( const in float alpha, const in float dotNH ) {\n\tfloat a2 = pow2( alpha );\n\tfloat denom = pow2( dotNH ) * ( a2 - 1.0 ) + 1.0;\n\treturn RECIPROCAL_PI * a2 / pow2( denom );\n}\nvec3 BRDF_Specular_GGX( const in IncidentLight incidentLight, const in GeometricContext geometry, const in vec3 specularColor, const in float roughness ) {\n\tfloat alpha = pow2( roughness );\n\tvec3 halfDir = normalize( incidentLight.direction + geometry.viewDir );\n\tfloat dotNL = saturate( dot( geometry.normal, incidentLight.direction ) );\n\tfloat dotNV = saturate( dot( geometry.normal, geometry.viewDir ) );\n\tfloat dotNH = saturate( dot( geometry.normal, halfDir ) );\n\tfloat dotLH = saturate( dot( incidentLight.direction, halfDir ) );\n\tvec3 F = F_Schlick( specularColor, dotLH );\n\tfloat G = G_GGX_SmithCorrelated( alpha, dotNL, dotNV );\n\tfloat D = D_GGX( alpha, dotNH );\n\treturn F * ( G * D );\n}\nvec2 LTC_Uv( const in vec3 N, const in vec3 V, const in float roughness ) {\n\tconst float LUT_SIZE = 64.0;\n\tconst float LUT_SCALE = ( LUT_SIZE - 1.0 ) / LUT_SIZE;\n\tconst float LUT_BIAS = 0.5 / LUT_SIZE;\n\tfloat dotNV = saturate( dot( N, V ) );\n\tvec2 uv = vec2( roughness, sqrt( 1.0 - dotNV ) );\n\tuv = uv * LUT_SCALE + LUT_BIAS;\n\treturn uv;\n}\nfloat LTC_ClippedSphereFormFactor( const in vec3 f ) {\n\tfloat l = length( f );\n\treturn max( ( l * l + f.z ) / ( l + 1.0 ), 0.0 );\n}\nvec3 LTC_EdgeVectorFormFactor( const in vec3 v1, const in vec3 v2 ) {\n\tfloat x = dot( v1, v2 );\n\tfloat y = abs( x );\n\tfloat a = 0.8543985 + ( 0.4965155 + 0.0145206 * y ) * y;\n\tfloat b = 3.4175940 + ( 4.1616724 + y ) * y;\n\tfloat v = a / b;\n\tfloat theta_sintheta = ( x > 0.0 ) ? v : 0.5 * inversesqrt( max( 1.0 - x * x, 1e-7 ) ) - v;\n\treturn cross( v1, v2 ) * theta_sintheta;\n}\nvec3 LTC_Evaluate( const in vec3 N, const in vec3 V, const in vec3 P, const in mat3 mInv, const in vec3 rectCoords[ 4 ] ) {\n\tvec3 v1 = rectCoords[ 1 ] - rectCoords[ 0 ];\n\tvec3 v2 = rectCoords[ 3 ] - rectCoords[ 0 ];\n\tvec3 lightNormal = cross( v1, v2 );\n\tif( dot( lightNormal, P - rectCoords[ 0 ] ) < 0.0 ) return vec3( 0.0 );\n\tvec3 T1, T2;\n\tT1 = normalize( V - N * dot( V, N ) );\n\tT2 = - cross( N, T1 );\n\tmat3 mat = mInv * transposeMat3( mat3( T1, T2, N ) );\n\tvec3 coords[ 4 ];\n\tcoords[ 0 ] = mat * ( rectCoords[ 0 ] - P );\n\tcoords[ 1 ] = mat * ( rectCoords[ 1 ] - P );\n\tcoords[ 2 ] = mat * ( rectCoords[ 2 ] - P );\n\tcoords[ 3 ] = mat * ( rectCoords[ 3 ] - P );\n\tcoords[ 0 ] = normalize( coords[ 0 ] );\n\tcoords[ 1 ] = normalize( coords[ 1 ] );\n\tcoords[ 2 ] = normalize( coords[ 2 ] );\n\tcoords[ 3 ] = normalize( coords[ 3 ] );\n\tvec3 vectorFormFactor = vec3( 0.0 );\n\tvectorFormFactor += LTC_EdgeVectorFormFactor( coords[ 0 ], coords[ 1 ] );\n\tvectorFormFactor += LTC_EdgeVectorFormFactor( coords[ 1 ], coords[ 2 ] );\n\tvectorFormFactor += LTC_EdgeVectorFormFactor( coords[ 2 ], coords[ 3 ] );\n\tvectorFormFactor += LTC_EdgeVectorFormFactor( coords[ 3 ], coords[ 0 ] );\n\tfloat result = LTC_ClippedSphereFormFactor( vectorFormFactor );\n\treturn vec3( result );\n}\nvec3 BRDF_Specular_GGX_Environment( const in GeometricContext geometry, const in vec3 specularColor, const in float roughness ) {\n\tfloat dotNV = saturate( dot( geometry.normal, geometry.viewDir ) );\n\tvec2 brdf = integrateSpecularBRDF( dotNV, roughness );\n\treturn specularColor * brdf.x + brdf.y;\n}\nvoid BRDF_Specular_Multiscattering_Environment( const in GeometricContext geometry, const in vec3 specularColor, const in float roughness, inout vec3 singleScatter, inout vec3 multiScatter ) {\n\tfloat dotNV = saturate( dot( geometry.normal, geometry.viewDir ) );\n\tvec3 F = F_Schlick( specularColor, dotNV );\n\tvec2 brdf = integrateSpecularBRDF( dotNV, roughness );\n\tvec3 FssEss = F * brdf.x + brdf.y;\n\tfloat Ess = brdf.x + brdf.y;\n\tfloat Ems = 1.0 - Ess;\n\tvec3 Favg = specularColor + ( 1.0 - specularColor ) * 0.047619;\tvec3 Fms = FssEss * Favg / ( 1.0 - Ems * Favg );\n\tsingleScatter += FssEss;\n\tmultiScatter += Fms * Ems;\n}\nfloat G_BlinnPhong_Implicit( ) {\n\treturn 0.25;\n}\nfloat D_BlinnPhong( const in float shininess, const in float dotNH ) {\n\treturn RECIPROCAL_PI * ( shininess * 0.5 + 1.0 ) * pow( dotNH, shininess );\n}\nvec3 BRDF_Specular_BlinnPhong( const in IncidentLight incidentLight, const in GeometricContext geometry, const in vec3 specularColor, const in float shininess ) {\n\tvec3 halfDir = normalize( incidentLight.direction + geometry.viewDir );\n\tfloat dotNH = saturate( dot( geometry.normal, halfDir ) );\n\tfloat dotLH = saturate( dot( incidentLight.direction, halfDir ) );\n\tvec3 F = F_Schlick( specularColor, dotLH );\n\tfloat G = G_BlinnPhong_Implicit( );\n\tfloat D = D_BlinnPhong( shininess, dotNH );\n\treturn F * ( G * D );\n}\nfloat GGXRoughnessToBlinnExponent( const in float ggxRoughness ) {\n\treturn ( 2.0 / pow2( ggxRoughness + 0.0001 ) - 2.0 );\n}\nfloat BlinnExponentToGGXRoughness( const in float blinnExponent ) {\n\treturn sqrt( 2.0 / ( blinnExponent + 2.0 ) );\n}";
-
- var bumpmap_pars_fragment = "#ifdef USE_BUMPMAP\n\tuniform sampler2D bumpMap;\n\tuniform float bumpScale;\n\tvec2 dHdxy_fwd() {\n\t\tvec2 dSTdx = dFdx( vUv );\n\t\tvec2 dSTdy = dFdy( vUv );\n\t\tfloat Hll = bumpScale * texture2D( bumpMap, vUv ).x;\n\t\tfloat dBx = bumpScale * texture2D( bumpMap, vUv + dSTdx ).x - Hll;\n\t\tfloat dBy = bumpScale * texture2D( bumpMap, vUv + dSTdy ).x - Hll;\n\t\treturn vec2( dBx, dBy );\n\t}\n\tvec3 perturbNormalArb( vec3 surf_pos, vec3 surf_norm, vec2 dHdxy ) {\n\t\tvec3 vSigmaX = vec3( dFdx( surf_pos.x ), dFdx( surf_pos.y ), dFdx( surf_pos.z ) );\n\t\tvec3 vSigmaY = vec3( dFdy( surf_pos.x ), dFdy( surf_pos.y ), dFdy( surf_pos.z ) );\n\t\tvec3 vN = surf_norm;\n\t\tvec3 R1 = cross( vSigmaY, vN );\n\t\tvec3 R2 = cross( vN, vSigmaX );\n\t\tfloat fDet = dot( vSigmaX, R1 );\n\t\tfDet *= ( float( gl_FrontFacing ) * 2.0 - 1.0 );\n\t\tvec3 vGrad = sign( fDet ) * ( dHdxy.x * R1 + dHdxy.y * R2 );\n\t\treturn normalize( abs( fDet ) * surf_norm - vGrad );\n\t}\n#endif";
-
- var clipping_planes_fragment = "#if NUM_CLIPPING_PLANES > 0\n\tvec4 plane;\n\t#pragma unroll_loop\n\tfor ( int i = 0; i < UNION_CLIPPING_PLANES; i ++ ) {\n\t\tplane = clippingPlanes[ i ];\n\t\tif ( dot( vViewPosition, plane.xyz ) > plane.w ) discard;\n\t}\n\t#if UNION_CLIPPING_PLANES < NUM_CLIPPING_PLANES\n\t\tbool clipped = true;\n\t\t#pragma unroll_loop\n\t\tfor ( int i = UNION_CLIPPING_PLANES; i < NUM_CLIPPING_PLANES; i ++ ) {\n\t\t\tplane = clippingPlanes[ i ];\n\t\t\tclipped = ( dot( vViewPosition, plane.xyz ) > plane.w ) && clipped;\n\t\t}\n\t\tif ( clipped ) discard;\n\t#endif\n#endif";
-
- var clipping_planes_pars_fragment = "#if NUM_CLIPPING_PLANES > 0\n\t#if ! defined( PHYSICAL ) && ! defined( PHONG ) && ! defined( MATCAP )\n\t\tvarying vec3 vViewPosition;\n\t#endif\n\tuniform vec4 clippingPlanes[ NUM_CLIPPING_PLANES ];\n#endif";
-
- var clipping_planes_pars_vertex = "#if NUM_CLIPPING_PLANES > 0 && ! defined( PHYSICAL ) && ! defined( PHONG ) && ! defined( MATCAP )\n\tvarying vec3 vViewPosition;\n#endif";
-
- var clipping_planes_vertex = "#if NUM_CLIPPING_PLANES > 0 && ! defined( PHYSICAL ) && ! defined( PHONG ) && ! defined( MATCAP )\n\tvViewPosition = - mvPosition.xyz;\n#endif";
-
- var color_fragment = "#ifdef USE_COLOR\n\tdiffuseColor.rgb *= vColor;\n#endif";
-
- var color_pars_fragment = "#ifdef USE_COLOR\n\tvarying vec3 vColor;\n#endif";
-
- var color_pars_vertex = "#ifdef USE_COLOR\n\tvarying vec3 vColor;\n#endif";
-
- var color_vertex = "#ifdef USE_COLOR\n\tvColor.xyz = color.xyz;\n#endif";
-
- var common = "#define PI 3.14159265359\n#define PI2 6.28318530718\n#define PI_HALF 1.5707963267949\n#define RECIPROCAL_PI 0.31830988618\n#define RECIPROCAL_PI2 0.15915494\n#define LOG2 1.442695\n#define EPSILON 1e-6\n#define saturate(a) clamp( a, 0.0, 1.0 )\n#define whiteCompliment(a) ( 1.0 - saturate( a ) )\nfloat pow2( const in float x ) { return x*x; }\nfloat pow3( const in float x ) { return x*x*x; }\nfloat pow4( const in float x ) { float x2 = x*x; return x2*x2; }\nfloat average( const in vec3 color ) { return dot( color, vec3( 0.3333 ) ); }\nhighp float rand( const in vec2 uv ) {\n\tconst highp float a = 12.9898, b = 78.233, c = 43758.5453;\n\thighp float dt = dot( uv.xy, vec2( a,b ) ), sn = mod( dt, PI );\n\treturn fract(sin(sn) * c);\n}\nstruct IncidentLight {\n\tvec3 color;\n\tvec3 direction;\n\tbool visible;\n};\nstruct ReflectedLight {\n\tvec3 directDiffuse;\n\tvec3 directSpecular;\n\tvec3 indirectDiffuse;\n\tvec3 indirectSpecular;\n};\nstruct GeometricContext {\n\tvec3 position;\n\tvec3 normal;\n\tvec3 viewDir;\n};\nvec3 transformDirection( in vec3 dir, in mat4 matrix ) {\n\treturn normalize( ( matrix * vec4( dir, 0.0 ) ).xyz );\n}\nvec3 inverseTransformDirection( in vec3 dir, in mat4 matrix ) {\n\treturn normalize( ( vec4( dir, 0.0 ) * matrix ).xyz );\n}\nvec3 projectOnPlane(in vec3 point, in vec3 pointOnPlane, in vec3 planeNormal ) {\n\tfloat distance = dot( planeNormal, point - pointOnPlane );\n\treturn - distance * planeNormal + point;\n}\nfloat sideOfPlane( in vec3 point, in vec3 pointOnPlane, in vec3 planeNormal ) {\n\treturn sign( dot( point - pointOnPlane, planeNormal ) );\n}\nvec3 linePlaneIntersect( in vec3 pointOnLine, in vec3 lineDirection, in vec3 pointOnPlane, in vec3 planeNormal ) {\n\treturn lineDirection * ( dot( planeNormal, pointOnPlane - pointOnLine ) / dot( planeNormal, lineDirection ) ) + pointOnLine;\n}\nmat3 transposeMat3( const in mat3 m ) {\n\tmat3 tmp;\n\ttmp[ 0 ] = vec3( m[ 0 ].x, m[ 1 ].x, m[ 2 ].x );\n\ttmp[ 1 ] = vec3( m[ 0 ].y, m[ 1 ].y, m[ 2 ].y );\n\ttmp[ 2 ] = vec3( m[ 0 ].z, m[ 1 ].z, m[ 2 ].z );\n\treturn tmp;\n}\nfloat linearToRelativeLuminance( const in vec3 color ) {\n\tvec3 weights = vec3( 0.2126, 0.7152, 0.0722 );\n\treturn dot( weights, color.rgb );\n}";
-
- var cube_uv_reflection_fragment = "#ifdef ENVMAP_TYPE_CUBE_UV\n#define cubeUV_textureSize (1024.0)\nint getFaceFromDirection(vec3 direction) {\n\tvec3 absDirection = abs(direction);\n\tint face = -1;\n\tif( absDirection.x > absDirection.z ) {\n\t\tif(absDirection.x > absDirection.y )\n\t\t\tface = direction.x > 0.0 ? 0 : 3;\n\t\telse\n\t\t\tface = direction.y > 0.0 ? 1 : 4;\n\t}\n\telse {\n\t\tif(absDirection.z > absDirection.y )\n\t\t\tface = direction.z > 0.0 ? 2 : 5;\n\t\telse\n\t\t\tface = direction.y > 0.0 ? 1 : 4;\n\t}\n\treturn face;\n}\n#define cubeUV_maxLods1 (log2(cubeUV_textureSize*0.25) - 1.0)\n#define cubeUV_rangeClamp (exp2((6.0 - 1.0) * 2.0))\nvec2 MipLevelInfo( vec3 vec, float roughnessLevel, float roughness ) {\n\tfloat scale = exp2(cubeUV_maxLods1 - roughnessLevel);\n\tfloat dxRoughness = dFdx(roughness);\n\tfloat dyRoughness = dFdy(roughness);\n\tvec3 dx = dFdx( vec * scale * dxRoughness );\n\tvec3 dy = dFdy( vec * scale * dyRoughness );\n\tfloat d = max( dot( dx, dx ), dot( dy, dy ) );\n\td = clamp(d, 1.0, cubeUV_rangeClamp);\n\tfloat mipLevel = 0.5 * log2(d);\n\treturn vec2(floor(mipLevel), fract(mipLevel));\n}\n#define cubeUV_maxLods2 (log2(cubeUV_textureSize*0.25) - 2.0)\n#define cubeUV_rcpTextureSize (1.0 / cubeUV_textureSize)\nvec2 getCubeUV(vec3 direction, float roughnessLevel, float mipLevel) {\n\tmipLevel = roughnessLevel > cubeUV_maxLods2 - 3.0 ? 0.0 : mipLevel;\n\tfloat a = 16.0 * cubeUV_rcpTextureSize;\n\tvec2 exp2_packed = exp2( vec2( roughnessLevel, mipLevel ) );\n\tvec2 rcp_exp2_packed = vec2( 1.0 ) / exp2_packed;\n\tfloat powScale = exp2_packed.x * exp2_packed.y;\n\tfloat scale = rcp_exp2_packed.x * rcp_exp2_packed.y * 0.25;\n\tfloat mipOffset = 0.75*(1.0 - rcp_exp2_packed.y) * rcp_exp2_packed.x;\n\tbool bRes = mipLevel == 0.0;\n\tscale = bRes && (scale < a) ? a : scale;\n\tvec3 r;\n\tvec2 offset;\n\tint face = getFaceFromDirection(direction);\n\tfloat rcpPowScale = 1.0 / powScale;\n\tif( face == 0) {\n\t\tr = vec3(direction.x, -direction.z, direction.y);\n\t\toffset = vec2(0.0+mipOffset,0.75 * rcpPowScale);\n\t\toffset.y = bRes && (offset.y < 2.0*a) ? a : offset.y;\n\t}\n\telse if( face == 1) {\n\t\tr = vec3(direction.y, direction.x, direction.z);\n\t\toffset = vec2(scale+mipOffset, 0.75 * rcpPowScale);\n\t\toffset.y = bRes && (offset.y < 2.0*a) ? a : offset.y;\n\t}\n\telse if( face == 2) {\n\t\tr = vec3(direction.z, direction.x, direction.y);\n\t\toffset = vec2(2.0*scale+mipOffset, 0.75 * rcpPowScale);\n\t\toffset.y = bRes && (offset.y < 2.0*a) ? a : offset.y;\n\t}\n\telse if( face == 3) {\n\t\tr = vec3(direction.x, direction.z, direction.y);\n\t\toffset = vec2(0.0+mipOffset,0.5 * rcpPowScale);\n\t\toffset.y = bRes && (offset.y < 2.0*a) ? 0.0 : offset.y;\n\t}\n\telse if( face == 4) {\n\t\tr = vec3(direction.y, direction.x, -direction.z);\n\t\toffset = vec2(scale+mipOffset, 0.5 * rcpPowScale);\n\t\toffset.y = bRes && (offset.y < 2.0*a) ? 0.0 : offset.y;\n\t}\n\telse {\n\t\tr = vec3(direction.z, -direction.x, direction.y);\n\t\toffset = vec2(2.0*scale+mipOffset, 0.5 * rcpPowScale);\n\t\toffset.y = bRes && (offset.y < 2.0*a) ? 0.0 : offset.y;\n\t}\n\tr = normalize(r);\n\tfloat texelOffset = 0.5 * cubeUV_rcpTextureSize;\n\tvec2 s = ( r.yz / abs( r.x ) + vec2( 1.0 ) ) * 0.5;\n\tvec2 base = offset + vec2( texelOffset );\n\treturn base + s * ( scale - 2.0 * texelOffset );\n}\n#define cubeUV_maxLods3 (log2(cubeUV_textureSize*0.25) - 3.0)\nvec4 textureCubeUV( sampler2D envMap, vec3 reflectedDirection, float roughness ) {\n\tfloat roughnessVal = roughness* cubeUV_maxLods3;\n\tfloat r1 = floor(roughnessVal);\n\tfloat r2 = r1 + 1.0;\n\tfloat t = fract(roughnessVal);\n\tvec2 mipInfo = MipLevelInfo(reflectedDirection, r1, roughness);\n\tfloat s = mipInfo.y;\n\tfloat level0 = mipInfo.x;\n\tfloat level1 = level0 + 1.0;\n\tlevel1 = level1 > 5.0 ? 5.0 : level1;\n\tlevel0 += min( floor( s + 0.5 ), 5.0 );\n\tvec2 uv_10 = getCubeUV(reflectedDirection, r1, level0);\n\tvec4 color10 = envMapTexelToLinear(texture2D(envMap, uv_10));\n\tvec2 uv_20 = getCubeUV(reflectedDirection, r2, level0);\n\tvec4 color20 = envMapTexelToLinear(texture2D(envMap, uv_20));\n\tvec4 result = mix(color10, color20, t);\n\treturn vec4(result.rgb, 1.0);\n}\n#endif";
-
- var defaultnormal_vertex = "vec3 transformedNormal = normalMatrix * objectNormal;\n#ifdef FLIP_SIDED\n\ttransformedNormal = - transformedNormal;\n#endif\n#ifdef USE_TANGENT\n\tvec3 transformedTangent = normalMatrix * objectTangent;\n\t#ifdef FLIP_SIDED\n\t\ttransformedTangent = - transformedTangent;\n\t#endif\n#endif";
-
- var displacementmap_pars_vertex = "#ifdef USE_DISPLACEMENTMAP\n\tuniform sampler2D displacementMap;\n\tuniform float displacementScale;\n\tuniform float displacementBias;\n#endif";
-
- var displacementmap_vertex = "#ifdef USE_DISPLACEMENTMAP\n\ttransformed += normalize( objectNormal ) * ( texture2D( displacementMap, uv ).x * displacementScale + displacementBias );\n#endif";
-
- var emissivemap_fragment = "#ifdef USE_EMISSIVEMAP\n\tvec4 emissiveColor = texture2D( emissiveMap, vUv );\n\temissiveColor.rgb = emissiveMapTexelToLinear( emissiveColor ).rgb;\n\ttotalEmissiveRadiance *= emissiveColor.rgb;\n#endif";
-
- var emissivemap_pars_fragment = "#ifdef USE_EMISSIVEMAP\n\tuniform sampler2D emissiveMap;\n#endif";
-
- var encodings_fragment = "gl_FragColor = linearToOutputTexel( gl_FragColor );";
-
- var encodings_pars_fragment = "\nvec4 LinearToLinear( in vec4 value ) {\n\treturn value;\n}\nvec4 GammaToLinear( in vec4 value, in float gammaFactor ) {\n\treturn vec4( pow( value.rgb, vec3( gammaFactor ) ), value.a );\n}\nvec4 LinearToGamma( in vec4 value, in float gammaFactor ) {\n\treturn vec4( pow( value.rgb, vec3( 1.0 / gammaFactor ) ), value.a );\n}\nvec4 sRGBToLinear( in vec4 value ) {\n\treturn vec4( mix( pow( value.rgb * 0.9478672986 + vec3( 0.0521327014 ), vec3( 2.4 ) ), value.rgb * 0.0773993808, vec3( lessThanEqual( value.rgb, vec3( 0.04045 ) ) ) ), value.a );\n}\nvec4 LinearTosRGB( in vec4 value ) {\n\treturn vec4( mix( pow( value.rgb, vec3( 0.41666 ) ) * 1.055 - vec3( 0.055 ), value.rgb * 12.92, vec3( lessThanEqual( value.rgb, vec3( 0.0031308 ) ) ) ), value.a );\n}\nvec4 RGBEToLinear( in vec4 value ) {\n\treturn vec4( value.rgb * exp2( value.a * 255.0 - 128.0 ), 1.0 );\n}\nvec4 LinearToRGBE( in vec4 value ) {\n\tfloat maxComponent = max( max( value.r, value.g ), value.b );\n\tfloat fExp = clamp( ceil( log2( maxComponent ) ), -128.0, 127.0 );\n\treturn vec4( value.rgb / exp2( fExp ), ( fExp + 128.0 ) / 255.0 );\n}\nvec4 RGBMToLinear( in vec4 value, in float maxRange ) {\n\treturn vec4( value.rgb * value.a * maxRange, 1.0 );\n}\nvec4 LinearToRGBM( in vec4 value, in float maxRange ) {\n\tfloat maxRGB = max( value.r, max( value.g, value.b ) );\n\tfloat M = clamp( maxRGB / maxRange, 0.0, 1.0 );\n\tM = ceil( M * 255.0 ) / 255.0;\n\treturn vec4( value.rgb / ( M * maxRange ), M );\n}\nvec4 RGBDToLinear( in vec4 value, in float maxRange ) {\n\treturn vec4( value.rgb * ( ( maxRange / 255.0 ) / value.a ), 1.0 );\n}\nvec4 LinearToRGBD( in vec4 value, in float maxRange ) {\n\tfloat maxRGB = max( value.r, max( value.g, value.b ) );\n\tfloat D = max( maxRange / maxRGB, 1.0 );\n\tD = min( floor( D ) / 255.0, 1.0 );\n\treturn vec4( value.rgb * ( D * ( 255.0 / maxRange ) ), D );\n}\nconst mat3 cLogLuvM = mat3( 0.2209, 0.3390, 0.4184, 0.1138, 0.6780, 0.7319, 0.0102, 0.1130, 0.2969 );\nvec4 LinearToLogLuv( in vec4 value ) {\n\tvec3 Xp_Y_XYZp = cLogLuvM * value.rgb;\n\tXp_Y_XYZp = max( Xp_Y_XYZp, vec3( 1e-6, 1e-6, 1e-6 ) );\n\tvec4 vResult;\n\tvResult.xy = Xp_Y_XYZp.xy / Xp_Y_XYZp.z;\n\tfloat Le = 2.0 * log2(Xp_Y_XYZp.y) + 127.0;\n\tvResult.w = fract( Le );\n\tvResult.z = ( Le - ( floor( vResult.w * 255.0 ) ) / 255.0 ) / 255.0;\n\treturn vResult;\n}\nconst mat3 cLogLuvInverseM = mat3( 6.0014, -2.7008, -1.7996, -1.3320, 3.1029, -5.7721, 0.3008, -1.0882, 5.6268 );\nvec4 LogLuvToLinear( in vec4 value ) {\n\tfloat Le = value.z * 255.0 + value.w;\n\tvec3 Xp_Y_XYZp;\n\tXp_Y_XYZp.y = exp2( ( Le - 127.0 ) / 2.0 );\n\tXp_Y_XYZp.z = Xp_Y_XYZp.y / value.y;\n\tXp_Y_XYZp.x = value.x * Xp_Y_XYZp.z;\n\tvec3 vRGB = cLogLuvInverseM * Xp_Y_XYZp.rgb;\n\treturn vec4( max( vRGB, 0.0 ), 1.0 );\n}";
-
- var envmap_fragment = "#ifdef USE_ENVMAP\n\t#if defined( USE_BUMPMAP ) || defined( USE_NORMALMAP ) || defined( PHONG )\n\t\tvec3 cameraToVertex = normalize( vWorldPosition - cameraPosition );\n\t\tvec3 worldNormal = inverseTransformDirection( normal, viewMatrix );\n\t\t#ifdef ENVMAP_MODE_REFLECTION\n\t\t\tvec3 reflectVec = reflect( cameraToVertex, worldNormal );\n\t\t#else\n\t\t\tvec3 reflectVec = refract( cameraToVertex, worldNormal, refractionRatio );\n\t\t#endif\n\t#else\n\t\tvec3 reflectVec = vReflect;\n\t#endif\n\t#ifdef ENVMAP_TYPE_CUBE\n\t\tvec4 envColor = textureCube( envMap, vec3( flipEnvMap * reflectVec.x, reflectVec.yz ) );\n\t#elif defined( ENVMAP_TYPE_EQUIREC )\n\t\tvec2 sampleUV;\n\t\treflectVec = normalize( reflectVec );\n\t\tsampleUV.y = asin( clamp( reflectVec.y, - 1.0, 1.0 ) ) * RECIPROCAL_PI + 0.5;\n\t\tsampleUV.x = atan( reflectVec.z, reflectVec.x ) * RECIPROCAL_PI2 + 0.5;\n\t\tvec4 envColor = texture2D( envMap, sampleUV );\n\t#elif defined( ENVMAP_TYPE_SPHERE )\n\t\treflectVec = normalize( reflectVec );\n\t\tvec3 reflectView = normalize( ( viewMatrix * vec4( reflectVec, 0.0 ) ).xyz + vec3( 0.0, 0.0, 1.0 ) );\n\t\tvec4 envColor = texture2D( envMap, reflectView.xy * 0.5 + 0.5 );\n\t#else\n\t\tvec4 envColor = vec4( 0.0 );\n\t#endif\n\tenvColor = envMapTexelToLinear( envColor );\n\t#ifdef ENVMAP_BLENDING_MULTIPLY\n\t\toutgoingLight = mix( outgoingLight, outgoingLight * envColor.xyz, specularStrength * reflectivity );\n\t#elif defined( ENVMAP_BLENDING_MIX )\n\t\toutgoingLight = mix( outgoingLight, envColor.xyz, specularStrength * reflectivity );\n\t#elif defined( ENVMAP_BLENDING_ADD )\n\t\toutgoingLight += envColor.xyz * specularStrength * reflectivity;\n\t#endif\n#endif";
-
- var envmap_pars_fragment = "#if defined( USE_ENVMAP ) || defined( PHYSICAL )\n\tuniform float reflectivity;\n\tuniform float envMapIntensity;\n#endif\n#ifdef USE_ENVMAP\n\t#if ! defined( PHYSICAL ) && ( defined( USE_BUMPMAP ) || defined( USE_NORMALMAP ) || defined( PHONG ) )\n\t\tvarying vec3 vWorldPosition;\n\t#endif\n\t#ifdef ENVMAP_TYPE_CUBE\n\t\tuniform samplerCube envMap;\n\t#else\n\t\tuniform sampler2D envMap;\n\t#endif\n\tuniform float flipEnvMap;\n\tuniform int maxMipLevel;\n\t#if defined( USE_BUMPMAP ) || defined( USE_NORMALMAP ) || defined( PHONG ) || defined( PHYSICAL )\n\t\tuniform float refractionRatio;\n\t#else\n\t\tvarying vec3 vReflect;\n\t#endif\n#endif";
-
- var envmap_pars_vertex = "#ifdef USE_ENVMAP\n\t#if defined( USE_BUMPMAP ) || defined( USE_NORMALMAP ) || defined( PHONG )\n\t\tvarying vec3 vWorldPosition;\n\t#else\n\t\tvarying vec3 vReflect;\n\t\tuniform float refractionRatio;\n\t#endif\n#endif";
-
- var envmap_vertex = "#ifdef USE_ENVMAP\n\t#if defined( USE_BUMPMAP ) || defined( USE_NORMALMAP ) || defined( PHONG )\n\t\tvWorldPosition = worldPosition.xyz;\n\t#else\n\t\tvec3 cameraToVertex = normalize( worldPosition.xyz - cameraPosition );\n\t\tvec3 worldNormal = inverseTransformDirection( transformedNormal, viewMatrix );\n\t\t#ifdef ENVMAP_MODE_REFLECTION\n\t\t\tvReflect = reflect( cameraToVertex, worldNormal );\n\t\t#else\n\t\t\tvReflect = refract( cameraToVertex, worldNormal, refractionRatio );\n\t\t#endif\n\t#endif\n#endif";
-
- var fog_vertex = "#ifdef USE_FOG\n\tfogDepth = -mvPosition.z;\n#endif";
-
- var fog_pars_vertex = "#ifdef USE_FOG\n\tvarying float fogDepth;\n#endif";
-
- var fog_fragment = "#ifdef USE_FOG\n\t#ifdef FOG_EXP2\n\t\tfloat fogFactor = whiteCompliment( exp2( - fogDensity * fogDensity * fogDepth * fogDepth * LOG2 ) );\n\t#else\n\t\tfloat fogFactor = smoothstep( fogNear, fogFar, fogDepth );\n\t#endif\n\tgl_FragColor.rgb = mix( gl_FragColor.rgb, fogColor, fogFactor );\n#endif";
-
- var fog_pars_fragment = "#ifdef USE_FOG\n\tuniform vec3 fogColor;\n\tvarying float fogDepth;\n\t#ifdef FOG_EXP2\n\t\tuniform float fogDensity;\n\t#else\n\t\tuniform float fogNear;\n\t\tuniform float fogFar;\n\t#endif\n#endif";
-
- var gradientmap_pars_fragment = "#ifdef TOON\n\tuniform sampler2D gradientMap;\n\tvec3 getGradientIrradiance( vec3 normal, vec3 lightDirection ) {\n\t\tfloat dotNL = dot( normal, lightDirection );\n\t\tvec2 coord = vec2( dotNL * 0.5 + 0.5, 0.0 );\n\t\t#ifdef USE_GRADIENTMAP\n\t\t\treturn texture2D( gradientMap, coord ).rgb;\n\t\t#else\n\t\t\treturn ( coord.x < 0.7 ) ? vec3( 0.7 ) : vec3( 1.0 );\n\t\t#endif\n\t}\n#endif";
-
- var lightmap_fragment = "#ifdef USE_LIGHTMAP\n\treflectedLight.indirectDiffuse += PI * texture2D( lightMap, vUv2 ).xyz * lightMapIntensity;\n#endif";
-
- var lightmap_pars_fragment = "#ifdef USE_LIGHTMAP\n\tuniform sampler2D lightMap;\n\tuniform float lightMapIntensity;\n#endif";
-
- var lights_lambert_vertex = "vec3 diffuse = vec3( 1.0 );\nGeometricContext geometry;\ngeometry.position = mvPosition.xyz;\ngeometry.normal = normalize( transformedNormal );\ngeometry.viewDir = normalize( -mvPosition.xyz );\nGeometricContext backGeometry;\nbackGeometry.position = geometry.position;\nbackGeometry.normal = -geometry.normal;\nbackGeometry.viewDir = geometry.viewDir;\nvLightFront = vec3( 0.0 );\nvIndirectFront = vec3( 0.0 );\n#ifdef DOUBLE_SIDED\n\tvLightBack = vec3( 0.0 );\n\tvIndirectBack = vec3( 0.0 );\n#endif\nIncidentLight directLight;\nfloat dotNL;\nvec3 directLightColor_Diffuse;\n#if NUM_POINT_LIGHTS > 0\n\t#pragma unroll_loop\n\tfor ( int i = 0; i < NUM_POINT_LIGHTS; i ++ ) {\n\t\tgetPointDirectLightIrradiance( pointLights[ i ], geometry, directLight );\n\t\tdotNL = dot( geometry.normal, directLight.direction );\n\t\tdirectLightColor_Diffuse = PI * directLight.color;\n\t\tvLightFront += saturate( dotNL ) * directLightColor_Diffuse;\n\t\t#ifdef DOUBLE_SIDED\n\t\t\tvLightBack += saturate( -dotNL ) * directLightColor_Diffuse;\n\t\t#endif\n\t}\n#endif\n#if NUM_SPOT_LIGHTS > 0\n\t#pragma unroll_loop\n\tfor ( int i = 0; i < NUM_SPOT_LIGHTS; i ++ ) {\n\t\tgetSpotDirectLightIrradiance( spotLights[ i ], geometry, directLight );\n\t\tdotNL = dot( geometry.normal, directLight.direction );\n\t\tdirectLightColor_Diffuse = PI * directLight.color;\n\t\tvLightFront += saturate( dotNL ) * directLightColor_Diffuse;\n\t\t#ifdef DOUBLE_SIDED\n\t\t\tvLightBack += saturate( -dotNL ) * directLightColor_Diffuse;\n\t\t#endif\n\t}\n#endif\n#if NUM_DIR_LIGHTS > 0\n\t#pragma unroll_loop\n\tfor ( int i = 0; i < NUM_DIR_LIGHTS; i ++ ) {\n\t\tgetDirectionalDirectLightIrradiance( directionalLights[ i ], geometry, directLight );\n\t\tdotNL = dot( geometry.normal, directLight.direction );\n\t\tdirectLightColor_Diffuse = PI * directLight.color;\n\t\tvLightFront += saturate( dotNL ) * directLightColor_Diffuse;\n\t\t#ifdef DOUBLE_SIDED\n\t\t\tvLightBack += saturate( -dotNL ) * directLightColor_Diffuse;\n\t\t#endif\n\t}\n#endif\n#if NUM_HEMI_LIGHTS > 0\n\t#pragma unroll_loop\n\tfor ( int i = 0; i < NUM_HEMI_LIGHTS; i ++ ) {\n\t\tvIndirectFront += getHemisphereLightIrradiance( hemisphereLights[ i ], geometry );\n\t\t#ifdef DOUBLE_SIDED\n\t\t\tvIndirectBack += getHemisphereLightIrradiance( hemisphereLights[ i ], backGeometry );\n\t\t#endif\n\t}\n#endif";
-
- var lights_pars_begin = "uniform vec3 ambientLightColor;\nvec3 getAmbientLightIrradiance( const in vec3 ambientLightColor ) {\n\tvec3 irradiance = ambientLightColor;\n\t#ifndef PHYSICALLY_CORRECT_LIGHTS\n\t\tirradiance *= PI;\n\t#endif\n\treturn irradiance;\n}\n#if NUM_DIR_LIGHTS > 0\n\tstruct DirectionalLight {\n\t\tvec3 direction;\n\t\tvec3 color;\n\t\tint shadow;\n\t\tfloat shadowBias;\n\t\tfloat shadowRadius;\n\t\tvec2 shadowMapSize;\n\t};\n\tuniform DirectionalLight directionalLights[ NUM_DIR_LIGHTS ];\n\tvoid getDirectionalDirectLightIrradiance( const in DirectionalLight directionalLight, const in GeometricContext geometry, out IncidentLight directLight ) {\n\t\tdirectLight.color = directionalLight.color;\n\t\tdirectLight.direction = directionalLight.direction;\n\t\tdirectLight.visible = true;\n\t}\n#endif\n#if NUM_POINT_LIGHTS > 0\n\tstruct PointLight {\n\t\tvec3 position;\n\t\tvec3 color;\n\t\tfloat distance;\n\t\tfloat decay;\n\t\tint shadow;\n\t\tfloat shadowBias;\n\t\tfloat shadowRadius;\n\t\tvec2 shadowMapSize;\n\t\tfloat shadowCameraNear;\n\t\tfloat shadowCameraFar;\n\t};\n\tuniform PointLight pointLights[ NUM_POINT_LIGHTS ];\n\tvoid getPointDirectLightIrradiance( const in PointLight pointLight, const in GeometricContext geometry, out IncidentLight directLight ) {\n\t\tvec3 lVector = pointLight.position - geometry.position;\n\t\tdirectLight.direction = normalize( lVector );\n\t\tfloat lightDistance = length( lVector );\n\t\tdirectLight.color = pointLight.color;\n\t\tdirectLight.color *= punctualLightIntensityToIrradianceFactor( lightDistance, pointLight.distance, pointLight.decay );\n\t\tdirectLight.visible = ( directLight.color != vec3( 0.0 ) );\n\t}\n#endif\n#if NUM_SPOT_LIGHTS > 0\n\tstruct SpotLight {\n\t\tvec3 position;\n\t\tvec3 direction;\n\t\tvec3 color;\n\t\tfloat distance;\n\t\tfloat decay;\n\t\tfloat coneCos;\n\t\tfloat penumbraCos;\n\t\tint shadow;\n\t\tfloat shadowBias;\n\t\tfloat shadowRadius;\n\t\tvec2 shadowMapSize;\n\t};\n\tuniform SpotLight spotLights[ NUM_SPOT_LIGHTS ];\n\tvoid getSpotDirectLightIrradiance( const in SpotLight spotLight, const in GeometricContext geometry, out IncidentLight directLight ) {\n\t\tvec3 lVector = spotLight.position - geometry.position;\n\t\tdirectLight.direction = normalize( lVector );\n\t\tfloat lightDistance = length( lVector );\n\t\tfloat angleCos = dot( directLight.direction, spotLight.direction );\n\t\tif ( angleCos > spotLight.coneCos ) {\n\t\t\tfloat spotEffect = smoothstep( spotLight.coneCos, spotLight.penumbraCos, angleCos );\n\t\t\tdirectLight.color = spotLight.color;\n\t\t\tdirectLight.color *= spotEffect * punctualLightIntensityToIrradianceFactor( lightDistance, spotLight.distance, spotLight.decay );\n\t\t\tdirectLight.visible = true;\n\t\t} else {\n\t\t\tdirectLight.color = vec3( 0.0 );\n\t\t\tdirectLight.visible = false;\n\t\t}\n\t}\n#endif\n#if NUM_RECT_AREA_LIGHTS > 0\n\tstruct RectAreaLight {\n\t\tvec3 color;\n\t\tvec3 position;\n\t\tvec3 halfWidth;\n\t\tvec3 halfHeight;\n\t};\n\tuniform sampler2D ltc_1;\tuniform sampler2D ltc_2;\n\tuniform RectAreaLight rectAreaLights[ NUM_RECT_AREA_LIGHTS ];\n#endif\n#if NUM_HEMI_LIGHTS > 0\n\tstruct HemisphereLight {\n\t\tvec3 direction;\n\t\tvec3 skyColor;\n\t\tvec3 groundColor;\n\t};\n\tuniform HemisphereLight hemisphereLights[ NUM_HEMI_LIGHTS ];\n\tvec3 getHemisphereLightIrradiance( const in HemisphereLight hemiLight, const in GeometricContext geometry ) {\n\t\tfloat dotNL = dot( geometry.normal, hemiLight.direction );\n\t\tfloat hemiDiffuseWeight = 0.5 * dotNL + 0.5;\n\t\tvec3 irradiance = mix( hemiLight.groundColor, hemiLight.skyColor, hemiDiffuseWeight );\n\t\t#ifndef PHYSICALLY_CORRECT_LIGHTS\n\t\t\tirradiance *= PI;\n\t\t#endif\n\t\treturn irradiance;\n\t}\n#endif";
-
- var envmap_physical_pars_fragment = "#if defined( USE_ENVMAP ) && defined( PHYSICAL )\n\tvec3 getLightProbeIndirectIrradiance( const in GeometricContext geometry, const in int maxMIPLevel ) {\n\t\tvec3 worldNormal = inverseTransformDirection( geometry.normal, viewMatrix );\n\t\t#ifdef ENVMAP_TYPE_CUBE\n\t\t\tvec3 queryVec = vec3( flipEnvMap * worldNormal.x, worldNormal.yz );\n\t\t\t#ifdef TEXTURE_LOD_EXT\n\t\t\t\tvec4 envMapColor = textureCubeLodEXT( envMap, queryVec, float( maxMIPLevel ) );\n\t\t\t#else\n\t\t\t\tvec4 envMapColor = textureCube( envMap, queryVec, float( maxMIPLevel ) );\n\t\t\t#endif\n\t\t\tenvMapColor.rgb = envMapTexelToLinear( envMapColor ).rgb;\n\t\t#elif defined( ENVMAP_TYPE_CUBE_UV )\n\t\t\tvec3 queryVec = vec3( flipEnvMap * worldNormal.x, worldNormal.yz );\n\t\t\tvec4 envMapColor = textureCubeUV( envMap, queryVec, 1.0 );\n\t\t#else\n\t\t\tvec4 envMapColor = vec4( 0.0 );\n\t\t#endif\n\t\treturn PI * envMapColor.rgb * envMapIntensity;\n\t}\n\tfloat getSpecularMIPLevel( const in float blinnShininessExponent, const in int maxMIPLevel ) {\n\t\tfloat maxMIPLevelScalar = float( maxMIPLevel );\n\t\tfloat desiredMIPLevel = maxMIPLevelScalar + 0.79248 - 0.5 * log2( pow2( blinnShininessExponent ) + 1.0 );\n\t\treturn clamp( desiredMIPLevel, 0.0, maxMIPLevelScalar );\n\t}\n\tvec3 getLightProbeIndirectRadiance( const in GeometricContext geometry, const in float blinnShininessExponent, const in int maxMIPLevel ) {\n\t\t#ifdef ENVMAP_MODE_REFLECTION\n\t\t\tvec3 reflectVec = reflect( -geometry.viewDir, geometry.normal );\n\t\t#else\n\t\t\tvec3 reflectVec = refract( -geometry.viewDir, geometry.normal, refractionRatio );\n\t\t#endif\n\t\treflectVec = inverseTransformDirection( reflectVec, viewMatrix );\n\t\tfloat specularMIPLevel = getSpecularMIPLevel( blinnShininessExponent, maxMIPLevel );\n\t\t#ifdef ENVMAP_TYPE_CUBE\n\t\t\tvec3 queryReflectVec = vec3( flipEnvMap * reflectVec.x, reflectVec.yz );\n\t\t\t#ifdef TEXTURE_LOD_EXT\n\t\t\t\tvec4 envMapColor = textureCubeLodEXT( envMap, queryReflectVec, specularMIPLevel );\n\t\t\t#else\n\t\t\t\tvec4 envMapColor = textureCube( envMap, queryReflectVec, specularMIPLevel );\n\t\t\t#endif\n\t\t\tenvMapColor.rgb = envMapTexelToLinear( envMapColor ).rgb;\n\t\t#elif defined( ENVMAP_TYPE_CUBE_UV )\n\t\t\tvec3 queryReflectVec = vec3( flipEnvMap * reflectVec.x, reflectVec.yz );\n\t\t\tvec4 envMapColor = textureCubeUV( envMap, queryReflectVec, BlinnExponentToGGXRoughness(blinnShininessExponent ));\n\t\t#elif defined( ENVMAP_TYPE_EQUIREC )\n\t\t\tvec2 sampleUV;\n\t\t\tsampleUV.y = asin( clamp( reflectVec.y, - 1.0, 1.0 ) ) * RECIPROCAL_PI + 0.5;\n\t\t\tsampleUV.x = atan( reflectVec.z, reflectVec.x ) * RECIPROCAL_PI2 + 0.5;\n\t\t\t#ifdef TEXTURE_LOD_EXT\n\t\t\t\tvec4 envMapColor = texture2DLodEXT( envMap, sampleUV, specularMIPLevel );\n\t\t\t#else\n\t\t\t\tvec4 envMapColor = texture2D( envMap, sampleUV, specularMIPLevel );\n\t\t\t#endif\n\t\t\tenvMapColor.rgb = envMapTexelToLinear( envMapColor ).rgb;\n\t\t#elif defined( ENVMAP_TYPE_SPHERE )\n\t\t\tvec3 reflectView = normalize( ( viewMatrix * vec4( reflectVec, 0.0 ) ).xyz + vec3( 0.0,0.0,1.0 ) );\n\t\t\t#ifdef TEXTURE_LOD_EXT\n\t\t\t\tvec4 envMapColor = texture2DLodEXT( envMap, reflectView.xy * 0.5 + 0.5, specularMIPLevel );\n\t\t\t#else\n\t\t\t\tvec4 envMapColor = texture2D( envMap, reflectView.xy * 0.5 + 0.5, specularMIPLevel );\n\t\t\t#endif\n\t\t\tenvMapColor.rgb = envMapTexelToLinear( envMapColor ).rgb;\n\t\t#endif\n\t\treturn envMapColor.rgb * envMapIntensity;\n\t}\n#endif";
-
- var lights_phong_fragment = "BlinnPhongMaterial material;\nmaterial.diffuseColor = diffuseColor.rgb;\nmaterial.specularColor = specular;\nmaterial.specularShininess = shininess;\nmaterial.specularStrength = specularStrength;";
-
- var lights_phong_pars_fragment = "varying vec3 vViewPosition;\n#ifndef FLAT_SHADED\n\tvarying vec3 vNormal;\n#endif\nstruct BlinnPhongMaterial {\n\tvec3\tdiffuseColor;\n\tvec3\tspecularColor;\n\tfloat\tspecularShininess;\n\tfloat\tspecularStrength;\n};\nvoid RE_Direct_BlinnPhong( const in IncidentLight directLight, const in GeometricContext geometry, const in BlinnPhongMaterial material, inout ReflectedLight reflectedLight ) {\n\t#ifdef TOON\n\t\tvec3 irradiance = getGradientIrradiance( geometry.normal, directLight.direction ) * directLight.color;\n\t#else\n\t\tfloat dotNL = saturate( dot( geometry.normal, directLight.direction ) );\n\t\tvec3 irradiance = dotNL * directLight.color;\n\t#endif\n\t#ifndef PHYSICALLY_CORRECT_LIGHTS\n\t\tirradiance *= PI;\n\t#endif\n\treflectedLight.directDiffuse += irradiance * BRDF_Diffuse_Lambert( material.diffuseColor );\n\treflectedLight.directSpecular += irradiance * BRDF_Specular_BlinnPhong( directLight, geometry, material.specularColor, material.specularShininess ) * material.specularStrength;\n}\nvoid RE_IndirectDiffuse_BlinnPhong( const in vec3 irradiance, const in GeometricContext geometry, const in BlinnPhongMaterial material, inout ReflectedLight reflectedLight ) {\n\treflectedLight.indirectDiffuse += irradiance * BRDF_Diffuse_Lambert( material.diffuseColor );\n}\n#define RE_Direct\t\t\t\tRE_Direct_BlinnPhong\n#define RE_IndirectDiffuse\t\tRE_IndirectDiffuse_BlinnPhong\n#define Material_LightProbeLOD( material )\t(0)";
-
- var lights_physical_fragment = "PhysicalMaterial material;\nmaterial.diffuseColor = diffuseColor.rgb * ( 1.0 - metalnessFactor );\nmaterial.specularRoughness = clamp( roughnessFactor, 0.04, 1.0 );\n#ifdef STANDARD\n\tmaterial.specularColor = mix( vec3( DEFAULT_SPECULAR_COEFFICIENT ), diffuseColor.rgb, metalnessFactor );\n#else\n\tmaterial.specularColor = mix( vec3( MAXIMUM_SPECULAR_COEFFICIENT * pow2( reflectivity ) ), diffuseColor.rgb, metalnessFactor );\n\tmaterial.clearCoat = saturate( clearCoat );\tmaterial.clearCoatRoughness = clamp( clearCoatRoughness, 0.04, 1.0 );\n#endif";
-
- var lights_physical_pars_fragment = "struct PhysicalMaterial {\n\tvec3\tdiffuseColor;\n\tfloat\tspecularRoughness;\n\tvec3\tspecularColor;\n\t#ifndef STANDARD\n\t\tfloat clearCoat;\n\t\tfloat clearCoatRoughness;\n\t#endif\n};\n#define MAXIMUM_SPECULAR_COEFFICIENT 0.16\n#define DEFAULT_SPECULAR_COEFFICIENT 0.04\nfloat clearCoatDHRApprox( const in float roughness, const in float dotNL ) {\n\treturn DEFAULT_SPECULAR_COEFFICIENT + ( 1.0 - DEFAULT_SPECULAR_COEFFICIENT ) * ( pow( 1.0 - dotNL, 5.0 ) * pow( 1.0 - roughness, 2.0 ) );\n}\n#if NUM_RECT_AREA_LIGHTS > 0\n\tvoid RE_Direct_RectArea_Physical( const in RectAreaLight rectAreaLight, const in GeometricContext geometry, const in PhysicalMaterial material, inout ReflectedLight reflectedLight ) {\n\t\tvec3 normal = geometry.normal;\n\t\tvec3 viewDir = geometry.viewDir;\n\t\tvec3 position = geometry.position;\n\t\tvec3 lightPos = rectAreaLight.position;\n\t\tvec3 halfWidth = rectAreaLight.halfWidth;\n\t\tvec3 halfHeight = rectAreaLight.halfHeight;\n\t\tvec3 lightColor = rectAreaLight.color;\n\t\tfloat roughness = material.specularRoughness;\n\t\tvec3 rectCoords[ 4 ];\n\t\trectCoords[ 0 ] = lightPos + halfWidth - halfHeight;\t\trectCoords[ 1 ] = lightPos - halfWidth - halfHeight;\n\t\trectCoords[ 2 ] = lightPos - halfWidth + halfHeight;\n\t\trectCoords[ 3 ] = lightPos + halfWidth + halfHeight;\n\t\tvec2 uv = LTC_Uv( normal, viewDir, roughness );\n\t\tvec4 t1 = texture2D( ltc_1, uv );\n\t\tvec4 t2 = texture2D( ltc_2, uv );\n\t\tmat3 mInv = mat3(\n\t\t\tvec3( t1.x, 0, t1.y ),\n\t\t\tvec3( 0, 1, 0 ),\n\t\t\tvec3( t1.z, 0, t1.w )\n\t\t);\n\t\tvec3 fresnel = ( material.specularColor * t2.x + ( vec3( 1.0 ) - material.specularColor ) * t2.y );\n\t\treflectedLight.directSpecular += lightColor * fresnel * LTC_Evaluate( normal, viewDir, position, mInv, rectCoords );\n\t\treflectedLight.directDiffuse += lightColor * material.diffuseColor * LTC_Evaluate( normal, viewDir, position, mat3( 1.0 ), rectCoords );\n\t}\n#endif\nvoid RE_Direct_Physical( const in IncidentLight directLight, const in GeometricContext geometry, const in PhysicalMaterial material, inout ReflectedLight reflectedLight ) {\n\tfloat dotNL = saturate( dot( geometry.normal, directLight.direction ) );\n\tvec3 irradiance = dotNL * directLight.color;\n\t#ifndef PHYSICALLY_CORRECT_LIGHTS\n\t\tirradiance *= PI;\n\t#endif\n\t#ifndef STANDARD\n\t\tfloat clearCoatDHR = material.clearCoat * clearCoatDHRApprox( material.clearCoatRoughness, dotNL );\n\t#else\n\t\tfloat clearCoatDHR = 0.0;\n\t#endif\n\treflectedLight.directSpecular += ( 1.0 - clearCoatDHR ) * irradiance * BRDF_Specular_GGX( directLight, geometry, material.specularColor, material.specularRoughness );\n\treflectedLight.directDiffuse += ( 1.0 - clearCoatDHR ) * irradiance * BRDF_Diffuse_Lambert( material.diffuseColor );\n\t#ifndef STANDARD\n\t\treflectedLight.directSpecular += irradiance * material.clearCoat * BRDF_Specular_GGX( directLight, geometry, vec3( DEFAULT_SPECULAR_COEFFICIENT ), material.clearCoatRoughness );\n\t#endif\n}\nvoid RE_IndirectDiffuse_Physical( const in vec3 irradiance, const in GeometricContext geometry, const in PhysicalMaterial material, inout ReflectedLight reflectedLight ) {\n\t#ifndef ENVMAP_TYPE_CUBE_UV\n\t\treflectedLight.indirectDiffuse += irradiance * BRDF_Diffuse_Lambert( material.diffuseColor );\n\t#endif\n}\nvoid RE_IndirectSpecular_Physical( const in vec3 radiance, const in vec3 irradiance, const in vec3 clearCoatRadiance, const in GeometricContext geometry, const in PhysicalMaterial material, inout ReflectedLight reflectedLight) {\n\t#ifndef STANDARD\n\t\tfloat dotNV = saturate( dot( geometry.normal, geometry.viewDir ) );\n\t\tfloat dotNL = dotNV;\n\t\tfloat clearCoatDHR = material.clearCoat * clearCoatDHRApprox( material.clearCoatRoughness, dotNL );\n\t#else\n\t\tfloat clearCoatDHR = 0.0;\n\t#endif\n\tfloat clearCoatInv = 1.0 - clearCoatDHR;\n\t#if defined( ENVMAP_TYPE_CUBE_UV )\n\t\tvec3 singleScattering = vec3( 0.0 );\n\t\tvec3 multiScattering = vec3( 0.0 );\n\t\tvec3 cosineWeightedIrradiance = irradiance * RECIPROCAL_PI;\n\t\tBRDF_Specular_Multiscattering_Environment( geometry, material.specularColor, material.specularRoughness, singleScattering, multiScattering );\n\t\tvec3 diffuse = material.diffuseColor;\n\t\treflectedLight.indirectSpecular += clearCoatInv * radiance * singleScattering;\n\t\treflectedLight.indirectDiffuse += multiScattering * cosineWeightedIrradiance;\n\t\treflectedLight.indirectDiffuse += diffuse * cosineWeightedIrradiance;\n\t#else\n\t\treflectedLight.indirectSpecular += clearCoatInv * radiance * BRDF_Specular_GGX_Environment( geometry, material.specularColor, material.specularRoughness );\n\t#endif\n\t#ifndef STANDARD\n\t\treflectedLight.indirectSpecular += clearCoatRadiance * material.clearCoat * BRDF_Specular_GGX_Environment( geometry, vec3( DEFAULT_SPECULAR_COEFFICIENT ), material.clearCoatRoughness );\n\t#endif\n}\n#define RE_Direct\t\t\t\tRE_Direct_Physical\n#define RE_Direct_RectArea\t\tRE_Direct_RectArea_Physical\n#define RE_IndirectDiffuse\t\tRE_IndirectDiffuse_Physical\n#define RE_IndirectSpecular\t\tRE_IndirectSpecular_Physical\n#define Material_BlinnShininessExponent( material ) GGXRoughnessToBlinnExponent( material.specularRoughness )\n#define Material_ClearCoat_BlinnShininessExponent( material ) GGXRoughnessToBlinnExponent( material.clearCoatRoughness )\nfloat computeSpecularOcclusion( const in float dotNV, const in float ambientOcclusion, const in float roughness ) {\n\treturn saturate( pow( dotNV + ambientOcclusion, exp2( - 16.0 * roughness - 1.0 ) ) - 1.0 + ambientOcclusion );\n}";
-
- var lights_fragment_begin = "\nGeometricContext geometry;\ngeometry.position = - vViewPosition;\ngeometry.normal = normal;\ngeometry.viewDir = normalize( vViewPosition );\nIncidentLight directLight;\n#if ( NUM_POINT_LIGHTS > 0 ) && defined( RE_Direct )\n\tPointLight pointLight;\n\t#pragma unroll_loop\n\tfor ( int i = 0; i < NUM_POINT_LIGHTS; i ++ ) {\n\t\tpointLight = pointLights[ i ];\n\t\tgetPointDirectLightIrradiance( pointLight, geometry, directLight );\n\t\t#ifdef USE_SHADOWMAP\n\t\tdirectLight.color *= all( bvec2( pointLight.shadow, directLight.visible ) ) ? getPointShadow( pointShadowMap[ i ], pointLight.shadowMapSize, pointLight.shadowBias, pointLight.shadowRadius, vPointShadowCoord[ i ], pointLight.shadowCameraNear, pointLight.shadowCameraFar ) : 1.0;\n\t\t#endif\n\t\tRE_Direct( directLight, geometry, material, reflectedLight );\n\t}\n#endif\n#if ( NUM_SPOT_LIGHTS > 0 ) && defined( RE_Direct )\n\tSpotLight spotLight;\n\t#pragma unroll_loop\n\tfor ( int i = 0; i < NUM_SPOT_LIGHTS; i ++ ) {\n\t\tspotLight = spotLights[ i ];\n\t\tgetSpotDirectLightIrradiance( spotLight, geometry, directLight );\n\t\t#ifdef USE_SHADOWMAP\n\t\tdirectLight.color *= all( bvec2( spotLight.shadow, directLight.visible ) ) ? getShadow( spotShadowMap[ i ], spotLight.shadowMapSize, spotLight.shadowBias, spotLight.shadowRadius, vSpotShadowCoord[ i ] ) : 1.0;\n\t\t#endif\n\t\tRE_Direct( directLight, geometry, material, reflectedLight );\n\t}\n#endif\n#if ( NUM_DIR_LIGHTS > 0 ) && defined( RE_Direct )\n\tDirectionalLight directionalLight;\n\t#pragma unroll_loop\n\tfor ( int i = 0; i < NUM_DIR_LIGHTS; i ++ ) {\n\t\tdirectionalLight = directionalLights[ i ];\n\t\tgetDirectionalDirectLightIrradiance( directionalLight, geometry, directLight );\n\t\t#ifdef USE_SHADOWMAP\n\t\tdirectLight.color *= all( bvec2( directionalLight.shadow, directLight.visible ) ) ? getShadow( directionalShadowMap[ i ], directionalLight.shadowMapSize, directionalLight.shadowBias, directionalLight.shadowRadius, vDirectionalShadowCoord[ i ] ) : 1.0;\n\t\t#endif\n\t\tRE_Direct( directLight, geometry, material, reflectedLight );\n\t}\n#endif\n#if ( NUM_RECT_AREA_LIGHTS > 0 ) && defined( RE_Direct_RectArea )\n\tRectAreaLight rectAreaLight;\n\t#pragma unroll_loop\n\tfor ( int i = 0; i < NUM_RECT_AREA_LIGHTS; i ++ ) {\n\t\trectAreaLight = rectAreaLights[ i ];\n\t\tRE_Direct_RectArea( rectAreaLight, geometry, material, reflectedLight );\n\t}\n#endif\n#if defined( RE_IndirectDiffuse )\n\tvec3 irradiance = getAmbientLightIrradiance( ambientLightColor );\n\t#if ( NUM_HEMI_LIGHTS > 0 )\n\t\t#pragma unroll_loop\n\t\tfor ( int i = 0; i < NUM_HEMI_LIGHTS; i ++ ) {\n\t\t\tirradiance += getHemisphereLightIrradiance( hemisphereLights[ i ], geometry );\n\t\t}\n\t#endif\n#endif\n#if defined( RE_IndirectSpecular )\n\tvec3 radiance = vec3( 0.0 );\n\tvec3 clearCoatRadiance = vec3( 0.0 );\n#endif";
-
- var lights_fragment_maps = "#if defined( RE_IndirectDiffuse )\n\t#ifdef USE_LIGHTMAP\n\t\tvec3 lightMapIrradiance = texture2D( lightMap, vUv2 ).xyz * lightMapIntensity;\n\t\t#ifndef PHYSICALLY_CORRECT_LIGHTS\n\t\t\tlightMapIrradiance *= PI;\n\t\t#endif\n\t\tirradiance += lightMapIrradiance;\n\t#endif\n\t#if defined( USE_ENVMAP ) && defined( PHYSICAL ) && defined( ENVMAP_TYPE_CUBE_UV )\n\t\tirradiance += getLightProbeIndirectIrradiance( geometry, maxMipLevel );\n\t#endif\n#endif\n#if defined( USE_ENVMAP ) && defined( RE_IndirectSpecular )\n\tradiance += getLightProbeIndirectRadiance( geometry, Material_BlinnShininessExponent( material ), maxMipLevel );\n\t#ifndef STANDARD\n\t\tclearCoatRadiance += getLightProbeIndirectRadiance( geometry, Material_ClearCoat_BlinnShininessExponent( material ), maxMipLevel );\n\t#endif\n#endif";
-
- var lights_fragment_end = "#if defined( RE_IndirectDiffuse )\n\tRE_IndirectDiffuse( irradiance, geometry, material, reflectedLight );\n#endif\n#if defined( RE_IndirectSpecular )\n\tRE_IndirectSpecular( radiance, irradiance, clearCoatRadiance, geometry, material, reflectedLight );\n#endif";
-
- var logdepthbuf_fragment = "#if defined( USE_LOGDEPTHBUF ) && defined( USE_LOGDEPTHBUF_EXT )\n\tgl_FragDepthEXT = log2( vFragDepth ) * logDepthBufFC * 0.5;\n#endif";
-
- var logdepthbuf_pars_fragment = "#if defined( USE_LOGDEPTHBUF ) && defined( USE_LOGDEPTHBUF_EXT )\n\tuniform float logDepthBufFC;\n\tvarying float vFragDepth;\n#endif";
-
- var logdepthbuf_pars_vertex = "#ifdef USE_LOGDEPTHBUF\n\t#ifdef USE_LOGDEPTHBUF_EXT\n\t\tvarying float vFragDepth;\n\t#else\n\t\tuniform float logDepthBufFC;\n\t#endif\n#endif";
-
- var logdepthbuf_vertex = "#ifdef USE_LOGDEPTHBUF\n\t#ifdef USE_LOGDEPTHBUF_EXT\n\t\tvFragDepth = 1.0 + gl_Position.w;\n\t#else\n\t\tgl_Position.z = log2( max( EPSILON, gl_Position.w + 1.0 ) ) * logDepthBufFC - 1.0;\n\t\tgl_Position.z *= gl_Position.w;\n\t#endif\n#endif";
-
- var map_fragment = "#ifdef USE_MAP\n\tvec4 texelColor = texture2D( map, vUv );\n\ttexelColor = mapTexelToLinear( texelColor );\n\tdiffuseColor *= texelColor;\n#endif";
-
- var map_pars_fragment = "#ifdef USE_MAP\n\tuniform sampler2D map;\n#endif";
-
- var map_particle_fragment = "#ifdef USE_MAP\n\tvec2 uv = ( uvTransform * vec3( gl_PointCoord.x, 1.0 - gl_PointCoord.y, 1 ) ).xy;\n\tvec4 mapTexel = texture2D( map, uv );\n\tdiffuseColor *= mapTexelToLinear( mapTexel );\n#endif";
-
- var map_particle_pars_fragment = "#ifdef USE_MAP\n\tuniform mat3 uvTransform;\n\tuniform sampler2D map;\n#endif";
-
- var metalnessmap_fragment = "float metalnessFactor = metalness;\n#ifdef USE_METALNESSMAP\n\tvec4 texelMetalness = texture2D( metalnessMap, vUv );\n\tmetalnessFactor *= texelMetalness.b;\n#endif";
-
- var metalnessmap_pars_fragment = "#ifdef USE_METALNESSMAP\n\tuniform sampler2D metalnessMap;\n#endif";
-
- var morphnormal_vertex = "#ifdef USE_MORPHNORMALS\n\tobjectNormal += ( morphNormal0 - normal ) * morphTargetInfluences[ 0 ];\n\tobjectNormal += ( morphNormal1 - normal ) * morphTargetInfluences[ 1 ];\n\tobjectNormal += ( morphNormal2 - normal ) * morphTargetInfluences[ 2 ];\n\tobjectNormal += ( morphNormal3 - normal ) * morphTargetInfluences[ 3 ];\n#endif";
-
- var morphtarget_pars_vertex = "#ifdef USE_MORPHTARGETS\n\t#ifndef USE_MORPHNORMALS\n\tuniform float morphTargetInfluences[ 8 ];\n\t#else\n\tuniform float morphTargetInfluences[ 4 ];\n\t#endif\n#endif";
-
- var morphtarget_vertex = "#ifdef USE_MORPHTARGETS\n\ttransformed += ( morphTarget0 - position ) * morphTargetInfluences[ 0 ];\n\ttransformed += ( morphTarget1 - position ) * morphTargetInfluences[ 1 ];\n\ttransformed += ( morphTarget2 - position ) * morphTargetInfluences[ 2 ];\n\ttransformed += ( morphTarget3 - position ) * morphTargetInfluences[ 3 ];\n\t#ifndef USE_MORPHNORMALS\n\ttransformed += ( morphTarget4 - position ) * morphTargetInfluences[ 4 ];\n\ttransformed += ( morphTarget5 - position ) * morphTargetInfluences[ 5 ];\n\ttransformed += ( morphTarget6 - position ) * morphTargetInfluences[ 6 ];\n\ttransformed += ( morphTarget7 - position ) * morphTargetInfluences[ 7 ];\n\t#endif\n#endif";
-
- var normal_fragment_begin = "#ifdef FLAT_SHADED\n\tvec3 fdx = vec3( dFdx( vViewPosition.x ), dFdx( vViewPosition.y ), dFdx( vViewPosition.z ) );\n\tvec3 fdy = vec3( dFdy( vViewPosition.x ), dFdy( vViewPosition.y ), dFdy( vViewPosition.z ) );\n\tvec3 normal = normalize( cross( fdx, fdy ) );\n#else\n\tvec3 normal = normalize( vNormal );\n\t#ifdef DOUBLE_SIDED\n\t\tnormal = normal * ( float( gl_FrontFacing ) * 2.0 - 1.0 );\n\t#endif\n\t#ifdef USE_TANGENT\n\t\tvec3 tangent = normalize( vTangent );\n\t\tvec3 bitangent = normalize( vBitangent );\n\t\t#ifdef DOUBLE_SIDED\n\t\t\ttangent = tangent * ( float( gl_FrontFacing ) * 2.0 - 1.0 );\n\t\t\tbitangent = bitangent * ( float( gl_FrontFacing ) * 2.0 - 1.0 );\n\t\t#endif\n\t#endif\n#endif";
-
- var normal_fragment_maps = "#ifdef USE_NORMALMAP\n\t#ifdef OBJECTSPACE_NORMALMAP\n\t\tnormal = texture2D( normalMap, vUv ).xyz * 2.0 - 1.0;\n\t\t#ifdef FLIP_SIDED\n\t\t\tnormal = - normal;\n\t\t#endif\n\t\t#ifdef DOUBLE_SIDED\n\t\t\tnormal = normal * ( float( gl_FrontFacing ) * 2.0 - 1.0 );\n\t\t#endif\n\t\tnormal = normalize( normalMatrix * normal );\n\t#else\n\t\t#ifdef USE_TANGENT\n\t\t\tmat3 vTBN = mat3( tangent, bitangent, normal );\n\t\t\tvec3 mapN = texture2D( normalMap, vUv ).xyz * 2.0 - 1.0;\n\t\t\tmapN.xy = normalScale * mapN.xy;\n\t\t\tnormal = normalize( vTBN * mapN );\n\t\t#else\n\t\t\tnormal = perturbNormal2Arb( -vViewPosition, normal );\n\t\t#endif\n\t#endif\n#elif defined( USE_BUMPMAP )\n\tnormal = perturbNormalArb( -vViewPosition, normal, dHdxy_fwd() );\n#endif";
-
- var normalmap_pars_fragment = "#ifdef USE_NORMALMAP\n\tuniform sampler2D normalMap;\n\tuniform vec2 normalScale;\n\t#ifdef OBJECTSPACE_NORMALMAP\n\t\tuniform mat3 normalMatrix;\n\t#else\n\t\tvec3 perturbNormal2Arb( vec3 eye_pos, vec3 surf_norm ) {\n\t\t\tvec3 q0 = vec3( dFdx( eye_pos.x ), dFdx( eye_pos.y ), dFdx( eye_pos.z ) );\n\t\t\tvec3 q1 = vec3( dFdy( eye_pos.x ), dFdy( eye_pos.y ), dFdy( eye_pos.z ) );\n\t\t\tvec2 st0 = dFdx( vUv.st );\n\t\t\tvec2 st1 = dFdy( vUv.st );\n\t\t\tfloat scale = sign( st1.t * st0.s - st0.t * st1.s );\n\t\t\tvec3 S = normalize( ( q0 * st1.t - q1 * st0.t ) * scale );\n\t\t\tvec3 T = normalize( ( - q0 * st1.s + q1 * st0.s ) * scale );\n\t\t\tvec3 N = normalize( surf_norm );\n\t\t\tmat3 tsn = mat3( S, T, N );\n\t\t\tvec3 mapN = texture2D( normalMap, vUv ).xyz * 2.0 - 1.0;\n\t\t\tmapN.xy *= normalScale;\n\t\t\tmapN.xy *= ( float( gl_FrontFacing ) * 2.0 - 1.0 );\n\t\t\treturn normalize( tsn * mapN );\n\t\t}\n\t#endif\n#endif";
-
- var packing = "vec3 packNormalToRGB( const in vec3 normal ) {\n\treturn normalize( normal ) * 0.5 + 0.5;\n}\nvec3 unpackRGBToNormal( const in vec3 rgb ) {\n\treturn 2.0 * rgb.xyz - 1.0;\n}\nconst float PackUpscale = 256. / 255.;const float UnpackDownscale = 255. / 256.;\nconst vec3 PackFactors = vec3( 256. * 256. * 256., 256. * 256., 256. );\nconst vec4 UnpackFactors = UnpackDownscale / vec4( PackFactors, 1. );\nconst float ShiftRight8 = 1. / 256.;\nvec4 packDepthToRGBA( const in float v ) {\n\tvec4 r = vec4( fract( v * PackFactors ), v );\n\tr.yzw -= r.xyz * ShiftRight8;\treturn r * PackUpscale;\n}\nfloat unpackRGBAToDepth( const in vec4 v ) {\n\treturn dot( v, UnpackFactors );\n}\nfloat viewZToOrthographicDepth( const in float viewZ, const in float near, const in float far ) {\n\treturn ( viewZ + near ) / ( near - far );\n}\nfloat orthographicDepthToViewZ( const in float linearClipZ, const in float near, const in float far ) {\n\treturn linearClipZ * ( near - far ) - near;\n}\nfloat viewZToPerspectiveDepth( const in float viewZ, const in float near, const in float far ) {\n\treturn (( near + viewZ ) * far ) / (( far - near ) * viewZ );\n}\nfloat perspectiveDepthToViewZ( const in float invClipZ, const in float near, const in float far ) {\n\treturn ( near * far ) / ( ( far - near ) * invClipZ - far );\n}";
-
- var premultiplied_alpha_fragment = "#ifdef PREMULTIPLIED_ALPHA\n\tgl_FragColor.rgb *= gl_FragColor.a;\n#endif";
-
- var project_vertex = "vec4 mvPosition = modelViewMatrix * vec4( transformed, 1.0 );\ngl_Position = projectionMatrix * mvPosition;";
-
- var dithering_fragment = "#if defined( DITHERING )\n\tgl_FragColor.rgb = dithering( gl_FragColor.rgb );\n#endif";
-
- var dithering_pars_fragment = "#if defined( DITHERING )\n\tvec3 dithering( vec3 color ) {\n\t\tfloat grid_position = rand( gl_FragCoord.xy );\n\t\tvec3 dither_shift_RGB = vec3( 0.25 / 255.0, -0.25 / 255.0, 0.25 / 255.0 );\n\t\tdither_shift_RGB = mix( 2.0 * dither_shift_RGB, -2.0 * dither_shift_RGB, grid_position );\n\t\treturn color + dither_shift_RGB;\n\t}\n#endif";
-
- var roughnessmap_fragment = "float roughnessFactor = roughness;\n#ifdef USE_ROUGHNESSMAP\n\tvec4 texelRoughness = texture2D( roughnessMap, vUv );\n\troughnessFactor *= texelRoughness.g;\n#endif";
-
- var roughnessmap_pars_fragment = "#ifdef USE_ROUGHNESSMAP\n\tuniform sampler2D roughnessMap;\n#endif";
-
- var shadowmap_pars_fragment = "#ifdef USE_SHADOWMAP\n\t#if NUM_DIR_LIGHTS > 0\n\t\tuniform sampler2D directionalShadowMap[ NUM_DIR_LIGHTS ];\n\t\tvarying vec4 vDirectionalShadowCoord[ NUM_DIR_LIGHTS ];\n\t#endif\n\t#if NUM_SPOT_LIGHTS > 0\n\t\tuniform sampler2D spotShadowMap[ NUM_SPOT_LIGHTS ];\n\t\tvarying vec4 vSpotShadowCoord[ NUM_SPOT_LIGHTS ];\n\t#endif\n\t#if NUM_POINT_LIGHTS > 0\n\t\tuniform sampler2D pointShadowMap[ NUM_POINT_LIGHTS ];\n\t\tvarying vec4 vPointShadowCoord[ NUM_POINT_LIGHTS ];\n\t#endif\n\tfloat texture2DCompare( sampler2D depths, vec2 uv, float compare ) {\n\t\treturn step( compare, unpackRGBAToDepth( texture2D( depths, uv ) ) );\n\t}\n\tfloat texture2DShadowLerp( sampler2D depths, vec2 size, vec2 uv, float compare ) {\n\t\tconst vec2 offset = vec2( 0.0, 1.0 );\n\t\tvec2 texelSize = vec2( 1.0 ) / size;\n\t\tvec2 centroidUV = floor( uv * size + 0.5 ) / size;\n\t\tfloat lb = texture2DCompare( depths, centroidUV + texelSize * offset.xx, compare );\n\t\tfloat lt = texture2DCompare( depths, centroidUV + texelSize * offset.xy, compare );\n\t\tfloat rb = texture2DCompare( depths, centroidUV + texelSize * offset.yx, compare );\n\t\tfloat rt = texture2DCompare( depths, centroidUV + texelSize * offset.yy, compare );\n\t\tvec2 f = fract( uv * size + 0.5 );\n\t\tfloat a = mix( lb, lt, f.y );\n\t\tfloat b = mix( rb, rt, f.y );\n\t\tfloat c = mix( a, b, f.x );\n\t\treturn c;\n\t}\n\tfloat getShadow( sampler2D shadowMap, vec2 shadowMapSize, float shadowBias, float shadowRadius, vec4 shadowCoord ) {\n\t\tfloat shadow = 1.0;\n\t\tshadowCoord.xyz /= shadowCoord.w;\n\t\tshadowCoord.z += shadowBias;\n\t\tbvec4 inFrustumVec = bvec4 ( shadowCoord.x >= 0.0, shadowCoord.x <= 1.0, shadowCoord.y >= 0.0, shadowCoord.y <= 1.0 );\n\t\tbool inFrustum = all( inFrustumVec );\n\t\tbvec2 frustumTestVec = bvec2( inFrustum, shadowCoord.z <= 1.0 );\n\t\tbool frustumTest = all( frustumTestVec );\n\t\tif ( frustumTest ) {\n\t\t#if defined( SHADOWMAP_TYPE_PCF )\n\t\t\tvec2 texelSize = vec2( 1.0 ) / shadowMapSize;\n\t\t\tfloat dx0 = - texelSize.x * shadowRadius;\n\t\t\tfloat dy0 = - texelSize.y * shadowRadius;\n\t\t\tfloat dx1 = + texelSize.x * shadowRadius;\n\t\t\tfloat dy1 = + texelSize.y * shadowRadius;\n\t\t\tshadow = (\n\t\t\t\ttexture2DCompare( shadowMap, shadowCoord.xy + vec2( dx0, dy0 ), shadowCoord.z ) +\n\t\t\t\ttexture2DCompare( shadowMap, shadowCoord.xy + vec2( 0.0, dy0 ), shadowCoord.z ) +\n\t\t\t\ttexture2DCompare( shadowMap, shadowCoord.xy + vec2( dx1, dy0 ), shadowCoord.z ) +\n\t\t\t\ttexture2DCompare( shadowMap, shadowCoord.xy + vec2( dx0, 0.0 ), shadowCoord.z ) +\n\t\t\t\ttexture2DCompare( shadowMap, shadowCoord.xy, shadowCoord.z ) +\n\t\t\t\ttexture2DCompare( shadowMap, shadowCoord.xy + vec2( dx1, 0.0 ), shadowCoord.z ) +\n\t\t\t\ttexture2DCompare( shadowMap, shadowCoord.xy + vec2( dx0, dy1 ), shadowCoord.z ) +\n\t\t\t\ttexture2DCompare( shadowMap, shadowCoord.xy + vec2( 0.0, dy1 ), shadowCoord.z ) +\n\t\t\t\ttexture2DCompare( shadowMap, shadowCoord.xy + vec2( dx1, dy1 ), shadowCoord.z )\n\t\t\t) * ( 1.0 / 9.0 );\n\t\t#elif defined( SHADOWMAP_TYPE_PCF_SOFT )\n\t\t\tvec2 texelSize = vec2( 1.0 ) / shadowMapSize;\n\t\t\tfloat dx0 = - texelSize.x * shadowRadius;\n\t\t\tfloat dy0 = - texelSize.y * shadowRadius;\n\t\t\tfloat dx1 = + texelSize.x * shadowRadius;\n\t\t\tfloat dy1 = + texelSize.y * shadowRadius;\n\t\t\tshadow = (\n\t\t\t\ttexture2DShadowLerp( shadowMap, shadowMapSize, shadowCoord.xy + vec2( dx0, dy0 ), shadowCoord.z ) +\n\t\t\t\ttexture2DShadowLerp( shadowMap, shadowMapSize, shadowCoord.xy + vec2( 0.0, dy0 ), shadowCoord.z ) +\n\t\t\t\ttexture2DShadowLerp( shadowMap, shadowMapSize, shadowCoord.xy + vec2( dx1, dy0 ), shadowCoord.z ) +\n\t\t\t\ttexture2DShadowLerp( shadowMap, shadowMapSize, shadowCoord.xy + vec2( dx0, 0.0 ), shadowCoord.z ) +\n\t\t\t\ttexture2DShadowLerp( shadowMap, shadowMapSize, shadowCoord.xy, shadowCoord.z ) +\n\t\t\t\ttexture2DShadowLerp( shadowMap, shadowMapSize, shadowCoord.xy + vec2( dx1, 0.0 ), shadowCoord.z ) +\n\t\t\t\ttexture2DShadowLerp( shadowMap, shadowMapSize, shadowCoord.xy + vec2( dx0, dy1 ), shadowCoord.z ) +\n\t\t\t\ttexture2DShadowLerp( shadowMap, shadowMapSize, shadowCoord.xy + vec2( 0.0, dy1 ), shadowCoord.z ) +\n\t\t\t\ttexture2DShadowLerp( shadowMap, shadowMapSize, shadowCoord.xy + vec2( dx1, dy1 ), shadowCoord.z )\n\t\t\t) * ( 1.0 / 9.0 );\n\t\t#else\n\t\t\tshadow = texture2DCompare( shadowMap, shadowCoord.xy, shadowCoord.z );\n\t\t#endif\n\t\t}\n\t\treturn shadow;\n\t}\n\tvec2 cubeToUV( vec3 v, float texelSizeY ) {\n\t\tvec3 absV = abs( v );\n\t\tfloat scaleToCube = 1.0 / max( absV.x, max( absV.y, absV.z ) );\n\t\tabsV *= scaleToCube;\n\t\tv *= scaleToCube * ( 1.0 - 2.0 * texelSizeY );\n\t\tvec2 planar = v.xy;\n\t\tfloat almostATexel = 1.5 * texelSizeY;\n\t\tfloat almostOne = 1.0 - almostATexel;\n\t\tif ( absV.z >= almostOne ) {\n\t\t\tif ( v.z > 0.0 )\n\t\t\t\tplanar.x = 4.0 - v.x;\n\t\t} else if ( absV.x >= almostOne ) {\n\t\t\tfloat signX = sign( v.x );\n\t\t\tplanar.x = v.z * signX + 2.0 * signX;\n\t\t} else if ( absV.y >= almostOne ) {\n\t\t\tfloat signY = sign( v.y );\n\t\t\tplanar.x = v.x + 2.0 * signY + 2.0;\n\t\t\tplanar.y = v.z * signY - 2.0;\n\t\t}\n\t\treturn vec2( 0.125, 0.25 ) * planar + vec2( 0.375, 0.75 );\n\t}\n\tfloat getPointShadow( sampler2D shadowMap, vec2 shadowMapSize, float shadowBias, float shadowRadius, vec4 shadowCoord, float shadowCameraNear, float shadowCameraFar ) {\n\t\tvec2 texelSize = vec2( 1.0 ) / ( shadowMapSize * vec2( 4.0, 2.0 ) );\n\t\tvec3 lightToPosition = shadowCoord.xyz;\n\t\tfloat dp = ( length( lightToPosition ) - shadowCameraNear ) / ( shadowCameraFar - shadowCameraNear );\t\tdp += shadowBias;\n\t\tvec3 bd3D = normalize( lightToPosition );\n\t\t#if defined( SHADOWMAP_TYPE_PCF ) || defined( SHADOWMAP_TYPE_PCF_SOFT )\n\t\t\tvec2 offset = vec2( - 1, 1 ) * shadowRadius * texelSize.y;\n\t\t\treturn (\n\t\t\t\ttexture2DCompare( shadowMap, cubeToUV( bd3D + offset.xyy, texelSize.y ), dp ) +\n\t\t\t\ttexture2DCompare( shadowMap, cubeToUV( bd3D + offset.yyy, texelSize.y ), dp ) +\n\t\t\t\ttexture2DCompare( shadowMap, cubeToUV( bd3D + offset.xyx, texelSize.y ), dp ) +\n\t\t\t\ttexture2DCompare( shadowMap, cubeToUV( bd3D + offset.yyx, texelSize.y ), dp ) +\n\t\t\t\ttexture2DCompare( shadowMap, cubeToUV( bd3D, texelSize.y ), dp ) +\n\t\t\t\ttexture2DCompare( shadowMap, cubeToUV( bd3D + offset.xxy, texelSize.y ), dp ) +\n\t\t\t\ttexture2DCompare( shadowMap, cubeToUV( bd3D + offset.yxy, texelSize.y ), dp ) +\n\t\t\t\ttexture2DCompare( shadowMap, cubeToUV( bd3D + offset.xxx, texelSize.y ), dp ) +\n\t\t\t\ttexture2DCompare( shadowMap, cubeToUV( bd3D + offset.yxx, texelSize.y ), dp )\n\t\t\t) * ( 1.0 / 9.0 );\n\t\t#else\n\t\t\treturn texture2DCompare( shadowMap, cubeToUV( bd3D, texelSize.y ), dp );\n\t\t#endif\n\t}\n#endif";
-
- var shadowmap_pars_vertex = "#ifdef USE_SHADOWMAP\n\t#if NUM_DIR_LIGHTS > 0\n\t\tuniform mat4 directionalShadowMatrix[ NUM_DIR_LIGHTS ];\n\t\tvarying vec4 vDirectionalShadowCoord[ NUM_DIR_LIGHTS ];\n\t#endif\n\t#if NUM_SPOT_LIGHTS > 0\n\t\tuniform mat4 spotShadowMatrix[ NUM_SPOT_LIGHTS ];\n\t\tvarying vec4 vSpotShadowCoord[ NUM_SPOT_LIGHTS ];\n\t#endif\n\t#if NUM_POINT_LIGHTS > 0\n\t\tuniform mat4 pointShadowMatrix[ NUM_POINT_LIGHTS ];\n\t\tvarying vec4 vPointShadowCoord[ NUM_POINT_LIGHTS ];\n\t#endif\n#endif";
-
- var shadowmap_vertex = "#ifdef USE_SHADOWMAP\n\t#if NUM_DIR_LIGHTS > 0\n\t#pragma unroll_loop\n\tfor ( int i = 0; i < NUM_DIR_LIGHTS; i ++ ) {\n\t\tvDirectionalShadowCoord[ i ] = directionalShadowMatrix[ i ] * worldPosition;\n\t}\n\t#endif\n\t#if NUM_SPOT_LIGHTS > 0\n\t#pragma unroll_loop\n\tfor ( int i = 0; i < NUM_SPOT_LIGHTS; i ++ ) {\n\t\tvSpotShadowCoord[ i ] = spotShadowMatrix[ i ] * worldPosition;\n\t}\n\t#endif\n\t#if NUM_POINT_LIGHTS > 0\n\t#pragma unroll_loop\n\tfor ( int i = 0; i < NUM_POINT_LIGHTS; i ++ ) {\n\t\tvPointShadowCoord[ i ] = pointShadowMatrix[ i ] * worldPosition;\n\t}\n\t#endif\n#endif";
-
- var shadowmask_pars_fragment = "float getShadowMask() {\n\tfloat shadow = 1.0;\n\t#ifdef USE_SHADOWMAP\n\t#if NUM_DIR_LIGHTS > 0\n\tDirectionalLight directionalLight;\n\t#pragma unroll_loop\n\tfor ( int i = 0; i < NUM_DIR_LIGHTS; i ++ ) {\n\t\tdirectionalLight = directionalLights[ i ];\n\t\tshadow *= bool( directionalLight.shadow ) ? getShadow( directionalShadowMap[ i ], directionalLight.shadowMapSize, directionalLight.shadowBias, directionalLight.shadowRadius, vDirectionalShadowCoord[ i ] ) : 1.0;\n\t}\n\t#endif\n\t#if NUM_SPOT_LIGHTS > 0\n\tSpotLight spotLight;\n\t#pragma unroll_loop\n\tfor ( int i = 0; i < NUM_SPOT_LIGHTS; i ++ ) {\n\t\tspotLight = spotLights[ i ];\n\t\tshadow *= bool( spotLight.shadow ) ? getShadow( spotShadowMap[ i ], spotLight.shadowMapSize, spotLight.shadowBias, spotLight.shadowRadius, vSpotShadowCoord[ i ] ) : 1.0;\n\t}\n\t#endif\n\t#if NUM_POINT_LIGHTS > 0\n\tPointLight pointLight;\n\t#pragma unroll_loop\n\tfor ( int i = 0; i < NUM_POINT_LIGHTS; i ++ ) {\n\t\tpointLight = pointLights[ i ];\n\t\tshadow *= bool( pointLight.shadow ) ? getPointShadow( pointShadowMap[ i ], pointLight.shadowMapSize, pointLight.shadowBias, pointLight.shadowRadius, vPointShadowCoord[ i ], pointLight.shadowCameraNear, pointLight.shadowCameraFar ) : 1.0;\n\t}\n\t#endif\n\t#endif\n\treturn shadow;\n}";
-
- var skinbase_vertex = "#ifdef USE_SKINNING\n\tmat4 boneMatX = getBoneMatrix( skinIndex.x );\n\tmat4 boneMatY = getBoneMatrix( skinIndex.y );\n\tmat4 boneMatZ = getBoneMatrix( skinIndex.z );\n\tmat4 boneMatW = getBoneMatrix( skinIndex.w );\n#endif";
-
- var skinning_pars_vertex = "#ifdef USE_SKINNING\n\tuniform mat4 bindMatrix;\n\tuniform mat4 bindMatrixInverse;\n\t#ifdef BONE_TEXTURE\n\t\tuniform sampler2D boneTexture;\n\t\tuniform int boneTextureSize;\n\t\tmat4 getBoneMatrix( const in float i ) {\n\t\t\tfloat j = i * 4.0;\n\t\t\tfloat x = mod( j, float( boneTextureSize ) );\n\t\t\tfloat y = floor( j / float( boneTextureSize ) );\n\t\t\tfloat dx = 1.0 / float( boneTextureSize );\n\t\t\tfloat dy = 1.0 / float( boneTextureSize );\n\t\t\ty = dy * ( y + 0.5 );\n\t\t\tvec4 v1 = texture2D( boneTexture, vec2( dx * ( x + 0.5 ), y ) );\n\t\t\tvec4 v2 = texture2D( boneTexture, vec2( dx * ( x + 1.5 ), y ) );\n\t\t\tvec4 v3 = texture2D( boneTexture, vec2( dx * ( x + 2.5 ), y ) );\n\t\t\tvec4 v4 = texture2D( boneTexture, vec2( dx * ( x + 3.5 ), y ) );\n\t\t\tmat4 bone = mat4( v1, v2, v3, v4 );\n\t\t\treturn bone;\n\t\t}\n\t#else\n\t\tuniform mat4 boneMatrices[ MAX_BONES ];\n\t\tmat4 getBoneMatrix( const in float i ) {\n\t\t\tmat4 bone = boneMatrices[ int(i) ];\n\t\t\treturn bone;\n\t\t}\n\t#endif\n#endif";
-
- var skinning_vertex = "#ifdef USE_SKINNING\n\tvec4 skinVertex = bindMatrix * vec4( transformed, 1.0 );\n\tvec4 skinned = vec4( 0.0 );\n\tskinned += boneMatX * skinVertex * skinWeight.x;\n\tskinned += boneMatY * skinVertex * skinWeight.y;\n\tskinned += boneMatZ * skinVertex * skinWeight.z;\n\tskinned += boneMatW * skinVertex * skinWeight.w;\n\ttransformed = ( bindMatrixInverse * skinned ).xyz;\n#endif";
-
- var skinnormal_vertex = "#ifdef USE_SKINNING\n\tmat4 skinMatrix = mat4( 0.0 );\n\tskinMatrix += skinWeight.x * boneMatX;\n\tskinMatrix += skinWeight.y * boneMatY;\n\tskinMatrix += skinWeight.z * boneMatZ;\n\tskinMatrix += skinWeight.w * boneMatW;\n\tskinMatrix = bindMatrixInverse * skinMatrix * bindMatrix;\n\tobjectNormal = vec4( skinMatrix * vec4( objectNormal, 0.0 ) ).xyz;\n\t#ifdef USE_TANGENT\n\t\tobjectTangent = vec4( skinMatrix * vec4( objectTangent, 0.0 ) ).xyz;\n\t#endif\n#endif";
-
- var specularmap_fragment = "float specularStrength;\n#ifdef USE_SPECULARMAP\n\tvec4 texelSpecular = texture2D( specularMap, vUv );\n\tspecularStrength = texelSpecular.r;\n#else\n\tspecularStrength = 1.0;\n#endif";
-
- var specularmap_pars_fragment = "#ifdef USE_SPECULARMAP\n\tuniform sampler2D specularMap;\n#endif";
-
- var tonemapping_fragment = "#if defined( TONE_MAPPING )\n\tgl_FragColor.rgb = toneMapping( gl_FragColor.rgb );\n#endif";
-
- var tonemapping_pars_fragment = "#ifndef saturate\n\t#define saturate(a) clamp( a, 0.0, 1.0 )\n#endif\nuniform float toneMappingExposure;\nuniform float toneMappingWhitePoint;\nvec3 LinearToneMapping( vec3 color ) {\n\treturn toneMappingExposure * color;\n}\nvec3 ReinhardToneMapping( vec3 color ) {\n\tcolor *= toneMappingExposure;\n\treturn saturate( color / ( vec3( 1.0 ) + color ) );\n}\n#define Uncharted2Helper( x ) max( ( ( x * ( 0.15 * x + 0.10 * 0.50 ) + 0.20 * 0.02 ) / ( x * ( 0.15 * x + 0.50 ) + 0.20 * 0.30 ) ) - 0.02 / 0.30, vec3( 0.0 ) )\nvec3 Uncharted2ToneMapping( vec3 color ) {\n\tcolor *= toneMappingExposure;\n\treturn saturate( Uncharted2Helper( color ) / Uncharted2Helper( vec3( toneMappingWhitePoint ) ) );\n}\nvec3 OptimizedCineonToneMapping( vec3 color ) {\n\tcolor *= toneMappingExposure;\n\tcolor = max( vec3( 0.0 ), color - 0.004 );\n\treturn pow( ( color * ( 6.2 * color + 0.5 ) ) / ( color * ( 6.2 * color + 1.7 ) + 0.06 ), vec3( 2.2 ) );\n}\nvec3 ACESFilmicToneMapping( vec3 color ) {\n\tcolor *= toneMappingExposure;\n\treturn saturate( ( color * ( 2.51 * color + 0.03 ) ) / ( color * ( 2.43 * color + 0.59 ) + 0.14 ) );\n}";
-
- var uv_pars_fragment = "#if defined( USE_MAP ) || defined( USE_BUMPMAP ) || defined( USE_NORMALMAP ) || defined( USE_SPECULARMAP ) || defined( USE_ALPHAMAP ) || defined( USE_EMISSIVEMAP ) || defined( USE_ROUGHNESSMAP ) || defined( USE_METALNESSMAP )\n\tvarying vec2 vUv;\n#endif";
-
- var uv_pars_vertex = "#if defined( USE_MAP ) || defined( USE_BUMPMAP ) || defined( USE_NORMALMAP ) || defined( USE_SPECULARMAP ) || defined( USE_ALPHAMAP ) || defined( USE_EMISSIVEMAP ) || defined( USE_ROUGHNESSMAP ) || defined( USE_METALNESSMAP )\n\tvarying vec2 vUv;\n\tuniform mat3 uvTransform;\n#endif";
-
- var uv_vertex = "#if defined( USE_MAP ) || defined( USE_BUMPMAP ) || defined( USE_NORMALMAP ) || defined( USE_SPECULARMAP ) || defined( USE_ALPHAMAP ) || defined( USE_EMISSIVEMAP ) || defined( USE_ROUGHNESSMAP ) || defined( USE_METALNESSMAP )\n\tvUv = ( uvTransform * vec3( uv, 1 ) ).xy;\n#endif";
-
- var uv2_pars_fragment = "#if defined( USE_LIGHTMAP ) || defined( USE_AOMAP )\n\tvarying vec2 vUv2;\n#endif";
-
- var uv2_pars_vertex = "#if defined( USE_LIGHTMAP ) || defined( USE_AOMAP )\n\tattribute vec2 uv2;\n\tvarying vec2 vUv2;\n#endif";
-
- var uv2_vertex = "#if defined( USE_LIGHTMAP ) || defined( USE_AOMAP )\n\tvUv2 = uv2;\n#endif";
-
- var worldpos_vertex = "#if defined( USE_ENVMAP ) || defined( DISTANCE ) || defined ( USE_SHADOWMAP )\n\tvec4 worldPosition = modelMatrix * vec4( transformed, 1.0 );\n#endif";
-
- var background_frag = "uniform sampler2D t2D;\nvarying vec2 vUv;\nvoid main() {\n\tvec4 texColor = texture2D( t2D, vUv );\n\tgl_FragColor = mapTexelToLinear( texColor );\n\t#include \n\t#include \n}";
-
- var background_vert = "varying vec2 vUv;\nuniform mat3 uvTransform;\nvoid main() {\n\tvUv = ( uvTransform * vec3( uv, 1 ) ).xy;\n\tgl_Position = vec4( position.xy, 1.0, 1.0 );\n}";
-
- var cube_frag = "uniform samplerCube tCube;\nuniform float tFlip;\nuniform float opacity;\nvarying vec3 vWorldDirection;\nvoid main() {\n\tvec4 texColor = textureCube( tCube, vec3( tFlip * vWorldDirection.x, vWorldDirection.yz ) );\n\tgl_FragColor = mapTexelToLinear( texColor );\n\tgl_FragColor.a *= opacity;\n\t#include \n\t#include \n}";
-
- var cube_vert = "varying vec3 vWorldDirection;\n#include \nvoid main() {\n\tvWorldDirection = transformDirection( position, modelMatrix );\n\t#include \n\t#include \n\tgl_Position.z = gl_Position.w;\n}";
-
- var depth_frag = "#if DEPTH_PACKING == 3200\n\tuniform float opacity;\n#endif\n#include \n#include \n#include \n#include \n#include \n#include \n#include \nvoid main() {\n\t#include \n\tvec4 diffuseColor = vec4( 1.0 );\n\t#if DEPTH_PACKING == 3200\n\t\tdiffuseColor.a = opacity;\n\t#endif\n\t#include \n\t#include \n\t#include \n\t#include \n\t#if DEPTH_PACKING == 3200\n\t\tgl_FragColor = vec4( vec3( 1.0 - gl_FragCoord.z ), opacity );\n\t#elif DEPTH_PACKING == 3201\n\t\tgl_FragColor = packDepthToRGBA( gl_FragCoord.z );\n\t#endif\n}";
-
- var depth_vert = "#include \n#include \n#include \n#include \n#include \n#include \n#include \nvoid main() {\n\t#include \n\t#include \n\t#ifdef USE_DISPLACEMENTMAP\n\t\t#include \n\t\t#include \n\t\t#include \n\t#endif\n\t#include \n\t#include \n\t#include \n\t#include \n\t#include \n\t#include \n\t#include \n}";
-
- var distanceRGBA_frag = "#define DISTANCE\nuniform vec3 referencePosition;\nuniform float nearDistance;\nuniform float farDistance;\nvarying vec3 vWorldPosition;\n#include \n#include \n#include \n#include \n#include \n#include \nvoid main () {\n\t#include \n\tvec4 diffuseColor = vec4( 1.0 );\n\t#include \n\t#include \n\t#include \n\tfloat dist = length( vWorldPosition - referencePosition );\n\tdist = ( dist - nearDistance ) / ( farDistance - nearDistance );\n\tdist = saturate( dist );\n\tgl_FragColor = packDepthToRGBA( dist );\n}";
-
- var distanceRGBA_vert = "#define DISTANCE\nvarying vec3 vWorldPosition;\n#include \n#include \n#include \n#include \n#include \n#include \nvoid main() {\n\t#include \n\t#include \n\t#ifdef USE_DISPLACEMENTMAP\n\t\t#include \n\t\t#include \n\t\t#include \n\t#endif\n\t#include \n\t#include \n\t#include \n\t#include \n\t#include \n\t#include \n\t#include \n\tvWorldPosition = worldPosition.xyz;\n}";
-
- var equirect_frag = "uniform sampler2D tEquirect;\nvarying vec3 vWorldDirection;\n#include \nvoid main() {\n\tvec3 direction = normalize( vWorldDirection );\n\tvec2 sampleUV;\n\tsampleUV.y = asin( clamp( direction.y, - 1.0, 1.0 ) ) * RECIPROCAL_PI + 0.5;\n\tsampleUV.x = atan( direction.z, direction.x ) * RECIPROCAL_PI2 + 0.5;\n\tvec4 texColor = texture2D( tEquirect, sampleUV );\n\tgl_FragColor = mapTexelToLinear( texColor );\n\t#include \n\t#include \n}";
-
- var equirect_vert = "varying vec3 vWorldDirection;\n#include \nvoid main() {\n\tvWorldDirection = transformDirection( position, modelMatrix );\n\t#include \n\t#include \n}";
-
- var linedashed_frag = "uniform vec3 diffuse;\nuniform float opacity;\nuniform float dashSize;\nuniform float totalSize;\nvarying float vLineDistance;\n#include \n#include \n#include \n#include \n#include \nvoid main() {\n\t#include \n\tif ( mod( vLineDistance, totalSize ) > dashSize ) {\n\t\tdiscard;\n\t}\n\tvec3 outgoingLight = vec3( 0.0 );\n\tvec4 diffuseColor = vec4( diffuse, opacity );\n\t#include \n\t#include \n\toutgoingLight = diffuseColor.rgb;\n\tgl_FragColor = vec4( outgoingLight, diffuseColor.a );\n\t#include \n\t#include \n\t#include \n\t#include \n}";
-
- var linedashed_vert = "uniform float scale;\nattribute float lineDistance;\nvarying float vLineDistance;\n#include \n#include \n#include \n#include \n#include \nvoid main() {\n\t#include \n\tvLineDistance = scale * lineDistance;\n\tvec4 mvPosition = modelViewMatrix * vec4( position, 1.0 );\n\tgl_Position = projectionMatrix * mvPosition;\n\t#include \n\t#include \n\t#include \n}";
-
- var meshbasic_frag = "uniform vec3 diffuse;\nuniform float opacity;\n#ifndef FLAT_SHADED\n\tvarying vec3 vNormal;\n#endif\n#include \n#include \n#include \n#include \n#include \n#include \n#include \n#include \n#include \n#include \n#include \n#include \n#include \nvoid main() {\n\t#include \n\tvec4 diffuseColor = vec4( diffuse, opacity );\n\t#include \n\t#include \n\t#include \n\t#include \n\t#include \n\t#include \n\tReflectedLight reflectedLight = ReflectedLight( vec3( 0.0 ), vec3( 0.0 ), vec3( 0.0 ), vec3( 0.0 ) );\n\t#ifdef USE_LIGHTMAP\n\t\treflectedLight.indirectDiffuse += texture2D( lightMap, vUv2 ).xyz * lightMapIntensity;\n\t#else\n\t\treflectedLight.indirectDiffuse += vec3( 1.0 );\n\t#endif\n\t#include \n\treflectedLight.indirectDiffuse *= diffuseColor.rgb;\n\tvec3 outgoingLight = reflectedLight.indirectDiffuse;\n\t#include \n\tgl_FragColor = vec4( outgoingLight, diffuseColor.a );\n\t#include \n\t#include \n\t#include \n\t#include \n}";
-
- var meshbasic_vert = "#include \n#include \n#include \n#include \n#include \n#include \n#include \n#include \n#include \n#include \nvoid main() {\n\t#include \n\t#include \n\t#include \n\t#include \n\t#ifdef USE_ENVMAP\n\t#include \n\t#include \n\t#include \n\t#include \n\t#endif\n\t#include \n\t#include \n\t#include \n\t#include \n\t#include \n\t#include \n\t#include \n\t#include \n\t#include \n}";
-
- var meshlambert_frag = "uniform vec3 diffuse;\nuniform vec3 emissive;\nuniform float opacity;\nvarying vec3 vLightFront;\nvarying vec3 vIndirectFront;\n#ifdef DOUBLE_SIDED\n\tvarying vec3 vLightBack;\n\tvarying vec3 vIndirectBack;\n#endif\n#include \n#include \n#include \n#include \n#include \n#include \n#include \n#include \n#include \n#include \n#include \n#include \n#include \n#include \n#include \n#include \n#include \n#include \n#include \n#include \nvoid main() {\n\t#include \n\tvec4 diffuseColor = vec4( diffuse, opacity );\n\tReflectedLight reflectedLight = ReflectedLight( vec3( 0.0 ), vec3( 0.0 ), vec3( 0.0 ), vec3( 0.0 ) );\n\tvec3 totalEmissiveRadiance = emissive;\n\t#include \n\t#include \n\t#include \n\t#include \n\t#include \n\t#include \n\t#include \n\treflectedLight.indirectDiffuse = getAmbientLightIrradiance( ambientLightColor );\n\t#ifdef DOUBLE_SIDED\n\t\treflectedLight.indirectDiffuse += ( gl_FrontFacing ) ? vIndirectFront : vIndirectBack;\n\t#else\n\t\treflectedLight.indirectDiffuse += vIndirectFront;\n\t#endif\n\t#include \n\treflectedLight.indirectDiffuse *= BRDF_Diffuse_Lambert( diffuseColor.rgb );\n\t#ifdef DOUBLE_SIDED\n\t\treflectedLight.directDiffuse = ( gl_FrontFacing ) ? vLightFront : vLightBack;\n\t#else\n\t\treflectedLight.directDiffuse = vLightFront;\n\t#endif\n\treflectedLight.directDiffuse *= BRDF_Diffuse_Lambert( diffuseColor.rgb ) * getShadowMask();\n\t#include \n\tvec3 outgoingLight = reflectedLight.directDiffuse + reflectedLight.indirectDiffuse + totalEmissiveRadiance;\n\t#include \n\tgl_FragColor = vec4( outgoingLight, diffuseColor.a );\n\t#include \n\t#include \n\t#include \n\t#include \n\t#include \n}";
-
- var meshlambert_vert = "#define LAMBERT\nvarying vec3 vLightFront;\nvarying vec3 vIndirectFront;\n#ifdef DOUBLE_SIDED\n\tvarying vec3 vLightBack;\n\tvarying vec3 vIndirectBack;\n#endif\n#include \n#include \n#include \n#include \n#include \n#include \n#include \n#include \n#include \n#include \n#include \n#include \n#include \nvoid main() {\n\t#include \n\t#include \n\t#include